hexsha
stringlengths 40
40
| size
int64 6
14.9M
| ext
stringclasses 1
value | lang
stringclasses 1
value | max_stars_repo_path
stringlengths 6
260
| max_stars_repo_name
stringlengths 6
119
| max_stars_repo_head_hexsha
stringlengths 40
41
| max_stars_repo_licenses
sequence | max_stars_count
int64 1
191k
⌀ | max_stars_repo_stars_event_min_datetime
stringlengths 24
24
⌀ | max_stars_repo_stars_event_max_datetime
stringlengths 24
24
⌀ | max_issues_repo_path
stringlengths 6
260
| max_issues_repo_name
stringlengths 6
119
| max_issues_repo_head_hexsha
stringlengths 40
41
| max_issues_repo_licenses
sequence | max_issues_count
int64 1
67k
⌀ | max_issues_repo_issues_event_min_datetime
stringlengths 24
24
⌀ | max_issues_repo_issues_event_max_datetime
stringlengths 24
24
⌀ | max_forks_repo_path
stringlengths 6
260
| max_forks_repo_name
stringlengths 6
119
| max_forks_repo_head_hexsha
stringlengths 40
41
| max_forks_repo_licenses
sequence | max_forks_count
int64 1
105k
⌀ | max_forks_repo_forks_event_min_datetime
stringlengths 24
24
⌀ | max_forks_repo_forks_event_max_datetime
stringlengths 24
24
⌀ | avg_line_length
float64 2
1.04M
| max_line_length
int64 2
11.2M
| alphanum_fraction
float64 0
1
| cells
sequence | cell_types
sequence | cell_type_groups
sequence |
---|---|---|---|---|---|---|---|---|---|---|---|---|---|---|---|---|---|---|---|---|---|---|---|---|---|---|---|---|---|---|
d0768341e30434bfc2c68d1a985bf22921bca8ab | 11,890 | ipynb | Jupyter Notebook | examples/Part 02 - Intro to Private Training with Remote Execution.ipynb | shubham3121/PySyft-TensorFlow | a8a6e47f206e324469dbeb995dc7117c09438ba0 | [
"Apache-2.0"
] | 39 | 2019-10-02T13:48:03.000Z | 2022-01-22T21:18:43.000Z | examples/Part 02 - Intro to Private Training with Remote Execution.ipynb | JEF-con/PySyft-TensorFlow | a8a6e47f206e324469dbeb995dc7117c09438ba0 | [
"Apache-2.0"
] | 19 | 2019-10-10T22:04:47.000Z | 2020-12-15T18:00:34.000Z | examples/Part 02 - Intro to Private Training with Remote Execution.ipynb | JEF-con/PySyft-TensorFlow | a8a6e47f206e324469dbeb995dc7117c09438ba0 | [
"Apache-2.0"
] | 12 | 2019-10-24T15:11:27.000Z | 2022-03-25T09:03:52.000Z | 33.587571 | 565 | 0.614634 | [
[
[
"# Part 2: Intro to Private Training with Remote Execution\n\nIn the last section, we learned about PointerTensors, which create the underlying infrastructure we need for privacy preserving Deep Learning. In this section, we're going to see how to use these basic tools to train our first deep learning model using remote execution.\n\nAuthors:\n- Yann Dupis - Twitter: [@YannDupis](https://twitter.com/YannDupis)\n- Andrew Trask - Twitter: [@iamtrask](https://twitter.com/iamtrask)\n\n### Why use remote execution?\n\nLet's say you are an AI startup who wants to build a deep learning model to detect [diabetic retinopathy (DR)](https://ai.googleblog.com/2016/11/deep-learning-for-detection-of-diabetic.html), which is the fastest growing cause of blindness. Before training your model, the first step would be to acquire a dataset of retinopathy images with signs of DR. One approach could be to work with a hospital and ask them to send you a copy of this dataset. However because of the sensitivity of the patients' data, the hospital might be exposed to liability risks.\n\n\nThat's where remote execution comes into the picture. Instead of bringing training data to the model (a central server), you bring the model to the training data (wherever it may live). In this case, it would be the hospital.\n\nThe idea is that this allows whoever is creating the data to own the only permanent copy, and thus maintain control over who ever has access to it. Pretty cool, eh?",
"_____no_output_____"
],
[
"# Section 2.1 - Private Training on MNIST\n\nFor this tutorial, we will train a model on the [MNIST dataset](http://yann.lecun.com/exdb/mnist/) to classify digits based on images.\n\nWe can assume that we have a remote worker named Bob who owns the data.",
"_____no_output_____"
]
],
[
[
"import tensorflow as tf\nimport syft as sy\n\nhook = sy.TensorFlowHook(tf)\nbob = sy.VirtualWorker(hook, id=\"bob\")",
"_____no_output_____"
]
],
[
[
"Let's download the MNIST data from `tf.keras.datasets`. Note that we are converting the data from numpy to `tf.Tensor` in order to have the PySyft functionalities.",
"_____no_output_____"
]
],
[
[
"mnist = tf.keras.datasets.mnist\n\n(x_train, y_train), (x_test, y_test) = mnist.load_data()\nx_train, x_test = x_train / 255.0, x_test / 255.0\n\nx_train, y_train = tf.convert_to_tensor(x_train), tf.convert_to_tensor(y_train)\nx_test, y_test = tf.convert_to_tensor(x_test), tf.convert_to_tensor(y_test)",
"_____no_output_____"
]
],
[
[
"As decribed in Part 1, we can send this data to Bob with the `send` method on the `tf.Tensor`. ",
"_____no_output_____"
]
],
[
[
"x_train_ptr = x_train.send(bob)\ny_train_ptr = y_train.send(bob)",
"_____no_output_____"
]
],
[
[
"Excellent! We have everything to start experimenting. To train our model on Bob's machine, we just have to perform the following steps:\n\n- Define a model, including optimizer and loss\n- Send the model to Bob\n- Start the training process\n- Get the trained model back\n\nLet's do it!",
"_____no_output_____"
]
],
[
[
"# Define the model\nmodel = tf.keras.models.Sequential([\n tf.keras.layers.Flatten(input_shape=(28, 28)),\n tf.keras.layers.Dense(128, activation='relu'),\n tf.keras.layers.Dropout(0.2),\n tf.keras.layers.Dense(10, activation='softmax')\n])\n\n# Compile with optimizer, loss and metrics\nmodel.compile(optimizer='adam',\n loss='sparse_categorical_crossentropy',\n metrics=['accuracy'])",
"_____no_output_____"
]
],
[
[
"Once you have defined your model, you can simply send it to Bob calling the `send` method. It's the exact same process as sending a tensor.",
"_____no_output_____"
]
],
[
[
"model_ptr = model.send(bob)",
"_____no_output_____"
],
[
"model_ptr",
"_____no_output_____"
]
],
[
[
"Now, we have a pointer pointing to the model on Bob's machine. We can validate that's the case by inspecting the attribute `_objects` on the virtual worker. ",
"_____no_output_____"
]
],
[
[
"bob._objects[model_ptr.id_at_location]",
"_____no_output_____"
]
],
[
[
"Everything is ready to start training our model on this remote dataset. You can call `fit` and pass `x_train_ptr` `y_train_ptr` which are pointing to Bob's data. Note that's the exact same interface as normal `tf.keras`.",
"_____no_output_____"
]
],
[
[
"model_ptr.fit(x_train_ptr, y_train_ptr, epochs=2, validation_split=0.2)",
"_____no_output_____"
]
],
[
[
"Fantastic! you have trained your model acheiving an accuracy greater than 95%.\n\nYou can get your trained model back by just calling `get` on it. ",
"_____no_output_____"
]
],
[
[
"model_gotten = model_ptr.get()\n\nmodel_gotten",
"_____no_output_____"
]
],
[
[
"It's good practice to see if your model can generalize by assessing its accuracy on an holdout dataset. You can simply call `evaluate`.",
"_____no_output_____"
]
],
[
[
"model_gotten.evaluate(x_test, y_test, verbose=2)",
"_____no_output_____"
]
],
[
[
"Boom! The model remotely trained on Bob's data is more than 95% accurate on this holdout dataset.",
"_____no_output_____"
],
[
"If your model doesn't fit into the Sequential paradigm, you can use Keras's functional API, or even subclass [tf.keras.Model](https://www.tensorflow.org/guide/keras/custom_layers_and_models#building_models) to create custom models.",
"_____no_output_____"
]
],
[
[
"class CustomModel(tf.keras.Model):\n\n def __init__(self, num_classes=10):\n super(CustomModel, self).__init__(name='custom_model')\n self.num_classes = num_classes\n \n self.flatten = tf.keras.layers.Flatten(input_shape=(28, 28))\n self.dense_1 = tf.keras.layers.Dense(128, activation='relu')\n self.dropout = tf.keras.layers.Dropout(0.2)\n self.dense_2 = tf.keras.layers.Dense(num_classes, activation='softmax')\n\n def call(self, inputs, training=False):\n x = self.flatten(inputs)\n x = self.dense_1(x)\n x = self.dropout(x, training=training)\n return self.dense_2(x)\n \nmodel = CustomModel(10)\n\n# need to call the model on dummy data before sending it\n# in order to set the input shape (required when saving to SavedModel)\nmodel.predict(tf.ones([1, 28, 28]))\n\nmodel.compile(optimizer='adam',\n loss='sparse_categorical_crossentropy',\n metrics=['accuracy'])\n\nmodel_ptr = model.send(bob)\n\nmodel_ptr.fit(x_train_ptr, y_train_ptr, epochs=2, validation_split=0.2)",
"_____no_output_____"
]
],
[
[
"## Well Done!\n\nAnd voilà! We have trained a Deep Learning model on Bob's data by sending the model to him. Never in this process do we ever see or request access to the underlying training data! We preserve the privacy of Bob!!!",
"_____no_output_____"
],
[
"# Congratulations!!! - Time to Join the Community!\n\nCongratulations on completing this notebook tutorial! If you enjoyed this and would like to join the movement toward privacy preserving, decentralized ownership of AI and the AI supply chain (data), you can do so in the following ways!\n\n### Star PySyft on GitHub\n\nThe easiest way to help our community is just by starring the Repos! This helps raise awareness of the cool tools we're building.\n\n- Star PySyft on GitHub! - [https://github.com/OpenMined/PySyft](https://github.com/OpenMined/PySyft)\n- Star PySyft-TensorFlow on GitHub! - [https://github.com/OpenMined/PySyft-TensorFlow]\n\n### Join our Slack!\n\nThe best way to keep up to date on the latest advancements is to join our community! You can do so by filling out the form at [http://slack.openmined.org](http://slack.openmined.org)\n\n### Join a Code Project!\n\nThe best way to contribute to our community is to become a code contributor! At any time you can go to PySyft GitHub Issues page and filter for \"Projects\". This will show you all the top level Tickets giving an overview of what projects you can join! If you don't want to join a project, but you would like to do a bit of coding, you can also look for more \"one off\" mini-projects by searching for GitHub issues marked \"good first issue\".\n\n- [PySyft Projects](https://github.com/OpenMined/PySyft/issues?q=is%3Aopen+is%3Aissue+label%3AProject)\n- [Good First Issue Tickets](https://github.com/OpenMined/PySyft/issues?q=is%3Aopen+is%3Aissue+label%3A%22good+first+issue%22)\n\n### Donate\n\nIf you don't have time to contribute to our codebase, but would still like to lend support, you can also become a Backer on our Open Collective. All donations go toward our web hosting and other community expenses such as hackathons and meetups!\n\n[OpenMined's Open Collective Page](https://opencollective.com/openmined)",
"_____no_output_____"
]
]
] | [
"markdown",
"code",
"markdown",
"code",
"markdown",
"code",
"markdown",
"code",
"markdown",
"code",
"markdown",
"code",
"markdown",
"code",
"markdown",
"code",
"markdown",
"code",
"markdown",
"code",
"markdown"
] | [
[
"markdown",
"markdown"
],
[
"code"
],
[
"markdown"
],
[
"code"
],
[
"markdown"
],
[
"code"
],
[
"markdown"
],
[
"code"
],
[
"markdown"
],
[
"code",
"code"
],
[
"markdown"
],
[
"code"
],
[
"markdown"
],
[
"code"
],
[
"markdown"
],
[
"code"
],
[
"markdown"
],
[
"code"
],
[
"markdown",
"markdown"
],
[
"code"
],
[
"markdown",
"markdown"
]
] |
d0768e3b42c06dd6d59665ebe9d619de6509245f | 11,468 | ipynb | Jupyter Notebook | courses/machine_learning/deepdive/07_structured/5_train.ipynb | varunsimhab/training-data-analyst | 7c99fbf2ef1310f4c1d42b543ac997c8120a2e83 | [
"Apache-2.0"
] | null | null | null | courses/machine_learning/deepdive/07_structured/5_train.ipynb | varunsimhab/training-data-analyst | 7c99fbf2ef1310f4c1d42b543ac997c8120a2e83 | [
"Apache-2.0"
] | null | null | null | courses/machine_learning/deepdive/07_structured/5_train.ipynb | varunsimhab/training-data-analyst | 7c99fbf2ef1310f4c1d42b543ac997c8120a2e83 | [
"Apache-2.0"
] | null | null | null | 28.176904 | 553 | 0.563655 | [
[
[
"<h1> Training on Cloud ML Engine </h1>\n\nThis notebook illustrates distributed training and hyperparameter tuning on Cloud ML Engine.",
"_____no_output_____"
]
],
[
[
"# change these to try this notebook out\nBUCKET = 'cloud-training-demos-ml'\nPROJECT = 'cloud-training-demos'\nREGION = 'us-central1'",
"_____no_output_____"
],
[
"import os\nos.environ['BUCKET'] = BUCKET\nos.environ['PROJECT'] = PROJECT\nos.environ['REGION'] = REGION",
"_____no_output_____"
],
[
"%%bash\nif ! gsutil ls | grep -q gs://${BUCKET}/; then\n gsutil mb -l ${REGION} gs://${BUCKET}\n # copy canonical set of preprocessed files if you didn't do previous notebook\n gsutil -m cp -R gs://cloud-training-demos/babyweight gs://${BUCKET}\nfi",
"_____no_output_____"
],
[
"%bash\ngsutil ls gs://${BUCKET}/babyweight/preproc/*-00000*",
"gs://cloud-training-demos-ml/babyweight/preproc/eval.csv-00000-of-00012\ngs://cloud-training-demos-ml/babyweight/preproc/train.csv-00000-of-00043\n"
]
],
[
[
"Now that we have the TensorFlow code working on a subset of the data, we can package the TensorFlow code up as a Python module and train it on Cloud ML Engine.\n<p>\n<h2> Train on Cloud ML Engine </h2>\n<p>\nTraining on Cloud ML Engine requires:\n<ol>\n<li> Making the code a Python package\n<li> Using gcloud to submit the training code to Cloud ML Engine\n</ol>\n<p>\nThe code in model.py is the same as in the TensorFlow notebook. I just moved it to a file so that I could package it up as a module.\n(explore the <a href=\"babyweight/trainer\">directory structure</a>).",
"_____no_output_____"
]
],
[
[
"%bash\ngrep \"^def\" babyweight/trainer/model.py",
"def read_dataset(prefix, pattern, batch_size=512):\ndef get_wide_deep():\ndef serving_input_fn():\ndef experiment_fn(output_dir):\ndef train_and_evaluate(output_dir):\n"
]
],
[
[
"After moving the code to a package, make sure it works standalone. (Note the --pattern and --train_examples lines so that I am not trying to boil the ocean on my laptop). Even then, this takes about <b>a minute</b> in which you won't see any output ...",
"_____no_output_____"
]
],
[
[
"%bash\necho \"bucket=${BUCKET}\"\nrm -rf babyweight_trained\nexport PYTHONPATH=${PYTHONPATH}:${PWD}/babyweight\npython -m trainer.task \\\n --bucket=${BUCKET} \\\n --output_dir=babyweight_trained \\\n --job-dir=./tmp \\\n --pattern=\"00000-of-\" --train_examples=500",
"_____no_output_____"
]
],
[
[
"Once the code works in standalone mode, you can run it on Cloud ML Engine. Because this is on the entire dataset, it will take a while. The training run took about <b> an hour </b> for me. You can monitor the job from the GCP console in the Cloud Machine Learning Engine section.",
"_____no_output_____"
]
],
[
[
"%bash\nOUTDIR=gs://${BUCKET}/babyweight/trained_model\nJOBNAME=babyweight_$(date -u +%y%m%d_%H%M%S)\necho $OUTDIR $REGION $JOBNAME\ngsutil -m rm -rf $OUTDIR\ngcloud ml-engine jobs submit training $JOBNAME \\\n --region=$REGION \\\n --module-name=trainer.task \\\n --package-path=$(pwd)/babyweight/trainer \\\n --job-dir=$OUTDIR \\\n --staging-bucket=gs://$BUCKET \\\n --scale-tier=STANDARD_1 \\\n --runtime-version=1.4 \\\n -- \\\n --bucket=${BUCKET} \\\n --output_dir=${OUTDIR} \\\n --train_examples=200000",
"_____no_output_____"
]
],
[
[
"When I ran it, training finished, and the evaluation happened three times (filter in Stackdriver on the word \"dict\"):\n<pre>\nSaving dict for global step 390632: average_loss = 1.06578, global_step = 390632, loss = 545.55\n</pre>\nThe final RMSE was 1.066 pounds.",
"_____no_output_____"
]
],
[
[
"from google.datalab.ml import TensorBoard\nTensorBoard().start('gs://{}/babyweight/trained_model'.format(BUCKET))",
"_____no_output_____"
],
[
"for pid in TensorBoard.list()['pid']:\n TensorBoard().stop(pid)\n print 'Stopped TensorBoard with pid {}'.format(pid)",
"Stopped TensorBoard with pid 10437\n"
]
],
[
[
"<h2> Hyperparameter tuning </h2>\n<p>\nAll of these are command-line parameters to my program. To do hyperparameter tuning, create hyperparam.xml and pass it as --configFile.\nThis step will take <b>1 hour</b> -- you can increase maxParallelTrials or reduce maxTrials to get it done faster. Since maxParallelTrials is the number of initial seeds to start searching from, you don't want it to be too large; otherwise, all you have is a random search.\n",
"_____no_output_____"
]
],
[
[
"%writefile hyperparam.yaml\ntrainingInput:\n scaleTier: STANDARD_1\n hyperparameters:\n hyperparameterMetricTag: average_loss\n goal: MINIMIZE\n maxTrials: 30\n maxParallelTrials: 3\n params:\n - parameterName: batch_size\n type: INTEGER\n minValue: 8\n maxValue: 512\n scaleType: UNIT_LOG_SCALE\n - parameterName: nembeds\n type: INTEGER\n minValue: 3\n maxValue: 30\n scaleType: UNIT_LINEAR_SCALE\n - parameterName: nnsize\n type: INTEGER\n minValue: 64\n maxValue: 512\n scaleType: UNIT_LOG_SCALE",
"Overwriting hyperparam.yaml\n"
]
],
[
[
"In reality, you would hyper-parameter tune over your entire dataset, and not on a smaller subset (see --pattern). But because this is a demo, I wanted it to finish quickly.",
"_____no_output_____"
]
],
[
[
"%bash\nOUTDIR=gs://${BUCKET}/babyweight/hyperparam\nJOBNAME=babyweight_$(date -u +%y%m%d_%H%M%S)\necho $OUTDIR $REGION $JOBNAME\ngsutil -m rm -rf $OUTDIR\ngcloud ml-engine jobs submit training $JOBNAME \\\n --region=$REGION \\\n --module-name=trainer.task \\\n --package-path=$(pwd)/babyweight/trainer \\\n --job-dir=$OUTDIR \\\n --staging-bucket=gs://$BUCKET \\\n --scale-tier=STANDARD_1 \\\n --config=hyperparam.yaml \\\n --runtime-version=1.4 \\\n -- \\\n --bucket=${BUCKET} \\\n --output_dir=${OUTDIR} \\\n --pattern=\"00000-of-\" --train_examples=5000",
"_____no_output_____"
],
[
"%bash\ngcloud ml-engine jobs describe babyweight_180123_202458",
"_____no_output_____"
]
],
[
[
"<h2> Repeat training </h2>\n<p>\nThis time with tuned parameters (note last line)",
"_____no_output_____"
]
],
[
[
"%bash\nOUTDIR=gs://${BUCKET}/babyweight/trained_model\nJOBNAME=babyweight_$(date -u +%y%m%d_%H%M%S)\necho $OUTDIR $REGION $JOBNAME\ngsutil -m rm -rf $OUTDIR\ngcloud ml-engine jobs submit training $JOBNAME \\\n --region=$REGION \\\n --module-name=trainer.task \\\n --package-path=$(pwd)/babyweight/trainer \\\n --job-dir=$OUTDIR \\\n --staging-bucket=gs://$BUCKET \\\n --scale-tier=STANDARD_1 \\\n -- \\\n --bucket=${BUCKET} \\\n --output_dir=${OUTDIR} \\\n --train_examples=200000 --batch_size=35 --nembeds=16 --nnsize=281",
"_____no_output_____"
]
],
[
[
"Copyright 2017 Google Inc. Licensed under the Apache License, Version 2.0 (the \"License\"); you may not use this file except in compliance with the License. You may obtain a copy of the License at http://www.apache.org/licenses/LICENSE-2.0 Unless required by applicable law or agreed to in writing, software distributed under the License is distributed on an \"AS IS\" BASIS, WITHOUT WARRANTIES OR CONDITIONS OF ANY KIND, either express or implied. See the License for the specific language governing permissions and limitations under the License",
"_____no_output_____"
]
]
] | [
"markdown",
"code",
"markdown",
"code",
"markdown",
"code",
"markdown",
"code",
"markdown",
"code",
"markdown",
"code",
"markdown",
"code",
"markdown",
"code",
"markdown"
] | [
[
"markdown"
],
[
"code",
"code",
"code",
"code"
],
[
"markdown"
],
[
"code"
],
[
"markdown"
],
[
"code"
],
[
"markdown"
],
[
"code"
],
[
"markdown"
],
[
"code",
"code"
],
[
"markdown"
],
[
"code"
],
[
"markdown"
],
[
"code",
"code"
],
[
"markdown"
],
[
"code"
],
[
"markdown"
]
] |
d076b32e55e2d7805343c7202e85425c3b2c8ba9 | 224,459 | ipynb | Jupyter Notebook | C4/W4/ungraded_labs/C4_W4_Lab_1_LSTM.ipynb | Mengxue12/tensorflow-1-public | 24012b7b87e3bcb8d3f6da586381f676cd231813 | [
"Apache-2.0"
] | null | null | null | C4/W4/ungraded_labs/C4_W4_Lab_1_LSTM.ipynb | Mengxue12/tensorflow-1-public | 24012b7b87e3bcb8d3f6da586381f676cd231813 | [
"Apache-2.0"
] | null | null | null | C4/W4/ungraded_labs/C4_W4_Lab_1_LSTM.ipynb | Mengxue12/tensorflow-1-public | 24012b7b87e3bcb8d3f6da586381f676cd231813 | [
"Apache-2.0"
] | null | null | null | 133.052164 | 65,206 | 0.708085 | [
[
[
"<a href=\"https://colab.research.google.com/github/Mengxue12/tensorflow-1-public/blob/main/C4/W4/ungraded_labs/C4_W4_Lab_1_LSTM.ipynb\" target=\"_parent\"><img src=\"https://colab.research.google.com/assets/colab-badge.svg\" alt=\"Open In Colab\"/></a>",
"_____no_output_____"
]
],
[
[
"#@title Licensed under the Apache License, Version 2.0 (the \"License\");\n# you may not use this file except in compliance with the License.\n# You may obtain a copy of the License at\n#\n# https://www.apache.org/licenses/LICENSE-2.0\n#\n# Unless required by applicable law or agreed to in writing, software\n# distributed under the License is distributed on an \"AS IS\" BASIS,\n# WITHOUT WARRANTIES OR CONDITIONS OF ANY KIND, either express or implied.\n# See the License for the specific language governing permissions and\n# limitations under the License.",
"_____no_output_____"
]
],
[
[
"**Note:** This notebook can run using TensorFlow 2.5.0",
"_____no_output_____"
]
],
[
[
"#!pip install tensorflow==2.5.0",
"_____no_output_____"
],
[
"import tensorflow as tf\nimport numpy as np\nimport matplotlib.pyplot as plt\nprint(tf.__version__)",
"2.8.0\n"
],
[
"def plot_series(time, series, format=\"-\", start=0, end=None):\n plt.plot(time[start:end], series[start:end], format)\n plt.xlabel(\"Time\")\n plt.ylabel(\"Value\")\n plt.grid(True)\n\ndef trend(time, slope=0):\n return slope * time\n\ndef seasonal_pattern(season_time):\n \"\"\"Just an arbitrary pattern, you can change it if you wish\"\"\"\n return np.where(season_time < 0.4,\n np.cos(season_time * 2 * np.pi),\n 1 / np.exp(3 * season_time))\n\ndef seasonality(time, period, amplitude=1, phase=0):\n \"\"\"Repeats the same pattern at each period\"\"\"\n season_time = ((time + phase) % period) / period\n return amplitude * seasonal_pattern(season_time)\n\ndef noise(time, noise_level=1, seed=None):\n rnd = np.random.RandomState(seed)\n return rnd.randn(len(time)) * noise_level\n\ntime = np.arange(4 * 365 + 1, dtype=\"float32\")\nbaseline = 10\nseries = trend(time, 0.1) \nbaseline = 10\namplitude = 40\nslope = 0.05\nnoise_level = 5\n\n# Create the series\nseries = baseline + trend(time, slope) + seasonality(time, period=365, amplitude=amplitude)\n# Update with noise\nseries += noise(time, noise_level, seed=42)\n\nsplit_time = 1000\ntime_train = time[:split_time]\nx_train = series[:split_time]\ntime_valid = time[split_time:]\nx_valid = series[split_time:]\n\nwindow_size = 20\nbatch_size = 32\nshuffle_buffer_size = 1000",
"_____no_output_____"
],
[
"def windowed_dataset(series, window_size, batch_size, shuffle_buffer):\n series = tf.expand_dims(series, axis=-1)\n ds = tf.data.Dataset.from_tensor_slices(series)\n ds = ds.window(window_size + 1, shift=1, drop_remainder=True)\n ds = ds.flat_map(lambda w: w.batch(window_size + 1))\n ds = ds.shuffle(shuffle_buffer)\n ds = ds.map(lambda w: (w[:-1], w[1:]))\n return ds.batch(batch_size).prefetch(1)",
"_____no_output_____"
],
[
"def model_forecast(model, series, window_size):\n ds = tf.data.Dataset.from_tensor_slices(series)\n ds = ds.window(window_size, shift=1, drop_remainder=True)\n ds = ds.flat_map(lambda w: w.batch(window_size))\n ds = ds.batch(32).prefetch(1)\n forecast = model.predict(ds)\n return forecast",
"_____no_output_____"
],
[
"tf.keras.backend.clear_session()\ntf.random.set_seed(51)\nnp.random.seed(51)\n\nwindow_size = 30\ntrain_set = windowed_dataset(x_train, window_size, batch_size=128, shuffle_buffer=shuffle_buffer_size)\n\nmodel = tf.keras.models.Sequential([\n tf.keras.layers.Conv1D(filters=32, kernel_size=5,\n strides=1, padding=\"causal\",\n activation=\"relu\",\n input_shape=[None, 1]),\n tf.keras.layers.Bidirectional(tf.keras.layers.LSTM(32, return_sequences=True)),\n tf.keras.layers.Bidirectional(tf.keras.layers.LSTM(32, return_sequences=True)),\n tf.keras.layers.Dense(1),\n tf.keras.layers.Lambda(lambda x: x * 200)\n])\nlr_schedule = tf.keras.callbacks.LearningRateScheduler(\n lambda epoch: 1e-8 * 10**(epoch / 20))\noptimizer = tf.keras.optimizers.SGD(learning_rate=1e-8, momentum=0.9)\nmodel.compile(loss=tf.keras.losses.Huber(),\n optimizer=optimizer,\n metrics=[\"mae\"])\nhistory = model.fit(train_set, epochs=100, callbacks=[lr_schedule])",
"Epoch 1/100\n8/8 [==============================] - 24s 86ms/step - loss: 73.1912 - mae: 73.6904 - lr: 1.0000e-08\nEpoch 2/100\n8/8 [==============================] - 0s 33ms/step - loss: 72.4799 - mae: 72.9791 - lr: 1.1220e-08\nEpoch 3/100\n8/8 [==============================] - 0s 34ms/step - loss: 71.3446 - mae: 71.8437 - lr: 1.2589e-08\nEpoch 4/100\n8/8 [==============================] - 0s 33ms/step - loss: 69.9230 - mae: 70.4223 - lr: 1.4125e-08\nEpoch 5/100\n8/8 [==============================] - 0s 31ms/step - loss: 68.2678 - mae: 68.7669 - lr: 1.5849e-08\nEpoch 6/100\n8/8 [==============================] - 0s 34ms/step - loss: 66.3621 - mae: 66.8609 - lr: 1.7783e-08\nEpoch 7/100\n8/8 [==============================] - 0s 33ms/step - loss: 64.2432 - mae: 64.7424 - lr: 1.9953e-08\nEpoch 8/100\n8/8 [==============================] - 0s 33ms/step - loss: 61.8749 - mae: 62.3738 - lr: 2.2387e-08\nEpoch 9/100\n8/8 [==============================] - 0s 33ms/step - loss: 59.2491 - mae: 59.7479 - lr: 2.5119e-08\nEpoch 10/100\n8/8 [==============================] - 0s 31ms/step - loss: 56.3267 - mae: 56.8254 - lr: 2.8184e-08\nEpoch 11/100\n8/8 [==============================] - 0s 32ms/step - loss: 53.0701 - mae: 53.5686 - lr: 3.1623e-08\nEpoch 12/100\n8/8 [==============================] - 0s 32ms/step - loss: 49.3867 - mae: 49.8849 - lr: 3.5481e-08\nEpoch 13/100\n8/8 [==============================] - 0s 33ms/step - loss: 45.1182 - mae: 45.6165 - lr: 3.9811e-08\nEpoch 14/100\n8/8 [==============================] - 0s 33ms/step - loss: 41.6538 - mae: 42.1518 - lr: 4.4668e-08\nEpoch 15/100\n8/8 [==============================] - 0s 33ms/step - loss: 40.6341 - mae: 41.1322 - lr: 5.0119e-08\nEpoch 16/100\n8/8 [==============================] - 1s 40ms/step - loss: 39.5119 - mae: 40.0100 - lr: 5.6234e-08\nEpoch 17/100\n8/8 [==============================] - 0s 31ms/step - loss: 38.1009 - mae: 38.5985 - lr: 6.3096e-08\nEpoch 18/100\n8/8 [==============================] - 0s 34ms/step - loss: 36.5304 - mae: 37.0279 - lr: 7.0795e-08\nEpoch 19/100\n8/8 [==============================] - 0s 32ms/step - loss: 34.9672 - mae: 35.4641 - lr: 7.9433e-08\nEpoch 20/100\n8/8 [==============================] - 0s 33ms/step - loss: 33.5379 - mae: 34.0347 - lr: 8.9125e-08\nEpoch 21/100\n8/8 [==============================] - 0s 33ms/step - loss: 32.2358 - mae: 32.7321 - lr: 1.0000e-07\nEpoch 22/100\n8/8 [==============================] - 0s 33ms/step - loss: 31.0509 - mae: 31.5469 - lr: 1.1220e-07\nEpoch 23/100\n8/8 [==============================] - 0s 33ms/step - loss: 29.9243 - mae: 30.4202 - lr: 1.2589e-07\nEpoch 24/100\n8/8 [==============================] - 0s 33ms/step - loss: 28.8317 - mae: 29.3274 - lr: 1.4125e-07\nEpoch 25/100\n8/8 [==============================] - 0s 32ms/step - loss: 27.7314 - mae: 28.2272 - lr: 1.5849e-07\nEpoch 26/100\n8/8 [==============================] - 0s 32ms/step - loss: 26.6107 - mae: 27.1066 - lr: 1.7783e-07\nEpoch 27/100\n8/8 [==============================] - 0s 32ms/step - loss: 25.4565 - mae: 25.9519 - lr: 1.9953e-07\nEpoch 28/100\n8/8 [==============================] - 0s 32ms/step - loss: 24.2668 - mae: 24.7617 - lr: 2.2387e-07\nEpoch 29/100\n8/8 [==============================] - 0s 33ms/step - loss: 23.0459 - mae: 23.5406 - lr: 2.5119e-07\nEpoch 30/100\n8/8 [==============================] - 0s 33ms/step - loss: 21.8057 - mae: 22.3000 - lr: 2.8184e-07\nEpoch 31/100\n8/8 [==============================] - 0s 32ms/step - loss: 20.5264 - mae: 21.0202 - lr: 3.1623e-07\nEpoch 32/100\n8/8 [==============================] - 0s 32ms/step - loss: 19.2341 - mae: 19.7269 - lr: 3.5481e-07\nEpoch 33/100\n8/8 [==============================] - 0s 33ms/step - loss: 17.9646 - mae: 18.4568 - lr: 3.9811e-07\nEpoch 34/100\n8/8 [==============================] - 0s 36ms/step - loss: 16.9362 - mae: 17.4279 - lr: 4.4668e-07\nEpoch 35/100\n8/8 [==============================] - 0s 34ms/step - loss: 16.2657 - mae: 16.7576 - lr: 5.0119e-07\nEpoch 36/100\n8/8 [==============================] - 0s 32ms/step - loss: 15.6281 - mae: 16.1192 - lr: 5.6234e-07\nEpoch 37/100\n8/8 [==============================] - 0s 33ms/step - loss: 15.0540 - mae: 15.5447 - lr: 6.3096e-07\nEpoch 38/100\n8/8 [==============================] - 0s 33ms/step - loss: 14.5219 - mae: 15.0126 - lr: 7.0795e-07\nEpoch 39/100\n8/8 [==============================] - 0s 33ms/step - loss: 13.9836 - mae: 14.4747 - lr: 7.9433e-07\nEpoch 40/100\n8/8 [==============================] - 0s 34ms/step - loss: 13.4414 - mae: 13.9318 - lr: 8.9125e-07\nEpoch 41/100\n8/8 [==============================] - 0s 32ms/step - loss: 12.8781 - mae: 13.3677 - lr: 1.0000e-06\nEpoch 42/100\n8/8 [==============================] - 0s 32ms/step - loss: 12.2855 - mae: 12.7749 - lr: 1.1220e-06\nEpoch 43/100\n8/8 [==============================] - 0s 32ms/step - loss: 11.6690 - mae: 12.1582 - lr: 1.2589e-06\nEpoch 44/100\n8/8 [==============================] - 0s 32ms/step - loss: 11.0181 - mae: 11.5063 - lr: 1.4125e-06\nEpoch 45/100\n8/8 [==============================] - 0s 32ms/step - loss: 10.3387 - mae: 10.8260 - lr: 1.5849e-06\nEpoch 46/100\n8/8 [==============================] - 0s 33ms/step - loss: 9.6894 - mae: 10.1757 - lr: 1.7783e-06\nEpoch 47/100\n8/8 [==============================] - 0s 34ms/step - loss: 9.0956 - mae: 9.5809 - lr: 1.9953e-06\nEpoch 48/100\n8/8 [==============================] - 0s 33ms/step - loss: 8.5688 - mae: 9.0536 - lr: 2.2387e-06\nEpoch 49/100\n8/8 [==============================] - 0s 32ms/step - loss: 8.1153 - mae: 8.5996 - lr: 2.5119e-06\nEpoch 50/100\n8/8 [==============================] - 0s 34ms/step - loss: 7.7040 - mae: 8.1884 - lr: 2.8184e-06\nEpoch 51/100\n8/8 [==============================] - 0s 33ms/step - loss: 7.3262 - mae: 7.8096 - lr: 3.1623e-06\nEpoch 52/100\n8/8 [==============================] - 0s 32ms/step - loss: 6.9725 - mae: 7.4548 - lr: 3.5481e-06\nEpoch 53/100\n8/8 [==============================] - 0s 33ms/step - loss: 6.6218 - mae: 7.1033 - lr: 3.9811e-06\nEpoch 54/100\n8/8 [==============================] - 0s 33ms/step - loss: 6.2898 - mae: 6.7712 - lr: 4.4668e-06\nEpoch 55/100\n8/8 [==============================] - 0s 32ms/step - loss: 5.9775 - mae: 6.4581 - lr: 5.0119e-06\nEpoch 56/100\n8/8 [==============================] - 0s 32ms/step - loss: 5.7096 - mae: 6.1898 - lr: 5.6234e-06\nEpoch 57/100\n8/8 [==============================] - 0s 33ms/step - loss: 5.4204 - mae: 5.8992 - lr: 6.3096e-06\nEpoch 58/100\n8/8 [==============================] - 0s 33ms/step - loss: 5.2126 - mae: 5.6904 - lr: 7.0795e-06\nEpoch 59/100\n8/8 [==============================] - 0s 33ms/step - loss: 4.9788 - mae: 5.4558 - lr: 7.9433e-06\nEpoch 60/100\n8/8 [==============================] - 0s 33ms/step - loss: 4.8674 - mae: 5.3438 - lr: 8.9125e-06\nEpoch 61/100\n8/8 [==============================] - 0s 32ms/step - loss: 4.7943 - mae: 5.2711 - lr: 1.0000e-05\nEpoch 62/100\n8/8 [==============================] - 0s 32ms/step - loss: 4.6056 - mae: 5.0814 - lr: 1.1220e-05\nEpoch 63/100\n8/8 [==============================] - 0s 33ms/step - loss: 4.7894 - mae: 5.2667 - lr: 1.2589e-05\nEpoch 64/100\n8/8 [==============================] - 0s 33ms/step - loss: 5.3932 - mae: 5.8745 - lr: 1.4125e-05\nEpoch 65/100\n8/8 [==============================] - 0s 33ms/step - loss: 5.8706 - mae: 6.3540 - lr: 1.5849e-05\nEpoch 66/100\n8/8 [==============================] - 0s 33ms/step - loss: 5.9400 - mae: 6.4234 - lr: 1.7783e-05\nEpoch 67/100\n8/8 [==============================] - 0s 33ms/step - loss: 4.9884 - mae: 5.4690 - lr: 1.9953e-05\nEpoch 68/100\n8/8 [==============================] - 0s 32ms/step - loss: 4.9481 - mae: 5.4273 - lr: 2.2387e-05\nEpoch 69/100\n8/8 [==============================] - 0s 33ms/step - loss: 5.5174 - mae: 5.9999 - lr: 2.5119e-05\nEpoch 70/100\n8/8 [==============================] - 0s 33ms/step - loss: 6.2505 - mae: 6.7357 - lr: 2.8184e-05\nEpoch 71/100\n8/8 [==============================] - 0s 34ms/step - loss: 5.7693 - mae: 6.2527 - lr: 3.1623e-05\nEpoch 72/100\n8/8 [==============================] - 0s 33ms/step - loss: 6.7677 - mae: 7.2529 - lr: 3.5481e-05\nEpoch 73/100\n8/8 [==============================] - 0s 31ms/step - loss: 7.3180 - mae: 7.8042 - lr: 3.9811e-05\nEpoch 74/100\n8/8 [==============================] - 0s 32ms/step - loss: 7.7811 - mae: 8.2695 - lr: 4.4668e-05\nEpoch 75/100\n8/8 [==============================] - 0s 32ms/step - loss: 7.6519 - mae: 8.1401 - lr: 5.0119e-05\nEpoch 76/100\n8/8 [==============================] - 0s 33ms/step - loss: 8.5045 - mae: 8.9957 - lr: 5.6234e-05\nEpoch 77/100\n8/8 [==============================] - 0s 32ms/step - loss: 9.3928 - mae: 9.8854 - lr: 6.3096e-05\nEpoch 78/100\n8/8 [==============================] - 0s 33ms/step - loss: 17.8352 - mae: 18.3274 - lr: 7.0795e-05\nEpoch 79/100\n8/8 [==============================] - 0s 33ms/step - loss: 32.4062 - mae: 32.9038 - lr: 7.9433e-05\nEpoch 80/100\n8/8 [==============================] - 0s 32ms/step - loss: 12.8659 - mae: 13.3580 - lr: 8.9125e-05\nEpoch 81/100\n8/8 [==============================] - 0s 33ms/step - loss: 9.9744 - mae: 10.4638 - lr: 1.0000e-04\nEpoch 82/100\n8/8 [==============================] - 0s 33ms/step - loss: 11.3594 - mae: 11.8533 - lr: 1.1220e-04\nEpoch 83/100\n8/8 [==============================] - 0s 33ms/step - loss: 10.1744 - mae: 10.6637 - lr: 1.2589e-04\nEpoch 84/100\n8/8 [==============================] - 0s 34ms/step - loss: 35.6556 - mae: 36.1541 - lr: 1.4125e-04\nEpoch 85/100\n8/8 [==============================] - 0s 37ms/step - loss: 23.5352 - mae: 24.0322 - lr: 1.5849e-04\nEpoch 86/100\n8/8 [==============================] - 1s 36ms/step - loss: 16.0095 - mae: 16.5044 - lr: 1.7783e-04\nEpoch 87/100\n8/8 [==============================] - 0s 38ms/step - loss: 14.1260 - mae: 14.6192 - lr: 1.9953e-04\nEpoch 88/100\n8/8 [==============================] - 1s 37ms/step - loss: 12.9790 - mae: 13.4720 - lr: 2.2387e-04\nEpoch 89/100\n8/8 [==============================] - 0s 38ms/step - loss: 15.1838 - mae: 15.6791 - lr: 2.5119e-04\nEpoch 90/100\n8/8 [==============================] - 1s 38ms/step - loss: 10.3792 - mae: 10.8697 - lr: 2.8184e-04\nEpoch 91/100\n8/8 [==============================] - 0s 33ms/step - loss: 9.4659 - mae: 9.9564 - lr: 3.1623e-04\nEpoch 92/100\n8/8 [==============================] - 0s 32ms/step - loss: 9.9145 - mae: 10.4040 - lr: 3.5481e-04\nEpoch 93/100\n8/8 [==============================] - 0s 33ms/step - loss: 20.9796 - mae: 21.4755 - lr: 3.9811e-04\nEpoch 94/100\n8/8 [==============================] - 0s 32ms/step - loss: 15.7421 - mae: 16.2352 - lr: 4.4668e-04\nEpoch 95/100\n8/8 [==============================] - 0s 34ms/step - loss: 21.3826 - mae: 21.8791 - lr: 5.0119e-04\nEpoch 96/100\n8/8 [==============================] - 0s 32ms/step - loss: 17.6574 - mae: 18.1528 - lr: 5.6234e-04\nEpoch 97/100\n8/8 [==============================] - 0s 32ms/step - loss: 19.3204 - mae: 19.8147 - lr: 6.3096e-04\nEpoch 98/100\n8/8 [==============================] - 0s 33ms/step - loss: 19.1665 - mae: 19.6608 - lr: 7.0795e-04\nEpoch 99/100\n8/8 [==============================] - 0s 34ms/step - loss: 18.3976 - mae: 18.8912 - lr: 7.9433e-04\nEpoch 100/100\n8/8 [==============================] - 0s 32ms/step - loss: 23.3824 - mae: 23.8778 - lr: 8.9125e-04\n"
],
[
"plt.semilogx(history.history[\"lr\"], history.history[\"loss\"])\nplt.axis([1e-8, 1e-4, 0, 30])",
"_____no_output_____"
],
[
"tf.keras.backend.clear_session()\ntf.random.set_seed(51)\nnp.random.seed(51)\n#batch_size = 16\ndataset = windowed_dataset(x_train, window_size, batch_size, shuffle_buffer_size)\n\nmodel = tf.keras.models.Sequential([\n tf.keras.layers.Conv1D(filters=32, kernel_size=3,\n strides=1, padding=\"causal\",\n activation=\"relu\",\n input_shape=[None, 1]),\n tf.keras.layers.LSTM(32, return_sequences=True),\n tf.keras.layers.LSTM(32, return_sequences=True),\n tf.keras.layers.Dense(1),\n tf.keras.layers.Lambda(lambda x: x * 200)\n])\n\noptimizer = tf.keras.optimizers.SGD(learning_rate=1e-5, momentum=0.9)\nmodel.compile(loss=tf.keras.losses.Huber(),\n optimizer=optimizer,\n metrics=[\"mae\"])\nhistory = model.fit(dataset,epochs=500)",
"Epoch 1/500\n31/31 [==============================] - 4s 18ms/step - loss: 20.0714 - mae: 20.5646\nEpoch 2/500\n31/31 [==============================] - 1s 16ms/step - loss: 7.9198 - mae: 8.4048\nEpoch 3/500\n31/31 [==============================] - 1s 16ms/step - loss: 6.6716 - mae: 7.1533\nEpoch 4/500\n31/31 [==============================] - 1s 17ms/step - loss: 6.1672 - mae: 6.6477\nEpoch 5/500\n31/31 [==============================] - 1s 16ms/step - loss: 5.7151 - mae: 6.1946\nEpoch 6/500\n31/31 [==============================] - 1s 16ms/step - loss: 5.6396 - mae: 6.1195\nEpoch 7/500\n31/31 [==============================] - 1s 16ms/step - loss: 5.4245 - mae: 5.9043\nEpoch 8/500\n31/31 [==============================] - 1s 17ms/step - loss: 5.3815 - mae: 5.8605\nEpoch 9/500\n31/31 [==============================] - 1s 17ms/step - loss: 5.3289 - mae: 5.8083\nEpoch 10/500\n31/31 [==============================] - 1s 16ms/step - loss: 5.2220 - mae: 5.7013\nEpoch 11/500\n31/31 [==============================] - 1s 17ms/step - loss: 5.1164 - mae: 5.5949\nEpoch 12/500\n31/31 [==============================] - 1s 17ms/step - loss: 5.0854 - mae: 5.5640\nEpoch 13/500\n31/31 [==============================] - 1s 17ms/step - loss: 5.0866 - mae: 5.5649\nEpoch 14/500\n31/31 [==============================] - 1s 17ms/step - loss: 5.1031 - mae: 5.5815\nEpoch 15/500\n31/31 [==============================] - 1s 17ms/step - loss: 5.0004 - mae: 5.4785\nEpoch 16/500\n31/31 [==============================] - 1s 18ms/step - loss: 5.1024 - mae: 5.5813\nEpoch 17/500\n31/31 [==============================] - 1s 17ms/step - loss: 4.8976 - mae: 5.3766\nEpoch 18/500\n31/31 [==============================] - 1s 17ms/step - loss: 4.8884 - mae: 5.3659\nEpoch 19/500\n31/31 [==============================] - 1s 17ms/step - loss: 4.9353 - mae: 5.4138\nEpoch 20/500\n31/31 [==============================] - 1s 17ms/step - loss: 4.8289 - mae: 5.3068\nEpoch 21/500\n31/31 [==============================] - 1s 17ms/step - loss: 4.7756 - mae: 5.2546\nEpoch 22/500\n31/31 [==============================] - 1s 16ms/step - loss: 4.8007 - mae: 5.2782\nEpoch 23/500\n31/31 [==============================] - 1s 16ms/step - loss: 4.7320 - mae: 5.2094\nEpoch 24/500\n31/31 [==============================] - 1s 16ms/step - loss: 4.7392 - mae: 5.2168\nEpoch 25/500\n31/31 [==============================] - 1s 17ms/step - loss: 4.6977 - mae: 5.1754\nEpoch 26/500\n31/31 [==============================] - 1s 17ms/step - loss: 4.6399 - mae: 5.1180\nEpoch 27/500\n31/31 [==============================] - 1s 17ms/step - loss: 4.6403 - mae: 5.1176\nEpoch 28/500\n31/31 [==============================] - 1s 17ms/step - loss: 4.6991 - mae: 5.1765\nEpoch 29/500\n31/31 [==============================] - 1s 17ms/step - loss: 4.6425 - mae: 5.1203\nEpoch 30/500\n31/31 [==============================] - 1s 17ms/step - loss: 4.6097 - mae: 5.0867\nEpoch 31/500\n31/31 [==============================] - 1s 17ms/step - loss: 4.5860 - mae: 5.0638\nEpoch 32/500\n31/31 [==============================] - 1s 17ms/step - loss: 4.6052 - mae: 5.0816\nEpoch 33/500\n31/31 [==============================] - 1s 16ms/step - loss: 4.5302 - mae: 5.0077\nEpoch 34/500\n31/31 [==============================] - 1s 17ms/step - loss: 4.5866 - mae: 5.0633\nEpoch 35/500\n31/31 [==============================] - 1s 16ms/step - loss: 4.5268 - mae: 5.0038\nEpoch 36/500\n31/31 [==============================] - 1s 17ms/step - loss: 4.5295 - mae: 5.0062\nEpoch 37/500\n31/31 [==============================] - 1s 17ms/step - loss: 4.5230 - mae: 5.0002\nEpoch 38/500\n31/31 [==============================] - 1s 17ms/step - loss: 4.5310 - mae: 5.0076\nEpoch 39/500\n31/31 [==============================] - 1s 17ms/step - loss: 4.5192 - mae: 4.9959\nEpoch 40/500\n31/31 [==============================] - 1s 18ms/step - loss: 4.4530 - mae: 4.9293\nEpoch 41/500\n31/31 [==============================] - 1s 17ms/step - loss: 4.5626 - mae: 5.0395\nEpoch 42/500\n31/31 [==============================] - 1s 17ms/step - loss: 4.4388 - mae: 4.9146\nEpoch 43/500\n31/31 [==============================] - 1s 17ms/step - loss: 4.4231 - mae: 4.8997\nEpoch 44/500\n31/31 [==============================] - 1s 17ms/step - loss: 4.4870 - mae: 4.9628\nEpoch 45/500\n31/31 [==============================] - 1s 17ms/step - loss: 4.5363 - mae: 5.0123\nEpoch 46/500\n31/31 [==============================] - 1s 17ms/step - loss: 4.4089 - mae: 4.8853\nEpoch 47/500\n31/31 [==============================] - 1s 17ms/step - loss: 4.4374 - mae: 4.9137\nEpoch 48/500\n31/31 [==============================] - 1s 17ms/step - loss: 4.4259 - mae: 4.9016\nEpoch 49/500\n31/31 [==============================] - 1s 17ms/step - loss: 4.4815 - mae: 4.9576\nEpoch 50/500\n31/31 [==============================] - 1s 17ms/step - loss: 4.4074 - mae: 4.8841\nEpoch 51/500\n31/31 [==============================] - 1s 17ms/step - loss: 4.4222 - mae: 4.8972\nEpoch 52/500\n31/31 [==============================] - 1s 16ms/step - loss: 4.6779 - mae: 5.1554\nEpoch 53/500\n31/31 [==============================] - 1s 17ms/step - loss: 4.4200 - mae: 4.8956\nEpoch 54/500\n31/31 [==============================] - 1s 17ms/step - loss: 4.3926 - mae: 4.8681\nEpoch 55/500\n31/31 [==============================] - 1s 17ms/step - loss: 4.5861 - mae: 5.0627\nEpoch 56/500\n31/31 [==============================] - 1s 17ms/step - loss: 4.3860 - mae: 4.8612\nEpoch 57/500\n31/31 [==============================] - 1s 17ms/step - loss: 4.4013 - mae: 4.8770\nEpoch 58/500\n31/31 [==============================] - 1s 17ms/step - loss: 4.3656 - mae: 4.8413\nEpoch 59/500\n31/31 [==============================] - 1s 18ms/step - loss: 4.3336 - mae: 4.8084\nEpoch 60/500\n31/31 [==============================] - 1s 17ms/step - loss: 4.3968 - mae: 4.8728\nEpoch 61/500\n31/31 [==============================] - 1s 17ms/step - loss: 4.3750 - mae: 4.8510\nEpoch 62/500\n31/31 [==============================] - 1s 17ms/step - loss: 4.3245 - mae: 4.7999\nEpoch 63/500\n31/31 [==============================] - 1s 17ms/step - loss: 4.3585 - mae: 4.8339\nEpoch 64/500\n31/31 [==============================] - 1s 17ms/step - loss: 4.3428 - mae: 4.8177\nEpoch 65/500\n31/31 [==============================] - 1s 17ms/step - loss: 4.3970 - mae: 4.8722\nEpoch 66/500\n31/31 [==============================] - 1s 17ms/step - loss: 4.3287 - mae: 4.8041\nEpoch 67/500\n31/31 [==============================] - 1s 17ms/step - loss: 4.4945 - mae: 4.9710\nEpoch 68/500\n31/31 [==============================] - 1s 17ms/step - loss: 4.5074 - mae: 4.9848\nEpoch 69/500\n31/31 [==============================] - 1s 17ms/step - loss: 4.2903 - mae: 4.7653\nEpoch 70/500\n31/31 [==============================] - 1s 17ms/step - loss: 4.2879 - mae: 4.7627\nEpoch 71/500\n31/31 [==============================] - 1s 17ms/step - loss: 4.3093 - mae: 4.7842\nEpoch 72/500\n31/31 [==============================] - 1s 17ms/step - loss: 4.3740 - mae: 4.8492\nEpoch 73/500\n31/31 [==============================] - 1s 16ms/step - loss: 4.3155 - mae: 4.7904\nEpoch 74/500\n31/31 [==============================] - 1s 17ms/step - loss: 4.2954 - mae: 4.7697\nEpoch 75/500\n31/31 [==============================] - 1s 17ms/step - loss: 4.2535 - mae: 4.7282\nEpoch 76/500\n31/31 [==============================] - 1s 17ms/step - loss: 4.2578 - mae: 4.7324\nEpoch 77/500\n31/31 [==============================] - 1s 17ms/step - loss: 4.2641 - mae: 4.7391\nEpoch 78/500\n31/31 [==============================] - 1s 16ms/step - loss: 4.3166 - mae: 4.7919\nEpoch 79/500\n31/31 [==============================] - 1s 16ms/step - loss: 4.3015 - mae: 4.7761\nEpoch 80/500\n31/31 [==============================] - 1s 16ms/step - loss: 4.3159 - mae: 4.7911\nEpoch 81/500\n31/31 [==============================] - 1s 17ms/step - loss: 4.2583 - mae: 4.7325\nEpoch 82/500\n31/31 [==============================] - 1s 17ms/step - loss: 4.3669 - mae: 4.8429\nEpoch 83/500\n31/31 [==============================] - 1s 17ms/step - loss: 4.3183 - mae: 4.7933\nEpoch 84/500\n31/31 [==============================] - 1s 17ms/step - loss: 4.4260 - mae: 4.9020\nEpoch 85/500\n31/31 [==============================] - 1s 17ms/step - loss: 4.3776 - mae: 4.8540\nEpoch 86/500\n31/31 [==============================] - 1s 17ms/step - loss: 4.2718 - mae: 4.7465\nEpoch 87/500\n31/31 [==============================] - 1s 16ms/step - loss: 4.2969 - mae: 4.7725\nEpoch 88/500\n31/31 [==============================] - 1s 17ms/step - loss: 4.2208 - mae: 4.6950\nEpoch 89/500\n31/31 [==============================] - 1s 17ms/step - loss: 4.2229 - mae: 4.6976\nEpoch 90/500\n31/31 [==============================] - 1s 17ms/step - loss: 4.2355 - mae: 4.7100\nEpoch 91/500\n31/31 [==============================] - 1s 17ms/step - loss: 4.2440 - mae: 4.7182\nEpoch 92/500\n31/31 [==============================] - 1s 17ms/step - loss: 4.2490 - mae: 4.7241\nEpoch 93/500\n31/31 [==============================] - 1s 17ms/step - loss: 4.2247 - mae: 4.6989\nEpoch 94/500\n31/31 [==============================] - 1s 17ms/step - loss: 4.3162 - mae: 4.7904\nEpoch 95/500\n31/31 [==============================] - 1s 17ms/step - loss: 4.2872 - mae: 4.7617\nEpoch 96/500\n31/31 [==============================] - 1s 17ms/step - loss: 4.2412 - mae: 4.7167\nEpoch 97/500\n31/31 [==============================] - 1s 17ms/step - loss: 4.2377 - mae: 4.7118\nEpoch 98/500\n31/31 [==============================] - 1s 17ms/step - loss: 4.3287 - mae: 4.8046\nEpoch 99/500\n31/31 [==============================] - 1s 17ms/step - loss: 4.2687 - mae: 4.7435\nEpoch 100/500\n31/31 [==============================] - 1s 17ms/step - loss: 4.1908 - mae: 4.6639\nEpoch 101/500\n31/31 [==============================] - 1s 17ms/step - loss: 4.2381 - mae: 4.7121\nEpoch 102/500\n31/31 [==============================] - 1s 16ms/step - loss: 4.2119 - mae: 4.6860\nEpoch 103/500\n31/31 [==============================] - 1s 17ms/step - loss: 4.1838 - mae: 4.6583\nEpoch 104/500\n31/31 [==============================] - 1s 17ms/step - loss: 4.1888 - mae: 4.6623\nEpoch 105/500\n31/31 [==============================] - 1s 17ms/step - loss: 4.1775 - mae: 4.6511\nEpoch 106/500\n31/31 [==============================] - 1s 17ms/step - loss: 4.2577 - mae: 4.7324\nEpoch 107/500\n31/31 [==============================] - 1s 17ms/step - loss: 4.2734 - mae: 4.7483\nEpoch 108/500\n31/31 [==============================] - 1s 17ms/step - loss: 4.2263 - mae: 4.7003\nEpoch 109/500\n31/31 [==============================] - 1s 17ms/step - loss: 4.2019 - mae: 4.6761\nEpoch 110/500\n31/31 [==============================] - 1s 17ms/step - loss: 4.2340 - mae: 4.7081\nEpoch 111/500\n31/31 [==============================] - 1s 18ms/step - loss: 4.2067 - mae: 4.6807\nEpoch 112/500\n31/31 [==============================] - 1s 17ms/step - loss: 4.2053 - mae: 4.6794\nEpoch 113/500\n31/31 [==============================] - 1s 17ms/step - loss: 4.1835 - mae: 4.6578\nEpoch 114/500\n31/31 [==============================] - 1s 17ms/step - loss: 4.2368 - mae: 4.7113\nEpoch 115/500\n31/31 [==============================] - 1s 17ms/step - loss: 4.2423 - mae: 4.7172\nEpoch 116/500\n31/31 [==============================] - 1s 17ms/step - loss: 4.1502 - mae: 4.6236\nEpoch 117/500\n31/31 [==============================] - 1s 17ms/step - loss: 4.1814 - mae: 4.6548\nEpoch 118/500\n31/31 [==============================] - 1s 18ms/step - loss: 4.1873 - mae: 4.6612\nEpoch 119/500\n31/31 [==============================] - 1s 17ms/step - loss: 4.2185 - mae: 4.6927\nEpoch 120/500\n31/31 [==============================] - 1s 16ms/step - loss: 4.2738 - mae: 4.7481\nEpoch 121/500\n31/31 [==============================] - 1s 17ms/step - loss: 4.1680 - mae: 4.6416\nEpoch 122/500\n31/31 [==============================] - 1s 17ms/step - loss: 4.2055 - mae: 4.6790\nEpoch 123/500\n31/31 [==============================] - 1s 17ms/step - loss: 4.1662 - mae: 4.6390\nEpoch 124/500\n31/31 [==============================] - 1s 17ms/step - loss: 4.1390 - mae: 4.6121\nEpoch 125/500\n31/31 [==============================] - 1s 17ms/step - loss: 4.1783 - mae: 4.6522\nEpoch 126/500\n31/31 [==============================] - 1s 17ms/step - loss: 4.1774 - mae: 4.6508\nEpoch 127/500\n31/31 [==============================] - 1s 17ms/step - loss: 4.1499 - mae: 4.6232\nEpoch 128/500\n31/31 [==============================] - 1s 17ms/step - loss: 4.2690 - mae: 4.7433\nEpoch 129/500\n31/31 [==============================] - 1s 17ms/step - loss: 4.2381 - mae: 4.7130\nEpoch 130/500\n31/31 [==============================] - 1s 17ms/step - loss: 4.2117 - mae: 4.6856\nEpoch 131/500\n31/31 [==============================] - 1s 17ms/step - loss: 4.2289 - mae: 4.7037\nEpoch 132/500\n31/31 [==============================] - 1s 18ms/step - loss: 4.3141 - mae: 4.7891\nEpoch 133/500\n31/31 [==============================] - 1s 17ms/step - loss: 4.3430 - mae: 4.8187\nEpoch 134/500\n31/31 [==============================] - 1s 17ms/step - loss: 4.1583 - mae: 4.6326\nEpoch 135/500\n31/31 [==============================] - 1s 17ms/step - loss: 4.1595 - mae: 4.6333\nEpoch 136/500\n31/31 [==============================] - 1s 17ms/step - loss: 4.1510 - mae: 4.6245\nEpoch 137/500\n31/31 [==============================] - 1s 17ms/step - loss: 4.1462 - mae: 4.6205\nEpoch 138/500\n31/31 [==============================] - 1s 17ms/step - loss: 4.2075 - mae: 4.6820\nEpoch 139/500\n31/31 [==============================] - 1s 17ms/step - loss: 4.2070 - mae: 4.6812\nEpoch 140/500\n31/31 [==============================] - 1s 17ms/step - loss: 4.1926 - mae: 4.6666\nEpoch 141/500\n31/31 [==============================] - 1s 17ms/step - loss: 4.1382 - mae: 4.6122\nEpoch 142/500\n31/31 [==============================] - 1s 17ms/step - loss: 4.1264 - mae: 4.6000\nEpoch 143/500\n31/31 [==============================] - 1s 17ms/step - loss: 4.1489 - mae: 4.6213\nEpoch 144/500\n31/31 [==============================] - 1s 17ms/step - loss: 4.2078 - mae: 4.6816\nEpoch 145/500\n31/31 [==============================] - 1s 17ms/step - loss: 4.1355 - mae: 4.6084\nEpoch 146/500\n31/31 [==============================] - 1s 17ms/step - loss: 4.1552 - mae: 4.6293\nEpoch 147/500\n31/31 [==============================] - 1s 17ms/step - loss: 4.1374 - mae: 4.6106\nEpoch 148/500\n31/31 [==============================] - 1s 17ms/step - loss: 4.2007 - mae: 4.6748\nEpoch 149/500\n31/31 [==============================] - 1s 17ms/step - loss: 4.1296 - mae: 4.6030\nEpoch 150/500\n31/31 [==============================] - 1s 17ms/step - loss: 4.1173 - mae: 4.5900\nEpoch 151/500\n31/31 [==============================] - 1s 17ms/step - loss: 4.1339 - mae: 4.6071\nEpoch 152/500\n31/31 [==============================] - 1s 17ms/step - loss: 4.3179 - mae: 4.7940\nEpoch 153/500\n31/31 [==============================] - 1s 18ms/step - loss: 4.1523 - mae: 4.6258\nEpoch 154/500\n31/31 [==============================] - 1s 17ms/step - loss: 4.2748 - mae: 4.7497\nEpoch 155/500\n31/31 [==============================] - 1s 17ms/step - loss: 4.2117 - mae: 4.6868\nEpoch 156/500\n31/31 [==============================] - 1s 17ms/step - loss: 4.2009 - mae: 4.6755\nEpoch 157/500\n31/31 [==============================] - 1s 17ms/step - loss: 4.1249 - mae: 4.5978\nEpoch 158/500\n31/31 [==============================] - 1s 16ms/step - loss: 4.2036 - mae: 4.6776\nEpoch 159/500\n31/31 [==============================] - 1s 17ms/step - loss: 4.1062 - mae: 4.5794\nEpoch 160/500\n31/31 [==============================] - 1s 18ms/step - loss: 4.1498 - mae: 4.6229\nEpoch 161/500\n31/31 [==============================] - 1s 17ms/step - loss: 4.1104 - mae: 4.5839\nEpoch 162/500\n31/31 [==============================] - 1s 17ms/step - loss: 4.1100 - mae: 4.5838\nEpoch 163/500\n31/31 [==============================] - 1s 16ms/step - loss: 4.1024 - mae: 4.5756\nEpoch 164/500\n31/31 [==============================] - 1s 17ms/step - loss: 4.1946 - mae: 4.6690\nEpoch 165/500\n31/31 [==============================] - 1s 17ms/step - loss: 4.1544 - mae: 4.6286\nEpoch 166/500\n31/31 [==============================] - 1s 17ms/step - loss: 4.1219 - mae: 4.5954\nEpoch 167/500\n31/31 [==============================] - 1s 17ms/step - loss: 4.1054 - mae: 4.5786\nEpoch 168/500\n31/31 [==============================] - 1s 17ms/step - loss: 4.1068 - mae: 4.5811\nEpoch 169/500\n31/31 [==============================] - 1s 17ms/step - loss: 4.1135 - mae: 4.5872\nEpoch 170/500\n31/31 [==============================] - 1s 17ms/step - loss: 4.2602 - mae: 4.7351\nEpoch 171/500\n31/31 [==============================] - 1s 17ms/step - loss: 4.1001 - mae: 4.5732\nEpoch 172/500\n31/31 [==============================] - 1s 17ms/step - loss: 4.1382 - mae: 4.6119\nEpoch 173/500\n31/31 [==============================] - 1s 17ms/step - loss: 4.1085 - mae: 4.5817\nEpoch 174/500\n31/31 [==============================] - 1s 17ms/step - loss: 4.1143 - mae: 4.5876\nEpoch 175/500\n31/31 [==============================] - 1s 17ms/step - loss: 4.1490 - mae: 4.6238\nEpoch 176/500\n31/31 [==============================] - 1s 17ms/step - loss: 4.1097 - mae: 4.5821\nEpoch 177/500\n31/31 [==============================] - 1s 17ms/step - loss: 4.1086 - mae: 4.5821\nEpoch 178/500\n31/31 [==============================] - 1s 17ms/step - loss: 4.1263 - mae: 4.6004\nEpoch 179/500\n31/31 [==============================] - 1s 17ms/step - loss: 4.2819 - mae: 4.7575\nEpoch 180/500\n31/31 [==============================] - 1s 17ms/step - loss: 4.1013 - mae: 4.5738\nEpoch 181/500\n31/31 [==============================] - 1s 17ms/step - loss: 4.1223 - mae: 4.5965\nEpoch 182/500\n31/31 [==============================] - 1s 17ms/step - loss: 4.1351 - mae: 4.6089\nEpoch 183/500\n31/31 [==============================] - 1s 17ms/step - loss: 4.2165 - mae: 4.6913\nEpoch 184/500\n31/31 [==============================] - 1s 18ms/step - loss: 4.0685 - mae: 4.5411\nEpoch 185/500\n31/31 [==============================] - 1s 18ms/step - loss: 4.0841 - mae: 4.5567\nEpoch 186/500\n31/31 [==============================] - 1s 17ms/step - loss: 4.1285 - mae: 4.6017\nEpoch 187/500\n31/31 [==============================] - 1s 17ms/step - loss: 4.2946 - mae: 4.7690\nEpoch 188/500\n31/31 [==============================] - 1s 17ms/step - loss: 4.1584 - mae: 4.6327\nEpoch 189/500\n31/31 [==============================] - 1s 17ms/step - loss: 4.1481 - mae: 4.6229\nEpoch 190/500\n31/31 [==============================] - 1s 17ms/step - loss: 4.1161 - mae: 4.5896\nEpoch 191/500\n31/31 [==============================] - 1s 17ms/step - loss: 4.1000 - mae: 4.5722\nEpoch 192/500\n31/31 [==============================] - 1s 17ms/step - loss: 4.1213 - mae: 4.5945\nEpoch 193/500\n31/31 [==============================] - 1s 17ms/step - loss: 4.0729 - mae: 4.5463\nEpoch 194/500\n31/31 [==============================] - 1s 17ms/step - loss: 4.1752 - mae: 4.6494\nEpoch 195/500\n31/31 [==============================] - 1s 17ms/step - loss: 4.1275 - mae: 4.6014\nEpoch 196/500\n31/31 [==============================] - 1s 17ms/step - loss: 4.2276 - mae: 4.7023\nEpoch 197/500\n31/31 [==============================] - 1s 17ms/step - loss: 4.0873 - mae: 4.5607\nEpoch 198/500\n31/31 [==============================] - 1s 17ms/step - loss: 4.0630 - mae: 4.5360\nEpoch 199/500\n31/31 [==============================] - 1s 17ms/step - loss: 4.0887 - mae: 4.5619\nEpoch 200/500\n31/31 [==============================] - 1s 17ms/step - loss: 4.1107 - mae: 4.5841\nEpoch 201/500\n31/31 [==============================] - 1s 17ms/step - loss: 4.0687 - mae: 4.5408\nEpoch 202/500\n31/31 [==============================] - 1s 17ms/step - loss: 4.0655 - mae: 4.5383\nEpoch 203/500\n31/31 [==============================] - 1s 17ms/step - loss: 4.1284 - mae: 4.6022\nEpoch 204/500\n31/31 [==============================] - 1s 17ms/step - loss: 4.1501 - mae: 4.6246\nEpoch 205/500\n31/31 [==============================] - 1s 17ms/step - loss: 4.0926 - mae: 4.5669\nEpoch 206/500\n31/31 [==============================] - 1s 17ms/step - loss: 4.1157 - mae: 4.5900\nEpoch 207/500\n31/31 [==============================] - 1s 17ms/step - loss: 4.0555 - mae: 4.5283\nEpoch 208/500\n31/31 [==============================] - 1s 17ms/step - loss: 4.1092 - mae: 4.5827\nEpoch 209/500\n31/31 [==============================] - 1s 17ms/step - loss: 4.0578 - mae: 4.5300\nEpoch 210/500\n31/31 [==============================] - 1s 17ms/step - loss: 4.0854 - mae: 4.5591\nEpoch 211/500\n31/31 [==============================] - 1s 17ms/step - loss: 4.0821 - mae: 4.5554\nEpoch 212/500\n31/31 [==============================] - 1s 18ms/step - loss: 4.0435 - mae: 4.5159\nEpoch 213/500\n31/31 [==============================] - 1s 17ms/step - loss: 4.0618 - mae: 4.5346\nEpoch 214/500\n31/31 [==============================] - 1s 18ms/step - loss: 4.0506 - mae: 4.5235\nEpoch 215/500\n31/31 [==============================] - 1s 17ms/step - loss: 4.0641 - mae: 4.5368\nEpoch 216/500\n31/31 [==============================] - 1s 17ms/step - loss: 4.0760 - mae: 4.5496\nEpoch 217/500\n31/31 [==============================] - 1s 17ms/step - loss: 4.0867 - mae: 4.5596\nEpoch 218/500\n31/31 [==============================] - 1s 17ms/step - loss: 4.0357 - mae: 4.5074\nEpoch 219/500\n31/31 [==============================] - 1s 17ms/step - loss: 4.0535 - mae: 4.5263\nEpoch 220/500\n31/31 [==============================] - 1s 17ms/step - loss: 4.0590 - mae: 4.5319\nEpoch 221/500\n31/31 [==============================] - 1s 17ms/step - loss: 4.0376 - mae: 4.5100\nEpoch 222/500\n31/31 [==============================] - 1s 17ms/step - loss: 4.0582 - mae: 4.5313\nEpoch 223/500\n31/31 [==============================] - 1s 17ms/step - loss: 4.1867 - mae: 4.6614\nEpoch 224/500\n31/31 [==============================] - 1s 17ms/step - loss: 4.0941 - mae: 4.5676\nEpoch 225/500\n31/31 [==============================] - 1s 17ms/step - loss: 4.1245 - mae: 4.5978\nEpoch 226/500\n31/31 [==============================] - 1s 17ms/step - loss: 4.0773 - mae: 4.5496\nEpoch 227/500\n31/31 [==============================] - 1s 17ms/step - loss: 4.0314 - mae: 4.5038\nEpoch 228/500\n31/31 [==============================] - 1s 17ms/step - loss: 4.0593 - mae: 4.5323\nEpoch 229/500\n31/31 [==============================] - 1s 17ms/step - loss: 4.0608 - mae: 4.5336\nEpoch 230/500\n31/31 [==============================] - 1s 18ms/step - loss: 4.1776 - mae: 4.6525\nEpoch 231/500\n31/31 [==============================] - 1s 17ms/step - loss: 4.3184 - mae: 4.7943\nEpoch 232/500\n31/31 [==============================] - 1s 17ms/step - loss: 4.0747 - mae: 4.5483\nEpoch 233/500\n31/31 [==============================] - 1s 17ms/step - loss: 4.0752 - mae: 4.5488\nEpoch 234/500\n31/31 [==============================] - 1s 17ms/step - loss: 4.1668 - mae: 4.6410\nEpoch 235/500\n31/31 [==============================] - 1s 18ms/step - loss: 4.1110 - mae: 4.5844\nEpoch 236/500\n31/31 [==============================] - 1s 17ms/step - loss: 4.0289 - mae: 4.5022\nEpoch 237/500\n31/31 [==============================] - 1s 17ms/step - loss: 4.0427 - mae: 4.5152\nEpoch 238/500\n31/31 [==============================] - 1s 18ms/step - loss: 4.1794 - mae: 4.6534\nEpoch 239/500\n31/31 [==============================] - 1s 17ms/step - loss: 4.1095 - mae: 4.5844\nEpoch 240/500\n31/31 [==============================] - 1s 17ms/step - loss: 4.1538 - mae: 4.6275\nEpoch 241/500\n31/31 [==============================] - 1s 17ms/step - loss: 4.1868 - mae: 4.6614\nEpoch 242/500\n31/31 [==============================] - 1s 17ms/step - loss: 4.0266 - mae: 4.4989\nEpoch 243/500\n31/31 [==============================] - 1s 17ms/step - loss: 4.1063 - mae: 4.5803\nEpoch 244/500\n31/31 [==============================] - 1s 17ms/step - loss: 4.0558 - mae: 4.5283\nEpoch 245/500\n31/31 [==============================] - 1s 17ms/step - loss: 4.0461 - mae: 4.5187\nEpoch 246/500\n31/31 [==============================] - 1s 17ms/step - loss: 4.0614 - mae: 4.5347\nEpoch 247/500\n31/31 [==============================] - 1s 18ms/step - loss: 4.0499 - mae: 4.5228\nEpoch 248/500\n31/31 [==============================] - 1s 17ms/step - loss: 4.0336 - mae: 4.5061\nEpoch 249/500\n31/31 [==============================] - 1s 18ms/step - loss: 4.0433 - mae: 4.5158\nEpoch 250/500\n31/31 [==============================] - 1s 18ms/step - loss: 4.0965 - mae: 4.5703\nEpoch 251/500\n31/31 [==============================] - 1s 17ms/step - loss: 4.0898 - mae: 4.5635\nEpoch 252/500\n31/31 [==============================] - 1s 17ms/step - loss: 4.0431 - mae: 4.5160\nEpoch 253/500\n31/31 [==============================] - 1s 17ms/step - loss: 4.0376 - mae: 4.5102\nEpoch 254/500\n31/31 [==============================] - 1s 18ms/step - loss: 4.0289 - mae: 4.5017\nEpoch 255/500\n31/31 [==============================] - 1s 17ms/step - loss: 4.1242 - mae: 4.5986\nEpoch 256/500\n31/31 [==============================] - 1s 18ms/step - loss: 4.1310 - mae: 4.6046\nEpoch 257/500\n31/31 [==============================] - 1s 17ms/step - loss: 4.1470 - mae: 4.6207\nEpoch 258/500\n31/31 [==============================] - 1s 17ms/step - loss: 4.0667 - mae: 4.5394\nEpoch 259/500\n31/31 [==============================] - 1s 17ms/step - loss: 4.0347 - mae: 4.5079\nEpoch 260/500\n31/31 [==============================] - 1s 18ms/step - loss: 4.0884 - mae: 4.5612\nEpoch 261/500\n31/31 [==============================] - 1s 17ms/step - loss: 4.1398 - mae: 4.6139\nEpoch 262/500\n31/31 [==============================] - 1s 17ms/step - loss: 4.0773 - mae: 4.5509\nEpoch 263/500\n31/31 [==============================] - 1s 17ms/step - loss: 4.0825 - mae: 4.5560\nEpoch 264/500\n31/31 [==============================] - 1s 17ms/step - loss: 4.0590 - mae: 4.5326\nEpoch 265/500\n31/31 [==============================] - 1s 17ms/step - loss: 4.0964 - mae: 4.5696\nEpoch 266/500\n31/31 [==============================] - 1s 18ms/step - loss: 4.0893 - mae: 4.5633\nEpoch 267/500\n31/31 [==============================] - 1s 17ms/step - loss: 4.1185 - mae: 4.5932\nEpoch 268/500\n31/31 [==============================] - 1s 17ms/step - loss: 4.0686 - mae: 4.5425\nEpoch 269/500\n31/31 [==============================] - 1s 18ms/step - loss: 4.0088 - mae: 4.4808\nEpoch 270/500\n31/31 [==============================] - 1s 17ms/step - loss: 4.0459 - mae: 4.5192\nEpoch 271/500\n31/31 [==============================] - 1s 17ms/step - loss: 4.0033 - mae: 4.4753\nEpoch 272/500\n31/31 [==============================] - 1s 17ms/step - loss: 4.0092 - mae: 4.4812\nEpoch 273/500\n31/31 [==============================] - 1s 17ms/step - loss: 4.0390 - mae: 4.5118\nEpoch 274/500\n31/31 [==============================] - 1s 17ms/step - loss: 4.0105 - mae: 4.4830\nEpoch 275/500\n31/31 [==============================] - 1s 17ms/step - loss: 4.0268 - mae: 4.4993\nEpoch 276/500\n31/31 [==============================] - 1s 17ms/step - loss: 4.0879 - mae: 4.5616\nEpoch 277/500\n31/31 [==============================] - 1s 18ms/step - loss: 4.0750 - mae: 4.5476\nEpoch 278/500\n31/31 [==============================] - 1s 18ms/step - loss: 4.0338 - mae: 4.5070\nEpoch 279/500\n31/31 [==============================] - 1s 17ms/step - loss: 4.0377 - mae: 4.5105\nEpoch 280/500\n31/31 [==============================] - 1s 17ms/step - loss: 4.0541 - mae: 4.5277\nEpoch 281/500\n31/31 [==============================] - 1s 17ms/step - loss: 4.0213 - mae: 4.4940\nEpoch 282/500\n31/31 [==============================] - 1s 17ms/step - loss: 4.0325 - mae: 4.5051\nEpoch 283/500\n31/31 [==============================] - 1s 17ms/step - loss: 4.1083 - mae: 4.5815\nEpoch 284/500\n31/31 [==============================] - 1s 17ms/step - loss: 4.0448 - mae: 4.5180\nEpoch 285/500\n31/31 [==============================] - 1s 17ms/step - loss: 4.0672 - mae: 4.5407\nEpoch 286/500\n31/31 [==============================] - 1s 18ms/step - loss: 4.0627 - mae: 4.5368\nEpoch 287/500\n31/31 [==============================] - 1s 18ms/step - loss: 4.0055 - mae: 4.4784\nEpoch 288/500\n31/31 [==============================] - 1s 18ms/step - loss: 4.0259 - mae: 4.4991\nEpoch 289/500\n31/31 [==============================] - 1s 18ms/step - loss: 4.0592 - mae: 4.5319\nEpoch 290/500\n31/31 [==============================] - 1s 18ms/step - loss: 4.1093 - mae: 4.5831\nEpoch 291/500\n31/31 [==============================] - 1s 17ms/step - loss: 4.1137 - mae: 4.5886\nEpoch 292/500\n31/31 [==============================] - 1s 17ms/step - loss: 4.0494 - mae: 4.5224\nEpoch 293/500\n31/31 [==============================] - 1s 18ms/step - loss: 4.0447 - mae: 4.5173\nEpoch 294/500\n31/31 [==============================] - 1s 17ms/step - loss: 4.0563 - mae: 4.5302\nEpoch 295/500\n31/31 [==============================] - 1s 17ms/step - loss: 4.0331 - mae: 4.5060\nEpoch 296/500\n31/31 [==============================] - 1s 18ms/step - loss: 4.1633 - mae: 4.6385\nEpoch 297/500\n31/31 [==============================] - 1s 17ms/step - loss: 4.1048 - mae: 4.5787\nEpoch 298/500\n31/31 [==============================] - 1s 17ms/step - loss: 4.0742 - mae: 4.5484\nEpoch 299/500\n31/31 [==============================] - 1s 18ms/step - loss: 4.2062 - mae: 4.6814\nEpoch 300/500\n31/31 [==============================] - 1s 17ms/step - loss: 4.1141 - mae: 4.5880\nEpoch 301/500\n31/31 [==============================] - 1s 17ms/step - loss: 4.0195 - mae: 4.4925\nEpoch 302/500\n31/31 [==============================] - 1s 17ms/step - loss: 4.0413 - mae: 4.5158\nEpoch 303/500\n31/31 [==============================] - 1s 17ms/step - loss: 4.0142 - mae: 4.4862\nEpoch 304/500\n31/31 [==============================] - 1s 18ms/step - loss: 3.9888 - mae: 4.4607\nEpoch 305/500\n31/31 [==============================] - 1s 17ms/step - loss: 4.0065 - mae: 4.4787\nEpoch 306/500\n31/31 [==============================] - 1s 17ms/step - loss: 3.9820 - mae: 4.4540\nEpoch 307/500\n31/31 [==============================] - 1s 17ms/step - loss: 4.0182 - mae: 4.4907\nEpoch 308/500\n31/31 [==============================] - 1s 18ms/step - loss: 3.9915 - mae: 4.4643\nEpoch 309/500\n31/31 [==============================] - 1s 17ms/step - loss: 3.9878 - mae: 4.4599\nEpoch 310/500\n31/31 [==============================] - 1s 17ms/step - loss: 3.9855 - mae: 4.4579\nEpoch 311/500\n31/31 [==============================] - 1s 17ms/step - loss: 4.1139 - mae: 4.5882\nEpoch 312/500\n31/31 [==============================] - 1s 17ms/step - loss: 4.0765 - mae: 4.5504\nEpoch 313/500\n31/31 [==============================] - 1s 17ms/step - loss: 4.0497 - mae: 4.5223\nEpoch 314/500\n31/31 [==============================] - 1s 18ms/step - loss: 3.9943 - mae: 4.4674\nEpoch 315/500\n31/31 [==============================] - 1s 17ms/step - loss: 3.9922 - mae: 4.4647\nEpoch 316/500\n31/31 [==============================] - 1s 18ms/step - loss: 3.9805 - mae: 4.4520\nEpoch 317/500\n31/31 [==============================] - 1s 17ms/step - loss: 4.0384 - mae: 4.5110\nEpoch 318/500\n31/31 [==============================] - 1s 17ms/step - loss: 4.0343 - mae: 4.5068\nEpoch 319/500\n31/31 [==============================] - 1s 18ms/step - loss: 3.9795 - mae: 4.4513\nEpoch 320/500\n31/31 [==============================] - 1s 18ms/step - loss: 4.0015 - mae: 4.4739\nEpoch 321/500\n31/31 [==============================] - 1s 18ms/step - loss: 4.0888 - mae: 4.5631\nEpoch 322/500\n31/31 [==============================] - 1s 18ms/step - loss: 4.0912 - mae: 4.5650\nEpoch 323/500\n31/31 [==============================] - 1s 18ms/step - loss: 4.0825 - mae: 4.5568\nEpoch 324/500\n31/31 [==============================] - 1s 17ms/step - loss: 3.9830 - mae: 4.4549\nEpoch 325/500\n31/31 [==============================] - 1s 18ms/step - loss: 3.9992 - mae: 4.4714\nEpoch 326/500\n31/31 [==============================] - 1s 18ms/step - loss: 3.9704 - mae: 4.4418\nEpoch 327/500\n31/31 [==============================] - 1s 18ms/step - loss: 3.9917 - mae: 4.4642\nEpoch 328/500\n31/31 [==============================] - 1s 17ms/step - loss: 4.0238 - mae: 4.4971\nEpoch 329/500\n31/31 [==============================] - 1s 18ms/step - loss: 4.0390 - mae: 4.5122\nEpoch 330/500\n31/31 [==============================] - 1s 18ms/step - loss: 3.9753 - mae: 4.4478\nEpoch 331/500\n31/31 [==============================] - 1s 18ms/step - loss: 3.9614 - mae: 4.4340\nEpoch 332/500\n31/31 [==============================] - 1s 17ms/step - loss: 3.9800 - mae: 4.4522\nEpoch 333/500\n31/31 [==============================] - 1s 18ms/step - loss: 3.9739 - mae: 4.4461\nEpoch 334/500\n31/31 [==============================] - 1s 17ms/step - loss: 3.9790 - mae: 4.4501\nEpoch 335/500\n31/31 [==============================] - 1s 17ms/step - loss: 4.0874 - mae: 4.5614\nEpoch 336/500\n31/31 [==============================] - 1s 18ms/step - loss: 4.0188 - mae: 4.4914\nEpoch 337/500\n31/31 [==============================] - 1s 18ms/step - loss: 4.0644 - mae: 4.5383\nEpoch 338/500\n31/31 [==============================] - 1s 18ms/step - loss: 3.9820 - mae: 4.4546\nEpoch 339/500\n31/31 [==============================] - 1s 18ms/step - loss: 3.9606 - mae: 4.4324\nEpoch 340/500\n31/31 [==============================] - 1s 18ms/step - loss: 4.0385 - mae: 4.5115\nEpoch 341/500\n31/31 [==============================] - 1s 18ms/step - loss: 4.0387 - mae: 4.5129\nEpoch 342/500\n31/31 [==============================] - 1s 18ms/step - loss: 4.0044 - mae: 4.4768\nEpoch 343/500\n31/31 [==============================] - 1s 18ms/step - loss: 3.9836 - mae: 4.4561\nEpoch 344/500\n31/31 [==============================] - 1s 18ms/step - loss: 4.0132 - mae: 4.4865\nEpoch 345/500\n31/31 [==============================] - 1s 18ms/step - loss: 4.0031 - mae: 4.4762\nEpoch 346/500\n31/31 [==============================] - 1s 18ms/step - loss: 3.9695 - mae: 4.4412\nEpoch 347/500\n31/31 [==============================] - 1s 18ms/step - loss: 3.9887 - mae: 4.4602\nEpoch 348/500\n31/31 [==============================] - 1s 18ms/step - loss: 4.0083 - mae: 4.4813\nEpoch 349/500\n31/31 [==============================] - 1s 17ms/step - loss: 4.0540 - mae: 4.5282\nEpoch 350/500\n31/31 [==============================] - 1s 18ms/step - loss: 3.9682 - mae: 4.4406\nEpoch 351/500\n31/31 [==============================] - 1s 18ms/step - loss: 3.9823 - mae: 4.4552\nEpoch 352/500\n31/31 [==============================] - 1s 18ms/step - loss: 3.9985 - mae: 4.4710\nEpoch 353/500\n31/31 [==============================] - 1s 17ms/step - loss: 3.9872 - mae: 4.4603\nEpoch 354/500\n31/31 [==============================] - 1s 18ms/step - loss: 3.9514 - mae: 4.4234\nEpoch 355/500\n31/31 [==============================] - 1s 17ms/step - loss: 3.9753 - mae: 4.4480\nEpoch 356/500\n31/31 [==============================] - 1s 18ms/step - loss: 3.9707 - mae: 4.4429\nEpoch 357/500\n31/31 [==============================] - 1s 18ms/step - loss: 4.0264 - mae: 4.4997\nEpoch 358/500\n31/31 [==============================] - 1s 17ms/step - loss: 3.9961 - mae: 4.4690\nEpoch 359/500\n31/31 [==============================] - 1s 17ms/step - loss: 3.9649 - mae: 4.4377\nEpoch 360/500\n31/31 [==============================] - 1s 17ms/step - loss: 3.9490 - mae: 4.4207\nEpoch 361/500\n31/31 [==============================] - 1s 17ms/step - loss: 3.9861 - mae: 4.4582\nEpoch 362/500\n31/31 [==============================] - 1s 17ms/step - loss: 4.0901 - mae: 4.5638\nEpoch 363/500\n31/31 [==============================] - 1s 18ms/step - loss: 4.0130 - mae: 4.4860\nEpoch 364/500\n31/31 [==============================] - 1s 18ms/step - loss: 4.0036 - mae: 4.4766\nEpoch 365/500\n31/31 [==============================] - 1s 17ms/step - loss: 4.0381 - mae: 4.5116\nEpoch 366/500\n31/31 [==============================] - 1s 17ms/step - loss: 4.0459 - mae: 4.5196\nEpoch 367/500\n31/31 [==============================] - 1s 18ms/step - loss: 3.9874 - mae: 4.4596\nEpoch 368/500\n31/31 [==============================] - 1s 18ms/step - loss: 3.9789 - mae: 4.4516\nEpoch 369/500\n31/31 [==============================] - 1s 18ms/step - loss: 4.0141 - mae: 4.4876\nEpoch 370/500\n31/31 [==============================] - 1s 18ms/step - loss: 3.9451 - mae: 4.4173\nEpoch 371/500\n31/31 [==============================] - 1s 17ms/step - loss: 4.0267 - mae: 4.5002\nEpoch 372/500\n31/31 [==============================] - 1s 18ms/step - loss: 4.0030 - mae: 4.4757\nEpoch 373/500\n31/31 [==============================] - 1s 17ms/step - loss: 3.9497 - mae: 4.4217\nEpoch 374/500\n31/31 [==============================] - 1s 18ms/step - loss: 3.9696 - mae: 4.4414\nEpoch 375/500\n31/31 [==============================] - 1s 18ms/step - loss: 3.9427 - mae: 4.4138\nEpoch 376/500\n31/31 [==============================] - 1s 18ms/step - loss: 3.9445 - mae: 4.4167\nEpoch 377/500\n31/31 [==============================] - 1s 18ms/step - loss: 3.9822 - mae: 4.4553\nEpoch 378/500\n31/31 [==============================] - 1s 18ms/step - loss: 3.9655 - mae: 4.4374\nEpoch 379/500\n31/31 [==============================] - 1s 18ms/step - loss: 3.9578 - mae: 4.4296\nEpoch 380/500\n31/31 [==============================] - 1s 17ms/step - loss: 3.9719 - mae: 4.4443\nEpoch 381/500\n31/31 [==============================] - 1s 18ms/step - loss: 3.9896 - mae: 4.4622\nEpoch 382/500\n31/31 [==============================] - 1s 18ms/step - loss: 3.9716 - mae: 4.4438\nEpoch 383/500\n31/31 [==============================] - 1s 18ms/step - loss: 3.9712 - mae: 4.4435\nEpoch 384/500\n31/31 [==============================] - 1s 18ms/step - loss: 3.9550 - mae: 4.4266\nEpoch 385/500\n31/31 [==============================] - 1s 18ms/step - loss: 3.9527 - mae: 4.4244\nEpoch 386/500\n31/31 [==============================] - 1s 18ms/step - loss: 3.9366 - mae: 4.4082\nEpoch 387/500\n31/31 [==============================] - 1s 18ms/step - loss: 4.0084 - mae: 4.4814\nEpoch 388/500\n31/31 [==============================] - 1s 18ms/step - loss: 3.9709 - mae: 4.4437\nEpoch 389/500\n31/31 [==============================] - 1s 18ms/step - loss: 3.9856 - mae: 4.4577\nEpoch 390/500\n31/31 [==============================] - 1s 18ms/step - loss: 4.0217 - mae: 4.4947\nEpoch 391/500\n31/31 [==============================] - 1s 18ms/step - loss: 3.9714 - mae: 4.4434\nEpoch 392/500\n31/31 [==============================] - 1s 18ms/step - loss: 3.9639 - mae: 4.4360\nEpoch 393/500\n31/31 [==============================] - 1s 18ms/step - loss: 3.9747 - mae: 4.4473\nEpoch 394/500\n31/31 [==============================] - 1s 18ms/step - loss: 4.0125 - mae: 4.4852\nEpoch 395/500\n31/31 [==============================] - 1s 18ms/step - loss: 3.9535 - mae: 4.4249\nEpoch 396/500\n31/31 [==============================] - 1s 18ms/step - loss: 4.0907 - mae: 4.5653\nEpoch 397/500\n31/31 [==============================] - 1s 18ms/step - loss: 3.9837 - mae: 4.4563\nEpoch 398/500\n31/31 [==============================] - 1s 18ms/step - loss: 4.1708 - mae: 4.6452\nEpoch 399/500\n31/31 [==============================] - 1s 18ms/step - loss: 3.9770 - mae: 4.4489\nEpoch 400/500\n31/31 [==============================] - 1s 18ms/step - loss: 4.0197 - mae: 4.4927\nEpoch 401/500\n31/31 [==============================] - 1s 19ms/step - loss: 3.9949 - mae: 4.4676\nEpoch 402/500\n31/31 [==============================] - 1s 17ms/step - loss: 3.9822 - mae: 4.4556\nEpoch 403/500\n31/31 [==============================] - 1s 18ms/step - loss: 3.9819 - mae: 4.4546\nEpoch 404/500\n31/31 [==============================] - 1s 17ms/step - loss: 3.9565 - mae: 4.4284\nEpoch 405/500\n31/31 [==============================] - 1s 18ms/step - loss: 4.0755 - mae: 4.5493\nEpoch 406/500\n31/31 [==============================] - 1s 18ms/step - loss: 3.9904 - mae: 4.4642\nEpoch 407/500\n31/31 [==============================] - 1s 18ms/step - loss: 3.9435 - mae: 4.4154\nEpoch 408/500\n31/31 [==============================] - 1s 17ms/step - loss: 3.9561 - mae: 4.4280\nEpoch 409/500\n31/31 [==============================] - 1s 18ms/step - loss: 3.9673 - mae: 4.4394\nEpoch 410/500\n31/31 [==============================] - 1s 18ms/step - loss: 4.0533 - mae: 4.5271\nEpoch 411/500\n31/31 [==============================] - 1s 18ms/step - loss: 3.9292 - mae: 4.4007\nEpoch 412/500\n31/31 [==============================] - 1s 18ms/step - loss: 3.9370 - mae: 4.4094\nEpoch 413/500\n31/31 [==============================] - 1s 18ms/step - loss: 3.9438 - mae: 4.4154\nEpoch 414/500\n31/31 [==============================] - 1s 18ms/step - loss: 3.9687 - mae: 4.4408\nEpoch 415/500\n31/31 [==============================] - 1s 18ms/step - loss: 3.9450 - mae: 4.4168\nEpoch 416/500\n31/31 [==============================] - 1s 18ms/step - loss: 3.9394 - mae: 4.4110\nEpoch 417/500\n31/31 [==============================] - 1s 18ms/step - loss: 4.0320 - mae: 4.5054\nEpoch 418/500\n31/31 [==============================] - 1s 18ms/step - loss: 4.0215 - mae: 4.4944\nEpoch 419/500\n31/31 [==============================] - 1s 18ms/step - loss: 3.9382 - mae: 4.4105\nEpoch 420/500\n31/31 [==============================] - 1s 18ms/step - loss: 3.9463 - mae: 4.4180\nEpoch 421/500\n31/31 [==============================] - 1s 18ms/step - loss: 3.9267 - mae: 4.3977\nEpoch 422/500\n31/31 [==============================] - 1s 18ms/step - loss: 3.9311 - mae: 4.4027\nEpoch 423/500\n31/31 [==============================] - 1s 18ms/step - loss: 3.9270 - mae: 4.3980\nEpoch 424/500\n31/31 [==============================] - 1s 18ms/step - loss: 3.9330 - mae: 4.4049\nEpoch 425/500\n31/31 [==============================] - 1s 18ms/step - loss: 3.9569 - mae: 4.4293\nEpoch 426/500\n31/31 [==============================] - 1s 18ms/step - loss: 3.9457 - mae: 4.4175\nEpoch 427/500\n31/31 [==============================] - 1s 18ms/step - loss: 3.9746 - mae: 4.4471\nEpoch 428/500\n31/31 [==============================] - 1s 18ms/step - loss: 3.9477 - mae: 4.4194\nEpoch 429/500\n31/31 [==============================] - 1s 18ms/step - loss: 3.9296 - mae: 4.4011\nEpoch 430/500\n31/31 [==============================] - 1s 18ms/step - loss: 4.0002 - mae: 4.4728\nEpoch 431/500\n31/31 [==============================] - 1s 18ms/step - loss: 3.9383 - mae: 4.4103\nEpoch 432/500\n31/31 [==============================] - 1s 18ms/step - loss: 3.9333 - mae: 4.4049\nEpoch 433/500\n31/31 [==============================] - 1s 18ms/step - loss: 3.9395 - mae: 4.4107\nEpoch 434/500\n31/31 [==============================] - 1s 18ms/step - loss: 3.9541 - mae: 4.4256\nEpoch 435/500\n31/31 [==============================] - 1s 18ms/step - loss: 4.0317 - mae: 4.5046\nEpoch 436/500\n31/31 [==============================] - 1s 18ms/step - loss: 3.9338 - mae: 4.4053\nEpoch 437/500\n31/31 [==============================] - 1s 18ms/step - loss: 4.0508 - mae: 4.5254\nEpoch 438/500\n31/31 [==============================] - 1s 18ms/step - loss: 3.9907 - mae: 4.4628\nEpoch 439/500\n31/31 [==============================] - 1s 18ms/step - loss: 3.9787 - mae: 4.4511\nEpoch 440/500\n31/31 [==============================] - 1s 18ms/step - loss: 4.0298 - mae: 4.5026\nEpoch 441/500\n31/31 [==============================] - 1s 19ms/step - loss: 3.9849 - mae: 4.4575\nEpoch 442/500\n31/31 [==============================] - 1s 18ms/step - loss: 3.9680 - mae: 4.4410\nEpoch 443/500\n31/31 [==============================] - 1s 18ms/step - loss: 3.9610 - mae: 4.4335\nEpoch 444/500\n31/31 [==============================] - 1s 18ms/step - loss: 3.9891 - mae: 4.4620\nEpoch 445/500\n31/31 [==============================] - 1s 18ms/step - loss: 3.9160 - mae: 4.3870\nEpoch 446/500\n31/31 [==============================] - 1s 18ms/step - loss: 3.9340 - mae: 4.4056\nEpoch 447/500\n31/31 [==============================] - 1s 18ms/step - loss: 3.9336 - mae: 4.4056\nEpoch 448/500\n31/31 [==============================] - 1s 18ms/step - loss: 3.9826 - mae: 4.4552\nEpoch 449/500\n31/31 [==============================] - 1s 18ms/step - loss: 4.0334 - mae: 4.5063\nEpoch 450/500\n31/31 [==============================] - 1s 18ms/step - loss: 3.9566 - mae: 4.4288\nEpoch 451/500\n31/31 [==============================] - 1s 18ms/step - loss: 3.9345 - mae: 4.4059\nEpoch 452/500\n31/31 [==============================] - 1s 18ms/step - loss: 3.9131 - mae: 4.3844\nEpoch 453/500\n31/31 [==============================] - 1s 18ms/step - loss: 3.9263 - mae: 4.3976\nEpoch 454/500\n31/31 [==============================] - 1s 18ms/step - loss: 4.0225 - mae: 4.4957\nEpoch 455/500\n31/31 [==============================] - 1s 18ms/step - loss: 3.9243 - mae: 4.3954\nEpoch 456/500\n31/31 [==============================] - 1s 18ms/step - loss: 3.9108 - mae: 4.3814\nEpoch 457/500\n31/31 [==============================] - 1s 19ms/step - loss: 3.9141 - mae: 4.3853\nEpoch 458/500\n31/31 [==============================] - 1s 19ms/step - loss: 3.9405 - mae: 4.4122\nEpoch 459/500\n31/31 [==============================] - 1s 18ms/step - loss: 3.9756 - mae: 4.4480\nEpoch 460/500\n31/31 [==============================] - 1s 18ms/step - loss: 3.9513 - mae: 4.4220\nEpoch 461/500\n31/31 [==============================] - 1s 19ms/step - loss: 3.9710 - mae: 4.4436\nEpoch 462/500\n31/31 [==============================] - 1s 18ms/step - loss: 3.9282 - mae: 4.3998\nEpoch 463/500\n31/31 [==============================] - 1s 18ms/step - loss: 3.9297 - mae: 4.4012\nEpoch 464/500\n31/31 [==============================] - 1s 18ms/step - loss: 3.9267 - mae: 4.3977\nEpoch 465/500\n31/31 [==============================] - 1s 18ms/step - loss: 3.9565 - mae: 4.4288\nEpoch 466/500\n31/31 [==============================] - 1s 18ms/step - loss: 3.9587 - mae: 4.4304\nEpoch 467/500\n31/31 [==============================] - 1s 19ms/step - loss: 3.9408 - mae: 4.4131\nEpoch 468/500\n31/31 [==============================] - 1s 18ms/step - loss: 3.9225 - mae: 4.3939\nEpoch 469/500\n31/31 [==============================] - 1s 18ms/step - loss: 3.9172 - mae: 4.3893\nEpoch 470/500\n31/31 [==============================] - 1s 18ms/step - loss: 3.9596 - mae: 4.4321\nEpoch 471/500\n31/31 [==============================] - 1s 18ms/step - loss: 3.9748 - mae: 4.4470\nEpoch 472/500\n31/31 [==============================] - 1s 18ms/step - loss: 3.9533 - mae: 4.4251\nEpoch 473/500\n31/31 [==============================] - 1s 18ms/step - loss: 3.9126 - mae: 4.3841\nEpoch 474/500\n31/31 [==============================] - 1s 18ms/step - loss: 3.9419 - mae: 4.4140\nEpoch 475/500\n31/31 [==============================] - 1s 19ms/step - loss: 3.9511 - mae: 4.4231\nEpoch 476/500\n31/31 [==============================] - 1s 18ms/step - loss: 3.9539 - mae: 4.4251\nEpoch 477/500\n31/31 [==============================] - 1s 18ms/step - loss: 3.9757 - mae: 4.4487\nEpoch 478/500\n31/31 [==============================] - 1s 18ms/step - loss: 3.9368 - mae: 4.4084\nEpoch 479/500\n31/31 [==============================] - 1s 18ms/step - loss: 3.9969 - mae: 4.4695\nEpoch 480/500\n31/31 [==============================] - 1s 19ms/step - loss: 3.9865 - mae: 4.4588\nEpoch 481/500\n31/31 [==============================] - 1s 19ms/step - loss: 3.9963 - mae: 4.4687\nEpoch 482/500\n31/31 [==============================] - 1s 19ms/step - loss: 3.9440 - mae: 4.4150\nEpoch 483/500\n31/31 [==============================] - 1s 18ms/step - loss: 3.9368 - mae: 4.4078\nEpoch 484/500\n31/31 [==============================] - 1s 18ms/step - loss: 3.9241 - mae: 4.3952\nEpoch 485/500\n31/31 [==============================] - 1s 18ms/step - loss: 3.9485 - mae: 4.4211\nEpoch 486/500\n31/31 [==============================] - 1s 19ms/step - loss: 4.0004 - mae: 4.4729\nEpoch 487/500\n31/31 [==============================] - 1s 18ms/step - loss: 3.9479 - mae: 4.4194\nEpoch 488/500\n31/31 [==============================] - 1s 19ms/step - loss: 3.9322 - mae: 4.4038\nEpoch 489/500\n31/31 [==============================] - 1s 18ms/step - loss: 3.9969 - mae: 4.4701\nEpoch 490/500\n31/31 [==============================] - 1s 19ms/step - loss: 3.9317 - mae: 4.4028\nEpoch 491/500\n31/31 [==============================] - 1s 19ms/step - loss: 3.9075 - mae: 4.3781\nEpoch 492/500\n31/31 [==============================] - 1s 18ms/step - loss: 3.9379 - mae: 4.4093\nEpoch 493/500\n31/31 [==============================] - 1s 18ms/step - loss: 3.9110 - mae: 4.3827\nEpoch 494/500\n31/31 [==============================] - 1s 18ms/step - loss: 4.0001 - mae: 4.4732\nEpoch 495/500\n31/31 [==============================] - 1s 18ms/step - loss: 3.9891 - mae: 4.4608\nEpoch 496/500\n31/31 [==============================] - 1s 18ms/step - loss: 3.9279 - mae: 4.3992\nEpoch 497/500\n31/31 [==============================] - 1s 18ms/step - loss: 3.9355 - mae: 4.4072\nEpoch 498/500\n31/31 [==============================] - 1s 18ms/step - loss: 3.9500 - mae: 4.4215\nEpoch 499/500\n31/31 [==============================] - 1s 18ms/step - loss: 3.9207 - mae: 4.3924\nEpoch 500/500\n31/31 [==============================] - 1s 18ms/step - loss: 3.9123 - mae: 4.3831\n"
],
[
"rnn_forecast = model_forecast(model, series[..., np.newaxis], window_size)\nrnn_forecast = rnn_forecast[split_time - window_size:-1, -1, 0]",
"_____no_output_____"
],
[
"plt.figure(figsize=(10, 6))\nplot_series(time_valid, x_valid)\nplot_series(time_valid, rnn_forecast)",
"_____no_output_____"
],
[
"tf.keras.metrics.mean_absolute_error(x_valid, rnn_forecast).numpy()",
"_____no_output_____"
],
[
"import matplotlib.image as mpimg\nimport matplotlib.pyplot as plt\n\n#-----------------------------------------------------------\n# Retrieve a list of list results on training and test data\n# sets for each training epoch\n#-----------------------------------------------------------\nmae=history.history['mae']\nloss=history.history['loss']\n\nepochs=range(len(loss)) # Get number of epochs\n\n#------------------------------------------------\n# Plot MAE and Loss\n#------------------------------------------------\nplt.plot(epochs, mae, 'r')\nplt.plot(epochs, loss, 'b')\nplt.title('MAE and Loss')\nplt.xlabel(\"Epochs\")\nplt.ylabel(\"Accuracy\")\nplt.legend([\"MAE\", \"Loss\"])\n\nplt.figure()\n\nepochs_zoom = epochs[200:]\nmae_zoom = mae[200:]\nloss_zoom = loss[200:]\n\n#------------------------------------------------\n# Plot Zoomed MAE and Loss\n#------------------------------------------------\nplt.plot(epochs_zoom, mae_zoom, 'r')\nplt.plot(epochs_zoom, loss_zoom, 'b')\nplt.title('MAE and Loss')\nplt.xlabel(\"Epochs\")\nplt.ylabel(\"Accuracy\")\nplt.legend([\"MAE\", \"Loss\"])\n\nplt.figure()",
"_____no_output_____"
]
]
] | [
"markdown",
"code",
"markdown",
"code"
] | [
[
"markdown"
],
[
"code"
],
[
"markdown"
],
[
"code",
"code",
"code",
"code",
"code",
"code",
"code",
"code",
"code",
"code",
"code",
"code"
]
] |
d076cab399d9fbf6deab0130e9c2bce56aabe838 | 314,559 | ipynb | Jupyter Notebook | examples/MIDASExamples.ipynb | QAQ-Ahuahuahuahua/midaspy-1 | 444a9a0f299b56f1f88f08a3a61a2e1400d9e99a | [
"MIT"
] | 23 | 2017-07-23T13:13:12.000Z | 2022-02-21T08:28:11.000Z | examples/MIDASExamples.ipynb | mikemull/pymidas | 444a9a0f299b56f1f88f08a3a61a2e1400d9e99a | [
"MIT"
] | 5 | 2017-08-19T14:41:51.000Z | 2019-08-06T14:51:41.000Z | examples/MIDASExamples.ipynb | mikemull/pymidas | 444a9a0f299b56f1f88f08a3a61a2e1400d9e99a | [
"MIT"
] | 13 | 2018-04-12T13:02:55.000Z | 2021-05-30T11:00:34.000Z | 208.317219 | 49,988 | 0.878891 | [
[
[
"# MIDAS Examples\n\nIf you're reading this you probably already know that MIDAS stands for Mixed Data Sampling, and it is a technique for creating time-series forecast models that allows you to mix series of different frequencies (ie, you can use monthly data as predictors for a quarterly series, or daily data as predictors for a monthly series, etc.). The general approach has been described in a series of papers by Ghysels, Santa-Clara, Valkanov and others.\n\nThis notebook attempts to recreate some of the examples from the paper [_Forecasting with Mixed Frequencies_](https://research.stlouisfed.org/publications/review/2010/11/01/forecasting-with-mixed-frequencies/) by Michelle T. Armesto, Kristie M. Engemann, and Michael T. Owyang.",
"_____no_output_____"
]
],
[
[
"%matplotlib inline\n\nimport datetime\nimport numpy as np\nimport pandas as pd\n\nfrom midas.mix import mix_freq\nfrom midas.adl import estimate, forecast, midas_adl, rmse",
"_____no_output_____"
]
],
[
[
"# MIDAS ADL\n\nThis package currently implements the MIDAS ADL (autoregressive distributed lag) method. We'll start with an example using quarterly GDP and monthly payroll data. We'll then show the basic steps in setting up and fitting this type of model, although in practice you'll probably used the top-level __midas_adl__ function to do forecasts.\n\nTODO: MIDAS equation and discussion\n\n\n# Example 1: GDP vs Non-Farm Payroll",
"_____no_output_____"
]
],
[
[
"gdp = pd.read_csv('../tests/data/gdp.csv', parse_dates=['DATE'], index_col='DATE')\npay = pd.read_csv('../tests/data/pay.csv', parse_dates=['DATE'], index_col='DATE')\ngdp.tail()",
"_____no_output_____"
],
[
"pay.tail()",
"_____no_output_____"
]
],
[
[
"## Figure 1\n\nThis is a variation of Figure 1 from the paper comparing year-over-year growth of GDP and employment.",
"_____no_output_____"
]
],
[
[
"gdp_yoy = ((1. + (np.log(gdp.GDP) - np.log(gdp.GDP.shift(3)))) ** 4) - 1.\nemp_yoy = ((1. + (np.log(pay.PAY) - np.log(pay.PAY.shift(1)))) ** 12) - 1.\ndf = pd.concat([gdp_yoy, emp_yoy], axis=1)\ndf.columns = ['gdp_yoy', 'emp_yoy']\ndf[['gdp_yoy','emp_yoy']].loc['1980-1-1':].plot(figsize=(15,4), style=['o','-'])",
"_____no_output_____"
]
],
[
[
"## Mixing Frequencies\n\nThe first step is to do the actual frequency mixing. In this case we're mixing monthly data (employment) with quarterly data (GDP). This may sometimes be useful to do directly, but again you'll probably used __midas_adl__ to do forecasting.",
"_____no_output_____"
]
],
[
[
"gdp['gdp_growth'] = (np.log(gdp.GDP) - np.log(gdp.GDP.shift(1))) * 100.\npay['emp_growth'] = (np.log(pay.PAY) - np.log(pay.PAY.shift(1))) * 100.\n\ny, yl, x, yf, ylf, xf = mix_freq(gdp.gdp_growth, pay.emp_growth, \"3m\", 1, 3,\n start_date=datetime.datetime(1985,1,1),\n end_date=datetime.datetime(2009,1,1))\nx.head()",
"_____no_output_____"
]
],
[
[
"The arguments here are as follows:\n- First, the dependent (low frequency) and independent (high-frequency) data are given as Pandas series, and they are assumed to be indexed by date.\n- xlag The number of lags for the high-frequency variable\n- ylag The number of lags for the low-frequency variable (the autoregressive part)\n- horizon: How much the high-frequency data is lagged before frequency mixing\n- start_date, end_date: The start and end date over which the model is fitted. If these are outside the range of the low-frequency data, they will be adjusted\n\n\nThe _horizon_ argument is a little tricky (the argument name was retained from the MatLab version). This is used both the align the data and to do _nowcasting_ (more on that later). For example, if it's September 2017 then the latest GDP data from FRED will be for Q2 and this will be dated 2017-04-01. The latest monthly data from non-farm payroll will be for August, which will be dated 2017-08-01. If we aligned just on dates, the payroll data for April (04-01), March (03-01), and February(02-01) would be aligned with Q2 (since xlag = \"3m\"), but what we want is June, May, and April, so here the horizon argument is 3 indicating that the high-frequency data should be lagged three months before being mixed with the quarterly data.",
"_____no_output_____"
],
[
"### Fitting the Model\n\nBecause of the form of the MIDAS model, fitting the model requires using non-linear least squares. For now, if you call the __estimate__ function directly, you'll get back a results of type scipy.optimize.optimize.OptimizeResult\n",
"_____no_output_____"
]
],
[
[
"res = estimate(y, yl, x, poly='beta')\nres.x",
"_____no_output_____"
]
],
[
[
"You can also call __forecast__ directly. This will use the optimization results returned from __eatimate__ to produce a forecast for every date in the index of the forecast inputs (here xf and ylf):",
"_____no_output_____"
]
],
[
[
"fc = forecast(xf, ylf, res, poly='beta')\nforecast_df = fc.join(yf)\nforecast_df['gap'] = forecast_df.yfh - forecast_df.gdp_growth\nforecast_df",
"_____no_output_____"
],
[
"gdp.join(fc)[['gdp_growth','yfh']].loc['2005-01-01':].plot(style=['-o','-+'], figsize=(12, 4))",
"_____no_output_____"
]
],
[
[
"### Comparison against univariate ARIMA model",
"_____no_output_____"
]
],
[
[
"import statsmodels.tsa.api as sm\n\nm = sm.AR(gdp['1975-01-01':'2011-01-01'].gdp_growth,)\nr = m.fit(maxlag=1)\nr.params\nfc_ar = r.predict(start='2005-01-01')\nfc_ar.name = 'xx'",
"_____no_output_____"
],
[
"df_p = gdp.join(fc)[['gdp_growth','yfh']]\ndf_p.join(fc_ar)[['gdp_growth','yfh','xx']].loc['2005-01-01':].plot(style=['-o','-+'], figsize=(12, 4))",
"_____no_output_____"
]
],
[
[
"## The midas_adl function\n\nThe __midas\\_adl__ function wraps up frequency-mixing, fitting, and forecasting into one process. The default mode of forecasting is _fixed_, which means that the data between start_date and end_date will be used to fit the model, and then any data in the input beyond end_date will be used for forecasting. For example, here we're fitting from the beginning of 1985 to the end of 2008, but the gdp data extends to Q1 of 2011 so we get nine forecast points. Three monthly lags of the high-frequency data are specified along with one quarterly lag of GDP.\n",
"_____no_output_____"
]
],
[
[
"rmse_fc, fc = midas_adl(gdp.gdp_growth, pay.emp_growth,\n start_date=datetime.datetime(1985,1,1),\n end_date=datetime.datetime(2009,1,1),\n xlag=\"3m\",\n ylag=1,\n horizon=3)\nrmse_fc",
"_____no_output_____"
]
],
[
[
"You can also change the polynomial used to weight the MIDAS coefficients. The default is 'beta', but you can also specify exponential Almom weighting ('expalmon') or beta with non-zero last term ('betann')",
"_____no_output_____"
]
],
[
[
"rmse_fc, fc = midas_adl(gdp.gdp_growth, pay.emp_growth,\n start_date=datetime.datetime(1985,1,1),\n end_date=datetime.datetime(2009,1,1),\n xlag=\"3m\",\n ylag=1,\n horizon=3,\n poly='expalmon')\nrmse_fc",
"_____no_output_____"
]
],
[
[
"### Rolling and Recursive Forecasting\n\nAs mentioned above the default forecasting method is fixed where the model is fit once and then all data after end_date is used for forecasting. Two other methods are supported _rolling window_ and _recursive_. The _rolling window_ method is just what it sounds like. The start_date and end_date are used for the initial window, and then each new forecast moves that window forward by one period so that you're always doing one step ahead forecasts. Of course, to do anything useful this also assumes that the date range of the dependent data extends beyond end_date accounting for the lags implied by _horizon_. Generally, you'll get lower RMSE values here since the forecasts are always one step ahead.",
"_____no_output_____"
]
],
[
[
"results = {h: midas_adl(gdp.gdp_growth, pay.emp_growth,\n start_date=datetime.datetime(1985,10,1),\n end_date=datetime.datetime(2009,1,1),\n xlag=\"3m\",\n ylag=1,\n horizon=3,\n forecast_horizon=h,\n poly='beta',\n method='rolling') for h in (1, 2, 5)}\nresults[1][0]",
"_____no_output_____"
]
],
[
[
"The _recursive_ method is similar except that the start date does not change, so the range over which the fitting happens increases for each new forecast.",
"_____no_output_____"
]
],
[
[
"results = {h: midas_adl(gdp.gdp_growth, pay.emp_growth,\n start_date=datetime.datetime(1985,10,1),\n end_date=datetime.datetime(2009,1,1),\n xlag=\"3m\",\n ylag=1,\n horizon=3,\n forecast_horizon=h,\n poly='beta',\n method='recursive') for h in (1, 2, 5)}\nresults[1][0]",
"_____no_output_____"
]
],
[
[
"## Nowcasting\n\nPer the manual for the MatLab Matlab Toolbox Version 1.0, you can do _nowcasting_ (or MIDAS with leads) basically by adjusting the _horizon_ parameter. For example, below we change the _horizon_ paremter to 1, we're now forecasting with a one month horizon rather than a one quarter horizon:",
"_____no_output_____"
]
],
[
[
"rmse_fc, fc = midas_adl(gdp.gdp_growth, pay.emp_growth,\n start_date=datetime.datetime(1985,1,1),\n end_date=datetime.datetime(2009,1,1),\n xlag=\"3m\",\n ylag=1,\n horizon=1)\nrmse_fc",
"_____no_output_____"
]
],
[
[
"Not surprisingly the RMSE drops considerably.",
"_____no_output_____"
],
[
"## CPI vs. Federal Funds Rate\n\n__UNDER CONSTRUCTION: Note that these models take considerably longer to fit__",
"_____no_output_____"
]
],
[
[
"cpi = pd.read_csv('CPIAUCSL.csv', parse_dates=['DATE'], index_col='DATE')\nffr = pd.read_csv('DFF_2_Vintages_Starting_2009_09_28.txt', sep='\\t', parse_dates=['observation_date'],\n index_col='observation_date')",
"_____no_output_____"
],
[
"cpi.head()",
"_____no_output_____"
],
[
"ffr.head(10)",
"_____no_output_____"
],
[
"cpi_yoy = ((1. + (np.log(cpi.CPIAUCSL) - np.log(cpi.CPIAUCSL.shift(1)))) ** 12) - 1.\ncpi_yoy.head()\ndf = pd.concat([cpi_yoy, ffr.DFF_20090928 / 100.], axis=1)\ndf.columns = ['cpi_growth', 'dff']\ndf.loc['1980-1-1':'2010-1-1'].plot(figsize=(15,4), style=['-+','-.'])",
"_____no_output_____"
],
[
"cpi_growth = (np.log(cpi.CPIAUCSL) - np.log(cpi.CPIAUCSL.shift(1))) * 100.\n\ny, yl, x, yf, ylf, xf = mix_freq(cpi_growth, ffr.DFF_20090928, \"1m\", 1, 1,\n start_date=datetime.datetime(1975,10,1),\n end_date=datetime.datetime(1991,1,1))",
"_____no_output_____"
],
[
"x.head()",
"_____no_output_____"
],
[
"res = estimate(y, yl, x)",
"_____no_output_____"
],
[
"fc = forecast(xf, ylf, res)\nfc.join(yf).head()",
"_____no_output_____"
],
[
"pd.concat([cpi_growth, fc],axis=1).loc['2008-01-01':'2010-01-01'].plot(style=['-o','-+'], figsize=(12, 4))",
"_____no_output_____"
],
[
"\nresults = {h: midas_adl(cpi_growth, ffr.DFF_20090928,\n start_date=datetime.datetime(1975,7,1),\n end_date=datetime.datetime(1990,11,1),\n xlag=\"1m\",\n ylag=1,\n horizon=1,\n forecast_horizon=h,\n method='rolling') for h in (1, 2, 5)}\n(results[1][0], results[2][0], results[5][0])",
"/Users/mikemull/Documents/Dev/midaspy/midas/weights.py:34: RuntimeWarning: overflow encountered in power\n beta_vals = u ** (self.theta1 - 1) * (1 - u) ** (self.theta2 - 1)\n/Users/mikemull/Documents/Dev/midaspy/midas/weights.py:36: RuntimeWarning: invalid value encountered in true_divide\n beta_vals = beta_vals / sum(beta_vals)\n"
],
[
"results[1][1].plot(figsize=(12,4))",
"_____no_output_____"
],
[
"results = {h: midas_adl(cpi_growth, ffr.DFF_20090928,\n start_date=datetime.datetime(1975,10,1),\n end_date=datetime.datetime(1991,1,1),\n xlag=\"1m\",\n ylag=1,\n horizon=1,\n forecast_horizon=h,\n method='recursive') for h in (1, 2, 5)}\nresults[1][0]",
"/Users/mikemull/Documents/Dev/midaspy/midas/weights.py:34: RuntimeWarning: overflow encountered in power\n beta_vals = u ** (self.theta1 - 1) * (1 - u) ** (self.theta2 - 1)\n/Users/mikemull/Documents/Dev/midaspy/midas/weights.py:36: RuntimeWarning: invalid value encountered in true_divide\n beta_vals = beta_vals / sum(beta_vals)\n"
],
[
"results[1][1].plot()",
"_____no_output_____"
]
]
] | [
"markdown",
"code",
"markdown",
"code",
"markdown",
"code",
"markdown",
"code",
"markdown",
"code",
"markdown",
"code",
"markdown",
"code",
"markdown",
"code",
"markdown",
"code",
"markdown",
"code",
"markdown",
"code",
"markdown",
"code",
"markdown",
"code"
] | [
[
"markdown"
],
[
"code"
],
[
"markdown"
],
[
"code",
"code"
],
[
"markdown"
],
[
"code"
],
[
"markdown"
],
[
"code"
],
[
"markdown",
"markdown"
],
[
"code"
],
[
"markdown"
],
[
"code",
"code"
],
[
"markdown"
],
[
"code",
"code"
],
[
"markdown"
],
[
"code"
],
[
"markdown"
],
[
"code"
],
[
"markdown"
],
[
"code"
],
[
"markdown"
],
[
"code"
],
[
"markdown"
],
[
"code"
],
[
"markdown",
"markdown"
],
[
"code",
"code",
"code",
"code",
"code",
"code",
"code",
"code",
"code",
"code",
"code",
"code",
"code"
]
] |
d076d8370069c8695b75ffe2a53f357d67709a57 | 36,304 | ipynb | Jupyter Notebook | Week1_Intro/gym_interface.ipynb | shih-chi-47/Practical_RL | ac2807d5c4d5f85781971126ba681122885f128c | [
"MIT"
] | 3 | 2021-12-09T11:02:25.000Z | 2022-02-15T16:37:29.000Z | Week1_Intro/gym_interface.ipynb | shih-chi-47/Practical_RL | ac2807d5c4d5f85781971126ba681122885f128c | [
"MIT"
] | null | null | null | Week1_Intro/gym_interface.ipynb | shih-chi-47/Practical_RL | ac2807d5c4d5f85781971126ba681122885f128c | [
"MIT"
] | 4 | 2020-10-28T18:55:08.000Z | 2022-01-17T15:09:55.000Z | 36,304 | 36,304 | 0.895191 | [
[
[
"import sys, os\nif 'google.colab' in sys.modules and not os.path.exists('.setup_complete'):\n !wget -q https://raw.githubusercontent.com/yandexdataschool/Practical_RL/spring20/setup_colab.sh -O- | bash\n\n !wget -q https://raw.githubusercontent.com/yandexdataschool/Practical_RL/coursera/grading.py -O ../grading.py\n !wget -q https://raw.githubusercontent.com/yandexdataschool/Practical_RL/coursera/week1_intro/submit.py\n\n !touch .setup_complete\n\n# This code creates a virtual display to draw game images on.\n# It will have no effect if your machine has a monitor.\nif type(os.environ.get(\"DISPLAY\")) is not str or len(os.environ.get(\"DISPLAY\")) == 0:\n !bash ../xvfb start\n os.environ['DISPLAY'] = ':1'",
"Selecting previously unselected package xvfb.\n(Reading database ... 144429 files and directories currently installed.)\nPreparing to unpack .../xvfb_2%3a1.19.6-1ubuntu4.4_amd64.deb ...\nUnpacking xvfb (2:1.19.6-1ubuntu4.4) ...\nSetting up xvfb (2:1.19.6-1ubuntu4.4) ...\nProcessing triggers for man-db (2.8.3-2ubuntu0.1) ...\nStarting virtual X frame buffer: Xvfb.\n"
],
[
"import numpy as np\nimport matplotlib.pyplot as plt\n%matplotlib inline",
"_____no_output_____"
]
],
[
[
"### OpenAI Gym\n\nWe're gonna spend several next weeks learning algorithms that solve decision processes. We are then in need of some interesting decision problems to test our algorithms.\n\nThat's where OpenAI gym comes into play. It's a python library that wraps many classical decision problems including robot control, videogames and board games.\n\nSo here's how it works:",
"_____no_output_____"
]
],
[
[
"import gym\n\nenv = gym.make(\"MountainCar-v0\")\nenv.reset()\n\nplt.imshow(env.render('rgb_array'))\nprint(\"Observation space:\", env.observation_space)\nprint(\"Action space:\", env.action_space)",
"Observation space: Box(2,)\nAction space: Discrete(3)\n"
]
],
[
[
"Note: if you're running this on your local machine, you'll see a window pop up with the image above. Don't close it, just alt-tab away.",
"_____no_output_____"
],
[
"### Gym interface\n\nThe three main methods of an environment are\n* __reset()__ - reset environment to initial state, _return first observation_\n* __render()__ - show current environment state (a more colorful version :) )\n* __step(a)__ - commit action __a__ and return (new observation, reward, is done, info)\n * _new observation_ - an observation right after commiting the action __a__\n * _reward_ - a number representing your reward for commiting action __a__\n * _is done_ - True if the MDP has just finished, False if still in progress\n * _info_ - some auxilary stuff about what just happened. Ignore it ~~for now~~.",
"_____no_output_____"
]
],
[
[
"obs0 = env.reset()\nprint(\"initial observation code:\", obs0)\n\n# Note: in MountainCar, observation is just two numbers: car position and velocity",
"initial observation code: [-0.51069213 0. ]\n"
],
[
"print(\"taking action 2 (right)\")\nnew_obs, reward, is_done, _ = env.step(2)\n\nprint(\"new observation code:\", new_obs)\nprint(\"reward:\", reward)\nprint(\"is game over?:\", is_done)\n\n# Note: as you can see, the car has moved to the right slightly (around 0.0005)",
"taking action 2 (right)\nnew observation code: [-0.50978891 0.00090322]\nreward: -1.0\nis game over?: False\n"
]
],
[
[
"### Play with it\n\nBelow is the code that drives the car to the right. However, if you simply use the default policy, the car will not reach the flag at the far right due to gravity.\n\n__Your task__ is to fix it. Find a strategy that reaches the flag. \n\nYou are not required to build any sophisticated algorithms for now, feel free to hard-code :)",
"_____no_output_____"
]
],
[
[
"from IPython import display\n\n# Create env manually to set time limit. Please don't change this.\nTIME_LIMIT = 250\nenv = gym.wrappers.TimeLimit(\n gym.envs.classic_control.MountainCarEnv(),\n max_episode_steps=TIME_LIMIT + 1,\n)\nactions = {'left': 0, 'stop': 1, 'right': 2}",
"_____no_output_____"
],
[
"def policy(obs, t):\n # Write the code for your policy here. You can use the observation\n # (a tuple of position and velocity), the current time step, or both,\n # if you want.\n position, velocity = obs\n \n if velocity > 0:\n a = actions['right']\n else:\n a = actions['left']\n\n # This is an example policy. You can try running it, but it will not work.\n # Your goal is to fix that.\n return a",
"_____no_output_____"
],
[
"plt.figure(figsize=(4, 3))\ndisplay.clear_output(wait=True)\n\nobs = env.reset()\nfor t in range(TIME_LIMIT):\n plt.gca().clear()\n \n action = policy(obs, t) # Call your policy\n obs, reward, done, _ = env.step(action) # Pass the action chosen by the policy to the environment\n \n # We don't do anything with reward here because MountainCar is a very simple environment,\n # and reward is a constant -1. Therefore, your goal is to end the episode as quickly as possible.\n\n # Draw game image on display.\n plt.imshow(env.render('rgb_array'))\n \n display.clear_output(wait=True)\n display.display(plt.gcf())\n print(obs)\n\n if done:\n print(\"Well done!\")\n break\nelse:\n print(\"Time limit exceeded. Try again.\")\n\ndisplay.clear_output(wait=True)",
"_____no_output_____"
],
[
"from submit import submit_interface\nsubmit_interface(policy, <EMAIL>, <TOKEN>)",
"_____no_output_____"
]
]
] | [
"code",
"markdown",
"code",
"markdown",
"code",
"markdown",
"code"
] | [
[
"code",
"code"
],
[
"markdown"
],
[
"code"
],
[
"markdown",
"markdown"
],
[
"code",
"code"
],
[
"markdown"
],
[
"code",
"code",
"code",
"code"
]
] |
d076e19c6bb31897345963b7a3096cc40f1ee879 | 15,484 | ipynb | Jupyter Notebook | tutorials/Certification_Trainings/Healthcare/25.Date_Normalizer.ipynb | Rock-ass/spark-nlp-workshop | dbcb1f4c504bd5d0e7ed85310307db0d24a9575e | [
"Apache-2.0"
] | 1 | 2022-02-22T20:52:58.000Z | 2022-02-22T20:52:58.000Z | tutorials/Certification_Trainings/Healthcare/25.Date_Normalizer.ipynb | Tommyhappy01/6-SPARK_NLP | 4081b67c1d7f857ba1853c001293917d30fe9c35 | [
"Apache-2.0"
] | null | null | null | tutorials/Certification_Trainings/Healthcare/25.Date_Normalizer.ipynb | Tommyhappy01/6-SPARK_NLP | 4081b67c1d7f857ba1853c001293917d30fe9c35 | [
"Apache-2.0"
] | null | null | null | 29.891892 | 267 | 0.431219 | [
[
[
"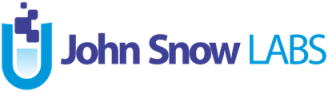",
"_____no_output_____"
],
[
"[](https://colab.research.google.com/github/JohnSnowLabs/spark-nlp-workshop/blob/master/tutorials/Certification_Trainings/Healthcare/25.Date_Normalizer.ipynb)",
"_____no_output_____"
],
[
"## Colab Setup",
"_____no_output_____"
]
],
[
[
"import json\n\nfrom google.colab import files\n\nlicense_keys = files.upload()\n\nwith open(list(license_keys.keys())[0]) as f:\n license_keys = json.load(f)",
"_____no_output_____"
],
[
"license_keys['JSL_VERSION']",
"_____no_output_____"
],
[
"\n%%capture\nfor k,v in license_keys.items(): \n %set_env $k=$v\n\n!wget https://raw.githubusercontent.com/JohnSnowLabs/spark-nlp-workshop/master/jsl_colab_setup.sh\n!bash jsl_colab_setup.sh\n\n",
"_____no_output_____"
],
[
"\nimport json\nimport os\nfrom pyspark.ml import Pipeline, PipelineModel\nfrom pyspark.sql import SparkSession\n\nfrom sparknlp.annotator import *\nfrom sparknlp_jsl.annotator import *\nfrom sparknlp.base import *\nimport sparknlp_jsl\nimport sparknlp\n\nfrom sparknlp.util import *\nfrom sparknlp.pretrained import ResourceDownloader\nfrom pyspark.sql import functions as F\n\nimport pandas as pd\n \n",
"_____no_output_____"
],
[
"spark = sparknlp_jsl.start(license_keys['SECRET'])",
"_____no_output_____"
],
[
"spark",
"_____no_output_____"
]
],
[
[
"# **Date Normalizer**\n\nNew Annotator that transforms chunks Dates to a normalized Date with format YYYY/MM/DD. This annotator identifies dates in chunk annotations and transforms those dates to the format YYYY/MM/DD. \n\n",
"_____no_output_____"
],
[
"We going to create a chunks dates with different formats:",
"_____no_output_____"
]
],
[
[
"dates = [\n'08/02/2018',\n'11/2018',\n'11/01/2018',\n'12Mar2021',\n'Jan 30, 2018',\n'13.04.1999', \n'3April 2020',\n'next monday',\n'today',\n'next week'\n]\n\n",
"_____no_output_____"
],
[
"from pyspark.sql.types import StringType\ndf_dates = spark.createDataFrame(dates,StringType()).toDF('ner_chunk')",
"_____no_output_____"
]
],
[
[
"We going to transform that text to documents in spark-nlp.",
"_____no_output_____"
]
],
[
[
"document_assembler = DocumentAssembler().setInputCol('ner_chunk').setOutputCol('document')\ndocuments_DF = document_assembler.transform(df_dates)",
"_____no_output_____"
]
],
[
[
"After that we going to transform that documents to chunks.",
"_____no_output_____"
]
],
[
[
"from sparknlp.functions import map_annotations_col\n\nchunks_df = map_annotations_col(documents_DF.select(\"document\",\"ner_chunk\"),\n lambda x: [Annotation('chunk', a.begin, a.end, a.result, a.metadata, a.embeddings) for a in x], \"document\",\n \"chunk_date\", \"chunk\")",
"_____no_output_____"
],
[
"chunks_df.select('chunk_date').show(truncate=False)",
"+---------------------------------------------------+\n|chunk_date |\n+---------------------------------------------------+\n|[{chunk, 0, 9, 08/02/2018, {sentence -> 0}, []}] |\n|[{chunk, 0, 6, 11/2018, {sentence -> 0}, []}] |\n|[{chunk, 0, 9, 11/01/2018, {sentence -> 0}, []}] |\n|[{chunk, 0, 8, 12Mar2021, {sentence -> 0}, []}] |\n|[{chunk, 0, 11, Jan 30, 2018, {sentence -> 0}, []}]|\n|[{chunk, 0, 9, 13.04.1999, {sentence -> 0}, []}] |\n|[{chunk, 0, 10, 3April 2020, {sentence -> 0}, []}] |\n|[{chunk, 0, 10, next monday, {sentence -> 0}, []}] |\n|[{chunk, 0, 4, today, {sentence -> 0}, []}] |\n|[{chunk, 0, 8, next week, {sentence -> 0}, []}] |\n+---------------------------------------------------+\n\n"
]
],
[
[
"Now we going to normalize that chunks using the DateNormalizer.",
"_____no_output_____"
]
],
[
[
"date_normalizer = DateNormalizer().setInputCols('chunk_date').setOutputCol('date')\n",
"_____no_output_____"
],
[
"date_normaliced_df = date_normalizer.transform(chunks_df)",
"_____no_output_____"
]
],
[
[
"We going to show how the date is normalized.",
"_____no_output_____"
]
],
[
[
"dateNormalizedClean = date_normaliced_df.selectExpr(\"ner_chunk\",\"date.result as dateresult\",\"date.metadata as metadata\")\n\ndateNormalizedClean.withColumn(\"dateresult\", dateNormalizedClean[\"dateresult\"]\n .getItem(0)).withColumn(\"metadata\", dateNormalizedClean[\"metadata\"]\n .getItem(0)['normalized']).show(truncate=False)",
"+------------+----------+--------+\n|ner_chunk |dateresult|metadata|\n+------------+----------+--------+\n|08/02/2018 |2018/08/02|true |\n|11/2018 |2018/11/DD|true |\n|11/01/2018 |2018/11/01|true |\n|12Mar2021 |2021/03/12|true |\n|Jan 30, 2018|2018/01/30|true |\n|13.04.1999 |1999/04/13|true |\n|3April 2020 |2020/04/03|true |\n|next monday |2021/06/19|true |\n|today |2021/06/13|true |\n|next week |2021/06/20|true |\n+------------+----------+--------+\n\n"
]
],
[
[
"We can configure the `anchorDateYear`,`anchorDateMonth` and `anchorDateDay` for the relatives dates.",
"_____no_output_____"
],
[
"In the following example we will use as a relative date 2021/02/22, to make that possible we need to set up the `anchorDateYear` to 2020, the `anchorDateMonth` to 2 and the `anchorDateDay` to 27. I will show you the configuration with the following example.",
"_____no_output_____"
]
],
[
[
"date_normalizer = DateNormalizer().setInputCols('chunk_date').setOutputCol('date')\\\n .setAnchorDateDay(27)\\\n .setAnchorDateMonth(2)\\\n .setAnchorDateYear(2021)",
"_____no_output_____"
],
[
"date_normaliced_df = date_normalizer.transform(chunks_df)\ndateNormalizedClean = date_normaliced_df.selectExpr(\"ner_chunk\",\"date.result as dateresult\",\"date.metadata as metadata\")\ndateNormalizedClean.withColumn(\"dateresult\", dateNormalizedClean[\"dateresult\"]\n .getItem(0)).withColumn(\"metadata\", dateNormalizedClean[\"metadata\"]\n .getItem(0)['normalized']).show(truncate=False)\n",
"+------------+----------+--------+\n|ner_chunk |dateresult|metadata|\n+------------+----------+--------+\n|08/02/2018 |2018/08/02|true |\n|11/2018 |2018/11/DD|true |\n|11/01/2018 |2018/11/01|true |\n|12Mar2021 |2021/03/12|true |\n|Jan 30, 2018|2018/01/30|true |\n|13.04.1999 |1999/04/13|true |\n|3April 2020 |2020/04/03|true |\n|next monday |2021/02/29|true |\n|today |2021/02/27|true |\n|next week |2021/03/03|true |\n+------------+----------+--------+\n\n"
]
],
[
[
"As you see the relatives dates like `next monday` , `today` and `next week` takes the `2021/02/22` as reference date.\n",
"_____no_output_____"
]
]
] | [
"markdown",
"code",
"markdown",
"code",
"markdown",
"code",
"markdown",
"code",
"markdown",
"code",
"markdown",
"code",
"markdown",
"code",
"markdown"
] | [
[
"markdown",
"markdown",
"markdown"
],
[
"code",
"code",
"code",
"code",
"code",
"code"
],
[
"markdown",
"markdown"
],
[
"code",
"code"
],
[
"markdown"
],
[
"code"
],
[
"markdown"
],
[
"code",
"code"
],
[
"markdown"
],
[
"code",
"code"
],
[
"markdown"
],
[
"code"
],
[
"markdown",
"markdown"
],
[
"code",
"code"
],
[
"markdown"
]
] |
d076e3b04264a788d4c8930be72db1e6fce71219 | 9,354 | ipynb | Jupyter Notebook | look_and_say.ipynb | AllenDowney/CompStats | dc459e04ba74613d533adae2550abefb18da002a | [
"MIT"
] | 226 | 2015-04-06T14:28:01.000Z | 2022-03-22T16:22:43.000Z | look_and_say.ipynb | defining/CompStats | dc459e04ba74613d533adae2550abefb18da002a | [
"MIT"
] | 6 | 2015-04-09T19:31:08.000Z | 2019-01-25T16:52:11.000Z | look_and_say.ipynb | defining/CompStats | dc459e04ba74613d533adae2550abefb18da002a | [
"MIT"
] | 218 | 2015-03-28T02:25:10.000Z | 2022-02-15T06:25:12.000Z | 31.18 | 85 | 0.440988 | [
[
[
"%config InteractiveShell.ast_node_interactivity='last_expr_or_assign'\n\nimport numpy as np",
"_____no_output_____"
],
[
"a = np.array([1,1])",
"_____no_output_____"
],
[
"diff = np.ediff1d(a, 1, 1)",
"_____no_output_____"
],
[
"index = np.nonzero(diff)[0]",
"_____no_output_____"
],
[
"counts = np.ediff1d(index)",
"_____no_output_____"
],
[
"vals = a[index[:-1]]",
"_____no_output_____"
],
[
"# best way to interleave arrays\n# https://stackoverflow.com/questions/5347065/interweaving-two-numpy-arrays\nb = np.empty((vals.size + counts.size,), dtype=vals.dtype)\nb[0::2] = counts\nb[1::2] = vals\nb",
"_____no_output_____"
],
[
"def look_and_say(a):\n diff = np.ediff1d(a, 1, 1)\n index = np.nonzero(diff)[0]\n counts = np.ediff1d(index)\n vals = a[index[:-1]]\n c = np.empty((vals.size + counts.size,), dtype=vals.dtype)\n c[0::2] = counts\n c[1::2] = vals\n return c",
"_____no_output_____"
],
[
"look_and_say(b)",
"_____no_output_____"
],
[
"c = a\nprint(c)\n\nfor i in range(20):\n c = look_and_say(c)\n print(c)",
"[1 1]\n[2 1]\n[1 2 1 1]\n[1 1 1 2 2 1]\n[3 1 2 2 1 1]\n[1 3 1 1 2 2 2 1]\n[1 1 1 3 2 1 3 2 1 1]\n[3 1 1 3 1 2 1 1 1 3 1 2 2 1]\n[1 3 2 1 1 3 1 1 1 2 3 1 1 3 1 1 2 2 1 1]\n[1 1 1 3 1 2 2 1 1 3 3 1 1 2 1 3 2 1 1 3 2 1 2 2 2 1]\n[3 1 1 3 1 1 2 2 2 1 2 3 2 1 1 2 1 1 1 3 1 2 2 1 1 3 1 2 1 1 3 2 1 1]\n[1 3 2 1 1 3 2 1 3 2 1 1 1 2 1 3 1 2 2 1 1 2 3 1 1 3 1 1 2 2 2 1 1 3 1 1 1\n 2 2 1 1 3 1 2 2 1]\n[1 1 1 3 1 2 2 1 1 3 1 2 1 1 1 3 1 2 3 1 1 2 1 1 1 3 1 1 2 2 2 1 1 2 1 3 2\n 1 1 3 2 1 3 2 2 1 1 3 3 1 2 2 2 1 1 3 1 1 2 2 1 1]\n[3 1 1 3 1 1 2 2 2 1 1 3 1 1 1 2 3 1 1 3 1 1 1 2 1 3 2 1 1 2 3 1 1 3 2 1 3\n 2 2 1 1 2 1 1 1 3 1 2 2 1 1 3 1 2 1 1 1 3 2 2 2 1 2 3 1 1 3 2 2 1 1 3 2 1\n 2 2 2 1]\n[1 3 2 1 1 3 2 1 3 2 2 1 1 3 3 1 1 2 1 3 2 1 1 3 3 1 1 2 1 1 1 3 1 2 2 1 1\n 2 1 3 2 1 1 3 1 2 1 1 1 3 2 2 2 1 1 2 3 1 1 3 1 1 2 2 2 1 1 3 1 1 1 2 3 1\n 1 3 3 2 1 1 1 2 1 3 2 1 1 3 2 2 2 1 1 3 1 2 1 1 3 2 1 1]\n[1 1 1 3 1 2 2 1 1 3 1 2 1 1 1 3 2 2 2 1 2 3 2 1 1 2 1 1 1 3 1 2 2 1 2 3 2\n 1 1 2 3 1 1 3 1 1 2 2 2 1 1 2 1 1 1 3 1 2 2 1 1 3 1 1 1 2 3 1 1 3 3 2 2 1\n 1 2 1 3 2 1 1 3 2 1 3 2 2 1 1 3 3 1 1 2 1 3 2 1 2 3 1 2 3 1 1 2 1 1 1 3 1\n 2 2 1 1 3 3 2 2 1 1 3 1 1 1 2 2 1 1 3 1 2 2 1]\n[3 1 1 3 1 1 2 2 2 1 1 3 1 1 1 2 3 1 1 3 3 2 1 1 1 2 1 3 1 2 2 1 1 2 3 1 1\n 3 1 1 2 2 1 1 1 2 1 3 1 2 2 1 1 2 1 3 2 1 1 3 2 1 3 2 2 1 1 2 3 1 1 3 1 1\n 2 2 2 1 1 3 3 1 1 2 1 3 2 1 2 3 2 2 2 1 1 2 1 1 1 3 1 2 2 1 1 3 1 2 1 1 1\n 3 2 2 2 1 2 3 2 1 1 2 1 1 1 3 1 2 1 1 1 2 1 3 1 1 1 2 1 3 2 1 1 2 3 1 1 3\n 1 1 2 2 2 1 2 3 2 2 2 1 1 3 3 1 2 2 2 1 1 3 1 1 2 2 1 1]\n[1 3 2 1 1 3 2 1 3 2 2 1 1 3 3 1 1 2 1 3 2 1 2 3 1 2 3 1 1 2 1 1 1 3 1 1 2\n 2 2 1 1 2 1 3 2 1 1 3 2 1 2 2 3 1 1 2 1 1 1 3 1 1 2 2 2 1 1 2 1 1 1 3 1 2\n 2 1 1 3 1 2 1 1 1 3 2 2 2 1 1 2 1 3 2 1 1 3 2 1 3 2 2 1 2 3 2 1 1 2 1 1 1\n 3 1 2 1 1 1 2 1 3 3 2 2 1 1 2 3 1 1 3 1 1 2 2 2 1 1 3 1 1 1 2 3 1 1 3 3 2\n 1 1 1 2 1 3 1 2 2 1 1 2 3 1 1 3 1 1 1 2 3 1 1 2 1 1 1 3 3 1 1 2 1 1 1 3 1\n 2 2 1 1 2 1 3 2 1 1 3 2 1 3 2 1 1 1 2 1 3 3 2 2 1 2 3 1 1 3 2 2 1 1 3 2 1\n 2 2 2 1]\n[1 1 1 3 1 2 2 1 1 3 1 2 1 1 1 3 2 2 2 1 2 3 2 1 1 2 1 1 1 3 1 2 1 1 1 2 1\n 3 1 1 1 2 1 3 2 1 1 2 3 1 1 3 2 1 3 2 2 1 1 2 1 1 1 3 1 2 2 1 1 3 1 2 1 1\n 2 2 1 3 2 1 1 2 3 1 1 3 2 1 3 2 2 1 1 2 3 1 1 3 1 1 2 2 2 1 1 3 1 1 1 2 3\n 1 1 3 3 2 2 1 1 2 1 1 1 3 1 2 2 1 1 3 1 2 1 1 1 3 2 2 1 1 1 2 1 3 1 2 2 1\n 1 2 3 1 1 3 1 1 1 2 3 1 1 2 1 1 2 3 2 2 2 1 1 2 1 3 2 1 1 3 2 1 3 2 2 1 1\n 3 3 1 1 2 1 3 2 1 2 3 1 2 3 1 1 2 1 1 1 3 1 1 2 2 2 1 1 2 1 3 2 1 1 3 3 1\n 1 2 1 3 2 1 1 2 3 1 2 3 2 1 1 2 3 1 1 3 1 1 2 2 2 1 1 2 1 1 1 3 1 2 2 1 1\n 3 1 2 1 1 1 3 1 2 3 1 1 2 1 1 2 3 2 2 1 1 1 2 1 3 2 1 1 3 2 2 2 1 1 3 1 2\n 1 1 3 2 1 1]\n[3 1 1 3 1 1 2 2 2 1 1 3 1 1 1 2 3 1 1 3 3 2 1 1 1 2 1 3 1 2 2 1 1 2 3 1 1\n 3 1 1 1 2 3 1 1 2 1 1 1 3 3 1 1 2 1 1 1 3 1 2 2 1 1 2 1 3 2 1 1 3 1 2 1 1\n 1 3 2 2 2 1 1 2 3 1 1 3 1 1 2 2 2 1 1 3 1 1 1 2 2 1 2 2 1 1 1 3 1 2 2 1 1\n 2 1 3 2 1 1 3 1 2 1 1 1 3 2 2 2 1 1 2 1 3 2 1 1 3 2 1 3 2 2 1 1 3 3 1 1 2\n 1 3 2 1 2 3 2 2 2 1 1 2 3 1 1 3 1 1 2 2 2 1 1 3 1 1 1 2 3 1 1 3 2 2 3 1 1\n 2 1 1 1 3 1 1 2 2 2 1 1 2 1 3 2 1 1 3 3 1 1 2 1 3 2 1 1 2 2 1 1 2 1 3 3 2\n 2 1 1 2 1 1 1 3 1 2 2 1 1 3 1 2 1 1 1 3 2 2 2 1 2 3 2 1 1 2 1 1 1 3 1 2 1\n 1 1 2 1 3 1 1 1 2 1 3 2 1 1 2 3 1 1 3 2 1 3 2 2 1 1 2 1 1 1 3 1 2 2 1 2 3\n 2 1 1 2 1 1 1 3 1 2 2 1 1 2 1 3 1 1 1 2 1 3 1 2 2 1 1 2 1 3 2 1 1 3 2 1 3\n 2 2 1 1 2 3 1 1 3 1 1 2 2 2 1 1 3 1 1 1 2 3 1 1 3 1 1 1 2 1 3 2 1 1 2 2 1\n 1 2 1 3 2 2 3 1 1 2 1 1 1 3 1 2 2 1 1 3 3 2 2 1 1 3 1 1 1 2 2 1 1 3 1 2 2\n 1]\n[1 3 2 1 1 3 2 1 3 2 2 1 1 3 3 1 1 2 1 3 2 1 2 3 1 2 3 1 1 2 1 1 1 3 1 1 2\n 2 2 1 1 2 1 3 2 1 1 3 3 1 1 2 1 3 2 1 1 2 3 1 2 3 2 1 1 2 3 1 1 3 1 1 2 2\n 2 1 1 2 1 1 1 3 1 2 2 1 1 3 1 1 1 2 3 1 1 3 3 2 2 1 1 2 1 3 2 1 1 3 2 1 3\n 2 2 1 1 3 3 1 2 2 1 1 2 2 3 1 1 3 1 1 2 2 2 1 1 2 1 1 1 3 1 2 2 1 1 3 1 1\n 1 2 3 1 1 3 3 2 2 1 1 2 1 1 1 3 1 2 2 1 1 3 1 2 1 1 1 3 2 2 2 1 2 3 2 1 1\n 2 1 1 1 3 1 2 1 1 1 2 1 3 3 2 2 1 1 2 1 3 2 1 1 3 2 1 3 2 2 1 1 3 3 1 1 2\n 1 3 2 1 1 3 2 2 1 3 2 1 1 2 3 1 1 3 2 1 3 2 2 1 1 2 1 1 1 3 1 2 2 1 2 3 2\n 1 1 2 1 1 1 3 1 2 2 1 2 2 2 1 1 2 1 1 2 3 2 2 2 1 1 2 3 1 1 3 1 1 2 2 2 1\n 1 3 1 1 1 2 3 1 1 3 3 2 1 1 1 2 1 3 1 2 2 1 1 2 3 1 1 3 1 1 1 2 3 1 1 2 1\n 1 1 3 3 1 1 2 1 1 1 3 1 2 2 1 1 2 1 3 2 1 1 3 1 2 1 1 1 3 2 2 2 1 1 2 3 1\n 1 3 1 1 2 2 1 1 1 2 1 3 1 2 2 1 1 2 3 1 1 3 1 1 2 2 2 1 1 2 1 1 1 3 3 1 1\n 2 1 1 1 3 1 1 2 2 2 1 1 2 1 1 1 3 1 2 2 1 1 3 1 2 1 1 1 3 2 2 2 1 1 2 1 3\n 2 1 1 3 2 1 3 2 2 1 1 3 3 1 1 2 1 3 2 1 1 3 3 1 1 2 1 1 1 3 1 2 2 1 2 2 2\n 1 1 2 1 1 1 3 2 2 1 3 2 1 1 2 3 1 1 3 1 1 2 2 2 1 2 3 2 2 2 1 1 3 3 1 2 2\n 2 1 1 3 1 1 2 2 1 1]\n"
]
]
] | [
"code"
] | [
[
"code",
"code",
"code",
"code",
"code",
"code",
"code",
"code",
"code",
"code"
]
] |
d076f21ef44ebb8dbd07c8fb22f0cf2c2cf8b14f | 80,779 | ipynb | Jupyter Notebook | Python_Project1.ipynb | gndede/python | 32340e55ba75c9bea83138647574f656a4c6202f | [
"MIT"
] | null | null | null | Python_Project1.ipynb | gndede/python | 32340e55ba75c9bea83138647574f656a4c6202f | [
"MIT"
] | null | null | null | Python_Project1.ipynb | gndede/python | 32340e55ba75c9bea83138647574f656a4c6202f | [
"MIT"
] | null | null | null | 41.984927 | 1,627 | 0.375927 | [
[
[
"<a href=\"https://colab.research.google.com/github/gndede/python/blob/main/Python_Project1.ipynb\" target=\"_parent\"><img src=\"https://colab.research.google.com/assets/colab-badge.svg\" alt=\"Open In Colab\"/></a>",
"_____no_output_____"
]
],
[
[
"import pandas as pd\n#data = pd.read_csv(filename, encoding= 'unicode_escape')\ndf = pd.read_csv(\"/content/test _123.csv\", encoding= 'unicode_escape')",
"_____no_output_____"
],
[
"df.head()",
"_____no_output_____"
],
[
"#del df[\"planType\"]",
"_____no_output_____"
],
[
"#del df[\"customField4\"]",
"_____no_output_____"
],
[
" #del\tdf[\"customField5\"]",
"_____no_output_____"
],
[
"#del df[\"pdfName\"]\n#del df[\"phoneNumber\"]\n",
"_____no_output_____"
],
[
"#del df[\"dropDate\"]",
"_____no_output_____"
],
[
"#df",
"_____no_output_____"
],
[
"#Group by the coordinatedMailingId\ngk = df.groupby(['coordinatedMailingId','asset1','addressLine1','isPrimary'])",
"_____no_output_____"
],
[
"gk.first()",
"_____no_output_____"
],
[
"#drop ALL duplicate values\n#df.drop_duplicates(subset = \"customerPersonId\", keep = False, inplace = True)",
"_____no_output_____"
],
[
"#drop ALL duplicate values\n#df.drop_duplicates(subset = \"asset1\", keep = False, inplace = True)",
"_____no_output_____"
],
[
"#drop ALL duplicate values\n#df.drop_duplicates(subset = \"asset2\", keep = False, inplace = True)",
"_____no_output_____"
],
[
"#df",
"_____no_output_____"
],
[
"df['fullName'] = df['firstName'].str.cat(df['lastName'],sep=\" \")\n#firstName\tlastName\tfullName",
"_____no_output_____"
],
[
"df",
"_____no_output_____"
],
[
"# Sorting by column \"isPrimary\"\ndf.sort_values(by=['isPrimary'], ascending=False)",
"_____no_output_____"
],
[
"df['phoneNumber']\n\nfor phone_no in df['phoneNumber']: \n contactphone = \"%c-%c%c%c-%c%c%c-%c%c%c%c\" % tuple(map(ord,list(str(phone_no)[:11])))\n\n print(contactphone)",
"1-888-598-7545\n1-855-872-6808\n1-855-872-6808\n1-855-872-6808\n1-855-872-6808\n1-855-872-6808\n1-855-238-0490\n1-855-238-0490\n1-855-832-0978\n1-844-448-7300\n1-844-448-7300\n1-844-596-0467\n1-844-809-5244\n1-844-596-0460\n1-866-715-4673\n1-844-620-5728\n1-866-715-4673\n1-888-812-2190\n1-855-832-0971\n1-855-832-0971\n1-855-233-5513\n1-855-233-5513\n1-855-832-0971\n1-855-832-0971\n1-844-353-0772\n1-844-638-4642\n1-855-832-0976\n1-855-832-0976\n1-855-832-0976\n1-844-596-0460\n1-855-832-0976\n1-855-832-0976\n1-866-202-9691\n1-888-812-2190\n1-844-889-9143\n1-844-889-9143\n1-844-889-9143\n1-844-889-9143\n1-855-832-0976\n1-855-832-0976\n1-855-238-0490\n1-844-596-0467\n1-855-671-6086\n1-855-832-0971\n1-855-832-0971\n1-844-596-0462\n1-855-238-0490\n1-866-202-9574\n1-844-287-9945\n1-888-586-0696\n1-888-586-0696\n1-888-586-0696\n1-855-671-6086\n1-844-596-0460\n1-866-202-9574\n1-855-832-0971\n1-855-832-0971\n1-888-427-8835\n1-888-427-8835\n1-866-202-9731\n1-866-202-9731\n1-866-202-9574\n1-844-596-0462\n1-844-596-0462\n1-866-202-9574\n1-866-202-9574\n1-855-671-6086\n1-855-238-0490\n1-844-809-5244\n1-844-809-5244\n1-844-809-5244\n1-844-809-5244\n1-855-238-0490\n1-844-809-5244\n1-844-809-5244\n1-844-809-5244\n1-844-809-5244\n1-844-809-5244\n1-844-809-5244\n1-844-809-5244\n1-855-832-0971\n1-866-202-9574\n1-855-671-6086\n1-855-232-5748\n1-855-359-7380\n1-855-359-7380\n1-866-202-9574\n1-844-266-1392\n1-844-266-1392\n1-844-266-1392\n1-844-266-1392\n1-855-238-0490\n1-888-427-8835\n1-844-686-0483\n1-855-663-4227\n1-855-241-5717\n1-855-671-6086\n1-866-202-9574\n1-866-202-9574\n1-855-232-5748\n1-866-844-4340\n1-866-844-4340\n1-844-596-0462\n1-844-596-0462\n1-844-596-0462\n1-866-202-9574\n1-855-259-2314\n1-888-651-7308\n1-888-651-7308\n1-888-651-7308\n1-855-832-0978\n1-888-794-1519\n1-855-671-6086\n1-888-651-7308\n1-888-812-2190\n1-855-359-7380\n1-866-451-4616\n1-866-451-4616\n1-866-451-4616\n1-866-451-4616\n1-866-451-4616\n1-866-451-4616\n1-866-451-4616\n1-855-832-0976\n1-855-535-7140\n1-866-202-9574\n1-866-202-9678\n1-855-832-0976\n1-866-202-9574\n1-844-889-9143\n1-855-241-5717\n1-844-287-9945\n1-855-241-5717\n1-866-451-4616\n1-844-596-0460\n1-855-241-5717\n1-855-241-5717\n1-866-202-9574\n1-833-945-1110\n1-866-451-4635\n1-855-671-6086\n1-855-671-6086\n1-855-671-6086\n1-888-429-8490\n1-866-202-9731\n1-855-241-5717\n1-855-241-5717\n1-855-241-5717\n1-855-359-7380\n1-855-359-7380\n1-855-238-0488\n1-855-671-6086\n1-844-266-1392\n1-855-241-5717\n1-855-359-7380\n1-855-359-7380\n1-855-359-7380\n1-855-671-6086\n1-866-202-9574\n1-866-202-9574\n1-866-202-9574\n1-866-202-9574\n1-866-202-9574\n1-866-202-9582\n1-866-202-9582\n1-844-686-0483\n1-844-686-0483\n1-844-686-0483\n1-844-686-0483\n1-844-686-0483\n1-844-686-0483\n1-844-686-0483\n1-844-686-0483\n1-844-686-0483\n1-844-686-0483\n1-844-686-0483\n1-844-686-0483\n1-844-686-0483\n1-844-686-0483\n1-844-686-0483\n1-844-686-0483\n1-844-686-0483\n1-844-686-0483\n1-844-686-0483\n1-844-686-0483\n1-844-686-0483\n1-844-686-0483\n1-844-686-0483\n1-844-686-0483\n1-844-686-0483\n1-844-686-0483\n1-844-686-0483\n1-844-686-0483\n1-844-686-0483\n1-844-686-0483\n1-844-686-0483\n1-844-686-0483\n1-844-686-0483\n1-844-686-0483\n1-844-686-0483\n1-844-686-0483\n1-844-686-0483\n1-844-686-0483\n1-844-686-0483\n1-844-686-0483\n1-844-596-0460\n1-844-596-0460\n1-844-638-4642\n1-844-596-0460\n1-844-596-0460\n1-844-596-0460\n1-888-812-2190\n1-844-287-9945\n1-844-287-9945\n1-844-596-0460\n1-866-259-2944\n1-844-596-0460\n1-855-535-7140\n1-844-596-0460\n1-888-354-7664\n1-844-809-5244\n1-844-809-5244\n1-844-809-5244\n1-844-809-5244\n1-844-809-5244\n1-888-812-2190\n1-888-429-8490\n1-866-356-0166\n1-844-596-0460\n1-844-596-0467\n1-844-287-9945\n1-844-287-9945\n1-844-596-0460\n1-844-596-0460\n1-888-429-8490\n1-888-598-7545\n1-888-598-7545\n1-866-451-4616\n1-866-451-4616\n1-855-241-5720\n1-855-832-0976\n1-844-448-7300\n1-844-448-7300\n1-844-596-0460\n1-877-495-6386\n1-844-448-7300\n1-844-448-7300\n1-855-671-6086\n1-844-256-0921\n1-855-651-5058\n1-855-241-5717\n1-844-596-0460\n1-866-202-9574\n1-866-356-1034\n1-844-596-0467\n1-855-832-0971\n1-844-448-7300\n1-844-448-7300\n1-888-794-1519\n1-844-596-0467\n1-844-448-7300\n1-844-686-0485\n1-888-864-0764\n1-888-864-0764\n1-844-596-0460\n1-844-596-0467\n1-855-671-6086\n1-888-264-0638\n1-844-448-7300\n1-866-259-2944\n1-844-596-0460\n1-866-766-6019\n1-855-835-3916\n1-855-241-5720\n1-844-596-0467\n1-844-596-0460\n1-844-596-0460\n1-844-448-7300\n1-866-682-4841\n1-866-682-4841\n1-855-241-5720\n1-844-287-9945\n1-888-812-2190\n1-844-596-0460\n1-866-766-6019\n1-855-881-7868\n1-844-596-0460\n1-844-596-0460\n1-844-596-0460\n1-844-596-0460\n1-844-596-0460\n1-844-596-0460\n1-844-596-0460\n1-844-596-0460\n1-844-596-0460\n1-844-596-0460\n1-844-596-0460\n1-844-596-0460\n1-844-596-0460\n1-844-596-0460\n1-844-596-0460\n1-844-596-0467\n1-844-889-9143\n1-844-889-9143\n1-844-889-9143\n1-866-508-5118\n1-855-323-8831\n1-855-323-8831\n1-855-323-8831\n1-855-323-8831\n1-855-323-8831\n1-855-323-8831\n1-855-323-8831\n1-844-809-5243\n1-844-809-5243\n1-866-249-7785\n1-888-429-8490\n1-844-287-9945\n1-844-287-9945\n1-844-287-9945\n1-844-287-9945\n1-844-287-9945\n1-844-287-9945\n1-844-287-9945\n1-844-287-9945\n1-844-287-9945\n1-844-287-9945\n1-844-287-9945\n1-855-835-3811\n1-855-835-3811\n1-855-241-5724\n1-866-844-4340\n1-866-844-4340\n1-888-230-0074\n1-888-230-0074\n1-888-230-0074\n1-888-230-0074\n1-844-287-9945\n1-844-287-9945\n1-844-287-9945\n1-844-287-9945\n1-844-287-9945\n1-844-287-9945\n1-844-287-9945\n1-844-287-9945\n1-844-287-9945\n1-844-287-9945\n1-844-287-9945\n1-844-287-9945\n1-844-287-9945\n1-844-287-9945\n1-844-287-9945\n1-844-287-9945\n1-844-287-9945\n1-866-682-4841\n1-855-835-3827\n1-855-835-3827\n1-855-835-3827\n1-855-835-3827\n1-855-663-4227\n1-855-238-0489\n1-866-682-4741\n1-844-448-7300\n1-844-448-7300\n1-844-448-7300\n1-844-448-7300\n1-844-448-7300\n1-844-448-7300\n1-844-448-7300\n1-844-448-7300\n1-844-448-7300\n1-844-448-7300\n1-844-448-7300\n1-844-448-7300\n1-844-448-7300\n1-844-448-7300\n1-844-448-7300\n1-844-448-7300\n1-844-448-7300\n1-855-663-4228\n1-855-663-4228\n1-855-663-4228\n1-855-663-4228\n1-855-238-0485\n1-844-686-0483\n1-844-686-0483\n1-844-686-0483\n1-844-686-0483\n1-844-686-0483\n1-844-686-0483\n1-844-686-0483\n1-844-686-0483\n1-844-686-0483\n1-844-686-0483\n1-866-201-0367\n1-866-201-0367\n1-866-201-0367\n1-888-812-2190\n1-866-766-6019\n1-855-835-3916\n1-855-241-5720\n1-844-596-0467\n1-844-596-0460\n"
],
[
"df['phoneNumber']\n\n\nfor phone_no in df['phoneNumber']:\n contactphone = \"%c-%c%c-%c%c%c-%c%c%c%c\" % tuple(map(ord,list(str(phone_no)[:11])))\n print(contactphone)",
"_____no_output_____"
],
[
"df = df.assign(phone=[2, 2, 4, 7, 4, 1],\n Identity=[0, 1, 1, 3, 2, 5])",
"_____no_output_____"
]
]
] | [
"markdown",
"code"
] | [
[
"markdown"
],
[
"code",
"code",
"code",
"code",
"code",
"code",
"code",
"code",
"code",
"code",
"code",
"code",
"code",
"code",
"code",
"code",
"code",
"code",
"code",
"code"
]
] |
d076f81cbee08f2600b2ea6070f90e48ad3878f7 | 11,966 | ipynb | Jupyter Notebook | python/The Guardian Data ingestion and wrangling/The_Guardian_JPMorgan.ipynb | georgetown-analytics/Economic-Events | 413765c40b8d7dcfcaae21e38dd5dc5c063f5c2c | [
"MIT"
] | 1 | 2021-03-26T15:51:31.000Z | 2021-03-26T15:51:31.000Z | python/The Guardian Data ingestion and wrangling/The_Guardian_JPMorgan.ipynb | georgetown-analytics/Economic-Events | 413765c40b8d7dcfcaae21e38dd5dc5c063f5c2c | [
"MIT"
] | null | null | null | python/The Guardian Data ingestion and wrangling/The_Guardian_JPMorgan.ipynb | georgetown-analytics/Economic-Events | 413765c40b8d7dcfcaae21e38dd5dc5c063f5c2c | [
"MIT"
] | null | null | null | 30.760925 | 151 | 0.587414 | [
[
[
"# Code to download The Guardian UK data and clean data for text analysis\n@Jorge de Leon \n\nThis script allows you to download news articles that match your parameters from the Guardian newspaper, https://www.theguardian.com/us.",
"_____no_output_____"
],
[
"## Set-up",
"_____no_output_____"
]
],
[
[
"import os\nimport re \nimport glob\nimport json\nimport requests\nimport pandas as pd \n\nfrom glob import glob\nfrom os import makedirs\nfrom textblob import TextBlob\nfrom os.path import join, exists\nfrom datetime import date, timedelta\n\nos.chdir(\"..\")\n\nimport nltk\nnltk.download('punkt')\nnltk.download('wordnet')\nnltk.download('stopwords')\nfrom nltk import sent_tokenize, word_tokenize\nfrom nltk.stem.snowball import SnowballStemmer\nfrom nltk.stem.wordnet import WordNetLemmatizer\nfrom nltk.corpus import stopwords",
"_____no_output_____"
]
],
[
[
"## API and news articles requests\n\nThis section contains the code that will be used to download articles from the Guardian website. \nthe initial variables will be determined as user-defined parameters.",
"_____no_output_____"
]
],
[
[
"#Enter API and parameters - these parameters can be obtained by playing around with the Guardian API tool:\n# https://open-platform.theguardian.com/explore/\n\n# Set up initial and end date \n\nstart_date_global = date(2000, 1, 1)\nend_date_global = date(2020, 5, 17)\nquery = \"JPMorgan\"\nterm = ('stock')\n\n#Enter API key, endpoint and parameters\nmy_api_key = open(\"..\\\\input files\\\\creds_guardian.txt\").read().strip()\napi_endpoint = \"http://content.guardianapis.com/search?\"\nmy_params = {\n 'from-date': '',\n 'to-date': '',\n 'show-fields': 'bodyText',\n 'q': query,\n 'page-size': 200,\n 'api-key': my_api_key\n}",
"_____no_output_____"
],
[
"articles_dir = join('theguardian','jpmorgan')\nmakedirs(articles_dir, exist_ok=True)",
"_____no_output_____"
],
[
"# day iteration from here:\n# http://stackoverflow.com/questions/7274267/print-all-day-dates-between-two-dates\nstart_date = start_date_global\nend_date = end_date_global\ndayrange = range((end_date - start_date).days + 1)\nfor daycount in dayrange:\n dt = start_date + timedelta(days=daycount)\n datestr = dt.strftime('%Y-%m-%d')\n fname = join(articles_dir, datestr + '.json')\n if not exists(fname):\n # then let's download it\n print(\"Downloading\", datestr)\n all_results = []\n my_params['from-date'] = datestr\n my_params['to-date'] = datestr\n current_page = 1\n total_pages = 1\n while current_page <= total_pages:\n print(\"...page\", current_page)\n my_params['page'] = current_page\n resp = requests.get(api_endpoint, my_params)\n data = resp.json()\n all_results.extend(data['response']['results'])\n # if there is more than one page\n current_page += 1\n total_pages = data['response']['pages']\n\n with open(fname, 'w') as f:\n print(\"Writing to\", fname)\n\n # re-serialize it for pretty indentation\n f.write(json.dumps(all_results, indent=2))",
"_____no_output_____"
],
[
"#Read all json files that will be concatenated\ntest_files = sorted(glob('theguardian/jpmorgan/*.json'))",
"_____no_output_____"
],
[
"#intialize empty list that we will append dataframes to\nall_files = []\n \n#write a for loop that will go through each of the file name through globbing and the end result will be the list \n#of dataframes\nfor file in test_files:\n try:\n articles = pd.read_json(file)\n all_files.append(articles)\n except pd.errors.EmptyDataError:\n print('Note: filename.csv ws empty. Skipping')\n continue #will skip the rest of the bloc and move to next file\n\n#create dataframe with data from json files\ntheguardian_rawdata = pd.concat(all_files, axis=0, ignore_index=True) ",
"_____no_output_____"
]
],
[
[
"## Text Analysis",
"_____no_output_____"
]
],
[
[
"#Drop empty columns\ntheguardian_rawdata = theguardian_rawdata.iloc[:,0:12]",
"_____no_output_____"
],
[
"#show types of media that was downloaded by type\ntheguardian_rawdata['type'].unique()",
"_____no_output_____"
],
[
"#filter only for articles\ntheguardian_rawdata = theguardian_rawdata[theguardian_rawdata['type'].str.match('article',na=False)]",
"_____no_output_____"
],
[
"#remove columns that do not contain relevant information for analysis\ntheguardian_dataset = theguardian_rawdata.drop(['apiUrl','id', 'isHosted', 'pillarId', 'pillarName',\n 'sectionId', 'sectionName', 'type','webTitle', 'webUrl'], axis=1)",
"_____no_output_____"
],
[
"#Modify the column webPublicationDate to Date and the fields to string and lower case\ntheguardian_dataset[\"date\"] = pd.to_datetime(theguardian_dataset[\"webPublicationDate\"]).dt.strftime('%Y-%m-%d')\ntheguardian_dataset['fields'] = theguardian_dataset['fields'].astype(str).str.lower()",
"_____no_output_____"
],
[
"#Clean the articles from URLS, remove punctuaction and numbers. \ntheguardian_dataset['fields'] = theguardian_dataset['fields'].str.replace('<.*?>','') # remove HTML tags\ntheguardian_dataset['fields'] = theguardian_dataset['fields'].str.replace('[^\\w\\s]','') # remove punc.",
"_____no_output_____"
],
[
"#Generate sentiment analysis for each article\n#Using TextBlob obtain polarity\ntheguardian_dataset['sentiment_polarity'] = theguardian_dataset['fields'].apply(lambda row: TextBlob(row).sentiment.polarity)\n#Using TextBlob obtain subjectivity\ntheguardian_dataset['sentiment_subjectivity'] = theguardian_dataset['fields'].apply(lambda row: TextBlob(row).sentiment.subjectivity)",
"_____no_output_____"
],
[
"#Remove numbers from text\ntheguardian_dataset['fields'] = theguardian_dataset['fields'].str.replace('\\d+','') # remove numbers\n\n#Then I will tokenize each word and remover stop words\ntheguardian_dataset['tokenized_fields'] = theguardian_dataset.apply(lambda row: nltk.word_tokenize(row['fields']), axis=1)",
"_____no_output_____"
],
[
"#Stop words\nstop_words=set(stopwords.words(\"english\"))",
"_____no_output_____"
],
[
"#Remove stop words\ntheguardian_dataset['tokenized_fields'] = theguardian_dataset['tokenized_fields'].apply(lambda x: [item for item in x if item not in stop_words])",
"_____no_output_____"
],
[
"#Count number of words and create a column with the most common 5 words per article\nfrom collections import Counter\ntheguardian_dataset['high_recurrence'] = theguardian_dataset['tokenized_fields'].apply(lambda x: [k for k, v in Counter(x).most_common(5)])",
"_____no_output_____"
],
[
"#Create a word count for the word \"stock\"\ntheguardian_dataset['word_ocurrence'] = theguardian_dataset['tokenized_fields'].apply(lambda x: [w for w in x if re.search(term, w)])\ntheguardian_dataset['word_count'] = theguardian_dataset['word_ocurrence'].apply(len)",
"_____no_output_____"
],
[
"#Create a count of the total number of words\ntheguardian_dataset['total_words'] = theguardian_dataset['tokenized_fields'].apply(len)",
"_____no_output_____"
],
[
"#Create new table with average polarity, subjectivity, count of the word \"stock\" per day\nguardian_microsoft = theguardian_dataset.groupby('date')['sentiment_polarity','sentiment_subjectivity','word_count','total_words'].agg('mean')",
"_____no_output_____"
],
[
"#Create a variable for the number of articles per day\ncount_articles = theguardian_dataset\ncount_articles['no_articles'] = count_articles.groupby(['date'])['fields'].transform('count')\ncount_articles = count_articles[[\"date\",\"no_articles\"]]\ncount_articles_df = count_articles.drop_duplicates(subset = \"date\", \n keep = \"first\", inplace=False) ",
"_____no_output_____"
],
[
"#Join tables by date\nguardian_microsoft = guardian_microsoft.merge(count_articles_df, on='date', how ='left')",
"_____no_output_____"
],
[
"#Save dataframes into CSV\ntheguardian_dataset.to_csv('theguardian/jpmorgan/theguardian_jpmorgan_text.csv', encoding='utf-8')\nguardian_microsoft.to_csv('theguardian/jpmorgan/theguardian_jpmorgan_data.csv', encoding='utf-8')",
"_____no_output_____"
]
]
] | [
"markdown",
"code",
"markdown",
"code",
"markdown",
"code"
] | [
[
"markdown",
"markdown"
],
[
"code"
],
[
"markdown"
],
[
"code",
"code",
"code",
"code",
"code"
],
[
"markdown"
],
[
"code",
"code",
"code",
"code",
"code",
"code",
"code",
"code",
"code",
"code",
"code",
"code",
"code",
"code",
"code",
"code",
"code"
]
] |
d0770e797dd802babcef03864713b40184fdec83 | 673,808 | ipynb | Jupyter Notebook | evaluation/trace_evaluation.ipynb | maSteinbach/TppFaaS | 28dfff53cdd835699f9f94a681b337feb9ce4b8f | [
"MIT"
] | 2 | 2022-02-08T10:35:53.000Z | 2022-02-08T12:39:46.000Z | evaluation/trace_evaluation.ipynb | maSteinbach/TppFaaS | 28dfff53cdd835699f9f94a681b337feb9ce4b8f | [
"MIT"
] | null | null | null | evaluation/trace_evaluation.ipynb | maSteinbach/TppFaaS | 28dfff53cdd835699f9f94a681b337feb9ce4b8f | [
"MIT"
] | null | null | null | 1,480.896703 | 184,878 | 0.953286 | [
[
[
"import torch\nimport matplotlib.pyplot as plt\nimport numpy as np\nimport seaborn as sns\nfrom pathlib import Path\n\nsns.color_palette(\"tab10\")\nsns.set(rc={\n \"figure.dpi\": 150,\n \"text.usetex\": True,\n \"xtick.labelsize\": \"small\",\n \"ytick.labelsize\": \"small\",\n \"axes.labelsize\": \"small\",\n \"axes.titlesize\": \"small\",\n \"figure.titlesize\": \"medium\",\n \"axes.titlepad\": 2.0,\n \"xtick.major.pad\": -4.0,\n #\"figure.subplot.hspace\": 0.0,\n \"figure.constrained_layout.use\": True,\n})",
"_____no_output_____"
],
[
"def attribute_to_matrix(sequences, attribute):\n attribute_list = []\n for seq in sequences:\n attribute_list.append(seq[attribute])\n return np.array(attribute_list)\n\ndef cold_starts(init_times):\n num_activations = init_times.size\n num_cold_starts = np.sum(init_times > 0)\n return num_activations, num_cold_starts\n\ndef app_name(filename: str) -> str:\n app_name = filename[filename.find(\":\")+4:filename.find(\"_fetched_\")]\n if \"_rand\" in filename:\n app_name += \"_rand\"\n return app_name\n\ndef get_index(applist: list, filename: str) -> int:\n return applist.index(app_name(filename))",
"_____no_output_____"
],
[
"# Number of activations vs number of cold starts.\n\n# Configuration\ndir = \"final_high_load_n_1000\"\n#dir = \"final_batched_high_load_n_400\"\n\nfiles = Path(f\"../data/{dir}\").glob('*.pkl')\n\nfor f in sorted(files):\n print(f)\n with open(f, \"rb\") as stream:\n data = torch.load(stream)\n num_activations, num_cold_starts = cold_starts(attribute_to_matrix(data[\"sequences\"], \"init_times\"))\n #print(f\"Number of activations: {num_activations}\")\n #print(f\"Number of cold starts: {num_cold_starts}\")\n print(f\"Cold start to activation ratio: {round(num_cold_starts / num_activations, 4)}\")",
"../data/final_high_load_n_1000/injected_20210610_23:14_fanout_large_fetched_397_n_400_l_0.01_u_0.01_b_50_w_120.pkl\nCold start to activation ratio: 0.3002\n../data/final_high_load_n_1000/injected_20210611_00:04_parallel_large_fetched_390_n_400_l_0.01_u_0.01_b_50_w_120.pkl\nCold start to activation ratio: 0.3008\n../data/final_high_load_n_1000/injected_20210611_00:53_tree_large_fetched_399_n_400_l_0.01_u_0.01_b_50_w_120.pkl\nCold start to activation ratio: 0.3007\n../data/final_high_load_n_1000/injected_20210611_01:43_fanout_large_fetched_391_n_400_l_0.01_u_0.01_b_50_w_120_rand.pkl\nCold start to activation ratio: 0.3002\n../data/final_high_load_n_1000/injected_20210611_02:33_parallel_large_fetched_383_n_400_l_0.01_u_0.01_b_50_w_120_rand.pkl\nCold start to activation ratio: 0.3005\n../data/final_high_load_n_1000/injected_20210611_03:23_tree_large_fetched_398_n_400_l_0.01_u_0.01_b_50_w_120_rand.pkl\nCold start to activation ratio: 0.3006\n../data/final_high_load_n_1000/injected_20210611_04:13_fanout_small_fetched_384_n_400_l_0.0001_u_0.0001_b_50_w_120.pkl\nCold start to activation ratio: 0.3008\n../data/final_high_load_n_1000/injected_20210611_07:23_fanout_small_fetched_394_n_400_l_0.0001_u_0.0001_b_50_w_120_rand.pkl\nCold start to activation ratio: 0.3002\n../data/final_high_load_n_1000/injected_20210611_11:32_parallel_small_fetched_397_n_400_l_0.0001_u_0.0001_b_50_w_120.pkl\nCold start to activation ratio: 0.3006\n../data/final_high_load_n_1000/injected_20210611_12:23_tree_small_fetched_399_n_400_l_0.0001_u_0.0001_b_50_w_120.pkl\nCold start to activation ratio: 0.3004\n../data/final_high_load_n_1000/injected_20210611_12:49_parallel_small_fetched_398_n_400_l_0.0001_u_0.0001_b_50_w_120_rand.pkl\nCold start to activation ratio: 0.3003\n../data/final_high_load_n_1000/injected_20210611_13:40_tree_small_fetched_394_n_400_l_0.0001_u_0.0001_b_50_w_120_rand.pkl\nCold start to activation ratio: 0.3002\n../data/final_high_load_n_1000/injected_20210611_16:55_sequence_fetched_500_n_500_l_0.0001_u_0.0001_b_50_w_120.pkl\nCold start to activation ratio: 0.3001\n../data/final_high_load_n_1000/injected_20210611_17:16_sequence_fetched_500_n_500_l_0.0001_u_0.0001_b_50_w_120_rand.pkl\nCold start to activation ratio: 0.3002\n"
],
[
"# Average inter-event time for each step.\n\n# Configuration\ndir = \"final_low_load_n_1000\"\n#dir = \"final_high_load_n_1000\"\nsave = False\n\ndef boxplot(applist: list, file2data: dict, title: str, ylabel: str) -> plt.Figure:\n fig, ax = plt.subplots(len(applist), 1)\n fig.set_size_inches(5.5, 6.8)\n fig.suptitle(title)\n for filename, data in file2data.items():\n if app_name(filename) in applist:\n sns.boxplot(ax=ax[get_index(applist, filename)], data=data, width=0.5, showfliers=False, linewidth=0.9)\n ax[get_index(applist, filename)].set_title(app_name(filename).replace(\"_\", \"\\_\"))\n ax[get_index(applist, filename)].set_ylabel(ylabel)\n ax[get_index(applist, filename)].set_xticklabels(list(range(1, data.shape[-1] + 1)))\n ax[-1].set_xlabel(\"i\")\n return fig\n\nprestr = \"ll_\"\nif \"high_load\" in dir: prestr = \"hl_\"\n\npoststr = \" (no cold starts)\"\nif \"high_load\" in dir: poststr = \" (30\\% cold starts)\"\n\napps = [\"sequence\", \"parallel_small\", \"tree_small\", \"fanout_small\", \"parallel_large\", \"tree_large\", \"fanout_large\"]\napps_rand = [\"sequence_rand\", \"parallel_small_rand\", \"tree_small_rand\", \"fanout_small_rand\", \"parallel_large_rand\", \"tree_large_rand\", \"fanout_large_rand\"]\n\ninter_dict = {}\ninit_dict = {}\nwait_dict = {}\n\nfiles = Path(f\"../data/{dir}\").glob('*.pkl')\nfor f in sorted(files):\n with open(f, \"rb\") as stream:\n data = torch.load(stream)\n inter_dict[str(f)] = np.diff(attribute_to_matrix(data[\"sequences\"], \"arrival_times\"), axis=-1)\n init_dict[str(f)] = attribute_to_matrix(data[\"sequences\"], \"init_times\")\n wait_dict[str(f)] = attribute_to_matrix(data[\"sequences\"], \"wait_times\") / 1000\n\ntitle = fr\"Distribution of inter-event time $\\tau_i${poststr}\"\nylabel = \"ms\"\nfig1 = boxplot(apps, inter_dict, title, ylabel)\nif save: plt.savefig(f\"data_plots/{prestr}dist_inter.pdf\")\nfig2 = boxplot(apps_rand, inter_dict, title, ylabel)\nif save: plt.savefig(f\"data_plots/{prestr}dist_inter_rand.pdf\")\n\nif \"high_load\" in dir:\n title = fr\"Distribution of initTime $i_i${poststr}\"\n ylabel = \"ms\"\n fig1 = boxplot(apps, init_dict, title, ylabel)\n if save: plt.savefig(f\"data_plots/{prestr}dist_init.pdf\")\n fig2 = boxplot(apps_rand, init_dict, title, ylabel)\n if save: plt.savefig(f\"data_plots/{prestr}dist_init_rand.pdf\")\n\n title = fr\"Distribution of waitTime $w_i${poststr}\"\n ylabel = \"sec\"\n fig1 = boxplot(apps, wait_dict, title, ylabel)\n if save: plt.savefig(f\"data_plots/{prestr}dist_wait.pdf\")\n fig2 = boxplot(apps_rand, wait_dict, title, ylabel)\n if save: plt.savefig(f\"data_plots/{prestr}dist_wait_rand.pdf\") ",
"_____no_output_____"
],
[
"# Distribution of inter-event, init and wait times.\n\n# Configuration\ndir = \"final_low_load_n_1000\"\n#dir = \"final_high_load_n_1000\"\n#dir = \"final_batched_high_load_n_400\"\nplot_init_times = True\nplot_wait_times = True\nsave = False\n\ndef compose_title(filename):\n def app_name(filename):\n app_name = filename[filename.find(\":\")+4:filename.find(\"_fetched_\")]\n return app_name.replace(\"_\", \"\\_\")\n title = app_name(filename)\n if \"_rand\" in filename:\n title += \"\\_rand\"\n if \"_b_\" in filename:\n title += \" (30% cold starts)\"\n return title\n\napps = [\"sequence\", \"parallel_small\", \"tree_small\", \"fanout_small\", \"parallel_large\", \n \"tree_large\", \"fanout_large\", \"sequence_rand\", \"parallel_small_rand\", \"tree_small_rand\",\n \"fanout_small_rand\", \"parallel_large_rand\", \"tree_large_rand\", \"fanout_large_rand\"]\nfiles = Path(f\"../data/{dir}\").glob('*.pkl')\n\nfig_inter, ax_inter = plt.subplots(14, 1)\nfig_inter.set_size_inches(6.4, 18)\nfig_init, ax_init = plt.subplots(14, 1)\nfig_init.set_size_inches(6.4, 18)\nfig_wait, ax_wait = plt.subplots(14, 1)\nfig_wait.set_size_inches(6.4, 18)\n\nfor i, f in enumerate(sorted(files)):\n with open(f, \"rb\") as stream:\n data = torch.load(stream)\n \n if \"small\" in str(f): \n numb_traces = 200\n else:\n numb_traces = 100\n \n inter_event_times = np.diff(attribute_to_matrix(data[\"sequences\"], \"arrival_times\"), axis=-1)[:numb_traces].reshape(-1)\n init_times = attribute_to_matrix(data[\"sequences\"], \"init_times\")[:numb_traces].reshape(-1)\n wait_times = attribute_to_matrix(data[\"sequences\"], \"wait_times\")[:numb_traces].reshape(-1) / 1000\n \n max_range = int(np.quantile(inter_event_times, 0.99))\n ax_inter[get_index(apps, str(f))].hist(inter_event_times, bins=300, range=(0.0, max_range), log=True)\n ax_inter[get_index(apps, str(f))].set_xlabel(\"milliseconds\")\n ax_inter[get_index(apps, str(f))].set_ylabel(\"count\")\n ax_inter[get_index(apps, str(f))].title.set_text(f\"Distribution over all inter-event times - {compose_title(str(f))}\")\n \n if plot_init_times:\n max_range = int(np.quantile(init_times, 0.99))\n ax_init[get_index(apps, str(f))].hist(init_times, bins=300, range=(0.0, max_range), log=True)\n ax_init[get_index(apps, str(f))].set_xlabel(\"milliseconds\")\n ax_init[get_index(apps, str(f))].set_ylabel(\"count\")\n ax_init[get_index(apps, str(f))].title.set_text(f\"Distribution over all initTimes - {compose_title(str(f))}\")\n \n if plot_wait_times:\n max_range = int(np.quantile(wait_times, 0.99))\n ax_wait[get_index(apps, str(f))].hist(wait_times, bins=300, range=(0.0, max_range), log=True)\n ax_wait[get_index(apps, str(f))].set_xlabel(\"seconds\")\n ax_wait[get_index(apps, str(f))].set_ylabel(\"count\")\n ax_wait[get_index(apps, str(f))].title.set_text(f\"Distribution over all waitTimes - {compose_title(str(f))}\")\n \n#fig_inter.tight_layout()\n#fig_init.tight_layout()\n#fig_wait.tight_layout()\n \nif save:\n filename = f\"{str(f)[str(f).rfind('/')+1:str(f).rfind('.pkl')]}.png\"\n plt.savefig(filename)",
"_____no_output_____"
],
[
"# Standard deviation of inter-event, init and wait time\n\n# Configuration\ndir = \"final_low_load_n_1000\"\n\nfiles = Path(f\"../data/{dir}\").glob('*.pkl')\n\ndef app_name(filename):\n app_name = filename[filename.find(\":\")+4:filename.find(\"_fetched_\")]\n if \"rand\" in filename:\n app_name += \"_rand\"\n return app_name\n\nfor f in sorted(files):\n with open(f, \"rb\") as stream:\n data = torch.load(stream)\n inter_event_times = np.diff(attribute_to_matrix(data[\"sequences\"], \"arrival_times\"), axis=-1)\n init_times = attribute_to_matrix(data[\"sequences\"], \"init_times\")\n wait_times = attribute_to_matrix(data[\"sequences\"], \"wait_times\") #/ 1000\n \n print(f\"app={app_name(str(f))}\\n\"\n f\"std_inter_event={np.std(inter_event_times)}\\n\"\n f\"std_init={np.std(init_times)}\\n\"\n f\"std_wait={np.std(wait_times)}\\n\")",
"app=fanout_large\nstd_inter_event=151.05177762236863\nstd_init=8.144257456732442\nstd_wait=114.77229044370371\n\napp=parallel_large\nstd_inter_event=109.44044903032881\nstd_init=9.297179170864\nstd_wait=127.57963467870893\n\napp=tree_large\nstd_inter_event=131.23154306078888\nstd_init=16.78905591489502\nstd_wait=291.31143757231473\n\napp=fanout_large_rand\nstd_inter_event=151.65812343240964\nstd_init=8.883851129119272\nstd_wait=130.1648441444701\n\napp=parallel_large_rand\nstd_inter_event=109.44652378874352\nstd_init=10.602357329970584\nstd_wait=138.1014849145728\n\napp=tree_large_rand\nstd_inter_event=97.52263492725262\nstd_init=11.27306997866161\nstd_wait=179.97986456871658\n\napp=fanout_small\nstd_inter_event=239.70282899756836\nstd_init=13.732588276619522\nstd_wait=234.60153714089307\n\napp=parallel_small\nstd_inter_event=161.9196585169324\nstd_init=11.742867522581214\nstd_wait=153.98688379668323\n\napp=sequence\nstd_inter_event=224.63991208489645\nstd_init=11.105719767848612\nstd_wait=139.50763483666051\n\napp=tree_small\nstd_inter_event=104.72267932207933\nstd_init=11.101776254275707\nstd_wait=157.67831093003883\n\napp=fanout_small_rand\nstd_inter_event=198.97358421057848\nstd_init=10.779445649697989\nstd_wait=167.92800845212872\n\napp=parallel_small_rand\nstd_inter_event=204.9385673453389\nstd_init=13.69195516467261\nstd_wait=199.91791532708436\n\napp=sequence_rand\nstd_inter_event=249.20868229207025\nstd_init=12.790173494387357\nstd_wait=164.10402365230425\n\napp=tree_small_rand\nstd_inter_event=111.61791923038606\nstd_init=9.332272280104133\nstd_wait=130.05539527063073\n\n"
]
]
] | [
"code"
] | [
[
"code",
"code",
"code",
"code",
"code",
"code"
]
] |
d0771d6e4ee67f9a3ab6ebdb74a7ad468577e14b | 223,068 | ipynb | Jupyter Notebook | old scripts/Time_synchronization.ipynb | pawelngei/sleep_project | f8f52d94a9a739bceaa7cc24dd6a6dee37a50c17 | [
"MIT"
] | 2 | 2019-06-09T08:13:09.000Z | 2020-11-02T03:03:13.000Z | old scripts/Time_synchronization.ipynb | pawelngei/sleep_project | f8f52d94a9a739bceaa7cc24dd6a6dee37a50c17 | [
"MIT"
] | null | null | null | old scripts/Time_synchronization.ipynb | pawelngei/sleep_project | f8f52d94a9a739bceaa7cc24dd6a6dee37a50c17 | [
"MIT"
] | 1 | 2020-11-02T16:25:10.000Z | 2020-11-02T16:25:10.000Z | 523.633803 | 114,034 | 0.925897 | [
[
[
"Neuroon cross-validation\n------------------------\n\n\nNeuroon and PSG recordings were simultanously collected over the course of two nights. This analysis will show whether Neuroon is able to accurately classify sleep stages. The PSG classification will be a benchmark against which Neuroon performance will be tested. \"The AASM Manual for te Scoring of Sleep ad Associated Events\" identifies 5 sleep stages: \n\n* Stage W (Wakefulness)\n* Stage N1 (NREM 1)\n* Stage N1 (NREM 2)\n* Stage N1 (NREM 3)\n* Stage R (REM)\n<img src=\"images/sleep_stages.png\">\n\n\nThese stages can be identified following the rules guidelines in [1] either visually or digitally using combined information from EEG, EOG and EMG. Extensive research is beeing conducted on developing automated and simpler methods for sleep stage classification suitable for everyday home use (for a review see [2]). Automatic methods based on single channel EEG, which is the Neuroon category, were shown to work accurately when compared to PSG scoring [3]. \n\n[1] Berry RB BR, Gamaldo CE, Harding SM, Lloyd RM, Marcus CL, Vaughn BV; for the American Academy of Sleep Medicine. The AASM Manual for the Scoring of Sleep and Associated Events: Rules, Terminology and Technical Specifications.,Version 2.0.3. Darien, IL: American Academy of Sleep Medicine; 2014.\n\n[2] Van De Water, A. T. M., Holmes, A., & Hurley, D. a. (2011). Objective measurements of sleep for non-laboratory settings as alternatives to polysomnography - a systematic review. Journal of Sleep Research, 20, 183–200. \n\n[3] Berthomier, C., Drouot, X., Herman-Stoïca, M., Berthomier, P., Prado, J., Bokar-Thire, D. d’Ortho, M.P. (2007). Automatic analysis of single-channel sleep EEG: validation in healthy individuals. Sleep, 30(11), 1587–1595.\n",
"_____no_output_____"
],
[
"Signals time-synchronization using crosscorelation\n--------------------------------------------------\n\nNeuroon and PSG were recorded on devices with (probably) unsycnhronized clocks. First we will use a cross-correlation method [4] to find the time offset between the two recordings.\n\n[4] Fridman, L., Brown, D. E., Angell, W., Abdić, I., Reimer, B., & Noh, H. Y. (2016). Automated synchronization of driving data using vibration and steering events. Pattern Recognition Letters, 75, 9-15.\n\nDefine cross correlation function - code from: (http://lexfridman.com/blogs/research/2015/09/18/fast-cross-correlation-and-time-series-synchronization-in-python/)\n\nfor other examlpes see: (http://stackoverflow.com/questions/4688715/find-time-shift-between-two-similar-waveforms)\n\n",
"_____no_output_____"
]
],
[
[
"\n%matplotlib inline\n%load_ext autoreload\n%autoreload 2\n\nimport numpy as np\nimport matplotlib.pyplot as plt\nfrom itertools import tee\nimport pandas as pd\nimport seaborn as sns\nfrom numpy.fft import fft, ifft, fft2, ifft2, fftshift\nfrom collections import OrderedDict \nfrom datetime import timedelta\n\nplt.rcParams['figure.figsize'] = (9.0, 5.0)\n\n\n\n",
"The autoreload extension is already loaded. To reload it, use:\n %reload_ext autoreload\n"
],
[
"from parse_signal import load_psg, load_neuroon\n\n# Cross-correlation function. Equivalent to numpy.correlate(x,y mode = 'full') but faster for large arrays \n# This function was tested against other cross correlation methods in -- LINK TO OTHER NOTEBOOK\ndef cross_correlation_using_fft(x, y):\n f1 = fft(x)\n f2 = fft(np.flipud(y))\n cc = np.real(ifft(f1 * f2))\n return fftshift(cc)\n \n# shift < 0 means that y starts 'shift' time steps before x # shift > 0 means that y starts 'shift' time steps after x\ndef compute_shift(x, y):\n assert len(x) == len(y)\n c = cross_correlation_using_fft(x, y)\n assert len(c) == len(x)\n zero_index = int(len(x) / 2) - 1\n shift = zero_index - np.argmax(c)\n return shift,c\n\n\ndef cross_correlate():\n # Load the signal from hdf database and parse it to pandas series with datetime index\n psg_signal = load_psg('F3-A2')\n neuroon_signal = load_neuroon()\n # Resample the signal to 100hz, to have the same length for cross correlation\n psg_10 = psg_signal.resample('10ms').mean()\n neuroon_10 = neuroon_signal.resample('10ms').mean()\n \n # Create ten minute intervals\n dates_range = pd.date_range(psg_signal.head(1).index.get_values()[0], neuroon_signal.tail(1).index.get_values()[0], freq=\"10min\")\n \n # Convert datetime interval boundaries to string with only hours, minutes and seconds\n dates_range = [d.strftime('%H:%M:%S') for d in dates_range]\n \n \n all_coefs = []\n \n # iterate over overlapping pairs of 10 minutes boundaries \n for start, end in pairwise(dates_range):\n # cut 10 minutes piece of signal\n neuroon_cut = neuroon_10.between_time(start, end)\n psg_cut = psg_10.between_time(start, end)\n # Compute the correlation using fft convolution\n shift, coeffs = compute_shift(neuroon_cut, psg_cut)\n #normalize the coefficients because they will be shown on the same heatmap and need a common color scale\n all_coefs.append((coeffs - coeffs.mean()) / coeffs.std())\n \n #print('max corr at shift %s is at sample %i'%(start, shift))\n\n all_coefs = np.array(all_coefs)\n return all_coefs, dates_range\n\n# This function is used to iterate over a list, taking two consecutive items at each iteration\ndef pairwise(iterable):\n \"s -> (s0,s1), (s1,s2), (s2, s3), ...\"\n a, b = tee(iterable)\n next(b, None)\n return zip(a, b)",
"_____no_output_____"
],
[
"# Construct a matrix where each row represents a 10 minute window from the recording\n# and each column represent correlation coefficient between neuroon and psg signals offset by samples number.\n# 0 samples offset coefficient is stored at the middle column -1. Negative offset and positive offset span left and right from the center.\n# offset < 0 means that psg starts 'shift' time steps before neuroon\n# offset > 0 means that psg starts 'shift' time steps after neuroon\n\ncoeffs_matrix, dates = cross_correlate()",
"_____no_output_____"
],
[
"from plotting_collection import plot_crosscorrelation_heatmap\n\n#Plot part of the coefficients matrix centered around the max average correlation for all 10 minute windows\nplot_crosscorrelation_heatmap(coeffs_matrix, dates)",
"_____no_output_____"
]
],
[
[
"Hipnogram time-delay\n--------------------\nFrom the crosscorrelation of the eeg signals we can see the two devices are off by 2 minutes 41 seconds. Now we'll see if there is a point in time where the hipnograms are most simmilar. The measure of hipnogram simmilarity will be the sum of times when two devices classified the same sleep stage.\n",
"_____no_output_____"
]
],
[
[
"import parse_hipnogram as ph\n\ndef get_hipnogram_intersection(neuroon_hipnogram, psg_hipnogram, time_shift):\n\n neuroon_hipnogram.index = neuroon_hipnogram.index + timedelta(seconds = int(time_shift))\n\n \n combined = psg_hipnogram.join(neuroon_hipnogram, how = 'outer', lsuffix = '_psg', rsuffix = '_neuro')\n \n combined.loc[:, ['stage_num_psg', 'stage_name_psg', 'stage_num_neuro', 'stage_name_neuro', 'event_number_psg', 'event_number_neuro']] = combined.loc[:, ['stage_num_psg', 'stage_name_psg', 'stage_num_neuro', 'stage_name_neuro', 'event_number_psg', 'event_number_neuro']].fillna( method = 'bfill') \n \n combined.loc[:, ['stage_shift_psg', 'stage_shift_neuro']] = combined.loc[:, ['stage_shift_psg', 'stage_shift_neuro']].fillna( value = 'inside') \n \n # From the occupied room number subtract the room occupied by another mouse.\n combined['overlap'] = combined['stage_num_psg'] - combined['stage_num_neuro']\n \n same_stage = combined.loc[combined['overlap'] == 0]\n same_stage.loc[:, 'event_union'] = same_stage['event_number_psg'] + same_stage['event_number_neuro']\n\n\n# common_window = np.array([neuroon_hipnogram.tail(1).index.get_values()[0] - psg_hipnogram.head(1).index.get_values()[0]],dtype='timedelta64[m]').astype(int)[0]\n\n all_durations = OrderedDict()\n\n for stage_name, intersection in same_stage.groupby('event_union'):\n # Subtract the first row timestamp from the last to get the duration. Store as the duration in milliseconds.\n duration = (intersection.index.to_series().iloc[-1]- intersection.index.to_series().iloc[0]).total_seconds()\n \n stage_id = intersection.iloc[0, intersection.columns.get_loc('stage_name_neuro')] \n # Keep appending results to a list stored in a dict. Check if the list exists, if not create it.\n if stage_id not in all_durations.keys():\n all_durations[stage_id] = [duration]\n \n else: \n all_durations[stage_id].append(duration)\n \n\n \n means = OrderedDict()\n stds = OrderedDict()\n sums = OrderedDict()\n stages_sum = 0\n #Adding it here so its first in ordered dict and leftmost on the plot\n sums['stages_sum'] = 0\n for key, value in all_durations.items():\n #if key != 'wake':\n means[key] = np.array(value).mean()\n stds[key] = np.array(value).std()\n sums[key] = np.array(value).sum()\n \n stages_sum += np.array(value).sum() \n \n sums['stages_sum'] = stages_sum\n # Divide total seconds by 60 to get minutes \n #return stages_sum\n return sums, means, stds",
"_____no_output_____"
],
[
"def intersect_with_shift():\n psg_hipnogram = ph.parse_psg_stages()\n neuroon_hipnogram = ph.parse_neuroon_stages()\n \n intersection = OrderedDict([('wake', []), ('rem',[]), ('N1',[]), ('N2',[]), ('N3', []), ('stages_sum', [])])\n\n shift_range = np.arange(-500, 100, 10)\n for shift in shift_range:\n sums, _, _ = get_hipnogram_intersection(neuroon_hipnogram.copy(), psg_hipnogram.copy(), shift)\n for stage, intersect_dur in sums.items():\n intersection[stage].append(intersect_dur)\n \n return intersection, shift_range\n",
"_____no_output_____"
],
[
"\ndef plot_intersection(intersection, shift_range):\n \n psg_hipnogram = ph.parse_psg_stages()\n neuroon_hipnogram = ph.parse_neuroon_stages()\n \n stage_color_dict = {'N1' : 'royalblue', 'N2' :'forestgreen', 'N3' : 'coral', 'rem' : 'plum', 'wake' : 'lightgrey', 'stages_sum': 'dodgerblue'}\n\n \n fig, axes = plt.subplots(2)\n zscore_ax = axes[0].twinx()\n \n for stage in ['rem', 'N2', 'N3', 'wake']:\n intersect_sum = np.array(intersection[stage])\n z_scored = (intersect_sum - intersect_sum.mean()) / intersect_sum.std()\n zscore_ax.plot(shift_range, z_scored, color = stage_color_dict[stage], label = stage, alpha = 0.5, linestyle = '--')\n \n\n max_overlap = shift_range[np.argmax(intersection['stages_sum'])]\n fig.suptitle('max overlap at %i seconds offset'%max_overlap)\n axes[0].plot(shift_range, intersection['stages_sum'], label = 'stages sum', color = 'dodgerblue')\n axes[0].axvline(max_overlap, color='k', linestyle='--')\n\n axes[0].set_ylabel('time in the same sleep stage')\n axes[0].set_xlabel('offset in seconds')\n \n axes[0].legend(loc = 'center right')\n zscore_ax.grid(b=False)\n zscore_ax.legend()\n \n sums0, means0, stds0 = get_hipnogram_intersection(neuroon_hipnogram.copy(), psg_hipnogram.copy(), 0)\n#\n width = 0.35 \n ind = np.arange(5)\n colors_inorder = ['dodgerblue', 'lightgrey', 'forestgreen', 'coral', 'plum']\n #Plot the non shifted overlaps \n axes[1].bar(left = ind, height = list(sums0.values()),width = width, alpha = 0.8, \n tick_label =list(sums0.keys()), edgecolor = 'black', color= colors_inorder)\n\n sumsMax, meansMax, stdsMax = get_hipnogram_intersection(neuroon_hipnogram.copy(), psg_hipnogram.copy(), max_overlap)\n # Plot the shifted overlaps\n axes[1].bar(left = ind +width, height = list(sumsMax.values()),width = width, alpha = 0.8,\n tick_label =list(sumsMax.keys()), edgecolor = 'black', color = colors_inorder)\n \n axes[1].set_xticks(ind + width)\n \n plt.tight_layout()\n",
"_____no_output_____"
],
[
"intersection, shift_range = intersect_with_shift()",
"_____no_output_____"
],
[
"plot_intersection(intersection, shift_range)",
"_____no_output_____"
]
],
[
[
"hipnogram analysis indicates the same direction of time delay - psg is 4 minutes 10 seconds before neuroon. The time delay is larger for the hipnograms than for the signals by 1 minute 30 seconds.\n\nTodo: \n\n* add second axis with percentages\n* see if the overlap increased in proportion with offset\n* plot parts of time corrected signals and hipnograms\n* add different correlation tests notebook\n* add spectral and pca analysis\n\n",
"_____no_output_____"
]
]
] | [
"markdown",
"code",
"markdown",
"code",
"markdown"
] | [
[
"markdown",
"markdown"
],
[
"code",
"code",
"code",
"code"
],
[
"markdown"
],
[
"code",
"code",
"code",
"code",
"code"
],
[
"markdown"
]
] |
d0771d9f63aa2d880552bc31c422d1288d1e4d31 | 3,417 | ipynb | Jupyter Notebook | python_and_data_analysis/numpy-sample.ipynb | krishansubudhi/krishanAI | 918f6cfb6cbd00b03550b13e4c15538260102ac7 | [
"Apache-2.0"
] | 1 | 2020-10-15T10:36:54.000Z | 2020-10-15T10:36:54.000Z | python_and_data_analysis/numpy-sample.ipynb | krishansubudhi/krishanAI | 918f6cfb6cbd00b03550b13e4c15538260102ac7 | [
"Apache-2.0"
] | null | null | null | python_and_data_analysis/numpy-sample.ipynb | krishansubudhi/krishanAI | 918f6cfb6cbd00b03550b13e4c15538260102ac7 | [
"Apache-2.0"
] | null | null | null | 17.890052 | 83 | 0.467076 | [
[
[
"@author : krishan subudhi",
"_____no_output_____"
],
[
"## create a list of 50 random numbers",
"_____no_output_____"
]
],
[
[
"import random\narr = [random.randint(0,1000) for i in range(50)]\narr[:10]",
"_____no_output_____"
]
],
[
[
"## Calculate square root\n",
"_____no_output_____"
]
],
[
[
"import math\n%timeit [math.sqrt(a) for a in arr]",
"20.9 µs ± 177 ns per loop (mean ± std. dev. of 7 runs, 10000 loops each)\n"
]
],
[
[
"# numpy \n1. Faster\n1. Easy to use\n2. Memory effecient\n3. Rich in mathematical functions\n4. Easy matrix operations",
"_____no_output_____"
]
],
[
[
"import numpy as np\nnumpy_arr = np.array(arr)\nnumpy_arr[:10]",
"_____no_output_____"
],
[
"%timeit np.sqrt(numpy_arr)",
"2.82 µs ± 196 ns per loop (mean ± std. dev. of 7 runs, 100000 loops each)\n"
]
],
[
[
"## Matrix operations",
"_____no_output_____"
]
],
[
[
"matrix_2d = np.random.randint(0,100,(2,3))\nmatrix_2d",
"_____no_output_____"
],
[
"matrix_2d.sum(axis = 0)",
"_____no_output_____"
]
]
] | [
"markdown",
"code",
"markdown",
"code",
"markdown",
"code",
"markdown",
"code"
] | [
[
"markdown",
"markdown"
],
[
"code"
],
[
"markdown"
],
[
"code"
],
[
"markdown"
],
[
"code",
"code"
],
[
"markdown"
],
[
"code",
"code"
]
] |
d077227dd38e1272f77da5e413eafcf74b2954f5 | 889,408 | ipynb | Jupyter Notebook | pi-icr-analysis.ipynb | jonas-ka/pi-icr-analysis | ab1d6dc8bf23ff45b415ff154039227ba3044df5 | [
"MIT"
] | 2 | 2020-09-12T19:35:23.000Z | 2020-10-20T08:56:11.000Z | pi-icr-analysis.ipynb | jonas-ka/pi-icr-analysis | ab1d6dc8bf23ff45b415ff154039227ba3044df5 | [
"MIT"
] | null | null | null | pi-icr-analysis.ipynb | jonas-ka/pi-icr-analysis | ab1d6dc8bf23ff45b415ff154039227ba3044df5 | [
"MIT"
] | 1 | 2020-09-12T19:35:29.000Z | 2020-09-12T19:35:29.000Z | 542.984127 | 471,864 | 0.929455 | [
[
[
"# PI-ICR analysis\n\nCreated on 17 July 2019 for the ISOLTRAP experiment\n- V1.1 (24 June 2020): Maximum likelihood estimation was simplified based on SciPy PDF's and the CERN-ROOT6 minimizer via the iminuit package (→ great performance)\n- V1.2 (20 February 2021): Preparations for scientific publication and iminuit v2 update integration\n\n@author: Jonas Karthein<br>\n@contact: [email protected]<br>\n@license: MIT license\n\n### References\n[1]: https://doi.org/10.1007/s00340-013-5621-0\n[2]: https://doi.org/10.1103/PhysRevLett.110.082501\n[3]: https://doi.org/10.1007/s10751-019-1601-z\n[4]: https://doi.org/10.1103/PhysRevLett.124.092502\n\n[1] S. Eliseev, _et al._ Appl. Phys. B (2014) 114: 107.<br>\n[2] S. Eliseev, _et al._ Phys. Rev. Lett. 110, 082501 (2013).<br>\n[3] J. Karthein, _et al._ Hyperfine Interact (2019) 240: 61.<br>\n\n### Application\n\nThe code was used to analyse data for the following publications:\n\n[3] J. Karthein, _et al._ Hyperfine Interact (2019) 240: 61.<br>\n[4] V. Manea and J. Karthein, _et al._ Phys. Rev. Lett. 124, 092502 (2020)<br>\n[5] M. Mougeot, _et al._ in preparation (2020)<br>\n\n### Introduction\n\nThe following code was written to reconstruct raw Phase-Imaging Ion-Cyclotron-Resonance (PI-ICR) data, to fit PI-ICR position information and calculate a frequency using the patter 1/2 scheme described in Ref. [1] and to determine a frequency ratio between a measurement ion and a reference ion. Additionally, the code allows to analyze isomeric states separated in pattern 2.\n data, to fit PI-ICR position information and calculate a frequency using the patter 1/2 scheme described in Ref. [1] and to determine a frequency ratio between a measurement ion and a reference ion. Additionally, the code allows to analyze isomeric states separated in pattern 2.\n\n### Required software and libraries\n\nThe following code was written in Python 3.7. The required libraries are listed below with a rough description for their task in the code. It doesn't claim to be a full description of the library.\n* pandas (data storage and calculation)\n* numpy (calculation)\n* matplotlib (plotting)\n* scipy (PDFs, least squares estimation)\n* configparser (configuration file processing)\n* jupyter (Python notebook environment)\n* iminuit (CERN-ROOT6 minimizer)\n\nAll packages can be fetched using pip:",
"_____no_output_____"
]
],
[
[
"!pip3 install --user pandas numpy matplotlib scipy configparser jupyter iminuit",
"_____no_output_____"
]
],
[
[
"Instead of the regular jupyter environment, one can also use CERN's SWAN service or Google Colab.",
"_____no_output_____"
]
],
[
[
"google_colab = False\n\nif google_colab:\n try:\n from google.colab import drive\n drive.mount('/content/drive')\n %cd /content/drive/My\\ Drive/Colab/pi-icr/\n except:\n %cd ~/cernbox/Documents/Colab/pi-icr/",
"_____no_output_____"
]
],
[
[
"### Data files\n\nSpecify, whether the analysis involves one or two states separated in pattern 2 by commenting out the not applicable case in lines 10 or 11. Then enter the file paths for all your data files without the `*.txt` extension. In the following, `ioi` represents the Ion of interest, and `ref` the reference ion.",
"_____no_output_____"
]
],
[
[
"%config InlineBackend.figure_format ='retina'\n\nimport pandas as pd\nimport numpy as np\nimport matplotlib.pyplot as plt\nimport matplotlib as mpl\nimport pickle, os\n\n# analysis = {'ioi_g': {},'ref': {}}\nanalysis = {'ioi_g': {},'ioi_m': {},'ref': {}}\n\nfiles_ioi_g = ['data/ioi_ground/85Rb_c_000',\n 'data/ioi_ground/85Rb_002',\n 'data/ioi_ground/85Rb_004',\n 'data/ioi_ground/85Rb_006']\n# files_ioi_m = ['data/ioi_isomer/101In_c_000',\n# 'data/ioi_isomer/101In_005']\nfiles_ref = ['data/ref/133Cs_c_000',\n 'data/ref/133Cs_003',\n 'data/ref/133Cs_005',\n 'data/ref/133Cs_007']\n\nlatex_ioi_g = '$^{88}$Rb'\n# latex_ioi_m = '$^{101}$In$^m$'\nlatex_ref = '$^{133}$Cs'",
"_____no_output_____"
]
],
[
[
"### Load pre-analyzed data from file or reconstruct raw data\n\nAll files are loaded and reconstructed in one big dictionary of dictionaries. It contains besides the positions and timestamps also information about the measurement conditions (excitation frequencies, rounds etc). One can load a whole beamtime at once. Center files must be indicated by a `_c_` in the name (e.g. regular name: `101In_001.txt` $\\rightarrow$ center name `101In_c_000.txt`). All the data is at later stages saved in a `pickle` file. This enables quick loading of the data dictionary without the need of re-reconstructing the data.\n\nThe reconstruction code is parallelized and can be found in the subfolder `bin/reconstruction.py`",
"_____no_output_____"
]
],
[
[
"from bin.reconstruction import PIICR\npiicr = PIICR()\n\nif os.path.isfile('data/data-save.p'):\n analysis = pickle.load(open('data/data-save.p','rb'))\n print('\\nLoading finished!')\nelse:\n for file in files_ioi_g:\n analysis['ioi_g'].update({file: piicr.prepare(file)})\n if analysis['ioi_m'] != {}:\n for file in files_ioi_m:\n analysis['ioi_m'].update({file: piicr.prepare(file)})\n for file in files_ref:\n analysis['ref'].update({file: piicr.prepare(file)})\n \n print('\\nReconstruction finished!')",
"\nLoading finished!\n"
]
],
[
[
"### Individual file selection\n\nThe analysis dictionary contains all files. The analysis however is intended to be performed on a file-by-file basis. Please select the individual files here in the variable `file_name`.",
"_____no_output_____"
]
],
[
[
"# load P1, P2 and C data in panda dataframes for selected file\n\n# file_name = files_ioi_g[1]\n# file_name = files_ioi_m[1]\n# file_name = files_ref[1]\n\nfile_name = files_ioi_g[3]\n\nprint('Selected file:',file_name)\n\nif 'ground' in file_name:\n df_p1 = pd.DataFrame(analysis['ioi_g'][file_name]['p1'], columns=['event','x','y','time'])\n df_p2 = pd.DataFrame(analysis['ioi_g'][file_name]['p2'], columns=['event','x','y','time'])\n df_c = pd.DataFrame(analysis['ioi_g'][file_name.split('_0', 1)[0]+'_c_000']['c'],\n columns=['event','x','y','time'])\nelif 'isomer' in file_name:\n df_p1 = pd.DataFrame(analysis['ioi_m'][file_name]['p1'], columns=['event','x','y','time'])\n df_p2 = pd.DataFrame(analysis['ioi_m'][file_name]['p2'], columns=['event','x','y','time'])\n df_c = pd.DataFrame(analysis['ioi_m'][file_name.split('_0', 1)[0]+'_c_000']['c'],\n columns=['event','x','y','time'])\nelse:\n df_p1 = pd.DataFrame(analysis['ref'][file_name]['p1'], columns=['event','x','y','time'])\n df_p2 = pd.DataFrame(analysis['ref'][file_name]['p2'], columns=['event','x','y','time'])\n df_c = pd.DataFrame(analysis['ref'][file_name.split('_0', 1)[0]+'_c_000']['c'],\n columns=['event','x','y','time'])",
"Selected file: data/ioi_ground/85Rb_006\n"
]
],
[
[
"### Manual space and time cut\n\nPlease perform a rough manual space cut for each file to improve results on the automatic space cutting tool. This is necessary if one deals with two states in pattern two or if there is a lot of background. This selection will be ellipsoidal. Additionally, please perform a rough time of flight (ToF) cut.",
"_____no_output_____"
]
],
[
[
"# manual_space_cut = [x_peak_pos, x_peak_spread, y_peak_pos, y_peak_spread]\nmanual_space_cut = {'data/ioi_ground/85Rb_002': [150, 150, 100, 150],\n 'data/ioi_ground/85Rb_004': [150, 150, 100, 150],\n 'data/ioi_ground/85Rb_006': [150, 150, 100, 150],\n 'data/ref/133Cs_003': [120, 150, 80, 150],\n 'data/ref/133Cs_005': [120, 150, 80, 150],\n 'data/ref/133Cs_007': [120, 150, 80, 150]}\n\n# manual_tof_cut = [tof_min, tof_max]\nmanual_tof_cut = [20, 50]\n\n# manual_z_cut <= number of ions in the trap\nmanual_z_cut = 5",
"_____no_output_____"
]
],
[
[
"### Automatic time and space cuts based on Gaussian distribution\n\nThis section contains all cuts in time and space in different steps. \n1. In the time domain contaminants are removed by fitting a gaussian distribution via maximum likelihood estimation to the largest peak in the ToF spectrum and cutting +/- 5 $\\sigma$ (change cut range in lines 70 & 71). The ToF distribution has to be binned first before the maximum can be found, but the fit is performed on the unbinned data set.\n2. Manual space cut is applied for pattern 1 and pattern 2 (not for the center spot)\n3. Outlyers/wrongly excited ions are removed +/- 3 $\\sigma$ by measures of a simple mean in x and y after applying the manual cut (change cut range in lines).\n4. Ejections with more than `manual_z_cut` number of ions in the trap (without taking into account the detector efficiency) are rejected (= z-class cut)",
"_____no_output_____"
]
],
[
[
"%config InlineBackend.figure_format ='retina'\n\nimport matplotlib as mpl\nfrom scipy.stats import norm\nfrom iminuit import Minuit\n\n# Utopia LaTeX font with greek letters\nmpl.rc('font', family='serif', serif='Linguistics Pro')\nmpl.rc('text', usetex=False)\nmpl.rc('mathtext', fontset='custom',\n rm='Linguistics Pro',\n it='Linguistics Pro:italic',\n bf='Linguistics Pro:bold')\nmpl.rcParams.update({'font.size': 18})\n\ncol = ['#FFCC00', '#FF2D55', '#00A2FF', '#61D935', 'k', 'grey', 'pink'] # yellow, red, blue, green\n\ndf_list = [df_p1, df_p2, df_c]\npattern = ['p1', 'p2', 'c']\nbin_time_df = [0,0,0] # [p1,p2,c] list of dataframes containing the time-binned data\nresult_t = [0,0,0] # [p1,p2,c] list of MLE fit result dicts\ncut_df = [0,0,0] # [p1,p2,c] list of dataframes containing the time- and space-cut data\nexcludes_df = [0,0,0] # [p1,p2,c] list of dataframes containing the time- and space-cut excluded data\nfig, axes = plt.subplots(nrows=3, ncols=2, figsize=(12, 15))\n\nfor df_nr in range(len(df_list)):\n \n \n ##############################\n ### BINNING, FITTING TOF DISTR\n ##############################\n \n \n bin_time_df[df_nr] = pd.DataFrame(pd.value_counts(pd.cut(df_list[df_nr].time, bins=np.arange(manual_tof_cut[0], manual_tof_cut[1],0.02))).sort_index()).rename(index=str, columns={'time': 'counts'}).reset_index(drop=True)\n bin_time_df[df_nr]['time'] = np.arange(manual_tof_cut[0]+0.01,manual_tof_cut[1]-0.01,0.02)\n\n # fit gaussian to time distribution using unbinned maximum likelihood estimation\n def NLL_1D(mean, sig):\n '''Negative log likelihood function for (n=1)-dimensional Gaussian distribution.'''\n return( -np.sum(norm.logpdf(x=data_t,\n loc=mean,\n scale=sig)) )\n\n def Start_Par(data):\n '''Starting parameter based on simple mean of 1D numpy array.'''\n return(np.array([data.mean(), # meanx\n data.std()])) #rho\n \n # minimize negative log likelihood function first for the symmetric case\n data_t = df_list[df_nr][(df_list[df_nr].time > bin_time_df[df_nr].time[bin_time_df[df_nr].counts.idxmax()] - 1.0) &\n (df_list[df_nr].time < bin_time_df[df_nr].time[bin_time_df[df_nr].counts.idxmax()] + 1.0)].time.to_numpy()\n\n result_t[df_nr] = Minuit(NLL_1D, mean=Start_Par(data_t)[0], sig=Start_Par(data_t)[1])\n result_t[df_nr].errors = (0.1, 0.1) # initital step size\n result_t[df_nr].limits =[(None, None), (None, None)] # fit ranges\n result_t[df_nr].errordef = Minuit.LIKELIHOOD # MLE definition (instead of Minuit.LEAST_SQUARES)\n result_t[df_nr].migrad() # finds minimum of mle function\n result_t[df_nr].hesse() # computes errors\n for p in result_t[df_nr].parameters:\n print(\"{} = {:3.5f} +/- {:3.5f}\".format(p, result_t[df_nr].values[p], result_t[df_nr].errors[p]))\n \n\n ##############################\n ### VISUALIZE TOF DISTRIBUTION # kind='bar' is VERY time consuming -> use kind='line' instead!\n ##############################\n \n \n # whole distribution\n bin_time_df[df_nr].plot(x='time', y='counts', kind='line', xticks=np.arange(manual_tof_cut[0],manual_tof_cut[1]+1,5), ax=axes[df_nr,0])\n\n # reduced peak plus fit\n bin_time_df[df_nr][bin_time_df[df_nr].counts.idxmax()-50:bin_time_df[df_nr].counts.idxmax()+50].plot(x='time', y='counts', kind='line', ax=axes[df_nr,1])\n pdf_x = np.arange(bin_time_df[df_nr].time[bin_time_df[df_nr].counts.idxmax()-50],\n bin_time_df[df_nr].time[bin_time_df[df_nr].counts.idxmax()+51],\n (bin_time_df[df_nr].time[bin_time_df[df_nr].counts.idxmax()+51]\n -bin_time_df[df_nr].time[bin_time_df[df_nr].counts.idxmax()-50])/100)\n pdf_y = norm.pdf(pdf_x, result_t[df_nr].values['mean'], result_t[df_nr].values['sig'])\n axes[df_nr,0].plot(pdf_x, pdf_y/pdf_y.max()*bin_time_df[df_nr].counts.max(), 'r', label='PDF')\n axes[df_nr,1].plot(pdf_x, pdf_y/pdf_y.max()*bin_time_df[df_nr].counts.max(), 'r', label='PDF')\n\n # mark events in t that will be cut away (+/- 3 sigma = 99.73% of data)\n bin_time_df[df_nr][(bin_time_df[df_nr].time < result_t[df_nr].values['mean'] - 3*result_t[df_nr].values['sig']) |\n (bin_time_df[df_nr].time > result_t[df_nr].values['mean'] + 3*result_t[df_nr].values['sig'])].plot(x='time', y='counts', kind='scatter', ax=axes[df_nr,0], c='y', marker='x', s=50, label='excluded')\n bin_time_df[df_nr][(bin_time_df[df_nr].time < result_t[df_nr].values['mean'] - 3*result_t[df_nr].values['sig']) |\n (bin_time_df[df_nr].time > result_t[df_nr].values['mean'] + 3*result_t[df_nr].values['sig'])].plot(x='time', y='counts', kind='scatter', ax=axes[df_nr,1], c='y', marker='x', s=50, label='excluded')\n \n # legend title shows total number of events and reduced number of events\n axes[df_nr,0].legend(title='total: {}'.format(bin_time_df[df_nr].counts.sum()),loc='upper right', fontsize=16)\n axes[df_nr,1].legend(title='considered: {}'.format(bin_time_df[df_nr].counts.sum()-bin_time_df[df_nr][(bin_time_df[df_nr].time < result_t[df_nr].values['mean'] - 3*result_t[df_nr].values['sig']) |\n (bin_time_df[df_nr].time > result_t[df_nr].values['mean'] + 3*result_t[df_nr].values['sig'])].counts.sum()),loc='upper left', fontsize=16)\n\n \n ##############################\n ### APPYING ALL CUTS\n ##############################\n \n \n # cutting in t: mean +/- 5 sigma\n cut_df[df_nr] = df_list[df_nr][(df_list[df_nr].time > (result_t[df_nr].values['mean'] - 5*result_t[df_nr].values['sig']))&\n (df_list[df_nr].time < (result_t[df_nr].values['mean'] + 5*result_t[df_nr].values['sig']))]\n len1 = cut_df[df_nr].shape[0]\n \n # applying manual cut in x and y:\n if df_nr < 2: # only for p1 and p2, not for c \n cut_df[df_nr] = cut_df[df_nr][((cut_df[df_nr].x-manual_space_cut[file_name][0])**2 + (cut_df[df_nr].y-manual_space_cut[file_name][2])**2) <\n manual_space_cut[file_name][1]*manual_space_cut[file_name][3]]\n len2 = cut_df[df_nr].shape[0]\n\n # applyig automatic cut in x and y: mean +/- 3 std in an ellipsoidal cut\n cut_df[df_nr] = cut_df[df_nr][((cut_df[df_nr].x-cut_df[df_nr].x.mean())**2 + (cut_df[df_nr].y-cut_df[df_nr].y.mean())**2) <\n 3*cut_df[df_nr].x.std()*3*cut_df[df_nr].y.std()]\n len3 = cut_df[df_nr].shape[0]\n\n # applying automatic z-class-cut (= cut by number of ions per event) for z>5 ions per event to reduce space-charge effects:\n cut_df[df_nr] = cut_df[df_nr][cut_df[df_nr].event.isin(cut_df[df_nr].event.value_counts()[cut_df[df_nr].event.value_counts() <= 6].index)]\n \n # printing the reduction of the number of ions per file in each of the cut steps\n print('\\n{}: data size: {} -> time cut: {} -> manual space cut: {} -> automatic space cut: {} -> z-class-cut: {}\\n'.format(pattern[df_nr], df_list[df_nr].shape[0], len1, len2, len3, cut_df[df_nr].shape[0]))\n\n # saves excluded data (allows visual checking later)\n excludes_df[df_nr] = pd.concat([df_list[df_nr], cut_df[df_nr]]).drop_duplicates(keep=False).reset_index(drop=True)\n\nplt.savefig('{}-tof.pdf'.format(file_name))\nplt.show()",
"mean = 25.54630 +/- 0.01769\nsig = 0.27570 +/- 0.01251\n\np1: data size: 248 -> time cut: 244 -> manual space cut: 239 -> automatic space cut: 235 -> z-class-cut: 235\n\nmean = 25.55908 +/- 0.01934\nsig = 0.32012 +/- 0.01367\n\np2: data size: 283 -> time cut: 281 -> manual space cut: 266 -> automatic space cut: 265 -> z-class-cut: 265\n\nmean = 25.54692 +/- 0.01284\nsig = 0.27694 +/- 0.00908\n\nc: data size: 480 -> time cut: 477 -> manual space cut: 477 -> automatic space cut: 474 -> z-class-cut: 359\n\n"
]
],
[
[
"### Spot fitting\n\n2D multivariate gaussian maximum likelihood estimations of the cleaned pattern 1, pattern 2 and center spot positions are performed SciPy PDF's and ROOT's minimizer. Displayed are all uncut data with a blue-transparent point. This allows displaying a density of points by the shade of blue without the need of binning the data (= reducing the information; also: binning is much more time-consuming). The cut data is displayed with a black \"x\" at the position of the blue point. These points are not considered in the fit (represented by the red (6-$\\sigma$ band) but allow for an additional check of the cutting functions. The scale of the MCP-position plots is given in the time unit of the position-sensitive MCP data. There is no need in converting it into a mm-unit since one is only interested in the angle. ",
"_____no_output_____"
]
],
[
[
"%config InlineBackend.figure_format ='retina'\n# activate interactive matplotlib plot -> uncomment line below!\n# %matplotlib notebook\n\nimport pickle, os\nfrom scipy.stats import multivariate_normal, linregress, pearsonr\nfrom scipy.optimize import minimize\nimport numpy as np\nfrom iminuit import Minuit\n\n# open preanalyzed dataset if existing\nif os.path.isfile('data/data-save.p'):\n analysis = pickle.load(open('data/data-save.p','rb'))\n\ndf_list = [df_p1, df_p2, df_c]\nresult = [{},{},{}]\nroot_res = [0,0,0]\nparameters = ['meanx', 'meany', 'sigx', 'sigy', 'theta']\nfig2, axes2 = plt.subplots(nrows=3, ncols=1, figsize=(7.5, 20))\npiicr_scheme_names = ['p1','p2','c']\n\n\n##############################\n### Prepare maximum likelihood estimation\n##############################\n\n\ndef Rot(theta):\n '''Rotation (matrix) of angle theta to cartesian coordinates.'''\n return np.array([[np.cos(theta), -np.sin(theta)],\n [np.sin(theta), np.cos(theta)]])\n\ndef NLL_2D(meanx, meany, sigx, sigy, theta):\n '''Negative log likelihood function for (n=2)-dimensional Gaussian distribution for Minuit.'''\n cov = Rot(theta) @ np.array([[np.power(sigx,2),0],[0,np.power(sigy,2)]]) @ Rot(theta).T\n return( -np.sum(multivariate_normal.logpdf(x=data,\n mean=np.array([meanx, meany]),\n cov=cov,\n allow_singular=True)) )\n\ndef NLL_2D_scipy(param):\n '''Negative log likelihood function for (n=2)-dimensional Gaussian distribution for SciPy.'''\n meanx, meany, sigx, sigy, theta = param\n cov = Rot(theta) @ np.array([[np.power(sigx,2),0],[0,np.power(sigy,2)]]) @ Rot(theta).T\n return( -np.sum(multivariate_normal.logpdf(x=data,\n mean=np.array([meanx, meany]),\n cov=cov,\n allow_singular=True)) )\n\ndef Start_Par(data):\n '''Starting parameter based on simple linear regression and 2D numpy array.'''\n # simple linear regression to guess the rotation angle based on slope\n slope, intercept, r_value, p_value, std_err = linregress(data[:, 0], data[:, 1])\n theta_guess = -np.arctan(slope)\n\n # data rotated based on theta guess\n data_rotated_guess = np.dot(Rot(theta_guess), [data[:,0], data[:,1]])\n \n first_guess = np.array([data[:,0].mean()+0.2, # meanx\n data[:,1].mean()+0.2, # meany\n data_rotated_guess[1].std(), # sigma-x\n data_rotated_guess[0].std(), # sigma-y\n theta_guess]) # rot. angle based on slope of lin. reg.\n \n # based on a first guess, a minimization based on a robust simplex is performed\n start_par = minimize(NLL_2D_scipy, first_guess, method='Nelder-Mead')\n return(start_par['x'])\n\n\n##############################\n### Fitting and visualization of P1, P2, C\n##############################\n\n\nfor df_nr in range(len(df_list)):\n \n # minimize negative log likelihood function first for the symmetric case\n data = cut_df[df_nr][['x', 'y']].to_numpy()\n root_res[df_nr] = Minuit(NLL_2D, meanx=Start_Par(data)[0], meany=Start_Par(data)[1],\n sigx=Start_Par(data)[2], sigy=Start_Par(data)[3],\n theta=Start_Par(data)[4])\n root_res[df_nr].errors = (0.1, 0.1, 0.1, 0.1, 0.1) # initital step size\n root_res[df_nr].limits =[(None, None), (None, None), (None, None), (None, None), (None, None)] # fit ranges\n root_res[df_nr].errordef = Minuit.LIKELIHOOD # MLE definition (instead of Minuit.LEAST_SQUARES)\n root_res[df_nr].migrad() # finds minimum of mle function\n root_res[df_nr].hesse() # computes errors\n \n # plotting of data, excluded data, reference MCP circle, and fit results\n axes2[df_nr].plot(df_list[df_nr].x.to_numpy(),df_list[df_nr].y.to_numpy(),'o',alpha=0.15,label='data',zorder=0)\n axes2[df_nr].plot(excludes_df[df_nr].x.to_numpy(), excludes_df[df_nr].y.to_numpy(), 'x k',\n label='excluded data',zorder=1)\n\n mcp_circ = mpl.patches.Ellipse((0,0), 1500, 1500, edgecolor='k', fc='None', lw=2)\n axes2[df_nr].add_patch(mcp_circ)\n axes2[df_nr].scatter(root_res[df_nr].values['meanx'], root_res[df_nr].values['meany'], marker='o', color=col[1], linewidth=0, zorder=2)\n sig = mpl.patches.Ellipse((root_res[df_nr].values['meanx'], root_res[df_nr].values['meany']),\n 3*root_res[df_nr].values['sigx'], 3*root_res[df_nr].values['sigy'],\n np.degrees(root_res[df_nr].values['theta']),\n edgecolor=col[1], fc='None', lw=2, label='6-$\\sigma$ band (fit)', zorder=2)\n axes2[df_nr].add_patch(sig)\n\n\n axes2[df_nr].legend(title='fit(x) = {:1.0f}({:1.0f})\\nfit(y) = {:1.0f}({:1.0f})'.format(root_res[df_nr].values['meanx'],root_res[df_nr].errors['meanx'],\n root_res[df_nr].values['meany'],root_res[df_nr].errors['meany']),\n loc='lower left', fontsize=14)\n axes2[df_nr].axis([-750,750,-750,750])\n axes2[df_nr].grid(True)\n axes2[df_nr].text(-730, 660, '{}: {}'.format(file_name.split('/',1)[-1], piicr_scheme_names[df_nr]))\n plt.tight_layout()\n\n \n # save fit information for each parameter:\n # 'parameter': [fitresult, fiterror, Hesse-covariance matrix]\n for i in range(len(parameters)):\n result[df_nr].update({'{}'.format(parameters[i]): [np.array(root_res[df_nr].values)[i],\n np.array(root_res[df_nr].errors)[i],\n root_res[df_nr].covariance]})\n if 'ground' in file_name:\n analysis['ioi_g'][file_name]['fit-{}'.format(piicr_scheme_names[df_nr])] = result[df_nr]\n elif 'isomer' in file_name:\n analysis['ioi_m'][file_name]['fit-{}'.format(piicr_scheme_names[df_nr])] = result[df_nr]\n else:\n analysis['ref'][file_name]['fit-{}'.format(piicr_scheme_names[df_nr])] = result[df_nr]\n\nplt.savefig('{}-fit.pdf'.format(file_name))\nplt.show()\n\n# save all data using pickle\npickle.dump(analysis, open('data/data-save.p','wb'))",
"'LinguisticsPro-Italic.otf' can not be subsetted into a Type 3 font. The entire font will be embedded in the output.\n'LinguisticsPro-Regular.otf' can not be subsetted into a Type 3 font. The entire font will be embedded in the output.\n"
]
],
[
[
"\n---\n# !!! <font color='red'>REPEAT</font> CODE ABOVE FOR ALL INDIVIDUAL FILES !!!\n---\n<br>\n<br>",
"_____no_output_____"
],
[
"### Save fit data to dataframe and *.csv file\n\n<br>Continue here after analyzing all files individually. The following command saves all necessary data and fit information in a `*.csv` file.",
"_____no_output_____"
]
],
[
[
"calc_df = pd.DataFrame()\nfor key in analysis.keys():\n for subkey in analysis[key].keys():\n if '_c_' not in subkey:\n calc_df = calc_df.append(pd.DataFrame({'file': subkey,\n 'p1_x': analysis[key][subkey]['fit-p1']['meanx'][0],\n 'p1_y': analysis[key][subkey]['fit-p1']['meany'][0],\n 'p2_x': analysis[key][subkey]['fit-p2']['meanx'][0],\n 'p2_y': analysis[key][subkey]['fit-p2']['meany'][0],\n 'c_x': analysis[key][subkey]['fit-c']['meanx'][0],\n 'c_y': analysis[key][subkey]['fit-c']['meany'][0],\n 'p1_x_unc': analysis[key][subkey]['fit-p1']['meanx'][1],\n 'p1_y_unc': analysis[key][subkey]['fit-p1']['meany'][1],\n 'p2_x_unc': analysis[key][subkey]['fit-p2']['meanx'][1],\n 'p2_y_unc': analysis[key][subkey]['fit-p2']['meany'][1],\n 'c_x_unc': analysis[key][subkey]['fit-c']['meanx'][1],\n 'c_y_unc': analysis[key][subkey]['fit-c']['meany'][1],\n 'cyc_freq_guess': analysis[key][subkey]['cyc_freq'],\n 'red_cyc_freq': analysis[key][subkey]['red_cyc_freq'],\n 'mag_freq': analysis[key][subkey]['mag_freq'],\n 'cyc_acc_time': analysis[key][subkey]['cyc_acc_time'],\n 'n_acc': analysis[key][subkey]['n_acc'],\n 'time_start': pd.to_datetime('{} {}'.format(analysis[key][subkey]['time-info'][0], analysis[key][subkey]['time-info'][1]), format='%m/%d/%Y %H:%M:%S', errors='ignore'),\n 'time_end': pd.to_datetime('{} {}'.format(analysis[key][subkey]['time-info'][2], analysis[key][subkey]['time-info'][3]), format='%m/%d/%Y %H:%M:%S', errors='ignore')}, index=[0]), ignore_index=True)\ncalc_df.to_csv('data/analysis-summary.csv')\ncalc_df",
"_____no_output_____"
]
],
[
[
"### Calculate $\\nu_c$ from position fits\n\n[1]: https://doi.org/10.1007/s00340-013-5621-0\n[2]: https://doi.org/10.1103/PhysRevLett.110.082501\n[3]: https://doi.org/10.1007/s10751-019-1601-z\n\nCan be run independently from everything above by loading the `analysis-summary.csv` file!<br> A detailed description of the $\\nu_c$ calculation can be found in Ref. [1], [2] and [3].",
"_____no_output_____"
]
],
[
[
"import pandas as pd\nimport numpy as np\n\n# load fit-data file, datetime has to be converted\ncalc_df = pd.read_csv('data/analysis-summary.csv', header=0, index_col=0)\n\n# calculate angle between the P1-vector (P1_x/y - C_x/y) and the P2-vector (P2_x/y - C_x/y)\ncalc_df['p1p2_angle'] = np.arctan2(calc_df.p1_y - calc_df.c_y, calc_df.p1_x - calc_df.c_x) \\\n - np.arctan2(calc_df.p2_y - calc_df.c_y, calc_df.p2_x - calc_df.c_x)\n \n# calculate the uncertainty on the angle between the P1/P2 vectors\n# see https://en.wikipedia.org/wiki/Atan2\ncalc_df['p1p2_angle_unc'] = np.sqrt(\n ( calc_df.p1_x_unc * (calc_df.c_y - calc_df.p1_y) / ( (calc_df.p1_x - calc_df.c_x)**2 + (calc_df.p1_y - calc_df.c_y)**2 ) )**2\n + ( calc_df.p1_y_unc * (calc_df.p1_x - calc_df.c_x) / ( (calc_df.p1_x - calc_df.c_x)**2 + (calc_df.p1_y - calc_df.c_y)**2 ) )**2\n + ( calc_df.p2_x_unc * (calc_df.c_y - calc_df.p2_y) / ( (calc_df.p2_x - calc_df.c_x)**2 + (calc_df.p2_y - calc_df.c_y)**2 ) )**2\n + ( calc_df.p2_y_unc * (calc_df.p2_x - calc_df.c_x) / ( (calc_df.p2_x - calc_df.c_x)**2 + (calc_df.p2_y - calc_df.c_y)**2 ) )**2\n + ( calc_df.c_x_unc *\n ( -(calc_df.c_y - calc_df.p1_y) / ( (calc_df.p1_x - calc_df.c_x)**2 + (calc_df.p1_y - calc_df.c_y)**2 ) \n -(calc_df.c_y - calc_df.p2_y) / ( (calc_df.p2_x - calc_df.c_x)**2 + (calc_df.p2_y - calc_df.c_y)**2 ) ) )**2\n + ( calc_df.c_y_unc *\n ( (calc_df.p1_x - calc_df.c_x) / ( (calc_df.p1_x - calc_df.c_x)**2 + (calc_df.p1_y - calc_df.c_y)**2 ) \n +(calc_df.p2_x - calc_df.c_x) / ( (calc_df.p2_x - calc_df.c_x)**2 + (calc_df.p2_y - calc_df.c_y)**2 ) ) )**2 )\n\n# calculate cyc freq: total phase devided by total time\ncalc_df['cyc_freq'] = (calc_df.p1p2_angle + 2*np.pi * calc_df.n_acc) / (2*np.pi * calc_df.cyc_acc_time * 0.000001)\ncalc_df['cyc_freq_unc'] = calc_df.p1p2_angle_unc / (2*np.pi * calc_df.cyc_acc_time * 0.000001)\n\ncalc_df.to_csv('data/analysis-summary.csv')",
"_____no_output_____"
],
[
"calc_df.head()",
"_____no_output_____"
]
],
[
[
"### Frequency-ratio calculation\n\n[1]: https://doi.org/10.1007/s00340-013-5621-0\n[2]: https://doi.org/10.1103/PhysRevLett.110.082501\n[3]: https://doi.org/10.1007/s10751-019-1601-z\n\nIn order to determine the frequency ratio between the ioi and the ref, simultaneous fits of all for the data set possible polynomial degrees are performed. The code calculates the reduced $\\chi^2_{red}$ for each fit and returns only the one with a $\\chi^2_{red}$ closest to 1. A detailed description of the procedure can be found in Ref. [3]. If problems in the fitting occur, please try to vary the starting parameter section in lines 125-135 of `~/bin/freq_ratio.py`",
"_____no_output_____"
]
],
[
[
"import pandas as pd\nimport numpy as np\nfrom bin.freq_ratio import Freq_ratio\nfreq = Freq_ratio()\n\n# load fit-data file\ncalc_df = pd.read_csv('data/analysis-summary.csv', header=0, index_col=0)\n\n# save average time of measurement: t_start+(t_end-t_start)/2\ncalc_df.time_start = pd.to_datetime(calc_df.time_start)\ncalc_df.time_end = pd.to_datetime(calc_df.time_end)\ncalc_df['time'] = calc_df.time_start + (calc_df.time_end - calc_df.time_start)/2\ncalc_df.to_csv('data/analysis-summary.csv')\n\n# convert avg.time to difference in minutes from first measurement -> allows fitting with small number as x value\ncalc_df['time_delta'] = ((calc_df['time']-calc_df['time'].min())/np.timedelta64(1, 's')/60)\n\n# selecting data for isotopes\ndf_ioi_g = calc_df[calc_df.file.str.contains('ground')][['time_delta','cyc_freq','cyc_freq_unc','time','file']]\ndf_ioi_m = calc_df[calc_df.file.str.contains('isomer')][['time_delta','cyc_freq','cyc_freq_unc','time','file']]\n\n# allows to define a subset of reference frequencies for ground and isomer\ndf_ref_g = calc_df[calc_df.file.str.contains('ref')][['time_delta','cyc_freq','cyc_freq_unc','time','file']]\ndf_ref_m = calc_df[calc_df.file.str.contains('ref')][['time_delta','cyc_freq','cyc_freq_unc','time','file']]\n\n# simultaneous polynomial fit, see https://doi.org/10.1007/s10751-019-1601-z\nfit1, fit2, ratio1, ratio_unc1, chi_sq1 = freq.ratio_sim_fit(['ref', 'ioi_g'],\n df_ref_g.time_delta.tolist(),\n df_ref_g.cyc_freq.tolist(),\n df_ref_g.cyc_freq_unc.tolist(),\n df_ioi_g.time_delta.tolist(),\n df_ioi_g.cyc_freq.tolist(),\n df_ioi_g.cyc_freq_unc.tolist())\nif len(df_ioi_m) > 0:\n fit3, fit4, ratio2, ratio_unc2, chi_sq2 = freq.ratio_sim_fit(['ref', 'ioi_m'],\n df_ref_m.time_delta.tolist(),\n df_ref_m.cyc_freq.tolist(),\n df_ref_m.cyc_freq_unc.tolist(),\n df_ioi_m.time_delta.tolist(),\n df_ioi_m.cyc_freq.tolist(),\n df_ioi_m.cyc_freq_unc.tolist())",
"[-10, 685181.1403450029, 1]\nPoly-degree: 2\n\nRed.Chi.Sq.: 0.6548141372439115\nCorellation: 2.7799756249708774e-12\nRatio fit parameter: 0.6388872125439365 +/- 2.1557485311110636e-09\n[1, -10, 685181.1403450029, 1]\nPoly-degree: 3\n\nRed.Chi.Sq.: 0.7550362063128235\n"
]
],
[
[
"### Frequency-ratio plotting",
"_____no_output_____"
]
],
[
[
"%config InlineBackend.figure_format ='retina'\nimport matplotlib.pyplot as plt\nimport matplotlib as mpl\nimport pandas as pd\nimport numpy as np\nmpl.rc('font', family='serif', serif='Linguistics Pro') # open source Utopia LaTeX font with greek letters\nmpl.rc('text', usetex=False)\nmpl.rc('mathtext', fontset='custom',\n rm='Linguistics Pro',\n it='Linguistics Pro:italic',\n bf='Linguistics Pro:bold')\nmpl.rcParams.update({'font.size': 18})\n\n# prepare fit data\nx1 = np.linspace(min([df_ioi_g.time_delta.min(),df_ref_g.time_delta.min()]),max([df_ioi_g.time_delta.max(),df_ref_g.time_delta.max()]),500)\nt1 = pd.date_range(pd.Series([df_ioi_g.time.min(),df_ref_g.time.min()]).min(),pd.Series([df_ioi_g.time.max(),df_ref_g.time.max()]).max(),periods=500)\nif len(df_ioi_m) > 0:\n x2 = np.linspace(min([df_ioi_m.time_delta.min(),df_ref_m.time_delta.min()]),max([df_ioi_m.time_delta.max(),df_ref_m.time_delta.max()]),500)\n t2 = pd.date_range(pd.Series([df_ioi_m.time.min(),df_ref_m.time.min()]).min(),pd.Series([df_ioi_m.time.max(),df_ref_m.time.max()]).max(),periods=500)\n\nfit1_y = [np.polyval(fit1, i) for i in x1]\nfit2_y = [np.polyval(fit2, i) for i in x1]\nif len(df_ioi_m) > 0:\n fit3_y = [np.polyval(fit3, i) for i in x2]\n fit4_y = [np.polyval(fit4, i) for i in x2]\n\n\n#########################\n### PLOTTING ground state\n#########################\n\nif len(df_ioi_m) > 0:\n fig, (ax1, ax3) = plt.subplots(figsize=(9,12),nrows=2, ncols=1)\nelse:\n fig, ax1 = plt.subplots(figsize=(9,6),nrows=1, ncols=1)\n\nax1.errorbar(df_ref_g.time, df_ref_g.cyc_freq, yerr=df_ref_g.cyc_freq_unc, fmt='o', label='{}'.format(latex_ref), marker='d', c='#1E77B4', ms=10, elinewidth=2.5)\nax1.set_xlabel('Time', fontsize=24, fontweight='bold')\n# Make the y-axis label, ticks and tick labels match the line color.\nax1.set_ylabel('Frequency (Hz)', fontsize=24, fontweight='bold')\nax1.tick_params('y', colors='#1E77B4')\nax1.plot(t1, fit1_y, ls=(5.5, (5, 1, 1, 1, 1, 1, 1, 1)),c='#1E77B4', label='poly-fit')\n# Allowing two axes in one subplot\nax2 = ax1.twinx()\nax2.errorbar(df_ioi_g.time, df_ioi_g.cyc_freq, yerr=df_ioi_g.cyc_freq_unc, fmt='o', color='#D62728', label='{}'.format(latex_ioi_g), fillstyle='none', ms=10, elinewidth=2.5) # green: #2ca02c\nax2.tick_params('y', colors='#D62728')\nax2.plot(t1, fit2_y, ls=(0, (5, 3, 1, 3)),c='#D62728', label='poly-fit')\n\n# adjust the y axes to be the same height\nmiddle_y1 = df_ref_g.cyc_freq.min() + (df_ref_g.cyc_freq.max() - df_ref_g.cyc_freq.min())/2\nmiddle_y2 = df_ioi_g.cyc_freq.min() + (df_ioi_g.cyc_freq.max() - df_ioi_g.cyc_freq.min())/2\nrange_y1 = df_ref_g.cyc_freq.max() - df_ref_g.cyc_freq.min() + 2 * df_ref_g.cyc_freq_unc.max()\nrange_y2 = df_ioi_g.cyc_freq.max() - df_ioi_g.cyc_freq.min() + 2 * df_ioi_g.cyc_freq_unc.max()\nax1.set_ylim(middle_y1 - 1.3 * max([range_y1, middle_y1*range_y2/middle_y2])/2, middle_y1 + 1.1 * max([range_y1, middle_y1*range_y2/middle_y2])/2) # outliers only\nax2.set_ylim(middle_y2 - 1.1 * max([middle_y2*range_y1/middle_y1, range_y2])/2, middle_y2 + 1.3 * max([middle_y2*range_y1/middle_y1, range_y2])/2) # most of the data\n\n# plotting only hours without the date\nax2.xaxis.set_major_formatter(mpl.dates.DateFormatter('%H:%M'))\nax2.xaxis.set_minor_locator(mpl.dates.HourLocator())\n\nhandles1, labels1 = ax1.get_legend_handles_labels()\nhandles2, labels2 = ax2.get_legend_handles_labels()\nhandles_g = [handles1[1], handles2[1], (handles1[0], handles2[0])]\nlabels_g = [labels1[1], labels2[1], labels1[0]]\nplt.legend(handles=handles_g, labels=labels_g,fontsize=18,title='Ratio: {:1.10f}\\n $\\\\pm${:1.10f}'.format(ratio1, ratio_unc1), loc='upper right')\nplt.text(0.03,0.03,'poly-{}: $\\chi^2_{{red}}$ {:3.2f}'.format(len(fit1)-1, chi_sq1),transform=ax1.transAxes)\n\n\n###########################\n### PLOTTING isomeric state\n###########################\n\nif len(df_ioi_m) > 0:\n ax3.errorbar(df_ref_m.time, df_ref_m.cyc_freq, yerr=df_ref_m.cyc_freq_unc, fmt='o', label='{}'.format(latex_ref), marker='d', c='#1E77B4', ms=10, elinewidth=2.5)\n ax3.set_xlabel('Time', fontsize=24, fontweight='bold')\n # Make the y-axis label, ticks and tick labels match the line color.\n ax3.set_ylabel('Frequency (Hz)', fontsize=24, fontweight='bold')\n ax3.tick_params('y', colors='#1E77B4')\n ax3.plot(t2, fit3_y, ls=(5.5, (5, 1, 1, 1, 1, 1, 1, 1)),c='#1E77B4', label='poly-fit')\n # Allowing two axes in one subplot\n ax4 = ax3.twinx()\n ax4.errorbar(df_ioi_m.time, df_ioi_m.cyc_freq, yerr=df_ioi_m.cyc_freq_unc, fmt='o', color='#D62728', label='{}'.format(latex_ioi_m), fillstyle='none', ms=10, elinewidth=2.5) # green: #2ca02c\n ax4.tick_params('y', colors='#D62728')\n ax4.plot(t2, fit4_y, ls=(0, (5, 3, 1, 3)),c='#D62728', label='poly-fit')\n\n # adjust the y axes to be the same height\n middle_y3 = df_ref_m.cyc_freq.min() + (df_ref_m.cyc_freq.max() - df_ref_m.cyc_freq.min())/2\n middle_y4 = df_ioi_m.cyc_freq.min() + (df_ioi_m.cyc_freq.max() - df_ioi_m.cyc_freq.min())/2\n range_y3 = df_ref_m.cyc_freq.max() - df_ref_m.cyc_freq.min() + 2 * df_ref_m.cyc_freq_unc.max()\n range_y4 = df_ioi_m.cyc_freq.max() - df_ioi_m.cyc_freq.min() + 2 * df_ioi_m.cyc_freq_unc.max()\n ax3.set_ylim(middle_y3 - 1.3 * max([range_y3, middle_y3*range_y4/middle_y4])/2, middle_y3 + 1.1 * max([range_y3, middle_y3*range_y4/middle_y4])/2) # outliers only\n ax4.set_ylim(middle_y4 - 1.1 * max([middle_y4*range_y3/middle_y3, range_y4])/2, middle_y4 + 1.3 * max([middle_y4*range_y3/middle_y3, range_y4])/2) # most of the data\n\n # plotting only hours without the date\n ax4.xaxis.set_major_formatter(mpl.dates.DateFormatter('%H:%M'))\n ax4.xaxis.set_minor_locator(mpl.dates.HourLocator())\n\n handles3, labels3 = ax3.get_legend_handles_labels()\n handles4, labels4 = ax4.get_legend_handles_labels()\n handles_m = [handles3[1], handles4[1], (handles3[0], handles4[0])]\n labels_m = [labels3[1], labels4[1], labels3[0]]\n plt.legend(handles=handles_m, labels=labels_m, fontsize=18,title='Ratio: {:1.10f}\\n $\\\\pm${:1.10f}'.format(ratio2, ratio_unc2), loc='upper right')\n plt.text(0.03,0.03,'poly-{}: $\\chi^2_{{red}}$ {:3.2f}'.format(len(fit3)-1, chi_sq2),transform=ax3.transAxes)\n\nplt.tight_layout()\nplt.savefig('data/freq-ratios.pdf')\nplt.show()",
"'LinguisticsPro-Bold.otf' can not be subsetted into a Type 3 font. The entire font will be embedded in the output.\n'LinguisticsPro-Italic.otf' can not be subsetted into a Type 3 font. The entire font will be embedded in the output.\n'LinguisticsPro-Regular.otf' can not be subsetted into a Type 3 font. The entire font will be embedded in the output.\n"
]
]
] | [
"markdown",
"code",
"markdown",
"code",
"markdown",
"code",
"markdown",
"code",
"markdown",
"code",
"markdown",
"code",
"markdown",
"code",
"markdown",
"code",
"markdown",
"code",
"markdown",
"code",
"markdown",
"code",
"markdown",
"code"
] | [
[
"markdown"
],
[
"code"
],
[
"markdown"
],
[
"code"
],
[
"markdown"
],
[
"code"
],
[
"markdown"
],
[
"code"
],
[
"markdown"
],
[
"code"
],
[
"markdown"
],
[
"code"
],
[
"markdown"
],
[
"code"
],
[
"markdown"
],
[
"code"
],
[
"markdown",
"markdown"
],
[
"code"
],
[
"markdown"
],
[
"code",
"code"
],
[
"markdown"
],
[
"code"
],
[
"markdown"
],
[
"code"
]
] |
d07722d9a7fcb092c667b4e601b0ef7978977e2b | 13,547 | ipynb | Jupyter Notebook | notebooks/1-intro-to-strings.ipynb | mchesterkadwell/bughunt-analysis | 0ba81243b7562a3c938eeed7b74edc9a5061bbcd | [
"MIT"
] | 5 | 2019-02-08T14:49:34.000Z | 2022-01-08T17:15:47.000Z | notebooks/1-intro-to-strings.ipynb | mchesterkadwell/bughunt-analysis | 0ba81243b7562a3c938eeed7b74edc9a5061bbcd | [
"MIT"
] | 9 | 2020-03-24T16:45:43.000Z | 2022-03-11T23:41:15.000Z | notebooks/1-intro-to-strings.ipynb | mchesterkadwell/bughunt-analysis | 0ba81243b7562a3c938eeed7b74edc9a5061bbcd | [
"MIT"
] | null | null | null | 24.234347 | 347 | 0.558721 | [
[
[
"# Introdution to Jupyter Notebooks and Text Processing in Python\nThis 'document' is a Jupyter notebook. It allows you to combine explanatory **text** and **code** that executes to produce results you can see on the same page.\n\n## Notebook Basics\n\n### Text cells\n\nThe box this text is written in is called a *cell*. It is a *text cell* written in a very simple markup language called 'Markdown'. Here is a useful [Markdown cheatsheet](https://github.com/adam-p/markdown-here/wiki/Markdown-Cheatsheet). You can edit and then run cells to produce a result. Running this text cell produces formatted text.\n\n### Code cells\n\nThe other main kind of cell is a *code cell*. The cell immediately below this one is a code cell. Running a code cell runs the code in the cell and produces a result.",
"_____no_output_____"
]
],
[
[
"# This is a comment in a code cell. Comments start with a # symbol. They are ignored and do not do anything.",
"_____no_output_____"
],
[
"# This box is a code cell. When this cell is run, the code below will execute and produce a result\n3 + 4",
"_____no_output_____"
]
],
[
[
"## Simple String Manipulation in Python\nThis section introduces some very basic things you can do in Python to create and manipulate *strings*. A string is a simple sequence of characters, like `flabbergast`. This introduction is limited to those things that may be useful to know in order to understand the *Bughunt!* data mining in the following two notebooks.",
"_____no_output_____"
],
[
"### Creating and Storing Strings in Variables\nStrings are simple to create in Python. You can simply write some characters in quote marks.",
"_____no_output_____"
]
],
[
[
"'Butterflies are important as pollinators.'",
"_____no_output_____"
]
],
[
[
"In order to do something useful with this string, other than print it out, we need to store in a *variable* by using the assignment operator `=` (equals sign). Whatever is on the right-hand side of the `=` is stored into a variable with the name on the left-hand side.",
"_____no_output_____"
]
],
[
[
"# my_variable is the variable on the left\n# 'manuscripts' is the string on the right that is stored in the variable my_variable\n\nmy_variable = 'Butterflies are important as pollinators.'",
"_____no_output_____"
]
],
[
[
"Notice that nothing is printing to the screen. That's because the string is stored in the variable `my_variable`. In order to see what is inside the variable `my_variable` we can simply write `my_variable` in a code cell, run it, and the interpreter will print it out for us.",
"_____no_output_____"
]
],
[
[
"my_variable",
"_____no_output_____"
]
],
[
[
"### Manipulating Bits of Strings\n\n#### Accessing Individual Characters\nA strings is just a sequence (or list) of characters. You can access **individual characters** in a string by specifying which ones you want in square brackets. If you want the first character you specify `1`.",
"_____no_output_____"
]
],
[
[
"my_variable[1]",
"_____no_output_____"
]
],
[
[
"Hang on a minute! Why did it give us `u` instead of `B`?\n\nIn programming, everything tends to be *zero indexed*, which means that things are counted from 0 rather than 1. Thus, in the example above, `1` gives us the *second* character in the string.\n\nIf you want the first character in the string, you need to specify the index `0`! ",
"_____no_output_____"
]
],
[
[
"my_variable[0]",
"_____no_output_____"
]
],
[
[
"#### Accessing a Range of Characters\n\nYou can also pick out a **range of characters** from within a string, by giving the *start index* followed by the *end index* with a semi-colon (`:`) in between.\n\nThe example below gives us the character at index `0` all the way up to, *but not including*, the character at index `20`.",
"_____no_output_____"
]
],
[
[
"my_variable[0:20]",
"_____no_output_____"
]
],
[
[
"### Changing Whole Strings with Functions\nPython has some built-in *functions* that allow you to change a whole string at once. You can change all characters to lowercase or uppercase:",
"_____no_output_____"
]
],
[
[
"my_variable.lower()",
"_____no_output_____"
],
[
"my_variable.upper()",
"_____no_output_____"
]
],
[
[
"NB: These functions do not change the original string but create a new one. Our original string is still the same as it was before:",
"_____no_output_____"
]
],
[
[
"my_variable",
"_____no_output_____"
]
],
[
[
"### Testing Strings\n\nYou can also test a string to see if it is passes some test, e.g. is the string all alphabetic characters only?",
"_____no_output_____"
]
],
[
[
"my_variable.isalpha()",
"_____no_output_____"
]
],
[
[
"Does the string have the letter `p` in it?",
"_____no_output_____"
]
],
[
[
"'p' in my_variable",
"_____no_output_____"
]
],
[
[
"### Lists of Strings\nAnother important thing we can do with strings is creating a list of strings by listing them inside square brackets `[]`:",
"_____no_output_____"
]
],
[
[
"my_list = ['Butterflies are important as pollinators',\n 'Butterflies feed primarily on nectar from flowers',\n 'Butterflies are widely used in objects of art']\nmy_list",
"_____no_output_____"
]
],
[
[
"### Manipulating Lists of Strings\nJust like with strings, we can access individual items inside a list by index number:",
"_____no_output_____"
]
],
[
[
"my_list[0]",
"_____no_output_____"
]
],
[
[
"And we can access a range of items inside a list by *slicing*:",
"_____no_output_____"
]
],
[
[
"my_list[0:2]",
"_____no_output_____"
]
],
[
[
"### Advanced: Creating Lists of Strings with List Comprehensions\nWe can create new lists in an elegant way by combining some of the things we have covered above. Here is an example where we have taken our original list `my_list` and created a new list `new_list` by going over each string in the list:",
"_____no_output_____"
]
],
[
[
"new_list = [string for string in my_list]\nnew_list",
"_____no_output_____"
]
],
[
[
"Why do this? If we combine it with a test, we can have a list that only contains strings with the letter `p` in them:",
"_____no_output_____"
]
],
[
[
"new_list_p = [string for string in my_list if 'p' in string]\nnew_list_p",
"_____no_output_____"
]
],
[
[
"This is a very powerful way to quickly create lists. We can even change all the strings to uppercase at the same time!",
"_____no_output_____"
]
],
[
[
"new_list_p_upper = [string.upper() for string in my_list if 'p' in string]\nnew_list_p_upper",
"_____no_output_____"
]
]
] | [
"markdown",
"code",
"markdown",
"code",
"markdown",
"code",
"markdown",
"code",
"markdown",
"code",
"markdown",
"code",
"markdown",
"code",
"markdown",
"code",
"markdown",
"code",
"markdown",
"code",
"markdown",
"code",
"markdown",
"code",
"markdown",
"code",
"markdown",
"code",
"markdown",
"code",
"markdown",
"code",
"markdown",
"code"
] | [
[
"markdown"
],
[
"code",
"code"
],
[
"markdown",
"markdown"
],
[
"code"
],
[
"markdown"
],
[
"code"
],
[
"markdown"
],
[
"code"
],
[
"markdown"
],
[
"code"
],
[
"markdown"
],
[
"code"
],
[
"markdown"
],
[
"code"
],
[
"markdown"
],
[
"code",
"code"
],
[
"markdown"
],
[
"code"
],
[
"markdown"
],
[
"code"
],
[
"markdown"
],
[
"code"
],
[
"markdown"
],
[
"code"
],
[
"markdown"
],
[
"code"
],
[
"markdown"
],
[
"code"
],
[
"markdown"
],
[
"code"
],
[
"markdown"
],
[
"code"
],
[
"markdown"
],
[
"code"
]
] |
d0772c50f07d2f4af5f0ae16167a67d6ff899913 | 3,158 | ipynb | Jupyter Notebook | codes/Untitled.ipynb | mert-kurttutan/qh_fm_01 | b5a56b2a671b3198b5517f0f50b9bb8f9a043df3 | [
"MIT"
] | null | null | null | codes/Untitled.ipynb | mert-kurttutan/qh_fm_01 | b5a56b2a671b3198b5517f0f50b9bb8f9a043df3 | [
"MIT"
] | null | null | null | codes/Untitled.ipynb | mert-kurttutan/qh_fm_01 | b5a56b2a671b3198b5517f0f50b9bb8f9a043df3 | [
"MIT"
] | null | null | null | 42.106667 | 1,304 | 0.620963 | [
[
[
"from src import helpers, utils, DMRG",
"_____no_output_____"
],
[
"DMRG.conv_FHH_SU2_n",
"_____no_output_____"
],
[
"helpers.mps_nm(p1)",
"_____no_output_____"
],
[
"utils.",
"_____no_output_____"
]
]
] | [
"code"
] | [
[
"code",
"code",
"code",
"code"
]
] |
d077336b8e731569184e6ab9eed65ba10af7db6e | 30,715 | ipynb | Jupyter Notebook | dlc/Example_DLC_access.ipynb | GaelleChapuis/iblapps | edfe7368b45480ce4a2307dd5d80ec2a38bb084d | [
"MIT"
] | 8 | 2020-03-26T12:13:22.000Z | 2021-12-20T20:10:55.000Z | dlc/Example_DLC_access.ipynb | GaelleChapuis/iblapps | edfe7368b45480ce4a2307dd5d80ec2a38bb084d | [
"MIT"
] | 21 | 2020-05-12T11:53:46.000Z | 2022-03-14T11:17:44.000Z | dlc/Example_DLC_access.ipynb | GaelleChapuis/iblapps | edfe7368b45480ce4a2307dd5d80ec2a38bb084d | [
"MIT"
] | 7 | 2020-11-02T10:28:26.000Z | 2022-03-17T16:31:49.000Z | 68.104213 | 16,772 | 0.78854 | [
[
[
"<font size=\"+1\">This notebook will illustrate how to access DeepLabCut(DLC) results for IBL sessions and how to create short videos with DLC labels printed onto, as well as wheel angle, starting by downloading data from the IBL flatiron server. It requires ibllib, a ONE account and the following script: https://github.com/int-brain-lab/iblapps/blob/master/DLC_labeled_video.py</font>",
"_____no_output_____"
]
],
[
[
"run '/home/mic/Dropbox/scripts/IBL/DLC_labeled_video.py'",
"_____no_output_____"
],
[
"one = ONE()",
"Connected to https://alyx.internationalbrainlab.org as michael.schartner\n"
]
],
[
[
"Let's first find IBL ephys sessions with DLC results:",
"_____no_output_____"
]
],
[
[
"eids= one.search(task_protocol='ephysChoiceworld', dataset_types=['camera.dlc'], details=False)",
"_____no_output_____"
],
[
"len(eids)",
"_____no_output_____"
]
],
[
[
"For a particular session, we can create a short labeled video by calling the function Viewer, specifying the eid of the desired session, the video type (there's 'left', 'right' and 'body' videos), and a range of trials for which the video should be created. Most sesions have around 700 trials. In the following, this is illustrated with session '3663d82b-f197-4e8b-b299-7b803a155b84', video type 'left', trials range [10,13] and without a zoom for the eye, such that nose, paw and tongue tracking is visible. The eye-zoom option shows only the four points delineating the pupil edges, which are too small to be visible in the normal view. Note that this automatically starts the download of the video from flatiron (in case it is not locally stored already), which may take a while since these videos are about 8 GB large. ",
"_____no_output_____"
]
],
[
[
"eid = eids[6]",
"_____no_output_____"
],
[
"Viewer(eid, 'left', [10,13], save_video=True, eye_zoom=False)",
"Connected to https://alyx.internationalbrainlab.org as michael.schartner\nConnected to https://alyx.internationalbrainlab.org as michael.schartner\n"
]
],
[
[
"As usual when downloading IBL data from flatiron, the dimensions are listed. Below is one frame of the video for illustration. One can see one point for each paw, two points for the edges of the tongue, one point for the nose and there are 4 points close together around the pupil edges. All points for which the DLC network had a confidence probability of below 0.9 are hidden. For instance when the mouse is not licking, there is no tongue and so the network cannot detect it, and no points are shown. \n\nThe script will display and save the short video in your local folder. ",
"_____no_output_____"
],
[
"",
"_____no_output_____"
],
[
"Sections of the script <code>DLC_labeled_video.py</code> can be recycled to analyse DLC traces. For example let's plot the x coordinate for the right paw in a <code>'left'</code> cam video for a given trial. ",
"_____no_output_____"
]
],
[
[
"one = ONE()",
"Connected to https://alyx.internationalbrainlab.org as michael.schartner\n"
],
[
"dataset_types = ['camera.times','trials.intervals','camera.dlc']\nvideo_type = 'left'",
"_____no_output_____"
],
[
"# get paths to load in data\nD = one.load('3663d82b-f197-4e8b-b299-7b803a155b84',dataset_types=dataset_types, dclass_output=True)\nalf_path = Path(D.local_path[0]).parent.parent / 'alf'\nvideo_data = alf_path.parent / 'raw_video_data'",
"_____no_output_____"
],
[
"# get trials start and end times, camera time stamps (one for each frame, synced with DLC trace)\ntrials = alf.io.load_object(alf_path, '_ibl_trials')\ncam0 = alf.io.load_object(alf_path, '_ibl_%sCamera' % video_type)\ncam1 = alf.io.load_object(video_data, '_ibl_%sCamera' % video_type)\ncam = {**cam0,**cam1}",
"Inconsistent dimensions for object:_ibl_trials\n(893,), itiDuration\n(767,), stimOn_times\n(767, 2), intervals\n(893,), feedbackType\n(893,), choice\n(893,), rewardVolume\n(767,), feedback_times\n(893,), contrastRight\n(893,), probabilityLeft\n(893,), contrastLeft\n(767,), goCue_times\nInconsistent dimensions for object:_ibl_leftCamera\n(379271,), times\n(379265,), nose_tip_x\n(379265,), nose_tip_y\n(379265,), nose_tip_likelihood\n(379265,), pupil_top_r_x\n(379265,), pupil_top_r_y\n(379265,), pupil_top_r_likelihood\n(379265,), pupil_right_r_x\n(379265,), pupil_right_r_y\n(379265,), pupil_right_r_likelihood\n(379265,), pupil_bottom_r_x\n(379265,), pupil_bottom_r_y\n(379265,), pupil_bottom_r_likelihood\n(379265,), pupil_left_r_x\n(379265,), pupil_left_r_y\n(379265,), pupil_left_r_likelihood\n(379265,), paw_l_x\n(379265,), paw_l_y\n(379265,), paw_l_likelihood\n(379265,), paw_r_x\n(379265,), paw_r_y\n(379265,), paw_r_likelihood\n(379265,), tube_top_x\n(379265,), tube_top_y\n(379265,), tube_top_likelihood\n(379265,), tube_bottom_x\n(379265,), tube_bottom_y\n(379265,), tube_bottom_likelihood\n(379265,), tongue_end_l_x\n(379265,), tongue_end_l_y\n(379265,), tongue_end_l_likelihood\n(379265,), tongue_end_r_x\n(379265,), tongue_end_r_y\n(379265,), tongue_end_r_likelihood\n"
],
[
"# for each tracked point there's x,y in [px] in the frame and a likelihood that indicates the network's confidence\ncam.keys()",
"_____no_output_____"
]
],
[
[
"There is also <code>'times'</code> in this dictionary, the time stamps for each frame that we'll use to sync it with other events in the experiment. Let's get rid of it briefly to have only DLC points and set coordinates to nan when the likelihood is below 0.9. ",
"_____no_output_____"
]
],
[
[
"Times = cam['times'] \ndel cam['times']\npoints = np.unique(['_'.join(x.split('_')[:-1]) for x in cam.keys()])\ncam['times'] = Times",
"_____no_output_____"
],
[
"# A helper function to find closest time stamps\ndef find_nearest(array, value):\n array = np.asarray(array)\n idx = (np.abs(array - value)).argmin()\n return idx",
"_____no_output_____"
]
],
[
[
"Let's pick say the 5th trial and find all DLC traces for it.",
"_____no_output_____"
]
],
[
[
"frame_start = find_nearest(cam['times'], trials['intervals'][4][0])\nframe_stop = find_nearest(cam['times'], trials['intervals'][4][1])",
"_____no_output_____"
],
[
"XYs = {}\nfor point in points:\n x = np.ma.masked_where(\n cam[point + '_likelihood'] < 0.9, cam[point + '_x'])\n x = x.filled(np.nan)\n y = np.ma.masked_where(\n cam[point + '_likelihood'] < 0.9, cam[point + '_y'])\n y = y.filled(np.nan)\n XYs[point] = np.array(\n [x[frame_start:frame_stop], y[frame_start:frame_stop]])",
"_____no_output_____"
],
[
"import matplotlib.pyplot as plt",
"_____no_output_____"
],
[
"plt.plot(cam['times'][frame_start:frame_stop],XYs['paw_r'][0])\nplt.xlabel('time [sec]')\nplt.ylabel('x location of right paw [px]')",
"_____no_output_____"
]
]
] | [
"markdown",
"code",
"markdown",
"code",
"markdown",
"code",
"markdown",
"code",
"markdown",
"code",
"markdown",
"code"
] | [
[
"markdown"
],
[
"code",
"code"
],
[
"markdown"
],
[
"code",
"code"
],
[
"markdown"
],
[
"code",
"code"
],
[
"markdown",
"markdown",
"markdown"
],
[
"code",
"code",
"code",
"code",
"code"
],
[
"markdown"
],
[
"code",
"code"
],
[
"markdown"
],
[
"code",
"code",
"code",
"code"
]
] |
d07735aa2ae55b53e29afba96e4ade0792ed5c69 | 10,059 | ipynb | Jupyter Notebook | Albert/pytorch_mnist_model/get_sample_image.ipynb | bharatnishant/UdacityOpenSource | abd641700f0878a2e083de7a392b837efdd244c4 | [
"Apache-2.0"
] | 2 | 2019-08-20T12:58:01.000Z | 2019-08-20T13:13:28.000Z | Albert/pytorch_mnist_model/get_sample_image.ipynb | bharatnishant/UdacityOpenSource | abd641700f0878a2e083de7a392b837efdd244c4 | [
"Apache-2.0"
] | null | null | null | Albert/pytorch_mnist_model/get_sample_image.ipynb | bharatnishant/UdacityOpenSource | abd641700f0878a2e083de7a392b837efdd244c4 | [
"Apache-2.0"
] | 1 | 2019-08-20T13:14:59.000Z | 2019-08-20T13:14:59.000Z | 65.745098 | 6,824 | 0.808828 | [
[
[
"import keras",
"Using TensorFlow backend.\n"
],
[
"from keras.datasets import fashion_mnist",
"_____no_output_____"
],
[
"(X_train, y_train), (X_test, y_test) = fashion_mnist.load_data()",
"Downloading data from http://fashion-mnist.s3-website.eu-central-1.amazonaws.com/train-labels-idx1-ubyte.gz\n32768/29515 [=================================] - 0s 6us/step\nDownloading data from http://fashion-mnist.s3-website.eu-central-1.amazonaws.com/train-images-idx3-ubyte.gz\n26427392/26421880 [==============================] - 21s 1us/step\nDownloading data from http://fashion-mnist.s3-website.eu-central-1.amazonaws.com/t10k-labels-idx1-ubyte.gz\n8192/5148 [===============================================] - 0s 0us/step\nDownloading data from http://fashion-mnist.s3-website.eu-central-1.amazonaws.com/t10k-images-idx3-ubyte.gz\n4423680/4422102 [==============================] - 4s 1us/step\n"
],
[
"type(X_train)",
"_____no_output_____"
],
[
"import matplotlib.pyplot as plt",
"_____no_output_____"
],
[
"plt.imshow(X_train[1,:,:])",
"_____no_output_____"
],
[
"plt.imsave('sample_tshirt.png', X_train[1,:,:])",
"_____no_output_____"
]
]
] | [
"code"
] | [
[
"code",
"code",
"code",
"code",
"code",
"code",
"code"
]
] |
d0773977672f48554f3e85df3bfc6b1dde39fdfa | 14,293 | ipynb | Jupyter Notebook | in-class/week-3-B-defined-functions-BLANKS.ipynb | jchapamalacara/fall21-students-practical-python | 3a1f4613b46933d3ab1d0741177f0d4ec6b6b600 | [
"MIT"
] | null | null | null | in-class/week-3-B-defined-functions-BLANKS.ipynb | jchapamalacara/fall21-students-practical-python | 3a1f4613b46933d3ab1d0741177f0d4ec6b6b600 | [
"MIT"
] | null | null | null | in-class/week-3-B-defined-functions-BLANKS.ipynb | jchapamalacara/fall21-students-practical-python | 3a1f4613b46933d3ab1d0741177f0d4ec6b6b600 | [
"MIT"
] | 1 | 2021-11-01T01:41:39.000Z | 2021-11-01T01:41:39.000Z | 20.477077 | 180 | 0.520395 | [
[
[
"# Week 3 - Functions",
"_____no_output_____"
],
[
"The real power in any programming language is the **Function**.\n\nA function is:\n\n* a little block of script (one line or many) that performs specific task or a series of tasks.\n* reusable and helps us make our code DRY.\n* triggered when something \"invokes\" or \"calls\" it.\n* ideally modular – it performs a narrow task and you call several functions to perform more complex tasks.\n",
"_____no_output_____"
],
[
"### What we'll cover today:\n\n* Simple function\n* Return statements\n*\n",
"_____no_output_____"
]
],
[
[
"## Build a function called myFunction that adds 2 numbers together\n## it should print \"The total is (whatever the number is)!\"",
"_____no_output_____"
],
[
"## build it here\n\n",
"_____no_output_____"
],
[
"## Call myFunction using 4 and 5 as the arguments\n",
"_____no_output_____"
],
[
"## Call myFunction using 10 and 2 as the arguments\n",
"_____no_output_____"
],
[
"## you might forget what arguments are needed for the function to work.\n## you can add notes that appear on shift-tab as you call the function.\n## write it here\n",
"_____no_output_____"
],
[
"## test it on 3 and 4\n",
"_____no_output_____"
]
],
[
[
"### To use or not use functions?\n\nLet's compare the two options with a simple example:",
"_____no_output_____"
]
],
[
[
"## You have a list of numbers. \nmylist1 = [1, -5, 22, -44.2, 33, -45]",
"_____no_output_____"
],
[
"## Turn each number into an absolute number.\n## a for loop works perfectly fine here.\n",
"_____no_output_____"
],
[
"## The problem is that your project keeps generating more lists.\n## Each list of numbers has to be turned into absolute numbers\nmylist2 = [-56, -34, -75, -111, -22]\nmylist3 = [-100, -200, 100, -300, -100]\nmylist4 = [-23, -89, -11, -45, -27]\nmylist5 = [0, 1, 2, 3, 4, 5]",
"_____no_output_____"
]
],
[
[
"## DRY\n\n\n### Do you keep writing for loops for each list?\n\n### No, that's a lot of repetition!\n### DRY stands for \"Don't Repeat Yourself\"",
"_____no_output_____"
]
],
[
[
"## Instead we write a function that takes a list,\n## converts each list item to an absolute number,\n## and prints out the number\n\n",
"_____no_output_____"
],
[
"## Try swapping out different lists into the function:\n",
"_____no_output_____"
]
],
[
[
"## Timesaver \n### Imagine for a moment that your editor tells you that the calculation needs to be updated. Instead of needing the absolute number, you need the absolute number minus 5.\n\n### Having used multiple for loops, you'd have to change each one. What if you miss one or two? Either way, it's a chore.\n\n### With functions, you just revise the function and the update runs everywhere.\n\n",
"_____no_output_____"
]
],
[
[
"## So if an editor says to actually multiply the absolute number by 1_000_000,\n\n",
"_____no_output_____"
],
[
"## Try swapping out different lists into the function:\n",
"_____no_output_____"
]
],
[
[
"## Return Statements\n\n### So far we have only printed out values processed by a function. \n\n### But we really want to retain the value the function creates. \n\n### We can then pass that value to other parts of our calculations and code.",
"_____no_output_____"
]
],
[
[
"## Simple example\n## A function that adds two numbers together and prints the value:\n",
"_____no_output_____"
],
[
"## call the function with the numbers 2 and 4\n",
"_____no_output_____"
],
[
"## let's try to save it in a variable called myCalc\n",
"_____no_output_____"
],
[
"## Print myCalc. What does it hold?\n",
"_____no_output_____"
]
],
[
[
"### The return Statement",
"_____no_output_____"
]
],
[
[
"## Tweak our function by adding return statement\n## instead of printing a value we want to return a value(or values).\n",
"_____no_output_____"
],
[
"## call the function add_numbers_ret \n## and store in variable called myCalc\n",
"_____no_output_____"
],
[
"## print myCalc\n",
"_____no_output_____"
],
[
"## What type is myCalc?\n",
"_____no_output_____"
]
],
[
[
"## Return multiple values",
"_____no_output_____"
]
],
[
[
"## demo function\n\n\n",
"_____no_output_____"
],
[
"name,age,country = getPerson(\"David\", 35, \"France\")\n",
"_____no_output_____"
]
],
[
[
"### Let's revise our earlier absolute values converter with a return statement\n#### Here is the earlier version:\n<img src=\"https://github.com/sandeepmj/fall20-student-practical-python/blob/master/support_files/abs-function.png?raw=true\" style=\"width: 100%;\">",
"_____no_output_____"
]
],
[
[
"## Here it is revised with a return statement\n\n \n \n ",
"_____no_output_____"
],
[
"## Let's actually make that a list comprehension version of the function:\n",
"_____no_output_____"
],
[
"## Let's test it by storing the return value in variable x\n",
"_____no_output_____"
],
[
"## What type of data object is it?\n",
"_____no_output_____"
]
],
[
[
"# Make a function more flexible and universal\n\n* Currently, we have a function that takes ONLY a list as an argument.\n* We'd have to write another one for a single number argument.",
"_____no_output_____"
]
],
[
[
"## try using return_absolutes_lc on a single number like -10\n## it will break\n\n",
"_____no_output_____"
]
],
[
[
"# Universalize our absolute numbers function",
"_____no_output_____"
]
],
[
[
"## call the function make_abs\n",
"_____no_output_____"
],
[
"## try it on -10\n",
"_____no_output_____"
],
[
"## Try it on mylist3 - it will break!\n",
"_____no_output_____"
]
],
[
[
"## We can use the ```map()``` function to tackle this problem.\n\n```map()``` takes 2 arguments: a ```function``` and ```iterable like a list```.",
"_____no_output_____"
]
],
[
[
"## try it on make_abs and mylist3\n",
"_____no_output_____"
],
[
"## save it into a list\n",
"_____no_output_____"
]
],
[
[
"## ```map()``` also works for multiple iterables\n\n#### remember our ```add_numbers_ret``` function.",
"_____no_output_____"
]
],
[
[
"## here it is again:\n\ndef add_numbers_ret(number1, number2):\n return (number1 + number2)",
"_____no_output_____"
],
[
"## two lists\na_even = [2, 4, 6, 8]\na_odd = [1, 3, 5, 7, 9] ## note this has one more item in the list.",
"_____no_output_____"
],
[
"## run map on a_even and a_odd\nb = list(map(add_numbers_ret, a_even, a_odd))\nb",
"_____no_output_____"
]
],
[
[
"## Functions that call other funcions",
"_____no_output_____"
]
],
[
[
"## let's create a function that returns the square of a number\n\n",
"_____no_output_____"
],
[
"## what is 9 squared?\n",
"_____no_output_____"
]
],
[
[
"### Making a point here with a simple example\nLet's say we want to add 2 numbers together and then square that result.\nInstead of writing one \"complex\" function, we can call on our modular functions.",
"_____no_output_____"
]
],
[
[
"## a function that calls our modular functions\n\n ",
"_____no_output_____"
],
[
"## call make_point() on 2 and 5\nmake_point(2,5)",
"_____no_output_____"
]
]
] | [
"markdown",
"code",
"markdown",
"code",
"markdown",
"code",
"markdown",
"code",
"markdown",
"code",
"markdown",
"code",
"markdown",
"code",
"markdown",
"code",
"markdown",
"code",
"markdown",
"code",
"markdown",
"code",
"markdown",
"code",
"markdown",
"code",
"markdown",
"code"
] | [
[
"markdown",
"markdown",
"markdown"
],
[
"code",
"code",
"code",
"code",
"code",
"code"
],
[
"markdown"
],
[
"code",
"code",
"code"
],
[
"markdown"
],
[
"code",
"code"
],
[
"markdown"
],
[
"code",
"code"
],
[
"markdown"
],
[
"code",
"code",
"code",
"code"
],
[
"markdown"
],
[
"code",
"code",
"code",
"code"
],
[
"markdown"
],
[
"code",
"code"
],
[
"markdown"
],
[
"code",
"code",
"code",
"code"
],
[
"markdown"
],
[
"code"
],
[
"markdown"
],
[
"code",
"code",
"code"
],
[
"markdown"
],
[
"code",
"code"
],
[
"markdown"
],
[
"code",
"code",
"code"
],
[
"markdown"
],
[
"code",
"code"
],
[
"markdown"
],
[
"code",
"code"
]
] |
d0773c617ead7966bdfd2d37ee11cbc6dd23a6ca | 257,635 | ipynb | Jupyter Notebook | piston_animation_euler.ipynb | MarkusLohmayer/master-thesis-code | b107d1b582064daf9ad4414e1c9f332ef0be8660 | [
"MIT"
] | 1 | 2020-11-14T15:56:07.000Z | 2020-11-14T15:56:07.000Z | piston_animation_euler.ipynb | MarkusLohmayer/master-thesis-code | b107d1b582064daf9ad4414e1c9f332ef0be8660 | [
"MIT"
] | null | null | null | piston_animation_euler.ipynb | MarkusLohmayer/master-thesis-code | b107d1b582064daf9ad4414e1c9f332ef0be8660 | [
"MIT"
] | null | null | null | 573.797327 | 152,308 | 0.94996 | [
[
[
"# piston example with explicit Euler scheme",
"_____no_output_____"
]
],
[
[
"%matplotlib inline\n\nimport matplotlib\nimport matplotlib.pyplot as plt\nimport matplotlib.animation as anim\n\nimport numpy as np\n\nimport sys\nsys.path.insert(0, './code')\n\nimport ideal_gas",
"_____no_output_____"
]
],
[
[
"### physical parameters",
"_____no_output_____"
]
],
[
[
"# length of cylinder\nl = 0.1\n\n# radius of cylinder\nr = 0.05 \n\n# thickness of wall\nw = 0.006\n\n# derived geometrical data\nr2 = 2 * r # diameter of cylinder\nw2 = w / 2 # halved thickness of wall\nl2 = l - w2\nA = r**2 * np.pi # cross-sectional area",
"_____no_output_____"
],
[
"def get_v_1(q):\n \"\"\"first volume\"\"\"\n return A * (q - w2)\n \ndef get_v_2(q):\n \"\"\"second volume\"\"\"\n return A * (l2 - q)",
"_____no_output_____"
],
[
"# density of aluminium\nm_Al = 2700.0\nm_Cu = 8960.0\n\n# mass of piston\nm = m_Cu * A * w\n\n# thermal conductivity of aluminium\nκ_Al = 237.0\nκ_Cu = 401.0\n\n# thermal conduction coefficient\nα = κ_Cu * A / w\n\nm_inv = 1 / m",
"_____no_output_____"
]
],
[
[
"### initial conditions\n\ndetermine $n_1$, $n_2$, $s_1$, $s_2$",
"_____no_output_____"
]
],
[
[
"# wanted conditions\nv_1 = v_2 = get_v_1(l/2)\nθ_1 = 273.15 + 25.0\nπ_1 = 1.5 * 1e5\nθ_2 = 273.15 + 20.0\nπ_2 = 1.0 * 1e5",
"_____no_output_____"
],
[
"from scipy.optimize import fsolve",
"_____no_output_____"
],
[
"n_1 = fsolve(lambda n : ideal_gas.S_π(ideal_gas.U2(θ_1, n), v_1, n) - π_1, x0=2e22)[0]\ns_1 = ideal_gas.S(ideal_gas.U2(θ_1, n_1), v_1, n_1)",
"_____no_output_____"
],
[
"# check temperature\nideal_gas.U_θ(s_1, v_1, n_1) - 273.15",
"_____no_output_____"
],
[
"# check pressure\nideal_gas.U_π(s_1, v_1, n_1) * 1e-5",
"_____no_output_____"
],
[
"n_2 = fsolve(lambda n : ideal_gas.S_π(ideal_gas.U2(θ_2, n), v_2, n) - π_2, x0=2e22)[0]\ns_2 = ideal_gas.S(ideal_gas.U2(θ_2, n_2), v_2, n_2)",
"_____no_output_____"
],
[
"# check temperature\nideal_gas.U_θ(s_2, v_2, n_2) - 273.15",
"_____no_output_____"
],
[
"# check pressure\nideal_gas.U_π(s_2, v_2, n_2) * 1e-5",
"_____no_output_____"
],
[
"x_0 = l/2, 0, s_1, s_2",
"_____no_output_____"
]
],
[
[
"### simulation",
"_____no_output_____"
]
],
[
[
"def set_state(data, i, x):\n q, p, s_1, s_2 = x\n\n data[i, 0] = q\n \n data[i, 1] = p\n data[i, 2] = v = m_inv * p\n \n data[i, 3] = v_1 = get_v_1(q)\n data[i, 4] = π_1 = ideal_gas.U_π(s_1, v_1, n_1)\n \n data[i, 5] = s_1\n data[i, 6] = θ_1 = ideal_gas.U_θ(s_1, v_1, n_1)\n \n data[i, 7] = v_2 = get_v_2(q)\n data[i, 8] = π_2 = ideal_gas.U_π(s_2, v_2, n_2)\n\n data[i, 9] = s_2\n data[i, 10] = θ_2 = ideal_gas.U_θ(s_2, v_2, n_2)\n \n data[i, 11] = E_kin = 0.5 * m_inv * p**2\n data[i, 12] = u_1 = ideal_gas.U(s_1, v_1, n_1)\n data[i, 13] = u_2 = ideal_gas.U(s_2, v_2, n_2)\n data[i, 14] = E = E_kin + u_1 + u_2\n data[i, 15] = S = s_1 + s_2\n\n \ndef get_state(data, i):\n return data[i, (0, 1, 5, 9)]",
"_____no_output_____"
],
[
"def rhs(x):\n \"\"\"right hand side of the explicit system\n of differential equations\n \"\"\"\n q, p, s_1, s_2 = x\n \n v_1 = get_v_1(q)\n v_2 = get_v_2(q)\n \n π_1 = ideal_gas.U_π(s_1, v_1, n_1)\n π_2 = ideal_gas.U_π(s_2, v_2, n_2)\n \n θ_1 = ideal_gas.U_θ(s_1, v_1, n_1)\n θ_2 = ideal_gas.U_θ(s_2, v_2, n_2)\n \n return np.array((m_inv*p, A*(π_1-π_2), α*(θ_2-θ_1)/θ_1, α*(θ_1-θ_2)/θ_2))",
"_____no_output_____"
],
[
"t_f = 1.0\ndt = 1e-4\n\nsteps = int(t_f // dt)\nprint(f'steps={steps}')\n\nt = np.linspace(0, t_f, num=steps)\ndt = t[1] - t[0]\n\ndata = np.empty((steps, 16), dtype=float)\nset_state(data, 0, x_0)\n\nx_old = get_state(data, 0)\nfor i in range(1, steps):\n x_new = x_old + dt * rhs(x_old)\n set_state(data, i, x_new)\n x_old = x_new\n\nθ_min = np.min(data[:, (6,10)])\nθ_max = np.max(data[:, (6,10)])",
"steps=9999\n"
],
[
"# plot transient\nfig, ax = plt.subplots(dpi=200)\nax.set_title(\"piston position q\")\nax.plot(t, data[:, 0]);",
"_____no_output_____"
],
[
"fig, ax = plt.subplots(dpi=200)\nax.set_title(\"total entropy S\")\nax.plot(t, data[:, 15]);",
"_____no_output_____"
],
[
"fig, ax = plt.subplots(dpi=200)\nax.set_title(\"total energy E\")\nax.plot(t, data[:, 14]);",
"_____no_output_____"
]
],
[
[
"the total energy is not conserved well",
"_____no_output_____"
]
]
] | [
"markdown",
"code",
"markdown",
"code",
"markdown",
"code",
"markdown",
"code",
"markdown"
] | [
[
"markdown"
],
[
"code"
],
[
"markdown"
],
[
"code",
"code",
"code"
],
[
"markdown"
],
[
"code",
"code",
"code",
"code",
"code",
"code",
"code",
"code",
"code"
],
[
"markdown"
],
[
"code",
"code",
"code",
"code",
"code",
"code"
],
[
"markdown"
]
] |
d0773f927393dcfde4a874a130e9e51983a75c43 | 40,810 | ipynb | Jupyter Notebook | notebooks/FunkSVD-lib.ipynb | HeegyuKim/RecSys-MovieLens100k | aa3a272e6045d8230ecbabbf94a6f68170a26c9e | [
"MIT"
] | null | null | null | notebooks/FunkSVD-lib.ipynb | HeegyuKim/RecSys-MovieLens100k | aa3a272e6045d8230ecbabbf94a6f68170a26c9e | [
"MIT"
] | null | null | null | notebooks/FunkSVD-lib.ipynb | HeegyuKim/RecSys-MovieLens100k | aa3a272e6045d8230ecbabbf94a6f68170a26c9e | [
"MIT"
] | null | null | null | 32.648 | 109 | 0.37236 | [
[
[
"설치하기",
"_____no_output_____"
]
],
[
[
"!pip install git+https://github.com/gbolmier/funk-svd",
"_____no_output_____"
],
[
"from funk_svd.dataset import fetch_ml_ratings\nfrom funk_svd import SVD\nfrom sklearn.metrics import mean_absolute_error\nimport pandas as pd",
"_____no_output_____"
],
[
"ds_ratings = pd.read_csv(\"../ml-latest-small/ratings.csv\")\nds_movies = pd.read_csv(\"../ml-latest-small/movies.csv\")\n# funk-svd 는 유저 칼럼이 u_id, 아이템 칼럼이 i_id여서 이름을 변경해줍니다\ndf = ds_ratings.rename(\n {\n \"userId\": \"u_id\",\n \"movieId\": \"i_id\"\n },\n axis=1\n)\ndf",
"_____no_output_____"
],
[
"# 80%를 학습에 사용하고 10%를 validation set, 10%를 test_set으로 사용합니다\ntrain = df.sample(frac=0.8, random_state=7)\nval = df.drop(train.index.tolist()).sample(frac=0.5, random_state=8)\ntest = df.drop(train.index.tolist()).drop(val.index.tolist())",
"_____no_output_____"
],
[
"# SVD를 학습합니다. n_factors가 SVD의 k를 의미합니다\nsvd = SVD(lr=0.001, reg=0.005, n_epochs=100, n_factors=15, early_stopping=True,\n shuffle=False, min_rating=1, max_rating=5)\nsvd.fit(X=train, X_val=val)\n\n# 학습 결과를 test set 으로 평가합니다.\npred = svd.predict(test)\nmae = mean_absolute_error(test['rating'], pred)\n\nprint(f'Test MAE: {mae:.2f}')",
"Preprocessing data...\n\nPreprocessing data...\n\nEpoch 1/100 | val_loss: 0.95 - val_rmse: 0.98 - val_mae: 0.78 - took 0.0 sec\nEpoch 2/100 | val_loss: 0.91 - val_rmse: 0.95 - val_mae: 0.76 - took 0.0 sec\nEpoch 3/100 | val_loss: 0.88 - val_rmse: 0.94 - val_mae: 0.75 - took 0.0 sec\nEpoch 4/100 | val_loss: 0.87 - val_rmse: 0.93 - val_mae: 0.74 - took 0.0 sec\nEpoch 5/100 | val_loss: 0.85 - val_rmse: 0.92 - val_mae: 0.73 - took 0.0 sec\nEpoch 6/100 | val_loss: 0.84 - val_rmse: 0.92 - val_mae: 0.72 - took 0.0 sec\nEpoch 7/100 | val_loss: 0.84 - val_rmse: 0.91 - val_mae: 0.72 - took 0.0 sec\nEpoch 8/100 | val_loss: 0.83 - val_rmse: 0.91 - val_mae: 0.71 - took 0.0 sec\nEpoch 9/100 | val_loss: 0.82 - val_rmse: 0.91 - val_mae: 0.71 - took 0.0 sec\nEpoch 10/100 | val_loss: 0.82 - val_rmse: 0.90 - val_mae: 0.71 - took 0.0 sec\nEpoch 11/100 | val_loss: 0.81 - val_rmse: 0.90 - val_mae: 0.70 - took 0.0 sec\nEpoch 12/100 | val_loss: 0.81 - val_rmse: 0.90 - val_mae: 0.70 - took 0.0 sec\nEpoch 13/100 | val_loss: 0.81 - val_rmse: 0.90 - val_mae: 0.70 - took 0.0 sec\nEpoch 14/100 | val_loss: 0.80 - val_rmse: 0.90 - val_mae: 0.70 - took 0.0 sec\nEpoch 15/100 | val_loss: 0.80 - val_rmse: 0.90 - val_mae: 0.70 - took 0.0 sec\nEpoch 16/100 | val_loss: 0.80 - val_rmse: 0.89 - val_mae: 0.69 - took 0.0 sec\nEpoch 17/100 | val_loss: 0.80 - val_rmse: 0.89 - val_mae: 0.69 - took 0.0 sec\nEpoch 18/100 | val_loss: 0.79 - val_rmse: 0.89 - val_mae: 0.69 - took 0.0 sec\nEpoch 19/100 | val_loss: 0.79 - val_rmse: 0.89 - val_mae: 0.69 - took 0.0 sec\nEpoch 20/100 | val_loss: 0.79 - val_rmse: 0.89 - val_mae: 0.69 - took 0.0 sec\n\nTraining took 0 sec\nTest MAE: 0.68\n"
],
[
"user_ratings = ds_ratings.pivot(index=\"userId\", columns=\"movieId\", values=\"rating\")\ndef get_user_real_score(user_id, item_id):\n return user_ratings.loc[user_id, item_id]",
"_____no_output_____"
],
[
"\ndef get_user_unseen_ranks(user_id, max_rank=100):\n # predict_pair 메서드를 이용해서\n # 유저의 모든 영화 예측 평점을 계산합니다.\n movie_ids = df.i_id.unique()\n rec = pd.DataFrame(\n [{\n \"id\": id, \n \"recommendation_score\": svd.predict_pair(user_id, id), \n \"real_score\": get_user_real_score(user_id, id)\n } \n for id in movie_ids\n ]\n )\n \n # 유저가 본 영화는 제외합니다\n user_seen_movies = train[train.u_id == user_id]\n rec = rec[~rec.id.isin(user_seen_movies.i_id)]\n rec.sort_values(\"recommendation_score\", ascending=False, inplace=True)\n \n # max_rank 개만 보여줍니다\n if max_rank is not None:\n rec = rec.head(max_rank)\n \n # 순위를 컬럼에 추가합니다\n rec[\"rank\"] = range(1, len(rec) + 1)\n \n # train 에는 포함되지 않았지만 실제로 유저가 봤던 영화 ID를 가져옵니다\n # 이후 추천 결과에서 해당 영화들만 필터링해서 몇 위로 추천됬는지 확인합니다\n user_unseen_movies = pd.concat([val, test], axis=0)\n user_unseen_movies = user_unseen_movies[user_unseen_movies.u_id == user_id].i_id\n rec = rec[rec.id.isin(user_unseen_movies)]\n rec.index = rec.id\n del rec[\"id\"]\n \n # 실제 추천할 영화 정보와 join합니다.\n rec = ds_movies.merge(rec, left_on=\"movieId\", right_index=True)\n rec.sort_values(\"rank\", inplace=True)\n \n top_k_accuracy = len(rec) / len(user_unseen_movies)\n return rec, top_k_accuracy\n\nfrom IPython.display import display\n\nuser_ids = df.u_id.unique()[:10]\ntotal_acc = 0\nfor uid in user_ids:\n top100, acc = get_user_unseen_ranks(uid)\n total_acc += acc\n print(\"User: \", uid, \"TOP 100 accuracy: \", round(acc, 2))\n display(top100)\n\ntotal_acc / len(user_ids)",
"User: 1 TOP 100 accuracy: 0.27\n"
]
]
] | [
"markdown",
"code"
] | [
[
"markdown"
],
[
"code",
"code",
"code",
"code",
"code",
"code",
"code"
]
] |
d0774eb3eebd36d45d8e3fd59c81aa4ece10f64a | 20,702 | ipynb | Jupyter Notebook | mlcc/lab4-mortality-prediction/mlcc_mortality_prediction.ipynb | dphelps/healthcare-demo | ee1546c101873649ac57addbade93772b5f715e6 | [
"MIT"
] | null | null | null | mlcc/lab4-mortality-prediction/mlcc_mortality_prediction.ipynb | dphelps/healthcare-demo | ee1546c101873649ac57addbade93772b5f715e6 | [
"MIT"
] | null | null | null | mlcc/lab4-mortality-prediction/mlcc_mortality_prediction.ipynb | dphelps/healthcare-demo | ee1546c101873649ac57addbade93772b5f715e6 | [
"MIT"
] | null | null | null | 65.929936 | 2,518 | 0.63646 | [
[
[
"# Import libraries\nimport numpy as np\nimport pandas as pd\nimport matplotlib.pyplot as plt\nimport sqlite3\nfrom sklearn.pipeline import Pipeline\n\n# used for train/test splits\nfrom sklearn.cross_validation import train_test_split\n\n# used to impute mean for data\nfrom sklearn.preprocessing import Imputer\n\n# logistic regression is our model of choice\nfrom sklearn.linear_model import LogisticRegression\nfrom sklearn.linear_model import LogisticRegressionCV\n\n# used to calculate AUROC/accuracy\nfrom sklearn import metrics\n\n# used to create confusion matrix\nfrom sklearn.metrics import confusion_matrix\n\nfrom sklearn.cross_validation import cross_val_score\n\n\n%matplotlib inline",
"/Users/danielcphelps/anaconda/lib/python2.7/site-packages/sklearn/cross_validation.py:44: DeprecationWarning: This module was deprecated in version 0.18 in favor of the model_selection module into which all the refactored classes and functions are moved. Also note that the interface of the new CV iterators are different from that of this module. This module will be removed in 0.20.\n \"This module will be removed in 0.20.\", DeprecationWarning)\n"
],
[
"# Connect to MIMIC\n# be sure to add the password as appropriate!\ncon = psycopg2.connect(dbname='MIMIC', user='workshop', password=''\n , host='<xxxxx>.amazonaws.com'\n , port=5432)\ncur = con.cursor()\ncur.execute('SET search_path to ''mimiciii_workshop''')",
"_____no_output_____"
],
[
"query = \"\"\"\nwith ce as\n(\n select\n icustay_id, charttime, itemid, valuenum\n from chartevents\n -- specify what data we want from chartevents\n where itemid in\n (\n 211, -- Heart Rate\n 618, --\tRespiratory Rate\n 615 --\tResp Rate (Total)\n )\n -- how did we know heart rates were stored using ITEMID 211? Simple, we looked in D_ITEMS!\n -- Try it for yourself: select * from d_items where lower(label) like '%heart rate%'\n)\nselect\n -- ICUSTAY_ID identifies each unique patient ICU stay\n -- note that if the same person stays in the ICU more than once, each stay would have a *different* ICUSTAY_ID\n -- however, since it's the same person, all those stays would have the same SUBJECT_ID\n ie.icustay_id\n\n -- this is the outcome of interest: in-hospital mortality\n , max(adm.HOSPITAL_EXPIRE_FLAG) as OUTCOME\n\n -- this is a case statement - essentially an \"if, else\" clause\n , min(\n case\n -- if the itemid is 211\n when itemid = 211\n -- then return the actual value stored in VALUENUM\n then valuenum\n -- otherwise, return 'null', which is SQL standard for an empty value\n else null\n -- end the case statement\n end\n ) as HeartRate_Min\n\n -- note we wrapped the above in \"min()\"\n -- this takes the minimum of all values inside, and *ignores* nulls\n -- by calling this on our case statement, we are ignoring all values except those with ITEMID = 211\n -- since ITEMID 211 are heart rates, we take the minimum of only heart rates\n\n , max(case when itemid = 211 then valuenum else null end) as HeartRate_Max\n , min(case when itemid in (615,618) then valuenum else null end) as RespRate_Min\n , max(case when itemid in (615,618) then valuenum else null end) as RespRate_Max\nfrom icustays ie\n\n-- join to the admissions table to get hospital outcome\ninner join admissions adm\n on ie.hadm_id = adm.hadm_id\n\n-- join to the chartevents table to get the observations\nleft join ce\n -- match the tables on the patient identifier\n on ie.icustay_id = ce.icustay_id\n -- and require that the observation be made after the patient is admitted to the ICU\n and ce.charttime >= ie.intime\n -- and *before* their admission time + 1 day, i.e. the observation must be made on their first day in the ICU\n and ce.charttime <= ie.intime + interval '1' day\ngroup by ie.icustay_id\norder by ie.icustay_id\n\"\"\"\nconn = sqlite3.connect('/Users/danielcphelps/Boxcryptor/Google Drive/daniel.c.phelps/Research/HealthcareAnalytics/mimic-workshop/data/mimicdata.sqlite')\ndata = pd.read_sql_query(query,conn)\nprint(data.head())",
"_____no_output_____"
],
[
"# close the connection as we are done loading data from server\ncur.close()\ncon.close()",
"_____no_output_____"
],
[
"# move from a data frame into a numpy array\nX = data.values\ny = X[:,1]\n\n# delete first 2 columns: the ID and the outcome\nX = np.delete(X,0,axis=1)\nX = np.delete(X,0,axis=1)",
"_____no_output_____"
],
[
"# evaluate a logistic regression model using an 80%-20% training/test split\nX_train, X_test, y_train, y_test = train_test_split(X, y, test_size=0.2)\n\n# impute mean for missing values\nimp = Imputer(missing_values='NaN', strategy='mean', axis=0)\nimp.fit(X_train)\n\nX_train = imp.transform(X_train)\nX_test = imp.transform(X_test)\n\nmodel = LogisticRegression(fit_intercept=True)\nmodel = model.fit(X_train, y_train)\n\n# predict class labels for the test set\ny_pred = model.predict(X_test)\n\n# generate class probabilities\ny_prob = model.predict_proba(X_test)\n\n# generate evaluation metrics\nprint('Accuracy = {}'.format(metrics.accuracy_score(y_test, y_pred)))\nprint('AUROC = {}'.format(metrics.roc_auc_score(y_test, y_prob[:, 1])))\n\nprint('\\nConfusion matrix')\nprint(metrics.confusion_matrix(y_test, y_pred))\nprint('\\nClassification report')\nprint(metrics.classification_report(y_test, y_pred))",
"Accuracy = 0.784267912773\nAUROC = 0.642288212031\n\nConfusion matrix\n[[977 17]\n [260 30]]\n\nClassification report\n precision recall f1-score support\n\n 0.0 0.79 0.98 0.88 994\n 1.0 0.64 0.10 0.18 290\n\navg / total 0.76 0.78 0.72 1284\n\n"
],
[
"# evaluate a logistic regression with L1 regularization\n\n# evaluate the model using 5-fold cross-validation\n# see: http://scikit-learn.org/stable/modules/model_evaluation.html#scoring-parameter\n# for list of scoring parameters\n\nestimator = Pipeline([(\"imputer\", Imputer(missing_values='NaN',\n strategy=\"mean\",\n axis=0)),\n (\"regression\", LogisticRegressionCV(penalty='l1',\n cv=5,\n scoring='roc_auc',\n solver='liblinear'))])\n\nscores = cross_val_score(estimator\n , X, y\n , scoring='roc_auc', cv=5)\n\n\nprint('AUROC for all folds:')\nprint(scores)\nprint('Average AUROC across folds:')\nprint(scores.mean())",
"AUROC for all folds:\n[ 0.632241 0.66711432 0.65462583 0.63505984 0.64856111]\nAverage AUROC across folds:\n0.647520418729\n"
]
]
] | [
"code"
] | [
[
"code",
"code",
"code",
"code",
"code",
"code",
"code"
]
] |
d0775377d71e56f21aecb59c8a1403cd63ba5dbf | 93,800 | ipynb | Jupyter Notebook | blog/black-lives-matter/Race_bias_A_synthetic_data_approach.ipynb | ydataai/academy | e5da7453385c3e8e2b1d753bb12ea9cbdb858284 | [
"MIT"
] | 16 | 2020-05-06T14:34:43.000Z | 2022-03-27T17:56:06.000Z | blog/black-lives-matter/Race_bias_A_synthetic_data_approach.ipynb | ydataai/academy | e5da7453385c3e8e2b1d753bb12ea9cbdb858284 | [
"MIT"
] | 2 | 2022-02-11T00:24:55.000Z | 2022-03-31T12:04:44.000Z | blog/black-lives-matter/Race_bias_A_synthetic_data_approach.ipynb | ydataai/academy | e5da7453385c3e8e2b1d753bb12ea9cbdb858284 | [
"MIT"
] | 1 | 2020-07-14T20:10:20.000Z | 2020-07-14T20:10:20.000Z | 91.333982 | 24,742 | 0.740267 | [
[
[
"#Using our synthetic data library for today's exercise\n#pip install ydata",
"/usr/local/lib/python3.6/dist-packages/statsmodels/tools/_testing.py:19: FutureWarning: pandas.util.testing is deprecated. Use the functions in the public API at pandas.testing instead.\n import pandas.util.testing as tm\n"
],
[
"#Loading the census dataset from kaggle\nimport logging\nimport os\nimport requests\nimport pandas as pd\nimport seaborn as sns\nfrom sklearn.model_selection import train_test_split\nfrom sklearn.preprocessing import OneHotEncoder\n#import ydata.synthetic.regular as synthetic\n\n#Dataset URL from kaggle\ndata_url = 'https://www.kaggle.com/uciml/adult-census-income/downloads/adult.csv'\n\n# The local path where the data set is saved.\nlocal_filename = \"adult.csv\"\n\n# Kaggle Username and Password\nkaggle_info = {'UserName': \"myusername\", 'Password': \"mypassword\"}\n\n# Attempts to download the CSV file. Gets rejected because we are not logged in.\nr = requests.get(data_url)\n\n# Login to Kaggle and retrieve the data.\nr = requests.post(r.url, data = kaggle_info)\n\n# Writes the data to a local file one chunk at a time.\nf = open(local_filename, 'wb')\nfor chunk in r.iter_content(chunk_size = 512 * 1024): # Reads 512KB at a time into memory\n\n if chunk: # filter out keep-alive new chunks\n f.write(chunk)\nf.close()",
"_____no_output_____"
],
[
"adult_census = pd.read_csv('adult.csv')\n\n#For the purpose of this exercise we will filter information regarding only black and white individuals. \nadult_census = adult_census[(adult_census['race']=='White') | (adult_census['race']=='Black')]\n\nincome = adult_census['income']\nadult_census = adult_census.drop('education.num', axis=1)\nadult_census = adult_census.drop('income', axis=1)\n\ntrain_adult, test_adult, income_train, income_test = train_test_split(adult_census, income, test_size=0.33, random_state=42)\n\ntrain_adult['income'] = income_train\n\ntrain_adult.head(10)",
"/usr/local/lib/python3.6/dist-packages/ipykernel_launcher.py:12: SettingWithCopyWarning: \nA value is trying to be set on a copy of a slice from a DataFrame.\nTry using .loc[row_indexer,col_indexer] = value instead\n\nSee the caveats in the documentation: https://pandas.pydata.org/pandas-docs/stable/user_guide/indexing.html#returning-a-view-versus-a-copy\n if sys.path[0] == '':\n"
],
[
"sns.set(style=\"dark\", rc={'figure.figsize':(11.7,8.27)})\n\nsns.countplot(x=\"race\",\n palette=\"Paired\", edgecolor=\".6\",\n data=train_adult)",
"_____no_output_____"
],
[
"#Let's tackling the bias present in the dataset.\n#For that purpose we will need to filter the records belonging to only the black individuals. \ndef filter_fn(row):\n if row['race'] == 'Black':\n return True\n else:\n return False\n\nfilt = train_adult.apply(filter_fn, axis=1)\ntrain_adult_black = train_adult[filt]",
"_____no_output_____"
]
],
[
[
"",
"_____no_output_____"
]
],
[
[
"print(\"Number of records belonging to black individuals: {}\".format(train_adult_black.shape[0]))\n\nsns.set(style=\"dark\", rc={'figure.figsize':(11.7,8.27)})\n\nsns.countplot(x=\"sex\",\n palette=\"Paired\", edgecolor=\".6\",\n data=train_adult_black)\n#In what concerns sex, we have an equal representation of women and man for the black population of the dataset",
"Number of records belonging to black individuals: 2097\n"
],
[
"#Using the YData synthetic data lib to generate new 3000 individuals for the black population\nsynth_model = synthetic.SynthTabular()\nsynth_model.fit(adult_black)\n\nsynth_data = synth_model.sample(n_samples=3000)",
"_____no_output_____"
],
[
"synth_data = pd.read_csv('synth_data.csv', index_col=[0])\nsynth_data = synth_data.drop('education.num', axis=1)\n\nsynth_data = pd.concat([synth_data[synth_data['income']=='>50K'],synth_data[synth_data['income']=='<=50K'][:1000]])\nsynth_data.describe()",
"_____no_output_____"
],
[
"#Now combining both the datasets\ntest_adult['income'] = income_test\nadult_combined = synth_data.append(test_adult).sample(frac=1)\n\n#Let's check again how are we regarding the balancing of our classes for the race variable\nsns.set(style=\"dark\", rc={'figure.figsize':(11.7,8.27)})\n\nsns.countplot(x=\"race\",\n palette=\"Paired\", edgecolor=\".6\",\n data=adult_combined)",
"/usr/local/lib/python3.6/dist-packages/ipykernel_launcher.py:2: SettingWithCopyWarning: \nA value is trying to be set on a copy of a slice from a DataFrame.\nTry using .loc[row_indexer,col_indexer] = value instead\n\nSee the caveats in the documentation: https://pandas.pydata.org/pandas-docs/stable/user_guide/indexing.html#returning-a-view-versus-a-copy\n \n"
],
[
"#Auxiliar function to encode the categorical variables\nimport numpy as np\nfrom sklearn.preprocessing import OneHotEncoder\nfrom sklearn.linear_model import LogisticRegression\nfrom sklearn.ensemble import RandomForestClassifier\nfrom sklearn.tree import DecisionTreeClassifier\nfrom sklearn.svm import SVC\nfrom sklearn.neighbors import KNeighborsClassifier\nfrom sklearn.metrics import f1_score, accuracy_score, average_precision_score\n\ndef numerical_encoding(df, cat_cols=[], ord_cols=[]):\n try:\n assert isinstance(df, pd.DataFrame)\n except AssertionError as e:\n logging.error('The df input object must a Pandas dataframe. This action will not be executed.')\n return\n ord_cols_val = None\n cat_cols_val = None\n dummies = None\n cont_cols = list(set(df.columns) - set(cat_cols+ord_cols))\n cont_vals = df[cont_cols].values\n\n if len(ord_cols) > 0:\n ord_cols_val = df[ord_cols].values\n label_encoder = LabelEncoder\n ord_encoded = label_encoder.fit_transform(ord_cols_val)\n\n if len(cat_cols) > 0:\n cat_cols_val = df[cat_cols].values\n hot_encoder = OneHotEncoder()\n cat_encoded = hot_encoder.fit_transform(cat_cols_val).toarray()\n\n dummies = []\n for i, cat in enumerate(hot_encoder.categories_):\n for j in cat:\n dummies.append(cat_cols[i]+'_'+str(j))\n\n if ord_cols_val is not None and cat_cols_val is not None:\n encoded = np.hstack([cont_vals, ord_encoded, cat_encoded])\n columns = cont_cols+ord_cols+dummies\n elif cat_cols_val is not None:\n encoded = np.hstack([cont_vals, cat_encoded])\n columns = cont_cols+ord_cols+dummies\n else:\n encoded = cont_vals\n columns = cont_cols\n\n return pd.DataFrame(encoded, columns=columns), dummies",
"_____no_output_____"
],
[
"#validation functions\ndef score_estimators(estimators, x_test, y_test):\n\n #f1_score average='micro'\n scores = {type(clf).__name__: f1_score(y_test, clf.predict(x_test), average='micro') for clf in estimators}\n\n return scores\n\n\ndef fit_estimators(estimators, data_train, y_train):\n estimators_fit = []\n for i, estimator in enumerate(estimators):\n estimators_fit.append(estimator.fit(data_train, y_train))\n return estimators_fit\n\ndef estimator_eval(data, y, cat_cols=[]):\n def order_cols(df):\n cols = sorted(df.columns.tolist())\n return df[cols]\n\n data,_ = numerical_encoding(data, cat_cols=cat_cols)\n\n y, uniques = pd.factorize(y)\n data = order_cols(data)\n\n x_train, x_test, y_train, y_test = train_test_split(data, y, test_size=0.33, random_state=42)\n\n # Prepare train and test datasets\n estimators = [\n LogisticRegression(multi_class='auto', solver='lbfgs', max_iter=500, random_state=42),\n RandomForestClassifier(n_estimators=10, random_state=42),\n DecisionTreeClassifier(random_state=42),\n SVC(gamma='auto'),\n KNeighborsClassifier(n_neighbors=5)\n ]\t\n \n estimators_names = [type(clf).__name__ for clf in estimators]\n\n for estimator in estimators:\n assert hasattr(estimator, 'fit')\n assert hasattr(estimator, 'score')\n\n estimators = fit_estimators(estimators, x_train, y_train) \n scores = score_estimators(estimators, x_test, y_test)\n\n return scores",
"_____no_output_____"
],
[
"real_scores = estimator_eval(data=test_adult.drop('income', axis=1),\n y=test_adult['income'], \n cat_cols=['workclass', 'education', 'marital.status', 'occupation', 'relationship','race', 'sex', 'native.country'])\n\nsynth_scores = estimator_eval(data=adult_combined.drop('income', axis=1),\n y=adult_combined['income'], \n cat_cols=['workclass', 'education', 'marital.status', 'occupation', 'relationship','race', 'sex', 'native.country'])",
"_____no_output_____"
],
[
"dict_results = {'original': real_scores, 'synthetic': synth_scores}\nresults = pd.DataFrame(dict_results).reset_index()\n\nprint(\"Mean average accuracy improvement: {}\".format((results['synthetic'] - results['original']).mean()))\n\nresults_graph = results.melt('index', var_name='data_source', value_name='accuracy')",
"Mean average accuracy improvement: 0.024448394946566386\n"
],
[
" pd.DataFrame(dict_results).transpose()",
"_____no_output_____"
],
[
"#Final results comparision\n\nsns.barplot(x=\"index\", y=\"accuracy\", hue=\"data_source\", data=results_graph, \n palette=\"Paired\", edgecolor=\".6\")",
"_____no_output_____"
]
]
] | [
"code",
"markdown",
"code"
] | [
[
"code",
"code",
"code",
"code",
"code"
],
[
"markdown"
],
[
"code",
"code",
"code",
"code",
"code",
"code",
"code",
"code",
"code",
"code"
]
] |
d077691ba7be4bc12592817e9e9aed49eaee5483 | 11,297 | ipynb | Jupyter Notebook | courses/machine_learning/deepdive2/structured/labs/5b_deploy_keras_ai_platform_babyweight.ipynb | Glairly/introduction_to_tensorflow | aa0a44d9c428a6eb86d1f79d73f54c0861b6358d | [
"Apache-2.0"
] | 2 | 2022-01-06T11:52:57.000Z | 2022-01-09T01:53:56.000Z | courses/machine_learning/deepdive2/structured/labs/5b_deploy_keras_ai_platform_babyweight.ipynb | Glairly/introduction_to_tensorflow | aa0a44d9c428a6eb86d1f79d73f54c0861b6358d | [
"Apache-2.0"
] | null | null | null | courses/machine_learning/deepdive2/structured/labs/5b_deploy_keras_ai_platform_babyweight.ipynb | Glairly/introduction_to_tensorflow | aa0a44d9c428a6eb86d1f79d73f54c0861b6358d | [
"Apache-2.0"
] | null | null | null | 33.032164 | 554 | 0.573781 | [
[
[
"# LAB 5b: Deploy and predict with Keras model on Cloud AI Platform.\n\n**Learning Objectives**\n\n1. Setup up the environment\n1. Deploy trained Keras model to Cloud AI Platform\n1. Online predict from model on Cloud AI Platform\n1. Batch predict from model on Cloud AI Platform\n\n\n## Introduction \nIn this notebook, we'll deploying our Keras model to Cloud AI Platform and creating predictions.\n\nWe will set up the environment, deploy a trained Keras model to Cloud AI Platform, online predict from deployed model on Cloud AI Platform, and batch predict from deployed model on Cloud AI Platform.\n\nEach learning objective will correspond to a __#TODO__ in this student lab notebook -- try to complete this notebook first and then review the [solution notebook](../solutions/5b_deploy_keras_ai_platform_babyweight.ipynb).",
"_____no_output_____"
],
[
"## Set up environment variables and load necessary libraries",
"_____no_output_____"
],
[
"Import necessary libraries.",
"_____no_output_____"
]
],
[
[
"import os",
"_____no_output_____"
]
],
[
[
"### Lab Task #1: Set environment variables.\n\nSet environment variables so that we can use them throughout the entire lab. We will be using our project name for our bucket, so you only need to change your project and region.",
"_____no_output_____"
]
],
[
[
"%%bash\nPROJECT=$(gcloud config list project --format \"value(core.project)\")\necho \"Your current GCP Project Name is: \"$PROJECT",
"_____no_output_____"
],
[
"# Change these to try this notebook out\nPROJECT = \"cloud-training-demos\" # TODO: Replace with your PROJECT\nBUCKET = PROJECT # defaults to PROJECT\nREGION = \"us-central1\" # TODO: Replace with your REGION",
"_____no_output_____"
],
[
"os.environ[\"BUCKET\"] = BUCKET\nos.environ[\"REGION\"] = REGION\nos.environ[\"TFVERSION\"] = \"2.1\"",
"_____no_output_____"
],
[
"%%bash\ngcloud config set compute/region $REGION\ngcloud config set ai_platform/region global",
"_____no_output_____"
]
],
[
[
"## Check our trained model files\n\nLet's check the directory structure of our outputs of our trained model in folder we exported the model to in our last [lab](../solutions/10_train_keras_ai_platform_babyweight.ipynb). We'll want to deploy the saved_model.pb within the timestamped directory as well as the variable values in the variables folder. Therefore, we need the path of the timestamped directory so that everything within it can be found by Cloud AI Platform's model deployment service.",
"_____no_output_____"
]
],
[
[
"%%bash\ngsutil ls gs://${BUCKET}/babyweight/trained_model",
"_____no_output_____"
],
[
"%%bash\nMODEL_LOCATION=$(gsutil ls -ld -- gs://${BUCKET}/babyweight/trained_model/2* \\\n | tail -1)\ngsutil ls ${MODEL_LOCATION}",
"_____no_output_____"
]
],
[
[
"## Lab Task #2: Deploy trained model.\n\nDeploying the trained model to act as a REST web service is a simple gcloud call. Complete __#TODO__ by providing location of saved_model.pb file to Cloud AI Platoform model deployment service. The deployment will take a few minutes.",
"_____no_output_____"
]
],
[
[
"%%bash\nMODEL_NAME=\"babyweight\"\nMODEL_VERSION=\"ml_on_gcp\"\nMODEL_LOCATION=# TODO: Add GCS path to saved_model.pb file.\necho \"Deleting and deploying $MODEL_NAME $MODEL_VERSION from $MODEL_LOCATION\"\n# gcloud ai-platform versions delete ${MODEL_VERSION} --model ${MODEL_NAME}\n# gcloud ai-platform models delete ${MODEL_NAME}\ngcloud ai-platform models create ${MODEL_NAME} --regions ${REGION}\ngcloud ai-platform versions create ${MODEL_VERSION} \\\n --model=${MODEL_NAME} \\\n --origin=${MODEL_LOCATION} \\\n --runtime-version=2.1 \\\n --python-version=3.7",
"_____no_output_____"
]
],
[
[
"## Lab Task #3: Use model to make online prediction.\n\nComplete __#TODO__s for both the Python and gcloud Shell API methods of calling our deployed model on Cloud AI Platform for online prediction.",
"_____no_output_____"
],
[
"### Python API\n\nWe can use the Python API to send a JSON request to the endpoint of the service to make it predict a baby's weight. The order of the responses are the order of the instances.",
"_____no_output_____"
]
],
[
[
"from oauth2client.client import GoogleCredentials\nimport requests\nimport json\n\nMODEL_NAME = # TODO: Add model name\nMODEL_VERSION = # TODO: Add model version\n\ntoken = GoogleCredentials.get_application_default().get_access_token().access_token\napi = \"https://ml.googleapis.com/v1/projects/{}/models/{}/versions/{}:predict\" \\\n .format(PROJECT, MODEL_NAME, MODEL_VERSION)\nheaders = {\"Authorization\": \"Bearer \" + token }\ndata = {\n \"instances\": [\n {\n \"is_male\": \"True\",\n \"mother_age\": 26.0,\n \"plurality\": \"Single(1)\",\n \"gestation_weeks\": 39\n },\n {\n \"is_male\": \"False\",\n \"mother_age\": 29.0,\n \"plurality\": \"Single(1)\",\n \"gestation_weeks\": 38\n },\n {\n \"is_male\": \"True\",\n \"mother_age\": 26.0,\n \"plurality\": \"Triplets(3)\",\n \"gestation_weeks\": 39\n },\n # TODO: Create another instance\n ]\n}\nresponse = requests.post(api, json=data, headers=headers)\nprint(response.content)",
"_____no_output_____"
]
],
[
[
"The predictions for the four instances were: 5.33, 6.09, 2.50, and 5.86 pounds respectively when I ran it (your results might be different).",
"_____no_output_____"
],
[
"### gcloud shell API\n\nInstead we could use the gcloud shell API. Create a newline delimited JSON file with one instance per line and submit using gcloud.",
"_____no_output_____"
]
],
[
[
"%%writefile inputs.json\n{\"is_male\": \"True\", \"mother_age\": 26.0, \"plurality\": \"Single(1)\", \"gestation_weeks\": 39}\n{\"is_male\": \"False\", \"mother_age\": 26.0, \"plurality\": \"Single(1)\", \"gestation_weeks\": 39}",
"_____no_output_____"
]
],
[
[
"Now call `gcloud ai-platform predict` using the JSON we just created and point to our deployed `model` and `version`.",
"_____no_output_____"
]
],
[
[
"%%bash\ngcloud ai-platform predict \\\n --model=babyweight \\\n --json-instances=inputs.json \\\n --version=# TODO: Add model version",
"_____no_output_____"
]
],
[
[
"## Lab Task #4: Use model to make batch prediction.\n\nBatch prediction is commonly used when you have thousands to millions of predictions. It will create an actual Cloud AI Platform job for prediction. Complete __#TODO__s so we can call our deployed model on Cloud AI Platform for batch prediction.",
"_____no_output_____"
]
],
[
[
"%%bash\nINPUT=gs://${BUCKET}/babyweight/batchpred/inputs.json\nOUTPUT=gs://${BUCKET}/babyweight/batchpred/outputs\ngsutil cp inputs.json $INPUT\ngsutil -m rm -rf $OUTPUT \ngcloud ai-platform jobs submit prediction babypred_$(date -u +%y%m%d_%H%M%S) \\\n --data-format=TEXT \\\n --region ${REGION} \\\n --input-paths=$INPUT \\\n --output-path=$OUTPUT \\\n --model=babyweight \\\n --version=# TODO: Add model version",
"_____no_output_____"
]
],
[
[
"## Lab Summary:\nIn this lab, we set up the environment, deployed a trained Keras model to Cloud AI Platform, online predicted from deployed model on Cloud AI Platform, and batch predicted from deployed model on Cloud AI Platform.",
"_____no_output_____"
],
[
"Copyright 2019 Google Inc. Licensed under the Apache License, Version 2.0 (the \"License\"); you may not use this file except in compliance with the License. You may obtain a copy of the License at http://www.apache.org/licenses/LICENSE-2.0 Unless required by applicable law or agreed to in writing, software distributed under the License is distributed on an \"AS IS\" BASIS, WITHOUT WARRANTIES OR CONDITIONS OF ANY KIND, either express or implied. See the License for the specific language governing permissions and limitations under the License",
"_____no_output_____"
]
]
] | [
"markdown",
"code",
"markdown",
"code",
"markdown",
"code",
"markdown",
"code",
"markdown",
"code",
"markdown",
"code",
"markdown",
"code",
"markdown",
"code",
"markdown"
] | [
[
"markdown",
"markdown",
"markdown"
],
[
"code"
],
[
"markdown"
],
[
"code",
"code",
"code",
"code"
],
[
"markdown"
],
[
"code",
"code"
],
[
"markdown"
],
[
"code"
],
[
"markdown",
"markdown"
],
[
"code"
],
[
"markdown",
"markdown"
],
[
"code"
],
[
"markdown"
],
[
"code"
],
[
"markdown"
],
[
"code"
],
[
"markdown",
"markdown"
]
] |
d0776ce09e716ed0c2ef765b8ed8f70300eca5eb | 67,301 | ipynb | Jupyter Notebook | notebooks/my_approach.ipynb | jimkon/adaptive-discretization | d5464917b1bef013787ccabf21ad2d8191194d20 | [
"MIT"
] | null | null | null | notebooks/my_approach.ipynb | jimkon/adaptive-discretization | d5464917b1bef013787ccabf21ad2d8191194d20 | [
"MIT"
] | null | null | null | notebooks/my_approach.ipynb | jimkon/adaptive-discretization | d5464917b1bef013787ccabf21ad2d8191194d20 | [
"MIT"
] | null | null | null | 373.894444 | 34,776 | 0.907936 | [
[
[
"#!/usr/bin/python3\nimport sys\nsys.path.insert(0, '../src/')\n\nfrom ntree import *\nfrom tree_vis import *\n\nimport numpy as np\nimport matplotlib.pyplot as plt",
"_____no_output_____"
]
],
[
[
"### Summary\nWe are given : \n* a positive integer $n$ which is the number of dimension of the space. \n* a positive integer $k$ which is the total number of discrete points we need to place inside that space. \n* each continuous point $x$ queried in that space that follows a probability density function $PDF$.\n\nAnd we are asked for: \n* the $k$ discrete points that are placed in a way that minimizes the average distance (or mean error $ME$) from those continuous points $x$ .\n\n# Proposed approach\n\nWe propose a method that: \n* is an almost optimal solution that provides a decent decrease of the $ME$.\n* works for almost any number $k$ of points.\n* works for almost any number $n$ of dimensions.\n* adapts very fast (close to $\\log k$ rate).\n* is always better than the $ME$ provided by a uniform discretization, even before it is adapted.\n* is \"model\" free. The $PDF$ of the appearance of $x$'s don't have to be known.\n\n## How it works\n\nThe core idea behind this method is the $2^n$-trees. Like quadtrees and octrees with $n$ equal to 2 and 3 respectively, n-trees have any positive integer number $n$ as spatial subdivision factor, or also known as branching factor. $n$ describes the dimensions of the space that these trees spread. The $2^n$ child branches of each parent node, expand to all the basic directions in order to fill up all the space as uniform as possible. \n\nEach node is a point in space that covers a specified region. This region is the corresponding fraction of its parent's region. In other words, the parent splits the region that is assigned to it to $2^n$ equal sub-regions with volume $\\frac{1}{2^n}$ times smaller than their parent's. The sub-regions that are produced are n-dimensional cubes too and have no overlapping except their touching surfaces. Of coursethey fully overlap with their parent because they are actually its sub-set. As the height of the tree is growing, the nodes that are created have smaller and smaller volumes. Each point is located in the middle of the cube so there will be no way for two or more points to be in the same location. So a tree with $k$ nodes will have $k$ different points inside the given space.\n\nWe assign to the root node the whole space we want to use, in our case the unit cube between the points $[0, 0, ..., 0]$ and $[1, 1, ..., 1]$. For example, lets take $n=2$. Root, the node in level zero, will have the point $[0.5,0.5]$ and determined by the vertices $[0,0],[1,1]$. Its $2^n=4$ branches, will split this area in 4 equal 2d-cubes (aka rectangles) with vertices and points: \n* cube 1 -> vertices $[0,0],[0.5,0.5]$ , point $[0.25,0.25]$ \n* cube 2 -> vertices $[0.5,0],[1,0.5]$ , point $[0.75,0.25]$ \n* cube 3 -> vertices $[0,0.5],[0.5,1]$ , point $[0.25,0.75]$ \n* cube 4 -> vertices $[0.5,0.5],[1,1]$ , point $[0.75,0.75]$ \n\nSo now we have 5 points to fill up this space. Those 4 branches can be extended to create 16 new sub-branches (4 more branches each) adding to a total of 21 points.",
"_____no_output_____"
]
],
[
[
"tree = Tree(2, 21)\ntree.plot()\n\npoints = tree.get_points()\n\nplt.figure()\nfor p in points:\n plt.plot(p[0], p[1], 'bo', label='Points')\nplt.plot([0, 0], [0, 1], 'g--', label='Space')\nplt.plot([0, 1], [0, 0], 'g--')\nplt.plot([1, 1], [0, 1], 'g--')\nplt.plot([1, 0], [1, 1], 'g--')\n\nplt.xticks(np.linspace(0, 1, 9))\nplt.yticks(np.linspace(0, 1, 9))\nplt.title('Points spread into space')\nplt.grid(True)\nplt.legend()\nplt.show()",
"_____no_output_____"
]
],
[
[
"Additionally, nodes keep some useful informations that help **evaluate** them. It's very important to know what _ME_ each node produces and what it would have produced if it wasn't there. When user queries a point $x$, we find the corresponding node by starting from root and recursively finding all the sub-branches until that one leaf, that is responsible for the region that this point belongs. This search will not necessarily give us the nearest point to $x$, but it is very useful for two other things.\n* First of all, the result may not be the nearest point, but it will be the node that is __responsible for this specific area__. This means that if we want to increase the resolution of points, near that particular $x$ we have to expand the node that will be returned from that search. Using our previous example, for $x=[0.4, 0.4]$ the result of the search will be the node with the point $[0.25, 0.25]$ even if root's point $[0.5, 0.5]$ is closer. But expanding this node will create a branch with point $[0.375, 0.375]$. \n* The second benefit is that while doing this search, __we can easily collect the information we need for the evaluation__, like the distance from the responsible point and the distance from its parent's point. Using again our previous example, searching for $[0.4, 0,4]$ will traverse root and its branch with the point $[0.25, 0.25]$. In the process we can find the Euclidean distance of $x$ to each node point, which is $dist([0.5, 0.5], [0.4, 0.4])=0.141421$ and $dist([0.25, 0.25], [0.4, 0.4])=0.212132$ respectively. This gives us useful information about each node's utility.\n\n\n## Adaption of the tree\nWe said before that the points of the nodes are have fixed locations, which make the unable to move and adapt by themselves. __By creating and deleting points we try to match the right set of points that match the PDF the most__. So the adaption is actually a fitting. And we have all the required components to do that. We can expand and prune nodes to increase and decrease resolution and we have a way of evaluating them to choose what is the best for each one of them. Also, in order to ensure that the adaption will produce stable results, and will always decrease the total %ME%, we make all the changes only to the outer level. The maximum difference between the old tree and the updated one is restricted to 1 level for each region.\n\n### Increase resolution\nIn order to decrease $ME$ we need to increase the resolution of points in regions that is needed. Obviously, the way to increase resolution in the desired regions is to expand the corresponding nodes. But there are two types of nodes. The expandable ones, that have unexpanded sub-branches, and the not expandable that are already fully expanded. \n\nTo expand an expandable node we just create all its sub-branches. New points are spread to all directions trying to fill up uniformly the space around the parent point. The goal is actually to search this region for a place of interest. Points that fall into this place will marked as useful by the evaluation process and will \"survive\" as the other will be deleted in the next update.\n\nExpanding a non-expandable node is trickier than expanding an expandable one. As we said before, this node is already fully expanded. But if our evaluation system says that we need to do that, we can't ignore it. Fully expand its closest expandable children would be a solution but it will create too many useless new nodes. We need a more targeted expansion. We can partly expand all the $2^n$ expandable nodes that are closest to the this node. By partly i mean only the node that is towards the desired point.\n\nTo generalize into a more compact solution, we create all the nodes that will approach the point we want independently of the current state of the corresponding node. This means that direct (if there are any) and more distant sub-branches will be created. So expansion always creates $2^n$ new nodes, but not necessary in the same levels. \n\n\n### Decrease resolution\nDecreasing resolution almost any time increases $ME$ but is necessary to maintain the right number of points. So we delete a node to free space for another node to be created. The goal of each prune is to increase the total $ME$ less than the amount that it will decreased by the following expansion. Pruning is done by simply deleting/cutting a leaf node from its parent. Because leaf nodes have no sub-branches, the total number of points is decreased by 1. \n\n\n### Decide which node to expand and which to cut\nOur goal is to decrease the $ME$ as much as possible so we get as close as possible to the optimal solution. The only criterion we can use is the evaluation of each node, which depends only on the distances collected while searching. We have available for each discrete point the sum of distances of each continuous point searched near it. Also, we store in every parent node, the total distance that will be collected if each of its child wasn't available. In the example we used earlier, for $x=[0.2, 0.2]$, we will add to the total distance counter of node $[0.25, 0.25]$ (which is the nearest neighbor) the number $dist([0.25, 0.25], [0.2, 0.2])=0.070711$. Also we will store on its parent node, $[0.5, 0.5]$, the information of the increase of the total distance that we would get if node $[0.25, 0.25]$ wasn't there. So the corresponding counter of node $[0.5, 0.5]$ for the node $[0.25, 0.25]$ will increased by $dist([0.5, 0.5], [0.2, 0.2])=0.424264$. With that information, we can predict how much the total $ME$ will increase if we cut node $[0.25, 0.25]$.\n\nNow that we have available the information we need, we make an update to our tree. Each update has 2 steps. One pass for pruning first to free some space and one for expanding.\n* On pruning, we take all the leaf nodes and we cut the ones that will not produce more $ME$ than the average $ME$ of all nodes.\n* On expanding, expand the nodes with the highest $ME$ until the tree reaches the desired size again. In case that there are not that many nodes to expand, the tree will stay incomplete until the next update.",
"_____no_output_____"
],
[
"## Comparison with other approaches\nThis solution contains many ideas and methods used to solve similar problem. Though, none of them have the same goals as this one.\n\nThe most similar approaches to this one, are quad and octrees used for spatial subdivision([AMR](https://en.wikipedia.org/wiki/Adaptive_mesh_refinement)) and collision detection. Except the fixed dimensions $n$ of these methods, there are differences on the adaption procedure too. First of all, almost none of them have a fixed number of points and they are all increasing resolution on the regions that is needed, while never decrease it on other regions to maintain their upper limit. The increase of the resolution is also different, while these methods use as points only the leaves of the tree and discard all the parent nodes inside it. This means that each single expansion results on an increase of $2^n-1$ (1 for the deleted parent) points, instead of $2^n$ of my approach. This seems like a disadvantage but it is not. When discarding the parent, you become unable (or you make it more difficult) to delete individual leaf nodes and can only merge the whole branch to recreate the parent. In the case that you need only one of the children, you are forced to keep all the other $2^n-1$ of them and this limits the maximum level that your tree can reach. In our method, the overhead is 1 node which means that the maximum level[\\*]() the tree can reach is the total number of points $k$. Of coursethis is the worst case scenario for their method and the best for ours, but in any case our method gives more freedom to the tree and reduces the overhead to minimum, as even the overheads are points in space that many times are required too.\n",
"_____no_output_____"
]
]
] | [
"code",
"markdown",
"code",
"markdown"
] | [
[
"code"
],
[
"markdown"
],
[
"code"
],
[
"markdown",
"markdown"
]
] |
d0776edb6f7bd22c02291a7461944cc157e39cc2 | 1,541 | ipynb | Jupyter Notebook | Fase 4 - Temas avanzados/Tema 12 - Manejo de ficheros/Leccion 04 - Catalogo de peliculas persistente/Leccion 04 - Catalogo de peliculas persistente.ipynb | ruben69695/python-course | a3d3532279510fa0315a7636c373016c7abe4f0a | [
"MIT"
] | 1 | 2019-01-27T20:44:53.000Z | 2019-01-27T20:44:53.000Z | Fase 4 - Temas avanzados/Tema 12 - Manejo de ficheros/Leccion 04 - Catalogo de peliculas persistente/Leccion 04 - Catalogo de peliculas persistente.ipynb | ruben69695/python-course | a3d3532279510fa0315a7636c373016c7abe4f0a | [
"MIT"
] | null | null | null | Fase 4 - Temas avanzados/Tema 12 - Manejo de ficheros/Leccion 04 - Catalogo de peliculas persistente/Leccion 04 - Catalogo de peliculas persistente.ipynb | ruben69695/python-course | a3d3532279510fa0315a7636c373016c7abe4f0a | [
"MIT"
] | null | null | null | 23.348485 | 71 | 0.480857 | [
[
[
"from io import open\nimport pickle\n\nclass Pelicula:\n \n # Constructor de clase\n def __init__(self, titulo, duracion, lanzamiento):\n self.titulo = titulo\n self.duracion = duracion\n self.lanzamiento = lanzamiento\n print('Se ha creado la película:',self.titulo)\n \n def __str__(self):\n return '{} ({})'.format(self.titulo, self.lanzamiento)\n\n\nclass Catalogo:\n \n peliculas = []\n \n # Constructor de clase\n def __init__(self, peliculas=[]):\n self.peliculas = peliculas\n \n def agregar(self,p):\n self.peliculas.append(p)\n \n def mostrar(self):\n for p in self.peliculas:\n print(p)",
"_____no_output_____"
]
]
] | [
"code"
] | [
[
"code"
]
] |
d0778b82dc5af7abe4480d5dc13e69dcb0c7ade4 | 18,283 | ipynb | Jupyter Notebook | docs/notebooks/xyz_pro_features_examples.ipynb | fangkun202303x/heremapsn | 67c0b670ef337d236761932a6fc4da4129d999ad | [
"Apache-2.0"
] | 28 | 2020-07-23T12:02:53.000Z | 2021-01-09T18:26:32.000Z | docs/notebooks/xyz_pro_features_examples.ipynb | fangkun202303x/heremapsn | 67c0b670ef337d236761932a6fc4da4129d999ad | [
"Apache-2.0"
] | 93 | 2020-07-24T10:45:40.000Z | 2021-08-18T16:14:10.000Z | docs/notebooks/xyz_pro_features_examples.ipynb | fangkun202303x/heremapsn | 67c0b670ef337d236761932a6fc4da4129d999ad | [
"Apache-2.0"
] | 9 | 2020-07-23T12:27:51.000Z | 2021-08-15T20:09:50.000Z | 32.474245 | 1,043 | 0.605426 | [
[
[
"## XYZ Pro Features\nThis notebook demonstrates some of the pro features for XYZ Hub API.\n\nXYZ paid features can be found here: [xyz pro features](https://www.here.xyz/xyz_pro/).\n\nXYZ plans can be found here: [xyz plans](https://developer.here.com/pricing).",
"_____no_output_____"
],
[
"### Virtual Space\nA virtual space is described by definition which references other existing spaces(the upstream spaces).\nQueries being done to a virtual space will return the features of its upstream spaces combined.\n\nBelow are different predefined operations of how to combine the features of the upstream spaces.\n\n- [group](#group_cell)\n- [merge](#merge_cell)\n- [override](#override_cell)\n- [custom](#custom_cell)",
"_____no_output_____"
]
],
[
[
"# Make necessary imports.\nimport os\nimport json\nimport warnings\n\nfrom xyzspaces.datasets import get_chicago_parks_data, get_countries_data\nfrom xyzspaces.exceptions import ApiError\nimport xyzspaces",
"_____no_output_____"
]
],
[
[
"<div class=\"alert alert-block alert-warning\">\n<b>Warning:</b> Before running below cells please make sure you have XYZ Token to interact with xyzspaces. \n Please see README.md in notebooks folder for more info on XYZ_TOKEN\n</div>",
"_____no_output_____"
]
],
[
[
"os.environ[\"XYZ_TOKEN\"] = \"MY-XYZ-TOKEN\" # Replace your token here.",
"_____no_output_____"
],
[
"xyz = xyzspaces.XYZ()",
"_____no_output_____"
],
[
"# create two spaces which will act as upstream spaces for virtual space created later.\n\ntitle1 = \"Testing xyzspaces\"\ndescription1 = \"Temporary space containing countries data.\"\nspace1 = xyz.spaces.new(title=title1, description=description1)\n\n# Add some data to it space1\ngj_countries = get_countries_data()\nspace1.add_features(features=gj_countries)\nspace_id1 = space1.info[\"id\"]\n\ntitle2 = \"Testing xyzspaces\"\ndescription2 = \"Temporary space containing Chicago parks data.\"\nspace2 = xyz.spaces.new(title=title2, description=description2)\n\n# Add some data to space2\nwith open(\"./data/chicago_parks.geo.json\", encoding=\"utf-8-sig\") as json_file:\n gj_chicago = json.load(json_file)\n\nspace2.add_features(features=gj_chicago)\nspace_id2 = space2.info[\"id\"]",
"_____no_output_____"
]
],
[
[
"<a id='group_cell'></a>\n#### Group\nGroup means to combine the content of the specified spaces. All objects of each space will be part of the response when the virtual space is queried by the user. The information about which object came from which space can be found in the XYZ-namespace in the properties of each feature. When writing back these objects to the virtual space they'll be written back to the upstream space from which they were actually coming.",
"_____no_output_____"
]
],
[
[
"# Create a new virtual space by grouping two spaces created above.\n\ntitle = \"Virtual Space for coutries and Chicago parks data\"\ndescription = \"Test group functionality of virtual space\"\n\nupstream_spaces = [space_id1, space_id2]\nkwargs = {\"virtualspace\": dict(group=upstream_spaces)}\n\nvspace = xyz.spaces.virtual(title=title, description=description, **kwargs)\nprint(json.dumps(vspace.info, indent=2))",
"_____no_output_____"
],
[
"# Reading a particular feature from space1 via virtual space.\n\nvfeature1 = vspace.get_feature(feature_id=\"FRA\")\nfeature1 = space1.get_feature(feature_id=\"FRA\")\nassert vfeature1 == feature1\n\n# Reading a particular feature from space2 via virtual space.\nvfeature2 = vspace.get_feature(feature_id=\"LP\")\nfeature2 = space2.get_feature(feature_id=\"LP\")\nassert vfeature2 == feature2",
"_____no_output_____"
],
[
"# Deleting a feature from virtual space deletes corresponding feature from upstream space.\n\nvspace.delete_feature(feature_id=\"FRA\")\ntry:\n space1.get_feature(\"FRA\")\nexcept ApiError as err:\n print(err)",
"_____no_output_____"
],
[
"# Delete temporary spaces created.\nvspace.delete()\nspace1.delete()\nspace2.delete()",
"_____no_output_____"
]
],
[
[
"<a id='merge_cell'></a>\n#### Merge\nMerge means that objects with the same ID will be merged together. If there are duplicate feature-IDs in the various data of the upstream spaces, the duplicates will be merged to build a single feature. The result will be a response that is guaranteed to have no features with duplicate IDs. The merge will happen in the order of the space-references in the specified array. That means objects coming from the second space will overwrite potentially existing property values of objects coming from the first space. The information about which object came from which space(s) can be found in the XYZ-namespace in the properties of each feature. When writing back these objects to the virtual space they'll be written back to the upstream space from which they were actually coming, or the last one in the list if none was specified.When deleting features from the virtual space a new pseudo-deleted feature is written to the last space in the list. Trying to read the feature with that ID from the virtual space is not possible afterward.",
"_____no_output_____"
]
],
[
[
"# create two spaces with duplicate data\n\ntitle1 = \"Testing xyzspaces\"\ndescription1 = \"Temporary space containing Chicago parks data.\"\nspace1 = xyz.spaces.new(title=title1, description=description1)\n\nwith open(\"./data/chicago_parks.geo.json\", encoding=\"utf-8-sig\") as json_file:\n gj_chicago = json.load(json_file)\n\n# Add some data to it space1\nspace1.add_features(features=gj_chicago)\nspace_id1 = space1.info[\"id\"]\n\ntitle2 = \"Testing xyzspaces duplicate\"\ndescription2 = \"Temporary space containing Chicago parks data duplicate\"\nspace2 = xyz.spaces.new(title=title1, description=description1)\n\n# Add some data to it space2\nspace2.add_features(features=gj_chicago)\nspace_id2 = space2.info[\"id\"]",
"_____no_output_____"
],
[
"# update a particular feature of second space so that post merge virtual space will have this feature merged\nlp = space2.get_feature(\"LP\")\nspace2.update_feature(feature_id=\"LP\", data=lp, add_tags=[\"foo\", \"bar\"])",
"_____no_output_____"
],
[
"# Create a new virtual space by merging two spaces created above.\n\ntitle = \"Virtual Space for coutries and Chicago parks data\"\ndescription = \"Test merge functionality of virtual space\"\n\nupstream_spaces = [space_id1, space_id2]\nkwargs = {\"virtualspace\": dict(merge=upstream_spaces)}\n\nvspace = xyz.spaces.virtual(title=title, description=description, **kwargs)\nprint(vspace.info)",
"_____no_output_____"
],
[
"vfeature1 = vspace.get_feature(feature_id=\"LP\")\nassert vfeature1[\"properties\"][\"@ns:com:here:xyz\"][\"tags\"] == [\"foo\", \"bar\"]",
"_____no_output_____"
],
[
"bp = space2.get_feature(\"BP\")\nspace2.update_feature(feature_id=\"BP\", data=lp, add_tags=[\"foo1\", \"bar1\"])",
"_____no_output_____"
],
[
"vfeature2 = vspace.get_feature(feature_id=\"BP\")\nassert vfeature2[\"properties\"][\"@ns:com:here:xyz\"][\"tags\"] == [\"foo1\", \"bar1\"]",
"_____no_output_____"
],
[
"space1.delete()\nspace2.delete()\nvspace.delete()",
"_____no_output_____"
]
],
[
[
"<a id='override_cell'></a>\n#### Override\nOverride means that objects with the same ID will be overridden completely. If there are duplicate feature-IDs in the various data of the upstream spaces, the duplicates will be overridden to result in a single feature. The result will be a response that is guaranteed to have no features with duplicate IDs. The override will happen in the order of the space-references in the specified array. That means objects coming from the second space one will override potentially existing features coming from the first space. The information about which object came from which space can be found in the XYZ-namespace in the properties of each feature. When writing back these objects to the virtual space they'll be written back to the upstream space from which they were actually coming. When deleting features from the virtual space the same rules as for merge apply.",
"_____no_output_____"
]
],
[
[
"# create two spaces with duplicate data\n\ntitle1 = \"Testing xyzspaces\"\ndescription1 = \"Temporary space containing Chicago parks data.\"\nspace1 = xyz.spaces.new(title=title1, description=description1)\n\nwith open(\"./data/chicago_parks.geo.json\", encoding=\"utf-8-sig\") as json_file:\n gj_chicago = json.load(json_file)\n\n# Add some data to it space1\nspace1.add_features(features=gj_chicago)\nspace_id1 = space1.info[\"id\"]\n\ntitle2 = \"Testing xyzspaces duplicate\"\ndescription2 = \"Temporary space containing Chicago parks data duplicate\"\nspace2 = xyz.spaces.new(title=title1, description=description1)\n\n# Add some data to it space2\nspace2.add_features(features=gj_chicago)\nspace_id2 = space2.info[\"id\"]",
"_____no_output_____"
],
[
"# Create a new virtual space by override operation.\n\ntitle = \"Virtual Space for coutries and Chicago parks data\"\ndescription = \"Test merge functionality of virtual space\"\n\nupstream_spaces = [space_id1, space_id2]\nkwargs = {\"virtualspace\": dict(override=upstream_spaces)}\n\nvspace = xyz.spaces.virtual(title=title, description=description, **kwargs)\nprint(vspace.info)",
"_____no_output_____"
],
[
"bp = space2.get_feature(\"BP\")\nspace2.update_feature(feature_id=\"BP\", data=bp, add_tags=[\"foo1\", \"bar1\"])",
"_____no_output_____"
],
[
"vfeature2 = vspace.get_feature(feature_id=\"BP\")\nassert vfeature2[\"properties\"][\"@ns:com:here:xyz\"][\"tags\"] == [\"foo1\", \"bar1\"]",
"_____no_output_____"
],
[
"space1.delete()\nspace2.delete()\nvspace.delete()",
"_____no_output_____"
]
],
[
[
"### Applying clustering in space",
"_____no_output_____"
]
],
[
[
"# create two spaces which will act as upstream spaces for virtual space created later.\n\ntitle1 = \"Testing xyzspaces\"\ndescription1 = \"Temporary space containing countries data.\"\nspace1 = xyz.spaces.new(title=title1, description=description1)\n\n# Add some data to it space1\ngj_countries = get_countries_data()\nspace1.add_features(features=gj_countries)\nspace_id1 = space1.info[\"id\"]",
"_____no_output_____"
],
[
"# Genereate clustering for the space\nspace1.cluster(clustering=\"hexbin\")",
"_____no_output_____"
],
[
"# Delete created space\nspace1.delete()",
"_____no_output_____"
]
],
[
[
"### Rule based Tagging\nRule based tagging makes tagging multiple features in space tagged to a particular tag, based in rules mentioned based on JSON-path expression. Users can update space with a map of rules where the key is the tag to be applied to all features matching the JSON-path expression being the value.\n\nIf multiple rules are matching, multiple tags will be applied to the according to matched sets of features. It could even happen that a feature is matched by multiple rules and thus multiple tags will get added to it.",
"_____no_output_____"
]
],
[
[
"# Create a new space\ntitle = \"Testing xyzspaces\"\ndescription = \"Temporary space containing Chicago parks data.\"\nspace = xyz.spaces.new(title=title, description=description)",
"_____no_output_____"
],
[
"# Add data to the space.\nwith open(\"./data/chicago_parks.geo.json\", encoding=\"utf-8-sig\") as json_file:\n gj_chicago = json.load(json_file)\n_ = space.add_features(features=gj_chicago)",
"_____no_output_____"
],
[
"# update space to add tagging rules to the above mentioned space.\ntagging_rules = {\n \"large\": \"$.features[?(@.properties.area>=500)]\",\n \"small\": \"$.features[?(@.properties.area<500)]\",\n}\n_ = space.update(tagging_rules=tagging_rules)",
"_____no_output_____"
],
[
"# verify that features are tagged correctly based on rules.\nlarge_parks = space.search(tags=[\"large\"])\nfor park in large_parks:\n assert park[\"id\"] in [\"LP\", \"BP\", \"JP\"]\nsmall_parks = space.search(tags=[\"small\"])\nfor park in small_parks:\n assert park[\"id\"] in [\"MP\", \"GP\", \"HP\", \"DP\", \"CP\", \"COP\"]",
"_____no_output_____"
],
[
"# Delete created space\nspace.delete()",
"_____no_output_____"
]
],
[
[
"### Activity Log\nThe Activity log will enable tracking of changes in your space.\nTo activate it, just create a space with the listener added and enable_uuid set to True.\nMore information on the activity log can be found [here](https://www.here.xyz/api/devguide/activitylogguide/).",
"_____no_output_____"
]
],
[
[
"title = \"Activity-Log Test\"\ndescription = \"Activity-Log Test\"\nlisteners = {\n \"id\": \"activity-log\",\n \"params\": {\"states\": 5, \"storageMode\": \"DIFF_ONLY\", \"writeInvalidatedAt\": \"true\"},\n \"eventTypes\": [\"ModifySpaceEvent.request\"],\n}\nspace = xyz.spaces.new(\n title=title,\n description=description,\n enable_uuid=True,\n listeners=listeners,\n)",
"_____no_output_____"
],
[
"from time import sleep\n\n# As activity log is async operation adding sleep to get info\nsleep(5)\nprint(json.dumps(space.info, indent=2))",
"_____no_output_____"
],
[
"space.delete()",
"_____no_output_____"
]
]
] | [
"markdown",
"code",
"markdown",
"code",
"markdown",
"code",
"markdown",
"code",
"markdown",
"code",
"markdown",
"code",
"markdown",
"code",
"markdown",
"code"
] | [
[
"markdown",
"markdown"
],
[
"code"
],
[
"markdown"
],
[
"code",
"code",
"code"
],
[
"markdown"
],
[
"code",
"code",
"code",
"code"
],
[
"markdown"
],
[
"code",
"code",
"code",
"code",
"code",
"code",
"code"
],
[
"markdown"
],
[
"code",
"code",
"code",
"code",
"code"
],
[
"markdown"
],
[
"code",
"code",
"code"
],
[
"markdown"
],
[
"code",
"code",
"code",
"code",
"code"
],
[
"markdown"
],
[
"code",
"code",
"code"
]
] |
d07795ac7906ec1a4d377d2870d403c3ee71c98d | 157,305 | ipynb | Jupyter Notebook | Pyber.ipynb | bmodie/Unit_5_Pyber | c9f16418df0b18bd76004ca7fa0214ebad722557 | [
"MIT"
] | null | null | null | Pyber.ipynb | bmodie/Unit_5_Pyber | c9f16418df0b18bd76004ca7fa0214ebad722557 | [
"MIT"
] | null | null | null | Pyber.ipynb | bmodie/Unit_5_Pyber | c9f16418df0b18bd76004ca7fa0214ebad722557 | [
"MIT"
] | null | null | null | 242.75463 | 49,134 | 0.896831 | [
[
[
"# Pyber Ride Sharing\n\n3 observations from the data:\n* Urban drivers typically drive more frequently yet charge on average (i.e., <30) less than rural drivers.\n* Roughly two-thirds of all rides occur in Urban cities, however, roughly 80% of all drivers work in Urban areas.\n* While less rides occur in rural cities, there are on average less drivers to manage the load, creating a more favorable driver to ride ratio. \n* Rural drivers have the greatest fare distribution (i.e., roughly 40 dollars/driver) among drivers of all 3 city types.",
"_____no_output_____"
]
],
[
[
"import pandas as pd\nimport matplotlib.pyplot as plt\nimport numpy as np",
"_____no_output_____"
],
[
"# Read in City Data csv file\ncity_df = pd.read_csv('city_data.csv')\n\n# Read in Ride Data csv file\nride_df = pd.read_csv('ride_data.csv')",
"_____no_output_____"
],
[
"# Combine the 2 dataframes\npyber_df = pd.merge(city_df, ride_df, on=\"city\", how='left')\npyber_df.head()",
"_____no_output_____"
],
[
"# Find the total fare per city\ncity_fare_total = pyber_df.groupby('city')['fare'].sum().to_frame()\n\n# Find the average fare ($) per city\ncity_fare_avg = pyber_df.groupby('city')['fare'].mean().to_frame()\n\n# Find the total number of rides per city\ncity_total_rides = pyber_df.groupby('city')['ride_id'].count().to_frame()\n\n# Find the total number of drivers per city\ncity_driver_count = pyber_df.groupby('city')['driver_count'].unique().to_frame()\ncity_driver_count['driver_count'] = city_driver_count['driver_count'].str.get(0)\n\n# Find the city type (urban, suburban, rural)\ncity_type = pyber_df.groupby('city')['type'].unique().to_frame()\ncity_type['type'] = city_type['type'].str.get(0)\n",
"_____no_output_____"
],
[
"# Combine each dataframe\ncity_fare_avg.columns=[\"city\"]\njoin_one = city_fare_avg.join(city_total_rides, how=\"left\")\njoin_one.columns=[\"Average Fare\", \"Total Rides\"]\n\njoin_two = join_one.join(city_fare_total, how=\"inner\")\njoin_two.columns=[\"Average Fare\", \"Total Rides\", \"City Fare Total\"]\n\njoin_three = join_two.join(city_driver_count, how=\"inner\")\njoin_three.columns=[\"Average Fare\", \"Total Rides\", \"City Fare Total\", \"Driver Count\"]\n\ncity_agg = join_three.join(city_type, how='inner')\ncity_agg.columns=[\"Average Fare\", \"Total Rides\", \"City Fare Total\", \"Driver Count\", \"City Type\"]\ncity_agg.head()",
"_____no_output_____"
],
[
"# Separate data by City Type\nurban_data = city_agg.loc[(city_agg['City Type']=='Urban'), :]\nsuburban_data = city_agg.loc[(city_agg['City Type']=='Suburban'), :]\nrural_data = city_agg.loc[(city_agg['City Type']=='Rural'), :]",
"_____no_output_____"
]
],
[
[
"## Bubble Plot",
"_____no_output_____"
]
],
[
[
"## Bubble Plot Data\nall_urban_rides = urban_data.groupby('city')['Total Rides'].sum()\navg_urban_fare = urban_data.groupby('city')['Average Fare'].mean()\n\nall_suburban_rides = suburban_data.groupby('city')['Total Rides'].sum()\navg_suburban_fare = suburban_data.groupby('city')['Average Fare'].mean()\n\nall_rural_rides = rural_data.groupby('city')['Total Rides'].sum()\navg_rural_fare = rural_data.groupby('city')['Average Fare'].mean()\n",
"_____no_output_____"
],
[
"## Bubble Plot\n\n# Store driver count as a Numpy Array\nnp_city_driver_count = np.array(city_driver_count)\nnp_city_driver_count = np_city_driver_count * 3\n\n# Add chart note\ntextstr = 'Note: Circle size corresponds to driver count/city'\n\nurban = plt.scatter(all_urban_rides, avg_urban_fare, s=np_city_driver_count, color='lightskyblue', alpha=0.65, edgecolors='none')\nsuburban = plt.scatter(all_suburban_rides, avg_suburban_fare, s=np_city_driver_count, color='gold', alpha=0.65, edgecolors='none')\nrural = plt.scatter(all_rural_rides, avg_rural_fare, s=np_city_driver_count, color='lightcoral', alpha=0.65, edgecolors='none')\n\nplt.grid(linestyle='dotted')\nplt.xlabel('Total Number of Rides (Per City)')\nplt.ylabel('Average Fare ($)')\nplt.title('Pyber Ride Sharing Data (2016)')\nplt.gcf().text(0.95, 0.50, textstr, fontsize=8)\nplt.legend((urban, suburban, rural),('Urban', 'Suburban', 'Rural'),scatterpoints=1,loc='upper right',ncol=1,\\\n fontsize=8, markerscale=0.75,title='City Type', edgecolor='none',framealpha=0.25)\n\nplt.show()\n",
"_____no_output_____"
]
],
[
[
"## Pie Charts",
"_____no_output_____"
],
[
"### Total Fares by City Type",
"_____no_output_____"
]
],
[
[
"## Find Total Fares By City Type\nurban_fare_total = urban_data['City Fare Total'].sum()\nsuburban_fare_total = suburban_data['City Fare Total'].sum()\nrural_fare_total = rural_data['City Fare Total'].sum()\n\n\n# Create a Pie Chart to Express the Above Date\ndriver_type = [\"Urban\", \"Suburban\", \"Rural\"]\ndriver_count = [urban_fare_total, suburban_fare_total, rural_fare_total]\ncolors = [\"lightskyblue\", \"gold\",\"lightcoral\"]\nexplode = (0.1,0,0)\nplt.pie(driver_count, explode=explode, labels=driver_type, colors=colors,\n \tautopct=\"%1.1f%%\", shadow=True, startangle=68)\nplt.title(\"% of Total Fares by City Type\")\nplt.axis(\"equal\")\nplt.show()",
"_____no_output_____"
]
],
[
[
"### Total Rides by City Type",
"_____no_output_____"
]
],
[
[
"## Find Total Rides By City Type\nurban_rides_count = urban_data['Total Rides'].sum()\nsuburban_rides_count = suburban_data['Total Rides'].sum()\nrural_rides_count = rural_data['Total Rides'].sum()\n\n\n# Create a Pie Chart to Express the Above Date\nride_type = [\"Urban\", \"Suburban\", \"Rural\"]\nride_count = [urban_rides_count, suburban_rides_count, rural_rides_count]\ncolors = [\"lightskyblue\", \"gold\",\"lightcoral\"]\nexplode = (0.1,0,0)\nplt.pie(ride_count, explode=explode, labels=ride_type, colors=colors,\n \tautopct=\"%1.1f%%\", shadow=True, startangle=60)\nplt.title(\"% of Total Rides by City Type\")\nplt.axis(\"equal\")\nplt.show()\n",
"_____no_output_____"
]
],
[
[
"### Total Drivers by City Type",
"_____no_output_____"
]
],
[
[
"## Find Total Drivers By City Type\nurban_driver_count = urban_data['Driver Count'].sum()\nsuburban_driver_count = suburban_data['Driver Count'].sum()\nrural_driver_count = rural_data['Driver Count'].sum()\n\n\n# Create a Pie Chart to Express the Above Date\ndriver_type = [\"Urban\", \"Suburban\", \"Rural\"]\ndriver_count = [urban_driver_count, suburban_driver_count, rural_driver_count]\ncolors = [\"lightskyblue\", \"gold\",\"lightcoral\"]\nexplode = (0.1,0,0)\nplt.pie(driver_count, explode=explode, labels=driver_type, colors=colors,\n \tautopct=\"%1.1f%%\", shadow=True, startangle=40)\nplt.title(\"% of Total Drivers by City Type\")\nplt.axis(\"equal\")\nplt.show()\n",
"_____no_output_____"
]
],
[
[
"## Average Ride Value Per Driver (by City Type)",
"_____no_output_____"
]
],
[
[
"# Identify the average fare for drivers in each city type\nurban_avg_driver_pay = urban_fare_total / urban_rides_count\nsuburban_avg_driver_pay = suburban_fare_total / suburban_rides_count\nrural_avg_driver_pay = rural_fare_total / rural_rides_count\n\n# Create a Bar Chart to Express the Above Date\ndriver_type = [\"Urban\", \"Suburban\", \"Rural\"]\navg_driver_pay = [urban_avg_driver_pay, suburban_avg_driver_pay, rural_avg_driver_pay]\nx_axis = np.arange(len(avg_driver_pay))\n\ncolors = [\"lightskyblue\", \"gold\",\"lightcoral\"]\nplt.bar(x_axis, avg_driver_pay, color=colors, align='edge')\ntick_locations = [value+0.4 for value in x_axis]\nplt.xticks(tick_locations, [\"Urban\", \"Suburban\", \"Rural\"])\nplt.ylim(0, max(avg_driver_pay)+1)\nplt.xlim(-0.25, len(driver_type))\n\n\nplt.title(\"Average Per Ride Value for Drivers\")\n\nplt.show()",
"_____no_output_____"
]
],
[
[
"## Average Fare Distribution Across All Drivers (by City Type)",
"_____no_output_____"
]
],
[
[
"urban_fare_dist = urban_fare_total / urban_driver_count\nsuburban_fare_dist = suburban_fare_total / suburban_driver_count\nrural_fare_dist = rural_fare_total / rural_driver_count\n\n# Create a Bar Chart to Express the Above Date\ndriver_type = [\"Urban\", \"Suburban\", \"Rural\"]\navg_fare_dist = [urban_fare_dist, suburban_fare_dist, rural_fare_dist]\nx_axis = np.arange(len(avg_fare_dist))\n\ncolors = [\"lightskyblue\", \"gold\",\"lightcoral\"]\nplt.bar(x_axis, avg_fare_dist, color=colors, align='edge')\ntick_locations = [value+0.4 for value in x_axis]\nplt.xticks(tick_locations, [\"Urban\", \"Suburban\", \"Rural\"])\nplt.ylim(0, max(avg_fare_dist)+1)\nplt.xlim(-0.25, len(driver_type))\n\n\nplt.title(\"Average Fare Distribution Across All Drivers\")\n\nplt.show()",
"_____no_output_____"
]
]
] | [
"markdown",
"code",
"markdown",
"code",
"markdown",
"code",
"markdown",
"code",
"markdown",
"code",
"markdown",
"code",
"markdown",
"code"
] | [
[
"markdown"
],
[
"code",
"code",
"code",
"code",
"code",
"code"
],
[
"markdown"
],
[
"code",
"code"
],
[
"markdown",
"markdown"
],
[
"code"
],
[
"markdown"
],
[
"code"
],
[
"markdown"
],
[
"code"
],
[
"markdown"
],
[
"code"
],
[
"markdown"
],
[
"code"
]
] |
d077a2290e869fb445d42f7b0883093d0dbb3ab1 | 1,601 | ipynb | Jupyter Notebook | regularization.ipynb | srujansurapaneni/ml-tensorflow | f421f7c1c972d2b99d39003ea990ce9db7f4b4b9 | [
"MIT"
] | null | null | null | regularization.ipynb | srujansurapaneni/ml-tensorflow | f421f7c1c972d2b99d39003ea990ce9db7f4b4b9 | [
"MIT"
] | null | null | null | regularization.ipynb | srujansurapaneni/ml-tensorflow | f421f7c1c972d2b99d39003ea990ce9db7f4b4b9 | [
"MIT"
] | null | null | null | 19.289157 | 83 | 0.52842 | [
[
[
"# TODO: Add import statements\n",
"_____no_output_____"
],
[
"# Assign the data to predictor and outcome variables\n# TODO: Load the data\ntrain_data = None\nX = None\ny = None",
"_____no_output_____"
],
[
"# TODO: Create the linear regression model with lasso regularization.\nlasso_reg = None",
"_____no_output_____"
],
[
"# TODO: Fit the model.",
"_____no_output_____"
],
[
"# TODO: Retrieve and print out the coefficients from the regression model.\nreg_coef = None\nprint(reg_coef)",
"_____no_output_____"
]
]
] | [
"code"
] | [
[
"code",
"code",
"code",
"code",
"code"
]
] |
d077a5eeba35d28ee0bb2d7cc5c6e033250b7efa | 26,333 | ipynb | Jupyter Notebook | notebooks/benchmark/torchserve.ipynb | frreiss/zero-copy-model-loading | d59c92544a9648b6ac059a2b4a472f9cacb9b535 | [
"Apache-2.0"
] | 1 | 2022-03-08T02:48:31.000Z | 2022-03-08T02:48:31.000Z | notebooks/benchmark/torchserve.ipynb | frreiss/zero-copy-model-loading | d59c92544a9648b6ac059a2b4a472f9cacb9b535 | [
"Apache-2.0"
] | null | null | null | notebooks/benchmark/torchserve.ipynb | frreiss/zero-copy-model-loading | d59c92544a9648b6ac059a2b4a472f9cacb9b535 | [
"Apache-2.0"
] | 1 | 2022-01-28T22:30:11.000Z | 2022-01-28T22:30:11.000Z | 30.337558 | 365 | 0.567463 | [
[
[
"# torchserve.ipynb\n\nThis notebook contains code for the portions of the benchmark in [the benchmark notebook](./benchmark.ipynb) that use [TorchServe](https://github.com/pytorch/serve).\n\n",
"_____no_output_____"
]
],
[
[
"# Imports go here\nimport json\nimport os\nimport requests\n\nimport scipy.special\nimport transformers\n\n# Fix silly warning messages about parallel tokenizers\nos.environ['TOKENIZERS_PARALLELISM'] = 'False'",
"_____no_output_____"
],
[
"# Constants go here\n\nINTENT_MODEL_NAME = 'mrm8488/t5-base-finetuned-e2m-intent'\nSENTIMENT_MODEL_NAME = 'cardiffnlp/twitter-roberta-base-sentiment'\nQA_MODEL_NAME = 'deepset/roberta-base-squad2'\nGENERATE_MODEL_NAME = 'gpt2'\n\n\nINTENT_INPUT = {\n 'context':\n (\"I came here to eat chips and beat you up, \"\n \"and I'm all out of chips.\")\n}\n\nSENTIMENT_INPUT = {\n 'context': \"We're not happy unless you're not happy.\"\n}\n\nQA_INPUT = {\n 'question': 'What is 1 + 1?',\n 'context': \n \"\"\"Addition (usually signified by the plus symbol +) is one of the four basic operations of \n arithmetic, the other three being subtraction, multiplication and division. The addition of two \n whole numbers results in the total amount or sum of those values combined. The example in the\n adjacent image shows a combination of three apples and two apples, making a total of five apples. \n This observation is equivalent to the mathematical expression \"3 + 2 = 5\" (that is, \"3 plus 2 \n is equal to 5\").\n \"\"\"\n}\n\nGENERATE_INPUT = {\n 'prompt_text': 'All your base are'\n}",
"_____no_output_____"
]
],
[
[
"## Model Packaging\n\nTorchServe requires models to be packaged up as model archive files. Documentation for this process (such as it is) is [here](https://github.com/pytorch/serve/blob/master/README.md#serve-a-model) and [here](https://github.com/pytorch/serve/blob/master/model-archiver/README.md).\n\n",
"_____no_output_____"
],
[
"### Intent Model\n\nThe intent model requires the caller to call the pre- and post-processing code manually. Only the model and tokenizer are provided on the model zoo.",
"_____no_output_____"
]
],
[
[
"# First we need to dump the model into a local directory.\nintent_model = transformers.AutoModelForSeq2SeqLM.from_pretrained(\n INTENT_MODEL_NAME)\nintent_tokenizer = transformers.AutoTokenizer.from_pretrained('t5-base')\n\nintent_model.save_pretrained('torchserve/intent')\nintent_tokenizer.save_pretrained('torchserve/intent')",
"_____no_output_____"
]
],
[
[
"Next we wrapped the model in a handler class, located at `./torchserve/handler_intent.py`, which \nneeds to be in its own separate Python file in order for the `torch-model-archiver`\nutility to work.\n\nThe following command turns this Python file, plus the data files created by the \nprevious cell, into a model archive (`.mar`) file at `torchserve/model_store/intent.mar`.",
"_____no_output_____"
]
],
[
[
"%%time\n!mkdir -p torchserve/model_store\n!torch-model-archiver --model-name intent --version 1.0 \\\n --serialized-file torchserve/intent/pytorch_model.bin \\\n --handler torchserve/handler_intent.py \\\n --extra-files \"torchserve/intent/config.json,torchserve/intent/special_tokens_map.json,torchserve/intent/tokenizer_config.json,torchserve/intent/tokenizer.json\" \\\n --export-path torchserve/model_store \\\n --force",
"CPU times: user 438 ms, sys: 116 ms, total: 553 ms\nWall time: 54 s\n"
]
],
[
[
"### Sentiment Model\n\nThe sentiment model operates similarly to the intent model.",
"_____no_output_____"
]
],
[
[
"sentiment_tokenizer = transformers.AutoTokenizer.from_pretrained(\n SENTIMENT_MODEL_NAME)\nsentiment_model = (\n transformers.AutoModelForSequenceClassification\n .from_pretrained(SENTIMENT_MODEL_NAME))\n\nsentiment_model.save_pretrained('torchserve/sentiment')\nsentiment_tokenizer.save_pretrained('torchserve/sentiment')",
"_____no_output_____"
],
[
"contexts = ['hello', 'world']\ninput_batch = sentiment_tokenizer(contexts, padding=True, \n return_tensors='pt')\n\ninference_output = sentiment_model(**input_batch)\n\nscores = inference_output.logits.detach().numpy()\nscores = scipy.special.softmax(scores, axis=1).tolist()\nscores = [{k: v for k, v in zip(['positive', 'neutral', 'negative'], row)}\n for row in scores]\n# return scores\n\nscores",
"_____no_output_____"
]
],
[
[
"As with the intent model, we created a handler class (located at `torchserve/handler_sentiment.py`), then\npass that class and the serialized model from two cells ago\nthrough the `torch-model-archiver` utility.",
"_____no_output_____"
]
],
[
[
"%%time\n!torch-model-archiver --model-name sentiment --version 1.0 \\\n --serialized-file torchserve/sentiment/pytorch_model.bin \\\n --handler torchserve/handler_sentiment.py \\\n --extra-files \"torchserve/sentiment/config.json,torchserve/sentiment/special_tokens_map.json,torchserve/sentiment/tokenizer_config.json,torchserve/sentiment/tokenizer.json\" \\\n --export-path torchserve/model_store \\\n --force",
"CPU times: user 210 ms, sys: 114 ms, total: 324 ms\nWall time: 24.2 s\n"
]
],
[
[
"### Question Answering Model\n\nThe QA model uses a `transformers` pipeline. We squeeze this model into the TorchServe APIs by telling the pipeline to serialize all of its parts to a single directory, then passing the parts that aren't `pytorch_model.bin` in as extra files. At runtime, our custom handler uses the model loading code from `transformers` on the reconstituted model directory.",
"_____no_output_____"
]
],
[
[
"qa_pipeline = transformers.pipeline('question-answering', model=QA_MODEL_NAME)\nqa_pipeline.save_pretrained('torchserve/qa')",
"_____no_output_____"
]
],
[
[
"As with the previous models, we wrote a class (located at `torchserve/handler_qa.py`), then\npass that wrapper class and the serialized model through the `torch-model-archiver` utility.",
"_____no_output_____"
]
],
[
[
"%%time\n!torch-model-archiver --model-name qa --version 1.0 \\\n --serialized-file torchserve/qa/pytorch_model.bin \\\n --handler torchserve/handler_qa.py \\\n --extra-files \"torchserve/qa/config.json,torchserve/qa/merges.txt,torchserve/qa/special_tokens_map.json,torchserve/qa/tokenizer_config.json,torchserve/qa/tokenizer.json,torchserve/qa/vocab.json\" \\\n --export-path torchserve/model_store \\\n --force",
"CPU times: user 287 ms, sys: 67.5 ms, total: 354 ms\nWall time: 24.7 s\n"
],
[
"data = [QA_INPUT, QA_INPUT]\n\n# Preprocessing\nsamples = [qa_pipeline.create_sample(**r) for r in data]\ngenerators = [qa_pipeline.preprocess(s) for s in samples]\n\n# Inference\ninference_outputs = ((qa_pipeline.forward(example) for example in batch) for batch in generators)\n\npost_results = [qa_pipeline.postprocess(o) for o in inference_outputs]\npost_results",
"_____no_output_____"
]
],
[
[
"### Natural Language Generation Model\n\nThe text generation model is roughly similar to the QA model, albeit with important differences in how the three stages of the pipeline operate. At least model loading is the same.",
"_____no_output_____"
]
],
[
[
"generate_pipeline = transformers.pipeline(\n 'text-generation', model=GENERATE_MODEL_NAME)\ngenerate_pipeline.save_pretrained('torchserve/generate')",
"_____no_output_____"
],
[
"data = [GENERATE_INPUT, GENERATE_INPUT]\n\n\npad_token_id = generate_pipeline.tokenizer.eos_token_id\n\njson_records = data\n\n# preprocess() takes a single input at a time, but we need to do \n# a batch at a time.\ninput_batch = [generate_pipeline.preprocess(**r) for r in json_records]\n\n# forward() takes a single input at a time, but we need to run a\n# batch at a time.\ninference_output = [\n generate_pipeline.forward(r, pad_token_id=pad_token_id)\n for r in input_batch]\n\n# postprocess() takes a single generation result at a time, but we\n# need to run a batch at a time.\ngenerate_result = [generate_pipeline.postprocess(i)\n for i in inference_output]\ngenerate_result",
"_____no_output_____"
]
],
[
[
"Once again, we wrote a class (located at `torchserve/handler_generate.py`), then\npass that wrapper class and the serialized model through the `torch-model-archiver` utility.",
"_____no_output_____"
]
],
[
[
"%%time\n!torch-model-archiver --model-name generate --version 1.0 \\\n --serialized-file torchserve/generate/pytorch_model.bin \\\n --handler torchserve/handler_generate.py \\\n --extra-files \"torchserve/generate/config.json,torchserve/generate/merges.txt,torchserve/generate/special_tokens_map.json,torchserve/generate/tokenizer_config.json,torchserve/generate/tokenizer.json,torchserve/generate/vocab.json\" \\\n --export-path torchserve/model_store \\\n --force",
"CPU times: user 198 ms, sys: 96 ms, total: 294 ms\nWall time: 24.5 s\n"
]
],
[
[
"## Testing\n\nNow we can fire up TorchServe and test our models.\n\nFor some reason, starting TorchServe needs to be done in a proper terminal window. Running the command from this notebook has no effect. The commands to run (from the root of the repository) are:\n\n```\n> conda activate ./env\n> cd notebooks/benchmark/torchserve\n> torchserve --start --ncs --model-store model_store --ts-config torchserve.properties\n```\n\nThen pick up a cup of coffee and a book and wait a while. The startup process is like cold-starting a gas turbine and takes about 10 minutes.\n\nOnce the server has started, we can test our deployed models by making POST requests.",
"_____no_output_____"
]
],
[
[
"# Probe the management API to verify that TorchServe is running.\nrequests.get('http://127.0.0.1:8081/models').json()",
"_____no_output_____"
],
[
"port = 8080\n\nintent_result = requests.put(\n f'http://127.0.0.1:{port}/predictions/intent_en', \n json.dumps(INTENT_INPUT)).json()\nprint(f'Intent result: {intent_result}')\n\nsentiment_result = requests.put(\n f'http://127.0.0.1:{port}/predictions/sentiment_en', \n json.dumps(SENTIMENT_INPUT)).json()\nprint(f'Sentiment result: {sentiment_result}')\n\nqa_result = requests.put(\n f'http://127.0.0.1:{port}/predictions/qa_en', \n json.dumps(QA_INPUT)).json()\nprint(f'Question answering result: {qa_result}')\n\ngenerate_result = requests.put(\n f'http://127.0.0.1:{port}/predictions/generate_en', \n json.dumps(GENERATE_INPUT)).json()\nprint(f'Natural language generation result: {generate_result}')",
"_____no_output_____"
]
],
[
[
"## Cleanup\n\nTorchServe consumes many resources even when it isn't doing anything. When you're done running the baseline portion of the benchmark, be sure to shut down the server by running:\n```\n> torchserve --stop\n```",
"_____no_output_____"
]
]
] | [
"markdown",
"code",
"markdown",
"code",
"markdown",
"code",
"markdown",
"code",
"markdown",
"code",
"markdown",
"code",
"markdown",
"code",
"markdown",
"code",
"markdown",
"code",
"markdown",
"code",
"markdown"
] | [
[
"markdown"
],
[
"code",
"code"
],
[
"markdown",
"markdown"
],
[
"code"
],
[
"markdown"
],
[
"code"
],
[
"markdown"
],
[
"code",
"code"
],
[
"markdown"
],
[
"code"
],
[
"markdown"
],
[
"code"
],
[
"markdown"
],
[
"code",
"code"
],
[
"markdown"
],
[
"code",
"code"
],
[
"markdown"
],
[
"code"
],
[
"markdown"
],
[
"code",
"code"
],
[
"markdown"
]
] |
d077a6cbfaa11f6d6ec5eaff0939c8b05ec7daae | 14,558 | ipynb | Jupyter Notebook | Lab 1/ Problem 3 genouadr.ipynb | AdrianGScorp/deeprlbootcamp | eb856f1dee90cd09395c40fa9d045f2149d5ea33 | [
"MIT"
] | null | null | null | Lab 1/ Problem 3 genouadr.ipynb | AdrianGScorp/deeprlbootcamp | eb856f1dee90cd09395c40fa9d045f2149d5ea33 | [
"MIT"
] | null | null | null | Lab 1/ Problem 3 genouadr.ipynb | AdrianGScorp/deeprlbootcamp | eb856f1dee90cd09395c40fa9d045f2149d5ea33 | [
"MIT"
] | null | null | null | 34.661905 | 314 | 0.538536 | [
[
[
"# Lab 1: Markov Decision Processes - Problem 3\n\n\n## Lab Instructions\nAll your answers should be written in this notebook. You shouldn't need to write or modify any other files.\n\n**You should execute every block of code to not miss any dependency.**\n\n*This project was developed by Peter Chen, Rocky Duan, Pieter Abbeel for the Berkeley Deep RL Bootcamp, August 2017. Bootcamp website with slides and lecture videos: https://sites.google.com/view/deep-rl-bootcamp/. It is adapted from CS188 project materials: http://ai.berkeley.edu/project_overview.html.*\n\n--------------------------",
"_____no_output_____"
]
],
[
[
"import numpy as np, numpy.random as nr, gym\nimport matplotlib.pyplot as plt\n%matplotlib inline\nnp.set_printoptions(precision=3)",
"_____no_output_____"
]
],
[
[
"### Problem 3: Sampling-based Tabular Q-Learning\n\nSo far we have implemented Value Iteration and Policy Iteration, both of which require access to an MDP's dynamics model. This requirement can sometimes be restrictive - for example, if the environment is given as a blackbox physics simulator, then we won't be able to read off the whole transition model.\n\nWe can however use sampling-based Q-Learning to learn from this type of environments. ",
"_____no_output_____"
],
[
"For this exercise, we will learn to control a Crawler robot. Let's first try some completely random actions to see how the robot moves and familiarize ourselves with Gym environment interface again.",
"_____no_output_____"
]
],
[
[
"from crawler_env import CrawlingRobotEnv\n\nenv = CrawlingRobotEnv()\n\nprint(\"We can inspect the observation space and action space of this Gym Environment\")\nprint(\"-----------------------------------------------------------------------------\")\nprint(\"Action space:\", env.action_space)\nprint(\"It's a discrete space with %i actions to take\" % env.action_space.n)\nprint(\"Each action corresponds to increasing/decreasing the angle of one of the joints\")\nprint(\"We can also sample from this action space:\", env.action_space.sample())\nprint(\"Another action sample:\", env.action_space.sample())\nprint(\"Another action sample:\", env.action_space.sample())\nprint(\"Observation space:\", env.observation_space, \", which means it's a 9x13 grid.\")\nprint(\"It's the discretized version of the robot's two joint angles\")",
"We can inspect the observation space and action space of this Gym Environment\n-----------------------------------------------------------------------------\nAction space: Discrete(4)\nIt's a discrete space with 4 actions to take\nEach action corresponds to increasing/decreasing the angle of one of the joints\nWe can also sample from this action space: 0\nAnother action sample: 3\nAnother action sample: 1\nObservation space: Tuple(Discrete(9), Discrete(13)) , which means it's a 9x13 grid.\nIt's the discretized version of the robot's two joint angles\n"
],
[
"env = CrawlingRobotEnv(\n render=True, # turn render mode on to visualize random motion\n)\n\n# standard procedure for interfacing with a Gym environment\ncur_state = env.reset() # reset environment and get initial state\nret = 0.\ndone = False\ni = 0\nwhile not done:\n action = env.action_space.sample() # sample an action randomly\n next_state, reward, done, info = env.step(action)\n ret += reward\n cur_state = next_state\n i += 1\n if i == 1500:\n break # for the purpose of this visualization, let's only run for 1500 steps\n # also note the GUI won't close automatically",
"_____no_output_____"
],
[
"# you can close the visualization GUI with the following method \nenv.close_gui()",
"_____no_output_____"
]
],
[
[
"You will see the random controller can sometimes make progress but it won't get very far. Let's implement Tabular Q-Learning with $\\epsilon$-greedy exploration to find a better policy piece by piece.\n",
"_____no_output_____"
]
],
[
[
"from collections import defaultdict\nimport random\n\n# dictionary that maps from state, s, to a numpy array of Q values [Q(s, a_1), Q(s, a_2) ... Q(s, a_n)]\n# and everything is initialized to 0.\nq_vals = defaultdict(lambda: np.array([0. for _ in range(env.action_space.n)]))\n\nprint(\"Q-values for state (0, 0): %s\" % q_vals[(0, 0)], \"which is a list of Q values for each action\")\nprint(\"As such, the Q value of taking action 3 in state (1,2), i.e. Q((1,2), 3), can be accessed by q_vals[(1,2)][3]:\", q_vals[(1,2)][3])",
"Q-values for state (0, 0): [ 0. 0. 0. 0.] which is a list of Q values for each action\nAs such, the Q value of taking action 3 in state (1,2), i.e. Q((1,2), 3), can be accessed by q_vals[(1,2)][3]: 0.0\n"
],
[
"def eps_greedy(q_vals, eps, state):\n \"\"\"\n Inputs:\n q_vals: q value tables\n eps: epsilon\n state: current state\n Outputs:\n random action with probability of eps; argmax Q(s, .) with probability of (1-eps)\n \"\"\"\n # you might want to use random.random() to implement random exploration\n # number of actions can be read off from len(q_vals[state])\n import random\n # YOUR CODE HERE\n \n # MY CODE -------------------------------------------------------------------\n if random.random() <= eps:\n return np.random.randint(0, len(q_vals[state]))\n return np.argmax(q_vals[state])\n #----------------------------------------------------------------------------\n\n# test 1\ndummy_q = defaultdict(lambda: np.array([0. for _ in range(env.action_space.n)]))\ntest_state = (0, 0)\ndummy_q[test_state][0] = 10.\ntrials = 100000\nsampled_actions = [\n int(eps_greedy(dummy_q, 0.3, test_state))\n for _ in range(trials)\n]\nfreq = np.sum(np.array(sampled_actions) == 0) / trials\ntgt_freq = 0.3 / env.action_space.n + 0.7\nif np.isclose(freq, tgt_freq, atol=1e-2):\n print(\"Test1 passed\")\nelse:\n print(\"Test1: Expected to select 0 with frequency %.2f but got %.2f\" % (tgt_freq, freq))\n \n# test 2\ndummy_q = defaultdict(lambda: np.array([0. for _ in range(env.action_space.n)]))\ntest_state = (0, 0)\ndummy_q[test_state][2] = 10.\ntrials = 100000\nsampled_actions = [\n int(eps_greedy(dummy_q, 0.5, test_state))\n for _ in range(trials)\n]\nfreq = np.sum(np.array(sampled_actions) == 2) / trials\ntgt_freq = 0.5 / env.action_space.n + 0.5\nif np.isclose(freq, tgt_freq, atol=1e-2):\n print(\"Test2 passed\")\nelse:\n print(\"Test2: Expected to select 2 with frequency %.2f but got %.2f\" % (tgt_freq, freq))",
"Test1 passed\nTest2 passed\n"
]
],
[
[
"Next we will implement Q learning update. After we observe a transition $s, a, s', r$,\n\n$$\\textrm{target}(s') = R(s,a,s') + \\gamma \\max_{a'} Q_{\\theta_k}(s',a')$$\n\n\n$$Q_{k+1}(s,a) \\leftarrow (1-\\alpha) Q_k(s,a) + \\alpha \\left[ \\textrm{target}(s') \\right]$$",
"_____no_output_____"
]
],
[
[
"def q_learning_update(gamma, alpha, q_vals, cur_state, action, next_state, reward):\n \"\"\"\n Inputs:\n gamma: discount factor\n alpha: learning rate\n q_vals: q value table\n cur_state: current state\n action: action taken in current state\n next_state: next state results from taking `action` in `cur_state`\n reward: reward received from this transition\n \n Performs in-place update of q_vals table to implement one step of Q-learning\n \"\"\"\n # YOUR CODE HERE\n \n # MY CODE -------------------------------------------------------------------\n target = reward + gamma*np.max(q_vals[next_state])\n q_vals[cur_state][action] -= alpha*(q_vals[cur_state][action] - target)\n #----------------------------------------------------------------------------\n\n# testing your q_learning_update implementation\ndummy_q = q_vals.copy()\ntest_state = (0, 0)\ntest_next_state = (0, 1)\ndummy_q[test_state][0] = 10.\ndummy_q[test_next_state][1] = 10.\nq_learning_update(0.9, 0.1, dummy_q, test_state, 0, test_next_state, 1.1)\ntgt = 10.01\nif np.isclose(dummy_q[test_state][0], tgt,):\n print(\"Test passed\")\nelse:\n print(\"Q(test_state, 0) is expected to be %.2f but got %.2f\" % (tgt, dummy_q[test_state][0]))",
"Test passed\n"
],
[
"# now with the main components tested, we can put everything together to create a complete q learning agent\n\nenv = CrawlingRobotEnv() \nq_vals = defaultdict(lambda: np.array([0. for _ in range(env.action_space.n)]))\ngamma = 0.9\nalpha = 0.1\neps = 0.5\ncur_state = env.reset()\n\ndef greedy_eval():\n \"\"\"evaluate greedy policy w.r.t current q_vals\"\"\"\n test_env = CrawlingRobotEnv(horizon=np.inf)\n prev_state = test_env.reset()\n ret = 0.\n done = False\n H = 100\n for i in range(H):\n action = np.argmax(q_vals[prev_state])\n state, reward, done, info = test_env.step(action)\n ret += reward\n prev_state = state\n return ret / H\n\nfor itr in range(300000):\n # YOUR CODE HERE\n # Hint: use eps_greedy & q_learning_update\n \n # MY CODE --------------------------------------------------------------------\n action = eps_greedy(q_vals, eps, cur_state)\n next_state, reward, done, info = env.step(action)\n q_learning_update(gamma, alpha, q_vals, cur_state, action, next_state, reward)\n cur_state = next_state\n #-----------------------------------------------------------------------------\n \n if itr % 50000 == 0: # evaluation\n print(\"Itr %i # Average speed: %.2f\" % (itr, greedy_eval()))\n\n# at the end of learning your crawler should reach a speed of >= 3",
"Itr 0 # Average speed: 0.05\nItr 50000 # Average speed: 2.03\nItr 100000 # Average speed: 3.37\nItr 150000 # Average speed: 3.37\nItr 200000 # Average speed: 3.37\nItr 250000 # Average speed: 3.37\n"
]
],
[
[
"After the learning is successful, we can visualize the learned robot controller. Remember we learn this just from interacting with the environment instead of peeking into the dynamics model!",
"_____no_output_____"
]
],
[
[
"env = CrawlingRobotEnv(render=True, horizon=500)\nprev_state = env.reset()\nret = 0.\ndone = False\nwhile not done:\n action = np.argmax(q_vals[prev_state])\n state, reward, done, info = env.step(action)\n ret += reward\n prev_state = state",
"_____no_output_____"
],
[
"# you can close the visualization GUI with the following method \nenv.close_gui()",
"_____no_output_____"
]
]
] | [
"markdown",
"code",
"markdown",
"code",
"markdown",
"code",
"markdown",
"code",
"markdown",
"code"
] | [
[
"markdown"
],
[
"code"
],
[
"markdown",
"markdown"
],
[
"code",
"code",
"code"
],
[
"markdown"
],
[
"code",
"code"
],
[
"markdown"
],
[
"code",
"code"
],
[
"markdown"
],
[
"code",
"code"
]
] |
d077aa983906c48252924329f661920e280b0383 | 172,221 | ipynb | Jupyter Notebook | week6/lstm.ipynb | changkun/ws-18-19-deep-learning-tutorial | 0bbd3d0a0c98a826795ea39d9bd19227731514ee | [
"MIT"
] | 16 | 2018-11-19T20:21:08.000Z | 2021-07-22T15:34:27.000Z | week6/lstm.ipynb | changkun/ws-18-19-deep-learning-tutorial | 0bbd3d0a0c98a826795ea39d9bd19227731514ee | [
"MIT"
] | null | null | null | week6/lstm.ipynb | changkun/ws-18-19-deep-learning-tutorial | 0bbd3d0a0c98a826795ea39d9bd19227731514ee | [
"MIT"
] | 1 | 2021-04-22T03:17:47.000Z | 2021-04-22T03:17:47.000Z | 714.609959 | 164,456 | 0.948642 | [
[
[
"# Exercise 6-3\n\n## LSTM\n\nThe following two cells will create a LSTM cell with one neuron.\nWe scale the output of the LSTM linear and add a bias.\nThen the output will be wrapped by a sigmoid activation.\nThe goal is to predict a time series where every $n^{th}$ ($5^{th}$ in the current example) element is 1 and all others are 0.\n\na) Please read and understand the source code below.\n\nb) Consult the output of the predictions. What do you observe? How does the LSTM manage to predict the next element in the sequence?",
"_____no_output_____"
]
],
[
[
"import tensorflow as tf\nimport numpy as np\nfrom matplotlib import pyplot as plt",
"_____no_output_____"
],
[
"tf.reset_default_graph()\ntf.set_random_seed(12314)\n\nepochs=50\nzero_steps = 5\nlearning_rate = 0.01\nlstm_neurons = 1\nout_dim = 1\n\nnum_features = 1\nbatch_size = zero_steps\nwindow_size = zero_steps*2\ntime_steps = 5\n\nx = tf.placeholder(tf.float32, [None, window_size, num_features], 'x')\ny = tf.placeholder(tf.float32, [None, out_dim], 'y')\n\nlstm = tf.nn.rnn_cell.LSTMCell(lstm_neurons)\nstate = lstm.zero_state(batch_size, dtype=tf.float32)\n\nregression_w = tf.Variable(tf.random_normal([lstm_neurons]))\nregression_b = tf.Variable(tf.random_normal([out_dim]))\n\noutputs, state = tf.contrib.rnn.static_rnn(lstm, tf.unstack(x, window_size, 1), state)\noutput = outputs[-1]\npredicted = tf.nn.sigmoid(output * regression_w + regression_b)\ncost = tf.reduce_mean(tf.losses.mean_squared_error(y, predicted))\noptimizer = tf.train.RMSPropOptimizer(learning_rate=learning_rate).minimize(cost)\n\n\nforget_gate = output.op.inputs[1].op.inputs[0].op.inputs[0].op.inputs[0]\ninput_gate = output.op.inputs[1].op.inputs[0].op.inputs[1].op.inputs[0]\ncell_candidates = output.op.inputs[1].op.inputs[0].op.inputs[1].op.inputs[1]\noutput_gate_sig = output.op.inputs[0]\noutput_gate_tanh = output.op.inputs[1]\n\n\nX = [\n [[ (shift-n) % zero_steps == 0 ] for n in range(window_size)\n ] for shift in range(batch_size)\n]\nY = [[ shift % zero_steps == 0 ] for shift in range(batch_size) ]\n\n\nwith tf.Session() as sess:\n sess.run(tf.initializers.global_variables())\n\n loss = 1\n epoch = 0\n while loss >= 1e-5:\n epoch += 1\n _, loss = sess.run([optimizer, cost], {x:X, y:Y})\n\n if epoch % (epochs//10) == 0:\n print(\"loss %.5f\" % (loss), end='\\t\\t\\r')\n print()\n \n outs, stat, pred, fg, inpg, cell_cands, outg_sig, outg_tanh = sess.run([outputs, state, predicted, forget_gate, input_gate, cell_candidates, output_gate_sig, output_gate_tanh], {x:X, y:Y})\n outs = np.asarray(outs)\n for batch in reversed(range(batch_size)):\n print(\"input:\")\n print(np.asarray(X)[batch].astype(int).reshape(-1))\n print(\"forget\\t\\t%.4f\\ninput gate\\t%.4f\\ncell cands\\t%.4f\\nout gate sig\\t%.4f\\nout gate tanh\\t%.4f\\nhidden state\\t%.4f\\ncell state\\t%.4f\\npred\\t\\t%.4f\\n\\n\" % (\n fg[batch,0], \n inpg[batch,0], \n cell_cands[batch,0], \n outg_sig[batch,0], \n outg_tanh[batch,0], \n stat.h[batch,0], \n stat.c[batch,0], \n pred[batch,0]))",
"loss 0.00001\t\t\ninput:\n[0 0 0 0 1 0 0 0 0 1]\nforget\t\t0.0135\ninput gate\t0.9997\ncell cands\t0.9994\nout gate sig\t1.0000\nout gate tanh\t0.7586\nhidden state\t0.7586\ncell state\t0.9928\npred\t\t0.0000\n\n\ninput:\n[0 0 0 1 0 0 0 0 1 0]\nforget\t\t0.8747\ninput gate\t0.9860\ncell cands\t-0.0476\nout gate sig\t1.0000\nout gate tanh\t0.6759\nhidden state\t0.6759\ncell state\t0.8215\npred\t\t0.0000\n\n\ninput:\n[0 0 1 0 0 0 0 1 0 0]\nforget\t\t0.8504\ninput gate\t0.9877\ncell cands\t-0.1311\nout gate sig\t0.9999\nout gate tanh\t0.5148\nhidden state\t0.5147\ncell state\t0.5692\npred\t\t0.0000\n\n\ninput:\n[0 1 0 0 0 0 1 0 0 0]\nforget\t\t0.7921\ninput gate\t0.9903\ncell cands\t-0.2875\nout gate sig\t0.9999\nout gate tanh\t0.1646\nhidden state\t0.1646\ncell state\t0.1661\npred\t\t0.0052\n\n\ninput:\n[1 0 0 0 0 1 0 0 0 0]\nforget\t\t0.6150\ninput gate\t0.9943\ncell cands\t-0.5732\nout gate sig\t0.9997\nout gate tanh\t-0.4363\nhidden state\t-0.4361\ncell state\t-0.4676\npred\t\t0.9953\n\n\n"
]
],
[
[
"LSTM gates:\n\n\n(image source: https://www.stratio.com/wp-content/uploads/2017/10/6-1.jpg)",
"_____no_output_____"
],
[
"### Answers\n\n* When the current element is 1, then the forget-gate tells \"forget\" (value is close to 0) $\\Rightarrow$ Reset cell state\n* The cell state (long term memory) decreases until it reached some certain point. Then the hidden state is activated and thus the prediction is close to 1.\n* The sigomoid output cell ($o_t$) is always close to 1 $\\Rightarrow$ the hidden layer directly dependent on the cell state (no short term memory is used).\n* The input gate ($i_t$) is always close to 1 thus the cell candidates ($c_t$) will always be accepted\n* The cell candidates ($c_t$) are mainly dependent on $x_t$. It is close to 1 when $x_t$ is one (resetting the counter) and negative if $x_t$ is 0 (decreasing the counter).\n\nNote that with other initial values (different seed) it may result in a different local minimum (the counter could increase, $h_t$ could be negative and be scaled negative, ...)",
"_____no_output_____"
]
]
] | [
"markdown",
"code",
"markdown"
] | [
[
"markdown"
],
[
"code",
"code"
],
[
"markdown",
"markdown"
]
] |
d077ac924c8bbc26d11087783338bdc4a7d54bb0 | 35,061 | ipynb | Jupyter Notebook | Prace_domowe/Praca_domowa2/Grupa1/EljasiakBartlomiej/main.ipynb | niladrem/2020L-WUM | ddccedd900e41de196612c517227e1348c7195df | [
"Apache-2.0"
] | null | null | null | Prace_domowe/Praca_domowa2/Grupa1/EljasiakBartlomiej/main.ipynb | niladrem/2020L-WUM | ddccedd900e41de196612c517227e1348c7195df | [
"Apache-2.0"
] | null | null | null | Prace_domowe/Praca_domowa2/Grupa1/EljasiakBartlomiej/main.ipynb | niladrem/2020L-WUM | ddccedd900e41de196612c517227e1348c7195df | [
"Apache-2.0"
] | 1 | 2020-06-01T23:23:16.000Z | 2020-06-01T23:23:16.000Z | 31.165333 | 487 | 0.490545 | [
[
[
"# 2020L-WUM Praca domowa 2",
"_____no_output_____"
],
[
"Kod: **Bartłomiej Eljasiak**",
"_____no_output_____"
],
[
"## Załadowanie bibliotek",
"_____no_output_____"
],
[
"Z tych bibliotek będziemy korzystać w wielu miejscach, jednak w niektórych fragmentach kodu znajdą się dodatkowe importowania, lecz w takich sytuacjach użytek załadowanej biblioteki jest ograniczony do 'chunku', w którym została załadowana.",
"_____no_output_____"
]
],
[
[
"import pandas as pd\nimport seaborn as sns\nimport numpy as np\nimport sklearn",
"_____no_output_____"
]
],
[
[
"## Wczytanie danych",
"_____no_output_____"
]
],
[
[
"# local version \n_data=pd.read_csv('allegro-api-transactions.csv')\n\n#online version\n#_data = pd.read_csv('https://www.dropbox.com/s/360xhh2d9lnaek3/allegro-api-transactions.csv?dl=1')\ncdata=_data.copy()",
"_____no_output_____"
]
],
[
[
"### Przyjrzenie się danym",
"_____no_output_____"
]
],
[
[
"cdata.head()",
"_____no_output_____"
]
],
[
[
"# Obróbka danych",
"_____no_output_____"
]
],
[
[
"len(cdata.it_location.unique())",
"_____no_output_____"
],
[
"cdata.info()",
"<class 'pandas.core.frame.DataFrame'>\nRangeIndex: 420020 entries, 0 to 420019\nData columns (total 14 columns):\nlp 420020 non-null int64\ndate 420020 non-null object\nitem_id 420020 non-null int64\ncategories 420020 non-null object\npay_option_on_delivery 420020 non-null int64\npay_option_transfer 420020 non-null int64\nseller 420020 non-null object\nprice 420020 non-null float64\nit_is_allegro_standard 420020 non-null int64\nit_quantity 420020 non-null int64\nit_is_brand_zone 420020 non-null int64\nit_seller_rating 420020 non-null int64\nit_location 420020 non-null object\nmain_category 420020 non-null object\ndtypes: float64(1), int64(8), object(5)\nmemory usage: 36.9+ MB\n"
]
],
[
[
"Na pierwszy rzut oka nie mamy żadnych braków w danych, co znacznie ułatwia nam prace. ",
"_____no_output_____"
],
[
"# Kodowanie zmiennych kategorycznych",
"_____no_output_____"
],
[
"Wiemy, że naszym targetem będzie `price`, chcemy więc w tym akapicie zamienić wszystkie zmienne kategoryczne, z których w dalszej części kodu będziemy korzystać, na zmienne liczbowe. Skuteczna zamiana przy użyciu odpowiednich metod takich jak **target encoding** oraz **on-hot encoding** pozwoli nam przekształcić obecne informacje, tak byśmy mogli je wykorzystać przy operacjach matematycznych. Pominiemy jednak w naszych przekształceniach kolumnę `categories`. ",
"_____no_output_____"
],
[
"## Target encoding dla `it_location`",
"_____no_output_____"
]
],
[
[
"import category_encoders\ny=cdata.price\nte = category_encoders.target_encoder.TargetEncoder(cdata.it_location, smoothing=100)\nencoded = te.fit_transform(cdata.it_location,y)\nencoded",
"_____no_output_____"
]
],
[
[
"## Różne rodzaje zakodowania kolumny `main_category`",
"_____no_output_____"
],
[
"## One-hot Coding",
"_____no_output_____"
]
],
[
[
"from sklearn.preprocessing import LabelEncoder\nfrom sklearn.preprocessing import OneHotEncoder\n\n# integer encode\nle = LabelEncoder()\ninteger_encoded = le.fit_transform(cdata.main_category)\nprint(integer_encoded)\n\n# binary encode\nonehot_encoder = OneHotEncoder(categories='auto',sparse=False)\ninteger_encoded = integer_encoded.reshape(len(integer_encoded), 1)\nonehot_encoded = onehot_encoder.fit_transform(integer_encoded)\nprint(onehot_encoded)",
"[12 18 6 ... 18 5 15]\n[[0. 0. 0. ... 0. 0. 0.]\n [0. 0. 0. ... 0. 0. 0.]\n [0. 0. 0. ... 0. 0. 0.]\n ...\n [0. 0. 0. ... 0. 0. 0.]\n [0. 0. 0. ... 0. 0. 0.]\n [0. 0. 0. ... 0. 0. 0.]]\n"
]
],
[
[
"Zamieniliśmy w ten sposób kolumnę kategoryczną na 26 kolumn o wartościach 0 lub 1. Nie jest to złe rozwiązanie, jednak stanowczo zwiększa rozmiar naszej ramki i wydłża czas uczenia się modelu. Prawdopodobnie może istnieć lepsze rozwiązanie.",
"_____no_output_____"
],
[
"## Helmert Coding\nDocumentation: [scikit-learn](http://contrib.scikit-learn.org/categorical-encoding/helmert.html)",
"_____no_output_____"
]
],
[
[
"# Pobieramy go z category_encoders\n\nhelmert_encoder = category_encoders.HelmertEncoder()\nhelmert_encoded = helmert_encoder.fit_transform(cdata.main_category)\n\n#showing only first 5 encoded rows\nprint(helmert_encoded.loc[1:5,:].transpose())",
" 1 2 3 4 5\nintercept 1.0 1.0 1.0 1.0 1.0\nmain_category_0 1.0 0.0 0.0 1.0 1.0\nmain_category_1 -1.0 2.0 0.0 -1.0 -1.0\nmain_category_2 -1.0 -1.0 3.0 -1.0 -1.0\nmain_category_3 -1.0 -1.0 -1.0 -1.0 -1.0\nmain_category_4 -1.0 -1.0 -1.0 -1.0 -1.0\nmain_category_5 -1.0 -1.0 -1.0 -1.0 -1.0\nmain_category_6 -1.0 -1.0 -1.0 -1.0 -1.0\nmain_category_7 -1.0 -1.0 -1.0 -1.0 -1.0\nmain_category_8 -1.0 -1.0 -1.0 -1.0 -1.0\nmain_category_9 -1.0 -1.0 -1.0 -1.0 -1.0\nmain_category_10 -1.0 -1.0 -1.0 -1.0 -1.0\nmain_category_11 -1.0 -1.0 -1.0 -1.0 -1.0\nmain_category_12 -1.0 -1.0 -1.0 -1.0 -1.0\nmain_category_13 -1.0 -1.0 -1.0 -1.0 -1.0\nmain_category_14 -1.0 -1.0 -1.0 -1.0 -1.0\nmain_category_15 -1.0 -1.0 -1.0 -1.0 -1.0\nmain_category_16 -1.0 -1.0 -1.0 -1.0 -1.0\nmain_category_17 -1.0 -1.0 -1.0 -1.0 -1.0\nmain_category_18 -1.0 -1.0 -1.0 -1.0 -1.0\nmain_category_19 -1.0 -1.0 -1.0 -1.0 -1.0\nmain_category_20 -1.0 -1.0 -1.0 -1.0 -1.0\nmain_category_21 -1.0 -1.0 -1.0 -1.0 -1.0\nmain_category_22 -1.0 -1.0 -1.0 -1.0 -1.0\nmain_category_23 -1.0 -1.0 -1.0 -1.0 -1.0\nmain_category_24 -1.0 -1.0 -1.0 -1.0 -1.0\nmain_category_25 -1.0 -1.0 -1.0 -1.0 -1.0\n"
]
],
[
[
"## Backward Difference Coding\nDocumentation: [scikit-learn](http://contrib.scikit-learn.org/categorical-encoding/backward_difference.html#backward-difference-coding)",
"_____no_output_____"
]
],
[
[
"# Pobieramy go z category_encoders\n\nback_diff_encoder = category_encoders.BackwardDifferenceEncoder()\nback_diff_encoded = back_diff_encoder.fit_transform(cdata.main_category)\n\n#showing only first 5 encoded rows\nprint(back_diff_encoded.loc[1:5,:].transpose())",
" 1 2 3 4 5\nintercept 1.000000 1.000000 1.000000 1.000000 1.000000\nmain_category_0 0.037037 0.037037 0.037037 0.037037 0.037037\nmain_category_1 -0.925926 0.074074 0.074074 -0.925926 -0.925926\nmain_category_2 -0.888889 -0.888889 0.111111 -0.888889 -0.888889\nmain_category_3 -0.851852 -0.851852 -0.851852 -0.851852 -0.851852\nmain_category_4 -0.814815 -0.814815 -0.814815 -0.814815 -0.814815\nmain_category_5 -0.777778 -0.777778 -0.777778 -0.777778 -0.777778\nmain_category_6 -0.740741 -0.740741 -0.740741 -0.740741 -0.740741\nmain_category_7 -0.703704 -0.703704 -0.703704 -0.703704 -0.703704\nmain_category_8 -0.666667 -0.666667 -0.666667 -0.666667 -0.666667\nmain_category_9 -0.629630 -0.629630 -0.629630 -0.629630 -0.629630\nmain_category_10 -0.592593 -0.592593 -0.592593 -0.592593 -0.592593\nmain_category_11 -0.555556 -0.555556 -0.555556 -0.555556 -0.555556\nmain_category_12 -0.518519 -0.518519 -0.518519 -0.518519 -0.518519\nmain_category_13 -0.481481 -0.481481 -0.481481 -0.481481 -0.481481\nmain_category_14 -0.444444 -0.444444 -0.444444 -0.444444 -0.444444\nmain_category_15 -0.407407 -0.407407 -0.407407 -0.407407 -0.407407\nmain_category_16 -0.370370 -0.370370 -0.370370 -0.370370 -0.370370\nmain_category_17 -0.333333 -0.333333 -0.333333 -0.333333 -0.333333\nmain_category_18 -0.296296 -0.296296 -0.296296 -0.296296 -0.296296\nmain_category_19 -0.259259 -0.259259 -0.259259 -0.259259 -0.259259\nmain_category_20 -0.222222 -0.222222 -0.222222 -0.222222 -0.222222\nmain_category_21 -0.185185 -0.185185 -0.185185 -0.185185 -0.185185\nmain_category_22 -0.148148 -0.148148 -0.148148 -0.148148 -0.148148\nmain_category_23 -0.111111 -0.111111 -0.111111 -0.111111 -0.111111\nmain_category_24 -0.074074 -0.074074 -0.074074 -0.074074 -0.074074\nmain_category_25 -0.037037 -0.037037 -0.037037 -0.037037 -0.037037\n"
]
],
[
[
"# Uzupełnianie braków",
"_____no_output_____"
],
[
"### Wybranie danych z ramki\nDalej będziemy pracowac tylko na 3 kolumnach danych, ograniczę więc je dla przejżystości poczynań.",
"_____no_output_____"
]
],
[
[
"data_selected= cdata.loc[:,['price','it_seller_rating','it_quantity']]\ndata_selected.head()",
"_____no_output_____"
]
],
[
[
"### Usunięcie danych z kolumny",
"_____no_output_____"
],
[
"Dane z kolumny będziemy usuwać funkcją `df.column.sample(frac)` gdzie `frac` będzie oznaczać procent danych, które chcemy zatrzymać. Gwarantuje nam to w miarę losowe usunięcie danych, które powinno być wystarczające do dalszych działań.",
"_____no_output_____"
]
],
[
[
"cdata.price.sample(frac=0.9)\n",
"_____no_output_____"
]
],
[
[
"### Ocena skutecznosci imputacji\nDo oceny skuteczności podanych algorytmów imputacji danych musimy przyjąć jakis sposób liczenia ich. Zgodnie z sugestią prowadzącej skorszystam z [RMSE](https://en.wikipedia.org/wiki/Root_mean_square) czyli root mean square error. Nazwa powinna przybliżyć sposób, którm RMSE jest wyznaczane, jednak ciekawskich zachęcam do wejścia w link.",
"_____no_output_____"
],
[
"## Imputacja danych\nNapiszmy więc funkcje, która pozwoli nam stestować wybrany sposób imputacji.",
"_____no_output_____"
]
],
[
[
"from sklearn.experimental import enable_iterative_imputer\nfrom sklearn.impute import IterativeImputer\nfrom sklearn.metrics import mean_squared_error\n\ndef test_imputation(imputer,iterations=10):\n _resoults=[]\n # we always use the same data, so it's taken globally \n for i in range(iterations):\n test_data = data_selected.copy()\n test_data.it_seller_rating = test_data.it_seller_rating.sample(frac = 0.9)\n data_imputed = pd.DataFrame(imputer.fit_transform(test_data))\n _resoults.append(np.sqrt(mean_squared_error(data_selected,data_imputed)))\n return _resoults",
"_____no_output_____"
]
],
[
[
"I to niech będzie przykład działania takiej funkcji",
"_____no_output_____"
]
],
[
[
"imputer = IterativeImputer(max_iter=10,random_state=0)\nRMSE_list = test_imputation(imputer,20)\n\n\nprint(\"Średnie RMSE wynosi\", round(np.mean(RMSE_list)))\nprint('Odchylenie standardowe RMSE wynosi: ', round(np.std(RMSE_list))) \nRMSE_list",
"Średnie RMSE wynosi 6659.0\nOdchylenie standardowe RMSE wynosi: 64.0\n"
]
],
[
[
"Odchylenie standardowe jest dosyć małe, więc metodę imputacji uważam za skuteczną. Chętnym polecam przetestowanie tej funkcji dla innych typów imputacji oraz zmiennej liczbie iteracji. ",
"_____no_output_____"
],
[
"### Usuwanie danych z wielu kolumn",
"_____no_output_____"
],
[
"Powtórzmy uprzedni przykład dodając drobną modyfikacje. Tym razem będziemy usuwali dane zarówno z `it_seller_rating` jak i `it_quantity`. Napiszmy do tego odpowiednią funkcję i zobaczmy wyniki.",
"_____no_output_____"
]
],
[
[
"def test_imputation2(imputer,iterations=10):\n _resoults=[]\n # we always use the same data, so it's taken globally \n for i in range(iterations):\n test_data = data_selected.copy()\n test_data.it_seller_rating = test_data.it_seller_rating.sample(frac = 0.9)\n test_data.it_quantity = test_data.it_quantity.sample(frac = 0.9)\n data_imputed = pd.DataFrame(imputer.fit_transform(test_data))\n _resoults.append(np.sqrt(mean_squared_error(data_selected,data_imputed)))\n return _resoults ",
"_____no_output_____"
],
[
"imputer = IterativeImputer(max_iter=10,random_state=0)\nRMSE_list = test_imputation2(imputer,20)\n\n \nprint(\"Średnie RMSE wynosi\", round(np.mean(RMSE_list)))\nprint('Odchylenie standardowe RMSE wynosi: ', round(np.std(RMSE_list))) \nRMSE_list",
"Średnie RMSE wynosi 7953.0\nOdchylenie standardowe RMSE wynosi: 60.0\n"
]
],
[
[
"Tak jak moglibyśmy się spodziewać, średni błąd jest większy w przypadku gdy usuneliśmy więcej danych. Ponownie zachęcam do powtórzenia obliczeń i sprawdzenia wyników. ",
"_____no_output_____"
],
[
"### Spojrzenie na imputacje typu `IterativeImputer`",
"_____no_output_____"
],
[
"Wykorzystałem pewien szczególny sposób imputacji danych tzn `IterativeImputer`, o którym nie wspomniałem za dużo podczas korzystania z niego. Chciałbym jednka w tym miejscu go bardziej szczegółowo przedstawić oraz zobaczyć jak liczba iteracji wpływa na jakość imputacji.",
"_____no_output_____"
],
[
"Nasz imputer będę chiał przetestować dla całego spektrum wartości `max_iter` i dokładnie to zrobię w poniższej pętli.",
"_____no_output_____"
],
[
"**uwaga poniższy kod wykonuję się dosyć długo**",
"_____no_output_____"
]
],
[
[
"upper_iter_limit = 30\nlower_iter_limit = 5\nimputation_iterations = 10\n\nmean_RMSE ={\n \"single\": [],\n \"multi\": [],\n}\n\nfor imputer_iterations in range(lower_iter_limit,upper_iter_limit,2):\n _resoults_single = []\n _resoults_multi = []\n imputer = IterativeImputer(max_iter=imputer_iterations,random_state=0)\n\n print(\"max_iter: \", imputer_iterations, \"/\",upper_iter_limit)\n # Data missing from single columns\n _resoults_multi.append(test_imputation(imputer,imputation_iterations))\n\n # Data missing from multiple column\n _resoults_single.append(test_imputation2(imputer,imputation_iterations))\n \n mean_RMSE['single'].append(np.mean(_resoults_single))\n mean_RMSE['multi'].append(np.mean(_resoults_multi))\n ",
"max_iter: 5 / 30\nmax_iter: 7 / 30\nmax_iter: 9 / 30\nmax_iter: 11 / 30\nmax_iter: 13 / 30\nmax_iter: 15 / 30\nmax_iter: 17 / 30\nmax_iter: 19 / 30\nmax_iter: 21 / 30\nmax_iter: 23 / 30\nmax_iter: 25 / 30\nmax_iter: 27 / 30\nmax_iter: 29 / 30\n"
]
],
[
[
"Przyjrzyjmy się wynikom.",
"_____no_output_____"
]
],
[
[
"mean_RMSE\n",
"_____no_output_____"
]
],
[
[
"### Komentarz\nCo ciekawe nie widać dużej różnicy w błędzie imputacji dla różnych współczynników imputacji. Co wiecej nie ma, żadnego typu korelacji, a więc nie opłaca się brać dużego współczynnika iteracji, ponieważ wcale nie daje on lepszych wyników. Pragnę jednak ograniczyć moje wnioski do tego zbioru, ponieważ nie dysponuję w tym momencie wystarczającą liczbą informacji by twierdzić, że jest to zjawisko globalne. Niech ten przykład posłuży jako pretekst do dalszych dyskusji na ten temat.",
"_____no_output_____"
]
]
] | [
"markdown",
"code",
"markdown",
"code",
"markdown",
"code",
"markdown",
"code",
"markdown",
"code",
"markdown",
"code",
"markdown",
"code",
"markdown",
"code",
"markdown",
"code",
"markdown",
"code",
"markdown",
"code",
"markdown",
"code",
"markdown",
"code",
"markdown",
"code",
"markdown",
"code",
"markdown"
] | [
[
"markdown",
"markdown",
"markdown",
"markdown"
],
[
"code"
],
[
"markdown"
],
[
"code"
],
[
"markdown"
],
[
"code"
],
[
"markdown"
],
[
"code",
"code"
],
[
"markdown",
"markdown",
"markdown",
"markdown"
],
[
"code"
],
[
"markdown",
"markdown"
],
[
"code"
],
[
"markdown",
"markdown"
],
[
"code"
],
[
"markdown"
],
[
"code"
],
[
"markdown",
"markdown"
],
[
"code"
],
[
"markdown",
"markdown"
],
[
"code"
],
[
"markdown",
"markdown"
],
[
"code"
],
[
"markdown"
],
[
"code"
],
[
"markdown",
"markdown",
"markdown"
],
[
"code",
"code"
],
[
"markdown",
"markdown",
"markdown",
"markdown",
"markdown"
],
[
"code"
],
[
"markdown"
],
[
"code"
],
[
"markdown"
]
] |
d077b996f83bc4e3b3ddadefa0fa3eb92ed51073 | 148,162 | ipynb | Jupyter Notebook | Men-Women Classification.ipynb | VanditGupta/Men_Women_Classification | dab26a1c2960727a8f7bd1852bbbf465af31b745 | [
"MIT"
] | null | null | null | Men-Women Classification.ipynb | VanditGupta/Men_Women_Classification | dab26a1c2960727a8f7bd1852bbbf465af31b745 | [
"MIT"
] | null | null | null | Men-Women Classification.ipynb | VanditGupta/Men_Women_Classification | dab26a1c2960727a8f7bd1852bbbf465af31b745 | [
"MIT"
] | null | null | null | 87.773697 | 285 | 0.658792 | [
[
[
"import os\nimport numpy as np\nimport matplotlib.pyplot as plt\nimport pandas as pd\nfrom keras.applications.resnet50 import ResNet50,preprocess_input,decode_predictions\nfrom keras.layers import Dense,Conv2D,Input,Dropout,MaxPooling2D,Flatten,GlobalAveragePooling2D\nfrom keras.optimizers import Adam\nfrom keras.preprocessing import image\nfrom keras.models import Sequential,Model\nfrom keras.utils import np_utils\nimport random\nimport cv2",
"Using TensorFlow backend.\n"
],
[
"dirs = os.listdir(\"./men-women-classification/\")\nprint(dirs)",
"['men', 'women']\n"
],
[
"path = \"./men-women-classification/\"",
"_____no_output_____"
],
[
"images = []\nlabels = []\n\nlabels_dict = {'men':0,'women':1}\ndict_labels = { 0:\"men\",1:\"women\"}",
"_____no_output_____"
],
[
"for ix in dirs:\n class_path = path + ix + \"/\"\n img_names = os.listdir(class_path)\n for im in img_names:\n im = image.load_img(class_path + im, target_size=(224,224))\n im_array = image.img_to_array(im)\n images.append(im_array)\n labels.append(labels_dict[ix])\n \nprint (len(images), len(labels))",
"1000 1000\n"
],
[
"combined = list(zip(images,labels))\nrandom.shuffle(combined)\n\nimages[:],labels[:] = zip(*combined) ",
"_____no_output_____"
],
[
"X_train = np.array(images)\ny_train = np.array(labels)\ny_train = np_utils.to_categorical(y_train)\nprint (X_train.shape, y_train.shape)",
"(1000, 224, 224, 3) (1000, 2)\n"
],
[
"res_model = ResNet50(include_top = False , weights = 'imagenet' , input_shape = (224,224,3))",
"WARNING: Logging before flag parsing goes to stderr.\nW0728 17:42:03.904074 140521947629376 deprecation_wrapper.py:119] From /usr/local/lib/python3.6/dist-packages/keras/backend/tensorflow_backend.py:74: The name tf.get_default_graph is deprecated. Please use tf.compat.v1.get_default_graph instead.\n\nW0728 17:42:04.210152 140521947629376 deprecation_wrapper.py:119] From /usr/local/lib/python3.6/dist-packages/keras/backend/tensorflow_backend.py:517: The name tf.placeholder is deprecated. Please use tf.compat.v1.placeholder instead.\n\nW0728 17:42:04.318412 140521947629376 deprecation_wrapper.py:119] From /usr/local/lib/python3.6/dist-packages/keras/backend/tensorflow_backend.py:4185: The name tf.truncated_normal is deprecated. Please use tf.random.truncated_normal instead.\n\nW0728 17:42:04.438638 140521947629376 deprecation_wrapper.py:119] From /usr/local/lib/python3.6/dist-packages/keras/backend/tensorflow_backend.py:174: The name tf.get_default_session is deprecated. Please use tf.compat.v1.get_default_session instead.\n\nW0728 17:42:04.439691 140521947629376 deprecation_wrapper.py:119] From /usr/local/lib/python3.6/dist-packages/keras/backend/tensorflow_backend.py:181: The name tf.ConfigProto is deprecated. Please use tf.compat.v1.ConfigProto instead.\n\nW0728 17:42:05.331743 140521947629376 deprecation_wrapper.py:119] From /usr/local/lib/python3.6/dist-packages/keras/backend/tensorflow_backend.py:1834: The name tf.nn.fused_batch_norm is deprecated. Please use tf.compat.v1.nn.fused_batch_norm instead.\n\nW0728 17:42:05.445978 140521947629376 deprecation_wrapper.py:119] From /usr/local/lib/python3.6/dist-packages/keras/backend/tensorflow_backend.py:3976: The name tf.nn.max_pool is deprecated. Please use tf.nn.max_pool2d instead.\n\n/usr/local/lib/python3.6/dist-packages/keras_applications/resnet50.py:265: UserWarning: The output shape of `ResNet50(include_top=False)` has been changed since Keras 2.2.0.\n warnings.warn('The output shape of `ResNet50(include_top=False)` '\n"
],
[
"res_model.summary()",
"__________________________________________________________________________________________________\nLayer (type) Output Shape Param # Connected to \n==================================================================================================\ninput_1 (InputLayer) (None, 224, 224, 3) 0 \n__________________________________________________________________________________________________\nconv1_pad (ZeroPadding2D) (None, 230, 230, 3) 0 input_1[0][0] \n__________________________________________________________________________________________________\nconv1 (Conv2D) (None, 112, 112, 64) 9472 conv1_pad[0][0] \n__________________________________________________________________________________________________\nbn_conv1 (BatchNormalization) (None, 112, 112, 64) 256 conv1[0][0] \n__________________________________________________________________________________________________\nactivation_1 (Activation) (None, 112, 112, 64) 0 bn_conv1[0][0] \n__________________________________________________________________________________________________\npool1_pad (ZeroPadding2D) (None, 114, 114, 64) 0 activation_1[0][0] \n__________________________________________________________________________________________________\nmax_pooling2d_1 (MaxPooling2D) (None, 56, 56, 64) 0 pool1_pad[0][0] \n__________________________________________________________________________________________________\nres2a_branch2a (Conv2D) (None, 56, 56, 64) 4160 max_pooling2d_1[0][0] \n__________________________________________________________________________________________________\nbn2a_branch2a (BatchNormalizati (None, 56, 56, 64) 256 res2a_branch2a[0][0] \n__________________________________________________________________________________________________\nactivation_2 (Activation) (None, 56, 56, 64) 0 bn2a_branch2a[0][0] \n__________________________________________________________________________________________________\nres2a_branch2b (Conv2D) (None, 56, 56, 64) 36928 activation_2[0][0] \n__________________________________________________________________________________________________\nbn2a_branch2b (BatchNormalizati (None, 56, 56, 64) 256 res2a_branch2b[0][0] \n__________________________________________________________________________________________________\nactivation_3 (Activation) (None, 56, 56, 64) 0 bn2a_branch2b[0][0] \n__________________________________________________________________________________________________\nres2a_branch2c (Conv2D) (None, 56, 56, 256) 16640 activation_3[0][0] \n__________________________________________________________________________________________________\nres2a_branch1 (Conv2D) (None, 56, 56, 256) 16640 max_pooling2d_1[0][0] \n__________________________________________________________________________________________________\nbn2a_branch2c (BatchNormalizati (None, 56, 56, 256) 1024 res2a_branch2c[0][0] \n__________________________________________________________________________________________________\nbn2a_branch1 (BatchNormalizatio (None, 56, 56, 256) 1024 res2a_branch1[0][0] \n__________________________________________________________________________________________________\nadd_1 (Add) (None, 56, 56, 256) 0 bn2a_branch2c[0][0] \n bn2a_branch1[0][0] \n__________________________________________________________________________________________________\nactivation_4 (Activation) (None, 56, 56, 256) 0 add_1[0][0] \n__________________________________________________________________________________________________\nres2b_branch2a (Conv2D) (None, 56, 56, 64) 16448 activation_4[0][0] \n__________________________________________________________________________________________________\nbn2b_branch2a (BatchNormalizati (None, 56, 56, 64) 256 res2b_branch2a[0][0] \n__________________________________________________________________________________________________\nactivation_5 (Activation) (None, 56, 56, 64) 0 bn2b_branch2a[0][0] \n__________________________________________________________________________________________________\nres2b_branch2b (Conv2D) (None, 56, 56, 64) 36928 activation_5[0][0] \n__________________________________________________________________________________________________\nbn2b_branch2b (BatchNormalizati (None, 56, 56, 64) 256 res2b_branch2b[0][0] \n__________________________________________________________________________________________________\nactivation_6 (Activation) (None, 56, 56, 64) 0 bn2b_branch2b[0][0] \n__________________________________________________________________________________________________\nres2b_branch2c (Conv2D) (None, 56, 56, 256) 16640 activation_6[0][0] \n__________________________________________________________________________________________________\nbn2b_branch2c (BatchNormalizati (None, 56, 56, 256) 1024 res2b_branch2c[0][0] \n__________________________________________________________________________________________________\nadd_2 (Add) (None, 56, 56, 256) 0 bn2b_branch2c[0][0] \n activation_4[0][0] \n__________________________________________________________________________________________________\nactivation_7 (Activation) (None, 56, 56, 256) 0 add_2[0][0] \n__________________________________________________________________________________________________\nres2c_branch2a (Conv2D) (None, 56, 56, 64) 16448 activation_7[0][0] \n__________________________________________________________________________________________________\nbn2c_branch2a (BatchNormalizati (None, 56, 56, 64) 256 res2c_branch2a[0][0] \n__________________________________________________________________________________________________\nactivation_8 (Activation) (None, 56, 56, 64) 0 bn2c_branch2a[0][0] \n__________________________________________________________________________________________________\nres2c_branch2b (Conv2D) (None, 56, 56, 64) 36928 activation_8[0][0] \n__________________________________________________________________________________________________\nbn2c_branch2b (BatchNormalizati (None, 56, 56, 64) 256 res2c_branch2b[0][0] \n__________________________________________________________________________________________________\nactivation_9 (Activation) (None, 56, 56, 64) 0 bn2c_branch2b[0][0] \n__________________________________________________________________________________________________\nres2c_branch2c (Conv2D) (None, 56, 56, 256) 16640 activation_9[0][0] \n__________________________________________________________________________________________________\nbn2c_branch2c (BatchNormalizati (None, 56, 56, 256) 1024 res2c_branch2c[0][0] \n__________________________________________________________________________________________________\nadd_3 (Add) (None, 56, 56, 256) 0 bn2c_branch2c[0][0] \n activation_7[0][0] \n__________________________________________________________________________________________________\nactivation_10 (Activation) (None, 56, 56, 256) 0 add_3[0][0] \n__________________________________________________________________________________________________\nres3a_branch2a (Conv2D) (None, 28, 28, 128) 32896 activation_10[0][0] \n__________________________________________________________________________________________________\nbn3a_branch2a (BatchNormalizati (None, 28, 28, 128) 512 res3a_branch2a[0][0] \n__________________________________________________________________________________________________\nactivation_11 (Activation) (None, 28, 28, 128) 0 bn3a_branch2a[0][0] \n__________________________________________________________________________________________________\nres3a_branch2b (Conv2D) (None, 28, 28, 128) 147584 activation_11[0][0] \n__________________________________________________________________________________________________\nbn3a_branch2b (BatchNormalizati (None, 28, 28, 128) 512 res3a_branch2b[0][0] \n__________________________________________________________________________________________________\nactivation_12 (Activation) (None, 28, 28, 128) 0 bn3a_branch2b[0][0] \n__________________________________________________________________________________________________\nres3a_branch2c (Conv2D) (None, 28, 28, 512) 66048 activation_12[0][0] \n__________________________________________________________________________________________________\nres3a_branch1 (Conv2D) (None, 28, 28, 512) 131584 activation_10[0][0] \n__________________________________________________________________________________________________\nbn3a_branch2c (BatchNormalizati (None, 28, 28, 512) 2048 res3a_branch2c[0][0] \n__________________________________________________________________________________________________\nbn3a_branch1 (BatchNormalizatio (None, 28, 28, 512) 2048 res3a_branch1[0][0] \n__________________________________________________________________________________________________\nadd_4 (Add) (None, 28, 28, 512) 0 bn3a_branch2c[0][0] \n bn3a_branch1[0][0] \n__________________________________________________________________________________________________\nactivation_13 (Activation) (None, 28, 28, 512) 0 add_4[0][0] \n__________________________________________________________________________________________________\nres3b_branch2a (Conv2D) (None, 28, 28, 128) 65664 activation_13[0][0] \n__________________________________________________________________________________________________\nbn3b_branch2a (BatchNormalizati (None, 28, 28, 128) 512 res3b_branch2a[0][0] \n__________________________________________________________________________________________________\nactivation_14 (Activation) (None, 28, 28, 128) 0 bn3b_branch2a[0][0] \n__________________________________________________________________________________________________\nres3b_branch2b (Conv2D) (None, 28, 28, 128) 147584 activation_14[0][0] \n__________________________________________________________________________________________________\nbn3b_branch2b (BatchNormalizati (None, 28, 28, 128) 512 res3b_branch2b[0][0] \n__________________________________________________________________________________________________\nactivation_15 (Activation) (None, 28, 28, 128) 0 bn3b_branch2b[0][0] \n__________________________________________________________________________________________________\nres3b_branch2c (Conv2D) (None, 28, 28, 512) 66048 activation_15[0][0] \n__________________________________________________________________________________________________\nbn3b_branch2c (BatchNormalizati (None, 28, 28, 512) 2048 res3b_branch2c[0][0] \n__________________________________________________________________________________________________\nadd_5 (Add) (None, 28, 28, 512) 0 bn3b_branch2c[0][0] \n activation_13[0][0] \n__________________________________________________________________________________________________\nactivation_16 (Activation) (None, 28, 28, 512) 0 add_5[0][0] \n__________________________________________________________________________________________________\nres3c_branch2a (Conv2D) (None, 28, 28, 128) 65664 activation_16[0][0] \n__________________________________________________________________________________________________\nbn3c_branch2a (BatchNormalizati (None, 28, 28, 128) 512 res3c_branch2a[0][0] \n__________________________________________________________________________________________________\nactivation_17 (Activation) (None, 28, 28, 128) 0 bn3c_branch2a[0][0] \n__________________________________________________________________________________________________\nres3c_branch2b (Conv2D) (None, 28, 28, 128) 147584 activation_17[0][0] \n__________________________________________________________________________________________________\nbn3c_branch2b (BatchNormalizati (None, 28, 28, 128) 512 res3c_branch2b[0][0] \n__________________________________________________________________________________________________\nactivation_18 (Activation) (None, 28, 28, 128) 0 bn3c_branch2b[0][0] \n__________________________________________________________________________________________________\nres3c_branch2c (Conv2D) (None, 28, 28, 512) 66048 activation_18[0][0] \n__________________________________________________________________________________________________\nbn3c_branch2c (BatchNormalizati (None, 28, 28, 512) 2048 res3c_branch2c[0][0] \n__________________________________________________________________________________________________\nadd_6 (Add) (None, 28, 28, 512) 0 bn3c_branch2c[0][0] \n activation_16[0][0] \n__________________________________________________________________________________________________\nactivation_19 (Activation) (None, 28, 28, 512) 0 add_6[0][0] \n__________________________________________________________________________________________________\nres3d_branch2a (Conv2D) (None, 28, 28, 128) 65664 activation_19[0][0] \n__________________________________________________________________________________________________\nbn3d_branch2a (BatchNormalizati (None, 28, 28, 128) 512 res3d_branch2a[0][0] \n__________________________________________________________________________________________________\nactivation_20 (Activation) (None, 28, 28, 128) 0 bn3d_branch2a[0][0] \n__________________________________________________________________________________________________\nres3d_branch2b (Conv2D) (None, 28, 28, 128) 147584 activation_20[0][0] \n__________________________________________________________________________________________________\nbn3d_branch2b (BatchNormalizati (None, 28, 28, 128) 512 res3d_branch2b[0][0] \n__________________________________________________________________________________________________\nactivation_21 (Activation) (None, 28, 28, 128) 0 bn3d_branch2b[0][0] \n__________________________________________________________________________________________________\nres3d_branch2c (Conv2D) (None, 28, 28, 512) 66048 activation_21[0][0] \n__________________________________________________________________________________________________\nbn3d_branch2c (BatchNormalizati (None, 28, 28, 512) 2048 res3d_branch2c[0][0] \n__________________________________________________________________________________________________\nadd_7 (Add) (None, 28, 28, 512) 0 bn3d_branch2c[0][0] \n activation_19[0][0] \n__________________________________________________________________________________________________\nactivation_22 (Activation) (None, 28, 28, 512) 0 add_7[0][0] \n__________________________________________________________________________________________________\nres4a_branch2a (Conv2D) (None, 14, 14, 256) 131328 activation_22[0][0] \n__________________________________________________________________________________________________\nbn4a_branch2a (BatchNormalizati (None, 14, 14, 256) 1024 res4a_branch2a[0][0] \n__________________________________________________________________________________________________\nactivation_23 (Activation) (None, 14, 14, 256) 0 bn4a_branch2a[0][0] \n__________________________________________________________________________________________________\nres4a_branch2b (Conv2D) (None, 14, 14, 256) 590080 activation_23[0][0] \n__________________________________________________________________________________________________\nbn4a_branch2b (BatchNormalizati (None, 14, 14, 256) 1024 res4a_branch2b[0][0] \n__________________________________________________________________________________________________\nactivation_24 (Activation) (None, 14, 14, 256) 0 bn4a_branch2b[0][0] \n__________________________________________________________________________________________________\nres4a_branch2c (Conv2D) (None, 14, 14, 1024) 263168 activation_24[0][0] \n__________________________________________________________________________________________________\nres4a_branch1 (Conv2D) (None, 14, 14, 1024) 525312 activation_22[0][0] \n__________________________________________________________________________________________________\nbn4a_branch2c (BatchNormalizati (None, 14, 14, 1024) 4096 res4a_branch2c[0][0] \n__________________________________________________________________________________________________\nbn4a_branch1 (BatchNormalizatio (None, 14, 14, 1024) 4096 res4a_branch1[0][0] \n__________________________________________________________________________________________________\nadd_8 (Add) (None, 14, 14, 1024) 0 bn4a_branch2c[0][0] \n bn4a_branch1[0][0] \n__________________________________________________________________________________________________\nactivation_25 (Activation) (None, 14, 14, 1024) 0 add_8[0][0] \n__________________________________________________________________________________________________\nres4b_branch2a (Conv2D) (None, 14, 14, 256) 262400 activation_25[0][0] \n__________________________________________________________________________________________________\nbn4b_branch2a (BatchNormalizati (None, 14, 14, 256) 1024 res4b_branch2a[0][0] \n__________________________________________________________________________________________________\nactivation_26 (Activation) (None, 14, 14, 256) 0 bn4b_branch2a[0][0] \n__________________________________________________________________________________________________\nres4b_branch2b (Conv2D) (None, 14, 14, 256) 590080 activation_26[0][0] \n__________________________________________________________________________________________________\nbn4b_branch2b (BatchNormalizati (None, 14, 14, 256) 1024 res4b_branch2b[0][0] \n__________________________________________________________________________________________________\nactivation_27 (Activation) (None, 14, 14, 256) 0 bn4b_branch2b[0][0] \n__________________________________________________________________________________________________\nres4b_branch2c (Conv2D) (None, 14, 14, 1024) 263168 activation_27[0][0] \n__________________________________________________________________________________________________\nbn4b_branch2c (BatchNormalizati (None, 14, 14, 1024) 4096 res4b_branch2c[0][0] \n__________________________________________________________________________________________________\nadd_9 (Add) (None, 14, 14, 1024) 0 bn4b_branch2c[0][0] \n activation_25[0][0] \n__________________________________________________________________________________________________\nactivation_28 (Activation) (None, 14, 14, 1024) 0 add_9[0][0] \n__________________________________________________________________________________________________\nres4c_branch2a (Conv2D) (None, 14, 14, 256) 262400 activation_28[0][0] \n__________________________________________________________________________________________________\nbn4c_branch2a (BatchNormalizati (None, 14, 14, 256) 1024 res4c_branch2a[0][0] \n__________________________________________________________________________________________________\nactivation_29 (Activation) (None, 14, 14, 256) 0 bn4c_branch2a[0][0] \n__________________________________________________________________________________________________\nres4c_branch2b (Conv2D) (None, 14, 14, 256) 590080 activation_29[0][0] \n__________________________________________________________________________________________________\nbn4c_branch2b (BatchNormalizati (None, 14, 14, 256) 1024 res4c_branch2b[0][0] \n__________________________________________________________________________________________________\nactivation_30 (Activation) (None, 14, 14, 256) 0 bn4c_branch2b[0][0] \n__________________________________________________________________________________________________\nres4c_branch2c (Conv2D) (None, 14, 14, 1024) 263168 activation_30[0][0] \n__________________________________________________________________________________________________\nbn4c_branch2c (BatchNormalizati (None, 14, 14, 1024) 4096 res4c_branch2c[0][0] \n__________________________________________________________________________________________________\nadd_10 (Add) (None, 14, 14, 1024) 0 bn4c_branch2c[0][0] \n activation_28[0][0] \n__________________________________________________________________________________________________\nactivation_31 (Activation) (None, 14, 14, 1024) 0 add_10[0][0] \n__________________________________________________________________________________________________\nres4d_branch2a (Conv2D) (None, 14, 14, 256) 262400 activation_31[0][0] \n__________________________________________________________________________________________________\nbn4d_branch2a (BatchNormalizati (None, 14, 14, 256) 1024 res4d_branch2a[0][0] \n__________________________________________________________________________________________________\nactivation_32 (Activation) (None, 14, 14, 256) 0 bn4d_branch2a[0][0] \n__________________________________________________________________________________________________\nres4d_branch2b (Conv2D) (None, 14, 14, 256) 590080 activation_32[0][0] \n__________________________________________________________________________________________________\nbn4d_branch2b (BatchNormalizati (None, 14, 14, 256) 1024 res4d_branch2b[0][0] \n__________________________________________________________________________________________________\nactivation_33 (Activation) (None, 14, 14, 256) 0 bn4d_branch2b[0][0] \n__________________________________________________________________________________________________\nres4d_branch2c (Conv2D) (None, 14, 14, 1024) 263168 activation_33[0][0] \n__________________________________________________________________________________________________\nbn4d_branch2c (BatchNormalizati (None, 14, 14, 1024) 4096 res4d_branch2c[0][0] \n__________________________________________________________________________________________________\nadd_11 (Add) (None, 14, 14, 1024) 0 bn4d_branch2c[0][0] \n activation_31[0][0] \n__________________________________________________________________________________________________\nactivation_34 (Activation) (None, 14, 14, 1024) 0 add_11[0][0] \n__________________________________________________________________________________________________\nres4e_branch2a (Conv2D) (None, 14, 14, 256) 262400 activation_34[0][0] \n__________________________________________________________________________________________________\nbn4e_branch2a (BatchNormalizati (None, 14, 14, 256) 1024 res4e_branch2a[0][0] \n__________________________________________________________________________________________________\nactivation_35 (Activation) (None, 14, 14, 256) 0 bn4e_branch2a[0][0] \n__________________________________________________________________________________________________\nres4e_branch2b (Conv2D) (None, 14, 14, 256) 590080 activation_35[0][0] \n__________________________________________________________________________________________________\nbn4e_branch2b (BatchNormalizati (None, 14, 14, 256) 1024 res4e_branch2b[0][0] \n__________________________________________________________________________________________________\nactivation_36 (Activation) (None, 14, 14, 256) 0 bn4e_branch2b[0][0] \n__________________________________________________________________________________________________\nres4e_branch2c (Conv2D) (None, 14, 14, 1024) 263168 activation_36[0][0] \n__________________________________________________________________________________________________\nbn4e_branch2c (BatchNormalizati (None, 14, 14, 1024) 4096 res4e_branch2c[0][0] \n__________________________________________________________________________________________________\nadd_12 (Add) (None, 14, 14, 1024) 0 bn4e_branch2c[0][0] \n activation_34[0][0] \n__________________________________________________________________________________________________\nactivation_37 (Activation) (None, 14, 14, 1024) 0 add_12[0][0] \n__________________________________________________________________________________________________\nres4f_branch2a (Conv2D) (None, 14, 14, 256) 262400 activation_37[0][0] \n__________________________________________________________________________________________________\nbn4f_branch2a (BatchNormalizati (None, 14, 14, 256) 1024 res4f_branch2a[0][0] \n__________________________________________________________________________________________________\nactivation_38 (Activation) (None, 14, 14, 256) 0 bn4f_branch2a[0][0] \n__________________________________________________________________________________________________\nres4f_branch2b (Conv2D) (None, 14, 14, 256) 590080 activation_38[0][0] \n__________________________________________________________________________________________________\nbn4f_branch2b (BatchNormalizati (None, 14, 14, 256) 1024 res4f_branch2b[0][0] \n__________________________________________________________________________________________________\nactivation_39 (Activation) (None, 14, 14, 256) 0 bn4f_branch2b[0][0] \n__________________________________________________________________________________________________\nres4f_branch2c (Conv2D) (None, 14, 14, 1024) 263168 activation_39[0][0] \n__________________________________________________________________________________________________\nbn4f_branch2c (BatchNormalizati (None, 14, 14, 1024) 4096 res4f_branch2c[0][0] \n__________________________________________________________________________________________________\nadd_13 (Add) (None, 14, 14, 1024) 0 bn4f_branch2c[0][0] \n activation_37[0][0] \n__________________________________________________________________________________________________\nactivation_40 (Activation) (None, 14, 14, 1024) 0 add_13[0][0] \n__________________________________________________________________________________________________\nres5a_branch2a (Conv2D) (None, 7, 7, 512) 524800 activation_40[0][0] \n__________________________________________________________________________________________________\nbn5a_branch2a (BatchNormalizati (None, 7, 7, 512) 2048 res5a_branch2a[0][0] \n__________________________________________________________________________________________________\nactivation_41 (Activation) (None, 7, 7, 512) 0 bn5a_branch2a[0][0] \n__________________________________________________________________________________________________\nres5a_branch2b (Conv2D) (None, 7, 7, 512) 2359808 activation_41[0][0] \n__________________________________________________________________________________________________\nbn5a_branch2b (BatchNormalizati (None, 7, 7, 512) 2048 res5a_branch2b[0][0] \n__________________________________________________________________________________________________\nactivation_42 (Activation) (None, 7, 7, 512) 0 bn5a_branch2b[0][0] \n__________________________________________________________________________________________________\nres5a_branch2c (Conv2D) (None, 7, 7, 2048) 1050624 activation_42[0][0] \n__________________________________________________________________________________________________\nres5a_branch1 (Conv2D) (None, 7, 7, 2048) 2099200 activation_40[0][0] \n__________________________________________________________________________________________________\nbn5a_branch2c (BatchNormalizati (None, 7, 7, 2048) 8192 res5a_branch2c[0][0] \n__________________________________________________________________________________________________\nbn5a_branch1 (BatchNormalizatio (None, 7, 7, 2048) 8192 res5a_branch1[0][0] \n__________________________________________________________________________________________________\nadd_14 (Add) (None, 7, 7, 2048) 0 bn5a_branch2c[0][0] \n bn5a_branch1[0][0] \n__________________________________________________________________________________________________\nactivation_43 (Activation) (None, 7, 7, 2048) 0 add_14[0][0] \n__________________________________________________________________________________________________\nres5b_branch2a (Conv2D) (None, 7, 7, 512) 1049088 activation_43[0][0] \n__________________________________________________________________________________________________\nbn5b_branch2a (BatchNormalizati (None, 7, 7, 512) 2048 res5b_branch2a[0][0] \n__________________________________________________________________________________________________\nactivation_44 (Activation) (None, 7, 7, 512) 0 bn5b_branch2a[0][0] \n__________________________________________________________________________________________________\nres5b_branch2b (Conv2D) (None, 7, 7, 512) 2359808 activation_44[0][0] \n__________________________________________________________________________________________________\nbn5b_branch2b (BatchNormalizati (None, 7, 7, 512) 2048 res5b_branch2b[0][0] \n__________________________________________________________________________________________________\nactivation_45 (Activation) (None, 7, 7, 512) 0 bn5b_branch2b[0][0] \n__________________________________________________________________________________________________\nres5b_branch2c (Conv2D) (None, 7, 7, 2048) 1050624 activation_45[0][0] \n__________________________________________________________________________________________________\nbn5b_branch2c (BatchNormalizati (None, 7, 7, 2048) 8192 res5b_branch2c[0][0] \n__________________________________________________________________________________________________\nadd_15 (Add) (None, 7, 7, 2048) 0 bn5b_branch2c[0][0] \n activation_43[0][0] \n__________________________________________________________________________________________________\nactivation_46 (Activation) (None, 7, 7, 2048) 0 add_15[0][0] \n__________________________________________________________________________________________________\nres5c_branch2a (Conv2D) (None, 7, 7, 512) 1049088 activation_46[0][0] \n__________________________________________________________________________________________________\nbn5c_branch2a (BatchNormalizati (None, 7, 7, 512) 2048 res5c_branch2a[0][0] \n__________________________________________________________________________________________________\nactivation_47 (Activation) (None, 7, 7, 512) 0 bn5c_branch2a[0][0] \n__________________________________________________________________________________________________\nres5c_branch2b (Conv2D) (None, 7, 7, 512) 2359808 activation_47[0][0] \n__________________________________________________________________________________________________\nbn5c_branch2b (BatchNormalizati (None, 7, 7, 512) 2048 res5c_branch2b[0][0] \n__________________________________________________________________________________________________\nactivation_48 (Activation) (None, 7, 7, 512) 0 bn5c_branch2b[0][0] \n__________________________________________________________________________________________________\nres5c_branch2c (Conv2D) (None, 7, 7, 2048) 1050624 activation_48[0][0] \n__________________________________________________________________________________________________\nbn5c_branch2c (BatchNormalizati (None, 7, 7, 2048) 8192 res5c_branch2c[0][0] \n__________________________________________________________________________________________________\nadd_16 (Add) (None, 7, 7, 2048) 0 bn5c_branch2c[0][0] \n activation_46[0][0] \n__________________________________________________________________________________________________\nactivation_49 (Activation) (None, 7, 7, 2048) 0 add_16[0][0] \n==================================================================================================\nTotal params: 23,587,712\nTrainable params: 23,534,592\nNon-trainable params: 53,120\n__________________________________________________________________________________________________\n"
],
[
"avg = GlobalAveragePooling2D()(res_model.output)\nfc1 = Dense(256, activation='relu')(avg)\nfc2 = Dense(2, activation='softmax')(fc1)\n\nmodel = Model(inputs=res_model.inputs, outputs=fc2)\nmodel.summary()\n",
"__________________________________________________________________________________________________\nLayer (type) Output Shape Param # Connected to \n==================================================================================================\ninput_1 (InputLayer) (None, 224, 224, 3) 0 \n__________________________________________________________________________________________________\nconv1_pad (ZeroPadding2D) (None, 230, 230, 3) 0 input_1[0][0] \n__________________________________________________________________________________________________\nconv1 (Conv2D) (None, 112, 112, 64) 9472 conv1_pad[0][0] \n__________________________________________________________________________________________________\nbn_conv1 (BatchNormalization) (None, 112, 112, 64) 256 conv1[0][0] \n__________________________________________________________________________________________________\nactivation_1 (Activation) (None, 112, 112, 64) 0 bn_conv1[0][0] \n__________________________________________________________________________________________________\npool1_pad (ZeroPadding2D) (None, 114, 114, 64) 0 activation_1[0][0] \n__________________________________________________________________________________________________\nmax_pooling2d_1 (MaxPooling2D) (None, 56, 56, 64) 0 pool1_pad[0][0] \n__________________________________________________________________________________________________\nres2a_branch2a (Conv2D) (None, 56, 56, 64) 4160 max_pooling2d_1[0][0] \n__________________________________________________________________________________________________\nbn2a_branch2a (BatchNormalizati (None, 56, 56, 64) 256 res2a_branch2a[0][0] \n__________________________________________________________________________________________________\nactivation_2 (Activation) (None, 56, 56, 64) 0 bn2a_branch2a[0][0] \n__________________________________________________________________________________________________\nres2a_branch2b (Conv2D) (None, 56, 56, 64) 36928 activation_2[0][0] \n__________________________________________________________________________________________________\nbn2a_branch2b (BatchNormalizati (None, 56, 56, 64) 256 res2a_branch2b[0][0] \n__________________________________________________________________________________________________\nactivation_3 (Activation) (None, 56, 56, 64) 0 bn2a_branch2b[0][0] \n__________________________________________________________________________________________________\nres2a_branch2c (Conv2D) (None, 56, 56, 256) 16640 activation_3[0][0] \n__________________________________________________________________________________________________\nres2a_branch1 (Conv2D) (None, 56, 56, 256) 16640 max_pooling2d_1[0][0] \n__________________________________________________________________________________________________\nbn2a_branch2c (BatchNormalizati (None, 56, 56, 256) 1024 res2a_branch2c[0][0] \n__________________________________________________________________________________________________\nbn2a_branch1 (BatchNormalizatio (None, 56, 56, 256) 1024 res2a_branch1[0][0] \n__________________________________________________________________________________________________\nadd_1 (Add) (None, 56, 56, 256) 0 bn2a_branch2c[0][0] \n bn2a_branch1[0][0] \n__________________________________________________________________________________________________\nactivation_4 (Activation) (None, 56, 56, 256) 0 add_1[0][0] \n__________________________________________________________________________________________________\nres2b_branch2a (Conv2D) (None, 56, 56, 64) 16448 activation_4[0][0] \n__________________________________________________________________________________________________\nbn2b_branch2a (BatchNormalizati (None, 56, 56, 64) 256 res2b_branch2a[0][0] \n__________________________________________________________________________________________________\nactivation_5 (Activation) (None, 56, 56, 64) 0 bn2b_branch2a[0][0] \n__________________________________________________________________________________________________\nres2b_branch2b (Conv2D) (None, 56, 56, 64) 36928 activation_5[0][0] \n__________________________________________________________________________________________________\nbn2b_branch2b (BatchNormalizati (None, 56, 56, 64) 256 res2b_branch2b[0][0] \n__________________________________________________________________________________________________\nactivation_6 (Activation) (None, 56, 56, 64) 0 bn2b_branch2b[0][0] \n__________________________________________________________________________________________________\nres2b_branch2c (Conv2D) (None, 56, 56, 256) 16640 activation_6[0][0] \n__________________________________________________________________________________________________\nbn2b_branch2c (BatchNormalizati (None, 56, 56, 256) 1024 res2b_branch2c[0][0] \n__________________________________________________________________________________________________\nadd_2 (Add) (None, 56, 56, 256) 0 bn2b_branch2c[0][0] \n activation_4[0][0] \n__________________________________________________________________________________________________\nactivation_7 (Activation) (None, 56, 56, 256) 0 add_2[0][0] \n__________________________________________________________________________________________________\nres2c_branch2a (Conv2D) (None, 56, 56, 64) 16448 activation_7[0][0] \n__________________________________________________________________________________________________\nbn2c_branch2a (BatchNormalizati (None, 56, 56, 64) 256 res2c_branch2a[0][0] \n__________________________________________________________________________________________________\nactivation_8 (Activation) (None, 56, 56, 64) 0 bn2c_branch2a[0][0] \n__________________________________________________________________________________________________\nres2c_branch2b (Conv2D) (None, 56, 56, 64) 36928 activation_8[0][0] \n__________________________________________________________________________________________________\nbn2c_branch2b (BatchNormalizati (None, 56, 56, 64) 256 res2c_branch2b[0][0] \n__________________________________________________________________________________________________\nactivation_9 (Activation) (None, 56, 56, 64) 0 bn2c_branch2b[0][0] \n__________________________________________________________________________________________________\nres2c_branch2c (Conv2D) (None, 56, 56, 256) 16640 activation_9[0][0] \n__________________________________________________________________________________________________\nbn2c_branch2c (BatchNormalizati (None, 56, 56, 256) 1024 res2c_branch2c[0][0] \n__________________________________________________________________________________________________\nadd_3 (Add) (None, 56, 56, 256) 0 bn2c_branch2c[0][0] \n activation_7[0][0] \n__________________________________________________________________________________________________\nactivation_10 (Activation) (None, 56, 56, 256) 0 add_3[0][0] \n__________________________________________________________________________________________________\nres3a_branch2a (Conv2D) (None, 28, 28, 128) 32896 activation_10[0][0] \n__________________________________________________________________________________________________\nbn3a_branch2a (BatchNormalizati (None, 28, 28, 128) 512 res3a_branch2a[0][0] \n__________________________________________________________________________________________________\nactivation_11 (Activation) (None, 28, 28, 128) 0 bn3a_branch2a[0][0] \n__________________________________________________________________________________________________\nres3a_branch2b (Conv2D) (None, 28, 28, 128) 147584 activation_11[0][0] \n__________________________________________________________________________________________________\nbn3a_branch2b (BatchNormalizati (None, 28, 28, 128) 512 res3a_branch2b[0][0] \n__________________________________________________________________________________________________\nactivation_12 (Activation) (None, 28, 28, 128) 0 bn3a_branch2b[0][0] \n__________________________________________________________________________________________________\nres3a_branch2c (Conv2D) (None, 28, 28, 512) 66048 activation_12[0][0] \n__________________________________________________________________________________________________\nres3a_branch1 (Conv2D) (None, 28, 28, 512) 131584 activation_10[0][0] \n__________________________________________________________________________________________________\nbn3a_branch2c (BatchNormalizati (None, 28, 28, 512) 2048 res3a_branch2c[0][0] \n__________________________________________________________________________________________________\nbn3a_branch1 (BatchNormalizatio (None, 28, 28, 512) 2048 res3a_branch1[0][0] \n__________________________________________________________________________________________________\nadd_4 (Add) (None, 28, 28, 512) 0 bn3a_branch2c[0][0] \n bn3a_branch1[0][0] \n__________________________________________________________________________________________________\nactivation_13 (Activation) (None, 28, 28, 512) 0 add_4[0][0] \n__________________________________________________________________________________________________\nres3b_branch2a (Conv2D) (None, 28, 28, 128) 65664 activation_13[0][0] \n__________________________________________________________________________________________________\nbn3b_branch2a (BatchNormalizati (None, 28, 28, 128) 512 res3b_branch2a[0][0] \n__________________________________________________________________________________________________\nactivation_14 (Activation) (None, 28, 28, 128) 0 bn3b_branch2a[0][0] \n__________________________________________________________________________________________________\nres3b_branch2b (Conv2D) (None, 28, 28, 128) 147584 activation_14[0][0] \n__________________________________________________________________________________________________\nbn3b_branch2b (BatchNormalizati (None, 28, 28, 128) 512 res3b_branch2b[0][0] \n__________________________________________________________________________________________________\nactivation_15 (Activation) (None, 28, 28, 128) 0 bn3b_branch2b[0][0] \n__________________________________________________________________________________________________\nres3b_branch2c (Conv2D) (None, 28, 28, 512) 66048 activation_15[0][0] \n__________________________________________________________________________________________________\nbn3b_branch2c (BatchNormalizati (None, 28, 28, 512) 2048 res3b_branch2c[0][0] \n__________________________________________________________________________________________________\nadd_5 (Add) (None, 28, 28, 512) 0 bn3b_branch2c[0][0] \n activation_13[0][0] \n__________________________________________________________________________________________________\nactivation_16 (Activation) (None, 28, 28, 512) 0 add_5[0][0] \n__________________________________________________________________________________________________\nres3c_branch2a (Conv2D) (None, 28, 28, 128) 65664 activation_16[0][0] \n__________________________________________________________________________________________________\nbn3c_branch2a (BatchNormalizati (None, 28, 28, 128) 512 res3c_branch2a[0][0] \n__________________________________________________________________________________________________\nactivation_17 (Activation) (None, 28, 28, 128) 0 bn3c_branch2a[0][0] \n__________________________________________________________________________________________________\nres3c_branch2b (Conv2D) (None, 28, 28, 128) 147584 activation_17[0][0] \n__________________________________________________________________________________________________\nbn3c_branch2b (BatchNormalizati (None, 28, 28, 128) 512 res3c_branch2b[0][0] \n__________________________________________________________________________________________________\nactivation_18 (Activation) (None, 28, 28, 128) 0 bn3c_branch2b[0][0] \n__________________________________________________________________________________________________\nres3c_branch2c (Conv2D) (None, 28, 28, 512) 66048 activation_18[0][0] \n__________________________________________________________________________________________________\nbn3c_branch2c (BatchNormalizati (None, 28, 28, 512) 2048 res3c_branch2c[0][0] \n__________________________________________________________________________________________________\nadd_6 (Add) (None, 28, 28, 512) 0 bn3c_branch2c[0][0] \n activation_16[0][0] \n__________________________________________________________________________________________________\nactivation_19 (Activation) (None, 28, 28, 512) 0 add_6[0][0] \n__________________________________________________________________________________________________\nres3d_branch2a (Conv2D) (None, 28, 28, 128) 65664 activation_19[0][0] \n__________________________________________________________________________________________________\nbn3d_branch2a (BatchNormalizati (None, 28, 28, 128) 512 res3d_branch2a[0][0] \n__________________________________________________________________________________________________\nactivation_20 (Activation) (None, 28, 28, 128) 0 bn3d_branch2a[0][0] \n__________________________________________________________________________________________________\nres3d_branch2b (Conv2D) (None, 28, 28, 128) 147584 activation_20[0][0] \n__________________________________________________________________________________________________\nbn3d_branch2b (BatchNormalizati (None, 28, 28, 128) 512 res3d_branch2b[0][0] \n__________________________________________________________________________________________________\nactivation_21 (Activation) (None, 28, 28, 128) 0 bn3d_branch2b[0][0] \n__________________________________________________________________________________________________\nres3d_branch2c (Conv2D) (None, 28, 28, 512) 66048 activation_21[0][0] \n__________________________________________________________________________________________________\nbn3d_branch2c (BatchNormalizati (None, 28, 28, 512) 2048 res3d_branch2c[0][0] \n__________________________________________________________________________________________________\nadd_7 (Add) (None, 28, 28, 512) 0 bn3d_branch2c[0][0] \n activation_19[0][0] \n__________________________________________________________________________________________________\nactivation_22 (Activation) (None, 28, 28, 512) 0 add_7[0][0] \n__________________________________________________________________________________________________\nres4a_branch2a (Conv2D) (None, 14, 14, 256) 131328 activation_22[0][0] \n__________________________________________________________________________________________________\nbn4a_branch2a (BatchNormalizati (None, 14, 14, 256) 1024 res4a_branch2a[0][0] \n__________________________________________________________________________________________________\nactivation_23 (Activation) (None, 14, 14, 256) 0 bn4a_branch2a[0][0] \n__________________________________________________________________________________________________\nres4a_branch2b (Conv2D) (None, 14, 14, 256) 590080 activation_23[0][0] \n__________________________________________________________________________________________________\nbn4a_branch2b (BatchNormalizati (None, 14, 14, 256) 1024 res4a_branch2b[0][0] \n__________________________________________________________________________________________________\nactivation_24 (Activation) (None, 14, 14, 256) 0 bn4a_branch2b[0][0] \n__________________________________________________________________________________________________\nres4a_branch2c (Conv2D) (None, 14, 14, 1024) 263168 activation_24[0][0] \n__________________________________________________________________________________________________\nres4a_branch1 (Conv2D) (None, 14, 14, 1024) 525312 activation_22[0][0] \n__________________________________________________________________________________________________\nbn4a_branch2c (BatchNormalizati (None, 14, 14, 1024) 4096 res4a_branch2c[0][0] \n__________________________________________________________________________________________________\nbn4a_branch1 (BatchNormalizatio (None, 14, 14, 1024) 4096 res4a_branch1[0][0] \n__________________________________________________________________________________________________\nadd_8 (Add) (None, 14, 14, 1024) 0 bn4a_branch2c[0][0] \n bn4a_branch1[0][0] \n__________________________________________________________________________________________________\nactivation_25 (Activation) (None, 14, 14, 1024) 0 add_8[0][0] \n__________________________________________________________________________________________________\nres4b_branch2a (Conv2D) (None, 14, 14, 256) 262400 activation_25[0][0] \n__________________________________________________________________________________________________\nbn4b_branch2a (BatchNormalizati (None, 14, 14, 256) 1024 res4b_branch2a[0][0] \n__________________________________________________________________________________________________\nactivation_26 (Activation) (None, 14, 14, 256) 0 bn4b_branch2a[0][0] \n__________________________________________________________________________________________________\nres4b_branch2b (Conv2D) (None, 14, 14, 256) 590080 activation_26[0][0] \n__________________________________________________________________________________________________\nbn4b_branch2b (BatchNormalizati (None, 14, 14, 256) 1024 res4b_branch2b[0][0] \n__________________________________________________________________________________________________\nactivation_27 (Activation) (None, 14, 14, 256) 0 bn4b_branch2b[0][0] \n__________________________________________________________________________________________________\nres4b_branch2c (Conv2D) (None, 14, 14, 1024) 263168 activation_27[0][0] \n__________________________________________________________________________________________________\nbn4b_branch2c (BatchNormalizati (None, 14, 14, 1024) 4096 res4b_branch2c[0][0] \n__________________________________________________________________________________________________\nadd_9 (Add) (None, 14, 14, 1024) 0 bn4b_branch2c[0][0] \n activation_25[0][0] \n__________________________________________________________________________________________________\nactivation_28 (Activation) (None, 14, 14, 1024) 0 add_9[0][0] \n__________________________________________________________________________________________________\nres4c_branch2a (Conv2D) (None, 14, 14, 256) 262400 activation_28[0][0] \n__________________________________________________________________________________________________\nbn4c_branch2a (BatchNormalizati (None, 14, 14, 256) 1024 res4c_branch2a[0][0] \n__________________________________________________________________________________________________\nactivation_29 (Activation) (None, 14, 14, 256) 0 bn4c_branch2a[0][0] \n__________________________________________________________________________________________________\nres4c_branch2b (Conv2D) (None, 14, 14, 256) 590080 activation_29[0][0] \n__________________________________________________________________________________________________\nbn4c_branch2b (BatchNormalizati (None, 14, 14, 256) 1024 res4c_branch2b[0][0] \n__________________________________________________________________________________________________\nactivation_30 (Activation) (None, 14, 14, 256) 0 bn4c_branch2b[0][0] \n__________________________________________________________________________________________________\nres4c_branch2c (Conv2D) (None, 14, 14, 1024) 263168 activation_30[0][0] \n__________________________________________________________________________________________________\nbn4c_branch2c (BatchNormalizati (None, 14, 14, 1024) 4096 res4c_branch2c[0][0] \n__________________________________________________________________________________________________\nadd_10 (Add) (None, 14, 14, 1024) 0 bn4c_branch2c[0][0] \n activation_28[0][0] \n__________________________________________________________________________________________________\nactivation_31 (Activation) (None, 14, 14, 1024) 0 add_10[0][0] \n__________________________________________________________________________________________________\nres4d_branch2a (Conv2D) (None, 14, 14, 256) 262400 activation_31[0][0] \n__________________________________________________________________________________________________\nbn4d_branch2a (BatchNormalizati (None, 14, 14, 256) 1024 res4d_branch2a[0][0] \n__________________________________________________________________________________________________\nactivation_32 (Activation) (None, 14, 14, 256) 0 bn4d_branch2a[0][0] \n__________________________________________________________________________________________________\nres4d_branch2b (Conv2D) (None, 14, 14, 256) 590080 activation_32[0][0] \n__________________________________________________________________________________________________\nbn4d_branch2b (BatchNormalizati (None, 14, 14, 256) 1024 res4d_branch2b[0][0] \n__________________________________________________________________________________________________\nactivation_33 (Activation) (None, 14, 14, 256) 0 bn4d_branch2b[0][0] \n__________________________________________________________________________________________________\nres4d_branch2c (Conv2D) (None, 14, 14, 1024) 263168 activation_33[0][0] \n__________________________________________________________________________________________________\nbn4d_branch2c (BatchNormalizati (None, 14, 14, 1024) 4096 res4d_branch2c[0][0] \n__________________________________________________________________________________________________\nadd_11 (Add) (None, 14, 14, 1024) 0 bn4d_branch2c[0][0] \n activation_31[0][0] \n__________________________________________________________________________________________________\nactivation_34 (Activation) (None, 14, 14, 1024) 0 add_11[0][0] \n__________________________________________________________________________________________________\nres4e_branch2a (Conv2D) (None, 14, 14, 256) 262400 activation_34[0][0] \n__________________________________________________________________________________________________\nbn4e_branch2a (BatchNormalizati (None, 14, 14, 256) 1024 res4e_branch2a[0][0] \n__________________________________________________________________________________________________\nactivation_35 (Activation) (None, 14, 14, 256) 0 bn4e_branch2a[0][0] \n__________________________________________________________________________________________________\nres4e_branch2b (Conv2D) (None, 14, 14, 256) 590080 activation_35[0][0] \n__________________________________________________________________________________________________\nbn4e_branch2b (BatchNormalizati (None, 14, 14, 256) 1024 res4e_branch2b[0][0] \n__________________________________________________________________________________________________\nactivation_36 (Activation) (None, 14, 14, 256) 0 bn4e_branch2b[0][0] \n__________________________________________________________________________________________________\nres4e_branch2c (Conv2D) (None, 14, 14, 1024) 263168 activation_36[0][0] \n__________________________________________________________________________________________________\nbn4e_branch2c (BatchNormalizati (None, 14, 14, 1024) 4096 res4e_branch2c[0][0] \n__________________________________________________________________________________________________\nadd_12 (Add) (None, 14, 14, 1024) 0 bn4e_branch2c[0][0] \n activation_34[0][0] \n__________________________________________________________________________________________________\nactivation_37 (Activation) (None, 14, 14, 1024) 0 add_12[0][0] \n__________________________________________________________________________________________________\nres4f_branch2a (Conv2D) (None, 14, 14, 256) 262400 activation_37[0][0] \n__________________________________________________________________________________________________\nbn4f_branch2a (BatchNormalizati (None, 14, 14, 256) 1024 res4f_branch2a[0][0] \n__________________________________________________________________________________________________\nactivation_38 (Activation) (None, 14, 14, 256) 0 bn4f_branch2a[0][0] \n__________________________________________________________________________________________________\nres4f_branch2b (Conv2D) (None, 14, 14, 256) 590080 activation_38[0][0] \n__________________________________________________________________________________________________\nbn4f_branch2b (BatchNormalizati (None, 14, 14, 256) 1024 res4f_branch2b[0][0] \n__________________________________________________________________________________________________\nactivation_39 (Activation) (None, 14, 14, 256) 0 bn4f_branch2b[0][0] \n__________________________________________________________________________________________________\nres4f_branch2c (Conv2D) (None, 14, 14, 1024) 263168 activation_39[0][0] \n__________________________________________________________________________________________________\nbn4f_branch2c (BatchNormalizati (None, 14, 14, 1024) 4096 res4f_branch2c[0][0] \n__________________________________________________________________________________________________\nadd_13 (Add) (None, 14, 14, 1024) 0 bn4f_branch2c[0][0] \n activation_37[0][0] \n__________________________________________________________________________________________________\nactivation_40 (Activation) (None, 14, 14, 1024) 0 add_13[0][0] \n__________________________________________________________________________________________________\nres5a_branch2a (Conv2D) (None, 7, 7, 512) 524800 activation_40[0][0] \n__________________________________________________________________________________________________\nbn5a_branch2a (BatchNormalizati (None, 7, 7, 512) 2048 res5a_branch2a[0][0] \n__________________________________________________________________________________________________\nactivation_41 (Activation) (None, 7, 7, 512) 0 bn5a_branch2a[0][0] \n__________________________________________________________________________________________________\nres5a_branch2b (Conv2D) (None, 7, 7, 512) 2359808 activation_41[0][0] \n__________________________________________________________________________________________________\nbn5a_branch2b (BatchNormalizati (None, 7, 7, 512) 2048 res5a_branch2b[0][0] \n__________________________________________________________________________________________________\nactivation_42 (Activation) (None, 7, 7, 512) 0 bn5a_branch2b[0][0] \n__________________________________________________________________________________________________\nres5a_branch2c (Conv2D) (None, 7, 7, 2048) 1050624 activation_42[0][0] \n__________________________________________________________________________________________________\nres5a_branch1 (Conv2D) (None, 7, 7, 2048) 2099200 activation_40[0][0] \n__________________________________________________________________________________________________\nbn5a_branch2c (BatchNormalizati (None, 7, 7, 2048) 8192 res5a_branch2c[0][0] \n__________________________________________________________________________________________________\nbn5a_branch1 (BatchNormalizatio (None, 7, 7, 2048) 8192 res5a_branch1[0][0] \n__________________________________________________________________________________________________\nadd_14 (Add) (None, 7, 7, 2048) 0 bn5a_branch2c[0][0] \n bn5a_branch1[0][0] \n__________________________________________________________________________________________________\nactivation_43 (Activation) (None, 7, 7, 2048) 0 add_14[0][0] \n__________________________________________________________________________________________________\nres5b_branch2a (Conv2D) (None, 7, 7, 512) 1049088 activation_43[0][0] \n__________________________________________________________________________________________________\nbn5b_branch2a (BatchNormalizati (None, 7, 7, 512) 2048 res5b_branch2a[0][0] \n__________________________________________________________________________________________________\nactivation_44 (Activation) (None, 7, 7, 512) 0 bn5b_branch2a[0][0] \n__________________________________________________________________________________________________\nres5b_branch2b (Conv2D) (None, 7, 7, 512) 2359808 activation_44[0][0] \n__________________________________________________________________________________________________\nbn5b_branch2b (BatchNormalizati (None, 7, 7, 512) 2048 res5b_branch2b[0][0] \n__________________________________________________________________________________________________\nactivation_45 (Activation) (None, 7, 7, 512) 0 bn5b_branch2b[0][0] \n__________________________________________________________________________________________________\nres5b_branch2c (Conv2D) (None, 7, 7, 2048) 1050624 activation_45[0][0] \n__________________________________________________________________________________________________\nbn5b_branch2c (BatchNormalizati (None, 7, 7, 2048) 8192 res5b_branch2c[0][0] \n__________________________________________________________________________________________________\nadd_15 (Add) (None, 7, 7, 2048) 0 bn5b_branch2c[0][0] \n activation_43[0][0] \n__________________________________________________________________________________________________\nactivation_46 (Activation) (None, 7, 7, 2048) 0 add_15[0][0] \n__________________________________________________________________________________________________\nres5c_branch2a (Conv2D) (None, 7, 7, 512) 1049088 activation_46[0][0] \n__________________________________________________________________________________________________\nbn5c_branch2a (BatchNormalizati (None, 7, 7, 512) 2048 res5c_branch2a[0][0] \n__________________________________________________________________________________________________\nactivation_47 (Activation) (None, 7, 7, 512) 0 bn5c_branch2a[0][0] \n__________________________________________________________________________________________________\nres5c_branch2b (Conv2D) (None, 7, 7, 512) 2359808 activation_47[0][0] \n__________________________________________________________________________________________________\nbn5c_branch2b (BatchNormalizati (None, 7, 7, 512) 2048 res5c_branch2b[0][0] \n__________________________________________________________________________________________________\nactivation_48 (Activation) (None, 7, 7, 512) 0 bn5c_branch2b[0][0] \n__________________________________________________________________________________________________\nres5c_branch2c (Conv2D) (None, 7, 7, 2048) 1050624 activation_48[0][0] \n__________________________________________________________________________________________________\nbn5c_branch2c (BatchNormalizati (None, 7, 7, 2048) 8192 res5c_branch2c[0][0] \n__________________________________________________________________________________________________\nadd_16 (Add) (None, 7, 7, 2048) 0 bn5c_branch2c[0][0] \n activation_46[0][0] \n__________________________________________________________________________________________________\nactivation_49 (Activation) (None, 7, 7, 2048) 0 add_16[0][0] \n__________________________________________________________________________________________________\nglobal_average_pooling2d_1 (Glo (None, 2048) 0 activation_49[0][0] \n__________________________________________________________________________________________________\ndense_1 (Dense) (None, 256) 524544 global_average_pooling2d_1[0][0] \n__________________________________________________________________________________________________\ndense_2 (Dense) (None, 2) 514 dense_1[0][0] \n==================================================================================================\nTotal params: 24,112,770\nTrainable params: 24,059,650\nNon-trainable params: 53,120\n__________________________________________________________________________________________________\n"
],
[
"for ix,layers in enumerate(model.layers):\n print (ix, layers)",
"0 <keras.engine.input_layer.InputLayer object at 0x7fcd75ec60f0>\n1 <keras.layers.convolutional.ZeroPadding2D object at 0x7fcd75ec66a0>\n2 <keras.layers.convolutional.Conv2D object at 0x7fcd75ec67b8>\n3 <keras.layers.normalization.BatchNormalization object at 0x7fcd75ec6be0>\n4 <keras.layers.core.Activation object at 0x7fcd75ec6dd8>\n5 <keras.layers.convolutional.ZeroPadding2D object at 0x7fcd51f5ee48>\n6 <keras.layers.pooling.MaxPooling2D object at 0x7fcd51efef28>\n7 <keras.layers.convolutional.Conv2D object at 0x7fcd75d99f28>\n8 <keras.layers.normalization.BatchNormalization object at 0x7fcd5062b3c8>\n9 <keras.layers.core.Activation object at 0x7fcd5062bcc0>\n10 <keras.layers.convolutional.Conv2D object at 0x7fcd5061b630>\n11 <keras.layers.normalization.BatchNormalization object at 0x7fcd505f8da0>\n12 <keras.layers.core.Activation object at 0x7fcd505bb748>\n13 <keras.layers.convolutional.Conv2D object at 0x7fcd50512fd0>\n14 <keras.layers.convolutional.Conv2D object at 0x7fcd504b5940>\n15 <keras.layers.normalization.BatchNormalization object at 0x7fcd504eef98>\n16 <keras.layers.normalization.BatchNormalization object at 0x7fcd503efbe0>\n17 <keras.layers.merge.Add object at 0x7fcd503b2eb8>\n18 <keras.layers.core.Activation object at 0x7fcd50331978>\n19 <keras.layers.convolutional.Conv2D object at 0x7fcd75ddd390>\n20 <keras.layers.normalization.BatchNormalization object at 0x7fcd5034d9b0>\n21 <keras.layers.core.Activation object at 0x7fcd75ec90f0>\n22 <keras.layers.convolutional.Conv2D object at 0x7fcd75e18630>\n23 <keras.layers.normalization.BatchNormalization object at 0x7fcd75e16908>\n24 <keras.layers.core.Activation object at 0x7fcd503097f0>\n25 <keras.layers.convolutional.Conv2D object at 0x7fcd50107cc0>\n26 <keras.layers.normalization.BatchNormalization object at 0x7fcd500e77f0>\n27 <keras.layers.merge.Add object at 0x7fcd500aeda0>\n28 <keras.layers.core.Activation object at 0x7fcd50050d30>\n29 <keras.layers.convolutional.Conv2D object at 0x7fcd50050d68>\n30 <keras.layers.normalization.BatchNormalization object at 0x7fcd387a0320>\n31 <keras.layers.core.Activation object at 0x7fcd386ff748>\n32 <keras.layers.convolutional.Conv2D object at 0x7fcd386f3e80>\n33 <keras.layers.normalization.BatchNormalization object at 0x7fcd386d2f28>\n34 <keras.layers.core.Activation object at 0x7fcd38696b00>\n35 <keras.layers.convolutional.Conv2D object at 0x7fcd38634860>\n36 <keras.layers.normalization.BatchNormalization object at 0x7fcd385af1d0>\n37 <keras.layers.merge.Add object at 0x7fcd38540860>\n38 <keras.layers.core.Activation object at 0x7fcd3852ae80>\n39 <keras.layers.convolutional.Conv2D object at 0x7fcd3852aba8>\n40 <keras.layers.normalization.BatchNormalization object at 0x7fcd384a5908>\n41 <keras.layers.core.Activation object at 0x7fcd38478ba8>\n42 <keras.layers.convolutional.Conv2D object at 0x7fcd38427dd8>\n43 <keras.layers.normalization.BatchNormalization object at 0x7fcd383da748>\n44 <keras.layers.core.Activation object at 0x7fcd3839c940>\n45 <keras.layers.convolutional.Conv2D object at 0x7fcd38292630>\n46 <keras.layers.convolutional.Conv2D object at 0x7fcd382b2748>\n47 <keras.layers.normalization.BatchNormalization object at 0x7fcd382eeda0>\n48 <keras.layers.normalization.BatchNormalization object at 0x7fcd381e7860>\n49 <keras.layers.merge.Add object at 0x7fcd381abb00>\n50 <keras.layers.core.Activation object at 0x7fcd3812e6d8>\n51 <keras.layers.convolutional.Conv2D object at 0x7fcd3812e630>\n52 <keras.layers.normalization.BatchNormalization object at 0x7fcd38096f98>\n53 <keras.layers.core.Activation object at 0x7fcd1a7ea208>\n54 <keras.layers.convolutional.Conv2D object at 0x7fcd1a7a1f28>\n55 <keras.layers.normalization.BatchNormalization object at 0x7fcd1a788e10>\n56 <keras.layers.core.Activation object at 0x7fcd1a788be0>\n57 <keras.layers.convolutional.Conv2D object at 0x7fcd1a654e48>\n58 <keras.layers.normalization.BatchNormalization object at 0x7fcd1a6b8908>\n59 <keras.layers.merge.Add object at 0x7fcd1a67cc50>\n60 <keras.layers.core.Activation object at 0x7fcd1a59fe48>\n61 <keras.layers.convolutional.Conv2D object at 0x7fcd1a59fe80>\n62 <keras.layers.normalization.BatchNormalization object at 0x7fcd1a515588>\n63 <keras.layers.core.Activation object at 0x7fcd1a4e7860>\n64 <keras.layers.convolutional.Conv2D object at 0x7fcd1a464f98>\n65 <keras.layers.normalization.BatchNormalization object at 0x7fcd1a446748>\n66 <keras.layers.core.Activation object at 0x7fcd1a38af60>\n67 <keras.layers.convolutional.Conv2D object at 0x7fcd1a3ad978>\n68 <keras.layers.normalization.BatchNormalization object at 0x7fcd1a3052e8>\n69 <keras.layers.merge.Add object at 0x7fcd1a325320>\n70 <keras.layers.core.Activation object at 0x7fcd1a2b9cf8>\n71 <keras.layers.convolutional.Conv2D object at 0x7fcd1a255518>\n72 <keras.layers.normalization.BatchNormalization object at 0x7fcd1a21a9e8>\n73 <keras.layers.core.Activation object at 0x7fcd1a1e8cc0>\n74 <keras.layers.convolutional.Conv2D object at 0x7fcd1a19ad68>\n75 <keras.layers.normalization.BatchNormalization object at 0x7fcd1a151c18>\n76 <keras.layers.core.Activation object at 0x7fcd1a10cf60>\n77 <keras.layers.convolutional.Conv2D object at 0x7fcd1a006748>\n78 <keras.layers.normalization.BatchNormalization object at 0x7fcd1a066e48>\n79 <keras.layers.merge.Add object at 0x7fcd1a028ef0>\n80 <keras.layers.core.Activation object at 0x7fcd19f5d978>\n81 <keras.layers.convolutional.Conv2D object at 0x7fcd19f5d898>\n82 <keras.layers.normalization.BatchNormalization object at 0x7fcd19ef5eb8>\n83 <keras.layers.core.Activation object at 0x7fcd19fa79e8>\n84 <keras.layers.convolutional.Conv2D object at 0x7fcd19e56d30>\n85 <keras.layers.normalization.BatchNormalization object at 0x7fcd19e42b70>\n86 <keras.layers.core.Activation object at 0x7fcd19e34668>\n87 <keras.layers.convolutional.Conv2D object at 0x7fcd19d0aeb8>\n88 <keras.layers.convolutional.Conv2D object at 0x7fcd19d2fc18>\n89 <keras.layers.normalization.BatchNormalization object at 0x7fcd19d67278>\n90 <keras.layers.normalization.BatchNormalization object at 0x7fcd19c6bcf8>\n91 <keras.layers.merge.Add object at 0x7fcd19c25e48>\n92 <keras.layers.core.Activation object at 0x7fcd19b44f60>\n93 <keras.layers.convolutional.Conv2D object at 0x7fcd19b7aba8>\n94 <keras.layers.normalization.BatchNormalization object at 0x7fcd19abe240>\n95 <keras.layers.core.Activation object at 0x7fcd19a3e048>\n96 <keras.layers.convolutional.Conv2D object at 0x7fcd19a75c18>\n97 <keras.layers.normalization.BatchNormalization object at 0x7fcd19a0ef60>\n98 <keras.layers.core.Activation object at 0x7fcd199d59b0>\n99 <keras.layers.convolutional.Conv2D object at 0x7fcd19972710>\n100 <keras.layers.normalization.BatchNormalization object at 0x7fcd1990c7b8>\n101 <keras.layers.merge.Add object at 0x7fcd198cef98>\n102 <keras.layers.core.Activation object at 0x7fcd19868d30>\n103 <keras.layers.convolutional.Conv2D object at 0x7fcd19868a58>\n104 <keras.layers.normalization.BatchNormalization object at 0x7fcd197e0748>\n105 <keras.layers.core.Activation object at 0x7fcd197b2f28>\n106 <keras.layers.convolutional.Conv2D object at 0x7fcd196d84e0>\n107 <keras.layers.normalization.BatchNormalization object at 0x7fcd19732f98>\n108 <keras.layers.core.Activation object at 0x7fcd196f35f8>\n109 <keras.layers.convolutional.Conv2D object at 0x7fcd19611cf8>\n110 <keras.layers.normalization.BatchNormalization object at 0x7fcd19629f60>\n111 <keras.layers.merge.Add object at 0x7fcd195ebba8>\n112 <keras.layers.core.Activation object at 0x7fcd19571e48>\n113 <keras.layers.convolutional.Conv2D object at 0x7fcd19571cf8>\n114 <keras.layers.normalization.BatchNormalization object at 0x7fcd194e4f28>\n115 <keras.layers.core.Activation object at 0x7fcd1945bb38>\n116 <keras.layers.convolutional.Conv2D object at 0x7fcd19405d68>\n117 <keras.layers.normalization.BatchNormalization object at 0x7fcd1937df60>\n118 <keras.layers.core.Activation object at 0x7fcd1937def0>\n119 <keras.layers.convolutional.Conv2D object at 0x7fcd192f05c0>\n120 <keras.layers.normalization.BatchNormalization object at 0x7fcd192cfd30>\n121 <keras.layers.merge.Add object at 0x7fcd192946d8>\n122 <keras.layers.core.Activation object at 0x7fcd19210f98>\n123 <keras.layers.convolutional.Conv2D object at 0x7fcd19210e10>\n124 <keras.layers.normalization.BatchNormalization object at 0x7fcd1918e240>\n125 <keras.layers.core.Activation object at 0x7fcd1910f5f8>\n126 <keras.layers.convolutional.Conv2D object at 0x7fcd190c7c18>\n127 <keras.layers.normalization.BatchNormalization object at 0x7fcd190ddf60>\n128 <keras.layers.core.Activation object at 0x7fcd190a59b0>\n129 <keras.layers.convolutional.Conv2D object at 0x7fcd19025cc0>\n130 <keras.layers.normalization.BatchNormalization object at 0x7fcd18fc2710>\n131 <keras.layers.merge.Add object at 0x7fcd18f9e0f0>\n132 <keras.layers.core.Activation object at 0x7fcd18f37d30>\n133 <keras.layers.convolutional.Conv2D object at 0x7fcd18f37a58>\n134 <keras.layers.normalization.BatchNormalization object at 0x7fcd18eb1780>\n135 <keras.layers.core.Activation object at 0x7fcd18dfeb38>\n136 <keras.layers.convolutional.Conv2D object at 0x7fcd18daa4e0>\n137 <keras.layers.normalization.BatchNormalization object at 0x7fcd18d82f98>\n138 <keras.layers.core.Activation object at 0x7fcd18d445f8>\n139 <keras.layers.convolutional.Conv2D object at 0x7fcd18ce3cf8>\n140 <keras.layers.normalization.BatchNormalization object at 0x7fcd18cfbf60>\n141 <keras.layers.merge.Add object at 0x7fcd18c3f908>\n142 <keras.layers.core.Activation object at 0x7fcd18bc34a8>\n143 <keras.layers.convolutional.Conv2D object at 0x7fcd18bc34e0>\n144 <keras.layers.normalization.BatchNormalization object at 0x7fcd18bb2da0>\n145 <keras.layers.core.Activation object at 0x7fcd18b28b38>\n146 <keras.layers.convolutional.Conv2D object at 0x7fcd18ad2eb8>\n147 <keras.layers.normalization.BatchNormalization object at 0x7fcd18a8b6d8>\n148 <keras.layers.core.Activation object at 0x7fcd18a4dd68>\n149 <keras.layers.convolutional.Conv2D object at 0x7fcd189425c0>\n150 <keras.layers.convolutional.Conv2D object at 0x7fcd189636d8>\n151 <keras.layers.normalization.BatchNormalization object at 0x7fcd1899dd30>\n152 <keras.layers.normalization.BatchNormalization object at 0x7fcd188987f0>\n153 <keras.layers.merge.Add object at 0x7fcd1885ca90>\n154 <keras.layers.core.Activation object at 0x7fcd187f8a90>\n155 <keras.layers.convolutional.Conv2D object at 0x7fcd187f8f60>\n156 <keras.layers.normalization.BatchNormalization object at 0x7fcd18771be0>\n157 <keras.layers.core.Activation object at 0x7fcd186c4e10>\n158 <keras.layers.convolutional.Conv2D object at 0x7fcd18669438>\n159 <keras.layers.normalization.BatchNormalization object at 0x7fcd18645f60>\n160 <keras.layers.core.Activation object at 0x7fcd1860b3c8>\n161 <keras.layers.convolutional.Conv2D object at 0x7fcd185a1c50>\n162 <keras.layers.normalization.BatchNormalization object at 0x7fcd185baf98>\n163 <keras.layers.merge.Add object at 0x7fcd18504860>\n164 <keras.layers.core.Activation object at 0x7fcd18483cf8>\n165 <keras.layers.convolutional.Conv2D object at 0x7fcd18483cc0>\n166 <keras.layers.normalization.BatchNormalization object at 0x7fcd1847ce80>\n167 <keras.layers.core.Activation object at 0x7fcd183eaa90>\n168 <keras.layers.convolutional.Conv2D object at 0x7fcd18367f28>\n169 <keras.layers.normalization.BatchNormalization object at 0x7fcd1834b978>\n170 <keras.layers.core.Activation object at 0x7fcd18311c88>\n171 <keras.layers.convolutional.Conv2D object at 0x7fcd181ff518>\n172 <keras.layers.normalization.BatchNormalization object at 0x7fcd18261fd0>\n173 <keras.layers.merge.Add object at 0x7fcd18222630>\n174 <keras.layers.core.Activation object at 0x7fcd18159748>\n175 <keras.layers.pooling.GlobalAveragePooling2D object at 0x7fcd137181d0>\n176 <keras.layers.core.Dense object at 0x7fcd13718e10>\n177 <keras.layers.core.Dense object at 0x7fcd13705a58>\n"
],
[
"for ix in range(171):\n model.layers[ix].trainable = False",
"_____no_output_____"
],
[
"model.summary()",
"__________________________________________________________________________________________________\nLayer (type) Output Shape Param # Connected to \n==================================================================================================\ninput_1 (InputLayer) (None, 224, 224, 3) 0 \n__________________________________________________________________________________________________\nconv1_pad (ZeroPadding2D) (None, 230, 230, 3) 0 input_1[0][0] \n__________________________________________________________________________________________________\nconv1 (Conv2D) (None, 112, 112, 64) 9472 conv1_pad[0][0] \n__________________________________________________________________________________________________\nbn_conv1 (BatchNormalization) (None, 112, 112, 64) 256 conv1[0][0] \n__________________________________________________________________________________________________\nactivation_1 (Activation) (None, 112, 112, 64) 0 bn_conv1[0][0] \n__________________________________________________________________________________________________\npool1_pad (ZeroPadding2D) (None, 114, 114, 64) 0 activation_1[0][0] \n__________________________________________________________________________________________________\nmax_pooling2d_1 (MaxPooling2D) (None, 56, 56, 64) 0 pool1_pad[0][0] \n__________________________________________________________________________________________________\nres2a_branch2a (Conv2D) (None, 56, 56, 64) 4160 max_pooling2d_1[0][0] \n__________________________________________________________________________________________________\nbn2a_branch2a (BatchNormalizati (None, 56, 56, 64) 256 res2a_branch2a[0][0] \n__________________________________________________________________________________________________\nactivation_2 (Activation) (None, 56, 56, 64) 0 bn2a_branch2a[0][0] \n__________________________________________________________________________________________________\nres2a_branch2b (Conv2D) (None, 56, 56, 64) 36928 activation_2[0][0] \n__________________________________________________________________________________________________\nbn2a_branch2b (BatchNormalizati (None, 56, 56, 64) 256 res2a_branch2b[0][0] \n__________________________________________________________________________________________________\nactivation_3 (Activation) (None, 56, 56, 64) 0 bn2a_branch2b[0][0] \n__________________________________________________________________________________________________\nres2a_branch2c (Conv2D) (None, 56, 56, 256) 16640 activation_3[0][0] \n__________________________________________________________________________________________________\nres2a_branch1 (Conv2D) (None, 56, 56, 256) 16640 max_pooling2d_1[0][0] \n__________________________________________________________________________________________________\nbn2a_branch2c (BatchNormalizati (None, 56, 56, 256) 1024 res2a_branch2c[0][0] \n__________________________________________________________________________________________________\nbn2a_branch1 (BatchNormalizatio (None, 56, 56, 256) 1024 res2a_branch1[0][0] \n__________________________________________________________________________________________________\nadd_1 (Add) (None, 56, 56, 256) 0 bn2a_branch2c[0][0] \n bn2a_branch1[0][0] \n__________________________________________________________________________________________________\nactivation_4 (Activation) (None, 56, 56, 256) 0 add_1[0][0] \n__________________________________________________________________________________________________\nres2b_branch2a (Conv2D) (None, 56, 56, 64) 16448 activation_4[0][0] \n__________________________________________________________________________________________________\nbn2b_branch2a (BatchNormalizati (None, 56, 56, 64) 256 res2b_branch2a[0][0] \n__________________________________________________________________________________________________\nactivation_5 (Activation) (None, 56, 56, 64) 0 bn2b_branch2a[0][0] \n__________________________________________________________________________________________________\nres2b_branch2b (Conv2D) (None, 56, 56, 64) 36928 activation_5[0][0] \n__________________________________________________________________________________________________\nbn2b_branch2b (BatchNormalizati (None, 56, 56, 64) 256 res2b_branch2b[0][0] \n__________________________________________________________________________________________________\nactivation_6 (Activation) (None, 56, 56, 64) 0 bn2b_branch2b[0][0] \n__________________________________________________________________________________________________\nres2b_branch2c (Conv2D) (None, 56, 56, 256) 16640 activation_6[0][0] \n__________________________________________________________________________________________________\nbn2b_branch2c (BatchNormalizati (None, 56, 56, 256) 1024 res2b_branch2c[0][0] \n__________________________________________________________________________________________________\nadd_2 (Add) (None, 56, 56, 256) 0 bn2b_branch2c[0][0] \n activation_4[0][0] \n__________________________________________________________________________________________________\nactivation_7 (Activation) (None, 56, 56, 256) 0 add_2[0][0] \n__________________________________________________________________________________________________\nres2c_branch2a (Conv2D) (None, 56, 56, 64) 16448 activation_7[0][0] \n__________________________________________________________________________________________________\nbn2c_branch2a (BatchNormalizati (None, 56, 56, 64) 256 res2c_branch2a[0][0] \n__________________________________________________________________________________________________\nactivation_8 (Activation) (None, 56, 56, 64) 0 bn2c_branch2a[0][0] \n__________________________________________________________________________________________________\nres2c_branch2b (Conv2D) (None, 56, 56, 64) 36928 activation_8[0][0] \n__________________________________________________________________________________________________\nbn2c_branch2b (BatchNormalizati (None, 56, 56, 64) 256 res2c_branch2b[0][0] \n__________________________________________________________________________________________________\nactivation_9 (Activation) (None, 56, 56, 64) 0 bn2c_branch2b[0][0] \n__________________________________________________________________________________________________\nres2c_branch2c (Conv2D) (None, 56, 56, 256) 16640 activation_9[0][0] \n__________________________________________________________________________________________________\nbn2c_branch2c (BatchNormalizati (None, 56, 56, 256) 1024 res2c_branch2c[0][0] \n__________________________________________________________________________________________________\nadd_3 (Add) (None, 56, 56, 256) 0 bn2c_branch2c[0][0] \n activation_7[0][0] \n__________________________________________________________________________________________________\nactivation_10 (Activation) (None, 56, 56, 256) 0 add_3[0][0] \n__________________________________________________________________________________________________\nres3a_branch2a (Conv2D) (None, 28, 28, 128) 32896 activation_10[0][0] \n__________________________________________________________________________________________________\nbn3a_branch2a (BatchNormalizati (None, 28, 28, 128) 512 res3a_branch2a[0][0] \n__________________________________________________________________________________________________\nactivation_11 (Activation) (None, 28, 28, 128) 0 bn3a_branch2a[0][0] \n__________________________________________________________________________________________________\nres3a_branch2b (Conv2D) (None, 28, 28, 128) 147584 activation_11[0][0] \n__________________________________________________________________________________________________\nbn3a_branch2b (BatchNormalizati (None, 28, 28, 128) 512 res3a_branch2b[0][0] \n__________________________________________________________________________________________________\nactivation_12 (Activation) (None, 28, 28, 128) 0 bn3a_branch2b[0][0] \n__________________________________________________________________________________________________\nres3a_branch2c (Conv2D) (None, 28, 28, 512) 66048 activation_12[0][0] \n__________________________________________________________________________________________________\nres3a_branch1 (Conv2D) (None, 28, 28, 512) 131584 activation_10[0][0] \n__________________________________________________________________________________________________\nbn3a_branch2c (BatchNormalizati (None, 28, 28, 512) 2048 res3a_branch2c[0][0] \n__________________________________________________________________________________________________\nbn3a_branch1 (BatchNormalizatio (None, 28, 28, 512) 2048 res3a_branch1[0][0] \n__________________________________________________________________________________________________\nadd_4 (Add) (None, 28, 28, 512) 0 bn3a_branch2c[0][0] \n bn3a_branch1[0][0] \n__________________________________________________________________________________________________\nactivation_13 (Activation) (None, 28, 28, 512) 0 add_4[0][0] \n__________________________________________________________________________________________________\nres3b_branch2a (Conv2D) (None, 28, 28, 128) 65664 activation_13[0][0] \n__________________________________________________________________________________________________\nbn3b_branch2a (BatchNormalizati (None, 28, 28, 128) 512 res3b_branch2a[0][0] \n__________________________________________________________________________________________________\nactivation_14 (Activation) (None, 28, 28, 128) 0 bn3b_branch2a[0][0] \n__________________________________________________________________________________________________\nres3b_branch2b (Conv2D) (None, 28, 28, 128) 147584 activation_14[0][0] \n__________________________________________________________________________________________________\nbn3b_branch2b (BatchNormalizati (None, 28, 28, 128) 512 res3b_branch2b[0][0] \n__________________________________________________________________________________________________\nactivation_15 (Activation) (None, 28, 28, 128) 0 bn3b_branch2b[0][0] \n__________________________________________________________________________________________________\nres3b_branch2c (Conv2D) (None, 28, 28, 512) 66048 activation_15[0][0] \n__________________________________________________________________________________________________\nbn3b_branch2c (BatchNormalizati (None, 28, 28, 512) 2048 res3b_branch2c[0][0] \n__________________________________________________________________________________________________\nadd_5 (Add) (None, 28, 28, 512) 0 bn3b_branch2c[0][0] \n activation_13[0][0] \n__________________________________________________________________________________________________\nactivation_16 (Activation) (None, 28, 28, 512) 0 add_5[0][0] \n__________________________________________________________________________________________________\nres3c_branch2a (Conv2D) (None, 28, 28, 128) 65664 activation_16[0][0] \n__________________________________________________________________________________________________\nbn3c_branch2a (BatchNormalizati (None, 28, 28, 128) 512 res3c_branch2a[0][0] \n__________________________________________________________________________________________________\nactivation_17 (Activation) (None, 28, 28, 128) 0 bn3c_branch2a[0][0] \n__________________________________________________________________________________________________\nres3c_branch2b (Conv2D) (None, 28, 28, 128) 147584 activation_17[0][0] \n__________________________________________________________________________________________________\nbn3c_branch2b (BatchNormalizati (None, 28, 28, 128) 512 res3c_branch2b[0][0] \n__________________________________________________________________________________________________\nactivation_18 (Activation) (None, 28, 28, 128) 0 bn3c_branch2b[0][0] \n__________________________________________________________________________________________________\nres3c_branch2c (Conv2D) (None, 28, 28, 512) 66048 activation_18[0][0] \n__________________________________________________________________________________________________\nbn3c_branch2c (BatchNormalizati (None, 28, 28, 512) 2048 res3c_branch2c[0][0] \n__________________________________________________________________________________________________\nadd_6 (Add) (None, 28, 28, 512) 0 bn3c_branch2c[0][0] \n activation_16[0][0] \n__________________________________________________________________________________________________\nactivation_19 (Activation) (None, 28, 28, 512) 0 add_6[0][0] \n__________________________________________________________________________________________________\nres3d_branch2a (Conv2D) (None, 28, 28, 128) 65664 activation_19[0][0] \n__________________________________________________________________________________________________\nbn3d_branch2a (BatchNormalizati (None, 28, 28, 128) 512 res3d_branch2a[0][0] \n__________________________________________________________________________________________________\nactivation_20 (Activation) (None, 28, 28, 128) 0 bn3d_branch2a[0][0] \n__________________________________________________________________________________________________\nres3d_branch2b (Conv2D) (None, 28, 28, 128) 147584 activation_20[0][0] \n__________________________________________________________________________________________________\nbn3d_branch2b (BatchNormalizati (None, 28, 28, 128) 512 res3d_branch2b[0][0] \n__________________________________________________________________________________________________\nactivation_21 (Activation) (None, 28, 28, 128) 0 bn3d_branch2b[0][0] \n__________________________________________________________________________________________________\nres3d_branch2c (Conv2D) (None, 28, 28, 512) 66048 activation_21[0][0] \n__________________________________________________________________________________________________\nbn3d_branch2c (BatchNormalizati (None, 28, 28, 512) 2048 res3d_branch2c[0][0] \n__________________________________________________________________________________________________\nadd_7 (Add) (None, 28, 28, 512) 0 bn3d_branch2c[0][0] \n activation_19[0][0] \n__________________________________________________________________________________________________\nactivation_22 (Activation) (None, 28, 28, 512) 0 add_7[0][0] \n__________________________________________________________________________________________________\nres4a_branch2a (Conv2D) (None, 14, 14, 256) 131328 activation_22[0][0] \n__________________________________________________________________________________________________\nbn4a_branch2a (BatchNormalizati (None, 14, 14, 256) 1024 res4a_branch2a[0][0] \n__________________________________________________________________________________________________\nactivation_23 (Activation) (None, 14, 14, 256) 0 bn4a_branch2a[0][0] \n__________________________________________________________________________________________________\nres4a_branch2b (Conv2D) (None, 14, 14, 256) 590080 activation_23[0][0] \n__________________________________________________________________________________________________\nbn4a_branch2b (BatchNormalizati (None, 14, 14, 256) 1024 res4a_branch2b[0][0] \n__________________________________________________________________________________________________\nactivation_24 (Activation) (None, 14, 14, 256) 0 bn4a_branch2b[0][0] \n__________________________________________________________________________________________________\nres4a_branch2c (Conv2D) (None, 14, 14, 1024) 263168 activation_24[0][0] \n__________________________________________________________________________________________________\nres4a_branch1 (Conv2D) (None, 14, 14, 1024) 525312 activation_22[0][0] \n__________________________________________________________________________________________________\nbn4a_branch2c (BatchNormalizati (None, 14, 14, 1024) 4096 res4a_branch2c[0][0] \n__________________________________________________________________________________________________\nbn4a_branch1 (BatchNormalizatio (None, 14, 14, 1024) 4096 res4a_branch1[0][0] \n__________________________________________________________________________________________________\nadd_8 (Add) (None, 14, 14, 1024) 0 bn4a_branch2c[0][0] \n bn4a_branch1[0][0] \n__________________________________________________________________________________________________\nactivation_25 (Activation) (None, 14, 14, 1024) 0 add_8[0][0] \n__________________________________________________________________________________________________\nres4b_branch2a (Conv2D) (None, 14, 14, 256) 262400 activation_25[0][0] \n__________________________________________________________________________________________________\nbn4b_branch2a (BatchNormalizati (None, 14, 14, 256) 1024 res4b_branch2a[0][0] \n__________________________________________________________________________________________________\nactivation_26 (Activation) (None, 14, 14, 256) 0 bn4b_branch2a[0][0] \n__________________________________________________________________________________________________\nres4b_branch2b (Conv2D) (None, 14, 14, 256) 590080 activation_26[0][0] \n__________________________________________________________________________________________________\nbn4b_branch2b (BatchNormalizati (None, 14, 14, 256) 1024 res4b_branch2b[0][0] \n__________________________________________________________________________________________________\nactivation_27 (Activation) (None, 14, 14, 256) 0 bn4b_branch2b[0][0] \n__________________________________________________________________________________________________\nres4b_branch2c (Conv2D) (None, 14, 14, 1024) 263168 activation_27[0][0] \n__________________________________________________________________________________________________\nbn4b_branch2c (BatchNormalizati (None, 14, 14, 1024) 4096 res4b_branch2c[0][0] \n__________________________________________________________________________________________________\nadd_9 (Add) (None, 14, 14, 1024) 0 bn4b_branch2c[0][0] \n activation_25[0][0] \n__________________________________________________________________________________________________\nactivation_28 (Activation) (None, 14, 14, 1024) 0 add_9[0][0] \n__________________________________________________________________________________________________\nres4c_branch2a (Conv2D) (None, 14, 14, 256) 262400 activation_28[0][0] \n__________________________________________________________________________________________________\nbn4c_branch2a (BatchNormalizati (None, 14, 14, 256) 1024 res4c_branch2a[0][0] \n__________________________________________________________________________________________________\nactivation_29 (Activation) (None, 14, 14, 256) 0 bn4c_branch2a[0][0] \n__________________________________________________________________________________________________\nres4c_branch2b (Conv2D) (None, 14, 14, 256) 590080 activation_29[0][0] \n__________________________________________________________________________________________________\nbn4c_branch2b (BatchNormalizati (None, 14, 14, 256) 1024 res4c_branch2b[0][0] \n__________________________________________________________________________________________________\nactivation_30 (Activation) (None, 14, 14, 256) 0 bn4c_branch2b[0][0] \n__________________________________________________________________________________________________\nres4c_branch2c (Conv2D) (None, 14, 14, 1024) 263168 activation_30[0][0] \n__________________________________________________________________________________________________\nbn4c_branch2c (BatchNormalizati (None, 14, 14, 1024) 4096 res4c_branch2c[0][0] \n__________________________________________________________________________________________________\nadd_10 (Add) (None, 14, 14, 1024) 0 bn4c_branch2c[0][0] \n activation_28[0][0] \n__________________________________________________________________________________________________\nactivation_31 (Activation) (None, 14, 14, 1024) 0 add_10[0][0] \n__________________________________________________________________________________________________\nres4d_branch2a (Conv2D) (None, 14, 14, 256) 262400 activation_31[0][0] \n__________________________________________________________________________________________________\nbn4d_branch2a (BatchNormalizati (None, 14, 14, 256) 1024 res4d_branch2a[0][0] \n__________________________________________________________________________________________________\nactivation_32 (Activation) (None, 14, 14, 256) 0 bn4d_branch2a[0][0] \n__________________________________________________________________________________________________\nres4d_branch2b (Conv2D) (None, 14, 14, 256) 590080 activation_32[0][0] \n__________________________________________________________________________________________________\nbn4d_branch2b (BatchNormalizati (None, 14, 14, 256) 1024 res4d_branch2b[0][0] \n__________________________________________________________________________________________________\nactivation_33 (Activation) (None, 14, 14, 256) 0 bn4d_branch2b[0][0] \n__________________________________________________________________________________________________\nres4d_branch2c (Conv2D) (None, 14, 14, 1024) 263168 activation_33[0][0] \n__________________________________________________________________________________________________\nbn4d_branch2c (BatchNormalizati (None, 14, 14, 1024) 4096 res4d_branch2c[0][0] \n__________________________________________________________________________________________________\nadd_11 (Add) (None, 14, 14, 1024) 0 bn4d_branch2c[0][0] \n activation_31[0][0] \n__________________________________________________________________________________________________\nactivation_34 (Activation) (None, 14, 14, 1024) 0 add_11[0][0] \n__________________________________________________________________________________________________\nres4e_branch2a (Conv2D) (None, 14, 14, 256) 262400 activation_34[0][0] \n__________________________________________________________________________________________________\nbn4e_branch2a (BatchNormalizati (None, 14, 14, 256) 1024 res4e_branch2a[0][0] \n__________________________________________________________________________________________________\nactivation_35 (Activation) (None, 14, 14, 256) 0 bn4e_branch2a[0][0] \n__________________________________________________________________________________________________\nres4e_branch2b (Conv2D) (None, 14, 14, 256) 590080 activation_35[0][0] \n__________________________________________________________________________________________________\nbn4e_branch2b (BatchNormalizati (None, 14, 14, 256) 1024 res4e_branch2b[0][0] \n__________________________________________________________________________________________________\nactivation_36 (Activation) (None, 14, 14, 256) 0 bn4e_branch2b[0][0] \n__________________________________________________________________________________________________\nres4e_branch2c (Conv2D) (None, 14, 14, 1024) 263168 activation_36[0][0] \n__________________________________________________________________________________________________\nbn4e_branch2c (BatchNormalizati (None, 14, 14, 1024) 4096 res4e_branch2c[0][0] \n__________________________________________________________________________________________________\nadd_12 (Add) (None, 14, 14, 1024) 0 bn4e_branch2c[0][0] \n activation_34[0][0] \n__________________________________________________________________________________________________\nactivation_37 (Activation) (None, 14, 14, 1024) 0 add_12[0][0] \n__________________________________________________________________________________________________\nres4f_branch2a (Conv2D) (None, 14, 14, 256) 262400 activation_37[0][0] \n__________________________________________________________________________________________________\nbn4f_branch2a (BatchNormalizati (None, 14, 14, 256) 1024 res4f_branch2a[0][0] \n__________________________________________________________________________________________________\nactivation_38 (Activation) (None, 14, 14, 256) 0 bn4f_branch2a[0][0] \n__________________________________________________________________________________________________\nres4f_branch2b (Conv2D) (None, 14, 14, 256) 590080 activation_38[0][0] \n__________________________________________________________________________________________________\nbn4f_branch2b (BatchNormalizati (None, 14, 14, 256) 1024 res4f_branch2b[0][0] \n__________________________________________________________________________________________________\nactivation_39 (Activation) (None, 14, 14, 256) 0 bn4f_branch2b[0][0] \n__________________________________________________________________________________________________\nres4f_branch2c (Conv2D) (None, 14, 14, 1024) 263168 activation_39[0][0] \n__________________________________________________________________________________________________\nbn4f_branch2c (BatchNormalizati (None, 14, 14, 1024) 4096 res4f_branch2c[0][0] \n__________________________________________________________________________________________________\nadd_13 (Add) (None, 14, 14, 1024) 0 bn4f_branch2c[0][0] \n activation_37[0][0] \n__________________________________________________________________________________________________\nactivation_40 (Activation) (None, 14, 14, 1024) 0 add_13[0][0] \n__________________________________________________________________________________________________\nres5a_branch2a (Conv2D) (None, 7, 7, 512) 524800 activation_40[0][0] \n__________________________________________________________________________________________________\nbn5a_branch2a (BatchNormalizati (None, 7, 7, 512) 2048 res5a_branch2a[0][0] \n__________________________________________________________________________________________________\nactivation_41 (Activation) (None, 7, 7, 512) 0 bn5a_branch2a[0][0] \n__________________________________________________________________________________________________\nres5a_branch2b (Conv2D) (None, 7, 7, 512) 2359808 activation_41[0][0] \n__________________________________________________________________________________________________\nbn5a_branch2b (BatchNormalizati (None, 7, 7, 512) 2048 res5a_branch2b[0][0] \n__________________________________________________________________________________________________\nactivation_42 (Activation) (None, 7, 7, 512) 0 bn5a_branch2b[0][0] \n__________________________________________________________________________________________________\nres5a_branch2c (Conv2D) (None, 7, 7, 2048) 1050624 activation_42[0][0] \n__________________________________________________________________________________________________\nres5a_branch1 (Conv2D) (None, 7, 7, 2048) 2099200 activation_40[0][0] \n__________________________________________________________________________________________________\nbn5a_branch2c (BatchNormalizati (None, 7, 7, 2048) 8192 res5a_branch2c[0][0] \n__________________________________________________________________________________________________\nbn5a_branch1 (BatchNormalizatio (None, 7, 7, 2048) 8192 res5a_branch1[0][0] \n__________________________________________________________________________________________________\nadd_14 (Add) (None, 7, 7, 2048) 0 bn5a_branch2c[0][0] \n bn5a_branch1[0][0] \n__________________________________________________________________________________________________\nactivation_43 (Activation) (None, 7, 7, 2048) 0 add_14[0][0] \n__________________________________________________________________________________________________\nres5b_branch2a (Conv2D) (None, 7, 7, 512) 1049088 activation_43[0][0] \n__________________________________________________________________________________________________\nbn5b_branch2a (BatchNormalizati (None, 7, 7, 512) 2048 res5b_branch2a[0][0] \n__________________________________________________________________________________________________\nactivation_44 (Activation) (None, 7, 7, 512) 0 bn5b_branch2a[0][0] \n__________________________________________________________________________________________________\nres5b_branch2b (Conv2D) (None, 7, 7, 512) 2359808 activation_44[0][0] \n__________________________________________________________________________________________________\nbn5b_branch2b (BatchNormalizati (None, 7, 7, 512) 2048 res5b_branch2b[0][0] \n__________________________________________________________________________________________________\nactivation_45 (Activation) (None, 7, 7, 512) 0 bn5b_branch2b[0][0] \n__________________________________________________________________________________________________\nres5b_branch2c (Conv2D) (None, 7, 7, 2048) 1050624 activation_45[0][0] \n__________________________________________________________________________________________________\nbn5b_branch2c (BatchNormalizati (None, 7, 7, 2048) 8192 res5b_branch2c[0][0] \n__________________________________________________________________________________________________\nadd_15 (Add) (None, 7, 7, 2048) 0 bn5b_branch2c[0][0] \n activation_43[0][0] \n__________________________________________________________________________________________________\nactivation_46 (Activation) (None, 7, 7, 2048) 0 add_15[0][0] \n__________________________________________________________________________________________________\nres5c_branch2a (Conv2D) (None, 7, 7, 512) 1049088 activation_46[0][0] \n__________________________________________________________________________________________________\nbn5c_branch2a (BatchNormalizati (None, 7, 7, 512) 2048 res5c_branch2a[0][0] \n__________________________________________________________________________________________________\nactivation_47 (Activation) (None, 7, 7, 512) 0 bn5c_branch2a[0][0] \n__________________________________________________________________________________________________\nres5c_branch2b (Conv2D) (None, 7, 7, 512) 2359808 activation_47[0][0] \n__________________________________________________________________________________________________\nbn5c_branch2b (BatchNormalizati (None, 7, 7, 512) 2048 res5c_branch2b[0][0] \n__________________________________________________________________________________________________\nactivation_48 (Activation) (None, 7, 7, 512) 0 bn5c_branch2b[0][0] \n__________________________________________________________________________________________________\nres5c_branch2c (Conv2D) (None, 7, 7, 2048) 1050624 activation_48[0][0] \n__________________________________________________________________________________________________\nbn5c_branch2c (BatchNormalizati (None, 7, 7, 2048) 8192 res5c_branch2c[0][0] \n__________________________________________________________________________________________________\nadd_16 (Add) (None, 7, 7, 2048) 0 bn5c_branch2c[0][0] \n activation_46[0][0] \n__________________________________________________________________________________________________\nactivation_49 (Activation) (None, 7, 7, 2048) 0 add_16[0][0] \n__________________________________________________________________________________________________\nglobal_average_pooling2d_1 (Glo (None, 2048) 0 activation_49[0][0] \n__________________________________________________________________________________________________\ndense_1 (Dense) (None, 256) 524544 global_average_pooling2d_1[0][0] \n__________________________________________________________________________________________________\ndense_2 (Dense) (None, 2) 514 dense_1[0][0] \n==================================================================================================\nTotal params: 24,112,770\nTrainable params: 1,579,778\nNon-trainable params: 22,532,992\n__________________________________________________________________________________________________\n"
],
[
"adam = Adam(lr=0.0003)",
"_____no_output_____"
],
[
"model.compile(loss='categorical_crossentropy',optimizer=adam,metrics=['accuracy'])",
"W0728 17:42:16.628257 140521947629376 deprecation_wrapper.py:119] From /usr/local/lib/python3.6/dist-packages/keras/optimizers.py:790: The name tf.train.Optimizer is deprecated. Please use tf.compat.v1.train.Optimizer instead.\n\n"
],
[
"model.fit(X_train,y_train,epochs=5,shuffle=True,batch_size=64,validation_split=.20) ",
"W0728 17:42:16.857084 140521947629376 deprecation.py:323] From /usr/local/lib/python3.6/dist-packages/tensorflow/python/ops/math_grad.py:1250: add_dispatch_support.<locals>.wrapper (from tensorflow.python.ops.array_ops) is deprecated and will be removed in a future version.\nInstructions for updating:\nUse tf.where in 2.0, which has the same broadcast rule as np.where\n"
],
[
"img_path = '/home/vandit/Desktop/My Projects/My_Submissions/Men_Women_Classification/men-women-classification/women/Sophie.jpg'\nimg = image.load_img(img_path, target_size=(224,224))\nx = image.img_to_array(img)\nx = np.expand_dims(x, axis=0)\nx = preprocess_input(x)\nprint(x.shape)\npreds = model.predict(x)\n# decode the results into a list of tuples (class, description, probability)\n# (one such list for each sample in the batch)\n# print('Predicted:', decode_predictions(preds, top=3)[0])\nans = model.predict(x)\nprint(dict_labels[np.argmax(ans)])",
"(1, 224, 224, 3)\nwomen\n"
]
]
] | [
"code"
] | [
[
"code",
"code",
"code",
"code",
"code",
"code",
"code",
"code",
"code",
"code",
"code",
"code",
"code",
"code",
"code",
"code",
"code"
]
] |
d077ca402390a58df486bf18e0620d2fc28b8f2b | 35,466 | ipynb | Jupyter Notebook | notebooks/scraping.ipynb | moviedatascience/startrek-dash-app | 47c85e3afc3ea7ac894ab2d8817e7fa4e40e7144 | [
"MIT"
] | null | null | null | notebooks/scraping.ipynb | moviedatascience/startrek-dash-app | 47c85e3afc3ea7ac894ab2d8817e7fa4e40e7144 | [
"MIT"
] | 4 | 2020-03-24T17:42:06.000Z | 2021-08-23T20:32:47.000Z | notebooks/scraping.ipynb | moviedatascience/startrek-dash-app | 47c85e3afc3ea7ac894ab2d8817e7fa4e40e7144 | [
"MIT"
] | null | null | null | 65.075229 | 19,488 | 0.763774 | [
[
[
"import requests\nimport pandas as pd\nfrom bs4 import BeautifulSoup\nimport string\nimport re\nimport nltk\nimport json\nimport matplotlib.pyplot as plt\nimport numpy as np",
"_____no_output_____"
],
[
"def get_text(url):\n response = requests.get(url)\n content = response.content\n parser = BeautifulSoup(content,'html.parser')\n return(parser.text)",
"_____no_output_____"
],
[
"def clean_text(script):\n script_clean=script.strip()\n script_clean=script_clean.replace(\"\\n\",\"\")\n script_clean=script_clean.replace(\"\\r\",\" \")\n script_clean=script_clean.replace(\"\\r\\n\",\"\")\n script_clean=re.sub(\"([\\(\\[]).*?([\\)\\]])\", \"\", script_clean)\n script_clean=re.sub(r'\\.([a-zA-Z])', r'. \\1', script_clean) #remove missing whitespace between character lines.\n script_clean=re.sub(r'\\!([a-zA-Z])', r'! \\1', script_clean)\n script_clean=re.sub(r'\\?([a-zA-Z])', r'? \\1', script_clean)\n return(script_clean)",
"_____no_output_____"
],
[
"def get_cast(script_clean):\n tokens=nltk.word_tokenize(script_clean)\n cast=[]\n for word in tokens:\n if re.search(\"\\\\b[A-Z]{3,}\\\\b\", word) is not None:\n cast.append(word)\n return(list(set(cast)))",
"_____no_output_____"
],
[
"script=get_text('http://www.chakoteya.net/DS9/575.htm')",
"_____no_output_____"
],
[
"script_clean=clean_text(script)",
"_____no_output_____"
],
[
"def get_lines(script_clean, cast):\n split_script=script_clean.split(':')\n lines_dict=dict.fromkeys(cast)\n for cast_member in cast:\n lines=[]\n for i in range(len(split_script)-1):\n if cast_member in split_script[i].strip().split(\" \"):\n line=split_script[i+1].strip().split(\" \")\n line=[word for word in line if word != '']\n for member in cast:\n if member in line:\n line.remove(member)\n line=' '.join(line)\n lines.append(line)\n lines_dict[cast_member]=lines\n\n return(lines_dict)",
"_____no_output_____"
],
[
"def get_page_links():\n top_links=[\"http://www.chakoteya.net/DS9/episodes.htm\", \n \"http://www.chakoteya.net/StarTrek/episodes.htm\", \n \"http://www.chakoteya.net/NextGen/episodes.htm\", \n \"http://www.chakoteya.net/Voyager/episode_listing.htm\", \n \"http://www.chakoteya.net/Enterprise/episodes.htm\"]\n short_links=[\"http://www.chakoteya.net/DS9/\", \n \"http://www.chakoteya.net/StarTrek/\", \n \"http://www.chakoteya.net/NextGen/\", \n \"http://www.chakoteya.net/Voyager/\", \n \"http://www.chakoteya.net/Enterprise/\"]\n links_list=[]\n names_list=[]\n for i, link in enumerate(top_links):\n response = requests.get(link)\n content = response.content\n parser = BeautifulSoup(content,'html.parser')\n urls = parser.find_all('a')\n for page in urls:\n links_list.append(short_links[i]+str(page.get('href')))\n name=page.text\n name=name.replace('\\r\\n',' ')\n names_list.append(name)\n \n \n links_to_remove=['http://www.chakoteya.net/Voyager/fortyseven.htm',\n 'http://www.chakoteya.net/Voyager/LineCountS1-S3.htm',\n 'http://www.chakoteya.net/Voyager/LineCountS4-S7.htm',\n 'http://www.chakoteya.net/Enterprise/fortyseven.htm',\n ]\n links_list=[link for link in links_list if (link.endswith('.htm')) & (link not in links_to_remove)]\n \n return(links_list)",
"_____no_output_____"
],
[
"# links_list",
"_____no_output_____"
],
[
"page_links=get_page_links()",
"_____no_output_____"
],
[
"len(page_links)",
"_____no_output_____"
],
[
"DS9_links = page_links[0:173]\nTOS_links = page_links[173:253]\nTAS_links = page_links[253:275]\nTNG_links = page_links[275:451]\nVOY_links = page_links[451:611]\nENT_links = page_links[611:708]\n\nlinks=[DS9_links, TOS_links, TAS_links, TNG_links, VOY_links, ENT_links]",
"_____no_output_____"
],
[
"links_names=['DS9', 'TOS', 'TAS', 'TNG', 'VOY', 'ENT']\nlinks=[DS9_links, TOS_links, TAS_links, TNG_links, VOY_links, ENT_links]\n\nall_series_scripts={}\nfor i,series in enumerate(links):\n series_name=str(links_names[i])\n print(series_name)\n all_series_scripts[series_name]={}\n episode_script={}\n all_cast=[]\n for j,link in enumerate(series):\n episode=\"episode \"+str(j)\n text=get_text(link)\n episode_script[episode]=text\n all_series_scripts[series_name]=episode_script\n\nprint(all_series_scripts)",
"DS9\nTOS\nTAS\nTNG\nVOY\nENT\n"
],
[
"with open('all_scripts_raw.json', 'w') as data:\n json.dump(all_series_scripts, data)",
"_____no_output_____"
],
[
"with open('all_scripts_raw.json', 'r') as data:\n all_scripts_raw = json.load(data)",
"_____no_output_____"
],
[
"\nlinks_names=['DS9', 'TOS', 'TAS', 'TNG', 'VOY', 'ENT']\n\nall_series_lines={}\nfor i,series in enumerate(links_names):\n print(series)\n series_name=str(links_names[i])\n all_series_lines[series_name]={}\n all_lines_dict={}\n all_cast=[]\n #for j,episode in enumerate(all_series_scripts[series]):\n for j,episode in enumerate(all_scripts_raw[series]):\n #script=all_series_scripts[series][episode]\n script=all_scripts_raw[series][episode]\n cleaned_script=clean_text(script)\n cast=get_cast(cleaned_script)\n for member in cast:\n if member not in all_cast:\n all_cast.append(member)\n lines_dict=get_lines(cleaned_script,all_cast)\n all_lines_dict[episode]=lines_dict\n all_series_lines[series]=all_lines_dict\n\nprint(all_series_lines)",
"DS9\nTOS\nTAS\nTNG\nVOY\nENT\n"
],
[
"with open('all_series_lines.json', 'w') as data:\n json.dump(all_series_lines, data)",
"_____no_output_____"
],
[
"with open('all_series_lines.json', 'r') as data:\n all_series_lines = json.load(data)",
"_____no_output_____"
],
[
"#checking against source to make sure the character lines\n#appear in the correct episode\nall_series_lines['TNG']['episode 30']['LAFORGE']",
"_____no_output_____"
],
[
"#writing the corrected df\n# all_series_lines = pd.DataFrame(data=all_series_lines)\n# all_series_lines.to_csv(r'C:\\Users\\Eric\\Desktop\\Star_Trek_Scripts-master\\Star_Trek_Scripts-master\\data\\all_series_lines.csv')\n#when I wrote it to a df spock ended up getting lines???????????????",
"_____no_output_____"
],
[
"episodes=all_series_lines['TNG'].keys()",
"_____no_output_____"
],
[
"total_lines_counts={}\nline_counts_by_episode={}\nfor i,ep in enumerate(episodes):\n if i == 0:\n episode=\"Episode 1 & 2\"\n else:\n episode=\"Episode \"+str(i+2)\n line_counts_by_episode[episode]={}\n if all_series_lines['TNG'][ep] is not np.NaN:\n for member in list(all_series_lines['TNG'][ep].keys()):\n line_counts_by_episode[episode][member]=len(all_series_lines['TNG'][ep][member])\n if member in total_lines_counts.keys():\n total_lines_counts[member]=total_lines_counts[member]+len(all_series_lines['TNG'][ep][member])\n else:\n total_lines_counts[member]=len(all_series_lines['TNG'][ep][member])",
"_____no_output_____"
],
[
"#checking to make sure Spock doesn't appear, since that was an issue before\nTNG_df_byep = pd.DataFrame(line_counts_by_episode)\n# TNG_df_byep.loc['SPOCK']\n\nTNG_df=pd.DataFrame(list(total_lines_counts.items()), columns=['Character','No. of Lines'])\nTop20=TNG_df.sort_values(by='No. of Lines', ascending=False).head(20)\n\nTop20.plot.bar(x='Character',y='No. of Lines')\nplt.show()\n\nTop20['Character']",
"_____no_output_____"
],
[
"export_TOP20 = Top20.to_csv(r'C:\\Users\\Eric\\startrek-dash-app\\assets\\top20')",
"_____no_output_____"
],
[
"export_vis_TNG = TNG_df_byep.to_csv(r'C:\\Users\\Eric\\startrek-dash-app\\assets\\bar_chart_TNG')",
"_____no_output_____"
]
]
] | [
"code"
] | [
[
"code",
"code",
"code",
"code",
"code",
"code",
"code",
"code",
"code",
"code",
"code",
"code",
"code",
"code",
"code",
"code",
"code",
"code",
"code",
"code",
"code",
"code",
"code",
"code",
"code"
]
] |
d077ddd034bc7947735187638a2f9f6b3e07aa7d | 36,008 | ipynb | Jupyter Notebook | intro-to-pytorch/Part 4 - Fashion-MNIST (Exercises).ipynb | GeoffKriston/deep-learning-v2-pytorch | 92f7b12e8afeb12753bc990829bfa8307b26ef6c | [
"MIT"
] | null | null | null | intro-to-pytorch/Part 4 - Fashion-MNIST (Exercises).ipynb | GeoffKriston/deep-learning-v2-pytorch | 92f7b12e8afeb12753bc990829bfa8307b26ef6c | [
"MIT"
] | null | null | null | intro-to-pytorch/Part 4 - Fashion-MNIST (Exercises).ipynb | GeoffKriston/deep-learning-v2-pytorch | 92f7b12e8afeb12753bc990829bfa8307b26ef6c | [
"MIT"
] | null | null | null | 144.032 | 24,796 | 0.877805 | [
[
[
"# Classifying Fashion-MNIST\n\nNow it's your turn to build and train a neural network. You'll be using the [Fashion-MNIST dataset](https://github.com/zalandoresearch/fashion-mnist), a drop-in replacement for the MNIST dataset. MNIST is actually quite trivial with neural networks where you can easily achieve better than 97% accuracy. Fashion-MNIST is a set of 28x28 greyscale images of clothes. It's more complex than MNIST, so it's a better representation of the actual performance of your network, and a better representation of datasets you'll use in the real world.\n\n<img src='assets/fashion-mnist-sprite.png' width=500px>\n\nIn this notebook, you'll build your own neural network. For the most part, you could just copy and paste the code from Part 3, but you wouldn't be learning. It's important for you to write the code yourself and get it to work. Feel free to consult the previous notebooks though as you work through this.\n\nFirst off, let's load the dataset through torchvision.",
"_____no_output_____"
]
],
[
[
"import torch\nfrom torchvision import datasets, transforms\nimport helper\n\n# Define a transform to normalize the data\ntransform = transforms.Compose([transforms.ToTensor(),\n transforms.Normalize((0.5, 0.5, 0.5), (0.5, 0.5, 0.5))])\n# Download and load the training data\ntrainset = datasets.FashionMNIST('~/.pytorch/F_MNIST_data/', download=True, train=True, transform=transform)\ntrainloader = torch.utils.data.DataLoader(trainset, batch_size=64, shuffle=True)\n\n# Download and load the test data\ntestset = datasets.FashionMNIST('~/.pytorch/F_MNIST_data/', download=True, train=False, transform=transform)\ntestloader = torch.utils.data.DataLoader(testset, batch_size=64, shuffle=True)",
"_____no_output_____"
]
],
[
[
"Here we can see one of the images.",
"_____no_output_____"
]
],
[
[
"image, label = next(iter(trainloader))\nhelper.imshow(image[0,:]);",
"_____no_output_____"
]
],
[
[
"## Building the network\n\nHere you should define your network. As with MNIST, each image is 28x28 which is a total of 784 pixels, and there are 10 classes. You should include at least one hidden layer. We suggest you use ReLU activations for the layers and to return the logits or log-softmax from the forward pass. It's up to you how many layers you add and the size of those layers.",
"_____no_output_____"
]
],
[
[
"# TODO: Define your network architecture here\nfrom torch import nn\nn_hidden1 = 1024\nn_hidden2 = 128\nn_hidden3 = 64\nmodel = nn.Sequential(nn.Linear(784,n_hidden1),\n nn.ReLU(),\n nn.Linear(n_hidden1,n_hidden2),\n nn.ReLU(),\n nn.Linear(n_hidden2,n_hidden3),\n nn.ReLU(),\n nn.Linear(n_hidden3, 10),\n nn.LogSoftmax(dim=1))",
"_____no_output_____"
]
],
[
[
"# Train the network\n\nNow you should create your network and train it. First you'll want to define [the criterion](http://pytorch.org/docs/master/nn.html#loss-functions) ( something like `nn.CrossEntropyLoss`) and [the optimizer](http://pytorch.org/docs/master/optim.html) (typically `optim.SGD` or `optim.Adam`).\n\nThen write the training code. Remember the training pass is a fairly straightforward process:\n\n* Make a forward pass through the network to get the logits \n* Use the logits to calculate the loss\n* Perform a backward pass through the network with `loss.backward()` to calculate the gradients\n* Take a step with the optimizer to update the weights\n\nBy adjusting the hyperparameters (hidden units, learning rate, etc), you should be able to get the training loss below 0.4.",
"_____no_output_____"
]
],
[
[
"# TODO: Create the network, define the criterion and optimizer\nfrom torch import optim\ncriterion = nn.NLLLoss()\noptimizer = optim.SGD(model.parameters(),lr=0.007)",
"_____no_output_____"
],
[
"# TODO: Train the network here\ndata = iter(trainloader)\nepochs = 10\nfor e in range(epochs):\n running_loss = 0\n for images,labels in trainloader:\n input=images.view(images.shape[0],784)\n optimizer.zero_grad()\n output = model(input)\n loss = criterion(output,labels)\n loss.backward()\n optimizer.step()\n running_loss+=loss.item()\n else:\n print \"Epoch: \", e, \"Training loss: \", running_loss/len(trainloader)",
"Epoch: 0 Training loss: 1.4089186108\nEpoch: 1 Training loss: 0.665717209008\nEpoch: 2 Training loss: 0.561825139182\nEpoch: 3 Training loss: 0.505677753738\nEpoch: 4 Training loss: 0.468876876572\nEpoch: 5 Training loss: 0.442242734071\nEpoch: 6 Training loss: 0.423429649252\nEpoch: 7 Training loss: 0.407437733559\nEpoch: 8 Training loss: 0.393918345239\nEpoch: 9 Training loss: 0.382098605384\n"
],
[
"%matplotlib inline\n%config InlineBackend.figure_format = 'retina'\n\nimport helper\n\n# Test out your network!\n\ndataiter = iter(testloader)\nimages, labels = dataiter.next()\nimg = images[0]\n# Convert 2D image to 1D vector\nimg = img.resize_(1, 784)\n\n# TODO: Calculate the class probabilities (softmax) for img\nwith torch.no_grad():\n logprobs = model(img)\nps = torch.exp(logprobs)\n\n# Plot the image and probabilities\nhelper.view_classify(img.resize_(1, 28, 28), ps, version='Fashion')",
"_____no_output_____"
]
]
] | [
"markdown",
"code",
"markdown",
"code",
"markdown",
"code",
"markdown",
"code"
] | [
[
"markdown"
],
[
"code"
],
[
"markdown"
],
[
"code"
],
[
"markdown"
],
[
"code"
],
[
"markdown"
],
[
"code",
"code",
"code"
]
] |
d077e4b946aa9bc5dde588abe55b1a84d5a2ef8a | 115,256 | ipynb | Jupyter Notebook | Advanced_DP_CGAN/AdvancedDPCGAN.ipynb | reihaneh-torkzadehmahani/DP-CGAN | 639ce4d261ee3202ab72ea5fe4ece916272bf524 | [
"Apache-2.0"
] | 22 | 2019-10-23T13:35:25.000Z | 2022-02-21T15:38:41.000Z | Advanced_DP_CGAN/AdvancedDPCGAN.ipynb | reihaneh-torkzadehmahani/DP-CGAN | 639ce4d261ee3202ab72ea5fe4ece916272bf524 | [
"Apache-2.0"
] | null | null | null | Advanced_DP_CGAN/AdvancedDPCGAN.ipynb | reihaneh-torkzadehmahani/DP-CGAN | 639ce4d261ee3202ab72ea5fe4ece916272bf524 | [
"Apache-2.0"
] | 7 | 2019-12-31T19:48:58.000Z | 2021-11-14T09:06:19.000Z | 47.062474 | 245 | 0.429427 | [
[
[
"<a href=\"https://colab.research.google.com/github/reihaneh-torkzadehmahani/MyDPGAN/blob/master/AdvancedDPCGAN.ipynb\" target=\"_parent\"><img src=\"https://colab.research.google.com/assets/colab-badge.svg\" alt=\"Open In Colab\"/></a>",
"_____no_output_____"
],
[
"## differential_privacy.analysis.rdp_accountant",
"_____no_output_____"
]
],
[
[
"# Copyright 2018 The TensorFlow Authors. All Rights Reserved.\n#\n# Licensed under the Apache License, Version 2.0 (the \"License\");\n# you may not use this file except in compliance with the License.\n# You may obtain a copy of the License at\n#\n# http://www.apache.org/licenses/LICENSE-2.0\n#\n# Unless required by applicable law or agreed to in writing, software\n# distributed under the License is distributed on an \"AS IS\" BASIS,\n# WITHOUT WARRANTIES OR CONDITIONS OF ANY KIND, either express or implied.\n# See the License for the specific language governing permissions and\n# limitations under the License.\n# ==============================================================================\n\"\"\"RDP analysis of the Sampled Gaussian Mechanism.\n\nFunctionality for computing Renyi differential privacy (RDP) of an additive\nSampled Gaussian Mechanism (SGM). Its public interface consists of two methods:\n compute_rdp(q, noise_multiplier, T, orders) computes RDP for SGM iterated\n T times.\n get_privacy_spent(orders, rdp, target_eps, target_delta) computes delta\n (or eps) given RDP at multiple orders and\n a target value for eps (or delta).\n\nExample use:\n\nSuppose that we have run an SGM applied to a function with l2-sensitivity 1.\nIts parameters are given as a list of tuples (q1, sigma1, T1), ...,\n(qk, sigma_k, Tk), and we wish to compute eps for a given delta.\nThe example code would be:\n\n max_order = 32\n orders = range(2, max_order + 1)\n rdp = np.zeros_like(orders, dtype=float)\n for q, sigma, T in parameters:\n rdp += rdp_accountant.compute_rdp(q, sigma, T, orders)\n eps, _, opt_order = rdp_accountant.get_privacy_spent(rdp, target_delta=delta)\n\"\"\"\nfrom __future__ import absolute_import\nfrom __future__ import division\nfrom __future__ import print_function\n\nimport math\nimport sys\n\nimport numpy as np\nfrom scipy import special\nimport six\n\n########################\n# LOG-SPACE ARITHMETIC #\n########################\n\n\ndef _log_add(logx, logy):\n \"\"\"Add two numbers in the log space.\"\"\"\n a, b = min(logx, logy), max(logx, logy)\n if a == -np.inf: # adding 0\n return b\n # Use exp(a) + exp(b) = (exp(a - b) + 1) * exp(b)\n return math.log1p(math.exp(a - b)) + b # log1p(x) = log(x + 1)\n\n\ndef _log_sub(logx, logy):\n \"\"\"Subtract two numbers in the log space. Answer must be non-negative.\"\"\"\n if logx < logy:\n raise ValueError(\"The result of subtraction must be non-negative.\")\n if logy == -np.inf: # subtracting 0\n return logx\n if logx == logy:\n return -np.inf # 0 is represented as -np.inf in the log space.\n\n try:\n # Use exp(x) - exp(y) = (exp(x - y) - 1) * exp(y).\n return math.log(\n math.expm1(logx - logy)) + logy # expm1(x) = exp(x) - 1\n except OverflowError:\n return logx\n\n\ndef _log_print(logx):\n \"\"\"Pretty print.\"\"\"\n if logx < math.log(sys.float_info.max):\n return \"{}\".format(math.exp(logx))\n else:\n return \"exp({})\".format(logx)\n\n\ndef _compute_log_a_int(q, sigma, alpha):\n \"\"\"Compute log(A_alpha) for integer alpha. 0 < q < 1.\"\"\"\n assert isinstance(alpha, six.integer_types)\n\n # Initialize with 0 in the log space.\n log_a = -np.inf\n\n for i in range(alpha + 1):\n log_coef_i = (math.log(special.binom(alpha, i)) + i * math.log(q) +\n (alpha - i) * math.log(1 - q))\n\n s = log_coef_i + (i * i - i) / (2 * (sigma**2))\n log_a = _log_add(log_a, s)\n\n return float(log_a)\n\n\ndef _compute_log_a_frac(q, sigma, alpha):\n \"\"\"Compute log(A_alpha) for fractional alpha. 0 < q < 1.\"\"\"\n # The two parts of A_alpha, integrals over (-inf,z0] and [z0, +inf), are\n # initialized to 0 in the log space:\n log_a0, log_a1 = -np.inf, -np.inf\n i = 0\n\n z0 = sigma**2 * math.log(1 / q - 1) + .5\n\n while True: # do ... until loop\n coef = special.binom(alpha, i)\n log_coef = math.log(abs(coef))\n j = alpha - i\n\n log_t0 = log_coef + i * math.log(q) + j * math.log(1 - q)\n log_t1 = log_coef + j * math.log(q) + i * math.log(1 - q)\n\n log_e0 = math.log(.5) + _log_erfc((i - z0) / (math.sqrt(2) * sigma))\n log_e1 = math.log(.5) + _log_erfc((z0 - j) / (math.sqrt(2) * sigma))\n\n log_s0 = log_t0 + (i * i - i) / (2 * (sigma**2)) + log_e0\n log_s1 = log_t1 + (j * j - j) / (2 * (sigma**2)) + log_e1\n\n if coef > 0:\n log_a0 = _log_add(log_a0, log_s0)\n log_a1 = _log_add(log_a1, log_s1)\n else:\n log_a0 = _log_sub(log_a0, log_s0)\n log_a1 = _log_sub(log_a1, log_s1)\n\n i += 1\n if max(log_s0, log_s1) < -30:\n break\n\n return _log_add(log_a0, log_a1)\n\n\ndef _compute_log_a(q, sigma, alpha):\n \"\"\"Compute log(A_alpha) for any positive finite alpha.\"\"\"\n if float(alpha).is_integer():\n return _compute_log_a_int(q, sigma, int(alpha))\n else:\n return _compute_log_a_frac(q, sigma, alpha)\n\n\ndef _log_erfc(x):\n \"\"\"Compute log(erfc(x)) with high accuracy for large x.\"\"\"\n try:\n return math.log(2) + special.log_ndtr(-x * 2**.5)\n except NameError:\n # If log_ndtr is not available, approximate as follows:\n r = special.erfc(x)\n if r == 0.0:\n # Using the Laurent series at infinity for the tail of the erfc function:\n # erfc(x) ~ exp(-x^2-.5/x^2+.625/x^4)/(x*pi^.5)\n # To verify in Mathematica:\n # Series[Log[Erfc[x]] + Log[x] + Log[Pi]/2 + x^2, {x, Infinity, 6}]\n return (-math.log(math.pi) / 2 - math.log(x) - x**2 - .5 * x**-2 +\n .625 * x**-4 - 37. / 24. * x**-6 + 353. / 64. * x**-8)\n else:\n return math.log(r)\n\n\ndef _compute_delta(orders, rdp, eps):\n \"\"\"Compute delta given a list of RDP values and target epsilon.\n\n Args:\n orders: An array (or a scalar) of orders.\n rdp: A list (or a scalar) of RDP guarantees.\n eps: The target epsilon.\n\n Returns:\n Pair of (delta, optimal_order).\n\n Raises:\n ValueError: If input is malformed.\n\n \"\"\"\n orders_vec = np.atleast_1d(orders)\n rdp_vec = np.atleast_1d(rdp)\n\n if len(orders_vec) != len(rdp_vec):\n raise ValueError(\"Input lists must have the same length.\")\n\n deltas = np.exp((rdp_vec - eps) * (orders_vec - 1))\n idx_opt = np.argmin(deltas)\n return min(deltas[idx_opt], 1.), orders_vec[idx_opt]\n\n\ndef _compute_eps(orders, rdp, delta):\n \"\"\"Compute epsilon given a list of RDP values and target delta.\n\n Args:\n orders: An array (or a scalar) of orders.\n rdp: A list (or a scalar) of RDP guarantees.\n delta: The target delta.\n\n Returns:\n Pair of (eps, optimal_order).\n\n Raises:\n ValueError: If input is malformed.\n\n \"\"\"\n orders_vec = np.atleast_1d(orders)\n rdp_vec = np.atleast_1d(rdp)\n\n if len(orders_vec) != len(rdp_vec):\n raise ValueError(\"Input lists must have the same length.\")\n\n eps = rdp_vec - math.log(delta) / (orders_vec - 1)\n\n idx_opt = np.nanargmin(eps) # Ignore NaNs\n return eps[idx_opt], orders_vec[idx_opt]\n\n\ndef _compute_rdp(q, sigma, alpha):\n \"\"\"Compute RDP of the Sampled Gaussian mechanism at order alpha.\n\n Args:\n q: The sampling rate.\n sigma: The std of the additive Gaussian noise.\n alpha: The order at which RDP is computed.\n\n Returns:\n RDP at alpha, can be np.inf.\n \"\"\"\n if q == 0:\n return 0\n\n if q == 1.:\n return alpha / (2 * sigma**2)\n\n if np.isinf(alpha):\n return np.inf\n\n return _compute_log_a(q, sigma, alpha) / (alpha - 1)\n\n\ndef compute_rdp(q, noise_multiplier, steps, orders):\n \"\"\"Compute RDP of the Sampled Gaussian Mechanism.\n\n Args:\n q: The sampling rate.\n noise_multiplier: The ratio of the standard deviation of the Gaussian noise\n to the l2-sensitivity of the function to which it is added.\n steps: The number of steps.\n orders: An array (or a scalar) of RDP orders.\n\n Returns:\n The RDPs at all orders, can be np.inf.\n \"\"\"\n if np.isscalar(orders):\n rdp = _compute_rdp(q, noise_multiplier, orders)\n else:\n rdp = np.array(\n [_compute_rdp(q, noise_multiplier, order) for order in orders])\n\n return rdp * steps\n\n\ndef get_privacy_spent(orders, rdp, target_eps=None, target_delta=None):\n \"\"\"Compute delta (or eps) for given eps (or delta) from RDP values.\n\n Args:\n orders: An array (or a scalar) of RDP orders.\n rdp: An array of RDP values. Must be of the same length as the orders list.\n target_eps: If not None, the epsilon for which we compute the corresponding\n delta.\n target_delta: If not None, the delta for which we compute the corresponding\n epsilon. Exactly one of target_eps and target_delta must be None.\n\n Returns:\n eps, delta, opt_order.\n\n Raises:\n ValueError: If target_eps and target_delta are messed up.\n \"\"\"\n if target_eps is None and target_delta is None:\n raise ValueError(\n \"Exactly one out of eps and delta must be None. (Both are).\")\n\n if target_eps is not None and target_delta is not None:\n raise ValueError(\n \"Exactly one out of eps and delta must be None. (None is).\")\n\n if target_eps is not None:\n delta, opt_order = _compute_delta(orders, rdp, target_eps)\n return target_eps, delta, opt_order\n else:\n eps, opt_order = _compute_eps(orders, rdp, target_delta)\n return eps, target_delta, opt_order\n",
"_____no_output_____"
]
],
[
[
"## dp query\n\n",
"_____no_output_____"
]
],
[
[
"# Copyright 2018, The TensorFlow Authors.\n#\n# Licensed under the Apache License, Version 2.0 (the \"License\");\n# you may not use this file except in compliance with the License.\n# You may obtain a copy of the License at\n#\n# http://www.apache.org/licenses/LICENSE-2.0\n#\n# Unless required by applicable law or agreed to in writing, software\n# distributed under the License is distributed on an \"AS IS\" BASIS,\n# WITHOUT WARRANTIES OR CONDITIONS OF ANY KIND, either express or implied.\n# See the License for the specific language governing permissions and\n# limitations under the License.\n\n\"\"\"An interface for differentially private query mechanisms.\n\"\"\"\n\nfrom __future__ import absolute_import\nfrom __future__ import division\nfrom __future__ import print_function\n\nimport abc\n\n\nclass DPQuery(object):\n \"\"\"Interface for differentially private query mechanisms.\"\"\"\n\n __metaclass__ = abc.ABCMeta\n\n @abc.abstractmethod\n def initial_global_state(self):\n \"\"\"Returns the initial global state for the DPQuery.\"\"\"\n pass\n\n @abc.abstractmethod\n def derive_sample_params(self, global_state):\n \"\"\"Given the global state, derives parameters to use for the next sample.\n\n Args:\n global_state: The current global state.\n\n Returns:\n Parameters to use to process records in the next sample.\n \"\"\"\n pass\n\n @abc.abstractmethod\n def initial_sample_state(self, global_state, tensors):\n \"\"\"Returns an initial state to use for the next sample.\n\n Args:\n global_state: The current global state.\n tensors: A structure of tensors used as a template to create the initial\n sample state.\n\n Returns: An initial sample state.\n \"\"\"\n pass\n\n @abc.abstractmethod\n def accumulate_record(self, params, sample_state, record):\n \"\"\"Accumulates a single record into the sample state.\n\n Args:\n params: The parameters for the sample.\n sample_state: The current sample state.\n record: The record to accumulate.\n\n Returns:\n The updated sample state.\n \"\"\"\n pass\n\n @abc.abstractmethod\n def get_noised_result(self, sample_state, global_state):\n \"\"\"Gets query result after all records of sample have been accumulated.\n\n Args:\n sample_state: The sample state after all records have been accumulated.\n global_state: The global state.\n\n Returns:\n A tuple (result, new_global_state) where \"result\" is the result of the\n query and \"new_global_state\" is the updated global state.\n \"\"\"\n pass\n",
"_____no_output_____"
]
],
[
[
"## gausian query",
"_____no_output_____"
]
],
[
[
"# Copyright 2018, The TensorFlow Authors.\n#\n# Licensed under the Apache License, Version 2.0 (the \"License\");\n# you may not use this file except in compliance with the License.\n# You may obtain a copy of the License at\n#\n# http://www.apache.org/licenses/LICENSE-2.0\n#\n# Unless required by applicable law or agreed to in writing, software\n# distributed under the License is distributed on an \"AS IS\" BASIS,\n# WITHOUT WARRANTIES OR CONDITIONS OF ANY KIND, either express or implied.\n# See the License for the specific language governing permissions and\n# limitations under the License.\n\n\"\"\"Implements DPQuery interface for Gaussian average queries.\n\"\"\"\n\nfrom __future__ import absolute_import\nfrom __future__ import division\nfrom __future__ import print_function\n\nimport collections\n\nimport tensorflow as tf\n\nnest = tf.contrib.framework.nest\n\n\nclass GaussianSumQuery(DPQuery):\n \"\"\"Implements DPQuery interface for Gaussian sum queries.\n\n Accumulates clipped vectors, then adds Gaussian noise to the sum.\n \"\"\"\n\n # pylint: disable=invalid-name\n _GlobalState = collections.namedtuple(\n '_GlobalState', ['l2_norm_clip', 'stddev'])\n\n def __init__(self, l2_norm_clip, stddev):\n \"\"\"Initializes the GaussianSumQuery.\n\n Args:\n l2_norm_clip: The clipping norm to apply to the global norm of each\n record.\n stddev: The stddev of the noise added to the sum.\n \"\"\"\n self._l2_norm_clip = l2_norm_clip\n self._stddev = stddev\n\n def initial_global_state(self):\n \"\"\"Returns the initial global state for the GaussianSumQuery.\"\"\"\n return self._GlobalState(float(self._l2_norm_clip), float(self._stddev))\n\n def derive_sample_params(self, global_state):\n \"\"\"Given the global state, derives parameters to use for the next sample.\n\n Args:\n global_state: The current global state.\n\n Returns:\n Parameters to use to process records in the next sample.\n \"\"\"\n return global_state.l2_norm_clip\n\n def initial_sample_state(self, global_state, tensors):\n \"\"\"Returns an initial state to use for the next sample.\n\n Args:\n global_state: The current global state.\n tensors: A structure of tensors used as a template to create the initial\n sample state.\n\n Returns: An initial sample state.\n \"\"\"\n del global_state # unused.\n return nest.map_structure(tf.zeros_like, tensors)\n\n def accumulate_record(self, params, sample_state, record):\n \"\"\"Accumulates a single record into the sample state.\n\n Args:\n params: The parameters for the sample.\n sample_state: The current sample state.\n record: The record to accumulate.\n\n Returns:\n The updated sample state.\n \"\"\"\n l2_norm_clip = params\n record_as_list = nest.flatten(record)\n clipped_as_list, _ = tf.clip_by_global_norm(record_as_list, l2_norm_clip)\n clipped = nest.pack_sequence_as(record, clipped_as_list)\n\n return nest.map_structure(tf.add, sample_state, clipped)\n\n def get_noised_result(self, sample_state, global_state, add_noise=True):\n \"\"\"Gets noised sum after all records of sample have been accumulated.\n\n Args:\n sample_state: The sample state after all records have been accumulated.\n global_state: The global state.\n\n Returns:\n A tuple (estimate, new_global_state) where \"estimate\" is the estimated\n sum of the records and \"new_global_state\" is the updated global state.\n \"\"\"\n def add_noise(v):\n if add_noise:\n return v + tf.random_normal(tf.shape(v), stddev=global_state.stddev)\n else:\n return v\n\n\n return nest.map_structure(add_noise, sample_state), global_state\n\n\nclass GaussianAverageQuery(DPQuery):\n \"\"\"Implements DPQuery interface for Gaussian average queries.\n\n Accumulates clipped vectors, adds Gaussian noise, and normalizes.\n\n Note that we use \"fixed-denominator\" estimation: the denominator should be\n specified as the expected number of records per sample. Accumulating the\n denominator separately would also be possible but would be produce a higher\n variance estimator.\n \"\"\"\n\n # pylint: disable=invalid-name\n _GlobalState = collections.namedtuple(\n '_GlobalState', ['sum_state', 'denominator'])\n\n def __init__(self, l2_norm_clip, sum_stddev, denominator):\n \"\"\"Initializes the GaussianAverageQuery.\n\n Args:\n l2_norm_clip: The clipping norm to apply to the global norm of each\n record.\n sum_stddev: The stddev of the noise added to the sum (before\n normalization).\n denominator: The normalization constant (applied after noise is added to\n the sum).\n \"\"\"\n self._numerator = GaussianSumQuery(l2_norm_clip, sum_stddev)\n self._denominator = denominator\n\n def initial_global_state(self):\n \"\"\"Returns the initial global state for the GaussianAverageQuery.\"\"\"\n sum_global_state = self._numerator.initial_global_state()\n return self._GlobalState(sum_global_state, float(self._denominator))\n\n def derive_sample_params(self, global_state):\n \"\"\"Given the global state, derives parameters to use for the next sample.\n\n Args:\n global_state: The current global state.\n\n Returns:\n Parameters to use to process records in the next sample.\n \"\"\"\n return self._numerator.derive_sample_params(global_state.sum_state)\n\n def initial_sample_state(self, global_state, tensors):\n \"\"\"Returns an initial state to use for the next sample.\n\n Args:\n global_state: The current global state.\n tensors: A structure of tensors used as a template to create the initial\n sample state.\n\n Returns: An initial sample state.\n \"\"\"\n # GaussianAverageQuery has no state beyond the sum state.\n return self._numerator.initial_sample_state(global_state.sum_state, tensors)\n\n def accumulate_record(self, params, sample_state, record):\n \"\"\"Accumulates a single record into the sample state.\n\n Args:\n params: The parameters for the sample.\n sample_state: The current sample state.\n record: The record to accumulate.\n\n Returns:\n The updated sample state.\n \"\"\"\n \n return self._numerator.accumulate_record(params, sample_state, record)\n\n def get_noised_result(self, sample_state, global_state, add_noise=True):\n \"\"\"Gets noised average after all records of sample have been accumulated.\n\n Args:\n sample_state: The sample state after all records have been accumulated.\n global_state: The global state.\n\n Returns:\n A tuple (estimate, new_global_state) where \"estimate\" is the estimated\n average of the records and \"new_global_state\" is the updated global state.\n \"\"\"\n noised_sum, new_sum_global_state = self._numerator.get_noised_result(\n sample_state, global_state.sum_state, add_noise)\n new_global_state = self._GlobalState(\n new_sum_global_state, global_state.denominator)\n def normalize(v):\n return tf.truediv(v, global_state.denominator)\n\n return nest.map_structure(normalize, noised_sum), new_global_state\n",
"\nWARNING: The TensorFlow contrib module will not be included in TensorFlow 2.0.\nFor more information, please see:\n * https://github.com/tensorflow/community/blob/master/rfcs/20180907-contrib-sunset.md\n * https://github.com/tensorflow/addons\nIf you depend on functionality not listed there, please file an issue.\n\n"
]
],
[
[
"## our_dp_optimizer",
"_____no_output_____"
]
],
[
[
"# Copyright 2018, The TensorFlow Authors.\n#\n# Licensed under the Apache License, Version 2.0 (the \"License\");\n# you may not use this file except in compliance with the License.\n# You may obtain a copy of the License at\n#\n# http://www.apache.org/licenses/LICENSE-2.0\n#\n# Unless required by applicable law or agreed to in writing, software\n# distributed under the License is distributed on an \"AS IS\" BASIS,\n# WITHOUT WARRANTIES OR CONDITIONS OF ANY KIND, either express or implied.\n# See the License for the specific language governing permissions and\n# limitations under the License.\n\"\"\"Differentially private optimizers for TensorFlow.\"\"\"\n\nfrom __future__ import absolute_import\nfrom __future__ import division\nfrom __future__ import print_function\n\nimport tensorflow as tf\n\n\ndef make_optimizer_class(cls):\n \"\"\"Constructs a DP optimizer class from an existing one.\"\"\"\n if (tf.train.Optimizer.compute_gradients.__code__ is\n not cls.compute_gradients.__code__):\n tf.logging.warning(\n 'WARNING: Calling make_optimizer_class() on class %s that overrides '\n 'method compute_gradients(). Check to ensure that '\n 'make_optimizer_class() does not interfere with overridden version.',\n cls.__name__)\n\n class DPOptimizerClass(cls):\n \"\"\"Differentially private subclass of given class cls.\"\"\"\n\n def __init__(\n self,\n l2_norm_clip,\n noise_multiplier,\n dp_average_query,\n num_microbatches,\n unroll_microbatches=False,\n *args, # pylint: disable=keyword-arg-before-vararg\n **kwargs):\n super(DPOptimizerClass, self).__init__(*args, **kwargs)\n self._dp_average_query = dp_average_query\n self._num_microbatches = num_microbatches\n self._global_state = self._dp_average_query.initial_global_state()\n\n # TODO(b/122613513): Set unroll_microbatches=True to avoid this bug.\n # Beware: When num_microbatches is large (>100), enabling this parameter\n # may cause an OOM error.\n self._unroll_microbatches = unroll_microbatches\n\n def dp_compute_gradients(self,\n loss,\n var_list,\n gate_gradients=tf.train.Optimizer.GATE_OP,\n aggregation_method=None,\n colocate_gradients_with_ops=False,\n grad_loss=None,\n add_noise=True):\n\n # Note: it would be closer to the correct i.i.d. sampling of records if\n # we sampled each microbatch from the appropriate binomial distribution,\n # although that still wouldn't be quite correct because it would be\n # sampling from the dataset without replacement.\n microbatches_losses = tf.reshape(loss,\n [self._num_microbatches, -1])\n sample_params = (self._dp_average_query.derive_sample_params(\n self._global_state))\n\n def process_microbatch(i, sample_state):\n \"\"\"Process one microbatch (record) with privacy helper.\"\"\"\n grads, _ = zip(*super(cls, self).compute_gradients(\n tf.gather(microbatches_losses, [i]), var_list,\n gate_gradients, aggregation_method,\n colocate_gradients_with_ops, grad_loss))\n\n # Converts tensor to list to replace None gradients with zero\n grads1 = list(grads)\n\n for inx in range(0, len(grads)):\n if (grads[inx] == None):\n grads1[inx] = tf.zeros_like(var_list[inx])\n\n grads_list = grads1\n sample_state = self._dp_average_query.accumulate_record(\n sample_params, sample_state, grads_list)\n\n return sample_state\n\n if var_list is None:\n var_list = (tf.trainable_variables() + tf.get_collection(\n tf.GraphKeys.TRAINABLE_RESOURCE_VARIABLES))\n sample_state = self._dp_average_query.initial_sample_state(\n self._global_state, var_list)\n\n if self._unroll_microbatches:\n for idx in range(self._num_microbatches):\n sample_state = process_microbatch(idx, sample_state)\n else:\n # Use of while_loop here requires that sample_state be a nested\n # structure of tensors. In general, we would prefer to allow it to be\n # an arbitrary opaque type.\n\n cond_fn = lambda i, _: tf.less(i, self._num_microbatches)\n body_fn = lambda i, state: [\n tf.add(i, 1), process_microbatch(i, state)\n ]\n\n idx = tf.constant(0)\n _, sample_state = tf.while_loop(cond_fn, body_fn,\n [idx, sample_state])\n\n final_grads, self._global_state = (\n self._dp_average_query.get_noised_result(\n sample_state, self._global_state, add_noise))\n\n return (final_grads)\n\n def minimize(self,\n d_loss_real,\n d_loss_fake,\n global_step=None,\n var_list=None,\n gate_gradients=tf.train.Optimizer.GATE_OP,\n aggregation_method=None,\n colocate_gradients_with_ops=False,\n name=None,\n grad_loss=None):\n \"\"\"Minimize using sanitized gradients\n\n Args:\n d_loss_real: the loss tensor for real data\n d_loss_fake: the loss tensor for fake data\n global_step: the optional global step.\n var_list: the optional variables.\n name: the optional name.\n Returns:\n the operation that runs one step of DP gradient descent.\n \"\"\"\n\n # First validate the var_list\n\n if var_list is None:\n var_list = tf.trainable_variables()\n for var in var_list:\n if not isinstance(var, tf.Variable):\n raise TypeError(\"Argument is not a variable.Variable: %s\" %\n var)\n\n # ------------------ OUR METHOD --------------------------------\n\n r_grads = self.dp_compute_gradients(\n d_loss_real,\n var_list=var_list,\n gate_gradients=gate_gradients,\n aggregation_method=aggregation_method,\n colocate_gradients_with_ops=colocate_gradients_with_ops,\n grad_loss=grad_loss, add_noise = True)\n\n f_grads = self.dp_compute_gradients(\n d_loss_fake,\n var_list=var_list,\n gate_gradients=gate_gradients,\n aggregation_method=aggregation_method,\n colocate_gradients_with_ops=colocate_gradients_with_ops,\n grad_loss=grad_loss,\n add_noise=False)\n\n # Compute the overall gradients \n s_grads = [(r_grads[idx] + f_grads[idx])\n for idx in range(len(r_grads))]\n\n sanitized_grads_and_vars = list(zip(s_grads, var_list))\n self._assert_valid_dtypes(\n [v for g, v in sanitized_grads_and_vars if g is not None])\n\n # Apply the overall gradients\n apply_grads = self.apply_gradients(sanitized_grads_and_vars,\n global_step=global_step,\n name=name)\n\n return apply_grads\n\n # -----------------------------------------------------------------\n\n return DPOptimizerClass\n\n\ndef make_gaussian_optimizer_class(cls):\n \"\"\"Constructs a DP optimizer with Gaussian averaging of updates.\"\"\"\n\n class DPGaussianOptimizerClass(make_optimizer_class(cls)):\n \"\"\"DP subclass of given class cls using Gaussian averaging.\"\"\"\n\n def __init__(\n self,\n l2_norm_clip,\n noise_multiplier,\n num_microbatches,\n unroll_microbatches=False,\n *args, # pylint: disable=keyword-arg-before-vararg\n **kwargs):\n dp_average_query = GaussianAverageQuery(\n l2_norm_clip, l2_norm_clip * noise_multiplier,\n num_microbatches)\n self.l2_norm_clip = l2_norm_clip\n self.noise_multiplier = noise_multiplier\n\n super(DPGaussianOptimizerClass,\n self).__init__(l2_norm_clip, noise_multiplier,\n dp_average_query, num_microbatches,\n unroll_microbatches, *args, **kwargs)\n\n return DPGaussianOptimizerClass\n\n\nDPAdagradOptimizer = make_optimizer_class(tf.train.AdagradOptimizer)\nDPAdamOptimizer = make_optimizer_class(tf.train.AdamOptimizer)\nDPGradientDescentOptimizer = make_optimizer_class(\n tf.train.GradientDescentOptimizer)\n\nDPAdagradGaussianOptimizer = make_gaussian_optimizer_class(\n tf.train.AdagradOptimizer)\nDPAdamGaussianOptimizer = make_gaussian_optimizer_class(tf.train.AdamOptimizer)\nDPGradientDescentGaussianOptimizer = make_gaussian_optimizer_class(\n tf.train.GradientDescentOptimizer)\n",
"_____no_output_____"
]
],
[
[
"## gan.ops",
"_____no_output_____"
]
],
[
[
"\"\"\"\nMost codes from https://github.com/carpedm20/DCGAN-tensorflow\n\"\"\"\nimport math\nimport numpy as np\nimport tensorflow as tf\n\n\nif \"concat_v2\" in dir(tf):\n\n def concat(tensors, axis, *args, **kwargs):\n return tf.concat_v2(tensors, axis, *args, **kwargs)\nelse:\n\n def concat(tensors, axis, *args, **kwargs):\n return tf.concat(tensors, axis, *args, **kwargs)\n\n\ndef bn(x, is_training, scope):\n return tf.contrib.layers.batch_norm(x,\n decay=0.9,\n updates_collections=None,\n epsilon=1e-5,\n scale=True,\n is_training=is_training,\n scope=scope)\n\n\ndef conv_out_size_same(size, stride):\n return int(math.ceil(float(size) / float(stride)))\n\n\ndef conv_cond_concat(x, y):\n \"\"\"Concatenate conditioning vector on feature map axis.\"\"\"\n x_shapes = x.get_shape()\n y_shapes = y.get_shape()\n return concat(\n [x, y * tf.ones([x_shapes[0], x_shapes[1], x_shapes[2], y_shapes[3]])],\n 3)\n\n\ndef conv2d(input_,\n output_dim,\n k_h=5,\n k_w=5,\n d_h=2,\n d_w=2,\n stddev=0.02,\n name=\"conv2d\"):\n with tf.variable_scope(name):\n w = tf.get_variable(\n 'w', [k_h, k_w, input_.get_shape()[-1], output_dim],\n initializer=tf.truncated_normal_initializer(stddev=stddev))\n conv = tf.nn.conv2d(input_,\n w,\n strides=[1, d_h, d_w, 1],\n padding='SAME')\n\n biases = tf.get_variable('biases', [output_dim],\n initializer=tf.constant_initializer(0.0))\n conv = tf.reshape(tf.nn.bias_add(conv, biases), conv.get_shape())\n\n return conv\n\n\ndef deconv2d(input_,\n output_shape,\n k_h=5,\n k_w=5,\n d_h=2,\n d_w=2,\n name=\"deconv2d\",\n stddev=0.02,\n with_w=False):\n with tf.variable_scope(name):\n # filter : [height, width, output_channels, in_channels]\n w = tf.get_variable(\n 'w', [k_h, k_w, output_shape[-1],\n input_.get_shape()[-1]],\n initializer=tf.random_normal_initializer(stddev=stddev))\n\n try:\n deconv = tf.nn.conv2d_transpose(input_,\n w,\n output_shape=output_shape,\n strides=[1, d_h, d_w, 1])\n\n # Support for verisons of TensorFlow before 0.7.0\n except AttributeError:\n deconv = tf.nn.deconv2d(input_,\n w,\n output_shape=output_shape,\n strides=[1, d_h, d_w, 1])\n\n biases = tf.get_variable('biases', [output_shape[-1]],\n initializer=tf.constant_initializer(0.0))\n deconv = tf.reshape(tf.nn.bias_add(deconv, biases), deconv.get_shape())\n\n if with_w:\n return deconv, w, biases\n else:\n return deconv\n\n\ndef lrelu(x, leak=0.2, name=\"lrelu\"):\n return tf.maximum(x, leak * x)\n\n\ndef linear(input_,\n output_size,\n scope=None,\n stddev=0.02,\n bias_start=0.0,\n with_w=False):\n shape = input_.get_shape().as_list()\n\n with tf.variable_scope(scope or \"Linear\"):\n matrix = tf.get_variable(\"Matrix\", [shape[1], output_size], tf.float32,\n tf.random_normal_initializer(stddev=stddev))\n bias = tf.get_variable(\"bias\", [output_size],\n initializer=tf.constant_initializer(bias_start))\n if with_w:\n return tf.matmul(input_, matrix) + bias, matrix, bias\n else:\n return tf.matmul(input_, matrix) + bias\n",
"_____no_output_____"
]
],
[
[
"## OUR DP CGAN",
"_____no_output_____"
]
],
[
[
"# -*- coding: utf-8 -*-\nfrom __future__ import division\nfrom keras.datasets import cifar10\n\nfrom mlxtend.data import loadlocal_mnist\nfrom sklearn.preprocessing import label_binarize\n\nfrom sklearn.multiclass import OneVsRestClassifier\nfrom sklearn.metrics import roc_curve, auc\nfrom sklearn.linear_model import LogisticRegression\nfrom sklearn.ensemble import RandomForestClassifier\nfrom sklearn.neural_network import MLPClassifier\n\n\nclass OUR_DP_CGAN(object):\n model_name = \"OUR_DP_CGAN\" # name for checkpoint\n\n def __init__(self, sess, epoch, batch_size, z_dim, epsilon, delta, sigma,\n clip_value, lr, dataset_name, base_dir, checkpoint_dir,\n result_dir, log_dir):\n self.sess = sess\n self.dataset_name = dataset_name\n self.base_dir = base_dir\n self.checkpoint_dir = checkpoint_dir\n self.result_dir = result_dir\n self.log_dir = log_dir\n self.epoch = epoch\n self.batch_size = batch_size\n self.epsilon = epsilon\n self.delta = delta\n self.noise_multiplier = sigma\n self.l2_norm_clip = clip_value\n self.lr = lr\n\n if dataset_name == 'mnist' or dataset_name == 'fashion-mnist':\n # parameters\n self.input_height = 28\n self.input_width = 28\n self.output_height = 28\n self.output_width = 28\n\n self.z_dim = z_dim # dimension of noise-vector\n self.y_dim = 10 # dimension of condition-vector (label)\n self.c_dim = 1\n\n # train\n self.learningRateD = self.lr\n self.learningRateG = self.learningRateD * 5\n self.beta1 = 0.5\n self.beta2 = 0.99\n # test\n self.sample_num = 64 # number of generated images to be saved\n\n # load mnist\n self.data_X, self.data_y = load_mnist(train = True)\n\n # get number of batches for a single epoch\n self.num_batches = len(self.data_X) // self.batch_size\n\n elif dataset_name == 'cifar10':\n # parameters\n self.input_height = 32\n self.input_width = 32\n self.output_height = 32\n self.output_width = 32\n\n self.z_dim = 100 # dimension of noise-vector\n self.y_dim = 10 # dimension of condition-vector (label)\n self.c_dim = 3 # color dimension\n\n # train\n # self.learning_rate = 0.0002 # 1e-3, 1e-4\n self.learningRateD = 1e-3\n self.learningRateG = 1e-4\n self.beta1 = 0.5\n self.beta2 = 0.99\n\n # test\n self.sample_num = 64 # number of generated images to be saved\n\n # load cifar10\n self.data_X, self.data_y = load_cifar10(train=True)\n\n self.num_batches = len(self.data_X) // self.batch_size\n\n else:\n raise NotImplementedError\n\n def discriminator(self, x, y, is_training=True, reuse=False):\n # Network Architecture is exactly same as in infoGAN (https://arxiv.org/abs/1606.03657)\n # Architecture : (64)4c2s-(128)4c2s_BL-FC1024_BL-FC1_S\n with tf.variable_scope(\"discriminator\", reuse=reuse):\n\n # merge image and label\n if (self.dataset_name == \"mnist\"):\n y = tf.reshape(y, [self.batch_size, 1, 1, self.y_dim])\n x = conv_cond_concat(x, y)\n\n net = lrelu(conv2d(x, 64, 4, 4, 2, 2, name='d_conv1'))\n net = lrelu(\n bn(conv2d(net, 128, 4, 4, 2, 2, name='d_conv2'),\n is_training=is_training,\n scope='d_bn2'))\n net = tf.reshape(net, [self.batch_size, -1])\n net = lrelu(\n bn(linear(net, 1024, scope='d_fc3'),\n is_training=is_training,\n scope='d_bn3'))\n out_logit = linear(net, 1, scope='d_fc4')\n out = tf.nn.sigmoid(out_logit)\n\n elif (self.dataset_name == \"cifar10\"):\n\n y = tf.reshape(y, [self.batch_size, 1, 1, self.y_dim])\n x = conv_cond_concat(x, y)\n lrelu_slope = 0.2\n kernel_size = 5\n w_init = tf.contrib.layers.xavier_initializer()\n\n net = lrelu(\n conv2d(x,\n 64,\n 5,\n 5,\n 2,\n 2,\n name='d_conv1' + '_' + self.dataset_name))\n \n net = lrelu(\n bn(conv2d(net,\n 128,\n 5,\n 5,\n 2,\n 2,\n name='d_conv2' + '_' + self.dataset_name),\n is_training=is_training,\n scope='d_bn2'))\n \n net = lrelu(\n bn(conv2d(net,\n 256,\n 5,\n 5,\n 2,\n 2,\n name='d_conv3' + '_' + self.dataset_name),\n is_training=is_training,\n scope='d_bn3'))\n \n net = lrelu(\n bn(conv2d(net,\n 512,\n 5,\n 5,\n 2,\n 2,\n name='d_conv4' + '_' + self.dataset_name),\n is_training=is_training,\n scope='d_bn4'))\n \n net = tf.reshape(net, [self.batch_size, -1])\n \n out_logit = linear(net,\n 1,\n scope='d_fc5' + '_' + self.dataset_name)\n \n out = tf.nn.sigmoid(out_logit)\n\n return out, out_logit\n\n def generator(self, z, y, is_training=True, reuse=False):\n # Network Architecture is exactly same as in infoGAN (https://arxiv.org/abs/1606.03657)\n # Architecture : FC1024_BR-FC7x7x128_BR-(64)4dc2s_BR-(1)4dc2s_S\n with tf.variable_scope(\"generator\", reuse=reuse):\n\n if (self.dataset_name == \"mnist\"):\n # merge noise and label\n z = concat([z, y], 1)\n\n net = tf.nn.relu(\n bn(linear(z, 1024, scope='g_fc1'),\n is_training=is_training,\n scope='g_bn1'))\n net = tf.nn.relu(\n bn(linear(net, 128 * 7 * 7, scope='g_fc2'),\n is_training=is_training,\n scope='g_bn2'))\n net = tf.reshape(net, [self.batch_size, 7, 7, 128])\n net = tf.nn.relu(\n bn(deconv2d(net, [self.batch_size, 14, 14, 64],\n 4,\n 4,\n 2,\n 2,\n name='g_dc3'),\n is_training=is_training,\n scope='g_bn3'))\n\n out = tf.nn.sigmoid(\n deconv2d(net, [self.batch_size, 28, 28, 1],\n 4,\n 4,\n 2,\n 2,\n name='g_dc4'))\n\n elif (self.dataset_name == \"cifar10\"):\n h_size = 32\n h_size_2 = 16\n h_size_4 = 8\n h_size_8 = 4\n h_size_16 = 2\n\n z = concat([z, y], 1)\n\n net = linear(z,\n 512 * h_size_16 * h_size_16,\n scope='g_fc1' + '_' + self.dataset_name)\n \n net = tf.nn.relu(\n bn(tf.reshape(\n net, [self.batch_size, h_size_16, h_size_16, 512]),\n is_training=is_training,\n scope='g_bn1'))\n \n net = tf.nn.relu(\n bn(deconv2d(net,\n [self.batch_size, h_size_8, h_size_8, 256],\n 5,\n 5,\n 2,\n 2,\n name='g_dc2' + '_' + self.dataset_name),\n is_training=is_training,\n scope='g_bn2'))\n \n net = tf.nn.relu(\n bn(deconv2d(net,\n [self.batch_size, h_size_4, h_size_4, 128],\n 5,\n 5,\n 2,\n 2,\n name='g_dc3' + '_' + self.dataset_name),\n is_training=is_training,\n scope='g_bn3'))\n \n net = tf.nn.relu(\n bn(deconv2d(net, [self.batch_size, h_size_2, h_size_2, 64],\n 5,\n 5,\n 2,\n 2,\n name='g_dc4' + '_' + self.dataset_name),\n is_training=is_training,\n scope='g_bn4'))\n \n out = tf.nn.tanh(\n deconv2d(net, [\n self.batch_size, self.output_height, self.output_width,\n self.c_dim\n ],\n 5,\n 5,\n 2,\n 2,\n name='g_dc5' + '_' + self.dataset_name))\n\n return out\n\n def build_model(self):\n # some parameters\n image_dims = [self.input_height, self.input_width, self.c_dim]\n bs = self.batch_size\n \n \"\"\" Graph Input \"\"\"\n # images\n self.inputs = tf.placeholder(tf.float32, [bs] + image_dims,\n name='real_images')\n\n # labels\n self.y = tf.placeholder(tf.float32, [bs, self.y_dim], name='y')\n\n # noises\n self.z = tf.placeholder(tf.float32, [bs, self.z_dim], name='z')\n \"\"\" Loss Function \"\"\"\n\n # output of D for real images\n D_real, D_real_logits = self.discriminator(self.inputs,\n self.y,\n is_training=True,\n reuse=False)\n\n # output of D for fake images\n G = self.generator(self.z, self.y, is_training=True, reuse=False)\n D_fake, D_fake_logits = self.discriminator(G,\n self.y,\n is_training=True,\n reuse=True)\n\n # get loss for discriminator\n d_loss_real = tf.reduce_mean(\n tf.nn.sigmoid_cross_entropy_with_logits(\n logits=D_real_logits, labels=tf.ones_like(D_real)))\n d_loss_fake = tf.reduce_mean(\n tf.nn.sigmoid_cross_entropy_with_logits(\n logits=D_fake_logits, labels=tf.zeros_like(D_fake)))\n\n self.d_loss_real_vec = tf.nn.sigmoid_cross_entropy_with_logits(\n logits=D_real_logits, labels=tf.ones_like(D_real))\n self.d_loss_fake_vec = tf.nn.sigmoid_cross_entropy_with_logits(\n logits=D_fake_logits, labels=tf.zeros_like(D_fake))\n\n self.d_loss = d_loss_real + d_loss_fake\n\n # get loss for generator\n self.g_loss = tf.reduce_mean(\n tf.nn.sigmoid_cross_entropy_with_logits(\n logits=D_fake_logits, labels=tf.ones_like(D_fake)))\n \n \"\"\" Training \"\"\"\n # divide trainable variables into a group for D and a group for G\n t_vars = tf.trainable_variables()\n d_vars = [\n var for var in t_vars if var.name.startswith('discriminator')\n ]\n g_vars = [var for var in t_vars if var.name.startswith('generator')]\n\n # optimizers\n with tf.control_dependencies(tf.get_collection(\n tf.GraphKeys.UPDATE_OPS)):\n\n d_optim_init = DPGradientDescentGaussianOptimizer(\n l2_norm_clip=self.l2_norm_clip,\n noise_multiplier=self.noise_multiplier,\n num_microbatches=self.batch_size,\n learning_rate=self.learningRateD)\n\n global_step = tf.train.get_global_step()\n\n self.d_optim = d_optim_init.minimize(\n d_loss_real=self.d_loss_real_vec,\n d_loss_fake=self.d_loss_fake_vec,\n global_step=global_step,\n var_list=d_vars)\n\n optimizer = DPGradientDescentGaussianOptimizer(\n l2_norm_clip=self.l2_norm_clip,\n noise_multiplier=self.noise_multiplier,\n num_microbatches=self.batch_size,\n learning_rate=self.learningRateD)\n\n self.g_optim = tf.train.GradientDescentOptimizer(self.learningRateG) \\\n .minimize(self.g_loss, var_list=g_vars)\n \n \"\"\"\" Testing \"\"\"\n self.fake_images = self.generator(self.z,\n self.y,\n is_training=False,\n reuse=True)\n \"\"\" Summary \"\"\"\n d_loss_real_sum = tf.summary.scalar(\"d_loss_real\", d_loss_real)\n d_loss_fake_sum = tf.summary.scalar(\"d_loss_fake\", d_loss_fake)\n d_loss_sum = tf.summary.scalar(\"d_loss\", self.d_loss)\n g_loss_sum = tf.summary.scalar(\"g_loss\", self.g_loss)\n\n # final summary operations\n self.g_sum = tf.summary.merge([d_loss_fake_sum, g_loss_sum])\n self.d_sum = tf.summary.merge([d_loss_real_sum, d_loss_sum])\n\n def train(self):\n\n # initialize all variables\n tf.global_variables_initializer().run()\n\n # graph inputs for visualize training results\n self.sample_z = np.random.uniform(-1,\n 1,\n size=(self.batch_size, self.z_dim))\n self.test_labels = self.data_y[0:self.batch_size]\n\n # saver to save model\n self.saver = tf.train.Saver()\n\n # summary writer\n self.writer = tf.summary.FileWriter(\n self.log_dir + '/' + self.model_name, self.sess.graph)\n\n # restore check-point if it exits\n could_load, checkpoint_counter = self.load(self.checkpoint_dir)\n if could_load:\n start_epoch = (int)(checkpoint_counter / self.num_batches)\n start_batch_id = checkpoint_counter - start_epoch * self.num_batches\n counter = checkpoint_counter\n print(\" [*] Load SUCCESS\")\n else:\n start_epoch = 0\n start_batch_id = 0\n counter = 1\n print(\" [!] Load failed...\")\n\n # loop for epoch\n epoch = start_epoch\n should_terminate = False\n \n while (epoch < self.epoch and not should_terminate):\n\n # get batch data\n for idx in range(start_batch_id, self.num_batches):\n batch_images = self.data_X[idx * self.batch_size:(idx + 1) *\n self.batch_size]\n batch_labels = self.data_y[idx * self.batch_size:(idx + 1) *\n self.batch_size]\n batch_z = np.random.uniform(\n -1, 1, [self.batch_size, self.z_dim]).astype(np.float32)\n\n # update D network\n\n _, summary_str, d_loss = self.sess.run(\n [self.d_optim, self.d_sum, self.d_loss],\n feed_dict={\n self.inputs: batch_images,\n self.y: batch_labels,\n self.z: batch_z\n })\n self.writer.add_summary(summary_str, counter)\n\n eps = self.compute_epsilon((epoch * self.num_batches) + idx)\n\n if (eps > self.epsilon):\n\n should_terminate = True\n print(\"TERMINATE !! Run out of Privacy Budget.....\")\n epoch = self.epoch\n break\n\n # update G network\n _, summary_str, g_loss = self.sess.run(\n [self.g_optim, self.g_sum, self.g_loss],\n feed_dict={\n self.inputs: batch_images,\n self.y: batch_labels,\n self.z: batch_z\n })\n\n self.writer.add_summary(summary_str, counter)\n\n # display training status\n counter += 1\n _ = self.sess.run(self.fake_images,\n feed_dict={\n self.z: self.sample_z,\n self.y: self.test_labels\n })\n\n # save training results for every 100 steps\n if np.mod(counter, 100) == 0:\n print(\"Iteration : \" + str(idx) + \" Eps: \" + str(eps))\n samples = self.sess.run(self.fake_images,\n feed_dict={\n self.z: self.sample_z,\n self.y: self.test_labels\n })\n tot_num_samples = min(self.sample_num, self.batch_size)\n manifold_h = int(np.floor(np.sqrt(tot_num_samples)))\n manifold_w = int(np.floor(np.sqrt(tot_num_samples)))\n save_images(\n samples[:manifold_h * manifold_w, :, :, :],\n [manifold_h, manifold_w],\n check_folder(self.result_dir + '/' + self.model_dir) +\n '/' + self.model_name +\n '_train_{:02d}_{:04d}.png'.format(epoch, idx))\n epoch = epoch + 1\n\n # After an epoch, start_batch_id is set to zero\n # non-zero value is only for the first epoch after loading pre-trained model\n start_batch_id = 0\n\n # save model\n self.save(self.checkpoint_dir, counter)\n\n # show temporal results\n if (self.dataset_name == 'mnist'):\n self.visualize_results_MNIST(epoch)\n elif (self.dataset_name == 'cifar10'):\n self.visualize_results_CIFAR(epoch)\n\n # save model for final step\n self.save(self.checkpoint_dir, counter)\n\n\n def compute_fpr_tpr_roc(Y_test, Y_score):\n n_classes = Y_score.shape[1]\n false_positive_rate = dict()\n true_positive_rate = dict()\n roc_auc = dict()\n for class_cntr in range(n_classes):\n false_positive_rate[class_cntr], true_positive_rate[\n class_cntr], _ = roc_curve(Y_test[:, class_cntr],\n Y_score[:, class_cntr])\n roc_auc[class_cntr] = auc(false_positive_rate[class_cntr],\n true_positive_rate[class_cntr])\n\n # Compute micro-average ROC curve and ROC area\n false_positive_rate[\"micro\"], true_positive_rate[\n \"micro\"], _ = roc_curve(Y_test.ravel(), Y_score.ravel())\n roc_auc[\"micro\"] = auc(false_positive_rate[\"micro\"],\n true_positive_rate[\"micro\"])\n\n return false_positive_rate, true_positive_rate, roc_auc\n\n def classify(X_train,\n Y_train,\n X_test,\n classiferName,\n random_state_value=0):\n if classiferName == \"lr\":\n classifier = OneVsRestClassifier(\n LogisticRegression(solver='lbfgs',\n multi_class='multinomial',\n random_state=random_state_value))\n elif classiferName == \"mlp\":\n classifier = OneVsRestClassifier(\n MLPClassifier(random_state=random_state_value, alpha=1))\n\n elif classiferName == \"rf\":\n classifier = OneVsRestClassifier(\n RandomForestClassifier(n_estimators=100,\n random_state=random_state_value))\n\n else:\n print(\"Classifier not in the list!\")\n exit()\n Y_score = classifier.fit(X_train, Y_train).predict_proba(X_test)\n return Y_score\n\n batch_size = int(self.batch_size)\n\n if (self.dataset_name == \"mnist\"):\n\n n_class = np.zeros(10)\n n_class[0] = 5923 - batch_size\n n_class[1] = 6742\n n_class[2] = 5958\n n_class[3] = 6131\n n_class[4] = 5842\n n_class[5] = 5421\n n_class[6] = 5918\n n_class[7] = 6265\n n_class[8] = 5851\n n_class[9] = 5949\n\n Z_sample = np.random.uniform(-1, 1, size=(batch_size, self.z_dim))\n y = np.zeros(batch_size, dtype=np.int64) + 0\n y_one_hot = np.zeros((batch_size, self.y_dim))\n y_one_hot[np.arange(batch_size), y] = 1\n images = self.sess.run(self.fake_images,\n feed_dict={\n self.z: Z_sample,\n self.y: y_one_hot\n })\n\n for classLabel in range(0, 10):\n for _ in range(0, int(n_class[classLabel]), batch_size):\n Z_sample = np.random.uniform(-1,\n 1,\n size=(batch_size, self.z_dim))\n y = np.zeros(batch_size, dtype=np.int64) + classLabel\n y_one_hot_init = np.zeros((batch_size, self.y_dim))\n y_one_hot_init[np.arange(batch_size), y] = 1\n\n images = np.append(images,\n self.sess.run(self.fake_images,\n feed_dict={\n self.z: Z_sample,\n self.y: y_one_hot_init\n }),\n axis=0)\n y_one_hot = np.append(y_one_hot, y_one_hot_init, axis=0)\n\n X_test, Y_test = load_mnist(train = False)\n\n Y_test = [int(y) for y in Y_test]\n\n classes = [0, 1, 2, 3, 4, 5, 6, 7, 8, 9]\n Y_test = label_binarize(Y_test, classes=classes)\n\n if (self.dataset_name == \"cifar10\"):\n n_class = np.zeros(10)\n for t in range(1, 10):\n n_class[t] = 1000\n \n Z_sample = np.random.uniform(-1, 1, size=(batch_size, self.z_dim))\n y = np.zeros(batch_size, dtype=np.int64) + 0\n y_one_hot = np.zeros((batch_size, self.y_dim))\n y_one_hot[np.arange(batch_size), y] = 1\n images = self.sess.run(self.fake_images,\n feed_dict={\n self.z: Z_sample,\n self.y: y_one_hot\n })\n\n for classLabel in range(0, 10):\n for _ in range(0, int(n_class[classLabel]), batch_size):\n Z_sample = np.random.uniform(-1,\n 1,\n size=(batch_size, self.z_dim))\n y = np.zeros(batch_size, dtype=np.int64) + classLabel\n y_one_hot_init = np.zeros((batch_size, self.y_dim))\n y_one_hot_init[np.arange(batch_size), y] = 1\n\n images = np.append(images,\n self.sess.run(self.fake_images,\n feed_dict={\n self.z: Z_sample,\n self.y: y_one_hot_init\n }),\n axis=0)\n y_one_hot = np.append(y_one_hot, y_one_hot_init, axis=0)\n\n X_test, Y_test = load_cifar10(train=False)\n\n classes = range(0, 10)\n Y_test = label_binarize(Y_test, classes=classes)\n\n print(\" Classifying - Logistic Regression...\")\n\n TwoDim_images = images.reshape(np.shape(images)[0], -2)\n \n X_test = X_test.reshape(np.shape(X_test)[0], -2)\n Y_score = classify(TwoDim_images,\n y_one_hot,\n X_test,\n \"lr\",\n random_state_value=30)\n\n false_positive_rate, true_positive_rate, roc_auc = compute_fpr_tpr_roc(\n Y_test, Y_score)\n\n classification_results_fname = self.base_dir + \"CGAN_AuROC.txt\"\n classification_results = open(classification_results_fname, \"w\")\n\n classification_results.write(\n \"\\nepsilon : {:.2f}, sigma: {:.2f}, clipping value: {:.2f}\".format(\n (self.epsilon), round(self.noise_multiplier, 2),\n round(self.l2_norm_clip, 2)))\n\n classification_results.write(\"\\nAuROC - logistic Regression: \" +\n str(roc_auc[\"micro\"]))\n classification_results.write(\n \"\\n--------------------------------------------------------------------\\n\"\n )\n \n print(\" Classifying - Random Forest...\")\n Y_score = classify(TwoDim_images,\n y_one_hot,\n X_test,\n \"rf\",\n random_state_value=30)\n\n print(\" Computing ROC - Random Forest ...\")\n false_positive_rate, true_positive_rate, roc_auc = compute_fpr_tpr_roc(\n Y_test, Y_score)\n\n classification_results.write(\n \"\\nepsilon : {:.2f}, sigma: {:.2f}, clipping value: {:.2f}\".format(\n (self.epsilon), round(self.noise_multiplier, 2),\n round(self.l2_norm_clip, 2)))\n\n classification_results.write(\"\\nAuROC - random Forest: \" +\n str(roc_auc[\"micro\"]))\n classification_results.write(\n \"\\n--------------------------------------------------------------------\\n\"\n )\n \n print(\" Classifying - multilayer Perceptron ...\")\n Y_score = classify(TwoDim_images,\n y_one_hot,\n X_test,\n \"mlp\",\n random_state_value=30)\n\n print(\" Computing ROC - Multilayer Perceptron ...\")\n false_positive_rate, true_positive_rate, roc_auc = compute_fpr_tpr_roc(\n Y_test, Y_score)\n\n classification_results.write(\n \"\\nepsilon : {:.2f}, sigma: {:.2f}, clipping value: {:.2f}\".format(\n (self.epsilon), round(self.noise_multiplier, 2),\n round(self.l2_norm_clip, 2)))\n\n classification_results.write(\"\\nAuROC - multilayer Perceptron: \" +\n str(roc_auc[\"micro\"]))\n classification_results.write(\n \"\\n--------------------------------------------------------------------\\n\"\n )\n\n # save model for final step\n self.save(self.checkpoint_dir, counter)\n\n def compute_epsilon(self, steps):\n \"\"\"Computes epsilon value for given hyperparameters.\"\"\"\n if self.noise_multiplier == 0.0:\n return float('inf')\n orders = [1 + x / 10. for x in range(1, 100)] + list(range(12, 64))\n sampling_probability = self.batch_size / 60000\n rdp = compute_rdp(q=sampling_probability,\n noise_multiplier=self.noise_multiplier,\n steps=steps,\n orders=orders)\n # Delta is set to 1e-5 because MNIST has 60000 training points.\n return get_privacy_spent(orders, rdp, target_delta=1e-5)[0]\n\n # CIFAR 10\n def visualize_results_CIFAR(self, epoch):\n tot_num_samples = min(self.sample_num, self.batch_size) # 64, 100\n image_frame_dim = int(np.floor(np.sqrt(tot_num_samples))) # 8\n \"\"\" random condition, random noise \"\"\"\n y = np.random.choice(self.y_dim, self.batch_size)\n y_one_hot = np.zeros((self.batch_size, self.y_dim))\n y_one_hot[np.arange(self.batch_size), y] = 1\n\n z_sample = np.random.uniform(-1, 1, size=(self.batch_size,\n self.z_dim)) # 100, 100\n\n samples = self.sess.run(self.fake_images,\n feed_dict={\n self.z: z_sample,\n self.y: y_one_hot\n })\n\n save_matplot_img(\n samples[:image_frame_dim * image_frame_dim, :, :, :],\n [image_frame_dim, image_frame_dim], self.result_dir + '/' +\n self.model_name + '_epoch%03d' % epoch + '_test_all_classes.png')\n\n # MNIST\n def visualize_results_MNIST(self, epoch):\n tot_num_samples = min(self.sample_num, self.batch_size)\n image_frame_dim = int(np.floor(np.sqrt(tot_num_samples)))\n \"\"\" random condition, random noise \"\"\"\n y = np.random.choice(self.y_dim, self.batch_size)\n y_one_hot = np.zeros((self.batch_size, self.y_dim))\n y_one_hot[np.arange(self.batch_size), y] = 1\n\n z_sample = np.random.uniform(-1, 1, size=(self.batch_size, self.z_dim))\n samples = self.sess.run(self.fake_images,\n feed_dict={\n self.z: z_sample,\n self.y: y_one_hot\n })\n\n save_images(\n samples[:image_frame_dim * image_frame_dim, :, :, :],\n [image_frame_dim, image_frame_dim],\n check_folder(self.result_dir + '/' + self.model_dir) + '/' +\n self.model_name + '_epoch%03d' % epoch + '_test_all_classes.png')\n \"\"\" specified condition, random noise \"\"\"\n n_styles = 10 # must be less than or equal to self.batch_size\n\n np.random.seed()\n si = np.random.choice(self.batch_size, n_styles)\n\n for l in range(self.y_dim):\n y = np.zeros(self.batch_size, dtype=np.int64) + l\n y_one_hot = np.zeros((self.batch_size, self.y_dim))\n y_one_hot[np.arange(self.batch_size), y] = 1\n\n samples = self.sess.run(self.fake_images,\n feed_dict={\n self.z: z_sample,\n self.y: y_one_hot\n })\n save_images(\n samples[:image_frame_dim * image_frame_dim, :, :, :],\n [image_frame_dim, image_frame_dim],\n check_folder(self.result_dir + '/' + self.model_dir) + '/' +\n self.model_name + '_epoch%03d' % epoch +\n '_test_class_%d.png' % l)\n\n samples = samples[si, :, :, :]\n\n if l == 0:\n all_samples = samples\n else:\n all_samples = np.concatenate((all_samples, samples), axis=0)\n \"\"\" save merged images to check style-consistency \"\"\"\n canvas = np.zeros_like(all_samples)\n for s in range(n_styles):\n for c in range(self.y_dim):\n canvas[s * self.y_dim +\n c, :, :, :] = all_samples[c * n_styles + s, :, :, :]\n\n save_images(\n canvas, [n_styles, self.y_dim],\n check_folder(self.result_dir + '/' + self.model_dir) + '/' +\n self.model_name + '_epoch%03d' % epoch +\n '_test_all_classes_style_by_style.png')\n\n @property\n def model_dir(self):\n return \"{}_{}_{}_{}\".format(self.model_name, self.dataset_name,\n self.batch_size, self.z_dim)\n\n def save(self, checkpoint_dir, step):\n checkpoint_dir = os.path.join(checkpoint_dir, self.model_dir,\n self.model_name)\n\n if not os.path.exists(checkpoint_dir):\n os.makedirs(checkpoint_dir)\n\n self.saver.save(self.sess,\n os.path.join(checkpoint_dir,\n self.model_name + '.model'),\n global_step=step)\n\n def load(self, checkpoint_dir):\n import re\n print(\" [*] Reading checkpoints...\")\n checkpoint_dir = os.path.join(checkpoint_dir, self.model_dir,\n self.model_name)\n\n ckpt = tf.train.get_checkpoint_state(checkpoint_dir)\n if ckpt and ckpt.model_checkpoint_path:\n ckpt_name = os.path.basename(ckpt.model_checkpoint_path)\n self.saver.restore(self.sess,\n os.path.join(checkpoint_dir, ckpt_name))\n counter = int(\n next(re.finditer(\"(\\d+)(?!.*\\d)\", ckpt_name)).group(0))\n print(\" [*] Success to read {}\".format(ckpt_name))\n return True, counter\n else:\n print(\" [*] Failed to find a checkpoint\")\n return False, 0\n",
"Using TensorFlow backend.\n"
]
],
[
[
"## gan.utils",
"_____no_output_____"
]
],
[
[
"\"\"\"\n Most codes from https://github.com/carpedm20/DCGAN-tensorflow\n\"\"\"\nfrom __future__ import division\n\nimport scipy.misc\nimport numpy as np\n\nfrom six.moves import xrange\nimport matplotlib.pyplot as plt\nimport os, gzip\n\nimport tensorflow as tf\nimport tensorflow.contrib.slim as slim\n\nfrom keras.datasets import cifar10\nfrom keras.datasets import mnist\n\n\ndef one_hot(x, n):\n \"\"\"\n convert index representation to one-hot representation\n \"\"\"\n x = np.array(x)\n assert x.ndim == 1\n return np.eye(n)[x]\n\n\ndef prepare_input(data=None, labels=None):\n image_height = 32\n image_width = 32\n image_depth = 3\n assert (data.shape[1] == image_height * image_width * image_depth)\n assert (data.shape[0] == labels.shape[0])\n # do mean normalization across all samples\n mu = np.mean(data, axis=0)\n mu = mu.reshape(1, -1)\n sigma = np.std(data, axis=0)\n sigma = sigma.reshape(1, -1)\n data = data - mu\n data = data / sigma\n is_nan = np.isnan(data)\n is_inf = np.isinf(data)\n if np.any(is_nan) or np.any(is_inf):\n print('data is not well-formed : is_nan {n}, is_inf: {i}'.format(\n n=np.any(is_nan), i=np.any(is_inf)))\n # data is transformed from (no_of_samples, 3072) to (no_of_samples , image_height, image_width, image_depth)\n # make sure the type of the data is no.float32\n data = data.reshape([-1, image_depth, image_height, image_width])\n data = data.transpose([0, 2, 3, 1])\n data = data.astype(np.float32)\n return data, labels\n\n\ndef read_cifar10(filename): # queue one element\n class CIFAR10Record(object):\n pass\n\n result = CIFAR10Record()\n\n label_bytes = 1 # 2 for CIFAR-100\n result.height = 32\n result.width = 32\n result.depth = 3\n\n data = np.load(filename, encoding='latin1')\n\n value = np.asarray(data['data']).astype(np.float32)\n labels = np.asarray(data['labels']).astype(np.int32)\n\n return prepare_input(value, labels)\n\n\ndef load_cifar10(train):\n (x_train, y_train), (x_test, y_test) = cifar10.load_data()\n\n if (train == True):\n\n dataX = x_train.reshape([-1, 32, 32, 3])\n dataY = y_train\n\n else:\n dataX = x_test.reshape([-1, 32, 32, 3])\n dataY = y_test\n\n seed = 547\n np.random.seed(seed)\n np.random.shuffle(dataX)\n np.random.seed(seed)\n np.random.shuffle(dataY)\n\n y_vec = np.zeros((len(dataY), 10), dtype=np.float)\n for i, label in enumerate(dataY):\n y_vec[i, dataY[i]] = 1.0\n\n return dataX / 255., y_vec\n\n\ndef load_mnist(train = True):\n\n def extract_data(filename, num_data, head_size, data_size):\n with gzip.open(filename) as bytestream:\n bytestream.read(head_size)\n buf = bytestream.read(data_size * num_data)\n data = np.frombuffer(buf, dtype=np.uint8).astype(np.float)\n return data\n\n (x_train, y_train), (x_test, y_test) = mnist.load_data()\n \n x_train = x_train.reshape((60000, 28, 28, 1))\n y_train = y_train.reshape((60000))\n\n x_test = x_test.reshape((10000, 28, 28, 1))\n y_test = y_test.reshape((10000))\n\n y_train = np.asarray(y_train)\n y_test = np.asarray(y_test)\n\n if (train == True):\n seed = 547\n np.random.seed(seed)\n np.random.shuffle(x_train)\n np.random.seed(seed)\n np.random.shuffle(y_train)\n\n y_vec = np.zeros((len(y_train), 10), dtype=np.float)\n for i, label in enumerate(y_train):\n y_vec[i, y_train[i]] = 1.0\n return x_train / 255., y_vec\n \n else:\n seed = 547\n np.random.seed(seed)\n np.random.shuffle(x_test)\n np.random.seed(seed)\n np.random.shuffle(y_test)\n\n y_vec = np.zeros((len(y_test), 10), dtype=np.float)\n for i, label in enumerate(y_test):\n y_vec[i, y_test[i]] = 1.0\n return x_test / 255., y_vec\n \n\n\ndef check_folder(log_dir):\n if not os.path.exists(log_dir):\n os.makedirs(log_dir)\n return log_dir\n\n\ndef show_all_variables():\n model_vars = tf.trainable_variables()\n slim.model_analyzer.analyze_vars(model_vars, print_info=True)\n\n\ndef get_image(image_path,\n input_height,\n input_width,\n resize_height=64,\n resize_width=64,\n crop=True,\n grayscale=False):\n image = imread(image_path, grayscale)\n return transform(image, input_height, input_width, resize_height,\n resize_width, crop)\n\n\ndef save_images(images, size, image_path):\n return imsave(inverse_transform(images), size, image_path)\n\n\ndef imread(path, grayscale=False):\n if (grayscale):\n return scipy.misc.imread(path, flatten=True).astype(np.float)\n else:\n return scipy.misc.imread(path).astype(np.float)\n\n\ndef merge_images(images, size):\n return inverse_transform(images)\n\n\ndef merge(images, size):\n h, w = images.shape[1], images.shape[2]\n if (images.shape[3] in (3, 4)):\n c = images.shape[3]\n img = np.zeros((h * size[0], w * size[1], c))\n for idx, image in enumerate(images):\n i = idx % size[1]\n j = idx // size[1]\n img[j * h:j * h + h, i * w:i * w + w, :] = image\n return img\n elif images.shape[3] == 1:\n img = np.zeros((h * size[0], w * size[1]))\n for idx, image in enumerate(images):\n i = idx % size[1]\n j = idx // size[1]\n img[j * h:j * h + h, i * w:i * w + w] = image[:, :, 0]\n return img\n else:\n raise ValueError('in merge(images,size) images parameter '\n 'must have dimensions: HxW or HxWx3 or HxWx4')\n\n\ndef imsave(images, size, path):\n image = np.squeeze(merge(images, size))\n return scipy.misc.imsave(path, image)\n\n\ndef center_crop(x, crop_h, crop_w, resize_h=64, resize_w=64):\n if crop_w is None:\n crop_w = crop_h\n h, w = x.shape[:2]\n j = int(round((h - crop_h) / 2.))\n i = int(round((w - crop_w) / 2.))\n return scipy.misc.imresize(x[j:j + crop_h, i:i + crop_w],\n [resize_h, resize_w])\n\n\ndef transform(image,\n input_height,\n input_width,\n resize_height=64,\n resize_width=64,\n crop=True):\n if crop:\n cropped_image = center_crop(image, input_height, input_width,\n resize_height, resize_width)\n else:\n cropped_image = scipy.misc.imresize(image,\n [resize_height, resize_width])\n return np.array(cropped_image) / 127.5 - 1.\n\n\ndef inverse_transform(images):\n return (images + 1.) / 2.\n\n\n\"\"\" Drawing Tools \"\"\"\n\n\n# borrowed from https://github.com/ykwon0407/variational_autoencoder/blob/master/variational_bayes.ipynb\ndef save_scattered_image(z,\n id,\n z_range_x,\n z_range_y,\n name='scattered_image.jpg'):\n N = 10\n plt.figure(figsize=(8, 6))\n plt.scatter(z[:, 0],\n z[:, 1],\n c=np.argmax(id, 1),\n marker='o',\n edgecolor='none',\n cmap=discrete_cmap(N, 'jet'))\n plt.colorbar(ticks=range(N))\n axes = plt.gca()\n axes.set_xlim([-z_range_x, z_range_x])\n axes.set_ylim([-z_range_y, z_range_y])\n plt.grid(True)\n plt.savefig(name)\n\n\n# borrowed from https://gist.github.com/jakevdp/91077b0cae40f8f8244a\ndef discrete_cmap(N, base_cmap=None):\n \"\"\"Create an N-bin discrete colormap from the specified input map\"\"\"\n\n # Note that if base_cmap is a string or None, you can simply do\n # return plt.cm.get_cmap(base_cmap, N)\n # The following works for string, None, or a colormap instance:\n\n base = plt.cm.get_cmap(base_cmap)\n color_list = base(np.linspace(0, 1, N))\n cmap_name = base.name + str(N)\n return base.from_list(cmap_name, color_list, N)\n\n\ndef save_matplot_img(images, size, image_path):\n # revice image data // M*N*3 // RGB float32 : value must set between 0. with 1.\n for idx in range(64):\n vMin = np.amin(images[idx])\n vMax = np.amax(images[idx])\n img_arr = images[idx].reshape(32 * 32 * 3, 1) # flatten\n for i, v in enumerate(img_arr):\n img_arr[i] = (v - vMin) / (vMax - vMin)\n img_arr = img_arr.reshape(32, 32, 3) # M*N*3\n\n plt.subplot(8, 8, idx + 1), plt.imshow(img_arr,\n interpolation='nearest')\n plt.axis(\"off\")\n\n\n plt.savefig(image_path)\n",
"_____no_output_____"
]
],
[
[
"## Main",
"_____no_output_____"
]
],
[
[
"import tensorflow as tf\nimport os\n\nbase_dir = \"./\"\n\nout_dir = base_dir + \"mnist_clip1_sigma0.6_lr0.55\"\n\nif not os.path.exists(out_dir):\n os.mkdir(out_dir)\n\ngpu_options = tf.GPUOptions(visible_device_list=\"0\")\nwith tf.Session(config=tf.ConfigProto(allow_soft_placement=True,\n gpu_options=gpu_options)) as sess:\n\n epoch = 100\n cgan = OUR_DP_CGAN(sess,\n epoch=epoch,\n batch_size=64,\n z_dim=100,\n epsilon=9.6,\n delta=1e-5,\n sigma=0.6,\n clip_value=1,\n lr=0.055,\n dataset_name='mnist',\n checkpoint_dir=out_dir + \"/checkpoint/\",\n result_dir=out_dir + \"/results/\",\n log_dir=out_dir + \"/logs/\",\n base_dir=base_dir)\n\n cgan.build_model()\n print(\" [*] Building model finished!\")\n\n show_all_variables()\n cgan.train()\n print(\" [*] Training finished!\")\n",
"Downloading data from https://s3.amazonaws.com/img-datasets/mnist.npz\n11493376/11490434 [==============================] - 1s 0us/step\nWARNING:tensorflow:From /usr/local/lib/python3.6/dist-packages/tensorflow/python/framework/op_def_library.py:263: colocate_with (from tensorflow.python.framework.ops) is deprecated and will be removed in a future version.\nInstructions for updating:\nColocations handled automatically by placer.\nWARNING:tensorflow:From /usr/local/lib/python3.6/dist-packages/tensorflow/python/ops/array_grad.py:425: to_int32 (from tensorflow.python.ops.math_ops) is deprecated and will be removed in a future version.\nInstructions for updating:\nUse tf.cast instead.\n [*] Building model finished!\n---------\nVariables: name (type shape) [size]\n---------\ndiscriminator/d_conv1/w:0 (float32_ref 4x4x11x64) [11264, bytes: 45056]\ndiscriminator/d_conv1/biases:0 (float32_ref 64) [64, bytes: 256]\ndiscriminator/d_conv2/w:0 (float32_ref 4x4x64x128) [131072, bytes: 524288]\ndiscriminator/d_conv2/biases:0 (float32_ref 128) [128, bytes: 512]\ndiscriminator/d_bn2/beta:0 (float32_ref 128) [128, bytes: 512]\ndiscriminator/d_bn2/gamma:0 (float32_ref 128) [128, bytes: 512]\ndiscriminator/d_fc3/Matrix:0 (float32_ref 6272x1024) [6422528, bytes: 25690112]\ndiscriminator/d_fc3/bias:0 (float32_ref 1024) [1024, bytes: 4096]\ndiscriminator/d_bn3/beta:0 (float32_ref 1024) [1024, bytes: 4096]\ndiscriminator/d_bn3/gamma:0 (float32_ref 1024) [1024, bytes: 4096]\ndiscriminator/d_fc4/Matrix:0 (float32_ref 1024x1) [1024, bytes: 4096]\ndiscriminator/d_fc4/bias:0 (float32_ref 1) [1, bytes: 4]\ngenerator/g_fc1/Matrix:0 (float32_ref 110x1024) [112640, bytes: 450560]\ngenerator/g_fc1/bias:0 (float32_ref 1024) [1024, bytes: 4096]\ngenerator/g_bn1/beta:0 (float32_ref 1024) [1024, bytes: 4096]\ngenerator/g_bn1/gamma:0 (float32_ref 1024) [1024, bytes: 4096]\ngenerator/g_fc2/Matrix:0 (float32_ref 1024x6272) [6422528, bytes: 25690112]\ngenerator/g_fc2/bias:0 (float32_ref 6272) [6272, bytes: 25088]\ngenerator/g_bn2/beta:0 (float32_ref 6272) [6272, bytes: 25088]\ngenerator/g_bn2/gamma:0 (float32_ref 6272) [6272, bytes: 25088]\ngenerator/g_dc3/w:0 (float32_ref 4x4x64x128) [131072, bytes: 524288]\ngenerator/g_dc3/biases:0 (float32_ref 64) [64, bytes: 256]\ngenerator/g_bn3/beta:0 (float32_ref 64) [64, bytes: 256]\ngenerator/g_bn3/gamma:0 (float32_ref 64) [64, bytes: 256]\ngenerator/g_dc4/w:0 (float32_ref 4x4x1x64) [1024, bytes: 4096]\ngenerator/g_dc4/biases:0 (float32_ref 1) [1, bytes: 4]\nTotal size of variables: 13258754\nTotal bytes of variables: 53035016\n [*] Reading checkpoints...\n [*] Failed to find a checkpoint\n [!] Load failed...\nIteration : 98 Eps: 5.750369579644922\n"
]
]
] | [
"markdown",
"code",
"markdown",
"code",
"markdown",
"code",
"markdown",
"code",
"markdown",
"code",
"markdown",
"code",
"markdown",
"code",
"markdown",
"code"
] | [
[
"markdown",
"markdown"
],
[
"code"
],
[
"markdown"
],
[
"code"
],
[
"markdown"
],
[
"code"
],
[
"markdown"
],
[
"code"
],
[
"markdown"
],
[
"code"
],
[
"markdown"
],
[
"code"
],
[
"markdown"
],
[
"code"
],
[
"markdown"
],
[
"code"
]
] |
d077ef8e01d4aa2d365962c446c9b468344ae797 | 2,606 | ipynb | Jupyter Notebook | examples/gallery/demos/bokeh/quiver_demo.ipynb | ppwadhwa/holoviews | e8e2ec08c669295479f98bb2f46bbd59782786bf | [
"BSD-3-Clause"
] | 864 | 2019-11-13T08:18:27.000Z | 2022-03-31T13:36:13.000Z | examples/gallery/demos/bokeh/quiver_demo.ipynb | ppwadhwa/holoviews | e8e2ec08c669295479f98bb2f46bbd59782786bf | [
"BSD-3-Clause"
] | 1,117 | 2019-11-12T16:15:59.000Z | 2022-03-30T22:57:59.000Z | examples/gallery/demos/bokeh/quiver_demo.ipynb | ppwadhwa/holoviews | e8e2ec08c669295479f98bb2f46bbd59782786bf | [
"BSD-3-Clause"
] | 180 | 2019-11-19T16:44:44.000Z | 2022-03-28T22:49:18.000Z | 23.267857 | 98 | 0.514198 | [
[
[
"URL: http://matplotlib.org/examples/pylab_examples/quiver_demo.html\n\nMost examples work across multiple plotting backends, this example is also available for:\n\n* [Matplotlib - quiver_demo](../matplotlib/quiver_demo.ipynb)",
"_____no_output_____"
]
],
[
[
"import numpy as np\nimport holoviews as hv\nfrom holoviews import opts\nhv.extension('bokeh')",
"_____no_output_____"
]
],
[
[
"## Define data",
"_____no_output_____"
]
],
[
[
"xs, ys = np.arange(0, 2 * np.pi, .2), np.arange(0, 2 * np.pi, .2)\nX, Y = np.meshgrid(xs, ys)\nU = np.cos(X)\nV = np.sin(Y)\n\n# Convert to magnitude and angle\nmag = np.sqrt(U**2 + V**2)\nangle = (np.pi/2.) - np.arctan2(U/mag, V/mag)",
"_____no_output_____"
]
],
[
[
"## Plot",
"_____no_output_____"
]
],
[
[
"label = 'Arrows scale with plot width, not view'\n\nopts.defaults(opts.VectorField(height=400, width=500))\n\nvectorfield = hv.VectorField((xs, ys, angle, mag))\nvectorfield.relabel(label)",
"_____no_output_____"
],
[
"label = \"pivot='mid'; every third arrow\"\n\nvf_mid = hv.VectorField((xs[::3], ys[::3], angle[::3, ::3], mag[::3, ::3], ))\npoints = hv.Points((X[::3, ::3].flat, Y[::3, ::3].flat))\n\nopts.defaults(opts.Points(color='red'))\n\n(vf_mid * points).relabel(label)",
"_____no_output_____"
],
[
"label = \"pivot='tip'; scales with x view\"\n\nvectorfield = hv.VectorField((xs, ys, angle, mag))\npoints = hv.Points((X.flat, Y.flat))\n\n(points * vectorfield).opts(\n opts.VectorField(magnitude='Magnitude', color='Magnitude',\n pivot='tip', line_width=2, title=label))",
"_____no_output_____"
]
]
] | [
"markdown",
"code",
"markdown",
"code",
"markdown",
"code"
] | [
[
"markdown"
],
[
"code"
],
[
"markdown"
],
[
"code"
],
[
"markdown"
],
[
"code",
"code",
"code"
]
] |
d077fde2f97718240fc5e587e53dda533b30241a | 415,352 | ipynb | Jupyter Notebook | codes/script5.ipynb | umairbinwaheed/PINN_eikonal | 9a2879d2b4f76a443fd260e72aeb90573d606d67 | [
"MIT"
] | 27 | 2021-03-12T18:47:48.000Z | 2022-03-16T13:34:46.000Z | codes/script5.ipynb | umairbinwaheed/PINN_eikonal | 9a2879d2b4f76a443fd260e72aeb90573d606d67 | [
"MIT"
] | 1 | 2022-03-02T18:37:35.000Z | 2022-03-02T18:37:35.000Z | codes/script5.ipynb | umairbinwaheed/PINN_eikonal | 9a2879d2b4f76a443fd260e72aeb90573d606d67 | [
"MIT"
] | 9 | 2020-09-23T08:50:28.000Z | 2022-03-29T07:46:52.000Z | 415,352 | 415,352 | 0.955341 | [
[
[
"### **PINN eikonal solver for a portion of the Marmousi model**",
"_____no_output_____"
]
],
[
[
"from google.colab import drive\ndrive.mount('/content/gdrive')",
"_____no_output_____"
],
[
"cd \"/content/gdrive/My Drive/Colab Notebooks/Codes/PINN_isotropic_eikonal_R1\"",
"_____no_output_____"
],
[
"!pip install sciann==0.5.4.0\n!pip install tensorflow==2.2.0\n#!pip install keras==2.3.1 ",
"_____no_output_____"
],
[
"import numpy as np\nimport pandas as pd\nimport matplotlib.pyplot as plt\nfrom mpl_toolkits.axes_grid1 import make_axes_locatable\nimport tensorflow as tf\nfrom sciann import Functional, Variable, SciModel, PDE\nfrom sciann.utils import *\nimport scipy.io \nimport time\nimport random\nfrom mpl_toolkits.axes_grid1.inset_locator import zoomed_inset_axes\nfrom mpl_toolkits.axes_grid1.inset_locator import mark_inset\n\ntf.config.threading.set_intra_op_parallelism_threads(1)\ntf.config.threading.set_inter_op_parallelism_threads(1)",
"---------------------- SCIANN 0.5.4.0 ---------------------- \nFor details, check out our review paper and the documentation at: \n + \"https://arxiv.org/abs/2005.08803\", \n + \"https://www.sciann.com\". \n\n"
],
[
"np.random.seed(123)\ntf.random.set_seed(123)",
"_____no_output_____"
],
[
"# Loading velocity model\n\nfilename=\"./inputs/marm/model/marm_vz.txt\"\nmarm = pd.read_csv(filename, index_col=None, header=None)\nvelmodel = np.reshape(np.array(marm), (101, 101)).T\n\n# Loading reference solution\n\nfilename=\"./inputs/marm/traveltimes/fmm_or2_marm_s(1,1).txt\"\nT_data = pd.read_csv(filename, index_col=None, header=None)\nT_data = np.reshape(np.array(T_data), (101, 101)).T",
"_____no_output_____"
],
[
"#Model specifications\n\nzmin = 0.; zmax = 2.; deltaz = 0.02;\nxmin = 0.; xmax = 2.; deltax = 0.02;\n\n\n# Point-source location\nsz = 1.0; sx = 1.0;\n\n# Number of training points\nnum_tr_pts = 3000",
"_____no_output_____"
],
[
"# Creating grid, calculating refrence traveltimes, and prepare list of grid points for training (X_star)\n\nz = np.arange(zmin,zmax+deltaz,deltaz)\nnz = z.size\n\nx = np.arange(xmin,xmax+deltax,deltax)\nnx = x.size\n\n\nZ,X = np.meshgrid(z,x,indexing='ij')\n\nX_star = [Z.reshape(-1,1), X.reshape(-1,1)]\n\nselected_pts = np.random.choice(np.arange(Z.size),num_tr_pts,replace=False)\nZf = Z.reshape(-1,1)[selected_pts]\nZf = np.append(Zf,sz)\nXf = X.reshape(-1,1)[selected_pts]\nXf = np.append(Xf,sx)\n\n\nX_starf = [Zf.reshape(-1,1), Xf.reshape(-1,1)]",
"_____no_output_____"
],
[
"# Plot the velocity model with the source location\n\nplt.style.use('default')\n\nplt.figure(figsize=(4,4))\n\nax = plt.gca()\nim = ax.imshow(velmodel, extent=[xmin,xmax,zmax,zmin], aspect=1, cmap=\"jet\")\n\nax.plot(sx,sz,'k*',markersize=8)\n\nplt.xlabel('Offset (km)', fontsize=14)\nplt.xticks(fontsize=10)\n\nplt.ylabel('Depth (km)', fontsize=14)\nplt.yticks(fontsize=10)\n\nax.xaxis.set_major_locator(plt.MultipleLocator(0.5))\nax.yaxis.set_major_locator(plt.MultipleLocator(0.5))\n\ndivider = make_axes_locatable(ax)\ncax = divider.append_axes(\"right\", size=\"6%\", pad=0.15)\n\ncbar = plt.colorbar(im, cax=cax)\n\ncbar.set_label('km/s',size=10)\ncbar.ax.tick_params(labelsize=10)\n\nplt.savefig(\"./figs/marm/velmodel.pdf\", format='pdf', bbox_inches=\"tight\")",
"_____no_output_____"
],
[
"# Analytical solution for the known traveltime part\nvel = velmodel[int(round(sz/deltaz)),int(round(sx/deltax))] # Velocity at the source location\n\nT0 = np.sqrt((Z-sz)**2 + (X-sx)**2)/vel; \n\npx0 = np.divide(X-sx, T0*vel**2, out=np.zeros_like(T0), where=T0!=0)\npz0 = np.divide(Z-sz, T0*vel**2, out=np.zeros_like(T0), where=T0!=0)",
"_____no_output_____"
],
[
"# Find source location id in X_star\n\nTOLX = 1e-6\nTOLZ = 1e-6\n\nsids,_ = np.where(np.logical_and(np.abs(X_starf[0]-sz)<TOLZ , np.abs(X_starf[1]-sx)<TOLX))\n\nprint(sids)\nprint(sids.shape)\nprint(X_starf[0][sids,0])\nprint(X_starf[1][sids,0])",
"[3000]\n(1,)\n[1.]\n[1.]\n"
],
[
"# Preparing the Sciann model object\n\nK.clear_session() \n\nlayers = [20]*10\n\n# Appending source values\nvelmodelf = velmodel.reshape(-1,1)[selected_pts]; velmodelf = np.append(velmodelf,vel)\npx0f = px0.reshape(-1,1)[selected_pts]; px0f = np.append(px0f,0.)\npz0f = pz0.reshape(-1,1)[selected_pts]; pz0f = np.append(pz0f,0.)\nT0f = T0.reshape(-1,1)[selected_pts]; T0f = np.append(T0f,0.)\n\nxt = Variable(\"xt\",dtype='float64')\nzt = Variable(\"zt\",dtype='float64')\nvt = Variable(\"vt\",dtype='float64')\npx0t = Variable(\"px0t\",dtype='float64')\npz0t = Variable(\"pz0t\",dtype='float64')\nT0t = Variable(\"T0t\",dtype='float64')\n\ntau = Functional(\"tau\", [zt, xt], layers, 'l-atan')\n\n# Loss function based on the factored isotropic eikonal equation\nL = (T0t*diff(tau, xt) + tau*px0t)**2 + (T0t*diff(tau, zt) + tau*pz0t)**2 - 1.0/vt**2\n\ntargets = [tau, 20*L, (1-sign(tau*T0t))*abs(tau*T0t)]\ntarget_vals = [(sids, np.ones(sids.shape).reshape(-1,1)), 'zeros', 'zeros']\n\nmodel = SciModel(\n [zt, xt, vt, pz0t, px0t, T0t], \n targets,\n load_weights_from='models/vofz_model-end.hdf5',\n optimizer='scipy-l-BFGS-B'\n)",
"_____no_output_____"
],
[
"#Model training\n\nstart_time = time.time()\nhist = model.train(\n X_starf + [velmodelf,pz0f,px0f,T0f],\n target_vals,\n batch_size = X_starf[0].size,\n epochs = 12000,\n learning_rate = 0.008,\n verbose=0\n )\nelapsed = time.time() - start_time\nprint('Training time: %.2f seconds' %(elapsed))\n",
"\nTotal samples: 3001 \nBatch size: 3001 \nTotal batches: 1 \n\nTraining time: 831.12 seconds\n"
],
[
"# Convergence history plot for verification\n\nfig = plt.figure(figsize=(5,3))\nax = plt.axes()\n#ax.semilogy(np.arange(0,300,0.001),hist.history['loss'],LineWidth=2)\nax.semilogy(hist.history['loss'],LineWidth=2)\n\nax.set_xlabel('Epochs (x $10^3$)',fontsize=16)\n\nplt.xticks(fontsize=12)\n#ax.xaxis.set_major_locator(plt.MultipleLocator(50))\n\nax.set_ylabel('Loss',fontsize=16)\nplt.yticks(fontsize=12);\nplt.grid()",
"_____no_output_____"
],
[
"# Predicting traveltime solution from the trained model\n\nL_pred = L.eval(model, X_star + [velmodel,pz0,px0,T0])\ntau_pred = tau.eval(model, X_star + [velmodel,pz0,px0,T0])\ntau_pred = tau_pred.reshape(Z.shape)\n\nT_pred = tau_pred*T0\n\nprint('Time at source: %.4f'%(tau_pred[int(round(sz/deltaz)),int(round(sx/deltax))]))",
"Time at source: 0.9995\n"
],
[
"# Plot the PINN solution error\n\nplt.style.use('default')\n\nplt.figure(figsize=(4,4))\n\nax = plt.gca()\nim = ax.imshow(np.abs(T_pred-T_data), extent=[xmin,xmax,zmax,zmin], aspect=1, cmap=\"jet\")\n\n\nplt.xlabel('Offset (km)', fontsize=14)\nplt.xticks(fontsize=10)\n\nplt.ylabel('Depth (km)', fontsize=14)\nplt.yticks(fontsize=10)\n\nax.xaxis.set_major_locator(plt.MultipleLocator(0.5))\nax.yaxis.set_major_locator(plt.MultipleLocator(0.5))\n\ndivider = make_axes_locatable(ax)\ncax = divider.append_axes(\"right\", size=\"6%\", pad=0.15)\n\ncbar = plt.colorbar(im, cax=cax)\n\ncbar.set_label('seconds',size=10)\ncbar.ax.tick_params(labelsize=10)\n\nplt.savefig(\"./figs/marm/pinnerror.pdf\", format='pdf', bbox_inches=\"tight\")",
"_____no_output_____"
],
[
"# Load fast sweeping traveltims for comparison\n\nT_fsm = np.load('./inputs/marm/traveltimes/Tcomp.npy')",
"_____no_output_____"
],
[
"# Plot the first order FMM solution error\n\nplt.style.use('default')\n\nplt.figure(figsize=(4,4))\n\nax = plt.gca()\nim = ax.imshow(np.abs(T_fsm-T_data), extent=[xmin,xmax,zmax,zmin], aspect=1, cmap=\"jet\")\n\n\nplt.xlabel('Offset (km)', fontsize=14)\nplt.xticks(fontsize=10)\n\nplt.ylabel('Depth (km)', fontsize=14)\nplt.yticks(fontsize=10)\n\nax.xaxis.set_major_locator(plt.MultipleLocator(0.5))\nax.yaxis.set_major_locator(plt.MultipleLocator(0.5))\n\ndivider = make_axes_locatable(ax)\ncax = divider.append_axes(\"right\", size=\"6%\", pad=0.15)\n\ncbar = plt.colorbar(im, cax=cax)\n\ncbar.set_label('seconds',size=10)\n\ncbar.ax.tick_params(labelsize=10)\n\nplt.savefig(\"./figs/marm/fmm1error.pdf\", format='pdf', bbox_inches=\"tight\")",
"_____no_output_____"
],
[
"# Traveltime contour plots\n\nfig = plt.figure(figsize=(5,5))\n\nax = plt.gca()\nim1 = ax.contour(T_data, 6, extent=[xmin,xmax,zmin,zmax], colors='r')\nim2 = ax.contour(T_pred, 6, extent=[xmin,xmax,zmin,zmax], colors='k',linestyles = 'dashed')\nim3 = ax.contour(T_fsm, 6, extent=[xmin,xmax,zmin,zmax], colors='b',linestyles = 'dotted')\n\nax.plot(sx,sz,'k*',markersize=8)\n\nplt.xlabel('Offset (km)', fontsize=14)\nplt.ylabel('Depth (km)', fontsize=14)\nax.tick_params(axis='both', which='major', labelsize=8)\nplt.gca().invert_yaxis()\nh1,_ = im1.legend_elements()\nh2,_ = im2.legend_elements()\nh3,_ = im3.legend_elements()\nax.legend([h1[0], h2[0], h3[0]], ['Reference', 'PINN', 'Fast sweeping'],fontsize=12)\n\nax.xaxis.set_major_locator(plt.MultipleLocator(0.5))\nax.yaxis.set_major_locator(plt.MultipleLocator(0.5))\n\nplt.xticks(fontsize=10)\nplt.yticks(fontsize=10)\n\n#ax.arrow(1.9, 1.7, -0.1, -0.1, head_width=0.05, head_length=0.075, fc='red', ec='red',width=0.02)\n\nplt.savefig(\"./figs/marm/contours.pdf\", format='pdf', bbox_inches=\"tight\")",
"_____no_output_____"
],
[
"print(np.linalg.norm(T_pred-T_data)/np.linalg.norm(T_data))\nprint(np.linalg.norm(T_pred-T_data))",
"0.005852164264049101\n0.19346281698941364\n"
]
]
] | [
"markdown",
"code"
] | [
[
"markdown"
],
[
"code",
"code",
"code",
"code",
"code",
"code",
"code",
"code",
"code",
"code",
"code",
"code",
"code",
"code",
"code",
"code",
"code",
"code",
"code",
"code"
]
] |
d0780288c6346c27abc4f4d49a5fc16116813cbc | 73,466 | ipynb | Jupyter Notebook | Task 1- Prediction using Supervised ML/Linear Regression.ipynb | smritig19/GRIP_Internship_Tasks | 7d5c7d7cd51163b8474fea725a9b684e3e557fa0 | [
"MIT"
] | null | null | null | Task 1- Prediction using Supervised ML/Linear Regression.ipynb | smritig19/GRIP_Internship_Tasks | 7d5c7d7cd51163b8474fea725a9b684e3e557fa0 | [
"MIT"
] | null | null | null | Task 1- Prediction using Supervised ML/Linear Regression.ipynb | smritig19/GRIP_Internship_Tasks | 7d5c7d7cd51163b8474fea725a9b684e3e557fa0 | [
"MIT"
] | null | null | null | 86.126612 | 19,276 | 0.811069 | [
[
[
"# GRIP June'21 - The Sparks Foundation\n\n## Data Science and Business Analytics \n\n## Author: Smriti Gupta\n\n### Task 1: **Prediction using Supervised ML** \n\n* Predict the percentage of an student based on the no. of study hours. \n* What will be predicted score if a student studies for 9.25 hrs/ day? \n* _LANGUAGE:_ Python\n* _DATASET:_ http://bit.ly/w-data\n",
"_____no_output_____"
]
],
[
[
"# Importing Libraries\n\nimport pandas as pd\nimport numpy as np\nimport matplotlib.pyplot as plt\nimport seaborn as sns\n%matplotlib inline\n%config Completer.use_jedi = False",
"_____no_output_____"
],
[
"# Reading data from remote link\n\nurl = \"https://raw.githubusercontent.com/AdiPersonalWorks/Random/master/student_scores%20-%20student_scores.csv\"\ndf = pd.read_csv(url)\n\n# Viewing the Data\n\ndf.head(10)",
"_____no_output_____"
],
[
"# Shape of the Dataset\n\ndf.shape",
"_____no_output_____"
],
[
"# Checking the information of Data\n\ndf.info()",
"<class 'pandas.core.frame.DataFrame'>\nRangeIndex: 25 entries, 0 to 24\nData columns (total 2 columns):\n # Column Non-Null Count Dtype \n--- ------ -------------- ----- \n 0 Hours 25 non-null float64\n 1 Scores 25 non-null int64 \ndtypes: float64(1), int64(1)\nmemory usage: 528.0 bytes\n"
],
[
"# Checking the statistical details of Data\n\ndf.describe()",
"_____no_output_____"
],
[
"# Checking the correlation between Hours and Scores\n\ncorr = df.corr()\ncorr",
"_____no_output_____"
],
[
"colors = ['#670067','#008080']",
"_____no_output_____"
]
],
[
[
"# Data Visualization",
"_____no_output_____"
]
],
[
[
"# 2-D graph to establish relationship between the Data and checking for linearity \n\nsns.set_style('darkgrid')\ndf.plot(x='Hours', y='Scores', style='o') \nplt.title('Hours vs Percentage') \nplt.xlabel('Hours Studied') \nplt.ylabel('Percentage Score') \nplt.show()",
"_____no_output_____"
]
],
[
[
"# Data Preprocessing",
"_____no_output_____"
]
],
[
[
"X = df.iloc[:, :-1].values \ny = df.iloc[:, 1].values ",
"_____no_output_____"
]
],
[
[
"# LINEAR REGRESSION MODEL",
"_____no_output_____"
],
[
"## Splitting Dataset into training and test sets:",
"_____no_output_____"
]
],
[
[
"from sklearn.model_selection import train_test_split\nX_train, X_test, y_train, y_test = train_test_split(X, y, test_size=0.2)",
"_____no_output_____"
]
],
[
[
"## Training the Model",
"_____no_output_____"
]
],
[
[
"from sklearn.linear_model import LinearRegression\nregressor = LinearRegression() \nregressor.fit(X_train, y_train) \n\nprint('TRAINING COMPLETED.')",
"TRAINING COMPLETED.\n"
]
],
[
[
"## Predicting the Score",
"_____no_output_____"
]
],
[
[
"y_predict = regressor.predict(X_test)\nprediction = pd.DataFrame({'Hours': [i[0] for i in X_test], 'Predicted Scores': [k for k in y_predict]})\nprediction",
"_____no_output_____"
],
[
"print(regressor.intercept_)",
"2.813097028256877\n"
],
[
"print(regressor.coef_)",
"[9.8272078]\n"
],
[
"# Plotting the regression line\n\nline = regressor.coef_*X+regressor.intercept_\n\n# Plotting for the test data\n\nplt.scatter(X, y, color = colors[1])\nplt.plot(X, line, color = colors[0]);\nplt.title('Hours vs Percentage') \nplt.xlabel('Hours Studied') \nplt.ylabel('Percentage Score')\nplt.show()",
"_____no_output_____"
]
],
[
[
"## Checking the Accuracy Scores for training and test set",
"_____no_output_____"
]
],
[
[
"print('Test Score')\nprint(regressor.score(X_test, y_test))\nprint('Training Score')\nprint(regressor.score(X_train, y_train))",
"Test Score\n0.9480612939203932\nTraining Score\n0.953103139564599\n"
]
],
[
[
"## Comparing Actual Scores and Predicted Scores",
"_____no_output_____"
]
],
[
[
"data= pd.DataFrame({'Actual': y_test,'Predicted': y_predict})\ndata",
"_____no_output_____"
],
[
"# Visualization comparing Actual Scores and Predicted Scores\n\nplt.scatter(X_test, y_test, color = colors[1]) \nplt.plot(X_test, y_predict, color = colors[0]) \nplt.title(\"Hours Studied Vs Percentage (Test Dataset)\") \nplt.xlabel(\"Hour\") \nplt.ylabel(\"Percentage\") \nplt.show()",
"_____no_output_____"
]
],
[
[
"## Model Evaluation Metrics",
"_____no_output_____"
]
],
[
[
"#Checking the efficiency of model\n\nfrom sklearn import metrics\nprint('Mean Absolute Error:', metrics.mean_absolute_error(y_test, y_predict))\nprint('Mean Squared Error:', metrics.mean_squared_error(y_test, y_predict))\nprint('Root Mean Squared Error:', np.sqrt(metrics.mean_squared_error(y_test, y_predict)))",
"Mean Absolute Error: 4.451593449522768\nMean Squared Error: 25.188194900366135\nRoot Mean Squared Error: 5.018784205399365\n"
]
],
[
[
"# What will be predicted score if a student studies for 9.25 hrs/ day?",
"_____no_output_____"
]
],
[
[
"hours = 9.25\nans = regressor.predict([[hours]])\nprint(\"No of Hours = {}\".format(hours))\nprint(\"Predicted Score = {}\".format(ans[0]))",
"No of Hours = 9.25\nPredicted Score = 93.71476919815156\n"
]
]
] | [
"markdown",
"code",
"markdown",
"code",
"markdown",
"code",
"markdown",
"code",
"markdown",
"code",
"markdown",
"code",
"markdown",
"code",
"markdown",
"code",
"markdown",
"code",
"markdown",
"code"
] | [
[
"markdown"
],
[
"code",
"code",
"code",
"code",
"code",
"code",
"code"
],
[
"markdown"
],
[
"code"
],
[
"markdown"
],
[
"code"
],
[
"markdown",
"markdown"
],
[
"code"
],
[
"markdown"
],
[
"code"
],
[
"markdown"
],
[
"code",
"code",
"code",
"code"
],
[
"markdown"
],
[
"code"
],
[
"markdown"
],
[
"code",
"code"
],
[
"markdown"
],
[
"code"
],
[
"markdown"
],
[
"code"
]
] |
d07814f72f59cfc45cbfb22ef0d360e4d0de41e8 | 284,560 | ipynb | Jupyter Notebook | kaggle/detecting-non-groundtruth-datasets.ipynb | fossabot/denmune-clustering-algorithm | aa815150bd3dc0dfddca70d7f75c207f92b5d3b0 | [
"BSD-3-Clause"
] | 9 | 2021-12-22T21:21:20.000Z | 2022-02-09T18:01:53.000Z | kaggle/detecting-non-groundtruth-datasets.ipynb | fossabot/denmune-clustering-algorithm | aa815150bd3dc0dfddca70d7f75c207f92b5d3b0 | [
"BSD-3-Clause"
] | 3 | 2022-01-01T00:45:12.000Z | 2022-02-05T01:21:34.000Z | kaggle/detecting-non-groundtruth-datasets.ipynb | fossabot/denmune-clustering-algorithm | aa815150bd3dc0dfddca70d7f75c207f92b5d3b0 | [
"BSD-3-Clause"
] | 2 | 2022-02-03T23:03:35.000Z | 2022-02-05T07:56:39.000Z | 284,560 | 284,560 | 0.952818 | [
[
[
"<a href=\"https://www.kaggle.com/egyfirst/detecting-non-groundtruth-datasets?scriptVersionId=86411863\" target=\"_blank\"><img align=\"left\" alt=\"Kaggle\" title=\"Open in Kaggle\" src=\"https://kaggle.com/static/images/open-in-kaggle.svg\"></a>",
"_____no_output_____"
]
],
[
[
"import pandas as pd\nimport time\nimport os.path\n\nimport warnings\nwarnings.filterwarnings('ignore')",
"_____no_output_____"
],
[
"# install DenMune clustering algorithm using pip command from the offecial Python repository, PyPi\n# from https://pypi.org/project/denmune/\n!pip install denmune\n\n# now import it\nfrom denmune import DenMune",
"Collecting denmune\r\n Downloading denmune-0.0.9.0-py3-none-any.whl (13 kB)\r\nRequirement already satisfied: pandas>=1.0.3 in /opt/conda/lib/python3.7/site-packages (from denmune) (1.3.5)\r\nRequirement already satisfied: seaborn>=0.10.1 in /opt/conda/lib/python3.7/site-packages (from denmune) (0.11.2)\r\nRequirement already satisfied: matplotlib>=3.2.1 in /opt/conda/lib/python3.7/site-packages (from denmune) (3.5.1)\r\nRequirement already satisfied: numpy>=1.18.5 in /opt/conda/lib/python3.7/site-packages (from denmune) (1.20.3)\r\nCollecting anytree>=2.8.0\r\n Downloading anytree-2.8.0-py2.py3-none-any.whl (41 kB)\r\n |████████████████████████████████| 41 kB 98 kB/s \r\n\u001b[?25hRequirement already satisfied: scikit-learn>=0.22.1 in /opt/conda/lib/python3.7/site-packages (from denmune) (0.23.2)\r\nCollecting ngt>=1.11.6\r\n Downloading ngt-1.12.2-cp37-cp37m-manylinux1_x86_64.whl (2.2 MB)\r\n |████████████████████████████████| 2.2 MB 1.5 MB/s \r\n\u001b[?25hCollecting treelib>=1.6.1\r\n Downloading treelib-1.6.1.tar.gz (24 kB)\r\n Preparing metadata (setup.py) ... \u001b[?25l-\b \bdone\r\n\u001b[?25hRequirement already satisfied: six>=1.9.0 in /opt/conda/lib/python3.7/site-packages (from anytree>=2.8.0->denmune) (1.16.0)\r\nRequirement already satisfied: pillow>=6.2.0 in /opt/conda/lib/python3.7/site-packages (from matplotlib>=3.2.1->denmune) (8.2.0)\r\nRequirement already satisfied: packaging>=20.0 in /opt/conda/lib/python3.7/site-packages (from matplotlib>=3.2.1->denmune) (21.3)\r\nRequirement already satisfied: pyparsing>=2.2.1 in /opt/conda/lib/python3.7/site-packages (from matplotlib>=3.2.1->denmune) (3.0.6)\r\nRequirement already satisfied: python-dateutil>=2.7 in /opt/conda/lib/python3.7/site-packages (from matplotlib>=3.2.1->denmune) (2.8.0)\r\nRequirement already satisfied: fonttools>=4.22.0 in /opt/conda/lib/python3.7/site-packages (from matplotlib>=3.2.1->denmune) (4.28.4)\r\nRequirement already satisfied: cycler>=0.10 in /opt/conda/lib/python3.7/site-packages (from matplotlib>=3.2.1->denmune) (0.11.0)\r\nRequirement already satisfied: kiwisolver>=1.0.1 in /opt/conda/lib/python3.7/site-packages (from matplotlib>=3.2.1->denmune) (1.3.2)\r\nRequirement already satisfied: pybind11 in /opt/conda/lib/python3.7/site-packages (from ngt>=1.11.6->denmune) (2.9.0)\r\nRequirement already satisfied: pytz>=2017.3 in /opt/conda/lib/python3.7/site-packages (from pandas>=1.0.3->denmune) (2021.3)\r\nRequirement already satisfied: scipy>=0.19.1 in /opt/conda/lib/python3.7/site-packages (from scikit-learn>=0.22.1->denmune) (1.7.3)\r\nRequirement already satisfied: threadpoolctl>=2.0.0 in /opt/conda/lib/python3.7/site-packages (from scikit-learn>=0.22.1->denmune) (3.0.0)\r\nRequirement already satisfied: joblib>=0.11 in /opt/conda/lib/python3.7/site-packages (from scikit-learn>=0.22.1->denmune) (1.1.0)\r\nRequirement already satisfied: future in /opt/conda/lib/python3.7/site-packages (from treelib>=1.6.1->denmune) (0.18.2)\r\nBuilding wheels for collected packages: treelib\r\n Building wheel for treelib (setup.py) ... \u001b[?25l-\b \b\\\b \bdone\r\n\u001b[?25h Created wheel for treelib: filename=treelib-1.6.1-py3-none-any.whl size=18386 sha256=c9fc88fcc62ccee93209a9c6ee2a4bbe17bb1537e6c3c43bc701bb73b4f3f75f\r\n Stored in directory: /root/.cache/pip/wheels/89/be/94/2c6d949ce599d1443426d83ba4dc93cd35c0f4638260930a53\r\nSuccessfully built treelib\r\nInstalling collected packages: treelib, ngt, anytree, denmune\r\nSuccessfully installed anytree-2.8.0 denmune-0.0.9.0 ngt-1.12.2 treelib-1.6.1\r\n\u001b[33mWARNING: Running pip as the 'root' user can result in broken permissions and conflicting behaviour with the system package manager. It is recommended to use a virtual environment instead: https://pip.pypa.io/warnings/venv\u001b[0m\r\n"
],
[
"# clone datasets from our repository datasets\nif not os.path.exists('datasets'):\n !git clone https://github.com/egy1st/datasets",
"Cloning into 'datasets'...\r\nremote: Enumerating objects: 52, done.\u001b[K\r\nremote: Counting objects: 100% (52/52), done.\u001b[K\r\nremote: Compressing objects: 100% (43/43), done.\u001b[K\r\nremote: Total 52 (delta 8), reused 49 (delta 8), pack-reused 0\u001b[K\r\nUnpacking objects: 100% (52/52), 20.40 MiB | 6.04 MiB/s, done.\r\n"
],
[
"data_path = 'datasets/denmune/chameleon/' \ndatasets = [\"t7.10k\", \"t4.8k\", \"t5.8k\", \"t8.8k\"]\n\nfor dataset in datasets:\n data_file = data_path + dataset + '.csv'\n X_train = pd.read_csv(data_file, sep=',', header=None)\n\n dm = DenMune(train_data=X_train, k_nearest=39, rgn_tsne=False)\n labels, validity = dm.fit_predict(show_noise=True, show_analyzer=True)\n",
"Plotting train data\n"
],
[
"dataset = \"clusterable\"\ndata_file = data_path + dataset + '.csv'\nX_train = pd.read_csv(data_file, sep=',', header=None)\n\ndm = DenMune(train_data=X_train, k_nearest=24, rgn_tsne=False)\nlabels, validity = dm.fit_predict(show_noise=True, show_analyzer=True)\n",
"Plotting train data\n"
]
]
] | [
"markdown",
"code"
] | [
[
"markdown"
],
[
"code",
"code",
"code",
"code",
"code"
]
] |
d078170ec347d15b50118a5b90862d2fa1da046f | 211,903 | ipynb | Jupyter Notebook | main/00_gundih_historical_production_data.ipynb | yohanesnuwara/bsc-thesis-carbon-capture-storage | 0be66ca14e1eb3787701541fd91d2d190c1e50b9 | [
"MIT"
] | null | null | null | main/00_gundih_historical_production_data.ipynb | yohanesnuwara/bsc-thesis-carbon-capture-storage | 0be66ca14e1eb3787701541fd91d2d190c1e50b9 | [
"MIT"
] | null | null | null | main/00_gundih_historical_production_data.ipynb | yohanesnuwara/bsc-thesis-carbon-capture-storage | 0be66ca14e1eb3787701541fd91d2d190c1e50b9 | [
"MIT"
] | null | null | null | 219.134436 | 81,358 | 0.843084 | [
[
[
"<a href=\"https://colab.research.google.com/github/yohanesnuwara/ccs-gundih/blob/master/main/gundih_historical_production_data.ipynb\" target=\"_parent\"><img src=\"https://colab.research.google.com/assets/colab-badge.svg\" alt=\"Open In Colab\"/></a>",
"_____no_output_____"
]
],
[
[
"import numpy as np\nimport matplotlib.pyplot as plt\nimport pandas as pd",
"_____no_output_____"
],
[
"!git clone https://github.com/yohanesnuwara/ccs-gundih",
"Cloning into 'ccs-gundih'...\nremote: Enumerating objects: 94, done.\u001b[K\nremote: Counting objects: 1% (1/94)\u001b[K\rremote: Counting objects: 2% (2/94)\u001b[K\rremote: Counting objects: 3% (3/94)\u001b[K\rremote: Counting objects: 4% (4/94)\u001b[K\rremote: Counting objects: 5% (5/94)\u001b[K\rremote: Counting objects: 6% (6/94)\u001b[K\rremote: Counting objects: 7% (7/94)\u001b[K\rremote: Counting objects: 8% (8/94)\u001b[K\rremote: Counting objects: 9% (9/94)\u001b[K\rremote: Counting objects: 10% (10/94)\u001b[K\rremote: Counting objects: 11% (11/94)\u001b[K\rremote: Counting objects: 12% (12/94)\u001b[K\rremote: Counting objects: 13% (13/94)\u001b[K\rremote: Counting objects: 14% (14/94)\u001b[K\rremote: Counting objects: 15% (15/94)\u001b[K\rremote: Counting objects: 17% (16/94)\u001b[K\rremote: Counting objects: 18% (17/94)\u001b[K\rremote: Counting objects: 19% (18/94)\u001b[K\rremote: Counting objects: 20% (19/94)\u001b[K\rremote: Counting objects: 21% (20/94)\u001b[K\rremote: Counting objects: 22% (21/94)\u001b[K\rremote: Counting objects: 23% (22/94)\u001b[K\rremote: Counting objects: 24% (23/94)\u001b[K\rremote: Counting objects: 25% (24/94)\u001b[K\rremote: Counting objects: 26% (25/94)\u001b[K\rremote: Counting objects: 27% (26/94)\u001b[K\rremote: Counting objects: 28% (27/94)\u001b[K\rremote: Counting objects: 29% (28/94)\u001b[K\rremote: Counting objects: 30% (29/94)\u001b[K\rremote: Counting objects: 31% (30/94)\u001b[K\rremote: Counting objects: 32% (31/94)\u001b[K\rremote: Counting objects: 34% (32/94)\u001b[K\rremote: Counting objects: 35% (33/94)\u001b[K\rremote: Counting objects: 36% (34/94)\u001b[K\rremote: Counting objects: 37% (35/94)\u001b[K\rremote: Counting objects: 38% (36/94)\u001b[K\rremote: Counting objects: 39% (37/94)\u001b[K\rremote: Counting objects: 40% (38/94)\u001b[K\rremote: Counting objects: 41% (39/94)\u001b[K\rremote: Counting objects: 42% (40/94)\u001b[K\rremote: Counting objects: 43% (41/94)\u001b[K\rremote: Counting objects: 44% (42/94)\u001b[K\rremote: Counting objects: 45% (43/94)\u001b[K\rremote: Counting objects: 46% (44/94)\u001b[K\rremote: Counting objects: 47% (45/94)\u001b[K\rremote: Counting objects: 48% (46/94)\u001b[K\rremote: Counting objects: 50% (47/94)\u001b[K\rremote: Counting objects: 51% (48/94)\u001b[K\rremote: Counting objects: 52% (49/94)\u001b[K\rremote: Counting objects: 53% (50/94)\u001b[K\rremote: Counting objects: 54% (51/94)\u001b[K\rremote: Counting objects: 55% (52/94)\u001b[K\rremote: Counting objects: 56% (53/94)\u001b[K\rremote: Counting objects: 57% (54/94)\u001b[K\rremote: Counting objects: 58% (55/94)\u001b[K\rremote: Counting objects: 59% (56/94)\u001b[K\rremote: Counting objects: 60% (57/94)\u001b[K\rremote: Counting objects: 61% (58/94)\u001b[K\rremote: Counting objects: 62% (59/94)\u001b[K\rremote: Counting objects: 63% (60/94)\u001b[K\rremote: Counting objects: 64% (61/94)\u001b[K\rremote: Counting objects: 65% (62/94)\u001b[K\rremote: Counting objects: 67% (63/94)\u001b[K\rremote: Counting objects: 68% (64/94)\u001b[K\rremote: Counting objects: 69% (65/94)\u001b[K\rremote: Counting objects: 70% (66/94)\u001b[K\rremote: Counting objects: 71% (67/94)\u001b[K\rremote: Counting objects: 72% (68/94)\u001b[K\rremote: Counting objects: 73% (69/94)\u001b[K\rremote: Counting objects: 74% (70/94)\u001b[K\rremote: Counting objects: 75% (71/94)\u001b[K\rremote: Counting objects: 76% (72/94)\u001b[K\rremote: Counting objects: 77% (73/94)\u001b[K\rremote: Counting objects: 78% (74/94)\u001b[K\rremote: Counting objects: 79% (75/94)\u001b[K\rremote: Counting objects: 80% (76/94)\u001b[K\rremote: Counting objects: 81% (77/94)\u001b[K\rremote: Counting objects: 82% (78/94)\u001b[K\rremote: Counting objects: 84% (79/94)\u001b[K\rremote: Counting objects: 85% (80/94)\u001b[K\rremote: Counting objects: 86% (81/94)\u001b[K\rremote: Counting objects: 87% (82/94)\u001b[K\rremote: Counting objects: 88% (83/94)\u001b[K\rremote: Counting objects: 89% (84/94)\u001b[K\rremote: Counting objects: 90% (85/94)\u001b[K\rremote: Counting objects: 91% (86/94)\u001b[K\rremote: Counting objects: 92% (87/94)\u001b[K\rremote: Counting objects: 93% (88/94)\u001b[K\rremote: Counting objects: 94% (89/94)\u001b[K\rremote: Counting objects: 95% (90/94)\u001b[K\rremote: Counting objects: 96% (91/94)\u001b[K\rremote: Counting objects: 97% (92/94)\u001b[K\rremote: Counting objects: 98% (93/94)\u001b[K\rremote: Counting objects: 100% (94/94)\u001b[K\rremote: Counting objects: 100% (94/94), done.\u001b[K\nremote: Compressing objects: 100% (94/94), done.\u001b[K\nremote: Total 259 (delta 55), reused 0 (delta 0), pack-reused 165\u001b[K\nReceiving objects: 100% (259/259), 14.37 MiB | 26.09 MiB/s, done.\nResolving deltas: 100% (136/136), done.\n"
]
],
[
[
"# Visualize the Historical Production Data",
"_____no_output_____"
]
],
[
[
"# Read simulation result\n\ncol = np.array(['Date', 'Days', 'WSAT', 'OSAT', 'GSAT', 'GMT', 'OMR', 'GMR', 'GCDI', 'GCDM', 'WCD',\n 'WGR', 'WCT', 'VPR', 'VPT', 'VIR', 'VIT', 'WPR', 'OPR', 'GPR', 'WPT', 'OPT', 'GPT',\n 'PR', 'GIR', 'GIT', 'GOR'])\n\ncase1 = pd.read_excel(r'/content/ccs-gundih/data/CASE_1.xlsx'); case1 = pd.DataFrame(case1, columns=col) #INJ-2, 15 MMSCFD, kv/kh = 0.1\ncase2 = pd.read_excel(r'/content/ccs-gundih/data/CASE_2.xlsx'); case2 = pd.DataFrame(case2, columns=col) #INJ-2, 15 MMSCFD, kv/kh = 0.5",
"_____no_output_____"
],
[
"case1.head(5)",
"_____no_output_____"
],
[
"case2.head(5)",
"_____no_output_____"
],
[
"# convert to Panda datetime\ndate = pd.to_datetime(case1['Date'])\n\n# gas production cumulative \nGPT1 = case1['GPT'] * 35.31 * 1E-06 # convert from m3 to ft3 then to mmscf\nGPT2 = case2['GPT'] * 35.31 * 1E-06\n\n# gas production rate\nGPR1 = case1['GPR'] * 35.31 * 1E-06\nGPR2 = case2['GPR'] * 35.31 * 1E-06\n\n# average pressure\nPR1 = case1['PR'] * 14.5038 # convert from bar to psi\nPR2 = case2['PR'] * 14.5038 ",
"_____no_output_____"
],
[
"# plot gas production and pressure data from 2014-01-01 to 2016-01-01\n\npd.plotting.register_matplotlib_converters()\n\nplt.figure(figsize=(12, 7))\nplt.plot(date, GPR1, color='blue')\nplt.plot(date, GPR2, color='red')\nplt.xlabel(\"Date\"); plt.ylabel(\"Cumulative Gas Production (MMscf)\")\nplt.title(\"Cumulative Gas Production from 01/01/2014 to 01/01/2019\", pad=20, size=15)\nplt.xlim('2014-01-01', '2019-01-01')\n# plt.ylim(0, 50000)",
"_____no_output_____"
],
[
"# plot gas production and pressure data from 2014-01-01 to 2016-01-01\n\npd.plotting.register_matplotlib_converters()\n\nfig = plt.figure()\nfig = plt.figure(figsize=(12,7))\nhost = fig.add_subplot(111)\n\npar1 = host.twinx()\npar2 = host.twinx()\n\nhost.set_xlabel(\"Year\")\nhost.set_ylabel(\"Cumulative Gas Production (MMscf)\")\npar1.set_ylabel(\"Gas Production Rate (MMscfd)\")\npar2.set_ylabel(\"Average Reservoir Pressure (psi)\")\n\nhost.set_title(\"Historical Production Data of Gundih Field from 2014 to 2019\", pad=20, size=15)\n\ncolor1 = plt.cm.viridis(0)\ncolor2 = plt.cm.viridis(.5)\ncolor3 = plt.cm.viridis(.8)\n\np1, = host.plot(date, GPR1, color=color1,label=\"Gas production rate (MMscfd)\")\np2, = par1.plot(date, GPT1, color=color2, label=\"Cumulative gas production (MMscf)\")\np3, = par2.plot(date, PR1, color=color3, label=\"Average Pressure (psi)\")\nhost.set_xlim('2014-05-01', '2019-01-01')\nhost.set_ylim(ymin=0)\npar1.set_ylim(0, 40000)\npar2.set_ylim(3400, 4100)\n\nlns = [p1, p2, p3]\nhost.legend(handles=lns, loc='best')\n\n# right, left, top, bottom\npar2.spines['right'].set_position(('outward', 60)) \n\nplt.savefig('/content/ccs-gundih/result/production_curve')",
"_____no_output_____"
]
],
[
[
"# Dry-Gas Reservoir Analysis",
"_____no_output_____"
]
],
[
[
"!git clone https://github.com/yohanesnuwara/reservoir-engineering",
"Cloning into 'reservoir-engineering'...\nremote: Enumerating objects: 32, done.\u001b[K\nremote: Counting objects: 3% (1/32)\u001b[K\rremote: Counting objects: 6% (2/32)\u001b[K\rremote: Counting objects: 9% (3/32)\u001b[K\rremote: Counting objects: 12% (4/32)\u001b[K\rremote: Counting objects: 15% (5/32)\u001b[K\rremote: Counting objects: 18% (6/32)\u001b[K\rremote: Counting objects: 21% (7/32)\u001b[K\rremote: Counting objects: 25% (8/32)\u001b[K\rremote: Counting objects: 28% (9/32)\u001b[K\rremote: Counting objects: 31% (10/32)\u001b[K\rremote: Counting objects: 34% (11/32)\u001b[K\rremote: Counting objects: 37% (12/32)\u001b[K\rremote: Counting objects: 40% (13/32)\u001b[K\rremote: Counting objects: 43% (14/32)\u001b[K\rremote: Counting objects: 46% (15/32)\u001b[K\rremote: Counting objects: 50% (16/32)\u001b[K\rremote: Counting objects: 53% (17/32)\u001b[K\rremote: Counting objects: 56% (18/32)\u001b[K\rremote: Counting objects: 59% (19/32)\u001b[K\rremote: Counting objects: 62% (20/32)\u001b[K\rremote: Counting objects: 65% (21/32)\u001b[K\rremote: Counting objects: 68% (22/32)\u001b[K\rremote: Counting objects: 71% (23/32)\u001b[K\rremote: Counting objects: 75% (24/32)\u001b[K\rremote: Counting objects: 78% (25/32)\u001b[K\rremote: Counting objects: 81% (26/32)\u001b[K\rremote: Counting objects: 84% (27/32)\u001b[K\rremote: Counting objects: 87% (28/32)\u001b[K\rremote: Counting objects: 90% (29/32)\u001b[K\rremote: Counting objects: 93% (30/32)\u001b[K\rremote: Counting objects: 96% (31/32)\u001b[K\rremote: Counting objects: 100% (32/32)\u001b[K\rremote: Counting objects: 100% (32/32), done.\u001b[K\nremote: Compressing objects: 3% (1/31)\u001b[K\rremote: Compressing objects: 6% (2/31)\u001b[K\rremote: Compressing objects: 9% (3/31)\u001b[K\rremote: Compressing objects: 12% (4/31)\u001b[K\rremote: Compressing objects: 16% (5/31)\u001b[K\rremote: Compressing objects: 19% (6/31)\u001b[K\rremote: Compressing objects: 22% (7/31)\u001b[K\rremote: Compressing objects: 25% (8/31)\u001b[K\rremote: Compressing objects: 29% (9/31)\u001b[K\rremote: Compressing objects: 32% (10/31)\u001b[K\rremote: Compressing objects: 35% (11/31)\u001b[K\rremote: Compressing objects: 38% (12/31)\u001b[K\rremote: Compressing objects: 41% (13/31)\u001b[K\rremote: Compressing objects: 45% (14/31)\u001b[K\rremote: Compressing objects: 48% (15/31)\u001b[K\rremote: Compressing objects: 51% (16/31)\u001b[K\rremote: Compressing objects: 54% (17/31)\u001b[K\rremote: Compressing objects: 58% (18/31)\u001b[K\rremote: Compressing objects: 61% (19/31)\u001b[K\rremote: Compressing objects: 64% (20/31)\u001b[K\rremote: Compressing objects: 67% (21/31)\u001b[K\rremote: Compressing objects: 70% (22/31)\u001b[K\rremote: Compressing objects: 74% (23/31)\u001b[K\rremote: Compressing objects: 77% (24/31)\u001b[K\rremote: Compressing objects: 80% (25/31)\u001b[K\rremote: Compressing objects: 83% (26/31)\u001b[K\rremote: Compressing objects: 87% (27/31)\u001b[K\rremote: Compressing objects: 90% (28/31)\u001b[K\rremote: Compressing objects: 93% (29/31)\u001b[K\rremote: Compressing objects: 96% (30/31)\u001b[K\rremote: Compressing objects: 100% (31/31)\u001b[K\rremote: Compressing objects: 100% (31/31), done.\u001b[K\nReceiving objects: 0% (1/941) \rReceiving objects: 1% (10/941) \rReceiving objects: 2% (19/941) \rReceiving objects: 3% (29/941) \rReceiving objects: 4% (38/941) \rReceiving objects: 5% (48/941) \rReceiving objects: 6% (57/941) \rReceiving objects: 7% (66/941) \rReceiving objects: 8% (76/941) \rReceiving objects: 9% (85/941) \rReceiving objects: 10% (95/941) \rReceiving objects: 11% (104/941) \rReceiving objects: 12% (113/941) \rReceiving objects: 13% (123/941) \rReceiving objects: 14% (132/941) \rReceiving objects: 15% (142/941) \rReceiving objects: 16% (151/941) \rReceiving objects: 17% (160/941) \rReceiving objects: 18% (170/941) \rReceiving objects: 19% (179/941) \rReceiving objects: 20% (189/941) \rReceiving objects: 21% (198/941) \rReceiving objects: 22% (208/941) \rReceiving objects: 23% (217/941) \rReceiving objects: 24% (226/941) \rReceiving objects: 25% (236/941) \rReceiving objects: 26% (245/941) \rReceiving objects: 27% (255/941) \rReceiving objects: 28% (264/941) \rReceiving objects: 29% (273/941) \rReceiving objects: 30% (283/941) \rReceiving objects: 31% (292/941) \rReceiving objects: 32% (302/941) \rReceiving objects: 33% (311/941) \rReceiving objects: 34% (320/941) \rReceiving objects: 35% (330/941) \rReceiving objects: 36% (339/941) \rReceiving objects: 37% (349/941) \rReceiving objects: 38% (358/941) \rReceiving objects: 39% (367/941) \rReceiving objects: 40% (377/941) \rReceiving objects: 41% (386/941) \rReceiving objects: 42% (396/941) \rReceiving objects: 43% (405/941) \rReceiving objects: 44% (415/941) \rReceiving objects: 45% (424/941) \rReceiving objects: 46% (433/941) \rReceiving objects: 47% (443/941) \rReceiving objects: 48% (452/941) \rReceiving objects: 49% (462/941) \rReceiving objects: 50% (471/941) \rReceiving objects: 51% (480/941) \rReceiving objects: 52% (490/941) \rReceiving objects: 53% (499/941) \rReceiving objects: 54% (509/941) \rReceiving objects: 55% (518/941) \rReceiving objects: 56% (527/941) \rReceiving objects: 57% (537/941) \rReceiving objects: 58% (546/941) \rReceiving objects: 59% (556/941) \rReceiving objects: 60% (565/941) \rReceiving objects: 61% (575/941) \rReceiving objects: 62% (584/941) \rReceiving objects: 63% (593/941) \rReceiving objects: 64% (603/941) \rReceiving objects: 65% (612/941) \rReceiving objects: 66% (622/941) \rReceiving objects: 67% (631/941) \rReceiving objects: 68% (640/941) \rReceiving objects: 69% (650/941) \rReceiving objects: 70% (659/941) \rReceiving objects: 71% (669/941) \rReceiving objects: 72% (678/941) \rReceiving objects: 73% (687/941) \rReceiving objects: 74% (697/941) \rReceiving objects: 75% (706/941) \rReceiving objects: 76% (716/941) \rReceiving objects: 77% (725/941) \rReceiving objects: 78% (734/941) \rReceiving objects: 79% (744/941) \rReceiving objects: 80% (753/941) \rReceiving objects: 81% (763/941) \rReceiving objects: 82% (772/941) \rReceiving objects: 83% (782/941) \rReceiving objects: 84% (791/941) \rReceiving objects: 85% (800/941) \rReceiving objects: 86% (810/941) \rReceiving objects: 87% (819/941) \rReceiving objects: 88% (829/941) \rReceiving objects: 89% (838/941) \rReceiving objects: 90% (847/941) \rReceiving objects: 91% (857/941) \rReceiving objects: 92% (866/941) \rReceiving objects: 93% (876/941) \rReceiving objects: 94% (885/941) \rReceiving objects: 95% (894/941) \rReceiving objects: 96% (904/941) \rReceiving objects: 97% (913/941) \rReceiving objects: 98% (923/941) \rremote: Total 941 (delta 15), reused 0 (delta 0), pack-reused 909\u001b[K\nReceiving objects: 99% (932/941) \rReceiving objects: 100% (941/941) \rReceiving objects: 100% (941/941), 12.23 MiB | 45.56 MiB/s, done.\nResolving deltas: 0% (0/410) \rResolving deltas: 1% (5/410) \rResolving deltas: 3% (16/410) \rResolving deltas: 6% (26/410) \rResolving deltas: 16% (69/410) \rResolving deltas: 18% (75/410) \rResolving deltas: 20% (85/410) \rResolving deltas: 27% (113/410) \rResolving deltas: 35% (147/410) \rResolving deltas: 41% (170/410) \rResolving deltas: 61% (252/410) \rResolving deltas: 63% (261/410) \rResolving deltas: 67% (276/410) \rResolving deltas: 68% (280/410) \rResolving deltas: 69% (285/410) \rResolving deltas: 71% (292/410) \rResolving deltas: 72% (296/410) \rResolving deltas: 73% (300/410) \rResolving deltas: 74% (304/410) \rResolving deltas: 75% (311/410) \rResolving deltas: 76% (313/410) \rResolving deltas: 77% (317/410) \rResolving deltas: 78% (320/410) \rResolving deltas: 79% (324/410) \rResolving deltas: 83% (342/410) \rResolving deltas: 84% (345/410) \rResolving deltas: 85% (352/410) \rResolving deltas: 86% (353/410) \rResolving deltas: 87% (357/410) \rResolving deltas: 88% (362/410) \rResolving deltas: 89% (368/410) \rResolving deltas: 90% (371/410) \rResolving deltas: 91% (375/410) \rResolving deltas: 92% (380/410) \rResolving deltas: 93% (382/410) \rResolving deltas: 94% (386/410) \rResolving deltas: 95% (390/410) \rResolving deltas: 96% (397/410) \rResolving deltas: 97% (398/410) \rResolving deltas: 98% (402/410) \rResolving deltas: 100% (410/410) \rResolving deltas: 100% (410/410), done.\n"
],
[
"# calculate gas z factor and FVF\n\nimport os, sys\nsys.path.append('/content/reservoir-engineering/Unit 2 Review of Rock and Fluid Properties/functions')\n\nfrom pseudoprops import pseudoprops\nfrom dranchuk_aboukassem import dranchuk\nfrom gasfvf import gasfvf\n\ntemp_f = (temp * 9/5) + 32 # Rankine\npressure = np.array(PR1)\n\nz_arr = []\nBg_arr = []\nfor i in range(len(pressure)):\n P_pr, T_pr = pseudoprops(temp_f, pressure[i], 0.8, 0.00467, 0.23)\n rho_pr, z = dranchuk(T_pr, P_pr)\n temp_r = temp_f + 459.67 \n Bg = 0.0282793 * z * temp_r / pressure[i] # Eq 2.2, temp in Rankine, p in psia, result in res ft3/scf\n z_arr.append(float(z))\n Bg_arr.append(float(Bg))",
"_____no_output_____"
],
[
"Bg_arr = np.array(Bg_arr)\nF = GPT1 * Bg_arr # MMscf\nEg = Bg_arr - Bg_arr[0]\nF_Eg = F / Eg\n\nplt.figure(figsize=(10,7))\nplt.plot(GPT1, (F_Eg / 1E+03), '.', color='red') # convert F_Eg from MMscf to Bscf\nplt.xlim(xmin=0); plt.ylim(ymin=0)\nplt.title(\"Waterdrive Diagnostic Plot of $F/E_g$ vs. $G_p$\", pad=20, size=15)\nplt.xlabel('Cumulative Gas Production (MMscf)')\nplt.ylabel('$F/E_g$ (Bscf)')\nplt.ylim(ymin=250)",
"_____no_output_____"
],
[
"date_hist = date.iloc[:1700]\nGPT1_hist = GPT1.iloc[:1700]\nBg_arr = np.array(Bg_arr)\nBg_arr_hist = Bg_arr[:1700]\n\nF_hist = GPT1_hist * Bg_arr_hist # MMscf\nEg_hist = Bg_arr_hist - Bg_arr_hist[0]\nF_Eg_hist = F_hist / Eg_hist\n\nplt.figure(figsize=(10,7))\nplt.plot(GPT1_hist, (F_Eg_hist / 1E+03), '.')\nplt.title(\"Waterdrive Diagnostic Plot of $F/E_g$ vs. $G_p$\", pad=20, size=15)\nplt.xlabel('Cumulative Gas Production (MMscf)')\nplt.ylabel('$F/E_g$ (Bscf)')\nplt.xlim(xmin=0); plt.ylim(300, 350)",
"_____no_output_____"
],
[
"p_z = PR1 / z_arr\nplt.plot(GPT1, p_z, '.')",
"_____no_output_____"
]
]
] | [
"markdown",
"code",
"markdown",
"code",
"markdown",
"code"
] | [
[
"markdown"
],
[
"code",
"code"
],
[
"markdown"
],
[
"code",
"code",
"code",
"code",
"code",
"code"
],
[
"markdown"
],
[
"code",
"code",
"code",
"code",
"code"
]
] |
d078248e4f9daf0ea215b7ba6a74577301c61a7c | 9,088 | ipynb | Jupyter Notebook | _doc/notebooks/td1a/pp_exo_deviner_un_nombre_correction.ipynb | Jerome-maker/ensae_teaching_cs | 43ea044361ee60c00c85aea354a7b25c21c0fd07 | [
"MIT"
] | 73 | 2015-05-12T13:12:11.000Z | 2021-12-21T11:44:29.000Z | _doc/notebooks/td1a/pp_exo_deviner_un_nombre_correction.ipynb | Pandinosaurus/ensae_teaching_cs | 3bc80f29d93c30de812e34c314bc96e6a4f0d025 | [
"MIT"
] | 90 | 2015-06-23T11:11:35.000Z | 2021-03-31T22:09:15.000Z | _doc/notebooks/td1a/pp_exo_deviner_un_nombre_correction.ipynb | Pandinosaurus/ensae_teaching_cs | 3bc80f29d93c30de812e34c314bc96e6a4f0d025 | [
"MIT"
] | 65 | 2015-01-13T08:23:55.000Z | 2022-02-11T22:42:07.000Z | 30.496644 | 221 | 0.422095 | [
[
[
"# 1A.1 - Deviner un nombre aléatoire (correction)\n\nOn reprend la fonction introduite dans l'énoncé et qui permet de saisir un nombre.",
"_____no_output_____"
]
],
[
[
"import random",
"_____no_output_____"
],
[
"nombre = input(\"Entrez un nombre\")",
"Entrez un nombre7\n"
],
[
"nombre",
"_____no_output_____"
]
],
[
[
"**Q1 :** Ecrire une jeu dans lequel python choisi aléatoirement un nombre entre 0 et 100, et essayer de trouver ce nombre en 10 étapes.",
"_____no_output_____"
]
],
[
[
"n = random.randint(0,100)\nappreciation = \"?\"\nwhile True:\n var = input(\"Entrez un nombre\")\n var = int(var)\n if var < n : \n appreciation = \"trop bas\"\n print(var, appreciation)\n \n else : \n appreciation = \"trop haut\"\n print(var, appreciation)\n if var == n:\n appreciation = \"bravo !\"\n print(var, appreciation)\n break",
"Entrez un nombre7\n7 trop bas\nEntrez un nombre10\n10 trop bas\nEntrez un nombre100\n100 trop haut\nEntrez un nombre50\n50 trop bas\nEntrez un nombre75\n75 trop haut\nEntrez un nombre60\n60 trop haut\nEntrez un nombre55\n55 trop haut\nEntrez un nombre53\n53 trop haut\nEntrez un nombre52\n52 trop haut\nEntrez un nombre51\n51 trop haut\n51 bravo !\n"
]
],
[
[
"**Q2 :** Transformer ce jeu en une fonction ``jeu(nVies)`` où ``nVies`` est le nombre d'itérations maximum.",
"_____no_output_____"
]
],
[
[
"import random\nn = random.randint(0,100)\nvies = 10\nappreciation = \"?\"\nwhile vies > 0:\n var = input(\"Entrez un nombre\")\n var = int(var)\n if var < n : \n appreciation = \"trop bas\"\n print(vies, var, appreciation)\n else : \n appreciation = \"trop haut\"\n print(vies, var, appreciation)\n if var == n:\n appreciation = \"bravo !\"\n print(vies, var, appreciation)\n break\n\n vies -= 1",
"Entrez un nombre50\n10 50 trop bas\nEntrez un nombre75\n9 75 trop haut\nEntrez un nombre60\n8 60 trop bas\nEntrez un nombre65\n7 65 trop bas\nEntrez un nombre70\n6 70 trop bas\nEntrez un nombre73\n5 73 trop haut\nEntrez un nombre72\n4 72 trop haut\nEntrez un nombre71\n3 71 trop haut\n3 71 bravo !\n"
]
],
[
[
"**Q3 :** Adapter le code pour faire une classe joueur avec une méthode jouer, où un joueur est défini par un pseudo et son nombre de vies. Faire jouer deux joueurs et déterminer le vainqueur.",
"_____no_output_____"
]
],
[
[
"class joueur:\n def __init__(self, vies, pseudo):\n self.vies = vies\n self.pseudo = pseudo\n \n def jouer(self):\n appreciation = \"?\"\n n = random.randint(0,100)\n while self.vies > 0:\n message = appreciation + \" -- \" + self.pseudo + \" : \" + str(self.vies) + \" vies restantes. Nombre choisi : \"\n var = input(message)\n var = int(var)\n if var < n : \n appreciation = \"trop bas\"\n print(vies, var, appreciation)\n else : \n appreciation = \"trop haut\"\n print(vies, var, appreciation)\n if var == n:\n appreciation = \"bravo !\"\n print(vies, var, appreciation)\n break\n\n self.vies -= 1\n\n# Initialisation des deux joueurs\nj1 = joueur(10, \"joueur 1\")\nj2 = joueur(10, \"joueur 2\")\n\n# j1 et j2 jouent\nj1.jouer()\nj2.jouer()\n\n# Nombre de vies restantes à chaque joueur\nprint(\"Nombre de vies restantes à chaque joueur\")\nprint(j1.pseudo + \" : \" + str(j1.vies) + \" restantes\")\nprint(j2.pseudo + \" : \" + str(j2.vies) + \" restantes\")\n\n# Résultat de la partie\nprint(\"Résultat de la partie\")\nif j1.vies < j2.vies:\n print(j1.pseudo + \"a gagné la partie\")\nelif j1.vies == j2.vies:\n print(\"match nul\")\nelse: print(j2.pseudo + \" a gagné la partie\")",
"? -- joueur 1 : 10 vies restantes. Nombre choisi : 50\n3 50 trop haut\ntrop haut -- joueur 1 : 9 vies restantes. Nombre choisi : 25\n3 25 trop haut\ntrop haut -- joueur 1 : 8 vies restantes. Nombre choisi : 10\n3 10 trop haut\ntrop haut -- joueur 1 : 7 vies restantes. Nombre choisi : 5\n3 5 trop bas\ntrop bas -- joueur 1 : 6 vies restantes. Nombre choisi : 7\n3 7 trop haut\n3 7 bravo !\n? -- joueur 2 : 10 vies restantes. Nombre choisi : 50\n3 50 trop haut\ntrop haut -- joueur 2 : 9 vies restantes. Nombre choisi : 25\n3 25 trop bas\ntrop bas -- joueur 2 : 8 vies restantes. Nombre choisi : 30\n3 30 trop haut\n3 30 bravo !\nNombre de vies restantes à chaque joueur\njoueur 1 : 6 restantes\njoueur 2 : 8 restantes\nRésultat de la partie\njoueur 1a gagné la partie\n"
]
]
] | [
"markdown",
"code",
"markdown",
"code",
"markdown",
"code",
"markdown",
"code"
] | [
[
"markdown"
],
[
"code",
"code",
"code"
],
[
"markdown"
],
[
"code"
],
[
"markdown"
],
[
"code"
],
[
"markdown"
],
[
"code"
]
] |
d07826664d0b41d37c30fca49a3a1ec75625f13b | 57,102 | ipynb | Jupyter Notebook | boqn/group_testing/notebooks/.ipynb_checkpoints/cornell_reopen_analysis_sensitivity_contact_tracing_effectiveness-checkpoint.ipynb | RaulAstudillo06/BOQN | c5b2bb9e547e2489f856ebf86c749fb24eba1022 | [
"MIT"
] | null | null | null | boqn/group_testing/notebooks/.ipynb_checkpoints/cornell_reopen_analysis_sensitivity_contact_tracing_effectiveness-checkpoint.ipynb | RaulAstudillo06/BOQN | c5b2bb9e547e2489f856ebf86c749fb24eba1022 | [
"MIT"
] | null | null | null | boqn/group_testing/notebooks/.ipynb_checkpoints/cornell_reopen_analysis_sensitivity_contact_tracing_effectiveness-checkpoint.ipynb | RaulAstudillo06/BOQN | c5b2bb9e547e2489f856ebf86c749fb24eba1022 | [
"MIT"
] | 1 | 2022-03-09T02:32:42.000Z | 2022-03-09T02:32:42.000Z | 239.92437 | 48,182 | 0.897499 | [
[
[
"import sys\nimport os\nimport numpy as np\nmodule_path = os.path.abspath(os.path.join('..'))\nif module_path not in sys.path:\n sys.path.append(module_path + \"/src/simulations_v2\")\n\nfrom analysis_helpers import poisson_waiting_function, \\\n run_multiple_trajectories, \\\n plot_aip_vs_t, \\\n plot_cip_vs_t, \\\n run_sensitivity_sims, \\\n extract_cips",
"_____no_output_____"
],
[
"# what percent of self-reports are from severe symptoms? \n# in reality I think this value will vary a lot in the first few days, \n# and then reach some kind of steady-state, and I'm not sure what makes the most\n# sense to use here. I am setting it to the very pessimistic value of 100% of\n# self-reporters are severe, which yields the smallest infectious window size\npct_self_reports_severe = 0.6\n\ndaily_self_report_severe = 0.85\ndaily_self_report_mild = 0.1\n\n# avg_infectious_window = (avg time in ID state) + (avg time in Sy state prior to self-reporting)\navg_infectious_window = 4 + pct_self_reports_severe * (1 / daily_self_report_severe) + \\\n (1-pct_self_reports_severe) * (1 / daily_self_report_mild)\nprint(avg_infectious_window)\npre_reopen_population = 1500\npre_reopen_daily_contacts = 7\n\nreopen_population = 2500\nreopen_daily_contacts = 10\n\npre_reopen_params = {\n 'max_time_exposed': 4,\n 'exposed_time_function': poisson_waiting_function(max_time=4, mean_time=1),\n \n 'max_time_pre_ID': 4,\n 'pre_ID_time_function': poisson_waiting_function(max_time=4, mean_time=1),\n \n 'max_time_ID': 8,\n 'ID_time_function': poisson_waiting_function(max_time=8, mean_time=4),\n \n 'max_time_SyID_mild': 14,\n 'SyID_mild_time_function': poisson_waiting_function(max_time=14, mean_time=10),\n \n 'max_time_SyID_severe': 14,\n 'SyID_severe_time_function': poisson_waiting_function(max_time=14, mean_time=10),\n \n 'sample_QI_exit_function': (lambda n: np.random.binomial(n, 0.05)),\n 'sample_QS_exit_function': (lambda n: np.random.binomial(n, 0.3)),\n \n 'exposed_infection_p': 0.026,\n 'expected_contacts_per_day': pre_reopen_daily_contacts,\n \n 'mild_symptoms_p': 0.4,\n 'mild_symptoms_daily_self_report_p': daily_self_report_mild,\n 'severe_symptoms_daily_self_report_p': daily_self_report_severe,\n \n 'days_between_tests': 300,\n 'test_population_fraction': 0,\n \n 'test_protocol_QFNR': 0.1,\n 'test_protocol_QFPR': 0.005,\n \n 'perform_contact_tracing': True,\n 'contact_tracing_constant': 0.5,\n 'contact_tracing_delay': 1,\n 'contact_trace_infectious_window': avg_infectious_window,\n \n 'pre_ID_state': 'detectable',\n \n 'population_size': pre_reopen_population,\n 'initial_E_count': 0,\n 'initial_pre_ID_count': 2,\n 'initial_ID_count': 0,\n 'initial_ID_prevalence': 0.001,\n 'initial_SyID_mild_count': 0,\n 'initial_SyID_severe_count': 0\n}\n\nreopen_params = pre_reopen_params.copy()\nreopen_params['population_size'] = reopen_population\nreopen_params['expected_contacts_per_day'] = reopen_daily_contacts",
"8.705882352941178\n"
]
],
[
[
"# Run sims to understand sensitivity of 'contact_tracing_constant'",
"_____no_output_____"
]
],
[
[
"ctc_range = [0.1 * x for x in range(11)]\ndfs_ctc_pre_reopen = run_sensitivity_sims(pre_reopen_params, param_to_vary='contact_tracing_constant',\n param_values = ctc_range, trajectories_per_config=250, time_horizon=100)\ndfs_ctc_post_reopen = run_sensitivity_sims(reopen_params, param_to_vary='contact_tracing_constant',\n param_values = ctc_range, trajectories_per_config=250, time_horizon=100)",
"Done simulating contact_tracing_constant equal to 0.0\nDone simulating contact_tracing_constant equal to 0.1\nDone simulating contact_tracing_constant equal to 0.2\nDone simulating contact_tracing_constant equal to 0.30000000000000004\nDone simulating contact_tracing_constant equal to 0.4\nDone simulating contact_tracing_constant equal to 0.5\nDone simulating contact_tracing_constant equal to 0.6000000000000001\nDone simulating contact_tracing_constant equal to 0.7000000000000001\nDone simulating contact_tracing_constant equal to 0.8\nDone simulating contact_tracing_constant equal to 0.9\nDone simulating contact_tracing_constant equal to 1.0\nDone simulating contact_tracing_constant equal to 0.0\nDone simulating contact_tracing_constant equal to 0.1\nDone simulating contact_tracing_constant equal to 0.2\nDone simulating contact_tracing_constant equal to 0.30000000000000004\nDone simulating contact_tracing_constant equal to 0.4\nDone simulating contact_tracing_constant equal to 0.5\nDone simulating contact_tracing_constant equal to 0.6000000000000001\nDone simulating contact_tracing_constant equal to 0.7000000000000001\nDone simulating contact_tracing_constant equal to 0.8\nDone simulating contact_tracing_constant equal to 0.9\nDone simulating contact_tracing_constant equal to 1.0\n"
],
[
"import matplotlib.pyplot as plt\ndef plot_many_dfs_threshold(dfs_dict, threshold=0.1, xlabel=\"\", title=\"\", figsize=(10,6)):\n plt.figure(figsize=figsize)\n for df_label, dfs_varied in dfs_dict.items():\n p_thresholds = []\n xs = sorted(list(dfs_varied.keys()))\n for x in xs:\n cips = extract_cips(dfs_varied[x])\n cip_exceed_thresh = [cip for cip in cips if cip >= threshold]\n p_thresholds.append(len(cip_exceed_thresh) / len(cips) * 100)\n plt.plot([x * 100 for x in xs], p_thresholds, marker='o', label=df_label)\n plt.xlabel(xlabel)\n plt.ylabel(\"Probability at least {:.0f}% infected (%)\".format(threshold * 100))\n plt.title(title)\n plt.legend(loc='best')\n plt.show()\n\ntitle = \"\"\"Outbreak Likelihood vs. Contact Tracing Effectiveness\"\"\"\nplot_many_dfs_threshold({'Post-Reopen (Population-size 2500, Contacts/person/day 10)': dfs_ctc_post_reopen,\n 'Pre-Reopen (Population-size 1500, Contacts/person/day 7)': dfs_ctc_pre_reopen, \n }, \n xlabel=\"Percentage of contacts recalled in contact tracing (%)\",\n title=title)",
"_____no_output_____"
]
]
] | [
"code",
"markdown",
"code"
] | [
[
"code",
"code"
],
[
"markdown"
],
[
"code",
"code"
]
] |
d078392e471192aeb28af40412867721a1e9dd73 | 29,431 | ipynb | Jupyter Notebook | notebooks/transformers_doc/tensorflow/quicktour.ipynb | yvr1037/transformers-of-Dian | 759a867e64c725e17a7aef37c78dd54b4c1fda81 | [
"Apache-2.0"
] | null | null | null | notebooks/transformers_doc/tensorflow/quicktour.ipynb | yvr1037/transformers-of-Dian | 759a867e64c725e17a7aef37c78dd54b4c1fda81 | [
"Apache-2.0"
] | null | null | null | notebooks/transformers_doc/tensorflow/quicktour.ipynb | yvr1037/transformers-of-Dian | 759a867e64c725e17a7aef37c78dd54b4c1fda81 | [
"Apache-2.0"
] | null | null | null | 38.572739 | 901 | 0.63073 | [
[
[
"empty"
]
]
] | [
"empty"
] | [
[
"empty"
]
] |
d0783cf82055d3d1b37ce539d6c91dfc9fcb4b80 | 47,239 | ipynb | Jupyter Notebook | machine_translation.ipynb | jay-thakur/machine_translation | 8f057e06bb307440d9345d506a5665f93ad4c830 | [
"MIT"
] | null | null | null | machine_translation.ipynb | jay-thakur/machine_translation | 8f057e06bb307440d9345d506a5665f93ad4c830 | [
"MIT"
] | null | null | null | machine_translation.ipynb | jay-thakur/machine_translation | 8f057e06bb307440d9345d506a5665f93ad4c830 | [
"MIT"
] | null | null | null | 44.776303 | 614 | 0.57842 | [
[
[
"# Artificial Intelligence Nanodegree\n## Machine Translation Project\nIn this notebook, sections that end with **'(IMPLEMENTATION)'** in the header indicate that the following blocks of code will require additional functionality which you must provide. Please be sure to read the instructions carefully!\n\n## Introduction\nIn this notebook, you will build a deep neural network that functions as part of an end-to-end machine translation pipeline. Your completed pipeline will accept English text as input and return the French translation.\n\n- **Preprocess** - You'll convert text to sequence of integers.\n- **Models** Create models which accepts a sequence of integers as input and returns a probability distribution over possible translations. After learning about the basic types of neural networks that are often used for machine translation, you will engage in your own investigations, to design your own model!\n- **Prediction** Run the model on English text.",
"_____no_output_____"
]
],
[
[
"%load_ext autoreload\n%aimport helper, tests\n%autoreload 1",
"_____no_output_____"
],
[
"import collections\n\nimport helper\nimport numpy as np\nimport project_tests as tests\n\nfrom keras.preprocessing.text import Tokenizer\nfrom keras.preprocessing.sequence import pad_sequences\nfrom keras.models import Model\nfrom keras.layers import GRU, Input, Dense, TimeDistributed, Activation, RepeatVector, Bidirectional\nfrom keras.layers.embeddings import Embedding\nfrom keras.optimizers import Adam\nfrom keras.losses import sparse_categorical_crossentropy",
"Using TensorFlow backend.\n"
]
],
[
[
"### Verify access to the GPU\nThe following test applies only if you expect to be using a GPU, e.g., while running in a Udacity Workspace or using an AWS instance with GPU support. Run the next cell, and verify that the device_type is \"GPU\".\n- If the device is not GPU & you are running from a Udacity Workspace, then save your workspace with the icon at the top, then click \"enable\" at the bottom of the workspace.\n- If the device is not GPU & you are running from an AWS instance, then refer to the cloud computing instructions in the classroom to verify your setup steps.",
"_____no_output_____"
]
],
[
[
"from tensorflow.python.client import device_lib\nprint(device_lib.list_local_devices())",
"[name: \"/cpu:0\"\ndevice_type: \"CPU\"\nmemory_limit: 268435456\nlocality {\n}\nincarnation: 15440441665772238793\n, name: \"/gpu:0\"\ndevice_type: \"GPU\"\nmemory_limit: 357433344\nlocality {\n bus_id: 1\n}\nincarnation: 3061658136091710780\nphysical_device_desc: \"device: 0, name: Tesla K80, pci bus id: 0000:00:04.0\"\n]\n"
]
],
[
[
"## Dataset\nWe begin by investigating the dataset that will be used to train and evaluate your pipeline. The most common datasets used for machine translation are from [WMT](http://www.statmt.org/). However, that will take a long time to train a neural network on. We'll be using a dataset we created for this project that contains a small vocabulary. You'll be able to train your model in a reasonable time with this dataset.\n### Load Data\nThe data is located in `data/small_vocab_en` and `data/small_vocab_fr`. The `small_vocab_en` file contains English sentences with their French translations in the `small_vocab_fr` file. Load the English and French data from these files from running the cell below.",
"_____no_output_____"
]
],
[
[
"# Load English data\nenglish_sentences = helper.load_data('data/small_vocab_en')\n# Load French data\nfrench_sentences = helper.load_data('data/small_vocab_fr')\n\nprint('Dataset Loaded')",
"Dataset Loaded\n"
]
],
[
[
"### Files\nEach line in `small_vocab_en` contains an English sentence with the respective translation in each line of `small_vocab_fr`. View the first two lines from each file.",
"_____no_output_____"
]
],
[
[
"for sample_i in range(2):\n print('small_vocab_en Line {}: {}'.format(sample_i + 1, english_sentences[sample_i]))\n print('small_vocab_fr Line {}: {}'.format(sample_i + 1, french_sentences[sample_i]))",
"small_vocab_en Line 1: new jersey is sometimes quiet during autumn , and it is snowy in april .\nsmall_vocab_fr Line 1: new jersey est parfois calme pendant l' automne , et il est neigeux en avril .\nsmall_vocab_en Line 2: the united states is usually chilly during july , and it is usually freezing in november .\nsmall_vocab_fr Line 2: les états-unis est généralement froid en juillet , et il gèle habituellement en novembre .\n"
]
],
[
[
"From looking at the sentences, you can see they have been preprocessed already. The puncuations have been delimited using spaces. All the text have been converted to lowercase. This should save you some time, but the text requires more preprocessing.\n### Vocabulary\nThe complexity of the problem is determined by the complexity of the vocabulary. A more complex vocabulary is a more complex problem. Let's look at the complexity of the dataset we'll be working with.",
"_____no_output_____"
]
],
[
[
"english_words_counter = collections.Counter([word for sentence in english_sentences for word in sentence.split()])\nfrench_words_counter = collections.Counter([word for sentence in french_sentences for word in sentence.split()])\n\nprint('{} English words.'.format(len([word for sentence in english_sentences for word in sentence.split()])))\nprint('{} unique English words.'.format(len(english_words_counter)))\nprint('10 Most common words in the English dataset:')\nprint('\"' + '\" \"'.join(list(zip(*english_words_counter.most_common(10)))[0]) + '\"')\nprint()\nprint('{} French words.'.format(len([word for sentence in french_sentences for word in sentence.split()])))\nprint('{} unique French words.'.format(len(french_words_counter)))\nprint('10 Most common words in the French dataset:')\nprint('\"' + '\" \"'.join(list(zip(*french_words_counter.most_common(10)))[0]) + '\"')",
"1823250 English words.\n227 unique English words.\n10 Most common words in the English dataset:\n\"is\" \",\" \".\" \"in\" \"it\" \"during\" \"the\" \"but\" \"and\" \"sometimes\"\n\n1961295 French words.\n355 unique French words.\n10 Most common words in the French dataset:\n\"est\" \".\" \",\" \"en\" \"il\" \"les\" \"mais\" \"et\" \"la\" \"parfois\"\n"
]
],
[
[
"For comparison, _Alice's Adventures in Wonderland_ contains 2,766 unique words of a total of 15,500 words.\n## Preprocess\nFor this project, you won't use text data as input to your model. Instead, you'll convert the text into sequences of integers using the following preprocess methods:\n1. Tokenize the words into ids\n2. Add padding to make all the sequences the same length.\n\nTime to start preprocessing the data...\n### Tokenize (IMPLEMENTATION)\nFor a neural network to predict on text data, it first has to be turned into data it can understand. Text data like \"dog\" is a sequence of ASCII character encodings. Since a neural network is a series of multiplication and addition operations, the input data needs to be number(s).\n\nWe can turn each character into a number or each word into a number. These are called character and word ids, respectively. Character ids are used for character level models that generate text predictions for each character. A word level model uses word ids that generate text predictions for each word. Word level models tend to learn better, since they are lower in complexity, so we'll use those.\n\nTurn each sentence into a sequence of words ids using Keras's [`Tokenizer`](https://keras.io/preprocessing/text/#tokenizer) function. Use this function to tokenize `english_sentences` and `french_sentences` in the cell below.\n\nRunning the cell will run `tokenize` on sample data and show output for debugging.",
"_____no_output_____"
]
],
[
[
"def tokenize(x):\n \"\"\"\n Tokenize x\n :param x: List of sentences/strings to be tokenized\n :return: Tuple of (tokenized x data, tokenizer used to tokenize x)\n \"\"\"\n # TODO: Implement\n tokenizer = Tokenizer()\n tokenizer.fit_on_texts(x)\n \n return tokenizer.texts_to_sequences(x), tokenizer\ntests.test_tokenize(tokenize)\n\n# Tokenize Example output\ntext_sentences = [\n 'The quick brown fox jumps over the lazy dog .',\n 'By Jove , my quick study of lexicography won a prize .',\n 'This is a short sentence .']\ntext_tokenized, text_tokenizer = tokenize(text_sentences)\nprint(text_tokenizer.word_index)\nprint()\nfor sample_i, (sent, token_sent) in enumerate(zip(text_sentences, text_tokenized)):\n print('Sequence {} in x'.format(sample_i + 1))\n print(' Input: {}'.format(sent))\n print(' Output: {}'.format(token_sent))",
"{'the': 1, 'quick': 2, 'a': 3, 'brown': 4, 'fox': 5, 'jumps': 6, 'over': 7, 'lazy': 8, 'dog': 9, 'by': 10, 'jove': 11, 'my': 12, 'study': 13, 'of': 14, 'lexicography': 15, 'won': 16, 'prize': 17, 'this': 18, 'is': 19, 'short': 20, 'sentence': 21}\n\nSequence 1 in x\n Input: The quick brown fox jumps over the lazy dog .\n Output: [1, 2, 4, 5, 6, 7, 1, 8, 9]\nSequence 2 in x\n Input: By Jove , my quick study of lexicography won a prize .\n Output: [10, 11, 12, 2, 13, 14, 15, 16, 3, 17]\nSequence 3 in x\n Input: This is a short sentence .\n Output: [18, 19, 3, 20, 21]\n"
]
],
[
[
"### Padding (IMPLEMENTATION)\nWhen batching the sequence of word ids together, each sequence needs to be the same length. Since sentences are dynamic in length, we can add padding to the end of the sequences to make them the same length.\n\nMake sure all the English sequences have the same length and all the French sequences have the same length by adding padding to the **end** of each sequence using Keras's [`pad_sequences`](https://keras.io/preprocessing/sequence/#pad_sequences) function.",
"_____no_output_____"
]
],
[
[
"def pad(x, length=None):\n \"\"\"\n Pad x\n :param x: List of sequences.\n :param length: Length to pad the sequence to. If None, use length of longest sequence in x.\n :return: Padded numpy array of sequences\n \"\"\"\n # TODO: Implement\n if length is None:\n length = max([len(sentence) for sentence in x])\n \n return pad_sequences(x, maxlen=length, padding='post')\ntests.test_pad(pad)\n\n# Pad Tokenized output\ntest_pad = pad(text_tokenized)\nfor sample_i, (token_sent, pad_sent) in enumerate(zip(text_tokenized, test_pad)):\n print('Sequence {} in x'.format(sample_i + 1))\n print(' Input: {}'.format(np.array(token_sent)))\n print(' Output: {}'.format(pad_sent))",
"Sequence 1 in x\n Input: [1 2 4 5 6 7 1 8 9]\n Output: [1 2 4 5 6 7 1 8 9 0]\nSequence 2 in x\n Input: [10 11 12 2 13 14 15 16 3 17]\n Output: [10 11 12 2 13 14 15 16 3 17]\nSequence 3 in x\n Input: [18 19 3 20 21]\n Output: [18 19 3 20 21 0 0 0 0 0]\n"
]
],
[
[
"### Preprocess Pipeline\nYour focus for this project is to build neural network architecture, so we won't ask you to create a preprocess pipeline. Instead, we've provided you with the implementation of the `preprocess` function.",
"_____no_output_____"
]
],
[
[
"def preprocess(x, y):\n \"\"\"\n Preprocess x and y\n :param x: Feature List of sentences\n :param y: Label List of sentences\n :return: Tuple of (Preprocessed x, Preprocessed y, x tokenizer, y tokenizer)\n \"\"\"\n preprocess_x, x_tk = tokenize(x)\n preprocess_y, y_tk = tokenize(y)\n\n preprocess_x = pad(preprocess_x)\n preprocess_y = pad(preprocess_y)\n\n # Keras's sparse_categorical_crossentropy function requires the labels to be in 3 dimensions\n preprocess_y = preprocess_y.reshape(*preprocess_y.shape, 1)\n\n return preprocess_x, preprocess_y, x_tk, y_tk\n\npreproc_english_sentences, preproc_french_sentences, english_tokenizer, french_tokenizer =\\\n preprocess(english_sentences, french_sentences)\n \nmax_english_sequence_length = preproc_english_sentences.shape[1]\nmax_french_sequence_length = preproc_french_sentences.shape[1]\nenglish_vocab_size = len(english_tokenizer.word_index)\nfrench_vocab_size = len(french_tokenizer.word_index)\n\nprint('Data Preprocessed')\nprint(\"Max English sentence length:\", max_english_sequence_length)\nprint(\"Max French sentence length:\", max_french_sequence_length)\nprint(\"English vocabulary size:\", english_vocab_size)\nprint(\"French vocabulary size:\", french_vocab_size)",
"Data Preprocessed\nMax English sentence length: 15\nMax French sentence length: 21\nEnglish vocabulary size: 199\nFrench vocabulary size: 344\n"
]
],
[
[
"## Models\nIn this section, you will experiment with various neural network architectures.\nYou will begin by training four relatively simple architectures.\n- Model 1 is a simple RNN\n- Model 2 is a RNN with Embedding\n- Model 3 is a Bidirectional RNN\n- Model 4 is an optional Encoder-Decoder RNN\n\nAfter experimenting with the four simple architectures, you will construct a deeper architecture that is designed to outperform all four models.\n### Ids Back to Text\nThe neural network will be translating the input to words ids, which isn't the final form we want. We want the French translation. The function `logits_to_text` will bridge the gab between the logits from the neural network to the French translation. You'll be using this function to better understand the output of the neural network.",
"_____no_output_____"
]
],
[
[
"def logits_to_text(logits, tokenizer):\n \"\"\"\n Turn logits from a neural network into text using the tokenizer\n :param logits: Logits from a neural network\n :param tokenizer: Keras Tokenizer fit on the labels\n :return: String that represents the text of the logits\n \"\"\"\n index_to_words = {id: word for word, id in tokenizer.word_index.items()}\n index_to_words[0] = '<PAD>'\n\n return ' '.join([index_to_words[prediction] for prediction in np.argmax(logits, 1)])\n\nprint('`logits_to_text` function loaded.')",
"`logits_to_text` function loaded.\n"
]
],
[
[
"### Model 1: RNN (IMPLEMENTATION)\n\nA basic RNN model is a good baseline for sequence data. In this model, you'll build a RNN that translates English to French.",
"_____no_output_____"
]
],
[
[
"def simple_model(input_shape, output_sequence_length, english_vocab_size, french_vocab_size):\n \"\"\"\n Build and train a basic RNN on x and y\n :param input_shape: Tuple of input shape\n :param output_sequence_length: Length of output sequence\n :param english_vocab_size: Number of unique English words in the dataset\n :param french_vocab_size: Number of unique French words in the dataset\n :return: Keras model built, but not trained\n \"\"\"\n # TODO: Build the layers\n inputs = Input(input_shape[1:])\n outputs = GRU(256, return_sequences=True)(inputs) \n outputs = TimeDistributed(Dense(french_vocab_size, activation=\"softmax\"))(outputs)\n \n model = Model(inputs, outputs)\n \n learning_rate = 0.001\n model.compile(loss=sparse_categorical_crossentropy,\n optimizer=Adam(learning_rate),\n metrics=['accuracy'])\n return model\ntests.test_simple_model(simple_model)\n\n# Reshaping the input to work with a basic RNN\ntmp_x = pad(preproc_english_sentences, max_french_sequence_length)\ntmp_x = tmp_x.reshape((-1, preproc_french_sentences.shape[-2], 1))\n\n# Train the neural network\nsimple_rnn_model = simple_model(\n tmp_x.shape,\n max_french_sequence_length,\n english_vocab_size,\n french_vocab_size)\nsimple_rnn_model.fit(tmp_x, preproc_french_sentences, batch_size=1024, epochs=10, validation_split=0.2)\n\n# Print prediction(s)\nprint(logits_to_text(simple_rnn_model.predict(tmp_x[:1])[0], french_tokenizer))",
"Train on 110288 samples, validate on 27573 samples\nEpoch 1/10\n110288/110288 [==============================] - 12s 109us/step - loss: 2.5907 - acc: 0.4812 - val_loss: nan - val_acc: 0.5582\nEpoch 2/10\n110288/110288 [==============================] - 10s 91us/step - loss: 1.6567 - acc: 0.5862 - val_loss: nan - val_acc: 0.6038\nEpoch 3/10\n110288/110288 [==============================] - 10s 91us/step - loss: 1.4347 - acc: 0.6124 - val_loss: nan - val_acc: 0.6220\nEpoch 4/10\n110288/110288 [==============================] - 10s 91us/step - loss: 1.3146 - acc: 0.6324 - val_loss: nan - val_acc: 0.6414\nEpoch 5/10\n110288/110288 [==============================] - 10s 91us/step - loss: 1.2245 - acc: 0.6483 - val_loss: nan - val_acc: 0.6516\nEpoch 6/10\n110288/110288 [==============================] - 10s 92us/step - loss: 1.1588 - acc: 0.6597 - val_loss: nan - val_acc: 0.6692\nEpoch 7/10\n110288/110288 [==============================] - 10s 92us/step - loss: 1.1090 - acc: 0.6685 - val_loss: nan - val_acc: 0.6745\nEpoch 8/10\n110288/110288 [==============================] - 10s 91us/step - loss: 1.0710 - acc: 0.6729 - val_loss: nan - val_acc: 0.6732\nEpoch 9/10\n110288/110288 [==============================] - 10s 91us/step - loss: 1.0381 - acc: 0.6766 - val_loss: nan - val_acc: 0.6822\nEpoch 10/10\n110288/110288 [==============================] - 10s 91us/step - loss: 1.0106 - acc: 0.6810 - val_loss: nan - val_acc: 0.6816\nnew jersey est parfois calme en mois et il est il est en en <PAD> <PAD> <PAD> <PAD> <PAD> <PAD> <PAD>\n"
]
],
[
[
"### Model 2: Embedding (IMPLEMENTATION)\n\nYou've turned the words into ids, but there's a better representation of a word. This is called word embeddings. An embedding is a vector representation of the word that is close to similar words in n-dimensional space, where the n represents the size of the embedding vectors.\n\nIn this model, you'll create a RNN model using embedding.",
"_____no_output_____"
]
],
[
[
"def embed_model(input_shape, output_sequence_length, english_vocab_size, french_vocab_size):\n \"\"\"\n Build and train a RNN model using word embedding on x and y\n :param input_shape: Tuple of input shape\n :param output_sequence_length: Length of output sequence\n :param english_vocab_size: Number of unique English words in the dataset\n :param french_vocab_size: Number of unique French words in the dataset\n :return: Keras model built, but not trained\n \"\"\"\n # TODO: Implement\n inputs = Input(input_shape[1:])\n \n outputs = Embedding(english_vocab_size, output_sequence_length)(inputs)\n outputs = GRU(256, return_sequences=True)(outputs) \n outputs = TimeDistributed(Dense(french_vocab_size, activation=\"softmax\"))(outputs)\n \n model = Model(inputs, outputs)\n \n learning_rate = 0.001\n model.compile(loss=sparse_categorical_crossentropy,\n optimizer=Adam(learning_rate),\n metrics=['accuracy'])\n return model\ntests.test_embed_model(embed_model)\n\n\n# TODO: Reshape the input\ntmp_x = pad(preproc_english_sentences, preproc_french_sentences.shape[1])\ntmp_x = tmp_x.reshape((-1, preproc_french_sentences.shape[-2]))\n\n# TODO: Train the neural network\nembed_rnn_model = embed_model(\n tmp_x.shape,\n preproc_french_sentences.shape[1],\n english_vocab_size,\n french_vocab_size)\n\nembed_rnn_model.fit(tmp_x, preproc_french_sentences, batch_size=1024, epochs=10, validation_split=0.2)\n\n# TODO: Print prediction(s)\nprint(logits_to_text(embed_rnn_model.predict(tmp_x[:1])[0], french_tokenizer))",
"Train on 110288 samples, validate on 27573 samples\nEpoch 1/10\n110288/110288 [==============================] - 12s 104us/step - loss: 3.3620 - acc: 0.4088 - val_loss: nan - val_acc: 0.4603\nEpoch 2/10\n110288/110288 [==============================] - 11s 102us/step - loss: 2.5504 - acc: 0.4842 - val_loss: nan - val_acc: 0.5128\nEpoch 3/10\n110288/110288 [==============================] - 11s 102us/step - loss: 2.0617 - acc: 0.5411 - val_loss: nan - val_acc: 0.5635\nEpoch 4/10\n110288/110288 [==============================] - 11s 101us/step - loss: 1.6021 - acc: 0.6006 - val_loss: nan - val_acc: 0.6337\nEpoch 5/10\n110288/110288 [==============================] - 11s 102us/step - loss: 1.3343 - acc: 0.6570 - val_loss: nan - val_acc: 0.6817\nEpoch 6/10\n110288/110288 [==============================] - 11s 102us/step - loss: 1.1285 - acc: 0.7028 - val_loss: nan - val_acc: 0.7282\nEpoch 7/10\n110288/110288 [==============================] - 11s 102us/step - loss: 0.9586 - acc: 0.7497 - val_loss: nan - val_acc: 0.7712\nEpoch 8/10\n110288/110288 [==============================] - 11s 101us/step - loss: 0.8094 - acc: 0.7879 - val_loss: nan - val_acc: 0.8058\nEpoch 9/10\n110288/110288 [==============================] - 11s 101us/step - loss: 0.6796 - acc: 0.8175 - val_loss: nan - val_acc: 0.8296\nEpoch 10/10\n110288/110288 [==============================] - 11s 101us/step - loss: 0.5832 - acc: 0.8390 - val_loss: nan - val_acc: 0.8477\nnew jersey est parfois calme en l' et il est il est en <PAD> <PAD> <PAD> <PAD> <PAD> <PAD> <PAD> <PAD>\n"
]
],
[
[
"### Model 3: Bidirectional RNNs (IMPLEMENTATION)\n\nOne restriction of a RNN is that it can't see the future input, only the past. This is where bidirectional recurrent neural networks come in. They are able to see the future data.",
"_____no_output_____"
]
],
[
[
"def bd_model(input_shape, output_sequence_length, english_vocab_size, french_vocab_size):\n \"\"\"\n Build and train a bidirectional RNN model on x and y\n :param input_shape: Tuple of input shape\n :param output_sequence_length: Length of output sequence\n :param english_vocab_size: Number of unique English words in the dataset\n :param french_vocab_size: Number of unique French words in the dataset\n :return: Keras model built, but not trained\n \"\"\"\n # TODO: Implement\n inputs = Input(input_shape[1:])\n outputs = Bidirectional(GRU(256, return_sequences=True))(inputs) \n outputs = TimeDistributed(Dense(french_vocab_size, activation=\"softmax\"))(outputs)\n \n model = Model(inputs, outputs)\n \n learning_rate = 0.001\n model.compile(loss=sparse_categorical_crossentropy,\n optimizer=Adam(learning_rate),\n metrics=['accuracy'])\n return model\ntests.test_bd_model(bd_model)\n\n\n# TODO: Train and Print prediction(s)\ntmp_x = pad(preproc_english_sentences, preproc_french_sentences.shape[1])\ntmp_x = tmp_x.reshape((-1, preproc_french_sentences.shape[-2]))\n\nbd_rnn_model = embed_model(\n tmp_x.shape,\n preproc_french_sentences.shape[1],\n english_vocab_size,\n french_vocab_size)\n\nbd_rnn_model.fit(tmp_x, preproc_french_sentences, batch_size=1024, epochs=10, validation_split=0.2)\n\nprint(logits_to_text(bd_rnn_model.predict(tmp_x[:1])[0], french_tokenizer))",
"Train on 110288 samples, validate on 27573 samples\nEpoch 1/10\n110288/110288 [==============================] - 12s 106us/step - loss: 3.3402 - acc: 0.4192 - val_loss: nan - val_acc: 0.4769\nEpoch 2/10\n110288/110288 [==============================] - 11s 101us/step - loss: 2.4795 - acc: 0.4908 - val_loss: nan - val_acc: 0.5142\nEpoch 3/10\n110288/110288 [==============================] - 11s 101us/step - loss: 1.9030 - acc: 0.5528 - val_loss: nan - val_acc: 0.5912\nEpoch 4/10\n110288/110288 [==============================] - 11s 101us/step - loss: 1.4347 - acc: 0.6258 - val_loss: nan - val_acc: 0.6628\nEpoch 5/10\n110288/110288 [==============================] - 11s 102us/step - loss: 1.1867 - acc: 0.6922 - val_loss: nan - val_acc: 0.7193\nEpoch 6/10\n110288/110288 [==============================] - 11s 102us/step - loss: 0.9938 - acc: 0.7419 - val_loss: nan - val_acc: 0.7685\nEpoch 7/10\n110288/110288 [==============================] - 11s 101us/step - loss: 0.8202 - acc: 0.7881 - val_loss: nan - val_acc: 0.8065\nEpoch 8/10\n110288/110288 [==============================] - 11s 101us/step - loss: 0.6827 - acc: 0.8198 - val_loss: nan - val_acc: 0.8325\nEpoch 9/10\n110288/110288 [==============================] - 11s 101us/step - loss: 0.5852 - acc: 0.8410 - val_loss: nan - val_acc: 0.8509\nEpoch 10/10\n110288/110288 [==============================] - 11s 101us/step - loss: 0.5119 - acc: 0.8583 - val_loss: nan - val_acc: 0.8654\nnew jersey est parfois calme en l' et et il et il avril <PAD> <PAD> <PAD> <PAD> <PAD> <PAD> <PAD> <PAD>\n"
]
],
[
[
"### Model 4: Encoder-Decoder (OPTIONAL)\nTime to look at encoder-decoder models. This model is made up of an encoder and decoder. The encoder creates a matrix representation of the sentence. The decoder takes this matrix as input and predicts the translation as output.\n\nCreate an encoder-decoder model in the cell below.",
"_____no_output_____"
]
],
[
[
"def encdec_model(input_shape, output_sequence_length, english_vocab_size, french_vocab_size):\n \"\"\"\n Build and train an encoder-decoder model on x and y\n :param input_shape: Tuple of input shape\n :param output_sequence_length: Length of output sequence\n :param english_vocab_size: Number of unique English words in the dataset\n :param french_vocab_size: Number of unique French words in the dataset\n :return: Keras model built, but not trained\n \"\"\"\n # OPTIONAL: Implement\n inputs = Input(input_shape[1:])\n encoded = GRU(256, return_sequences=False)(inputs)\n \n decoded = RepeatVector(output_sequence_length)(encoded)\n \n outputs = GRU(256, return_sequences=True)(decoded)\n outputs = Dense(french_vocab_size, activation=\"softmax\")(outputs)\n \n model = Model(inputs, outputs)\n \n learning_rate = 0.001\n model.compile(loss=sparse_categorical_crossentropy,\n optimizer=Adam(learning_rate),\n metrics=['accuracy'])\n return model\ntests.test_encdec_model(encdec_model)\n\n\n# OPTIONAL: Train and Print prediction(s)\ntmp_x = pad(preproc_english_sentences, preproc_french_sentences.shape[1])\ntmp_x = tmp_x.reshape((-1, preproc_french_sentences.shape[-2]))\n\nencdec_rnn_model = embed_model(\n tmp_x.shape,\n preproc_french_sentences.shape[1],\n english_vocab_size,\n french_vocab_size)\n\nencdec_rnn_model.fit(tmp_x, preproc_french_sentences, batch_size=1024, epochs=10, validation_split=0.2)\n\nprint(logits_to_text(encdec_rnn_model.predict(tmp_x[:1])[0], french_tokenizer))",
"Train on 110288 samples, validate on 27573 samples\nEpoch 1/10\n110288/110288 [==============================] - 12s 106us/step - loss: 3.3761 - acc: 0.4076 - val_loss: nan - val_acc: 0.4515\nEpoch 2/10\n110288/110288 [==============================] - 11s 100us/step - loss: 2.5353 - acc: 0.4798 - val_loss: nan - val_acc: 0.5145\nEpoch 3/10\n110288/110288 [==============================] - 11s 101us/step - loss: 2.0491 - acc: 0.5406 - val_loss: nan - val_acc: 0.5683\nEpoch 4/10\n110288/110288 [==============================] - 11s 101us/step - loss: 1.5999 - acc: 0.5997 - val_loss: nan - val_acc: 0.6365\nEpoch 5/10\n110288/110288 [==============================] - 11s 101us/step - loss: 1.3258 - acc: 0.6664 - val_loss: nan - val_acc: 0.6911\nEpoch 6/10\n110288/110288 [==============================] - 11s 101us/step - loss: 1.1065 - acc: 0.7156 - val_loss: nan - val_acc: 0.7447\nEpoch 7/10\n110288/110288 [==============================] - 11s 101us/step - loss: 0.9247 - acc: 0.7625 - val_loss: nan - val_acc: 0.7824\nEpoch 8/10\n110288/110288 [==============================] - 11s 101us/step - loss: 0.7729 - acc: 0.7968 - val_loss: nan - val_acc: 0.8140\nEpoch 9/10\n110288/110288 [==============================] - 11s 101us/step - loss: 0.6530 - acc: 0.8240 - val_loss: nan - val_acc: 0.8355\nEpoch 10/10\n110288/110288 [==============================] - 11s 101us/step - loss: 0.5646 - acc: 0.8437 - val_loss: nan - val_acc: 0.8511\nnew jersey est parfois calme en l' et il est neigeux en avril <PAD> <PAD> <PAD> <PAD> <PAD> <PAD> <PAD> <PAD>\n"
]
],
[
[
"### Model 5: Custom (IMPLEMENTATION)\nUse everything you learned from the previous models to create a model that incorporates embedding and a bidirectional rnn into one model.",
"_____no_output_____"
]
],
[
[
"def model_final(input_shape, output_sequence_length, english_vocab_size, french_vocab_size):\n \"\"\"\n Build and train a model that incorporates embedding, encoder-decoder, and bidirectional RNN on x and y\n :param input_shape: Tuple of input shape\n :param output_sequence_length: Length of output sequence\n :param english_vocab_size: Number of unique English words in the dataset\n :param french_vocab_size: Number of unique French words in the dataset\n :return: Keras model built, but not trained\n \"\"\"\n # TODO: Implement\n learning_rate = 0.001\n \n inputs = Input(shape=input_shape[1:])\n \n encoded = Embedding(english_vocab_size, 300)(inputs)\n encoded = Bidirectional(GRU(512, dropout=0.2))(encoded)\n encoded = Dense(512, activation='relu')(encoded)\n \n decoded = RepeatVector(output_sequence_length)(encoded)\n decoded = Bidirectional(GRU(512, dropout=0.2, return_sequences=True))(decoded)\n decoded = TimeDistributed(Dense(french_vocab_size))(decoded)\n \n predictions = Activation('softmax')(decoded)\n model = Model(inputs=inputs, outputs=predictions)\n \n model.compile(loss=sparse_categorical_crossentropy,\n optimizer=Adam(learning_rate),\n metrics=['accuracy'])\n return model\ntests.test_model_final(model_final)\n\n\nprint('Final Model Loaded')\n# TODO: Train the final model\ntmp_x = pad(preproc_english_sentences, preproc_french_sentences.shape[1])\ntmp_x = tmp_x.reshape((-1, preproc_french_sentences.shape[-2]))\n\nfinal_model = model_final(\n tmp_x.shape,\n preproc_french_sentences.shape[1],\n english_vocab_size,\n french_vocab_size)\n\nfinal_model.fit(tmp_x, preproc_french_sentences, batch_size=1024, epochs=10, validation_split=0.2)\nprint(logits_to_text(encdec_rnn_model.predict(tmp_x[:1])[0], french_tokenizer))",
"Final Model Loaded\nTrain on 110288 samples, validate on 27573 samples\nEpoch 1/10\n110288/110288 [==============================] - 91s 824us/step - loss: 2.4410 - acc: 0.4865 - val_loss: nan - val_acc: 0.5841\nEpoch 2/10\n110288/110288 [==============================] - 89s 807us/step - loss: 1.4055 - acc: 0.6253 - val_loss: nan - val_acc: 0.6683\nEpoch 3/10\n110288/110288 [==============================] - 89s 806us/step - loss: 1.0897 - acc: 0.6953 - val_loss: nan - val_acc: 0.7250\nEpoch 4/10\n110288/110288 [==============================] - 89s 806us/step - loss: 0.9146 - acc: 0.7333 - val_loss: nan - val_acc: 0.7512\nEpoch 5/10\n110288/110288 [==============================] - 89s 806us/step - loss: 0.7888 - acc: 0.7662 - val_loss: nan - val_acc: 0.7899\nEpoch 6/10\n110288/110288 [==============================] - 89s 806us/step - loss: 0.6854 - acc: 0.7937 - val_loss: nan - val_acc: 0.8163\nEpoch 7/10\n110288/110288 [==============================] - 89s 806us/step - loss: 0.5770 - acc: 0.8241 - val_loss: nan - val_acc: 0.8468\nEpoch 8/10\n110288/110288 [==============================] - 89s 805us/step - loss: 0.4818 - acc: 0.8538 - val_loss: nan - val_acc: 0.8753\nEpoch 9/10\n110288/110288 [==============================] - 89s 805us/step - loss: 0.3899 - acc: 0.8836 - val_loss: nan - val_acc: 0.9093\nEpoch 10/10\n110288/110288 [==============================] - 89s 805us/step - loss: 0.3099 - acc: 0.9085 - val_loss: nan - val_acc: 0.9293\nnew jersey est parfois calme en l' et il est neigeux en avril <PAD> <PAD> <PAD> <PAD> <PAD> <PAD> <PAD> <PAD>\n"
]
],
[
[
"## Prediction (IMPLEMENTATION)",
"_____no_output_____"
]
],
[
[
"def final_predictions(x, y, x_tk, y_tk):\n \"\"\"\n Gets predictions using the final model\n :param x: Preprocessed English data\n :param y: Preprocessed French data\n :param x_tk: English tokenizer\n :param y_tk: French tokenizer\n \"\"\"\n # TODO: Train neural network using model_final\n model = model_final(x.shape, \n y.shape[1], \n len(x_tk.word_index), \n len(y_tk.word_index))\n \n model.fit(x, y, batch_size=1024, epochs=12, validation_split=0.2)\n\n \n ## DON'T EDIT ANYTHING BELOW THIS LINE\n y_id_to_word = {value: key for key, value in y_tk.word_index.items()}\n y_id_to_word[0] = '<PAD>'\n\n sentence = 'he saw a old yellow truck'\n sentence = [x_tk.word_index[word] for word in sentence.split()]\n sentence = pad_sequences([sentence], maxlen=x.shape[-1], padding='post')\n sentences = np.array([sentence[0], x[0]])\n predictions = model.predict(sentences, len(sentences))\n\n print('Sample 1:')\n print(' '.join([y_id_to_word[np.argmax(x)] for x in predictions[0]]))\n print('Il a vu un vieux camion jaune')\n print('Sample 2:')\n print(' '.join([y_id_to_word[np.argmax(x)] for x in predictions[1]]))\n print(' '.join([y_id_to_word[np.max(x)] for x in y[0]]))\n\n\nfinal_predictions(preproc_english_sentences, preproc_french_sentences, english_tokenizer, french_tokenizer)",
"Train on 110288 samples, validate on 27573 samples\nEpoch 1/12\n110288/110288 [==============================] - 81s 733us/step - loss: 2.3220 - acc: 0.5011 - val_loss: nan - val_acc: 0.5999\nEpoch 2/12\n110288/110288 [==============================] - 79s 713us/step - loss: 1.3477 - acc: 0.6375 - val_loss: nan - val_acc: 0.6822\nEpoch 3/12\n110288/110288 [==============================] - 79s 713us/step - loss: 1.0208 - acc: 0.7100 - val_loss: nan - val_acc: 0.7388\nEpoch 4/12\n110288/110288 [==============================] - 79s 713us/step - loss: 0.8375 - acc: 0.7518 - val_loss: nan - val_acc: 0.7783\nEpoch 5/12\n110288/110288 [==============================] - 79s 713us/step - loss: 0.7114 - acc: 0.7849 - val_loss: nan - val_acc: 0.8114\nEpoch 6/12\n110288/110288 [==============================] - 79s 714us/step - loss: 0.5968 - acc: 0.8179 - val_loss: nan - val_acc: 0.8416\nEpoch 7/12\n110288/110288 [==============================] - 79s 713us/step - loss: 0.4868 - acc: 0.8513 - val_loss: nan - val_acc: 0.8810\nEpoch 8/12\n110288/110288 [==============================] - 79s 713us/step - loss: 0.3818 - acc: 0.8846 - val_loss: nan - val_acc: 0.9105\nEpoch 9/12\n110288/110288 [==============================] - 79s 713us/step - loss: 0.2995 - acc: 0.9113 - val_loss: nan - val_acc: 0.9290\nEpoch 10/12\n110288/110288 [==============================] - 79s 713us/step - loss: 0.2387 - acc: 0.9299 - val_loss: nan - val_acc: 0.9451\nEpoch 11/12\n110288/110288 [==============================] - 79s 713us/step - loss: 0.1893 - acc: 0.9444 - val_loss: nan - val_acc: 0.9505\nEpoch 12/12\n110288/110288 [==============================] - 79s 713us/step - loss: 0.1621 - acc: 0.9521 - val_loss: nan - val_acc: 0.9566\nSample 1:\nil a vu un vieux camion jaune <PAD> <PAD> <PAD> <PAD> <PAD> <PAD> <PAD> <PAD> <PAD> <PAD> <PAD> <PAD> <PAD> <PAD>\nIl a vu un vieux camion jaune\nSample 2:\nnew jersey est parfois calme pendant l' automne et il est neigeux en avril <PAD> <PAD> <PAD> <PAD> <PAD> <PAD> <PAD>\nnew jersey est parfois calme pendant l' automne et il est neigeux en avril <PAD> <PAD> <PAD> <PAD> <PAD> <PAD> <PAD>\n"
]
],
[
[
"## Submission\nWhen you're ready to submit, complete the following steps:\n1. Review the [rubric](https://review.udacity.com/#!/rubrics/1004/view) to ensure your submission meets all requirements to pass\n2. Generate an HTML version of this notebook\n\n - Run the next cell to attempt automatic generation (this is the recommended method in Workspaces)\n - Navigate to **FILE -> Download as -> HTML (.html)**\n - Manually generate a copy using `nbconvert` from your shell terminal\n```\n$ pip install nbconvert\n$ python -m nbconvert machine_translation.ipynb\n```\n \n3. Submit the project\n\n - If you are in a Workspace, simply click the \"Submit Project\" button (bottom towards the right)\n \n - Otherwise, add the following files into a zip archive and submit them \n - `helper.py`\n - `machine_translation.ipynb`\n - `machine_translation.html`\n - You can export the notebook by navigating to **File -> Download as -> HTML (.html)**.",
"_____no_output_____"
],
[
"### Generate the html\n\n**Save your notebook before running the next cell to generate the HTML output.** Then submit your project.",
"_____no_output_____"
]
],
[
[
"# Save before you run this cell!\n!!jupyter nbconvert *.ipynb",
"_____no_output_____"
]
],
[
[
"## Optional Enhancements\n\nThis project focuses on learning various network architectures for machine translation, but we don't evaluate the models according to best practices by splitting the data into separate test & training sets -- so the model accuracy is overstated. Use the [`sklearn.model_selection.train_test_split()`](http://scikit-learn.org/stable/modules/generated/sklearn.model_selection.train_test_split.html) function to create separate training & test datasets, then retrain each of the models using only the training set and evaluate the prediction accuracy using the hold out test set. Does the \"best\" model change?",
"_____no_output_____"
]
]
] | [
"markdown",
"code",
"markdown",
"code",
"markdown",
"code",
"markdown",
"code",
"markdown",
"code",
"markdown",
"code",
"markdown",
"code",
"markdown",
"code",
"markdown",
"code",
"markdown",
"code",
"markdown",
"code",
"markdown",
"code",
"markdown",
"code",
"markdown",
"code",
"markdown",
"code",
"markdown",
"code",
"markdown"
] | [
[
"markdown"
],
[
"code",
"code"
],
[
"markdown"
],
[
"code"
],
[
"markdown"
],
[
"code"
],
[
"markdown"
],
[
"code"
],
[
"markdown"
],
[
"code"
],
[
"markdown"
],
[
"code"
],
[
"markdown"
],
[
"code"
],
[
"markdown"
],
[
"code"
],
[
"markdown"
],
[
"code"
],
[
"markdown"
],
[
"code"
],
[
"markdown"
],
[
"code"
],
[
"markdown"
],
[
"code"
],
[
"markdown"
],
[
"code"
],
[
"markdown"
],
[
"code"
],
[
"markdown"
],
[
"code"
],
[
"markdown",
"markdown"
],
[
"code"
],
[
"markdown"
]
] |
d07842e5fbc29a2dfae53e679737feb19056714f | 606,287 | ipynb | Jupyter Notebook | Scratch Folder/financial-planner-v4.ipynb | HassanAlam55/ColumbiaFintechHW5API | d123e61c4b2dc83aaf6266e52c64822079856dee | [
"Apache-2.0"
] | null | null | null | Scratch Folder/financial-planner-v4.ipynb | HassanAlam55/ColumbiaFintechHW5API | d123e61c4b2dc83aaf6266e52c64822079856dee | [
"Apache-2.0"
] | null | null | null | Scratch Folder/financial-planner-v4.ipynb | HassanAlam55/ColumbiaFintechHW5API | d123e61c4b2dc83aaf6266e52c64822079856dee | [
"Apache-2.0"
] | null | null | null | 86.736338 | 145,872 | 0.720867 | [
[
[
"# Unit 5 - Financial Planning\n",
"_____no_output_____"
]
],
[
[
"# Initial imports\nimport os\nimport requests\nimport pandas as pd\nfrom dotenv import load_dotenv\nimport alpaca_trade_api as tradeapi\nfrom MCForecastTools import MCSimulation\n\n# date here\nfrom datetime import date\n\n\n%matplotlib inline",
"_____no_output_____"
],
[
"# Load .env enviroment variables\nload_dotenv()",
"_____no_output_____"
]
],
[
[
"## Part 1 - Personal Finance Planner",
"_____no_output_____"
],
[
"## Collect Crypto Prices Using the `requests` Library",
"_____no_output_____"
]
],
[
[
"# Set current amount of crypto assets\n# YOUR CODE HERE!\nmy_btc = 1.2\nmy_eth = 5.3",
"_____no_output_____"
],
[
"# Crypto API URLs\nbtc_url = \"https://api.alternative.me/v2/ticker/Bitcoin/?convert=CAD\"\neth_url = \"https://api.alternative.me/v2/ticker/Ethereum/?convert=CAD\"",
"_____no_output_____"
],
[
"# response_data = requests.get(create_deck_url).json()\n# response_data\nbtc_resp = requests.get(btc_url).json()\nbtc_price = btc_resp['data']['1']['quotes']['USD']['price']\nmy_btc_value =my_btc * btc_price\n\neth_resp = requests.get(eth_url).json()\neth_price = eth_resp['data']['1027']['quotes']['USD']['price']\nmy_eth_value = my_eth * eth_price\n\nprint(f\"The current value of your {my_btc} BTC is ${my_btc_value:0.2f}\")\nprint(f\"The current value of your {my_eth} ETH is ${my_eth_value:0.2f}\")",
"The current value of your 1.2 BTC is $60097.20\nThe current value of your 5.3 ETH is $11897.07\n"
]
],
[
[
"### Collect Investments Data Using Alpaca: `SPY` (stocks) and `AGG` (bonds)",
"_____no_output_____"
]
],
[
[
"# Current amount of shares\n# Create two variables named my_agg and my_spy and set them equal to 200 and 50, respectively.\n# YOUR CODE HERE!\nmy_agg = 200\nmy_spy = 50",
"_____no_output_____"
],
[
"# Set Alpaca API key and secret\n# YOUR CODE HERE!\nalpaca_api_key = os.getenv(\"ALPACA_API_KEY\")\nalpaca_secret_key = os.getenv(\"ALPACA_SECRET_KEY\")\n\n# Create the Alpaca API object\n# YOUR CODE HERE!\napi = tradeapi.REST(\n alpaca_api_key,\n alpaca_secret_key,\n api_version = \"v2\"\n)",
"_____no_output_____"
],
[
"# Format current date as ISO format\n# YOUR CODE HERE!\n# use \"2021-04-16\" so weekend gives actual data\nstart_date = pd.Timestamp(\"2021-04-16\", tz=\"America/New_York\").isoformat()\ntoday_date = pd.Timestamp(date.today(), tz=\"America/New_York\").isoformat()\n\n# Set the tickers\ntickers = [\"AGG\", \"SPY\"]\n\n# Set timeframe to '1D' for Alpaca API\ntimeframe = \"1D\"\n\n# Get current closing prices for SPY and AGG\n# YOUR CODE HERE!\nticker_data = api.get_barset(\n tickers,\n timeframe,\n start=start_date,\n end=start_date,\n).df\n# Preview DataFrame\n# YOUR CODE HERE!\nticker_data",
"_____no_output_____"
],
[
"# Pick AGG and SPY close prices\n# YOUR CODE HERE!\nagg_close_price = ticker_data['AGG']['close'][0]\nspy_close_price = ticker_data['SPY']['close'][0]\n\n# Print AGG and SPY close prices\nprint(f\"Current AGG closing price: ${agg_close_price}\")\nprint(f\"Current SPY closing price: ${spy_close_price}\")\n",
"Current AGG closing price: $114.54\nCurrent SPY closing price: $417.31\n"
],
[
"# Compute the current value of shares\n# YOUR CODE HERE!\nmy_spy_value = spy_close_price * my_spy\nmy_agg_value = agg_close_price * my_agg\n\n# Print current value of share\nprint(f\"The current value of your {my_spy} SPY shares is ${my_spy_value:0.2f}\")\nprint(f\"The current value of your {my_agg} AGG shares is ${my_agg_value:0.2f}\")",
"The current value of your 50 SPY shares is $20865.50\nThe current value of your 200 AGG shares is $22908.00\n"
]
],
[
[
"### Savings Health Analysis",
"_____no_output_____"
]
],
[
[
"# Set monthly household income\n# YOUR CODE HERE!\nmonthly_income = 12000\n\n# Create savings DataFrame\n# YOUR CODE HERE!\ndf_savings = pd.DataFrame([my_btc_value+ my_eth_value, my_spy_value + my_agg_value], columns = ['amount'], index = ['crypto', 'shares'])\n\n# Display savings DataFrame\ndisplay(df_savings)",
"_____no_output_____"
],
[
"# Plot savings pie chart\n# YOUR CODE HERE!",
"_____no_output_____"
],
[
"df_savings['amount'].plot.pie(y= ['crypto', 'shares'])\n# how to put label",
"_____no_output_____"
],
[
"# Set ideal emergency fund\nemergency_fund = monthly_income * 3\n\n# Calculate total amount of savings\n# YOUR CODE HERE!\ntotal_saving = df_savings['amount'].sum()\n\n# Validate saving health\n# YOUR CODE HERE!\n\n# If total savings are greater than the emergency fund, display a message congratulating the person for having enough money in this fund.\n# If total savings are equal to the emergency fund, display a message congratulating the person on reaching this financial goal.\n# If total savings are less than the emergency fund, display a message showing how many dollars away the person is from reaching the goal.\n\nif total_saving > emergency_fund:\n print ('Congratulations! You have enough money in your emergency fund.')\nelif total_saving == emergency_fund:\n print ('Contratulations you reached your financial goals ')\nelse:\n print ('You are making great progress. You need to save $ {round((emergency_fund - total_saving), 2)}')",
"Congratulations! You have enough money in your emergency fund.\n"
]
],
[
[
"## Part 2 - Retirement Planning\n\n### Monte Carlo Simulation\n\n#### Hassan's Note for some reason AlPaca would not let me get more than 1000 records. To get 5 years data (252 * 5), I had to break it up into two reads and concat the data. ",
"_____no_output_____"
]
],
[
[
"# Set start and end dates of five years back from today.\n# Sample results may vary from the solution based on the time frame chosen\nstart_date1 = pd.Timestamp('2015-08-07', tz='America/New_York').isoformat()\nend_date1 = pd.Timestamp('2017-08-07', tz='America/New_York').isoformat()\nstart_date2 = pd.Timestamp('2017-08-08', tz='America/New_York').isoformat()\nend_date2 = pd.Timestamp('2020-08-07', tz='America/New_York').isoformat()\n# end_date1 = pd.Timestamp('2019-08-07', tz='America/New_York').isoformat()\n# hits 1000 item limit, have to do in two batches and pringt",
"_____no_output_____"
],
[
"# Get 5 years' worth of historical data for SPY and AGG\n# YOUR CODE HERE!\n\n# Display sample data\ndf_stock_data.head()",
"_____no_output_____"
],
[
"# Get 5 years' worth of historical data for SPY and AGG\n# create two dataframes and concaternate them \n# first period data frame\ndf_stock_data1 = api.get_barset(\n tickers,\n timeframe,\n start = start_date1,\n end = end_date1,\n limit = 1000\n).df\n\n# second period dataframe \ndf_stock_data2 = api.get_barset(\n tickers,\n timeframe,\n start = start_date2,\n end = end_date2,\n limit = 1000\n).df\n\ndf_stock_data = pd.concat ([df_stock_data1, df_stock_data2], axis = 0, join = 'inner')\nprint (f'stock data head: ')\nprint (df_stock_data.head(5))\nprint (f'\\nstock data 1 tail: ')\nprint (df_stock_data.tail(5))\n# print (f'stock data 1 head: {start_date1}')\n# print (df_stock_data1.head(5))\n# print (f'\\nstock data 1 tail: {end_date1}')\n# print (df_stock_data1.tail(5))\n# print (f'\\nstock data 2 head: {start_date2}')\n# print (df_stock_data2.head(5))\n# print (f'\\nstock data 2 tail: {end_date2}')\n# print (df_stock_data2.tail(5))\n",
"stock data head: \n AGG \\\n open high low close volume \ntime \n2015-08-07 00:00:00-04:00 109.14 109.2750 109.035 109.21 2041167.0 \n2015-08-10 00:00:00-04:00 109.15 109.1700 108.920 109.06 1149778.0 \n2015-08-11 00:00:00-04:00 109.42 109.5765 109.284 109.42 1420907.0 \n2015-08-12 00:00:00-04:00 109.55 109.7100 109.350 109.36 1468979.0 \n2015-08-13 00:00:00-04:00 109.36 109.3651 109.110 109.15 1465173.0 \n\n SPY \n open high low close volume \ntime \n2015-08-07 00:00:00-04:00 208.16 208.34 206.87 207.93 87669782 \n2015-08-10 00:00:00-04:00 209.28 210.67 209.28 210.58 66755890 \n2015-08-11 00:00:00-04:00 208.98 209.47 207.76 208.63 88424557 \n2015-08-12 00:00:00-04:00 207.11 209.14 205.36 208.89 136171450 \n2015-08-13 00:00:00-04:00 208.73 209.55 208.01 208.63 77197796 \n\nstock data 1 tail: \n AGG \\\n open high low close volume \ntime \n2020-08-03 00:00:00-04:00 119.37 119.40 119.1903 119.400 17837420.0 \n2020-08-04 00:00:00-04:00 119.42 119.63 119.4200 119.630 21512268.0 \n2020-08-05 00:00:00-04:00 119.39 119.49 119.3100 119.400 34175883.0 \n2020-08-06 00:00:00-04:00 119.62 119.73 119.5300 119.580 9009216.0 \n2020-08-07 00:00:00-04:00 119.66 119.73 119.3950 119.445 8830420.0 \n\n SPY \n open high low close volume \ntime \n2020-08-03 00:00:00-04:00 328.3200 329.62 327.73 328.76 71741125 \n2020-08-04 00:00:00-04:00 327.8600 330.06 327.86 330.03 73684427 \n2020-08-05 00:00:00-04:00 331.4700 332.39 331.18 332.06 72846458 \n2020-08-06 00:00:00-04:00 331.4799 334.46 331.13 334.31 76900649 \n2020-08-07 00:00:00-04:00 333.2800 334.88 332.30 334.55 98710236 \n"
],
[
"# delete\n# # Get 5 years' worth of historical data for SPY and AGG\n# # YOUR CODE HERE!\n# df_stock_data = api.get_barset(\n# tickers,\n# timeframe,\n# start = start_date,\n# end = end_date,\n# limit = 1000\n# ).df\n\n# # Display sample data\n\n# # to fix.\n# df_stock_data.head()",
"_____no_output_____"
],
[
"# Configuring a Monte Carlo simulation to forecast 30 years cumulative returns\n# YOUR CODE HERE!\nMC_even_dist = MCSimulation(\n portfolio_data = df_stock_data,\n weights = [.4, .6],\n num_simulation = 500,\n num_trading_days = 252*30\n)",
"_____no_output_____"
],
[
"# Printing the simulation input data\n# YOUR CODE HERE!",
"_____no_output_____"
],
[
"# Printing the simulation input data\n# YOUR CODE HERE!\nMC_even_dist.portfolio_data.head()",
"_____no_output_____"
],
[
"# Running a Monte Carlo simulation to forecast 30 years cumulative returns\n# YOUR CODE HERE!",
"_____no_output_____"
],
[
"# Running a Monte Carlo simulation to forecast 30 years cumulative returns\n# YOUR CODE HERE!\nMC_even_dist.calc_cumulative_return()",
"Running Monte Carlo simulation number 0.\nRunning Monte Carlo simulation number 10.\nRunning Monte Carlo simulation number 20.\nRunning Monte Carlo simulation number 30.\nRunning Monte Carlo simulation number 40.\nRunning Monte Carlo simulation number 50.\nRunning Monte Carlo simulation number 60.\nRunning Monte Carlo simulation number 70.\nRunning Monte Carlo simulation number 80.\nRunning Monte Carlo simulation number 90.\nRunning Monte Carlo simulation number 100.\nRunning Monte Carlo simulation number 110.\nRunning Monte Carlo simulation number 120.\nRunning Monte Carlo simulation number 130.\nRunning Monte Carlo simulation number 140.\nRunning Monte Carlo simulation number 150.\nRunning Monte Carlo simulation number 160.\nRunning Monte Carlo simulation number 170.\nRunning Monte Carlo simulation number 180.\nRunning Monte Carlo simulation number 190.\nRunning Monte Carlo simulation number 200.\nRunning Monte Carlo simulation number 210.\nRunning Monte Carlo simulation number 220.\nRunning Monte Carlo simulation number 230.\nRunning Monte Carlo simulation number 240.\nRunning Monte Carlo simulation number 250.\nRunning Monte Carlo simulation number 260.\nRunning Monte Carlo simulation number 270.\nRunning Monte Carlo simulation number 280.\nRunning Monte Carlo simulation number 290.\nRunning Monte Carlo simulation number 300.\nRunning Monte Carlo simulation number 310.\nRunning Monte Carlo simulation number 320.\nRunning Monte Carlo simulation number 330.\nRunning Monte Carlo simulation number 340.\nRunning Monte Carlo simulation number 350.\nRunning Monte Carlo simulation number 360.\nRunning Monte Carlo simulation number 370.\nRunning Monte Carlo simulation number 380.\nRunning Monte Carlo simulation number 390.\nRunning Monte Carlo simulation number 400.\nRunning Monte Carlo simulation number 410.\nRunning Monte Carlo simulation number 420.\nRunning Monte Carlo simulation number 430.\nRunning Monte Carlo simulation number 440.\nRunning Monte Carlo simulation number 450.\nRunning Monte Carlo simulation number 460.\nRunning Monte Carlo simulation number 470.\nRunning Monte Carlo simulation number 480.\nRunning Monte Carlo simulation number 490.\n"
],
[
"# Plot simulation outcomes\n# YOUR CODE HERE!",
"_____no_output_____"
],
[
"# Plot simulation outcomes\nline_plot = MC_even_dist.plot_simulation()",
"_____no_output_____"
],
[
"# delete\n# Plot probability distribution and confidence intervals\n# YOUR CODE HERE!",
"_____no_output_____"
],
[
"# Plot probability distribution and confidence intervals\n# YOUR CODE HERE!\ndist_plot = MC_even_dist.plot_distribution()",
"_____no_output_____"
]
],
[
[
"### Retirement Analysis",
"_____no_output_____"
]
],
[
[
"# delete\n# Fetch summary statistics from the Monte Carlo simulation results\n# YOUR CODE HERE!\n\n# Print summary statistics\n# YOUR CODE HERE!",
"_____no_output_____"
],
[
"# Fetch summary statistics from the Monte Carlo simulation results\n# YOUR CODE HERE!\ntbl = MC_even_dist.summarize_cumulative_return()\n\n# Print summary statistics\nprint (tbl)",
"count 500.000000\nmean 9.683146\nstd 6.514698\nmin 1.320640\n25% 5.124632\n50% 8.047245\n75% 12.513634\nmax 51.017155\n95% CI Lower 2.421887\n95% CI Upper 25.440141\nName: 7560, dtype: float64\n"
]
],
[
[
"### Calculate the expected portfolio return at the 95% lower and upper confidence intervals based on a `$20,000` initial investment.",
"_____no_output_____"
]
],
[
[
"# delete\n# # Set initial investment\n# initial_investment = 20000\n\n# # Use the lower and upper `95%` confidence intervals to calculate the range of the possible outcomes of our $20,000\n# # YOUR CODE HERE!\n\n# # Print results\n# print(f\"There is a 95% chance that an initial investment of ${initial_investment} in the portfolio\"\n# f\" over the next 30 years will end within in the range of\"\n# f\" ${ci_lower} and ${ci_upper}\")",
"_____no_output_____"
],
[
"# Set initial investment\ninitial_investment = 20000\n\n# Use the lower and upper `95%` confidence intervals to calculate the range of the possible outcomes of our $20,000\n# YOUR CODE HERE!\nci_lower = round(tbl[8]*initial_investment,2)\nci_upper = round(tbl[9]*initial_investment,2)\n\n\n# Print results\nprint(f\"There is a 95% chance that an initial investment of ${initial_investment} in the portfolio\"\n f\" over the next 30 years will end within in the range of\"\n f\" ${ci_lower} and ${ci_upper}\")",
"There is a 95% chance that an initial investment of $20000 in the portfolio over the next 30 years will end within in the range of $48437.75 and $508802.81\n"
]
],
[
[
"### Calculate the expected portfolio return at the `95%` lower and upper confidence intervals based on a `50%` increase in the initial investment.",
"_____no_output_____"
]
],
[
[
"# delete\n# # Set initial investment\n# initial_investment = 20000 * 1.5\n\n# # Use the lower and upper `95%` confidence intervals to calculate the range of the possible outcomes of our $30,000\n# # YOUR CODE HERE!\n\n# # Print results\n# print(f\"There is a 95% chance that an initial investment of ${initial_investment} in the portfolio\"\n# f\" over the next 30 years will end within in the range of\"\n# f\" ${ci_lower} and ${ci_upper}\")",
"_____no_output_____"
],
[
"# Set initial investment\ninitial_investment = 20000 * 1.5\n\n# Use the lower and upper `95%` confidence intervals to calculate the range of the possible outcomes of our $30,000\n# YOUR CODE HERE!\nci_lower = round(tbl[8]*initial_investment,2)\nci_upper = round(tbl[9]*initial_investment,2)\n\n# Print results\nprint(f\"There is a 95% chance that an initial investment of ${initial_investment} in the portfolio\"\n f\" over the next 30 years will end within in the range of\"\n f\" ${ci_lower} and ${ci_upper}\")",
"There is a 95% chance that an initial investment of $30000.0 in the portfolio over the next 30 years will end within in the range of $72656.62 and $763204.22\n"
]
],
[
[
"## Optional Challenge - Early Retirement\n\n\n### Five Years Retirement Option",
"_____no_output_____"
]
],
[
[
"# Configuring a Monte Carlo simulation to forecast 5 years cumulative returns\n# YOUR CODE HERE!\nMC_even_dist = MCSimulation(\n portfolio_data = df_stock_data,\n weights = [.4, .6],\n num_simulation = 500,\n num_trading_days = 252*5\n)",
"_____no_output_____"
],
[
"# Running a Monte Carlo simulation to forecast 5 years cumulative returns\n# YOUR CODE HERE!",
"_____no_output_____"
],
[
"# Running a Monte Carlo simulation to forecast 5 years cumulative returns\nMC_even_dist.calc_cumulative_return()",
"Running Monte Carlo simulation number 0.\nRunning Monte Carlo simulation number 10.\nRunning Monte Carlo simulation number 20.\nRunning Monte Carlo simulation number 30.\nRunning Monte Carlo simulation number 40.\nRunning Monte Carlo simulation number 50.\nRunning Monte Carlo simulation number 60.\nRunning Monte Carlo simulation number 70.\nRunning Monte Carlo simulation number 80.\nRunning Monte Carlo simulation number 90.\nRunning Monte Carlo simulation number 100.\nRunning Monte Carlo simulation number 110.\nRunning Monte Carlo simulation number 120.\nRunning Monte Carlo simulation number 130.\nRunning Monte Carlo simulation number 140.\nRunning Monte Carlo simulation number 150.\nRunning Monte Carlo simulation number 160.\nRunning Monte Carlo simulation number 170.\nRunning Monte Carlo simulation number 180.\nRunning Monte Carlo simulation number 190.\nRunning Monte Carlo simulation number 200.\nRunning Monte Carlo simulation number 210.\nRunning Monte Carlo simulation number 220.\nRunning Monte Carlo simulation number 230.\nRunning Monte Carlo simulation number 240.\nRunning Monte Carlo simulation number 250.\nRunning Monte Carlo simulation number 260.\nRunning Monte Carlo simulation number 270.\nRunning Monte Carlo simulation number 280.\nRunning Monte Carlo simulation number 290.\nRunning Monte Carlo simulation number 300.\nRunning Monte Carlo simulation number 310.\nRunning Monte Carlo simulation number 320.\nRunning Monte Carlo simulation number 330.\nRunning Monte Carlo simulation number 340.\nRunning Monte Carlo simulation number 350.\nRunning Monte Carlo simulation number 360.\nRunning Monte Carlo simulation number 370.\nRunning Monte Carlo simulation number 380.\nRunning Monte Carlo simulation number 390.\nRunning Monte Carlo simulation number 400.\nRunning Monte Carlo simulation number 410.\nRunning Monte Carlo simulation number 420.\nRunning Monte Carlo simulation number 430.\nRunning Monte Carlo simulation number 440.\nRunning Monte Carlo simulation number 450.\nRunning Monte Carlo simulation number 460.\nRunning Monte Carlo simulation number 470.\nRunning Monte Carlo simulation number 480.\nRunning Monte Carlo simulation number 490.\n"
],
[
"# Plot simulation outcomes\n# YOUR CODE HERE!",
"_____no_output_____"
],
[
"# Plot simulation outcomes\nline_plot = MC_even_dist.plot_simulation()",
"_____no_output_____"
],
[
"# Plot probability distribution and confidence intervals\n# YOUR CODE HERE!",
"_____no_output_____"
],
[
"# Plot probability distribution and confidence intervals\n# YOUR CODE HERE!\ndist_plot = MC_even_dist.plot_distribution()",
"_____no_output_____"
],
[
"# Fetch summary statistics from the Monte Carlo simulation results\n# YOUR CODE HERE!\n\n# Print summary statistics\n# YOUR CODE HERE!",
"_____no_output_____"
],
[
"# Fetch summary statistics from the Monte Carlo simulation results\n# YOUR CODE HERE!\ntbl = MC_even_dist.summarize_cumulative_return()\n\n# Print summary statistics\nprint (tbl)",
"count 500.000000\nmean 1.469826\nstd 0.393313\nmin 0.630926\n25% 1.172070\n50% 1.420760\n75% 1.721414\nmax 2.939185\n95% CI Lower 0.854639\n95% CI Upper 2.365985\nName: 1260, dtype: float64\n"
],
[
"# Set initial investment\n# YOUR CODE HERE!\n\n# Use the lower and upper `95%` confidence intervals to calculate the range of the possible outcomes of our $60,000\n# YOUR CODE HERE!\n\n# Print results\nprint(f\"There is a 95% chance that an initial investment of ${initial_investment} in the portfolio\"\n f\" over the next 5 years will end within in the range of\"\n f\" ${ci_lower_five} and ${ci_upper_five}\")",
"There is a 95% chance that an initial investment of $30000.0 in the portfolio over the next 5 years will end within in the range of $50062.36 and $148745.63\n"
],
[
"# Set initial investment\n# YOUR CODE HERE!\ninitial_investment = 60000\n# Use the lower and upper `95%` confidence intervals to calculate the range of the possible outcomes of our $60,000\n# YOUR CODE HERE!\nci_lower_five = round(tbl[8]*initial_investment,2)\nci_upper_five = round(tbl[9]*initial_investment,2)\n# Print results\nprint(f\"There is a 95% chance that an initial investment of ${initial_investment} in the portfolio\"\n f\" over the next 5 years will end within in the range of\"\n f\" ${ci_lower_five} and ${ci_upper_five}\")",
"There is a 95% chance that an initial investment of $60000 in the portfolio over the next 5 years will end within in the range of $51278.36 and $141959.07\n"
]
],
[
[
"### Ten Years Retirement Option",
"_____no_output_____"
]
],
[
[
"# Configuring a Monte Carlo simulation to forecast 10 years cumulative returns\n# YOUR CODE HERE!\nMC_even_dist = MCSimulation(\n portfolio_data = df_stock_data,\n weights = [.4, .6],\n num_simulation = 500,\n num_trading_days = 252*10\n)",
"_____no_output_____"
],
[
"# Running a Monte Carlo simulation to forecast 10 years cumulative returns\n# YOUR CODE HERE!",
"_____no_output_____"
],
[
"MC_even_dist.calc_cumulative_return()",
"Running Monte Carlo simulation number 0.\nRunning Monte Carlo simulation number 10.\nRunning Monte Carlo simulation number 20.\nRunning Monte Carlo simulation number 30.\nRunning Monte Carlo simulation number 40.\nRunning Monte Carlo simulation number 50.\nRunning Monte Carlo simulation number 60.\nRunning Monte Carlo simulation number 70.\nRunning Monte Carlo simulation number 80.\nRunning Monte Carlo simulation number 90.\nRunning Monte Carlo simulation number 100.\nRunning Monte Carlo simulation number 110.\nRunning Monte Carlo simulation number 120.\nRunning Monte Carlo simulation number 130.\nRunning Monte Carlo simulation number 140.\nRunning Monte Carlo simulation number 150.\nRunning Monte Carlo simulation number 160.\nRunning Monte Carlo simulation number 170.\nRunning Monte Carlo simulation number 180.\nRunning Monte Carlo simulation number 190.\nRunning Monte Carlo simulation number 200.\nRunning Monte Carlo simulation number 210.\nRunning Monte Carlo simulation number 220.\nRunning Monte Carlo simulation number 230.\nRunning Monte Carlo simulation number 240.\nRunning Monte Carlo simulation number 250.\nRunning Monte Carlo simulation number 260.\nRunning Monte Carlo simulation number 270.\nRunning Monte Carlo simulation number 280.\nRunning Monte Carlo simulation number 290.\nRunning Monte Carlo simulation number 300.\nRunning Monte Carlo simulation number 310.\nRunning Monte Carlo simulation number 320.\nRunning Monte Carlo simulation number 330.\nRunning Monte Carlo simulation number 340.\nRunning Monte Carlo simulation number 350.\nRunning Monte Carlo simulation number 360.\nRunning Monte Carlo simulation number 370.\nRunning Monte Carlo simulation number 380.\nRunning Monte Carlo simulation number 390.\nRunning Monte Carlo simulation number 400.\nRunning Monte Carlo simulation number 410.\nRunning Monte Carlo simulation number 420.\nRunning Monte Carlo simulation number 430.\nRunning Monte Carlo simulation number 440.\nRunning Monte Carlo simulation number 450.\nRunning Monte Carlo simulation number 460.\nRunning Monte Carlo simulation number 470.\nRunning Monte Carlo simulation number 480.\nRunning Monte Carlo simulation number 490.\n"
],
[
"# Plot simulation outcomes\n# YOUR CODE HERE!",
"_____no_output_____"
],
[
"# Plot simulation outcomes\nline_plot = MC_even_dist.plot_simulation()",
"_____no_output_____"
],
[
"# Plot probability distribution and confidence intervals\n# YOUR CODE HERE!",
"_____no_output_____"
],
[
"# Plot probability distribution and confidence intervals\ndist_plot = MC_even_dist.plot_distribution()",
"_____no_output_____"
],
[
"# Fetch summary statistics from the Monte Carlo simulation results\n# YOUR CODE HERE!\n\n# Print summary statistics\n# YOUR CODE HERE!",
"_____no_output_____"
],
[
"# Fetch summary statistics from the Monte Carlo simulation results\n# YOUR CODE HERE!\ntbl = MC_even_dist.summarize_cumulative_return()\n\n# Print summary statistics\nprint (tbl)",
"count 500.000000\nmean 2.134640\nstd 0.847886\nmin 0.587560\n25% 1.573795\n50% 1.988818\n75% 2.559716\nmax 8.737952\n95% CI Lower 0.919582\n95% CI Upper 4.016863\nName: 2520, dtype: float64\n"
],
[
"# Set initial investment\n# YOUR CODE HERE!\n\n# Use the lower and upper `95%` confidence intervals to calculate the range of the possible outcomes of our $60,000\n# YOUR CODE HERE!\n\n# Print results\nprint(f\"There is a 95% chance that an initial investment of ${initial_investment} in the portfolio\"\n f\" over the next 10 years will end within in the range of\"\n f\" ${ci_lower_ten} and ${ci_upper_ten}\")",
"There is a 95% chance that an initial investment of $60000 in the portfolio over the next 10 years will end within in the range of $53013.83 and $246126.55\n"
],
[
"# Set initial investment\n# YOUR CODE HERE!\ninitial_investment = 60000\n\n# Use the lower and upper `95%` confidence intervals to calculate the range of the possible outcomes of our $60,000\n# YOUR CODE HERE!\nci_lower_ten = round(tbl[8]*initial_investment,2)\nci_upper_ten = round(tbl[9]*initial_investment,2)\n\n# Print results\nprint(f\"There is a 95% chance that an initial investment of ${initial_investment} in the portfolio\"\n f\" over the next 10 years will end within in the range of\"\n f\" ${ci_lower_ten} and ${ci_upper_ten}\")",
"There is a 95% chance that an initial investment of $60000 in the portfolio over the next 10 years will end within in the range of $55174.9 and $241011.77\n"
]
]
] | [
"markdown",
"code",
"markdown",
"code",
"markdown",
"code",
"markdown",
"code",
"markdown",
"code",
"markdown",
"code",
"markdown",
"code",
"markdown",
"code",
"markdown",
"code",
"markdown",
"code"
] | [
[
"markdown"
],
[
"code",
"code"
],
[
"markdown",
"markdown"
],
[
"code",
"code",
"code"
],
[
"markdown"
],
[
"code",
"code",
"code",
"code",
"code"
],
[
"markdown"
],
[
"code",
"code",
"code",
"code"
],
[
"markdown"
],
[
"code",
"code",
"code",
"code",
"code",
"code",
"code",
"code",
"code",
"code",
"code",
"code",
"code"
],
[
"markdown"
],
[
"code",
"code"
],
[
"markdown"
],
[
"code",
"code"
],
[
"markdown"
],
[
"code",
"code"
],
[
"markdown"
],
[
"code",
"code",
"code",
"code",
"code",
"code",
"code",
"code",
"code",
"code",
"code"
],
[
"markdown"
],
[
"code",
"code",
"code",
"code",
"code",
"code",
"code",
"code",
"code",
"code",
"code"
]
] |
d07844266a3c7ee4f7722e8fd210c57380aeabed | 34,134 | ipynb | Jupyter Notebook | mBSUS_XRay_Test_Program.ipynb | rjthompson22/bMSUS_XRay_Model | 073f5cad5c189a832185f00bb580deceec94b1e4 | [
"Apache-2.0"
] | null | null | null | mBSUS_XRay_Test_Program.ipynb | rjthompson22/bMSUS_XRay_Model | 073f5cad5c189a832185f00bb580deceec94b1e4 | [
"Apache-2.0"
] | null | null | null | mBSUS_XRay_Test_Program.ipynb | rjthompson22/bMSUS_XRay_Model | 073f5cad5c189a832185f00bb580deceec94b1e4 | [
"Apache-2.0"
] | null | null | null | 81.660287 | 6,937 | 0.673522 | [
[
[
"<a href=\"https://colab.research.google.com/github/rjthompson22/bMSUS_XRay_Model/blob/master/mBSUS_XRay_Test_Program.ipynb\" target=\"_parent\"><img src=\"https://colab.research.google.com/assets/colab-badge.svg\" alt=\"Open In Colab\"/></a>",
"_____no_output_____"
]
],
[
[
"!pip install h5py pyyaml\nimport tensorflow as tf\nfrom tensorflow import keras\n",
"Requirement already satisfied: h5py in /usr/local/lib/python3.6/dist-packages (2.10.0)\nRequirement already satisfied: pyyaml in /usr/local/lib/python3.6/dist-packages (3.13)\nRequirement already satisfied: six in /usr/local/lib/python3.6/dist-packages (from h5py) (1.15.0)\nRequirement already satisfied: numpy>=1.7 in /usr/local/lib/python3.6/dist-packages (from h5py) (1.18.5)\n"
],
[
"import urllib.request\n\nprint('Beginning file download with urllib2...')\n\nurl = 'https://drive.google.com/file/d/1Z0dlwhtS03lYhHv-f3ZT7nf_PgbIXBMB/view?usp=sharing'\nurllib.request.urlretrieve(url, 'basic_CNN.h5')\nbasic_CNN = keras.models.load_model('basic_CNN.h5')",
"Beginning file download with urllib2...\n"
],
[
"from google.colab import drive\ndrive.mount('/content/gdrive')\n\n%cd /content/gdrive/My Drive/Colab Notebooks/mBSUS",
"/root/.keras/datasets/basic_CNN.h5\n"
],
[
"#basic_CNN = keras.models.load_model(\"basic_CNN.h5\")",
"_____no_output_____"
],
[
"import numpy as np\nfrom google.colab import files\nfrom tensorflow.keras.preprocessing import image\n\nos.chdir('/content/')\n\nuploaded = files.upload()\n\nfor fn in uploaded.keys():\n path = '/content/' + fn\n img = image.load_img(path, target_size = (300,300))\n x = image.img_to_array(img)\n x = np.expand_dims(x, axis=0)\n\n images = np.vstack([x])\n \n classes = basic_CNN.predict(images, batch_size = 10)\n\n print(classes[0])\n\n if classes[0] > 0.5:\n print (fn + \" is pneumonia: \" + str(classes[0]))\n else:\n print(fn + \" is not pneumonia: \" + str(classes[0]))\n",
"_____no_output_____"
]
]
] | [
"markdown",
"code"
] | [
[
"markdown"
],
[
"code",
"code",
"code",
"code",
"code"
]
] |
d078464c2e4d2f94e129b21119fac1ea8c454dc5 | 11,667 | ipynb | Jupyter Notebook | Other/Creational Design Pattern - Abstract Factory.ipynb | deepaksood619/Python-Competitive-Programming | c8353d732a372c2bc62f5f12169acc421e802d0c | [
"MIT"
] | null | null | null | Other/Creational Design Pattern - Abstract Factory.ipynb | deepaksood619/Python-Competitive-Programming | c8353d732a372c2bc62f5f12169acc421e802d0c | [
"MIT"
] | null | null | null | Other/Creational Design Pattern - Abstract Factory.ipynb | deepaksood619/Python-Competitive-Programming | c8353d732a372c2bc62f5f12169acc421e802d0c | [
"MIT"
] | null | null | null | 30.865079 | 399 | 0.532099 | [
[
[
"### Abstract Factory Design Pattern",
"_____no_output_____"
],
[
">An abstract factory is a generative design pattern that allows you to create families of related objects without getting attached to specific classes of created objects. The pattern is being implemented by creating an abstract class (for example - Factory), which is represented as an interface for creating system components. Then the classes that implement this interface are being written.",
"_____no_output_____"
],
[
"https://py.checkio.org/blog/design-patterns-part-1/",
"_____no_output_____"
]
],
[
[
"class AbstractFactory:\n def create_chair(self):\n raise NotImplementedError()\n \n def create_sofa(self):\n raise NotImplementedError()\n \n def create_table(self):\n raise NotImplementedError()",
"_____no_output_____"
],
[
"class Chair:\n def __init__(self, name):\n self._name = name\n \n def __str__(self):\n return self._name\n \nclass Sofa:\n def __init__(self, name):\n self._name = name\n \n def __str__(self):\n return self._name\n \nclass Table:\n def __init__(self, name):\n self._name = name\n \n def __str__(self):\n return self._name",
"_____no_output_____"
],
[
"class VictorianFactory(AbstractFactory):\n def create_chair(self):\n return Chair('victorian chair')\n \n def create_sofa(self):\n return Sofa('victorian sofa')\n \n def create_table(self):\n return Table('victorian table')",
"_____no_output_____"
],
[
"class ModernFactory(AbstractFactory):\n def create_chair(self):\n return Chair('modern chair')\n\n def create_sofa(self):\n return Sofa('modern sofa')\n\n def create_table(self):\n return Table('modern table')",
"_____no_output_____"
],
[
"class FuturisticFactory(AbstractFactory):\n def create_chair(self):\n return Chair('futuristic chair')\n\n def create_sofa(self):\n return Sofa('futuristic sofa')\n\n def create_table(self):\n return Table('futuristic table')",
"_____no_output_____"
],
[
"factory_1 = VictorianFactory()\nfactory_2 = ModernFactory()\nfactory_3 = FuturisticFactory()\nprint(factory_1.create_chair())\nprint(factory_1.create_sofa())\nprint(factory_1.create_table())\nprint(factory_2.create_chair())\nprint(factory_2.create_sofa())\nprint(factory_2.create_table())\nprint(factory_3.create_chair())\nprint(factory_3.create_sofa())\nprint(factory_3.create_table())",
"victorian chair\nvictorian sofa\nvictorian table\nmodern chair\nmodern sofa\nmodern table\nfuturistic chair\nfuturistic sofa\nfuturistic table\n"
]
],
[
[
"Example - https://py.checkio.org/mission/army-units/solve/",
"_____no_output_____"
]
],
[
[
"class Army:\n def train_swordsman(self, name):\n return NotImplementedError()\n \n def train_lancer(self, name):\n return NotImplementedError()\n \n def train_archer(self, name):\n return NotImplementedError()\n\nclass Swordsman:\n def __init__(self, soldier_type, name, army_type):\n self.army_type = army_type + ' swordsman'\n self.name = name\n self.soldier_type = soldier_type\n \n def introduce(self):\n return '{} {}, {}'.format(self.soldier_type, self.name, self.army_type)\n\nclass Lancer:\n def __init__(self, soldier_type, name, army_type):\n self.army_type = army_type + ' lancer'\n self.name = name\n self.soldier_type = soldier_type\n \n def introduce(self):\n return '{} {}, {}'.format(self.soldier_type, self.name, self.army_type)\n\nclass Archer:\n def __init__(self, soldier_type, name, army_type):\n self.army_type = army_type + ' archer'\n self.name = name\n self.soldier_type = soldier_type\n \n def introduce(self):\n return '{} {}, {}'.format(self.soldier_type, self.name, self.army_type)\n\nclass AsianArmy(Army):\n def __init__(self):\n self.army_type = 'Asian'\n \n def train_swordsman(self, name):\n return Swordsman('Samurai', name, self.army_type)\n \n def train_lancer(self, name):\n return Lancer('Ronin', name, self.army_type)\n \n def train_archer(self, name):\n return Archer('Shinobi', name, self.army_type)\n\nclass EuropeanArmy(Army):\n def __init__(self):\n self.army_type = 'European'\n \n def train_swordsman(self, name):\n return Swordsman('Knight', name, self.army_type)\n \n def train_lancer(self, name):\n return Lancer('Raubritter', name, self.army_type)\n \n def train_archer(self, name):\n return Archer('Ranger', name, self.army_type)\n\n\nif __name__ == '__main__':\n #These \"asserts\" using only for self-checking and not necessary for auto-testing\n\n my_army = EuropeanArmy()\n enemy_army = AsianArmy()\n\n soldier_1 = my_army.train_swordsman(\"Jaks\")\n soldier_2 = my_army.train_lancer(\"Harold\")\n soldier_3 = my_army.train_archer(\"Robin\")\n\n soldier_4 = enemy_army.train_swordsman(\"Kishimoto\")\n soldier_5 = enemy_army.train_lancer(\"Ayabusa\")\n soldier_6 = enemy_army.train_archer(\"Kirigae\")\n\n assert soldier_1.introduce() == \"Knight Jaks, European swordsman\"\n assert soldier_2.introduce() == \"Raubritter Harold, European lancer\"\n assert soldier_3.introduce() == \"Ranger Robin, European archer\"\n \n assert soldier_4.introduce() == \"Samurai Kishimoto, Asian swordsman\"\n assert soldier_5.introduce() == \"Ronin Ayabusa, Asian lancer\"\n assert soldier_6.introduce() == \"Shinobi Kirigae, Asian archer\"\n\n print(\"Coding complete? Let's try tests!\")\n",
"Coding complete? Let's try tests!\n"
],
[
"class Army:\n def train_swordsman(self, name):\n return Swordsman(self, name)\n\n def train_lancer(self, name):\n return Lancer(self, name)\n\n def train_archer(self, name):\n return Archer(self, name)\n\n def introduce(self, name, army_type):\n return f'{self.title[army_type]} {name}, {self.region} {army_type}'\n\n\nclass Fighter:\n def __init__(self, army, name):\n self.army = army\n self.name = name\n\n def introduce(self):\n return self.army.introduce(self.name, self.army_type)\n\n\nclass Swordsman(Fighter):\n army_type = 'swordsman'\n\nclass Lancer(Fighter):\n army_type = 'lancer'\n\nclass Archer(Fighter):\n army_type = 'archer'\n\nclass AsianArmy(Army):\n title = {'swordsman': 'Samurai', 'lancer': 'Ronin', 'archer': 'Shinobi'}\n region = 'Asian'\n\nclass EuropeanArmy(Army):\n title = {'swordsman': 'Knight', 'lancer': 'Raubritter', 'archer': 'Ranger'}\n region = 'European'\n\n\n\nif __name__ == '__main__':\n #These \"asserts\" using only for self-checking and not necessary for auto-testing\n\n my_army = EuropeanArmy()\n enemy_army = AsianArmy()\n\n soldier_1 = my_army.train_swordsman(\"Jaks\")\n soldier_2 = my_army.train_lancer(\"Harold\")\n soldier_3 = my_army.train_archer(\"Robin\")\n\n soldier_4 = enemy_army.train_swordsman(\"Kishimoto\")\n soldier_5 = enemy_army.train_lancer(\"Ayabusa\")\n soldier_6 = enemy_army.train_archer(\"Kirigae\")\n\n print(soldier_1.introduce())\n print(\"Knight Jaks, European swordsman\")\n print(soldier_2.introduce())\n print(soldier_3.introduce())\n assert soldier_1.introduce() == \"Knight Jaks, European swordsman\"\n assert soldier_2.introduce() == \"Raubritter Harold, European lancer\"\n assert soldier_3.introduce() == \"Ranger Robin, European archer\"\n \n assert soldier_4.introduce() == \"Samurai Kishimoto, Asian swordsman\"\n assert soldier_5.introduce() == \"Ronin Ayabusa, Asian lancer\"\n assert soldier_6.introduce() == \"Shinobi Kirigae, Asian archer\"\n\n print(\"Coding complete? Let's try tests!\")",
"Knight Jaks, European swordsman\nKnight Jaks, European swordsman\nRaubritter Harold, European lancer\nRanger Robin, European archer\nCoding complete? Let's try tests!\n"
]
]
] | [
"markdown",
"code",
"markdown",
"code"
] | [
[
"markdown",
"markdown",
"markdown"
],
[
"code",
"code",
"code",
"code",
"code",
"code"
],
[
"markdown"
],
[
"code",
"code"
]
] |
d078615d08d289d0dcebe334f26d3994934311db | 211,594 | ipynb | Jupyter Notebook | examples/1. Cosine prediction.ipynb | meng-zha/FOST | 2fc32ded470cc465a64f46a2c06c2a081e02f3e5 | [
"MIT"
] | 181 | 2021-11-12T09:17:54.000Z | 2022-03-22T05:53:35.000Z | examples/1. Cosine prediction.ipynb | meng-zha/FOST | 2fc32ded470cc465a64f46a2c06c2a081e02f3e5 | [
"MIT"
] | 12 | 2021-11-12T16:50:47.000Z | 2022-03-07T09:22:16.000Z | examples/1. Cosine prediction.ipynb | meng-zha/FOST | 2fc32ded470cc465a64f46a2c06c2a081e02f3e5 | [
"MIT"
] | 41 | 2021-11-13T14:33:49.000Z | 2022-03-11T04:19:58.000Z | 142.104768 | 38,274 | 0.746368 | [
[
[
"## Cosine prediction\n\n### Data preparation\nIn this notebook, we will use fost to predict a cosine curve. Let's import `pandas` and `numpy` first.",
"_____no_output_____"
]
],
[
[
"import pandas as pd\nimport numpy as np",
"_____no_output_____"
]
],
[
[
"Next, generate cosine data.",
"_____no_output_____"
]
],
[
[
"train_df = pd.DataFrame()\n#we don't have actual timestamp in this case, use a default one\ntrain_df['Date'] = [pd.datetime(year=2000,month=1,day=1)+pd.Timedelta(days=i) for i in range(2000)] \ntrain_df['TARGET'] = [np.cos(i/2) for i in range(2000)]\n#there is not a 'Node' concept in this dataset, thus all the Node are 0\ntrain_df.loc[:, 'Node'] = 0",
"/usr/local/lib/python3.6/dist-packages/ipykernel_launcher.py:4: FutureWarning: The pandas.datetime class is deprecated and will be removed from pandas in a future version. Import from datetime instead.\n after removing the cwd from sys.path.\n"
],
[
"#show first 40 data\ntrain_df.iloc[:40].set_index('Date')['TARGET'].plot()",
"_____no_output_____"
]
],
[
[
"FOST only supports file path as input, save the data to `train.csv`",
"_____no_output_____"
]
],
[
[
"train_df.to_csv('train.csv',index=False)",
"_____no_output_____"
]
],
[
[
"### Load fost to predict future",
"_____no_output_____"
]
],
[
[
"import fostool\nfrom fostool.pipeline import Pipeline",
"_____no_output_____"
],
[
"#predict future 10 steps\nlookahead = 10\nfost = Pipeline(lookahead=lookahead, train_path='train.csv')",
"2021-11-08 09:46:43 fostool/task/config_handler.py 26 \\ - INFO - yaml handler load path: /root/HierST/src/config/default.yaml\n2021-11-08 09:46:43 fostool/dataset/data_utils.py 402 \\ - INFO - Detected Sample Frequency: <Day>.\n2021-11-08 09:46:43 fostool/dataset/data_utils.py 426 \\ - INFO - 2000 Rows Before Time Reindex.\n2021-11-08 09:46:43 fostool/dataset/data_utils.py 428 \\ - INFO - 2000 Rows After Time Reindex.\n2021-11-08 09:46:43 fostool/dataset/data_utils.py 429 \\ - INFO - --------------------\n2021-11-08 09:46:43 fostool/dataset/data_utils.py 457 \\ - INFO - 2000 Rows Before Fill Missing.\n2021-11-08 09:46:43 fostool/dataset/data_utils.py 461 \\ - INFO - 2000 Rows After Fill Missing.\n2021-11-08 09:46:43 fostool/dataset/data_utils.py 462 \\ - INFO - --------------------\n"
],
[
"#fit in one line\nfost.fit()",
"2021-11-08 09:46:59 fostool/tools/trainer.py 129 \\ - INFO - On epoch 0, train loss 0.9837532142798106, val loss 1.1207878589630127\n2021-11-08 09:46:59 fostool/tools/trainer.py 130 \\ - INFO - ------------\n2021-11-08 09:46:59 fostool/tools/trainer.py 137 \\ - INFO - For model KRNNModel_290a_10, current best val loss 1.1207878589630127\n2021-11-08 09:46:59 fostool/tools/trainer.py 129 \\ - INFO - On epoch 1, train loss 0.8563873469829559, val loss 1.0255659818649292\n2021-11-08 09:46:59 fostool/tools/trainer.py 130 \\ - INFO - ------------\n2021-11-08 09:46:59 fostool/tools/trainer.py 137 \\ - INFO - For model KRNNModel_290a_10, current best val loss 1.0255659818649292\n2021-11-08 09:46:59 fostool/tools/trainer.py 129 \\ - INFO - On epoch 2, train loss 0.7682058115800222, val loss 0.9656972289085388\n2021-11-08 09:46:59 fostool/tools/trainer.py 130 \\ - INFO - ------------\n2021-11-08 09:46:59 fostool/tools/trainer.py 137 \\ - INFO - For model KRNNModel_290a_10, current best val loss 0.9656972289085388\n2021-11-08 09:46:59 fostool/tools/trainer.py 129 \\ - INFO - On epoch 3, train loss 0.6545088986555735, val loss 0.8323457837104797\n2021-11-08 09:46:59 fostool/tools/trainer.py 130 \\ - INFO - ------------\n2021-11-08 09:46:59 fostool/tools/trainer.py 137 \\ - INFO - For model KRNNModel_290a_10, current best val loss 0.8323457837104797\n2021-11-08 09:46:59 fostool/tools/trainer.py 129 \\ - INFO - On epoch 4, train loss 0.5395551969607671, val loss 0.7392954230308533\n2021-11-08 09:46:59 fostool/tools/trainer.py 130 \\ - INFO - ------------\n2021-11-08 09:46:59 fostool/tools/trainer.py 137 \\ - INFO - For model KRNNModel_290a_10, current best val loss 0.7392954230308533\n2021-11-08 09:46:59 fostool/tools/trainer.py 129 \\ - INFO - On epoch 5, train loss 0.41803252696990967, val loss 0.6145674586296082\n2021-11-08 09:46:59 fostool/tools/trainer.py 130 \\ - INFO - ------------\n2021-11-08 09:46:59 fostool/tools/trainer.py 137 \\ - INFO - For model KRNNModel_290a_10, current best val loss 0.6145674586296082\n2021-11-08 09:47:00 fostool/tools/trainer.py 129 \\ - INFO - On epoch 6, train loss 0.32809186975161236, val loss 0.4214208722114563\n2021-11-08 09:47:00 fostool/tools/trainer.py 130 \\ - INFO - ------------\n2021-11-08 09:47:00 fostool/tools/trainer.py 137 \\ - INFO - For model KRNNModel_290a_10, current best val loss 0.4214208722114563\n2021-11-08 09:47:00 fostool/tools/trainer.py 129 \\ - INFO - On epoch 7, train loss 0.20533942927916846, val loss 0.18617308139801025\n2021-11-08 09:47:00 fostool/tools/trainer.py 130 \\ - INFO - ------------\n2021-11-08 09:47:00 fostool/tools/trainer.py 137 \\ - INFO - For model KRNNModel_290a_10, current best val loss 0.18617308139801025\n2021-11-08 09:47:00 fostool/tools/trainer.py 129 \\ - INFO - On epoch 8, train loss 0.12697207058469454, val loss 0.09616108983755112\n2021-11-08 09:47:00 fostool/tools/trainer.py 130 \\ - INFO - ------------\n2021-11-08 09:47:00 fostool/tools/trainer.py 137 \\ - INFO - For model KRNNModel_290a_10, current best val loss 0.09616108983755112\n2021-11-08 09:47:00 fostool/tools/trainer.py 129 \\ - INFO - On epoch 9, train loss 0.05325042083859444, val loss 0.05969296023249626\n2021-11-08 09:47:00 fostool/tools/trainer.py 130 \\ - INFO - ------------\n2021-11-08 09:47:00 fostool/tools/trainer.py 137 \\ - INFO - For model KRNNModel_290a_10, current best val loss 0.05969296023249626\n2021-11-08 09:47:00 fostool/tools/trainer.py 129 \\ - INFO - On epoch 10, train loss 0.0261272427936395, val loss 0.011045633815228939\n2021-11-08 09:47:00 fostool/tools/trainer.py 130 \\ - INFO - ------------\n2021-11-08 09:47:00 fostool/tools/trainer.py 137 \\ - INFO - For model KRNNModel_290a_10, current best val loss 0.011045633815228939\n2021-11-08 09:47:00 fostool/tools/trainer.py 129 \\ - INFO - On epoch 11, train loss 0.012711387127637863, val loss 0.003730224911123514\n2021-11-08 09:47:00 fostool/tools/trainer.py 130 \\ - INFO - ------------\n2021-11-08 09:47:00 fostool/tools/trainer.py 137 \\ - INFO - For model KRNNModel_290a_10, current best val loss 0.003730224911123514\n2021-11-08 09:47:00 fostool/tools/trainer.py 129 \\ - INFO - On epoch 12, train loss 0.008007198184107741, val loss 0.004111938178539276\n2021-11-08 09:47:00 fostool/tools/trainer.py 130 \\ - INFO - ------------\n2021-11-08 09:47:00 fostool/tools/trainer.py 129 \\ - INFO - On epoch 13, train loss 0.006259295002867778, val loss 0.0038592375349253416\n2021-11-08 09:47:00 fostool/tools/trainer.py 130 \\ - INFO - ------------\n2021-11-08 09:47:01 fostool/tools/trainer.py 129 \\ - INFO - On epoch 14, train loss 0.005420396104454994, val loss 0.0015936356503516436\n2021-11-08 09:47:01 fostool/tools/trainer.py 130 \\ - INFO - ------------\n2021-11-08 09:47:01 fostool/tools/trainer.py 137 \\ - INFO - For model KRNNModel_290a_10, current best val loss 0.0015936356503516436\n2021-11-08 09:47:01 fostool/tools/trainer.py 129 \\ - INFO - On epoch 15, train loss 0.0032053233978028097, val loss 0.0009960811585187912\n2021-11-08 09:47:01 fostool/tools/trainer.py 130 \\ - INFO - ------------\n2021-11-08 09:47:01 fostool/tools/trainer.py 137 \\ - INFO - For model KRNNModel_290a_10, current best val loss 0.0009960811585187912\n2021-11-08 09:47:01 fostool/tools/trainer.py 129 \\ - INFO - On epoch 16, train loss 0.0030816590103010335, val loss 0.000641592894680798\n2021-11-08 09:47:01 fostool/tools/trainer.py 130 \\ - INFO - ------------\n2021-11-08 09:47:01 fostool/tools/trainer.py 137 \\ - INFO - For model KRNNModel_290a_10, current best val loss 0.000641592894680798\n2021-11-08 09:47:01 fostool/tools/trainer.py 129 \\ - INFO - On epoch 17, train loss 0.0024648708446572223, val loss 0.0006953171687200665\n2021-11-08 09:47:01 fostool/tools/trainer.py 130 \\ - INFO - ------------\n2021-11-08 09:47:01 fostool/tools/trainer.py 129 \\ - INFO - On epoch 18, train loss 0.0018245108852473397, val loss 0.0006199749186635017\n2021-11-08 09:47:01 fostool/tools/trainer.py 130 \\ - INFO - ------------\n2021-11-08 09:47:01 fostool/tools/trainer.py 137 \\ - INFO - For model KRNNModel_290a_10, current best val loss 0.0006199749186635017\n2021-11-08 09:47:01 fostool/tools/trainer.py 129 \\ - INFO - On epoch 19, train loss 0.0013166117617705215, val loss 0.0006043225876055658\n2021-11-08 09:47:01 fostool/tools/trainer.py 130 \\ - INFO - ------------\n2021-11-08 09:47:01 fostool/tools/trainer.py 137 \\ - INFO - For model KRNNModel_290a_10, current best val loss 0.0006043225876055658\n2021-11-08 09:47:01 fostool/tools/trainer.py 129 \\ - INFO - On epoch 20, train loss 0.0011962133382136624, val loss 0.00043523628846742213\n2021-11-08 09:47:01 fostool/tools/trainer.py 130 \\ - INFO - ------------\n2021-11-08 09:47:01 fostool/tools/trainer.py 137 \\ - INFO - For model KRNNModel_290a_10, current best val loss 0.00043523628846742213\n2021-11-08 09:47:01 fostool/tools/trainer.py 129 \\ - INFO - On epoch 21, train loss 0.0010729129620206852, val loss 0.00041648070327937603\n2021-11-08 09:47:01 fostool/tools/trainer.py 130 \\ - INFO - ------------\n2021-11-08 09:47:01 fostool/tools/trainer.py 137 \\ - INFO - For model KRNNModel_290a_10, current best val loss 0.00041648070327937603\n2021-11-08 09:47:01 fostool/tools/trainer.py 129 \\ - INFO - On epoch 22, train loss 0.0009700010802286366, val loss 0.00017646198102738708\n2021-11-08 09:47:01 fostool/tools/trainer.py 130 \\ - INFO - ------------\n2021-11-08 09:47:01 fostool/tools/trainer.py 137 \\ - INFO - For model KRNNModel_290a_10, current best val loss 0.00017646198102738708\n2021-11-08 09:47:02 fostool/tools/trainer.py 129 \\ - INFO - On epoch 23, train loss 0.0008185463472424696, val loss 0.0001829482353059575\n2021-11-08 09:47:02 fostool/tools/trainer.py 130 \\ - INFO - ------------\n2021-11-08 09:47:02 fostool/tools/trainer.py 129 \\ - INFO - On epoch 24, train loss 0.0008309520975065728, val loss 0.00022877224546391517\n2021-11-08 09:47:02 fostool/tools/trainer.py 130 \\ - INFO - ------------\n2021-11-08 09:47:02 fostool/tools/trainer.py 129 \\ - INFO - On epoch 25, train loss 0.0007969176222104579, val loss 0.00020417450286913663\n2021-11-08 09:47:02 fostool/tools/trainer.py 130 \\ - INFO - ------------\n2021-11-08 09:47:02 fostool/tools/trainer.py 129 \\ - INFO - On epoch 26, train loss 0.0007901331215786437, val loss 0.0003080877650063485\n2021-11-08 09:47:02 fostool/tools/trainer.py 130 \\ - INFO - ------------\n"
],
[
"#get predict result\nres = fost.predict()",
"2021-11-08 09:48:53 fostool/task/fusion.py 67 \\ - INFO - val_loss model_name\n0 0.000035 KRNNModel_290a_10\n1 0.000038 KRNNModel_3bf8_20\n2 0.000005 KRNNModel_d82f_30\n7 0.000031 SandwichModel_3f7a_30\n"
],
[
"#plot prediction\nfost.plot(res, lookback_size=lookahead)",
"_____no_output_____"
]
]
] | [
"markdown",
"code",
"markdown",
"code",
"markdown",
"code",
"markdown",
"code"
] | [
[
"markdown"
],
[
"code"
],
[
"markdown"
],
[
"code",
"code"
],
[
"markdown"
],
[
"code"
],
[
"markdown"
],
[
"code",
"code",
"code",
"code",
"code"
]
] |
d0786efd07508fe4cf0443d075317f89f5155308 | 12,790 | ipynb | Jupyter Notebook | my_notebook/1_basic_forward.ipynb | jjeamin/pytorch-tutorial | c5ff713278295d992645f1aa12948a4bb38c562f | [
"MIT"
] | 1 | 2019-06-16T08:46:54.000Z | 2019-06-16T08:46:54.000Z | my_notebook/1_basic_forward.ipynb | jjeamin/pytorch-tutorial | c5ff713278295d992645f1aa12948a4bb38c562f | [
"MIT"
] | null | null | null | my_notebook/1_basic_forward.ipynb | jjeamin/pytorch-tutorial | c5ff713278295d992645f1aa12948a4bb38c562f | [
"MIT"
] | null | null | null | 37.840237 | 5,148 | 0.666145 | [
[
[
"# Pytorch Basic",
"_____no_output_____"
]
],
[
[
"import torch\nimport torch.nn as nn\nimport torchvision\nimport torchvision.transforms as transforms\nimport matplotlib.pyplot as plt\nfrom IPython.display import clear_output",
"_____no_output_____"
],
[
"torch.cuda.is_available()",
"_____no_output_____"
]
],
[
[
"## Device",
"_____no_output_____"
]
],
[
[
"device = torch.device('cuda' if torch.cuda.is_available() else 'cpu')",
"_____no_output_____"
]
],
[
[
"## Hyper Parameter",
"_____no_output_____"
]
],
[
[
"input_size = 784\nhidden_size = 500\nnum_class = 10\nepochs = 5\nbatch_size = 100\nlr = 0.001",
"_____no_output_____"
]
],
[
[
"## Load MNIST Dataset",
"_____no_output_____"
]
],
[
[
"train_dataset = torchvision.datasets.MNIST(root='../data', \n train=True, \n transform=transforms.ToTensor(), \n download=True)\ntest_dataset = torchvision.datasets.MNIST(root='../data', \n train=False, \n transform=transforms.ToTensor())",
"_____no_output_____"
],
[
"print('train dataset shape : ',train_dataset.data.shape)\nprint('test dataset shape : ',test_dataset.data.shape)\nplt.imshow(train_dataset.data[0])",
"train dataset shape : torch.Size([60000, 28, 28])\ntest dataset shape : torch.Size([10000, 28, 28])\n"
],
[
"train_loader = torch.utils.data.DataLoader(dataset=train_dataset, \n batch_size=batch_size, \n shuffle=True)\ntest_loader = torch.utils.data.DataLoader(dataset=test_dataset, \n batch_size=batch_size, \n shuffle=False)",
"_____no_output_____"
]
],
[
[
"## Simple Model",
"_____no_output_____"
]
],
[
[
"class NeuralNet(nn.Module):\n def __init__(self, input_size, hidden_size, num_class):\n super(NeuralNet, self).__init__()\n self.fc1 = nn.Linear(input_size,hidden_size)\n self.relu = nn.ReLU()\n self.fc2 = nn.Linear(hidden_size, num_class)\n \n def forward(self, x):\n out = self.fc1(x)\n out = self.relu(out)\n out = self.fc2(out)\n return out",
"_____no_output_____"
],
[
"model = NeuralNet(input_size,hidden_size,num_class).to(device)",
"_____no_output_____"
]
],
[
[
"## Loss and Optimizer",
"_____no_output_____"
]
],
[
[
"criterion = nn.CrossEntropyLoss()\noptimizer = torch.optim.Adam(model.parameters(), lr=lr) ",
"_____no_output_____"
]
],
[
[
"## Train",
"_____no_output_____"
]
],
[
[
"total_step = len(train_loader)\n\nfor epoch in range(epochs):\n for i, (images, labels) in enumerate(train_loader):\n images = images.reshape(-1,28*28).to(device)\n labels = labels.to(device)\n \n outputs = model(images)\n loss = criterion(outputs, labels)\n \n optimizer.zero_grad()\n loss.backward()\n optimizer.step()\n \n if (i+1) % 100 == 0:\n clear_output()\n print('EPOCH [{}/{}] STEP [{}/{}] Loss {: .4f})'\n .format(epoch+1, epochs, i+1, total_step, loss.item()))",
"EPOCH [5/5] STEP [600/600] Loss 0.0343)\n"
]
],
[
[
"## Test",
"_____no_output_____"
]
],
[
[
"with torch.no_grad():\n correct = 0\n total = 0\n for images, labels in test_loader:\n images = images.reshape(-1, 28*28).to(device)\n labels = labels.to(device)\n outputs = model(images)\n _, predicted = torch.max(outputs.data, 1)\n total += labels.size(0)\n correct += (predicted == labels).sum().item()\n\n print('Accuracy of the network on the 10000 test images: {} %'.format(100 * correct / total))",
"Accuracy of the network on the 10000 test images: 97.85 %\n"
]
],
[
[
"## save",
"_____no_output_____"
]
],
[
[
"torch.save(model.state_dict(), 'model.ckpt')",
"_____no_output_____"
]
],
[
[
"---",
"_____no_output_____"
]
]
] | [
"markdown",
"code",
"markdown",
"code",
"markdown",
"code",
"markdown",
"code",
"markdown",
"code",
"markdown",
"code",
"markdown",
"code",
"markdown",
"code",
"markdown",
"code",
"markdown"
] | [
[
"markdown"
],
[
"code",
"code"
],
[
"markdown"
],
[
"code"
],
[
"markdown"
],
[
"code"
],
[
"markdown"
],
[
"code",
"code",
"code"
],
[
"markdown"
],
[
"code",
"code"
],
[
"markdown"
],
[
"code"
],
[
"markdown"
],
[
"code"
],
[
"markdown"
],
[
"code"
],
[
"markdown"
],
[
"code"
],
[
"markdown"
]
] |
d0787760e8bc8ea83e5920526d177c4c4fdac5aa | 1,254 | ipynb | Jupyter Notebook | csv_to_html.ipynb | sboehm86/web_design_challenge | cc3dae22cbd249bfbca323fb4e6c724c7a6f3b61 | [
"ADSL"
] | null | null | null | csv_to_html.ipynb | sboehm86/web_design_challenge | cc3dae22cbd249bfbca323fb4e6c724c7a6f3b61 | [
"ADSL"
] | null | null | null | csv_to_html.ipynb | sboehm86/web_design_challenge | cc3dae22cbd249bfbca323fb4e6c724c7a6f3b61 | [
"ADSL"
] | null | null | null | 19.292308 | 102 | 0.5311 | [
[
[
"# import dependencies \nimport os\nimport pandas as pd",
"_____no_output_____"
],
[
"# generate path to csv\npath = '../web_design_challenge/Resources/cities.csv'\ncities= pd.read_csv(path)",
"_____no_output_____"
],
[
"# create html table and save to file \ncities_csv.to_html('table.html', index=False, classes=['table', 'table-striped', 'table-hover'])",
"_____no_output_____"
]
]
] | [
"code"
] | [
[
"code",
"code",
"code"
]
] |
d07887d3e3a7ae6f6d0e3e7dcacdfb139c30028b | 462,714 | ipynb | Jupyter Notebook | Efecto Arima en final.ipynb | ramirezdiana/Forecast-with-fourier | cfbafff0df3419bda7c7c6dce03613157a562181 | [
"MIT"
] | null | null | null | Efecto Arima en final.ipynb | ramirezdiana/Forecast-with-fourier | cfbafff0df3419bda7c7c6dce03613157a562181 | [
"MIT"
] | null | null | null | Efecto Arima en final.ipynb | ramirezdiana/Forecast-with-fourier | cfbafff0df3419bda7c7c6dce03613157a562181 | [
"MIT"
] | null | null | null | 374.667206 | 64,732 | 0.9304 | [
[
[
"### Importar librerías y series de datos",
"_____no_output_____"
]
],
[
[
"import time\nstart = time.time()\n\n#importar datos y librerias\nimport numpy as np\nimport pandas as pd\nfrom datetime import datetime\nimport matplotlib.pyplot as plt\nfrom scipy import signal\nfrom sklearn.linear_model import LinearRegression\nfrom statsmodels.tsa.seasonal import seasonal_decompose\nfrom scipy.stats import boxcox\nfrom scipy import special\n#leer excel de datos y de dias especiales\ngeneral = pd.read_excel (r'C:\\Users\\Diana\\PAP\\Data\\Data1.xlsx')\nspecial_days= pd.read_excel (r'C:\\Users\\Diana\\PAP\\Data\\Christmas.xlsx')\n#convertir dias especiales a fechas en python\nfor column in special_days.columns:\n special_days[column] = pd.to_datetime(special_days[column])\ngeneral = general.set_index('fecha')",
"_____no_output_____"
]
],
[
[
"### Establecer las funciones a utilizar",
"_____no_output_____"
]
],
[
[
"def kronecker(data1:'Dataframe 1',data2:'Dataframe 2'):\n x=0\n data1_kron=data1[x:x+1]\n data2_kron=data2[x:x+1]\n Combinacion=np.kron(data1_kron,data2_kron)\n Combinacion=pd.DataFrame(Combinacion)\n for x in range(1,len(data1)):\n data1_kron=data1[x:x+1]\n data2_kron=data2[x:x+1]\n kron=np.kron(data1_kron,data2_kron)\n Kron=pd.DataFrame(kron)\n Combinacion=Combinacion.append(Kron)\n return Combinacion",
"_____no_output_____"
],
[
"def regresion_linear(X:'variables para regresion',y:'datos'):\n global model\n model.fit(X, y)\n coefficients=model.coef_\n return model.predict(X)",
"_____no_output_____"
],
[
"def comparacion(real,pred):\n comparacion=pd.DataFrame(columns=['real','prediccion','error'])\n comparacion.real=real\n comparacion.prediccion=pred\n comparacion.error=np.abs((comparacion.real.values-comparacion.prediccion)/comparacion.real)*100\n return comparacion",
"_____no_output_____"
]
],
[
[
"### Hacer variables dummies",
"_____no_output_____"
]
],
[
[
"n=-10\nfinal=general.MWh.tail(-n)\nonlyMWh=pd.DataFrame(general.MWh)\ngeneral['Month'] = general.index.month\ngeneral['Weekday_Name'] = general.index.weekday_name\ndates=general.index\ndummies = pd.get_dummies(general['Weekday_Name']).astype(int)\ndummies2 = pd.get_dummies(general['Month']).astype(int)\nDum=pd.DataFrame(dummies.join(dummies2))\nt=np.arange(0,len(onlyMWh))\nDum[\"t\"]= np.arange(0,len(onlyMWh))\nDum[\"tiempo\"]= np.arange(1,len(onlyMWh)+1)\nDum[\"ones\"]=np.ones(len(t))\nDum= Dum.set_index('t')",
"_____no_output_____"
],
[
"Dum[\"Dom santo\"]=0\nDum[\"NewYear\"]=0\nDum[\"Constitucion\"]=0\nDum[\"Benito\"]=0\nDum[\"Jue santo\"]=0\nDum[\"Vie santo\"]=0\nDum[\"Trabajo\"]=0\nDum[\"Madre\"]=0\nDum[\"Grito\"]=0\nDum[\"virgen\"]=0\nDum[\"muertos\"]=0\nDum[\"Virgen2\"]=0\nDum[\"Navidad\"]=0\nDum[\"elecciones\"]=0\nDum[\"toma\"]=0\nDum[\"sab santo\"]=0\nDum[\"rev\"]=0\n\nind=0 \nfor date in general.index:\n for date2 in special_days[\"Dom santo\"]:\n if date ==date2:\n Dum.iloc[ind,21]=1\n for date2 in special_days[\"NewYear\"]:\n if date ==date2:\n Dum.iloc[ind,22]=1\n for date2 in special_days[\"Constitucion\"]:\n if date ==date2:\n Dum.iloc[ind,23]=1\n for date2 in special_days[\"Benito\"]:\n if date ==date2:\n Dum.iloc[ind,24]=1\n for date2 in special_days[\"Jue santo\"]:\n if date ==date2:\n Dum.iloc[ind,25]=1\n for date2 in special_days[\"Vie santo\"]:\n if date ==date2:\n Dum.iloc[ind,26]=1\n for date2 in special_days[\"Trabajo\"]:\n if date ==date2:\n Dum.iloc[ind,27]=1\n for date2 in special_days[\"Madre\"]:\n if date ==date2:\n Dum.iloc[ind,28]=1\n for date2 in special_days[\"Grito\"]:\n if date ==date2:\n Dum.iloc[ind,29]=1\n for date2 in special_days[\"virgen\"]:\n if date ==date2:\n Dum.iloc[ind,30]=1\n for date2 in special_days[\"muertos\"]:\n if date ==date2:\n Dum.iloc[ind,31]=1\n for date2 in special_days[\"Virgen2\"]:\n if date ==date2:\n Dum.iloc[ind,32]=1\n for date2 in special_days[\"Navidad\"]:\n if date ==date2:\n Dum.iloc[ind,33]=1\n for date2 in special_days[\"elecciones\"]:\n if date ==date2:\n Dum.iloc[ind,34]=1\n for date2 in special_days[\"toma\"]:\n if date ==date2:\n Dum.iloc[ind,35]=1\n for date2 in special_days[\"sab santo\"]:\n if date ==date2:\n Dum.iloc[ind,36]=1\n for date2 in special_days[\"rev\"]:\n if date ==date2:\n Dum.iloc[ind,37]=1\n ind+=1\ndel Dum[\"Friday\"]\nDum.drop(Dum.columns[[15]], axis=1,inplace=True)",
"_____no_output_____"
]
],
[
[
"### Observar descomposición",
"_____no_output_____"
]
],
[
[
"part=general.MWh.tail(100)\nresult=seasonal_decompose(part, model='multiplicative')\nfig = result.seasonal.plot(figsize=(20,5))",
"_____no_output_____"
]
],
[
[
"Al ver la decomposición, se puede ver por la forma que fourier debe estblecerse en senos y cosenos absolutos, para que se parezca a la estacionalidad de la serie. Se agrega a las variables dummies esta estacionalidad semanal, que parece ser fundamental en los datos",
"_____no_output_____"
],
[
"### Detectar efecto de las variables dummies",
"_____no_output_____"
]
],
[
[
"t=np.arange(1,len(onlyMWh)+1)\nTiempo=pd.DataFrame(t)\nTiempo[\"one\"]=np.ones(len(onlyMWh))\nTiempo['sen']=np.abs(np.sin(((2*np.pi)/14)*t))\nTiempo['cos']=np.abs(np.cos(((2*np.pi)/14)*t))\nCombinacion=kronecker(Dum,Tiempo)",
"_____no_output_____"
],
[
"model = LinearRegression()\nprediction=regresion_linear(Combinacion[:n],general.MWh.values[:n])\nplt.figure(figsize=(10,5))\nplt.plot(onlyMWh.MWh.values[:n],label =\"Datos\")\nplt.plot(prediction,label=\"Predicción\")\nplt.ylabel(\"demanda en MWh\")\nplt.xlabel(\"días\")\nplt.legend()\n#plt.axis([1630,1650,120000,160000])\nplt.show()\ncomp=comparacion(onlyMWh.MWh.values[:n],prediction)\nMAPE=comp.error.mean()\nprint(\"MAPE = \",round(MAPE,4),\"%\")",
"_____no_output_____"
]
],
[
[
"### Obtener error de datos con variables dummies vs datos reales",
"_____no_output_____"
]
],
[
[
"Tabla=pd.DataFrame(columns=['regresion','datos','resta'])\nTabla[\"regresion\"]=prediction\nTabla[\"datos\"]=onlyMWh.MWh.values[:n]\nTabla[\"resta\"]=Tabla.datos-Tabla.regresion\nplt.plot(Tabla.resta)\nplt.show()",
"_____no_output_____"
]
],
[
[
"### Establecer las frecuencias que se debe considerar en la serie de fourier",
"_____no_output_____"
]
],
[
[
"f, Pxx_den = signal.periodogram(Tabla.resta, 1)\nplt.plot(1/f, Pxx_den)\nplt.xlabel('periodo')\nplt.ylabel('PSD')\nplt.show()",
"C:\\Users\\Diana\\Anaconda3\\lib\\site-packages\\ipykernel_launcher.py:2: RuntimeWarning: divide by zero encountered in true_divide\n \n"
],
[
"top_50_periods = {}\n# get indices for 3 highest Pxx values\ntop50_freq_indices = np.flip(np.argsort(Pxx_den), 0)[2:12]\n\nfreqs = f[top50_freq_indices]\npower = Pxx_den[top50_freq_indices]\nperiods = 1 / np.array(freqs)\nmatrix=pd.DataFrame(columns=[\"power\",\"periods\"])\nmatrix.power=power\nmatrix.periods=periods\nprint(matrix)",
" power periods\n0 8.749328e+09 1911.333333\n1 5.225123e+09 45.507937\n2 4.883263e+09 819.142857\n3 4.626260e+09 1146.800000\n4 4.413181e+09 36.522293\n5 4.324008e+09 955.666667\n6 4.069662e+09 573.400000\n7 4.064267e+09 521.272727\n8 3.968931e+09 61.000000\n9 3.914966e+09 91.015873\n"
]
],
[
[
"### Hacer la regresión del efecto cruzado de variables dummies y senos/cosenos absolutos de frecuencia de error",
"_____no_output_____"
]
],
[
[
"sencos = pd.DataFrame()\nsencos[\"t\"]=np.arange(1,len(onlyMWh)+1)\nfor i in matrix.periods:\n sencos[\"{}_sen\".format(i)] = np.abs(np.sin(((2*np.pi)/i)*t))\n sencos[\"{}_cos\".format(i)] = np.abs(np.cos(((2*np.pi)/i)*t))\nsencos[\"unos\"] = 1\nsencos['sen']=np.abs(np.sin(((2*np.pi)/14)*t))\nsencos['cos']=np.abs(np.cos(((2*np.pi)/14)*t))\nsencos['sen1']=np.abs(np.sin(((2*np.pi)/365)*t))\nsencos['cos1']=np.abs(np.cos(((2*np.pi)/365)*t))\nsencos['sen2']=np.abs(np.sin(((2*np.pi)/28)*t))\nsencos['cos2']=np.abs(np.cos(((2*np.pi)/28)*t))",
"_____no_output_____"
],
[
"sencos_test=sencos[n:]\nsencos_train=sencos[0:n]\nDum_test=Dum[n:]\nDum_train=Dum[0:n]\nCombinacion=kronecker(Dum_train,sencos_train)",
"_____no_output_____"
],
[
"model = LinearRegression()\nprediction=regresion_linear(Combinacion,general.MWh.values[0:n])",
"_____no_output_____"
]
],
[
[
"### MAPE de la regresion",
"_____no_output_____"
]
],
[
[
"plt.figure(figsize=(10,5))\nplt.plot(onlyMWh.MWh[0:n].values,label =\"Datos\")\nplt.plot(prediction,label=\"Predicción\")\nplt.ylabel(\"demanda en MWh\")\nplt.xlabel(\"días\")\nplt.legend()\nplt.show()\n#%%obtener mape de regresión\ncomp=comparacion(onlyMWh.MWh.values[:n],prediction)\nMAPE=comp.error.mean()\nprint(\"MAPE = \",round(MAPE,4),\"%\")",
"_____no_output_____"
]
],
[
[
"### Graficar residuales de la regresión",
"_____no_output_____"
]
],
[
[
"Tabla=pd.DataFrame(columns=['regresion','datos','resta'])\nTabla[\"regresion\"]=prediction\nTabla[\"datos\"]=onlyMWh.MWh[0:n].values\nTabla[\"resta\"]=Tabla.datos-Tabla.regresion\nplt.plot(Tabla.resta)\nplt.show()",
"_____no_output_____"
],
[
"plt.hist(Tabla[\"resta\"],bins=50)\nplt.show()",
"_____no_output_____"
],
[
"resta=pd.DataFrame(Tabla[\"resta\"])",
"_____no_output_____"
],
[
"from statsmodels.tsa.arima_model import ARIMA\nmod = ARIMA(resta, order=(1,0,4))\nresults = mod.fit()\nplt.plot(resta)\nplt.plot(results.fittedvalues, color='red')",
"_____no_output_____"
],
[
"T=pd.DataFrame(columns=['regresion','datos','nuevo'])\nT[\"regresion\"]=results.fittedvalues\nT[\"datos\"]=resta\nT[\"nuevo\"]=T.datos-T.regresion\nplt.plot(T.nuevo)\nplt.show()\n",
"_____no_output_____"
],
[
"plt.figure(figsize=(10,5))\nplt.plot(onlyMWh.MWh[0:n].values,label=\"Reales\")\nplt.plot(prediction+results.fittedvalues,label=\"Predicción\")\n#plt.axis([1630,1650,120000,160000])\nplt.ylabel(\"demanda en MWh\")\nplt.xlabel(\"días\")\nplt.legend()\nplt.show()\n#%%obtener mape de regresión\ncomp=comparacion(onlyMWh.MWh[0:n].values,prediction+results.fittedvalues)\nMAPE=comp.error.mean()\nprint(\"MAPE = \",round(MAPE,4),\"%\")",
"_____no_output_____"
]
],
[
[
"### Gráfica de manera dinámica",
"_____no_output_____"
]
],
[
[
"extra=results.predict(len(onlyMWh.MWh[0:n]),len(onlyMWh.MWh[0:n])-n)\nextra=extra.iloc[1:]",
"_____no_output_____"
],
[
"from sklearn.linear_model import Lasso\nCombinaciontest=kronecker(Dum_test,sencos_test)\n#Initializing the Lasso Regressor with Normalization Factor as True\nlasso_reg = Lasso(normalize=True)\n#Fitting the Training data to the Lasso regressor\nlasso_reg.fit(Combinacion,onlyMWh.MWh[0:n])\ncoeff = lasso_reg.coef_\n#coeff\n#Predicting for X_test\ny_pred_lass =lasso_reg.predict(Combinaciontest)",
"_____no_output_____"
],
[
"coeff = np.sum(abs(lasso_reg.coef_)==0)\ncoeff",
"_____no_output_____"
],
[
"len(lasso_reg.coef_)",
"_____no_output_____"
],
[
"#comb=Combinacion\n#comb2=Combinaciontest\n#x=np.where(lasso_reg.coef_==0)",
"_____no_output_____"
],
[
"#comb=comb.drop(comb.columns[x], axis=1)\n#comb2=comb2.drop(comb2.columns[x], axis=1)",
"_____no_output_____"
],
[
"#from sklearn.linear_model import HuberRegressor\n#huber = HuberRegressor().fit(comb,onlyMWh.MWh[0:n])\n#hubpredict=huber.predict(comb2)",
"_____no_output_____"
]
],
[
[
"### todo para pronóstico",
"_____no_output_____"
]
],
[
[
"comp_pronostico=comparacion(final,y_pred_lass+extra.values)\n#comp_pronostico=comparacion(final,hubpredict+extra.values)\nMAPE=comp_pronostico.error.mean()\nplt.figure(figsize=(10,5))\nplt.plot(final,label=\"Real\")\nplt.plot(comp_pronostico.prediccion,label=\"Pronóstico\")\nplt.ylabel(\"demanda en MWh\")\nplt.xlabel(\"días\")\nplt.legend()\nplt.show()\nprint(\"MAPE = \",round(MAPE,4),\"%\")",
"_____no_output_____"
],
[
"comp_pronostico",
"_____no_output_____"
],
[
"end = time.time()\nprint((end - start)/60)",
"6.8972692886988325\n"
],
[
"model =LinearRegression()\nmodel.fit(comb,onlyMWh.MWh[0:n])\nprediction=model.predict(comb2)\ncomp_pronostico=comparacion(final,prediction+extra.values)\nMAPE=comp_pronostico.error.mean()\nplt.figure(figsize=(10,5))\nplt.plot(final,label=\"Real\")\nplt.plot(comp_pronostico.prediccion,label=\"Pronóstico\")\nplt.ylabel(\"demanda en MWh\")\nplt.xlabel(\"días\")\nplt.legend()\nplt.show()\nprint(\"MAPE = \",round(MAPE,4),\"%\")",
"_____no_output_____"
],
[
"comp_pronostico 799,39.39 58.39 13.01",
"_____no_output_____"
],
[
"lasso_reg = Lasso(normalize=True)\n#Fitting the Training data to the Lasso regressor\nlasso_reg.fit(comb,onlyMWh.MWh[0:n])\ncoeff = lasso_reg.coef_\n#coeff\n#Predicting for X_test\ny_pred_lass =lasso_reg.predict(comb2)\ncomp_pronostico=comparacion(final,y_pred_lass+extra.values)\nMAPE=comp_pronostico.error.mean()\nplt.figure(figsize=(10,5))\nplt.plot(final,label=\"Real\")\nplt.plot(comp_pronostico.prediccion,label=\"Pronóstico\")\nplt.ylabel(\"demanda en MWh\")\nplt.xlabel(\"días\")\nplt.legend()\nplt.show()\nprint(\"MAPE = \",round(MAPE,4),\"%\")",
"_____no_output_____"
],
[
"#coeff = lasso_reg.coef_\n#coeff",
"_____no_output_____"
]
]
] | [
"markdown",
"code",
"markdown",
"code",
"markdown",
"code",
"markdown",
"code",
"markdown",
"code",
"markdown",
"code",
"markdown",
"code",
"markdown",
"code",
"markdown",
"code",
"markdown",
"code",
"markdown",
"code",
"markdown",
"code"
] | [
[
"markdown"
],
[
"code"
],
[
"markdown"
],
[
"code",
"code",
"code"
],
[
"markdown"
],
[
"code",
"code"
],
[
"markdown"
],
[
"code"
],
[
"markdown",
"markdown"
],
[
"code",
"code"
],
[
"markdown"
],
[
"code"
],
[
"markdown"
],
[
"code",
"code"
],
[
"markdown"
],
[
"code",
"code",
"code"
],
[
"markdown"
],
[
"code"
],
[
"markdown"
],
[
"code",
"code",
"code",
"code",
"code",
"code"
],
[
"markdown"
],
[
"code",
"code",
"code",
"code",
"code",
"code",
"code"
],
[
"markdown"
],
[
"code",
"code",
"code",
"code",
"code",
"code",
"code"
]
] |
d0788fdd5affe165e1e5aebf8d597e0d7f166ae9 | 12,541 | ipynb | Jupyter Notebook | Students/Tunitskaya/Hometask_1(1).ipynb | Alken1/Sberbank_ML | 20086d2568bed9444790eac221cda7bcad9f7538 | [
"MIT"
] | null | null | null | Students/Tunitskaya/Hometask_1(1).ipynb | Alken1/Sberbank_ML | 20086d2568bed9444790eac221cda7bcad9f7538 | [
"MIT"
] | null | null | null | Students/Tunitskaya/Hometask_1(1).ipynb | Alken1/Sberbank_ML | 20086d2568bed9444790eac221cda7bcad9f7538 | [
"MIT"
] | null | null | null | 24.833663 | 445 | 0.516625 | [
[
[
"# МАДМО\n\n<a href=\"https://mipt.ru/science/labs/laboratoriya-neyronnykh-sistem-i-glubokogo-obucheniya/\"><img align=\"right\" src=\"https://avatars1.githubusercontent.com/u/29918795?v=4&s=200\" alt=\"DeepHackLab\" style=\"position:relative;top:-40px;right:10px;height:100px;\" /></a>\n\n\n\n### Физтех-Школа Прикладной математики и информатики МФТИ \n### Лаборатория нейронных сетей и глубокого обучения (DeepHackLab) \nДомашнее задание необходимо загрузить в общий репозиторий с именной папкой \n",
"_____no_output_____"
],
[
"## Домашнее задание 1\n### Основы Python и пакет NumPy\n---\n",
"_____no_output_____"
]
],
[
[
"import numpy as np\nimport random\nimport scipy.stats as sps",
"_____no_output_____"
]
],
[
[
"### Задача 1\nВ первой задаче вам предлагается перемножить две квадратные матрицы двумя способами -- без использования пакета ***numpy*** и с ним.",
"_____no_output_____"
]
],
[
[
"# Для генерации матриц используем фукнцию random -- она используется для генерации случайных объектов \n# функция sample создает случайную выборку. В качестве аргумента ей передается кортеж (i,j), здесь i -- число строк,\n# j -- число столбцов.\na = np.random.sample((1000,1000))\nb = np.random.sample((1000,1000))\n# выведите ранг каждой матрицы с помощью функции np.linalg.rank.\n# Используйте функцию shape, что она вывела?\n# ========\nrank_a = np.linalg.matrix_rank(a)\nprint(rank_a)\nprint(a.shape)\nprint(np.linalg.matrix_rank(b))\nprint(b.shape)\n# ========\n#print(a)\n#print(b)",
"1000\n(1000, 1000)\n1000\n(1000, 1000)\n"
],
[
" # здесь напишите перемножение матриц без \n # использования NumPy и выведите результат \n\ndef mult(a, b):\n rows_a = len(a)\n cols_a = len(a[0])\n rows_b = len(b)\n cols_b = len(b[0])\n if cols_a != rows_b:\n return 'Error'\n c = [[0 for row in range(cols_b)] for col in range(rows_a)] \n for i in range(rows_a):\n for j in range(cols_b):\n for k in range(cols_a):\n c[i][j] += a[i][k] * b[k][j]\n #return c \n\n",
"_____no_output_____"
],
[
"def np_mult(a, b):\n # здесь напишите перемножение матриц с\n # использованием NumPy и выведите результат\n return np.dot(a,b)",
"_____no_output_____"
],
[
"%%time\n# засечем время работы функции без NumPy\nmult(a,b)\n",
"Wall time: 18min 9s\n"
],
[
"%%time\n# засечем время работы функции с NumPy\nnp_mult(a,b)",
"Wall time: 46.8 ms\n"
]
],
[
[
"### Задача 2\nНапишите функцию, которая по данной последовательности $\\{A_i\\}_{i=1}^n$ строит последовательность $S_n$, где $S_k = \\frac{A_1 + ... + A_k}{k}$. \nАналогично -- с помощью библиотеки **NumPy** и без нее. Сравните скорость, объясните результат.",
"_____no_output_____"
]
],
[
[
"\n# функция, решающая задачу с помощью NumPy\ndef sec_av(A):\n return np.cumsum(A)/list(range(1,len(A)+1))\n pass",
"_____no_output_____"
],
[
"# функция без NumPy\ndef stupid_sec_av(A):\n S = [0 for i in range(len(A))]\n S[0] = A[0]\n \n for i in range(len(A)-1):\n S[i+1] = A[i+1] + S[i]\n \n numb = list(range(1,len(A)+1))\n \n for i in range(len(A)):\n S[i] = S[i] / numb[i]\n \n return S\n\n# зададим некоторую последовательность и проверим ее на ваших функциях. \n# Первая функция должна работать ~ в 50 раз быстрее\nA = sps.uniform.rvs(size=10 ** 7) \n\n%time S1 = sec_av(A)\n%time S2 = stupid_sec_av(A)\n#проверим корректность:\nnp.abs(S1 - S2).sum()",
"Wall time: 1.39 s\nWall time: 10.7 s\n"
]
],
[
[
"### Задача 3\n\nПусть задан некоторый массив $X$. Надо построить новый массив, где все элементы с нечетными индексами требуется заменить на число $a$ (если оно не указано, то на 1). Все элементы с четными индексами исходного массива нужно возвести в куб и записать в обратном порядке относительно позиций этих элементов. Массив $X$ при этом должен остаться без изменений. В конце требуется слить массив X с преобразованным X и вывести в обратном порядке. ",
"_____no_output_____"
]
],
[
[
"# функция, решающая задачу с помощью NumPy\n\ndef transformation(X, a = 1):\n \n X[1::2] = a\n \n X[::2] **= 3\n \n X[::2] = X[::2][::-1]\n \n \n return X",
"_____no_output_____"
],
[
"# функция, решающая задачу без NumPy\n\ndef stupid_transformation(X):\n t_odd = []\n t_ev = []\n t_ev_inv = []\n Y = []\n\n t_odd = int(round(len(X)/2)) * [1] \n\n for i in range(0,len(X),2):\n t_ev = t_ev + [round(X[i]**3,8)] \n \n for i in range(len(t_ev),0,-1):\n t_ev_inv = t_ev_inv + [temp_ev[i-1]]\n \n for i in range(min(len(t_ev_inv), len(t_odd))):\n Y = Y + [t_ev_inv[i]] + [t_odd[i]]\n\n if len(t_ev_inv) > len(t_odd):\n Y = Y + [t_ev_inv[-1]]\n \n if len(t_ev_inv) < len(t_odd):\n Y = Y + [t_odd[-1]]\n \n \n return Y",
"_____no_output_____"
],
[
"X = sps.uniform.rvs(size=10 ** 7) \n# здесь код эффективнее примерно в 20 раз. \n# если Вы вдруг соберетесь печатать массив без np -- лучше сначала посмотрите на его размер\n%time S1 = transformation(X)\n%time S2 = stupid_transformation(X)\n# проверим корректность:\nnp.abs(S1 - S2).sum()",
"Wall time: 172 ms\n"
]
],
[
[
"Почему методы ***numpy*** оказываются эффективнее?",
"_____no_output_____"
]
],
[
[
"# Написаны на C",
"_____no_output_____"
]
],
[
[
"## Дополнительные задачи",
"_____no_output_____"
],
[
"Дополнительные задачи подразумевают, что Вы самостоятельно разберётесь в некоторых функциях ***numpy***, чтобы их сделать. \n\nЭти задачи не являются обязательными, но могут повлиять на Ваш рейтинг в лучшую сторону (точные правила учёта доп. задач будут оглашены позже).",
"_____no_output_____"
],
[
"### Задача 4*",
"_____no_output_____"
],
[
"Дана функция двух переменных: $f(x, y) = sin(x)cos(y)$ (это просто такой красивый 3D-график), а также дана функция для отрисовки $f(x, y)$ (`draw_f()`), которая принимает на вход двумерную сетку, на которой будет вычисляться функция. \n\nВам нужно разобраться в том, как строить такие сетки (подсказка - это одна конкретная функция ***numpy***), и подать такую сетку на вход функции отрисовки.",
"_____no_output_____"
]
],
[
[
"from matplotlib import pyplot as plt\nfrom mpl_toolkits.mplot3d import Axes3D\n%matplotlib inline\n\ndef f(x, y):\n '''Функция двух переменных'''\n return np.sin(x) * np.cos(y)\n\ndef draw_f(grid_x, grid_y):\n '''Функция отрисовки функции f(x, y)'''\n fig = plt.figure(figsize=(10, 8))\n ax = Axes3D(fig)\n ax.plot_surface(grid_x, grid_y, f(grid_x, grid_y), cmap='inferno')\n plt.show()",
"_____no_output_____"
],
[
"\n\ni = np.arange(-1, 1, 0.02)\n\ngrid_x, grid_y = np.meshgrid(i, i)\n\ndraw_f(grid_x, grid_y)",
"_____no_output_____"
]
],
[
[
"### Задача 5*",
"_____no_output_____"
],
[
"Выберите любую картинку и загрузите ее в папку с кодом. При загрузке её размерность равна 3: **(w, h, num_channels)**, где **w** - ширина картинки в пикселях, **h** - высота картинки в пикселях, **num_channels** - количество каналов *(R, G, B, alpha)*.\n\nВам нужно \"развернуть\" картинку в одномерный массив размера w \\* h \\* num_channels, написав **одну строку кода**.",
"_____no_output_____"
]
],
[
[
"from matplotlib import pyplot as plt\n%matplotlib inline",
"_____no_output_____"
],
[
"path_to_image = './image.png'\nimage_array = plt.imread(path_to_image)\nplt.imshow(image_array);\n",
"_____no_output_____"
],
[
"flat_image_array = = image_array.flatten()",
"_____no_output_____"
],
[
"print(len(flat_image_array))",
"_____no_output_____"
]
]
] | [
"markdown",
"code",
"markdown",
"code",
"markdown",
"code",
"markdown",
"code",
"markdown",
"code",
"markdown",
"code",
"markdown",
"code"
] | [
[
"markdown",
"markdown"
],
[
"code"
],
[
"markdown"
],
[
"code",
"code",
"code",
"code",
"code"
],
[
"markdown"
],
[
"code",
"code"
],
[
"markdown"
],
[
"code",
"code",
"code"
],
[
"markdown"
],
[
"code"
],
[
"markdown",
"markdown",
"markdown",
"markdown"
],
[
"code",
"code"
],
[
"markdown",
"markdown"
],
[
"code",
"code",
"code",
"code"
]
] |
d0789986eed8e7aef633cb4c95593727cce20271 | 50,764 | ipynb | Jupyter Notebook | 9_SqueezeNet.ipynb | styler00dollar/Colab-image-classification | b17bbd17defe04522c12a92289a98563fa0a27cc | [
"MIT"
] | 5 | 2021-03-26T14:34:46.000Z | 2021-11-07T12:34:24.000Z | 9_SqueezeNet.ipynb | styler00dollar/Colab-image-classification | b17bbd17defe04522c12a92289a98563fa0a27cc | [
"MIT"
] | null | null | null | 9_SqueezeNet.ipynb | styler00dollar/Colab-image-classification | b17bbd17defe04522c12a92289a98563fa0a27cc | [
"MIT"
] | null | null | null | 40.097946 | 330 | 0.458258 | [
[
[
"# Colab-pytorch-image-classification\r\nOriginal repo: [bentrevett/pytorch-image-classification](https://github.com/bentrevett/pytorch-image-classification)\r\n\r\n[SqueezeNet code](https://github.com/pytorch/vision/blob/master/torchvision/models/squeezenet.py): [pytorch/vision](https://github.com/pytorch/vision)\r\n\r\nMy fork: [styler00dollar/Colab-image-classification](https://github.com/styler00dollar/Colab-image-classification)\r\n\r\nThis colab is a combination of [this Colab](https://colab.research.google.com/github/bentrevett/pytorch-image-classification/blob/master/5_resnet.ipynb) and [my other Colab](https://colab.research.google.com/github/styler00dollar/Colab-image-classification/blob/master/5_(small)_ResNet.ipynb) to do SqueezeNet training. ",
"_____no_output_____"
]
],
[
[
"!nvidia-smi",
"_____no_output_____"
]
],
[
[
"# DATASET CREATION",
"_____no_output_____"
]
],
[
[
"#@title Mount Google Drive\r\nfrom google.colab import drive\r\ndrive.mount('/content/drive')\r\nprint('Google Drive connected.')",
"_____no_output_____"
],
[
"# copy data somehow\r\n!mkdir '/content/classification'\r\n!mkdir '/content/classification/images'\r\n!cp \"/content/drive/MyDrive/classification_v2.7z\" \"/content/classification/images/classification.7z\"\r\n%cd /content/classification/images\r\n!7z x \"classification.7z\"\r\n!rm -rf /content/classification/images/classification.7z",
"_____no_output_____"
],
[
"#@title dataset creation\nTRAIN_RATIO = 0.90 #@param {type:\"number\"}\nimport os\nimport shutil\nfrom tqdm import tqdm\n#data_dir = os.path.join(ROOT, 'CUB_200_2011')\ndata_dir = '/content/classification' #@param {type:\"string\"}\nimages_dir = os.path.join(data_dir, 'images')\ntrain_dir = os.path.join(data_dir, 'train')\ntest_dir = os.path.join(data_dir, 'test')\n\nif os.path.exists(train_dir):\n shutil.rmtree(train_dir) \nif os.path.exists(test_dir):\n shutil.rmtree(test_dir)\n \nos.makedirs(train_dir)\nos.makedirs(test_dir)\n\nclasses = os.listdir(images_dir)\n\nfor c in classes:\n \n class_dir = os.path.join(images_dir, c)\n \n images = os.listdir(class_dir)\n \n n_train = int(len(images) * TRAIN_RATIO)\n \n train_images = images[:n_train]\n test_images = images[n_train:]\n \n os.makedirs(os.path.join(train_dir, c), exist_ok = True)\n os.makedirs(os.path.join(test_dir, c), exist_ok = True)\n \n for image in tqdm(train_images):\n image_src = os.path.join(class_dir, image)\n image_dst = os.path.join(train_dir, c, image) \n shutil.copyfile(image_src, image_dst)\n \n for image in tqdm(test_images):\n image_src = os.path.join(class_dir, image)\n image_dst = os.path.join(test_dir, c, image) \n shutil.copyfile(image_src, image_dst)",
"_____no_output_____"
]
],
[
[
"# CALC MEANS & STDS",
"_____no_output_____"
]
],
[
[
"#@title print means and stds\nimport torch\nimport torchvision.transforms as transforms\nimport torchvision.datasets as datasets\nfrom tqdm import tqdm\ntrain_data = datasets.ImageFolder(root = train_dir, \n transform = transforms.ToTensor())\n\nmeans = torch.zeros(3)\nstds = torch.zeros(3)\n\nfor img, label in tqdm(train_data):\n means += torch.mean(img, dim = (1,2))\n stds += torch.std(img, dim = (1,2))\n\nmeans /= len(train_data)\nstds /= len(train_data)\n\nprint(\"\\n\")\nprint(f'Calculated means: {means}')\nprint(f'Calculated stds: {stds}')",
"_____no_output_____"
]
],
[
[
"# TRAIN",
"_____no_output_____"
]
],
[
[
"#@title import, seed, transforms, dataloader, functions, plot, model, parameter\n%cd /content/\nfrom tqdm import tqdm\nimport torch\nimport torch.nn as nn\nimport torch.nn.functional as F\nimport torch.optim as optim\nimport torch.optim.lr_scheduler as lr_scheduler\nfrom torch.optim.lr_scheduler import _LRScheduler\nimport torch.utils.data as data\n\nimport torchvision.transforms as transforms\nimport torchvision.datasets as datasets\nimport torchvision.models as models\n\nfrom sklearn import decomposition\nfrom sklearn import manifold\nfrom sklearn.metrics import confusion_matrix\nfrom sklearn.metrics import ConfusionMatrixDisplay\nimport matplotlib.pyplot as plt\nimport numpy as np\n\nimport copy\nfrom collections import namedtuple\nimport os\nimport random\nimport shutil\n\nSEED = 1234 #@param {type:\"number\"}\n\nrandom.seed(SEED)\nnp.random.seed(SEED)\ntorch.manual_seed(SEED)\ntorch.cuda.manual_seed(SEED)\ntorch.backends.cudnn.deterministic = True\n\ntrain_dir = '/content/classification/train' #@param {type:\"string\"}\ntest_dir = '/content/classification/test' #@param {type:\"string\"}\npretrained_size = 256 #@param {type:\"number\"}\npretrained_means = [0.6838, 0.6086, 0.6063] #@param {type:\"raw\"}\npretrained_stds= [0.2411, 0.2403, 0.2306] #@param {type:\"raw\"}\n\n\n#https://github.com/mit-han-lab/data-efficient-gans/blob/master/DiffAugment_pytorch.py\nimport torch\nimport torch.nn.functional as F\n\n\ndef DiffAugment(x, policy='', channels_first=True):\n if policy:\n if not channels_first:\n x = x.permute(0, 3, 1, 2)\n for p in policy.split(','):\n for f in AUGMENT_FNS[p]:\n x = f(x)\n if not channels_first:\n x = x.permute(0, 2, 3, 1)\n x = x.contiguous()\n return x\n\n\ndef rand_brightness(x):\n x = x + (torch.rand(x.size(0), 1, 1, 1, dtype=x.dtype, device=x.device) - 0.5)\n return x\n\n\ndef rand_saturation(x):\n x_mean = x.mean(dim=1, keepdim=True)\n x = (x - x_mean) * (torch.rand(x.size(0), 1, 1, 1, dtype=x.dtype, device=x.device) * 2) + x_mean\n return x\n\n\ndef rand_contrast(x):\n x_mean = x.mean(dim=[1, 2, 3], keepdim=True)\n x = (x - x_mean) * (torch.rand(x.size(0), 1, 1, 1, dtype=x.dtype, device=x.device) + 0.5) + x_mean\n return x\n\n\ndef rand_translation(x, ratio=0.125):\n shift_x, shift_y = int(x.size(2) * ratio + 0.5), int(x.size(3) * ratio + 0.5)\n translation_x = torch.randint(-shift_x, shift_x + 1, size=[x.size(0), 1, 1], device=x.device)\n translation_y = torch.randint(-shift_y, shift_y + 1, size=[x.size(0), 1, 1], device=x.device)\n grid_batch, grid_x, grid_y = torch.meshgrid(\n torch.arange(x.size(0), dtype=torch.long, device=x.device),\n torch.arange(x.size(2), dtype=torch.long, device=x.device),\n torch.arange(x.size(3), dtype=torch.long, device=x.device),\n )\n grid_x = torch.clamp(grid_x + translation_x + 1, 0, x.size(2) + 1)\n grid_y = torch.clamp(grid_y + translation_y + 1, 0, x.size(3) + 1)\n x_pad = F.pad(x, [1, 1, 1, 1, 0, 0, 0, 0])\n x = x_pad.permute(0, 2, 3, 1).contiguous()[grid_batch, grid_x, grid_y].permute(0, 3, 1, 2)\n return x\n\n\ndef rand_cutout(x, ratio=0.5):\n cutout_size = int(x.size(2) * ratio + 0.5), int(x.size(3) * ratio + 0.5)\n offset_x = torch.randint(0, x.size(2) + (1 - cutout_size[0] % 2), size=[x.size(0), 1, 1], device=x.device)\n offset_y = torch.randint(0, x.size(3) + (1 - cutout_size[1] % 2), size=[x.size(0), 1, 1], device=x.device)\n grid_batch, grid_x, grid_y = torch.meshgrid(\n torch.arange(x.size(0), dtype=torch.long, device=x.device),\n torch.arange(cutout_size[0], dtype=torch.long, device=x.device),\n torch.arange(cutout_size[1], dtype=torch.long, device=x.device),\n )\n grid_x = torch.clamp(grid_x + offset_x - cutout_size[0] // 2, min=0, max=x.size(2) - 1)\n grid_y = torch.clamp(grid_y + offset_y - cutout_size[1] // 2, min=0, max=x.size(3) - 1)\n mask = torch.ones(x.size(0), x.size(2), x.size(3), dtype=x.dtype, device=x.device)\n mask[grid_batch, grid_x, grid_y] = 0\n x = x * mask.unsqueeze(1)\n return x\n\n\nAUGMENT_FNS = {\n 'color': [rand_brightness, rand_saturation, rand_contrast],\n 'translation': [rand_translation],\n 'cutout': [rand_cutout],\n}\n\n\ntrain_transforms = transforms.Compose([\n transforms.Resize(pretrained_size),\n transforms.RandomRotation(5),\n transforms.RandomHorizontalFlip(0.5),\n transforms.RandomCrop(pretrained_size, padding = 10),\n transforms.ToTensor(),\n transforms.Normalize(mean = pretrained_means, \n std = pretrained_stds)\n ])\n\ntest_transforms = transforms.Compose([\n transforms.Resize(pretrained_size),\n transforms.CenterCrop(pretrained_size),\n transforms.ToTensor(),\n transforms.Normalize(mean = pretrained_means, \n std = pretrained_stds)\n ])\n\ntrain_data = datasets.ImageFolder(root = train_dir, \n transform = train_transforms)\n\ntest_data = datasets.ImageFolder(root = test_dir, \n transform = test_transforms) \n\nVALID_RATIO = 0.90 #@param {type:\"number\"}\n\nn_train_examples = int(len(train_data) * VALID_RATIO)\nn_valid_examples = len(train_data) - n_train_examples\n\ntrain_data, valid_data = data.random_split(train_data, \n [n_train_examples, n_valid_examples])\n\nvalid_data = copy.deepcopy(valid_data)\nvalid_data.dataset.transform = test_transforms\n\nprint(f'Number of training examples: {len(train_data)}')\nprint(f'Number of validation examples: {len(valid_data)}')\nprint(f'Number of testing examples: {len(test_data)}')\n\nBATCH_SIZE = 32 #@param {type:\"number\"}\n\ntrain_iterator = data.DataLoader(train_data, \n shuffle = True, \n batch_size = BATCH_SIZE)\n\nvalid_iterator = data.DataLoader(valid_data, \n batch_size = BATCH_SIZE)\n\ntest_iterator = data.DataLoader(test_data, \n batch_size = BATCH_SIZE)\n\ndef normalize_image(image):\n image_min = image.min()\n image_max = image.max()\n image.clamp_(min = image_min, max = image_max)\n image.add_(-image_min).div_(image_max - image_min + 1e-5)\n return image \ndef plot_images(images, labels, classes, normalize = True):\n\n n_images = len(images)\n\n rows = int(np.sqrt(n_images))\n cols = int(np.sqrt(n_images))\n\n fig = plt.figure(figsize = (15, 15))\n\n for i in range(rows*cols):\n\n ax = fig.add_subplot(rows, cols, i+1)\n \n image = images[i]\n\n if normalize:\n image = normalize_image(image)\n\n ax.imshow(image.permute(1, 2, 0).cpu().numpy())\n label = classes[labels[i]]\n ax.set_title(label)\n ax.axis('off')\n\nN_IMAGES = 25 #@param {type:\"number\"}\n\nimages, labels = zip(*[(image, label) for image, label in \n [train_data[i] for i in range(N_IMAGES)]])\n\nclasses = test_data.classes\n\nplot_images(images, labels, classes)\n\ndef format_label(label):\n label = label.split('.')[-1]\n label = label.replace('_', ' ')\n label = label.title()\n label = label.replace(' ', '')\n return label\n\ntest_data.classes = [format_label(c) for c in test_data.classes]\n\nclasses = test_data.classes\n\nplot_images(images, labels, classes)\n\n\n#https://github.com/pytorch/vision/blob/master/torchvision/models/squeezenet.py\nimport torch\nimport torch.nn as nn\nimport torch.nn.init as init\n#from .utils import load_state_dict_from_url\nfrom typing import Any\n\n#__all__ = ['SqueezeNet', 'squeezenet1_0', 'squeezenet1_1']\n\nmodel_urls = {\n '1_0': 'https://download.pytorch.org/models/squeezenet1_0-a815701f.pth',\n '1_1': 'https://download.pytorch.org/models/squeezenet1_1-f364aa15.pth',\n}\n\n\nclass Fire(nn.Module):\n\n def __init__(\n self,\n inplanes: int,\n squeeze_planes: int,\n expand1x1_planes: int,\n expand3x3_planes: int\n ) -> None:\n super(Fire, self).__init__()\n self.inplanes = inplanes\n self.squeeze = nn.Conv2d(inplanes, squeeze_planes, kernel_size=1)\n self.squeeze_activation = nn.ReLU(inplace=True)\n self.expand1x1 = nn.Conv2d(squeeze_planes, expand1x1_planes,\n kernel_size=1)\n self.expand1x1_activation = nn.ReLU(inplace=True)\n self.expand3x3 = nn.Conv2d(squeeze_planes, expand3x3_planes,\n kernel_size=3, padding=1)\n self.expand3x3_activation = nn.ReLU(inplace=True)\n\n def forward(self, x: torch.Tensor) -> torch.Tensor:\n x = self.squeeze_activation(self.squeeze(x))\n return torch.cat([\n self.expand1x1_activation(self.expand1x1(x)),\n self.expand3x3_activation(self.expand3x3(x))\n ], 1)\n\n\nclass SqueezeNet(nn.Module):\n\n def __init__(\n self,\n version: str = '1_0',\n num_classes: int = 1000\n ) -> None:\n super(SqueezeNet, self).__init__()\n self.num_classes = num_classes\n if version == '1_0':\n self.features = nn.Sequential(\n nn.Conv2d(3, 96, kernel_size=7, stride=2),\n nn.ReLU(inplace=True),\n nn.MaxPool2d(kernel_size=3, stride=2, ceil_mode=True),\n Fire(96, 16, 64, 64),\n Fire(128, 16, 64, 64),\n Fire(128, 32, 128, 128),\n nn.MaxPool2d(kernel_size=3, stride=2, ceil_mode=True),\n Fire(256, 32, 128, 128),\n Fire(256, 48, 192, 192),\n Fire(384, 48, 192, 192),\n Fire(384, 64, 256, 256),\n nn.MaxPool2d(kernel_size=3, stride=2, ceil_mode=True),\n Fire(512, 64, 256, 256),\n )\n elif version == '1_1':\n self.features = nn.Sequential(\n nn.Conv2d(3, 64, kernel_size=3, stride=2),\n nn.ReLU(inplace=True),\n nn.MaxPool2d(kernel_size=3, stride=2, ceil_mode=True),\n Fire(64, 16, 64, 64),\n Fire(128, 16, 64, 64),\n nn.MaxPool2d(kernel_size=3, stride=2, ceil_mode=True),\n Fire(128, 32, 128, 128),\n Fire(256, 32, 128, 128),\n nn.MaxPool2d(kernel_size=3, stride=2, ceil_mode=True),\n Fire(256, 48, 192, 192),\n Fire(384, 48, 192, 192),\n Fire(384, 64, 256, 256),\n Fire(512, 64, 256, 256),\n )\n else:\n # FIXME: Is this needed? SqueezeNet should only be called from the\n # FIXME: squeezenet1_x() functions\n # FIXME: This checking is not done for the other models\n raise ValueError(\"Unsupported SqueezeNet version {version}:\"\n \"1_0 or 1_1 expected\".format(version=version))\n\n # Final convolution is initialized differently from the rest\n final_conv = nn.Conv2d(512, self.num_classes, kernel_size=1)\n self.classifier = nn.Sequential(\n nn.Dropout(p=0.5),\n final_conv,\n nn.ReLU(inplace=True),\n nn.AdaptiveAvgPool2d((1, 1))\n )\n\n for m in self.modules():\n if isinstance(m, nn.Conv2d):\n if m is final_conv:\n init.normal_(m.weight, mean=0.0, std=0.01)\n else:\n init.kaiming_uniform_(m.weight)\n if m.bias is not None:\n init.constant_(m.bias, 0)\n\n def forward(self, x: torch.Tensor) -> torch.Tensor:\n x = self.features(x)\n x = self.classifier(x)\n return torch.flatten(x, 1)\n\n\ndef _squeezenet(version: str, pretrained: bool, progress: bool, **kwargs: Any) -> SqueezeNet:\n model = SqueezeNet(version, **kwargs)\n if pretrained:\n arch = 'squeezenet' + version\n state_dict = load_state_dict_from_url(model_urls[arch],\n progress=progress)\n model.load_state_dict(state_dict)\n return model\n\n\ndef squeezenet1_0(pretrained: bool = False, progress: bool = True, **kwargs: Any) -> SqueezeNet:\n r\"\"\"SqueezeNet model architecture from the `\"SqueezeNet: AlexNet-level\n accuracy with 50x fewer parameters and <0.5MB model size\"\n <https://arxiv.org/abs/1602.07360>`_ paper.\n\n Args:\n pretrained (bool): If True, returns a model pre-trained on ImageNet\n progress (bool): If True, displays a progress bar of the download to stderr\n \"\"\"\n return _squeezenet('1_0', pretrained, progress, **kwargs)\n\n\ndef squeezenet1_1(pretrained: bool = False, progress: bool = True, **kwargs: Any) -> SqueezeNet:\n r\"\"\"SqueezeNet 1.1 model from the `official SqueezeNet repo\n <https://github.com/DeepScale/SqueezeNet/tree/master/SqueezeNet_v1.1>`_.\n SqueezeNet 1.1 has 2.4x less computation and slightly fewer parameters\n than SqueezeNet 1.0, without sacrificing accuracy.\n\n Args:\n pretrained (bool): If True, returns a model pre-trained on ImageNet\n progress (bool): If True, displays a progress bar of the download to stderr\n \"\"\"\n return _squeezenet('1_1', pretrained, progress, **kwargs)\n\n\"\"\"\n#https://github.com/pytorch/vision/blob/master/torchvision/models/utils.py\ntry:\n from torch.hub import load_state_dict_from_url\nexcept ImportError:\n from torch.utils.model_zoo import load_url as load_state_dict_from_url\n\"\"\"\n\nmodel_train = '1_1' #@param [\"1_0\", \"1_1\"] {type:\"string\"}\nif model_train == '1_0':\n model = SqueezeNet(num_classes=len(test_data.classes), version='1_0')\n #state_dict = load_state_dict_from_url(model_urls[model_train],\n # progress=True)\n #model.load_state_dict(state_dict)\nelif model_train == '1_1':\n model = SqueezeNet(num_classes=len(test_data.classes), version='1_1')\n #state_dict = load_state_dict_from_url(model_urls[model_train],\n # progress=True)\n #model.load_state_dict(state_dict)\n\n\ndef count_parameters(model):\n return sum(p.numel() for p in model.parameters() if p.requires_grad)\n\nprint(f'The model has {count_parameters(model):,} trainable parameters')\n\nSTART_LR = 1e-7 #@param {type:\"number\"}\n\noptimizer = optim.Adam(model.parameters(), lr=START_LR)\n\ndevice = torch.device('cuda' if torch.cuda.is_available() else 'cpu')\n\ncriterion = nn.CrossEntropyLoss()\n\nmodel = model.to(device)\ncriterion = criterion.to(device)\n\nclass LRFinder:\n def __init__(self, model, optimizer, criterion, device):\n \n self.optimizer = optimizer\n self.model = model\n self.criterion = criterion\n self.device = device\n \n torch.save(model.state_dict(), 'init_params.pt')\n\n def range_test(self, iterator, end_lr = 10, num_iter = 100, \n smooth_f = 0.05, diverge_th = 5):\n \n lrs = []\n losses = []\n best_loss = float('inf')\n\n lr_scheduler = ExponentialLR(self.optimizer, end_lr, num_iter)\n \n iterator = IteratorWrapper(iterator)\n \n for iteration in tqdm(range(num_iter)):\n\n loss = self._train_batch(iterator)\n\n #update lr\n lr_scheduler.step()\n \n lrs.append(lr_scheduler.get_lr()[0])\n\n if iteration > 0:\n loss = smooth_f * loss + (1 - smooth_f) * losses[-1]\n \n if loss < best_loss:\n best_loss = loss\n\n losses.append(loss)\n \n if loss > diverge_th * best_loss:\n print(\"Stopping early, the loss has diverged\")\n break\n \n #reset model to initial parameters\n model.load_state_dict(torch.load('init_params.pt'))\n \n return lrs, losses\n\n def _train_batch(self, iterator):\n \n self.model.train()\n \n self.optimizer.zero_grad()\n \n x, y = iterator.get_batch()\n \n x = x.to(self.device)\n y = y.to(self.device)\n \n y_pred, _ = self.model(x)\n \n loss = self.criterion(y_pred, y)\n \n loss.backward()\n \n self.optimizer.step()\n \n return loss.item()\n\nclass ExponentialLR(_LRScheduler):\n def __init__(self, optimizer, end_lr, num_iter, last_epoch=-1):\n self.end_lr = end_lr\n self.num_iter = num_iter\n super(ExponentialLR, self).__init__(optimizer, last_epoch)\n\n def get_lr(self):\n curr_iter = self.last_epoch + 1\n r = curr_iter / self.num_iter\n return [base_lr * (self.end_lr / base_lr) ** r for base_lr in self.base_lrs]\n\nclass IteratorWrapper:\n def __init__(self, iterator):\n self.iterator = iterator\n self._iterator = iter(iterator)\n\n def __next__(self):\n try:\n inputs, labels = next(self._iterator)\n except StopIteration:\n self._iterator = iter(self.iterator)\n inputs, labels, *_ = next(self._iterator)\n\n return inputs, labels\n\n def get_batch(self):\n return next(self)\n\n\ndef calculate_topk_accuracy(y_pred, y, k = 5):\n with torch.no_grad():\n batch_size = y.shape[0]\n\n _, top_pred = y_pred.topk(k=1)\n \n top_pred = top_pred.t()\n correct = top_pred.eq(y.view(1, -1).expand_as(top_pred))\n correct_1 = correct[:1].view(-1).float().sum(0, keepdim = True)\n #correct_k = correct[:k].view(-1).float().sum(0, keepdim = True)\n acc_1 = correct_1 / batch_size\n #acc_k = correct_k / batch_size\n acc_k = 0\n return acc_1, acc_k\n\n\ndef train(model, iterator, optimizer, criterion, scheduler, device, current_epoch):\n \n epoch_loss = 0\n epoch_acc_1 = 0\n epoch_acc_5 = 0\n \n model.train()\n policy = 'color,translation,cutout' #@param {type:\"string\"}\n diffaug_activate = True #@param [\"False\", \"True\"] {type:\"raw\"}\n #https://stackoverflow.com/questions/45465031/printing-text-below-tqdm-progress-bar\n with tqdm(iterator, position=1, bar_format='{desc}') as desc:\n\n for (x, y) in tqdm(iterator, position=0):\n x = x.to(device)\n y = y.to(device)\n \n optimizer.zero_grad()\n \n if diffaug_activate == False:\n y_pred = model(x)\n else:\n y_pred = model(DiffAugment(x, policy=policy))\n\n loss = criterion(y_pred, y)\n \n acc_1, acc_5 = calculate_topk_accuracy(y_pred, y)\n\n \n loss.backward()\n \n optimizer.step()\n \n scheduler.step()\n \n epoch_loss += loss.item()\n epoch_acc_1 += acc_1.item()\n #epoch_acc_5 += acc_5.item()\n \n epoch_loss /= len(iterator)\n epoch_acc_1 /= len(iterator)\n desc.set_description(f'Epoch: {current_epoch+1}')\n desc.set_description(f'\\tTrain Loss: {epoch_loss:.3f} | Train Acc @1: {epoch_acc_1*100:6.2f}% | ' \\\n f'Train Acc @5: {epoch_acc_5*100:6.2f}%')\n\n\n return epoch_loss, epoch_acc_1, epoch_acc_5\n\ndef evaluate(model, iterator, criterion, device):\n \n epoch_loss = 0\n epoch_acc_1 = 0\n epoch_acc_5 = 0\n \n model.eval()\n \n with torch.no_grad():\n with tqdm(iterator, position=0, bar_format='{desc}', leave=True) as desc:\n for (x, y) in iterator:\n\n x = x.to(device)\n y = y.to(device)\n\n y_pred = model(x)\n\n loss = criterion(y_pred, y)\n\n acc_1, acc_5 = calculate_topk_accuracy(y_pred, y)\n\n epoch_loss += loss.item()\n epoch_acc_1 += acc_1.item()\n #epoch_acc_5 += acc_5.item()\n \n epoch_loss /= len(iterator)\n epoch_acc_1 /= len(iterator)\n #epoch_acc_5 /= len(iterator)\n \n desc.set_description(f'\\tValid Loss: {epoch_loss:.3f} | Valid Acc @1: {epoch_acc_1*100:6.2f}% | ' \\\n f'Valid Acc @5: {epoch_acc_5*100:6.2f}%')\n return epoch_loss, epoch_acc_1, epoch_acc_5\n\ndef epoch_time(start_time, end_time):\n elapsed_time = end_time - start_time\n elapsed_mins = int(elapsed_time / 60)\n elapsed_secs = int(elapsed_time - (elapsed_mins * 60))\n return elapsed_mins, elapsed_secs",
"_____no_output_____"
],
[
"#@title lr_finder\nEND_LR = 10 #@param {type:\"number\"}\nNUM_ITER = 100#@param {type:\"number\"} #100\n\nlr_finder = LRFinder(model, optimizer, criterion, device)\nlrs, losses = lr_finder.range_test(train_iterator, END_LR, NUM_ITER)",
"_____no_output_____"
],
[
"#@title plot_lr_finder\ndef plot_lr_finder(lrs, losses, skip_start = 5, skip_end = 5):\n \n if skip_end == 0:\n lrs = lrs[skip_start:]\n losses = losses[skip_start:]\n else:\n lrs = lrs[skip_start:-skip_end]\n losses = losses[skip_start:-skip_end]\n \n fig = plt.figure(figsize = (16,8))\n ax = fig.add_subplot(1,1,1)\n ax.plot(lrs, losses)\n ax.set_xscale('log')\n ax.set_xlabel('Learning rate')\n ax.set_ylabel('Loss')\n ax.grid(True, 'both', 'x')\n plt.show()\n\nplot_lr_finder(lrs, losses, skip_start = 30, skip_end = 30)",
"_____no_output_____"
],
[
"#@title config\nFOUND_LR = 2e-4 #@param {type:\"number\"}\n\"\"\"\nparams = [\n {'params': model.conv1.parameters(), 'lr': FOUND_LR / 10},\n {'params': model.bn1.parameters(), 'lr': FOUND_LR / 10},\n {'params': model.layer1.parameters(), 'lr': FOUND_LR / 8},\n {'params': model.layer2.parameters(), 'lr': FOUND_LR / 6},\n {'params': model.layer3.parameters(), 'lr': FOUND_LR / 4},\n {'params': model.layer4.parameters(), 'lr': FOUND_LR / 2},\n {'params': model.fc.parameters()}\n ]\n\n\"\"\"\n#optimizer = optim.Adam(params, lr = FOUND_LR)\noptimizer = optim.Adam(model.parameters(), lr = FOUND_LR)\n\nEPOCHS = 100 #@param {type:\"number\"}\nSTEPS_PER_EPOCH = len(train_iterator)\nTOTAL_STEPS = EPOCHS * STEPS_PER_EPOCH\n\nMAX_LRS = [p['lr'] for p in optimizer.param_groups]\n\nscheduler = lr_scheduler.OneCycleLR(optimizer,\n max_lr = MAX_LRS,\n total_steps = TOTAL_STEPS)",
"_____no_output_____"
],
[
"#@title training without topk\nimport time\nbest_valid_loss = float('inf')\nbest_valid_accuracy = 0\n\nfor epoch in range(EPOCHS):\n start_time = time.monotonic()\n \n train_loss, train_acc_1, train_acc_5 = train(model, train_iterator, optimizer, criterion, scheduler, device, epoch)\n valid_loss, valid_acc_1, valid_acc_5 = evaluate(model, valid_iterator, criterion, device)\n \n if valid_loss < best_valid_loss:\n best_valid_loss = valid_loss\n torch.save(model.state_dict(), 'best-validation-loss.pt')\n if best_valid_accuracy < valid_acc_1:\n best_valid_accuracy = valid_acc_1\n torch.save(model.state_dict(), 'best-validation-accuracy.pt')\n\n end_time = time.monotonic()\n\n epoch_mins, epoch_secs = epoch_time(start_time, end_time)",
"_____no_output_____"
]
],
[
[
"#####################################################################################################",
"_____no_output_____"
],
[
"# TESTING",
"_____no_output_____"
]
],
[
[
"#@title Calc test loss\nmodel.load_state_dict(torch.load('best-validation-accuracy.pt'))\nprint(\"best-validation-accuracy.pt\")\ntest_loss, test_acc_1, test_acc_5 = evaluate(model, test_iterator, criterion, device)\nprint(\"-----------------------------\")\nmodel.load_state_dict(torch.load('best-validation-loss.pt'))\nprint(\"best-validation-loss.pt\")\ntest_loss, test_acc_1, test_acc_5 = evaluate(model, test_iterator, criterion, device)",
"_____no_output_____"
],
[
"#@title plot_confusion_matrix\ndef get_predictions(model, iterator):\n\n model.eval()\n\n images = []\n labels = []\n probs = []\n\n with torch.no_grad():\n\n for (x, y) in iterator:\n\n x = x.to(device)\n\n y_pred = model(x)\n\n y_prob = F.softmax(y_pred, dim = -1)\n top_pred = y_prob.argmax(1, keepdim = True)\n\n images.append(x.cpu())\n labels.append(y.cpu())\n probs.append(y_prob.cpu())\n\n images = torch.cat(images, dim = 0)\n labels = torch.cat(labels, dim = 0)\n probs = torch.cat(probs, dim = 0)\n\n return images, labels, probs\n\nimages, labels, probs = get_predictions(model, test_iterator)\npred_labels = torch.argmax(probs, 1)\n\ndef plot_confusion_matrix(labels, pred_labels, classes):\n \n fig = plt.figure(figsize = (50, 50));\n ax = fig.add_subplot(1, 1, 1);\n cm = confusion_matrix(labels, pred_labels);\n cm = ConfusionMatrixDisplay(cm, display_labels = classes);\n cm.plot(values_format = 'd', cmap = 'Blues', ax = ax)\n fig.delaxes(fig.axes[1]) #delete colorbar\n plt.xticks(rotation = 90)\n plt.xlabel('Predicted Label', fontsize = 50)\n plt.ylabel('True Label', fontsize = 50)\n\nplot_confusion_matrix(labels, pred_labels, classes)",
"_____no_output_____"
],
[
"#@title plot\r\ncorrects = torch.eq(labels, pred_labels)\r\n\r\nincorrect_examples = []\r\n\r\nfor image, label, prob, correct in zip(images, labels, probs, corrects):\r\n if not correct:\r\n incorrect_examples.append((image, label, prob))\r\n\r\nincorrect_examples.sort(reverse = True, key = lambda x: torch.max(x[2], dim = 0).values)\r\n\r\ndef plot_most_incorrect(incorrect, classes, n_images, normalize = True):\r\n\r\n rows = int(np.sqrt(n_images))\r\n cols = int(np.sqrt(n_images))\r\n\r\n fig = plt.figure(figsize = (25, 20))\r\n\r\n for i in range(rows*cols):\r\n\r\n ax = fig.add_subplot(rows, cols, i+1)\r\n \r\n image, true_label, probs = incorrect[i]\r\n image = image.permute(1, 2, 0)\r\n true_prob = probs[true_label]\r\n incorrect_prob, incorrect_label = torch.max(probs, dim = 0)\r\n true_class = classes[true_label]\r\n incorrect_class = classes[incorrect_label]\r\n\r\n if normalize:\r\n image = normalize_image(image)\r\n\r\n ax.imshow(image.cpu().numpy())\r\n ax.set_title(f'true label: {true_class} ({true_prob:.3f})\\n' \\\r\n f'pred label: {incorrect_class} ({incorrect_prob:.3f})')\r\n ax.axis('off')\r\n \r\n fig.subplots_adjust(hspace=0.4)\r\n\r\nN_IMAGES = 36\r\n\r\nplot_most_incorrect(incorrect_examples, classes, N_IMAGES)",
"_____no_output_____"
],
[
"#@title plot_representations\ndef get_representations(model, iterator):\n\n model.eval()\n\n outputs = []\n intermediates = []\n labels = []\n\n with torch.no_grad():\n \n for (x, y) in iterator:\n\n x = x.to(device)\n\n y_pred, _ = model(x)\n\n outputs.append(y_pred.cpu())\n labels.append(y)\n \n outputs = torch.cat(outputs, dim = 0)\n labels = torch.cat(labels, dim = 0)\n\n return outputs, labels\n\noutputs, labels = get_representations(model, train_iterator)\n\ndef get_pca(data, n_components = 2):\n pca = decomposition.PCA()\n pca.n_components = n_components\n pca_data = pca.fit_transform(data)\n return pca_data\n \ndef plot_representations(data, labels, classes, n_images = None):\n \n if n_images is not None:\n data = data[:n_images]\n labels = labels[:n_images]\n \n fig = plt.figure(figsize = (15, 15))\n ax = fig.add_subplot(111)\n scatter = ax.scatter(data[:, 0], data[:, 1], c = labels, cmap = 'hsv')\n #handles, _ = scatter.legend_elements(num = None)\n #legend = plt.legend(handles = handles, labels = classes)\n\noutput_pca_data = get_pca(outputs)\nplot_representations(output_pca_data, labels, classes)",
"_____no_output_____"
],
[
"#@title get_tsne\ndef get_tsne(data, n_components = 2, n_images = None):\n \n if n_images is not None:\n data = data[:n_images]\n \n tsne = manifold.TSNE(n_components = n_components, random_state = 0)\n tsne_data = tsne.fit_transform(data)\n return tsne_data\n\noutput_tsne_data = get_tsne(outputs)\nplot_representations(output_tsne_data, labels, classes)",
"_____no_output_____"
],
[
"#@title plot_filtered_images\ndef plot_filtered_images(images, filters, n_filters = None, normalize = True):\n\n images = torch.cat([i.unsqueeze(0) for i in images], dim = 0).cpu()\n filters = filters.cpu()\n\n if n_filters is not None:\n filters = filters[:n_filters]\n\n n_images = images.shape[0]\n n_filters = filters.shape[0]\n\n filtered_images = F.conv2d(images, filters)\n\n fig = plt.figure(figsize = (30, 30))\n\n for i in range(n_images):\n\n image = images[i]\n\n if normalize:\n image = normalize_image(image)\n\n ax = fig.add_subplot(n_images, n_filters+1, i+1+(i*n_filters))\n ax.imshow(image.permute(1,2,0).numpy())\n ax.set_title('Original')\n ax.axis('off')\n\n for j in range(n_filters):\n image = filtered_images[i][j]\n\n if normalize:\n image = normalize_image(image)\n\n ax = fig.add_subplot(n_images, n_filters+1, i+1+(i*n_filters)+j+1)\n ax.imshow(image.numpy(), cmap = 'bone')\n ax.set_title(f'Filter {j+1}')\n ax.axis('off');\n\n fig.subplots_adjust(hspace = -0.7)\n\nN_IMAGES = 5\nN_FILTERS = 7\n\nimages = [image for image, label in [train_data[i] for i in range(N_IMAGES)]]\nfilters = model.conv1.weight.data\n\nplot_filtered_images(images, filters, N_FILTERS)",
"_____no_output_____"
],
[
"#@title plot_filters\n#filters = model.conv1.weight.data\n\ndef plot_filters(filters, normalize = True):\n\n filters = filters.cpu()\n\n n_filters = filters.shape[0]\n\n rows = int(np.sqrt(n_filters))\n cols = int(np.sqrt(n_filters))\n\n fig = plt.figure(figsize = (30, 15))\n\n for i in range(rows*cols):\n\n image = filters[i]\n\n if normalize:\n image = normalize_image(image)\n\n ax = fig.add_subplot(rows, cols, i+1)\n ax.imshow(image.permute(1, 2, 0))\n ax.axis('off')\n \n fig.subplots_adjust(wspace = -0.9)\n\nplot_filters(filters)",
"_____no_output_____"
]
]
] | [
"markdown",
"code",
"markdown",
"code",
"markdown",
"code",
"markdown",
"code",
"markdown",
"code"
] | [
[
"markdown"
],
[
"code"
],
[
"markdown"
],
[
"code",
"code",
"code"
],
[
"markdown"
],
[
"code"
],
[
"markdown"
],
[
"code",
"code",
"code",
"code",
"code"
],
[
"markdown",
"markdown"
],
[
"code",
"code",
"code",
"code",
"code",
"code",
"code"
]
] |
d078d0336c903e713be58cc3d497be3bb54472e5 | 987 | ipynb | Jupyter Notebook | String/1228/557. Reverse Words in a String III.ipynb | YuHe0108/Leetcode | 90d904dde125dd35ee256a7f383961786f1ada5d | [
"Apache-2.0"
] | 1 | 2020-08-05T11:47:47.000Z | 2020-08-05T11:47:47.000Z | String/1228/557. Reverse Words in a String III.ipynb | YuHe0108/LeetCode | b9e5de69b4e4d794aff89497624f558343e362ad | [
"Apache-2.0"
] | null | null | null | String/1228/557. Reverse Words in a String III.ipynb | YuHe0108/LeetCode | b9e5de69b4e4d794aff89497624f558343e362ad | [
"Apache-2.0"
] | null | null | null | 18.622642 | 51 | 0.489362 | [
[
[
"class Solution:\n def reverseWords(self, s: str) -> str:\n s_list = s.split(' ')\n res = [w[::-1] for w in s_list]\n return ' '.join(res)",
"_____no_output_____"
]
]
] | [
"code"
] | [
[
"code"
]
] |
d078d992b9e7c4c12e814c50451d65ff5368f330 | 55,630 | ipynb | Jupyter Notebook | 00_Variables_to_Classes.ipynb | darius74/geothermics | e7b1d0a9ecb2efb28f74f328e6d45c89ee6c09da | [
"MIT"
] | 2 | 2018-09-20T13:17:37.000Z | 2020-03-22T19:51:37.000Z | 00_Variables_to_Classes.ipynb | darius74/geothermics | e7b1d0a9ecb2efb28f74f328e6d45c89ee6c09da | [
"MIT"
] | null | null | null | 00_Variables_to_Classes.ipynb | darius74/geothermics | e7b1d0a9ecb2efb28f74f328e6d45c89ee6c09da | [
"MIT"
] | 1 | 2020-03-22T23:02:59.000Z | 2020-03-22T23:02:59.000Z | 99.695341 | 26,472 | 0.848966 | [
[
[
"# From Variables to Classes\n## A short Introduction \n\nPython - as any programming language - has many extensions and libraries at its disposal. Basically, there are libraries for everything. \n\n<center>But what are **libraries**? </center> \n\nBasically, **libraries** are a collection of methods (_small pieces of code where you put sth in and get sth else out_) which you can use to analyse your data, visualise your data, run models ... do anything you like. \n\nAs said, methods usually take _something_ as input. That _something_ is usually a **variable**. \n\nIn the following, we will work our way from **variables** to **libraries**.",
"_____no_output_____"
],
[
"## Variables \n\nVariables are one of the simplest types of objects in a programming language. An [object](https://en.wikipedia.org/wiki/Object_(computer_science) is a value stored in the memory of your computer, marked by a specific identifyer. Variables can have different types, such as [strings, numbers, and booleans](https://www.learnpython.org/en/Variables_and_Types). Differently to other programming languages, you do not need to declare the type of a variable, as variables are handled as objects in Python. \n\n```python\nx = 4.2 # floating point number\ny = 'Hello World!' # string\nz = True # boolean\n```",
"_____no_output_____"
]
],
[
[
"x = 4.2 \nprint(type(x))\n\ny = 'Hello World!'\nprint(type(y))\n\nz = True\nprint(type(z))",
"<class 'float'>\n<class 'str'>\n<class 'bool'>\n"
]
],
[
[
"We can use operations (normal arithmetic operations) to use variables for getting results we want. With numbers, you can add, substract, multiply, divide, basically taking the values from the memory assigned to the variable name and performing calculations. \n\nLet's have a look at operations with numbers and strings. We leave booleans to the side for the moment. We will simply add the variables below. \n\n```python \nn1 = 7 \nn2 = 42\n\ns1 = 'Looking good, '\ns2 = 'you are.'\n```",
"_____no_output_____"
]
],
[
[
"n1 = 7\nn2 = 42\n\ns1 = 'Looking good, ' \ns2 = 'you are.'\n\nfirst_sum = n1 + n2\nprint(first_sum)\nfirst_conc = s1 + s2\nprint(first_conc)",
"49\nLooking good, you are.\n"
]
],
[
[
"Variables can be more than just a number. If you think of an Excel-Spreadsheet, a variable can be the content of a single cell, or multiple cells can be combined in one variable (e.g. one column of an Excel table). \nSo let's create a list -_a collection of variables_ - from `x`, `n1`, and `n2`. Lists in python are created using [ ]. \nNow, if you want to calculate the sum of this list, it is really exhausting to sum up every item of this list manually. \n\n```python\nfirst_list = [x, n1, n2] \n# a sum of a list could look like\nsecond_sum = some_list[0] + some_list[1] + ... + some_list[n] # where n is the last item of the list, e.g. 2 for first_list. \n```",
"_____no_output_____"
],
[
"Actually, writing the second sum like this is the same as before. It would be great, if this step of calculating the sum could be used many times without writing it out. And this is, what functions are for. For example, there already exists a sum function: \n\n```python \nsum(first_list)```",
"_____no_output_____"
]
],
[
[
"first_list = [x, n1, n2]\nsecond_sum = first_list[0] + first_list[1] + first_list[2]\nprint('manual sum {}'.format(second_sum))\n\n# This can also be done with a function \nprint('sum function {}'.format(sum(first_list)))",
"manual sum 53.2\nsum function 53.2\n"
]
],
[
[
"## Functions \nThe `sum()` method we used above is a **function**. \nFunctions (later we will call them methods) are pieces of code, which take an input, perform some kind of operation, and (_optionally_) return an output. ",
"_____no_output_____"
],
[
"In Python, functions are written like: \n\n```python\ndef func(input):\n \"\"\"\n Description of the functions content # called the function header\n \"\"\"\n some kind of operation on input # called the function body\n \n return output\n```\nAs an example, we write a `sumup` function which sums up a list.",
"_____no_output_____"
]
],
[
[
"def sumup(inp):\n \"\"\"\n input: inp - list/array with floating point or integer numbers\n return: sumd - scalar value of the summed up list\n \"\"\"\n val = 0\n for i in inp:\n val = val + i\n return val\n\n# let's compare the implemented standard sum function with the new sumup function\nsum1 = sum(first_list)\nsum2 = sumup(first_list)\nprint(\"The python sum function yields {}, \\nand our sumup function yields {}.\".format(*(sum1,sum2)))",
"The python sum function yields 53.2, \nand our sumup function yields 53.2.\n"
],
[
"# summing up the numbers from 1 to 100\nimport numpy as np\nar_2_sum = np.linspace(1,100,100, dtype='i')\n\nprint(\"the sum of the array is: {}\".format(sumup(ar_2_sum)))",
"the sum of the array is: 5050\n"
]
],
[
[
"As we see above, functions are quite practical and save a lot of time. Further, they help structuring your code. Some functions are directly available in python without any libraries or other external software. In the example above however, you might have noticed, that we `import`ed a library called `numpy`. \nIn those libraries, functions are merged to one package, having the advantage that you don't need to import each single function at a time. \nImagine you move and have to pack all your belongings. You can think of libraries as packing things with similar purpose in the same box (= library).",
"_____no_output_____"
],
[
"## Functions to Methods as part of classes\nWhen we talk about functions in the environment of classes, we usually call them methods. But what are **classes**? \n[Classes](https://docs.python.org/3/tutorial/classes.html) are ways to bundle functionality together. Logically, functionality with similar purpose (or different kind of similarity). \nOne example could be: think of **apples**. \n\nApples are now a class. You can apply methods to this class, such as `eat()` or `cut()`. Or more sophisticated methods including various recipes using apples comprised in a cookbook. \nThe `eat()` method is straight forward. But the `cut()` method may be more interesting, since there are various ways to cut an apple.\n",
"_____no_output_____"
],
[
"Let's assume there are two apples to be cut differently. In python, once you have assigned a class to a variable, you have created an **instance** of that class. Then, methods of are applied to that instance by using a . notation.\n```python\nGolden_Delicious = apple()\nYoya = apple()\n\nGolden_Delicious.cut(4)\nYoya.cut(8)\n```\nThe two apples Golden Delicious and Yoya are _instances_ of the class apple. Real _incarnations_ of the abstract concept _apple_. The Golden Delicious is cut into 4 pieces, while the Yoya is cut into 8 pieces. ",
"_____no_output_____"
],
[
"This is similar to more complex libraries, such as the `scikit-learn`. In one exercise, you used the command: \n```python\nfrom sklearn.cluster import KMeans\n```\nwhich simply imports the **class** `KMeans` from the library part `sklearn.cluster`. `KMeans` comprises several methods for clustering, which you can use by calling them similar to the apple example before.",
"_____no_output_____"
],
[
" For this, you need to create an _instance_ of the `KMeans` class. \n```python\n...\nkmeans_inst = KMeans(n_clusters=n_clusters) # first we create the instance of the KMeans class called kmeans_inst\nkmeans_inst.fit(data) # then we apply a method to the instance kmeans_inst\n...\n```\nAn example:",
"_____no_output_____"
]
],
[
[
"# here we just create the data for clustering\nfrom sklearn.datasets.samples_generator import make_blobs\nimport matplotlib.pyplot as plt\n%matplotlib inline\nX, y = make_blobs(n_samples=100, centers=3, cluster_std= 0.5,\n random_state=0)\n\nplt.scatter(X[:,0], X[:,1], s=70)",
"_____no_output_____"
],
[
"# now we create an instance of the KMeans class\nfrom sklearn.cluster import KMeans\nnr_of_clusters = 3 # because we see 3 clusters in the plot above\nkmeans_inst = KMeans(n_clusters= nr_of_clusters) # create the instance kmeans_inst\nkmeans_inst.fit(X) # apply a method to the instance\ny_predict = kmeans_inst.predict(X) # apply another method to the instance and save it in another variable",
"_____no_output_____"
],
[
"# lets plot the predicted cluster centers colored in the cluster color\nplt.scatter(X[:, 0], X[:, 1], c=y_predict, s=50, cmap='Accent')\ncenters = kmeans_inst.cluster_centers_ # apply the method to find the new centers of the determined clusters\nplt.scatter(centers[:, 0], centers[:, 1], c='red', s=200, alpha=0.6); # plot the cluster centers",
"_____no_output_____"
]
],
[
[
"## Summary \nThis short presentation is meant to make you familiar with the concept of variables, functions, methods and classes. All of which are objects! \n* Variables are normally declared by the user and link a value stored in the memory of your pc to a variable name. They are usually the input of functions \n* Functions are pieces of code taking an input and performing some operation on said input. Optionally, they return directly an output value \n* To facilitate the use of functions, they are sometimes bundled as methods within classes. Classes in turn can build up whole libraries in python. ",
"_____no_output_____"
],
[
"* Similar to real book libraries, python libraries contain a collection of _recipes_ which can be applied to your data. \n* In terms of apples: You own different kinds of apples. A book about apple dishes (_class_) from the library contains different recipes (_methods_) which can be used for your different apples (_instances of the class_).",
"_____no_output_____"
],
[
"## Further links \n* [Data Science Handbook](https://jakevdp.github.io/PythonDataScienceHandbook/)\n* [Python for Geosciences](https://github.com/koldunovn/python_for_geosciences) \n* [Introduction to Python for Geoscientists](http://ggorman.github.io/Introduction-to-programming-for-geoscientists/) \n* [Full Video course on Object Oriented Programming](https://www.youtube.com/watch?v=ZDa-Z5JzLYM&list=PL-osiE80TeTsqhIuOqKhwlXsIBIdSeYtc)",
"_____no_output_____"
]
]
] | [
"markdown",
"code",
"markdown",
"code",
"markdown",
"code",
"markdown",
"code",
"markdown",
"code",
"markdown"
] | [
[
"markdown",
"markdown"
],
[
"code"
],
[
"markdown"
],
[
"code"
],
[
"markdown",
"markdown"
],
[
"code"
],
[
"markdown",
"markdown"
],
[
"code",
"code"
],
[
"markdown",
"markdown",
"markdown",
"markdown",
"markdown"
],
[
"code",
"code",
"code"
],
[
"markdown",
"markdown",
"markdown"
]
] |
d078da8ffd55b306eafa9d7c19857448a34cb548 | 428,822 | ipynb | Jupyter Notebook | dementia_optima/preprocessing/.ipynb_checkpoints/dataAnalysis_and_preparation-checkpoint.ipynb | SDM-TIB/dementia_mmse | bf22947aa06350edd454794eb2b926f082d2dcf2 | [
"MIT"
] | null | null | null | dementia_optima/preprocessing/.ipynb_checkpoints/dataAnalysis_and_preparation-checkpoint.ipynb | SDM-TIB/dementia_mmse | bf22947aa06350edd454794eb2b926f082d2dcf2 | [
"MIT"
] | null | null | null | dementia_optima/preprocessing/.ipynb_checkpoints/dataAnalysis_and_preparation-checkpoint.ipynb | SDM-TIB/dementia_mmse | bf22947aa06350edd454794eb2b926f082d2dcf2 | [
"MIT"
] | null | null | null | 41.669614 | 629 | 0.262459 | [
[
[
"import pandas as pd\nimport numpy as np\n\npd.set_option('display.max_columns', None) \npd.set_option('display.max_rows', None)\npd.set_option('display.max_colwidth', -1)\n\n%matplotlib inline\n\nfrom sidecar import Sidecar\nfrom ipywidgets import IntSlider\n\nsc = Sidecar(title='Sidecar Output')\nsl = IntSlider(description='Some slider')",
"_____no_output_____"
],
[
"# Remove unrelated columns form data and get their name\n\nfolder_path = '../../../datalcdem/data/optima/dementia_18July/'\n\npatient_df = pd.read_csv(folder_path + 'optima_patients.csv')\ndisplay(patient_df.head(5))\n\n#patient_df[['MMS1', 'MMS2']].hist()\n\npatient_com_df = pd.read_csv(folder_path + 'optima_patients_comorbidities.csv').groupby(by=['patient_id', 'EPISODE_DATE'], as_index=False).agg(lambda x: x.tolist())[['patient_id', 'EPISODE_DATE', 'Comorbidity_cui']]\ndisplay(patient_com_df.head(10))\n\npatient_filt_df = pd.read_csv(folder_path + 'optima_patients_filtered.csv')\n#display(patient_filt_df.head(5))\n\n\npatient_treat_df = pd.read_csv(folder_path + 'optima_patients_treatments.csv').groupby(by=['patient_id', 'EPISODE_DATE'], as_index=False).agg(lambda x: x.tolist())[['patient_id', 'EPISODE_DATE', 'Medication_cui']]\ndisplay(patient_treat_df.head(5))",
"_____no_output_____"
],
[
"len(set(patient_com_df['patient_id'].tolist())), len(set(patient_treat_df['patient_id'].tolist()))",
"_____no_output_____"
],
[
"patient_treat_df['EPISODE_DATE'] = pd.to_datetime(patient_treat_df['EPISODE_DATE'])\npatient_com_df['EPISODE_DATE'] = pd.to_datetime(patient_com_df['EPISODE_DATE'])\n\npatient_com_treat_df = pd.merge(patient_com_df, patient_treat_df,on=['patient_id', 'EPISODE_DATE'], how='outer')\n#pd.concat([patient_com_df, patient_treat_df], keys=['patient_id', 'EPISODE_DATE'], ignore_index=True, sort=False) #patient_com_df.append(patient_treat_df, sort=False) #pd.concat([patient_com_df, patient_treat_df], axis=0, sort=False)\n#patient_treat_com_df = patient_treat_df.join(patient_com_df, on=['patient_id', 'EPISODE_DATE'], how='outer')\nprint (patient_com_treat_df.shape)\nprint (len(set(patient_com_treat_df['patient_id'].tolist())))\npatient_com_treat_df.sort_values(by=['patient_id', 'EPISODE_DATE'],axis=0, inplace=True, ascending=True)\npatient_com_treat_df.reset_index(drop=True, inplace=True)\npatient_com_treat_df.head(10)",
"(3690, 4)\n820\n"
],
[
"patient_com_treat_df.to_csv('../../../datalcdem/data/optima/optima_ahmad/patient_com_treat_df.csv')",
"_____no_output_____"
],
[
"folder_path = '../../../datalcdem/data/optima/dementia_18July/'",
"_____no_output_____"
],
[
"df_datarequest = pd.read_excel(folder_path+'Data_Request_Jan_2019_final.xlsx')\ndf_datarequest.head(5)\ndf_datarequest_mmse = df_datarequest[['GLOBAL_PATIENT_DB_ID', 'Age At Episode', 'EPISODE_DATE', 'CAMDEX SCORES: MINI MENTAL SCORE']]\ndf_datarequest_mmse_1 = df_datarequest_mmse.rename(columns={'GLOBAL_PATIENT_DB_ID':'patient_id'})\ndf_datarequest_mmse_1.head(10)",
"_____no_output_____"
],
[
"#patient_com_treat_df.astype('datetime')\npatient_com_treat_df['EPISODE_DATE'] = pd.to_datetime(patient_com_treat_df['EPISODE_DATE'])\nprint (df_datarequest_mmse_1.dtypes, patient_com_treat_df.dtypes)\npatient_com_treat_df = pd.merge(patient_com_treat_df,df_datarequest_mmse_1,on=['patient_id', 'EPISODE_DATE'], how='left')\npatient_com_treat_df.shape, patient_com_treat_df.head(10)",
"patient_id int64 \nAge At Episode int64 \nEPISODE_DATE datetime64[ns]\nCAMDEX SCORES: MINI MENTAL SCORE float64 \ndtype: object patient_id int64 \nEPISODE_DATE datetime64[ns]\nComorbidity_cui object \nMedication_cui object \ndtype: object\n"
],
[
"len(set (patient_com_treat_df['patient_id'].tolist()))\npatient_com_treat_df.sort_values(by=['patient_id', 'EPISODE_DATE'],axis=0, inplace=True, ascending=True)\npatient_com_treat_df.head(20)\npatient_com_treat_df.reset_index(inplace=True, drop=True)\npatient_com_treat_df.head(5)",
"_____no_output_____"
],
[
"def setLineNumber(lst):\n lst_dict = {ide:0 for ide in lst}\n lineNumber_list = []\n \n for idx in lst:\n if idx in lst_dict:\n lst_dict[idx] = lst_dict[idx] + 1 \n lineNumber_list.append(lst_dict[idx])\n \n return lineNumber_list\n \n\npatient_com_treat_df['lineNumber'] = setLineNumber(patient_com_treat_df['patient_id'].tolist())\npatient_com_treat_df.tail(20)",
"_____no_output_____"
],
[
"df = patient_com_treat_df\n\nid_dict = {i:0 for i in df['patient_id'].tolist()}\nfor x in df['patient_id'].tolist():\n if x in id_dict:\n id_dict[x]=id_dict[x]+1\n\nline_updated = [int(j) for i in id_dict.values() for j in range(1,i+1)]\nprint (line_updated[0:10])\ndf.update(pd.Series(line_updated, name='lineNumber'),errors='ignore')\ndisplay(df.head(20))\n\n\n#patients merging based on id and creating new columns\nr = df['lineNumber'].max()\nprint ('Max line:',r)\nl = [df[df['lineNumber']==i] for i in range(1, int(r+1))]\nprint('Number of Dfs to merge: ',len(l))\ndf_new = pd.DataFrame()\ntmp_id = []\nfor i, df_l in enumerate(l):\n df_l = df_l[~df_l['patient_id'].isin(tmp_id)]\n for j, df_ll in enumerate(l[i+1:]):\n #df_l = df_l.merge(df_ll, on='id', how='left', suffix=(str(j), str(j+1))) #suffixe is not working\n #print (j)\n df_l = df_l.join(df_ll.set_index('patient_id'), on='patient_id', rsuffix='_'+str(j+1))\n tmp_id = tmp_id + df_l['patient_id'].tolist()\n #display(df_l)\n df_new = df_new.append(df_l, ignore_index=True, sort=False)\ndisplay(df_new.head(20))\ndisplay(df_new[['patient_id']+[col for col in df_new.columns if 'line' in col or 'DATE' in col]].head(10))",
"[1, 2, 3, 1, 2, 3, 4, 5, 6, 7]\n"
],
[
"fltr_linnum = ['_'+str(i) for i in range(10, 27)]\nprint (fltr_linnum)\ndf_new.drop(columns=[col for col in df_new.columns for i in fltr_linnum if i in col],inplace=True)",
"['_10', '_11', '_12', '_13', '_14', '_15', '_16', '_17', '_18', '_19', '_20', '_21', '_22', '_23', '_24', '_25', '_26']\n"
],
[
"df_new.to_csv(folder_path+'dementialTreatmentLine_preData_line_episode.csv', index=False)\ndf_new = df_new.drop([col for col in df_new.columns if 'lineNumber' in col or 'EPISODE_DATE' in col], axis=1).reset_index(drop=True)\ndf_new.to_csv(folder_path+'dementialTreatmentLine_preData.csv', index=False)",
"_____no_output_____"
],
[
"# Calculate matching intial episode in the data\n\n''' df_episode= pd.read_csv('../../../datalcdem/data/optima/dementialTreatmentLine_preData_line_episode.csv')\ndf_patients = pd.read_csv('../../../datalcdem/data/optima/patients.csv')\ndisplay(df_episode.columns, df_patients.columns)\n\ndf_pat_ep = pd.merge(df_episode[['patient_id', 'EPISODE_DATE']], df_patients[['patient_id', 'epDateInicial', 'mmseInicial']])\n\ndf_episode.shape, df_patients.shape, df_pat_ep.shape\n\ndf_pat_ep['dateEqual']=df_pat_ep['EPISODE_DATE']==df_pat_ep['epDateInicial']\ndisplay(sum(df_pat_ep['dateEqual'].tolist()))\ndf_pat_ep.head(10)\ndisplay(sum(df_pat_ep['mmseInicial']<24))'''",
"_____no_output_____"
],
[
"df_new.head(10)",
"_____no_output_____"
],
[
"# Take Some other features from API\ndf_patient_api = pd.read_csv(folder_path+'patients.csv')\ndisplay(df_patient_api.head(10))\ndf_patient_api = df_patient_api[['patient_id', 'gender', 'dementia', 'smoker', 'alcohol', 'education',\n 'bmi', 'weight', 'apoe']]\ndisplay(df_patient_api.head(10))\ndisplay(df_new.head(10))\ndf_patient_new = df_patient_api.merge(df_new, on=['patient_id'], how='inner')\ndf_patient_new.head(10)",
"_____no_output_____"
],
[
"df_patient_new.to_csv(folder_path+'patients_new.csv', index=False)",
"_____no_output_____"
],
[
"def removeNANvalues(lst):\n return lst[~numpy.isnan(lst)]\n\ncomorbidity_cui_lst = df_patient_new[[col for col in df_patient_new.columns if 'Comorbidity_cui' in col]].values.flatten()\nmedication_cui_lst = df_patient_new[[col for col in df_patient_new.columns if 'Medication_cui' in col]].values.flatten()\nx = x[~numpy.isnan(x)]\n\nmedication_cui_lst",
"_____no_output_____"
],
[
"[val for lst_1 in medication_cui.flatten() for val in lst_1]",
"_____no_output_____"
]
]
] | [
"code"
] | [
[
"code",
"code",
"code",
"code",
"code",
"code",
"code",
"code",
"code",
"code",
"code",
"code",
"code",
"code",
"code",
"code",
"code",
"code",
"code"
]
] |
d078dbca6f8740469c8369b528c6adbd76d77d77 | 95,338 | ipynb | Jupyter Notebook | AI506/hw1/hw1-20194331/hw1_problem.ipynb | sungnyun/AI-assignments | 6451fd6db33fd8671ca362b4ad4c190979a98c22 | [
"MIT"
] | null | null | null | AI506/hw1/hw1-20194331/hw1_problem.ipynb | sungnyun/AI-assignments | 6451fd6db33fd8671ca362b4ad4c190979a98c22 | [
"MIT"
] | null | null | null | AI506/hw1/hw1-20194331/hw1_problem.ipynb | sungnyun/AI-assignments | 6451fd6db33fd8671ca362b4ad4c190979a98c22 | [
"MIT"
] | null | null | null | 83.629825 | 14,088 | 0.719241 | [
[
[
"import numpy as np\nimport matplotlib.pyplot as plt\nfrom sklearn import datasets\nfrom tqdm import tqdm\n\nfrom collections import defaultdict\nfrom itertools import combinations\nimport re\nimport time",
"_____no_output_____"
],
[
"### You may import any Python's standard library here (Do not import other external libraries) ###\n",
"_____no_output_____"
],
[
"pass_test1_1_1 = False\npass_test1_1_2 = False\npass_test1_2 = False\npass_test2_1 = False\npass_test2_2 = False",
"_____no_output_____"
]
],
[
[
"## Implementing LSH algorithm",
"_____no_output_____"
],
[
"### 0. Dataset",
"_____no_output_____"
],
[
"#### 0.1 Import 20-news dataset",
"_____no_output_____"
]
],
[
[
"newsgroup_dataset = datasets.fetch_20newsgroups(data_home='./dataset/', subset='train', \n remove=('headers', 'footers', 'quotes'), download_if_missing=True)",
"_____no_output_____"
],
[
"raw_documents = newsgroup_dataset['data'][:]\nlen(raw_documents)",
"_____no_output_____"
],
[
"raw_documents[0]",
"_____no_output_____"
]
],
[
[
"#### 0.2 Preprocess the documents",
"_____no_output_____"
]
],
[
[
"K = 5 # number of word tokens to shinlge",
"_____no_output_____"
],
[
"def preprocess(documents):\n processed_words = defaultdict(list)\n cnt = 0\n \n for doc in documents:\n # first, filter out some uncesseary symbols like punctuations\n doc = re.sub('\\/|\\-|\\'|\\@|\\%|\\$|\\#|\\,|\\(|\\)|\\}|\\\"|\\{|\\?|\\.|\\!|\\;|\\:', '', doc)\n \n # second, split the document into the words\n for word in doc.split():\n \n # third, let word to be the lower-case\n if word.isalpha():\n processed_words[cnt].append(word.lower())\n \n # fourth, filter out the articles that has less than k shingles\n if len(processed_words[cnt]) < K:\n continue\n else:\n processed_words[cnt] = ' '.join(processed_words[cnt])\n cnt += 1\n\n return list(processed_words.values())",
"_____no_output_____"
],
[
"documents = preprocess(raw_documents)\ndel raw_documents\nlen(documents)",
"_____no_output_____"
],
[
"documents[0]",
"_____no_output_____"
]
],
[
[
"### 1. Shingling",
"_____no_output_____"
]
],
[
[
"########################################################################################################################\n# Programming 1 [15pt] #\n# In this section, you will implement the shingling algorithm to convert the document into the characteristic matrix. #\n# However, since storing the whole characteristic matrix in the form of a dense matrix is expensivein terms of space, #\n# your implementation should store the characteristic matrix in the form of a dictionary. #\n# #\n# i) get the all unique shingles from the documents [10pt] #\n# ii) create the dictionary that maps each document to the list of shingles [5pt] #\n# #\n# Note that, shingling is divided into 2-steps just for the readability of the algorithm #\n# #\n########################################################################################################################",
"_____no_output_____"
]
],
[
[
"#### 1.1 Get Shingles from the documents",
"_____no_output_____"
]
],
[
[
"def get_shingles(documents):\n ######################################################################################\n # Programming 1.1 [10pt] #\n # Implement 'get_shingles' function to get 1-singles from the preprocessed documents #\n # You should especially be take care of your algorithm's computational efficiency #\n # #\n # Parameters: #\n # documents (dict) #\n # #\n # Returns: #\n # shingles (set) set of tuples where each element is a k-shingle #\n # ex) shingles = {('its', 'hard', 'to', 'say', 'whether'), #\n # ('known', 'bugs', 'in', 'the', 'warning') ...} #\n ######################################################################################\n shingles = set()\n for doc in documents:\n doc_split = doc.split()\n for i in range(len(doc_split) - (K-1)):\n shingles.add(tuple(doc_split[i:i+K]))\n \n return shingles",
"_____no_output_____"
],
[
"start = time.time()\nshingles = get_shingles(documents)\nend = time.time()\n\n# Check whether your implementation is correct [5pt]\nif len(shingles) == 1766049:\n pass_test1_1_1 = True\n print('Test1 passed')\n \n # Check whether your implementation is efficient enough [5pt]\n # With 4-lines of my implementations, it took 4.8 seconds with i7-8700 cpu\n if (end - start) < 20:\n pass_test1_1_2 = True\n print('Test2 passed')\n ",
"Test1 passed\nTest2 passed\n"
],
[
"print(end - start)",
"1.0299334526062012\n"
]
],
[
[
"#### 1.2 Build document to shingles dictionary",
"_____no_output_____"
]
],
[
[
"def build_doc_to_shingle_dictionary(documents, shingles):\n ################################################################################################################################\n # Programming 1.2 [5pt] #\n # Implement 'build_doc_to_shingle_dictionary' function to convert documents into shingle dictionary with documents & shingles #\n # You need to construct and utilize a shingle2idx dictionary that maps each shingle into the uniuqe integer index. #\n # #\n # Parameters: #\n # documents (dict) # \n # shingles (set) # \n # #\n # Returns: #\n # doc_to_shingles (dict) #\n # key: index of the documents #\n # value: list of the shingle indexes #\n # ex) doc_to_shingles = {0: [1705196, 422880, 491967, ...], #\n # 1: [863922, 1381606, 1524066, ...], #\n # ... } #\n ################################################################################################################################\n doc_to_shingles = {}\n shingle2idx = {}\n for idx, shingle in enumerate(shingles):\n shingle2idx[shingle] = idx\n for idx, doc in enumerate(documents):\n shingle_list = [shingle2idx[s] for s in get_shingles([doc])]\n doc_to_shingles[idx] = shingle_list\n \n return doc_to_shingles",
"_____no_output_____"
],
[
"doc_to_shingles = build_doc_to_shingle_dictionary(documents, shingles)\n\n# Check whether your implementation is correct [5pt]\nif len(doc_to_shingles) == 10882 and len(doc_to_shingles[0]) == 84:\n pass_test1_2 = True\n print('Test passed')",
"Test passed\n"
]
],
[
[
"### 2. Min-Hashing",
"_____no_output_____"
]
],
[
[
"############################################################################################################################\n# Programming 2 [25pt] #\n# In this section, you will implement the min-hashing algorithm to convert the characteristic matrix into the signatures. #\n# #\n# i) implement the jaccard-similarity algorithm [5pt] #\n# ii) implement the min-hash algorithm to create the signatures for the documents [20pt] #\n# #\n############################################################################################################################",
"_____no_output_____"
]
],
[
[
"#### 2.1 Generate Prime numbers for Universal Hashing",
"_____no_output_____"
]
],
[
[
"def is_prime(n):\n for i in range(2,int(np.sqrt(n))+1):\n if not n % i:\n return False\n return True\n\ndef generate_prime_numbers(M, N):\n # this function generate the M prime numbers where each prime number is greater than N\n primes = []\n cnt = 0\n n = N + 1\n \n while cnt < M:\n if is_prime(n):\n primes.append(n)\n cnt += 1\n n += 1\n return primes",
"_____no_output_____"
],
[
"# Test prime number generation\ngenerate_prime_numbers(M = 3, N = 3)",
"_____no_output_____"
]
],
[
[
"#### 2.2 Jaccard Similarity",
"_____no_output_____"
]
],
[
[
"def jaccard_similarity(s1, s2):\n ##################################################################################\n # Programming 2.2 [5pt] # \n # Implement the jaccard similarity algorithm to get the similarity of two sets #\n # #\n # Parameters #\n # s1 (set) #\n # s2 (set) #\n # Returns #\n # similarity (float) #\n ##################################################################################\n similarity = len(s1&s2) / len(s1|s2)\n return similarity",
"_____no_output_____"
],
[
"s1 = {1, 3, 4}\ns2 = {3, 4, 6}\n\nif (jaccard_similarity(s1, s2) - 0.5) < 1e-3:\n pass_test2_1 = True\n print('Test passed')",
"Test passed\n"
]
],
[
[
"#### 2.3 Min Hash",
"_____no_output_____"
]
],
[
[
"M = 100 # Number of Hash functions to use\nN = len(shingles)\n\n# First we will create M universal hashing functions\n# You can also modify or implement your own hash functions for implementing min_hash function\n\nclass Hash():\n def __init__(self, M, N):\n self.M = M\n self.N = N\n self.p = generate_prime_numbers(M, N)\n \n self.a = np.random.choice(9999, M)\n self.b = np.random.choice(9999, M)\n \n def __call__(self, x):\n return np.mod(np.mod((self.a * x + self.b), self.p), self.N)\n \n def __len__(self):\n return M\n \n#primes = generate_prime_numbers(M, N)\nhash_functions = Hash(M, N)",
"_____no_output_____"
],
[
"def min_hash(doc_to_shingles, hash_functions):\n ###########################################################################################\n # Programming 2.3 [20pt] # \n # Implement the min-hash algorithm to create the signatures for the documents #\n # It would take about ~10 minutes to finish computation, #\n # while would take ~20 seconds if you parallelize your hash functions # \n # #\n # Parameters #\n # doc_to_shingles: (dict) dictionary that maps each document to the list of shingles #\n # hash_functions: [list] list of hash functions #\n # Returns #\n # signatures (np.array) numpy array of size (M, C) where C is the number of documents #\n # #\n ###########################################################################################\n \n C = len(doc_to_shingles)\n M = len(hash_functions)\n signatures = np.array(np.ones((M, C)) * 999999999999, dtype = np.int)\n \n for doc_id in range(C):\n shingles = doc_to_shingles[doc_id]\n for shingle in shingles:\n hash_shingle = hash_functions(shingle)\n signatures[:,doc_id] = np.where(hash_shingle < signatures[:,doc_id], hash_shingle, signatures[:,doc_id])\n \n return signatures",
"_____no_output_____"
],
[
"def compare(signatures, doc_to_shingles, trials = 10000):\n M, C = signatures.shape\n diff_list = []\n \n for t in tqdm(range(trials)):\n doc1, doc2 = np.random.choice(C, 2, replace = False)\n \n shingle1, shingle2 = set(doc_to_shingles[doc1]), set(doc_to_shingles[doc2])\n sig1, sig2 = signatures[:,doc1], signatures[:,doc2]\n \n true_sim = jaccard_similarity(shingle1, shingle2)\n approx_sim = sum(np.equal(sig1, sig2)) / M\n \n diff_list.append(abs(true_sim - approx_sim))\n \n return diff_list",
"_____no_output_____"
],
[
"start = time.time()\nsignatures = min_hash(doc_to_shingles, hash_functions)\nend = time.time()\n\ndiff_list = compare(signatures, doc_to_shingles)\n\n# Check whether your implementation is correct [20pt]\n# Average difference of document's jaccard similarity between characteristic matrix and signatures should be at most 1%\n# With 10 random seeds, difference was around 1e-5 ~ 1e-6%\nif np.mean(diff_list) < 0.01:\n pass_test2_2 = True\n print('Test passed')",
"100%|██████████| 10000/10000 [00:04<00:00, 2437.12it/s]"
]
],
[
[
"#### 2.4 Qualitive Analysis",
"_____no_output_____"
]
],
[
[
"print('Document 3542')\nprint(documents[3542])\nprint('-------------')\nprint('Document 8033')\nprint(documents[8033])\nprint('-------------')\n\nprint('true jaccard similarity:' ,jaccard_similarity(set(doc_to_shingles[3542]), set(doc_to_shingles[8033])))\nprint('approx jaccard similarity:',sum(np.equal(signatures[:,3542], signatures[:,8033])) / M)\nprint('Do you think signature well reflects the characteristic matrix?')",
"Document 3542\ni have one complaint for the cameramen doing the jerseypitt series show the shots not the hits on more than one occassion the camera zoomed in on a check along the boards while the puck was in the slot they panned back to show the rebound maybe moms camera people were a little more experienced\n-------------\nDocument 8033\ni have one complaint for the cameramen doing the jerseypitt series show the shots not the hits on more than one occassion the camera zoomed in on a check along the boards while the puck was in the slot they panned back to show the rebound maybe moms camera people were a little more experienced joseph stiehm exactly that is my biggest complaint about the coverage so far follow that damn puck ravi shah\n-------------\ntrue jaccard similarity: 0.7285714285714285\napprox jaccard similarity: 0.74\nDo you think signature well reflects the characteristic matrix?\n"
]
],
[
[
"### 3. Locality Sensitive Hashing",
"_____no_output_____"
]
],
[
[
"########################################################################################################################\n# Programming 3 [35pt] #\n# In this section, you will implement the Min-Hash based Locality Sensitive Hashing algorithm to convert signatures #\n# into the similar document pair candidates #\n# Finally, we will test our results based on the precision, recall and F1 score #\n# #\n# 1) get the similar document pair candidates [20pt] #\n# 2) calculate precision, recall, and f1 score [10pt] #\n# #\n########################################################################################################################",
"_____no_output_____"
]
],
[
[
"#### 3.1 Min-Hash based LSH",
"_____no_output_____"
]
],
[
[
"def lsh(signatures, b, r):\n #########################################################################################################\n # Programming 3.1 [20pt] #\n # Implement the min-hash based LSH algorithm to find the candidate pairs of the similar documents. #\n # In the implementation, use python's dictionary to make your hash table, #\n # where each column is hashed into a bucket. #\n # Convert each column vector (within a band) into the tuple and use it as a key of the dictionary. #\n # #\n # Parameters #\n # signatures: (np.array) numpy array of size (M, C) where #\n # M is the number of min-hash functions, C is the number of documents #\n # b: (int) the number of bands #\n # r: (int) the number of rows per each band #\n # #\n # Requirements #\n # 1) M should be equivalent to b * r #\n # #\n # Returns #\n # candidatePairs (Set[Tuple[int, int]]) set of the pairs of indexes of candidate document pairs #\n # #\n #########################################################################################################\n M = signatures.shape[0] # The number of min-hash functions\n C = signatures.shape[1] # The number of documents\n\n assert M == b * r\n\n candidatePairs = set()\n\n # TODO: Write down your code here \n for num_b in range(b):\n bucket = {}\n bands = signatures[num_b*r:(num_b+1)*r]\n for col in range(C):\n if tuple(bands[:,col]) in bucket.keys():\n bucket[tuple(bands[:,col])].append(col)\n else:\n bucket[tuple(bands[:,col])] = [col]\n\n for value in bucket.values():\n if len(value) >= 2:\n combi = combinations(value, 2)\n candidatePairs.update(list(combi))\n #import ipdb; ipdb.set_trace()\n ### Implementation End ###\n\n return candidatePairs",
"_____no_output_____"
],
[
"# You can test your implementation here\nb = 10\nn = 0\ntmpPairs = list(lsh(signatures, b, M // b))\nprint(f\"b={b}\")\nprint(f\"# of candidate pairs = {len(tmpPairs)}\")\nsamplePair = tmpPairs[n]\nshingle1, shingle2 = set(doc_to_shingles[samplePair[0]]), set(doc_to_shingles[samplePair[1]])\nprint(f\"{n}th sample pair: {samplePair}\")\nprint(f\"Jaccard similarity: {jaccard_similarity(shingle1, shingle2)}\")\nprint('-------------')\nprint(documents[samplePair[0]])\nprint('-------------')\nprint(documents[samplePair[1]])\nprint('-------------')",
"b=10\n# of candidate pairs = 162\n0th sample pair: (1658, 5780)\nJaccard similarity: 0.8108108108108109\n-------------\nfrom paynecrldeccom andrew payne messageid organization dec cambridge research lab date tue apr gmt does anyone know if a source for the modem chips as used in the baycom and my pmp modems ideally something that is geared toward hobbyists small quantity mail order etc for years weve been buying them from a distributor marshall by the hundreds for pmp kits but orders have dropped to the point where we can no longer afford to offer this service and all of the distributors ive checked have some crazy minimum order or so id like to find a source for those still interested in building pmp kits any suggestions andrew c payne dec cambridge research lab\n-------------\ndoes anyone know if a source for the modem chips as used in the baycom and my pmp modems ideally something that is geared toward hobbyists small quantity mail order etc for years weve been buying them from a distributor marshall by the hundreds for pmp kits but orders have dropped to the point where we can no longer afford to offer this service and all of the distributors ive checked have some crazy minimum order or so id like to find a source for those still interested in building pmp kits any suggestions\n-------------\n"
]
],
[
[
"#### 3.2 Compute the precision, recall, and F1-score",
"_____no_output_____"
]
],
[
[
"# Compute the number of condition positives, which is the number of every document pair whose Jaccard similarity is greater than or equal to the threshold\n\ns = 0.8 # similarity threshold for checking condition positives\nnumConditionPositives = 151 # This is the computed result when s=0.8, but I gave it to you to save your time.\n\ncomputeConditionPositives = False # If you want to calculate it, then change it to True. It will take 30 minutes to compute.\nif computeConditionPositives:\n numConditionPositives = 0\n\n numDocs = len(documents)\n for i in tqdm(range(numDocs)):\n shingle1 = set(doc_to_shingles[i])\n\n for j in range(i+1, numDocs):\n shingle2 = set(doc_to_shingles[j])\n true_sim = jaccard_similarity(shingle1, shingle2)\n if true_sim >= s:\n numConditionPositives += 1\n\nprint(f\"The number of condition positives: {numConditionPositives} when s={s}\")",
"The number of condition positives: 151 when s=0.8\n"
],
[
"def query_analysis(signatures, b, s, numConditionPositives):\n ###########################################################################################################\n # Programming 3.2 [10pt] #\n # Calculate the query time, precision, recall, and F1 score for the given configuration #\n # #\n # Parameters #\n # signatures: (np.array) numpy array of size (M, C) where #\n # M is the number of min-hash functions, C is the number of documents #\n # b: (int) the number of bands #\n # s: (float) similarity threshold for checking condition positives #\n # numConditionPositives: (int) the number of condition positives #\n # #\n # Requirements #\n # 1) b should be the divisor of M #\n # 2) 0 <= s <= 1 #\n # #\n # Returns #\n # query time: (float) the execution time of the codes which find the similar document candidate pairs #\n # precision: (float) #\n # recall: (float) #\n # f1: (float) F1-Score #\n # #\n ###########################################################################################################\n M = signatures.shape[0] # The number of min-hash functions\n assert M % b == 0\n\n # TODO: Write down your code here\n TP = 0\n t = time.time()\n candidatePairs = lsh(signatures, b, M // b)\n query_time = time.time() - t\n\n for pair in candidatePairs:\n shingle1, shingle2 = set(doc_to_shingles[pair[0]]), set(doc_to_shingles[pair[1]])\n if jaccard_similarity(shingle1, shingle2) >= s:\n TP += 1\n \n precision = TP / len(candidatePairs)\n recall = TP / numConditionPositives\n f1 = 2 * precision * recall / (precision + recall)\n ### Implementation End ###\n\n return query_time, precision, recall, f1",
"_____no_output_____"
],
[
"# Return the list of every divisor of given integer\ndef find_divisors(x):\n divisors = list()\n for i in range(1, x + 1):\n if x % i == 0:\n divisors.append(i)\n return divisors",
"_____no_output_____"
],
[
"b_list = find_divisors(M)\n\nquery_time_list = list()\nprecision_list = list()\nrecall_list = list()\nf1_list = list()\n\nfor b in tqdm(b_list):\n query_time, precision, recall, f1 = query_analysis(signatures, b, s, numConditionPositives)\n \n query_time_list.append(query_time)\n precision_list.append(precision)\n recall_list.append(recall)\n f1_list.append(f1)",
"100%|██████████| 9/9 [00:24<00:00, 2.67s/it]\n"
],
[
"print(\"b: \", b_list)\nprint(\"Query times: \", query_time_list)\nprint(\"Precisions: \", precision_list)\nprint(\"Recalls: \", recall_list)\nprint(\"F1 scores: \", f1_list)",
"b: [1, 2, 4, 5, 10, 20, 25, 50, 100]\nQuery times: [0.4562802314758301, 0.284761905670166, 0.5345544815063477, 0.5704622268676758, 0.9000422954559326, 1.581861972808838, 1.9276137351989746, 3.863903522491455, 7.186660051345825]\nPrecisions: [1.0, 1.0, 1.0, 1.0, 0.9197530864197531, 0.5571955719557196, 0.3511627906976744, 0.050333333333333334, 0.002708908901725808]\nRecalls: [0.6556291390728477, 0.6688741721854304, 0.7549668874172185, 0.8145695364238411, 0.9867549668874173, 1.0, 1.0, 1.0, 1.0]\nF1 scores: [0.792, 0.8015873015873015, 0.8603773584905661, 0.8978102189781023, 0.952076677316294, 0.7156398104265402, 0.5197934595524957, 0.09584258965407808, 0.005403181078131429]\n"
],
[
"plt.title(f\"Query time (s={s})\")\nplt.xlabel(\"b\")\nplt.ylabel(\"Query time [sec]\")\nplt.plot(b_list, query_time_list)\nplt.show()",
"_____no_output_____"
],
[
"plt.title(f\"Precision (s={s})\")\nplt.xlabel(\"b\")\nplt.ylabel(\"Precision\")\nplt.plot(b_list, precision_list)\nplt.show()",
"_____no_output_____"
],
[
"plt.title(f\"Recall (s={s})\")\nplt.xlabel(\"b\")\nplt.ylabel(\"Recall\")\nplt.plot(b_list, recall_list)\nplt.show()",
"_____no_output_____"
],
[
"plt.title(f\"F1 Score (s={s})\")\nplt.xlabel(\"b\")\nplt.ylabel(\"F1 Score\")\nplt.plot(b_list, f1_list)\nplt.show()",
"_____no_output_____"
],
[
"# Check whether the test passed\ntest_msg = {True: \"Passed\", False: \"Failed\"}\n\nprint(\"-----Test results-----\")\nprint(f\"[Test 1.1 (1)]: {test_msg[pass_test1_1_1]}\")\nprint(f\"[Test 1.1 (2)]: {test_msg[pass_test1_1_2]}\")\nprint(f\"[Test 1.2]: {test_msg[pass_test1_2]}\")\nprint(f\"[Test 2.1]: {test_msg[pass_test2_1]}\")\nprint(f\"[Test 2.2]: {test_msg[pass_test2_2]}\")\nprint(\"----------------------\")",
"-----Test results-----\n[Test 1.1 (1)]: Passed\n[Test 1.1 (2)]: Passed\n[Test 1.2]: Passed\n[Test 2.1]: Passed\n[Test 2.2]: Passed\n----------------------\n"
]
]
] | [
"code",
"markdown",
"code",
"markdown",
"code",
"markdown",
"code",
"markdown",
"code",
"markdown",
"code",
"markdown",
"code",
"markdown",
"code",
"markdown",
"code",
"markdown",
"code",
"markdown",
"code",
"markdown",
"code",
"markdown",
"code",
"markdown",
"code"
] | [
[
"code",
"code",
"code"
],
[
"markdown",
"markdown",
"markdown"
],
[
"code",
"code",
"code"
],
[
"markdown"
],
[
"code",
"code",
"code",
"code"
],
[
"markdown"
],
[
"code"
],
[
"markdown"
],
[
"code",
"code",
"code"
],
[
"markdown"
],
[
"code",
"code"
],
[
"markdown"
],
[
"code"
],
[
"markdown"
],
[
"code",
"code"
],
[
"markdown"
],
[
"code",
"code"
],
[
"markdown"
],
[
"code",
"code",
"code",
"code"
],
[
"markdown"
],
[
"code"
],
[
"markdown"
],
[
"code"
],
[
"markdown"
],
[
"code",
"code"
],
[
"markdown"
],
[
"code",
"code",
"code",
"code",
"code",
"code",
"code",
"code",
"code",
"code"
]
] |
d078f50494fa20885536fecd879f8cc67f016979 | 716,829 | ipynb | Jupyter Notebook | notebooks/04-jet-study.ipynb | glouppe/recnn | 55821d8ded10a08315349285134d9800ab8f1b41 | [
"BSD-3-Clause"
] | 50 | 2017-02-03T08:23:32.000Z | 2020-05-26T20:03:43.000Z | notebooks/04-jet-study.ipynb | fdreyer/recnn | bca5b297eeba10881ab6868cf378b73fe7b8735c | [
"BSD-3-Clause"
] | 3 | 2018-10-07T05:02:00.000Z | 2020-08-18T15:18:42.000Z | notebooks/04-jet-study.ipynb | fdreyer/recnn | bca5b297eeba10881ab6868cf378b73fe7b8735c | [
"BSD-3-Clause"
] | 16 | 2017-02-04T15:14:00.000Z | 2021-11-28T06:25:27.000Z | 396.476217 | 121,958 | 0.910754 | [
[
[
"import sys\nsys.path.append(\"..\")",
"_____no_output_____"
],
[
"import numpy as np\nnp.seterr(divide=\"ignore\")\nimport logging\nimport pickle\nimport glob\n\nfrom sklearn.metrics import roc_curve\nfrom sklearn.metrics import roc_auc_score\nfrom sklearn.preprocessing import RobustScaler\nfrom sklearn.utils import check_random_state\n\nfrom scipy import interp\n\nfrom recnn.preprocessing import rewrite_content\nfrom recnn.preprocessing import permute_by_pt\nfrom recnn.preprocessing import extract\nfrom recnn.preprocessing import sequentialize_by_pt\nfrom recnn.preprocessing import randomize\n\n%matplotlib inline\nimport matplotlib.pyplot as plt\nplt.rcParams[\"figure.figsize\"] = (6, 6)",
"_____no_output_____"
]
],
[
[
"# Plotting functions",
"_____no_output_____"
]
],
[
[
"from recnn.preprocessing import sequentialize_by_pt\n\ndef load_tf(filename_train, preprocess=None, n_events_train=-1):\n # Make training data\n print(\"Loading training data...\")\n\n fd = open(filename_train, \"rb\")\n X, y = pickle.load(fd)\n fd.close()\n \n y = np.array(y)\n \n if n_events_train > 0:\n indices = check_random_state(123).permutation(len(X))[:n_events_train]\n X = [X[i] for i in indices]\n y = y[indices]\n\n print(\"\\tfilename = %s\" % filename_train)\n print(\"\\tX size = %d\" % len(X))\n print(\"\\ty size = %d\" % len(y))\n\n # Preprocessing \n print(\"Preprocessing...\")\n X = [rewrite_content(jet) for jet in X]\n \n if preprocess:\n X = [preprocess(jet) for jet in X]\n \n X = [extract(permute_by_pt(jet)) for jet in X]\n tf = RobustScaler().fit(np.vstack([jet[\"content\"] for jet in X]))\n \n return tf\n\ndef load_test(tf, filename_test, preprocess=None, cropping=True):\n # Make test data \n print(\"Loading test data...\")\n\n fd = open(filename_test, \"rb\")\n X, y = pickle.load(fd)\n fd.close()\n y = np.array(y)\n\n print(\"\\tfilename = %s\" % filename_test)\n print(\"\\tX size = %d\" % len(X))\n print(\"\\ty size = %d\" % len(y))\n\n # Preprocessing \n print(\"Preprocessing...\")\n X = [rewrite_content(jet) for jet in X]\n \n if preprocess:\n X = [preprocess(jet) for jet in X]\n \n X = [extract(permute_by_pt(jet)) for jet in X]\n\n for jet in X:\n jet[\"content\"] = tf.transform(jet[\"content\"])\n \n if not cropping:\n return X, y\n \n # Cropping\n X_ = [j for j in X if 250 < j[\"pt\"] < 300 and 50 < j[\"mass\"] < 110]\n y_ = [y[i] for i, j in enumerate(X) if 250 < j[\"pt\"] < 300 and 50 < j[\"mass\"] < 110]\n\n X = X_\n y = y_\n y = np.array(y)\n \n print(\"\\tX size = %d\" % len(X))\n print(\"\\ty size = %d\" % len(y))\n \n # Weights for flatness in pt\n w = np.zeros(len(y)) \n \n X0 = [X[i] for i in range(len(y)) if y[i] == 0]\n pdf, edges = np.histogram([j[\"pt\"] for j in X0], density=True, range=[250, 300], bins=50)\n pts = [j[\"pt\"] for j in X0]\n indices = np.searchsorted(edges, pts) - 1\n inv_w = 1. / pdf[indices]\n inv_w /= inv_w.sum()\n w[y==0] = inv_w\n \n X1 = [X[i] for i in range(len(y)) if y[i] == 1]\n pdf, edges = np.histogram([j[\"pt\"] for j in X1], density=True, range=[250, 300], bins=50)\n pts = [j[\"pt\"] for j in X1]\n indices = np.searchsorted(edges, pts) - 1\n inv_w = 1. / pdf[indices]\n inv_w /= inv_w.sum()\n w[y==1] = inv_w\n \n return X, y, w",
"_____no_output_____"
],
[
"from recnn.recnn import grnn_transform_simple\nfrom recnn.recnn import grnn_predict_simple\nfrom recnn.recnn import grnn_predict_gated\nfrom recnn.recnn import grnn_predict_simple_join\n\n\ndef predict(X, filename, func=grnn_predict_simple):\n fd = open(filename, \"rb\")\n params = pickle.load(fd)\n fd.close()\n y_pred = func(params, X)\n return y_pred\n\n\ndef evaluate_models(X, y, w, pattern, func=grnn_predict_simple):\n rocs = []\n fprs = []\n tprs = []\n \n for filename in glob.glob(pattern):\n print(\"Loading %s\" % filename),\n \n y_pred = predict(X, filename, func=func)\n \n # Roc\n rocs.append(roc_auc_score(y, y_pred, sample_weight=w))\n fpr, tpr, _ = roc_curve(y, y_pred, sample_weight=w)\n \n fprs.append(fpr)\n tprs.append(tpr)\n \n print(\"ROC AUC = %.4f\" % rocs[-1])\n \n print(\"Mean ROC AUC = %.4f\" % np.mean(rocs))\n \n return rocs, fprs, tprs\n\ndef build_rocs(prefix_train, prefix_test, model_pattern, preprocess=None, gated=False):\n tf = load_tf(\"../data/w-vs-qcd/final/%s-train.pickle\" % prefix_train, preprocess=preprocess)\n X, y, w = load_test(tf, \"../data/w-vs-qcd/final/%s-test.pickle\" % prefix_test, preprocess=preprocess) \n \n if not gated:\n rocs, fprs, tprs = evaluate_models(X, y, w, \n \"../models/jet-study-2/model-w-s-%s-[0-9]*.pickle\" % model_pattern)\n else:\n rocs, fprs, tprs = evaluate_models(X, y, w, \n \"../models/jet-study-2/model-w-g-%s-[0-9]*.pickle\" % model_pattern, func=grnn_predict_gated)\n \n return rocs, fprs, tprs",
"_____no_output_____"
],
[
"def remove_outliers(rocs, fprs, tprs):\n inv_fprs = []\n base_tpr = np.linspace(0.05, 1, 476)\n\n for fpr, tpr in zip(fprs, tprs):\n inv_fpr = interp(base_tpr, tpr, 1. / fpr)\n inv_fprs.append(inv_fpr)\n\n inv_fprs = np.array(inv_fprs)\n scores = inv_fprs[:, 225]\n \n p25 = np.percentile(scores, 1 / 6. * 100.)\n p75 = np.percentile(scores, 5 / 6. * 100)\n \n robust_mean = np.mean([scores[i] for i in range(len(scores)) if p25 <= scores[i] <= p75])\n robust_std = np.std([scores[i] for i in range(len(scores)) if p25 <= scores[i] <= p75])\n \n indices = [i for i in range(len(scores)) if robust_mean - 3*robust_std <= scores[i] <= robust_mean + 3*robust_std]\n \n new_r, new_f, new_t = [], [], []\n \n for i in indices:\n new_r.append(rocs[i])\n new_f.append(fprs[i])\n new_t.append(tprs[i])\n \n return new_r, new_f, new_t\n\n\ndef report_score(rocs, fprs, tprs, label, latex=False, input=\"particles\", short=False): \n inv_fprs = []\n base_tpr = np.linspace(0.05, 1, 476)\n \n for fpr, tpr in zip(fprs, tprs):\n inv_fpr = interp(base_tpr, tpr, 1. / fpr)\n inv_fprs.append(inv_fpr)\n \n inv_fprs = np.array(inv_fprs)\n mean_inv_fprs = inv_fprs.mean(axis=0)\n \n if not latex:\n print(\"%32s\\tROC AUC=%.4f+-%.2f\\t1/FPR@TPR=0.5=%.2f+-%.2f\" % (label, \n np.mean(rocs), \n np.std(rocs),\n np.mean(inv_fprs[:, 225]),\n np.std(inv_fprs[:, 225])))\n else:\n if not short:\n print(\"%10s \\t& %30s \\t& %.4f $\\pm$ %.4f \\t& %.1f $\\pm$ %.1f \\\\\\\\\" % \n (input,\n label,\n np.mean(rocs), \n np.std(rocs),\n np.mean(inv_fprs[:, 225]),\n np.std(inv_fprs[:, 225])))\n else:\n print(\"%30s \\t& %.4f $\\pm$ %.4f \\t& %.1f $\\pm$ %.1f \\\\\\\\\" % \n (label,\n np.mean(rocs), \n np.std(rocs),\n np.mean(inv_fprs[:, 225]),\n np.std(inv_fprs[:, 225])))\n \ndef plot_rocs(rocs, fprs, tprs, label=\"\", color=\"r\", show_all=False):\n inv_fprs = []\n base_tpr = np.linspace(0.05, 1, 476)\n \n for fpr, tpr in zip(fprs, tprs):\n inv_fpr = interp(base_tpr, tpr, 1. / fpr)\n inv_fprs.append(inv_fpr)\n if show_all:\n plt.plot(base_tpr, inv_fpr, alpha=0.1, color=color)\n \n inv_fprs = np.array(inv_fprs)\n mean_inv_fprs = inv_fprs.mean(axis=0)\n\n\n plt.plot(base_tpr, mean_inv_fprs, color, \n label=\"%s\" % label)\n \ndef plot_show(filename=None):\n plt.xlabel(\"Signal efficiency\")\n plt.ylabel(\"1 / Background efficiency\")\n plt.xlim([0.1, 1.0])\n plt.ylim(1, 500)\n plt.yscale(\"log\")\n plt.legend(loc=\"best\")\n plt.grid()\n \n if filename:\n plt.savefig(filename)\n \n plt.show()",
"_____no_output_____"
]
],
[
[
"# Count parameters",
"_____no_output_____"
]
],
[
[
"def count(params):\n def _count(thing):\n if isinstance(thing, list):\n c = 0\n for stuff in thing:\n c += _count(stuff)\n return c \n\n elif isinstance(thing, np.ndarray):\n return np.prod(thing.shape)\n \n c = 0\n for k, v in params.items():\n c += _count(v)\n return c\n \n# Simple vs gated\nfd = open(\"../models/jet-study-2/model-w-s-antikt-kt-1.pickle\", \"rb\")\nparams = pickle.load(fd)\nfd.close()\nprint(\"Simple =\", count(params)) \n\nfd = open(\"../models/jet-study-2/model-w-g-antikt-kt-1.pickle\", \"rb\")\nparams = pickle.load(fd)\nfd.close()\nprint(\"Gated =\", count(params))",
"('Simple =', 8481)\n('Gated =', 48761)\n"
],
[
"# double\n# Simple vs gated\nfd = open(\"../models/jet-study-2/model-w-sd-antikt-kt-1.pickle\", \"rb\")\nparams = pickle.load(fd)\nfd.close()\nprint(\"Simple =\", count(params)) \n\nfd = open(\"../models/jet-study-2/model-w-gd-antikt-kt-1.pickle\", \"rb\")\nparams = pickle.load(fd)\nfd.close()\nprint(\"Gated =\", count(params))",
"('Simple =', 10081)\n('Gated =', 50361)\n"
]
],
[
[
"# Embedding visualization",
"_____no_output_____"
]
],
[
[
"prefix_train = \"antikt-kt\"\nprefix_test = prefix_train\ntf = load_tf(\"../data/w-vs-qcd/final/%s-train.pickle\" % prefix_train)\nX, y, w = load_test(tf, \"../data/w-vs-qcd/final/%s-test.pickle\" % prefix_test) ",
"_____no_output_____"
],
[
"fd = open(\"../models/jet-study-2/model-w-s-antikt-kt-1.pickle\", \"rb\")\nparams = pickle.load(fd)\nfd.close()",
"_____no_output_____"
],
[
"Xt = grnn_transform_simple(params, X[:5000])",
"_____no_output_____"
],
[
"from sklearn.manifold import TSNE\nXtt = TSNE(n_components=2).fit_transform(Xt)",
"_____no_output_____"
],
[
"for i in range(5000):\n plt.scatter(Xtt[i, 0], Xtt[i, 1], color=\"b\" if y[i] == 1 else \"r\", alpha=0.5)\n\nplt.show()",
"_____no_output_____"
],
[
"from sklearn.decomposition import PCA\nXtt = PCA(n_components=2).fit_transform(Xt)\n\nfor i in range(5000):\n plt.scatter(Xtt[i, 0], Xtt[i, 1], color=\"b\" if y[i] == 1 else \"r\", alpha=0.5)\n\nplt.show()",
"_____no_output_____"
]
],
[
[
"# Generate all ROCs",
"_____no_output_____"
]
],
[
[
"for pattern, gated in [\n # Simple\n ## Particles\n (\"antikt-kt\", False),\n (\"antikt-cambridge\", False),\n (\"antikt-antikt\", False),\n (\"antikt-random\", False),\n (\"antikt-seqpt\", False),\n (\"antikt-seqpt-reversed\", False),\n ## Towers\n (\"antikt-kt-delphes\", False),\n (\"antikt-cambridge-delphes\", False),\n (\"antikt-antikt-delphes\", False),\n (\"antikt-random-delphes\", False),\n (\"antikt-seqpt-delphes\", False),\n (\"antikt-seqpt-reversed-delphes\", False),\n ## Images\n (\"antikt-kt-images\", False),\n\n # Gated\n ## Particles\n (\"antikt-kt\", True),\n (\"antikt-antikt\", True),\n (\"antikt-seqpt\", True),\n (\"antikt-seqpt-reversed\", True),\n (\"antikt-cambridge\", True),\n (\"antikt-random\", True),\n ## Towers\n (\"antikt-kt-delphes\", True),\n (\"antikt-antikt-delphes\", True),\n (\"antikt-seqpt-delphes\", True),\n (\"antikt-seqpt-reversed-delphes\", True),\n (\"antikt-cambridge-delphes\", True),\n (\"antikt-random-delphes\", True),\n ## Images\n (\"antikt-kt-images\", True)\n ]:\n r, f, t = build_rocs(pattern, pattern, pattern, gated=gated)\n \n # Save\n fd = open(\"../models/jet-study-2/rocs/rocs-%s-%s.pickle\" % (\"s\" if not gated else \"g\", pattern), \"wb\")\n pickle.dump((r, f, t), fd)\n fd.close()",
"_____no_output_____"
],
[
"# sd/gd == contatenate embeddings of h1_L + h1_R\nfor pattern, gated in [\n # Simple\n ## Particles\n (\"antikt-kt\", False),\n ## Towers\n (\"antikt-kt-delphes\", False),\n ## Images\n (\"antikt-kt-images\", False),\n\n # Gated\n ## Particles\n (\"antikt-kt\", True),\n ## Towers\n (\"antikt-kt-delphes\", True),\n ## Images\n (\"antikt-kt-images\", True)\n ]:\n r, f, t = build_rocs(pattern, pattern, pattern, gated=gated)\n \n # Save\n fd = open(\"../models/jet-study-2/rocs/rocs-%s-%s.pickle\" % (\"sd\" if not gated else \"gd\", pattern), \"wb\")\n pickle.dump((r, f, t), fd)\n fd.close()",
"_____no_output_____"
]
],
[
[
"# Table",
"_____no_output_____"
]
],
[
[
"for pattern, gated, label in [\n # Simple\n ## Particles\n (\"antikt-kt\", False, \"RNN $k_t$\"),\n (\"antikt-cambridge\", False, \"RNN C/A\"),\n (\"antikt-antikt\", False, \"RNN anti-$k_t$\"),\n (\"antikt-random\", False, \"RNN random\"),\n (\"antikt-seqpt\", False, \"RNN asc-$p_T$\"),\n (\"antikt-seqpt-reversed\", False, \"RNN desc-$p_T$\"),\n ## Towers\n (\"antikt-kt-delphes\", False, \"RNN $k_t$\"),\n (\"antikt-cambridge-delphes\", False, \"RNN C/A\"),\n (\"antikt-antikt-delphes\", False, \"RNN anti-$k_t$\"),\n (\"antikt-random-delphes\", False, \"RNN random\"),\n (\"antikt-seqpt-delphes\", False, \"RNN asc-$p_T$\"),\n (\"antikt-seqpt-reversed-delphes\", False, \"RNN desc-$p_T$\"),\n ## Images\n (\"antikt-kt-images\", False, \"RNN $k_t$\"),\n\n # Gated\n ## Particles\n (\"antikt-kt\", True, \"RNN $k_t$ (gated)\"),\n (\"antikt-cambridge\", True, \"RNN C/A (gated)\"),\n (\"antikt-antikt\", True, \"RNN anti-$k_t$ (gated)\"),\n (\"antikt-random\", True, \"RNN random (gated)\"),\n (\"antikt-seqpt\", True, \"RNN asc-$p_T$ (gated)\"),\n (\"antikt-seqpt-reversed\", True, \"RNN desc-$p_T$ (gated)\"),\n ## Towers\n (\"antikt-kt-delphes\", True, \"RNN $k_t$ (gated)\"),\n (\"antikt-cambridge-delphes\", True, \"RNN C/A (gated)\"),\n (\"antikt-antikt-delphes\", True, \"RNN anti-$k_t$ (gated)\"),\n (\"antikt-random-delphes\", True, \"RNN random (gated)\"),\n (\"antikt-seqpt-delphes\", True, \"RNN asc-$p_T$ (gated)\"),\n (\"antikt-seqpt-reversed-delphes\", True, \"RNN desc-$p_T$ (gated)\"),\n \n # Images\n (\"antikt-kt-images\", False, \"RNN $k_t$\"),\n (\"antikt-kt-images\", True, \"RNN $k_t$ (gated)\")\n ]:\n \n fd = open(\"../models/jet-study-2/rocs/rocs-%s-%s.pickle\" % (\"s\" if not gated else \"g\", pattern), \"rb\")\n r, f, t = pickle.load(fd)\n fd.close()\n \n r, f, t = remove_outliers(r, f, t)\n \n report_score(r, f, t, label=label, \n latex=True, \n input=\"particles\" if \"delphes\" not in pattern and \"images\" not in pattern else \"towers\")",
" particles \t& RNN $k_t$ \t& 0.9185 $\\pm$ 0.0006 \t& 68.3 $\\pm$ 1.8 \\\\\n particles \t& RNN C/A \t& 0.9192 $\\pm$ 0.0008 \t& 68.3 $\\pm$ 3.6 \\\\\n particles \t& RNN anti-$k_t$ \t& 0.9096 $\\pm$ 0.0013 \t& 51.7 $\\pm$ 3.5 \\\\\n particles \t& RNN random \t& 0.9121 $\\pm$ 0.0008 \t& 51.1 $\\pm$ 2.0 \\\\\n particles \t& RNN asc-$p_T$ \t& 0.9130 $\\pm$ 0.0031 \t& 52.5 $\\pm$ 7.3 \\\\\n particles \t& RNN desc-$p_T$ \t& 0.9189 $\\pm$ 0.0009 \t& 70.4 $\\pm$ 3.6 \\\\\n towers \t& RNN $k_t$ \t& 0.8807 $\\pm$ 0.0010 \t& 24.1 $\\pm$ 0.6 \\\\\n towers \t& RNN C/A \t& 0.8831 $\\pm$ 0.0010 \t& 24.2 $\\pm$ 0.7 \\\\\n towers \t& RNN anti-$k_t$ \t& 0.8737 $\\pm$ 0.0017 \t& 22.3 $\\pm$ 0.8 \\\\\n towers \t& RNN random \t& 0.8704 $\\pm$ 0.0011 \t& 20.4 $\\pm$ 0.3 \\\\\n towers \t& RNN asc-$p_T$ \t& 0.8835 $\\pm$ 0.0009 \t& 26.2 $\\pm$ 0.7 \\\\\n towers \t& RNN desc-$p_T$ \t& 0.8838 $\\pm$ 0.0010 \t& 25.1 $\\pm$ 0.6 \\\\\n towers \t& RNN $k_t$ \t& 0.8321 $\\pm$ 0.0025 \t& 12.7 $\\pm$ 0.4 \\\\\n particles \t& RNN $k_t$ (gated) \t& 0.9195 $\\pm$ 0.0009 \t& 74.3 $\\pm$ 2.4 \\\\\n particles \t& RNN C/A (gated) \t& 0.9222 $\\pm$ 0.0007 \t& 81.8 $\\pm$ 3.1 \\\\\n particles \t& RNN anti-$k_t$ (gated) \t& 0.9156 $\\pm$ 0.0012 \t& 68.3 $\\pm$ 3.2 \\\\\n particles \t& RNN random (gated) \t& 0.9106 $\\pm$ 0.0035 \t& 50.7 $\\pm$ 6.7 \\\\\n particles \t& RNN asc-$p_T$ (gated) \t& 0.9137 $\\pm$ 0.0046 \t& 54.8 $\\pm$ 11.7 \\\\\n particles \t& RNN desc-$p_T$ (gated) \t& 0.9212 $\\pm$ 0.0005 \t& 83.3 $\\pm$ 3.1 \\\\\n towers \t& RNN $k_t$ (gated) \t& 0.8822 $\\pm$ 0.0006 \t& 25.4 $\\pm$ 0.4 \\\\\n towers \t& RNN C/A (gated) \t& 0.8861 $\\pm$ 0.0014 \t& 26.2 $\\pm$ 0.8 \\\\\n towers \t& RNN anti-$k_t$ (gated) \t& 0.8804 $\\pm$ 0.0010 \t& 24.4 $\\pm$ 0.4 \\\\\n towers \t& RNN random (gated) \t& 0.8751 $\\pm$ 0.0029 \t& 22.8 $\\pm$ 1.2 \\\\\n towers \t& RNN asc-$p_T$ (gated) \t& 0.8849 $\\pm$ 0.0012 \t& 27.2 $\\pm$ 0.8 \\\\\n towers \t& RNN desc-$p_T$ (gated) \t& 0.8864 $\\pm$ 0.0007 \t& 27.5 $\\pm$ 0.6 \\\\\n towers \t& RNN $k_t$ \t& 0.8321 $\\pm$ 0.0025 \t& 12.7 $\\pm$ 0.4 \\\\\n towers \t& RNN $k_t$ (gated) \t& 0.8277 $\\pm$ 0.0028 \t& 12.4 $\\pm$ 0.3 \\\\\n"
],
[
"for pattern, gated, label in [\n # Simple\n ## Particles\n (\"antikt-kt\", False, \"RNN $k_t$\"),\n ## Towers\n (\"antikt-kt-delphes\", False, \"RNN $k_t$\"),\n ## Images\n (\"antikt-kt-images\", False, \"RNN $k_t$\"),\n\n # Gated\n ## Particles\n (\"antikt-kt\", True, \"RNN $k_t$ (gated)\"),\n ## Towers\n (\"antikt-kt-delphes\", True, \"RNN $k_t$ (gated)\"),\n # Images\n (\"antikt-kt-images\", True, \"RNN $k_t$ (gated)\")\n ]:\n \n fd = open(\"../models/jet-study-2/rocs/rocs-%s-%s.pickle\" % (\"sd\" if not gated else \"gd\", pattern), \"rb\")\n r, f, t = pickle.load(fd)\n fd.close()\n \n r, f, t = remove_outliers(r, f, t)\n \n report_score(r, f, t, label=label, \n latex=True, \n input=\"particles\" if \"delphes\" not in pattern and \"images\" not in pattern else \"towers\")",
" particles \t& RNN $k_t$ \t& 0.9178 $\\pm$ 0.0008 \t& 67.0 $\\pm$ 2.6 \\\\\n towers \t& RNN $k_t$ \t& 0.8801 $\\pm$ 0.0012 \t& 24.1 $\\pm$ 0.6 \\\\\n towers \t& RNN $k_t$ \t& 0.8332 $\\pm$ 0.0029 \t& 12.6 $\\pm$ 0.4 \\\\\n particles \t& RNN $k_t$ (gated) \t& 0.9187 $\\pm$ 0.0011 \t& 70.2 $\\pm$ 3.8 \\\\\n towers \t& RNN $k_t$ (gated) \t& 0.8810 $\\pm$ 0.0012 \t& 24.5 $\\pm$ 0.5 \\\\\n towers \t& RNN $k_t$ (gated) \t& 0.8255 $\\pm$ 0.0050 \t& 12.2 $\\pm$ 0.6 \\\\\n"
]
],
[
[
"# Plots",
"_____no_output_____"
]
],
[
[
"# Simple vs gated\nfor pattern, gated, label, color in [\n (\"antikt-kt\", False, \"RNN $k_t$ (simple)\", \"r\"),\n (\"antikt-kt\", True, \"RNN $k_t$ (gated)\", \"b\")\n ]:\n \n fd = open(\"../models/jet-study-2/rocs/rocs-%s-%s.pickle\" % (\"s\" if not gated else \"g\", pattern), \"rb\")\n r, f, t = pickle.load(fd)\n fd.close()\n \n r, f, t = remove_outliers(r, f, t)\n \n plot_rocs(r, f, t, label=label, color=color)\n report_score(r, f, t, label=label)\n \nplot_show()",
" RNN $k_t$ (simple)\tROC AUC=0.9185+-0.00\t1/FPR@TPR=0.5=68.32+-1.80\n RNN $k_t$ (gated)\tROC AUC=0.9195+-0.00\t1/FPR@TPR=0.5=74.34+-2.40\n"
],
[
"# Topologies (particles, simple)\nfor pattern, gated, label, color in [\n (\"antikt-kt\", False, \"$k_t$\", \"r\"), \n (\"antikt-cambridge\", False, \"C/A\", \"g\"),\n (\"antikt-antikt\", False, \"anti-$k_t$\", \"b\"), \n (\"antikt-seqpt\", False, \"asc-$p_T$\", \"c\"),\n (\"antikt-seqpt-reversed\", False, \"desc-$p_T$\", \"m\"),\n (\"antikt-random\", False, \"random\", \"orange\")\n ]:\n \n fd = open(\"../models/jet-study-2/rocs/rocs-%s-%s.pickle\" % (\"s\" if not gated else \"g\", pattern), \"rb\")\n r, f, t = pickle.load(fd)\n fd.close()\n \n r, f, t = remove_outliers(r, f, t)\n \n plot_rocs(r, f, t, label=label, color=color)\n report_score(r, f, t, label=label)\n \nplot_show()",
" $k_t$\tROC AUC=0.9185+-0.00\t1/FPR@TPR=0.5=68.32+-1.80\n C/A\tROC AUC=0.9192+-0.00\t1/FPR@TPR=0.5=68.29+-3.62\n anti-$k_t$\tROC AUC=0.9096+-0.00\t1/FPR@TPR=0.5=51.66+-3.49\n asc-$p_T$\tROC AUC=0.9130+-0.00\t1/FPR@TPR=0.5=52.51+-7.27\n desc-$p_T$\tROC AUC=0.9189+-0.00\t1/FPR@TPR=0.5=70.38+-3.65\n random\tROC AUC=0.9121+-0.00\t1/FPR@TPR=0.5=51.06+-2.00\n"
],
[
"# Topologies (towers, simple)\nfor pattern, gated, label, color in [\n (\"antikt-kt-delphes\", False, \"RNN $k_t$\", \"r\"), \n (\"antikt-cambridge-delphes\", False, \"RNN C/A\", \"g\"),\n (\"antikt-antikt-delphes\", False, \"RNN anti-$k_t$\", \"b\"), \n (\"antikt-seqpt-delphes\", False, \"RNN asc-$p_T$\", \"c\"),\n (\"antikt-seqpt-reversed-delphes\", False, \"RNN desc-$p_T$\", \"m\"),\n (\"antikt-random-delphes\", False, \"RNN random\", \"orange\")\n ]:\n \n fd = open(\"../models/jet-study-2/rocs/rocs-%s-%s.pickle\" % (\"s\" if not gated else \"g\", pattern), \"rb\")\n r, f, t = pickle.load(fd)\n fd.close()\n \n r, f, t = remove_outliers(r, f, t)\n \n plot_rocs(r, f, t, label=label, color=color)\n report_score(r, f, t, label=label)\n \nplot_show()",
" RNN $k_t$\tROC AUC=0.8807+-0.00\t1/FPR@TPR=0.5=24.07+-0.55\n RNN C/A\tROC AUC=0.8831+-0.00\t1/FPR@TPR=0.5=24.15+-0.69\n RNN anti-$k_t$\tROC AUC=0.8737+-0.00\t1/FPR@TPR=0.5=22.33+-0.85\n RNN asc-$p_T$\tROC AUC=0.8835+-0.00\t1/FPR@TPR=0.5=26.15+-0.70\n RNN desc-$p_T$\tROC AUC=0.8838+-0.00\t1/FPR@TPR=0.5=25.13+-0.58\n RNN random\tROC AUC=0.8704+-0.00\t1/FPR@TPR=0.5=20.35+-0.34\n"
],
[
"# Topologies (particles, gated)\nfor pattern, gated, label, color in [\n (\"antikt-kt\", True, \"RNN $k_t$\", \"r\"), \n (\"antikt-antikt\", True, \"RNN anti-$k_t$\", \"b\"), \n (\"antikt-seqpt\", True, \"RNN asc-$p_T$\", \"c\"),\n (\"antikt-seqpt-reversed\", True, \"RNN desc-$p_T$\", \"m\"),\n ]:\n \n fd = open(\"../models/jet-study-2/rocs/rocs-%s-%s.pickle\" % (\"s\" if not gated else \"g\", pattern), \"rb\")\n r, f, t = pickle.load(fd)\n fd.close()\n \n r, f, t = remove_outliers(r, f, t)\n \n plot_rocs(r, f, t, label=label, color=color)\n report_score(r, f, t, label=label)\n \nplot_show()",
" RNN $k_t$\tROC AUC=0.9195+-0.00\t1/FPR@TPR=0.5=74.34+-2.40\n RNN anti-$k_t$\tROC AUC=0.9156+-0.00\t1/FPR@TPR=0.5=68.25+-3.18\n RNN asc-$p_T$\tROC AUC=0.9137+-0.00\t1/FPR@TPR=0.5=54.79+-11.70\n RNN desc-$p_T$\tROC AUC=0.9212+-0.00\t1/FPR@TPR=0.5=83.27+-3.08\n"
],
[
"# Topologies (towers, gated)\nfor pattern, gated, label, color in [\n (\"antikt-kt-delphes\", True, \"RNN $k_t$\", \"r\"), \n (\"antikt-antikt-delphes\", True, \"RNN anti-$k_t$\", \"b\"), \n (\"antikt-seqpt-delphes\", True, \"RNN asc-$p_T$\", \"c\"),\n (\"antikt-seqpt-reversed-delphes\", True, \"RNN desc-$p_T$\", \"m\"),\n ]:\n \n fd = open(\"../models/jet-study-2/rocs/rocs-%s-%s.pickle\" % (\"s\" if not gated else \"g\", pattern), \"rb\")\n r, f, t = pickle.load(fd)\n fd.close()\n \n r, f, t = remove_outliers(r, f, t)\n \n plot_rocs(r, f, t, label=label, color=color)\n report_score(r, f, t, label=label)\n \nplot_show()",
" RNN $k_t$\tROC AUC=0.8822+-0.00\t1/FPR@TPR=0.5=25.41+-0.36\n RNN anti-$k_t$\tROC AUC=0.8804+-0.00\t1/FPR@TPR=0.5=24.37+-0.42\n RNN asc-$p_T$\tROC AUC=0.8849+-0.00\t1/FPR@TPR=0.5=27.16+-0.82\n RNN desc-$p_T$\tROC AUC=0.8864+-0.00\t1/FPR@TPR=0.5=27.50+-0.55\n"
],
[
"# Particles vs towers vs images (simple)\nfor pattern, gated, label, color in [\n (\"antikt-kt\", False, \"particles\", \"r\"), \n (\"antikt-kt-delphes\", False, \"towers\", \"g\"),\n (\"antikt-kt-images\", False, \"images\", \"b\"), \n ]:\n \n fd = open(\"../models/jet-study-2/rocs/rocs-%s-%s.pickle\" % (\"s\" if not gated else \"g\", pattern), \"rb\")\n r, f, t = pickle.load(fd)\n fd.close()\n \n r, f, t = remove_outliers(r, f, t)\n \n plot_rocs(r, f, t, label=label, color=color)\n report_score(r, f, t, label=label)\n \nplot_show(filename=\"particles-towers-images.pdf\")",
" particles\tROC AUC=0.9185+-0.00\t1/FPR@TPR=0.5=68.32+-1.80\n towers\tROC AUC=0.8807+-0.00\t1/FPR@TPR=0.5=24.07+-0.55\n images\tROC AUC=0.8321+-0.00\t1/FPR@TPR=0.5=12.67+-0.43\n"
],
[
"# Particles vs towers vs images (gated)\nfor pattern, gated, label, color in [\n (\"antikt-kt\", True, \"particles\", \"r\"), \n (\"antikt-kt-delphes\", True, \"towers\", \"g\"),\n (\"antikt-kt-images\", True, \"images\", \"b\"), \n ]:\n \n fd = open(\"../models/jet-study-2/rocs/rocs-%s-%s.pickle\" % (\"s\" if not gated else \"g\", pattern), \"rb\")\n r, f, t = pickle.load(fd)\n fd.close()\n \n r, f, t = remove_outliers(r, f, t)\n \n plot_rocs(r, f, t, label=label, color=color)\n report_score(r, f, t, label=label)\n \nplot_show()",
" particles\tROC AUC=0.9195+-0.00\t1/FPR@TPR=0.5=74.34+-2.40\n towers\tROC AUC=0.8822+-0.00\t1/FPR@TPR=0.5=25.41+-0.36\n images\tROC AUC=0.8277+-0.00\t1/FPR@TPR=0.5=12.36+-0.30\n"
]
],
[
[
"# Trimming",
"_____no_output_____"
]
],
[
[
"for pattern_train, pattern_test, gated in [\n (\"antikt-kt\", \"antikt-kt\", False),\n (\"antikt-kt\", \"antikt-kt-trimmed\", False),\n (\"antikt-kt-trimmed\", \"antikt-kt-trimmed\", False),\n (\"antikt-kt-trimmed\", \"antikt-kt\", False),\n ]:\n r, f, t = build_rocs(pattern_train, pattern_test, pattern_train, gated=gated)\n \n # Save\n fd = open(\"../models/jet-study-2/rocs/rocs-%s-%s-%s.pickle\" % \n (\"s\" if not gated else \"g\", pattern_train, pattern_test), \"wb\")\n pickle.dump((r, f, t), fd)\n fd.close()",
"_____no_output_____"
],
[
"for pattern_train, pattern_test, gated, label, color in [\n (\"antikt-kt\", \"antikt-kt\", False, \"$k_t$ on $k_t$\", \"b\"),\n (\"antikt-kt\", \"antikt-kt-trimmed\", False, \"$k_t$ on $k_t$-trimmed\", \"c\"),\n (\"antikt-kt-trimmed\", \"antikt-kt-trimmed\", False, \"$k_t$-trimmed on $k_t$-trimmed\", \"r\"),\n (\"antikt-kt-trimmed\", \"antikt-kt\", False, \"$k_t$-trimmed on $k_t$\", \"orange\"),\n ]:\n \n fd = open(\"../models/jet-study-2/rocs/rocs-%s-%s-%s.pickle\" % \n (\"s\" if not gated else \"g\", pattern_train, pattern_test), \"rb\")\n r, f, t = pickle.load(fd)\n fd.close()\n \n r, f, t = remove_outliers(r, f, t)\n \n plot_rocs(r, f, t, label=label, color=color)\n report_score(r, f, t, label=label)\n \nplot_show()",
" $k_t$ on $k_t$\tROC AUC=0.9185+-0.00\t1/FPR@TPR=0.5=68.32+-1.80\n $k_t$ on $k_t$-trimmed\tROC AUC=0.8952+-0.00\t1/FPR@TPR=0.5=28.70+-1.30\n $k_t$-trimmed on $k_t$-trimmed\tROC AUC=0.9034+-0.00\t1/FPR@TPR=0.5=33.05+-1.85\n $k_t$-trimmed on $k_t$\tROC AUC=0.8893+-0.01\t1/FPR@TPR=0.5=36.78+-5.88\n"
]
],
[
[
"# Colinear splits",
"_____no_output_____"
]
],
[
[
"from functools import partial\nfrom recnn.preprocessing import sequentialize_by_pt\n\npreprocess_seqpt = partial(sequentialize_by_pt, reverse=False)\npreprocess_seqpt_rev = partial(sequentialize_by_pt, reverse=True)\n\nfor pattern_train, pattern_test, gated, preprocess in [\n # kt\n (\"antikt-kt\", \"antikt-kt-colinear1\", False, None),\n (\"antikt-kt\", \"antikt-kt-colinear10\", False, None),\n (\"antikt-kt\", \"antikt-kt-colinear1-max\", False, None),\n (\"antikt-kt\", \"antikt-kt-colinear10-max\", False, None),\n \n # asc-pt\n (\"antikt-seqpt\", \"antikt-kt-colinear1\", False, preprocess_seqpt),\n (\"antikt-seqpt\", \"antikt-kt-colinear10\", False, preprocess_seqpt),\n (\"antikt-seqpt\", \"antikt-kt-colinear1-max\", False, preprocess_seqpt),\n (\"antikt-seqpt\", \"antikt-kt-colinear10-max\", False, preprocess_seqpt),\n \n # desc-pt\n (\"antikt-seqpt-reversed\", \"antikt-kt-colinear1\", False, preprocess_seqpt_rev),\n (\"antikt-seqpt-reversed\", \"antikt-kt-colinear10\", False, preprocess_seqpt_rev),\n (\"antikt-seqpt-reversed\", \"antikt-kt-colinear1-max\", False, preprocess_seqpt_rev),\n (\"antikt-seqpt-reversed\", \"antikt-kt-colinear10-max\", False, preprocess_seqpt_rev),\n ]:\n \n r, f, t = build_rocs(pattern_train, pattern_test, pattern_train, gated=gated, preprocess=preprocess)\n \n # Save\n fd = open(\"../models/jet-study-2/rocs/rocs-%s-%s-%s.pickle\" % \n (\"s\" if not gated else \"g\", pattern_train, pattern_test), \"wb\")\n pickle.dump((r, f, t), fd)\n fd.close()",
"_____no_output_____"
],
[
"for pattern_train, pattern_test, gated, label in [\n # kt\n (\"antikt-kt\", \"antikt-kt-colinear1\", False, \"$k_t$ colinear1\"),\n (\"antikt-kt\", \"antikt-kt-colinear10\", False, \"$k_t$ colinear10\"),\n (\"antikt-kt\", \"antikt-kt-colinear1-max\", False, \"$k_t$ colinear1-max\"),\n (\"antikt-kt\", \"antikt-kt-colinear10-max\", False, \"$k_t$ colinear10-max\"),\n \n # asc-pt\n (\"antikt-seqpt\", \"antikt-kt-colinear1\", False, \"asc-$p_T$ colinear1\"),\n (\"antikt-seqpt\", \"antikt-kt-colinear10\", False, \"asc-$p_T$ colinear10\"),\n (\"antikt-seqpt\", \"antikt-kt-colinear1-max\", False, \"asc-$p_T$ colinear1-max\"),\n (\"antikt-seqpt\", \"antikt-kt-colinear10-max\", False, \"asc-$p_T$ colinear10-max\"),\n \n # desc-pt\n (\"antikt-seqpt-reversed\", \"antikt-kt-colinear1\", False, \"desc-$p_T$ colinear1\"),\n (\"antikt-seqpt-reversed\", \"antikt-kt-colinear10\", False, \"desc-$p_T$ colinear10\"),\n (\"antikt-seqpt-reversed\", \"antikt-kt-colinear1-max\", False, \"desc-$p_T$ colinear1-max\"),\n (\"antikt-seqpt-reversed\", \"antikt-kt-colinear10-max\", False, \"desc-$p_T$ colinear10-max\"),\n ]:\n \n fd = open(\"../models/jet-study-2/rocs/rocs-%s-%s-%s.pickle\" % \n (\"s\" if not gated else \"g\", pattern_train, pattern_test), \"rb\")\n r, f, t = pickle.load(fd)\n fd.close()\n \n r, f, t = remove_outliers(r, f, t)\n \n report_score(r, f, t, label=label,\n latex=True, short=True)",
" $k_t$ colinear1 \t& 0.9183 $\\pm$ 0.0006 \t& 68.7 $\\pm$ 2.0 \\\\\n $k_t$ colinear10 \t& 0.9174 $\\pm$ 0.0006 \t& 67.5 $\\pm$ 2.6 \\\\\n $k_t$ colinear1-max \t& 0.9184 $\\pm$ 0.0006 \t& 68.5 $\\pm$ 2.8 \\\\\n $k_t$ colinear10-max \t& 0.9159 $\\pm$ 0.0009 \t& 65.7 $\\pm$ 2.7 \\\\\n asc-$p_T$ colinear1 \t& 0.9129 $\\pm$ 0.0031 \t& 53.0 $\\pm$ 7.5 \\\\\n asc-$p_T$ colinear10 \t& 0.9126 $\\pm$ 0.0033 \t& 53.6 $\\pm$ 7.2 \\\\\n asc-$p_T$ colinear1-max \t& 0.9129 $\\pm$ 0.0032 \t& 54.2 $\\pm$ 7.9 \\\\\n asc-$p_T$ colinear10-max \t& 0.9090 $\\pm$ 0.0039 \t& 50.2 $\\pm$ 8.2 \\\\\n desc-$p_T$ colinear1 \t& 0.9188 $\\pm$ 0.0010 \t& 70.7 $\\pm$ 4.0 \\\\\n desc-$p_T$ colinear10 \t& 0.9178 $\\pm$ 0.0011 \t& 67.9 $\\pm$ 4.3 \\\\\n desc-$p_T$ colinear1-max \t& 0.9191 $\\pm$ 0.0010 \t& 72.4 $\\pm$ 4.3 \\\\\n desc-$p_T$ colinear10-max \t& 0.9140 $\\pm$ 0.0016 \t& 63.5 $\\pm$ 5.2 \\\\\n"
]
],
[
[
"# Soft particles",
"_____no_output_____"
]
],
[
[
"from functools import partial\nfrom recnn.preprocessing import sequentialize_by_pt\n\npreprocess_seqpt = partial(sequentialize_by_pt, reverse=False)\npreprocess_seqpt_rev = partial(sequentialize_by_pt, reverse=True)\n\nfor pattern_train, pattern_test, gated, preprocess in [\n (\"antikt-kt\", \"antikt-kt-soft\", False, None),\n (\"antikt-seqpt\", \"antikt-kt-soft\", False, preprocess_seqpt),\n (\"antikt-seqpt-reversed\", \"antikt-kt-soft\", False, preprocess_seqpt_rev),\n ]:\n \n r, f, t = build_rocs(pattern_train, pattern_test, pattern_train, gated=gated, preprocess=preprocess)\n \n # Save\n fd = open(\"../models/jet-study-2/rocs/rocs-%s-%s-%s.pickle\" % \n (\"s\" if not gated else \"g\", pattern_train, pattern_test), \"wb\")\n pickle.dump((r, f, t), fd)\n fd.close()",
"_____no_output_____"
],
[
"for pattern_train, pattern_test, gated, label in [\n (\"antikt-kt\", \"antikt-kt-soft\", False, \"$k_t$ soft\"),\n (\"antikt-seqpt\", \"antikt-kt-soft\", False, \"asc-$p_T$ soft\"),\n (\"antikt-seqpt-reversed\", \"antikt-kt-soft\", False, \"desc-$p_T$ soft\"),\n ]:\n \n fd = open(\"../models/jet-study-2/rocs/rocs-%s-%s-%s.pickle\" % \n (\"s\" if not gated else \"g\", pattern_train, pattern_test), \"rb\")\n r, f, t = pickle.load(fd)\n fd.close()\n \n r, f, t = remove_outliers(r, f, t)\n \n report_score(r, f, t, label=label, latex=True, short=True)",
" $k_t$ soft \t& 0.9179 $\\pm$ 0.0006 \t& 68.2 $\\pm$ 2.3 \\\\\n asc-$p_T$ soft \t& 0.9121 $\\pm$ 0.0032 \t& 51.3 $\\pm$ 6.0 \\\\\n desc-$p_T$ soft \t& 0.9188 $\\pm$ 0.0009 \t& 70.2 $\\pm$ 3.7 \\\\\n"
]
],
[
[
"# Learning curve",
"_____no_output_____"
]
],
[
[
"for pattern, gated, n_events in [\n# (\"antikt-kt\", False, 6000),\n# (\"antikt-seqpt-reversed\", False, 6000),\n (\"antikt-kt\", True, 6000),\n (\"antikt-seqpt-reversed\", True, 6000),\n# (\"antikt-kt\", False, 15000),\n# (\"antikt-seqpt-reversed\", False, 15000),\n (\"antikt-kt\", True, 15000),\n (\"antikt-seqpt-reversed\", True, 15000),\n ]:\n \n tf = load_tf(\"../data/w-vs-qcd/final/%s-train.pickle\" % pattern, n_events_train=n_events)\n X, y, w = load_test(tf, \"../data/w-vs-qcd/final/%s-test.pickle\" % pattern) \n \n if not gated:\n rocs, fprs, tprs = evaluate_models(X, y, w, \n \"../models/jet-study-2/model-w-s-%s-%d-[0-9]*.pickle\" % (pattern, n_events))\n else:\n rocs, fprs, tprs = evaluate_models(X, y, w, \n \"../models/jet-study-2/model-w-g-%s-%d-[0-9]*.pickle\" % (pattern, n_events), func=grnn_predict_gated)\n \n # Save\n fd = open(\"../models/jet-study-2/rocs/rocs-%s-%s-%d.pickle\" % (\"s\" if not gated else \"g\", pattern, n_events), \"wb\")\n pickle.dump((rocs, fprs, tprs), fd)\n fd.close()",
"_____no_output_____"
],
[
"for pattern, label, color in [\n (\"s-antikt-kt\", \"$k_t$ 100k\", \"r\"),\n (\"s-antikt-kt-15000\", \"$k_t$ 10k\", \"g\"),\n (\"s-antikt-kt-6000\", \"$k_t$ 1k\", \"b\"),\n (\"s-antikt-seqpt-reversed\", \"desc-$p_T$ 100k\", \"r--\"),\n (\"s-antikt-seqpt-reversed-15000\", \"desc-$p_T$ 10k\", \"g--\"),\n (\"s-antikt-seqpt-reversed-6000\", \"desc-$p_T$ 1k\", \"b--\"),\n ]:\n \n fd = open(\"../models/jet-study-2/rocs/rocs-%s.pickle\" % pattern, \"rb\")\n r, f, t = pickle.load(fd)\n fd.close()\n \n r, f, t = remove_outliers(r, f, t)\n plot_rocs(r, f, t, label=label, color=color)\n report_score(r, f, t, label=label)\n \nplot_show()",
" $k_t$ 100k\tROC AUC=0.9185+-0.00\t1/FPR@TPR=0.5=68.32+-1.80\n $k_t$ 10k\tROC AUC=0.9038+-0.00\t1/FPR@TPR=0.5=40.66+-4.58\n $k_t$ 1k\tROC AUC=0.8758+-0.01\t1/FPR@TPR=0.5=23.74+-1.95\n desc-$p_T$ 100k\tROC AUC=0.9189+-0.00\t1/FPR@TPR=0.5=70.38+-3.65\n desc-$p_T$ 10k\tROC AUC=0.9030+-0.00\t1/FPR@TPR=0.5=36.71+-4.00\n desc-$p_T$ 1k\tROC AUC=0.8768+-0.00\t1/FPR@TPR=0.5=22.04+-2.38\n"
],
[
"for pattern, label, color in [\n (\"g-antikt-kt\", \"$k_t$ 100k\", \"r\"),\n (\"g-antikt-kt-15000\", \"$k_t$ 10k\", \"g\"),\n (\"g-antikt-kt-6000\", \"$k_t$ 1k\", \"b\"),\n (\"g-antikt-seqpt-reversed\", \"desc-$p_T$ 100k\", \"r--\"),\n (\"g-antikt-seqpt-reversed-15000\", \"desc-$p_T$ 10k\", \"g--\"),\n (\"g-antikt-seqpt-reversed-6000\", \"desc-$p_T$ 1k\", \"b--\"),\n ]:\n \n fd = open(\"../models/jet-study-2/rocs/rocs-%s.pickle\" % pattern, \"rb\")\n r, f, t = pickle.load(fd)\n fd.close()\n \n r, f, t = remove_outliers(r, f, t)\n plot_rocs(r, f, t, label=label, color=color)\n report_score(r, f, t, label=label)\n \nplot_show()",
" $k_t$ 100k\tROC AUC=0.9195+-0.00\t1/FPR@TPR=0.5=74.34+-2.40\n $k_t$ 10k\tROC AUC=0.9042+-0.00\t1/FPR@TPR=0.5=46.42+-5.09\n $k_t$ 1k\tROC AUC=0.8726+-0.01\t1/FPR@TPR=0.5=22.63+-2.97\n desc-$p_T$ 100k\tROC AUC=0.9212+-0.00\t1/FPR@TPR=0.5=83.27+-3.08\n desc-$p_T$ 10k\tROC AUC=0.9123+-0.00\t1/FPR@TPR=0.5=56.12+-4.75\n desc-$p_T$ 1k\tROC AUC=0.8860+-0.00\t1/FPR@TPR=0.5=27.35+-2.51\n"
]
],
[
[
"# Tau21",
"_____no_output_____"
]
],
[
[
"import h5py",
"_____no_output_____"
],
[
"f = h5py.File(\"../data/w-vs-qcd/h5/w_100000_j1p0_sj0p30_delphes_jets_images.h5\", \"r\")[\"auxvars\"]\ntau1 = f[\"tau_1\"]\ntau2 = f[\"tau_2\"]\ntau21 = np.true_divide(tau2, tau1)\npt = f[\"pt_trimmed\"]\nmass = f[\"mass_trimmed\"]\nmask = (f[\"mass_trimmed\"] < 110) & (f[\"mass_trimmed\"] > 50) & (f[\"pt_trimmed\"] < 300) & (f[\"pt_trimmed\"] > 250)\n#mask = mask & np.isfinite(tau21) & (tau21 != 0.)\nsignal_tau21 = tau21[mask]\nsignal_pt = pt[mask]\nsignal_mass = mass[mask]\n\nf = h5py.File(\"../data/w-vs-qcd/h5/qcd_100000_j1p0_sj0p30_delphes_jets_images.h5\", \"r\")[\"auxvars\"]\ntau1 = f[\"tau_1\"]\ntau2 = f[\"tau_2\"]\ntau21 = np.true_divide(tau2, tau1)\npt = f[\"pt_trimmed\"]\nmass = f[\"mass_trimmed\"]\nmask = (f[\"mass_trimmed\"] < 110) & (f[\"mass_trimmed\"] > 50) & (f[\"pt_trimmed\"] < 300) & (f[\"pt_trimmed\"] > 250)\n#mask = mask & np.isfinite(tau21) & (tau21 != 0.)\nbkg_tau21 = tau21[mask]\nbkg_pt = pt[mask]\nbkg_mass = mass[mask]",
"/home/gilles/anaconda3/envs/hep/lib/python2.7/site-packages/ipykernel/__main__.py:4: RuntimeWarning: invalid value encountered in true_divide\n/home/gilles/anaconda3/envs/hep/lib/python2.7/site-packages/ipykernel/__main__.py:16: RuntimeWarning: invalid value encountered in true_divide\n"
],
[
"plt.hist(bkg_mass, histtype=\"step\", bins=40, normed=1)\nplt.hist(signal_mass, histtype=\"step\", bins=40, normed=1)",
"_____no_output_____"
],
[
"tau21 = np.concatenate((signal_tau21, bkg_tau21))\npts = np.concatenate((signal_pt, bkg_pt))\nmasss = np.concatenate((signal_mass, bkg_mass))\n\nX = np.hstack([tau21.reshape(-1,1), masss.reshape(-1,1)])\ny = np.concatenate((np.ones(len(signal_tau21)), np.zeros(len(bkg_tau21))))\n\nw = np.zeros(len(y)) \n\npdf, edges = np.histogram(pts[y == 0], density=True, range=[250, 300], bins=50)\nindices = np.searchsorted(edges, pts[y == 0]) - 1\ninv_w = 1. / pdf[indices]\ninv_w /= inv_w.sum()\nw[y==0] = inv_w\n\npdf, edges = np.histogram(pts[y == 1], density=True, range=[250, 300], bins=50)\nindices = np.searchsorted(edges, pts[y == 1]) - 1\ninv_w = 1. / pdf[indices]\ninv_w /= inv_w.sum()\nw[y==1] = inv_w",
"_____no_output_____"
],
[
"X_train, X_test, y_train, y_test, w_train, w_test = train_test_split(X, y, w, train_size=0.5)",
"_____no_output_____"
],
[
"def evaluate_models(X, y, w):\n rocs = []\n fprs = []\n tprs = []\n \n y_pred = X\n\n # Roc\n rocs.append(roc_auc_score(y, y_pred, sample_weight=w))\n fpr, tpr, _ = roc_curve(y, y_pred, sample_weight=w)\n\n fprs.append(fpr)\n tprs.append(tpr)\n \n return rocs, fprs, tprs\n\nr, f, t = evaluate_models(-tau21, y, w)\nplot_rocs(r, f, t, label=\"tau21\")\nreport_score(r, f, t, label=\"tau21\")\n\nr, f, t = evaluate_models(masss, y, w)\nplot_rocs(r, f, t, label=\"mass\")\nreport_score(r, f, t, label=\"mass\")\n\nplot_show()",
" tau21\tROC AUC=0.7644+-0.00\t1/FPR@TPR=0.5=6.79+-0.00\n mass\tROC AUC=0.6194+-0.00\t1/FPR@TPR=0.5=2.80+-0.00\n"
],
[
"clf = ExtraTreesClassifier(n_estimators=1000, min_samples_leaf=100, max_features=1)\nclf.fit(X_train, y_train)",
"_____no_output_____"
],
[
"r, f, t = evaluate_models(-clf.predict_proba(X_test)[:, 0], y_test, w_test)\nplot_rocs(r, f, t, label=\"tau21+mass\")\nreport_score(r, f, t, label=\"tau21+mass\")\n\nplot_show()",
" tau21+mass\tROC AUC=0.8207+-0.00\t1/FPR@TPR=0.5=11.06+-0.00\n"
]
]
] | [
"code",
"markdown",
"code",
"markdown",
"code",
"markdown",
"code",
"markdown",
"code",
"markdown",
"code",
"markdown",
"code",
"markdown",
"code",
"markdown",
"code",
"markdown",
"code",
"markdown",
"code",
"markdown",
"code"
] | [
[
"code",
"code"
],
[
"markdown"
],
[
"code",
"code",
"code"
],
[
"markdown"
],
[
"code",
"code"
],
[
"markdown"
],
[
"code",
"code",
"code",
"code",
"code",
"code"
],
[
"markdown"
],
[
"code",
"code"
],
[
"markdown"
],
[
"code",
"code"
],
[
"markdown"
],
[
"code",
"code",
"code",
"code",
"code",
"code",
"code"
],
[
"markdown"
],
[
"code",
"code"
],
[
"markdown"
],
[
"code",
"code"
],
[
"markdown"
],
[
"code",
"code"
],
[
"markdown"
],
[
"code",
"code",
"code"
],
[
"markdown"
],
[
"code",
"code",
"code",
"code",
"code",
"code",
"code",
"code"
]
] |
d079023a7fef4becae51f50bcae895d20c5f96bb | 151,888 | ipynb | Jupyter Notebook | 01-Lesson-Plans/19-Supervised-Machine-Learning/1/Extra-Activities/01-Ins_Hyperparameters/Solved/Ins_Hyperparameters.ipynb | anirudhmungre/sneaky-lessons | 8e48015c50865059db96f8cd369bcc15365d66c7 | [
"ADSL"
] | null | null | null | 01-Lesson-Plans/19-Supervised-Machine-Learning/1/Extra-Activities/01-Ins_Hyperparameters/Solved/Ins_Hyperparameters.ipynb | anirudhmungre/sneaky-lessons | 8e48015c50865059db96f8cd369bcc15365d66c7 | [
"ADSL"
] | null | null | null | 01-Lesson-Plans/19-Supervised-Machine-Learning/1/Extra-Activities/01-Ins_Hyperparameters/Solved/Ins_Hyperparameters.ipynb | anirudhmungre/sneaky-lessons | 8e48015c50865059db96f8cd369bcc15365d66c7 | [
"ADSL"
] | null | null | null | 79.60587 | 25,400 | 0.492969 | [
[
[
"import numpy as np\nimport matplotlib.pyplot as plt",
"_____no_output_____"
],
[
"from sklearn.datasets import make_blobs\nX, y = make_blobs(n_samples=100, centers=2, random_state=0, cluster_std=.95)\nplt.scatter(X[:, 0], X[:, 1], c=y, s=100, cmap=\"bwr\");\nplt.show()",
"_____no_output_____"
],
[
"# Split the data into training and testing sets\nfrom sklearn.model_selection import train_test_split\nX_train, X_test, y_train, y_test = train_test_split(X, y, random_state=42)",
"_____no_output_____"
],
[
"# Create the logistic model\nfrom sklearn.linear_model import LogisticRegression\nmodel = LogisticRegression()\nmodel",
"_____no_output_____"
],
[
"param_grid = {\n 'C': [0.001, 0.01, 0.1, 1, 10, 100],\n 'tol': [0.00001, 0.0001, 0.001]\n}\nparam_grid",
"_____no_output_____"
],
[
"from sklearn.model_selection import GridSearchCV\ngrid_clf = GridSearchCV(model, param_grid, verbose=3)",
"_____no_output_____"
],
[
"# Fit the model by using the grid search classifier. \n# This will take the LogisticRegression model and try each combination of parameters.\ngrid_clf.fit(X_train, y_train)",
"Fitting 5 folds for each of 18 candidates, totalling 90 fits\n[CV] C=0.001, tol=1e-05 ..............................................\n[CV] .................. C=0.001, tol=1e-05, score=0.733, total= 0.0s\n[CV] C=0.001, tol=1e-05 ..............................................\n[CV] .................. C=0.001, tol=1e-05, score=0.600, total= 0.0s\n[CV] C=0.001, tol=1e-05 ..............................................\n[CV] .................. C=0.001, tol=1e-05, score=0.600, total= 0.0s\n[CV] C=0.001, tol=1e-05 ..............................................\n[CV] .................. C=0.001, tol=1e-05, score=0.600, total= 0.0s\n[CV] C=0.001, tol=1e-05 ..............................................\n[CV] .................. C=0.001, tol=1e-05, score=0.533, total= 0.0s\n[CV] C=0.001, tol=0.0001 .............................................\n[CV] ................. C=0.001, tol=0.0001, score=0.733, total= 0.0s\n[CV] C=0.001, tol=0.0001 .............................................\n[CV] ................. C=0.001, tol=0.0001, score=0.600, total= 0.0s\n[CV] C=0.001, tol=0.0001 .............................................\n[CV] ................. C=0.001, tol=0.0001, score=0.600, total= 0.0s\n[CV] C=0.001, tol=0.0001 .............................................\n[CV] ................. C=0.001, tol=0.0001, score=0.600, total= 0.0s\n[CV] C=0.001, tol=0.0001 .............................................\n[CV] ................. C=0.001, tol=0.0001, score=0.533, total= 0.0s\n[CV] C=0.001, tol=0.001 ..............................................\n[CV] .................. C=0.001, tol=0.001, score=0.733, total= 0.0s\n[CV] C=0.001, tol=0.001 ..............................................\n[CV] .................. C=0.001, tol=0.001, score=0.600, total= 0.0s\n[CV] C=0.001, tol=0.001 ..............................................\n[CV] .................. C=0.001, tol=0.001, score=0.600, total= 0.0s\n[CV] C=0.001, tol=0.001 ..............................................\n[CV] .................. C=0.001, tol=0.001, score=0.600, total= 0.0s\n[CV] C=0.001, tol=0.001 ..............................................\n[CV] .................. C=0.001, tol=0.001, score=0.533, total= 0.0s\n[CV] C=0.01, tol=1e-05 ...............................................\n[CV] ................... C=0.01, tol=1e-05, score=1.000, total= 0.0s\n[CV] C=0.01, tol=1e-05 ...............................................\n[CV] ................... C=0.01, tol=1e-05, score=0.867, total= 0.0s\n[CV] C=0.01, tol=1e-05 ...............................................\n[CV] ................... C=0.01, tol=1e-05, score=0.933, total= 0.0s\n[CV] C=0.01, tol=1e-05 ...............................................\n[CV] ................... C=0.01, tol=1e-05, score=0.933, total= 0.0s\n[CV] C=0.01, tol=1e-05 ...............................................\n[CV] ................... C=0.01, tol=1e-05, score=1.000, total= 0.0s\n[CV] C=0.01, tol=0.0001 ..............................................\n[CV] .................. C=0.01, tol=0.0001, score=1.000, total= 0.0s\n[CV] C=0.01, tol=0.0001 ..............................................\n[CV] .................. C=0.01, tol=0.0001, score=0.867, total= 0.0s\n[CV] C=0.01, tol=0.0001 ..............................................\n[CV] .................. C=0.01, tol=0.0001, score=0.933, total= 0.0s\n[CV] C=0.01, tol=0.0001 ..............................................\n[CV] .................. C=0.01, tol=0.0001, score=0.933, total= 0.0s\n[CV] C=0.01, tol=0.0001 ..............................................\n[CV] .................. C=0.01, tol=0.0001, score=1.000, total= 0.0s\n[CV] C=0.01, tol=0.001 ...............................................\n[CV] ................... C=0.01, tol=0.001, score=1.000, total= 0.0s\n[CV] C=0.01, tol=0.001 ...............................................\n[CV] ................... C=0.01, tol=0.001, score=0.867, total= 0.0s\n[CV] C=0.01, tol=0.001 ...............................................\n[CV] ................... C=0.01, tol=0.001, score=0.933, total= 0.0s\n[CV] C=0.01, tol=0.001 ...............................................\n[CV] ................... C=0.01, tol=0.001, score=0.933, total= 0.0s\n[CV] C=0.01, tol=0.001 ...............................................\n[CV] ................... C=0.01, tol=0.001, score=1.000, total= 0.0s\n[CV] C=0.1, tol=1e-05 ................................................\n[CV] .................... C=0.1, tol=1e-05, score=1.000, total= 0.0s\n[CV] C=0.1, tol=1e-05 ................................................\n[CV] .................... C=0.1, tol=1e-05, score=0.867, total= 0.0s\n[CV] C=0.1, tol=1e-05 ................................................\n[CV] .................... C=0.1, tol=1e-05, score=0.867, total= 0.0s\n[CV] C=0.1, tol=1e-05 ................................................\n[CV] .................... C=0.1, tol=1e-05, score=1.000, total= 0.0s\n[CV] C=0.1, tol=1e-05 ................................................\n[CV] .................... C=0.1, tol=1e-05, score=1.000, total= 0.0s\n[CV] C=0.1, tol=0.0001 ...............................................\n[CV] ................... C=0.1, tol=0.0001, score=1.000, total= 0.0s\n[CV] C=0.1, tol=0.0001 ...............................................\n[CV] ................... C=0.1, tol=0.0001, score=0.867, total= 0.0s\n[CV] C=0.1, tol=0.0001 ...............................................\n[CV] ................... C=0.1, tol=0.0001, score=0.867, total= 0.0s\n[CV] C=0.1, tol=0.0001 ...............................................\n[CV] ................... C=0.1, tol=0.0001, score=1.000, total= 0.0s\n[CV] C=0.1, tol=0.0001 ...............................................\n[CV] ................... C=0.1, tol=0.0001, score=1.000, total= 0.0s\n[CV] C=0.1, tol=0.001 ................................................\n[CV] .................... C=0.1, tol=0.001, score=1.000, total= 0.0s\n[CV] C=0.1, tol=0.001 ................................................\n[CV] .................... C=0.1, tol=0.001, score=0.867, total= 0.0s\n[CV] C=0.1, tol=0.001 ................................................\n[CV] .................... C=0.1, tol=0.001, score=0.867, total= 0.0s"
],
[
"# List the best parameters for this dataset\nprint(grid_clf.best_params_)",
"{'C': 0.01, 'tol': 1e-05}\n"
],
[
"# List the best score\nprint(grid_clf.best_score_)",
"0.9466666666666667\n"
],
[
"# Make predictions with the hypertuned model\npredictions = grid_clf.predict(X_test)\npredictions",
"_____no_output_____"
],
[
"# Score the hypertuned model on the test dataset\ngrid_clf.score(X_test, y_test)",
"_____no_output_____"
]
],
[
[
"# RandomizedSearchCV",
"_____no_output_____"
]
],
[
[
"big_param_grid = {\n 'C' : np.arange(0, 10, 0.01),\n 'tol': np.arange(0, 0.001, 1e-5),\n}\nbig_param_grid",
"_____no_output_____"
],
[
"# Create the RandomizedSearch estimator along with a parameter object containing the values to adjust\nfrom sklearn.model_selection import RandomizedSearchCV\nrandom_clf = RandomizedSearchCV(model, big_param_grid, n_iter=100, random_state=1, verbose=3)\nrandom_clf",
"_____no_output_____"
],
[
"# Fit the model by using the randomized search estimator. \n# This will take the LogisticRegression model and try a random sample of combinations of parameters\nrandom_clf.fit(X_train, y_train)",
"Fitting 5 folds for each of 100 candidates, totalling 500 fits\n[CV] tol=0.00039000000000000005, C=9.85 ..............................\n[CV] .. tol=0.00039000000000000005, C=9.85, score=1.000, total= 0.0s\n[CV] tol=0.00039000000000000005, C=9.85 ..............................\n[CV] .. tol=0.00039000000000000005, C=9.85, score=0.867, total= 0.0s\n[CV] tol=0.00039000000000000005, C=9.85 ..............................\n[CV] .. tol=0.00039000000000000005, C=9.85, score=0.800, total= 0.0s\n[CV] tol=0.00039000000000000005, C=9.85 ..............................\n[CV] .. tol=0.00039000000000000005, C=9.85, score=1.000, total= 0.0s\n[CV] tol=0.00039000000000000005, C=9.85 ..............................\n[CV] .. tol=0.00039000000000000005, C=9.85, score=1.000, total= 0.0s\n[CV] tol=8e-05, C=7.7700000000000005 .................................\n[CV] ..... tol=8e-05, C=7.7700000000000005, score=1.000, total= 0.0s\n[CV] tol=8e-05, C=7.7700000000000005 .................................\n[CV] ..... tol=8e-05, C=7.7700000000000005, score=0.867, total= 0.0s\n[CV] tol=8e-05, C=7.7700000000000005 .................................\n[CV] ..... tol=8e-05, C=7.7700000000000005, score=0.800, total= 0.0s\n[CV] tol=8e-05, C=7.7700000000000005 .................................\n[CV] ..... tol=8e-05, C=7.7700000000000005, score=1.000, total= 0.0s\n[CV] tol=8e-05, C=7.7700000000000005 .................................\n[CV] ..... tol=8e-05, C=7.7700000000000005, score=1.000, total= 0.0s\n[CV] tol=0.00092, C=0.51 .............................................\n[CV] ................. tol=0.00092, C=0.51, score=1.000, total= 0.0s\n[CV] tol=0.00092, C=0.51 .............................................\n[CV] ................. tol=0.00092, C=0.51, score=0.867, total= 0.0s\n[CV] tol=0.00092, C=0.51 .............................................\n[CV] ................. tol=0.00092, C=0.51, score=0.867, total= 0.0s\n[CV] tol=0.00092, C=0.51 .............................................\n[CV] ................. tol=0.00092, C=0.51, score=1.000, total= 0.0s\n[CV] tol=0.00092, C=0.51 .............................................\n[CV] ................. tol=0.00092, C=0.51, score=1.000, total= 0.0s\n[CV] tol=0.00047000000000000004, C=9.8 ...............................\n[CV] ... tol=0.00047000000000000004, C=9.8, score=1.000, total= 0.0s\n[CV] tol=0.00047000000000000004, C=9.8 ...............................\n[CV] ... tol=0.00047000000000000004, C=9.8, score=0.867, total= 0.0s\n[CV] tol=0.00047000000000000004, C=9.8 ...............................\n[CV] ... tol=0.00047000000000000004, C=9.8, score=0.800, total= 0.0s\n[CV] tol=0.00047000000000000004, C=9.8 ...............................\n[CV] ... tol=0.00047000000000000004, C=9.8, score=1.000, total= 0.0s\n[CV] tol=0.00047000000000000004, C=9.8 ...............................\n[CV] ... tol=0.00047000000000000004, C=9.8, score=1.000, total= 0.0s\n[CV] tol=0.0005700000000000001, C=5.0 ................................\n[CV] .... tol=0.0005700000000000001, C=5.0, score=1.000, total= 0.0s\n[CV] tol=0.0005700000000000001, C=5.0 ................................\n[CV] .... tol=0.0005700000000000001, C=5.0, score=0.867, total= 0.0s\n[CV] tol=0.0005700000000000001, C=5.0 ................................\n[CV] .... tol=0.0005700000000000001, C=5.0, score=0.800, total= 0.0s\n[CV] tol=0.0005700000000000001, C=5.0 ................................\n[CV] .... tol=0.0005700000000000001, C=5.0, score=1.000, total= 0.0s\n[CV] tol=0.0005700000000000001, C=5.0 ................................\n[CV] .... tol=0.0005700000000000001, C=5.0, score=1.000, total= 0.0s\n[CV] tol=0.0004900000000000001, C=7.33 ...............................\n[CV] ... tol=0.0004900000000000001, C=7.33, score=1.000, total= 0.0s\n[CV] tol=0.0004900000000000001, C=7.33 ...............................\n[CV] ... tol=0.0004900000000000001, C=7.33, score=0.867, total= 0.0s\n[CV] tol=0.0004900000000000001, C=7.33 ...............................\n[CV] ... tol=0.0004900000000000001, C=7.33, score=0.800, total= 0.0s\n[CV] tol=0.0004900000000000001, C=7.33 ...............................\n[CV] ... tol=0.0004900000000000001, C=7.33, score=1.000, total= 0.0s\n[CV] tol=0.0004900000000000001, C=7.33 ...............................\n[CV] ... tol=0.0004900000000000001, C=7.33, score=1.000, total= 0.0s\n[CV] tol=0.0004, C=2.14 ..............................................\n[CV] .................. tol=0.0004, C=2.14, score=1.000, total= 0.0s\n[CV] tol=0.0004, C=2.14 ..............................................\n[CV] .................. tol=0.0004, C=2.14, score=0.867, total= 0.0s\n[CV] tol=0.0004, C=2.14 ..............................................\n[CV] .................. tol=0.0004, C=2.14, score=0.800, total= 0.0s\n[CV] tol=0.0004, C=2.14 ..............................................\n[CV] .................. tol=0.0004, C=2.14, score=1.000, total= 0.0s\n[CV] tol=0.0004, C=2.14 ..............................................\n[CV] .................. tol=0.0004, C=2.14, score=1.000, total= 0.0s\n[CV] tol=0.00048000000000000007, C=9.84 ..............................\n[CV] .. tol=0.00048000000000000007, C=9.84, score=1.000, total= 0.0s\n[CV] tol=0.00048000000000000007, C=9.84 ..............................\n[CV] .. tol=0.00048000000000000007, C=9.84, score=0.867, total= 0.0s"
],
[
"# List the best parameters for this dataset\nprint(random_clf.best_params_)",
"{'tol': 0.00092, 'C': 0.51}\n"
],
[
"# List the best score\nprint(random_clf.best_score_)",
"0.9466666666666667\n"
],
[
"# Make predictions with the hypertuned model\npredictions = random_clf.predict(X_test)",
"_____no_output_____"
],
[
"# Calculate the classification report\nfrom sklearn.metrics import classification_report\nprint(classification_report(y_test, predictions,\n target_names=[\"blue\", \"red\"]))",
" precision recall f1-score support\n\n blue 0.83 1.00 0.91 10\n red 1.00 0.87 0.93 15\n\n accuracy 0.92 25\n macro avg 0.92 0.93 0.92 25\nweighted avg 0.93 0.92 0.92 25\n\n"
]
]
] | [
"code",
"markdown",
"code"
] | [
[
"code",
"code",
"code",
"code",
"code",
"code",
"code",
"code",
"code",
"code",
"code"
],
[
"markdown"
],
[
"code",
"code",
"code",
"code",
"code",
"code",
"code"
]
] |
d07929a487d2b2f8c29af990da723f5b3e6cb23c | 67,648 | ipynb | Jupyter Notebook | notebooks/layers/pooling/AveragePooling3D.ipynb | GTDev87/tpt-hackathon | c6ce8bb970d59a8cac8e55137829cf014c98124a | [
"MIT"
] | 2 | 2018-07-02T17:20:35.000Z | 2021-02-12T16:30:35.000Z | notebooks/layers/pooling/AveragePooling3D.ipynb | qinwf-nuan/keras-js | dafc91e6f58bd663656872014cf1d1bd5359c97d | [
"MIT"
] | 1 | 2017-09-27T18:41:18.000Z | 2017-09-27T18:41:18.000Z | notebooks/layers/pooling/AveragePooling3D.ipynb | GTDev87/tpt-hackathon | c6ce8bb970d59a8cac8e55137829cf014c98124a | [
"MIT"
] | 1 | 2018-02-02T17:35:01.000Z | 2018-02-02T17:35:01.000Z | 86.951157 | 23,064 | 0.620344 | [
[
[
"import numpy as np\nfrom keras.models import Model\nfrom keras.layers import Input\nfrom keras.layers.pooling import AveragePooling3D\nfrom keras import backend as K\nimport json\nfrom collections import OrderedDict",
"Using TensorFlow backend.\n"
],
[
"def format_decimal(arr, places=6):\n return [round(x * 10**places) / 10**places for x in arr]",
"_____no_output_____"
],
[
"DATA = OrderedDict()",
"_____no_output_____"
]
],
[
[
"### AveragePooling3D",
"_____no_output_____"
],
[
"**[pooling.AveragePooling3D.0] input 4x4x4x2, pool_size=(2, 2, 2), strides=None, padding='valid', data_format='channels_last'**",
"_____no_output_____"
]
],
[
[
"data_in_shape = (4, 4, 4, 2)\nL = AveragePooling3D(pool_size=(2, 2, 2), strides=None, padding='valid', data_format='channels_last')\n\nlayer_0 = Input(shape=data_in_shape)\nlayer_1 = L(layer_0)\nmodel = Model(inputs=layer_0, outputs=layer_1)\n\n# set weights to random (use seed for reproducibility)\nnp.random.seed(290)\ndata_in = 2 * np.random.random(data_in_shape) - 1\nresult = model.predict(np.array([data_in]))\ndata_out_shape = result[0].shape\ndata_in_formatted = format_decimal(data_in.ravel().tolist())\ndata_out_formatted = format_decimal(result[0].ravel().tolist())\nprint('')\nprint('in shape:', data_in_shape)\nprint('in:', data_in_formatted)\nprint('out shape:', data_out_shape)\nprint('out:', data_out_formatted)\n\nDATA['pooling.AveragePooling3D.0'] = {\n 'input': {'data': data_in_formatted, 'shape': data_in_shape},\n 'expected': {'data': data_out_formatted, 'shape': data_out_shape}\n}",
"\nin shape: (4, 4, 4, 2)\nin: [-0.453175, -0.475078, 0.486234, -0.949643, -0.349099, -0.837108, 0.933439, 0.167853, -0.995191, 0.459466, -0.788337, -0.120985, 0.06215, 0.138625, -0.102201, 0.976605, -0.591051, -0.592066, 0.469334, -0.435067, 0.621416, 0.817698, 0.790015, 0.485862, 0.469679, -0.611443, 0.582845, -0.503885, -0.379174, -0.451035, 0.052289, 0.131836, -0.609312, -0.828722, -0.422428, -0.648754, -0.339801, -0.758017, 0.754244, -0.544823, 0.691656, 0.076848, -0.32539, 0.306448, -0.662415, 0.334329, 0.030666, -0.414111, -0.757096, -0.20427, -0.893088, -0.681919, -0.619269, -0.640749, 0.867436, 0.971453, -0.42039, -0.574905, -0.34642, 0.588678, -0.247265, 0.436084, 0.220126, 0.114202, 0.613623, 0.401452, -0.270262, -0.591146, -0.872383, 0.818368, 0.336808, 0.338197, -0.275646, 0.375308, -0.928722, -0.836727, -0.504007, -0.503397, -0.636099, 0.948482, -0.639661, -0.026878, -0.122643, -0.634018, -0.247016, 0.517246, -0.398639, 0.752174, -0.014633, -0.170534, -0.463453, -0.289716, 0.837207, 0.769962, -0.401357, 0.076406, 0.270433, -0.036538, -0.05766, 0.625256, 0.626847, -0.27321, -0.217219, -0.775553, -0.182939, 0.327385, -0.976376, -0.337729, -0.178467, -0.1545, -0.334259, -0.537949, 0.499229, 0.775269, -0.657598, 0.921864, 0.376821, 0.420375, -0.937835, -0.176425, -0.516753, 0.737286, 0.867807, -0.515893, 0.710035, 0.680377, -0.350561, -0.28645]\nout shape: (2, 2, 2, 2)\nout: [-0.301993, -0.272552, 0.040873, -0.117081, -0.185773, -0.37686, 0.163197, 0.233169, -0.225944, -0.009092, -0.222347, -0.017445, -0.130963, 0.099673, -0.051418, 0.344208]\n"
]
],
[
[
"**[pooling.AveragePooling3D.1] input 4x4x4x2, pool_size=(2, 2, 2), strides=(1, 1, 1), padding='valid', data_format='channels_last'**",
"_____no_output_____"
]
],
[
[
"data_in_shape = (4, 4, 4, 2)\nL = AveragePooling3D(pool_size=(2, 2, 2), strides=(1, 1, 1), padding='valid', data_format='channels_last')\n\nlayer_0 = Input(shape=data_in_shape)\nlayer_1 = L(layer_0)\nmodel = Model(inputs=layer_0, outputs=layer_1)\n\n# set weights to random (use seed for reproducibility)\nnp.random.seed(291)\ndata_in = 2 * np.random.random(data_in_shape) - 1\nresult = model.predict(np.array([data_in]))\ndata_out_shape = result[0].shape\ndata_in_formatted = format_decimal(data_in.ravel().tolist())\ndata_out_formatted = format_decimal(result[0].ravel().tolist())\nprint('')\nprint('in shape:', data_in_shape)\nprint('in:', data_in_formatted)\nprint('out shape:', data_out_shape)\nprint('out:', data_out_formatted)\n\nDATA['pooling.AveragePooling3D.1'] = {\n 'input': {'data': data_in_formatted, 'shape': data_in_shape},\n 'expected': {'data': data_out_formatted, 'shape': data_out_shape}\n}",
"\nin shape: (4, 4, 4, 2)\nin: [-0.803361, 0.348731, 0.30124, -0.168638, 0.516406, -0.258765, 0.297839, 0.993235, 0.958465, -0.273175, -0.704992, 0.261477, -0.301255, 0.263104, 0.678631, -0.644936, -0.029034, -0.320266, 0.307733, -0.479016, 0.608177, 0.034951, 0.456908, -0.929353, 0.594982, -0.243058, -0.524918, 0.455339, 0.034216, 0.356824, 0.63906, -0.259773, -0.084724, 0.248472, -0.608134, 0.0077, 0.400591, -0.960703, -0.247926, -0.774509, 0.496174, -0.319044, -0.324046, -0.616632, -0.322142, -0.472846, 0.171825, -0.030013, 0.992861, -0.645264, 0.524886, 0.673229, 0.883122, 0.25346, -0.706988, -0.654436, 0.918349, -0.139113, 0.742737, 0.338472, -0.812719, 0.860081, 0.003489, 0.667897, 0.362284, -0.283972, 0.995162, 0.67962, -0.700244, -0.137142, 0.045695, -0.450433, 0.929977, 0.157542, -0.720517, -0.939063, 0.295004, 0.308728, -0.094057, -0.374756, -0.400976, -0.539654, 0.27965, 0.977688, -0.361264, -0.027757, -0.67149, 0.57064, -0.861888, 0.616985, -0.027436, 0.40181, -0.30391, 0.92268, -0.486416, -0.335828, 0.138558, -0.445691, 0.156253, -0.967633, 0.127471, -0.783301, -0.691353, -0.76759, 0.618771, -0.377474, 0.50152, 0.126867, -0.872708, 0.649418, 0.697987, -0.33456, 0.906767, 0.604333, -0.129366, 0.579445, -0.033479, -0.22433, -0.979529, -0.251153, 0.839734, 0.550506, -0.599678, 0.584326, 0.211245, -0.66845, 0.948298, -0.004521]\nout shape: (3, 3, 3, 2)\nout: [-0.096172, -0.063889, -0.130291, -0.243163, 0.149246, -0.235679, 0.277756, -0.214836, 0.083935, -0.010284, 0.183535, -0.272509, 0.440949, -0.04496, 0.220404, 0.311668, 0.138158, 0.041206, 0.130772, -0.133172, -0.123041, -0.266292, -0.056407, -0.361459, 0.222251, -0.1564, 0.031836, 0.019601, -0.100749, -0.053372, 0.271023, 0.210519, 0.115633, 0.549958, -0.307022, 0.282092, 0.372751, -0.256226, -0.027257, -0.132813, -0.149026, -0.236204, 0.248228, 0.07371, -0.130145, 0.181375, -0.252442, 0.039529, 0.000851, 0.47193, -0.12053, 0.318177, -0.209568, -0.00234]\n"
]
],
[
[
"**[pooling.AveragePooling3D.2] input 4x5x2x3, pool_size=(2, 2, 2), strides=(2, 1, 1), padding='valid', data_format='channels_last'**",
"_____no_output_____"
]
],
[
[
"data_in_shape = (4, 5, 2, 3)\nL = AveragePooling3D(pool_size=(2, 2, 2), strides=(2, 1, 1), padding='valid', data_format='channels_last')\n\nlayer_0 = Input(shape=data_in_shape)\nlayer_1 = L(layer_0)\nmodel = Model(inputs=layer_0, outputs=layer_1)\n\n# set weights to random (use seed for reproducibility)\nnp.random.seed(282)\ndata_in = 2 * np.random.random(data_in_shape) - 1\nresult = model.predict(np.array([data_in]))\ndata_out_shape = result[0].shape\ndata_in_formatted = format_decimal(data_in.ravel().tolist())\ndata_out_formatted = format_decimal(result[0].ravel().tolist())\nprint('')\nprint('in shape:', data_in_shape)\nprint('in:', data_in_formatted)\nprint('out shape:', data_out_shape)\nprint('out:', data_out_formatted)\n\nDATA['pooling.AveragePooling3D.2'] = {\n 'input': {'data': data_in_formatted, 'shape': data_in_shape},\n 'expected': {'data': data_out_formatted, 'shape': data_out_shape}\n}",
"\nin shape: (4, 5, 2, 3)\nin: [-0.263147, -0.216555, -0.75766, -0.396007, 0.85243, 0.98415, -0.230197, -0.979579, 0.117628, -0.66833, 0.714058, -0.907302, -0.574249, 0.299573, 0.101165, 0.655872, -0.104788, 0.242064, -0.409262, -0.124059, 0.105687, -0.969325, -0.167941, 0.382377, 0.710487, 0.793042, 0.180663, -0.80231, 0.684253, -0.516992, 0.471203, -0.152325, 0.509501, 0.613742, -0.877379, 0.755416, 0.427677, 0.931956, 0.827636, -0.860685, 0.562326, -0.716081, 0.028046, 0.594422, -0.862333, 0.336131, 0.713855, 0.386247, -0.986659, 0.242413, 0.753777, -0.159358, 0.166548, -0.437388, 0.291152, -0.775555, 0.796086, -0.592021, -0.251661, 0.187174, 0.899283, 0.431861, -0.685273, -0.085991, -0.629026, -0.478334, 0.714983, 0.53745, -0.310438, 0.973848, -0.675219, 0.422743, -0.992263, 0.374017, -0.687462, -0.190455, -0.560081, 0.22484, -0.079631, 0.815275, 0.338641, -0.538279, -0.10891, -0.929005, 0.514762, 0.322038, 0.702195, -0.697122, 0.925468, -0.274158, 0.148379, 0.333239, 0.63072, -0.652956, -0.356451, -0.71114, 0.111465, 0.31787, 0.242578, 0.8926, -0.60807, 0.218759, 0.42079, 0.71253, -0.082496, 0.272704, 0.277213, -0.099807, -0.899322, -0.175367, -0.642213, -0.661032, 0.730145, 0.37799, 0.935939, -0.448007, -0.320652, -0.352629, 0.258139, 0.30254]\nout shape: (2, 4, 1, 3)\nout: [-0.113218, 0.104366, 0.101661, -0.110717, 0.341478, -0.101372, -0.259851, 0.202503, 0.083949, -0.364662, 0.07088, 0.181423, 0.375201, -0.081043, -0.083798, 0.275459, 0.046964, -0.00891, -0.333436, 0.258103, -0.187439, -0.222164, 0.289848, -0.055583]\n"
]
],
[
[
"**[pooling.AveragePooling3D.3] input 4x4x4x2, pool_size=(3, 3, 3), strides=None, padding='valid', data_format='channels_last'**",
"_____no_output_____"
]
],
[
[
"data_in_shape = (4, 4, 4, 2)\nL = AveragePooling3D(pool_size=(3, 3, 3), strides=None, padding='valid', data_format='channels_last')\n\nlayer_0 = Input(shape=data_in_shape)\nlayer_1 = L(layer_0)\nmodel = Model(inputs=layer_0, outputs=layer_1)\n\n# set weights to random (use seed for reproducibility)\nnp.random.seed(283)\ndata_in = 2 * np.random.random(data_in_shape) - 1\nresult = model.predict(np.array([data_in]))\ndata_out_shape = result[0].shape\ndata_in_formatted = format_decimal(data_in.ravel().tolist())\ndata_out_formatted = format_decimal(result[0].ravel().tolist())\nprint('')\nprint('in shape:', data_in_shape)\nprint('in:', data_in_formatted)\nprint('out shape:', data_out_shape)\nprint('out:', data_out_formatted)\n\nDATA['pooling.AveragePooling3D.3'] = {\n 'input': {'data': data_in_formatted, 'shape': data_in_shape},\n 'expected': {'data': data_out_formatted, 'shape': data_out_shape}\n}",
"\nin shape: (4, 4, 4, 2)\nin: [0.19483, -0.346754, 0.281648, -0.656271, 0.588328, 0.864284, -0.661556, 0.344578, 0.534692, 0.187914, -0.172976, 0.100575, 0.287857, 0.151936, 0.679748, 0.137527, 0.726773, -0.503042, -0.902524, -0.895315, 0.870645, 0.792427, -0.102238, -0.748643, -0.048728, -0.025835, 0.358631, 0.804295, -0.300104, -0.99179, -0.699454, -0.943476, -0.448011, 0.628611, 0.060595, 0.716813, -0.33607, 0.549002, 0.810379, 0.074881, -0.689823, 0.17513, -0.975426, 0.961779, -0.030624, -0.914643, -0.735591, 0.031988, -0.554272, 0.253033, 0.73405, 0.426412, -0.361457, 0.787875, -0.266747, -0.166595, 0.922155, -0.04597, -0.465312, 0.157074, -0.201136, -0.004584, -0.158067, 0.244864, -0.495687, 0.416834, -0.583545, 0.654634, -0.318258, -0.709804, -0.393463, 0.589381, -0.900991, 0.266171, 0.955916, -0.6571, 0.990855, -0.078764, 0.609356, -0.526011, -0.902476, 0.040574, -0.045497, -0.110604, 0.035908, -0.91532, -0.170028, -0.02148, -0.994139, 0.020418, 0.989168, -0.802385, 0.353583, -0.981395, -0.959128, 0.785969, -0.325003, -0.541583, -0.929888, 0.40832, 0.565713, 0.449217, -0.21377, -0.491438, -0.352481, 0.469042, 0.272024, 0.101279, -0.70562, -0.296457, 0.210789, -0.049051, -0.002596, 0.630726, 0.023403, -0.216062, 0.510835, -0.446393, -0.075211, 0.4807, 0.581605, 0.705887, 0.741715, -0.448041, 0.88241, -0.866934, -0.341714, -0.245376]\nout shape: (1, 1, 1, 2)\nout: [-0.053909, 0.080977]\n"
]
],
[
[
"**[pooling.AveragePooling3D.4] input 4x4x4x2, pool_size=(3, 3, 3), strides=(3, 3, 3), padding='valid', data_format='channels_last'**",
"_____no_output_____"
]
],
[
[
"data_in_shape = (4, 4, 4, 2)\nL = AveragePooling3D(pool_size=(3, 3, 3), strides=(3, 3, 3), padding='valid', data_format='channels_last')\n\nlayer_0 = Input(shape=data_in_shape)\nlayer_1 = L(layer_0)\nmodel = Model(inputs=layer_0, outputs=layer_1)\n\n# set weights to random (use seed for reproducibility)\nnp.random.seed(284)\ndata_in = 2 * np.random.random(data_in_shape) - 1\nresult = model.predict(np.array([data_in]))\ndata_out_shape = result[0].shape\ndata_in_formatted = format_decimal(data_in.ravel().tolist())\ndata_out_formatted = format_decimal(result[0].ravel().tolist())\nprint('')\nprint('in shape:', data_in_shape)\nprint('in:', data_in_formatted)\nprint('out shape:', data_out_shape)\nprint('out:', data_out_formatted)\n\nDATA['pooling.AveragePooling3D.4'] = {\n 'input': {'data': data_in_formatted, 'shape': data_in_shape},\n 'expected': {'data': data_out_formatted, 'shape': data_out_shape}\n}",
"\nin shape: (4, 4, 4, 2)\nin: [0.691755, -0.79282, -0.953135, 0.756956, -0.736874, 0.171061, -0.801845, 0.588236, -0.884749, 0.06721, -0.585121, -0.546211, -0.605281, -0.998989, 0.309413, -0.260604, -0.123585, 0.168908, -0.179496, 0.657412, -0.973664, 0.146258, -0.851615, -0.320588, 0.375102, -0.048494, 0.822789, 0.063572, -0.956466, 0.083595, 0.121146, 0.789353, -0.815498, -0.056454, -0.472042, -0.423572, 0.460752, 0.784129, -0.964421, -0.02912, -0.194265, 0.17147, -0.336383, -0.785223, 0.978845, 0.88826, -0.498649, -0.958507, 0.055052, -0.991654, -0.027882, 0.079693, 0.901998, 0.036266, -0.73015, -0.472116, 0.651073, 0.821196, 0.562183, 0.42342, -0.236111, 0.661076, -0.983951, -0.116893, -0.179815, 0.375962, -0.018703, -0.242038, -0.561415, 0.322072, 0.468695, 0.768235, -0.354887, 0.528139, 0.796988, -0.976979, 0.279858, -0.790546, 0.485339, 0.693701, -0.130412, 0.211269, -0.346429, 0.06497, 0.932512, -0.675758, -0.636085, 0.065187, -0.720225, -0.060809, -0.783716, -0.1708, 0.256143, 0.365727, -0.458241, 0.515217, -0.269055, 0.378065, 0.066507, 0.207271, -0.303131, 0.632455, 0.147251, 0.35156, -0.852052, -0.382054, 0.42108, -0.350071, 0.092818, 0.516404, 0.448487, 0.503722, -0.86555, 0.747871, 0.320894, -0.163714, 0.107681, 0.562623, 0.757089, 0.85338, -0.875069, 0.324594, -0.093024, 0.016279, -0.507882, -0.549638, -0.913588, 0.078328]\nout shape: (1, 1, 1, 2)\nout: [-0.125255, -0.068526]\n"
]
],
[
[
"**[pooling.AveragePooling3D.5] input 4x4x4x2, pool_size=(2, 2, 2), strides=None, padding='same', data_format='channels_last'**",
"_____no_output_____"
]
],
[
[
"data_in_shape = (4, 4, 4, 2)\nL = AveragePooling3D(pool_size=(2, 2, 2), strides=None, padding='same', data_format='channels_last')\n\nlayer_0 = Input(shape=data_in_shape)\nlayer_1 = L(layer_0)\nmodel = Model(inputs=layer_0, outputs=layer_1)\n\n# set weights to random (use seed for reproducibility)\nnp.random.seed(285)\ndata_in = 2 * np.random.random(data_in_shape) - 1\nresult = model.predict(np.array([data_in]))\ndata_out_shape = result[0].shape\ndata_in_formatted = format_decimal(data_in.ravel().tolist())\ndata_out_formatted = format_decimal(result[0].ravel().tolist())\nprint('')\nprint('in shape:', data_in_shape)\nprint('in:', data_in_formatted)\nprint('out shape:', data_out_shape)\nprint('out:', data_out_formatted)\n\nDATA['pooling.AveragePooling3D.5'] = {\n 'input': {'data': data_in_formatted, 'shape': data_in_shape},\n 'expected': {'data': data_out_formatted, 'shape': data_out_shape}\n}",
"\nin shape: (4, 4, 4, 2)\nin: [-0.495196, -0.886872, 0.220815, 0.126844, 0.168234, -0.640849, 0.457897, -0.375014, 0.001134, -0.486501, -0.819617, -0.468351, 0.15859, 0.39238, -0.590545, -0.402922, 0.821619, -0.208255, -0.512219, -0.586151, -0.365648, -0.195611, -0.280978, -0.08818, -0.449229, 0.169082, 0.075074, -0.719751, 0.657827, -0.060862, -0.217533, 0.907503, 0.902317, 0.613945, 0.670047, -0.808346, 0.060215, -0.446612, -0.710328, -0.018744, 0.348018, -0.294409, 0.623986, -0.216504, 0.270099, -0.216285, -0.433193, -0.197968, -0.829926, -0.93864, -0.901724, -0.388869, -0.658339, -0.931401, -0.654674, -0.469503, 0.970661, 0.008063, -0.751014, 0.519043, 0.197895, 0.959095, 0.875405, 0.700615, 0.301314, -0.980157, 0.275373, -0.082646, 0.100727, -0.027273, -0.322366, 0.26563, 0.668139, 0.890289, 0.854229, -0.85773, -0.07833, -0.319645, -0.948873, 0.403526, 0.683097, 0.174958, 0.926944, -0.418256, -0.406667, -0.333808, 0.102223, -0.00576, 0.182281, 0.979655, 0.230246, 0.422968, -0.381217, 0.146697, 0.660828, 0.060741, -0.201812, 0.587619, 0.188211, -0.652713, -0.937225, -0.814998, 0.277993, -0.539363, -0.665425, 0.72739, 0.919326, 0.710163, -0.819091, -0.089805, -0.778517, -0.593048, 0.945303, -0.078936, 0.303422, 0.206755, -0.899923, -0.868598, 0.249905, -0.47891, 0.006871, -0.263386, -0.484493, -0.75917, 0.857292, 0.401094, -0.077826, -0.44546]\nout shape: (2, 2, 2, 2)\nout: [0.181438, -0.302524, -0.077379, -0.238252, -0.197095, -0.268185, -0.055756, 0.102707, 0.292419, 0.042777, -0.43821, -0.214372, 0.349209, 0.033074, 0.013077, -0.1905]\n"
]
],
[
[
"**[pooling.AveragePooling3D.6] input 4x4x4x2, pool_size=(2, 2, 2), strides=(1, 1, 1), padding='same', data_format='channels_last'**",
"_____no_output_____"
]
],
[
[
"data_in_shape = (4, 4, 4, 2)\nL = AveragePooling3D(pool_size=(2, 2, 2), strides=(1, 1, 1), padding='same', data_format='channels_last')\n\nlayer_0 = Input(shape=data_in_shape)\nlayer_1 = L(layer_0)\nmodel = Model(inputs=layer_0, outputs=layer_1)\n\n# set weights to random (use seed for reproducibility)\nnp.random.seed(286)\ndata_in = 2 * np.random.random(data_in_shape) - 1\nresult = model.predict(np.array([data_in]))\ndata_out_shape = result[0].shape\ndata_in_formatted = format_decimal(data_in.ravel().tolist())\ndata_out_formatted = format_decimal(result[0].ravel().tolist())\nprint('')\nprint('in shape:', data_in_shape)\nprint('in:', data_in_formatted)\nprint('out shape:', data_out_shape)\nprint('out:', data_out_formatted)\n\nDATA['pooling.AveragePooling3D.6'] = {\n 'input': {'data': data_in_formatted, 'shape': data_in_shape},\n 'expected': {'data': data_out_formatted, 'shape': data_out_shape}\n}",
"\nin shape: (4, 4, 4, 2)\nin: [-0.709952, -0.532913, -0.169956, -0.391538, 0.729034, -0.2004, -0.67324, -0.973672, 0.879975, -0.981827, -0.4828, -0.887985, 0.843364, 0.710745, -0.260613, 0.20082, 0.309563, 0.721671, -0.967848, -0.976471, -0.13058, 0.052684, 0.666494, -0.319759, -0.060338, 0.359151, -0.795562, 0.70488, 0.100816, 0.466479, 0.992415, 0.066527, -0.690663, -0.741365, -0.251801, -0.479328, 0.62187, 0.578729, 0.598481, 0.817115, -0.913801, -0.694569, 0.397726, -0.31274, 0.163147, 0.087004, -0.744957, -0.920201, 0.440377, -0.191648, -0.227724, -0.562736, -0.484598, -0.230876, 0.019055, 0.988723, 0.656988, 0.185623, -0.629304, -0.321252, 0.329452, 0.355461, 0.734458, 0.496983, 0.181439, 0.414232, 0.776873, 0.68191, -0.846744, -0.442164, -0.526272, 0.92696, -0.704629, -0.800248, 0.643923, 0.775996, -0.203863, -0.756864, -0.398058, -0.914275, 0.980404, 0.329099, -0.576086, 0.851052, -0.74133, -0.23673, -0.001628, 0.972916, -0.571033, 0.669151, -0.977945, -0.707472, 0.371069, -0.772292, -0.207482, -0.094619, -0.604913, 0.111706, -0.123427, 0.284132, 0.292284, -0.490954, -0.873365, 0.109881, -0.40172, 0.103223, 0.396366, -0.415444, 0.766823, -0.057373, 0.619422, 0.30151, -0.126582, 0.862041, -0.083425, -0.018503, 0.744106, -0.681409, 0.556506, -0.628066, -0.697587, -0.201239, 0.051677, -0.585768, 0.202332, -0.634928, -0.410351, 0.005911]\nout shape: (4, 4, 4, 2)\nout: [-0.242659, -0.627783, 0.231323, -0.111939, 0.159636, 0.037518, -0.270082, -0.218984, -0.070567, -0.485788, -0.111164, -0.265047, 0.008914, 0.071142, -0.080005, -0.012604, -0.159231, -0.010098, -0.350668, -0.063979, 0.278439, 0.234528, 0.603106, 0.308118, -0.207054, 0.232101, -0.248649, 0.301392, 0.539285, 0.346362, 0.863437, 0.281755, -0.070117, -0.144514, 0.162641, 0.016568, -0.167049, -0.077962, -0.267701, -0.0226, 0.005024, -0.075724, -0.128601, -0.048237, -0.299029, -0.126288, -0.281397, 0.031791, -0.11304, 0.031477, -0.367058, -0.203106, 0.002375, 0.184946, 0.136101, 0.591001, -0.380324, -0.043487, -0.226682, -0.361389, 0.306874, -0.003617, 0.263488, 0.201182, 0.020489, 0.144438, 0.212779, -0.052595, -0.146222, -0.16541, -0.294568, 0.106019, 0.016031, 0.210902, 0.118314, -0.067409, 0.167747, -0.250036, 0.194061, -0.066979, -0.250072, 0.149795, -0.1262, -0.348256, 0.064153, -0.258652, -0.015739, 0.064035, -0.548722, -0.206332, -0.088217, -0.675115, -0.011108, -0.373982, -0.308916, -0.044354, -0.183423, 0.020904, 0.333012, -0.16991, 0.201291, -0.034234, -0.126972, 0.205695, -0.05384, 0.132829, 0.455967, -0.293182, 0.671714, -0.266335, 0.587964, -0.163278, -0.213979, 0.014133, 0.228672, -0.480152, 0.273148, -0.484623, 0.073078, -0.311078, -0.322955, -0.393504, 0.127005, -0.610348, -0.10401, -0.314508, -0.410351, 0.005911]\n"
]
],
[
[
"**[pooling.AveragePooling3D.7] input 4x5x4x2, pool_size=(2, 2, 2), strides=(1, 2, 1), padding='same', data_format='channels_last'**",
"_____no_output_____"
]
],
[
[
"data_in_shape = (4, 5, 4, 2)\nL = AveragePooling3D(pool_size=(2, 2, 2), strides=(1, 2, 1), padding='same', data_format='channels_last')\n\nlayer_0 = Input(shape=data_in_shape)\nlayer_1 = L(layer_0)\nmodel = Model(inputs=layer_0, outputs=layer_1)\n\n# set weights to random (use seed for reproducibility)\nnp.random.seed(287)\ndata_in = 2 * np.random.random(data_in_shape) - 1\nresult = model.predict(np.array([data_in]))\ndata_out_shape = result[0].shape\ndata_in_formatted = format_decimal(data_in.ravel().tolist())\ndata_out_formatted = format_decimal(result[0].ravel().tolist())\nprint('')\nprint('in shape:', data_in_shape)\nprint('in:', data_in_formatted)\nprint('out shape:', data_out_shape)\nprint('out:', data_out_formatted)\n\nDATA['pooling.AveragePooling3D.7'] = {\n 'input': {'data': data_in_formatted, 'shape': data_in_shape},\n 'expected': {'data': data_out_formatted, 'shape': data_out_shape}\n}",
"\nin shape: (4, 5, 4, 2)\nin: [-0.71103, 0.421506, 0.752321, 0.542455, -0.557162, -0.963774, 0.910303, -0.933284, 0.67521, 0.588709, -0.782848, -0.108964, -0.767069, 0.338318, -0.660374, -0.967294, -0.501079, -0.917532, -0.087991, -0.160473, 0.520493, 0.612007, -0.955448, -0.809749, -0.627003, 0.494441, 0.985405, 0.99813, -0.278165, 0.090068, 0.803872, 0.287682, 0.162199, 0.1796, -0.630223, 0.044743, 0.9092, 0.023879, -0.403203, -0.005329, -0.29237, -0.510033, -0.190427, 0.149011, 0.873547, -0.58793, -0.302525, 0.102122, -0.804112, 0.965834, 0.302039, -0.806929, 0.627682, 0.876256, 0.176245, 0.051969, 0.005712, -0.877694, -0.776877, -0.360984, 0.172577, 0.953108, 0.755911, 0.973515, 0.745292, 0.765506, 0.119956, 0.378346, 0.425789, 0.048668, 0.363691, -0.499862, 0.315721, 0.243267, 0.333434, -0.001645, -0.007235, -0.463152, -0.002048, 0.862117, -0.575785, 0.594789, 0.068012, 0.165267, 0.081581, 0.128645, 0.559305, -0.494595, 0.10207, 0.278472, -0.815856, 0.817863, 0.101417, -0.432774, -0.36832, 0.682055, -0.852236, 0.756063, 0.741739, 0.403911, 0.363444, -0.853088, 0.429379, 0.95063, 0.19365, 0.707334, 0.883575, 0.037535, 0.735855, -0.597979, -0.328964, -0.63363, 0.533345, 0.628204, -0.831273, -0.475492, -0.120719, -0.049689, 0.474126, -0.534385, 0.898272, -0.060213, 0.134975, -0.81603, -0.09329, -0.56951, 0.744421, 0.561587, -0.094, 0.780616, -0.206093, 0.992174, 0.563806, 0.562612, -0.754387, -0.36159, -0.288989, 0.195871, -0.156575, 0.108674, 0.037465, 0.115865, -0.313897, -0.290763, -0.920174, -0.943574, 0.610507, -0.749795, -0.802955, 0.296183, -0.862404, 0.174227, 0.6721, 0.674769, -0.198663, 0.163696, -0.572753, 0.149323, 0.456671, 0.162098]\nout shape: (4, 3, 4, 2)\nout: [-0.131402, 0.155199, 0.03226, -0.070195, 0.037581, -0.260452, 0.030912, -0.436622, -0.017073, 0.039968, 0.135148, 0.319859, 0.22609, 0.20693, 0.242007, -0.012103, 0.045283, 0.116491, 0.151294, -0.099044, 0.124178, 0.104379, -0.202626, 0.428394, -0.275804, 0.206784, 0.130999, 0.038676, 0.218617, 0.040718, 0.016176, 0.085388, 0.132601, 0.226252, 0.333257, 0.00119, 0.36471, 0.04267, 0.305004, 0.197663, 0.087807, 0.098584, -0.156448, -0.247495, 0.086031, -0.046277, 0.236039, 0.163866, -0.061051, 0.344117, -0.020681, 0.106031, 0.104317, 0.009554, 0.045255, 0.096864, 0.026437, 0.064502, 0.301632, -0.154837, -0.09276, -0.104819, -0.268972, 0.050116, 0.043877, 0.247794, -0.430852, -0.053041, 0.059331, -0.068163, 0.465398, -0.186144, 0.183288, 0.224137, 0.099849, 0.042311, 0.115137, 0.048274, -0.004983, 0.099998, -0.188808, -0.347206, -0.07789, -0.057268, -0.485448, 0.073878, -0.588151, -0.058268, 0.236719, 0.419233, -0.385708, 0.156509, -0.058041, 0.15571, 0.456671, 0.162098]\n"
]
],
[
[
"**[pooling.AveragePooling3D.8] input 4x4x4x2, pool_size=(3, 3, 3), strides=None, padding='same', data_format='channels_last'**",
"_____no_output_____"
]
],
[
[
"data_in_shape = (4, 4, 4, 2)\nL = AveragePooling3D(pool_size=(3, 3, 3), strides=None, padding='same', data_format='channels_last')\n\nlayer_0 = Input(shape=data_in_shape)\nlayer_1 = L(layer_0)\nmodel = Model(inputs=layer_0, outputs=layer_1)\n\n# set weights to random (use seed for reproducibility)\nnp.random.seed(288)\ndata_in = 2 * np.random.random(data_in_shape) - 1\nresult = model.predict(np.array([data_in]))\ndata_out_shape = result[0].shape\ndata_in_formatted = format_decimal(data_in.ravel().tolist())\ndata_out_formatted = format_decimal(result[0].ravel().tolist())\nprint('')\nprint('in shape:', data_in_shape)\nprint('in:', data_in_formatted)\nprint('out shape:', data_out_shape)\nprint('out:', data_out_formatted)\n\nDATA['pooling.AveragePooling3D.8'] = {\n 'input': {'data': data_in_formatted, 'shape': data_in_shape},\n 'expected': {'data': data_out_formatted, 'shape': data_out_shape}\n}",
"\nin shape: (4, 4, 4, 2)\nin: [0.106539, 0.430065, 0.625063, -0.956042, 0.681684, 0.345995, -0.589061, 0.186737, 0.535452, -0.125905, -0.396262, -0.44893, 0.39021, 0.253402, -0.238515, 0.337141, 0.178107, 0.244331, -0.93179, -0.081267, 0.895223, 0.820023, 0.365435, -0.738456, 0.893031, -0.787916, -0.518813, 0.661518, -0.464144, -0.639165, -0.252917, 0.784083, 0.577398, 0.769552, 0.036096, 0.847521, -0.171916, 0.07536, -0.830068, 0.734205, -0.437818, 0.295701, 0.252657, -0.859452, -0.425833, -0.650296, -0.584695, 0.163986, 0.43905, -0.521755, 0.620616, 0.066707, -0.101702, 0.941175, 0.479202, 0.624312, -0.372154, 0.625845, 0.980521, -0.834695, -0.40269, 0.784157, 0.814068, -0.485038, -0.150738, 0.682911, 0.406096, -0.405868, -0.337905, 0.803583, -0.764964, 0.96897, -0.057235, 0.403604, -0.605392, 0.389273, 0.235543, -0.095585, -0.860692, 0.937457, -0.928888, 0.702073, -0.18066, 0.033968, -0.082046, -0.237205, 0.922919, 0.064731, -0.026908, -0.865491, 0.881128, 0.265603, -0.132321, -0.701801, 0.490064, 0.718745, -0.884446, 0.538162, -0.086979, -0.734317, 0.089006, 0.349945, -0.415428, -0.621358, 0.892372, 0.090398, 0.883604, 0.772612, 0.589633, 0.187399, 0.807184, -0.128627, -0.534439, 0.258966, 0.141399, -0.777263, 0.911318, -0.359087, 0.789361, 0.470019, 0.836149, 0.415029, 0.920315, -0.916153, 0.645573, -0.446523, -0.899169, 0.78844]\nout shape: (2, 2, 2, 2)\nout: [0.162391, -0.005936, -0.221024, 0.180816, 0.161071, -0.078404, 0.166559, 0.261386, 0.04966, 0.217097, -0.082203, 0.300223, 0.138512, -0.110408, 0.330712, 0.037165]\n"
]
],
[
[
"**[pooling.AveragePooling3D.9] input 4x4x4x2, pool_size=(3, 3, 3), strides=(3, 3, 3), padding='same', data_format='channels_last'**",
"_____no_output_____"
]
],
[
[
"data_in_shape = (4, 4, 4, 2)\nL = AveragePooling3D(pool_size=(3, 3, 3), strides=(3, 3, 3), padding='same', data_format='channels_last')\n\nlayer_0 = Input(shape=data_in_shape)\nlayer_1 = L(layer_0)\nmodel = Model(inputs=layer_0, outputs=layer_1)\n\n# set weights to random (use seed for reproducibility)\nnp.random.seed(289)\ndata_in = 2 * np.random.random(data_in_shape) - 1\nresult = model.predict(np.array([data_in]))\ndata_out_shape = result[0].shape\ndata_in_formatted = format_decimal(data_in.ravel().tolist())\ndata_out_formatted = format_decimal(result[0].ravel().tolist())\nprint('')\nprint('in shape:', data_in_shape)\nprint('in:', data_in_formatted)\nprint('out shape:', data_out_shape)\nprint('out:', data_out_formatted)\n\nDATA['pooling.AveragePooling3D.9'] = {\n 'input': {'data': data_in_formatted, 'shape': data_in_shape},\n 'expected': {'data': data_out_formatted, 'shape': data_out_shape}\n}",
"\nin shape: (4, 4, 4, 2)\nin: [0.454263, 0.047178, -0.644362, 0.432654, 0.776147, -0.088086, -0.16527, -0.152361, -0.723283, 0.119471, -0.020663, 0.230897, 0.249349, -0.825224, 0.809245, 0.37136, 0.649976, 0.690981, -0.5766, 0.750394, -0.777363, -0.359006, 0.398419, -0.851015, -0.479232, -0.924962, -0.898893, 0.135445, 0.819369, -0.867218, 0.039715, 0.304805, -0.865872, -0.891635, 0.730554, 0.178083, 0.981329, 0.047786, -0.466968, -0.89441, -0.037018, -0.880158, 0.635061, 0.108217, 0.405675, 0.242025, 0.524396, -0.46013, -0.98454, 0.227442, -0.159924, -0.396205, -0.843265, 0.181395, -0.743803, 0.445469, 0.05215, 0.837067, -0.756402, -0.959109, -0.580594, -0.677936, -0.929683, -0.165592, -0.870784, 0.91887, 0.542361, 0.46359, -0.521332, 0.778263, 0.662447, 0.692057, 0.224535, -0.087731, 0.904644, 0.207457, -0.564079, -0.389642, 0.590403, -0.861828, -0.280471, -0.593786, -0.542645, 0.788946, -0.808773, -0.334536, -0.973711, 0.68675, 0.383992, -0.38838, 0.278601, -0.89188, -0.582918, -0.190511, -0.493528, 0.635115, -0.375152, 0.586508, -0.986557, -0.449484, 0.216757, 0.746825, -0.144795, 0.448144, -0.828083, 0.224525, -0.958965, -0.566069, -0.850394, -0.261458, -0.589888, 0.75667, 0.531888, 0.146437, -0.877887, -0.575355, 0.06156, -0.714865, 0.710365, -0.439259, -0.084566, -0.854224, 0.467254, 0.59934, 0.527409, 0.791222, -0.66992, 0.644258]\nout shape: (2, 2, 2, 2)\nout: [-0.058915, -0.081912, 0.389238, -0.21988, -0.394183, 0.045132, -0.327151, -0.248637, -0.2935, 0.162208, -0.15011, 0.238629, -0.015479, -0.221113, -0.27869, 0.134772]\n"
]
],
[
[
"**[pooling.AveragePooling3D.10] input 2x3x3x4, pool_size=(3, 3, 3), strides=(2, 2, 2), padding='valid', data_format='channels_first'**",
"_____no_output_____"
]
],
[
[
"data_in_shape = (2, 3, 3, 4)\nL = AveragePooling3D(pool_size=(3, 3, 3), strides=(2, 2, 2), padding='valid', data_format='channels_first')\n\nlayer_0 = Input(shape=data_in_shape)\nlayer_1 = L(layer_0)\nmodel = Model(inputs=layer_0, outputs=layer_1)\n\n# set weights to random (use seed for reproducibility)\nnp.random.seed(290)\ndata_in = 2 * np.random.random(data_in_shape) - 1\nresult = model.predict(np.array([data_in]))\ndata_out_shape = result[0].shape\ndata_in_formatted = format_decimal(data_in.ravel().tolist())\ndata_out_formatted = format_decimal(result[0].ravel().tolist())\nprint('')\nprint('in shape:', data_in_shape)\nprint('in:', data_in_formatted)\nprint('out shape:', data_out_shape)\nprint('out:', data_out_formatted)\n\nDATA['pooling.AveragePooling3D.10'] = {\n 'input': {'data': data_in_formatted, 'shape': data_in_shape},\n 'expected': {'data': data_out_formatted, 'shape': data_out_shape}\n}",
"\nin shape: (2, 3, 3, 4)\nin: [-0.453175, -0.475078, 0.486234, -0.949643, -0.349099, -0.837108, 0.933439, 0.167853, -0.995191, 0.459466, -0.788337, -0.120985, 0.06215, 0.138625, -0.102201, 0.976605, -0.591051, -0.592066, 0.469334, -0.435067, 0.621416, 0.817698, 0.790015, 0.485862, 0.469679, -0.611443, 0.582845, -0.503885, -0.379174, -0.451035, 0.052289, 0.131836, -0.609312, -0.828722, -0.422428, -0.648754, -0.339801, -0.758017, 0.754244, -0.544823, 0.691656, 0.076848, -0.32539, 0.306448, -0.662415, 0.334329, 0.030666, -0.414111, -0.757096, -0.20427, -0.893088, -0.681919, -0.619269, -0.640749, 0.867436, 0.971453, -0.42039, -0.574905, -0.34642, 0.588678, -0.247265, 0.436084, 0.220126, 0.114202, 0.613623, 0.401452, -0.270262, -0.591146, -0.872383, 0.818368, 0.336808, 0.338197]\nout shape: (2, 1, 1, 1)\nout: [-0.096379, -0.08704]\n"
]
],
[
[
"**[pooling.AveragePooling3D.11] input 2x3x3x4, pool_size=(3, 3, 3), strides=(1, 1, 1), padding='same', data_format='channels_first'**",
"_____no_output_____"
]
],
[
[
"data_in_shape = (2, 3, 3, 4)\nL = AveragePooling3D(pool_size=(3, 3, 3), strides=(1, 1, 1), padding='same', data_format='channels_first')\n\nlayer_0 = Input(shape=data_in_shape)\nlayer_1 = L(layer_0)\nmodel = Model(inputs=layer_0, outputs=layer_1)\n\n# set weights to random (use seed for reproducibility)\nnp.random.seed(291)\ndata_in = 2 * np.random.random(data_in_shape) - 1\nresult = model.predict(np.array([data_in]))\ndata_out_shape = result[0].shape\ndata_in_formatted = format_decimal(data_in.ravel().tolist())\ndata_out_formatted = format_decimal(result[0].ravel().tolist())\nprint('')\nprint('in shape:', data_in_shape)\nprint('in:', data_in_formatted)\nprint('out shape:', data_out_shape)\nprint('out:', data_out_formatted)\n\nDATA['pooling.AveragePooling3D.11'] = {\n 'input': {'data': data_in_formatted, 'shape': data_in_shape},\n 'expected': {'data': data_out_formatted, 'shape': data_out_shape}\n}",
"\nin shape: (2, 3, 3, 4)\nin: [-0.803361, 0.348731, 0.30124, -0.168638, 0.516406, -0.258765, 0.297839, 0.993235, 0.958465, -0.273175, -0.704992, 0.261477, -0.301255, 0.263104, 0.678631, -0.644936, -0.029034, -0.320266, 0.307733, -0.479016, 0.608177, 0.034951, 0.456908, -0.929353, 0.594982, -0.243058, -0.524918, 0.455339, 0.034216, 0.356824, 0.63906, -0.259773, -0.084724, 0.248472, -0.608134, 0.0077, 0.400591, -0.960703, -0.247926, -0.774509, 0.496174, -0.319044, -0.324046, -0.616632, -0.322142, -0.472846, 0.171825, -0.030013, 0.992861, -0.645264, 0.524886, 0.673229, 0.883122, 0.25346, -0.706988, -0.654436, 0.918349, -0.139113, 0.742737, 0.338472, -0.812719, 0.860081, 0.003489, 0.667897, 0.362284, -0.283972, 0.995162, 0.67962, -0.700244, -0.137142, 0.045695, -0.450433]\nout shape: (2, 3, 3, 4)\nout: [-0.073055, 0.083417, 0.109908, 0.160761, 0.061998, 0.11563, 0.00915, 0.030844, 0.154595, 0.132854, -0.051119, 0.025479, 0.01321, 0.103228, 0.096798, 0.132983, 0.091705, 0.092372, 0.008749, 0.004411, 0.149296, 0.121109, -0.012738, -0.001443, 0.044439, 0.121335, 0.01906, 0.021515, 0.096866, 0.117315, -0.031152, -0.075063, 0.106077, 0.137015, -0.045408, -0.108109, 0.13765, 0.028927, -0.316498, -0.265803, 0.090454, 0.069218, -0.177051, -0.075283, 0.162245, 0.098457, -0.146385, -0.134885, 0.102239, 0.081747, -0.04865, 0.018312, 0.020763, 0.058465, -0.029871, 0.057668, 0.044907, 0.081293, -0.050427, 0.015913, 0.201232, 0.2022, 0.197264, 0.272857, 0.129309, 0.175371, 0.153743, 0.238277, 0.144593, 0.186112, 0.056922, 0.123728]\n"
]
],
[
[
"**[pooling.AveragePooling3D.12] input 3x4x4x3, pool_size=(2, 2, 2), strides=None, padding='valid', data_format='channels_first'**",
"_____no_output_____"
]
],
[
[
"data_in_shape = (3, 4, 4, 3)\nL = AveragePooling3D(pool_size=(2, 2, 2), strides=None, padding='valid', data_format='channels_first')\n\nlayer_0 = Input(shape=data_in_shape)\nlayer_1 = L(layer_0)\nmodel = Model(inputs=layer_0, outputs=layer_1)\n\n# set weights to random (use seed for reproducibility)\nnp.random.seed(292)\ndata_in = 2 * np.random.random(data_in_shape) - 1\nresult = model.predict(np.array([data_in]))\ndata_out_shape = result[0].shape\ndata_in_formatted = format_decimal(data_in.ravel().tolist())\ndata_out_formatted = format_decimal(result[0].ravel().tolist())\nprint('')\nprint('in shape:', data_in_shape)\nprint('in:', data_in_formatted)\nprint('out shape:', data_out_shape)\nprint('out:', data_out_formatted)\n\nDATA['pooling.AveragePooling3D.12'] = {\n 'input': {'data': data_in_formatted, 'shape': data_in_shape},\n 'expected': {'data': data_out_formatted, 'shape': data_out_shape}\n}",
"\nin shape: (3, 4, 4, 3)\nin: [-0.497409, -0.250345, 0.196124, -0.044334, -0.324906, 0.560065, 0.220435, -0.167776, -0.923771, 0.77337, -0.862909, -0.584756, -0.70451, 0.870272, 0.841773, -0.312016, 0.599915, 0.073955, 0.944336, -0.4175, 0.865698, 0.609184, 0.033839, -0.72494, -0.239473, 0.514968, -0.318523, -0.244443, 0.275468, -0.85993, -0.262732, 0.026767, -0.937574, 0.872647, 0.540013, 0.055422, 0.322167, 0.972206, 0.92596, -0.82368, -0.63508, 0.671616, -0.678809, 0.202761, -0.260164, -0.241878, 0.188534, -0.47291, -0.077436, -0.016304, 0.548747, -0.236224, -0.780147, -0.013071, -0.67362, -0.807763, -0.351361, 0.533701, 0.274553, -0.933379, -0.49029, 0.928012, -0.719924, 0.453519, 0.173223, 0.030778, 0.12229, 0.547074, -0.860491, 0.206434, 0.248515, -0.189106, -0.393127, -0.152128, -0.822508, -0.361768, -0.702917, 0.998304, -0.011396, -0.644766, -0.150506, -0.153633, -0.772981, -0.470261, -0.056372, 0.082635, 0.017418, 0.26302, 0.730468, 0.268813, -0.163174, 0.332229, -0.698119, -0.397122, -0.426552, -0.931893, -0.6652, -0.986456, -0.062208, -0.90263, -0.12278, -0.277462, 0.072233, 0.466157, -0.917268, 0.053668, -0.45609, 0.072386, 0.376642, 0.133363, -0.799663, -0.984724, 0.337956, -0.088779, -0.04311, 0.520989, -0.611655, -0.456082, -0.662569, 0.09705, 0.256941, -0.987104, -0.939188, -0.296892, 0.123336, 0.710366, -0.675095, -0.037022, -0.616184, -0.925359, -0.734003, -0.629605, 0.071318, 0.6548, -0.019787, -0.435663, -0.412123, 0.967997, -0.80066, -0.337085, 0.471011, -0.945095, -0.74401, 0.924175]\nout shape: (3, 2, 2, 1)\nout: [-0.082917, 0.141622, 0.017767, 0.080913, -0.005706, 0.056398, -0.073774, -0.279674, -0.351729, -0.0631, -0.128173, -0.649791]\n"
]
],
[
[
"### export for Keras.js tests",
"_____no_output_____"
]
],
[
[
"print(json.dumps(DATA))",
"{\"pooling.AveragePooling3D.0\": {\"input\": {\"data\": [-0.453175, -0.475078, 0.486234, -0.949643, -0.349099, -0.837108, 0.933439, 0.167853, -0.995191, 0.459466, -0.788337, -0.120985, 0.06215, 0.138625, -0.102201, 0.976605, -0.591051, -0.592066, 0.469334, -0.435067, 0.621416, 0.817698, 0.790015, 0.485862, 0.469679, -0.611443, 0.582845, -0.503885, -0.379174, -0.451035, 0.052289, 0.131836, -0.609312, -0.828722, -0.422428, -0.648754, -0.339801, -0.758017, 0.754244, -0.544823, 0.691656, 0.076848, -0.32539, 0.306448, -0.662415, 0.334329, 0.030666, -0.414111, -0.757096, -0.20427, -0.893088, -0.681919, -0.619269, -0.640749, 0.867436, 0.971453, -0.42039, -0.574905, -0.34642, 0.588678, -0.247265, 0.436084, 0.220126, 0.114202, 0.613623, 0.401452, -0.270262, -0.591146, -0.872383, 0.818368, 0.336808, 0.338197, -0.275646, 0.375308, -0.928722, -0.836727, -0.504007, -0.503397, -0.636099, 0.948482, -0.639661, -0.026878, -0.122643, -0.634018, -0.247016, 0.517246, -0.398639, 0.752174, -0.014633, -0.170534, -0.463453, -0.289716, 0.837207, 0.769962, -0.401357, 0.076406, 0.270433, -0.036538, -0.05766, 0.625256, 0.626847, -0.27321, -0.217219, -0.775553, -0.182939, 0.327385, -0.976376, -0.337729, -0.178467, -0.1545, -0.334259, -0.537949, 0.499229, 0.775269, -0.657598, 0.921864, 0.376821, 0.420375, -0.937835, -0.176425, -0.516753, 0.737286, 0.867807, -0.515893, 0.710035, 0.680377, -0.350561, -0.28645], \"shape\": [4, 4, 4, 2]}, \"expected\": {\"data\": [-0.301993, -0.272552, 0.040873, -0.117081, -0.185773, -0.37686, 0.163197, 0.233169, -0.225944, -0.009092, -0.222347, -0.017445, -0.130963, 0.099673, -0.051418, 0.344208], \"shape\": [2, 2, 2, 2]}}, \"pooling.AveragePooling3D.1\": {\"input\": {\"data\": [-0.803361, 0.348731, 0.30124, -0.168638, 0.516406, -0.258765, 0.297839, 0.993235, 0.958465, -0.273175, -0.704992, 0.261477, -0.301255, 0.263104, 0.678631, -0.644936, -0.029034, -0.320266, 0.307733, -0.479016, 0.608177, 0.034951, 0.456908, -0.929353, 0.594982, -0.243058, -0.524918, 0.455339, 0.034216, 0.356824, 0.63906, -0.259773, -0.084724, 0.248472, -0.608134, 0.0077, 0.400591, -0.960703, -0.247926, -0.774509, 0.496174, -0.319044, -0.324046, -0.616632, -0.322142, -0.472846, 0.171825, -0.030013, 0.992861, -0.645264, 0.524886, 0.673229, 0.883122, 0.25346, -0.706988, -0.654436, 0.918349, -0.139113, 0.742737, 0.338472, -0.812719, 0.860081, 0.003489, 0.667897, 0.362284, -0.283972, 0.995162, 0.67962, -0.700244, -0.137142, 0.045695, -0.450433, 0.929977, 0.157542, -0.720517, -0.939063, 0.295004, 0.308728, -0.094057, -0.374756, -0.400976, -0.539654, 0.27965, 0.977688, -0.361264, -0.027757, -0.67149, 0.57064, -0.861888, 0.616985, -0.027436, 0.40181, -0.30391, 0.92268, -0.486416, -0.335828, 0.138558, -0.445691, 0.156253, -0.967633, 0.127471, -0.783301, -0.691353, -0.76759, 0.618771, -0.377474, 0.50152, 0.126867, -0.872708, 0.649418, 0.697987, -0.33456, 0.906767, 0.604333, -0.129366, 0.579445, -0.033479, -0.22433, -0.979529, -0.251153, 0.839734, 0.550506, -0.599678, 0.584326, 0.211245, -0.66845, 0.948298, -0.004521], \"shape\": [4, 4, 4, 2]}, \"expected\": {\"data\": [-0.096172, -0.063889, -0.130291, -0.243163, 0.149246, -0.235679, 0.277756, -0.214836, 0.083935, -0.010284, 0.183535, -0.272509, 0.440949, -0.04496, 0.220404, 0.311668, 0.138158, 0.041206, 0.130772, -0.133172, -0.123041, -0.266292, -0.056407, -0.361459, 0.222251, -0.1564, 0.031836, 0.019601, -0.100749, -0.053372, 0.271023, 0.210519, 0.115633, 0.549958, -0.307022, 0.282092, 0.372751, -0.256226, -0.027257, -0.132813, -0.149026, -0.236204, 0.248228, 0.07371, -0.130145, 0.181375, -0.252442, 0.039529, 0.000851, 0.47193, -0.12053, 0.318177, -0.209568, -0.00234], \"shape\": [3, 3, 3, 2]}}, \"pooling.AveragePooling3D.2\": {\"input\": {\"data\": [-0.263147, -0.216555, -0.75766, -0.396007, 0.85243, 0.98415, -0.230197, -0.979579, 0.117628, -0.66833, 0.714058, -0.907302, -0.574249, 0.299573, 0.101165, 0.655872, -0.104788, 0.242064, -0.409262, -0.124059, 0.105687, -0.969325, -0.167941, 0.382377, 0.710487, 0.793042, 0.180663, -0.80231, 0.684253, -0.516992, 0.471203, -0.152325, 0.509501, 0.613742, -0.877379, 0.755416, 0.427677, 0.931956, 0.827636, -0.860685, 0.562326, -0.716081, 0.028046, 0.594422, -0.862333, 0.336131, 0.713855, 0.386247, -0.986659, 0.242413, 0.753777, -0.159358, 0.166548, -0.437388, 0.291152, -0.775555, 0.796086, -0.592021, -0.251661, 0.187174, 0.899283, 0.431861, -0.685273, -0.085991, -0.629026, -0.478334, 0.714983, 0.53745, -0.310438, 0.973848, -0.675219, 0.422743, -0.992263, 0.374017, -0.687462, -0.190455, -0.560081, 0.22484, -0.079631, 0.815275, 0.338641, -0.538279, -0.10891, -0.929005, 0.514762, 0.322038, 0.702195, -0.697122, 0.925468, -0.274158, 0.148379, 0.333239, 0.63072, -0.652956, -0.356451, -0.71114, 0.111465, 0.31787, 0.242578, 0.8926, -0.60807, 0.218759, 0.42079, 0.71253, -0.082496, 0.272704, 0.277213, -0.099807, -0.899322, -0.175367, -0.642213, -0.661032, 0.730145, 0.37799, 0.935939, -0.448007, -0.320652, -0.352629, 0.258139, 0.30254], \"shape\": [4, 5, 2, 3]}, \"expected\": {\"data\": [-0.113218, 0.104366, 0.101661, -0.110717, 0.341478, -0.101372, -0.259851, 0.202503, 0.083949, -0.364662, 0.07088, 0.181423, 0.375201, -0.081043, -0.083798, 0.275459, 0.046964, -0.00891, -0.333436, 0.258103, -0.187439, -0.222164, 0.289848, -0.055583], \"shape\": [2, 4, 1, 3]}}, \"pooling.AveragePooling3D.3\": {\"input\": {\"data\": [0.19483, -0.346754, 0.281648, -0.656271, 0.588328, 0.864284, -0.661556, 0.344578, 0.534692, 0.187914, -0.172976, 0.100575, 0.287857, 0.151936, 0.679748, 0.137527, 0.726773, -0.503042, -0.902524, -0.895315, 0.870645, 0.792427, -0.102238, -0.748643, -0.048728, -0.025835, 0.358631, 0.804295, -0.300104, -0.99179, -0.699454, -0.943476, -0.448011, 0.628611, 0.060595, 0.716813, -0.33607, 0.549002, 0.810379, 0.074881, -0.689823, 0.17513, -0.975426, 0.961779, -0.030624, -0.914643, -0.735591, 0.031988, -0.554272, 0.253033, 0.73405, 0.426412, -0.361457, 0.787875, -0.266747, -0.166595, 0.922155, -0.04597, -0.465312, 0.157074, -0.201136, -0.004584, -0.158067, 0.244864, -0.495687, 0.416834, -0.583545, 0.654634, -0.318258, -0.709804, -0.393463, 0.589381, -0.900991, 0.266171, 0.955916, -0.6571, 0.990855, -0.078764, 0.609356, -0.526011, -0.902476, 0.040574, -0.045497, -0.110604, 0.035908, -0.91532, -0.170028, -0.02148, -0.994139, 0.020418, 0.989168, -0.802385, 0.353583, -0.981395, -0.959128, 0.785969, -0.325003, -0.541583, -0.929888, 0.40832, 0.565713, 0.449217, -0.21377, -0.491438, -0.352481, 0.469042, 0.272024, 0.101279, -0.70562, -0.296457, 0.210789, -0.049051, -0.002596, 0.630726, 0.023403, -0.216062, 0.510835, -0.446393, -0.075211, 0.4807, 0.581605, 0.705887, 0.741715, -0.448041, 0.88241, -0.866934, -0.341714, -0.245376], \"shape\": [4, 4, 4, 2]}, \"expected\": {\"data\": [-0.053909, 0.080977], \"shape\": [1, 1, 1, 2]}}, \"pooling.AveragePooling3D.4\": {\"input\": {\"data\": [0.691755, -0.79282, -0.953135, 0.756956, -0.736874, 0.171061, -0.801845, 0.588236, -0.884749, 0.06721, -0.585121, -0.546211, -0.605281, -0.998989, 0.309413, -0.260604, -0.123585, 0.168908, -0.179496, 0.657412, -0.973664, 0.146258, -0.851615, -0.320588, 0.375102, -0.048494, 0.822789, 0.063572, -0.956466, 0.083595, 0.121146, 0.789353, -0.815498, -0.056454, -0.472042, -0.423572, 0.460752, 0.784129, -0.964421, -0.02912, -0.194265, 0.17147, -0.336383, -0.785223, 0.978845, 0.88826, -0.498649, -0.958507, 0.055052, -0.991654, -0.027882, 0.079693, 0.901998, 0.036266, -0.73015, -0.472116, 0.651073, 0.821196, 0.562183, 0.42342, -0.236111, 0.661076, -0.983951, -0.116893, -0.179815, 0.375962, -0.018703, -0.242038, -0.561415, 0.322072, 0.468695, 0.768235, -0.354887, 0.528139, 0.796988, -0.976979, 0.279858, -0.790546, 0.485339, 0.693701, -0.130412, 0.211269, -0.346429, 0.06497, 0.932512, -0.675758, -0.636085, 0.065187, -0.720225, -0.060809, -0.783716, -0.1708, 0.256143, 0.365727, -0.458241, 0.515217, -0.269055, 0.378065, 0.066507, 0.207271, -0.303131, 0.632455, 0.147251, 0.35156, -0.852052, -0.382054, 0.42108, -0.350071, 0.092818, 0.516404, 0.448487, 0.503722, -0.86555, 0.747871, 0.320894, -0.163714, 0.107681, 0.562623, 0.757089, 0.85338, -0.875069, 0.324594, -0.093024, 0.016279, -0.507882, -0.549638, -0.913588, 0.078328], \"shape\": [4, 4, 4, 2]}, \"expected\": {\"data\": [-0.125255, -0.068526], \"shape\": [1, 1, 1, 2]}}, \"pooling.AveragePooling3D.5\": {\"input\": {\"data\": [-0.495196, -0.886872, 0.220815, 0.126844, 0.168234, -0.640849, 0.457897, -0.375014, 0.001134, -0.486501, -0.819617, -0.468351, 0.15859, 0.39238, -0.590545, -0.402922, 0.821619, -0.208255, -0.512219, -0.586151, -0.365648, -0.195611, -0.280978, -0.08818, -0.449229, 0.169082, 0.075074, -0.719751, 0.657827, -0.060862, -0.217533, 0.907503, 0.902317, 0.613945, 0.670047, -0.808346, 0.060215, -0.446612, -0.710328, -0.018744, 0.348018, -0.294409, 0.623986, -0.216504, 0.270099, -0.216285, -0.433193, -0.197968, -0.829926, -0.93864, -0.901724, -0.388869, -0.658339, -0.931401, -0.654674, -0.469503, 0.970661, 0.008063, -0.751014, 0.519043, 0.197895, 0.959095, 0.875405, 0.700615, 0.301314, -0.980157, 0.275373, -0.082646, 0.100727, -0.027273, -0.322366, 0.26563, 0.668139, 0.890289, 0.854229, -0.85773, -0.07833, -0.319645, -0.948873, 0.403526, 0.683097, 0.174958, 0.926944, -0.418256, -0.406667, -0.333808, 0.102223, -0.00576, 0.182281, 0.979655, 0.230246, 0.422968, -0.381217, 0.146697, 0.660828, 0.060741, -0.201812, 0.587619, 0.188211, -0.652713, -0.937225, -0.814998, 0.277993, -0.539363, -0.665425, 0.72739, 0.919326, 0.710163, -0.819091, -0.089805, -0.778517, -0.593048, 0.945303, -0.078936, 0.303422, 0.206755, -0.899923, -0.868598, 0.249905, -0.47891, 0.006871, -0.263386, -0.484493, -0.75917, 0.857292, 0.401094, -0.077826, -0.44546], \"shape\": [4, 4, 4, 2]}, \"expected\": {\"data\": [0.181438, -0.302524, -0.077379, -0.238252, -0.197095, -0.268185, -0.055756, 0.102707, 0.292419, 0.042777, -0.43821, -0.214372, 0.349209, 0.033074, 0.013077, -0.1905], \"shape\": [2, 2, 2, 2]}}, \"pooling.AveragePooling3D.6\": {\"input\": {\"data\": [-0.709952, -0.532913, -0.169956, -0.391538, 0.729034, -0.2004, -0.67324, -0.973672, 0.879975, -0.981827, -0.4828, -0.887985, 0.843364, 0.710745, -0.260613, 0.20082, 0.309563, 0.721671, -0.967848, -0.976471, -0.13058, 0.052684, 0.666494, -0.319759, -0.060338, 0.359151, -0.795562, 0.70488, 0.100816, 0.466479, 0.992415, 0.066527, -0.690663, -0.741365, -0.251801, -0.479328, 0.62187, 0.578729, 0.598481, 0.817115, -0.913801, -0.694569, 0.397726, -0.31274, 0.163147, 0.087004, -0.744957, -0.920201, 0.440377, -0.191648, -0.227724, -0.562736, -0.484598, -0.230876, 0.019055, 0.988723, 0.656988, 0.185623, -0.629304, -0.321252, 0.329452, 0.355461, 0.734458, 0.496983, 0.181439, 0.414232, 0.776873, 0.68191, -0.846744, -0.442164, -0.526272, 0.92696, -0.704629, -0.800248, 0.643923, 0.775996, -0.203863, -0.756864, -0.398058, -0.914275, 0.980404, 0.329099, -0.576086, 0.851052, -0.74133, -0.23673, -0.001628, 0.972916, -0.571033, 0.669151, -0.977945, -0.707472, 0.371069, -0.772292, -0.207482, -0.094619, -0.604913, 0.111706, -0.123427, 0.284132, 0.292284, -0.490954, -0.873365, 0.109881, -0.40172, 0.103223, 0.396366, -0.415444, 0.766823, -0.057373, 0.619422, 0.30151, -0.126582, 0.862041, -0.083425, -0.018503, 0.744106, -0.681409, 0.556506, -0.628066, -0.697587, -0.201239, 0.051677, -0.585768, 0.202332, -0.634928, -0.410351, 0.005911], \"shape\": [4, 4, 4, 2]}, \"expected\": {\"data\": [-0.242659, -0.627783, 0.231323, -0.111939, 0.159636, 0.037518, -0.270082, -0.218984, -0.070567, -0.485788, -0.111164, -0.265047, 0.008914, 0.071142, -0.080005, -0.012604, -0.159231, -0.010098, -0.350668, -0.063979, 0.278439, 0.234528, 0.603106, 0.308118, -0.207054, 0.232101, -0.248649, 0.301392, 0.539285, 0.346362, 0.863437, 0.281755, -0.070117, -0.144514, 0.162641, 0.016568, -0.167049, -0.077962, -0.267701, -0.0226, 0.005024, -0.075724, -0.128601, -0.048237, -0.299029, -0.126288, -0.281397, 0.031791, -0.11304, 0.031477, -0.367058, -0.203106, 0.002375, 0.184946, 0.136101, 0.591001, -0.380324, -0.043487, -0.226682, -0.361389, 0.306874, -0.003617, 0.263488, 0.201182, 0.020489, 0.144438, 0.212779, -0.052595, -0.146222, -0.16541, -0.294568, 0.106019, 0.016031, 0.210902, 0.118314, -0.067409, 0.167747, -0.250036, 0.194061, -0.066979, -0.250072, 0.149795, -0.1262, -0.348256, 0.064153, -0.258652, -0.015739, 0.064035, -0.548722, -0.206332, -0.088217, -0.675115, -0.011108, -0.373982, -0.308916, -0.044354, -0.183423, 0.020904, 0.333012, -0.16991, 0.201291, -0.034234, -0.126972, 0.205695, -0.05384, 0.132829, 0.455967, -0.293182, 0.671714, -0.266335, 0.587964, -0.163278, -0.213979, 0.014133, 0.228672, -0.480152, 0.273148, -0.484623, 0.073078, -0.311078, -0.322955, -0.393504, 0.127005, -0.610348, -0.10401, -0.314508, -0.410351, 0.005911], \"shape\": [4, 4, 4, 2]}}, \"pooling.AveragePooling3D.7\": {\"input\": {\"data\": [-0.71103, 0.421506, 0.752321, 0.542455, -0.557162, -0.963774, 0.910303, -0.933284, 0.67521, 0.588709, -0.782848, -0.108964, -0.767069, 0.338318, -0.660374, -0.967294, -0.501079, -0.917532, -0.087991, -0.160473, 0.520493, 0.612007, -0.955448, -0.809749, -0.627003, 0.494441, 0.985405, 0.99813, -0.278165, 0.090068, 0.803872, 0.287682, 0.162199, 0.1796, -0.630223, 0.044743, 0.9092, 0.023879, -0.403203, -0.005329, -0.29237, -0.510033, -0.190427, 0.149011, 0.873547, -0.58793, -0.302525, 0.102122, -0.804112, 0.965834, 0.302039, -0.806929, 0.627682, 0.876256, 0.176245, 0.051969, 0.005712, -0.877694, -0.776877, -0.360984, 0.172577, 0.953108, 0.755911, 0.973515, 0.745292, 0.765506, 0.119956, 0.378346, 0.425789, 0.048668, 0.363691, -0.499862, 0.315721, 0.243267, 0.333434, -0.001645, -0.007235, -0.463152, -0.002048, 0.862117, -0.575785, 0.594789, 0.068012, 0.165267, 0.081581, 0.128645, 0.559305, -0.494595, 0.10207, 0.278472, -0.815856, 0.817863, 0.101417, -0.432774, -0.36832, 0.682055, -0.852236, 0.756063, 0.741739, 0.403911, 0.363444, -0.853088, 0.429379, 0.95063, 0.19365, 0.707334, 0.883575, 0.037535, 0.735855, -0.597979, -0.328964, -0.63363, 0.533345, 0.628204, -0.831273, -0.475492, -0.120719, -0.049689, 0.474126, -0.534385, 0.898272, -0.060213, 0.134975, -0.81603, -0.09329, -0.56951, 0.744421, 0.561587, -0.094, 0.780616, -0.206093, 0.992174, 0.563806, 0.562612, -0.754387, -0.36159, -0.288989, 0.195871, -0.156575, 0.108674, 0.037465, 0.115865, -0.313897, -0.290763, -0.920174, -0.943574, 0.610507, -0.749795, -0.802955, 0.296183, -0.862404, 0.174227, 0.6721, 0.674769, -0.198663, 0.163696, -0.572753, 0.149323, 0.456671, 0.162098], \"shape\": [4, 5, 4, 2]}, \"expected\": {\"data\": [-0.131402, 0.155199, 0.03226, -0.070195, 0.037581, -0.260452, 0.030912, -0.436622, -0.017073, 0.039968, 0.135148, 0.319859, 0.22609, 0.20693, 0.242007, -0.012103, 0.045283, 0.116491, 0.151294, -0.099044, 0.124178, 0.104379, -0.202626, 0.428394, -0.275804, 0.206784, 0.130999, 0.038676, 0.218617, 0.040718, 0.016176, 0.085388, 0.132601, 0.226252, 0.333257, 0.00119, 0.36471, 0.04267, 0.305004, 0.197663, 0.087807, 0.098584, -0.156448, -0.247495, 0.086031, -0.046277, 0.236039, 0.163866, -0.061051, 0.344117, -0.020681, 0.106031, 0.104317, 0.009554, 0.045255, 0.096864, 0.026437, 0.064502, 0.301632, -0.154837, -0.09276, -0.104819, -0.268972, 0.050116, 0.043877, 0.247794, -0.430852, -0.053041, 0.059331, -0.068163, 0.465398, -0.186144, 0.183288, 0.224137, 0.099849, 0.042311, 0.115137, 0.048274, -0.004983, 0.099998, -0.188808, -0.347206, -0.07789, -0.057268, -0.485448, 0.073878, -0.588151, -0.058268, 0.236719, 0.419233, -0.385708, 0.156509, -0.058041, 0.15571, 0.456671, 0.162098], \"shape\": [4, 3, 4, 2]}}, \"pooling.AveragePooling3D.8\": {\"input\": {\"data\": [0.106539, 0.430065, 0.625063, -0.956042, 0.681684, 0.345995, -0.589061, 0.186737, 0.535452, -0.125905, -0.396262, -0.44893, 0.39021, 0.253402, -0.238515, 0.337141, 0.178107, 0.244331, -0.93179, -0.081267, 0.895223, 0.820023, 0.365435, -0.738456, 0.893031, -0.787916, -0.518813, 0.661518, -0.464144, -0.639165, -0.252917, 0.784083, 0.577398, 0.769552, 0.036096, 0.847521, -0.171916, 0.07536, -0.830068, 0.734205, -0.437818, 0.295701, 0.252657, -0.859452, -0.425833, -0.650296, -0.584695, 0.163986, 0.43905, -0.521755, 0.620616, 0.066707, -0.101702, 0.941175, 0.479202, 0.624312, -0.372154, 0.625845, 0.980521, -0.834695, -0.40269, 0.784157, 0.814068, -0.485038, -0.150738, 0.682911, 0.406096, -0.405868, -0.337905, 0.803583, -0.764964, 0.96897, -0.057235, 0.403604, -0.605392, 0.389273, 0.235543, -0.095585, -0.860692, 0.937457, -0.928888, 0.702073, -0.18066, 0.033968, -0.082046, -0.237205, 0.922919, 0.064731, -0.026908, -0.865491, 0.881128, 0.265603, -0.132321, -0.701801, 0.490064, 0.718745, -0.884446, 0.538162, -0.086979, -0.734317, 0.089006, 0.349945, -0.415428, -0.621358, 0.892372, 0.090398, 0.883604, 0.772612, 0.589633, 0.187399, 0.807184, -0.128627, -0.534439, 0.258966, 0.141399, -0.777263, 0.911318, -0.359087, 0.789361, 0.470019, 0.836149, 0.415029, 0.920315, -0.916153, 0.645573, -0.446523, -0.899169, 0.78844], \"shape\": [4, 4, 4, 2]}, \"expected\": {\"data\": [0.162391, -0.005936, -0.221024, 0.180816, 0.161071, -0.078404, 0.166559, 0.261386, 0.04966, 0.217097, -0.082203, 0.300223, 0.138512, -0.110408, 0.330712, 0.037165], \"shape\": [2, 2, 2, 2]}}, \"pooling.AveragePooling3D.9\": {\"input\": {\"data\": [0.454263, 0.047178, -0.644362, 0.432654, 0.776147, -0.088086, -0.16527, -0.152361, -0.723283, 0.119471, -0.020663, 0.230897, 0.249349, -0.825224, 0.809245, 0.37136, 0.649976, 0.690981, -0.5766, 0.750394, -0.777363, -0.359006, 0.398419, -0.851015, -0.479232, -0.924962, -0.898893, 0.135445, 0.819369, -0.867218, 0.039715, 0.304805, -0.865872, -0.891635, 0.730554, 0.178083, 0.981329, 0.047786, -0.466968, -0.89441, -0.037018, -0.880158, 0.635061, 0.108217, 0.405675, 0.242025, 0.524396, -0.46013, -0.98454, 0.227442, -0.159924, -0.396205, -0.843265, 0.181395, -0.743803, 0.445469, 0.05215, 0.837067, -0.756402, -0.959109, -0.580594, -0.677936, -0.929683, -0.165592, -0.870784, 0.91887, 0.542361, 0.46359, -0.521332, 0.778263, 0.662447, 0.692057, 0.224535, -0.087731, 0.904644, 0.207457, -0.564079, -0.389642, 0.590403, -0.861828, -0.280471, -0.593786, -0.542645, 0.788946, -0.808773, -0.334536, -0.973711, 0.68675, 0.383992, -0.38838, 0.278601, -0.89188, -0.582918, -0.190511, -0.493528, 0.635115, -0.375152, 0.586508, -0.986557, -0.449484, 0.216757, 0.746825, -0.144795, 0.448144, -0.828083, 0.224525, -0.958965, -0.566069, -0.850394, -0.261458, -0.589888, 0.75667, 0.531888, 0.146437, -0.877887, -0.575355, 0.06156, -0.714865, 0.710365, -0.439259, -0.084566, -0.854224, 0.467254, 0.59934, 0.527409, 0.791222, -0.66992, 0.644258], \"shape\": [4, 4, 4, 2]}, \"expected\": {\"data\": [-0.058915, -0.081912, 0.389238, -0.21988, -0.394183, 0.045132, -0.327151, -0.248637, -0.2935, 0.162208, -0.15011, 0.238629, -0.015479, -0.221113, -0.27869, 0.134772], \"shape\": [2, 2, 2, 2]}}, \"pooling.AveragePooling3D.10\": {\"input\": {\"data\": [-0.453175, -0.475078, 0.486234, -0.949643, -0.349099, -0.837108, 0.933439, 0.167853, -0.995191, 0.459466, -0.788337, -0.120985, 0.06215, 0.138625, -0.102201, 0.976605, -0.591051, -0.592066, 0.469334, -0.435067, 0.621416, 0.817698, 0.790015, 0.485862, 0.469679, -0.611443, 0.582845, -0.503885, -0.379174, -0.451035, 0.052289, 0.131836, -0.609312, -0.828722, -0.422428, -0.648754, -0.339801, -0.758017, 0.754244, -0.544823, 0.691656, 0.076848, -0.32539, 0.306448, -0.662415, 0.334329, 0.030666, -0.414111, -0.757096, -0.20427, -0.893088, -0.681919, -0.619269, -0.640749, 0.867436, 0.971453, -0.42039, -0.574905, -0.34642, 0.588678, -0.247265, 0.436084, 0.220126, 0.114202, 0.613623, 0.401452, -0.270262, -0.591146, -0.872383, 0.818368, 0.336808, 0.338197], \"shape\": [2, 3, 3, 4]}, \"expected\": {\"data\": [-0.096379, -0.08704], \"shape\": [2, 1, 1, 1]}}, \"pooling.AveragePooling3D.11\": {\"input\": {\"data\": [-0.803361, 0.348731, 0.30124, -0.168638, 0.516406, -0.258765, 0.297839, 0.993235, 0.958465, -0.273175, -0.704992, 0.261477, -0.301255, 0.263104, 0.678631, -0.644936, -0.029034, -0.320266, 0.307733, -0.479016, 0.608177, 0.034951, 0.456908, -0.929353, 0.594982, -0.243058, -0.524918, 0.455339, 0.034216, 0.356824, 0.63906, -0.259773, -0.084724, 0.248472, -0.608134, 0.0077, 0.400591, -0.960703, -0.247926, -0.774509, 0.496174, -0.319044, -0.324046, -0.616632, -0.322142, -0.472846, 0.171825, -0.030013, 0.992861, -0.645264, 0.524886, 0.673229, 0.883122, 0.25346, -0.706988, -0.654436, 0.918349, -0.139113, 0.742737, 0.338472, -0.812719, 0.860081, 0.003489, 0.667897, 0.362284, -0.283972, 0.995162, 0.67962, -0.700244, -0.137142, 0.045695, -0.450433], \"shape\": [2, 3, 3, 4]}, \"expected\": {\"data\": [-0.073055, 0.083417, 0.109908, 0.160761, 0.061998, 0.11563, 0.00915, 0.030844, 0.154595, 0.132854, -0.051119, 0.025479, 0.01321, 0.103228, 0.096798, 0.132983, 0.091705, 0.092372, 0.008749, 0.004411, 0.149296, 0.121109, -0.012738, -0.001443, 0.044439, 0.121335, 0.01906, 0.021515, 0.096866, 0.117315, -0.031152, -0.075063, 0.106077, 0.137015, -0.045408, -0.108109, 0.13765, 0.028927, -0.316498, -0.265803, 0.090454, 0.069218, -0.177051, -0.075283, 0.162245, 0.098457, -0.146385, -0.134885, 0.102239, 0.081747, -0.04865, 0.018312, 0.020763, 0.058465, -0.029871, 0.057668, 0.044907, 0.081293, -0.050427, 0.015913, 0.201232, 0.2022, 0.197264, 0.272857, 0.129309, 0.175371, 0.153743, 0.238277, 0.144593, 0.186112, 0.056922, 0.123728], \"shape\": [2, 3, 3, 4]}}, \"pooling.AveragePooling3D.12\": {\"input\": {\"data\": [-0.497409, -0.250345, 0.196124, -0.044334, -0.324906, 0.560065, 0.220435, -0.167776, -0.923771, 0.77337, -0.862909, -0.584756, -0.70451, 0.870272, 0.841773, -0.312016, 0.599915, 0.073955, 0.944336, -0.4175, 0.865698, 0.609184, 0.033839, -0.72494, -0.239473, 0.514968, -0.318523, -0.244443, 0.275468, -0.85993, -0.262732, 0.026767, -0.937574, 0.872647, 0.540013, 0.055422, 0.322167, 0.972206, 0.92596, -0.82368, -0.63508, 0.671616, -0.678809, 0.202761, -0.260164, -0.241878, 0.188534, -0.47291, -0.077436, -0.016304, 0.548747, -0.236224, -0.780147, -0.013071, -0.67362, -0.807763, -0.351361, 0.533701, 0.274553, -0.933379, -0.49029, 0.928012, -0.719924, 0.453519, 0.173223, 0.030778, 0.12229, 0.547074, -0.860491, 0.206434, 0.248515, -0.189106, -0.393127, -0.152128, -0.822508, -0.361768, -0.702917, 0.998304, -0.011396, -0.644766, -0.150506, -0.153633, -0.772981, -0.470261, -0.056372, 0.082635, 0.017418, 0.26302, 0.730468, 0.268813, -0.163174, 0.332229, -0.698119, -0.397122, -0.426552, -0.931893, -0.6652, -0.986456, -0.062208, -0.90263, -0.12278, -0.277462, 0.072233, 0.466157, -0.917268, 0.053668, -0.45609, 0.072386, 0.376642, 0.133363, -0.799663, -0.984724, 0.337956, -0.088779, -0.04311, 0.520989, -0.611655, -0.456082, -0.662569, 0.09705, 0.256941, -0.987104, -0.939188, -0.296892, 0.123336, 0.710366, -0.675095, -0.037022, -0.616184, -0.925359, -0.734003, -0.629605, 0.071318, 0.6548, -0.019787, -0.435663, -0.412123, 0.967997, -0.80066, -0.337085, 0.471011, -0.945095, -0.74401, 0.924175], \"shape\": [3, 4, 4, 3]}, \"expected\": {\"data\": [-0.082917, 0.141622, 0.017767, 0.080913, -0.005706, 0.056398, -0.073774, -0.279674, -0.351729, -0.0631, -0.128173, -0.649791], \"shape\": [3, 2, 2, 1]}}}\n"
]
]
] | [
"code",
"markdown",
"code",
"markdown",
"code",
"markdown",
"code",
"markdown",
"code",
"markdown",
"code",
"markdown",
"code",
"markdown",
"code",
"markdown",
"code",
"markdown",
"code",
"markdown",
"code",
"markdown",
"code",
"markdown",
"code",
"markdown",
"code",
"markdown",
"code"
] | [
[
"code",
"code",
"code"
],
[
"markdown",
"markdown"
],
[
"code"
],
[
"markdown"
],
[
"code"
],
[
"markdown"
],
[
"code"
],
[
"markdown"
],
[
"code"
],
[
"markdown"
],
[
"code"
],
[
"markdown"
],
[
"code"
],
[
"markdown"
],
[
"code"
],
[
"markdown"
],
[
"code"
],
[
"markdown"
],
[
"code"
],
[
"markdown"
],
[
"code"
],
[
"markdown"
],
[
"code"
],
[
"markdown"
],
[
"code"
],
[
"markdown"
],
[
"code"
],
[
"markdown"
],
[
"code"
]
] |
d079354de09178062fb01c6302622afa3d98b82f | 7,538 | ipynb | Jupyter Notebook | reference/Debugging strategies.ipynb | ireapps/cfj-2018 | 0813137f1507c4d64dfa51ec799bdc883a9446cd | [
"MIT"
] | 6 | 2018-12-25T09:16:15.000Z | 2022-01-05T14:35:32.000Z | reference/Debugging strategies.ipynb | ireapps/cfj-2018 | 0813137f1507c4d64dfa51ec799bdc883a9446cd | [
"MIT"
] | null | null | null | reference/Debugging strategies.ipynb | ireapps/cfj-2018 | 0813137f1507c4d64dfa51ec799bdc883a9446cd | [
"MIT"
] | null | null | null | 33.954955 | 561 | 0.584373 | [
[
[
"# Debugging strategies\n\nIn this notebook, we'll talk about what happens when you get an error message (it will happen often!) and some steps you can take to resolve them.\n\nRun the code in the next cell.",
"_____no_output_____"
]
],
[
[
"x = 10\n\nif x > 20\n print(f'{x} is greater than 20!')",
"_____no_output_____"
]
],
[
[
"The \"traceback\" message shows you a couple of useful things:\n\n- What line the error is on: `line 3`\n- The class of error: `SyntaxError` (v common)\n- Exactly _where_ the error occured -- see where the `^` symbol is pointing?\n\nWhat's the problem?\n\n#### Googling\n\nIf it's not immediately clear what's wrong -- if you're not even sure what a `SyntaxError` even is -- I might start by Googling the error messsage, the word \"python\" and maybe some keywords for what I was trying to do when I got the error. Something like [`\"SyntaxError: invalid syntax\" python if statement`](https://www.google.com/search?q=%22SyntaxError%3A+invalid+syntax%22+python+if+statement)\n\nClick through the first couple of links -- you'll become _very_ familiar with StackOverflow -- and see if you spot the problem.\n\nIf you're still stuck, maybe it's time to ...\n\n#### Read the docs\n\nMy next stop would be the Python documentation to find some examples of the thing I'm trying to do. [Here's the page outlining how to write an `if` statement in Python](https://docs.python.org/3/tutorial/controlflow.html). From there, I would copy the example code, run it, compare it line by line with my code and see what's different.\n\nIf I'm _still_ stuck, I might see if there are other keywords to search on and take another run at Google.\n\n#### Use `print()` liberally\n\nThe `print()` function can be a lifesaver -- it can show you _what_ a value is before you try to do something to it, and whether it matches up with your expectations of what that value should be, and thereby give you a clue about why your script is failing. An example can help clarify this idea.\n\n**Scenario:** Your newsroom is handing out longevity bonuses. (Congratulations!) Each employee's bonus will be the number of years they've been with the company, times 50.\n\nSo we're going to loop over our staff data, held in a list of dictionaries, and calculate each person's bonus.",
"_____no_output_____"
]
],
[
[
"staff = [\n {'name': 'Fran', 'years_of_service': 2, 'job': 'Reporter'},\n {'name': 'Graham', 'years_of_service': 7, 'job': 'Reporter'},\n {'name': 'Pat', 'years_of_service': 4, 'job': 'Web Producer'},\n {'name': 'John', 'years_of_service': '26', 'job': 'Managing Editor'},\n {'name': 'Sue', 'years_of_service': 33, 'job': 'Executive Editor'}\n]\n\nfor person in staff:\n name = person['name']\n bonus = person['years_of_service'] * 50\n print(f'{name} is getting a bonus of {bonus}')",
"_____no_output_____"
]
],
[
[
"We didn't get an exception, but something is _clearly_ wrong with John's bonus. What's going on?\n\nMaybe you spot the error already. If not, we might Google something like [\"python multiply numbers repeating\"](https://www.google.com/search?q=python+multiply+numbers+repeating) -- which leads us to [this StackOverflow answer](https://stackoverflow.com/questions/20401871/want-to-multiply-not-repeat-variable). Is that what's going on here? Let's add a `print()` statement before we do the multiplication and use the [`type()`](https://docs.python.org/3/library/functions.html#type) function to check the value that we're pulling out of each dictionary.",
"_____no_output_____"
]
],
[
[
"for person in staff:\n name = person['name']\n bonus = person['years_of_service'] * 50\n print(name, type(person['years_of_service']))\n print(f'{name} is getting a bonus of {bonus}')",
"_____no_output_____"
]
],
[
[
"Aha! John's value for `years_of_service` has been stored as a string, not an integer. Let's fix that by using the [`int()`](https://docs.python.org/3/library/functions.html#int) function to coerce the value to an integer.",
"_____no_output_____"
]
],
[
[
"for person in staff:\n name = person['name']\n bonus = int(person['years_of_service']) ** 2\n print(f'{name} is getting a bonus of {bonus}')",
"_____no_output_____"
]
],
[
[
"Winner winner, chicken dinner.\n\nHere are some more debugging exercises for you to work through. See if you can figure out what's wrong and fix them.",
"_____no_output_____"
]
],
[
[
"print(Hello, Pittsburgh!)",
"_____no_output_____"
],
[
"desk = {\n 'wood': 'fir',\n 'color': 'black',\n 'height_in': 36,\n 'width_in': 48,\n 'length_in': 68\n}\n\nprint(desk['drawer_count'])",
"_____no_output_____"
],
[
"students = ['Kelly', 'Larry', 'José', 'Frank', 'Sarah', 'Sue']\n\nfor student in students:\n if student = 'Kelly':\n print('It's Kelly!')\n elif student == 'José':\n print(\"It's José!\")",
"_____no_output_____"
],
[
"import cvs\n\nwith open('../../../data/eels.csv', 'r') as o:\n reader = csv.DictReader(o)\n for row in Reader:\n print(row)",
"_____no_output_____"
]
],
[
[
"### Further reading\n\n- [Python's tutorial on errors and exceptions](https://docs.python.org/3/tutorial/errors.html)\n- [Software Carpentry post on understanding Python errors](https://anenadic.github.io/2014-11-10-manchester/novice/python/07-errors.html)\n- [How to read a traceback](http://cs.franklin.edu/~ansaria/traceback.html)",
"_____no_output_____"
]
]
] | [
"markdown",
"code",
"markdown",
"code",
"markdown",
"code",
"markdown",
"code",
"markdown",
"code",
"markdown"
] | [
[
"markdown"
],
[
"code"
],
[
"markdown"
],
[
"code"
],
[
"markdown"
],
[
"code"
],
[
"markdown"
],
[
"code"
],
[
"markdown"
],
[
"code",
"code",
"code",
"code"
],
[
"markdown"
]
] |
d0794d14d49660a6b85df205465d16119da1b7ac | 30,432 | ipynb | Jupyter Notebook | conda_notebooks/09_Numpy_Stats.ipynb | t4d-classes/python-data-analysis_10252021 | 0bb21d566f1962e0f5190bd746c6f76ec3ab9ac2 | [
"MIT"
] | null | null | null | conda_notebooks/09_Numpy_Stats.ipynb | t4d-classes/python-data-analysis_10252021 | 0bb21d566f1962e0f5190bd746c6f76ec3ab9ac2 | [
"MIT"
] | null | null | null | conda_notebooks/09_Numpy_Stats.ipynb | t4d-classes/python-data-analysis_10252021 | 0bb21d566f1962e0f5190bd746c6f76ec3ab9ac2 | [
"MIT"
] | null | null | null | 60.500994 | 8,880 | 0.804318 | [
[
[
"import math\nimport statistics as stats2\n\nfrom matplotlib import pyplot as plt\nfrom matplotlib.ticker import MaxNLocator\nfrom scipy import stats\nimport numpy as np\n\n%matplotlib inline",
"_____no_output_____"
],
[
"population = np.arange(0, 20)\n\npopulation",
"_____no_output_____"
],
[
"np.mean(population)",
"_____no_output_____"
],
[
"population.mean()",
"_____no_output_____"
],
[
"sample1 = np.random.choice(population, size=15, replace=False)\n\nprint(sample1)\nprint(np.mean(sample1))\n\nsample2 = np.random.choice(population, size=15, replace=False)\n\nprint(sample2)\nprint(np.mean(sample2))",
"[18 4 5 11 9 6 10 14 0 1 16 8 15 7 2]\n8.4\n[10 15 11 13 9 2 18 14 1 0 3 19 4 6 5]\n8.666666666666666\n"
],
[
"population = np.arange(0, 21)\n\nprint(np.median(population))",
"10.0\n"
],
[
"sample1 = np.random.choice(population, size=15, replace=False)\n\nprint(sample1)\nprint(np.median(sample1))\n\nsample2 = np.random.choice(population, size=15, replace=False)\n\nprint(sample2)\nprint(np.median(sample2))",
"[ 6 17 14 8 1 15 13 5 0 3 7 19 10 18 12]\n10.0\n[15 12 8 19 13 5 16 0 9 7 10 11 20 14 2]\n11.0\n"
],
[
"nums = np.array([3,5,1,7,5,2,7,1,2,2,3,5,6,5])\nprint(stats2.mode(nums))",
"5\n"
],
[
"population = np.arange(0, 100)\n\nprint(stats2.pvariance(population))\n\nsample1 = np.random.choice(population, size=80)\nprint(stats2.variance(sample1))\n\nsample2 = np.random.choice(population, size=80)\nprint(stats2.variance(sample2))",
"833\n876\n1035\n"
],
[
"population = np.arange(0, 100)\n\nprint(stats2.pstdev(population))\n\nsample1 = np.random.choice(population, size=80)\nprint(stats2.stdev(sample1))\n\nsample2 = np.random.choice(population, size=80)\nprint(stats2.stdev(sample2))",
"28.861739379323623\n27.784887978899608\n26.40075756488817\n"
],
[
"nums = np.array([10,20,30,40,50])\n\nprint(np.percentile(nums, 25))\n\nprint(np.percentile(nums, 75))",
"20.0\n40.0\n"
],
[
"print(np.percentile(nums, 15))",
"16.0\n"
],
[
"print(np.percentile(nums, 65))",
"36.0\n"
],
[
"nums = np.concatenate([\n np.random.randint(\n (x-1)*10, x*10, size=x*100)\n for x in np.arange(10, 0, -1)\n])\n\nprint(stats.skew(nums))\n\nplt.hist(nums, bins=100)\nplt.show()",
"-0.5563593022672454\n"
],
[
"nums = np.concatenate([\n np.random.randint(\n x*10, (x+1)*10, size=(10-x)*100)\n for x in np.arange(0, 10, 1)\n])\n\nprint(stats.skew(nums))\n\nplt.hist(nums, bins=100)\nplt.show()",
"0.5674587717688663\n"
],
[
"nums = np.arange(0,20)\n\nprint(stats.describe(nums))",
"DescribeResult(nobs=20, minmax=(0, 19), mean=9.5, variance=35.0, skewness=0.0, kurtosis=-1.206015037593985)\n"
],
[
"display(np.random.choice(5, 10))\n\ndisplay(np.random.choice(5, 5, replace=False))\n\narr = np.array([1,3,5,7,9])\n\ndisplay(np.random.choice(arr, 10))",
"_____no_output_____"
],
[
"first_semester = np.array([65, 67, 68, 72, 72, 80, 81, 82, 82, 91, 91, 91, 93, 94, 91, 91, 91, 93, 94, 100, 100, 100])\nsecond_semester = np.array([75, 77, 78, 72, 72, 70, 71, 72, 82, 91, 91, 91, 93, 94, 91, 91, 91, 93, 94, 100, 100, 100])\n\nplt.title('Scores by Semester')\nplt.xlabel('Semester')\nplt.ylabel('Scores')\n\nplt.boxplot([first_semester, second_semester], labels=[\"First\", \"Second\"])\nplt.show()",
"_____no_output_____"
]
]
] | [
"code"
] | [
[
"code",
"code",
"code",
"code",
"code",
"code",
"code",
"code",
"code",
"code",
"code",
"code",
"code",
"code",
"code",
"code",
"code",
"code"
]
] |
d0795dd6144893b82f0a006e8c8b57d434b8007f | 769,249 | ipynb | Jupyter Notebook | notebooks/synthetic_envelope_waveform_matching_signalGPs.ipynb | davmre/sigvisa | 91a1f163b8f3a258dfb78d88a07f2a11da41bd04 | [
"BSD-3-Clause"
] | null | null | null | notebooks/synthetic_envelope_waveform_matching_signalGPs.ipynb | davmre/sigvisa | 91a1f163b8f3a258dfb78d88a07f2a11da41bd04 | [
"BSD-3-Clause"
] | null | null | null | notebooks/synthetic_envelope_waveform_matching_signalGPs.ipynb | davmre/sigvisa | 91a1f163b8f3a258dfb78d88a07f2a11da41bd04 | [
"BSD-3-Clause"
] | null | null | null | 60.863122 | 61,269 | 0.716615 | [
[
[
"empty"
]
]
] | [
"empty"
] | [
[
"empty"
]
] |
d079668b309925215478d725d48b35068ff49e2d | 59,499 | ipynb | Jupyter Notebook | Notebooks/GCN_cuda_Implement_speed.ipynb | oqbrady/Sentinel2_Traffic | ca1f10a0001d409081950f2794a3b4a4e7490bc2 | [
"MIT"
] | 1 | 2021-06-04T23:40:27.000Z | 2021-06-04T23:40:27.000Z | Notebooks/GCN_cuda_Implement_speed.ipynb | oqbrady/Sentinel2_Traffic | ca1f10a0001d409081950f2794a3b4a4e7490bc2 | [
"MIT"
] | null | null | null | Notebooks/GCN_cuda_Implement_speed.ipynb | oqbrady/Sentinel2_Traffic | ca1f10a0001d409081950f2794a3b4a4e7490bc2 | [
"MIT"
] | 1 | 2021-06-04T23:40:08.000Z | 2021-06-04T23:40:08.000Z | 59,499 | 59,499 | 0.725407 | [
[
[
"import subprocess\ntry:\n import dgl\nexcept:\n subprocess.check_call([\"python\", '-m', 'pip', 'install', 'dgl-cu110'])\n import dgl",
"DGL backend not selected or invalid. Assuming PyTorch for now.\n"
],
[
"import os\nimport dgl.data\nfrom dgl.data import DGLDataset\nimport torch\nimport torch.nn as nn\nimport torch.nn.functional as F\nimport pandas as pd\nimport numpy as np\nimport tqdm\nfrom sklearn.linear_model import LinearRegression\nfrom dgl.data.utils import save_graphs\nfrom dgl.data.utils import load_graphs\nfrom dgl.nn.pytorch.conv import ChebConv\nimport copy\nimport matplotlib.pyplot as plt",
"_____no_output_____"
],
[
"os.chdir(\"/content/drive/MyDrive/Winter_Research\")",
"_____no_output_____"
],
[
"max_pixs = 128309",
"_____no_output_____"
]
],
[
[
"## Make the Dataset",
"_____no_output_____"
]
],
[
[
"CA_x, CA_y = [], []\nKS_x, KS_y = [], []\nMT_x, MT_y = [], []\nTX_x, TX_y = [], []\nOH_x, OH_y = [], []\nstates = {\"CA\" : [CA_x, CA_y, \"Roi_1\"],\n \"KS\" : [KS_x, KS_y, \"Roi_2\"],\n \"MT\" : [MT_x, MT_y, \"Roi_3\"],\n \"TX\" : [TX_x, TX_y, \"Roi_4\"],\n \"OH\" : [OH_x, OH_y, \"Roi_5\"]}",
"_____no_output_____"
]
],
[
[
"#### Load into RAM",
"_____no_output_____"
]
],
[
[
"master_df = pd.read_csv(\"Sentinel2_Traffic/Traffic_Data/5_state_traffic.csv\")\nmaster_df = master_df.set_index(\"Unnamed: 0\")\nCA_x, CA_y = [], []\nKS_x, KS_y = [], []\nMT_x, MT_y = [], []\nTX_x, TX_y = [], []\nOH_x, OH_y = [], []\nstates = {\"Cali\" : [CA_x, CA_y, \"Roi_1\"],\n \"KS\" : [KS_x, KS_y, \"Roi_2\"],\n \"MT\" : [MT_x, MT_y, \"Roi_3\"],\n \"TX\" : [TX_x, TX_y, \"Roi_4\"],\n \"Ohio\" : [OH_x, OH_y, \"Roi_5\"]}\nj = 0\nfor st in [\"Cali\", \"KS\", \"MT\", \"TX\", \"Ohio\"]:\n# for st in [\"TX\"]:\n # path_check = \"R/\" + states[st][2] + \"/greedy_a/\"\n path = \"new_roi/\" + st # + \"/sent_cloud_90p_raw/\"\n # imgs_check = os.listdir(path_check)\n imgs = os.listdir(path)\n # for img, img_check in zip(imgs, imgs_check):\n for img in imgs:\n date = img[len(st):len(st) + 10]\n # print(date)\n # break\n try:\n photo = pd.read_csv(path + '/' + img)\n except:\n continue\n # photo_check = np.loadtxt(path_check + img_check).reshape(-1, 7, 3)\n# cali_pixs = 72264 #\n# kansas_pixs = 69071 #\n# mont_pixs = 72099 #\n# texas_pixs = 71764\n# ohio_pixs = 62827\n if photo.shape[0] < 50000:\n continue\n if date in list(master_df.index):\n if st == \"Cali\":\n lookup_st = \"CA\"\n elif st == \"Ohio\":\n lookup_st = \"OH\"\n else:\n lookup_st = st\n if not pd.isna(master_df.loc[date][lookup_st]):\n states[st][0].append(photo)\n states[st][1].append(master_df.loc[date][lookup_st])\n print(j, st, photo.shape)\n j += 1",
"0 Cali (67690, 7)\n1 Cali (52888, 7)\n2 Cali (70689, 7)\n3 Cali (70162, 7)\n4 Cali (71523, 7)\n5 Cali (71058, 7)\n6 Cali (61338, 7)\n7 Cali (70828, 7)\n8 Cali (69707, 7)\n9 Cali (69819, 7)\n10 Cali (118378, 7)\n11 Cali (68459, 7)\n12 Cali (71375, 7)\n13 Cali (70410, 7)\n14 Cali (118378, 7)\n15 Cali (70102, 7)\n16 Cali (118378, 7)\n17 Cali (70043, 7)\n18 Cali (69951, 7)\n19 Cali (117484, 7)\n20 Cali (69912, 7)\n21 Cali (118378, 7)\n22 Cali (69639, 7)\n23 Cali (70370, 7)\n24 Cali (118378, 7)\n25 Cali (71215, 7)\n26 Cali (71215, 7)\n27 Cali (113338, 7)\n28 Cali (71335, 7)\n29 Cali (95720, 7)\n30 Cali (113977, 7)\n31 Cali (52690, 7)\n32 Cali (69626, 7)\n33 Cali (52084, 7)\n34 Cali (117246, 7)\n35 Cali (117797, 7)\n36 Cali (109498, 7)\n37 Cali (70828, 7)\n38 Cali (70881, 7)\n39 Cali (70787, 7)\n40 Cali (61416, 7)\n41 Cali (69661, 7)\n42 Cali (63068, 7)\n43 Cali (106988, 7)\n44 Cali (69716, 7)\n45 Cali (118378, 7)\n46 Cali (70917, 7)\n47 Cali (118378, 7)\n48 Cali (71335, 7)\n49 Cali (69906, 7)\n50 Cali (70827, 7)\n51 Cali (111410, 7)\n52 Cali (63106, 7)\n53 Cali (104238, 7)\n54 Cali (70370, 7)\n55 Cali (69573, 7)\n56 Cali (70044, 7)\n57 Cali (118378, 7)\n58 Cali (68604, 7)\n59 Cali (69767, 7)\n60 Cali (52004, 7)\n61 Cali (69573, 7)\n62 Cali (117626, 7)\n63 Cali (118378, 7)\n64 Cali (62653, 7)\n65 Cali (69906, 7)\n66 Cali (85843, 7)\n67 Cali (57312, 7)\n68 Cali (117049, 7)\n69 Cali (69587, 7)\n70 Cali (70606, 7)\n71 Cali (91237, 7)\n72 Cali (70149, 7)\n73 Cali (60694, 7)\n74 Cali (69995, 7)\n75 Cali (117120, 7)\n76 Cali (71633, 7)\n77 Cali (117713, 7)\n78 Cali (58230, 7)\n79 Cali (118378, 7)\n80 Cali (69906, 7)\n81 Cali (71566, 7)\n82 Cali (70093, 7)\n83 Cali (118378, 7)\n84 Cali (118378, 7)\n85 Cali (116697, 7)\n86 Cali (117041, 7)\n87 Cali (68868, 7)\n88 Cali (84267, 7)\n89 Cali (70531, 7)\n90 Cali (103804, 7)\n91 Cali (70729, 7)\n92 Cali (67493, 7)\n93 Cali (115934, 7)\n94 Cali (70271, 7)\n95 Cali (117224, 7)\n96 Cali (70299, 7)\n97 Cali (118366, 7)\n98 Cali (70360, 7)\n99 Cali (75325, 7)\n100 Cali (118266, 7)\n101 Cali (63426, 7)\n102 Cali (118239, 7)\n103 Cali (66583, 7)\n104 Cali (68753, 7)\n105 Cali (107472, 7)\n106 Cali (71190, 7)\n107 Cali (118378, 7)\n108 Cali (70200, 7)\n109 Cali (118378, 7)\n110 Cali (50151, 7)\n111 Cali (109499, 7)\n112 Cali (63740, 7)\n113 Cali (70267, 7)\n114 Cali (118378, 7)\n115 Cali (69882, 7)\n116 Cali (118378, 7)\n117 Cali (118378, 7)\n118 Cali (118378, 7)\n119 Cali (118378, 7)\n120 Cali (69953, 7)\n121 Cali (118378, 7)\n122 Cali (58597, 7)\n123 Cali (118378, 7)\n124 Cali (118378, 7)\n125 Cali (118378, 7)\n126 Cali (70549, 7)\n127 Cali (115539, 7)\n128 Cali (118378, 7)\n129 Cali (71046, 7)\n130 Cali (118378, 7)\n131 Cali (70549, 7)\n132 Cali (118378, 7)\n133 Cali (70642, 7)\n134 Cali (118378, 7)\n135 Cali (70397, 7)\n136 Cali (70344, 7)\n137 Cali (118378, 7)\n138 Cali (70359, 7)\n139 Cali (118326, 7)\n140 Cali (63121, 7)\n141 Cali (118378, 7)\n142 Cali (70360, 7)\n143 Cali (61377, 7)\n144 Cali (70074, 7)\n145 Cali (117761, 7)\n146 Cali (70510, 7)\n147 Cali (113370, 7)\n148 Cali (69995, 7)\n149 Cali (118378, 7)\n150 Cali (68064, 7)\n151 Cali (106656, 7)\n152 Cali (70043, 7)\n153 Cali (118378, 7)\n154 Cali (71015, 7)\n155 Cali (118346, 7)\n156 Cali (69816, 7)\n157 Cali (105039, 7)\n158 Cali (71356, 7)\n159 Cali (83575, 7)\n160 Cali (69504, 7)\n161 Cali (118057, 7)\n162 Cali (70804, 7)\n163 Cali (118378, 7)\n164 Cali (69349, 7)\n165 Cali (117553, 7)\n166 Cali (70834, 7)\n167 Cali (70029, 7)\n168 Cali (118378, 7)\n169 Cali (98579, 7)\n170 Cali (114871, 7)\n171 Cali (53594, 7)\n172 Cali (70359, 7)\n173 Cali (118378, 7)\n174 Cali (118378, 7)\n175 Cali (69834, 7)\n176 Cali (69975, 7)\n177 Cali (118378, 7)\n178 Cali (70043, 7)\n179 Cali (63552, 7)\n180 Cali (115502, 7)\n181 Cali (117723, 7)\n182 Cali (69811, 7)\n183 Cali (65026, 7)\n184 Cali (117838, 7)\n185 Cali (63189, 7)\n186 Cali (75125, 7)\n187 Cali (114896, 7)\n188 Cali (70317, 7)\n189 Cali (113343, 7)\n190 Cali (112886, 7)\n191 Cali (59053, 7)\n192 Cali (70631, 7)\n193 Cali (115934, 7)\n194 KS (80636, 7)\n195 KS (72536, 7)\n196 KS (92220, 7)\n197 KS (90166, 7)\n198 KS (92589, 7)\n199 KS (92589, 7)\n200 KS (92589, 7)\n201 KS (68415, 7)\n202 KS (78303, 7)\n203 KS (50211, 7)\n204 KS (71097, 7)\n205 KS (92262, 7)\n206 KS (92589, 7)\n207 KS (92589, 7)\n208 KS (92589, 7)\n209 KS (92589, 7)\n210 KS (83981, 7)\n211 KS (92589, 7)\n212 KS (81079, 7)\n213 KS (87803, 7)\n214 KS (92248, 7)\n215 KS (85460, 7)\n216 KS (92589, 7)\n217 KS (92589, 7)\n218 KS (50406, 7)\n219 KS (92589, 7)\n220 KS (50136, 7)\n221 KS (92589, 7)\n222 KS (92026, 7)\n223 KS (74379, 7)\n224 KS (76223, 7)\n225 KS (92402, 7)\n226 KS (85322, 7)\n227 KS (72945, 7)\n228 KS (92181, 7)\n229 KS (92589, 7)\n230 KS (92487, 7)\n231 KS (92589, 7)\n232 KS (92589, 7)\n233 KS (92589, 7)\n234 KS (91249, 7)\n235 KS (92515, 7)\n236 KS (79417, 7)\n237 KS (92589, 7)\n238 KS (85730, 7)\n239 KS (92589, 7)\n240 KS (92589, 7)\n241 KS (90592, 7)\n242 KS (55291, 7)\n243 KS (92589, 7)\n244 KS (90834, 7)\n245 KS (83424, 7)\n246 KS (92589, 7)\n247 KS (92490, 7)\n248 KS (81547, 7)\n249 KS (92589, 7)\n250 KS (92589, 7)\n251 KS (92589, 7)\n252 KS (92589, 7)\n253 KS (92589, 7)\n254 KS (92589, 7)\n255 KS (77843, 7)\n256 KS (80254, 7)\n257 KS (88232, 7)\n258 KS (74681, 7)\n259 KS (92589, 7)\n260 KS (78816, 7)\n261 MT (81399, 7)\n262 MT (85179, 7)\n263 MT (59320, 7)\n264 MT (82582, 7)\n265 MT (85255, 7)\n266 MT (84656, 7)\n267 MT (85255, 7)\n268 MT (62568, 7)\n269 MT (71907, 7)\n270 MT (85255, 7)\n271 MT (54501, 7)\n272 MT (85255, 7)\n273 MT (84981, 7)\n274 MT (83900, 7)\n275 MT (82106, 7)\n276 MT (60749, 7)\n277 MT (65469, 7)\n278 MT (80322, 7)\n279 MT (77895, 7)\n280 MT (84900, 7)\n281 MT (83018, 7)\n282 MT (52297, 7)\n283 MT (54600, 7)\n284 MT (56119, 7)\n285 MT (85255, 7)\n286 MT (85255, 7)\n287 MT (85223, 7)\n288 MT (55322, 7)\n289 MT (85048, 7)\n290 MT (85255, 7)\n291 MT (85239, 7)\n292 MT (63209, 7)\n293 MT (83094, 7)\n294 MT (85213, 7)\n295 MT (53792, 7)\n296 MT (53897, 7)\n297 MT (70065, 7)\n298 MT (55357, 7)\n299 MT (79968, 7)\n300 MT (85255, 7)\n301 MT (82496, 7)\n302 MT (70127, 7)\n303 MT (84908, 7)\n304 MT (84790, 7)\n305 MT (67375, 7)\n306 MT (85255, 7)\n307 MT (85205, 7)\n308 MT (85255, 7)\n309 MT (75393, 7)\n310 MT (85255, 7)\n311 MT (85255, 7)\n312 MT (85255, 7)\n313 MT (85255, 7)\n314 MT (79655, 7)\n315 MT (85255, 7)\n316 MT (78480, 7)\n317 MT (85255, 7)\n318 MT (83588, 7)\n319 MT (58018, 7)\n320 MT (65410, 7)\n321 MT (60054, 7)\n322 MT (56884, 7)\n323 MT (85255, 7)\n324 MT (85112, 7)\n325 MT (85255, 7)\n326 MT (82531, 7)\n327 MT (84659, 7)\n328 MT (76809, 7)\n329 MT (80951, 7)\n330 MT (77545, 7)\n331 MT (62328, 7)\n332 MT (60665, 7)\n333 MT (85255, 7)\n334 MT (84204, 7)\n335 MT (84831, 7)\n336 MT (84681, 7)\n337 MT (85255, 7)\n338 MT (59544, 7)\n339 MT (62871, 7)\n340 MT (56895, 7)\n341 MT (85255, 7)\n342 MT (85255, 7)\n343 MT (84389, 7)\n344 MT (77994, 7)\n345 MT (85255, 7)\n346 MT (84134, 7)\n347 MT (83239, 7)\n348 MT (63750, 7)\n349 MT (65272, 7)\n350 TX (128309, 7)\n351 TX (122052, 7)\n352 TX (128309, 7)\n353 TX (128277, 7)\n354 TX (118967, 7)\n355 TX (66831, 7)\n356 TX (125091, 7)\n357 TX (128309, 7)\n358 TX (128309, 7)\n359 TX (111848, 7)\n360 TX (128309, 7)\n361 TX (128309, 7)\n362 TX (128309, 7)\n363 TX (128309, 7)\n364 TX (128309, 7)\n365 TX (107770, 7)\n366 TX (93374, 7)\n367 TX (128309, 7)\n368 TX (62716, 7)\n369 TX (128309, 7)\n370 TX (96915, 7)\n371 TX (93156, 7)\n372 TX (114888, 7)\n373 TX (128309, 7)\n374 TX (106970, 7)\n375 TX (128241, 7)\n376 TX (128309, 7)\n377 TX (128309, 7)\n378 TX (128309, 7)\n379 TX (128309, 7)\n380 TX (128309, 7)\n381 TX (119248, 7)\n382 TX (125738, 7)\n383 TX (128309, 7)\n384 TX (71383, 7)\n385 TX (128309, 7)\n386 TX (124717, 7)\n387 TX (128309, 7)\n388 TX (128309, 7)\n389 TX (59049, 7)\n390 TX (109676, 7)\n391 TX (105957, 7)\n392 TX (122679, 7)\n393 TX (128305, 7)\n394 TX (119583, 7)\n395 TX (126320, 7)\n396 TX (64089, 7)\n397 TX (128309, 7)\n398 TX (127224, 7)\n399 TX (124644, 7)\n400 TX (105941, 7)\n401 TX (125279, 7)\n402 TX (125484, 7)\n403 TX (127613, 7)\n404 TX (127486, 7)\n405 TX (74019, 7)\n406 TX (117343, 7)\n407 TX (79323, 7)\n408 TX (127919, 7)\n409 TX (107286, 7)\n410 TX (112966, 7)\n411 TX (70734, 7)\n412 TX (128196, 7)\n413 TX (54655, 7)\n414 TX (106354, 7)\n415 TX (128309, 7)\n416 TX (60271, 7)\n417 TX (96603, 7)\n418 TX (128309, 7)\n419 TX (128309, 7)\n420 TX (128309, 7)\n421 TX (128309, 7)\n422 TX (128309, 7)\n423 Ohio (53514, 7)\n424 Ohio (83527, 7)\n425 Ohio (82668, 7)\n426 Ohio (85705, 7)\n427 Ohio (63568, 7)\n428 Ohio (70939, 7)\n429 Ohio (70836, 7)\n430 Ohio (84270, 7)\n431 Ohio (67092, 7)\n432 Ohio (63922, 7)\n433 Ohio (69212, 7)\n434 Ohio (85481, 7)\n435 Ohio (73164, 7)\n436 Ohio (67113, 7)\n437 Ohio (85699, 7)\n438 Ohio (75682, 7)\n439 Ohio (51339, 7)\n440 Ohio (81503, 7)\n441 Ohio (64298, 7)\n442 Ohio (77875, 7)\n443 Ohio (85705, 7)\n444 Ohio (85705, 7)\n445 Ohio (70803, 7)\n446 Ohio (52200, 7)\n447 Ohio (70545, 7)\n448 Ohio (85347, 7)\n449 Ohio (70111, 7)\n450 Ohio (69700, 7)\n451 Ohio (69664, 7)\n452 Ohio (85705, 7)\n453 Ohio (84508, 7)\n454 Ohio (81231, 7)\n455 Ohio (85211, 7)\n456 Ohio (70290, 7)\n457 Ohio (85705, 7)\n458 Ohio (85705, 7)\n459 Ohio (70340, 7)\n460 Ohio (61167, 7)\n461 Ohio (85655, 7)\n462 Ohio (85098, 7)\n463 Ohio (63348, 7)\n464 Ohio (85654, 7)\n465 Ohio (54411, 7)\n466 Ohio (83807, 7)\n467 Ohio (55784, 7)\n468 Ohio (70178, 7)\n469 Ohio (66478, 7)\n470 Ohio (69559, 7)\n471 Ohio (65693, 7)\n472 Ohio (84674, 7)\n473 Ohio (60217, 7)\n474 Ohio (57744, 7)\n475 Ohio (60551, 7)\n476 Ohio (63741, 7)\n477 Ohio (85641, 7)\n478 Ohio (62570, 7)\n479 Ohio (82207, 7)\n"
],
[
"def gen_around(x, y):\n return [(x, y), (x, y + 10), (x, y - 10), (x + 10, y), (x - 10, y), (x + 10, y + 10), (x + 10, y - 10), (x - 10, y + 10), (x - 10, y - 10)]\n\ndef gen_around_strict(x, y):\n return [(x, y), (x, y + 10), (x, y - 10), (x + 10, y), (x - 10, y)]\n\ndef neighbors(road, coords, x, y, diagonal=True):\n neigh = []\n if diagonal:\n cand = gen_around(x, y)\n else:\n cand = gen_around_strict(x, y)\n for pix in cand:\n if pix[0] in coords:\n if pix[1] in coords[pix[0]]:\n neigh.append(coords[pix[0]][pix[1]]['idx'])\n return neigh\n\ndef src_dst(road, coords, diagonal=True):\n src, dst, values = [], [] , []\n for row in range(road.shape[0]):\n x = road[\"x\"][row]\n y = road[\"y\"][row]\n idx = coords[x][y]['idx']\n val = coords[x][y]['val']\n # if val[0] != road[row][:3][0]:\n # assert(False)\n for c in neighbors(road, coords, x, y, diagonal):\n src.append(idx)\n dst.append(c)\n values.append(val)\n return src, dst #, values",
"_____no_output_____"
],
[
"device = torch.cuda.current_device()",
"_____no_output_____"
],
[
"class RoadDataset(DGLDataset):\n def __init__(self, states):\n self.states = states\n super().__init__(name='road_graphs')\n\n def process(self):\n self.graphs = []\n self.labels = []\n self.state = []\n for st in self.states.keys():\n # for st in [\"TX\"]:\n print(st)\n for i in range(len(self.states[st][0])):\n print(i)\n img = states[st][0][i]\n coords = {}\n vals = []\n print(img.shape[0])\n for j in range(img.shape[0]):\n # print(img[j].shape)\n lon = img[\"x\"][j].astype(int)\n # print(lon)\n lat = img[\"y\"][j].astype(int)\n val = [img[\"B2\"][j], img[\"B3\"][j], img[\"B4\"][j]]\n vals.append(val)\n if lon not in coords:\n coords[lon] = {}\n coords[lon][lat] = {'idx' : j, 'val' : val}\n src, dst = src_dst(img, coords)\n #src, dst, values = src_dst(img, coords)\n # print(np.mean(src), np.mean(dst), np.mean(values))\n graph = dgl.graph((src, dst), num_nodes=img.shape[0])\n graph.ndata['feat'] = torch.from_numpy(np.array(vals))\n #graph = graph.add_self_loop(graph)\n graph = graph.to(device)\n self.graphs.append(graph)\n self.labels.append(self.states[st][1][i])\n self.state.append(st)\n # assert(False)\n\n def __getitem__(self, i):\n return self.graphs[i], self.labels[i], self.state[i]\n\n def __len__(self):\n return len(self.graphs)\n",
"_____no_output_____"
],
[
"class RoadDatasetLoad(DGLDataset):\n def __init__(self, states):\n self.states = states\n super().__init__(name='road_graphs')\n\n def process(self):\n self.graphs = load_graphs(\"graphs/data_new.bin\")[0]\n self.labels = np.loadtxt(\"graphs/labels_new.csv\")\n self.state = np.loadtxt(\"graphs/states_new.csv\", dtype=np.str)\n\n\n def __getitem__(self, i):\n return self.graphs[i], self.labels[i]#, self.state[i]\n\n def __len__(self):\n return len(self.graphs)",
"_____no_output_____"
],
[
"Road_Graphs = RoadDataset(states)",
"_____no_output_____"
],
[
"dataset = Road_Graphs",
"_____no_output_____"
],
[
"dataset[100]",
"_____no_output_____"
],
[
"# Road_Graphs = RoadDataset(states)\nsave_graphs('graphs/data_new.bin', dataset.graphs)\nlabels = np.array(dataset.labels)\nstates = np.array(dataset.state)\nnp.savetxt(\"graphs/labels_new.csv\", labels)\nnp.savetxt('graphs/states_new.csv', states, fmt=\"%s\")",
"_____no_output_____"
],
[
"Road_Load = RoadDatasetLoad(states)",
"_____no_output_____"
],
[
"dataset_save = dataset",
"_____no_output_____"
],
[
"# Generate a synthetic dataset with 10000 graphs, ranging from 10 to 500 nodes.\n# dataset = dgl.data.GINDataset('PROTEINS', self_loop=True)\ndataset = Road_Load",
"_____no_output_____"
]
],
[
[
"## Train the Model",
"_____no_output_____"
]
],
[
[
"# X = dataset[:][0]\n# y = dataset[:][1]",
"_____no_output_____"
],
[
"print(dataset.state[0:37])\nprint(dataset.state[37:64])\nprint(dataset.state[64:88])\nprint(dataset.state[88:119])\nprint(dataset.state[119:124])",
"_____no_output_____"
],
[
"from dgl.dataloading import GraphDataLoader\nfrom torch.utils.data.sampler import SubsetRandomSampler\nfrom torch.utils.data import DataLoader",
"_____no_output_____"
],
[
"state_val = False\none_sample = False\nstate = \"TX\"\nlookup_state = {\"CA\" : 0, \"KS\" : 1, \"MT\" : 2, \"TX\" : 3, \"OH\" : 4}\nstate_idxs = [(0, 37), (37, 64), (64, 88), (88, 119), (119, 124)]",
"_____no_output_____"
],
[
"num_examples = len(dataset)\nif state_val:\n x = torch.arange(num_examples)\n start = state_idxs[lookup_state[state]][0]\n end = state_idxs[lookup_state[state]][1]\n test_sample = x[start + 3: end]\n val_sample = x[start : start + 3]\n train_sample = torch.cat((x[:start], x[end:]))\n train_sample = train_sample[torch.randperm(train_sample.shape[0])]\n print(train_sample)\nelse:\n num_train = int(num_examples * 0.7)\n num_val = int(num_examples * 0.85)\n x = torch.randperm(num_examples)\n train_sample = x[:num_train]\n val_sample = x[num_train: num_val]\n test_sample = x[num_val:]\n\ntrain_sampler = SubsetRandomSampler(train_sample)\nval_sampler = SubsetRandomSampler(val_sample)\ntest_sampler = SubsetRandomSampler(test_sample)\n\ntrain_dataloader = GraphDataLoader(\n dataset, sampler=train_sampler, batch_size=16, drop_last=False)\nval_dataloader = GraphDataLoader(\n dataset, sampler=val_sampler, batch_size=16, drop_last=False)\ntest_dataloader = GraphDataLoader(\n dataset, sampler=test_sampler, batch_size=16, drop_last=False)",
"_____no_output_____"
],
[
"# print(train_sample, val_sample, test_sample)",
"_____no_output_____"
],
[
"it = iter(test_dataloader)",
"_____no_output_____"
],
[
"batch = next(it)\nprint(batch)\nbatched_graph, labels = batch\nprint('Number of nodes for each graph element in the batch:', batched_graph.batch_num_nodes())\nprint('Number of edges for each graph element in the batch:', batched_graph.batch_num_edges())\n\n# Recover the original graph elements from the minibatch\ngraphs = dgl.unbatch(batched_graph)\nprint('The original graphs in the minibatch:')\nprint(graphs)\nprint(labels)",
"[Graph(num_nodes=1391195, num_edges=11304515,\n ndata_schemes={'feat': Scheme(shape=(3,), dtype=torch.int64)}\n edata_schemes={}), tensor([1965., 1639., 3670., 826., 3139., 2746., 2037., 590., 1769., 484.,\n 2131., 311., 934., 2246., 2940., 2316.], dtype=torch.float64)]\nNumber of nodes for each graph element in the batch: tensor([ 69951, 63068, 69906, 127224, 52690, 118378, 66583, 70803, 118378,\n 84674, 70549, 84389, 92248, 113370, 70606, 118378])\nNumber of edges for each graph element in the batch: tensor([ 571747, 514596, 571380, 1029080, 429136, 963178, 542709, 572075,\n 963178, 683436, 576661, 677637, 750020, 919372, 577132, 963178])\nThe original graphs in the minibatch:\n[Graph(num_nodes=69951, num_edges=571747,\n ndata_schemes={'feat': Scheme(shape=(3,), dtype=torch.int64)}\n edata_schemes={}), Graph(num_nodes=63068, num_edges=514596,\n ndata_schemes={'feat': Scheme(shape=(3,), dtype=torch.int64)}\n edata_schemes={}), Graph(num_nodes=69906, num_edges=571380,\n ndata_schemes={'feat': Scheme(shape=(3,), dtype=torch.int64)}\n edata_schemes={}), Graph(num_nodes=127224, num_edges=1029080,\n ndata_schemes={'feat': Scheme(shape=(3,), dtype=torch.int64)}\n edata_schemes={}), Graph(num_nodes=52690, num_edges=429136,\n ndata_schemes={'feat': Scheme(shape=(3,), dtype=torch.int64)}\n edata_schemes={}), Graph(num_nodes=118378, num_edges=963178,\n ndata_schemes={'feat': Scheme(shape=(3,), dtype=torch.int64)}\n edata_schemes={}), Graph(num_nodes=66583, num_edges=542709,\n ndata_schemes={'feat': Scheme(shape=(3,), dtype=torch.int64)}\n edata_schemes={}), Graph(num_nodes=70803, num_edges=572075,\n ndata_schemes={'feat': Scheme(shape=(3,), dtype=torch.int64)}\n edata_schemes={}), Graph(num_nodes=118378, num_edges=963178,\n ndata_schemes={'feat': Scheme(shape=(3,), dtype=torch.int64)}\n edata_schemes={}), Graph(num_nodes=84674, num_edges=683436,\n ndata_schemes={'feat': Scheme(shape=(3,), dtype=torch.int64)}\n edata_schemes={}), Graph(num_nodes=70549, num_edges=576661,\n ndata_schemes={'feat': Scheme(shape=(3,), dtype=torch.int64)}\n edata_schemes={}), Graph(num_nodes=84389, num_edges=677637,\n ndata_schemes={'feat': Scheme(shape=(3,), dtype=torch.int64)}\n edata_schemes={}), Graph(num_nodes=92248, num_edges=750020,\n ndata_schemes={'feat': Scheme(shape=(3,), dtype=torch.int64)}\n edata_schemes={}), Graph(num_nodes=113370, num_edges=919372,\n ndata_schemes={'feat': Scheme(shape=(3,), dtype=torch.int64)}\n edata_schemes={}), Graph(num_nodes=70606, num_edges=577132,\n ndata_schemes={'feat': Scheme(shape=(3,), dtype=torch.int64)}\n edata_schemes={}), Graph(num_nodes=118378, num_edges=963178,\n ndata_schemes={'feat': Scheme(shape=(3,), dtype=torch.int64)}\n edata_schemes={})]\ntensor([1965., 1639., 3670., 826., 3139., 2746., 2037., 590., 1769., 484.,\n 2131., 311., 934., 2246., 2940., 2316.], dtype=torch.float64)\n"
],
[
"from dgl.nn import GraphConv, DenseGraphConv, GATConv\n\nclass GCN(nn.Module):\n def __init__(self, in_feats, conv_hidden, lin_hidden):\n super(GCN, self).__init__()\n self.conv_layers = nn.ModuleList()\n self.LR = nn.LeakyReLU(0.2)\n self.lin_layers = nn.ModuleList()\n self.conv_layers.append(GraphConv(in_feats, conv_hidden[0]))\n for i in range(1, len(conv_hidden)):\n self.conv_layers.append(GraphConv(conv_hidden[i - 1], conv_hidden[i]))\n for i in range(1, len(lin_hidden) - 1):\n self.lin_layers.append(nn.Linear(lin_hidden[i - 1], lin_hidden[i]))\n #self.lin_layers.append(nn.BatchNorm1d(lin_hidden[i]))\n self.lin_layers.append(nn.Linear(lin_hidden[-2], lin_hidden[-1]))\n \n\n def forward(self, g, in_feat):\n output = in_feat\n for layer in self.conv_layers:\n output = self.LR(layer(g, output))\n # print(torch.mean(output))\n graphs = dgl.unbatch(g)\n flat_arr = torch.zeros((g.batch_size, max_pixs))\n prev = 0\n # print(\"Before\", torch.mean(output))\n for i in range(len(batched_graph.batch_num_nodes())):\n end = prev + int(batched_graph.batch_num_nodes()[i].item())\n entry = output[prev: end]\n entry = entry / int(g.batch_num_nodes()[i].item())\n pad_val = int(torch.mean(entry).item())\n pad_length = (max_pixs - entry.shape[0]) // 2\n entry = torch.nn.functional.pad(entry.flatten(), (pad_length, pad_length), value=pad_val)\n flat_arr[i][:entry.shape[0]] = entry\n prev = end\n flat_arr = flat_arr.to(device)\n #print(\"After\", torch.mean(flat_arr))\n output = flat_arr\n for i, layer in enumerate(self.lin_layers):\n output = layer(output)\n if i != (len(self.lin_layers) - 1):\n output = self.LR(output)\n #print(flat_arr.shape)\n # g.ndata['h'] = h\n # print(dgl.mean_nodes(g, 'h'))\n # assert(False)\n return output #dgl.mean_nodes(g, 'h')",
"_____no_output_____"
],
[
"# # Create the model with given dimensions\nmodel = GCN(3, [10, 10, 1], [max_pixs,1000, 500, 100, 50, 10, 1])\n# model = GCN(3, 16, 1)\nmodel.cuda()\ncriterion = nn.MSELoss()\n#model.to('cuda:0')\noptimizer = torch.optim.Adam(model.parameters(), lr=0.01)",
"_____no_output_____"
],
[
"del criterion\ndel optimizer\ndel model\ntorch.cuda.empty_cache()",
"_____no_output_____"
],
[
"def init_weights(m):\n if type(m) == nn.Linear:\n torch.nn.init.xavier_uniform(m.weight)\n m.bias.data.fill_(0.01)\nmodel.apply(init_weights)",
"/usr/local/lib/python3.7/dist-packages/ipykernel_launcher.py:3: UserWarning: nn.init.xavier_uniform is now deprecated in favor of nn.init.xavier_uniform_.\n This is separate from the ipykernel package so we can avoid doing imports until\n"
],
[
"best_model = model\nmin_val = 1e9",
"_____no_output_____"
],
[
"j = 0\nfor epoch in range(100):\n loss_tot = 0\n loss = 0\n batches = 0\n model.train()\n for batched_graph, labels in train_dataloader:\n batched_graph = batched_graph.to(device)\n labels = labels.to(device)\n pred = model(batched_graph, batched_graph.ndata['feat'].float()) \n # print(pred, labels)\n labels = labels.to(device)\n loss = criterion(pred, labels.reshape(labels.shape[0], 1).float())\n loss_tot += loss.item()\n batches += 1\n optimizer.zero_grad()\n loss.backward()\n optimizer.step()\n if j % 10 == 0:\n print(\"Train Loss:\", loss_tot / batches)\n num_tests = 0\n loss_i = 0\n with torch.no_grad():\n model.eval()\n for batched_graph, labels in val_dataloader:\n batched_graph = batched_graph.to(device)\n labels = labels.to(device)\n pred = model(batched_graph, batched_graph.ndata['feat'].float())\n loss_i += criterion(pred, labels.reshape(labels.shape[0], 1).float()).item()\n # x.extend([x[0] for x in pred.cpu().detach().numpy().tolist()])\n # y.extend([x[0] for x in labels.reshape(labels.shape[0], 1).cpu().detach().numpy().tolist()])\n # print(type(pred))\n num_tests += 1\n val_loss = loss_i / num_tests\n if j % 10 == 0:\n print('Val loss:', val_loss)\n # val_loss.append(loss_v.item())\n if val_loss < min_val:\n print(\"new_best:\", val_loss)\n min_val = val_loss\n best_model = copy.deepcopy(model)\n j += 1",
"Train Loss: 76757.93666992188\nVal loss: 694629.7125\nTrain Loss: 12493.833142089843\nVal loss: 768211.99375\nTrain Loss: 8094.956567382813\nVal loss: 809729.95\nTrain Loss: 58567.025073242185\nVal loss: 682895.2\nTrain Loss: 6346.937719726562\nVal loss: 677687.85\nTrain Loss: 6815.377615356445\nVal loss: 633869.85625\nTrain Loss: 2691.4429260253905\nVal loss: 727192.43125\nTrain Loss: 2097.0757019042967\nVal loss: 683974.8625\nTrain Loss: 1544.1816467285157\nVal loss: 667326.5125\nTrain Loss: 1331830.2625\nVal loss: 1113972.8125\n"
],
[
"# num_correct = 0\nnum_tests = 0\nx = []\ny = []\nloss = 0\nwith torch.no_grad():\n for batched_graph, labels in test_dataloader:\n # print(batched_graph)\n batched_graph = batched_graph.to(device)\n labels = labels.to(device)\n pred = best_model(batched_graph, batched_graph.ndata['feat'].float())\n loss += criterion(pred, labels.reshape(labels.shape[0], 1).float()).item()\n x.extend([x[0] for x in pred.cpu().detach().numpy().tolist()])\n y.extend([x[0] for x in labels.reshape(labels.shape[0], 1).cpu().detach().numpy().tolist()])\n num_tests += 1\n\nprint('Test loss:', loss / num_tests)",
"Test loss: 543203.75625\n"
],
[
"x_temp = y\ny_temp = x\n# print(y_temp)\n# for i in range(len(y_temp)):\n# if y_temp[i] < 600:\n# y_temp.pop(i)\n# x_temp.pop(i)\n# break\nx_plot = np.array(y_temp)\ny_plot = np.array(x_temp)\nnew_x = np.array(x_plot).reshape(-1,1)\nnew_y = np.array(y_plot)\nfit = LinearRegression().fit(new_x, new_y)\nscore = fit.score(new_x, new_y)\nplt.xlabel(\"Prediction\")\nplt.ylabel(\"Actual Traffic\")\nprint(score)\nplt.scatter(new_x, new_y)\naxes = plt.gca()\nx_vals = np.array(axes.get_xlim())\ny_vals = x_vals\nplt.plot(x_vals, y_vals, '--')\npre_y = fit.predict(new_x)\n# plt.plot\nplt.plot(new_x, pre_y)\nplt.plot(x_vals, y_vals, '--')\n# plt.savefig(\"GCN_MSE_143_r_881.png\")\nplt.show()",
"0.4706106732383747\n"
],
[
"y",
"_____no_output_____"
],
[
"labels",
"_____no_output_____"
],
[
"class ChebNet(nn.Module):\n def __init__(self,\n k,\n in_feats,\n hiddens,\n out_feats):\n super(ChebNet, self).__init__()\n self.pool = nn.MaxPool1d(2)\n self.layers = nn.ModuleList()\n self.readout = MaxPooling()\n\n # Input layer\n self.layers.append(\n ChebConv(in_feats, hiddens[0], k))\n\n for i in range(1, len(hiddens)):\n self.layers.append(\n ChebConv(hiddens[i - 1], hiddens[i], k))\n\n self.cls = nn.Sequential(\n nn.Linear(hiddens[-1], out_feats),\n nn.LogSoftmax()\n )\n\n def forward(self, g_arr, feat):\n for g, layer in zip(g_arr, self.layers):\n feat = self.pool(layer(g, feat, [2] * g.batch_size).transpose(-1, -2).unsqueeze(0))\\\n .squeeze(0).transpose(-1, -2)\n return self.cls(self.readout(g_arr[-1], feat))",
"_____no_output_____"
],
[
"",
"_____no_output_____"
]
]
] | [
"code",
"markdown",
"code",
"markdown",
"code",
"markdown",
"code"
] | [
[
"code",
"code",
"code",
"code"
],
[
"markdown"
],
[
"code"
],
[
"markdown"
],
[
"code",
"code",
"code",
"code",
"code",
"code",
"code",
"code",
"code",
"code",
"code",
"code"
],
[
"markdown"
],
[
"code",
"code",
"code",
"code",
"code",
"code",
"code",
"code",
"code",
"code",
"code",
"code",
"code",
"code",
"code",
"code",
"code",
"code",
"code",
"code"
]
] |
d0796ac96932f25ba952abba514e38826cc3f3ab | 45,048 | ipynb | Jupyter Notebook | ChatBot.ipynb | DEK11/Chatbot-RNN | c7aeff22b5884c1a5365a334dd7ed81a75b06c22 | [
"Apache-2.0"
] | null | null | null | ChatBot.ipynb | DEK11/Chatbot-RNN | c7aeff22b5884c1a5365a334dd7ed81a75b06c22 | [
"Apache-2.0"
] | null | null | null | ChatBot.ipynb | DEK11/Chatbot-RNN | c7aeff22b5884c1a5365a334dd7ed81a75b06c22 | [
"Apache-2.0"
] | null | null | null | 38.37138 | 233 | 0.532721 | [
[
[
"!wget http://www.cs.cornell.edu/~cristian/data/cornell_movie_dialogs_corpus.zip",
"--2018-12-09 17:15:59-- http://www.cs.cornell.edu/~cristian/data/cornell_movie_dialogs_corpus.zip\nResolving www.cs.cornell.edu (www.cs.cornell.edu)... 132.236.207.20\nConnecting to www.cs.cornell.edu (www.cs.cornell.edu)|132.236.207.20|:80... connected.\nHTTP request sent, awaiting response... 200 OK\nLength: 9916637 (9.5M) [application/zip]\nSaving to: ‘cornell_movie_dialogs_corpus.zip’\n\ncornell_movie_dialo 100%[===================>] 9.46M 6.89MB/s in 1.4s \n\n2018-12-09 17:16:00 (6.89 MB/s) - ‘cornell_movie_dialogs_corpus.zip’ saved [9916637/9916637]\n\n"
],
[
"!unzip cornell_movie_dialogs_corpus.zip -d dialogues",
"Archive: cornell_movie_dialogs_corpus.zip\n creating: dialogues/cornell movie-dialogs corpus/\n inflating: dialogues/cornell movie-dialogs corpus/.DS_Store \n creating: dialogues/__MACOSX/\n creating: dialogues/__MACOSX/cornell movie-dialogs corpus/\n inflating: dialogues/__MACOSX/cornell movie-dialogs corpus/._.DS_Store \n inflating: dialogues/cornell movie-dialogs corpus/chameleons.pdf \n inflating: dialogues/__MACOSX/cornell movie-dialogs corpus/._chameleons.pdf \n inflating: dialogues/cornell movie-dialogs corpus/movie_characters_metadata.txt \n inflating: dialogues/cornell movie-dialogs corpus/movie_conversations.txt \n inflating: dialogues/cornell movie-dialogs corpus/movie_lines.txt \n inflating: dialogues/cornell movie-dialogs corpus/movie_titles_metadata.txt \n inflating: dialogues/cornell movie-dialogs corpus/raw_script_urls.txt \n inflating: dialogues/cornell movie-dialogs corpus/README.txt \n inflating: dialogues/__MACOSX/cornell movie-dialogs corpus/._README.txt \n"
],
[
"import torch\nfrom torch.jit import script, trace\nimport torch.nn as nn\nfrom torch import optim\nimport torch.nn.functional as F\nimport csv\nimport random\nimport re\nimport os\nimport unicodedata\nimport codecs\nfrom io import open\nimport itertools\nimport math",
"_____no_output_____"
],
[
"device = torch.device(\"cuda\" if torch.cuda.is_available() else \"cpu\")",
"_____no_output_____"
],
[
"device",
"_____no_output_____"
],
[
"corpus_name = \"cornell movie-dialogs corpus\"\ncorpus = os.path.join(\"dialogues\", corpus_name)\n\ndef printLines(file, n=10):\n with open(file, 'rb') as datafile:\n lines = datafile.readlines()\n for line in lines[:n]:\n print(line)\n\nprintLines(os.path.join(corpus, \"movie_lines.txt\"))",
"b'L1045 +++$+++ u0 +++$+++ m0 +++$+++ BIANCA +++$+++ They do not!\\n'\nb'L1044 +++$+++ u2 +++$+++ m0 +++$+++ CAMERON +++$+++ They do to!\\n'\nb'L985 +++$+++ u0 +++$+++ m0 +++$+++ BIANCA +++$+++ I hope so.\\n'\nb'L984 +++$+++ u2 +++$+++ m0 +++$+++ CAMERON +++$+++ She okay?\\n'\nb\"L925 +++$+++ u0 +++$+++ m0 +++$+++ BIANCA +++$+++ Let's go.\\n\"\nb'L924 +++$+++ u2 +++$+++ m0 +++$+++ CAMERON +++$+++ Wow\\n'\nb\"L872 +++$+++ u0 +++$+++ m0 +++$+++ BIANCA +++$+++ Okay -- you're gonna need to learn how to lie.\\n\"\nb'L871 +++$+++ u2 +++$+++ m0 +++$+++ CAMERON +++$+++ No\\n'\nb'L870 +++$+++ u0 +++$+++ m0 +++$+++ BIANCA +++$+++ I\\'m kidding. You know how sometimes you just become this \"persona\"? And you don\\'t know how to quit?\\n'\nb'L869 +++$+++ u0 +++$+++ m0 +++$+++ BIANCA +++$+++ Like my fear of wearing pastels?\\n'\n"
],
[
"printLines(os.path.join(corpus, \"movie_conversations.txt\"))",
"b\"u0 +++$+++ u2 +++$+++ m0 +++$+++ ['L194', 'L195', 'L196', 'L197']\\n\"\nb\"u0 +++$+++ u2 +++$+++ m0 +++$+++ ['L198', 'L199']\\n\"\nb\"u0 +++$+++ u2 +++$+++ m0 +++$+++ ['L200', 'L201', 'L202', 'L203']\\n\"\nb\"u0 +++$+++ u2 +++$+++ m0 +++$+++ ['L204', 'L205', 'L206']\\n\"\nb\"u0 +++$+++ u2 +++$+++ m0 +++$+++ ['L207', 'L208']\\n\"\nb\"u0 +++$+++ u2 +++$+++ m0 +++$+++ ['L271', 'L272', 'L273', 'L274', 'L275']\\n\"\nb\"u0 +++$+++ u2 +++$+++ m0 +++$+++ ['L276', 'L277']\\n\"\nb\"u0 +++$+++ u2 +++$+++ m0 +++$+++ ['L280', 'L281']\\n\"\nb\"u0 +++$+++ u2 +++$+++ m0 +++$+++ ['L363', 'L364']\\n\"\nb\"u0 +++$+++ u2 +++$+++ m0 +++$+++ ['L365', 'L366']\\n\"\n"
],
[
"# load movie_lines.txt\ndef loadLines(fileName, fields):\n lines = {}\n with open(fileName, 'r', encoding='iso-8859-1') as f:\n for line in f:\n values = line.split(\" +++$+++ \")\n lineObj = {}\n for i, field in enumerate(fields):\n lineObj[field] = values[i]\n lines[lineObj['lineID']] = lineObj\n return lines",
"_____no_output_____"
],
[
"# # load movie_conversations.txt\ndef loadConversations(fileName, lines, fields):\n conversations = []\n with open(fileName, 'r', encoding='iso-8859-1') as f:\n for line in f:\n values = line.split(\" +++$+++ \")\n convObj = {}\n for i, field in enumerate(fields):\n convObj[field] = values[i]\n lineIds = eval(convObj[\"utteranceIDs\"])\n convObj[\"lines\"] = []\n for lineId in lineIds:\n convObj[\"lines\"].append(lines[lineId])\n conversations.append(convObj)\n return conversations",
"_____no_output_____"
],
[
"def extractSentencePairs(conversations):\n qa_pairs = []\n for conversation in conversations:\n for i in range(len(conversation[\"lines\"]) - 1): # We ignore the last line (no answer for it)\n inputLine = conversation[\"lines\"][i][\"text\"].strip()\n targetLine = conversation[\"lines\"][i+1][\"text\"].strip()\n if inputLine and targetLine:\n qa_pairs.append([inputLine, targetLine])\n return qa_pairs",
"_____no_output_____"
],
[
"MOVIE_LINES_FIELDS = [\"lineID\", \"characterID\", \"movieID\", \"character\", \"text\"]\nMOVIE_CONVERSATIONS_FIELDS = [\"character1ID\", \"character2ID\", \"movieID\", \"utteranceIDs\"]\n\nlines = loadLines(os.path.join(corpus, \"movie_lines.txt\"), MOVIE_LINES_FIELDS)\nconversations = loadConversations(os.path.join(corpus, \"movie_conversations.txt\"), lines, MOVIE_CONVERSATIONS_FIELDS)",
"_____no_output_____"
],
[
"lines['L194']",
"_____no_output_____"
],
[
"conversations[:1]",
"_____no_output_____"
],
[
"datafile = os.path.join(corpus, \"formatted_movie_lines.txt\")\n\ndelimiter = '\\t'\ndelimiter = str(codecs.decode(delimiter, \"unicode_escape\"))\n\n\nwith open(datafile, 'w', encoding='utf-8') as outputfile:\n writer = csv.writer(outputfile, delimiter=delimiter, lineterminator='\\n')\n for pair in extractSentencePairs(conversations):\n writer.writerow(pair)\n\nprint(\"\\nSample lines from file:\")\nprintLines(datafile)",
"\nWriting newly formatted file...\n\nSample lines from file:\nb\"Can we make this quick? Roxanne Korrine and Andrew Barrett are having an incredibly horrendous public break- up on the quad. Again.\\tWell, I thought we'd start with pronunciation, if that's okay with you.\\n\"\nb\"Well, I thought we'd start with pronunciation, if that's okay with you.\\tNot the hacking and gagging and spitting part. Please.\\n\"\nb\"Not the hacking and gagging and spitting part. Please.\\tOkay... then how 'bout we try out some French cuisine. Saturday? Night?\\n\"\nb\"You're asking me out. That's so cute. What's your name again?\\tForget it.\\n\"\nb\"No, no, it's my fault -- we didn't have a proper introduction ---\\tCameron.\\n\"\nb\"Cameron.\\tThe thing is, Cameron -- I'm at the mercy of a particularly hideous breed of loser. My sister. I can't date until she does.\\n\"\nb\"The thing is, Cameron -- I'm at the mercy of a particularly hideous breed of loser. My sister. I can't date until she does.\\tSeems like she could get a date easy enough...\\n\"\nb'Why?\\tUnsolved mystery. She used to be really popular when she started high school, then it was just like she got sick of it or something.\\n'\nb\"Unsolved mystery. She used to be really popular when she started high school, then it was just like she got sick of it or something.\\tThat's a shame.\\n\"\nb'Gosh, if only we could find Kat a boyfriend...\\tLet me see what I can do.\\n'\n"
],
[
"PAD_token = 0 # padding short sentences\nSOS_token = 1 # Start-of-sentence \nEOS_token = 2 # End-of-sentence \n\nclass Voc:\n def __init__(self, name):\n self.name = name\n self.trimmed = False\n self.word2index = {}\n self.word2count = {}\n self.index2word = {PAD_token: \"PAD\", SOS_token: \"SOS\", EOS_token: \"EOS\"}\n self.num_words = 3 # Count SOS, EOS, PAD\n\n def addSentence(self, sentence):\n for word in sentence.split(' '):\n self.addWord(word)\n\n def addWord(self, word):\n if word not in self.word2index:\n self.word2index[word] = self.num_words\n self.word2count[word] = 1\n self.index2word[self.num_words] = word\n self.num_words += 1\n else:\n self.word2count[word] += 1\n\n def trim(self, min_count):\n if self.trimmed:\n return\n self.trimmed = True\n\n keep_words = []\n\n for k, v in self.word2count.items():\n if v >= min_count:\n keep_words.append(k)\n\n print('keep_words {} / {} = {:.4f}'.format(\n len(keep_words), len(self.word2index), len(keep_words) / len(self.word2index)\n ))\n\n # Reinitialize\n self.word2index = {}\n self.word2count = {}\n self.index2word = {PAD_token: \"PAD\", SOS_token: \"SOS\", EOS_token: \"EOS\"}\n self.num_words = 3 \n\n for word in keep_words:\n self.addWord(word)",
"_____no_output_____"
],
[
"MAX_LENGTH = 10 \ndef unicodeToAscii(s):\n return ''.join(\n c for c in unicodedata.normalize('NFD', s)\n if unicodedata.category(c) != 'Mn'\n )\n\ndef normalizeString(s):\n s = unicodeToAscii(s.lower().strip())\n s = re.sub(r\"([.!?])\", r\" \\1\", s)\n s = re.sub(r\"[^a-zA-Z.!?]+\", r\" \", s)\n s = re.sub(r\"\\s+\", r\" \", s).strip()\n return s",
"_____no_output_____"
],
[
"def readFile(datafile):\n lines = open(datafile, encoding='utf-8').read().strip().split('\\n')\n pairs = [[normalizeString(s) for s in l.split('\\t')] for l in lines]\n return pairs\n\ndef filterPair(p):\n return len(p[0].split(' ')) < MAX_LENGTH and len(p[1].split(' ')) < MAX_LENGTH\n\ndef filterPairs(pairs):\n return [pair for pair in pairs if filterPair(pair)]",
"_____no_output_____"
],
[
"def loadPrepareData(corpus_name, datafile):\n pairs = readFile(datafile)\n print(\"Read {!s} sentence pairs\".format(len(pairs)))\n pairs = filterPairs(pairs)\n print(\"Trimmed to {!s} sentence pairs\".format(len(pairs)))\n print(\"Counting words...\")\n voc = Voc(corpus_name)\n for pair in pairs:\n voc.addSentence(pair[0])\n voc.addSentence(pair[1])\n print(\"Counted words:\", voc.num_words)\n return voc, pairs",
"_____no_output_____"
],
[
"voc, pairs = loadPrepareData(corpus_name, datafile)\nprint(\"\\nsanity check\\npairs:\")\nfor pair in pairs[:10]:\n print(pair)",
"Read 221282 sentence pairs\nTrimmed to 64271 sentence pairs\nCounting words...\nCounted words: 18008\n\nsanity check\npairs:\n['there .', 'where ?']\n['you have my word . as a gentleman', 'you re sweet .']\n['hi .', 'looks like things worked out tonight huh ?']\n['you know chastity ?', 'i believe we share an art instructor']\n['have fun tonight ?', 'tons']\n['well no . . .', 'then that s all you had to say .']\n['then that s all you had to say .', 'but']\n['but', 'you always been this selfish ?']\n['do you listen to this crap ?', 'what crap ?']\n['what good stuff ?', 'the real you .']\n"
],
[
"MIN_COUNT = 3\n\ndef trimRareWords(voc, pairs, MIN_COUNT):\n voc.trim(MIN_COUNT)\n # Filter pairs with trimmed words\n keep_pairs = []\n for pair in pairs:\n input_sentence = pair[0]\n output_sentence = pair[1]\n keep_input = True\n keep_output = True\n for word in input_sentence.split(' '):\n if word not in voc.word2index:\n keep_input = False\n break\n for word in output_sentence.split(' '):\n if word not in voc.word2index:\n keep_output = False\n break\n\n if keep_input and keep_output:\n keep_pairs.append(pair)\n\n print(\"Trimmed from {} pairs to {}, {:.4f} of total\".format(len(pairs), len(keep_pairs), len(keep_pairs) / len(pairs)))\n return keep_pairs\n\n\npairs = trimRareWords(voc, pairs, MIN_COUNT)",
"keep_words 7823 / 18005 = 0.4345\nTrimmed from 64271 pairs to 53165, 0.8272 of total\n"
],
[
"def indexesFromSentence(voc, sentence):\n return [voc.word2index[word] for word in sentence.split(' ')] + [EOS_token]\n\ndef zeroPadding(l, fillvalue=PAD_token):\n return list(itertools.zip_longest(*l, fillvalue=fillvalue))\n\ndef binaryMatrix(l, value=PAD_token):\n m = []\n for i, seq in enumerate(l):\n m.append([])\n for token in seq:\n if token == PAD_token:\n m[i].append(0)\n else:\n m[i].append(1)\n return m",
"_____no_output_____"
],
[
"def inputVar(l, voc):\n indexes_batch = [indexesFromSentence(voc, sentence) for sentence in l]\n lengths = torch.tensor([len(indexes) for indexes in indexes_batch])\n padList = zeroPadding(indexes_batch)\n padVar = torch.LongTensor(padList)\n return padVar, lengths\n\ndef outputVar(l, voc):\n indexes_batch = [indexesFromSentence(voc, sentence) for sentence in l]\n max_target_len = max([len(indexes) for indexes in indexes_batch])\n padList = zeroPadding(indexes_batch)\n mask = binaryMatrix(padList)\n mask = torch.ByteTensor(mask)\n padVar = torch.LongTensor(padList)\n return padVar, mask, max_target_len",
"_____no_output_____"
],
[
"def batch2TrainData(voc, pair_batch):\n pair_batch.sort(key=lambda x: len(x[0].split(\" \")), reverse=True)\n input_batch, output_batch = [], []\n for pair in pair_batch:\n input_batch.append(pair[0])\n output_batch.append(pair[1])\n inp, lengths = inputVar(input_batch, voc)\n output, mask, max_target_len = outputVar(output_batch, voc)\n return inp, lengths, output, mask, max_target_len",
"_____no_output_____"
],
[
"small_batch_size = 5\nbatches = batch2TrainData(voc, [random.choice(pairs) for _ in range(small_batch_size)])\ninput_variable, lengths, target_variable, mask, max_target_len = batches\n\nprint(\"input_variable:\", input_variable)\nprint(\"lengths:\", lengths)\nprint(\"target_variable:\", target_variable)\nprint(\"mask:\", mask)\nprint(\"max_target_len:\", max_target_len)",
"input_variable: tensor([[ 51, 680, 158, 25, 266],\n [ 4, 680, 53, 296, 4],\n [ 411, 4, 654, 7, 7],\n [ 83, 4, 653, 153, 14],\n [ 21, 4, 159, 7148, 266],\n [ 159, 571, 66, 2474, 4],\n [ 4, 66, 2, 2, 2],\n [ 2, 2, 0, 0, 0]])\nlengths: tensor([ 8, 8, 7, 7, 7])\ntarget_variable: tensor([[ 169, 25, 199, 319, 1084],\n [ 50, 450, 76, 18, 266],\n [ 6, 542, 37, 36, 25],\n [ 2, 40, 112, 2, 200],\n [ 0, 9, 18, 0, 266],\n [ 0, 3347, 3004, 0, 4],\n [ 0, 66, 4, 0, 2],\n [ 0, 2, 2, 0, 0]])\nmask: tensor([[ 1, 1, 1, 1, 1],\n [ 1, 1, 1, 1, 1],\n [ 1, 1, 1, 1, 1],\n [ 1, 1, 1, 1, 1],\n [ 0, 1, 1, 0, 1],\n [ 0, 1, 1, 0, 1],\n [ 0, 1, 1, 0, 1],\n [ 0, 1, 1, 0, 0]], dtype=torch.uint8)\nmax_target_len: 8\n"
]
],
[
[
"## Bi directional GRU encoder",
"_____no_output_____"
]
],
[
[
"class EncoderRNN(nn.Module):\n def __init__(self, hidden_size, embedding, n_layers=1, dropout=0):\n super(EncoderRNN, self).__init__()\n self.n_layers = n_layers\n self.hidden_size = hidden_size\n self.embedding = embedding\n self.gru = nn.GRU(hidden_size, hidden_size, n_layers,\n dropout=(0 if n_layers == 1 else dropout), bidirectional=True)\n\n def forward(self, input_seq, input_lengths, hidden=None):\n embedded = self.embedding(input_seq)\n packed = torch.nn.utils.rnn.pack_padded_sequence(embedded, input_lengths)\n outputs, hidden = self.gru(packed, hidden)\n outputs, _ = torch.nn.utils.rnn.pad_packed_sequence(outputs)\n outputs = outputs[:, :, :self.hidden_size] + outputs[:, : ,self.hidden_size:]\n return outputs, hidden",
"_____no_output_____"
]
],
[
[
"### Attention module",
"_____no_output_____"
]
],
[
[
"class Attn(torch.nn.Module):\n def __init__(self, method, hidden_size):\n super(Attn, self).__init__()\n self.method = method\n if self.method not in ['dot', 'general', 'concat']:\n raise ValueError(self.method, \"is not an appropriate attention method.\")\n self.hidden_size = hidden_size\n if self.method == 'general':\n self.attn = torch.nn.Linear(self.hidden_size, hidden_size)\n elif self.method == 'concat':\n self.attn = torch.nn.Linear(self.hidden_size * 2, hidden_size)\n self.v = torch.nn.Parameter(torch.FloatTensor(hidden_size))\n\n def dot_score(self, hidden, encoder_output):\n return torch.sum(hidden * encoder_output, dim=2)\n\n def general_score(self, hidden, encoder_output):\n energy = self.attn(encoder_output)\n return torch.sum(hidden * energy, dim=2)\n\n def concat_score(self, hidden, encoder_output):\n energy = self.attn(torch.cat((hidden.expand(encoder_output.size(0), -1, -1), encoder_output), 2)).tanh()\n return torch.sum(self.v * energy, dim=2)\n\n def forward(self, hidden, encoder_outputs):\n if self.method == 'general':\n attn_energies = self.general_score(hidden, encoder_outputs)\n elif self.method == 'concat':\n attn_energies = self.concat_score(hidden, encoder_outputs)\n elif self.method == 'dot':\n attn_energies = self.dot_score(hidden, encoder_outputs)\n\n attn_energies = attn_energies.t()\n\n return F.softmax(attn_energies, dim=1).unsqueeze(1)",
"_____no_output_____"
],
[
"class AttnDecoderRNN(nn.Module):\n def __init__(self, attn_model, embedding, hidden_size, output_size, n_layers=1, dropout=0.1):\n super(AttnDecoderRNN, self).__init__()\n\n self.attn_model = attn_model\n self.hidden_size = hidden_size\n self.output_size = output_size\n self.n_layers = n_layers\n self.dropout = dropout\n\n self.embedding = embedding\n self.embedding_dropout = nn.Dropout(dropout)\n self.gru = nn.GRU(hidden_size, hidden_size, n_layers, dropout=(0 if n_layers == 1 else dropout))\n self.concat = nn.Linear(hidden_size * 2, hidden_size)\n self.out = nn.Linear(hidden_size, output_size)\n self.attn = Attn(attn_model, hidden_size)\n\n def forward(self, input_step, last_hidden, encoder_outputs):\n embedded = self.embedding(input_step)\n embedded = self.embedding_dropout(embedded)\n rnn_output, hidden = self.gru(embedded, last_hidden)\n attn_weights = self.attn(rnn_output, encoder_outputs)\n context = attn_weights.bmm(encoder_outputs.transpose(0, 1))\n rnn_output = rnn_output.squeeze(0)\n context = context.squeeze(1)\n concat_input = torch.cat((rnn_output, context), 1)\n concat_output = torch.tanh(self.concat(concat_input))\n output = self.out(concat_output)\n output = F.softmax(output, dim=1)\n return output, hidden",
"_____no_output_____"
],
[
"def maskNLLLoss(inp, target, mask):\n nTotal = mask.sum()\n crossEntropy = -torch.log(torch.gather(inp, 1, target.view(-1, 1)))\n loss = crossEntropy.masked_select(mask).mean()\n loss = loss.to(device)\n return loss, nTotal.item()",
"_____no_output_____"
],
[
"def train(input_variable, lengths, target_variable, mask, max_target_len, encoder, decoder,\n encoder_optimizer, decoder_optimizer, batch_size, clip):\n\n encoder_optimizer.zero_grad()\n decoder_optimizer.zero_grad()\n\n input_variable = input_variable.to(device)\n lengths = lengths.to(device)\n target_variable = target_variable.to(device)\n mask = mask.to(device)\n\n loss = 0\n print_losses = []\n n_totals = 0\n\n encoder_outputs, encoder_hidden = encoder(input_variable, lengths)\n\n decoder_input = torch.LongTensor([[SOS_token for _ in range(batch_size)]])\n decoder_input = decoder_input.to(device)\n\n decoder_hidden = encoder_hidden[:decoder.n_layers]\n\n use_teacher_forcing = True if random.random() < teacher_forcing_ratio else False\n\n if use_teacher_forcing:\n for t in range(max_target_len):\n decoder_output, decoder_hidden = decoder(decoder_input, decoder_hidden, encoder_outputs)\n decoder_input = target_variable[t].view(1, -1)\n mask_loss, nTotal = maskNLLLoss(decoder_output, target_variable[t], mask[t])\n loss += mask_loss\n print_losses.append(mask_loss.item() * nTotal)\n n_totals += nTotal\n else:\n for t in range(max_target_len):\n decoder_output, decoder_hidden = decoder(decoder_input, decoder_hidden, encoder_outputs)\n _, topi = decoder_output.topk(1)\n decoder_input = torch.LongTensor([[topi[i][0] for i in range(batch_size)]])\n decoder_input = decoder_input.to(device)\n mask_loss, nTotal = maskNLLLoss(decoder_output, target_variable[t], mask[t])\n loss += mask_loss\n print_losses.append(mask_loss.item() * nTotal)\n n_totals += nTotal\n\n loss.backward()\n\n _ = torch.nn.utils.clip_grad_norm_(encoder.parameters(), clip)\n _ = torch.nn.utils.clip_grad_norm_(decoder.parameters(), clip)\n\n encoder_optimizer.step()\n decoder_optimizer.step()\n\n return sum(print_losses) / n_totals",
"_____no_output_____"
],
[
"def trainIters(model_name, voc, pairs, encoder, decoder, encoder_optimizer, decoder_optimizer, encoder_n_layers, decoder_n_layers, save_dir, n_iteration, batch_size, print_every, save_every, clip, corpus_name, loadFilename):\n\n training_batches = [batch2TrainData(voc, [random.choice(pairs) for _ in range(batch_size)])\n for _ in range(n_iteration)]\n\n print('Initializing ...')\n start_iteration = 1\n print_loss = 0\n if loadFilename:\n start_iteration = checkpoint['iteration'] + 1\n\n print(\"Training...\")\n for iteration in range(start_iteration, n_iteration + 1):\n training_batch = training_batches[iteration - 1]\n input_variable, lengths, target_variable, mask, max_target_len = training_batch\n\n # Run a training iteration with batch\n loss = train(input_variable, lengths, target_variable, mask, max_target_len, encoder,\n decoder, encoder_optimizer, decoder_optimizer, batch_size, clip)\n print_loss += loss\n\n if iteration % print_every == 0:\n print_loss_avg = print_loss / print_every\n print(\"Iteration: {}; Percent complete: {:.1f}%; Average loss: {:.4f}\".format(iteration, iteration / n_iteration * 100, print_loss_avg))\n print_loss = 0\n\n if (iteration % save_every == 0):\n directory = os.path.join(save_dir, model_name, corpus_name, '{}-{}_{}'.format(encoder_n_layers, decoder_n_layers, hidden_size))\n if not os.path.exists(directory):\n os.makedirs(directory)\n torch.save({\n 'iteration': iteration,\n 'en': encoder.state_dict(),\n 'de': decoder.state_dict(),\n 'en_opt': encoder_optimizer.state_dict(),\n 'de_opt': decoder_optimizer.state_dict(),\n 'loss': loss,\n 'voc_dict': voc.__dict__,\n 'embedding': embedding.state_dict()\n }, os.path.join(directory, '{}_{}.tar'.format(iteration, 'checkpoint')))",
"_____no_output_____"
],
[
"class GreedySearchDecoder(nn.Module):\n def __init__(self, encoder, decoder):\n super(GreedySearchDecoder, self).__init__()\n self.encoder = encoder\n self.decoder = decoder\n\n def forward(self, input_seq, input_length, max_length):\n encoder_outputs, encoder_hidden = self.encoder(input_seq, input_length)\n decoder_hidden = encoder_hidden[:decoder.n_layers]\n decoder_input = torch.ones(1, 1, device=device, dtype=torch.long) * SOS_token\n all_tokens = torch.zeros([0], device=device, dtype=torch.long)\n all_scores = torch.zeros([0], device=device)\n for _ in range(max_length):\n decoder_output, decoder_hidden = self.decoder(decoder_input, decoder_hidden, encoder_outputs)\n decoder_scores, decoder_input = torch.max(decoder_output, dim=1)\n all_tokens = torch.cat((all_tokens, decoder_input), dim=0)\n all_scores = torch.cat((all_scores, decoder_scores), dim=0)\n decoder_input = torch.unsqueeze(decoder_input, 0)\n return all_tokens, all_scores\n",
"_____no_output_____"
],
[
"def evaluate(encoder, decoder, searcher, voc, sentence, max_length=MAX_LENGTH):\n indexes_batch = [indexesFromSentence(voc, sentence)]\n lengths = torch.tensor([len(indexes) for indexes in indexes_batch])\n input_batch = torch.LongTensor(indexes_batch).transpose(0, 1)\n input_batch = input_batch.to(device)\n lengths = lengths.to(device)\n tokens, scores = searcher(input_batch, lengths, max_length)\n decoded_words = [voc.index2word[token.item()] for token in tokens]\n return decoded_words\n\n\ndef evaluateInput(encoder, decoder, searcher, voc):\n input_sentence = ''\n while(1):\n try:\n input_sentence = input('> ')\n # Check if it is quit case\n if input_sentence == 'q' or input_sentence == 'quit': break\n input_sentence = normalizeString(input_sentence)\n output_words = evaluate(encoder, decoder, searcher, voc, input_sentence)\n output_words[:] = [x for x in output_words if not (x == 'EOS' or x == 'PAD')]\n print('Bot:', ' '.join(output_words))\n except KeyError:\n print(\"Error: Encountered unknown word.\")",
"_____no_output_____"
],
[
"model_name = 'cb_model'\nattn_model = 'dot'\n#attn_model = 'general'\n#attn_model = 'concat'\nhidden_size = 500\nencoder_n_layers = 2\ndecoder_n_layers = 2\ndropout = 0.1\nbatch_size = 64\n\n# Set checkpoint to load from; set to None if starting from scratch\nloadFilename = None\ncheckpoint_iter = 4000\n#loadFilename = os.path.join(save_dir, model_name, corpus_name,\n# '{}-{}_{}'.format(encoder_n_layers, decoder_n_layers, hidden_size),\n# '{}_checkpoint.tar'.format(checkpoint_iter))\n\n\n# Load model if a loadFilename is provided\nif loadFilename:\n # If loading on same machine the model was trained on\n checkpoint = torch.load(loadFilename)\n # If loading a model trained on GPU to CPU\n #checkpoint = torch.load(loadFilename, map_location=torch.device('cpu'))\n encoder_sd = checkpoint['en']\n decoder_sd = checkpoint['de']\n encoder_optimizer_sd = checkpoint['en_opt']\n decoder_optimizer_sd = checkpoint['de_opt']\n embedding_sd = checkpoint['embedding']\n voc.__dict__ = checkpoint['voc_dict']\n\nsave_dir = os.path.join(\"dialogues\", \"save\")\n\nprint('Building encoder and decoder ...')\nembedding = nn.Embedding(voc.num_words, hidden_size)\nif loadFilename:\n embedding.load_state_dict(embedding_sd)\n# Initialize encoder & decoder models\nencoder = EncoderRNN(hidden_size, embedding, encoder_n_layers, dropout)\ndecoder = AttnDecoderRNN(attn_model, embedding, hidden_size, voc.num_words, decoder_n_layers, dropout)\nif loadFilename:\n encoder.load_state_dict(encoder_sd)\n decoder.load_state_dict(decoder_sd)\n# Use appropriate device\nencoder = encoder.to(device)\ndecoder = decoder.to(device)\nprint('Models built and ready to go!')",
"Building encoder and decoder ...\nModels built and ready to go!\n"
],
[
"clip = 50.0\nteacher_forcing_ratio = 1.0\nlearning_rate = 0.0001\ndecoder_learning_ratio = 5.0\nn_iteration = 4000\nprint_every = 100\nsave_every = 500\n\nencoder.train()\ndecoder.train()\n\nprint('Building optimizers ...')\nencoder_optimizer = optim.Adam(encoder.parameters(), lr=learning_rate)\ndecoder_optimizer = optim.Adam(decoder.parameters(), lr=learning_rate * decoder_learning_ratio)\nif loadFilename:\n encoder_optimizer.load_state_dict(encoder_optimizer_sd)\n decoder_optimizer.load_state_dict(decoder_optimizer_sd)\n\nprint(\"Starting Training!\")\ntrainIters(model_name, voc, pairs, encoder, decoder, encoder_optimizer, decoder_optimizer, encoder_n_layers, decoder_n_layers, save_dir, n_iteration, batch_size, print_every, save_every, clip, corpus_name, loadFilename)",
"Building optimizers ...\nStarting Training!\nInitializing ...\nTraining...\nIteration: 100; Percent complete: 2.5%; Average loss: 4.4373\nIteration: 200; Percent complete: 5.0%; Average loss: 3.6450\nIteration: 300; Percent complete: 7.5%; Average loss: 3.3875\nIteration: 400; Percent complete: 10.0%; Average loss: 3.2737\nIteration: 500; Percent complete: 12.5%; Average loss: 3.1978\nIteration: 600; Percent complete: 15.0%; Average loss: 3.1109\nIteration: 700; Percent complete: 17.5%; Average loss: 3.0324\nIteration: 800; Percent complete: 20.0%; Average loss: 2.9989\nIteration: 900; Percent complete: 22.5%; Average loss: 2.9784\nIteration: 1000; Percent complete: 25.0%; Average loss: 2.9273\nIteration: 1100; Percent complete: 27.5%; Average loss: 2.8988\nIteration: 1200; Percent complete: 30.0%; Average loss: 2.9030\nIteration: 1300; Percent complete: 32.5%; Average loss: 2.8583\nIteration: 1400; Percent complete: 35.0%; Average loss: 2.8254\nIteration: 1500; Percent complete: 37.5%; Average loss: 2.7930\nIteration: 1600; Percent complete: 40.0%; Average loss: 2.7583\nIteration: 1700; Percent complete: 42.5%; Average loss: 2.7559\nIteration: 1800; Percent complete: 45.0%; Average loss: 2.7137\nIteration: 1900; Percent complete: 47.5%; Average loss: 2.6843\nIteration: 2000; Percent complete: 50.0%; Average loss: 2.6595\nIteration: 2100; Percent complete: 52.5%; Average loss: 2.6381\nIteration: 2200; Percent complete: 55.0%; Average loss: 2.6101\nIteration: 2300; Percent complete: 57.5%; Average loss: 2.5657\nIteration: 2400; Percent complete: 60.0%; Average loss: 2.5367\nIteration: 2500; Percent complete: 62.5%; Average loss: 2.5185\nIteration: 2600; Percent complete: 65.0%; Average loss: 2.5145\nIteration: 2700; Percent complete: 67.5%; Average loss: 2.4713\nIteration: 2800; Percent complete: 70.0%; Average loss: 2.4500\nIteration: 2900; Percent complete: 72.5%; Average loss: 2.4370\nIteration: 3000; Percent complete: 75.0%; Average loss: 2.3958\nIteration: 3100; Percent complete: 77.5%; Average loss: 2.3685\nIteration: 3200; Percent complete: 80.0%; Average loss: 2.3411\nIteration: 3300; Percent complete: 82.5%; Average loss: 2.3139\nIteration: 3400; Percent complete: 85.0%; Average loss: 2.3081\nIteration: 3500; Percent complete: 87.5%; Average loss: 2.2935\nIteration: 3600; Percent complete: 90.0%; Average loss: 2.2415\nIteration: 3700; Percent complete: 92.5%; Average loss: 2.2259\nIteration: 3800; Percent complete: 95.0%; Average loss: 2.2153\nIteration: 3900; Percent complete: 97.5%; Average loss: 2.1855\nIteration: 4000; Percent complete: 100.0%; Average loss: 2.1638\n"
],
[
"encoder.eval()\ndecoder.eval()\n\nsearcher = GreedySearchDecoder(encoder, decoder)\n\nevaluateInput(encoder, decoder, searcher, voc)",
"> Hi\nBot: hi .\n> How are you?\nBot: i m fine .\n> Are you in love?\nBot: i m not .\n> What are you thinking?\nBot: i m thinking of a little .\n> Do you watch movies?\nBot: sure .\n> which is your favourite?\nBot: the big one .\n> Okay, bye now!\nBot: okay .\n> q\n"
]
]
] | [
"code",
"markdown",
"code",
"markdown",
"code"
] | [
[
"code",
"code",
"code",
"code",
"code",
"code",
"code",
"code",
"code",
"code",
"code",
"code",
"code",
"code",
"code",
"code",
"code",
"code",
"code",
"code",
"code",
"code",
"code",
"code"
],
[
"markdown"
],
[
"code"
],
[
"markdown"
],
[
"code",
"code",
"code",
"code",
"code",
"code",
"code",
"code",
"code",
"code"
]
] |
d0797f992c1036b026665939d270644cabd63983 | 130,509 | ipynb | Jupyter Notebook | examples/.ipynb_checkpoints/Greedy pirates-checkpoint.ipynb | sportsracer48/ThinkBayes2 | e488b68eae16a8b656039bc48bb84164a50614e2 | [
"MIT"
] | null | null | null | examples/.ipynb_checkpoints/Greedy pirates-checkpoint.ipynb | sportsracer48/ThinkBayes2 | e488b68eae16a8b656039bc48bb84164a50614e2 | [
"MIT"
] | null | null | null | examples/.ipynb_checkpoints/Greedy pirates-checkpoint.ipynb | sportsracer48/ThinkBayes2 | e488b68eae16a8b656039bc48bb84164a50614e2 | [
"MIT"
] | null | null | null | 303.509302 | 24,260 | 0.923461 | [
[
[
"# When can we start watching?\n---\nHenry Rachootin - December 2018\n\nMIT License: https://opensource.org/licenses/MIT\n\n---\n\nBitTorrent allows people to download movies without staying strictly within the confines of the law, but because of the peer to peer nature of the download, the file will not download sequentially. The VLC player can play the incomplete movie, but if it encounters a missing piece while streaming it will fail.\n\nOur pirate pirate friend is downloading _Avengers: Infinity War_, which is 149 minutes long and 12.91 GB. The torrent downloads in 4 MB pieces. If we start watching the movie when their torrent client says it is $x$ percent downloaded, What is the probability that we can get $t$ seconds into the movie without VLC failing on a missing piece?",
"_____no_output_____"
]
],
[
[
"# Configure Jupyter so figures appear in the notebook\n%matplotlib inline\n\n# Configure Jupyter to display the assigned value after an assignment\n%config InteractiveShell.ast_node_interactivity='last_expr_or_assign'\n\nimport numpy as np\nfrom scipy.stats import poisson\nfrom math import ceil,exp,floor\nfrom thinkbayes2 import Suite\nimport thinkplot\nimport pandas as pd\nfrom itertools import product",
"_____no_output_____"
]
],
[
[
"First we will just define some values.\n\nLet's define $T$ to be the runtime of the movie in seconds and $N$ to be the number of 4 MB pieces in the movie. From these, we can define $t_p$, the runtime of a single 4 MB piece as $\\frac{T}{N}$.",
"_____no_output_____"
]
],
[
[
"T = 149*60 #movie runtime in seconds\nN = ceil(12.91*1000/4) #number of 4MB pieces in the whole movie\nt_p = T/N #runtime of a single 4MB piece\n\nprint(f\"The runtime of a single piece is {t_p:.2f} seconds\")",
"The runtime of a single piece is 2.77 seconds\n"
]
],
[
[
"Let's now consider where we are going with this calculation. When watching the movie, we need to have the next piece every 2.77 seconds. If we assume that each piece is equally likely to be downloaded, we can define a function $P_p(t)$ which tells us the probability of having a specific piece after $t$ seconds, and that will be the probability of having the next piece. We will find the actual form of $P_p(t)$ later.\n\nWe want to find $P(t)$, the probability of making it $t$ seconds into the movie without missing a piece. Let's define $n(t)=\\lceil\\frac{t}{t_p}\\rceil$ to be the number of pieces needed to get $t$ seconds into the movie. We need to have each of those $n$ pieces at the time that they are played, and we have a function to tell us the probability that we will have them at that time. We can then say that\n\n$$P(t)=\\prod_{i=0}^{n(t)} P_p(i~t_p).$$\n\nAs for the actual form of $P_p(t)$, we will first find the distribution of the number of pieces downloaded at time $t$. Let's define the probability distribution $P_n(n,t)$, the probability of having $n$ pieces downloaded at time $t$. If we model piece arrival as a Poisson process, we can define $P_n(n,t)$ as\n\n$$P_n(n,t)=\\text{poisson}(n;\\lambda t)$$\n\nwhere $\\lambda$ is the unknown mean piece arrival rate in pieces per second. We will find a distribution for $\\lambda$ using real data. If we further assume that each piece is equally likely to be downloaded at any time, we can define $P_p(t)$ by the law of total probability as\n\n$$P_p(t)=\\sum_{n=n_0}^{N} \\frac{n}{N}P_n(n-n_0,t)$$\n\nwhere $n_0$ is the number of pieces downloaded when we start watching the movie, which we can just approximate as $\\left\\lfloor\\frac{xN}{100}\\right\\rfloor$, were $x$ is still the percent downloaded at the start.\n\nOf course, whatever probabilities we get out of that will be dependent on $\\lambda$, so we will have to sum them over our probability distribution for $\\lambda$, once we have that. We will use a grid algorithm to find that $\\lambda$ distribution, by starting with a uniform prior for a number of sample $\\lambda$ values and updating it with measured interarrival times, remembering that the likelihood of an interarrival time $t$ is $\\lambda e^{\\lambda t}$ for a poisson process.",
"_____no_output_____"
]
],
[
[
"#wireshark dump\ndata = pd.read_csv('torrent pieces.csv')\n\n#this finds the piece packets \ndata = data[data.Info==\"Piece[Malformed Packet]\"] \n\n#extract the time each piece arrived at\ntimes = np.array(data.Time)\n\n#dump the initial times, they don't represent the long term behavior\ntimes = times[45:] \ninterTimes = np.diff(times)\n\nclass Lambda(Suite):\n def Likelihood(self, inter, lam):\n #poisson process interarrival likelihood\n return lam*exp(-lam*inter)\n\n#start with a uniform distribution for lambda\nlamPrior = np.linspace(0.5,1.8,25) \nlam = Lambda(lamPrior)\nthinkplot.Pdf(lam,label='prior')\nlam.UpdateSet(interTimes)\nthinkplot.Pdf(lam,label='posterior')\nthinkplot.decorate(title=\"PMF for $\\lambda$\",xlabel=\"$\\lambda$ (pieces/s)\",ylabel=\"PMF\")",
"_____no_output_____"
]
],
[
[
"And we can implement all the functions we defined above:",
"_____no_output_____"
]
],
[
[
"def P_n(n,t,lam): \n \"\"\"probability of having exactly n pieces at time t for rate lambda\"\"\"\n return poisson.pmf(n,lam*t)\n\ndef P_p(t,n_0,lam): \n \"\"\"probability of having a specific piece at time t for rate lambda\"\"\"\n #all the numbers of pieces there could be\n ns = np.array(range(n_0,N+1))\n \n #the probabilities of having them\n ps = P_n(ns-n_0,t,lam)\n \n #the total probability \n #(since we are cutting off the poisson distribution at N\n #this is not always 1)\n P = np.sum(ps) \n if(P==0):\n #if lam*t is so large that we have cut off the whole poisson distribution, we can\n #just assume that we will have downloaded the whole movie\n return 1 \n return np.sum(ns*ps)/(N*P)\n\ndef P(t,n_0,lam):\n \"\"\"probability of getting to time t without missing a piece\"\"\"\n #total pieces we will need\n nt = ceil(t/t_p)\n \n #times we need each piece at\n ts = np.array(range(nt))*t_p\n \n #probabilitis of having each piece in time\n ps = np.array([P_p(t,n_0,lam) for t in ts])\n \n #total probability\n return np.product(ps) ",
"_____no_output_____"
]
],
[
[
"With those done, we can make our final $P(t,x)$ function, which will give us the probability of getting to time $t$ if we start at $x$ percent downloaded with our derived distribution for $\\lambda$.",
"_____no_output_____"
]
],
[
[
"def PWatch(t,x):\n \"\"\"Probability of getting to time t with initial download percentage x\"\"\"\n #intial piece number approximation\n n0 = floor(x*N/100)\n Ptot = 0\n \n #law of total probability\n for l,p in lam.Items(): \n Ptot += p*P(t,n0,l)\n return Ptot",
"_____no_output_____"
]
],
[
[
"Unfortunately that function is prohibitively slow. We can speed it up quite a lot by improving our $P_p$ function to be less accurate but much faster. We will approximate it by\n\n$$P_p(t)=\\frac{\\min(\\lambda t+n_0,N)}{N}$$\n\nwhich is just assuming that we get one piece every $\\lambda$ seconds. This ignores the uncertanty of the poisson distribution, but is much faster to calculate since it does not involve a sum.",
"_____no_output_____"
]
],
[
[
"def P_p_fast(t,n_0,lam):\n return min(lam*t+n_0,N)/N\n\ntestLam = lam.Mean()\nts = np.linspace(0,4000)\nps = np.array([P_p(t,0,testLam) for t in ts])\npsFast = np.array([P_p_fast(t,0,testLam) for t in ts])\nthinkplot.plot(ts,ps,label='Correct')\nthinkplot.plot(ts,psFast,label='Fast')\nthinkplot.decorate(title='Probability of having a specific piece over time',\n xlabel='time (s)',\n ylabel='probability')",
"_____no_output_____"
]
],
[
[
"From the graph we can see that this is an ok approximation.\n\nWith that done, we can start making graphs and answering the original question.",
"_____no_output_____"
]
],
[
[
"P_p = P_p_fast #use the fast function from now on\n\nts = np.linspace(0,500)\nxs = [50,90,95,99]\n\nfor x in xs:\n ps = [PWatch(t,x) for t in ts]\n thinkplot.plot(ts,ps,label=f'start at {x}%')\n thinkplot.decorate(title='Probability of getting to different times in the movie',\n xlabel='Time (s)',\n ylabel='Probability')",
"_____no_output_____"
]
],
[
[
"That graph is zoomed in near the start of the movie, but here's what it looks like over the whole runtime:",
"_____no_output_____"
]
],
[
[
"ts = np.linspace(0,T)\nxs = [50,90,95,99]\n\nfor x in xs:\n ps = [PWatch(t,x) for t in ts]\n thinkplot.plot(ts,ps,label=f'start at {x}%')\n thinkplot.decorate(title='Probability of getting to different times in the movie',\n xlabel='Time (s)',\n ylabel='Probability')",
"_____no_output_____"
]
],
[
[
"So we can see there is a definite falling off period, and after that we will probably finish the movie. With that in mind, we can ask what the probability of finishing the movie will be for different starting percentages.",
"_____no_output_____"
]
],
[
[
"xs = np.linspace(0,100)\nps = [PWatch(T,x) for x in xs]\nthinkplot.plot(xs,ps)\nthinkplot.decorate(title='Probability of finishing movie',\n xlabel='Starting percent downloaded',\n ylabel='Probability of finishing movie')",
"_____no_output_____"
]
],
[
[
"Here's the nonzero portion of that graph:",
"_____no_output_____"
]
],
[
[
"xs = np.linspace(90,100)\nps = [PWatch(T,x) for x in xs]\nthinkplot.plot(xs,ps)\nthinkplot.decorate(title='Probability of finishing movie',\n xlabel='Starting percent downloaded',\n ylabel='Probability of finishing movie')",
"_____no_output_____"
]
],
[
[
"So we can see that you need to wait until about 90% has downloaded before we can expect to have any chance of finishing, and then the probability picks up rather quickly between 95% and 100%.",
"_____no_output_____"
]
]
] | [
"markdown",
"code",
"markdown",
"code",
"markdown",
"code",
"markdown",
"code",
"markdown",
"code",
"markdown",
"code",
"markdown",
"code",
"markdown",
"code",
"markdown",
"code",
"markdown",
"code",
"markdown"
] | [
[
"markdown"
],
[
"code"
],
[
"markdown"
],
[
"code"
],
[
"markdown"
],
[
"code"
],
[
"markdown"
],
[
"code"
],
[
"markdown"
],
[
"code"
],
[
"markdown"
],
[
"code"
],
[
"markdown"
],
[
"code"
],
[
"markdown"
],
[
"code"
],
[
"markdown"
],
[
"code"
],
[
"markdown"
],
[
"code"
],
[
"markdown"
]
] |
d079824e564f36feee427de25dbae32eef24566a | 9,902 | ipynb | Jupyter Notebook | Python/file .ipynb | vikramdevraj/python-programming | a8077994a1959dbfc63ff2ca51492c8d7675587a | [
"MIT"
] | null | null | null | Python/file .ipynb | vikramdevraj/python-programming | a8077994a1959dbfc63ff2ca51492c8d7675587a | [
"MIT"
] | null | null | null | Python/file .ipynb | vikramdevraj/python-programming | a8077994a1959dbfc63ff2ca51492c8d7675587a | [
"MIT"
] | null | null | null | 19.685885 | 266 | 0.48677 | [
[
[
"test.txt # nums=emptylist(2,7,11,15) target=9 index= kisko add krne se 9 aaega",
"_____no_output_____"
],
[
"%%writefile myfile.txt\nHello this is a text file\nthis is the second line\nthis is the third line",
"Overwriting myfile.txt\n"
],
[
"myfile=open('myfile.txt')",
"_____no_output_____"
],
[
"pwd",
"_____no_output_____"
],
[
" myfile= open('myfile.txt')",
"_____no_output_____"
],
[
"myfile.read()",
"_____no_output_____"
],
[
"myfile.read() # only one time read,when we read it the cursor goes to the end of the file,if we wanna see again then seek zero reset the cursor",
"_____no_output_____"
],
[
"myfile.seek(0)",
"_____no_output_____"
],
[
"myfile.read()",
"_____no_output_____"
],
[
"myfile.read()",
"_____no_output_____"
],
[
"myfile.seek(0)",
"_____no_output_____"
],
[
"contents=myfile.read()",
"_____no_output_____"
],
[
"contents",
"_____no_output_____"
],
[
"myfile.seek(0)",
"_____no_output_____"
],
[
"myfile.readlines()",
"_____no_output_____"
],
[
"pwd",
"_____no_output_____"
],
[
"myfile.close()",
"_____no_output_____"
],
[
"with open('myfile.txt') as my_new_file:\n contents = my_new_file.read()",
"_____no_output_____"
],
[
"contents",
"_____no_output_____"
],
[
"with open('myfile.txt',mode='r') as myfile:\n contents= myfile.read()",
"_____no_output_____"
],
[
"# reading,writing,appending modes\n# mode='r' is read only,\n# mode='w' write only\n# mode ='a' append only\n# mode='r+' read and write\n#mode='w+' writing and read(overwrites existing file or creates a new file)",
"_____no_output_____"
],
[
"%%writefile my_new_file.text\nONE ON FIRST\nTWO ON SECOND\nTHREE ON THIRE",
"Overwriting my_new_file.text\n"
],
[
"with open('my_new_file.txt',mode='a') as f:\n f.write('\\nFOUR ON FOURTH')",
"_____no_output_____"
],
[
"with open('my_new_file.txt',mode='r')as f:\n print(f.read())",
"\nFOUR ON FOURTH\nFOUR ON FOURTH\nFOUR ON FOURTH\n"
],
[
"with open ('bdkjbfkbkjbfbfeb.txt',mode='w')as f:\n f.write('I CREATED THUS FILE!')",
"_____no_output_____"
],
[
"with open ('bdkjbfkbkjbfbfeb.txt',mode='r')as f:\n print(f.read())",
"I CREATED THUS FILE!\n"
],
[
"# Basic Practice:\n# http:// codingbat.com/python",
"_____no_output_____"
]
]
] | [
"code"
] | [
[
"code",
"code",
"code",
"code",
"code",
"code",
"code",
"code",
"code",
"code",
"code",
"code",
"code",
"code",
"code",
"code",
"code",
"code",
"code",
"code",
"code",
"code",
"code",
"code",
"code",
"code",
"code"
]
] |
d079a01e8a7464cedaf5aff7dde80ebd65098114 | 139,012 | ipynb | Jupyter Notebook | 2018/PAN_AA_2018-char-simplified.ipynb | jeleandro/PANAA2018 | aa681fcb4e2f90841cf30f53265fecbb111123e1 | [
"Apache-2.0"
] | null | null | null | 2018/PAN_AA_2018-char-simplified.ipynb | jeleandro/PANAA2018 | aa681fcb4e2f90841cf30f53265fecbb111123e1 | [
"Apache-2.0"
] | null | null | null | 2018/PAN_AA_2018-char-simplified.ipynb | jeleandro/PANAA2018 | aa681fcb4e2f90841cf30f53265fecbb111123e1 | [
"Apache-2.0"
] | null | null | null | 38.614444 | 113 | 0.369047 | [
[
[
"# Notebook para o PAN - Atribuição Autoral - 2018",
"_____no_output_____"
]
],
[
[
"%matplotlib inline\n#python basic libs\nfrom __future__ import print_function\n\nfrom tempfile import mkdtemp\nfrom shutil import rmtree\nimport os;\nfrom os.path import join as pathjoin;\n\nimport re;\nimport glob;\nimport json;\nimport codecs;\nfrom collections import defaultdict;\nimport pprint;\n\n\nfrom pprint import pprint\nfrom time import time\nimport logging\n\n\n#data analysis libs\nimport numpy as np;\nimport pandas as pd;\nimport matplotlib.pyplot as plt;\nimport random;\n\n#machine learning libs\n#feature extraction\nfrom sklearn.feature_extraction.text import CountVectorizer, TfidfVectorizer\n\n#preprocessing and transformation\nfrom sklearn.preprocessing import normalize, MaxAbsScaler, MinMaxScaler;\nfrom sklearn.preprocessing import LabelBinarizer;\nfrom sklearn.decomposition import PCA;\nfrom sklearn.metrics.pairwise import cosine_similarity;\n\n\nfrom sklearn.base import BaseEstimator, ClassifierMixin\n\n#classifiers\nfrom sklearn.svm import LinearSVC, SVC\nfrom sklearn.multiclass import OneVsOneClassifier, OneVsRestClassifier\nfrom sklearn.linear_model import LogisticRegression\nfrom sklearn.neural_network import MLPClassifier\n\nfrom sklearn.feature_selection import RFE,SelectFpr,SelectPercentile, chi2;\n\n#\nfrom sklearn.ensemble import AdaBoostClassifier, BaggingClassifier\nfrom sklearn.ensemble import VotingClassifier\n\nfrom sklearn.model_selection import GridSearchCV\nfrom sklearn.pipeline import Pipeline\n\n#model valuation\nfrom sklearn.model_selection import train_test_split;\nfrom sklearn.metrics import roc_auc_score, f1_score, precision_score, recall_score, accuracy_score;\n",
"_____no_output_____"
],
[
"import seaborn as sns;\nsns.set(color_codes=True);\nfrom pandas.plotting import scatter_matrix",
"_____no_output_____"
],
[
"import platform; print(platform.platform())\nprint(\"NumPy\", np.__version__)\nimport scipy; print(\"SciPy\", scipy.__version__)\nimport sklearn; print(\"Scikit-Learn\", sklearn.__version__)\nprint(\"seaborn\", sns.__version__)",
"Darwin-17.5.0-x86_64-i386-64bit\nNumPy 1.14.2\nSciPy 1.0.1\nScikit-Learn 0.19.1\nseaborn 0.8.1\n"
]
],
[
[
"### paths configuration",
"_____no_output_____"
]
],
[
[
"baseDir = '/Users/joseeleandrocustodio/Dropbox/mestrado/02 - Pesquisa/code';\n\ninputDir= pathjoin(baseDir,'pan18aa');\noutputDir= pathjoin(baseDir,'out',\"oficial\");\nif not os.path.exists(outputDir):\n os.mkdir(outputDir);",
"_____no_output_____"
]
],
[
[
"## loading the dataset",
"_____no_output_____"
]
],
[
[
"def readCollectionsOfProblems(path):\n # Reading information about the collection\n infocollection = path+os.sep+'collection-info.json'\n with open(infocollection, 'r') as f:\n problems = [\n {\n 'problem': attrib['problem-name'],\n 'language': attrib['language'],\n 'encoding': attrib['encoding'],\n }\n for attrib in json.load(f)\n \n ]\n return problems;",
"_____no_output_____"
],
[
"problems = readCollectionsOfProblems(inputDir);",
"_____no_output_____"
],
[
"problems[0]",
"_____no_output_____"
],
[
"def readProblem(path, problem):\n # Reading information about the problem\n infoproblem = path+os.sep+problem+os.sep+'problem-info.json'\n candidates = []\n with open(infoproblem, 'r') as f:\n fj = json.load(f)\n unk_folder = fj['unknown-folder']\n for attrib in fj['candidate-authors']:\n candidates.append(attrib['author-name'])\n return unk_folder, candidates;",
"_____no_output_____"
],
[
"def read_files(path,label):\n # Reads all text files located in the 'path' and assigns them to 'label' class\n files = glob.glob(pathjoin(path,label,'*.txt'))\n texts=[]\n for i,v in enumerate(files):\n f=codecs.open(v,'r',encoding='utf-8')\n texts.append((f.read(),label, os.path.basename(v)))\n f.close()\n return texts",
"_____no_output_____"
],
[
"for index,problem in enumerate(problems):\n unk_folder, candidates_folder = readProblem(inputDir, problem['problem']); \n problem['candidates_folder_count'] = len(candidates_folder);\n problem['candidates'] = [];\n for candidate in candidates_folder:\n problem['candidates'].extend(read_files(pathjoin(inputDir, problem['problem']),candidate));\n \n problem['unknown'] = read_files(pathjoin(inputDir, problem['problem']),unk_folder); ",
"_____no_output_____"
],
[
"pd.DataFrame(problems)",
"_____no_output_____"
],
[
"#*******************************************************************************************************\nimport warnings\nfrom sklearn.metrics import f1_score, precision_score, recall_score, accuracy_score\nfrom sklearn.preprocessing import LabelEncoder\n\n\ndef eval_measures(gt, pred):\n \"\"\"Compute macro-averaged F1-scores, macro-averaged precision, \n macro-averaged recall, and micro-averaged accuracy according the ad hoc\n rules discussed at the top of this file.\n Parameters\n ----------\n gt : dict\n Ground truth, where keys indicate text file names\n (e.g. `unknown00002.txt`), and values represent\n author labels (e.g. `candidate00003`)\n pred : dict\n Predicted attribution, where keys indicate text file names\n (e.g. `unknown00002.txt`), and values represent\n author labels (e.g. `candidate00003`)\n Returns\n -------\n f1 : float\n Macro-averaged F1-score\n precision : float\n Macro-averaged precision\n recall : float\n Macro-averaged recall\n accuracy : float\n Micro-averaged F1-score\n \"\"\"\n\n actual_authors = list(gt.values())\n encoder = LabelEncoder().fit(['<UNK>'] + actual_authors)\n\n text_ids, gold_authors, silver_authors = [], [], []\n for text_id in sorted(gt):\n text_ids.append(text_id)\n gold_authors.append(gt[text_id])\n try:\n silver_authors.append(pred[text_id])\n except KeyError:\n # missing attributions get <UNK>:\n silver_authors.append('<UNK>')\n\n assert len(text_ids) == len(gold_authors)\n assert len(text_ids) == len(silver_authors)\n\n # replace non-existent silver authors with '<UNK>':\n silver_authors = [a if a in encoder.classes_ else '<UNK>' \n for a in silver_authors]\n\n gold_author_ints = encoder.transform(gold_authors)\n silver_author_ints = encoder.transform(silver_authors)\n\n # get F1 for individual classes (and suppress warnings):\n with warnings.catch_warnings():\n warnings.simplefilter('ignore')\n f1 = f1_score(gold_author_ints,\n silver_author_ints,\n labels=list(set(gold_author_ints)),\n average='macro')\n precision = precision_score(gold_author_ints,\n silver_author_ints,\n labels=list(set(gold_author_ints)),\n average='macro')\n recall = recall_score(gold_author_ints,\n silver_author_ints,\n labels=list(set(gold_author_ints)),\n average='macro')\n accuracy = accuracy_score(gold_author_ints,\n silver_author_ints)\n\n return f1,precision,recall,accuracy\n",
"_____no_output_____"
],
[
"def evaluate(ground_truth_file,predictions_file):\n # Calculates evaluation measures for a single attribution problem\n gt = {}\n with open(ground_truth_file, 'r') as f:\n for attrib in json.load(f)['ground_truth']:\n gt[attrib['unknown-text']] = attrib['true-author']\n\n pred = {}\n with open(predictions_file, 'r') as f:\n for attrib in json.load(f):\n if attrib['unknown-text'] not in pred:\n pred[attrib['unknown-text']] = attrib['predicted-author']\n f1,precision,recall,accuracy = eval_measures(gt,pred)\n return f1, precision, recall, accuracy",
"_____no_output_____"
],
[
"from sklearn.base import BaseEstimator\nfrom scipy.sparse import issparse\n\n\nclass DenseTransformer(BaseEstimator):\n \"\"\"Convert a sparse array into a dense array.\"\"\"\n\n def __init__(self, return_copy=True):\n self.return_copy = return_copy\n self.is_fitted = False\n\n def transform(self, X, y=None):\n \"\"\" Return a dense version of the input array.\n Parameters\n ----------\n X : {array-like, sparse matrix}, shape = [n_samples, n_features]\n Training vectors, where n_samples is the number of samples and\n n_features is the number of features.\n y : array-like, shape = [n_samples] (default: None)\n Returns\n ---------\n X_dense : dense version of the input X array.\n \"\"\"\n if issparse(X):\n return X.toarray()\n elif self.return_copy:\n return X.copy()\n else:\n return X\n\n def fit(self, X, y=None):\n \"\"\" Mock method. Does nothing.\n Parameters\n ----------\n X : {array-like, sparse matrix}, shape = [n_samples, n_features]\n Training vectors, where n_samples is the number of samples and\n n_features is the number of features.\n y : array-like, shape = [n_samples] (default: None)\n Returns\n ---------\n self\n \"\"\"\n self.is_fitted = True\n return self\n\n def fit_transform(self, X, y=None):\n \"\"\" Return a dense version of the input array.\n Parameters\n ----------\n X : {array-like, sparse matrix}, shape = [n_samples, n_features]\n Training vectors, where n_samples is the number of samples and\n n_features is the number of features.\n y : array-like, shape = [n_samples] (default: None)\n Returns\n ---------\n X_dense : dense version of the input X array.\n \"\"\"\n return self.transform(X=X, y=y)",
"_____no_output_____"
]
],
[
[
"### examinando o parametro min_df isoladamente",
"_____no_output_____"
]
],
[
[
"def runML(problem):\n print (\"\\nProblem: %s, language: %s, \" %(problem['problem'],problem['language']))\n \n train_docs, train_labels, _ = zip(*problem['candidates'])\n problem['training_docs_size'] = len(train_docs);\n test_docs, _, test_filename = zip(*problem['unknown'])\n \n pipeline = Pipeline([\n ('vect', TfidfVectorizer(analyzer='char',\n min_df=0.05,\n max_df=1.0,\n norm='l1',\n ngram_range=(3,5),\n sublinear_tf=True,\n smooth_idf=True,\n lowercase =False)),\n ('dense', DenseTransformer()),\n ('scaler', MaxAbsScaler()),\n ('transf', PCA(0.999)),\n ('clf', LogisticRegression(random_state=0,multi_class='multinomial', solver='newton-cg')),\n ])\n \n \n # uncommenting more parameters will give better exploring power but will\n # increase processing time in a combinatorial way\n parameters = {\n 'vect__min_df':(2,0.01,0.05,0.1)\n }\n \n grid_search = GridSearchCV(pipeline,\n parameters,\n cv=5,\n n_jobs=-1,\n verbose=False,\n scoring='f1_macro')\n \n print(\"Performing grid search...\")\n t0 = time()\n grid_search.fit(train_docs, train_labels)\n print(\"done in %0.3fs\" % (time() - t0))\n\n print(\"Best score: %0.3f\" % grid_search.best_score_)\n print(\"Best parameters set:\")\n best_parameters = grid_search.best_estimator_.get_params()\n for param_name in sorted(parameters.keys()):\n print(\"\\t%s: %r\" % (param_name, best_parameters[param_name]))\n \n train_pred=grid_search.predict(train_docs);\n test_pred=grid_search.predict(test_docs);\n \n \n # Writing output file\n out_data=[]\n for i,v in enumerate(test_pred):\n out_data.append({'unknown-text': test_filename[i],'predicted-author': v})\n answerFile = pathjoin(outputDir,'answers-'+problem['problem']+'.json');\n with open(answerFile, 'w') as f:\n json.dump(out_data, f, indent=4)\n \n \n #evaluation train\n f1,precision,recall,accuracy=evaluate(\n pathjoin(inputDir, problem['problem'], 'ground-truth.json'),\n answerFile)\n \n return {\n 'problem-name' : problem['problem'],\n \"language\" : problem['language'],\n 'AuthorCount' : len(set(train_labels)),\n \"train_doc_size\": len(train_docs),\n \"train_caract_per_doc\": sum([len(l) for l in train_docs])/len(train_docs),\n \"test_doc_size\" : len(test_docs),\n \"test_caract_per_doc\": sum([len(l) for l in test_docs])/len(test_docs),\n \n 'macro-f1' : round(f1,3),\n 'macro-precision': round(precision,3),\n 'macro-recall' : round(recall,3),\n 'micro-accuracy' : round(accuracy,3),\n \n }, grid_search.cv_results_, best_parameters;",
"_____no_output_____"
],
[
"result = [];\ncv_result = [];\nbest_parameters = [];\nfor problem in problems:\n r, c, b = runML(problem);\n result.append(r);\n cv_result.append(c);\n b['problem'] = problem['problem'];\n best_parameters.append(b);",
"\nProblem: problem00001, language: en, \nPerforming grid search...\ndone in 38.968s\nBest score: 0.769\nBest parameters set:\n\tvect__min_df: 0.01\n\nProblem: problem00002, language: en, \nPerforming grid search...\ndone in 29.814s\nBest score: 0.874\nBest parameters set:\n\tvect__min_df: 0.1\n\nProblem: problem00003, language: fr, \nPerforming grid search...\ndone in 89.584s\nBest score: 0.775\nBest parameters set:\n\tvect__min_df: 0.01\n\nProblem: problem00004, language: fr, \nPerforming grid search...\ndone in 31.481s\nBest score: 0.903\nBest parameters set:\n\tvect__min_df: 0.01\n\nProblem: problem00005, language: it, \nPerforming grid search...\ndone in 91.047s\nBest score: 0.743\nBest parameters set:\n\tvect__min_df: 2\n\nProblem: problem00006, language: it, \nPerforming grid search...\ndone in 33.172s\nBest score: 0.970\nBest parameters set:\n\tvect__min_df: 2\n\nProblem: problem00007, language: pl, \nPerforming grid search...\ndone in 135.618s\nBest score: 0.811\nBest parameters set:\n\tvect__min_df: 0.01\n\nProblem: problem00008, language: pl, \nPerforming grid search...\ndone in 49.869s\nBest score: 0.851\nBest parameters set:\n\tvect__min_df: 0.1\n\nProblem: problem00009, language: sp, \nPerforming grid search...\ndone in 104.835s\nBest score: 0.917\nBest parameters set:\n\tvect__min_df: 0.01\n\nProblem: problem00010, language: sp, \nPerforming grid search...\ndone in 37.666s\nBest score: 0.893\nBest parameters set:\n\tvect__min_df: 2\n"
],
[
"pd.DataFrame(best_parameters)[['problem','vect__min_df']]",
"_____no_output_____"
]
],
[
[
"### analisando os demais parametros",
"_____no_output_____"
]
],
[
[
"def runML(problem):\n print (\"\\nProblem: %s, language: %s, \" %(problem['problem'],problem['language']))\n \n train_docs, train_labels, _ = zip(*problem['candidates'])\n problem['training_docs_size'] = len(train_docs);\n test_docs, _, test_filename = zip(*problem['unknown'])\n \n pipeline = Pipeline([\n ('vect', TfidfVectorizer(analyzer='char',\n min_df=0.01,\n max_df=1.0,\n norm='l1',\n lowercase =False,\n sublinear_tf=True)),\n ('dense', DenseTransformer()),\n ('scaler', MaxAbsScaler()),\n ('transf', PCA()),\n ('clf', LogisticRegression(random_state=0,multi_class='multinomial', solver='newton-cg')),\n ])\n \n \n # uncommenting more parameters will give better exploring power but will\n # increase processing time in a combinatorial way\n parameters = {\n 'vect__ngram_range':((2,3),(2,4),(2,5),(3,5)),\n 'vect__sublinear_tf':(True, False),\n 'vect__norm':('l1','l2'),\n 'transf__n_components': (0.1,0.25,0.5,0.75,0.9,0.99),\n }\n \n grid_search = GridSearchCV(pipeline,\n parameters,\n cv=3,\n n_jobs=-1,\n verbose=False,\n scoring='f1_macro')\n \n print(\"Performing grid search...\")\n t0 = time()\n grid_search.fit(train_docs, train_labels)\n print(\"done in %0.3fs\" % (time() - t0))\n\n print(\"Best score: %0.3f\" % grid_search.best_score_)\n print(\"Best parameters set:\")\n best_parameters = grid_search.best_estimator_.get_params()\n for param_name in sorted(parameters.keys()):\n print(\"\\t%s: %r\" % (param_name, best_parameters[param_name]))\n \n train_pred=grid_search.predict(train_docs);\n test_pred=grid_search.predict(test_docs);\n \n \n # Writing output file\n out_data=[]\n for i,v in enumerate(test_pred):\n out_data.append({'unknown-text': test_filename[i],'predicted-author': v})\n answerFile = pathjoin(outputDir,'answers-'+problem['problem']+'.json');\n with open(answerFile, 'w') as f:\n json.dump(out_data, f, indent=4)\n \n \n #evaluation train\n f1,precision,recall,accuracy=evaluate(\n pathjoin(inputDir, problem['problem'], 'ground-truth.json'),\n answerFile)\n \n return {\n 'problem-name' : problem['problem'],\n \"language\" : problem['language'],\n 'AuthorCount' : len(set(train_labels)),\n \"train_doc_size\": len(train_docs),\n \"train_caract_per_doc\": sum([len(l) for l in train_docs])/len(train_docs),\n \"test_doc_size\" : len(test_docs),\n \"test_caract_per_doc\": sum([len(l) for l in test_docs])/len(test_docs),\n \n 'macro-f1' : round(f1,3),\n 'macro-precision': round(precision,3),\n 'macro-recall' : round(recall,3),\n 'micro-accuracy' : round(accuracy,3),\n \n }, grid_search.cv_results_,best_parameters;",
"_____no_output_____"
],
[
"result = [];\ncv_result = [];\nbest_parameters = [];\nfor problem in problems:\n r, c, b = runML(problem);\n result.append(r);\n cv_result.append(c);\n b['problem'] = problem['problem'];\n best_parameters.append(b);",
"\nProblem: problem00001, language: en, \nPerforming grid search...\ndone in 658.937s\nBest score: 0.833\nBest parameters set:\n\ttransf__n_components: 0.99\n\tvect__ngram_range: (2, 5)\n\tvect__norm: 'l1'\n\tvect__sublinear_tf: True\n\nProblem: problem00002, language: en, \nPerforming grid search...\ndone in 123.030s\nBest score: 0.971\nBest parameters set:\n\ttransf__n_components: 0.75\n\tvect__ngram_range: (2, 4)\n\tvect__norm: 'l1'\n\tvect__sublinear_tf: True\n\nProblem: problem00003, language: fr, \nPerforming grid search...\ndone in 675.776s\nBest score: 0.800\nBest parameters set:\n\ttransf__n_components: 0.99\n\tvect__ngram_range: (2, 3)\n\tvect__norm: 'l1'\n\tvect__sublinear_tf: False\n\nProblem: problem00004, language: fr, \nPerforming grid search...\ndone in 143.218s\nBest score: 0.854\nBest parameters set:\n\ttransf__n_components: 0.75\n\tvect__ngram_range: (2, 4)\n\tvect__norm: 'l2'\n\tvect__sublinear_tf: True\n\nProblem: problem00005, language: it, \nPerforming grid search...\ndone in 837.817s\nBest score: 0.701\nBest parameters set:\n\ttransf__n_components: 0.75\n\tvect__ngram_range: (2, 3)\n\tvect__norm: 'l2'\n\tvect__sublinear_tf: True\n\nProblem: problem00006, language: it, \nPerforming grid search...\ndone in 237.214s\nBest score: 0.971\nBest parameters set:\n\ttransf__n_components: 0.9\n\tvect__ngram_range: (2, 4)\n\tvect__norm: 'l1'\n\tvect__sublinear_tf: True\n\nProblem: problem00007, language: pl, \nPerforming grid search...\ndone in 1587.061s\nBest score: 0.816\nBest parameters set:\n\ttransf__n_components: 0.99\n\tvect__ngram_range: (2, 4)\n\tvect__norm: 'l1'\n\tvect__sublinear_tf: True\n\nProblem: problem00008, language: pl, \nPerforming grid search...\ndone in 188.265s\nBest score: 0.845\nBest parameters set:\n\ttransf__n_components: 0.9\n\tvect__ngram_range: (2, 4)\n\tvect__norm: 'l2'\n\tvect__sublinear_tf: True\n\nProblem: problem00009, language: sp, \nPerforming grid search...\ndone in 866.830s\nBest score: 0.894\nBest parameters set:\n\ttransf__n_components: 0.99\n\tvect__ngram_range: (2, 5)\n\tvect__norm: 'l1'\n\tvect__sublinear_tf: True\n\nProblem: problem00010, language: sp, \nPerforming grid search...\ndone in 171.587s\nBest score: 0.939\nBest parameters set:\n\ttransf__n_components: 0.99\n\tvect__ngram_range: (3, 5)\n\tvect__norm: 'l2'\n\tvect__sublinear_tf: False\n"
],
[
"df=pd.DataFrame(result)[['problem-name',\n \"language\",\n 'AuthorCount',\n \"train_doc_size\",\"train_caract_per_doc\",\n \"test_doc_size\", \"test_caract_per_doc\",\n 'macro-f1','macro-precision','macro-recall' ,'micro-accuracy']]",
"_____no_output_____"
],
[
"df",
"_____no_output_____"
],
[
"print(df[[\"macro-f1\"]].reset_index().to_latex(index=False).replace(\" \",\" \"))",
"\\begin{tabular}{rr}\n\\toprule\n index & macro-f1 \\\\\n\\midrule\n 0 & 0.643 \\\\\n 1 & 0.477 \\\\\n 2 & 0.641 \\\\\n 3 & 0.747 \\\\\n 4 & 0.481 \\\\\n 5 & 0.596 \\\\\n 6 & 0.465 \\\\\n 7 & 0.822 \\\\\n 8 & 0.787 \\\\\n 9 & 0.832 \\\\\n\\bottomrule\n\\end{tabular}\n\n"
],
[
"languages={\n 'en':'inglesa',\n 'sp':'espanhola',\n 'it':'italiana',\n 'pl':'polonesa',\n 'fr':'francesa'\n}",
"_____no_output_____"
],
[
"cv_result2 = [];\ndfCV = pd.DataFrame();\nfor i, c in enumerate(cv_result):\n temp = pd.DataFrame(c);\n temp['problem'] = i+1;\n temp['language'] = languages[problems[i]['language']]\n dfCV = dfCV.append(temp);\n\nfor p in ['param_transf__n_components',\n 'mean_test_score','std_test_score','mean_train_score', \n 'split0_test_score','split0_train_score',\n 'split1_test_score','split1_train_score',\n 'split2_test_score','split2_train_score']:\n dfCV[p]=dfCV[p].astype(np.float32);\n\n \ndfCV =dfCV[[\n 'problem',\n 'language',\n 'rank_test_score',\n 'param_transf__n_components',\n 'param_vect__ngram_range',\n 'param_vect__sublinear_tf',\n 'param_vect__norm',\n 'mean_test_score', \n 'std_test_score',\n 'mean_train_score', \n\n 'split0_test_score','split0_train_score',\n 'split1_test_score','split1_train_score',\n 'split2_test_score','split2_train_score',\n\n 'mean_score_time',\n 'mean_fit_time',\n 'std_fit_time',\n 'std_score_time',\n 'std_train_score',\n]];\n\ndfCV.rename(columns={\n 'param_transf__n_components':'PCA_componentes',\n 'param_vect__ngram_range':'ngram_range',\n 'param_vect__sublinear_tf':'sublinear_tf',\n 'param_vect__smooth_idf':'smooth_idf',\n 'param_vect__norm':'norm'\n},inplace=True);\n\n#print('\\',\\n\\''.join(dfCV.columns))",
"_____no_output_____"
],
[
"dfCV.to_csv('PANAA2018_CHAR.csv', index=False)",
"_____no_output_____"
],
[
"dfCV = pd.read_csv('PANAA2018_CHAR.csv', na_values='')",
"_____no_output_____"
],
[
"(dfCV[dfCV.rank_test_score == 1])[\n ['problem',\n 'language',\n 'rank_test_score',\n 'mean_test_score',\n 'std_test_score',\n 'ngram_range',\n 'sublinear_tf',\n 'norm',\n 'PCA_componentes']\n].sort_values(by=[\n 'problem',\n 'mean_test_score',\n 'ngram_range',\n 'sublinear_tf',\n 'PCA_componentes'\n], ascending=[True, False,False,False,False])",
"_____no_output_____"
],
[
"dfCV.pivot_table(\n index=['problem','language','PCA_componentes'],\n columns=['norm','sublinear_tf', 'ngram_range'],\n values='mean_test_score'\n )",
"_____no_output_____"
],
[
"pd.options.display.precision = 3 \nprint(u\"\\\\begin{table}[h]\\n\\\\centering\\n\\\\caption{Medida F1 para os parâmetros }\")\n\nprint(re.sub(r'[ ]{2,}',' ',dfCV[dfCV.PCA_componentes >= 0.99].pivot_table(\n index=['problem','language','sublinear_tf','norm'],\n columns=['ngram_range'],\n values='mean_test_score'\n ).to_latex()))\nprint (\"\\label{tab:modelocaracter}\")\nprint(r\"\\end{table}\")",
"\\begin{table}[h]\n\\centering\n\\caption{Medida F1 para os parâmetros }\n\\begin{tabular}{llllrrrr}\n\\toprule\n & & & ngram\\_range & (2, 3) & (2, 4) & (2, 5) & (3, 5) \\\\\nproblem & language & sublinear\\_tf & norm & & & & \\\\\n\\midrule\n1 & inglesa & False & l1 & 0.680 & 0.787 & 0.804 & 0.803 \\\\\n & & & l2 & 0.670 & 0.760 & 0.715 & 0.734 \\\\\n & & True & l1 & 0.791 & 0.827 & 0.833 & 0.827 \\\\\n & & & l2 & 0.816 & 0.818 & 0.819 & 0.826 \\\\\n2 & inglesa & False & l1 & 0.883 & 0.940 & 0.940 & 0.971 \\\\\n & & & l2 & 0.883 & 0.879 & 0.940 & 0.940 \\\\\n & & True & l1 & 0.883 & 0.971 & 0.971 & 0.971 \\\\\n & & & l2 & 0.910 & 0.971 & 0.971 & 0.971 \\\\\n3 & francesa & False & l1 & 0.800 & 0.782 & 0.772 & 0.772 \\\\\n & & & l2 & 0.794 & 0.761 & 0.732 & 0.724 \\\\\n & & True & l1 & 0.778 & 0.788 & 0.762 & 0.775 \\\\\n & & & l2 & 0.786 & 0.769 & 0.763 & 0.776 \\\\\n4 & francesa & False & l1 & 0.775 & 0.800 & 0.825 & 0.825 \\\\\n & & & l2 & 0.744 & 0.800 & 0.854 & 0.854 \\\\\n & & True & l1 & 0.744 & 0.800 & 0.854 & 0.854 \\\\\n & & & l2 & 0.799 & 0.828 & 0.854 & 0.854 \\\\\n5 & italiana & False & l1 & 0.654 & 0.629 & 0.643 & 0.651 \\\\\n & & & l2 & 0.628 & 0.550 & 0.393 & 0.409 \\\\\n & & True & l1 & 0.681 & 0.665 & 0.677 & 0.666 \\\\\n & & & l2 & 0.682 & 0.688 & 0.659 & 0.660 \\\\\n6 & italiana & False & l1 & 0.940 & 0.971 & 0.940 & 0.940 \\\\\n & & & l2 & 0.880 & 0.971 & 0.940 & 0.940 \\\\\n & & True & l1 & 0.911 & 0.940 & 0.910 & 0.910 \\\\\n & & & l2 & 0.911 & 0.971 & 0.940 & 0.940 \\\\\n7 & polonesa & False & l1 & 0.782 & 0.793 & 0.770 & 0.770 \\\\\n & & & l2 & 0.748 & 0.723 & 0.713 & 0.707 \\\\\n & & True & l1 & 0.784 & 0.816 & 0.814 & 0.799 \\\\\n & & & l2 & 0.784 & 0.810 & 0.814 & 0.799 \\\\\n8 & polonesa & False & l1 & 0.712 & 0.810 & 0.810 & 0.810 \\\\\n & & & l2 & 0.760 & 0.810 & 0.845 & 0.845 \\\\\n & & True & l1 & 0.810 & 0.810 & 0.810 & 0.760 \\\\\n & & & l2 & 0.810 & 0.810 & 0.810 & 0.810 \\\\\n9 & espanhola & False & l1 & 0.823 & 0.848 & 0.871 & 0.848 \\\\\n & & & l2 & 0.831 & 0.823 & 0.784 & 0.740 \\\\\n & & True & l1 & 0.862 & 0.881 & 0.894 & 0.886 \\\\\n & & & l2 & 0.879 & 0.888 & 0.879 & 0.879 \\\\\n10 & espanhola & False & l1 & 0.912 & 0.909 & 0.882 & 0.882 \\\\\n & & & l2 & 0.882 & 0.909 & 0.882 & 0.939 \\\\\n & & True & l1 & 0.882 & 0.909 & 0.882 & 0.882 \\\\\n & & & l2 & 0.882 & 0.909 & 0.882 & 0.882 \\\\\n\\bottomrule\n\\end{tabular}\n\n\\label{tab:modelocaracter}\n\\end{table}\n"
]
]
] | [
"markdown",
"code",
"markdown",
"code",
"markdown",
"code",
"markdown",
"code",
"markdown",
"code"
] | [
[
"markdown"
],
[
"code",
"code",
"code"
],
[
"markdown"
],
[
"code"
],
[
"markdown"
],
[
"code",
"code",
"code",
"code",
"code",
"code",
"code",
"code",
"code",
"code"
],
[
"markdown"
],
[
"code",
"code",
"code"
],
[
"markdown"
],
[
"code",
"code",
"code",
"code",
"code",
"code",
"code",
"code",
"code",
"code",
"code",
"code"
]
] |
d079aa8c2fb9430b2ef3bd1d3316626b7ca85bcf | 788 | ipynb | Jupyter Notebook | docs/example.ipynb | ekimz/webtoons_data | ae7516f69671ddd746d663facc59ff07c4a09460 | [
"MIT"
] | null | null | null | docs/example.ipynb | ekimz/webtoons_data | ae7516f69671ddd746d663facc59ff07c4a09460 | [
"MIT"
] | null | null | null | docs/example.ipynb | ekimz/webtoons_data | ae7516f69671ddd746d663facc59ff07c4a09460 | [
"MIT"
] | null | null | null | 17.511111 | 41 | 0.522843 | [
[
[
"# Example usage\n\nTo use `webtoon_data` in a project:",
"_____no_output_____"
]
],
[
[
"import webtoon_data\n\nprint(webtoon_data.__version__)",
"_____no_output_____"
]
]
] | [
"markdown",
"code"
] | [
[
"markdown"
],
[
"code"
]
] |
d079b678269df319281ccf66a0bb96238337b746 | 23,200 | ipynb | Jupyter Notebook | site/en/2/tutorials/keras/save_and_restore_models.ipynb | allenlavoie/docs | 2feaa86b54ad96b53bec5851e89203b201b8cbb4 | [
"Apache-2.0"
] | 1 | 2019-06-06T22:49:00.000Z | 2019-06-06T22:49:00.000Z | site/en/2/tutorials/keras/save_and_restore_models.ipynb | allenlavoie/docs | 2feaa86b54ad96b53bec5851e89203b201b8cbb4 | [
"Apache-2.0"
] | 1 | 2021-02-28T07:14:03.000Z | 2021-02-28T07:14:03.000Z | site/en/2/tutorials/keras/save_and_restore_models.ipynb | allenlavoie/docs | 2feaa86b54ad96b53bec5851e89203b201b8cbb4 | [
"Apache-2.0"
] | null | null | null | 33.095578 | 517 | 0.535862 | [
[
[
"##### Copyright 2018 The TensorFlow Authors.",
"_____no_output_____"
]
],
[
[
"#@title Licensed under the Apache License, Version 2.0 (the \"License\");\n# you may not use this file except in compliance with the License.\n# You may obtain a copy of the License at\n#\n# https://www.apache.org/licenses/LICENSE-2.0\n#\n# Unless required by applicable law or agreed to in writing, software\n# distributed under the License is distributed on an \"AS IS\" BASIS,\n# WITHOUT WARRANTIES OR CONDITIONS OF ANY KIND, either express or implied.\n# See the License for the specific language governing permissions and\n# limitations under the License.",
"_____no_output_____"
],
[
"#@title MIT License\n#\n# Copyright (c) 2017 François Chollet\n#\n# Permission is hereby granted, free of charge, to any person obtaining a\n# copy of this software and associated documentation files (the \"Software\"),\n# to deal in the Software without restriction, including without limitation\n# the rights to use, copy, modify, merge, publish, distribute, sublicense,\n# and/or sell copies of the Software, and to permit persons to whom the\n# Software is furnished to do so, subject to the following conditions:\n#\n# The above copyright notice and this permission notice shall be included in\n# all copies or substantial portions of the Software.\n#\n# THE SOFTWARE IS PROVIDED \"AS IS\", WITHOUT WARRANTY OF ANY KIND, EXPRESS OR\n# IMPLIED, INCLUDING BUT NOT LIMITED TO THE WARRANTIES OF MERCHANTABILITY,\n# FITNESS FOR A PARTICULAR PURPOSE AND NONINFRINGEMENT. IN NO EVENT SHALL\n# THE AUTHORS OR COPYRIGHT HOLDERS BE LIABLE FOR ANY CLAIM, DAMAGES OR OTHER\n# LIABILITY, WHETHER IN AN ACTION OF CONTRACT, TORT OR OTHERWISE, ARISING\n# FROM, OUT OF OR IN CONNECTION WITH THE SOFTWARE OR THE USE OR OTHER\n# DEALINGS IN THE SOFTWARE.",
"_____no_output_____"
]
],
[
[
"# Save and restore models",
"_____no_output_____"
],
[
"<table class=\"tfo-notebook-buttons\" align=\"left\">\n <td>\n <a target=\"_blank\" href=\"https://www.tensorflow.org/tutorials/keras/save_and_restore_models\"><img src=\"https://www.tensorflow.org/images/tf_logo_32px.png\" />View on TensorFlow.org</a>\n </td>\n <td>\n <a target=\"_blank\" href=\"https://colab.research.google.com/github/tensorflow/docs/blob/master/site/en/tutorials/keras/save_and_restore_models.ipynb\"><img src=\"https://www.tensorflow.org/images/colab_logo_32px.png\" />Run in Google Colab</a>\n </td>\n <td>\n <a target=\"_blank\" href=\"https://github.com/tensorflow/docs/blob/master/site/en/tutorials/keras/save_and_restore_models.ipynb\"><img src=\"https://www.tensorflow.org/images/GitHub-Mark-32px.png\" />View source on GitHub</a>\n </td>\n</table>",
"_____no_output_____"
],
[
"Model progress can be saved during—and after—training. This means a model can resume where it left off and avoid long training times. Saving also means you can share your model and others can recreate your work. When publishing research models and techniques, most machine learning practitioners share:\n\n* code to create the model, and\n* the trained weights, or parameters, for the model\n\nSharing this data helps others understand how the model works and try it themselves with new data.\n\nCaution: Be careful with untrusted code—TensorFlow models are code. See [Using TensorFlow Securely](https://github.com/tensorflow/tensorflow/blob/master/SECURITY.md) for details.\n\n### Options\n\nThere are different ways to save TensorFlow models—depending on the API you're using. This guide uses [tf.keras](https://www.tensorflow.org/guide/keras), a high-level API to build and train models in TensorFlow. For other approaches, see the TensorFlow [Save and Restore](https://www.tensorflow.org/guide/saved_model) guide or [Saving in eager](https://www.tensorflow.org/guide/eager#object_based_saving).\n",
"_____no_output_____"
],
[
"## Setup\n\n### Installs and imports",
"_____no_output_____"
],
[
"Install and import TensorFlow and dependencies:",
"_____no_output_____"
]
],
[
[
"!pip install h5py pyyaml ",
"_____no_output_____"
]
],
[
[
"### Get an example dataset\n\nWe'll use the [MNIST dataset](http://yann.lecun.com/exdb/mnist/) to train our model to demonstrate saving weights. To speed up these demonstration runs, only use the first 1000 examples:",
"_____no_output_____"
]
],
[
[
"from __future__ import absolute_import, division, print_function\n\nimport os\n\n!pip install tf-nightly-2.0-preview\nimport tensorflow as tf\nkeras = tf.keras\n\ntf.__version__",
"_____no_output_____"
],
[
"(train_images, train_labels), (test_images, test_labels) = tf.keras.datasets.mnist.load_data()\n\ntrain_labels = train_labels[:1000]\ntest_labels = test_labels[:1000]\n\ntrain_images = train_images[:1000].reshape(-1, 28 * 28) / 255.0\ntest_images = test_images[:1000].reshape(-1, 28 * 28) / 255.0",
"_____no_output_____"
]
],
[
[
"### Define a model",
"_____no_output_____"
],
[
"Let's build a simple model we'll use to demonstrate saving and loading weights.",
"_____no_output_____"
]
],
[
[
"# Returns a short sequential model\ndef create_model():\n model = tf.keras.models.Sequential([\n keras.layers.Dense(512, activation=tf.keras.activations.relu, input_shape=(784,)),\n keras.layers.Dropout(0.2),\n keras.layers.Dense(10, activation=tf.keras.activations.softmax)\n ])\n \n model.compile(optimizer='adam', \n loss=tf.keras.losses.sparse_categorical_crossentropy,\n metrics=['accuracy'])\n \n return model\n\n\n# Create a basic model instance\nmodel = create_model()\nmodel.summary()",
"_____no_output_____"
]
],
[
[
"## Save checkpoints during training",
"_____no_output_____"
],
[
"The primary use case is to automatically save checkpoints *during* and at *the end* of training. This way you can use a trained model without having to retrain it, or pick-up training where you left of—in case the training process was interrupted.\n\n`tf.keras.callbacks.ModelCheckpoint` is a callback that performs this task. The callback takes a couple of arguments to configure checkpointing.\n\n### Checkpoint callback usage\n\nTrain the model and pass it the `ModelCheckpoint` callback:",
"_____no_output_____"
]
],
[
[
"checkpoint_path = \"training_1/cp.ckpt\"\ncheckpoint_dir = os.path.dirname(checkpoint_path)\n\n# Create checkpoint callback\ncp_callback = tf.keras.callbacks.ModelCheckpoint(checkpoint_path, \n save_weights_only=True,\n verbose=1)\n\nmodel = create_model()\n\nmodel.fit(train_images, train_labels, epochs = 10, \n validation_data = (test_images,test_labels),\n callbacks = [cp_callback]) # pass callback to training",
"_____no_output_____"
]
],
[
[
"This creates a single collection of TensorFlow checkpoint files that are updated at the end of each epoch:",
"_____no_output_____"
]
],
[
[
"!ls {checkpoint_dir}",
"_____no_output_____"
]
],
[
[
"Create a new, untrained model. When restoring a model from only weights, you must have a model with the same architecture as the original model. Since it's the same model architecture, we can share weights despite that it's a different *instance* of the model.\n\nNow rebuild a fresh, untrained model, and evaluate it on the test set. An untrained model will perform at chance levels (~10% accuracy):",
"_____no_output_____"
]
],
[
[
"model = create_model()\n\nloss, acc = model.evaluate(test_images, test_labels)\nprint(\"Untrained model, accuracy: {:5.2f}%\".format(100*acc))",
"_____no_output_____"
]
],
[
[
"Then load the weights from the checkpoint, and re-evaluate:",
"_____no_output_____"
]
],
[
[
"model.load_weights(checkpoint_path)\nloss,acc = model.evaluate(test_images, test_labels)\nprint(\"Restored model, accuracy: {:5.2f}%\".format(100*acc))",
"_____no_output_____"
]
],
[
[
"### Checkpoint callback options\n\nThe callback provides several options to give the resulting checkpoints unique names, and adjust the checkpointing frequency.\n\nTrain a new model, and save uniquely named checkpoints once every 5-epochs:\n",
"_____no_output_____"
]
],
[
[
"# include the epoch in the file name. (uses `str.format`)\ncheckpoint_path = \"training_2/cp-{epoch:04d}.ckpt\"\ncheckpoint_dir = os.path.dirname(checkpoint_path)\n\ncp_callback = tf.keras.callbacks.ModelCheckpoint(\n checkpoint_path, verbose=1, save_weights_only=True,\n # Save weights, every 5-epochs.\n period=5)\n\nmodel = create_model()\nmodel.fit(train_images, train_labels,\n epochs = 50, callbacks = [cp_callback],\n validation_data = (test_images,test_labels),\n verbose=0)",
"_____no_output_____"
]
],
[
[
"Now, look at the resulting checkpoints and choose the latest one:",
"_____no_output_____"
]
],
[
[
"! ls {checkpoint_dir}",
"_____no_output_____"
],
[
"latest = tf.train.latest_checkpoint(checkpoint_dir)\nlatest",
"_____no_output_____"
]
],
[
[
"Note: the default tensorflow format only saves the 5 most recent checkpoints.\n\nTo test, reset the model and load the latest checkpoint:",
"_____no_output_____"
]
],
[
[
"model = create_model()\nmodel.load_weights(latest)\nloss, acc = model.evaluate(test_images, test_labels)\nprint(\"Restored model, accuracy: {:5.2f}%\".format(100*acc))",
"_____no_output_____"
]
],
[
[
"## What are these files?",
"_____no_output_____"
],
[
"The above code stores the weights to a collection of [checkpoint](https://www.tensorflow.org/guide/saved_model#save_and_restore_variables)-formatted files that contain only the trained weights in a binary format. Checkpoints contain:\n* One or more shards that contain your model's weights. \n* An index file that indicates which weights are stored in a which shard. \n\nIf you are only training a model on a single machine, you'll have one shard with the suffix: `.data-00000-of-00001`",
"_____no_output_____"
],
[
"## Manually save weights\n\nAbove you saw how to load the weights into a model.\n\nManually saving the weights is just as simple, use the `Model.save_weights` method.",
"_____no_output_____"
]
],
[
[
"# Save the weights\nmodel.save_weights('./checkpoints/my_checkpoint')\n\n# Restore the weights\nmodel = create_model()\nmodel.load_weights('./checkpoints/my_checkpoint')\n\nloss,acc = model.evaluate(test_images, test_labels)\nprint(\"Restored model, accuracy: {:5.2f}%\".format(100*acc))",
"_____no_output_____"
]
],
[
[
"## Save the entire model\n\nThe entire model can be saved to a file that contains the weight values, the model's configuration, and even the optimizer's configuration (depends on set up). This allows you to checkpoint a model and resume training later—from the exact same state—without access to the original code.\n\nSaving a fully-functional model is very useful—you can load them in TensorFlow.js ([HDF5](https://js.tensorflow.org/tutorials/import-keras.html), [Saved Model](https://js.tensorflow.org/tutorials/import-saved-model.html)) and then train and run them in web browsers, or convert them to run on mobile devices using TensorFlow Lite ([HDF5](https://www.tensorflow.org/lite/convert/python_api#exporting_a_tfkeras_file_), [Saved Model](https://www.tensorflow.org/lite/convert/python_api#exporting_a_savedmodel_))",
"_____no_output_____"
],
[
"### As an HDF5 file\n\nKeras provides a basic save format using the [HDF5](https://en.wikipedia.org/wiki/Hierarchical_Data_Format) standard. For our purposes, the saved model can be treated as a single binary blob.",
"_____no_output_____"
]
],
[
[
"model = create_model()\n\n# You need to use a keras.optimizer to restore the optimizer state from an HDF5 file.\nmodel.compile(optimizer='adam', \n loss=tf.keras.losses.sparse_categorical_crossentropy,\n metrics=['accuracy'])\n\nmodel.fit(train_images, train_labels, epochs=5)\n\n# Save entire model to a HDF5 file\nmodel.save('my_model.h5')",
"_____no_output_____"
]
],
[
[
"Now recreate the model from that file:",
"_____no_output_____"
]
],
[
[
"# Recreate the exact same model, including weights and optimizer.\nnew_model = keras.models.load_model('my_model.h5')\nnew_model.summary()",
"_____no_output_____"
]
],
[
[
"Check its accuracy:",
"_____no_output_____"
]
],
[
[
"loss, acc = new_model.evaluate(test_images, test_labels)\nprint(\"Restored model, accuracy: {:5.2f}%\".format(100*acc))",
"_____no_output_____"
]
],
[
[
"This technique saves everything:\n\n* The weight values\n* The model's configuration(architecture)\n* The optimizer configuration\n\nKeras saves models by inspecting the architecture. Currently, it is not able to save TensorFlow optimizers (from `tf.train`). When using those you will need to re-compile the model after loading, and you will loose the state of the optimizer.\n",
"_____no_output_____"
],
[
"## What's Next\n\nThat was a quick guide to saving and loading in with `tf.keras`.\n\n* The [tf.keras guide](https://www.tensorflow.org/guide/keras) shows more about saving and loading models with `tf.keras`.\n\n* See [Saving in eager](https://www.tensorflow.org/guide/eager#object_based_saving) for saving during eager execution.\n\n* The [Save and Restore](https://www.tensorflow.org/guide/saved_model) guide has low-level details about TensorFlow saving.",
"_____no_output_____"
]
]
] | [
"markdown",
"code",
"markdown",
"code",
"markdown",
"code",
"markdown",
"code",
"markdown",
"code",
"markdown",
"code",
"markdown",
"code",
"markdown",
"code",
"markdown",
"code",
"markdown",
"code",
"markdown",
"code",
"markdown",
"code",
"markdown",
"code",
"markdown",
"code",
"markdown",
"code",
"markdown"
] | [
[
"markdown"
],
[
"code",
"code"
],
[
"markdown",
"markdown",
"markdown",
"markdown",
"markdown"
],
[
"code"
],
[
"markdown"
],
[
"code",
"code"
],
[
"markdown",
"markdown"
],
[
"code"
],
[
"markdown",
"markdown"
],
[
"code"
],
[
"markdown"
],
[
"code"
],
[
"markdown"
],
[
"code"
],
[
"markdown"
],
[
"code"
],
[
"markdown"
],
[
"code"
],
[
"markdown"
],
[
"code",
"code"
],
[
"markdown"
],
[
"code"
],
[
"markdown",
"markdown",
"markdown"
],
[
"code"
],
[
"markdown",
"markdown"
],
[
"code"
],
[
"markdown"
],
[
"code"
],
[
"markdown"
],
[
"code"
],
[
"markdown",
"markdown"
]
] |
d079bc6d0db51713fb337b1999bacf90b42fef94 | 14,252 | ipynb | Jupyter Notebook | ipython/home/tutorials/3. Stream Analytics Tutorial.ipynb | samarth-bhutani/epidata-community | 581c947377ce6166a2ef27507e8bf788176ace4d | [
"Apache-2.0"
] | 8 | 2017-08-20T18:57:21.000Z | 2021-02-22T02:16:19.000Z | ipython/home/tutorials/3. Stream Analytics Tutorial.ipynb | rohithn1/epidata-community | b528fc3f99b6ef3c427bcd3899339f10c2a8d5e1 | [
"Apache-2.0"
] | 2 | 2021-01-03T02:06:24.000Z | 2021-01-25T07:25:18.000Z | ipython/home/tutorials/3. Stream Analytics Tutorial.ipynb | legendaryfu/epidata-community | 48a4dd8a67f361ac9d50771f693e33985b10dbbf | [
"Apache-2.0"
] | 7 | 2019-08-17T14:58:53.000Z | 2021-02-15T18:01:08.000Z | 39.370166 | 418 | 0.594022 | [
[
[
"<h1 style=\"text-align:center;text-decoration: underline\">Stream Analytics Tutorial</h1>\n<h1>Overview</h1>\n<p>Welcome to the stream analytics tutorial for EpiData. In this tutorial we will perform near real-time stream analytics on sample weather data acquired from a simulated wireless sensor network.</p>",
"_____no_output_____"
],
[
"<h2>Package and Module Imports</h2>\n<p>As a first step, we will import packages and modules required for this tutorial. Since <i>EpiData Context (ec)</i> is required to use the application, it is implicitly imported. Sample functions for near real-time analytics are avaialable in <i>EpiData Analytics</i> package. Other packages and modules, such as <i>datetime</i>, <i>pandas</i> and <i>matplotlib</i>, can also be imported at this time.</p>",
"_____no_output_____"
]
],
[
[
"#from epidata.context import ec\nfrom epidata.analytics import *\n\n%matplotlib inline\nfrom datetime import datetime, timedelta\nimport pandas as pd\nimport time\nimport pylab as pl\nfrom IPython import display\nimport json",
"_____no_output_____"
]
],
[
[
"<h2>Stream Analysis</h2>\n<h3>Function Definition</h3>\n<p>EpiData supports development and deployment of custom algorithms via Jupyter Notebook. Below, we define python functions for substituting extreme outliers and aggregating temperature measurements. These functions can be operated on near real-time and historic data. In this tutorial, we will apply the functions on near real-time data available from Kafka 'measurements' and 'measurements_cleansed' topics</p>",
"_____no_output_____"
]
],
[
[
"import pandas as pd\nimport math, numbers\n\ndef substitute_demo(df, meas_names, method=\"rolling\", size=3):\n \"\"\"\n Substitute missing measurement values within a data frame, using the specified method.\n \"\"\"\n\n df[\"meas_value\"].replace(250, np.nan, inplace=True)\n \n for meas_name in meas_names:\n\n if (method == \"rolling\"):\n if ((size % 2 == 0) and (size != 0)): size += 1 \n if df.loc[df[\"meas_name\"]==meas_name].size > 0:\n indices = df.loc[df[\"meas_name\"] == meas_name].index[df.loc[df[\"meas_name\"] == meas_name][\"meas_value\"].apply(\n lambda x: not isinstance(x, basestring) and (x == None or np.isnan(x)))]\n substitutes = df.loc[df[\"meas_name\"]==meas_name][\"meas_value\"].rolling( window=size, min_periods=1, center=True).mean()\n \n df[\"meas_value\"].fillna(substitutes, inplace=True)\n df.loc[indices, \"meas_flag\"] = \"substituted\"\n df.loc[indices, \"meas_method\"] = \"rolling average\"\n else:\n raise ValueError(\"Unsupported substitution method: \", repr(method))\n \n return df\n",
"_____no_output_____"
],
[
"import pandas as pd\nimport numpy as np\nimport json\n\ndef subgroup_statistics(row):\n row['start_time'] = np.min(row[\"ts\"])\n row[\"stop_time\"] = np.max(row[\"ts\"])\n row[\"meas_summary_name\"] = \"statistics\"\n row[\"meas_summary_value\"] = json.dumps({'count': row[\"meas_value\"].count(), 'mean': row[\"meas_value\"].mean(),\n 'std': row[\"meas_value\"].std(), 'min': row[\"meas_value\"].min(), \n 'max': row[\"meas_value\"].max()})\n row[\"meas_summary_description\"] = \"descriptive statistics\"\n return row\n\ndef meas_statistics_demo(df, meas_names, method=\"standard\"):\n \"\"\"\n Compute statistics on measurement values within a data frame, using the specified method.\n \"\"\"\n \n if (method == \"standard\"):\n df_grouped = df.loc[df[\"meas_name\"].isin(meas_names)].groupby([\"company\", \"site\", \"station\", \"sensor\"], \n as_index=False)\n df_summary = df_grouped.apply(subgroup_statistics).loc[:, [\"company\", \"site\", \"station\", \"sensor\",\n \"start_time\", \"stop_time\", \"event\", \"meas_name\", \"meas_summary_name\", \"meas_summary_value\", \n \"meas_summary_description\"]].drop_duplicates()\n else:\n raise ValueError(\"Unsupported summary method: \", repr(method))\n \n return df_summary",
"_____no_output_____"
]
],
[
[
"<h3>Transformations and Streams</h3>\n<p>The analytics algorithms are executed on near real-time data through transformations. A transformation specifies the function, its parameters and destination. The destination can be one of the database tables, namely <i>'measurements_cleansed'</i> or <i>'measurements_summary'</i>, or another Kafka topic.</p>\n<p>Once the transformations are defined, they are initiated via <i>ec.create_stream(transformations, data_source, batch_duration)</i> function call.</p>",
"_____no_output_____"
]
],
[
[
"#Stop current near real-time processing\nec.stop_streaming()\n",
"_____no_output_____"
],
[
"# Define tranformations and steam operations\nop1 = ec.create_transformation(substitute_demo, [[\"Temperature\", \"Wind_Speed\"], \"rolling\", 3], \"measurements_substituted\")\nec.create_stream([op1], \"measurements\")\n\nop2 = ec.create_transformation(identity, [], \"measurements_cleansed\")\nop3 = ec.create_transformation(meas_statistics, [[\"Temperature\", \"Wind_Speed\"], \"standard\"], \"measurements_summary\")\nec.create_stream([op2, op3],\"measurements_substituted\")\n\n# Start near real-time processing\nec.start_streaming()",
"_____no_output_____"
]
],
[
[
"<h3>Data Ingestion</h3>\n<p>We can now start data ingestion from simulated wireless sensor network. To do so, you can download and run the <i>sensor_data_with_outliers.py</i> example shown in the image below.</p>\n<img src=\"./static/jupyter_tree.png\">",
"_____no_output_____"
],
[
"<h3>Data Query and Visualization</h3>\n<p>We query the original and processed data from Kafka queue using Kafka Consumer. The data obtained from the quey is visualized using Bokeh charts.</p>",
"_____no_output_____"
]
],
[
[
"from bokeh.io import push_notebook, show, output_notebook\nfrom bokeh.layouts import row, column\nfrom bokeh.plotting import figure\nfrom bokeh.models import ColumnDataSource\n\nfrom kafka import KafkaConsumer\nimport json\nfrom pandas.io.json import json_normalize\n\noutput_notebook()",
"_____no_output_____"
],
[
"plot1 = figure(plot_width=750, plot_height=200, x_axis_type='datetime', y_range=(30, 300))\nplot2 = figure(plot_width=750, plot_height=200, x_axis_type='datetime', y_range=(30, 300))\ndf_kafka_init = pd.DataFrame(columns = [\"ts\", \"meas_value\"])\ntest_data_1 = ColumnDataSource(data=df_kafka_init.to_dict(orient='list'))\ntest_data_2 = ColumnDataSource(data=df_kafka_init.to_dict(orient='list'))\nmeas_name = \"Temperature\"\n\nplot1.circle(\"ts\", \"meas_value\", source=test_data_1, legend=meas_name, line_color='orangered', line_width=1.5)\nline1 = plot1.line(\"ts\", \"meas_value\", source=test_data_1, legend=meas_name, line_color='orangered', line_width=1.5)\nplot1.legend.location = \"top_right\"\nplot2.circle(\"ts\", \"meas_value\", source=test_data_2, legend=meas_name, line_color='blue', line_width=1.5)\nline2 = plot2.line(\"ts\", \"meas_value\", source=test_data_2, legend=meas_name, line_color='blue', line_width=1.5)\nplot2.legend.location = \"top_right\"",
"_____no_output_____"
],
[
"consumer = KafkaConsumer()\nconsumer.subscribe(['measurements', 'measurements_substituted'])\ndelay = .1\n\nhandle = show(column(plot1, plot2), notebook_handle=True) ",
"_____no_output_____"
],
[
"for message in consumer:\n topic = message.topic\n measurements = json.loads(message.value)\n df_kafka = json_normalize(measurements)\n df_kafka[\"meas_value\"] = np.nan if \"meas_value\" not in measurements else measurements[\"meas_value\"]\n df_kafka = df_kafka.loc[df_kafka[\"meas_name\"]==meas_name] \n df_kafka = df_kafka[[\"ts\", \"meas_value\"]]\n df_kafka[\"ts\"] = df_kafka[\"ts\"].apply(lambda x: pd.to_datetime(x, unit='ms').tz_localize('UTC').tz_convert('US/Pacific')) \n \n if (not df_kafka.empty):\n if (topic == 'measurements'):\n test_data_1.stream(df_kafka.to_dict(orient='list'))\n if (topic == 'measurements_substituted'):\n test_data_2.stream(df_kafka.to_dict(orient='list'))\n push_notebook(handle=handle)\n \n time.sleep(delay)",
"_____no_output_____"
]
],
[
[
"<p>Another way to query and visualize processed data is using <i>ec.query_measurements_cleansed(..) and ec.query_measurements_summary(..)</i> functions. For our example, we specify paramaters that match sample data set, and query the aggregated values using <i>ec.query_measurements_summary(..)</i> function call.</p>",
"_____no_output_____"
]
],
[
[
"# QUERY MEASUREMENTS_CLEANSED TABLE\n\nprimary_key={\"company\": \"EpiData\", \"site\": \"San_Jose\", \"station\":\"WSN-1\", \n \"sensor\": [\"Temperature_Probe\", \"RH_Probe\", \"Anemometer\"]}\nstart_time = datetime.strptime('8/19/2017 00:00:00', '%m/%d/%Y %H:%M:%S')\nstop_time = datetime.strptime('8/20/2017 00:00:00', '%m/%d/%Y %H:%M:%S')\ndf_cleansed = ec.query_measurements_cleansed(primary_key, start_time, stop_time)\nprint \"Number of records:\", df_cleansed.count()\n\ndf_cleansed_local = df_cleansed.toPandas()\ndf_cleansed_local[df_cleansed_local[\"meas_name\"]==\"Temperature\"].tail(10).sort_values(by=\"ts\",ascending=False)",
"_____no_output_____"
],
[
"# QUERY MEASUREMNTS_SUMMARY TABLE\n\nprimary_key={\"company\": \"EpiData\", \"site\": \"San_Jose\", \"station\":\"WSN-1\", \"sensor\": [\"Temperature_Probe\"]}\nstart_time = datetime.strptime('8/19/2017 00:00:00', '%m/%d/%Y %H:%M:%S')\nstop_time = datetime.strptime('8/20/2017 00:00:00', '%m/%d/%Y %H:%M:%S')\nlast_index = -1\nsummary_result = pd.DataFrame()\n\ndf_summary = ec.query_measurements_summary(primary_key, start_time, stop_time)\ndf_summary_local = df_summary.toPandas()\nsummary_keys = df_summary_local[[\"company\", \"site\", \"station\", \"sensor\", \"start_time\", \"stop_time\", \"meas_name\", \"meas_summary_name\"]]\nsummary_result = df_summary_local[\"meas_summary_value\"].apply(json.loads).apply(pd.Series)\nsummary_combined = pd.concat([summary_keys, summary_result], axis=1)\n\nsummary_combined.tail(5)",
"_____no_output_____"
]
],
[
[
"<h3>Stop Stream Analytics</h3>\n<p>The transformations can be stopped at any time via <i>ec.stop_streaming()</i> function call<p>",
"_____no_output_____"
]
],
[
[
"#Stop current near real-time processing\nec.stop_streaming()",
"_____no_output_____"
]
],
[
[
"<h2>Next Steps</h2>\n<p>Congratulations, you have successfully perfomed near real-time analytics on sample data aquired by a simulated wireless sensor network. The next step is to explore various capabilities of EpiData by creating your own custom analytics application!</p>",
"_____no_output_____"
]
]
] | [
"markdown",
"code",
"markdown",
"code",
"markdown",
"code",
"markdown",
"code",
"markdown",
"code",
"markdown",
"code",
"markdown"
] | [
[
"markdown",
"markdown"
],
[
"code"
],
[
"markdown"
],
[
"code",
"code"
],
[
"markdown"
],
[
"code",
"code"
],
[
"markdown",
"markdown"
],
[
"code",
"code",
"code",
"code"
],
[
"markdown"
],
[
"code",
"code"
],
[
"markdown"
],
[
"code"
],
[
"markdown"
]
] |
d079c55e531c1ba8ac1fb12022e4d06f338dddc1 | 5,446 | ipynb | Jupyter Notebook | YEAR_WISE_PYTHON_CODE/ntt_arima_year_himachal.ipynb | rajesh016/rain_prediction | 50432fdb867ceea003f3270baf2b0865d28ed848 | [
"MIT"
] | null | null | null | YEAR_WISE_PYTHON_CODE/ntt_arima_year_himachal.ipynb | rajesh016/rain_prediction | 50432fdb867ceea003f3270baf2b0865d28ed848 | [
"MIT"
] | null | null | null | YEAR_WISE_PYTHON_CODE/ntt_arima_year_himachal.ipynb | rajesh016/rain_prediction | 50432fdb867ceea003f3270baf2b0865d28ed848 | [
"MIT"
] | null | null | null | 45.008264 | 364 | 0.664157 | [
[
[
"import pandas\nimport matplotlib.mlab as mlab\nimport matplotlib.pyplot as plt\nimport numpy as np\nimport math\nfrom statsmodels.tsa.stattools import acf, pacf\nimport statsmodels.tsa.stattools as ts\nfrom statsmodels.tsa.arima_model import ARIMA\n\nvariables = pandas.read_csv('STATEWISE_ DATASET1/data_HIMACHAL_PRADESH.csv')\nrain1 = variables['ANNUAL']\n#rain1 = (rain[0:90,:])\nrain1=rain1.replace(0,rain1.mean())\nrain1.fillna((rain1.mean()), inplace=True)\nrain1=rain1.head(110)\nlnrain1=np.log(rain1)\nrain_matrix=lnrain1.as_matrix()\nmodel = ARIMA(rain_matrix, order=(5,1,0))\nmodel_fit = model.fit(disp=0)\nmodel.dates=None\nmodel.freq=None\nmodel.missing=None\nmodel_fit.save('model_arima_year_himachal_pradesh.pkl')\n",
"/home/rajesh/anaconda3/envs/virtual_platform/lib/python3.5/site-packages/statsmodels/compat/pandas.py:56: FutureWarning: The pandas.core.datetools module is deprecated and will be removed in a future version. Please use the pandas.tseries module instead.\n from pandas.core import datetools\n/home/rajesh/.local/lib/python3.5/site-packages/scipy/signal/signaltools.py:1341: FutureWarning: Using a non-tuple sequence for multidimensional indexing is deprecated; use `arr[tuple(seq)]` instead of `arr[seq]`. In the future this will be interpreted as an array index, `arr[np.array(seq)]`, which will result either in an error or a different result.\n out_full[ind] += zi\n/home/rajesh/.local/lib/python3.5/site-packages/scipy/signal/signaltools.py:1344: FutureWarning: Using a non-tuple sequence for multidimensional indexing is deprecated; use `arr[tuple(seq)]` instead of `arr[seq]`. In the future this will be interpreted as an array index, `arr[np.array(seq)]`, which will result either in an error or a different result.\n out = out_full[ind]\n/home/rajesh/.local/lib/python3.5/site-packages/scipy/signal/signaltools.py:1350: FutureWarning: Using a non-tuple sequence for multidimensional indexing is deprecated; use `arr[tuple(seq)]` instead of `arr[seq]`. In the future this will be interpreted as an array index, `arr[np.array(seq)]`, which will result either in an error or a different result.\n zf = out_full[ind]\n/home/rajesh/anaconda3/envs/virtual_platform/lib/python3.5/site-packages/statsmodels/tsa/kalmanf/kalmanfilter.py:646: FutureWarning: Conversion of the second argument of issubdtype from `float` to `np.floating` is deprecated. In future, it will be treated as `np.float64 == np.dtype(float).type`.\n if issubdtype(paramsdtype, float):\n/home/rajesh/anaconda3/envs/virtual_platform/lib/python3.5/site-packages/statsmodels/tsa/kalmanf/kalmanfilter.py:650: FutureWarning: Conversion of the second argument of issubdtype from `complex` to `np.complexfloating` is deprecated. In future, it will be treated as `np.complex128 == np.dtype(complex).type`.\n elif issubdtype(paramsdtype, complex):\n"
],
[
"from statsmodels.tsa.arima_model import ARIMAResults\nloaded=ARIMAResults.load('model_arima_year_himachal_pradesh.pkl')\nprediction_year_HIMACHAL_PRADESH=loaded.predict(115,130 ,typ='levels')\nprediction_year_HIMACHAL_PRADESH\npredictionsadjusted_year_HIMACHAL_PRADESH=np.exp(prediction_year_HIMACHAL_PRADESH)\npredictionsadjusted_year_HIMACHAL_PRADESH",
"/home/rajesh/anaconda3/envs/virtual_platform/lib/python3.5/site-packages/statsmodels/tsa/kalmanf/kalmanfilter.py:577: FutureWarning: Conversion of the second argument of issubdtype from `float` to `np.floating` is deprecated. In future, it will be treated as `np.float64 == np.dtype(float).type`.\n if issubdtype(paramsdtype, float):\n"
]
]
] | [
"code"
] | [
[
"code",
"code"
]
] |
d079cd0832fdea909e142476a3170f32b8d1ec1a | 1,705 | ipynb | Jupyter Notebook | Data/Processes/.ipynb_checkpoints/Suma_TodasInteracciones-checkpoint.ipynb | JonnHenry/Deeds_Machine-Learning | c230222a0d1acac2fac92b33718c6d2d9f73fb96 | [
"MIT"
] | 1 | 2021-07-13T00:20:58.000Z | 2021-07-13T00:20:58.000Z | Data/Processes/Suma_TodasSesiones.ipynb | JonnHenry/Deeds_Machine-Learning | c230222a0d1acac2fac92b33718c6d2d9f73fb96 | [
"MIT"
] | null | null | null | Data/Processes/Suma_TodasSesiones.ipynb | JonnHenry/Deeds_Machine-Learning | c230222a0d1acac2fac92b33718c6d2d9f73fb96 | [
"MIT"
] | null | null | null | 21.858974 | 98 | 0.556012 | [
[
[
"import pandas as pd",
"_____no_output_____"
],
[
"#sesion1=pd.read_csv('sesion1.csv')\nsesion2=pd.read_csv('./Suma/Suma_sesion2.csv')\nsesion3=pd.read_csv('./Suma/Suma_sesion3.csv')\nsesion4=pd.read_csv('./Suma/Suma_sesion4.csv')\nsesion5=pd.read_csv('./Suma/Suma_sesion5.csv')\nsesion6=pd.read_csv('./Suma/Suma_sesion6.csv')\n",
"_____no_output_____"
],
[
"sumaSesiones =len(sesion2)+len(sesion3)+len(sesion4)+len(sesion5)+len(sesion6)\nprint(sumaSesiones)\n\ntodasSesiones = pd.concat([sesion2,sesion3,sesion4,sesion5,sesion6])\ntodasSesiones.fillna(0,inplace=True)\ntodasSesiones.to_csv('./Suma/Suma_todasLasSesiones.csv', index=False, header=True,sep=',')\n",
"443\n"
]
]
] | [
"code"
] | [
[
"code",
"code",
"code"
]
] |
d079f10f124f2b91e9cc31fc659fcd5c590f61b0 | 32,337 | ipynb | Jupyter Notebook | T-CNN.ipynb | Kwongrf/B-ALL | f190b3d0d3c47ffb05be7c6b33e5d8e71a6cfdf1 | [
"Apache-2.0"
] | null | null | null | T-CNN.ipynb | Kwongrf/B-ALL | f190b3d0d3c47ffb05be7c6b33e5d8e71a6cfdf1 | [
"Apache-2.0"
] | null | null | null | T-CNN.ipynb | Kwongrf/B-ALL | f190b3d0d3c47ffb05be7c6b33e5d8e71a6cfdf1 | [
"Apache-2.0"
] | null | null | null | 50.84434 | 876 | 0.579305 | [
[
[
"# %load train.py\n#!/usr/bin/env python\n\n# In[1]:\nfrom t_cnn import tcnn\n\nmodel = tcnn(num_classes = 2,pretrained = True, model_root = '/home/krf/model/BALL/')\n\nimport senet\nimport os\nimport numpy as np\nimport torch\nfrom torchvision.datasets import ImageFolder\nfrom utils import TransformImage\nimport shutil\nimport time\n\nimport torch\nimport torch.nn as nn\nimport torch.nn.parallel\nimport torch.backends.cudnn as cudnn\nimport torch.optim as optim\nfrom torch.optim import lr_scheduler\nimport torch.utils.data\nimport torchvision.transforms as transforms\nimport torchvision.datasets as datasets\nfrom tensorboardX import SummaryWriter\n\nDATA_DIR = \"/home/krf/dataset/BALL/\"\ntraindir = DATA_DIR + \"train\"\nvaldir = DATA_DIR +\"val\"\nos.environ[\"CUDA_VISIBLE_DEVICES\"] = \"2\"\nBATCH_SIZE = 32\nWORKERS = 4\nSTART = 0\nEPOCHS = 1200\nPRINT_FREQ = 20\n\n\n# In[31]:\n\n\n#model = senet.se_resnext50_32x4d(num_classes = 2)\n#通过随机变化来进行数据增强\ntrain_tf = TransformImage(\n model,\n \n random_crop=False,\n random_hflip=True,\n random_vflip=True,\n random_rotate=True,\n preserve_aspect_ratio=True\n)\ntrain_loader = torch.utils.data.DataLoader(\n# datasets.ImageFolder(traindir, transforms.Compose([\n# # transforms.RandomSizedCrop(max(model.input_size)),\n# transforms.RandomHorizontalFlip(),\n# transforms.ToTensor(),\n# normalize,\n# ])),\n datasets.ImageFolder(traindir,train_tf),\n batch_size=BATCH_SIZE, shuffle=True,\n num_workers=WORKERS, pin_memory=True)\n\n\nval_tf = TransformImage(\n model,\n \n preserve_aspect_ratio=True)\nval_loader = torch.utils.data.DataLoader(\n datasets.ImageFolder(valdir,val_tf),\n batch_size=BATCH_SIZE, shuffle=False,\n num_workers=WORKERS, pin_memory=True)\n\n\n# In[29]:\n\n\ndef train(train_loader, model, criterion, optimizer, epoch,scheduler):\n # switch to train mode\n model.train()\n# end = time.time()\n for i, (input, target) in enumerate(train_loader):\n # measure data loading time\n# data_time.update(time.time() - end)\n input = input.cuda()\n target = target.cuda(async=True)\n input_var = torch.autograd.Variable(input.float())\n target_var = torch.autograd.Variable(target)\n #print(input_var.type())\n # compute output\n output = model(input_var)\n loss = criterion(output, target_var)\n #print(output.data)\n\n\n\n# # compute gradient and do SGD step\n optimizer.zero_grad()\n loss.backward()\n optimizer.step()\n\n# # measure elapsed time\n# batch_time.update(time.time() - end)\n# end = time.time()\n meters = trainMeter.update(output,target,loss,input.size(0))\n\n if i % PRINT_FREQ == 0:\n print('Epoch: [{0}][{1}/{2}]\\t'\n 'Loss {loss:.5f}\\t'\n 'Acc {Acc:.5f}\\t'\n 'Precision {P:.5f}\\t'\n 'Recall {R:.5f}\\t'\n 'F1 {F1:.5f}'.format(\n epoch,i, len(train_loader), loss=meters[4],\n Acc=meters[3],P=meters[0],R=meters[1],F1=meters[2]))\n \n step = epoch*len(train_loader) + i\n \n writer.add_scalar('TRAIN/Precision', meters[0], step)\n writer.add_scalar('TRAIN/Recall', meters[1], step)\n writer.add_scalar('TRAIN/F1', meters[2], step)\n writer.add_scalar('TRAIN/Acc', meters[3], step)\n writer.add_scalar('TRAIN/loss',meters[4], step)\n \n scheduler.step(meters[4])\n\n\ndef validate(val_loader, model, criterion,epoch):\n # switch to evaluate mode\n model.eval()\n \n# end = time.time()\n meters = []\n for i, (input, target) in enumerate(val_loader):\n target = target.cuda()\n input = input.cuda()\n input_var = torch.autograd.Variable(input, volatile=True)\n target_var = torch.autograd.Variable(target, volatile=True)\n # compute output\n output = model(input_var)\n loss = criterion(output, target_var)\n meters = valMeter.update(output,target,loss,input.size(0))\n if i % PRINT_FREQ == 0:\n print('Test: [{0}/{1}]\\t'\n 'Loss {loss:.5f}\\t'\n 'Acc {Acc:.5f}\\t'\n 'Precision {P:.5f}\\t'\n 'Recall {R:.5f}\\t'\n 'F1 {F1:.5f}'.format(\n i, len(val_loader), loss=meters[4],\n Acc=meters[3],P=meters[0],R=meters[1],F1=meters[2]))\n \n step = epoch * len(val_loader) + i\n writer.add_scalar('VAL/Precision', meters[0], step)\n writer.add_scalar('VAL/Recall', meters[1], step)\n writer.add_scalar('VAL/F1', meters[2], step)\n writer.add_scalar('VAL/Acc', meters[3], step)\n writer.add_scalar('VAL/loss',meters[4], step)\n print(' * Acc {Acc:.5f} F1 {F1:.5f}'\n .format(Acc=meters[3],F1=meters[2]))\n writer.add_scalar('VAL/EPOCH_F1', meters[2], epoch)\n return meters[2]\n\n\ndef save_checkpoint(state, is_best, filename='checkpoint.pth.tar'):\n torch.save(state, filename)\n if is_best:\n shutil.copyfile(filename, 'tcnn5_model_best.pth.tar')\n\nclass ModelMeter(object):\n def __init__(self):\n self.reset()\n \n def reset(self):\n self.losses = AverageMeter()\n self.top1 = AverageMeter()\n self.TP = 1e-8\n self.TN = 1e-8\n self.FN = 1e-8\n self.FP = 1e-8\n self.P=1e-8\n self.R=1e-8\n self.F1=1e-8\n self.Acc=1e-8\n\n def update(self, output,target,loss, n=1):\n _, pred = output.data.topk(1, 1, True, True)\n pred = pred.t()\n# print(pred,target.data)\n # TP predict 和 label 同时为1\n self.TP += ((pred == 1) & (target.data == 1)).cpu().numpy().sum()\n # TN predict 和 label 同时为0\n self.TN += ((pred == 0) & (target.data == 0)).cpu().numpy().sum()\n # FN predict 0 label 1\n self.FN += ((pred == 0) & (target.data == 1)).cpu().numpy().sum()\n # FP predict 1 label 0\n self.FP += ((pred == 1) & (target.data == 0)).cpu().numpy().sum()\n# print(self.TP,self.TN,self.FN,self.FP)\n# zes=torch.autograd.Variable(torch.zeros(target.size()).type(torch.cuda.LongTensor))#全0变量\n\n# ons=torch.autograd.Variable(torch.ones(target.size()).type(torch.cuda.LongTensor))#全1变量\n# print(zes,ons)\n# train_correct01 = ((pred==zes)&(target.data.squeeze(1)==ons)).sum()#原标签为1,预测为 0 的总数\n\n# train_correct10 = ((pred==ons)&(target.data.squeeze(1)==zes)).sum()#原标签为0,预测为1 的总数\n\n# train_correct11 = ((pred_y==ons)&(target.data.squeeze(1)==ons)).sum()\n# train_correct00 = ((pred_y==zes)&(target.data.squeeze(1)==zes)).sum()\n# self.FN += train_correct01.data[0] \n\n# self.FP += train_correct10.data[0]\n\n# self.TP += train_correct11.data[0] \n# self.TN += train_correct00.data[0]\n \n self.P = self.TP / (self.TP + self.FP)\n self.R = self.TP / (self.TP + self.FN)\n self.F1 = 2 * self.R * self.P / (self.R + self.P)\n \n self.Acc = (self.TP + self.TN) / (self.TP + self.TN + self.FP + self.FN)\n \n self.losses.update(loss.data[0],n)\n\n return [self.P,self.R,self.F1,self.Acc,self.losses.avg]\n\nclass AverageMeter(object):\n \"\"\"Computes and stores the average and current value\"\"\"\n\n def __init__(self):\n self.reset()\n\n def reset(self):\n self.val = 1e-8\n self.avg = 1e-8\n self.sum = 1e-8\n self.count = 0\n\n def update(self, val, n=1):\n self.val = val\n self.sum += val * n\n self.count += n\n self.avg = self.sum / self.count\n\n\ndef adjust_learning_rate(lr,optimizer, epoch):\n if epoch >= 300 and epoch % 100 == 0 :\n lr /= 10\n for param_group in optimizer.param_groups:\n param_group['lr'] = lr\n print(\"adjuct learning rate to {}\".format(lr))\n return lr\n\n\ndef accuracy(output, target, topk=(1,)):\n \"\"\"Computes the precision@k for the specified values of k\"\"\"\n maxk = max(topk)\n batch_size = target.size(0)\n\n _, pred = output.topk(maxk, 1, True, True)\n pred = pred.t()\n correct = pred.eq(target.view(1, -1).expand_as(pred))\n\n res = []\n for k in topk:\n correct_k = correct[:k].view(-1).float().sum(0)\n res.append(correct_k.mul_(100.0 / batch_size))\n return res\n\n\n# In[ ]:\n\n# 加载模型,解决命名和维度不匹配问题,解决多个gpu并行\ndef load_state_keywise(model, model_path):\n model_dict = model.state_dict()\n \n print(\"=> loading checkpoint '{}'\".format(model_path))\n checkpoint = torch.load(model_path,map_location='cpu')\n START = checkpoint['epoch']\n best_F1 = checkpoint['best_prec1']\n #model.load_state_dict(checkpoint['state_dict'])\n \n pretrained_dict = checkpoint['state_dict']#torch.load(model_path, map_location='cpu')\n key = list(pretrained_dict.keys())[0]\n # 1. filter out unnecessary keys\n # 1.1 multi-GPU ->CPU\n if (str(key).startswith('module.')):\n pretrained_dict = {k[7:]: v for k, v in pretrained_dict.items() if\n k[7:] in model_dict and v.size() == model_dict[k[7:]].size()}\n else:\n pretrained_dict = {k: v for k, v in pretrained_dict.items() if\n k in model_dict and v.size() == model_dict[k].size()}\n # 2. overwrite entries in the existing state dict\n model_dict.update(pretrained_dict)\n # 3. load the new state dict\n model.load_state_dict(model_dict)\n print(\"start at epoch {}, best_f1={}\".format(START,best_F1))\n return model,START,best_F1\n\n\n\ncriterion = nn.CrossEntropyLoss().cuda()\nlr = 1e-4\noptimizer = torch.optim.SGD(model.parameters(), lr = lr,momentum = 0.9,weight_decay=1e-6)\n\nscheduler = optim.lr_scheduler.ReduceLROnPlateau(optimizer, patience=10, verbose=True)\nbest_f1 = 0\nmodel,START,best_f1 = load_state_keywise(model,'tcnn5_checkpoint-Copy1.pth.tar')\n# model = model.cuda()\nmodel = torch.nn.DataParallel(model).cuda()\n\n# TP = 0,TN = 0,FN = 0, FP = 0\nwriter = SummaryWriter()\n\ntrainMeter = ModelMeter()\nvalMeter = ModelMeter()\nfor epoch in range(START,EPOCHS):\n # train for one epoch\n lr = optimizer.param_groups[0]['lr']\n writer.add_scalar('LR', lr, epoch)\n train(train_loader, model, criterion, optimizer, epoch, scheduler)\n # evaluate on validation set\n F1 = validate(val_loader, model, criterion,epoch)\n \n # remember best prec@1 and save checkpoint\n is_best = F1 > best_f1\n best_f1 = max(F1, best_f1)\n save_checkpoint({\n 'epoch': epoch + 1,\n 'arch': \"T-CNN\",\n 'state_dict': model.state_dict(),\n 'best_prec1': best_f1,\n }, is_best,filename='tcnn5_checkpoint.pth.tar')\n# export scalar data to JSON for external processing\nwriter.export_scalars_to_json(\"./test.json\")\nwriter.close()\n\n\n\n\n\n",
"TCNN_Alex(\n (conv1): Sequential(\n (0): Conv2d(3, 64, kernel_size=(11, 11), stride=(4, 4), padding=(2, 2))\n (1): ReLU(inplace)\n (2): MaxPool2d(kernel_size=3, stride=2, padding=0, dilation=1, ceil_mode=False)\n )\n (conv2): Sequential(\n (0): Conv2d(64, 192, kernel_size=(5, 5), stride=(1, 1), padding=(2, 2))\n (1): ReLU(inplace)\n (2): MaxPool2d(kernel_size=3, stride=2, padding=0, dilation=1, ceil_mode=False)\n )\n (conv3): Sequential(\n (0): Conv2d(192, 384, kernel_size=(3, 3), stride=(1, 1), padding=(1, 1))\n (1): ReLU(inplace)\n )\n (conv4): Sequential(\n (0): Conv2d(384, 256, kernel_size=(3, 3), stride=(1, 1), padding=(1, 1))\n (1): ReLU(inplace)\n )\n (conv5): Sequential(\n (0): Conv2d(256, 256, kernel_size=(3, 3), stride=(1, 1), padding=(1, 1))\n (1): ReLU(inplace)\n )\n (maxpool): MaxPool2d(kernel_size=3, stride=2, padding=0, dilation=1, ceil_mode=False)\n (fc1): Linear(in_features=9216, out_features=4096, bias=True)\n (classifier): Sequential(\n (0): ReLU(inplace)\n (1): Linear(in_features=4096, out_features=4096, bias=True)\n (2): ReLU(inplace)\n (3): Linear(in_features=4096, out_features=2, bias=True)\n )\n)\n=> loading checkpoint 'tcnn5_checkpoint-Copy1.pth.tar'\nstart at epoch 800, best_f1=0.765144861579079\n"
],
[
"# import torch.utils.model_zoo as model_zoo\n# model_dict = model_zoo.load_url('https://download.pytorch.org/models/alexnet-owt-4df8aa71.pth', '/home/krf/model/BALL/')",
"_____no_output_____"
],
[
"# print(model_dict.keys())",
"odict_keys(['features.0.weight', 'features.0.bias', 'features.3.weight', 'features.3.bias', 'features.6.weight', 'features.6.bias', 'features.8.weight', 'features.8.bias', 'features.10.weight', 'features.10.bias', 'classifier.1.weight', 'classifier.1.bias', 'classifier.4.weight', 'classifier.4.bias', 'classifier.6.weight', 'classifier.6.bias'])\n"
],
[
"# model = tcnn(2)\n# pre_dict = model.state_dict()\n# print(pre_dict.keys())",
"_____no_output_____"
],
[
"# k1 = ['features.0.weight', 'features.0.bias', 'features.3.weight', 'features.3.bias', 'features.6.weight', 'features.6.bias', 'features.8.weight', 'features.8.bias', 'features.10.weight', 'features.10.bias', 'classifier.1.weight', 'classifier.1.bias', 'classifier.4.weight', 'classifier.4.bias', 'classifier.6.weight', 'classifier.6.bias']\n# k2 = ['conv1.0.weight', 'conv1.0.bias', 'conv2.0.weight', 'conv2.0.bias', 'conv3.0.weight', 'conv3.0.bias', 'conv4.0.weight', 'conv4.0.bias', 'conv5.0.weight', 'conv5.0.bias', 'fc1.weight', 'fc1.bias', 'classifier.1.weight', 'classifier.1.bias', 'classifier.3.weight', 'classifier.3.bias']\n# for i in range(len(k1)):\n# pretrained_dict[k2[i]] = model_dict[k1[i]]\n\n# # 2. overwrite entries in the existing state dict\n# model_dict.update(pretrained_dict)",
"_____no_output_____"
]
]
] | [
"code"
] | [
[
"code",
"code",
"code",
"code",
"code"
]
] |
d079fb5202af1e76ef4a49fa73dfe6761aaf3ab6 | 7,312 | ipynb | Jupyter Notebook | examples/notebooks/18_create_landsat_timelapse.ipynb | jitendra-kumar/geemap | 8b5948f9a890416ed749530360d553f84dc8146a | [
"MIT"
] | 1 | 2020-12-15T17:49:04.000Z | 2020-12-15T17:49:04.000Z | examples/notebooks/18_create_landsat_timelapse.ipynb | TinchoBay/geemap | 0af4b82e19e6355bac6c0e068f890f707b5bbea5 | [
"MIT"
] | null | null | null | examples/notebooks/18_create_landsat_timelapse.ipynb | TinchoBay/geemap | 0af4b82e19e6355bac6c0e068f890f707b5bbea5 | [
"MIT"
] | 1 | 2020-12-13T13:49:06.000Z | 2020-12-13T13:49:06.000Z | 21.569322 | 197 | 0.528993 | [
[
[
"import geemap",
"_____no_output_____"
],
[
"geemap.show_youtube('OwjSJnGWKJs')",
"_____no_output_____"
]
],
[
[
"## Update the geemap package\n\nIf you run into errors with this notebook, please uncomment the line below to update the [geemap](https://github.com/giswqs/geemap#installation) package to the latest version from GitHub. \nRestart the Kernel (Menu -> Kernel -> Restart) to take effect.",
"_____no_output_____"
]
],
[
[
"# geemap.update_package()",
"_____no_output_____"
]
],
[
[
"## Create an interactive map\n\n### Use the Drawing tool to draw a rectangle on the map",
"_____no_output_____"
]
],
[
[
"Map = geemap.Map()\nMap",
"_____no_output_____"
]
],
[
[
"## Generate a Landsat timelapse animation",
"_____no_output_____"
]
],
[
[
"import os\nout_dir = os.path.join(os.path.expanduser(\"~\"), 'Downloads')\nif not os.path.exists(out_dir):\n os.makedirs(out_dir)",
"_____no_output_____"
],
[
"label = 'Urban Growth in Las Vegas'\nMap.add_landsat_ts_gif(label=label, start_year=1985, bands=['Red', 'Green', 'Blue'], font_color='white', frames_per_second=10, progress_bar_color='blue')",
"_____no_output_____"
]
],
[
[
"## Create Landsat timeseries",
"_____no_output_____"
]
],
[
[
"import os\nimport ee\nimport geemap",
"_____no_output_____"
],
[
"Map = geemap.Map()\nMap",
"_____no_output_____"
]
],
[
[
"You and define an roi or draw a rectangle on the map",
"_____no_output_____"
]
],
[
[
"roi = ee.Geometry.Polygon(\n [[[-115.471773, 35.892718],\n [-115.471773, 36.409454],\n [-114.271283, 36.409454],\n [-114.271283, 35.892718],\n [-115.471773, 35.892718]]], None, False)",
"_____no_output_____"
],
[
"# roi = Map.draw_last_feature",
"_____no_output_____"
],
[
"collection = geemap.landsat_timeseries(roi=roi, start_year=1985, end_year=2019, start_date='06-10', end_date='09-20')",
"_____no_output_____"
],
[
"print(collection.size().getInfo())",
"_____no_output_____"
],
[
"first_image = collection.first()\n\nvis = {\n 'bands': ['NIR', 'Red', 'Green'],\n 'min': 0,\n 'max': 4000,\n 'gamma': [1, 1, 1]\n}\n\nMap.addLayer(first_image, vis, 'First image')",
"_____no_output_____"
]
],
[
[
"## Download ImageCollection as a GIF",
"_____no_output_____"
]
],
[
[
"# Define arguments for animation function parameters.\nvideo_args = {\n 'dimensions': 768,\n 'region': roi,\n 'framesPerSecond': 10,\n 'bands': ['NIR', 'Red', 'Green'],\n 'min': 0,\n 'max': 4000,\n 'gamma': [1, 1, 1]\n}",
"_____no_output_____"
],
[
"work_dir = os.path.join(os.path.expanduser(\"~\"), 'Downloads')\nif not os.path.exists(work_dir):\n os.makedirs(work_dir)\nout_gif = os.path.join(work_dir, \"landsat_ts.gif\")",
"_____no_output_____"
],
[
"geemap.download_ee_video(collection, video_args, out_gif)",
"_____no_output_____"
]
],
[
[
"## Add animated text to GIF",
"_____no_output_____"
]
],
[
[
"geemap.show_image(out_gif)",
"_____no_output_____"
],
[
"texted_gif = os.path.join(work_dir, \"landsat_ts_text.gif\")\ngeemap.add_text_to_gif(out_gif, texted_gif, xy=('3%', '5%'), text_sequence=1985, font_size=30, font_color='#ffffff', add_progress_bar=False)",
"_____no_output_____"
],
[
"label = 'Urban Growth in Las Vegas'\ngeemap.add_text_to_gif(texted_gif, texted_gif, xy=('2%', '88%'), text_sequence=label, font_size=30, font_color='#ffffff', progress_bar_color='cyan')",
"_____no_output_____"
],
[
"geemap.show_image(texted_gif)",
"_____no_output_____"
]
]
] | [
"code",
"markdown",
"code",
"markdown",
"code",
"markdown",
"code",
"markdown",
"code",
"markdown",
"code",
"markdown",
"code",
"markdown",
"code"
] | [
[
"code",
"code"
],
[
"markdown"
],
[
"code"
],
[
"markdown"
],
[
"code"
],
[
"markdown"
],
[
"code",
"code"
],
[
"markdown"
],
[
"code",
"code"
],
[
"markdown"
],
[
"code",
"code",
"code",
"code",
"code"
],
[
"markdown"
],
[
"code",
"code",
"code"
],
[
"markdown"
],
[
"code",
"code",
"code",
"code"
]
] |
d079fe2e1d1ca3dd5138caa3c6892331e6862703 | 254,925 | ipynb | Jupyter Notebook | main_PACT/data_processing.ipynb | jvonk/pact | 2775b732e15244a2bb3374b656b0d61982fe020e | [
"MIT"
] | 2 | 2020-07-04T01:16:05.000Z | 2020-08-06T04:38:19.000Z | main_PACT/data_processing.ipynb | jvonk/pact | 2775b732e15244a2bb3374b656b0d61982fe020e | [
"MIT"
] | null | null | null | main_PACT/data_processing.ipynb | jvonk/pact | 2775b732e15244a2bb3374b656b0d61982fe020e | [
"MIT"
] | null | null | null | 72.196262 | 34,848 | 0.741104 | [
[
[
"# Copyright © 2020, Johan Vonk\n# SPDX-License-Identifier: MIT",
"_____no_output_____"
],
[
"%matplotlib inline\nimport numpy as np\nimport pandas as pd\nimport math\nimport matplotlib.pyplot as plt\nfrom sklearn.manifold import MDS\nfrom sklearn.metrics import pairwise_distances\nimport paho.mqtt.client as mqtt\nfrom threading import Timer\nimport json\nfrom config import username, password\nimport seaborn as sns",
"_____no_output_____"
],
[
"measured=np.array([\n [0, 37.9, 92.2, 95.2, 56.6, 95.5, 73.5, 56.7, 121.2, 73.9],\n [0, 0, 54.7, 71.8, 44.4, 59.4, 41.6, 21.9, 89.5, 46.8],\n [0, 0, 0, 60.3, 67.6, 27.3, 45.8, 42.3, 65.1, 43.5],\n [0, 0, 0, 0, 40.4, 87.1, 94.8, 78.9, 125.4, 25.4],\n [0, 0, 0, 0, 0, 86.9, 81.3, 61.5, 123.0, 28.0],\n [0, 0, 0, 0, 0, 0, 29.1, 39.1, 28.3, 67.2],\n [0, 0, 0, 0, 0, 0, 0, 20.6, 48.6, 70.0],\n [0, 0, 0, 0, 0, 0, 0, 0, 67.6, 53.5],\n [0, 0, 0, 0, 0, 0, 0, 0, 0, 105.5],\n [0, 0, 0, 0, 0, 0, 0, 0, 0, 0],\n])\nmeasured*=0.0254\nmeasured+=measured.T\nmodel = MDS(n_components=2, metric=True, dissimilarity='precomputed', random_state=1, n_init=1000, max_iter=1000)\npositions = model.fit_transform(measured)\npositions -= positions[8]\npositions[:, 1]*=-1\ntheta=np.radians(221)+math.atan2(positions[5,1],positions[5,0])\npositions=positions.dot([[np.cos(theta), -np.sin(theta)],\n [np.sin(theta), np.cos(theta)]])\npositions[:,0]-=positions[3,0]\nangles=np.radians([18,9,-18,135,156,-59,-23,77,-90,62])\nplt.quiver(positions[:,0], positions[:,1], np.cos(angles), np.sin(angles))",
"_____no_output_____"
],
[
"devices=pd.DataFrame(columns=(\"name\", \"address\", \"version\", \"date\"))\ndf=pd.DataFrame(columns=(\"TIMESTAMP\",\"SCANNER\",\"ADVERTISER\",\"TX POWER\",\"RSSI\",\"DISTANCE\",\"ANGLE\"))\nclass RepeatTimer(Timer):\n def run(self):\n while not self.finished.wait(self.interval):\n self.function(*self.args, **self.kwargs)\n\ndef switch_devices(client, devices):\n for device,payload in zip(devices[\"name\"],np.random.choice(['scan', 'adv'],len(devices))):\n client.publish(\"blescan/ctrl/\"+device, payload=payload)\n\ndef on_connect(client, userdata, flags, rc):\n client.subscribe(\"blescan/data/#\")\n client.publish(\"blescan/ctrl\", payload=\"who\")\n client.publish(\"blescan/ctrl\", payload=\"int 2\")\n\ndef on_message(client, userdata, msg):\n source=msg.topic.rsplit('/', 1)[-1]\n data = json.loads(msg.payload.decode('ASCII').replace('\"\"','\"'))\n if \"name\" in data and data[\"name\"] not in devices[\"name\"].values:\n devices.loc[len(devices)]=[data[\"name\"],data[\"address\"],data[\"version\"],data[\"date\"]]\n elif \"RSSI\" in data and data[\"address\"] in devices[\"address\"].values and source in devices[\"name\"].values:\n sc_pos=positions[int(source.replace(\"esp32-\",\"\"))-1]\n advertiser=devices[devices['address']==data['address']]['name'].values[0]\n ad_pos=positions[int(advertiser.replace(\"esp32-\",\"\"))-1]\n dx=sc_pos[0]-ad_pos[0]\n dy=sc_pos[1]-ad_pos[1]\n df.loc[len(df)]=[pd.Timestamp.now(),source,advertiser,data[\"txPwr\"],data[\"RSSI\"],math.sqrt(dx**2+dy**2),(math.atan2(dy,dx)-angles[int(advertiser.replace(\"esp32-\",\"\"))-1]+2*np.pi)%(2*np.pi)]\n\nclient=mqtt.Client(\"reader\")\nclient.on_connect = on_connect\nclient.on_message = on_message\nclient.connect('mqtt.vonk', 1883)\nclient.username_pw_set(username=username,password=password)\ntimer = RepeatTimer(60, switch_devices, args=(client,devices))\ntry:\n client.loop_start()\n timer.start()\nexcept KeyboardInterrupt:\n client.loop_stop()\n timer.cancel()",
"_____no_output_____"
],
[
"d=df.copy()\nd['TIMESTAMP']=pd.to_datetime(d['TIMESTAMP'],errors='coerce')\nd['SCANNER']=d['SCANNER'].astype(str)\nd['ADVERTISER']=d['ADVERTISER'].astype(str)\nd['TX POWER']=pd.to_numeric(d['TX POWER'],errors='coerce').astype('int8')\nd['RSSI']=pd.to_numeric(d['RSSI'],errors='coerce').astype('int8')\nd['DISTANCE']=pd.to_numeric(d['DISTANCE'],errors='coerce')\nd['ANGLE']=pd.to_numeric(d['ANGLE'],errors='coerce')\nangle_shift=(1-np.cos(2*d['ANGLE']))/d['ANGLE']*3-0.855\nd['HUMAN PREDICTION']=10**((11.5511+d['TX POWER']-d['RSSI']-angle_shift)/10/2)\nd['HUMAN PREDICTION']=pd.to_numeric(d['HUMAN PREDICTION'],errors='coerce')\nd['HUMAN SLE']=np.log((d['DISTANCE']+1)/(d['HUMAN PREDICTION']+1))**2\nd['HUMAN SLE']=pd.to_numeric(d['HUMAN SLE'],errors='coerce')",
"_____no_output_____"
],
[
"print('Received {0:.5} messages per second.'.format(len(df)/(df.iloc[-1][\"TIMESTAMP\"]-df.iloc[0][\"TIMESTAMP\"]).total_seconds()))\nprint(\"Human distance and angle mean squared log error is {0:.5}.\".format(np.sum(d['HUMAN SLE'])/len(d)))\nplot_data=d.query('`HUMAN PREDICTION`>0 and `HUMAN PREDICTION`<4')\nsns.jointplot(x=\"DISTANCE\", y=\"HUMAN PREDICTION\", data=plot_data, kind=\"hex\")",
"Received 5.3641 messages per second.\nHuman distance and angle mean squared log error is 0.29687.\n"
],
[
"d['DISTANCE PREDICTION']=10**((11.5511+d['TX POWER']-d['RSSI'])/10/2)\nd['DISTANCE PREDICTION']=pd.to_numeric(d['DISTANCE PREDICTION'],errors='coerce')\nd['DISTANCE SLE']=np.log((d['DISTANCE']+1)/(d['DISTANCE PREDICTION']+1))**2\nd['DISTANCE SLE']=pd.to_numeric(d['DISTANCE SLE'],errors='coerce')",
"_____no_output_____"
],
[
"print(\"Distance-only mean squared log error is {0:.5}.\".format(np.sum(d['DISTANCE SLE'])/len(d)))\nplot_data=d.query('`DISTANCE PREDICTION`>0 and `DISTANCE PREDICTION`<4')\nsns.jointplot(x=\"DISTANCE\", y=\"DISTANCE PREDICTION\", data=plot_data, kind=\"hex\")",
"Distance-only mean squared log error is 0.34482.\n"
],
[
"import tensorflow as tf\nfrom tensorflow.keras.models import Sequential\nfrom tensorflow.keras.layers import Dense\nfrom sklearn.model_selection import train_test_split\nfrom sklearn.preprocessing import StandardScaler",
"_____no_output_____"
],
[
"power=10**((11.5511+d['TX POWER']-d['RSSI'])/20)\ncos_2angle=np.cos(2*d['ANGLE'])\nsin_2angle=np.sin(2*d['ANGLE'])\ncos_angle=np.cos(d['ANGLE'])\nsin_angle=np.sin(d['ANGLE'])\nX = pd.DataFrame([power,cos_2angle,sin_2angle,cos_angle,sin_angle]).T\ny = np.ravel(d['DISTANCE'])\nX_train, X_test, y_train, y_test = train_test_split(X, y, test_size=0.33, random_state=42)",
"_____no_output_____"
],
[
"scaler = StandardScaler().fit(X_train)\nX_train = scaler.transform(X_train)\nX_test = scaler.transform(X_test)",
"_____no_output_____"
],
[
"model = Sequential()\nmodel.add(Dense(8, kernel_initializer='normal', activation='relu', input_shape=(5,)))\nmodel.add(Dense(8, kernel_initializer='normal', activation='relu'))\nmodel.add(Dense(1, kernel_initializer='normal'))\nmodel.compile(loss='mean_squared_logarithmic_error',\n optimizer='sgd',\n metrics=['mse'])\nmodel.summary()",
"Model: \"sequential_19\"\n_________________________________________________________________\nLayer (type) Output Shape Param # \n=================================================================\ndense_57 (Dense) (None, 8) 48 \n_________________________________________________________________\ndense_58 (Dense) (None, 8) 72 \n_________________________________________________________________\ndense_59 (Dense) (None, 1) 9 \n=================================================================\nTotal params: 129\nTrainable params: 129\nNon-trainable params: 0\n_________________________________________________________________\n"
],
[
"history = model.fit(X_train, y_train, epochs=36, batch_size=32, verbose=1, validation_data=(X_test, y_test))",
"Train on 466235 samples, validate on 229639 samples\nEpoch 1/36\n466235/466235 [==============================] - 494s 1ms/sample - loss: 0.0600 - mse: 0.3272 - val_loss: 0.0475 - val_mse: 0.2613\nEpoch 2/36\n466235/466235 [==============================] - 513s 1ms/sample - loss: 0.0376 - mse: 0.2126 - val_loss: 0.0341 - val_mse: 0.1920\nEpoch 3/36\n466235/466235 [==============================] - 528s 1ms/sample - loss: 0.0320 - mse: 0.1839 - val_loss: 0.0301 - val_mse: 0.1693\nEpoch 4/36\n466235/466235 [==============================] - 618s 1ms/sample - loss: 0.0280 - mse: 0.1592 - val_loss: 0.0263 - val_mse: 0.1511\nEpoch 5/36\n466235/466235 [==============================] - 604s 1ms/sample - loss: 0.0251 - mse: 0.1445 - val_loss: 0.0243 - val_mse: 0.1406\nEpoch 6/36\n466235/466235 [==============================] - 489s 1ms/sample - loss: 0.0239 - mse: 0.1393 - val_loss: 0.0237 - val_mse: 0.1376\nEpoch 7/36\n466235/466235 [==============================] - 437s 938us/sample - loss: 0.0233 - mse: 0.1347 - val_loss: 0.0230 - val_mse: 0.1334\nEpoch 8/36\n466235/466235 [==============================] - 411s 882us/sample - loss: 0.0227 - mse: 0.1317 - val_loss: 0.0226 - val_mse: 0.1299\nEpoch 9/36\n466235/466235 [==============================] - 403s 865us/sample - loss: 0.0222 - mse: 0.1289 - val_loss: 0.0219 - val_mse: 0.1275\nEpoch 10/36\n466235/466235 [==============================] - 436s 935us/sample - loss: 0.0217 - mse: 0.1263 - val_loss: 0.0217 - val_mse: 0.1251\nEpoch 11/36\n466235/466235 [==============================] - 415s 889us/sample - loss: 0.0213 - mse: 0.1247 - val_loss: 0.0215 - val_mse: 0.1246\nEpoch 12/36\n466235/466235 [==============================] - 428s 917us/sample - loss: 0.0209 - mse: 0.1228 - val_loss: 0.0206 - val_mse: 0.1223\nEpoch 13/36\n466235/466235 [==============================] - 432s 926us/sample - loss: 0.0204 - mse: 0.1202 - val_loss: 0.0201 - val_mse: 0.1193\nEpoch 14/36\n466235/466235 [==============================] - 430s 921us/sample - loss: 0.0199 - mse: 0.1173 - val_loss: 0.0197 - val_mse: 0.1173\nEpoch 15/36\n466235/466235 [==============================] - 458s 981us/sample - loss: 0.0193 - mse: 0.1139 - val_loss: 0.0189 - val_mse: 0.1107\nEpoch 16/36\n466235/466235 [==============================] - 33164s 71ms/sample - loss: 0.0184 - mse: 0.1083 - val_loss: 0.0179 - val_mse: 0.1046\nEpoch 17/36\n466235/466235 [==============================] - 41s 88us/sample - loss: 0.0174 - mse: 0.1016 - val_loss: 0.0170 - val_mse: 0.1002\nEpoch 18/36\n466235/466235 [==============================] - 36s 77us/sample - loss: 0.0163 - mse: 0.0956 - val_loss: 0.0161 - val_mse: 0.0922\nEpoch 19/36\n466235/466235 [==============================] - 41s 88us/sample - loss: 0.0155 - mse: 0.0911 - val_loss: 0.0152 - val_mse: 0.0883\nEpoch 20/36\n466235/466235 [==============================] - 45s 97us/sample - loss: 0.0149 - mse: 0.0879 - val_loss: 0.0147 - val_mse: 0.0877\nEpoch 21/36\n466235/466235 [==============================] - 149s 320us/sample - loss: 0.0142 - mse: 0.0841 - val_loss: 0.0137 - val_mse: 0.0833\nEpoch 22/36\n466235/466235 [==============================] - 46s 98us/sample - loss: 0.0135 - mse: 0.0805 - val_loss: 0.0133 - val_mse: 0.0797\nEpoch 23/36\n466235/466235 [==============================] - 270s 580us/sample - loss: 0.0132 - mse: 0.0784 - val_loss: 0.0129 - val_mse: 0.0771\nEpoch 24/36\n466235/466235 [==============================] - 372s 798us/sample - loss: 0.0129 - mse: 0.0764 - val_loss: 0.0127 - val_mse: 0.0748\nEpoch 25/36\n466235/466235 [==============================] - 231s 496us/sample - loss: 0.0126 - mse: 0.0746 - val_loss: 0.0125 - val_mse: 0.0743\nEpoch 26/36\n466235/466235 [==============================] - 46s 98us/sample - loss: 0.0123 - mse: 0.0724 - val_loss: 0.0133 - val_mse: 0.0805\nEpoch 27/36\n466235/466235 [==============================] - 46s 99us/sample - loss: 0.0119 - mse: 0.0694 - val_loss: 0.0118 - val_mse: 0.0695\nEpoch 28/36\n466235/466235 [==============================] - 46s 100us/sample - loss: 0.0116 - mse: 0.0676 - val_loss: 0.0115 - val_mse: 0.0658\nEpoch 29/36\n466235/466235 [==============================] - 206s 442us/sample - loss: 0.0115 - mse: 0.0666 - val_loss: 0.0113 - val_mse: 0.0661\nEpoch 30/36\n466235/466235 [==============================] - 367s 787us/sample - loss: 0.0113 - mse: 0.0655 - val_loss: 0.0112 - val_mse: 0.0656\nEpoch 31/36\n466235/466235 [==============================] - 364s 781us/sample - loss: 0.0112 - mse: 0.0647 - val_loss: 0.0111 - val_mse: 0.0644\nEpoch 32/36\n466235/466235 [==============================] - 424s 909us/sample - loss: 0.0111 - mse: 0.0642 - val_loss: 0.0108 - val_mse: 0.0631\nEpoch 33/36\n466235/466235 [==============================] - 418s 897us/sample - loss: 0.0110 - mse: 0.0636 - val_loss: 0.0109 - val_mse: 0.0634\nEpoch 34/36\n466235/466235 [==============================] - 418s 896us/sample - loss: 0.0109 - mse: 0.0630 - val_loss: 0.0119 - val_mse: 0.0707\nEpoch 35/36\n466235/466235 [==============================] - 445s 953us/sample - loss: 0.0109 - mse: 0.0625 - val_loss: 0.0108 - val_mse: 0.0615\nEpoch 36/36\n466235/466235 [==============================] - 431s 924us/sample - loss: 0.0108 - mse: 0.0621 - val_loss: 0.0108 - val_mse: 0.0610\n"
],
[
"model.save('model')",
"INFO:tensorflow:Assets written to: model\\assets\n"
],
[
"X_predict=scaler.transform(X)\nd['PREDICTION']=model.predict(X_predict, verbose=1)",
"695874/695874 [==============================] - 302s 434us/sample\n"
],
[
"d['SLE']=np.log((d['DISTANCE']+1)/(d['PREDICTION']+1))**2\nd.to_csv(f\"pact_{d.iloc[0]['TIMESTAMP']:%Y%m%dT%H%M%S}.csv\")",
"_____no_output_____"
],
[
"plt.plot(history.history['loss'])\nplt.plot(history.history['val_loss'])\nplt.title('model loss (msle)')\nplt.ylabel('loss')\nplt.xlabel('epoch')\nplt.legend(['train', 'test'], loc='upper left')",
"_____no_output_____"
],
[
"print(\"ML model mean squared log error is {0:.5}.\".format(np.sum(d['SLE'])/len(d)))\nprint(\"False positive rate is {0:.3%}.\".format(len(d.query('DISTANCE>0.9144 and PREDICTION<=0.9144'))/len(d)))\nprint(\"False negative rate is {0:.3%}.\".format(len(d.query('DISTANCE<=0.9144 and PREDICTION>0.9144'))/len(d)))\nprint(\"True positive rate is {0:.3%}.\".format(len(d.query('DISTANCE<=0.9144 and PREDICTION<=0.9144'))/len(d)))\nprint(\"True negative rate is {0:.3%}.\".format(len(d.query('DISTANCE>0.9144 and PREDICTION>0.9144'))/len(d)))\nplot_data=d.query('`PREDICTION`>0 and `PREDICTION`<4')\nsns.jointplot(x=\"DISTANCE\", y=\"PREDICTION\", data=plot_data, kind=\"hex\")",
"ML model mean squared log error is 0.010761.\nFalse positive rate is 0.990%.\nFalse negative rate is 3.323%.\nTrue positive rate is 16.360%.\nTrue negative rate is 79.327%.\n"
],
[
"yard_power=0.9144\nn_points=1000\nangles=2*np.pi/n_points*np.arange(0, n_points)\nX_angles = scaler.transform(pd.DataFrame([np.full(len(angles),yard_power),np.cos(2*angles),np.sin(2*angles),np.cos(angles),np.sin(angles)]).T)\nresult_angles=np.log10(model.predict(X_angles, verbose=1).flatten())*20\nresult_angles-=result_angles.max()-30\nimport plotly.express as px\npx.line_polar(r=result_angles, theta=angles*180/np.pi, line_close=True)",
"1000/1000 [==============================] - 0s 433us/sample\n"
],
[
"#df[df['TIMESTAMP'] <= df['TIMESTAMP'].iloc[0]+pd.Timedelta(2,'D')]\ngraph=df.sample(n=100)\npx.line(graph['TIMESTAMP'],graph['RSSI'])",
"_____no_output_____"
],
[
"df",
"_____no_output_____"
]
]
] | [
"code"
] | [
[
"code",
"code",
"code",
"code",
"code",
"code",
"code",
"code",
"code",
"code",
"code",
"code",
"code",
"code",
"code",
"code",
"code",
"code",
"code",
"code",
"code"
]
] |
d07a0153f3ff4c58ba017e53bf762eac180f1950 | 15,130 | ipynb | Jupyter Notebook | site/en-snapshot/hub/tutorials/retrieval_with_tf_hub_universal_encoder_qa.ipynb | ilyaspiridonov/docs-l10n | a061a44e40d25028d0a4458094e48ab717d3565c | [
"Apache-2.0"
] | 1 | 2021-09-23T09:56:29.000Z | 2021-09-23T09:56:29.000Z | site/en-snapshot/hub/tutorials/retrieval_with_tf_hub_universal_encoder_qa.ipynb | ilyaspiridonov/docs-l10n | a061a44e40d25028d0a4458094e48ab717d3565c | [
"Apache-2.0"
] | null | null | null | site/en-snapshot/hub/tutorials/retrieval_with_tf_hub_universal_encoder_qa.ipynb | ilyaspiridonov/docs-l10n | a061a44e40d25028d0a4458094e48ab717d3565c | [
"Apache-2.0"
] | 1 | 2020-08-03T21:04:29.000Z | 2020-08-03T21:04:29.000Z | 40.346667 | 680 | 0.559352 | [
[
[
"##### Copyright 2019 The TensorFlow Hub Authors.\n\nLicensed under the Apache License, Version 2.0 (the \"License\");",
"_____no_output_____"
]
],
[
[
"# Copyright 2019 The TensorFlow Hub Authors. All Rights Reserved.\n#\n# Licensed under the Apache License, Version 2.0 (the \"License\");\n# you may not use this file except in compliance with the License.\n# You may obtain a copy of the License at\n#\n# http://www.apache.org/licenses/LICENSE-2.0\n#\n# Unless required by applicable law or agreed to in writing, software\n# distributed under the License is distributed on an \"AS IS\" BASIS,\n# WITHOUT WARRANTIES OR CONDITIONS OF ANY KIND, either express or implied.\n# See the License for the specific language governing permissions and\n# limitations under the License.\n# ==============================================================================",
"_____no_output_____"
]
],
[
[
"# Multilingual Universal Sentence Encoder Q&A Retrieval\n",
"_____no_output_____"
],
[
"<table class=\"tfo-notebook-buttons\" align=\"left\">\n <td>\n <a target=\"_blank\" href=\"https://www.tensorflow.org/hub/tutorials/retrieval_with_tf_hub_universal_encoder_qa\"><img src=\"https://www.tensorflow.org/images/tf_logo_32px.png\" />View on TensorFlow.org</a>\n </td>\n <td>\n <a target=\"_blank\" href=\"https://colab.research.google.com/github/tensorflow/hub/blob/master/examples/colab/retrieval_with_tf_hub_universal_encoder_qa.ipynb\"><img src=\"https://www.tensorflow.org/images/colab_logo_32px.png\" />Run in Google Colab</a>\n </td>\n <td>\n <a target=\"_blank\" href=\"https://github.com/tensorflow/hub/blob/master/examples/colab/retrieval_with_tf_hub_universal_encoder_qa.ipynb\"><img src=\"https://www.tensorflow.org/images/GitHub-Mark-32px.png\" />View source on GitHub</a>\n </td>\n <td>\n <a href=\"https://storage.googleapis.com/tensorflow_docs/hub/examples/colab/retrieval_with_tf_hub_universal_encoder_qa.ipynb\"><img src=\"https://www.tensorflow.org/images/download_logo_32px.png\" />Download notebook</a>\n </td>\n</table>",
"_____no_output_____"
],
[
"This is a demo for using [Univeral Encoder Multilingual Q&A model](https://tfhub.dev/google/universal-sentence-encoder-multilingual-qa/3) for question-answer retrieval of text, illustrating the use of **question_encoder** and **response_encoder** of the model. We use sentences from [SQuAD](https://rajpurkar.github.io/SQuAD-explorer/) paragraphs as the demo dataset, each sentence and its context (the text surrounding the sentence) is encoded into high dimension embeddings with the **response_encoder**. These embeddings are stored in an index built using the [simpleneighbors](https://pypi.org/project/simpleneighbors/) library for question-answer retrieval.\n\nOn retrieval a random question is selected from the [SQuAD](https://rajpurkar.github.io/SQuAD-explorer/) dataset and encoded into high dimension embedding with the **question_encoder** and query the simpleneighbors index returning a list of approximate nearest neighbors in semantic space.",
"_____no_output_____"
],
[
"## Setup\n",
"_____no_output_____"
]
],
[
[
"%%capture\n#@title Setup Environment\n# Install the latest Tensorflow version.\n!pip install -q tensorflow_text\n!pip install -q simpleneighbors[annoy]\n!pip install -q nltk\n!pip install -q tqdm",
"_____no_output_____"
],
[
"#@title Setup common imports and functions\nimport json\nimport nltk\nimport os\nimport pprint\nimport random\nimport simpleneighbors\nimport urllib\nfrom IPython.display import HTML, display\nfrom tqdm.notebook import tqdm\n\nimport tensorflow.compat.v2 as tf\nimport tensorflow_hub as hub\nfrom tensorflow_text import SentencepieceTokenizer\n\nnltk.download('punkt')\n\n\ndef download_squad(url):\n return json.load(urllib.request.urlopen(url))\n\ndef extract_sentences_from_squad_json(squad):\n all_sentences = []\n for data in squad['data']:\n for paragraph in data['paragraphs']:\n sentences = nltk.tokenize.sent_tokenize(paragraph['context'])\n all_sentences.extend(zip(sentences, [paragraph['context']] * len(sentences)))\n return list(set(all_sentences)) # remove duplicates\n\ndef extract_questions_from_squad_json(squad):\n questions = []\n for data in squad['data']:\n for paragraph in data['paragraphs']:\n for qas in paragraph['qas']:\n if qas['answers']:\n questions.append((qas['question'], qas['answers'][0]['text']))\n return list(set(questions))\n\ndef output_with_highlight(text, highlight):\n output = \"<li> \"\n i = text.find(highlight)\n while True:\n if i == -1:\n output += text\n break\n output += text[0:i]\n output += '<b>'+text[i:i+len(highlight)]+'</b>'\n text = text[i+len(highlight):]\n i = text.find(highlight)\n return output + \"</li>\\n\"\n\ndef display_nearest_neighbors(query_text, answer_text=None):\n query_embedding = model.signatures['question_encoder'](tf.constant([query_text]))['outputs'][0]\n search_results = index.nearest(query_embedding, n=num_results)\n\n if answer_text:\n result_md = '''\n <p>Random Question from SQuAD:</p>\n <p> <b>%s</b></p>\n <p>Answer:</p>\n <p> <b>%s</b></p>\n ''' % (query_text , answer_text)\n else:\n result_md = '''\n <p>Question:</p>\n <p> <b>%s</b></p>\n ''' % query_text\n\n result_md += '''\n <p>Retrieved sentences :\n <ol>\n '''\n\n if answer_text:\n for s in search_results:\n result_md += output_with_highlight(s, answer_text)\n else:\n for s in search_results:\n result_md += '<li>' + s + '</li>\\n'\n\n result_md += \"</ol>\"\n display(HTML(result_md))",
"_____no_output_____"
]
],
[
[
"Run the following code block to download and extract the SQuAD dataset into:\n\n* **sentences** is a list of (text, context) tuples - each paragraph from the SQuAD dataset are splitted into sentences using nltk library and the sentence and paragraph text forms the (text, context) tuple.\n* **questions** is a list of (question, answer) tuples.\n\nNote: You can use this demo to index the SQuAD train dataset or the smaller dev dataset (1.1 or 2.0) by selecting the **squad_url** below.\n",
"_____no_output_____"
]
],
[
[
"#@title Download and extract SQuAD data\nsquad_url = 'https://rajpurkar.github.io/SQuAD-explorer/dataset/dev-v1.1.json' #@param [\"https://rajpurkar.github.io/SQuAD-explorer/dataset/train-v2.0.json\", \"https://rajpurkar.github.io/SQuAD-explorer/dataset/dev-v2.0.json\", \"https://rajpurkar.github.io/SQuAD-explorer/dataset/train-v1.1.json\", \"https://rajpurkar.github.io/SQuAD-explorer/dataset/dev-v1.1.json\"]\n\nsquad_json = download_squad(squad_url)\nsentences = extract_sentences_from_squad_json(squad_json)\nquestions = extract_questions_from_squad_json(squad_json)\nprint(\"%s sentences, %s questions extracted from SQuAD %s\" % (len(sentences), len(questions), squad_url))\n\nprint(\"\\nExample sentence and context:\\n\")\nsentence = random.choice(sentences)\nprint(\"sentence:\\n\")\npprint.pprint(sentence[0])\nprint(\"\\ncontext:\\n\")\npprint.pprint(sentence[1])\nprint()",
"_____no_output_____"
]
],
[
[
"The following code block setup the tensorflow graph **g** and **session** with the [Univeral Encoder Multilingual Q&A model](https://tfhub.dev/google/universal-sentence-encoder-multilingual-qa/3)'s **question_encoder** and **response_encoder** signatures.",
"_____no_output_____"
]
],
[
[
"#@title Load model from tensorflow hub\nmodule_url = \"https://tfhub.dev/google/universal-sentence-encoder-multilingual-qa/3\" #@param [\"https://tfhub.dev/google/universal-sentence-encoder-multilingual-qa/3\", \"https://tfhub.dev/google/universal-sentence-encoder-qa/3\"]\nmodel = hub.load(module_url)\n",
"_____no_output_____"
]
],
[
[
"The following code block compute the embeddings for all the text, context tuples and store them in a [simpleneighbors](https://pypi.org/project/simpleneighbors/) index using the **response_encoder**.\n",
"_____no_output_____"
]
],
[
[
"#@title Compute embeddings and build simpleneighbors index\nbatch_size = 100\n\nencodings = model.signatures['response_encoder'](\n input=tf.constant([sentences[0][0]]),\n context=tf.constant([sentences[0][1]]))\nindex = simpleneighbors.SimpleNeighbors(\n len(encodings['outputs'][0]), metric='angular')\n\nprint('Computing embeddings for %s sentences' % len(sentences))\nslices = zip(*(iter(sentences),) * batch_size)\nnum_batches = int(len(sentences) / batch_size)\nfor s in tqdm(slices, total=num_batches):\n response_batch = list([r for r, c in s])\n context_batch = list([c for r, c in s])\n encodings = model.signatures['response_encoder'](\n input=tf.constant(response_batch),\n context=tf.constant(context_batch)\n )\n for batch_index, batch in enumerate(response_batch):\n index.add_one(batch, encodings['outputs'][batch_index])\n\nindex.build()\nprint('simpleneighbors index for %s sentences built.' % len(sentences))\n",
"_____no_output_____"
]
],
[
[
"On retrieval, the question is encoded using the **question_encoder** and the question embedding is used to query the simpleneighbors index.",
"_____no_output_____"
]
],
[
[
"#@title Retrieve nearest neighbors for a random question from SQuAD\nnum_results = 25 #@param {type:\"slider\", min:5, max:40, step:1}\n\nquery = random.choice(questions)\ndisplay_nearest_neighbors(query[0], query[1])",
"_____no_output_____"
]
]
] | [
"markdown",
"code",
"markdown",
"code",
"markdown",
"code",
"markdown",
"code",
"markdown",
"code",
"markdown",
"code"
] | [
[
"markdown"
],
[
"code"
],
[
"markdown",
"markdown",
"markdown",
"markdown"
],
[
"code",
"code"
],
[
"markdown"
],
[
"code"
],
[
"markdown"
],
[
"code"
],
[
"markdown"
],
[
"code"
],
[
"markdown"
],
[
"code"
]
] |
d07a04d99b679b1bb3fe50e929714738bd8d6f97 | 39,836 | ipynb | Jupyter Notebook | tb_models/Basic_MNIST-tb.ipynb | KiranArun/Neural_Networks-demo | 5996afb9933553c4523780fd9a7dba08acf6f755 | [
"MIT"
] | null | null | null | tb_models/Basic_MNIST-tb.ipynb | KiranArun/Neural_Networks-demo | 5996afb9933553c4523780fd9a7dba08acf6f755 | [
"MIT"
] | null | null | null | tb_models/Basic_MNIST-tb.ipynb | KiranArun/Neural_Networks-demo | 5996afb9933553c4523780fd9a7dba08acf6f755 | [
"MIT"
] | null | null | null | 39,836 | 39,836 | 0.847625 | [
[
[
"COPYRIGHT © 2018 Kiran Arun <[email protected]>",
"_____no_output_____"
],
[
"### Setup",
"_____no_output_____"
]
],
[
[
"# install dependencies\n!rm -r Neural_Networks-101-demo\n!git clone -b explanations https://github.com/KiranArun/Neural_Networks-101-demo.git\n!python3 /content/Neural_Networks-101-demo/scripts/setup.py helper_funcs tensorboard",
"Cloning into 'Neural_Networks-101-demo'...\nremote: Counting objects: 352, done.\u001b[K\nremote: Compressing objects: 100% (67/67), done.\u001b[K\nremote: Total 352 (delta 30), reused 57 (delta 14), pack-reused 271\u001b[K\nReceiving objects: 100% (352/352), 7.53 MiB | 19.26 MiB/s, done.\nResolving deltas: 100% (153/153), done.\nGetting helper functions...\n"
],
[
"# run tensorboard\nget_ipython().system_raw('tensorboard --logdir=/content/logdir/ --host=0.0.0.0 --port=6006 &')\nget_ipython().system_raw('./ngrok http 6006 &')\n! curl -s http://localhost:4040/api/tunnels | python3 -c \"import sys, json; print('Tensorboard Link:', json.load(sys.stdin)['tunnels'][0]['public_url'])\"",
"Tensorboard Link: http://bdb495ac.ngrok.io\r\n"
]
],
[
[
"# MNIST Handwriten Digits Classifier",
"_____no_output_____"
]
],
[
[
"import tensorflow as tf\nimport numpy as np\nimport matplotlib.pyplot as plt\nimport os\n\nfrom math import ceil,floor\nimport helper_funcs as helper",
"_____no_output_____"
],
[
"# this is the directory where we will keep and external files, eg. data, logs\nmodel_root_dir = '/content/'\n\n# get data\nmnist = helper.MNIST_data(model_root_dir+'MNIST_data/',shuffle=False)",
"Download complete.\nSave complete.\n"
],
[
"image_dims = (28,28)\ninput_size = 28**2\nnum_classes = 10",
"_____no_output_____"
],
[
"batch_size = 100\nlearning_rate = 0.1\n\nepochs = 2\niterations = ceil(mnist.number_train_samples/batch_size)\n\nhidden_size = 256\nembedding_size = 10",
"_____no_output_____"
],
[
"model_logdir = model_root_dir+'logdir/'\n\nLABELS = os.path.join(os.getcwd(), model_logdir+\"labels_1024.tsv\")\nSPRITES = os.path.join(os.getcwd(), model_logdir+\"sprite_1024.png\")\n\nhparam_str = 'fc2,lr_%f' % (learning_rate)\nprevious_runs = list(f for f in os.listdir(model_logdir) if f.startswith('run'))\n\nif len(previous_runs) == 0:\n run_number = 1 \nelse:\n run_number = max([int(s[4:6]) for s in previous_runs]) + 1\n\nLOGDIR = '%srun_%02d,' % (model_logdir, run_number)+hparam_str",
"_____no_output_____"
],
[
"tf.reset_default_graph()",
"_____no_output_____"
],
[
"with tf.name_scope('input'):\n X_placeholder = tf.placeholder(shape=[None, input_size], dtype=tf.float32, name='X_placeholder')\n Y_placeholder = tf.placeholder(shape=[None, num_classes], dtype=tf.int64, name='Y_placeholder')\n\nwith tf.name_scope('input_reshaped'):\n X_image = tf.reshape(X_placeholder, shape=[-1,*image_dims, 1])\n tf.summary.image('input', X_image, 3)",
"_____no_output_____"
],
[
"def variable_summaries(var):\n with tf.name_scope('summaries'):\n mean = tf.reduce_mean(var)\n tf.summary.scalar('mean', mean)\n stddev = tf.sqrt(tf.reduce_mean(tf.square(var - mean)))\n tf.summary.scalar('stddev', stddev)\n tf.summary.scalar('max', tf.reduce_max(var))\n tf.summary.scalar('min', tf.reduce_min(var))\n tf.summary.histogram('histogram', var)",
"_____no_output_____"
],
[
"with tf.name_scope('hidden_layer'):\n \n with tf.name_scope('Weights'):\n W1 = tf.Variable(tf.truncated_normal(shape=[input_size, hidden_size]), dtype=tf.float32, name='W1')\n variable_summaries(W1)\n \n with tf.name_scope('biases'):\n b1 = tf.Variable(tf.constant(0.1,shape=[hidden_size]), dtype=tf.float32, name='b1')\n variable_summaries(b1)\n \n with tf.name_scope('output'):\n hidden_output = tf.nn.relu(tf.matmul(X_placeholder, W1) + b1)",
"_____no_output_____"
],
[
"with tf.name_scope('output_layer'):\n \n with tf.name_scope('Weights'):\n W2 = tf.Variable(tf.truncated_normal(shape=[hidden_size, num_classes]), dtype=tf.float32, name='W2')\n variable_summaries(W2)\n \n with tf.name_scope('biases'):\n b2 = tf.Variable(tf.constant(0.1,shape=[num_classes]), dtype=tf.float32, name='b2')\n variable_summaries(b2)\n \n with tf.name_scope('output'):\n Y_predictions = tf.matmul(hidden_output, W2) + b2\n \n embedding_input = Y_predictions",
"_____no_output_____"
],
[
"with tf.name_scope('loss'):\n cross_entropy = tf.nn.softmax_cross_entropy_with_logits_v2(labels=Y_placeholder,\n logits=Y_predictions,\n name='cross_entropy')\n \n loss = tf.reduce_mean(cross_entropy)\n tf.summary.scalar('loss', loss)\n\nwith tf.name_scope('train'):\n train_step = tf.train.GradientDescentOptimizer(learning_rate).minimize(loss)",
"_____no_output_____"
],
[
"with tf.name_scope('accuracy'):\n with tf.name_scope('correct_predictions'):\n correct_prediction = tf.equal(tf.argmax(Y_predictions, 1), tf.argmax(Y_placeholder, 1))\n accuracy = tf.reduce_mean(tf.cast(correct_prediction, tf.float32))\n tf.summary.scalar('accuracy', accuracy)",
"_____no_output_____"
],
[
"sess = tf.InteractiveSession()",
"_____no_output_____"
],
[
"summ = tf.summary.merge_all()\n\nembedding = tf.Variable(tf.zeros([1024, embedding_size]), name=\"embedding\")\nassignment = embedding.assign(embedding_input)\n\nsaver = tf.train.Saver()\nsess.run(tf.global_variables_initializer())\n\nwriter = tf.summary.FileWriter(LOGDIR)\nwriter.add_graph(sess.graph)\n\nconfig = tf.contrib.tensorboard.plugins.projector.ProjectorConfig()\nembedding_config = config.embeddings.add()\nembedding_config.tensor_name = embedding.name\nembedding_config.sprite.image_path = SPRITES\nembedding_config.metadata_path = LABELS\nembedding_config.sprite.single_image_dim.extend([*image_dims])\ntf.contrib.tensorboard.plugins.projector.visualize_embeddings(writer, config)",
"_____no_output_____"
],
[
"losses = np.array([])\n\nfor epoch in range(epochs):\n \n print('New epoch', str(epoch+1)+'/'+str(epochs))\n\n for iteration in range(iterations):\n\n batch_xs, batch_ys = mnist.get_batch(iteration, batch_size)\n\n _, _loss, _summary = sess.run([train_step, loss, summ], \n feed_dict={\n X_placeholder: batch_xs,\n Y_placeholder: batch_ys\n })\n\n if (iteration+1) % (iterations/5) == 0:\n\n _accuracy = sess.run(accuracy, feed_dict={X_placeholder : mnist.validation_images,\n Y_placeholder : mnist.validation_labels\n })\n\n print('step', str(iteration+1)+'/'+str(iterations), 'loss', _loss, 'accuracy', str(round(100*_accuracy,2))+'%')\n\n if iteration % 10 == 0:\n writer.add_summary(_summary, (epoch*iterations)+iteration)\n \n losses = np.append(losses, _loss)\n\n sess.run(assignment, feed_dict={X_placeholder: mnist.test_images[:1024], Y_placeholder: mnist.test_labels[:1024]})\n saver.save(sess, os.path.join(LOGDIR, \"model.ckpt\"), (epoch*iterations)+iteration)",
"New epoch 1/2\nstep 110/550 loss 3.44714 accuracy 86.6%\nstep 220/550 loss 2.7567492 accuracy 89.56%\nstep 330/550 loss 1.7258672 accuracy 90.24%\nstep 440/550 loss 2.7518563 accuracy 91.08%\nstep 550/550 loss 3.2196543 accuracy 91.7%\nNew epoch 2/2\nstep 110/550 loss 1.7953887 accuracy 91.36%\nstep 220/550 loss 0.86466604 accuracy 92.64%\nstep 330/550 loss 0.92905486 accuracy 92.78%\nstep 440/550 loss 1.6599205 accuracy 92.7%\nstep 550/550 loss 1.464082 accuracy 92.78%\n"
],
[
"fig, ax = plt.subplots(figsize=(10,6))\nax.plot(losses)\nax.grid(True)",
"_____no_output_____"
],
[
"_accuracy = sess.run(accuracy, feed_dict={X_placeholder : mnist.test_images,\n Y_placeholder : mnist.test_labels\n })\n\nprint(str(round(100*_accuracy,2))+'%')",
"91.62%\n"
],
[
"",
"_____no_output_____"
]
]
] | [
"markdown",
"code",
"markdown",
"code"
] | [
[
"markdown",
"markdown"
],
[
"code",
"code"
],
[
"markdown"
],
[
"code",
"code",
"code",
"code",
"code",
"code",
"code",
"code",
"code",
"code",
"code",
"code",
"code",
"code",
"code",
"code",
"code",
"code"
]
] |
d07a2043f452c50617ce92aecdad66d5c993e382 | 702,470 | ipynb | Jupyter Notebook | 16_reinforcement_learning.ipynb | fsv20/Sergey | a5c93fc1dc84bd884392ed9578deca26e0af1119 | [
"Apache-2.0"
] | 99 | 2018-01-02T22:16:08.000Z | 2022-03-03T04:35:37.000Z | 16_reinforcement_learning.ipynb | fsv20/Sergey | a5c93fc1dc84bd884392ed9578deca26e0af1119 | [
"Apache-2.0"
] | 13 | 2019-12-16T20:58:43.000Z | 2022-03-11T23:24:45.000Z | 16_reinforcement_learning.ipynb | fsv20/Sergey | a5c93fc1dc84bd884392ed9578deca26e0af1119 | [
"Apache-2.0"
] | 65 | 2018-06-26T19:46:49.000Z | 2022-02-22T14:25:45.000Z | 64.482284 | 84,769 | 0.665116 | [
[
[
"**Chapter 16 – Reinforcement Learning**",
"_____no_output_____"
],
[
"This notebook contains all the sample code and solutions to the exercices in chapter 16.",
"_____no_output_____"
],
[
"# Setup",
"_____no_output_____"
],
[
"First, let's make sure this notebook works well in both python 2 and 3, import a few common modules, ensure MatplotLib plots figures inline and prepare a function to save the figures:",
"_____no_output_____"
]
],
[
[
"# To support both python 2 and python 3\nfrom __future__ import division, print_function, unicode_literals\n\n# Common imports\nimport numpy as np\nimport numpy.random as rnd\nimport os\nimport sys\n\n# to make this notebook's output stable across runs\nrnd.seed(42)\n\n# To plot pretty figures and animations\n%matplotlib nbagg\nimport matplotlib\nimport matplotlib.animation as animation\nimport matplotlib.pyplot as plt\nplt.rcParams['axes.labelsize'] = 14\nplt.rcParams['xtick.labelsize'] = 12\nplt.rcParams['ytick.labelsize'] = 12\n\n# Where to save the figures\nPROJECT_ROOT_DIR = \".\"\nCHAPTER_ID = \"rl\"\n\ndef save_fig(fig_id, tight_layout=True):\n path = os.path.join(PROJECT_ROOT_DIR, \"images\", CHAPTER_ID, fig_id + \".png\")\n print(\"Saving figure\", fig_id)\n if tight_layout:\n plt.tight_layout()\n plt.savefig(path, format='png', dpi=300)",
"_____no_output_____"
]
],
[
[
"# Introduction to OpenAI gym",
"_____no_output_____"
],
[
"In this notebook we will be using [OpenAI gym](https://gym.openai.com/), a great toolkit for developing and comparing Reinforcement Learning algorithms. It provides many environments for your learning *agents* to interact with. Let's start by importing `gym`:",
"_____no_output_____"
]
],
[
[
"import gym",
"_____no_output_____"
]
],
[
[
"Next we will load the MsPacman environment, version 0.",
"_____no_output_____"
]
],
[
[
"env = gym.make('MsPacman-v0')",
"[2017-02-17 10:57:41,836] Making new env: MsPacman-v0\n"
]
],
[
[
"Let's initialize the environment by calling is `reset()` method. This returns an observation:",
"_____no_output_____"
]
],
[
[
"obs = env.reset()",
"_____no_output_____"
]
],
[
[
"Observations vary depending on the environment. In this case it is an RGB image represented as a 3D NumPy array of shape [width, height, channels] (with 3 channels: Red, Green and Blue). In other environments it may return different objects, as we will see later.",
"_____no_output_____"
]
],
[
[
"obs.shape",
"_____no_output_____"
]
],
[
[
"An environment can be visualized by calling its `render()` method, and you can pick the rendering mode (the rendering options depend on the environment). In this example we will set `mode=\"rgb_array\"` to get an image of the environment as a NumPy array:",
"_____no_output_____"
]
],
[
[
"img = env.render(mode=\"rgb_array\")",
"_____no_output_____"
]
],
[
[
"Let's plot this image:",
"_____no_output_____"
]
],
[
[
"plt.figure(figsize=(5,4))\nplt.imshow(img)\nplt.axis(\"off\")\nsave_fig(\"MsPacman\")\nplt.show()",
"_____no_output_____"
]
],
[
[
"Welcome back to the 1980s! :)",
"_____no_output_____"
],
[
"In this environment, the rendered image is simply equal to the observation (but in many environments this is not the case):",
"_____no_output_____"
]
],
[
[
"(img == obs).all()",
"_____no_output_____"
]
],
[
[
"Let's create a little helper function to plot an environment:",
"_____no_output_____"
]
],
[
[
"def plot_environment(env, figsize=(5,4)):\n plt.close() # or else nbagg sometimes plots in the previous cell\n plt.figure(figsize=figsize)\n img = env.render(mode=\"rgb_array\")\n plt.imshow(img)\n plt.axis(\"off\")\n plt.show()",
"_____no_output_____"
]
],
[
[
"Let's see how to interact with an environment. Your agent will need to select an action from an \"action space\" (the set of possible actions). Let's see what this environment's action space looks like:",
"_____no_output_____"
]
],
[
[
"env.action_space",
"_____no_output_____"
]
],
[
[
"`Discrete(9)` means that the possible actions are integers 0 through 8, which represents the 9 possible positions of the joystick (0=center, 1=up, 2=right, 3=left, 4=down, 5=upper-right, 6=upper-left, 7=lower-right, 8=lower-left).",
"_____no_output_____"
],
[
"Next we need to tell the environment which action to play, and it will compute the next step of the game. Let's go left for 110 steps, then lower left for 40 steps:",
"_____no_output_____"
]
],
[
[
"env.reset()\nfor step in range(110):\n env.step(3) #left\nfor step in range(40):\n env.step(8) #lower-left",
"_____no_output_____"
]
],
[
[
"Where are we now?",
"_____no_output_____"
]
],
[
[
"plot_environment(env)",
"_____no_output_____"
]
],
[
[
"The `step()` function actually returns several important objects:",
"_____no_output_____"
]
],
[
[
"obs, reward, done, info = env.step(0)",
"_____no_output_____"
]
],
[
[
"The observation tells the agent what the environment looks like, as discussed earlier. This is a 210x160 RGB image:",
"_____no_output_____"
]
],
[
[
"obs.shape",
"_____no_output_____"
]
],
[
[
"The environment also tells the agent how much reward it got during the last step:",
"_____no_output_____"
]
],
[
[
"reward",
"_____no_output_____"
]
],
[
[
"When the game is over, the environment returns `done=True`:",
"_____no_output_____"
]
],
[
[
"done",
"_____no_output_____"
]
],
[
[
"Finally, `info` is an environment-specific dictionary that can provide some extra information about the internal state of the environment. This is useful for debugging, but your agent should not use this information for learning (it would be cheating).",
"_____no_output_____"
]
],
[
[
"info",
"_____no_output_____"
]
],
[
[
"Let's play one full game (with 3 lives), by moving in random directions for 10 steps at a time, recording each frame:",
"_____no_output_____"
]
],
[
[
"frames = []\n\nn_max_steps = 1000\nn_change_steps = 10\n\nobs = env.reset()\nfor step in range(n_max_steps):\n img = env.render(mode=\"rgb_array\")\n frames.append(img)\n if step % n_change_steps == 0:\n action = env.action_space.sample() # play randomly\n obs, reward, done, info = env.step(action)\n if done:\n break",
"_____no_output_____"
]
],
[
[
"Now show the animation (it's a bit jittery within Jupyter):",
"_____no_output_____"
]
],
[
[
"def update_scene(num, frames, patch):\n patch.set_data(frames[num])\n return patch,\n\ndef plot_animation(frames, repeat=False, interval=40):\n plt.close() # or else nbagg sometimes plots in the previous cell\n fig = plt.figure()\n patch = plt.imshow(frames[0])\n plt.axis('off')\n return animation.FuncAnimation(fig, update_scene, fargs=(frames, patch), frames=len(frames), repeat=repeat, interval=interval)",
"_____no_output_____"
],
[
"video = plot_animation(frames)\nplt.show()",
"_____no_output_____"
]
],
[
[
"Once you have finished playing with an environment, you should close it to free up resources:",
"_____no_output_____"
]
],
[
[
"env.close()",
"_____no_output_____"
]
],
[
[
"To code our first learning agent, we will be using a simpler environment: the Cart-Pole. ",
"_____no_output_____"
],
[
"# A simple environment: the Cart-Pole",
"_____no_output_____"
],
[
"The Cart-Pole is a very simple environment composed of a cart that can move left or right, and pole placed vertically on top of it. The agent must move the cart left or right to keep the pole upright.",
"_____no_output_____"
]
],
[
[
"env = gym.make(\"CartPole-v0\")",
"[2017-02-17 10:58:12,166] Making new env: CartPole-v0\n"
],
[
"obs = env.reset()",
"_____no_output_____"
],
[
"obs",
"_____no_output_____"
]
],
[
[
"The observation is a 1D NumPy array composed of 4 floats: they represent the cart's horizontal position, its velocity, the angle of the pole (0 = vertical), and the angular velocity. Let's render the environment... unfortunately we need to fix an annoying rendering issue first.",
"_____no_output_____"
],
[
"## Fixing the rendering issue",
"_____no_output_____"
],
[
"Some environments (including the Cart-Pole) require access to your display, which opens up a separate window, even if you specify the `rgb_array` mode. In general you can safely ignore that window. However, if Jupyter is running on a headless server (ie. without a screen) it will raise an exception. One way to avoid this is to install a fake X server like Xvfb. You can start Jupyter using the `xvfb-run` command:\n\n $ xvfb-run -s \"-screen 0 1400x900x24\" jupyter notebook\n\nIf Jupyter is running on a headless server but you don't want to worry about Xvfb, then you can just use the following rendering function for the Cart-Pole:",
"_____no_output_____"
]
],
[
[
"from PIL import Image, ImageDraw\n\ntry:\n from pyglet.gl import gl_info\n openai_cart_pole_rendering = True # no problem, let's use OpenAI gym's rendering function\nexcept Exception:\n openai_cart_pole_rendering = False # probably no X server available, let's use our own rendering function\n\ndef render_cart_pole(env, obs):\n if openai_cart_pole_rendering:\n # use OpenAI gym's rendering function\n return env.render(mode=\"rgb_array\")\n else:\n # rendering for the cart pole environment (in case OpenAI gym can't do it)\n img_w = 600\n img_h = 400\n cart_w = img_w // 12\n cart_h = img_h // 15\n pole_len = img_h // 3.5\n pole_w = img_w // 80 + 1\n x_width = 2\n max_ang = 0.2\n bg_col = (255, 255, 255)\n cart_col = 0x000000 # Blue Green Red\n pole_col = 0x669acc # Blue Green Red\n\n pos, vel, ang, ang_vel = obs\n img = Image.new('RGB', (img_w, img_h), bg_col)\n draw = ImageDraw.Draw(img)\n cart_x = pos * img_w // x_width + img_w // x_width\n cart_y = img_h * 95 // 100\n top_pole_x = cart_x + pole_len * np.sin(ang)\n top_pole_y = cart_y - cart_h // 2 - pole_len * np.cos(ang)\n draw.line((0, cart_y, img_w, cart_y), fill=0)\n draw.rectangle((cart_x - cart_w // 2, cart_y - cart_h // 2, cart_x + cart_w // 2, cart_y + cart_h // 2), fill=cart_col) # draw cart\n draw.line((cart_x, cart_y - cart_h // 2, top_pole_x, top_pole_y), fill=pole_col, width=pole_w) # draw pole\n return np.array(img)\n\ndef plot_cart_pole(env, obs):\n plt.close() # or else nbagg sometimes plots in the previous cell\n img = render_cart_pole(env, obs)\n plt.imshow(img)\n plt.axis(\"off\")\n plt.show()",
"_____no_output_____"
],
[
"plot_cart_pole(env, obs)",
"_____no_output_____"
]
],
[
[
"Now let's look at the action space:",
"_____no_output_____"
]
],
[
[
"env.action_space",
"_____no_output_____"
]
],
[
[
"Yep, just two possible actions: accelerate towards the left or towards the right. Let's push the cart left until the pole falls:",
"_____no_output_____"
]
],
[
[
"obs = env.reset()\nwhile True:\n obs, reward, done, info = env.step(0)\n if done:\n break",
"_____no_output_____"
],
[
"plt.close() # or else nbagg sometimes plots in the previous cell\nimg = render_cart_pole(env, obs)\nplt.imshow(img)\nplt.axis(\"off\")\nsave_fig(\"cart_pole_plot\")",
"_____no_output_____"
]
],
[
[
"Notice that the game is over when the pole tilts too much, not when it actually falls. Now let's reset the environment and push the cart to right instead:",
"_____no_output_____"
]
],
[
[
"obs = env.reset()\nwhile True:\n obs, reward, done, info = env.step(1)\n if done:\n break",
"_____no_output_____"
],
[
"plot_cart_pole(env, obs)",
"_____no_output_____"
]
],
[
[
"Looks like it's doing what we're telling it to do. Now how can we make the poll remain upright? We will need to define a _policy_ for that. This is the strategy that the agent will use to select an action at each step. It can use all the past actions and observations to decide what to do.",
"_____no_output_____"
],
[
"# A simple hard-coded policy",
"_____no_output_____"
],
[
"Let's hard code a simple strategy: if the pole is tilting to the left, then push the cart to the left, and _vice versa_. Let's see if that works:",
"_____no_output_____"
]
],
[
[
"frames = []\n\nn_max_steps = 1000\nn_change_steps = 10\n\nobs = env.reset()\nfor step in range(n_max_steps):\n img = render_cart_pole(env, obs)\n frames.append(img)\n\n # hard-coded policy\n position, velocity, angle, angular_velocity = obs\n if angle < 0:\n action = 0\n else:\n action = 1\n\n obs, reward, done, info = env.step(action)\n if done:\n break",
"_____no_output_____"
],
[
"video = plot_animation(frames)\nplt.show()",
"_____no_output_____"
]
],
[
[
"Nope, the system is unstable and after just a few wobbles, the pole ends up too tilted: game over. We will need to be smarter than that!",
"_____no_output_____"
],
[
"# Neural Network Policies",
"_____no_output_____"
],
[
"Let's create a neural network that will take observations as inputs, and output the action to take for each observation. To choose an action, the network will first estimate a probability for each action, then select an action randomly according to the estimated probabilities. In the case of the Cart-Pole environment, there are just two possible actions (left or right), so we only need one output neuron: it will output the probability `p` of the action 0 (left), and of course the probability of action 1 (right) will be `1 - p`.",
"_____no_output_____"
]
],
[
[
"import tensorflow as tf\nfrom tensorflow.contrib.layers import fully_connected\n\n# 1. Specify the network architecture\nn_inputs = 4 # == env.observation_space.shape[0]\nn_hidden = 4 # it's a simple task, we don't need more than this\nn_outputs = 1 # only outputs the probability of accelerating left\ninitializer = tf.contrib.layers.variance_scaling_initializer()\n\n# 2. Build the neural network\nX = tf.placeholder(tf.float32, shape=[None, n_inputs])\nhidden = fully_connected(X, n_hidden, activation_fn=tf.nn.elu,\n weights_initializer=initializer)\noutputs = fully_connected(hidden, n_outputs, activation_fn=tf.nn.sigmoid,\n weights_initializer=initializer)\n\n# 3. Select a random action based on the estimated probabilities\np_left_and_right = tf.concat(axis=1, values=[outputs, 1 - outputs])\naction = tf.multinomial(tf.log(p_left_and_right), num_samples=1)\n\ninit = tf.global_variables_initializer()",
"_____no_output_____"
]
],
[
[
"In this particular environment, the past actions and observations can safely be ignored, since each observation contains the environment's full state. If there were some hidden state then you may need to consider past actions and observations in order to try to infer the hidden state of the environment. For example, if the environment only revealed the position of the cart but not its velocity, you would have to consider not only the current observation but also the previous observation in order to estimate the current velocity. Another example is if the observations are noisy: you may want to use the past few observations to estimate the most likely current state. Our problem is thus as simple as can be: the current observation is noise-free and contains the environment's full state.",
"_____no_output_____"
],
[
"You may wonder why we are picking a random action based on the probability given by the policy network, rather than just picking the action with the highest probability. This approach lets the agent find the right balance between _exploring_ new actions and _exploiting_ the actions that are known to work well. Here's an analogy: suppose you go to a restaurant for the first time, and all the dishes look equally appealing so you randomly pick one. If it turns out to be good, you can increase the probability to order it next time, but you shouldn't increase that probability to 100%, or else you will never try out the other dishes, some of which may be even better than the one you tried.",
"_____no_output_____"
],
[
"Let's randomly initialize this policy neural network and use it to play one game:",
"_____no_output_____"
]
],
[
[
"n_max_steps = 1000\nframes = []\n\nwith tf.Session() as sess:\n init.run()\n obs = env.reset()\n for step in range(n_max_steps):\n img = render_cart_pole(env, obs)\n frames.append(img)\n action_val = action.eval(feed_dict={X: obs.reshape(1, n_inputs)})\n obs, reward, done, info = env.step(action_val[0][0])\n if done:\n break\n\nenv.close()",
"_____no_output_____"
]
],
[
[
"Now let's look at how well this randomly initialized policy network performed:",
"_____no_output_____"
]
],
[
[
"video = plot_animation(frames)\nplt.show()",
"_____no_output_____"
]
],
[
[
"Yeah... pretty bad. The neural network will have to learn to do better. First let's see if it is capable of learning the basic policy we used earlier: go left if the pole is tilting left, and go right if it is tilting right. The following code defines the same neural network but we add the target probabilities `y`, and the training operations (`cross_entropy`, `optimizer` and `training_op`):",
"_____no_output_____"
]
],
[
[
"import tensorflow as tf\nfrom tensorflow.contrib.layers import fully_connected\n\ntf.reset_default_graph()\n\nn_inputs = 4\nn_hidden = 4\nn_outputs = 1\n\nlearning_rate = 0.01\n\ninitializer = tf.contrib.layers.variance_scaling_initializer()\n\nX = tf.placeholder(tf.float32, shape=[None, n_inputs])\ny = tf.placeholder(tf.float32, shape=[None, n_outputs])\n\nhidden = fully_connected(X, n_hidden, activation_fn=tf.nn.elu, weights_initializer=initializer)\nlogits = fully_connected(hidden, n_outputs, activation_fn=None)\noutputs = tf.nn.sigmoid(logits) # probability of action 0 (left)\np_left_and_right = tf.concat(axis=1, values=[outputs, 1 - outputs])\naction = tf.multinomial(tf.log(p_left_and_right), num_samples=1)\n\ncross_entropy = tf.nn.sigmoid_cross_entropy_with_logits(labels=y, logits=logits)\noptimizer = tf.train.AdamOptimizer(learning_rate)\ntraining_op = optimizer.minimize(cross_entropy)\n\ninit = tf.global_variables_initializer()\nsaver = tf.train.Saver()",
"_____no_output_____"
]
],
[
[
"We can make the same net play in 10 different environments in parallel, and train for 1000 iterations. We also reset environments when they are done.",
"_____no_output_____"
]
],
[
[
"n_environments = 10\nn_iterations = 1000\n\nenvs = [gym.make(\"CartPole-v0\") for _ in range(n_environments)]\nobservations = [env.reset() for env in envs]\n\nwith tf.Session() as sess:\n init.run()\n for iteration in range(n_iterations):\n target_probas = np.array([([1.] if obs[2] < 0 else [0.]) for obs in observations]) # if angle<0 we want proba(left)=1., or else proba(left)=0.\n action_val, _ = sess.run([action, training_op], feed_dict={X: np.array(observations), y: target_probas})\n for env_index, env in enumerate(envs):\n obs, reward, done, info = env.step(action_val[env_index][0])\n observations[env_index] = obs if not done else env.reset()\n saver.save(sess, \"./my_policy_net_basic.ckpt\")\n\nfor env in envs:\n env.close()",
"[2017-02-17 10:58:53,509] Making new env: CartPole-v0\n[2017-02-17 10:58:53,511] Making new env: CartPole-v0\n[2017-02-17 10:58:53,513] Making new env: CartPole-v0\n[2017-02-17 10:58:53,514] Making new env: CartPole-v0\n[2017-02-17 10:58:53,516] Making new env: CartPole-v0\n[2017-02-17 10:58:53,517] Making new env: CartPole-v0\n[2017-02-17 10:58:53,518] Making new env: CartPole-v0\n[2017-02-17 10:58:53,520] Making new env: CartPole-v0\n[2017-02-17 10:58:53,521] Making new env: CartPole-v0\n[2017-02-17 10:58:53,522] Making new env: CartPole-v0\n"
],
[
"def render_policy_net(model_path, action, X, n_max_steps = 1000):\n frames = []\n env = gym.make(\"CartPole-v0\")\n obs = env.reset()\n with tf.Session() as sess:\n saver.restore(sess, model_path)\n for step in range(n_max_steps):\n img = render_cart_pole(env, obs)\n frames.append(img)\n action_val = action.eval(feed_dict={X: obs.reshape(1, n_inputs)})\n obs, reward, done, info = env.step(action_val[0][0])\n if done:\n break\n env.close()\n return frames ",
"_____no_output_____"
],
[
"frames = render_policy_net(\"./my_policy_net_basic.ckpt\", action, X)\nvideo = plot_animation(frames)\nplt.show()",
"[2017-02-17 10:58:55,704] Making new env: CartPole-v0\n"
]
],
[
[
"Looks like it learned the policy correctly. Now let's see if it can learn a better policy on its own.",
"_____no_output_____"
],
[
"# Policy Gradients",
"_____no_output_____"
],
[
"To train this neural network we will need to define the target probabilities `y`. If an action is good we should increase its probability, and conversely if it is bad we should reduce it. But how do we know whether an action is good or bad? The problem is that most actions have delayed effects, so when you win or lose points in a game, it is not clear which actions contributed to this result: was it just the last action? Or the last 10? Or just one action 50 steps earlier? This is called the _credit assignment problem_.\n\nThe _Policy Gradients_ algorithm tackles this problem by first playing multiple games, then making the actions in good games slightly more likely, while actions in bad games are made slightly less likely. First we play, then we go back and think about what we did.",
"_____no_output_____"
]
],
[
[
"import tensorflow as tf\nfrom tensorflow.contrib.layers import fully_connected\n\ntf.reset_default_graph()\n\nn_inputs = 4\nn_hidden = 4\nn_outputs = 1\n\nlearning_rate = 0.01\n\ninitializer = tf.contrib.layers.variance_scaling_initializer()\n\nX = tf.placeholder(tf.float32, shape=[None, n_inputs])\n\nhidden = fully_connected(X, n_hidden, activation_fn=tf.nn.elu, weights_initializer=initializer)\nlogits = fully_connected(hidden, n_outputs, activation_fn=None)\noutputs = tf.nn.sigmoid(logits) # probability of action 0 (left)\np_left_and_right = tf.concat(axis=1, values=[outputs, 1 - outputs])\naction = tf.multinomial(tf.log(p_left_and_right), num_samples=1)\n\ny = 1. - tf.to_float(action)\ncross_entropy = tf.nn.sigmoid_cross_entropy_with_logits(labels=y, logits=logits)\noptimizer = tf.train.AdamOptimizer(learning_rate)\ngrads_and_vars = optimizer.compute_gradients(cross_entropy)\ngradients = [grad for grad, variable in grads_and_vars]\ngradient_placeholders = []\ngrads_and_vars_feed = []\nfor grad, variable in grads_and_vars:\n gradient_placeholder = tf.placeholder(tf.float32, shape=grad.get_shape())\n gradient_placeholders.append(gradient_placeholder)\n grads_and_vars_feed.append((gradient_placeholder, variable))\ntraining_op = optimizer.apply_gradients(grads_and_vars_feed)\n\ninit = tf.global_variables_initializer()\nsaver = tf.train.Saver()",
"_____no_output_____"
],
[
"def discount_rewards(rewards, discount_rate):\n discounted_rewards = np.zeros(len(rewards))\n cumulative_rewards = 0\n for step in reversed(range(len(rewards))):\n cumulative_rewards = rewards[step] + cumulative_rewards * discount_rate\n discounted_rewards[step] = cumulative_rewards\n return discounted_rewards\n\ndef discount_and_normalize_rewards(all_rewards, discount_rate):\n all_discounted_rewards = [discount_rewards(rewards, discount_rate) for rewards in all_rewards]\n flat_rewards = np.concatenate(all_discounted_rewards)\n reward_mean = flat_rewards.mean()\n reward_std = flat_rewards.std()\n return [(discounted_rewards - reward_mean)/reward_std for discounted_rewards in all_discounted_rewards]",
"_____no_output_____"
],
[
"discount_rewards([10, 0, -50], discount_rate=0.8)",
"_____no_output_____"
],
[
"discount_and_normalize_rewards([[10, 0, -50], [10, 20]], discount_rate=0.8)",
"_____no_output_____"
],
[
"env = gym.make(\"CartPole-v0\")\n\nn_games_per_update = 10\nn_max_steps = 1000\nn_iterations = 250\nsave_iterations = 10\ndiscount_rate = 0.95\n\nwith tf.Session() as sess:\n init.run()\n for iteration in range(n_iterations):\n print(\"\\rIteration: {}\".format(iteration), end=\"\")\n all_rewards = []\n all_gradients = []\n for game in range(n_games_per_update):\n current_rewards = []\n current_gradients = []\n obs = env.reset()\n for step in range(n_max_steps):\n action_val, gradients_val = sess.run([action, gradients], feed_dict={X: obs.reshape(1, n_inputs)})\n obs, reward, done, info = env.step(action_val[0][0])\n current_rewards.append(reward)\n current_gradients.append(gradients_val)\n if done:\n break\n all_rewards.append(current_rewards)\n all_gradients.append(current_gradients)\n\n all_rewards = discount_and_normalize_rewards(all_rewards, discount_rate=discount_rate)\n feed_dict = {}\n for var_index, gradient_placeholder in enumerate(gradient_placeholders):\n mean_gradients = np.mean([reward * all_gradients[game_index][step][var_index]\n for game_index, rewards in enumerate(all_rewards)\n for step, reward in enumerate(rewards)], axis=0)\n feed_dict[gradient_placeholder] = mean_gradients\n sess.run(training_op, feed_dict=feed_dict)\n if iteration % save_iterations == 0:\n saver.save(sess, \"./my_policy_net_pg.ckpt\")",
"[2017-02-17 11:03:24,353] Making new env: CartPole-v0\n"
],
[
"env.close()",
"_____no_output_____"
],
[
"frames = render_policy_net(\"./my_policy_net_pg.ckpt\", action, X, n_max_steps=1000)\nvideo = plot_animation(frames)\nplt.show()",
"[2017-02-17 11:06:16,047] Making new env: CartPole-v0\n"
]
],
[
[
"# Markov Chains",
"_____no_output_____"
]
],
[
[
"transition_probabilities = [\n [0.7, 0.2, 0.0, 0.1], # from s0 to s0, s1, s2, s3\n [0.0, 0.0, 0.9, 0.1], # from s1 to ...\n [0.0, 1.0, 0.0, 0.0], # from s2 to ...\n [0.0, 0.0, 0.0, 1.0], # from s3 to ...\n ]\n\nn_max_steps = 50\n\ndef print_sequence(start_state=0):\n current_state = start_state\n print(\"States:\", end=\" \")\n for step in range(n_max_steps):\n print(current_state, end=\" \")\n if current_state == 3:\n break\n current_state = rnd.choice(range(4), p=transition_probabilities[current_state])\n else:\n print(\"...\", end=\"\")\n print()\n\nfor _ in range(10):\n print_sequence()",
"States: 0 0 3 \nStates: 0 1 2 1 2 1 2 1 2 1 3 \nStates: 0 1 2 1 2 1 2 1 2 1 2 1 2 1 2 1 2 1 2 1 2 1 3 \nStates: 0 3 \nStates: 0 1 2 1 2 1 2 1 2 1 2 1 2 1 2 1 3 \nStates: 0 1 3 \nStates: 0 1 2 1 2 1 2 1 2 1 2 1 2 1 2 1 2 1 2 1 2 1 2 1 2 1 2 1 2 1 2 1 2 1 2 1 2 1 2 1 2 1 2 1 2 1 2 1 2 1 ...\nStates: 0 0 3 \nStates: 0 0 0 1 2 1 2 1 3 \nStates: 0 1 2 1 2 1 2 1 2 1 2 1 2 1 2 1 2 1 2 1 2 1 3 \n"
]
],
[
[
"# Markov Decision Process",
"_____no_output_____"
]
],
[
[
"transition_probabilities = [\n [[0.7, 0.3, 0.0], [1.0, 0.0, 0.0], [0.8, 0.2, 0.0]], # in s0, if action a0 then proba 0.7 to state s0 and 0.3 to state s1, etc.\n [[0.0, 1.0, 0.0], None, [0.0, 0.0, 1.0]],\n [None, [0.8, 0.1, 0.1], None],\n ]\n\nrewards = [\n [[+10, 0, 0], [0, 0, 0], [0, 0, 0]],\n [[0, 0, 0], [0, 0, 0], [0, 0, -50]],\n [[0, 0, 0], [+40, 0, 0], [0, 0, 0]],\n ]\n\npossible_actions = [[0, 1, 2], [0, 2], [1]]\n\ndef policy_fire(state):\n return [0, 2, 1][state]\n\ndef policy_random(state):\n return rnd.choice(possible_actions[state])\n\ndef policy_safe(state):\n return [0, 0, 1][state]\n\nclass MDPEnvironment(object):\n def __init__(self, start_state=0):\n self.start_state=start_state\n self.reset()\n def reset(self):\n self.total_rewards = 0\n self.state = self.start_state\n def step(self, action):\n next_state = rnd.choice(range(3), p=transition_probabilities[self.state][action])\n reward = rewards[self.state][action][next_state]\n self.state = next_state\n self.total_rewards += reward\n return self.state, reward\n\ndef run_episode(policy, n_steps, start_state=0, display=True):\n env = MDPEnvironment()\n if display:\n print(\"States (+rewards):\", end=\" \")\n for step in range(n_steps):\n if display:\n if step == 10:\n print(\"...\", end=\" \")\n elif step < 10:\n print(env.state, end=\" \")\n action = policy(env.state)\n state, reward = env.step(action)\n if display and step < 10:\n if reward:\n print(\"({})\".format(reward), end=\" \")\n if display:\n print(\"Total rewards =\", env.total_rewards)\n return env.total_rewards\n\nfor policy in (policy_fire, policy_random, policy_safe):\n all_totals = []\n print(policy.__name__)\n for episode in range(1000):\n all_totals.append(run_episode(policy, n_steps=100, display=(episode<5)))\n print(\"Summary: mean={:.1f}, std={:1f}, min={}, max={}\".format(np.mean(all_totals), np.std(all_totals), np.min(all_totals), np.max(all_totals)))\n print()",
"policy_fire\nStates (+rewards): 0 (10) 0 (10) 0 1 (-50) 2 2 2 (40) 0 (10) 0 (10) 0 (10) ... Total rewards = 210\nStates (+rewards): 0 1 (-50) 2 (40) 0 (10) 0 (10) 0 1 (-50) 2 2 (40) 0 (10) ... Total rewards = 70\nStates (+rewards): 0 (10) 0 1 (-50) 2 (40) 0 (10) 0 (10) 0 (10) 0 (10) 0 (10) 0 (10) ... Total rewards = 70\nStates (+rewards): 0 1 (-50) 2 1 (-50) 2 (40) 0 (10) 0 1 (-50) 2 (40) 0 ... Total rewards = -10\nStates (+rewards): 0 1 (-50) 2 (40) 0 (10) 0 (10) 0 1 (-50) 2 (40) 0 (10) 0 (10) ... Total rewards = 290\nSummary: mean=121.1, std=129.333766, min=-330, max=470\n\npolicy_random\nStates (+rewards): 0 1 (-50) 2 1 (-50) 2 (40) 0 1 (-50) 2 2 (40) 0 ... Total rewards = -60\nStates (+rewards): 0 (10) 0 0 0 0 0 (10) 0 0 0 (10) 0 ... Total rewards = -30\nStates (+rewards): 0 1 1 (-50) 2 (40) 0 0 1 1 1 1 ... Total rewards = 10\nStates (+rewards): 0 (10) 0 (10) 0 0 0 0 1 (-50) 2 (40) 0 0 ... Total rewards = 0\nStates (+rewards): 0 0 (10) 0 1 (-50) 2 (40) 0 0 0 0 (10) 0 (10) ... Total rewards = 40\nSummary: mean=-22.1, std=88.152740, min=-380, max=200\n\npolicy_safe\nStates (+rewards): 0 1 1 1 1 1 1 1 1 1 ... Total rewards = 0\nStates (+rewards): 0 1 1 1 1 1 1 1 1 1 ... Total rewards = 0\nStates (+rewards): 0 (10) 0 (10) 0 (10) 0 1 1 1 1 1 1 ... Total rewards = 30\nStates (+rewards): 0 (10) 0 1 1 1 1 1 1 1 1 ... Total rewards = 10\nStates (+rewards): 0 1 1 1 1 1 1 1 1 1 ... Total rewards = 0\nSummary: mean=22.3, std=26.244312, min=0, max=170\n\n"
]
],
[
[
"# Q-Learning",
"_____no_output_____"
],
[
"Q-Learning will learn the optimal policy by watching the random policy play.",
"_____no_output_____"
]
],
[
[
"n_states = 3\nn_actions = 3\nn_steps = 20000\nalpha = 0.01\ngamma = 0.99\nexploration_policy = policy_random\nq_values = np.full((n_states, n_actions), -np.inf)\nfor state, actions in enumerate(possible_actions):\n q_values[state][actions]=0\n\nenv = MDPEnvironment()\nfor step in range(n_steps):\n action = exploration_policy(env.state)\n state = env.state\n next_state, reward = env.step(action)\n next_value = np.max(q_values[next_state]) # greedy policy\n q_values[state, action] = (1-alpha)*q_values[state, action] + alpha*(reward + gamma * next_value)",
"_____no_output_____"
],
[
"def optimal_policy(state):\n return np.argmax(q_values[state])",
"_____no_output_____"
],
[
"q_values",
"_____no_output_____"
],
[
"all_totals = []\nfor episode in range(1000):\n all_totals.append(run_episode(optimal_policy, n_steps=100, display=(episode<5)))\nprint(\"Summary: mean={:.1f}, std={:1f}, min={}, max={}\".format(np.mean(all_totals), np.std(all_totals), np.min(all_totals), np.max(all_totals)))\nprint()",
"States (+rewards): 0 (10) 0 (10) 0 1 (-50) 2 (40) 0 (10) 0 1 (-50) 2 (40) 0 (10) ... Total rewards = 230\nStates (+rewards): 0 (10) 0 (10) 0 (10) 0 1 (-50) 2 2 1 (-50) 2 (40) 0 (10) ... Total rewards = 90\nStates (+rewards): 0 1 (-50) 2 (40) 0 (10) 0 (10) 0 (10) 0 (10) 0 (10) 0 (10) 0 (10) ... Total rewards = 170\nStates (+rewards): 0 1 (-50) 2 (40) 0 (10) 0 (10) 0 (10) 0 (10) 0 (10) 0 (10) 0 (10) ... Total rewards = 220\nStates (+rewards): 0 1 (-50) 2 (40) 0 (10) 0 1 (-50) 2 (40) 0 (10) 0 (10) 0 (10) ... Total rewards = -50\nSummary: mean=125.6, std=127.363464, min=-290, max=500\n\n"
]
],
[
[
"# Learning to play MsPacman using Deep Q-Learning",
"_____no_output_____"
]
],
[
[
"env = gym.make(\"MsPacman-v0\")\nobs = env.reset()",
"[2017-02-17 11:06:47,254] Making new env: MsPacman-v0\n"
],
[
"obs.shape",
"_____no_output_____"
],
[
"env.action_space",
"_____no_output_____"
]
],
[
[
"## Preprocessing",
"_____no_output_____"
],
[
"Preprocessing the images is optional but greatly speeds up training.",
"_____no_output_____"
]
],
[
[
"mspacman_color = np.array([210, 164, 74]).mean()\n\ndef preprocess_observation(obs):\n img = obs[1:176:2, ::2] # crop and downsize\n img = img.mean(axis=2) # to greyscale\n img[img==mspacman_color] = 0 # Improve contrast\n img = (img - 128) / 128 - 1 # normalize from -1. to 1.\n return img.reshape(88, 80, 1)\n\nimg = preprocess_observation(obs)",
"_____no_output_____"
],
[
"plt.figure(figsize=(11, 7))\nplt.subplot(121)\nplt.title(\"Original observation (160×210 RGB)\")\nplt.imshow(obs)\nplt.axis(\"off\")\nplt.subplot(122)\nplt.title(\"Preprocessed observation (88×80 greyscale)\")\nplt.imshow(img.reshape(88, 80), interpolation=\"nearest\", cmap=\"gray\")\nplt.axis(\"off\")\nsave_fig(\"preprocessing_plot\")\nplt.show()",
"_____no_output_____"
]
],
[
[
"## Build DQN",
"_____no_output_____"
]
],
[
[
"tf.reset_default_graph()\n\nfrom tensorflow.contrib.layers import convolution2d, fully_connected\n\ninput_height = 88\ninput_width = 80\ninput_channels = 1\nconv_n_maps = [32, 64, 64]\nconv_kernel_sizes = [(8,8), (4,4), (3,3)]\nconv_strides = [4, 2, 1]\nconv_paddings = [\"SAME\"]*3 \nconv_activation = [tf.nn.relu]*3\nn_hidden_inputs = 64 * 11 * 10 # conv3 has 64 maps of 11x10 each\nn_hidden = 512\nhidden_activation = tf.nn.relu\nn_outputs = env.action_space.n\ninitializer = tf.contrib.layers.variance_scaling_initializer()\n\nlearning_rate = 0.01\n\ndef q_network(X_state, scope):\n prev_layer = X_state\n conv_layers = []\n with tf.variable_scope(scope) as scope:\n for n_maps, kernel_size, stride, padding, activation in zip(conv_n_maps, conv_kernel_sizes, conv_strides, conv_paddings, conv_activation):\n prev_layer = convolution2d(prev_layer, num_outputs=n_maps, kernel_size=kernel_size, stride=stride, padding=padding, activation_fn=activation, weights_initializer=initializer)\n conv_layers.append(prev_layer)\n last_conv_layer_flat = tf.reshape(prev_layer, shape=[-1, n_hidden_inputs])\n hidden = fully_connected(last_conv_layer_flat, n_hidden, activation_fn=hidden_activation, weights_initializer=initializer)\n outputs = fully_connected(hidden, n_outputs, activation_fn=None)\n trainable_vars = {var.name[len(scope.name):]: var for var in tf.get_collection(tf.GraphKeys.TRAINABLE_VARIABLES, scope=scope.name)}\n return outputs, trainable_vars\n\nX_state = tf.placeholder(tf.float32, shape=[None, input_height, input_width, input_channels])\nactor_q_values, actor_vars = q_network(X_state, scope=\"q_networks/actor\") # acts\ncritic_q_values, critic_vars = q_network(X_state, scope=\"q_networks/critic\") # learns\n\ncopy_ops = [actor_var.assign(critic_vars[var_name])\n for var_name, actor_var in actor_vars.items()]\ncopy_critic_to_actor = tf.group(*copy_ops)\n\nwith tf.variable_scope(\"train\"):\n X_action = tf.placeholder(tf.int32, shape=[None])\n y = tf.placeholder(tf.float32, shape=[None, 1])\n q_value = tf.reduce_sum(critic_q_values * tf.one_hot(X_action, n_outputs),\n axis=1, keep_dims=True)\n cost = tf.reduce_mean(tf.square(y - q_value))\n global_step = tf.Variable(0, trainable=False, name='global_step')\n optimizer = tf.train.AdamOptimizer(learning_rate)\n training_op = optimizer.minimize(cost, global_step=global_step)\n \ninit = tf.global_variables_initializer()\nsaver = tf.train.Saver()",
"_____no_output_____"
],
[
"actor_vars",
"_____no_output_____"
],
[
"from collections import deque\n\nreplay_memory_size = 10000\nreplay_memory = deque([], maxlen=replay_memory_size)\n\ndef sample_memories(batch_size):\n indices = rnd.permutation(len(replay_memory))[:batch_size]\n cols = [[], [], [], [], []] # state, action, reward, next_state, continue\n for idx in indices:\n memory = replay_memory[idx]\n for col, value in zip(cols, memory):\n col.append(value)\n cols = [np.array(col) for col in cols]\n return cols[0], cols[1], cols[2].reshape(-1, 1), cols[3], cols[4].reshape(-1, 1)",
"_____no_output_____"
],
[
"eps_min = 0.05\neps_max = 1.0\neps_decay_steps = 50000\nimport sys\n\ndef epsilon_greedy(q_values, step):\n epsilon = max(eps_min, eps_max - (eps_max-eps_min) * step/eps_decay_steps)\n if rnd.rand() < epsilon:\n return rnd.randint(n_outputs) # random action\n else:\n return np.argmax(q_values) # optimal action",
"_____no_output_____"
],
[
"n_steps = 100000 # total number of training steps\ntraining_start = 1000 # start training after 1,000 game iterations\ntraining_interval = 3 # run a training step every 3 game iterations\nsave_steps = 50 # save the model every 50 training steps\ncopy_steps = 25 # copy the critic to the actor every 25 training steps\ndiscount_rate = 0.95\nskip_start = 90 # Skip the start of every game (it's just waiting time).\nbatch_size = 50\niteration = 0 # game iterations\ncheckpoint_path = \"./my_dqn.ckpt\"\ndone = True # env needs to be reset\n\nwith tf.Session() as sess:\n if os.path.isfile(checkpoint_path):\n saver.restore(sess, checkpoint_path)\n else:\n init.run()\n while True:\n step = global_step.eval()\n if step >= n_steps:\n break\n iteration += 1\n print(\"\\rIteration {}\\tTraining step {}/{} ({:.1f}%)\".format(iteration, step, n_steps, step * 100 / n_steps), end=\"\")\n if done: # game over, start again\n obs = env.reset()\n for skip in range(skip_start): # skip boring game iterations at the start of each game\n obs, reward, done, info = env.step(0)\n state = preprocess_observation(obs)\n\n # Actor evaluates what to do\n q_values = actor_q_values.eval(feed_dict={X_state: [state]})\n action = epsilon_greedy(q_values, step)\n\n # Actor plays\n obs, reward, done, info = env.step(action)\n next_state = preprocess_observation(obs)\n\n # Let's memorize what happened\n replay_memory.append((state, action, reward, next_state, 1.0 - done))\n state = next_state\n\n if iteration < training_start or iteration % training_interval != 0:\n continue\n \n # Critic learns\n X_state_val, X_action_val, rewards, X_next_state_val, continues = sample_memories(batch_size)\n next_q_values = actor_q_values.eval(feed_dict={X_state: X_next_state_val})\n y_val = rewards + continues * discount_rate * np.max(next_q_values, axis=1, keepdims=True)\n training_op.run(feed_dict={X_state: X_state_val, X_action: X_action_val, y: y_val})\n\n # Regularly copy critic to actor\n if step % copy_steps == 0:\n copy_critic_to_actor.run()\n\n # And save regularly\n if step % save_steps == 0:\n saver.save(sess, checkpoint_path)",
"Iteration 328653\tTraining step 100000/100000 (100.0%)"
]
],
[
[
"# Exercise solutions",
"_____no_output_____"
],
[
"Coming soon...",
"_____no_output_____"
]
]
] | [
"markdown",
"code",
"markdown",
"code",
"markdown",
"code",
"markdown",
"code",
"markdown",
"code",
"markdown",
"code",
"markdown",
"code",
"markdown",
"code",
"markdown",
"code",
"markdown",
"code",
"markdown",
"code",
"markdown",
"code",
"markdown",
"code",
"markdown",
"code",
"markdown",
"code",
"markdown",
"code",
"markdown",
"code",
"markdown",
"code",
"markdown",
"code",
"markdown",
"code",
"markdown",
"code",
"markdown",
"code",
"markdown",
"code",
"markdown",
"code",
"markdown",
"code",
"markdown",
"code",
"markdown",
"code",
"markdown",
"code",
"markdown",
"code",
"markdown",
"code",
"markdown",
"code",
"markdown",
"code",
"markdown",
"code",
"markdown",
"code",
"markdown",
"code",
"markdown",
"code",
"markdown",
"code",
"markdown",
"code",
"markdown"
] | [
[
"markdown",
"markdown",
"markdown",
"markdown"
],
[
"code"
],
[
"markdown",
"markdown"
],
[
"code"
],
[
"markdown"
],
[
"code"
],
[
"markdown"
],
[
"code"
],
[
"markdown"
],
[
"code"
],
[
"markdown"
],
[
"code"
],
[
"markdown"
],
[
"code"
],
[
"markdown",
"markdown"
],
[
"code"
],
[
"markdown"
],
[
"code"
],
[
"markdown"
],
[
"code"
],
[
"markdown",
"markdown"
],
[
"code"
],
[
"markdown"
],
[
"code"
],
[
"markdown"
],
[
"code"
],
[
"markdown"
],
[
"code"
],
[
"markdown"
],
[
"code"
],
[
"markdown"
],
[
"code"
],
[
"markdown"
],
[
"code"
],
[
"markdown"
],
[
"code"
],
[
"markdown"
],
[
"code",
"code"
],
[
"markdown"
],
[
"code"
],
[
"markdown",
"markdown",
"markdown"
],
[
"code",
"code",
"code"
],
[
"markdown",
"markdown",
"markdown"
],
[
"code",
"code"
],
[
"markdown"
],
[
"code"
],
[
"markdown"
],
[
"code",
"code"
],
[
"markdown"
],
[
"code",
"code"
],
[
"markdown",
"markdown",
"markdown"
],
[
"code",
"code"
],
[
"markdown",
"markdown",
"markdown"
],
[
"code"
],
[
"markdown",
"markdown",
"markdown"
],
[
"code"
],
[
"markdown"
],
[
"code"
],
[
"markdown"
],
[
"code"
],
[
"markdown"
],
[
"code",
"code",
"code"
],
[
"markdown",
"markdown",
"markdown"
],
[
"code",
"code",
"code",
"code",
"code",
"code",
"code"
],
[
"markdown"
],
[
"code"
],
[
"markdown"
],
[
"code"
],
[
"markdown",
"markdown"
],
[
"code",
"code",
"code",
"code"
],
[
"markdown"
],
[
"code",
"code",
"code"
],
[
"markdown",
"markdown"
],
[
"code",
"code"
],
[
"markdown"
],
[
"code",
"code",
"code",
"code",
"code"
],
[
"markdown",
"markdown"
]
] |
d07a39381e447c4a1b41ec3665a92d7be98852e5 | 3,593 | ipynb | Jupyter Notebook | data_processing/toco.ipynb | flyingdutchman23/step-detector | 07d3a94917cc646cc3bb6e98c3284bd4725654e7 | [
"Apache-2.0"
] | null | null | null | data_processing/toco.ipynb | flyingdutchman23/step-detector | 07d3a94917cc646cc3bb6e98c3284bd4725654e7 | [
"Apache-2.0"
] | null | null | null | data_processing/toco.ipynb | flyingdutchman23/step-detector | 07d3a94917cc646cc3bb6e98c3284bd4725654e7 | [
"Apache-2.0"
] | null | null | null | 30.709402 | 113 | 0.563318 | [
[
[
"import numpy as np\nimport tensorflow as tf\nfrom tensorflow.python.saved_model import loader\nimport os\n\ntf.__version__\nimport tempfile\nimport subprocess\ntf.contrib.lite.tempfile = tempfile\ntf.contrib.lite.subprocess = subprocess",
"_____no_output_____"
],
[
"saved_model_dir = 'saved_models/no_maxpool_10-5--10-5_120_1_0.01_0.95/1519994594'\nloaded_graph = tf.Graph()\nwith tf.Session(graph=loaded_graph) as sess:\n with loaded_graph.as_default():\n loader.load(sess, ['serve'], saved_model_dir)\n# print(loaded_graph.get_operations())\n frozen_graph_def = tf.graph_util.convert_variables_to_constants(sess,\n loaded_graph.as_graph_def(),\n [\"softmax_tensor\"])\n# frozen_graph = tf.Graph()\n# with frozen_graph.as_default():\n tf.import_graph_def(frozen_graph_def)\n# print(loaded_graph.get_operations())\n graph_input = loaded_graph.get_operation_by_name('accelerometer_input')\n graph_output = loaded_graph.get_operation_by_name('softmax_tensor')\n input_tensor = graph_input.values()[0]\n input_shape = list(input_tensor.get_shape())\n print(input_shape)\n input_shape[0] = 1\n input_tensor.set_shape(input_shape)\n tflite_model = tf.contrib.lite.toco_convert(frozen_graph_def, graph_input.values(), [graph_output])",
"_____no_output_____"
],
[
"saved_model_dir_head, _ = os.path.split(saved_model_dir)\n_, name = os.path.split(saved_model_dir_head)\nwith open(os.path.join(saved_model_dir, '{}.tflite'.format(name)), 'wb') as f:\n f.write(tflite_model)",
"_____no_output_____"
],
[
"with tf.Session() as sess:\n tf.global_variables_initializer()\n tf.import_graph_def(frozen_graph_def)\n graph_input = sess.graph.get_operation_by_name('accelerometer_input')\n input_tensor = graph_input.values()[0]\n graph_output = sess.graph.get_operation_by_name('softmax_tensor')\n \n rand_data = np.random.rand(1, 120, 3)\n result = sess.run(graph_output, feed_dict={input_tensor: rand_data})\n print(result)",
"_____no_output_____"
]
]
] | [
"code"
] | [
[
"code",
"code",
"code",
"code"
]
] |
d07a39788436bbfafab578699b86bf8215ce799a | 123,575 | ipynb | Jupyter Notebook | site/_build/jupyter_execute/notebooks/08-intro-nlp/01-titanic-features.ipynb | rpi-techfundamentals/spring2020_website | b4b208ce7555f5574054ff5ff5d79b9e0e825499 | [
"MIT"
] | 2 | 2020-10-18T23:05:09.000Z | 2021-11-14T08:09:11.000Z | site/_build/jupyter_execute/notebooks/08-intro-nlp/01-titanic-features.ipynb | rpi-techfundamentals/spring2020_website | b4b208ce7555f5574054ff5ff5d79b9e0e825499 | [
"MIT"
] | 2 | 2020-12-31T14:33:02.000Z | 2020-12-31T14:38:26.000Z | site/_build/jupyter_execute/notebooks/08-intro-nlp/01-titanic-features.ipynb | rpi-techfundamentals/spring2020_website | b4b208ce7555f5574054ff5ff5d79b9e0e825499 | [
"MIT"
] | 3 | 2021-01-05T20:26:15.000Z | 2021-02-15T14:54:44.000Z | 35.469288 | 156 | 0.299955 | [
[
[
"[](http://rpi.analyticsdojo.com)\n<center><h1>Basic Text Feature Creation in Python</h1></center>\n<center><h3><a href = 'http://rpi.analyticsdojo.com'>rpi.analyticsdojo.com</a></h3></center>",
"_____no_output_____"
],
[
"# Basic Text Feature Creation in Python",
"_____no_output_____"
]
],
[
[
"!wget https://raw.githubusercontent.com/rpi-techfundamentals/spring2019-materials/master/input/train.csv\n!wget https://raw.githubusercontent.com/rpi-techfundamentals/spring2019-materials/master/input/test.csv",
"--2019-03-11 14:58:22-- https://raw.githubusercontent.com/rpi-techfundamentals/spring2019-materials/master/input/train.csv\nResolving raw.githubusercontent.com (raw.githubusercontent.com)... 151.101.0.133, 151.101.64.133, 151.101.128.133, ...\nConnecting to raw.githubusercontent.com (raw.githubusercontent.com)|151.101.0.133|:443... connected.\nHTTP request sent, awaiting response... 200 OK\nLength: 61194 (60K) [text/plain]\nSaving to: ‘train.csv.1’\n\ntrain.csv.1 100%[===================>] 59.76K --.-KB/s in 0.03s \n\n2019-03-11 14:58:23 (2.32 MB/s) - ‘train.csv.1’ saved [61194/61194]\n\n--2019-03-11 14:58:23-- https://raw.githubusercontent.com/rpi-techfundamentals/spring2019-materials/master/input/test.csv\nResolving raw.githubusercontent.com (raw.githubusercontent.com)... 151.101.0.133, 151.101.64.133, 151.101.128.133, ...\nConnecting to raw.githubusercontent.com (raw.githubusercontent.com)|151.101.0.133|:443... connected.\nHTTP request sent, awaiting response... 200 OK\nLength: 28629 (28K) [text/plain]\nSaving to: ‘test.csv.1’\n\ntest.csv.1 100%[===================>] 27.96K --.-KB/s in 0.01s \n\n2019-03-11 14:58:24 (2.27 MB/s) - ‘test.csv.1’ saved [28629/28629]\n\n"
],
[
"import numpy as np\nimport pandas as pd\nimport pandas as pd\n\ntrain= pd.read_csv('train.csv')\ntest = pd.read_csv('test.csv')\n\n",
"_____no_output_____"
],
[
"#Print to standard output, and see the results in the \"log\" section below after running your script\ntrain.head()",
"_____no_output_____"
],
[
"#Print to standard output, and see the results in the \"log\" section below after running your script\ntrain.describe()",
"_____no_output_____"
],
[
"train.dtypes",
"_____no_output_____"
],
[
"#Let's look at the age field. We can see \"NaN\" (which indicates missing values).s\ntrain[\"Age\"]",
"_____no_output_____"
],
[
"#Now let's recode. \nmedianAge=train[\"Age\"].median()\nprint (\"The Median age is:\", medianAge, \" years old.\")\ntrain[\"Age\"] = train[\"Age\"].fillna(medianAge)\n\n#Option 2 all in one shot! \ntrain[\"Age\"] = train[\"Age\"].fillna(train[\"Age\"].median())\ntrain[\"Age\"] ",
"The Median age is: 28.0 years old.\n"
],
[
"#For Recoding Data, we can use what we know of selecting rows and columns\ntrain[\"Embarked\"] = train[\"Embarked\"].fillna(\"S\")\ntrain.loc[train[\"Embarked\"] == \"S\", \"EmbarkedRecode\"] = 0\ntrain.loc[train[\"Embarked\"] == \"C\", \"EmbarkedRecode\"] = 1\ntrain.loc[train[\"Embarked\"] == \"Q\", \"EmbarkedRecode\"] = 2",
"_____no_output_____"
],
[
"# We can also use something called a lambda function \n# You can read more about the lambda function here.\n#http://www.python-course.eu/lambda.php \ngender_fn = lambda x: 0 if x == 'male' else 1\ntrain['Gender'] = train['Sex'].map(gender_fn)",
"_____no_output_____"
],
[
"#or we can do in one shot\ntrain['NameLength'] = train['Name'].map(lambda x: len(x))\ntrain['Age2'] = train['Age'].map(lambda x: x*x)\ntrain",
"_____no_output_____"
],
[
"\n#We can start to create little small functions that will find a string.\ndef has_title(name):\n for s in ['Mr.', 'Mrs.', 'Miss.', 'Dr.', 'Sir.']:\n if name.find(s) >= 0:\n return True\n return False\n\n#Now we are using that separate function in another function. \ntitle_fn = lambda x: 1 if has_title(x) else 0\n#Finally, we call the function for name\ntrain['Title'] = train['Name'].map(title_fn)\ntest['Title']= train['Name'].map(title_fn)\n",
"_____no_output_____"
],
[
"test",
"_____no_output_____"
],
[
"#Writing to File\nsubmission=pd.DataFrame(test.loc[:,['PassengerId','Survived']])\n\n#Any files you save will be available in the output tab below\nsubmission.to_csv('submission.csv', index=False)",
"/usr/local/lib/python3.6/dist-packages/ipykernel_launcher.py:1: FutureWarning: \nPassing list-likes to .loc or [] with any missing label will raise\nKeyError in the future, you can use .reindex() as an alternative.\n\nSee the documentation here:\nhttp://pandas.pydata.org/pandas-docs/stable/indexing.html#deprecate-loc-reindex-listlike\n \"\"\"Entry point for launching an IPython kernel.\n/usr/local/lib/python3.6/dist-packages/pandas/core/indexing.py:1367: FutureWarning: \nPassing list-likes to .loc or [] with any missing label will raise\nKeyError in the future, you can use .reindex() as an alternative.\n\nSee the documentation here:\nhttp://pandas.pydata.org/pandas-docs/stable/indexing.html#deprecate-loc-reindex-listlike\n return self._getitem_tuple(key)\n"
]
]
] | [
"markdown",
"code"
] | [
[
"markdown",
"markdown"
],
[
"code",
"code",
"code",
"code",
"code",
"code",
"code",
"code",
"code",
"code",
"code",
"code",
"code"
]
] |
d07a3e6835d39b1e27fe804df4628ab554aecc6a | 28,935 | ipynb | Jupyter Notebook | 04_Extract_Organize_Objectives.ipynb | NadimKawwa/DOTAWardFinder | 96529aa8e6667242b74db959e72c8d9fbba7ba9f | [
"MIT"
] | 2 | 2021-10-03T05:43:58.000Z | 2021-10-03T23:50:29.000Z | 04_Extract_Organize_Objectives.ipynb | NadimKawwa/DOTAWardFinder | 96529aa8e6667242b74db959e72c8d9fbba7ba9f | [
"MIT"
] | null | null | null | 04_Extract_Organize_Objectives.ipynb | NadimKawwa/DOTAWardFinder | 96529aa8e6667242b74db959e72c8d9fbba7ba9f | [
"MIT"
] | null | null | null | 32.221604 | 162 | 0.39879 | [
[
[
"# Warding Tied to Objectives \nRepear previous exercise but add status of towers at time of ward.\nCode here is incorporated into a class to make things smoother",
"_____no_output_____"
]
],
[
[
"import pandas as pd\nimport numpy as np\nimport matplotlib.pyplot as plt\nimport json\nimport matplotlib.patches as patches\nimport os\n\n\n",
"_____no_output_____"
],
[
"data_path = os.path.join('data_obj', 'data_April_09_to_May_01.json')\ndf = pd.read_json(data_path)\ndf.head()",
"_____no_output_____"
],
[
"os.listdir('data_obj')",
"_____no_output_____"
],
[
"df.shape",
"_____no_output_____"
],
[
"df['match_id'].nunique()",
"_____no_output_____"
]
],
[
[
"#### We have more entries that number of unique match IDs. We can reduce work and avoid redundancy by parsing through unique match IDs to extract objectives",
"_____no_output_____"
]
],
[
[
"df['match_id'][52]",
"_____no_output_____"
],
[
"#look inside a obs log column\ndf['objectives'][52]",
"_____no_output_____"
],
[
"for item in df['objectives'][52]:\n print(item['type'])",
"CHAT_MESSAGE_FIRSTBLOOD\nCHAT_MESSAGE_COURIER_LOST\nbuilding_kill\nbuilding_kill\nbuilding_kill\nCHAT_MESSAGE_ROSHAN_KILL\nCHAT_MESSAGE_AEGIS\nbuilding_kill\nbuilding_kill\nbuilding_kill\nbuilding_kill\nbuilding_kill\nbuilding_kill\nbuilding_kill\nbuilding_kill\nCHAT_MESSAGE_COURIER_LOST\nbuilding_kill\nCHAT_MESSAGE_ROSHAN_KILL\nCHAT_MESSAGE_AEGIS\nbuilding_kill\n"
],
[
"for item in df['objectives'][0]:\n if item['type']== 'building_kill':\n print(item['key'])",
"npc_dota_goodguys_tower1_bot\nnpc_dota_goodguys_tower1_top\nnpc_dota_badguys_tower1_top\nnpc_dota_goodguys_tower1_mid\nnpc_dota_badguys_tower1_bot\nnpc_dota_badguys_tower1_mid\nnpc_dota_badguys_tower2_bot\nnpc_dota_goodguys_tower2_top\nnpc_dota_badguys_tower3_bot\nnpc_dota_goodguys_tower2_bot\nnpc_dota_badguys_tower2_top\nnpc_dota_goodguys_tower3_bot\nnpc_dota_badguys_tower3_top\nnpc_dota_goodguys_melee_rax_bot\nnpc_dota_goodguys_range_rax_bot\nnpc_dota_goodguys_tower2_mid\nnpc_dota_goodguys_tower3_top\nnpc_dota_goodguys_tower4\nnpc_dota_goodguys_tower4\nnpc_dota_goodguys_fort\n"
],
[
"arr = [{'bot1': 999, 'bot2': 55}, {'bot2': 100, 'bot3': 300}]",
"_____no_output_____"
],
[
"pd.DataFrame(arr)",
"_____no_output_____"
],
[
"def getObjectiveTimes(match_id, log):\n \"\"\"\n Reads a log associated with a match and returns the\n \"\"\"\n #empty dict\n d = {}\n \n #keep track of how many rosh kills\n i = 0\n \n #store the match id\n d['match_id'] = match_id\n \n #Extract buildings\n for item in log:\n #check if objective tied to buildings\n if item['type']== 'building_kill':\n d[item['key']] = item['time']\n #check for ROSHAN time killed\n elif item['type'] == 'CHAT_MESSAGE_ROSHAN_KILL':\n #has rosh been killed before?\n if 'ROSHAN_0' not in d:\n d['ROSHAN_0'] = item['time']\n else:\n i += 1\n name= 'ROSHAN_' + str(i)\n d[name] = item['time']\n \n return d\n \n ",
"_____no_output_____"
],
[
"#test it out\ngetObjectiveTimes(123, #arbitrary match ID \n df['objectives'][52])",
"_____no_output_____"
],
[
"def getObjectiveDataframe(df):\n #filter by keeping unique match ids\n df_match = df.drop_duplicates(subset='match_id', \n keep='first')\n \n obj_arr = []\n for row in df_match.itertuples(index=False):\n d = getObjectiveTimes(row.match_id, \n row.objectives)\n obj_arr.append(d)\n \n return pd.DataFrame(obj_arr)",
"_____no_output_____"
],
[
"a = getObjectiveDataframe(df)\na.head()",
"_____no_output_____"
],
[
"a.shape",
"_____no_output_____"
]
]
] | [
"markdown",
"code",
"markdown",
"code"
] | [
[
"markdown"
],
[
"code",
"code",
"code",
"code",
"code"
],
[
"markdown"
],
[
"code",
"code",
"code",
"code",
"code",
"code",
"code",
"code",
"code",
"code",
"code"
]
] |
d07a447b1481bd161f9e333b0b97f08c47b9ade5 | 687,471 | ipynb | Jupyter Notebook | tutorials/W1D5_DimensionalityReduction/W1D5_Tutorial3.ipynb | simpleParadox/course-content | 84672ceae4b7674416df4b1c19ef58ba4b0c2885 | [
"CC-BY-4.0"
] | 1 | 2020-07-13T08:30:37.000Z | 2020-07-13T08:30:37.000Z | tutorials/W1D5_DimensionalityReduction/W1D5_Tutorial3.ipynb | iirubio/course-content | cd7c79a920a5937329f14d7ef3f05868f686bc64 | [
"CC-BY-4.0"
] | null | null | null | tutorials/W1D5_DimensionalityReduction/W1D5_Tutorial3.ipynb | iirubio/course-content | cd7c79a920a5937329f14d7ef3f05868f686bc64 | [
"CC-BY-4.0"
] | null | null | null | 405.109605 | 114,752 | 0.931539 | [
[
[
"<a href=\"https://colab.research.google.com/github/NeuromatchAcademy/course-content/blob/master/tutorials/W1D5_DimensionalityReduction/W1D5_Tutorial3.ipynb\" target=\"_parent\"><img src=\"https://colab.research.google.com/assets/colab-badge.svg\" alt=\"Open In Colab\"/></a>",
"_____no_output_____"
],
[
"# Neuromatch Academy: Week 1, Day 5, Tutorial 3\n# Dimensionality Reduction and reconstruction\n\n__Content creators:__ Alex Cayco Gajic, John Murray\n\n__Content reviewers:__ Roozbeh Farhoudi, Matt Krause, Spiros Chavlis, Richard Gao, Michael Waskom",
"_____no_output_____"
],
[
"---\n# Tutorial Objectives\n\nIn this notebook we'll learn to apply PCA for dimensionality reduction, using a classic dataset that is often used to benchmark machine learning algorithms: MNIST. We'll also learn how to use PCA for reconstruction and denoising.\n\nOverview:\n- Perform PCA on MNIST\n- Calculate the variance explained\n- Reconstruct data with different numbers of PCs\n- (Bonus) Examine denoising using PCA\n\nYou can learn more about MNIST dataset [here](https://en.wikipedia.org/wiki/MNIST_database).",
"_____no_output_____"
]
],
[
[
"# @title Video 1: PCA for dimensionality reduction\nfrom IPython.display import YouTubeVideo\nvideo = YouTubeVideo(id=\"oO0bbInoO_0\", width=854, height=480, fs=1)\nprint(\"Video available at https://youtube.com/watch?v=\" + video.id)\nvideo",
"Video available at https://youtube.com/watch?v=oO0bbInoO_0\n"
]
],
[
[
"---\n# Setup\nRun these cells to get the tutorial started.",
"_____no_output_____"
]
],
[
[
"# Imports\nimport numpy as np\nimport matplotlib.pyplot as plt",
"_____no_output_____"
],
[
"# @title Figure Settings\nimport ipywidgets as widgets # interactive display\n%config InlineBackend.figure_format = 'retina'\nplt.style.use(\"https://raw.githubusercontent.com/NeuromatchAcademy/course-content/master/nma.mplstyle\")",
"_____no_output_____"
],
[
"# @title Helper Functions\n\n\ndef plot_variance_explained(variance_explained):\n \"\"\"\n Plots eigenvalues.\n\n Args:\n variance_explained (numpy array of floats) : Vector of variance explained\n for each PC\n\n Returns:\n Nothing.\n\n \"\"\"\n\n plt.figure()\n plt.plot(np.arange(1, len(variance_explained) + 1), variance_explained,\n '--k')\n plt.xlabel('Number of components')\n plt.ylabel('Variance explained')\n plt.show()\n\n\ndef plot_MNIST_reconstruction(X, X_reconstructed):\n \"\"\"\n Plots 9 images in the MNIST dataset side-by-side with the reconstructed\n images.\n\n Args:\n X (numpy array of floats) : Data matrix each column\n corresponds to a different\n random variable\n X_reconstructed (numpy array of floats) : Data matrix each column\n corresponds to a different\n random variable\n\n Returns:\n Nothing.\n \"\"\"\n\n plt.figure()\n ax = plt.subplot(121)\n k = 0\n for k1 in range(3):\n for k2 in range(3):\n k = k + 1\n plt.imshow(np.reshape(X[k, :], (28, 28)),\n extent=[(k1 + 1) * 28, k1 * 28, (k2 + 1) * 28, k2 * 28],\n vmin=0, vmax=255)\n plt.xlim((3 * 28, 0))\n plt.ylim((3 * 28, 0))\n plt.tick_params(axis='both', which='both', bottom=False, top=False,\n labelbottom=False)\n ax.set_xticks([])\n ax.set_yticks([])\n plt.title('Data')\n plt.clim([0, 250])\n ax = plt.subplot(122)\n k = 0\n for k1 in range(3):\n for k2 in range(3):\n k = k + 1\n plt.imshow(np.reshape(np.real(X_reconstructed[k, :]), (28, 28)),\n extent=[(k1 + 1) * 28, k1 * 28, (k2 + 1) * 28, k2 * 28],\n vmin=0, vmax=255)\n plt.xlim((3 * 28, 0))\n plt.ylim((3 * 28, 0))\n plt.tick_params(axis='both', which='both', bottom=False, top=False,\n labelbottom=False)\n ax.set_xticks([])\n ax.set_yticks([])\n plt.clim([0, 250])\n plt.title('Reconstructed')\n plt.tight_layout()\n\n\ndef plot_MNIST_sample(X):\n \"\"\"\n Plots 9 images in the MNIST dataset.\n\n Args:\n X (numpy array of floats) : Data matrix each column corresponds to a\n different random variable\n\n Returns:\n Nothing.\n\n \"\"\"\n\n fig, ax = plt.subplots()\n k = 0\n for k1 in range(3):\n for k2 in range(3):\n k = k + 1\n plt.imshow(np.reshape(X[k, :], (28, 28)),\n extent=[(k1 + 1) * 28, k1 * 28, (k2+1) * 28, k2 * 28],\n vmin=0, vmax=255)\n plt.xlim((3 * 28, 0))\n plt.ylim((3 * 28, 0))\n plt.tick_params(axis='both', which='both', bottom=False, top=False,\n labelbottom=False)\n plt.clim([0, 250])\n ax.set_xticks([])\n ax.set_yticks([])\n plt.show()\n\n\ndef plot_MNIST_weights(weights):\n \"\"\"\n Visualize PCA basis vector weights for MNIST. Red = positive weights,\n blue = negative weights, white = zero weight.\n\n Args:\n weights (numpy array of floats) : PCA basis vector\n\n Returns:\n Nothing.\n \"\"\"\n\n fig, ax = plt.subplots()\n cmap = plt.cm.get_cmap('seismic')\n plt.imshow(np.real(np.reshape(weights, (28, 28))), cmap=cmap)\n plt.tick_params(axis='both', which='both', bottom=False, top=False,\n labelbottom=False)\n plt.clim(-.15, .15)\n plt.colorbar(ticks=[-.15, -.1, -.05, 0, .05, .1, .15])\n ax.set_xticks([])\n ax.set_yticks([])\n plt.show()\n\n\ndef add_noise(X, frac_noisy_pixels):\n \"\"\"\n Randomly corrupts a fraction of the pixels by setting them to random values.\n\n Args:\n X (numpy array of floats) : Data matrix\n frac_noisy_pixels (scalar) : Fraction of noisy pixels\n\n Returns:\n (numpy array of floats) : Data matrix + noise\n\n \"\"\"\n\n X_noisy = np.reshape(X, (X.shape[0] * X.shape[1]))\n N_noise_ixs = int(X_noisy.shape[0] * frac_noisy_pixels)\n noise_ixs = np.random.choice(X_noisy.shape[0], size=N_noise_ixs,\n replace=False)\n X_noisy[noise_ixs] = np.random.uniform(0, 255, noise_ixs.shape)\n X_noisy = np.reshape(X_noisy, (X.shape[0], X.shape[1]))\n\n return X_noisy\n\n\ndef change_of_basis(X, W):\n \"\"\"\n Projects data onto a new basis.\n\n Args:\n X (numpy array of floats) : Data matrix each column corresponding to a\n different random variable\n W (numpy array of floats) : new orthonormal basis columns correspond to\n basis vectors\n\n Returns:\n (numpy array of floats) : Data matrix expressed in new basis\n \"\"\"\n\n Y = np.matmul(X, W)\n\n return Y\n\n\ndef get_sample_cov_matrix(X):\n \"\"\"\n Returns the sample covariance matrix of data X.\n\n Args:\n X (numpy array of floats) : Data matrix each column corresponds to a\n different random variable\n\n Returns:\n (numpy array of floats) : Covariance matrix\n\"\"\"\n\n X = X - np.mean(X, 0)\n cov_matrix = 1 / X.shape[0] * np.matmul(X.T, X)\n return cov_matrix\n\n\ndef sort_evals_descending(evals, evectors):\n \"\"\"\n Sorts eigenvalues and eigenvectors in decreasing order. Also aligns first two\n eigenvectors to be in first two quadrants (if 2D).\n\n Args:\n evals (numpy array of floats) : Vector of eigenvalues\n evectors (numpy array of floats) : Corresponding matrix of eigenvectors\n each column corresponds to a different\n eigenvalue\n\n Returns:\n (numpy array of floats) : Vector of eigenvalues after sorting\n (numpy array of floats) : Matrix of eigenvectors after sorting\n \"\"\"\n\n index = np.flip(np.argsort(evals))\n evals = evals[index]\n evectors = evectors[:, index]\n if evals.shape[0] == 2:\n if np.arccos(np.matmul(evectors[:, 0],\n 1 / np.sqrt(2) * np.array([1, 1]))) > np.pi / 2:\n evectors[:, 0] = -evectors[:, 0]\n if np.arccos(np.matmul(evectors[:, 1],\n 1 / np.sqrt(2)*np.array([-1, 1]))) > np.pi / 2:\n evectors[:, 1] = -evectors[:, 1]\n\n return evals, evectors\n\n\ndef pca(X):\n \"\"\"\n Performs PCA on multivariate data. Eigenvalues are sorted in decreasing order\n\n Args:\n X (numpy array of floats) : Data matrix each column corresponds to a\n different random variable\n\n Returns:\n (numpy array of floats) : Data projected onto the new basis\n (numpy array of floats) : Vector of eigenvalues\n (numpy array of floats) : Corresponding matrix of eigenvectors\n\n \"\"\"\n\n X = X - np.mean(X, 0)\n cov_matrix = get_sample_cov_matrix(X)\n evals, evectors = np.linalg.eigh(cov_matrix)\n evals, evectors = sort_evals_descending(evals, evectors)\n score = change_of_basis(X, evectors)\n\n return score, evectors, evals\n\n\ndef plot_eigenvalues(evals, limit=True):\n \"\"\"\n Plots eigenvalues.\n\n Args:\n (numpy array of floats) : Vector of eigenvalues\n\n Returns:\n Nothing.\n\n \"\"\"\n\n plt.figure()\n plt.plot(np.arange(1, len(evals) + 1), evals, 'o-k')\n plt.xlabel('Component')\n plt.ylabel('Eigenvalue')\n plt.title('Scree plot')\n if limit:\n plt.show()",
"_____no_output_____"
]
],
[
[
"---\n# Section 1: Perform PCA on MNIST\n\nThe MNIST dataset consists of a 70,000 images of individual handwritten digits. Each image is a 28x28 pixel grayscale image. For convenience, each 28x28 pixel image is often unravelled into a single 784 (=28*28) element vector, so that the whole dataset is represented as a 70,000 x 784 matrix. Each row represents a different image, and each column represents a different pixel.\n \nEnter the following cell to load the MNIST dataset and plot the first nine images.",
"_____no_output_____"
]
],
[
[
"from sklearn.datasets import fetch_openml\nmnist = fetch_openml(name='mnist_784')\nX = mnist.data\nplot_MNIST_sample(X)",
"_____no_output_____"
]
],
[
[
"The MNIST dataset has an extrinsic dimensionality of 784, much higher than the 2-dimensional examples used in the previous tutorials! To make sense of this data, we'll use dimensionality reduction. But first, we need to determine the intrinsic dimensionality $K$ of the data. One way to do this is to look for an \"elbow\" in the scree plot, to determine which eigenvalues are signficant.",
"_____no_output_____"
],
[
"## Exercise 1: Scree plot of MNIST\n\nIn this exercise you will examine the scree plot in the MNIST dataset.\n\n**Steps:**\n- Perform PCA on the dataset and examine the scree plot. \n- When do the eigenvalues appear (by eye) to reach zero? (**Hint:** use `plt.xlim` to zoom into a section of the plot).\n",
"_____no_output_____"
]
],
[
[
"help(pca)\nhelp(plot_eigenvalues)",
"Help on function pca in module __main__:\n\npca(X)\n Performs PCA on multivariate data. Eigenvalues are sorted in decreasing order\n \n Args:\n X (numpy array of floats) : Data matrix each column corresponds to a\n different random variable\n \n Returns:\n (numpy array of floats) : Data projected onto the new basis\n (numpy array of floats) : Vector of eigenvalues\n (numpy array of floats) : Corresponding matrix of eigenvectors\n\nHelp on function plot_eigenvalues in module __main__:\n\nplot_eigenvalues(evals, limit=True)\n Plots eigenvalues.\n \n Args:\n (numpy array of floats) : Vector of eigenvalues\n \n Returns:\n Nothing.\n\n"
],
[
"#################################################\n## TO DO for students: perform PCA and plot the eigenvalues\n#################################################\n\n# perform PCA\n# score, evectors, evals = ...\n# plot the eigenvalues\n# plot_eigenvalues(evals, limit=False)\n# plt.xlim(...) # limit x-axis up to 100 for zooming",
"_____no_output_____"
],
[
"# to_remove solution\n# perform PCA\nscore, evectors, evals = pca(X)\n\n# plot the eigenvalues\nwith plt.xkcd():\n plot_eigenvalues(evals, limit=False)\n plt.xlim([0, 100]) # limit x-axis up to 100 for zooming",
"_____no_output_____"
]
],
[
[
"---\n# Section 2: Calculate the variance explained\n\nThe scree plot suggests that most of the eigenvalues are near zero, with fewer than 100 having large values. Another common way to determine the intrinsic dimensionality is by considering the variance explained. This can be examined with a cumulative plot of the fraction of the total variance explained by the top $K$ components, i.e.,\n\n\\begin{equation}\n\\text{var explained} = \\frac{\\sum_{i=1}^K \\lambda_i}{\\sum_{i=1}^N \\lambda_i}\n\\end{equation}\n\nThe intrinsic dimensionality is often quantified by the $K$ necessary to explain a large proportion of the total variance of the data (often a defined threshold, e.g., 90%).",
"_____no_output_____"
],
[
"## Exercise 2: Plot the explained variance\n\nIn this exercise you will plot the explained variance.\n\n**Steps:**\n- Fill in the function below to calculate the fraction variance explained as a function of the number of principal componenets. **Hint:** use `np.cumsum`.\n- Plot the variance explained using `plot_variance_explained`.\n\n**Questions:**\n- How many principal components are required to explain 90% of the variance?\n- How does the intrinsic dimensionality of this dataset compare to its extrinsic dimensionality?\n",
"_____no_output_____"
]
],
[
[
"help(plot_variance_explained)",
"Help on function plot_variance_explained in module __main__:\n\nplot_variance_explained(variance_explained)\n Plots eigenvalues.\n \n Args:\n variance_explained (numpy array of floats) : Vector of variance explained\n for each PC\n \n Returns:\n Nothing.\n\n"
],
[
"def get_variance_explained(evals):\n \"\"\"\n Calculates variance explained from the eigenvalues.\n\n Args:\n evals (numpy array of floats) : Vector of eigenvalues\n\n Returns:\n (numpy array of floats) : Vector of variance explained\n\n \"\"\"\n\n #################################################\n ## TO DO for students: calculate the explained variance using the equation\n ## from Section 2.\n # Comment once you've filled in the function\n raise NotImplementedError(\"Student excercise: calculate explaine variance!\")\n #################################################\n\n # cumulatively sum the eigenvalues\n csum = ...\n # normalize by the sum of eigenvalues\n variance_explained = ...\n\n return variance_explained\n\n\n#################################################\n## TO DO for students: call the function and plot the variance explained\n#################################################\n\n# calculate the variance explained\nvariance_explained = ...\n\n# Uncomment to plot the variance explained\n# plot_variance_explained(variance_explained)",
"_____no_output_____"
],
[
"# to_remove solution\ndef get_variance_explained(evals):\n \"\"\"\n Plots eigenvalues.\n\n Args:\n (numpy array of floats) : Vector of eigenvalues\n\n Returns:\n Nothing.\n\n \"\"\"\n\n # cumulatively sum the eigenvalues\n csum = np.cumsum(evals)\n # normalize by the sum of eigenvalues\n variance_explained = csum / np.sum(evals)\n\n return variance_explained\n\n\n# calculate the variance explained\nvariance_explained = get_variance_explained(evals)\nwith plt.xkcd():\n plot_variance_explained(variance_explained)",
"_____no_output_____"
]
],
[
[
"---\n# Section 3: Reconstruct data with different numbers of PCs\n",
"_____no_output_____"
],
[
"Now we have seen that the top 100 or so principal components of the data can explain most of the variance. We can use this fact to perform *dimensionality reduction*, i.e., by storing the data using only 100 components rather than the samples of all 784 pixels. Remarkably, we will be able to reconstruct much of the structure of the data using only the top 100 components. To see this, recall that to perform PCA we projected the data $\\bf X$ onto the eigenvectors of the covariance matrix:\n\\begin{equation}\n\\bf S = X W\n\\end{equation}\nSince $\\bf W$ is an orthogonal matrix, ${\\bf W}^{-1} = {\\bf W}^T$. So by multiplying by ${\\bf W}^T$ on each side we can rewrite this equation as \n\\begin{equation}\n{\\bf X = S W}^T.\n\\end{equation}\nThis now gives us a way to reconstruct the data matrix from the scores and loadings. To reconstruct the data from a low-dimensional approximation, we just have to truncate these matrices. Let's call ${\\bf S}_{1:K}$ and ${\\bf W}_{1:K}$ as keeping only the first $K$ columns of this matrix. Then our reconstruction is:\n\\begin{equation}\n{\\bf \\hat X = S}_{1:K} ({\\bf W}_{1:K})^T.\n\\end{equation}\n",
"_____no_output_____"
],
[
"## Exercise 3: Data reconstruction\n\nFill in the function below to reconstruct the data using different numbers of principal components. \n\n**Steps:**\n\n* Fill in the following function to reconstruct the data based on the weights and scores. Don't forget to add the mean!\n* Make sure your function works by reconstructing the data with all $K=784$ components. The two images should look identical.",
"_____no_output_____"
]
],
[
[
"help(plot_MNIST_reconstruction)",
"Help on function plot_MNIST_reconstruction in module __main__:\n\nplot_MNIST_reconstruction(X, X_reconstructed)\n Plots 9 images in the MNIST dataset side-by-side with the reconstructed\n images.\n \n Args:\n X (numpy array of floats) : Data matrix each column\n corresponds to a different\n random variable\n X_reconstructed (numpy array of floats) : Data matrix each column\n corresponds to a different\n random variable\n \n Returns:\n Nothing.\n\n"
],
[
"def reconstruct_data(score, evectors, X_mean, K):\n \"\"\"\n Reconstruct the data based on the top K components.\n\n Args:\n score (numpy array of floats) : Score matrix\n evectors (numpy array of floats) : Matrix of eigenvectors\n X_mean (numpy array of floats) : Vector corresponding to data mean\n K (scalar) : Number of components to include\n\n Returns:\n (numpy array of floats) : Matrix of reconstructed data\n\n \"\"\"\n\n #################################################\n ## TO DO for students: Reconstruct the original data in X_reconstructed\n # Comment once you've filled in the function\n raise NotImplementedError(\"Student excercise: reconstructing data function!\")\n #################################################\n\n # Reconstruct the data from the score and eigenvectors\n # Don't forget to add the mean!!\n X_reconstructed = ...\n\n return X_reconstructed\n\n\nK = 784\n\n#################################################\n## TO DO for students: Calculate the mean and call the function, then plot\n## the original and the recostructed data\n#################################################\n\n# Reconstruct the data based on all components\nX_mean = ...\nX_reconstructed = ...\n\n# Plot the data and reconstruction\n# plot_MNIST_reconstruction(X, X_reconstructed)",
"_____no_output_____"
],
[
"# to_remove solution\ndef reconstruct_data(score, evectors, X_mean, K):\n \"\"\"\n Reconstruct the data based on the top K components.\n\n Args:\n score (numpy array of floats) : Score matrix\n evectors (numpy array of floats) : Matrix of eigenvectors\n X_mean (numpy array of floats) : Vector corresponding to data mean\n K (scalar) : Number of components to include\n\n Returns:\n (numpy array of floats) : Matrix of reconstructed data\n\n \"\"\"\n\n # Reconstruct the data from the score and eigenvectors\n # Don't forget to add the mean!!\n X_reconstructed = np.matmul(score[:, :K], evectors[:, :K].T) + X_mean\n\n return X_reconstructed\n\n\nK = 784\n\n# Reconstruct the data based on all components\nX_mean = np.mean(X, 0)\nX_reconstructed = reconstruct_data(score, evectors, X_mean, K)\n\n# Plot the data and reconstruction\nwith plt.xkcd():\n plot_MNIST_reconstruction(X, X_reconstructed)",
"_____no_output_____"
]
],
[
[
"## Interactive Demo: Reconstruct the data matrix using different numbers of PCs\n\nNow run the code below and experiment with the slider to reconstruct the data matrix using different numbers of principal components.\n\n**Steps**\n* How many principal components are necessary to reconstruct the numbers (by eye)? How does this relate to the intrinsic dimensionality of the data?\n* Do you see any information in the data with only a single principal component?",
"_____no_output_____"
]
],
[
[
"# @title\n\n# @markdown Make sure you execute this cell to enable the widget!\n\n\ndef refresh(K=100):\n X_reconstructed = reconstruct_data(score, evectors, X_mean, K)\n plot_MNIST_reconstruction(X, X_reconstructed)\n plt.title('Reconstructed, K={}'.format(K))\n\n\n_ = widgets.interact(refresh, K=(1, 784, 10))",
"_____no_output_____"
]
],
[
[
"## Exercise 4: Visualization of the weights\n\nNext, let's take a closer look at the first principal component by visualizing its corresponding weights. \n\n**Steps:**\n\n* Enter `plot_MNIST_weights` to visualize the weights of the first basis vector.\n* What structure do you see? Which pixels have a strong positive weighting? Which have a strong negative weighting? What kinds of images would this basis vector differentiate?\n* Try visualizing the second and third basis vectors. Do you see any structure? What about the 100th basis vector? 500th? 700th?",
"_____no_output_____"
]
],
[
[
"help(plot_MNIST_weights)",
"Help on function plot_MNIST_weights in module __main__:\n\nplot_MNIST_weights(weights)\n Visualize PCA basis vector weights for MNIST. Red = positive weights,\n blue = negative weights, white = zero weight.\n \n Args:\n weights (numpy array of floats) : PCA basis vector\n \n Returns:\n Nothing.\n\n"
],
[
"#################################################\n## TO DO for students: plot the weights calling the plot_MNIST_weights function\n#################################################\n\n# Plot the weights of the first principal component\n# plot_MNIST_weights(...)",
"_____no_output_____"
],
[
"# to_remove solution\n# Plot the weights of the first principal component\nwith plt.xkcd():\n plot_MNIST_weights(evectors[:, 0])",
"_____no_output_____"
]
],
[
[
"---\n# Summary\n* In this tutorial, we learned how to use PCA for dimensionality reduction by selecting the top principal components. This can be useful as the intrinsic dimensionality ($K$) is often less than the extrinsic dimensionality ($N$) in neural data. $K$ can be inferred by choosing the number of eigenvalues necessary to capture some fraction of the variance.\n* We also learned how to reconstruct an approximation of the original data using the top $K$ principal components. In fact, an alternate formulation of PCA is to find the $K$ dimensional space that minimizes the reconstruction error.\n* Noise tends to inflate the apparent intrinsic dimensionality, however the higher components reflect noise rather than new structure in the data. PCA can be used for denoising data by removing noisy higher components.\n* In MNIST, the weights corresponding to the first principal component appear to discriminate between a 0 and 1. We will discuss the implications of this for data visualization in the following tutorial.",
"_____no_output_____"
],
[
"---\n# Bonus: Examine denoising using PCA\n\nIn this lecture, we saw that PCA finds an optimal low-dimensional basis to minimize the reconstruction error. Because of this property, PCA can be useful for denoising corrupted samples of the data.",
"_____no_output_____"
],
[
"## Exercise 5: Add noise to the data\nIn this exercise you will add salt-and-pepper noise to the original data and see how that affects the eigenvalues. \n\n**Steps:**\n- Use the function `add_noise` to add noise to 20% of the pixels.\n- Then, perform PCA and plot the variance explained. How many principal components are required to explain 90% of the variance? How does this compare to the original data? \n",
"_____no_output_____"
]
],
[
[
"help(add_noise)",
"Help on function add_noise in module __main__:\n\nadd_noise(X, frac_noisy_pixels)\n Randomly corrupts a fraction of the pixels by setting them to random values.\n \n Args:\n X (numpy array of floats) : Data matrix\n frac_noisy_pixels (scalar) : Fraction of noisy pixels\n \n Returns:\n (numpy array of floats) : Data matrix + noise\n\n"
],
[
"###################################################################\n# Insert your code here to:\n# Add noise to the data\n# Plot noise-corrupted data\n# Perform PCA on the noisy data\n# Calculate and plot the variance explained\n###################################################################\nnp.random.seed(2020) # set random seed\nX_noisy = ...\n# score_noisy, evectors_noisy, evals_noisy = ...\n# variance_explained_noisy = ...\n# plot_MNIST_sample(X_noisy)\n# plot_variance_explained(variance_explained_noisy)",
"_____no_output_____"
],
[
"# to_remove solution\n\nnp.random.seed(2020) # set random seed\nX_noisy = add_noise(X, .2)\nscore_noisy, evectors_noisy, evals_noisy = pca(X_noisy)\nvariance_explained_noisy = get_variance_explained(evals_noisy)\n\nwith plt.xkcd():\n plot_MNIST_sample(X_noisy)\n plot_variance_explained(variance_explained_noisy)",
"_____no_output_____"
]
],
[
[
"## Exercise 6: Denoising\n\nNext, use PCA to perform denoising by projecting the noise-corrupted data onto the basis vectors found from the original dataset. By taking the top K components of this projection, we can reduce noise in dimensions orthogonal to the K-dimensional latent space. \n\n**Steps:**\n- Subtract the mean of the noise-corrupted data.\n- Project the data onto the basis found with the original dataset (`evectors`, not `evectors_noisy`) and take the top $K$ components. \n- Reconstruct the data as normal, using the top 50 components. \n- Play around with the amount of noise and K to build intuition.\n",
"_____no_output_____"
]
],
[
[
"###################################################################\n# Insert your code here to:\n# Subtract the mean of the noise-corrupted data\n# Project onto the original basis vectors evectors\n# Reconstruct the data using the top 50 components\n# Plot the result\n###################################################################\n\nX_noisy_mean = ...\nprojX_noisy = ...\nX_reconstructed = ...\n# plot_MNIST_reconstruction(X_noisy, X_reconstructed)",
"_____no_output_____"
],
[
"# to_remove solution\n\nX_noisy_mean = np.mean(X_noisy, 0)\nprojX_noisy = np.matmul(X_noisy - X_noisy_mean, evectors)\nX_reconstructed = reconstruct_data(projX_noisy, evectors, X_noisy_mean, 50)\n\nwith plt.xkcd():\n plot_MNIST_reconstruction(X_noisy, X_reconstructed)",
"_____no_output_____"
]
]
] | [
"markdown",
"code",
"markdown",
"code",
"markdown",
"code",
"markdown",
"code",
"markdown",
"code",
"markdown",
"code",
"markdown",
"code",
"markdown",
"code",
"markdown",
"code",
"markdown",
"code"
] | [
[
"markdown",
"markdown",
"markdown"
],
[
"code"
],
[
"markdown"
],
[
"code",
"code",
"code"
],
[
"markdown"
],
[
"code"
],
[
"markdown",
"markdown"
],
[
"code",
"code",
"code"
],
[
"markdown",
"markdown"
],
[
"code",
"code",
"code"
],
[
"markdown",
"markdown",
"markdown"
],
[
"code",
"code",
"code"
],
[
"markdown"
],
[
"code"
],
[
"markdown"
],
[
"code",
"code",
"code"
],
[
"markdown",
"markdown",
"markdown"
],
[
"code",
"code",
"code"
],
[
"markdown"
],
[
"code",
"code"
]
] |
d07a67c30112dbd536431c1e2ef65982152aefa0 | 336,437 | ipynb | Jupyter Notebook | quick-start.ipynb | XudongWang97/rmi | 46349c19a413a89be6a02adc57255eea0ddd0bc0 | [
"MIT"
] | 15 | 2020-06-04T10:11:59.000Z | 2022-01-11T06:12:35.000Z | quick-start.ipynb | XudongWang97/rmi | 46349c19a413a89be6a02adc57255eea0ddd0bc0 | [
"MIT"
] | 1 | 2021-03-08T11:31:32.000Z | 2021-03-08T11:31:32.000Z | quick-start.ipynb | XudongWang97/rmi | 46349c19a413a89be6a02adc57255eea0ddd0bc0 | [
"MIT"
] | 3 | 2020-07-19T14:57:20.000Z | 2021-06-24T07:58:48.000Z | 233.961752 | 62,376 | 0.917025 | [
[
[
"# Quick Start",
"_____no_output_____"
],
[
"**A tutorial on Renormalized Mutual Information**\n\nWe describe in detail the implementation of RMI estimation in the very simple case of a Gaussian distribution.\nOf course, in this case the optimal feature is given by the Principal Component Analysis",
"_____no_output_____"
]
],
[
[
"import numpy as np\n\n# parameters of the Gaussian distribution\nmu = [0,0]\nsigma = [[1, 0.5],[0.5,2]]\n\n# extract the samples\nN_samples = 100000\nsamples = np.random.multivariate_normal(mu, sigma, N_samples )",
"_____no_output_____"
]
],
[
[
"Visualize the distribution with a 2D histogram",
"_____no_output_____"
]
],
[
[
"import matplotlib.pyplot as plt\nplt.figure()\nplt.hist2d(*samples.T, bins=100, cmap=plt.cm.binary)\nplt.gca().set_aspect(\"equal\")\nplt.xlabel(\"$x_1$\")\nplt.ylabel(\"$x_2$\")\nplt.title(\"$P_x(x)$\")\nplt.show()",
"_____no_output_____"
]
],
[
[
"## Estimate Renormalized Mutual Information of a feature\n\nNow we would like to find a one-dimensional function $f(x_1,x_2)$ to describe this 2d distribution.\n\n### Simplest feature\nFor example, we could consider ignoring one of the variables:",
"_____no_output_____"
]
],
[
[
"def f(x):\n # feature\n # shape [N_samples, N_features=1]\n return x[:,0][...,None]\n\ndef grad_f(x):\n # gradient\n # shape [N_samples, N_features=1, N_x=2]\n grad_f = np.zeros([len(x),1,2])\n grad_f[...,0] = 1\n return grad_f\n\ndef feat_and_grad(x):\n return f(x), grad_f(x)",
"_____no_output_____"
]
],
[
[
"Let's plot it on top of the distribution",
"_____no_output_____"
]
],
[
[
"# Range of the plot\nxmin = -4\nxmax = 4\n# Number of points in the grid\nN = 100\n\n# We evaluate the feature on a grid\nx_linspace = np.linspace(xmin, xmax, N)\nx1_grid, x2_grid = np.meshgrid(x_linspace, x_linspace, indexing='ij')\nx_points = np.array([x1_grid.flatten(), x2_grid.flatten()]).T\n\nfeature = f(x_points)\ngradient = grad_f(x_points)",
"_____no_output_____"
],
[
"plt.figure()\nplt.title(\"Feature contours\")\nplt.xlabel(r\"$x_1$\")\nplt.ylabel(r\"$x_2$\")\n\nplt.gca().set_aspect('equal')\n# Draw the input distribution on the background\nplt.hist2d(*samples.T, bins=100, cmap=plt.cm.binary)\n\n# Draw the contour lines of the extracted feature\nplt.xlim([-4,4])\nplt.ylim([-4,4])\nplt.contour(x1_grid, x2_grid, feature.reshape([N,N]),15, \n linewidths=4, cmap=plt.cm.Blues)\nplt.colorbar()\nplt.show()",
"_____no_output_____"
]
],
[
[
"$f(x)=x_1$ is clearly a linear function that ignores $x_2$ and increases in the $x_1$ direction\n\n**How much information does it give us on $x$?**\nIf we used common mutual information, it would be $\\infty$, because $f$ is a deterministic function, and $H(y|x) = -\\log \\delta(0)$.\n\nLet's estimate the renormalized mutual information",
"_____no_output_____"
]
],
[
[
"import rmi.estimation as inf\n\nsamples = np.random.multivariate_normal(mu, sigma, N_samples )\n\nfeature = f(samples)\ngradient = grad_f(samples)\nRMI = inf.RenormalizedMutualInformation(feature, gradient)\nprint(\"Renormalized Mutual Information (x,f(x)): %2.2f\" % RMI)",
"Renormalized Mutual Information (x,f(x)): 1.42\n"
]
],
[
[
"Please note that we perform the plot by calculating the feature on a uniform grid. But, to estimate RMI, the feature should be calculated on x **sampled** from the $x$ distribution.",
"_____no_output_____"
],
[
"In particular, we have",
"_____no_output_____"
]
],
[
[
"p_y, delta_y = inf.produce_P(feature)\nentropy = inf.Entropy(p_y, delta_y)\n\nfterm = inf.RegTerm(gradient)\n\nprint(\"Entropy\\t %2.2f\" % entropy)\nprint(\"Fterm\\t %2.2f\" % fterm)\n\nprint(\"Renormalized Mutual Information (x,f(x)): %2.2f\" % \n (entropy + fterm))",
"Entropy\t 1.42\nFterm\t -0.00\nRenormalized Mutual Information (x,f(x)): 1.42\n"
]
],
[
[
"Renormalized Mutual Information is the sum of the two terms\n- Entropy\n- RegTerm",
"_____no_output_____"
],
[
"### Reparametrization invariance\n\nDo we gain information if we increase the variance of the feature?\n\nFor example, let's rescale our feature. Clearly the information on $x$ should remain the same",
"_____no_output_____"
]
],
[
[
"scale_factor = 4\nfeature *= scale_factor\ngradient *= scale_factor\n\nRMI = inf.RenormalizedMutualInformation(feature, gradient)\nprint(\"Renormalized Mutual Information (x,f(x)): %2.2f\" % RMI)\n\np_y, delta_y = inf.produce_P(feature)\nentropy = inf.Entropy(p_y, delta_y)\nfterm = inf.RegTerm(gradient)\n\nprint(\"Entropy\\t %2.2f\" % entropy)\nprint(\"Fterm\\t %2.2f\" % fterm)",
"Renormalized Mutual Information (x,f(x)): 1.42\nEntropy\t 2.80\nFterm\t -1.39\n"
]
],
[
[
"Let's try even a non-linear transformation. As long as it is invertible, we will get the same RMI",
"_____no_output_____"
]
],
[
[
"# For example\ny_lin = np.linspace(-4,4,100)\nf_lin = y_lin**3 + 5*y_lin\nplt.figure()\nplt.title(\"Reparametrization function\")\nplt.plot(y_lin, f_lin)\nplt.show()",
"_____no_output_____"
],
[
"feature_new = feature**3 + 5*feature\ngradient_new = 3*feature[...,None]**2*gradient +5*gradient# chain rule...\n\nRMI = inf.RenormalizedMutualInformation(feature_new, gradient_new, 2000)\nprint(\"Renormalized Mutual Information (x,f(x)): %2.2f\" % RMI)\n\np_y, delta_y = inf.produce_P(feature_new)\nentropy = inf.Entropy(p_y, delta_y)\nfterm = inf.RegTerm(gradient_new)\n\nprint(\"Entropy\\t %2.2f\" % entropy)\nprint(\"Fterm\\t %2.2f\" % fterm)\n",
"Renormalized Mutual Information (x,f(x)): 1.42\nEntropy\t 6.24\nFterm\t -4.70\n"
]
],
[
[
"In this case, we have to increase the number of bins \nto calculate the Entropy with reasonable accuracy.\nThe reason is that the feature now spans a quite larger range but changes very rapidly in the few bins around zero (but we use a uniform binning when estimating the entropy).",
"_____no_output_____"
]
],
[
[
"plt.hist(feature_new,1000)\nplt.show()",
"_____no_output_____"
]
],
[
[
"And if we instead appliead a **non-invertible** transformation? The consequence is clear: we will **lose information**.\nConsider for example:",
"_____no_output_____"
]
],
[
[
"feature_new = feature**2\ngradient_new = 2*feature[...,None]*gradient # chain rule...\n\nRMI_2 = inf.RenormalizedMutualInformation(feature_new, gradient_new, 2000)\nprint(\"Renormalized Mutual Information (x,f(x)): %2.2f\" % RMI_2)\n\np_y, delta_y = inf.produce_P(feature_new)\nentropy = inf.Entropy(p_y, delta_y)\nfterm = inf.RegTerm(gradient_new)\n\nprint(\"Entropy\\t %2.2f\" % entropy)\nprint(\"Fterm\\t %2.2f\" % fterm)\n",
"Renormalized Mutual Information (x,f(x)): 0.74\nEntropy\t 3.64\nFterm\t -2.83\n"
],
[
"plt.hist(feature_new,1000)\nplt.show()",
"_____no_output_____"
]
],
[
[
"The careful observer will be able to guess how much information we have lost in this case: \nour feature is centered in zero and we squared it. We lose the sign, and on average the half of the samples have one sign and the half the other sign. One bit of information is lost. The difference is $\\log 2$!",
"_____no_output_____"
]
],
[
[
"deltaRMI = RMI - RMI_2\nprint(\"delta RMI %2.3f\" %deltaRMI)\nprint(\"log 2 = %2.3f\" % np.log(2))",
"delta RMI 0.672\nlog 2 = 0.693\n"
]
],
[
[
"### Another feature\n\nLet's take another linear feature, for example, this time in the other direction ",
"_____no_output_____"
]
],
[
[
"def f(x):\n # feature\n # shape [N_samples, N_features=1]\n return x[:,1][...,None]\n\ndef grad_f(x):\n # gradient\n # shape [N_samples, N_features=1, N_x=2]\n grad_f = np.zeros([len(x),1,2])\n grad_f[...,1] = 1\n return grad_f\n\ndef feat_and_grad(x):\n return f(x), grad_f(x)\n\nfeature = f(x_points)\ngradient = grad_f(x_points)\n\nplt.figure()\nplt.title(\"Feature contours\")\nplt.xlabel(r\"$x_1$\")\nplt.ylabel(r\"$x_2$\")\n\nplt.gca().set_aspect('equal')\n# Draw the input distribution on the background\nsamples = np.random.multivariate_normal(mu, sigma, N_samples )\nplt.hist2d(*samples.T, bins=100, cmap=plt.cm.binary)\n\n# Draw the contour lines of the extracted feature\nplt.xlim([-4,4])\nplt.ylim([-4,4])\nplt.contour(x1_grid, x2_grid, feature.reshape([N,N]),15, \n linewidths=4, cmap=plt.cm.Blues)\nplt.colorbar()\nplt.show()\n\n\nfeature = f(samples)\ngradient = grad_f(samples)\nRMI = inf.RenormalizedMutualInformation(feature, gradient)\nprint(\"Renormalized Mutual Information (x,f(x)): %2.2f\" % RMI)",
"_____no_output_____"
]
],
[
[
"This feature seems to better describe our input. This is reasonable: it lies closer to the direction of larger fluctuation of the distribution.",
"_____no_output_____"
],
[
"What is the best linear feature that we can take?",
"_____no_output_____"
]
],
[
[
"# Let's define a linear feature\ndef linear(x, th):\n \"\"\" linear increasing in the direction given by angle th.\n\n Args:\n x (array_like): [N_samples, 2] array of samples\n th (float): direction of the feature in which it increases\n\n Returns:\n feature (array_like): [N_samples, 1] feature\n grad_feature (array_like): [N_samples, 1, N_x] gradient of the feature\n \"\"\"\n\n Feature = x[:, 0]*np.cos(th) + x[:, 1]*np.sin(th)\n Grad1 = np.full(np.shape(x)[0], np.cos(th))\n Grad2 = np.full(np.shape(x)[0], np.sin(th))\n return Feature, np.array([Grad1, Grad2]).T\n\nsamples = np.random.multivariate_normal(mu, sigma, N_samples )\n\nth_lin = np.linspace(0,np.pi, 30)\nrmis = []\nfor th in th_lin:\n feature, grad = linear(samples, th)\n rmi = inf.RenormalizedMutualInformation(feature,grad)\n rmis.append([th,rmi])\nrmis = np.array(rmis)",
"_____no_output_____"
],
[
"plt.figure()\nplt.title(\"Best linear feature\")\nplt.xlabel(\"$\\theta$\")\nplt.ylabel(r\"$RMI(x,f_\\theta(x))$\")\nplt.plot(rmis[:,0], rmis[:,1])\nplt.show()\n\nbest_theta = th_lin[np.argmax(rmis[:,1])]",
"_____no_output_____"
]
],
[
[
"Let's plot the feature with the parameter that gives the largest Renormalized Mutual Information",
"_____no_output_____"
]
],
[
[
"\nfeature, gradient = linear(x_points,best_theta)\n\nplt.figure()\nplt.title(\"Feature contours\")\nplt.xlabel(r\"$x_1$\")\nplt.ylabel(r\"$x_2$\")\n\nplt.gca().set_aspect('equal')\n# Draw the input distribution on the background\nsamples = np.random.multivariate_normal(mu, sigma, N_samples )\nplt.hist2d(*samples.T, bins=100, cmap=plt.cm.binary)\n\n# Draw the contour lines of the extracted feature\nplt.xlim([-4,4])\nplt.ylim([-4,4])\nplt.contour(x1_grid, x2_grid, feature.reshape([N,N]),15, \n linewidths=4, cmap=plt.cm.Blues)\nplt.colorbar()\nplt.show()\n\n\nfeature, gradient = linear(samples,best_theta)\nRMI = inf.RenormalizedMutualInformation(feature, gradient)\nprint(\"Renormalized Mutual Information (x,f(x)): %2.2f\" % RMI)",
"_____no_output_____"
]
],
[
[
"This is the same feature that we would get if we considered the first Principal Component of PCA. This is the only case in which this is possible: PCA can only extract linear features, and in particular, since it only takes into account the covariance matrix of the distribution, it can provide the best feature only for a Gaussian (which is identified by its mean and covariance matrix)",
"_____no_output_____"
]
],
[
[
"import rmi.pca\nsamples = np.random.multivariate_normal(mu, sigma, N_samples )\ng_pca = rmi.pca.pca(samples,1)\neigenv = g_pca.w[0]\nangle_pca = np.arctan(eigenv[1]/eigenv[0])\n\nfeature, gradient = linear(samples,angle_pca)\nRMI = inf.RenormalizedMutualInformation(feature, gradient)\nprint(\"Renormalized Mutual Information (x,f(x)): %2.2f\" % RMI)",
"Renormalized Mutual Information (x,f(x)): 1.81\n"
],
[
"print(\"best found angle %2.2f\" %best_theta)\nprint(\"pca direction %2.2f\" %angle_pca)",
"best found angle 1.19\npca direction 1.18\n"
]
],
[
[
"We recall that in this very special case, and as long as the proposed feature is only rotated (without changing the scale), the simple maximization of the Feature Entropy would have given the same result.\n\nAgain, this only holds for linear features, and in particular for those whose gradient vector is not affected by a change of parameters).\n\nAs soon as we use a non-linear feature, just looking at the entropy of the feature is not enough anymore - entropy is not reparametrization invariant.\n\nAlso, given an arbitrary deterministic feature function, RMI is the only quantity that allows to estimate it's dependence with its arguments",
"_____no_output_____"
],
[
"## Feature Optimization",
"_____no_output_____"
],
[
"Let's try now to optimize a neural network to extract a feature. In this case, as we already discussed, we will still get a linear feature",
"_____no_output_____"
]
],
[
[
"import rmi.neuralnets as nn\n\n# Define the layout of the neural network\n# The cost function is implicit when choosing the model RMIOptimizer\nrmi_optimizer = nn.RMIOptimizer(\n layers=[\n nn.K.layers.Dense(30, activation=\"relu\",input_shape=(2,)),\n nn.K.layers.Dense(1)\n])\n\n# Compile the network === choose the optimizer to use during the training\nrmi_optimizer.compile(optimizer=nn.tf.optimizers.Adam(1e-3))\n\n# Print the table with the structure of the network\nrmi_optimizer.summary()\n\n# Define an objects that handles the training\nrmi_net = nn.Net(rmi_optimizer)",
"Model: \"rmi_optimizer\"\n_________________________________________________________________\nLayer (type) Output Shape Param # \n=================================================================\ndense (Dense) (None, 30) 90 \n_________________________________________________________________\ndense_1 (Dense) (None, 1) 31 \n=================================================================\nTotal params: 121\nTrainable params: 121\nNon-trainable params: 0\n_________________________________________________________________\n"
],
[
"# Perform the training of the neural network\nbatchsize = 1000\nN_train = 5000\ndef get_batch():\n return np.random.multivariate_normal(mu, sigma, batchsize)\nrmi_net.fit_generator(get_batch, N_train)",
" 0%| | 0/5000 [00:00<?, ?it/s]"
],
[
"# Plot the training history (value of RMI)\n# The large fluctuations can be reduced by increasing the batchsize\nrmi_net.plot_history()",
"_____no_output_____"
]
],
[
[
"Calculate the feature on the input points: just apply the object `rmi_net`!",
"_____no_output_____"
]
],
[
[
"feature = rmi_net(x_points)\n\nplt.figure()\nplt.title(\"Feature contours\")\nplt.xlabel(r\"$x_1$\")\nplt.ylabel(r\"$x_2$\")\n\nplt.gca().set_aspect('equal')\n# Draw the input distribution on the background\nsamples = np.random.multivariate_normal(mu, sigma, N_samples )\nplt.hist2d(*samples.T, bins=100, cmap=plt.cm.binary)\n\n# Draw the contour lines of the extracted feature\nplt.xlim([-4,4])\nplt.ylim([-4,4])\nplt.contour(x1_grid, x2_grid, feature.reshape([N,N]),15, \n linewidths=4, cmap=plt.cm.Blues)\nplt.colorbar()\nplt.show()",
"_____no_output_____"
]
],
[
[
"To calculate also the gradient of the feature, one can use the function `get_feature_and_grad`",
"_____no_output_____"
]
],
[
[
"feature, gradient = rmi_net.get_feature_and_grad(samples)\nRMI = inf.RenormalizedMutualInformation(feature, gradient)\nprint(\"Renormalized Mutual Information (x,f(x)): %2.2f\" % RMI)",
"Renormalized Mutual Information (x,f(x)): 1.81\n"
]
],
[
[
"## Tradeoff between simplicity and compression",
"_____no_output_____"
],
[
"When optimizing renormalized mutual information to obtain a **meaningful feature** (in the sense of representation learning), one should avoid to employ too powerful networks.\n\nA good feature should set a convenient tradeoff between its **\"simplicity\"** (i.e. number of parameters, or how \"smooth\" the feature is) and its **information content** (i.e. how much the input space is compressed in a smaller dimension). \n\nIn other words, useful representations should be \"well-behaved\", even at the price of reducing their renormalized mutual information. We can show this idea in a straight forward example\n",
"_____no_output_____"
]
],
[
[
"# Let's define a linear feature\ndef cheating_feature(x):\n Feature = x[:, 0]*np.cos(best_theta) + x[:, 1]*np.sin(best_theta) \n \n step_size = 3\n step_width = 1/12\n step_argument = x[:, 0]*np.cos(best_theta+np.pi/2) + x[:, 1]*np.sin(best_theta+np.pi/2)\n Feature +=step_size*np.tanh(step_argument/step_width)\n \n Grad1 = np.full(x.shape[0], np.cos(best_theta))\n Grad2 = np.full(x.shape[0], np.sin(best_theta))\n \n Grad1 += step_size/step_width*np.cos(best_theta+np.pi/2)/np.cosh(step_argument/step_width)**2\n Grad2 += step_size/step_width*np.sin(best_theta+np.pi/2)/np.cosh(step_argument/step_width)**2\n return Feature, np.array([Grad1, Grad2]).T\n\nsamples = np.random.multivariate_normal(mu, sigma, N_samples )\n\nfeature, gradient = cheating_feature(x_points)\n\nplt.figure()\nplt.title(\"Feature contours\")\nplt.xlabel(r\"$x_1$\")\nplt.ylabel(r\"$x_2$\")\n\nplt.gca().set_aspect('equal')\n# Draw the input distribution on the background\nsamples = np.random.multivariate_normal(mu, sigma, N_samples )\nplt.hist2d(*samples.T, bins=100, cmap=plt.cm.binary)\n\n# Draw the contour lines of the extracted feature\nplt.xlim([-4,4])\nplt.ylim([-4,4])\nplt.contour(x1_grid, x2_grid, feature.reshape([N,N]),15, \n linewidths=4, cmap=plt.cm.Blues)\nplt.colorbar()\nplt.show()\n\nfeature, gradient = cheating_feature(samples)\nRMI = inf.RenormalizedMutualInformation(feature, gradient)\nprint(\"Renormalized Mutual Information (x,f(x)): %2.2f\" % RMI)\n\np_y, delta_y = inf.produce_P(feature)\nentropy = inf.Entropy(p_y, delta_y)\nfterm = inf.RegTerm(gradient)\n\nprint(\"Entropy\\t %2.2f\" % entropy)\nprint(\"Fterm\\t %2.2f\" % fterm)\n",
"_____no_output_____"
]
],
[
[
"This feature has a larger mutual information than the linear one. It is still increasing in the direction of largest variance of $x$.\n\nHowever, it contains a _jump_ in the orthogonal direction. This jump allows to encode a \"bit\" of additional information (about the orthogonal coordinate), allowing to unambiguously distinguish whether $x$ was extracted on the left or right side of the Gaussian.\n\nIn principle, one can add an arbitrary number of jumps until the missing coordinate can be identified with arbitrary precision. This feature would have an arbitrary high renormalized mutual information (as it should be, since it contains more information on $x$). However, such a non-smooth feature is definitely not useful for feature extraction!\n\nOne can avoid these extremely compressed representations by encouraging simpler features (like smooth, or a neural network with a limited number of layers for example).",
"_____no_output_____"
]
],
[
[
"# Histogram of the feature\n# The continuous value of x encodes one coordinate, \n# the two peaks of the distribution provide additional information \n# on the second coordinate!\nplt.hist(feature,1000)\nplt.show()",
"_____no_output_____"
]
],
[
[
"## Conclusions",
"_____no_output_____"
],
[
"This technique can be applied to \n- estimate the information that a deterministic feature $f(x)$ carries about a (higher-dimensional) $x$\n - in other words, to estimate how useful is a given \"macroscopic\" quantity to describe a system?\n- extract non-linear representations in an unsupervised way, by optimizinng Renormalized Mutual Information. \n\nFor more examples: \n- see the notebooks with the spiral-shaped distribution for an example with a non-Gaussian input distribution\n- see the Wave Packet and Liquid Drop notebooks for proof-of-concept applications in physics (or in general for higher-dimensional input spaces and extraction of a two-dimensional feature)\n\nAt the moment, only one-dimensional or two-dimensional features can be extracted with the neural network class. This is due to the implementation of the Entropy estimation, which currently is based on a histogram - which is not efficient in larger dimensions. An alternative (differentiable) way to estimate the Entropy will allow to extend this technique to also extract features with more than 2 dimensions.",
"_____no_output_____"
]
]
] | [
"markdown",
"code",
"markdown",
"code",
"markdown",
"code",
"markdown",
"code",
"markdown",
"code",
"markdown",
"code",
"markdown",
"code",
"markdown",
"code",
"markdown",
"code",
"markdown",
"code",
"markdown",
"code",
"markdown",
"code",
"markdown",
"code",
"markdown",
"code",
"markdown",
"code",
"markdown",
"code",
"markdown",
"code",
"markdown",
"code",
"markdown",
"code",
"markdown",
"code",
"markdown"
] | [
[
"markdown",
"markdown"
],
[
"code"
],
[
"markdown"
],
[
"code"
],
[
"markdown"
],
[
"code"
],
[
"markdown"
],
[
"code",
"code"
],
[
"markdown"
],
[
"code"
],
[
"markdown",
"markdown"
],
[
"code"
],
[
"markdown",
"markdown"
],
[
"code"
],
[
"markdown"
],
[
"code",
"code"
],
[
"markdown"
],
[
"code"
],
[
"markdown"
],
[
"code",
"code"
],
[
"markdown"
],
[
"code"
],
[
"markdown"
],
[
"code"
],
[
"markdown",
"markdown"
],
[
"code",
"code"
],
[
"markdown"
],
[
"code"
],
[
"markdown"
],
[
"code",
"code"
],
[
"markdown",
"markdown",
"markdown"
],
[
"code",
"code",
"code"
],
[
"markdown"
],
[
"code"
],
[
"markdown"
],
[
"code"
],
[
"markdown",
"markdown"
],
[
"code"
],
[
"markdown"
],
[
"code"
],
[
"markdown",
"markdown"
]
] |
d07a70b8835c0d4dcbd3bda23698f8c76c2a61f9 | 233,788 | ipynb | Jupyter Notebook | OldFiles/NLSS.ipynb | AndrewRyan95/Twitch_Sentiment_Analysis | 18e6acd92322865e4c9eaab9a4aa3c5e564bbf0f | [
"MIT"
] | 1 | 2017-10-01T17:14:29.000Z | 2017-10-01T17:14:29.000Z | OldFiles/NLSS.ipynb | AndrewRyan95/Twitch_Sentiment_Analysis | 18e6acd92322865e4c9eaab9a4aa3c5e564bbf0f | [
"MIT"
] | null | null | null | OldFiles/NLSS.ipynb | AndrewRyan95/Twitch_Sentiment_Analysis | 18e6acd92322865e4c9eaab9a4aa3c5e564bbf0f | [
"MIT"
] | null | null | null | 48.736294 | 29,199 | 0.461966 | [
[
[
"# UPDATE\n\nThis notebook is no longer being used. Please look at the most recent version, NLSS_V2 found in the same directory.",
"_____no_output_____"
],
[
"My project looks at the Northernlion Live Super Show, a thrice a week Twitch stream which has been running since 2013. Unlike a video service like Youtube, the live nature of Twitch allows for a more conversational 'live comment stream' to accompany a video. My goal is to gather statistics about the episodes and pair this with a list of all the comments corrosponding to a video. Using this I will attempt to recognize patterns in Twitch comments based on the video statistics.",
"_____no_output_____"
]
],
[
[
"# Every returned Out[] is displayed, not just the last one. \nfrom IPython.core.interactiveshell import InteractiveShell\nInteractiveShell.ast_node_interactivity = \"all\"",
"_____no_output_____"
],
[
"import nltk\nimport pandas as pd\nimport numpy as np",
"_____no_output_____"
]
],
[
[
"A text file containing basic information about every NLSS episode must be organized into something usable",
"_____no_output_____"
]
],
[
[
"with open(r'data\\NLSS_Dockets.txt') as f:\n file = f.read()\nshows = file.split('\\n\\n') #split into every show\nshows[:5]",
"_____no_output_____"
]
],
[
[
"This text file was taken from a webpage and so it contains links to Nick's livestream. Let's get rid of this since it's not needed.",
"_____no_output_____"
]
],
[
[
"index = 0\nfor s in shows:\n shows[index] = s.replace(' Nick View', '')\n index+=1\nshows[-10:]",
"_____no_output_____"
]
],
[
[
"Now I need to split up each show into their meaningful parts. Let's start with the games played on each episode.",
"_____no_output_____"
]
],
[
[
"games = []\nfor s in shows:\n g = s.split('\\n') #Text files has games on second line\n games.append(g[1])\ngames",
"_____no_output_____"
]
],
[
[
"I'll have to clean these up later to make sure all the games are spelled consistantly.",
"_____no_output_____"
]
],
[
[
"#Number of dockets, not individual games\nlen(games)",
"_____no_output_____"
]
],
[
[
"Now lets take a look at the first lines of the text file which contain the date of the show and the people who joined the show that day. They are seperated in the file by ().",
"_____no_output_____"
]
],
[
[
"date_crew = []\nfor s in shows:\n dc = s.split('\\n')[0]\n date_crew.append(dc)\nprint(date_crew)",
"['(August 24, 2017) (NL, RLS, CS, rob)', '(August 23, 2017) (NL, RLS, rob w/ Baer, LGW, HCJ)', '(August 21, 2017) (NL, RLS, JS, rob)', '(August 17, 2017) (NL w/ Sin, RLS, LGW, HCJ, Baer)', '(August 16, 2017) (NL, RLS w/ rob, Baer, LGW, Dan)', '(August 14, 2017) (NL, JS, MALF, LGW w/ Baer, HCJ)', '(August 10, 2017) (NL, RLS, rob, LGW)', '(August 7, 2017) (NL, RLS, JS, rob w/ Baer)', '(August 3, 2017) (NL, RLS, CS w/ rob, MALF)', '(August 2, 2017) (NL, RLS, LGW w/ Baer, Kory)', '(July 31, 2017) (NL, RLS, JS, rob w/ Sin, Baer, TB)', '(July 27, 2017) (NL, RLS, CS w/ MALF)', '(July 26, 2017) (NL, RLS w/ LGW, Sin, Baer, Dan)', '(July 24, 2017) (NL, RLS, JS w/ LGW)', '(July 20, 2017) (NL, RLS, CS, LGW w/ Baer)', '(July 19, 2017) (NL, RLS, LGW w/ MALF, rob, Baer)', '(July 13, 2017) (NL, RLS, rob w/ LGW, Baer)', '(July 12, 2017) (NL, RLS, rob w/ Baer)', '(July 10, 2017) part 1, part 2 (NL, RLS, JS w/ rob, Sin)', '(July 6, 2017) (NL, RLS, CS, rob w/ Baer)', '(July 5, 2017) (NL, RLS, rob w/ LGW, Baer)', '(July 3, 2017) (NL, RLS, rob w/ Sin, LGW, Baer)', '(June 22, 2017) (NL, RLS, CS, rob w/ JS)', '(June 21, 2017) Nick view (NL, RLS, rob w/ LGW, Baer)', '(June 19, 2017) Nick view (NL, RLS, JS w/ rob, Kate, Baer)', 'Solo (June 15, 2017) (NL w/ MALF, rob)', 'Solo (June 14, 2017) (NL w/ MALF, LGW, JS, Baer)', 'NLSS Masters (June 12, 2017) (NL, RLS, JS, MALF)', '(June 8, 2017) (NL, RLS w/ rob, MALF, Baer)', '(June 7, 2017) (NL, RLS, rob w/ Dan, Baer, Sin, Blueman)', '(June 5, 2017) Nick view (NL, RLS, JS w/ MALF)', '(June 1, 2017) Nick view (NL, RLS, CS w/ rob, Baer)', '(May 31, 2017) (NL, rob, LGW, Baer)', '(May 29, 2017) (NL, RLS, JS w/ rob, MALF)', '(May 25, 2017) (NL, RLS, rob w/ MALF, Baer)', '(May 24, 2017) (NL, RLS, rob, LGW w/ MALF, Dan)', '(May 22, 2017) (NL, RLS, JS w/ MALF)', '(May 18, 2017) (NL, RLS, CS, rob w/ Kate, Baer)', '(May 17, 2017) (NL, RLS w/ Dan, LGW)', '(May 15, 2017) (NL, RLS, JS w/ rob, LGW, MALF)', '(May 11, 2017) (NL, RLS, rob, Baer w/ LGW, Sin)', '(May 10, 2017) (NL, RLS, rob w/ Baer, LGW, Dan)', '(May 8, 2017) (NL, RLS w/ rob, MALF)', '(May 4, 2017) (NL, RLS, rob w/ MALF, Baer)', '(May 3, 2017) (NL, RLS, rob w/ MALF)', '(May 1, 2017) (NL, RLS, JS w/ rob, MALF)', '(April 27, 2017) (NL, RLS w/ JS, MALF, Baer, LGW, Kate)', '(April 26, 2017) (NL, RLS, rob, LGW w/ Baer)', '(April 24, 2017) (NL, RLS, rob, LGW w/ Baer)', '(April 20, 2017) Nick view (NL, RLS, JS, CS w/ rob)', '(April 19, 2017) Nick view (NL, RLS, LGW w/ rob, Baer)', '(April 6, 2017) part 1 part 2 Nick view (NL, RLS, CS w/ LGW)', '(April 5, 2017) (NL, RLS, rob w/ Baer)', '(April 3, 2017) (NL, JS w/ MALF, LGW, rob, Baer, Sin)', '(March 30, 2017) (NL, RLS, CS w/ BaerBaer, MALF)', '(March 29, 2017) Nick Video (NL, RLS, rob, LGW w/ Dan)', '(March 27, 2017) (NL, RLS, JS w/ rob)', '(March 23, 2017) (NL, RLS, CS w/ MALF)', '(March 22, 2017) Nick Video (NL, RLS, LGW w/ MALF, Baer)', '(March 20, 2017) (NL, RLS, LGW w/ Baer, rob)', '(March 16, 2017) (NL, CS, LGW w/ MALF, Baer)', '(March 15, 2017) (NL, RLS, LGW w/ Kate)', '(March 8, 2017) (NL, RLS, rob w/ LGW, Baer, Sin)', '(March 6, 2017) (NL, RLS, JS w/ LGW, Baer, Sin)', '(March 1, 2017) (NL, rob, LGW w/ MALF, Baer)', '(February 27, 2017) (NL, RLS, JS w/ rob, LGW, Baer)', '(February 23, 2017) (NL, RLS, MALF w/ LGW, Baer)', '(February 22, 2017) (NL, RLS, rob, LGW w/ Dan)', '(February 20, 2017) Nick view (NL, RLS, JS, LGW w/ rob, Baer, Sin)', '(February 16, 2017) (NL, RLS, LGW w/ rob, Baer, MALF)', '(February 15, 2017) (NL, RLS, rob, LGW w/ Mathas)', '(February 13, 2017) (NL, JS w/ rob, LGW)', '(February 9, 2017) (NL, RLS, rob, LGW w/ MALF, Baer)', '(February 8, 2017) part 1 part 2 (NL, RLS, CS w/ rob, LGW, MALF, Baer)', '(February 6, 2017) (NL, RLS, JS w/ MALF)', '(February 2, 2017) (NL, RLS, rob, LGW w/ Baer, MALF)', '(February 1, 2017) (NL, RLS, rob, LGW)', '(January 31, 2017) (NL, JS, MALF, rob w/ Sin)', '(January 26, 2017) (NL, MALF, rob, LGW)', '(January 25, 2017) (NL, rob, LGW w/ MALF, Dan, Sin, Kate)', '(January 23, 2017) part 1 part 2 (NL, JS, MALF)', '(January 19, 2017) (NL, MALF, rob, LGW)', '(January 18, 2017) (NL, rob, LGW w/ MALF)', '(January 16, 2017) part 1 part 2 (NL, JS, MALF w/ LGW, rob, Baer, Sin, Dan)', '(January 12, 2017) (NL, RLS, MALF w/ LGW, Crendor)', '(January 11, 2017) (NL, RLS w/ rob, LGW, Dan)', '(January 9, 2017) (NL, RLS, JS w/ Baer, rob, LGW)', '(January 5, 2017) (NL, RLS, rob w/ MALF, Baer, rob)', '(January 4, 2017) part 1 part 2 (NL, RLS, rob w/ MALF, LGW)', '(January 2, 2017) (NL, RLS, JS w/ LGW)', '(December 29, 2016) (NL, RLS, LGW w/ rob, Baer, JS)', '(December 28, 2016) (NL, RLS, LGW w/ rob, Baer)', '(December 26, 2016) (NL, RLS, JS w/ rob, Sin)', 'Bootleg (December 22, 2016) part 1 part 2 part 3 (NL, LGW w/ Kate)', '(December 21, 2016) (NL, RLS, CS w/ Baer, rob, LGW, Dan)', '(December 19, 2016) (NL, RLS, JS, rob w/ LGW)', '(December 15, 2016) (NL, RLS, MALF, rob w/ Kate, LGW)', '(December 14, 2016) (NL, RLS, CS w/ LGW)', '(December 12, 2016) (NL, RLS, JS, LGW)', '(December 8, 2016) (NL, RLS, MALF, rob w/ LGW)', '(December 7, 2016) (NL, RLS, rob, LGW w/ MALF)', '(December 6, 2016) (NL, RLS, MALF, LGW w/ rob)', '(December 5, 2016) (NL, RLS, JS, rob w/ LGW, Baer)', '(November 30, 2016) (NL, RLS, rob, LGW)', '(November 28, 2016) (NL, RLS, rob, LGW w/ Baer)', '(November 24, 2016) (NL, MALF, rob, LGW)', '(November 23, 2016) (NL, RLS, CS w/ rob, LGW, Dan, MALF, Baer)', '(November 21, 2016) (NL, RLS, rob, LGW w/ Baer, Sin)', '(November 17, 2016) (NL, RLS, MALF, rob w/ Baer, LGW)', '(November 16, 2016) (NL, RLS, CS w/ Mathas, rob)', '(November 14, 2016) (NL, RLS, JS, rob w/ LGW, BRex)', '(November 10, 2016) (NL, RLS, rob, LGW w/ JS, MALF)', '(November 9, 2016) (NL, JS, MALF, rob, LGW)', '(November 7, 2016) (NL, RLS, JS, LGW)', '(November 3, 2016) (NL, RLS w/ rob, LGW)', '(November 2, 2016) Nick view (NL, RLS w/ MALF, rob, LGW, Dan)', '(October 31, 2016) (NL, RLS, JS, Sin w/ rob, LGW)', '(October 27, 2016) (NL, RLS, MALF w/ LGW, rob)', '(October 26, 2016) Nick view (NL, RLS, CS, rob w/ LGW)', '(October 24, 2016) (NL, RLS, JS, LGW w/ rob, Baer)', '(October 20, 2016) (NL, RLS, MALF w/ rob, Baer, LGW)', '(October 19, 2016)(NL, RLS, CS, rob w/ Baer)', '(October 17, 2016) (NL, RLS, JS, MALF w/ rob, LGW, Baer)', '(October 13, 2016) (NL, RLS w/ rob, LGW)', '(October 12, 2016) (NL, RLS, CS, LGW w/ MALF, rob, Baer)', '(October 10, 2016) (NL, RLS, JS, rob w/ LGW, GhostBill)', '(October 6, 2016) (NL, RLS, MALF, LGW w/ rob, Baer)', '(October 5, 2016) (NL, RLS, rob, LGW w/ Baer, Sin)', '(October 3, 2016) (NL, RLS, JS w/ rob)', '(September 29, 2016) (NL, RLS, rob, LGW)', '(September 28, 2016) (NL, RLS, CS w/ rob, LGW)', '(September 26, 2016) (NL, RLS, JS w/ rob, Kate)', '(September 22, 2016) (NL, RLS, MALF w/ rob, LGW)', '(September 12, 2016) (NL, RLS, JS w/ MALF, rob, LGW)', '(September 10, 2016) (NL, RLS, MALF w/ rob, Baer)', '(September 8, 2016) (NL, RLS, MALF w/ alpacapatrol, LGW, Sin)', 'Solo (September 7, 2016) (NL w/ Sin, MALF, LGW, Baer, Dan, rob)', '(August 31, 2016) (NL, RLS w/ rob, LGW, Dan)', '(August 29, 2016) Nick view (NL, RLS, JS w/ rob, LGW)', '(August 25, 2016) (NL, RLS w/ rob, MALF, LGW)', '(August 24, 2016) (NL, MALF w/ rob, LGW, dan)', '(August 22, 2016) (NL, MALF w/ rob, LGW)', '(August 18, 2016) (NL, RLS w/ JS, MALF, rob, LGW)', '(August 17, 2016) (NL, RLS, CS w/ rob, Baer, LGW, Dan)', '(August 15, 2016) part 1 part 2 Nick view (NL, RLS, JS w/ rob, Baer, LGW)', '(August 10, 2016) (NL, RLS w/ rob, LGW)', '(August 8, 2016) (NL, RLS, JS w/ LGW, Baer)', '(August 4, 2016) Nick view (NL, RLS, MALF w/ rob, LGW, Sin, Baer)', '(August 3, 2016) part 1 part 2 (NL, RLS, CS w/ rob, LGW)', '(August 1, 2016) (NL, RLS, JS w/ Baer, LGW)', 'Bootleg (July 28, 2016) (NL, MALF w/ JS, LGW)', 'Solo (July 27, 2016) (NL, MALF)', 'Solo (July 25, 2016) (NL)', '(July 21, 2016) (NL, RLS w/ LGW, Sin, Mathas)', '(July 20, 2016) (NL, RLS, CS w/ Baer, LGW)', '(July 18, 2016) Nick view (NL, RLS w/ MALF, LGW)', '(July 14, 2016) (NL, RLS w/ LGW, Sin)', '(July 13, 2016) (NL, RLS, CS w/ Sin)', '(July 11, 2016) (NL, RLS w/ Baer, rob, LGW)', 'Solo (July 7, 2016) (NL w/ rob)', '(July 6, 2016) (NL, RLS, CS w/ LGW)', '(July 4, 2016) (NL, RLS, JS w/ rob, LGW)', '(June 30, 2016) (NL, RLS w/ MALF, rob, Baer, LGW)', '(June 29, 2016) (NL, RLS, CS w/ rob, LGW)', '(June 27, 2016) (NL, RLS, JS w/ rob, LGW)', '(June 23, 2016) (NL, RLS w/ rob, MALF, Dan)', '(June 20, 2016) (NL, RLS, JS w/ rob, Mathas)', '(June 9, 2016) Nick view (NL, RLS, rob w/ LGW)', '(June 8, 2016) (NL, RLS, CS w/ rob, LGW)', '(June 6, 2016) (NL, RLS, JS w/ LGW, MALF, Dan)', '(June 2, 2016) (NL, RLS w/ rob, LGW)', '(June 1, 2016) (NL, RLS, CS w/ Baer, LGW)', '(May 30, 2016) (NL, RLS, JS w/ rob, Baer, LGW)', '(May 26, 2016) (NL, RLS w/ rob, LGW, Baer)', '(May 25, 2016) (NL, RLS, CS w/ rob, MALF)', '(May 23, 2016) (NL, JS w/ rob, Dan, Sin, LGW)', 'Bootleg Solo (May 19, 2016) (NL w/ Mathas, rob, LGW, Sin)', 'Bootleg Solo (May 18, 2016) (NL w/ Sin, rob, LGW)', 'Bootleg (May 16, 2016) (NL, RLS, JS w/ rob, LGW)', '(May 12, 2016) Nick view (NL, RLS w/ rob, LGW)', '(May 11, 2016) (NL, RLS, CS w/ rob)', 'Solo (May 9, 2016) (NL w/ Sin, rob, LGW)', '(May 5, 2016) (NL, RLS w/ JS, rob)', '(May 4, 2016) (NL, RLS, rob w/ MALF)', '(May 2, 2016)(NL, RLS, JS w/ MALF)', 'Solo (April 21, 2016) (NL w/ Sin, rob, LGW)', 'Solo (April 20, 2016) (NL w/ Sin)', '(April 18, 2016) (NL, RLS, JS)', 'Bootleg (April 14, 2016) (NL, RLS w/ MALF, rob, LGW)', '(April 13, 2016) (NL, RLS w/ rob, MALF, Dan, LGW)', '(April 11, 2016) (NL, RLS, JS)', '(April 7, 2016) (NL, RLS w/ MALF, rob, LGW)', '(April 6, 2016) (NL, RLS w/ LGW)', '(April 4, 2016) (NL, RLS, JS w/ rob)', '(March 31, 2016) (NL, RLS w/ MALF, rob, LGW)', '(March 30, 2016) (NL, RLS, CS w/ JS, rob)', '(March 28, 2016) (NL, RLS w/ MALF, rob)', '(March 24, 2016) part 1 part 2 Nick view (NL, RLS w/ LGW)', '(March 23, 2016) part 1 part 2 (NL, RLS, CS w/ MALF, rob)', 'Bootleg Solo (March 3, 2016) (NL, RLS w/ rob, Dan, LGW)', '(March 2, 2016) (NL, RLS, CS w/ MALF)', '(February 29, 2016) (NL, RLS, JS w/ rob)', '[3 year NLversary!] (February 25, 2016) (NL, RLS, dan)', '(February 24, 2016) (NL, RLS, CS)', '(February 22, 2016) (NL, RLS, JS w/ rob)', '(February 18, 2016) (NL, RLS)', 'Bootleg Solo (February 17, 2016) (NL)', '(February 15, 2016) (NL, RLS, JS w/ MALF)', '(February 10, 2016) (NL, RLS, CS w/ MALF)', '(February 8, 2016) (NL, RLS, JS)', '(February 4, 2016) (NL, RLS w/ MALF)', '(February 3, 2016) (NL, RLS, CS w/ MALF)', '(February 1, 2016) (NL, RLS, JS)', '(January 28, 2016) (NL, RLS, MALF)', '(January 27, 2016) (NL, RLS)', '(January 25, 2016) (NL, RLS, JS, MALF)', '(January 21, 2016) (NL, RLS, MALF w/ rob)', '(January 20, 2016) (NL, RLS w/ rob)', '(January 18, 2016) (NL, RLS, JS)', '(January 13, 2016) (NL, RLS, CS w/ rob)', '(January 11, 2016) (NL, RLS, JS w/ rob)', '(January 7, 2016) (NL, RLS w/ MALF, rob)', '(January 6, 2016) (NL, RLS, CS w/ rob)', '(January 4, 2016) Nick view (NL, RLS, JS w/ rob)', '(December 31, 2015) (NL, RLS w/ rob)', '(December 30, 2015) (NL, RLS, CS)', '(December 28, 2015)(NL, RLS, JS)', 'Solo (December 24, 2015) (NL w/ Kate)', '(December 23, 2015) part 1 part 2 (NL, RLS, CS)', '(December 21, 2015) (NL, RLS, JS, w/ MALF)', 'Solo (December 19, 2015) (NL w/ Kate, Baer)', 'Solo (December 18, 2015) (NL w/ Kate)', '(December 10, 2015) (NL, RLS, MALF)', '(December 9, 2015) (NL, RLS, CS w/ rob)', '(December 7, 2015) part 1 part 2 (NL, RLS)', '(December 2, 2015) (NL, RLS, CS w/ rob)', '(November 30, 2015) part 1 part 2 (NL, RLS, MALF w/ rob)', 'Solo (November 26, 2015) (NL)', '(November 25, 2015) (NL, RLS, CS w/ rob)', '(November 23, 2015) (NL, RLS, JS w/ rob)', '(November 19, 2015) (NL, RLS, MALF)', '(November 18, 2015) part 1 part 2 (NL, RLS w/ Baer, rob)', '(November 16, 2015) (NL, RLS, JS)', '(November 12, 2015) (NL, RLS w/ rob, Baer)', '(November 11, 2015) (NL, RLS, CS w/ rob)', '(November 9, 2015) (NL, RLS, JS w/ Baer)', '(November 5, 2015) (NL, RLS, MALF)', '(November 4, 2015) (NL, RLS, CS)', '(November 2, 2015) part 1 part 2 (NL, RLS, JS)', '(October 29, 2015) (NL, RLS)', 'Bootleg Solo (October 28, 2015) part 1, part 2, part 3 (NL)', 'Bootleg (October 26, 2015) part 1 part 2 part 3 (NL, JS, MALF)', 'Bootleg Solo (October 22, 2015) part 1, part 2, part 3 (NL)', 'Solo (October 21, 2015) part 1 part 2 (NL)', '(October 19, 2015) part 1 part 2 (NL, JS, MALF)', '(October 15, 2015) part 1 part 2 (NL, MALF)', '(October 14, 2015) part 1 part 2 (NL, MALF)', 'October 7, 2015 (NL, RLS, CS w/ rob)', '(October 5, 2015) part 1 part 2 (NL, RLS, JS w/ rob)', '(October 1, 2015) part 1 part 2 (NL, RLS w/ rob, Dan)', '(September 30, 2015) part 1 part 2 (NL, RLS, CS w/ rob)', '(September 28, 2015) part 1 part 2 (NL, RLS, JS)', '(September 24, 2015) (NL, RLS)', '(September 23, 2015) (NL, RLS, CS w/ rob)', '(September 21, 2015) (NL, RLS, JS w/ rob)', '(September 17, 2015) part 1 part 2 Nick view (NL, RLS)', '(September 16, 2015) part 1 part 2 (NL, RLS, CS)', '(September 14, 2015) part 1 part 2 (NL, RLS, JS)', '(September 10, 2015) (NL, RLS)', '(September 9, 2015) part 1 part 2 (NL, RLS, CS w/ rob)', '(September 7, 2015) part 1 part 2 (NL, RLS, JS)', '(September 3, 2015) part 1 part 2 (NL, RLS w/ Baer, MALF)', '(September 2, 2015) part 1 part 2 Nick view (NL, RLS, CS w/ rob)', '(August 24, 2015) (NL, RLS, JS)', '(August 20, 2015) part 1 part 2 (NL, RLS)', '(August 19, 2015) part 1 part 2 Nick view (NL, RLS, CS w/ rob)', '(August 17, 2015) part 1 part 2 Nick view (NL, RLS, JS)', '(August 13, 2015) part 1 part 2 Nick view (NL, RLS, rob)', '(August 12, 2015) part 1 part 2 Nick view (NL, RLS, CS w/ JS)', '(August 10, 2015) part 1 part 2 part 3 Nick view (NL, RLS, JS)', 'Bootleg (August 6, 2015) part 1, part 2 (NL, RLS w/ rob, Baer, MALF)', '(August 5, 2015) part 1 part 2 Nick view (NL, RLS, CS)', '(August 3, 2015) part 1 part 2 (NL, RLS, JS w/ rob)', '(July 30, 2015) part 1 part 2 Nick view (NL, RLS)', '(July 29, 2015) part 1 part 2 part 3 (NL, RLS w/ rob, Mathas)', '(July 27, 2015) part 1 part 2 (NL, RLS, JS)', '(July 16, 2015) part 1 part 2 Nick view (NL, RLS w/ Brex)', '(July 15, 2015) part 1 part 2 Nick view (NL, RLS, CS)', '(July 13, 2015) part 1 part 2 Nick view (NL, RLS, JS w/ Baer)', '(July 9, 2015) part 1 part 2 part 3 Nick view (NL, RLS w/ Baer, MALF)', '(July 8, 2015) Part 1 Part 2 (NL, RLS, CS)', '(July 6, 2015) part 1 part 2 (NL, RLS, JS)', '(July 2, 2015) part 1 part 2 Nick view (NL, RLS w/ rob)', '(July 1, 2015) Nick view (NL, RLS, CS w/ rob)', '(June 29, 2015) part 1 part 2 Nick view (NL, RLS, JS)', '(June 25, 2015) Nick view (NL, RLS w/ rob)', '(June 24, 2015) Nick view (NL, RLS, CS w/ rob)', '(June 22, 2015) part 1 part 2 Nick view (NL, RLS, JS)', '(June 18, 2015) part 1 part 2 (NL, RLS)', '(June 17, 2015) part 1 part 2 (NL, RLS)', '(June 15, 2015) part 1 part 2 (NL, RLS, JS w/ MALF)', '(June 11, 2015) part 1 part 2 (NL, RLS w/ rob, MALF)', '(June 10, 2015) part 1 part 2 (NL, RLS w/ rob, Baer)', '(June 8, 2015) part 1 part 2 (NL, RLS w/ rob)', '(May 28, 2015) part 1 part 2 (NL w/ Baer, Fox)', '(May 27, 2015) part 1 part 2 (NL)', '(May 25, 2015) part 1 part 2 (NL, Arumba)', '(May 21, 2015) part 1 part 2 (NL, RLS)', '(May 20, 2015) part 1 part 2 (NL, RLS)', 'Bootleg (May 18, 2015) part 1 part 2 part 3 (NL, RLS w/ rob, Baer)', 'Bootleg (May 14, 2015) part 1 part 2 part 3 (NL, RLS)', 'Bootleg (May 13, 2015) part 1 part 2 part 3 (NL, RLS w/ rob, Baer)', 'Bootleg (May 11, 2015) part 1 part 2 part 3 (NL, RLS)', '(May 7, 2015) part 1 part 2 (NL, RLS w/ rob, Baer)', '(May 6, 2015) part 1 part 2 (NL, RLS w/ rob, Baer)', '(May 4, 2015) Part 1 part 2 (NL, RLS w/ rob, Baer)', 'Bootleg Solo (April 23, 2015) part 2 part 3 (NL)', 'Bootleg (April 22, 2015) part 1 part 2 part 3 (NL, RLS w/ rob, Baer)', '(April 20, 2015) part 1 part 2 (NL, RLS w/ rob, Baer)', 'Bootleg (April 16, 2015) part 1 part 2 part 3 (NL, RLS w/ rob, Baer)', '(April 15, 2015) part 1 part 2 (NL, RLS w/ cobaltstreak, baer, rob)', 'Bootleg (April 13, 2015) part 1 part 2 part 3 (NL, RLS w/ rob, Baer)', '(April 9, 2015) part 1 part 2 (NL, RLS w/ rob, Baer)', '(April 8, 2015) part 1 part 2 part 3 (NL, RLS w/ rob)', '(April 6, 2015) part 1 part 2 (NL, RLS)', '(April 2, 2015) part 1 part 2 (NL, RLS w/ Baer)', '(April 1, 2015) part 1 part 2 (NL, RLS w/ rob, Baer)', '(March 26, 2015) part 1 part 2 (NL, RLS w/ rob, Baer)', 'Bootleg (March 25, 2015) part 1 part 2 part 3 (NL, RLS)', '(March 23, 2015) part 1 part 2 (NL, RLS)', '(March 19, 2015) part 1 part 2 (NL, RLS w/ rob, Baer)', '(March 18, 2015) part 1 part 2 (NL, RLS)', 'Solo (March 12, 2015) part 1 part 2 (NL)', '(March 11, 2015) part 1 part 2 (NL, RLS w/ rob, Baer)', 'Bootleg (February 26, 2015) part 1 part 2 part 3 (NL, RLS w/ rob, fox)', '[2 year NLversary!] Bootleg (February 25, 2015) part 1 part 2 part 3 (NL, RLS w/ JS, rob)', '(February 23, 2015) part 1 part 2 (NL, RLS w/ JS, rob)', 'Bootleg (February 19, 2015) part 1 part 2 part 3 (NL, RLS w/ rob)', '(February 18, 2015) part 1 part 2 (NL, RLS w/ JS, rob)', '(February 16, 2015) part 1 part 2 (NL, RLS w/ JS, rob)', '(February 12, 2015) part 1 part 2 (NL, RLS)', '(February 11, 2015) part 1 part 2 (NL w/ Baer)', '(February 9, 2015) part 1 part 2 (NL, RLS)', '(February 5, 2015) part 1 part 2 part 3 (NL, RLS w/ JS, rob)', '(February 2, 2015) part 1 part 2 (NL, RLS w/ rob)', '(January 29, 2015) part 1 part 2 (NL, RLS w/ rob)', '(January 28, 2015) part 1 part 2 (NL, RLS)', 'Bootleg (January 8, 2015) part 1, part 2, part 3 (NL, RLS)', '(January 7, 2015) part 1 part 2 (NL, RLS w/ JS, rob)', '(January 5, 2015) part 1 part 2 (NL, RLS w/ rob)', '(January 1, 2015) part 1 part 2 (NL, RLS)', '(December 31, 2014) part 1 part 2 (NL, RLS)', '(December 29, 2014) part 1, part 2 (NL, RLS w/ fox)', 'Bootleg (December 22, 2014) part 1, part 2 (NL, RLS)', '(December 18, 2014) part 1, part 2 (NL)', '(December 15, 2014) part 1, part 2 (NL, RLS)', 'Bootleg (December 11, 2014) part 1 part 2 (NL, RLS w/ Kate, Baer)', '(December 10, 2014) part 1, part 2 (NL, RLS)', '(December 8, 2014) part 1, part 2 (cat cam!) (NL, RLS w/ rob, Mag)', '(December 4,2014) part 1, part 2 (NL, RLS)', '(December 3, 2014) part 1, part 2 (NL, RLS)', '(November 27, 2014) part 1, part 2 (NL)', '(November 26, 2014) part 1, part 2 (NL)', '(November 24, 2014) part 1, part 2 (NL, RLS)', '(November 20, 2014) part 1, part 2 (NL, RLS)', 'Bootleg (November 19, 2014) part 1, part 2 (NLS, RLS, JS!)', '(November 17, 2014) part 1, part 2 (NL, RLS w/ rob, Baer)', '(November 13, 2014) part 1, part 2 (NL, RLS)', \"(November 12, 2014) part 1 Bootleg Nick's view part 2 (NL, RLS w/ rob, Baer)\", 'Bootleg (November 6, 2014) part 1 part 2 (NL, RLS w/ rob, Baer)', '(November 5, 2014) part 1, part 2 (NL, RLS)', '(November 3, 2014) part 1, part 2 (NL, RLS w/ rob)', '(October 30, 2014) part 1, part 2 (NL, RLS)', '(October 29, 2014) part 1, part 2 (NL, RLS)', '(October 27, 2014) part 1, part 2 (NL, RLS w/rob, Baer)', 'Bootleg (October 23, 2014) part 1, part 2 (NL, RLS)', '(October 22, 2014) Part 1, Part 2 (NL, RLS w/ rob, MALF)', '(October 20, 2014) Part 1, Part 2 (NL, RLS w/ rob, Baer)', '(October 16, 2014) part 1, part 2 (NL, RLS)', '(October 15, 2014) part 1, part 2, part 3 (NL, RLS w/ rob, Baer)', '(October 13, 2014) part 1, part 2 (NL, RLS)', '(October 9, 2014) part 1, part 2 (NL, RLS w/ rob, Baer)', '(October 8, 2014) part 1, part 2 (NL, RLS)', '(October 6, 2014) part 1, part 2, part 3 (NL, RLS w/ rob)', '(October 2, 2014) part 1, part 2 (NL, RLS w/ rob, Baer)', '(October 1, 2014) part 1, part 2 (NL, RLS)', '(September 29, 2014) part 1, part 2 (NL)', '(September 25, 2014) part 1, part 2, part 3 (NL, RLS w/ rob, Mag)', '(September 24, 2014) part 1, part 2 (NL, RLS w/ rob, Baer)', '(September 22, 2014) part 1, part 2 (NL, RLS w/ rob, Mag)', '(September 18, 2014) part 1 part 2 (NL, RLS)', '(September 17, 2014) part 1 part 2 (NL, RLS w/ JS, rob)', '(September 15, 2014) part 1, part 2 (NL, RLS w/ JS, rob)', '(September 11, 2014) part 1, part 2 (NL, RLS)', '(September 10, 2014) part 1, part 2 (NL, RLS w/ JS, Mag)', '(September 8, 2014) part 1, Part 2 (NL, RLS w/ JS)', '(August 27, 2014) part 1, part 2 (NL, RLS in person)', '(August 25, 2014) part 1, part 2 (NL, RLS, Kate in person)', '(August 13, 2014) part 1, part 2 (NL, RLS)', '(August 12, 2014) part 1, part 2 (NL, RLS w/ rob)', '(August 7, 2014) part 1, part 2 (NL, RLS w/ rob)', '(August 6, 2014) part 1, part 2 (NL, RLS)', '(August 4, 2014) part 1, part 2 (NL, RLS w/ rob)', '(July 31, 2014) part 1, part 2 (NL, RLS)', '(July 30, 2014) part 1, part 2 (NL, RLS)', '(July 28, 2014) part 1, part 2 (NL, RLS w/ Kate, Baer, rob)', '(July 24, 2014) part 1, part 2 (NL, RLS w/ rob, Baer)', '(July 21, 2014) part 1, part 2 (NL, RLS)', '(July 16, 2014) part 1, part 2, part 3 (NL, RLS)', '(July 14, 2014) part 1, part 2 (NL, RLS)', '(July 10, 2014) part 1, part 2 (NL, RLS w/ Baer)', '(July 9, 2014) part 1, part 2 (NL, RLS)', '(July 7, 2014) part 1, part 2 (NL, RLS w/ Baer)', '(July 2, 2014) part 1, part 2 (NL, RLS)', '(June 30, 2014) part 1, part 2 (NL, RLS w/ Kate, rob)', '(June 26, 2014) part 1, part 2 (NL, RLS)', '(June 25, 2014) part 1, part 2 (NL, RLS)', '(June 19, 2014) part 1, part 2 (NL, RLS w/ rob)', '(June 18, 2014) part 1, part 2 (NL, RLS w/ Kate, rob, Baer, Mathas)', '(June 16, 2014) part 1, part 2 (NL, RLS, JS)', '(June 12, 2014) part 1, part 2 (NL, RLS, JS)', '(June 11, 2014) part 1, part 2 (NL, RLS, JS w/ Mathas)', '(June 9, 2014) part 1, part 2 (NL, RLS, JS)', '(June 5, 2014) part 1, part 2 (NL, RLS, JS)', '(June 4, 2014) part 1, part 2 (NL, RLS, JS)', '(June 2, 2014) part 1, part 2 (NL, RLS, JS)', '(May 29, 2014) part 1, part 2 (NL, RLS, JS)', '(May 28, 2014) part 1, part 2 (NL, RLS, JS)', '(May 26, 2014) part 1, part 2 (NL, RLS, JS)', '(May 15, 2014) part 1, part 2 (NL, RLS)', '(May 14, 2014) part 1, part 2 (NL, RLS)', '(May 12, 2014) part 1, part 2 (NL, RLS)', '(May 8, 2014) part 1, part 2 (NL, RLS, JS)', '(May 5, 2014) part 1, part 2 (NL, RLS, JS w/ Mike Bithell)', '(May 1, 2014) part 1, part 2 (NL, RLS, JS)', '(April 30, 2014) part 1, part 2 (NL, RLS, JS)', '(April 28, 2014) part 1, part 2 (NL, RLS, JS)', '(April 24, 2014) part 1, part 2 (NL, RLS, JS)', '(April 23, 2014) part 1, part 2 (NL, RLS, JS)', '(April 21, 2014) part 1, part 2 (NL, RLS, JS)', '(April 17, 2014) part 1, part 2 (NL, RLS, JS)', '(April 16, 2014) part 1, part 2 (NL, RLS, JS)', '(April 7, 2014) part 1, part 2 (NL, RLS, JS)', '(April 3, 2014) part 1, part 2 (NL, RLS, JS)', '(April 2, 2014) part 1, part 2 (NL, RLS, JS)', '(March 31, 2014) part 1, part 2 (NL, RLS, JS)', '(March 27, 2014) part 1, part 2 (NL, RLS, JS)', '(March 26, 2014) part 1, part 2 (NL, RLS, JS)', '(March 24, 2014) part 1, part 2 (NL, RLS, JS)', '(March 13, 2014) part 1, part 2 (NL, RLS, JS)', '(March 12, 2014) part 1, part 2 (NL, RLS, JS)', '(March 10, 2014) part 1, part 2 (NL, RLS, JS)', '(March 6, 2014) part 1, part 2 (NL, RLS, JS)', '(March 5, 2014) part 1, part 2 (NL, RLS, JS)', '(March 3, 2014) part 1, part 2 (NL, RLS, JS)', '(February 27, 2014) part 1, part 2 (NL, RLS, JS)', '(February 26, 2014) part 1, part 2 (NL, RLS, JS)', '(February 24, 2014) part 1, part 2 (NL, RLS, JS)', '(February 20, 2014) part 1, part 2 (NL, RLS)', '(February 19, 2014) part 1, part 2 (NL, RLS, JS)', '(February 17, 2014) part 1, part 2 (NL, RLS, JS)', '(February 13, 2014) part 1, part 2 (NL, RLS)', '(February 12, 2014) part 1, part 2, part 3, part 4, part 5 (NL, RLS)', '(February 10, 2014) part 1, part 2 (NL, RLS, JS)', '(February 6, 2014) part 1, part 2 (NL, RLS, JS)', '(February 5, 2014) part 1, part 2 (NL, RLS, JS, MALF)', '(February 3, 2014) part 1, part 2 (NL, RLS w/ rob, MALF)', '(January 30, 2014) part 1, part 2 (NL, RLS, JS w/ Mike Bithell)', '(January 29, 2014) part 1, part 2 (NL, RLS, JS)', '(January 27, 2014) (NL, RLS, JS w/ Crendor)', '(January 20, 2014) part 1, part 2 (NL, RLS, JS)', '(January 16, 2014) part 1, part 2 (NL, RLS, JS)', '(January 15, 2014) part 1, part 2 (NL, RLS, JS)', '(January 13, 2014) part 1, part 2 (NL, RLS, MALF)', '(January 9, 2014) part 1, part 2 (NL, RLS, MALF w/ rob)', '(January 8, 2014) part 1, part 2 (NL, RLS, JS)', '(January 6, 2014) part 1, part 2 (NL, RLS, JS)', '(December 19, 2013), part 1, part 2 (NL, RLS, JS)', '(December 18, 2013), part 1, part 2 (NL, JS)', '(December 16, 2013), part 1, part 2 (NL, RLS, JS)', '(December 12, 2013), part 1, part 2 (NL, RLS, JS)', '(December 11, 2013), part 1, part 2 (NL, RLS, JS)', '(December 9, 2013), part 1, part 2 (NL, RLS, JS)', '(December 5, 2013), part 1, part 2 (NL, RLS, JS)', '(December 4, 2013), part 1, part 2 (NL, RLS, JS)', '(December 2, 2013), part 1, part 2 (NL, RLS, JS)', '(November 28, 2013), part 1, part 2 (NL, JS)', '(November 27, 2013), part 1, part 2 (NL, RLS, JS)', '(November 25, 2013), part 1, Part 2 (NL, RLS, JS)', '(November 21, 2013), part 1, part 2 (NL, RLS, JS)', '(November 20, 2013), part 1, part 2 (NL, RLS, JS)', '(November 18, 2013), part 1, part 2 (NL, RLS, JS)', '(November 14, 2013), part 1, part 2 (NL, RLS, JS)', '(November 13, 2013), part 1, part 2 (NL, RLS, MALF)', '(November 11, 2013), part 1, part 2 (NL, RLS, MALF)', '(November 7, 2013), part 1, part 2 (NL, RLS, JS, MALF)', '(November 6, 2013), part 1, part 2 (NL, RLS, JS)', '(November 4, 2013), part 1, part 2 (NL, RLS, MALF)', '(October 31, 2013) part 1 part 2 (NL, RLS, JS)', '(October 30, 2013), part 1, part 2 (NL, RLS, JS)', '(October 28, 2013) (NL, RLS, JS)', '(October 24, 2013) Part 1, Part 2 (NL, RLS, JS)', '(October 23, 2013) (NL, RLS, JS w/ rob)', '(October 21, 2013) (NL, RLS, JS)', '(October 17, 2013) (NL, RLS, JS w/ rob)', '(October 16, 2013) (NL, RLS, JS w/ rob)', '(October 14, 2013) (NL, RLS, JS)', '(October 10, 2013) (NL, RLS, JS w/ RPG)', '(October 9, 2013) (NL, RLS, JS)', '(October 7, 2013) (NL, RLS, JS)', '(October 3, 2013) (NL, RLS, JS)', '(October 2, 2013) (NL, RLS, JS)', '(September 30, 2013) Part 1, Part 2 (NL, RLS, JS)', '(September 26, 2013) (NL, RLS, JS)', '(September 25, 2013) Part 1, Part 2 (NL, RLS, JS)', '(September 23, 2013) (NL, RLS, JS)', '(September 19, 2013) (NL, RLS, JS)', '(September 18, 2013) (NL, RLS, JS, MALF)', '(September 16, 2013) (NL, RLS, JS)', '(September 12, 2013) (NL, RLS, JS)', '(September 11, 2013) (NL, RLS, JS)', '(September 9, 2013) (NL, RLS, JS)', '(September 5, 2013) (NL, RLS, JS)', '(September 4, 2013) (NL, RLS, JS w/ Ohm)', '(August 26, 2013) part 1, part 2 (NL, RLS, JS)', '(August 22, 2013) (NL, RLS, JS w/ Ohm)', '(August 21, 2013) (NL, RLS, JS w/ Ohm)', '(August 19, 2013) (NL, RLS, JS)', '(August 15, 2013) (NL, RLS, JS)', '(August 14, 2013) (NL, RLS, JS)', '(August 12, 2013) (NL, RLS, JS)', '(August 1, 2013) (NL, RLS, JS w/ Kate)', '(July 31, 2013) (NL, RLS, JS w/ Kate)', '(July 29, 2013) (NL, RLS, JS w/ Ohm)', '(July 25, 2013) (NL, RLS, JS w/ Kate)', '(July 24, 2013) (NL, RLS, JS w/ Kate, MALF)', '(July 22, 2013) (NL, RLS, JS w/ Mike Bithell)', '(July 18, 2013) (NL, RLS, JS w/ Kate)', '(July 17, 2013) part 1, part 2 (NL, RLS, JS w/ Kate)', '(July 15, 2013) (NL, RLS, JS)', '(July 11, 2013) (NL, RLS, JS)', '(July 8, 2013) (NL, RLS, JS)', '(July 4, 2013) (NL, RLS, JS w/ Ohm)', '(July 3, 2013) (NL, RLS, JS w/ Kate, Ohm, rob)', '(July 1, 2013) (NL, RLS, JS)', '(June 20, 2013) (NL, RLS, JS w/ Ohm, rob, Pixel)', '(June 19, 2013) (NL, RLS, JS w/ Ohm, rob)', '(June 17, 2013) (NL, RLS, JS w/ Ohm, Green)', '(June 13, 2013) (NL, RLS, JS w/ Ohm, rob, LGW)', '(June 12, 2013) (NL, RLS, JS w/ Ohm, rob, RPG, Mathas)', '(June 10, 2013) (NL, RLS, JS w/ Ohm, rob)', '(June 5, 2013) (NL, RLS, JS w/ Ohm)', '(June 3, 2013) (NL, RLS, JS w/ Green, rob)', '(May 30, 2013) (NL, RLS, JS w/ Ohm, rob, Green)', '(May 29, 2013) (NL, RLS, JS)', '(May 27, 2013) (NL, RLS, JS w/ Ohm, rob)', '(May 23, 2013) (NL, RLS, JS w/ Kate, Ohm)', '(May 22, 2013) (NL, RLS, JS w/ Kate, Ohm, rob, Green)', '(May 20, 2013) (NL, RLS, JS w/ Kate, Ohm, Green)', '(May 16, 2013) (NL, RLS w/ Ohm, rob, Pixel)', '(May 15, 2013) (NL, RLS, Ohm)', '(May 13, 2013) (NL, RLS w/ Ohm, Mathas, rob)', '(May 2, 2013) (NL, RLS, JS w/ Ohm, RPG, Green)', '(May 1, 2013) (NL, RLS, JS w/ Ohm)', '(April 29, 2013) (NL, RLS, JS w/ Ohm, rob, Mathas, Green)', '(April 25, 2013) (NL, RLS, JS w/ rob, Green)', '(April 24, 2013) (NL, RLS, JS w/ Ohm)', '(April 22, 2013) (NL, RLS, JS w/ Ohm, rob, Green, RPG)', '(April 18, 2013) (NL, RLS w/ RPG, Green, Ohm, rob)', '(April 17, 2013) (NL, RLS, JS w/ Green, Ohm)', '(April 15, 2013) (NL, RLS, JS w/ Ohm, Green, rob, Mathas, MALF)', '(April 11, 2013) (NL, RLS, JS w/ Green, rob)', '(April 10, 2013) (NL, RLS, JS w/ Green, Ohm)', '(April 8, 2013) (NL, RLS, JS w/ Ohm, RPG, MALF)', '(April 4, 2013) (NL, RLS, JS w/ Green, Ohm)', '(April 3, 2013) (NL, RLS, JS w/ MALF, Ohm)', '(April 1, 2013) (NL, RLS, JS w/ Ohm)', '(March 28, 2013) (NL, RLS, JS w/ RPG, Ohm)', '(March 27, 2013) (NL, RLS, JS w/ Ohm)', '(March 18, 2013) (NL, RLS, JS)', '(March 14, 2013) (NL, RLS, JS w/ MALF)', '(March 13, 2013) (NL, RLS, JS w/ Ohm)', '(March 11, 2013) (NL, RLS, JS w/ Ohm)', '(March 6, 2013) (NL, RLS, JS)', '(March 4, 2013) (NL, RLS, JS)', '(February 28, 2013) (NL, RLS, JS)', '(February 27, 2013) (NL, Kate)', '(February 25, 2013) (NL)']\n"
]
],
[
[
"I'm going to use regex to split these up.",
"_____no_output_____"
]
],
[
[
"import re\ndate = []\ncrew = []\nfor entry in date_crew:\n foo = re.search(r'\\((.*)\\)', entry).group(1)\n d = foo.split(r')')[0]\n date.append(d)\n c = foo.split(r'(')[-1]\n crew.append(c)",
"_____no_output_____"
]
],
[
[
"Now I'll start creating a data frame of this information",
"_____no_output_____"
]
],
[
[
"date_df = pd.DataFrame(date, columns = [\"Date\"])\ndate_df.head()",
"_____no_output_____"
],
[
"games_df = pd.DataFrame(games, columns = [\"Docket\"])\ngames_df.head()",
"_____no_output_____"
],
[
"crew_df = pd.DataFrame(crew, columns = [\"Crew\"])\ncrew_df.head()",
"_____no_output_____"
]
],
[
[
"Now combine them",
"_____no_output_____"
]
],
[
[
"nlss_df = pd.DataFrame()\nnlss_df['Date'] = date_df['Date']\nnlss_df['Crew'] = crew_df['Crew']\nnlss_df['Docket'] = games_df['Docket']\nnlss_df.head()",
"_____no_output_____"
],
[
"nlss_df.describe()",
"_____no_output_____"
]
],
[
[
"I noticed that some lines had a link called \"(continued)\" in the games list. I want to get rid of these. While I'm at it, let's make the games docket contain the games as lists.",
"_____no_output_____"
]
],
[
[
"improved = []\n#For each docket\nfor d in nlss_df['Docket']:\n #Split docket into list of games\n d = d.split(r',')\n #For each game\n for g in d:\n #If game matches string to remove\n if g == r\" (continued)\" or g == r\" (Continued)\":\n #Remove game\n d.remove(g)\n improved.append(d)\nnlss_df['Docket'] = improved",
"_____no_output_____"
],
[
"nlss_df.head()",
"_____no_output_____"
],
[
"nlss_df['Crew']",
"_____no_output_____"
]
],
[
[
"I want to split on \"w/\" so each crew member is individual item. I'm also going to put them into a list.",
"_____no_output_____"
]
],
[
[
"improved = []\n#For each cast of crew\nfor e in nlss_df['Crew']:\n #Split cast into list of members\n e = e.split(r',')\n #For each member\n for m in e:\n #If member contains a /w\n if r'w/' in m:\n both = m.split(r'w/')\n e.remove(m)\n e.extend(both)\n improved.append(e)\nimproved[:20]",
"_____no_output_____"
]
],
[
[
"Strip extra spaces",
"_____no_output_____"
]
],
[
[
"fullstripped = []\nfor entry in improved:\n stripped = []\n for member in entry:\n member = member.strip(' ')\n stripped.append(member)\n fullstripped.append(stripped)\nfullstripped[:10]",
"_____no_output_____"
]
],
[
[
"Let's make the names consistant. Luckily I know the aliases that are used. Let's see what we're working with.",
"_____no_output_____"
]
],
[
[
"names = []\nfor entry in fullstripped:\n for user in entry:\n if user not in names:\n names.append(user)\nprint(names)",
"['NL', 'RLS', 'CS', 'rob', 'LGW', 'HCJ', 'Baer', 'JS', 'Sin', 'Dan', 'MALF', 'Kory', 'TB', 'Kate', 'Blueman', 'BaerBaer', 'Mathas', 'Crendor', 'BRex', 'GhostBill', 'alpacapatrol', 'dan', '', 'Brex', 'Fox', 'Arumba', 'baer', 'cobaltstreak', 'fox', 'Mag', 'NLS', 'JS!', 'RLS in person', 'Kate in person', 'Mike Bithell', 'RPG', 'Ohm', 'Pixel', 'Green']\n"
]
],
[
[
"Translated: Northernlion, RockLeeSmile, CobaltStreak, AlpacaPatrol, LastGreyWolf, HCJustin, BaerTaffy, JSmithOTI, Sinvicta, DanGheesling, MALF, FlackBlag, TotalBiscuit, LovelyMomo, Blueman, BaerTaffy, MathasGames, Crendor, BananasaurusRex, NOTREAL, AlpacaPatrol, DanGheesling, BananasaurusRex, MALF, Arumba, BaerTaffy, CobaltStreak, MALF, Magresta, Northernlion, JSmithOTI, RockLeeSmile, LovelyMomo, MikeBithell, RedPandaGamer, OhmWrecker, PrescriptionPixel, Green9090",
"_____no_output_____"
]
],
[
[
"foo = \"Northernlion, RockLeeSmile, CobaltStreak, AlpacaPatrol, LastGreyWolf, HCJustin, BaerTaffy, JSmithOTI, Sinvicta, DanGheesling, MALF, FlackBlag, TotalBiscuit, LovelyMomo, Blueman, BaerTaffy, MathasGames, Crendor, BananasaurusRex, NOTREAL, AlpacaPatrol, DanGheesling, NOTREAL, BananasaurusRex, MALF, Arumba, BaerTaffy, CobaltStreak, MALF, Magresta, Northernlion, JSmithOTI, RockLeeSmile, LovelyMomo, MikeBithell, RedPandaGamer, OhmWrecker, PrescriptionPixel, Green9090\"\ntranslated = foo.split(\", \")\ntranslated",
"_____no_output_____"
],
[
"guests = []\nfor cast in fullstripped:\n guests.append([translated[names.index(user)] for user in cast])",
"_____no_output_____"
],
[
"#Replace first names with second names\nguests[0]",
"_____no_output_____"
]
],
[
[
"Looking better. Let's swap it into our DF.",
"_____no_output_____"
]
],
[
[
"nlss_df['Crew'] = guests\nnlss_df.head()",
"_____no_output_____"
]
],
[
[
"# Adding more stats",
"_____no_output_____"
],
[
"File from https://sullygnome.com/channel/Northernlion/365/streams\n\nThis version can only go back 365 days. Can we create a column for date that matches nlss_df format? If so, we can combine overlapping stats. I also have a larger CSV which I'm working on in FullCSV.ipynb. I will combine these once formated correctly.",
"_____no_output_____"
]
],
[
[
"import os\nimport glob\nprint(os.getcwd())\n\nallFiles = glob.glob(r\"data\\*.csv\")\nstream_df = pd.DataFrame()\nl = []\nfor foo in allFiles:\n stream_df = pd.read_csv(foo,index_col=None, header=0)\n l.append(stream_df)\nstream_df = pd.concat(l)\n\n#stream_df = pd.read_csv(r'StreamStats365.csv')\nstream_df",
"C:\\Users\\sonofabrat\\Documents\\Data_Science\\NLSS_Project\n"
],
[
"formatted = []\norder = [1,0,2]\nfor date in stream_df['Stream start time']:\n dmy = date.split(' ')[1:-1] #Date/Month/Year\n dmy[0] = dmy[0][:-2] #Remove day suffixes\n mdy = [dmy[i] for i in order]\n formatted.append(str(mdy[0] + \" \" + mdy[1] + \", \" + mdy[2]))\nformatted[:15]",
"_____no_output_____"
],
[
"stream_df[\"Date\"] = formatted\nstream_df = stream_df.reset_index()\nstream_df.index = stream_df[\"index\"]\nstream_df = stream_df.drop('index', axis=1)\nstream_df.head()",
"_____no_output_____"
]
],
[
[
"There was a day where an extra non-NLSS stream happened. It messes up our ordering so let's remove it.",
"_____no_output_____"
]
],
[
[
"stream_df[stream_df[\"Date\"]=='January 4, 2017']",
"_____no_output_____"
],
[
"stream_df = stream_df[stream_df['Unnamed: 0'] != 0]\nstream_df[stream_df[\"Date\"]=='January 4, 2017']",
"_____no_output_____"
],
[
"combined = nlss_df.merge(stream_df)\n#drop eronious columns\ncombined = combined.drop('Games', 1)\ncombined = combined.drop('Unnamed: 0', 1)",
"_____no_output_____"
],
[
"nlss_df.head()",
"_____no_output_____"
],
[
"combined.head()",
"_____no_output_____"
],
[
"result = pd.concat([nlss_df, combined], axis=1)\n#Removes repeat columns\nresult = result.T.groupby(level=0).first().T\n#Reorder columns\nresult = result[['Date','Crew','Docket','Stream start time','End time','Stream length','Avg Viewers','Peak viewers','Followers gained','Followers per hour','Views','Views per hour']]",
"_____no_output_____"
],
[
"nlss_df = result\nnlss_df.loc[50]",
"_____no_output_____"
],
[
"nlss_df[70:85]",
"_____no_output_____"
],
[
"nlss_df.loc[nlss_df['Date']==\"Wednesday 8th February 2017 22:15\"]",
"_____no_output_____"
]
],
[
[
"# Let's Explore",
"_____no_output_____"
],
[
"Our stats have been compiled. Now let's look around. Which show had most peak viewers?",
"_____no_output_____"
]
],
[
[
"mpv = nlss_df.loc[nlss_df['Peak viewers'].idxmax()]\nprint(\"Date:\", mpv[\"Date\"])\nprint(\"Peak viewers:\", mpv['Peak viewers'])\nprint(\"Peak percentage:\", (mpv['Peak viewers']/mpv['Views'])*100)\nprint(\"Total viewers:\", mpv['Views'])\nprint(\"Games:\", mpv['Docket'])\nnlss_df.loc[nlss_df['Peak viewers'].idxmax()]",
"Date: December 6, 2016\nPeak viewers: 9752.0\nPeak percentage: 81.37516688918558\nTotal viewers: 11984.0\nGames: ['Ultimate Chicken Horse', ' Move or Die', ' Quiplash']\n"
],
[
"nlss_df.head()",
"_____no_output_____"
]
],
[
[
"# NLSS Dataframe",
"_____no_output_____"
]
],
[
[
"len(nlss_df)\nnlss_df.head()\nnlss_df.tail()",
"_____no_output_____"
]
],
[
[
"8/25/2017 - 2/25/2013",
"_____no_output_____"
],
[
"# Current Goals",
"_____no_output_____"
],
[
"I'm working on an ipynb called FullCSV. I got this by contacting the owner of Sullygnome.com. This CSV goes back further than the ones I've been working with and so will be helpful to use. However, there are differences in how it is formated compared to the CSVs used here. I'm currently working to set the dates up in the same format so I can add in the stats from FullCSV not found here.\n\nMy new major issue is rather recent. Twitch recently (earlier this week as of writing) [changed their API](https://blog.twitch.tv/the-new-twitch-api-be3fb2b078e6), breaking most existing apps using it. This new update requires new types of authenication. I'm working on learning the new API, however my formerly working Twitch comment downloader is not in working order yet. As far as I can tell, none of the comment downloaders I found on Github currently work, but a few developers are working on updating them to the newest API.\n\nI converted a file of Twitch emote commands from Twitch's API site. I will attempt to find user channel specific emote commands and combine it with list. I can then use this master list to search for or omit emotes from analysis.",
"_____no_output_____"
],
[
"# Sharability of Data",
"_____no_output_____"
],
[
"I've been in contact with the people who created the NLSS Docket list and the CSVs. They both gave me permission to use and publicly release the data in my project. I will work on organizing a cleaned up folder of my data to publish on Github.",
"_____no_output_____"
]
]
] | [
"markdown",
"code",
"markdown",
"code",
"markdown",
"code",
"markdown",
"code",
"markdown",
"code",
"markdown",
"code",
"markdown",
"code",
"markdown",
"code",
"markdown",
"code",
"markdown",
"code",
"markdown",
"code",
"markdown",
"code",
"markdown",
"code",
"markdown",
"code",
"markdown",
"code",
"markdown",
"code",
"markdown",
"code",
"markdown",
"code",
"markdown",
"code",
"markdown"
] | [
[
"markdown",
"markdown"
],
[
"code",
"code"
],
[
"markdown"
],
[
"code"
],
[
"markdown"
],
[
"code"
],
[
"markdown"
],
[
"code"
],
[
"markdown"
],
[
"code"
],
[
"markdown"
],
[
"code"
],
[
"markdown"
],
[
"code"
],
[
"markdown"
],
[
"code",
"code",
"code"
],
[
"markdown"
],
[
"code",
"code"
],
[
"markdown"
],
[
"code",
"code",
"code"
],
[
"markdown"
],
[
"code"
],
[
"markdown"
],
[
"code"
],
[
"markdown"
],
[
"code"
],
[
"markdown"
],
[
"code",
"code",
"code"
],
[
"markdown"
],
[
"code"
],
[
"markdown",
"markdown"
],
[
"code",
"code",
"code"
],
[
"markdown"
],
[
"code",
"code",
"code",
"code",
"code",
"code",
"code",
"code",
"code"
],
[
"markdown",
"markdown"
],
[
"code",
"code"
],
[
"markdown"
],
[
"code"
],
[
"markdown",
"markdown",
"markdown",
"markdown",
"markdown"
]
] |
d07a7607dff090670ca181cc26ea8a42d0cc1719 | 40,440 | ipynb | Jupyter Notebook | notebook/taruma_udemy_boltzmann.ipynb | taruma/hidrokit-nb | 5f2ddfe1240b3f30fd6b94954628aba75e0e08d7 | [
"MIT"
] | 1 | 2020-07-20T23:52:46.000Z | 2020-07-20T23:52:46.000Z | notebook/taruma_udemy_boltzmann.ipynb | taruma/hidrokit-nb | 5f2ddfe1240b3f30fd6b94954628aba75e0e08d7 | [
"MIT"
] | 45 | 2019-06-21T02:42:47.000Z | 2020-02-18T00:53:43.000Z | notebook/taruma_udemy_boltzmann.ipynb | taruma/hidrokit-nb | 5f2ddfe1240b3f30fd6b94954628aba75e0e08d7 | [
"MIT"
] | 8 | 2019-06-21T02:31:33.000Z | 2020-04-06T23:20:18.000Z | 40.359281 | 3,683 | 0.326657 | [
[
[
"# Boltzmann Machines\n\nNotebook ini berdasarkan kursus __Deep Learning A-Z™: Hands-On Artificial Neural Networks__ di Udemy. [Lihat Kursus](https://www.udemy.com/deeplearning/).\n\n## Informasi Notebook\n- __notebook name__: `taruma_udemy_boltzmann`\n- __notebook version/date__: `1.0.0`/`20190730`\n- __notebook server__: Google Colab\n- __python version__: `3.6`\n- __pytorch version__: `1.1.0`",
"_____no_output_____"
]
],
[
[
"#### NOTEBOOK DESCRIPTION\n\nfrom datetime import datetime\n\nNOTEBOOK_TITLE = 'taruma_udemy_boltzmann'\nNOTEBOOK_VERSION = '1.0.0'\nNOTEBOOK_DATE = 1 # Set 1, if you want add date classifier\n\nNOTEBOOK_NAME = \"{}_{}\".format(\n NOTEBOOK_TITLE, \n NOTEBOOK_VERSION.replace('.','_')\n)\nPROJECT_NAME = \"{}_{}{}\".format(\n NOTEBOOK_TITLE, \n NOTEBOOK_VERSION.replace('.','_'), \n \"_\" + datetime.utcnow().strftime(\"%Y%m%d_%H%M\") if NOTEBOOK_DATE else \"\"\n)\n\nprint(f\"Nama Notebook: {NOTEBOOK_NAME}\")\nprint(f\"Nama Proyek: {PROJECT_NAME}\")",
"Nama Notebook: taruma_udemy_boltzmann_1_0_0\nNama Proyek: taruma_udemy_boltzmann_1_0_0_20190730_0822\n"
],
[
"#### System Version\nimport sys, torch\nprint(\"versi python: {}\".format(sys.version))\nprint(\"versi pytorch: {}\".format(torch.__version__))",
"versi python: 3.6.8 (default, Jan 14 2019, 11:02:34) \n[GCC 8.0.1 20180414 (experimental) [trunk revision 259383]]\nversi pytorch: 1.1.0\n"
],
[
"#### Load Notebook Extensions\n%load_ext google.colab.data_table",
"_____no_output_____"
],
[
"#### Download dataset\n# ref: https://grouplens.org/datasets/movielens/\n!wget -O boltzmann.zip \"https://sds-platform-private.s3-us-east-2.amazonaws.com/uploads/P16-Boltzmann-Machines.zip\"\n!unzip boltzmann.zip",
"_____no_output_____"
],
[
"#### Atur dataset path\nDATASET_DIRECTORY = 'Boltzmann_Machines/'",
"_____no_output_____"
],
[
"def showdata(dataframe):\n print('Dataframe Size: {}'.format(dataframe.shape))\n return dataframe",
"_____no_output_____"
]
],
[
[
"# STEP 1-5 DATA PREPROCESSING",
"_____no_output_____"
]
],
[
[
"# Importing the libraries\nimport numpy as np\nimport pandas as pd\nimport torch\nimport torch.nn as nn\nimport torch.nn.parallel\nimport torch.optim as optim\nimport torch.utils.data\nfrom torch.autograd import Variable",
"_____no_output_____"
],
[
"movies = pd.read_csv(DATASET_DIRECTORY + 'ml-1m/movies.dat', sep='::', header=None, engine='python', encoding='latin-1')\nshowdata(movies).head(10)",
"Dataframe Size: (3883, 3)\n"
],
[
"users = pd.read_csv(DATASET_DIRECTORY + 'ml-1m/users.dat', sep='::', header=None, engine='python', encoding='latin-1')\nshowdata(users).head(10)",
"Dataframe Size: (6040, 5)\n"
],
[
"ratings = pd.read_csv(DATASET_DIRECTORY + 'ml-1m/ratings.dat', sep='::', header=None, engine='python', encoding='latin-1')\nshowdata(ratings).head(10)",
"Dataframe Size: (1000209, 4)\n"
],
[
"# Preparing the training set and the test set\ntraining_set = pd.read_csv(DATASET_DIRECTORY + 'ml-100k/u1.base', delimiter='\\t')\ntraining_set = np.array(training_set, dtype='int')\ntest_set = pd.read_csv(DATASET_DIRECTORY + 'ml-100k/u1.test', delimiter='\\t')\ntest_set = np.array(test_set, dtype='int')",
"_____no_output_____"
],
[
"# Getting the number of users and movies\nnb_users = int(max(max(training_set[:, 0]), max(test_set[:, 0])))\nnb_movies = int(max(max(training_set[:, 1]), max(test_set[:, 1])))",
"_____no_output_____"
],
[
"# Converting the data into an array with users in lines and movies in columns\ndef convert(data):\n new_data = []\n for id_users in range(1, nb_users+1):\n id_movies = data[:, 1][data[:, 0] == id_users]\n id_ratings = data[:, 2][data[:, 0] == id_users]\n ratings = np.zeros(nb_movies)\n ratings[id_movies - 1] = id_ratings\n new_data.append(list(ratings))\n return new_data\n\ntraining_set = convert(training_set)\ntest_set = convert(test_set)",
"_____no_output_____"
],
[
"# Converting the data into Torch tensors\ntraining_set = torch.FloatTensor(training_set)\ntest_set = torch.FloatTensor(test_set)",
"_____no_output_____"
],
[
"training_set.",
"_____no_output_____"
]
],
[
[
"# STEP 6",
"_____no_output_____"
]
],
[
[
"# Converting the ratings into binary ratings 1 (Liked) or 0 (Not Liked)\ntraining_set[training_set == 0] = -1\ntraining_set[training_set == 1] = 0\ntraining_set[training_set == 2] = 0\ntraining_set[training_set >= 3] = 1\n\ntest_set[test_set == 0] = -1\ntest_set[test_set == 1] = 0\ntest_set[test_set == 2] = 0\ntest_set[test_set >= 3] = 1",
"_____no_output_____"
],
[
"training_set",
"_____no_output_____"
]
],
[
[
"# STEP 7 - 10 Building RBM Object",
"_____no_output_____"
]
],
[
[
"# Creating the architecture of the Neural Network\n# nv = number visible nodes, nh = number hidden nodes\nclass RBM():\n def __init__(self, nv, nh):\n self.W = torch.randn(nh, nv)\n self.a = torch.randn(1, nh)\n self.b = torch.randn(1, nv)\n def sample_h(self, x):\n wx = torch.mm(x, self.W.t())\n activation = wx + self.a.expand_as(wx)\n p_h_given_v = torch.sigmoid(activation)\n return p_h_given_v, torch.bernoulli(p_h_given_v)\n def sample_v(self, y):\n wy = torch.mm(y, self.W)\n activation = wy + self.b.expand_as(wy)\n p_v_given_h = torch.sigmoid(activation)\n return p_v_given_h, torch.bernoulli(p_v_given_h)\n def train(self, v0, vk, ph0, phk):\n self.W += (torch.mm(v0.t(), ph0) - torch.mm(vk.t(), phk)).t()\n self.b += torch.sum((v0 - vk), 0)\n self.a += torch.sum((ph0 - phk), 0)",
"_____no_output_____"
]
],
[
[
"# STEP 11",
"_____no_output_____"
]
],
[
[
"nv = len(training_set[0])\nnh = 100\nbatch_size = 100\nrbm = RBM(nv, nh)",
"_____no_output_____"
]
],
[
[
"# STEP 12-13",
"_____no_output_____"
]
],
[
[
"# Training the RBM\nnb_epochs = 10\nfor epoch in range(1, nb_epochs + 1):\n train_loss = 0\n s = 0.\n for id_user in range(0, nb_users - batch_size, batch_size):\n vk = training_set[id_user:id_user+batch_size]\n v0 = training_set[id_user:id_user+batch_size]\n ph0,_ = rbm.sample_h(v0)\n for k in range(10):\n _,hk = rbm.sample_h(vk)\n _,vk = rbm.sample_v(hk)\n vk[v0<0] = v0[v0<0]\n phk,_ = rbm.sample_h(vk)\n rbm.train(v0, vk, ph0, phk)\n train_loss += torch.mean(torch.abs(v0[v0>=0] - vk[v0>=0]))\n s += 1.\n print('epoch: '+str(epoch)+' loss: '+str(train_loss/s))",
"epoch: 1 loss: tensor(0.3424)\nepoch: 2 loss: tensor(0.2527)\nepoch: 3 loss: tensor(0.2509)\nepoch: 4 loss: tensor(0.2483)\nepoch: 5 loss: tensor(0.2474)\nepoch: 6 loss: tensor(0.2478)\nepoch: 7 loss: tensor(0.2467)\nepoch: 8 loss: tensor(0.2461)\nepoch: 9 loss: tensor(0.2482)\nepoch: 10 loss: tensor(0.2491)\n"
]
],
[
[
"# STEP 14",
"_____no_output_____"
]
],
[
[
"# Testing the RBM\ntest_loss = 0\ns = 0.\nfor id_user in range(nb_users):\n v = training_set[id_user:id_user+1]\n vt = test_set[id_user:id_user+1]\n if len(vt[vt>=0]) > 0:\n _,h = rbm.sample_h(v)\n _,v = rbm.sample_v(h)\n test_loss += torch.mean(torch.abs(vt[vt>=0] - v[vt>=0]))\n s += 1.\nprint('test loss: '+str(test_loss/s))",
"test loss: tensor(0.2403)\n"
]
]
] | [
"markdown",
"code",
"markdown",
"code",
"markdown",
"code",
"markdown",
"code",
"markdown",
"code",
"markdown",
"code",
"markdown",
"code"
] | [
[
"markdown"
],
[
"code",
"code",
"code",
"code",
"code",
"code"
],
[
"markdown"
],
[
"code",
"code",
"code",
"code",
"code",
"code",
"code",
"code",
"code"
],
[
"markdown"
],
[
"code",
"code"
],
[
"markdown"
],
[
"code"
],
[
"markdown"
],
[
"code"
],
[
"markdown"
],
[
"code"
],
[
"markdown"
],
[
"code"
]
] |
d07a957192475aea1189fa16709df113b7038199 | 138,437 | ipynb | Jupyter Notebook | Python_Stock/Technical_Indicators/Volume_Weighted_Moving_Average.ipynb | chunsj/Stock_Analysis_For_Quant | 5f28ef9537885a695245d26f3010592a29d45a34 | [
"MIT"
] | 962 | 2019-07-17T09:57:41.000Z | 2022-03-29T01:55:20.000Z | Python_Stock/Technical_Indicators/Volume_Weighted_Moving_Average.ipynb | chunsj/Stock_Analysis_For_Quant | 5f28ef9537885a695245d26f3010592a29d45a34 | [
"MIT"
] | 5 | 2020-04-29T16:54:30.000Z | 2022-02-10T02:57:30.000Z | Python_Stock/Technical_Indicators/Volume_Weighted_Moving_Average.ipynb | chunsj/Stock_Analysis_For_Quant | 5f28ef9537885a695245d26f3010592a29d45a34 | [
"MIT"
] | 286 | 2019-08-04T10:37:58.000Z | 2022-03-28T06:31:56.000Z | 202.09781 | 66,650 | 0.85912 | [
[
[
"# Volume-Weighted Moving Average (VWMA) ",
"_____no_output_____"
],
[
"https://www.tradingsetupsreview.com/volume-weighted-moving-average-vwma/",
"_____no_output_____"
]
],
[
[
"import numpy as np\nimport pandas as pd\nimport matplotlib.pyplot as plt\n\nimport warnings\nwarnings.filterwarnings(\"ignore\")\n\n# fix_yahoo_finance is used to fetch data \nimport fix_yahoo_finance as yf\nyf.pdr_override()",
"_____no_output_____"
],
[
"# input\nsymbol = 'AAPL'\nstart = '2018-12-01'\nend = '2019-02-01'\n\n# Read data \ndf = yf.download(symbol,start,end)\n\n# View Columns\ndf.head()",
"[*********************100%***********************] 1 of 1 downloaded\n"
],
[
"import talib as ta",
"_____no_output_____"
],
[
"df['SMA'] = ta.SMA(df['Adj Close'], timeperiod=3)",
"_____no_output_____"
],
[
"df['VWMA'] = ((df['Adj Close']*df['Volume'])+(df['Adj Close'].shift(1)*df['Volume'].shift(1))+(df['Adj Close'].shift(2)*df['Volume'].shift(2))) / (df['Volume'].rolling(3).sum())\ndf.head()",
"_____no_output_____"
],
[
"def VWMA(close,volume, n):\n cv =pd.Series(close.shift(n) * volume.shift(n))\n tv = volume.rolling(n).sum()\n vwma = cv/tv\n return vwma\n\nVWMA(df['Adj Close'],df['Volume'], 3)",
"_____no_output_____"
],
[
"plt.figure(figsize=(14,8))\nplt.plot(df['Adj Close'])\nplt.plot(df['VWMA'], label='Volume Weighted Moving Average')\nplt.plot(df['SMA'], label='Simple Moving Average')\nplt.legend(loc='best')\nplt.title('Stock of Midpoint Method')\nplt.xlabel('Date')\nplt.ylabel('Price')\nplt.show()",
"_____no_output_____"
]
],
[
[
"## Candlestick with VWMA",
"_____no_output_____"
]
],
[
[
"from matplotlib import dates as mdates\nimport datetime as dt\n\ndfc = df.copy()\ndfc['VolumePositive'] = dfc['Open'] < dfc['Adj Close']\n#dfc = dfc.dropna()\ndfc = dfc.reset_index()\ndfc['Date'] = mdates.date2num(dfc['Date'].astype(dt.date))\ndfc.head()",
"_____no_output_____"
],
[
"from mpl_finance import candlestick_ohlc\n\nfig = plt.figure(figsize=(14,10))\nax1 = plt.subplot(2, 1, 1)\ncandlestick_ohlc(ax1,dfc.values, width=0.5, colorup='g', colordown='r', alpha=1.0)\nax1.plot(df['VWMA'], label='Volume Weighted Moving Average')\nax1.plot(df['SMA'], label='Simple Moving Average')\nax1.set_title('Stock '+ symbol +' Closing Price')\nax1.set_ylabel('Price')\nax1.xaxis_date()\nax1.xaxis.set_major_formatter(mdates.DateFormatter('%d-%m-%Y'))\nax1.grid(True, which='both')\nax1.minorticks_on()\nax1v = ax1.twinx()\ncolors = dfc.VolumePositive.map({True: 'g', False: 'r'})\nax1v.bar(dfc.Date, dfc['Volume'], color=colors, alpha=0.4)\nax1v.axes.yaxis.set_ticklabels([])\nax1v.set_ylim(0, 3*df.Volume.max())\nax1.set_title('Stock '+ symbol +' Closing Price')\nax1.set_ylabel('Price')\nax1.legend(loc='best')",
"_____no_output_____"
]
]
] | [
"markdown",
"code",
"markdown",
"code"
] | [
[
"markdown",
"markdown"
],
[
"code",
"code",
"code",
"code",
"code",
"code",
"code"
],
[
"markdown"
],
[
"code",
"code"
]
] |
d07aa72d408df0a807ac376e417a920f575dfa52 | 2,819 | ipynb | Jupyter Notebook | pelajaran/.ipynb_checkpoints/01_Bab_1_Pendahuluan-checkpoint.ipynb | psychohaxer/tutorial-python-bahasa-indonesia | 7a6cae9cee116e44208a54c335c0d88260428a49 | [
"MIT"
] | 2 | 2021-02-03T01:46:48.000Z | 2021-12-27T08:06:00.000Z | pelajaran/.ipynb_checkpoints/01_Bab_1_Pendahuluan-checkpoint.ipynb | psychohaxer/tutorial-python-bahasa-indonesia | 7a6cae9cee116e44208a54c335c0d88260428a49 | [
"MIT"
] | null | null | null | pelajaran/.ipynb_checkpoints/01_Bab_1_Pendahuluan-checkpoint.ipynb | psychohaxer/tutorial-python-bahasa-indonesia | 7a6cae9cee116e44208a54c335c0d88260428a49 | [
"MIT"
] | null | null | null | 39.704225 | 502 | 0.687123 | [
[
[
"# Modul Python Bahasa Indonesia\n## Seri Pertama\n___\nCoded by psychohaxer | Version 1.8 (2020.12.13)\n___\nNotebook ini berisi contoh kode dalam Python sekaligus outputnya sebagai referensi dalam coding. Notebook ini boleh disebarluaskan dan diedit tanpa mengubah atau menghilangkan nama pembuatnya. Selamat belajar dan semoga waktu Anda menyenangkan.\n\nCatatan: Modul ini menggunakan Python 3\n\nNotebook ini dilisensikan dibawah [MIT License](https://opensource.org/licenses/MIT).\n___",
"_____no_output_____"
],
[
"## Bab 1 Pendahuluan\n### Python\n<img src=\"img/python-logo.png\">\n\nPython adalah bahasa pemrograman tingkat tinggi yang mengutamakan keterbacaan kode. Bahasa pemrograman serbaguna ini dapat digunakan pada banyak hal seperti pemrograman berorientasi objek, pemrograman imperatif, dan pemrograman fungsional. Tapi tidak terbatas pada itu saja. Python sering digunakan untuk pengolahan data karena banyaknya library yang tersedia.\nSingkatnya, Python adalah bahasa pemrograman serbaguna yang mudah dipahami penulisannya. Notebook ini membahas pembelajaran Python versi 3.\n\n### Jupyter Notebook\n<img src=\"img/jupyter-logo.png\">\n\nJupyter Notebook (sebelumnya IPython Notebooks) adalah aplikasi untuk mengembangkan program berbahasa Python. Yang membedakan Jupyter dari IDE lainnya adalah kemampuannya membuat dokumentasi dengan baik, menampilkan dan menyimpan output (termasuk gambar), dan bisa disimpan ke berbagai macam format (PDF, HTML, Latex, juga tentunya file Python). File Jupyter menggunakan ekstensi .ipynb yang didalamnya memuat dokumentasi dan tentu saja kode program termasuk output jika ada dalam bentuk JSON.\n\nKode dalam Jupyter disimpan dalam _cell_ dimana masing-masing cell akan menampilkan outputnya setelah dijalankan jika tersedia. Ini memudahkan proses debugging karena output dapat langsung dilihat, mengingat Python adalah _intepreted language_.",
"_____no_output_____"
],
[
"___\ncoded with ❤ by [psychohaxer](http://github.com/psychohaxer)\n___",
"_____no_output_____"
]
]
] | [
"markdown"
] | [
[
"markdown",
"markdown",
"markdown"
]
] |
d07aa8e4473bc13323fb91c09616d73fe09c33da | 157,288 | ipynb | Jupyter Notebook | notebooks/One1DExample.ipynb | enthought/sandia-blusky | bbefd799fa3e4215896006f8de51ce057e47e23e | [
"BSD-3-Clause"
] | 3 | 2020-03-26T15:10:25.000Z | 2020-11-11T22:13:53.000Z | notebooks/One1DExample.ipynb | enthought/sandia-blusky | bbefd799fa3e4215896006f8de51ce057e47e23e | [
"BSD-3-Clause"
] | 42 | 2019-06-24T15:56:12.000Z | 2020-01-15T21:42:21.000Z | notebooks/One1DExample.ipynb | enthought/sandia-blusky | bbefd799fa3e4215896006f8de51ce057e47e23e | [
"BSD-3-Clause"
] | 4 | 2020-07-22T11:33:54.000Z | 2021-03-02T21:16:23.000Z | 406.428941 | 52,676 | 0.93126 | [
[
[
"As a demonstration, create an ARMA22 model drawing innovations from there different distributions, a bernoulli, normal and inverse normal. Then build a keras/tensorflow model for the 1-d scattering transform to create \"features\", use these features to classify which model for the innovations was used.",
"_____no_output_____"
]
],
[
[
"from blusky.blusky_models import build_model_1d",
"_____no_output_____"
],
[
"import matplotlib.pylab as plt\nimport numpy as np\nfrom scipy.stats import bernoulli, norm, norminvgauss\n\ndef arma22(N, alpha, beta, rnd, eps=0.5):\n inov = rnd.rvs(2*N)\n x = np.zeros(2*N)\n\n # arma22 mode\n for i in range(2,N*2):\n x[i] = (alpha[0] * x[i-1] + alpha[1]*x[i-2] + \n beta[0] * inov[i-1] + beta[1] * inov[i-2] + eps * inov[i])\n\n return x[N:]\n\nN = 512\nk = 10\n\nalpha = [0.99, -0.1]\nbeta = [0.2, 0.0]\neps = 1\n\nseries = np.zeros((24*k, N))\ny = np.zeros(24*k)\nfor i in range(8*k):\n series[i, :] = arma22(N, alpha, beta, norm(1.0), eps=eps)\n y[i] = 0\n \nfor i in range(8*k, 16*k):\n series[i, :] = arma22(N, alpha, beta, norminvgauss(1,0.5), eps=eps)\n y[i] = 1\n \nfor i in range(16*k, 24*k):\n series[i, :] = arma22(N, alpha, beta, bernoulli(0.5), eps=eps)*2\n y[i] = 2\n\nplt.plot(series[3*k,:200], '-r')\nplt.plot(series[8*k,:200])\nplt.plot(series[-3*k,:200])\nplt.legend(['normal', 'inverse normal', 'bernoulli'])",
"_____no_output_____"
],
[
"#Hold out data:\nk = 8\nhodl_series = np.zeros((24*k, N))\nhodl_y = np.zeros(24*k)\nfor i in range(8*k):\n hodl_series[i, :] = arma22(N, alpha, beta, norm(1.0), eps=eps)\n hodl_y[i] = 0\n \nfor i in range(8*k, 16*k):\n hodl_series[i, :] = arma22(N, alpha, beta, norminvgauss(1,0.5), eps=eps)\n hodl_y[i] = 1\n \nfor i in range(16*k, 24*k):\n hodl_series[i, :] = arma22(N, alpha, beta, bernoulli(0.5), eps=eps)*2\n hodl_y[i] = 2\n \n\n# hold out data\nplt.plot(hodl_series[0,:200], '-r')\nplt.plot(hodl_series[8*k,:200])\nplt.plot(hodl_series[16*k,:200])\nplt.legend(['normal', 'inverse normal', 'bernoulli'])",
"_____no_output_____"
]
],
[
[
"The scattering transform reduces the timeseries to a set of features, which we use for classification. The seperation between the series is more obvious looking at the log- of the features (see below). A support vector machine has an easy time classifying these processes.",
"_____no_output_____"
]
],
[
[
"base_model = build_model_1d(N, 7,6, concatenate=True)\nresult = base_model.predict(hodl_series)\n\nplt.semilogy(np.mean(result[:,0,:], axis=0), '-r')\nplt.semilogy(np.mean(result[8*k:16*k,0,:], axis=0), '-b')\nplt.semilogy(np.mean(result[16*k:,0,:], axis=0), '-g')",
"_____no_output_____"
],
[
"from sklearn.svm import SVC\nfrom sklearn.metrics import classification_report\n\nmodel = build_model_1d(N, 7, 6, concatenate=True)\nresult = np.log(model.predict(series))\nX = result[:,0,:]\nrdf = SVC()\nrdf.fit(X,y)\n\nhodl_result = np.log(model.predict(hodl_series))\nhodl_X = hodl_result[:,0,:]\ny_pred = rdf.predict(hodl_X)\n\ncls1 = classification_report(hodl_y, y_pred)\nprint(cls1)",
" precision recall f1-score support\n\n 0.0 0.95 0.91 0.93 64\n 1.0 0.97 0.95 0.96 64\n 2.0 0.94 1.00 0.97 64\n\n accuracy 0.95 192\n macro avg 0.95 0.95 0.95 192\nweighted avg 0.95 0.95 0.95 192\n\n"
]
],
[
[
"Blusky build_model_1d creates a regular old keras model, which you can use like another, think VGG16 etc. The order (order < J) defines the depth of the network. If you want a deeper network, increase this parameter. Here we attach a set of fully connected layers to classify like we did previously with the SVM.\n\nDropping in a batch normalization here, seeems to be important for regularizong the problem.",
"_____no_output_____"
]
],
[
[
"from tensorflow.keras import Input, Model\nimport tensorflow.keras.backend as K\nfrom tensorflow.keras.callbacks import EarlyStopping\nfrom tensorflow.keras.layers import BatchNormalization, Dense, Flatten, Lambda\nfrom tensorflow.keras.utils import to_categorical\n\nearly_stopping = EarlyStopping(monitor=\"val_loss\", patience=50, verbose=True, \n restore_best_weights=True)\n\nJ = 7\norder = 6\nbase_model = build_model_1d(N, J, order, concatenate=True)\n\ndnn = Flatten()(base_model.output)\n\n# let's add the \"log\" here like we did above\ndnn = Lambda(lambda x : K.log(x))(dnn)\n\ndnn = BatchNormalization()(dnn)\n\ndnn = Dense(32, activation='linear', name='dnn1')(dnn)\ndnn = Dense(3, activation='softmax', name='softmax')(dnn)\n\ndeep_model_1 = Model(inputs=base_model.input, outputs=dnn)\ndeep_model_1.compile(optimizer='rmsprop', loss='categorical_crossentropy')\n\nhistory_1 = deep_model_1.fit(series, to_categorical(y), \n validation_data=(hodl_series, to_categorical(hodl_y)), \n callbacks=[early_stopping],\n epochs=200)\n\ny_pred = deep_model_1.predict(hodl_series)\n\ncls_2 = classification_report(hodl_y, np.argmax(y_pred, axis=1))",
"_____no_output_____"
],
[
"base_model.output",
"_____no_output_____"
],
[
"plt.plot(history_1.history['loss'][-100:])\nplt.plot(history_1.history['val_loss'][-100:])",
"_____no_output_____"
],
[
"print(cls_2)",
" precision recall f1-score support\n\n 0.0 0.92 0.84 0.88 64\n 1.0 0.94 0.95 0.95 64\n 2.0 0.91 0.97 0.94 64\n\n accuracy 0.92 192\n macro avg 0.92 0.92 0.92 192\nweighted avg 0.92 0.92 0.92 192\n\n"
]
]
] | [
"markdown",
"code",
"markdown",
"code",
"markdown",
"code"
] | [
[
"markdown"
],
[
"code",
"code",
"code"
],
[
"markdown"
],
[
"code",
"code"
],
[
"markdown"
],
[
"code",
"code",
"code",
"code"
]
] |
d07ab040c7419cee18e41e5fbd1187b380f64381 | 701,404 | ipynb | Jupyter Notebook | 3.feature-differences/1.apply-signatures.ipynb | DavidStirling/profiling-resistance-mechanisms | 5848c9c8605e3b6ff4f7331906cbf2d956b8b9ab | [
"BSD-3-Clause"
] | null | null | null | 3.feature-differences/1.apply-signatures.ipynb | DavidStirling/profiling-resistance-mechanisms | 5848c9c8605e3b6ff4f7331906cbf2d956b8b9ab | [
"BSD-3-Clause"
] | null | null | null | 3.feature-differences/1.apply-signatures.ipynb | DavidStirling/profiling-resistance-mechanisms | 5848c9c8605e3b6ff4f7331906cbf2d956b8b9ab | [
"BSD-3-Clause"
] | null | null | null | 445.618806 | 189,448 | 0.900023 | [
[
[
"# Apply Signature Analysis to Cell Morphology Features\n\nGregory Way, 2020\n\nHere, I apply [`singscore`](https://bioconductor.org/packages/devel/bioc/vignettes/singscore/inst/doc/singscore.html) ([Foroutan et al. 2018](https://doi.org/10.1186/s12859-018-2435-4)) to our Cell Painting profiles.\nThis notebook largely follows the [package vignette](https://bioconductor.org/packages/devel/bioc/vignettes/singscore/inst/doc/singscore.html).\n\nI generate two distinct signatures.\n\n1. Comparing Clone A and E resistant clones to sensitive wildtype cell lines.\n * Clones A and E both have a confirmed _PSMB5_ mutation which is known to cause bortezomib resistance.\n2. Derived from comparing four other resistant clones to four other sensitive wildtype clones.\n * We do not know the resistance mechanism in these four resistant clones.\n\nHowever, we can hypothesize that the mechanisms are similar based on single sample enrichment using the potential PSMB5 signature.\n\nTo review how I derived these signatures see `0.build-morphology-signatures.ipynb`.",
"_____no_output_____"
]
],
[
[
"suppressPackageStartupMessages(library(singscore))\nsuppressPackageStartupMessages(library(dplyr))\nsuppressPackageStartupMessages(library(ggplot2))",
"_____no_output_____"
],
[
"seed <- 1234\nnum_permutations <- 1000",
"_____no_output_____"
],
[
"set.seed(seed)",
"_____no_output_____"
]
],
[
[
"## Load Clone A/E (_PSMB5_ Mutations) Signature",
"_____no_output_____"
]
],
[
[
"sig_cols <- readr::cols(\n feature = readr::col_character(),\n estimate = readr::col_double(),\n adj.p.value = readr::col_double()\n)\n\nsig_file <- file.path(\"results\", \"cloneAE_signature_tukey.tsv\")\npsmb_signature_scores <- readr::read_tsv(sig_file, col_types=sig_cols)\n\nhead(psmb_signature_scores, 2)",
"_____no_output_____"
],
[
"# Extract features that are up and down in the signature\nup_features <- psmb_signature_scores %>% dplyr::filter(estimate > 0) %>% dplyr::pull(feature)\ndown_features <- psmb_signature_scores %>% dplyr::filter(estimate < 0) %>% dplyr::pull(feature)",
"_____no_output_____"
]
],
[
[
"## Load Four Clone Dataset",
"_____no_output_____"
]
],
[
[
"col_types <- readr::cols(\n .default = readr::col_double(),\n Metadata_Plate = readr::col_character(),\n Metadata_Well = readr::col_character(),\n Metadata_plate_map_name = readr::col_character(),\n Metadata_clone_number = readr::col_character(),\n Metadata_clone_type = readr::col_character(),\n Metadata_plate_ID = readr::col_character(),\n Metadata_plate_filename = readr::col_character(),\n Metadata_treatment = readr::col_character(),\n Metadata_batch = readr::col_character()\n)\n\n# Do not load the feature selected data\nprofile_dir <- file.path(\"..\", \"2.describe-data\", \"data\", \"merged\")\nprofile_file <- file.path(profile_dir, \"combined_four_clone_dataset.csv\")\n\nfourclone_data_df <- readr::read_csv(profile_file, col_types = col_types)\n\nprint(dim(fourclone_data_df))\nhead(fourclone_data_df, 2)",
"[1] 300 3537\n"
],
[
"# Generate unique sample names (for downstream merging of results)\nsample_names <- paste(\n fourclone_data_df$Metadata_clone_number,\n fourclone_data_df$Metadata_Plate,\n fourclone_data_df$Metadata_Well,\n fourclone_data_df$Metadata_batch,\n sep = \"_\"\n)\n\nfourclone_data_df <- fourclone_data_df %>%\n dplyr::mutate(Metadata_unique_sample_name = sample_names)",
"_____no_output_____"
]
],
[
[
"## Apply `singscore`",
"_____no_output_____"
]
],
[
[
"# Convert the four clone dataset into a feature x sample matrix without metadata\nfeatures_only_df <- t(fourclone_data_df %>% dplyr::select(!starts_with(\"Metadata_\")))\n\n# Apply the `rankGenes()` method to get feature rankings per feature for each sample\nrankData <- rankGenes(features_only_df)\ncolnames(rankData) <- fourclone_data_df$Metadata_unique_sample_name\n\nprint(dim(rankData))\nhead(rankData, 3)",
"[1] 3528 300\n"
],
[
"# Using the rank dataframe, up, and down features, get the sample scores\nscoredf <- simpleScore(rankData, upSet = up_features, downSet = down_features)\n\n# Merge scores with metadata features\nfull_result_df <- dplyr::bind_cols(\n fourclone_data_df %>% dplyr::select(starts_with(\"Metadata_\")),\n scoredf\n )\n\nprint(dim(full_result_df))\nhead(full_result_df, 2)",
"[1] 300 16\n"
]
],
[
[
"## Perform Permutation Testing to Determine Significance of Observation",
"_____no_output_____"
]
],
[
[
"# Generate a null distribution of scores by randomly shuffling ranks\npermuteResult <- generateNull(\n upSet = up_features,\n downSet = down_features, \n rankData = rankData,\n centerScore = TRUE,\n knownDirection = TRUE,\n B = num_permutations,\n seed = seed,\n useBPPARAM = NULL\n)\n\n# Calculate p values and add to list\npvals <- getPvals(permuteResult, scoredf)\npval_tidy <- broom::tidy(pvals)\ncolnames(pval_tidy) <- c(\"names\", \"Metadata_permuted_p_value\")\n\nfull_result_df <- full_result_df %>%\n dplyr::left_join(\n pval_tidy,\n by = c(\"Metadata_unique_sample_name\" = \"names\")\n )",
"Warning message:\n“'tidy.numeric' is deprecated.\nSee help(\"Deprecated\")”"
],
[
"# Are there differences in quantiles across batch?\nbatch_info <- gsub(\"^.*_\", \"\", rownames(t(permuteResult)))\nbatch_permute <- t(permuteResult) %>%\n dplyr::as_tibble() %>%\n dplyr::mutate(batch = batch_info)\n\npermute_bounds <- list()\nfor (batch_id in unique(batch_permute$batch)) {\n subset_permute <- batch_permute %>% dplyr::filter(batch == !!batch_id) %>% dplyr::select(!batch)\n min_val <- quantile(as.vector(as.matrix(subset_permute)), 0.005)\n max_val <- quantile(as.vector(as.matrix(subset_permute)), 0.995)\n permute_bounds[[batch_id]] <- c(batch_id, min_val, max_val)\n}\n\ndo.call(rbind, permute_bounds)",
"Warning message:\n“`as_tibble.matrix()` requires a matrix with column names or a `.name_repair` argument. Using compatibility `.name_repair`.\n\u001b[90mThis warning is displayed once per session.\u001b[39m”"
]
],
[
[
"## Visualize Results",
"_____no_output_____"
]
],
[
[
"min_val <- quantile(as.vector(as.matrix(permuteResult)), 0.05)\nmax_val <- quantile(as.vector(as.matrix(permuteResult)), 0.95)",
"_____no_output_____"
],
[
"apply_psmb_signature_gg <- ggplot(full_result_df,\n aes(y = TotalScore,\n x = Metadata_clone_number)) +\n geom_boxplot(aes(fill = Metadata_treatment), outlier.alpha = 0) +\n geom_point(\n aes(fill = Metadata_treatment, group = Metadata_treatment),\n position = position_dodge(width=0.75),\n size = 0.9,\n alpha = 0.7,\n shape = 21) +\n scale_fill_manual(name = \"Treatment\",\n labels = c(\"bortezomib\" = \"Bortezomib\", \"DMSO\" = \"DMSO\"),\n values = c(\"bortezomib\" = \"#9e0ba3\", \"DMSO\" = \"#fcba03\")) +\n theme_bw() +\n annotate(\"rect\", ymin = min_val,\n ymax = max_val,\n xmin = 0,\n xmax = length(unique(full_result_df$Metadata_clone_number)) + 1,\n alpha = 0.2,\n color = \"red\",\n linetype = \"dashed\",\n fill = \"grey\") +\n xlab(\"\") +\n ylab(\"PSMB5 Signature Score\") +\n theme(axis.text.x = element_text(angle=90)) +\n facet_wrap(\"Metadata_batch~Metadata_plate_ID\", nrow=3) +\n theme(strip.text = element_text(size = 8, color = \"black\"),\n strip.background = element_rect(colour = \"black\", fill = \"#fdfff4\"))\n\noutput_fig <- file.path(\"figures\", \"signature\", \"psmb5_signature_apply_fourclone.png\")\nggsave(output_fig, dpi = 500, height = 5, width = 10)\napply_psmb_signature_gg",
"_____no_output_____"
],
[
"summarized_mean_result_df <- full_result_df %>%\n dplyr::group_by(\n Metadata_batch, Metadata_plate_map_name, Metadata_clone_number, Metadata_treatment, Metadata_clone_type\n ) %>%\n dplyr::mutate(mean_score = mean(TotalScore)) %>%\n dplyr::select(\n Metadata_batch, Metadata_plate_map_name, Metadata_clone_number, Metadata_clone_type, Metadata_treatment, mean_score\n ) %>%\n dplyr::distinct() %>%\n tidyr::spread(key = \"Metadata_treatment\", value = \"mean_score\") %>%\n dplyr::mutate(treatment_score_diff = DMSO - bortezomib)\n\nhead(summarized_mean_result_df)",
"_____no_output_____"
],
[
"apply_psmb_signature_diff_gg <- ggplot(summarized_mean_result_df,\n aes(y = treatment_score_diff,\n x = Metadata_clone_number,\n fill = Metadata_clone_type)) +\n geom_boxplot(outlier.alpha = 0) +\n geom_jitter(\n width = 0.2,\n size = 2,\n alpha = 0.7,\n shape = 21) +\n\n scale_fill_manual(name = \"Clone Type\",\n labels = c(\"resistant\" = \"Resistant\", \"wildtype\" = \"Wildtype\"),\n values = c(\"resistant\" = \"#9e0ba3\", \"wildtype\" = \"#fcba03\")) +\n theme_bw() +\n xlab(\"\") +\n ylab(\"Difference PSMB5 Signature Score\\nDMSO - Bortezomib\") +\n theme(axis.text.x = element_text(angle=90)) +\n theme(strip.text = element_text(size = 8, color = \"black\"),\n strip.background = element_rect(colour = \"black\", fill = \"#fdfff4\"))\n\noutput_fig <- file.path(\"figures\", \"signature\", \"psmb5_signature_apply_fourclone_difference.png\")\nggsave(output_fig, dpi = 500, height = 4.5, width = 6)\n\napply_psmb_signature_diff_gg",
"_____no_output_____"
]
],
[
[
"## Load Four Clone Signature (Generic Resistance)",
"_____no_output_____"
]
],
[
[
"sig_file <- file.path(\"results\", \"fourclone_signature_tukey.tsv\")\nresistance_signature_scores <- readr::read_tsv(sig_file, col_types=sig_cols)\n\nhead(resistance_signature_scores, 2)",
"_____no_output_____"
],
[
"# Extract features that are up and down in the signature\nup_resistance_features <- resistance_signature_scores %>%\n dplyr::filter(estimate > 0) %>%\n dplyr::pull(feature)\n\ndown_resistance_features <- resistance_signature_scores %>%\n dplyr::filter(estimate < 0) %>%\n dplyr::pull(feature)",
"_____no_output_____"
]
],
[
[
"## Load Clone A/E Dataset",
"_____no_output_____"
]
],
[
[
"# Do not load the feature selected data\nprofile_file <- file.path(profile_dir, \"combined_cloneAcloneE_dataset.csv\")\n\ncloneae_cols <- readr::cols(\n .default = readr::col_double(),\n Metadata_CellLine = readr::col_character(),\n Metadata_Plate = readr::col_character(),\n Metadata_Well = readr::col_character(),\n Metadata_batch = readr::col_character(),\n Metadata_plate_map_name = readr::col_character(),\n Metadata_clone_type = readr::col_character()\n)\n\n\ncloneAE_data_df <- readr::read_csv(profile_file, col_types = cloneae_cols)\n\nprint(dim(cloneAE_data_df))\nhead(cloneAE_data_df, 2)",
"[1] 72 3535\n"
],
[
"# Generate unique sample names (for downstream merging of results)\ncloneae_sample_names <- paste(\n cloneAE_data_df$Metadata_CellLine,\n cloneAE_data_df$Metadata_Plate,\n cloneAE_data_df$Metadata_Well,\n cloneAE_data_df$Metadata_batch,\n sep = \"_\"\n)\n\ncloneAE_data_df <- cloneAE_data_df %>%\n dplyr::mutate(Metadata_unique_sample_name = cloneae_sample_names)",
"_____no_output_____"
]
],
[
[
"## Apply `singscore`",
"_____no_output_____"
]
],
[
[
"# Convert the four clone dataset into a feature x sample matrix without metadata\nfeatures_only_res_df <- t(cloneAE_data_df %>% dplyr::select(!starts_with(\"Metadata_\")))\n\n# Apply the `rankGenes()` method to get feature rankings per feature for each sample\nrankData_res <- rankGenes(features_only_res_df)\ncolnames(rankData_res) <- cloneAE_data_df$Metadata_unique_sample_name\n\nprint(dim(rankData_res))\nhead(rankData_res, 3)",
"[1] 3528 72\n"
],
[
"# Using the rank dataframe, up, and down features, get the sample scores\nscoredf_res <- simpleScore(rankData_res,\n upSet = up_resistance_features,\n downSet = down_resistance_features)\n\n# Merge scores with metadata features\nfull_res_result_df <- dplyr::bind_cols(\n cloneAE_data_df %>% dplyr::select(starts_with(\"Metadata_\")),\n scoredf_res\n )\n\nprint(dim(full_res_result_df))\nhead(full_res_result_df, 2)",
"[1] 72 14\n"
]
],
[
[
"## Perform Permutation Testing",
"_____no_output_____"
]
],
[
[
"# Generate a null distribution of scores by randomly shuffling ranks\npermuteResult_res <- generateNull(\n upSet = up_resistance_features,\n downSet = down_resistance_features, \n rankData = rankData_res,\n centerScore = TRUE,\n knownDirection = TRUE,\n B = num_permutations,\n seed = seed,\n useBPPARAM = NULL\n)\n\n# Calculate p values and add to list\npvals_res <- getPvals(permuteResult_res, scoredf_res)\npval_res_tidy <- broom::tidy(pvals)\ncolnames(pval_res_tidy) <- c(\"names\", \"Metadata_permuted_p_value\")\n\nfull_res_result_df <- full_res_result_df %>%\n dplyr::left_join(\n pval_res_tidy,\n by = c(\"Metadata_unique_sample_name\" = \"names\")\n )",
"Warning message:\n“'tidy.numeric' is deprecated.\nSee help(\"Deprecated\")”"
]
],
[
[
"## Visualize Signature Results",
"_____no_output_____"
]
],
[
[
"min_val <- quantile(as.vector(as.matrix(permuteResult_res)), 0.05)\nmax_val <- quantile(as.vector(as.matrix(permuteResult_res)), 0.95)",
"_____no_output_____"
],
[
"append_dose <- function(string) paste0(\"Dose: \", string, \"nM\")\n\napply_res_signature_gg <- ggplot(full_res_result_df,\n aes(y = TotalScore,\n x = Metadata_CellLine)) +\n geom_boxplot(aes(fill = Metadata_clone_type), outlier.alpha = 0) +\n geom_point(\n aes(fill = Metadata_clone_type, group = Metadata_clone_type),\n position = position_dodge(width=0.75),\n size = 0.9,\n alpha = 0.7,\n shape = 21) +\n scale_fill_manual(name = \"Clone Type\",\n labels = c(\"resistant\" = \"Resistant\", \"wildtype\" = \"WildType\"),\n values = c(\"resistant\" = \"#f5b222\", \"wildtype\" = \"#4287f5\")) +\n theme_bw() +\n annotate(\"rect\", ymin = min_val,\n ymax = max_val,\n xmin = 0,\n xmax = length(unique(full_res_result_df$Metadata_CellLine)) + 1,\n alpha = 0.2,\n color = \"red\",\n linetype = \"dashed\",\n fill = \"grey\") +\n xlab(\"\") +\n ylab(\"Generic Resistance Signature Score\") +\n theme(axis.text.x = element_text(angle=90)) +\n facet_grid(\"Metadata_Dosage~Metadata_batch\",\n labeller = labeller(Metadata_Dosage = as_labeller(append_dose))) +\n theme(strip.text = element_text(size = 8, color = \"black\"),\n strip.background = element_rect(colour = \"black\", fill = \"#fdfff4\"))\n\n\noutput_fig <- file.path(\"figures\", \"signature\", \"generic_resistance_signature_apply_cloneAE.png\")\nggsave(output_fig, dpi = 500, height = 5, width = 5)\napply_res_signature_gg",
"_____no_output_____"
],
[
"full_res_result_df$Metadata_Dosage <- factor(\n full_res_result_df$Metadata_Dosage, levels = unique(sort(full_res_result_df$Metadata_Dosage))\n)\nfull_res_result_df <- full_res_result_df %>%\n dplyr::mutate(Metadata_group = paste0(Metadata_batch, Metadata_CellLine))",
"_____no_output_____"
],
[
"ggplot(full_res_result_df, aes(x = Metadata_Dosage, y = TotalScore, color = Metadata_CellLine, group = Metadata_group)) +\n geom_point(size = 1) +\n geom_smooth(aes(fill = Metadata_clone_type), method = \"loess\", lwd = 0.5) +\n facet_wrap(\"~Metadata_batch\", nrow = 2) +\n theme_bw() +\n scale_fill_manual(name = \"Clone Type\",\n labels = c(\"resistant\" = \"Resistant\", \"wildtype\" = \"WildType\"),\n values = c(\"resistant\" = \"#f5b222\", \"wildtype\" = \"#4287f5\")) +\n ylab(\"Generic Resistance Signature Score\") +\n annotate(\"rect\", ymin = min_val,\n ymax = max_val,\n xmin = 0,\n xmax = length(unique(full_res_result_df$Metadata_CellLine)) + 2,\n alpha = 0.2,\n color = \"red\",\n linetype = \"dashed\",\n fill = \"grey\") +\n theme(strip.text = element_text(size = 8, color = \"black\"),\n strip.background = element_rect(colour = \"black\", fill = \"#fdfff4\"))\n\noutput_fig <- file.path(\"figures\", \"signature\", \"generic_resistance_signature_apply_cloneAE_xaxis_dosage.png\")\nggsave(output_fig, dpi = 500, height = 5, width = 5)",
"Warning message in simpleLoess(y, x, w, span, degree = degree, parametric = parametric, :\n“pseudoinverse used at 0.985”Warning message in simpleLoess(y, x, w, span, degree = degree, parametric = parametric, :\n“neighborhood radius 2.015”Warning message in simpleLoess(y, x, w, span, degree = degree, parametric = parametric, :\n“reciprocal condition number 4.2401e-17”Warning message in simpleLoess(y, x, w, span, degree = degree, parametric = parametric, :\n“There are other near singularities as well. 4.0602”Warning message in predLoess(object$y, object$x, newx = if (is.null(newdata)) object$x else if (is.data.frame(newdata)) as.matrix(model.frame(delete.response(terms(object)), :\n“pseudoinverse used at 0.985”Warning message in predLoess(object$y, object$x, newx = if (is.null(newdata)) object$x else if (is.data.frame(newdata)) as.matrix(model.frame(delete.response(terms(object)), :\n“neighborhood radius 2.015”Warning message in predLoess(object$y, object$x, newx = if (is.null(newdata)) object$x else if (is.data.frame(newdata)) as.matrix(model.frame(delete.response(terms(object)), :\n“reciprocal condition number 4.2401e-17”Warning message in predLoess(object$y, object$x, newx = if (is.null(newdata)) object$x else if (is.data.frame(newdata)) as.matrix(model.frame(delete.response(terms(object)), :\n“There are other near singularities as well. 4.0602”Warning message in simpleLoess(y, x, w, span, degree = degree, parametric = parametric, :\n“pseudoinverse used at 0.985”Warning message in simpleLoess(y, x, w, span, degree = degree, parametric = parametric, :\n“neighborhood radius 2.015”Warning message in simpleLoess(y, x, w, span, degree = degree, parametric = parametric, :\n“reciprocal condition number 4.2401e-17”Warning message in simpleLoess(y, x, w, span, degree = degree, parametric = parametric, :\n“There are other near singularities as well. 4.0602”Warning message in predLoess(object$y, object$x, newx = if (is.null(newdata)) object$x else if (is.data.frame(newdata)) as.matrix(model.frame(delete.response(terms(object)), :\n“pseudoinverse used at 0.985”Warning message in predLoess(object$y, object$x, newx = if (is.null(newdata)) object$x else if (is.data.frame(newdata)) as.matrix(model.frame(delete.response(terms(object)), :\n“neighborhood radius 2.015”Warning message in predLoess(object$y, object$x, newx = if (is.null(newdata)) object$x else if (is.data.frame(newdata)) as.matrix(model.frame(delete.response(terms(object)), :\n“reciprocal condition number 4.2401e-17”Warning message in predLoess(object$y, object$x, newx = if (is.null(newdata)) object$x else if (is.data.frame(newdata)) as.matrix(model.frame(delete.response(terms(object)), :\n“There are other near singularities as well. 4.0602”Warning message in simpleLoess(y, x, w, span, degree = degree, parametric = parametric, :\n“pseudoinverse used at 0.985”Warning message in simpleLoess(y, x, w, span, degree = degree, parametric = parametric, :\n“neighborhood radius 2.015”Warning message in simpleLoess(y, x, w, span, degree = degree, parametric = parametric, :\n“reciprocal condition number 4.2401e-17”Warning message in simpleLoess(y, x, w, span, degree = degree, parametric = parametric, :\n“There are other near singularities as well. 4.0602”Warning message in predLoess(object$y, object$x, newx = if (is.null(newdata)) object$x else if (is.data.frame(newdata)) as.matrix(model.frame(delete.response(terms(object)), :\n“pseudoinverse used at 0.985”Warning message in predLoess(object$y, object$x, newx = if (is.null(newdata)) object$x else if (is.data.frame(newdata)) as.matrix(model.frame(delete.response(terms(object)), :\n“neighborhood radius 2.015”Warning message in predLoess(object$y, object$x, newx = if (is.null(newdata)) object$x else if (is.data.frame(newdata)) as.matrix(model.frame(delete.response(terms(object)), :\n“reciprocal condition number 4.2401e-17”Warning message in predLoess(object$y, object$x, newx = if (is.null(newdata)) object$x else if (is.data.frame(newdata)) as.matrix(model.frame(delete.response(terms(object)), :\n“There are other near singularities as well. 4.0602”Warning message in simpleLoess(y, x, w, span, degree = degree, parametric = parametric, :\n“pseudoinverse used at 0.985”Warning message in simpleLoess(y, x, w, span, degree = degree, parametric = parametric, :\n“neighborhood radius 2.015”Warning message in simpleLoess(y, x, w, span, degree = degree, parametric = parametric, :\n“reciprocal condition number 4.2401e-17”Warning message in simpleLoess(y, x, w, span, degree = degree, parametric = parametric, :\n“There are other near singularities as well. 4.0602”Warning message in predLoess(object$y, object$x, newx = if (is.null(newdata)) object$x else if (is.data.frame(newdata)) as.matrix(model.frame(delete.response(terms(object)), :\n“pseudoinverse used at 0.985”Warning message in predLoess(object$y, object$x, newx = if (is.null(newdata)) object$x else if (is.data.frame(newdata)) as.matrix(model.frame(delete.response(terms(object)), :\n“neighborhood radius 2.015”Warning message in predLoess(object$y, object$x, newx = if (is.null(newdata)) object$x else if (is.data.frame(newdata)) as.matrix(model.frame(delete.response(terms(object)), :\n“reciprocal condition number 4.2401e-17”Warning message in predLoess(object$y, object$x, newx = if (is.null(newdata)) object$x else if (is.data.frame(newdata)) as.matrix(model.frame(delete.response(terms(object)), :\n“There are other near singularities as well. 4.0602”Warning message in simpleLoess(y, x, w, span, degree = degree, parametric = parametric, :\n“pseudoinverse used at 0.985”Warning message in simpleLoess(y, x, w, span, degree = degree, parametric = parametric, :\n“neighborhood radius 2.015”Warning message in simpleLoess(y, x, w, span, degree = degree, parametric = parametric, :\n“reciprocal condition number 4.2401e-17”Warning message in simpleLoess(y, x, w, span, degree = degree, parametric = parametric, :\n“There are other near singularities as well. 4.0602”Warning message in predLoess(object$y, object$x, newx = if (is.null(newdata)) object$x else if (is.data.frame(newdata)) as.matrix(model.frame(delete.response(terms(object)), :\n“pseudoinverse used at 0.985”Warning message in predLoess(object$y, object$x, newx = if (is.null(newdata)) object$x else if (is.data.frame(newdata)) as.matrix(model.frame(delete.response(terms(object)), :\n“neighborhood radius 2.015”Warning message in predLoess(object$y, object$x, newx = if (is.null(newdata)) object$x else if (is.data.frame(newdata)) as.matrix(model.frame(delete.response(terms(object)), :\n“reciprocal condition number 4.2401e-17”Warning message in predLoess(object$y, object$x, newx = if (is.null(newdata)) object$x else if (is.data.frame(newdata)) as.matrix(model.frame(delete.response(terms(object)), :\n“There are other near singularities as well. 4.0602”Warning message in simpleLoess(y, x, w, span, degree = degree, parametric = parametric, :\n“pseudoinverse used at 0.985”Warning message in simpleLoess(y, x, w, span, degree = degree, parametric = parametric, :\n“neighborhood radius 2.015”Warning message in simpleLoess(y, x, w, span, degree = degree, parametric = parametric, :\n“reciprocal condition number 4.2401e-17”Warning message in simpleLoess(y, x, w, span, degree = degree, parametric = parametric, :\n“There are other near singularities as well. 4.0602”Warning message in predLoess(object$y, object$x, newx = if (is.null(newdata)) object$x else if (is.data.frame(newdata)) as.matrix(model.frame(delete.response(terms(object)), :\n“pseudoinverse used at 0.985”Warning message in predLoess(object$y, object$x, newx = if (is.null(newdata)) object$x else if (is.data.frame(newdata)) as.matrix(model.frame(delete.response(terms(object)), :\n“neighborhood radius 2.015”Warning message in predLoess(object$y, object$x, newx = if (is.null(newdata)) object$x else if (is.data.frame(newdata)) as.matrix(model.frame(delete.response(terms(object)), :\n“reciprocal condition number 4.2401e-17”Warning message in predLoess(object$y, object$x, newx = if (is.null(newdata)) object$x else if (is.data.frame(newdata)) as.matrix(model.frame(delete.response(terms(object)), :\n“There are other near singularities as well. 4.0602”Warning message in simpleLoess(y, x, w, span, degree = degree, parametric = parametric, :\n"
]
]
] | [
"markdown",
"code",
"markdown",
"code",
"markdown",
"code",
"markdown",
"code",
"markdown",
"code",
"markdown",
"code",
"markdown",
"code",
"markdown",
"code",
"markdown",
"code",
"markdown",
"code",
"markdown",
"code"
] | [
[
"markdown"
],
[
"code",
"code",
"code"
],
[
"markdown"
],
[
"code",
"code"
],
[
"markdown"
],
[
"code",
"code"
],
[
"markdown"
],
[
"code",
"code"
],
[
"markdown"
],
[
"code",
"code"
],
[
"markdown"
],
[
"code",
"code",
"code",
"code"
],
[
"markdown"
],
[
"code",
"code"
],
[
"markdown"
],
[
"code",
"code"
],
[
"markdown"
],
[
"code",
"code"
],
[
"markdown"
],
[
"code"
],
[
"markdown"
],
[
"code",
"code",
"code",
"code"
]
] |
d07ab8196a553af917e9455bf19b3180decab6f2 | 5,692 | ipynb | Jupyter Notebook | notebooks/ROC-Example.ipynb | gditzler/UA-ECE-523-Sp2018 | fa2aefe3a6a2dad798ca8575c371616e51570380 | [
"MIT"
] | 14 | 2018-01-10T06:15:01.000Z | 2022-03-21T22:08:36.000Z | notebooks/ROC-Example.ipynb | gditzler/UA-ECE-523-Sp2018 | fa2aefe3a6a2dad798ca8575c371616e51570380 | [
"MIT"
] | 1 | 2018-01-23T04:12:01.000Z | 2018-01-26T05:05:46.000Z | notebooks/ROC-Example.ipynb | gditzler/UA-ECE-523-Sp2018 | fa2aefe3a6a2dad798ca8575c371616e51570380 | [
"MIT"
] | 15 | 2018-01-29T05:49:48.000Z | 2022-03-21T22:09:53.000Z | 33.880952 | 355 | 0.534786 | [
[
[
"# Generating an ROC Curve \n\n\nThis notebook is meant to be be an introduction to generating an ROC curve for multi-class prediction problems and the code comes directly from an [Scikit-Learn demo](http://scikit-learn.org/stable/auto_examples/model_selection/plot_roc.html). Please issue a comment on my Github account if you would like to suggest any changes to this notebook. \n",
"_____no_output_____"
]
],
[
[
"%matplotlib inline\nimport numpy as np\nimport matplotlib.pyplot as plt\nfrom sklearn import svm, datasets\nfrom sklearn.metrics import roc_curve, auc\nfrom sklearn.cross_validation import train_test_split\nfrom sklearn.preprocessing import label_binarize\nfrom sklearn.multiclass import OneVsRestClassifier\nfrom scipy import interp",
"_____no_output_____"
],
[
"# Import some data to play with\niris = datasets.load_iris()\nX = iris.data\ny = iris.target\n\n# Binarize the output\ny = label_binarize(y, classes=[0, 1, 2])\nn_classes = y.shape[1]\n\n# Add noisy features to make the problem harder\nrandom_state = np.random.RandomState(0)\nn_samples, n_features = X.shape\nX = np.c_[X, random_state.randn(n_samples, 200 * n_features)]\n\n# shuffle and split training and test sets\nX_train, X_test, y_train, y_test = train_test_split(X, y, test_size=.5,\n random_state=0)\n\n# Learn to predict each class against the other\nclassifier = OneVsRestClassifier(svm.SVC(kernel='linear', probability=True,\n random_state=random_state))\ny_score = classifier.fit(X_train, y_train).decision_function(X_test)\n\n# Compute ROC curve and ROC area for each class\nfpr = dict()\ntpr = dict()\nroc_auc = dict()\nfor i in range(n_classes):\n fpr[i], tpr[i], _ = roc_curve(y_test[:, i], y_score[:, i])\n roc_auc[i] = auc(fpr[i], tpr[i])\n\n# Compute micro-average ROC curve and ROC area\nfpr[\"micro\"], tpr[\"micro\"], _ = roc_curve(y_test.ravel(), y_score.ravel())\nroc_auc[\"micro\"] = auc(fpr[\"micro\"], tpr[\"micro\"])\n\n\n##############################################################################\n# Plot of a ROC curve for a specific class\nplt.figure()\nplt.plot(fpr[2], tpr[2], label='ROC curve (area = %0.2f)' % roc_auc[2])\nplt.plot([0, 1], [0, 1], 'k--')\nplt.xlim([0.0, 1.0])\nplt.ylim([0.0, 1.05])\nplt.xlabel('False Positive Rate')\nplt.ylabel('True Positive Rate')\nplt.title('Receiver operating characteristic example')\nplt.legend(loc=\"lower right\")\nplt.show()\n\n\n##############################################################################\n# Plot ROC curves for the multiclass problem\n\n# Compute macro-average ROC curve and ROC area\n\n# First aggregate all false positive rates\nall_fpr = np.unique(np.concatenate([fpr[i] for i in range(n_classes)]))\n\n# Then interpolate all ROC curves at this points\nmean_tpr = np.zeros_like(all_fpr)\nfor i in range(n_classes):\n mean_tpr += interp(all_fpr, fpr[i], tpr[i])\n\n# Finally average it and compute AUC\nmean_tpr /= n_classes\n\nfpr[\"macro\"] = all_fpr\ntpr[\"macro\"] = mean_tpr\nroc_auc[\"macro\"] = auc(fpr[\"macro\"], tpr[\"macro\"])\n\n# Plot all ROC curves\nplt.figure()\nplt.plot(fpr[\"micro\"], tpr[\"micro\"],\n label='micro-average ROC curve (area = {0:0.2f})'\n ''.format(roc_auc[\"micro\"]),\n linewidth=2)\n\nplt.plot(fpr[\"macro\"], tpr[\"macro\"],\n label='macro-average ROC curve (area = {0:0.2f})'\n ''.format(roc_auc[\"macro\"]),\n linewidth=2)\n\nfor i in range(n_classes):\n plt.plot(fpr[i], tpr[i], label='ROC curve of class {0} (area = {1:0.2f})'\n ''.format(i, roc_auc[i]))\n\nplt.plot([0, 1], [0, 1], 'k--')\nplt.xlim([0.0, 1.0])\nplt.ylim([0.0, 1.05])\nplt.xlabel('False Positive Rate')\nplt.ylabel('True Positive Rate')\nplt.title('Some extension of Receiver operating characteristic to multi-class')\nplt.legend(loc=\"lower right\")\nplt.show()",
"_____no_output_____"
]
]
] | [
"markdown",
"code"
] | [
[
"markdown"
],
[
"code",
"code"
]
] |
d07abf38c9f97aac25a743a6cd2111882be551cb | 2,651 | ipynb | Jupyter Notebook | pandas/pandas_01_content.ipynb | whyAndBetter/pandas--tutorial | 907ff76e35d298ba55a035e4bb33ae3b03b72724 | [
"MIT"
] | 1 | 2022-03-24T12:21:03.000Z | 2022-03-24T12:21:03.000Z | pandas/pandas_01_content.ipynb | whyAndBetter/pandas-tutorial | daffbe76075f581db61061d7d4452a12857940fc | [
"MIT"
] | null | null | null | pandas/pandas_01_content.ipynb | whyAndBetter/pandas-tutorial | daffbe76075f581db61061d7d4452a12857940fc | [
"MIT"
] | null | null | null | 21.729508 | 148 | 0.473029 | [
[
[
"'''\n@Author: Haihui Pan\n@Date: 2021-12-06\n@Ref:\n[1]:https://pandas.pydata.org/docs/user_guide/10min.html\n'''\nimport pandas",
"_____no_output_____"
],
[
"%%html\n<img src=\"pd_01.png\",width=\"10%\",height=\"10%\">",
"_____no_output_____"
]
],
[
[
"* Pandas是一个用于数据处理和数据分析的Python三方库。Pandas可以从各种文件(txt,csv,excel,json...)中导入数据,并对数据进行运算操作(选择,拼接,统计...)。Pandas最主要的数据结构为Series(一维数据)和DataFrame(二维数据)",
"_____no_output_____"
],
[
"* pandas_02_dataframe & series\n - DataFrame & Series说明\n - DataFrame初始化\n - 通过dict初始化\n - 读取文件数据初始化(excel,csv,txt,json)\n - DataFrame保存\n - 保存为指定文件(excel,csv,txt,json)\n \n * pandas_03_常用基础方法\n - 查看数据: df.head(),df.tail()\n - 数据大小: df.shape\n - 字段的基础统信息: df.describe()\n - 字段取值的统计: df.value_counts()\n - 新增列 \n - 字段非空信息: df.info()\n - 缺失值处理\n - 缺失值填充\n - 删除缺失数据\n - 遍历DataFrame\n - 行列处理函数: df.apply \n - 类别编码(将类别列映射为one-hot)\n\n * pandas_04_数据选取&分组&修改\n - 数据选取\n - 选取行&列数据\n - 布尔索引(按指定条件索引)\n - 位置索引(df.icol)\n - 数据分组(Group)\n - sum,average\n - max,min\n - count\n - 数据修改\n - 修改选取数据(df.loc)\n\n * pandas_05_多DataFrame处理\n - 垂直拼接(pd.concat)\n - 水平拼接(pd.merge)\n \n",
"_____no_output_____"
]
]
] | [
"code",
"markdown"
] | [
[
"code",
"code"
],
[
"markdown",
"markdown"
]
] |
d07ac05c37465f70c63ad2ce080337140f4ca849 | 17,146 | ipynb | Jupyter Notebook | content/lessons/07/Class-Coding-Lab/CCL-Strings.ipynb | jvrecca-su/ist256project | 255ee5ae91e6f1d0a56519804701633443b75bc6 | [
"MIT"
] | null | null | null | content/lessons/07/Class-Coding-Lab/CCL-Strings.ipynb | jvrecca-su/ist256project | 255ee5ae91e6f1d0a56519804701633443b75bc6 | [
"MIT"
] | null | null | null | content/lessons/07/Class-Coding-Lab/CCL-Strings.ipynb | jvrecca-su/ist256project | 255ee5ae91e6f1d0a56519804701633443b75bc6 | [
"MIT"
] | null | null | null | 27.789303 | 931 | 0.538085 | [
[
[
"# In-Class Coding Lab: Strings\n\nThe goals of this lab are to help you to understand:\n\n- String slicing for substrings\n- How to use Python's built-in String functions in the standard library.\n- Tokenizing and Parsing Data\n- How to create user-defined functions to parse and tokenize strings\n\n\n# Strings\n\n## Strings are immutable sequences\n\nPython strings are immutable sequences.This means we cannot change them \"in part\" and there is impicit ordering. \n\nThe characters in a string are zero-based. Meaning the index of the first character is 0.\n\nWe can leverage this in a variety of ways.\n\nFor example:",
"_____no_output_____"
]
],
[
[
"x = input(\"Enter something: \")\nprint (\"You typed:\", x)\nprint (\"number of characters:\", len(x) )\nprint (\"First character is:\", x[0])\nprint (\"Last character is:\", x[-1])\n\n## They're sequences, so you can loop definately:\nprint(\"Printing one character at a time: \")\nfor ch in x:\n print(ch) # print a character at a time!",
"Enter something: tony\nYou typed: tony\nnumber of characters: 4\nFirst character is: t\nLast character is: y\nPrinting one character at a time: \nt\no\nn\ny\n"
]
],
[
[
"## Slices as substrings\n\nPython lists and sequences use **slice notation** which is a clever way to get substring from a given string.\n\nSlice notation requires two values: A start index and the end index. The substring returned starts at the start index, and *ends at the position before the end index*. It ends at the position *before* so that when you slice a string into parts you know where you've \"left off\". \n\nFor example:",
"_____no_output_____"
]
],
[
[
"state = \"Mississippi\"\nprint (state[0:4]) # Miss\nprint (state[4:len(state)]) # issippi",
"Miss\nissippi\n"
]
],
[
[
"In this next example, play around with the variable `split` adjusting it to how you want the string to be split up. Re run the cell several times with different values to get a feel for what happens.",
"_____no_output_____"
]
],
[
[
"state = \"Mississippi\"\nsplit = 4 # TODO: play around with this number\nleft = state[0:split]\nright = state[split:len(state)]\nprint(left, right)",
"Miss issippi\n"
],
[
"state = \"Mississippi\"\nsplit = 2 # TODO: play around with this number\nleft = state[0:split]\nright = state[split:len(state)]\nprint(left, right)",
"Mi ssissippi\n"
],
[
"state = \"Mississippi\"\nsplit = 8 # TODO: play around with this number\nleft = state[0:split]\nright = state[split:len(state)]\nprint(left, right)",
"Mississi ppi\n"
],
[
"state = \"Mississippi\"\nsplit = 5 # TODO: play around with this number\nleft = state[0:split]\nright = state[split:len(state)]\nprint(left, right)",
"Missi ssippi\n"
]
],
[
[
"### Slicing from the beginning or to the end\n\nIf you omit the begin or end slice, Python will slice from the beginnning of the string or all the way to the end. So if you say `x[:5]` its the same as `x[0:5]`\n\nFor example:",
"_____no_output_____"
]
],
[
[
"state = \"Ohio\"\nprint(state[0:2], state[:2]) # same!\nprint(state[2:len(state)], state[2:]) # same\n",
"Oh Oh\nio io\n"
]
],
[
[
"### Now Try It!\n\nSplit the string `\"New Hampshire\"` into two sub-strings one containing `\"New\"` the other containing `\"Hampshire\"` (without the space).",
"_____no_output_____"
]
],
[
[
"## TODO: Write code here\nstate = \"NewHampshire\"\nsplit = 3 \nleft = state[0:split]\nright = state[split:len(state)]\nprint(left, right)",
"New Hampshire\n"
]
],
[
[
"## Python's built in String Functions\n\nPython includes several handy built-in string functions (also known as *methods* in object-oriented parlance). To get a list of available functions, use the `dir()` function on any string variable, or on the type `str` itself.\n",
"_____no_output_____"
]
],
[
[
"print ( dir(str))",
"['__add__', '__class__', '__contains__', '__delattr__', '__dir__', '__doc__', '__eq__', '__format__', '__ge__', '__getattribute__', '__getitem__', '__getnewargs__', '__gt__', '__hash__', '__init__', '__init_subclass__', '__iter__', '__le__', '__len__', '__lt__', '__mod__', '__mul__', '__ne__', '__new__', '__reduce__', '__reduce_ex__', '__repr__', '__rmod__', '__rmul__', '__setattr__', '__sizeof__', '__str__', '__subclasshook__', 'capitalize', 'casefold', 'center', 'count', 'encode', 'endswith', 'expandtabs', 'find', 'format', 'format_map', 'index', 'isalnum', 'isalpha', 'isdecimal', 'isdigit', 'isidentifier', 'islower', 'isnumeric', 'isprintable', 'isspace', 'istitle', 'isupper', 'join', 'ljust', 'lower', 'lstrip', 'maketrans', 'partition', 'replace', 'rfind', 'rindex', 'rjust', 'rpartition', 'rsplit', 'rstrip', 'split', 'splitlines', 'startswith', 'strip', 'swapcase', 'title', 'translate', 'upper', 'zfill']\n"
]
],
[
[
"Let's suppose you want to learn how to use the `count` function. There are 2 ways you can do this.\n\n1. search the web for `python 3 str count` or\n1. bring up internal help `help(str.count)` \n\nBoth have their advantages and disadvanges. I would start with the second one, and only fall back to a web search when you can't figure it out from the Python documenation. \n\nHere's the documentation for `count`",
"_____no_output_____"
]
],
[
[
"help(str.count)",
"Help on method_descriptor:\n\ncount(...)\n S.count(sub[, start[, end]]) -> int\n \n Return the number of non-overlapping occurrences of substring sub in\n string S[start:end]. Optional arguments start and end are\n interpreted as in slice notation.\n\n"
]
],
[
[
"You'll notice in the help output it says S.count() this indicates this function is a method function. this means you invoke it like this `variable.count()`.\n\n### Now Try It\n\nTry to use the count() function method to count the number of `'i'`'s in the string `'Mississippi`:",
"_____no_output_____"
]
],
[
[
"state = 'Mississippi'\n#TODO: use state.count\nstate.count(\"i\")\nprint(state.count('i'))",
"4\n"
]
],
[
[
"### TANGENT: The Subtle difference between function and method.\n\nYou'll notice sometimes we call our function alone, other times it's attached to a variable, as was the case in the example above. when we say `state.count('i')` the period (`.`) between the variable and function indicates this function is a *method function*. The key difference between a the two is a method is attached to a variable. To call a method function you must say `variable.function()` whereas when you call a function its just `function()`. The variable associated with the method call is usually part of the function's context.\n\nHere's an example:",
"_____no_output_____"
]
],
[
[
"name = \"Larry\"\nprint( len(name) ) # a function call len(name) stands on its own. Gets length of 'Larry'\nprint( name.__len__() ) # a method call name.__len__() does the name thing for its variable 'Larry'",
"5\n2\n"
]
],
[
[
"### Now Try It\n\nTry to figure out which built in string function to use to accomplish this task.\n\nWrite some code to find the text `'is'` in some text. The program shoud output the first position of `'is'` in the text. \n\nExamples:\n\n```\nWhen: text = 'Mississippi' then position = 1\nWhen: text = \"This is great\" then position = 2\nWhen: text = \"Burger\" then position = -1\n```",
"_____no_output_____"
]
],
[
[
"print(dir(str))",
"['__add__', '__class__', '__contains__', '__delattr__', '__dir__', '__doc__', '__eq__', '__format__', '__ge__', '__getattribute__', '__getitem__', '__getnewargs__', '__gt__', '__hash__', '__init__', '__init_subclass__', '__iter__', '__le__', '__len__', '__lt__', '__mod__', '__mul__', '__ne__', '__new__', '__reduce__', '__reduce_ex__', '__repr__', '__rmod__', '__rmul__', '__setattr__', '__sizeof__', '__str__', '__subclasshook__', 'capitalize', 'casefold', 'center', 'count', 'encode', 'endswith', 'expandtabs', 'find', 'format', 'format_map', 'index', 'isalnum', 'isalpha', 'isdecimal', 'isdigit', 'isidentifier', 'islower', 'isnumeric', 'isprintable', 'isspace', 'istitle', 'isupper', 'join', 'ljust', 'lower', 'lstrip', 'maketrans', 'partition', 'replace', 'rfind', 'rindex', 'rjust', 'rpartition', 'rsplit', 'rstrip', 'split', 'splitlines', 'startswith', 'strip', 'swapcase', 'title', 'translate', 'upper', 'zfill']\n"
],
[
"help(str.find)",
"Help on built-in function find:\n\nfind(...) method of builtins.str instance\n S.find(sub[, start[, end]]) -> int\n \n Return the lowest index in S where substring sub is found,\n such that sub is contained within S[start:end]. Optional\n arguments start and end are interpreted as in slice notation.\n \n Return -1 on failure.\n\n"
],
[
"# TODO: Write your code here\ntext = input(\"Enter some text: \")\ntext.find('is')\nprint(\"when text =\", text,\"then position =\",text.find('is'))",
"Enter some text: This is fun\nwhen text = This is fun then position = 2\n"
],
[
"text = input(\"Enter some text: \")\ntext.find('is')\nprint(\"when text =\", text,\"then position =\",text.find('is'))",
"Enter some text: Here is my food\nwhen text = Here is my food then position = 5\n"
],
[
"text = input(\"Enter some text: \")\ntext.find('is')\nprint(\"when text =\", text,\"then position =\",text.find('is'))",
"Enter some text: fries\nwhen text = fries then position = -1\n"
]
],
[
[
"### Now Try It\n\n**Is that a URL?**\n\nTry to write a rudimentary URL checker. The program should input a text string and then use the `startswith` function to check if the string begins with `\"http://\"` or `\"https://\"` If it does we can assume it is a URL. ",
"_____no_output_____"
]
],
[
[
"## TODO: write code here:\nurl = input(\"Enter a URL: \")\nif url.startswith('http://'):\n print(\"We can assume this is a URL\")\nelif url.startswith('https://'):\n print(\"We can assume this is a URL\")\nelse:\n print(\"This is not a URL\")",
"Enter a URL: https://www.bing.com/search?q=google&FORM=EDGENA&PC=DCTS&refig=efcfbd660ffc4b5ad464ee97d0f0d3da\nWe can assume this is a URL\n"
],
[
"url = input(\"Enter a URL: \")\nif url.startswith('http://'):\n print(\"We can assume this is a URL\")\nelif url.startswith('https://'):\n print(\"We can assume this is a URL\")\nelse:\n print(\"This is not a URL\")",
"Enter a URL: http://www.google.com\nWe can assume this is a URL\n"
],
[
"url = input(\"Enter a URL: \")\nif url.startswith('http://'):\n print(\"We can assume this is a URL\")\nelif url.startswith('https://'):\n print(\"We can assume this is a URL\")\nelse:\n print(\"This is not a URL\")",
"Enter a URL: jfksdlfjaldskl\nThis is not a URL\n"
]
]
] | [
"markdown",
"code",
"markdown",
"code",
"markdown",
"code",
"markdown",
"code",
"markdown",
"code",
"markdown",
"code",
"markdown",
"code",
"markdown",
"code",
"markdown",
"code",
"markdown",
"code",
"markdown",
"code"
] | [
[
"markdown"
],
[
"code"
],
[
"markdown"
],
[
"code"
],
[
"markdown"
],
[
"code",
"code",
"code",
"code"
],
[
"markdown"
],
[
"code"
],
[
"markdown"
],
[
"code"
],
[
"markdown"
],
[
"code"
],
[
"markdown"
],
[
"code"
],
[
"markdown"
],
[
"code"
],
[
"markdown"
],
[
"code"
],
[
"markdown"
],
[
"code",
"code",
"code",
"code",
"code"
],
[
"markdown"
],
[
"code",
"code",
"code"
]
] |
d07ac9300c0cd65aeeeec0e5a1e3836c0708b4d1 | 113,368 | ipynb | Jupyter Notebook | Chapter08/Activity8.01-Activity8.03/Activity8.01-Activity8.03.ipynb | PacktWorkshops/Applied-Unsupervised-Learning-with-Python | ddafe8ed6917e1e0020489170dacdc820e4800bb | [
"MIT"
] | 17 | 2020-04-06T09:26:34.000Z | 2022-03-08T04:38:27.000Z | Chapter08/Activity8.01-Activity8.03/Activity8.01-Activity8.03.ipynb | PacktWorkshops/The-Unsupervised-Learning-Workshop | ddafe8ed6917e1e0020489170dacdc820e4800bb | [
"MIT"
] | null | null | null | Chapter08/Activity8.01-Activity8.03/Activity8.01-Activity8.03.ipynb | PacktWorkshops/The-Unsupervised-Learning-Workshop | ddafe8ed6917e1e0020489170dacdc820e4800bb | [
"MIT"
] | 22 | 2020-02-25T07:20:56.000Z | 2022-03-09T01:03:58.000Z | 97.899827 | 31,480 | 0.745431 | [
[
[
"# CH. 8 - Market Basket Analysis\n## Activities",
"_____no_output_____"
],
[
"#### Activity 8.01: Load and Prep Full Online Retail Data",
"_____no_output_____"
]
],
[
[
"import matplotlib.pyplot as plt\nimport mlxtend.frequent_patterns\nimport mlxtend.preprocessing\nimport numpy\nimport pandas",
"_____no_output_____"
],
[
"online = pandas.read_excel(\n io=\"./Online Retail.xlsx\", \n sheet_name=\"Online Retail\", \n header=0\n)",
"_____no_output_____"
],
[
"online['IsCPresent'] = (\n online['InvoiceNo']\n .astype(str)\n .apply(lambda x: 1 if x.find('C') != -1 else 0)\n)",
"_____no_output_____"
],
[
"online1 = (\n online\n .loc[online[\"Quantity\"] > 0]\n .loc[online['IsCPresent'] != 1]\n .loc[:, [\"InvoiceNo\", \"Description\"]]\n .dropna()\n)",
"_____no_output_____"
],
[
"invoice_item_list = []\nfor num in list(set(online1.InvoiceNo.tolist())):\n tmp_df = online1.loc[online1['InvoiceNo'] == num]\n tmp_items = tmp_df.Description.tolist()\n invoice_item_list.append(tmp_items)",
"_____no_output_____"
],
[
"online_encoder = mlxtend.preprocessing.TransactionEncoder()\nonline_encoder_array = online_encoder.fit_transform(invoice_item_list)",
"_____no_output_____"
],
[
"online_encoder_df = pandas.DataFrame(\n online_encoder_array, \n columns=online_encoder.columns_\n)",
"_____no_output_____"
],
[
"## COL in different order\nonline_encoder_df.loc[\n 20125:20135, \n online_encoder_df.columns.tolist()[100:110]\n]",
"_____no_output_____"
]
],
[
[
"#### Activity 8.02: Apriori on the Complete Online Retail Data Set",
"_____no_output_____"
]
],
[
[
"mod_colnames_minsupport = mlxtend.frequent_patterns.apriori(\n online_encoder_df, \n min_support=0.01,\n use_colnames=True\n)\nmod_colnames_minsupport.loc[0:6]",
"_____no_output_____"
],
[
"mod_colnames_minsupport[\n mod_colnames_minsupport['itemsets'] == frozenset(\n {'10 COLOUR SPACEBOY PEN'}\n )\n]",
"_____no_output_____"
],
[
"mod_colnames_minsupport['length'] = (\n mod_colnames_minsupport['itemsets'].apply(lambda x: len(x))\n)",
"_____no_output_____"
],
[
"## item set order different\n\nmod_colnames_minsupport[\n (mod_colnames_minsupport['length'] == 2) & \n (mod_colnames_minsupport['support'] >= 0.02) &\n (mod_colnames_minsupport['support'] < 0.021)\n]",
"_____no_output_____"
],
[
"mod_colnames_minsupport.hist(\"support\", grid=False, bins=30)\nplt.xlabel(\"Support of item\")\nplt.ylabel(\"Number of items\")\nplt.title(\"Frequency distribution of Support\")\nplt.show() ",
"_____no_output_____"
]
],
[
[
"#### Activity 8.03: Find the Association Rules on the Complete Online Retail Data Set",
"_____no_output_____"
]
],
[
[
"rules = mlxtend.frequent_patterns.association_rules(\n mod_colnames_minsupport, \n metric=\"confidence\",\n min_threshold=0.6, \n support_only=False\n)\n\nrules.loc[0:6]",
"_____no_output_____"
],
[
"print(\"Number of Associations: {}\".format(rules.shape[0]))",
"Number of Associations: 498\n"
],
[
"rules.plot.scatter(\"support\", \"confidence\", alpha=0.5, marker=\"*\")\nplt.xlabel(\"Support\")\nplt.ylabel(\"Confidence\")\nplt.title(\"Association Rules\")\nplt.show()",
"_____no_output_____"
],
[
"rules.hist(\"lift\", grid=False, bins=30)\nplt.xlabel(\"Lift of item\")\nplt.ylabel(\"Number of items\")\nplt.title(\"Frequency distribution of Lift\")\nplt.show() ",
"_____no_output_____"
],
[
"rules.hist(\"leverage\", grid=False, bins=30)\nplt.xlabel(\"Leverage of item\")\nplt.ylabel(\"Number of items\")\nplt.title(\"Frequency distribution of Leverage\")\nplt.show() ",
"_____no_output_____"
],
[
"plt.hist(rules[numpy.isfinite(rules['conviction'])].conviction.values, bins = 30)\nplt.xlabel(\"Conviction of item\")\nplt.ylabel(\"Number of items\")\nplt.title(\"Frequency distribution of Conviction\")\nplt.show() ",
"_____no_output_____"
],
[
"",
"_____no_output_____"
]
]
] | [
"markdown",
"code",
"markdown",
"code",
"markdown",
"code"
] | [
[
"markdown",
"markdown"
],
[
"code",
"code",
"code",
"code",
"code",
"code",
"code",
"code"
],
[
"markdown"
],
[
"code",
"code",
"code",
"code",
"code"
],
[
"markdown"
],
[
"code",
"code",
"code",
"code",
"code",
"code",
"code"
]
] |
d07aca788cb7b4d42dc62b90ba38dc73a6cdbb0e | 380,641 | ipynb | Jupyter Notebook | lessons/jupyter/general_jupyter_notebook_tutorial.ipynb | huxiaoni/espin | 78791e1c91a9ec7a0f059652e8b7c9df6aba9617 | [
"MIT"
] | 27 | 2020-08-07T23:16:44.000Z | 2022-03-30T15:59:16.000Z | lessons/jupyter/general_jupyter_notebook_tutorial.ipynb | KarstModel/espin | 8ad941c2798653000382a66656d3ae09f105db81 | [
"CC-BY-4.0"
] | 28 | 2020-07-09T21:28:49.000Z | 2022-03-11T16:49:24.000Z | lessons/jupyter/general_jupyter_notebook_tutorial.ipynb | KarstModel/espin | 8ad941c2798653000382a66656d3ae09f105db81 | [
"CC-BY-4.0"
] | 48 | 2020-08-09T23:03:15.000Z | 2021-06-18T20:50:11.000Z | 498.221204 | 363,801 | 0.955015 | [
[
[
"# Welcome to Jupyter Notebooks!",
"_____no_output_____"
],
[
"Author: Shelley Knuth \nDate: 23 August 2019 \nPurpose: This is a general purpose tutorial to designed to provide basic information about Jupyter notebooks",
"_____no_output_____"
],
[
"## Outline\n\n1. General information about notebooks\n1. Formatting text in notebooks\n1. Formatting mathematics in notebooks\n1. Importing graphics\n1. Plotting",
"_____no_output_____"
],
[
"## General Information about Notebooks\n\n\n### What is a Jupyter Notebook?\nIt's an interactive web platform that allows one to create and edit live code, add text descriptions, and visualizations in a document that can be easily shared and displayed. ",
"_____no_output_____"
],
[
"### How to work with a Notebook\n\nTo run a cell, hit \"shift\" and \"enter\" at the same time \n\nDon't be alarmed if your notebook runs for awhile - indicated by [*] \nSometimes takes awhile",
"_____no_output_____"
],
[
"### Different cell types\n\nCode, Markdown are the two I use most frequently",
"_____no_output_____"
],
[
"### Exercise\n\nWrite one sentence on what you are planning to do this weekend in a cell.",
"_____no_output_____"
],
[
"### Opening, saving notebooks\n\nOpening: File -> New Notebook -> Python 3 \nSaving: File -> Save as -> Save and Checkpoint (Ctrl + S) \nPrinting: File -> Print Preview\nDownload: File -> Download as PDF (or others)",
"_____no_output_____"
],
[
"## Keyboard shortcuts\n\nToggle between edit and command mode with Esc and Enter, respectively. \n\nOnce in command mode: \nScroll up and down your cells with your Up and Down keys. \nPress A or B to insert a new cell above or below the active cell. \nM will transform the active cell to a Markdown cell. \nY will set the active cell to a code cell. \nD + D (D twice) will delete the active cell. \nZ will undo cell deletion. \nHold Shift and press Up or Down to select multiple cells at once. \nWith multiple cells selected, Shift + M will merge your selection. \nCtrl + Shift + -, in edit mode, will split the active cell at the cursor. \nYou can also click and Shift + Click in the margin to the left of your cells to select them. \n\n(from https://www.dataquest.io/blog/jupyter-notebook-tutorial/)",
"_____no_output_____"
],
[
"## Formatting text in notebooks",
"_____no_output_____"
]
],
[
[
"__Bold__ or **bold** \n_italics_ or *italics*",
"_____no_output_____"
]
],
[
[
"Jupyter notebooks are __really__ cool! \nJupyter notebooks are _really_ cool!",
"_____no_output_____"
],
[
"Two spaces after text gives you a newline!",
"_____no_output_____"
],
[
"### Headings",
"_____no_output_____"
]
],
[
[
"# title \n## major headings \n### subheadings \n#### 4th level subheadings",
"_____no_output_____"
]
],
[
[
"# Jupyter notebooks are really cool!\n## Do you know what else is cool?\n### Turtles!\n#### And Bon Jovi!",
"_____no_output_____"
],
[
"### Code\nThe best program to use for this is the `grep` command",
"_____no_output_____"
]
],
[
[
"The best program to use for this is the `grep` command",
"_____no_output_____"
]
],
[
[
"### Text color and size\nThe sky is <font color = blue, size = 30>blue!</font> \nSometimes the <font color = blue>color</font> doesn't turn out <font size=30> WELL</font>",
"_____no_output_____"
]
],
[
[
"The sky is <font color = blue, size = 30>blue!</font> \nSometimes the <font color = blue>color</font> doesn't turn out <font size=30> WELL</font>",
"_____no_output_____"
]
],
[
[
"### Indent or list your text\n> This is how!\n- This is how!\n - This is how!\n1. This is how!",
"_____no_output_____"
]
],
[
[
"> This is how!\n- This is how!\n - This is how!\n1. This is how!",
"_____no_output_____"
]
],
[
[
"* This is also how!\n * This is also how!",
"_____no_output_____"
]
],
[
[
"* This is also how!\n * This is also how!",
"_____no_output_____"
]
],
[
[
"### Hyperlinks\n\nSometimes copy and paste is just fine too! https://github.com/adam-p/markdown-here/wiki/Markdown-Cheatsheet \n\n[I'm an inline-style link](https://www.google.com)\n\n[I'm a reference-style link][Arbitrary case-insensitive reference text]\n\n[I'm a relative reference to a repository file](../blob/master/LICENSE)\n\n[You can use numbers for reference-style link definitions][1]\n\nOr leave it empty and use the [link text itself].\n\nURLs and URLs in angle brackets will automatically get turned into links. \nhttp://www.example.com or <http://www.example.com> and sometimes \nexample.com (but not on Github, for example).\n\nSome text to show that the reference links can follow later.\n\n[arbitrary case-insensitive reference text]: https://www.mozilla.org\n[1]: http://slashdot.org\n[link text itself]: http://www.reddit.com\n\n(from https://github.com/adam-p/markdown-here/wiki/Markdown-Cheatsheet)",
"_____no_output_____"
]
],
[
[
"[I'm an inline-style link](https://www.google.com)\n\n[I'm a reference-style link][Arbitrary case-insensitive reference text]\n\n[I'm a relative reference to a repository file](../blob/master/LICENSE)\n\n[You can use numbers for reference-style link definitions][1]\n\nOr leave it empty and use the [link text itself].\n\nURLs and URLs in angle brackets will automatically get turned into links. \nhttp://www.example.com or <http://www.example.com> and sometimes \nexample.com (but not on Github, for example).\n\nSome text to show that the reference links can follow later.\n\n[arbitrary case-insensitive reference text]: https://www.mozilla.org\n[1]: http://slashdot.org\n[link text itself]: http://www.reddit.com\n\n(from https://github.com/adam-p/markdown-here/wiki/Markdown-Cheatsheet) ",
"_____no_output_____"
]
],
[
[
"## Mathematical Equations in Notebooks\n\n$F=ma$\n\nThis is an equation, $x=y+z$, where $y=10$ and $z=20$ ",
"_____no_output_____"
]
],
[
[
"$F = ma$\nThis is an equation, $x=y+z$, where $y=10$ and $z=20$",
"_____no_output_____"
]
],
[
[
"### Superscripts and Subscripts\n$y = x^3 + x^2 + 3x$ \n$F_g = m g$ ",
"_____no_output_____"
]
],
[
[
"$a^2 = b^2 + c^2$\n$F_g = m g$ ",
"_____no_output_____"
]
],
[
[
"### Grouping\n$6.022\\times 10^{23}$ . ",
"_____no_output_____"
]
],
[
[
"$6.022\\times 10^{23}$ . ",
"_____no_output_____"
]
],
[
[
"### Greek Letters\n\n$\\pi = 3.1415926$ \n$\\Omega = 10$ \n$\\delta$",
"_____no_output_____"
]
],
[
[
"$\\pi = 3.1415926$ \n$\\Omega = 10$\n$\\delta$",
"_____no_output_____"
]
],
[
[
"### Special Symbols\n\n$\\pm$, $\\gg$, $\\ll$, $\\infty$ \n$i = \\sqrt{-1}$ \n$\\int_a^b$",
"_____no_output_____"
]
],
[
[
"$\\pm$, $\\gg$, $\\ll$, $\\infty$\n$i = \\sqrt{-1}$ \n$\\int_a^b$",
"_____no_output_____"
]
],
[
[
"## Fractions and Derivatives\n\nFractions \n$\\frac{1}{2}$\n\nDerivatives \n$\\frac{dm}{dt}$, $\\frac{\\partial m}{\\partial t}$\n",
"_____no_output_____"
]
],
[
[
"Fractions \n$\\frac{1}{2}$\n\nDerivative \n$\\frac{dm}{dt}$\n$\\frac{\\partial m}{\\partial t}$",
"_____no_output_____"
]
],
[
[
"### Matrices\n\n$$\\begin{matrix} a & b \\\\ c & d \\end{matrix}$$",
"_____no_output_____"
]
],
[
[
"$$\\begin{matrix} a & b \\\\ c & d \\end{matrix}$$",
"_____no_output_____"
]
],
[
[
"### Exercise\n\nWrite out an equation where the total derivative of x over y is equal to the square root of 10 added to 7/8 pi",
"_____no_output_____"
],
[
"$\\frac{dx}{dy} = \\sqrt{10} + \\pi$\n",
"_____no_output_____"
],
[
"### Exercise \n\nWrite out an equation where x sub j is equal to a 2x2 matrix containing 10, 20, 30, and 40",
"_____no_output_____"
],
[
"$x_j = \\begin{matrix} 10 & 20 \\\\ 30 & 40 \\end{matrix}$",
"_____no_output_____"
],
[
"## Importing Graphics",
"_____no_output_____"
],
[
"Easy way: Drag and drop! \nOr \"Edit -> Insert image\" when in Markdown\n\nHarder ways:",
"_____no_output_____"
],
[
"Python:",
"_____no_output_____"
]
],
[
[
"from IPython.display import Image\nImage(\"bonjovi.jpg\")\n",
"_____no_output_____"
]
],
[
[
"HTML:",
"_____no_output_____"
],
[
"<img src=\"bonjovi.jpg\">",
"_____no_output_____"
]
],
[
[
"<img src=\"bonjovi.jpg\">",
"_____no_output_____"
]
],
[
[
"## Basic Programming with Python\n### Print statements",
"_____no_output_____"
]
],
[
[
"print(\"Hello, World!\")",
"Hello, World!\n"
]
],
[
[
"Look at how the input changed (to the left of the cell). Look at the output! \n(This and several cells from https://www.dataquest.io/blog/jupyter-notebook-tutorial/)",
"_____no_output_____"
],
[
"Anything run in the kernal persists in the notebook\nCan run code and import libraries in the cells",
"_____no_output_____"
],
[
"### Variables in Python\n\n* Variables are not declared\n* Variables are created at assignment time\n* Variable type determined implicitly via assignment\n\nx=2 Int \nx=2.0 . Float \nZ=\"hello\" str (single or double quotes) \nz=True Boolean Note capital \"T\" or \"F\" \n\n* Can convert types using conversion functions: int(), float(), str(), bool()\n\n* Python is case sensitive \n* Check variable type using type function \n\n(from https://github.com/ResearchComputing/Python_Spring_2019/blob/master/session1_overview/session1_slides.pdf)",
"_____no_output_____"
]
],
[
[
"z=10.0\nprint('z is: ', type(z) )\n\nx=int(43.4)\nprint(x)",
"z is: <class 'float'>\n43\n"
]
],
[
[
"Arithmetic in Python respects the order of operations \n\n* Addition: +\n* Subtraction: -\n* Multiplication: * \n* Division: / (returns float) \n* Floor Division: // (returns int or float; rounds down) \n* Mod: % (3%2 -> 1) \n* Exponentiation: ** 2**4 -> 16) \n\nCan concatenate strings using \"+\"\n\n(from https://github.com/ResearchComputing/Python_Spring_2019/blob/master/session1_overview/session1_slides.pdf)",
"_____no_output_____"
]
],
[
[
"x='hello '+'there'\nprint(x)",
"hello there\n"
]
],
[
[
"### Lists\n",
"_____no_output_____"
],
[
"Multiple Values can be grouped into a list",
"_____no_output_____"
]
],
[
[
"- lists\n- basic plotting with matplotlib\n- arrays and numpy; doing calculations\n- plotting using numpy\n- importing data from csv files",
"_____no_output_____"
],
[
"mylist=[1, 2, 10]\nprint(mylist)",
"[1, 2, 10]\n"
]
],
[
[
"* List elements accessed with [] notation\n* Element numbering starts at 0",
"_____no_output_____"
]
],
[
[
"print(mylist[1])",
"2\n"
]
],
[
[
"* Lists can contain different variable types",
"_____no_output_____"
]
],
[
[
"mylist=[1, 'two', 10.0]\nprint(mylist)",
"[1, 'two', 10.0]\n"
]
]
] | [
"markdown",
"raw",
"markdown",
"raw",
"markdown",
"raw",
"markdown",
"raw",
"markdown",
"raw",
"markdown",
"raw",
"markdown",
"raw",
"markdown",
"raw",
"markdown",
"raw",
"markdown",
"raw",
"markdown",
"raw",
"markdown",
"raw",
"markdown",
"raw",
"markdown",
"raw",
"markdown",
"code",
"markdown",
"raw",
"markdown",
"code",
"markdown",
"code",
"markdown",
"code",
"markdown",
"code",
"markdown",
"code",
"markdown",
"code"
] | [
[
"markdown",
"markdown",
"markdown",
"markdown",
"markdown",
"markdown",
"markdown",
"markdown",
"markdown",
"markdown"
],
[
"raw"
],
[
"markdown",
"markdown",
"markdown"
],
[
"raw"
],
[
"markdown",
"markdown"
],
[
"raw"
],
[
"markdown"
],
[
"raw"
],
[
"markdown"
],
[
"raw"
],
[
"markdown"
],
[
"raw"
],
[
"markdown"
],
[
"raw"
],
[
"markdown"
],
[
"raw"
],
[
"markdown"
],
[
"raw"
],
[
"markdown"
],
[
"raw"
],
[
"markdown"
],
[
"raw"
],
[
"markdown"
],
[
"raw"
],
[
"markdown"
],
[
"raw"
],
[
"markdown"
],
[
"raw"
],
[
"markdown",
"markdown",
"markdown",
"markdown",
"markdown",
"markdown",
"markdown"
],
[
"code"
],
[
"markdown",
"markdown"
],
[
"raw"
],
[
"markdown"
],
[
"code"
],
[
"markdown",
"markdown",
"markdown"
],
[
"code"
],
[
"markdown"
],
[
"code"
],
[
"markdown",
"markdown"
],
[
"code",
"code"
],
[
"markdown"
],
[
"code"
],
[
"markdown"
],
[
"code"
]
] |
d07acde1a5766b57968ba3ea6d514dcf4857e9e6 | 3,093 | ipynb | Jupyter Notebook | jupyter/notebooks/prod_db/create users.ipynb | kafonek/fastapi_tdd | 32dbd53e3b63e30fe5a694ee5a02d8fc4de99b47 | [
"MIT"
] | null | null | null | jupyter/notebooks/prod_db/create users.ipynb | kafonek/fastapi_tdd | 32dbd53e3b63e30fe5a694ee5a02d8fc4de99b47 | [
"MIT"
] | null | null | null | jupyter/notebooks/prod_db/create users.ipynb | kafonek/fastapi_tdd | 32dbd53e3b63e30fe5a694ee5a02d8fc4de99b47 | [
"MIT"
] | null | null | null | 20.483444 | 80 | 0.537666 | [
[
[
"%load_ext nb_black",
"_____no_output_____"
],
[
"import sys\n\nsys.path.append(\"/home/jovyan/src\")",
"_____no_output_____"
],
[
"from app.config import settings\n\nsettings",
"_____no_output_____"
],
[
"settings.DB_ECHO = True\nsettings.DATABASE_URL = 'sqlite:////home/jovyan/src/db/prod.db'",
"_____no_output_____"
],
[
"from app import database\n\nsession = database.SessionLocal()\nsession",
"_____no_output_____"
],
[
"from app.models import User\nfrom app.security import pwd_context\n\nuser = User(username=\"jovyan\", password=pwd_context.hash(\"jovyan\"))\n\nsession.add(user)\nsession.commit()",
"_____no_output_____"
]
]
] | [
"code"
] | [
[
"code",
"code",
"code",
"code",
"code",
"code"
]
] |
d07add1aa86158d32cd196922428ac30e1bcb9f5 | 45,113 | ipynb | Jupyter Notebook | Case Studies/R/wage_data_analysis/.ipynb_checkpoints/analysis-checkpoint.ipynb | AnanduR32/Data_Science_R | 17a0b3056f558d917cec222b2d27a9d16920a9b6 | [
"MIT"
] | 1 | 2021-07-03T06:38:12.000Z | 2021-07-03T06:38:12.000Z | Case Studies/R/wage_data_analysis/.ipynb_checkpoints/analysis-checkpoint.ipynb | AnanduR32/Data_Science_R | 17a0b3056f558d917cec222b2d27a9d16920a9b6 | [
"MIT"
] | null | null | null | Case Studies/R/wage_data_analysis/.ipynb_checkpoints/analysis-checkpoint.ipynb | AnanduR32/Data_Science_R | 17a0b3056f558d917cec222b2d27a9d16920a9b6 | [
"MIT"
] | 1 | 2021-08-12T17:55:43.000Z | 2021-08-12T17:55:43.000Z | 87.768482 | 15,594 | 0.797619 | [
[
[
"empty"
]
]
] | [
"empty"
] | [
[
"empty"
]
] |
d07ae5badc4d1bdc01de546711894445bf72e024 | 3,104 | ipynb | Jupyter Notebook | GA Lesson 8 TSP/GA Lesson 8 TSP.ipynb | 404nofound/Genetic-Algorithm | affc86494fa878c8d5ba6733d985f3a84b0c3fe2 | [
"MIT"
] | null | null | null | GA Lesson 8 TSP/GA Lesson 8 TSP.ipynb | 404nofound/Genetic-Algorithm | affc86494fa878c8d5ba6733d985f3a84b0c3fe2 | [
"MIT"
] | null | null | null | GA Lesson 8 TSP/GA Lesson 8 TSP.ipynb | 404nofound/Genetic-Algorithm | affc86494fa878c8d5ba6733d985f3a84b0c3fe2 | [
"MIT"
] | null | null | null | 26.305085 | 106 | 0.402706 | [
[
[
"import numpy as np\nfrom numpy.random import randint, rand, shuffle\nfrom matplotlib.pyplot import plot, show, rc\n\na = np.loadtxt(\"Pdata17_2.txt\")\n\nxy, d = a[:, :2], a[:, 2:]\nN = len(xy)\n#w为种群的个数\nw = 50\n#g为进化的代数\ng = 100\nJ = []\n\nfor i in np.arange(w):\n c = np.arange(1, N-1)\n shuffle(c)\n c1 = np.r_[0, c, 101]\n flag = 1\n \n while flag > 0:\n flag = 0\n for m in np.arange(1, N - 3):\n for n in np.arange(m + 1, N - 2):\n if d[c1[m], c1[n]] + d[c1[m+1], c1[n+1]] < d[c1[m], c1[m+1]] + d[c1[n], c1[n+1]]:\n c1[m+1:n+1] = c1[n:m:-1]\n flag = 1\n c1[c1] = np.arange(N)\n J.append(c1)\nJ = np.array(J) / (N-1)\n#print('JJJ',J.shape)\n#print(J)\nfor k in np.arange(g):\n #print('JJJ',J.shape)\n A = J.copy()\n c1 = np.arange(w)\n #交叉操作的染色体配对组\n shuffle(c1)\n #交叉点的数据\n c2 = randint(2, 100, w)\n for i in np.arange(0, w, 2):\n #保存中介变量\n temp = A[c1[i], c2[i]:N-1]\n A[c1[i], c2[i]:N-1] = A[c1[i+1],c2[i]:N-1]\n A[c1[i+1],c2[i]:N-1]=temp\n B = A.copy()\n #初始化变异染色体的序号\n by = []\n while len(by) < 1:\n by = np.where(rand(w) < 0.1)\n #print(by)\n by = by[0]\n B = B[by,:]\n #print('AAAA', A.shape)\n #print('BBBB', B.shape)\n G = np.r_[J,A,B]\n #print(G.shape)\n #把染色体翻译成0,1,...,101\n ind = np.argsort(G, axis=1)\n #print(ind)\n NN = G.shape[0]\n #print(NN)\n L = np.zeros(NN)\n for j in np.arange(NN):\n for i in np.arange(101):\n L[j] = L[j] + d[ind[j,i],ind[j,i+1]]\n ind2 = np.argsort(L)\n J = G[ind2,:]\npath = ind[ind2[0],:]\nzL = L[ind2[0]]\nxx = xy[path,0]\nyy = xy[path, 1]\nrc('font', size=16)\nplot(xx,yy,'-*')\nshow()\nprint('所求的巡航路径长度为:', zL)\n ",
"_____no_output_____"
]
]
] | [
"code"
] | [
[
"code"
]
] |
d07ae753445a1f8f00e845ebd238f8b85126f73a | 113,220 | ipynb | Jupyter Notebook | pipelining/pdp-exp1/pdp-exp1_cslg-rand-5000_plotting.ipynb | ZeruiW/s2search | cb0539b9594d7afe12e64c0b4ada4fb29c793060 | [
"Apache-2.0"
] | 2 | 2022-02-07T16:08:04.000Z | 2022-03-27T19:29:33.000Z | pipelining/pdp-exp1/pdp-exp1_cslg-rand-5000_plotting.ipynb | youyinnn/s2search | f965a595386b24ffab0385b860a1028e209fde86 | [
"Apache-2.0"
] | 1 | 2022-03-30T17:50:32.000Z | 2022-03-30T17:50:32.000Z | pipelining/pdp-exp1/pdp-exp1_cslg-rand-5000_plotting.ipynb | ZeruiW/s2search | cb0539b9594d7afe12e64c0b4ada4fb29c793060 | [
"Apache-2.0"
] | 1 | 2022-03-14T19:44:47.000Z | 2022-03-14T19:44:47.000Z | 400.070671 | 64,358 | 0.926471 | [
[
[
"<a href=\"https://colab.research.google.com/github/DingLi23/s2search/blob/pipelining/pipelining/pdp-exp1/pdp-exp1_cslg-rand-5000_plotting.ipynb\" target=\"_blank\"><img src=\"https://colab.research.google.com/assets/colab-badge.svg\" alt=\"Open In Colab\"/></a>",
"_____no_output_____"
],
[
"### Experiment Description\n\nProduce PDP for a randomly picked data from cslg.\n\n> This notebook is for experiment \\<pdp-exp1\\> and data sample \\<cslg-rand-5000\\>.",
"_____no_output_____"
],
[
"### Initialization",
"_____no_output_____"
]
],
[
[
"%load_ext autoreload\n%autoreload 2\nimport numpy as np, sys, os\nin_colab = 'google.colab' in sys.modules\n# fetching code and data(if you are using colab\nif in_colab:\n !rm -rf s2search\n !git clone --branch pipelining https://github.com/youyinnn/s2search.git\n sys.path.insert(1, './s2search')\n %cd s2search/pipelining/pdp-exp1/\n\npic_dir = os.path.join('.', 'plot')\nif not os.path.exists(pic_dir):\n os.mkdir(pic_dir)\n",
"The autoreload extension is already loaded. To reload it, use:\n %reload_ext autoreload\n"
]
],
[
[
"### Loading data",
"_____no_output_____"
]
],
[
[
"sys.path.insert(1, '../../')\nimport numpy as np, sys, os, pandas as pd\nfrom s2search_score_pdp import pdp_based_importance, apply_order\n\nsample_name = 'cslg-rand-5000'\n\nf_list = ['title', 'abstract', 'venue', 'authors', 'year', 'n_citations']\n\npdp_xy = {}\npdp_metric = pd.DataFrame(columns=['feature_name', 'pdp_range', 'pdp_importance'])\n\nfor f in f_list:\n file = os.path.join('.', 'scores', f'{sample_name}_pdp_{f}.npz')\n if os.path.exists(file):\n data = np.load(file)\n sorted_pdp_data = apply_order(data)\n feature_pdp_data = [np.mean(pdps) for pdps in sorted_pdp_data]\n \n pdp_xy[f] = {\n 'y': feature_pdp_data,\n 'numerical': True\n }\n if f == 'year' or f == 'n_citations':\n pdp_xy[f]['x'] = np.sort(data['arr_1'])\n else:\n pdp_xy[f]['y'] = feature_pdp_data\n pdp_xy[f]['x'] = list(range(len(feature_pdp_data)))\n pdp_xy[f]['numerical'] = False\n \n pdp_metric.loc[len(pdp_metric.index)] = [f, np.max(feature_pdp_data) - np.min(feature_pdp_data), pdp_based_importance(feature_pdp_data, f)]\n \n pdp_xy[f]['weird'] = feature_pdp_data[len(feature_pdp_data) - 1] > 30\n \n\nprint(pdp_metric.sort_values(by=['pdp_importance'], ascending=False))\n",
" feature_name pdp_range pdp_importance\n1 abstract 17.129012 6.155994\n0 title 15.760054 3.788171\n2 venue 12.542338 0.971027\n4 year 2.190717 0.470416\n5 n_citations 0.977882 0.193581\n3 authors 0.000000 0.000000\n"
]
],
[
[
"### PDP",
"_____no_output_____"
]
],
[
[
"import matplotlib.pyplot as plt\n\ncategorical_plot_conf = [\n {\n 'xlabel': 'Title',\n 'ylabel': 'Scores',\n 'pdp_xy': pdp_xy['title']\n },\n {\n 'xlabel': 'Abstract',\n 'pdp_xy': pdp_xy['abstract']\n }, \n {\n 'xlabel': 'Authors',\n 'pdp_xy': pdp_xy['authors']\n },\n {\n 'xlabel': 'Venue',\n 'pdp_xy': pdp_xy['venue'],\n 'zoom': {\n 'inset_axes': [0.15, 0.45, 0.47, 0.47],\n 'x_limit': [4900, 5050],\n 'y_limit': [-9, 7],\n 'connects': [True, True, False, False]\n }\n },\n]\n\nnumerical_plot_conf = [\n {\n 'xlabel': 'Year',\n 'ylabel': 'Scores',\n 'pdp_xy': pdp_xy['year']\n },\n {\n 'xlabel': 'Citation Count',\n 'pdp_xy': pdp_xy['n_citations'],\n 'zoom': {\n 'inset_axes': [0.4, 0.2, 0.47, 0.47],\n 'x_limit': [-100, 500],\n 'y_limit': [-7.5, -6.2],\n 'connects': [True, False, False, True]\n }\n }\n]\n\ndef pdp_plot(confs, title):\n fig, axes = plt.subplots(nrows=1, ncols=len(confs), figsize=(20, 5), dpi=100)\n subplot_idx = 0\n # plt.suptitle(title, fontsize=20, fontweight='bold')\n # plt.autoscale(False)\n for conf in confs:\n axess = axes if len(confs) == 1 else axes[subplot_idx]\n\n axess.plot(conf['pdp_xy']['x'], conf['pdp_xy']['y'])\n axess.grid(alpha = 0.4)\n\n if ('ylabel' in conf):\n axess.set_ylabel(conf.get('ylabel'), fontsize=20, labelpad=10)\n \n axess.set_xlabel(conf['xlabel'], fontsize=16, labelpad=10)\n \n if not (conf['pdp_xy']['weird']):\n if (conf['pdp_xy']['numerical']):\n axess.set_ylim([-9, -6])\n pass\n else:\n axess.set_ylim([-15, 10])\n pass\n \n if 'zoom' in conf:\n axins = axess.inset_axes(conf['zoom']['inset_axes']) \n axins.plot(conf['pdp_xy']['x'], conf['pdp_xy']['y'])\n axins.set_xlim(conf['zoom']['x_limit'])\n axins.set_ylim(conf['zoom']['y_limit'])\n axins.grid(alpha=0.3)\n rectpatch, connects = axess.indicate_inset_zoom(axins)\n connects[0].set_visible(conf['zoom']['connects'][0])\n connects[1].set_visible(conf['zoom']['connects'][1])\n connects[2].set_visible(conf['zoom']['connects'][2])\n connects[3].set_visible(conf['zoom']['connects'][3])\n \n subplot_idx += 1\n\npdp_plot(categorical_plot_conf, \"PDPs for four categorical features\")\nplt.savefig(os.path.join('.', 'plot', f'{sample_name}-categorical.png'), facecolor='white', transparent=False, bbox_inches='tight')\n\n# second fig\npdp_plot(numerical_plot_conf, \"PDPs for two numerical features\")\nplt.savefig(os.path.join('.', 'plot', f'{sample_name}-numerical.png'), facecolor='white', transparent=False, bbox_inches='tight')\n",
"_____no_output_____"
]
]
] | [
"markdown",
"code",
"markdown",
"code",
"markdown",
"code"
] | [
[
"markdown",
"markdown",
"markdown"
],
[
"code"
],
[
"markdown"
],
[
"code"
],
[
"markdown"
],
[
"code"
]
] |
Subsets and Splits
No community queries yet
The top public SQL queries from the community will appear here once available.