modelId
stringlengths 4
112
| sha
stringlengths 40
40
| lastModified
stringlengths 24
24
| tags
sequence | pipeline_tag
stringclasses 29
values | private
bool 1
class | author
stringlengths 2
38
⌀ | config
null | id
stringlengths 4
112
| downloads
float64 0
36.8M
⌀ | likes
float64 0
712
⌀ | library_name
stringclasses 17
values | __index_level_0__
int64 0
38.5k
| readme
stringlengths 0
186k
|
---|---|---|---|---|---|---|---|---|---|---|---|---|---|
huggingtweets/o_strunz | 16607e9f8e61e684ff9764283a8c0abab075440b | 2022-07-01T21:57:48.000Z | [
"pytorch",
"gpt2",
"text-generation",
"en",
"transformers",
"huggingtweets"
] | text-generation | false | huggingtweets | null | huggingtweets/o_strunz | 0 | null | transformers | 38,400 | ---
language: en
thumbnail: http://www.huggingtweets.com/o_strunz/1656712663617/predictions.png
tags:
- huggingtweets
widget:
- text: "My dream is"
---
<div class="inline-flex flex-col" style="line-height: 1.5;">
<div class="flex">
<div
style="display:inherit; margin-left: 4px; margin-right: 4px; width: 92px; height:92px; border-radius: 50%; background-size: cover; background-image: url('https://pbs.twimg.com/profile_images/1013878708539666439/FqgS0pMK_400x400.jpg')">
</div>
<div
style="display:none; margin-left: 4px; margin-right: 4px; width: 92px; height:92px; border-radius: 50%; background-size: cover; background-image: url('')">
</div>
<div
style="display:none; margin-left: 4px; margin-right: 4px; width: 92px; height:92px; border-radius: 50%; background-size: cover; background-image: url('')">
</div>
</div>
<div style="text-align: center; margin-top: 3px; font-size: 16px; font-weight: 800">🤖 AI BOT 🤖</div>
<div style="text-align: center; font-size: 16px; font-weight: 800">'O Strunz</div>
<div style="text-align: center; font-size: 14px;">@o_strunz</div>
</div>
I was made with [huggingtweets](https://github.com/borisdayma/huggingtweets).
Create your own bot based on your favorite user with [the demo](https://colab.research.google.com/github/borisdayma/huggingtweets/blob/master/huggingtweets-demo.ipynb)!
## How does it work?
The model uses the following pipeline.
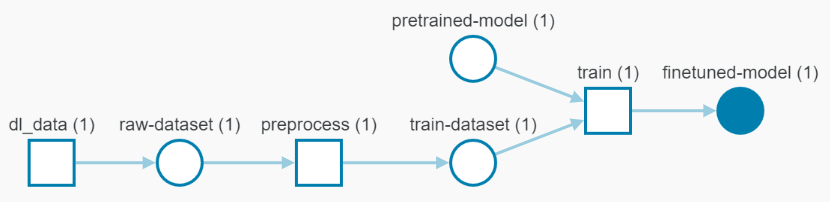
To understand how the model was developed, check the [W&B report](https://wandb.ai/wandb/huggingtweets/reports/HuggingTweets-Train-a-Model-to-Generate-Tweets--VmlldzoxMTY5MjI).
## Training data
The model was trained on tweets from 'O Strunz.
| Data | 'O Strunz |
| --- | --- |
| Tweets downloaded | 3250 |
| Retweets | 9 |
| Short tweets | 306 |
| Tweets kept | 2935 |
[Explore the data](https://wandb.ai/wandb/huggingtweets/runs/dn48t762/artifacts), which is tracked with [W&B artifacts](https://docs.wandb.com/artifacts) at every step of the pipeline.
## Training procedure
The model is based on a pre-trained [GPT-2](https://huggingface.co/gpt2) which is fine-tuned on @o_strunz's tweets.
Hyperparameters and metrics are recorded in the [W&B training run](https://wandb.ai/wandb/huggingtweets/runs/1essqqbv) for full transparency and reproducibility.
At the end of training, [the final model](https://wandb.ai/wandb/huggingtweets/runs/1essqqbv/artifacts) is logged and versioned.
## How to use
You can use this model directly with a pipeline for text generation:
```python
from transformers import pipeline
generator = pipeline('text-generation',
model='huggingtweets/o_strunz')
generator("My dream is", num_return_sequences=5)
```
## Limitations and bias
The model suffers from [the same limitations and bias as GPT-2](https://huggingface.co/gpt2#limitations-and-bias).
In addition, the data present in the user's tweets further affects the text generated by the model.
## About
*Built by Boris Dayma*
[](https://twitter.com/intent/follow?screen_name=borisdayma)
For more details, visit the project repository.
[](https://github.com/borisdayma/huggingtweets)
|
jdeboever/xlm-roberta-base-finetuned-panx-de | 788128771a069512052def7f2e6ce02631105cdf | 2022-07-02T02:05:26.000Z | [
"pytorch",
"tensorboard",
"xlm-roberta",
"token-classification",
"dataset:xtreme",
"transformers",
"generated_from_trainer",
"license:mit",
"model-index",
"autotrain_compatible"
] | token-classification | false | jdeboever | null | jdeboever/xlm-roberta-base-finetuned-panx-de | 0 | null | transformers | 38,401 | ---
license: mit
tags:
- generated_from_trainer
datasets:
- xtreme
metrics:
- f1
model-index:
- name: xlm-roberta-base-finetuned-panx-de
results:
- task:
name: Token Classification
type: token-classification
dataset:
name: xtreme
type: xtreme
args: PAN-X.de
metrics:
- name: F1
type: f1
value: 0.8627004891366169
---
<!-- This model card has been generated automatically according to the information the Trainer had access to. You
should probably proofread and complete it, then remove this comment. -->
# xlm-roberta-base-finetuned-panx-de
This model is a fine-tuned version of [xlm-roberta-base](https://huggingface.co/xlm-roberta-base) on the xtreme dataset.
It achieves the following results on the evaluation set:
- Loss: 0.1363
- F1: 0.8627
## Model description
More information needed
## Intended uses & limitations
More information needed
## Training and evaluation data
More information needed
## Training procedure
### Training hyperparameters
The following hyperparameters were used during training:
- learning_rate: 5e-05
- train_batch_size: 24
- eval_batch_size: 24
- seed: 42
- optimizer: Adam with betas=(0.9,0.999) and epsilon=1e-08
- lr_scheduler_type: linear
- num_epochs: 3
### Training results
| Training Loss | Epoch | Step | Validation Loss | F1 |
|:-------------:|:-----:|:----:|:---------------:|:------:|
| 0.2539 | 1.0 | 525 | 0.1697 | 0.8179 |
| 0.1317 | 2.0 | 1050 | 0.1327 | 0.8516 |
| 0.0819 | 3.0 | 1575 | 0.1363 | 0.8627 |
### Framework versions
- Transformers 4.11.3
- Pytorch 1.11.0
- Datasets 1.16.1
- Tokenizers 0.10.3
|
markrogersjr/summarization | 07ab905a213a8ad96776a2d94c0ef988e212dbc6 | 2022-07-02T00:00:45.000Z | [
"pytorch",
"mt5",
"text2text-generation",
"transformers",
"autotrain_compatible"
] | text2text-generation | false | markrogersjr | null | markrogersjr/summarization | 0 | null | transformers | 38,402 | Entry not found |
huggingtweets/pldroneoperator-superpiss | 8c8afa7db3ed6ec08a9c68d2b9d5879f56954a6e | 2022-07-02T06:21:02.000Z | [
"pytorch",
"gpt2",
"text-generation",
"en",
"transformers",
"huggingtweets"
] | text-generation | false | huggingtweets | null | huggingtweets/pldroneoperator-superpiss | 0 | null | transformers | 38,403 | ---
language: en
thumbnail: http://www.huggingtweets.com/pldroneoperator-superpiss/1656742858038/predictions.png
tags:
- huggingtweets
widget:
- text: "My dream is"
---
<div class="inline-flex flex-col" style="line-height: 1.5;">
<div class="flex">
<div
style="display:inherit; margin-left: 4px; margin-right: 4px; width: 92px; height:92px; border-radius: 50%; background-size: cover; background-image: url('https://pbs.twimg.com/profile_images/1458465489425158144/WQBM7dy1_400x400.jpg')">
</div>
<div
style="display:inherit; margin-left: 4px; margin-right: 4px; width: 92px; height:92px; border-radius: 50%; background-size: cover; background-image: url('https://pbs.twimg.com/profile_images/1077258168315473920/b8-3h6l4_400x400.jpg')">
</div>
<div
style="display:none; margin-left: 4px; margin-right: 4px; width: 92px; height:92px; border-radius: 50%; background-size: cover; background-image: url('')">
</div>
</div>
<div style="text-align: center; margin-top: 3px; font-size: 16px; font-weight: 800">🤖 AI CYBORG 🤖</div>
<div style="text-align: center; font-size: 16px; font-weight: 800">Peter🦍🍌 & xbox 720</div>
<div style="text-align: center; font-size: 14px;">@pldroneoperator-superpiss</div>
</div>
I was made with [huggingtweets](https://github.com/borisdayma/huggingtweets).
Create your own bot based on your favorite user with [the demo](https://colab.research.google.com/github/borisdayma/huggingtweets/blob/master/huggingtweets-demo.ipynb)!
## How does it work?
The model uses the following pipeline.
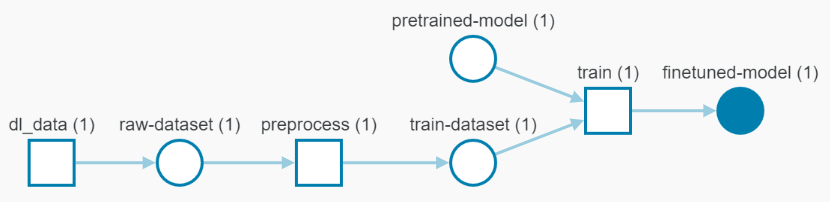
To understand how the model was developed, check the [W&B report](https://wandb.ai/wandb/huggingtweets/reports/HuggingTweets-Train-a-Model-to-Generate-Tweets--VmlldzoxMTY5MjI).
## Training data
The model was trained on tweets from Peter🦍🍌 & xbox 720.
| Data | Peter🦍🍌 | xbox 720 |
| --- | --- | --- |
| Tweets downloaded | 3236 | 206 |
| Retweets | 111 | 0 |
| Short tweets | 617 | 7 |
| Tweets kept | 2508 | 199 |
[Explore the data](https://wandb.ai/wandb/huggingtweets/runs/vbt632lx/artifacts), which is tracked with [W&B artifacts](https://docs.wandb.com/artifacts) at every step of the pipeline.
## Training procedure
The model is based on a pre-trained [GPT-2](https://huggingface.co/gpt2) which is fine-tuned on @pldroneoperator-superpiss's tweets.
Hyperparameters and metrics are recorded in the [W&B training run](https://wandb.ai/wandb/huggingtweets/runs/nmy5gsm5) for full transparency and reproducibility.
At the end of training, [the final model](https://wandb.ai/wandb/huggingtweets/runs/nmy5gsm5/artifacts) is logged and versioned.
## How to use
You can use this model directly with a pipeline for text generation:
```python
from transformers import pipeline
generator = pipeline('text-generation',
model='huggingtweets/pldroneoperator-superpiss')
generator("My dream is", num_return_sequences=5)
```
## Limitations and bias
The model suffers from [the same limitations and bias as GPT-2](https://huggingface.co/gpt2#limitations-and-bias).
In addition, the data present in the user's tweets further affects the text generated by the model.
## About
*Built by Boris Dayma*
[](https://twitter.com/intent/follow?screen_name=borisdayma)
For more details, visit the project repository.
[](https://github.com/borisdayma/huggingtweets)
|
openclimatefix/graph-weather-forecaster-0.5deg-nolandsea | 0263dfbe7043f051639a5e7d805eea03fff8be97 | 2022-07-02T13:17:37.000Z | [
"pytorch"
] | null | false | openclimatefix | null | openclimatefix/graph-weather-forecaster-0.5deg-nolandsea | 0 | null | null | 38,404 | Entry not found |
gaunernst/bert-L2-H256-uncased | d44a2b538f42e05e72be36fd6139dcc88dba1d97 | 2022-07-02T08:09:26.000Z | [
"pytorch",
"bert",
"transformers",
"license:apache-2.0"
] | null | false | gaunernst | null | gaunernst/bert-L2-H256-uncased | 0 | null | transformers | 38,405 | ---
license: apache-2.0
---
|
gaunernst/bert-L6-H768-uncased | 92b543d7caeed13f47358448eac3c4cd6650acf3 | 2022-07-02T08:26:39.000Z | [
"pytorch",
"bert",
"transformers",
"license:apache-2.0"
] | null | false | gaunernst | null | gaunernst/bert-L6-H768-uncased | 0 | null | transformers | 38,406 | ---
license: apache-2.0
---
|
gaunernst/bert-L10-H128-uncased | 88372cf07803f662df3165b37e63a3413b631e93 | 2022-07-02T08:42:07.000Z | [
"pytorch",
"bert",
"transformers",
"license:apache-2.0"
] | null | false | gaunernst | null | gaunernst/bert-L10-H128-uncased | 0 | null | transformers | 38,407 | ---
license: apache-2.0
---
|
gaunernst/bert-L10-H256-uncased | 9ef1495eb67efd61347fbe0c84fb4a27496d281d | 2022-07-02T08:42:45.000Z | [
"pytorch",
"bert",
"transformers",
"license:apache-2.0"
] | null | false | gaunernst | null | gaunernst/bert-L10-H256-uncased | 0 | null | transformers | 38,408 | ---
license: apache-2.0
---
|
gaunernst/bert-L10-H768-uncased | 9af19fb436ee9d71b7ba90f7e3395f5683f30f3a | 2022-07-02T08:47:25.000Z | [
"pytorch",
"bert",
"transformers",
"license:apache-2.0"
] | null | false | gaunernst | null | gaunernst/bert-L10-H768-uncased | 0 | null | transformers | 38,409 | ---
license: apache-2.0
---
|
gaunernst/bert-L12-H512-uncased | f6c8e79ecffa6ec5bf68f0adc17fe8a66103720e | 2022-07-02T08:55:43.000Z | [
"pytorch",
"bert",
"transformers",
"license:apache-2.0"
] | null | false | gaunernst | null | gaunernst/bert-L12-H512-uncased | 0 | null | transformers | 38,410 | ---
license: apache-2.0
---
|
jdang/xlm-roberta-base-finetuned-panx-de | c24b3019522a4d151b5c74e0f8a243748253fc60 | 2022-07-03T14:37:39.000Z | [
"pytorch",
"tensorboard",
"xlm-roberta",
"token-classification",
"dataset:xtreme",
"transformers",
"generated_from_trainer",
"license:mit",
"model-index",
"autotrain_compatible"
] | token-classification | false | jdang | null | jdang/xlm-roberta-base-finetuned-panx-de | 0 | null | transformers | 38,411 | ---
license: mit
tags:
- generated_from_trainer
datasets:
- xtreme
metrics:
- f1
model-index:
- name: xlm-roberta-base-finetuned-panx-de
results:
- task:
name: Token Classification
type: token-classification
dataset:
name: xtreme
type: xtreme
args: PAN-X.de
metrics:
- name: F1
type: f1
value: 0.8648740833380706
---
<!-- This model card has been generated automatically according to the information the Trainer had access to. You
should probably proofread and complete it, then remove this comment. -->
# xlm-roberta-base-finetuned-panx-de
This model is a fine-tuned version of [xlm-roberta-base](https://huggingface.co/xlm-roberta-base) on the xtreme dataset.
It achieves the following results on the evaluation set:
- Loss: 0.1365
- F1: 0.8649
## Model description
More information needed
## Intended uses & limitations
More information needed
## Training and evaluation data
More information needed
## Training procedure
### Training hyperparameters
The following hyperparameters were used during training:
- learning_rate: 5e-05
- train_batch_size: 24
- eval_batch_size: 24
- seed: 42
- optimizer: Adam with betas=(0.9,0.999) and epsilon=1e-08
- lr_scheduler_type: linear
- num_epochs: 3
### Training results
| Training Loss | Epoch | Step | Validation Loss | F1 |
|:-------------:|:-----:|:----:|:---------------:|:------:|
| 0.2553 | 1.0 | 525 | 0.1575 | 0.8279 |
| 0.1284 | 2.0 | 1050 | 0.1386 | 0.8463 |
| 0.0813 | 3.0 | 1575 | 0.1365 | 0.8649 |
### Framework versions
- Transformers 4.11.3
- Pytorch 1.12.0
- Datasets 1.16.1
- Tokenizers 0.10.3
|
jdang/xlm-roberta-base-finetuned-panx-de-fr | 7ed48dd458c9c178ca6e882c96e53ea7c6e47645 | 2022-07-02T16:40:04.000Z | [
"pytorch",
"xlm-roberta",
"token-classification",
"transformers",
"generated_from_trainer",
"license:mit",
"model-index",
"autotrain_compatible"
] | token-classification | false | jdang | null | jdang/xlm-roberta-base-finetuned-panx-de-fr | 0 | null | transformers | 38,412 | ---
license: mit
tags:
- generated_from_trainer
metrics:
- f1
model-index:
- name: xlm-roberta-base-finetuned-panx-de-fr
results: []
---
<!-- This model card has been generated automatically according to the information the Trainer had access to. You
should probably proofread and complete it, then remove this comment. -->
# xlm-roberta-base-finetuned-panx-de-fr
This model is a fine-tuned version of [xlm-roberta-base](https://huggingface.co/xlm-roberta-base) on the None dataset.
It achieves the following results on the evaluation set:
- Loss: 0.1608
- F1: 0.8593
## Model description
More information needed
## Intended uses & limitations
More information needed
## Training and evaluation data
More information needed
## Training procedure
### Training hyperparameters
The following hyperparameters were used during training:
- learning_rate: 5e-05
- train_batch_size: 24
- eval_batch_size: 24
- seed: 42
- optimizer: Adam with betas=(0.9,0.999) and epsilon=1e-08
- lr_scheduler_type: linear
- num_epochs: 3
### Training results
| Training Loss | Epoch | Step | Validation Loss | F1 |
|:-------------:|:-----:|:----:|:---------------:|:------:|
| 0.2888 | 1.0 | 715 | 0.1779 | 0.8233 |
| 0.1437 | 2.0 | 1430 | 0.1570 | 0.8497 |
| 0.0931 | 3.0 | 2145 | 0.1608 | 0.8593 |
### Framework versions
- Transformers 4.11.3
- Pytorch 1.12.0
- Datasets 1.16.1
- Tokenizers 0.10.3
|
tner/roberta-large-tweetner-selflabel2020 | 2d662e4368e11b3194b33864633d1384a0495f17 | 2022-07-02T19:14:34.000Z | [
"pytorch",
"roberta",
"token-classification",
"transformers",
"autotrain_compatible"
] | token-classification | false | tner | null | tner/roberta-large-tweetner-selflabel2020 | 0 | null | transformers | 38,413 | Entry not found |
tner/roberta-large-tweetner-2020-selflabel2020-concat | 0f322d544cdf105d85a58a0c1925ba33faff121e | 2022-07-02T19:19:06.000Z | [
"pytorch",
"roberta",
"token-classification",
"transformers",
"autotrain_compatible"
] | token-classification | false | tner | null | tner/roberta-large-tweetner-2020-selflabel2020-concat | 0 | null | transformers | 38,414 | Entry not found |
tner/roberta-large-tweetner-2020-selflabel2021-concat | 5884ac382ea1df9c52a57b7ba633c0b25fa18270 | 2022-07-02T19:19:21.000Z | [
"pytorch",
"roberta",
"token-classification",
"transformers",
"autotrain_compatible"
] | token-classification | false | tner | null | tner/roberta-large-tweetner-2020-selflabel2021-concat | 0 | null | transformers | 38,415 | Entry not found |
tner/roberta-large-tweetner-2020-selflabel2020-continuous | c5a5024d26224064f9500e52e4c5e10f10d2e78e | 2022-07-02T19:23:35.000Z | [
"pytorch",
"roberta",
"token-classification",
"transformers",
"autotrain_compatible"
] | token-classification | false | tner | null | tner/roberta-large-tweetner-2020-selflabel2020-continuous | 0 | null | transformers | 38,416 | Entry not found |
tner/roberta-large-tweetner-2020-selflabel2021-continuous | 0d4a771964856d0ad803933d12852fecfd5e79ad | 2022-07-02T19:23:48.000Z | [
"pytorch",
"roberta",
"token-classification",
"transformers",
"autotrain_compatible"
] | token-classification | false | tner | null | tner/roberta-large-tweetner-2020-selflabel2021-continuous | 0 | null | transformers | 38,417 | Entry not found |
xzhang/distilgpt2-finetuned-wikitext2 | 1b1c36c20899b917657856262833c673c7fdb437 | 2022-07-03T18:48:46.000Z | [
"pytorch",
"tensorboard",
"gpt2",
"text-generation",
"transformers",
"generated_from_trainer",
"license:apache-2.0",
"model-index"
] | text-generation | false | xzhang | null | xzhang/distilgpt2-finetuned-wikitext2 | 0 | null | transformers | 38,418 | ---
license: apache-2.0
tags:
- generated_from_trainer
model-index:
- name: distilgpt2-finetuned-wikitext2
results: []
---
<!-- This model card has been generated automatically according to the information the Trainer had access to. You
should probably proofread and complete it, then remove this comment. -->
# distilgpt2-finetuned-wikitext2
This model is a fine-tuned version of [distilgpt2](https://huggingface.co/distilgpt2) on the None dataset.
It achieves the following results on the evaluation set:
- Loss: 3.6421
## Model description
More information needed
## Intended uses & limitations
More information needed
## Training and evaluation data
More information needed
## Training procedure
### Training hyperparameters
The following hyperparameters were used during training:
- learning_rate: 2e-05
- train_batch_size: 8
- eval_batch_size: 8
- seed: 42
- optimizer: Adam with betas=(0.9,0.999) and epsilon=1e-08
- lr_scheduler_type: linear
- num_epochs: 3.0
### Training results
| Training Loss | Epoch | Step | Validation Loss |
|:-------------:|:-----:|:----:|:---------------:|
| 3.7602 | 1.0 | 2334 | 3.6669 |
| 3.653 | 2.0 | 4668 | 3.6472 |
| 3.6006 | 3.0 | 7002 | 3.6421 |
### Framework versions
- Transformers 4.20.1
- Pytorch 1.11.0+cu113
- Datasets 2.3.2
- Tokenizers 0.12.1
|
Li-Tang/test_model | 9312795e328350594047dec6b108ec8f16c5206b | 2022-07-13T07:43:08.000Z | [
"pytorch",
"license:apache-2.0"
] | null | false | Li-Tang | null | Li-Tang/test_model | 0 | null | null | 38,419 | ---
license: apache-2.0
---
|
BlinkDL/rwkv-3-pile-169m | e1d36bf249b6acdb97a86ed1283a9e433358c907 | 2022-07-20T01:50:57.000Z | [
"en",
"dataset:The Pile",
"pytorch",
"text-generation",
"causal-lm",
"rwkv",
"license:bsd-2-clause"
] | text-generation | false | BlinkDL | null | BlinkDL/rwkv-3-pile-169m | 0 | 1 | null | 38,420 | ---
language:
- en
tags:
- pytorch
- text-generation
- causal-lm
- rwkv
license: bsd-2-clause
datasets:
- The Pile
---
# RWKV-3 169M
## Model Description
RWKV-3 169M is a L12-D768 causal language model trained on the Pile. See https://github.com/BlinkDL/RWKV-LM for details.
At this moment you have to use my Github code (https://github.com/BlinkDL/RWKV-v2-RNN-Pile) to run it.
ctx_len = 768
n_layer = 12
n_embd = 768
Final checkpoint:
RWKV-3-Pile-20220720-10704.pth : Trained on the Pile for 328B tokens.
* Pile loss 2.5596
* LAMBADA ppl 28.82, acc 32.33%
* PIQA acc 64.15%
* SC2016 acc 57.88%
* Hellaswag acc_norm 32.45%
Preview checkpoint:
20220703-1652.pth : Trained on the Pile for 50B tokens. Pile loss 2.6375, LAMBADA ppl 33.30, acc 31.24%. |
samayl24/test-cifar-10 | 5a1e06f22ffc401b07ed07ae103ca717695128e4 | 2022-07-06T17:34:36.000Z | [
"pytorch"
] | null | false | samayl24 | null | samayl24/test-cifar-10 | 0 | null | null | 38,421 | Entry not found |
loicmagne/pr_dataset_metadata | 6495d76b2f8681d1fa7b1d00056d6101d9438da9 | 2022-07-07T19:06:41.000Z | [
"pytorch",
"tensorboard",
"dataset:imdb",
"generated_from_trainer",
"license:apache-2.0",
"model-index"
] | null | false | loicmagne | null | loicmagne/pr_dataset_metadata | 0 | null | null | 38,422 | ---
license: apache-2.0
tags:
- generated_from_trainer
datasets:
- imdb
metrics:
- accuracy
model-index:
- name: pr_dataset_metadata
results:
- task:
name: Text Classification
type: text-classification
dataset:
name: imdb
type: imdb
config: plain_text
split: test
args: plain_text
metrics:
- name: eval_accuracy
type: accuracy
value: 1.0
---
<!-- This model card has been generated automatically according to the information the Trainer had access to. You
should probably proofread and complete it, then remove this comment. -->
# pr_dataset_metadata
This model is a fine-tuned version of [distilbert-base-uncased](https://huggingface.co/distilbert-base-uncased) on the imdb dataset.
It achieves the following results on the evaluation set:
- eval_loss: 0.6216
- eval_accuracy: 1.0
- eval_runtime: 0.4472
- eval_samples_per_second: 2.236
- eval_steps_per_second: 2.236
- step: 0
## Model description
More information needed
## Intended uses & limitations
More information needed
## Training and evaluation data
More information needed
## Training procedure
### Training hyperparameters
The following hyperparameters were used during training:
- learning_rate: 5e-05
- train_batch_size: 16
- eval_batch_size: 16
- seed: 42
- distributed_type: not_parallel
- optimizer: Adam with betas=(0.9,0.999) and epsilon=1e-08
- lr_scheduler_type: linear
- num_epochs: 3.0
### Framework versions
- Transformers 4.21.0.dev0
- Pytorch 1.11.0+cu102
- Datasets 2.0.0
- Tokenizers 0.12.1
|
nateraw/yolov6n | 1c1f377c2300c7dec9cac72548bb532155e2c7c6 | 2022-07-12T02:01:10.000Z | [
"en",
"arxiv:1910.09700",
"pytorch",
"object-detection",
"yolo",
"autogenerated-modelcard",
"license:gpl-3.0"
] | object-detection | false | nateraw | null | nateraw/yolov6n | 0 | null | pytorch | 38,423 | ---
language: en
license: gpl-3.0
library_name: pytorch
tags:
- object-detection
- yolo
- autogenerated-modelcard
model_name: yolov6n
---
# Model Card for yolov6n
<!-- Provide a quick summary of what the model is/does. -->
# Table of Contents
1. [Model Details](#model-details)
2. [Uses](#uses)
3. [Bias, Risks, and Limitations](#bias-risks-and-limitations)
4. [Training Details](#training-details)
5. [Evaluation](#evaluation)
6. [Model Examination](#model-examination)
7. [Environmental Impact](#environmental-impact)
8. [Technical Specifications](#technical-specifications-optional)
9. [Citation](#citation)
10. [Glossary](#glossary-optional)
11. [More Information](#more-information-optional)
12. [Model Card Authors](#model-card-authors-optional)
13. [Model Card Contact](#model-card-contact)
14. [How To Get Started With the Model](#how-to-get-started-with-the-model)
# Model Details
## Model Description
<!-- Provide a longer summary of what this model is. -->
YOLOv6 is a single-stage object detection framework dedicated to industrial applications, with hardware-friendly efficient design and high performance.
- **Developed by:** [More Information Needed]
- **Shared by [Optional]:** [@nateraw](https://hf.co/nateraw)
- **Model type:** [More Information Needed]
- **Language(s) (NLP):** [More Information Needed]
- **License:** [More Information Needed]
- **Related Models:** [yolov6t](https://hf.co/nateraw/yolov6t), [yolov6s](https://hf.co/nateraw/yolov6s)
- **Parent Model:** N/A
- **Resources for more information:** The [official GitHub Repository](https://github.com/meituan/YOLOv6)
# Uses
<!-- Address questions around how the model is intended to be used, including the foreseeable users of the model and those affected by the model. -->
## Direct Use
<!-- This section is for the model use without fine-tuning or plugging into a larger ecosystem/app. -->
This model is meant to be used as a general object detector.
## Downstream Use [Optional]
<!-- This section is for the model use when fine-tuned for a task, or when plugged into a larger ecosystem/app -->
You can fine-tune this model for your specific task
## Out-of-Scope Use
<!-- This section addresses misuse, malicious use, and uses that the model will not work well for. -->
Don't be evil.
# Bias, Risks, and Limitations
<!-- This section is meant to convey both technical and sociotechnical limitations. -->
This model often classifies objects incorrectly, especially when applied to videos. It does not handle crowds very well.
## Recommendations
<!-- This section is meant to convey recommendations with respect to the bias, risk, and technical limitations. -->
Users (both direct and downstream) should be made aware of the risks, biases and limitations of the model. More information needed for further recomendations.
# Training Details
## Training Data
<!-- This should link to a Data Card, perhaps with a short stub of information on what the training data is all about as well as documentation related to data pre-processing or additional filtering. -->
[More Information Needed]
## Training Procedure
<!-- This relates heavily to the Technical Specifications. Content here should link to that section when it is relevant to the training procedure. -->
### Preprocessing
[More Information Needed]
### Speeds, Sizes, Times
<!-- This section provides information about throughput, start/end time, checkpoint size if relevant, etc. -->
[More Information Needed]
# Evaluation
<!-- This section describes the evaluation protocols and provides the results. -->
## Testing Data, Factors & Metrics
### Testing Data
<!-- This should link to a Data Card if possible. -->
[More Information Needed]
### Factors
<!-- These are the things the evaluation is disaggregating by, e.g., subpopulations or domains. -->
[More Information Needed]
### Metrics
<!-- These are the evaluation metrics being used, ideally with a description of why. -->
[More Information Needed]
## Results
[More Information Needed]
# Model Examination
[More Information Needed]
# Environmental Impact
<!-- Total emissions (in grams of CO2eq) and additional considerations, such as electricity usage, go here. Edit the suggested text below accordingly -->
Carbon emissions can be estimated using the [Machine Learning Impact calculator](https://mlco2.github.io/impact#compute) presented in [Lacoste et al. (2019)](https://arxiv.org/abs/1910.09700).
- **Hardware Type:** [More Information Needed]
- **Hours used:** [More Information Needed]
- **Cloud Provider:** [More Information Needed]
- **Compute Region:** [More Information Needed]
- **Carbon Emitted:** [More Information Needed]
# Technical Specifications [optional]
## Model Architecture and Objective
[More Information Needed]
## Compute Infrastructure
[More Information Needed]
### Hardware
[More Information Needed]
### Software
[More Information Needed]
# Citation
<!-- If there is a paper or blog post introducing the model, the APA and Bibtex information for that should go in this section. -->
**BibTeX:**
[More Information Needed]
**APA:**
[More Information Needed]
# Glossary [optional]
<!-- If relevant, include terms and calculations in this section that can help readers understand the model or model card. -->
[More Information Needed]
# More Information [optional]
Please refer to the [official GitHub Repository](https://github.com/meituan/YOLOv6)
# Model Card Authors [optional]
[@nateraw](https://hf.co/nateraw)
# Model Card Contact
[@nateraw](https://hf.co/nateraw) - please leave a note in the discussions tab here
# How to Get Started with the Model
Use the code below to get started with the model.
<details>
<summary> Click to expand </summary>
[More Information Needed]
</details> |
nateraw/yolov6s | 7d80ec834b4117d1af91266506bdc9ec488e8632 | 2022-07-12T02:01:18.000Z | [
"en",
"arxiv:1910.09700",
"pytorch",
"object-detection",
"yolo",
"autogenerated-modelcard",
"license:gpl-3.0"
] | object-detection | false | nateraw | null | nateraw/yolov6s | 0 | null | pytorch | 38,424 | ---
language: en
license: gpl-3.0
library_name: pytorch
tags:
- object-detection
- yolo
- autogenerated-modelcard
model_name: yolov6s
---
# Model Card for yolov6s
<!-- Provide a quick summary of what the model is/does. -->
# Table of Contents
1. [Model Details](#model-details)
2. [Uses](#uses)
3. [Bias, Risks, and Limitations](#bias-risks-and-limitations)
4. [Training Details](#training-details)
5. [Evaluation](#evaluation)
6. [Model Examination](#model-examination)
7. [Environmental Impact](#environmental-impact)
8. [Technical Specifications](#technical-specifications-optional)
9. [Citation](#citation)
10. [Glossary](#glossary-optional)
11. [More Information](#more-information-optional)
12. [Model Card Authors](#model-card-authors-optional)
13. [Model Card Contact](#model-card-contact)
14. [How To Get Started With the Model](#how-to-get-started-with-the-model)
# Model Details
## Model Description
<!-- Provide a longer summary of what this model is. -->
YOLOv6 is a single-stage object detection framework dedicated to industrial applications, with hardware-friendly efficient design and high performance.
- **Developed by:** [More Information Needed]
- **Shared by [Optional]:** [@nateraw](https://hf.co/nateraw)
- **Model type:** [More Information Needed]
- **Language(s) (NLP):** [More Information Needed]
- **License:** [More Information Needed]
- **Related Models:** [yolov6t](https://hf.co/nateraw/yolov6t), [yolov6n](https://hf.co/nateraw/yolov6n)
- **Parent Model:** N/A
- **Resources for more information:** The [official GitHub Repository](https://github.com/meituan/YOLOv6)
# Uses
<!-- Address questions around how the model is intended to be used, including the foreseeable users of the model and those affected by the model. -->
## Direct Use
<!-- This section is for the model use without fine-tuning or plugging into a larger ecosystem/app. -->
This model is meant to be used as a general object detector.
## Downstream Use [Optional]
<!-- This section is for the model use when fine-tuned for a task, or when plugged into a larger ecosystem/app -->
You can fine-tune this model for your specific task
## Out-of-Scope Use
<!-- This section addresses misuse, malicious use, and uses that the model will not work well for. -->
Don't be evil.
# Bias, Risks, and Limitations
<!-- This section is meant to convey both technical and sociotechnical limitations. -->
This model often classifies objects incorrectly, especially when applied to videos. It does not handle crowds very well.
## Recommendations
<!-- This section is meant to convey recommendations with respect to the bias, risk, and technical limitations. -->
Users (both direct and downstream) should be made aware of the risks, biases and limitations of the model. More information needed for further recomendations.
# Training Details
## Training Data
<!-- This should link to a Data Card, perhaps with a short stub of information on what the training data is all about as well as documentation related to data pre-processing or additional filtering. -->
[More Information Needed]
## Training Procedure
<!-- This relates heavily to the Technical Specifications. Content here should link to that section when it is relevant to the training procedure. -->
### Preprocessing
[More Information Needed]
### Speeds, Sizes, Times
<!-- This section provides information about throughput, start/end time, checkpoint size if relevant, etc. -->
[More Information Needed]
# Evaluation
<!-- This section describes the evaluation protocols and provides the results. -->
## Testing Data, Factors & Metrics
### Testing Data
<!-- This should link to a Data Card if possible. -->
[More Information Needed]
### Factors
<!-- These are the things the evaluation is disaggregating by, e.g., subpopulations or domains. -->
[More Information Needed]
### Metrics
<!-- These are the evaluation metrics being used, ideally with a description of why. -->
[More Information Needed]
## Results
[More Information Needed]
# Model Examination
[More Information Needed]
# Environmental Impact
<!-- Total emissions (in grams of CO2eq) and additional considerations, such as electricity usage, go here. Edit the suggested text below accordingly -->
Carbon emissions can be estimated using the [Machine Learning Impact calculator](https://mlco2.github.io/impact#compute) presented in [Lacoste et al. (2019)](https://arxiv.org/abs/1910.09700).
- **Hardware Type:** [More Information Needed]
- **Hours used:** [More Information Needed]
- **Cloud Provider:** [More Information Needed]
- **Compute Region:** [More Information Needed]
- **Carbon Emitted:** [More Information Needed]
# Technical Specifications [optional]
## Model Architecture and Objective
[More Information Needed]
## Compute Infrastructure
[More Information Needed]
### Hardware
[More Information Needed]
### Software
[More Information Needed]
# Citation
<!-- If there is a paper or blog post introducing the model, the APA and Bibtex information for that should go in this section. -->
**BibTeX:**
[More Information Needed]
**APA:**
[More Information Needed]
# Glossary [optional]
<!-- If relevant, include terms and calculations in this section that can help readers understand the model or model card. -->
[More Information Needed]
# More Information [optional]
Please refer to the [official GitHub Repository](https://github.com/meituan/YOLOv6)
# Model Card Authors [optional]
[@nateraw](https://hf.co/nateraw)
# Model Card Contact
[@nateraw](https://hf.co/nateraw) - please leave a note in the discussions tab here
# How to Get Started with the Model
Use the code below to get started with the model.
<details>
<summary> Click to expand </summary>
[More Information Needed]
</details> |
nateraw/yolov6t | ee7378eb5023cdd334003dff505d8ee18ea608cb | 2022-07-12T02:01:04.000Z | [
"en",
"arxiv:1910.09700",
"pytorch",
"object-detection",
"yolo",
"autogenerated-modelcard",
"license:gpl-3.0"
] | object-detection | false | nateraw | null | nateraw/yolov6t | 0 | null | pytorch | 38,425 | ---
language: en
license: gpl-3.0
library_name: pytorch
tags:
- object-detection
- yolo
- autogenerated-modelcard
model_name: yolov6t
---
# Model Card for yolov6t
<!-- Provide a quick summary of what the model is/does. -->
# Table of Contents
1. [Model Details](#model-details)
2. [Uses](#uses)
3. [Bias, Risks, and Limitations](#bias-risks-and-limitations)
4. [Training Details](#training-details)
5. [Evaluation](#evaluation)
6. [Model Examination](#model-examination)
7. [Environmental Impact](#environmental-impact)
8. [Technical Specifications](#technical-specifications-optional)
9. [Citation](#citation)
10. [Glossary](#glossary-optional)
11. [More Information](#more-information-optional)
12. [Model Card Authors](#model-card-authors-optional)
13. [Model Card Contact](#model-card-contact)
14. [How To Get Started With the Model](#how-to-get-started-with-the-model)
# Model Details
## Model Description
<!-- Provide a longer summary of what this model is. -->
YOLOv6 is a single-stage object detection framework dedicated to industrial applications, with hardware-friendly efficient design and high performance.
- **Developed by:** [More Information Needed]
- **Shared by [Optional]:** [@nateraw](https://hf.co/nateraw)
- **Model type:** [More Information Needed]
- **Language(s) (NLP):** [More Information Needed]
- **License:** [More Information Needed]
- **Related Models:** [yolov6s](https://hf.co/nateraw/yolov6s), [yolov6n](https://hf.co/nateraw/yolov6n)
- **Parent Model:** N/A
- **Resources for more information:** The [official GitHub Repository](https://github.com/meituan/YOLOv6)
# Uses
<!-- Address questions around how the model is intended to be used, including the foreseeable users of the model and those affected by the model. -->
## Direct Use
<!-- This section is for the model use without fine-tuning or plugging into a larger ecosystem/app. -->
This model is meant to be used as a general object detector.
## Downstream Use [Optional]
<!-- This section is for the model use when fine-tuned for a task, or when plugged into a larger ecosystem/app -->
You can fine-tune this model for your specific task
## Out-of-Scope Use
<!-- This section addresses misuse, malicious use, and uses that the model will not work well for. -->
Don't be evil.
# Bias, Risks, and Limitations
<!-- This section is meant to convey both technical and sociotechnical limitations. -->
This model often classifies objects incorrectly, especially when applied to videos. It does not handle crowds very well.
## Recommendations
<!-- This section is meant to convey recommendations with respect to the bias, risk, and technical limitations. -->
Users (both direct and downstream) should be made aware of the risks, biases and limitations of the model. More information needed for further recomendations.
# Training Details
## Training Data
<!-- This should link to a Data Card, perhaps with a short stub of information on what the training data is all about as well as documentation related to data pre-processing or additional filtering. -->
[More Information Needed]
## Training Procedure
<!-- This relates heavily to the Technical Specifications. Content here should link to that section when it is relevant to the training procedure. -->
### Preprocessing
[More Information Needed]
### Speeds, Sizes, Times
<!-- This section provides information about throughput, start/end time, checkpoint size if relevant, etc. -->
[More Information Needed]
# Evaluation
<!-- This section describes the evaluation protocols and provides the results. -->
## Testing Data, Factors & Metrics
### Testing Data
<!-- This should link to a Data Card if possible. -->
[More Information Needed]
### Factors
<!-- These are the things the evaluation is disaggregating by, e.g., subpopulations or domains. -->
[More Information Needed]
### Metrics
<!-- These are the evaluation metrics being used, ideally with a description of why. -->
[More Information Needed]
## Results
[More Information Needed]
# Model Examination
[More Information Needed]
# Environmental Impact
<!-- Total emissions (in grams of CO2eq) and additional considerations, such as electricity usage, go here. Edit the suggested text below accordingly -->
Carbon emissions can be estimated using the [Machine Learning Impact calculator](https://mlco2.github.io/impact#compute) presented in [Lacoste et al. (2019)](https://arxiv.org/abs/1910.09700).
- **Hardware Type:** [More Information Needed]
- **Hours used:** [More Information Needed]
- **Cloud Provider:** [More Information Needed]
- **Compute Region:** [More Information Needed]
- **Carbon Emitted:** [More Information Needed]
# Technical Specifications [optional]
## Model Architecture and Objective
[More Information Needed]
## Compute Infrastructure
[More Information Needed]
### Hardware
[More Information Needed]
### Software
[More Information Needed]
# Citation
<!-- If there is a paper or blog post introducing the model, the APA and Bibtex information for that should go in this section. -->
**BibTeX:**
[More Information Needed]
**APA:**
[More Information Needed]
# Glossary [optional]
<!-- If relevant, include terms and calculations in this section that can help readers understand the model or model card. -->
[More Information Needed]
# More Information [optional]
Please refer to the [official GitHub Repository](https://github.com/meituan/YOLOv6)
# Model Card Authors [optional]
[@nateraw](https://hf.co/nateraw)
# Model Card Contact
[@nateraw](https://hf.co/nateraw) - please leave a note in the discussions tab here
# How to Get Started with the Model
Use the code below to get started with the model.
<details>
<summary> Click to expand </summary>
[More Information Needed]
</details> |
Nitika/distilbert-base-uncased-finetuned-squad-d5716d28 | 6aa88e71e3070a2c66fcb071a2f5369717292139 | 2022-07-08T16:36:38.000Z | [
"pytorch",
"en",
"dataset:squad",
"arxiv:1910.01108",
"question-answering",
"license:apache-2.0"
] | question-answering | false | Nitika | null | Nitika/distilbert-base-uncased-finetuned-squad-d5716d28 | 0 | null | null | 38,426 | ---
language:
- en
thumbnail: https://github.com/karanchahal/distiller/blob/master/distiller.jpg
tags:
- question-answering
license: apache-2.0
datasets:
- squad
metrics:
- squad
---
# DistilBERT with a second step of distillation
## Model description
This model replicates the "DistilBERT (D)" model from Table 2 of the [DistilBERT paper](https://arxiv.org/pdf/1910.01108.pdf). In this approach, a DistilBERT student is fine-tuned on SQuAD v1.1, but with a BERT model (also fine-tuned on SQuAD v1.1) acting as a teacher for a second step of task-specific distillation.
In this version, the following pre-trained models were used:
* Student: `distilbert-base-uncased`
* Teacher: `lewtun/bert-base-uncased-finetuned-squad-v1`
## Training data
This model was trained on the SQuAD v1.1 dataset which can be obtained from the `datasets` library as follows:
```python
from datasets import load_dataset
squad = load_dataset('squad')
```
## Training procedure
## Eval results
| | Exact Match | F1 |
|------------------|-------------|------|
| DistilBERT paper | 79.1 | 86.9 |
| Ours | 78.4 | 86.5 |
The scores were calculated using the `squad` metric from `datasets`.
### BibTeX entry and citation info
```bibtex
@misc{sanh2020distilbert,
title={DistilBERT, a distilled version of BERT: smaller, faster, cheaper and lighter},
author={Victor Sanh and Lysandre Debut and Julien Chaumond and Thomas Wolf},
year={2020},
eprint={1910.01108},
archivePrefix={arXiv},
primaryClass={cs.CL}
}
``` |
bmichele/poetry-generation-nextline-mbart-gut-en-multi-75k | 9ee6f297c1f512718f86b237cc52aa69de22674d | 2022-07-08T23:46:02.000Z | [
"pytorch"
] | null | false | bmichele | null | bmichele/poetry-generation-nextline-mbart-gut-en-multi-75k | 0 | null | null | 38,427 | Entry not found |
maurya/clay__2__gc | 6c4950569aa8796adc9f01b440eec6ee0cb6510b | 2022-07-09T14:24:06.000Z | [
"pytorch"
] | null | false | maurya | null | maurya/clay__2__gc | 0 | null | null | 38,428 | Entry not found |
hugginglearners/fastai-style-transfer | d6f6735b37cf8684cdfa3fec88b56286c0d12bc4 | 2022-07-13T00:15:26.000Z | [
"fastai",
"pytorch",
"image-to-image"
] | image-to-image | false | hugginglearners | null | hugginglearners/fastai-style-transfer | 0 | 3 | fastai | 38,429 | ---
tags:
- fastai
- pytorch
- image-to-image
---
## Model description
This repo contains the trained model for Style transfer using vgg16 as the backbone.
Full credits go to [Nhu Hoang](https://www.linkedin.com/in/nhu-hoang/)
Motivation: Style transfer is an interesting task with an amazing outcome.
## Training and evaluation data
### Training hyperparameters
The following hyperparameters were used during training:
| Hyperparameters | Value |
| :-- | :-- |
| name | Adam |
| learning_rate | 3e-5 |
| training_precision | float16 | |
nateraw/image-2-line-drawing | 37428caf161345535a4601b5abda150bd8b82d52 | 2022-07-11T01:10:30.000Z | [
"pytorch",
"license:mit"
] | null | false | nateraw | null | nateraw/image-2-line-drawing | 0 | null | null | 38,430 | ---
license: mit
---
|
sejalchopra/brio-legal-data | cb55c48957b4de880b911f6ed5ec508fbad13d4b | 2022-07-12T19:38:42.000Z | [
"pytorch"
] | null | false | sejalchopra | null | sejalchopra/brio-legal-data | 0 | null | null | 38,431 | Entry not found |
nickcpk/distilbert-base-uncased-finetuned-squad-d5716d28 | cbb2142419608f6588a395ea4f378a195b3d068b | 2022-07-13T09:51:40.000Z | [
"pytorch",
"en",
"dataset:squad",
"arxiv:1910.01108",
"question-answering",
"license:apache-2.0"
] | question-answering | false | nickcpk | null | nickcpk/distilbert-base-uncased-finetuned-squad-d5716d28 | 0 | null | null | 38,432 | ---
language:
- en
thumbnail: https://github.com/karanchahal/distiller/blob/master/distiller.jpg
tags:
- question-answering
license: apache-2.0
datasets:
- squad
metrics:
- squad
---
# DistilBERT with a second step of distillation
## Model description
This model replicates the "DistilBERT (D)" model from Table 2 of the [DistilBERT paper](https://arxiv.org/pdf/1910.01108.pdf). In this approach, a DistilBERT student is fine-tuned on SQuAD v1.1, but with a BERT model (also fine-tuned on SQuAD v1.1) acting as a teacher for a second step of task-specific distillation.
In this version, the following pre-trained models were used:
* Student: `distilbert-base-uncased`
* Teacher: `lewtun/bert-base-uncased-finetuned-squad-v1`
## Training data
This model was trained on the SQuAD v1.1 dataset which can be obtained from the `datasets` library as follows:
```python
from datasets import load_dataset
squad = load_dataset('squad')
```
## Training procedure
## Eval results
| | Exact Match | F1 |
|------------------|-------------|------|
| DistilBERT paper | 79.1 | 86.9 |
| Ours | 78.4 | 86.5 |
The scores were calculated using the `squad` metric from `datasets`.
### BibTeX entry and citation info
```bibtex
@misc{sanh2020distilbert,
title={DistilBERT, a distilled version of BERT: smaller, faster, cheaper and lighter},
author={Victor Sanh and Lysandre Debut and Julien Chaumond and Thomas Wolf},
year={2020},
eprint={1910.01108},
archivePrefix={arXiv},
primaryClass={cs.CL}
}
``` |
rajnishrajput12/finber | d8985b84d9565eee18f7df8274c0bacad011f214 | 2022-07-14T10:40:20.000Z | [
"pytorch",
"license:other"
] | null | false | rajnishrajput12 | null | rajnishrajput12/finber | 0 | null | null | 38,433 | ---
license: other
---
|
gossminn/pp-fcd-bert-base-multilingual-cased | 0c8dde8706d1c0a747a50aaa90a6109057138071 | 2022-07-15T06:55:42.000Z | [
"pytorch",
"tensorboard"
] | null | false | gossminn | null | gossminn/pp-fcd-bert-base-multilingual-cased | 0 | null | null | 38,434 | Entry not found |
CompVis/ldm-celebahq-256 | 03978f22272a3c2502da709c3940e227c9714bdd | 2022-07-28T08:12:07.000Z | [
"diffusers",
"arxiv:2112.10752",
"pytorch",
"unconditional-image-generation",
"license:apache-2.0"
] | unconditional-image-generation | false | CompVis | null | CompVis/ldm-celebahq-256 | 0 | 6 | diffusers | 38,435 | ---
license: apache-2.0
tags:
- pytorch
- diffusers
- unconditional-image-generation
---
# Latent Diffusion Models (LDM)
**Paper**: [High-Resolution Image Synthesis with Latent Diffusion Models](https://arxiv.org/abs/2112.10752)
**Abstract**:
*By decomposing the image formation process into a sequential application of denoising autoencoders, diffusion models (DMs) achieve state-of-the-art synthesis results on image data and beyond. Additionally, their formulation allows for a guiding mechanism to control the image generation process without retraining. However, since these models typically operate directly in pixel space, optimization of powerful DMs often consumes hundreds of GPU days and inference is expensive due to sequential evaluations. To enable DM training on limited computational resources while retaining their quality and flexibility, we apply them in the latent space of powerful pretrained autoencoders. In contrast to previous work, training diffusion models on such a representation allows for the first time to reach a near-optimal point between complexity reduction and detail preservation, greatly boosting visual fidelity. By introducing cross-attention layers into the model architecture, we turn diffusion models into powerful and flexible generators for general conditioning inputs such as text or bounding boxes and high-resolution synthesis becomes possible in a convolutional manner. Our latent diffusion models (LDMs) achieve a new state of the art for image inpainting and highly competitive performance on various tasks, including unconditional image generation, semantic scene synthesis, and super-resolution, while significantly reducing computational requirements compared to pixel-based DMs.*
**Authors**
*Robin Rombach, Andreas Blattmann, Dominik Lorenz, Patrick Esser, Björn Ommer*
## Usage
### Inference with a pipeline
```python
!pip install diffusers
from diffusers import DiffusionPipeline
model_id = "CompVis/ldm-celebahq-256"
# load model and scheduler
pipeline = DiffusionPipeline.from_pretrained(model_id)
# run pipeline in inference (sample random noise and denoise)
image = pipeline(num_inference_steps=200)["sample"]
# save image
image[0].save("ldm_generated_image.png")
```
### Inference with an unrolled loop
```python
!pip install diffusers
from diffusers import UNet2DModel, DDIMScheduler, VQModel
import torch
import PIL.Image
import numpy as np
import tqdm
seed = 3
# load all models
unet = UNet2DModel.from_pretrained("CompVis/ldm-celebahq-256", subfolder="unet")
vqvae = VQModel.from_pretrained("CompVis/ldm-celebahq-256", subfolder="vqvae")
scheduler = DDIMScheduler.from_config("CompVis/ldm-celebahq-256", subfolder="scheduler")
# set to cuda
torch_device = "cuda" if torch.cuda.is_available() else "cpu"
unet.to(torch_device)
vqvae.to(torch_device)
# generate gaussian noise to be decoded
generator = torch.manual_seed(seed)
noise = torch.randn(
(1, unet.in_channels, unet.sample_size, unet.sample_size),
generator=generator,
).to(torch_device)
# set inference steps for DDIM
scheduler.set_timesteps(num_inference_steps=200)
image = noise
for t in tqdm.tqdm(scheduler.timesteps):
# predict noise residual of previous image
with torch.no_grad():
residual = unet(image, t)["sample"]
# compute previous image x_t according to DDIM formula
prev_image = scheduler.step(residual, t, image, eta=0.0)["prev_sample"]
# x_t-1 -> x_t
image = prev_image
# decode image with vae
with torch.no_grad():
image = vqvae.decode(image)
# process image
image_processed = image.cpu().permute(0, 2, 3, 1)
image_processed = (image_processed + 1.0) * 127.5
image_processed = image_processed.clamp(0, 255).numpy().astype(np.uint8)
image_pil = PIL.Image.fromarray(image_processed[0])
image_pil.save(f"generated_image_{seed}.png")
```
## Samples
1. 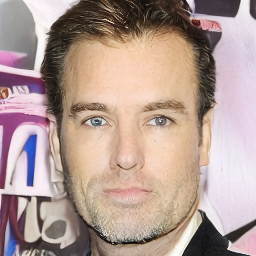
2. 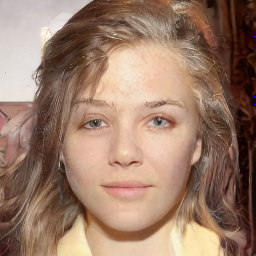
3. 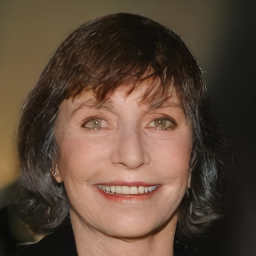
4. 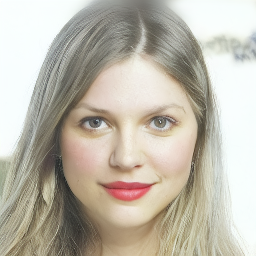
|
CompVis/ldm-text2im-large-256 | 9bd2b48d2d45e6deb6fb5a03eb2a601e4b95bd91 | 2022-07-28T08:11:31.000Z | [
"diffusers",
"arxiv:2112.10752",
"pytorch",
"text-to-image",
"license:apache-2.0"
] | text-to-image | false | CompVis | null | CompVis/ldm-text2im-large-256 | 0 | 3 | diffusers | 38,436 | ---
license: apache-2.0
tags:
- pytorch
- diffusers
- text-to-image
---
# High-Resolution Image Synthesis with Latent Diffusion Models (LDM)
**Paper**: [High-Resolution Image Synthesis with Latent Diffusion Models (LDM)s](https://arxiv.org/abs/2112.10752239)
**Abstract**:
*By decomposing the image formation process into a sequential application of denoising autoencoders, diffusion models (DMs) achieve state-of-the-art synthesis results on image data and beyond. Additionally, their formulation allows for a guiding mechanism to control the image generation process without retraining. However, since these models typically operate directly in pixel space, optimization of powerful DMs often consumes hundreds of GPU days and inference is expensive due to sequential evaluations. To enable DM training on limited computational resources while retaining their quality and flexibility, we apply them in the latent space of powerful pretrained autoencoders. In contrast to previous work, training diffusion models on such a representation allows for the first time to reach a near-optimal point between complexity reduction and detail preservation, greatly boosting visual fidelity. By introducing cross-attention layers into the model architecture, we turn diffusion models into powerful and flexible generators for general conditioning inputs such as text or bounding boxes and high-resolution synthesis becomes possible in a convolutional manner. Our latent diffusion models (LDMs) achieve a new state of the art for image inpainting and highly competitive performance on various tasks, including unconditional image generation, semantic scene synthesis, and super-resolution, while significantly reducing computational requirements compared to pixel-based DMs.*
## Safety
Please note that text-to-image models are known to at times produce harmful content.
Please raise any concerns you may have.
## Usage
```python
# !pip install diffusers transformers
from diffusers import DiffusionPipeline
model_id = "CompVis/ldm-text2im-large-256"
# load model and scheduler
ldm = DiffusionPipeline.from_pretrained(model_id)
# run pipeline in inference (sample random noise and denoise)
prompt = "A painting of a squirrel eating a burger"
images = ldm([prompt], num_inference_steps=50, eta=0.3, guidance_scale=6)["sample"]
# save images
for idx, image in enumerate(images):
image.save(f"squirrel-{idx}.png")
```
## Demo
[Hugging Face Spaces](https://huggingface.co/spaces/CompVis/ldm-text2im-large-256-diffusers)
## Samples
1. 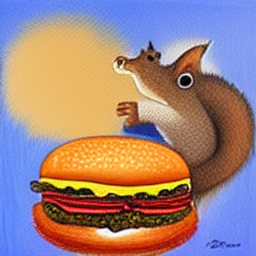
2. 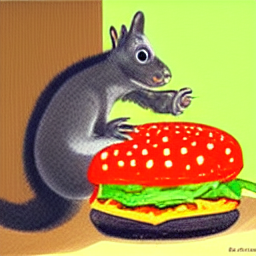
3. 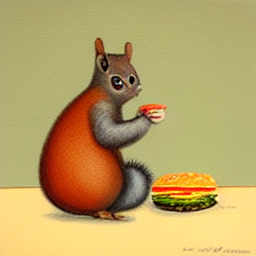
4. 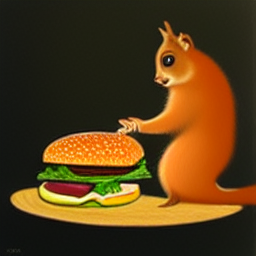
|
BlinkDL/rwkv-3-pile-430m | 23b0eb672c557631a651be8e49bb09a766201466 | 2022-07-22T11:17:06.000Z | [
"en",
"dataset:The Pile",
"pytorch",
"text-generation",
"causal-lm",
"rwkv",
"license:bsd-2-clause"
] | text-generation | false | BlinkDL | null | BlinkDL/rwkv-3-pile-430m | 0 | 2 | null | 38,437 | ---
language:
- en
tags:
- pytorch
- text-generation
- causal-lm
- rwkv
license: bsd-2-clause
datasets:
- The Pile
---
# RWKV-3 430M
## Model Description
RWKV-3 430M is a L24-D1024 causal language model trained on the Pile. See https://github.com/BlinkDL/RWKV-LM for details.
At this moment you have to use my Github code (https://github.com/BlinkDL/RWKV-v2-RNN-Pile) to run it.
ctx_len = 768
n_layer = 24
n_embd = 1024
Preview checkpoint: RWKV-3-Pile-20220721-3029.pth : Trained on the Pile for 93B tokens.
* Pile loss 2.341
* LAMBADA ppl 14.18, acc 44.25%
* PIQA acc 67.95%
* SC2016 acc 63.39%
* Hellaswag acc_norm 39.06%
(I am still training it) |
miazhao/test | 62266320e588f4af4dd2f5c8e29c62308444885e | 2022-07-27T05:30:00.000Z | [
"pytorch",
"xlm-roberta",
"fill-mask",
"transformers",
"autotrain_compatible"
] | fill-mask | false | miazhao | null | miazhao/test | 0 | null | transformers | 38,438 | Entry not found |
shonenkov-AI/RuDOLPH-350M-v2 | 720e8c60ffe6720ac452378a5b2f80d0c15695a4 | 2022-07-26T05:58:33.000Z | [
"pytorch"
] | null | false | shonenkov-AI | null | shonenkov-AI/RuDOLPH-350M-v2 | 0 | null | null | 38,439 | Entry not found |
kaisugi/BERTRanker_CiRec_ACL200 | 741cdedbd34a3b773d6d382041fbb06550fe5d65 | 2022-07-26T07:52:46.000Z | [
"pytorch"
] | null | false | kaisugi | null | kaisugi/BERTRanker_CiRec_ACL200 | 0 | null | null | 38,440 | Entry not found |
kaisugi/BERTRanker_CiRec_ACL200_global | c1a785c834d5cadda7602cce93167e14c4270423 | 2022-07-26T08:49:07.000Z | [
"pytorch"
] | null | false | kaisugi | null | kaisugi/BERTRanker_CiRec_ACL200_global | 0 | null | null | 38,441 | Entry not found |
kaisugi/BERTRanker_CiRec_ACL600 | 2608f55dde4667111d728e37b85fc97f95329e81 | 2022-07-26T08:51:49.000Z | [
"pytorch"
] | null | false | kaisugi | null | kaisugi/BERTRanker_CiRec_ACL600 | 0 | null | null | 38,442 | Entry not found |
kaisugi/BERTRanker_CiRec_ACL600_global | ab10001fe4b7f8892ab65e22524f67d3074b69ff | 2022-07-26T08:54:48.000Z | [
"pytorch"
] | null | false | kaisugi | null | kaisugi/BERTRanker_CiRec_ACL600_global | 0 | null | null | 38,443 | Entry not found |
kaisugi/BERTRanker_CiRec_RefSeer | 87b78b1062664ac793067fffa3c79839e8c5ff5d | 2022-07-26T10:50:59.000Z | [
"pytorch"
] | null | false | kaisugi | null | kaisugi/BERTRanker_CiRec_RefSeer | 0 | null | null | 38,444 | Entry not found |
kaisugi/BERTRanker_CiRec_RefSeer_global | 22084da469321e8afdc59423983364bf5ad78bae | 2022-07-26T10:53:20.000Z | [
"pytorch"
] | null | false | kaisugi | null | kaisugi/BERTRanker_CiRec_RefSeer_global | 0 | null | null | 38,445 | Entry not found |
olemeyer/zero_shot_issue_classification_bart-large-16 | 8ea616276aa8dac370f0f4d296d4c13f51594a10 | 2022-07-26T14:00:41.000Z | [
"pytorch",
"tensorboard",
"bart",
"text2text-generation",
"transformers",
"autotrain_compatible"
] | text2text-generation | false | olemeyer | null | olemeyer/zero_shot_issue_classification_bart-large-16 | 0 | null | transformers | 38,446 | Entry not found |
BlinkDL/rwkv-4-pile-169m | 2a4dd69a7600696bb7c5ba4c9f24765e0a2d5a3a | 2022-07-28T08:36:41.000Z | [
"en",
"dataset:The Pile",
"pytorch",
"text-generation",
"causal-lm",
"rwkv",
"license:bsd-2-clause"
] | text-generation | false | BlinkDL | null | BlinkDL/rwkv-4-pile-169m | 0 | 1 | null | 38,447 | ---
language:
- en
tags:
- pytorch
- text-generation
- causal-lm
- rwkv
license: bsd-2-clause
datasets:
- The Pile
---
# RWKV-4 169M
## Model Description
RWKV-4 169M is a L12-D768 causal language model trained on the Pile. See https://github.com/BlinkDL/RWKV-LM for details.
At this moment you have to use my Github code (https://github.com/BlinkDL/RWKV-v2-RNN-Pile) to run it.
|
DouglasPontes/29jul | d3f7a7cf8e33f1b7bb82088d2a63a95fc0c5e9e5 | 2022-07-30T05:35:37.000Z | [
"pytorch",
"tensorboard",
"roberta",
"fill-mask",
"transformers",
"autotrain_compatible"
] | fill-mask | false | DouglasPontes | null | DouglasPontes/29jul | 0 | null | transformers | 38,448 | Entry not found |
davidcechak/DNADebertaK8b | f8b8c9c1f6ced830b96945383cc58269672c32e9 | 2022-07-30T06:31:32.000Z | [
"pytorch",
"tensorboard",
"deberta",
"fill-mask",
"transformers",
"autotrain_compatible"
] | fill-mask | false | davidcechak | null | davidcechak/DNADebertaK8b | 0 | null | transformers | 38,449 | Entry not found |
DrY/dummy-model | 0d0809eb3c179b5f1c51c3c3f254d06f69e4afa3 | 2022-07-30T06:03:39.000Z | [
"pytorch",
"camembert",
"fill-mask",
"transformers",
"autotrain_compatible"
] | fill-mask | false | DrY | null | DrY/dummy-model | null | null | transformers | 38,450 | Entry not found |
mesolitica/t5-tiny-finetuned-noisy-en-ms | b05520dcbac799aaa5c0df4fe4272f8963fc8b47 | 2022-07-30T06:11:02.000Z | [
"pytorch",
"tf",
"tensorboard",
"t5",
"text2text-generation",
"transformers",
"generated_from_keras_callback",
"model-index",
"autotrain_compatible"
] | text2text-generation | false | mesolitica | null | mesolitica/t5-tiny-finetuned-noisy-en-ms | null | null | transformers | 38,451 | ---
tags:
- generated_from_keras_callback
model-index:
- name: t5-tiny-finetuned-noisy-en-ms
results: []
---
<!-- This model card has been generated automatically according to the information Keras had access to. You should
probably proofread and complete it, then remove this comment. -->
# t5-tiny-finetuned-noisy-en-ms
This model was trained from scratch on an unknown dataset.
It achieves the following results on the evaluation set:
## Model description
More information needed
## Intended uses & limitations
More information needed
## Training and evaluation data
More information needed
## Training procedure
### Training hyperparameters
The following hyperparameters were used during training:
- optimizer: None
- training_precision: float32
### Training results
### Framework versions
- Transformers 4.21.0.dev0
- TensorFlow 2.6.0
- Datasets 2.1.0
- Tokenizers 0.12.1
|
fzwd6666/NLTbert | 7b158dbefd741abde2fb09277e18e78dab4016db | 2022-07-30T06:11:52.000Z | [
"pytorch",
"bert",
"text-classification",
"transformers"
] | text-classification | false | fzwd6666 | null | fzwd6666/NLTbert | null | null | transformers | 38,452 | Entry not found |
DrY/marian-finetuned-kde4-en-to-zh | d0cb17f484de1084f5a56dbcfdc543b8bc8bca56 | 2022-07-30T08:05:06.000Z | [
"pytorch",
"tensorboard",
"marian",
"text2text-generation",
"dataset:kde4",
"transformers",
"translation",
"generated_from_trainer",
"license:apache-2.0",
"model-index",
"autotrain_compatible"
] | translation | false | DrY | null | DrY/marian-finetuned-kde4-en-to-zh | null | null | transformers | 38,453 | ---
license: apache-2.0
tags:
- translation
- generated_from_trainer
datasets:
- kde4
metrics:
- bleu
model-index:
- name: marian-finetuned-kde4-en-to-zh
results:
- task:
name: Sequence-to-sequence Language Modeling
type: text2text-generation
dataset:
name: kde4
type: kde4
config: en-zh_CN
split: train
args: en-zh_CN
metrics:
- name: Bleu
type: bleu
value: 40.66579724271391
---
<!-- This model card has been generated automatically according to the information the Trainer had access to. You
should probably proofread and complete it, then remove this comment. -->
# marian-finetuned-kde4-en-to-zh
This model is a fine-tuned version of [Helsinki-NLP/opus-mt-en-zh](https://huggingface.co/Helsinki-NLP/opus-mt-en-zh) on the kde4 dataset.
It achieves the following results on the evaluation set:
- Loss: 0.9338
- Bleu: 40.6658
## Model description
More information needed
## Intended uses & limitations
More information needed
## Training and evaluation data
More information needed
## Training procedure
### Training hyperparameters
The following hyperparameters were used during training:
- learning_rate: 2e-05
- train_batch_size: 32
- eval_batch_size: 64
- seed: 42
- optimizer: Adam with betas=(0.9,0.999) and epsilon=1e-08
- lr_scheduler_type: linear
- num_epochs: 3
- mixed_precision_training: Native AMP
### Training results
### Framework versions
- Transformers 4.21.0
- Pytorch 1.12.0+cu113
- Datasets 2.4.0
- Tokenizers 0.12.1
|
abdulmatinomotoso/t5_headline_generator_testing | 782d3d34e91593dfd8156a3dd42d268612a3af9f | 2022-07-30T07:59:01.000Z | [
"pytorch",
"tensorboard",
"t5",
"text2text-generation",
"transformers",
"generated_from_trainer",
"license:apache-2.0",
"model-index",
"autotrain_compatible"
] | text2text-generation | false | abdulmatinomotoso | null | abdulmatinomotoso/t5_headline_generator_testing | null | null | transformers | 38,454 | ---
license: apache-2.0
tags:
- generated_from_trainer
model-index:
- name: t5_headline_generator_testing
results: []
---
<!-- This model card has been generated automatically according to the information the Trainer had access to. You
should probably proofread and complete it, then remove this comment. -->
# t5_headline_generator_testing
This model is a fine-tuned version of [t5-base](https://huggingface.co/t5-base) on an unknown dataset.
It achieves the following results on the evaluation set:
- Loss: 1.2394
## Model description
More information needed
## Intended uses & limitations
More information needed
## Training and evaluation data
More information needed
## Training procedure
### Training hyperparameters
The following hyperparameters were used during training:
- learning_rate: 5e-05
- train_batch_size: 1
- eval_batch_size: 1
- seed: 42
- gradient_accumulation_steps: 16
- total_train_batch_size: 16
- optimizer: Adam with betas=(0.9,0.999) and epsilon=1e-08
- lr_scheduler_type: linear
- lr_scheduler_warmup_steps: 500
- num_epochs: 1
### Training results
| Training Loss | Epoch | Step | Validation Loss |
|:-------------:|:-----:|:----:|:---------------:|
| 1.4003 | 0.82 | 500 | 1.2394 |
### Framework versions
- Transformers 4.18.0
- Pytorch 1.11.0
- Datasets 2.1.0
- Tokenizers 0.12.1
|
pete/distilbert-base-uncased-finetuned-emotion | 489ca4dfc89eb97e4629b50f5c6dfcf5fa33d406 | 2022-07-30T08:19:56.000Z | [
"pytorch",
"tensorboard",
"distilbert",
"text-classification",
"dataset:emotion",
"transformers",
"generated_from_trainer",
"license:apache-2.0",
"model-index"
] | text-classification | false | pete | null | pete/distilbert-base-uncased-finetuned-emotion | null | null | transformers | 38,455 | ---
license: apache-2.0
tags:
- generated_from_trainer
datasets:
- emotion
metrics:
- accuracy
- f1
model-index:
- name: distilbert-base-uncased-finetuned-emotion
results:
- task:
name: Text Classification
type: text-classification
dataset:
name: emotion
type: emotion
config: default
split: train
args: default
metrics:
- name: Accuracy
type: accuracy
value: 0.9265
- name: F1
type: f1
value: 0.9265114997421897
---
<!-- This model card has been generated automatically according to the information the Trainer had access to. You
should probably proofread and complete it, then remove this comment. -->
# distilbert-base-uncased-finetuned-emotion
This model is a fine-tuned version of [distilbert-base-uncased](https://huggingface.co/distilbert-base-uncased) on the emotion dataset.
It achieves the following results on the evaluation set:
- Loss: 0.2142
- Accuracy: 0.9265
- F1: 0.9265
## Model description
More information needed
## Intended uses & limitations
More information needed
## Training and evaluation data
More information needed
## Training procedure
### Training hyperparameters
The following hyperparameters were used during training:
- learning_rate: 2e-05
- train_batch_size: 64
- eval_batch_size: 64
- seed: 42
- optimizer: Adam with betas=(0.9,0.999) and epsilon=1e-08
- lr_scheduler_type: linear
- num_epochs: 2
### Training results
| Training Loss | Epoch | Step | Validation Loss | Accuracy | F1 |
|:-------------:|:-----:|:----:|:---------------:|:--------:|:------:|
| 0.8365 | 1.0 | 250 | 0.3209 | 0.9035 | 0.8993 |
| 0.2479 | 2.0 | 500 | 0.2142 | 0.9265 | 0.9265 |
### Framework versions
- Transformers 4.21.0
- Pytorch 1.12.0+cu113
- Datasets 2.4.0
- Tokenizers 0.12.1
|
alex-apostolo/distilbert-base-uncased-finetuned-squad | c6cac3416e5564e1e0ab6bd9a3bfba25dbb5b198 | 2022-07-30T09:57:49.000Z | [
"pytorch",
"tensorboard",
"distilbert",
"question-answering",
"dataset:squad",
"transformers",
"generated_from_trainer",
"license:apache-2.0",
"model-index",
"autotrain_compatible"
] | question-answering | false | alex-apostolo | null | alex-apostolo/distilbert-base-uncased-finetuned-squad | null | null | transformers | 38,456 | ---
license: apache-2.0
tags:
- generated_from_trainer
datasets:
- squad
model-index:
- name: distilbert-base-uncased-finetuned-squad
results: []
---
<!-- This model card has been generated automatically according to the information the Trainer had access to. You
should probably proofread and complete it, then remove this comment. -->
# distilbert-base-uncased-finetuned-squad
This model is a fine-tuned version of [distilbert-base-uncased](https://huggingface.co/distilbert-base-uncased) on the squad dataset.
It achieves the following results on the evaluation set:
- Loss: 1.1573
## Model description
More information needed
## Intended uses & limitations
More information needed
## Training and evaluation data
More information needed
## Training procedure
### Training hyperparameters
The following hyperparameters were used during training:
- learning_rate: 2e-05
- train_batch_size: 16
- eval_batch_size: 16
- seed: 42
- optimizer: Adam with betas=(0.9,0.999) and epsilon=1e-08
- lr_scheduler_type: linear
- num_epochs: 3
### Training results
| Training Loss | Epoch | Step | Validation Loss |
|:-------------:|:-----:|:-----:|:---------------:|
| 1.2216 | 1.0 | 5533 | 1.1506 |
| 0.9484 | 2.0 | 11066 | 1.1197 |
| 0.7474 | 3.0 | 16599 | 1.1573 |
### Framework versions
- Transformers 4.21.0
- Pytorch 1.12.0+cu113
- Datasets 2.4.0
- Tokenizers 0.12.1
|
mmmmmmd/setting_1 | 606397293aacab0a034263ef0f7cb9ff577ccb26 | 2022-07-30T08:59:18.000Z | [
"pytorch",
"bert",
"text-classification",
"transformers"
] | text-classification | false | mmmmmmd | null | mmmmmmd/setting_1 | null | null | transformers | 38,457 | Entry not found |
SummerChiam/pond_image_classification_10 | 492b1bad1623aad3c83fb78a8fbb6e207a8e6118 | 2022-07-30T08:57:50.000Z | [
"pytorch",
"tensorboard",
"vit",
"image-classification",
"transformers",
"huggingpics",
"model-index"
] | image-classification | false | SummerChiam | null | SummerChiam/pond_image_classification_10 | null | null | transformers | 38,458 | ---
tags:
- image-classification
- pytorch
- huggingpics
metrics:
- accuracy
model-index:
- name: pond_image_classification_10
results:
- task:
name: Image Classification
type: image-classification
metrics:
- name: Accuracy
type: accuracy
value: 0.9948979616165161
---
# pond_image_classification_10
Autogenerated by HuggingPics🤗🖼️
Create your own image classifier for **anything** by running [the demo on Google Colab](https://colab.research.google.com/github/nateraw/huggingpics/blob/main/HuggingPics.ipynb).
Report any issues with the demo at the [github repo](https://github.com/nateraw/huggingpics).
## Example Images
#### Algae

#### Boiling

#### BoilingNight

#### Normal

#### NormalCement

#### NormalNight

#### NormalRain
 |
clefourrier/nystromformer-cf-artificial-balanced-max500-490000-1 | 876a7c9320e096d2658d510a9dcfa365fb06cfbe | 2022-07-30T09:01:58.000Z | [
"pytorch",
"graph_nystromformer",
"text-classification",
"transformers"
] | text-classification | false | clefourrier | null | clefourrier/nystromformer-cf-artificial-balanced-max500-490000-1 | null | null | transformers | 38,459 | Entry not found |
SummerChiam/rust_image_classification_2 | 41ffe762c74bdf1b51bb88cc1e481d6f591597fb | 2022-07-30T10:05:44.000Z | [
"pytorch",
"tensorboard",
"vit",
"image-classification",
"transformers",
"huggingpics",
"model-index"
] | image-classification | false | SummerChiam | null | SummerChiam/rust_image_classification_2 | null | null | transformers | 38,460 | ---
tags:
- image-classification
- pytorch
- huggingpics
metrics:
- accuracy
model-index:
- name: rust_image_classification_2
results:
- task:
name: Image Classification
type: image-classification
metrics:
- name: Accuracy
type: accuracy
value: 0.853164553642273
---
# rust_image_classification_2
Autogenerated by HuggingPics🤗🖼️
Create your own image classifier for **anything** by running [the demo on Google Colab](https://colab.research.google.com/github/nateraw/huggingpics/blob/main/HuggingPics.ipynb).
Report any issues with the demo at the [github repo](https://github.com/nateraw/huggingpics).
## Example Images
#### nonrust

#### rust
 |