sha
stringlengths 40
40
| text
stringlengths 1
13.4M
| id
stringlengths 2
117
| tags
sequencelengths 1
7.91k
| created_at
stringlengths 25
25
| metadata
stringlengths 2
875k
| last_modified
stringlengths 25
25
| arxiv
sequencelengths 0
25
| languages
sequencelengths 0
7.91k
| tags_str
stringlengths 17
159k
| text_str
stringlengths 1
447k
| text_lists
sequencelengths 0
352
| processed_texts
sequencelengths 1
353
|
---|---|---|---|---|---|---|---|---|---|---|---|---|
a8cc9be686d49bec5783b9556531620875d05eba |
# Dataset Card for CoFiF
## Dataset Description
- **Repository:** https://github.com/CoFiF/Corpus
- **Paper:** https://aclanthology.org/W19-5504/
- **Point of Contact:** Sina Ahmadi ([email protected]) and Tobias Daudert ([email protected])
### Dataset Summary
CoFiF is the first corpus comprising company reports in the French language. It contains over **188 million** tokens in **2655** reports, covering four types of documents:
- Reference documents (documents de référence) published annually, usually in the months following the end of the calendar year, and contain information regarding the financial situation and perspectives of a company
- Annual report (résultats annuels) which summarises a company’s business and activities throughout the previous year
- Semestrial (résultats semestriels): similar to annual reports in content but published every 6 months
- Trimestrial reports (résultats trimestriels): similar to annual reports but published every 3 months
These documents are collected from the 60 largest French companies listed in France’s main stock indices [CAC40](https://en.wikipedia.org/wiki/CAC_40) and [CAC Next 20](https://en.wikipedia.org/wiki/CAC_Next_20). The corpus spans over 20 years, ranging from 1995 to 2018.
### Supported Tasks and Leaderboards
Language modeling : can be used to train a French model (CamemBERT, FlauBERT, BARTHez, etc.) on data of financial types
### Languages
French
## Dataset Structure
Raw text
## Dataset Creation
### Curation Rationale
No French language datasets related to finance were available.
### Source Data
- Reference documents (documents de référence) published annually, usually in the months following the end of the calendar year, and contain information regarding the financial situation and perspectives of a company
- Annual report (résultats annuels) which summarises a company’s business and activities throughout the previous year
- Semestrial (résultats semestriels): similar to annual reports in content but published every 6 months
- Trimestrial reports (résultats trimestriels): similar to annual reports but published every 3 months
These documents are collected from the 60 largest French companies listed in France’s main stock indices CAC40 and CAC Next 20. The corpus spans over 20 years, ranging from 1995 to 2018.
#### Initial Data Collection and Normalization
The authors have done a cleanup but have not detailed it in their publication or on their GitHub directory.
#### Who are the source language producers?
Public administrative reports written by humans.
### Annotations
No annotations.
### Personal and Sensitive Information
[More Information Needed]
## Considerations for Using the Data
### Social Impact of Dataset
[More Information Needed]
### Discussion of Biases
[More Information Needed]
### Other Known Limitations
[More Information Needed]
## Additional Information
### Dataset Curators
This publication has emanated from research conducted with the financial support of Science Foundation Ireland (SFI) under Grant Number SFI/12/RC/2289, co-funded by the European Regional Development Fund.
### Licensing Information
The authors of the publication (Tobias Daudert and Sina Ahmadi) do not acknowledge any third party, so it appears that they were the only ones involved in the data collection.
This corpus is openly available for non-commercial use under the [Attribution-NonCommercial-ShareAlike 4.0 International](https://creativecommons.org/licenses/by-nc-sa/4.0/).
### Citation Information
If you're using CoFiF in your researches, please don't forget to cite [this paper](https://www.aclweb.org/anthology/papers/W/W19/W19-5504/):
~~~
@inproceedings{daudert-ahmadi-2019-cofif,
title = "{C}o{F}i{F}: A Corpus of Financial Reports in {F}rench Language",
author = "Daudert, Tobias and Ahmadi, Sina",
booktitle = "Proceedings of the First Workshop on Financial Technology and Natural Language Processing",
month = "12 " # aug,
year = "2019",
address = "Macao, China",
url = "https://www.aclweb.org/anthology/W19-5504",
pages = "21--26",
}
~~~ | FrancophonIA/CoFiF | [
"task_categories:fill-mask",
"annotations_creators:no-annotation",
"language_creators:found",
"multilinguality:monolingual",
"source_datasets:original",
"language:fr",
"license:cc-by-nc-4.0",
"region:us"
] | 2022-03-02T23:29:22+00:00 | {"annotations_creators": ["no-annotation"], "language_creators": ["found"], "language": ["fr"], "license": ["cc-by-nc-4.0"], "multilinguality": ["monolingual"], "source_datasets": ["original"], "task_categories": ["fill-mask"]} | 2023-10-25T15:34:56+00:00 | [] | [
"fr"
] | TAGS
#task_categories-fill-mask #annotations_creators-no-annotation #language_creators-found #multilinguality-monolingual #source_datasets-original #language-French #license-cc-by-nc-4.0 #region-us
|
# Dataset Card for CoFiF
## Dataset Description
- Repository: URL
- Paper: URL
- Point of Contact: Sina Ahmadi (URL@URL) and Tobias Daudert (tobias.daudert@URL)
### Dataset Summary
CoFiF is the first corpus comprising company reports in the French language. It contains over 188 million tokens in 2655 reports, covering four types of documents:
- Reference documents (documents de référence) published annually, usually in the months following the end of the calendar year, and contain information regarding the financial situation and perspectives of a company
- Annual report (résultats annuels) which summarises a company’s business and activities throughout the previous year
- Semestrial (résultats semestriels): similar to annual reports in content but published every 6 months
- Trimestrial reports (résultats trimestriels): similar to annual reports but published every 3 months
These documents are collected from the 60 largest French companies listed in France’s main stock indices CAC40 and CAC Next 20. The corpus spans over 20 years, ranging from 1995 to 2018.
### Supported Tasks and Leaderboards
Language modeling : can be used to train a French model (CamemBERT, FlauBERT, BARTHez, etc.) on data of financial types
### Languages
French
## Dataset Structure
Raw text
## Dataset Creation
### Curation Rationale
No French language datasets related to finance were available.
### Source Data
- Reference documents (documents de référence) published annually, usually in the months following the end of the calendar year, and contain information regarding the financial situation and perspectives of a company
- Annual report (résultats annuels) which summarises a company’s business and activities throughout the previous year
- Semestrial (résultats semestriels): similar to annual reports in content but published every 6 months
- Trimestrial reports (résultats trimestriels): similar to annual reports but published every 3 months
These documents are collected from the 60 largest French companies listed in France’s main stock indices CAC40 and CAC Next 20. The corpus spans over 20 years, ranging from 1995 to 2018.
#### Initial Data Collection and Normalization
The authors have done a cleanup but have not detailed it in their publication or on their GitHub directory.
#### Who are the source language producers?
Public administrative reports written by humans.
### Annotations
No annotations.
### Personal and Sensitive Information
## Considerations for Using the Data
### Social Impact of Dataset
### Discussion of Biases
### Other Known Limitations
## Additional Information
### Dataset Curators
This publication has emanated from research conducted with the financial support of Science Foundation Ireland (SFI) under Grant Number SFI/12/RC/2289, co-funded by the European Regional Development Fund.
### Licensing Information
The authors of the publication (Tobias Daudert and Sina Ahmadi) do not acknowledge any third party, so it appears that they were the only ones involved in the data collection.
This corpus is openly available for non-commercial use under the Attribution-NonCommercial-ShareAlike 4.0 International.
If you're using CoFiF in your researches, please don't forget to cite this paper:
~~~
@inproceedings{daudert-ahmadi-2019-cofif,
title = "{C}o{F}i{F}: A Corpus of Financial Reports in {F}rench Language",
author = "Daudert, Tobias and Ahmadi, Sina",
booktitle = "Proceedings of the First Workshop on Financial Technology and Natural Language Processing",
month = "12 " # aug,
year = "2019",
address = "Macao, China",
url = "URL
pages = "21--26",
}
~~~ | [
"# Dataset Card for CoFiF",
"## Dataset Description\n\n- Repository: URL\n\n- Paper: URL\n\n- Point of Contact: Sina Ahmadi (URL@URL) and Tobias Daudert (tobias.daudert@URL)",
"### Dataset Summary\n\nCoFiF is the first corpus comprising company reports in the French language. It contains over 188 million tokens in 2655 reports, covering four types of documents:\n\n- Reference documents (documents de référence) published annually, usually in the months following the end of the calendar year, and contain information regarding the financial situation and perspectives of a company\n- Annual report (résultats annuels) which summarises a company’s business and activities throughout the previous year\n- Semestrial (résultats semestriels): similar to annual reports in content but published every 6 months\n- Trimestrial reports (résultats trimestriels): similar to annual reports but published every 3 months\n\nThese documents are collected from the 60 largest French companies listed in France’s main stock indices CAC40 and CAC Next 20. The corpus spans over 20 years, ranging from 1995 to 2018.",
"### Supported Tasks and Leaderboards\nLanguage modeling : can be used to train a French model (CamemBERT, FlauBERT, BARTHez, etc.) on data of financial types",
"### Languages\nFrench",
"## Dataset Structure\nRaw text",
"## Dataset Creation",
"### Curation Rationale\nNo French language datasets related to finance were available.",
"### Source Data\n- Reference documents (documents de référence) published annually, usually in the months following the end of the calendar year, and contain information regarding the financial situation and perspectives of a company\n- Annual report (résultats annuels) which summarises a company’s business and activities throughout the previous year\n- Semestrial (résultats semestriels): similar to annual reports in content but published every 6 months\n- Trimestrial reports (résultats trimestriels): similar to annual reports but published every 3 months\n\nThese documents are collected from the 60 largest French companies listed in France’s main stock indices CAC40 and CAC Next 20. The corpus spans over 20 years, ranging from 1995 to 2018.",
"#### Initial Data Collection and Normalization\nThe authors have done a cleanup but have not detailed it in their publication or on their GitHub directory.",
"#### Who are the source language producers?\nPublic administrative reports written by humans.",
"### Annotations\nNo annotations.",
"### Personal and Sensitive Information",
"## Considerations for Using the Data",
"### Social Impact of Dataset",
"### Discussion of Biases",
"### Other Known Limitations",
"## Additional Information",
"### Dataset Curators\nThis publication has emanated from research conducted with the financial support of Science Foundation Ireland (SFI) under Grant Number SFI/12/RC/2289, co-funded by the European Regional Development Fund.",
"### Licensing Information\nThe authors of the publication (Tobias Daudert and Sina Ahmadi) do not acknowledge any third party, so it appears that they were the only ones involved in the data collection.\n\nThis corpus is openly available for non-commercial use under the Attribution-NonCommercial-ShareAlike 4.0 International.\n\n\n\n\nIf you're using CoFiF in your researches, please don't forget to cite this paper:\n\n~~~\n@inproceedings{daudert-ahmadi-2019-cofif,\n title = \"{C}o{F}i{F}: A Corpus of Financial Reports in {F}rench Language\",\n author = \"Daudert, Tobias and Ahmadi, Sina\",\n booktitle = \"Proceedings of the First Workshop on Financial Technology and Natural Language Processing\",\n month = \"12 \" # aug,\n year = \"2019\",\n address = \"Macao, China\",\n url = \"URL\n pages = \"21--26\",\n}\n~~~"
] | [
"TAGS\n#task_categories-fill-mask #annotations_creators-no-annotation #language_creators-found #multilinguality-monolingual #source_datasets-original #language-French #license-cc-by-nc-4.0 #region-us \n",
"# Dataset Card for CoFiF",
"## Dataset Description\n\n- Repository: URL\n\n- Paper: URL\n\n- Point of Contact: Sina Ahmadi (URL@URL) and Tobias Daudert (tobias.daudert@URL)",
"### Dataset Summary\n\nCoFiF is the first corpus comprising company reports in the French language. It contains over 188 million tokens in 2655 reports, covering four types of documents:\n\n- Reference documents (documents de référence) published annually, usually in the months following the end of the calendar year, and contain information regarding the financial situation and perspectives of a company\n- Annual report (résultats annuels) which summarises a company’s business and activities throughout the previous year\n- Semestrial (résultats semestriels): similar to annual reports in content but published every 6 months\n- Trimestrial reports (résultats trimestriels): similar to annual reports but published every 3 months\n\nThese documents are collected from the 60 largest French companies listed in France’s main stock indices CAC40 and CAC Next 20. The corpus spans over 20 years, ranging from 1995 to 2018.",
"### Supported Tasks and Leaderboards\nLanguage modeling : can be used to train a French model (CamemBERT, FlauBERT, BARTHez, etc.) on data of financial types",
"### Languages\nFrench",
"## Dataset Structure\nRaw text",
"## Dataset Creation",
"### Curation Rationale\nNo French language datasets related to finance were available.",
"### Source Data\n- Reference documents (documents de référence) published annually, usually in the months following the end of the calendar year, and contain information regarding the financial situation and perspectives of a company\n- Annual report (résultats annuels) which summarises a company’s business and activities throughout the previous year\n- Semestrial (résultats semestriels): similar to annual reports in content but published every 6 months\n- Trimestrial reports (résultats trimestriels): similar to annual reports but published every 3 months\n\nThese documents are collected from the 60 largest French companies listed in France’s main stock indices CAC40 and CAC Next 20. The corpus spans over 20 years, ranging from 1995 to 2018.",
"#### Initial Data Collection and Normalization\nThe authors have done a cleanup but have not detailed it in their publication or on their GitHub directory.",
"#### Who are the source language producers?\nPublic administrative reports written by humans.",
"### Annotations\nNo annotations.",
"### Personal and Sensitive Information",
"## Considerations for Using the Data",
"### Social Impact of Dataset",
"### Discussion of Biases",
"### Other Known Limitations",
"## Additional Information",
"### Dataset Curators\nThis publication has emanated from research conducted with the financial support of Science Foundation Ireland (SFI) under Grant Number SFI/12/RC/2289, co-funded by the European Regional Development Fund.",
"### Licensing Information\nThe authors of the publication (Tobias Daudert and Sina Ahmadi) do not acknowledge any third party, so it appears that they were the only ones involved in the data collection.\n\nThis corpus is openly available for non-commercial use under the Attribution-NonCommercial-ShareAlike 4.0 International.\n\n\n\n\nIf you're using CoFiF in your researches, please don't forget to cite this paper:\n\n~~~\n@inproceedings{daudert-ahmadi-2019-cofif,\n title = \"{C}o{F}i{F}: A Corpus of Financial Reports in {F}rench Language\",\n author = \"Daudert, Tobias and Ahmadi, Sina\",\n booktitle = \"Proceedings of the First Workshop on Financial Technology and Natural Language Processing\",\n month = \"12 \" # aug,\n year = \"2019\",\n address = \"Macao, China\",\n url = \"URL\n pages = \"21--26\",\n}\n~~~"
] |
f8bf264495f105aaae53aa559a1af98875c9f10c | # Dataset Card for `lbox_open`
## Dataset Description
- **Homepage:** `https://lbox.kr`
- **Repository:** `https://github.com/lbox-kr/lbox_open`
- **Point of Contact:** [Wonseok Hwang](mailto:[email protected])
### Dataset Summary
A Legal AI Benchmark Dataset from Korean Legal Cases.
### Languages
Korean
### How to use
```python
from datasets import load_dataset
# casename classficiation task
data_cn = load_dataset("lbox/lbox_open", "casename_classification")
data_cn_plus = load_dataset("lbox/lbox_open", "casename_classification_plus")
# statutes classification task
data_st = load_dataset("lbox/lbox_open", "statute_classification")
data_st_plus = load_dataset("lbox/lbox_open", "statute_classification_plus")
# Legal judgement prediction tasks
data_ljp_criminal = load_dataset("lbox/lbox_open", "ljp_criminal")
data_ljp_civil = load_dataset("lbox/lbox_open", "ljp_civil")
# case summarization task
data_summ = load_dataset("lbox/lbox_open", "summarization")
data_summ_plus = load_dataset("lbox/lbox_open", "summarization_plus")
# precedent corpus
data_corpus = load_dataset("lbox/lbox_open", "precedent_corpus")
```
For more information about the dataset, please visit <https://github.com/lbox-kr/lbox_open>.
## Licensing Information
Copyright 2022-present [LBox Co. Ltd.](https://lbox.kr/)
Licensed under the [CC BY-NC 4.0](https://creativecommons.org/licenses/by-nc/4.0/) | lbox/lbox_open | [
"license:cc-by-nc-4.0",
"region:us"
] | 2022-03-02T23:29:22+00:00 | {"license": "cc-by-nc-4.0"} | 2022-11-09T06:41:26+00:00 | [] | [] | TAGS
#license-cc-by-nc-4.0 #region-us
| # Dataset Card for 'lbox_open'
## Dataset Description
- Homepage: 'URL'
- Repository: 'URL
- Point of Contact: Wonseok Hwang
### Dataset Summary
A Legal AI Benchmark Dataset from Korean Legal Cases.
### Languages
Korean
### How to use
For more information about the dataset, please visit <URL
## Licensing Information
Copyright 2022-present LBox Co. Ltd.
Licensed under the CC BY-NC 4.0 | [
"# Dataset Card for 'lbox_open'",
"## Dataset Description\n\n- Homepage: 'URL'\n- Repository: 'URL\n- Point of Contact: Wonseok Hwang",
"### Dataset Summary\n\nA Legal AI Benchmark Dataset from Korean Legal Cases.",
"### Languages\n\nKorean",
"### How to use\n\n\nFor more information about the dataset, please visit <URL",
"## Licensing Information\nCopyright 2022-present LBox Co. Ltd.\n\nLicensed under the CC BY-NC 4.0"
] | [
"TAGS\n#license-cc-by-nc-4.0 #region-us \n",
"# Dataset Card for 'lbox_open'",
"## Dataset Description\n\n- Homepage: 'URL'\n- Repository: 'URL\n- Point of Contact: Wonseok Hwang",
"### Dataset Summary\n\nA Legal AI Benchmark Dataset from Korean Legal Cases.",
"### Languages\n\nKorean",
"### How to use\n\n\nFor more information about the dataset, please visit <URL",
"## Licensing Information\nCopyright 2022-present LBox Co. Ltd.\n\nLicensed under the CC BY-NC 4.0"
] |
e82279f58c20065502e66ca496ca54a11fdb57cb | The AMI Meeting Corpus is a multi-modal data set consisting of 100 hours of meeting recordings. For a gentle introduction to the corpus, see the corpus overview. To access the data, follow the directions given there. Around two-thirds of the data has been elicited using a scenario in which the participants play different roles in a design team, taking a design project from kick-off to completion over the course of a day. The rest consists of naturally occurring meetings in a range of domains. Detailed information can be found in the documentation section.
https://groups.inf.ed.ac.uk/ami/corpus/ | leoapolonio/AMI_Meeting_Corpus | [
"region:us"
] | 2022-03-02T23:29:22+00:00 | {} | 2021-09-27T12:16:24+00:00 | [] | [] | TAGS
#region-us
| The AMI Meeting Corpus is a multi-modal data set consisting of 100 hours of meeting recordings. For a gentle introduction to the corpus, see the corpus overview. To access the data, follow the directions given there. Around two-thirds of the data has been elicited using a scenario in which the participants play different roles in a design team, taking a design project from kick-off to completion over the course of a day. The rest consists of naturally occurring meetings in a range of domains. Detailed information can be found in the documentation section.
URL | [] | [
"TAGS\n#region-us \n"
] |
317fdc0bede6f6ef0c941c30547395966f87f82a | 轴承故障诊断领域的NER语料,按BIO规则标注。 | leonadase/fdner | [
"region:us"
] | 2022-03-02T23:29:22+00:00 | {} | 2022-05-26T11:44:15+00:00 | [] | [] | TAGS
#region-us
| 轴承故障诊断领域的NER语料,按BIO规则标注。 | [] | [
"TAGS\n#region-us \n"
] |
e39b708dcfb727f0092341fd471c83de9c73864b |
# Dummy dataset to test evaluation framework for SUPERB. | lewtun/asr-preds-test | [
"benchmark:superb",
"region:us"
] | 2022-03-02T23:29:22+00:00 | {"benchmark": "superb", "task": "asr"} | 2021-07-28T00:22:53+00:00 | [] | [] | TAGS
#benchmark-superb #region-us
|
# Dummy dataset to test evaluation framework for SUPERB. | [
"# Dummy dataset to test evaluation framework for SUPERB."
] | [
"TAGS\n#benchmark-superb #region-us \n",
"# Dummy dataset to test evaluation framework for SUPERB."
] |
75cac4251eb0dbc3282eaa5ff95c608032df6628 |
# Batch job
model_id: lewtun/superb-s3prl-osanseviero__hubert_base-asr-cbcd177a
dataset_name: superb
dataset_config: asr
dataset_split: test
dataset_column: file | lewtun/bulk-superb-s3p-superb-49606 | [
"benchmark:superb",
"region:us"
] | 2022-03-02T23:29:22+00:00 | {"benchmark": "superb", "task": "asr", "type": "prediction"} | 2021-08-02T15:38:29+00:00 | [] | [] | TAGS
#benchmark-superb #region-us
|
# Batch job
model_id: lewtun/superb-s3prl-osanseviero__hubert_base-asr-cbcd177a
dataset_name: superb
dataset_config: asr
dataset_split: test
dataset_column: file | [
"# Batch job\n\nmodel_id: lewtun/superb-s3prl-osanseviero__hubert_base-asr-cbcd177a\ndataset_name: superb\ndataset_config: asr\ndataset_split: test\ndataset_column: file"
] | [
"TAGS\n#benchmark-superb #region-us \n",
"# Batch job\n\nmodel_id: lewtun/superb-s3prl-osanseviero__hubert_base-asr-cbcd177a\ndataset_name: superb\ndataset_config: asr\ndataset_split: test\ndataset_column: file"
] |
3d61f200cf73279e51fb903b58f80de3fb344769 |
# GEM submissions for gem-sub-03
## Submitting to the benchmark
FILL ME IN
### Submission file format
Please follow this format for your `submission.json` file:
```json
{
"submission_name": "An identifying name of your system",
"param_count": 123, # the number of parameters your system has.
"description": "An optional brief description of the system that will be shown on the website",
"tasks":
{
"dataset_identifier": {
"values": ["output1", "output2", "..."], # A list of system outputs
# Optionally, you can add the keys which are part of an example to ensure that there is no shuffling mistakes.
"keys": ["key-0", "key-1", ...]
}
}
}
```
In this case, `dataset_identifier` is the identifier of the dataset followed by an identifier of the set the outputs were created from, for example `_validation` or `_test`. That means, the `mlsum_de` test set would have the identifier `mlsum_de_test`.
The `keys` field can be set to avoid accidental shuffling to impact your metrics. Simply add a list of the `gem_id` for each output example in the same order as your values.
### Validate your submission
To ensure that your submission files are correctly formatted, run the following command from the root of the repository:
```
python cli.py validate
```
If everything is correct, you should see the following message:
```
All submission files validated! ✨ 🚀 ✨
Now you can make a submission 🤗
```
### Push your submission to the Hugging Face Hub!
The final step is to commit your files and push them to the Hub:
```
python cli.py submit
```
If there are no errors, you should see the following message:
```
Submission successful! 🎉 🥳 🎉
Your submission will be evaulated on Sunday 05 September 2021 ⏳
```
where the evaluation is run every Sunday and your results will be visible on the leaderboard. | lewtun/gem-sub-03 | [
"benchmark:gem",
"region:us"
] | 2022-03-02T23:29:22+00:00 | {"benchmark": "gem", "type": "prediction", "submission_name": "T5-base (Baseline)"} | 2021-12-15T14:34:30+00:00 | [] | [] | TAGS
#benchmark-gem #region-us
|
# GEM submissions for gem-sub-03
## Submitting to the benchmark
FILL ME IN
### Submission file format
Please follow this format for your 'URL' file:
In this case, 'dataset_identifier' is the identifier of the dataset followed by an identifier of the set the outputs were created from, for example '_validation' or '_test'. That means, the 'mlsum_de' test set would have the identifier 'mlsum_de_test'.
The 'keys' field can be set to avoid accidental shuffling to impact your metrics. Simply add a list of the 'gem_id' for each output example in the same order as your values.
### Validate your submission
To ensure that your submission files are correctly formatted, run the following command from the root of the repository:
If everything is correct, you should see the following message:
### Push your submission to the Hugging Face Hub!
The final step is to commit your files and push them to the Hub:
If there are no errors, you should see the following message:
where the evaluation is run every Sunday and your results will be visible on the leaderboard. | [
"# GEM submissions for gem-sub-03",
"## Submitting to the benchmark\n\nFILL ME IN",
"### Submission file format\n\nPlease follow this format for your 'URL' file:\n\n\n\nIn this case, 'dataset_identifier' is the identifier of the dataset followed by an identifier of the set the outputs were created from, for example '_validation' or '_test'. That means, the 'mlsum_de' test set would have the identifier 'mlsum_de_test'.\n\nThe 'keys' field can be set to avoid accidental shuffling to impact your metrics. Simply add a list of the 'gem_id' for each output example in the same order as your values.",
"### Validate your submission\n\nTo ensure that your submission files are correctly formatted, run the following command from the root of the repository:\n\n\n\nIf everything is correct, you should see the following message:",
"### Push your submission to the Hugging Face Hub!\n\nThe final step is to commit your files and push them to the Hub:\n\n\n\nIf there are no errors, you should see the following message:\n\n\n\nwhere the evaluation is run every Sunday and your results will be visible on the leaderboard."
] | [
"TAGS\n#benchmark-gem #region-us \n",
"# GEM submissions for gem-sub-03",
"## Submitting to the benchmark\n\nFILL ME IN",
"### Submission file format\n\nPlease follow this format for your 'URL' file:\n\n\n\nIn this case, 'dataset_identifier' is the identifier of the dataset followed by an identifier of the set the outputs were created from, for example '_validation' or '_test'. That means, the 'mlsum_de' test set would have the identifier 'mlsum_de_test'.\n\nThe 'keys' field can be set to avoid accidental shuffling to impact your metrics. Simply add a list of the 'gem_id' for each output example in the same order as your values.",
"### Validate your submission\n\nTo ensure that your submission files are correctly formatted, run the following command from the root of the repository:\n\n\n\nIf everything is correct, you should see the following message:",
"### Push your submission to the Hugging Face Hub!\n\nThe final step is to commit your files and push them to the Hub:\n\n\n\nIf there are no errors, you should see the following message:\n\n\n\nwhere the evaluation is run every Sunday and your results will be visible on the leaderboard."
] |
e3e3c81a4d7cc98d61d3b63b16b25e92008a9ba3 | # GitHub Issues Dataset | lewtun/github-issues-test | [
"region:us"
] | 2022-03-02T23:29:22+00:00 | {} | 2021-08-12T22:55:28+00:00 | [] | [] | TAGS
#region-us
| # GitHub Issues Dataset | [
"# GitHub Issues Dataset"
] | [
"TAGS\n#region-us \n",
"# GitHub Issues Dataset"
] |
3bb24dcad2b45b45e20fc0accc93058dcbe8087d | # Dataset Card for GitHub Issues
## Dataset Description
- **Point of Contact:** [Lewis Tunstall]([email protected])
### Dataset Summary
GitHub Issues is a dataset consisting of GitHub issues and pull requests associated with the 🤗 Datasets [repository](https://github.com/huggingface/datasets). It is intended for educational purposes and can be used for semantic search or multilabel text classification. The contents of each GitHub issue are in English and concern the domain of datasets for NLP, computer vision, and beyond.
### Supported Tasks and Leaderboards
For each of the tasks tagged for this dataset, give a brief description of the tag, metrics, and suggested models (with a link to their HuggingFace implementation if available). Give a similar description of tasks that were not covered by the structured tag set (repace the `task-category-tag` with an appropriate `other:other-task-name`).
- `task-category-tag`: The dataset can be used to train a model for [TASK NAME], which consists in [TASK DESCRIPTION]. Success on this task is typically measured by achieving a *high/low* [metric name](https://huggingface.co/metrics/metric_name). The ([model name](https://huggingface.co/model_name) or [model class](https://huggingface.co/transformers/model_doc/model_class.html)) model currently achieves the following score. *[IF A LEADERBOARD IS AVAILABLE]:* This task has an active leaderboard which can be found at [leaderboard url]() and ranks models based on [metric name](https://huggingface.co/metrics/metric_name) while also reporting [other metric name](https://huggingface.co/metrics/other_metric_name).
### Languages
Provide a brief overview of the languages represented in the dataset. Describe relevant details about specifics of the language such as whether it is social media text, African American English,...
When relevant, please provide [BCP-47 codes](https://tools.ietf.org/html/bcp47), which consist of a [primary language subtag](https://tools.ietf.org/html/bcp47#section-2.2.1), with a [script subtag](https://tools.ietf.org/html/bcp47#section-2.2.3) and/or [region subtag](https://tools.ietf.org/html/bcp47#section-2.2.4) if available.
## Dataset Structure
### Data Instances
Provide an JSON-formatted example and brief description of a typical instance in the dataset. If available, provide a link to further examples.
```
{
'example_field': ...,
...
}
```
Provide any additional information that is not covered in the other sections about the data here. In particular describe any relationships between data points and if these relationships are made explicit.
### Data Fields
List and describe the fields present in the dataset. Mention their data type, and whether they are used as input or output in any of the tasks the dataset currently supports. If the data has span indices, describe their attributes, such as whether they are at the character level or word level, whether they are contiguous or not, etc. If the datasets contains example IDs, state whether they have an inherent meaning, such as a mapping to other datasets or pointing to relationships between data points.
- `example_field`: description of `example_field`
Note that the descriptions can be initialized with the **Show Markdown Data Fields** output of the [tagging app](https://github.com/huggingface/datasets-tagging), you will then only need to refine the generated descriptions.
### Data Splits
Describe and name the splits in the dataset if there are more than one.
Describe any criteria for splitting the data, if used. If their are differences between the splits (e.g. if the training annotations are machine-generated and the dev and test ones are created by humans, or if different numbers of annotators contributed to each example), describe them here.
Provide the sizes of each split. As appropriate, provide any descriptive statistics for the features, such as average length. For example:
| | Tain | Valid | Test |
| ----- | ------ | ----- | ---- |
| Input Sentences | | | |
| Average Sentence Length | | | |
## Dataset Creation
### Curation Rationale
What need motivated the creation of this dataset? What are some of the reasons underlying the major choices involved in putting it together?
### Source Data
This section describes the source data (e.g. news text and headlines, social media posts, translated sentences,...)
#### Initial Data Collection and Normalization
Describe the data collection process. Describe any criteria for data selection or filtering. List any key words or search terms used. If possible, include runtime information for the collection process.
If data was collected from other pre-existing datasets, link to source here and to their [Hugging Face version](https://huggingface.co/datasets/dataset_name).
If the data was modified or normalized after being collected (e.g. if the data is word-tokenized), describe the process and the tools used.
#### Who are the source language producers?
State whether the data was produced by humans or machine generated. Describe the people or systems who originally created the data.
If available, include self-reported demographic or identity information for the source data creators, but avoid inferring this information. Instead state that this information is unknown. See [Larson 2017](https://www.aclweb.org/anthology/W17-1601.pdf) for using identity categories as a variables, particularly gender.
Describe the conditions under which the data was created (for example, if the producers were crowdworkers, state what platform was used, or if the data was found, what website the data was found on). If compensation was provided, include that information here.
Describe other people represented or mentioned in the data. Where possible, link to references for the information.
### Annotations
If the dataset contains annotations which are not part of the initial data collection, describe them in the following paragraphs.
#### Annotation process
If applicable, describe the annotation process and any tools used, or state otherwise. Describe the amount of data annotated, if not all. Describe or reference annotation guidelines provided to the annotators. If available, provide interannotator statistics. Describe any annotation validation processes.
#### Who are the annotators?
If annotations were collected for the source data (such as class labels or syntactic parses), state whether the annotations were produced by humans or machine generated.
Describe the people or systems who originally created the annotations and their selection criteria if applicable.
If available, include self-reported demographic or identity information for the annotators, but avoid inferring this information. Instead state that this information is unknown. See [Larson 2017](https://www.aclweb.org/anthology/W17-1601.pdf) for using identity categories as a variables, particularly gender.
Describe the conditions under which the data was annotated (for example, if the annotators were crowdworkers, state what platform was used, or if the data was found, what website the data was found on). If compensation was provided, include that information here.
### Personal and Sensitive Information
State whether the dataset uses identity categories and, if so, how the information is used. Describe where this information comes from (i.e. self-reporting, collecting from profiles, inferring, etc.). See [Larson 2017](https://www.aclweb.org/anthology/W17-1601.pdf) for using identity categories as a variables, particularly gender. State whether the data is linked to individuals and whether those individuals can be identified in the dataset, either directly or indirectly (i.e., in combination with other data).
State whether the dataset contains other data that might be considered sensitive (e.g., data that reveals racial or ethnic origins, sexual orientations, religious beliefs, political opinions or union memberships, or locations; financial or health data; biometric or genetic data; forms of government identification, such as social security numbers; criminal history).
If efforts were made to anonymize the data, describe the anonymization process.
## Considerations for Using the Data
### Social Impact of Dataset
Please discuss some of the ways you believe the use of this dataset will impact society.
The statement should include both positive outlooks, such as outlining how technologies developed through its use may improve people's lives, and discuss the accompanying risks. These risks may range from making important decisions more opaque to people who are affected by the technology, to reinforcing existing harmful biases (whose specifics should be discussed in the next section), among other considerations.
Also describe in this section if the proposed dataset contains a low-resource or under-represented language. If this is the case or if this task has any impact on underserved communities, please elaborate here.
### Discussion of Biases
Provide descriptions of specific biases that are likely to be reflected in the data, and state whether any steps were taken to reduce their impact.
For Wikipedia text, see for example [Dinan et al 2020 on biases in Wikipedia (esp. Table 1)](https://arxiv.org/abs/2005.00614), or [Blodgett et al 2020](https://www.aclweb.org/anthology/2020.acl-main.485/) for a more general discussion of the topic.
If analyses have been run quantifying these biases, please add brief summaries and links to the studies here.
### Other Known Limitations
If studies of the datasets have outlined other limitations of the dataset, such as annotation artifacts, please outline and cite them here.
## Additional Information
### Dataset Curators
List the people involved in collecting the dataset and their affiliation(s). If funding information is known, include it here.
### Licensing Information
Provide the license and link to the license webpage if available.
### Citation Information
Provide the [BibTex](http://www.bibtex.org/)-formatted reference for the dataset. For example:
```
@article{article_id,
author = {Author List},
title = {Dataset Paper Title},
journal = {Publication Venue},
year = {2525}
}
```
If the dataset has a [DOI](https://www.doi.org/), please provide it here.
### Contributions
Thanks to [@lewtun](https://github.com/lewtun) for adding this dataset. | lewtun/github-issues | [
"arxiv:2005.00614",
"region:us"
] | 2022-03-02T23:29:22+00:00 | {} | 2021-10-04T14:49:55+00:00 | [
"2005.00614"
] | [] | TAGS
#arxiv-2005.00614 #region-us
| Dataset Card for GitHub Issues
==============================
Dataset Description
-------------------
* Point of Contact: Lewis Tunstall
### Dataset Summary
GitHub Issues is a dataset consisting of GitHub issues and pull requests associated with the Datasets repository. It is intended for educational purposes and can be used for semantic search or multilabel text classification. The contents of each GitHub issue are in English and concern the domain of datasets for NLP, computer vision, and beyond.
### Supported Tasks and Leaderboards
For each of the tasks tagged for this dataset, give a brief description of the tag, metrics, and suggested models (with a link to their HuggingFace implementation if available). Give a similar description of tasks that were not covered by the structured tag set (repace the 'task-category-tag' with an appropriate 'other:other-task-name').
* 'task-category-tag': The dataset can be used to train a model for [TASK NAME], which consists in [TASK DESCRIPTION]. Success on this task is typically measured by achieving a *high/low* metric name. The (model name or model class) model currently achieves the following score. *[IF A LEADERBOARD IS AVAILABLE]:* This task has an active leaderboard which can be found at leaderboard url and ranks models based on metric name while also reporting other metric name.
### Languages
Provide a brief overview of the languages represented in the dataset. Describe relevant details about specifics of the language such as whether it is social media text, African American English,...
When relevant, please provide BCP-47 codes, which consist of a primary language subtag, with a script subtag and/or region subtag if available.
Dataset Structure
-----------------
### Data Instances
Provide an JSON-formatted example and brief description of a typical instance in the dataset. If available, provide a link to further examples.
Provide any additional information that is not covered in the other sections about the data here. In particular describe any relationships between data points and if these relationships are made explicit.
### Data Fields
List and describe the fields present in the dataset. Mention their data type, and whether they are used as input or output in any of the tasks the dataset currently supports. If the data has span indices, describe their attributes, such as whether they are at the character level or word level, whether they are contiguous or not, etc. If the datasets contains example IDs, state whether they have an inherent meaning, such as a mapping to other datasets or pointing to relationships between data points.
* 'example\_field': description of 'example\_field'
Note that the descriptions can be initialized with the Show Markdown Data Fields output of the tagging app, you will then only need to refine the generated descriptions.
### Data Splits
Describe and name the splits in the dataset if there are more than one.
Describe any criteria for splitting the data, if used. If their are differences between the splits (e.g. if the training annotations are machine-generated and the dev and test ones are created by humans, or if different numbers of annotators contributed to each example), describe them here.
Provide the sizes of each split. As appropriate, provide any descriptive statistics for the features, such as average length. For example:
Dataset Creation
----------------
### Curation Rationale
What need motivated the creation of this dataset? What are some of the reasons underlying the major choices involved in putting it together?
### Source Data
This section describes the source data (e.g. news text and headlines, social media posts, translated sentences,...)
#### Initial Data Collection and Normalization
Describe the data collection process. Describe any criteria for data selection or filtering. List any key words or search terms used. If possible, include runtime information for the collection process.
If data was collected from other pre-existing datasets, link to source here and to their Hugging Face version.
If the data was modified or normalized after being collected (e.g. if the data is word-tokenized), describe the process and the tools used.
#### Who are the source language producers?
State whether the data was produced by humans or machine generated. Describe the people or systems who originally created the data.
If available, include self-reported demographic or identity information for the source data creators, but avoid inferring this information. Instead state that this information is unknown. See Larson 2017 for using identity categories as a variables, particularly gender.
Describe the conditions under which the data was created (for example, if the producers were crowdworkers, state what platform was used, or if the data was found, what website the data was found on). If compensation was provided, include that information here.
Describe other people represented or mentioned in the data. Where possible, link to references for the information.
### Annotations
If the dataset contains annotations which are not part of the initial data collection, describe them in the following paragraphs.
#### Annotation process
If applicable, describe the annotation process and any tools used, or state otherwise. Describe the amount of data annotated, if not all. Describe or reference annotation guidelines provided to the annotators. If available, provide interannotator statistics. Describe any annotation validation processes.
#### Who are the annotators?
If annotations were collected for the source data (such as class labels or syntactic parses), state whether the annotations were produced by humans or machine generated.
Describe the people or systems who originally created the annotations and their selection criteria if applicable.
If available, include self-reported demographic or identity information for the annotators, but avoid inferring this information. Instead state that this information is unknown. See Larson 2017 for using identity categories as a variables, particularly gender.
Describe the conditions under which the data was annotated (for example, if the annotators were crowdworkers, state what platform was used, or if the data was found, what website the data was found on). If compensation was provided, include that information here.
### Personal and Sensitive Information
State whether the dataset uses identity categories and, if so, how the information is used. Describe where this information comes from (i.e. self-reporting, collecting from profiles, inferring, etc.). See Larson 2017 for using identity categories as a variables, particularly gender. State whether the data is linked to individuals and whether those individuals can be identified in the dataset, either directly or indirectly (i.e., in combination with other data).
State whether the dataset contains other data that might be considered sensitive (e.g., data that reveals racial or ethnic origins, sexual orientations, religious beliefs, political opinions or union memberships, or locations; financial or health data; biometric or genetic data; forms of government identification, such as social security numbers; criminal history).
If efforts were made to anonymize the data, describe the anonymization process.
Considerations for Using the Data
---------------------------------
### Social Impact of Dataset
Please discuss some of the ways you believe the use of this dataset will impact society.
The statement should include both positive outlooks, such as outlining how technologies developed through its use may improve people's lives, and discuss the accompanying risks. These risks may range from making important decisions more opaque to people who are affected by the technology, to reinforcing existing harmful biases (whose specifics should be discussed in the next section), among other considerations.
Also describe in this section if the proposed dataset contains a low-resource or under-represented language. If this is the case or if this task has any impact on underserved communities, please elaborate here.
### Discussion of Biases
Provide descriptions of specific biases that are likely to be reflected in the data, and state whether any steps were taken to reduce their impact.
For Wikipedia text, see for example Dinan et al 2020 on biases in Wikipedia (esp. Table 1), or Blodgett et al 2020 for a more general discussion of the topic.
If analyses have been run quantifying these biases, please add brief summaries and links to the studies here.
### Other Known Limitations
If studies of the datasets have outlined other limitations of the dataset, such as annotation artifacts, please outline and cite them here.
Additional Information
----------------------
### Dataset Curators
List the people involved in collecting the dataset and their affiliation(s). If funding information is known, include it here.
### Licensing Information
Provide the license and link to the license webpage if available.
Provide the BibTex-formatted reference for the dataset. For example:
If the dataset has a DOI, please provide it here.
### Contributions
Thanks to @lewtun for adding this dataset.
| [
"### Dataset Summary\n\n\nGitHub Issues is a dataset consisting of GitHub issues and pull requests associated with the Datasets repository. It is intended for educational purposes and can be used for semantic search or multilabel text classification. The contents of each GitHub issue are in English and concern the domain of datasets for NLP, computer vision, and beyond.",
"### Supported Tasks and Leaderboards\n\n\nFor each of the tasks tagged for this dataset, give a brief description of the tag, metrics, and suggested models (with a link to their HuggingFace implementation if available). Give a similar description of tasks that were not covered by the structured tag set (repace the 'task-category-tag' with an appropriate 'other:other-task-name').\n\n\n* 'task-category-tag': The dataset can be used to train a model for [TASK NAME], which consists in [TASK DESCRIPTION]. Success on this task is typically measured by achieving a *high/low* metric name. The (model name or model class) model currently achieves the following score. *[IF A LEADERBOARD IS AVAILABLE]:* This task has an active leaderboard which can be found at leaderboard url and ranks models based on metric name while also reporting other metric name.",
"### Languages\n\n\nProvide a brief overview of the languages represented in the dataset. Describe relevant details about specifics of the language such as whether it is social media text, African American English,...\n\n\nWhen relevant, please provide BCP-47 codes, which consist of a primary language subtag, with a script subtag and/or region subtag if available.\n\n\nDataset Structure\n-----------------",
"### Data Instances\n\n\nProvide an JSON-formatted example and brief description of a typical instance in the dataset. If available, provide a link to further examples.\n\n\nProvide any additional information that is not covered in the other sections about the data here. In particular describe any relationships between data points and if these relationships are made explicit.",
"### Data Fields\n\n\nList and describe the fields present in the dataset. Mention their data type, and whether they are used as input or output in any of the tasks the dataset currently supports. If the data has span indices, describe their attributes, such as whether they are at the character level or word level, whether they are contiguous or not, etc. If the datasets contains example IDs, state whether they have an inherent meaning, such as a mapping to other datasets or pointing to relationships between data points.\n\n\n* 'example\\_field': description of 'example\\_field'\n\n\nNote that the descriptions can be initialized with the Show Markdown Data Fields output of the tagging app, you will then only need to refine the generated descriptions.",
"### Data Splits\n\n\nDescribe and name the splits in the dataset if there are more than one.\n\n\nDescribe any criteria for splitting the data, if used. If their are differences between the splits (e.g. if the training annotations are machine-generated and the dev and test ones are created by humans, or if different numbers of annotators contributed to each example), describe them here.\n\n\nProvide the sizes of each split. As appropriate, provide any descriptive statistics for the features, such as average length. For example:\n\n\n\nDataset Creation\n----------------",
"### Curation Rationale\n\n\nWhat need motivated the creation of this dataset? What are some of the reasons underlying the major choices involved in putting it together?",
"### Source Data\n\n\nThis section describes the source data (e.g. news text and headlines, social media posts, translated sentences,...)",
"#### Initial Data Collection and Normalization\n\n\nDescribe the data collection process. Describe any criteria for data selection or filtering. List any key words or search terms used. If possible, include runtime information for the collection process.\n\n\nIf data was collected from other pre-existing datasets, link to source here and to their Hugging Face version.\n\n\nIf the data was modified or normalized after being collected (e.g. if the data is word-tokenized), describe the process and the tools used.",
"#### Who are the source language producers?\n\n\nState whether the data was produced by humans or machine generated. Describe the people or systems who originally created the data.\n\n\nIf available, include self-reported demographic or identity information for the source data creators, but avoid inferring this information. Instead state that this information is unknown. See Larson 2017 for using identity categories as a variables, particularly gender.\n\n\nDescribe the conditions under which the data was created (for example, if the producers were crowdworkers, state what platform was used, or if the data was found, what website the data was found on). If compensation was provided, include that information here.\n\n\nDescribe other people represented or mentioned in the data. Where possible, link to references for the information.",
"### Annotations\n\n\nIf the dataset contains annotations which are not part of the initial data collection, describe them in the following paragraphs.",
"#### Annotation process\n\n\nIf applicable, describe the annotation process and any tools used, or state otherwise. Describe the amount of data annotated, if not all. Describe or reference annotation guidelines provided to the annotators. If available, provide interannotator statistics. Describe any annotation validation processes.",
"#### Who are the annotators?\n\n\nIf annotations were collected for the source data (such as class labels or syntactic parses), state whether the annotations were produced by humans or machine generated.\n\n\nDescribe the people or systems who originally created the annotations and their selection criteria if applicable.\n\n\nIf available, include self-reported demographic or identity information for the annotators, but avoid inferring this information. Instead state that this information is unknown. See Larson 2017 for using identity categories as a variables, particularly gender.\n\n\nDescribe the conditions under which the data was annotated (for example, if the annotators were crowdworkers, state what platform was used, or if the data was found, what website the data was found on). If compensation was provided, include that information here.",
"### Personal and Sensitive Information\n\n\nState whether the dataset uses identity categories and, if so, how the information is used. Describe where this information comes from (i.e. self-reporting, collecting from profiles, inferring, etc.). See Larson 2017 for using identity categories as a variables, particularly gender. State whether the data is linked to individuals and whether those individuals can be identified in the dataset, either directly or indirectly (i.e., in combination with other data).\n\n\nState whether the dataset contains other data that might be considered sensitive (e.g., data that reveals racial or ethnic origins, sexual orientations, religious beliefs, political opinions or union memberships, or locations; financial or health data; biometric or genetic data; forms of government identification, such as social security numbers; criminal history).\n\n\nIf efforts were made to anonymize the data, describe the anonymization process.\n\n\nConsiderations for Using the Data\n---------------------------------",
"### Social Impact of Dataset\n\n\nPlease discuss some of the ways you believe the use of this dataset will impact society.\n\n\nThe statement should include both positive outlooks, such as outlining how technologies developed through its use may improve people's lives, and discuss the accompanying risks. These risks may range from making important decisions more opaque to people who are affected by the technology, to reinforcing existing harmful biases (whose specifics should be discussed in the next section), among other considerations.\n\n\nAlso describe in this section if the proposed dataset contains a low-resource or under-represented language. If this is the case or if this task has any impact on underserved communities, please elaborate here.",
"### Discussion of Biases\n\n\nProvide descriptions of specific biases that are likely to be reflected in the data, and state whether any steps were taken to reduce their impact.\n\n\nFor Wikipedia text, see for example Dinan et al 2020 on biases in Wikipedia (esp. Table 1), or Blodgett et al 2020 for a more general discussion of the topic.\n\n\nIf analyses have been run quantifying these biases, please add brief summaries and links to the studies here.",
"### Other Known Limitations\n\n\nIf studies of the datasets have outlined other limitations of the dataset, such as annotation artifacts, please outline and cite them here.\n\n\nAdditional Information\n----------------------",
"### Dataset Curators\n\n\nList the people involved in collecting the dataset and their affiliation(s). If funding information is known, include it here.",
"### Licensing Information\n\n\nProvide the license and link to the license webpage if available.\n\n\nProvide the BibTex-formatted reference for the dataset. For example:\n\n\nIf the dataset has a DOI, please provide it here.",
"### Contributions\n\n\nThanks to @lewtun for adding this dataset."
] | [
"TAGS\n#arxiv-2005.00614 #region-us \n",
"### Dataset Summary\n\n\nGitHub Issues is a dataset consisting of GitHub issues and pull requests associated with the Datasets repository. It is intended for educational purposes and can be used for semantic search or multilabel text classification. The contents of each GitHub issue are in English and concern the domain of datasets for NLP, computer vision, and beyond.",
"### Supported Tasks and Leaderboards\n\n\nFor each of the tasks tagged for this dataset, give a brief description of the tag, metrics, and suggested models (with a link to their HuggingFace implementation if available). Give a similar description of tasks that were not covered by the structured tag set (repace the 'task-category-tag' with an appropriate 'other:other-task-name').\n\n\n* 'task-category-tag': The dataset can be used to train a model for [TASK NAME], which consists in [TASK DESCRIPTION]. Success on this task is typically measured by achieving a *high/low* metric name. The (model name or model class) model currently achieves the following score. *[IF A LEADERBOARD IS AVAILABLE]:* This task has an active leaderboard which can be found at leaderboard url and ranks models based on metric name while also reporting other metric name.",
"### Languages\n\n\nProvide a brief overview of the languages represented in the dataset. Describe relevant details about specifics of the language such as whether it is social media text, African American English,...\n\n\nWhen relevant, please provide BCP-47 codes, which consist of a primary language subtag, with a script subtag and/or region subtag if available.\n\n\nDataset Structure\n-----------------",
"### Data Instances\n\n\nProvide an JSON-formatted example and brief description of a typical instance in the dataset. If available, provide a link to further examples.\n\n\nProvide any additional information that is not covered in the other sections about the data here. In particular describe any relationships between data points and if these relationships are made explicit.",
"### Data Fields\n\n\nList and describe the fields present in the dataset. Mention their data type, and whether they are used as input or output in any of the tasks the dataset currently supports. If the data has span indices, describe their attributes, such as whether they are at the character level or word level, whether they are contiguous or not, etc. If the datasets contains example IDs, state whether they have an inherent meaning, such as a mapping to other datasets or pointing to relationships between data points.\n\n\n* 'example\\_field': description of 'example\\_field'\n\n\nNote that the descriptions can be initialized with the Show Markdown Data Fields output of the tagging app, you will then only need to refine the generated descriptions.",
"### Data Splits\n\n\nDescribe and name the splits in the dataset if there are more than one.\n\n\nDescribe any criteria for splitting the data, if used. If their are differences between the splits (e.g. if the training annotations are machine-generated and the dev and test ones are created by humans, or if different numbers of annotators contributed to each example), describe them here.\n\n\nProvide the sizes of each split. As appropriate, provide any descriptive statistics for the features, such as average length. For example:\n\n\n\nDataset Creation\n----------------",
"### Curation Rationale\n\n\nWhat need motivated the creation of this dataset? What are some of the reasons underlying the major choices involved in putting it together?",
"### Source Data\n\n\nThis section describes the source data (e.g. news text and headlines, social media posts, translated sentences,...)",
"#### Initial Data Collection and Normalization\n\n\nDescribe the data collection process. Describe any criteria for data selection or filtering. List any key words or search terms used. If possible, include runtime information for the collection process.\n\n\nIf data was collected from other pre-existing datasets, link to source here and to their Hugging Face version.\n\n\nIf the data was modified or normalized after being collected (e.g. if the data is word-tokenized), describe the process and the tools used.",
"#### Who are the source language producers?\n\n\nState whether the data was produced by humans or machine generated. Describe the people or systems who originally created the data.\n\n\nIf available, include self-reported demographic or identity information for the source data creators, but avoid inferring this information. Instead state that this information is unknown. See Larson 2017 for using identity categories as a variables, particularly gender.\n\n\nDescribe the conditions under which the data was created (for example, if the producers were crowdworkers, state what platform was used, or if the data was found, what website the data was found on). If compensation was provided, include that information here.\n\n\nDescribe other people represented or mentioned in the data. Where possible, link to references for the information.",
"### Annotations\n\n\nIf the dataset contains annotations which are not part of the initial data collection, describe them in the following paragraphs.",
"#### Annotation process\n\n\nIf applicable, describe the annotation process and any tools used, or state otherwise. Describe the amount of data annotated, if not all. Describe or reference annotation guidelines provided to the annotators. If available, provide interannotator statistics. Describe any annotation validation processes.",
"#### Who are the annotators?\n\n\nIf annotations were collected for the source data (such as class labels or syntactic parses), state whether the annotations were produced by humans or machine generated.\n\n\nDescribe the people or systems who originally created the annotations and their selection criteria if applicable.\n\n\nIf available, include self-reported demographic or identity information for the annotators, but avoid inferring this information. Instead state that this information is unknown. See Larson 2017 for using identity categories as a variables, particularly gender.\n\n\nDescribe the conditions under which the data was annotated (for example, if the annotators were crowdworkers, state what platform was used, or if the data was found, what website the data was found on). If compensation was provided, include that information here.",
"### Personal and Sensitive Information\n\n\nState whether the dataset uses identity categories and, if so, how the information is used. Describe where this information comes from (i.e. self-reporting, collecting from profiles, inferring, etc.). See Larson 2017 for using identity categories as a variables, particularly gender. State whether the data is linked to individuals and whether those individuals can be identified in the dataset, either directly or indirectly (i.e., in combination with other data).\n\n\nState whether the dataset contains other data that might be considered sensitive (e.g., data that reveals racial or ethnic origins, sexual orientations, religious beliefs, political opinions or union memberships, or locations; financial or health data; biometric or genetic data; forms of government identification, such as social security numbers; criminal history).\n\n\nIf efforts were made to anonymize the data, describe the anonymization process.\n\n\nConsiderations for Using the Data\n---------------------------------",
"### Social Impact of Dataset\n\n\nPlease discuss some of the ways you believe the use of this dataset will impact society.\n\n\nThe statement should include both positive outlooks, such as outlining how technologies developed through its use may improve people's lives, and discuss the accompanying risks. These risks may range from making important decisions more opaque to people who are affected by the technology, to reinforcing existing harmful biases (whose specifics should be discussed in the next section), among other considerations.\n\n\nAlso describe in this section if the proposed dataset contains a low-resource or under-represented language. If this is the case or if this task has any impact on underserved communities, please elaborate here.",
"### Discussion of Biases\n\n\nProvide descriptions of specific biases that are likely to be reflected in the data, and state whether any steps were taken to reduce their impact.\n\n\nFor Wikipedia text, see for example Dinan et al 2020 on biases in Wikipedia (esp. Table 1), or Blodgett et al 2020 for a more general discussion of the topic.\n\n\nIf analyses have been run quantifying these biases, please add brief summaries and links to the studies here.",
"### Other Known Limitations\n\n\nIf studies of the datasets have outlined other limitations of the dataset, such as annotation artifacts, please outline and cite them here.\n\n\nAdditional Information\n----------------------",
"### Dataset Curators\n\n\nList the people involved in collecting the dataset and their affiliation(s). If funding information is known, include it here.",
"### Licensing Information\n\n\nProvide the license and link to the license webpage if available.\n\n\nProvide the BibTex-formatted reference for the dataset. For example:\n\n\nIf the dataset has a DOI, please provide it here.",
"### Contributions\n\n\nThanks to @lewtun for adding this dataset."
] |
be8eb418f71d209bd05f3f1be13e916c283c6540 |
# Dataset Card for RAFT Submission | lewtun/mnist-preds | [
"benchmark:test",
"region:us"
] | 2022-03-02T23:29:22+00:00 | {"benchmark": "test"} | 2021-07-16T08:00:01+00:00 | [] | [] | TAGS
#benchmark-test #region-us
|
# Dataset Card for RAFT Submission | [
"# Dataset Card for RAFT Submission"
] | [
"TAGS\n#benchmark-test #region-us \n",
"# Dataset Card for RAFT Submission"
] |
b66c0539f6b2df8daab58de1edb5371b19db5486 |
# Dataset Card for Demo
## Table of Contents
- [Table of Contents](#table-of-contents)
- [Dataset Description](#dataset-description)
- [Dataset Summary](#dataset-summary)
- [Supported Tasks and Leaderboards](#supported-tasks-and-leaderboards)
- [Languages](#languages)
- [Dataset Structure](#dataset-structure)
- [Data Instances](#data-instances)
- [Data Fields](#data-fields)
- [Data Splits](#data-splits)
- [Dataset Creation](#dataset-creation)
- [Curation Rationale](#curation-rationale)
- [Source Data](#source-data)
- [Annotations](#annotations)
- [Personal and Sensitive Information](#personal-and-sensitive-information)
- [Considerations for Using the Data](#considerations-for-using-the-data)
- [Social Impact of Dataset](#social-impact-of-dataset)
- [Discussion of Biases](#discussion-of-biases)
- [Other Known Limitations](#other-known-limitations)
- [Additional Information](#additional-information)
- [Dataset Curators](#dataset-curators)
- [Licensing Information](#licensing-information)
- [Citation Information](#citation-information)
- [Contributions](#contributions)
## Dataset Description
- **Homepage:**
- **Repository:**
- **Paper:**
- **Leaderboard:**
- **Point of Contact:**
### Dataset Summary
This is a demo dataset with two files `train.csv` and `test.csv`.
Load it by:
```python
from datasets import load_dataset
data_files = {"train": "train.csv", "test": "test.csv"}
demo = load_dataset("stevhliu/demo", data_files=data_files)
```
### Supported Tasks and Leaderboards
[More Information Needed]
### Languages
[More Information Needed]
## Dataset Structure
### Data Instances
[More Information Needed]
### Data Fields
[More Information Needed]
### Data Splits
[More Information Needed]
## Dataset Creation
### Curation Rationale
[More Information Needed]
### Source Data
#### Initial Data Collection and Normalization
[More Information Needed]
#### Who are the source language producers?
[More Information Needed]
### Annotations
#### Annotation process
[More Information Needed]
#### Who are the annotators?
[More Information Needed]
### Personal and Sensitive Information
[More Information Needed]
## Considerations for Using the Data
### Social Impact of Dataset
[More Information Needed]
### Discussion of Biases
[More Information Needed]
### Other Known Limitations
[More Information Needed]
## Additional Information
### Dataset Curators
[More Information Needed]
### Licensing Information
[More Information Needed]
### Citation Information
[More Information Needed]
### Contributions
Thanks to [@github-username](https://github.com/<github-username>) for adding this dataset. | lewtun/my-awesome-dataset | [
"annotations_creators:no-annotation",
"language_creators:found",
"multilinguality:monolingual",
"size_categories:100K<n<1M",
"source_datasets:original",
"language:en",
"license:apache-2.0",
"region:us"
] | 2022-03-02T23:29:22+00:00 | {"annotations_creators": ["no-annotation"], "language_creators": ["found"], "language": ["en"], "license": ["apache-2.0"], "multilinguality": ["monolingual"], "size_categories": ["100K<n<1M"], "source_datasets": ["original"], "task_categories": ["conditional-text-generation"], "task_ids": ["summarization"]} | 2022-07-03T04:16:07+00:00 | [] | [
"en"
] | TAGS
#annotations_creators-no-annotation #language_creators-found #multilinguality-monolingual #size_categories-100K<n<1M #source_datasets-original #language-English #license-apache-2.0 #region-us
|
# Dataset Card for Demo
## Table of Contents
- Table of Contents
- Dataset Description
- Dataset Summary
- Supported Tasks and Leaderboards
- Languages
- Dataset Structure
- Data Instances
- Data Fields
- Data Splits
- Dataset Creation
- Curation Rationale
- Source Data
- Annotations
- Personal and Sensitive Information
- Considerations for Using the Data
- Social Impact of Dataset
- Discussion of Biases
- Other Known Limitations
- Additional Information
- Dataset Curators
- Licensing Information
- Citation Information
- Contributions
## Dataset Description
- Homepage:
- Repository:
- Paper:
- Leaderboard:
- Point of Contact:
### Dataset Summary
This is a demo dataset with two files 'URL' and 'URL'.
Load it by:
### Supported Tasks and Leaderboards
### Languages
## Dataset Structure
### Data Instances
### Data Fields
### Data Splits
## Dataset Creation
### Curation Rationale
### Source Data
#### Initial Data Collection and Normalization
#### Who are the source language producers?
### Annotations
#### Annotation process
#### Who are the annotators?
### Personal and Sensitive Information
## Considerations for Using the Data
### Social Impact of Dataset
### Discussion of Biases
### Other Known Limitations
## Additional Information
### Dataset Curators
### Licensing Information
### Contributions
Thanks to @github-username for adding this dataset. | [
"# Dataset Card for Demo",
"## Table of Contents\n- Table of Contents\n- Dataset Description\n - Dataset Summary\n - Supported Tasks and Leaderboards\n - Languages\n- Dataset Structure\n - Data Instances\n - Data Fields\n - Data Splits\n- Dataset Creation\n - Curation Rationale\n - Source Data\n - Annotations\n - Personal and Sensitive Information\n- Considerations for Using the Data\n - Social Impact of Dataset\n - Discussion of Biases\n - Other Known Limitations\n- Additional Information\n - Dataset Curators\n - Licensing Information\n - Citation Information\n - Contributions",
"## Dataset Description\n\n- Homepage:\n- Repository:\n- Paper:\n- Leaderboard:\n- Point of Contact:",
"### Dataset Summary\n\nThis is a demo dataset with two files 'URL' and 'URL'.\n\nLoad it by:",
"### Supported Tasks and Leaderboards",
"### Languages",
"## Dataset Structure",
"### Data Instances",
"### Data Fields",
"### Data Splits",
"## Dataset Creation",
"### Curation Rationale",
"### Source Data",
"#### Initial Data Collection and Normalization",
"#### Who are the source language producers?",
"### Annotations",
"#### Annotation process",
"#### Who are the annotators?",
"### Personal and Sensitive Information",
"## Considerations for Using the Data",
"### Social Impact of Dataset",
"### Discussion of Biases",
"### Other Known Limitations",
"## Additional Information",
"### Dataset Curators",
"### Licensing Information",
"### Contributions\n\nThanks to @github-username for adding this dataset."
] | [
"TAGS\n#annotations_creators-no-annotation #language_creators-found #multilinguality-monolingual #size_categories-100K<n<1M #source_datasets-original #language-English #license-apache-2.0 #region-us \n",
"# Dataset Card for Demo",
"## Table of Contents\n- Table of Contents\n- Dataset Description\n - Dataset Summary\n - Supported Tasks and Leaderboards\n - Languages\n- Dataset Structure\n - Data Instances\n - Data Fields\n - Data Splits\n- Dataset Creation\n - Curation Rationale\n - Source Data\n - Annotations\n - Personal and Sensitive Information\n- Considerations for Using the Data\n - Social Impact of Dataset\n - Discussion of Biases\n - Other Known Limitations\n- Additional Information\n - Dataset Curators\n - Licensing Information\n - Citation Information\n - Contributions",
"## Dataset Description\n\n- Homepage:\n- Repository:\n- Paper:\n- Leaderboard:\n- Point of Contact:",
"### Dataset Summary\n\nThis is a demo dataset with two files 'URL' and 'URL'.\n\nLoad it by:",
"### Supported Tasks and Leaderboards",
"### Languages",
"## Dataset Structure",
"### Data Instances",
"### Data Fields",
"### Data Splits",
"## Dataset Creation",
"### Curation Rationale",
"### Source Data",
"#### Initial Data Collection and Normalization",
"#### Who are the source language producers?",
"### Annotations",
"#### Annotation process",
"#### Who are the annotators?",
"### Personal and Sensitive Information",
"## Considerations for Using the Data",
"### Social Impact of Dataset",
"### Discussion of Biases",
"### Other Known Limitations",
"## Additional Information",
"### Dataset Curators",
"### Licensing Information",
"### Contributions\n\nThanks to @github-username for adding this dataset."
] |
49e6a3b37d4666b3554ea90a6c76e02d07505fec |
Open Minuscule
==============
A little small wee corpus to train little small wee models.
## Dataset Description
### Dataset Summary
This is a raw text corpus, mainly intended for testing purposes.
### Languages
- French
- English
## Dataset Structure
### Data Instances
[More Information Needed]
### Data Fields
[More Information Needed]
### Data Splits
[More Information Needed]
## Dataset Creation
### Source Data
It is a mashup including the following [CC-BY-SA 4.0](https://creativecommons.org/licenses/by-sa/4.0/) licenced texts
- [*Rayons émis par les composés de l’uranium et du
thorium*](https://fr.wikisource.org/wiki/Rayons_%C3%A9mis_par_les_compos%C3%A9s_de_l%E2%80%99uranium_et_du_thorium),
Maria Skłodowska Curie
- [*Frankenstein, or the Modern
Prometheus*](https://en.wikisource.org/wiki/Frankenstein,_or_the_Modern_Prometheus_(Revised_Edition,_1831)),
Mary Wollstonecraft Shelley
- [*Les maîtres sonneurs*](https://fr.wikisource.org/wiki/Les_Ma%C3%AEtres_sonneurs), George Sand
It also includes the text of *Sketch of The Analytical Engine Invented by Charles Babbage With
notes upon the Memoir by the Translator* by Luigi Menabrea and Ada Lovelace, which to the best of
my knowledge should be public domain.
## Considerations for Using the Data
This really should not be used for anything but testing purposes
## Licence
This corpus is available under the Creative Commons Attribution-ShareAlike 4.0 License | lgrobol/openminuscule | [
"task_categories:text-generation",
"task_ids:language-modeling",
"language_creators:crowdsourced",
"multilinguality:multilingual",
"size_categories:100k<n<1M",
"source_datasets:original",
"language:en",
"language:fr",
"license:cc-by-4.0",
"region:us"
] | 2022-03-02T23:29:22+00:00 | {"language_creators": ["crowdsourced"], "language": ["en", "fr"], "license": ["cc-by-4.0"], "multilinguality": ["multilingual"], "size_categories": ["100k<n<1M"], "source_datasets": ["original"], "task_categories": ["text-generation"], "task_ids": ["language-modeling"], "pretty_name": "Open Minuscule", "language_bcp47": ["en-GB", "fr-FR"]} | 2022-10-23T08:28:36+00:00 | [] | [
"en",
"fr"
] | TAGS
#task_categories-text-generation #task_ids-language-modeling #language_creators-crowdsourced #multilinguality-multilingual #size_categories-100k<n<1M #source_datasets-original #language-English #language-French #license-cc-by-4.0 #region-us
|
Open Minuscule
==============
A little small wee corpus to train little small wee models.
## Dataset Description
### Dataset Summary
This is a raw text corpus, mainly intended for testing purposes.
### Languages
- French
- English
## Dataset Structure
### Data Instances
### Data Fields
### Data Splits
## Dataset Creation
### Source Data
It is a mashup including the following CC-BY-SA 4.0 licenced texts
- *Rayons émis par les composés de l’uranium et du
thorium*,
Maria Skłodowska Curie
- *Frankenstein, or the Modern
Prometheus*),
Mary Wollstonecraft Shelley
- *Les maîtres sonneurs*, George Sand
It also includes the text of *Sketch of The Analytical Engine Invented by Charles Babbage With
notes upon the Memoir by the Translator* by Luigi Menabrea and Ada Lovelace, which to the best of
my knowledge should be public domain.
## Considerations for Using the Data
This really should not be used for anything but testing purposes
## Licence
This corpus is available under the Creative Commons Attribution-ShareAlike 4.0 License | [
"## Dataset Description",
"### Dataset Summary\n\nThis is a raw text corpus, mainly intended for testing purposes.",
"### Languages\n\n- French\n- English",
"## Dataset Structure",
"### Data Instances",
"### Data Fields",
"### Data Splits",
"## Dataset Creation",
"### Source Data\n\nIt is a mashup including the following CC-BY-SA 4.0 licenced texts\n\n - *Rayons émis par les composés de l’uranium et du\n thorium*,\n Maria Skłodowska Curie\n - *Frankenstein, or the Modern\n Prometheus*),\n Mary Wollstonecraft Shelley\n - *Les maîtres sonneurs*, George Sand\n\n It also includes the text of *Sketch of The Analytical Engine Invented by Charles Babbage With\n notes upon the Memoir by the Translator* by Luigi Menabrea and Ada Lovelace, which to the best of\n my knowledge should be public domain.",
"## Considerations for Using the Data\n\nThis really should not be used for anything but testing purposes",
"## Licence\n\nThis corpus is available under the Creative Commons Attribution-ShareAlike 4.0 License"
] | [
"TAGS\n#task_categories-text-generation #task_ids-language-modeling #language_creators-crowdsourced #multilinguality-multilingual #size_categories-100k<n<1M #source_datasets-original #language-English #language-French #license-cc-by-4.0 #region-us \n",
"## Dataset Description",
"### Dataset Summary\n\nThis is a raw text corpus, mainly intended for testing purposes.",
"### Languages\n\n- French\n- English",
"## Dataset Structure",
"### Data Instances",
"### Data Fields",
"### Data Splits",
"## Dataset Creation",
"### Source Data\n\nIt is a mashup including the following CC-BY-SA 4.0 licenced texts\n\n - *Rayons émis par les composés de l’uranium et du\n thorium*,\n Maria Skłodowska Curie\n - *Frankenstein, or the Modern\n Prometheus*),\n Mary Wollstonecraft Shelley\n - *Les maîtres sonneurs*, George Sand\n\n It also includes the text of *Sketch of The Analytical Engine Invented by Charles Babbage With\n notes upon the Memoir by the Translator* by Luigi Menabrea and Ada Lovelace, which to the best of\n my knowledge should be public domain.",
"## Considerations for Using the Data\n\nThis really should not be used for anything but testing purposes",
"## Licence\n\nThis corpus is available under the Creative Commons Attribution-ShareAlike 4.0 License"
] |
6bb129c79cbc02860807e12dd09bf9e152c3f73d |
# Dataset Card for "squad"
## Table of Contents
- [Dataset Description](#dataset-description)
- [Dataset Summary](#dataset-summary)
- [Supported Tasks](#supported-tasks)
- [Languages](#languages)
- [Dataset Structure](#dataset-structure)
- [Data Instances](#data-instances)
- [Data Fields](#data-fields)
- [Data Splits Sample Size](#data-splits-sample-size)
- [Dataset Creation](#dataset-creation)
- [Curation Rationale](#curation-rationale)
- [Source Data](#source-data)
- [Annotations](#annotations)
- [Personal and Sensitive Information](#personal-and-sensitive-information)
- [Considerations for Using the Data](#considerations-for-using-the-data)
- [Social Impact of Dataset](#social-impact-of-dataset)
- [Discussion of Biases](#discussion-of-biases)
- [Other Known Limitations](#other-known-limitations)
- [Additional Information](#additional-information)
- [Dataset Curators](#dataset-curators)
- [Licensing Information](#licensing-information)
- [Citation Information](#citation-information)
- [Contributions](#contributions)
## Dataset Description
- **Homepage:** [https://rajpurkar.github.io/SQuAD-explorer/](https://rajpurkar.github.io/SQuAD-explorer/)
- **Repository:** [More Information Needed](https://github.com/huggingface/datasets/blob/master/CONTRIBUTING.md#how-to-contribute-to-the-dataset-cards)
- **Paper:** [More Information Needed](https://github.com/huggingface/datasets/blob/master/CONTRIBUTING.md#how-to-contribute-to-the-dataset-cards)
- **Point of Contact:** [More Information Needed](https://github.com/huggingface/datasets/blob/master/CONTRIBUTING.md#how-to-contribute-to-the-dataset-cards)
- **Size of downloaded dataset files:** 33.51 MB
- **Size of the generated dataset:** 85.75 MB
- **Total amount of disk used:** 119.27 MB
### Dataset Summary
This dataset is a custom copy of the original SQuAD dataset. It is used to showcase dataset repositories. Data are the same as the original dataset.
Stanford Question Answering Dataset (SQuAD) is a reading comprehension dataset, consisting of questions posed by crowdworkers on a set of Wikipedia articles, where the answer to every question is a segment of text, or span, from the corresponding reading passage, or the question might be unanswerable.
### Supported Tasks
[More Information Needed](https://github.com/huggingface/datasets/blob/master/CONTRIBUTING.md#how-to-contribute-to-the-dataset-cards)
### Languages
[More Information Needed](https://github.com/huggingface/datasets/blob/master/CONTRIBUTING.md#how-to-contribute-to-the-dataset-cards)
## Dataset Structure
We show detailed information for up to 5 configurations of the dataset.
### Data Instances
#### plain_text
- **Size of downloaded dataset files:** 33.51 MB
- **Size of the generated dataset:** 85.75 MB
- **Total amount of disk used:** 119.27 MB
An example of 'train' looks as follows.
```
{
"answers": {
"answer_start": [1],
"text": ["This is a test text"]
},
"context": "This is a test context.",
"id": "1",
"question": "Is this a test?",
"title": "train test"
}
```
### Data Fields
The data fields are the same among all splits.
#### plain_text
- `id`: a `string` feature.
- `title`: a `string` feature.
- `context`: a `string` feature.
- `question`: a `string` feature.
- `answers`: a dictionary feature containing:
- `text`: a `string` feature.
- `answer_start`: a `int32` feature.
### Data Splits Sample Size
| name |train|validation|
|----------|----:|---------:|
|plain_text|87599| 10570|
## Dataset Creation
### Curation Rationale
[More Information Needed]
### Source Data
[More Information Needed]
### Annotations
[More Information Needed]
### Personal and Sensitive Information
[More Information Needed]
## Considerations for Using the Data
### Social Impact of Dataset
[More Information Needed]
### Discussion of Biases
[More Information Needed]
### Other Known Limitations
[More Information Needed]
## Additional Information
### Dataset Curators
[More Information Needed]
### Licensing Information
[More Information Needed]
### Citation Information
```
@article{2016arXiv160605250R,
author = {{Rajpurkar}, Pranav and {Zhang}, Jian and {Lopyrev},
Konstantin and {Liang}, Percy},
title = "{SQuAD: 100,000+ Questions for Machine Comprehension of Text}",
journal = {arXiv e-prints},
year = 2016,
eid = {arXiv:1606.05250},
pages = {arXiv:1606.05250},
archivePrefix = {arXiv},
eprint = {1606.05250},
}
```
### Contributions
Thanks to [@lewtun](https://github.com/lewtun), [@albertvillanova](https://github.com/albertvillanova), [@patrickvonplaten](https://github.com/patrickvonplaten), [@thomwolf](https://github.com/thomwolf) for adding this dataset. | lhoestq/custom_squad | [
"task_categories:question-answering",
"task_ids:extractive-qa",
"annotations_creators:crowdsourced",
"language_creators:crowdsourced",
"language_creators:found",
"multilinguality:monolingual",
"size_categories:10K<n<100K",
"source_datasets:extended|wikipedia",
"language:en",
"license:cc-by-4.0",
"arxiv:1606.05250",
"region:us"
] | 2022-03-02T23:29:22+00:00 | {"annotations_creators": ["crowdsourced"], "language_creators": ["crowdsourced", "found"], "language": ["en"], "license": ["cc-by-4.0"], "multilinguality": ["monolingual"], "size_categories": ["10K<n<100K"], "source_datasets": ["extended|wikipedia"], "task_categories": ["question-answering"], "task_ids": ["extractive-qa"]} | 2022-10-25T08:50:53+00:00 | [
"1606.05250"
] | [
"en"
] | TAGS
#task_categories-question-answering #task_ids-extractive-qa #annotations_creators-crowdsourced #language_creators-crowdsourced #language_creators-found #multilinguality-monolingual #size_categories-10K<n<100K #source_datasets-extended|wikipedia #language-English #license-cc-by-4.0 #arxiv-1606.05250 #region-us
| Dataset Card for "squad"
========================
Table of Contents
-----------------
* Dataset Description
+ Dataset Summary
+ Supported Tasks
+ Languages
* Dataset Structure
+ Data Instances
+ Data Fields
+ Data Splits Sample Size
* Dataset Creation
+ Curation Rationale
+ Source Data
+ Annotations
+ Personal and Sensitive Information
* Considerations for Using the Data
+ Social Impact of Dataset
+ Discussion of Biases
+ Other Known Limitations
* Additional Information
+ Dataset Curators
+ Licensing Information
+ Citation Information
+ Contributions
Dataset Description
-------------------
* Homepage: URL
* Repository:
* Paper:
* Point of Contact:
* Size of downloaded dataset files: 33.51 MB
* Size of the generated dataset: 85.75 MB
* Total amount of disk used: 119.27 MB
### Dataset Summary
This dataset is a custom copy of the original SQuAD dataset. It is used to showcase dataset repositories. Data are the same as the original dataset.
Stanford Question Answering Dataset (SQuAD) is a reading comprehension dataset, consisting of questions posed by crowdworkers on a set of Wikipedia articles, where the answer to every question is a segment of text, or span, from the corresponding reading passage, or the question might be unanswerable.
### Supported Tasks
### Languages
Dataset Structure
-----------------
We show detailed information for up to 5 configurations of the dataset.
### Data Instances
#### plain\_text
* Size of downloaded dataset files: 33.51 MB
* Size of the generated dataset: 85.75 MB
* Total amount of disk used: 119.27 MB
An example of 'train' looks as follows.
### Data Fields
The data fields are the same among all splits.
#### plain\_text
* 'id': a 'string' feature.
* 'title': a 'string' feature.
* 'context': a 'string' feature.
* 'question': a 'string' feature.
* 'answers': a dictionary feature containing:
+ 'text': a 'string' feature.
+ 'answer\_start': a 'int32' feature.
### Data Splits Sample Size
Dataset Creation
----------------
### Curation Rationale
### Source Data
### Annotations
### Personal and Sensitive Information
Considerations for Using the Data
---------------------------------
### Social Impact of Dataset
### Discussion of Biases
### Other Known Limitations
Additional Information
----------------------
### Dataset Curators
### Licensing Information
### Contributions
Thanks to @lewtun, @albertvillanova, @patrickvonplaten, @thomwolf for adding this dataset.
| [
"### Dataset Summary\n\n\nThis dataset is a custom copy of the original SQuAD dataset. It is used to showcase dataset repositories. Data are the same as the original dataset.\nStanford Question Answering Dataset (SQuAD) is a reading comprehension dataset, consisting of questions posed by crowdworkers on a set of Wikipedia articles, where the answer to every question is a segment of text, or span, from the corresponding reading passage, or the question might be unanswerable.",
"### Supported Tasks",
"### Languages\n\n\nDataset Structure\n-----------------\n\n\nWe show detailed information for up to 5 configurations of the dataset.",
"### Data Instances",
"#### plain\\_text\n\n\n* Size of downloaded dataset files: 33.51 MB\n* Size of the generated dataset: 85.75 MB\n* Total amount of disk used: 119.27 MB\n\n\nAn example of 'train' looks as follows.",
"### Data Fields\n\n\nThe data fields are the same among all splits.",
"#### plain\\_text\n\n\n* 'id': a 'string' feature.\n* 'title': a 'string' feature.\n* 'context': a 'string' feature.\n* 'question': a 'string' feature.\n* 'answers': a dictionary feature containing:\n\t+ 'text': a 'string' feature.\n\t+ 'answer\\_start': a 'int32' feature.",
"### Data Splits Sample Size\n\n\n\nDataset Creation\n----------------",
"### Curation Rationale",
"### Source Data",
"### Annotations",
"### Personal and Sensitive Information\n\n\nConsiderations for Using the Data\n---------------------------------",
"### Social Impact of Dataset",
"### Discussion of Biases",
"### Other Known Limitations\n\n\nAdditional Information\n----------------------",
"### Dataset Curators",
"### Licensing Information",
"### Contributions\n\n\nThanks to @lewtun, @albertvillanova, @patrickvonplaten, @thomwolf for adding this dataset."
] | [
"TAGS\n#task_categories-question-answering #task_ids-extractive-qa #annotations_creators-crowdsourced #language_creators-crowdsourced #language_creators-found #multilinguality-monolingual #size_categories-10K<n<100K #source_datasets-extended|wikipedia #language-English #license-cc-by-4.0 #arxiv-1606.05250 #region-us \n",
"### Dataset Summary\n\n\nThis dataset is a custom copy of the original SQuAD dataset. It is used to showcase dataset repositories. Data are the same as the original dataset.\nStanford Question Answering Dataset (SQuAD) is a reading comprehension dataset, consisting of questions posed by crowdworkers on a set of Wikipedia articles, where the answer to every question is a segment of text, or span, from the corresponding reading passage, or the question might be unanswerable.",
"### Supported Tasks",
"### Languages\n\n\nDataset Structure\n-----------------\n\n\nWe show detailed information for up to 5 configurations of the dataset.",
"### Data Instances",
"#### plain\\_text\n\n\n* Size of downloaded dataset files: 33.51 MB\n* Size of the generated dataset: 85.75 MB\n* Total amount of disk used: 119.27 MB\n\n\nAn example of 'train' looks as follows.",
"### Data Fields\n\n\nThe data fields are the same among all splits.",
"#### plain\\_text\n\n\n* 'id': a 'string' feature.\n* 'title': a 'string' feature.\n* 'context': a 'string' feature.\n* 'question': a 'string' feature.\n* 'answers': a dictionary feature containing:\n\t+ 'text': a 'string' feature.\n\t+ 'answer\\_start': a 'int32' feature.",
"### Data Splits Sample Size\n\n\n\nDataset Creation\n----------------",
"### Curation Rationale",
"### Source Data",
"### Annotations",
"### Personal and Sensitive Information\n\n\nConsiderations for Using the Data\n---------------------------------",
"### Social Impact of Dataset",
"### Discussion of Biases",
"### Other Known Limitations\n\n\nAdditional Information\n----------------------",
"### Dataset Curators",
"### Licensing Information",
"### Contributions\n\n\nThanks to @lewtun, @albertvillanova, @patrickvonplaten, @thomwolf for adding this dataset."
] |
87ecf163bedca9d80598b528940a9c4f99e14c11 |
# Dataset Card for Demo1
## Table of Contents
- [Table of Contents](#table-of-contents)
- [Dataset Description](#dataset-description)
- [Dataset Summary](#dataset-summary)
- [Supported Tasks and Leaderboards](#supported-tasks-and-leaderboards)
- [Languages](#languages)
- [Dataset Structure](#dataset-structure)
- [Data Instances](#data-instances)
- [Data Fields](#data-fields)
- [Data Splits](#data-splits)
- [Dataset Creation](#dataset-creation)
- [Curation Rationale](#curation-rationale)
- [Source Data](#source-data)
- [Annotations](#annotations)
- [Personal and Sensitive Information](#personal-and-sensitive-information)
- [Considerations for Using the Data](#considerations-for-using-the-data)
- [Social Impact of Dataset](#social-impact-of-dataset)
- [Discussion of Biases](#discussion-of-biases)
- [Other Known Limitations](#other-known-limitations)
- [Additional Information](#additional-information)
- [Dataset Curators](#dataset-curators)
- [Licensing Information](#licensing-information)
- [Citation Information](#citation-information)
- [Contributions](#contributions)
## Dataset Description
- **Homepage:**
- **Repository:**
- **Paper:**
- **Leaderboard:**
- **Point of Contact:**
### Dataset Summary
This is a demo dataset. It consists in two files `data/train.csv` and `data/test.csv`
You can load it with
```python
from datasets import load_dataset
demo1 = load_dataset("lhoestq/demo1")
```
### Supported Tasks and Leaderboards
[More Information Needed]
### Languages
[More Information Needed]
## Dataset Structure
### Data Instances
[More Information Needed]
### Data Fields
[More Information Needed]
### Data Splits
[More Information Needed]
## Dataset Creation
### Curation Rationale
[More Information Needed]
### Source Data
#### Initial Data Collection and Normalization
[More Information Needed]
#### Who are the source language producers?
[More Information Needed]
### Annotations
#### Annotation process
[More Information Needed]
#### Who are the annotators?
[More Information Needed]
### Personal and Sensitive Information
[More Information Needed]
## Considerations for Using the Data
### Social Impact of Dataset
[More Information Needed]
### Discussion of Biases
[More Information Needed]
### Other Known Limitations
[More Information Needed]
## Additional Information
### Dataset Curators
[More Information Needed]
### Licensing Information
[More Information Needed]
### Citation Information
[More Information Needed]
### Contributions
Thanks to [@github-username](https://github.com/<github-username>) for adding this dataset.
| lhoestq/demo1 | [
"region:us"
] | 2022-03-02T23:29:22+00:00 | {"type": "demo"} | 2021-11-08T14:36:41+00:00 | [] | [] | TAGS
#region-us
|
# Dataset Card for Demo1
## Table of Contents
- Table of Contents
- Dataset Description
- Dataset Summary
- Supported Tasks and Leaderboards
- Languages
- Dataset Structure
- Data Instances
- Data Fields
- Data Splits
- Dataset Creation
- Curation Rationale
- Source Data
- Annotations
- Personal and Sensitive Information
- Considerations for Using the Data
- Social Impact of Dataset
- Discussion of Biases
- Other Known Limitations
- Additional Information
- Dataset Curators
- Licensing Information
- Citation Information
- Contributions
## Dataset Description
- Homepage:
- Repository:
- Paper:
- Leaderboard:
- Point of Contact:
### Dataset Summary
This is a demo dataset. It consists in two files 'data/URL' and 'data/URL'
You can load it with
### Supported Tasks and Leaderboards
### Languages
## Dataset Structure
### Data Instances
### Data Fields
### Data Splits
## Dataset Creation
### Curation Rationale
### Source Data
#### Initial Data Collection and Normalization
#### Who are the source language producers?
### Annotations
#### Annotation process
#### Who are the annotators?
### Personal and Sensitive Information
## Considerations for Using the Data
### Social Impact of Dataset
### Discussion of Biases
### Other Known Limitations
## Additional Information
### Dataset Curators
### Licensing Information
### Contributions
Thanks to @github-username for adding this dataset.
| [
"# Dataset Card for Demo1",
"## Table of Contents\n- Table of Contents\n- Dataset Description\n - Dataset Summary\n - Supported Tasks and Leaderboards\n - Languages\n- Dataset Structure\n - Data Instances\n - Data Fields\n - Data Splits\n- Dataset Creation\n - Curation Rationale\n - Source Data\n - Annotations\n - Personal and Sensitive Information\n- Considerations for Using the Data\n - Social Impact of Dataset\n - Discussion of Biases\n - Other Known Limitations\n- Additional Information\n - Dataset Curators\n - Licensing Information\n - Citation Information\n - Contributions",
"## Dataset Description\n\n- Homepage:\n- Repository:\n- Paper:\n- Leaderboard:\n- Point of Contact:",
"### Dataset Summary\n\nThis is a demo dataset. It consists in two files 'data/URL' and 'data/URL'\n\nYou can load it with",
"### Supported Tasks and Leaderboards",
"### Languages",
"## Dataset Structure",
"### Data Instances",
"### Data Fields",
"### Data Splits",
"## Dataset Creation",
"### Curation Rationale",
"### Source Data",
"#### Initial Data Collection and Normalization",
"#### Who are the source language producers?",
"### Annotations",
"#### Annotation process",
"#### Who are the annotators?",
"### Personal and Sensitive Information",
"## Considerations for Using the Data",
"### Social Impact of Dataset",
"### Discussion of Biases",
"### Other Known Limitations",
"## Additional Information",
"### Dataset Curators",
"### Licensing Information",
"### Contributions\n\nThanks to @github-username for adding this dataset."
] | [
"TAGS\n#region-us \n",
"# Dataset Card for Demo1",
"## Table of Contents\n- Table of Contents\n- Dataset Description\n - Dataset Summary\n - Supported Tasks and Leaderboards\n - Languages\n- Dataset Structure\n - Data Instances\n - Data Fields\n - Data Splits\n- Dataset Creation\n - Curation Rationale\n - Source Data\n - Annotations\n - Personal and Sensitive Information\n- Considerations for Using the Data\n - Social Impact of Dataset\n - Discussion of Biases\n - Other Known Limitations\n- Additional Information\n - Dataset Curators\n - Licensing Information\n - Citation Information\n - Contributions",
"## Dataset Description\n\n- Homepage:\n- Repository:\n- Paper:\n- Leaderboard:\n- Point of Contact:",
"### Dataset Summary\n\nThis is a demo dataset. It consists in two files 'data/URL' and 'data/URL'\n\nYou can load it with",
"### Supported Tasks and Leaderboards",
"### Languages",
"## Dataset Structure",
"### Data Instances",
"### Data Fields",
"### Data Splits",
"## Dataset Creation",
"### Curation Rationale",
"### Source Data",
"#### Initial Data Collection and Normalization",
"#### Who are the source language producers?",
"### Annotations",
"#### Annotation process",
"#### Who are the annotators?",
"### Personal and Sensitive Information",
"## Considerations for Using the Data",
"### Social Impact of Dataset",
"### Discussion of Biases",
"### Other Known Limitations",
"## Additional Information",
"### Dataset Curators",
"### Licensing Information",
"### Contributions\n\nThanks to @github-username for adding this dataset."
] |
8af5b3fc20bfa28cc0f09ddc1a0c0bcddf906e3a |
This is a test dataset | lhoestq/test | [
"annotations_creators:expert-generated",
"language_creators:found",
"multilinguality:monolingual",
"size_categories:n<1K",
"source_datasets:original",
"language:en",
"license:mit",
"region:us"
] | 2022-03-02T23:29:22+00:00 | {"annotations_creators": ["expert-generated"], "language_creators": ["found"], "language": ["en"], "license": ["mit"], "multilinguality": ["monolingual"], "size_categories": ["n<1K"], "source_datasets": ["original"], "task_categories": ["other-test"], "task_ids": ["other-test"], "pretty_name": "Test Dataset", "type": "test"} | 2022-07-01T14:26:34+00:00 | [] | [
"en"
] | TAGS
#annotations_creators-expert-generated #language_creators-found #multilinguality-monolingual #size_categories-n<1K #source_datasets-original #language-English #license-mit #region-us
|
This is a test dataset | [] | [
"TAGS\n#annotations_creators-expert-generated #language_creators-found #multilinguality-monolingual #size_categories-n<1K #source_datasets-original #language-English #license-mit #region-us \n"
] |
dd797fcf8beacd44987048d5e2606edf1fe0a230 | This is a readme
| lhoestq/test2 | [
"region:us"
] | 2022-03-02T23:29:22+00:00 | {} | 2021-07-23T13:21:45+00:00 | [] | [] | TAGS
#region-us
| This is a readme
| [] | [
"TAGS\n#region-us \n"
] |
7f14f05cd0effd0d847886ede953e6808c3e3a27 | 带情感标注 新浪微博({0: '喜悦', 1: '愤怒', 2: '厌恶', 3: '低落'}) | liam168/nlp_c4_sentiment | [
"region:us"
] | 2022-03-02T23:29:22+00:00 | {} | 2021-07-30T03:05:45+00:00 | [] | [] | TAGS
#region-us
| 带情感标注 新浪微博({0: '喜悦', 1: '愤怒', 2: '厌恶', 3: '低落'}) | [] | [
"TAGS\n#region-us \n"
] |
7b78af8a83bdeebb85f2d78f883acdb9c947c655 | 仅自用
出自:https://github.com/junzeng-pluto/ChineseSquad
感谢! | lijingxin/squad_zen | [
"region:us"
] | 2022-03-02T23:29:22+00:00 | {} | 2022-02-09T03:05:31+00:00 | [] | [] | TAGS
#region-us
| 仅自用
出自:URL
感谢! | [] | [
"TAGS\n#region-us \n"
] |
6aca57928d2edaffa6f9a29bdecaa789c28d0391 |
# Dataset Card for newsquadfr
## Table of Contents
- [Dataset Description](#dataset-description)
- [Dataset Summary](#dataset-summary)
- [Supported Tasks](#supported-tasks-and-leaderboards)
- [Languages](#languages)
- [Dataset Structure](#dataset-structure)
- [Data Instances](#data-instances)
- [Data Fields](#data-instances)
- [Data Splits](#data-instances)
- [Dataset Creation](#dataset-creation)
- [Curation Rationale](#curation-rationale)
- [Source Data](#source-data)
- [Annotations](#annotations)
- [Personal and Sensitive Information](#personal-and-sensitive-information)
- [Considerations for Using the Data](#considerations-for-using-the-data)
- [Social Impact of Dataset](#social-impact-of-dataset)
- [Discussion of Biases](#discussion-of-biases)
- [Other Known Limitations](#other-known-limitations)
- [Additional Information](#additional-information)
- [Dataset Curators](#dataset-curators)
- [Licensing Information](#licensing-information)
- [Citation Information](#citation-information)
## Dataset Description
- **Homepage:** [lincoln.fr](https://www.lincoln.fr/)
- **Repository:** [github/Lincoln-France](https://github.com/Lincoln-France)
- **Paper:** [Needs More Information]
- **Leaderboard:** [Needs More Information]
- **Point of Contact:** [email]([email protected])
### Dataset Summary
newsquadfr is a small dataset created for Question Answering task. Contexts are paragraphs of articles extracted from nine online french newspaper during year 2020/2021. newsquadfr stands for Newspaper question answering dataset in french. inspired by Piaf and Squad dataset. 2 520 triplets context - question - answer.
```py
from datasets import load_dataset
ds_name = 'lincoln/newsquadfr'
# exemple 1
ds_newsquad = load_dataset(ds_name)
# exemple 2
data_files = {'train': 'train.json', 'test': 'test.json', 'valid': 'valid.json'}
ds_newsquad = load_dataset(ds_name, data_files=data_files)
# exemple 3
ds_newsquad = load_dataset(ds_name, data_files=data_files, split="valid+test")
```
(train set)
| website | Nb |
|---------------|-----|
| cnews | 20 |
| francetvinfo | 40 |
| la-croix | 375 |
| lefigaro | 160 |
| lemonde | 325 |
| lesnumeriques | 70 |
| numerama | 140 |
| sudouest | 475 |
| usinenouvelle | 45 |
### Supported Tasks and Leaderboards
- extractive-qa
- open-domain-qa
### Languages
Fr-fr
## Dataset Structure
### Data Instances
```json
{'answers': {'answer_start': [53], 'text': ['manSuvre "agressive']},
'article_id': 34138,
'article_title': 'Caricatures, Libye, Haut-Karabakh... Les six dossiers qui '
'opposent Emmanuel Macron et Recep Tayyip Erdogan.',
'article_url': 'https://www.francetvinfo.fr/monde/turquie/caricatures-libye-haut-karabakh-les-six-dossiers-qui-opposent-emmanuel-macron-et-recep-tayyip-erdogan_4155611.html#xtor=RSS-3-[france]',
'context': 'Dans ce contexte déjà tendu, la France a dénoncé une manSuvre '
'"agressive" de la part de frégates turques à l\'encontre de l\'un '
"de ses navires engagés dans une mission de l'Otan, le 10 juin. "
'Selon Paris, la frégate Le Courbet cherchait à identifier un '
'cargo suspecté de transporter des armes vers la Libye quand elle '
'a été illuminée à trois reprises par le radar de conduite de tir '
"de l'escorte turque.",
'id': '2261',
'paragraph_id': 201225,
'question': "Qu'est ce que la France reproche à la Turquie?",
'website': 'francetvinfo'}
```
### Data Fields
- `answers`: a dictionary feature containing:
- `text`: a `string` feature.
- `answer_start`: a `int64` feature.
- `article_id`: a `int64` feature.
- `article_title`: a string feature.
- `article_url`: a string feature.
- `context`: a `string` feature.
- `id`: a `string` feature.
- `paragraph_id`: a `int64` feature.
- `question`: a `string` feature.
- `website`: a `string` feature.
### Data Splits
| Split | Nb |
|-------|----|
| train |1650|
| test |415 |
| valid |455 |
## Dataset Creation
### Curation Rationale
[Needs More Information]
### Source Data
#### Initial Data Collection and Normalization
Paragraphs were chosen according to theses rules:
- parent article must have more than 71% ASCII characters
- paragraphs size must be between 170 and 670 characters
- paragraphs shouldn't contain "A LIRE" or "A VOIR AUSSI"
Then, we stratified our original dataset to create this dataset according to :
- website
- number of named entities
- paragraph size
#### Who are the source language producers?
[Needs More Information]
### Annotations
#### Annotation process
Using Piaf annotation tools. Three different persons mostly.
#### Who are the annotators?
Lincoln
### Personal and Sensitive Information
[Needs More Information]
## Considerations for Using the Data
### Social Impact of Dataset
[Needs More Information]
### Discussion of Biases
- Annotation is not well controlled
- asking question on news is biaised
### Other Known Limitations
[Needs More Information]
## Additional Information
### Dataset Curators
[Needs More Information]
### Licensing Information
https://creativecommons.org/licenses/by-nc-sa/4.0/deed.fr
### Citation Information
[Needs More Information] | lincoln/newsquadfr | [
"task_categories:question-answering",
"task_ids:extractive-qa",
"task_ids:open-domain-qa",
"annotations_creators:private",
"multilinguality:monolingual",
"size_categories:1K<n<10K",
"source_datasets:original",
"source_datasets:newspaper",
"source_datasets:online",
"license:cc-by-nc-sa-4.0",
"region:us"
] | 2022-03-02T23:29:22+00:00 | {"annotations_creators": ["private"], "language": ["fr-FR"], "license": ["cc-by-nc-sa-4.0"], "multilinguality": ["monolingual"], "size_categories": ["1K<n<10K"], "source_datasets": ["original", "newspaper", "online"], "task_categories": ["question-answering"], "task_ids": ["extractive-qa", "open-domain-qa"]} | 2022-08-05T11:05:24+00:00 | [] | [
"fr-FR"
] | TAGS
#task_categories-question-answering #task_ids-extractive-qa #task_ids-open-domain-qa #annotations_creators-private #multilinguality-monolingual #size_categories-1K<n<10K #source_datasets-original #source_datasets-newspaper #source_datasets-online #license-cc-by-nc-sa-4.0 #region-us
| Dataset Card for newsquadfr
===========================
Table of Contents
-----------------
* Dataset Description
+ Dataset Summary
+ Supported Tasks
+ Languages
* Dataset Structure
+ Data Instances
+ Data Fields
+ Data Splits
* Dataset Creation
+ Curation Rationale
+ Source Data
+ Annotations
+ Personal and Sensitive Information
* Considerations for Using the Data
+ Social Impact of Dataset
+ Discussion of Biases
+ Other Known Limitations
* Additional Information
+ Dataset Curators
+ Licensing Information
+ Citation Information
Dataset Description
-------------------
* Homepage: URL
* Repository: github/Lincoln-France
* Paper:
* Leaderboard:
* Point of Contact: email
### Dataset Summary
newsquadfr is a small dataset created for Question Answering task. Contexts are paragraphs of articles extracted from nine online french newspaper during year 2020/2021. newsquadfr stands for Newspaper question answering dataset in french. inspired by Piaf and Squad dataset. 2 520 triplets context - question - answer.
(train set)
### Supported Tasks and Leaderboards
* extractive-qa
* open-domain-qa
### Languages
Fr-fr
Dataset Structure
-----------------
### Data Instances
### Data Fields
* 'answers': a dictionary feature containing:
+ 'text': a 'string' feature.
+ 'answer\_start': a 'int64' feature.
* 'article\_id': a 'int64' feature.
* 'article\_title': a string feature.
* 'article\_url': a string feature.
* 'context': a 'string' feature.
* 'id': a 'string' feature.
* 'paragraph\_id': a 'int64' feature.
* 'question': a 'string' feature.
* 'website': a 'string' feature.
### Data Splits
Dataset Creation
----------------
### Curation Rationale
### Source Data
#### Initial Data Collection and Normalization
Paragraphs were chosen according to theses rules:
* parent article must have more than 71% ASCII characters
* paragraphs size must be between 170 and 670 characters
* paragraphs shouldn't contain "A LIRE" or "A VOIR AUSSI"
Then, we stratified our original dataset to create this dataset according to :
* website
* number of named entities
* paragraph size
#### Who are the source language producers?
### Annotations
#### Annotation process
Using Piaf annotation tools. Three different persons mostly.
#### Who are the annotators?
Lincoln
### Personal and Sensitive Information
Considerations for Using the Data
---------------------------------
### Social Impact of Dataset
### Discussion of Biases
* Annotation is not well controlled
* asking question on news is biaised
### Other Known Limitations
Additional Information
----------------------
### Dataset Curators
### Licensing Information
URL
| [
"### Dataset Summary\n\n\nnewsquadfr is a small dataset created for Question Answering task. Contexts are paragraphs of articles extracted from nine online french newspaper during year 2020/2021. newsquadfr stands for Newspaper question answering dataset in french. inspired by Piaf and Squad dataset. 2 520 triplets context - question - answer.\n\n\n(train set)",
"### Supported Tasks and Leaderboards\n\n\n* extractive-qa\n* open-domain-qa",
"### Languages\n\n\nFr-fr\n\n\nDataset Structure\n-----------------",
"### Data Instances",
"### Data Fields\n\n\n* 'answers': a dictionary feature containing:\n\t+ 'text': a 'string' feature.\n\t+ 'answer\\_start': a 'int64' feature.\n* 'article\\_id': a 'int64' feature.\n* 'article\\_title': a string feature.\n* 'article\\_url': a string feature.\n* 'context': a 'string' feature.\n* 'id': a 'string' feature.\n* 'paragraph\\_id': a 'int64' feature.\n* 'question': a 'string' feature.\n* 'website': a 'string' feature.",
"### Data Splits\n\n\n\nDataset Creation\n----------------",
"### Curation Rationale",
"### Source Data",
"#### Initial Data Collection and Normalization\n\n\nParagraphs were chosen according to theses rules:\n\n\n* parent article must have more than 71% ASCII characters\n* paragraphs size must be between 170 and 670 characters\n* paragraphs shouldn't contain \"A LIRE\" or \"A VOIR AUSSI\"\n\n\nThen, we stratified our original dataset to create this dataset according to :\n\n\n* website\n* number of named entities\n* paragraph size",
"#### Who are the source language producers?",
"### Annotations",
"#### Annotation process\n\n\nUsing Piaf annotation tools. Three different persons mostly.",
"#### Who are the annotators?\n\n\nLincoln",
"### Personal and Sensitive Information\n\n\nConsiderations for Using the Data\n---------------------------------",
"### Social Impact of Dataset",
"### Discussion of Biases\n\n\n* Annotation is not well controlled\n* asking question on news is biaised",
"### Other Known Limitations\n\n\nAdditional Information\n----------------------",
"### Dataset Curators",
"### Licensing Information\n\n\nURL"
] | [
"TAGS\n#task_categories-question-answering #task_ids-extractive-qa #task_ids-open-domain-qa #annotations_creators-private #multilinguality-monolingual #size_categories-1K<n<10K #source_datasets-original #source_datasets-newspaper #source_datasets-online #license-cc-by-nc-sa-4.0 #region-us \n",
"### Dataset Summary\n\n\nnewsquadfr is a small dataset created for Question Answering task. Contexts are paragraphs of articles extracted from nine online french newspaper during year 2020/2021. newsquadfr stands for Newspaper question answering dataset in french. inspired by Piaf and Squad dataset. 2 520 triplets context - question - answer.\n\n\n(train set)",
"### Supported Tasks and Leaderboards\n\n\n* extractive-qa\n* open-domain-qa",
"### Languages\n\n\nFr-fr\n\n\nDataset Structure\n-----------------",
"### Data Instances",
"### Data Fields\n\n\n* 'answers': a dictionary feature containing:\n\t+ 'text': a 'string' feature.\n\t+ 'answer\\_start': a 'int64' feature.\n* 'article\\_id': a 'int64' feature.\n* 'article\\_title': a string feature.\n* 'article\\_url': a string feature.\n* 'context': a 'string' feature.\n* 'id': a 'string' feature.\n* 'paragraph\\_id': a 'int64' feature.\n* 'question': a 'string' feature.\n* 'website': a 'string' feature.",
"### Data Splits\n\n\n\nDataset Creation\n----------------",
"### Curation Rationale",
"### Source Data",
"#### Initial Data Collection and Normalization\n\n\nParagraphs were chosen according to theses rules:\n\n\n* parent article must have more than 71% ASCII characters\n* paragraphs size must be between 170 and 670 characters\n* paragraphs shouldn't contain \"A LIRE\" or \"A VOIR AUSSI\"\n\n\nThen, we stratified our original dataset to create this dataset according to :\n\n\n* website\n* number of named entities\n* paragraph size",
"#### Who are the source language producers?",
"### Annotations",
"#### Annotation process\n\n\nUsing Piaf annotation tools. Three different persons mostly.",
"#### Who are the annotators?\n\n\nLincoln",
"### Personal and Sensitive Information\n\n\nConsiderations for Using the Data\n---------------------------------",
"### Social Impact of Dataset",
"### Discussion of Biases\n\n\n* Annotation is not well controlled\n* asking question on news is biaised",
"### Other Known Limitations\n\n\nAdditional Information\n----------------------",
"### Dataset Curators",
"### Licensing Information\n\n\nURL"
] |
464a2f8d553fa88728761e7a29bc83ac8ebebcbe | ## PULPO
PULPO, the Prolific Unannotated Literary Poetry Corpus, is a set of multilingual corpora of verses and stanzas with over 95M words.
See https://arxiv.org/abs/2307.01387.
The following corpora has been downloaded using the [Averell](https://github.com/linhd-postdata/averell/) tool, developed by the [POSTDATA](https://postdata.linhd.uned.es/) team:
### Spanish
- [Disco v3](https://github.com/pruizf/disco)
- [Corpus of Spanish Golden-Age Sonnets](https://github.com/bncolorado/CorpusSonetosSigloDeOro)
- [Corpus general de poesía lírica castellana del Siglo de Oro](https://github.com/bncolorado/CorpusGeneralPoesiaLiricaCastellanaDelSigloDeOro)
- [Gongocorpus](https://github.com/linhd-postdata/gongocorpus) - [source](http://obvil.sorbonne-universite.site/corpus/gongora/gongora_obra-poetica)
### English
- [Eighteenth-Century Poetry Archive (ECPA)](https://github.com/alhuber1502/ECPA)
- [For better for verse](https://github.com/waynegraham/for_better_for_verse)
### French
- [Métrique en Ligne](https://crisco2.unicaen.fr/verlaine/index.php?navigation=accueil) - [source](https://github.com/linhd-postdata/metrique-en-ligne)
### Italian
- [Biblioteca italiana](https://github.com/linhd-postdata/biblioteca_italiana) - [source](http://www.bibliotecaitaliana.it/)
### Czech
- [Corpus of Czech Verse](https://github.com/versotym/corpusCzechVerse)
### Portuguese
- [Stichotheque](https://gitlab.com/stichotheque/stichotheque-pt)
Also, we obtained the following corpora from these sources:
### Spanish
- [Poesi.as](https://github.com/linhd-postdata/poesi.as) - [source](http://www.poesi.as/)
### English
- [A Gutenberg Poetry Corpus](https://github.com/aparrish/gutenberg-poetry-corpus)
### Arabic
- [Arabic Poetry dataset](https://www.kaggle.com/ahmedabelal/arabic-poetry)
### Chinese
- [THU Chinese Classical Poetry Corpus](https://github.com/THUNLP-AIPoet/Datasets/tree/master/CCPC)
### Finnish
- [SKVR](https://github.com/sks190/SKVR)
### German
- [TextGrid Poetry Corpus](https://github.com/linhd-postdata/textgrid-poetry) - [source](https://textgrid.de/en/digitale-bibliothek)
- [German Rhyme Corpus](https://github.com/tnhaider/german-rhyme-corpus)
### Hungarian
- [verskorpusz](https://github.com/ELTE-DH/verskorpusz)
### Portuguese
- [Poems in Portuguese](https://www.kaggle.com/oliveirasp6/poems-in-portuguese)
### Russian
- [19 000 Russian poems](https://www.kaggle.com/grafstor/19-000-russian-poems) | linhd-postdata/pulpo | [
"size_categories:10M<n<100M",
"language:es",
"language:en",
"language:fr",
"language:it",
"language:cs",
"language:pt",
"language:ar",
"language:zh",
"language:fi",
"language:de",
"language:hu",
"language:ru",
"poetry",
"arxiv:2307.01387",
"region:us"
] | 2022-03-02T23:29:22+00:00 | {"language": ["es", "en", "fr", "it", "cs", "pt", "ar", "zh", "fi", "de", "hu", "ru"], "size_categories": ["10M<n<100M"], "pretty_name": "Prolific Unannotated Literary Poetry Corpus", "tags": ["poetry"]} | 2023-07-10T12:38:07+00:00 | [
"2307.01387"
] | [
"es",
"en",
"fr",
"it",
"cs",
"pt",
"ar",
"zh",
"fi",
"de",
"hu",
"ru"
] | TAGS
#size_categories-10M<n<100M #language-Spanish #language-English #language-French #language-Italian #language-Czech #language-Portuguese #language-Arabic #language-Chinese #language-Finnish #language-German #language-Hungarian #language-Russian #poetry #arxiv-2307.01387 #region-us
| ## PULPO
PULPO, the Prolific Unannotated Literary Poetry Corpus, is a set of multilingual corpora of verses and stanzas with over 95M words.
See URL
The following corpora has been downloaded using the Averell tool, developed by the POSTDATA team:
### Spanish
- Disco v3
- Corpus of Spanish Golden-Age Sonnets
- Corpus general de poesía lírica castellana del Siglo de Oro
- Gongocorpus - source
### English
- Eighteenth-Century Poetry Archive (ECPA)
- For better for verse
### French
- Métrique en Ligne - source
### Italian
- Biblioteca italiana - source
### Czech
- Corpus of Czech Verse
### Portuguese
- Stichotheque
Also, we obtained the following corpora from these sources:
### Spanish
- URL - source
### English
- A Gutenberg Poetry Corpus
### Arabic
- Arabic Poetry dataset
### Chinese
- THU Chinese Classical Poetry Corpus
### Finnish
- SKVR
### German
- TextGrid Poetry Corpus - source
- German Rhyme Corpus
### Hungarian
- verskorpusz
### Portuguese
- Poems in Portuguese
### Russian
- 19 000 Russian poems | [
"## PULPO\n\nPULPO, the Prolific Unannotated Literary Poetry Corpus, is a set of multilingual corpora of verses and stanzas with over 95M words.\n\nSee URL\n\nThe following corpora has been downloaded using the Averell tool, developed by the POSTDATA team:",
"### Spanish\n- Disco v3\n- Corpus of Spanish Golden-Age Sonnets\n- Corpus general de poesía lírica castellana del Siglo de Oro\n- Gongocorpus - source",
"### English\n- Eighteenth-Century Poetry Archive (ECPA)\n- For better for verse",
"### French\n- Métrique en Ligne - source",
"### Italian\n- Biblioteca italiana - source",
"### Czech\n- Corpus of Czech Verse",
"### Portuguese\n- Stichotheque\n\nAlso, we obtained the following corpora from these sources:",
"### Spanish \n- URL - source",
"### English\n- A Gutenberg Poetry Corpus",
"### Arabic\n- Arabic Poetry dataset",
"### Chinese\n- THU Chinese Classical Poetry Corpus",
"### Finnish\n- SKVR",
"### German\n- TextGrid Poetry Corpus - source\n- German Rhyme Corpus",
"### Hungarian\n- verskorpusz",
"### Portuguese\n- Poems in Portuguese",
"### Russian\n- 19 000 Russian poems"
] | [
"TAGS\n#size_categories-10M<n<100M #language-Spanish #language-English #language-French #language-Italian #language-Czech #language-Portuguese #language-Arabic #language-Chinese #language-Finnish #language-German #language-Hungarian #language-Russian #poetry #arxiv-2307.01387 #region-us \n",
"## PULPO\n\nPULPO, the Prolific Unannotated Literary Poetry Corpus, is a set of multilingual corpora of verses and stanzas with over 95M words.\n\nSee URL\n\nThe following corpora has been downloaded using the Averell tool, developed by the POSTDATA team:",
"### Spanish\n- Disco v3\n- Corpus of Spanish Golden-Age Sonnets\n- Corpus general de poesía lírica castellana del Siglo de Oro\n- Gongocorpus - source",
"### English\n- Eighteenth-Century Poetry Archive (ECPA)\n- For better for verse",
"### French\n- Métrique en Ligne - source",
"### Italian\n- Biblioteca italiana - source",
"### Czech\n- Corpus of Czech Verse",
"### Portuguese\n- Stichotheque\n\nAlso, we obtained the following corpora from these sources:",
"### Spanish \n- URL - source",
"### English\n- A Gutenberg Poetry Corpus",
"### Arabic\n- Arabic Poetry dataset",
"### Chinese\n- THU Chinese Classical Poetry Corpus",
"### Finnish\n- SKVR",
"### German\n- TextGrid Poetry Corpus - source\n- German Rhyme Corpus",
"### Hungarian\n- verskorpusz",
"### Portuguese\n- Poems in Portuguese",
"### Russian\n- 19 000 Russian poems"
] |
1b0382449b4273d9de8e6d6ad15ca6873884758a | # C4 200M
# Dataset Summary
c4_200m is a collection of 185 million sentence pairs generated from the cleaned English dataset from C4. This dataset can be used in grammatical error correction (GEC) tasks.
The corruption edits and scripts used to synthesize this dataset is referenced from: [C4_200M Synthetic Dataset](https://github.com/google-research-datasets/C4_200M-synthetic-dataset-for-grammatical-error-correction)
# Description
As discussed before, this dataset contains 185 million sentence pairs. Each article has these two attributes: `input` and `output`. Here is a sample of dataset:
```
{
"input": "Bitcoin is for $7,094 this morning, which CoinDesk says."
"output": "Bitcoin goes for $7,094 this morning, according to CoinDesk."
}
``` | liweili/c4_200m | [
"task_categories:text-generation",
"source_datasets:allenai/c4",
"language:en",
"grammatical-error-correction",
"region:us"
] | 2022-03-02T23:29:22+00:00 | {"language": ["en"], "source_datasets": ["allenai/c4"], "task_categories": ["text-generation"], "pretty_name": "C4 200M Grammatical Error Correction Dataset", "tags": ["grammatical-error-correction"]} | 2022-10-23T10:00:46+00:00 | [] | [
"en"
] | TAGS
#task_categories-text-generation #source_datasets-allenai/c4 #language-English #grammatical-error-correction #region-us
| # C4 200M
# Dataset Summary
c4_200m is a collection of 185 million sentence pairs generated from the cleaned English dataset from C4. This dataset can be used in grammatical error correction (GEC) tasks.
The corruption edits and scripts used to synthesize this dataset is referenced from: C4_200M Synthetic Dataset
# Description
As discussed before, this dataset contains 185 million sentence pairs. Each article has these two attributes: 'input' and 'output'. Here is a sample of dataset:
| [
"# C4 200M",
"# Dataset Summary\nc4_200m is a collection of 185 million sentence pairs generated from the cleaned English dataset from C4. This dataset can be used in grammatical error correction (GEC) tasks.\n\nThe corruption edits and scripts used to synthesize this dataset is referenced from: C4_200M Synthetic Dataset",
"# Description\nAs discussed before, this dataset contains 185 million sentence pairs. Each article has these two attributes: 'input' and 'output'. Here is a sample of dataset:"
] | [
"TAGS\n#task_categories-text-generation #source_datasets-allenai/c4 #language-English #grammatical-error-correction #region-us \n",
"# C4 200M",
"# Dataset Summary\nc4_200m is a collection of 185 million sentence pairs generated from the cleaned English dataset from C4. This dataset can be used in grammatical error correction (GEC) tasks.\n\nThe corruption edits and scripts used to synthesize this dataset is referenced from: C4_200M Synthetic Dataset",
"# Description\nAs discussed before, this dataset contains 185 million sentence pairs. Each article has these two attributes: 'input' and 'output'. Here is a sample of dataset:"
] |
5b55bbc6818bccd55b604d71bd1b288b46a050a2 | ## Data Description
Long-COVID related articles have been manually collected by information specialists.
Please find further information [here](https://doi.org/10.1093/database/baac048).
## Size
||Training|Development|Test|Total|
|--|--|--|--|--|
Positive Examples|215|76|70|345|
Negative Examples|199|62|68|345|
Total|414|238|138|690|
## Citation
@article{10.1093/database/baac048,
author = {Langnickel, Lisa and Darms, Johannes and Heldt, Katharina and Ducks, Denise and Fluck, Juliane},
title = "{Continuous development of the semantic search engine preVIEW: from COVID-19 to long COVID}",
journal = {Database},
volume = {2022},
year = {2022},
month = {07},
issn = {1758-0463},
doi = {10.1093/database/baac048},
url = {https://doi.org/10.1093/database/baac048},
note = {baac048},
eprint = {https://academic.oup.com/database/article-pdf/doi/10.1093/database/baac048/44371817/baac048.pdf},
} | llangnickel/long-covid-classification-data | [
"task_categories:text-classification",
"annotations_creators:expert-generated",
"language_creators:expert-generated",
"multilinguality:monolingual",
"size_categories:unknown",
"source_datasets:original",
"language:en",
"license:cc-by-4.0",
"region:us"
] | 2022-03-02T23:29:22+00:00 | {"annotations_creators": ["expert-generated"], "language_creators": ["expert-generated"], "language": ["en"], "license": ["cc-by-4.0"], "multilinguality": ["monolingual"], "size_categories": ["unknown"], "source_datasets": ["original"], "task_categories": ["text-classification"], "pretty_name": "Dataset containing abstracts from PubMed, either related to long COVID or not. "} | 2022-11-24T10:29:58+00:00 | [] | [
"en"
] | TAGS
#task_categories-text-classification #annotations_creators-expert-generated #language_creators-expert-generated #multilinguality-monolingual #size_categories-unknown #source_datasets-original #language-English #license-cc-by-4.0 #region-us
| Data Description
----------------
Long-COVID related articles have been manually collected by information specialists.
Please find further information here.
Size
----
@article{10.1093/database/baac048,
author = {Langnickel, Lisa and Darms, Johannes and Heldt, Katharina and Ducks, Denise and Fluck, Juliane},
title = "{Continuous development of the semantic search engine preVIEW: from COVID-19 to long COVID}",
journal = {Database},
volume = {2022},
year = {2022},
month = {07},
issn = {1758-0463},
doi = {10.1093/database/baac048},
url = {URL
note = {baac048},
eprint = {URL
}
| [] | [
"TAGS\n#task_categories-text-classification #annotations_creators-expert-generated #language_creators-expert-generated #multilinguality-monolingual #size_categories-unknown #source_datasets-original #language-English #license-cc-by-4.0 #region-us \n"
] |
936ce94b2393ccff9d8ab5e37c17c3cba70075f4 | # spoken-punctuation
Spoken punctuation for Speech-to-Text by language and locale.
## Disclaimer
Data collected from Google Cloud Speech-to-Text "Supported spoken punctuation" documentation: https://cloud.google.com/speech-to-text/docs/spoken-punctuation
| loretoparisi/spoken-punctuation | [
"region:us"
] | 2022-03-02T23:29:22+00:00 | {} | 2022-02-24T21:12:49+00:00 | [] | [] | TAGS
#region-us
| # spoken-punctuation
Spoken punctuation for Speech-to-Text by language and locale.
## Disclaimer
Data collected from Google Cloud Speech-to-Text "Supported spoken punctuation" documentation: URL
| [
"# spoken-punctuation\nSpoken punctuation for Speech-to-Text by language and locale.",
"## Disclaimer\nData collected from Google Cloud Speech-to-Text \"Supported spoken punctuation\" documentation: URL"
] | [
"TAGS\n#region-us \n",
"# spoken-punctuation\nSpoken punctuation for Speech-to-Text by language and locale.",
"## Disclaimer\nData collected from Google Cloud Speech-to-Text \"Supported spoken punctuation\" documentation: URL"
] |
ebad3013a8a015074a69a9826d06c38b750e1bce |
# Dataset Card for MeLiSA (Mercado Libre for Sentiment Analysis)
** **NOTE: THIS CARD IS UNDER CONSTRUCTION** **
** **NOTE 2: THE RELEASED VERSION OF THIS DATASET IS A DEMO VERSION.** **
## Table of Contents
- [Dataset Description](#dataset-description)
- [Dataset Summary](#dataset-summary)
- [Supported Tasks and Leaderboards](#supported-tasks-and-leaderboards)
- [Languages](#languages)
- [Dataset Structure](#dataset-structure)
- [Data Instances](#data-instances)
- [Data Fields](#data-fields)
- [Data Splits](#data-splits)
- [Dataset Creation](#dataset-creation)
- [Curation Rationale](#curation-rationale)
- [Source Data](#source-data)
- [Annotations](#annotations)
- [Personal and Sensitive Information](#personal-and-sensitive-information)
- [Considerations for Using the Data](#considerations-for-using-the-data)
- [Social Impact of Dataset](#social-impact-of-dataset)
- [Discussion of Biases](#discussion-of-biases)
- [Other Known Limitations](#other-known-limitations)
- [Additional Information](#additional-information)
- [Dataset Curators](#dataset-curators)
- [Licensing Information](#licensing-information)
- [Citation Information](#citation-information)
- [Contributions](#contributions)
## Dataset Description
- **Webpage:** https://github.com/lpsc-fiuba/MeLiSA
- **Paper:**
- **Point of Contact:** [email protected]
[More Information Needed]
### Dataset Summary
We provide a Mercado Libre product reviews dataset for spanish and portuguese text classification. The dataset contains reviews in these two languages collected between August 2020 and January 2021. Each record in the dataset contains the review content and title, the star rating, the country where it was pubilshed and the product category (arts, technology, etc.). The corpus is roughly balanced across stars, so each star rating constitutes approximately 20% of the reviews in each language.
| || Spanish ||| Portugese ||
|---|:------:|:----------:|:-----:|:------:|:----------:|:-----:|
| | Train | Validation | Test | Train | Validation | Test |
| 1 | 88.425 | 4.052 | 5.000 | 50.801 | 4.052 | 5.000 |
| 2 | 88.397 | 4.052 | 5.000 | 50.782 | 4.052 | 5.000 |
| 3 | 88.435 | 4.052 | 5.000 | 50.797 | 4.052 | 5.000 |
| 4 | 88.449 | 4.052 | 5.000 | 50.794 | 4.052 | 5.000 |
| 5 | 88.402 | 4.052 | 5.000 | 50.781 | 4.052 | 5.000 |
Table shows the number of samples per star rate in each split. There is a total of 442.108 training samples in spanish and 253.955 in portuguese. We limited the number of reviews per product to 30 and we perform a ranked inclusion of the downloaded reviews to include those with rich semantic content. In these ranking, the lenght of the review content and the valorization (difference between likes and dislikes) was prioritized. For more details on this process, see (CITATION).
Reviews in spanish were obtained from 8 different Latin Amercian countries (Argentina, Colombia, Peru, Uruguay, Chile, Venezuela and Mexico), and portuguese reviews were extracted from Brasil. To match the language with its respective country, we applied a language detection algorithm based on the works of Joulin et al. (2016a and 2016b) to determine the language of the review text and we removed reviews that were not written in the expected language.
[More Information Needed]
### Languages
The dataset contains reviews in Latin American Spanish and Portuguese.
## Dataset Structure
### Data Instances
Each data instance corresponds to a review. Each split is stored in a separated `.csv` file, so every row in each file consists on a review. For example, here we show a snippet of the spanish training split:
```csv
country,category,review_content,review_title,review_rate
...
MLA,Tecnología y electrónica / Tecnologia e electronica,Todo bien me fue muy util.,Muy bueno,2
MLU,"Salud, ropa y cuidado personal / Saúde, roupas e cuidado pessoal",No fue lo que esperaba. El producto no me sirvió.,No fue el producto que esperé ,2
MLM,Tecnología y electrónica / Tecnologia e electronica,No fue del todo lo que se esperaba.,No me fue muy funcional ahí que hacer ajustes,2
...
```
### Data Fields
- `country`: The string identifier of the country. It could be one of the following: `MLA` (Argentina), `MCO` (Colombia), `MPE` (Peru), `MLU` (Uruguay), `MLC` (Chile), `MLV` (Venezuela), `MLM` (Mexico) or `MLB` (Brasil).
- `category`: String representation of the product's category. It could be one of the following:
- Hogar / Casa
- Tecnologı́a y electrónica / Tecnologia e electronica
- Salud, ropa y cuidado personal / Saúde, roupas e cuidado pessoal
- Arte y entretenimiento / Arte e Entretenimiento
- Alimentos y Bebidas / Alimentos e Bebidas
- `review_content`: The text content of the review.
- `review_title`: The text title of the review.
- `review_rate`: An int between 1-5 indicating the number of stars.
### Data Splits
Each language configuration comes with it's own `train`, `validation`, and `test` splits. The `all_languages` split is simply a concatenation of the corresponding split across all languages. That is, the `train` split for `all_languages` is a concatenation of the `train` splits for each of the languages and likewise for `validation` and `test`.
## Dataset Creation
### Curation Rationale
The dataset is motivated by the desire to advance sentiment analysis and text classification in Latin American Spanish and Portuguese.
### Source Data
#### Initial Data Collection and Normalization
The authors gathered the reviews from the marketplaces in Argentina, Colombia, Peru, Uruguay, Chile, Venezuela and Mexico for the Spanish language and from Brasil for Portuguese. They prioritized reviews that contained relevant semantic content by applying a ranking filter based in the lenght and the valorization (difference betweent the number of likes and dislikes) of the review. They then ensured the correct language by applying a semi-automatic language detection algorithm, only retaining those of the target language. No normalization was applied to the review content or title.
Original products categories were grouped in higher level categories, resulting in five different types of products: "Home" (Hogar / Casa), "Technology and electronics" (Tecnologı́a y electrónica
/ Tecnologia e electronica), "Health, Dress and Personal Care" (Salud, ropa y cuidado personal / Saúde, roupas e cuidado pessoal) and "Arts and Entertainment" (Arte y entretenimiento / Arte e Entretenimiento).
#### Who are the source language producers?
The original text comes from Mercado Libre customers reviewing products on the marketplace across a variety of product categories.
### Annotations
#### Annotation process
Each of the fields included are submitted by the user with the review or otherwise associated with the review. No manual or machine-driven annotation was necessary.
#### Who are the annotators?
N/A
### Personal and Sensitive Information
Mercado Libre Reviews are submitted by users with the knowledge and attention of being public. The reviewer ID's included in this dataset are anonymized, meaning that they are disassociated from the original user profiles. However, these fields would likely be easy to deannoymize given the public and identifying nature of free-form text responses.
## Considerations for Using the Data
### Social Impact of Dataset
Although Spanish and Portuguese languages are relatively high resource, most of the data is collected from European or United State users. This dataset is part of an effort to encourage text classification research in languages other than English and European Spanish and Portuguese. Such work increases the accessibility of natural language technology to more regions and cultures.
### Discussion of Biases
The data included here are from unverified consumers. Some percentage of these reviews may be fake or contain misleading or offensive language.
### Other Known Limitations
The dataset is constructed so that the distribution of star ratings is roughly balanced. This feature has some advantages for purposes of classification, but some types of language may be over or underrepresented relative to the original distribution of reviews to acheive this balance.
[More Information Needed]
## Additional Information
### Dataset Curators
Published by Lautaro Estienne, Matías Vera and Leonardo Rey Vega. Managed by the Signal Processing in Comunications Laboratory of the Electronic Department at the Engeneering School of the Buenos Aires University (UBA).
### Licensing Information
Amazon has licensed this dataset under its own agreement, to be found at the dataset webpage here:
https://docs.opendata.aws/amazon-reviews-ml/license.txt
### Citation Information
Please cite the following paper if you found this dataset useful:
(CITATION)
[More Information Needed]
### Contributions
[More Information Needed]
| lpsc-fiuba/melisa | [
"task_categories:text-classification",
"task_ids:language-modeling",
"task_ids:sentiment-classification",
"task_ids:sentiment-scoring",
"task_ids:topic-classification",
"annotations_creators:found",
"language_creators:found",
"source_datasets:original",
"language:es",
"language:pt",
"license:other",
"region:us"
] | 2022-03-02T23:29:22+00:00 | {"annotations_creators": ["found"], "language_creators": ["found"], "language": ["es", "pt"], "license": ["other"], "multilinguality": {"all_languages": ["multilingual"], "es": ["monolingual"], "pt": ["monolingual"]}, "size_categories": {"all_languages": ["100K<n<1M"], "es": ["100K<n<1M"], "pt": ["100K<n<1M"]}, "source_datasets": ["original"], "task_categories": ["conditional-text-generation", "sequence-modeling", "text-classification", "text-scoring"], "task_ids": ["language-modeling", "sentiment-classification", "sentiment-scoring", "summarization", "topic-classification"]} | 2022-10-22T07:52:56+00:00 | [] | [
"es",
"pt"
] | TAGS
#task_categories-text-classification #task_ids-language-modeling #task_ids-sentiment-classification #task_ids-sentiment-scoring #task_ids-topic-classification #annotations_creators-found #language_creators-found #source_datasets-original #language-Spanish #language-Portuguese #license-other #region-us
| Dataset Card for MeLiSA (Mercado Libre for Sentiment Analysis)
==============================================================
NOTE: THIS CARD IS UNDER CONSTRUCTION
NOTE 2: THE RELEASED VERSION OF THIS DATASET IS A DEMO VERSION.
Table of Contents
-----------------
* Dataset Description
+ Dataset Summary
+ Supported Tasks and Leaderboards
+ Languages
* Dataset Structure
+ Data Instances
+ Data Fields
+ Data Splits
* Dataset Creation
+ Curation Rationale
+ Source Data
+ Annotations
+ Personal and Sensitive Information
* Considerations for Using the Data
+ Social Impact of Dataset
+ Discussion of Biases
+ Other Known Limitations
* Additional Information
+ Dataset Curators
+ Licensing Information
+ Citation Information
+ Contributions
Dataset Description
-------------------
* Webpage: URL
* Paper:
* Point of Contact: lestienne@URL
### Dataset Summary
We provide a Mercado Libre product reviews dataset for spanish and portuguese text classification. The dataset contains reviews in these two languages collected between August 2020 and January 2021. Each record in the dataset contains the review content and title, the star rating, the country where it was pubilshed and the product category (arts, technology, etc.). The corpus is roughly balanced across stars, so each star rating constitutes approximately 20% of the reviews in each language.
Table shows the number of samples per star rate in each split. There is a total of 442.108 training samples in spanish and 253.955 in portuguese. We limited the number of reviews per product to 30 and we perform a ranked inclusion of the downloaded reviews to include those with rich semantic content. In these ranking, the lenght of the review content and the valorization (difference between likes and dislikes) was prioritized. For more details on this process, see (CITATION).
Reviews in spanish were obtained from 8 different Latin Amercian countries (Argentina, Colombia, Peru, Uruguay, Chile, Venezuela and Mexico), and portuguese reviews were extracted from Brasil. To match the language with its respective country, we applied a language detection algorithm based on the works of Joulin et al. (2016a and 2016b) to determine the language of the review text and we removed reviews that were not written in the expected language.
### Languages
The dataset contains reviews in Latin American Spanish and Portuguese.
Dataset Structure
-----------------
### Data Instances
Each data instance corresponds to a review. Each split is stored in a separated '.csv' file, so every row in each file consists on a review. For example, here we show a snippet of the spanish training split:
### Data Fields
* 'country': The string identifier of the country. It could be one of the following: 'MLA' (Argentina), 'MCO' (Colombia), 'MPE' (Peru), 'MLU' (Uruguay), 'MLC' (Chile), 'MLV' (Venezuela), 'MLM' (Mexico) or 'MLB' (Brasil).
* 'category': String representation of the product's category. It could be one of the following:
+ Hogar / Casa
+ Tecnologı́a y electrónica / Tecnologia e electronica
+ Salud, ropa y cuidado personal / Saúde, roupas e cuidado pessoal
+ Arte y entretenimiento / Arte e Entretenimiento
+ Alimentos y Bebidas / Alimentos e Bebidas
* 'review\_content': The text content of the review.
* 'review\_title': The text title of the review.
* 'review\_rate': An int between 1-5 indicating the number of stars.
### Data Splits
Each language configuration comes with it's own 'train', 'validation', and 'test' splits. The 'all\_languages' split is simply a concatenation of the corresponding split across all languages. That is, the 'train' split for 'all\_languages' is a concatenation of the 'train' splits for each of the languages and likewise for 'validation' and 'test'.
Dataset Creation
----------------
### Curation Rationale
The dataset is motivated by the desire to advance sentiment analysis and text classification in Latin American Spanish and Portuguese.
### Source Data
#### Initial Data Collection and Normalization
The authors gathered the reviews from the marketplaces in Argentina, Colombia, Peru, Uruguay, Chile, Venezuela and Mexico for the Spanish language and from Brasil for Portuguese. They prioritized reviews that contained relevant semantic content by applying a ranking filter based in the lenght and the valorization (difference betweent the number of likes and dislikes) of the review. They then ensured the correct language by applying a semi-automatic language detection algorithm, only retaining those of the target language. No normalization was applied to the review content or title.
Original products categories were grouped in higher level categories, resulting in five different types of products: "Home" (Hogar / Casa), "Technology and electronics" (Tecnologı́a y electrónica
/ Tecnologia e electronica), "Health, Dress and Personal Care" (Salud, ropa y cuidado personal / Saúde, roupas e cuidado pessoal) and "Arts and Entertainment" (Arte y entretenimiento / Arte e Entretenimiento).
#### Who are the source language producers?
The original text comes from Mercado Libre customers reviewing products on the marketplace across a variety of product categories.
### Annotations
#### Annotation process
Each of the fields included are submitted by the user with the review or otherwise associated with the review. No manual or machine-driven annotation was necessary.
#### Who are the annotators?
N/A
### Personal and Sensitive Information
Mercado Libre Reviews are submitted by users with the knowledge and attention of being public. The reviewer ID's included in this dataset are anonymized, meaning that they are disassociated from the original user profiles. However, these fields would likely be easy to deannoymize given the public and identifying nature of free-form text responses.
Considerations for Using the Data
---------------------------------
### Social Impact of Dataset
Although Spanish and Portuguese languages are relatively high resource, most of the data is collected from European or United State users. This dataset is part of an effort to encourage text classification research in languages other than English and European Spanish and Portuguese. Such work increases the accessibility of natural language technology to more regions and cultures.
### Discussion of Biases
The data included here are from unverified consumers. Some percentage of these reviews may be fake or contain misleading or offensive language.
### Other Known Limitations
The dataset is constructed so that the distribution of star ratings is roughly balanced. This feature has some advantages for purposes of classification, but some types of language may be over or underrepresented relative to the original distribution of reviews to acheive this balance.
Additional Information
----------------------
### Dataset Curators
Published by Lautaro Estienne, Matías Vera and Leonardo Rey Vega. Managed by the Signal Processing in Comunications Laboratory of the Electronic Department at the Engeneering School of the Buenos Aires University (UBA).
### Licensing Information
Amazon has licensed this dataset under its own agreement, to be found at the dataset webpage here:
URL
Please cite the following paper if you found this dataset useful:
(CITATION)
### Contributions
| [
"### Dataset Summary\n\n\nWe provide a Mercado Libre product reviews dataset for spanish and portuguese text classification. The dataset contains reviews in these two languages collected between August 2020 and January 2021. Each record in the dataset contains the review content and title, the star rating, the country where it was pubilshed and the product category (arts, technology, etc.). The corpus is roughly balanced across stars, so each star rating constitutes approximately 20% of the reviews in each language.\n\n\n\nTable shows the number of samples per star rate in each split. There is a total of 442.108 training samples in spanish and 253.955 in portuguese. We limited the number of reviews per product to 30 and we perform a ranked inclusion of the downloaded reviews to include those with rich semantic content. In these ranking, the lenght of the review content and the valorization (difference between likes and dislikes) was prioritized. For more details on this process, see (CITATION).\n\n\nReviews in spanish were obtained from 8 different Latin Amercian countries (Argentina, Colombia, Peru, Uruguay, Chile, Venezuela and Mexico), and portuguese reviews were extracted from Brasil. To match the language with its respective country, we applied a language detection algorithm based on the works of Joulin et al. (2016a and 2016b) to determine the language of the review text and we removed reviews that were not written in the expected language.",
"### Languages\n\n\nThe dataset contains reviews in Latin American Spanish and Portuguese.\n\n\nDataset Structure\n-----------------",
"### Data Instances\n\n\nEach data instance corresponds to a review. Each split is stored in a separated '.csv' file, so every row in each file consists on a review. For example, here we show a snippet of the spanish training split:",
"### Data Fields\n\n\n* 'country': The string identifier of the country. It could be one of the following: 'MLA' (Argentina), 'MCO' (Colombia), 'MPE' (Peru), 'MLU' (Uruguay), 'MLC' (Chile), 'MLV' (Venezuela), 'MLM' (Mexico) or 'MLB' (Brasil).\n* 'category': String representation of the product's category. It could be one of the following:\n\t+ Hogar / Casa\n\t+ Tecnologı́a y electrónica / Tecnologia e electronica\n\t+ Salud, ropa y cuidado personal / Saúde, roupas e cuidado pessoal\n\t+ Arte y entretenimiento / Arte e Entretenimiento\n\t+ Alimentos y Bebidas / Alimentos e Bebidas\n* 'review\\_content': The text content of the review.\n* 'review\\_title': The text title of the review.\n* 'review\\_rate': An int between 1-5 indicating the number of stars.",
"### Data Splits\n\n\nEach language configuration comes with it's own 'train', 'validation', and 'test' splits. The 'all\\_languages' split is simply a concatenation of the corresponding split across all languages. That is, the 'train' split for 'all\\_languages' is a concatenation of the 'train' splits for each of the languages and likewise for 'validation' and 'test'.\n\n\nDataset Creation\n----------------",
"### Curation Rationale\n\n\nThe dataset is motivated by the desire to advance sentiment analysis and text classification in Latin American Spanish and Portuguese.",
"### Source Data",
"#### Initial Data Collection and Normalization\n\n\nThe authors gathered the reviews from the marketplaces in Argentina, Colombia, Peru, Uruguay, Chile, Venezuela and Mexico for the Spanish language and from Brasil for Portuguese. They prioritized reviews that contained relevant semantic content by applying a ranking filter based in the lenght and the valorization (difference betweent the number of likes and dislikes) of the review. They then ensured the correct language by applying a semi-automatic language detection algorithm, only retaining those of the target language. No normalization was applied to the review content or title.\n\n\nOriginal products categories were grouped in higher level categories, resulting in five different types of products: \"Home\" (Hogar / Casa), \"Technology and electronics\" (Tecnologı́a y electrónica\n/ Tecnologia e electronica), \"Health, Dress and Personal Care\" (Salud, ropa y cuidado personal / Saúde, roupas e cuidado pessoal) and \"Arts and Entertainment\" (Arte y entretenimiento / Arte e Entretenimiento).",
"#### Who are the source language producers?\n\n\nThe original text comes from Mercado Libre customers reviewing products on the marketplace across a variety of product categories.",
"### Annotations",
"#### Annotation process\n\n\nEach of the fields included are submitted by the user with the review or otherwise associated with the review. No manual or machine-driven annotation was necessary.",
"#### Who are the annotators?\n\n\nN/A",
"### Personal and Sensitive Information\n\n\nMercado Libre Reviews are submitted by users with the knowledge and attention of being public. The reviewer ID's included in this dataset are anonymized, meaning that they are disassociated from the original user profiles. However, these fields would likely be easy to deannoymize given the public and identifying nature of free-form text responses.\n\n\nConsiderations for Using the Data\n---------------------------------",
"### Social Impact of Dataset\n\n\nAlthough Spanish and Portuguese languages are relatively high resource, most of the data is collected from European or United State users. This dataset is part of an effort to encourage text classification research in languages other than English and European Spanish and Portuguese. Such work increases the accessibility of natural language technology to more regions and cultures.",
"### Discussion of Biases\n\n\nThe data included here are from unverified consumers. Some percentage of these reviews may be fake or contain misleading or offensive language.",
"### Other Known Limitations\n\n\nThe dataset is constructed so that the distribution of star ratings is roughly balanced. This feature has some advantages for purposes of classification, but some types of language may be over or underrepresented relative to the original distribution of reviews to acheive this balance.\n\n\nAdditional Information\n----------------------",
"### Dataset Curators\n\n\nPublished by Lautaro Estienne, Matías Vera and Leonardo Rey Vega. Managed by the Signal Processing in Comunications Laboratory of the Electronic Department at the Engeneering School of the Buenos Aires University (UBA).",
"### Licensing Information\n\n\nAmazon has licensed this dataset under its own agreement, to be found at the dataset webpage here:\nURL\n\n\nPlease cite the following paper if you found this dataset useful:\n\n\n(CITATION)",
"### Contributions"
] | [
"TAGS\n#task_categories-text-classification #task_ids-language-modeling #task_ids-sentiment-classification #task_ids-sentiment-scoring #task_ids-topic-classification #annotations_creators-found #language_creators-found #source_datasets-original #language-Spanish #language-Portuguese #license-other #region-us \n",
"### Dataset Summary\n\n\nWe provide a Mercado Libre product reviews dataset for spanish and portuguese text classification. The dataset contains reviews in these two languages collected between August 2020 and January 2021. Each record in the dataset contains the review content and title, the star rating, the country where it was pubilshed and the product category (arts, technology, etc.). The corpus is roughly balanced across stars, so each star rating constitutes approximately 20% of the reviews in each language.\n\n\n\nTable shows the number of samples per star rate in each split. There is a total of 442.108 training samples in spanish and 253.955 in portuguese. We limited the number of reviews per product to 30 and we perform a ranked inclusion of the downloaded reviews to include those with rich semantic content. In these ranking, the lenght of the review content and the valorization (difference between likes and dislikes) was prioritized. For more details on this process, see (CITATION).\n\n\nReviews in spanish were obtained from 8 different Latin Amercian countries (Argentina, Colombia, Peru, Uruguay, Chile, Venezuela and Mexico), and portuguese reviews were extracted from Brasil. To match the language with its respective country, we applied a language detection algorithm based on the works of Joulin et al. (2016a and 2016b) to determine the language of the review text and we removed reviews that were not written in the expected language.",
"### Languages\n\n\nThe dataset contains reviews in Latin American Spanish and Portuguese.\n\n\nDataset Structure\n-----------------",
"### Data Instances\n\n\nEach data instance corresponds to a review. Each split is stored in a separated '.csv' file, so every row in each file consists on a review. For example, here we show a snippet of the spanish training split:",
"### Data Fields\n\n\n* 'country': The string identifier of the country. It could be one of the following: 'MLA' (Argentina), 'MCO' (Colombia), 'MPE' (Peru), 'MLU' (Uruguay), 'MLC' (Chile), 'MLV' (Venezuela), 'MLM' (Mexico) or 'MLB' (Brasil).\n* 'category': String representation of the product's category. It could be one of the following:\n\t+ Hogar / Casa\n\t+ Tecnologı́a y electrónica / Tecnologia e electronica\n\t+ Salud, ropa y cuidado personal / Saúde, roupas e cuidado pessoal\n\t+ Arte y entretenimiento / Arte e Entretenimiento\n\t+ Alimentos y Bebidas / Alimentos e Bebidas\n* 'review\\_content': The text content of the review.\n* 'review\\_title': The text title of the review.\n* 'review\\_rate': An int between 1-5 indicating the number of stars.",
"### Data Splits\n\n\nEach language configuration comes with it's own 'train', 'validation', and 'test' splits. The 'all\\_languages' split is simply a concatenation of the corresponding split across all languages. That is, the 'train' split for 'all\\_languages' is a concatenation of the 'train' splits for each of the languages and likewise for 'validation' and 'test'.\n\n\nDataset Creation\n----------------",
"### Curation Rationale\n\n\nThe dataset is motivated by the desire to advance sentiment analysis and text classification in Latin American Spanish and Portuguese.",
"### Source Data",
"#### Initial Data Collection and Normalization\n\n\nThe authors gathered the reviews from the marketplaces in Argentina, Colombia, Peru, Uruguay, Chile, Venezuela and Mexico for the Spanish language and from Brasil for Portuguese. They prioritized reviews that contained relevant semantic content by applying a ranking filter based in the lenght and the valorization (difference betweent the number of likes and dislikes) of the review. They then ensured the correct language by applying a semi-automatic language detection algorithm, only retaining those of the target language. No normalization was applied to the review content or title.\n\n\nOriginal products categories were grouped in higher level categories, resulting in five different types of products: \"Home\" (Hogar / Casa), \"Technology and electronics\" (Tecnologı́a y electrónica\n/ Tecnologia e electronica), \"Health, Dress and Personal Care\" (Salud, ropa y cuidado personal / Saúde, roupas e cuidado pessoal) and \"Arts and Entertainment\" (Arte y entretenimiento / Arte e Entretenimiento).",
"#### Who are the source language producers?\n\n\nThe original text comes from Mercado Libre customers reviewing products on the marketplace across a variety of product categories.",
"### Annotations",
"#### Annotation process\n\n\nEach of the fields included are submitted by the user with the review or otherwise associated with the review. No manual or machine-driven annotation was necessary.",
"#### Who are the annotators?\n\n\nN/A",
"### Personal and Sensitive Information\n\n\nMercado Libre Reviews are submitted by users with the knowledge and attention of being public. The reviewer ID's included in this dataset are anonymized, meaning that they are disassociated from the original user profiles. However, these fields would likely be easy to deannoymize given the public and identifying nature of free-form text responses.\n\n\nConsiderations for Using the Data\n---------------------------------",
"### Social Impact of Dataset\n\n\nAlthough Spanish and Portuguese languages are relatively high resource, most of the data is collected from European or United State users. This dataset is part of an effort to encourage text classification research in languages other than English and European Spanish and Portuguese. Such work increases the accessibility of natural language technology to more regions and cultures.",
"### Discussion of Biases\n\n\nThe data included here are from unverified consumers. Some percentage of these reviews may be fake or contain misleading or offensive language.",
"### Other Known Limitations\n\n\nThe dataset is constructed so that the distribution of star ratings is roughly balanced. This feature has some advantages for purposes of classification, but some types of language may be over or underrepresented relative to the original distribution of reviews to acheive this balance.\n\n\nAdditional Information\n----------------------",
"### Dataset Curators\n\n\nPublished by Lautaro Estienne, Matías Vera and Leonardo Rey Vega. Managed by the Signal Processing in Comunications Laboratory of the Electronic Department at the Engeneering School of the Buenos Aires University (UBA).",
"### Licensing Information\n\n\nAmazon has licensed this dataset under its own agreement, to be found at the dataset webpage here:\nURL\n\n\nPlease cite the following paper if you found this dataset useful:\n\n\n(CITATION)",
"### Contributions"
] |
01646b472299e7f15ee59772d329eb5da3646a9a | # Million English Numbers
A list of a million American English numbers, under a AGPL 3.0 license. This datasheet is inspired by [Datasheets for Datasets](https://arxiv.org/abs/1803.09010).
## Sample
```
$ tail -n 5 million-english-numbers
nine hundred ninety nine thousand nine hundred ninety five
nine hundred ninety nine thousand nine hundred ninety six
nine hundred ninety nine thousand nine hundred ninety seven
nine hundred ninety nine thousand nine hundred ninety eight
nine hundred ninety nine thousand nine hundred ninety nine
```
## Motivation
This dataset was created as a toy sample of text for use in natural language processing, in machine learning.
The goal was to create small samples of text with minimal variation and results that could be easily audited (observe how often the model predicts "eighty twenty hundred three ten forty").
This is original research, produced by the linguistic model in the NodeJS package `written-number` by Pedro Tacla Yamada, freely available on npm.
The estimated cost of creating the dataset is minimal, and subsidized with private funds.
## Composition
The instances that comprise the dataset are spelled-out integers, in colloquial Mid-Atlantic American English, identifiable to a speaker born around the year 2000.
There are one million instances, from 0 to 999999 consecutively.
The instances consist of ASCII text, delimited by line feeds.
Counting lines from zero, the line number of each instance is its integer value.
No information is missing from each instance.
In the related _fast.ai_ `HUMAN_NUMBERS` dataset, the split is between 1-7999, and 8001-9999. A user may elect to split this dataset similarly, with the last percentages of lines used for validation or testing.
There are no known errors or sources of noise or redundancies in the dataset.
The dataset is self-contained.
The dataset is not confidential, and its method of generation is public as well.
The dataset will probably not be offensive / insulting / threatening / anxiety-inducing to many people. The numerologically-minded may wish to exercise discernment when choosing which numbers to use: all of the auspicious numbers, all of the inauspicious numbers, all of the meaningful numbers, for all numerological traditions, are included in this dataset, without any emphasis or warnings besides sequential ordering.
The dataset does not relate to people, except by using human language to express integers.
## Collection
The data was directly observed from the `written-number` npm package.
To rebuild this dataset, run `docker run -e MAXINT=1000000 -e WN=written-number -w /x node sh -c 'npm i $WN 2>1 >/dev/null; node -e "const w=require(process.env.WN);for(i=0;i<process.env.MAXINT;i++) console.log(w(i,{noAnd: true}))" | tr "-" " "'` on any x86 machine with Docker. Manual spot-checking confirmed the results.
This is a subset of the set of integers, in increasing order, with no omissions, starting from zero.
This was collected by one individual, writing minimal code, using free time donated to the project.
The data was collected at one point in time, using colloquial Mid-Atlantic American English. The idea of integers including zero is long-standing, and dates back to Babylonians in 700 BCE, the Olmec and Maya in 100 BCE, Brahmagupta in 628 CE.
There was no IRB involved in the making of this data product.
The instances individually do not relate to people.
## Preprocessing
The default output of the version of `written-number` puts a hyphen between the tens and ones place, and this hyphen was translated into a space in the output.
Further, the default conjunction _`and`_ between the hundreds and tens place was removed, as visible above in the sample (_`nine hundred ninety`_).
This raw data was not saved.
The code to regenerate the raw data, and the code to run the preprocessing, is available in this datasheet.
## Uses
This dataset has not been used for any tasks already. It has been inspired by a smaller _fast.ai_ dataset used pedagogically for training NLP models.
The dataset could also be used in place of the original code that generated it, if someone desired a list of human-readable numbers in this dialect of English.
The dataset could also be used as a normative spelling of integers (to correct someone writing "fourty" for instance).
The dataset could also be used, as an artifact of language, could be used to establish normative language for reading integers.
The dataset is composed of only one of the many languages and dialects that `written-number` produces.
A native user of another dialect might elect to change language or dialect, for easier auditing of the output of the language model trained on the numbers.
Specifically, someone might expect to see _`nine lakh ninety nine thousand nine hundred ninety nine`_ instead of _`nine hundred ninety nine thousand nine hundred ninety nine`_ as the last line of the sample above.
It is important to not use this dataset as a normative spelling of integers, especially to impose American English readings of integers on speakers of other dialects of English.
## Distribution
This dataset is distributed worldwide.
It is available on Huggingface, at https://huggingface.co/datasets/lsb/million-english-numbers .
It is currently available.
The license is AGPL 3.0.
The library `written-number` is available under the MIT license, and its output is not currently restricted by license.
No third parties have imposed any restrictions on the data associated with these instance of written numbers.
No export controls or other regulatory restrictions currently apply to the dataset or to individual instances in the dataset.
## Maintenance
Huggingface is currently hosting the dataset, and @lsb is maintaining the dataset.
Contact is available via pull-request, and via email at `[email protected]` .
There are currently no errata, and the full edit history of the dataset is available in the `git` repository in which this datasheet is included.
This dataset is not expected to frequently update. Any users of the dataset may elect to `git pull` any updates.
The data does not relate to people, and there are no limits on the retention of the data associated with the instances.
Older versions of the dataset continue to be supported and hosted and maintained, through the `git` repository that includes the full edit history of the dataset.
If others wish to extend or augment or build on or contribute to the dataset, a mechanism available is to upload additional datasets to Huggingface.
| lsb/million-english-numbers | [
"arxiv:1803.09010",
"region:us"
] | 2022-03-02T23:29:22+00:00 | {} | 2022-01-31T07:17:04+00:00 | [
"1803.09010"
] | [] | TAGS
#arxiv-1803.09010 #region-us
| # Million English Numbers
A list of a million American English numbers, under a AGPL 3.0 license. This datasheet is inspired by Datasheets for Datasets.
## Sample
## Motivation
This dataset was created as a toy sample of text for use in natural language processing, in machine learning.
The goal was to create small samples of text with minimal variation and results that could be easily audited (observe how often the model predicts "eighty twenty hundred three ten forty").
This is original research, produced by the linguistic model in the NodeJS package 'written-number' by Pedro Tacla Yamada, freely available on npm.
The estimated cost of creating the dataset is minimal, and subsidized with private funds.
## Composition
The instances that comprise the dataset are spelled-out integers, in colloquial Mid-Atlantic American English, identifiable to a speaker born around the year 2000.
There are one million instances, from 0 to 999999 consecutively.
The instances consist of ASCII text, delimited by line feeds.
Counting lines from zero, the line number of each instance is its integer value.
No information is missing from each instance.
In the related _fast.ai_ 'HUMAN_NUMBERS' dataset, the split is between 1-7999, and 8001-9999. A user may elect to split this dataset similarly, with the last percentages of lines used for validation or testing.
There are no known errors or sources of noise or redundancies in the dataset.
The dataset is self-contained.
The dataset is not confidential, and its method of generation is public as well.
The dataset will probably not be offensive / insulting / threatening / anxiety-inducing to many people. The numerologically-minded may wish to exercise discernment when choosing which numbers to use: all of the auspicious numbers, all of the inauspicious numbers, all of the meaningful numbers, for all numerological traditions, are included in this dataset, without any emphasis or warnings besides sequential ordering.
The dataset does not relate to people, except by using human language to express integers.
## Collection
The data was directly observed from the 'written-number' npm package.
To rebuild this dataset, run 'docker run -e MAXINT=1000000 -e WN=written-number -w /x node sh -c 'npm i $WN 2>1 >/dev/null; node -e "const w=require(URL.WN);for(i=0;i<URL.MAXINT;i++) URL(w(i,{noAnd: true}))" | tr "-" " "'' on any x86 machine with Docker. Manual spot-checking confirmed the results.
This is a subset of the set of integers, in increasing order, with no omissions, starting from zero.
This was collected by one individual, writing minimal code, using free time donated to the project.
The data was collected at one point in time, using colloquial Mid-Atlantic American English. The idea of integers including zero is long-standing, and dates back to Babylonians in 700 BCE, the Olmec and Maya in 100 BCE, Brahmagupta in 628 CE.
There was no IRB involved in the making of this data product.
The instances individually do not relate to people.
## Preprocessing
The default output of the version of 'written-number' puts a hyphen between the tens and ones place, and this hyphen was translated into a space in the output.
Further, the default conjunction _'and'_ between the hundreds and tens place was removed, as visible above in the sample (_'nine hundred ninety'_).
This raw data was not saved.
The code to regenerate the raw data, and the code to run the preprocessing, is available in this datasheet.
## Uses
This dataset has not been used for any tasks already. It has been inspired by a smaller _fast.ai_ dataset used pedagogically for training NLP models.
The dataset could also be used in place of the original code that generated it, if someone desired a list of human-readable numbers in this dialect of English.
The dataset could also be used as a normative spelling of integers (to correct someone writing "fourty" for instance).
The dataset could also be used, as an artifact of language, could be used to establish normative language for reading integers.
The dataset is composed of only one of the many languages and dialects that 'written-number' produces.
A native user of another dialect might elect to change language or dialect, for easier auditing of the output of the language model trained on the numbers.
Specifically, someone might expect to see _'nine lakh ninety nine thousand nine hundred ninety nine'_ instead of _'nine hundred ninety nine thousand nine hundred ninety nine'_ as the last line of the sample above.
It is important to not use this dataset as a normative spelling of integers, especially to impose American English readings of integers on speakers of other dialects of English.
## Distribution
This dataset is distributed worldwide.
It is available on Huggingface, at URL .
It is currently available.
The license is AGPL 3.0.
The library 'written-number' is available under the MIT license, and its output is not currently restricted by license.
No third parties have imposed any restrictions on the data associated with these instance of written numbers.
No export controls or other regulatory restrictions currently apply to the dataset or to individual instances in the dataset.
## Maintenance
Huggingface is currently hosting the dataset, and @lsb is maintaining the dataset.
Contact is available via pull-request, and via email at 'hi@URL' .
There are currently no errata, and the full edit history of the dataset is available in the 'git' repository in which this datasheet is included.
This dataset is not expected to frequently update. Any users of the dataset may elect to 'git pull' any updates.
The data does not relate to people, and there are no limits on the retention of the data associated with the instances.
Older versions of the dataset continue to be supported and hosted and maintained, through the 'git' repository that includes the full edit history of the dataset.
If others wish to extend or augment or build on or contribute to the dataset, a mechanism available is to upload additional datasets to Huggingface.
| [
"# Million English Numbers\nA list of a million American English numbers, under a AGPL 3.0 license. This datasheet is inspired by Datasheets for Datasets.",
"## Sample",
"## Motivation\n\nThis dataset was created as a toy sample of text for use in natural language processing, in machine learning.\n\nThe goal was to create small samples of text with minimal variation and results that could be easily audited (observe how often the model predicts \"eighty twenty hundred three ten forty\").\n\nThis is original research, produced by the linguistic model in the NodeJS package 'written-number' by Pedro Tacla Yamada, freely available on npm.\n\nThe estimated cost of creating the dataset is minimal, and subsidized with private funds.",
"## Composition\n\nThe instances that comprise the dataset are spelled-out integers, in colloquial Mid-Atlantic American English, identifiable to a speaker born around the year 2000.\n\nThere are one million instances, from 0 to 999999 consecutively.\n\nThe instances consist of ASCII text, delimited by line feeds.\n\nCounting lines from zero, the line number of each instance is its integer value.\n\nNo information is missing from each instance.\n\nIn the related _fast.ai_ 'HUMAN_NUMBERS' dataset, the split is between 1-7999, and 8001-9999. A user may elect to split this dataset similarly, with the last percentages of lines used for validation or testing.\n\nThere are no known errors or sources of noise or redundancies in the dataset.\n\nThe dataset is self-contained.\n\nThe dataset is not confidential, and its method of generation is public as well.\n\nThe dataset will probably not be offensive / insulting / threatening / anxiety-inducing to many people. The numerologically-minded may wish to exercise discernment when choosing which numbers to use: all of the auspicious numbers, all of the inauspicious numbers, all of the meaningful numbers, for all numerological traditions, are included in this dataset, without any emphasis or warnings besides sequential ordering.\n\nThe dataset does not relate to people, except by using human language to express integers.",
"## Collection\n\nThe data was directly observed from the 'written-number' npm package.\n\nTo rebuild this dataset, run 'docker run -e MAXINT=1000000 -e WN=written-number -w /x node sh -c 'npm i $WN 2>1 >/dev/null; node -e \"const w=require(URL.WN);for(i=0;i<URL.MAXINT;i++) URL(w(i,{noAnd: true}))\" | tr \"-\" \" \"'' on any x86 machine with Docker. Manual spot-checking confirmed the results.\n\nThis is a subset of the set of integers, in increasing order, with no omissions, starting from zero.\n\nThis was collected by one individual, writing minimal code, using free time donated to the project.\n\nThe data was collected at one point in time, using colloquial Mid-Atlantic American English. The idea of integers including zero is long-standing, and dates back to Babylonians in 700 BCE, the Olmec and Maya in 100 BCE, Brahmagupta in 628 CE.\n\nThere was no IRB involved in the making of this data product.\n\nThe instances individually do not relate to people.",
"## Preprocessing\n\nThe default output of the version of 'written-number' puts a hyphen between the tens and ones place, and this hyphen was translated into a space in the output.\nFurther, the default conjunction _'and'_ between the hundreds and tens place was removed, as visible above in the sample (_'nine hundred ninety'_).\n\nThis raw data was not saved.\n\nThe code to regenerate the raw data, and the code to run the preprocessing, is available in this datasheet.",
"## Uses\n\nThis dataset has not been used for any tasks already. It has been inspired by a smaller _fast.ai_ dataset used pedagogically for training NLP models.\n\nThe dataset could also be used in place of the original code that generated it, if someone desired a list of human-readable numbers in this dialect of English.\nThe dataset could also be used as a normative spelling of integers (to correct someone writing \"fourty\" for instance).\nThe dataset could also be used, as an artifact of language, could be used to establish normative language for reading integers.\n\nThe dataset is composed of only one of the many languages and dialects that 'written-number' produces.\nA native user of another dialect might elect to change language or dialect, for easier auditing of the output of the language model trained on the numbers.\nSpecifically, someone might expect to see _'nine lakh ninety nine thousand nine hundred ninety nine'_ instead of _'nine hundred ninety nine thousand nine hundred ninety nine'_ as the last line of the sample above.\n\nIt is important to not use this dataset as a normative spelling of integers, especially to impose American English readings of integers on speakers of other dialects of English.",
"## Distribution\n\nThis dataset is distributed worldwide.\n\nIt is available on Huggingface, at URL .\n\nIt is currently available.\n\nThe license is AGPL 3.0.\n\nThe library 'written-number' is available under the MIT license, and its output is not currently restricted by license.\n\nNo third parties have imposed any restrictions on the data associated with these instance of written numbers.\n\nNo export controls or other regulatory restrictions currently apply to the dataset or to individual instances in the dataset.",
"## Maintenance\n\nHuggingface is currently hosting the dataset, and @lsb is maintaining the dataset.\n\nContact is available via pull-request, and via email at 'hi@URL' .\n\nThere are currently no errata, and the full edit history of the dataset is available in the 'git' repository in which this datasheet is included.\n\nThis dataset is not expected to frequently update. Any users of the dataset may elect to 'git pull' any updates.\n\nThe data does not relate to people, and there are no limits on the retention of the data associated with the instances.\n\nOlder versions of the dataset continue to be supported and hosted and maintained, through the 'git' repository that includes the full edit history of the dataset.\n\nIf others wish to extend or augment or build on or contribute to the dataset, a mechanism available is to upload additional datasets to Huggingface."
] | [
"TAGS\n#arxiv-1803.09010 #region-us \n",
"# Million English Numbers\nA list of a million American English numbers, under a AGPL 3.0 license. This datasheet is inspired by Datasheets for Datasets.",
"## Sample",
"## Motivation\n\nThis dataset was created as a toy sample of text for use in natural language processing, in machine learning.\n\nThe goal was to create small samples of text with minimal variation and results that could be easily audited (observe how often the model predicts \"eighty twenty hundred three ten forty\").\n\nThis is original research, produced by the linguistic model in the NodeJS package 'written-number' by Pedro Tacla Yamada, freely available on npm.\n\nThe estimated cost of creating the dataset is minimal, and subsidized with private funds.",
"## Composition\n\nThe instances that comprise the dataset are spelled-out integers, in colloquial Mid-Atlantic American English, identifiable to a speaker born around the year 2000.\n\nThere are one million instances, from 0 to 999999 consecutively.\n\nThe instances consist of ASCII text, delimited by line feeds.\n\nCounting lines from zero, the line number of each instance is its integer value.\n\nNo information is missing from each instance.\n\nIn the related _fast.ai_ 'HUMAN_NUMBERS' dataset, the split is between 1-7999, and 8001-9999. A user may elect to split this dataset similarly, with the last percentages of lines used for validation or testing.\n\nThere are no known errors or sources of noise or redundancies in the dataset.\n\nThe dataset is self-contained.\n\nThe dataset is not confidential, and its method of generation is public as well.\n\nThe dataset will probably not be offensive / insulting / threatening / anxiety-inducing to many people. The numerologically-minded may wish to exercise discernment when choosing which numbers to use: all of the auspicious numbers, all of the inauspicious numbers, all of the meaningful numbers, for all numerological traditions, are included in this dataset, without any emphasis or warnings besides sequential ordering.\n\nThe dataset does not relate to people, except by using human language to express integers.",
"## Collection\n\nThe data was directly observed from the 'written-number' npm package.\n\nTo rebuild this dataset, run 'docker run -e MAXINT=1000000 -e WN=written-number -w /x node sh -c 'npm i $WN 2>1 >/dev/null; node -e \"const w=require(URL.WN);for(i=0;i<URL.MAXINT;i++) URL(w(i,{noAnd: true}))\" | tr \"-\" \" \"'' on any x86 machine with Docker. Manual spot-checking confirmed the results.\n\nThis is a subset of the set of integers, in increasing order, with no omissions, starting from zero.\n\nThis was collected by one individual, writing minimal code, using free time donated to the project.\n\nThe data was collected at one point in time, using colloquial Mid-Atlantic American English. The idea of integers including zero is long-standing, and dates back to Babylonians in 700 BCE, the Olmec and Maya in 100 BCE, Brahmagupta in 628 CE.\n\nThere was no IRB involved in the making of this data product.\n\nThe instances individually do not relate to people.",
"## Preprocessing\n\nThe default output of the version of 'written-number' puts a hyphen between the tens and ones place, and this hyphen was translated into a space in the output.\nFurther, the default conjunction _'and'_ between the hundreds and tens place was removed, as visible above in the sample (_'nine hundred ninety'_).\n\nThis raw data was not saved.\n\nThe code to regenerate the raw data, and the code to run the preprocessing, is available in this datasheet.",
"## Uses\n\nThis dataset has not been used for any tasks already. It has been inspired by a smaller _fast.ai_ dataset used pedagogically for training NLP models.\n\nThe dataset could also be used in place of the original code that generated it, if someone desired a list of human-readable numbers in this dialect of English.\nThe dataset could also be used as a normative spelling of integers (to correct someone writing \"fourty\" for instance).\nThe dataset could also be used, as an artifact of language, could be used to establish normative language for reading integers.\n\nThe dataset is composed of only one of the many languages and dialects that 'written-number' produces.\nA native user of another dialect might elect to change language or dialect, for easier auditing of the output of the language model trained on the numbers.\nSpecifically, someone might expect to see _'nine lakh ninety nine thousand nine hundred ninety nine'_ instead of _'nine hundred ninety nine thousand nine hundred ninety nine'_ as the last line of the sample above.\n\nIt is important to not use this dataset as a normative spelling of integers, especially to impose American English readings of integers on speakers of other dialects of English.",
"## Distribution\n\nThis dataset is distributed worldwide.\n\nIt is available on Huggingface, at URL .\n\nIt is currently available.\n\nThe license is AGPL 3.0.\n\nThe library 'written-number' is available under the MIT license, and its output is not currently restricted by license.\n\nNo third parties have imposed any restrictions on the data associated with these instance of written numbers.\n\nNo export controls or other regulatory restrictions currently apply to the dataset or to individual instances in the dataset.",
"## Maintenance\n\nHuggingface is currently hosting the dataset, and @lsb is maintaining the dataset.\n\nContact is available via pull-request, and via email at 'hi@URL' .\n\nThere are currently no errata, and the full edit history of the dataset is available in the 'git' repository in which this datasheet is included.\n\nThis dataset is not expected to frequently update. Any users of the dataset may elect to 'git pull' any updates.\n\nThe data does not relate to people, and there are no limits on the retention of the data associated with the instances.\n\nOlder versions of the dataset continue to be supported and hosted and maintained, through the 'git' repository that includes the full edit history of the dataset.\n\nIf others wish to extend or augment or build on or contribute to the dataset, a mechanism available is to upload additional datasets to Huggingface."
] |
c435ecfd98f198f2ea0e741591d347423ff056e7 |
# Dataset Card for World Bank Project Documents
## Table of Contents
- [Table of Contents](#table-of-contents)
- [Dataset Description](#dataset-description)
- [Dataset Summary](#dataset-summary)
- [Supported Tasks and Leaderboards](#supported-tasks-and-leaderboards)
- [Languages](#languages)
- [Dataset Structure](#dataset-structure)
- [Data Instances](#data-instances)
- [Data Fields](#data-fields)
- [Data Splits](#data-splits)
- [Dataset Creation](#dataset-creation)
- [Curation Rationale](#curation-rationale)
- [Source Data](#source-data)
- [Annotations](#annotations)
- [Personal and Sensitive Information](#personal-and-sensitive-information)
- [Considerations for Using the Data](#considerations-for-using-the-data)
- [Social Impact of Dataset](#social-impact-of-dataset)
- [Discussion of Biases](#discussion-of-biases)
- [Other Known Limitations](#other-known-limitations)
- [Additional Information](#additional-information)
- [Dataset Curators](#dataset-curators)
- [Licensing Information](#licensing-information)
- [Citation Information](#citation-information)
- [Contributions](#contributions)
## Dataset Description
- **Homepage:**
- **Repository:** https://github.com/luke-grassroot/aid-outcomes-ml
- **Paper:** Forthcoming
- **Point of Contact:** Luke Jordan (lukej at mit)
### Dataset Summary
This is a dataset of documents related to World Bank development projects in the period 1947-2020. The dataset includes
the documents used to propose or describe projects when they are launched, and those in the review. The documents are indexed
by the World Bank project ID, which can be used to obtain features from multiple publicly available tabular datasets.
### Supported Tasks and Leaderboards
No leaderboard yet. A wide range of possible supported tasks, including varieties of summarization, QA, and language modelling. To date, the datasets have been used primarily in conjunction with tabular data (via BERT embeddings) to predict project outcomes.
### Languages
English
## Dataset Structure
### Data Instances
### Data Fields
* World Bank project ID
* Document text
* Document type: "APPROVAL" for documents written at the beginning of a project, when it is approved; and "REVIEW" for documents written at the end of a project
### Data Splits
To allow for open exploration, and since different applications will want to do splits based on different sampling weights, we have not done a train test split but left all files in the train branch.
## Dataset Creation
### Source Data
Documents were scraped from the World Bank's public project archive, following links through to specific project pages and then collecting the text files made available by the [World Bank](https://projects.worldbank.org/en/projects-operations/projects-home).
### Annotations
This dataset is not annotated.
### Personal and Sensitive Information
None.
## Considerations for Using the Data
### Social Impact of Dataset
Affects development projects, which can have large-scale consequences for many millions of people.
### Discussion of Biases
The documents reflect the history of development, which has well-documented and well-studied issues with the imposition of developed world ideas on developing world countries. The documents provide a way to study those in the field of development, but should not be used for their description of the recipient countries, since that language will reflect a multitude of biases, especially in the earlier reaches of the historical projects.
## Additional Information
### Dataset Curators
Luke Jordan, Busani Ndlovu.
### Licensing Information
MIT +no-false-attribs license (MITNFA).
### Citation Information
@dataset{world-bank-project-documents,
author = {Jordan, Luke and Ndlovu, Busani and Shenk, Justin},
title = {World Bank Project Documents Dataset},
year = {2021}
}
### Contributions
Thanks to [@luke-grassroot](https://github.com/luke-grassroot), [@FRTNX](https://github.com/FRTNX/) and [@justinshenk](https://github.com/justinshenk) for adding this dataset. | lukesjordan/worldbank-project-documents | [
"task_categories:table-to-text",
"task_categories:question-answering",
"task_categories:summarization",
"task_categories:text-generation",
"task_ids:abstractive-qa",
"task_ids:closed-domain-qa",
"task_ids:extractive-qa",
"task_ids:language-modeling",
"task_ids:named-entity-recognition",
"task_ids:text-simplification",
"annotations_creators:no-annotation",
"language_creators:found",
"multilinguality:monolingual",
"size_categories:unknown",
"source_datasets:original",
"language:en",
"license:other",
"conditional-text-generation",
"structure-prediction",
"region:us"
] | 2022-03-02T23:29:22+00:00 | {"annotations_creators": ["no-annotation"], "language_creators": ["found"], "language": ["en"], "license": ["other"], "multilinguality": ["monolingual"], "size_categories": ["unknown"], "source_datasets": ["original"], "task_categories": ["table-to-text", "question-answering", "summarization", "text-generation"], "task_ids": ["abstractive-qa", "closed-domain-qa", "extractive-qa", "language-modeling", "named-entity-recognition", "text-simplification"], "pretty_name": "worldbank_project_documents", "language_bcp47": ["en-US"], "tags": ["conditional-text-generation", "structure-prediction"]} | 2022-10-24T19:10:40+00:00 | [] | [
"en"
] | TAGS
#task_categories-table-to-text #task_categories-question-answering #task_categories-summarization #task_categories-text-generation #task_ids-abstractive-qa #task_ids-closed-domain-qa #task_ids-extractive-qa #task_ids-language-modeling #task_ids-named-entity-recognition #task_ids-text-simplification #annotations_creators-no-annotation #language_creators-found #multilinguality-monolingual #size_categories-unknown #source_datasets-original #language-English #license-other #conditional-text-generation #structure-prediction #region-us
|
# Dataset Card for World Bank Project Documents
## Table of Contents
- Table of Contents
- Dataset Description
- Dataset Summary
- Supported Tasks and Leaderboards
- Languages
- Dataset Structure
- Data Instances
- Data Fields
- Data Splits
- Dataset Creation
- Curation Rationale
- Source Data
- Annotations
- Personal and Sensitive Information
- Considerations for Using the Data
- Social Impact of Dataset
- Discussion of Biases
- Other Known Limitations
- Additional Information
- Dataset Curators
- Licensing Information
- Citation Information
- Contributions
## Dataset Description
- Homepage:
- Repository: URL
- Paper: Forthcoming
- Point of Contact: Luke Jordan (lukej at mit)
### Dataset Summary
This is a dataset of documents related to World Bank development projects in the period 1947-2020. The dataset includes
the documents used to propose or describe projects when they are launched, and those in the review. The documents are indexed
by the World Bank project ID, which can be used to obtain features from multiple publicly available tabular datasets.
### Supported Tasks and Leaderboards
No leaderboard yet. A wide range of possible supported tasks, including varieties of summarization, QA, and language modelling. To date, the datasets have been used primarily in conjunction with tabular data (via BERT embeddings) to predict project outcomes.
### Languages
English
## Dataset Structure
### Data Instances
### Data Fields
* World Bank project ID
* Document text
* Document type: "APPROVAL" for documents written at the beginning of a project, when it is approved; and "REVIEW" for documents written at the end of a project
### Data Splits
To allow for open exploration, and since different applications will want to do splits based on different sampling weights, we have not done a train test split but left all files in the train branch.
## Dataset Creation
### Source Data
Documents were scraped from the World Bank's public project archive, following links through to specific project pages and then collecting the text files made available by the World Bank.
### Annotations
This dataset is not annotated.
### Personal and Sensitive Information
None.
## Considerations for Using the Data
### Social Impact of Dataset
Affects development projects, which can have large-scale consequences for many millions of people.
### Discussion of Biases
The documents reflect the history of development, which has well-documented and well-studied issues with the imposition of developed world ideas on developing world countries. The documents provide a way to study those in the field of development, but should not be used for their description of the recipient countries, since that language will reflect a multitude of biases, especially in the earlier reaches of the historical projects.
## Additional Information
### Dataset Curators
Luke Jordan, Busani Ndlovu.
### Licensing Information
MIT +no-false-attribs license (MITNFA).
@dataset{world-bank-project-documents,
author = {Jordan, Luke and Ndlovu, Busani and Shenk, Justin},
title = {World Bank Project Documents Dataset},
year = {2021}
}
### Contributions
Thanks to @luke-grassroot, @FRTNX and @justinshenk for adding this dataset. | [
"# Dataset Card for World Bank Project Documents",
"## Table of Contents\n- Table of Contents\n- Dataset Description\n - Dataset Summary\n - Supported Tasks and Leaderboards\n - Languages\n- Dataset Structure\n - Data Instances\n - Data Fields\n - Data Splits\n- Dataset Creation\n - Curation Rationale\n - Source Data\n - Annotations\n - Personal and Sensitive Information\n- Considerations for Using the Data\n - Social Impact of Dataset\n - Discussion of Biases\n - Other Known Limitations\n- Additional Information\n - Dataset Curators\n - Licensing Information\n - Citation Information\n - Contributions",
"## Dataset Description\n\n- Homepage:\n- Repository: URL\n- Paper: Forthcoming\n- Point of Contact: Luke Jordan (lukej at mit)",
"### Dataset Summary\n\nThis is a dataset of documents related to World Bank development projects in the period 1947-2020. The dataset includes\nthe documents used to propose or describe projects when they are launched, and those in the review. The documents are indexed\nby the World Bank project ID, which can be used to obtain features from multiple publicly available tabular datasets.",
"### Supported Tasks and Leaderboards\n\nNo leaderboard yet. A wide range of possible supported tasks, including varieties of summarization, QA, and language modelling. To date, the datasets have been used primarily in conjunction with tabular data (via BERT embeddings) to predict project outcomes.",
"### Languages\n\nEnglish",
"## Dataset Structure",
"### Data Instances",
"### Data Fields\n\n* World Bank project ID\n* Document text\n* Document type: \"APPROVAL\" for documents written at the beginning of a project, when it is approved; and \"REVIEW\" for documents written at the end of a project",
"### Data Splits\n\nTo allow for open exploration, and since different applications will want to do splits based on different sampling weights, we have not done a train test split but left all files in the train branch.",
"## Dataset Creation",
"### Source Data\n\nDocuments were scraped from the World Bank's public project archive, following links through to specific project pages and then collecting the text files made available by the World Bank.",
"### Annotations\n\nThis dataset is not annotated.",
"### Personal and Sensitive Information\n\nNone.",
"## Considerations for Using the Data",
"### Social Impact of Dataset\n\nAffects development projects, which can have large-scale consequences for many millions of people.",
"### Discussion of Biases\n\nThe documents reflect the history of development, which has well-documented and well-studied issues with the imposition of developed world ideas on developing world countries. The documents provide a way to study those in the field of development, but should not be used for their description of the recipient countries, since that language will reflect a multitude of biases, especially in the earlier reaches of the historical projects.",
"## Additional Information",
"### Dataset Curators\n\nLuke Jordan, Busani Ndlovu.",
"### Licensing Information\n\nMIT +no-false-attribs license (MITNFA).\n\n\n\n@dataset{world-bank-project-documents,\n author = {Jordan, Luke and Ndlovu, Busani and Shenk, Justin},\n title = {World Bank Project Documents Dataset},\n year = {2021}\n}",
"### Contributions\n\nThanks to @luke-grassroot, @FRTNX and @justinshenk for adding this dataset."
] | [
"TAGS\n#task_categories-table-to-text #task_categories-question-answering #task_categories-summarization #task_categories-text-generation #task_ids-abstractive-qa #task_ids-closed-domain-qa #task_ids-extractive-qa #task_ids-language-modeling #task_ids-named-entity-recognition #task_ids-text-simplification #annotations_creators-no-annotation #language_creators-found #multilinguality-monolingual #size_categories-unknown #source_datasets-original #language-English #license-other #conditional-text-generation #structure-prediction #region-us \n",
"# Dataset Card for World Bank Project Documents",
"## Table of Contents\n- Table of Contents\n- Dataset Description\n - Dataset Summary\n - Supported Tasks and Leaderboards\n - Languages\n- Dataset Structure\n - Data Instances\n - Data Fields\n - Data Splits\n- Dataset Creation\n - Curation Rationale\n - Source Data\n - Annotations\n - Personal and Sensitive Information\n- Considerations for Using the Data\n - Social Impact of Dataset\n - Discussion of Biases\n - Other Known Limitations\n- Additional Information\n - Dataset Curators\n - Licensing Information\n - Citation Information\n - Contributions",
"## Dataset Description\n\n- Homepage:\n- Repository: URL\n- Paper: Forthcoming\n- Point of Contact: Luke Jordan (lukej at mit)",
"### Dataset Summary\n\nThis is a dataset of documents related to World Bank development projects in the period 1947-2020. The dataset includes\nthe documents used to propose or describe projects when they are launched, and those in the review. The documents are indexed\nby the World Bank project ID, which can be used to obtain features from multiple publicly available tabular datasets.",
"### Supported Tasks and Leaderboards\n\nNo leaderboard yet. A wide range of possible supported tasks, including varieties of summarization, QA, and language modelling. To date, the datasets have been used primarily in conjunction with tabular data (via BERT embeddings) to predict project outcomes.",
"### Languages\n\nEnglish",
"## Dataset Structure",
"### Data Instances",
"### Data Fields\n\n* World Bank project ID\n* Document text\n* Document type: \"APPROVAL\" for documents written at the beginning of a project, when it is approved; and \"REVIEW\" for documents written at the end of a project",
"### Data Splits\n\nTo allow for open exploration, and since different applications will want to do splits based on different sampling weights, we have not done a train test split but left all files in the train branch.",
"## Dataset Creation",
"### Source Data\n\nDocuments were scraped from the World Bank's public project archive, following links through to specific project pages and then collecting the text files made available by the World Bank.",
"### Annotations\n\nThis dataset is not annotated.",
"### Personal and Sensitive Information\n\nNone.",
"## Considerations for Using the Data",
"### Social Impact of Dataset\n\nAffects development projects, which can have large-scale consequences for many millions of people.",
"### Discussion of Biases\n\nThe documents reflect the history of development, which has well-documented and well-studied issues with the imposition of developed world ideas on developing world countries. The documents provide a way to study those in the field of development, but should not be used for their description of the recipient countries, since that language will reflect a multitude of biases, especially in the earlier reaches of the historical projects.",
"## Additional Information",
"### Dataset Curators\n\nLuke Jordan, Busani Ndlovu.",
"### Licensing Information\n\nMIT +no-false-attribs license (MITNFA).\n\n\n\n@dataset{world-bank-project-documents,\n author = {Jordan, Luke and Ndlovu, Busani and Shenk, Justin},\n title = {World Bank Project Documents Dataset},\n year = {2021}\n}",
"### Contributions\n\nThanks to @luke-grassroot, @FRTNX and @justinshenk for adding this dataset."
] |
904b9cef2f649654deef43f0eecb44986395f1bc | # dureader
数据来自千言DuReader数据集,这里是原始地址 [千言数据集:阅读理解](https://aistudio.baidu.com/aistudio/competition/detail/49/0/task-definition)。
> 本数据集只用作学术研究使用。如果本仓库涉及侵权行为,会立即删除。
目前包含以下两个子集:
* DuReader-robust
* DuReader-checklist
```python
from datasets import load_dataset
robust = load_dataset("luozhouyang/dureader", "robust")
checklist = load_dataset("luozhouyang/dureader", "checklist")
``` | luozhouyang/dureader | [
"region:us"
] | 2022-03-02T23:29:22+00:00 | {} | 2021-11-29T04:44:53+00:00 | [] | [] | TAGS
#region-us
| # dureader
数据来自千言DuReader数据集,这里是原始地址 千言数据集:阅读理解。
> 本数据集只用作学术研究使用。如果本仓库涉及侵权行为,会立即删除。
目前包含以下两个子集:
* DuReader-robust
* DuReader-checklist
| [
"# dureader\n\n数据来自千言DuReader数据集,这里是原始地址 千言数据集:阅读理解。\n\n> 本数据集只用作学术研究使用。如果本仓库涉及侵权行为,会立即删除。\n\n目前包含以下两个子集:\n\n* DuReader-robust\n* DuReader-checklist"
] | [
"TAGS\n#region-us \n",
"# dureader\n\n数据来自千言DuReader数据集,这里是原始地址 千言数据集:阅读理解。\n\n> 本数据集只用作学术研究使用。如果本仓库涉及侵权行为,会立即删除。\n\n目前包含以下两个子集:\n\n* DuReader-robust\n* DuReader-checklist"
] |
acc2f0223357b039635bd616443e53c609eff279 | # KgCLUE-Knowledge
The original data is from [CLUEbenchmark/KgCLUE](https://github.com/CLUEbenchmark/KgCLUE).
Here is a JSON version of the original knowledge base.
## Usage
```bash
from datasets import load_dataset
dataset = load_dataset("luozhouyang/kgclue-knowledge")
# or select files
dataset = load_dataset("luozhouyang/kgclue-knowledge", data_files=["kgclue.knowledge00.jsonl"])
```
| luozhouyang/kgclue-knowledge | [
"region:us"
] | 2022-03-02T23:29:22+00:00 | {} | 2021-12-24T03:30:49+00:00 | [] | [] | TAGS
#region-us
| # KgCLUE-Knowledge
The original data is from CLUEbenchmark/KgCLUE.
Here is a JSON version of the original knowledge base.
## Usage
| [
"# KgCLUE-Knowledge\n\nThe original data is from CLUEbenchmark/KgCLUE.\n\nHere is a JSON version of the original knowledge base.",
"## Usage"
] | [
"TAGS\n#region-us \n",
"# KgCLUE-Knowledge\n\nThe original data is from CLUEbenchmark/KgCLUE.\n\nHere is a JSON version of the original knowledge base.",
"## Usage"
] |
02dc192e9908ab6186e86689cd1a948c9771eefd | # question-answering-datasets
Datasets for Question Answering task!
```yaml
annotations_creators:
- found
language_creators:
- found
languages:
- zh-CN
licenses:
- unknown
multilinguality:
- monolingual
pretty_name: question-answering-datasets
size_categories:
- unknown
source_datasets:
- original
task_categories:
- question-answering
task_ids:
- open-domain-qa
- extractive-qa
```
| luozhouyang/question-answering-datasets | [
"region:us"
] | 2022-03-02T23:29:22+00:00 | {} | 2021-11-26T11:09:10+00:00 | [] | [] | TAGS
#region-us
| # question-answering-datasets
Datasets for Question Answering task!
| [
"# question-answering-datasets\n\n\nDatasets for Question Answering task!"
] | [
"TAGS\n#region-us \n",
"# question-answering-datasets\n\n\nDatasets for Question Answering task!"
] |
3e6ab65f2864931e041f6a82db9b5a6ec2b71ab4 | # CodeParrot 🦜 Dataset Cleaned (train)
Train split of [CodeParrot 🦜 Dataset Cleaned](https://huggingface.co/datasets/lvwerra/codeparrot-clean).
## Dataset structure
```python
DatasetDict({
train: Dataset({
features: ['repo_name', 'path', 'copies', 'size', 'content', 'license', 'hash', 'line_mean', 'line_max', 'alpha_frac', 'autogenerated'],
num_rows: 5300000
})
})
``` | codeparrot/codeparrot-clean-train | [
"region:us"
] | 2022-03-02T23:29:22+00:00 | {} | 2022-10-10T14:27:50+00:00 | [] | [] | TAGS
#region-us
| # CodeParrot Dataset Cleaned (train)
Train split of CodeParrot Dataset Cleaned.
## Dataset structure
| [
"# CodeParrot Dataset Cleaned (train)\n\nTrain split of CodeParrot Dataset Cleaned.",
"## Dataset structure"
] | [
"TAGS\n#region-us \n",
"# CodeParrot Dataset Cleaned (train)\n\nTrain split of CodeParrot Dataset Cleaned.",
"## Dataset structure"
] |
4db92d2ec0c1b4c41eeb439cfae16854511d9dcd | # CodeParrot 🦜 Dataset Cleaned (valid)
Train split of [CodeParrot 🦜 Dataset Cleaned](https://huggingface.co/datasets/lvwerra/codeparrot-clean).
## Dataset structure
```python
DatasetDict({
train: Dataset({
features: ['repo_name', 'path', 'copies', 'size', 'content', 'license', 'hash', 'line_mean', 'line_max', 'alpha_frac', 'autogenerated'],
num_rows: 61373
})
})
``` | codeparrot/codeparrot-clean-valid | [
"region:us"
] | 2022-03-02T23:29:22+00:00 | {} | 2022-10-10T14:28:51+00:00 | [] | [] | TAGS
#region-us
| # CodeParrot Dataset Cleaned (valid)
Train split of CodeParrot Dataset Cleaned.
## Dataset structure
| [
"# CodeParrot Dataset Cleaned (valid)\n\nTrain split of CodeParrot Dataset Cleaned.",
"## Dataset structure"
] | [
"TAGS\n#region-us \n",
"# CodeParrot Dataset Cleaned (valid)\n\nTrain split of CodeParrot Dataset Cleaned.",
"## Dataset structure"
] |
35a59fb025bc0a102f7d96eac09d145b896d487b |
# CodeParrot 🦜 Dataset Cleaned
## What is it?
A dataset of Python files from Github. This is the deduplicated version of the [codeparrot](https://huggingface.co/datasets/transformersbook/codeparrot).
## Processing
The original dataset contains a lot of duplicated and noisy data. Therefore, the dataset was cleaned with the following steps:
- Deduplication
- Remove exact matches
- Filtering
- Average line length < 100
- Maximum line length < 1000
- Alpha numeric characters fraction > 0.25
- Remove auto-generated files (keyword search)
For more details see the preprocessing script in the transformers repository [here](https://github.com/huggingface/transformers/tree/master/examples/research_projects/codeparrot).
## Splits
The dataset is split in a [train](https://huggingface.co/datasets/codeparrot/codeparrot-clean-train) and [validation](https://huggingface.co/datasets/codeparrot/codeparrot-clean-valid) split used for training and evaluation.
## Structure
This dataset has ~50GB of code and 5361373 files.
```python
DatasetDict({
train: Dataset({
features: ['repo_name', 'path', 'copies', 'size', 'content', 'license', 'hash', 'line_mean', 'line_max', 'alpha_frac', 'autogenerated'],
num_rows: 5361373
})
})
``` | codeparrot/codeparrot-clean | [
"python",
"code",
"region:us"
] | 2022-03-02T23:29:22+00:00 | {"tags": ["python", "code"]} | 2022-10-10T14:23:51+00:00 | [] | [] | TAGS
#python #code #region-us
|
# CodeParrot Dataset Cleaned
## What is it?
A dataset of Python files from Github. This is the deduplicated version of the codeparrot.
## Processing
The original dataset contains a lot of duplicated and noisy data. Therefore, the dataset was cleaned with the following steps:
- Deduplication
- Remove exact matches
- Filtering
- Average line length < 100
- Maximum line length < 1000
- Alpha numeric characters fraction > 0.25
- Remove auto-generated files (keyword search)
For more details see the preprocessing script in the transformers repository here.
## Splits
The dataset is split in a train and validation split used for training and evaluation.
## Structure
This dataset has ~50GB of code and 5361373 files.
| [
"# CodeParrot Dataset Cleaned",
"## What is it?\n\nA dataset of Python files from Github. This is the deduplicated version of the codeparrot.",
"## Processing\nThe original dataset contains a lot of duplicated and noisy data. Therefore, the dataset was cleaned with the following steps:\n- Deduplication \n - Remove exact matches\n- Filtering\n - Average line length < 100\n - Maximum line length < 1000\n - Alpha numeric characters fraction > 0.25\n - Remove auto-generated files (keyword search)\n \nFor more details see the preprocessing script in the transformers repository here.",
"## Splits\nThe dataset is split in a train and validation split used for training and evaluation.",
"## Structure\nThis dataset has ~50GB of code and 5361373 files."
] | [
"TAGS\n#python #code #region-us \n",
"# CodeParrot Dataset Cleaned",
"## What is it?\n\nA dataset of Python files from Github. This is the deduplicated version of the codeparrot.",
"## Processing\nThe original dataset contains a lot of duplicated and noisy data. Therefore, the dataset was cleaned with the following steps:\n- Deduplication \n - Remove exact matches\n- Filtering\n - Average line length < 100\n - Maximum line length < 1000\n - Alpha numeric characters fraction > 0.25\n - Remove auto-generated files (keyword search)\n \nFor more details see the preprocessing script in the transformers repository here.",
"## Splits\nThe dataset is split in a train and validation split used for training and evaluation.",
"## Structure\nThis dataset has ~50GB of code and 5361373 files."
] |
b5661e6b17396364b2bcf8e68977b0d28e1ebd19 |
# GitHub Code Dataset
## Dataset Description
The GitHub Code dataset consists of 115M code files from GitHub in 32 programming languages with 60 extensions totaling in 1TB of data. The dataset was created from the public GitHub dataset on Google BiqQuery.
### How to use it
The GitHub Code dataset is a very large dataset so for most use cases it is recommended to make use of the streaming API of `datasets`. You can load and iterate through the dataset with the following two lines of code:
```python
from datasets import load_dataset
ds = load_dataset("codeparrot/github-code", streaming=True, split="train")
print(next(iter(ds)))
#OUTPUT:
{
'code': "import mod189 from './mod189';\nvar value=mod189+1;\nexport default value;\n",
'repo_name': 'MirekSz/webpack-es6-ts',
'path': 'app/mods/mod190.js',
'language': 'JavaScript',
'license': 'isc',
'size': 73
}
```
You can see that besides the code, repo name, and path also the programming language, license, and the size of the file are part of the dataset. You can also filter the dataset for any subset of the 30 included languages (see the full list below) in the dataset. Just pass the list of languages as a list. E.g. if your dream is to build a Codex model for Dockerfiles use the following configuration:
```python
ds = load_dataset("codeparrot/github-code", streaming=True, split="train", languages=["Dockerfile"])
print(next(iter(ds))["code"])
#OUTPUT:
"""\
FROM rockyluke/ubuntu:precise
ENV DEBIAN_FRONTEND="noninteractive" \
TZ="Europe/Amsterdam"
...
"""
```
We also have access to the license of the origin repo of a file so we can filter for licenses in the same way we filtered for languages:
```python
ds = load_dataset("codeparrot/github-code", streaming=True, split="train", licenses=["mit", "isc"])
licenses = []
for element in iter(ds).take(10_000):
licenses.append(element["license"])
print(Counter(licenses))
#OUTPUT:
Counter({'mit': 9896, 'isc': 104})
```
Naturally, you can also download the full dataset. Note that this will download ~300GB compressed text data and the uncompressed dataset will take up ~1TB of storage:
```python
ds = load_dataset("codeparrot/github-code", split="train")
```
## Data Structure
### Data Instances
```python
{
'code': "import mod189 from './mod189';\nvar value=mod189+1;\nexport default value;\n",
'repo_name': 'MirekSz/webpack-es6-ts',
'path': 'app/mods/mod190.js',
'language': 'JavaScript',
'license': 'isc',
'size': 73
}
```
### Data Fields
|Field|Type|Description|
|---|---|---|
|code|string|content of source file|
|repo_name|string|name of the GitHub repository|
|path|string|path of file in GitHub repository|
|language|string|programming language as inferred by extension|
|license|string|license of GitHub repository|
|size|int|size of source file in bytes|
### Data Splits
The dataset only contains a train split.
## Languages
The dataset contains 30 programming languages with over 60 extensions:
```python
{
"Assembly": [".asm"],
"Batchfile": [".bat", ".cmd"],
"C": [".c", ".h"],
"C#": [".cs"],
"C++": [".cpp", ".hpp", ".c++", ".h++", ".cc", ".hh", ".C", ".H"],
"CMake": [".cmake"],
"CSS": [".css"],
"Dockerfile": [".dockerfile", "Dockerfile"],
"FORTRAN": ['.f90', '.f', '.f03', '.f08', '.f77', '.f95', '.for', '.fpp'],
"GO": [".go"],
"Haskell": [".hs"],
"HTML":[".html"],
"Java": [".java"],
"JavaScript": [".js"],
"Julia": [".jl"],
"Lua": [".lua"],
"Makefile": ["Makefile"],
"Markdown": [".md", ".markdown"],
"PHP": [".php", ".php3", ".php4", ".php5", ".phps", ".phpt"],
"Perl": [".pl", ".pm", ".pod", ".perl"],
"PowerShell": ['.ps1', '.psd1', '.psm1'],
"Python": [".py"],
"Ruby": [".rb"],
"Rust": [".rs"],
"SQL": [".sql"],
"Scala": [".scala"],
"Shell": [".sh", ".bash", ".command", ".zsh"],
"TypeScript": [".ts", ".tsx"],
"TeX": [".tex"],
"Visual Basic": [".vb"]
}
```
## Licenses
Each example is also annotated with the license of the associated repository. There are in total 15 licenses:
```python
[
'mit',
'apache-2.0',
'gpl-3.0',
'gpl-2.0',
'bsd-3-clause',
'agpl-3.0',
'lgpl-3.0',
'lgpl-2.1',
'bsd-2-clause',
'cc0-1.0',
'epl-1.0',
'mpl-2.0',
'unlicense',
'isc',
'artistic-2.0'
]
```
## Dataset Statistics
The dataset contains 115M files and the sum of all the source code file sizes is 873 GB (note that the size of the dataset is larger due to the extra fields). A breakdown per language is given in the plot and table below:
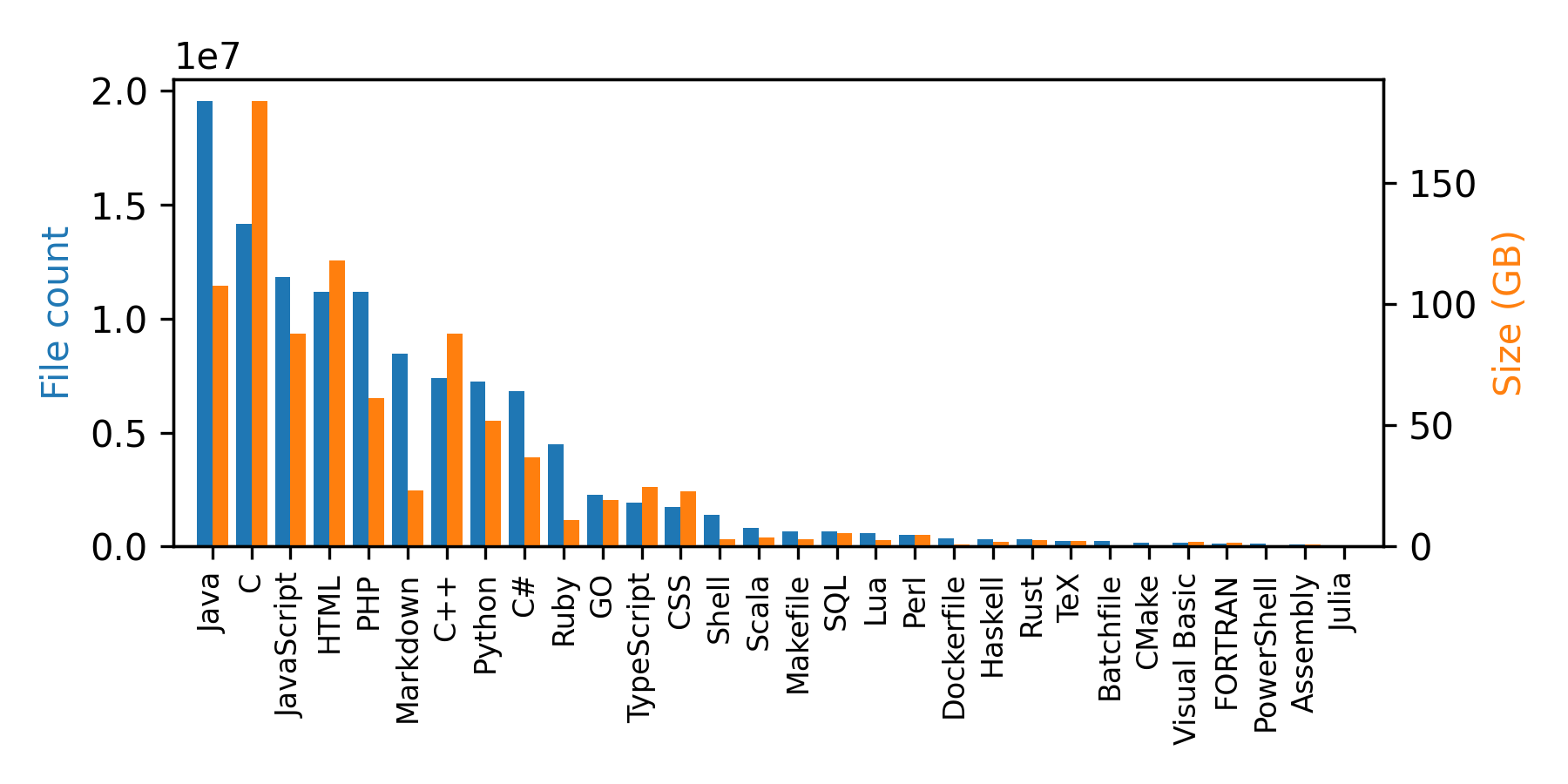
| | Language |File Count| Size (GB)|
|---:|:-------------|---------:|-------:|
| 0 | Java | 19548190 | 107.70 |
| 1 | C | 14143113 | 183.83 |
| 2 | JavaScript | 11839883 | 87.82 |
| 3 | HTML | 11178557 | 118.12 |
| 4 | PHP | 11177610 | 61.41 |
| 5 | Markdown | 8464626 | 23.09 |
| 6 | C++ | 7380520 | 87.73 |
| 7 | Python | 7226626 | 52.03 |
| 8 | C# | 6811652 | 36.83 |
| 9 | Ruby | 4473331 | 10.95 |
| 10 | GO | 2265436 | 19.28 |
| 11 | TypeScript | 1940406 | 24.59 |
| 12 | CSS | 1734406 | 22.67 |
| 13 | Shell | 1385648 | 3.01 |
| 14 | Scala | 835755 | 3.87 |
| 15 | Makefile | 679430 | 2.92 |
| 16 | SQL | 656671 | 5.67 |
| 17 | Lua | 578554 | 2.81 |
| 18 | Perl | 497949 | 4.70 |
| 19 | Dockerfile | 366505 | 0.71 |
| 20 | Haskell | 340623 | 1.85 |
| 21 | Rust | 322431 | 2.68 |
| 22 | TeX | 251015 | 2.15 |
| 23 | Batchfile | 236945 | 0.70 |
| 24 | CMake | 175282 | 0.54 |
| 25 | Visual Basic | 155652 | 1.91 |
| 26 | FORTRAN | 142038 | 1.62 |
| 27 | PowerShell | 136846 | 0.69 |
| 28 | Assembly | 82905 | 0.78 |
| 29 | Julia | 58317 | 0.29 |
## Dataset Creation
The dataset was created in two steps:
1. Files of with the extensions given in the list above were retrieved from the GitHub dataset on BigQuery (full query [here](https://huggingface.co/datasets/codeparrot/github-code/blob/main/query.sql)). The query was executed on _Mar 16, 2022, 6:23:39 PM UTC+1_.
2. Files with lines longer than 1000 characters and duplicates (exact duplicates ignoring whitespaces) were dropped (full preprocessing script [here](https://huggingface.co/datasets/codeparrot/github-code/blob/main/github_preprocessing.py)).
## Considerations for Using the Data
The dataset consists of source code from a wide range of repositories. As such they can potentially include harmful or biased code as well as sensitive information like passwords or usernames.
## Releases
You can load any older version of the dataset with the `revision` argument:
```Python
ds = load_dataset("codeparrot/github-code", revision="v1.0")
```
### v1.0
- Initial release of dataset
- The query was executed on _Feb 14, 2022, 12:03:16 PM UTC+1_
### v1.1
- Fix missing Scala/TypeScript
- Fix deduplication issue with inconsistent Python `hash`
- The query was executed on _Mar 16, 2022, 6:23:39 PM UTC+1_
| codeparrot/github-code | [
"task_categories:text-generation",
"task_ids:language-modeling",
"language_creators:crowdsourced",
"language_creators:expert-generated",
"multilinguality:multilingual",
"size_categories:unknown",
"language:code",
"license:other",
"region:us"
] | 2022-03-02T23:29:22+00:00 | {"annotations_creators": [], "language_creators": ["crowdsourced", "expert-generated"], "language": ["code"], "license": ["other"], "multilinguality": ["multilingual"], "size_categories": ["unknown"], "source_datasets": [], "task_categories": ["text-generation"], "task_ids": ["language-modeling"], "pretty_name": "github-code"} | 2022-10-20T14:01:14+00:00 | [] | [
"code"
] | TAGS
#task_categories-text-generation #task_ids-language-modeling #language_creators-crowdsourced #language_creators-expert-generated #multilinguality-multilingual #size_categories-unknown #language-code #license-other #region-us
| GitHub Code Dataset
===================
Dataset Description
-------------------
The GitHub Code dataset consists of 115M code files from GitHub in 32 programming languages with 60 extensions totaling in 1TB of data. The dataset was created from the public GitHub dataset on Google BiqQuery.
### How to use it
The GitHub Code dataset is a very large dataset so for most use cases it is recommended to make use of the streaming API of 'datasets'. You can load and iterate through the dataset with the following two lines of code:
You can see that besides the code, repo name, and path also the programming language, license, and the size of the file are part of the dataset. You can also filter the dataset for any subset of the 30 included languages (see the full list below) in the dataset. Just pass the list of languages as a list. E.g. if your dream is to build a Codex model for Dockerfiles use the following configuration:
We also have access to the license of the origin repo of a file so we can filter for licenses in the same way we filtered for languages:
Naturally, you can also download the full dataset. Note that this will download ~300GB compressed text data and the uncompressed dataset will take up ~1TB of storage:
Data Structure
--------------
### Data Instances
### Data Fields
Field: code, Type: string, Description: content of source file
Field: repo\_name, Type: string, Description: name of the GitHub repository
Field: path, Type: string, Description: path of file in GitHub repository
Field: language, Type: string, Description: programming language as inferred by extension
Field: license, Type: string, Description: license of GitHub repository
Field: size, Type: int, Description: size of source file in bytes
### Data Splits
The dataset only contains a train split.
Languages
---------
The dataset contains 30 programming languages with over 60 extensions:
Licenses
--------
Each example is also annotated with the license of the associated repository. There are in total 15 licenses:
Dataset Statistics
------------------
The dataset contains 115M files and the sum of all the source code file sizes is 873 GB (note that the size of the dataset is larger due to the extra fields). A breakdown per language is given in the plot and table below:
!dataset-statistics
Dataset Creation
----------------
The dataset was created in two steps:
1. Files of with the extensions given in the list above were retrieved from the GitHub dataset on BigQuery (full query here). The query was executed on *Mar 16, 2022, 6:23:39 PM UTC+1*.
2. Files with lines longer than 1000 characters and duplicates (exact duplicates ignoring whitespaces) were dropped (full preprocessing script here).
Considerations for Using the Data
---------------------------------
The dataset consists of source code from a wide range of repositories. As such they can potentially include harmful or biased code as well as sensitive information like passwords or usernames.
Releases
--------
You can load any older version of the dataset with the 'revision' argument:
### v1.0
* Initial release of dataset
* The query was executed on *Feb 14, 2022, 12:03:16 PM UTC+1*
### v1.1
* Fix missing Scala/TypeScript
* Fix deduplication issue with inconsistent Python 'hash'
* The query was executed on *Mar 16, 2022, 6:23:39 PM UTC+1*
| [
"### How to use it\n\n\nThe GitHub Code dataset is a very large dataset so for most use cases it is recommended to make use of the streaming API of 'datasets'. You can load and iterate through the dataset with the following two lines of code:\n\n\nYou can see that besides the code, repo name, and path also the programming language, license, and the size of the file are part of the dataset. You can also filter the dataset for any subset of the 30 included languages (see the full list below) in the dataset. Just pass the list of languages as a list. E.g. if your dream is to build a Codex model for Dockerfiles use the following configuration:\n\n\nWe also have access to the license of the origin repo of a file so we can filter for licenses in the same way we filtered for languages:\n\n\nNaturally, you can also download the full dataset. Note that this will download ~300GB compressed text data and the uncompressed dataset will take up ~1TB of storage:\n\n\nData Structure\n--------------",
"### Data Instances",
"### Data Fields\n\n\nField: code, Type: string, Description: content of source file\nField: repo\\_name, Type: string, Description: name of the GitHub repository\nField: path, Type: string, Description: path of file in GitHub repository\nField: language, Type: string, Description: programming language as inferred by extension\nField: license, Type: string, Description: license of GitHub repository\nField: size, Type: int, Description: size of source file in bytes",
"### Data Splits\n\n\nThe dataset only contains a train split.\n\n\nLanguages\n---------\n\n\nThe dataset contains 30 programming languages with over 60 extensions:\n\n\nLicenses\n--------\n\n\nEach example is also annotated with the license of the associated repository. There are in total 15 licenses:\n\n\nDataset Statistics\n------------------\n\n\nThe dataset contains 115M files and the sum of all the source code file sizes is 873 GB (note that the size of the dataset is larger due to the extra fields). A breakdown per language is given in the plot and table below:\n\n\n!dataset-statistics\n\n\n\nDataset Creation\n----------------\n\n\nThe dataset was created in two steps:\n\n\n1. Files of with the extensions given in the list above were retrieved from the GitHub dataset on BigQuery (full query here). The query was executed on *Mar 16, 2022, 6:23:39 PM UTC+1*.\n2. Files with lines longer than 1000 characters and duplicates (exact duplicates ignoring whitespaces) were dropped (full preprocessing script here).\n\n\nConsiderations for Using the Data\n---------------------------------\n\n\nThe dataset consists of source code from a wide range of repositories. As such they can potentially include harmful or biased code as well as sensitive information like passwords or usernames.\n\n\nReleases\n--------\n\n\nYou can load any older version of the dataset with the 'revision' argument:",
"### v1.0\n\n\n* Initial release of dataset\n* The query was executed on *Feb 14, 2022, 12:03:16 PM UTC+1*",
"### v1.1\n\n\n* Fix missing Scala/TypeScript\n* Fix deduplication issue with inconsistent Python 'hash'\n* The query was executed on *Mar 16, 2022, 6:23:39 PM UTC+1*"
] | [
"TAGS\n#task_categories-text-generation #task_ids-language-modeling #language_creators-crowdsourced #language_creators-expert-generated #multilinguality-multilingual #size_categories-unknown #language-code #license-other #region-us \n",
"### How to use it\n\n\nThe GitHub Code dataset is a very large dataset so for most use cases it is recommended to make use of the streaming API of 'datasets'. You can load and iterate through the dataset with the following two lines of code:\n\n\nYou can see that besides the code, repo name, and path also the programming language, license, and the size of the file are part of the dataset. You can also filter the dataset for any subset of the 30 included languages (see the full list below) in the dataset. Just pass the list of languages as a list. E.g. if your dream is to build a Codex model for Dockerfiles use the following configuration:\n\n\nWe also have access to the license of the origin repo of a file so we can filter for licenses in the same way we filtered for languages:\n\n\nNaturally, you can also download the full dataset. Note that this will download ~300GB compressed text data and the uncompressed dataset will take up ~1TB of storage:\n\n\nData Structure\n--------------",
"### Data Instances",
"### Data Fields\n\n\nField: code, Type: string, Description: content of source file\nField: repo\\_name, Type: string, Description: name of the GitHub repository\nField: path, Type: string, Description: path of file in GitHub repository\nField: language, Type: string, Description: programming language as inferred by extension\nField: license, Type: string, Description: license of GitHub repository\nField: size, Type: int, Description: size of source file in bytes",
"### Data Splits\n\n\nThe dataset only contains a train split.\n\n\nLanguages\n---------\n\n\nThe dataset contains 30 programming languages with over 60 extensions:\n\n\nLicenses\n--------\n\n\nEach example is also annotated with the license of the associated repository. There are in total 15 licenses:\n\n\nDataset Statistics\n------------------\n\n\nThe dataset contains 115M files and the sum of all the source code file sizes is 873 GB (note that the size of the dataset is larger due to the extra fields). A breakdown per language is given in the plot and table below:\n\n\n!dataset-statistics\n\n\n\nDataset Creation\n----------------\n\n\nThe dataset was created in two steps:\n\n\n1. Files of with the extensions given in the list above were retrieved from the GitHub dataset on BigQuery (full query here). The query was executed on *Mar 16, 2022, 6:23:39 PM UTC+1*.\n2. Files with lines longer than 1000 characters and duplicates (exact duplicates ignoring whitespaces) were dropped (full preprocessing script here).\n\n\nConsiderations for Using the Data\n---------------------------------\n\n\nThe dataset consists of source code from a wide range of repositories. As such they can potentially include harmful or biased code as well as sensitive information like passwords or usernames.\n\n\nReleases\n--------\n\n\nYou can load any older version of the dataset with the 'revision' argument:",
"### v1.0\n\n\n* Initial release of dataset\n* The query was executed on *Feb 14, 2022, 12:03:16 PM UTC+1*",
"### v1.1\n\n\n* Fix missing Scala/TypeScript\n* Fix deduplication issue with inconsistent Python 'hash'\n* The query was executed on *Mar 16, 2022, 6:23:39 PM UTC+1*"
] |
5a76387bbac31d8574fd3f977cd0003cf5cf8519 | # Red Wine Dataset 🍷
This dataset contains the red wine dataset found [here](https://github.com/suvoooo/Machine_Learning). See also [this](https://huggingface.co/julien-c/wine-quality) example of a Scikit-Learn model trained on this dataset. | lvwerra/red-wine | [
"region:us"
] | 2022-03-02T23:29:22+00:00 | {} | 2022-02-15T15:55:52+00:00 | [] | [] | TAGS
#region-us
| # Red Wine Dataset
This dataset contains the red wine dataset found here. See also this example of a Scikit-Learn model trained on this dataset. | [
"# Red Wine Dataset \n\nThis dataset contains the red wine dataset found here. See also this example of a Scikit-Learn model trained on this dataset."
] | [
"TAGS\n#region-us \n",
"# Red Wine Dataset \n\nThis dataset contains the red wine dataset found here. See also this example of a Scikit-Learn model trained on this dataset."
] |
d91ce8bd583c4cbaad3420e16e1d112e3b5c9113 | # RecipeNLG: A Cooking Recipes Dataset
RecipeNLG: A Cooking Recipes Dataset for Semi-Structured Text Generation - Lite version
The dataset contains `7,198` cooking recipes (`>7K`).
It's processed in more careful way and provides more samples than any other dataset in the area.
## How to use
```bash
pip install git+https://github.com/huggingface/datasets.git
```
Load `m3hrdadfi/recipe_nlg_lite` dataset using `load_dataset`:
```python
from datasets import load_dataset
dataset = load_dataset("m3hrdadfi/recipe_nlg_lite")
print(dataset)
```
Output:
```text
DatasetDict({
train: Dataset({
features: ['uid', 'name', 'description', 'link', 'ner', 'ingredients', 'steps'],
num_rows: 6118
})
test: Dataset({
features: ['uid', 'name', 'description', 'link', 'ner', 'ingredients', 'steps'],
num_rows: 1080
})
})
```
## Examples
```json
{
"description": "we all know how satisfying it is to make great pork tenderloin, ribs, or a roast but the end of the meal creates a new quandary what do you do with the leftover pork contrary to what you might think, it's not that difficult . how to repurpose your meal is where real cooking creativity comes into play, so let us present to you our favorite pork chop soup recipe . with this recipe, you'll discover how the natural bold flavor of pork gives this hearty soup a lift that a vegetable soup or chicken noodle soup just can't get . it's a dinner recipe to warm you up on a cold winter night or a midday restorative for a long work week . throw all the ingredients in a large pot and let it simmer on the stove for a couple hours, or turn it into a slow cooker recipe and let it percolate for an afternoon . this foolproof recipe transforms your favorite comfort food into an easy meal to warm you up again and again . the health benefits of pork pork is a great option if you're on a low carb diet or trying to up your protein intake . the protein percentage of leaner cuts of pork can be as high as 89 percent pork also provides valuable vitamins and minerals that make pork recipes worthy endeavors . pork has high levels of thiamin and niacin, which other types of meat like beef and lamb lack . they are both b vitamins that aid in several body functions such as metabolism and cell function . pork also delivers a healthy amount of zinc, which aids in brain and immune system function . that makes digging into this pork chop noodle soup all the more alluring . recipe variations this pork soup recipe can be adapted to many diets . if you're following a low carb or ketogenic diet, you can modify the recipe to suit you by leaving out the noodles . if you like, you can add a little crunch by topping it with french fried onions . for cheese lovers, a sprinkle of parmesan cheese can give the soup more body and extra umami flavors . if you're not a noodle lover, this soup recipe works equally well as a potato soup with diced potatoes . if you want to make a southwestern or mexican version, add a can of diced tomatoes and bell peppers for a little extra depth . if you have a penchant for spicy soups, add a little chili powder or red pepper flakes . it's up to you this recipe is great for using up leftover pork chops, but you can make this soup using fresh chops however you decide to do it, you won't be disappointed.",
"ingredients": "3.0 bone in pork chops, salt, pepper, 2.0 tablespoon vegetable oil, 2.0 cup chicken broth, 4.0 cup vegetable broth, 1.0 red onion, 4.0 carrots, 2.0 clove garlic, 1.0 teaspoon dried thyme, 0.5 teaspoon dried basil, 1.0 cup rotini pasta, 2.0 stalk celery",
"link": "https://www.yummly.com/private/recipe/Pork-Chop-Noodle-Soup-2249011?layout=prep-steps",
"name": "pork chop noodle soup",
"ner": "bone in pork chops, salt, pepper, vegetable oil, chicken broth, vegetable broth, red onion, carrots, garlic, dried thyme, dried basil, rotini pasta, celery",
"steps": "season pork chops with salt and pepper . heat oil in a dutch oven over medium high heat . add chops and cook for about 4 minutes, until golden brown . flip and cook 4 minutes more, until golden brown . transfer chops to a plate and set aside . pour half of chicken broth into pot, scraping all browned bits from bottom . add remaining chicken broth, vegetable broth, onion, carrots, celery and garlic . mix well and bring to a simmer . add 1 quart water, thyme, basil, 2 teaspoons salt and 1 teaspoon pepper . mix well and bring to a simmer . add chops back to pot and return to simmer . reduce heat and simmer for 90 minutes, stirring occasionally, being careful not to break up chops . transfer chops to plate, trying not to break them up . set aside to cool . raise the heat and bring the soup to a boil . add pasta and cook for about 12 minutes, until tender . when the chops are cool, pull them apart, discarding all the bones and fat . add the meat back to soup and stir well . taste for salt and pepper, and add if needed, before serving.",
"uid": "dab8b7d0-e0f6-4bb0-aed9-346e80dace1f"
}
```
## Citation
```bibtex
@misc{RecipeNLGLite,
author = {Mehrdad Farahani},
title = {RecipeNLG: A Cooking Recipes Dataset for Semi-Structured Text Generation (Lite)},
year = 2021,
publisher = {GitHub},
journal = {GitHub repository},
howpublished = {url{https://github.com/m3hrdadfi/recipe-nlg-lite}},
}
```
| m3hrdadfi/recipe_nlg_lite | [
"region:us"
] | 2022-03-02T23:29:22+00:00 | {} | 2021-07-03T08:34:56+00:00 | [] | [] | TAGS
#region-us
| # RecipeNLG: A Cooking Recipes Dataset
RecipeNLG: A Cooking Recipes Dataset for Semi-Structured Text Generation - Lite version
The dataset contains '7,198' cooking recipes ('>7K').
It's processed in more careful way and provides more samples than any other dataset in the area.
## How to use
Load 'm3hrdadfi/recipe_nlg_lite' dataset using 'load_dataset':
Output:
## Examples
| [
"# RecipeNLG: A Cooking Recipes Dataset\nRecipeNLG: A Cooking Recipes Dataset for Semi-Structured Text Generation - Lite version\n\nThe dataset contains '7,198' cooking recipes ('>7K'). \nIt's processed in more careful way and provides more samples than any other dataset in the area.",
"## How to use\n\n\n\nLoad 'm3hrdadfi/recipe_nlg_lite' dataset using 'load_dataset':\n\n\n\nOutput:",
"## Examples"
] | [
"TAGS\n#region-us \n",
"# RecipeNLG: A Cooking Recipes Dataset\nRecipeNLG: A Cooking Recipes Dataset for Semi-Structured Text Generation - Lite version\n\nThe dataset contains '7,198' cooking recipes ('>7K'). \nIt's processed in more careful way and provides more samples than any other dataset in the area.",
"## How to use\n\n\n\nLoad 'm3hrdadfi/recipe_nlg_lite' dataset using 'load_dataset':\n\n\n\nOutput:",
"## Examples"
] |
cf4949dc173b1216a9ddc5f2b0d225259a202f17 | # Indonesia News Dataset
## Source
- [Kompas](https://www.kompas.com/)
- [Detik](https://www.detik.com/) (Cooming Soon)
- [Liputan6](https://www.liputan6.com/) (Cooming Soon)
- [Tempo](https://www.tempo.co/) (Cooming Soon)
- [Okezone](https://www.okezone.com/) (Cooming Soon)
- [CNN Indonesia](https://www.cnnindonesia.com/) (Cooming Soon)
- [CNBC Indonesia](https://www.cnbcindonesia.com/) (Cooming Soon)
## Roadmap
1. Web Data Crawling and Indexing
2. NER Model and Sentiment Analysis Model
3. Data Visualization
## Contact
- You Can Contact Me at [twitter](https://twitter.com/skidipapGUY) | mad/IndonesiaNewsDataset | [
"region:us"
] | 2022-03-02T23:29:22+00:00 | {} | 2021-06-24T11:42:33+00:00 | [] | [] | TAGS
#region-us
| # Indonesia News Dataset
## Source
- Kompas
- Detik (Cooming Soon)
- Liputan6 (Cooming Soon)
- Tempo (Cooming Soon)
- Okezone (Cooming Soon)
- CNN Indonesia (Cooming Soon)
- CNBC Indonesia (Cooming Soon)
## Roadmap
1. Web Data Crawling and Indexing
2. NER Model and Sentiment Analysis Model
3. Data Visualization
## Contact
- You Can Contact Me at twitter | [
"# Indonesia News Dataset",
"## Source \n\n- Kompas\n- Detik (Cooming Soon)\n- Liputan6 (Cooming Soon)\n- Tempo (Cooming Soon)\n- Okezone (Cooming Soon)\n- CNN Indonesia (Cooming Soon)\n- CNBC Indonesia (Cooming Soon)",
"## Roadmap \n\n1. Web Data Crawling and Indexing \n2. NER Model and Sentiment Analysis Model \n3. Data Visualization",
"## Contact \n\n- You Can Contact Me at twitter"
] | [
"TAGS\n#region-us \n",
"# Indonesia News Dataset",
"## Source \n\n- Kompas\n- Detik (Cooming Soon)\n- Liputan6 (Cooming Soon)\n- Tempo (Cooming Soon)\n- Okezone (Cooming Soon)\n- CNN Indonesia (Cooming Soon)\n- CNBC Indonesia (Cooming Soon)",
"## Roadmap \n\n1. Web Data Crawling and Indexing \n2. NER Model and Sentiment Analysis Model \n3. Data Visualization",
"## Contact \n\n- You Can Contact Me at twitter"
] |
b2c20f00d794fcc32a597182ef23f64382cb8aae |
# mammut-corpus-venezuela
HuggingFace Dataset for testing purposes. The train dataset is `mammut/mammut-corpus-venezuela`.
## 1. How to use
How to load this dataset directly with the datasets library:
`>>> from datasets import load_dataset`
`>>> dataset = load_dataset("mammut/mammut-corpus-venezuela")`
## 2. Dataset Summary
**mammut-corpus-venezuela** is a dataset for Spanish language modeling. This dataset comprises a large number of Venezuelan and Latin-American Spanish texts, manually selected and collected in 2021. The data was collected by a process of web scraping from different portals, downloading of Telegram group chats' history, and selecting of Venezuelan and Latin-American Spanish corpus available online. The texts come from Venezuelan Spanish speakers, subtitlers, journalists, politicians, doctors, writers, and online sellers. Social biases may be present, and a percentage of the texts may be fake or contain misleading or offensive language.
Each record in the dataset contains the author of the text (anonymized for conversation authors), the date on which the text entered in the corpus, the text which was automatically tokenized at sentence level for sources other than conversations, the source of the text, the title of the text, the number of tokens (excluding punctuation marks) of the text, and the linguistic register of the text.
This is the test set for `mammut/mammut-corpus-venezuela` dataset.
## 3. Supported Tasks and Leaderboards
This dataset can be used for language modeling testing.
## 4. Languages
The dataset contains Venezuelan and Latin-American Spanish.
## 5. Dataset Structure
Dataset structure features.
### 5.1 Data Instances
An example from the dataset:
"AUTHOR":"author in title",
"TITLE":"Luis Alberto Buttó: Hecho en socialismo",
"SENTENCE":"Históricamente, siempre fue así.",
"DATE":"2021-07-04 07:18:46.918253",
"SOURCE":"la patilla",
"TOKENS":"4",
"TYPE":"opinion/news",
The average word token count are provided below:
### 5.2 Total of tokens (no spelling marks)
Test: 4,876,739.
### 5.3 Data Fields
The data have several fields:
AUTHOR: author of the text. It is anonymized for conversation authors.
DATE: date on which the text was entered in the corpus.
SENTENCE: text. It was automatically tokenized for sources other than conversations.
SOURCE: source of the texts.
TITLE: title of the text from which SENTENCE originates.
TOKENS: number of tokens (excluding punctuation marks) of SENTENCE.
TYPE: linguistic register of the text.
### 5.4 Data Splits
The mammut-corpus-venezuela dataset has 2 splits: train and test. Below are the statistics:
Number of Instances in Split.
Test: 157,011.
## 6. Dataset Creation
### 6.1 Curation Rationale
The purpose of the mammut-corpus-venezuela dataset is language modeling. It can be used for pre-training a model from scratch or for fine-tuning on another pre-trained model.
### 6.2 Source Data
**6.2.1 Initial Data Collection and Normalization**
The data consists of opinion articles and text messages. It was collected by a process of web scraping from different portals, downloading of Telegram group chats’ history and selecting of Venezuelan and Latin-American Spanish corpus available online.
The text from the web scraping process was separated in sentences and was automatically tokenized for sources other than conversations.
An arrow parquet file was created.
Text sources: El Estímulo (website), cinco8 (website), csm-1990 (oral speaking corpus), "El atajo más largo" (blog), El Pitazo (website), La Patilla (website), Venezuelan movies subtitles, Preseea Mérida (oral speaking corpus), Prodavinci (website), Runrunes (website), and Telegram group chats.
**6.2.2 Who are the source language producers?**
The texts come from Venezuelan Spanish speakers, subtitlers, journalists, politicians, doctors, writers, and online sellers.
## 6.3 Annotations
**6.3.1 Annotation process**
At the moment the dataset does not contain any additional annotations.
**6.3.2 Who are the annotators?**
Not applicable.
### 6.4 Personal and Sensitive Information
The data is partially anonymized. Also, there are messages from Telegram selling chats, some percentage of these messages may be fake or contain misleading or offensive language.
## 7. Considerations for Using the Data
### 7.1 Social Impact of Dataset
The purpose of this dataset is to help the development of language modeling models (pre-training or fine-tuning) in Venezuelan Spanish.
### 7.2 Discussion of Biases
Most of the content comes from political, economical and sociological opinion articles. Social biases may be present.
### 7.3 Other Known Limitations
(If applicable, description of the other limitations in the data.)
Not applicable.
## 8. Additional Information
### 8.1 Dataset Curators
The data was originally collected by Lino Urdaneta and Miguel Riveros from Mammut.io.
### 8.2 Licensing Information
Not applicable.
### 8.3 Citation Information
Not applicable.
### 8.4 Contributions
Not applicable.
| mammut/mammut-corpus-venezuela-test-set | [
"task_ids:language-modeling",
"annotations_creators:no-annotation",
"language_creators:expert-generated",
"multilinguality:monolingual",
"size_categories:unknown",
"source_datasets:original",
"language:es",
"license:cc-by-nc-nd-4.0",
"region:us"
] | 2022-03-02T23:29:22+00:00 | {"annotations_creators": ["no-annotation"], "language_creators": ["expert-generated"], "language": ["es"], "license": ["cc-by-nc-nd-4.0"], "multilinguality": ["monolingual"], "size_categories": ["unknown"], "source_datasets": ["original"], "task_categories": ["sequence-modeling"], "task_ids": ["language-modeling"], "pretty_name": "mammut-corpus-venezuela", "language_bcp47": ["es-VE"]} | 2022-10-22T07:58:48+00:00 | [] | [
"es"
] | TAGS
#task_ids-language-modeling #annotations_creators-no-annotation #language_creators-expert-generated #multilinguality-monolingual #size_categories-unknown #source_datasets-original #language-Spanish #license-cc-by-nc-nd-4.0 #region-us
|
# mammut-corpus-venezuela
HuggingFace Dataset for testing purposes. The train dataset is 'mammut/mammut-corpus-venezuela'.
## 1. How to use
How to load this dataset directly with the datasets library:
'>>> from datasets import load_dataset'
'>>> dataset = load_dataset("mammut/mammut-corpus-venezuela")'
## 2. Dataset Summary
mammut-corpus-venezuela is a dataset for Spanish language modeling. This dataset comprises a large number of Venezuelan and Latin-American Spanish texts, manually selected and collected in 2021. The data was collected by a process of web scraping from different portals, downloading of Telegram group chats' history, and selecting of Venezuelan and Latin-American Spanish corpus available online. The texts come from Venezuelan Spanish speakers, subtitlers, journalists, politicians, doctors, writers, and online sellers. Social biases may be present, and a percentage of the texts may be fake or contain misleading or offensive language.
Each record in the dataset contains the author of the text (anonymized for conversation authors), the date on which the text entered in the corpus, the text which was automatically tokenized at sentence level for sources other than conversations, the source of the text, the title of the text, the number of tokens (excluding punctuation marks) of the text, and the linguistic register of the text.
This is the test set for 'mammut/mammut-corpus-venezuela' dataset.
## 3. Supported Tasks and Leaderboards
This dataset can be used for language modeling testing.
## 4. Languages
The dataset contains Venezuelan and Latin-American Spanish.
## 5. Dataset Structure
Dataset structure features.
### 5.1 Data Instances
An example from the dataset:
"AUTHOR":"author in title",
"TITLE":"Luis Alberto Buttó: Hecho en socialismo",
"SENTENCE":"Históricamente, siempre fue así.",
"DATE":"2021-07-04 07:18:46.918253",
"SOURCE":"la patilla",
"TOKENS":"4",
"TYPE":"opinion/news",
The average word token count are provided below:
### 5.2 Total of tokens (no spelling marks)
Test: 4,876,739.
### 5.3 Data Fields
The data have several fields:
AUTHOR: author of the text. It is anonymized for conversation authors.
DATE: date on which the text was entered in the corpus.
SENTENCE: text. It was automatically tokenized for sources other than conversations.
SOURCE: source of the texts.
TITLE: title of the text from which SENTENCE originates.
TOKENS: number of tokens (excluding punctuation marks) of SENTENCE.
TYPE: linguistic register of the text.
### 5.4 Data Splits
The mammut-corpus-venezuela dataset has 2 splits: train and test. Below are the statistics:
Number of Instances in Split.
Test: 157,011.
## 6. Dataset Creation
### 6.1 Curation Rationale
The purpose of the mammut-corpus-venezuela dataset is language modeling. It can be used for pre-training a model from scratch or for fine-tuning on another pre-trained model.
### 6.2 Source Data
6.2.1 Initial Data Collection and Normalization
The data consists of opinion articles and text messages. It was collected by a process of web scraping from different portals, downloading of Telegram group chats’ history and selecting of Venezuelan and Latin-American Spanish corpus available online.
The text from the web scraping process was separated in sentences and was automatically tokenized for sources other than conversations.
An arrow parquet file was created.
Text sources: El Estímulo (website), cinco8 (website), csm-1990 (oral speaking corpus), "El atajo más largo" (blog), El Pitazo (website), La Patilla (website), Venezuelan movies subtitles, Preseea Mérida (oral speaking corpus), Prodavinci (website), Runrunes (website), and Telegram group chats.
6.2.2 Who are the source language producers?
The texts come from Venezuelan Spanish speakers, subtitlers, journalists, politicians, doctors, writers, and online sellers.
## 6.3 Annotations
6.3.1 Annotation process
At the moment the dataset does not contain any additional annotations.
6.3.2 Who are the annotators?
Not applicable.
### 6.4 Personal and Sensitive Information
The data is partially anonymized. Also, there are messages from Telegram selling chats, some percentage of these messages may be fake or contain misleading or offensive language.
## 7. Considerations for Using the Data
### 7.1 Social Impact of Dataset
The purpose of this dataset is to help the development of language modeling models (pre-training or fine-tuning) in Venezuelan Spanish.
### 7.2 Discussion of Biases
Most of the content comes from political, economical and sociological opinion articles. Social biases may be present.
### 7.3 Other Known Limitations
(If applicable, description of the other limitations in the data.)
Not applicable.
## 8. Additional Information
### 8.1 Dataset Curators
The data was originally collected by Lino Urdaneta and Miguel Riveros from URL.
### 8.2 Licensing Information
Not applicable.
### 8.3 Citation Information
Not applicable.
### 8.4 Contributions
Not applicable.
| [
"# mammut-corpus-venezuela\n\nHuggingFace Dataset for testing purposes. The train dataset is 'mammut/mammut-corpus-venezuela'.",
"## 1. How to use\n\nHow to load this dataset directly with the datasets library:\n\n'>>> from datasets import load_dataset' \n'>>> dataset = load_dataset(\"mammut/mammut-corpus-venezuela\")'",
"## 2. Dataset Summary\n\nmammut-corpus-venezuela is a dataset for Spanish language modeling. This dataset comprises a large number of Venezuelan and Latin-American Spanish texts, manually selected and collected in 2021. The data was collected by a process of web scraping from different portals, downloading of Telegram group chats' history, and selecting of Venezuelan and Latin-American Spanish corpus available online. The texts come from Venezuelan Spanish speakers, subtitlers, journalists, politicians, doctors, writers, and online sellers. Social biases may be present, and a percentage of the texts may be fake or contain misleading or offensive language.\n\nEach record in the dataset contains the author of the text (anonymized for conversation authors), the date on which the text entered in the corpus, the text which was automatically tokenized at sentence level for sources other than conversations, the source of the text, the title of the text, the number of tokens (excluding punctuation marks) of the text, and the linguistic register of the text.\n\nThis is the test set for 'mammut/mammut-corpus-venezuela' dataset.",
"## 3. Supported Tasks and Leaderboards\n\nThis dataset can be used for language modeling testing.",
"## 4. Languages\n\nThe dataset contains Venezuelan and Latin-American Spanish.",
"## 5. Dataset Structure\n\nDataset structure features.",
"### 5.1 Data Instances\n\nAn example from the dataset:\n \n \n \"AUTHOR\":\"author in title\", \n \"TITLE\":\"Luis Alberto Buttó: Hecho en socialismo\", \n \"SENTENCE\":\"Históricamente, siempre fue así.\",\n \"DATE\":\"2021-07-04 07:18:46.918253\", \n \"SOURCE\":\"la patilla\", \n \"TOKENS\":\"4\", \n \"TYPE\":\"opinion/news\", \n\n\nThe average word token count are provided below:",
"### 5.2 Total of tokens (no spelling marks)\n\nTest: 4,876,739.",
"### 5.3 Data Fields\n\nThe data have several fields:\n\n AUTHOR: author of the text. It is anonymized for conversation authors. \n DATE: date on which the text was entered in the corpus. \n SENTENCE: text. It was automatically tokenized for sources other than conversations. \n SOURCE: source of the texts. \n TITLE: title of the text from which SENTENCE originates. \n TOKENS: number of tokens (excluding punctuation marks) of SENTENCE. \n TYPE: linguistic register of the text.",
"### 5.4 Data Splits\n\nThe mammut-corpus-venezuela dataset has 2 splits: train and test. Below are the statistics:\n\nNumber of Instances in Split. \n\nTest: 157,011.",
"## 6. Dataset Creation",
"### 6.1 Curation Rationale\n\nThe purpose of the mammut-corpus-venezuela dataset is language modeling. It can be used for pre-training a model from scratch or for fine-tuning on another pre-trained model.",
"### 6.2 Source Data\n\n6.2.1 Initial Data Collection and Normalization\n\nThe data consists of opinion articles and text messages. It was collected by a process of web scraping from different portals, downloading of Telegram group chats’ history and selecting of Venezuelan and Latin-American Spanish corpus available online.\n\nThe text from the web scraping process was separated in sentences and was automatically tokenized for sources other than conversations.\n\nAn arrow parquet file was created.\n\nText sources: El Estímulo (website), cinco8 (website), csm-1990 (oral speaking corpus), \"El atajo más largo\" (blog), El Pitazo (website), La Patilla (website), Venezuelan movies subtitles, Preseea Mérida (oral speaking corpus), Prodavinci (website), Runrunes (website), and Telegram group chats.\n\n6.2.2 Who are the source language producers?\n\nThe texts come from Venezuelan Spanish speakers, subtitlers, journalists, politicians, doctors, writers, and online sellers.",
"## 6.3 Annotations\n\n6.3.1 Annotation process\n\nAt the moment the dataset does not contain any additional annotations.\n\n6.3.2 Who are the annotators?\n\nNot applicable.",
"### 6.4 Personal and Sensitive Information\n\nThe data is partially anonymized. Also, there are messages from Telegram selling chats, some percentage of these messages may be fake or contain misleading or offensive language.",
"## 7. Considerations for Using the Data",
"### 7.1 Social Impact of Dataset\n\nThe purpose of this dataset is to help the development of language modeling models (pre-training or fine-tuning) in Venezuelan Spanish.",
"### 7.2 Discussion of Biases\n\nMost of the content comes from political, economical and sociological opinion articles. Social biases may be present.",
"### 7.3 Other Known Limitations\n\n(If applicable, description of the other limitations in the data.)\n\nNot applicable.",
"## 8. Additional Information",
"### 8.1 Dataset Curators\n\nThe data was originally collected by Lino Urdaneta and Miguel Riveros from URL.",
"### 8.2 Licensing Information\n\nNot applicable.",
"### 8.3 Citation Information\n\nNot applicable.",
"### 8.4 Contributions\n\nNot applicable."
] | [
"TAGS\n#task_ids-language-modeling #annotations_creators-no-annotation #language_creators-expert-generated #multilinguality-monolingual #size_categories-unknown #source_datasets-original #language-Spanish #license-cc-by-nc-nd-4.0 #region-us \n",
"# mammut-corpus-venezuela\n\nHuggingFace Dataset for testing purposes. The train dataset is 'mammut/mammut-corpus-venezuela'.",
"## 1. How to use\n\nHow to load this dataset directly with the datasets library:\n\n'>>> from datasets import load_dataset' \n'>>> dataset = load_dataset(\"mammut/mammut-corpus-venezuela\")'",
"## 2. Dataset Summary\n\nmammut-corpus-venezuela is a dataset for Spanish language modeling. This dataset comprises a large number of Venezuelan and Latin-American Spanish texts, manually selected and collected in 2021. The data was collected by a process of web scraping from different portals, downloading of Telegram group chats' history, and selecting of Venezuelan and Latin-American Spanish corpus available online. The texts come from Venezuelan Spanish speakers, subtitlers, journalists, politicians, doctors, writers, and online sellers. Social biases may be present, and a percentage of the texts may be fake or contain misleading or offensive language.\n\nEach record in the dataset contains the author of the text (anonymized for conversation authors), the date on which the text entered in the corpus, the text which was automatically tokenized at sentence level for sources other than conversations, the source of the text, the title of the text, the number of tokens (excluding punctuation marks) of the text, and the linguistic register of the text.\n\nThis is the test set for 'mammut/mammut-corpus-venezuela' dataset.",
"## 3. Supported Tasks and Leaderboards\n\nThis dataset can be used for language modeling testing.",
"## 4. Languages\n\nThe dataset contains Venezuelan and Latin-American Spanish.",
"## 5. Dataset Structure\n\nDataset structure features.",
"### 5.1 Data Instances\n\nAn example from the dataset:\n \n \n \"AUTHOR\":\"author in title\", \n \"TITLE\":\"Luis Alberto Buttó: Hecho en socialismo\", \n \"SENTENCE\":\"Históricamente, siempre fue así.\",\n \"DATE\":\"2021-07-04 07:18:46.918253\", \n \"SOURCE\":\"la patilla\", \n \"TOKENS\":\"4\", \n \"TYPE\":\"opinion/news\", \n\n\nThe average word token count are provided below:",
"### 5.2 Total of tokens (no spelling marks)\n\nTest: 4,876,739.",
"### 5.3 Data Fields\n\nThe data have several fields:\n\n AUTHOR: author of the text. It is anonymized for conversation authors. \n DATE: date on which the text was entered in the corpus. \n SENTENCE: text. It was automatically tokenized for sources other than conversations. \n SOURCE: source of the texts. \n TITLE: title of the text from which SENTENCE originates. \n TOKENS: number of tokens (excluding punctuation marks) of SENTENCE. \n TYPE: linguistic register of the text.",
"### 5.4 Data Splits\n\nThe mammut-corpus-venezuela dataset has 2 splits: train and test. Below are the statistics:\n\nNumber of Instances in Split. \n\nTest: 157,011.",
"## 6. Dataset Creation",
"### 6.1 Curation Rationale\n\nThe purpose of the mammut-corpus-venezuela dataset is language modeling. It can be used for pre-training a model from scratch or for fine-tuning on another pre-trained model.",
"### 6.2 Source Data\n\n6.2.1 Initial Data Collection and Normalization\n\nThe data consists of opinion articles and text messages. It was collected by a process of web scraping from different portals, downloading of Telegram group chats’ history and selecting of Venezuelan and Latin-American Spanish corpus available online.\n\nThe text from the web scraping process was separated in sentences and was automatically tokenized for sources other than conversations.\n\nAn arrow parquet file was created.\n\nText sources: El Estímulo (website), cinco8 (website), csm-1990 (oral speaking corpus), \"El atajo más largo\" (blog), El Pitazo (website), La Patilla (website), Venezuelan movies subtitles, Preseea Mérida (oral speaking corpus), Prodavinci (website), Runrunes (website), and Telegram group chats.\n\n6.2.2 Who are the source language producers?\n\nThe texts come from Venezuelan Spanish speakers, subtitlers, journalists, politicians, doctors, writers, and online sellers.",
"## 6.3 Annotations\n\n6.3.1 Annotation process\n\nAt the moment the dataset does not contain any additional annotations.\n\n6.3.2 Who are the annotators?\n\nNot applicable.",
"### 6.4 Personal and Sensitive Information\n\nThe data is partially anonymized. Also, there are messages from Telegram selling chats, some percentage of these messages may be fake or contain misleading or offensive language.",
"## 7. Considerations for Using the Data",
"### 7.1 Social Impact of Dataset\n\nThe purpose of this dataset is to help the development of language modeling models (pre-training or fine-tuning) in Venezuelan Spanish.",
"### 7.2 Discussion of Biases\n\nMost of the content comes from political, economical and sociological opinion articles. Social biases may be present.",
"### 7.3 Other Known Limitations\n\n(If applicable, description of the other limitations in the data.)\n\nNot applicable.",
"## 8. Additional Information",
"### 8.1 Dataset Curators\n\nThe data was originally collected by Lino Urdaneta and Miguel Riveros from URL.",
"### 8.2 Licensing Information\n\nNot applicable.",
"### 8.3 Citation Information\n\nNot applicable.",
"### 8.4 Contributions\n\nNot applicable."
] |
f02d7037b1c83ba041f36da8d299553488dd6924 |
# mammut-corpus-venezuela
HuggingFace Dataset
## 1. How to use
How to load this dataset directly with the datasets library:
`>>> from datasets import load_dataset`
`>>> dataset = load_dataset("mammut-corpus-venezuela")`
## 2. Dataset Summary
**mammut-corpus-venezuela** is a dataset for Spanish language modeling. This dataset comprises a large number of Venezuelan and Latin-American Spanish texts, manually selected and collected in 2021. The data was collected by a process of web scraping from different portals, downloading of Telegram group chats' history, and selecting of Venezuelan and Latin-American Spanish corpus available online. The texts come from Venezuelan Spanish speakers, subtitlers, journalists, politicians, doctors, writers, and online sellers. Social biases may be present, and a percentage of the texts may be fake or contain misleading or offensive language.
Each record in the dataset contains the author of the text (anonymized for conversation authors), the date on which the text entered in the corpus, the text which was automatically tokenized at sentence level for sources other than conversations, the source of the text, the title of the text, the number of tokens (excluding punctuation marks) of the text, and the linguistic register of the text.
The dataset counts with a train split and a test split.
## 3. Supported Tasks and Leaderboards
This dataset can be used for language modeling.
## 4. Languages
The dataset contains Venezuelan and Latin-American Spanish.
## 5. Dataset Structure
Dataset structure features.
### 5.1 Data Instances
An example from the dataset:
"AUTHOR":"author in title",
"TITLE":"Luis Alberto Buttó: Hecho en socialismo",
"SENTENCE":"Históricamente, siempre fue así.",
"DATE":"2021-07-04 07:18:46.918253",
"SOURCE":"la patilla",
"TOKENS":"4",
"TYPE":"opinion/news",
The average word token count are provided below:
### 5.2 Total of tokens (no spelling marks)
Train: 92,431,194.
Test: 4,876,739 (in another file).
### 5.3 Data Fields
The data have several fields:
AUTHOR: author of the text. It is anonymized for conversation authors.
DATE: date on which the text was entered in the corpus.
SENTENCE: text. It was automatically tokenized for sources other than conversations.
SOURCE: source of the texts.
TITLE: title of the text from which SENTENCE originates.
TOKENS: number of tokens (excluding punctuation marks) of SENTENCE.
TYPE: linguistic register of the text.
### 5.4 Data Splits
The mammut-corpus-venezuela dataset has 2 splits: train and test. Below are the statistics:
Number of Instances in Split.
Train: 2,983,302.
Test: 157,011.
## 6. Dataset Creation
### 6.1 Curation Rationale
The purpose of the mammut-corpus-venezuela dataset is language modeling. It can be used for pre-training a model from scratch or for fine-tuning on another pre-trained model.
### 6.2 Source Data
**6.2.1 Initial Data Collection and Normalization**
The data consists of opinion articles and text messages. It was collected by a process of web scraping from different portals, downloading of Telegram group chats’ history and selecting of Venezuelan and Latin-American Spanish corpus available online.
The text from the web scraping process was separated in sentences and was automatically tokenized for sources other than conversations.
An arrow parquet file was created.
Text sources: El Estímulo (website), cinco8 (website), csm-1990 (oral speaking corpus), "El atajo más largo" (blog), El Pitazo (website), La Patilla (website), Venezuelan movies subtitles, Preseea Mérida (oral speaking corpus), Prodavinci (website), Runrunes (website), and Telegram group chats.
**6.2.2 Who are the source language producers?**
The texts come from Venezuelan Spanish speakers, subtitlers, journalists, politicians, doctors, writers, and online sellers.
## 6.3 Annotations
**6.3.1 Annotation process**
At the moment the dataset does not contain any additional annotations.
**6.3.2 Who are the annotators?**
Not applicable.
### 6.4 Personal and Sensitive Information
The data is partially anonymized. Also, there are messages from Telegram selling chats, some percentage of these messages may be fake or contain misleading or offensive language.
## 7. Considerations for Using the Data
### 7.1 Social Impact of Dataset
The purpose of this dataset is to help the development of language modeling models (pre-training or fine-tuning) in Venezuelan Spanish.
### 7.2 Discussion of Biases
Most of the content comes from political, economical and sociological opinion articles. Social biases may be present.
### 7.3 Other Known Limitations
(If applicable, description of the other limitations in the data.)
Not applicable.
## 8. Additional Information
### 8.1 Dataset Curators
The data was originally collected by Lino Urdaneta and Miguel Riveros from Mammut.io.
### 8.2 Licensing Information
Not applicable.
### 8.3 Citation Information
Not applicable.
### 8.4 Contributions
Not applicable.
| mammut/mammut-corpus-venezuela | [
"task_ids:language-modeling",
"annotations_creators:no-annotation",
"language_creators:expert-generated",
"multilinguality:monolingual",
"size_categories:unknown",
"source_datasets:original",
"language:es",
"license:cc-by-nc-nd-4.0",
"region:us"
] | 2022-03-02T23:29:22+00:00 | {"annotations_creators": ["no-annotation"], "language_creators": ["expert-generated"], "language": ["es"], "license": ["cc-by-nc-nd-4.0"], "multilinguality": ["monolingual"], "size_categories": ["unknown"], "source_datasets": ["original"], "task_categories": ["sequence-modeling"], "task_ids": ["language-modeling"], "pretty_name": "mammut-corpus-venezuela", "language_bcp47": ["es-VE"]} | 2022-10-22T08:00:04+00:00 | [] | [
"es"
] | TAGS
#task_ids-language-modeling #annotations_creators-no-annotation #language_creators-expert-generated #multilinguality-monolingual #size_categories-unknown #source_datasets-original #language-Spanish #license-cc-by-nc-nd-4.0 #region-us
|
# mammut-corpus-venezuela
HuggingFace Dataset
## 1. How to use
How to load this dataset directly with the datasets library:
'>>> from datasets import load_dataset'
'>>> dataset = load_dataset("mammut-corpus-venezuela")'
## 2. Dataset Summary
mammut-corpus-venezuela is a dataset for Spanish language modeling. This dataset comprises a large number of Venezuelan and Latin-American Spanish texts, manually selected and collected in 2021. The data was collected by a process of web scraping from different portals, downloading of Telegram group chats' history, and selecting of Venezuelan and Latin-American Spanish corpus available online. The texts come from Venezuelan Spanish speakers, subtitlers, journalists, politicians, doctors, writers, and online sellers. Social biases may be present, and a percentage of the texts may be fake or contain misleading or offensive language.
Each record in the dataset contains the author of the text (anonymized for conversation authors), the date on which the text entered in the corpus, the text which was automatically tokenized at sentence level for sources other than conversations, the source of the text, the title of the text, the number of tokens (excluding punctuation marks) of the text, and the linguistic register of the text.
The dataset counts with a train split and a test split.
## 3. Supported Tasks and Leaderboards
This dataset can be used for language modeling.
## 4. Languages
The dataset contains Venezuelan and Latin-American Spanish.
## 5. Dataset Structure
Dataset structure features.
### 5.1 Data Instances
An example from the dataset:
"AUTHOR":"author in title",
"TITLE":"Luis Alberto Buttó: Hecho en socialismo",
"SENTENCE":"Históricamente, siempre fue así.",
"DATE":"2021-07-04 07:18:46.918253",
"SOURCE":"la patilla",
"TOKENS":"4",
"TYPE":"opinion/news",
The average word token count are provided below:
### 5.2 Total of tokens (no spelling marks)
Train: 92,431,194.
Test: 4,876,739 (in another file).
### 5.3 Data Fields
The data have several fields:
AUTHOR: author of the text. It is anonymized for conversation authors.
DATE: date on which the text was entered in the corpus.
SENTENCE: text. It was automatically tokenized for sources other than conversations.
SOURCE: source of the texts.
TITLE: title of the text from which SENTENCE originates.
TOKENS: number of tokens (excluding punctuation marks) of SENTENCE.
TYPE: linguistic register of the text.
### 5.4 Data Splits
The mammut-corpus-venezuela dataset has 2 splits: train and test. Below are the statistics:
Number of Instances in Split.
Train: 2,983,302.
Test: 157,011.
## 6. Dataset Creation
### 6.1 Curation Rationale
The purpose of the mammut-corpus-venezuela dataset is language modeling. It can be used for pre-training a model from scratch or for fine-tuning on another pre-trained model.
### 6.2 Source Data
6.2.1 Initial Data Collection and Normalization
The data consists of opinion articles and text messages. It was collected by a process of web scraping from different portals, downloading of Telegram group chats’ history and selecting of Venezuelan and Latin-American Spanish corpus available online.
The text from the web scraping process was separated in sentences and was automatically tokenized for sources other than conversations.
An arrow parquet file was created.
Text sources: El Estímulo (website), cinco8 (website), csm-1990 (oral speaking corpus), "El atajo más largo" (blog), El Pitazo (website), La Patilla (website), Venezuelan movies subtitles, Preseea Mérida (oral speaking corpus), Prodavinci (website), Runrunes (website), and Telegram group chats.
6.2.2 Who are the source language producers?
The texts come from Venezuelan Spanish speakers, subtitlers, journalists, politicians, doctors, writers, and online sellers.
## 6.3 Annotations
6.3.1 Annotation process
At the moment the dataset does not contain any additional annotations.
6.3.2 Who are the annotators?
Not applicable.
### 6.4 Personal and Sensitive Information
The data is partially anonymized. Also, there are messages from Telegram selling chats, some percentage of these messages may be fake or contain misleading or offensive language.
## 7. Considerations for Using the Data
### 7.1 Social Impact of Dataset
The purpose of this dataset is to help the development of language modeling models (pre-training or fine-tuning) in Venezuelan Spanish.
### 7.2 Discussion of Biases
Most of the content comes from political, economical and sociological opinion articles. Social biases may be present.
### 7.3 Other Known Limitations
(If applicable, description of the other limitations in the data.)
Not applicable.
## 8. Additional Information
### 8.1 Dataset Curators
The data was originally collected by Lino Urdaneta and Miguel Riveros from URL.
### 8.2 Licensing Information
Not applicable.
### 8.3 Citation Information
Not applicable.
### 8.4 Contributions
Not applicable.
| [
"# mammut-corpus-venezuela\n\nHuggingFace Dataset",
"## 1. How to use\n\nHow to load this dataset directly with the datasets library:\n\n'>>> from datasets import load_dataset' \n'>>> dataset = load_dataset(\"mammut-corpus-venezuela\")'",
"## 2. Dataset Summary\n\nmammut-corpus-venezuela is a dataset for Spanish language modeling. This dataset comprises a large number of Venezuelan and Latin-American Spanish texts, manually selected and collected in 2021. The data was collected by a process of web scraping from different portals, downloading of Telegram group chats' history, and selecting of Venezuelan and Latin-American Spanish corpus available online. The texts come from Venezuelan Spanish speakers, subtitlers, journalists, politicians, doctors, writers, and online sellers. Social biases may be present, and a percentage of the texts may be fake or contain misleading or offensive language.\n\nEach record in the dataset contains the author of the text (anonymized for conversation authors), the date on which the text entered in the corpus, the text which was automatically tokenized at sentence level for sources other than conversations, the source of the text, the title of the text, the number of tokens (excluding punctuation marks) of the text, and the linguistic register of the text.\n\nThe dataset counts with a train split and a test split.",
"## 3. Supported Tasks and Leaderboards\n\nThis dataset can be used for language modeling.",
"## 4. Languages\n\nThe dataset contains Venezuelan and Latin-American Spanish.",
"## 5. Dataset Structure\n\nDataset structure features.",
"### 5.1 Data Instances\n\nAn example from the dataset:\n \n \n \"AUTHOR\":\"author in title\", \n \"TITLE\":\"Luis Alberto Buttó: Hecho en socialismo\", \n \"SENTENCE\":\"Históricamente, siempre fue así.\",\n \"DATE\":\"2021-07-04 07:18:46.918253\", \n \"SOURCE\":\"la patilla\", \n \"TOKENS\":\"4\", \n \"TYPE\":\"opinion/news\", \n\n\nThe average word token count are provided below:",
"### 5.2 Total of tokens (no spelling marks)\n\nTrain: 92,431,194. \nTest: 4,876,739 (in another file).",
"### 5.3 Data Fields\n\nThe data have several fields:\n\n AUTHOR: author of the text. It is anonymized for conversation authors. \n DATE: date on which the text was entered in the corpus. \n SENTENCE: text. It was automatically tokenized for sources other than conversations. \n SOURCE: source of the texts. \n TITLE: title of the text from which SENTENCE originates. \n TOKENS: number of tokens (excluding punctuation marks) of SENTENCE. \n TYPE: linguistic register of the text.",
"### 5.4 Data Splits\n\nThe mammut-corpus-venezuela dataset has 2 splits: train and test. Below are the statistics:\n\nNumber of Instances in Split. \n\nTrain: 2,983,302. \nTest: 157,011.",
"## 6. Dataset Creation",
"### 6.1 Curation Rationale\n\nThe purpose of the mammut-corpus-venezuela dataset is language modeling. It can be used for pre-training a model from scratch or for fine-tuning on another pre-trained model.",
"### 6.2 Source Data\n\n6.2.1 Initial Data Collection and Normalization\n\nThe data consists of opinion articles and text messages. It was collected by a process of web scraping from different portals, downloading of Telegram group chats’ history and selecting of Venezuelan and Latin-American Spanish corpus available online.\n\nThe text from the web scraping process was separated in sentences and was automatically tokenized for sources other than conversations.\n\nAn arrow parquet file was created.\n\nText sources: El Estímulo (website), cinco8 (website), csm-1990 (oral speaking corpus), \"El atajo más largo\" (blog), El Pitazo (website), La Patilla (website), Venezuelan movies subtitles, Preseea Mérida (oral speaking corpus), Prodavinci (website), Runrunes (website), and Telegram group chats.\n\n6.2.2 Who are the source language producers?\n\nThe texts come from Venezuelan Spanish speakers, subtitlers, journalists, politicians, doctors, writers, and online sellers.",
"## 6.3 Annotations\n\n6.3.1 Annotation process\n\nAt the moment the dataset does not contain any additional annotations.\n\n6.3.2 Who are the annotators?\n\nNot applicable.",
"### 6.4 Personal and Sensitive Information\n\nThe data is partially anonymized. Also, there are messages from Telegram selling chats, some percentage of these messages may be fake or contain misleading or offensive language.",
"## 7. Considerations for Using the Data",
"### 7.1 Social Impact of Dataset\n\nThe purpose of this dataset is to help the development of language modeling models (pre-training or fine-tuning) in Venezuelan Spanish.",
"### 7.2 Discussion of Biases\n\nMost of the content comes from political, economical and sociological opinion articles. Social biases may be present.",
"### 7.3 Other Known Limitations\n\n(If applicable, description of the other limitations in the data.)\n\nNot applicable.",
"## 8. Additional Information",
"### 8.1 Dataset Curators\n\nThe data was originally collected by Lino Urdaneta and Miguel Riveros from URL.",
"### 8.2 Licensing Information\n\nNot applicable.",
"### 8.3 Citation Information\n\nNot applicable.",
"### 8.4 Contributions\n\nNot applicable."
] | [
"TAGS\n#task_ids-language-modeling #annotations_creators-no-annotation #language_creators-expert-generated #multilinguality-monolingual #size_categories-unknown #source_datasets-original #language-Spanish #license-cc-by-nc-nd-4.0 #region-us \n",
"# mammut-corpus-venezuela\n\nHuggingFace Dataset",
"## 1. How to use\n\nHow to load this dataset directly with the datasets library:\n\n'>>> from datasets import load_dataset' \n'>>> dataset = load_dataset(\"mammut-corpus-venezuela\")'",
"## 2. Dataset Summary\n\nmammut-corpus-venezuela is a dataset for Spanish language modeling. This dataset comprises a large number of Venezuelan and Latin-American Spanish texts, manually selected and collected in 2021. The data was collected by a process of web scraping from different portals, downloading of Telegram group chats' history, and selecting of Venezuelan and Latin-American Spanish corpus available online. The texts come from Venezuelan Spanish speakers, subtitlers, journalists, politicians, doctors, writers, and online sellers. Social biases may be present, and a percentage of the texts may be fake or contain misleading or offensive language.\n\nEach record in the dataset contains the author of the text (anonymized for conversation authors), the date on which the text entered in the corpus, the text which was automatically tokenized at sentence level for sources other than conversations, the source of the text, the title of the text, the number of tokens (excluding punctuation marks) of the text, and the linguistic register of the text.\n\nThe dataset counts with a train split and a test split.",
"## 3. Supported Tasks and Leaderboards\n\nThis dataset can be used for language modeling.",
"## 4. Languages\n\nThe dataset contains Venezuelan and Latin-American Spanish.",
"## 5. Dataset Structure\n\nDataset structure features.",
"### 5.1 Data Instances\n\nAn example from the dataset:\n \n \n \"AUTHOR\":\"author in title\", \n \"TITLE\":\"Luis Alberto Buttó: Hecho en socialismo\", \n \"SENTENCE\":\"Históricamente, siempre fue así.\",\n \"DATE\":\"2021-07-04 07:18:46.918253\", \n \"SOURCE\":\"la patilla\", \n \"TOKENS\":\"4\", \n \"TYPE\":\"opinion/news\", \n\n\nThe average word token count are provided below:",
"### 5.2 Total of tokens (no spelling marks)\n\nTrain: 92,431,194. \nTest: 4,876,739 (in another file).",
"### 5.3 Data Fields\n\nThe data have several fields:\n\n AUTHOR: author of the text. It is anonymized for conversation authors. \n DATE: date on which the text was entered in the corpus. \n SENTENCE: text. It was automatically tokenized for sources other than conversations. \n SOURCE: source of the texts. \n TITLE: title of the text from which SENTENCE originates. \n TOKENS: number of tokens (excluding punctuation marks) of SENTENCE. \n TYPE: linguistic register of the text.",
"### 5.4 Data Splits\n\nThe mammut-corpus-venezuela dataset has 2 splits: train and test. Below are the statistics:\n\nNumber of Instances in Split. \n\nTrain: 2,983,302. \nTest: 157,011.",
"## 6. Dataset Creation",
"### 6.1 Curation Rationale\n\nThe purpose of the mammut-corpus-venezuela dataset is language modeling. It can be used for pre-training a model from scratch or for fine-tuning on another pre-trained model.",
"### 6.2 Source Data\n\n6.2.1 Initial Data Collection and Normalization\n\nThe data consists of opinion articles and text messages. It was collected by a process of web scraping from different portals, downloading of Telegram group chats’ history and selecting of Venezuelan and Latin-American Spanish corpus available online.\n\nThe text from the web scraping process was separated in sentences and was automatically tokenized for sources other than conversations.\n\nAn arrow parquet file was created.\n\nText sources: El Estímulo (website), cinco8 (website), csm-1990 (oral speaking corpus), \"El atajo más largo\" (blog), El Pitazo (website), La Patilla (website), Venezuelan movies subtitles, Preseea Mérida (oral speaking corpus), Prodavinci (website), Runrunes (website), and Telegram group chats.\n\n6.2.2 Who are the source language producers?\n\nThe texts come from Venezuelan Spanish speakers, subtitlers, journalists, politicians, doctors, writers, and online sellers.",
"## 6.3 Annotations\n\n6.3.1 Annotation process\n\nAt the moment the dataset does not contain any additional annotations.\n\n6.3.2 Who are the annotators?\n\nNot applicable.",
"### 6.4 Personal and Sensitive Information\n\nThe data is partially anonymized. Also, there are messages from Telegram selling chats, some percentage of these messages may be fake or contain misleading or offensive language.",
"## 7. Considerations for Using the Data",
"### 7.1 Social Impact of Dataset\n\nThe purpose of this dataset is to help the development of language modeling models (pre-training or fine-tuning) in Venezuelan Spanish.",
"### 7.2 Discussion of Biases\n\nMost of the content comes from political, economical and sociological opinion articles. Social biases may be present.",
"### 7.3 Other Known Limitations\n\n(If applicable, description of the other limitations in the data.)\n\nNot applicable.",
"## 8. Additional Information",
"### 8.1 Dataset Curators\n\nThe data was originally collected by Lino Urdaneta and Miguel Riveros from URL.",
"### 8.2 Licensing Information\n\nNot applicable.",
"### 8.3 Citation Information\n\nNot applicable.",
"### 8.4 Contributions\n\nNot applicable."
] |
530b6fdc040b2f9286024f546f5d6a63572fc3b2 | import data from dataset | manishk31/Demo | [
"region:us"
] | 2022-03-02T23:29:22+00:00 | {} | 2021-11-29T08:23:08+00:00 | [] | [] | TAGS
#region-us
| import data from dataset | [] | [
"TAGS\n#region-us \n"
] |
f7332122c1a298c8b46313e1c5b97358de30b46b | "This database was created by Nordic Language Technology for the development of automatic speech recognition and dictation in Swedish. In this updated version, the organization of the data have been altered to improve the usefulness of the database.
In the original version of the material, the files were organized in a specific folder structure where the folder names were meaningful. However, the file names were not meaningful, and there were also cases of files with identical names in different folders. This proved to be impractical, since users had to keep the original folder structure in order to use the data. The files have been renamed, such that the file names are unique and meaningful regardless of the folder structure. The original metadata files were in spl format. These have been converted to JSON format. The converted metadata files are also anonymized and the text encoding has been converted from ANSI to UTF-8.
See the documentation file for a full description of the data and the changes made to the database." - dataset originally available at https://www.nb.no/sprakbanken/en/resource-catalogue/oai-nb-no-sbr-54/
Full documentation in english available at https://www.nb.no/sbfil/talegjenkjenning/16kHz_2020/no_2020/no-16khz_reorganized_english.pdf
In 🤗 datasets, this dataset will have a structure similar to common_voice. TO BE UPDATED. | marinone94/nst_no | [
"region:us"
] | 2022-03-02T23:29:22+00:00 | {} | 2022-02-04T23:04:16+00:00 | [] | [] | TAGS
#region-us
| "This database was created by Nordic Language Technology for the development of automatic speech recognition and dictation in Swedish. In this updated version, the organization of the data have been altered to improve the usefulness of the database.
In the original version of the material, the files were organized in a specific folder structure where the folder names were meaningful. However, the file names were not meaningful, and there were also cases of files with identical names in different folders. This proved to be impractical, since users had to keep the original folder structure in order to use the data. The files have been renamed, such that the file names are unique and meaningful regardless of the folder structure. The original metadata files were in spl format. These have been converted to JSON format. The converted metadata files are also anonymized and the text encoding has been converted from ANSI to UTF-8.
See the documentation file for a full description of the data and the changes made to the database." - dataset originally available at URL
Full documentation in english available at URL
In datasets, this dataset will have a structure similar to common_voice. TO BE UPDATED. | [] | [
"TAGS\n#region-us \n"
] |
81fb7d117be7ffeffc660de0b33f7a4614e13ac0 | "This database was created by Nordic Language Technology for the development of automatic speech recognition and dictation in Swedish. In this updated version, the organization of the data have been altered to improve the usefulness of the database.
In the original version of the material, the files were organized in a specific folder structure where the folder names were meaningful. However, the file names were not meaningful, and there were also cases of files with identical names in different folders. This proved to be impractical, since users had to keep the original folder structure in order to use the data. The files have been renamed, such that the file names are unique and meaningful regardless of the folder structure. The original metadata files were in spl format. These have been converted to JSON format. The converted metadata files are also anonymized and the text encoding has been converted from ANSI to UTF-8.
See the documentation file for a full description of the data and the changes made to the database." - dataset originally available at https://www.nb.no/sprakbanken/en/resource-catalogue/oai-nb-no-sbr-56/
In 🤗 datasets, this dataset will have a structure similar to common_voice. TO BE UPDATED. | marinone94/nst_sv | [
"region:us"
] | 2022-03-02T23:29:22+00:00 | {} | 2022-05-22T15:25:46+00:00 | [] | [] | TAGS
#region-us
| "This database was created by Nordic Language Technology for the development of automatic speech recognition and dictation in Swedish. In this updated version, the organization of the data have been altered to improve the usefulness of the database.
In the original version of the material, the files were organized in a specific folder structure where the folder names were meaningful. However, the file names were not meaningful, and there were also cases of files with identical names in different folders. This proved to be impractical, since users had to keep the original folder structure in order to use the data. The files have been renamed, such that the file names are unique and meaningful regardless of the folder structure. The original metadata files were in spl format. These have been converted to JSON format. The converted metadata files are also anonymized and the text encoding has been converted from ANSI to UTF-8.
See the documentation file for a full description of the data and the changes made to the database." - dataset originally available at URL
In datasets, this dataset will have a structure similar to common_voice. TO BE UPDATED. | [] | [
"TAGS\n#region-us \n"
] |
44b28e1e4a5213852da5fcbdf41b8ba29a085d20 | # Dataset Card for "dummy_test"
[More Information needed](https://github.com/huggingface/datasets/blob/main/CONTRIBUTING.md#how-to-contribute-to-the-dataset-cards) | mariosasko/dummy_test | [
"region:us"
] | 2022-03-02T23:29:22+00:00 | {"dataset_info": {"features": [{"name": "a", "dtype": "int64"}, {"name": "b", "dtype": "bool"}], "splits": [{"name": "train", "num_bytes": 81250, "num_examples": 10000}], "download_size": 58757, "dataset_size": 81250}, "configs": [{"config_name": "default", "data_files": [{"split": "train", "path": "data/train-*"}]}]} | 2023-11-02T18:23:02+00:00 | [] | [] | TAGS
#region-us
| # Dataset Card for "dummy_test"
More Information needed | [
"# Dataset Card for \"dummy_test\"\n\nMore Information needed"
] | [
"TAGS\n#region-us \n",
"# Dataset Card for \"dummy_test\"\n\nMore Information needed"
] |
8127852d5534bf35a46d9353a055c8ee5dbb46e9 | annotations_creators:
- machine-generated
language_creators:
- machine-generated
languages:
- english
licenses:
- unknown
multilinguality:
- monolingual
pretty_name: This dataset contains issues from Hugging Face Github
size_categories:
- unknown
source_datasets:
- original
task_categories:
- text-classification
- text-retrieval
task_ids:
- multi-class-classification
- multi-label-classification
- document-retrieval | matteopilotto/github-issues | [
"region:us"
] | 2022-03-02T23:29:22+00:00 | {} | 2022-02-12T18:39:01+00:00 | [] | [] | TAGS
#region-us
| annotations_creators:
- machine-generated
language_creators:
- machine-generated
languages:
- english
licenses:
- unknown
multilinguality:
- monolingual
pretty_name: This dataset contains issues from Hugging Face Github
size_categories:
- unknown
source_datasets:
- original
task_categories:
- text-classification
- text-retrieval
task_ids:
- multi-class-classification
- multi-label-classification
- document-retrieval | [] | [
"TAGS\n#region-us \n"
] |
319ff9de80c03bdea535b4838c6041161e35b84b | TRSAv1 (Turkish Sentiment Analysis Version 1) Dataset
This data set has been produced to contribute to Turkish NLP studies.
The dataset consists of a total of 150 thousand samples, 50 thousand negative, 50 thousand positive and 50 thousand neutral.
It can be used in text classification and sentiment analysis studies by citing the related study.
Related Work
Aydoğan M, Kocaman V. TRSAv1: A new benchmark dataset for classifying user reviews on Turkish e-commerce websites. Journal of Information Science. February 2022. doi:10.1177/01655515221074328 | maydogan/TRSAv1 | [
"region:us"
] | 2022-03-02T23:29:22+00:00 | {} | 2022-02-24T12:36:52+00:00 | [] | [] | TAGS
#region-us
| TRSAv1 (Turkish Sentiment Analysis Version 1) Dataset
This data set has been produced to contribute to Turkish NLP studies.
The dataset consists of a total of 150 thousand samples, 50 thousand negative, 50 thousand positive and 50 thousand neutral.
It can be used in text classification and sentiment analysis studies by citing the related study.
Related Work
Aydoğan M, Kocaman V. TRSAv1: A new benchmark dataset for classifying user reviews on Turkish e-commerce websites. Journal of Information Science. February 2022. doi:10.1177/01655515221074328 | [] | [
"TAGS\n#region-us \n"
] |
92ceb811a149c3f0d306fa2a184fd82859556060 | # Dataset Card for GitHub Issues
## Dataset Description
- **Point of Contact:** [Michael Bateman]([email protected])
### Dataset Summary
GitHub Issues is a dataset consisting of GitHub issues and pull requests associated with the 🤗 Datasets [repository](https://github.com/huggingface/datasets). It is intended for educational purposes and can be used for semantic search or multilabel text classification. The contents of each GitHub issue are in English and concern the domain of datasets for NLP, computer vision, and beyond.
### Supported Tasks and Leaderboards
For each of the tasks tagged for this dataset, give a brief description of the tag, metrics, and suggested models (with a link to their HuggingFace implementation if available). Give a similar description of tasks that were not covered by the structured tag set (repace the `task-category-tag` with an appropriate `other:other-task-name`).
- `task-category-tag`: The dataset can be used to train a model for [TASK NAME], which consists in [TASK DESCRIPTION]. Success on this task is typically measured by achieving a *high/low* [metric name](https://huggingface.co/metrics/metric_name). The ([model name](https://huggingface.co/model_name) or [model class](https://huggingface.co/transformers/model_doc/model_class.html)) model currently achieves the following score. *[IF A LEADERBOARD IS AVAILABLE]:* This task has an active leaderboard which can be found at [leaderboard url]() and ranks models based on [metric name](https://huggingface.co/metrics/metric_name) while also reporting [other metric name](https://huggingface.co/metrics/other_metric_name).
### Languages
Provide a brief overview of the languages represented in the dataset. Describe relevant details about specifics of the language such as whether it is social media text, African American English,...
When relevant, please provide [BCP-47 codes](https://tools.ietf.org/html/bcp47), which consist of a [primary language subtag](https://tools.ietf.org/html/bcp47#section-2.2.1), with a [script subtag](https://tools.ietf.org/html/bcp47#section-2.2.3) and/or [region subtag](https://tools.ietf.org/html/bcp47#section-2.2.4) if available.
## Dataset Structure
### Data Instances
Provide an JSON-formatted example and brief description of a typical instance in the dataset. If available, provide a link to further examples.
```
{
'example_field': ...,
...
}
```
Provide any additional information that is not covered in the other sections about the data here. In particular describe any relationships between data points and if these relationships are made explicit.
### Data Fields
List and describe the fields present in the dataset. Mention their data type, and whether they are used as input or output in any of the tasks the dataset currently supports. If the data has span indices, describe their attributes, such as whether they are at the character level or word level, whether they are contiguous or not, etc. If the datasets contains example IDs, state whether they have an inherent meaning, such as a mapping to other datasets or pointing to relationships between data points.
- `example_field`: description of `example_field`
Note that the descriptions can be initialized with the **Show Markdown Data Fields** output of the [tagging app](https://github.com/huggingface/datasets-tagging), you will then only need to refine the generated descriptions.
### Data Splits
Describe and name the splits in the dataset if there are more than one.
Describe any criteria for splitting the data, if used. If their are differences between the splits (e.g. if the training annotations are machine-generated and the dev and test ones are created by humans, or if different numbers of annotators contributed to each example), describe them here.
Provide the sizes of each split. As appropriate, provide any descriptive statistics for the features, such as average length. For example:
| | Tain | Valid | Test |
| ----- | ------ | ----- | ---- |
| Input Sentences | | | |
| Average Sentence Length | | | |
## Dataset Creation
### Curation Rationale
What need motivated the creation of this dataset? What are some of the reasons underlying the major choices involved in putting it together?
### Source Data
This section describes the source data (e.g. news text and headlines, social media posts, translated sentences,...)
#### Initial Data Collection and Normalization
Describe the data collection process. Describe any criteria for data selection or filtering. List any key words or search terms used. If possible, include runtime information for the collection process.
If data was collected from other pre-existing datasets, link to source here and to their [Hugging Face version](https://huggingface.co/datasets/dataset_name).
If the data was modified or normalized after being collected (e.g. if the data is word-tokenized), describe the process and the tools used.
#### Who are the source language producers?
State whether the data was produced by humans or machine generated. Describe the people or systems who originally created the data.
If available, include self-reported demographic or identity information for the source data creators, but avoid inferring this information. Instead state that this information is unknown. See [Larson 2017](https://www.aclweb.org/anthology/W17-1601.pdf) for using identity categories as a variables, particularly gender.
Describe the conditions under which the data was created (for example, if the producers were crowdworkers, state what platform was used, or if the data was found, what website the data was found on). If compensation was provided, include that information here.
Describe other people represented or mentioned in the data. Where possible, link to references for the information.
### Annotations
If the dataset contains annotations which are not part of the initial data collection, describe them in the following paragraphs.
#### Annotation process
If applicable, describe the annotation process and any tools used, or state otherwise. Describe the amount of data annotated, if not all. Describe or reference annotation guidelines provided to the annotators. If available, provide interannotator statistics. Describe any annotation validation processes.
#### Who are the annotators?
If annotations were collected for the source data (such as class labels or syntactic parses), state whether the annotations were produced by humans or machine generated.
Describe the people or systems who originally created the annotations and their selection criteria if applicable.
If available, include self-reported demographic or identity information for the annotators, but avoid inferring this information. Instead state that this information is unknown. See [Larson 2017](https://www.aclweb.org/anthology/W17-1601.pdf) for using identity categories as a variables, particularly gender.
Describe the conditions under which the data was annotated (for example, if the annotators were crowdworkers, state what platform was used, or if the data was found, what website the data was found on). If compensation was provided, include that information here.
### Personal and Sensitive Information
State whether the dataset uses identity categories and, if so, how the information is used. Describe where this information comes from (i.e. self-reporting, collecting from profiles, inferring, etc.). See [Larson 2017](https://www.aclweb.org/anthology/W17-1601.pdf) for using identity categories as a variables, particularly gender. State whether the data is linked to individuals and whether those individuals can be identified in the dataset, either directly or indirectly (i.e., in combination with other data).
State whether the dataset contains other data that might be considered sensitive (e.g., data that reveals racial or ethnic origins, sexual orientations, religious beliefs, political opinions or union memberships, or locations; financial or health data; biometric or genetic data; forms of government identification, such as social security numbers; criminal history).
If efforts were made to anonymize the data, describe the anonymization process.
## Considerations for Using the Data
### Social Impact of Dataset
Please discuss some of the ways you believe the use of this dataset will impact society.
The statement should include both positive outlooks, such as outlining how technologies developed through its use may improve people's lives, and discuss the accompanying risks. These risks may range from making important decisions more opaque to people who are affected by the technology, to reinforcing existing harmful biases (whose specifics should be discussed in the next section), among other considerations.
Also describe in this section if the proposed dataset contains a low-resource or under-represented language. If this is the case or if this task has any impact on underserved communities, please elaborate here.
### Discussion of Biases
Provide descriptions of specific biases that are likely to be reflected in the data, and state whether any steps were taken to reduce their impact.
For Wikipedia text, see for example [Dinan et al 2020 on biases in Wikipedia (esp. Table 1)](https://arxiv.org/abs/2005.00614), or [Blodgett et al 2020](https://www.aclweb.org/anthology/2020.acl-main.485/) for a more general discussion of the topic.
If analyses have been run quantifying these biases, please add brief summaries and links to the studies here.
### Other Known Limitations
If studies of the datasets have outlined other limitations of the dataset, such as annotation artifacts, please outline and cite them here.
## Additional Information
### Dataset Curators
List the people involved in collecting the dataset and their affiliation(s). If funding information is known, include it here.
### Licensing Information
Provide the license and link to the license webpage if available.
### Citation Information
Provide the [BibTex](http://www.bibtex.org/)-formatted reference for the dataset. For example:
```
@article{article_id,
author = {Author List},
title = {Dataset Paper Title},
journal = {Publication Venue},
year = {2525}
}
```
If the dataset has a [DOI](https://www.doi.org/), please provide it here.
### Contributions
Thanks to [@mbateman](https://github.com/mbateman) for adding this dataset. | mbateman/github-issues | [
"arxiv:2005.00614",
"region:us"
] | 2022-03-02T23:29:22+00:00 | {} | 2021-12-09T17:56:11+00:00 | [
"2005.00614"
] | [] | TAGS
#arxiv-2005.00614 #region-us
| Dataset Card for GitHub Issues
==============================
Dataset Description
-------------------
* Point of Contact: Michael Bateman
### Dataset Summary
GitHub Issues is a dataset consisting of GitHub issues and pull requests associated with the 🤗 Datasets repository. It is intended for educational purposes and can be used for semantic search or multilabel text classification. The contents of each GitHub issue are in English and concern the domain of datasets for NLP, computer vision, and beyond.
### Supported Tasks and Leaderboards
For each of the tasks tagged for this dataset, give a brief description of the tag, metrics, and suggested models (with a link to their HuggingFace implementation if available). Give a similar description of tasks that were not covered by the structured tag set (repace the 'task-category-tag' with an appropriate 'other:other-task-name').
* 'task-category-tag': The dataset can be used to train a model for [TASK NAME], which consists in [TASK DESCRIPTION]. Success on this task is typically measured by achieving a *high/low* metric name. The (model name or model class) model currently achieves the following score. *[IF A LEADERBOARD IS AVAILABLE]:* This task has an active leaderboard which can be found at leaderboard url and ranks models based on metric name while also reporting other metric name.
### Languages
Provide a brief overview of the languages represented in the dataset. Describe relevant details about specifics of the language such as whether it is social media text, African American English,...
When relevant, please provide BCP-47 codes, which consist of a primary language subtag, with a script subtag and/or region subtag if available.
Dataset Structure
-----------------
### Data Instances
Provide an JSON-formatted example and brief description of a typical instance in the dataset. If available, provide a link to further examples.
Provide any additional information that is not covered in the other sections about the data here. In particular describe any relationships between data points and if these relationships are made explicit.
### Data Fields
List and describe the fields present in the dataset. Mention their data type, and whether they are used as input or output in any of the tasks the dataset currently supports. If the data has span indices, describe their attributes, such as whether they are at the character level or word level, whether they are contiguous or not, etc. If the datasets contains example IDs, state whether they have an inherent meaning, such as a mapping to other datasets or pointing to relationships between data points.
* 'example\_field': description of 'example\_field'
Note that the descriptions can be initialized with the Show Markdown Data Fields output of the tagging app, you will then only need to refine the generated descriptions.
### Data Splits
Describe and name the splits in the dataset if there are more than one.
Describe any criteria for splitting the data, if used. If their are differences between the splits (e.g. if the training annotations are machine-generated and the dev and test ones are created by humans, or if different numbers of annotators contributed to each example), describe them here.
Provide the sizes of each split. As appropriate, provide any descriptive statistics for the features, such as average length. For example:
Dataset Creation
----------------
### Curation Rationale
What need motivated the creation of this dataset? What are some of the reasons underlying the major choices involved in putting it together?
### Source Data
This section describes the source data (e.g. news text and headlines, social media posts, translated sentences,...)
#### Initial Data Collection and Normalization
Describe the data collection process. Describe any criteria for data selection or filtering. List any key words or search terms used. If possible, include runtime information for the collection process.
If data was collected from other pre-existing datasets, link to source here and to their Hugging Face version.
If the data was modified or normalized after being collected (e.g. if the data is word-tokenized), describe the process and the tools used.
#### Who are the source language producers?
State whether the data was produced by humans or machine generated. Describe the people or systems who originally created the data.
If available, include self-reported demographic or identity information for the source data creators, but avoid inferring this information. Instead state that this information is unknown. See Larson 2017 for using identity categories as a variables, particularly gender.
Describe the conditions under which the data was created (for example, if the producers were crowdworkers, state what platform was used, or if the data was found, what website the data was found on). If compensation was provided, include that information here.
Describe other people represented or mentioned in the data. Where possible, link to references for the information.
### Annotations
If the dataset contains annotations which are not part of the initial data collection, describe them in the following paragraphs.
#### Annotation process
If applicable, describe the annotation process and any tools used, or state otherwise. Describe the amount of data annotated, if not all. Describe or reference annotation guidelines provided to the annotators. If available, provide interannotator statistics. Describe any annotation validation processes.
#### Who are the annotators?
If annotations were collected for the source data (such as class labels or syntactic parses), state whether the annotations were produced by humans or machine generated.
Describe the people or systems who originally created the annotations and their selection criteria if applicable.
If available, include self-reported demographic or identity information for the annotators, but avoid inferring this information. Instead state that this information is unknown. See Larson 2017 for using identity categories as a variables, particularly gender.
Describe the conditions under which the data was annotated (for example, if the annotators were crowdworkers, state what platform was used, or if the data was found, what website the data was found on). If compensation was provided, include that information here.
### Personal and Sensitive Information
State whether the dataset uses identity categories and, if so, how the information is used. Describe where this information comes from (i.e. self-reporting, collecting from profiles, inferring, etc.). See Larson 2017 for using identity categories as a variables, particularly gender. State whether the data is linked to individuals and whether those individuals can be identified in the dataset, either directly or indirectly (i.e., in combination with other data).
State whether the dataset contains other data that might be considered sensitive (e.g., data that reveals racial or ethnic origins, sexual orientations, religious beliefs, political opinions or union memberships, or locations; financial or health data; biometric or genetic data; forms of government identification, such as social security numbers; criminal history).
If efforts were made to anonymize the data, describe the anonymization process.
Considerations for Using the Data
---------------------------------
### Social Impact of Dataset
Please discuss some of the ways you believe the use of this dataset will impact society.
The statement should include both positive outlooks, such as outlining how technologies developed through its use may improve people's lives, and discuss the accompanying risks. These risks may range from making important decisions more opaque to people who are affected by the technology, to reinforcing existing harmful biases (whose specifics should be discussed in the next section), among other considerations.
Also describe in this section if the proposed dataset contains a low-resource or under-represented language. If this is the case or if this task has any impact on underserved communities, please elaborate here.
### Discussion of Biases
Provide descriptions of specific biases that are likely to be reflected in the data, and state whether any steps were taken to reduce their impact.
For Wikipedia text, see for example Dinan et al 2020 on biases in Wikipedia (esp. Table 1), or Blodgett et al 2020 for a more general discussion of the topic.
If analyses have been run quantifying these biases, please add brief summaries and links to the studies here.
### Other Known Limitations
If studies of the datasets have outlined other limitations of the dataset, such as annotation artifacts, please outline and cite them here.
Additional Information
----------------------
### Dataset Curators
List the people involved in collecting the dataset and their affiliation(s). If funding information is known, include it here.
### Licensing Information
Provide the license and link to the license webpage if available.
Provide the BibTex-formatted reference for the dataset. For example:
If the dataset has a DOI, please provide it here.
### Contributions
Thanks to @mbateman for adding this dataset.
| [
"### Dataset Summary\n\n\nGitHub Issues is a dataset consisting of GitHub issues and pull requests associated with the 🤗 Datasets repository. It is intended for educational purposes and can be used for semantic search or multilabel text classification. The contents of each GitHub issue are in English and concern the domain of datasets for NLP, computer vision, and beyond.",
"### Supported Tasks and Leaderboards\n\n\nFor each of the tasks tagged for this dataset, give a brief description of the tag, metrics, and suggested models (with a link to their HuggingFace implementation if available). Give a similar description of tasks that were not covered by the structured tag set (repace the 'task-category-tag' with an appropriate 'other:other-task-name').\n\n\n* 'task-category-tag': The dataset can be used to train a model for [TASK NAME], which consists in [TASK DESCRIPTION]. Success on this task is typically measured by achieving a *high/low* metric name. The (model name or model class) model currently achieves the following score. *[IF A LEADERBOARD IS AVAILABLE]:* This task has an active leaderboard which can be found at leaderboard url and ranks models based on metric name while also reporting other metric name.",
"### Languages\n\n\nProvide a brief overview of the languages represented in the dataset. Describe relevant details about specifics of the language such as whether it is social media text, African American English,...\n\n\nWhen relevant, please provide BCP-47 codes, which consist of a primary language subtag, with a script subtag and/or region subtag if available.\n\n\nDataset Structure\n-----------------",
"### Data Instances\n\n\nProvide an JSON-formatted example and brief description of a typical instance in the dataset. If available, provide a link to further examples.\n\n\nProvide any additional information that is not covered in the other sections about the data here. In particular describe any relationships between data points and if these relationships are made explicit.",
"### Data Fields\n\n\nList and describe the fields present in the dataset. Mention their data type, and whether they are used as input or output in any of the tasks the dataset currently supports. If the data has span indices, describe their attributes, such as whether they are at the character level or word level, whether they are contiguous or not, etc. If the datasets contains example IDs, state whether they have an inherent meaning, such as a mapping to other datasets or pointing to relationships between data points.\n\n\n* 'example\\_field': description of 'example\\_field'\n\n\nNote that the descriptions can be initialized with the Show Markdown Data Fields output of the tagging app, you will then only need to refine the generated descriptions.",
"### Data Splits\n\n\nDescribe and name the splits in the dataset if there are more than one.\n\n\nDescribe any criteria for splitting the data, if used. If their are differences between the splits (e.g. if the training annotations are machine-generated and the dev and test ones are created by humans, or if different numbers of annotators contributed to each example), describe them here.\n\n\nProvide the sizes of each split. As appropriate, provide any descriptive statistics for the features, such as average length. For example:\n\n\n\nDataset Creation\n----------------",
"### Curation Rationale\n\n\nWhat need motivated the creation of this dataset? What are some of the reasons underlying the major choices involved in putting it together?",
"### Source Data\n\n\nThis section describes the source data (e.g. news text and headlines, social media posts, translated sentences,...)",
"#### Initial Data Collection and Normalization\n\n\nDescribe the data collection process. Describe any criteria for data selection or filtering. List any key words or search terms used. If possible, include runtime information for the collection process.\n\n\nIf data was collected from other pre-existing datasets, link to source here and to their Hugging Face version.\n\n\nIf the data was modified or normalized after being collected (e.g. if the data is word-tokenized), describe the process and the tools used.",
"#### Who are the source language producers?\n\n\nState whether the data was produced by humans or machine generated. Describe the people or systems who originally created the data.\n\n\nIf available, include self-reported demographic or identity information for the source data creators, but avoid inferring this information. Instead state that this information is unknown. See Larson 2017 for using identity categories as a variables, particularly gender.\n\n\nDescribe the conditions under which the data was created (for example, if the producers were crowdworkers, state what platform was used, or if the data was found, what website the data was found on). If compensation was provided, include that information here.\n\n\nDescribe other people represented or mentioned in the data. Where possible, link to references for the information.",
"### Annotations\n\n\nIf the dataset contains annotations which are not part of the initial data collection, describe them in the following paragraphs.",
"#### Annotation process\n\n\nIf applicable, describe the annotation process and any tools used, or state otherwise. Describe the amount of data annotated, if not all. Describe or reference annotation guidelines provided to the annotators. If available, provide interannotator statistics. Describe any annotation validation processes.",
"#### Who are the annotators?\n\n\nIf annotations were collected for the source data (such as class labels or syntactic parses), state whether the annotations were produced by humans or machine generated.\n\n\nDescribe the people or systems who originally created the annotations and their selection criteria if applicable.\n\n\nIf available, include self-reported demographic or identity information for the annotators, but avoid inferring this information. Instead state that this information is unknown. See Larson 2017 for using identity categories as a variables, particularly gender.\n\n\nDescribe the conditions under which the data was annotated (for example, if the annotators were crowdworkers, state what platform was used, or if the data was found, what website the data was found on). If compensation was provided, include that information here.",
"### Personal and Sensitive Information\n\n\nState whether the dataset uses identity categories and, if so, how the information is used. Describe where this information comes from (i.e. self-reporting, collecting from profiles, inferring, etc.). See Larson 2017 for using identity categories as a variables, particularly gender. State whether the data is linked to individuals and whether those individuals can be identified in the dataset, either directly or indirectly (i.e., in combination with other data).\n\n\nState whether the dataset contains other data that might be considered sensitive (e.g., data that reveals racial or ethnic origins, sexual orientations, religious beliefs, political opinions or union memberships, or locations; financial or health data; biometric or genetic data; forms of government identification, such as social security numbers; criminal history).\n\n\nIf efforts were made to anonymize the data, describe the anonymization process.\n\n\nConsiderations for Using the Data\n---------------------------------",
"### Social Impact of Dataset\n\n\nPlease discuss some of the ways you believe the use of this dataset will impact society.\n\n\nThe statement should include both positive outlooks, such as outlining how technologies developed through its use may improve people's lives, and discuss the accompanying risks. These risks may range from making important decisions more opaque to people who are affected by the technology, to reinforcing existing harmful biases (whose specifics should be discussed in the next section), among other considerations.\n\n\nAlso describe in this section if the proposed dataset contains a low-resource or under-represented language. If this is the case or if this task has any impact on underserved communities, please elaborate here.",
"### Discussion of Biases\n\n\nProvide descriptions of specific biases that are likely to be reflected in the data, and state whether any steps were taken to reduce their impact.\n\n\nFor Wikipedia text, see for example Dinan et al 2020 on biases in Wikipedia (esp. Table 1), or Blodgett et al 2020 for a more general discussion of the topic.\n\n\nIf analyses have been run quantifying these biases, please add brief summaries and links to the studies here.",
"### Other Known Limitations\n\n\nIf studies of the datasets have outlined other limitations of the dataset, such as annotation artifacts, please outline and cite them here.\n\n\nAdditional Information\n----------------------",
"### Dataset Curators\n\n\nList the people involved in collecting the dataset and their affiliation(s). If funding information is known, include it here.",
"### Licensing Information\n\n\nProvide the license and link to the license webpage if available.\n\n\nProvide the BibTex-formatted reference for the dataset. For example:\n\n\nIf the dataset has a DOI, please provide it here.",
"### Contributions\n\n\nThanks to @mbateman for adding this dataset."
] | [
"TAGS\n#arxiv-2005.00614 #region-us \n",
"### Dataset Summary\n\n\nGitHub Issues is a dataset consisting of GitHub issues and pull requests associated with the 🤗 Datasets repository. It is intended for educational purposes and can be used for semantic search or multilabel text classification. The contents of each GitHub issue are in English and concern the domain of datasets for NLP, computer vision, and beyond.",
"### Supported Tasks and Leaderboards\n\n\nFor each of the tasks tagged for this dataset, give a brief description of the tag, metrics, and suggested models (with a link to their HuggingFace implementation if available). Give a similar description of tasks that were not covered by the structured tag set (repace the 'task-category-tag' with an appropriate 'other:other-task-name').\n\n\n* 'task-category-tag': The dataset can be used to train a model for [TASK NAME], which consists in [TASK DESCRIPTION]. Success on this task is typically measured by achieving a *high/low* metric name. The (model name or model class) model currently achieves the following score. *[IF A LEADERBOARD IS AVAILABLE]:* This task has an active leaderboard which can be found at leaderboard url and ranks models based on metric name while also reporting other metric name.",
"### Languages\n\n\nProvide a brief overview of the languages represented in the dataset. Describe relevant details about specifics of the language such as whether it is social media text, African American English,...\n\n\nWhen relevant, please provide BCP-47 codes, which consist of a primary language subtag, with a script subtag and/or region subtag if available.\n\n\nDataset Structure\n-----------------",
"### Data Instances\n\n\nProvide an JSON-formatted example and brief description of a typical instance in the dataset. If available, provide a link to further examples.\n\n\nProvide any additional information that is not covered in the other sections about the data here. In particular describe any relationships between data points and if these relationships are made explicit.",
"### Data Fields\n\n\nList and describe the fields present in the dataset. Mention their data type, and whether they are used as input or output in any of the tasks the dataset currently supports. If the data has span indices, describe their attributes, such as whether they are at the character level or word level, whether they are contiguous or not, etc. If the datasets contains example IDs, state whether they have an inherent meaning, such as a mapping to other datasets or pointing to relationships between data points.\n\n\n* 'example\\_field': description of 'example\\_field'\n\n\nNote that the descriptions can be initialized with the Show Markdown Data Fields output of the tagging app, you will then only need to refine the generated descriptions.",
"### Data Splits\n\n\nDescribe and name the splits in the dataset if there are more than one.\n\n\nDescribe any criteria for splitting the data, if used. If their are differences between the splits (e.g. if the training annotations are machine-generated and the dev and test ones are created by humans, or if different numbers of annotators contributed to each example), describe them here.\n\n\nProvide the sizes of each split. As appropriate, provide any descriptive statistics for the features, such as average length. For example:\n\n\n\nDataset Creation\n----------------",
"### Curation Rationale\n\n\nWhat need motivated the creation of this dataset? What are some of the reasons underlying the major choices involved in putting it together?",
"### Source Data\n\n\nThis section describes the source data (e.g. news text and headlines, social media posts, translated sentences,...)",
"#### Initial Data Collection and Normalization\n\n\nDescribe the data collection process. Describe any criteria for data selection or filtering. List any key words or search terms used. If possible, include runtime information for the collection process.\n\n\nIf data was collected from other pre-existing datasets, link to source here and to their Hugging Face version.\n\n\nIf the data was modified or normalized after being collected (e.g. if the data is word-tokenized), describe the process and the tools used.",
"#### Who are the source language producers?\n\n\nState whether the data was produced by humans or machine generated. Describe the people or systems who originally created the data.\n\n\nIf available, include self-reported demographic or identity information for the source data creators, but avoid inferring this information. Instead state that this information is unknown. See Larson 2017 for using identity categories as a variables, particularly gender.\n\n\nDescribe the conditions under which the data was created (for example, if the producers were crowdworkers, state what platform was used, or if the data was found, what website the data was found on). If compensation was provided, include that information here.\n\n\nDescribe other people represented or mentioned in the data. Where possible, link to references for the information.",
"### Annotations\n\n\nIf the dataset contains annotations which are not part of the initial data collection, describe them in the following paragraphs.",
"#### Annotation process\n\n\nIf applicable, describe the annotation process and any tools used, or state otherwise. Describe the amount of data annotated, if not all. Describe or reference annotation guidelines provided to the annotators. If available, provide interannotator statistics. Describe any annotation validation processes.",
"#### Who are the annotators?\n\n\nIf annotations were collected for the source data (such as class labels or syntactic parses), state whether the annotations were produced by humans or machine generated.\n\n\nDescribe the people or systems who originally created the annotations and their selection criteria if applicable.\n\n\nIf available, include self-reported demographic or identity information for the annotators, but avoid inferring this information. Instead state that this information is unknown. See Larson 2017 for using identity categories as a variables, particularly gender.\n\n\nDescribe the conditions under which the data was annotated (for example, if the annotators were crowdworkers, state what platform was used, or if the data was found, what website the data was found on). If compensation was provided, include that information here.",
"### Personal and Sensitive Information\n\n\nState whether the dataset uses identity categories and, if so, how the information is used. Describe where this information comes from (i.e. self-reporting, collecting from profiles, inferring, etc.). See Larson 2017 for using identity categories as a variables, particularly gender. State whether the data is linked to individuals and whether those individuals can be identified in the dataset, either directly or indirectly (i.e., in combination with other data).\n\n\nState whether the dataset contains other data that might be considered sensitive (e.g., data that reveals racial or ethnic origins, sexual orientations, religious beliefs, political opinions or union memberships, or locations; financial or health data; biometric or genetic data; forms of government identification, such as social security numbers; criminal history).\n\n\nIf efforts were made to anonymize the data, describe the anonymization process.\n\n\nConsiderations for Using the Data\n---------------------------------",
"### Social Impact of Dataset\n\n\nPlease discuss some of the ways you believe the use of this dataset will impact society.\n\n\nThe statement should include both positive outlooks, such as outlining how technologies developed through its use may improve people's lives, and discuss the accompanying risks. These risks may range from making important decisions more opaque to people who are affected by the technology, to reinforcing existing harmful biases (whose specifics should be discussed in the next section), among other considerations.\n\n\nAlso describe in this section if the proposed dataset contains a low-resource or under-represented language. If this is the case or if this task has any impact on underserved communities, please elaborate here.",
"### Discussion of Biases\n\n\nProvide descriptions of specific biases that are likely to be reflected in the data, and state whether any steps were taken to reduce their impact.\n\n\nFor Wikipedia text, see for example Dinan et al 2020 on biases in Wikipedia (esp. Table 1), or Blodgett et al 2020 for a more general discussion of the topic.\n\n\nIf analyses have been run quantifying these biases, please add brief summaries and links to the studies here.",
"### Other Known Limitations\n\n\nIf studies of the datasets have outlined other limitations of the dataset, such as annotation artifacts, please outline and cite them here.\n\n\nAdditional Information\n----------------------",
"### Dataset Curators\n\n\nList the people involved in collecting the dataset and their affiliation(s). If funding information is known, include it here.",
"### Licensing Information\n\n\nProvide the license and link to the license webpage if available.\n\n\nProvide the BibTex-formatted reference for the dataset. For example:\n\n\nIf the dataset has a DOI, please provide it here.",
"### Contributions\n\n\nThanks to @mbateman for adding this dataset."
] |
ba7ba09357e05b2d2f1cc0d45dcb92c9005b9264 |
# Dataset Card for [Dataset Name]
## Table of Contents
- [Table of Contents](#table-of-contents)
- [Dataset Description](#dataset-description)
- [Dataset Summary](#dataset-summary)
- [Supported Tasks and Leaderboards](#supported-tasks-and-leaderboards)
- [Languages](#languages)
- [Dataset Structure](#dataset-structure)
- [Data Instances](#data-instances)
- [Data Fields](#data-fields)
- [Data Splits](#data-splits)
## Dataset Description
- **Homepage:**
- **Repository:**
- **Paper:**
- **Leaderboard:**
- **Point of Contact:**
### Dataset Summary
[More Information Needed]
### Supported Tasks and Leaderboards
[More Information Needed]
### Languages
[More Information Needed]
## Dataset Structure
### Data Instances
[More Information Needed]
### Data Fields
[More Information Needed]
### Data Splits
[More Information Needed]
## Licensing information
Academic Free License v1.2. | meghanabhange/hilm141021 | [
"annotations_creators:other",
"language_creators:other",
"multilinguality:monolingual",
"size_categories:unknown",
"source_datasets:original",
"language:hi",
"license:other",
"region:us"
] | 2022-03-02T23:29:22+00:00 | {"annotations_creators": ["other"], "language_creators": ["other"], "language": ["hi"], "license": ["other"], "multilinguality": ["monolingual"], "size_categories": ["unknown"], "source_datasets": ["original"], "task_categories": ["conditional-text-generation"], "task_ids": ["conditional-text-generation-other-next-word-prediction"], "pretty_name": "Hindi Language Modelling"} | 2022-10-20T17:37:30+00:00 | [] | [
"hi"
] | TAGS
#annotations_creators-other #language_creators-other #multilinguality-monolingual #size_categories-unknown #source_datasets-original #language-Hindi #license-other #region-us
|
# Dataset Card for [Dataset Name]
## Table of Contents
- Table of Contents
- Dataset Description
- Dataset Summary
- Supported Tasks and Leaderboards
- Languages
- Dataset Structure
- Data Instances
- Data Fields
- Data Splits
## Dataset Description
- Homepage:
- Repository:
- Paper:
- Leaderboard:
- Point of Contact:
### Dataset Summary
### Supported Tasks and Leaderboards
### Languages
## Dataset Structure
### Data Instances
### Data Fields
### Data Splits
## Licensing information
Academic Free License v1.2. | [
"# Dataset Card for [Dataset Name]",
"## Table of Contents\n- Table of Contents\n- Dataset Description\n - Dataset Summary\n - Supported Tasks and Leaderboards\n - Languages\n- Dataset Structure\n - Data Instances\n - Data Fields\n - Data Splits",
"## Dataset Description\n\n- Homepage:\n- Repository:\n- Paper:\n- Leaderboard:\n- Point of Contact:",
"### Dataset Summary",
"### Supported Tasks and Leaderboards",
"### Languages",
"## Dataset Structure",
"### Data Instances",
"### Data Fields",
"### Data Splits",
"## Licensing information\n\nAcademic Free License v1.2."
] | [
"TAGS\n#annotations_creators-other #language_creators-other #multilinguality-monolingual #size_categories-unknown #source_datasets-original #language-Hindi #license-other #region-us \n",
"# Dataset Card for [Dataset Name]",
"## Table of Contents\n- Table of Contents\n- Dataset Description\n - Dataset Summary\n - Supported Tasks and Leaderboards\n - Languages\n- Dataset Structure\n - Data Instances\n - Data Fields\n - Data Splits",
"## Dataset Description\n\n- Homepage:\n- Repository:\n- Paper:\n- Leaderboard:\n- Point of Contact:",
"### Dataset Summary",
"### Supported Tasks and Leaderboards",
"### Languages",
"## Dataset Structure",
"### Data Instances",
"### Data Fields",
"### Data Splits",
"## Licensing information\n\nAcademic Free License v1.2."
] |
42ae7d0b95cafd6594877fa555000b8708ea1706 |
# Dataset Card for [Dataset Name]
## Table of Contents
- [Table of Contents](#table-of-contents)
- [Dataset Description](#dataset-description)
- [Dataset Summary](#dataset-summary)
- [Supported Tasks and Leaderboards](#supported-tasks-and-leaderboards)
- [Languages](#languages)
- [Dataset Structure](#dataset-structure)
- [Data Instances](#data-instances)
- [Data Fields](#data-fields)
- [Data Splits](#data-splits)
## Dataset Description
- **Homepage:**
- **Repository:**
- **Paper:**
- **Leaderboard:**
- **Point of Contact:**
### Dataset Summary
[More Information Needed]
### Supported Tasks and Leaderboards
[More Information Needed]
### Languages
[More Information Needed]
## Dataset Structure
### Data Instances
[More Information Needed]
### Data Fields
[More Information Needed]
### Data Splits
[More Information Needed]
# Licensing information
Academic Free License v1.2.
| meghanabhange/hitalm141021 | [
"annotations_creators:other",
"language_creators:other",
"multilinguality:multilingual",
"size_categories:unknown",
"source_datasets:original",
"language:hi",
"language:ta",
"license:other",
"region:us"
] | 2022-03-02T23:29:22+00:00 | {"annotations_creators": ["other"], "language_creators": ["other"], "language": ["hi", "ta"], "license": ["other"], "multilinguality": ["multilingual"], "size_categories": ["unknown"], "source_datasets": ["original"], "task_categories": ["conditional-text-generation"], "task_ids": ["conditional-text-generation-other-next-word-prediction"], "pretty_name": "Hindi Language Modelling"} | 2022-10-20T17:39:07+00:00 | [] | [
"hi",
"ta"
] | TAGS
#annotations_creators-other #language_creators-other #multilinguality-multilingual #size_categories-unknown #source_datasets-original #language-Hindi #language-Tamil #license-other #region-us
|
# Dataset Card for [Dataset Name]
## Table of Contents
- Table of Contents
- Dataset Description
- Dataset Summary
- Supported Tasks and Leaderboards
- Languages
- Dataset Structure
- Data Instances
- Data Fields
- Data Splits
## Dataset Description
- Homepage:
- Repository:
- Paper:
- Leaderboard:
- Point of Contact:
### Dataset Summary
### Supported Tasks and Leaderboards
### Languages
## Dataset Structure
### Data Instances
### Data Fields
### Data Splits
# Licensing information
Academic Free License v1.2.
| [
"# Dataset Card for [Dataset Name]",
"## Table of Contents\n- Table of Contents\n- Dataset Description\n - Dataset Summary\n - Supported Tasks and Leaderboards\n - Languages\n- Dataset Structure\n - Data Instances\n - Data Fields\n - Data Splits",
"## Dataset Description\n\n- Homepage:\n- Repository:\n- Paper:\n- Leaderboard:\n- Point of Contact:",
"### Dataset Summary",
"### Supported Tasks and Leaderboards",
"### Languages",
"## Dataset Structure",
"### Data Instances",
"### Data Fields",
"### Data Splits",
"# Licensing information\n\nAcademic Free License v1.2."
] | [
"TAGS\n#annotations_creators-other #language_creators-other #multilinguality-multilingual #size_categories-unknown #source_datasets-original #language-Hindi #language-Tamil #license-other #region-us \n",
"# Dataset Card for [Dataset Name]",
"## Table of Contents\n- Table of Contents\n- Dataset Description\n - Dataset Summary\n - Supported Tasks and Leaderboards\n - Languages\n- Dataset Structure\n - Data Instances\n - Data Fields\n - Data Splits",
"## Dataset Description\n\n- Homepage:\n- Repository:\n- Paper:\n- Leaderboard:\n- Point of Contact:",
"### Dataset Summary",
"### Supported Tasks and Leaderboards",
"### Languages",
"## Dataset Structure",
"### Data Instances",
"### Data Fields",
"### Data Splits",
"# Licensing information\n\nAcademic Free License v1.2."
] |
753f1cdf7b14f086c7c4b9e2e5f9df829a5545fe |
# Dataset Card for [Dataset Name]
## Table of Contents
- [Table of Contents](#table-of-contents)
- [Dataset Description](#dataset-description)
- [Dataset Summary](#dataset-summary)
- [Supported Tasks and Leaderboards](#supported-tasks-and-leaderboards)
- [Languages](#languages)
- [Dataset Structure](#dataset-structure)
- [Data Instances](#data-instances)
- [Data Fields](#data-fields)
- [Data Splits](#data-splits)
## Dataset Description
- **Homepage:**
- **Repository:**
- **Paper:**
- **Leaderboard:**
- **Point of Contact:**
### Dataset Summary
[More Information Needed]
### Supported Tasks and Leaderboards
[More Information Needed]
### Languages
[More Information Needed]
## Dataset Structure
### Data Instances
[More Information Needed]
### Data Fields
[More Information Needed]
### Data Splits
[More Information Needed]
## Licensing information
Academic Free License v1.2. | meghanabhange/talm141021 | [
"annotations_creators:other",
"language_creators:other",
"multilinguality:monolingual",
"size_categories:unknown",
"source_datasets:original",
"language:ta",
"license:other",
"region:us"
] | 2022-03-02T23:29:22+00:00 | {"annotations_creators": ["other"], "language_creators": ["other"], "language": ["ta"], "license": ["other"], "multilinguality": ["monolingual"], "size_categories": ["unknown"], "source_datasets": ["original"], "task_categories": ["conditional-text-generation"], "task_ids": ["conditional-text-generation-other-next-word-prediction"], "pretty_name": "Hindi Language Modelling"} | 2022-10-20T17:40:30+00:00 | [] | [
"ta"
] | TAGS
#annotations_creators-other #language_creators-other #multilinguality-monolingual #size_categories-unknown #source_datasets-original #language-Tamil #license-other #region-us
|
# Dataset Card for [Dataset Name]
## Table of Contents
- Table of Contents
- Dataset Description
- Dataset Summary
- Supported Tasks and Leaderboards
- Languages
- Dataset Structure
- Data Instances
- Data Fields
- Data Splits
## Dataset Description
- Homepage:
- Repository:
- Paper:
- Leaderboard:
- Point of Contact:
### Dataset Summary
### Supported Tasks and Leaderboards
### Languages
## Dataset Structure
### Data Instances
### Data Fields
### Data Splits
## Licensing information
Academic Free License v1.2. | [
"# Dataset Card for [Dataset Name]",
"## Table of Contents\n- Table of Contents\n- Dataset Description\n - Dataset Summary\n - Supported Tasks and Leaderboards\n - Languages\n- Dataset Structure\n - Data Instances\n - Data Fields\n - Data Splits",
"## Dataset Description\n\n- Homepage:\n- Repository:\n- Paper:\n- Leaderboard:\n- Point of Contact:",
"### Dataset Summary",
"### Supported Tasks and Leaderboards",
"### Languages",
"## Dataset Structure",
"### Data Instances",
"### Data Fields",
"### Data Splits",
"## Licensing information\n\nAcademic Free License v1.2."
] | [
"TAGS\n#annotations_creators-other #language_creators-other #multilinguality-monolingual #size_categories-unknown #source_datasets-original #language-Tamil #license-other #region-us \n",
"# Dataset Card for [Dataset Name]",
"## Table of Contents\n- Table of Contents\n- Dataset Description\n - Dataset Summary\n - Supported Tasks and Leaderboards\n - Languages\n- Dataset Structure\n - Data Instances\n - Data Fields\n - Data Splits",
"## Dataset Description\n\n- Homepage:\n- Repository:\n- Paper:\n- Leaderboard:\n- Point of Contact:",
"### Dataset Summary",
"### Supported Tasks and Leaderboards",
"### Languages",
"## Dataset Structure",
"### Data Instances",
"### Data Fields",
"### Data Splits",
"## Licensing information\n\nAcademic Free License v1.2."
] |
1b80bcb297e13e5582c37dd38d6c74de07a5f22d | Link to original dataset is [https://sites.pitt.edu/~dash/folktexts.html](here).
Link to merged and cleaned version is [https://www.kaggle.com/cuddlefish/fairy-tales?select=merged_clean.txt](here)
annotations_creators:
- found
language_creators:
- found
languages:
- en
licenses:
- cc0-1.0
multilinguality:
- monolingual
pretty_name: Folklore and Mythology Electronic Texts
size_categories:
- unknown
# Dataset Card for folk-mythology-tales
## Table of Contents
- [Dataset Description](#dataset-description)
- [Dataset Summary](#dataset-summary)
- [Supported Tasks](#supported-tasks-and-leaderboards)
- [Languages](#languages)
- [Dataset Structure](#dataset-structure)
- [Data Instances](#data-instances)
- [Data Fields](#data-instances)
- [Data Splits](#data-instances)
- [Dataset Creation](#dataset-creation)
- [Curation Rationale](#curation-rationale)
- [Source Data](#source-data)
- [Annotations](#annotations)
- [Personal and Sensitive Information](#personal-and-sensitive-information)
- [Considerations for Using the Data](#considerations-for-using-the-data)
- [Social Impact of Dataset](#social-impact-of-dataset)
- [Discussion of Biases](#discussion-of-biases)
- [Other Known Limitations](#other-known-limitations)
- [Additional Information](#additional-information)
- [Dataset Curators](#dataset-curators)
- [Licensing Information](#licensing-information)
- [Citation Information](#citation-information)
## Dataset Description
- **Homepage:** https://sites.pitt.edu/~dash/folktexts.html
- **Repository:** https://www.kaggle.com/cuddlefish/fairy-tales?select=merged_clean.txt
- **Paper:** [Needs More Information]
- **Leaderboard:** [Needs More Information]
- **Point of Contact:** [Needs More Information]
### Dataset Summary
Folklore and Mythology Electronic Texts dataset, original dataset is found [https://sites.pitt.edu/~dash/folktexts.html](here)
### Supported Tasks and Leaderboards
[Needs More Information]
### Languages
en
## Dataset Structure
### Data Instances
Plain text with no json structure
### Data Fields
No fields
### Data Splits
Only training set
## Dataset Creation
### Curation Rationale
[Needs More Information]
### Source Data
#### Initial Data Collection and Normalization
[Needs More Information]
#### Who are the source language producers?
[Needs More Information]
### Annotations
#### Annotation process
[Needs More Information]
#### Who are the annotators?
[Needs More Information]
### Personal and Sensitive Information
[Needs More Information]
## Considerations for Using the Data
### Social Impact of Dataset
[Needs More Information]
### Discussion of Biases
[Needs More Information]
### Other Known Limitations
[Needs More Information]
## Additional Information
### Dataset Curators
[Needs More Information]
### Licensing Information
[Needs More Information]
### Citation Information
[Needs More Information]---
annotations_creators:
- found
language_creators:
- found
languages:
- en
licenses:
- cc0-1.0
multilinguality:
- monolingual
pretty_name: Folklore and Mythology Electronic Texts
size_categories:
- unknown
source_datasets: []
task_categories: []
task_ids: []
--- | merve/folk-mythology-tales | [
"region:us"
] | 2022-03-02T23:29:22+00:00 | {} | 2021-09-27T09:10:03+00:00 | [] | [] | TAGS
#region-us
| Link to original dataset is URL
Link to merged and cleaned version is URL
annotations_creators:
- found
language_creators:
- found
languages:
- en
licenses:
- cc0-1.0
multilinguality:
- monolingual
pretty_name: Folklore and Mythology Electronic Texts
size_categories:
- unknown
# Dataset Card for folk-mythology-tales
## Table of Contents
- Dataset Description
- Dataset Summary
- Supported Tasks
- Languages
- Dataset Structure
- Data Instances
- Data Fields
- Data Splits
- Dataset Creation
- Curation Rationale
- Source Data
- Annotations
- Personal and Sensitive Information
- Considerations for Using the Data
- Social Impact of Dataset
- Discussion of Biases
- Other Known Limitations
- Additional Information
- Dataset Curators
- Licensing Information
- Citation Information
## Dataset Description
- Homepage: URL
- Repository: URL
- Paper:
- Leaderboard:
- Point of Contact:
### Dataset Summary
Folklore and Mythology Electronic Texts dataset, original dataset is found URL
### Supported Tasks and Leaderboards
### Languages
en
## Dataset Structure
### Data Instances
Plain text with no json structure
### Data Fields
No fields
### Data Splits
Only training set
## Dataset Creation
### Curation Rationale
### Source Data
#### Initial Data Collection and Normalization
#### Who are the source language producers?
### Annotations
#### Annotation process
#### Who are the annotators?
### Personal and Sensitive Information
## Considerations for Using the Data
### Social Impact of Dataset
### Discussion of Biases
### Other Known Limitations
## Additional Information
### Dataset Curators
### Licensing Information
---
annotations_creators:
- found
language_creators:
- found
languages:
- en
licenses:
- cc0-1.0
multilinguality:
- monolingual
pretty_name: Folklore and Mythology Electronic Texts
size_categories:
- unknown
source_datasets: []
task_categories: []
task_ids: []
--- | [
"# Dataset Card for folk-mythology-tales",
"## Table of Contents\n- Dataset Description\n - Dataset Summary\n - Supported Tasks\n - Languages\n- Dataset Structure\n - Data Instances\n - Data Fields\n - Data Splits\n- Dataset Creation\n - Curation Rationale\n - Source Data\n - Annotations\n - Personal and Sensitive Information\n- Considerations for Using the Data\n - Social Impact of Dataset\n - Discussion of Biases\n - Other Known Limitations\n- Additional Information\n - Dataset Curators\n - Licensing Information\n - Citation Information",
"## Dataset Description\n\n- Homepage: URL\n- Repository: URL\n- Paper: \n- Leaderboard: \n- Point of Contact:",
"### Dataset Summary\n\nFolklore and Mythology Electronic Texts dataset, original dataset is found URL",
"### Supported Tasks and Leaderboards",
"### Languages\n\nen",
"## Dataset Structure",
"### Data Instances\n\nPlain text with no json structure",
"### Data Fields\n\nNo fields",
"### Data Splits\n\nOnly training set",
"## Dataset Creation",
"### Curation Rationale",
"### Source Data",
"#### Initial Data Collection and Normalization",
"#### Who are the source language producers?",
"### Annotations",
"#### Annotation process",
"#### Who are the annotators?",
"### Personal and Sensitive Information",
"## Considerations for Using the Data",
"### Social Impact of Dataset",
"### Discussion of Biases",
"### Other Known Limitations",
"## Additional Information",
"### Dataset Curators",
"### Licensing Information\n\n\n\n\n\n---\nannotations_creators:\n- found\nlanguage_creators:\n- found\nlanguages:\n- en\nlicenses:\n- cc0-1.0\nmultilinguality:\n- monolingual\npretty_name: Folklore and Mythology Electronic Texts\nsize_categories:\n- unknown\nsource_datasets: []\ntask_categories: []\ntask_ids: []\n---"
] | [
"TAGS\n#region-us \n",
"# Dataset Card for folk-mythology-tales",
"## Table of Contents\n- Dataset Description\n - Dataset Summary\n - Supported Tasks\n - Languages\n- Dataset Structure\n - Data Instances\n - Data Fields\n - Data Splits\n- Dataset Creation\n - Curation Rationale\n - Source Data\n - Annotations\n - Personal and Sensitive Information\n- Considerations for Using the Data\n - Social Impact of Dataset\n - Discussion of Biases\n - Other Known Limitations\n- Additional Information\n - Dataset Curators\n - Licensing Information\n - Citation Information",
"## Dataset Description\n\n- Homepage: URL\n- Repository: URL\n- Paper: \n- Leaderboard: \n- Point of Contact:",
"### Dataset Summary\n\nFolklore and Mythology Electronic Texts dataset, original dataset is found URL",
"### Supported Tasks and Leaderboards",
"### Languages\n\nen",
"## Dataset Structure",
"### Data Instances\n\nPlain text with no json structure",
"### Data Fields\n\nNo fields",
"### Data Splits\n\nOnly training set",
"## Dataset Creation",
"### Curation Rationale",
"### Source Data",
"#### Initial Data Collection and Normalization",
"#### Who are the source language producers?",
"### Annotations",
"#### Annotation process",
"#### Who are the annotators?",
"### Personal and Sensitive Information",
"## Considerations for Using the Data",
"### Social Impact of Dataset",
"### Discussion of Biases",
"### Other Known Limitations",
"## Additional Information",
"### Dataset Curators",
"### Licensing Information\n\n\n\n\n\n---\nannotations_creators:\n- found\nlanguage_creators:\n- found\nlanguages:\n- en\nlicenses:\n- cc0-1.0\nmultilinguality:\n- monolingual\npretty_name: Folklore and Mythology Electronic Texts\nsize_categories:\n- unknown\nsource_datasets: []\ntask_categories: []\ntask_ids: []\n---"
] |
956ae34eaf5d5086454b0667c7aa441fdd1fe0f7 | # Dataset Card for poetry
## Table of Contents
- [Dataset Description](#dataset-description)
- [Dataset Summary](#dataset-summary)
- [Supported Tasks](#supported-tasks-and-leaderboards)
- [Languages](#languages)
- [Dataset Structure](#dataset-structure)
- [Data Instances](#data-instances)
- [Data Fields](#data-instances)
- [Data Splits](#data-instances)
- [Dataset Creation](#dataset-creation)
- [Curation Rationale](#curation-rationale)
- [Source Data](#source-data)
- [Annotations](#annotations)
- [Personal and Sensitive Information](#personal-and-sensitive-information)
- [Considerations for Using the Data](#considerations-for-using-the-data)
- [Social Impact of Dataset](#social-impact-of-dataset)
- [Discussion of Biases](#discussion-of-biases)
- [Other Known Limitations](#other-known-limitations)
- [Additional Information](#additional-information)
- [Dataset Curators](#dataset-curators)
- [Licensing Information](#licensing-information)
- [Citation Information](#citation-information)
## Dataset Description
- **Homepage:** poetryfoundation.com
- **Repository:** https://www.kaggle.com/ishnoor/poetry-analysis-with-machine-learning
- **Paper:** [Needs More Information]
- **Leaderboard:** [Needs More Information]
- **Point of Contact:** [Needs More Information]
### Dataset Summary
It contains poems from subjects: Love, Nature and Mythology & Folklore that belong to two periods namely Renaissance and Modern
### Supported Tasks and Leaderboards
[Needs More Information]
### Languages
[Needs More Information]
## Dataset Structure
### Data Instances
[Needs More Information]
### Data Fields
Has 5 columns:
- Content
- Author
- Poem name
- Age
- Type
### Data Splits
Only training set
## Dataset Creation
### Curation Rationale
[Needs More Information]
### Source Data
#### Initial Data Collection and Normalization
[Needs More Information]
#### Who are the source language producers?
[Needs More Information]
### Annotations
#### Annotation process
[Needs More Information]
#### Who are the annotators?
[Needs More Information]
### Personal and Sensitive Information
[Needs More Information]
## Considerations for Using the Data
### Social Impact of Dataset
[Needs More Information]
### Discussion of Biases
[Needs More Information]
### Other Known Limitations
[Needs More Information]
## Additional Information
### Dataset Curators
[Needs More Information]
### Licensing Information
[Needs More Information]
### Citation Information
[Needs More Information]
---
annotations_creators:
- found
language_creators:
- found
language:
- en
license:
- unknown
multilinguality:
- monolingual
pretty_name: poetry
size_categories:
- unknown
source_datasets:
- original
task_categories:
- text-classification
task_ids: []
--- | merve/poetry | [
"region:us"
] | 2022-03-02T23:29:22+00:00 | {} | 2022-10-25T08:50:55+00:00 | [] | [] | TAGS
#region-us
| # Dataset Card for poetry
## Table of Contents
- Dataset Description
- Dataset Summary
- Supported Tasks
- Languages
- Dataset Structure
- Data Instances
- Data Fields
- Data Splits
- Dataset Creation
- Curation Rationale
- Source Data
- Annotations
- Personal and Sensitive Information
- Considerations for Using the Data
- Social Impact of Dataset
- Discussion of Biases
- Other Known Limitations
- Additional Information
- Dataset Curators
- Licensing Information
- Citation Information
## Dataset Description
- Homepage: URL
- Repository: URL
- Paper:
- Leaderboard:
- Point of Contact:
### Dataset Summary
It contains poems from subjects: Love, Nature and Mythology & Folklore that belong to two periods namely Renaissance and Modern
### Supported Tasks and Leaderboards
### Languages
## Dataset Structure
### Data Instances
### Data Fields
Has 5 columns:
- Content
- Author
- Poem name
- Age
- Type
### Data Splits
Only training set
## Dataset Creation
### Curation Rationale
### Source Data
#### Initial Data Collection and Normalization
#### Who are the source language producers?
### Annotations
#### Annotation process
#### Who are the annotators?
### Personal and Sensitive Information
## Considerations for Using the Data
### Social Impact of Dataset
### Discussion of Biases
### Other Known Limitations
## Additional Information
### Dataset Curators
### Licensing Information
---
annotations_creators:
- found
language_creators:
- found
language:
- en
license:
- unknown
multilinguality:
- monolingual
pretty_name: poetry
size_categories:
- unknown
source_datasets:
- original
task_categories:
- text-classification
task_ids: []
--- | [
"# Dataset Card for poetry",
"## Table of Contents\n- Dataset Description\n - Dataset Summary\n - Supported Tasks\n - Languages\n- Dataset Structure\n - Data Instances\n - Data Fields\n - Data Splits\n- Dataset Creation\n - Curation Rationale\n - Source Data\n - Annotations\n - Personal and Sensitive Information\n- Considerations for Using the Data\n - Social Impact of Dataset\n - Discussion of Biases\n - Other Known Limitations\n- Additional Information\n - Dataset Curators\n - Licensing Information\n - Citation Information",
"## Dataset Description\n\n- Homepage: URL\n- Repository: URL\n- Paper: \n- Leaderboard: \n- Point of Contact:",
"### Dataset Summary\n\nIt contains poems from subjects: Love, Nature and Mythology & Folklore that belong to two periods namely Renaissance and Modern",
"### Supported Tasks and Leaderboards",
"### Languages",
"## Dataset Structure",
"### Data Instances",
"### Data Fields\n\nHas 5 columns:\n- Content\n- Author\n- Poem name\n- Age\n- Type",
"### Data Splits\n\nOnly training set",
"## Dataset Creation",
"### Curation Rationale",
"### Source Data",
"#### Initial Data Collection and Normalization",
"#### Who are the source language producers?",
"### Annotations",
"#### Annotation process",
"#### Who are the annotators?",
"### Personal and Sensitive Information",
"## Considerations for Using the Data",
"### Social Impact of Dataset",
"### Discussion of Biases",
"### Other Known Limitations",
"## Additional Information",
"### Dataset Curators",
"### Licensing Information\n\n\n\n\n\n\n\n---\nannotations_creators:\n- found\nlanguage_creators:\n- found\nlanguage:\n- en\nlicense:\n- unknown\nmultilinguality:\n- monolingual\npretty_name: poetry\nsize_categories:\n- unknown\nsource_datasets:\n- original\ntask_categories:\n- text-classification\ntask_ids: []\n---"
] | [
"TAGS\n#region-us \n",
"# Dataset Card for poetry",
"## Table of Contents\n- Dataset Description\n - Dataset Summary\n - Supported Tasks\n - Languages\n- Dataset Structure\n - Data Instances\n - Data Fields\n - Data Splits\n- Dataset Creation\n - Curation Rationale\n - Source Data\n - Annotations\n - Personal and Sensitive Information\n- Considerations for Using the Data\n - Social Impact of Dataset\n - Discussion of Biases\n - Other Known Limitations\n- Additional Information\n - Dataset Curators\n - Licensing Information\n - Citation Information",
"## Dataset Description\n\n- Homepage: URL\n- Repository: URL\n- Paper: \n- Leaderboard: \n- Point of Contact:",
"### Dataset Summary\n\nIt contains poems from subjects: Love, Nature and Mythology & Folklore that belong to two periods namely Renaissance and Modern",
"### Supported Tasks and Leaderboards",
"### Languages",
"## Dataset Structure",
"### Data Instances",
"### Data Fields\n\nHas 5 columns:\n- Content\n- Author\n- Poem name\n- Age\n- Type",
"### Data Splits\n\nOnly training set",
"## Dataset Creation",
"### Curation Rationale",
"### Source Data",
"#### Initial Data Collection and Normalization",
"#### Who are the source language producers?",
"### Annotations",
"#### Annotation process",
"#### Who are the annotators?",
"### Personal and Sensitive Information",
"## Considerations for Using the Data",
"### Social Impact of Dataset",
"### Discussion of Biases",
"### Other Known Limitations",
"## Additional Information",
"### Dataset Curators",
"### Licensing Information\n\n\n\n\n\n\n\n---\nannotations_creators:\n- found\nlanguage_creators:\n- found\nlanguage:\n- en\nlicense:\n- unknown\nmultilinguality:\n- monolingual\npretty_name: poetry\nsize_categories:\n- unknown\nsource_datasets:\n- original\ntask_categories:\n- text-classification\ntask_ids: []\n---"
] |
340b7b6ca58a930b5d2ffa0c4f25f05055085168 |
Blimp with the coarse categories and recasted as a classification task (Cola format). | tasksource/blimp_classification | [
"task_categories:text-classification",
"task_ids:acceptability-classification",
"size_categories:10K<n<100K",
"language:en",
"license:apache-2.0",
"cola",
"region:us"
] | 2022-03-02T23:29:22+00:00 | {"language": ["en"], "license": "apache-2.0", "size_categories": ["10K<n<100K"], "task_categories": ["text-classification"], "task_ids": ["acceptability-classification"], "tags": ["cola"]} | 2023-01-09T10:50:25+00:00 | [] | [
"en"
] | TAGS
#task_categories-text-classification #task_ids-acceptability-classification #size_categories-10K<n<100K #language-English #license-apache-2.0 #cola #region-us
|
Blimp with the coarse categories and recasted as a classification task (Cola format). | [] | [
"TAGS\n#task_categories-text-classification #task_ids-acceptability-classification #size_categories-10K<n<100K #language-English #license-apache-2.0 #cola #region-us \n"
] |
6d2c046fd8032a11c5779deb27d2c1880297dac9 |
```
@inproceedings{van2012designing,
title={Designing a scalable crowdsourcing platform},
author={Van Pelt, Chris and Sorokin, Alex},
booktitle={Proceedings of the 2012 ACM SIGMOD International Conference on Management of Data},
pages={765--766},
year={2012}
}
``` | tasksource/crowdflower | [
"task_categories:text-classification",
"task_ids:sentiment-classification",
"task_ids:fact-checking",
"annotations_creators:crowdsourced",
"language_creators:crowdsourced",
"multilinguality:monolingual",
"size_categories:unknown",
"language:en",
"region:us"
] | 2022-03-02T23:29:22+00:00 | {"annotations_creators": ["crowdsourced"], "language_creators": ["crowdsourced"], "language": ["en"], "license": [], "multilinguality": ["monolingual"], "size_categories": ["unknown"], "source_datasets": [], "task_categories": ["text-classification"], "task_ids": ["sentiment-classification", "fact-checking"], "pretty_name": "ethics", "tags": []} | 2023-06-21T11:50:08+00:00 | [] | [
"en"
] | TAGS
#task_categories-text-classification #task_ids-sentiment-classification #task_ids-fact-checking #annotations_creators-crowdsourced #language_creators-crowdsourced #multilinguality-monolingual #size_categories-unknown #language-English #region-us
| [] | [
"TAGS\n#task_categories-text-classification #task_ids-sentiment-classification #task_ids-fact-checking #annotations_creators-crowdsourced #language_creators-crowdsourced #multilinguality-monolingual #size_categories-unknown #language-English #region-us \n"
] |
|
1d762d62f0b8f28d1699af28efd177131935e472 | https://github.com/hendrycks/ethics | metaeval/ethics | [
"task_categories:text-classification",
"annotations_creators:crowdsourced",
"language_creators:crowdsourced",
"multilinguality:monolingual",
"size_categories:unknown",
"language:en",
"region:us"
] | 2022-03-02T23:29:22+00:00 | {"annotations_creators": ["crowdsourced"], "language_creators": ["crowdsourced"], "language": ["en"], "license": [], "multilinguality": ["monolingual"], "size_categories": ["unknown"], "source_datasets": [], "task_categories": ["text-classification"], "task_ids": [], "pretty_name": "ethics", "tags": []} | 2023-06-02T13:45:34+00:00 | [] | [
"en"
] | TAGS
#task_categories-text-classification #annotations_creators-crowdsourced #language_creators-crowdsourced #multilinguality-monolingual #size_categories-unknown #language-English #region-us
| URL | [] | [
"TAGS\n#task_categories-text-classification #annotations_creators-crowdsourced #language_creators-crowdsourced #multilinguality-monolingual #size_categories-unknown #language-English #region-us \n"
] |
aec774df2787114414cc592d17e3c7953d1e4a56 |
http://decomp.io/ | tasksource/recast | [
"task_categories:text-classification",
"task_ids:natural-language-inference",
"annotations_creators:expert-generated",
"language_creators:crowdsourced",
"multilinguality:monolingual",
"size_categories:1K<n<10K",
"source_datasets:original",
"language:en",
"license:apache-2.0",
"nli",
"natural-language-inference",
"region:us"
] | 2022-03-02T23:29:22+00:00 | {"annotations_creators": ["expert-generated"], "language_creators": ["crowdsourced"], "language": ["en"], "license": ["apache-2.0"], "multilinguality": ["monolingual"], "size_categories": ["1K<n<10K"], "source_datasets": ["original"], "task_categories": ["text-classification"], "task_ids": ["natural-language-inference"], "pretty_name": "recast_nli", "tags": ["nli", "natural-language-inference"]} | 2023-06-02T13:40:17+00:00 | [] | [
"en"
] | TAGS
#task_categories-text-classification #task_ids-natural-language-inference #annotations_creators-expert-generated #language_creators-crowdsourced #multilinguality-monolingual #size_categories-1K<n<10K #source_datasets-original #language-English #license-apache-2.0 #nli #natural-language-inference #region-us
|
URL | [] | [
"TAGS\n#task_categories-text-classification #task_ids-natural-language-inference #annotations_creators-expert-generated #language_creators-crowdsourced #multilinguality-monolingual #size_categories-1K<n<10K #source_datasets-original #language-English #license-apache-2.0 #nli #natural-language-inference #region-us \n"
] |
cfc353d7b4acc4bdd16759b076d1176758454460 | ## Dataset Summary
A dataset for benchmarking keyphrase extraction and generation techniques from long document english scientific articles. For more details about the dataset please refer the original paper - [https://aclanthology.org/D09-1137/](https://aclanthology.org/D09-1137/)
Original source of the data - []()
## Dataset Structure
### Data Fields
- **id**: unique identifier of the document.
- **document**: Whitespace separated list of words in the document.
- **doc_bio_tags**: BIO tags for each word in the document. B stands for the beginning of a keyphrase and I stands for inside the keyphrase. O stands for outside the keyphrase and represents the word that isn't a part of the keyphrase at all.
- **extractive_keyphrases**: List of all the present keyphrases.
- **abstractive_keyphrase**: List of all the absent keyphrases.
### Data Splits
|Split| #datapoints |
|--|--|
| Test | 182 |
## Usage
### Full Dataset
```python
from datasets import load_dataset
# get entire dataset
dataset = load_dataset("midas/citeulike180", "raw")
# sample from the test split
print("Sample from test dataset split")
test_sample = dataset["test"][0]
print("Fields in the sample: ", [key for key in test_sample.keys()])
print("Tokenized Document: ", test_sample["document"])
print("Document BIO Tags: ", test_sample["doc_bio_tags"])
print("Extractive/present Keyphrases: ", test_sample["extractive_keyphrases"])
print("Abstractive/absent Keyphrases: ", test_sample["abstractive_keyphrases"])
print("\n-----------\n")
```
**Output**
```bash
Sample from test data split
Fields in the sample: ['id', 'document', 'doc_bio_tags', 'extractive_keyphrases', 'abstractive_keyphrases', 'other_metadata']
Tokenized Document: ['Vol', '450', '|', '8', 'November', '2007', '|', 'doi', ':', '10.1038', '/', 'nature06341', 'ARTICLES', 'Evolution', 'of', 'genes', 'and', 'genomes', 'on', 'the', 'Drosophila', 'phylogeny', 'Drosophila', '12', 'Genomes', 'Consortium', '*', 'Comparative', 'analysis', 'of', 'multiple', 'genomes', 'in', 'a', 'phylogenetic', 'framework', 'dramatically', 'improves', 'the', 'precision', 'and', 'sensitivity', 'of', 'evolutionary', 'inference', ',', 'producing', 'more', 'robust', 'results', 'than', 'single-genome', 'analyses', 'can', 'provide', '.', 'The', 'genomes', 'of', '12', 'Drosophila', 'species', ',', 'ten', 'of', 'which', 'are', 'presented', 'here', 'for', 'the', 'first', 'time', '-LRB-', 'sechellia', ',', 'simulans', ',', 'yakuba', ',', 'erecta', ',', 'ananassae', ',', 'persimilis', ',', 'willistoni', ',', 'mojavensis', ',', 'virilis', 'and', 'grimshawi', '-RRB-', ',', 'illustrate', 'how', 'rates', 'and', 'patterns', 'of', 'sequence', 'divergence', 'across', 'taxa', 'can', 'illuminate', 'evolutionary', 'processes', 'on', 'a', 'genomic', 'scale', '.', 'These', 'genome', 'sequences', 'augment', 'the', 'formidable', 'genetic', 'tools', 'that', 'have', 'made', 'Drosophila', 'melanogaster', 'a', 'pre-eminent', 'model', 'for', 'animal', 'genetics', ',', 'and', 'will', 'further', 'catalyse', 'fundamental', 'research', 'on', 'mechanisms', 'of', 'development', ',', 'cell', 'biology', ',', 'genetics', ',', 'disease', ',', 'neurobiology', ',', 'behaviour', ',', 'physiology', 'and', 'evolution', '.', 'Despite', 'remarkable', 'similarities', 'among', 'these', 'Drosophila', 'species', ',', 'we', 'identified', 'many', 'putatively', 'non-neutral', 'changes', 'in', 'protein-coding', 'genes', ',', 'non-coding', 'RNA', 'genes', ',', 'and', 'cis-regulatory', 'regions', '.', 'These', 'may', 'prove', 'to', 'underlie', 'differences', 'in', 'the', 'ecology', 'and', 'behaviour', 'of', 'these', 'diverse', 'species', '.', 'As', 'one', 'might', 'expect', 'from', 'a', 'genus', 'with', 'species', 'living', 'in', 'deserts', ',', 'in', 'the', 'tropics', ',', 'on', 'chains', 'of', 'volcanic', 'islands', 'and', ',', 'often', ',', 'commensally', 'with', 'humans', ',', 'Drosophila', 'species', 'vary', 'considerably', 'in', 'their', 'morphology', ',', 'ecology', 'and', 'behaviour1', '.', 'Species', 'in', 'this', 'genus', 'span', 'a', 'wide', 'range', 'of', 'global', 'distributions', ':', 'the', '12', 'sequenced', 'species', 'originate', 'from', 'Africa', ',', 'Asia', ',', 'the', 'Americas', 'and', 'the', 'Pacific', 'Islands', ',', 'and', 'also', 'include', 'cosmopolitan', 'species', 'that', 'have', 'colonized', 'the', 'planet', '-LRB-', 'D.', 'melanogaster', 'and', 'D.', 'simulans', '-RRB-', 'as', 'well', 'as', 'closely', 'related', 'species', 'that', 'live', 'on', 'single', 'islands', '-LRB-', 'D.', 'sechellia', '-RRB-', '2', '.', 'A', 'variety', 'of', 'behavioural', 'strategies', 'is', 'also', 'encompassed', 'by', 'the', 'sequenced', 'species', ',', 'ranging', 'in', 'feeding', 'habit', 'from', 'generalist', ',', 'such', 'as', 'D.', 'ananassae', ',', 'to', 'specialist', ',', 'such', 'as', 'D.', 'sechellia', ',', 'which', 'feeds', 'on', 'the', 'fruit', 'of', 'a', 'single', 'plant', 'species', '.', 'Despite', 'this', 'wealth', 'of', 'phenotypic', 'diversity', ',', 'Drosophila', 'species', 'share', 'a', 'distinctive', 'body', 'plan', 'and', 'life', 'cycle', '.', 'Although', 'only', 'D.', 'melanogaster', 'has', 'been', 'extensively', 'characterized', ',', 'it', 'seems', 'that', 'the', 'most', 'important', 'aspects', 'of', 'the', 'cellular', ',', 'molecular', 'and', 'developmental', 'biology', 'of', 'these', 'species', 'are', 'well', 'conserved', '.', 'Thus', ',', 'in', 'addition', 'to', 'providing', 'an', 'extensive', 'resource', 'for', 'the', 'study', 'of', 'the', 'relationship', 'between', 'sequence', 'and', 'phenotypic', 'diversity', ',', 'the', 'genomes', 'of', 'these', 'species', 'provide', 'an', 'excellent', 'model', 'for', 'studying', 'how', 'conserved', 'functions', 'are', 'maintained', 'in', 'the', 'face', 'of', 'sequence', 'divergence', '.', 'These', 'genome', 'sequences', 'provide', 'an', 'unprecedented', 'dataset', 'to', 'contrast', 'genome', 'structure', ',', 'genome', 'content', ',', 'and', 'evolutionary', 'dynamics', 'across', 'the', 'well-defined', 'phylogeny', 'of', 'the', 'sequenced', 'species', '-LRB-', 'Fig.', '1', '-RRB-', '.', 'Genome', 'assembly', ',', 'annotation', 'and', 'alignment', 'Genome', 'sequencing', 'and', 'assembly', '.', 'We', 'used', 'the', 'previously', 'published', 'sequence', 'and', 'updated', 'assemblies', 'for', 'two', 'Drosophila', 'species', ',', 'D.', 'melanogaster3', ',4', '-LRB-', 'release', '4', '-RRB-', 'and', 'D.', 'pseudoobscura5', '-LRB-', 'release', '2', '-RRB-', ',', 'and', 'generated', 'DNA', 'sequence', 'data', 'for', '10', 'additional', 'Drosophila', 'genomes', 'by', 'whole-genome', 'shotgun', 'sequencing6', ',7', '.', 'These', 'species', 'were', 'chosen', 'to', 'span', 'a', 'wide', 'variety', 'of', 'evolutionary', 'distances', ',', 'from', 'closely', 'related', 'pairs', 'such', 'as', 'D.', 'sechellia/D', '.', 'simulans', 'and', 'D.', 'persimilis/D', '.', 'pseudoobscura', 'to', 'the', 'distantly', 'related', 'species', 'of', 'the', 'Drosophila', 'and', 'Sophophora', 'subgenera', '.', 'Whereas', 'the', 'time', 'to', 'the', 'most', 'recent', 'common', 'ancestor', 'of', 'the', 'sequenced', 'species', 'may', 'seem', 'small', 'on', 'an', 'evolutionary', 'timescale', ',', 'the', 'evolutionary', 'divergence', 'spanned', 'by', 'the', 'genus', 'Drosophila', 'exceeds', '*', 'A', 'list', 'of', 'participants', 'and', 'affiliations', 'appears', 'at', 'the', 'end', 'of', 'the', 'paper', '.', 'that', 'of', 'the', 'entire', 'mammalian', 'radiation', 'when', 'generation', 'time', 'is', 'taken', 'into', 'account', ',', 'as', 'discussed', 'further', 'in', 'ref', '.', '8', '.', 'We', 'sequenced', 'seven', 'of', 'the', 'new', 'species', '-LRB-', 'D.', 'yakuba', ',', 'D.', 'erecta', ',', 'D.', 'ananassae', ',', 'D.', 'willistoni', ',', 'D.', 'virilis', ',', 'D.', 'mojavensis', 'and', 'D.', 'grimshawi', '-RRB-', 'to', 'deep', 'coverage', '-LRB-', '8.43', 'to', '11.03', '-RRB-', 'to', 'produce', 'high', 'quality', 'draft', 'sequences', '.', 'We', 'sequenced', 'two', 'species', ',', 'D.', 'sechellia', 'and', 'D.', 'persimilis', ',', 'to', 'intermediate', 'coverage', '-LRB-', '4.93', 'and', '4.13', ',', 'respectively', '-RRB-', 'under', 'the', 'assumption', 'that', 'the', 'availability', 'of', 'a', 'sister', 'species', 'sequenced', 'to', 'high', 'coverage', 'would', 'obviate', 'the', 'need', 'for', 'deep', 'sequencing', 'without', 'sacrificing', 'draft', 'genome', 'quality', '.', 'Finally', ',', 'seven', 'inbred', 'strains', 'of', 'D.', 'simulans', 'were', 'sequenced', 'to', 'low', 'coverage', '-LRB-', '2.93', 'coverage', 'from', 'w501', 'and', ',13', 'coverage', 'of', 'six', 'other', 'strains', '-RRB-', 'to', 'provide', 'population', 'variation', 'data9', '.', 'Further', 'details', 'of', 'the', 'sequencing', 'strategy', 'can', 'be', 'found', 'in', 'Table', '1', ',', 'Supplementary', 'Table', '1', 'and', 'section', '1', 'in', 'Supplementary', 'Information', '.', 'We', 'generated', 'an', 'initial', 'draft', 'assembly', 'for', 'each', 'species', 'using', 'one', 'of', 'three', 'different', 'whole-genome', 'shotgun', 'assembly', 'programs', '-LRB-', 'Table', '1', '-RRB-', '.', 'For', 'D.', 'ananassae', ',', 'D.', 'erecta', ',', 'D.', 'grimshawi', ',', 'D.', 'mojavensis', ',', 'D.', 'virilis', 'and', 'D.', 'willistoni', ',', 'we', 'also', 'generated', 'secondary', 'assemblies', ';', 'reconciliation', 'of', 'these', 'with', 'the', 'primary', 'assemblies', 'resulted', 'in', 'a', '7', '30', '%', 'decrease', 'in', 'the', 'estimated', 'number', 'of', 'misassembled', 'regions', 'and', 'a', '12', '23', '%', 'increase', 'in', 'the', 'N50', 'contig', 'size10', '-LRB-', 'Supplementary', 'Table', '2', '-RRB-', '.', 'For', 'D.', 'yakuba', ',', 'we', 'generated', '52,000', 'targeted', 'reads', 'across', 'low-quality', 'regions', 'and', 'gaps', 'to', 'improve', 'the', 'assembly', '.', 'This', 'doubled', 'the', 'mean', 'contig', 'and', 'scaffold', 'sizes', 'and', 'increased', 'the', 'total', 'fraction', 'of', 'high', 'quality', 'bases', '-LRB-', 'quality', 'score', '-LRB-', 'Q', '-RRB-', '.', '40', '-RRB-', 'from', '96.5', '%', 'to', '98.5', '%', '.', 'We', 'improved', 'the', 'initial', '2.93', 'D.', 'simulans', 'w501', 'whole-genome', 'shotgun', 'assembly', 'by', 'filling', 'assembly', 'gaps', 'with', 'contigs', 'and', 'unplaced', 'reads', 'from', 'the', ',13', 'assemblies', 'of', 'the', 'six', 'other', 'D.', 'simulans', 'strains', ',', 'generating', 'a', '`', 'mosaic', "'", 'assembly', '-LRB-', 'Supplementary', 'Table', '3', '-RRB-', '.', 'This', 'integration', 'markedly', 'improved', 'the', 'D.', 'simulans', 'assembly', ':', 'the', 'N50', 'contig', 'size', 'of', 'the', 'mosaic', 'assembly', ',', 'for', 'instance', ',', 'is', 'more', 'than', 'twice', 'that', 'of', 'the', 'initial', 'w501', 'assembly', '-LRB-', '17']
Document BIO Tags: ['O', 'O', 'O', 'O', 'O', 'O', 'O', 'O', 'O', 'O', 'O', 'O', 'O', 'B', 'O', 'O', 'O', 'O', 'O', 'O', 'B', 'B', 'B', 'O', 'O', 'O', 'O', 'O', 'O', 'O', 'O', 'O', 'O', 'O', 'O', 'O', 'O', 'O', 'O', 'O', 'O', 'O', 'O', 'O', 'O', 'O', 'O', 'O', 'O', 'O', 'O', 'O', 'O', 'O', 'O', 'O', 'O', 'O', 'O', 'O', 'B', 'O', 'O', 'O', 'O', 'O', 'O', 'O', 'O', 'O', 'O', 'O', 'O', 'O', 'O', 'O', 'O', 'O', 'O', 'O', 'O', 'O', 'O', 'O', 'O', 'O', 'O', 'O', 'O', 'O', 'O', 'O', 'O', 'O', 'O', 'O', 'O', 'O', 'O', 'O', 'O', 'O', 'O', 'O', 'O', 'O', 'O', 'O', 'O', 'O', 'O', 'O', 'O', 'O', 'O', 'O', 'O', 'O', 'O', 'O', 'O', 'O', 'O', 'O', 'O', 'B', 'O', 'O', 'O', 'O', 'O', 'O', 'O', 'O', 'O', 'O', 'O', 'O', 'O', 'O', 'O', 'O', 'O', 'O', 'O', 'O', 'O', 'O', 'O', 'O', 'O', 'O', 'O', 'O', 'O', 'O', 'O', 'O', 'B', 'O', 'O', 'O', 'O', 'O', 'O', 'B', 'O', 'O', 'O', 'O', 'O', 'O', 'O', 'O', 'O', 'O', 'O', 'O', 'O', 'O', 'O', 'O', 'O', 'O', 'O', 'O', 'O', 'O', 'O', 'O', 'O', 'O', 'O', 'O', 'O', 'O', 'O', 'O', 'O', 'O', 'O', 'O', 'O', 'O', 'O', 'O', 'O', 'O', 'O', 'O', 'O', 'O', 'O', 'O', 'O', 'O', 'O', 'O', 'O', 'O', 'O', 'O', 'O', 'O', 'O', 'O', 'O', 'O', 'O', 'O', 'O', 'O', 'B', 'O', 'O', 'O', 'O', 'O', 'O', 'O', 'O', 'O', 'O', 'O', 'O', 'O', 'O', 'O', 'O', 'O', 'O', 'O', 'O', 'O', 'O', 'O', 'O', 'O', 'O', 'O', 'O', 'O', 'O', 'O', 'O', 'O', 'O', 'O', 'O', 'O', 'O', 'O', 'O', 'O', 'O', 'O', 'O', 'O', 'O', 'O', 'O', 'O', 'O', 'O', 'O', 'O', 'O', 'O', 'O', 'O', 'O', 'O', 'O', 'O', 'O', 'O', 'O', 'O', 'O', 'O', 'O', 'O', 'O', 'O', 'O', 'O', 'O', 'O', 'O', 'O', 'O', 'O', 'O', 'O', 'O', 'O', 'O', 'O', 'O', 'O', 'O', 'O', 'O', 'O', 'O', 'O', 'O', 'O', 'O', 'O', 'O', 'O', 'O', 'O', 'O', 'O', 'O', 'O', 'O', 'O', 'O', 'O', 'O', 'O', 'O', 'O', 'O', 'O', 'O', 'O', 'O', 'O', 'O', 'O', 'O', 'O', 'O', 'O', 'B', 'O', 'O', 'O', 'O', 'O', 'O', 'O', 'O', 'O', 'O', 'O', 'O', 'O', 'O', 'O', 'O', 'O', 'O', 'O', 'O', 'O', 'O', 'O', 'O', 'O', 'O', 'O', 'O', 'O', 'O', 'O', 'O', 'O', 'O', 'O', 'O', 'O', 'O', 'O', 'O', 'O', 'O', 'O', 'O', 'O', 'O', 'O', 'O', 'O', 'O', 'O', 'O', 'O', 'O', 'O', 'O', 'O', 'O', 'O', 'O', 'O', 'O', 'O', 'O', 'O', 'O', 'O', 'O', 'O', 'O', 'O', 'O', 'O', 'O', 'O', 'O', 'O', 'O', 'O', 'O', 'O', 'O', 'O', 'O', 'O', 'O', 'O', 'O', 'O', 'O', 'O', 'O', 'O', 'O', 'O', 'O', 'O', 'O', 'O', 'O', 'O', 'O', 'O', 'O', 'O', 'O', 'B', 'O', 'O', 'O', 'O', 'O', 'O', 'O', 'O', 'O', 'O', 'O', 'O', 'O', 'O', 'O', 'O', 'O', 'O', 'O', 'O', 'O', 'O', 'O', 'O', 'O', 'O', 'O', 'O', 'O', 'O', 'O', 'B', 'O', 'O', 'O', 'O', 'O', 'O', 'O', 'O', 'O', 'O', 'O', 'O', 'O', 'O', 'O', 'O', 'O', 'O', 'O', 'O', 'O', 'O', 'O', 'O', 'O', 'B', 'O', 'O', 'O', 'O', 'O', 'O', 'O', 'O', 'O', 'O', 'O', 'O', 'O', 'O', 'O', 'O', 'O', 'O', 'O', 'O', 'O', 'O', 'O', 'O', 'O', 'O', 'O', 'O', 'O', 'O', 'O', 'O', 'O', 'O', 'O', 'O', 'O', 'O', 'O', 'O', 'O', 'O', 'B', 'O', 'O', 'O', 'O', 'O', 'O', 'O', 'O', 'O', 'O', 'O', 'O', 'O', 'O', 'O', 'O', 'O', 'O', 'O', 'O', 'O', 'O', 'O', 'O', 'O', 'O', 'O', 'O', 'O', 'O', 'O', 'O', 'B', 'O', 'O', 'O', 'O', 'O', 'O', 'O', 'O', 'O', 'O', 'O', 'O', 'O', 'O', 'O', 'O', 'O', 'O', 'O', 'O', 'O', 'O', 'O', 'O', 'O', 'O', 'O', 'O', 'O', 'O', 'O', 'O', 'O', 'O', 'O', 'O', 'O', 'O', 'O', 'O', 'O', 'O', 'O', 'O', 'O', 'O', 'O', 'O', 'O', 'O', 'O', 'O', 'O', 'O', 'O', 'O', 'O', 'O', 'O', 'O', 'O', 'O', 'O', 'O', 'O', 'O', 'O', 'O', 'O', 'O', 'O', 'O', 'O', 'O', 'O', 'O', 'O', 'O', 'O', 'O', 'O', 'O', 'O', 'O', 'O', 'O', 'O', 'O', 'O', 'O', 'O', 'O', 'O', 'O', 'O', 'O', 'O', 'O', 'O', 'O', 'O', 'O', 'O', 'O', 'O', 'O', 'O', 'O', 'O', 'O', 'O', 'O', 'O', 'O', 'O', 'O', 'O', 'O', 'O', 'O', 'O', 'O', 'O', 'O', 'O', 'O', 'O', 'O', 'O', 'O', 'O', 'O', 'O', 'O', 'O', 'O', 'O', 'O', 'O', 'O', 'O', 'O', 'O', 'O', 'O', 'O', 'O', 'O', 'O', 'O', 'O', 'O', 'O', 'O', 'O', 'O', 'O', 'O', 'O', 'O', 'O', 'O', 'O', 'O', 'O', 'O', 'O', 'O', 'O', 'O', 'O', 'O', 'O', 'O', 'O', 'O', 'O', 'O', 'O', 'O', 'O', 'O', 'O', 'O', 'O', 'O', 'O', 'O', 'O', 'O', 'O', 'O', 'O', 'O', 'O', 'O', 'O', 'O', 'O', 'O', 'O', 'O', 'O', 'O', 'O', 'O', 'O', 'O', 'O', 'O', 'O', 'O', 'O', 'O', 'O', 'O', 'O', 'O', 'O', 'O', 'O', 'O', 'O', 'O', 'O', 'O', 'O', 'O', 'O', 'O', 'O', 'O', 'O', 'O', 'O', 'O', 'O', 'O', 'O', 'O', 'O', 'O', 'O', 'O', 'O', 'O', 'O', 'O', 'O', 'O', 'O', 'O', 'O', 'O', 'O', 'O', 'O', 'O', 'O', 'O', 'O', 'O', 'O', 'O', 'O', 'O', 'O', 'O', 'O', 'O', 'O', 'O', 'O', 'O', 'O', 'O', 'O', 'O', 'O', 'O', 'O', 'O', 'O', 'O', 'O', 'O', 'O', 'O', 'O', 'O', 'O', 'O', 'O', 'O', 'O', 'O', 'O', 'O', 'O', 'O', 'O', 'O', 'O', 'O', 'O', 'O', 'O', 'O', 'O', 'O', 'O', 'O', 'O', 'O', 'O', 'O', 'O', 'O', 'O', 'O', 'O', 'O', 'O', 'O', 'O', 'O', 'O', 'O', 'O', 'O', 'O', 'O', 'O', 'O', 'O', 'O', 'O', 'O', 'O', 'O', 'O', 'O', 'O', 'O', 'O', 'O', 'O', 'O', 'O', 'O', 'O', 'O', 'O', 'O', 'O', 'O', 'O', 'O', 'O', 'O', 'O', 'O', 'O', 'O', 'O', 'O', 'O', 'O', 'O', 'O', 'O', 'O', 'O', 'O', 'O', 'O', 'O', 'O', 'O', 'O', 'O', 'O', 'O', 'O', 'O', 'O', 'O', 'O', 'O', 'O', 'O', 'O', 'O', 'O', 'O', 'O', 'O', 'O', 'O', 'O']
Extractive/present Keyphrases: ['phylogeny', 'drosophila', 'evolution', 'fly', 'genomics']
Abstractive/absent Keyphrases: ['droso', 'comparative genomics']
-----------
```
### Keyphrase Extraction
```python
from datasets import load_dataset
# get the dataset only for keyphrase extraction
dataset = load_dataset("midas/citeulike180", "extraction")
print("Samples for Keyphrase Extraction")
# sample from the test split
print("Sample from test data split")
test_sample = dataset["test"][0]
print("Fields in the sample: ", [key for key in test_sample.keys()])
print("Tokenized Document: ", test_sample["document"])
print("Document BIO Tags: ", test_sample["doc_bio_tags"])
print("\n-----------\n")
```
### Keyphrase Generation
```python
# get the dataset only for keyphrase generation
dataset = load_dataset("midas/citeulike180", "generation")
print("Samples for Keyphrase Generation")
# sample from the test split
print("Sample from test data split")
test_sample = dataset["test"][0]
print("Fields in the sample: ", [key for key in test_sample.keys()])
print("Tokenized Document: ", test_sample["document"])
print("Extractive/present Keyphrases: ", test_sample["extractive_keyphrases"])
print("Abstractive/absent Keyphrases: ", test_sample["abstractive_keyphrases"])
print("\n-----------\n")
```
## Citation Information
```
@inproceedings{medelyan-etal-2009-human,
title = "Human-competitive tagging using automatic keyphrase extraction",
author = "Medelyan, Olena and
Frank, Eibe and
Witten, Ian H.",
booktitle = "Proceedings of the 2009 Conference on Empirical Methods in Natural Language Processing",
month = aug,
year = "2009",
address = "Singapore",
publisher = "Association for Computational Linguistics",
url = "https://aclanthology.org/D09-1137",
pages = "1318--1327",
}
```
## Contributions
Thanks to [@debanjanbhucs](https://github.com/debanjanbhucs), [@dibyaaaaax](https://github.com/dibyaaaaax) and [@ad6398](https://github.com/ad6398) for adding this dataset
| midas/citeulike180 | [
"region:us"
] | 2022-03-02T23:29:22+00:00 | {} | 2022-01-23T06:52:23+00:00 | [] | [] | TAGS
#region-us
| Dataset Summary
---------------
A dataset for benchmarking keyphrase extraction and generation techniques from long document english scientific articles. For more details about the dataset please refer the original paper - URL
Original source of the data -
Dataset Structure
-----------------
### Data Fields
* id: unique identifier of the document.
* document: Whitespace separated list of words in the document.
* doc\_bio\_tags: BIO tags for each word in the document. B stands for the beginning of a keyphrase and I stands for inside the keyphrase. O stands for outside the keyphrase and represents the word that isn't a part of the keyphrase at all.
* extractive\_keyphrases: List of all the present keyphrases.
* abstractive\_keyphrase: List of all the absent keyphrases.
### Data Splits
Usage
-----
### Full Dataset
Output
### Keyphrase Extraction
### Keyphrase Generation
Contributions
-------------
Thanks to @debanjanbhucs, @dibyaaaaax and @ad6398 for adding this dataset
| [
"### Data Fields\n\n\n* id: unique identifier of the document.\n* document: Whitespace separated list of words in the document.\n* doc\\_bio\\_tags: BIO tags for each word in the document. B stands for the beginning of a keyphrase and I stands for inside the keyphrase. O stands for outside the keyphrase and represents the word that isn't a part of the keyphrase at all.\n* extractive\\_keyphrases: List of all the present keyphrases.\n* abstractive\\_keyphrase: List of all the absent keyphrases.",
"### Data Splits\n\n\n\nUsage\n-----",
"### Full Dataset\n\n\nOutput",
"### Keyphrase Extraction",
"### Keyphrase Generation\n\n\nContributions\n-------------\n\n\nThanks to @debanjanbhucs, @dibyaaaaax and @ad6398 for adding this dataset"
] | [
"TAGS\n#region-us \n",
"### Data Fields\n\n\n* id: unique identifier of the document.\n* document: Whitespace separated list of words in the document.\n* doc\\_bio\\_tags: BIO tags for each word in the document. B stands for the beginning of a keyphrase and I stands for inside the keyphrase. O stands for outside the keyphrase and represents the word that isn't a part of the keyphrase at all.\n* extractive\\_keyphrases: List of all the present keyphrases.\n* abstractive\\_keyphrase: List of all the absent keyphrases.",
"### Data Splits\n\n\n\nUsage\n-----",
"### Full Dataset\n\n\nOutput",
"### Keyphrase Extraction",
"### Keyphrase Generation\n\n\nContributions\n-------------\n\n\nThanks to @debanjanbhucs, @dibyaaaaax and @ad6398 for adding this dataset"
] |
3fcd68096f6029dfefbe7f2b28c3038456e9d689 | ## Dataset Summary
A dataset for benchmarking keyphrase extraction and generation techniques from english scientific papers. For more details about the dataset please refer the original paper - [https://dl.acm.org/doi/abs/10.1145/313238.313437](https://dl.acm.org/doi/abs/10.1145/313238.313437)
Original source of the data - []()
## Dataset Structure
### Data Fields
- **id**: unique identifier of the document.
- **document**: Whitespace separated list of words in the document.
- **doc_bio_tags**: BIO tags for each word in the document. B stands for the beginning of a keyphrase and I stands for inside the keyphrase. O stands for outside the keyphrase and represents the word that isn't a part of the keyphrase at all.
- **extractive_keyphrases**: List of all the present keyphrases.
- **abstractive_keyphrase**: List of all the absent keyphrases.
### Data Splits
|Split| #datapoints |
|--|--|
| Train | 130 |
| Test | 500 |
Train
- Percentage of keyphrases that are named entities: 69.49% (named entities detected using scispacy - en-core-sci-lg model)
- Percentage of keyphrases that are noun phrases: 81.26% (noun phrases detected using spacy en-core-web-lg after removing determiners)
Test
- Percentage of keyphrases that are named entities: 70.79% (named entities detected using scispacy - en-core-sci-lg model)
- Percentage of keyphrases that are noun phrases: 82.74% (noun phrases detected using spacy en-core-web-lg after removing determiners)
## Usage
### Full Dataset
```python
from datasets import load_dataset
# get entire dataset
dataset = load_dataset("midas/cstr", "raw")
# sample from the train split
print("Sample from train dataset split")
test_sample = dataset["train"][0]
print("Fields in the sample: ", [key for key in test_sample.keys()])
print("Tokenized Document: ", test_sample["document"])
print("Document BIO Tags: ", test_sample["doc_bio_tags"])
print("Extractive/present Keyphrases: ", test_sample["extractive_keyphrases"])
print("Abstractive/absent Keyphrases: ", test_sample["abstractive_keyphrases"])
print("\n-----------\n")
# sample from the test split
print("Sample from test dataset split")
test_sample = dataset["test"][0]
print("Fields in the sample: ", [key for key in test_sample.keys()])
print("Tokenized Document: ", test_sample["document"])
print("Document BIO Tags: ", test_sample["doc_bio_tags"])
print("Extractive/present Keyphrases: ", test_sample["extractive_keyphrases"])
print("Abstractive/absent Keyphrases: ", test_sample["abstractive_keyphrases"])
print("\n-----------\n")
```
**Output**
```bash
Sample from training data split
Fields in the sample: ['id', 'document', 'doc_bio_tags', 'extractive_keyphrases', 'abstractive_keyphrases', 'other_metadata']
Tokenized Document: ['Reasoning', 'with', 'Non-Atomic', 'Memories', 'Manhoi', 'Choy', '?', 'and', 'Ambuj', 'K.', 'Singh', '?', 'Department', 'of', 'Computer', 'Science', 'University', 'of', 'California', 'at', 'Santa', 'Barbara', 'Santa', 'Barbara', ',', 'CA', '93106', 'August', '3', ',', '1993', 'Abstract', 'A', 'method', 'for', 'reasoning', 'with', 'non-atomic', 'memory', 'is', 'developed', '.', 'A', 'program', 'using', 'non-atomic', 'memory', 'is', 'transformed', 'into', 'an', 'equivalent', 'one', 'that', 'uses', 'atomic', 'memory', '.', 'A', 'number', 'of', 'non-atomic', 'memories', 'including', 'pipelined', 'RAM', ',', 'causal', 'memory', ',', 'and', 'hybrid', 'consistency', 'are', 'examined', '.', 'The', 'approach', 'is', 'illustrated', 'with', 'some', 'examples', '.', '1', 'Introduction', 'The', 'traditional', 'abstraction', 'of', 'shared', 'memory', 'which', 'supported', 'atomic', 'reads', 'and', 'writes', 'has', 'come', 'under', 'increasing', 'scrutiny', '.', 'Hardware', 'architects', 'seem', 'to', 'agree', 'that', 'atomic', 'memory', 'leads', 'to', 'a', 'large', 'latency', 'that', 'is', 'unacceptable', 'for', 'efficient', 'programming', '.', 'Based', 'on', 'this', 'observation', ',', 'non-atomic', 'abstractions', 'of', 'shared', 'memory', 'have', 'been', 'proposed', 'in', 'the', 'literature', '.', 'These', 'definitions', 'are', 'usually', 'motivated', 'by', 'hardware', 'and', 'their', 'semantics', 'and', 'usefulness', 'from', 'the', 'point', 'of', 'view', 'of', 'a', 'user', 'are', 'far', 'from', 'clear', '.', 'Coupled', 'with', 'the', 'problems', 'of', 'concurrency', 'and', 'non-determinism', ',', 'these', 'definitions', 'have', 'the', 'potential', 'of', 'making', 'the', 'programming', 'of', 'concurrent', 'systems', 'very', 'difficult', '.', 'This', 'paper', 'examines', 'some', 'existing', 'definitions', 'of', 'non-atomic', 'memories', 'and', 'provides', 'a', 'mechanism', 'for', 'reasoning', 'about', 'them', '.', 'Instead', 'of', 'designing', 'a', 'new', 'proof', 'system', 'for', 'each', 'kind', 'of', 'non-atomic', 'memory', ',', 'our', 'approach', 'is', 'to', 'define', 'rules', 'for', 'transforming', 'a', 'program', 'that', 'uses', 'non-atomic', 'memory', 'into', 'an', 'equivalent', 'program', 'that', 'uses', 'atomic', 'memory', '.', 'The', 'transformed', 'program', 'can', 'then', 'be', 'proved', 'using', 'any', 'of', 'the', 'existing', 'proof', 'systems', 'such', 'as', 'Temporal', 'logic', '-LSB-', '15', '-RSB-', 'and', 'Unity', '-LSB-', '6', '-RSB-', '.', 'Besides', 'providing', 'a', 'technique', 'for', 'reasoning', 'about', 'non-atomic', 'memory', ',', 'the', 'approach', 'also', 'provides', 'a', 'clear', 'uniform', 'semantics', 'for', 'the', 'non-atomic', 'memories', '.', 'Traditional', 'approaches', 'toward', 'defining', 'non-atomic', 'memories', 'are', 'based', 'on', 'histories', '.', 'Systemwide', 'execution', 'histories', 'are', 'considered', 'and', 'those', 'that', 'satisfy', 'the', 'specification', 'are', 'isolated', 'by', 'considering', 'interleavings', 'of', 'events', '.', 'It', 'may', 'be', 'difficult', 'for', 'users', 'to', 'understand', 'the', 'semantics', 'of', 'non-atomic', 'operations', 'in', 'such', 'an', 'approach', '.', 'In', 'contrast', ',', 'the', 'technique', 'proposed', 'here', 'is', 'based', 'on', 'the', 'idea', 'of', 'transforming', 'each', 'non-atomic', 'operation', 'as', 'a', 'set', 'of', 'atomic', 'operations', '.', 'The', 'motivation', 'is', 'to', 'show', 'that', 'understanding', 'non-atomic', 'memories', 'is', ',', 'in', 'principle', ',', '?', 'Work', 'supported', 'in', 'part', 'by', 'NSF', 'grant', 'CCR-9008628', '.', 'no', 'harder', 'that', 'understanding', 'atomic', 'memories', '.', 'This', 'approach', 'of', 'transforming', 'a', 'program', 'that', 'uses', 'non-atomic', 'variables', 'into', 'one', 'that', 'uses', 'atomic', 'variables', 'was', 'used', 'earlier', 'by', 'Anderson', 'and', 'Gouda', '-LSB-', '3', '-RSB-', 'to', 'prove', 'the', 'correctness', 'of', 'programs', 'that', 'use', 'safe', 'and', 'regular', 'variables', '-LSB-', '11', '-RSB-', '.', 'The', 'specific', 'abstractions', 'of', 'non-atomic', 'memory', 'that', 'we', 'examine', 'include', 'pipelined', 'RAM', '-LSB-', '12', '-RSB-', ',', 'causal', 'memory', '-LSB-', '2', '-RSB-', ',', 'TSO', 'and', 'PSO', 'memory', 'models', 'of', 'Sparc', '-LSB-', '9', '-RSB-', ',', 'and', 'hybrid', 'consistency', '-LSB-', '5', '-RSB-', '.', 'In', 'each', 'of', 'these', 'cases', ',', 'suitable', 'auxiliary', 'variables', 'are', 'defined', 'in', 'the', 'process', 'of', 'transformation', '.', 'These', 'auxiliary', 'variables', 'may', 'be', 'viewed', 'as', 'an', 'abstract', 'implementation', 'of', 'the', 'corresponding', 'kind', 'of', 'memory', '.', 'The', 'rest', 'of', 'the', 'paper', 'is', 'organized', 'as', 'follows', '.', 'Sections', '2', 'through', '6', 'examine', 'the', 'different', 'kinds', 'of', 'memory', '.', 'In', 'each', 'case', ',', 'rules', 'for', 'transforming', 'each', 'non-atomic', 'read', 'and', 'write', 'are', 'included', '.', 'In', 'some', 'cases', ',', 'the', 'transformations', 'are', 'also', 'illustrated', 'with', 'small', 'examples', '.', 'Section', '7', 'includes', 'a', 'brief', 'discussion', '.', '2', 'Pipelined', 'RAM', 'In', 'this', 'kind', 'of', 'non-atomic', 'memory', 'introduced', 'by', 'Lipton', 'and', 'Sandberg', '-LSB-', '12', '-RSB-', ',', 'every', 'process', 'has', 'its', 'own', 'copy', 'of', 'the', 'shared', 'memory', '.', 'A', 'read', 'operation', 'is', 'performed', 'by', 'reading', 'this', 'local', 'copy', 'and', 'a', 'write', 'operation', 'is', 'performed', 'by', 'updating', 'the', 'local', 'copy', 'and', 'sending', 'the', 'update', 'to', 'all', 'other', 'processes', 'on', 'FIFO', 'channels', '.', 'These', 'updates', 'are', 'then', 'executed', 'asynchronously', 'at', 'the', 'remote', 'processes', '.', 'In', 'order', 'to', 'model', 'this', 'memory', ',', 'we', 'introduce', 'the', 'following', 'auxiliary', 'variables', 'for', 'each', 'shared', 'variable', 'x', 'and', 'each', 'process', 'p', ':', 'ffl', 'xp', ',', 'a', 'local', 'copy', 'of', 'variable', 'x', 'at', 'process', 'p', '.', 'It', 'is', 'initialized', 'to', 'the', 'initial', 'value', 'of', 'x.', 'ffl', 'Xp', ',', 'a', 'set', 'containing', 'the', 'updates', 'performed', 'by', 'remote', 'processes', 'on', 'variable', 'x.', 'Each', 'tuple', 'in', 'Xp', 'consists', 'of', 'three', 'fields', ':', 'the', 'updated', 'value', ',', 'the', 'timestamp', 'of', 'the', 'updating', 'process', ',', 'and', 'the', 'identity', 'of', 'the', 'updating', 'process', '.', 'It', 'is', 'initialized', 'to', 'an', 'empty', 'set', '.', 'ffl', 'tsp', ',', 'a', 'counter', 'that', 'is', 'used', 'for', 'distinguishing', 'updates', 'by', 'process', 'p', '.', 'It', 'is', 'initialized', 'to', '0', '.', 'Each', 'read', 'and', 'write', 'operation', 'of', 'process', 'p', 'is', 'now', 'translated', 'as', 'follows', '.', '1', '.', 'A', 'read', 'statement', 'v', ':', '=', 'x', 'is', 'translated', 'to', 'v', ':', '=', 'xp', ',', 'i.e.', ',', 'the', 'local', 'copy', 'is', 'read', '.', '2', '.', 'A', 'write', 'statement', 'x', ':', '=', 'm', 'is', 'translated', 'to', 'an', 'update', 'of', 'the', 'local', 'copy', 'along', 'with', 'an', 'increment', 'of', 'the', 'local', 'counter', ',', 'followed', 'by', 'a', 'transmittal', 'of', 'the', 'update', 'to', 'all', 'other', 'processes', ':', 'xp', ';', 'tsp', ':', '=', 'm', ';', 'tsp', '+', '1', ';', 'h8q', ':', 'q', '<', '>', 'p', ':', 'Xq', ':', '=', 'Xq', '-LSB-', 'f', '-LRB-', 'm', ';', 'tsp', ';', 'p', '-RRB-', 'gi', '.', '3', '.', 'Finally', ',', 'for', 'each', 'shared', 'variable', 'x', ',', 'we', 'add', 'a', 'process', 'Mp', ';', 'x', 'that', 'services', 'the', 'remote', 'updates', 'for', 'variable', 'x', 'at', 'process', 'p.', 'Process', 'Mp', ';', 'x', 'examines', 'the', 'contents', 'of', 'set', 'Xp', 'and', 'assigns', 'the', 'values', 'existing', 'there', 'to', 'xp', 'in', 'a', 'FIFO', 'order', ':', 'repeat', 'if', 'Xp', '<', '>', 'fg', 'then', 'xp', ';', 'Xp', ':', '=', 'm', ';', 'Xp', '?', 'f', '-LRB-', 'm', ';', 'ts', ';', 'q', '-RRB-', 'g', 'where', 'Min', '-LRB-', 'Xp', ';', '-LRB-', 'm', ';', 'ts', ';', 'q', '-RRB-', '-RRB-', 'forever', 'Predicate', 'Min', '-LRB-', 'S', ';', 't', '-RRB-', 'denotes', 'that', 'tuple', 't', 'is', 'a', 'minimal', 'element', 'in', 'set', 'S', 'in', 'a', 'specified', 'ordering', '.', 'In', 'this', 'case', ',', 'tuple', 't', 'is', 'less', 'than', 'tuple', 't0', 'provided', 'they', 'mention', 'the', 'same']
Document BIO Tags: ['O', 'O', 'O', 'O', 'O', 'O', 'O', 'O', 'O', 'O', 'O', 'O', 'O', 'O', 'O', 'O', 'O', 'O', 'O', 'O', 'O', 'O', 'O', 'O', 'O', 'O', 'O', 'O', 'O', 'O', 'O', 'O', 'O', 'O', 'O', 'O', 'O', 'O', 'O', 'O', 'O', 'O', 'O', 'O', 'O', 'O', 'O', 'O', 'O', 'O', 'O', 'O', 'O', 'O', 'O', 'O', 'O', 'O', 'O', 'O', 'O', 'O', 'O', 'O', 'O', 'O', 'O', 'O', 'O', 'O', 'O', 'O', 'O', 'O', 'O', 'O', 'O', 'O', 'O', 'O', 'O', 'O', 'O', 'O', 'O', 'O', 'O', 'O', 'O', 'O', 'O', 'O', 'O', 'O', 'O', 'O', 'O', 'O', 'O', 'O', 'O', 'O', 'O', 'O', 'O', 'O', 'O', 'O', 'O', 'O', 'O', 'O', 'O', 'O', 'O', 'O', 'O', 'O', 'O', 'O', 'O', 'O', 'O', 'O', 'O', 'O', 'O', 'O', 'O', 'O', 'O', 'O', 'O', 'O', 'O', 'O', 'O', 'O', 'O', 'O', 'O', 'O', 'O', 'O', 'O', 'O', 'O', 'O', 'O', 'O', 'O', 'O', 'O', 'O', 'O', 'O', 'O', 'O', 'O', 'O', 'O', 'O', 'O', 'O', 'O', 'O', 'O', 'O', 'O', 'O', 'O', 'B', 'O', 'O', 'O', 'O', 'O', 'O', 'O', 'O', 'O', 'O', 'O', 'O', 'O', 'O', 'O', 'O', 'O', 'O', 'O', 'O', 'O', 'O', 'O', 'O', 'O', 'O', 'O', 'O', 'O', 'O', 'O', 'O', 'O', 'O', 'O', 'O', 'O', 'O', 'O', 'O', 'O', 'O', 'O', 'O', 'O', 'O', 'O', 'O', 'O', 'O', 'O', 'O', 'O', 'O', 'O', 'O', 'O', 'O', 'O', 'O', 'O', 'O', 'O', 'O', 'O', 'O', 'O', 'O', 'O', 'O', 'O', 'O', 'O', 'O', 'O', 'O', 'O', 'O', 'O', 'O', 'O', 'O', 'O', 'O', 'O', 'O', 'O', 'O', 'O', 'O', 'O', 'O', 'O', 'O', 'O', 'O', 'O', 'O', 'O', 'O', 'O', 'O', 'O', 'O', 'O', 'O', 'O', 'O', 'O', 'O', 'O', 'O', 'O', 'O', 'O', 'O', 'O', 'O', 'O', 'O', 'O', 'O', 'O', 'O', 'O', 'O', 'O', 'O', 'O', 'O', 'O', 'O', 'O', 'O', 'O', 'O', 'O', 'O', 'O', 'O', 'O', 'O', 'O', 'O', 'O', 'O', 'O', 'O', 'O', 'O', 'O', 'O', 'O', 'O', 'O', 'O', 'O', 'O', 'O', 'O', 'O', 'O', 'O', 'O', 'O', 'O', 'O', 'O', 'O', 'O', 'O', 'O', 'O', 'O', 'O', 'O', 'O', 'O', 'O', 'O', 'O', 'O', 'O', 'O', 'O', 'O', 'O', 'O', 'O', 'O', 'O', 'O', 'O', 'O', 'O', 'O', 'O', 'O', 'O', 'O', 'O', 'O', 'O', 'O', 'O', 'O', 'O', 'O', 'O', 'O', 'O', 'O', 'O', 'O', 'O', 'O', 'O', 'O', 'O', 'O', 'O', 'O', 'O', 'O', 'O', 'O', 'O', 'O', 'O', 'O', 'O', 'O', 'O', 'O', 'O', 'O', 'O', 'O', 'O', 'O', 'O', 'O', 'O', 'O', 'O', 'O', 'O', 'O', 'O', 'O', 'O', 'O', 'O', 'O', 'O', 'O', 'O', 'O', 'O', 'O', 'O', 'O', 'O', 'O', 'O', 'O', 'O', 'O', 'O', 'O', 'O', 'O', 'O', 'O', 'O', 'O', 'O', 'O', 'O', 'O', 'O', 'O', 'O', 'O', 'O', 'O', 'O', 'O', 'O', 'O', 'O', 'O', 'O', 'O', 'O', 'O', 'O', 'O', 'O', 'O', 'O', 'O', 'O', 'O', 'O', 'O', 'O', 'O', 'O', 'O', 'O', 'O', 'O', 'O', 'O', 'O', 'O', 'O', 'O', 'O', 'O', 'O', 'O', 'O', 'O', 'O', 'O', 'O', 'O', 'O', 'O', 'O', 'O', 'O', 'O', 'O', 'O', 'O', 'O', 'O', 'O', 'O', 'O', 'O', 'O', 'O', 'O', 'O', 'O', 'O', 'O', 'O', 'O', 'O', 'O', 'O', 'O', 'O', 'O', 'O', 'O', 'O', 'O', 'O', 'O', 'O', 'O', 'O', 'O', 'O', 'O', 'O', 'O', 'O', 'O', 'O', 'O', 'O', 'O', 'O', 'O', 'O', 'O', 'O', 'O', 'O', 'O', 'O', 'O', 'O', 'O', 'O', 'O', 'O', 'O', 'O', 'O', 'O', 'O', 'O', 'O', 'O', 'O', 'O', 'O', 'O', 'O', 'O', 'O', 'O', 'O', 'O', 'O', 'O', 'O', 'O', 'O', 'O', 'O', 'O', 'O', 'O', 'O', 'O', 'O', 'O', 'O', 'O', 'O', 'O', 'O', 'O', 'O', 'O', 'O', 'O', 'O', 'O', 'O', 'O', 'O', 'O', 'O', 'O', 'O', 'O', 'O', 'O', 'O', 'O', 'O', 'O', 'O', 'O', 'O', 'O', 'O', 'O', 'O', 'O', 'O', 'O', 'O', 'O', 'O', 'O', 'O', 'O', 'O', 'O', 'O', 'O', 'O', 'O', 'O', 'O', 'O', 'O', 'O', 'O', 'O', 'O', 'O', 'O', 'O', 'O', 'O', 'O', 'O', 'O', 'O', 'O', 'O', 'O', 'O', 'O', 'O', 'O', 'O', 'O', 'O', 'O', 'O', 'O', 'O', 'O', 'O', 'O', 'O', 'O', 'O', 'O', 'O', 'O', 'O', 'O', 'O', 'O', 'O', 'O', 'O', 'O', 'O', 'O', 'O', 'O', 'O', 'O', 'O', 'O', 'O', 'O', 'O', 'O', 'O', 'O', 'O', 'O', 'O', 'O', 'O', 'O', 'O', 'O', 'O', 'O', 'O', 'O', 'O', 'O', 'O', 'O', 'O', 'O', 'O', 'O', 'O', 'O', 'O', 'O', 'O', 'O', 'O', 'O', 'O', 'O', 'O', 'O', 'O', 'O', 'O', 'O', 'O', 'O', 'O', 'O', 'O', 'O', 'O', 'O', 'O', 'O', 'O', 'O', 'O', 'O', 'O', 'O', 'O', 'O', 'O', 'O', 'O', 'O', 'O', 'O', 'O', 'O', 'O', 'O', 'O', 'O', 'O', 'O', 'O', 'O', 'O', 'O', 'O', 'O', 'O', 'O', 'O', 'O', 'O', 'O', 'O', 'O', 'O', 'O', 'O', 'O', 'O', 'O', 'O', 'O', 'O', 'O', 'O', 'O', 'O', 'O', 'O', 'O', 'O', 'O', 'O', 'O', 'O', 'O', 'O', 'O', 'O', 'O', 'O', 'O', 'O', 'O', 'O', 'O', 'O', 'O', 'O', 'O', 'O', 'O', 'O', 'O', 'O', 'O', 'O', 'O', 'O', 'O', 'O', 'O', 'O', 'O', 'O', 'O', 'O', 'O', 'O', 'O', 'O', 'O', 'O', 'O', 'O', 'O', 'O', 'O', 'O', 'O', 'O', 'O', 'O', 'O', 'O', 'O', 'O', 'O', 'O', 'O', 'O', 'O', 'O', 'O', 'O', 'O', 'O', 'O', 'O', 'O', 'O', 'O', 'O', 'O', 'O', 'O', 'O', 'O', 'O', 'O', 'O', 'O', 'O', 'O', 'O', 'O', 'O', 'O', 'O', 'O', 'O', 'O', 'O', 'O', 'O', 'O', 'O', 'O', 'O', 'O', 'O', 'O', 'O', 'O', 'O', 'O', 'O', 'O', 'O', 'O', 'O', 'O', 'O', 'O', 'O', 'O', 'O', 'O', 'O', 'O', 'O', 'O', 'O', 'O', 'O', 'O', 'O', 'O', 'O', 'O', 'O', 'O', 'O', 'O', 'O', 'O', 'O', 'O', 'O', 'O', 'O', 'O', 'O', 'O', 'O', 'O', 'O', 'O', 'O', 'O', 'O', 'O', 'O', 'O', 'O', 'O', 'O', 'O', 'O', 'O', 'O', 'O', 'O', 'O', 'O', 'O', 'O', 'O', 'O', 'O', 'O', 'O', 'O', 'O', 'O', 'O', 'O', 'O', 'O', 'O', 'O', 'O', 'O', 'O', 'O', 'O', 'O', 'O', 'O', 'O', 'O', 'O', 'O', 'O']
Extractive/present Keyphrases: ['concurrency']
Abstractive/absent Keyphrases: ['memory consistency conditions', 'program correctness']
-----------
Sample from test data split
Fields in the sample: ['id', 'document', 'doc_bio_tags', 'extractive_keyphrases', 'abstractive_keyphrases', 'other_metadata']
Tokenized Document: ['Dynamic', 'analysis', 'of', 'some', 'Relational', 'Data', 'Bases', 'parameters', 'I', ':', 'Projections', 'Dani?ele', 'GARDY', '?', 'Guy', 'LOUCHARDy', 'January', '1994', 'Abstract', 'We', 'present', 'a', 'dynamic', 'study', 'of', 'a', 'data', 'structure', 'related', 'to', 'relational', 'databases', '.', 'We', 'show', 'that', 'some', 'parameters', 'of', 'relational', 'databases', '-LRB-', 'sizes', 'of', 'projections', '-RRB-', ',', 'related', 'to', 'an', 'occupancy', 'problem', 'in', 'urn', 'models', ',', 'behave', 'asymptotically', 'as', 'gaussian', 'stochastic', 'processes', 'under', 'a', 'sequence', 'of', 'updates', 'and', 'queries', '.', 'As', 'a', 'consequence', ',', 'we', 'analyze', 'the', 'distribution', 'of', 'the', 'maximum', 'size', 'of', 'the', 'projection', '.', '1', 'Introduction', 'We', 'consider', 'dynamic', 'objects', ',', 'obtained', 'by', 'updating', 'and', 'querying', 'a', 'data', 'structure', ',', 'on', 'which', 'we', 'want', 'to', 'study', 'a', 'parameter', ',', 'most', 'often', 'defining', 'some', 'size', '.', 'This', 'parameter', 'defines', 'a', 'random', 'variable', ',', 'and', 'we', 'study', 'its', 'behaviour', 'when', 'the', 'initial', 'object', 'is', 'submitted', 'to', 'a', 'sequence', 'of', 'insertions', ',', 'deletions', 'and', 'queries', ',', 'characterizing', 'it', '-LRB-', 'when', 'possible', '-RRB-', 'as', 'a', 'gaussian', 'stochastic', 'process', '.', 'The', 'dynamic', 'objects', 'are', 'here', 'relations', 'in', 'a', 'relational', 'database', ',', 'and', 'the', 'parameter', 'we', 'want', 'to', 'study', 'is', 'the', 'size', 'of', 'their', 'projection', 'on', 'a', 'set', 'of', 'attributes', '.', 'We', 'gave', 'in', 'a', 'former', 'paper', 'conditions', 'which', 'ensure', 'that', ',', 'in', 'the', 'static', 'case', '-LRB-', 'i.e.', 'at', 'a', 'given', 'time', '-RRB-', ',', 'the', 'size', 'of', 'the', 'projection', 'of', 'a', 'relation', 'follows', 'a', 'normal', 'limiting', 'distribution', '.', 'Our', 'goal', 'here', 'is', 'to', 'study', 'the', 'variation', 'of', 'the', 'size', 'of', 'the', 'projection', 'under', 'a', 'sequence', 'of', 'queries', 'and', 'updates', '.', 'We', 'shall', 'show', 'that', 'it', 'is', 'a', 'gaussian', 'process', ',', 'and', 'analyze', 'its', 'maximum', '.', '?', 'Laboratoire', 'PRISM', ',', 'Universit?e', 'de', 'Versailles', 'Saint-Quentin', ',', '78035', 'Versailles', '-LRB-', 'France', '-RRB-', '.', 'This', 'research', 'was', 'partly', 'supported', 'by', 'ESPRIT', 'III-Basic', 'Research', 'Action', 'ALCOM', 'II', '-LRB-', 'no.', '7141', '-RRB-', ',', 'by', 'the', 'CNRS', 'PRC', 'Math?ematique', '-', 'Informatique', 'and', 'by', 'a', 'cooperation', 'between', 'the', 'CNRS', 'and', 'the', 'FNRS', '.', 'yD?epartement', "d'Informatique", ',', 'Universit?e', 'Libre', 'de', 'Bruxelles', ',', 'Bruxelles', '-LRB-', 'Belgique', '-RRB-', '.', 'This', 'research', 'was', 'partially', 'supported', 'by', 'a', 'cooperation', 'between', 'the', 'FNRS', 'and', 'the', 'CNRS', '.', 'R', 'X', 'Y', 'x0', 'y0', 'x1', 'y1', 'x1', 'y2', 'ssX', '-LRB-', 'R', '-RRB-', 'X', 'x0', 'x1', 'Figure', '1', ':', 'Projection', 'of', 'the', 'relation', 'R', '-LSB-', 'X', ',', 'Y', '-RSB-', 'on', 'the', 'attribute', 'X', 'The', 'paper', 'is', 'organized', 'as', 'follows', '.', 'Section', '2', 'presents', 'the', 'database', 'parameters', 'that', 'we', 'shall', 'study', 'and', 'gives', 'a', 'modelization', 'in', 'terms', 'of', 'urn', 'models', ',', 'then', 'briefly', 'recalls', 'the', 'sequences', 'of', 'operations', 'which', 'may', 'be', 'considered', '.', 'Section', '3', 'gives', 'our', 'main', 'results', '-LRB-', 'characterization', 'of', 'the', 'parameter', 'we', 'study', 'as', 'a', 'gaussian', 'process', 'and', 'distribution', 'of', 'its', 'maximum', '-RRB-', 'and', 'presents', 'an', 'overview', 'of', 'our', 'method', ',', 'with', 'a', 'sketch', 'of', 'the', 'proof', '.', 'Section', '4', 'introduces', 'our', 'notations', ',', 'then', 'Section', '5', 'presents', 'the', 'basic', 'processes', '-LRB-', 'number', 'of', 'tuples', 'in', 'a', 'relation', '-RRB-', 'corresponding', 'to', 'different', 'update', 'models', 'and', 'to', 'several', 'constraints', 'on', 'the', 'initial', 'objects', '-LRB-', 'relations', '-RRB-', '.', 'Sections', '6', 'to', '10', 'are', 'devoted', 'to', 'the', 'detailed', 'proofs', '.', '2', 'Databases', 'and', 'urn', 'models', '2.1', 'Projections', 'and', 'the', 'occupancy', 'problem', 'in', 'urn', 'models', 'We', 'briefly', 'recall', 'here', 'some', 'definitions', 'relative', 'to', 'relational', 'databases', 'and', 'to', 'the', 'modelization', 'of', 'relations', ';', 'we', 'refer', 'the', 'reader', 'to', '-LSB-', '7', '-RSB-', 'for', 'a', 'detailed', 'presentation', '.', 'The', 'basic', 'objects', 'we', 'consider', 'are', 'relations', ',', 'which', 'are', 'sets', 'of', '-LRB-', 'distinct', '-RRB-', 'tuples', '.', 'They', 'can', 'be', 'seen', 'as', 'tables', ':', 'a', 'row', 'represents', 'a', 'tuple', ',', 'and', 'the', 'number', 'of', 'lines', 'is', 'the', 'number', 'of', 'elements', 'of', 'the', 'relation', '-LRB-', 'its', 'size', '-RRB-', ';', 'the', 'columns', 'are', 'called', 'the', 'attributes', '.', 'The', 'projection', 'of', 'a', 'relation', 'on', 'a', 'subset', 'of', 'the', 'set', 'of', 'attributes', 'is', 'a', 'new', 'relation', ',', 'obtained', 'by', 'suppressing', 'the', 'corresponding', 'columns', ',', 'then', 'all', 'the', 'duplicate', 'rows', 'in', 'the', 'resulting', 'table', ':', 'We', 'keep', 'only', 'one', 'instance', 'of', 'each', 'tuple', '.', 'We', 'give', 'in', 'Figure', '1', 'an', 'instance', 'of', 'a', 'relation', 'R', '-LSB-', 'X', ',', 'Y', '-RSB-', 'and', 'of', 'its', 'projection', '-LRB-', 'noted', 'ssX', '-LRB-', 'R', '-RRB-', '-RRB-', 'on', 'the', 'attribute', 'X.', 'For', 'ease', 'of', 'presentation', ',', 'and', 'without', 'loss', 'of', 'generality', ',', 'we', 'shall', 'restrict', 'ourselves', 'to', 'the', 'case', 'of', 'a', 'relation', 'R', 'with', 'two', 'attributes', 'X', 'and', 'Y', ',', 'and', 'of', 'its', 'projection', 'on', 'X', '.', 'We', 'shall', 'use', 'the', 'terms', 'initial', 'relation', 'for', 'the', 'relation', 'R', ',', 'and', 'derived', 'relation', 'for', 'its', 'projection', '.', 'Let', 'd', 'be', 'the', 'number', 'of', 'distinct', 'possible', 'values', 'for', 'the', 'attribute', 'X', ';', 'we', 'assume', 'that', ',', 'although', 'it', 'may', 'become', 'large', ',', 'd', 'is', 'finite', '.', 'The', 'projection', 'of', 'the', 'relation', 'R', 'can', 'be', 'modelized', 'with', 'urns', 'and', 'balls', ',', 'according', 'to', 'a', 'well-known', 'occupancy', 'model', ',', 'as', 'follows', '.', 'We', 'consider', 'a', 'sequence', 'of', 'd', 'urns', ',', 'each', 'urn', 'being', 'labelled', 'with', 'a', 'distinct', 'value', 'of', 'the', 'attribute', 'X.', 'To', 'each', 'tuple', 'of', 'the', 'relation', 'R', ',', 'we', 'associate', 'a', 'ball', 'labelled', 'by', 'the', 'value', 'of', 'the', 'tuple', 'on', 'the', 'column', 'X', ';', 'this', 'ball', 'falls', 'into', 'the', 'corresponding', 'urn', '.', 'An', 'equivalent', 'way', 'of', 'seeing', 'this', 'phenomenon', 'is', 'to', 'consider', 'instead', 'that', 'we', 'have', 'a', 'finite', 'supply', 'of', 'balls', ',', 'and', 'that', 'we', 'allocate', 'them', 'at', 'random', 'among', 'the', 'd', 'urns', ',', 'each', 'trial', 'being', 'independent', 'of', 'the', 'others', '.', 'Each', 'ball', 'then', 'receives', 'the', 'label', 'of', 'the', 'urn', 'it', 'falls', 'into', '.', 'After', 'coupling', 'all', 'the', 'tuples', 'of', 'the', 'initial', 'relation', 'R', 'with', 'urns', ',', 'some', 'urns', 'are', 'empty', 'and', 'some', 'contain', 'at', 'least', 'one', 'ball', '.', 'The', 'number', 'of', 'urns', 'with', 'at', 'least', 'one', 'ball', 'is', 'exactly', 'the', 'number', 'of', 'tuples', 'in', 'the', 'projection', 'of', 'the', 'relation', 'R.', 'If', ',', 'instead', 'of', 'the', 'number', 'of', 'urns', 'with', 'at', 'least', 'one', 'ball', ',', 'we', 'consider', 'the', 'number', 'of', 'empty', 'urns', ',', 'and', 'if', 'we', 'assume', 'that', 'each', 'urn', 'can', 'receive', 'an', 'unbounded', 'number', 'of', 'balls', ',', 'then', 'we', 'have', 'the', 'classical', 'occupancy', 'problem', 'presented', 'for', 'example', 'in', '-LSB-', '8', '-RSB-', '.', 'Assuming', 'that', 'the', 'urn', 'size', 'is', 'infinite', 'corresponds', ',', 'in', 'terms', 'of', 'relational', 'databases', ',', 'to', 'a']
Document BIO Tags: ['O', 'O', 'O', 'O', 'O', 'O', 'O', 'O', 'O', 'O', 'O', 'O', 'O', 'O', 'O', 'O', 'O', 'O', 'O', 'O', 'O', 'O', 'O', 'O', 'O', 'O', 'O', 'O', 'O', 'O', 'O', 'O', 'O', 'O', 'O', 'O', 'O', 'O', 'O', 'O', 'O', 'O', 'O', 'O', 'O', 'O', 'O', 'O', 'O', 'O', 'B', 'I', 'O', 'O', 'O', 'O', 'O', 'O', 'O', 'O', 'O', 'O', 'O', 'O', 'O', 'O', 'O', 'O', 'O', 'O', 'O', 'O', 'O', 'O', 'O', 'O', 'O', 'O', 'O', 'O', 'O', 'O', 'O', 'O', 'O', 'O', 'O', 'O', 'O', 'O', 'O', 'O', 'O', 'O', 'O', 'O', 'O', 'O', 'O', 'O', 'O', 'O', 'O', 'O', 'O', 'O', 'O', 'O', 'O', 'O', 'O', 'O', 'O', 'O', 'O', 'O', 'O', 'O', 'O', 'O', 'O', 'O', 'O', 'O', 'O', 'O', 'O', 'O', 'O', 'O', 'O', 'O', 'O', 'O', 'O', 'O', 'O', 'O', 'O', 'O', 'O', 'O', 'O', 'O', 'O', 'O', 'O', 'O', 'O', 'O', 'O', 'O', 'O', 'O', 'O', 'O', 'O', 'O', 'O', 'O', 'O', 'O', 'O', 'O', 'O', 'B', 'I', 'O', 'O', 'O', 'O', 'O', 'O', 'O', 'O', 'O', 'O', 'O', 'O', 'O', 'O', 'O', 'O', 'O', 'O', 'O', 'O', 'O', 'O', 'O', 'O', 'O', 'O', 'O', 'O', 'O', 'O', 'O', 'O', 'O', 'O', 'O', 'O', 'O', 'O', 'O', 'O', 'O', 'O', 'O', 'O', 'O', 'O', 'O', 'O', 'O', 'O', 'O', 'O', 'O', 'O', 'O', 'O', 'O', 'O', 'O', 'O', 'O', 'O', 'O', 'O', 'O', 'O', 'O', 'O', 'O', 'O', 'O', 'O', 'O', 'O', 'O', 'O', 'O', 'O', 'O', 'O', 'O', 'O', 'O', 'O', 'O', 'O', 'B', 'I', 'O', 'O', 'O', 'O', 'O', 'O', 'O', 'O', 'O', 'O', 'O', 'O', 'O', 'O', 'O', 'O', 'O', 'O', 'O', 'O', 'O', 'O', 'O', 'O', 'O', 'O', 'O', 'O', 'O', 'O', 'O', 'O', 'O', 'O', 'O', 'O', 'O', 'O', 'O', 'O', 'O', 'O', 'O', 'O', 'O', 'O', 'O', 'O', 'O', 'O', 'O', 'O', 'O', 'O', 'O', 'O', 'O', 'O', 'O', 'O', 'O', 'O', 'O', 'O', 'O', 'O', 'O', 'O', 'O', 'O', 'O', 'O', 'O', 'O', 'O', 'O', 'O', 'O', 'O', 'O', 'O', 'O', 'O', 'O', 'O', 'O', 'O', 'O', 'O', 'O', 'O', 'O', 'O', 'O', 'O', 'O', 'O', 'O', 'O', 'O', 'O', 'O', 'O', 'O', 'O', 'O', 'O', 'O', 'O', 'O', 'O', 'O', 'O', 'O', 'O', 'O', 'O', 'O', 'O', 'O', 'O', 'O', 'O', 'O', 'O', 'O', 'O', 'O', 'O', 'O', 'O', 'O', 'O', 'O', 'O', 'O', 'O', 'O', 'O', 'O', 'O', 'O', 'O', 'O', 'O', 'O', 'O', 'O', 'O', 'O', 'O', 'O', 'O', 'O', 'O', 'O', 'O', 'O', 'O', 'O', 'O', 'O', 'O', 'O', 'O', 'O', 'O', 'O', 'O', 'O', 'O', 'B', 'I', 'O', 'O', 'O', 'O', 'O', 'O', 'O', 'O', 'O', 'O', 'O', 'O', 'O', 'O', 'O', 'O', 'O', 'O', 'O', 'O', 'O', 'O', 'O', 'O', 'O', 'O', 'O', 'O', 'O', 'O', 'O', 'O', 'O', 'O', 'O', 'O', 'O', 'O', 'O', 'O', 'O', 'O', 'O', 'O', 'O', 'O', 'O', 'O', 'O', 'O', 'O', 'O', 'O', 'O', 'O', 'O', 'O', 'O', 'O', 'O', 'O', 'O', 'O', 'O', 'O', 'O', 'O', 'O', 'O', 'O', 'O', 'O', 'O', 'O', 'O', 'O', 'O', 'O', 'O', 'B', 'I', 'O', 'O', 'O', 'O', 'O', 'O', 'O', 'O', 'O', 'O', 'O', 'O', 'O', 'O', 'O', 'O', 'O', 'O', 'O', 'O', 'O', 'O', 'O', 'O', 'O', 'O', 'O', 'O', 'O', 'O', 'O', 'O', 'O', 'O', 'O', 'O', 'O', 'O', 'O', 'O', 'O', 'O', 'O', 'O', 'O', 'O', 'O', 'O', 'O', 'O', 'O', 'O', 'O', 'O', 'O', 'O', 'O', 'O', 'O', 'O', 'O', 'O', 'O', 'O', 'O', 'O', 'O', 'O', 'O', 'O', 'O', 'O', 'O', 'O', 'O', 'O', 'O', 'O', 'O', 'O', 'O', 'O', 'O', 'O', 'O', 'O', 'O', 'O', 'O', 'O', 'O', 'O', 'O', 'O', 'O', 'O', 'O', 'O', 'O', 'O', 'O', 'O', 'O', 'O', 'O', 'O', 'O', 'O', 'O', 'O', 'O', 'O', 'O', 'O', 'O', 'O', 'O', 'O', 'O', 'O', 'O', 'O', 'O', 'O', 'O', 'O', 'O', 'O', 'O', 'O', 'O', 'O', 'O', 'O', 'O', 'O', 'O', 'O', 'O', 'O', 'O', 'O', 'O', 'O', 'O', 'O', 'O', 'O', 'O', 'O', 'O', 'O', 'O', 'O', 'O', 'O', 'O', 'O', 'O', 'O', 'O', 'O', 'O', 'O', 'O', 'O', 'O', 'O', 'O', 'O', 'O', 'O', 'O', 'O', 'O', 'O', 'O', 'O', 'O', 'O', 'O', 'O', 'O', 'O', 'O', 'O', 'O', 'O', 'O', 'O', 'O', 'O', 'O', 'O', 'O', 'O', 'O', 'O', 'O', 'O', 'O', 'O', 'O', 'O', 'O', 'O', 'O', 'O', 'O', 'O', 'O', 'O', 'B', 'I', 'O', 'O', 'O', 'O', 'O', 'O', 'O', 'O', 'O', 'O', 'O', 'O', 'O', 'O', 'O', 'O', 'O', 'O', 'O', 'O', 'O', 'O', 'O', 'O', 'O', 'O', 'O', 'O', 'O', 'O', 'O', 'O', 'O', 'O', 'O', 'O', 'O', 'O', 'O', 'O', 'O', 'O', 'O', 'O', 'O', 'O', 'O', 'O', 'O', 'O', 'O', 'O', 'O', 'O', 'O', 'O', 'O', 'O', 'O', 'O', 'O', 'O', 'O', 'O', 'O', 'O', 'O', 'O', 'O', 'O', 'O', 'O', 'O', 'O', 'O', 'O', 'O', 'O', 'O', 'O', 'O', 'O', 'O', 'O', 'O', 'O', 'O', 'O', 'O', 'O', 'O', 'O', 'O', 'O', 'O', 'O', 'O', 'O', 'O', 'O', 'O', 'O', 'O', 'O', 'O', 'O', 'O', 'O', 'O', 'O', 'O', 'O', 'O', 'O', 'O', 'O', 'O', 'O', 'O', 'O', 'O', 'O', 'O', 'O', 'O', 'O', 'O', 'O', 'O', 'O', 'O', 'O', 'O', 'O', 'O', 'O', 'O', 'O', 'O', 'O', 'O', 'O', 'O', 'O', 'O', 'O', 'O', 'O', 'O', 'O', 'O', 'O', 'O', 'O', 'O', 'O', 'O', 'O', 'O', 'O', 'O', 'O', 'O', 'O', 'O', 'O', 'O', 'O', 'O', 'O', 'O', 'O', 'O', 'O', 'O', 'O', 'O', 'O', 'O', 'O', 'O', 'O', 'O', 'O', 'O', 'O', 'O', 'O', 'O', 'O', 'O', 'O', 'O', 'O', 'O', 'O', 'O', 'O', 'O', 'O', 'O', 'O', 'O', 'O', 'O', 'O', 'O', 'O', 'O', 'O', 'O', 'O', 'O', 'O', 'O', 'O', 'O', 'O', 'O', 'O', 'O', 'O', 'O', 'O', 'O', 'O', 'O', 'O', 'O', 'O', 'O', 'O', 'O', 'O', 'O', 'O', 'O', 'O', 'O', 'O', 'O', 'O', 'O', 'O', 'O', 'O', 'O', 'O', 'O', 'O', 'B', 'I', 'O', 'O', 'O', 'O', 'O', 'O', 'O', 'O', 'O', 'O', 'O', 'O', 'O', 'O', 'O', 'O', 'O', 'O', 'O', 'O', 'O', 'O', 'O', 'O', 'O']
Extractive/present Keyphrases: ['derived relation', 'gaussian process', 'occupancy problem', 'relational database', 'urn model']
Abstractive/absent Keyphrases: []
-----------
```
### Keyphrase Extraction
```python
from datasets import load_dataset
# get the dataset only for keyphrase extraction
dataset = load_dataset("midas/cstr", "extraction")
print("Samples for Keyphrase Extraction")
# sample from the train split
print("Sample from train data split")
test_sample = dataset["train"][0]
print("Fields in the sample: ", [key for key in test_sample.keys()])
print("Tokenized Document: ", test_sample["document"])
print("Document BIO Tags: ", test_sample["doc_bio_tags"])
print("\n-----------\n")
# sample from the test split
print("Sample from test data split")
test_sample = dataset["test"][0]
print("Fields in the sample: ", [key for key in test_sample.keys()])
print("Tokenized Document: ", test_sample["document"])
print("Document BIO Tags: ", test_sample["doc_bio_tags"])
print("\n-----------\n")
```
### Keyphrase Generation
```python
# get the dataset only for keyphrase generation
dataset = load_dataset("midas/cstr", "generation")
print("Samples for Keyphrase Generation")
# sample from the train split
print("Sample from train data split")
test_sample = dataset["train"][0]
print("Fields in the sample: ", [key for key in test_sample.keys()])
print("Tokenized Document: ", test_sample["document"])
print("Extractive/present Keyphrases: ", test_sample["extractive_keyphrases"])
print("Abstractive/absent Keyphrases: ", test_sample["abstractive_keyphrases"])
print("\n-----------\n")
# sample from the test split
print("Sample from test data split")
test_sample = dataset["test"][0]
print("Fields in the sample: ", [key for key in test_sample.keys()])
print("Tokenized Document: ", test_sample["document"])
print("Extractive/present Keyphrases: ", test_sample["extractive_keyphrases"])
print("Abstractive/absent Keyphrases: ", test_sample["abstractive_keyphrases"])
print("\n-----------\n")
```
## Citation Information
```
@inproceedings{10.1145/313238.313437,
author = {Witten, Ian H. and Paynter, Gordon W. and Frank, Eibe and Gutwin, Carl and Nevill-Manning, Craig G.},
title = {KEA: Practical Automatic Keyphrase Extraction},
year = {1999},
isbn = {1581131453},
publisher = {Association for Computing Machinery},
address = {New York, NY, USA},
url = {https://doi.org/10.1145/313238.313437},
doi = {10.1145/313238.313437},
booktitle = {Proceedings of the Fourth ACM Conference on Digital Libraries},
pages = {254–255},
numpages = {2},
location = {Berkeley, California, USA},
series = {DL '99}
}
```
## Contributions
Thanks to [@debanjanbhucs](https://github.com/debanjanbhucs), [@dibyaaaaax](https://github.com/dibyaaaaax) and [@ad6398](https://github.com/ad6398) for adding this dataset
| midas/cstr | [
"region:us"
] | 2022-03-02T23:29:22+00:00 | {} | 2022-03-05T04:36:34+00:00 | [] | [] | TAGS
#region-us
| Dataset Summary
---------------
A dataset for benchmarking keyphrase extraction and generation techniques from english scientific papers. For more details about the dataset please refer the original paper - URL
Original source of the data -
Dataset Structure
-----------------
### Data Fields
* id: unique identifier of the document.
* document: Whitespace separated list of words in the document.
* doc\_bio\_tags: BIO tags for each word in the document. B stands for the beginning of a keyphrase and I stands for inside the keyphrase. O stands for outside the keyphrase and represents the word that isn't a part of the keyphrase at all.
* extractive\_keyphrases: List of all the present keyphrases.
* abstractive\_keyphrase: List of all the absent keyphrases.
### Data Splits
Train
* Percentage of keyphrases that are named entities: 69.49% (named entities detected using scispacy - en-core-sci-lg model)
* Percentage of keyphrases that are noun phrases: 81.26% (noun phrases detected using spacy en-core-web-lg after removing determiners)
Test
* Percentage of keyphrases that are named entities: 70.79% (named entities detected using scispacy - en-core-sci-lg model)
* Percentage of keyphrases that are noun phrases: 82.74% (noun phrases detected using spacy en-core-web-lg after removing determiners)
Usage
-----
### Full Dataset
Output
### Keyphrase Extraction
### Keyphrase Generation
Contributions
-------------
Thanks to @debanjanbhucs, @dibyaaaaax and @ad6398 for adding this dataset
| [
"### Data Fields\n\n\n* id: unique identifier of the document.\n* document: Whitespace separated list of words in the document.\n* doc\\_bio\\_tags: BIO tags for each word in the document. B stands for the beginning of a keyphrase and I stands for inside the keyphrase. O stands for outside the keyphrase and represents the word that isn't a part of the keyphrase at all.\n* extractive\\_keyphrases: List of all the present keyphrases.\n* abstractive\\_keyphrase: List of all the absent keyphrases.",
"### Data Splits\n\n\n\nTrain\n\n\n* Percentage of keyphrases that are named entities: 69.49% (named entities detected using scispacy - en-core-sci-lg model)\n* Percentage of keyphrases that are noun phrases: 81.26% (noun phrases detected using spacy en-core-web-lg after removing determiners)\n\n\nTest\n\n\n* Percentage of keyphrases that are named entities: 70.79% (named entities detected using scispacy - en-core-sci-lg model)\n* Percentage of keyphrases that are noun phrases: 82.74% (noun phrases detected using spacy en-core-web-lg after removing determiners)\n\n\nUsage\n-----",
"### Full Dataset\n\n\nOutput",
"### Keyphrase Extraction",
"### Keyphrase Generation\n\n\nContributions\n-------------\n\n\nThanks to @debanjanbhucs, @dibyaaaaax and @ad6398 for adding this dataset"
] | [
"TAGS\n#region-us \n",
"### Data Fields\n\n\n* id: unique identifier of the document.\n* document: Whitespace separated list of words in the document.\n* doc\\_bio\\_tags: BIO tags for each word in the document. B stands for the beginning of a keyphrase and I stands for inside the keyphrase. O stands for outside the keyphrase and represents the word that isn't a part of the keyphrase at all.\n* extractive\\_keyphrases: List of all the present keyphrases.\n* abstractive\\_keyphrase: List of all the absent keyphrases.",
"### Data Splits\n\n\n\nTrain\n\n\n* Percentage of keyphrases that are named entities: 69.49% (named entities detected using scispacy - en-core-sci-lg model)\n* Percentage of keyphrases that are noun phrases: 81.26% (noun phrases detected using spacy en-core-web-lg after removing determiners)\n\n\nTest\n\n\n* Percentage of keyphrases that are named entities: 70.79% (named entities detected using scispacy - en-core-sci-lg model)\n* Percentage of keyphrases that are noun phrases: 82.74% (noun phrases detected using spacy en-core-web-lg after removing determiners)\n\n\nUsage\n-----",
"### Full Dataset\n\n\nOutput",
"### Keyphrase Extraction",
"### Keyphrase Generation\n\n\nContributions\n-------------\n\n\nThanks to @debanjanbhucs, @dibyaaaaax and @ad6398 for adding this dataset"
] |
e5e10a8cd8896bc9d9899625b7af430c062a58b0 | ## Dataset Summary
A dataset for benchmarking keyphrase extraction and generation techniques from english news articles. For more details about the dataset please refer the original paper - [https://dl.acm.org/doi/10.5555/1620163.1620205](https://dl.acm.org/doi/10.5555/1620163.1620205)
Original source of the data - []()
## Dataset Structure
### Data Fields
- **id**: unique identifier of the document.
- **document**: Whitespace separated list of words in the document.
- **doc_bio_tags**: BIO tags for each word in the document. B stands for the beginning of a keyphrase and I stands for inside the keyphrase. O stands for outside the keyphrase and represents the word that isn't a part of the keyphrase at all.
- **extractive_keyphrases**: List of all the present keyphrases.
- **abstractive_keyphrase**: List of all the absent keyphrases.
### Data Splits
|Split| #datapoints |
|--|--|
| Test | 308 |
## Usage
### Full Dataset
```python
from datasets import load_dataset
# get entire dataset
dataset = load_dataset("midas/duc2001", "raw")
# sample from the test split
print("Sample from test dataset split")
test_sample = dataset["test"][0]
print("Fields in the sample: ", [key for key in test_sample.keys()])
print("Tokenized Document: ", test_sample["document"])
print("Document BIO Tags: ", test_sample["doc_bio_tags"])
print("Extractive/present Keyphrases: ", test_sample["extractive_keyphrases"])
print("Abstractive/absent Keyphrases: ", test_sample["abstractive_keyphrases"])
print("\n-----------\n")
```
**Output**
```bash
Sample from test data split
Fields in the sample: ['id', 'document', 'doc_bio_tags', 'extractive_keyphrases', 'abstractive_keyphrases', 'other_metadata']
Tokenized Document: ['Here', ',', 'at', 'a', 'glance', ',', 'are', 'developments', 'today', 'involving', 'the', 'crash', 'of', 'Pan', 'American', 'World', 'Airways', 'Flight', '103', 'Wednesday', 'night', 'in', 'Lockerbie', ',', 'Scotland', ',', 'that', 'killed', 'all', '259', 'people', 'aboard', 'and', 'more', 'than', '20', 'people', 'on', 'the', 'ground', ':']
Document BIO Tags: ['O', 'O', 'O', 'O', 'O', 'O', 'O', 'O', 'O', 'O', 'O', 'B', 'O', 'B', 'I', 'I', 'I', 'I', 'I', 'O', 'O', 'O', 'B', 'O', 'O', 'O', 'O', 'O', 'O', 'O', 'O', 'O', 'O', 'O', 'O', 'O', 'O', 'O', 'O', 'O', 'O']
Extractive/present Keyphrases: ['pan american world airways flight 103', 'crash', 'lockerbie']
Abstractive/absent Keyphrases: ['terrorist threats', 'widespread wreckage', 'radical palestinian faction', 'terrorist bombing', 'bomb threat', 'sabotage']
-----------
```
### Keyphrase Extraction
```python
from datasets import load_dataset
# get the dataset only for keyphrase extraction
dataset = load_dataset("midas/duc2001", "extraction")
print("Samples for Keyphrase Extraction")
# sample from the test split
print("Sample from test data split")
test_sample = dataset["test"][0]
print("Fields in the sample: ", [key for key in test_sample.keys()])
print("Tokenized Document: ", test_sample["document"])
print("Document BIO Tags: ", test_sample["doc_bio_tags"])
print("\n-----------\n")
```
### Keyphrase Generation
```python
# get the dataset only for keyphrase generation
dataset = load_dataset("midas/duc2001", "generation")
print("Samples for Keyphrase Generation")
# sample from the test split
print("Sample from test data split")
test_sample = dataset["test"][0]
print("Fields in the sample: ", [key for key in test_sample.keys()])
print("Tokenized Document: ", test_sample["document"])
print("Extractive/present Keyphrases: ", test_sample["extractive_keyphrases"])
print("Abstractive/absent Keyphrases: ", test_sample["abstractive_keyphrases"])
print("\n-----------\n")
```
## Citation Information
```
@inproceedings{10.5555/1620163.1620205,
author = {Wan, Xiaojun and Xiao, Jianguo},
title = {Single Document Keyphrase Extraction Using Neighborhood Knowledge},
year = {2008},
isbn = {9781577353683},
publisher = {AAAI Press},
booktitle = {Proceedings of the 23rd National Conference on Artificial Intelligence - Volume 2},
pages = {855–860},
numpages = {6},
location = {Chicago, Illinois},
series = {AAAI'08}
}
```
## Contributions
Thanks to [@debanjanbhucs](https://github.com/debanjanbhucs), [@dibyaaaaax](https://github.com/dibyaaaaax) and [@ad6398](https://github.com/ad6398) for adding this dataset
| midas/duc2001 | [
"region:us"
] | 2022-03-02T23:29:22+00:00 | {} | 2022-01-23T06:13:06+00:00 | [] | [] | TAGS
#region-us
| Dataset Summary
---------------
A dataset for benchmarking keyphrase extraction and generation techniques from english news articles. For more details about the dataset please refer the original paper - URL
Original source of the data -
Dataset Structure
-----------------
### Data Fields
* id: unique identifier of the document.
* document: Whitespace separated list of words in the document.
* doc\_bio\_tags: BIO tags for each word in the document. B stands for the beginning of a keyphrase and I stands for inside the keyphrase. O stands for outside the keyphrase and represents the word that isn't a part of the keyphrase at all.
* extractive\_keyphrases: List of all the present keyphrases.
* abstractive\_keyphrase: List of all the absent keyphrases.
### Data Splits
Usage
-----
### Full Dataset
Output
### Keyphrase Extraction
### Keyphrase Generation
Contributions
-------------
Thanks to @debanjanbhucs, @dibyaaaaax and @ad6398 for adding this dataset
| [
"### Data Fields\n\n\n* id: unique identifier of the document.\n* document: Whitespace separated list of words in the document.\n* doc\\_bio\\_tags: BIO tags for each word in the document. B stands for the beginning of a keyphrase and I stands for inside the keyphrase. O stands for outside the keyphrase and represents the word that isn't a part of the keyphrase at all.\n* extractive\\_keyphrases: List of all the present keyphrases.\n* abstractive\\_keyphrase: List of all the absent keyphrases.",
"### Data Splits\n\n\n\nUsage\n-----",
"### Full Dataset\n\n\nOutput",
"### Keyphrase Extraction",
"### Keyphrase Generation\n\n\nContributions\n-------------\n\n\nThanks to @debanjanbhucs, @dibyaaaaax and @ad6398 for adding this dataset"
] | [
"TAGS\n#region-us \n",
"### Data Fields\n\n\n* id: unique identifier of the document.\n* document: Whitespace separated list of words in the document.\n* doc\\_bio\\_tags: BIO tags for each word in the document. B stands for the beginning of a keyphrase and I stands for inside the keyphrase. O stands for outside the keyphrase and represents the word that isn't a part of the keyphrase at all.\n* extractive\\_keyphrases: List of all the present keyphrases.\n* abstractive\\_keyphrase: List of all the absent keyphrases.",
"### Data Splits\n\n\n\nUsage\n-----",
"### Full Dataset\n\n\nOutput",
"### Keyphrase Extraction",
"### Keyphrase Generation\n\n\nContributions\n-------------\n\n\nThanks to @debanjanbhucs, @dibyaaaaax and @ad6398 for adding this dataset"
] |
9617780e99705df88a4cb239174c86b9d8d8300f | A dataset for benchmarking keyphrase extraction and generation techniques from abstracts of English scientific papers. For more details about the dataset please refer the original paper - [https://dl.acm.org/doi/pdf/10.3115/1119355.1119383](https://dl.acm.org/doi/pdf/10.3115/1119355.1119383).
Data source - [https://github.com/boudinfl/ake-datasets/tree/master/datasets/Inspec](https://github.com/boudinfl/ake-datasets/tree/master/datasets/Inspec)
## Dataset Summary
The Inspec dataset was originally proposed by *Hulth* in the paper titled - [Improved automatic keyword extraction given more linguistic knowledge](https://aclanthology.org/W03-1028.pdf) in the year 2003. The dataset consists of abstracts of 2,000 English scientific papers from the [Inspec database](https://clarivate.com/webofsciencegroup/solutions/webofscience-inspec/). The abstracts are from papers belonging to the scientific domains of *Computers and Control* and *Information Technology* published between 1998 to 2002. Each abstract has two sets of keyphrases annotated by professional indexers - *controlled* and *uncontrolled*. The *controlled* keyphrases are obtained from the Inspec thesaurus and therefore are often not present in the abstract's text. Only 18.1% of the *controlled* keyphrases are actually present in the abstract's text. The *uncontrolled* keyphrases are those selected by the indexers after reading the full-length scientific articles and 76.2% of them are present in the abstract's text. There is no information in the original paper about how these 2,000 scientific papers were selected. It is unknown whether the papers were randomly selected out of all the papers published between 1998-2002 in the *Computers and Control* and *Information Technology* domains or were there only 2,000 papers in this domain that were indexed by Inspec. The train, dev and test splits of the data were arbitrarily chosen.
One of the key aspect of this dataset which makes it unique is that it provides keyphrases assigned by professional indexers, which is uncommon in the keyphrase literature. Most of the datasets in this domain have author assigned keyphrases as the ground truth. The dataset shared over here does not explicitly presents the *controlled* and *uncontrolled* keyphrases instead it only categorizes the keyphrases into *extractive* and *abstractive*. **Extractive keyphrases** are those that could be found in the input text and the **abstractive keyphrases** are those that are not present in the input text. In order to get all the meta-data about the documents and keyphrases please refer to the [original source](https://github.com/boudinfl/ake-datasets/tree/master/datasets/Inspec) from which the dataset was taken from. The main motivation behind making this dataset available in the form as presented over here is to make it easy for the researchers to programmatically download it and evaluate their models for the tasks of keyphrase extraction and generation. As keyphrase extraction by treating it as a sequence tagging task and using contextual language models has become popular - [Keyphrase extraction from scholarly articles as sequence labeling using contextualized embeddings](https://arxiv.org/pdf/1910.08840.pdf), we have also made the token tags available in the BIO tagging format.
## Dataset Structure
## Dataset Statistics
Table 1: Statistics on the length of the abstractive keyphrases for Train, Test, and Validation splits of Inspec dataset.
| | Train | Test | Validation |
|:---------------:|:-----------------------:|:----------------------:|:------------------------:|
| Single word | 9.0% | 9.5% | 10.1% |
| Two words | 50.4% | 48.2% | 45.7% |
| Three words | 27.6% | 28.6% | 29.8% |
| Four words | 9.3% | 10.3% | 10.3% |
| Five words | 2.4% | 2.0% | 3.2% |
| Six words | 0.9% | 1.2% | 0.7% |
| Seven words | 0.3% | 0.2% | 0.2% |
| Eight words | 0.1% | 0% | 0.1% |
| Nine words | 0% | 0.1% | 0% |
Table 2: Statistics on the length of the extractive keyphrases for Train, Test, and Validation splits of Inspec dataset.
| | Train | Test | Validation |
|:------------:|:-----------------------:|:----------------------:|:------------------------:|
| Single word | 16.2% | 15.4% | 17.0% |
| Two words | 52.4% | 54.8% | 51.6% |
| Three words | 24.3% | 22.99% | 24.3% |
| Four words | 5.6% | 4.96% | 5.8% |
| Five words | 1.2% | 1.3% | 1.1% |
| Six words | 0.2% | 0.36% | 0.2% |
| Seven words | 0.1% | 0.06% | 0.1% |
| Eight words | 0% | 0% | 0.03% |
Table 3: General statistics of the Inspec dataset.
| Type of Analysis | Train | Test | Validation |
|:----------------------------------------------:|:------------------------------:|:------------------------------:|:------------------------------:|
| Annotator Type | Professional Indexers | Professional Indexers | Professional Indexers |
| Document Type | Abstracts from Inspec Database | Abstracts from Inspec Database | Abstracts from Inspec Database |
| No. of Documents | 1000 | 500 | 500 |
| Avg. Document length (words) | 141.5 | 134.6 | 132.6 |
| Max Document length (words) | 557 | 384 | 330 |
| Max no. of abstractive keyphrases in a document | 17 | 20 | 14 |
| Min no. of abstractive keyphrases in a document | 0 | 0 | 0 |
| Avg. no. of abstractive keyphrases per document | 3.39 | 3.26 | 3.12 |
| Max no. of extractive keyphrases in a document | 24 | 27 | 22 |
| Min no. of extractive keyphrases in a document | 0 | 0 | 0 |
| Avg. no. of extractive keyphrases per document | 6.39 | 6.56 | 5.95 |
- Percentage of keyphrases that are named entities: 55.25% (named entities detected using scispacy - en-core-sci-lg model)
- Percentage of keyphrases that are noun phrases: 73.59% (noun phrases detected using spacy after removing determiners)
### Data Fields
- **id**: unique identifier of the document.
- **document**: Whitespace separated list of words in the document.
- **doc_bio_tags**: BIO tags for each word in the document. B stands for the beginning of a keyphrase and I stands for inside the keyphrase. O stands for outside the keyphrase and represents the word that isn't a part of the keyphrase at all.
- **extractive_keyphrases**: List of all the present keyphrases.
- **abstractive_keyphrase**: List of all the absent keyphrases.
### Data Splits
|Split| No. of datapoints |
|--|--|
| Train | 1,000 |
| Test | 500 |
| Validation | 500 |
## Usage
### Full Dataset
```python
from datasets import load_dataset
# get entire dataset
dataset = load_dataset("midas/inspec", "raw")
# sample from the train split
print("Sample from training dataset split")
train_sample = dataset["train"][0]
print("Fields in the sample: ", [key for key in train_sample.keys()])
print("Tokenized Document: ", train_sample["document"])
print("Document BIO Tags: ", train_sample["doc_bio_tags"])
print("Extractive/present Keyphrases: ", train_sample["extractive_keyphrases"])
print("Abstractive/absent Keyphrases: ", train_sample["abstractive_keyphrases"])
print("\n-----------\n")
# sample from the validation split
print("Sample from validation dataset split")
validation_sample = dataset["validation"][0]
print("Fields in the sample: ", [key for key in validation_sample.keys()])
print("Tokenized Document: ", validation_sample["document"])
print("Document BIO Tags: ", validation_sample["doc_bio_tags"])
print("Extractive/present Keyphrases: ", validation_sample["extractive_keyphrases"])
print("Abstractive/absent Keyphrases: ", validation_sample["abstractive_keyphrases"])
print("\n-----------\n")
# sample from the test split
print("Sample from test dataset split")
test_sample = dataset["test"][0]
print("Fields in the sample: ", [key for key in test_sample.keys()])
print("Tokenized Document: ", test_sample["document"])
print("Document BIO Tags: ", test_sample["doc_bio_tags"])
print("Extractive/present Keyphrases: ", test_sample["extractive_keyphrases"])
print("Abstractive/absent Keyphrases: ", test_sample["abstractive_keyphrases"])
print("\n-----------\n")
```
**Output**
```bash
Sample from training data split
Fields in the sample: ['id', 'document', 'doc_bio_tags', 'extractive_keyphrases', 'abstractive_keyphrases', 'other_metadata']
Tokenized Document: ['A', 'conflict', 'between', 'language', 'and', 'atomistic', 'information', 'Fred', 'Dretske', 'and', 'Jerry', 'Fodor', 'are', 'responsible', 'for', 'popularizing', 'three', 'well-known', 'theses', 'in', 'contemporary', 'philosophy', 'of', 'mind', ':', 'the', 'thesis', 'of', 'Information-Based', 'Semantics', '-LRB-', 'IBS', '-RRB-', ',', 'the', 'thesis', 'of', 'Content', 'Atomism', '-LRB-', 'Atomism', '-RRB-', 'and', 'the', 'thesis', 'of', 'the', 'Language', 'of', 'Thought', '-LRB-', 'LOT', '-RRB-', '.', 'LOT', 'concerns', 'the', 'semantically', 'relevant', 'structure', 'of', 'representations', 'involved', 'in', 'cognitive', 'states', 'such', 'as', 'beliefs', 'and', 'desires', '.', 'It', 'maintains', 'that', 'all', 'such', 'representations', 'must', 'have', 'syntactic', 'structures', 'mirroring', 'the', 'structure', 'of', 'their', 'contents', '.', 'IBS', 'is', 'a', 'thesis', 'about', 'the', 'nature', 'of', 'the', 'relations', 'that', 'connect', 'cognitive', 'representations', 'and', 'their', 'parts', 'to', 'their', 'contents', '-LRB-', 'semantic', 'relations', '-RRB-', '.', 'It', 'holds', 'that', 'these', 'relations', 'supervene', 'solely', 'on', 'relations', 'of', 'the', 'kind', 'that', 'support', 'information', 'content', ',', 'perhaps', 'with', 'some', 'help', 'from', 'logical', 'principles', 'of', 'combination', '.', 'Atomism', 'is', 'a', 'thesis', 'about', 'the', 'nature', 'of', 'the', 'content', 'of', 'simple', 'symbols', '.', 'It', 'holds', 'that', 'each', 'substantive', 'simple', 'symbol', 'possesses', 'its', 'content', 'independently', 'of', 'all', 'other', 'symbols', 'in', 'the', 'representational', 'system', '.', 'I', 'argue', 'that', 'Dretske', "'s", 'and', 'Fodor', "'s", 'theories', 'are', 'false', 'and', 'that', 'their', 'falsehood', 'results', 'from', 'a', 'conflict', 'IBS', 'and', 'Atomism', ',', 'on', 'the', 'one', 'hand', ',', 'and', 'LOT', ',', 'on', 'the', 'other']
Document BIO Tags: ['O', 'O', 'O', 'O', 'O', 'O', 'O', 'O', 'O', 'O', 'O', 'O', 'O', 'O', 'O', 'O', 'O', 'O', 'O', 'O', 'O', 'B', 'I', 'I', 'O', 'O', 'O', 'O', 'O', 'O', 'O', 'B', 'O', 'O', 'O', 'O', 'O', 'B', 'I', 'O', 'O', 'O', 'O', 'O', 'O', 'O', 'O', 'B', 'I', 'I', 'O', 'B', 'O', 'O', 'B', 'O', 'O', 'O', 'O', 'O', 'O', 'O', 'O', 'O', 'B', 'I', 'O', 'O', 'B', 'O', 'B', 'O', 'O', 'O', 'O', 'O', 'O', 'O', 'O', 'O', 'O', 'O', 'O', 'O', 'O', 'O', 'O', 'O', 'O', 'B', 'O', 'O', 'O', 'O', 'O', 'O', 'O', 'O', 'O', 'O', 'O', 'O', 'O', 'O', 'O', 'O', 'O', 'O', 'O', 'O', 'O', 'O', 'O', 'O', 'O', 'O', 'O', 'O', 'O', 'O', 'O', 'O', 'O', 'O', 'O', 'O', 'O', 'O', 'O', 'O', 'O', 'O', 'O', 'O', 'O', 'O', 'O', 'O', 'O', 'O', 'O', 'O', 'O', 'O', 'O', 'O', 'O', 'O', 'O', 'O', 'O', 'O', 'O', 'O', 'O', 'O', 'O', 'O', 'O', 'O', 'O', 'O', 'O', 'O', 'O', 'O', 'O', 'O', 'O', 'O', 'O', 'O', 'O', 'O', 'O', 'O', 'O', 'O', 'O', 'O', 'O', 'O', 'O', 'O', 'O', 'O', 'O', 'O', 'O', 'O', 'O', 'O', 'O', 'O', 'B', 'O', 'O', 'O', 'O', 'O', 'O', 'O', 'O', 'O', 'B', 'O', 'O', 'O', 'O']
Extractive/present Keyphrases: ['philosophy of mind', 'content atomism', 'ibs', 'language of thought', 'lot', 'cognitive states', 'beliefs', 'desires']
Abstractive/absent Keyphrases: ['information-based semantics']
-----------
Sample from validation data split
Fields in the sample: ['id', 'document', 'doc_bio_tags', 'extractive_keyphrases', 'abstractive_keyphrases', 'other_metadata']
Tokenized Document: ['Impact', 'of', 'aviation', 'highway-in-the-sky', 'displays', 'on', 'pilot', 'situation', 'awareness', 'Thirty-six', 'pilots', '-LRB-', '31', 'men', ',', '5', 'women', '-RRB-', 'were', 'tested', 'in', 'a', 'flight', 'simulator', 'on', 'their', 'ability', 'to', 'intercept', 'a', 'pathway', 'depicted', 'on', 'a', 'highway-in-the-sky', '-LRB-', 'HITS', '-RRB-', 'display', '.', 'While', 'intercepting', 'and', 'flying', 'the', 'pathway', ',', 'pilots', 'were', 'required', 'to', 'watch', 'for', 'traffic', 'outside', 'the', 'cockpit', '.', 'Additionally', ',', 'pilots', 'were', 'tested', 'on', 'their', 'awareness', 'of', 'speed', ',', 'altitude', ',', 'and', 'heading', 'during', 'the', 'flight', '.', 'Results', 'indicated', 'that', 'the', 'presence', 'of', 'a', 'flight', 'guidance', 'cue', 'significantly', 'improved', 'flight', 'path', 'awareness', 'while', 'intercepting', 'the', 'pathway', ',', 'but', 'significant', 'practice', 'effects', 'suggest', 'that', 'a', 'guidance', 'cue', 'might', 'be', 'unnecessary', 'if', 'pilots', 'are', 'given', 'proper', 'training', '.', 'The', 'amount', 'of', 'time', 'spent', 'looking', 'outside', 'the', 'cockpit', 'while', 'using', 'the', 'HITS', 'display', 'was', 'significantly', 'less', 'than', 'when', 'using', 'conventional', 'aircraft', 'instruments', '.', 'Additionally', ',', 'awareness', 'of', 'flight', 'information', 'present', 'on', 'the', 'HITS', 'display', 'was', 'poor', '.', 'Actual', 'or', 'potential', 'applications', 'of', 'this', 'research', 'include', 'guidance', 'for', 'the', 'development', 'of', 'perspective', 'flight', 'display', 'standards', 'and', 'as', 'a', 'basis', 'for', 'flight', 'training', 'requirements']
Document BIO Tags: ['O', 'O', 'O', 'O', 'O', 'O', 'O', 'B', 'I', 'O', 'B', 'O', 'O', 'O', 'O', 'O', 'O', 'O', 'O', 'O', 'O', 'O', 'B', 'I', 'O', 'O', 'O', 'O', 'O', 'O', 'O', 'O', 'O', 'O', 'O', 'O', 'O', 'O', 'O', 'O', 'O', 'O', 'O', 'O', 'O', 'O', 'O', 'B', 'O', 'O', 'O', 'O', 'O', 'O', 'O', 'O', 'B', 'O', 'O', 'O', 'B', 'O', 'O', 'O', 'O', 'O', 'O', 'O', 'O', 'O', 'O', 'O', 'O', 'O', 'O', 'O', 'O', 'O', 'O', 'O', 'O', 'O', 'O', 'O', 'B', 'I', 'O', 'O', 'O', 'B', 'I', 'I', 'O', 'O', 'O', 'O', 'O', 'O', 'O', 'O', 'O', 'O', 'O', 'O', 'O', 'O', 'O', 'O', 'O', 'O', 'B', 'O', 'O', 'O', 'O', 'O', 'O', 'O', 'O', 'O', 'O', 'O', 'O', 'O', 'B', 'O', 'O', 'O', 'O', 'O', 'O', 'O', 'O', 'O', 'O', 'O', 'O', 'O', 'O', 'O', 'O', 'O', 'O', 'O', 'O', 'O', 'O', 'O', 'O', 'O', 'O', 'O', 'O', 'O', 'O', 'O', 'O', 'O', 'O', 'O', 'O', 'O', 'O', 'O', 'O', 'O', 'O', 'O', 'O', 'O', 'O', 'O', 'O', 'O', 'O', 'O', 'O', 'O', 'O']
Extractive/present Keyphrases: ['flight simulator', 'pilots', 'cockpit', 'flight guidance', 'situation awareness', 'flight path awareness']
Abstractive/absent Keyphrases: ['highway-in-the-sky display', 'human factors', 'aircraft display']
-----------
Sample from test data split
Fields in the sample: ['id', 'document', 'doc_bio_tags', 'extractive_keyphrases', 'abstractive_keyphrases', 'other_metadata']
Tokenized Document: ['A', 'new', 'graphical', 'user', 'interface', 'for', 'fast', 'construction', 'of', 'computation', 'phantoms', 'and', 'MCNP', 'calculations', ':', 'application', 'to', 'calibration', 'of', 'in', 'vivo', 'measurement', 'systems', 'Reports', 'on', 'a', 'new', 'utility', 'for', 'development', 'of', 'computational', 'phantoms', 'for', 'Monte', 'Carlo', 'calculations', 'and', 'data', 'analysis', 'for', 'in', 'vivo', 'measurements', 'of', 'radionuclides', 'deposited', 'in', 'tissues', '.', 'The', 'individual', 'properties', 'of', 'each', 'worker', 'can', 'be', 'acquired', 'for', 'a', 'rather', 'precise', 'geometric', 'representation', 'of', 'his', '-LRB-', 'her', '-RRB-', 'anatomy', ',', 'which', 'is', 'particularly', 'important', 'for', 'low', 'energy', 'gamma', 'ray', 'emitting', 'sources', 'such', 'as', 'thorium', ',', 'uranium', ',', 'plutonium', 'and', 'other', 'actinides', '.', 'The', 'software', 'enables', 'automatic', 'creation', 'of', 'an', 'MCNP', 'input', 'data', 'file', 'based', 'on', 'scanning', 'data', '.', 'The', 'utility', 'includes', 'segmentation', 'of', 'images', 'obtained', 'with', 'either', 'computed', 'tomography', 'or', 'magnetic', 'resonance', 'imaging', 'by', 'distinguishing', 'tissues', 'according', 'to', 'their', 'signal', '-LRB-', 'brightness', '-RRB-', 'and', 'specification', 'of', 'the', 'source', 'and', 'detector', '.', 'In', 'addition', ',', 'a', 'coupling', 'of', 'individual', 'voxels', 'within', 'the', 'tissue', 'is', 'used', 'to', 'reduce', 'the', 'memory', 'demand', 'and', 'to', 'increase', 'the', 'calculational', 'speed', '.', 'The', 'utility', 'was', 'tested', 'for', 'low', 'energy', 'emitters', 'in', 'plastic', 'and', 'biological', 'tissues', 'as', 'well', 'as', 'for', 'computed', 'tomography', 'and', 'magnetic', 'resonance', 'imaging', 'scanning', 'information']
Document BIO Tags: ['O', 'O', 'B', 'I', 'I', 'O', 'O', 'O', 'O', 'B', 'I', 'O', 'O', 'O', 'O', 'O', 'O', 'B', 'O', 'B', 'I', 'I', 'I', 'O', 'O', 'O', 'O', 'O', 'O', 'O', 'O', 'B', 'I', 'O', 'B', 'I', 'I', 'O', 'O', 'O', 'O', 'B', 'I', 'I', 'O', 'B', 'O', 'O', 'B', 'O', 'O', 'O', 'O', 'O', 'O', 'B', 'O', 'O', 'O', 'O', 'O', 'O', 'B', 'I', 'I', 'O', 'O', 'O', 'O', 'O', 'B', 'O', 'O', 'O', 'O', 'O', 'O', 'B', 'I', 'I', 'I', 'I', 'I', 'O', 'O', 'O', 'O', 'O', 'O', 'O', 'O', 'O', 'B', 'O', 'O', 'B', 'O', 'B', 'I', 'O', 'O', 'B', 'I', 'I', 'I', 'O', 'O', 'B', 'I', 'O', 'O', 'O', 'O', 'O', 'O', 'O', 'O', 'O', 'O', 'B', 'I', 'O', 'O', 'O', 'O', 'O', 'O', 'B', 'O', 'O', 'O', 'B', 'O', 'B', 'O', 'O', 'O', 'O', 'O', 'O', 'O', 'B', 'O', 'O', 'O', 'O', 'O', 'O', 'O', 'B', 'I', 'O', 'O', 'O', 'O', 'O', 'O', 'O', 'O', 'B', 'I', 'O', 'O', 'O', 'O', 'B', 'I', 'O', 'O', 'O', 'O', 'O', 'O', 'O', 'O', 'O', 'O', 'B', 'O', 'O', 'B', 'O', 'O', 'O', 'O', 'B', 'I', 'O', 'B', 'I', 'I', 'I', 'I']
Extractive/present Keyphrases: ['computational phantoms', 'monte carlo calculations', 'in vivo measurements', 'radionuclides', 'tissues', 'worker', 'precise geometric representation', 'mcnp input data file', 'scanning data', 'computed tomography', 'brightness', 'graphical user interface', 'computation phantoms', 'calibration', 'in vivo measurement systems', 'signal', 'detector', 'individual voxels', 'memory demand', 'calculational speed', 'plastic', 'magnetic resonance imaging scanning information', 'anatomy', 'low energy gamma ray emitting sources', 'actinides', 'software', 'automatic creation']
Abstractive/absent Keyphrases: ['th', 'u', 'pu', 'biological tissues']
-----------
```
### Keyphrase Extraction
```python
from datasets import load_dataset
# get the dataset only for keyphrase extraction
dataset = load_dataset("midas/inspec", "extraction")
print("Samples for Keyphrase Extraction")
# sample from the train split
print("Sample from training data split")
train_sample = dataset["train"][0]
print("Fields in the sample: ", [key for key in train_sample.keys()])
print("Tokenized Document: ", train_sample["document"])
print("Document BIO Tags: ", train_sample["doc_bio_tags"])
print("\n-----------\n")
# sample from the validation split
print("Sample from validation data split")
validation_sample = dataset["validation"][0]
print("Fields in the sample: ", [key for key in validation_sample.keys()])
print("Tokenized Document: ", validation_sample["document"])
print("Document BIO Tags: ", validation_sample["doc_bio_tags"])
print("\n-----------\n")
# sample from the test split
print("Sample from test data split")
test_sample = dataset["test"][0]
print("Fields in the sample: ", [key for key in test_sample.keys()])
print("Tokenized Document: ", test_sample["document"])
print("Document BIO Tags: ", test_sample["doc_bio_tags"])
print("\n-----------\n")
```
### Keyphrase Generation
```python
# get the dataset only for keyphrase generation
dataset = load_dataset("midas/inspec", "generation")
print("Samples for Keyphrase Generation")
# sample from the train split
print("Sample from training data split")
train_sample = dataset["train"][0]
print("Fields in the sample: ", [key for key in train_sample.keys()])
print("Tokenized Document: ", train_sample["document"])
print("Extractive/present Keyphrases: ", train_sample["extractive_keyphrases"])
print("Abstractive/absent Keyphrases: ", train_sample["abstractive_keyphrases"])
print("\n-----------\n")
# sample from the validation split
print("Sample from validation data split")
validation_sample = dataset["validation"][0]
print("Fields in the sample: ", [key for key in validation_sample.keys()])
print("Tokenized Document: ", validation_sample["document"])
print("Extractive/present Keyphrases: ", validation_sample["extractive_keyphrases"])
print("Abstractive/absent Keyphrases: ", validation_sample["abstractive_keyphrases"])
print("\n-----------\n")
# sample from the test split
print("Sample from test data split")
test_sample = dataset["test"][0]
print("Fields in the sample: ", [key for key in test_sample.keys()])
print("Tokenized Document: ", test_sample["document"])
print("Extractive/present Keyphrases: ", test_sample["extractive_keyphrases"])
print("Abstractive/absent Keyphrases: ", test_sample["abstractive_keyphrases"])
print("\n-----------\n")
```
## Citation Information
Please cite the works below if you use this dataset in your work.
```
@inproceedings{hulth2003improved,
title={Improved automatic keyword extraction given more linguistic knowledge},
author={Hulth, Anette},
booktitle={Proceedings of the 2003 conference on Empirical methods in natural language processing},
pages={216--223},
year={2003}
}
```
and
```
@InProceedings{10.1007/978-3-030-45442-5_41,
author="Sahrawat, Dhruva
and Mahata, Debanjan
and Zhang, Haimin
and Kulkarni, Mayank
and Sharma, Agniv
and Gosangi, Rakesh
and Stent, Amanda
and Kumar, Yaman
and Shah, Rajiv Ratn
and Zimmermann, Roger",
editor="Jose, Joemon M.
and Yilmaz, Emine
and Magalh{\~a}es, Jo{\~a}o
and Castells, Pablo
and Ferro, Nicola
and Silva, M{\'a}rio J.
and Martins, Fl{\'a}vio",
title="Keyphrase Extraction as Sequence Labeling Using Contextualized Embeddings",
booktitle="Advances in Information Retrieval",
year="2020",
publisher="Springer International Publishing",
address="Cham",
pages="328--335",
abstract="In this paper, we formulate keyphrase extraction from scholarly articles as a sequence labeling task solved using a BiLSTM-CRF, where the words in the input text are represented using deep contextualized embeddings. We evaluate the proposed architecture using both contextualized and fixed word embedding models on three different benchmark datasets, and compare with existing popular unsupervised and supervised techniques. Our results quantify the benefits of: (a) using contextualized embeddings over fixed word embeddings; (b) using a BiLSTM-CRF architecture with contextualized word embeddings over fine-tuning the contextualized embedding model directly; and (c) using domain-specific contextualized embeddings (SciBERT). Through error analysis, we also provide some insights into why particular models work better than the others. Lastly, we present a case study where we analyze different self-attention layers of the two best models (BERT and SciBERT) to better understand their predictions.",
isbn="978-3-030-45442-5"
}
```
and
```
@article{kulkarni2021learning,
title={Learning Rich Representation of Keyphrases from Text},
author={Kulkarni, Mayank and Mahata, Debanjan and Arora, Ravneet and Bhowmik, Rajarshi},
journal={arXiv preprint arXiv:2112.08547},
year={2021}
}
```
## Contributions
Thanks to [@debanjanbhucs](https://github.com/debanjanbhucs), [@dibyaaaaax](https://github.com/dibyaaaaax), [@UmaGunturi](https://github.com/UmaGunturi) and [@ad6398](https://github.com/ad6398) for adding this dataset
| midas/inspec | [
"arxiv:1910.08840",
"region:us"
] | 2022-03-02T23:29:22+00:00 | {} | 2022-03-05T03:08:37+00:00 | [
"1910.08840"
] | [] | TAGS
#arxiv-1910.08840 #region-us
| A dataset for benchmarking keyphrase extraction and generation techniques from abstracts of English scientific papers. For more details about the dataset please refer the original paper - URL
Data source - URL
Dataset Summary
---------------
The Inspec dataset was originally proposed by *Hulth* in the paper titled - Improved automatic keyword extraction given more linguistic knowledge in the year 2003. The dataset consists of abstracts of 2,000 English scientific papers from the Inspec database. The abstracts are from papers belonging to the scientific domains of *Computers and Control* and *Information Technology* published between 1998 to 2002. Each abstract has two sets of keyphrases annotated by professional indexers - *controlled* and *uncontrolled*. The *controlled* keyphrases are obtained from the Inspec thesaurus and therefore are often not present in the abstract's text. Only 18.1% of the *controlled* keyphrases are actually present in the abstract's text. The *uncontrolled* keyphrases are those selected by the indexers after reading the full-length scientific articles and 76.2% of them are present in the abstract's text. There is no information in the original paper about how these 2,000 scientific papers were selected. It is unknown whether the papers were randomly selected out of all the papers published between 1998-2002 in the *Computers and Control* and *Information Technology* domains or were there only 2,000 papers in this domain that were indexed by Inspec. The train, dev and test splits of the data were arbitrarily chosen.
One of the key aspect of this dataset which makes it unique is that it provides keyphrases assigned by professional indexers, which is uncommon in the keyphrase literature. Most of the datasets in this domain have author assigned keyphrases as the ground truth. The dataset shared over here does not explicitly presents the *controlled* and *uncontrolled* keyphrases instead it only categorizes the keyphrases into *extractive* and *abstractive*. Extractive keyphrases are those that could be found in the input text and the abstractive keyphrases are those that are not present in the input text. In order to get all the meta-data about the documents and keyphrases please refer to the original source from which the dataset was taken from. The main motivation behind making this dataset available in the form as presented over here is to make it easy for the researchers to programmatically download it and evaluate their models for the tasks of keyphrase extraction and generation. As keyphrase extraction by treating it as a sequence tagging task and using contextual language models has become popular - Keyphrase extraction from scholarly articles as sequence labeling using contextualized embeddings, we have also made the token tags available in the BIO tagging format.
Dataset Structure
-----------------
Dataset Statistics
------------------
Table 1: Statistics on the length of the abstractive keyphrases for Train, Test, and Validation splits of Inspec dataset.
Table 2: Statistics on the length of the extractive keyphrases for Train, Test, and Validation splits of Inspec dataset.
Table 3: General statistics of the Inspec dataset.
* Percentage of keyphrases that are named entities: 55.25% (named entities detected using scispacy - en-core-sci-lg model)
* Percentage of keyphrases that are noun phrases: 73.59% (noun phrases detected using spacy after removing determiners)
### Data Fields
* id: unique identifier of the document.
* document: Whitespace separated list of words in the document.
* doc\_bio\_tags: BIO tags for each word in the document. B stands for the beginning of a keyphrase and I stands for inside the keyphrase. O stands for outside the keyphrase and represents the word that isn't a part of the keyphrase at all.
* extractive\_keyphrases: List of all the present keyphrases.
* abstractive\_keyphrase: List of all the absent keyphrases.
### Data Splits
Usage
-----
### Full Dataset
Output
### Keyphrase Extraction
### Keyphrase Generation
Please cite the works below if you use this dataset in your work.
and
and
Contributions
-------------
Thanks to @debanjanbhucs, @dibyaaaaax, @UmaGunturi and @ad6398 for adding this dataset
| [
"### Data Fields\n\n\n* id: unique identifier of the document.\n* document: Whitespace separated list of words in the document.\n* doc\\_bio\\_tags: BIO tags for each word in the document. B stands for the beginning of a keyphrase and I stands for inside the keyphrase. O stands for outside the keyphrase and represents the word that isn't a part of the keyphrase at all.\n* extractive\\_keyphrases: List of all the present keyphrases.\n* abstractive\\_keyphrase: List of all the absent keyphrases.",
"### Data Splits\n\n\n\nUsage\n-----",
"### Full Dataset\n\n\nOutput",
"### Keyphrase Extraction",
"### Keyphrase Generation\n\n\nPlease cite the works below if you use this dataset in your work.\n\n\nand\n\n\nand\n\n\nContributions\n-------------\n\n\nThanks to @debanjanbhucs, @dibyaaaaax, @UmaGunturi and @ad6398 for adding this dataset"
] | [
"TAGS\n#arxiv-1910.08840 #region-us \n",
"### Data Fields\n\n\n* id: unique identifier of the document.\n* document: Whitespace separated list of words in the document.\n* doc\\_bio\\_tags: BIO tags for each word in the document. B stands for the beginning of a keyphrase and I stands for inside the keyphrase. O stands for outside the keyphrase and represents the word that isn't a part of the keyphrase at all.\n* extractive\\_keyphrases: List of all the present keyphrases.\n* abstractive\\_keyphrase: List of all the absent keyphrases.",
"### Data Splits\n\n\n\nUsage\n-----",
"### Full Dataset\n\n\nOutput",
"### Keyphrase Extraction",
"### Keyphrase Generation\n\n\nPlease cite the works below if you use this dataset in your work.\n\n\nand\n\n\nand\n\n\nContributions\n-------------\n\n\nThanks to @debanjanbhucs, @dibyaaaaax, @UmaGunturi and @ad6398 for adding this dataset"
] |
0dbf0783978f44aa6a03acaf28868757bcbac0a4 | ## Dataset Summary
A dataset for benchmarking keyphrase extraction and generation techniques from abstracts of english scientific papers. For more details about the dataset please refer the original paper - [https://aclanthology.org/D14-1150.pdf](https://aclanthology.org/D14-1150.pdf)
Original source of the data - []()
## Dataset Structure
### Data Fields
- **id**: unique identifier of the document.
- **document**: Whitespace separated list of words in the document.
- **doc_bio_tags**: BIO tags for each word in the document. B stands for the beginning of a keyphrase and I stands for inside the keyphrase. O stands for outside the keyphrase and represents the word that isn't a part of the keyphrase at all.
- **extractive_keyphrases**: List of all the present keyphrases.
- **abstractive_keyphrase**: List of all the absent keyphrases.
### Data Splits
|Split| #datapoints |
|--|--|
| Test | 755 |
- Percentage of keyphrases that are named entities: 56.99% (named entities detected using scispacy - en-core-sci-lg model)
- Percentage of keyphrases that are noun phrases: 54.99% (noun phrases detected using spacy en-core-web-lg after removing determiners)
## Usage
### Full Dataset
```python
from datasets import load_dataset
# get entire dataset
dataset = load_dataset("midas/kdd", "raw")
# sample from the test split
print("Sample from test dataset split")
test_sample = dataset["test"][0]
print("Fields in the sample: ", [key for key in test_sample.keys()])
print("Tokenized Document: ", test_sample["document"])
print("Document BIO Tags: ", test_sample["doc_bio_tags"])
print("Extractive/present Keyphrases: ", test_sample["extractive_keyphrases"])
print("Abstractive/absent Keyphrases: ", test_sample["abstractive_keyphrases"])
print("\n-----------\n")
```
**Output**
```bash
Sample from test data split
Fields in the sample: ['id', 'document', 'doc_bio_tags', 'extractive_keyphrases', 'abstractive_keyphrases', 'other_metadata']
Tokenized Document: ['Discovering', 'roll-up', 'dependencies']
Document BIO Tags: ['O', 'O', 'O']
Extractive/present Keyphrases: []
Abstractive/absent Keyphrases: ['logical design']
-----------
```
### Keyphrase Extraction
```python
from datasets import load_dataset
# get the dataset only for keyphrase extraction
dataset = load_dataset("midas/kdd", "extraction")
print("Samples for Keyphrase Extraction")
# sample from the test split
print("Sample from test data split")
test_sample = dataset["test"][0]
print("Fields in the sample: ", [key for key in test_sample.keys()])
print("Tokenized Document: ", test_sample["document"])
print("Document BIO Tags: ", test_sample["doc_bio_tags"])
print("\n-----------\n")
```
### Keyphrase Generation
```python
# get the dataset only for keyphrase generation
dataset = load_dataset("midas/kdd", "generation")
print("Samples for Keyphrase Generation")
# sample from the test split
print("Sample from test data split")
test_sample = dataset["test"][0]
print("Fields in the sample: ", [key for key in test_sample.keys()])
print("Tokenized Document: ", test_sample["document"])
print("Extractive/present Keyphrases: ", test_sample["extractive_keyphrases"])
print("Abstractive/absent Keyphrases: ", test_sample["abstractive_keyphrases"])
print("\n-----------\n")
```
## Citation Information
```
@inproceedings{caragea-etal-2014-citation,
title = "Citation-Enhanced Keyphrase Extraction from Research Papers: A Supervised Approach",
author = "Caragea, Cornelia and
Bulgarov, Florin Adrian and
Godea, Andreea and
Das Gollapalli, Sujatha",
booktitle = "Proceedings of the 2014 Conference on Empirical Methods in Natural Language Processing ({EMNLP})",
month = oct,
year = "2014",
address = "Doha, Qatar",
publisher = "Association for Computational Linguistics",
url = "https://aclanthology.org/D14-1150",
doi = "10.3115/v1/D14-1150",
pages = "1435--1446",
}
```
## Contributions
Thanks to [@debanjanbhucs](https://github.com/debanjanbhucs), [@dibyaaaaax](https://github.com/dibyaaaaax) and [@ad6398](https://github.com/ad6398) for adding this dataset
| midas/kdd | [
"region:us"
] | 2022-03-02T23:29:22+00:00 | {} | 2022-03-05T04:06:21+00:00 | [] | [] | TAGS
#region-us
| Dataset Summary
---------------
A dataset for benchmarking keyphrase extraction and generation techniques from abstracts of english scientific papers. For more details about the dataset please refer the original paper - URL
Original source of the data -
Dataset Structure
-----------------
### Data Fields
* id: unique identifier of the document.
* document: Whitespace separated list of words in the document.
* doc\_bio\_tags: BIO tags for each word in the document. B stands for the beginning of a keyphrase and I stands for inside the keyphrase. O stands for outside the keyphrase and represents the word that isn't a part of the keyphrase at all.
* extractive\_keyphrases: List of all the present keyphrases.
* abstractive\_keyphrase: List of all the absent keyphrases.
### Data Splits
* Percentage of keyphrases that are named entities: 56.99% (named entities detected using scispacy - en-core-sci-lg model)
* Percentage of keyphrases that are noun phrases: 54.99% (noun phrases detected using spacy en-core-web-lg after removing determiners)
Usage
-----
### Full Dataset
Output
### Keyphrase Extraction
### Keyphrase Generation
Contributions
-------------
Thanks to @debanjanbhucs, @dibyaaaaax and @ad6398 for adding this dataset
| [
"### Data Fields\n\n\n* id: unique identifier of the document.\n* document: Whitespace separated list of words in the document.\n* doc\\_bio\\_tags: BIO tags for each word in the document. B stands for the beginning of a keyphrase and I stands for inside the keyphrase. O stands for outside the keyphrase and represents the word that isn't a part of the keyphrase at all.\n* extractive\\_keyphrases: List of all the present keyphrases.\n* abstractive\\_keyphrase: List of all the absent keyphrases.",
"### Data Splits\n\n\n\n* Percentage of keyphrases that are named entities: 56.99% (named entities detected using scispacy - en-core-sci-lg model)\n* Percentage of keyphrases that are noun phrases: 54.99% (noun phrases detected using spacy en-core-web-lg after removing determiners)\n\n\nUsage\n-----",
"### Full Dataset\n\n\nOutput",
"### Keyphrase Extraction",
"### Keyphrase Generation\n\n\nContributions\n-------------\n\n\nThanks to @debanjanbhucs, @dibyaaaaax and @ad6398 for adding this dataset"
] | [
"TAGS\n#region-us \n",
"### Data Fields\n\n\n* id: unique identifier of the document.\n* document: Whitespace separated list of words in the document.\n* doc\\_bio\\_tags: BIO tags for each word in the document. B stands for the beginning of a keyphrase and I stands for inside the keyphrase. O stands for outside the keyphrase and represents the word that isn't a part of the keyphrase at all.\n* extractive\\_keyphrases: List of all the present keyphrases.\n* abstractive\\_keyphrase: List of all the absent keyphrases.",
"### Data Splits\n\n\n\n* Percentage of keyphrases that are named entities: 56.99% (named entities detected using scispacy - en-core-sci-lg model)\n* Percentage of keyphrases that are noun phrases: 54.99% (noun phrases detected using spacy en-core-web-lg after removing determiners)\n\n\nUsage\n-----",
"### Full Dataset\n\n\nOutput",
"### Keyphrase Extraction",
"### Keyphrase Generation\n\n\nContributions\n-------------\n\n\nThanks to @debanjanbhucs, @dibyaaaaax and @ad6398 for adding this dataset"
] |
a871c01a55b2421ecb94fc6569c341746f673220 | A dataset for benchmarking keyphrase extraction and generation techniques from abstracts of English scientific papers. For more details about the dataset please refer the original paper - [http://memray.me/uploads/acl17-keyphrase-generation.pdf](http://memray.me/uploads/acl17-keyphrase-generation.pdf).
Data source - [https://github.com/memray/seq2seq-keyphrase](https://github.com/memray/seq2seq-keyphrase)
## Dataset Summary
## Dataset Structure
## Dataset Statistics
### Data Fields
- **id**: unique identifier of the document.
- **document**: Whitespace separated list of words in the document.
- **doc_bio_tags**: BIO tags for each word in the document. B stands for the beginning of a keyphrase and I stands for inside the keyphrase. O stands for outside the keyphrase and represents the word that isn't a part of the keyphrase at all.
- **extractive_keyphrases**: List of all the present keyphrases.
- **abstractive_keyphrase**: List of all the absent keyphrases.
### Data Splits
|Split| No. of datapoints |
|--|--|
| Train | 530,809 |
| Test | 20,000|
| Validation | 20,000|
## Usage
### Full Dataset
```python
from datasets import load_dataset
# get entire dataset
dataset = load_dataset("midas/kp20k", "raw")
# sample from the train split
print("Sample from training dataset split")
train_sample = dataset["train"][0]
print("Fields in the sample: ", [key for key in train_sample.keys()])
print("Tokenized Document: ", train_sample["document"])
print("Document BIO Tags: ", train_sample["doc_bio_tags"])
print("Extractive/present Keyphrases: ", train_sample["extractive_keyphrases"])
print("Abstractive/absent Keyphrases: ", train_sample["abstractive_keyphrases"])
print("\n-----------\n")
# sample from the validation split
print("Sample from validation dataset split")
validation_sample = dataset["validation"][0]
print("Fields in the sample: ", [key for key in validation_sample.keys()])
print("Tokenized Document: ", validation_sample["document"])
print("Document BIO Tags: ", validation_sample["doc_bio_tags"])
print("Extractive/present Keyphrases: ", validation_sample["extractive_keyphrases"])
print("Abstractive/absent Keyphrases: ", validation_sample["abstractive_keyphrases"])
print("\n-----------\n")
# sample from the test split
print("Sample from test dataset split")
test_sample = dataset["test"][0]
print("Fields in the sample: ", [key for key in test_sample.keys()])
print("Tokenized Document: ", test_sample["document"])
print("Document BIO Tags: ", test_sample["doc_bio_tags"])
print("Extractive/present Keyphrases: ", test_sample["extractive_keyphrases"])
print("Abstractive/absent Keyphrases: ", test_sample["abstractive_keyphrases"])
print("\n-----------\n")
```
**Output**
```bash
```
### Keyphrase Extraction
```python
from datasets import load_dataset
# get the dataset only for keyphrase extraction
dataset = load_dataset("midas/kp20k", "extraction")
print("Samples for Keyphrase Extraction")
# sample from the train split
print("Sample from training data split")
train_sample = dataset["train"][0]
print("Fields in the sample: ", [key for key in train_sample.keys()])
print("Tokenized Document: ", train_sample["document"])
print("Document BIO Tags: ", train_sample["doc_bio_tags"])
print("\n-----------\n")
# sample from the validation split
print("Sample from validation data split")
validation_sample = dataset["validation"][0]
print("Fields in the sample: ", [key for key in validation_sample.keys()])
print("Tokenized Document: ", validation_sample["document"])
print("Document BIO Tags: ", validation_sample["doc_bio_tags"])
print("\n-----------\n")
# sample from the test split
print("Sample from test data split")
test_sample = dataset["test"][0]
print("Fields in the sample: ", [key for key in test_sample.keys()])
print("Tokenized Document: ", test_sample["document"])
print("Document BIO Tags: ", test_sample["doc_bio_tags"])
print("\n-----------\n")
```
### Keyphrase Generation
```python
# get the dataset only for keyphrase generation
dataset = load_dataset("midas/kp20k", "generation")
print("Samples for Keyphrase Generation")
# sample from the train split
print("Sample from training data split")
train_sample = dataset["train"][0]
print("Fields in the sample: ", [key for key in train_sample.keys()])
print("Tokenized Document: ", train_sample["document"])
print("Extractive/present Keyphrases: ", train_sample["extractive_keyphrases"])
print("Abstractive/absent Keyphrases: ", train_sample["abstractive_keyphrases"])
print("\n-----------\n")
# sample from the validation split
print("Sample from validation data split")
validation_sample = dataset["validation"][0]
print("Fields in the sample: ", [key for key in validation_sample.keys()])
print("Tokenized Document: ", validation_sample["document"])
print("Extractive/present Keyphrases: ", validation_sample["extractive_keyphrases"])
print("Abstractive/absent Keyphrases: ", validation_sample["abstractive_keyphrases"])
print("\n-----------\n")
# sample from the test split
print("Sample from test data split")
test_sample = dataset["test"][0]
print("Fields in the sample: ", [key for key in test_sample.keys()])
print("Tokenized Document: ", test_sample["document"])
print("Extractive/present Keyphrases: ", test_sample["extractive_keyphrases"])
print("Abstractive/absent Keyphrases: ", test_sample["abstractive_keyphrases"])
print("\n-----------\n")
```
## Citation Information
Please cite the works below if you use this dataset in your work.
```
@InProceedings{meng-EtAl:2017:Long,
author = {Meng, Rui and Zhao, Sanqiang and Han, Shuguang and He, Daqing and Brusilovsky, Peter and Chi, Yu},
title = {Deep Keyphrase Generation},
booktitle = {Proceedings of the 55th Annual Meeting of the Association for Computational Linguistics (Volume 1: Long Papers)},
month = {July},
year = {2017},
address = {Vancouver, Canada},
publisher = {Association for Computational Linguistics},
pages = {582--592},
url = {http://aclweb.org/anthology/P17-1054}
}
@article{mahata2022ldkp,
title={LDKP: A Dataset for Identifying Keyphrases from Long Scientific Documents},
author={Mahata, Debanjan and Agarwal, Navneet and Gautam, Dibya and Kumar, Amardeep and Parekh, Swapnil and Singla, Yaman Kumar and Acharya, Anish and Shah, Rajiv Ratn},
journal={arXiv preprint arXiv:2203.15349},
year={2022}
}
```
## Contributions
Thanks to [@debanjanbhucs](https://github.com/debanjanbhucs), [@dibyaaaaax](https://github.com/dibyaaaaax), [@UmaGunturi](https://github.com/UmaGunturi) and [@ad6398](https://github.com/ad6398) for adding this dataset
| midas/kp20k | [
"region:us"
] | 2022-03-02T23:29:22+00:00 | {} | 2023-09-25T04:14:59+00:00 | [] | [] | TAGS
#region-us
| A dataset for benchmarking keyphrase extraction and generation techniques from abstracts of English scientific papers. For more details about the dataset please refer the original paper - URL
Data source - URL
Dataset Summary
---------------
Dataset Structure
-----------------
Dataset Statistics
------------------
### Data Fields
* id: unique identifier of the document.
* document: Whitespace separated list of words in the document.
* doc\_bio\_tags: BIO tags for each word in the document. B stands for the beginning of a keyphrase and I stands for inside the keyphrase. O stands for outside the keyphrase and represents the word that isn't a part of the keyphrase at all.
* extractive\_keyphrases: List of all the present keyphrases.
* abstractive\_keyphrase: List of all the absent keyphrases.
### Data Splits
Usage
-----
### Full Dataset
Output
### Keyphrase Extraction
### Keyphrase Generation
Please cite the works below if you use this dataset in your work.
Contributions
-------------
Thanks to @debanjanbhucs, @dibyaaaaax, @UmaGunturi and @ad6398 for adding this dataset
| [
"### Data Fields\n\n\n* id: unique identifier of the document.\n* document: Whitespace separated list of words in the document.\n* doc\\_bio\\_tags: BIO tags for each word in the document. B stands for the beginning of a keyphrase and I stands for inside the keyphrase. O stands for outside the keyphrase and represents the word that isn't a part of the keyphrase at all.\n* extractive\\_keyphrases: List of all the present keyphrases.\n* abstractive\\_keyphrase: List of all the absent keyphrases.",
"### Data Splits\n\n\n\nUsage\n-----",
"### Full Dataset\n\n\nOutput",
"### Keyphrase Extraction",
"### Keyphrase Generation\n\n\nPlease cite the works below if you use this dataset in your work.\n\n\nContributions\n-------------\n\n\nThanks to @debanjanbhucs, @dibyaaaaax, @UmaGunturi and @ad6398 for adding this dataset"
] | [
"TAGS\n#region-us \n",
"### Data Fields\n\n\n* id: unique identifier of the document.\n* document: Whitespace separated list of words in the document.\n* doc\\_bio\\_tags: BIO tags for each word in the document. B stands for the beginning of a keyphrase and I stands for inside the keyphrase. O stands for outside the keyphrase and represents the word that isn't a part of the keyphrase at all.\n* extractive\\_keyphrases: List of all the present keyphrases.\n* abstractive\\_keyphrase: List of all the absent keyphrases.",
"### Data Splits\n\n\n\nUsage\n-----",
"### Full Dataset\n\n\nOutput",
"### Keyphrase Extraction",
"### Keyphrase Generation\n\n\nPlease cite the works below if you use this dataset in your work.\n\n\nContributions\n-------------\n\n\nThanks to @debanjanbhucs, @dibyaaaaax, @UmaGunturi and @ad6398 for adding this dataset"
] |
0532a67451ae1be349ee6a6c62b17c81f6cefab2 | ## Dataset Summary
A dataset for benchmarking keyphrase extraction and generation techniques from english news articles. For more details about the dataset please refer the original paper - [https://arxiv.org/abs/1306.4886](https://arxiv.org/abs/1306.4886)
Original source of the data - []()
## Dataset Structure
### Dataset Statistics
Table 1: Statistics on the length of the extractive keyphrases for Train, Test splits of kpcrowd dataset.
| | Train | Test |
|:-----------:|:------:|:------:|
| Single word | 81.62% | 80.27% |
| Two words | 14.41% | 15.44% |
| Three words | 2.79% | 3.36% |
| Four words | 0.78% | 0.56% |
| Five words | 0.20% | 0.25% |
| Six words | 0.12% | 0.05% |
| Seven words | 0% | 0.05% |
| Eight words | 0.01% | 0% |
Table 2: Statistics on the length of the abstractive keyphrases for Train, Test splits of kpcrowd dataset.
| | Train | Test |
|:-----------:|:--------:|:--------:|
| Zero words | 0.24% | 0% |
| Single word | 22.38 % | 21.81% |
| Two words | 45.14% | 43.03% |
| Three words | 18.35% | 19.69% |
| Four words | 7.71% | 7.28% |
| Five words | 3.09% | 3.94% |
| Six words | 1.51% | 3.33% |
| Seven words | 0.82% | 0.61%% |
| Eight words | 0.55% | 0.30% |
| Nine words | 0.17% | 0% |
Table 3: General statistics of the kpcrowd dataset.
| Type of Analysis | Train | Test |
|:------------------------------------------------:|:-------------:|:-------------:|
| Annotator Type | Authors | Authors |
| Document Type | News Articles | News Articles |
| No. of Documents | 450 | 50 |
| Avg. Document length (words) | 511.89 | 465.3 |
| Max Document length (words) | 7006 | 1609 |
| Max no. of abstractive keyphrases in a document | 66 | 30 |
| Min no. of abstractive keyphrases in a document | 0 | 0 |
| Avg. no. of abstractive keyphrases per document | 6.45 | 6.6 |
| Max no. of extractive keyphrases in a document | 231 | 86 |
| Min no. of extractive keyphrases in a document | 5 | 9 |
| Avg. no. of extractive keyphrases per document | 42.81 | 39.24 |
### Data Fields
- **id**: unique identifier of the document.
- **document**: Whitespace separated list of words in the document.
- **doc_bio_tags**: BIO tags for each word in the document. B stands for the beginning of a keyphrase and I stands for inside the keyphrase. O stands for outside the keyphrase and represents the word that isn't a part of the keyphrase at all.
- **extractive_keyphrases**: List of all the present keyphrases.
- **abstractive_keyphrase**: List of all the absent keyphrases.
### Data Splits
|Split| #datapoints |
|--|--|
| Train | 450 |
| Test | 50 |
## Usage
### Full Dataset
```python
from datasets import load_dataset
# get entire dataset
dataset = load_dataset("midas/kpcrowd", "raw")
# sample from the train split
print("Sample from train dataset split")
test_sample = dataset["train"][0]
print("Fields in the sample: ", [key for key in test_sample.keys()])
print("Tokenized Document: ", test_sample["document"])
print("Document BIO Tags: ", test_sample["doc_bio_tags"])
print("Extractive/present Keyphrases: ", test_sample["extractive_keyphrases"])
print("Abstractive/absent Keyphrases: ", test_sample["abstractive_keyphrases"])
print("\n-----------\n")
# sample from the test split
print("Sample from test dataset split")
test_sample = dataset["test"][0]
print("Fields in the sample: ", [key for key in test_sample.keys()])
print("Tokenized Document: ", test_sample["document"])
print("Document BIO Tags: ", test_sample["doc_bio_tags"])
print("Extractive/present Keyphrases: ", test_sample["extractive_keyphrases"])
print("Abstractive/absent Keyphrases: ", test_sample["abstractive_keyphrases"])
print("\n-----------\n")
```
**Output**
```bash
Sample from training data split
Fields in the sample: ['id', 'document', 'doc_bio_tags', 'extractive_keyphrases', 'abstractive_keyphrases', 'other_metadata']
Tokenized Document: ['James', 'Cameron', 'and', 'the', 'Future', 'of', 'Cinema', 'This', 'past', 'week', 'at', 'Cinemacon', ',', 'which', 'is', 'known', 'as', 'the', "''", '``', 'official', 'convention', 'of', 'the', 'National', 'Organization', 'of', 'Theatre', 'Owners', "''", "''", 'or', 'NATO', '-LRB-', 'really', '?', '-RRB-', ',', 'industry', 'professionals', 'of', 'all', 'sorts', 'gathered', 'at', 'Caesar', "'s", 'Palace', 'in', 'Las', 'Vegas', '.', 'The', 'convention', ',', 'previously', 'known', 'as', 'ShoWest', 'is', "''", '``', 'the', 'largest', 'cinema', 'trade', 'show', 'in', 'the', 'world', "''", "''", '-LRB-', 'www.cinemacon.com', '-RRB-', 'It', 'was', 'at', 'this', 'convention', 'that', 'filmmaker', 'James', 'Cameron', '-LRB-', 'Titanic', ',', 'Avatar', '-RRB-', 'delivered', 'a', 'presentation', 'entitled', "''", '``', 'A', 'Demonstration', 'and', 'Exclusive', 'Look', 'at', 'the', 'Future', 'of', 'Cinema', '.', "''", "''", 'The', 'last', 'time', 'Cameron', 'spoke', 'at', 'ShoWest', ',', 'he', 'and', 'George', 'Lucas', 'had', 'presented', 'a', 'plea', 'to', 'the', 'movie', 'industry', 'to', 'begin', 'its', 'huge', 'investment', 'in', 'digital', 'filmmaking', 'technology', 'in', 'preparation', 'of', 'the', '3D', 'revolution', 'that', 'was', 'bound', 'to', 'take', 'over', 'cinema', '.', 'One', 'year', 'removed', 'from', 'the', 'release', 'of', 'Cameron', "'s", 'technologically', 'groundbreaking', 'and', 'box', 'office', 'titan', 'Avatar', ',', 'the', 'film', 'industry', 'seems', 'to', 'have', 'done', 'exactly', 'what', 'Cameron', 'and', 'Lucas', 'predicted', '.', 'With', 'the', 'addition', 'of', 'digital', 'projection', 'systems', 'to', 'nearly', 'every', 'major', 'cineplex', 'or', 'theater', 'around', 'the', 'nation', 'and', 'of', 'course', 'the', 'overwhelming', 'use', 'of', '3D', ',', 'one', 'can', 'not', 'help', 'but', 'trust', 'that', 'Cameron', 'knows', 'what', 'he', 'is', 'talking', 'about.When', 'he', 'spoke', 'this', 'year', 'at', 'Cinemacon', ',', 'he', ',', 'once', 'again', ',', 'spoke', 'of', 'a', 'revolution', '.', 'Instead', 'of', 'promoting', '3D', 'cinema', ',', 'this', 'time', 'around', 'Cameron', 'talked', 'framerates', '.', 'Framerates', ',', 'for', 'those', 'not', 'fluent', 'in', 'film', 'jargon', ',', 'is', 'the', 'term', 'used', 'to', 'describe', 'the', 'speed', 'at', 'which', 'a', 'camera', 'shoots', 'and', 'subsequently', 'plays', 'back', 'individual', 'frames', 'on', 'a', 'film', 'strip', '.', 'The', 'industry', 'standard', 'has', 'been', '24', 'frames', 'per', 'second', '-LRB-', 'fps', '-RRB-', 'since', 'around', 'the', 'mid-20', "''", '``', 's', ',', 'as', 'it', 'is', 'believed', 'to', 'be', 'the', 'closest', 'to', 'mimicking', 'reality', '.', 'However', ',', 'filmmakers', 'have', 'always', 'experimented', 'with', 'framerates', 'whether', 'it', 'be', 'shooting', 'at', 'slower', 'frame', 'rates', 'to', 'produce', 'a', 'sensation', 'of', 'fast', 'motion', '-LRB-', 'think', ':', 'the', 'this', 'scene', 'in', 'Stanley', 'Kubrick', "'s", 'A', 'Clockwork', 'Orange', '-RRB-', 'or', 'shooting', 'at', 'faster', 'framerates', 'like', '48', 'fps', 'to', 'produce', 'what', 'is', 'known', 'as', 'slow', 'motion', '-LRB-', 'think', ':', 'sports', 'instant', 'replays', ',', 'or', 'this', 'funny', 'video', '.', "''", "''", 'Advertisement', 'Cameron', 'wants', 'the', 'industry', 'standard', 'to', 'change', '.', 'He', 'believes', 'that', 'by', 'making', 'the', 'industry', 'standard', 'something', 'like', '48', 'fps', ',', 'not', 'only', 'does', 'the', 'clarity', 'of', 'the', 'image', 'go', 'from', "''", '``', 'Good', "''", "''", 'to', "''", '``', 'Holy', 'S%@#!,', "''", "''", 'he', 'believes', 'it', 'will', 'improve', 'and', 'smooth', 'out', 'any', 'movement', 'that', 'the', 'camera', 'utilizes', '.', 'With', 'handheld', 'footage', 'practically', 'being', 'an', 'independent', 'film', 'standard', ',', 'it', 'will', 'help', 'translate', 'to', 'a', 'smoother', ',', 'more', 'pleasurable', 'film', 'experience', '.', 'His', 'argument', 'is', 'an', 'interesting', 'one', 'and', 'one', 'that', 'is', 'technically', 'relevant', 'and', 'affordable', 'for', 'all', 'kinds', 'of', 'filmmakers', '.', 'With', 'the', 'almost', 'overwhelming', 'transition', 'from', 'film', 'to', 'digital', ',', 'the', 'cost', 'of', 'shooting', 'at', 'higher', 'framerates', 'is', 'almost', 'null', 'and', 'void', '.', 'Most', 'of', 'the', 'newest', 'digital', 'video', 'cameras', 'like', 'Canon', "'s", '5D', 'and', '7D', 'already', 'shoot', 'at', 'a', 'standard', 'close', 'to', '30', 'fps', '.', 'So', ',', 'shooting', 'digitally', ',', 'one', 'does', "n't", 'have', 'to', 'empty', 'their', 'wallet', 'too', 'much', 'to', 'afford', 'to', 'shoot', 'at', 'higher', 'framerates', '.', 'That', 'being', 'said', ',', 'Cameron', "'s", 'proposal', 'presents', 'an', 'interesting', 'direction', 'for', 'the', 'future', 'of', 'cinema', '.', 'Many', 'filmmakers', 'like', 'Peter', 'Jackson', 'and', 'of', 'course', 'James', 'Cameron', 'have', 'already', 'experimented', 'with', 'increased', 'framerates', ',', 'and', 'their', 'arument', 'is', 'surely', 'a', 'compelling', 'one', ',', 'one', 'the', 'industry', 'will', 'have', 'to', 'keep', 'an', 'eye', 'on', '.']
Document BIO Tags: ['B', 'I', 'O', 'O', 'B', 'I', 'I', 'O', 'O', 'O', 'O', 'B', 'O', 'O', 'O', 'O', 'O', 'O', 'O', 'O', 'O', 'O', 'O', 'O', 'B', 'I', 'I', 'I', 'I', 'O', 'O', 'O', 'B', 'O', 'O', 'O', 'O', 'O', 'B', 'O', 'O', 'O', 'O', 'O', 'O', 'O', 'O', 'O', 'O', 'B', 'I', 'O', 'O', 'O', 'O', 'O', 'O', 'O', 'B', 'O', 'O', 'O', 'O', 'O', 'B', 'O', 'O', 'O', 'O', 'O', 'O', 'O', 'O', 'B', 'O', 'O', 'O', 'O', 'O', 'O', 'O', 'O', 'B', 'I', 'O', 'O', 'O', 'O', 'O', 'O', 'O', 'O', 'O', 'O', 'O', 'O', 'B', 'O', 'B', 'I', 'O', 'O', 'B', 'I', 'I', 'O', 'O', 'O', 'O', 'O', 'O', 'B', 'O', 'O', 'B', 'O', 'O', 'O', 'B', 'I', 'O', 'O', 'O', 'O', 'O', 'O', 'O', 'B', 'O', 'O', 'O', 'B', 'I', 'O', 'B', 'O', 'B', 'O', 'B', 'O', 'O', 'O', 'B', 'O', 'O', 'O', 'O', 'O', 'O', 'B', 'O', 'O', 'O', 'O', 'O', 'O', 'O', 'O', 'B', 'O', 'B', 'O', 'O', 'B', 'I', 'O', 'O', 'O', 'O', 'O', 'B', 'O', 'O', 'O', 'O', 'O', 'O', 'B', 'O', 'O', 'O', 'O', 'O', 'O', 'O', 'O', 'B', 'O', 'O', 'O', 'O', 'O', 'O', 'O', 'O', 'O', 'O', 'O', 'B', 'O', 'O', 'O', 'O', 'O', 'O', 'O', 'O', 'O', 'O', 'O', 'O', 'O', 'O', 'O', 'O', 'B', 'O', 'O', 'O', 'O', 'O', 'O', 'O', 'O', 'O', 'O', 'O', 'B', 'O', 'O', 'O', 'O', 'O', 'O', 'O', 'O', 'O', 'B', 'O', 'O', 'O', 'O', 'O', 'B', 'O', 'O', 'O', 'O', 'B', 'O', 'B', 'O', 'B', 'O', 'O', 'O', 'O', 'B', 'O', 'O', 'B', 'O', 'O', 'O', 'O', 'O', 'O', 'O', 'O', 'O', 'O', 'O', 'O', 'B', 'O', 'O', 'B', 'O', 'O', 'O', 'O', 'O', 'O', 'O', 'O', 'O', 'O', 'B', 'I', 'O', 'O', 'O', 'O', 'O', 'O', 'O', 'O', 'O', 'O', 'O', 'O', 'O', 'O', 'O', 'O', 'O', 'O', 'O', 'O', 'O', 'O', 'O', 'O', 'B', 'O', 'O', 'O', 'O', 'O', 'O', 'B', 'O', 'O', 'O', 'O', 'B', 'O', 'O', 'O', 'B', 'O', 'O', 'O', 'O', 'O', 'B', 'O', 'B', 'O', 'O', 'B', 'O', 'O', 'O', 'O', 'O', 'B', 'O', 'O', 'O', 'O', 'O', 'B', 'O', 'O', 'O', 'B', 'O', 'O', 'B', 'O', 'B', 'I', 'O', 'B', 'O', 'O', 'O', 'O', 'O', 'B', 'O', 'O', 'O', 'O', 'O', 'O', 'O', 'O', 'O', 'O', 'O', 'O', 'O', 'O', 'B', 'B', 'O', 'O', 'B', 'I', 'O', 'O', 'O', 'O', 'O', 'O', 'O', 'O', 'O', 'B', 'I', 'O', 'O', 'B', 'I', 'O', 'O', 'O', 'O', 'O', 'B', 'O', 'O', 'O', 'O', 'O', 'O', 'O', 'O', 'O', 'O', 'O', 'O', 'O', 'B', 'I', 'O', 'O', 'O', 'O', 'O', 'O', 'B', 'O', 'O', 'O', 'O', 'B', 'O', 'O', 'B', 'O', 'O', 'O', 'O', 'B', 'O', 'O', 'O', 'B', 'O', 'B', 'O', 'O', 'O', 'O', 'B', 'O', 'O', 'B', 'O', 'O', 'B', 'O', 'B', 'O', 'O', 'B', 'O', 'O', 'B', 'O', 'O', 'O', 'O', 'O', 'B', 'I', 'O', 'B', 'O', 'O', 'O', 'O', 'B', 'O', 'O', 'O', 'O', 'O', 'O', 'O', 'O', 'O', 'B', 'O', 'O', 'O', 'O', 'B', 'O', 'B', 'B', 'O', 'O', 'O', 'O', 'O', 'O', 'O', 'O', 'O', 'B', 'I', 'I', 'I', 'O', 'O', 'O', 'O', 'O', 'B', 'O', 'O', 'O', 'O', 'B', 'O', 'O', 'B', 'I', 'O', 'O', 'O', 'B', 'O', 'O', 'O', 'O', 'O', 'O', 'O', 'O', 'O', 'B', 'O', 'O', 'O', 'B', 'O', 'O', 'O', 'B', 'B', 'O', 'O', 'O', 'O', 'O', 'B', 'O', 'B', 'O', 'O', 'B', 'O', 'O', 'O', 'B', 'I', 'I', 'O', 'O', 'B', 'O', 'B', 'I', 'O', 'O', 'O', 'B', 'I', 'O', 'O', 'O', 'O', 'O', 'B', 'O', 'O', 'O', 'B', 'O', 'O', 'O', 'O', 'O', 'O', 'O', 'O', 'B', 'O', 'O', 'O', 'B', 'O', 'O', 'O', 'O']
Extractive/present Keyphrases: ['technically relevant', 'framerates', 'interesting', 'las vegas', 'shooting', '7d', 'cinemacon', 'exclusive look', 'nato', 'clockwork', 'future of cinema', 'showest', 'george lucas', 'cinema', 'newest digital video cameras', 'peter jackson', 'holy s', 'advertisement', '30 fps', '48 fps', 'wwwcinemaconcom', 'national organization of theatre owners', 'james cameron', 'filmmakers', 'higher', 'movement', 'digital', 'jargon', 'independent', 'afford', 'keep', 'arument', 'wallet', 'subsequently', 'closest', 'motion', 'nation', 'sensation', 'pleasurable', 'experience', 'fluent', 'camera', 'cameron', 'clarity', 'revolution', 'industry standard', 'industry', 'preparation', 'scene', 'smoother', 'demonstration', 'huge investment', 'proposal', 'translate', 'produce', 'technology', 'footage', 'technologically', 'argument', 'affordable', 'box office', 'improve', 'standard']
Abstractive/absent Keyphrases: ['increased framerates', "canon's 5d", "stanley kubrick's", 'future', 'titanic avatar', "caesar's palace", 'mid20s', "cameron's", 'exclusive', 'theatre owners', 'largest cinema', 'faster framerates', 'interesting direction', 'like peter jackson', 'newest', 'fast motion', 'official convention of the national organization', 'relevant', 'digital projection', 'movie industry']
-----------
Sample from test data split
Fields in the sample: ['id', 'document', 'doc_bio_tags', 'extractive_keyphrases', 'abstractive_keyphrases', 'other_metadata']
Tokenized Document: ['&', 'lsquo', ';', 'Miral', '&', 'rsquo', ';', ':', 'Director', 'has', 'conflict', 'of', 'interest', '``', 'Miral', "''", 'Rated', 'PG', '-', '13', '.', 'At', 'Kendall', 'Square', 'Cinema', ':', 'C', '+', 'Painter-turned-director', 'Julian', 'Schnabel', '-LRB-', 'Oscar-nominated', 'for', 'his', 'exquisite', '``', 'The', 'Diving', 'Bell', 'and', 'the', 'Butterfly', "''", '-RRB-', 'has', 'built', 'a', 'terrific', 'second', 'career', 'from', 'filmed', 'biographies', '-LRB-', 'also', 'in-cluding', '``', 'Before', 'Night', 'Falls', "''", 'and', '``', 'Basquiat', "''", '-RRB-', 'that', 'deal', 'with', 'people', 'confined', 'by', 'circumstance', 'yearning', 'to', 'break', 'free', '.', 'I', "'d", 'love', 'to', 'report', 'that', 'his', 'fourth', 'film', ',', '``', 'Miral', ',', "''", 'continues', 'the', 'upward', 'trend', ',', 'but', 'the', 'screenplay', 'by', 'Schnabel', "'s", 'girlfriend', ',', 'Palestinian', 'journalist', 'Rula', 'Jebreal', '-LRB-', 'based', 'on', 'her', 'semiautobiographical', 'novel', '-RRB-', ',', 'contains', 'too', 'many', 'earnest', 'platitudes', 'in', 'its', 'one-sided', 'look', 'at', 'four', 'women', "'s", 'intertwining', 'lives', 'during', 'the', 'first', 'intifada', 'of', 'the', '1980s', '.', '-LRB-', 'Some', 'musical', 'choices', ',', 'such', 'as', 'Tom', 'Waits', "'", '``', 'All', 'the', 'World', 'Is', 'Green', "''", 'playing', 'over', 'a', 'climactic', 'funeral', ',', 'also', 'stand', 'out', 'in', 'a', 'bad', 'way', '.', '-RRB-', 'Miral', '-LRB-', 'Freida', 'Pinto', ',', '``', 'Slumdog', 'Millionaire', "''", '-RRB-', ',', 'the', 'young', 'Arab', 'woman', 'growing', 'up', 'in', 'Jerusalem', 'during', 'this', 'period', ',', 'does', "n't", 'enter', 'the', 'picture', 'immediately', ',', 'and', 'when', 'she', 'does', ',', 'she', 'does', "n't", 'have', 'much', 'to', 'say', '--', 'at', 'first', '.', '-LRB-', 'A', 'bit', 'of', 'a', 'good', 'thing', ',', 'because', 'Pinto', "'s", 'Indian-accented', 'English', 'does', "n't", 'quite', 'jibe', 'with', 'the', 'Arabic-tinged', 'tongues', 'of', 'her', 'co-stars', '.', '-RRB-', 'Beginning', 'in', 'war-torn', 'Jerusalem', 'circa', '1948', ',', 'when', '``', 'Mama', "''", 'Hind', 'Husseini', '-LRB-', 'Hiam', 'Abbass', ',', '``', 'The', 'Visitor', "''", '-RRB-', 'established', 'an', 'orphanage', 'for', 'refugees', 'that', 'quickly', 'becomes', 'home', 'to', '2,000', ',', 'the', 'movie', 'spans', 'the', 'next', '50', 'years', ',', 'and', 'though', 'Schnabel', "'s", 'artist', "'s", 'eye', 'is', 'on', 'display', ',', 'the', 'Israel', '/', 'Palestine', 'conflict', 'is', 'a', 'subject', 'that', 'he', 'never', 'brings', 'into', 'clear', 'focus', '--', 'at', 'least', 'with', 'regard', 'to', 'Israelis', '.', 'And', 'when', 'he', 'presents', 'what', 'some', 'would', 'believe', 'terrorist', 'actions', 'of', 'his', 'protagonists', ',', 'he', 'sidesteps', 'the', 'potentially', 'horrible', 'consequences', ':', 'a', 'disgraced', 'former', 'nurse', '--', 'a', 'lifesaver', '--', 'plants', 'a', 'bomb', 'in', 'a', 'crowded', 'movie', 'theater', '-LRB-', 'playing', ',', 'without', 'a', 'hint', 'of', 'subtlety', ',', 'Roman', 'Polanski', "'s", '``', 'Repulsion', "''", '-RRB-', 'but', 'the', 'device', 'fails', 'to', 'explode', ';', 'a', 'car', 'bomb', 'is', 'set', 'off', 'by', 'Miral', "'s", 'political', 'activist', 'boyfriend', '--', 'though', 'there', 'are', 'seemingly', 'no', 'casualties', '.', 'So', 'much', 'for', 'the', 'horrors', 'of', 'war', '.', '-LRB-', '``', 'Miral', "''", 'contains', 'anger-inducing', 'violent', 'themes', ',', 'particularly', 'for', 'those', 'sympathetic', 'to', 'Israel', '.', '-RRB-']
Document BIO Tags: ['O', 'O', 'O', 'B', 'O', 'O', 'O', 'O', 'B', 'O', 'B', 'I', 'I', 'O', 'B', 'O', 'O', 'O', 'O', 'O', 'O', 'O', 'B', 'I', 'I', 'O', 'O', 'O', 'O', 'O', 'O', 'O', 'O', 'O', 'O', 'O', 'O', 'O', 'O', 'O', 'O', 'O', 'B', 'O', 'O', 'O', 'O', 'O', 'B', 'I', 'O', 'O', 'O', 'B', 'O', 'O', 'O', 'O', 'B', 'I', 'I', 'O', 'O', 'O', 'B', 'O', 'O', 'O', 'O', 'O', 'O', 'O', 'O', 'O', 'O', 'O', 'O', 'O', 'O', 'O', 'O', 'O', 'O', 'O', 'O', 'O', 'O', 'O', 'O', 'O', 'B', 'O', 'O', 'O', 'O', 'O', 'O', 'O', 'O', 'O', 'O', 'O', 'O', 'O', 'O', 'O', 'O', 'O', 'O', 'O', 'O', 'O', 'O', 'O', 'O', 'O', 'O', 'O', 'O', 'O', 'O', 'B', 'I', 'O', 'O', 'O', 'O', 'O', 'O', 'O', 'O', 'O', 'O', 'O', 'O', 'O', 'O', 'O', 'O', 'O', 'O', 'O', 'O', 'B', 'I', 'O', 'O', 'O', 'B', 'I', 'O', 'O', 'O', 'O', 'O', 'O', 'O', 'O', 'O', 'O', 'O', 'O', 'O', 'O', 'O', 'O', 'O', 'O', 'O', 'O', 'O', 'O', 'O', 'B', 'O', 'O', 'O', 'O', 'O', 'B', 'I', 'O', 'O', 'O', 'O', 'O', 'O', 'O', 'O', 'O', 'O', 'O', 'O', 'O', 'O', 'O', 'O', 'O', 'O', 'O', 'O', 'O', 'O', 'O', 'O', 'O', 'O', 'O', 'O', 'O', 'O', 'O', 'O', 'O', 'O', 'O', 'O', 'O', 'O', 'O', 'O', 'O', 'O', 'O', 'O', 'O', 'O', 'O', 'O', 'O', 'O', 'O', 'O', 'O', 'O', 'O', 'O', 'O', 'B', 'I', 'O', 'O', 'O', 'O', 'O', 'O', 'O', 'O', 'B', 'I', 'O', 'O', 'O', 'O', 'O', 'O', 'O', 'O', 'O', 'O', 'O', 'O', 'O', 'O', 'O', 'O', 'O', 'O', 'O', 'O', 'O', 'O', 'O', 'O', 'O', 'O', 'O', 'O', 'O', 'O', 'O', 'O', 'O', 'O', 'O', 'O', 'O', 'O', 'O', 'O', 'O', 'O', 'O', 'O', 'O', 'O', 'O', 'O', 'O', 'O', 'O', 'O', 'B', 'O', 'O', 'O', 'O', 'O', 'O', 'O', 'O', 'O', 'O', 'O', 'O', 'O', 'O', 'O', 'O', 'O', 'O', 'O', 'O', 'O', 'O', 'O', 'O', 'O', 'O', 'O', 'O', 'O', 'O', 'O', 'O', 'O', 'O', 'O', 'O', 'O', 'O', 'O', 'O', 'O', 'O', 'O', 'O', 'O', 'O', 'O', 'O', 'O', 'O', 'O', 'O', 'O', 'O', 'O', 'O', 'O', 'O', 'O', 'O', 'O', 'O', 'O', 'O', 'O', 'O', 'O', 'O', 'O', 'O', 'O', 'O', 'O', 'O', 'O', 'O', 'O', 'O', 'O', 'O', 'O', 'O', 'O', 'O', 'O', 'B', 'O', 'O', 'O', 'O', 'O', 'O', 'O', 'O', 'O', 'O', 'O', 'O', 'O', 'O', 'O', 'O', 'O', 'O', 'O', 'O', 'O', 'O', 'B', 'O', 'O', 'O', 'O', 'O', 'O', 'O', 'O', 'O', 'O', 'O', 'O', 'O', 'O']
Extractive/present Keyphrases: ['conflict of interest', 'arabictinged tongues', 'slumdog millionaire', 'kendall square cinema', 'before night falls', 'earnest platitudes', 'basquiat', 'biographies', 'jerusalem circa', 'butterfly', 'musical choices', 'terrific second', 'tom waits', 'miral', 'director', 'conflict']
Abstractive/absent Keyphrases: ['lsquomiralrsquo director', 'mama hind husseini', 'miral rated pg 13', 'painterturneddirector julian schnabel oscarnominated', 'schnabels girlfriend palestinian journalist rula jebreal', 'exquisite the diving bell', 'interest']
-----------
```
### Keyphrase Extraction
```python
from datasets import load_dataset
# get the dataset only for keyphrase extraction
dataset = load_dataset("midas/kpcrowd", "extraction")
print("Samples for Keyphrase Extraction")
# sample from the train split
print("Sample from train data split")
test_sample = dataset["train"][0]
print("Fields in the sample: ", [key for key in test_sample.keys()])
print("Tokenized Document: ", test_sample["document"])
print("Document BIO Tags: ", test_sample["doc_bio_tags"])
print("\n-----------\n")
# sample from the test split
print("Sample from test data split")
test_sample = dataset["test"][0]
print("Fields in the sample: ", [key for key in test_sample.keys()])
print("Tokenized Document: ", test_sample["document"])
print("Document BIO Tags: ", test_sample["doc_bio_tags"])
print("\n-----------\n")
```
### Keyphrase Generation
```python
# get the dataset only for keyphrase generation
dataset = load_dataset("midas/kpcrowd", "generation")
print("Samples for Keyphrase Generation")
# sample from the train split
print("Sample from train data split")
test_sample = dataset["train"][0]
print("Fields in the sample: ", [key for key in test_sample.keys()])
print("Tokenized Document: ", test_sample["document"])
print("Extractive/present Keyphrases: ", test_sample["extractive_keyphrases"])
print("Abstractive/absent Keyphrases: ", test_sample["abstractive_keyphrases"])
print("\n-----------\n")
# sample from the test split
print("Sample from test data split")
test_sample = dataset["test"][0]
print("Fields in the sample: ", [key for key in test_sample.keys()])
print("Tokenized Document: ", test_sample["document"])
print("Extractive/present Keyphrases: ", test_sample["extractive_keyphrases"])
print("Abstractive/absent Keyphrases: ", test_sample["abstractive_keyphrases"])
print("\n-----------\n")
```
## Citation Information
```
@misc{marujo2013supervised,
title={Supervised Topical Key Phrase Extraction of News Stories using Crowdsourcing, Light Filtering and Co-reference Normalization},
author={Luis Marujo and Anatole Gershman and Jaime Carbonell and Robert Frederking and João P. Neto},
year={2013},
eprint={1306.4886},
archivePrefix={arXiv},
primaryClass={cs.CL}
}
```
## Contributions
Thanks to [@debanjanbhucs](https://github.com/debanjanbhucs), [@dibyaaaaax](https://github.com/dibyaaaaax) and [@ad6398](https://github.com/ad6398) for adding this dataset
| midas/kpcrowd | [
"arxiv:1306.4886",
"region:us"
] | 2022-03-02T23:29:22+00:00 | {} | 2022-02-12T05:52:48+00:00 | [
"1306.4886"
] | [] | TAGS
#arxiv-1306.4886 #region-us
| Dataset Summary
---------------
A dataset for benchmarking keyphrase extraction and generation techniques from english news articles. For more details about the dataset please refer the original paper - URL
Original source of the data -
Dataset Structure
-----------------
### Dataset Statistics
Table 1: Statistics on the length of the extractive keyphrases for Train, Test splits of kpcrowd dataset.
Table 2: Statistics on the length of the abstractive keyphrases for Train, Test splits of kpcrowd dataset.
Table 3: General statistics of the kpcrowd dataset.
### Data Fields
* id: unique identifier of the document.
* document: Whitespace separated list of words in the document.
* doc\_bio\_tags: BIO tags for each word in the document. B stands for the beginning of a keyphrase and I stands for inside the keyphrase. O stands for outside the keyphrase and represents the word that isn't a part of the keyphrase at all.
* extractive\_keyphrases: List of all the present keyphrases.
* abstractive\_keyphrase: List of all the absent keyphrases.
### Data Splits
Usage
-----
### Full Dataset
Output
### Keyphrase Extraction
### Keyphrase Generation
Contributions
-------------
Thanks to @debanjanbhucs, @dibyaaaaax and @ad6398 for adding this dataset
| [
"### Dataset Statistics\n\n\nTable 1: Statistics on the length of the extractive keyphrases for Train, Test splits of kpcrowd dataset.\n\n\n\nTable 2: Statistics on the length of the abstractive keyphrases for Train, Test splits of kpcrowd dataset.\n\n\n\nTable 3: General statistics of the kpcrowd dataset.",
"### Data Fields\n\n\n* id: unique identifier of the document.\n* document: Whitespace separated list of words in the document.\n* doc\\_bio\\_tags: BIO tags for each word in the document. B stands for the beginning of a keyphrase and I stands for inside the keyphrase. O stands for outside the keyphrase and represents the word that isn't a part of the keyphrase at all.\n* extractive\\_keyphrases: List of all the present keyphrases.\n* abstractive\\_keyphrase: List of all the absent keyphrases.",
"### Data Splits\n\n\n\nUsage\n-----",
"### Full Dataset\n\n\nOutput",
"### Keyphrase Extraction",
"### Keyphrase Generation\n\n\nContributions\n-------------\n\n\nThanks to @debanjanbhucs, @dibyaaaaax and @ad6398 for adding this dataset"
] | [
"TAGS\n#arxiv-1306.4886 #region-us \n",
"### Dataset Statistics\n\n\nTable 1: Statistics on the length of the extractive keyphrases for Train, Test splits of kpcrowd dataset.\n\n\n\nTable 2: Statistics on the length of the abstractive keyphrases for Train, Test splits of kpcrowd dataset.\n\n\n\nTable 3: General statistics of the kpcrowd dataset.",
"### Data Fields\n\n\n* id: unique identifier of the document.\n* document: Whitespace separated list of words in the document.\n* doc\\_bio\\_tags: BIO tags for each word in the document. B stands for the beginning of a keyphrase and I stands for inside the keyphrase. O stands for outside the keyphrase and represents the word that isn't a part of the keyphrase at all.\n* extractive\\_keyphrases: List of all the present keyphrases.\n* abstractive\\_keyphrase: List of all the absent keyphrases.",
"### Data Splits\n\n\n\nUsage\n-----",
"### Full Dataset\n\n\nOutput",
"### Keyphrase Extraction",
"### Keyphrase Generation\n\n\nContributions\n-------------\n\n\nThanks to @debanjanbhucs, @dibyaaaaax and @ad6398 for adding this dataset"
] |
e8dc9af01653631fad51515b77dd39166445b732 |
A dataset for benchmarking keyphrase extraction and generation techniques from news. For more details about the dataset please refer the original paper - [https://aclanthology.org/W19-8617.pdf](https://aclanthology.org/W19-8617.pdf)
Original source of the data - [https://github.com/ygorg/KPTimes](https://github.com/ygorg/KPTimes)
## Dataset Summary
<br>
<p align="center">
<img src="https://huggingface.co/datasets/midas/kptimes/resolve/main/kptimes-details.png" alt="KPTimes dataset summary" width="90%"/>
<br>
</p>
<br>
KPTimes is a large scale dataset comprising of 279,923 news articles from NY Times and 10K from JPTimes. It is one of the datasets which has annotations of keyphrases curated by the editors who can be considered as experts. The motivation of the authors behind producing this dataset was to have a large dataset for training neural models for keyphrase generation in a domain other than the scientific domain, and to understand the differences between keyphrases annotated by experts and non-experts. The authors show that the editors tend to assign generic keyphrases that are not present in the actual news article's text, with 55% of them being abstractive keyphrases. The keyphrases in the news domain as presented in this work were also on an average shorter (1.4 words) than those in the scientific datasets (2.4 words).
The dataset is randomly divided into train (92.8%), validation (3.6%) and test (3.6%) splits. In order to enable the models trained on this dataset to generalize well the authors did not want to have the entire data taken from a single source (NY Times), and therefore added 10K more articles from JPTimes dataset. The authors collected free to read article URLs from NY Times spanning from 2006 to 2017, and obtained their corresponding HTML pages from the Internet Archive. They cleaned the HTML tags and extracted the title, and the main content of the articles using heuristics. The gold keyphrases were obtained from the metadata fields - *news_keywords* and *keywords*. The documents in the dataset are full-length news articles, which also makes it a suitable dataset for developing models for identifying keyphrases from long documents.
<br>
<p align="center">
<img src="https://huggingface.co/datasets/midas/kptimes/resolve/main/KPTimesExample.png" alt="KPTimes sample" width="90%"/>
<br>
</p>
<br>
## Dataset Structure
## Dataset Statistics
Table 1: Statistics on the length of the abstractive keyphrases for Train, Test, and Validation splits of Inspec dataset.
| | Train | Test | Validation |
|:------------------: |:-------: |:-------: |:----------: |
| Single word | 15.6% | 29.59% | 15.52% |
| Two words | 36.7% | 36.88% | 12.38% |
| Three words | 29.5% | 20.86% | 29.29% |
| Four words | 12.5% | 8.88% | 0% |
| Five words | 3.4% | 2.33% | 3.50% |
| Six words | 1.4% | 0.93% | 1.38% |
| Seven words | 0.4% | 0.27% | 0.37% |
| Eight words | 0.24% | 0.13% | 0.21% |
| Nine words | 0.14% | 0.013% | 0.10% |
| Ten words | 0.02% | 0.0007% | 0.03% |
| Eleven words | 0.01% | 0.01% | 0.003% |
| Twelve words | 0.008% | 0.011% | 0.007% |
| Thirteen words | 0.01% | 0.02% | 0.02% |
| Fourteen words | 0.001% | 0% | 0% |
| Fifteen words | 0.001% | 0.004% | 0.003% |
| Sixteen words | 0.0004% | 0% | 0% |
| Seventeen words | 0.0005% | 0% | 0% |
| Eighteen words | 0.0004% | 0% | 0% |
| Nineteen words | 0.0001% | 0% | 0% |
| Twenty words | 0.0001% | 0% | 0% |
| Twenty-three words | 0.0001% | 0% | 0% |
Table 2: Statistics on the length of the extractive keyphrases for Train, Test, and Validation splits of Inspec dataset.
| | Train | Test | Validation |
|:--------------: |:-------: |:------: |:----------: |
| Single word | 54.2% | 60.0% | 54.38% |
| Two words | 33.9% | 32.4% | 33.73% |
| Three words | 8.8% | 5.5% | 8.70% |
| Four words | 1.9% | 1.04% | 1.97% |
| Five words | 0.5% | 0.25% | 0.53% |
| Six words | 0.4% | 0.16% | 0.44% |
| Seven words | 0.12% | 0.06% | 0.15% |
| Eight words | 0.05% | 0.03% | 0.08% |
| Nine words | 0.009% | 0% | 0% |
| Ten words | 0.0007% | 0.001% | 0% |
| Eleven words | 0.0002% | 0% | 0% |
| Twelve words | 0.0002% | 0% | 0% |
| Thirteen words | 0.0002% | 0% | 0% ||
Table 3: General statistics of the Inspec dataset.
| Type of Analysis | Train | Test | Validation |
|:------------------------------------------------: |:---------------------: |:---------------------: |:---------------------: |
| Annotator Type | Professional Indexers | Professional Indexers | Professional Indexers |
| Document Type | News Articles | News Articles | News articles |
| No. of Documents | 259,923 | 20,000 | 10,000 |
| Avg. Document length (words) | 783.32 | 643.2 | 784.65 |
| Max Document length (words) | 7278 | 5503 | 5627 |
| Max no. of abstractive keyphrases in a document | 10 | 10 | 10 |
| Min no. of abstractive keyphrases in a document | 0 | 0 | 0 |
| Avg. no. of abstractive keyphrases per document | 2.87 | 2.30 | 2.89 |
| Max no. of extractive keyphrases in a document | 10 | 10 | 9 |
| Min no. of extractive keyphrases in a document | 0 | 0 | 0 |
| Avg. no. of extractive keyphrases per document | 2.15 | 2.72 | 2.13 |
### Data Fields
- **id**: unique identifier of the document.
- **document**: Whitespace separated list of words in the document.
- **doc_bio_tags**: BIO tags for each word in the document. B stands for the beginning of a keyphrase and I stands for inside the keyphrase. O stands for outside the keyphrase and represents the word that isn't a part of the keyphrase at all.
- **extractive_keyphrases**: List of all the present keyphrases.
- **abstractive_keyphrase**: List of all the absent keyphrases.
- **other metadata**: Additional information present in the original dataset.
- **id** : unique identifier for the document
- **date** : publishing date (YYYY/MM/DD)
- **categories** : categories of the article (1 or 2 categories)
- **title** : title of the document
- **abstract** : content of the article
- **keyword** : list of keywords
### Data Splits
|Split| #datapoints |
|--|--|
| Train | 259923 |
| Test | 20000 |
| Validation | 10000 |
## Usage
### Full Dataset
```python
from datasets import load_dataset
# get entire dataset
dataset = load_dataset("midas/kptimes", "raw")
# sample from the train split
print("Sample from training data split")
train_sample = dataset["train"][0]
print("Fields in the sample: ", [key for key in train_sample.keys()])
print("Tokenized Document: ", train_sample["document"])
print("Document BIO Tags: ", train_sample["doc_bio_tags"])
print("Extractive/present Keyphrases: ", train_sample["extractive_keyphrases"])
print("Abstractive/absent Keyphrases: ", train_sample["abstractive_keyphrases"])
print("Other Metadata: ", train_sample["other_metadata"])
print("\n-----------\n")
# sample from the validation split
print("Sample from validation data split")
validation_sample = dataset["validation"][0]
print("Fields in the sample: ", [key for key in validation_sample.keys()])
print("Tokenized Document: ", validation_sample["document"])
print("Document BIO Tags: ", validation_sample["doc_bio_tags"])
print("Extractive/present Keyphrases: ", validation_sample["extractive_keyphrases"])
print("Abstractive/absent Keyphrases: ", validation_sample["abstractive_keyphrases"])
print("Other Metadata: ", validation_sample["other_metadata"])
print("\n-----------\n")
# sample from the test split
print("Sample from test data split")
test_sample = dataset["test"][0]
print("Fields in the sample: ", [key for key in test_sample.keys()])
print("Tokenized Document: ", test_sample["document"])
print("Document BIO Tags: ", test_sample["doc_bio_tags"])
print("Extractive/present Keyphrases: ", test_sample["extractive_keyphrases"])
print("Abstractive/absent Keyphrases: ", test_sample["abstractive_keyphrases"])
print("Other Metadata: ", test_sample["other_metadata"])
print("\n-----------\n")
```
**Output**
```bash
Sample from training data split
Fields in the sample: ['id', 'document', 'doc_bio_tags', 'extractive_keyphrases', 'abstractive_keyphrases', 'other_metadata']
Tokenized Document: ['For', 'Donald', 'Trump’s', 'Big', 'Speech,', 'an', 'Added', 'Pressure:', 'No', 'Echoes', 'CLEVELAND', '—', 'Until', 'Monday', 'night,', 'Donald', 'J.', 'Trump’s', 'biggest', 'concern', 'about', 'his', 'convention', 'speech', 'was', 'how', 'much', 'to', 'reveal', 'about', 'himself', 'and', 'his', 'family', 'in', 'an', 'address', 'that', 'is', 'often', 'the', 'most', 'personal', 'one', 'a', 'presidential', 'candidate', 'delivers.', 'But', 'the', 'political', 'firestorm', 'over', 'his', 'wife’s', 'speech', ',', 'which', 'borrowed', 'passages', 'from', 'Michelle', 'Obama’s', 'convention', 'remarks', 'in', '2008,', 'raised', 'the', 'stakes', 'exponentially.', 'Mr.', 'Trump’s', 'speech', 'on', 'Thursday', 'night', 'cannot', 'merely', 'be', 'his', 'best', 'ever.', 'It', 'also', 'has', 'to', 'be', 'bulletproof.', 'By', 'Tuesday', 'morning,', 'word', 'had', 'spread', 'throughout', 'his', 'campaign', 'that', 'any', 'language', 'in', 'Mr.', 'Trump’s', 'address', 'even', 'loosely', 'inspired', 'by', 'speeches,', 'essays,', 'books', 'or', 'Twitter', 'posts', 'had', 'to', 'be', 'either', 'rewritten', 'or', 'attributed.', 'Mr.', 'Trump’s', 'chief', 'speechwriter,', 'Stephen', 'Miller,', 'reassured', 'colleagues', 'that', 'the', 'acceptance', 'speech', 'was', 'wholly', 'original,', 'according', 'to', 'two', 'staff', 'members', 'who', 'spoke', 'with', 'him', 'and', 'described', 'those', 'conversations', 'on', 'the', 'condition', 'of', 'anonymity.', 'Mr.', 'Miller', 'also', 'told', 'campaign', 'aides', 'that', 'he', 'had', 'looked', 'closely', 'at', 'passages', 'that', 'Mr.', 'Trump', 'had', 'contributed', '—', 'handwritten', 'on', 'unlined', 'white', 'pages', '—', 'and', 'was', 'confident', 'they', 'contained', 'no', 'problems.', '(Mr.', 'Miller', 'declined', 'an', 'interview', 'request.)', 'Even', 'so,', 'one', 'of', 'the', 'staff', 'members', 'downloaded', 'plagiarism-detection', 'software', 'and', 'ran', 'a', 'draft', 'of', 'the', 'speech', 'through', 'the', 'program.', 'No', 'red', 'flags', 'came', 'up.', 'The', 'intense', 'scrutiny', 'of', 'Mr.', 'Trump’s', 'words', 'added', 'new', 'pressure', 'to', 'a', 'speechwriting', 'process', 'that', 'has', 'been', 'one', 'of', 'the', 'most', 'unpredictable', 'and', 'free-form', 'in', 'modern', 'presidential', 'campaigns.', 'A', 'month', 'ago,', 'Mr.', 'Trump', 'began', 'giving', 'dictation', 'on', 'themes', 'for', 'the', 'speech,', 'and', 'he', 'tossed', 'ideas', 'and', 'phrases', 'to', 'Mr.', 'Miller', 'or', 'other', 'advisers', 'on', 'a', 'daily', 'basis.', 'On', 'printed', 'copies', 'of', 'each', 'draft,', 'he', 'circled', 'passages', 'he', 'liked,', 'crossed', 'out', 'or', 'put', 'question', 'marks', 'beside', 'lines', 'that', 'he', 'did', 'not', 'favor', 'and', 'frequently', 'suggested', 'new', 'words', 'or', 'phrases.', 'Image', 'Stephen', 'Miller,', 'left,', 'Mr.', 'Trump’s', 'chief', 'speechwriter,', 'and', 'Paul', 'Manafort,', 'the', 'campaign', 'chairman,', 'before', 'an', 'event', 'for', 'the', 'candidate', 'at', 'the', 'Trump', 'SoHo', 'hotel', 'in', 'New', 'York', 'last', 'month.', 'Credit', 'Damon', 'Winter/The', 'New', 'York', 'Times', '“I’ve', 'been', 'amending', 'the', 'drafts', 'big-league,”', 'Mr.', 'Trump', 'said', 'in', 'an', 'interview', 'in', 'his', 'Manhattan', 'office', 'before', 'the', 'convention.', '“I', 'get', 'ideas', 'from', 'a', 'lot', 'of', 'different', 'places,', 'a', 'lot', 'of', 'smart', 'people,', 'but', 'mostly', 'I', 'like', 'language', 'that', 'sounds', 'like', 'me.”', 'Yet', 'in', 'the', 'aftermath', 'of', 'Melania', 'Trump’s', 'speech,', 'campaign', 'advisers', 'have', 'fretted', 'that', 'they', 'do', 'not', 'know', 'for', 'sure', 'where', 'Mr.', 'Trump', 'gets', 'his', 'ideas', 'and', 'language', '—', 'whether', 'they', 'are', 'his', 'own,', 'in', 'other', 'words,', 'or', 'are', 'picked', 'up', 'from', 'Twitter,', 'television,', 'or,', 'say,', 'a', 'best', 'seller', 'by', 'Bill', 'O’Reilly', 'of', 'Fox', 'News,', 'a', 'commentator', 'whom', 'Mr.', 'Trump', 'likes.', 'Borrowing', 'or', 'adapting', 'may', 'not', 'always', 'be', 'tantamount', 'to', 'plagiarism,', 'but', 'several', 'Trump', 'advisers,', 'who', 'also', 'insisted', 'on', 'anonymity,', 'said', 'that', 'after', 'the', 'furor', 'over', 'Ms.', 'Trump’s', 'remarks,', 'the', 'campaign', 'cannot', 'allow', 'a', 'similar', 'blowup.', 'Ed', 'Rollins,', 'a', 'Republican', 'strategist', 'who', 'is', 'advising', 'a', '“super', 'PAC”', 'supporting', 'Mr.', 'Trump,', 'said', 'that', 'the', 'candidate', 'could', 'not', 'afford', 'any', 'mistakes.', '“His', 'speech', 'is', 'the', 'whole', 'game,”', 'Mr.', 'Rollins', 'said.', '“Viewers', 'have', 'to', 'watch', 'it', 'and', 'say,', '‘There', 'is', 'the', 'next', 'president', 'of', 'the', 'United', 'States.’”', 'In', 'the', 'interview,', 'Mr.', 'Trump', 'said', 'his', 'speech', 'would', 'center', 'on', 'his', 'vision', 'of', 'a', 'strong', 'and', 'secure', 'America', 'that', '“once', 'existed', 'and', 'no', 'longer', 'does,', 'but', 'can', 'again', 'under', 'a', 'Trump', 'administration.”', 'Latest', 'Election', 'Polls', '2016', 'Get', 'the', 'latest', 'national', 'and', 'state', 'polls', 'on', 'the', 'presidential', 'election', 'between', 'Hillary', 'Clinton', 'and', 'Donald', 'J.', 'Trump.', 'His', 'greatest', 'challenge,', 'he', 'said,', 'was', '“putting', 'myself', 'in', 'the', 'speech”', '—', 'discussing', 'his', 'upbringing', 'and', 'early', 'experiences', 'and', 'relating', 'them', 'to', 'the', 'hopes', 'and', 'aspirations', 'of', 'other', 'Americans.', '“I', 'was', 'never', 'comfortable', 'getting', 'personal', 'about', 'my', 'family', 'because', 'I', 'thought', 'it', 'was', 'special', 'territory,”', 'Mr.', 'Trump', 'said,', 'glancing', 'at', 'a', 'picture', 'of', 'his', 'father', 'on', 'his', 'desk.', '“It', 'can', 'feel', 'exploitative', 'to', 'use', 'family', 'stories', 'to', 'win', 'votes.', 'And', 'I', 'had', 'a', 'very', 'happy', 'and', 'comfortable', 'life', 'growing', 'up.', 'I', 'had', 'a', 'great', 'relationship', 'with', 'my', 'father.', 'But', 'my', 'focus', 'needs', 'to', 'be', 'on', 'all', 'the', 'Americans', 'who', 'are', 'struggling.”', 'He', 'said', 'he', 'was', 'unsure', 'if', 'he', 'would', 'discuss', 'his', 'older', 'brother', 'Fred,', 'who', 'died', 'as', 'an', 'alcoholic', 'in', '1981', 'at', '43', '—', 'and', 'whom', 'he', 'has', 'described', 'as', 'an', 'example', 'of', 'how', 'destructive', 'choices', 'can', 'damage', 'lives', 'that', 'seem', 'golden.', '“Without', 'my', 'brother', 'Fred', 'I', 'might', 'not', 'be', 'here,”', 'Mr.', 'Trump', 'said.', '“He', 'was', 'really', 'smart,', 'great-looking.', 'I', 'don’t', 'drink', 'or', 'smoke', 'because', 'of', 'what', 'happened', 'to', 'him.', 'I', 'focused', 'on', 'building', 'my', 'business', 'and', 'making', 'good', 'choices.', 'I', 'may', 'talk', 'about', 'that,', 'but', 'I', 'don’t', 'know', 'if', 'I', 'should.”', 'Acceptance', 'speeches', 'seldom', 'seem', 'complete', 'without', 'anecdotes', 'about', 'personal', 'trials', 'and', 'triumphs:', 'Mitt', 'Romney,', 'trying', 'to', 'persuade', 'voters', 'to', 'see', 'him', 'as', 'more', 'than', 'a', 'rich', 'businessman,', 'devoted', 'about', 'a', 'fourth', 'of', 'his', '2012', 'address', 'to', 'his', 'parents’', 'unconditional', 'love,', 'his', 'Mormon', 'faith', 'and', 'reminiscences', 'about', 'watching', 'the', 'moon', 'landing.', 'In', '2008', ',', 'Barack', 'Obama', 'described', 'how', 'his', 'grandfather', 'benefited', 'from', 'the', 'G.I.', 'Bill', 'and', 'how', 'his', 'mother', 'and', 'grandmother', 'taught', 'him', 'the', 'value', 'of', 'hard', 'work.', 'And', 'Bill', 'Clinton’s', '1992', 'speech', 'vividly', 'recalled', 'the', 'life', 'lessons', 'he', 'learned', 'from', 'his', 'mother', 'about', 'fighting', 'and', 'working', 'hard,', 'from', 'his', 'grandfather', 'about', 'racial', 'equality', '—', 'and', 'from', 'his', 'wife,', 'Hillary,', 'who,', 'Mr.', 'Clinton', 'said,', 'taught', 'him', 'that', 'every', 'child', 'could', 'learn.', 'Mr.', 'Clinton', 'finished', 'his', 'speech', 'with', 'a', 'now-famous', 'line', 'tying', 'his', 'Arkansas', 'hometown', 'to', 'the', 'American', 'dream.', '“I', 'end', 'tonight', 'where', 'it', 'all', 'began', 'for', 'me,”', 'he', 'said.', '“I', 'still', 'believe', 'in', 'a', 'place', 'called', 'Hope.”', 'James', 'Carville,', 'a', 'senior', 'strategist', 'for', 'Mr.', 'Clinton’s', '1992', 'campaign,', 'said', 'that', 'if', 'Mr.', 'Trump', 'hoped', 'to', 'change', 'the', 'minds', 'of', 'those', 'who', 'see', 'him', 'as', 'divisive', 'or', 'bigoted,', 'he', 'would', 'need', 'to', 'open', 'himself', 'up', 'to', 'voters', 'in', 'meaningfully', 'personal', 'ways', 'in', 'his', 'speech.', '“If', 'he’s', 'really', 'different', 'than', 'the', 'way', 'he', 'seems', 'in', 'television', 'interviews', 'or', 'at', 'his', 'rallies,', 'Thursday’s', 'speech', 'will', 'be', 'his', 'single', 'greatest', 'opportunity', 'to', 'show', 'voters', 'who', 'he', 'really', 'is,”', 'Mr.', 'Carville', 'said.', 'Paul', 'Manafort,', 'the', 'Trump', 'campaign', 'chairman,', 'said', 'that', 'Thursday’s', 'speech', 'would', 'be', '“very', 'much', 'a', 'reflection', 'of', 'Mr.', 'Trump’s', 'own', 'words,', 'as', 'opposed', 'to', 'remarks', 'that', 'others', 'create', 'and', 'the', 'campaign', 'puts', 'in', 'his', 'mouth.”', '“He’s', 'not', 'an', 'editor', '—', 'he', 'is', 'actually', 'the', 'creator', 'of', 'the', 'speech,”', 'Mr.', 'Manafort', 'said.', '“Mr.', 'Trump', 'has', 'given', 'Steve', 'Miller', 'and', 'I', 'very', 'specific', 'directions', 'about', 'how', 'he', 'views', 'the', 'speech,', 'what', 'he', 'wants', 'to', 'communicate,', 'and', 'ways', 'to', 'tie', 'together', 'things', 'that', 'he', 'has', 'been', 'talking', 'about', 'in', 'the', 'campaign.', 'The', 'speech', 'will', 'end', 'up', 'being', 'tone-perfect', 'because', 'the', 'speech’s', 'words', 'will', 'be', 'his', 'words.”', 'Mr.', 'Trump', 'prefers', 'speaking', 'off', 'the', 'cuff', 'with', 'handwritten', 'notes,', 'a', 'style', 'that', 'has', 'proved', 'successful', 'at', 'his', 'rallies,', 'where', 'he', 'has', 'shown', 'a', 'talent', 'for', 'connecting', 'with', 'and', 'electrifying', 'crowds.', 'But', 'his', 'adjustment', 'to', 'formal', 'speeches', 'remains', 'a', 'work', 'in', 'progress:', 'He', 'does', 'not', 'always', 'sound', 'like', 'himself,', 'and', 'reading', 'from', 'a', 'text', 'can', 'detract', 'from', 'the', 'sense', 'of', 'authenticity', 'that', 'his', 'supporters', 'prize.', 'One', 'question', 'is', 'whether,', 'or', 'how', 'much,', 'he', 'will', 'ad-lib.', 'He', 'has', 'sometimes', 'seemed', 'unable', 'to', 'resist', 'deviating', 'from', 'prepared', 'remarks,', 'often', 'to', 'ill', 'effect', '—', 'ranting', 'about', 'a', 'mosquito', ',', 'or', 'joking', 'that', 'a', 'passing', 'airplane', 'was', 'from', 'Mexico', 'and', 'was', '“', 'getting', 'ready', 'to', 'attack', '.”', '“Ad-libbing', 'is', 'instinct,', 'all', 'instinct,”', 'Mr.', 'Trump', 'said.', '“I', 'thought', 'maybe', 'about', 'doing', 'a', 'freewheeling', 'speech', 'for', 'the', 'convention,', 'but', 'that', 'really', 'wouldn’t', 'work.', 'But', 'even', 'with', 'a', 'teleprompter,', 'the', 'speech', 'will', 'be', 'me', '—', 'my', 'ideas,', 'my', 'beliefs,', 'my', 'words.”']
Document BIO Tags: ['O', 'O', 'O', 'O', 'O', 'O', 'O', 'O', 'O', 'O', 'O', 'O', 'O', 'O', 'O', 'O', 'O', 'O', 'O', 'O', 'O', 'O', 'O', 'O', 'O', 'O', 'O', 'O', 'O', 'O', 'O', 'O', 'O', 'O', 'O', 'O', 'O', 'O', 'O', 'O', 'O', 'O', 'O', 'O', 'O', 'O', 'O', 'O', 'O', 'O', 'O', 'O', 'O', 'O', 'O', 'O', 'O', 'O', 'O', 'O', 'O', 'O', 'O', 'O', 'O', 'O', 'O', 'O', 'O', 'O', 'O', 'O', 'O', 'O', 'O', 'O', 'O', 'O', 'O', 'O', 'O', 'O', 'O', 'O', 'O', 'O', 'O', 'O', 'O', 'O', 'O', 'O', 'O', 'O', 'O', 'O', 'O', 'O', 'O', 'O', 'O', 'O', 'O', 'O', 'O', 'O', 'O', 'O', 'O', 'B', 'O', 'O', 'O', 'O', 'O', 'O', 'O', 'O', 'O', 'O', 'O', 'O', 'O', 'O', 'O', 'O', 'O', 'O', 'O', 'O', 'O', 'O', 'O', 'O', 'O', 'O', 'O', 'O', 'O', 'O', 'O', 'O', 'O', 'O', 'O', 'O', 'O', 'O', 'O', 'O', 'O', 'O', 'O', 'O', 'O', 'O', 'O', 'O', 'O', 'O', 'O', 'O', 'O', 'O', 'O', 'O', 'O', 'O', 'O', 'O', 'O', 'O', 'O', 'O', 'O', 'O', 'O', 'O', 'O', 'O', 'O', 'O', 'O', 'O', 'O', 'O', 'O', 'O', 'O', 'O', 'O', 'O', 'O', 'O', 'O', 'O', 'O', 'O', 'O', 'O', 'O', 'O', 'O', 'O', 'O', 'O', 'O', 'O', 'O', 'O', 'O', 'O', 'O', 'O', 'O', 'O', 'O', 'O', 'O', 'O', 'O', 'O', 'O', 'O', 'O', 'O', 'O', 'O', 'O', 'O', 'O', 'O', 'O', 'O', 'O', 'O', 'O', 'O', 'O', 'O', 'O', 'O', 'O', 'O', 'O', 'O', 'O', 'O', 'O', 'O', 'O', 'O', 'O', 'O', 'O', 'O', 'O', 'O', 'O', 'O', 'O', 'O', 'O', 'O', 'O', 'O', 'O', 'O', 'O', 'O', 'O', 'O', 'O', 'O', 'O', 'O', 'O', 'O', 'O', 'O', 'O', 'O', 'O', 'O', 'O', 'O', 'O', 'O', 'O', 'O', 'O', 'O', 'O', 'O', 'O', 'O', 'O', 'O', 'O', 'O', 'O', 'O', 'O', 'O', 'O', 'O', 'O', 'O', 'O', 'O', 'O', 'O', 'O', 'O', 'O', 'O', 'O', 'O', 'O', 'O', 'O', 'O', 'O', 'O', 'O', 'O', 'O', 'O', 'O', 'O', 'O', 'O', 'O', 'O', 'O', 'O', 'O', 'O', 'O', 'O', 'O', 'O', 'O', 'O', 'O', 'O', 'O', 'O', 'O', 'O', 'O', 'O', 'O', 'O', 'O', 'O', 'O', 'O', 'O', 'O', 'O', 'O', 'O', 'O', 'O', 'O', 'O', 'O', 'O', 'O', 'O', 'O', 'O', 'O', 'O', 'O', 'O', 'O', 'O', 'O', 'O', 'O', 'O', 'O', 'O', 'O', 'O', 'O', 'O', 'O', 'O', 'O', 'O', 'O', 'O', 'O', 'O', 'O', 'O', 'O', 'O', 'O', 'O', 'O', 'O', 'O', 'O', 'O', 'O', 'O', 'O', 'O', 'O', 'O', 'O', 'O', 'O', 'O', 'O', 'O', 'O', 'O', 'O', 'O', 'O', 'O', 'O', 'O', 'O', 'O', 'O', 'O', 'O', 'O', 'O', 'O', 'O', 'O', 'O', 'O', 'O', 'O', 'O', 'O', 'O', 'O', 'O', 'O', 'O', 'O', 'O', 'O', 'O', 'B', 'O', 'O', 'O', 'O', 'O', 'O', 'O', 'O', 'O', 'O', 'O', 'O', 'O', 'O', 'O', 'O', 'O', 'O', 'O', 'O', 'O', 'O', 'O', 'O', 'O', 'O', 'O', 'O', 'O', 'O', 'O', 'O', 'O', 'O', 'O', 'O', 'O', 'O', 'O', 'O', 'O', 'O', 'O', 'O', 'O', 'O', 'O', 'O', 'O', 'O', 'O', 'O', 'O', 'O', 'O', 'O', 'O', 'O', 'O', 'O', 'O', 'O', 'O', 'O', 'O', 'O', 'O', 'O', 'O', 'O', 'O', 'O', 'O', 'O', 'O', 'O', 'O', 'O', 'O', 'O', 'O', 'O', 'O', 'O', 'O', 'O', 'O', 'O', 'O', 'O', 'O', 'O', 'O', 'O', 'O', 'O', 'O', 'O', 'O', 'O', 'O', 'O', 'O', 'O', 'O', 'O', 'O', 'O', 'O', 'O', 'O', 'O', 'O', 'O', 'O', 'O', 'O', 'O', 'O', 'O', 'O', 'O', 'O', 'O', 'O', 'O', 'O', 'O', 'O', 'O', 'O', 'O', 'O', 'O', 'O', 'O', 'O', 'O', 'O', 'O', 'O', 'O', 'O', 'O', 'O', 'O', 'O', 'O', 'O', 'O', 'O', 'O', 'O', 'O', 'O', 'O', 'O', 'O', 'O', 'O', 'O', 'O', 'O', 'O', 'O', 'O', 'O', 'O', 'O', 'O', 'O', 'O', 'O', 'O', 'O', 'O', 'O', 'O', 'O', 'O', 'O', 'O', 'O', 'O', 'O', 'O', 'O', 'O', 'O', 'O', 'O', 'O', 'O', 'O', 'O', 'O', 'O', 'O', 'O', 'O', 'O', 'O', 'O', 'O', 'O', 'O', 'O', 'O', 'O', 'O', 'O', 'O', 'O', 'O', 'O', 'O', 'O', 'O', 'O', 'O', 'O', 'O', 'O', 'O', 'O', 'O', 'O', 'O', 'O', 'O', 'O', 'O', 'O', 'O', 'O', 'O', 'O', 'O', 'O', 'O', 'O', 'O', 'O', 'O', 'O', 'O', 'O', 'O', 'O', 'O', 'O', 'O', 'O', 'O', 'O', 'O', 'O', 'O', 'O', 'O', 'O', 'O', 'O', 'O', 'O', 'O', 'O', 'O', 'O', 'O', 'O', 'O', 'O', 'O', 'O', 'O', 'O', 'O', 'O', 'O', 'O', 'O', 'O', 'O', 'O', 'O', 'O', 'O', 'O', 'O', 'O', 'O', 'O', 'O', 'O', 'O', 'O', 'O', 'O', 'O', 'O', 'O', 'O', 'O', 'O', 'O', 'O', 'O', 'O', 'O', 'O', 'O', 'O', 'O', 'O', 'O', 'O', 'O', 'O', 'O', 'O', 'B', 'O', 'O', 'O', 'O', 'O', 'O', 'O', 'O', 'O', 'O', 'O', 'O', 'O', 'O', 'O', 'O', 'O', 'O', 'O', 'O', 'O', 'O', 'O', 'O', 'O', 'O', 'O', 'O', 'O', 'O', 'O', 'O', 'O', 'O', 'O', 'O', 'O', 'O', 'O', 'O', 'O', 'O', 'O', 'O', 'O', 'O', 'O', 'O', 'O', 'O', 'O', 'O', 'O', 'O', 'O', 'O', 'O', 'O', 'O', 'O', 'O', 'O', 'O', 'O', 'O', 'O', 'O', 'O', 'O', 'O', 'O', 'O', 'O', 'O', 'O', 'O', 'O', 'O', 'O', 'O', 'O', 'O', 'O', 'O', 'O', 'O', 'O', 'O', 'O', 'O', 'O', 'O', 'O', 'O', 'O', 'O', 'O', 'O', 'O', 'O', 'O', 'O', 'O', 'O', 'O', 'O', 'O', 'O', 'O', 'O', 'O', 'O', 'O', 'O', 'O', 'O', 'O', 'O', 'O', 'O', 'O', 'O', 'O', 'O', 'O', 'O', 'O', 'O', 'O', 'O', 'O', 'O', 'O', 'O', 'O', 'O', 'O', 'O', 'O', 'O', 'O', 'O', 'O', 'O', 'O', 'O', 'O', 'O', 'O', 'O', 'O', 'O', 'O', 'O', 'O', 'O', 'O', 'O', 'O', 'O', 'O', 'O', 'O', 'O', 'O', 'O', 'O', 'O', 'O', 'O', 'O', 'O', 'O', 'O', 'O', 'O', 'O', 'O', 'O', 'O', 'O', 'O', 'O', 'O', 'O', 'O', 'O', 'O', 'O', 'O', 'O', 'O', 'O', 'O', 'O', 'O', 'O', 'O', 'O', 'O', 'O', 'O', 'O', 'O', 'O', 'O', 'O', 'O', 'O', 'O', 'O', 'O', 'O', 'O', 'O', 'O', 'O', 'O', 'O', 'O', 'O', 'O', 'O', 'O', 'O', 'O', 'O', 'O', 'O', 'O', 'O', 'O', 'O', 'O', 'O', 'O', 'O', 'O', 'O', 'O', 'O', 'O', 'O', 'O', 'O', 'O', 'O', 'O', 'O', 'O', 'O', 'O', 'O', 'O', 'O', 'O', 'O', 'O', 'O', 'O', 'O', 'O', 'O', 'O', 'O', 'O', 'O', 'O', 'O', 'O', 'O', 'O', 'O', 'O', 'O', 'O', 'O', 'O', 'O', 'O', 'O', 'O', 'O', 'O', 'O', 'O', 'O', 'O', 'O', 'O', 'O', 'O', 'O', 'O', 'O', 'O', 'O', 'O', 'O', 'O', 'O', 'O', 'O', 'O', 'O', 'O', 'O', 'O', 'O', 'O', 'O', 'O', 'O', 'O', 'O', 'O', 'O', 'O', 'O', 'O', 'O', 'O', 'O', 'O', 'O', 'O', 'O', 'O', 'O', 'O', 'O', 'O', 'O', 'O', 'O', 'O', 'O', 'O', 'O', 'O', 'O', 'O', 'O', 'O', 'O', 'O', 'O', 'O', 'O', 'O', 'O', 'O', 'O', 'O', 'O', 'O', 'O', 'O', 'O', 'O', 'O', 'O', 'O', 'O', 'O', 'O', 'O', 'O', 'O', 'O', 'O', 'O', 'B', 'O', 'O', 'O', 'O', 'O', 'O', 'O', 'O', 'O', 'O', 'O', 'O', 'O', 'O', 'O', 'O', 'O', 'O', 'O', 'O', 'O', 'O', 'O', 'O', 'O', 'O', 'O', 'O', 'O', 'O', 'O', 'O', 'O', 'O', 'O', 'O', 'O', 'O', 'O', 'O', 'O', 'O', 'O', 'O', 'O', 'O', 'O', 'O', 'O', 'O', 'O', 'O', 'O', 'O', 'O', 'O', 'O', 'O', 'O', 'O', 'O', 'O', 'O', 'O', 'O', 'O', 'O', 'O', 'O', 'O', 'O', 'O', 'O', 'O', 'O', 'O', 'O', 'O', 'O', 'O', 'O', 'O', 'O', 'O', 'O', 'O', 'O', 'O', 'O', 'O', 'O', 'O', 'O', 'O', 'O', 'O', 'O', 'O', 'O', 'O', 'O', 'O', 'O', 'O', 'O', 'O', 'O', 'O', 'O', 'O', 'O', 'O', 'O', 'O', 'O', 'O', 'O']
Extractive/present Keyphrases: ['speeches', 'plagiarism']
Abstractive/absent Keyphrases: ['2016 presidential election', 'donald trump', 'republican national convention,rnc', 'melania trump']
Other Metadata: {'id': 'ny0282969', 'categories': ['us', 'politics'], 'date': '2016/07/21', 'title': 'For Donald Trump’s Big Speech, an Added Pressure: No Echoes', 'abstract': 'CLEVELAND — Until Monday night, Donald J. Trump’s biggest concern about his convention speech was how much to reveal about himself and his family in an address that is often the most personal one a presidential candidate delivers. But the political firestorm over his wife’s speech , which borrowed passages from Michelle Obama’s convention remarks in 2008, raised the stakes exponentially. Mr. Trump’s speech on Thursday night cannot merely be his best ever. It also has to be bulletproof. By Tuesday morning, word had spread throughout his campaign that any language in Mr. Trump’s address even loosely inspired by speeches, essays, books or Twitter posts had to be either rewritten or attributed. Mr. Trump’s chief speechwriter, Stephen Miller, reassured colleagues that the acceptance speech was wholly original, according to two staff members who spoke with him and described those conversations on the condition of anonymity. Mr. Miller also told campaign aides that he had looked closely at passages that Mr. Trump had contributed — handwritten on unlined white pages — and was confident they contained no problems. (Mr. Miller declined an interview request.) Even so, one of the staff members downloaded plagiarism-detection software and ran a draft of the speech through the program. No red flags came up. The intense scrutiny of Mr. Trump’s words added new pressure to a speechwriting process that has been one of the most unpredictable and free-form in modern presidential campaigns. A month ago, Mr. Trump began giving dictation on themes for the speech, and he tossed ideas and phrases to Mr. Miller or other advisers on a daily basis. On printed copies of each draft, he circled passages he liked, crossed out or put question marks beside lines that he did not favor and frequently suggested new words or phrases. Image Stephen Miller, left, Mr. Trump’s chief speechwriter, and Paul Manafort, the campaign chairman, before an event for the candidate at the Trump SoHo hotel in New York last month. Credit Damon Winter/The New York Times “I’ve been amending the drafts big-league,” Mr. Trump said in an interview in his Manhattan office before the convention. “I get ideas from a lot of different places, a lot of smart people, but mostly I like language that sounds like me.” Yet in the aftermath of Melania Trump’s speech, campaign advisers have fretted that they do not know for sure where Mr. Trump gets his ideas and language — whether they are his own, in other words, or are picked up from Twitter, television, or, say, a best seller by Bill O’Reilly of Fox News, a commentator whom Mr. Trump likes. Borrowing or adapting may not always be tantamount to plagiarism, but several Trump advisers, who also insisted on anonymity, said that after the furor over Ms. Trump’s remarks, the campaign cannot allow a similar blowup. Ed Rollins, a Republican strategist who is advising a “super PAC” supporting Mr. Trump, said that the candidate could not afford any mistakes. “His speech is the whole game,” Mr. Rollins said. “Viewers have to watch it and say, ‘There is the next president of the United States.’” In the interview, Mr. Trump said his speech would center on his vision of a strong and secure America that “once existed and no longer does, but can again under a Trump administration.” Latest Election Polls 2016 Get the latest national and state polls on the presidential election between Hillary Clinton and Donald J. Trump. His greatest challenge, he said, was “putting myself in the speech” — discussing his upbringing and early experiences and relating them to the hopes and aspirations of other Americans. “I was never comfortable getting personal about my family because I thought it was special territory,” Mr. Trump said, glancing at a picture of his father on his desk. “It can feel exploitative to use family stories to win votes. And I had a very happy and comfortable life growing up. I had a great relationship with my father. But my focus needs to be on all the Americans who are struggling.” He said he was unsure if he would discuss his older brother Fred, who died as an alcoholic in 1981 at 43 — and whom he has described as an example of how destructive choices can damage lives that seem golden. “Without my brother Fred I might not be here,” Mr. Trump said. “He was really smart, great-looking. I don’t drink or smoke because of what happened to him. I focused on building my business and making good choices. I may talk about that, but I don’t know if I should.” Acceptance speeches seldom seem complete without anecdotes about personal trials and triumphs: Mitt Romney, trying to persuade voters to see him as more than a rich businessman, devoted about a fourth of his 2012 address to his parents’ unconditional love, his Mormon faith and reminiscences about watching the moon landing. In 2008 , Barack Obama described how his grandfather benefited from the G.I. Bill and how his mother and grandmother taught him the value of hard work. And Bill Clinton’s 1992 speech vividly recalled the life lessons he learned from his mother about fighting and working hard, from his grandfather about racial equality — and from his wife, Hillary, who, Mr. Clinton said, taught him that every child could learn. Mr. Clinton finished his speech with a now-famous line tying his Arkansas hometown to the American dream. “I end tonight where it all began for me,” he said. “I still believe in a place called Hope.” James Carville, a senior strategist for Mr. Clinton’s 1992 campaign, said that if Mr. Trump hoped to change the minds of those who see him as divisive or bigoted, he would need to open himself up to voters in meaningfully personal ways in his speech. “If he’s really different than the way he seems in television interviews or at his rallies, Thursday’s speech will be his single greatest opportunity to show voters who he really is,” Mr. Carville said. Paul Manafort, the Trump campaign chairman, said that Thursday’s speech would be “very much a reflection of Mr. Trump’s own words, as opposed to remarks that others create and the campaign puts in his mouth.” “He’s not an editor — he is actually the creator of the speech,” Mr. Manafort said. “Mr. Trump has given Steve Miller and I very specific directions about how he views the speech, what he wants to communicate, and ways to tie together things that he has been talking about in the campaign. The speech will end up being tone-perfect because the speech’s words will be his words.” Mr. Trump prefers speaking off the cuff with handwritten notes, a style that has proved successful at his rallies, where he has shown a talent for connecting with and electrifying crowds. But his adjustment to formal speeches remains a work in progress: He does not always sound like himself, and reading from a text can detract from the sense of authenticity that his supporters prize. One question is whether, or how much, he will ad-lib. He has sometimes seemed unable to resist deviating from prepared remarks, often to ill effect — ranting about a mosquito , or joking that a passing airplane was from Mexico and was “ getting ready to attack .” “Ad-libbing is instinct, all instinct,” Mr. Trump said. “I thought maybe about doing a freewheeling speech for the convention, but that really wouldn’t work. But even with a teleprompter, the speech will be me — my ideas, my beliefs, my words.”', 'keyword': '2016 Presidential Election;Donald Trump;Republican National Convention,RNC;Speeches;Plagiarism;Melania Trump'}
-----------
Sample from validation data split
Fields in the sample: ['id', 'document', 'doc_bio_tags', 'extractive_keyphrases', 'abstractive_keyphrases', 'other_metadata']
Tokenized Document: ['Jack', 'Sock', 'Picks', 'Up', 'Where', 'He', 'Left', 'Off', 'at', 'Last', 'Year’s', 'U.S.', 'Open', 'When', 'we', 'last', 'saw', 'Jack', 'Sock', 'at', 'the', 'United', 'States', 'Open', ',', 'a', 'year', 'ago', 'September,', 'he', 'was', 'holding', 'a', 'trophy', 'over', 'his', 'head', 'and', '—', 'not', 'yet', '19', 'and', 'a', 'newly', 'declared', 'professional', '—', 'being', 'hailed', 'a', 'Grand', 'Slam', 'champion.', 'Granted,', 'as', 'major', 'titles', 'go,', 'mixed', 'doubles', '(with', 'Melanie', 'Oudin)', 'was', 'akin', 'to', 'a', 'serving', 'of', 'cheese', 'and', 'crackers,', 'with', 'the', 'steak,', 'or', 'singles', 'title,', 'still', 'lodged', 'in', 'the', 'freezer.', 'But', 'as', 'Sock', 'had', 'the', 'previous', 'year', 'also', 'won', 'the', 'junior', 'boys', 'title', 'in', 'Flushing', 'Meadows', 'and,', 'with', 'legend', 'holding', 'that', 'he', 'had', 'never', 'lost', 'a', 'high', 'school', 'match,', 'it', 'was', 'natural', '—', 'at', 'least', 'hopeful', '—', 'to', 'think', 'he', 'might', 'have', 'a', 'healthy', 'share', 'of', 'winning', 'genes', 'to', 'go', 'with', 'his', 'booming', 'serve.', 'And', 'his', 'name,', 'for', 'goodness', 'sakes,', 'is', 'Jack', 'Sock;', 'of', 'Lincoln,', 'Neb.,', 'a', 'proud', 'Cornhusker.', 'Does', 'it', 'get', 'any', 'more', 'wholesome', 'and', 'hearty', 'for', 'a', 'country', 'in', 'a', 'continuous', 'search', 'for', 'its', 'next', 'men’s', 'star', 'in', 'this', 'athletically', 'enhanced', 'smash-mouth', 'era?', 'So', 'after', 'Sock', 'introduced', 'himself', 'to', 'Florian', 'Mayer,', 'a', 'German', 'seeded', '22nd,', 'with', 'a', 'sizzling', 'ace', 'down', 'the', 'T', 'and', 'held', 'serve', 'to', 'begin', 'a', 'first-round', 'match', 'Monday', 'on', 'the', 'grandstand', 'court,', 'fans', 'responded', 'with', 'a', 'chant', 'of', '“Let’s', 'Go', 'Sock!”', 'Forgetting', 'for', 'the', 'moment', 'that', 'New', 'York', 'is', 'a', 'Yankees', 'town,', 'it', 'was', 'better', 'than', 'one', 'alternative', '—', 'Sock', 'it', 'to', 'him', '—', 'and', 'completely', 'understandable', 'as', 'Sock', 'was', 'in', 'the', 'process', 'of', 'feeding', 'America’s', 'slam', 'its', 'first', 'helping', 'of', 'nationalistic', 'fervor', 'by', 'overpowering', 'Mayer,', 'who', 'retired', 'while', 'trailing,', '6-3,', '6-2,', '3-2.', 'One', 'or', 'two', 'more', 'performances', 'like', 'this', 'and', 'we', 'can', 'expect', 'a', 'slew', 'of', 'word', 'play', 'headlines,', 'beginning', 'with', 'Sock', 'and', 'Awe.', 'It', 'doesn’t', 'take', 'much', 'to', 'fire', 'up', 'the', 'Next', 'Great', 'American', 'news', 'media', 'machine,', 'not', 'that', 'Sock', 'is', 'lacking', 'in', 'confidence', 'or', 'ambition.', '“I', 'feel', 'like', 'my', 'game', 'is', 'right', 'on', 'the', 'verge', 'of', 'going', 'to', 'the', 'next', 'level,”', 'he', 'said', 'after', 'winning', 'his', 'fourth', 'tour', 'match', 'of', '2012', 'against', 'six', 'losses.', 'To', 'explain', 'what', 'he', 'meant', 'of', 'taking', 'his', 'game', 'to', 'the', '“next', 'level,”', 'put', 'it', 'this', 'way:', 'from', 'his', 'current', 'ranking,', '243,', 'there', 'are', 'many', 'stops', 'to', 'make', 'on', 'the', 'ride', 'to', 'the', 'dizzying', 'heights', 'where', 'Roger', 'Federer', 'and', 'elite', 'company', 'reside', '—', 'beginning', 'with', 'leaping', 'into', 'position', 'near', 'another', 'young', 'and', 'hopeful', 'Yank,', 'Ryan', 'Harrison,', 'currently', 'No.', '61.', 'On', 'the', 'scale', 'of', 'youthful', 'and', 'potential', 'men’s', 'tour', 'heirs,', 'the', '21-year-old', 'Milos', 'Raonic', 'of', 'Canada', 'is', 'the', 'closest', 'to', 'a', 'major', 'breakthrough,', 'though', 'it', 'is', 'also', 'difficult', 'to', 'define', 'what', 'even', 'that', 'means', 'when', 'three', 'players', '—', 'Federer,', 'Rafael', 'Nadal', 'and', 'Novak', 'Djokovic', '—', 'have', 'won', '29', 'of', 'the', 'last', '30', 'slam', 'titles', 'and', 'show', 'little', 'inclination', 'of', 'easing', 'their', 'chokehold.', 'Compared', 'with', 'what', 'the', 'more', 'promising', 'newbies', 'face', 'these', 'days,', 'the', 'emergent', 'superstars', 'of', 'yore', 'practically', 'took', 'their', 'Grand', 'Slam', 'treats', 'by', 'merely', 'growing', 'tall', 'enough', 'to', 'reach', 'into', 'the', 'cookie', 'jar.', 'Boris', 'Becker', 'won', 'Wimbledon', 'as', 'a', '17-year-old', 'mop-haired', 'redhead.', 'John', 'McEnroe', 'and', 'Pete', 'Sampras', 'broke', 'through', 'in', 'New', 'York', 'at', '20', 'and', '19.', 'Into', 'the', '21st', 'century,', 'Nadal', 'began', 'his', 'domination', 'of', 'the', 'French', 'Open', 'at', '19,', 'Djokovic', 'won', 'the', 'Australian', 'Open', 'at', '21', 'and', 'Federer', 'sank', 'to', 'his', 'knees', 'at', 'Wimbledon', 'weeks', 'before', 'turning', '22.', 'These', 'days,', 'it', 'is', 'unfathomable', 'to', 'think', 'of', 'a', 'skinny', 'and', 'moon-balling', 'Michael', 'Chang', 'winning', 'the', 'French', 'Open', 'at', '17,', 'as', 'he', 'did', 'in', '1989,', 'or', 'a', 'teenager', 'winning', 'any', 'of', 'the', 'slams.', '“I', 'don’t', 'think', 'that’s', 'going', 'to', 'be', 'the', 'case', 'any', 'time', 'soon', 'because', 'this', 'game', 'is', 'so', 'physical', 'now', 'and', 'people', 'need', 'to', 'grow', 'into', 'their', 'body,”', 'said', 'John', 'Isner,', 'who', 'at', '27', 'has', 'reason', 'to', 'believe', 'that', 'his', 'best', 'results,', 'whatever', 'they', 'may', 'be,', 'are', 'still', 'ahead', 'of', 'him.', 'At', '31,', 'Federer,', 'who', 'absurdly', 'has', 'not', 'missed', 'a', 'Grand', 'Slam', 'tournament', 'in', '13', 'years,', 'may', 'be', 'the', 'best-conditioned', 'of', 'all.', 'Andy', 'Murray,', 'at', '25,', 'is', 'thought', 'to', 'be', 'on', 'the', 'verge', 'of', 'his', 'prime.', 'It', 'is', 'mind-boggling', 'to', 'think', 'that', 'Bjorn', 'Borg,', 'McEnroe,', 'Becker', 'and', 'others', 'were', 'playing', 'on', 'fumes,', 'their', 'best', 'matches', 'behind', 'them,', 'by', 'their', 'mid-20s.', 'A', 'no-kidding', 'adult’s', 'tour', 'that', 'provides', 'longevity', 'and', 'personal', 'context', 'is', 'so', 'much', 'richer', 'than', 'the', 'alternative.', 'But', 'given', 'such', 'dramatic', 'career', 'clock', 'changes,', 'patience', 'may', 'be', 'a', 'most', 'valuable', 'virtue', 'for', 'players', 'like', 'Raonic,', 'Harrison', 'and', 'Bernard', 'Tomic', 'of', 'Australia.', '“Those', 'guys,', 'it', 'might', 'take', 'them', 'a', 'little', 'while', 'to', 'see', 'their', 'very,', 'very', 'best', 'results,', 'but', 'they’re', 'certainly', 'not', 'doing', 'so', 'bad', 'right', 'now,”', 'said', 'Isner,', 'who', 'didn’t', 'hesitate', 'to', 'include', 'Sock,', 'calling', 'him', '“a', 'very', 'good', 'player.”', 'Sock', 'is', 'a', 'strapping', '’Husker,', '6', 'feet', '1', 'inch,', '180', 'pounds,', 'but', 'he', 'was', 'set', 'back', 'physically', 'in', 'March', 'by', 'surgery', 'to', 'repair', 'a', 'torn', 'abdominal', 'muscle.', 'In', 'a', 'brilliant', 'stroke,', 'he', 'has', 'been', 'working', 'in', 'Las', 'Vegas', 'with', 'the', 'trainer', 'Gil', 'Reyes,', 'who', 'whipped', 'the', 'once-profligate', 'Andre', 'Agassi', 'into', 'shape.', 'He', 'has', 'hired', 'the', 'former', 'Swedish', 'player,', 'Joakim', 'Nystrom,', 'to', 'help', 'him', 'play', 'a', 'more', 'patient', 'game.', 'On', 'today’s', 'altered', 'career', 'time', 'clock,', 'there', 'is', 'no', 'choice', 'but', 'to', 'wait', 'one’s', 'turn', 'and', 'see', 'what', 'happens.', 'In', 'a', 'microcosm', 'of', 'that', 'strategy,', 'Sock', 'fell', 'behind,', '0-40,', 'while', 'serving', 'at', '4-2', 'in', 'the', 'first', 'set,', 'rallied', 'to', 'deuce,', 'kept', 'his', 'cool', 'as', 'Mayer', 'challenged', 'two', 'line', 'calls', 'and', 'won', 'both,', 'and', 'wound', 'up', 'winning', 'the', 'long', 'game', 'with', 'the', 'help', 'of', 'his', 'own', 'challenge', 'of', 'an', 'out', 'call.', 'He', 'was', 'never', 'threatened', 'after', 'that,', 'cranking', 'his', 'first', 'serve', 'as', 'high', 'as', '134', 'miles', 'per', 'hour,', 'winning', '17', 'of', '25', 'second-service', 'points', 'and', 'shrugging', 'off', 'the', 'question', 'of', 'when', 'the', 'Next', 'Great', 'American', 'will', 'arrive', 'as', 'easily', 'as', 'he', 'did', 'Mayer.', '“Until', 'the', 'results', 'are', 'there,', 'until', 'the', 'rankings', 'and', 'everything', 'is', 'there,', 'not', 'a', 'different', 'answer', 'to', 'give,”', 'he', 'said.', 'Give', 'him', 'time,', 'in', 'other', 'words.', 'By', 'today’s', 'standards,', 'he’s', 'got', 'a', 'few', 'years', 'before', 'we', 'have', 'to', 'stop', 'asking.']
Document BIO Tags: ['O', 'O', 'O', 'O', 'O', 'O', 'O', 'O', 'O', 'O', 'O', 'O', 'O', 'O', 'O', 'O', 'O', 'O', 'O', 'O', 'O', 'O', 'O', 'O', 'O', 'O', 'O', 'O', 'O', 'O', 'O', 'O', 'O', 'O', 'O', 'O', 'O', 'O', 'O', 'O', 'O', 'O', 'O', 'O', 'O', 'O', 'O', 'O', 'O', 'O', 'O', 'O', 'O', 'O', 'O', 'O', 'O', 'O', 'O', 'O', 'O', 'O', 'O', 'O', 'O', 'O', 'O', 'O', 'O', 'O', 'O', 'O', 'O', 'O', 'O', 'O', 'O', 'O', 'O', 'O', 'O', 'O', 'O', 'O', 'O', 'O', 'O', 'O', 'O', 'O', 'O', 'O', 'O', 'O', 'O', 'O', 'O', 'O', 'O', 'O', 'O', 'O', 'O', 'O', 'O', 'O', 'O', 'O', 'O', 'O', 'O', 'O', 'O', 'O', 'O', 'O', 'O', 'O', 'O', 'O', 'O', 'O', 'O', 'O', 'O', 'O', 'O', 'O', 'O', 'O', 'O', 'O', 'O', 'O', 'O', 'O', 'O', 'O', 'O', 'O', 'O', 'O', 'O', 'O', 'O', 'O', 'O', 'O', 'O', 'O', 'O', 'O', 'O', 'O', 'O', 'O', 'O', 'O', 'O', 'O', 'O', 'O', 'O', 'O', 'O', 'O', 'O', 'O', 'O', 'O', 'O', 'O', 'O', 'O', 'O', 'O', 'O', 'O', 'O', 'O', 'O', 'O', 'O', 'O', 'O', 'O', 'O', 'O', 'O', 'O', 'O', 'O', 'O', 'O', 'O', 'O', 'O', 'O', 'O', 'O', 'O', 'O', 'O', 'O', 'O', 'O', 'O', 'O', 'O', 'O', 'O', 'O', 'O', 'O', 'O', 'O', 'O', 'O', 'O', 'O', 'O', 'O', 'O', 'O', 'O', 'O', 'O', 'O', 'O', 'O', 'O', 'O', 'O', 'O', 'O', 'O', 'O', 'O', 'O', 'O', 'O', 'O', 'O', 'O', 'O', 'O', 'O', 'O', 'O', 'O', 'O', 'O', 'O', 'O', 'O', 'O', 'O', 'O', 'O', 'O', 'O', 'O', 'O', 'O', 'O', 'O', 'O', 'O', 'O', 'O', 'O', 'O', 'O', 'O', 'O', 'O', 'O', 'O', 'O', 'O', 'O', 'O', 'O', 'O', 'O', 'O', 'O', 'O', 'O', 'O', 'O', 'O', 'O', 'O', 'O', 'O', 'O', 'O', 'O', 'O', 'O', 'O', 'O', 'O', 'O', 'O', 'O', 'O', 'O', 'O', 'O', 'O', 'O', 'O', 'O', 'O', 'O', 'O', 'O', 'O', 'O', 'O', 'O', 'O', 'O', 'O', 'O', 'O', 'O', 'O', 'O', 'O', 'O', 'O', 'O', 'O', 'O', 'O', 'O', 'O', 'O', 'O', 'O', 'O', 'O', 'O', 'O', 'O', 'O', 'O', 'O', 'O', 'O', 'O', 'O', 'O', 'O', 'O', 'O', 'O', 'O', 'O', 'O', 'O', 'O', 'O', 'O', 'O', 'O', 'O', 'O', 'O', 'O', 'O', 'O', 'O', 'O', 'O', 'O', 'O', 'O', 'O', 'O', 'O', 'O', 'O', 'O', 'O', 'O', 'O', 'O', 'O', 'O', 'O', 'O', 'O', 'O', 'O', 'O', 'O', 'O', 'O', 'O', 'O', 'O', 'O', 'O', 'O', 'O', 'O', 'O', 'O', 'O', 'O', 'O', 'O', 'O', 'O', 'O', 'O', 'O', 'O', 'O', 'O', 'O', 'O', 'O', 'O', 'O', 'O', 'O', 'O', 'O', 'O', 'O', 'O', 'O', 'O', 'O', 'O', 'O', 'O', 'O', 'O', 'O', 'O', 'O', 'O', 'O', 'O', 'O', 'O', 'O', 'O', 'O', 'O', 'O', 'O', 'O', 'O', 'O', 'O', 'O', 'O', 'O', 'O', 'O', 'O', 'O', 'O', 'O', 'O', 'O', 'O', 'O', 'O', 'O', 'O', 'O', 'O', 'O', 'O', 'O', 'O', 'O', 'O', 'O', 'O', 'O', 'O', 'O', 'O', 'O', 'O', 'O', 'O', 'O', 'O', 'O', 'O', 'O', 'O', 'O', 'O', 'O', 'O', 'O', 'O', 'O', 'O', 'O', 'O', 'O', 'O', 'O', 'O', 'O', 'O', 'O', 'O', 'O', 'O', 'O', 'O', 'O', 'O', 'O', 'O', 'O', 'O', 'O', 'O', 'O', 'O', 'O', 'O', 'O', 'O', 'O', 'O', 'O', 'O', 'O', 'O', 'O', 'O', 'O', 'O', 'O', 'O', 'O', 'O', 'O', 'O', 'O', 'O', 'O', 'O', 'O', 'O', 'O', 'O', 'O', 'O', 'O', 'O', 'O', 'O', 'O', 'O', 'O', 'O', 'O', 'O', 'O', 'O', 'O', 'O', 'O', 'O', 'O', 'O', 'O', 'O', 'O', 'O', 'O', 'O', 'O', 'O', 'O', 'O', 'O', 'O', 'O', 'O', 'O', 'O', 'O', 'O', 'O', 'O', 'O', 'O', 'O', 'O', 'O', 'O', 'O', 'O', 'O', 'O', 'O', 'O', 'O', 'O', 'O', 'O', 'O', 'O', 'O', 'O', 'O', 'O', 'O', 'O', 'O', 'O', 'O', 'O', 'O', 'O', 'O', 'O', 'O', 'O', 'O', 'O', 'O', 'O', 'O', 'O', 'O', 'O', 'O', 'O', 'O', 'O', 'O', 'O', 'O', 'O', 'O', 'O', 'O', 'O', 'O', 'O', 'O', 'O', 'O', 'O', 'O', 'O', 'O', 'O', 'O', 'O', 'O', 'O', 'O', 'O', 'O', 'O', 'O', 'O', 'O', 'O', 'O', 'O', 'O', 'O', 'O', 'O', 'O', 'O', 'O', 'O', 'O', 'O', 'O', 'O', 'O', 'O', 'O', 'O', 'O', 'O', 'O', 'O', 'O', 'O', 'O', 'O', 'O', 'O', 'O', 'O', 'O', 'O', 'O', 'O', 'O', 'O', 'O', 'O', 'O', 'O', 'O', 'O', 'O', 'O', 'O', 'O', 'O', 'O', 'O', 'O', 'O', 'O', 'O', 'O', 'O', 'O', 'O', 'O', 'O', 'O', 'O', 'O', 'O', 'O', 'O', 'O', 'O', 'O', 'O', 'O', 'O', 'O', 'O', 'O', 'O', 'O', 'O', 'O', 'O', 'O', 'O', 'O', 'O', 'O', 'O', 'O', 'O', 'O', 'O', 'O', 'O', 'O', 'O', 'O', 'O', 'O', 'O', 'O', 'O', 'O', 'O', 'O', 'O', 'O', 'O', 'O', 'O', 'O', 'O', 'O', 'O', 'O', 'O', 'O', 'O', 'O', 'O', 'O', 'O', 'O', 'O', 'O', 'O', 'O', 'O', 'O', 'O', 'O', 'O', 'O', 'O', 'O', 'O', 'O', 'O', 'O', 'O', 'O', 'O', 'O', 'O', 'O', 'O', 'O', 'O', 'O', 'O', 'O', 'O', 'O', 'O', 'O', 'O', 'O', 'O', 'O', 'O', 'O', 'O', 'O', 'O', 'O', 'O', 'O', 'O', 'O', 'O', 'O', 'O', 'O', 'O', 'O', 'O', 'O', 'O', 'O', 'O', 'O', 'O', 'O', 'O', 'O', 'O', 'O', 'O', 'O', 'O', 'O', 'O', 'O', 'O', 'O', 'O', 'O', 'O', 'O', 'O', 'O', 'O', 'O', 'O', 'O', 'O', 'O', 'O', 'O', 'O', 'O', 'O', 'O', 'O', 'O', 'O', 'O', 'O', 'O', 'O', 'O', 'O', 'O', 'O', 'O', 'O', 'O', 'O', 'O', 'O', 'O', 'O', 'O', 'O', 'O', 'O', 'O', 'O', 'O', 'O', 'O', 'O', 'O', 'O', 'O', 'O', 'O', 'O', 'O', 'O', 'O', 'O', 'O', 'O', 'O', 'O', 'O', 'O', 'O', 'O', 'O', 'O', 'O', 'O', 'O', 'O', 'O', 'O', 'O', 'O', 'O', 'O', 'O', 'O', 'O', 'O', 'O', 'O', 'O', 'O', 'O', 'O', 'O', 'O', 'O', 'O', 'O', 'O', 'O', 'O', 'O', 'O', 'O', 'O', 'O', 'O', 'O', 'O', 'O', 'O', 'O', 'O', 'O', 'O', 'O', 'O', 'O', 'O', 'O', 'O', 'O', 'O', 'O', 'O', 'O', 'O', 'O', 'O', 'O', 'O', 'O', 'O']
Extractive/present Keyphrases: []
Abstractive/absent Keyphrases: ['tennis', 'united states open (tennis)', 'sock jack']
Other Metadata: {'id': 'ny0125215', 'categories': ['sports', 'tennis'], 'date': '2012/08/28', 'title': 'Jack Sock Picks Up Where He Left Off at Last Year’s U.S. Open', 'abstract': 'When we last saw Jack Sock at the United States Open , a year ago September, he was holding a trophy over his head and — not yet 19 and a newly declared professional — being hailed a Grand Slam champion. Granted, as major titles go, mixed doubles (with Melanie Oudin) was akin to a serving of cheese and crackers, with the steak, or singles title, still lodged in the freezer. But as Sock had the previous year also won the junior boys title in Flushing Meadows and, with legend holding that he had never lost a high school match, it was natural — at least hopeful — to think he might have a healthy share of winning genes to go with his booming serve. And his name, for goodness sakes, is Jack Sock; of Lincoln, Neb., a proud Cornhusker. Does it get any more wholesome and hearty for a country in a continuous search for its next men’s star in this athletically enhanced smash-mouth era? So after Sock introduced himself to Florian Mayer, a German seeded 22nd, with a sizzling ace down the T and held serve to begin a first-round match Monday on the grandstand court, fans responded with a chant of “Let’s Go Sock!” Forgetting for the moment that New York is a Yankees town, it was better than one alternative — Sock it to him — and completely understandable as Sock was in the process of feeding America’s slam its first helping of nationalistic fervor by overpowering Mayer, who retired while trailing, 6-3, 6-2, 3-2. One or two more performances like this and we can expect a slew of word play headlines, beginning with Sock and Awe. It doesn’t take much to fire up the Next Great American news media machine, not that Sock is lacking in confidence or ambition. “I feel like my game is right on the verge of going to the next level,” he said after winning his fourth tour match of 2012 against six losses. To explain what he meant of taking his game to the “next level,” put it this way: from his current ranking, 243, there are many stops to make on the ride to the dizzying heights where Roger Federer and elite company reside — beginning with leaping into position near another young and hopeful Yank, Ryan Harrison, currently No. 61. On the scale of youthful and potential men’s tour heirs, the 21-year-old Milos Raonic of Canada is the closest to a major breakthrough, though it is also difficult to define what even that means when three players — Federer, Rafael Nadal and Novak Djokovic — have won 29 of the last 30 slam titles and show little inclination of easing their chokehold. Compared with what the more promising newbies face these days, the emergent superstars of yore practically took their Grand Slam treats by merely growing tall enough to reach into the cookie jar. Boris Becker won Wimbledon as a 17-year-old mop-haired redhead. John McEnroe and Pete Sampras broke through in New York at 20 and 19. Into the 21st century, Nadal began his domination of the French Open at 19, Djokovic won the Australian Open at 21 and Federer sank to his knees at Wimbledon weeks before turning 22. These days, it is unfathomable to think of a skinny and moon-balling Michael Chang winning the French Open at 17, as he did in 1989, or a teenager winning any of the slams. “I don’t think that’s going to be the case any time soon because this game is so physical now and people need to grow into their body,” said John Isner, who at 27 has reason to believe that his best results, whatever they may be, are still ahead of him. At 31, Federer, who absurdly has not missed a Grand Slam tournament in 13 years, may be the best-conditioned of all. Andy Murray, at 25, is thought to be on the verge of his prime. It is mind-boggling to think that Bjorn Borg, McEnroe, Becker and others were playing on fumes, their best matches behind them, by their mid-20s. A no-kidding adult’s tour that provides longevity and personal context is so much richer than the alternative. But given such dramatic career clock changes, patience may be a most valuable virtue for players like Raonic, Harrison and Bernard Tomic of Australia. “Those guys, it might take them a little while to see their very, very best results, but they’re certainly not doing so bad right now,” said Isner, who didn’t hesitate to include Sock, calling him “a very good player.” Sock is a strapping ’Husker, 6 feet 1 inch, 180 pounds, but he was set back physically in March by surgery to repair a torn abdominal muscle. In a brilliant stroke, he has been working in Las Vegas with the trainer Gil Reyes, who whipped the once-profligate Andre Agassi into shape. He has hired the former Swedish player, Joakim Nystrom, to help him play a more patient game. On today’s altered career time clock, there is no choice but to wait one’s turn and see what happens. In a microcosm of that strategy, Sock fell behind, 0-40, while serving at 4-2 in the first set, rallied to deuce, kept his cool as Mayer challenged two line calls and won both, and wound up winning the long game with the help of his own challenge of an out call. He was never threatened after that, cranking his first serve as high as 134 miles per hour, winning 17 of 25 second-service points and shrugging off the question of when the Next Great American will arrive as easily as he did Mayer. “Until the results are there, until the rankings and everything is there, not a different answer to give,” he said. Give him time, in other words. By today’s standards, he’s got a few years before we have to stop asking.', 'keyword': 'Tennis;United States Open (Tennis);Sock Jack'}
-----------
Sample from test data split
Fields in the sample: ['id', 'document', 'doc_bio_tags', 'extractive_keyphrases', 'abstractive_keyphrases', 'other_metadata']
Tokenized Document: ['World', 'records', 'no', 'joke', 'to', 'frustrated', 'Pakistanis', 'ISLAMABAD', '-', 'One', 'young', 'contender', 'created', 'the', 'world’s', 'largest', 'sequin', 'mosaic', 'using', '325,000', 'of', 'the', 'sparkly', 'discs.', 'Two', 'other', 'youths', 'achieved', '123', 'consecutive', 'badminton', 'passes', 'in', 'one', 'minute.', 'And', '1,450', 'participants', 'broke', 'the', 'record', 'for', 'the', 'most', 'people', 'arm', 'wrestling.', 'Such', 'are', 'the', 'skills', 'that', 'Guinness', 'World', 'Records', 'are', 'made', 'of', 'in', 'Pakistan,', 'where', 'thousands', 'of', 'young', 'people', 'are', 'groomed', 'to', 'establish', 'their', 'unique', 'feats', 'for', 'posterity.', 'Last', 'week,', 'the', 'contestants', 'came', 'together', 'for', 'the', 'annual', 'Punjab', 'Youth', 'Festival', 'to', 'show', 'their', 'stuff', '—', 'many', 'in', 'athletics,', 'but', 'others', 'in', 'downright', 'quirky', 'displays,', 'including', 'one', 'young', 'boy', 'who', 'achieved', 'fame', 'by', 'kicking', '50', 'coconuts', 'from', 'on', 'top', 'of', 'the', 'heads', 'of', 'a', 'row', 'of', 'people.', 'It', 'seems', 'Pakistan', 'has', 'become', 'a', 'world', 'record-creating', 'machine,', 'with', 'the', 'coordinated', 'effort', 'reaping', 'an', 'impressive', '23', 'world', 'records,', 'event', 'organizers', 'boasted.', 'The', 'push', 'for', 'inclusion', 'of', 'Pakistanis', 'in', 'the', 'venerable', 'Guinness', 'World', 'Records', 'entries', '(which', 'began', 'in', 'book', 'form', 'in', '1955)', 'stems', 'in', 'part', 'from', 'festival', 'organizers’', 'desire', 'to', 'boost', 'the', 'image', 'of', 'a', 'country', 'often', 'associated', 'with', 'militancy,', 'religious', 'strife', 'and', 'economic', 'decline.', 'There', 'is', 'a', 'patriotic', 'element,', 'as', 'well:', 'Last', 'October,', 'for', 'instance,', '42,813', 'Pakistanis', 'got', 'together', 'in', 'a', 'Lahore', 'hockey', 'stadium', 'to', 'belt', 'out', 'the', 'national', 'anthem', 'and', 'create', 'yet', 'another', 'world', 'record', 'for', 'the', 'most', 'people', 'singing', 'their', 'country’s', 'anthem.', 'Days', 'later,', 'another', '24,200', 'people', 'held', 'green', 'and', 'white', 'boxes', '—', 'the', 'colors', 'of', 'the', 'national', 'flag', 'of', 'Pakistan', '—', 'to', 'set', 'the', 'world', 'record', 'for', 'creating', 'the', 'largest', 'human', 'flag.', 'Although', 'some', 'of', 'the', 'records', 'might', 'seem', 'amusing', 'to', 'others', '—', 'coconut', 'kicking', 'champ', 'Mohammad', 'Rashid', 'of', 'Karachi', 'last', 'week', 'claimed', 'his', 'fourth', 'world', 'record', 'by', 'breaking', '34', 'pine', 'boards', 'in', '32', 'seconds', 'with', 'his', 'head', '—', 'the', 'competitions', 'were', 'no', 'laughing', 'matter', 'to', 'participants.', 'Usman', 'Anwar,', 'director', 'of', 'the', 'Punjab', 'Youth', 'Festival,', 'explained', 'that', 'the', 'kids', 'have', 'been', 'training', 'for', 'eight', 'months.', '“We', 'started', 'at', 'the', 'neighborhood', 'and', 'village', 'level', 'so', 'that', 'children', 'could', 'come', 'out', 'and', 'participate,”', 'said', 'Anwar.', '“Our', 'main', 'objective', 'was', 'to', 'inculcate', 'interest', 'for', 'sports', 'in', 'the', 'public.”', 'Young', 'people', 'from', 'over', '55,000', 'neighborhood', 'and', 'village', 'councils', 'vied', 'for', 'a', 'chance', 'to', 'compete', 'in', 'the', 'games.', '“We', 'were', 'able', 'to', 'select', 'the', 'best', 'of', 'the', 'best', 'to', 'train', 'for', 'the', 'world', 'records,”', 'said', 'Anwar.', 'Because', 'of', 'terrorism,', 'political', 'upheaval', 'and', 'widespread', 'unemployment,', 'many', 'young', 'people', 'appear', 'to', 'have', 'little', 'hope', 'for', 'the', 'future,', 'says', 'Hafeez', 'Rehman,', 'a', 'professor', 'in', 'the', 'anthropology', 'department', 'at', 'Quaid-i-Azam', 'University', 'in', 'the', 'capital,', 'Islamabad.', 'Sports', 'competitions,', 'Rehman', 'said,', 'create', 'an', 'opportunity', 'for', 'youth', 'to', 'excel', 'personally', 'and', 'also', 'to', 'improve', 'Pakistan’s', 'image.', '“We', 'have', 'energetic', 'youth.', 'Pakistan', 'has', 'more', 'than', '55', 'million', 'young', 'people.', 'It', 'becomes', 'an', 'asset', 'for', 'the', 'country,”', 'he', 'added.', 'The', 'festival', 'itself', 'has', 'become', 'part', 'of', 'the', 'record-setting', 'mania.', 'It', 'was', 'recognized', 'for', 'having', 'more', 'participants', '—', '3.3', 'million,', 'most', 'of', 'whom', 'registered', 'online,', 'according', 'to', 'Anwar', '—', 'constituting', 'a', 'world', 'record', 'for', 'sporting', 'events.']
Document BIO Tags: ['O', 'O', 'O', 'O', 'O', 'O', 'O', 'O', 'O', 'O', 'O', 'O', 'O', 'O', 'O', 'O', 'O', 'O', 'O', 'O', 'O', 'O', 'O', 'O', 'O', 'O', 'O', 'O', 'O', 'O', 'O', 'O', 'O', 'O', 'O', 'O', 'O', 'O', 'O', 'O', 'O', 'O', 'O', 'O', 'O', 'O', 'O', 'O', 'O', 'O', 'O', 'O', 'B', 'O', 'O', 'O', 'O', 'O', 'O', 'B', 'O', 'O', 'O', 'O', 'O', 'O', 'O', 'O', 'O', 'O', 'O', 'O', 'O', 'O', 'O', 'O', 'O', 'O', 'O', 'O', 'O', 'O', 'O', 'O', 'O', 'O', 'O', 'O', 'O', 'O', 'O', 'O', 'O', 'O', 'O', 'O', 'O', 'O', 'O', 'O', 'O', 'O', 'O', 'O', 'O', 'O', 'O', 'O', 'O', 'O', 'O', 'O', 'O', 'O', 'O', 'O', 'O', 'O', 'O', 'O', 'O', 'O', 'O', 'O', 'B', 'O', 'O', 'O', 'O', 'O', 'O', 'O', 'O', 'O', 'O', 'O', 'O', 'O', 'O', 'O', 'O', 'O', 'O', 'O', 'O', 'O', 'O', 'O', 'O', 'O', 'O', 'O', 'O', 'B', 'O', 'O', 'O', 'O', 'O', 'O', 'O', 'O', 'O', 'O', 'O', 'O', 'O', 'O', 'O', 'O', 'O', 'O', 'O', 'O', 'O', 'O', 'O', 'O', 'O', 'O', 'O', 'O', 'O', 'O', 'O', 'O', 'O', 'O', 'O', 'O', 'O', 'O', 'O', 'O', 'O', 'O', 'O', 'O', 'O', 'O', 'O', 'O', 'O', 'O', 'O', 'O', 'O', 'O', 'O', 'O', 'O', 'O', 'O', 'O', 'O', 'O', 'O', 'O', 'O', 'O', 'O', 'O', 'O', 'O', 'O', 'O', 'O', 'O', 'O', 'O', 'O', 'O', 'O', 'O', 'O', 'O', 'O', 'O', 'O', 'O', 'O', 'O', 'O', 'O', 'O', 'B', 'O', 'O', 'O', 'O', 'O', 'O', 'O', 'O', 'O', 'O', 'O', 'O', 'O', 'O', 'O', 'O', 'O', 'O', 'O', 'O', 'O', 'O', 'O', 'O', 'O', 'O', 'O', 'O', 'O', 'O', 'O', 'O', 'O', 'O', 'O', 'O', 'O', 'O', 'O', 'O', 'O', 'O', 'O', 'O', 'O', 'O', 'O', 'O', 'O', 'O', 'O', 'O', 'O', 'O', 'O', 'O', 'O', 'O', 'O', 'O', 'O', 'O', 'O', 'O', 'O', 'O', 'O', 'O', 'O', 'O', 'O', 'O', 'O', 'O', 'O', 'O', 'O', 'O', 'O', 'O', 'O', 'O', 'O', 'O', 'O', 'O', 'O', 'O', 'O', 'O', 'O', 'O', 'O', 'O', 'O', 'O', 'O', 'O', 'O', 'O', 'O', 'O', 'O', 'O', 'O', 'O', 'O', 'O', 'O', 'O', 'O', 'O', 'O', 'O', 'O', 'O', 'O', 'O', 'O', 'O', 'O', 'O', 'O', 'O', 'O', 'O', 'O', 'O', 'O', 'O', 'O', 'O', 'O', 'O', 'O', 'O', 'O', 'O', 'O', 'O', 'O', 'O', 'O', 'O', 'O', 'O', 'O', 'O', 'O', 'O', 'O', 'O', 'O', 'O', 'O', 'O', 'O', 'O', 'O', 'O', 'O', 'O', 'O', 'O', 'O', 'O', 'O', 'O', 'O', 'O', 'O', 'O', 'O', 'O', 'O', 'O', 'O', 'O', 'O', 'O', 'O', 'O', 'O', 'O', 'O', 'O', 'O', 'O', 'O', 'O', 'O', 'O', 'O', 'O', 'O', 'O', 'O', 'O', 'B', 'O', 'O', 'O', 'O', 'O', 'O', 'O', 'O', 'O', 'O', 'O', 'O', 'O', 'O', 'O', 'O', 'O', 'O', 'O', 'O', 'O', 'O', 'O', 'O', 'O', 'O', 'O', 'O', 'O', 'O', 'O', 'O', 'O', 'O', 'O', 'O', 'O', 'O', 'O', 'O', 'O', 'O', 'O', 'O', 'O', 'O', 'O', 'O', 'O', 'O', 'O', 'O']
Extractive/present Keyphrases: ['pakistan', 'guinness']
Abstractive/absent Keyphrases: ['india']
Other Metadata: {'id': 'jp0000001', 'categories': ['asia-pacific', 'offbeat-asia-pacific'], 'date': '2013/03/17', 'title': 'World records no joke to frustrated Pakistanis ', 'abstract': 'ISLAMABAD - One young contender created the world’s largest sequin mosaic using 325,000 of the sparkly discs. Two other youths achieved 123 consecutive badminton passes in one minute. And 1,450 participants broke the record for the most people arm wrestling. Such are the skills that Guinness World Records are made of in Pakistan, where thousands of young people are groomed to establish their unique feats for posterity. Last week, the contestants came together for the annual Punjab Youth Festival to show their stuff — many in athletics, but others in downright quirky displays, including one young boy who achieved fame by kicking 50 coconuts from on top of the heads of a row of people. It seems Pakistan has become a world record-creating machine, with the coordinated effort reaping an impressive 23 world records, event organizers boasted. The push for inclusion of Pakistanis in the venerable Guinness World Records entries (which began in book form in 1955) stems in part from festival organizers’ desire to boost the image of a country often associated with militancy, religious strife and economic decline. There is a patriotic element, as well: Last October, for instance, 42,813 Pakistanis got together in a Lahore hockey stadium to belt out the national anthem and create yet another world record for the most people singing their country’s anthem. Days later, another 24,200 people held green and white boxes — the colors of the national flag of Pakistan — to set the world record for creating the largest human flag. Although some of the records might seem amusing to others — coconut kicking champ Mohammad Rashid of Karachi last week claimed his fourth world record by breaking 34 pine boards in 32 seconds with his head — the competitions were no laughing matter to participants. Usman Anwar, director of the Punjab Youth Festival, explained that the kids have been training for eight months. “We started at the neighborhood and village level so that children could come out and participate,” said Anwar. “Our main objective was to inculcate interest for sports in the public.” Young people from over 55,000 neighborhood and village councils vied for a chance to compete in the games. “We were able to select the best of the best to train for the world records,” said Anwar. Because of terrorism, political upheaval and widespread unemployment, many young people appear to have little hope for the future, says Hafeez Rehman, a professor in the anthropology department at Quaid-i-Azam University in the capital, Islamabad. Sports competitions, Rehman said, create an opportunity for youth to excel personally and also to improve Pakistan’s image. “We have energetic youth. Pakistan has more than 55 million young people. It becomes an asset for the country,” he added. The festival itself has become part of the record-setting mania. It was recognized for having more participants — 3.3 million, most of whom registered online, according to Anwar — constituting a world record for sporting events.', 'keyword': 'india;pakistan;guinness'}
-----------
```
### Keyphrase Extraction
```python
from datasets import load_dataset
# get the dataset only for keyphrase extraction
dataset = load_dataset("midas/kptimes", "extraction")
print("Samples for Keyphrase Extraction")
# sample from the train split
print("Sample from training data split")
train_sample = dataset["train"][0]
print("Fields in the sample: ", [key for key in train_sample.keys()])
print("Tokenized Document: ", train_sample["document"])
print("Document BIO Tags: ", train_sample["doc_bio_tags"])
print("\n-----------\n")
# sample from the validation split
print("Sample from validation data split")
validation_sample = dataset["validation"][0]
print("Fields in the sample: ", [key for key in validation_sample.keys()])
print("Tokenized Document: ", validation_sample["document"])
print("Document BIO Tags: ", validation_sample["doc_bio_tags"])
print("\n-----------\n")
# sample from the test split
print("Sample from test data split")
test_sample = dataset["test"][0]
print("Fields in the sample: ", [key for key in test_sample.keys()])
print("Tokenized Document: ", test_sample["document"])
print("Document BIO Tags: ", test_sample["doc_bio_tags"])
print("\n-----------\n")
```
### Keyphrase Generation
```python
# get the dataset only for keyphrase generation
dataset = load_dataset("midas/kptimes", "generation")
print("Samples for Keyphrase Generation")
# sample from the train split
print("Sample from training data split")
train_sample = dataset["train"][0]
print("Fields in the sample: ", [key for key in train_sample.keys()])
print("Tokenized Document: ", train_sample["document"])
print("Extractive/present Keyphrases: ", train_sample["extractive_keyphrases"])
print("Abstractive/absent Keyphrases: ", train_sample["abstractive_keyphrases"])
print("\n-----------\n")
# sample from the validation split
print("Sample from validation data split")
validation_sample = dataset["validation"][0]
print("Fields in the sample: ", [key for key in validation_sample.keys()])
print("Tokenized Document: ", validation_sample["document"])
print("Extractive/present Keyphrases: ", validation_sample["extractive_keyphrases"])
print("Abstractive/absent Keyphrases: ", validation_sample["abstractive_keyphrases"])
print("\n-----------\n")
# sample from the test split
print("Sample from test data split")
test_sample = dataset["test"][0]
print("Fields in the sample: ", [key for key in test_sample.keys()])
print("Tokenized Document: ", test_sample["document"])
print("Extractive/present Keyphrases: ", test_sample["extractive_keyphrases"])
print("Abstractive/absent Keyphrases: ", test_sample["abstractive_keyphrases"])
print("\n-----------\n")
```
## Citation Information
```
@inproceedings{gallina2019kptimes,
title={KPTimes: A Large-Scale Dataset for Keyphrase Generation on News Documents},
author={Gallina, Ygor and Boudin, Florian and Daille, B{\'e}atrice},
booktitle={Proceedings of the 12th International Conference on Natural Language Generation},
pages={130--135},
year={2019}
}
```
## Contributions
Thanks to [@debanjanbhucs](https://github.com/debanjanbhucs), [@dibyaaaaax](https://github.com/dibyaaaaax), [@UmaGunturi](https://github.com/UmaGunturi) and [@ad6398](https://github.com/ad6398) for adding this dataset
| midas/kptimes | [
"region:us"
] | 2022-03-02T23:29:22+00:00 | {} | 2022-02-06T06:21:58+00:00 | [] | [] | TAGS
#region-us
| A dataset for benchmarking keyphrase extraction and generation techniques from news. For more details about the dataset please refer the original paper - URL
Original source of the data - URL
Dataset Summary
---------------

KPTimes is a large scale dataset comprising of 279,923 news articles from NY Times and 10K from JPTimes. It is one of the datasets which has annotations of keyphrases curated by the editors who can be considered as experts. The motivation of the authors behind producing this dataset was to have a large dataset for training neural models for keyphrase generation in a domain other than the scientific domain, and to understand the differences between keyphrases annotated by experts and non-experts. The authors show that the editors tend to assign generic keyphrases that are not present in the actual news article's text, with 55% of them being abstractive keyphrases. The keyphrases in the news domain as presented in this work were also on an average shorter (1.4 words) than those in the scientific datasets (2.4 words).
The dataset is randomly divided into train (92.8%), validation (3.6%) and test (3.6%) splits. In order to enable the models trained on this dataset to generalize well the authors did not want to have the entire data taken from a single source (NY Times), and therefore added 10K more articles from JPTimes dataset. The authors collected free to read article URLs from NY Times spanning from 2006 to 2017, and obtained their corresponding HTML pages from the Internet Archive. They cleaned the HTML tags and extracted the title, and the main content of the articles using heuristics. The gold keyphrases were obtained from the metadata fields - *news\_keywords* and *keywords*. The documents in the dataset are full-length news articles, which also makes it a suitable dataset for developing models for identifying keyphrases from long documents.

Dataset Structure
-----------------
Dataset Statistics
------------------
Table 1: Statistics on the length of the abstractive keyphrases for Train, Test, and Validation splits of Inspec dataset.
Table 2: Statistics on the length of the extractive keyphrases for Train, Test, and Validation splits of Inspec dataset.
Table 3: General statistics of the Inspec dataset.
### Data Fields
* id: unique identifier of the document.
* document: Whitespace separated list of words in the document.
* doc\_bio\_tags: BIO tags for each word in the document. B stands for the beginning of a keyphrase and I stands for inside the keyphrase. O stands for outside the keyphrase and represents the word that isn't a part of the keyphrase at all.
* extractive\_keyphrases: List of all the present keyphrases.
* abstractive\_keyphrase: List of all the absent keyphrases.
* other metadata: Additional information present in the original dataset.
+ id : unique identifier for the document
+ date : publishing date (YYYY/MM/DD)
+ categories : categories of the article (1 or 2 categories)
+ title : title of the document
+ abstract : content of the article
+ keyword : list of keywords
### Data Splits
Usage
-----
### Full Dataset
Output
### Keyphrase Extraction
### Keyphrase Generation
Contributions
-------------
Thanks to @debanjanbhucs, @dibyaaaaax, @UmaGunturi and @ad6398 for adding this dataset
| [
"### Data Fields\n\n\n* id: unique identifier of the document.\n* document: Whitespace separated list of words in the document.\n* doc\\_bio\\_tags: BIO tags for each word in the document. B stands for the beginning of a keyphrase and I stands for inside the keyphrase. O stands for outside the keyphrase and represents the word that isn't a part of the keyphrase at all.\n* extractive\\_keyphrases: List of all the present keyphrases.\n* abstractive\\_keyphrase: List of all the absent keyphrases.\n* other metadata: Additional information present in the original dataset.\n\t+ id : unique identifier for the document\n\t+ date : publishing date (YYYY/MM/DD)\n\t+ categories : categories of the article (1 or 2 categories)\n\t+ title : title of the document\n\t+ abstract : content of the article\n\t+ keyword : list of keywords",
"### Data Splits\n\n\n\nUsage\n-----",
"### Full Dataset\n\n\nOutput",
"### Keyphrase Extraction",
"### Keyphrase Generation\n\n\nContributions\n-------------\n\n\nThanks to @debanjanbhucs, @dibyaaaaax, @UmaGunturi and @ad6398 for adding this dataset"
] | [
"TAGS\n#region-us \n",
"### Data Fields\n\n\n* id: unique identifier of the document.\n* document: Whitespace separated list of words in the document.\n* doc\\_bio\\_tags: BIO tags for each word in the document. B stands for the beginning of a keyphrase and I stands for inside the keyphrase. O stands for outside the keyphrase and represents the word that isn't a part of the keyphrase at all.\n* extractive\\_keyphrases: List of all the present keyphrases.\n* abstractive\\_keyphrase: List of all the absent keyphrases.\n* other metadata: Additional information present in the original dataset.\n\t+ id : unique identifier for the document\n\t+ date : publishing date (YYYY/MM/DD)\n\t+ categories : categories of the article (1 or 2 categories)\n\t+ title : title of the document\n\t+ abstract : content of the article\n\t+ keyword : list of keywords",
"### Data Splits\n\n\n\nUsage\n-----",
"### Full Dataset\n\n\nOutput",
"### Keyphrase Extraction",
"### Keyphrase Generation\n\n\nContributions\n-------------\n\n\nThanks to @debanjanbhucs, @dibyaaaaax, @UmaGunturi and @ad6398 for adding this dataset"
] |
6657c24632b70981f244b7f3d0abfa1d07b62dbe | ## Dataset Summary
A dataset for benchmarking keyphrase extraction and generation techniques from long document english scientific papers. For more details about the dataset please refer the original paper - [https://www.semanticscholar.org/paper/Large-Dataset-for-Keyphrases-Extraction-Krapivin-Autaeu/2c56421ff3c2a69894d28b09a656b7157df8eb83](https://www.semanticscholar.org/paper/Large-Dataset-for-Keyphrases-Extraction-Krapivin-Autaeu/2c56421ff3c2a69894d28b09a656b7157df8eb83)
Original source of the data - []()
## Dataset Structure
### Data Fields
- **id**: unique identifier of the document.
- **document**: Whitespace separated list of words in the document.
- **doc_bio_tags**: BIO tags for each word in the document. B stands for the beginning of a keyphrase and I stands for inside the keyphrase. O stands for outside the keyphrase and represents the word that isn't a part of the keyphrase at all.
- **extractive_keyphrases**: List of all the present keyphrases.
- **abstractive_keyphrase**: List of all the absent keyphrases.
### Data Splits
|Split| #datapoints |
|--|--|
| Test | 2305 |
## Usage
### Full Dataset
```python
from datasets import load_dataset
# get entire dataset
dataset = load_dataset("midas/krapivin", "raw")
# sample from the test split
print("Sample from test dataset split")
test_sample = dataset["test"][0]
print("Fields in the sample: ", [key for key in test_sample.keys()])
print("Tokenized Document: ", test_sample["document"])
print("Document BIO Tags: ", test_sample["doc_bio_tags"])
print("Extractive/present Keyphrases: ", test_sample["extractive_keyphrases"])
print("Abstractive/absent Keyphrases: ", test_sample["abstractive_keyphrases"])
print("\n-----------\n")
```
**Output**
```bash
```
### Keyphrase Extraction
```python
from datasets import load_dataset
# get the dataset only for keyphrase extraction
dataset = load_dataset("midas/krapivin", "extraction")
print("Samples for Keyphrase Extraction")
# sample from the test split
print("Sample from test data split")
test_sample = dataset["test"][0]
print("Fields in the sample: ", [key for key in test_sample.keys()])
print("Tokenized Document: ", test_sample["document"])
print("Document BIO Tags: ", test_sample["doc_bio_tags"])
print("\n-----------\n")
```
### Keyphrase Generation
```python
# get the dataset only for keyphrase generation
dataset = load_dataset("midas/krapivin", "generation")
print("Samples for Keyphrase Generation")
# sample from the test split
print("Sample from test data split")
test_sample = dataset["test"][0]
print("Fields in the sample: ", [key for key in test_sample.keys()])
print("Tokenized Document: ", test_sample["document"])
print("Extractive/present Keyphrases: ", test_sample["extractive_keyphrases"])
print("Abstractive/absent Keyphrases: ", test_sample["abstractive_keyphrases"])
print("\n-----------\n")
```
## Citation Information
```
@inproceedings{Krapivin2009LargeDF,
title={Large Dataset for Keyphrases Extraction},
author={Mikalai Krapivin and Aliaksandr Autaeu and Maurizio Marchese},
year={2009}
}
```
## Contributions
Thanks to [@debanjanbhucs](https://github.com/debanjanbhucs), [@dibyaaaaax](https://github.com/dibyaaaaax) and [@ad6398](https://github.com/ad6398) for adding this dataset
| midas/krapivin | [
"region:us"
] | 2022-03-02T23:29:22+00:00 | {} | 2022-01-10T06:52:51+00:00 | [] | [] | TAGS
#region-us
| Dataset Summary
---------------
A dataset for benchmarking keyphrase extraction and generation techniques from long document english scientific papers. For more details about the dataset please refer the original paper - URL
Original source of the data -
Dataset Structure
-----------------
### Data Fields
* id: unique identifier of the document.
* document: Whitespace separated list of words in the document.
* doc\_bio\_tags: BIO tags for each word in the document. B stands for the beginning of a keyphrase and I stands for inside the keyphrase. O stands for outside the keyphrase and represents the word that isn't a part of the keyphrase at all.
* extractive\_keyphrases: List of all the present keyphrases.
* abstractive\_keyphrase: List of all the absent keyphrases.
### Data Splits
Usage
-----
### Full Dataset
Output
### Keyphrase Extraction
### Keyphrase Generation
Contributions
-------------
Thanks to @debanjanbhucs, @dibyaaaaax and @ad6398 for adding this dataset
| [
"### Data Fields\n\n\n* id: unique identifier of the document.\n* document: Whitespace separated list of words in the document.\n* doc\\_bio\\_tags: BIO tags for each word in the document. B stands for the beginning of a keyphrase and I stands for inside the keyphrase. O stands for outside the keyphrase and represents the word that isn't a part of the keyphrase at all.\n* extractive\\_keyphrases: List of all the present keyphrases.\n* abstractive\\_keyphrase: List of all the absent keyphrases.",
"### Data Splits\n\n\n\nUsage\n-----",
"### Full Dataset\n\n\nOutput",
"### Keyphrase Extraction",
"### Keyphrase Generation\n\n\nContributions\n-------------\n\n\nThanks to @debanjanbhucs, @dibyaaaaax and @ad6398 for adding this dataset"
] | [
"TAGS\n#region-us \n",
"### Data Fields\n\n\n* id: unique identifier of the document.\n* document: Whitespace separated list of words in the document.\n* doc\\_bio\\_tags: BIO tags for each word in the document. B stands for the beginning of a keyphrase and I stands for inside the keyphrase. O stands for outside the keyphrase and represents the word that isn't a part of the keyphrase at all.\n* extractive\\_keyphrases: List of all the present keyphrases.\n* abstractive\\_keyphrase: List of all the absent keyphrases.",
"### Data Splits\n\n\n\nUsage\n-----",
"### Full Dataset\n\n\nOutput",
"### Keyphrase Extraction",
"### Keyphrase Generation\n\n\nContributions\n-------------\n\n\nThanks to @debanjanbhucs, @dibyaaaaax and @ad6398 for adding this dataset"
] |
5e73606ea32a9456e235015e11241a5dfd5da7d6 | A dataset for benchmarking keyphrase extraction and generation techniques from long document English scientific papers. For more details about the dataset please refer the original paper - []().
Data source - []()
## Dataset Summary
## Dataset Structure
### Data Fields
- **id**: unique identifier of the document.
- **sections**: list of all the sections present in the document.
- **sec_text**: list of white space separated list of words present in each section.
- **sec_bio_tags**: list of BIO tags of white space separated list of words present in each section.
- **extractive_keyphrases**: List of all the present keyphrases.
- **abstractive_keyphrase**: List of all the absent keyphrases.
### Data Splits
|Split| #datapoints |
|--|--|
| Train-Small | 20,000 |
| Train-Medium | 50,000 |
| Train-Large | 1,296,613 |
| Test | 10,000 |
| Validation | 10,000 |
## Usage
### Small Dataset
```python
from datasets import load_dataset
# get small dataset
dataset = load_dataset("midas/ldkp10k", "small")
def order_sections(sample):
"""
corrects the order in which different sections appear in the document.
resulting order is: title, abstract, other sections in the body
"""
sections = []
sec_text = []
sec_bio_tags = []
if "title" in sample["sections"]:
title_idx = sample["sections"].index("title")
sections.append(sample["sections"].pop(title_idx))
sec_text.append(sample["sec_text"].pop(title_idx))
sec_bio_tags.append(sample["sec_bio_tags"].pop(title_idx))
if "abstract" in sample["sections"]:
abstract_idx = sample["sections"].index("abstract")
sections.append(sample["sections"].pop(abstract_idx))
sec_text.append(sample["sec_text"].pop(abstract_idx))
sec_bio_tags.append(sample["sec_bio_tags"].pop(abstract_idx))
sections += sample["sections"]
sec_text += sample["sec_text"]
sec_bio_tags += sample["sec_bio_tags"]
return sections, sec_text, sec_bio_tags
# sample from the train split
print("Sample from train data split")
train_sample = dataset["train"][0]
sections, sec_text, sec_bio_tags = order_sections(train_sample)
print("Fields in the sample: ", [key for key in train_sample.keys()])
print("Section names: ", sections)
print("Tokenized Document: ", sec_text)
print("Document BIO Tags: ", sec_bio_tags)
print("Extractive/present Keyphrases: ", train_sample["extractive_keyphrases"])
print("Abstractive/absent Keyphrases: ", train_sample["abstractive_keyphrases"])
print("\n-----------\n")
# sample from the validation split
print("Sample from validation data split")
validation_sample = dataset["validation"][0]
sections, sec_text, sec_bio_tags = order_sections(validation_sample)
print("Fields in the sample: ", [key for key in validation_sample.keys()])
print("Section names: ", sections)
print("Tokenized Document: ", sec_text)
print("Document BIO Tags: ", sec_bio_tags)
print("Extractive/present Keyphrases: ", validation_sample["extractive_keyphrases"])
print("Abstractive/absent Keyphrases: ", validation_sample["abstractive_keyphrases"])
print("\n-----------\n")
# sample from the test split
print("Sample from test data split")
test_sample = dataset["test"][0]
sections, sec_text, sec_bio_tags = order_sections(test_sample)
print("Fields in the sample: ", [key for key in test_sample.keys()])
print("Section names: ", sections)
print("Tokenized Document: ", sec_text)
print("Document BIO Tags: ", sec_bio_tags)
print("Extractive/present Keyphrases: ", test_sample["extractive_keyphrases"])
print("Abstractive/absent Keyphrases: ", test_sample["abstractive_keyphrases"])
print("\n-----------\n")
```
**Output**
```bash
```
### Medium Dataset
```python
from datasets import load_dataset
# get medium dataset
dataset = load_dataset("midas/ldkp10k", "medium")
```
### Large Dataset
```python
from datasets import load_dataset
# get large dataset
dataset = load_dataset("midas/ldkp10k", "large")
```
## Citation Information
Please cite the works below if you use this dataset in your work.
```
@article{mahata2022ldkp,
title={LDKP: A Dataset for Identifying Keyphrases from Long Scientific Documents},
author={Mahata, Debanjan and Agarwal, Naveen and Gautam, Dibya and Kumar, Amardeep and Parekh, Swapnil and Singla, Yaman Kumar and Acharya, Anish and Shah, Rajiv Ratn},
journal={arXiv preprint arXiv:2203.15349},
year={2022}
}
```
```
@article{lo2019s2orc,
title={S2ORC: The semantic scholar open research corpus},
author={Lo, Kyle and Wang, Lucy Lu and Neumann, Mark and Kinney, Rodney and Weld, Dan S},
journal={arXiv preprint arXiv:1911.02782},
year={2019}
}
```
```
@inproceedings{ccano2019keyphrase,
title={Keyphrase generation: A multi-aspect survey},
author={{\c{C}}ano, Erion and Bojar, Ond{\v{r}}ej},
booktitle={2019 25th Conference of Open Innovations Association (FRUCT)},
pages={85--94},
year={2019},
organization={IEEE}
}
```
```
@article{meng2017deep,
title={Deep keyphrase generation},
author={Meng, Rui and Zhao, Sanqiang and Han, Shuguang and He, Daqing and Brusilovsky, Peter and Chi, Yu},
journal={arXiv preprint arXiv:1704.06879},
year={2017}
}
```
## Contributions
Thanks to [@debanjanbhucs](https://github.com/debanjanbhucs), [@dibyaaaaax](https://github.com/dibyaaaaax), [@UmaGunturi](https://github.com/UmaGunturi) and [@ad6398](https://github.com/ad6398) for adding this dataset
| midas/ldkp10k | [
"region:us"
] | 2022-03-02T23:29:22+00:00 | {} | 2022-04-02T15:49:45+00:00 | [] | [] | TAGS
#region-us
| A dataset for benchmarking keyphrase extraction and generation techniques from long document English scientific papers. For more details about the dataset please refer the original paper - .
Data source -
Dataset Summary
---------------
Dataset Structure
-----------------
### Data Fields
* id: unique identifier of the document.
* sections: list of all the sections present in the document.
* sec\_text: list of white space separated list of words present in each section.
* sec\_bio\_tags: list of BIO tags of white space separated list of words present in each section.
* extractive\_keyphrases: List of all the present keyphrases.
* abstractive\_keyphrase: List of all the absent keyphrases.
### Data Splits
Usage
-----
### Small Dataset
Output
### Medium Dataset
### Large Dataset
Please cite the works below if you use this dataset in your work.
Contributions
-------------
Thanks to @debanjanbhucs, @dibyaaaaax, @UmaGunturi and @ad6398 for adding this dataset
| [
"### Data Fields\n\n\n* id: unique identifier of the document.\n* sections: list of all the sections present in the document.\n* sec\\_text: list of white space separated list of words present in each section.\n* sec\\_bio\\_tags: list of BIO tags of white space separated list of words present in each section.\n* extractive\\_keyphrases: List of all the present keyphrases.\n* abstractive\\_keyphrase: List of all the absent keyphrases.",
"### Data Splits\n\n\n\nUsage\n-----",
"### Small Dataset\n\n\nOutput",
"### Medium Dataset",
"### Large Dataset\n\n\nPlease cite the works below if you use this dataset in your work.\n\n\nContributions\n-------------\n\n\nThanks to @debanjanbhucs, @dibyaaaaax, @UmaGunturi and @ad6398 for adding this dataset"
] | [
"TAGS\n#region-us \n",
"### Data Fields\n\n\n* id: unique identifier of the document.\n* sections: list of all the sections present in the document.\n* sec\\_text: list of white space separated list of words present in each section.\n* sec\\_bio\\_tags: list of BIO tags of white space separated list of words present in each section.\n* extractive\\_keyphrases: List of all the present keyphrases.\n* abstractive\\_keyphrase: List of all the absent keyphrases.",
"### Data Splits\n\n\n\nUsage\n-----",
"### Small Dataset\n\n\nOutput",
"### Medium Dataset",
"### Large Dataset\n\n\nPlease cite the works below if you use this dataset in your work.\n\n\nContributions\n-------------\n\n\nThanks to @debanjanbhucs, @dibyaaaaax, @UmaGunturi and @ad6398 for adding this dataset"
] |
6f86fdd8ffcf1635ee7d47c65cf25ac7efd51f75 | A dataset for benchmarking keyphrase extraction and generation techniques from long document English scientific papers. For more details about the dataset please refer the original paper - []().
Data source - []()
## Dataset Summary
## Dataset Structure
### Data Fields
- **id**: unique identifier of the document.
- **sections**: list of all the sections present in the document.
- **sec_text**: list of white space separated list of words present in each section.
- **sec_bio_tags**: list of BIO tags of white space separated list of words present in each section.
- **extractive_keyphrases**: List of all the present keyphrases.
- **abstractive_keyphrase**: List of all the absent keyphrases.
### Data Splits
|Split| #datapoints |
|--|--|
| Train-Small | 20,000 |
| Train-Medium | 50,000 |
| Train-Large | 90,019 |
| Test | 3413 |
| Validation | 3339 |
## Usage
### Small Dataset
```python
from datasets import load_dataset
# get small dataset
dataset = load_dataset("midas/ldkp3k", "small")
def order_sections(sample):
"""
corrects the order in which different sections appear in the document.
resulting order is: title, abstract, other sections in the body
"""
sections = []
sec_text = []
sec_bio_tags = []
if "title" in sample["sections"]:
title_idx = sample["sections"].index("title")
sections.append(sample["sections"].pop(title_idx))
sec_text.append(sample["sec_text"].pop(title_idx))
sec_bio_tags.append(sample["sec_bio_tags"].pop(title_idx))
if "abstract" in sample["sections"]:
abstract_idx = sample["sections"].index("abstract")
sections.append(sample["sections"].pop(abstract_idx))
sec_text.append(sample["sec_text"].pop(abstract_idx))
sec_bio_tags.append(sample["sec_bio_tags"].pop(abstract_idx))
sections += sample["sections"]
sec_text += sample["sec_text"]
sec_bio_tags += sample["sec_bio_tags"]
return sections, sec_text, sec_bio_tags
# sample from the train split
print("Sample from train data split")
train_sample = dataset["train"][0]
sections, sec_text, sec_bio_tags = order_sections(train_sample)
print("Fields in the sample: ", [key for key in train_sample.keys()])
print("Section names: ", sections)
print("Tokenized Document: ", sec_text)
print("Document BIO Tags: ", sec_bio_tags)
print("Extractive/present Keyphrases: ", train_sample["extractive_keyphrases"])
print("Abstractive/absent Keyphrases: ", train_sample["abstractive_keyphrases"])
print("\n-----------\n")
# sample from the validation split
print("Sample from validation data split")
validation_sample = dataset["validation"][0]
sections, sec_text, sec_bio_tags = order_sections(validation_sample)
print("Fields in the sample: ", [key for key in validation_sample.keys()])
print("Section names: ", sections)
print("Tokenized Document: ", sec_text)
print("Document BIO Tags: ", sec_bio_tags)
print("Extractive/present Keyphrases: ", validation_sample["extractive_keyphrases"])
print("Abstractive/absent Keyphrases: ", validation_sample["abstractive_keyphrases"])
print("\n-----------\n")
# sample from the test split
print("Sample from test data split")
test_sample = dataset["test"][0]
sections, sec_text, sec_bio_tags = order_sections(test_sample)
print("Fields in the sample: ", [key for key in test_sample.keys()])
print("Section names: ", sections)
print("Tokenized Document: ", sec_text)
print("Document BIO Tags: ", sec_bio_tags)
print("Extractive/present Keyphrases: ", test_sample["extractive_keyphrases"])
print("Abstractive/absent Keyphrases: ", test_sample["abstractive_keyphrases"])
print("\n-----------\n")
```
**Output**
```bash
```
### Medium Dataset
```python
from datasets import load_dataset
# get medium dataset
dataset = load_dataset("midas/ldkp3k", "medium")
```
### Large Dataset
```python
from datasets import load_dataset
# get large dataset
dataset = load_dataset("midas/ldkp3k", "large")
```
## Citation Information
Please cite the works below if you use this dataset in your work.
```
@article{dl4srmahata2022ldkp,
title={LDKP - A Dataset for Identifying Keyphrases from Long Scientific Documents},
author={Mahata, Debanjan and Agarwal, Naveen and Gautam, Dibya and Kumar, Amardeep and Parekh, Swapnil and Singla, Yaman Kumar and Acharya, Anish and Shah, Rajiv Ratn},
journal={DL4SR-22: Workshop on Deep Learning for Search and Recommendation, co-located with the 31st ACM International Conference on Information and Knowledge Management (CIKM)},
address={Atlanta, USA},
month={October},
year={2022}
}
```
```
@article{mahata2022ldkp,
title={LDKP: A Dataset for Identifying Keyphrases from Long Scientific Documents},
author={Mahata, Debanjan and Agarwal, Naveen and Gautam, Dibya and Kumar, Amardeep and Parekh, Swapnil and Singla, Yaman Kumar and Acharya, Anish and Shah, Rajiv Ratn},
journal={arXiv preprint arXiv:2203.15349},
year={2022}
}
```
```
@article{lo2019s2orc,
title={S2ORC: The semantic scholar open research corpus},
author={Lo, Kyle and Wang, Lucy Lu and Neumann, Mark and Kinney, Rodney and Weld, Dan S},
journal={arXiv preprint arXiv:1911.02782},
year={2019}
}
```
```
@inproceedings{ccano2019keyphrase,
title={Keyphrase generation: A multi-aspect survey},
author={{\c{C}}ano, Erion and Bojar, Ond{\v{r}}ej},
booktitle={2019 25th Conference of Open Innovations Association (FRUCT)},
pages={85--94},
year={2019},
organization={IEEE}
}
```
```
@article{meng2017deep,
title={Deep keyphrase generation},
author={Meng, Rui and Zhao, Sanqiang and Han, Shuguang and He, Daqing and Brusilovsky, Peter and Chi, Yu},
journal={arXiv preprint arXiv:1704.06879},
year={2017}
}
```
## Contributions
Thanks to [@debanjanbhucs](https://github.com/debanjanbhucs), [@dibyaaaaax](https://github.com/dibyaaaaax), [@UmaGunturi](https://github.com/UmaGunturi) and [@ad6398](https://github.com/ad6398) for adding this dataset
| midas/ldkp3k | [
"region:us"
] | 2022-03-02T23:29:22+00:00 | {} | 2022-09-27T17:29:25+00:00 | [] | [] | TAGS
#region-us
| A dataset for benchmarking keyphrase extraction and generation techniques from long document English scientific papers. For more details about the dataset please refer the original paper - .
Data source -
Dataset Summary
---------------
Dataset Structure
-----------------
### Data Fields
* id: unique identifier of the document.
* sections: list of all the sections present in the document.
* sec\_text: list of white space separated list of words present in each section.
* sec\_bio\_tags: list of BIO tags of white space separated list of words present in each section.
* extractive\_keyphrases: List of all the present keyphrases.
* abstractive\_keyphrase: List of all the absent keyphrases.
### Data Splits
Usage
-----
### Small Dataset
Output
### Medium Dataset
### Large Dataset
Please cite the works below if you use this dataset in your work.
Contributions
-------------
Thanks to @debanjanbhucs, @dibyaaaaax, @UmaGunturi and @ad6398 for adding this dataset
| [
"### Data Fields\n\n\n* id: unique identifier of the document.\n* sections: list of all the sections present in the document.\n* sec\\_text: list of white space separated list of words present in each section.\n* sec\\_bio\\_tags: list of BIO tags of white space separated list of words present in each section.\n* extractive\\_keyphrases: List of all the present keyphrases.\n* abstractive\\_keyphrase: List of all the absent keyphrases.",
"### Data Splits\n\n\n\nUsage\n-----",
"### Small Dataset\n\n\nOutput",
"### Medium Dataset",
"### Large Dataset\n\n\nPlease cite the works below if you use this dataset in your work.\n\n\nContributions\n-------------\n\n\nThanks to @debanjanbhucs, @dibyaaaaax, @UmaGunturi and @ad6398 for adding this dataset"
] | [
"TAGS\n#region-us \n",
"### Data Fields\n\n\n* id: unique identifier of the document.\n* sections: list of all the sections present in the document.\n* sec\\_text: list of white space separated list of words present in each section.\n* sec\\_bio\\_tags: list of BIO tags of white space separated list of words present in each section.\n* extractive\\_keyphrases: List of all the present keyphrases.\n* abstractive\\_keyphrase: List of all the absent keyphrases.",
"### Data Splits\n\n\n\nUsage\n-----",
"### Small Dataset\n\n\nOutput",
"### Medium Dataset",
"### Large Dataset\n\n\nPlease cite the works below if you use this dataset in your work.\n\n\nContributions\n-------------\n\n\nThanks to @debanjanbhucs, @dibyaaaaax, @UmaGunturi and @ad6398 for adding this dataset"
] |
690ccba1cd830e0343e7eaec3a0c22bfd88dd949 | ## Dataset Summary
A dataset for benchmarking keyphrase extraction and generation techniques from long document english scientific papers. For more details about the dataset please refer the original paper - [https://www.comp.nus.edu.sg/~kanmy/papers/icadl2007.pdf](https://www.comp.nus.edu.sg/~kanmy/papers/icadl2007.pdf)
Original source of the data - []()
## Dataset Structure
### Data Fields
- **id**: unique identifier of the document.
- **document**: Whitespace separated list of words in the document.
- **doc_bio_tags**: BIO tags for each word in the document. B stands for the beginning of a keyphrase and I stands for inside the keyphrase. O stands for outside the keyphrase and represents the word that isn't a part of the keyphrase at all.
- **extractive_keyphrases**: List of all the present keyphrases.
- **abstractive_keyphrase**: List of all the absent keyphrases.
### Data Splits
|Split| #datapoints |
|--|--|
| Test | 211 |
- Percentage of keyphrases that are named entities: 67.95% (named entities detected using scispacy - en-core-sci-lg model)
- Percentage of keyphrases that are noun phrases: 82.16% (noun phrases detected using spacy en-core-web-lg after removing determiners)
## Usage
### Full Dataset
```python
from datasets import load_dataset
# get entire dataset
dataset = load_dataset("midas/nus", "raw")
# sample from the test split
print("Sample from test dataset split")
test_sample = dataset["test"][0]
print("Fields in the sample: ", [key for key in test_sample.keys()])
print("Tokenized Document: ", test_sample["document"])
print("Document BIO Tags: ", test_sample["doc_bio_tags"])
print("Extractive/present Keyphrases: ", test_sample["extractive_keyphrases"])
print("Abstractive/absent Keyphrases: ", test_sample["abstractive_keyphrases"])
print("\n-----------\n")
```
**Output**
```bash
Sample from test data split
Fields in the sample: ['id', 'document', 'doc_bio_tags', 'extractive_keyphrases', 'abstractive_keyphrases', 'other_metadata']
Tokenized Document: ['Learning', 'Spatially', 'Variant', 'Dissimilarity', '-LRB-', 'Svad', '-RRB-', 'Measures', 'Clustering', 'algorithms', 'typically', 'operate', 'on', 'a', 'feature', 'vector', 'representation', 'of', 'the', 'data', 'and', 'find', 'clusters', 'that', 'are', 'compact', 'with', 'respect', 'to', 'an', 'assumed', '-LRB-', 'dis', '-RRB-', 'similarity', 'measure', 'between', 'the', 'data', 'points', 'in', 'feature', 'space', '.', 'This', 'makes', 'the', 'type', 'of', 'clusters', 'identified', 'highly', 'dependent', 'on', 'the', 'assumed', 'similarity', 'measure', '.', 'Building', 'on', 'recent', 'work', 'in', 'this', 'area', ',', 'we', 'formally', 'define', 'a', 'class', 'of', 'spatially', 'varying', 'dissimilarity', 'measures', 'and', 'propose', 'algorithms', 'to', 'learn', 'the', 'dissimilarity', 'measure', 'automatically', 'from', 'the', 'data', '.', 'The', 'idea', 'is', 'to', 'identify', 'clusters', 'that', 'are', 'compact', 'with', 'respect', 'to', 'the', 'unknown', 'spatially', 'varying', 'dissimilarity', 'measure', '.', 'Our', 'experiments', 'show', 'that', 'the', 'proposed', 'algorithms', 'are', 'more', 'stable', 'and', 'achieve', 'better', 'accuracy', 'on', 'various', 'textual', 'data', 'sets', 'when', 'compared', 'with', 'similar', 'algorithms', 'proposed', 'in', 'the', 'literature', '.', 'H.', '2.8', '-LSB-', 'Database', 'Management', '-RSB-', ':', 'Database', 'Applications-Data', 'Mining', 'Algorithms', 'Clustering', 'plays', 'a', 'major', 'role', 'in', 'data', 'mining', 'as', 'a', 'tool', 'to', 'discover', 'structure', 'in', 'data', '.', 'Object', 'clustering', 'algorithms', 'operate', 'on', 'a', 'feature', 'vector', 'representation', 'of', 'the', 'data', 'and', 'find', 'clusters', 'that', 'are', 'compact', 'with', 'respect', 'to', 'an', 'assumed', '-LRB-', 'dis', '-RRB-', 'similarity', 'measure', 'between', 'the', 'data', 'points', 'in', 'feature', 'space', '.', 'As', 'a', 'consequence', ',', 'the', 'nature', 'of', 'clusters', 'identified', 'by', 'a', 'clustering', 'algorithm', 'is', 'highly', 'dependent', 'on', 'the', 'assumed', 'similarity', 'measure', '.', 'The', 'most', 'commonly', 'used', 'dissimilarity', 'measure', ',', 'namely', 'the', 'Euclidean', 'metric', ',', 'assumes', 'that', 'the', 'dissimilarity', 'measure', 'is', 'isotropic', 'and', 'spatially', 'invariant', ',', 'and', 'Permission', 'to', 'make', 'digital', 'or', 'hard', 'copies', 'of', 'all', 'or', 'part', 'of', 'this', 'work', 'for', 'personal', 'or', 'classroom', 'use', 'is', 'granted', 'without', 'fee', 'provided', 'that', 'copies', 'are', 'not', 'made', 'or', 'distributed', 'for', 'profit', 'or', 'commercial', 'advantage', 'and', 'that', 'copies', 'bear', 'this', 'notice', 'and', 'the', 'full', 'citation', 'on', 'the', 'first', 'page', '.', 'To', 'copy', 'otherwise', ',', 'to', 'republish', ',', 'to', 'post', 'on', 'servers', 'or', 'to', 'redistribute', 'to', 'lists', ',', 'requires', 'prior', 'specific', 'permission', 'and/or', 'a', 'fee', '.', 'KDD', "'", '04', ',', 'August', '22', '25', ',', '2004', ',', 'Seattle', ',', 'Washington', ',', 'USA', '.', 'Copyright', '2004', 'ACM', '1-58113-888-1', '/', '04/0008', '...', '$', '5.00', '.', 'it', 'is', 'effective', 'only', 'when', 'the', 'clusters', 'are', 'roughly', 'spherical', 'and', 'all', 'of', 'them', 'have', 'approximately', 'the', 'same', 'size', ',', 'which', 'is', 'rarely', 'the', 'case', 'in', 'practice', '-LSB-', '8', '-RSB-', '.', 'The', 'problem', 'of', 'finding', 'non-spherical', 'clusters', 'is', 'often', 'addressed', 'by', 'utilizing', 'a', 'feature', 'weighting', 'technique', '.', 'These', 'techniques', 'discover', 'a', 'single', 'set', 'of', 'weights', 'such', 'that', 'relevant', 'features', 'are', 'given', 'more', 'importance', 'than', 'irrelevant', 'features', '.', 'However', ',', 'in', 'practice', ',', 'each', 'cluster', 'may', 'have', 'a', 'different', 'set', 'of', 'relevant', 'features', '.', 'We', 'consider', 'Spatially', 'Varying', 'Dissimilarity', '-LRB-', 'SVaD', '-RRB-', 'measures', 'to', 'address', 'this', 'problem', '.', 'Diday', 'et', '.', 'al.', '-LSB-', '4', '-RSB-', 'proposed', 'the', 'adaptive', 'distance', 'dynamic', 'clusters', '-LRB-', 'ADDC', '-RRB-', 'algorithm', 'in', 'this', 'vain', '.', 'A', 'fuzzified', 'version', 'of', 'ADDC', ',', 'popularly', 'known', 'as', 'the', 'Gustafson-Kessel', '-LRB-', 'GK', '-RRB-', 'algorithm', '-LSB-', '7', '-RSB-', 'uses', 'a', 'dynamically', 'updated', 'covariance', 'matrix', 'so', 'that', 'each', 'cluster', 'can', 'have', 'its', 'own', 'norm', 'matrix', '.', 'These', 'algorithms', 'can', 'deal', 'with', 'hyperelliposoidal', 'clusters', 'of', 'various', 'sizes', 'and', 'orientations', '.', 'The', 'EM', 'algorithm', '-LSB-', '2', '-RSB-', 'with', 'Gaussian', 'probability', 'distributions', 'can', 'also', 'be', 'used', 'to', 'achieve', 'similar', 'results', '.', 'However', ',', 'the', 'above', 'algorithms', 'are', 'computationally', 'expensive', 'for', 'high-dimensional', 'data', 'since', 'they', 'invert', 'covariance', 'matrices', 'in', 'every', 'iteration', '.', 'Moreover', ',', 'matrix', 'inversion', 'can', 'be', 'unstable', 'when', 'the', 'data', 'is', 'sparse', 'in', 'relation', 'to', 'the', 'dimensionality', '.', 'One', 'possible', 'solution', 'to', 'the', 'problems', 'of', 'high', 'computation', 'and', 'instability', 'arising', 'out', 'of', 'using', 'covariance', 'matrices', 'is', 'to', 'force', 'the', 'matrices', 'to', 'be', 'diagonal', ',', 'which', 'amounts', 'to', 'weighting', 'each', 'feature', 'differently', 'in', 'different', 'clusters', '.', 'While', 'this', 'restricts', 'the', 'dissimilarity', 'measures', 'to', 'have', 'axis', 'parallel', 'isometry', ',', 'the', 'weights', 'also', 'provide', 'a', 'simple', 'interpretation', 'of', 'the', 'clusters', 'in', 'terms', 'of', 'relevant', 'features', ',', 'which', 'is', 'important', 'in', 'knowledge', 'discovery', '.', 'Examples', 'of', 'such', 'algorithms', 'are', 'SCAD', 'and', 'Fuzzy-SKWIC', '-LSB-', '5', ',', '6', '-RSB-', ',', 'which', 'perform', 'fuzzy', 'clustering', 'of', 'data', 'while', 'simultaneously', 'finding', 'feature', 'weights', 'in', 'individual', 'clusters', '.', 'In', 'this', 'paper', ',', 'we', 'generalize', 'the', 'idea', 'of', 'the', 'feature', 'weighting', 'approach', 'to', 'define', 'a', 'class', 'of', 'spatially', 'varying', 'dissimilarity', 'measures', 'and', 'propose', 'algorithms', 'that', 'learn', 'the', 'dissimilarity', 'measure', 'automatically', 'from', 'the', 'given', 'data', 'while', 'performing', 'the', 'clustering', '.', 'The', 'idea', 'is', 'to', 'identify', 'clusters', 'inherent', 'in', 'the', 'data', 'that', 'are', 'compact', 'with', 'respect', 'to', 'the', 'unknown', 'spatially', 'varying', 'dissimilarity', 'measure', '.', 'We', 'compare', 'the', 'proposed', 'algorithms', 'with', 'a', 'diagonal', 'version', 'of', 'GK', '-LRB-', 'DGK', '-RRB-', 'and', 'a', 'crisp', 'version', 'of', 'SCAD', '-LRB-', 'CSCAD', '-RRB-', 'on', 'a', 'variety', 'of', 'data', 'sets', '.', 'Our', 'algorithms', 'perform', 'better', 'than', 'DGK', 'and', 'CSCAD', ',', 'and', 'use', 'more', 'stable', 'update', 'equations', 'for', 'weights', 'than', 'CSCAD', '.', 'The', 'rest', 'of', 'the', 'paper', 'is', 'organized', 'as', 'follows', '.', 'In', 'the', 'next', 'section', ',', 'we', 'define', 'a', 'general', 'class', 'of', 'dissimilarity', 'measures', '611', 'Research', 'Track', 'Poster', 'and', 'formulate', 'two', 'objective', 'functions', 'based', 'on', 'them', '.', 'In', 'Section', '3', ',', 'we', 'derive', 'learning', 'algorithms', 'that', 'optimize', 'the', 'objective', 'functions', '.', 'We', 'present', 'an', 'experimental', 'study', 'of', 'the', 'proposed', 'algorithms', 'in', 'Section', '4', '.', 'We', 'compare', 'the', 'performance', 'of', 'the', 'proposed', 'algorithms', 'with', 'that', 'of', 'DGK', 'and', 'CSCAD', '.', 'These', 'two', 'algorithms', 'are', 'explained', 'in', 'Appendix', 'A.', 'Finally', ',', 'we', 'summarize', 'our', 'contributions', 'and', 'conclude', 'with', 'some', 'future', 'directions', 'in', 'Section', '5', '.', 'We', 'first', 'define', 'a', 'general', 'class', 'of', 'dissimilarity', 'measures', 'and', 'formulate', 'a', 'few', 'objective', 'functions', 'in', 'terms', 'of', 'the', 'given', 'data', 'set', '.', 'Optimization', 'of', 'the', 'objective', 'functions', 'would', 'result', 'in', 'learning', 'the', 'underlying', 'dissimilarity', 'measure', '.', '2.1', 'SVaD', 'Measures', 'In', 'the', 'following', 'definition', ',', 'we', 'generalize', 'the', 'concept', 'of', 'dissimilarity', 'measures', 'in', 'which', 'the', 'weights', 'associated', 'with', 'features', 'change', 'over', 'feature', 'space', '.', 'Definition', '2.1', 'We', 'define', 'the', 'measure', 'of', 'dissimilarity', 'of', 'x', 'from', 'y', '1', 'to', 'be', 'a', 'weighted', 'sum', 'of', 'M', 'dissimilarity', 'measures', 'between', 'x', 'and', 'y', 'where', 'the', 'values', 'of', 'the', 'weights', 'depend', 'on', 'the', 'region', 'from', 'which', 'the', 'dissimilarity', 'is', 'being', 'measured', '.', 'Let', 'P', '=', '-LCB-', 'R', '1', ',', '...', ',', 'R', 'K', '-RCB-', 'be', 'a', 'collection', 'of', 'K', 'regions', 'that', 'partition', 'the', 'feature', 'space', ',', 'and', 'w', '1', ',', 'w', '2', ',', '...', ',', 'and', 'w', 'K', 'be', 'the', 'weights', 'associated', 'with', 'R', '1', ',', 'R', '2', ',', '...', ',', 'and', 'R', 'K', ',', 'respectively', '.', 'Let', 'g', '1', ',', 'g', '2', ',', '...', ',', 'and', 'g', 'M', 'be', 'M', 'dissimilarity', 'measures', '.', 'Then', ',', 'each', 'w', 'j', ',', 'j', '=', '1', ',', '...', ',', 'K', ',', 'is', 'an', 'M', '-', 'dimensional', 'vector', 'where', 'its', 'l-th', 'component', ',', 'w', 'jl', 'is', 'associated', 'with', 'g', 'l', '.', 'Let', 'W', 'denote', 'the', 'K-tuple', '-LRB-', 'w', '1', ',', '...', ',', 'w', 'K', '-RRB-', 'and', 'let', 'r', 'be', 'a', 'real', 'number', '.', 'Then', ',', 'the', 'dissimilarity', 'of', 'x', 'from', 'y', 'is', 'given', 'by', ':', 'f', 'W', '-LRB-', 'x', ',', 'y', '-RRB-', '=', 'M', 'l', '=', '1', 'w', 'r', 'jl', 'g', 'l', '-LRB-', 'x', ',', 'y', '-RRB-', ',', 'if', 'y', 'R', 'j', '.', '-LRB-', '1', '-RRB-', 'We', 'refer', 'to', 'f', 'W', 'as', 'a', 'Spatially', 'Variant', 'Dissimilarity', '-LRB-', 'SVaD', '-RRB-', 'measure', '.', 'Note', 'that', 'f', 'W', 'need', 'not', 'be', 'symmetric', 'even', 'if', 'g', 'i', 'are', 'symmetric', '.', 'Hence', ',', 'f', 'W', 'is', 'not', 'a', 'metric', '.', 'Moreover', ',', 'the', 'behavior', 'of', 'f', 'W', 'depends', 'on', 'the', 'behavior', 'of', 'g', 'i', '.', 'There', 'are', 'many', 'ways', 'to', 'define', 'g', 'i', '.', 'We', 'list', 'two', 'instances', 'of', 'f', 'W', '.', 'Example', '2.1', '-LRB-', 'Minkowski', '-RRB-', 'Let', 'd', 'be', 'the', 'feature', 'space', 'and', 'M', '=', 'd.', 'Let', 'a', 'point', 'x', 'd', 'be', 'represented', 'as', '-LRB-', 'x', '1', ',', '...', ',', 'x', 'd', '-RRB-', '.', 'Then', ',', 'when', 'g', 'i', '-LRB-', 'x', ',', 'y', '-RRB-', '=', '|', 'x', 'i', '-', 'y', 'i', '|', 'p', 'for', 'i', '=', '1', ',', '...', ',', 'd', ',', 'and', 'p', '1', ',', 'the', 'resulting', 'SVaD', 'measure', ',', 'f', 'M', 'W', 'is', 'called', 'Minkowski', 'SVaD', '-LRB-', 'MSVaD', '-RRB-', 'measure', '.', 'That', 'is', ',', 'f', 'M', 'W', '-LRB-', 'x', ',', 'y', '-RRB-', '=', 'd', 'l', '=', '1', 'w', 'r', 'jl', '|', 'x', 'l', '-', 'y', 'l', '|', 'p', ',', 'if', 'y', 'R', 'j', '.', '-LRB-', '2', '-RRB-', 'One', 'may', 'note', 'that', 'when', 'w', '1', '=', '=', 'w', 'K', 'and', 'p', '=', '2', ',', 'f', 'M', 'W', 'is', 'the', 'weighted', 'Euclidean', 'distance', '.', 'When', 'p', '=', '2', ',', 'we', 'call', 'f', 'M', 'W', 'a', 'Euclidean', 'SVaD', '-LRB-', 'ESVaD', '-RRB-', 'measure', 'and', 'denote', 'it', 'by', 'f', 'E', 'W', '.', '1', 'We', 'use', 'the', 'phrase', '``', 'dissimilarity', 'of', 'x', 'from', 'y', "''", 'rather', 'than', '``', 'dissimilarity', 'between', 'x', 'and', 'y', "''", 'because', 'we', 'consider', 'a', 'general', 'situation', 'where', 'the', 'dissimilarity', 'measure', 'depends', 'on', 'the', 'location', 'of', 'y', '.', 'As', 'an', 'example', 'of', 'this', 'situation', 'in', 'text', 'mining', ',', 'when', 'the', 'dissimilarity', 'is', 'measured', 'from', 'a', 'document', 'on', '`', 'terrorism', "'", 'to', 'a', 'document', 'x', ',', 'a', 'particular', 'set', 'of', 'keywords', 'may', 'be', 'weighted', 'heavily', 'whereas', 'when', 'the', 'dissimilarity', 'is', 'measured', 'from', 'a', 'document', 'on', '`', 'football', "'", 'to', 'x', ',', 'a', 'different', 'set', 'of', 'keywords', 'may', 'be', 'weighted', 'heavily', '.', 'Example', '2.2', '-LRB-', 'Cosine', '-RRB-', 'Let', 'the', 'feature', 'space', 'be', 'the', 'set', 'of', 'points', 'with', 'l', '2', 'norm', 'equal', 'to', 'one', '.', 'That', 'is', ',', 'x', '2', '=', '1', 'for', 'all', 'points', 'x', 'in', 'feature', 'space', '.', 'Then', ',', 'when', 'g', 'l', '-LRB-', 'x', ',', 'y', '-RRB-', '=', '-LRB-', '1/d', '-', 'x', 'l', 'y', 'l', '-RRB-', 'for', 'l', '=', '1', ',', '...', ',', 'd', ',', 'the', 'resulting', 'SVaD', 'measure', 'f', 'C', 'W', 'is', 'called', 'a', 'Cosine', 'SVaD', '-LRB-', 'CSVaD', '-RRB-', 'measure', ':', 'f', 'C', 'W', '-LRB-', 'x', ',', 'y', '-RRB-', '=', 'd', 'i', '=', '1', 'w', 'r', 'jl', '-LRB-', '1/d', '-', 'x', 'l', 'y', 'l', '-RRB-', ',', 'if', 'y', 'R', 'j', '.', '-LRB-', '3', '-RRB-', 'In', 'the', 'formulation', 'of', 'the', 'objective', 'function', 'below', ',', 'we', 'use', 'a', 'set', 'of', 'parameters', 'to', 'represent', 'the', 'regions', 'R', '1', ',', 'R', '2', ',', '...', ',', 'and', 'R', 'K', '.', 'Let', 'c', '1', ',', 'c', '2', ',', '...', ',', 'and', 'c', 'K', 'be', 'K', 'points', 'in', 'feature', 'space', '.', 'Then', 'y', 'R', 'j', 'iff', 'f', 'W', '-LRB-', 'y', ',', 'c', 'j', '-RRB-', '<', 'f', 'W', '-LRB-', 'y', ',', 'c', 'i', '-RRB-', 'for', 'i', '=', 'j.', '-LRB-', '4', '-RRB-', 'In', 'the', 'case', 'of', 'ties', ',', 'y', 'is', 'assigned', 'to', 'the', 'region', 'with', 'the', 'lowest', 'index', '.', 'Thus', ',', 'the', 'K-tuple', 'of', 'points', 'C', '=', '-LRB-', 'c', '1', ',', 'c', '2', ',', '...', ',', 'c', 'K', '-RRB-', 'defines', 'a', 'partition', 'in', 'feature', 'space', '.', 'The', 'partition', 'induced', 'by', 'the', 'points', 'in', 'C', 'is', 'similar', 'in', 'nature', 'to', 'a', 'Voronoi', 'tessellation', '.', 'We', 'use', 'the', 'notation', 'f', 'W', ',', 'C', 'whenever', 'we', 'use', 'the', 'set', 'C', 'to', 'parameterize', 'the', 'regions', 'used', 'in', 'the', 'dissimilarity', 'measure', '.', '2.2', 'Objective', 'Function', 'for', 'Clustering', 'The', 'goal', 'of', 'the', 'present', 'work', 'is', 'to', 'identify', 'the', 'spatially', 'varying', 'dissimilarity', 'measure', 'and', 'the', 'associated', 'compact', 'clusters', 'simultaneously', '.', 'It', 'is', 'worth', 'mentioning', 'here', 'that', ',', 'as', 'in', 'the', 'case', 'of', 'any', 'clustering', 'algorithm', ',', 'the', 'underlying', 'assumption', 'in', 'this', 'paper', 'is', 'the', 'existence', 'of', 'such', 'a', 'dissimilarity', 'measure', 'and', 'clusters', 'for', 'a', 'given', 'data', 'set', '.', 'Let', 'x', '1', ',', 'x', '2', ',', '...', ',', 'and', 'x', 'n', 'be', 'n', 'given', 'data', 'points', '.', 'Let', 'K', 'be', 'a', 'given', 'positive', 'integer', '.', 'Assuming', 'that', 'C', 'represents', 'the', 'cluster', 'centers', ',', 'let', 'us', 'assign', 'each', 'data', 'point', 'x', 'i', 'to', 'a', 'cluster', 'R', 'j', 'with', 'the', 'closest', 'c', 'j', 'as', 'the', 'cluster', 'center', '2', ',', 'i.e.', ',', 'j', '=', 'arg', 'min', 'l', 'f', 'W', ',', 'C', '-LRB-', 'x', 'i', ',', 'c', 'l', '-RRB-', '.', '-LRB-', '5', '-RRB-', 'Then', ',', 'the', 'within-cluster', 'dissimilarity', 'is', 'given', 'by', 'J', '-LRB-', 'W', ',', 'C', '-RRB-', '=', 'K', 'j', '=', '1', 'x', 'i', 'R', 'j', 'M', 'l', '=', '1', 'w', 'r', 'jl', 'g', 'l', '-LRB-', 'x', 'i', ',', 'c', 'j', '-RRB-', '.', '-LRB-', '6', '-RRB-', 'J', '-LRB-', 'W', ',', 'C', '-RRB-', 'represents', 'the', 'sum', 'of', 'the', 'dissimilarity', 'measures', 'of', 'all', 'the', 'data', 'points', 'from', 'their', 'closest', 'centroids', '.', 'The', 'objective', 'is', 'to', 'find', 'W', 'and', 'C', 'that', 'minimize', 'J', '-LRB-', 'W', ',', 'C', '-RRB-', '.', 'To', 'avoid', 'the', 'trivial', 'solution', 'to', 'J', '-LRB-', 'W', ',', 'C', '-RRB-', ',', 'we', 'consider', 'a', 'normalization', 'condition', 'on', 'w', 'j', ',', 'viz.', ',', 'M', 'l', '=', '1', 'w', 'jl', '=', '1', '.', '-LRB-', '7', '-RRB-', 'Note', 'that', 'even', 'with', 'this', 'condition', ',', 'J', '-LRB-', 'W', ',', 'C', '-RRB-', 'has', 'a', 'trivial', 'solution', ':', 'w', 'jp', '=', '1', 'where', 'p', '=', 'arg', 'min', 'l', 'x', 'i', 'R', 'j', 'g', 'l', '-LRB-', 'x', 'i', ',', 'c', 'j', '-RRB-', ',', 'and', 'the', 'remaining', 'weights', 'are', 'zero', '.', 'One', 'way', 'to', 'avoid', 'convergence', 'of', 'w', 'j', 'to', 'unit', 'vectors', 'is', 'to', 'impose', 'a', 'regularization', 'condition', 'on', 'w', 'j', '.', 'We', 'consider', 'the', 'following', 'two', 'regularization', 'measures', 'in', 'this', 'paper', ':', '-LRB-', '1', '-RRB-', 'Entropy', 'measure', ':', 'M', 'l', '=', '1', 'w', 'jl', 'log', '-LRB-', 'w', 'jl', '-RRB-', 'and', '-LRB-', '2', '-RRB-', 'Gini', 'measure', ':', 'M', 'l', '=', '1', 'w', '2', 'jl', '.', '2', 'We', 'use', 'P', '=', '-LCB-', 'R', '1', ',', 'R', '2', ',', '...', ',', 'R', 'K', '-RCB-', 'to', 'represent', 'the', 'corresponding', 'partition', 'of', 'the', 'data', 'set', 'as', 'well', '.', 'The', 'intended', 'interpretation', '-LRB-', 'cluster', 'or', 'region', '-RRB-', 'would', 'be', 'evident', 'from', 'the', 'context', '.', '612', 'Research', 'Track', 'Poster', 'The', 'problem', 'of', 'determining', 'the', 'optimal', 'W', 'and', 'C', 'is', 'similar', 'to', 'the', 'traditional', 'clustering', 'problem', 'that', 'is', 'solved', 'by', 'the', 'K-Means', 'Algorithm', '-LRB-', 'KMA', '-RRB-', 'except', 'for', 'the', 'additional', 'W', 'matrix', '.', 'We', 'propose', 'a', 'class', 'of', 'iterative', 'algorithms', 'similar', 'to', 'KMA', '.', 'These', 'algorithms', 'start', 'with', 'a', 'random', 'partition', 'of', 'the', 'data', 'set', 'and', 'iteratively', 'update', 'C', ',', 'W', 'and', 'P', 'so', 'that', 'J', '-LRB-', 'W', ',', 'C', '-RRB-', 'is', 'minimized', '.', 'These', 'iterative', 'algorithms', 'are', 'instances', 'of', 'Alternating', 'Optimization', '-LRB-', 'AO', '-RRB-', 'algorithms', '.', 'In', '-LSB-', '1', '-RSB-', ',', 'it', 'is', 'shown', 'that', 'AO', 'algorithms', 'converge', 'to', 'a', 'local', 'optimum', 'under', 'some', 'conditions', '.', 'We', 'outline', 'the', 'algorithm', 'below', 'before', 'actually', 'describing', 'how', 'to', 'update', 'C', ',', 'W', 'and', 'P', 'in', 'every', 'iteration', '.', 'Randomly', 'assign', 'the', 'data', 'points', 'to', 'K', 'clusters', '.', 'REPEAT', 'Update', 'C', ':', 'Compute', 'the', 'centroid', 'of', 'each', 'cluster', 'c', 'j', '.', 'Update', 'W', ':', 'Compute', 'the', 'w', 'jl', 'j', ',', 'l.', 'Update', 'P', ':', 'Reassign', 'the', 'data', 'points', 'to', 'the', 'clusters', '.', 'UNTIL', '-LRB-', 'termination', 'condition', 'is', 'reached', '-RRB-', '.', 'In', 'the', 'above', 'algorithm', ',', 'the', 'update', 'of', 'C', 'depends', 'on', 'the', 'definition', 'of', 'g', 'i', ',', 'and', 'the', 'update', 'of', 'W', 'on', 'the', 'regularization', 'terms', '.', 'The', 'update', 'of', 'P', 'is', 'done', 'by', 'reassigning', 'the', 'data', 'points', 'according', 'to', '-LRB-', '5', '-RRB-', '.', 'Before', 'explaining', 'the', 'computation', 'of', 'C', 'in', 'every', 'iteration', 'for', 'various', 'g', 'i', ',', 'we', 'first', 'derive', 'update', 'equations', 'for', 'W', 'for', 'various', 'regularization', 'measures', '.', '3.1', 'Update', 'of', 'Weights', 'While', 'updating', 'weights', ',', 'we', 'need', 'to', 'find', 'the', 'values', 'of', 'weights', 'that', 'minimize', 'the', 'objective', 'function', 'for', 'a', 'given', 'C', 'and', 'P', '.', 'As', 'mentioned', 'above', ',', 'we', 'consider', 'the', 'two', 'regularization', 'measures', 'for', 'w', 'jl', 'and', 'derive', 'update', 'equations', '.', 'If', 'we', 'consider', 'the', 'entropy', 'regularization', 'with', 'r', '=', '1', ',', 'the', 'objective', 'function', 'becomes', ':', 'J', 'EN', 'T', '-LRB-', 'W', ',', 'C', '-RRB-', '=', 'K', 'j', '=', '1', 'x', 'i', 'R', 'j', 'M', 'l', '=', '1', 'w', 'jl', 'g', 'l', '-LRB-', 'x', 'i', ',', 'c', 'j', '-RRB-', '+', 'K', 'j', '=', '1', 'j', 'M', 'l', '=', '1', 'w', 'jl', 'log', '-LRB-', 'w', 'jl', '-RRB-', '+', 'K', 'j', '=', '1', 'j', 'M', 'l', '=', '1', 'w', 'jl', '-', '1', '.', '-LRB-', '8', '-RRB-', 'Note', 'that', 'j', 'are', 'the', 'Lagrange', 'multipliers', 'corresponding', 'to', 'the', 'normalization', 'constraints', 'in', '-LRB-', '7', '-RRB-', ',', 'and', 'j', 'represent', 'the', 'relative', 'importance', 'given', 'to', 'the', 'regularization', 'term', 'relative', 'to', 'the', 'within-cluster', 'dissimilarity', '.', 'Differentiating', 'J', 'EN', 'T', '-LRB-', 'W', ',', 'C', '-RRB-', 'with', 'respect', 'to', 'w', 'jl', 'and', 'equating', 'it', 'to', 'zero', ',', 'we', 'obtain', 'w', 'jl', '=', 'exp', '-', '-LRB-', 'j', '+', 'x', 'i', 'Rj', 'g', 'l', '-LRB-', 'x', 'i', ',', 'c', 'j', '-RRB-', '-RRB-', 'j', '-', '1', '.', 'Solving', 'for', 'j', 'by', 'substituting', 'the', 'above', 'value', 'of', 'w', 'jl', 'in', '-LRB-', '7', '-RRB-', 'and', 'substituting', 'the', 'value', 'of', 'j', 'back', 'in', 'the', 'above', 'equation', ',', 'we', 'obtain', 'w', 'jl', '=', 'exp', 'x', 'i', 'R', 'j', 'g', 'l', '-LRB-', 'x', 'i', ',', 'c', 'j', '-RRB-', '/', 'j', 'M', 'n', '=', '1', 'exp', 'x', 'i', 'R', 'j', 'g', 'n', '-LRB-', 'x', 'i', ',', 'c', 'j', '-RRB-', '/', 'j', '.', '-LRB-', '9', '-RRB-', 'If', 'we', 'consider', 'the', 'Gini', 'measure', 'for', 'regularization', 'with', 'r', '=', '2', ',', 'the', 'corresponding', 'w', 'jl', 'that', 'minimizes', 'the', 'objective', 'function', 'can', 'be', 'shown', 'to', 'be', 'w', 'jl', '=', '1', '/', '-LRB-', 'j', '+', 'x', 'i', 'R', 'j', 'g', 'l', '-LRB-', 'x', 'i', ',', 'c', 'j', '-RRB-', '-RRB-', 'M', 'n', '=', '1', '-LRB-', '1', '/', '-LRB-', 'j', '+', 'x', 'i', 'R', 'j', 'g', 'n', '-LRB-', 'x', 'i', ',', 'c', 'j', '-RRB-', '-RRB-', '-RRB-', '.', '-LRB-', '10', '-RRB-', 'In', 'both', 'cases', ',', 'the', 'updated', 'value', 'of', 'w', 'jl', 'is', 'inversely', 'related', 'Algorithm', 'Update', 'Equations', 'Acronyms', 'P', 'C', 'W', 'EEnt', '-LRB-', '5', '-RRB-', '-LRB-', '11', '-RRB-', '-LRB-', '9', '-RRB-', 'EsGini', '-LRB-', '5', '-RRB-', '-LRB-', '11', '-RRB-', '-LRB-', '10', '-RRB-', 'CEnt', '-LRB-', '5', '-RRB-', '-LRB-', '12', '-RRB-', '-LRB-', '9', '-RRB-', 'CsGini', '-LRB-', '5', '-RRB-', '-LRB-', '12', '-RRB-', '-LRB-', '10', '-RRB-', 'Table', '1', ':', 'Summary', 'of', 'algorithms', '.', 'to', 'x', 'i', 'R', 'j', 'g', 'l', '-LRB-', 'x', 'i', ',', 'c', 'j', '-RRB-', '.', 'This', 'has', 'various', 'interpretations', 'based', 'on', 'the', 'nature', 'of', 'g', 'l', '.', 'For', 'example', ',', 'when', 'we', 'consider', 'the', 'ESVaD', 'measure', ',', 'w', 'jl', 'is', 'inversely', 'related', 'to', 'the', 'variance', 'of', 'l-th', 'element', 'of', 'the', 'data', 'vectors', 'in', 'the', 'j-th', 'cluster', '.', 'In', 'other', 'words', ',', 'when', 'the', 'variance', 'along', 'a', 'particular', 'dimension', 'is', 'high', 'in', 'a', 'cluster', ',', 'then', 'the', 'dimension', 'is', 'less', 'important', 'to', 'the', 'cluster', '.', 'This', 'popular', 'heuristic', 'has', 'been', 'used', 'in', 'various', 'contexts', '-LRB-', 'such', 'as', 'relevance', 'feedback', '-RRB-', 'in', 'the', 'literature', '-LSB-', '9', '-RSB-', '.', 'Similarly', ',', 'when', 'we', 'consider', 'the', 'CSVaD', 'measure', ',', 'w', 'jl', 'is', 'directly', 'proportional', 'to', 'the', 'correlation', 'of', 'the', 'j-th', 'dimension', 'in', 'the', 'l-th', 'cluster', '.', '3.2', 'Update', 'of', 'Centroids', 'Learning', 'ESVaD', 'Measures', ':', 'Substituting', 'the', 'ESVaD', 'measure', 'in', 'the', 'objective', 'function', 'and', 'solving', 'the', 'first', 'order', 'necessary', 'conditions', ',', 'we', 'observe', 'that', 'c', 'jl', '=', '1', '|', 'R', 'j', '|', 'x', 'i', 'R', 'j', 'x', 'il', '-LRB-', '11', '-RRB-', 'minimizes', 'J', 'ESV', 'AD', '-LRB-', 'W', ',', 'C', '-RRB-', '.', 'Learning', 'CSVaD', 'Measures', ':', 'Let', 'x', 'il', '=', 'w', 'jl', 'x', 'il', ',', 'then', 'using', 'the', 'Cauchy-Swartz', 'inequality', ',', 'it', 'can', 'be', 'shown', 'that', 'c', 'jl', '=', '1', '|', 'R', 'j', '|', 'x', 'i', 'R', 'j', 'x', 'il', '-LRB-', '12', '-RRB-', 'maximizes', 'x', 'i', 'R', 'j', 'd', 'l', '=', '1', 'w', 'jl', 'x', 'il', 'c', 'jl', '.', 'Hence', ',', '-LRB-', '12', '-RRB-', 'also', 'minimizes', 'the', 'objective', 'function', 'when', 'CSVaD', 'is', 'used', 'as', 'the', 'dissimilarity', 'measure', '.', 'Table', '1', 'summarizes', 'the', 'update', 'equations', 'used', 'in', 'various', 'algorithms', '.', 'We', 'refer', 'to', 'this', 'set', 'of', 'algorithms', 'as', 'SVaD', 'learning', 'algorithms', '.', 'In', 'this', 'section', ',', 'we', 'present', 'an', 'experimental', 'study', 'of', 'the', 'algorithms', 'described', 'in', 'the', 'previous', 'sections', '.', 'We', 'applied', 'the', 'proposed', 'algorithms', 'on', 'various', 'text', 'data', 'sets', 'and', 'compared', 'the', 'performance', 'of', 'EEnt', 'and', 'EsGini', 'with', 'that', 'of', 'K-Means', ',', 'CSCAD', 'and', 'DGK', 'algorithms', '.', 'The', 'reason', 'for', 'choosing', 'the', 'K-Means', 'algorithm', '-LRB-', 'KMA', '-RRB-', 'apart', 'from', 'CSCAD', 'and', 'DGK', 'is', 'that', 'it', 'provides', 'a', 'baseline', 'for', 'assessing', 'the', 'advantages', 'of', 'feature', 'weighting', '.', 'KMA', 'is', 'also', 'a', 'popular', 'algorithm', 'for', 'text', 'clustering', '.', 'We', 'have', 'included', 'a', 'brief', 'description', 'of', 'CSCAD', 'and', 'DGK', 'algorithms', 'in', 'Appendix', 'A.', 'Text', 'data', 'sets', 'are', 'sparse', 'and', 'high', 'dimensional', '.', 'We', 'consider', 'standard', 'labeled', 'document', 'collections', 'and', 'test', 'the', 'proposed', 'algorithms', 'for', 'their', 'ability', 'to', 'discover', 'dissimilarity', 'measures', 'that', 'distinguish', 'one', 'class', 'from', 'another', 'without', 'actually', 'considering', 'the', 'class', 'labels', 'of', 'the', 'documents', '.', 'We', 'measure', 'the', 'success', 'of', 'the', 'algorithms', 'by', 'the', 'purity', 'of', 'the', 'regions', 'that', 'they', 'discover', '.', '613', 'Research', 'Track', 'Poster', '4.1', 'Data', 'Sets', 'We', 'performed', 'our', 'experiments', 'on', 'three', 'standard', 'data', 'sets', ':', '20', 'News', 'Group', ',', 'Yahoo', 'K1', ',', 'and', 'Classic', '3', '.', 'These', 'data', 'sets', 'are', 'described', 'below', '.', '20', 'News', 'Group', '3', ':', 'We', 'considered', 'different', 'subsets', 'of', '20', 'News', 'Group', 'data', 'that', 'are', 'known', 'to', 'contain', 'clusters', 'of', 'varying', 'degrees', 'of', 'separation', '-LSB-', '10', '-RSB-', '.', 'As', 'in', '-LSB-', '10', '-RSB-', ',', 'we', 'considered', 'three', 'random', 'samples', 'of', 'three', 'subsets', 'of', 'the', '20', 'News', 'Group', 'data', '.', 'The', 'subsets', 'denoted', 'by', 'Binary', 'has', '250', 'documents', 'each', 'from', 'talk.politics.mideast', 'and', 'talk.politics.misc', '.', 'Multi5', 'has', '100', 'documents', 'each', 'from', 'comp.graphics', ',', 'rec.motorcycles', ',', 'rec.sport.baseball', ',', 'sci.space', ',', 'and', 'talk.politics.mideast', '.', 'Finally', ',', 'Multi10', 'has', '50', 'documents', 'each', 'from', 'alt.atheism', ',', 'comp', '.', 'sys.mac.hardware', ',', 'misc.forsale', ',', 'rec.autos', ',', 'rec.sport.hockey', ',', 'sci.crypt', ',', 'sci.electronics', ',', 'sci.med', ',', 'sci.space', ',', 'and', 'talk.politics', '.', 'gun', '.', 'It', 'may', 'be', 'noted', 'that', 'Binary', 'data', 'sets', 'have', 'two', 'highly', 'overlapping', 'classes', '.', 'Each', 'of', 'Multi5', 'data', 'sets', 'has', 'samples', 'from', '5', 'distinct', 'classes', ',', 'whereas', 'Multi10', 'data', 'sets', 'have', 'only', 'a', 'few', 'samples', 'from', '10', 'different', 'classes', '.', 'The', 'size', 'of', 'the', 'vocabulary', 'used', 'to', 'represent', 'the', 'documents', 'in', 'Binary', 'data', 'set', 'is', 'about', '4000', ',', 'Multi5', 'about', '3200', 'and', 'Multi10', 'about', '2800', '.', 'We', 'observed', 'that', 'the', 'relative', 'performance', 'of', 'the', 'algorithms', 'on', 'various', 'samples', 'of', 'Binary', ',', 'Multi5', 'and', 'Multi10', 'data', 'sets', 'was', 'similar', '.', 'Hence', ',', 'we', 'report', 'results', 'on', 'only', 'one', 'of', 'them', '.', 'Yahoo', 'K1', '4', ':', 'This', 'data', 'set', 'contains', '2340', 'Reuters', 'news', 'articles', 'downloaded', 'from', 'Yahoo', 'in', '1997', '.', 'There', 'are', '494', 'from', 'Health', ',', '1389', 'from', 'Entertainment', ',', '141', 'from', 'Sports', ',', '114', 'from', 'Politics', ',', '60', 'from', 'Technology', 'and', '142', 'from', 'Business', '.', 'After', 'preprocessing', ',', 'the', 'documents', 'from', 'this', 'data', 'set', 'are', 'represented', 'using', '12015', 'words', '.', 'Note', 'that', 'this', 'data', 'set', 'has', 'samples', 'from', '6', 'different', 'classes', '.', 'Here', ',', 'the', 'distribution', 'of', 'data', 'points', 'across', 'the', 'class', 'is', 'uneven', ',', 'ranging', 'from', '60', 'to', '1389', '.', 'Classic', '3', '5', ':', 'Classic', '3', 'data', 'set', 'contains', '1400', 'aerospace', 'systems', 'abstracts', 'from', 'the', 'Cranfield', 'collection', ',', '1033', 'medical', 'abstracts', 'from', 'the', 'Medline', 'collection', 'and', '1460', 'information', 'retrieval', 'abstracts', 'from', 'the', 'Cisi', 'collection', ',', 'making', 'up', '3893', 'documents', 'in', 'all', '.', 'After', 'preprocessing', ',', 'this', 'data', 'set', 'has', '4301', 'words', '.', 'The', 'points', 'are', 'almost', 'equally', 'distributed', 'among', 'the', 'three', 'distinct', 'classes', '.', 'The', 'data', 'sets', 'were', 'preprocessed', 'using', 'two', 'major', 'steps', '.', 'First', ',', 'a', 'set', 'of', 'words', '-LRB-', 'vocabulary', '-RRB-', 'is', 'extracted', 'and', 'then', 'each', 'document', 'is', 'represented', 'with', 'respect', 'to', 'this', 'vocabulary', '.', 'Finding', 'the', 'vocabulary', 'includes', ':', '-LRB-', '1', '-RRB-', 'elimination', 'of', 'the', 'standard', 'list', 'of', 'stop', 'words', 'from', 'the', 'documents', ',', '-LRB-', '2', '-RRB-', 'application', 'of', 'Porter', 'stemming', '6', 'for', 'term', 'normalization', ',', 'and', '-LRB-', '3', '-RRB-', 'keeping', 'only', 'the', 'words', 'which', 'appear', 'in', 'at', 'least', '3', 'documents', '.', 'We', 'represent', 'each', 'document', 'by', 'the', 'unitized', 'frequency', 'vector', '.', '4.2', 'Evaluation', 'of', 'Algorithms', 'We', 'use', 'the', 'accuracy', 'measure', 'to', 'compare', 'the', 'performance', 'of', 'various', 'algorithms', '.', 'Let', 'a', 'ij', 'represent', 'the', 'number', 'of', 'data', 'points', 'from', 'class', 'i', 'that', 'are', 'in', 'cluster', 'j', '.', 'Then', 'the', 'accuracy', 'of', 'the', 'partition', 'is', 'given', 'by', 'j', 'max', 'i', 'a', 'ij', '/', 'n', 'where', 'n', 'is', 'the', 'total', 'number', 'of', 'data', 'points', '.', 'It', 'is', 'to', 'be', 'noted', 'that', 'points', 'coming', 'from', 'a', 'single', 'class', 'need', 'not', 'form', 'a', 'single', 'cluster', '.', 'There', 'could', 'be', 'multiple', '3', 'http://www-2.cs.cmu.edu/afs/cs.cmu.edu/project/theo-20/www/data/news20', '.', 'tar.gz', '4', 'ftp://ftp.cs.umn.edu/dept/users/boley/PDDPdata/doc-K', '5', 'ftp://ftp.cs.cornell.edu/pub/smart', '6', 'http://www.tartarus.org/~martin/PorterStemmer/', 'Iteration', '0', '1', '2', '3', '4', '5', 'J', '-LRB-', 'W', ',', 'C', '-RRB-', '334.7', '329.5', '328.3', '328.1', '327.8', 'Accuracy', '73.8', '80.2', '81.4', '81.6', '82', '82', 'Table', '2', ':', 'Evolution', 'of', 'J', '-LRB-', 'W', ',', 'C', '-RRB-', 'and', 'Accuracies', 'with', 'iterations', 'when', 'EEnt', 'applied', 'on', 'a', 'Multi5', 'data', '.', 'clusters', 'in', 'a', 'class', 'that', 'represent', 'sub-classes', '.', 'We', 'study', 'the', 'performance', 'of', 'SVaD', 'learning', 'algorithms', 'for', 'various', 'values', 'of', 'K', ',', 'i.e.', ',', 'the', 'number', 'of', 'clusters', '.', '4.3', 'Experimental', 'Setup', 'In', 'our', 'implementations', ',', 'we', 'have', 'observed', 'that', 'the', 'proposed', 'algorithms', ',', 'if', 'applied', 'on', 'randomly', 'initialized', 'centroids', ',', 'show', 'unstable', 'behavior', '.', 'One', 'reason', 'for', 'this', 'behavior', 'is', 'that', 'the', 'number', 'of', 'parameters', 'that', 'are', 'estimated', 'in', 'feature-weighting', 'clustering', 'algorithms', 'is', 'twice', 'as', 'large', 'as', 'that', 'estimated', 'by', 'the', 'traditional', 'KMA', '.', 'We', ',', 'therefore', ',', 'first', 'estimate', 'the', 'cluster', 'centers', 'giving', 'equal', 'weights', 'to', 'all', 'the', 'dimensions', 'using', 'KMA', 'and', 'then', 'fine-tune', 'the', 'cluster', 'centers', 'and', 'the', 'weights', 'using', 'the', 'feature-weighting', 'clustering', 'algorithms', '.', 'In', 'every', 'iteration', ',', 'the', 'new', 'sets', 'of', 'weights', 'are', 'updated', 'as', 'follows', '.', 'Let', 'w', 'n', '-LRB-', 't', '+1', '-RRB-', 'represent', 'the', 'weights', 'com-puted', 'using', 'one', 'of', '-LRB-', '9', '-RRB-', ',', '-LRB-', '10', '-RRB-', ',', '-LRB-', '14', '-RRB-', 'or', '-LRB-', '15', '-RRB-', 'in', 'iteration', '-LRB-', 't', '+', '1', '-RRB-', 'and', 'w', '-LRB-', 't', '-RRB-', 'the', 'weights', 'in', 'iteration', 't.', 'Then', ',', 'the', 'weights', 'in', 'iteration', '-LRB-', 't', '+', '1', '-RRB-', 'are', 'w', '-LRB-', 't', '+', '1', '-RRB-', '=', '-LRB-', '1', '-', '-LRB-', 't', '-RRB-', '-RRB-', 'w', '-LRB-', 't', '-RRB-', '+', '-LRB-', 't', '-RRB-', 'w', 'n', '-LRB-', 't', '+', '1', '-RRB-', ',', '-LRB-', '13', '-RRB-', 'where', '-LRB-', 't', '-RRB-', '-LSB-', '0', ',', '1', '-RSB-', 'decreases', 'with', 't', '.', 'That', 'is', ',', '-LRB-', 't', '-RRB-', '=', '-LRB-', 't', '1', '-RRB-', ',', 'for', 'a', 'given', 'constant', '-LSB-', '0', ',', '1', '-RSB-', '.', 'In', 'our', 'experiments', ',', 'we', 'observed', 'that', 'the', 'variance', 'of', 'purity', 'values', 'for', 'different', 'initial', 'values', 'of', '-LRB-', '0', '-RRB-', 'and', 'above', '0.5', 'is', 'very', 'small', '.', 'Hence', ',', 'we', 'report', 'the', 'results', 'for', '-LRB-', '0', '-RRB-', '=', '0.5', 'and', '=', '0.5', '.', 'We', 'set', 'the', 'value', 'of', 'j', '=', '1', '.', 'It', 'may', 'be', 'noted', 'that', 'when', 'the', 'documents', 'are', 'represented', 'as', 'unit', 'vectors', ',', 'KMA', 'with', 'the', 'cosine', 'dissimilarity', 'measure', 'and', 'Euclidean', 'distance', 'measure', 'would', 'yield', 'the', 'same', 'clusters', '.', 'This', 'is', 'essentially', 'the', 'same', 'as', 'Spherical', 'K-Means', 'algorithms', 'described', 'in', '-LSB-', '3', '-RSB-', '.', 'Therefore', ',', 'we', 'consider', 'only', 'the', 'weighted', 'Euclidean', 'measure', 'and', 'restrict', 'our', 'comparisons', 'to', 'EEnt', 'and', 'EsGini', 'in', 'the', 'experiments', '.', 'Since', 'the', 'clusters', 'obtained', 'by', 'KMA', 'are', 'used', 'to', 'initialize', 'all', 'other', 'algorithms', 'considered', 'here', ',', 'and', 'since', 'the', 'results', 'of', 'KMA', 'are', 'sensitive', 'to', 'initialization', ',', 'the', 'accuracy', 'numbers', 'reported', 'in', 'this', 'section', 'are', 'averages', 'over', '10', 'random', 'initializations', 'of', 'KMA', '.', '4.4', 'Results', 'and', 'Observations', '4.4.1', 'Effect', 'of', 'SVaD', 'Measures', 'on', 'Accuracies', 'In', 'Table', '2', ',', 'we', 'show', 'a', 'sample', 'run', 'of', 'EEnt', 'algorithm', 'on', 'one', 'of', 'the', 'Multi5', 'data', 'sets', '.', 'This', 'table', 'shows', 'the', 'evolution', 'of', 'J', '-LRB-', 'W', ',', 'C', '-RRB-', 'and', 'the', 'corresponding', 'accuracies', 'of', 'the', 'clusters', 'with', 'the', 'iterations', '.', 'The', 'accuracy', ',', 'shown', 'at', 'iteration', '0', ',', 'is', 'that', 'of', 'the', 'clusters', 'obtained', 'by', 'KMA', '.', 'The', 'purity', 'of', 'clusters', 'increases', 'with', 'decrease', 'in', 'the', 'value', 'of', 'the', 'objective', 'function', 'defined', 'using', 'SVaD', 'measures', '.', 'We', 'have', 'observed', 'a', 'similar', 'behavior', 'of', 'EEnt', 'and', 'EsGini', 'on', 'other', 'data', 'sets', 'also', '.', 'This', 'validates', 'our', 'hypothesis', 'that', 'SVaD', 'measures', 'capture', 'the', 'underlying', 'structure', 'in', 'the', 'data', 'sets', 'more', 'accurately', '.', '614', 'Research', 'Track', 'Poster', '4.4.2', 'Comparison', 'with', 'Other', 'Algorithms', 'Figure', '1', 'to', 'Figure', '5', 'show', 'average', 'accuracies', 'of', 'various', 'algorithms', 'on', 'the', '5', 'data', 'sets', 'for', 'various', 'number', 'of', 'clusters', '.', 'The', 'accuracies', 'of', 'KMA', 'and', 'DGK', 'are', 'very', 'close', 'to', 'each', 'other', 'and', 'hence', ',', 'in', 'the', 'figures', ',', 'the', 'lines', 'corresponding', 'to', 'these', 'algorithms', 'are', 'indistinguishable', '.', 'The', 'lines', 'corresponding', 'to', 'CSCAD', 'are', 'also', 'close', 'to', 'that', 'of', 'KMA', 'in', 'all', 'the', 'cases', 'except', 'Class', '3', '.', 'General', 'observations', ':', 'The', 'accuracies', 'of', 'SVaD', 'algorithms', 'follow', 'the', 'trend', 'of', 'the', 'accuracies', 'of', 'other', 'algorithms', '.', 'In', 'all', 'our', 'experiments', ',', 'both', 'SVaD', 'learning', 'algorithms', 'improve', 'the', 'accuracies', 'of', 'clusters', 'obtained', 'by', 'KMA', '.', 'It', 'is', 'observed', 'in', 'our', 'experiments', 'that', 'the', 'improvement', 'could', 'be', 'as', 'large', 'as', '8', '%', 'in', 'some', 'instances', '.', 'EEnt', 'and', 'EsGini', 'consis-tently', 'perform', 'better', 'than', 'DGK', 'on', 'all', 'data', 'sets', 'and', 'for', 'all', 'values', 'of', 'K.', 'EEnt', 'and', 'EsGini', 'perform', 'better', 'than', 'CSCAD', 'on', 'all', 'data', 'sets', 'excepts', 'in', 'the', 'case', 'of', 'Classic', '3', 'and', 'for', 'a', 'few', 'values', 'of', 'K.', 'Note', 'that', 'the', 'weight', 'update', 'equation', 'of', 'CSCAD', '-LRB-', '15', '-RRB-', 'may', 'result', 'in', 'negative', 'values', 'of', 'w', 'jl', '.', 'Our', 'experience', 'with', 'CSCAD', 'shows', 'that', 'it', 'is', 'quite', 'sensitive', 'to', 'initialization', 'and', 'it', 'may', 'have', 'convergence', 'problems', '.', 'In', 'contrast', ',', 'it', 'may', 'be', 'observed', 'that', 'w', 'jl', 'in', '-LRB-', '9', '-RRB-', 'and', '-LRB-', '10', '-RRB-', 'are', 'always', 'positive', '.', 'Moreover', ',', 'in', 'our', 'experience', ',', 'these', 'two', 'versions', 'are', 'much', 'less', 'sensitive', 'to', 'the', 'choice', 'of', 'j', '.', 'Data', 'specific', 'observations', ':', 'When', 'K', '=', '2', ',', 'EEnt', 'and', 'EsGini', 'could', 'not', 'further', 'improve', 'the', 'results', 'of', 'KMA', 'on', 'the', 'Binary', 'data', 'set', '.', 'The', 'reason', 'is', 'that', 'the', 'data', 'set', 'contains', 'two', 'highly', 'overlapping', 'classes', '.', 'However', ',', 'for', 'other', 'values', 'of', 'K', ',', 'they', 'marginally', 'improve', 'the', 'accuracies', '.', 'In', 'the', 'case', 'of', 'Multi5', ',', 'the', 'accuracies', 'of', 'the', 'algorithms', 'are', 'non-monotonic', 'with', 'K', '.', 'The', 'improvement', 'of', 'accuracies', 'is', 'large', 'for', 'intermediate', 'values', 'of', 'K', 'and', 'small', 'for', 'extreme', 'values', 'of', 'K', '.', 'When', 'K', '=', '5', ',', 'KMA', 'finds', 'relatively', 'stable', 'clusters', '.', 'Hence', ',', 'SVaD', 'algorithms', 'are', 'unable', 'to', 'improve', 'the', 'accuracies', 'as', 'much', 'as', 'they', 'did', 'for', 'intermediate', 'values', 'of', 'K.', 'For', 'larger', 'values', 'of', 'K', ',', 'the', 'clusters', 'are', 'closely', 'spaced', 'and', 'hence', 'there', 'is', 'little', 'scope', 'for', 'improvement', 'by', 'the', 'SVaD', 'algorithms', '.', 'Multi10', 'data', 'sets', 'are', 'the', 'toughest', 'to', 'cluster', 'because', 'of', 'the', 'large', 'number', 'of', 'classes', 'present', 'in', 'the', 'data', '.', 'In', 'this', 'case', ',', 'the', 'accuracies', 'of', 'the', 'algorithms', 'are', 'monotonically', 'increasing', 'with', 'the', 'number', 'of', 'clusters', '.', 'The', 'extent', 'of', 'improvement', 'of', 'accuracies', 'of', 'SVaD', 'algorithms', 'over', 'KMA', 'is', 'almost', 'constant', 'over', 'the', 'entire', 'range', 'of', 'K', '.', 'This', 'reflects', 'the', 'fact', 'that', 'the', 'documents', 'in', 'Multi10', 'data', 'set', 'are', 'uniformly', 'distributed', 'over', 'feature', 'space', '.', 'The', 'distribution', 'of', 'documents', 'in', 'Yahoo', 'K1', 'data', 'set', 'is', 'highly', 'skewed', '.', 'The', 'extent', 'of', 'improvements', 'that', 'the', 'SVaD', 'algorithms', 'could', 'achieve', 'decrease', 'with', 'K.', 'For', 'higher', 'values', 'of', 'K', ',', 'KMA', 'is', 'able', 'to', 'find', 'almost', 'pure', 'sub-clusters', ',', 'resulting', 'in', 'accuracies', 'of', 'about', '90', '%', '.', 'This', 'leaves', 'little', 'scope', 'for', 'improvement', '.', 'The', 'performance', 'of', 'CSCAD', 'differs', 'noticeably', 'in', 'the', 'case', 'of', 'Classic', '3', '.', 'It', 'performs', 'better', 'than', 'the', 'SVaD', 'algorithms', 'for', 'K', '=', '3', 'and', 'better', 'than', 'EEnt', 'for', 'K', '=', '9', '.', 'However', ',', 'for', 'larger', 'values', 'of', 'K', ',', 'the', 'SVaD', 'algorithms', 'perform', 'better', 'than', 'the', 'rest', '.', 'As', 'in', 'the', 'case', 'of', 'Multi5', ',', 'the', 'improvements', 'of', 'SVaD', 'algorithms', 'over', 'others', 'are', 'significant', 'and', 'consistent', '.', 'One', 'may', 'recall', 'that', 'Multi5', 'and', 'Classic', '3', 'consist', 'of', 'documents', 'from', 'distinct', 'classes', '.', 'Therefore', ',', 'this', 'observation', 'implies', 'that', 'when', 'there', 'are', 'distinct', 'clusters', 'in', 'the', 'data', 'set', ',', 'KMA', 'yields', 'confusing', 'clusters', 'when', 'the', 'number', 'of', 'clusters', 'is', 'over-Figure', '1', ':', 'Accuracy', 'results', 'on', 'Binary', 'data', '.', 'Figure', '2', ':', 'Accuracy', 'results', 'on', 'Multi5', 'data', '.', 'specified', '.', 'In', 'this', 'scenario', ',', 'EEnt', 'and', 'EsGini', 'can', 'fine-tune', 'the', 'clusters', 'to', 'improve', 'their', 'purity', '.', 'We', 'have', 'defined', 'a', 'general', 'class', 'of', 'spatially', 'variant', 'dissimilarity', 'measures', 'and', 'proposed', 'algorithms', 'to', 'learn', 'the', 'measure', 'underlying', 'a', 'given', 'data', 'set', 'in', 'an', 'unsupervised', 'learning', 'framework', '.', 'Through', 'our', 'experiments', 'on', 'various', 'textual', 'data', 'sets', ',', 'we', 'have', 'shown', 'that', 'such', 'a', 'formulation', 'of', 'dissimilarity', 'measure', 'can', 'more', 'accurately', 'capture', 'the', 'hidden', 'structure', 'in', 'the', 'data', 'than', 'a', 'standard', 'Euclidean', 'measure', 'that', 'does', 'not', 'vary', 'over', 'feature', 'space', '.', 'We', 'have', 'also', 'shown', 'that', 'the', 'proposed', 'learning', 'algorithms', 'perform', 'better', 'than', 'other', 'similar', 'algorithms', 'in', 'the', 'literature', ',', 'and', 'have', 'better', 'stability', 'properties', '.', 'Even', 'though', 'we', 'have', 'applied', 'these', 'algorithms', 'only', 'to', 'text', 'data', 'sets', ',', 'the', 'algorithms', 'derived', 'here', 'do', 'not', 'assume', 'any', 'specific', 'characteristics', 'of', 'textual', 'data', 'sets', '.', 'Hence', ',', 'they', 'Figure', '3', ':', 'Accuracy', 'results', 'on', 'Multi10', 'data', '.', '615', 'Research', 'Track', 'Poster', 'Figure', '4', ':', 'Accuracy', 'results', 'on', 'Yahoo', 'K1', 'data', '.', 'Figure', '5', ':', 'Accuracy', 'results', 'on', 'Classic', '3', 'data', '.', 'are', 'applicable', 'to', 'general', 'data', 'sets', '.', 'Since', 'the', 'algorithms', 'perform', 'better', 'for', 'larger', 'K', ',', 'it', 'would', 'be', 'interesting', 'to', 'investigate', 'whether', 'they', 'can', 'be', 'used', 'to', 'find', 'subtopics', 'of', 'a', 'topic', '.', 'Finally', ',', 'it', 'will', 'be', 'interesting', 'to', 'learn', 'SVaD', 'measures', 'for', 'labeled', 'data', 'sets', '.', '-LSB-', '1', '-RSB-', 'J.', 'C.', 'Bezdek', 'and', 'R.', 'J.', 'Hathaway', '.', 'Some', 'notes', 'on', 'alternating', 'optimization', '.', 'In', 'Proceedings', 'of', 'the', '2002', 'AFSS', 'International', 'Conference', 'on', 'Fuzzy', 'Systems', '.', 'Calcutta', ',', 'pages', '288', '300', '.', 'Springer-Verlag', ',', '2002', '.', '-LSB-', '2', '-RSB-', 'A.', 'P.', 'Dempster', ',', 'N.', 'M.', 'Laird', ',', 'and', 'Rubin', '.', 'Maximum', 'likelihood', 'from', 'incomplete', 'data', 'via', 'the', 'EM', 'algorithm', '.', 'Journal', 'Royal', 'Statistical', 'Society', 'B', ',', '39', '-LRB-', '2', '-RRB-', ':', '1', '38', ',', '1977', '.', '-LSB-', '3', '-RSB-', 'I.', 'S.', 'Dhillon', 'and', 'D.', 'S.', 'Modha', '.', 'Concept', 'decompositions', 'for', 'large', 'sparse', 'text', 'data', 'using', 'clustering', '.', 'Machine', 'Learning', ',', '42', '-LRB-', '1', '-RRB-', ':', '143', '175', ',', 'January', '2001', '.', '-LSB-', '4', '-RSB-', 'E.', 'Diday', 'and', 'J.', 'C.', 'Simon', '.', 'Cluster', 'analysis', '.', 'In', 'K.', 'S.', 'Fu', ',', 'editor', ',', 'Pattern', 'Recognition', ',', 'pages', '47', '94', '.', 'Springer-Verlag', ',', '1976', '.', '-LSB-', '5', '-RSB-', 'H.', 'Frigui', 'and', 'O.', 'Nasraoui', '.', 'Simultaneous', 'clustering', 'and', 'attribute', 'discrimination', '.', 'In', 'Proceedings', 'of', 'FUZZIEEE', ',', 'pages', '158', '163', ',', 'San', 'Antonio', ',', '2000', '.', '-LSB-', '6', '-RSB-', 'H.', 'Frigui', 'and', 'O.', 'Nasraoui', '.', 'Simultaneous', 'categorization', 'of', 'text', 'documents', 'and', 'identification', 'of', 'cluster-dependent', 'keywords', '.', 'In', 'Proceedings', 'of', 'FUZZIEEE', ',', 'pages', '158', '163', ',', 'Honolulu', ',', 'Hawaii', ',', '2001', '.', '-LSB-', '7', '-RSB-', 'D.', 'E.', 'Gustafson', 'and', 'W.', 'C.', 'Kessel', '.', 'Fuzzy', 'clustering', 'with', 'the', 'fuzzy', 'covariance', 'matrix', '.', 'In', 'Proccedings', 'of', 'IEEE', 'CDC', ',', 'pages', '761', '766', ',', 'San', 'Diego', ',', 'California', ',', '1979', '.', '-LSB-', '8', '-RSB-', 'R.', 'Krishnapuram', 'and', 'J.', 'Kim', '.', 'A', 'note', 'on', 'fuzzy', 'clustering', 'algorithms', 'for', 'Gaussian', 'clusters', '.', 'IEEE', 'Transactions', 'on', 'Fuzzy', 'Systems', ',', '7', '-LRB-', '4', '-RRB-', ':', '453', '461', ',', 'Aug', '1999', '.', '-LSB-', '9', '-RSB-', 'Y.', 'Rui', ',', 'T.', 'S.', 'Huang', ',', 'and', 'S.', 'Mehrotra', '.', 'Relevance', 'feedback', 'techniques', 'in', 'interactive', 'content-based', 'image', 'retrieval', '.', 'In', 'Storage', 'and', 'Retrieval', 'for', 'Image', 'and', 'Video', 'Databases', '-LRB-', 'SPIE', '-RRB-', ',', 'pages', '25', '36', ',', '1998', '.', '-LSB-', '10', '-RSB-', 'N.', 'Slonim', 'and', 'N.', 'Tishby', '.', 'Document', 'clustering', 'using', 'word', 'clusters', 'via', 'the', 'information', 'bottleneck', 'method', '.', 'In', 'Proceedings', 'of', 'SIGIR', ',', 'pages', '208', '215', ',', '2000', '.', 'APPENDIX', 'A', '.', 'OTHER', 'FEATURE', 'WEIGHTING', 'CLUSTERING', 'TECHNIQUES', 'A.', '1', 'Diagonal', 'Gustafson-Kessel', '-LRB-', 'DGK', '-RRB-', 'Gustafson', 'and', 'Kessel', '-LSB-', '7', '-RSB-', 'associate', 'each', 'cluster', 'with', 'a', 'different', 'norm', 'matrix', '.', 'Let', 'A', '=', '-LRB-', 'A', '1', ',', '...', ',', 'A', 'k', '-RRB-', 'be', 'the', 'set', 'of', 'k', 'norm', 'matrices', 'associated', 'with', 'k', 'clusters', '.', 'Let', 'u', 'ji', 'is', 'the', 'fuzzy', 'membership', 'of', 'x', 'i', 'in', 'cluster', 'j', 'and', 'U', '=', '-LSB-', 'u', 'ji', '-RSB-', '.', 'By', 'restricting', 'A', 'j', 's', 'to', 'be', 'diagonal', 'and', 'u', 'ji', '-LCB-', '0', ',', '1', '-RCB-', ',', 'we', 'can', 'reformulate', 'the', 'original', 'optimization', 'problem', 'in', 'terms', 'of', 'SVaD', 'measures', 'as', 'follows', ':', 'min', 'C', ',', 'W', 'J', 'DGK', '-LRB-', 'C', ',', 'W', '-RRB-', '=', 'k', 'j', '=', '1', 'x', 'i', 'R', 'j', 'M', 'l', '=', '1', 'w', 'jl', 'g', 'l', '-LRB-', 'x', 'i', ',', 'c', 'j', '-RRB-', ',', 'subject', 'to', 'l', 'w', 'jl', '=', 'j', '.', 'Note', 'that', 'this', 'problem', 'can', 'be', 'solved', 'using', 'the', 'same', 'AO', 'algorithms', 'described', 'in', 'Section', '3', '.', 'Here', ',', 'the', 'update', 'for', 'C', 'and', 'P', 'would', 'remain', 'the', 'same', 'as', 'that', 'discussed', 'in', 'Section', '3', '.', 'It', 'can', 'be', 'easily', 'shown', 'that', 'when', 'j', '=', '1', ',', 'j', ',', 'w', 'jl', '=', 'M', 'm', '=', '1', 'x', 'i', 'R', 'j', 'g', 'm', '-LRB-', 'x', 'i', ',', 'c', 'j', '-RRB-', '1/M', 'x', 'i', 'R', 'j', 'g', 'l', '-LRB-', 'x', 'i', ',', 'c', 'j', '-RRB-', '-LRB-', '14', '-RRB-', 'minimize', 'J', 'DGK', 'for', 'a', 'given', 'C.', 'A.', '2', 'Crisp', 'Simultaneous', 'Clustering', 'and', 'Attribute', 'Discrimination', '-LRB-', 'CSCAD', '-RRB-', 'Frigui', 'et', '.', 'al.', 'in', '-LSB-', '5', ',', '6', '-RSB-', ',', 'considered', 'a', 'fuzzy', 'version', 'of', 'the', 'feature-weighting', 'based', 'clustering', 'problem', '-LRB-', 'SCAD', '-RRB-', '.', 'To', 'make', 'a', 'fair', 'comparison', 'of', 'our', 'algorithms', 'with', 'SCAD', ',', 'we', 'derive', 'its', 'crisp', 'version', 'and', 'refer', 'to', 'it', 'as', 'Crisp', 'SCAD', '-LRB-', 'CSCAD', '-RRB-', '.', 'In', '-LSB-', '5', ',', '6', '-RSB-', ',', 'the', 'Gini', 'measure', 'is', 'used', 'for', 'regularization', '.', 'If', 'the', 'Gini', 'measure', 'is', 'considered', 'with', 'r', '=', '1', ',', 'the', 'weights', 'w', 'jl', 'that', 'minimize', 'the', 'corresponding', 'objective', 'function', 'for', 'a', 'given', 'C', 'and', 'P', ',', 'are', 'given', 'by', 'w', 'jl', '=', '1', 'M', '+', '1', '2', 'j', '1', 'M', 'M', 'n', '=', '1', 'x', 'i', 'R', 'j', 'g', 'n', '-LRB-', 'x', 'i', ',', 'c', 'j', '-RRB-', 'x', 'i', 'R', 'j', 'g', 'l', '-LRB-', 'x', 'i', ',', 'c', 'j', '-RRB-', '.', '-LRB-', '15', '-RRB-', 'Since', 'SCAD', 'uses', 'the', 'weighted', 'Euclidean', 'measure', ',', 'the', 'update', 'equations', 'of', 'centroids', 'in', 'CSCAD', 'remain', 'the', 'same', 'as', 'in', '-LRB-', '11', '-RRB-', '.', 'The', 'update', 'equation', 'for', 'w', 'jl', 'in', 'SCAD', 'is', 'quite', 'similar', 'to', '-LRB-', '15', '-RRB-', '.', 'One', 'may', 'note', 'that', ',', 'in', '-LRB-', '15', '-RRB-', ',', 'the', 'value', 'of', 'w', 'jl', 'can', 'become', 'negative', '.', 'In', '-LSB-', '5', '-RSB-', ',', 'a', 'heuristic', 'is', 'used', 'to', 'estimate', 'the', 'value', 'j', 'in', 'every', 'iteration', 'and', 'set', 'the', 'negative', 'values', 'of', 'w', 'jl', 'to', 'zero', 'before', 'normalizing', 'the', 'weights', '.', '616', 'Research', 'Track', 'Poster']
Document BIO Tags: ['O', 'O', 'O', 'O', 'O', 'O', 'O', 'O', 'B', 'O', 'O', 'O', 'O', 'O', 'O', 'O', 'O', 'O', 'O', 'O', 'O', 'O', 'O', 'O', 'O', 'O', 'O', 'O', 'O', 'O', 'O', 'O', 'O', 'O', 'O', 'O', 'O', 'O', 'O', 'O', 'O', 'O', 'O', 'O', 'O', 'O', 'O', 'O', 'O', 'O', 'O', 'O', 'O', 'O', 'O', 'O', 'O', 'O', 'O', 'O', 'O', 'O', 'O', 'O', 'O', 'O', 'O', 'O', 'O', 'O', 'O', 'O', 'O', 'O', 'O', 'O', 'O', 'O', 'O', 'O', 'O', 'O', 'O', 'B', 'I', 'O', 'O', 'O', 'O', 'O', 'O', 'O', 'O', 'O', 'O', 'O', 'O', 'O', 'O', 'O', 'O', 'O', 'O', 'O', 'O', 'O', 'B', 'I', 'O', 'O', 'O', 'O', 'O', 'O', 'O', 'O', 'O', 'O', 'O', 'O', 'O', 'O', 'O', 'O', 'O', 'O', 'O', 'O', 'O', 'O', 'O', 'O', 'O', 'O', 'O', 'O', 'O', 'O', 'O', 'O', 'O', 'O', 'O', 'O', 'O', 'O', 'O', 'O', 'O', 'B', 'O', 'O', 'O', 'O', 'O', 'O', 'O', 'O', 'O', 'O', 'O', 'O', 'O', 'O', 'O', 'O', 'O', 'B', 'O', 'O', 'O', 'O', 'O', 'O', 'O', 'O', 'O', 'O', 'O', 'O', 'O', 'O', 'O', 'O', 'O', 'O', 'O', 'O', 'O', 'O', 'O', 'O', 'O', 'O', 'O', 'O', 'O', 'O', 'O', 'O', 'O', 'O', 'O', 'O', 'O', 'O', 'O', 'O', 'O', 'O', 'O', 'O', 'O', 'B', 'O', 'O', 'O', 'O', 'O', 'O', 'O', 'O', 'O', 'O', 'O', 'O', 'O', 'O', 'B', 'I', 'O', 'O', 'O', 'O', 'O', 'O', 'O', 'O', 'O', 'B', 'I', 'O', 'O', 'O', 'O', 'O', 'O', 'O', 'O', 'O', 'O', 'O', 'O', 'O', 'O', 'O', 'O', 'O', 'O', 'O', 'O', 'O', 'O', 'O', 'O', 'O', 'O', 'O', 'O', 'O', 'O', 'O', 'O', 'O', 'O', 'O', 'O', 'O', 'O', 'O', 'O', 'O', 'O', 'O', 'O', 'O', 'O', 'O', 'O', 'O', 'O', 'O', 'O', 'O', 'O', 'O', 'O', 'O', 'O', 'O', 'O', 'O', 'O', 'O', 'O', 'O', 'O', 'O', 'O', 'O', 'O', 'O', 'O', 'O', 'O', 'O', 'O', 'O', 'O', 'O', 'O', 'O', 'O', 'O', 'O', 'O', 'O', 'O', 'O', 'O', 'O', 'O', 'O', 'O', 'O', 'O', 'O', 'O', 'O', 'O', 'O', 'O', 'O', 'O', 'O', 'O', 'O', 'O', 'O', 'O', 'O', 'O', 'O', 'O', 'O', 'O', 'O', 'O', 'O', 'O', 'O', 'O', 'O', 'O', 'O', 'O', 'O', 'O', 'O', 'O', 'O', 'O', 'O', 'O', 'O', 'O', 'O', 'O', 'O', 'O', 'O', 'O', 'O', 'O', 'O', 'O', 'O', 'O', 'O', 'O', 'O', 'O', 'O', 'B', 'I', 'O', 'O', 'O', 'O', 'O', 'O', 'O', 'O', 'O', 'O', 'O', 'O', 'O', 'O', 'O', 'O', 'O', 'O', 'O', 'O', 'O', 'O', 'O', 'O', 'O', 'O', 'O', 'O', 'O', 'O', 'O', 'O', 'O', 'O', 'O', 'O', 'O', 'O', 'O', 'O', 'O', 'O', 'O', 'O', 'O', 'O', 'O', 'O', 'O', 'O', 'O', 'O', 'O', 'O', 'O', 'O', 'O', 'O', 'O', 'O', 'O', 'O', 'O', 'O', 'O', 'O', 'O', 'O', 'O', 'O', 'O', 'O', 'O', 'O', 'O', 'O', 'O', 'O', 'O', 'O', 'O', 'O', 'O', 'O', 'O', 'O', 'O', 'O', 'O', 'O', 'O', 'O', 'O', 'O', 'O', 'O', 'O', 'O', 'O', 'O', 'O', 'O', 'O', 'O', 'O', 'O', 'O', 'O', 'O', 'O', 'O', 'O', 'O', 'O', 'O', 'O', 'O', 'O', 'O', 'O', 'O', 'O', 'O', 'O', 'O', 'O', 'O', 'O', 'O', 'O', 'O', 'O', 'O', 'O', 'O', 'O', 'O', 'O', 'O', 'O', 'O', 'O', 'O', 'O', 'O', 'O', 'O', 'O', 'O', 'O', 'O', 'O', 'O', 'O', 'O', 'O', 'O', 'O', 'O', 'O', 'O', 'O', 'O', 'O', 'O', 'O', 'O', 'O', 'O', 'O', 'O', 'O', 'O', 'O', 'O', 'O', 'O', 'O', 'O', 'O', 'O', 'O', 'O', 'O', 'O', 'O', 'O', 'O', 'O', 'O', 'O', 'O', 'O', 'O', 'O', 'O', 'O', 'O', 'O', 'O', 'O', 'O', 'O', 'O', 'O', 'O', 'O', 'O', 'O', 'O', 'O', 'O', 'O', 'O', 'O', 'O', 'O', 'O', 'O', 'O', 'O', 'O', 'O', 'O', 'O', 'O', 'O', 'O', 'O', 'O', 'O', 'O', 'O', 'O', 'O', 'O', 'O', 'O', 'O', 'O', 'O', 'O', 'O', 'O', 'O', 'O', 'O', 'O', 'O', 'O', 'O', 'O', 'O', 'O', 'O', 'O', 'O', 'O', 'O', 'O', 'O', 'O', 'O', 'O', 'O', 'O', 'O', 'B', 'O', 'O', 'O', 'O', 'O', 'O', 'O', 'O', 'O', 'O', 'O', 'O', 'O', 'O', 'O', 'O', 'O', 'O', 'O', 'O', 'O', 'B', 'I', 'O', 'O', 'O', 'O', 'O', 'O', 'O', 'O', 'O', 'O', 'O', 'O', 'O', 'O', 'O', 'O', 'B', 'I', 'O', 'O', 'O', 'O', 'O', 'O', 'O', 'O', 'B', 'O', 'O', 'O', 'O', 'O', 'O', 'O', 'O', 'O', 'O', 'O', 'O', 'O', 'O', 'O', 'O', 'O', 'O', 'O', 'O', 'O', 'B', 'I', 'O', 'O', 'O', 'O', 'O', 'O', 'O', 'O', 'O', 'O', 'O', 'O', 'O', 'O', 'O', 'O', 'O', 'O', 'O', 'O', 'O', 'O', 'O', 'O', 'O', 'O', 'O', 'O', 'O', 'O', 'O', 'O', 'O', 'O', 'O', 'O', 'O', 'O', 'O', 'O', 'O', 'O', 'O', 'O', 'O', 'O', 'O', 'O', 'O', 'O', 'O', 'O', 'O', 'O', 'O', 'O', 'O', 'O', 'O', 'O', 'O', 'O', 'O', 'O', 'O', 'O', 'O', 'O', 'O', 'O', 'O', 'O', 'O', 'O', 'O', 'O', 'O', 'O', 'O', 'O', 'O', 'O', 'O', 'O', 'O', 'O', 'O', 'O', 'O', 'O', 'O', 'O', 'O', 'O', 'O', 'O', 'O', 'O', 'O', 'O', 'O', 'O', 'O', 'O', 'O', 'O', 'O', 'O', 'O', 'O', 'O', 'O', 'O', 'O', 'O', 'O', 'O', 'O', 'O', 'O', 'O', 'O', 'O', 'O', 'O', 'O', 'O', 'O', 'O', 'O', 'O', 'O', 'O', 'O', 'O', 'O', 'O', 'O', 'O', 'O', 'O', 'O', 'O', 'O', 'O', 'O', 'O', 'O', 'O', 'O', 'O', 'O', 'O', 'O', 'O', 'O', 'O', 'O', 'O', 'O', 'O', 'O', 'O', 'O', 'O', 'O', 'O', 'O', 'O', 'O', 'O', 'O', 'O', 'O', 'O', 'O', 'O', 'O', 'O', 'O', 'O', 'O', 'O', 'O', 'O', 'O', 'O', 'B', 'I', 'O', 'O', 'O', 'O', 'O', 'O', 'O', 'O', 'O', 'O', 'O', 'O', 'O', 'O', 'O', 'O', 'O', 'O', 'O', 'O', 'O', 'O', 'O', 'O', 'O', 'O', 'O', 'O', 'O', 'O', 'O', 'O', 'O', 'O', 'O', 'O', 'O', 'O', 'O', 'O', 'O', 'O', 'O', 'O', 'O', 'O', 'O', 'O', 'O', 'O', 'O', 'O', 'O', 'O', 'O', 'O', 'O', 'O', 'O', 'O', 'O', 'O', 'O', 'O', 'O', 'O', 'O', 'O', 'O', 'O', 'O', 'O', 'O', 'O', 'O', 'O', 'O', 'O', 'O', 'O', 'O', 'O', 'O', 'O', 'O', 'O', 'O', 'O', 'O', 'O', 'O', 'O', 'O', 'O', 'O', 'O', 'O', 'O', 'O', 'O', 'O', 'O', 'O', 'O', 'O', 'O', 'O', 'O', 'O', 'O', 'O', 'O', 'O', 'O', 'O', 'O', 'O', 'O', 'O', 'O', 'O', 'O', 'O', 'O', 'O', 'O', 'O', 'O', 'O', 'O', 'O', 'O', 'O', 'O', 'O', 'O', 'O', 'O', 'O', 'O', 'O', 'O', 'O', 'O', 'O', 'O', 'O', 'O', 'O', 'O', 'O', 'O', 'O', 'O', 'O', 'O', 'O', 'O', 'O', 'O', 'O', 'O', 'O', 'O', 'O', 'O', 'O', 'O', 'O', 'O', 'O', 'O', 'O', 'O', 'O', 'O', 'O', 'O', 'O', 'O', 'O', 'O', 'O', 'O', 'O', 'O', 'O', 'O', 'O', 'O', 'O', 'O', 'O', 'O', 'O', 'O', 'O', 'O', 'O', 'O', 'O', 'O', 'O', 'O', 'O', 'O', 'O', 'O', 'O', 'O', 'O', 'O', 'O', 'O', 'O', 'O', 'O', 'O', 'O', 'O', 'O', 'O', 'O', 'O', 'O', 'O', 'O', 'O', 'O', 'O', 'O', 'O', 'O', 'O', 'O', 'O', 'O', 'O', 'O', 'O', 'O', 'O', 'O', 'O', 'O', 'O', 'O', 'O', 'O', 'O', 'O', 'O', 'O', 'O', 'O', 'O', 'O', 'O', 'O', 'O', 'O', 'O', 'O', 'O', 'O', 'O', 'O', 'O', 'O', 'O', 'O', 'O', 'O', 'O', 'O', 'O', 'O', 'O', 'O', 'O', 'O', 'O', 'O', 'O', 'O', 'O', 'O', 'O', 'O', 'O', 'O', 'O', 'O', 'O', 'O', 'O', 'O', 'O', 'O', 'O', 'O', 'O', 'O', 'O', 'O', 'O', 'O', 'O', 'O', 'O', 'O', 'O', 'O', 'O', 'O', 'O', 'O', 'O', 'O', 'O', 'O', 'O', 'O', 'O', 'O', 'O', 'O', 'O', 'O', 'O', 'O', 'O', 'O', 'O', 'O', 'O', 'O', 'O', 'O', 'O', 'O', 'O', 'O', 'O', 'O', 'O', 'O', 'O', 'O', 'O', 'O', 'O', 'O', 'O', 'O', 'O', 'O', 'O', 'O', 'O', 'O', 'O', 'O', 'O', 'O', 'O', 'O', 'O', 'O', 'O', 'O', 'O', 'O', 'O', 'O', 'O', 'O', 'O', 'O', 'O', 'O', 'O', 'O', 'O', 'O', 'O', 'O', 'O', 'O', 'O', 'O', 'O', 'O', 'O', 'O', 'O', 'O', 'O', 'O', 'O', 'O', 'O', 'O', 'O', 'O', 'O', 'O', 'O', 'O', 'O', 'O', 'O', 'O', 'O', 'O', 'O', 'O', 'O', 'O', 'O', 'O', 'O', 'O', 'O', 'O', 'O', 'O', 'O', 'O', 'O', 'O', 'O', 'O', 'O', 'O', 'O', 'O', 'O', 'O', 'O', 'O', 'O', 'O', 'O', 'O', 'O', 'O', 'O', 'O', 'O', 'O', 'O', 'O', 'O', 'O', 'O', 'O', 'O', 'O', 'O', 'O', 'O', 'O', 'O', 'O', 'O', 'O', 'O', 'O', 'O', 'O', 'O', 'O', 'O', 'O', 'O', 'O', 'O', 'O', 'O', 'O', 'O', 'O', 'O', 'O', 'O', 'O', 'O', 'O', 'O', 'O', 'O', 'O', 'O', 'O', 'O', 'O', 'O', 'O', 'O', 'O', 'O', 'O', 'O', 'O', 'O', 'O', 'O', 'O', 'O', 'B', 'I', 'O', 'O', 'O', 'O', 'O', 'O', 'O', 'O', 'O', 'O', 'O', 'O', 'O', 'O', 'O', 'O', 'O', 'O', 'O', 'O', 'O', 'O', 'O', 'O', 'O', 'O', 'O', 'O', 'O', 'O', 'O', 'O', 'O', 'O', 'O', 'O', 'O', 'O', 'O', 'O', 'O', 'O', 'O', 'O', 'O', 'O', 'O', 'O', 'O', 'O', 'O', 'O', 'O', 'O', 'O', 'O', 'O', 'O', 'O', 'O', 'O', 'O', 'O', 'O', 'O', 'O', 'O', 'O', 'O', 'O', 'O', 'O', 'O', 'O', 'O', 'O', 'O', 'O', 'O', 'O', 'O', 'O', 'O', 'O', 'O', 'O', 'O', 'O', 'O', 'O', 'O', 'O', 'O', 'O', 'O', 'O', 'O', 'O', 'O', 'O', 'O', 'O', 'O', 'O', 'O', 'O', 'O', 'O', 'O', 'O', 'O', 'O', 'O', 'O', 'O', 'O', 'O', 'O', 'O', 'O', 'O', 'O', 'O', 'O', 'O', 'O', 'O', 'O', 'O', 'O', 'O', 'O', 'O', 'O', 'O', 'O', 'O', 'O', 'O', 'O', 'O', 'O', 'O', 'O', 'O', 'O', 'O', 'O', 'O', 'O', 'O', 'O', 'O', 'O', 'O', 'O', 'O', 'O', 'O', 'O', 'O', 'O', 'O', 'O', 'O', 'O', 'O', 'O', 'O', 'O', 'O', 'O', 'O', 'O', 'O', 'O', 'O', 'O', 'O', 'O', 'O', 'O', 'O', 'O', 'O', 'O', 'O', 'O', 'O', 'O', 'O', 'O', 'O', 'O', 'O', 'O', 'O', 'O', 'O', 'O', 'O', 'O', 'O', 'O', 'O', 'O', 'O', 'O', 'O', 'O', 'O', 'O', 'O', 'O', 'O', 'O', 'O', 'O', 'O', 'O', 'O', 'O', 'O', 'O', 'O', 'O', 'O', 'O', 'O', 'O', 'O', 'O', 'O', 'O', 'O', 'O', 'O', 'O', 'O', 'O', 'O', 'O', 'O', 'O', 'O', 'O', 'O', 'O', 'O', 'O', 'O', 'O', 'O', 'O', 'O', 'O', 'O', 'O', 'O', 'O', 'O', 'O', 'O', 'O', 'O', 'O', 'O', 'O', 'O', 'O', 'O', 'O', 'O', 'O', 'O', 'O', 'O', 'O', 'O', 'O', 'O', 'O', 'O', 'O', 'O', 'O', 'O', 'O', 'O', 'O', 'O', 'O', 'O', 'O', 'O', 'O', 'O', 'O', 'O', 'O', 'O', 'O', 'O', 'O', 'O', 'O', 'O', 'O', 'O', 'O', 'O', 'O', 'O', 'O', 'O', 'O', 'O', 'O', 'O', 'O', 'O', 'O', 'O', 'O', 'O', 'O', 'O', 'O', 'O', 'O', 'O', 'O', 'O', 'O', 'O', 'O', 'O', 'O', 'O', 'O', 'O', 'O', 'O', 'O', 'O', 'B', 'I', 'O', 'O', 'O', 'O', 'O', 'B', 'O', 'O', 'O', 'O', 'O', 'O', 'O', 'O', 'O', 'O', 'O', 'O', 'B', 'I', 'O', 'O', 'O', 'O', 'O', 'O', 'O', 'O', 'O', 'O', 'O', 'O', 'O', 'O', 'O', 'O', 'O', 'O', 'O', 'O', 'B', 'O', 'O', 'O', 'O', 'O', 'O', 'O', 'O', 'O', 'O', 'O', 'O', 'O', 'O', 'B', 'I', 'O', 'O', 'O', 'O', 'O', 'O', 'O', 'O', 'O', 'O', 'O', 'O', 'O', 'O', 'O', 'O', 'O', 'O', 'O', 'O', 'O', 'O', 'O', 'O', 'O', 'O', 'O', 'O', 'O', 'O', 'O', 'O', 'O', 'O', 'O', 'O', 'O', 'O', 'O', 'O', 'O', 'O', 'O', 'O', 'O', 'O', 'O', 'O', 'O', 'O', 'O', 'O', 'O', 'O', 'O', 'O', 'O', 'O', 'O', 'O', 'O', 'O', 'O', 'O', 'O', 'O', 'O', 'O', 'O', 'O', 'O', 'O', 'O', 'O', 'O', 'O', 'O', 'O', 'O', 'O', 'O', 'O', 'O', 'O', 'O', 'O', 'O', 'O', 'O', 'O', 'O', 'O', 'O', 'O', 'O', 'O', 'O', 'O', 'O', 'O', 'O', 'O', 'O', 'O', 'O', 'O', 'O', 'O', 'O', 'O', 'O', 'O', 'O', 'O', 'O', 'O', 'O', 'O', 'O', 'O', 'O', 'O', 'O', 'O', 'O', 'O', 'O', 'O', 'O', 'O', 'O', 'O', 'O', 'O', 'O', 'O', 'O', 'O', 'O', 'O', 'O', 'O', 'O', 'O', 'O', 'O', 'O', 'O', 'O', 'O', 'O', 'O', 'O', 'O', 'O', 'O', 'O', 'O', 'O', 'O', 'O', 'O', 'O', 'O', 'O', 'O', 'O', 'O', 'O', 'O', 'O', 'O', 'O', 'O', 'O', 'O', 'O', 'O', 'O', 'O', 'O', 'O', 'O', 'O', 'O', 'O', 'O', 'O', 'O', 'O', 'O', 'O', 'O', 'O', 'O', 'O', 'O', 'O', 'O', 'O', 'O', 'O', 'O', 'O', 'O', 'O', 'O', 'O', 'O', 'O', 'O', 'O', 'O', 'O', 'O', 'O', 'O', 'O', 'O', 'O', 'O', 'O', 'O', 'O', 'O', 'O', 'O', 'O', 'O', 'O', 'O', 'O', 'O', 'O', 'O', 'O', 'O', 'O', 'O', 'O', 'O', 'O', 'O', 'O', 'O', 'O', 'O', 'O', 'O', 'O', 'O', 'O', 'O', 'O', 'O', 'O', 'O', 'O', 'O', 'O', 'O', 'O', 'O', 'O', 'O', 'O', 'O', 'O', 'O', 'O', 'O', 'O', 'O', 'O', 'O', 'O', 'O', 'O', 'O', 'O', 'O', 'O', 'O', 'O', 'O', 'O', 'O', 'O', 'O', 'O', 'O', 'O', 'O', 'O', 'O', 'O', 'O', 'O', 'O', 'O', 'O', 'O', 'O', 'O', 'O', 'O', 'O', 'O', 'O', 'O', 'O', 'O', 'O', 'O', 'O', 'O', 'O', 'O', 'O', 'O', 'O', 'O', 'O', 'O', 'O', 'O', 'O', 'O', 'O', 'O', 'O', 'O', 'O', 'O', 'O', 'O', 'O', 'O', 'O', 'O', 'O', 'O', 'O', 'O', 'O', 'O', 'O', 'O', 'O', 'O', 'O', 'O', 'O', 'O', 'O', 'O', 'O', 'O', 'O', 'O', 'O', 'O', 'O', 'O', 'O', 'O', 'O', 'O', 'O', 'O', 'O', 'O', 'O', 'O', 'O', 'O', 'O', 'O', 'O', 'O', 'O', 'O', 'B', 'O', 'O', 'O', 'O', 'O', 'O', 'O', 'O', 'O', 'O', 'O', 'O', 'O', 'O', 'O', 'O', 'O', 'O', 'O', 'O', 'O', 'O', 'O', 'O', 'O', 'O', 'O', 'O', 'O', 'O', 'O', 'O', 'O', 'O', 'O', 'O', 'O', 'O', 'O', 'O', 'O', 'O', 'O', 'O', 'O', 'O', 'O', 'O', 'O', 'O', 'O', 'O', 'O', 'O', 'O', 'O', 'O', 'O', 'O', 'O', 'O', 'O', 'O', 'O', 'O', 'O', 'O', 'O', 'O', 'O', 'O', 'O', 'O', 'O', 'O', 'O', 'O', 'O', 'O', 'O', 'O', 'O', 'O', 'O', 'O', 'O', 'O', 'O', 'O', 'O', 'O', 'O', 'O', 'O', 'O', 'O', 'O', 'O', 'O', 'O', 'O', 'O', 'O', 'O', 'O', 'O', 'O', 'O', 'O', 'O', 'O', 'O', 'O', 'O', 'O', 'O', 'O', 'O', 'O', 'O', 'O', 'O', 'O', 'O', 'O', 'O', 'O', 'O', 'O', 'O', 'O', 'O', 'O', 'O', 'O', 'O', 'O', 'O', 'O', 'O', 'O', 'O', 'O', 'O', 'O', 'O', 'O', 'O', 'O', 'O', 'O', 'O', 'O', 'O', 'O', 'O', 'O', 'O', 'O', 'O', 'O', 'O', 'O', 'O', 'O', 'O', 'O', 'O', 'O', 'O', 'O', 'O', 'O', 'O', 'O', 'O', 'O', 'O', 'O', 'O', 'O', 'O', 'O', 'O', 'O', 'O', 'O', 'O', 'O', 'O', 'O', 'O', 'O', 'O', 'O', 'O', 'O', 'O', 'O', 'O', 'O', 'O', 'O', 'O', 'O', 'O', 'O', 'O', 'O', 'O', 'O', 'O', 'O', 'O', 'O', 'O', 'O', 'O', 'O', 'O', 'O', 'O', 'O', 'O', 'O', 'O', 'O', 'O', 'O', 'O', 'O', 'O', 'O', 'O', 'O', 'O', 'O', 'O', 'O', 'O', 'O', 'O', 'O', 'O', 'O', 'O', 'O', 'O', 'O', 'O', 'O', 'O', 'O', 'O', 'O', 'O', 'O', 'O', 'O', 'O', 'O', 'O', 'O', 'O', 'O', 'O', 'O', 'O', 'O', 'O', 'O', 'O', 'O', 'O', 'O', 'O', 'O', 'O', 'O', 'O', 'O', 'O', 'O', 'O', 'O', 'O', 'O', 'O', 'O', 'O', 'O', 'O', 'O', 'O', 'O', 'O', 'O', 'O', 'O', 'O', 'O', 'O', 'O', 'O', 'O', 'O', 'O', 'O', 'O', 'O', 'O', 'O', 'O', 'O', 'O', 'O', 'O', 'O', 'O', 'O', 'O', 'O', 'O', 'O', 'O', 'O', 'O', 'O', 'O', 'O', 'O', 'O', 'O', 'O', 'O', 'O', 'O', 'O', 'O', 'O', 'O', 'O', 'O', 'O', 'O', 'O', 'O', 'O', 'O', 'O', 'O', 'O', 'O', 'O', 'O', 'O', 'O', 'O', 'O', 'O', 'O', 'O', 'O', 'O', 'O', 'O', 'O', 'O', 'O', 'O', 'O', 'O', 'O', 'O', 'O', 'O', 'O', 'O', 'O', 'O', 'O', 'O', 'O', 'O', 'O', 'O', 'O', 'O', 'O', 'O', 'O', 'O', 'O', 'O', 'O', 'O', 'O', 'O', 'O', 'O', 'O', 'O', 'O', 'O', 'O', 'O', 'O', 'O', 'O', 'O', 'O', 'O', 'O', 'O', 'O', 'O', 'O', 'O', 'O', 'O', 'O', 'O', 'O', 'O', 'O', 'O', 'O', 'O', 'O', 'O', 'O', 'O', 'O', 'O', 'O', 'O', 'O', 'O', 'O', 'O', 'O', 'O', 'O', 'O', 'O', 'O', 'O', 'O', 'O', 'O', 'O', 'O', 'O', 'O', 'O', 'O', 'O', 'O', 'O', 'O', 'O', 'O', 'O', 'O', 'O', 'O', 'O', 'O', 'O', 'O', 'O', 'O', 'O', 'O', 'O', 'O', 'O', 'O', 'O', 'O', 'O', 'O', 'O', 'O', 'O', 'O', 'O', 'O', 'O', 'O', 'O', 'O', 'O', 'O', 'O', 'O', 'O', 'O', 'O', 'O', 'O', 'O', 'O', 'O', 'O', 'O', 'O', 'O', 'O', 'O', 'O', 'O', 'O', 'O', 'O', 'O', 'O', 'O', 'O', 'O', 'O', 'O', 'O', 'O', 'O', 'O', 'O', 'O', 'O', 'O', 'O', 'O', 'O', 'O', 'O', 'O', 'O', 'O', 'O', 'O', 'O', 'O', 'O', 'O', 'O', 'O', 'O', 'O', 'O', 'O', 'O', 'O', 'O', 'O', 'O', 'O', 'O', 'O', 'O', 'O', 'O', 'O', 'O', 'O', 'O', 'O', 'O', 'O', 'O', 'O', 'O', 'O', 'O', 'O', 'O', 'O', 'O', 'O', 'O', 'O', 'O', 'O', 'O', 'O', 'O', 'O', 'O', 'O', 'O', 'O', 'O', 'O', 'O', 'O', 'O', 'O', 'O', 'O', 'O', 'O', 'O', 'O', 'O', 'O', 'O', 'O', 'O', 'O', 'O', 'O', 'O', 'O', 'O', 'O', 'O', 'O', 'O', 'O', 'O', 'O', 'O', 'O', 'O', 'O', 'O', 'O', 'O', 'O', 'O', 'O', 'O', 'O', 'O', 'O', 'O', 'O', 'O', 'O', 'O', 'O', 'O', 'O', 'O', 'O', 'O', 'O', 'O', 'O', 'O', 'O', 'O', 'O', 'O', 'O', 'O', 'O', 'O', 'O', 'O', 'O', 'O', 'O', 'O', 'O', 'O', 'O', 'O', 'O', 'O', 'O', 'O', 'O', 'O', 'O', 'O', 'O', 'O', 'O', 'O', 'O', 'O', 'O', 'O', 'O', 'O', 'O', 'O', 'O', 'O', 'O', 'O', 'O', 'O', 'O', 'O', 'O', 'O', 'O', 'O', 'O', 'O', 'O', 'O', 'O', 'O', 'O', 'O', 'O', 'O', 'O', 'O', 'O', 'O', 'O', 'O', 'O', 'O', 'O', 'O', 'O', 'O', 'O', 'O', 'O', 'O', 'O', 'O', 'O', 'O', 'O', 'O', 'O', 'O', 'O', 'O', 'O', 'O', 'O', 'O', 'O', 'O', 'O', 'O', 'O', 'O', 'O', 'O', 'O', 'O', 'O', 'O', 'O', 'O', 'O', 'O', 'O', 'O', 'O', 'O', 'O', 'O', 'O', 'O', 'O', 'O', 'O', 'O', 'O', 'O', 'O', 'O', 'O', 'O', 'O', 'O', 'O', 'O', 'O', 'O', 'O', 'O', 'O', 'O', 'O', 'O', 'O', 'O', 'O', 'O', 'O', 'O', 'O', 'O', 'O', 'O', 'O', 'O', 'O', 'O', 'O', 'O', 'O', 'O', 'O', 'O', 'O', 'O', 'O', 'O', 'O', 'O', 'O', 'O', 'O', 'O', 'O', 'O', 'O', 'O', 'O', 'O', 'O', 'O', 'O', 'O', 'O', 'O', 'O', 'O', 'O', 'O', 'O', 'O', 'O', 'O', 'O', 'O', 'O', 'O', 'O', 'O', 'O', 'O', 'O', 'O', 'O', 'O', 'O', 'O', 'O', 'O', 'O', 'O', 'O', 'O', 'O', 'O', 'O', 'O', 'O', 'O', 'O', 'O', 'O', 'O', 'O', 'O', 'O', 'O', 'O', 'O', 'O', 'O', 'O', 'O', 'O', 'O', 'O', 'O', 'O', 'O', 'O', 'O', 'O', 'O', 'O', 'O', 'O', 'O', 'O', 'O', 'O', 'O', 'O', 'O', 'O', 'O', 'O', 'O', 'O', 'O', 'O', 'O', 'O', 'O', 'O', 'O', 'O', 'O', 'O', 'O', 'O', 'O', 'O', 'O', 'O', 'O', 'O', 'O', 'B', 'I', 'O', 'O', 'O', 'O', 'O', 'O', 'O', 'O', 'O', 'O', 'O', 'O', 'O', 'O', 'O', 'O', 'O', 'O', 'O', 'O', 'O', 'O', 'O', 'O', 'O', 'O', 'O', 'O', 'O', 'O', 'O', 'O', 'O', 'O', 'O', 'O', 'O', 'O', 'O', 'O', 'O', 'O', 'O', 'O', 'O', 'O', 'O', 'O', 'O', 'O', 'O', 'O', 'O', 'O', 'O', 'O', 'O', 'O', 'O', 'O', 'O', 'O', 'O', 'O', 'O', 'O', 'O', 'O', 'O', 'O', 'O', 'O', 'O', 'O', 'O', 'O', 'O', 'O', 'O', 'O', 'O', 'O', 'O', 'O', 'O', 'O', 'O', 'O', 'O', 'O', 'O', 'O', 'O', 'O', 'O', 'O', 'B', 'I', 'O', 'O', 'O', 'O', 'O', 'O', 'O', 'O', 'O', 'B', 'O', 'O', 'O', 'O', 'O', 'O', 'O', 'O', 'O', 'O', 'O', 'O', 'O', 'O', 'O', 'O', 'O', 'O', 'O', 'O', 'O', 'O', 'O', 'O', 'O', 'O', 'O', 'O', 'O', 'O', 'O', 'O', 'O', 'O', 'O', 'O', 'O', 'O', 'O', 'O', 'O', 'O', 'O', 'O', 'O', 'O', 'O', 'O', 'O', 'O', 'O', 'O', 'O', 'O', 'O', 'O', 'O', 'O', 'O', 'O', 'O', 'O', 'O', 'O', 'O', 'O', 'O', 'O', 'O', 'O', 'O', 'O', 'O', 'O', 'O', 'O', 'O', 'O', 'O', 'O', 'O', 'O', 'O', 'O', 'O', 'O', 'O', 'O', 'O', 'O', 'O', 'O', 'O', 'O', 'O', 'O', 'O', 'O', 'O', 'O', 'O', 'O', 'O', 'O', 'O', 'O', 'O', 'O', 'O', 'O', 'O', 'O', 'O', 'O', 'O', 'O', 'O', 'O', 'O', 'O', 'O', 'O', 'O', 'O', 'O', 'O', 'O', 'O', 'O', 'O', 'O', 'O', 'O', 'O', 'O', 'O', 'O', 'O', 'O', 'O', 'O', 'O', 'O', 'O', 'O', 'O', 'O', 'O', 'O', 'O', 'O', 'O', 'O', 'O', 'O', 'O', 'O', 'O', 'O', 'O', 'O', 'O', 'O', 'O', 'O', 'O', 'O', 'O', 'O', 'O', 'O', 'O', 'O', 'O', 'O', 'O', 'O', 'O', 'O', 'O', 'O', 'O', 'O', 'O', 'O', 'O', 'O', 'O', 'O', 'O', 'O', 'O', 'O', 'O', 'O', 'O', 'O', 'O', 'O', 'O', 'O', 'O', 'O', 'O', 'O', 'O', 'O', 'O', 'O', 'O', 'O', 'O', 'O', 'O', 'O', 'O', 'O', 'O', 'O', 'O', 'O', 'O', 'O', 'O', 'O', 'O', 'O', 'O', 'O', 'O', 'O', 'O', 'O', 'O', 'O', 'O', 'O', 'O', 'O', 'O', 'O', 'O', 'O', 'O', 'O', 'O', 'O', 'O', 'O', 'O', 'O', 'O', 'O', 'O', 'O', 'O', 'O', 'O', 'O', 'O', 'O', 'O', 'O', 'O', 'O', 'O', 'O', 'O', 'O', 'O', 'O', 'O', 'O', 'O', 'O', 'O', 'O', 'O', 'O', 'O', 'O', 'O', 'O', 'O', 'O', 'O', 'O', 'O', 'O', 'O', 'O', 'O', 'O', 'O', 'O', 'O', 'O', 'O', 'O', 'O', 'O', 'O', 'O', 'O', 'O', 'O', 'O', 'O', 'O', 'O', 'O', 'O', 'O', 'O', 'O', 'O', 'O', 'O', 'O', 'O', 'O', 'O', 'O', 'O', 'O', 'O', 'O', 'O', 'O', 'O', 'O', 'O', 'O', 'O', 'O', 'O', 'O', 'O', 'O', 'O', 'O', 'O', 'O', 'O', 'O', 'O', 'O', 'O', 'O', 'O', 'O', 'O', 'O', 'O', 'O', 'O', 'O', 'O', 'O', 'O', 'O', 'O', 'O', 'O', 'O', 'O', 'O', 'O', 'O', 'O', 'O', 'O', 'O', 'O', 'O', 'O', 'O', 'O', 'O', 'O', 'O', 'O', 'O', 'O', 'O', 'O', 'O', 'O', 'O', 'O', 'O', 'O', 'O', 'O', 'O', 'O', 'O', 'O', 'O', 'O', 'O', 'O', 'O', 'O', 'O', 'O', 'O', 'O', 'O', 'O', 'O', 'O', 'O', 'O', 'O', 'O', 'O', 'O', 'O', 'O', 'O', 'O', 'O', 'O', 'O', 'O', 'O', 'O', 'O', 'O', 'O', 'O', 'O', 'O', 'O', 'O', 'O', 'O', 'O', 'O', 'O', 'O', 'O', 'O', 'O', 'O', 'O', 'O', 'O', 'O', 'O', 'O', 'O', 'O', 'O', 'O', 'O', 'O', 'O', 'O', 'O', 'O', 'O', 'O', 'O', 'O', 'O', 'O', 'O', 'O', 'O', 'O', 'O', 'O', 'O', 'O', 'O', 'O', 'O', 'O', 'O', 'O', 'O', 'O', 'O', 'O', 'O', 'O', 'O', 'O', 'O', 'O', 'O', 'O', 'O', 'O', 'O', 'O', 'O', 'O', 'O', 'O', 'O', 'O', 'O', 'O', 'O', 'O', 'O', 'O', 'O', 'O', 'O', 'O', 'O', 'O', 'O', 'O', 'O', 'O', 'O', 'O', 'O', 'O', 'O', 'O', 'O', 'O', 'O', 'O', 'O', 'O', 'O', 'O', 'O', 'O', 'O', 'O', 'O', 'O', 'O', 'O', 'O', 'O', 'O', 'O', 'O', 'O', 'O', 'O', 'O', 'O', 'O', 'O', 'O', 'O', 'O', 'O', 'O', 'O', 'O', 'O', 'O', 'O', 'O', 'O', 'O', 'O', 'O', 'O', 'O', 'O', 'O', 'O', 'O', 'O', 'O', 'O', 'O', 'O', 'O', 'O', 'O', 'O', 'O', 'O', 'O', 'O', 'O', 'O', 'O', 'O', 'O', 'O', 'O', 'O', 'O', 'O', 'O', 'O', 'O', 'O', 'O', 'O', 'O', 'O', 'O', 'O', 'O', 'O', 'O', 'O', 'O', 'O', 'O', 'O', 'O', 'O', 'O', 'O', 'O', 'O', 'O', 'O', 'O', 'O', 'O', 'O', 'O', 'O', 'O', 'O', 'O', 'O', 'O', 'O', 'O', 'O', 'O', 'O', 'O', 'O', 'O', 'O', 'O', 'O', 'O', 'O', 'O', 'O', 'O', 'O', 'O', 'O', 'O', 'O', 'O', 'O', 'O', 'O', 'O', 'O', 'O', 'O', 'O', 'O', 'O', 'O', 'O', 'O', 'O', 'O', 'O', 'O', 'O', 'O', 'O', 'O', 'O', 'O', 'O', 'O', 'O', 'O', 'O', 'O', 'O', 'O', 'O', 'O', 'O', 'O', 'O', 'O', 'O', 'O', 'O', 'O', 'O', 'O', 'O', 'O', 'O', 'O', 'O', 'O', 'O', 'O', 'O', 'O', 'O', 'O', 'O', 'O', 'O', 'O', 'O', 'O', 'O', 'O', 'O', 'O', 'O', 'O', 'O', 'O', 'O', 'O', 'O', 'O', 'O', 'O', 'O', 'O', 'O', 'O', 'O', 'O', 'O', 'O', 'O', 'O', 'O', 'O', 'O', 'O', 'O', 'O', 'O', 'O', 'O', 'O', 'O', 'O', 'O', 'O', 'O', 'O', 'O', 'O', 'O', 'O', 'O', 'O', 'O', 'O', 'O', 'O', 'O', 'O', 'O', 'O', 'O', 'O', 'O', 'O', 'O', 'O', 'O', 'O', 'O', 'O', 'B', 'O', 'O', 'O', 'O', 'O', 'O', 'O', 'O', 'O', 'O', 'O', 'O', 'O', 'O', 'O', 'O', 'O', 'O', 'O', 'O', 'O', 'O', 'O', 'O', 'O', 'O', 'O', 'O', 'O', 'O', 'O', 'O', 'O', 'O', 'O', 'O', 'O', 'O', 'O', 'O', 'O', 'O', 'O', 'B', 'O', 'O', 'O', 'O', 'O', 'O', 'O', 'O', 'O', 'O', 'O', 'O', 'O', 'O', 'O', 'O', 'O', 'O', 'O', 'O', 'O', 'O', 'O', 'O', 'O', 'O', 'O', 'O', 'O', 'O', 'O', 'O', 'O', 'O', 'O', 'O', 'O', 'O', 'O', 'O', 'O', 'O', 'O', 'O', 'O', 'O', 'O', 'O', 'O', 'O', 'O', 'O', 'O', 'O', 'O', 'O', 'O', 'O', 'O', 'O', 'O', 'O', 'O', 'O', 'O', 'O', 'O', 'O', 'O', 'O', 'O', 'O', 'O', 'O', 'O', 'O', 'O', 'O', 'O', 'O', 'O', 'O', 'O', 'O', 'O', 'O', 'O', 'O', 'O', 'O', 'O', 'O', 'O', 'O', 'O', 'O', 'O', 'O', 'O', 'O', 'O', 'O', 'O', 'O', 'O', 'O', 'O', 'O', 'O', 'O', 'O', 'O', 'O', 'O', 'O', 'O', 'O', 'O', 'O', 'O', 'O', 'O', 'O', 'O', 'O', 'O', 'O', 'O', 'O', 'O', 'O', 'O', 'O', 'O', 'O', 'O', 'O', 'O', 'O', 'O', 'O', 'O', 'O', 'O', 'O', 'O', 'O', 'O', 'O', 'O', 'O', 'O', 'O', 'O', 'O', 'O', 'O', 'O', 'O', 'O', 'O', 'O', 'O', 'O', 'O', 'O', 'O', 'O', 'O', 'O', 'O', 'O', 'O', 'O', 'O', 'O', 'O', 'O', 'O', 'O', 'O', 'O', 'O', 'O', 'O', 'O', 'O', 'O', 'O', 'O', 'O', 'O', 'O', 'O', 'O', 'O', 'O', 'O', 'O', 'O', 'O', 'O', 'O', 'O', 'O', 'O', 'O', 'O', 'O', 'O', 'O', 'O', 'B', 'I', 'O', 'O', 'O', 'O', 'O', 'O', 'O', 'O', 'O', 'O', 'O', 'O', 'O', 'O', 'O', 'O', 'O', 'O', 'O', 'O', 'O', 'O', 'O', 'O', 'O', 'O', 'O', 'O', 'O', 'O', 'O', 'O', 'O', 'O', 'O', 'O', 'O', 'O', 'O', 'O', 'O', 'O', 'O', 'O', 'O', 'O', 'O', 'O', 'O', 'O', 'O', 'O', 'O', 'O', 'O', 'O', 'O', 'O', 'O', 'O', 'O', 'O', 'O', 'O', 'O', 'O', 'O', 'O', 'O', 'O', 'O', 'O', 'O', 'O', 'O', 'O', 'O', 'O', 'O', 'O', 'O', 'O', 'O', 'O', 'O', 'O', 'O', 'O', 'O', 'O', 'O', 'O', 'O', 'O', 'O', 'O', 'O', 'O', 'O', 'O', 'O', 'O', 'O', 'O', 'O', 'O', 'O', 'O', 'O', 'O', 'O', 'O', 'O', 'O', 'O', 'O', 'O', 'O', 'O', 'O', 'O', 'O', 'O', 'O', 'O', 'O', 'O', 'O', 'O', 'O', 'O', 'O', 'O', 'O', 'O', 'O', 'O', 'O', 'O', 'O', 'O', 'O', 'O', 'O', 'O', 'O', 'O', 'O', 'O', 'O', 'O', 'O', 'O', 'O', 'O', 'O', 'O', 'O', 'O', 'O', 'O', 'O', 'O', 'O', 'O', 'O', 'O', 'O', 'O', 'O', 'O', 'O', 'O', 'O', 'O', 'O', 'O', 'O', 'O', 'O', 'O', 'O', 'O', 'O', 'O', 'O', 'O', 'O', 'O', 'O', 'O', 'O', 'O', 'O', 'O', 'O', 'O', 'O', 'O', 'O', 'O', 'O', 'O', 'O', 'O', 'O', 'O', 'O', 'O', 'O', 'O', 'O', 'O', 'O', 'O', 'O', 'O', 'O', 'O', 'O', 'O', 'O', 'O', 'O', 'O', 'O', 'O', 'O', 'O', 'O', 'O', 'O', 'O', 'O', 'O', 'O', 'O', 'O', 'O', 'O', 'O', 'O', 'O', 'O', 'O', 'O', 'O', 'O', 'O', 'O', 'O', 'O', 'O', 'O', 'O', 'O', 'O', 'O', 'O', 'O', 'O', 'O', 'O', 'O', 'O', 'O', 'O', 'O', 'O', 'O', 'O', 'O', 'O', 'O', 'O', 'O', 'O', 'O', 'O', 'O', 'O', 'O', 'O', 'O', 'O', 'O', 'O', 'O', 'O', 'O', 'O', 'O', 'O', 'O', 'O', 'O', 'O', 'O', 'O', 'O', 'O', 'O', 'O', 'O', 'O', 'O', 'O', 'O', 'O', 'O', 'O', 'O', 'O', 'O', 'O', 'O', 'O', 'O', 'O', 'O', 'O', 'O', 'O', 'O', 'O', 'O', 'O', 'O', 'O', 'O', 'O', 'O', 'O', 'O', 'O', 'O', 'O', 'O', 'O', 'O', 'O', 'O', 'O', 'O', 'O', 'O', 'O', 'O', 'O', 'O', 'O', 'O', 'O', 'O', 'O', 'O', 'O', 'O', 'O', 'O', 'O', 'O', 'O', 'O', 'O', 'O', 'O', 'O', 'O', 'O', 'O', 'O', 'O', 'O', 'O', 'O', 'O', 'O', 'O', 'O', 'O', 'O', 'O', 'O', 'O', 'O', 'O', 'O', 'O', 'O', 'O', 'O', 'O', 'O', 'O', 'O', 'O', 'O', 'O', 'O', 'O', 'O', 'O', 'O', 'O', 'O', 'O', 'O', 'O', 'O', 'O', 'O', 'O', 'O', 'O', 'O', 'O', 'O', 'O', 'O', 'O', 'O', 'O', 'O', 'O', 'O', 'O', 'O', 'O', 'O', 'O', 'O', 'O', 'O', 'O', 'O', 'O', 'O', 'O', 'O', 'O', 'O', 'O', 'O', 'O', 'O', 'O', 'O', 'O', 'O', 'O', 'O', 'O', 'O', 'O', 'O', 'O', 'O', 'O', 'O', 'O', 'O', 'O', 'O', 'O', 'O', 'O', 'O', 'O', 'O', 'O', 'O', 'O', 'O', 'O', 'O', 'O', 'O', 'O', 'O', 'O', 'O', 'O', 'O', 'O', 'O', 'O', 'O', 'O', 'O', 'O', 'O', 'O', 'O', 'O', 'O', 'O', 'O', 'O', 'O', 'O', 'O', 'O', 'O', 'O', 'O', 'O', 'O', 'O', 'O', 'O', 'O', 'O', 'O', 'O', 'O', 'O', 'O', 'O', 'O', 'O', 'O', 'O', 'O', 'O', 'O', 'O', 'O', 'O', 'O', 'O', 'O', 'O', 'O', 'O', 'O', 'O', 'O', 'O', 'O', 'O', 'O', 'O', 'O', 'O', 'O', 'O', 'O', 'O', 'O', 'O', 'O', 'O', 'O', 'O', 'O', 'O', 'O', 'O', 'O', 'O', 'O', 'O', 'O', 'O', 'O', 'O', 'O', 'O', 'O', 'O', 'O', 'O', 'O', 'O', 'O', 'O', 'O', 'O', 'O', 'O', 'O', 'O', 'O', 'O', 'O', 'O', 'O', 'O', 'O', 'O', 'O', 'O', 'O', 'O', 'O', 'O', 'O', 'O', 'O', 'O', 'O', 'O', 'O', 'O', 'O', 'O', 'O', 'O', 'O', 'O', 'O', 'O', 'O', 'O', 'O', 'O', 'O', 'O', 'O', 'O', 'O', 'O', 'O', 'O', 'O', 'O', 'O', 'O', 'O', 'O', 'O', 'O', 'O', 'O', 'O', 'O', 'O', 'O', 'O', 'O', 'O', 'O', 'O', 'O', 'O', 'O', 'O', 'O', 'O', 'O', 'O', 'O', 'O', 'O', 'O', 'O', 'O', 'O', 'O', 'O', 'O', 'O', 'O', 'O', 'O', 'O', 'O', 'O', 'O', 'O', 'O', 'O', 'O', 'O', 'O', 'O', 'O', 'O', 'O', 'O', 'O', 'O', 'O', 'O', 'O', 'O', 'O', 'O', 'O', 'O', 'O', 'O', 'O', 'O', 'O', 'O', 'O', 'O', 'O', 'O', 'O', 'O', 'O', 'O', 'O', 'O', 'O', 'O', 'O', 'O', 'O', 'O', 'O', 'O', 'O', 'O', 'O', 'O', 'O', 'O', 'O', 'O', 'O', 'O', 'O', 'O', 'O', 'O', 'O', 'O', 'O', 'O', 'O', 'O', 'O', 'O', 'O', 'O', 'O', 'O', 'O', 'O', 'O', 'O', 'O', 'O', 'O', 'O', 'O', 'O', 'O', 'O', 'O', 'O', 'O', 'O', 'O', 'O', 'O', 'O', 'O', 'O', 'O', 'O', 'O', 'O', 'O', 'O', 'O', 'O', 'O', 'O', 'O', 'O', 'O', 'O', 'O', 'O', 'O', 'O', 'O', 'O', 'O', 'O', 'O', 'O', 'O', 'O', 'O', 'O', 'O', 'O', 'O', 'O', 'O', 'O', 'O', 'O', 'O', 'O', 'O', 'O', 'O', 'O', 'O', 'O', 'O', 'O', 'O', 'O', 'O', 'O', 'O', 'O', 'O', 'O', 'O', 'O', 'O', 'O', 'O', 'O', 'O', 'O', 'O', 'O', 'O', 'O', 'O', 'O', 'O', 'O', 'O', 'O', 'O', 'O', 'O', 'O', 'O', 'O', 'O', 'O', 'O', 'O', 'O', 'O', 'O', 'O', 'O', 'O', 'O', 'O', 'O', 'O', 'O', 'O', 'O', 'O', 'O', 'O', 'O', 'O', 'O', 'O', 'O', 'O', 'O', 'O', 'O', 'O', 'O', 'O', 'O', 'O', 'O', 'O', 'O', 'O', 'O', 'O', 'O', 'O', 'O', 'O', 'O', 'O', 'O', 'O', 'O', 'O', 'O', 'O', 'O', 'O', 'O', 'O', 'O', 'O', 'O', 'O', 'O', 'O', 'O', 'O', 'O', 'O', 'O', 'O', 'O', 'O', 'O', 'O', 'O', 'O', 'O', 'O', 'O', 'O', 'O', 'O', 'O', 'O', 'O', 'O', 'O', 'O', 'O', 'O', 'O', 'O', 'O', 'O', 'O', 'O', 'O', 'O', 'O', 'O', 'B', 'I', 'O', 'O', 'O', 'O', 'O', 'O', 'O', 'O', 'O', 'O', 'O', 'O', 'O', 'O', 'O', 'O', 'O', 'O', 'O', 'O', 'O', 'O', 'O', 'O', 'O', 'O', 'O', 'O', 'O', 'O', 'O', 'O', 'O', 'O', 'O', 'O', 'O', 'O', 'O', 'O', 'O', 'O', 'O', 'O', 'O', 'O', 'O', 'O', 'O', 'O', 'O', 'O', 'O', 'O', 'O', 'O', 'O', 'O', 'O', 'O', 'O', 'O', 'O', 'O', 'O', 'O', 'O', 'O', 'O', 'O', 'O', 'O', 'O', 'O', 'O', 'O', 'O', 'O', 'O', 'O', 'O', 'O', 'O', 'O', 'O', 'O', 'O', 'O', 'O', 'O', 'O', 'O', 'O', 'O', 'O', 'O', 'O', 'O', 'O', 'O', 'O', 'O', 'O', 'O', 'O', 'O', 'O', 'O', 'O', 'O', 'O', 'O', 'O', 'O', 'O', 'O', 'O', 'O', 'O', 'O', 'O', 'O', 'O', 'O', 'O', 'O', 'O', 'O', 'O', 'O', 'O', 'O', 'O', 'O', 'O', 'O', 'O', 'O', 'O', 'O', 'O', 'O', 'O', 'O', 'O', 'O', 'O', 'O', 'O', 'O', 'O', 'O', 'O', 'O', 'O', 'O', 'O', 'O', 'O', 'O', 'O', 'O', 'O', 'O', 'O', 'O', 'O', 'O', 'O', 'O', 'O', 'O', 'O', 'O', 'O', 'O', 'O', 'O', 'O', 'O', 'O', 'O', 'O', 'O', 'O', 'O', 'O', 'O', 'O', 'O', 'O', 'O', 'O', 'O', 'O', 'O', 'O', 'O', 'O', 'O', 'O', 'O', 'O', 'O', 'O', 'O', 'O', 'O', 'O', 'O', 'O', 'O', 'O', 'O', 'O', 'O', 'O', 'O', 'O', 'O', 'O', 'O', 'O', 'O', 'O', 'O', 'O', 'O', 'O', 'O', 'O', 'O', 'O', 'O', 'O', 'O', 'O', 'O', 'O', 'O', 'O', 'O', 'O', 'O', 'O', 'O', 'O', 'O', 'O', 'O', 'O', 'O', 'O', 'O', 'O', 'O', 'O', 'O', 'O', 'B', 'O', 'O', 'O', 'O', 'O', 'O', 'O', 'O', 'O', 'O', 'O', 'O', 'O', 'O', 'O', 'O', 'O', 'O', 'O', 'O', 'O', 'O', 'O', 'O', 'O', 'O', 'O', 'O', 'O', 'O', 'O', 'O', 'O', 'O', 'O', 'O', 'O', 'O', 'O', 'O', 'O', 'O', 'O', 'O', 'O', 'O', 'O', 'O', 'O', 'O', 'O', 'O', 'O', 'O', 'O', 'O', 'B', 'O', 'O', 'O', 'O', 'O', 'O', 'O', 'O', 'O', 'O', 'O', 'O', 'O', 'O', 'O', 'O', 'O', 'O', 'O', 'O', 'O', 'O', 'O', 'O', 'O', 'O', 'O', 'O', 'O', 'O', 'O', 'O', 'O', 'O', 'O', 'O', 'O', 'O', 'O', 'O', 'O', 'O', 'O', 'O', 'O', 'O', 'O', 'O', 'O', 'O', 'O', 'O', 'O', 'O', 'O', 'O', 'O', 'O', 'O', 'O', 'O', 'O', 'O', 'O', 'O', 'B', 'O', 'O', 'O', 'O', 'O', 'O', 'O', 'O', 'O', 'O', 'O', 'O', 'O', 'O', 'O', 'O', 'O', 'O', 'O', 'O', 'O', 'O', 'O', 'O', 'O', 'O', 'O', 'O', 'O', 'O', 'O', 'O', 'O', 'O', 'O', 'O', 'B', 'O', 'O', 'O', 'O', 'O', 'O', 'O', 'O', 'O', 'O', 'O', 'O', 'O', 'O', 'O', 'O', 'O', 'O', 'O', 'O', 'O', 'O', 'O', 'O', 'O', 'O', 'O', 'O', 'O', 'O', 'O', 'O', 'O', 'O', 'O', 'O', 'O', 'O', 'O', 'O', 'O', 'O', 'O', 'O', 'O', 'O', 'O', 'O', 'O', 'O', 'O', 'O', 'O', 'O', 'O', 'O', 'O', 'O', 'O', 'O', 'O', 'O', 'O', 'O', 'O', 'O', 'O', 'O', 'O', 'O', 'O', 'O', 'O', 'O', 'B', 'O', 'O', 'O', 'O', 'O', 'O', 'O', 'O', 'O', 'O', 'O', 'O', 'O', 'O', 'O', 'O', 'O', 'O', 'O', 'O', 'O', 'O', 'O', 'O', 'B', 'I', 'B', 'O', 'O', 'O', 'O', 'O', 'O', 'O', 'O', 'O', 'O', 'O', 'O', 'O', 'O', 'O', 'O', 'O', 'O', 'O', 'O', 'O', 'O', 'O', 'O', 'O', 'O', 'O', 'O', 'O', 'O', 'O', 'O', 'O', 'O', 'O', 'O', 'O', 'O', 'O', 'O', 'O', 'O', 'O', 'O', 'O', 'O', 'O', 'O', 'O', 'O', 'O', 'O', 'O', 'O', 'O', 'O', 'O', 'O', 'O', 'O', 'O', 'O', 'O', 'O', 'O', 'O', 'O', 'O', 'O', 'O', 'O', 'O', 'O', 'O', 'O', 'O', 'O', 'O', 'O', 'O', 'O', 'O', 'O', 'O', 'O', 'O', 'O', 'O', 'O', 'O', 'O', 'O', 'O', 'O', 'O', 'O', 'O', 'O', 'O', 'O', 'O', 'O', 'O', 'O', 'O', 'O', 'O', 'O', 'O', 'O', 'O', 'O', 'O', 'O', 'O', 'O', 'O', 'O', 'O', 'O', 'O', 'O', 'O', 'O', 'O', 'O', 'O', 'O', 'O', 'O', 'O', 'O', 'O', 'O', 'O', 'O', 'O', 'O', 'O', 'O', 'O', 'O', 'O', 'O', 'O', 'O', 'O', 'O', 'O', 'O', 'O', 'O', 'O', 'O', 'O', 'O', 'O', 'O', 'O', 'O', 'O', 'O', 'O', 'O', 'O', 'O', 'O', 'O', 'O', 'O', 'O', 'O', 'O', 'O', 'O', 'O', 'O', 'O', 'O', 'O', 'O', 'O', 'O', 'O', 'O', 'O', 'O', 'O', 'O', 'O', 'O', 'O', 'O', 'O', 'O', 'O', 'O', 'O', 'O', 'O', 'O', 'O', 'O', 'O', 'O', 'O', 'O', 'O', 'O', 'O', 'O', 'O', 'O', 'O', 'O', 'O', 'O', 'O', 'O', 'O', 'O', 'O', 'O', 'O', 'O', 'O', 'O', 'O', 'O', 'O', 'O', 'O', 'O', 'O', 'O', 'O', 'O', 'O', 'O', 'O', 'O', 'B', 'O', 'O', 'O', 'O', 'O', 'O', 'O', 'O', 'O', 'O', 'O', 'O', 'O', 'O', 'O', 'O', 'O', 'O', 'O', 'O', 'O', 'O', 'O', 'O', 'O', 'B', 'O', 'O', 'O', 'O', 'O', 'O', 'O', 'O', 'O', 'O', 'O', 'O', 'O', 'O', 'O', 'O', 'O', 'O', 'O', 'O', 'O', 'O', 'O', 'O', 'O', 'O', 'O', 'O', 'O', 'O', 'O', 'O', 'O', 'O', 'O', 'O', 'O', 'O', 'O', 'O', 'O', 'O', 'O', 'O', 'O', 'O', 'O', 'O', 'O', 'O', 'O', 'O', 'O', 'O', 'O', 'O', 'O', 'O', 'O', 'O', 'O', 'O', 'O', 'O', 'O', 'O', 'O', 'O', 'O', 'O', 'O', 'O', 'O', 'O', 'O', 'O', 'O', 'O', 'O', 'O', 'O', 'O', 'O', 'O', 'O', 'O', 'O', 'O', 'O', 'O', 'O', 'O', 'O', 'O', 'O', 'O', 'O', 'O', 'O', 'O', 'O', 'O', 'O', 'O', 'O', 'O', 'O', 'O', 'O', 'O', 'O', 'O', 'O', 'O', 'O', 'O', 'O', 'O', 'O', 'O', 'O', 'O', 'O', 'O', 'O', 'O', 'O', 'O', 'O', 'O', 'O', 'O', 'O', 'O', 'O', 'O', 'O', 'O', 'O', 'O', 'O', 'O', 'O', 'O', 'O', 'O', 'O', 'O', 'O', 'O', 'O', 'O', 'O', 'O', 'O', 'O', 'O', 'O', 'O', 'O', 'O', 'O', 'O', 'O', 'O', 'O', 'O', 'O', 'O', 'O', 'O', 'O', 'O', 'O', 'O', 'O', 'O', 'O', 'O', 'O', 'O', 'O', 'O', 'O', 'O', 'O', 'O', 'O', 'O', 'O', 'O', 'O', 'O', 'O', 'O', 'O', 'O', 'O', 'O', 'O', 'O', 'O', 'O', 'O', 'O', 'O', 'O', 'O', 'O', 'O', 'O', 'O', 'O', 'O', 'O', 'O', 'O', 'O']
Extractive/present Keyphrases: ['dissimilarity measure', 'clustering', 'feature weighting']
Abstractive/absent Keyphrases: ['spatially varying dissimilarity (svad)', 'learning dissimilarity measures']
-----------
```
### Keyphrase Extraction
```python
from datasets import load_dataset
# get the dataset only for keyphrase extraction
dataset = load_dataset("midas/nus", "extraction")
print("Samples for Keyphrase Extraction")
# sample from the test split
print("Sample from test data split")
test_sample = dataset["test"][0]
print("Fields in the sample: ", [key for key in test_sample.keys()])
print("Tokenized Document: ", test_sample["document"])
print("Document BIO Tags: ", test_sample["doc_bio_tags"])
print("\n-----------\n")
```
### Keyphrase Generation
```python
# get the dataset only for keyphrase generation
dataset = load_dataset("midas/nus", "generation")
print("Samples for Keyphrase Generation")
# sample from the test split
print("Sample from test data split")
test_sample = dataset["test"][0]
print("Fields in the sample: ", [key for key in test_sample.keys()])
print("Tokenized Document: ", test_sample["document"])
print("Extractive/present Keyphrases: ", test_sample["extractive_keyphrases"])
print("Abstractive/absent Keyphrases: ", test_sample["abstractive_keyphrases"])
print("\n-----------\n")
```
## Citation Information
```
@InProceedings{10.1007/978-3-540-77094-7_41,
author="Nguyen, Thuy Dung
and Kan, Min-Yen",
editor="Goh, Dion Hoe-Lian
and Cao, Tru Hoang
and Solvberg, Ingeborg Torvik
and Rasmussen, Edie",
title="Keyphrase Extraction in Scientific Publications",
booktitle="Asian Digital Libraries. Looking Back 10 Years and Forging New Frontiers",
year="2007",
publisher="Springer Berlin Heidelberg",
address="Berlin, Heidelberg",
pages="317--326",
isbn="978-3-540-77094-7"
}
```
## Contributions
Thanks to [@debanjanbhucs](https://github.com/debanjanbhucs), [@dibyaaaaax](https://github.com/dibyaaaaax) and [@ad6398](https://github.com/ad6398) for adding this dataset
| midas/nus | [
"region:us"
] | 2022-03-02T23:29:22+00:00 | {} | 2022-03-05T03:35:59+00:00 | [] | [] | TAGS
#region-us
| Dataset Summary
---------------
A dataset for benchmarking keyphrase extraction and generation techniques from long document english scientific papers. For more details about the dataset please refer the original paper - URL
Original source of the data -
Dataset Structure
-----------------
### Data Fields
* id: unique identifier of the document.
* document: Whitespace separated list of words in the document.
* doc\_bio\_tags: BIO tags for each word in the document. B stands for the beginning of a keyphrase and I stands for inside the keyphrase. O stands for outside the keyphrase and represents the word that isn't a part of the keyphrase at all.
* extractive\_keyphrases: List of all the present keyphrases.
* abstractive\_keyphrase: List of all the absent keyphrases.
### Data Splits
* Percentage of keyphrases that are named entities: 67.95% (named entities detected using scispacy - en-core-sci-lg model)
* Percentage of keyphrases that are noun phrases: 82.16% (noun phrases detected using spacy en-core-web-lg after removing determiners)
Usage
-----
### Full Dataset
Output
### Keyphrase Extraction
### Keyphrase Generation
Contributions
-------------
Thanks to @debanjanbhucs, @dibyaaaaax and @ad6398 for adding this dataset
| [
"### Data Fields\n\n\n* id: unique identifier of the document.\n* document: Whitespace separated list of words in the document.\n* doc\\_bio\\_tags: BIO tags for each word in the document. B stands for the beginning of a keyphrase and I stands for inside the keyphrase. O stands for outside the keyphrase and represents the word that isn't a part of the keyphrase at all.\n* extractive\\_keyphrases: List of all the present keyphrases.\n* abstractive\\_keyphrase: List of all the absent keyphrases.",
"### Data Splits\n\n\n\n* Percentage of keyphrases that are named entities: 67.95% (named entities detected using scispacy - en-core-sci-lg model)\n* Percentage of keyphrases that are noun phrases: 82.16% (noun phrases detected using spacy en-core-web-lg after removing determiners)\n\n\nUsage\n-----",
"### Full Dataset\n\n\nOutput",
"### Keyphrase Extraction",
"### Keyphrase Generation\n\n\nContributions\n-------------\n\n\nThanks to @debanjanbhucs, @dibyaaaaax and @ad6398 for adding this dataset"
] | [
"TAGS\n#region-us \n",
"### Data Fields\n\n\n* id: unique identifier of the document.\n* document: Whitespace separated list of words in the document.\n* doc\\_bio\\_tags: BIO tags for each word in the document. B stands for the beginning of a keyphrase and I stands for inside the keyphrase. O stands for outside the keyphrase and represents the word that isn't a part of the keyphrase at all.\n* extractive\\_keyphrases: List of all the present keyphrases.\n* abstractive\\_keyphrase: List of all the absent keyphrases.",
"### Data Splits\n\n\n\n* Percentage of keyphrases that are named entities: 67.95% (named entities detected using scispacy - en-core-sci-lg model)\n* Percentage of keyphrases that are noun phrases: 82.16% (noun phrases detected using spacy en-core-web-lg after removing determiners)\n\n\nUsage\n-----",
"### Full Dataset\n\n\nOutput",
"### Keyphrase Extraction",
"### Keyphrase Generation\n\n\nContributions\n-------------\n\n\nThanks to @debanjanbhucs, @dibyaaaaax and @ad6398 for adding this dataset"
] |
8982466c7faa3c4aab9d117b6f238bc62d523c2f | ## Dataset Summary
Original source - [https://github.com/microsoft/OpenKP](https://github.com/microsoft/OpenKP)
## Dataset Structure
### Data Fields
- **id**: unique identifier of the document.
- **document**: Whitespace separated list of words in the document.
- **doc_bio_tags**: BIO tags for each word in the document. B stands for the beginning of a keyphrase and I stands for inside the keyphrase. O stands for outside the keyphrase and represents the word that isn't a part of the keyphrase at all.
- **extractive_keyphrases**: List of all the present keyphrases.
- **abstractive_keyphrase**: List of all the absent keyphrases.
### Data Splits
|Split| #datapoints |
|--|--|
| Train | 134894 |
| Test | 6614 |
| Validation | 6616 |
## Usage
### Full Dataset
```python
from datasets import load_dataset
# get entire dataset
dataset = load_dataset("midas/openkp", "raw")
# sample from the train split
print("Sample from training data split")
train_sample = dataset["train"][0]
print("Fields in the sample: ", [key for key in train_sample.keys()])
print("Tokenized Document: ", train_sample["document"])
print("Document BIO Tags: ", train_sample["doc_bio_tags"])
print("Extractive/present Keyphrases: ", train_sample["extractive_keyphrases"])
print("Abstractive/absent Keyphrases: ", train_sample["abstractive_keyphrases"])
print("\n-----------\n")
# sample from the validation split
print("Sample from validation data split")
validation_sample = dataset["validation"][0]
print("Fields in the sample: ", [key for key in validation_sample.keys()])
print("Tokenized Document: ", validation_sample["document"])
print("Document BIO Tags: ", validation_sample["doc_bio_tags"])
print("Extractive/present Keyphrases: ", validation_sample["extractive_keyphrases"])
print("Abstractive/absent Keyphrases: ", validation_sample["abstractive_keyphrases"])
print("\n-----------\n")
# sample from the test split
print("Sample from test data split")
test_sample = dataset["test"][0]
print("Fields in the sample: ", [key for key in test_sample.keys()])
print("Tokenized Document: ", test_sample["document"])
print("Document BIO Tags: ", test_sample["doc_bio_tags"])
print("Extractive/present Keyphrases: ", test_sample["extractive_keyphrases"])
print("Abstractive/absent Keyphrases: ", test_sample["abstractive_keyphrases"])
print("\n-----------\n")
```
**Output**
```bash
Sample from training data split
Fields in the sample: ['id', 'document', 'doc_bio_tags', 'extractive_keyphrases', 'abstractive_keyphrases', 'other_metadata']
Tokenized Document: ['Star', 'Trek', 'Discovery', 'Season', '1', 'Director', 'NA', 'Actors', 'Jason', 'Isaacs', 'Doug', 'Jones', 'Shazad', 'Latif', 'Sonequa', 'MartinGreen', 'Genres', 'SciFi', 'Country', 'USA', 'Release', 'Year', '2017', 'Duration', 'NA', 'Synopsis', 'Ten', 'years', 'before', 'Kirk', 'Spock', 'and', 'the', 'Enterprise', 'the', 'USS', 'Discovery', 'discovers', 'new', 'worlds', 'and', 'lifeforms', 'as', 'one', 'Starfleet', 'officer', 'learns', 'to', 'understand', 'all', 'things', 'alien', 'YOU', 'ARE', 'WATCHING', 'Star', 'Trek', 'Discovery', 'Season', '1', '000', '000', 'Loaded', 'Progress', 'The', 'video', 'keeps', 'buffering', 'Just', 'pause', 'it', 'for', '510', 'minutes', 'then', 'continue', 'playing', 'Share', 'Star', 'Trek', 'Discovery', 'Season', '1', 'movie', 'to', 'your', 'friends', 'Share', 'to', 'support', 'Putlocker', '1', '2', '3', '4', '5', '6', '7', '8', '9', '10', '11', '12', '13', '14', '15', 'Version', '1', 'Server', 'Mega', 'Play', 'Movie', 'Version', '2', 'Server', 'TheVideo', 'Link', '1', 'Play', 'Movie', 'Version', '3', 'Server', 'TheVideo', 'Link', '2', 'Play', 'Movie', 'Version', '4', 'Server', 'TheVideo', 'Link', '3', 'Play', 'Movie', 'Version', '5', 'Server', 'TheVideo', 'Link', '4', 'Play', 'Movie', 'Version', '6', 'Server', 'NowVideo', 'Play', 'Movie', 'Version', '7', 'Server', 'NovaMov', 'Play', 'Movie', 'Version', '8', 'Server', 'VideoWeed', 'Play', 'Movie', 'Version', '9', 'Server', 'MovShare', 'Play', 'Movie', 'Version', '10', 'Server', 'CloudTime', 'Play', 'Movie', 'Version', '11', 'Server', 'VShare', 'Link', '1', 'Play', 'Movie', 'Version', '12', 'Server', 'VShare', 'Link', '2', 'Play', 'Movie', 'Version', '13', 'Server', 'VShare', 'Link', '3', 'Play', 'Movie', 'Version', '14', 'Server', 'VShare', 'Link', '4', 'Play', 'Movie', 'Version', '15', 'Other', 'Link', '1', 'Play', 'Movie', 'Version', '16', 'Other', 'Link', '2', 'Play', 'Movie', 'Version', '17', 'Other', 'Link', '3', 'Play', 'Movie', 'Version', '18', 'Other', 'Link', '4', 'Play', 'Movie', 'Version', '19', 'Other', 'Link', '5', 'Play', 'Movie', 'Version', '20', 'Other', 'Link', '6', 'Play', 'Movie', 'Version', '21', 'Other', 'Link', '7', 'Play', 'Movie', 'Version', '22', 'Other', 'Link', '8', 'Play', 'Movie', 'Version', '23', 'Other', 'Link', '9', 'Play', 'Movie', 'Version', '24', 'Other', 'Link', '10', 'Play', 'Movie', 'Version', '25', 'Other', 'Link', '11', 'Play', 'Movie', 'Version', '26', 'Other', 'Link', '12', 'Play', 'Movie', 'Version', '27', 'Other', 'Link', '13', 'Play', 'Movie', 'Version', '28', 'Other', 'Link', '14', 'Play', 'Movie', 'Version', '29', 'Other', 'Link', '15', 'Play', 'Movie']
Document BIO Tags: ['B', 'I', 'O', 'O', 'O', 'O', 'O', 'O', 'B', 'I', 'B', 'I', 'O', 'O', 'O', 'O', 'O', 'O', 'O', 'O', 'O', 'O', 'O', 'O', 'O', 'O', 'O', 'O', 'O', 'O', 'O', 'O', 'O', 'O', 'O', 'O', 'O', 'O', 'O', 'O', 'O', 'O', 'O', 'O', 'O', 'O', 'O', 'O', 'O', 'O', 'O', 'O', 'O', 'O', 'O', 'B', 'I', 'O', 'O', 'O', 'O', 'O', 'O', 'O', 'O', 'O', 'O', 'O', 'O', 'O', 'O', 'O', 'O', 'O', 'O', 'O', 'O', 'O', 'B', 'I', 'O', 'O', 'O', 'O', 'O', 'O', 'O', 'O', 'O', 'O', 'O', 'O', 'O', 'O', 'O', 'O', 'O', 'O', 'O', 'O', 'O', 'O', 'O', 'O', 'O', 'O', 'O', 'O', 'O', 'O', 'O', 'O', 'O', 'O', 'O', 'O', 'O', 'O', 'O', 'O', 'O', 'O', 'O', 'O', 'O', 'O', 'O', 'O', 'O', 'O', 'O', 'O', 'O', 'O', 'O', 'O', 'O', 'O', 'O', 'O', 'O', 'O', 'O', 'O', 'O', 'O', 'O', 'O', 'O', 'O', 'O', 'O', 'O', 'O', 'O', 'O', 'O', 'O', 'O', 'O', 'O', 'O', 'O', 'O', 'O', 'O', 'O', 'O', 'O', 'O', 'O', 'O', 'O', 'O', 'O', 'O', 'O', 'O', 'O', 'O', 'O', 'O', 'O', 'O', 'O', 'O', 'O', 'O', 'O', 'O', 'O', 'O', 'O', 'O', 'O', 'O', 'O', 'O', 'O', 'O', 'O', 'O', 'O', 'O', 'O', 'O', 'O', 'O', 'O', 'O', 'O', 'O', 'O', 'O', 'O', 'O', 'O', 'O', 'O', 'O', 'O', 'O', 'O', 'O', 'O', 'O', 'O', 'O', 'O', 'O', 'O', 'O', 'O', 'O', 'O', 'O', 'O', 'O', 'O', 'O', 'O', 'O', 'O', 'O', 'O', 'O', 'O', 'O', 'O', 'O', 'O', 'O', 'O', 'O', 'O', 'O', 'O', 'O', 'O', 'O', 'O', 'O', 'O', 'O', 'O', 'O', 'O', 'O', 'O', 'O', 'O', 'O', 'O', 'O', 'O', 'O', 'O', 'O', 'O', 'O', 'O', 'O', 'O', 'O', 'O', 'O', 'O', 'O', 'O', 'O', 'O', 'O', 'O', 'O', 'O', 'O', 'O', 'O', 'O', 'O', 'O', 'O', 'O', 'O', 'O', 'O', 'O', 'O', 'O', 'O', 'O']
Extractive/present Keyphrases: ['star trek', 'jason isaacs', 'doug jones']
Abstractive/absent Keyphrases: []
-----------
Sample from validation data split
Fields in the sample: ['id', 'document', 'doc_bio_tags', 'extractive_keyphrases', 'abstractive_keyphrases', 'other_metadata']
Tokenized Document: ['Home', 'Keygen', 'NBA', '2K17', 'nba', '2k17', 'activation', 'key', 'generator', 'nba', '2k17', 'beta', 'keygen', 'free', 'nba', '2k17', 'cd', 'codes', 'free', 'nba', '2k17', 'cd', 'download', 'nba', '2k17', 'cd', 'generator', 'no', 'survey', 'nba', '2k17', 'cd', 'key', 'download', 'without', 'survey', 'NBA', '2K17', 'CD', 'Key', 'Generator', 'No', 'Survey', 'PC', 'PS34', 'Xbox', '360ONE', 'NBA', '2K17', 'CD', 'Key', 'Generator', 'No', 'Survey', 'PC', 'PS34', 'Xbox', '360ONE', 'Penulis', 'Hacker', 'Stock', 'on', 'Friday', '9', 'September', '2016', '1253', 'NBA', '2K17', 'CD', 'Key', 'Generator', 'No', 'Survey', 'PC', 'PS34', 'Xbox', '360ONE', 'Hello', 'everybody', 'welcome', 'on', 'our', 'web', 'site', 'HackerStockcom', 'these', 'days', 'weve', 'a', 'replacement', 'Key', 'Generator', 'for', 'you', 'that', 'is', 'known', 'as', 'NBA', '2K17', 'CD', 'Key', 'Generator', 'No', 'Survey', 'PC', 'PS34', 'Xbox', '360ONE', 'youll', 'be', 'ready', 'to', 'get', 'the', 'game', 'without', 'charge', 'this', 'keygen', 'will', 'find', 'unlimited', 'Activation', 'Codes', 'for', 'you', 'on', 'any', 'platform', 'Steam', 'or', 'Origin', 'on', 'computer', 'or', 'why', 'not', 'PlayStation', 'and', 'Xbox', 'Weve', 'ready', 'one', 'thing', 'special', 'for', 'all', 'NBA', 'fans', 'and', 'players', 'a', 'special', 'tool', 'that', 'were', 'certain', 'that', 'you', 'just', 'will', 'agree', 'Our', 'tool', 'may', 'generate', 'tons', 'of', 'key', 'codes', 'for', 'laptop', 'PlayStation', '3', 'PlayStation', '4', 'Xbox', '360', 'and', 'Xbox', 'ONE', 'So', 'youll', 'get', 'early', 'access', 'to', 'the', 'current', 'game', 'through', 'our', 'key', 'generator', 'for', 'NBA', '2K17', 'simply', 'with', 'few', 'clicks', 'This', 'tool', 'will', 'generate', 'over', '800', '000', 'key', 'codes', 'for', 'various', 'platforms', 'The', 'key', 'code', 'is', 'valid', 'and', 'youll', 'be', 'ready', 'to', 'try', 'it', 'and', 'be', 'able', 'to', 'play', 'NBA', '2K17', 'without', 'charge', 'Our', 'serial', 'key', 'generator', 'tool', 'is', 'NBA', '2K17', 'CD', 'Key', 'Generator', 'No', 'Survey', 'PC', 'PS34', 'Xbox', '360ONE', 'Instructions', 'using', 'the', 'NBA', '2K17', 'CD', 'Key', 'Generator', '2017', 'is', 'quick', 'and', 'easy', 'First', 'just', 'download', 'the', 'exe', 'file', 'and', 'install', 'it', 'on', 'your', 'computer', 'After', 'running', 'the', 'program', 'select', 'the', 'platform', 'on', 'which', 'you', 'want', 'to', 'play', 'NBA', '2K17', 'Next', 'click', 'the', 'GENERATE', 'button', 'This', 'will', 'produce', 'an', 'alphanumeric', 'code', 'also', 'known', 'as', 'your', 'product', 'key', 'You', 'will', 'use', 'it', 'to', 'validate', 'the', 'authenticity', 'of', 'your', 'NBA', '2K17', 'game', 'Now', 'copy', 'and', 'paste', 'the', 'product', 'key', 'onto', 'the', 'serial', 'number', 'window', 'prompt', 'of', 'your', 'NBA', '2K17', 'software', 'You', 'will', 'gain', 'access', 'to', 'NBA', '2K17', 'Finally', 'enjoy', 'your', 'game', 'We', 'designed', 'this', 'NBA', '2K17', 'game', 'key', 'generator', 'to', 'the', 'best', 'of', 'our', 'abilities', 'We', 'truly', 'hope', 'that', 'you', 'take', 'advantage', 'of', 'its', 'features', 'to', 'fully', 'enjoy', 'your', 'NBA', '2K17', 'Please', 'let', 'us', 'know', 'if', 'you', 'encounter', 'any', 'problems', 'with', 'our', 'software', 'We', 'would', 'love', 'to', 'help', 'you', 'out', 'NBA', '2K17', 'nba', '2k17', 'activation', 'key', 'generator', 'nba', '2k17', 'beta', 'keygen', 'free', 'nba', '2k17', 'cd', 'codes', 'free', 'nba', '2k17', 'cd', 'download', 'nba', '2k17', 'cd', 'generator', 'no', 'survey', 'nba', '2k17', 'cd', 'key', 'download', 'without', 'survey', 'nba', '2k17', 'cd', 'key', 'free', 'download', 'nba', '2k17', 'cd', 'key', 'free', 'online', 'nba', '2k17', 'cd', 'key', 'generator', 'no', 'survey', 'nba', '2k17', 'cd', 'key', 'pc', 'download', 'nba', '2k17', 'cd', 'key', 'ps4', 'free', 'download', 'nba', '2k17', 'cd', 'key', 'xbox', 'free', 'download', 'nba', '2k17', 'cd', 'keyexe', 'no', 'survey', 'nba', '2k17', 'crack', 'version', 'download', 'nba', '2k17', 'download', '2016', 'nba', '2k17', 'download', 'for', 'pc', '2016', 'nba', '2k17', 'download', 'full', 'crack', 'nba', 'Posted', 'by', 'Hacker', 'Stock', 'at', '1253', 'Email', 'This', 'BlogThis', 'Labels', 'Keygen', 'NBA', '2K17', 'nba', '2k17', 'activation', 'key', 'generator', 'nba', '2k17', 'beta', 'keygen', 'free', 'nba', '2k17', 'cd', 'codes', 'free', 'nba', '2k17', 'cd', 'download', 'nba', '2k17', 'cd', 'generator', 'no', 'survey', 'nba', '2k17', 'cd', 'key', 'download', 'without', 'survey', 'Older', 'Post', 'Home', 'Subscribe', 'to', 'Post', 'Comments', 'Atom']
Document BIO Tags: ['O', 'O', 'B', 'I', 'B', 'I', 'O', 'B', 'I', 'B', 'I', 'O', 'O', 'O', 'B', 'I', 'O', 'O', 'O', 'B', 'I', 'O', 'O', 'B', 'I', 'O', 'O', 'O', 'O', 'B', 'I', 'O', 'O', 'O', 'O', 'O', 'B', 'I', 'O', 'B', 'I', 'O', 'O', 'O', 'O', 'B', 'O', 'B', 'I', 'O', 'B', 'I', 'O', 'O', 'O', 'O', 'B', 'O', 'O', 'O', 'O', 'O', 'O', 'O', 'O', 'O', 'O', 'B', 'I', 'O', 'B', 'I', 'O', 'O', 'O', 'O', 'B', 'O', 'O', 'O', 'O', 'O', 'O', 'O', 'O', 'O', 'O', 'O', 'O', 'O', 'O', 'B', 'I', 'O', 'O', 'O', 'O', 'O', 'O', 'B', 'I', 'O', 'B', 'I', 'O', 'O', 'O', 'O', 'B', 'O', 'O', 'O', 'O', 'O', 'O', 'O', 'O', 'O', 'O', 'O', 'O', 'O', 'O', 'O', 'O', 'O', 'O', 'O', 'O', 'O', 'O', 'O', 'O', 'O', 'O', 'O', 'O', 'O', 'O', 'O', 'O', 'B', 'O', 'O', 'O', 'O', 'O', 'O', 'O', 'O', 'O', 'O', 'O', 'O', 'O', 'O', 'O', 'O', 'O', 'O', 'O', 'O', 'O', 'O', 'O', 'O', 'O', 'O', 'O', 'O', 'O', 'O', 'O', 'O', 'O', 'O', 'O', 'O', 'B', 'O', 'O', 'B', 'O', 'O', 'O', 'O', 'O', 'O', 'O', 'O', 'O', 'O', 'O', 'O', 'B', 'I', 'O', 'B', 'I', 'O', 'O', 'O', 'O', 'O', 'O', 'O', 'O', 'O', 'O', 'O', 'O', 'O', 'O', 'O', 'O', 'O', 'O', 'O', 'O', 'O', 'O', 'O', 'O', 'O', 'O', 'O', 'O', 'O', 'O', 'O', 'O', 'O', 'B', 'I', 'O', 'O', 'O', 'O', 'B', 'I', 'O', 'O', 'B', 'I', 'O', 'B', 'I', 'O', 'O', 'O', 'O', 'B', 'O', 'O', 'O', 'O', 'B', 'I', 'O', 'B', 'I', 'O', 'O', 'O', 'O', 'O', 'O', 'O', 'O', 'O', 'O', 'O', 'O', 'O', 'O', 'O', 'O', 'O', 'O', 'O', 'O', 'O', 'O', 'O', 'O', 'O', 'O', 'O', 'O', 'O', 'O', 'B', 'I', 'O', 'O', 'O', 'O', 'O', 'O', 'O', 'O', 'O', 'O', 'O', 'O', 'O', 'O', 'O', 'O', 'O', 'O', 'O', 'O', 'O', 'O', 'O', 'O', 'O', 'O', 'O', 'B', 'I', 'O', 'O', 'O', 'O', 'O', 'O', 'O', 'O', 'O', 'O', 'O', 'O', 'O', 'O', 'O', 'O', 'B', 'I', 'O', 'O', 'O', 'O', 'O', 'O', 'B', 'I', 'O', 'O', 'O', 'O', 'O', 'O', 'O', 'B', 'I', 'O', 'B', 'I', 'O', 'O', 'O', 'O', 'O', 'O', 'O', 'O', 'O', 'O', 'O', 'O', 'O', 'O', 'O', 'O', 'O', 'O', 'O', 'O', 'B', 'I', 'O', 'O', 'O', 'O', 'O', 'O', 'O', 'O', 'O', 'O', 'O', 'O', 'O', 'O', 'O', 'O', 'O', 'O', 'O', 'B', 'I', 'B', 'I', 'O', 'B', 'I', 'B', 'I', 'O', 'O', 'O', 'B', 'I', 'O', 'O', 'O', 'B', 'I', 'O', 'O', 'B', 'I', 'O', 'O', 'O', 'O', 'B', 'I', 'O', 'O', 'O', 'O', 'O', 'B', 'I', 'O', 'O', 'O', 'O', 'B', 'I', 'O', 'O', 'O', 'O', 'B', 'I', 'O', 'B', 'I', 'O', 'O', 'B', 'I', 'O', 'O', 'O', 'O', 'B', 'I', 'O', 'O', 'O', 'O', 'O', 'B', 'I', 'O', 'O', 'B', 'O', 'O', 'B', 'I', 'O', 'O', 'O', 'O', 'B', 'I', 'O', 'O', 'O', 'B', 'I', 'O', 'O', 'B', 'I', 'O', 'O', 'O', 'O', 'B', 'I', 'O', 'O', 'O', 'O', 'O', 'O', 'O', 'O', 'O', 'O', 'O', 'O', 'O', 'O', 'O', 'B', 'I', 'B', 'I', 'O', 'B', 'I', 'B', 'I', 'O', 'O', 'O', 'B', 'I', 'O', 'O', 'O', 'B', 'I', 'O', 'O', 'B', 'I', 'O', 'O', 'O', 'O', 'B', 'I', 'O', 'O', 'O', 'O', 'O', 'O', 'O', 'O', 'O', 'O', 'O', 'O', 'O']
Extractive/present Keyphrases: ['nba 2k17', 'key generator', 'xbox']
Abstractive/absent Keyphrases: []
-----------
Sample from test data split
Fields in the sample: ['id', 'document', 'doc_bio_tags', 'extractive_keyphrases', 'abstractive_keyphrases', 'other_metadata']
Tokenized Document: ['KSLI', '1280', 'AM', 'LATEST', 'POSTS', 'Scotty', 'McCreery', 'and', 'Wife', 'Welcome', 'New', 'Addition', 'to', 'the', 'Family', 'The', 'McCreerys', 'have', 'an', 'announcement', 'to', 'share', 'with', 'fansthe', 'family', 'is', 'getting', 'bigger', 'Wendy', 'Hermanson', '13', 'hours', 'ago', 'Kane', 'Brown', 'Serenades', 'Fans', 'With', 'Michael', 'Jackson', 'Hit', 'Watch', 'Brown', 'dusted', 'off', 'an', '80s', 'gem', 'to', 'post', 'on', 'social', 'media', 'and', 'put', 'smiles', 'on', 'the', 'faces', 'of', 'his', 'followers', 'Wendy', 'Hermanson', '20', 'hours', 'ago', 'Dolly', 'Parton', 'Scores', 'Golden', 'Globe', 'Nod', 'for', 'Girl', 'in', 'the', 'Movies', 'Congratulations', 'This', 'is', 'her', 'sixth', 'nomination', 'Sterling', 'Whitaker', '21', 'hours', 'ago', 'Remember', 'When', 'Johnny', 'Cash', 'Attacked', 'Homer', 'Simpson', 'It', 'was', 'one', 'of', 'the', 'coolest', 'guest', 'appearances', 'in', 'the', 'history', 'of', 'the', 'show', 'Sterling', 'Whitaker', '2', 'days', 'ago', 'Remember', 'Which', 'Country', 'Star', 'Murdered', 'His', 'Wife', 'The', 'career', 'of', 'one', 'of', 'country', 'musics', 'most', 'successful', 'early', 'stars', 'was', 'derailed', 'after', 'he', 'was', 'convicted', 'of', 'murdering', 'his', 'wife', 'Sterling', 'Whitaker', '2', 'days', 'ago', 'William', 'Shatner', 'to', 'Make', 'Grand', 'Ole', 'Opry', 'Debut', 'Hes', 'appearing', 'alongside', 'a', 'legendary', 'country', 'musician', 'Sterling', 'Whitaker', '2', 'days', 'ago', 'Remember', 'Who', 'First', 'Recorded', 'Garths', 'The', 'Thunder', 'Rolls', 'Have', 'you', 'ever', 'heard', 'the', 'extra', 'verse', 'Sterling', 'Whitaker', '2', 'days', 'ago', 'Danielle', 'Bradberys', 'Cover', 'of', 'Post', 'Malones', 'Psycho', 'Is', 'a', 'Stunner', 'Danielle', 'Bradbery', 'is', 'rounding', 'out', 'her', 'Yours', 'Truly', '2018', 'covers', 'project', 'by', 'sharing', 'her', 'take', 'on', 'rapper', 'Post', 'Malones', 'hit', 'Psycho', 'Angela', 'Stefano', '2', 'days', 'ago', 'Enjoy', 'Wild', 'Game', 'at', 'the', 'Texas', 'Wild', 'Bunch', 'Bonanza', 'Cook', 'Off', 'Its', 'time', 'for', 'the', 'Texas', 'Wild', 'Bunch', 'Bonanza', 'Cook', 'Off', 'and', 'Auction', 'All', 'attendees', 'get', 'to', 'sample', 'everything', 'from', 'deer', 'to', 'elk', 'to', 'bacon', 'wrapped', 'jalapeno', 'poppers', 'Rudy', 'Fernandez', '2', 'days', 'ago', 'Kid', 'Rocks', '20Foot', 'Butt', 'Bar', 'Sign', 'Gets', 'Approved', 'in', 'Nashville', 'The', 'crazy', 'sign', 'featuring', 'a', 'womans', 'rear', 'end', 'caused', 'a', 'swirl', 'of', 'discussion', 'Wendy', 'Hermanson', '2', 'days', 'ago', 'Remember', 'When', 'Dolly', 'Parton', 'Surprised', 'Reba', 'McEntire', 'on', 'the', 'Opry', 'Shes', 'made', 'so', 'many', 'special', 'memories', 'on', 'the', 'Opry', 'stage', 'Sterling', 'Whitaker', '3', 'days', 'ago', 'Chris', 'Young', 'Takes', 'on', 'the', 'Hag', 'With', 'Silver', 'Wings', 'Cover', 'Watch', 'In', 'his', 'new', 'single', 'Chris', 'Young', 'proudly', 'proclaims', 'that', 'he', 'was', 'raised', 'on', 'country', 'and', 'he', 'can', 'prove', 'it', 'Angela', 'Stefano', '3', 'days', 'ago', 'The', 'Tractors', 'Guitarist', 'Steve', 'Ripley', 'Dead', 'at', '69', 'Rest', 'in', 'peace', 'Steve', 'Carena', 'Liptak', '3', 'days', 'ago', 'Danielle', 'Bradbery', 'Rounds', 'Out', 'Yours', 'Truly', '2018', 'With', 'Psycho', 'The', 'final', 'third', 'of', 'Bradberys', 'Yours', 'Truly', '2018', 'tribute', 'project', 'is', 'here', 'Carena', 'Liptak', '3', 'days', 'ago', 'Load', 'More', 'Articles', 'Country', 'Music', 'News', 'Kane', 'Brown', 'Serenades', 'Fans', 'With', 'Michael', 'Jackson', 'Hit', 'Watch', 'Scotty', 'McCreery', 'and', 'Wife', 'Welcome', 'New', 'Addition', 'to', 'the', 'Family', 'Meet', 'the', 'Staff', 'Rudy', 'Fernandez', 'Shay', 'Hill', 'Chaz', 'Frank', 'Pain', 'Classic', 'Country', '1280', 'on', 'Facebook', 'Abilene', 'TX', 'January', '7', '2019', '70000', 'AM', 'PST', 'January', '7', '2019', '70000', 'AM', 'PST', 'January', '7', '2019', '70000', 'AM', 'PSTth', '62', 'Clear', '71', '42', 'view', 'forecast', 'VIP', 'Contests', 'New', 'Year', 'New', 'You', '100', 'Amazon', 'Gift', 'Card', 'Small', 'Business', 'Solutions', 'Devote', 'more', 'time', 'to', 'running', 'your', 'business', 'Engage', 'your', 'clients', 'across', 'multiple', 'platforms', 'Reach', 'more', 'customers', 'than', 'ever', 'before', 'Get', 'an', 'Edge', 'on', 'the', 'Competition', 'Today', 'KSLIs', 'Daily', 'Deal', 'Certificate', 'for', 'a', 'Rhythm', 'USA', 'Clock', 'From', 'Jewels', 'of', 'Time']
Document BIO Tags: ['B', 'I', 'I', 'O', 'O', 'O', 'O', 'O', 'O', 'O', 'O', 'O', 'O', 'O', 'O', 'O', 'O', 'O', 'O', 'O', 'O', 'O', 'O', 'O', 'O', 'O', 'O', 'O', 'O', 'O', 'O', 'O', 'O', 'O', 'O', 'O', 'O', 'O', 'O', 'O', 'O', 'O', 'O', 'O', 'O', 'O', 'O', 'O', 'O', 'O', 'O', 'O', 'O', 'O', 'O', 'O', 'O', 'O', 'O', 'O', 'O', 'O', 'O', 'O', 'O', 'O', 'O', 'O', 'O', 'O', 'O', 'O', 'O', 'O', 'O', 'O', 'O', 'O', 'O', 'O', 'O', 'O', 'O', 'O', 'O', 'O', 'O', 'O', 'O', 'O', 'O', 'O', 'O', 'O', 'O', 'O', 'O', 'O', 'O', 'O', 'O', 'O', 'O', 'O', 'O', 'O', 'O', 'O', 'O', 'O', 'O', 'O', 'O', 'O', 'O', 'O', 'O', 'O', 'O', 'O', 'O', 'O', 'O', 'O', 'O', 'O', 'O', 'O', 'O', 'O', 'O', 'O', 'O', 'O', 'O', 'O', 'O', 'O', 'O', 'O', 'O', 'O', 'O', 'O', 'O', 'O', 'O', 'O', 'O', 'O', 'O', 'O', 'O', 'O', 'O', 'O', 'O', 'O', 'O', 'O', 'O', 'O', 'O', 'O', 'O', 'O', 'O', 'O', 'O', 'O', 'O', 'O', 'O', 'O', 'O', 'O', 'O', 'O', 'O', 'O', 'O', 'O', 'O', 'O', 'O', 'O', 'O', 'O', 'O', 'O', 'O', 'O', 'O', 'O', 'O', 'O', 'O', 'O', 'O', 'O', 'O', 'O', 'O', 'O', 'O', 'O', 'O', 'O', 'O', 'O', 'O', 'O', 'O', 'O', 'O', 'O', 'O', 'O', 'O', 'O', 'O', 'O', 'O', 'O', 'O', 'O', 'O', 'O', 'O', 'O', 'O', 'O', 'O', 'O', 'O', 'O', 'O', 'O', 'O', 'O', 'O', 'O', 'O', 'O', 'O', 'O', 'O', 'O', 'O', 'O', 'O', 'O', 'O', 'O', 'O', 'O', 'O', 'O', 'O', 'O', 'O', 'O', 'O', 'O', 'O', 'O', 'O', 'O', 'O', 'O', 'O', 'O', 'O', 'O', 'O', 'O', 'O', 'O', 'O', 'O', 'O', 'O', 'O', 'O', 'O', 'O', 'O', 'O', 'O', 'O', 'O', 'O', 'O', 'O', 'O', 'O', 'O', 'O', 'O', 'O', 'O', 'O', 'O', 'O', 'O', 'O', 'O', 'O', 'O', 'O', 'O', 'O', 'O', 'O', 'O', 'O', 'O', 'O', 'O', 'O', 'O', 'O', 'O', 'O', 'O', 'O', 'O', 'O', 'O', 'O', 'O', 'O', 'O', 'O', 'O', 'O', 'O', 'O', 'O', 'O', 'O', 'O', 'O', 'O', 'O', 'O', 'O', 'O', 'O', 'O', 'O', 'O', 'O', 'O', 'O', 'O', 'O', 'O', 'O', 'O', 'O', 'O', 'O', 'O', 'O', 'O', 'O', 'O', 'O', 'O', 'O', 'O', 'O', 'O', 'O', 'O', 'O', 'O', 'O', 'O', 'O', 'O', 'O', 'O', 'O', 'O', 'O', 'O', 'O', 'O', 'O', 'O', 'O', 'O', 'O', 'O', 'O', 'O', 'O', 'O', 'O', 'O', 'O', 'O', 'O', 'O', 'O', 'O', 'O', 'O', 'O', 'O', 'O', 'O', 'O', 'O', 'O', 'O', 'O', 'O', 'O', 'O', 'O', 'O', 'O', 'O', 'O', 'O', 'O', 'O', 'O', 'O', 'O', 'O', 'O', 'O', 'O', 'O', 'O', 'O', 'O', 'O', 'O', 'O', 'O', 'O', 'O', 'O', 'O', 'O', 'O', 'O', 'O', 'O', 'O', 'O', 'O', 'O', 'O', 'O', 'O', 'O', 'O', 'O', 'O', 'O', 'O', 'O', 'O', 'O', 'O', 'O', 'O', 'O', 'O', 'O', 'O', 'O', 'O', 'O', 'O', 'O', 'O', 'O', 'O', 'O', 'O', 'O', 'O', 'O', 'O', 'O', 'O', 'O', 'O', 'O', 'O', 'O', 'O', 'O', 'O', 'O', 'O', 'O', 'O', 'O', 'O', 'O', 'O', 'O', 'O', 'O', 'O', 'O', 'O', 'O']
Extractive/present Keyphrases: ['ksli 1280 am']
Abstractive/absent Keyphrases: []
-----------
```
### Keyphrase Extraction
```python
from datasets import load_dataset
# get the dataset only for keyphrase extraction
dataset = load_dataset("midas/openkp", "extraction")
print("Samples for Keyphrase Extraction")
# sample from the train split
print("Sample from training data split")
train_sample = dataset["train"][0]
print("Fields in the sample: ", [key for key in train_sample.keys()])
print("Tokenized Document: ", train_sample["document"])
print("Document BIO Tags: ", train_sample["doc_bio_tags"])
print("\n-----------\n")
# sample from the validation split
print("Sample from validation data split")
validation_sample = dataset["validation"][0]
print("Fields in the sample: ", [key for key in validation_sample.keys()])
print("Tokenized Document: ", validation_sample["document"])
print("Document BIO Tags: ", validation_sample["doc_bio_tags"])
print("\n-----------\n")
# sample from the test split
print("Sample from test data split")
test_sample = dataset["test"][0]
print("Fields in the sample: ", [key for key in test_sample.keys()])
print("Tokenized Document: ", test_sample["document"])
print("Document BIO Tags: ", test_sample["doc_bio_tags"])
print("\n-----------\n")
```
### Keyphrase Generation
```python
# get the dataset only for keyphrase generation
dataset = load_dataset("midas/openkp", "generation")
print("Samples for Keyphrase Generation")
# sample from the train split
print("Sample from training data split")
train_sample = dataset["train"][0]
print("Fields in the sample: ", [key for key in train_sample.keys()])
print("Tokenized Document: ", train_sample["document"])
print("Extractive/present Keyphrases: ", train_sample["extractive_keyphrases"])
print("Abstractive/absent Keyphrases: ", train_sample["abstractive_keyphrases"])
print("\n-----------\n")
# sample from the validation split
print("Sample from validation data split")
validation_sample = dataset["validation"][0]
print("Fields in the sample: ", [key for key in validation_sample.keys()])
print("Tokenized Document: ", validation_sample["document"])
print("Extractive/present Keyphrases: ", validation_sample["extractive_keyphrases"])
print("Abstractive/absent Keyphrases: ", validation_sample["abstractive_keyphrases"])
print("\n-----------\n")
# sample from the test split
print("Sample from test data split")
test_sample = dataset["test"][0]
print("Fields in the sample: ", [key for key in test_sample.keys()])
print("Tokenized Document: ", test_sample["document"])
print("Extractive/present Keyphrases: ", test_sample["extractive_keyphrases"])
print("Abstractive/absent Keyphrases: ", test_sample["abstractive_keyphrases"])
print("\n-----------\n")
```
## Citation Information
```
@inproceedings{Xiong2019OpenDW,
title={Open Domain Web Keyphrase Extraction Beyond Language Modeling},
author={Lee Xiong and Chuan Hu and Chenyan Xiong and Daniel Fernando Campos and Arnold Overwijk},
booktitle={EMNLP},
year={2019}
}
```
## Contributions
Thanks to [@debanjanbhucs](https://github.com/debanjanbhucs), [@dibyaaaaax](https://github.com/dibyaaaaax) and [@ad6398](https://github.com/ad6398) for adding this dataset
| midas/openkp | [
"region:us"
] | 2022-03-02T23:29:22+00:00 | {} | 2022-01-09T17:01:43+00:00 | [] | [] | TAGS
#region-us
| Dataset Summary
---------------
Original source - URL
Dataset Structure
-----------------
### Data Fields
* id: unique identifier of the document.
* document: Whitespace separated list of words in the document.
* doc\_bio\_tags: BIO tags for each word in the document. B stands for the beginning of a keyphrase and I stands for inside the keyphrase. O stands for outside the keyphrase and represents the word that isn't a part of the keyphrase at all.
* extractive\_keyphrases: List of all the present keyphrases.
* abstractive\_keyphrase: List of all the absent keyphrases.
### Data Splits
Usage
-----
### Full Dataset
Output
### Keyphrase Extraction
### Keyphrase Generation
Contributions
-------------
Thanks to @debanjanbhucs, @dibyaaaaax and @ad6398 for adding this dataset
| [
"### Data Fields\n\n\n* id: unique identifier of the document.\n* document: Whitespace separated list of words in the document.\n* doc\\_bio\\_tags: BIO tags for each word in the document. B stands for the beginning of a keyphrase and I stands for inside the keyphrase. O stands for outside the keyphrase and represents the word that isn't a part of the keyphrase at all.\n* extractive\\_keyphrases: List of all the present keyphrases.\n* abstractive\\_keyphrase: List of all the absent keyphrases.",
"### Data Splits\n\n\n\nUsage\n-----",
"### Full Dataset\n\n\nOutput",
"### Keyphrase Extraction",
"### Keyphrase Generation\n\n\nContributions\n-------------\n\n\nThanks to @debanjanbhucs, @dibyaaaaax and @ad6398 for adding this dataset"
] | [
"TAGS\n#region-us \n",
"### Data Fields\n\n\n* id: unique identifier of the document.\n* document: Whitespace separated list of words in the document.\n* doc\\_bio\\_tags: BIO tags for each word in the document. B stands for the beginning of a keyphrase and I stands for inside the keyphrase. O stands for outside the keyphrase and represents the word that isn't a part of the keyphrase at all.\n* extractive\\_keyphrases: List of all the present keyphrases.\n* abstractive\\_keyphrase: List of all the absent keyphrases.",
"### Data Splits\n\n\n\nUsage\n-----",
"### Full Dataset\n\n\nOutput",
"### Keyphrase Extraction",
"### Keyphrase Generation\n\n\nContributions\n-------------\n\n\nThanks to @debanjanbhucs, @dibyaaaaax and @ad6398 for adding this dataset"
] |
f2dbc6b1aea3c53dfe27b6471158c3ccaaaeb091 | ## Dataset Summary
A dataset for benchmarking keyphrase extraction and generation techniques from long document english scientific papers. For more details about the dataset please refer the original paper - [https://www.semanticscholar.org/paper/Keyphrase-Extraction-from-Single-Documents-in-the-Schutz/08b75d31a90f206b36e806a7ec372f6f0d12457e](https://www.semanticscholar.org/paper/Keyphrase-Extraction-from-Single-Documents-in-the-Schutz/08b75d31a90f206b36e806a7ec372f6f0d12457e)
Original source of the data - []()
## Dataset Structure
### Data Fields
- **id**: unique identifier of the document.
- **document**: Whitespace separated list of words in the document.
- **doc_bio_tags**: BIO tags for each word in the document. B stands for the beginning of a keyphrase and I stands for inside the keyphrase. O stands for outside the keyphrase and represents the word that isn't a part of the keyphrase at all.
- **extractive_keyphrases**: List of all the present keyphrases.
- **abstractive_keyphrase**: List of all the absent keyphrases.
### Data Splits
|Split| #datapoints |
|--|--|
| Test | 1320 |
- Percentage of keyphrases that are named entities: 84.94% (named entities detected using scispacy - en-core-sci-lg model)
- Percentage of keyphrases that are noun phrases: 81.54% (noun phrases detected using spacy en-core-web-lg after removing determiners)
## Usage
### Full Dataset
```python
from datasets import load_dataset
# get entire dataset
dataset = load_dataset("midas/pubmed", "raw")
# sample from the test split
print("Sample from test dataset split")
test_sample = dataset["test"][0]
print("Fields in the sample: ", [key for key in test_sample.keys()])
print("Tokenized Document: ", test_sample["document"])
print("Document BIO Tags: ", test_sample["doc_bio_tags"])
print("Extractive/present Keyphrases: ", test_sample["extractive_keyphrases"])
print("Abstractive/absent Keyphrases: ", test_sample["abstractive_keyphrases"])
print("\n-----------\n")
```
**Output**
```bash
Sample from test data split
Fields in the sample: ['id', 'document', 'doc_bio_tags', 'extractive_keyphrases', 'abstractive_keyphrases', 'other_metadata']
Tokenized Document: ['Impact', 'of', 'Solitary', 'Involved', 'Lymph', 'Node', 'on', 'Outcome', 'in', 'Localized', 'Cancer', 'of', 'the', 'Esophagus', 'and', 'Esophagogastric', 'Junction', 'Node-positive', 'esophageal', 'cancer', 'is', 'associated', 'with', 'a', 'dismal', 'prognosis', '.', 'The', 'impact', 'of', 'a', 'solitary', 'involved', 'node', ',', 'however', ',', 'is', 'unclear', ',', 'and', 'this', 'study', 'examined', 'the', 'implications', 'of', 'a', 'solitary', 'node', 'compared', 'with', 'greater', 'nodal', 'involvement', 'and', 'node-negative', 'disease', '.', 'The', 'clinical', 'and', 'pathologic', 'details', 'of', '604', 'patients', 'were', 'entered', 'prospectively', 'into', 'a', 'database', 'from1993', 'and', '2005', '.', 'Four', 'pathologic', 'groups', 'were', 'analyzed', ':', 'node-negative', ',', 'one', 'lymph', 'node', 'positive', ',', 'two', 'or', 'three', 'lymph', 'nodes', 'positive', ',', 'and', 'greater', 'than', 'three', 'lymph', 'nodes', 'positive', '.', 'Three', 'hundred', 'and', 'fifteen', 'patients', '-LRB-', '52', '%', '-RRB-', 'were', 'node-positive', 'and', '289', 'were', 'node-negative', '.', 'The', 'median', 'survival', 'was', '26', 'months', 'in', 'the', 'node-negative', 'group', '.', 'Patients', '-LRB-', 'n', '=', '84', '-RRB-', 'who', 'had', 'one', 'node', 'positive', 'had', 'a', 'median', 'survival', 'of', '16', 'months', '-LRB-', 'p', '=', '0.03', 'vs', 'node-negative', '-RRB-', '.', 'Eighty-four', 'patients', 'who', 'had', 'two', 'or', 'three', 'nodes', 'positive', 'had', 'a', 'median', 'survival', 'of', '11', 'months', 'compared', 'with', 'a', 'median', 'survival', 'of', '8', 'months', 'in', 'the', '146', 'patients', 'who', 'had', 'greater', 'than', 'three', 'nodes', 'positive', '-LRB-', 'p', '=', '0.01', '-RRB-', '.', 'The', 'survival', 'of', 'patients', 'with', 'one', 'node', 'positive', '-LSB-', 'number', 'of', 'nodes', '-LRB-', 'N', '-RRB-', '=', '1', '-RSB-', 'was', 'also', 'significantly', 'greater', 'than', 'the', 'survival', 'of', 'patients', 'with', '2', '--', '3', 'nodes', 'positive', '-LRB-', 'N', '=', '2', '--', '3', '-RRB-', '-LRB-', 'p', '=', '0.049', '-RRB-', 'and', 'greater', 'than', 'three', 'nodes', 'positive', '-LRB-', 'p', '<', '0001', '-RRB-', '.', 'The', 'presence', 'of', 'a', 'solitary', 'involved', 'lymph', 'node', 'has', 'a', 'negative', 'impact', 'on', 'survival', 'compared', 'with', 'node-negative', 'disease', ',', 'but', 'it', 'is', 'associated', 'with', 'significantly', 'improved', 'overall', 'survival', 'compared', 'with', 'all', 'other', 'nodal', 'groups', '.', 'Introduction', 'Carcinoma', 'of', 'the', 'esophagus', 'carries', 'a', 'dismal', 'prognosis', ',', 'and', 'for', 'patients', 'presenting', 'with', 'localized', 'resectable', 'disease', ',', 'multivariate', 'analysis', 'has', 'established', 'that', 'the', 'presence', 'or', 'absence', 'of', 'involved', 'lymph', 'nodes', 'confers', 'the', 'greatest', 'prognostic', 'significance', '.1', 'In', 'surgical', 'management', ',', 'the', 'extent', 'and', 'type', 'of', 'lymphadenectomy', 'undertaken', 'varies', 'from', 'no', 'formal', 'lymphadenectomy', 'to', 'two', 'and', 'three', 'field', 'dissection', '.2', '--', '5', 'The', 'presence', 'and', 'extent', 'of', 'lymph', 'node', 'involvement', 'is', 'important', 'as', 'selective', 'approaches', 'may', 'be', 'considered', 'depending', 'on', 'the', 'nodal', 'stage', 'at', 'presentation', '.', 'In', 'early', 'tumors', ',', 'for', 'instance', ',', 'the', 'sentinel', 'node', 'concept', 'initially', 'developed', 'in', 'melanoma', 'and', 'breast', 'cancer', 'was', 'explored', 'to', 'help', 'identify', 'patients', 'who', 'may', 'not', 'require', 'lymph', 'node', 'dissection', '.6', '--', '8', 'The', 'advent', 'of', 'minimally', 'invasive', 'esophagectomy', 'may', 'also', 'highlight', 'the', 'need', 'to', 'subselect', 'patients', 'for', 'lymphadenectomy', '.9', 'In', 'the', 'observations', 'of', 'the', 'senior', 'author', '-LRB-', 'JVR', '-RRB-', ',', 'patients', 'with', 'solitary', 'involved', 'lymph', 'nodes', 'may', 'achieve', 'good', 'outcomes', ',', 'and', 'this', 'hypothesis', 'was', 'evaluated', 'in', 'this', 'analysis', 'of', 'a', 'large', 'prospective', 'database', '.', 'We', 'report', 'herein', 'that', 'the', 'cohort', 'with', 'a', 'solitary', 'node', 'involved', 'had', 'cancer', 'outcomes', 'closer', 'to', 'node-negative', 'disease', 'than', 'other', 'node-positive', 'subgroups', ',', 'and', 'suggest', 'that', 'this', 'represents', 'a', 'distinct', 'prognostic', 'subgroup', '.', 'Patients', 'and', 'Methods', 'The', 'study', 'population', 'consisted', 'of', 'all', 'patients', 'with', 'tumors', 'of', 'the', 'esophagus', 'and', 'esophagogastric', 'junction', 'who', 'underwent', 'surgical', 'resection', ',', 'either', 'alone', 'or', 'preceded', 'by', 'neoadjuvant', 'chemoradiation', ',', 'between', '1993', 'and', '2005', '.', 'Patients', 'receiving', 'multimodal', 'therapy', 'received', 'cisplatin', ',', '5-fluorouracil', ',', 'and', 'external', 'beam', 'radiotherapy', '-LRB-', '40', '--', '44', 'Gy', ',', '2', '--', '2.67', 'Gy/fraction', '-RRB-', 'as', 'previously', 'described', '.10', 'Data', 'concerning', 'the', 'clinical', 'and', 'pathologic', 'parameters', 'for', 'all', 'patients', 'was', 'obtained', 'from', 'a', 'detailed', 'prospective', 'database', 'maintained', 'by', 'a', 'full-time', 'data', 'manager', '.', 'Pathologic', 'parameters', 'analyzed', 'included', 'the', 'location', 'of', 'the', 'tumor', ',', 'tumor', 'morphology', ',', 'i.e.', ',', 'adenocarcinoma', 'or', 'squamous', 'cell', 'carcinoma', ',', 'histological', 'differentiation', '-LRB-', 'grade', '-RRB-', ',', 'TNM', 'staging', ',', 'number', 'and', 'site', 'of', 'involved', 'lymph', 'nodes', ',', 'and', 'R', 'classification', 'after', 'surgical', 'resection', '.', 'Staging', 'of', 'tumors', 'was', 'performed', 'according', 'to', 'the', 'American', 'Joint', 'Committee', 'on', 'Cancer', 'TNM', 'system', '.11', 'A', 'subtotal', 'esophagectomy', 'was', 'performed', 'with', 'a', 'sutured', 'anastomosis', 'either', 'in', 'the', 'right', 'thorax', '-LRB-', 'two-stage', '-RRB-', 'or', 'neck', '-LRB-', 'three-stage', '-RRB-', '.', 'All', 'cases', 'underwent', 'a', 'formal', 'abdominal', 'lymphadenectomy', 'and', 'mediastinal', 'lymph', 'node', 'dissection', 'up', 'to', 'and', 'including', 'the', 'subcarinal', 'nodes', '.', 'Thoracic', 'nodes', 'were', 'submitted', 'separately', 'to', 'abdominal', 'nodes', '.', 'Statistical', 'Analysis', 'Data', 'are', 'presented', 'as', 'frequencies', ',', 'means', ',', 'and', 'percentages', '.', 'ANOVA', 'was', 'used', 'for', 'comparison', 'of', 'the', 'four', 'demographic', 'groups', '.', 'Survival', 'probability', 'was', 'estimated', 'using', 'the', 'Kaplan', '--', 'Meier', 'method', '.', 'Survival', 'was', 'calculated', 'from', 'the', 'date', 'of', 'clinical', 'diagnosis', 'to', 'date', 'of', 'death', 'or', 'date', 'last', 'seen', '.', 'In', 'the', 'multivariate', 'analysis', ',', 'independent', 'prognostic', 'factors', 'for', 'survival', 'were', 'determined', 'by', 'using', 'a', 'Cox', 'regression', 'hazard', 'model', '.', 'Two', 'analyses', 'were', 'performed', ',', 'one', 'for', 'all', 'patients', 'and', 'the', 'other', 'exclusive', 'to', 'node-positive', 'patients', '.', 'All', 'statistical', 'analyses', 'were', 'performed', 'using', 'Stata', 'software', '-LRB-', 'version', '9.1', 'for', 'Windows', ',', 'Statcorp', ',', 'TX', '-RRB-', '.', 'A', 'p', 'value', '<', '0.05', 'was', 'considered', 'statistically', 'significant', '.12', 'Results', 'Patients/histology', 'Six', 'hundred', 'and', 'four', 'patients', 'underwent', 'surgery', 'for', 'localized', 'malignancy', 'of', 'the', 'esophagus', 'or', 'esophagogastric', 'junction', '.', 'The', 'mean', 'age', 'was', '62', '±', '10.4', '-LRB-', 'median', '=', '64', ',', 'range', '56', 'to', '70', '-RRB-', '.', 'Four', 'hundred', 'and', 'twelve', '-LRB-', '68', '%', '-RRB-', 'patients', 'were', 'men', '.', 'The', 'mean', 'number', 'of', 'lymph', 'nodes', 'examined', 'per', 'specimen', 'was', '12', '±', '6', '-LRB-', 'median', '=', '10', ',', 'range', '=', '6', 'to', '55', '-RRB-', '.', 'Two', 'hundred', 'and', 'eighty-nine', 'patients', '-LRB-', '48', '%', '-RRB-', 'had', 'node-negative', 'disease', '-LSB-', 'number', 'of', 'nodes', '-LRB-', 'N', '-RRB-', '=', '0', '-RSB-', ',', '84', '-LRB-', '14', '%', '-RRB-', 'had', 'one', 'node', 'positive', '-LRB-', 'N', '=', '1', '-RRB-', ',', '84', 'had', 'two', 'or', 'three', 'nodes', 'positive', ',', 'and', '147', '-LRB-', '24', '%', '-RRB-', 'had', 'greater', 'than', 'three', 'nodes', 'positive', '-LRB-', 'N', '>', '3', '-RRB-', '.', 'In', 'patients', 'with', 'one', 'involved', 'node', ',', 'in', 'all', 'cases', 'the', 'node', 'was', 'adjacent', 'to', 'the', 'tumor', ',', 'mediastinal', 'for', 'esophageal', 'tumors', ',', 'and', 'periesophageal', 'or', 'along', 'the', 'left', 'gastric', 'artery', 'for', 'junctional', 'tumors', '-LRB-', 'Tables', '1', 'and', '2', '-RRB-', '.', 'Table', '1Demographics', 'of', 'Nodal', 'SubgroupsHistologic', 'DataN', '=', '0', '-LRB-', 'n', '=', '289', '-RRB-', 'N', '=', '1', '-LRB-', 'n', '=', '84', '-RRB-', 'N', '=', '2', '--', '3', '-LRB-', 'n', '=', '84', '-RRB-', 'N', '>', '3', '-LRB-', 'n', '=', '147', '-RRB-', 'Tumor', 'site', '-LRB-', '%', '-RRB-', 'Lower', 'esophagus138', '-LRB-', '47', '-RRB-', '39', '-LRB-', '46', '-RRB-', '37', '-LRB-', '44', '-RRB-', '57', '-LRB-', '39', '-RRB-', 'EG', 'junction80', '-LRB-', '28', '-RRB-', '35', '-LRB-', '42', '-RRB-', '33', '-LRB-', '39', '-RRB-', '75', '-LRB-', '51', '-RRB-', 'Middle', 'esophagus55', '-LRB-', '19', '-RRB-', '10', '-LRB-', '12', '-RRB-', '12', '-LRB-', '14', '-RRB-', '11', '-LRB-', '7', '-RRB-', 'Upper', 'esophagus16', '-LRB-', '6', '-RRB-', '02', '-LRB-', '3', '-RRB-', '4', '-LRB-', '3', '-RRB-', 'Morphology', '-LRB-', '%', '-RRB-', 'Adenocarcinoma140', '-LRB-', '48', '-RRB-', '51', '-LRB-', '61', '-RRB-', '57', '-LRB-', '68', '-RRB-', '113', '-LRB-', '77', '-RRB-', 'Squamous', 'cell', 'carcinoma140', '-LRB-', '48', '-RRB-', '29', '-LRB-', '35', '-RRB-', '25', '-LRB-', '30', '-RRB-', '32', '-LRB-', '22', '-RRB-', 'Others9', '-LRB-', '4', '-RRB-', '4', '-LRB-', '5', '-RRB-', '2', '-LRB-', '1', '-RRB-', '2', '-LRB-', '1', '-RRB-', 'Treatment', '-LRB-', '%', '-RRB-', 'Multimodal', 'therapy129', '-LRB-', '44', '-RRB-', '28', '-LRB-', '33', '-RRB-', '24', '-LRB-', '29', '-RRB-', '21', '-LRB-', '14', '-RRB-', 'Surgery', 'alone161', '-LRB-', '56', '-RRB-', '56', '-LRB-', '76', '-RRB-', '60', '-LRB-', '71', '-RRB-', '125', '-LRB-', '86', '-RRB-', 'Residual', 'tumor', '-LRB-', '%', '-RRB-', 'R0', ':', 'no', 'residual', 'tumor250', '-LRB-', '86', '-RRB-', '71', '-LRB-', '85', '-RRB-', '64', '-LRB-', '76', '-RRB-', '108', '-LRB-', '73', '-RRB-', 'R1', ':', 'residual', 'tumor', 'found39', '-LRB-', '13', '-RRB-', '13', '-LRB-', '15', '-RRB-', '19', '-LRB-', '23', '-RRB-', '39', '-LRB-', '27', '-RRB-', 'Rx', ':', 'unknown1', '-LRB-', '1', '-RRB-', '--', '1', '-LRB-', '1', '-RRB-', '--', 'Pathological', 'stage', '-LRB-', '%', '-RRB-', 'Stage', '053', '-LRB-', '18', '-RRB-', '--', '--', '--', 'Stage', 'I59', '-LRB-', '20', '-RRB-', '1', '-LRB-', '1', '-RRB-', '--', '--', 'Stage', 'II170', '-LRB-', '59', '-RRB-', '21', '-LRB-', '25', '-RRB-', '25', '-LRB-', '30', '-RRB-', '16', '-LRB-', '11', '-RRB-', 'Stage', 'III5', '-LRB-', '2', '-RRB-', '58', '-LRB-', '29', '-RRB-', '53', '-LRB-', '63', '-RRB-', '110', '-LRB-', '76', '-RRB-', 'Stage', 'IV1', '-LRB-', '1', '-RRB-', '4', '-LRB-', '5', '-RRB-', '6', '-LRB-', '7', '-RRB-', '20', '-LRB-', '13', '-RRB-', 'pT', 'stage', '-LRB-', '%', '-RRB-', 'Tx3', '-LRB-', '1', '-RRB-', '02', '-LRB-', '3', '-RRB-', '1', '-LRB-', '0.5', '-RRB-', 'Tis12', '-LRB-', '4', '-RRB-', '000T040', '-LRB-', '14', '-RRB-', '1', '-LRB-', '1', '-RRB-', '2', '-LRB-', '3', '-RRB-', '2', '-LRB-', '1', '-RRB-', 'T156', '-LRB-', '19', '-RRB-', '5', '-LRB-', '6', '-RRB-', '4', '-LRB-', '5', '-RRB-', '3', '-LRB-', '2', '-RRB-', 'T235', '-LRB-', '12', '-RRB-', '16', '-LRB-', '19', '-RRB-', '18', '-LRB-', '21', '-RRB-', '12', '-LRB-', '8', '-RRB-', 'T3138', '-LRB-', '48', '-RRB-', '60', '-LRB-', '71', '-RRB-', '54', '-LRB-', '64', '-RRB-', '120', '-LRB-', '82', '-RRB-', 'T46', '-LRB-', '2', '-RRB-', '2', '-LRB-', '3', '-RRB-', '4', '-LRB-', '5', '-RRB-', '8', '-LRB-', '5', '-RRB-', 'EG', '=', 'esophagogastricTable', '2Histology', 'of', 'Nodal', 'SubgroupsHistologic', 'DataN', '=', '0N', '=', '1N', '=', '2', '--', '3N', '>', '3AdenoSCCAdenoSCCAdenoSCCAdenoSCCn', '=', '140N', '=', '140n', '=', '51n', '=', '29n', '=', '57n', '=', '25n', '=', '113n', '=', '32No', '%', 'No', '%', 'No', '%', 'No', '%', 'No', '%', 'No', '%', 'No', '%', 'No', '%', 'Tumor', 'siteLower', 'Esophagus64', '-LRB-', '46', '-RRB-', '66', '-LRB-', '47', '-RRB-', '19', '-LRB-', '52', '-RRB-', '15', '-LRB-', '52', '-RRB-', '23', '-LRB-', '40', '-RRB-', '13', '-LRB-', '52', '-RRB-', '39', '-LRB-', '35', '-RRB-', '17', '-LRB-', '53', '-RRB-', 'EG', 'Junction73', '-LRB-', '52', '-RRB-', '6', '-LRB-', '4', '-RRB-', '31', '-LRB-', '10', '-RRB-', '3', '-LRB-', '10', '-RRB-', '33', '-LRB-', '58', '-RRB-', '0074', '-LRB-', '65', '-RRB-', '1', '-LRB-', '3', '-RRB-', 'Middle', 'Esophagus3', '-LRB-', '2', '-RRB-', '52', '-LRB-', '37', '-RRB-', '1', '-LRB-', '28', '-RRB-', '8', '-LRB-', '28', '-RRB-', '1', '-LRB-', '2', '-RRB-', '10', '-LRB-', '40', '-RRB-', '0010', '-LRB-', '31', '-RRB-', 'Upper', 'Esophagus0016', '-LRB-', '11', '-RRB-', '0', '-LRB-', '10', '-RRB-', '3', '-LRB-', '10', '-RRB-', '002', '-LRB-', '8', '-RRB-', '004', '-LRB-', '13', '-RRB-', 'TreatmentMultimodal80', '-LRB-', '57', '-RRB-', '46', '-LRB-', '34', '-RRB-', '23', '-LRB-', '45', '-RRB-', '5', '-LRB-', '17', '-RRB-', '20', '-LRB-', '35', '-RRB-', '4', '-LRB-', '16', '-RRB-', '19', '-LRB-', '13', '-RRB-', '3', '-LRB-', '10', '-RRB-', 'Surgery', 'alone60', '-LRB-', '43', '-RRB-', '93', '-LRB-', '66', '-RRB-', '28', '-LRB-', '55', '-RRB-', '24', '-LRB-', '83', '-RRB-', '37', '-LRB-', '65', '-RRB-', '21', '-LRB-', '84', '-RRB-', '94', '-LRB-', '87', '-RRB-', '28', '-LRB-', '90', '-RRB-', 'Path', 'stageStage', '029', '-LRB-', '21', '-RRB-', '18', '-LRB-13-RRB-000000000', '000Stage', '142', '-LRB-', '30', '-RRB-', '15', '-LRB-', '10', '-RRB-', '1', '-LRB-', '2', '-RRB-', '0000000000Stage', '266', '-LRB-', '47', '-RRB-', '102', '-LRB-', '73', '-RRB-', '15', '-LRB-', '29', '-RRB-', '4', '-LRB-', '14', '-RRB-', '19', '-LRB-', '33', '-RRB-', '5', '-LRB-', '20', '-RRB-', '15', '-LRB-', '13', '-RRB-', '1', '-LRB-', '3', '-RRB-', 'Stage', '32', '-LRB-', '1', '-RRB-', '4', '-LRB-', '3', '-RRB-', '32', '-LRB-', '63', '-RRB-', '24', '-LRB-', '83', '-RRB-', '35', '-LRB-', '61', '-RRB-', '17', '-LRB-', '68', '-RRB-', '82', '-LRB-', '73', '-RRB-', '27', '-LRB-', '84', '-RRB-', 'Stage', '4001', '-LRB-', '1', '-RRB-', '3', '-LRB-', '6', '-RRB-', '1', '-LRB-', '3', '-RRB-', '3', '-LRB-', '6', '-RRB-', '3', '-LRB-', '12', '-RRB-', '16914', '-RRB-', '3', '-LRB-', '10', '-RRB-', 'Unknown1', '-LRB-', '1', '-RRB-', '0000000000001', '-LRB-', '3', '-RRB-', 'pT', 'stageTx2', '-LRB-', '1', '-RRB-', '1', '-LRB-', '1', '-RRB-', '0000002', '-LRB-', '8', '-RRB-', '001', '-LRB-', '3', '-RRB-', 'Tis9', '-LRB-', '6', '-RRB-', '0000002', '-LRB-', '4', '-RRB-', '000000T019', '-LRB-', '14', '-RRB-', '17', '-LRB-', '12', '-RRB-', '1', '-LRB-', '2', '-RRB-', '003', '-LRB-', '5', '-RRB-', '002', '-LRB-', '2', '-RRB-', '00T139', '-LRB-', '29', '-RRB-', '15', '-LRB-', '11', '-RRB-', '4', '-LRB-', '8', '-RRB-', '0014', '-LRB-', '24', '-RRB-', '003', '-LRB-', '3', '-RRB-', '00T216', '-LRB-', '11', '-RRB-', '19', '-LRB-', '14', '-RRB-', '12', '-LRB-', '23', '-RRB-', '3', '-LRB-', '10', '-RRB-', '36', '-LRB-', '63', '-RRB-', '4', '-LRB-', '16', '-RRB-', '11', '-LRB-', '10', '-RRB-', '1', '-LRB-', '3', '-RRB-', 'T353', '-LRB-', '38', '-RRB-', '84', '-LRB-', '60', '-RRB-', '33', '-LRB-', '65', '-RRB-', '26', '-LRB-', '90', '-RRB-', '2', '-LRB-', '4', '-RRB-', '17', '-LRB-', '68', '-RRB-', '91', '-LRB-', '80', '-RRB-', '28', '-LRB-', '88', '-RRB-', 'T42', '-LRB-', '1', '-RRB-', '4', '-LRB-', '2', '-RRB-', '1', '-LRB-', '2', '-RRB-', '00002', '-LRB-', '8', '-RRB-', '6', '-LRB-', '5', '-RRB-', '2', '-LRB-', '6', '-RRB-', 'Adeno', '=', 'adenocarcinoma', ',', 'SCC', '=', 'small', 'cell', 'carcinoma', ',', 'EG', '=', 'esophagogastric', 'Two', 'hundred', 'and', 'two', 'patients', '-LRB-', '33', '%', '-RRB-', 'had', 'multimodal', 'therapy', 'and', '402', 'patients', '-LRB-', '67', '%', '-RRB-', 'had', 'surgery', 'alone', '.', 'Of', 'the', 'multimodal', 'cohort', ',', '129', '-LRB-', '64', '%', '-RRB-', 'were', 'ypN0', 'on', 'histopathologic', 'assessment', ',', '28', '-LRB-', '14', '%', '-RRB-', 'had', 'one', 'node', 'positive', ',', '24', '-LRB-', '12', '%', '-RRB-', 'had', 'two', 'to', 'three', 'positive', 'nodes', ',', 'and', '21', '-LRB-', '10', '%', '-RRB-', 'had', 'greater', 'than', 'three', 'positive', 'nodes', '.', 'The', 'attainment', 'of', 'an', 'R0', 'resection', 'was', 'significantly', 'greater', 'in', 'patients', 'with', 'none', 'or', 'one', 'node', 'involved', 'compared', 'with', 'both', 'other', 'groups', '-LRB-', 'p', '<', '0.05', '-RRB-', '.', 'The', 'majority', 'of', 'patients', 'in', 'all', 'groups', 'had', 'pT3', 'tumors', ',', '48', '%', 'in', 'the', 'pN0', 'group', 'compared', 'with', '71', ',', '64', ',', 'and', '82', '%', 'in', 'the', 'N', '=', '1', ',', 'N', '=', '2', '--', '3', ',', 'and', 'N', '>', '3', 'groups', ',', 'respectively', '-LRB-', 'p', '<', '0.05', '-RRB-', '.', 'One', 'hundred', 'and', 'forty', '-LRB-', '62', '%', '-RRB-', 'of', 'the', 'squamous', 'cell', 'carcinoma', 'cohort', 'were', 'node-negative', '-LRB-', 'N', '=', '0', '-RRB-', 'compared', 'with', '140', '-LRB-', '39', '%', '-RRB-', 'of', 'cases', 'with', 'adenocarcinoma', '-LRB-', '39', '%', '-RRB-', '-LRB-', 'p', '<', '0.05', '-RRB-', '.', 'Survival', 'The', 'median', 'survival', 'for', 'all', 'patients', 'was', '20', 'months', 'at', 'a', 'median', 'follow-up', 'of', '19', 'months', '-LRB-', '3', '--', '167', '-RRB-', '.', 'Patients', 'who', 'were', 'node-negative', '-LRB-', 'N', '=', '0', '-RRB-', 'had', 'a', 'median', 'survival', 'of', '26', 'months', '-LRB-', 'Table', '3', '-RRB-', ',', 'compared', 'with', '16', 'months', 'when', 'one', 'node', 'was', 'positive', '-LRB-', 'p', '=', '0.03', '-RRB-', '.', 'Patients', 'who', 'had', 'two', 'to', 'three', 'nodes', 'positive', 'had', 'a', 'median', 'survival', 'of', '11', 'months', ',', 'and', '8', 'months', 'in', 'patients', 'who', 'had', 'greater', 'than', 'three', 'nodes', 'positive', '-LRB-', 'p', '=', '0.01', ';', 'N', '=', '2', '--', '3', 'vs', 'N', '>', '3', '-RRB-', '.', 'The', 'survival', 'of', 'patients', 'with', 'one', 'node', 'positive', '-LRB-', 'N', '=', '1', '-RRB-', 'was', 'significantly', 'greater', 'than', 'the', 'survival', 'of', 'patients', 'with', '2', '--', '3', 'nodes', 'positive', '-LRB-', 'p', '=', '0.04', '-RRB-', 'and', 'the', 'cohort', 'with', 'greater', 'than', 'three', 'involved', 'nodes', '-LRB-', 'p', '<', '0.0001', '-RRB-', '.', 'Table', '3Univariate', 'and', 'Multivariate', 'Analysis', ':', 'All', 'PatientsVariablesNo', '.', 'of', 'PatientsMedian', 'Survival', '-LRB-', 'moths', '-RRB-', 'p', 'Valuea', '-LRB-', 'Univariate', '-RRB-', 'HR95', '%', 'CIap', 'valueb', '-LRB-', 'Multivariate', '-RRB-', 'HR95', '%', 'CITreatmentSurgery', 'only401130', '.0771', '--', '--', '--', 'Multimodal203190', '.840.69', '--', '1.02', 'Tumor', 'siteUpper', 'esophagus25160', '.3711', '--', '--', '--', 'Middle', 'esophagus87140', '.9460.980.58', '--', '1.66', 'Lower', 'esophagus268140', '.6581.160.69', '--', '1.81', 'EG', 'junction224140', '.6241.130.69', '--', '1.84', 'Depth', 'of', 'invasionT05755', '<', '0.00110.6521', 'T168260', '.5371.160.73', '--', '1.830.4720.710.21', '--', '2.3', 'T281260', '.4191.200.77', '--', '1.850.5731.110.31', '--', '3.94', 'T337311', '<', '0.0012.281.60', '--', '3.260.8711.400.79', '--', '2.41', 'T4197', '<', '0.0014.342.46', '--', '7.680.6492.591.42', '--', '4.08', 'No', '.', 'of', 'nodes028926', '<', '0.0011', '<', '0.00110.63', '--', '1.87184160.0381.361.02', '--', '1.820.7741.080.83', '--', '2.432', '--', '38411', '<', '0.0011.911.45', '--', '2.520.2021.421.07', '--', '3.18', '>', '31478', '<', '0.0012.612.08', '--', '3.290.0271.84', 'HistologySquamous361140', '.9161', 'Adenocarcinoma224130', '.5961.050.87', '--', '1.28', '--', '--', '--', 'Other19260', '.4830.800.44', '--', '1.48', 'Stage05355', '<', '0.00110.1181', 'I63550', '.7470.920.56', '--', '1.510.5760.680.18', '--', '2.59', 'II230200', '.0371.491.02', '--', '2.170.5081.550.42', '--', '4.69', 'III22510', '<', '0.0012.711.86', '--', '3.950.5271.680.34', '--', '5.58', 'IV316', '<', '0.0016.163.72', '--', '10.20.1823.141.14', '--', '7.76', 'Residual', 'tumorR049217', '<', '0.00110.0521', 'R111081', '.701.37', '--', '2.121.250.99', '--', '1.58', 'aχ2bCox', 'regressionHR', '=', 'hazard', 'ratio', ',', 'CI', '=', '95', '%', 'confidence', 'intervals', ',', 'EG', '=', 'esophagogastric', 'The', '1', '-', ',', '3', '-', ',', 'and', '5-year', 'survival', 'of', 'the', 'pN0', 'group', 'was', '78', ',', '51', ',', 'and', '44', '%', ',', 'respectively', '-LRB-', 'Fig.', '1', '-RRB-', '.', 'Where', 'one', 'node', 'was', 'involved', ',', 'survival', 'was', '67', ',', '41', ',', 'and', '35', '%', ',', 'respectively', '.', 'Where', 'two', 'to', 'three', 'nodes', 'were', 'involved', ',', 'the', '1', '-', ',', '3', '-', ',', 'and', '5-year', 'survival', 'was', '57', ',', '25', ',', 'and', '13', '%', ',', 'respectively', ',', 'and', 'where', 'greater', 'than', 'three', 'nodes', 'were', 'involved', ',', 'this', 'was', '40', ',', '14', ',', 'and', '8', '%', ',', 'respectively', '.', 'Figure', '1Overall', 'survival', 'by', 'number', 'of', 'nodes', 'positive', '.', 'Univariate', 'analysis', '-LRB-', 'Table', '3', '-RRB-', 'revealed', 'nodal', 'status', ',', 'pT', 'stage', ',', 'pathologic', 'stage', ',', 'and', 'R', 'status', 'as', 'predictors', 'of', 'survival', '.', 'Multivariate', 'analysis', 'revealed', 'nodal', 'status', 'alone', 'to', 'significantly', '-LRB-', 'p', '<', '0.0001', '-RRB-', 'impact', 'on', 'survival', '.', 'By', 'this', 'analysis', 'the', 'hazards', 'ratio', 'increased', 'from', '1.08', 'for', 'one', 'involved', 'node', 'to', '1.42', 'for', 'two', 'to', 'three', 'involved', 'nodes', ',', 'and', '1.84', 'for', 'greater', 'than', 'three', 'nodes', '.', 'Excluding', 'node-negative', 'patients', ',', 'univariate', 'analysis', '-LRB-', 'Table', '4', '-RRB-', 'revealed', 'pT', 'stage', ',', 'pathologic', 'stage', ',', 'R', 'status', ',', 'and', 'number', 'of', 'nodes', 'as', 'predictive', 'of', 'survival', '.', 'By', 'multivariate', 'analysis', '-LRB-', 'Table', '5', '-RRB-', ',', 'pathologic', 'stage', '-LRB-', 'p', '=', '0.010', '-RRB-', 'and', 'number', 'of', 'nodes', 'were', 'significant', 'determinants', 'of', 'survival', '.', 'Compared', 'with', 'the', 'cohort', 'with', 'one', 'involved', 'node', ',', 'the', 'hazard', 'ratio', 'for', 'two', 'to', 'three', 'nodes', 'was', '1.56', '-LRB-', 'p', '=', '0.049', '-RRB-', 'and', '2.06', '-LRB-', 'p', '=', '0.007', '-RRB-', 'for', 'greater', 'than', 'three', 'nodes', '.', 'Table', '4Univariate', 'Analysis', ':', 'Node-positive', 'AloneVariablesNo', '.', 'of', 'PatientsMedian', 'Survival', '-LRB-', 'moths', '-RRB-', 'p', 'valuea', '-LRB-', 'Univariate', '-RRB-', 'HR95', '%', 'CITreatmentSurgery', 'only241110', '.23410.63', '--', '1.11', 'Multimodal74110', '.84', 'Tumor', 'siteUpper', 'esophagus9180', '.6501', 'Middle', 'esophagus32100', '.5561.310.54', '--', '3.18', 'Lower', 'esophagus130100', '.1831.750.77', '--', '3.98', 'OG', 'junction144120', '.3501.480.65', '--', '3.36', 'Depth', 'of', 'invasionT05110', '.0011', 'T11280', '.9171.060.33', '--', '3.41', 'T246240', '.1761.120.43', '--', '1.78', 'T3235110', '.7571.430.74', '--', '2.14', 'T41450', '.1572.230.74', '--', '6.78', 'HistologySquamous86110', '.6381', 'Adenocarcinoma221110', '.6381.070.81', '--', '1.40', 'Other830', '.8481.070.49', '--', '2.35', 'Stage1', '--', 'II6319', '<', '0.0011', 'III', '--', 'IV251102', '.011.43', '--', '2.83', 'Residual', 'tumorR0259120', '.0351', 'R16191', '.331.02', '--', '1.73', 'No', '.', 'of', 'nodes18417', '<', '0.00112', '--', '384130.0211.671.06', '--', '2.29', '>', '31479', '<', '0.0012.531.50', '--', '3.62', 'aχ2HR', '=', 'hazard', 'ratio', ',', 'CI', '=', '95', '%', 'confidence', 'intervalsTable', '5Mutivariate', 'Analysis', ':', 'Node-positive', 'OnlyVariablesp', 'valuea', '-LRB-', 'Multivariate', '-RRB-', 'HR95', '%', 'CIDepth', 'of', 'invasionT01T10', '.5440.820.31', '--', '1.75', 'T20', '.6791.230.74', '--', '1.81', 'T30', '.3131.490.99', '--', '2.21', 'T40', '.2021.831.39', '--', '3.24', 'StageI', '--', 'II0', '.0101', 'III', '--', 'IV1', '.590.82', '--', '3.06', 'No', '.', 'of', 'nodes112', '--', '30.0491.561.21', '--', '2.35', '>', '30.0072.061.51', '--', '2.82', 'Residual', 'tumorR00', '.2831', 'R11', '.220.80', '--', '1.79', 'aCox', 'regressionHR', '=', 'hazard', 'ratio', ',', 'CI', '=', '95', '%', 'confidence', 'intervals', 'Discussion', 'Cancers', 'of', 'the', 'esophagus', 'and', 'esophagogastric', 'junction', 'are', 'aggressive', 'tumors', ',', 'which', 'are', 'typically', 'diagnosed', 'at', 'an', 'advanced', 'stage', 'of', 'disease', 'progression', '.13', 'This', 'large', 'retrospective', 'review', 'of', 'a', 'tertiary', 'center', "'s", 'experiences', 'over', '12', 'years', 'highlights', 'the', 'importance', 'of', 'lymph', 'node', 'involvement', 'in', 'the', 'prognosis', 'of', 'these', 'tumors', '.', 'The', 'study', 'shows', 'that', 'the', 'presence', 'of', 'a', 'solitary', 'node', ',', 'although', 'a', 'significantly', 'negative', 'factor', 'compared', 'with', 'pN0', 'disease', ',', 'is', 'associated', 'with', 'significantly', 'improved', 'median', 'and', '1', '-', ',', '3', '-', ',', 'and', '5-year', 'survival', 'compared', 'with', 'cohorts', 'of', 'patients', 'with', 'greater', 'nodal', 'involvement', '.', 'The', '5-year', 'survival', ',', 'for', 'instance', ',', 'was', '35', '%', 'compared', 'with', '13', 'and', '8', '%', ',', 'respectively', ',', 'for', 'cohorts', 'with', 'two', 'to', 'three', 'positive', 'nodes', 'and', 'greater', 'than', 'three', 'positive', 'nodes', '.', 'There', 'is', 'no', 'uniform', 'consensus', 'on', 'the', 'number', 'of', 'lymph', 'nodes', 'that', 'must', 'be', 'sampled', '.', 'In', 'a', 'study', 'by', 'Ito', 'et', 'al.', ',3', 'the', 'median', 'number', 'of', 'lymph', 'nodes', 'examined', 'per', 'specimen', 'was', '6', '-LRB-', 'range', '0', 'to', '35', '-RRB-', 'and', 'only', '20', '%', 'of', 'patients', 'had', 'at', 'least', '15', 'lymph', 'nodes', 'examined', '.', 'In', 'this', 'study', ',', 'the', 'median', 'number', 'of', 'lymph', 'nodes', 'examined', 'per', 'specimen', 'was', '12', '-LRB-', 'range', '6', 'to', '55', '-RRB-', ',', 'and', '24', '%', 'of', 'the', 'patients', 'had', 'at', 'least', '15', 'lymph', 'nodes', 'examined', '.', 'These', 'results', 'appear', 'consistent', 'with', 'practice', 'in', 'the', 'United', 'States', 'where', 'an', 'analysis', 'of', 'the', 'National', 'Cancer', 'Database', 'indicated', 'that', 'only', '18', '%', 'of', 'patients', 'undergoing', 'surgery', 'for', 'gastric', 'cancer', 'have', 'more', 'than', '15', 'lymph', 'nodes', 'analyzed', '.14', 'In', 'this', 'Unit', ',', 'lymph', 'node', 'clearance', 'involves', 'a', 'D2', 'dissection', 'of', 'abdominal', 'nodes', ',', 'and', 'wide', 'mediastinal', 'clearance', 'to', 'the', 'carina', 'and', 'paratracheal', 'node', 'dissection', 'if', 'they', 'appear', 'involved', '.', 'No', 'cervical', 'dissection', 'is', 'performed', ',', 'consistent', 'with', 'recommendations', 'from', 'another', 'group', '.15', 'It', 'is', 'acknowledged', 'that', 'variation', 'in', 'lymph', 'node', 'yield', 'may', 'mask', 'stage', 'migration', ',', 'particularly', 'in', 'a', 'retrospective', 'analysis', ',', 'but', 'the', 'standardization', 'of', 'lymphadenectomy', 'is', 'likely', 'to', 'minimize', 'the', 'impact', 'of', 'this', 'potential', 'bias', '.', 'The', 'association', 'between', 'extent', 'of', 'nodal', 'involvement', 'and', 'outcome', 'is', 'well', 'described', '.16', '--', '18', 'No', 'study', 'to', 'our', 'knowledge', 'has', 'previously', 'focused', 'on', 'the', 'impact', 'of', 'one', 'positive', 'node', 'on', 'outcome', 'in', 'esophageal', 'cancer', '.', 'The', 'observation', ',', 'however', ',', 'of', 'the', 'unique', 'prognostic', 'significance', 'of', 'a', 'solitary', 'involved', 'node', 'was', 'recently', 'reported', '.19', 'In', 'a', 'study', 'of', '187', 'patients', 'with', 'esophageal', 'adenocarcinoma', 'treated', 'with', 'neoadjuvant', 'chemoradiotherapy', ',', 'Gu', 'et', 'al.', '19', 'at', 'the', 'MD', 'Anderson', 'observed', 'from', 'their', 'analysis', 'that', 'patients', 'with', 'a', 'solitary', 'involved', 'node', 'had', 'better', 'overall', 'and', 'relapse-free', 'survival', 'compared', 'with', 'other', 'nodal', 'groups', '.', 'Moreover', ',', 'the', '5-year', 'survival', 'outcomes', 'and', '2-year', 'relapse-free', 'survival', 'was', 'not', 'significantly', 'different', 'from', 'the', 'node-negative', 'cohort', '.', 'Although', 'in', 'our', 'series', 'survival', 'figures', 'were', 'better', 'for', 'node-negative', 'patients', 'than', 'patients', 'with', 'a', 'solitary', 'involved', 'node', ',', 'the', 'overall', 'pattern', 'of', 'outcome', 'data', 'in', 'our', 'series', 'is', 'consistent', 'with', 'the', 'report', 'from', 'the', 'Anderson', 'group', ',', 'with', 'prognosis', 'in', 'this', 'cohort', 'closer', 'to', 'node-negative', 'than', 'other', 'node-positive', 'subgroups', '.', 'The', 'clinical', 'implication', 'of', 'this', 'finding', 'is', 'not', 'clear', 'at', 'this', 'time', ',', 'but', 'it', 'should', ',', 'at', 'minimum', ',', 'encourage', 'a', 'more', 'optimistic', 'view', 'of', 'patients', 'who', 'have', 'a', 'solitary', 'lymph', 'node', 'identified', 'after', 'adequate', 'lymphadenectomy', ',', 'as', 'approximately', '35', '%', 'of', 'patients', 'with', 'this', 'pathologic', 'stage', 'may', 'be', 'cured', '.', 'In', 'the', 'future', ',', 'it', 'is', 'possible', 'that', 'advances', 'in', 'endoscopic', 'US', 'staging', ',', 'fluorodeoxyglucose', 'PET', ',', 'and', 'sentinel', 'node', 'assessment', 'may', 'improve', 'pre', '-', 'and', 'intraoperative', 'assessment', 'of', 'nodal', 'involvement', ',', 'defining', 'node-negative', ',', 'solitary', 'involved', 'node', 'and', 'micrometastatic-involved', 'subgroups', ',', 'and', 'selective', 'lymphadenectomy', 'and', 'minimally', 'invasive', 'approaches', 'may', 'be', 'evaluated', 'in', 'these', 'situations', '.', 'This', 'demands', 'prospective', 'evaluation', ',', 'but', 'it', 'may', 'be', 'noteworthy', 'that', 'all', 'involved', 'nodes', 'in', 'the', 'solitary', 'involved', 'node', 'cohort', 'were', 'close', 'to', 'the', 'primary', 'site', 'and', 'may', 'possibly', 'have', 'been', 'identified', 'as', 'sentinel', 'nodes', '.', 'In', 'conclusion', ',', 'this', 'study', 'shows', 'that', 'in', 'a', 'large', 'cohort', 'of', 'patients', ',', 'lymph', 'node', 'status', 'and', 'the', 'number', 'of', 'lymph', 'nodes', 'positive', 'at', 'the', 'time', 'of', 'surgical', 'resection', 'is', 'directly', 'linked', 'to', 'survival', '.', 'Extensive', 'nodal', 'involvement', 'is', 'confirmed', 'as', 'carrying', 'a', 'dismal', 'prognosis', ',', 'but', 'greater', 'optimism', 'is', 'justified', 'where', 'a', 'solitary', 'involved', 'lymph', 'gland', 'defines', 'the', 'pN', 'stage', 'after', 'an', 'adequate', 'lymphadenectomy', '.']
Document BIO Tags: ['O', 'O', 'O', 'O', 'B', 'I', 'O', 'O', 'O', 'O', 'O', 'O', 'O', 'O', 'O', 'O', 'O', 'O', 'O', 'O', 'O', 'O', 'O', 'O', 'O', 'O', 'O', 'O', 'O', 'O', 'O', 'O', 'O', 'O', 'O', 'O', 'O', 'O', 'O', 'O', 'O', 'O', 'O', 'O', 'O', 'O', 'O', 'O', 'O', 'O', 'O', 'O', 'O', 'O', 'O', 'O', 'O', 'O', 'O', 'O', 'O', 'O', 'O', 'O', 'O', 'O', 'O', 'O', 'O', 'O', 'O', 'O', 'O', 'O', 'O', 'O', 'O', 'O', 'O', 'O', 'O', 'O', 'O', 'O', 'O', 'O', 'B', 'I', 'O', 'O', 'O', 'O', 'O', 'O', 'O', 'O', 'O', 'O', 'O', 'O', 'O', 'O', 'O', 'O', 'O', 'O', 'O', 'O', 'O', 'O', 'O', 'O', 'O', 'O', 'O', 'O', 'O', 'O', 'O', 'O', 'O', 'O', 'O', 'B', 'O', 'O', 'O', 'O', 'O', 'O', 'O', 'O', 'O', 'O', 'O', 'O', 'O', 'O', 'O', 'O', 'O', 'O', 'O', 'O', 'O', 'O', 'B', 'O', 'O', 'O', 'O', 'O', 'O', 'O', 'O', 'O', 'O', 'O', 'O', 'O', 'O', 'O', 'O', 'O', 'O', 'O', 'O', 'O', 'O', 'O', 'B', 'O', 'O', 'O', 'O', 'O', 'O', 'O', 'B', 'O', 'O', 'O', 'O', 'O', 'O', 'O', 'O', 'O', 'O', 'O', 'O', 'O', 'O', 'O', 'O', 'O', 'O', 'O', 'O', 'O', 'B', 'O', 'O', 'O', 'O', 'O', 'O', 'O', 'O', 'O', 'O', 'O', 'O', 'O', 'O', 'O', 'O', 'O', 'O', 'O', 'O', 'O', 'O', 'B', 'O', 'O', 'O', 'O', 'O', 'O', 'O', 'O', 'O', 'O', 'O', 'O', 'O', 'O', 'O', 'O', 'O', 'O', 'O', 'O', 'O', 'O', 'O', 'O', 'O', 'O', 'O', 'O', 'O', 'O', 'O', 'O', 'O', 'O', 'O', 'O', 'O', 'O', 'B', 'I', 'O', 'O', 'O', 'O', 'O', 'B', 'O', 'O', 'O', 'O', 'O', 'O', 'O', 'O', 'O', 'O', 'O', 'O', 'O', 'B', 'O', 'O', 'O', 'O', 'O', 'O', 'O', 'O', 'O', 'O', 'O', 'O', 'O', 'O', 'O', 'O', 'O', 'O', 'O', 'O', 'O', 'O', 'O', 'O', 'O', 'O', 'O', 'O', 'O', 'O', 'O', 'O', 'O', 'O', 'O', 'O', 'O', 'O', 'O', 'O', 'O', 'O', 'O', 'O', 'O', 'O', 'O', 'O', 'O', 'O', 'O', 'O', 'O', 'O', 'B', 'O', 'O', 'O', 'O', 'O', 'B', 'O', 'O', 'O', 'O', 'O', 'O', 'O', 'O', 'O', 'O', 'O', 'O', 'O', 'O', 'B', 'I', 'O', 'O', 'O', 'O', 'O', 'O', 'O', 'O', 'O', 'O', 'O', 'O', 'O', 'O', 'O', 'O', 'O', 'O', 'O', 'O', 'O', 'O', 'O', 'O', 'O', 'O', 'O', 'O', 'O', 'O', 'O', 'O', 'O', 'O', 'O', 'O', 'O', 'O', 'O', 'O', 'O', 'O', 'O', 'O', 'O', 'B', 'I', 'O', 'O', 'O', 'O', 'O', 'O', 'O', 'O', 'O', 'B', 'O', 'O', 'O', 'O', 'O', 'O', 'O', 'O', 'O', 'B', 'O', 'O', 'O', 'O', 'O', 'O', 'O', 'O', 'O', 'O', 'O', 'O', 'O', 'O', 'O', 'O', 'O', 'O', 'O', 'O', 'O', 'O', 'O', 'O', 'O', 'O', 'O', 'O', 'O', 'O', 'O', 'O', 'O', 'O', 'O', 'O', 'O', 'O', 'O', 'O', 'O', 'O', 'O', 'O', 'O', 'O', 'O', 'O', 'O', 'O', 'O', 'O', 'O', 'O', 'O', 'O', 'O', 'O', 'O', 'O', 'O', 'O', 'O', 'O', 'O', 'O', 'O', 'O', 'O', 'O', 'O', 'O', 'O', 'O', 'O', 'O', 'O', 'O', 'O', 'O', 'O', 'O', 'O', 'O', 'O', 'O', 'O', 'O', 'O', 'O', 'O', 'O', 'O', 'O', 'O', 'O', 'O', 'O', 'O', 'O', 'O', 'O', 'O', 'O', 'O', 'O', 'O', 'O', 'O', 'O', 'O', 'O', 'O', 'O', 'O', 'O', 'O', 'O', 'O', 'O', 'O', 'O', 'O', 'O', 'O', 'O', 'O', 'O', 'O', 'O', 'O', 'O', 'O', 'O', 'O', 'O', 'O', 'O', 'O', 'O', 'O', 'O', 'O', 'O', 'O', 'O', 'O', 'O', 'O', 'O', 'O', 'O', 'O', 'O', 'O', 'O', 'O', 'O', 'O', 'O', 'O', 'O', 'O', 'O', 'O', 'O', 'O', 'O', 'O', 'O', 'O', 'O', 'O', 'O', 'O', 'O', 'O', 'O', 'O', 'O', 'O', 'O', 'O', 'O', 'O', 'O', 'O', 'O', 'O', 'O', 'O', 'O', 'O', 'O', 'O', 'O', 'O', 'O', 'O', 'O', 'O', 'O', 'O', 'O', 'O', 'O', 'O', 'O', 'O', 'O', 'O', 'O', 'O', 'O', 'O', 'O', 'O', 'O', 'O', 'O', 'O', 'B', 'O', 'O', 'O', 'O', 'O', 'O', 'O', 'O', 'O', 'O', 'O', 'O', 'O', 'O', 'O', 'O', 'O', 'O', 'O', 'O', 'O', 'O', 'O', 'O', 'O', 'O', 'B', 'O', 'O', 'B', 'I', 'O', 'O', 'O', 'O', 'O', 'O', 'O', 'O', 'O', 'O', 'O', 'O', 'O', 'O', 'O', 'O', 'O', 'O', 'O', 'O', 'O', 'O', 'O', 'O', 'O', 'O', 'O', 'O', 'O', 'O', 'O', 'O', 'O', 'O', 'O', 'O', 'O', 'O', 'O', 'O', 'O', 'O', 'B', 'O', 'O', 'O', 'O', 'O', 'O', 'O', 'O', 'O', 'O', 'B', 'O', 'O', 'O', 'O', 'O', 'O', 'O', 'O', 'O', 'O', 'O', 'O', 'O', 'O', 'O', 'O', 'O', 'O', 'O', 'O', 'O', 'O', 'O', 'O', 'O', 'O', 'B', 'O', 'O', 'O', 'O', 'O', 'O', 'O', 'O', 'O', 'O', 'O', 'O', 'O', 'O', 'O', 'O', 'O', 'O', 'O', 'O', 'O', 'O', 'O', 'O', 'O', 'O', 'O', 'O', 'O', 'O', 'O', 'O', 'O', 'O', 'O', 'O', 'O', 'O', 'O', 'O', 'O', 'O', 'O', 'O', 'O', 'O', 'O', 'O', 'O', 'O', 'O', 'O', 'O', 'O', 'O', 'O', 'O', 'O', 'O', 'O', 'O', 'O', 'O', 'O', 'O', 'O', 'O', 'O', 'O', 'O', 'O', 'O', 'O', 'O', 'O', 'O', 'O', 'O', 'O', 'O', 'O', 'O', 'O', 'O', 'O', 'O', 'O', 'O', 'O', 'O', 'O', 'O', 'O', 'O', 'O', 'O', 'O', 'O', 'O', 'O', 'O', 'O', 'O', 'O', 'O', 'O', 'O', 'O', 'O', 'O', 'O', 'O', 'O', 'O', 'O', 'O', 'O', 'O', 'O', 'O', 'O', 'O', 'O', 'O', 'O', 'O', 'O', 'O', 'O', 'O', 'O', 'O', 'O', 'O', 'O', 'O', 'O', 'O', 'O', 'O', 'O', 'O', 'O', 'O', 'O', 'O', 'O', 'O', 'O', 'O', 'O', 'O', 'O', 'O', 'O', 'O', 'O', 'O', 'O', 'O', 'O', 'O', 'O', 'O', 'O', 'O', 'O', 'O', 'O', 'O', 'O', 'O', 'O', 'O', 'O', 'O', 'O', 'O', 'O', 'O', 'O', 'O', 'O', 'O', 'O', 'O', 'O', 'O', 'O', 'O', 'O', 'O', 'O', 'O', 'O', 'O', 'O', 'O', 'O', 'O', 'O', 'O', 'O', 'O', 'O', 'O', 'O', 'O', 'O', 'O', 'O', 'O', 'O', 'O', 'O', 'O', 'O', 'O', 'O', 'O', 'O', 'O', 'O', 'O', 'O', 'O', 'O', 'O', 'O', 'O', 'O', 'O', 'O', 'O', 'O', 'O', 'O', 'O', 'O', 'O', 'O', 'O', 'O', 'O', 'O', 'O', 'O', 'O', 'O', 'O', 'O', 'O', 'O', 'O', 'O', 'O', 'O', 'O', 'O', 'O', 'O', 'O', 'O', 'O', 'O', 'O', 'O', 'O', 'O', 'O', 'O', 'O', 'O', 'O', 'O', 'O', 'O', 'O', 'O', 'O', 'O', 'O', 'O', 'O', 'O', 'O', 'O', 'O', 'O', 'O', 'O', 'O', 'O', 'O', 'O', 'O', 'O', 'O', 'O', 'O', 'O', 'O', 'O', 'O', 'O', 'O', 'O', 'O', 'O', 'O', 'O', 'O', 'O', 'O', 'O', 'O', 'O', 'O', 'O', 'O', 'O', 'O', 'O', 'O', 'O', 'O', 'O', 'O', 'O', 'O', 'O', 'O', 'O', 'O', 'O', 'O', 'O', 'O', 'O', 'O', 'O', 'O', 'O', 'O', 'O', 'O', 'O', 'O', 'O', 'O', 'O', 'O', 'O', 'O', 'O', 'O', 'O', 'O', 'O', 'O', 'O', 'O', 'O', 'O', 'O', 'O', 'O', 'O', 'O', 'O', 'O', 'O', 'O', 'O', 'O', 'O', 'O', 'O', 'O', 'O', 'O', 'O', 'O', 'O', 'O', 'O', 'O', 'O', 'O', 'O', 'O', 'O', 'O', 'O', 'O', 'O', 'O', 'O', 'O', 'O', 'O', 'O', 'O', 'O', 'O', 'O', 'O', 'O', 'O', 'O', 'O', 'O', 'O', 'O', 'O', 'O', 'O', 'O', 'O', 'O', 'O', 'O', 'O', 'O', 'O', 'O', 'O', 'O', 'O', 'O', 'O', 'O', 'O', 'O', 'O', 'O', 'O', 'O', 'O', 'O', 'O', 'O', 'O', 'O', 'O', 'O', 'O', 'O', 'O', 'O', 'O', 'O', 'O', 'O', 'O', 'O', 'O', 'O', 'O', 'O', 'O', 'O', 'O', 'O', 'O', 'O', 'O', 'O', 'O', 'O', 'O', 'O', 'O', 'O', 'O', 'O', 'O', 'O', 'O', 'O', 'O', 'O', 'O', 'O', 'O', 'O', 'O', 'O', 'O', 'O', 'O', 'O', 'O', 'O', 'O', 'O', 'O', 'O', 'O', 'O', 'O', 'O', 'O', 'O', 'O', 'O', 'O', 'O', 'O', 'O', 'O', 'O', 'O', 'O', 'O', 'O', 'O', 'O', 'O', 'O', 'O', 'O', 'O', 'O', 'O', 'O', 'O', 'O', 'O', 'O', 'O', 'O', 'O', 'O', 'O', 'O', 'O', 'O', 'O', 'O', 'O', 'O', 'O', 'O', 'O', 'O', 'O', 'O', 'O', 'O', 'O', 'O', 'O', 'O', 'O', 'O', 'O', 'O', 'O', 'O', 'O', 'O', 'O', 'O', 'O', 'O', 'O', 'O', 'O', 'O', 'O', 'O', 'O', 'O', 'O', 'O', 'O', 'O', 'O', 'O', 'O', 'O', 'O', 'O', 'O', 'O', 'O', 'O', 'O', 'O', 'O', 'O', 'O', 'O', 'O', 'O', 'O', 'O', 'O', 'O', 'O', 'O', 'O', 'O', 'O', 'O', 'O', 'O', 'O', 'O', 'O', 'O', 'O', 'O', 'O', 'O', 'O', 'O', 'O', 'O', 'O', 'O', 'O', 'O', 'O', 'O', 'O', 'O', 'O', 'O', 'O', 'O', 'O', 'O', 'O', 'O', 'O', 'O', 'O', 'O', 'O', 'O', 'O', 'O', 'O', 'O', 'O', 'O', 'O', 'O', 'O', 'O', 'O', 'O', 'O', 'O', 'O', 'O', 'O', 'O', 'O', 'O', 'O', 'O', 'O', 'O', 'O', 'O', 'O', 'O', 'O', 'O', 'O', 'O', 'O', 'O', 'O', 'O', 'O', 'O', 'O', 'O', 'O', 'O', 'O', 'O', 'O', 'O', 'O', 'O', 'O', 'O', 'O', 'O', 'O', 'O', 'O', 'O', 'O', 'O', 'O', 'O', 'O', 'O', 'O', 'O', 'O', 'O', 'O', 'O', 'O', 'O', 'O', 'O', 'O', 'O', 'O', 'O', 'O', 'O', 'O', 'O', 'O', 'O', 'O', 'O', 'O', 'O', 'O', 'O', 'O', 'O', 'O', 'O', 'O', 'O', 'O', 'O', 'O', 'O', 'O', 'O', 'O', 'O', 'O', 'O', 'O', 'O', 'O', 'O', 'O', 'O', 'O', 'O', 'O', 'O', 'O', 'O', 'O', 'O', 'O', 'O', 'O', 'O', 'O', 'O', 'O', 'O', 'O', 'O', 'O', 'O', 'O', 'O', 'O', 'O', 'O', 'O', 'O', 'O', 'O', 'O', 'O', 'O', 'O', 'O', 'O', 'O', 'O', 'O', 'O', 'O', 'O', 'O', 'O', 'O', 'O', 'O', 'O', 'O', 'O', 'O', 'O', 'O', 'O', 'O', 'O', 'O', 'O', 'O', 'O', 'O', 'O', 'O', 'O', 'O', 'O', 'O', 'O', 'O', 'O', 'O', 'O', 'O', 'O', 'O', 'O', 'O', 'O', 'O', 'O', 'O', 'O', 'O', 'O', 'O', 'O', 'O', 'O', 'O', 'O', 'O', 'O', 'O', 'O', 'O', 'O', 'O', 'O', 'O', 'O', 'O', 'O', 'O', 'O', 'O', 'O', 'O', 'O', 'O', 'O', 'O', 'O', 'O', 'O', 'O', 'O', 'O', 'O', 'O', 'O', 'O', 'O', 'O', 'O', 'O', 'O', 'O', 'O', 'O', 'O', 'O', 'O', 'O', 'O', 'O', 'O', 'O', 'O', 'O', 'O', 'O', 'O', 'O', 'O', 'O', 'O', 'O', 'O', 'O', 'O', 'O', 'O', 'O', 'O', 'O', 'O', 'O', 'O', 'O', 'O', 'O', 'O', 'O', 'O', 'O', 'O', 'O', 'O', 'O', 'O', 'O', 'O', 'O', 'O', 'O', 'O', 'O', 'O', 'O', 'O', 'O', 'O', 'O', 'O', 'O', 'O', 'O', 'O', 'O', 'O', 'O', 'O', 'O', 'O', 'O', 'O', 'O', 'O', 'O', 'O', 'O', 'O', 'O', 'O', 'O', 'O', 'O', 'O', 'O', 'O', 'O', 'O', 'O', 'O', 'O', 'O', 'O', 'O', 'O', 'O', 'O', 'O', 'O', 'O', 'O', 'O', 'O', 'O', 'O', 'O', 'O', 'O', 'O', 'O', 'O', 'O', 'O', 'O', 'O', 'O', 'O', 'O', 'O', 'O', 'O', 'O', 'O', 'O', 'O', 'O', 'O', 'O', 'O', 'O', 'O', 'O', 'O', 'O', 'O', 'O', 'O', 'O', 'O', 'O', 'O', 'O', 'O', 'O', 'O', 'O', 'O', 'O', 'O', 'O', 'O', 'O', 'O', 'O', 'O', 'O', 'O', 'O', 'O', 'O', 'O', 'O', 'O', 'O', 'O', 'O', 'O', 'O', 'O', 'O', 'O', 'O', 'O', 'O', 'O', 'O', 'O', 'O', 'O', 'O', 'O', 'O', 'O', 'O', 'O', 'O', 'O', 'O', 'O', 'O', 'O', 'O', 'O', 'O', 'O', 'O', 'O', 'O', 'O', 'O', 'O', 'O', 'O', 'O', 'O', 'O', 'O', 'O', 'O', 'O', 'O', 'O', 'O', 'O', 'O', 'O', 'O', 'O', 'O', 'O', 'O', 'O', 'O', 'O', 'O', 'O', 'O', 'O', 'O', 'O', 'O', 'O', 'O', 'O', 'O', 'O', 'O', 'O', 'O', 'O', 'O', 'O', 'O', 'O', 'O', 'O', 'O', 'O', 'O', 'O', 'O', 'O', 'O', 'O', 'O', 'O', 'O', 'O', 'O', 'O', 'O', 'O', 'O', 'O', 'O', 'O', 'O', 'O', 'O', 'O', 'O', 'O', 'O', 'O', 'O', 'O', 'O', 'O', 'O', 'O', 'O', 'O', 'O', 'O', 'O', 'O', 'O', 'O', 'O', 'O', 'O', 'O', 'O', 'O', 'O', 'O', 'O', 'O', 'O', 'O', 'O', 'O', 'O', 'O', 'O', 'O', 'O', 'O', 'O', 'O', 'O', 'O', 'O', 'O', 'O', 'O', 'O', 'O', 'O', 'O', 'O', 'O', 'O', 'O', 'O', 'O', 'O', 'O', 'O', 'O', 'O', 'O', 'O', 'O', 'O', 'O', 'O', 'O', 'O', 'O', 'O', 'O', 'O', 'O', 'O', 'O', 'O', 'O', 'O', 'O', 'O', 'O', 'O', 'O', 'O', 'O', 'O', 'O', 'O', 'O', 'O', 'O', 'O', 'O', 'O', 'O', 'O', 'O', 'O', 'O', 'O', 'O', 'O', 'O', 'O', 'O', 'O', 'O', 'O', 'O', 'O', 'O', 'O', 'O', 'O', 'O', 'O', 'O', 'O', 'O', 'O', 'O', 'O', 'O', 'O', 'O', 'O', 'O', 'O', 'O', 'O', 'O', 'O', 'O', 'O', 'O', 'O', 'O', 'O', 'O', 'O', 'O', 'O', 'O', 'O', 'O', 'O', 'O', 'O', 'O', 'O', 'O', 'O', 'O', 'O', 'O', 'O', 'O', 'O', 'O', 'O', 'O', 'O', 'O', 'O', 'O', 'O', 'O', 'O', 'O', 'O', 'O', 'O', 'O', 'O', 'O', 'O', 'O', 'O', 'O', 'O', 'O', 'O', 'O', 'O', 'O', 'O', 'O', 'O', 'O', 'O', 'O', 'O', 'O', 'O', 'O', 'O', 'O', 'O', 'O', 'O', 'O', 'O', 'O', 'O', 'O', 'O', 'O', 'O', 'O', 'O', 'O', 'O', 'O', 'O', 'O', 'O', 'O', 'O', 'O', 'O', 'O', 'O', 'O', 'O', 'O', 'O', 'O', 'O', 'O', 'O', 'O', 'O', 'B', 'O', 'O', 'B', 'O', 'O', 'O', 'O', 'O', 'O', 'O', 'O', 'O', 'O', 'O', 'O', 'O', 'O', 'O', 'O', 'O', 'O', 'O', 'O', 'O', 'O', 'O', 'O', 'O', 'O', 'O', 'O', 'O', 'O', 'O', 'B', 'O', 'O', 'O', 'O', 'O', 'O', 'O', 'O', 'O', 'O', 'O', 'O', 'O', 'O', 'O', 'O', 'O', 'O', 'O', 'O', 'O', 'O', 'O', 'O', 'O', 'O', 'O', 'O', 'O', 'O', 'O', 'O', 'O', 'O', 'B', 'O', 'O', 'O', 'O', 'O', 'O', 'O', 'O', 'O', 'O', 'O', 'O', 'O', 'O', 'O', 'O', 'O', 'O', 'O', 'O', 'O', 'O', 'O', 'O', 'O', 'O', 'O', 'O', 'O', 'O', 'O', 'O', 'O', 'B', 'O', 'O', 'O', 'O', 'O', 'O', 'O', 'O', 'O', 'O', 'O', 'O', 'O', 'O', 'O', 'O', 'B', 'O', 'O', 'O', 'O', 'O', 'O', 'O', 'O', 'O', 'O', 'O', 'O', 'O', 'O', 'O', 'O', 'O', 'O', 'O', 'O', 'O', 'O', 'O', 'O', 'O', 'O', 'O', 'O', 'O', 'O', 'O', 'O', 'O', 'O', 'O', 'O', 'O', 'O', 'O', 'B', 'O', 'O', 'O', 'O', 'O', 'O', 'O', 'O', 'O', 'O', 'O', 'O', 'O', 'O', 'O', 'O', 'O', 'O', 'O', 'O', 'O', 'O', 'O', 'O', 'O', 'O', 'O', 'O', 'O', 'O', 'O', 'O', 'O', 'O', 'O', 'O', 'O', 'O', 'O', 'O', 'O', 'O', 'O', 'O', 'O', 'O', 'O', 'O', 'O', 'O', 'O', 'O', 'O', 'O', 'O', 'O', 'O', 'O', 'O', 'O', 'O', 'O', 'O', 'O', 'O', 'O', 'O', 'O', 'O', 'O', 'O', 'O', 'O', 'O', 'O', 'O', 'O', 'O', 'O', 'O', 'O', 'O', 'O', 'O', 'O', 'O', 'O', 'O', 'O', 'O', 'O', 'O', 'O', 'O', 'O', 'O', 'O', 'O', 'O', 'O', 'O', 'O', 'O', 'O', 'O', 'O', 'O', 'O', 'O', 'O', 'O', 'O', 'O', 'O', 'O', 'O', 'O', 'O', 'O', 'O', 'O', 'O', 'O', 'O', 'O', 'O', 'O', 'O', 'O', 'O', 'O', 'O', 'O', 'O', 'O', 'O', 'O', 'O', 'O', 'O', 'O', 'O', 'O', 'O', 'O', 'O', 'O', 'O', 'O', 'O', 'O', 'O', 'O', 'O', 'O', 'O', 'O', 'O', 'O', 'O', 'O', 'O', 'O', 'O', 'O', 'O', 'O', 'O', 'O', 'O', 'O', 'O', 'O', 'O', 'O', 'O', 'O', 'O', 'O', 'O', 'O', 'O', 'O', 'O', 'O', 'B', 'O', 'O', 'O', 'O', 'O', 'O', 'O', 'O', 'O', 'O', 'O', 'O', 'O', 'O', 'O', 'O', 'O', 'O', 'O', 'O', 'O', 'O', 'O', 'O', 'O', 'B', 'O', 'O', 'O', 'O', 'O', 'O', 'O', 'O', 'O', 'O', 'O', 'O', 'O', 'O', 'O', 'O', 'O', 'O', 'O', 'O', 'O', 'O', 'O', 'O', 'O', 'O', 'O', 'O', 'B', 'O', 'O', 'O', 'O', 'O', 'O', 'O', 'O', 'O', 'O', 'O', 'O', 'O', 'O', 'O', 'O', 'O', 'O', 'O', 'O', 'O', 'O', 'O', 'O', 'O', 'O', 'O', 'O', 'O', 'O', 'O', 'O', 'O', 'O', 'B', 'O', 'O', 'O', 'O', 'O', 'O', 'O', 'O', 'O', 'O', 'O', 'O', 'O', 'O', 'O', 'O', 'O', 'O', 'O', 'O', 'O', 'O', 'O', 'O', 'O', 'O', 'O', 'O', 'B', 'O', 'O', 'O', 'O', 'O', 'O', 'O', 'O', 'O', 'O', 'O', 'O', 'O', 'O', 'O', 'O', 'B', 'O', 'O', 'O', 'O', 'O', 'O', 'O', 'O', 'O', 'O', 'O', 'O', 'O', 'O', 'O', 'O', 'O', 'O', 'O', 'O', 'O', 'O', 'O', 'O', 'O', 'O', 'O', 'O', 'O', 'O', 'O', 'O', 'O', 'O', 'O', 'O', 'O', 'O', 'O', 'O', 'O', 'O', 'O', 'O', 'O', 'O', 'O', 'O', 'O', 'O', 'O', 'O', 'O', 'O', 'O', 'O', 'O', 'O', 'B', 'O', 'O', 'O', 'O', 'O', 'O', 'O', 'O', 'O', 'O', 'O', 'O', 'O', 'O', 'O', 'O', 'O', 'O', 'O', 'O', 'O', 'O', 'O', 'O', 'B', 'O', 'O', 'O', 'O', 'O', 'O', 'O', 'O', 'O', 'O', 'O', 'O', 'O', 'O', 'O', 'O', 'O', 'O', 'O', 'O', 'O', 'O', 'O', 'O', 'O', 'O', 'O', 'O', 'O', 'O', 'O', 'O', 'O', 'O', 'O', 'O', 'O', 'O', 'O', 'O', 'O', 'O', 'O', 'O', 'O', 'O', 'O', 'B', 'O', 'O', 'O', 'O', 'O', 'O', 'O', 'O', 'O', 'O', 'O', 'O', 'O', 'O', 'O', 'O', 'O', 'O', 'O', 'O', 'O', 'O', 'O', 'O', 'O', 'O', 'O', 'O', 'O', 'O', 'O', 'O', 'O', 'O', 'O', 'O', 'O', 'O', 'O', 'O', 'O', 'O', 'O', 'O', 'O', 'O', 'O', 'O', 'O', 'O', 'O', 'O', 'O', 'O', 'O', 'O', 'O', 'O', 'O', 'O', 'O', 'O', 'O', 'O', 'O', 'O', 'O', 'O', 'O', 'O', 'O', 'O', 'O', 'O', 'O', 'O', 'O', 'O', 'O', 'O', 'O', 'O', 'O', 'O', 'O', 'O', 'O', 'O', 'O', 'O', 'O', 'O', 'O', 'O', 'O', 'O', 'O', 'O', 'O', 'O', 'O', 'O', 'O', 'O', 'O', 'O', 'O', 'O', 'O', 'O', 'O', 'O', 'O', 'O', 'O', 'O', 'O', 'O', 'O', 'O', 'O', 'O', 'O', 'O', 'O', 'O', 'O', 'O', 'O', 'O', 'O', 'O', 'O', 'O', 'O', 'O', 'O', 'O', 'O', 'O', 'O', 'O', 'O', 'O', 'O', 'O', 'O', 'O', 'O', 'O', 'O', 'O', 'O', 'O', 'O', 'O', 'O', 'O', 'O', 'O', 'O', 'O', 'O', 'O', 'O', 'O', 'O', 'O', 'O', 'O', 'O', 'O', 'O', 'O', 'O', 'O', 'O', 'O', 'O', 'O', 'O', 'O', 'O', 'O', 'O', 'O', 'O', 'O', 'O', 'O', 'O', 'O', 'O', 'O', 'O', 'O', 'O', 'O', 'O', 'O', 'O', 'O', 'O', 'O', 'O', 'O', 'O', 'O', 'O', 'O', 'O', 'O', 'O', 'O', 'O', 'O', 'O', 'O', 'O', 'O', 'O', 'O', 'B', 'I', 'O', 'O', 'O', 'O', 'O', 'O', 'O', 'O', 'O', 'O', 'O', 'O', 'O', 'O', 'O', 'O', 'O', 'O', 'O', 'O', 'O', 'O', 'O', 'O', 'O', 'O', 'O', 'O', 'O', 'O', 'O', 'O', 'O', 'O', 'O', 'O', 'O', 'O', 'O', 'O', 'O', 'O', 'O', 'O', 'B', 'O', 'O', 'O', 'O', 'O', 'O', 'O', 'O', 'O', 'O', 'O', 'O', 'B', 'O', 'O', 'O', 'O', 'O', 'O', 'O', 'O', 'O', 'O', 'O', 'O', 'O', 'O', 'O', 'O', 'O', 'O', 'O', 'O', 'O', 'O', 'O', 'O', 'O', 'O', 'O', 'O', 'O', 'O', 'O', 'O', 'O', 'O', 'O', 'O', 'O', 'O', 'O', 'O', 'O', 'O', 'O', 'O', 'O', 'O', 'O', 'O', 'O', 'O', 'O', 'O', 'O', 'O', 'O', 'O', 'O', 'O', 'O', 'O', 'O', 'O', 'O', 'O', 'O', 'O', 'O', 'O', 'O', 'O', 'O', 'O', 'O', 'O', 'O', 'O', 'O', 'O', 'O', 'O', 'O', 'O', 'O', 'O', 'O', 'O', 'O', 'O', 'O', 'O', 'O', 'O', 'O', 'O', 'O', 'O', 'O', 'O', 'O', 'O', 'O', 'O', 'O', 'O', 'O', 'O', 'O', 'O', 'O', 'O', 'O', 'O', 'O', 'O', 'O', 'O', 'O', 'O', 'O', 'O', 'O', 'O', 'O', 'O', 'O', 'O', 'O', 'O', 'O', 'O', 'O', 'O', 'O', 'O', 'O', 'O', 'O', 'O', 'O', 'O', 'O', 'O', 'O', 'O', 'O', 'O', 'O', 'O', 'O', 'O', 'O', 'O', 'O', 'O', 'O', 'O', 'O', 'O', 'O', 'O', 'O', 'O', 'O', 'O', 'B', 'I', 'O', 'O', 'O', 'O', 'O', 'O', 'O', 'O', 'O', 'O', 'O', 'O', 'O', 'O', 'O', 'O', 'O', 'O', 'O', 'O', 'O', 'O', 'O', 'O', 'O', 'O', 'O', 'O', 'O', 'O', 'O', 'O', 'O', 'O', 'O', 'O', 'O', 'O', 'O', 'O', 'O', 'O', 'O', 'O', 'B', 'I', 'O', 'O', 'O', 'O', 'O', 'O', 'O', 'O', 'O', 'O', 'O', 'O', 'O', 'O', 'O', 'O', 'B', 'O', 'O', 'O', 'O', 'O', 'O', 'O', 'O', 'O', 'O', 'O', 'O', 'O', 'O', 'O', 'O', 'O', 'O', 'O', 'O', 'O', 'O', 'O', 'O', 'O', 'O', 'O', 'O', 'O', 'O', 'O', 'O', 'O', 'O', 'O', 'O', 'O', 'O', 'O', 'O', 'O', 'O', 'O', 'O', 'O', 'O', 'O', 'O', 'O', 'O', 'O', 'O', 'O', 'O', 'O', 'O', 'O', 'O', 'O', 'O', 'O', 'O', 'O', 'O', 'O', 'O', 'O', 'O', 'O', 'O', 'O', 'O', 'O', 'O', 'O', 'O', 'O', 'O', 'O', 'O', 'O', 'O', 'O', 'O', 'O', 'O', 'O', 'O', 'O', 'O', 'O', 'O', 'O', 'O', 'O', 'O', 'O', 'O', 'O', 'O', 'O', 'O', 'O', 'O', 'B', 'O', 'O', 'O', 'O', 'O', 'O', 'O', 'O', 'O', 'O', 'B', 'O', 'O', 'O', 'O', 'B', 'O', 'O', 'O', 'O', 'O', 'O', 'O', 'O', 'O', 'O', 'O', 'O', 'O', 'B', 'O', 'O', 'O', 'O', 'O', 'O', 'O', 'O', 'O', 'O', 'O', 'O', 'O', 'O', 'O', 'O', 'O', 'O', 'O', 'O', 'O', 'O', 'O', 'O', 'O', 'O', 'O', 'O', 'O', 'O', 'O', 'O', 'O', 'O', 'O', 'O', 'O', 'O', 'O', 'O', 'O', 'O', 'O', 'O', 'O', 'O', 'O', 'O', 'O', 'O', 'O', 'O', 'O', 'O', 'O', 'O', 'O', 'O', 'O', 'O', 'O', 'O', 'O', 'O', 'O', 'O', 'O', 'O', 'O', 'O', 'O', 'O', 'O', 'O', 'O', 'O', 'O', 'B', 'I', 'O', 'O', 'O', 'B', 'O', 'O', 'O', 'O', 'O', 'O', 'O', 'O', 'O', 'O', 'O', 'O', 'O', 'O', 'O', 'O', 'O', 'O', 'O', 'O', 'O', 'O', 'O', 'O', 'O', 'O', 'O', 'O', 'O', 'O', 'O', 'O', 'O', 'O', 'O', 'O', 'O', 'O', 'O', 'O', 'O', 'O', 'O', 'O', 'O', 'O', 'O', 'O', 'O', 'O', 'O', 'O', 'O', 'O', 'O', 'O', 'O', 'O', 'O', 'B', 'O', 'O', 'O', 'O', 'O', 'O', 'O', 'O', 'O', 'O', 'O', 'O', 'O', 'O', 'O', 'O', 'O', 'O', 'O', 'O', 'O', 'O', 'O', 'O', 'O', 'O', 'O', 'O', 'O', 'O', 'O', 'O', 'O', 'O', 'O', 'O', 'O', 'O', 'O', 'O', 'O', 'O', 'O', 'O', 'O', 'O', 'O', 'O', 'O', 'O', 'O', 'O', 'O', 'O', 'O', 'O', 'O', 'O', 'O', 'O', 'O', 'B', 'I', 'O', 'O', 'O', 'O', 'O', 'O', 'O', 'O', 'O', 'O', 'O', 'O', 'O', 'O', 'O', 'O', 'O', 'O', 'B', 'O', 'O', 'O', 'O', 'O', 'O', 'O', 'O', 'O', 'O', 'O', 'O', 'O', 'O', 'O', 'O', 'O', 'O', 'O', 'O', 'O', 'O', 'O', 'O', 'O', 'O', 'O', 'O', 'O', 'O', 'B', 'O']
Extractive/present Keyphrases: ['lymph node', 'esophagectomy', 'lymphadenectomy', 'survival']
Abstractive/absent Keyphrases: []
-----------
```
### Keyphrase Extraction
```python
from datasets import load_dataset
# get the dataset only for keyphrase extraction
dataset = load_dataset("midas/pubmed", "extraction")
print("Samples for Keyphrase Extraction")
# sample from the test split
print("Sample from test data split")
test_sample = dataset["test"][0]
print("Fields in the sample: ", [key for key in test_sample.keys()])
print("Tokenized Document: ", test_sample["document"])
print("Document BIO Tags: ", test_sample["doc_bio_tags"])
print("\n-----------\n")
```
### Keyphrase Generation
```python
# get the dataset only for keyphrase generation
dataset = load_dataset("midas/pubmed", "generation")
print("Samples for Keyphrase Generation")
# sample from the test split
print("Sample from test data split")
test_sample = dataset["test"][0]
print("Fields in the sample: ", [key for key in test_sample.keys()])
print("Tokenized Document: ", test_sample["document"])
print("Extractive/present Keyphrases: ", test_sample["extractive_keyphrases"])
print("Abstractive/absent Keyphrases: ", test_sample["abstractive_keyphrases"])
print("\n-----------\n")
```
## Citation Information
```
@inproceedings{Schutz2008KeyphraseEF,
title={Keyphrase Extraction from Single Documents in the Open Domain Exploiting Linguistic and Statistical Methods},
author={Alexander Schutz},
year={2008}
}
```
## Contributions
Thanks to [@debanjanbhucs](https://github.com/debanjanbhucs), [@dibyaaaaax](https://github.com/dibyaaaaax) and [@ad6398](https://github.com/ad6398) for adding this dataset
| midas/pubmed | [
"region:us"
] | 2022-03-02T23:29:22+00:00 | {} | 2022-03-05T03:59:56+00:00 | [] | [] | TAGS
#region-us
| Dataset Summary
---------------
A dataset for benchmarking keyphrase extraction and generation techniques from long document english scientific papers. For more details about the dataset please refer the original paper - URL
Original source of the data -
Dataset Structure
-----------------
### Data Fields
* id: unique identifier of the document.
* document: Whitespace separated list of words in the document.
* doc\_bio\_tags: BIO tags for each word in the document. B stands for the beginning of a keyphrase and I stands for inside the keyphrase. O stands for outside the keyphrase and represents the word that isn't a part of the keyphrase at all.
* extractive\_keyphrases: List of all the present keyphrases.
* abstractive\_keyphrase: List of all the absent keyphrases.
### Data Splits
* Percentage of keyphrases that are named entities: 84.94% (named entities detected using scispacy - en-core-sci-lg model)
* Percentage of keyphrases that are noun phrases: 81.54% (noun phrases detected using spacy en-core-web-lg after removing determiners)
Usage
-----
### Full Dataset
Output
### Keyphrase Extraction
### Keyphrase Generation
Contributions
-------------
Thanks to @debanjanbhucs, @dibyaaaaax and @ad6398 for adding this dataset
| [
"### Data Fields\n\n\n* id: unique identifier of the document.\n* document: Whitespace separated list of words in the document.\n* doc\\_bio\\_tags: BIO tags for each word in the document. B stands for the beginning of a keyphrase and I stands for inside the keyphrase. O stands for outside the keyphrase and represents the word that isn't a part of the keyphrase at all.\n* extractive\\_keyphrases: List of all the present keyphrases.\n* abstractive\\_keyphrase: List of all the absent keyphrases.",
"### Data Splits\n\n\n\n* Percentage of keyphrases that are named entities: 84.94% (named entities detected using scispacy - en-core-sci-lg model)\n* Percentage of keyphrases that are noun phrases: 81.54% (noun phrases detected using spacy en-core-web-lg after removing determiners)\n\n\nUsage\n-----",
"### Full Dataset\n\n\nOutput",
"### Keyphrase Extraction",
"### Keyphrase Generation\n\n\nContributions\n-------------\n\n\nThanks to @debanjanbhucs, @dibyaaaaax and @ad6398 for adding this dataset"
] | [
"TAGS\n#region-us \n",
"### Data Fields\n\n\n* id: unique identifier of the document.\n* document: Whitespace separated list of words in the document.\n* doc\\_bio\\_tags: BIO tags for each word in the document. B stands for the beginning of a keyphrase and I stands for inside the keyphrase. O stands for outside the keyphrase and represents the word that isn't a part of the keyphrase at all.\n* extractive\\_keyphrases: List of all the present keyphrases.\n* abstractive\\_keyphrase: List of all the absent keyphrases.",
"### Data Splits\n\n\n\n* Percentage of keyphrases that are named entities: 84.94% (named entities detected using scispacy - en-core-sci-lg model)\n* Percentage of keyphrases that are noun phrases: 81.54% (noun phrases detected using spacy en-core-web-lg after removing determiners)\n\n\nUsage\n-----",
"### Full Dataset\n\n\nOutput",
"### Keyphrase Extraction",
"### Keyphrase Generation\n\n\nContributions\n-------------\n\n\nThanks to @debanjanbhucs, @dibyaaaaax and @ad6398 for adding this dataset"
] |
7933201e69f12fa015074ec28bc6c6721880c299 |
A dataset for benchmarking keyphrase extraction and generation techniques from long document english scientific articles. For more details about the dataset please refer the original paper - [https://dl.acm.org/doi/10.5555/1859664.1859668](https://dl.acm.org/doi/10.5555/1859664.1859668)
Original source of the data - [https://github.com/boudinfl/semeval-2010-pre](https://github.com/boudinfl/semeval-2010-pre)
## Dataset Summary
The Semeval-2010 dataset was originally proposed by *Su Nam Kim et al* in the paper titled - [SemEval-2010 Task 5: Automatic Keyphrase Extraction from Scientific Articles](https://aclanthology.org/S10-1004.pdf) in the year 2010. The dataset consists of a set of 284 English scientific papers from the ACM Digital Library (conference and work-shop papers). The selected articles belong to the
following four 1998 ACM classifications: C2.4 (Distributed Systems), H3.3 (Information Search and Retrieval), I2.11 (Distributed Artificial Intelligence – Multiagent Systems) and J4 (Socialand Behavioral Sciences – Economics). Each paper has two sets of keyphrases annotated by readers and author. The original dataset was divided into trail, training and test splits, evenly distributed across four domains. The trial, training and test splits had 40, 144 and 100 articles respectively, and the trial split was a subset of training split. We provide test and train splits with 100 and 144 articles respectively.
The dataset shared over here categorizes the keyphrases into *extractive* and *abstractive*. **Extractive keyphrases** are those that could be found in the input text and the **abstractive keyphrases** are those that are not present in the input text. In order to get all the meta-data about the documents and keyphrases please refer to the [original source](https://github.com/boudinfl/semeval-2010-pre) from which the dataset was taken from. The main motivation behind making this dataset available in the form as presented over here is to make it easy for the researchers to programmatically download it and evaluate their models for the tasks of keyphrase extraction and generation. As keyphrase extraction by treating it as a sequence tagging task and using contextual language models has become popular - [Keyphrase extraction from scholarly articles as sequence labeling using contextualized embeddings](https://arxiv.org/pdf/1910.08840.pdf), we have also made the token tags available in the BIO tagging format.
## Dataset Structure
### Data Fields
- **id**: unique identifier of the document.
- **document**: Whitespace separated list of words in the document.
- **doc_bio_tags**: BIO tags for each word in the document. B stands for the beginning of a keyphrase and I stands for inside the keyphrase. O stands for outside the keyphrase and represents the word that isn't a part of the keyphrase at all.
- **extractive_keyphrases**: List of all the present keyphrases.
- **abstractive_keyphrase**: List of all the absent keyphrases.
### Data Splits
|Split| #datapoints |
|--|--|
| Test | 100 |
| Train | 144 |
Train
- Percentage of keyphrases that are named entities: 63.01% (named entities detected using scispacy - en-core-sci-lg model)
- Percentage of keyphrases that are noun phrases: 82.50% (noun phrases detected using spacy en-core-web-lg after removing determiners)
Test
- Percentage of keyphrases that are named entities: 62.06% (named entities detected using scispacy - en-core-sci-lg model)
- Percentage of keyphrases that are noun phrases: 78.36% (noun phrases detected using spacy after removing determiners)
## Usage
### Full Dataset
```python
from datasets import load_dataset
# get entire dataset
dataset = load_dataset("midas/semeval2010", "raw")
# sample from the train split
print("Sample from train dataset split")
test_sample = dataset["train"][0]
print("Fields in the sample: ", [key for key in test_sample.keys()])
print("Tokenized Document: ", test_sample["document"])
print("Document BIO Tags: ", test_sample["doc_bio_tags"])
print("Extractive/present Keyphrases: ", test_sample["extractive_keyphrases"])
print("Abstractive/absent Keyphrases: ", test_sample["abstractive_keyphrases"])
print("\n-----------\n")
# sample from the test split
print("Sample from test dataset split")
test_sample = dataset["test"][0]
print("Fields in the sample: ", [key for key in test_sample.keys()])
print("Tokenized Document: ", test_sample["document"])
print("Document BIO Tags: ", test_sample["doc_bio_tags"])
print("Extractive/present Keyphrases: ", test_sample["extractive_keyphrases"])
print("Abstractive/absent Keyphrases: ", test_sample["abstractive_keyphrases"])
print("\n-----------\n")
```
**Output**
```bash
Sample from train dataset split
Fields in the sample: ['id', 'document', 'doc_bio_tags', 'extractive_keyphrases', 'abstractive_keyphrases', 'other_metadata']
Tokenized Document: ['HITS', 'on', 'the', 'Web:', 'How', 'does', 'it', 'Compare?', 'Marc', 'Najork', 'Microsoft', 'Research', '1065', 'La', 'Avenida', 'Mountain', 'View,', 'CA,', 'USA', '[email protected]', 'Hugo', 'Zaragoza', '∗', 'Yahoo!', 'Research', 'Barcelona', 'Ocata', '1', 'Barcelona', '08003,', 'Spain', '[email protected]', 'Michael', 'Taylor', 'Microsoft', 'Research', '7', 'J', 'J', 'Thompson', 'Ave', 'Cambridge', 'CB3', '0FB,', 'UK', '[email protected]', 'ABSTRACT', 'This', 'paper', 'describes', 'a', 'large-scale', 'evaluation', 'of', 'the', 'effectiveness', 'of', 'HITS', 'in', 'comparison', 'with', 'other', 'link-based', 'ranking', 'algorithms,', 'when', 'used', 'in', 'combination', 'with', 'a', 'state-ofthe-art', 'text', 'retrieval', 'algorithm', 'exploiting', 'anchor', 'text.', 'We', 'quantified', 'their', 'effectiveness', 'using', 'three', 'common', 'performance', 'measures:', 'the', 'mean', 'reciprocal', 'rank,', 'the', 'mean', 'average', 'precision,', 'and', 'the', 'normalized', 'discounted', 'cumulative', 'gain', 'measurements.', 'The', 'evaluation', 'is', 'based', 'on', 'two', 'large', 'data', 'sets:', 'a', 'breadth-first', 'search', 'crawl', 'of', '463', 'million', 'web', 'pages', 'containing', '17.6', 'billion', 'hyperlinks', 'and', 'referencing', '2.9', 'billion', 'distinct', 'URLs;', 'and', 'a', 'set', 'of', '28,043', 'queries', 'sampled', 'from', 'a', 'query', 'log,', 'each', 'query', 'having', 'on', 'average', '2,383', 'results,', 'about', '17', 'of', 'which', 'were', 'labeled', 'by', 'judges.', 'We', 'found', 'that', 'HITS', 'outperforms', 'PageRank,', 'but', 'is', 'about', 'as', 'effective', 'as', 'web-page', 'in-degree.', 'The', 'same', 'holds', 'true', 'when', 'any', 'of', 'the', 'link-based', 'features', 'are', 'combined', 'with', 'the', 'text', 'retrieval', 'algorithm.', 'Finally,', 'we', 'studied', 'the', 'relationship', 'between', 'query', 'specificity', 'and', 'the', 'effectiveness', 'of', 'selected', 'features,', 'and', 'found', 'that', 'link-based', 'features', 'perform', 'better', 'for', 'general', 'queries,', 'whereas', 'BM25F', 'performs', 'better', 'for', 'specific', 'queries.', 'Categories', 'and', 'Subject', 'Descriptors', 'H.3.3', '[Information', 'Search', 'and', 'Retrieval]:', 'Information', 'Storage', 'and', 'Retrieval-search', 'process,', 'selection', 'process', 'General', 'Terms', 'Algorithms,', 'Measurement,', 'Experimentation', '1.', 'INTRODUCTION', 'Link', 'graph', 'features', 'such', 'as', 'in-degree', 'and', 'PageRank', 'have', 'been', 'shown', 'to', 'significantly', 'improve', 'the', 'performance', 'of', 'text', 'retrieval', 'algorithms', 'on', 'the', 'web.', 'The', 'HITS', 'algorithm', 'is', 'also', 'believed', 'to', 'be', 'of', 'interest', 'for', 'web', 'search;', 'to', 'some', 'degree,', 'one', 'may', 'expect', 'HITS', 'to', 'be', 'more', 'informative', 'that', 'other', 'link-based', 'features', 'because', 'it', 'is', 'query-dependent:', 'it', 'tries', 'to', 'measure', 'the', 'interest', 'of', 'pages', 'with', 'respect', 'to', 'a', 'given', 'query.', 'However,', 'it', 'remains', 'unclear', 'today', 'whether', 'there', 'are', 'practical', 'benefits', 'of', 'HITS', 'over', 'other', 'link', 'graph', 'measures.', 'This', 'is', 'even', 'more', 'true', 'when', 'we', 'consider', 'that', 'modern', 'retrieval', 'algorithms', 'used', 'on', 'the', 'web', 'use', 'a', 'document', 'representation', 'which', 'incorporates', 'the', 'document"s', 'anchor', 'text,', 'i.e.', 'the', 'text', 'of', 'incoming', 'links.', 'This,', 'at', 'least', 'to', 'some', 'degree,', 'takes', 'the', 'link', 'graph', 'into', 'account,', 'in', 'a', 'query-dependent', 'manner.', 'Comparing', 'HITS', 'to', 'PageRank', 'or', 'in-degree', 'empirically', 'is', 'no', 'easy', 'task.', 'There', 'are', 'two', 'main', 'difficulties:', 'scale', 'and', 'relevance.', 'Scale', 'is', 'important', 'because', 'link-based', 'features', 'are', 'known', 'to', 'improve', 'in', 'quality', 'as', 'the', 'document', 'graph', 'grows.', 'If', 'we', 'carry', 'out', 'a', 'small', 'experiment,', 'our', 'conclusions', 'won"t', 'carry', 'over', 'to', 'large', 'graphs', 'such', 'as', 'the', 'web.', 'However,', 'computing', 'HITS', 'efficiently', 'on', 'a', 'graph', 'the', 'size', 'of', 'a', 'realistic', 'web', 'crawl', 'is', 'extraordinarily', 'difficult.', 'Relevance', 'is', 'also', 'crucial', 'because', 'we', 'cannot', 'measure', 'the', 'performance', 'of', 'a', 'feature', 'in', 'the', 'absence', 'of', 'human', 'judgments:', 'what', 'is', 'crucial', 'is', 'ranking', 'at', 'the', 'top', 'of', 'the', 'ten', 'or', 'so', 'documents', 'that', 'a', 'user', 'will', 'peruse.', 'To', 'our', 'knowledge,', 'this', 'paper', 'is', 'the', 'first', 'attempt', 'to', 'evaluate', 'HITS', 'at', 'a', 'large', 'scale', 'and', 'compare', 'it', 'to', 'other', 'link-based', 'features', 'with', 'respect', 'to', 'human', 'evaluated', 'judgment.', 'Our', 'results', 'confirm', 'many', 'of', 'the', 'intuitions', 'we', 'have', 'about', 'link-based', 'features', 'and', 'their', 'relationship', 'to', 'text', 'retrieval', 'methods', 'exploiting', 'anchor', 'text.', 'This', 'is', 'reassuring:', 'in', 'the', 'absence', 'of', 'a', 'theoretical', 'model', 'capable', 'of', 'tying', 'these', 'measures', 'with', 'relevance,', 'the', 'only', 'way', 'to', 'validate', 'our', 'intuitions', 'is', 'to', 'carry', 'out', 'realistic', 'experiments.', 'However,', 'we', 'were', 'quite', 'surprised', 'to', 'find', 'that', 'HITS,', 'a', 'query-dependent', 'feature,', 'is', 'about', 'as', 'effective', 'as', 'web', 'page', 'in-degree,', 'the', 'most', 'simpleminded', 'query-independent', 'link-based', 'feature.', 'This', 'continues', 'to', 'be', 'true', 'when', 'the', 'link-based', 'features', 'are', 'combined', 'with', 'a', 'text', 'retrieval', 'algorithm', 'exploiting', 'anchor', 'text.', 'The', 'remainder', 'of', 'this', 'paper', 'is', 'structured', 'as', 'follows:', 'Section', '2', 'surveys', 'related', 'work.', 'Section', '3', 'describes', 'the', 'data', 'sets', 'we', 'used', 'in', 'our', 'study.', 'Section', '4', 'reviews', 'the', 'performance', 'measures', 'we', 'used.', 'Sections', '5', 'and', '6', 'describe', 'the', 'PageRank', 'and', 'HITS', 'algorithms', 'in', 'more', 'detail,', 'and', 'sketch', 'the', 'computational', 'infrastructure', 'we', 'employed', 'to', 'carry', 'out', 'large', 'scale', 'experiments.', 'Section', '7', 'presents', 'the', 'results', 'of', 'our', 'evaluations,', 'and', 'Section', '8', 'offers', 'concluding', 'remarks.', '2.', 'RELATED', 'WORK', 'The', 'idea', 'of', 'using', 'hyperlink', 'analysis', 'for', 'ranking', 'web', 'search', 'results', 'arose', 'around', '1997,', 'and', 'manifested', 'itself', 'in', 'the', 'HITS', '[16,', '17]', 'and', 'PageRank', '[5,', '21]', 'algorithms.', 'The', 'popularity', 'of', 'these', 'two', 'algorithms', 'and', 'the', 'phenomenal', 'success', 'of', 'the', 'Google', 'search', 'engine,', 'which', 'uses', 'PageRank,', 'have', 'spawned', 'a', 'large', 'amount', 'of', 'subsequent', 'research.', 'There', 'are', 'numerous', 'attempts', 'at', 'improving', 'the', 'effectiveness', 'of', 'HITS', 'and', 'PageRank.', 'Query-dependent', 'link-based', 'ranking', 'algorithms', 'inspired', 'by', 'HITS', 'include', 'SALSA', '[19],', 'Randomized', 'HITS', '[20],', 'and', 'PHITS', '[7],', 'to', 'name', 'a', 'few.', 'Query-independent', 'link-based', 'ranking', 'algorithms', 'inspired', 'by', 'PageRank', 'include', 'TrafficRank', '[22],', 'BlockRank', '[14],', 'and', 'TrustRank', '[11],', 'and', 'many', 'others.', 'Another', 'line', 'of', 'research', 'is', 'concerned', 'with', 'analyzing', 'the', 'mathematical', 'properties', 'of', 'HITS', 'and', 'PageRank.', 'For', 'example,', 'Borodin', 'et', 'al.', '[3]', 'investigated', 'various', 'theoretical', 'properties', 'of', 'PageRank,', 'HITS,', 'SALSA,', 'and', 'PHITS,', 'including', 'their', 'similarity', 'and', 'stability,', 'while', 'Bianchini', 'et', 'al.', '[2]', 'studied', 'the', 'relationship', 'between', 'the', 'structure', 'of', 'the', 'web', 'graph', 'and', 'the', 'distribution', 'of', 'PageRank', 'scores,', 'and', 'Langville', 'and', 'Meyer', 'examined', 'basic', 'properties', 'of', 'PageRank', 'such', 'as', 'existence', 'and', 'uniqueness', 'of', 'an', 'eigenvector', 'and', 'convergence', 'of', 'power', 'iteration', '[18].', 'Given', 'the', 'attention', 'that', 'has', 'been', 'paid', 'to', 'improving', 'the', 'effectiveness', 'of', 'PageRank', 'and', 'HITS,', 'and', 'the', 'thorough', 'studies', 'of', 'the', 'mathematical', 'properties', 'of', 'these', 'algorithms,', 'it', 'is', 'somewhat', 'surprising', 'that', 'very', 'few', 'evaluations', 'of', 'their', 'effectiveness', 'have', 'been', 'published.', 'We', 'are', 'aware', 'of', 'two', 'studies', 'that', 'have', 'attempted', 'to', 'formally', 'evaluate', 'the', 'effectiveness', 'of', 'HITS', 'and', 'of', 'PageRank.', 'Amento', 'et', 'al.', '[1]', 'employed', 'quantitative', 'measures,', 'but', 'based', 'their', 'experiments', 'on', 'the', 'result', 'sets', 'of', 'just', '5', 'queries', 'and', 'the', 'web-graph', 'induced', 'by', 'topical', 'crawls', 'around', 'the', 'result', 'set', 'of', 'each', 'query.', 'A', 'more', 'recent', 'study', 'by', 'Borodin', 'et', 'al.', '[4]', 'is', 'based', 'on', '34', 'queries,', 'result', 'sets', 'of', '200', 'pages', 'per', 'query', 'obtained', 'from', 'Google,', 'and', 'a', 'neighborhood', 'graph', 'derived', 'by', 'retrieving', '50', 'in-links', 'per', 'result', 'from', 'Google.', 'By']
Document BIO Tags: ['O', 'O', 'O', 'O', 'O', 'O', 'O', 'O', 'O', 'O', 'O', 'O', 'O', 'O', 'O', 'O', 'O', 'O', 'O', 'O', 'O', 'O', 'O', 'O', 'O', 'O', 'O', 'O', 'O', 'O', 'O', 'O', 'O', 'O', 'O', 'O', 'O', 'O', 'O', 'O', 'O', 'O', 'O', 'O', 'O', 'O', 'O', 'O', 'O', 'O', 'O', 'O', 'O', 'O', 'O', 'O', 'O', 'O', 'O', 'O', 'O', 'O', 'O', 'B', 'O', 'O', 'O', 'O', 'O', 'O', 'O', 'O', 'O', 'O', 'O', 'O', 'O', 'O', 'O', 'O', 'O', 'O', 'O', 'O', 'O', 'O', 'O', 'O', 'B', 'I', 'I', 'O', 'B', 'I', 'I', 'O', 'O', 'O', 'O', 'O', 'O', 'O', 'O', 'O', 'O', 'O', 'O', 'O', 'O', 'O', 'O', 'O', 'O', 'O', 'O', 'O', 'O', 'O', 'O', 'O', 'O', 'O', 'O', 'O', 'O', 'O', 'O', 'O', 'O', 'O', 'O', 'O', 'O', 'O', 'O', 'O', 'O', 'O', 'O', 'O', 'O', 'O', 'O', 'O', 'O', 'O', 'O', 'O', 'O', 'O', 'O', 'O', 'O', 'O', 'O', 'O', 'O', 'O', 'O', 'O', 'O', 'B', 'O', 'O', 'O', 'O', 'O', 'O', 'O', 'O', 'O', 'O', 'O', 'O', 'O', 'O', 'O', 'O', 'O', 'O', 'O', 'O', 'O', 'O', 'O', 'O', 'O', 'O', 'O', 'O', 'O', 'O', 'O', 'B', 'I', 'O', 'O', 'O', 'O', 'O', 'O', 'O', 'O', 'O', 'O', 'O', 'O', 'O', 'O', 'O', 'O', 'O', 'B', 'O', 'O', 'O', 'O', 'O', 'O', 'O', 'O', 'O', 'O', 'O', 'O', 'O', 'O', 'O', 'O', 'O', 'O', 'O', 'O', 'O', 'O', 'O', 'O', 'O', 'O', 'O', 'O', 'B', 'I', 'O', 'O', 'O', 'O', 'O', 'B', 'O', 'O', 'O', 'O', 'O', 'O', 'O', 'O', 'O', 'O', 'O', 'O', 'O', 'O', 'O', 'O', 'O', 'O', 'O', 'O', 'O', 'O', 'O', 'O', 'O', 'O', 'O', 'O', 'O', 'O', 'O', 'O', 'O', 'O', 'O', 'O', 'O', 'O', 'O', 'O', 'O', 'O', 'O', 'O', 'O', 'O', 'O', 'O', 'O', 'O', 'O', 'O', 'O', 'O', 'O', 'O', 'O', 'O', 'O', 'O', 'O', 'O', 'O', 'O', 'O', 'O', 'O', 'O', 'O', 'O', 'O', 'O', 'O', 'O', 'O', 'B', 'I', 'O', 'O', 'O', 'O', 'O', 'O', 'O', 'O', 'O', 'O', 'O', 'O', 'O', 'O', 'O', 'O', 'O', 'O', 'O', 'O', 'O', 'O', 'O', 'O', 'O', 'O', 'O', 'O', 'O', 'O', 'O', 'O', 'O', 'O', 'O', 'O', 'O', 'O', 'O', 'O', 'O', 'B', 'I', 'O', 'O', 'O', 'O', 'O', 'O', 'O', 'O', 'O', 'B', 'O', 'O', 'O', 'O', 'O', 'O', 'O', 'O', 'O', 'O', 'O', 'O', 'B', 'I', 'I', 'O', 'O', 'O', 'O', 'O', 'O', 'O', 'O', 'O', 'O', 'O', 'O', 'O', 'O', 'O', 'O', 'O', 'O', 'O', 'O', 'O', 'O', 'O', 'O', 'O', 'O', 'O', 'O', 'O', 'O', 'O', 'O', 'O', 'O', 'O', 'O', 'O', 'O', 'O', 'O', 'O', 'O', 'O', 'O', 'O', 'O', 'O', 'O', 'O', 'O', 'O', 'O', 'O', 'O', 'O', 'O', 'O', 'O', 'O', 'O', 'O', 'O', 'O', 'O', 'O', 'O', 'O', 'O', 'O', 'O', 'O', 'O', 'O', 'O', 'O', 'O', 'B', 'O', 'O', 'O', 'O', 'O', 'O', 'O', 'O', 'O', 'O', 'O', 'O', 'O', 'O', 'O', 'O', 'O', 'O', 'O', 'O', 'O', 'O', 'O', 'O', 'O', 'O', 'O', 'O', 'O', 'O', 'O', 'O', 'O', 'O', 'O', 'O', 'O', 'O', 'O', 'O', 'O', 'O', 'O', 'O', 'O', 'O', 'O', 'O', 'O', 'O', 'O', 'O', 'O', 'O', 'O', 'O', 'O', 'O', 'O', 'O', 'O', 'O', 'O', 'O', 'O', 'O', 'O', 'O', 'O', 'O', 'O', 'O', 'O', 'O', 'O', 'O', 'O', 'O', 'O', 'O', 'O', 'O', 'O', 'O', 'O', 'O', 'O', 'O', 'O', 'O', 'O', 'O', 'O', 'O', 'O', 'O', 'O', 'O', 'O', 'O', 'O', 'O', 'O', 'O', 'O', 'O', 'O', 'O', 'O', 'O', 'O', 'O', 'O', 'O', 'O', 'O', 'O', 'O', 'O', 'O', 'O', 'O', 'O', 'O', 'O', 'O', 'O', 'O', 'O', 'O', 'O', 'O', 'O', 'O', 'O', 'O', 'O', 'O', 'O', 'O', 'O', 'O', 'O', 'O', 'O', 'O', 'O', 'O', 'O', 'O', 'O', 'O', 'O', 'O', 'O', 'O', 'O', 'O', 'O', 'O', 'O', 'O', 'O', 'O', 'O', 'O', 'O', 'O', 'O', 'O', 'O', 'O', 'O', 'O', 'O', 'O', 'O', 'O', 'O', 'B', 'O', 'O', 'O', 'O', 'O', 'O', 'O', 'O', 'O', 'O', 'O', 'O', 'O', 'O', 'O', 'O', 'O', 'O', 'O', 'O', 'O', 'O', 'O', 'O', 'O', 'O', 'O', 'O', 'O', 'O', 'O', 'O', 'O', 'O', 'O', 'O', 'O', 'O', 'O', 'O', 'B', 'I', 'O', 'B', 'O', 'O', 'O', 'O', 'O', 'O', 'O', 'O', 'O', 'O', 'O', 'O', 'O', 'O', 'O', 'B', 'O', 'O', 'O', 'O', 'O', 'O', 'O', 'O', 'O', 'O', 'O', 'O', 'O', 'O', 'O', 'O', 'O', 'O', 'O', 'O', 'B', 'O', 'O', 'O', 'O', 'O', 'O', 'O', 'O', 'O', 'O', 'O', 'O', 'O', 'O', 'O', 'O', 'O', 'O', 'O', 'B', 'O', 'O', 'B', 'O', 'O', 'O', 'O', 'O', 'O', 'O', 'O', 'O', 'O', 'O', 'O', 'O', 'O', 'O', 'O', 'O', 'O', 'O', 'B', 'O', 'O', 'O', 'B', 'O', 'O', 'O', 'O', 'O', 'O', 'O', 'O', 'O', 'O', 'O', 'O', 'O', 'O', 'O', 'O', 'O', 'O', 'O', 'O', 'O', 'O', 'O', 'O', 'O', 'B', 'O', 'O', 'O', 'O', 'O', 'O', 'O', 'O', 'O', 'O', 'O', 'B', 'O', 'O', 'O', 'O', 'O', 'O', 'O', 'O', 'O', 'O', 'O', 'O', 'O', 'O', 'O', 'O', 'O', 'O', 'O', 'O', 'O', 'O', 'O', 'O', 'O', 'O', 'O', 'O', 'B', 'O', 'O', 'O', 'O', 'O', 'O', 'O', 'O', 'O', 'B', 'O', 'O', 'O', 'O', 'O', 'O', 'O', 'O', 'O', 'O', 'O', 'O', 'O', 'O', 'O', 'O', 'O', 'O', 'O', 'O', 'O', 'O', 'O', 'O', 'O', 'O', 'B', 'O', 'O', 'O', 'O', 'O', 'O', 'O', 'O', 'O', 'O', 'O', 'O', 'O', 'O', 'O', 'O', 'O', 'O', 'O', 'O', 'O', 'O', 'O', 'O', 'O', 'O', 'O', 'O', 'O', 'O', 'O', 'O', 'O', 'O', 'O', 'O', 'O', 'O', 'O', 'O', 'O', 'O', 'O', 'O', 'O', 'B', 'O', 'O', 'O', 'O', 'O', 'O', 'O', 'O', 'O', 'O', 'O', 'O', 'O', 'O', 'O', 'O', 'O', 'O', 'O', 'O', 'O', 'O', 'O', 'O', 'O', 'O', 'O', 'O', 'O', 'O', 'O', 'O', 'O', 'O', 'O', 'O', 'O', 'O', 'O', 'O', 'O', 'O', 'O', 'O', 'O', 'O', 'O', 'O', 'O', 'O', 'O', 'O', 'O', 'O', 'O', 'O', 'O', 'O', 'O', 'O', 'O', 'O', 'O', 'O', 'O', 'O', 'O', 'O', 'O', 'O', 'O']
Extractive/present Keyphrases: ['ranking', 'pagerank', 'mean reciprocal rank', 'mean average precision', 'query specificity', 'link graph', 'scale and relevance', 'hyperlink analysis', 'rank', 'bm25f', 'mrr', 'map', 'ndcg']
Abstractive/absent Keyphrases: ['normalized discounted cumulative gain measurement', 'breadth-first search crawl', 'feature selection', 'link-based feature', 'quantitative measure', 'crawled web page', 'hit']
-----------
Sample from test dataset split
Fields in the sample: ['id', 'document', 'doc_bio_tags', 'extractive_keyphrases', 'abstractive_keyphrases', 'other_metadata']
Tokenized Document: ['Live', 'Data', 'Center', 'Migration', 'across', 'WANs:', 'A', 'Robust', 'Cooperative', 'Context', 'Aware', 'Approach', 'K.K.', 'Ramakrishnan,', 'Prashant', 'Shenoy', ',', 'Jacobus', 'Van', 'der', 'Merwe', 'AT&T', 'Labs-Research', '/', 'University', 'of', 'Massachusetts', 'ABSTRACT', 'A', 'significant', 'concern', 'for', 'Internet-based', 'service', 'providers', 'is', 'the', 'continued', 'operation', 'and', 'availability', 'of', 'services', 'in', 'the', 'face', 'of', 'outages,', 'whether', 'planned', 'or', 'unplanned.', 'In', 'this', 'paper', 'we', 'advocate', 'a', 'cooperative,', 'context-aware', 'approach', 'to', 'data', 'center', 'migration', 'across', 'WANs', 'to', 'deal', 'with', 'outages', 'in', 'a', 'non-disruptive', 'manner.', 'We', 'specifically', 'seek', 'to', 'achieve', 'high', 'availability', 'of', 'data', 'center', 'services', 'in', 'the', 'face', 'of', 'both', 'planned', 'and', 'unanticipated', 'outages', 'of', 'data', 'center', 'facilities.', 'We', 'make', 'use', 'of', 'server', 'virtualization', 'technologies', 'to', 'enable', 'the', 'replication', 'and', 'migration', 'of', 'server', 'functions.', 'We', 'propose', 'new', 'network', 'functions', 'to', 'enable', 'server', 'migration', 'and', 'replication', 'across', 'wide', 'area', 'networks', '(e.g.,', 'the', 'Internet),', 'and', 'finally', 'show', 'the', 'utility', 'of', 'intelligent', 'and', 'dynamic', 'storage', 'replication', 'technology', 'to', 'ensure', 'applications', 'have', 'access', 'to', 'data', 'in', 'the', 'face', 'of', 'outages', 'with', 'very', 'tight', 'recovery', 'point', 'objectives.', 'Categories', 'and', 'Subject', 'Descriptors', 'C.2.4', '[Computer-Communication', 'Networks]:', 'Distributed', 'Systems', 'General', 'Terms', 'Design,', 'Reliability', '1.', 'INTRODUCTION', 'A', 'significant', 'concern', 'for', 'Internet-based', 'service', 'providers', 'is', 'the', 'continued', 'operation', 'and', 'availability', 'of', 'services', 'in', 'the', 'face', 'of', 'outages,', 'whether', 'planned', 'or', 'unplanned.', 'These', 'concerns', 'are', 'exacerbated', 'by', 'the', 'increased', 'use', 'of', 'the', 'Internet', 'for', 'mission', 'critical', 'business', 'and', 'real-time', 'entertainment', 'applications.', 'A', 'relatively', 'minor', 'outage', 'can', 'disrupt', 'and', 'inconvenience', 'a', 'large', 'number', 'of', 'users.', 'Today', 'these', 'services', 'are', 'almost', 'exclusively', 'hosted', 'in', 'data', 'centers.', 'Recent', 'advances', 'in', 'server', 'virtualization', 'technologies', '[8,', '14,', '22]', 'allow', 'for', 'the', 'live', 'migration', 'of', 'services', 'within', 'a', 'local', 'area', 'network', '(LAN)', 'environment.', 'In', 'the', 'LAN', 'environment,', 'these', 'technologies', 'have', 'proven', 'to', 'be', 'a', 'very', 'effective', 'tool', 'to', 'enable', 'data', 'center', 'management', 'in', 'a', 'non-disruptive', 'fashion.', 'Not', 'only', 'can', 'it', 'support', 'planned', 'maintenance', 'events', '[8],', 'but', 'it', 'can', 'also', 'be', 'used', 'in', 'a', 'more', 'dynamic', 'fashion', 'to', 'automatically', 'balance', 'load', 'between', 'the', 'physical', 'servers', 'in', 'a', 'data', 'center', '[22].', 'When', 'using', 'these', 'technologies', 'in', 'a', 'LAN', 'environment,', 'services', 'execute', 'in', 'a', 'virtual', 'server,', 'and', 'the', 'migration', 'services', 'provided', 'by', 'the', 'underlying', 'virtualization', 'framework', 'allows', 'for', 'a', 'virtual', 'server', 'to', 'be', 'migrated', 'from', 'one', 'physical', 'server', 'to', 'another,', 'without', 'any', 'significant', 'downtime', 'for', 'the', 'service', 'or', 'application.', 'In', 'particular,', 'since', 'the', 'virtual', 'server', 'retains', 'the', 'same', 'network', 'address', 'as', 'before,', 'any', 'ongoing', 'network', 'level', 'interactions', 'are', 'not', 'disrupted.', 'Similarly,', 'in', 'a', 'LAN', 'environment,', 'storage', 'requirements', 'are', 'normally', 'met', 'via', 'either', 'network', 'attached', 'storage', '(NAS)', 'or', 'via', 'a', 'storage', 'area', 'network', '(SAN)', 'which', 'is', 'still', 'reachable', 'from', 'the', 'new', 'physical', 'server', 'location', 'to', 'allow', 'for', 'continued', 'storage', 'access.', 'Unfortunately', 'in', 'a', 'wide', 'area', 'environment', '(WAN),', 'live', 'server', 'migration', 'is', 'not', 'as', 'easily', 'achievable', 'for', 'two', 'reasons:', 'First,', 'live', 'migration', 'requires', 'the', 'virtual', 'server', 'to', 'maintain', 'the', 'same', 'network', 'address', 'so', 'that', 'from', 'a', 'network', 'connectivity', 'viewpoint', 'the', 'migrated', 'server', 'is', 'indistinguishable', 'from', 'the', 'original.', 'While', 'this', 'is', 'fairly', 'easily', 'achieved', 'in', 'a', 'shared', 'LAN', 'environment,', 'no', 'current', 'mechanisms', 'are', 'available', 'to', 'efficiently', 'achieve', 'the', 'same', 'feat', 'in', 'a', 'WAN', 'environment.', 'Second,', 'while', 'fairly', 'sophisticated', 'remote', 'replication', 'mechanisms', 'have', 'been', 'developed', 'in', 'the', 'context', 'of', 'disaster', 'recovery', '[20,', '7,', '11],', 'these', 'mechanisms', 'are', 'ill', 'suited', 'to', 'live', 'data', 'center', 'migration,', 'because', 'in', 'general', 'the', 'available', 'technologies', 'are', 'unaware', 'of', 'application/service', 'level', 'semantics.', 'In', 'this', 'paper', 'we', 'outline', 'a', 'design', 'for', 'live', 'service', 'migration', 'across', 'WANs.', 'Our', 'design', 'makes', 'use', 'of', 'existing', 'server', 'virtualization', 'technologies', 'and', 'propose', 'network', 'and', 'storage', 'mechanisms', 'to', 'facilitate', 'migration', 'across', 'a', 'WAN.', 'The', 'essence', 'of', 'our', 'approach', 'is', 'cooperative,', 'context', 'aware', 'migration,', 'where', 'a', 'migration', 'management', 'system', 'orchestrates', 'the', 'data', 'center', 'migration', 'across', 'all', 'three', 'subsystems', 'involved,', 'namely', 'the', 'server', 'platforms,', 'the', 'wide', 'area', 'network', 'and', 'the', 'disk', 'storage', 'system.', 'While', 'conceptually', 'similar', 'in', 'nature', 'to', 'the', 'LAN', 'based', 'work', 'described', 'above,', 'using', 'migration', 'technologies', 'across', 'a', 'wide', 'area', 'network', 'presents', 'unique', 'challenges', 'and', 'has', 'to', 'our', 'knowledge', 'not', 'been', 'achieved.', 'Our', 'main', 'contribution', 'is', 'the', 'design', 'of', 'a', 'framework', 'that', 'will', 'allow', 'the', 'migration', 'across', 'a', 'WAN', 'of', 'all', 'subsystems', 'involved', 'with', 'enabling', 'data', 'center', 'services.', 'We', 'describe', 'new', 'mechanisms', 'as', 'well', 'as', 'extensions', 'to', 'existing', 'technologies', 'to', 'enable', 'this', 'and', 'outline', 'the', 'cooperative,', 'context', 'aware', 'functionality', 'needed', 'across', 'the', 'different', 'subsystems', 'to', 'enable', 'this.', '262', '2.', 'LIVE', 'DATA', 'CENTER', 'MIGRATION', 'ACROSS', 'WANS', 'Three', 'essential', 'subsystems', 'are', 'involved', 'with', 'hosting', 'services', 'in', 'a', 'data', 'center:', 'First,', 'the', 'servers', 'host', 'the', 'application', 'or', 'service', 'logic.', 'Second,', 'services', 'are', 'normally', 'hosted', 'in', 'a', 'data', 'center', 'to', 'provide', 'shared', 'access', 'through', 'a', 'network,', 'either', 'the', 'Internet', 'or', 'virtual', 'private', 'networks', '(VPNs).', 'Finally,', 'most', 'applications', 'require', 'disk', 'storage', 'for', 'storing', 'data', 'and', 'the', 'amount', 'of', 'disk', 'space', 'and', 'the', 'frequency', 'of', 'access', 'varies', 'greatly', 'between', 'different', 'services/applications.', 'Disruptions,', 'failures,', 'or', 'in', 'general,', 'outages', 'of', 'any', 'kind', 'of', 'any', 'of', 'these', 'components', 'will', 'cause', 'service', 'disruption.', 'For', 'this', 'reason,', 'prior', 'work', 'and', 'current', 'practices', 'have', 'addressed', 'the', 'robustness', 'of', 'individual', 'components.', 'For', 'example,', 'data', 'centers', 'typically', 'have', 'multiple', 'network', 'connections', 'and', 'redundant', 'LAN', 'devices', 'to', 'ensure', 'redundancy', 'at', 'the', 'networking', 'level.', 'Similarly,', 'physical', 'servers', 'are', 'being', 'designed', 'with', 'redundant', 'hot-swappable', 'components', '(disks,', 'processor', 'blades,', 'power', 'supplies', 'etc).', 'Finally,', 'redundancy', 'at', 'the', 'storage', 'level', 'can', 'be', 'provided', 'through', 'sophisticated', 'data', 'mirroring', 'technologies.', 'The', 'focus', 'of', 'our', 'work,', 'however,', 'is', 'on', 'the', 'case', 'where', 'such', 'local', 'redundancy', 'mechanisms', 'are', 'not', 'sufficient.', 'Specifically,', 'we', 'are', 'interested', 'in', 'providing', 'service', 'availability', 'when', 'the', 'data', 'center', 'as', 'a', 'whole', 'becomes', 'unavailable,', 'for', 'example', 'because', 'of', 'data', 'center', 'wide', 'maintenance', 'operations,', 'or', 'because', 'of', 'catastrophic', 'events.', 'As', 'such,', 'our', 'basic', 'approach', 'is', 'to', 'migrate', 'services', 'between', 'data', 'centers', 'across', 'the', 'wide', 'are', 'network', '(WAN).', 'By', 'necessity,', 'moving', 'or', 'migrating', 'services', 'from', 'one', 'data', 'center', 'to', 'another', 'needs', 'to', 'consider', 'all', 'three', 'of', 'these', 'components.', 'Historically,', 'such', 'migration', 'has', 'been', 'disruptive', 'in', 'nature,', 'requiring', 'downtime', 'of', 'the', 'actual', 'services', 'involved,', 'or', 'requiring', 'heavy', 'weight', 'replication', 'techniques.', 'In', 'the', 'latter', 'case', 'concurrently', 'running', 'replicas', 'of', 'a', 'service', 'can', 'be', 'made', 'available', 'thus', 'allowing', 'a', 'subset', 'of', 'the', 'service', 'to', 'be', 'migrated', 'or', 'maintained', 'without', 'impacting', 'the', 'service']
Document BIO Tags: ['O', 'B', 'I', 'I', 'O', 'O', 'O', 'O', 'O', 'O', 'O', 'O', 'O', 'O', 'O', 'O', 'O', 'O', 'O', 'O', 'O', 'O', 'O', 'O', 'O', 'O', 'O', 'O', 'O', 'O', 'O', 'O', 'O', 'O', 'O', 'O', 'O', 'O', 'O', 'O', 'O', 'O', 'O', 'O', 'O', 'O', 'O', 'O', 'O', 'O', 'O', 'O', 'O', 'O', 'O', 'O', 'O', 'O', 'O', 'O', 'O', 'O', 'B', 'I', 'I', 'O', 'O', 'O', 'O', 'O', 'O', 'O', 'O', 'O', 'O', 'O', 'O', 'O', 'O', 'O', 'O', 'O', 'O', 'O', 'O', 'O', 'O', 'O', 'O', 'O', 'O', 'O', 'O', 'O', 'O', 'O', 'O', 'O', 'O', 'O', 'O', 'O', 'O', 'O', 'O', 'O', 'O', 'O', 'O', 'O', 'O', 'O', 'O', 'O', 'O', 'O', 'O', 'O', 'O', 'O', 'O', 'O', 'O', 'O', 'O', 'O', 'O', 'O', 'O', 'O', 'O', 'O', 'O', 'O', 'O', 'O', 'O', 'O', 'O', 'O', 'O', 'O', 'B', 'I', 'O', 'O', 'O', 'O', 'O', 'O', 'O', 'O', 'O', 'O', 'O', 'O', 'O', 'O', 'O', 'O', 'O', 'O', 'O', 'O', 'O', 'O', 'O', 'O', 'O', 'O', 'O', 'O', 'O', 'O', 'O', 'O', 'O', 'O', 'O', 'O', 'O', 'O', 'O', 'O', 'O', 'O', 'O', 'O', 'O', 'O', 'O', 'O', 'O', 'O', 'O', 'O', 'O', 'O', 'O', 'O', 'O', 'O', 'O', 'O', 'O', 'O', 'O', 'O', 'O', 'O', 'O', 'O', 'O', 'O', 'O', 'O', 'O', 'O', 'O', 'O', 'O', 'O', 'O', 'O', 'O', 'O', 'O', 'O', 'O', 'O', 'O', 'O', 'O', 'O', 'O', 'O', 'O', 'O', 'O', 'O', 'O', 'O', 'O', 'O', 'O', 'O', 'O', 'O', 'O', 'O', 'O', 'O', 'O', 'O', 'O', 'O', 'O', 'O', 'O', 'O', 'O', 'O', 'O', 'O', 'O', 'B', 'O', 'O', 'O', 'B', 'O', 'O', 'O', 'O', 'O', 'O', 'O', 'O', 'O', 'O', 'O', 'O', 'O', 'O', 'O', 'O', 'O', 'O', 'O', 'O', 'O', 'O', 'O', 'O', 'O', 'O', 'O', 'O', 'O', 'O', 'O', 'O', 'O', 'O', 'O', 'O', 'O', 'O', 'O', 'O', 'O', 'O', 'O', 'O', 'O', 'O', 'O', 'O', 'O', 'O', 'O', 'O', 'O', 'O', 'O', 'O', 'O', 'O', 'O', 'B', 'O', 'O', 'O', 'O', 'O', 'B', 'I', 'O', 'O', 'O', 'O', 'O', 'O', 'O', 'O', 'O', 'O', 'O', 'O', 'O', 'B', 'I', 'O', 'O', 'O', 'O', 'O', 'O', 'O', 'O', 'O', 'O', 'O', 'O', 'O', 'O', 'O', 'O', 'O', 'O', 'O', 'O', 'O', 'O', 'B', 'I', 'O', 'O', 'O', 'O', 'O', 'O', 'O', 'O', 'O', 'O', 'O', 'O', 'O', 'O', 'O', 'O', 'O', 'O', 'B', 'O', 'B', 'O', 'O', 'O', 'O', 'O', 'O', 'O', 'O', 'B', 'O', 'O', 'O', 'O', 'B', 'O', 'O', 'O', 'O', 'O', 'O', 'O', 'O', 'O', 'O', 'O', 'O', 'O', 'O', 'O', 'O', 'O', 'B', 'O', 'O', 'O', 'O', 'O', 'O', 'O', 'B', 'O', 'O', 'O', 'O', 'O', 'O', 'O', 'O', 'O', 'O', 'O', 'O', 'O', 'O', 'O', 'O', 'B', 'I', 'O', 'O', 'O', 'O', 'O', 'O', 'O', 'O', 'O', 'O', 'O', 'O', 'O', 'O', 'O', 'O', 'O', 'O', 'O', 'O', 'O', 'O', 'O', 'O', 'O', 'O', 'O', 'O', 'O', 'O', 'B', 'O', 'O', 'O', 'O', 'O', 'O', 'O', 'O', 'O', 'O', 'O', 'O', 'O', 'O', 'B', 'O', 'O', 'O', 'O', 'O', 'O', 'O', 'O', 'O', 'O', 'O', 'O', 'O', 'O', 'O', 'O', 'O', 'O', 'O', 'O', 'O', 'O', 'O', 'O', 'O', 'O', 'O', 'B', 'I', 'I', 'O', 'O', 'O', 'O', 'O', 'O', 'O', 'O', 'O', 'O', 'O', 'O', 'O', 'O', 'O', 'O', 'O', 'O', 'O', 'O', 'O', 'O', 'O', 'O', 'O', 'O', 'O', 'O', 'O', 'O', 'O', 'O', 'O', 'O', 'O', 'O', 'O', 'O', 'B', 'O', 'O', 'O', 'O', 'O', 'O', 'B', 'O', 'O', 'O', 'O', 'O', 'O', 'O', 'O', 'O', 'O', 'O', 'O', 'O', 'O', 'O', 'O', 'O', 'B', 'I', 'I', 'O', 'O', 'O', 'O', 'O', 'O', 'O', 'O', 'O', 'O', 'O', 'O', 'O', 'O', 'O', 'O', 'B', 'O', 'O', 'O', 'O', 'O', 'O', 'O', 'O', 'B', 'O', 'O', 'O', 'O', 'O', 'O', 'O', 'O', 'O', 'O', 'O', 'O', 'O', 'O', 'O', 'O', 'O', 'O', 'O', 'O', 'O', 'O', 'O', 'O', 'O', 'O', 'O', 'O', 'O', 'O', 'O', 'O', 'O', 'O', 'O', 'O', 'O', 'O', 'O', 'B', 'O', 'O', 'O', 'O', 'O', 'O', 'O', 'O', 'O', 'O', 'O', 'O', 'O', 'O', 'O', 'O', 'O', 'O', 'O', 'O', 'O', 'O', 'O', 'O', 'O', 'O', 'O', 'O', 'O', 'O', 'O', 'O', 'O', 'O', 'O', 'O', 'O', 'O', 'O', 'O', 'O', 'B', 'I', 'I', 'O', 'O', 'O', 'O', 'O', 'O', 'O', 'O', 'O', 'O', 'O', 'O', 'O', 'O', 'O', 'O', 'O', 'O', 'O', 'O', 'O', 'O', 'O', 'O', 'O', 'O', 'O', 'O', 'O', 'O', 'O', 'O', 'O', 'O', 'O', 'O', 'O', 'O', 'O', 'O', 'O', 'O', 'O', 'O', 'O', 'O', 'O', 'O', 'O', 'O', 'O', 'O', 'B', 'O', 'O', 'O', 'O', 'O', 'O', 'O', 'O', 'O', 'O', 'O', 'O', 'O', 'O', 'O', 'O', 'O', 'O', 'O', 'O', 'O', 'O', 'O', 'O', 'O', 'O', 'O', 'O', 'O', 'O', 'O', 'O', 'O', 'O', 'O', 'O', 'O', 'O', 'O', 'O', 'O', 'O', 'O', 'O', 'O', 'O', 'O', 'O', 'O', 'O', 'O', 'O', 'O', 'O', 'O', 'O', 'O', 'O', 'O', 'O', 'O', 'O', 'O', 'B', 'O', 'O', 'O', 'O', 'O', 'O', 'O', 'O', 'O', 'O', 'O', 'O', 'O', 'O', 'O', 'O', 'O', 'O', 'O', 'O', 'O', 'O', 'O', 'O', 'O', 'O', 'O', 'O', 'B', 'O', 'O', 'O', 'O', 'O', 'O', 'O', 'O', 'O', 'O', 'O', 'O', 'O', 'O', 'O', 'O', 'O', 'O', 'O', 'O', 'O', 'O', 'O', 'O', 'O', 'O', 'O', 'O', 'O', 'O', 'O', 'O', 'O', 'O', 'O', 'O', 'O', 'O', 'O', 'O', 'O', 'O', 'O', 'O', 'O', 'O', 'O', 'O', 'O', 'O', 'O', 'O', 'O', 'O', 'O', 'O', 'O', 'O', 'O', 'O', 'O', 'O', 'O', 'O', 'O', 'O', 'O', 'O', 'O', 'O', 'O', 'O', 'O', 'O', 'O', 'B', 'O', 'O', 'O', 'O', 'O', 'O', 'O', 'O', 'O', 'O', 'O', 'O', 'O', 'O', 'O', 'O', 'O', 'O', 'O', 'O', 'O', 'O', 'O', 'O', 'O', 'O', 'O', 'O', 'O', 'O', 'O', 'O', 'O', 'O', 'O', 'O', 'O', 'O', 'O', 'O', 'O', 'O', 'O', 'O', 'O', 'O', 'O', 'O', 'O', 'O', 'O', 'O', 'O', 'O', 'O', 'O', 'O', 'O', 'O', 'O', 'O', 'O', 'O', 'O', 'O', 'O', 'O', 'O', 'O', 'O', 'O']
Extractive/present Keyphrases: ['data center migration', 'wan', 'lan', 'virtual server', 'storage replication', 'synchronous replication', 'asynchronous replication', 'network support', 'storage', 'voip']
Abstractive/absent Keyphrases: ['internet-based service', 'voice-over-ip', 'database']
-----------
```
### Keyphrase Extraction
```python
from datasets import load_dataset
# get the dataset only for keyphrase extraction
dataset = load_dataset("midas/semeval2010", "extraction")
print("Samples for Keyphrase Extraction")
# sample from the train split
print("Sample from train data split")
test_sample = dataset["train"][0]
print("Fields in the sample: ", [key for key in test_sample.keys()])
print("Tokenized Document: ", test_sample["document"])
print("Document BIO Tags: ", test_sample["doc_bio_tags"])
print("\n-----------\n")
# sample from the test split
print("Sample from test data split")
test_sample = dataset["test"][0]
print("Fields in the sample: ", [key for key in test_sample.keys()])
print("Tokenized Document: ", test_sample["document"])
print("Document BIO Tags: ", test_sample["doc_bio_tags"])
print("\n-----------\n")
```
### Keyphrase Generation
```python
# get the dataset only for keyphrase generation
dataset = load_dataset("midas/semeval2010", "generation")
print("Samples for Keyphrase Generation")
# sample from the train split
print("Sample from train data split")
test_sample = dataset["train"][0]
print("Fields in the sample: ", [key for key in test_sample.keys()])
print("Tokenized Document: ", test_sample["document"])
print("Extractive/present Keyphrases: ", test_sample["extractive_keyphrases"])
print("Abstractive/absent Keyphrases: ", test_sample["abstractive_keyphrases"])
print("\n-----------\n")
# sample from the test split
print("Sample from test data split")
test_sample = dataset["test"][0]
print("Fields in the sample: ", [key for key in test_sample.keys()])
print("Tokenized Document: ", test_sample["document"])
print("Extractive/present Keyphrases: ", test_sample["extractive_keyphrases"])
print("Abstractive/absent Keyphrases: ", test_sample["abstractive_keyphrases"])
print("\n-----------\n")
```
## Citation Information
```
@inproceedings{10.5555/1859664.1859668,
author = {Kim, Su Nam and Medelyan, Olena and Kan, Min-Yen and Baldwin, Timothy},
title = {SemEval-2010 Task 5: Automatic Keyphrase Extraction from Scientific Articles},
year = {2010},
publisher = {Association for Computational Linguistics},
address = {USA},
abstract = {This paper describes Task 5 of the Workshop on Semantic Evaluation 2010 (SemEval-2010). Systems are to automatically assign keyphrases or keywords to given scientific articles. The participating systems were evaluated by matching their extracted keyphrases against manually assigned ones. We present the overall ranking of the submitted systems and discuss our findings to suggest future directions for this task.},
booktitle = {Proceedings of the 5th International Workshop on Semantic Evaluation},
pages = {21–26},
numpages = {6},
location = {Los Angeles, California},
series = {SemEval '10}
}
```
## Contributions
Thanks to [@debanjanbhucs](https://github.com/debanjanbhucs), [@dibyaaaaax](https://github.com/dibyaaaaax) and [@ad6398](https://github.com/ad6398) for adding this dataset
| midas/semeval2010 | [
"arxiv:1910.08840",
"region:us"
] | 2022-03-02T23:29:22+00:00 | {} | 2022-03-05T03:24:16+00:00 | [
"1910.08840"
] | [] | TAGS
#arxiv-1910.08840 #region-us
| A dataset for benchmarking keyphrase extraction and generation techniques from long document english scientific articles. For more details about the dataset please refer the original paper - URL
Original source of the data - URL
Dataset Summary
---------------
The Semeval-2010 dataset was originally proposed by *Su Nam Kim et al* in the paper titled - SemEval-2010 Task 5: Automatic Keyphrase Extraction from Scientific Articles in the year 2010. The dataset consists of a set of 284 English scientific papers from the ACM Digital Library (conference and work-shop papers). The selected articles belong to the
following four 1998 ACM classifications: C2.4 (Distributed Systems), H3.3 (Information Search and Retrieval), I2.11 (Distributed Artificial Intelligence – Multiagent Systems) and J4 (Socialand Behavioral Sciences – Economics). Each paper has two sets of keyphrases annotated by readers and author. The original dataset was divided into trail, training and test splits, evenly distributed across four domains. The trial, training and test splits had 40, 144 and 100 articles respectively, and the trial split was a subset of training split. We provide test and train splits with 100 and 144 articles respectively.
The dataset shared over here categorizes the keyphrases into *extractive* and *abstractive*. Extractive keyphrases are those that could be found in the input text and the abstractive keyphrases are those that are not present in the input text. In order to get all the meta-data about the documents and keyphrases please refer to the original source from which the dataset was taken from. The main motivation behind making this dataset available in the form as presented over here is to make it easy for the researchers to programmatically download it and evaluate their models for the tasks of keyphrase extraction and generation. As keyphrase extraction by treating it as a sequence tagging task and using contextual language models has become popular - Keyphrase extraction from scholarly articles as sequence labeling using contextualized embeddings, we have also made the token tags available in the BIO tagging format.
Dataset Structure
-----------------
### Data Fields
* id: unique identifier of the document.
* document: Whitespace separated list of words in the document.
* doc\_bio\_tags: BIO tags for each word in the document. B stands for the beginning of a keyphrase and I stands for inside the keyphrase. O stands for outside the keyphrase and represents the word that isn't a part of the keyphrase at all.
* extractive\_keyphrases: List of all the present keyphrases.
* abstractive\_keyphrase: List of all the absent keyphrases.
### Data Splits
Train
* Percentage of keyphrases that are named entities: 63.01% (named entities detected using scispacy - en-core-sci-lg model)
* Percentage of keyphrases that are noun phrases: 82.50% (noun phrases detected using spacy en-core-web-lg after removing determiners)
Test
* Percentage of keyphrases that are named entities: 62.06% (named entities detected using scispacy - en-core-sci-lg model)
* Percentage of keyphrases that are noun phrases: 78.36% (noun phrases detected using spacy after removing determiners)
Usage
-----
### Full Dataset
Output
### Keyphrase Extraction
### Keyphrase Generation
Contributions
-------------
Thanks to @debanjanbhucs, @dibyaaaaax and @ad6398 for adding this dataset
| [
"### Data Fields\n\n\n* id: unique identifier of the document.\n* document: Whitespace separated list of words in the document.\n* doc\\_bio\\_tags: BIO tags for each word in the document. B stands for the beginning of a keyphrase and I stands for inside the keyphrase. O stands for outside the keyphrase and represents the word that isn't a part of the keyphrase at all.\n* extractive\\_keyphrases: List of all the present keyphrases.\n* abstractive\\_keyphrase: List of all the absent keyphrases.",
"### Data Splits\n\n\n\nTrain\n\n\n* Percentage of keyphrases that are named entities: 63.01% (named entities detected using scispacy - en-core-sci-lg model)\n* Percentage of keyphrases that are noun phrases: 82.50% (noun phrases detected using spacy en-core-web-lg after removing determiners)\n\n\nTest\n\n\n* Percentage of keyphrases that are named entities: 62.06% (named entities detected using scispacy - en-core-sci-lg model)\n* Percentage of keyphrases that are noun phrases: 78.36% (noun phrases detected using spacy after removing determiners)\n\n\nUsage\n-----",
"### Full Dataset\n\n\nOutput",
"### Keyphrase Extraction",
"### Keyphrase Generation\n\n\nContributions\n-------------\n\n\nThanks to @debanjanbhucs, @dibyaaaaax and @ad6398 for adding this dataset"
] | [
"TAGS\n#arxiv-1910.08840 #region-us \n",
"### Data Fields\n\n\n* id: unique identifier of the document.\n* document: Whitespace separated list of words in the document.\n* doc\\_bio\\_tags: BIO tags for each word in the document. B stands for the beginning of a keyphrase and I stands for inside the keyphrase. O stands for outside the keyphrase and represents the word that isn't a part of the keyphrase at all.\n* extractive\\_keyphrases: List of all the present keyphrases.\n* abstractive\\_keyphrase: List of all the absent keyphrases.",
"### Data Splits\n\n\n\nTrain\n\n\n* Percentage of keyphrases that are named entities: 63.01% (named entities detected using scispacy - en-core-sci-lg model)\n* Percentage of keyphrases that are noun phrases: 82.50% (noun phrases detected using spacy en-core-web-lg after removing determiners)\n\n\nTest\n\n\n* Percentage of keyphrases that are named entities: 62.06% (named entities detected using scispacy - en-core-sci-lg model)\n* Percentage of keyphrases that are noun phrases: 78.36% (noun phrases detected using spacy after removing determiners)\n\n\nUsage\n-----",
"### Full Dataset\n\n\nOutput",
"### Keyphrase Extraction",
"### Keyphrase Generation\n\n\nContributions\n-------------\n\n\nThanks to @debanjanbhucs, @dibyaaaaax and @ad6398 for adding this dataset"
] |
d0e60069bbcd0c0bdd4127eb52494e928b765103 | A dataset for benchmarking keyphrase extraction and generation techniques from abstracts of english scientific articles. For more details about the dataset please refer the original paper - [https://arxiv.org/abs/1704.02853](https://arxiv.org/abs/1704.02853)
Original source of the data - [https://scienceie.github.io/](https://scienceie.github.io/)
## Dataset Summary
The Semeval-2017 dataset was originally proposed by *Isabelle Augenstein et al.* in the paper titled - [SemEval 2017 Task 10: ScienceIE - Extracting Keyphrases and Relations from Scientific Publications](https://arxiv.org/abs/1704.02853) in the year 2017. The dataset consists of a abstracts of 500 English scientific papers from the ScienceDirect open access publications. The selected articles were evenly distributed among the domains of Computer Science, Material Sciences and Physics. Each paper has a set of keyphrases annotated by student volunteers. Each paper was double-annotated, where the second annotation was done by an expert annotator. In case of disagreement, the annotations done by expert annotators were chosen. The original dataset was divided into train, dev and test splits, evenly distributed across the three domains. The train, dev and test splits had 350, 50 and 100 articles respectively.
The dataset shared over here categorizes the keyphrases into *extractive* and *abstractive*. **Extractive keyphrases** are those that could be found in the input text and the **abstractive keyphrases** are those that are not present in the input text. In order to get all the meta-data about the documents and keyphrases please refer to the [original source](https://scienceie.github.io/) from which the dataset was taken from. The main motivation behind making this dataset available in the form as presented over here is to make it easy for the researchers to programmatically download it and evaluate their models for the tasks of keyphrase extraction and generation. As keyphrase extraction by treating it as a sequence tagging task and using contextual language models has become popular - [Keyphrase extraction from scholarly articles as sequence labeling using contextualized embeddings](https://arxiv.org/pdf/1910.08840.pdf), we have also made the token tags available in the BIO tagging format.
## Dataset Structure
Table 1: Statistics on the length of the abstractive keyphrases for Train, Test, and Validation splits of SemEval 2017 dataset.
| | Train | Test | Validation |
|:-----------------:|:------:|:------:|:----------:|
| Single word | 11.59% | 12.47% | 12.89% |
| Two words | 30.69% | 40.92% | 33.45% |
| Three words | 19.20% | 17.50% | 19.16% |
| Four words | 10.25% | 10.94% | 9.41% |
| Five words | 7.43% | 4.60% | 8.36% |
| Six words | 5.96% | 4.37% | 6.27% |
| Seven words | 4.28% | 2.40% | 3.14% |
| Eight words | 2.59% | 1.75% | 1.34% |
| Nine words | 2.19% | 1.75% | 1.74% |
| Ten words | 1.35% | 1.31% | 0.69% |
| Eleven words | 0.96% | 0.44% | 1.04% |
| Twelve words | 1.13% | 0.44% | 1.04% |
| Thirteen words | 0% | 0.44% | 0.34% |
| Fourteen words | 0.45% | 0.22% | 0.348% |
| Fifteen words | 0.39% | 0% | 0% |
| Sixteen words | 0.17% | 0% | 0% |
| Seventeen words | 0.11% | 0.22% | 0.34% |
| Eighteen words | 0.11% | 0% | 0% |
| Nineteen words | 0.11% | 0.22% | 0.34% |
| Twenty words | 0.06% | 0% | 0% |
| Twenty-two words | 0.06% | 0% | 0% |
| Twenty-five words | 0% | 0% | 0% |
Table 2: Statistics on the length of the extractive keyphrases for Train, Test, and Validation splits of SemEval 2017 dataset.
| | Train | Test | Validation |
|:-----------------:|:------:|:------:|:----------:|
| Single word | 27.94% | 34.50% | 36.56% |
| Two words | 33.04% | 39.64% | 31.72% |
| Three words | 17.85% | 13.45% | 15.50% |
| Four words | 8.75% | 6.19% | 7.11% |
| Five words | 4.72% | 2.44% | 4.27% |
| Six words | 2.24% | 0.89% | 1.85% |
| Seven words | 1.66% | 0.73% | 1.28% |
| Eight words | 1.33% | 0.48% | 0.43% |
| Nine words | 0.54% | 0.97% | 0.14% |
| Ten words | 0.21% | 0.24% | 0.57% |
| Eleven words | 0.38% | 0.081% | 0.28% |
| Twelve words | 0% | 0.16% | 0.14% |
| Thirteen words | 0.28% | 0% | 0% |
| Fourteen words | 0.21% | 0% | 0% |
| Fifteen words | 0.071% | 0% | 0% |
| Sixteen words | 0.02% | 0.081% | 0% |
| Eighteen words | 0% | 0.081% | 0.14 |
| Nineteen words | 0.02% | 0% | 0% |
| Twenty-five words | 0.04% | 0% | 0% |
Table 3: General statistics of the Semeval 2017 dataset.
| Type of Analysis | Train | Test | Validation |
|:------------------------------------------------:|:-------------------:|:-------------------:|:-------------------:|
| Annotator Type | Authors and Readers | Authors and Readers | Authors and Readers |
| Document Type | Scientific Papers | Scientific Papers | Scientific Papers |
| No. of Documents | 350 | 100 | 50 |
| Avg. Document length (words) | 160.5 | 190.4 | 380.8 |
| Max Document length (words) | 355 | 297 | 355 |
| Max no. of abstractive keyphrases in a document | 23 | 13 | 22 |
| Min no. of abstractive keyphrases in a document | 0 | 0 | 0 |
| Avg. no. of abstractive keyphrases per document | 5.07 | 4.57 | 5.74 |
| Max no. of extractive keyphrases in a document | 29 | 27 | 30 |
| Min no. of extractive keyphrases in a document | 2 | 4 | 2 |
| Avg. no. of extractive keyphrases per document | 11.9 | 12.26 | 14.06 |
Train
- Percentage of keyphrases that are named entities: 50.09% (named entities detected using scispacy - en-core-sci-lg model)
- Percentage of keyphrases that are noun phrases: 57.65% (noun phrases detected using spacy en-core-web-lg after removing determiners)
Validation
- Percentage of keyphrases that are named entities: 60.02% (named entities detected using scispacy - en-core-sci-lg model)
- Percentage of keyphrases that are noun phrases: 62.87% (noun phrases detected using spacy en-core-web-lg after removing determiners)
Test
- Percentage of keyphrases that are named entities: 59.78% (named entities detected using scispacy - en-core-sci-lg model)
- Percentage of keyphrases that are noun phrases: 66.39% (noun phrases detected using spacy en-core-web-lg after removing determiners)
### Data Fields
- **id**: unique identifier of the document.
- **document**: Whitespace separated list of words in the document.
- **doc_bio_tags**: BIO tags for each word in the document. B stands for the beginning of a keyphrase and I stands for inside the keyphrase. O stands for outside the keyphrase and represents the word that isn't a part of the keyphrase at all.
- **extractive_keyphrases**: List of all the present keyphrases.
- **abstractive_keyphrase**: List of all the absent keyphrases.
### Data Splits
|Split| #datapoints |
|--|--|
| Train | 350 |
| Test | 100 |
| Validation | 50 |
## Usage
### Full Dataset
```python
from datasets import load_dataset
# get entire dataset
dataset = load_dataset("midas/semeval2017", "raw")
# sample from the train split
print("Sample from train dataset split")
test_sample = dataset["train"][0]
print("Fields in the sample: ", [key for key in test_sample.keys()])
print("Tokenized Document: ", test_sample["document"])
print("Document BIO Tags: ", test_sample["doc_bio_tags"])
print("Extractive/present Keyphrases: ", test_sample["extractive_keyphrases"])
print("Abstractive/absent Keyphrases: ", test_sample["abstractive_keyphrases"])
print("\n-----------\n")
# sample from the validation split
print("Sample from validation dataset split")
validation_sample = dataset["validation"][0]
print("Fields in the sample: ", [key for key in validation_sample.keys()])
print("Tokenized Document: ", validation_sample["document"])
print("Document BIO Tags: ", validation_sample["doc_bio_tags"])
print("Extractive/present Keyphrases: ", validation_sample["extractive_keyphrases"])
print("Abstractive/absent Keyphrases: ", validation_sample["abstractive_keyphrases"])
print("\n-----------\n")
# sample from the test split
print("Sample from test dataset split")
test_sample = dataset["test"][0]
print("Fields in the sample: ", [key for key in test_sample.keys()])
print("Tokenized Document: ", test_sample["document"])
print("Document BIO Tags: ", test_sample["doc_bio_tags"])
print("Extractive/present Keyphrases: ", test_sample["extractive_keyphrases"])
print("Abstractive/absent Keyphrases: ", test_sample["abstractive_keyphrases"])
print("\n-----------\n")
```
**Output**
```bash
Sample from train dataset split
Fields in the sample: ['id', 'document', 'doc_bio_tags', 'extractive_keyphrases', 'abstractive_keyphrases', 'other_metadata']
Tokenized Document: ['It', 'is', 'well', 'known', 'that', 'one', 'of', 'the', 'long', 'standing', 'problems', 'in', 'physics', 'is', 'understanding', 'the', 'confinement', 'physics', 'from', 'first', 'principles.', 'Hence', 'the', 'challenge', 'is', 'to', 'develop', 'analytical', 'approaches', 'which', 'provide', 'valuable', 'insight', 'and', 'theoretical', 'guidance.', 'According', 'to', 'this', 'viewpoint,', 'an', 'effective', 'theory', 'in', 'which', 'confining', 'potentials', 'are', 'obtained', 'as', 'a', 'consequence', 'of', 'spontaneous', 'symmetry', 'breaking', 'of', 'scale', 'invariance', 'has', 'been', 'developed', '[1].', 'In', 'particular,', 'it', 'was', 'shown', 'that', 'a', 'such', 'theory', 'relies', 'on', 'a', 'scale-invariant', 'Lagrangian', 'of', 'the', 'type', '[2]', '(1)L=14w2−12w−FμνaFaμν,', 'where', 'Fμνa=∂μAνa−∂νAμa+gfabcAμbAνc,', 'and', 'w', 'is', 'not', 'a', 'fundamental', 'field', 'but', 'rather', 'is', 'a', 'function', 'of', '4-index', 'field', 'strength,', 'that', 'is,', '(2)w=εμναβ∂μAναβ.', 'The', 'Aναβ', 'equation', 'of', 'motion', 'leads', 'to', '(3)εμναβ∂βw−−FγδaFaγδ=0,', 'which', 'is', 'then', 'integrated', 'to', '(4)w=−FμνaFaμν+M.', 'It', 'is', 'easy', 'to', 'verify', 'that', 'the', 'Aaμ', 'equation', 'of', 'motion', 'leads', 'us', 'to', '(5)∇μFaμν+MFaμν−FαβbFbαβ=0.', 'It', 'is', 'worth', 'stressing', 'at', 'this', 'stage', 'that', 'the', 'above', 'equation', 'can', 'be', 'obtained', 'from', 'the', 'effective', 'Lagrangian', '(6)Leff=−14FμνaFaμν+M2−FμνaFaμν.', 'Spherically', 'symmetric', 'solutions', 'of', 'Eq.', '(5)', 'display,', 'even', 'in', 'the', 'Abelian', 'case,', 'a', 'Coulomb', 'piece', 'and', 'a', 'confining', 'part.', 'Also,', 'the', 'quantum', 'theory', 'calculation', 'of', 'the', 'static', 'energy', 'between', 'two', 'charges', 'displays', 'the', 'same', 'behavior', '[1].', 'It', 'is', 'well', 'known', 'that', 'the', 'square', 'root', 'part', 'describes', 'string', 'like', 'solutions', '[3,4].']
Document BIO Tags: ['O', 'O', 'O', 'O', 'O', 'O', 'O', 'O', 'O', 'O', 'O', 'O', 'O', 'O', 'B', 'I', 'I', 'I', 'I', 'I', 'I', 'O', 'O', 'O', 'O', 'O', 'B', 'I', 'I', 'O', 'O', 'O', 'O', 'O', 'O', 'O', 'O', 'O', 'O', 'O', 'O', 'O', 'O', 'O', 'O', 'O', 'O', 'O', 'O', 'O', 'O', 'O', 'O', 'B', 'I', 'I', 'I', 'I', 'I', 'O', 'O', 'O', 'O', 'O', 'O', 'O', 'O', 'O', 'O', 'O', 'O', 'O', 'O', 'O', 'O', 'O', 'O', 'O', 'O', 'O', 'O', 'O', 'O', 'O', 'O', 'O', 'O', 'O', 'O', 'O', 'O', 'O', 'O', 'O', 'O', 'O', 'O', 'O', 'O', 'O', 'O', 'O', 'O', 'O', 'B', 'I', 'I', 'I', 'I', 'O', 'O', 'O', 'O', 'O', 'O', 'O', 'O', 'O', 'O', 'O', 'O', 'O', 'O', 'O', 'B', 'I', 'I', 'I', 'O', 'O', 'O', 'O', 'O', 'O', 'O', 'O', 'O', 'O', 'O', 'O', 'O', 'O', 'O', 'O', 'O', 'O', 'O', 'B', 'I', 'I', 'O', 'B', 'I', 'I', 'O', 'O', 'O', 'O', 'O', 'O', 'O', 'O', 'O', 'O', 'B', 'I', 'O', 'O', 'B', 'I', 'O', 'O', 'B', 'I', 'I', 'I', 'I', 'I', 'I', 'I', 'I', 'I', 'O', 'O', 'O', 'O', 'O', 'O', 'O', 'O', 'O', 'O', 'O', 'O', 'O', 'O', 'O', 'B', 'I', 'I', 'O']
Extractive/present Keyphrases: ['aaμ equation of motion', 'aναβ equation of motion leads', 'confining part', 'coulomb piece', 'develop analytical approaches', 'quantum theory calculation of the static energy between two charges', 'spherically symmetric solutions', 'spontaneous symmetry breaking of scale invariance', 'string like solutions', 'the effective lagrangian', 'understanding the confinement physics from first principles']
Abstractive/absent Keyphrases: ['(2)w=εμναβ∂μaναβ', 'function of 4-index field strength', 'integrated to (4)w=−fμνafaμν+m', 'leff=−14fμνafaμν+m2−fμνafaμν', 'scale-invariant lagrangian', 'εμναβ∂βw−−fγδafaγδ=0']
-----------
Sample from validation dataset split
Fields in the sample: ['id', 'document', 'doc_bio_tags', 'extractive_keyphrases', 'abstractive_keyphrases', 'other_metadata']
Tokenized Document: ['In', 'the', 'current', 'CLSVOF', 'method,', 'the', 'normal', 'vector', 'is', 'calculated', 'directly', 'by', 'discretising', 'the', 'LS', 'gradient', 'using', 'a', 'finite', 'difference', 'scheme.', 'By', 'appropriately', 'choosing', 'one', 'of', 'three', 'finite', 'difference', 'schemes', '(central,', 'forward,', 'or', 'backward', 'differencing),', 'it', 'has', 'been', 'demonstrated', 'that', 'thin', 'liquid', 'ligaments', 'can', 'be', 'well', 'resolved', 'see', 'Xiao', '(2012).', 'Although', 'a', 'high', 'order', 'discretisation', 'scheme', '(e.g.', '5th', 'order', 'WENO)', 'has', 'been', 'found', 'necessary', 'for', 'LS', 'evolution', 'in', 'pure', 'LS', 'methods', 'to', 'reduce', 'mass', 'error,', 'low', 'order', 'LS', 'discretisation', 'schemes', '(2nd', 'order', 'is', 'used', 'here)', 'can', 'produce', 'accurate', 'results', 'when', 'the', 'LS', 'equation', 'is', 'solved', 'and', 'constrained', 'as', 'indicated', 'above', 'in', 'a', 'CLSVOF', 'method', '(see', 'Xiao,', '2012),', 'since', 'the', 'VOF', 'method', 'maintains', '2nd', 'order', 'accuracy.', 'This', 'is', 'a', 'further', 'reason', 'to', 'adopt', 'the', 'CLSVOF', 'method,', 'which', 'has', 'been', 'used', 'for', 'all', 'the', 'following', 'simulations', 'of', 'liquid', 'jet', 'primary', 'breakup.']
Document BIO Tags: ['O', 'O', 'O', 'B', 'I', 'O', 'O', 'O', 'O', 'O', 'O', 'O', 'O', 'O', 'B', 'O', 'O', 'O', 'B', 'I', 'I', 'O', 'O', 'O', 'O', 'O', 'O', 'B', 'I', 'I', 'O', 'O', 'O', 'O', 'O', 'O', 'O', 'O', 'O', 'O', 'O', 'B', 'O', 'O', 'O', 'O', 'O', 'O', 'O', 'O', 'O', 'O', 'B', 'I', 'I', 'I', 'O', 'B', 'I', 'I', 'O', 'O', 'O', 'O', 'O', 'B', 'O', 'O', 'O', 'B', 'O', 'O', 'B', 'I', 'I', 'B', 'I', 'I', 'I', 'I', 'O', 'O', 'O', 'O', 'O', 'O', 'O', 'O', 'O', 'O', 'O', 'B', 'O', 'O', 'O', 'O', 'O', 'O', 'O', 'O', 'O', 'O', 'B', 'I', 'O', 'O', 'O', 'O', 'O', 'B', 'I', 'O', 'O', 'O', 'O', 'O', 'O', 'O', 'O', 'O', 'O', 'O', 'O', 'B', 'I', 'O', 'O', 'O', 'O', 'O', 'O', 'O', 'O', 'O', 'O', 'B', 'O', 'O', 'O']
Extractive/present Keyphrases: ['5th order weno', 'clsvof method', 'finite difference scheme', 'finite difference schemes', 'high order discretisation scheme', 'liquid', 'low order ls discretisation schemes', 'ls', 'reduce mass error', 'vof method']
Abstractive/absent Keyphrases: ['central, forward, or backward differencing', 'ls methods', 'simulations of liquid jet primary breakup', 'thin liquid ligaments']
-----------
Sample from test dataset split
Fields in the sample: ['id', 'document', 'doc_bio_tags', 'extractive_keyphrases', 'abstractive_keyphrases', 'other_metadata']
Tokenized Document: ['Traditionally,', 'archaeologists', 'have', 'recorded', 'sites', 'and', 'artefacts', 'via', 'a', 'combination', 'of', 'ordinary', 'still', 'photographs,', '2D', 'line', 'drawings', 'and', 'occasional', 'cross-sections.', 'Given', 'these', 'constraints,', 'the', 'attractions', 'of', '3D', 'models', 'have', 'been', 'obvious', 'for', 'some', 'time,', 'with', 'digital', 'photogrammetry', 'and', 'laser', 'scanners', 'offering', 'two', 'well-known', 'methods', 'for', 'data', 'capture', 'at', 'close', 'range', '(e.g.', 'Bates', 'et', 'al.,', '2010;', 'Hess', 'and', 'Robson,', '2010).', 'The', 'highest', 'specification', 'laser', 'scanners', 'still', 'boast', 'better', 'positional', 'accuracy', 'and', 'greater', 'true', 'colour', 'fidelity', 'than', 'SfM–MVS', 'methods', '(James', 'and', 'Robson,', '2012),', 'but', 'the', 'latter', 'produce', 'very', 'good', 'quality', 'models', 'nonetheless', 'and', 'have', 'many', 'unique', 'selling', 'points.', 'Unlike', 'traditional', 'digital', 'photogrammetry,', 'little', 'or', 'no', 'prior', 'control', 'of', 'camera', 'position', 'is', 'necessary,', 'and', 'unlike', 'laser', 'scanning,', 'no', 'major', 'equipment', 'costs', 'or', 'setup', 'are', 'involved.', 'However,', 'the', 'key', 'attraction', 'of', 'SfM–MVS', 'is', 'that', 'the', 'required', 'input', 'can', 'be', 'taken', 'by', 'anyone', 'with', 'a', 'digital', 'camera', 'and', 'modest', 'prior', 'training', 'about', 'the', 'required', 'number', 'and', 'overlap', 'of', 'photographs.', 'A', 'whole', 'series', 'of', 'traditional', 'bottlenecks', 'are', 'thereby', 'removed', 'from', 'the', 'recording', 'process', 'and', 'large', 'numbers', 'of', 'archaeological', 'landscapes,', 'sites', 'or', 'artefacts', 'can', 'now', 'be', 'captured', 'rapidly,', 'in', 'the', 'field,', 'in', 'the', 'laboratory', 'or', 'in', 'the', 'museum.', 'Fig.', '2a–c', 'shows', 'examples', 'of', 'terracotta', 'warrior', 'models', 'for', 'which', 'the', 'level', 'of', 'surface', 'detail', 'is', 'considerable.']
Document BIO Tags: ['O', 'O', 'O', 'O', 'B', 'O', 'B', 'O', 'O', 'O', 'O', 'B', 'I', 'I', 'B', 'I', 'I', 'O', 'O', 'O', 'O', 'O', 'O', 'O', 'O', 'O', 'B', 'I', 'O', 'O', 'O', 'O', 'O', 'O', 'O', 'B', 'I', 'O', 'B', 'I', 'O', 'O', 'O', 'O', 'O', 'B', 'I', 'I', 'I', 'I', 'O', 'O', 'O', 'O', 'O', 'O', 'O', 'O', 'O', 'O', 'O', 'O', 'B', 'I', 'O', 'O', 'O', 'O', 'O', 'O', 'O', 'O', 'O', 'O', 'O', 'B', 'O', 'O', 'O', 'O', 'O', 'O', 'O', 'O', 'O', 'O', 'O', 'O', 'O', 'O', 'O', 'O', 'O', 'O', 'O', 'O', 'O', 'O', 'B', 'I', 'O', 'O', 'O', 'O', 'B', 'I', 'I', 'I', 'O', 'O', 'O', 'O', 'B', 'I', 'O', 'O', 'O', 'O', 'O', 'O', 'O', 'O', 'O', 'O', 'O', 'O', 'O', 'B', 'O', 'O', 'O', 'O', 'O', 'O', 'O', 'O', 'O', 'O', 'O', 'O', 'B', 'I', 'O', 'O', 'B', 'I', 'I', 'I', 'I', 'I', 'I', 'I', 'I', 'I', 'O', 'O', 'O', 'O', 'O', 'O', 'O', 'O', 'O', 'O', 'O', 'B', 'I', 'O', 'O', 'O', 'O', 'B', 'I', 'B', 'O', 'B', 'O', 'O', 'O', 'O', 'O', 'O', 'O', 'O', 'O', 'O', 'O', 'O', 'O', 'O', 'O', 'O', 'O', 'O', 'O', 'O', 'B', 'I', 'I', 'O', 'O', 'O', 'O', 'O', 'O', 'O', 'O', 'O']
Extractive/present Keyphrases: ['2d line drawings', '3d models', 'archaeological landscapes', 'artefacts', 'control of camera position', 'data capture at close range', 'digital camera', 'digital photogrammetry', 'laser scanners', 'laser scanning', 'ordinary still photographs', 'prior training about the required number and overlap of photographs', 'recording process', 'sfm–mvs', 'sites', 'terracotta warrior models']
Abstractive/absent Keyphrases: ['occasional cross-sections', 'recorded sites and artefacts', 'sfm–mvs methods', 'traditional digital photogrammetry']
-----------
```
### Keyphrase Extraction
```python
from datasets import load_dataset
# get the dataset only for keyphrase extraction
dataset = load_dataset("midas/semeval2017", "extraction")
print("Samples for Keyphrase Extraction")
# sample from the train split
print("Sample from train data split")
test_sample = dataset["train"][0]
print("Fields in the sample: ", [key for key in test_sample.keys()])
print("Tokenized Document: ", test_sample["document"])
print("Document BIO Tags: ", test_sample["doc_bio_tags"])
print("\n-----------\n")
# sample from the test split
print("Sample from test data split")
test_sample = dataset["test"][0]
print("Fields in the sample: ", [key for key in test_sample.keys()])
print("Tokenized Document: ", test_sample["document"])
print("Document BIO Tags: ", test_sample["doc_bio_tags"])
print("\n-----------\n")
```
### Keyphrase Generation
```python
# get the dataset only for keyphrase generation
dataset = load_dataset("midas/semeval2017", "generation")
print("Samples for Keyphrase Generation")
# sample from the train split
print("Sample from train data split")
test_sample = dataset["train"][0]
print("Fields in the sample: ", [key for key in test_sample.keys()])
print("Tokenized Document: ", test_sample["document"])
print("Extractive/present Keyphrases: ", test_sample["extractive_keyphrases"])
print("Abstractive/absent Keyphrases: ", test_sample["abstractive_keyphrases"])
print("\n-----------\n")
# sample from the test split
print("Sample from test data split")
test_sample = dataset["test"][0]
print("Fields in the sample: ", [key for key in test_sample.keys()])
print("Tokenized Document: ", test_sample["document"])
print("Extractive/present Keyphrases: ", test_sample["extractive_keyphrases"])
print("Abstractive/absent Keyphrases: ", test_sample["abstractive_keyphrases"])
print("\n-----------\n")
```
## Citation Information
```
@article{DBLP:journals/corr/AugensteinDRVM17,
author = {Isabelle Augenstein and
Mrinal Das and
Sebastian Riedel and
Lakshmi Vikraman and
Andrew McCallum},
title = {SemEval 2017 Task 10: ScienceIE - Extracting Keyphrases and Relations
from Scientific Publications},
journal = {CoRR},
volume = {abs/1704.02853},
year = {2017},
url = {http://arxiv.org/abs/1704.02853},
eprinttype = {arXiv},
eprint = {1704.02853},
timestamp = {Mon, 13 Aug 2018 16:46:36 +0200},
biburl = {https://dblp.org/rec/journals/corr/AugensteinDRVM17.bib},
bibsource = {dblp computer science bibliography, https://dblp.org}
}
```
## Contributions
Thanks to [@debanjanbhucs](https://github.com/debanjanbhucs), [@dibyaaaaax](https://github.com/dibyaaaaax) and [@ad6398](https://github.com/ad6398) for adding this dataset
| midas/semeval2017 | [
"arxiv:1704.02853",
"arxiv:1910.08840",
"region:us"
] | 2022-03-02T23:29:22+00:00 | {} | 2022-03-05T03:27:44+00:00 | [
"1704.02853",
"1910.08840"
] | [] | TAGS
#arxiv-1704.02853 #arxiv-1910.08840 #region-us
| A dataset for benchmarking keyphrase extraction and generation techniques from abstracts of english scientific articles. For more details about the dataset please refer the original paper - URL
Original source of the data - URL
Dataset Summary
---------------
The Semeval-2017 dataset was originally proposed by *Isabelle Augenstein et al.* in the paper titled - SemEval 2017 Task 10: ScienceIE - Extracting Keyphrases and Relations from Scientific Publications in the year 2017. The dataset consists of a abstracts of 500 English scientific papers from the ScienceDirect open access publications. The selected articles were evenly distributed among the domains of Computer Science, Material Sciences and Physics. Each paper has a set of keyphrases annotated by student volunteers. Each paper was double-annotated, where the second annotation was done by an expert annotator. In case of disagreement, the annotations done by expert annotators were chosen. The original dataset was divided into train, dev and test splits, evenly distributed across the three domains. The train, dev and test splits had 350, 50 and 100 articles respectively.
The dataset shared over here categorizes the keyphrases into *extractive* and *abstractive*. Extractive keyphrases are those that could be found in the input text and the abstractive keyphrases are those that are not present in the input text. In order to get all the meta-data about the documents and keyphrases please refer to the original source from which the dataset was taken from. The main motivation behind making this dataset available in the form as presented over here is to make it easy for the researchers to programmatically download it and evaluate their models for the tasks of keyphrase extraction and generation. As keyphrase extraction by treating it as a sequence tagging task and using contextual language models has become popular - Keyphrase extraction from scholarly articles as sequence labeling using contextualized embeddings, we have also made the token tags available in the BIO tagging format.
Dataset Structure
-----------------
Table 1: Statistics on the length of the abstractive keyphrases for Train, Test, and Validation splits of SemEval 2017 dataset.
Table 2: Statistics on the length of the extractive keyphrases for Train, Test, and Validation splits of SemEval 2017 dataset.
Table 3: General statistics of the Semeval 2017 dataset.
Train
* Percentage of keyphrases that are named entities: 50.09% (named entities detected using scispacy - en-core-sci-lg model)
* Percentage of keyphrases that are noun phrases: 57.65% (noun phrases detected using spacy en-core-web-lg after removing determiners)
Validation
* Percentage of keyphrases that are named entities: 60.02% (named entities detected using scispacy - en-core-sci-lg model)
* Percentage of keyphrases that are noun phrases: 62.87% (noun phrases detected using spacy en-core-web-lg after removing determiners)
Test
* Percentage of keyphrases that are named entities: 59.78% (named entities detected using scispacy - en-core-sci-lg model)
* Percentage of keyphrases that are noun phrases: 66.39% (noun phrases detected using spacy en-core-web-lg after removing determiners)
### Data Fields
* id: unique identifier of the document.
* document: Whitespace separated list of words in the document.
* doc\_bio\_tags: BIO tags for each word in the document. B stands for the beginning of a keyphrase and I stands for inside the keyphrase. O stands for outside the keyphrase and represents the word that isn't a part of the keyphrase at all.
* extractive\_keyphrases: List of all the present keyphrases.
* abstractive\_keyphrase: List of all the absent keyphrases.
### Data Splits
Usage
-----
### Full Dataset
Output
### Keyphrase Extraction
### Keyphrase Generation
Contributions
-------------
Thanks to @debanjanbhucs, @dibyaaaaax and @ad6398 for adding this dataset
| [
"### Data Fields\n\n\n* id: unique identifier of the document.\n* document: Whitespace separated list of words in the document.\n* doc\\_bio\\_tags: BIO tags for each word in the document. B stands for the beginning of a keyphrase and I stands for inside the keyphrase. O stands for outside the keyphrase and represents the word that isn't a part of the keyphrase at all.\n* extractive\\_keyphrases: List of all the present keyphrases.\n* abstractive\\_keyphrase: List of all the absent keyphrases.",
"### Data Splits\n\n\n\nUsage\n-----",
"### Full Dataset\n\n\nOutput",
"### Keyphrase Extraction",
"### Keyphrase Generation\n\n\nContributions\n-------------\n\n\nThanks to @debanjanbhucs, @dibyaaaaax and @ad6398 for adding this dataset"
] | [
"TAGS\n#arxiv-1704.02853 #arxiv-1910.08840 #region-us \n",
"### Data Fields\n\n\n* id: unique identifier of the document.\n* document: Whitespace separated list of words in the document.\n* doc\\_bio\\_tags: BIO tags for each word in the document. B stands for the beginning of a keyphrase and I stands for inside the keyphrase. O stands for outside the keyphrase and represents the word that isn't a part of the keyphrase at all.\n* extractive\\_keyphrases: List of all the present keyphrases.\n* abstractive\\_keyphrase: List of all the absent keyphrases.",
"### Data Splits\n\n\n\nUsage\n-----",
"### Full Dataset\n\n\nOutput",
"### Keyphrase Extraction",
"### Keyphrase Generation\n\n\nContributions\n-------------\n\n\nThanks to @debanjanbhucs, @dibyaaaaax and @ad6398 for adding this dataset"
] |
e1242dcb068c73051541188b911aa0ea2f297e02 | ## Dataset Summary
A dataset for benchmarking keyphrase extraction and generation techniques from abstract of english scientific articles. For more details about the dataset please refer the original paper - [https://aclanthology.org/D14-1150/](https://aclanthology.org/D14-1150/)
Original source of the data - []()
## Dataset Structure
Table 1: Statistics on the length of the abstractive keyphrases for Test split of www dataset.
| | Test |
|:-----------:|:------:|
| Single word | 28.21% |
| Two words | 47.65% |
| Three words | 15.20% |
| Four words | 8.04% |
| Five words | 0.65% |
| Six words | 0.12% |
| Seven words | 0.05% |
| Eight words | 0.05% |
Table 2: Statistics on the length of the extractive keyphrases for Test split of www dataset.
| | Test |
|:-----------:|:------:|
| Single word | 44.09% |
| Two words | 48.07% |
| Three words | 7.20% |
| Four words | 0.45% |
| Five words | 0.16% |
Table 3: General statistics about www dataset.
| Type of Analysis | Test |
|:------------------------------------------------:|:-------------------:|
| Annotator Type | Authors and Readers |
| Document Type | Scientific Articles |
| No. of Documents | 1330 |
| Avg. Document length (words) | 163.51 |
| Max Document length (words) | 587 |
| Max no. of abstractive keyphrases in a document | 13 |
| Min no. of abstractive keyphrases in a document | 0 |
| Avg. no. of abstractive keyphrases per document | 2.98 |
| Max no. of extractive keyphrases in a document | 9 |
| Min no. of extractive keyphrases in a document | 0 |
| Avg. no. of extractive keyphrases per document | 1.81 |
### Data Fields
- **id**: unique identifier of the document.
- **document**: Whitespace separated list of words in the document.
- **doc_bio_tags**: BIO tags for each word in the document. B stands for the beginning of a keyphrase and I stands for inside the keyphrase. O stands for outside the keyphrase and represents the word that isn't a part of the keyphrase at all.
- **extractive_keyphrases**: List of all the present keyphrases.
- **abstractive_keyphrase**: List of all the absent keyphrases.
### Data Splits
|Split| #datapoints |
|--|--|
| Test | 1330 |
## Usage
### Full Dataset
```python
from datasets import load_dataset
# get entire dataset
dataset = load_dataset("midas/www", "raw")
# sample from the test split
print("Sample from test dataset split")
test_sample = dataset["test"][0]
print("Fields in the sample: ", [key for key in test_sample.keys()])
print("Tokenized Document: ", test_sample["document"])
print("Document BIO Tags: ", test_sample["doc_bio_tags"])
print("Extractive/present Keyphrases: ", test_sample["extractive_keyphrases"])
print("Abstractive/absent Keyphrases: ", test_sample["abstractive_keyphrases"])
print("\n-----------\n")
```
**Output**
```bash
Sample from test data split
Fields in the sample: ['id', 'document', 'doc_bio_tags', 'extractive_keyphrases', 'abstractive_keyphrases', 'other_metadata']
Tokenized Document: ['The', 'web', 'of', 'nations', 'In', 'this', 'paper', ',', 'we', 'report', 'on', 'a', 'large-scale', 'study', 'of', 'structural', 'differences', 'among', 'the', 'national', 'webs', '.', 'The', 'study', 'is', 'based', 'on', 'a', 'web-scale', 'crawl', 'conducted', 'in', 'the', 'summer', '2008', '.', 'More', 'specifically', ',', 'we', 'study', 'two', 'graphs', 'derived', 'from', 'this', 'crawl', ',', 'the', 'nation', 'graph', ',', 'with', 'nodes', 'corresponding', 'to', 'nations', 'and', 'edges', '-', 'to', 'links', 'among', 'nations', ',', 'and', 'the', 'host', 'graph', ',', 'with', 'nodes', 'corresponding', 'to', 'hosts', 'and', 'edges', '-', 'to', 'hyperlinks', 'among', 'pages', 'on', 'the', 'hosts', '.', 'Contrary', 'to', 'some', 'of', 'the', 'previous', 'work', '(', '2', ')', ',', 'our', 'results', 'show', 'that', 'webs', 'of', 'different', 'nations', 'are', 'often', 'very', 'different', 'from', 'each', 'other', ',', 'both', 'in', 'terms', 'of', 'their', 'internal', 'structure', ',', 'and', 'in', 'terms', 'of', 'their', 'connectivity', 'with', 'other', 'nations', '.']
Document BIO Tags: ['O', 'O', 'O', 'O', 'O', 'O', 'O', 'O', 'O', 'O', 'O', 'O', 'O', 'O', 'O', 'O', 'O', 'O', 'O', 'O', 'O', 'O', 'O', 'O', 'O', 'O', 'O', 'O', 'O', 'O', 'O', 'O', 'O', 'O', 'O', 'O', 'O', 'O', 'O', 'O', 'O', 'O', 'O', 'O', 'O', 'O', 'O', 'O', 'O', 'B', 'I', 'O', 'O', 'O', 'O', 'O', 'O', 'O', 'O', 'O', 'O', 'O', 'O', 'O', 'O', 'O', 'O', 'B', 'I', 'O', 'O', 'O', 'O', 'O', 'O', 'O', 'O', 'O', 'O', 'O', 'O', 'O', 'O', 'O', 'O', 'O', 'O', 'O', 'O', 'O', 'O', 'O', 'O', 'O', 'O', 'O', 'O', 'O', 'O', 'O', 'O', 'O', 'O', 'O', 'O', 'O', 'O', 'O', 'O', 'O', 'O', 'O', 'O', 'O', 'O', 'O', 'O', 'O', 'O', 'O', 'O', 'O', 'O', 'O', 'O', 'O', 'O', 'O', 'O', 'O', 'O']
Extractive/present Keyphrases: ['host graph', 'nation graph']
Abstractive/absent Keyphrases: ['web graph', 'web structure']
-----------
```
### Keyphrase Extraction
```python
from datasets import load_dataset
# get the dataset only for keyphrase extraction
dataset = load_dataset("midas/www", "extraction")
print("Samples for Keyphrase Extraction")
# sample from the test split
print("Sample from test data split")
test_sample = dataset["test"][0]
print("Fields in the sample: ", [key for key in test_sample.keys()])
print("Tokenized Document: ", test_sample["document"])
print("Document BIO Tags: ", test_sample["doc_bio_tags"])
print("\n-----------\n")
```
### Keyphrase Generation
```python
# get the dataset only for keyphrase generation
dataset = load_dataset("midas/www", "generation")
print("Samples for Keyphrase Generation")
# sample from the test split
print("Sample from test data split")
test_sample = dataset["test"][0]
print("Fields in the sample: ", [key for key in test_sample.keys()])
print("Tokenized Document: ", test_sample["document"])
print("Extractive/present Keyphrases: ", test_sample["extractive_keyphrases"])
print("Abstractive/absent Keyphrases: ", test_sample["abstractive_keyphrases"])
print("\n-----------\n")
```
## Citation Information
```
@inproceedings{caragea-etal-2014-citation,
title = "Citation-Enhanced Keyphrase Extraction from Research Papers: A Supervised Approach",
author = "Caragea, Cornelia and
Bulgarov, Florin Adrian and
Godea, Andreea and
Das Gollapalli, Sujatha",
booktitle = "Proceedings of the 2014 Conference on Empirical Methods in Natural Language Processing ({EMNLP})",
month = oct,
year = "2014",
address = "Doha, Qatar",
publisher = "Association for Computational Linguistics",
url = "https://aclanthology.org/D14-1150",
doi = "10.3115/v1/D14-1150",
pages = "1435--1446",
}
```
## Contributions
Thanks to [@debanjanbhucs](https://github.com/debanjanbhucs), [@dibyaaaaax](https://github.com/dibyaaaaax) and [@ad6398](https://github.com/ad6398) for adding this dataset
| midas/www | [
"region:us"
] | 2022-03-02T23:29:22+00:00 | {} | 2022-02-11T22:49:09+00:00 | [] | [] | TAGS
#region-us
| Dataset Summary
---------------
A dataset for benchmarking keyphrase extraction and generation techniques from abstract of english scientific articles. For more details about the dataset please refer the original paper - URL
Original source of the data -
Dataset Structure
-----------------
Table 1: Statistics on the length of the abstractive keyphrases for Test split of www dataset.
Table 2: Statistics on the length of the extractive keyphrases for Test split of www dataset.
Table 3: General statistics about www dataset.
### Data Fields
* id: unique identifier of the document.
* document: Whitespace separated list of words in the document.
* doc\_bio\_tags: BIO tags for each word in the document. B stands for the beginning of a keyphrase and I stands for inside the keyphrase. O stands for outside the keyphrase and represents the word that isn't a part of the keyphrase at all.
* extractive\_keyphrases: List of all the present keyphrases.
* abstractive\_keyphrase: List of all the absent keyphrases.
### Data Splits
Usage
-----
### Full Dataset
Output
### Keyphrase Extraction
### Keyphrase Generation
Contributions
-------------
Thanks to @debanjanbhucs, @dibyaaaaax and @ad6398 for adding this dataset
| [
"### Data Fields\n\n\n* id: unique identifier of the document.\n* document: Whitespace separated list of words in the document.\n* doc\\_bio\\_tags: BIO tags for each word in the document. B stands for the beginning of a keyphrase and I stands for inside the keyphrase. O stands for outside the keyphrase and represents the word that isn't a part of the keyphrase at all.\n* extractive\\_keyphrases: List of all the present keyphrases.\n* abstractive\\_keyphrase: List of all the absent keyphrases.",
"### Data Splits\n\n\n\nUsage\n-----",
"### Full Dataset\n\n\nOutput",
"### Keyphrase Extraction",
"### Keyphrase Generation\n\n\nContributions\n-------------\n\n\nThanks to @debanjanbhucs, @dibyaaaaax and @ad6398 for adding this dataset"
] | [
"TAGS\n#region-us \n",
"### Data Fields\n\n\n* id: unique identifier of the document.\n* document: Whitespace separated list of words in the document.\n* doc\\_bio\\_tags: BIO tags for each word in the document. B stands for the beginning of a keyphrase and I stands for inside the keyphrase. O stands for outside the keyphrase and represents the word that isn't a part of the keyphrase at all.\n* extractive\\_keyphrases: List of all the present keyphrases.\n* abstractive\\_keyphrase: List of all the absent keyphrases.",
"### Data Splits\n\n\n\nUsage\n-----",
"### Full Dataset\n\n\nOutput",
"### Keyphrase Extraction",
"### Keyphrase Generation\n\n\nContributions\n-------------\n\n\nThanks to @debanjanbhucs, @dibyaaaaax and @ad6398 for adding this dataset"
] |
a9bbb2728beebe8168ae226c60459cdf5a39342a |
This is the Icelandic Common Crawl Corpus (IC3).
| mideind/icelandic-common-crawl-corpus-IC3 | [
"task_categories:text-generation",
"task_ids:language-modeling",
"annotations_creators:no-annotation",
"language_creators:found",
"multilinguality:monolingual",
"size_categories:unknown",
"source_datasets:original",
"language:is",
"license:unknown",
"region:us"
] | 2022-03-02T23:29:22+00:00 | {"annotations_creators": ["no-annotation"], "language_creators": ["found"], "language": ["is"], "license": ["unknown"], "multilinguality": ["monolingual"], "size_categories": ["unknown"], "source_datasets": ["original"], "task_categories": ["text-generation"], "task_ids": ["language-modeling"], "pretty_name": "Icelandic Common Crawl Corpus - IC3"} | 2022-10-22T14:44:37+00:00 | [] | [
"is"
] | TAGS
#task_categories-text-generation #task_ids-language-modeling #annotations_creators-no-annotation #language_creators-found #multilinguality-monolingual #size_categories-unknown #source_datasets-original #language-Icelandic #license-unknown #region-us
|
This is the Icelandic Common Crawl Corpus (IC3).
| [] | [
"TAGS\n#task_categories-text-generation #task_ids-language-modeling #annotations_creators-no-annotation #language_creators-found #multilinguality-monolingual #size_categories-unknown #source_datasets-original #language-Icelandic #license-unknown #region-us \n"
] |
6f1df59ddca5d65f3bd6c822f384e2ea8b5f7c3b |
# Icelandic Error Corpus
Refer to [https://github.com/antonkarl/iceErrorCorpus](https://github.com/antonkarl/iceErrorCorpus) for a description of the dataset.
Please cite the dataset as follows if you use it.
```
Anton Karl Ingason, Lilja Björk Stefánsdóttir, Þórunn Arnardóttir, and Xindan Xu. 2021. The Icelandic Error Corpus (IceEC). Version 1.1. (https://github.com/antonkarl/iceErrorCorpus)
``` | mideind/icelandic-error-corpus-IceEC | [
"annotations_creators:expert-generated",
"multilinguality:monolingual",
"size_categories:10K<n<100K",
"source_datasets:original",
"language:is",
"license:cc-by-4.0",
"region:us"
] | 2022-03-02T23:29:22+00:00 | {"annotations_creators": ["expert-generated"], "language": ["is"], "license": ["cc-by-4.0"], "multilinguality": ["monolingual"], "size_categories": ["10K<n<100K"], "source_datasets": ["original"], "pretty_name": "Icelandic Error Corpus"} | 2022-10-25T08:51:04+00:00 | [] | [
"is"
] | TAGS
#annotations_creators-expert-generated #multilinguality-monolingual #size_categories-10K<n<100K #source_datasets-original #language-Icelandic #license-cc-by-4.0 #region-us
|
# Icelandic Error Corpus
Refer to URL for a description of the dataset.
Please cite the dataset as follows if you use it.
| [
"# Icelandic Error Corpus\n\nRefer to URL for a description of the dataset.\n\nPlease cite the dataset as follows if you use it."
] | [
"TAGS\n#annotations_creators-expert-generated #multilinguality-monolingual #size_categories-10K<n<100K #source_datasets-original #language-Icelandic #license-cc-by-4.0 #region-us \n",
"# Icelandic Error Corpus\n\nRefer to URL for a description of the dataset.\n\nPlease cite the dataset as follows if you use it."
] |
eccff1c84ba55f542a1f003ef9a621da692e1380 | # Dataset Card for Dutch CNN Dailymail Dataset
## Dataset Description
- **Repository:** [CNN / DailyMail Dataset NL repository](https://huggingface.co/datasets/ml6team/cnn_dailymail_nl)
### Dataset Summary
The Dutch CNN / DailyMail Dataset is a machine-translated version of the English CNN / Dailymail dataset containing just over 300k unique news aticles as written by journalists at CNN and the Daily Mail.
Most information about the dataset can be found on the [HuggingFace page](https://huggingface.co/datasets/cnn_dailymail) of the original English version.
These are the basic steps used to create this dataset (+ some chunking):
```
load_dataset("cnn_dailymail", '3.0.0')
```
And this is the HuggingFace translation pipeline:
```
pipeline(
task='translation_en_to_nl',
model='Helsinki-NLP/opus-mt-en-nl',
tokenizer='Helsinki-NLP/opus-mt-en-nl')
```
### Data Fields
- `id`: a string containing the heximal formated SHA1 hash of the url where the story was retrieved from
- `article`: a string containing the body of the news article
- `highlights`: a string containing the highlight of the article as written by the article author
### Data Splits
The Dutch CNN/DailyMail dataset follows the same splits as the original English version and has 3 splits: _train_, _validation_, and _test_.
| Dataset Split | Number of Instances in Split |
| ------------- | ------------------------------------------- |
| Train | 287,113 |
| Validation | 13,368 |
| Test | 11,490 |
| ml6team/cnn_dailymail_nl | [
"annotations_creators:no-annotation",
"language_creators:found",
"multilinguality:monolingual",
"size_categories:100K<n<1M",
"source_datasets:https://github.com/huggingface/datasets/tree/master/datasets/cnn_dailymail",
"language:nl",
"license:mit",
"region:us"
] | 2022-03-02T23:29:22+00:00 | {"annotations_creators": ["no-annotation"], "language_creators": ["found"], "language": ["nl"], "license": ["mit"], "multilinguality": ["monolingual"], "size_categories": ["100K<n<1M"], "source_datasets": ["https://github.com/huggingface/datasets/tree/master/datasets/cnn_dailymail"], "task_categories": ["conditional-text-generation"], "task_ids": ["summarization"]} | 2022-10-22T13:03:06+00:00 | [] | [
"nl"
] | TAGS
#annotations_creators-no-annotation #language_creators-found #multilinguality-monolingual #size_categories-100K<n<1M #source_datasets-https-//github.com/huggingface/datasets/tree/master/datasets/cnn_dailymail #language-Dutch #license-mit #region-us
| Dataset Card for Dutch CNN Dailymail Dataset
============================================
Dataset Description
-------------------
* Repository: CNN / DailyMail Dataset NL repository
### Dataset Summary
The Dutch CNN / DailyMail Dataset is a machine-translated version of the English CNN / Dailymail dataset containing just over 300k unique news aticles as written by journalists at CNN and the Daily Mail.
Most information about the dataset can be found on the HuggingFace page of the original English version.
These are the basic steps used to create this dataset (+ some chunking):
And this is the HuggingFace translation pipeline:
### Data Fields
* 'id': a string containing the heximal formated SHA1 hash of the url where the story was retrieved from
* 'article': a string containing the body of the news article
* 'highlights': a string containing the highlight of the article as written by the article author
### Data Splits
The Dutch CNN/DailyMail dataset follows the same splits as the original English version and has 3 splits: *train*, *validation*, and *test*.
| [
"### Dataset Summary\n\n\nThe Dutch CNN / DailyMail Dataset is a machine-translated version of the English CNN / Dailymail dataset containing just over 300k unique news aticles as written by journalists at CNN and the Daily Mail.\n\n\nMost information about the dataset can be found on the HuggingFace page of the original English version.\n\n\nThese are the basic steps used to create this dataset (+ some chunking):\n\n\nAnd this is the HuggingFace translation pipeline:",
"### Data Fields\n\n\n* 'id': a string containing the heximal formated SHA1 hash of the url where the story was retrieved from\n* 'article': a string containing the body of the news article\n* 'highlights': a string containing the highlight of the article as written by the article author",
"### Data Splits\n\n\nThe Dutch CNN/DailyMail dataset follows the same splits as the original English version and has 3 splits: *train*, *validation*, and *test*."
] | [
"TAGS\n#annotations_creators-no-annotation #language_creators-found #multilinguality-monolingual #size_categories-100K<n<1M #source_datasets-https-//github.com/huggingface/datasets/tree/master/datasets/cnn_dailymail #language-Dutch #license-mit #region-us \n",
"### Dataset Summary\n\n\nThe Dutch CNN / DailyMail Dataset is a machine-translated version of the English CNN / Dailymail dataset containing just over 300k unique news aticles as written by journalists at CNN and the Daily Mail.\n\n\nMost information about the dataset can be found on the HuggingFace page of the original English version.\n\n\nThese are the basic steps used to create this dataset (+ some chunking):\n\n\nAnd this is the HuggingFace translation pipeline:",
"### Data Fields\n\n\n* 'id': a string containing the heximal formated SHA1 hash of the url where the story was retrieved from\n* 'article': a string containing the body of the news article\n* 'highlights': a string containing the highlight of the article as written by the article author",
"### Data Splits\n\n\nThe Dutch CNN/DailyMail dataset follows the same splits as the original English version and has 3 splits: *train*, *validation*, and *test*."
] |
fc98c1ee39d3a8d02483d10d0b08e3e2d591ed1b | # Dataset Card for XSum NL
## Table of Contents
- [Table of Contents](#table-of-contents)
- [Dataset Description](#dataset-description)
- [Dataset Summary](#dataset-summary)
- [Supported Tasks and Leaderboards](#supported-tasks-and-leaderboards)
- [Languages](#languages)
- [Dataset Structure](#dataset-structure)
- [Data Instances](#data-instances)
- [Data Fields](#data-fields)
- [Data Splits](#data-splits)
- [Dataset Creation](#dataset-creation)
- [Curation Rationale](#curation-rationale)
- [Source Data](#source-data)
- [Annotations](#annotations)
- [Personal and Sensitive Information](#personal-and-sensitive-information)
- [Considerations for Using the Data](#considerations-for-using-the-data)
- [Social Impact of Dataset](#social-impact-of-dataset)
- [Discussion of Biases](#discussion-of-biases)
- [Other Known Limitations](#other-known-limitations)
- [Additional Information](#additional-information)
- [Dataset Curators](#dataset-curators)
- [Licensing Information](#licensing-information)
- [Citation Information](#citation-information)
- [Contributions](#contributions)
## Dataset Description
- **Homepage:**
- **Repository:**
- **Paper:**
- **Leaderboard:**
- **Point of Contact:**
### Dataset Summary
This dataset is a machine translated dataset. It's the [XSum dataset](https://huggingface.co/datasets/xsum) translated with [this model](https://huggingface.co/Helsinki-NLP/opus-mt-en-nl) from English to Dutch.
See the [Hugginface page of the original dataset](https://huggingface.co/datasets/xsum) for more information on the format of this dataset.
Use with:
```python
from datasets import load_dataset
load_dataset("csv", "ml6team/xsum_nl")
```
### Languages
Dutch
## Dataset Structure
### Data Instances
[More Information Needed]
### Data Fields
- `id`: BBC ID of the article.
- `document`: a string containing the body of the news article
- `summary`: a string containing a one sentence summary of the article.
### Data Splits
- `train`
- `test`
- `validation`
## Dataset Creation
### Curation Rationale
[More Information Needed]
### Source Data
#### Initial Data Collection and Normalization
[More Information Needed]
#### Who are the source language producers?
[More Information Needed]
### Annotations
#### Annotation process
[More Information Needed]
#### Who are the annotators?
[More Information Needed]
### Personal and Sensitive Information
[More Information Needed]
## Considerations for Using the Data
### Social Impact of Dataset
[More Information Needed]
### Discussion of Biases
[More Information Needed]
### Other Known Limitations
[More Information Needed]
## Additional Information
### Dataset Curators
[More Information Needed]
### Licensing Information
[More Information Needed]
### Citation Information
[More Information Needed]
### Contributions
Thanks to [@github-username](https://github.com/<github-username>) for adding this dataset. | ml6team/xsum_nl | [
"annotations_creators:machine-generated",
"language_creators:machine-generated",
"multilinguality:monolingual",
"size_categories:unknown",
"source_datasets:extended|xsum",
"language:nl",
"license:unknown",
"region:us"
] | 2022-03-02T23:29:22+00:00 | {"annotations_creators": ["machine-generated"], "language_creators": ["machine-generated"], "language": ["nl"], "license": ["unknown"], "multilinguality": ["monolingual"], "size_categories": ["unknown"], "source_datasets": ["extended|xsum"], "task_categories": ["conditional-text-generation"], "task_ids": ["summarization"], "pretty_name": "XSum NL", "language_bcp47": ["nl-BE"]} | 2022-10-22T13:47:41+00:00 | [] | [
"nl"
] | TAGS
#annotations_creators-machine-generated #language_creators-machine-generated #multilinguality-monolingual #size_categories-unknown #source_datasets-extended|xsum #language-Dutch #license-unknown #region-us
| # Dataset Card for XSum NL
## Table of Contents
- Table of Contents
- Dataset Description
- Dataset Summary
- Supported Tasks and Leaderboards
- Languages
- Dataset Structure
- Data Instances
- Data Fields
- Data Splits
- Dataset Creation
- Curation Rationale
- Source Data
- Annotations
- Personal and Sensitive Information
- Considerations for Using the Data
- Social Impact of Dataset
- Discussion of Biases
- Other Known Limitations
- Additional Information
- Dataset Curators
- Licensing Information
- Citation Information
- Contributions
## Dataset Description
- Homepage:
- Repository:
- Paper:
- Leaderboard:
- Point of Contact:
### Dataset Summary
This dataset is a machine translated dataset. It's the XSum dataset translated with this model from English to Dutch.
See the Hugginface page of the original dataset for more information on the format of this dataset.
Use with:
### Languages
Dutch
## Dataset Structure
### Data Instances
### Data Fields
- 'id': BBC ID of the article.
- 'document': a string containing the body of the news article
- 'summary': a string containing a one sentence summary of the article.
### Data Splits
- 'train'
- 'test'
- 'validation'
## Dataset Creation
### Curation Rationale
### Source Data
#### Initial Data Collection and Normalization
#### Who are the source language producers?
### Annotations
#### Annotation process
#### Who are the annotators?
### Personal and Sensitive Information
## Considerations for Using the Data
### Social Impact of Dataset
### Discussion of Biases
### Other Known Limitations
## Additional Information
### Dataset Curators
### Licensing Information
### Contributions
Thanks to @github-username for adding this dataset. | [
"# Dataset Card for XSum NL",
"## Table of Contents\n- Table of Contents\n- Dataset Description\n - Dataset Summary\n - Supported Tasks and Leaderboards\n - Languages\n- Dataset Structure\n - Data Instances\n - Data Fields\n - Data Splits\n- Dataset Creation\n - Curation Rationale\n - Source Data\n - Annotations\n - Personal and Sensitive Information\n- Considerations for Using the Data\n - Social Impact of Dataset\n - Discussion of Biases\n - Other Known Limitations\n- Additional Information\n - Dataset Curators\n - Licensing Information\n - Citation Information\n - Contributions",
"## Dataset Description\n\n- Homepage:\n- Repository:\n- Paper:\n- Leaderboard:\n- Point of Contact:",
"### Dataset Summary\n\nThis dataset is a machine translated dataset. It's the XSum dataset translated with this model from English to Dutch.\n\nSee the Hugginface page of the original dataset for more information on the format of this dataset.\n\nUse with:",
"### Languages\n\nDutch",
"## Dataset Structure",
"### Data Instances",
"### Data Fields\n\n- 'id': BBC ID of the article.\n- 'document': a string containing the body of the news article \n- 'summary': a string containing a one sentence summary of the article.",
"### Data Splits\n\n- 'train'\n- 'test'\n- 'validation'",
"## Dataset Creation",
"### Curation Rationale",
"### Source Data",
"#### Initial Data Collection and Normalization",
"#### Who are the source language producers?",
"### Annotations",
"#### Annotation process",
"#### Who are the annotators?",
"### Personal and Sensitive Information",
"## Considerations for Using the Data",
"### Social Impact of Dataset",
"### Discussion of Biases",
"### Other Known Limitations",
"## Additional Information",
"### Dataset Curators",
"### Licensing Information",
"### Contributions\n\nThanks to @github-username for adding this dataset."
] | [
"TAGS\n#annotations_creators-machine-generated #language_creators-machine-generated #multilinguality-monolingual #size_categories-unknown #source_datasets-extended|xsum #language-Dutch #license-unknown #region-us \n",
"# Dataset Card for XSum NL",
"## Table of Contents\n- Table of Contents\n- Dataset Description\n - Dataset Summary\n - Supported Tasks and Leaderboards\n - Languages\n- Dataset Structure\n - Data Instances\n - Data Fields\n - Data Splits\n- Dataset Creation\n - Curation Rationale\n - Source Data\n - Annotations\n - Personal and Sensitive Information\n- Considerations for Using the Data\n - Social Impact of Dataset\n - Discussion of Biases\n - Other Known Limitations\n- Additional Information\n - Dataset Curators\n - Licensing Information\n - Citation Information\n - Contributions",
"## Dataset Description\n\n- Homepage:\n- Repository:\n- Paper:\n- Leaderboard:\n- Point of Contact:",
"### Dataset Summary\n\nThis dataset is a machine translated dataset. It's the XSum dataset translated with this model from English to Dutch.\n\nSee the Hugginface page of the original dataset for more information on the format of this dataset.\n\nUse with:",
"### Languages\n\nDutch",
"## Dataset Structure",
"### Data Instances",
"### Data Fields\n\n- 'id': BBC ID of the article.\n- 'document': a string containing the body of the news article \n- 'summary': a string containing a one sentence summary of the article.",
"### Data Splits\n\n- 'train'\n- 'test'\n- 'validation'",
"## Dataset Creation",
"### Curation Rationale",
"### Source Data",
"#### Initial Data Collection and Normalization",
"#### Who are the source language producers?",
"### Annotations",
"#### Annotation process",
"#### Who are the annotators?",
"### Personal and Sensitive Information",
"## Considerations for Using the Data",
"### Social Impact of Dataset",
"### Discussion of Biases",
"### Other Known Limitations",
"## Additional Information",
"### Dataset Curators",
"### Licensing Information",
"### Contributions\n\nThanks to @github-username for adding this dataset."
] |
765eb565ac8ae91267817425017ad7b81829f06f | Reuters model for demo | mmcquade11-test/reuters-for-summarization-two | [
"region:us"
] | 2022-03-02T23:29:22+00:00 | {} | 2021-11-30T16:49:22+00:00 | [] | [] | TAGS
#region-us
| Reuters model for demo | [] | [
"TAGS\n#region-us \n"
] |
a13e63b05aa133aae304a4f190cbe680412dbe30 |
# Dataset Card for "Widdd"
## Dataset Description
WiDDD stands for WIkiData Disambig with Descriptions. The former dataset comes from [Cetoli & al](https://arxiv.org/pdf/1810.09164.pdf) paper, and is aimed at solving Named Entity Disambiguation. This datasets tries to extract relevant information from entities descriptions only, instead of working with graphs. In order to do so, we mapped every Wikidata id (correct id and wrong id) in the original paper with its WikiData description. If not found, row is discarded for the 1.+ versions.
### Supported Tasks and Leaderboards
[More Information Needed](https://github.com/huggingface/datasets/blob/master/CONTRIBUTING.md#how-to-contribute-to-the-dataset-cards)
### Languages
english
## Dataset Structure
We show detailed information for up to 5 configurations of the dataset.
### Data Instances
#### plain_text
- **Size of downloaded dataset files:** 46.64 MB
An example of 'train' looks as follows.
```
{'example_id': 11,
'string': 'pausanias',
'text': ' mention the spear, which he would indeed have touched with excitement. But it was being shown in the time of Pausanias in the second century AD. Achilles and ',
'correct_id': 'Q192931',
'wrong_id': 'Q941521',
'correct_description': 'ancient Greek geographer, travel writer and mythographer',
'wrong_description': 'Wikimedia disambiguation page'}
```
### Data Fields
The data fields are the same among all splits.
#### plain_text
- `example_id`: an `int32` feature,
- `string`: a `string` feature,
- `text`: a `string` feature,
- `correct_id`: a `string` feature,
- `wrong_id`: a `string` feature,
- `correct_description`: a `string` feature,
- `wrong_description`: a `string` feature,
### Data Splits
| name |train|validation|test|
|----------|----:|-----:|-----:|
|plain_text|96523|9609|9584|
## Dataset Creation
### Curation Rationale
[More Information Needed](https://github.com/huggingface/datasets/blob/master/CONTRIBUTING.md#how-to-contribute-to-the-dataset-cards)
### Source Data
#### Initial Data Collection and Normalization
[More Information Needed](https://github.com/huggingface/datasets/blob/master/CONTRIBUTING.md#how-to-contribute-to-the-dataset-cards)
#### Who are the source language producers?
[More Information Needed](https://github.com/huggingface/datasets/blob/master/CONTRIBUTING.md#how-to-contribute-to-the-dataset-cards)
### Annotations
#### Annotation process
[More Information Needed](https://github.com/huggingface/datasets/blob/master/CONTRIBUTING.md#how-to-contribute-to-the-dataset-cards)
#### Who are the annotators?
[More Information Needed](https://github.com/huggingface/datasets/blob/master/CONTRIBUTING.md#how-to-contribute-to-the-dataset-cards)
### Personal and Sensitive Information
[More Information Needed](https://github.com/huggingface/datasets/blob/master/CONTRIBUTING.md#how-to-contribute-to-the-dataset-cards)
## Considerations for Using the Data
### Social Impact of Dataset
[More Information Needed](https://github.com/huggingface/datasets/blob/master/CONTRIBUTING.md#how-to-contribute-to-the-dataset-cards)
### Discussion of Biases
[More Information Needed](https://github.com/huggingface/datasets/blob/master/CONTRIBUTING.md#how-to-contribute-to-the-dataset-cards)
### Other Known Limitations
[More Information Needed](https://github.com/huggingface/datasets/blob/master/CONTRIBUTING.md#how-to-contribute-to-the-dataset-cards)
## Additional Information
### Dataset Curators
[More Information Needed](https://github.com/huggingface/datasets/blob/master/CONTRIBUTING.md#how-to-contribute-to-the-dataset-cards)
### Licensing Information
[More Information Needed](https://github.com/huggingface/datasets/blob/master/CONTRIBUTING.md#how-to-contribute-to-the-dataset-cards)
### Citation Information
### Contributions
| mnemlaghi/widdd | [
"annotations_creators:machine-generated",
"language_creators:machine-generated",
"multilinguality:monolingual",
"size_categories:100K<n<1M",
"language:en",
"license:apache-2.0",
"arxiv:1810.09164",
"region:us"
] | 2022-03-02T23:29:22+00:00 | {"annotations_creators": ["machine-generated"], "language_creators": ["machine-generated"], "language": ["en"], "license": ["apache-2.0"], "multilinguality": ["monolingual"], "size_categories": ["100K<n<1M"], "source_datasets": [], "task_categories": ["named-entity-disambiguation"], "task_ids": ["wikidata-disambiguation"], "pretty_name": "Wikidisamb Dataset with Descriptions"} | 2022-10-22T14:02:03+00:00 | [
"1810.09164"
] | [
"en"
] | TAGS
#annotations_creators-machine-generated #language_creators-machine-generated #multilinguality-monolingual #size_categories-100K<n<1M #language-English #license-apache-2.0 #arxiv-1810.09164 #region-us
| Dataset Card for "Widdd"
========================
Dataset Description
-------------------
WiDDD stands for WIkiData Disambig with Descriptions. The former dataset comes from Cetoli & al paper, and is aimed at solving Named Entity Disambiguation. This datasets tries to extract relevant information from entities descriptions only, instead of working with graphs. In order to do so, we mapped every Wikidata id (correct id and wrong id) in the original paper with its WikiData description. If not found, row is discarded for the 1.+ versions.
### Supported Tasks and Leaderboards
### Languages
english
Dataset Structure
-----------------
We show detailed information for up to 5 configurations of the dataset.
### Data Instances
#### plain\_text
* Size of downloaded dataset files: 46.64 MB
An example of 'train' looks as follows.
### Data Fields
The data fields are the same among all splits.
#### plain\_text
* 'example\_id': an 'int32' feature,
* 'string': a 'string' feature,
* 'text': a 'string' feature,
* 'correct\_id': a 'string' feature,
* 'wrong\_id': a 'string' feature,
* 'correct\_description': a 'string' feature,
* 'wrong\_description': a 'string' feature,
### Data Splits
Dataset Creation
----------------
### Curation Rationale
### Source Data
#### Initial Data Collection and Normalization
#### Who are the source language producers?
### Annotations
#### Annotation process
#### Who are the annotators?
### Personal and Sensitive Information
Considerations for Using the Data
---------------------------------
### Social Impact of Dataset
### Discussion of Biases
### Other Known Limitations
Additional Information
----------------------
### Dataset Curators
### Licensing Information
### Contributions
| [
"### Supported Tasks and Leaderboards",
"### Languages\n\n\nenglish\n\n\nDataset Structure\n-----------------\n\n\nWe show detailed information for up to 5 configurations of the dataset.",
"### Data Instances",
"#### plain\\_text\n\n\n* Size of downloaded dataset files: 46.64 MB\n\n\nAn example of 'train' looks as follows.",
"### Data Fields\n\n\nThe data fields are the same among all splits.",
"#### plain\\_text\n\n\n* 'example\\_id': an 'int32' feature,\n* 'string': a 'string' feature,\n* 'text': a 'string' feature,\n* 'correct\\_id': a 'string' feature,\n* 'wrong\\_id': a 'string' feature,\n* 'correct\\_description': a 'string' feature,\n* 'wrong\\_description': a 'string' feature,",
"### Data Splits\n\n\n\nDataset Creation\n----------------",
"### Curation Rationale",
"### Source Data",
"#### Initial Data Collection and Normalization",
"#### Who are the source language producers?",
"### Annotations",
"#### Annotation process",
"#### Who are the annotators?",
"### Personal and Sensitive Information\n\n\nConsiderations for Using the Data\n---------------------------------",
"### Social Impact of Dataset",
"### Discussion of Biases",
"### Other Known Limitations\n\n\nAdditional Information\n----------------------",
"### Dataset Curators",
"### Licensing Information",
"### Contributions"
] | [
"TAGS\n#annotations_creators-machine-generated #language_creators-machine-generated #multilinguality-monolingual #size_categories-100K<n<1M #language-English #license-apache-2.0 #arxiv-1810.09164 #region-us \n",
"### Supported Tasks and Leaderboards",
"### Languages\n\n\nenglish\n\n\nDataset Structure\n-----------------\n\n\nWe show detailed information for up to 5 configurations of the dataset.",
"### Data Instances",
"#### plain\\_text\n\n\n* Size of downloaded dataset files: 46.64 MB\n\n\nAn example of 'train' looks as follows.",
"### Data Fields\n\n\nThe data fields are the same among all splits.",
"#### plain\\_text\n\n\n* 'example\\_id': an 'int32' feature,\n* 'string': a 'string' feature,\n* 'text': a 'string' feature,\n* 'correct\\_id': a 'string' feature,\n* 'wrong\\_id': a 'string' feature,\n* 'correct\\_description': a 'string' feature,\n* 'wrong\\_description': a 'string' feature,",
"### Data Splits\n\n\n\nDataset Creation\n----------------",
"### Curation Rationale",
"### Source Data",
"#### Initial Data Collection and Normalization",
"#### Who are the source language producers?",
"### Annotations",
"#### Annotation process",
"#### Who are the annotators?",
"### Personal and Sensitive Information\n\n\nConsiderations for Using the Data\n---------------------------------",
"### Social Impact of Dataset",
"### Discussion of Biases",
"### Other Known Limitations\n\n\nAdditional Information\n----------------------",
"### Dataset Curators",
"### Licensing Information",
"### Contributions"
] |
534086adc3aec801687316b3fe162e4231ab0a6b |
# RAFT submissions for my_raft
## Submitting to the leaderboard
To make a submission to the [leaderboard](https://huggingface.co/spaces/ought/raft-leaderboard), there are three main steps:
1. Generate predictions on the unlabeled test set of each task
2. Validate the predictions are compatible with the evaluation framework
3. Push the predictions to the Hub!
See the instructions below for more details.
### Rules
1. To prevent overfitting to the public leaderboard, we only evaluate **one submission per week**. You can push predictions to the Hub as many times as you wish, but we will only evaluate the most recent commit in a given week.
2. Transfer or meta-learning using other datasets, including further pre-training on other corpora, is allowed.
3. Use of unlabeled test data is allowed, as is it always available in the applied setting. For example, further pre-training using the unlabeled data for a task would be permitted.
4. Systems may be augmented with information retrieved from the internet, e.g. via automated web searches.
### Submission file format
For each task in RAFT, you should create a CSV file called `predictions.csv` with your model's predictions on the unlabeled test set. Each file should have exactly 2 columns:
* ID (int)
* Label (string)
See the dummy predictions in the `data` folder for examples with the expected format. Here is a simple example that creates a majority-class baseline:
```python
from pathlib import Path
import pandas as pd
from collections import Counter
from datasets import load_dataset, get_dataset_config_names
tasks = get_dataset_config_names("ought/raft")
for task in tasks:
# Load dataset
raft_subset = load_dataset("ought/raft", task)
# Compute majority class over training set
counter = Counter(raft_subset["train"]["Label"])
majority_class = counter.most_common(1)[0][0]
# Load predictions file
preds = pd.read_csv(f"data/{task}/predictions.csv")
# Convert label IDs to label names
preds["Label"] = raft_subset["train"].features["Label"].int2str(majority_class)
# Save predictions
preds.to_csv(f"data/{task}/predictions.csv", index=False)
```
As you can see in the example, each `predictions.csv` file should be stored in the task's subfolder in `data` and at the end you should have something like the following:
```
data
├── ade_corpus_v2
│ ├── predictions.csv
│ └── task.json
├── banking_77
│ ├── predictions.csv
│ └── task.json
├── neurips_impact_statement_risks
│ ├── predictions.csv
│ └── task.json
├── one_stop_english
│ ├── predictions.csv
│ └── task.json
├── overruling
│ ├── predictions.csv
│ └── task.json
├── semiconductor_org_types
│ ├── predictions.csv
│ └── task.json
├── systematic_review_inclusion
│ ├── predictions.csv
│ └── task.json
├── tai_safety_research
│ ├── predictions.csv
│ └── task.json
├── terms_of_service
│ ├── predictions.csv
│ └── task.json
├── tweet_eval_hate
│ ├── predictions.csv
│ └── task.json
└── twitter_complaints
├── predictions.csv
└── task.json
```
### Validate your submission
To ensure that your submission files are correctly formatted, run the following command from the root of the repository:
```
python cli.py validate
```
If everything is correct, you should see the following message:
```
All submission files validated! ✨ 🚀 ✨
Now you can make a submission 🤗
```
### Push your submission to the Hugging Face Hub!
The final step is to commit your files and push them to the Hub:
```
python cli.py submit
```
If there are no errors, you should see the following message:
```
Submission successful! 🎉 🥳 🎉
Your submission will be evaulated on Sunday 05 September 2021 ⏳
```
where the evaluation is run every Sunday and your results will be visible on the leaderboard. | moshew/my_raft | [
"benchmark:raft",
"region:us"
] | 2022-03-02T23:29:22+00:00 | {"benchmark": "raft", "type": "prediction", "submission_name": "SetFit300"} | 2022-07-16T16:01:04+00:00 | [] | [] | TAGS
#benchmark-raft #region-us
|
# RAFT submissions for my_raft
## Submitting to the leaderboard
To make a submission to the leaderboard, there are three main steps:
1. Generate predictions on the unlabeled test set of each task
2. Validate the predictions are compatible with the evaluation framework
3. Push the predictions to the Hub!
See the instructions below for more details.
### Rules
1. To prevent overfitting to the public leaderboard, we only evaluate one submission per week. You can push predictions to the Hub as many times as you wish, but we will only evaluate the most recent commit in a given week.
2. Transfer or meta-learning using other datasets, including further pre-training on other corpora, is allowed.
3. Use of unlabeled test data is allowed, as is it always available in the applied setting. For example, further pre-training using the unlabeled data for a task would be permitted.
4. Systems may be augmented with information retrieved from the internet, e.g. via automated web searches.
### Submission file format
For each task in RAFT, you should create a CSV file called 'URL' with your model's predictions on the unlabeled test set. Each file should have exactly 2 columns:
* ID (int)
* Label (string)
See the dummy predictions in the 'data' folder for examples with the expected format. Here is a simple example that creates a majority-class baseline:
As you can see in the example, each 'URL' file should be stored in the task's subfolder in 'data' and at the end you should have something like the following:
### Validate your submission
To ensure that your submission files are correctly formatted, run the following command from the root of the repository:
If everything is correct, you should see the following message:
### Push your submission to the Hugging Face Hub!
The final step is to commit your files and push them to the Hub:
If there are no errors, you should see the following message:
where the evaluation is run every Sunday and your results will be visible on the leaderboard. | [
"# RAFT submissions for my_raft",
"## Submitting to the leaderboard\n\nTo make a submission to the leaderboard, there are three main steps:\n\n1. Generate predictions on the unlabeled test set of each task\n2. Validate the predictions are compatible with the evaluation framework\n3. Push the predictions to the Hub!\n\nSee the instructions below for more details.",
"### Rules\n\n1. To prevent overfitting to the public leaderboard, we only evaluate one submission per week. You can push predictions to the Hub as many times as you wish, but we will only evaluate the most recent commit in a given week. \n2. Transfer or meta-learning using other datasets, including further pre-training on other corpora, is allowed.\n3. Use of unlabeled test data is allowed, as is it always available in the applied setting. For example, further pre-training using the unlabeled data for a task would be permitted.\n4. Systems may be augmented with information retrieved from the internet, e.g. via automated web searches.",
"### Submission file format\n\nFor each task in RAFT, you should create a CSV file called 'URL' with your model's predictions on the unlabeled test set. Each file should have exactly 2 columns:\n\n* ID (int)\n* Label (string)\n\nSee the dummy predictions in the 'data' folder for examples with the expected format. Here is a simple example that creates a majority-class baseline:\n\n\n\nAs you can see in the example, each 'URL' file should be stored in the task's subfolder in 'data' and at the end you should have something like the following:",
"### Validate your submission\n\nTo ensure that your submission files are correctly formatted, run the following command from the root of the repository:\n\n\n\nIf everything is correct, you should see the following message:",
"### Push your submission to the Hugging Face Hub!\n\nThe final step is to commit your files and push them to the Hub:\n\n\n\nIf there are no errors, you should see the following message:\n\n\n\nwhere the evaluation is run every Sunday and your results will be visible on the leaderboard."
] | [
"TAGS\n#benchmark-raft #region-us \n",
"# RAFT submissions for my_raft",
"## Submitting to the leaderboard\n\nTo make a submission to the leaderboard, there are three main steps:\n\n1. Generate predictions on the unlabeled test set of each task\n2. Validate the predictions are compatible with the evaluation framework\n3. Push the predictions to the Hub!\n\nSee the instructions below for more details.",
"### Rules\n\n1. To prevent overfitting to the public leaderboard, we only evaluate one submission per week. You can push predictions to the Hub as many times as you wish, but we will only evaluate the most recent commit in a given week. \n2. Transfer or meta-learning using other datasets, including further pre-training on other corpora, is allowed.\n3. Use of unlabeled test data is allowed, as is it always available in the applied setting. For example, further pre-training using the unlabeled data for a task would be permitted.\n4. Systems may be augmented with information retrieved from the internet, e.g. via automated web searches.",
"### Submission file format\n\nFor each task in RAFT, you should create a CSV file called 'URL' with your model's predictions on the unlabeled test set. Each file should have exactly 2 columns:\n\n* ID (int)\n* Label (string)\n\nSee the dummy predictions in the 'data' folder for examples with the expected format. Here is a simple example that creates a majority-class baseline:\n\n\n\nAs you can see in the example, each 'URL' file should be stored in the task's subfolder in 'data' and at the end you should have something like the following:",
"### Validate your submission\n\nTo ensure that your submission files are correctly formatted, run the following command from the root of the repository:\n\n\n\nIf everything is correct, you should see the following message:",
"### Push your submission to the Hugging Face Hub!\n\nThe final step is to commit your files and push them to the Hub:\n\n\n\nIf there are no errors, you should see the following message:\n\n\n\nwhere the evaluation is run every Sunday and your results will be visible on the leaderboard."
] |
12d1c4b52a0e7468d8c740398f7960dcd7f3eae1 | Pronunciation information pulled from wiktionary.org. | mostol/wiktionary-ipa | [
"region:us"
] | 2022-03-02T23:29:22+00:00 | {} | 2022-02-02T17:30:11+00:00 | [] | [] | TAGS
#region-us
| Pronunciation information pulled from URL. | [] | [
"TAGS\n#region-us \n"
] |
746c4f3e740f7b3dc6b58fc1ae109656fc464ca0 |
# Dataset Card for Common Voice Corpus 1
## Table of Contents
- [Dataset Description](#dataset-description)
- [Dataset Summary](#dataset-summary)
- [Supported Tasks and Leaderboards](#supported-tasks-and-leaderboards)
- [Languages](#languages)
- [Dataset Structure](#dataset-structure)
- [Data Instances](#data-instances)
- [Data Fields](#data-fields)
- [Data Splits](#data-splits)
- [Dataset Creation](#dataset-creation)
- [Curation Rationale](#curation-rationale)
- [Source Data](#source-data)
- [Annotations](#annotations)
- [Personal and Sensitive Information](#personal-and-sensitive-information)
- [Considerations for Using the Data](#considerations-for-using-the-data)
- [Social Impact of Dataset](#social-impact-of-dataset)
- [Discussion of Biases](#discussion-of-biases)
- [Other Known Limitations](#other-known-limitations)
- [Additional Information](#additional-information)
- [Dataset Curators](#dataset-curators)
- [Licensing Information](#licensing-information)
- [Citation Information](#citation-information)
- [Contributions](#contributions)
## Dataset Description
- **Homepage:** https://commonvoice.mozilla.org/en/datasets
- **Repository:** https://github.com/common-voice/common-voice
- **Paper:** https://arxiv.org/abs/1912.06670
- **Leaderboard:** https://paperswithcode.com/dataset/common-voice
- **Point of Contact:** [Anton Lozhkov](mailto:[email protected])
### Dataset Summary
The Common Voice dataset consists of a unique MP3 and corresponding text file.
Many of the 1368 recorded hours in the dataset also include demographic metadata like age, sex, and accent
that can help improve the accuracy of speech recognition engines.
The dataset currently consists of 1096 validated hours in 19 languages, but more voices and languages are always added.
Take a look at the [Languages](https://commonvoice.mozilla.org/en/languages) page to request a language or start contributing.
### Supported Tasks and Leaderboards
The results for models trained on the Common Voice datasets are available via the
[🤗 Speech Bench](https://huggingface.co/spaces/huggingface/hf-speech-bench)
### Languages
```
Breton, Catalan, Chinese (Taiwan), Chuvash, Dutch, English, Esperanto, Estonian, French, German, Hakha Chin, Irish, Italian, Kabyle, Kyrgyz, Slovenian, Tatar, Turkish, Welsh
```
## Dataset Structure
### Data Instances
A typical data point comprises the `path` to the audio file and its `sentence`.
Additional fields include `accent`, `age`, `client_id`, `up_votes`, `down_votes`, `gender`, `locale` and `segment`.
```python
{
'client_id': 'd59478fbc1ee646a28a3c652a119379939123784d99131b865a89f8b21c81f69276c48bd574b81267d9d1a77b83b43e6d475a6cfc79c232ddbca946ae9c7afc5',
'path': 'et/clips/common_voice_et_18318995.mp3',
'audio': {
'path': 'et/clips/common_voice_et_18318995.mp3',
'array': array([-0.00048828, -0.00018311, -0.00137329, ..., 0.00079346, 0.00091553, 0.00085449], dtype=float32),
'sampling_rate': 48000
},
'sentence': 'Tasub kokku saada inimestega, keda tunned juba ammust ajast saati.',
'up_votes': 2,
'down_votes': 0,
'age': 'twenties',
'gender': 'male',
'accent': '',
'locale': 'et',
'segment': ''
}
```
### Data Fields
`client_id` (`string`): An id for which client (voice) made the recording
`path` (`string`): The path to the audio file
`audio` (`dict`): A dictionary containing the path to the downloaded audio file, the decoded audio array, and the sampling rate. Note that when accessing the audio column: `dataset[0]["audio"]` the audio file is automatically decoded and resampled to `dataset.features["audio"].sampling_rate`. Decoding and resampling of a large number of audio files might take a significant amount of time. Thus it is important to first query the sample index before the `"audio"` column, *i.e.* `dataset[0]["audio"]` should **always** be preferred over `dataset["audio"][0]`.
`sentence` (`string`): The sentence the user was prompted to speak
`up_votes` (`int64`): How many upvotes the audio file has received from reviewers
`down_votes` (`int64`): How many downvotes the audio file has received from reviewers
`age` (`string`): The age of the speaker (e.g. `teens`, `twenties`, `fifties`)
`gender` (`string`): The gender of the speaker
`accent` (`string`): Accent of the speaker
`locale` (`string`): The locale of the speaker
`segment` (`string`): Usually an empty field
### Data Splits
The speech material has been subdivided into portions for dev, train, test, validated, invalidated, reported and other.
The validated data is data that has been validated with reviewers and received upvotes that the data is of high quality.
The invalidated data is data has been invalidated by reviewers
and received downvotes indicating that the data is of low quality.
The reported data is data that has been reported, for different reasons.
The other data is data that has not yet been reviewed.
The dev, test, train are all data that has been reviewed, deemed of high quality and split into dev, test and train.
## Data Preprocessing Recommended by Hugging Face
The following are data preprocessing steps advised by the Hugging Face team. They are accompanied by an example code snippet that shows how to put them to practice.
Many examples in this dataset have trailing quotations marks, e.g _“the cat sat on the mat.“_. These trailing quotation marks do not change the actual meaning of the sentence, and it is near impossible to infer whether a sentence is a quotation or not a quotation from audio data alone. In these cases, it is advised to strip the quotation marks, leaving: _the cat sat on the mat_.
In addition, the majority of training sentences end in punctuation ( . or ? or ! ), whereas just a small proportion do not. In the dev set, **almost all** sentences end in punctuation. Thus, it is recommended to append a full-stop ( . ) to the end of the small number of training examples that do not end in punctuation.
```python
from datasets import load_dataset
ds = load_dataset("mozilla-foundation/common_voice_1_0", "en", use_auth_token=True)
def prepare_dataset(batch):
"""Function to preprocess the dataset with the .map method"""
transcription = batch["sentence"]
if transcription.startswith('"') and transcription.endswith('"'):
# we can remove trailing quotation marks as they do not affect the transcription
transcription = transcription[1:-1]
if transcription[-1] not in [".", "?", "!"]:
# append a full-stop to sentences that do not end in punctuation
transcription = transcription + "."
batch["sentence"] = transcription
return batch
ds = ds.map(prepare_dataset, desc="preprocess dataset")
```
## Dataset Creation
### Curation Rationale
[Needs More Information]
### Source Data
#### Initial Data Collection and Normalization
[Needs More Information]
#### Who are the source language producers?
[Needs More Information]
### Annotations
#### Annotation process
[Needs More Information]
#### Who are the annotators?
[Needs More Information]
### Personal and Sensitive Information
The dataset consists of people who have donated their voice online. You agree to not attempt to determine the identity of speakers in the Common Voice dataset.
## Considerations for Using the Data
### Social Impact of Dataset
The dataset consists of people who have donated their voice online. You agree to not attempt to determine the identity of speakers in the Common Voice dataset.
### Discussion of Biases
[More Information Needed]
### Other Known Limitations
[More Information Needed]
## Additional Information
### Dataset Curators
[More Information Needed]
### Licensing Information
Public Domain, [CC-0](https://creativecommons.org/share-your-work/public-domain/cc0/)
### Citation Information
```
@inproceedings{commonvoice:2020,
author = {Ardila, R. and Branson, M. and Davis, K. and Henretty, M. and Kohler, M. and Meyer, J. and Morais, R. and Saunders, L. and Tyers, F. M. and Weber, G.},
title = {Common Voice: A Massively-Multilingual Speech Corpus},
booktitle = {Proceedings of the 12th Conference on Language Resources and Evaluation (LREC 2020)},
pages = {4211--4215},
year = 2020
}
```
| mozilla-foundation/common_voice_1_0 | [
"task_categories:automatic-speech-recognition",
"annotations_creators:crowdsourced",
"language_creators:crowdsourced",
"multilinguality:multilingual",
"source_datasets:extended|common_voice",
"license:cc0-1.0",
"arxiv:1912.06670",
"region:us"
] | 2022-03-02T23:29:22+00:00 | {"annotations_creators": ["crowdsourced"], "language_creators": ["crowdsourced"], "license": ["cc0-1.0"], "multilinguality": ["multilingual"], "size_categories": {"br": ["1K<n<10K"], "ca": ["10K<n<100K"], "cnh": ["1K<n<10K"], "cv": ["1K<n<10K"], "cy": ["10K<n<100K"], "de": ["100K<n<1M"], "en": ["100K<n<1M"], "eo": ["1K<n<10K"], "et": ["n<1K"], "fr": ["10K<n<100K"], "ga-IE": ["1K<n<10K"], "it": ["10K<n<100K"], "kab": ["100K<n<1M"], "ky": ["1K<n<10K"], "nl": ["10K<n<100K"], "sl": ["1K<n<10K"], "tr": ["1K<n<10K"], "tt": ["10K<n<100K"], "zh-TW": ["10K<n<100K"]}, "source_datasets": ["extended|common_voice"], "task_categories": ["automatic-speech-recognition"], "paperswithcode_id": "common-voice", "pretty_name": "Common Voice Corpus 1", "language_bcp47": ["br", "ca", "cnh", "cv", "cy", "de", "en", "eo", "et", "fr", "ga-IE", "it", "kab", "ky", "nl", "sl", "tr", "tt", "zh-TW"], "extra_gated_prompt": "By clicking on \u201cAccess repository\u201d below, you also agree to not attempt to determine the identity of speakers in the Common Voice dataset."} | 2023-07-29T14:59:56+00:00 | [
"1912.06670"
] | [] | TAGS
#task_categories-automatic-speech-recognition #annotations_creators-crowdsourced #language_creators-crowdsourced #multilinguality-multilingual #source_datasets-extended|common_voice #license-cc0-1.0 #arxiv-1912.06670 #region-us
|
# Dataset Card for Common Voice Corpus 1
## Table of Contents
- Dataset Description
- Dataset Summary
- Supported Tasks and Leaderboards
- Languages
- Dataset Structure
- Data Instances
- Data Fields
- Data Splits
- Dataset Creation
- Curation Rationale
- Source Data
- Annotations
- Personal and Sensitive Information
- Considerations for Using the Data
- Social Impact of Dataset
- Discussion of Biases
- Other Known Limitations
- Additional Information
- Dataset Curators
- Licensing Information
- Citation Information
- Contributions
## Dataset Description
- Homepage: URL
- Repository: URL
- Paper: URL
- Leaderboard: URL
- Point of Contact: Anton Lozhkov
### Dataset Summary
The Common Voice dataset consists of a unique MP3 and corresponding text file.
Many of the 1368 recorded hours in the dataset also include demographic metadata like age, sex, and accent
that can help improve the accuracy of speech recognition engines.
The dataset currently consists of 1096 validated hours in 19 languages, but more voices and languages are always added.
Take a look at the Languages page to request a language or start contributing.
### Supported Tasks and Leaderboards
The results for models trained on the Common Voice datasets are available via the
Speech Bench
### Languages
## Dataset Structure
### Data Instances
A typical data point comprises the 'path' to the audio file and its 'sentence'.
Additional fields include 'accent', 'age', 'client_id', 'up_votes', 'down_votes', 'gender', 'locale' and 'segment'.
### Data Fields
'client_id' ('string'): An id for which client (voice) made the recording
'path' ('string'): The path to the audio file
'audio' ('dict'): A dictionary containing the path to the downloaded audio file, the decoded audio array, and the sampling rate. Note that when accessing the audio column: 'dataset[0]["audio"]' the audio file is automatically decoded and resampled to 'dataset.features["audio"].sampling_rate'. Decoding and resampling of a large number of audio files might take a significant amount of time. Thus it is important to first query the sample index before the '"audio"' column, *i.e.* 'dataset[0]["audio"]' should always be preferred over 'dataset["audio"][0]'.
'sentence' ('string'): The sentence the user was prompted to speak
'up_votes' ('int64'): How many upvotes the audio file has received from reviewers
'down_votes' ('int64'): How many downvotes the audio file has received from reviewers
'age' ('string'): The age of the speaker (e.g. 'teens', 'twenties', 'fifties')
'gender' ('string'): The gender of the speaker
'accent' ('string'): Accent of the speaker
'locale' ('string'): The locale of the speaker
'segment' ('string'): Usually an empty field
### Data Splits
The speech material has been subdivided into portions for dev, train, test, validated, invalidated, reported and other.
The validated data is data that has been validated with reviewers and received upvotes that the data is of high quality.
The invalidated data is data has been invalidated by reviewers
and received downvotes indicating that the data is of low quality.
The reported data is data that has been reported, for different reasons.
The other data is data that has not yet been reviewed.
The dev, test, train are all data that has been reviewed, deemed of high quality and split into dev, test and train.
## Data Preprocessing Recommended by Hugging Face
The following are data preprocessing steps advised by the Hugging Face team. They are accompanied by an example code snippet that shows how to put them to practice.
Many examples in this dataset have trailing quotations marks, e.g _“the cat sat on the mat.“_. These trailing quotation marks do not change the actual meaning of the sentence, and it is near impossible to infer whether a sentence is a quotation or not a quotation from audio data alone. In these cases, it is advised to strip the quotation marks, leaving: _the cat sat on the mat_.
In addition, the majority of training sentences end in punctuation ( . or ? or ! ), whereas just a small proportion do not. In the dev set, almost all sentences end in punctuation. Thus, it is recommended to append a full-stop ( . ) to the end of the small number of training examples that do not end in punctuation.
## Dataset Creation
### Curation Rationale
### Source Data
#### Initial Data Collection and Normalization
#### Who are the source language producers?
### Annotations
#### Annotation process
#### Who are the annotators?
### Personal and Sensitive Information
The dataset consists of people who have donated their voice online. You agree to not attempt to determine the identity of speakers in the Common Voice dataset.
## Considerations for Using the Data
### Social Impact of Dataset
The dataset consists of people who have donated their voice online. You agree to not attempt to determine the identity of speakers in the Common Voice dataset.
### Discussion of Biases
### Other Known Limitations
## Additional Information
### Dataset Curators
### Licensing Information
Public Domain, CC-0
| [
"# Dataset Card for Common Voice Corpus 1",
"## Table of Contents\n- Dataset Description\n - Dataset Summary\n - Supported Tasks and Leaderboards\n - Languages\n- Dataset Structure\n - Data Instances\n - Data Fields\n - Data Splits\n- Dataset Creation\n - Curation Rationale\n - Source Data\n - Annotations\n - Personal and Sensitive Information\n- Considerations for Using the Data\n - Social Impact of Dataset\n - Discussion of Biases\n - Other Known Limitations\n- Additional Information\n - Dataset Curators\n - Licensing Information\n - Citation Information\n - Contributions",
"## Dataset Description\n\n- Homepage: URL\n- Repository: URL\n- Paper: URL\n- Leaderboard: URL\n- Point of Contact: Anton Lozhkov",
"### Dataset Summary\n\nThe Common Voice dataset consists of a unique MP3 and corresponding text file. \nMany of the 1368 recorded hours in the dataset also include demographic metadata like age, sex, and accent \nthat can help improve the accuracy of speech recognition engines.\n\nThe dataset currently consists of 1096 validated hours in 19 languages, but more voices and languages are always added. \nTake a look at the Languages page to request a language or start contributing.",
"### Supported Tasks and Leaderboards\n\nThe results for models trained on the Common Voice datasets are available via the \n Speech Bench",
"### Languages",
"## Dataset Structure",
"### Data Instances\n\nA typical data point comprises the 'path' to the audio file and its 'sentence'. \nAdditional fields include 'accent', 'age', 'client_id', 'up_votes', 'down_votes', 'gender', 'locale' and 'segment'.",
"### Data Fields\n\n'client_id' ('string'): An id for which client (voice) made the recording\n\n'path' ('string'): The path to the audio file\n\n'audio' ('dict'): A dictionary containing the path to the downloaded audio file, the decoded audio array, and the sampling rate. Note that when accessing the audio column: 'dataset[0][\"audio\"]' the audio file is automatically decoded and resampled to 'dataset.features[\"audio\"].sampling_rate'. Decoding and resampling of a large number of audio files might take a significant amount of time. Thus it is important to first query the sample index before the '\"audio\"' column, *i.e.* 'dataset[0][\"audio\"]' should always be preferred over 'dataset[\"audio\"][0]'.\n\n'sentence' ('string'): The sentence the user was prompted to speak\n\n'up_votes' ('int64'): How many upvotes the audio file has received from reviewers\n\n'down_votes' ('int64'): How many downvotes the audio file has received from reviewers\n\n'age' ('string'): The age of the speaker (e.g. 'teens', 'twenties', 'fifties')\n\n'gender' ('string'): The gender of the speaker\n\n'accent' ('string'): Accent of the speaker\n\n'locale' ('string'): The locale of the speaker\n\n'segment' ('string'): Usually an empty field",
"### Data Splits\n\nThe speech material has been subdivided into portions for dev, train, test, validated, invalidated, reported and other.\n\nThe validated data is data that has been validated with reviewers and received upvotes that the data is of high quality.\n\nThe invalidated data is data has been invalidated by reviewers\nand received downvotes indicating that the data is of low quality.\n\nThe reported data is data that has been reported, for different reasons.\n\nThe other data is data that has not yet been reviewed.\n\nThe dev, test, train are all data that has been reviewed, deemed of high quality and split into dev, test and train.",
"## Data Preprocessing Recommended by Hugging Face\n\nThe following are data preprocessing steps advised by the Hugging Face team. They are accompanied by an example code snippet that shows how to put them to practice. \n\nMany examples in this dataset have trailing quotations marks, e.g _“the cat sat on the mat.“_. These trailing quotation marks do not change the actual meaning of the sentence, and it is near impossible to infer whether a sentence is a quotation or not a quotation from audio data alone. In these cases, it is advised to strip the quotation marks, leaving: _the cat sat on the mat_.\n\nIn addition, the majority of training sentences end in punctuation ( . or ? or ! ), whereas just a small proportion do not. In the dev set, almost all sentences end in punctuation. Thus, it is recommended to append a full-stop ( . ) to the end of the small number of training examples that do not end in punctuation.",
"## Dataset Creation",
"### Curation Rationale",
"### Source Data",
"#### Initial Data Collection and Normalization",
"#### Who are the source language producers?",
"### Annotations",
"#### Annotation process",
"#### Who are the annotators?",
"### Personal and Sensitive Information\n\nThe dataset consists of people who have donated their voice online. You agree to not attempt to determine the identity of speakers in the Common Voice dataset.",
"## Considerations for Using the Data",
"### Social Impact of Dataset\n\nThe dataset consists of people who have donated their voice online. You agree to not attempt to determine the identity of speakers in the Common Voice dataset.",
"### Discussion of Biases",
"### Other Known Limitations",
"## Additional Information",
"### Dataset Curators",
"### Licensing Information\n\nPublic Domain, CC-0"
] | [
"TAGS\n#task_categories-automatic-speech-recognition #annotations_creators-crowdsourced #language_creators-crowdsourced #multilinguality-multilingual #source_datasets-extended|common_voice #license-cc0-1.0 #arxiv-1912.06670 #region-us \n",
"# Dataset Card for Common Voice Corpus 1",
"## Table of Contents\n- Dataset Description\n - Dataset Summary\n - Supported Tasks and Leaderboards\n - Languages\n- Dataset Structure\n - Data Instances\n - Data Fields\n - Data Splits\n- Dataset Creation\n - Curation Rationale\n - Source Data\n - Annotations\n - Personal and Sensitive Information\n- Considerations for Using the Data\n - Social Impact of Dataset\n - Discussion of Biases\n - Other Known Limitations\n- Additional Information\n - Dataset Curators\n - Licensing Information\n - Citation Information\n - Contributions",
"## Dataset Description\n\n- Homepage: URL\n- Repository: URL\n- Paper: URL\n- Leaderboard: URL\n- Point of Contact: Anton Lozhkov",
"### Dataset Summary\n\nThe Common Voice dataset consists of a unique MP3 and corresponding text file. \nMany of the 1368 recorded hours in the dataset also include demographic metadata like age, sex, and accent \nthat can help improve the accuracy of speech recognition engines.\n\nThe dataset currently consists of 1096 validated hours in 19 languages, but more voices and languages are always added. \nTake a look at the Languages page to request a language or start contributing.",
"### Supported Tasks and Leaderboards\n\nThe results for models trained on the Common Voice datasets are available via the \n Speech Bench",
"### Languages",
"## Dataset Structure",
"### Data Instances\n\nA typical data point comprises the 'path' to the audio file and its 'sentence'. \nAdditional fields include 'accent', 'age', 'client_id', 'up_votes', 'down_votes', 'gender', 'locale' and 'segment'.",
"### Data Fields\n\n'client_id' ('string'): An id for which client (voice) made the recording\n\n'path' ('string'): The path to the audio file\n\n'audio' ('dict'): A dictionary containing the path to the downloaded audio file, the decoded audio array, and the sampling rate. Note that when accessing the audio column: 'dataset[0][\"audio\"]' the audio file is automatically decoded and resampled to 'dataset.features[\"audio\"].sampling_rate'. Decoding and resampling of a large number of audio files might take a significant amount of time. Thus it is important to first query the sample index before the '\"audio\"' column, *i.e.* 'dataset[0][\"audio\"]' should always be preferred over 'dataset[\"audio\"][0]'.\n\n'sentence' ('string'): The sentence the user was prompted to speak\n\n'up_votes' ('int64'): How many upvotes the audio file has received from reviewers\n\n'down_votes' ('int64'): How many downvotes the audio file has received from reviewers\n\n'age' ('string'): The age of the speaker (e.g. 'teens', 'twenties', 'fifties')\n\n'gender' ('string'): The gender of the speaker\n\n'accent' ('string'): Accent of the speaker\n\n'locale' ('string'): The locale of the speaker\n\n'segment' ('string'): Usually an empty field",
"### Data Splits\n\nThe speech material has been subdivided into portions for dev, train, test, validated, invalidated, reported and other.\n\nThe validated data is data that has been validated with reviewers and received upvotes that the data is of high quality.\n\nThe invalidated data is data has been invalidated by reviewers\nand received downvotes indicating that the data is of low quality.\n\nThe reported data is data that has been reported, for different reasons.\n\nThe other data is data that has not yet been reviewed.\n\nThe dev, test, train are all data that has been reviewed, deemed of high quality and split into dev, test and train.",
"## Data Preprocessing Recommended by Hugging Face\n\nThe following are data preprocessing steps advised by the Hugging Face team. They are accompanied by an example code snippet that shows how to put them to practice. \n\nMany examples in this dataset have trailing quotations marks, e.g _“the cat sat on the mat.“_. These trailing quotation marks do not change the actual meaning of the sentence, and it is near impossible to infer whether a sentence is a quotation or not a quotation from audio data alone. In these cases, it is advised to strip the quotation marks, leaving: _the cat sat on the mat_.\n\nIn addition, the majority of training sentences end in punctuation ( . or ? or ! ), whereas just a small proportion do not. In the dev set, almost all sentences end in punctuation. Thus, it is recommended to append a full-stop ( . ) to the end of the small number of training examples that do not end in punctuation.",
"## Dataset Creation",
"### Curation Rationale",
"### Source Data",
"#### Initial Data Collection and Normalization",
"#### Who are the source language producers?",
"### Annotations",
"#### Annotation process",
"#### Who are the annotators?",
"### Personal and Sensitive Information\n\nThe dataset consists of people who have donated their voice online. You agree to not attempt to determine the identity of speakers in the Common Voice dataset.",
"## Considerations for Using the Data",
"### Social Impact of Dataset\n\nThe dataset consists of people who have donated their voice online. You agree to not attempt to determine the identity of speakers in the Common Voice dataset.",
"### Discussion of Biases",
"### Other Known Limitations",
"## Additional Information",
"### Dataset Curators",
"### Licensing Information\n\nPublic Domain, CC-0"
] |
47c28d1f638d66bbf0688901e12d379117b0818d |
# Dataset Card for Common Voice Corpus 2
## Table of Contents
- [Dataset Description](#dataset-description)
- [Dataset Summary](#dataset-summary)
- [Supported Tasks and Leaderboards](#supported-tasks-and-leaderboards)
- [Languages](#languages)
- [Dataset Structure](#dataset-structure)
- [Data Instances](#data-instances)
- [Data Fields](#data-fields)
- [Data Splits](#data-splits)
- [Dataset Creation](#dataset-creation)
- [Curation Rationale](#curation-rationale)
- [Source Data](#source-data)
- [Annotations](#annotations)
- [Personal and Sensitive Information](#personal-and-sensitive-information)
- [Considerations for Using the Data](#considerations-for-using-the-data)
- [Social Impact of Dataset](#social-impact-of-dataset)
- [Discussion of Biases](#discussion-of-biases)
- [Other Known Limitations](#other-known-limitations)
- [Additional Information](#additional-information)
- [Dataset Curators](#dataset-curators)
- [Licensing Information](#licensing-information)
- [Citation Information](#citation-information)
- [Contributions](#contributions)
## Dataset Description
- **Homepage:** https://commonvoice.mozilla.org/en/datasets
- **Repository:** https://github.com/common-voice/common-voice
- **Paper:** https://arxiv.org/abs/1912.06670
- **Leaderboard:** https://paperswithcode.com/dataset/common-voice
- **Point of Contact:** [Anton Lozhkov](mailto:[email protected])
### Dataset Summary
The Common Voice dataset consists of a unique MP3 and corresponding text file.
Many of the 2366 recorded hours in the dataset also include demographic metadata like age, sex, and accent
that can help improve the accuracy of speech recognition engines.
The dataset currently consists of 1872 validated hours in 28 languages, but more voices and languages are always added.
Take a look at the [Languages](https://commonvoice.mozilla.org/en/languages) page to request a language or start contributing.
### Supported Tasks and Leaderboards
The results for models trained on the Common Voice datasets are available via the
[🤗 Speech Bench](https://huggingface.co/spaces/huggingface/hf-speech-bench)
### Languages
```
Basque, Breton, Catalan, Chinese (China), Chinese (Taiwan), Chuvash, Dhivehi, Dutch, English, Esperanto, Estonian, French, German, Hakha Chin, Irish, Italian, Kabyle, Kinyarwanda, Kyrgyz, Mongolian, Russian, Sakha, Slovenian, Spanish, Swedish, Tatar, Turkish, Welsh
```
## Dataset Structure
### Data Instances
A typical data point comprises the `path` to the audio file and its `sentence`.
Additional fields include `accent`, `age`, `client_id`, `up_votes`, `down_votes`, `gender`, `locale` and `segment`.
```python
{
'client_id': 'd59478fbc1ee646a28a3c652a119379939123784d99131b865a89f8b21c81f69276c48bd574b81267d9d1a77b83b43e6d475a6cfc79c232ddbca946ae9c7afc5',
'path': 'et/clips/common_voice_et_18318995.mp3',
'audio': {
'path': 'et/clips/common_voice_et_18318995.mp3',
'array': array([-0.00048828, -0.00018311, -0.00137329, ..., 0.00079346, 0.00091553, 0.00085449], dtype=float32),
'sampling_rate': 48000
},
'sentence': 'Tasub kokku saada inimestega, keda tunned juba ammust ajast saati.',
'up_votes': 2,
'down_votes': 0,
'age': 'twenties',
'gender': 'male',
'accent': '',
'locale': 'et',
'segment': ''
}
```
### Data Fields
`client_id` (`string`): An id for which client (voice) made the recording
`path` (`string`): The path to the audio file
`audio` (`dict`): A dictionary containing the path to the downloaded audio file, the decoded audio array, and the sampling rate. Note that when accessing the audio column: `dataset[0]["audio"]` the audio file is automatically decoded and resampled to `dataset.features["audio"].sampling_rate`. Decoding and resampling of a large number of audio files might take a significant amount of time. Thus it is important to first query the sample index before the `"audio"` column, *i.e.* `dataset[0]["audio"]` should **always** be preferred over `dataset["audio"][0]`.
`sentence` (`string`): The sentence the user was prompted to speak
`up_votes` (`int64`): How many upvotes the audio file has received from reviewers
`down_votes` (`int64`): How many downvotes the audio file has received from reviewers
`age` (`string`): The age of the speaker (e.g. `teens`, `twenties`, `fifties`)
`gender` (`string`): The gender of the speaker
`accent` (`string`): Accent of the speaker
`locale` (`string`): The locale of the speaker
`segment` (`string`): Usually an empty field
### Data Splits
The speech material has been subdivided into portions for dev, train, test, validated, invalidated, reported and other.
The validated data is data that has been validated with reviewers and received upvotes that the data is of high quality.
The invalidated data is data has been invalidated by reviewers
and received downvotes indicating that the data is of low quality.
The reported data is data that has been reported, for different reasons.
The other data is data that has not yet been reviewed.
The dev, test, train are all data that has been reviewed, deemed of high quality and split into dev, test and train.
## Data Preprocessing Recommended by Hugging Face
The following are data preprocessing steps advised by the Hugging Face team. They are accompanied by an example code snippet that shows how to put them to practice.
Many examples in this dataset have trailing quotations marks, e.g _“the cat sat on the mat.“_. These trailing quotation marks do not change the actual meaning of the sentence, and it is near impossible to infer whether a sentence is a quotation or not a quotation from audio data alone. In these cases, it is advised to strip the quotation marks, leaving: _the cat sat on the mat_.
In addition, the majority of training sentences end in punctuation ( . or ? or ! ), whereas just a small proportion do not. In the dev set, **almost all** sentences end in punctuation. Thus, it is recommended to append a full-stop ( . ) to the end of the small number of training examples that do not end in punctuation.
```python
from datasets import load_dataset
ds = load_dataset("mozilla-foundation/common_voice_2_0", "en", use_auth_token=True)
def prepare_dataset(batch):
"""Function to preprocess the dataset with the .map method"""
transcription = batch["sentence"]
if transcription.startswith('"') and transcription.endswith('"'):
# we can remove trailing quotation marks as they do not affect the transcription
transcription = transcription[1:-1]
if transcription[-1] not in [".", "?", "!"]:
# append a full-stop to sentences that do not end in punctuation
transcription = transcription + "."
batch["sentence"] = transcription
return batch
ds = ds.map(prepare_dataset, desc="preprocess dataset")
```
## Dataset Creation
### Curation Rationale
[Needs More Information]
### Source Data
#### Initial Data Collection and Normalization
[Needs More Information]
#### Who are the source language producers?
[Needs More Information]
### Annotations
#### Annotation process
[Needs More Information]
#### Who are the annotators?
[Needs More Information]
### Personal and Sensitive Information
The dataset consists of people who have donated their voice online. You agree to not attempt to determine the identity of speakers in the Common Voice dataset.
## Considerations for Using the Data
### Social Impact of Dataset
The dataset consists of people who have donated their voice online. You agree to not attempt to determine the identity of speakers in the Common Voice dataset.
### Discussion of Biases
[More Information Needed]
### Other Known Limitations
[More Information Needed]
## Additional Information
### Dataset Curators
[More Information Needed]
### Licensing Information
Public Domain, [CC-0](https://creativecommons.org/share-your-work/public-domain/cc0/)
### Citation Information
```
@inproceedings{commonvoice:2020,
author = {Ardila, R. and Branson, M. and Davis, K. and Henretty, M. and Kohler, M. and Meyer, J. and Morais, R. and Saunders, L. and Tyers, F. M. and Weber, G.},
title = {Common Voice: A Massively-Multilingual Speech Corpus},
booktitle = {Proceedings of the 12th Conference on Language Resources and Evaluation (LREC 2020)},
pages = {4211--4215},
year = 2020
}
```
| mozilla-foundation/common_voice_2_0 | [
"task_categories:automatic-speech-recognition",
"annotations_creators:crowdsourced",
"language_creators:crowdsourced",
"multilinguality:multilingual",
"source_datasets:extended|common_voice",
"license:cc0-1.0",
"arxiv:1912.06670",
"region:us"
] | 2022-03-02T23:29:22+00:00 | {"annotations_creators": ["crowdsourced"], "language_creators": ["crowdsourced"], "license": ["cc0-1.0"], "multilinguality": ["multilingual"], "size_categories": {"br": ["10K<n<100K"], "ca": ["10K<n<100K"], "cnh": ["1K<n<10K"], "cv": ["1K<n<10K"], "cy": ["10K<n<100K"], "de": ["100K<n<1M"], "dv": ["1K<n<10K"], "en": ["100K<n<1M"], "eo": ["10K<n<100K"], "es": ["10K<n<100K"], "et": ["1K<n<10K"], "eu": ["10K<n<100K"], "fr": ["100K<n<1M"], "ga-IE": ["1K<n<10K"], "it": ["10K<n<100K"], "kab": ["100K<n<1M"], "ky": ["10K<n<100K"], "mn": ["1K<n<10K"], "nl": ["10K<n<100K"], "ru": ["10K<n<100K"], "rw": ["1K<n<10K"], "sah": ["1K<n<10K"], "sl": ["1K<n<10K"], "sv-SE": ["1K<n<10K"], "tr": ["1K<n<10K"], "tt": ["10K<n<100K"], "zh-CN": ["1K<n<10K"], "zh-TW": ["10K<n<100K"]}, "source_datasets": ["extended|common_voice"], "task_categories": ["automatic-speech-recognition"], "paperswithcode_id": "common-voice", "pretty_name": "Common Voice Corpus 2", "language_bcp47": ["br", "ca", "cnh", "cv", "cy", "de", "dv", "en", "eo", "es", "et", "eu", "fr", "ga-IE", "it", "kab", "ky", "mn", "nl", "ru", "rw", "sah", "sl", "sv-SE", "tr", "tt", "zh-CN", "zh-TW"], "extra_gated_prompt": "By clicking on \u201cAccess repository\u201d below, you also agree to not attempt to determine the identity of speakers in the Common Voice dataset."} | 2023-07-29T14:59:58+00:00 | [
"1912.06670"
] | [] | TAGS
#task_categories-automatic-speech-recognition #annotations_creators-crowdsourced #language_creators-crowdsourced #multilinguality-multilingual #source_datasets-extended|common_voice #license-cc0-1.0 #arxiv-1912.06670 #region-us
|
# Dataset Card for Common Voice Corpus 2
## Table of Contents
- Dataset Description
- Dataset Summary
- Supported Tasks and Leaderboards
- Languages
- Dataset Structure
- Data Instances
- Data Fields
- Data Splits
- Dataset Creation
- Curation Rationale
- Source Data
- Annotations
- Personal and Sensitive Information
- Considerations for Using the Data
- Social Impact of Dataset
- Discussion of Biases
- Other Known Limitations
- Additional Information
- Dataset Curators
- Licensing Information
- Citation Information
- Contributions
## Dataset Description
- Homepage: URL
- Repository: URL
- Paper: URL
- Leaderboard: URL
- Point of Contact: Anton Lozhkov
### Dataset Summary
The Common Voice dataset consists of a unique MP3 and corresponding text file.
Many of the 2366 recorded hours in the dataset also include demographic metadata like age, sex, and accent
that can help improve the accuracy of speech recognition engines.
The dataset currently consists of 1872 validated hours in 28 languages, but more voices and languages are always added.
Take a look at the Languages page to request a language or start contributing.
### Supported Tasks and Leaderboards
The results for models trained on the Common Voice datasets are available via the
Speech Bench
### Languages
## Dataset Structure
### Data Instances
A typical data point comprises the 'path' to the audio file and its 'sentence'.
Additional fields include 'accent', 'age', 'client_id', 'up_votes', 'down_votes', 'gender', 'locale' and 'segment'.
### Data Fields
'client_id' ('string'): An id for which client (voice) made the recording
'path' ('string'): The path to the audio file
'audio' ('dict'): A dictionary containing the path to the downloaded audio file, the decoded audio array, and the sampling rate. Note that when accessing the audio column: 'dataset[0]["audio"]' the audio file is automatically decoded and resampled to 'dataset.features["audio"].sampling_rate'. Decoding and resampling of a large number of audio files might take a significant amount of time. Thus it is important to first query the sample index before the '"audio"' column, *i.e.* 'dataset[0]["audio"]' should always be preferred over 'dataset["audio"][0]'.
'sentence' ('string'): The sentence the user was prompted to speak
'up_votes' ('int64'): How many upvotes the audio file has received from reviewers
'down_votes' ('int64'): How many downvotes the audio file has received from reviewers
'age' ('string'): The age of the speaker (e.g. 'teens', 'twenties', 'fifties')
'gender' ('string'): The gender of the speaker
'accent' ('string'): Accent of the speaker
'locale' ('string'): The locale of the speaker
'segment' ('string'): Usually an empty field
### Data Splits
The speech material has been subdivided into portions for dev, train, test, validated, invalidated, reported and other.
The validated data is data that has been validated with reviewers and received upvotes that the data is of high quality.
The invalidated data is data has been invalidated by reviewers
and received downvotes indicating that the data is of low quality.
The reported data is data that has been reported, for different reasons.
The other data is data that has not yet been reviewed.
The dev, test, train are all data that has been reviewed, deemed of high quality and split into dev, test and train.
## Data Preprocessing Recommended by Hugging Face
The following are data preprocessing steps advised by the Hugging Face team. They are accompanied by an example code snippet that shows how to put them to practice.
Many examples in this dataset have trailing quotations marks, e.g _“the cat sat on the mat.“_. These trailing quotation marks do not change the actual meaning of the sentence, and it is near impossible to infer whether a sentence is a quotation or not a quotation from audio data alone. In these cases, it is advised to strip the quotation marks, leaving: _the cat sat on the mat_.
In addition, the majority of training sentences end in punctuation ( . or ? or ! ), whereas just a small proportion do not. In the dev set, almost all sentences end in punctuation. Thus, it is recommended to append a full-stop ( . ) to the end of the small number of training examples that do not end in punctuation.
## Dataset Creation
### Curation Rationale
### Source Data
#### Initial Data Collection and Normalization
#### Who are the source language producers?
### Annotations
#### Annotation process
#### Who are the annotators?
### Personal and Sensitive Information
The dataset consists of people who have donated their voice online. You agree to not attempt to determine the identity of speakers in the Common Voice dataset.
## Considerations for Using the Data
### Social Impact of Dataset
The dataset consists of people who have donated their voice online. You agree to not attempt to determine the identity of speakers in the Common Voice dataset.
### Discussion of Biases
### Other Known Limitations
## Additional Information
### Dataset Curators
### Licensing Information
Public Domain, CC-0
| [
"# Dataset Card for Common Voice Corpus 2",
"## Table of Contents\n- Dataset Description\n - Dataset Summary\n - Supported Tasks and Leaderboards\n - Languages\n- Dataset Structure\n - Data Instances\n - Data Fields\n - Data Splits\n- Dataset Creation\n - Curation Rationale\n - Source Data\n - Annotations\n - Personal and Sensitive Information\n- Considerations for Using the Data\n - Social Impact of Dataset\n - Discussion of Biases\n - Other Known Limitations\n- Additional Information\n - Dataset Curators\n - Licensing Information\n - Citation Information\n - Contributions",
"## Dataset Description\n\n- Homepage: URL\n- Repository: URL\n- Paper: URL\n- Leaderboard: URL\n- Point of Contact: Anton Lozhkov",
"### Dataset Summary\n\nThe Common Voice dataset consists of a unique MP3 and corresponding text file. \nMany of the 2366 recorded hours in the dataset also include demographic metadata like age, sex, and accent \nthat can help improve the accuracy of speech recognition engines.\n\nThe dataset currently consists of 1872 validated hours in 28 languages, but more voices and languages are always added. \nTake a look at the Languages page to request a language or start contributing.",
"### Supported Tasks and Leaderboards\n\nThe results for models trained on the Common Voice datasets are available via the \n Speech Bench",
"### Languages",
"## Dataset Structure",
"### Data Instances\n\nA typical data point comprises the 'path' to the audio file and its 'sentence'. \nAdditional fields include 'accent', 'age', 'client_id', 'up_votes', 'down_votes', 'gender', 'locale' and 'segment'.",
"### Data Fields\n\n'client_id' ('string'): An id for which client (voice) made the recording\n\n'path' ('string'): The path to the audio file\n\n'audio' ('dict'): A dictionary containing the path to the downloaded audio file, the decoded audio array, and the sampling rate. Note that when accessing the audio column: 'dataset[0][\"audio\"]' the audio file is automatically decoded and resampled to 'dataset.features[\"audio\"].sampling_rate'. Decoding and resampling of a large number of audio files might take a significant amount of time. Thus it is important to first query the sample index before the '\"audio\"' column, *i.e.* 'dataset[0][\"audio\"]' should always be preferred over 'dataset[\"audio\"][0]'.\n\n'sentence' ('string'): The sentence the user was prompted to speak\n\n'up_votes' ('int64'): How many upvotes the audio file has received from reviewers\n\n'down_votes' ('int64'): How many downvotes the audio file has received from reviewers\n\n'age' ('string'): The age of the speaker (e.g. 'teens', 'twenties', 'fifties')\n\n'gender' ('string'): The gender of the speaker\n\n'accent' ('string'): Accent of the speaker\n\n'locale' ('string'): The locale of the speaker\n\n'segment' ('string'): Usually an empty field",
"### Data Splits\n\nThe speech material has been subdivided into portions for dev, train, test, validated, invalidated, reported and other.\n\nThe validated data is data that has been validated with reviewers and received upvotes that the data is of high quality.\n\nThe invalidated data is data has been invalidated by reviewers\nand received downvotes indicating that the data is of low quality.\n\nThe reported data is data that has been reported, for different reasons.\n\nThe other data is data that has not yet been reviewed.\n\nThe dev, test, train are all data that has been reviewed, deemed of high quality and split into dev, test and train.",
"## Data Preprocessing Recommended by Hugging Face\n\nThe following are data preprocessing steps advised by the Hugging Face team. They are accompanied by an example code snippet that shows how to put them to practice. \n\nMany examples in this dataset have trailing quotations marks, e.g _“the cat sat on the mat.“_. These trailing quotation marks do not change the actual meaning of the sentence, and it is near impossible to infer whether a sentence is a quotation or not a quotation from audio data alone. In these cases, it is advised to strip the quotation marks, leaving: _the cat sat on the mat_.\n\nIn addition, the majority of training sentences end in punctuation ( . or ? or ! ), whereas just a small proportion do not. In the dev set, almost all sentences end in punctuation. Thus, it is recommended to append a full-stop ( . ) to the end of the small number of training examples that do not end in punctuation.",
"## Dataset Creation",
"### Curation Rationale",
"### Source Data",
"#### Initial Data Collection and Normalization",
"#### Who are the source language producers?",
"### Annotations",
"#### Annotation process",
"#### Who are the annotators?",
"### Personal and Sensitive Information\n\nThe dataset consists of people who have donated their voice online. You agree to not attempt to determine the identity of speakers in the Common Voice dataset.",
"## Considerations for Using the Data",
"### Social Impact of Dataset\n\nThe dataset consists of people who have donated their voice online. You agree to not attempt to determine the identity of speakers in the Common Voice dataset.",
"### Discussion of Biases",
"### Other Known Limitations",
"## Additional Information",
"### Dataset Curators",
"### Licensing Information\n\nPublic Domain, CC-0"
] | [
"TAGS\n#task_categories-automatic-speech-recognition #annotations_creators-crowdsourced #language_creators-crowdsourced #multilinguality-multilingual #source_datasets-extended|common_voice #license-cc0-1.0 #arxiv-1912.06670 #region-us \n",
"# Dataset Card for Common Voice Corpus 2",
"## Table of Contents\n- Dataset Description\n - Dataset Summary\n - Supported Tasks and Leaderboards\n - Languages\n- Dataset Structure\n - Data Instances\n - Data Fields\n - Data Splits\n- Dataset Creation\n - Curation Rationale\n - Source Data\n - Annotations\n - Personal and Sensitive Information\n- Considerations for Using the Data\n - Social Impact of Dataset\n - Discussion of Biases\n - Other Known Limitations\n- Additional Information\n - Dataset Curators\n - Licensing Information\n - Citation Information\n - Contributions",
"## Dataset Description\n\n- Homepage: URL\n- Repository: URL\n- Paper: URL\n- Leaderboard: URL\n- Point of Contact: Anton Lozhkov",
"### Dataset Summary\n\nThe Common Voice dataset consists of a unique MP3 and corresponding text file. \nMany of the 2366 recorded hours in the dataset also include demographic metadata like age, sex, and accent \nthat can help improve the accuracy of speech recognition engines.\n\nThe dataset currently consists of 1872 validated hours in 28 languages, but more voices and languages are always added. \nTake a look at the Languages page to request a language or start contributing.",
"### Supported Tasks and Leaderboards\n\nThe results for models trained on the Common Voice datasets are available via the \n Speech Bench",
"### Languages",
"## Dataset Structure",
"### Data Instances\n\nA typical data point comprises the 'path' to the audio file and its 'sentence'. \nAdditional fields include 'accent', 'age', 'client_id', 'up_votes', 'down_votes', 'gender', 'locale' and 'segment'.",
"### Data Fields\n\n'client_id' ('string'): An id for which client (voice) made the recording\n\n'path' ('string'): The path to the audio file\n\n'audio' ('dict'): A dictionary containing the path to the downloaded audio file, the decoded audio array, and the sampling rate. Note that when accessing the audio column: 'dataset[0][\"audio\"]' the audio file is automatically decoded and resampled to 'dataset.features[\"audio\"].sampling_rate'. Decoding and resampling of a large number of audio files might take a significant amount of time. Thus it is important to first query the sample index before the '\"audio\"' column, *i.e.* 'dataset[0][\"audio\"]' should always be preferred over 'dataset[\"audio\"][0]'.\n\n'sentence' ('string'): The sentence the user was prompted to speak\n\n'up_votes' ('int64'): How many upvotes the audio file has received from reviewers\n\n'down_votes' ('int64'): How many downvotes the audio file has received from reviewers\n\n'age' ('string'): The age of the speaker (e.g. 'teens', 'twenties', 'fifties')\n\n'gender' ('string'): The gender of the speaker\n\n'accent' ('string'): Accent of the speaker\n\n'locale' ('string'): The locale of the speaker\n\n'segment' ('string'): Usually an empty field",
"### Data Splits\n\nThe speech material has been subdivided into portions for dev, train, test, validated, invalidated, reported and other.\n\nThe validated data is data that has been validated with reviewers and received upvotes that the data is of high quality.\n\nThe invalidated data is data has been invalidated by reviewers\nand received downvotes indicating that the data is of low quality.\n\nThe reported data is data that has been reported, for different reasons.\n\nThe other data is data that has not yet been reviewed.\n\nThe dev, test, train are all data that has been reviewed, deemed of high quality and split into dev, test and train.",
"## Data Preprocessing Recommended by Hugging Face\n\nThe following are data preprocessing steps advised by the Hugging Face team. They are accompanied by an example code snippet that shows how to put them to practice. \n\nMany examples in this dataset have trailing quotations marks, e.g _“the cat sat on the mat.“_. These trailing quotation marks do not change the actual meaning of the sentence, and it is near impossible to infer whether a sentence is a quotation or not a quotation from audio data alone. In these cases, it is advised to strip the quotation marks, leaving: _the cat sat on the mat_.\n\nIn addition, the majority of training sentences end in punctuation ( . or ? or ! ), whereas just a small proportion do not. In the dev set, almost all sentences end in punctuation. Thus, it is recommended to append a full-stop ( . ) to the end of the small number of training examples that do not end in punctuation.",
"## Dataset Creation",
"### Curation Rationale",
"### Source Data",
"#### Initial Data Collection and Normalization",
"#### Who are the source language producers?",
"### Annotations",
"#### Annotation process",
"#### Who are the annotators?",
"### Personal and Sensitive Information\n\nThe dataset consists of people who have donated their voice online. You agree to not attempt to determine the identity of speakers in the Common Voice dataset.",
"## Considerations for Using the Data",
"### Social Impact of Dataset\n\nThe dataset consists of people who have donated their voice online. You agree to not attempt to determine the identity of speakers in the Common Voice dataset.",
"### Discussion of Biases",
"### Other Known Limitations",
"## Additional Information",
"### Dataset Curators",
"### Licensing Information\n\nPublic Domain, CC-0"
] |
4ce95388c69e4b64ed37b5184c3002f4a4c11e3d |
# Dataset Card for Common Voice Corpus 3
## Table of Contents
- [Dataset Description](#dataset-description)
- [Dataset Summary](#dataset-summary)
- [Supported Tasks and Leaderboards](#supported-tasks-and-leaderboards)
- [Languages](#languages)
- [Dataset Structure](#dataset-structure)
- [Data Instances](#data-instances)
- [Data Fields](#data-fields)
- [Data Splits](#data-splits)
- [Dataset Creation](#dataset-creation)
- [Curation Rationale](#curation-rationale)
- [Source Data](#source-data)
- [Annotations](#annotations)
- [Personal and Sensitive Information](#personal-and-sensitive-information)
- [Considerations for Using the Data](#considerations-for-using-the-data)
- [Social Impact of Dataset](#social-impact-of-dataset)
- [Discussion of Biases](#discussion-of-biases)
- [Other Known Limitations](#other-known-limitations)
- [Additional Information](#additional-information)
- [Dataset Curators](#dataset-curators)
- [Licensing Information](#licensing-information)
- [Citation Information](#citation-information)
- [Contributions](#contributions)
## Dataset Description
- **Homepage:** https://commonvoice.mozilla.org/en/datasets
- **Repository:** https://github.com/common-voice/common-voice
- **Paper:** https://arxiv.org/abs/1912.06670
- **Leaderboard:** https://paperswithcode.com/dataset/common-voice
- **Point of Contact:** [Anton Lozhkov](mailto:[email protected])
### Dataset Summary
The Common Voice dataset consists of a unique MP3 and corresponding text file.
Many of the 2454 recorded hours in the dataset also include demographic metadata like age, sex, and accent
that can help improve the accuracy of speech recognition engines.
The dataset currently consists of 1979 validated hours in 29 languages, but more voices and languages are always added.
Take a look at the [Languages](https://commonvoice.mozilla.org/en/languages) page to request a language or start contributing.
### Supported Tasks and Leaderboards
The results for models trained on the Common Voice datasets are available via the
[🤗 Speech Bench](https://huggingface.co/spaces/huggingface/hf-speech-bench)
### Languages
```
Basque, Breton, Catalan, Chinese (China), Chinese (Taiwan), Chuvash, Dhivehi, Dutch, English, Esperanto, Estonian, French, German, Hakha Chin, Irish, Italian, Kabyle, Kinyarwanda, Kyrgyz, Mongolian, Persian, Russian, Sakha, Slovenian, Spanish, Swedish, Tatar, Turkish, Welsh
```
## Dataset Structure
### Data Instances
A typical data point comprises the `path` to the audio file and its `sentence`.
Additional fields include `accent`, `age`, `client_id`, `up_votes`, `down_votes`, `gender`, `locale` and `segment`.
```python
{
'client_id': 'd59478fbc1ee646a28a3c652a119379939123784d99131b865a89f8b21c81f69276c48bd574b81267d9d1a77b83b43e6d475a6cfc79c232ddbca946ae9c7afc5',
'path': 'et/clips/common_voice_et_18318995.mp3',
'audio': {
'path': 'et/clips/common_voice_et_18318995.mp3',
'array': array([-0.00048828, -0.00018311, -0.00137329, ..., 0.00079346, 0.00091553, 0.00085449], dtype=float32),
'sampling_rate': 48000
},
'sentence': 'Tasub kokku saada inimestega, keda tunned juba ammust ajast saati.',
'up_votes': 2,
'down_votes': 0,
'age': 'twenties',
'gender': 'male',
'accent': '',
'locale': 'et',
'segment': ''
}
```
### Data Fields
`client_id` (`string`): An id for which client (voice) made the recording
`path` (`string`): The path to the audio file
`audio` (`dict`): A dictionary containing the path to the downloaded audio file, the decoded audio array, and the sampling rate. Note that when accessing the audio column: `dataset[0]["audio"]` the audio file is automatically decoded and resampled to `dataset.features["audio"].sampling_rate`. Decoding and resampling of a large number of audio files might take a significant amount of time. Thus it is important to first query the sample index before the `"audio"` column, *i.e.* `dataset[0]["audio"]` should **always** be preferred over `dataset["audio"][0]`.
`sentence` (`string`): The sentence the user was prompted to speak
`up_votes` (`int64`): How many upvotes the audio file has received from reviewers
`down_votes` (`int64`): How many downvotes the audio file has received from reviewers
`age` (`string`): The age of the speaker (e.g. `teens`, `twenties`, `fifties`)
`gender` (`string`): The gender of the speaker
`accent` (`string`): Accent of the speaker
`locale` (`string`): The locale of the speaker
`segment` (`string`): Usually an empty field
### Data Splits
The speech material has been subdivided into portions for dev, train, test, validated, invalidated, reported and other.
The validated data is data that has been validated with reviewers and received upvotes that the data is of high quality.
The invalidated data is data has been invalidated by reviewers
and received downvotes indicating that the data is of low quality.
The reported data is data that has been reported, for different reasons.
The other data is data that has not yet been reviewed.
The dev, test, train are all data that has been reviewed, deemed of high quality and split into dev, test and train.
## Data Preprocessing Recommended by Hugging Face
The following are data preprocessing steps advised by the Hugging Face team. They are accompanied by an example code snippet that shows how to put them to practice.
Many examples in this dataset have trailing quotations marks, e.g _“the cat sat on the mat.“_. These trailing quotation marks do not change the actual meaning of the sentence, and it is near impossible to infer whether a sentence is a quotation or not a quotation from audio data alone. In these cases, it is advised to strip the quotation marks, leaving: _the cat sat on the mat_.
In addition, the majority of training sentences end in punctuation ( . or ? or ! ), whereas just a small proportion do not. In the dev set, **almost all** sentences end in punctuation. Thus, it is recommended to append a full-stop ( . ) to the end of the small number of training examples that do not end in punctuation.
```python
from datasets import load_dataset
ds = load_dataset("mozilla-foundation/common_voice_3_0", "en", use_auth_token=True)
def prepare_dataset(batch):
"""Function to preprocess the dataset with the .map method"""
transcription = batch["sentence"]
if transcription.startswith('"') and transcription.endswith('"'):
# we can remove trailing quotation marks as they do not affect the transcription
transcription = transcription[1:-1]
if transcription[-1] not in [".", "?", "!"]:
# append a full-stop to sentences that do not end in punctuation
transcription = transcription + "."
batch["sentence"] = transcription
return batch
ds = ds.map(prepare_dataset, desc="preprocess dataset")
```
## Dataset Creation
### Curation Rationale
[Needs More Information]
### Source Data
#### Initial Data Collection and Normalization
[Needs More Information]
#### Who are the source language producers?
[Needs More Information]
### Annotations
#### Annotation process
[Needs More Information]
#### Who are the annotators?
[Needs More Information]
### Personal and Sensitive Information
The dataset consists of people who have donated their voice online. You agree to not attempt to determine the identity of speakers in the Common Voice dataset.
## Considerations for Using the Data
### Social Impact of Dataset
The dataset consists of people who have donated their voice online. You agree to not attempt to determine the identity of speakers in the Common Voice dataset.
### Discussion of Biases
[More Information Needed]
### Other Known Limitations
[More Information Needed]
## Additional Information
### Dataset Curators
[More Information Needed]
### Licensing Information
Public Domain, [CC-0](https://creativecommons.org/share-your-work/public-domain/cc0/)
### Citation Information
```
@inproceedings{commonvoice:2020,
author = {Ardila, R. and Branson, M. and Davis, K. and Henretty, M. and Kohler, M. and Meyer, J. and Morais, R. and Saunders, L. and Tyers, F. M. and Weber, G.},
title = {Common Voice: A Massively-Multilingual Speech Corpus},
booktitle = {Proceedings of the 12th Conference on Language Resources and Evaluation (LREC 2020)},
pages = {4211--4215},
year = 2020
}
```
| mozilla-foundation/common_voice_3_0 | [
"task_categories:automatic-speech-recognition",
"annotations_creators:crowdsourced",
"language_creators:crowdsourced",
"multilinguality:multilingual",
"source_datasets:extended|common_voice",
"license:cc0-1.0",
"arxiv:1912.06670",
"region:us"
] | 2022-03-02T23:29:22+00:00 | {"annotations_creators": ["crowdsourced"], "language_creators": ["crowdsourced"], "license": ["cc0-1.0"], "multilinguality": ["multilingual"], "size_categories": {"br": ["10K<n<100K"], "ca": ["10K<n<100K"], "cnh": ["1K<n<10K"], "cv": ["1K<n<10K"], "cy": ["10K<n<100K"], "de": ["100K<n<1M"], "dv": ["1K<n<10K"], "en": ["100K<n<1M"], "eo": ["10K<n<100K"], "es": ["10K<n<100K"], "et": ["1K<n<10K"], "eu": ["10K<n<100K"], "fa": ["10K<n<100K"], "fr": ["100K<n<1M"], "ga-IE": ["1K<n<10K"], "it": ["10K<n<100K"], "kab": ["100K<n<1M"], "ky": ["10K<n<100K"], "mn": ["1K<n<10K"], "nl": ["10K<n<100K"], "ru": ["10K<n<100K"], "rw": ["1K<n<10K"], "sah": ["1K<n<10K"], "sl": ["1K<n<10K"], "sv-SE": ["1K<n<10K"], "tr": ["1K<n<10K"], "tt": ["10K<n<100K"], "zh-CN": ["1K<n<10K"], "zh-TW": ["10K<n<100K"]}, "source_datasets": ["extended|common_voice"], "task_categories": ["automatic-speech-recognition"], "paperswithcode_id": "common-voice", "pretty_name": "Common Voice Corpus 3", "language_bcp47": ["br", "ca", "cnh", "cv", "cy", "de", "dv", "en", "eo", "es", "et", "eu", "fa", "fr", "ga-IE", "it", "kab", "ky", "mn", "nl", "ru", "rw", "sah", "sl", "sv-SE", "tr", "tt", "zh-CN", "zh-TW"], "extra_gated_prompt": "By clicking on \u201cAccess repository\u201d below, you also agree to not attempt to determine the identity of speakers in the Common Voice dataset."} | 2023-07-29T14:59:59+00:00 | [
"1912.06670"
] | [] | TAGS
#task_categories-automatic-speech-recognition #annotations_creators-crowdsourced #language_creators-crowdsourced #multilinguality-multilingual #source_datasets-extended|common_voice #license-cc0-1.0 #arxiv-1912.06670 #region-us
|
# Dataset Card for Common Voice Corpus 3
## Table of Contents
- Dataset Description
- Dataset Summary
- Supported Tasks and Leaderboards
- Languages
- Dataset Structure
- Data Instances
- Data Fields
- Data Splits
- Dataset Creation
- Curation Rationale
- Source Data
- Annotations
- Personal and Sensitive Information
- Considerations for Using the Data
- Social Impact of Dataset
- Discussion of Biases
- Other Known Limitations
- Additional Information
- Dataset Curators
- Licensing Information
- Citation Information
- Contributions
## Dataset Description
- Homepage: URL
- Repository: URL
- Paper: URL
- Leaderboard: URL
- Point of Contact: Anton Lozhkov
### Dataset Summary
The Common Voice dataset consists of a unique MP3 and corresponding text file.
Many of the 2454 recorded hours in the dataset also include demographic metadata like age, sex, and accent
that can help improve the accuracy of speech recognition engines.
The dataset currently consists of 1979 validated hours in 29 languages, but more voices and languages are always added.
Take a look at the Languages page to request a language or start contributing.
### Supported Tasks and Leaderboards
The results for models trained on the Common Voice datasets are available via the
Speech Bench
### Languages
## Dataset Structure
### Data Instances
A typical data point comprises the 'path' to the audio file and its 'sentence'.
Additional fields include 'accent', 'age', 'client_id', 'up_votes', 'down_votes', 'gender', 'locale' and 'segment'.
### Data Fields
'client_id' ('string'): An id for which client (voice) made the recording
'path' ('string'): The path to the audio file
'audio' ('dict'): A dictionary containing the path to the downloaded audio file, the decoded audio array, and the sampling rate. Note that when accessing the audio column: 'dataset[0]["audio"]' the audio file is automatically decoded and resampled to 'dataset.features["audio"].sampling_rate'. Decoding and resampling of a large number of audio files might take a significant amount of time. Thus it is important to first query the sample index before the '"audio"' column, *i.e.* 'dataset[0]["audio"]' should always be preferred over 'dataset["audio"][0]'.
'sentence' ('string'): The sentence the user was prompted to speak
'up_votes' ('int64'): How many upvotes the audio file has received from reviewers
'down_votes' ('int64'): How many downvotes the audio file has received from reviewers
'age' ('string'): The age of the speaker (e.g. 'teens', 'twenties', 'fifties')
'gender' ('string'): The gender of the speaker
'accent' ('string'): Accent of the speaker
'locale' ('string'): The locale of the speaker
'segment' ('string'): Usually an empty field
### Data Splits
The speech material has been subdivided into portions for dev, train, test, validated, invalidated, reported and other.
The validated data is data that has been validated with reviewers and received upvotes that the data is of high quality.
The invalidated data is data has been invalidated by reviewers
and received downvotes indicating that the data is of low quality.
The reported data is data that has been reported, for different reasons.
The other data is data that has not yet been reviewed.
The dev, test, train are all data that has been reviewed, deemed of high quality and split into dev, test and train.
## Data Preprocessing Recommended by Hugging Face
The following are data preprocessing steps advised by the Hugging Face team. They are accompanied by an example code snippet that shows how to put them to practice.
Many examples in this dataset have trailing quotations marks, e.g _“the cat sat on the mat.“_. These trailing quotation marks do not change the actual meaning of the sentence, and it is near impossible to infer whether a sentence is a quotation or not a quotation from audio data alone. In these cases, it is advised to strip the quotation marks, leaving: _the cat sat on the mat_.
In addition, the majority of training sentences end in punctuation ( . or ? or ! ), whereas just a small proportion do not. In the dev set, almost all sentences end in punctuation. Thus, it is recommended to append a full-stop ( . ) to the end of the small number of training examples that do not end in punctuation.
## Dataset Creation
### Curation Rationale
### Source Data
#### Initial Data Collection and Normalization
#### Who are the source language producers?
### Annotations
#### Annotation process
#### Who are the annotators?
### Personal and Sensitive Information
The dataset consists of people who have donated their voice online. You agree to not attempt to determine the identity of speakers in the Common Voice dataset.
## Considerations for Using the Data
### Social Impact of Dataset
The dataset consists of people who have donated their voice online. You agree to not attempt to determine the identity of speakers in the Common Voice dataset.
### Discussion of Biases
### Other Known Limitations
## Additional Information
### Dataset Curators
### Licensing Information
Public Domain, CC-0
| [
"# Dataset Card for Common Voice Corpus 3",
"## Table of Contents\n- Dataset Description\n - Dataset Summary\n - Supported Tasks and Leaderboards\n - Languages\n- Dataset Structure\n - Data Instances\n - Data Fields\n - Data Splits\n- Dataset Creation\n - Curation Rationale\n - Source Data\n - Annotations\n - Personal and Sensitive Information\n- Considerations for Using the Data\n - Social Impact of Dataset\n - Discussion of Biases\n - Other Known Limitations\n- Additional Information\n - Dataset Curators\n - Licensing Information\n - Citation Information\n - Contributions",
"## Dataset Description\n\n- Homepage: URL\n- Repository: URL\n- Paper: URL\n- Leaderboard: URL\n- Point of Contact: Anton Lozhkov",
"### Dataset Summary\n\nThe Common Voice dataset consists of a unique MP3 and corresponding text file. \nMany of the 2454 recorded hours in the dataset also include demographic metadata like age, sex, and accent \nthat can help improve the accuracy of speech recognition engines.\n\nThe dataset currently consists of 1979 validated hours in 29 languages, but more voices and languages are always added. \nTake a look at the Languages page to request a language or start contributing.",
"### Supported Tasks and Leaderboards\n\nThe results for models trained on the Common Voice datasets are available via the \n Speech Bench",
"### Languages",
"## Dataset Structure",
"### Data Instances\n\nA typical data point comprises the 'path' to the audio file and its 'sentence'. \nAdditional fields include 'accent', 'age', 'client_id', 'up_votes', 'down_votes', 'gender', 'locale' and 'segment'.",
"### Data Fields\n\n'client_id' ('string'): An id for which client (voice) made the recording\n\n'path' ('string'): The path to the audio file\n\n'audio' ('dict'): A dictionary containing the path to the downloaded audio file, the decoded audio array, and the sampling rate. Note that when accessing the audio column: 'dataset[0][\"audio\"]' the audio file is automatically decoded and resampled to 'dataset.features[\"audio\"].sampling_rate'. Decoding and resampling of a large number of audio files might take a significant amount of time. Thus it is important to first query the sample index before the '\"audio\"' column, *i.e.* 'dataset[0][\"audio\"]' should always be preferred over 'dataset[\"audio\"][0]'.\n\n'sentence' ('string'): The sentence the user was prompted to speak\n\n'up_votes' ('int64'): How many upvotes the audio file has received from reviewers\n\n'down_votes' ('int64'): How many downvotes the audio file has received from reviewers\n\n'age' ('string'): The age of the speaker (e.g. 'teens', 'twenties', 'fifties')\n\n'gender' ('string'): The gender of the speaker\n\n'accent' ('string'): Accent of the speaker\n\n'locale' ('string'): The locale of the speaker\n\n'segment' ('string'): Usually an empty field",
"### Data Splits\n\nThe speech material has been subdivided into portions for dev, train, test, validated, invalidated, reported and other.\n\nThe validated data is data that has been validated with reviewers and received upvotes that the data is of high quality.\n\nThe invalidated data is data has been invalidated by reviewers\nand received downvotes indicating that the data is of low quality.\n\nThe reported data is data that has been reported, for different reasons.\n\nThe other data is data that has not yet been reviewed.\n\nThe dev, test, train are all data that has been reviewed, deemed of high quality and split into dev, test and train.",
"## Data Preprocessing Recommended by Hugging Face\n\nThe following are data preprocessing steps advised by the Hugging Face team. They are accompanied by an example code snippet that shows how to put them to practice. \n\nMany examples in this dataset have trailing quotations marks, e.g _“the cat sat on the mat.“_. These trailing quotation marks do not change the actual meaning of the sentence, and it is near impossible to infer whether a sentence is a quotation or not a quotation from audio data alone. In these cases, it is advised to strip the quotation marks, leaving: _the cat sat on the mat_.\n\nIn addition, the majority of training sentences end in punctuation ( . or ? or ! ), whereas just a small proportion do not. In the dev set, almost all sentences end in punctuation. Thus, it is recommended to append a full-stop ( . ) to the end of the small number of training examples that do not end in punctuation.",
"## Dataset Creation",
"### Curation Rationale",
"### Source Data",
"#### Initial Data Collection and Normalization",
"#### Who are the source language producers?",
"### Annotations",
"#### Annotation process",
"#### Who are the annotators?",
"### Personal and Sensitive Information\n\nThe dataset consists of people who have donated their voice online. You agree to not attempt to determine the identity of speakers in the Common Voice dataset.",
"## Considerations for Using the Data",
"### Social Impact of Dataset\n\nThe dataset consists of people who have donated their voice online. You agree to not attempt to determine the identity of speakers in the Common Voice dataset.",
"### Discussion of Biases",
"### Other Known Limitations",
"## Additional Information",
"### Dataset Curators",
"### Licensing Information\n\nPublic Domain, CC-0"
] | [
"TAGS\n#task_categories-automatic-speech-recognition #annotations_creators-crowdsourced #language_creators-crowdsourced #multilinguality-multilingual #source_datasets-extended|common_voice #license-cc0-1.0 #arxiv-1912.06670 #region-us \n",
"# Dataset Card for Common Voice Corpus 3",
"## Table of Contents\n- Dataset Description\n - Dataset Summary\n - Supported Tasks and Leaderboards\n - Languages\n- Dataset Structure\n - Data Instances\n - Data Fields\n - Data Splits\n- Dataset Creation\n - Curation Rationale\n - Source Data\n - Annotations\n - Personal and Sensitive Information\n- Considerations for Using the Data\n - Social Impact of Dataset\n - Discussion of Biases\n - Other Known Limitations\n- Additional Information\n - Dataset Curators\n - Licensing Information\n - Citation Information\n - Contributions",
"## Dataset Description\n\n- Homepage: URL\n- Repository: URL\n- Paper: URL\n- Leaderboard: URL\n- Point of Contact: Anton Lozhkov",
"### Dataset Summary\n\nThe Common Voice dataset consists of a unique MP3 and corresponding text file. \nMany of the 2454 recorded hours in the dataset also include demographic metadata like age, sex, and accent \nthat can help improve the accuracy of speech recognition engines.\n\nThe dataset currently consists of 1979 validated hours in 29 languages, but more voices and languages are always added. \nTake a look at the Languages page to request a language or start contributing.",
"### Supported Tasks and Leaderboards\n\nThe results for models trained on the Common Voice datasets are available via the \n Speech Bench",
"### Languages",
"## Dataset Structure",
"### Data Instances\n\nA typical data point comprises the 'path' to the audio file and its 'sentence'. \nAdditional fields include 'accent', 'age', 'client_id', 'up_votes', 'down_votes', 'gender', 'locale' and 'segment'.",
"### Data Fields\n\n'client_id' ('string'): An id for which client (voice) made the recording\n\n'path' ('string'): The path to the audio file\n\n'audio' ('dict'): A dictionary containing the path to the downloaded audio file, the decoded audio array, and the sampling rate. Note that when accessing the audio column: 'dataset[0][\"audio\"]' the audio file is automatically decoded and resampled to 'dataset.features[\"audio\"].sampling_rate'. Decoding and resampling of a large number of audio files might take a significant amount of time. Thus it is important to first query the sample index before the '\"audio\"' column, *i.e.* 'dataset[0][\"audio\"]' should always be preferred over 'dataset[\"audio\"][0]'.\n\n'sentence' ('string'): The sentence the user was prompted to speak\n\n'up_votes' ('int64'): How many upvotes the audio file has received from reviewers\n\n'down_votes' ('int64'): How many downvotes the audio file has received from reviewers\n\n'age' ('string'): The age of the speaker (e.g. 'teens', 'twenties', 'fifties')\n\n'gender' ('string'): The gender of the speaker\n\n'accent' ('string'): Accent of the speaker\n\n'locale' ('string'): The locale of the speaker\n\n'segment' ('string'): Usually an empty field",
"### Data Splits\n\nThe speech material has been subdivided into portions for dev, train, test, validated, invalidated, reported and other.\n\nThe validated data is data that has been validated with reviewers and received upvotes that the data is of high quality.\n\nThe invalidated data is data has been invalidated by reviewers\nand received downvotes indicating that the data is of low quality.\n\nThe reported data is data that has been reported, for different reasons.\n\nThe other data is data that has not yet been reviewed.\n\nThe dev, test, train are all data that has been reviewed, deemed of high quality and split into dev, test and train.",
"## Data Preprocessing Recommended by Hugging Face\n\nThe following are data preprocessing steps advised by the Hugging Face team. They are accompanied by an example code snippet that shows how to put them to practice. \n\nMany examples in this dataset have trailing quotations marks, e.g _“the cat sat on the mat.“_. These trailing quotation marks do not change the actual meaning of the sentence, and it is near impossible to infer whether a sentence is a quotation or not a quotation from audio data alone. In these cases, it is advised to strip the quotation marks, leaving: _the cat sat on the mat_.\n\nIn addition, the majority of training sentences end in punctuation ( . or ? or ! ), whereas just a small proportion do not. In the dev set, almost all sentences end in punctuation. Thus, it is recommended to append a full-stop ( . ) to the end of the small number of training examples that do not end in punctuation.",
"## Dataset Creation",
"### Curation Rationale",
"### Source Data",
"#### Initial Data Collection and Normalization",
"#### Who are the source language producers?",
"### Annotations",
"#### Annotation process",
"#### Who are the annotators?",
"### Personal and Sensitive Information\n\nThe dataset consists of people who have donated their voice online. You agree to not attempt to determine the identity of speakers in the Common Voice dataset.",
"## Considerations for Using the Data",
"### Social Impact of Dataset\n\nThe dataset consists of people who have donated their voice online. You agree to not attempt to determine the identity of speakers in the Common Voice dataset.",
"### Discussion of Biases",
"### Other Known Limitations",
"## Additional Information",
"### Dataset Curators",
"### Licensing Information\n\nPublic Domain, CC-0"
] |
69aa649b97d1798e6aa67f05980379570f920d29 |
# Dataset Card for Common Voice Corpus 4
## Table of Contents
- [Dataset Description](#dataset-description)
- [Dataset Summary](#dataset-summary)
- [Supported Tasks and Leaderboards](#supported-tasks-and-leaderboards)
- [Languages](#languages)
- [Dataset Structure](#dataset-structure)
- [Data Instances](#data-instances)
- [Data Fields](#data-fields)
- [Data Splits](#data-splits)
- [Dataset Creation](#dataset-creation)
- [Curation Rationale](#curation-rationale)
- [Source Data](#source-data)
- [Annotations](#annotations)
- [Personal and Sensitive Information](#personal-and-sensitive-information)
- [Considerations for Using the Data](#considerations-for-using-the-data)
- [Social Impact of Dataset](#social-impact-of-dataset)
- [Discussion of Biases](#discussion-of-biases)
- [Other Known Limitations](#other-known-limitations)
- [Additional Information](#additional-information)
- [Dataset Curators](#dataset-curators)
- [Licensing Information](#licensing-information)
- [Citation Information](#citation-information)
- [Contributions](#contributions)
## Dataset Description
- **Homepage:** https://commonvoice.mozilla.org/en/datasets
- **Repository:** https://github.com/common-voice/common-voice
- **Paper:** https://arxiv.org/abs/1912.06670
- **Leaderboard:** https://paperswithcode.com/dataset/common-voice
- **Point of Contact:** [Anton Lozhkov](mailto:[email protected])
### Dataset Summary
The Common Voice dataset consists of a unique MP3 and corresponding text file.
Many of the 4257 recorded hours in the dataset also include demographic metadata like age, sex, and accent
that can help improve the accuracy of speech recognition engines.
The dataset currently consists of 3401 validated hours in 40 languages, but more voices and languages are always added.
Take a look at the [Languages](https://commonvoice.mozilla.org/en/languages) page to request a language or start contributing.
### Supported Tasks and Leaderboards
The results for models trained on the Common Voice datasets are available via the
[🤗 Speech Bench](https://huggingface.co/spaces/huggingface/hf-speech-bench)
### Languages
```
Abkhaz, Arabic, Basque, Breton, Catalan, Chinese (China), Chinese (Hong Kong), Chinese (Taiwan), Chuvash, Dhivehi, Dutch, English, Esperanto, Estonian, French, German, Hakha Chin, Indonesian, Interlingua, Irish, Italian, Japanese, Kabyle, Kinyarwanda, Kyrgyz, Latvian, Mongolian, Persian, Portuguese, Romansh Sursilvan, Russian, Sakha, Slovenian, Spanish, Swedish, Tamil, Tatar, Turkish, Votic, Welsh
```
## Dataset Structure
### Data Instances
A typical data point comprises the `path` to the audio file and its `sentence`.
Additional fields include `accent`, `age`, `client_id`, `up_votes`, `down_votes`, `gender`, `locale` and `segment`.
```python
{
'client_id': 'd59478fbc1ee646a28a3c652a119379939123784d99131b865a89f8b21c81f69276c48bd574b81267d9d1a77b83b43e6d475a6cfc79c232ddbca946ae9c7afc5',
'path': 'et/clips/common_voice_et_18318995.mp3',
'audio': {
'path': 'et/clips/common_voice_et_18318995.mp3',
'array': array([-0.00048828, -0.00018311, -0.00137329, ..., 0.00079346, 0.00091553, 0.00085449], dtype=float32),
'sampling_rate': 48000
},
'sentence': 'Tasub kokku saada inimestega, keda tunned juba ammust ajast saati.',
'up_votes': 2,
'down_votes': 0,
'age': 'twenties',
'gender': 'male',
'accent': '',
'locale': 'et',
'segment': ''
}
```
### Data Fields
`client_id` (`string`): An id for which client (voice) made the recording
`path` (`string`): The path to the audio file
`audio` (`dict`): A dictionary containing the path to the downloaded audio file, the decoded audio array, and the sampling rate. Note that when accessing the audio column: `dataset[0]["audio"]` the audio file is automatically decoded and resampled to `dataset.features["audio"].sampling_rate`. Decoding and resampling of a large number of audio files might take a significant amount of time. Thus it is important to first query the sample index before the `"audio"` column, *i.e.* `dataset[0]["audio"]` should **always** be preferred over `dataset["audio"][0]`.
`sentence` (`string`): The sentence the user was prompted to speak
`up_votes` (`int64`): How many upvotes the audio file has received from reviewers
`down_votes` (`int64`): How many downvotes the audio file has received from reviewers
`age` (`string`): The age of the speaker (e.g. `teens`, `twenties`, `fifties`)
`gender` (`string`): The gender of the speaker
`accent` (`string`): Accent of the speaker
`locale` (`string`): The locale of the speaker
`segment` (`string`): Usually an empty field
### Data Splits
The speech material has been subdivided into portions for dev, train, test, validated, invalidated, reported and other.
The validated data is data that has been validated with reviewers and received upvotes that the data is of high quality.
The invalidated data is data has been invalidated by reviewers
and received downvotes indicating that the data is of low quality.
The reported data is data that has been reported, for different reasons.
The other data is data that has not yet been reviewed.
The dev, test, train are all data that has been reviewed, deemed of high quality and split into dev, test and train.
## Data Preprocessing Recommended by Hugging Face
The following are data preprocessing steps advised by the Hugging Face team. They are accompanied by an example code snippet that shows how to put them to practice.
Many examples in this dataset have trailing quotations marks, e.g _“the cat sat on the mat.“_. These trailing quotation marks do not change the actual meaning of the sentence, and it is near impossible to infer whether a sentence is a quotation or not a quotation from audio data alone. In these cases, it is advised to strip the quotation marks, leaving: _the cat sat on the mat_.
In addition, the majority of training sentences end in punctuation ( . or ? or ! ), whereas just a small proportion do not. In the dev set, **almost all** sentences end in punctuation. Thus, it is recommended to append a full-stop ( . ) to the end of the small number of training examples that do not end in punctuation.
```python
from datasets import load_dataset
ds = load_dataset("mozilla-foundation/common_voice_4_0", "en", use_auth_token=True)
def prepare_dataset(batch):
"""Function to preprocess the dataset with the .map method"""
transcription = batch["sentence"]
if transcription.startswith('"') and transcription.endswith('"'):
# we can remove trailing quotation marks as they do not affect the transcription
transcription = transcription[1:-1]
if transcription[-1] not in [".", "?", "!"]:
# append a full-stop to sentences that do not end in punctuation
transcription = transcription + "."
batch["sentence"] = transcription
return batch
ds = ds.map(prepare_dataset, desc="preprocess dataset")
```
## Dataset Creation
### Curation Rationale
[Needs More Information]
### Source Data
#### Initial Data Collection and Normalization
[Needs More Information]
#### Who are the source language producers?
[Needs More Information]
### Annotations
#### Annotation process
[Needs More Information]
#### Who are the annotators?
[Needs More Information]
### Personal and Sensitive Information
The dataset consists of people who have donated their voice online. You agree to not attempt to determine the identity of speakers in the Common Voice dataset.
## Considerations for Using the Data
### Social Impact of Dataset
The dataset consists of people who have donated their voice online. You agree to not attempt to determine the identity of speakers in the Common Voice dataset.
### Discussion of Biases
[More Information Needed]
### Other Known Limitations
[More Information Needed]
## Additional Information
### Dataset Curators
[More Information Needed]
### Licensing Information
Public Domain, [CC-0](https://creativecommons.org/share-your-work/public-domain/cc0/)
### Citation Information
```
@inproceedings{commonvoice:2020,
author = {Ardila, R. and Branson, M. and Davis, K. and Henretty, M. and Kohler, M. and Meyer, J. and Morais, R. and Saunders, L. and Tyers, F. M. and Weber, G.},
title = {Common Voice: A Massively-Multilingual Speech Corpus},
booktitle = {Proceedings of the 12th Conference on Language Resources and Evaluation (LREC 2020)},
pages = {4211--4215},
year = 2020
}
```
| mozilla-foundation/common_voice_4_0 | [
"task_categories:automatic-speech-recognition",
"annotations_creators:crowdsourced",
"language_creators:crowdsourced",
"multilinguality:multilingual",
"source_datasets:extended|common_voice",
"license:cc0-1.0",
"arxiv:1912.06670",
"region:us"
] | 2022-03-02T23:29:22+00:00 | {"annotations_creators": ["crowdsourced"], "language_creators": ["crowdsourced"], "license": ["cc0-1.0"], "multilinguality": ["multilingual"], "size_categories": {"ab": ["n<1K"], "ar": ["10K<n<100K"], "br": ["10K<n<100K"], "ca": ["100K<n<1M"], "cnh": ["1K<n<10K"], "cv": ["1K<n<10K"], "cy": ["10K<n<100K"], "de": ["100K<n<1M"], "dv": ["1K<n<10K"], "en": ["1M<n<10M"], "eo": ["10K<n<100K"], "es": ["100K<n<1M"], "et": ["1K<n<10K"], "eu": ["10K<n<100K"], "fa": ["100K<n<1M"], "fr": ["100K<n<1M"], "ga-IE": ["1K<n<10K"], "ia": ["1K<n<10K"], "id": ["1K<n<10K"], "it": ["10K<n<100K"], "ja": ["1K<n<10K"], "kab": ["100K<n<1M"], "ky": ["10K<n<100K"], "lv": ["1K<n<10K"], "mn": ["1K<n<10K"], "nl": ["10K<n<100K"], "pt": ["10K<n<100K"], "rm-sursilv": ["n<1K"], "ru": ["10K<n<100K"], "rw": ["10K<n<100K"], "sah": ["1K<n<10K"], "sl": ["1K<n<10K"], "sv-SE": ["1K<n<10K"], "ta": ["1K<n<10K"], "tr": ["10K<n<100K"], "tt": ["10K<n<100K"], "vot": ["n<1K"], "zh-CN": ["10K<n<100K"], "zh-HK": ["n<1K"], "zh-TW": ["10K<n<100K"]}, "source_datasets": ["extended|common_voice"], "task_categories": ["automatic-speech-recognition"], "paperswithcode_id": "common-voice", "pretty_name": "Common Voice Corpus 4", "language_bcp47": ["ab", "ar", "br", "ca", "cnh", "cv", "cy", "de", "dv", "en", "eo", "es", "et", "eu", "fa", "fr", "ga-IE", "ia", "id", "it", "ja", "kab", "ky", "lv", "mn", "nl", "pt", "rm-sursilv", "ru", "rw", "sah", "sl", "sv-SE", "ta", "tr", "tt", "vot", "zh-CN", "zh-HK", "zh-TW"], "extra_gated_prompt": "By clicking on \u201cAccess repository\u201d below, you also agree to not attempt to determine the identity of speakers in the Common Voice dataset."} | 2023-07-29T15:00:01+00:00 | [
"1912.06670"
] | [] | TAGS
#task_categories-automatic-speech-recognition #annotations_creators-crowdsourced #language_creators-crowdsourced #multilinguality-multilingual #source_datasets-extended|common_voice #license-cc0-1.0 #arxiv-1912.06670 #region-us
|
# Dataset Card for Common Voice Corpus 4
## Table of Contents
- Dataset Description
- Dataset Summary
- Supported Tasks and Leaderboards
- Languages
- Dataset Structure
- Data Instances
- Data Fields
- Data Splits
- Dataset Creation
- Curation Rationale
- Source Data
- Annotations
- Personal and Sensitive Information
- Considerations for Using the Data
- Social Impact of Dataset
- Discussion of Biases
- Other Known Limitations
- Additional Information
- Dataset Curators
- Licensing Information
- Citation Information
- Contributions
## Dataset Description
- Homepage: URL
- Repository: URL
- Paper: URL
- Leaderboard: URL
- Point of Contact: Anton Lozhkov
### Dataset Summary
The Common Voice dataset consists of a unique MP3 and corresponding text file.
Many of the 4257 recorded hours in the dataset also include demographic metadata like age, sex, and accent
that can help improve the accuracy of speech recognition engines.
The dataset currently consists of 3401 validated hours in 40 languages, but more voices and languages are always added.
Take a look at the Languages page to request a language or start contributing.
### Supported Tasks and Leaderboards
The results for models trained on the Common Voice datasets are available via the
Speech Bench
### Languages
## Dataset Structure
### Data Instances
A typical data point comprises the 'path' to the audio file and its 'sentence'.
Additional fields include 'accent', 'age', 'client_id', 'up_votes', 'down_votes', 'gender', 'locale' and 'segment'.
### Data Fields
'client_id' ('string'): An id for which client (voice) made the recording
'path' ('string'): The path to the audio file
'audio' ('dict'): A dictionary containing the path to the downloaded audio file, the decoded audio array, and the sampling rate. Note that when accessing the audio column: 'dataset[0]["audio"]' the audio file is automatically decoded and resampled to 'dataset.features["audio"].sampling_rate'. Decoding and resampling of a large number of audio files might take a significant amount of time. Thus it is important to first query the sample index before the '"audio"' column, *i.e.* 'dataset[0]["audio"]' should always be preferred over 'dataset["audio"][0]'.
'sentence' ('string'): The sentence the user was prompted to speak
'up_votes' ('int64'): How many upvotes the audio file has received from reviewers
'down_votes' ('int64'): How many downvotes the audio file has received from reviewers
'age' ('string'): The age of the speaker (e.g. 'teens', 'twenties', 'fifties')
'gender' ('string'): The gender of the speaker
'accent' ('string'): Accent of the speaker
'locale' ('string'): The locale of the speaker
'segment' ('string'): Usually an empty field
### Data Splits
The speech material has been subdivided into portions for dev, train, test, validated, invalidated, reported and other.
The validated data is data that has been validated with reviewers and received upvotes that the data is of high quality.
The invalidated data is data has been invalidated by reviewers
and received downvotes indicating that the data is of low quality.
The reported data is data that has been reported, for different reasons.
The other data is data that has not yet been reviewed.
The dev, test, train are all data that has been reviewed, deemed of high quality and split into dev, test and train.
## Data Preprocessing Recommended by Hugging Face
The following are data preprocessing steps advised by the Hugging Face team. They are accompanied by an example code snippet that shows how to put them to practice.
Many examples in this dataset have trailing quotations marks, e.g _“the cat sat on the mat.“_. These trailing quotation marks do not change the actual meaning of the sentence, and it is near impossible to infer whether a sentence is a quotation or not a quotation from audio data alone. In these cases, it is advised to strip the quotation marks, leaving: _the cat sat on the mat_.
In addition, the majority of training sentences end in punctuation ( . or ? or ! ), whereas just a small proportion do not. In the dev set, almost all sentences end in punctuation. Thus, it is recommended to append a full-stop ( . ) to the end of the small number of training examples that do not end in punctuation.
## Dataset Creation
### Curation Rationale
### Source Data
#### Initial Data Collection and Normalization
#### Who are the source language producers?
### Annotations
#### Annotation process
#### Who are the annotators?
### Personal and Sensitive Information
The dataset consists of people who have donated their voice online. You agree to not attempt to determine the identity of speakers in the Common Voice dataset.
## Considerations for Using the Data
### Social Impact of Dataset
The dataset consists of people who have donated their voice online. You agree to not attempt to determine the identity of speakers in the Common Voice dataset.
### Discussion of Biases
### Other Known Limitations
## Additional Information
### Dataset Curators
### Licensing Information
Public Domain, CC-0
| [
"# Dataset Card for Common Voice Corpus 4",
"## Table of Contents\n- Dataset Description\n - Dataset Summary\n - Supported Tasks and Leaderboards\n - Languages\n- Dataset Structure\n - Data Instances\n - Data Fields\n - Data Splits\n- Dataset Creation\n - Curation Rationale\n - Source Data\n - Annotations\n - Personal and Sensitive Information\n- Considerations for Using the Data\n - Social Impact of Dataset\n - Discussion of Biases\n - Other Known Limitations\n- Additional Information\n - Dataset Curators\n - Licensing Information\n - Citation Information\n - Contributions",
"## Dataset Description\n\n- Homepage: URL\n- Repository: URL\n- Paper: URL\n- Leaderboard: URL\n- Point of Contact: Anton Lozhkov",
"### Dataset Summary\n\nThe Common Voice dataset consists of a unique MP3 and corresponding text file. \nMany of the 4257 recorded hours in the dataset also include demographic metadata like age, sex, and accent \nthat can help improve the accuracy of speech recognition engines.\n\nThe dataset currently consists of 3401 validated hours in 40 languages, but more voices and languages are always added. \nTake a look at the Languages page to request a language or start contributing.",
"### Supported Tasks and Leaderboards\n\nThe results for models trained on the Common Voice datasets are available via the \n Speech Bench",
"### Languages",
"## Dataset Structure",
"### Data Instances\n\nA typical data point comprises the 'path' to the audio file and its 'sentence'. \nAdditional fields include 'accent', 'age', 'client_id', 'up_votes', 'down_votes', 'gender', 'locale' and 'segment'.",
"### Data Fields\n\n'client_id' ('string'): An id for which client (voice) made the recording\n\n'path' ('string'): The path to the audio file\n\n'audio' ('dict'): A dictionary containing the path to the downloaded audio file, the decoded audio array, and the sampling rate. Note that when accessing the audio column: 'dataset[0][\"audio\"]' the audio file is automatically decoded and resampled to 'dataset.features[\"audio\"].sampling_rate'. Decoding and resampling of a large number of audio files might take a significant amount of time. Thus it is important to first query the sample index before the '\"audio\"' column, *i.e.* 'dataset[0][\"audio\"]' should always be preferred over 'dataset[\"audio\"][0]'.\n\n'sentence' ('string'): The sentence the user was prompted to speak\n\n'up_votes' ('int64'): How many upvotes the audio file has received from reviewers\n\n'down_votes' ('int64'): How many downvotes the audio file has received from reviewers\n\n'age' ('string'): The age of the speaker (e.g. 'teens', 'twenties', 'fifties')\n\n'gender' ('string'): The gender of the speaker\n\n'accent' ('string'): Accent of the speaker\n\n'locale' ('string'): The locale of the speaker\n\n'segment' ('string'): Usually an empty field",
"### Data Splits\n\nThe speech material has been subdivided into portions for dev, train, test, validated, invalidated, reported and other.\n\nThe validated data is data that has been validated with reviewers and received upvotes that the data is of high quality.\n\nThe invalidated data is data has been invalidated by reviewers\nand received downvotes indicating that the data is of low quality.\n\nThe reported data is data that has been reported, for different reasons.\n\nThe other data is data that has not yet been reviewed.\n\nThe dev, test, train are all data that has been reviewed, deemed of high quality and split into dev, test and train.",
"## Data Preprocessing Recommended by Hugging Face\n\nThe following are data preprocessing steps advised by the Hugging Face team. They are accompanied by an example code snippet that shows how to put them to practice. \n\nMany examples in this dataset have trailing quotations marks, e.g _“the cat sat on the mat.“_. These trailing quotation marks do not change the actual meaning of the sentence, and it is near impossible to infer whether a sentence is a quotation or not a quotation from audio data alone. In these cases, it is advised to strip the quotation marks, leaving: _the cat sat on the mat_.\n\nIn addition, the majority of training sentences end in punctuation ( . or ? or ! ), whereas just a small proportion do not. In the dev set, almost all sentences end in punctuation. Thus, it is recommended to append a full-stop ( . ) to the end of the small number of training examples that do not end in punctuation.",
"## Dataset Creation",
"### Curation Rationale",
"### Source Data",
"#### Initial Data Collection and Normalization",
"#### Who are the source language producers?",
"### Annotations",
"#### Annotation process",
"#### Who are the annotators?",
"### Personal and Sensitive Information\n\nThe dataset consists of people who have donated their voice online. You agree to not attempt to determine the identity of speakers in the Common Voice dataset.",
"## Considerations for Using the Data",
"### Social Impact of Dataset\n\nThe dataset consists of people who have donated their voice online. You agree to not attempt to determine the identity of speakers in the Common Voice dataset.",
"### Discussion of Biases",
"### Other Known Limitations",
"## Additional Information",
"### Dataset Curators",
"### Licensing Information\n\nPublic Domain, CC-0"
] | [
"TAGS\n#task_categories-automatic-speech-recognition #annotations_creators-crowdsourced #language_creators-crowdsourced #multilinguality-multilingual #source_datasets-extended|common_voice #license-cc0-1.0 #arxiv-1912.06670 #region-us \n",
"# Dataset Card for Common Voice Corpus 4",
"## Table of Contents\n- Dataset Description\n - Dataset Summary\n - Supported Tasks and Leaderboards\n - Languages\n- Dataset Structure\n - Data Instances\n - Data Fields\n - Data Splits\n- Dataset Creation\n - Curation Rationale\n - Source Data\n - Annotations\n - Personal and Sensitive Information\n- Considerations for Using the Data\n - Social Impact of Dataset\n - Discussion of Biases\n - Other Known Limitations\n- Additional Information\n - Dataset Curators\n - Licensing Information\n - Citation Information\n - Contributions",
"## Dataset Description\n\n- Homepage: URL\n- Repository: URL\n- Paper: URL\n- Leaderboard: URL\n- Point of Contact: Anton Lozhkov",
"### Dataset Summary\n\nThe Common Voice dataset consists of a unique MP3 and corresponding text file. \nMany of the 4257 recorded hours in the dataset also include demographic metadata like age, sex, and accent \nthat can help improve the accuracy of speech recognition engines.\n\nThe dataset currently consists of 3401 validated hours in 40 languages, but more voices and languages are always added. \nTake a look at the Languages page to request a language or start contributing.",
"### Supported Tasks and Leaderboards\n\nThe results for models trained on the Common Voice datasets are available via the \n Speech Bench",
"### Languages",
"## Dataset Structure",
"### Data Instances\n\nA typical data point comprises the 'path' to the audio file and its 'sentence'. \nAdditional fields include 'accent', 'age', 'client_id', 'up_votes', 'down_votes', 'gender', 'locale' and 'segment'.",
"### Data Fields\n\n'client_id' ('string'): An id for which client (voice) made the recording\n\n'path' ('string'): The path to the audio file\n\n'audio' ('dict'): A dictionary containing the path to the downloaded audio file, the decoded audio array, and the sampling rate. Note that when accessing the audio column: 'dataset[0][\"audio\"]' the audio file is automatically decoded and resampled to 'dataset.features[\"audio\"].sampling_rate'. Decoding and resampling of a large number of audio files might take a significant amount of time. Thus it is important to first query the sample index before the '\"audio\"' column, *i.e.* 'dataset[0][\"audio\"]' should always be preferred over 'dataset[\"audio\"][0]'.\n\n'sentence' ('string'): The sentence the user was prompted to speak\n\n'up_votes' ('int64'): How many upvotes the audio file has received from reviewers\n\n'down_votes' ('int64'): How many downvotes the audio file has received from reviewers\n\n'age' ('string'): The age of the speaker (e.g. 'teens', 'twenties', 'fifties')\n\n'gender' ('string'): The gender of the speaker\n\n'accent' ('string'): Accent of the speaker\n\n'locale' ('string'): The locale of the speaker\n\n'segment' ('string'): Usually an empty field",
"### Data Splits\n\nThe speech material has been subdivided into portions for dev, train, test, validated, invalidated, reported and other.\n\nThe validated data is data that has been validated with reviewers and received upvotes that the data is of high quality.\n\nThe invalidated data is data has been invalidated by reviewers\nand received downvotes indicating that the data is of low quality.\n\nThe reported data is data that has been reported, for different reasons.\n\nThe other data is data that has not yet been reviewed.\n\nThe dev, test, train are all data that has been reviewed, deemed of high quality and split into dev, test and train.",
"## Data Preprocessing Recommended by Hugging Face\n\nThe following are data preprocessing steps advised by the Hugging Face team. They are accompanied by an example code snippet that shows how to put them to practice. \n\nMany examples in this dataset have trailing quotations marks, e.g _“the cat sat on the mat.“_. These trailing quotation marks do not change the actual meaning of the sentence, and it is near impossible to infer whether a sentence is a quotation or not a quotation from audio data alone. In these cases, it is advised to strip the quotation marks, leaving: _the cat sat on the mat_.\n\nIn addition, the majority of training sentences end in punctuation ( . or ? or ! ), whereas just a small proportion do not. In the dev set, almost all sentences end in punctuation. Thus, it is recommended to append a full-stop ( . ) to the end of the small number of training examples that do not end in punctuation.",
"## Dataset Creation",
"### Curation Rationale",
"### Source Data",
"#### Initial Data Collection and Normalization",
"#### Who are the source language producers?",
"### Annotations",
"#### Annotation process",
"#### Who are the annotators?",
"### Personal and Sensitive Information\n\nThe dataset consists of people who have donated their voice online. You agree to not attempt to determine the identity of speakers in the Common Voice dataset.",
"## Considerations for Using the Data",
"### Social Impact of Dataset\n\nThe dataset consists of people who have donated their voice online. You agree to not attempt to determine the identity of speakers in the Common Voice dataset.",
"### Discussion of Biases",
"### Other Known Limitations",
"## Additional Information",
"### Dataset Curators",
"### Licensing Information\n\nPublic Domain, CC-0"
] |
4865862f7798fce8ade9ecf7624307eb642fcaba |
# Dataset Card for Common Voice Corpus 5
## Table of Contents
- [Dataset Description](#dataset-description)
- [Dataset Summary](#dataset-summary)
- [Supported Tasks and Leaderboards](#supported-tasks-and-leaderboards)
- [Languages](#languages)
- [Dataset Structure](#dataset-structure)
- [Data Instances](#data-instances)
- [Data Fields](#data-fields)
- [Data Splits](#data-splits)
- [Dataset Creation](#dataset-creation)
- [Curation Rationale](#curation-rationale)
- [Source Data](#source-data)
- [Annotations](#annotations)
- [Personal and Sensitive Information](#personal-and-sensitive-information)
- [Considerations for Using the Data](#considerations-for-using-the-data)
- [Social Impact of Dataset](#social-impact-of-dataset)
- [Discussion of Biases](#discussion-of-biases)
- [Other Known Limitations](#other-known-limitations)
- [Additional Information](#additional-information)
- [Dataset Curators](#dataset-curators)
- [Licensing Information](#licensing-information)
- [Citation Information](#citation-information)
- [Contributions](#contributions)
## Dataset Description
- **Homepage:** https://commonvoice.mozilla.org/en/datasets
- **Repository:** https://github.com/common-voice/common-voice
- **Paper:** https://arxiv.org/abs/1912.06670
- **Leaderboard:** https://paperswithcode.com/dataset/common-voice
- **Point of Contact:** [Anton Lozhkov](mailto:[email protected])
### Dataset Summary
The Common Voice dataset consists of a unique MP3 and corresponding text file.
Many of the 7226 recorded hours in the dataset also include demographic metadata like age, sex, and accent
that can help improve the accuracy of speech recognition engines.
The dataset currently consists of 5591 validated hours in 54 languages, but more voices and languages are always added.
Take a look at the [Languages](https://commonvoice.mozilla.org/en/languages) page to request a language or start contributing.
### Supported Tasks and Leaderboards
The results for models trained on the Common Voice datasets are available via the
[🤗 Speech Bench](https://huggingface.co/spaces/huggingface/hf-speech-bench)
### Languages
```
Abkhaz, Arabic, Assamese, Basque, Breton, Catalan, Chinese (China), Chinese (Hong Kong), Chinese (Taiwan), Chuvash, Czech, Dhivehi, Dutch, English, Esperanto, Estonian, French, Frisian, Georgian, German, Greek, Hakha Chin, Indonesian, Interlingua, Irish, Italian, Japanese, Kabyle, Kinyarwanda, Kyrgyz, Latvian, Maltese, Mongolian, Odia, Persian, Polish, Portuguese, Punjabi, Romanian, Romansh Sursilvan, Romansh Vallader, Russian, Sakha, Slovenian, Sorbian, Upper, Spanish, Swedish, Tamil, Tatar, Turkish, Ukrainian, Vietnamese, Votic, Welsh
```
## Dataset Structure
### Data Instances
A typical data point comprises the `path` to the audio file and its `sentence`.
Additional fields include `accent`, `age`, `client_id`, `up_votes`, `down_votes`, `gender`, `locale` and `segment`.
```python
{
'client_id': 'd59478fbc1ee646a28a3c652a119379939123784d99131b865a89f8b21c81f69276c48bd574b81267d9d1a77b83b43e6d475a6cfc79c232ddbca946ae9c7afc5',
'path': 'et/clips/common_voice_et_18318995.mp3',
'audio': {
'path': 'et/clips/common_voice_et_18318995.mp3',
'array': array([-0.00048828, -0.00018311, -0.00137329, ..., 0.00079346, 0.00091553, 0.00085449], dtype=float32),
'sampling_rate': 48000
},
'sentence': 'Tasub kokku saada inimestega, keda tunned juba ammust ajast saati.',
'up_votes': 2,
'down_votes': 0,
'age': 'twenties',
'gender': 'male',
'accent': '',
'locale': 'et',
'segment': ''
}
```
### Data Fields
`client_id` (`string`): An id for which client (voice) made the recording
`path` (`string`): The path to the audio file
`audio` (`dict`): A dictionary containing the path to the downloaded audio file, the decoded audio array, and the sampling rate. Note that when accessing the audio column: `dataset[0]["audio"]` the audio file is automatically decoded and resampled to `dataset.features["audio"].sampling_rate`. Decoding and resampling of a large number of audio files might take a significant amount of time. Thus it is important to first query the sample index before the `"audio"` column, *i.e.* `dataset[0]["audio"]` should **always** be preferred over `dataset["audio"][0]`.
`sentence` (`string`): The sentence the user was prompted to speak
`up_votes` (`int64`): How many upvotes the audio file has received from reviewers
`down_votes` (`int64`): How many downvotes the audio file has received from reviewers
`age` (`string`): The age of the speaker (e.g. `teens`, `twenties`, `fifties`)
`gender` (`string`): The gender of the speaker
`accent` (`string`): Accent of the speaker
`locale` (`string`): The locale of the speaker
`segment` (`string`): Usually an empty field
### Data Splits
The speech material has been subdivided into portions for dev, train, test, validated, invalidated, reported and other.
The validated data is data that has been validated with reviewers and received upvotes that the data is of high quality.
The invalidated data is data has been invalidated by reviewers
and received downvotes indicating that the data is of low quality.
The reported data is data that has been reported, for different reasons.
The other data is data that has not yet been reviewed.
The dev, test, train are all data that has been reviewed, deemed of high quality and split into dev, test and train.
## Data Preprocessing Recommended by Hugging Face
The following are data preprocessing steps advised by the Hugging Face team. They are accompanied by an example code snippet that shows how to put them to practice.
Many examples in this dataset have trailing quotations marks, e.g _“the cat sat on the mat.“_. These trailing quotation marks do not change the actual meaning of the sentence, and it is near impossible to infer whether a sentence is a quotation or not a quotation from audio data alone. In these cases, it is advised to strip the quotation marks, leaving: _the cat sat on the mat_.
In addition, the majority of training sentences end in punctuation ( . or ? or ! ), whereas just a small proportion do not. In the dev set, **almost all** sentences end in punctuation. Thus, it is recommended to append a full-stop ( . ) to the end of the small number of training examples that do not end in punctuation.
```python
from datasets import load_dataset
ds = load_dataset("mozilla-foundation/common_voice_5_0", "en", use_auth_token=True)
def prepare_dataset(batch):
"""Function to preprocess the dataset with the .map method"""
transcription = batch["sentence"]
if transcription.startswith('"') and transcription.endswith('"'):
# we can remove trailing quotation marks as they do not affect the transcription
transcription = transcription[1:-1]
if transcription[-1] not in [".", "?", "!"]:
# append a full-stop to sentences that do not end in punctuation
transcription = transcription + "."
batch["sentence"] = transcription
return batch
ds = ds.map(prepare_dataset, desc="preprocess dataset")
```
## Dataset Creation
### Curation Rationale
[Needs More Information]
### Source Data
#### Initial Data Collection and Normalization
[Needs More Information]
#### Who are the source language producers?
[Needs More Information]
### Annotations
#### Annotation process
[Needs More Information]
#### Who are the annotators?
[Needs More Information]
### Personal and Sensitive Information
The dataset consists of people who have donated their voice online. You agree to not attempt to determine the identity of speakers in the Common Voice dataset.
## Considerations for Using the Data
### Social Impact of Dataset
The dataset consists of people who have donated their voice online. You agree to not attempt to determine the identity of speakers in the Common Voice dataset.
### Discussion of Biases
[More Information Needed]
### Other Known Limitations
[More Information Needed]
## Additional Information
### Dataset Curators
[More Information Needed]
### Licensing Information
Public Domain, [CC-0](https://creativecommons.org/share-your-work/public-domain/cc0/)
### Citation Information
```
@inproceedings{commonvoice:2020,
author = {Ardila, R. and Branson, M. and Davis, K. and Henretty, M. and Kohler, M. and Meyer, J. and Morais, R. and Saunders, L. and Tyers, F. M. and Weber, G.},
title = {Common Voice: A Massively-Multilingual Speech Corpus},
booktitle = {Proceedings of the 12th Conference on Language Resources and Evaluation (LREC 2020)},
pages = {4211--4215},
year = 2020
}
```
| mozilla-foundation/common_voice_5_0 | [
"task_categories:automatic-speech-recognition",
"annotations_creators:crowdsourced",
"language_creators:crowdsourced",
"multilinguality:multilingual",
"source_datasets:extended|common_voice",
"license:cc0-1.0",
"arxiv:1912.06670",
"region:us"
] | 2022-03-02T23:29:22+00:00 | {"annotations_creators": ["crowdsourced"], "language_creators": ["crowdsourced"], "license": ["cc0-1.0"], "multilinguality": ["multilingual"], "size_categories": {"ab": ["n<1K"], "ar": ["10K<n<100K"], "as": ["n<1K"], "br": ["10K<n<100K"], "ca": ["100K<n<1M"], "cnh": ["1K<n<10K"], "cs": ["10K<n<100K"], "cv": ["1K<n<10K"], "cy": ["10K<n<100K"], "de": ["100K<n<1M"], "dv": ["1K<n<10K"], "el": ["10K<n<100K"], "en": ["1M<n<10M"], "eo": ["10K<n<100K"], "es": ["100K<n<1M"], "et": ["10K<n<100K"], "eu": ["10K<n<100K"], "fa": ["100K<n<1M"], "fr": ["100K<n<1M"], "fy-NL": ["10K<n<100K"], "ga-IE": ["1K<n<10K"], "hsb": ["1K<n<10K"], "ia": ["1K<n<10K"], "id": ["10K<n<100K"], "it": ["100K<n<1M"], "ja": ["1K<n<10K"], "ka": ["1K<n<10K"], "kab": ["100K<n<1M"], "ky": ["10K<n<100K"], "lv": ["1K<n<10K"], "mn": ["10K<n<100K"], "mt": ["10K<n<100K"], "nl": ["10K<n<100K"], "or": ["1K<n<10K"], "pa-IN": ["n<1K"], "pl": ["100K<n<1M"], "pt": ["10K<n<100K"], "rm-sursilv": ["1K<n<10K"], "rm-vallader": ["1K<n<10K"], "ro": ["1K<n<10K"], "ru": ["10K<n<100K"], "rw": ["100K<n<1M"], "sah": ["1K<n<10K"], "sl": ["1K<n<10K"], "sv-SE": ["10K<n<100K"], "ta": ["10K<n<100K"], "tr": ["10K<n<100K"], "tt": ["10K<n<100K"], "uk": ["10K<n<100K"], "vi": ["n<1K"], "vot": ["n<1K"], "zh-CN": ["10K<n<100K"], "zh-HK": ["10K<n<100K"], "zh-TW": ["10K<n<100K"]}, "source_datasets": ["extended|common_voice"], "task_categories": ["automatic-speech-recognition"], "paperswithcode_id": "common-voice", "pretty_name": "Common Voice Corpus 5", "language_bcp47": ["ab", "ar", "as", "br", "ca", "cnh", "cs", "cv", "cy", "de", "dv", "el", "en", "eo", "es", "et", "eu", "fa", "fr", "fy-NL", "ga-IE", "hsb", "ia", "id", "it", "ja", "ka", "kab", "ky", "lv", "mn", "mt", "nl", "or", "pa-IN", "pl", "pt", "rm-sursilv", "rm-vallader", "ro", "ru", "rw", "sah", "sl", "sv-SE", "ta", "tr", "tt", "uk", "vi", "vot", "zh-CN", "zh-HK", "zh-TW"], "extra_gated_prompt": "By clicking on \u201cAccess repository\u201d below, you also agree to not attempt to determine the identity of speakers in the Common Voice dataset."} | 2023-07-29T15:00:03+00:00 | [
"1912.06670"
] | [] | TAGS
#task_categories-automatic-speech-recognition #annotations_creators-crowdsourced #language_creators-crowdsourced #multilinguality-multilingual #source_datasets-extended|common_voice #license-cc0-1.0 #arxiv-1912.06670 #region-us
|
# Dataset Card for Common Voice Corpus 5
## Table of Contents
- Dataset Description
- Dataset Summary
- Supported Tasks and Leaderboards
- Languages
- Dataset Structure
- Data Instances
- Data Fields
- Data Splits
- Dataset Creation
- Curation Rationale
- Source Data
- Annotations
- Personal and Sensitive Information
- Considerations for Using the Data
- Social Impact of Dataset
- Discussion of Biases
- Other Known Limitations
- Additional Information
- Dataset Curators
- Licensing Information
- Citation Information
- Contributions
## Dataset Description
- Homepage: URL
- Repository: URL
- Paper: URL
- Leaderboard: URL
- Point of Contact: Anton Lozhkov
### Dataset Summary
The Common Voice dataset consists of a unique MP3 and corresponding text file.
Many of the 7226 recorded hours in the dataset also include demographic metadata like age, sex, and accent
that can help improve the accuracy of speech recognition engines.
The dataset currently consists of 5591 validated hours in 54 languages, but more voices and languages are always added.
Take a look at the Languages page to request a language or start contributing.
### Supported Tasks and Leaderboards
The results for models trained on the Common Voice datasets are available via the
Speech Bench
### Languages
## Dataset Structure
### Data Instances
A typical data point comprises the 'path' to the audio file and its 'sentence'.
Additional fields include 'accent', 'age', 'client_id', 'up_votes', 'down_votes', 'gender', 'locale' and 'segment'.
### Data Fields
'client_id' ('string'): An id for which client (voice) made the recording
'path' ('string'): The path to the audio file
'audio' ('dict'): A dictionary containing the path to the downloaded audio file, the decoded audio array, and the sampling rate. Note that when accessing the audio column: 'dataset[0]["audio"]' the audio file is automatically decoded and resampled to 'dataset.features["audio"].sampling_rate'. Decoding and resampling of a large number of audio files might take a significant amount of time. Thus it is important to first query the sample index before the '"audio"' column, *i.e.* 'dataset[0]["audio"]' should always be preferred over 'dataset["audio"][0]'.
'sentence' ('string'): The sentence the user was prompted to speak
'up_votes' ('int64'): How many upvotes the audio file has received from reviewers
'down_votes' ('int64'): How many downvotes the audio file has received from reviewers
'age' ('string'): The age of the speaker (e.g. 'teens', 'twenties', 'fifties')
'gender' ('string'): The gender of the speaker
'accent' ('string'): Accent of the speaker
'locale' ('string'): The locale of the speaker
'segment' ('string'): Usually an empty field
### Data Splits
The speech material has been subdivided into portions for dev, train, test, validated, invalidated, reported and other.
The validated data is data that has been validated with reviewers and received upvotes that the data is of high quality.
The invalidated data is data has been invalidated by reviewers
and received downvotes indicating that the data is of low quality.
The reported data is data that has been reported, for different reasons.
The other data is data that has not yet been reviewed.
The dev, test, train are all data that has been reviewed, deemed of high quality and split into dev, test and train.
## Data Preprocessing Recommended by Hugging Face
The following are data preprocessing steps advised by the Hugging Face team. They are accompanied by an example code snippet that shows how to put them to practice.
Many examples in this dataset have trailing quotations marks, e.g _“the cat sat on the mat.“_. These trailing quotation marks do not change the actual meaning of the sentence, and it is near impossible to infer whether a sentence is a quotation or not a quotation from audio data alone. In these cases, it is advised to strip the quotation marks, leaving: _the cat sat on the mat_.
In addition, the majority of training sentences end in punctuation ( . or ? or ! ), whereas just a small proportion do not. In the dev set, almost all sentences end in punctuation. Thus, it is recommended to append a full-stop ( . ) to the end of the small number of training examples that do not end in punctuation.
## Dataset Creation
### Curation Rationale
### Source Data
#### Initial Data Collection and Normalization
#### Who are the source language producers?
### Annotations
#### Annotation process
#### Who are the annotators?
### Personal and Sensitive Information
The dataset consists of people who have donated their voice online. You agree to not attempt to determine the identity of speakers in the Common Voice dataset.
## Considerations for Using the Data
### Social Impact of Dataset
The dataset consists of people who have donated their voice online. You agree to not attempt to determine the identity of speakers in the Common Voice dataset.
### Discussion of Biases
### Other Known Limitations
## Additional Information
### Dataset Curators
### Licensing Information
Public Domain, CC-0
| [
"# Dataset Card for Common Voice Corpus 5",
"## Table of Contents\n- Dataset Description\n - Dataset Summary\n - Supported Tasks and Leaderboards\n - Languages\n- Dataset Structure\n - Data Instances\n - Data Fields\n - Data Splits\n- Dataset Creation\n - Curation Rationale\n - Source Data\n - Annotations\n - Personal and Sensitive Information\n- Considerations for Using the Data\n - Social Impact of Dataset\n - Discussion of Biases\n - Other Known Limitations\n- Additional Information\n - Dataset Curators\n - Licensing Information\n - Citation Information\n - Contributions",
"## Dataset Description\n\n- Homepage: URL\n- Repository: URL\n- Paper: URL\n- Leaderboard: URL\n- Point of Contact: Anton Lozhkov",
"### Dataset Summary\n\nThe Common Voice dataset consists of a unique MP3 and corresponding text file. \nMany of the 7226 recorded hours in the dataset also include demographic metadata like age, sex, and accent \nthat can help improve the accuracy of speech recognition engines.\n\nThe dataset currently consists of 5591 validated hours in 54 languages, but more voices and languages are always added. \nTake a look at the Languages page to request a language or start contributing.",
"### Supported Tasks and Leaderboards\n\nThe results for models trained on the Common Voice datasets are available via the \n Speech Bench",
"### Languages",
"## Dataset Structure",
"### Data Instances\n\nA typical data point comprises the 'path' to the audio file and its 'sentence'. \nAdditional fields include 'accent', 'age', 'client_id', 'up_votes', 'down_votes', 'gender', 'locale' and 'segment'.",
"### Data Fields\n\n'client_id' ('string'): An id for which client (voice) made the recording\n\n'path' ('string'): The path to the audio file\n\n'audio' ('dict'): A dictionary containing the path to the downloaded audio file, the decoded audio array, and the sampling rate. Note that when accessing the audio column: 'dataset[0][\"audio\"]' the audio file is automatically decoded and resampled to 'dataset.features[\"audio\"].sampling_rate'. Decoding and resampling of a large number of audio files might take a significant amount of time. Thus it is important to first query the sample index before the '\"audio\"' column, *i.e.* 'dataset[0][\"audio\"]' should always be preferred over 'dataset[\"audio\"][0]'.\n\n'sentence' ('string'): The sentence the user was prompted to speak\n\n'up_votes' ('int64'): How many upvotes the audio file has received from reviewers\n\n'down_votes' ('int64'): How many downvotes the audio file has received from reviewers\n\n'age' ('string'): The age of the speaker (e.g. 'teens', 'twenties', 'fifties')\n\n'gender' ('string'): The gender of the speaker\n\n'accent' ('string'): Accent of the speaker\n\n'locale' ('string'): The locale of the speaker\n\n'segment' ('string'): Usually an empty field",
"### Data Splits\n\nThe speech material has been subdivided into portions for dev, train, test, validated, invalidated, reported and other.\n\nThe validated data is data that has been validated with reviewers and received upvotes that the data is of high quality.\n\nThe invalidated data is data has been invalidated by reviewers\nand received downvotes indicating that the data is of low quality.\n\nThe reported data is data that has been reported, for different reasons.\n\nThe other data is data that has not yet been reviewed.\n\nThe dev, test, train are all data that has been reviewed, deemed of high quality and split into dev, test and train.",
"## Data Preprocessing Recommended by Hugging Face\n\nThe following are data preprocessing steps advised by the Hugging Face team. They are accompanied by an example code snippet that shows how to put them to practice. \n\nMany examples in this dataset have trailing quotations marks, e.g _“the cat sat on the mat.“_. These trailing quotation marks do not change the actual meaning of the sentence, and it is near impossible to infer whether a sentence is a quotation or not a quotation from audio data alone. In these cases, it is advised to strip the quotation marks, leaving: _the cat sat on the mat_.\n\nIn addition, the majority of training sentences end in punctuation ( . or ? or ! ), whereas just a small proportion do not. In the dev set, almost all sentences end in punctuation. Thus, it is recommended to append a full-stop ( . ) to the end of the small number of training examples that do not end in punctuation.",
"## Dataset Creation",
"### Curation Rationale",
"### Source Data",
"#### Initial Data Collection and Normalization",
"#### Who are the source language producers?",
"### Annotations",
"#### Annotation process",
"#### Who are the annotators?",
"### Personal and Sensitive Information\n\nThe dataset consists of people who have donated their voice online. You agree to not attempt to determine the identity of speakers in the Common Voice dataset.",
"## Considerations for Using the Data",
"### Social Impact of Dataset\n\nThe dataset consists of people who have donated their voice online. You agree to not attempt to determine the identity of speakers in the Common Voice dataset.",
"### Discussion of Biases",
"### Other Known Limitations",
"## Additional Information",
"### Dataset Curators",
"### Licensing Information\n\nPublic Domain, CC-0"
] | [
"TAGS\n#task_categories-automatic-speech-recognition #annotations_creators-crowdsourced #language_creators-crowdsourced #multilinguality-multilingual #source_datasets-extended|common_voice #license-cc0-1.0 #arxiv-1912.06670 #region-us \n",
"# Dataset Card for Common Voice Corpus 5",
"## Table of Contents\n- Dataset Description\n - Dataset Summary\n - Supported Tasks and Leaderboards\n - Languages\n- Dataset Structure\n - Data Instances\n - Data Fields\n - Data Splits\n- Dataset Creation\n - Curation Rationale\n - Source Data\n - Annotations\n - Personal and Sensitive Information\n- Considerations for Using the Data\n - Social Impact of Dataset\n - Discussion of Biases\n - Other Known Limitations\n- Additional Information\n - Dataset Curators\n - Licensing Information\n - Citation Information\n - Contributions",
"## Dataset Description\n\n- Homepage: URL\n- Repository: URL\n- Paper: URL\n- Leaderboard: URL\n- Point of Contact: Anton Lozhkov",
"### Dataset Summary\n\nThe Common Voice dataset consists of a unique MP3 and corresponding text file. \nMany of the 7226 recorded hours in the dataset also include demographic metadata like age, sex, and accent \nthat can help improve the accuracy of speech recognition engines.\n\nThe dataset currently consists of 5591 validated hours in 54 languages, but more voices and languages are always added. \nTake a look at the Languages page to request a language or start contributing.",
"### Supported Tasks and Leaderboards\n\nThe results for models trained on the Common Voice datasets are available via the \n Speech Bench",
"### Languages",
"## Dataset Structure",
"### Data Instances\n\nA typical data point comprises the 'path' to the audio file and its 'sentence'. \nAdditional fields include 'accent', 'age', 'client_id', 'up_votes', 'down_votes', 'gender', 'locale' and 'segment'.",
"### Data Fields\n\n'client_id' ('string'): An id for which client (voice) made the recording\n\n'path' ('string'): The path to the audio file\n\n'audio' ('dict'): A dictionary containing the path to the downloaded audio file, the decoded audio array, and the sampling rate. Note that when accessing the audio column: 'dataset[0][\"audio\"]' the audio file is automatically decoded and resampled to 'dataset.features[\"audio\"].sampling_rate'. Decoding and resampling of a large number of audio files might take a significant amount of time. Thus it is important to first query the sample index before the '\"audio\"' column, *i.e.* 'dataset[0][\"audio\"]' should always be preferred over 'dataset[\"audio\"][0]'.\n\n'sentence' ('string'): The sentence the user was prompted to speak\n\n'up_votes' ('int64'): How many upvotes the audio file has received from reviewers\n\n'down_votes' ('int64'): How many downvotes the audio file has received from reviewers\n\n'age' ('string'): The age of the speaker (e.g. 'teens', 'twenties', 'fifties')\n\n'gender' ('string'): The gender of the speaker\n\n'accent' ('string'): Accent of the speaker\n\n'locale' ('string'): The locale of the speaker\n\n'segment' ('string'): Usually an empty field",
"### Data Splits\n\nThe speech material has been subdivided into portions for dev, train, test, validated, invalidated, reported and other.\n\nThe validated data is data that has been validated with reviewers and received upvotes that the data is of high quality.\n\nThe invalidated data is data has been invalidated by reviewers\nand received downvotes indicating that the data is of low quality.\n\nThe reported data is data that has been reported, for different reasons.\n\nThe other data is data that has not yet been reviewed.\n\nThe dev, test, train are all data that has been reviewed, deemed of high quality and split into dev, test and train.",
"## Data Preprocessing Recommended by Hugging Face\n\nThe following are data preprocessing steps advised by the Hugging Face team. They are accompanied by an example code snippet that shows how to put them to practice. \n\nMany examples in this dataset have trailing quotations marks, e.g _“the cat sat on the mat.“_. These trailing quotation marks do not change the actual meaning of the sentence, and it is near impossible to infer whether a sentence is a quotation or not a quotation from audio data alone. In these cases, it is advised to strip the quotation marks, leaving: _the cat sat on the mat_.\n\nIn addition, the majority of training sentences end in punctuation ( . or ? or ! ), whereas just a small proportion do not. In the dev set, almost all sentences end in punctuation. Thus, it is recommended to append a full-stop ( . ) to the end of the small number of training examples that do not end in punctuation.",
"## Dataset Creation",
"### Curation Rationale",
"### Source Data",
"#### Initial Data Collection and Normalization",
"#### Who are the source language producers?",
"### Annotations",
"#### Annotation process",
"#### Who are the annotators?",
"### Personal and Sensitive Information\n\nThe dataset consists of people who have donated their voice online. You agree to not attempt to determine the identity of speakers in the Common Voice dataset.",
"## Considerations for Using the Data",
"### Social Impact of Dataset\n\nThe dataset consists of people who have donated their voice online. You agree to not attempt to determine the identity of speakers in the Common Voice dataset.",
"### Discussion of Biases",
"### Other Known Limitations",
"## Additional Information",
"### Dataset Curators",
"### Licensing Information\n\nPublic Domain, CC-0"
] |
bc45ee4e29cffd942f529a6e00cc524b802926f7 |
# Dataset Card for Common Voice Corpus 5.1
## Table of Contents
- [Dataset Description](#dataset-description)
- [Dataset Summary](#dataset-summary)
- [Supported Tasks and Leaderboards](#supported-tasks-and-leaderboards)
- [Languages](#languages)
- [Dataset Structure](#dataset-structure)
- [Data Instances](#data-instances)
- [Data Fields](#data-fields)
- [Data Splits](#data-splits)
- [Dataset Creation](#dataset-creation)
- [Curation Rationale](#curation-rationale)
- [Source Data](#source-data)
- [Annotations](#annotations)
- [Personal and Sensitive Information](#personal-and-sensitive-information)
- [Considerations for Using the Data](#considerations-for-using-the-data)
- [Social Impact of Dataset](#social-impact-of-dataset)
- [Discussion of Biases](#discussion-of-biases)
- [Other Known Limitations](#other-known-limitations)
- [Additional Information](#additional-information)
- [Dataset Curators](#dataset-curators)
- [Licensing Information](#licensing-information)
- [Citation Information](#citation-information)
- [Contributions](#contributions)
## Dataset Description
- **Homepage:** https://commonvoice.mozilla.org/en/datasets
- **Repository:** https://github.com/common-voice/common-voice
- **Paper:** https://arxiv.org/abs/1912.06670
- **Leaderboard:** https://paperswithcode.com/dataset/common-voice
- **Point of Contact:** [Anton Lozhkov](mailto:[email protected])
### Dataset Summary
The Common Voice dataset consists of a unique MP3 and corresponding text file.
Many of the 7226 recorded hours in the dataset also include demographic metadata like age, sex, and accent
that can help improve the accuracy of speech recognition engines.
The dataset currently consists of 5671 validated hours in 54 languages, but more voices and languages are always added.
Take a look at the [Languages](https://commonvoice.mozilla.org/en/languages) page to request a language or start contributing.
### Supported Tasks and Leaderboards
The results for models trained on the Common Voice datasets are available via the
[🤗 Speech Bench](https://huggingface.co/spaces/huggingface/hf-speech-bench)
### Languages
```
Abkhaz, Arabic, Assamese, Basque, Breton, Catalan, Chinese (China), Chinese (Hong Kong), Chinese (Taiwan), Chuvash, Czech, Dhivehi, Dutch, English, Esperanto, Estonian, French, Frisian, Georgian, German, Greek, Hakha Chin, Indonesian, Interlingua, Irish, Italian, Japanese, Kabyle, Kinyarwanda, Kyrgyz, Latvian, Maltese, Mongolian, Odia, Persian, Polish, Portuguese, Punjabi, Romanian, Romansh Sursilvan, Romansh Vallader, Russian, Sakha, Slovenian, Sorbian, Upper, Spanish, Swedish, Tamil, Tatar, Turkish, Ukrainian, Vietnamese, Votic, Welsh
```
## Dataset Structure
### Data Instances
A typical data point comprises the `path` to the audio file and its `sentence`.
Additional fields include `accent`, `age`, `client_id`, `up_votes`, `down_votes`, `gender`, `locale` and `segment`.
```python
{
'client_id': 'd59478fbc1ee646a28a3c652a119379939123784d99131b865a89f8b21c81f69276c48bd574b81267d9d1a77b83b43e6d475a6cfc79c232ddbca946ae9c7afc5',
'path': 'et/clips/common_voice_et_18318995.mp3',
'audio': {
'path': 'et/clips/common_voice_et_18318995.mp3',
'array': array([-0.00048828, -0.00018311, -0.00137329, ..., 0.00079346, 0.00091553, 0.00085449], dtype=float32),
'sampling_rate': 48000
},
'sentence': 'Tasub kokku saada inimestega, keda tunned juba ammust ajast saati.',
'up_votes': 2,
'down_votes': 0,
'age': 'twenties',
'gender': 'male',
'accent': '',
'locale': 'et',
'segment': ''
}
```
### Data Fields
`client_id` (`string`): An id for which client (voice) made the recording
`path` (`string`): The path to the audio file
`audio` (`dict`): A dictionary containing the path to the downloaded audio file, the decoded audio array, and the sampling rate. Note that when accessing the audio column: `dataset[0]["audio"]` the audio file is automatically decoded and resampled to `dataset.features["audio"].sampling_rate`. Decoding and resampling of a large number of audio files might take a significant amount of time. Thus it is important to first query the sample index before the `"audio"` column, *i.e.* `dataset[0]["audio"]` should **always** be preferred over `dataset["audio"][0]`.
`sentence` (`string`): The sentence the user was prompted to speak
`up_votes` (`int64`): How many upvotes the audio file has received from reviewers
`down_votes` (`int64`): How many downvotes the audio file has received from reviewers
`age` (`string`): The age of the speaker (e.g. `teens`, `twenties`, `fifties`)
`gender` (`string`): The gender of the speaker
`accent` (`string`): Accent of the speaker
`locale` (`string`): The locale of the speaker
`segment` (`string`): Usually an empty field
### Data Splits
The speech material has been subdivided into portions for dev, train, test, validated, invalidated, reported and other.
The validated data is data that has been validated with reviewers and received upvotes that the data is of high quality.
The invalidated data is data has been invalidated by reviewers
and received downvotes indicating that the data is of low quality.
The reported data is data that has been reported, for different reasons.
The other data is data that has not yet been reviewed.
The dev, test, train are all data that has been reviewed, deemed of high quality and split into dev, test and train.
## Data Preprocessing Recommended by Hugging Face
The following are data preprocessing steps advised by the Hugging Face team. They are accompanied by an example code snippet that shows how to put them to practice.
Many examples in this dataset have trailing quotations marks, e.g _“the cat sat on the mat.“_. These trailing quotation marks do not change the actual meaning of the sentence, and it is near impossible to infer whether a sentence is a quotation or not a quotation from audio data alone. In these cases, it is advised to strip the quotation marks, leaving: _the cat sat on the mat_.
In addition, the majority of training sentences end in punctuation ( . or ? or ! ), whereas just a small proportion do not. In the dev set, **almost all** sentences end in punctuation. Thus, it is recommended to append a full-stop ( . ) to the end of the small number of training examples that do not end in punctuation.
```python
from datasets import load_dataset
ds = load_dataset("mozilla-foundation/common_voice_5_1", "en", use_auth_token=True)
def prepare_dataset(batch):
"""Function to preprocess the dataset with the .map method"""
transcription = batch["sentence"]
if transcription.startswith('"') and transcription.endswith('"'):
# we can remove trailing quotation marks as they do not affect the transcription
transcription = transcription[1:-1]
if transcription[-1] not in [".", "?", "!"]:
# append a full-stop to sentences that do not end in punctuation
transcription = transcription + "."
batch["sentence"] = transcription
return batch
ds = ds.map(prepare_dataset, desc="preprocess dataset")
```
## Dataset Creation
### Curation Rationale
[Needs More Information]
### Source Data
#### Initial Data Collection and Normalization
[Needs More Information]
#### Who are the source language producers?
[Needs More Information]
### Annotations
#### Annotation process
[Needs More Information]
#### Who are the annotators?
[Needs More Information]
### Personal and Sensitive Information
The dataset consists of people who have donated their voice online. You agree to not attempt to determine the identity of speakers in the Common Voice dataset.
## Considerations for Using the Data
### Social Impact of Dataset
The dataset consists of people who have donated their voice online. You agree to not attempt to determine the identity of speakers in the Common Voice dataset.
### Discussion of Biases
[More Information Needed]
### Other Known Limitations
[More Information Needed]
## Additional Information
### Dataset Curators
[More Information Needed]
### Licensing Information
Public Domain, [CC-0](https://creativecommons.org/share-your-work/public-domain/cc0/)
### Citation Information
```
@inproceedings{commonvoice:2020,
author = {Ardila, R. and Branson, M. and Davis, K. and Henretty, M. and Kohler, M. and Meyer, J. and Morais, R. and Saunders, L. and Tyers, F. M. and Weber, G.},
title = {Common Voice: A Massively-Multilingual Speech Corpus},
booktitle = {Proceedings of the 12th Conference on Language Resources and Evaluation (LREC 2020)},
pages = {4211--4215},
year = 2020
}
```
| mozilla-foundation/common_voice_5_1 | [
"task_categories:automatic-speech-recognition",
"annotations_creators:crowdsourced",
"language_creators:crowdsourced",
"multilinguality:multilingual",
"source_datasets:extended|common_voice",
"license:cc0-1.0",
"arxiv:1912.06670",
"region:us"
] | 2022-03-02T23:29:22+00:00 | {"annotations_creators": ["crowdsourced"], "language_creators": ["crowdsourced"], "license": ["cc0-1.0"], "multilinguality": ["multilingual"], "size_categories": {"ab": ["n<1K"], "ar": ["10K<n<100K"], "as": ["n<1K"], "br": ["10K<n<100K"], "ca": ["100K<n<1M"], "cnh": ["1K<n<10K"], "cs": ["10K<n<100K"], "cv": ["1K<n<10K"], "cy": ["10K<n<100K"], "de": ["100K<n<1M"], "dv": ["1K<n<10K"], "el": ["10K<n<100K"], "en": ["1M<n<10M"], "eo": ["10K<n<100K"], "es": ["100K<n<1M"], "et": ["10K<n<100K"], "eu": ["10K<n<100K"], "fa": ["100K<n<1M"], "fr": ["100K<n<1M"], "fy-NL": ["10K<n<100K"], "ga-IE": ["1K<n<10K"], "hsb": ["1K<n<10K"], "ia": ["1K<n<10K"], "id": ["10K<n<100K"], "it": ["100K<n<1M"], "ja": ["1K<n<10K"], "ka": ["1K<n<10K"], "kab": ["100K<n<1M"], "ky": ["10K<n<100K"], "lv": ["1K<n<10K"], "mn": ["10K<n<100K"], "mt": ["10K<n<100K"], "nl": ["10K<n<100K"], "or": ["1K<n<10K"], "pa-IN": ["n<1K"], "pl": ["100K<n<1M"], "pt": ["10K<n<100K"], "rm-sursilv": ["1K<n<10K"], "rm-vallader": ["1K<n<10K"], "ro": ["1K<n<10K"], "ru": ["10K<n<100K"], "rw": ["100K<n<1M"], "sah": ["1K<n<10K"], "sl": ["1K<n<10K"], "sv-SE": ["10K<n<100K"], "ta": ["10K<n<100K"], "tr": ["10K<n<100K"], "tt": ["10K<n<100K"], "uk": ["10K<n<100K"], "vi": ["n<1K"], "vot": ["n<1K"], "zh-CN": ["10K<n<100K"], "zh-HK": ["10K<n<100K"], "zh-TW": ["10K<n<100K"]}, "source_datasets": ["extended|common_voice"], "task_categories": ["automatic-speech-recognition"], "paperswithcode_id": "common-voice", "pretty_name": "Common Voice Corpus 5.1", "language_bcp47": ["ab", "ar", "as", "br", "ca", "cnh", "cs", "cv", "cy", "de", "dv", "el", "en", "eo", "es", "et", "eu", "fa", "fr", "fy-NL", "ga-IE", "hsb", "ia", "id", "it", "ja", "ka", "kab", "ky", "lv", "mn", "mt", "nl", "or", "pa-IN", "pl", "pt", "rm-sursilv", "rm-vallader", "ro", "ru", "rw", "sah", "sl", "sv-SE", "ta", "tr", "tt", "uk", "vi", "vot", "zh-CN", "zh-HK", "zh-TW"], "extra_gated_prompt": "By clicking on \u201cAccess repository\u201d below, you also agree to not attempt to determine the identity of speakers in the Common Voice dataset."} | 2023-07-29T15:00:04+00:00 | [
"1912.06670"
] | [] | TAGS
#task_categories-automatic-speech-recognition #annotations_creators-crowdsourced #language_creators-crowdsourced #multilinguality-multilingual #source_datasets-extended|common_voice #license-cc0-1.0 #arxiv-1912.06670 #region-us
|
# Dataset Card for Common Voice Corpus 5.1
## Table of Contents
- Dataset Description
- Dataset Summary
- Supported Tasks and Leaderboards
- Languages
- Dataset Structure
- Data Instances
- Data Fields
- Data Splits
- Dataset Creation
- Curation Rationale
- Source Data
- Annotations
- Personal and Sensitive Information
- Considerations for Using the Data
- Social Impact of Dataset
- Discussion of Biases
- Other Known Limitations
- Additional Information
- Dataset Curators
- Licensing Information
- Citation Information
- Contributions
## Dataset Description
- Homepage: URL
- Repository: URL
- Paper: URL
- Leaderboard: URL
- Point of Contact: Anton Lozhkov
### Dataset Summary
The Common Voice dataset consists of a unique MP3 and corresponding text file.
Many of the 7226 recorded hours in the dataset also include demographic metadata like age, sex, and accent
that can help improve the accuracy of speech recognition engines.
The dataset currently consists of 5671 validated hours in 54 languages, but more voices and languages are always added.
Take a look at the Languages page to request a language or start contributing.
### Supported Tasks and Leaderboards
The results for models trained on the Common Voice datasets are available via the
Speech Bench
### Languages
## Dataset Structure
### Data Instances
A typical data point comprises the 'path' to the audio file and its 'sentence'.
Additional fields include 'accent', 'age', 'client_id', 'up_votes', 'down_votes', 'gender', 'locale' and 'segment'.
### Data Fields
'client_id' ('string'): An id for which client (voice) made the recording
'path' ('string'): The path to the audio file
'audio' ('dict'): A dictionary containing the path to the downloaded audio file, the decoded audio array, and the sampling rate. Note that when accessing the audio column: 'dataset[0]["audio"]' the audio file is automatically decoded and resampled to 'dataset.features["audio"].sampling_rate'. Decoding and resampling of a large number of audio files might take a significant amount of time. Thus it is important to first query the sample index before the '"audio"' column, *i.e.* 'dataset[0]["audio"]' should always be preferred over 'dataset["audio"][0]'.
'sentence' ('string'): The sentence the user was prompted to speak
'up_votes' ('int64'): How many upvotes the audio file has received from reviewers
'down_votes' ('int64'): How many downvotes the audio file has received from reviewers
'age' ('string'): The age of the speaker (e.g. 'teens', 'twenties', 'fifties')
'gender' ('string'): The gender of the speaker
'accent' ('string'): Accent of the speaker
'locale' ('string'): The locale of the speaker
'segment' ('string'): Usually an empty field
### Data Splits
The speech material has been subdivided into portions for dev, train, test, validated, invalidated, reported and other.
The validated data is data that has been validated with reviewers and received upvotes that the data is of high quality.
The invalidated data is data has been invalidated by reviewers
and received downvotes indicating that the data is of low quality.
The reported data is data that has been reported, for different reasons.
The other data is data that has not yet been reviewed.
The dev, test, train are all data that has been reviewed, deemed of high quality and split into dev, test and train.
## Data Preprocessing Recommended by Hugging Face
The following are data preprocessing steps advised by the Hugging Face team. They are accompanied by an example code snippet that shows how to put them to practice.
Many examples in this dataset have trailing quotations marks, e.g _“the cat sat on the mat.“_. These trailing quotation marks do not change the actual meaning of the sentence, and it is near impossible to infer whether a sentence is a quotation or not a quotation from audio data alone. In these cases, it is advised to strip the quotation marks, leaving: _the cat sat on the mat_.
In addition, the majority of training sentences end in punctuation ( . or ? or ! ), whereas just a small proportion do not. In the dev set, almost all sentences end in punctuation. Thus, it is recommended to append a full-stop ( . ) to the end of the small number of training examples that do not end in punctuation.
## Dataset Creation
### Curation Rationale
### Source Data
#### Initial Data Collection and Normalization
#### Who are the source language producers?
### Annotations
#### Annotation process
#### Who are the annotators?
### Personal and Sensitive Information
The dataset consists of people who have donated their voice online. You agree to not attempt to determine the identity of speakers in the Common Voice dataset.
## Considerations for Using the Data
### Social Impact of Dataset
The dataset consists of people who have donated their voice online. You agree to not attempt to determine the identity of speakers in the Common Voice dataset.
### Discussion of Biases
### Other Known Limitations
## Additional Information
### Dataset Curators
### Licensing Information
Public Domain, CC-0
| [
"# Dataset Card for Common Voice Corpus 5.1",
"## Table of Contents\n- Dataset Description\n - Dataset Summary\n - Supported Tasks and Leaderboards\n - Languages\n- Dataset Structure\n - Data Instances\n - Data Fields\n - Data Splits\n- Dataset Creation\n - Curation Rationale\n - Source Data\n - Annotations\n - Personal and Sensitive Information\n- Considerations for Using the Data\n - Social Impact of Dataset\n - Discussion of Biases\n - Other Known Limitations\n- Additional Information\n - Dataset Curators\n - Licensing Information\n - Citation Information\n - Contributions",
"## Dataset Description\n\n- Homepage: URL\n- Repository: URL\n- Paper: URL\n- Leaderboard: URL\n- Point of Contact: Anton Lozhkov",
"### Dataset Summary\n\nThe Common Voice dataset consists of a unique MP3 and corresponding text file. \nMany of the 7226 recorded hours in the dataset also include demographic metadata like age, sex, and accent \nthat can help improve the accuracy of speech recognition engines.\n\nThe dataset currently consists of 5671 validated hours in 54 languages, but more voices and languages are always added. \nTake a look at the Languages page to request a language or start contributing.",
"### Supported Tasks and Leaderboards\n\nThe results for models trained on the Common Voice datasets are available via the \n Speech Bench",
"### Languages",
"## Dataset Structure",
"### Data Instances\n\nA typical data point comprises the 'path' to the audio file and its 'sentence'. \nAdditional fields include 'accent', 'age', 'client_id', 'up_votes', 'down_votes', 'gender', 'locale' and 'segment'.",
"### Data Fields\n\n'client_id' ('string'): An id for which client (voice) made the recording\n\n'path' ('string'): The path to the audio file\n\n'audio' ('dict'): A dictionary containing the path to the downloaded audio file, the decoded audio array, and the sampling rate. Note that when accessing the audio column: 'dataset[0][\"audio\"]' the audio file is automatically decoded and resampled to 'dataset.features[\"audio\"].sampling_rate'. Decoding and resampling of a large number of audio files might take a significant amount of time. Thus it is important to first query the sample index before the '\"audio\"' column, *i.e.* 'dataset[0][\"audio\"]' should always be preferred over 'dataset[\"audio\"][0]'.\n\n'sentence' ('string'): The sentence the user was prompted to speak\n\n'up_votes' ('int64'): How many upvotes the audio file has received from reviewers\n\n'down_votes' ('int64'): How many downvotes the audio file has received from reviewers\n\n'age' ('string'): The age of the speaker (e.g. 'teens', 'twenties', 'fifties')\n\n'gender' ('string'): The gender of the speaker\n\n'accent' ('string'): Accent of the speaker\n\n'locale' ('string'): The locale of the speaker\n\n'segment' ('string'): Usually an empty field",
"### Data Splits\n\nThe speech material has been subdivided into portions for dev, train, test, validated, invalidated, reported and other.\n\nThe validated data is data that has been validated with reviewers and received upvotes that the data is of high quality.\n\nThe invalidated data is data has been invalidated by reviewers\nand received downvotes indicating that the data is of low quality.\n\nThe reported data is data that has been reported, for different reasons.\n\nThe other data is data that has not yet been reviewed.\n\nThe dev, test, train are all data that has been reviewed, deemed of high quality and split into dev, test and train.",
"## Data Preprocessing Recommended by Hugging Face\n\nThe following are data preprocessing steps advised by the Hugging Face team. They are accompanied by an example code snippet that shows how to put them to practice. \n\nMany examples in this dataset have trailing quotations marks, e.g _“the cat sat on the mat.“_. These trailing quotation marks do not change the actual meaning of the sentence, and it is near impossible to infer whether a sentence is a quotation or not a quotation from audio data alone. In these cases, it is advised to strip the quotation marks, leaving: _the cat sat on the mat_.\n\nIn addition, the majority of training sentences end in punctuation ( . or ? or ! ), whereas just a small proportion do not. In the dev set, almost all sentences end in punctuation. Thus, it is recommended to append a full-stop ( . ) to the end of the small number of training examples that do not end in punctuation.",
"## Dataset Creation",
"### Curation Rationale",
"### Source Data",
"#### Initial Data Collection and Normalization",
"#### Who are the source language producers?",
"### Annotations",
"#### Annotation process",
"#### Who are the annotators?",
"### Personal and Sensitive Information\n\nThe dataset consists of people who have donated their voice online. You agree to not attempt to determine the identity of speakers in the Common Voice dataset.",
"## Considerations for Using the Data",
"### Social Impact of Dataset\n\nThe dataset consists of people who have donated their voice online. You agree to not attempt to determine the identity of speakers in the Common Voice dataset.",
"### Discussion of Biases",
"### Other Known Limitations",
"## Additional Information",
"### Dataset Curators",
"### Licensing Information\n\nPublic Domain, CC-0"
] | [
"TAGS\n#task_categories-automatic-speech-recognition #annotations_creators-crowdsourced #language_creators-crowdsourced #multilinguality-multilingual #source_datasets-extended|common_voice #license-cc0-1.0 #arxiv-1912.06670 #region-us \n",
"# Dataset Card for Common Voice Corpus 5.1",
"## Table of Contents\n- Dataset Description\n - Dataset Summary\n - Supported Tasks and Leaderboards\n - Languages\n- Dataset Structure\n - Data Instances\n - Data Fields\n - Data Splits\n- Dataset Creation\n - Curation Rationale\n - Source Data\n - Annotations\n - Personal and Sensitive Information\n- Considerations for Using the Data\n - Social Impact of Dataset\n - Discussion of Biases\n - Other Known Limitations\n- Additional Information\n - Dataset Curators\n - Licensing Information\n - Citation Information\n - Contributions",
"## Dataset Description\n\n- Homepage: URL\n- Repository: URL\n- Paper: URL\n- Leaderboard: URL\n- Point of Contact: Anton Lozhkov",
"### Dataset Summary\n\nThe Common Voice dataset consists of a unique MP3 and corresponding text file. \nMany of the 7226 recorded hours in the dataset also include demographic metadata like age, sex, and accent \nthat can help improve the accuracy of speech recognition engines.\n\nThe dataset currently consists of 5671 validated hours in 54 languages, but more voices and languages are always added. \nTake a look at the Languages page to request a language or start contributing.",
"### Supported Tasks and Leaderboards\n\nThe results for models trained on the Common Voice datasets are available via the \n Speech Bench",
"### Languages",
"## Dataset Structure",
"### Data Instances\n\nA typical data point comprises the 'path' to the audio file and its 'sentence'. \nAdditional fields include 'accent', 'age', 'client_id', 'up_votes', 'down_votes', 'gender', 'locale' and 'segment'.",
"### Data Fields\n\n'client_id' ('string'): An id for which client (voice) made the recording\n\n'path' ('string'): The path to the audio file\n\n'audio' ('dict'): A dictionary containing the path to the downloaded audio file, the decoded audio array, and the sampling rate. Note that when accessing the audio column: 'dataset[0][\"audio\"]' the audio file is automatically decoded and resampled to 'dataset.features[\"audio\"].sampling_rate'. Decoding and resampling of a large number of audio files might take a significant amount of time. Thus it is important to first query the sample index before the '\"audio\"' column, *i.e.* 'dataset[0][\"audio\"]' should always be preferred over 'dataset[\"audio\"][0]'.\n\n'sentence' ('string'): The sentence the user was prompted to speak\n\n'up_votes' ('int64'): How many upvotes the audio file has received from reviewers\n\n'down_votes' ('int64'): How many downvotes the audio file has received from reviewers\n\n'age' ('string'): The age of the speaker (e.g. 'teens', 'twenties', 'fifties')\n\n'gender' ('string'): The gender of the speaker\n\n'accent' ('string'): Accent of the speaker\n\n'locale' ('string'): The locale of the speaker\n\n'segment' ('string'): Usually an empty field",
"### Data Splits\n\nThe speech material has been subdivided into portions for dev, train, test, validated, invalidated, reported and other.\n\nThe validated data is data that has been validated with reviewers and received upvotes that the data is of high quality.\n\nThe invalidated data is data has been invalidated by reviewers\nand received downvotes indicating that the data is of low quality.\n\nThe reported data is data that has been reported, for different reasons.\n\nThe other data is data that has not yet been reviewed.\n\nThe dev, test, train are all data that has been reviewed, deemed of high quality and split into dev, test and train.",
"## Data Preprocessing Recommended by Hugging Face\n\nThe following are data preprocessing steps advised by the Hugging Face team. They are accompanied by an example code snippet that shows how to put them to practice. \n\nMany examples in this dataset have trailing quotations marks, e.g _“the cat sat on the mat.“_. These trailing quotation marks do not change the actual meaning of the sentence, and it is near impossible to infer whether a sentence is a quotation or not a quotation from audio data alone. In these cases, it is advised to strip the quotation marks, leaving: _the cat sat on the mat_.\n\nIn addition, the majority of training sentences end in punctuation ( . or ? or ! ), whereas just a small proportion do not. In the dev set, almost all sentences end in punctuation. Thus, it is recommended to append a full-stop ( . ) to the end of the small number of training examples that do not end in punctuation.",
"## Dataset Creation",
"### Curation Rationale",
"### Source Data",
"#### Initial Data Collection and Normalization",
"#### Who are the source language producers?",
"### Annotations",
"#### Annotation process",
"#### Who are the annotators?",
"### Personal and Sensitive Information\n\nThe dataset consists of people who have donated their voice online. You agree to not attempt to determine the identity of speakers in the Common Voice dataset.",
"## Considerations for Using the Data",
"### Social Impact of Dataset\n\nThe dataset consists of people who have donated their voice online. You agree to not attempt to determine the identity of speakers in the Common Voice dataset.",
"### Discussion of Biases",
"### Other Known Limitations",
"## Additional Information",
"### Dataset Curators",
"### Licensing Information\n\nPublic Domain, CC-0"
] |
c31398ea2f97ed4530762c47150207e9faa2f8d6 |
# Dataset Card for Common Voice Corpus 6.0
## Table of Contents
- [Dataset Description](#dataset-description)
- [Dataset Summary](#dataset-summary)
- [Supported Tasks and Leaderboards](#supported-tasks-and-leaderboards)
- [Languages](#languages)
- [Dataset Structure](#dataset-structure)
- [Data Instances](#data-instances)
- [Data Fields](#data-fields)
- [Data Splits](#data-splits)
- [Dataset Creation](#dataset-creation)
- [Curation Rationale](#curation-rationale)
- [Source Data](#source-data)
- [Annotations](#annotations)
- [Personal and Sensitive Information](#personal-and-sensitive-information)
- [Considerations for Using the Data](#considerations-for-using-the-data)
- [Social Impact of Dataset](#social-impact-of-dataset)
- [Discussion of Biases](#discussion-of-biases)
- [Other Known Limitations](#other-known-limitations)
- [Additional Information](#additional-information)
- [Dataset Curators](#dataset-curators)
- [Licensing Information](#licensing-information)
- [Citation Information](#citation-information)
- [Contributions](#contributions)
## Dataset Description
- **Homepage:** https://commonvoice.mozilla.org/en/datasets
- **Repository:** https://github.com/common-voice/common-voice
- **Paper:** https://arxiv.org/abs/1912.06670
- **Leaderboard:** https://paperswithcode.com/dataset/common-voice
- **Point of Contact:** [Anton Lozhkov](mailto:[email protected])
### Dataset Summary
The Common Voice dataset consists of a unique MP3 and corresponding text file.
Many of the 9261 recorded hours in the dataset also include demographic metadata like age, sex, and accent
that can help improve the accuracy of speech recognition engines.
The dataset currently consists of 7327 validated hours in 60 languages, but more voices and languages are always added.
Take a look at the [Languages](https://commonvoice.mozilla.org/en/languages) page to request a language or start contributing.
### Supported Tasks and Leaderboards
The results for models trained on the Common Voice datasets are available via the
[🤗 Speech Bench](https://huggingface.co/spaces/huggingface/hf-speech-bench)
### Languages
```
Abkhaz, Arabic, Assamese, Basque, Breton, Catalan, Chinese (China), Chinese (Hong Kong), Chinese (Taiwan), Chuvash, Czech, Dhivehi, Dutch, English, Esperanto, Estonian, Finnish, French, Frisian, Georgian, German, Greek, Hakha Chin, Hindi, Hungarian, Indonesian, Interlingua, Irish, Italian, Japanese, Kabyle, Kinyarwanda, Kyrgyz, Latvian, Lithuanian, Luganda, Maltese, Mongolian, Odia, Persian, Polish, Portuguese, Punjabi, Romanian, Romansh Sursilvan, Romansh Vallader, Russian, Sakha, Slovenian, Sorbian, Upper, Spanish, Swedish, Tamil, Tatar, Thai, Turkish, Ukrainian, Vietnamese, Votic, Welsh
```
## Dataset Structure
### Data Instances
A typical data point comprises the `path` to the audio file and its `sentence`.
Additional fields include `accent`, `age`, `client_id`, `up_votes`, `down_votes`, `gender`, `locale` and `segment`.
```python
{
'client_id': 'd59478fbc1ee646a28a3c652a119379939123784d99131b865a89f8b21c81f69276c48bd574b81267d9d1a77b83b43e6d475a6cfc79c232ddbca946ae9c7afc5',
'path': 'et/clips/common_voice_et_18318995.mp3',
'audio': {
'path': 'et/clips/common_voice_et_18318995.mp3',
'array': array([-0.00048828, -0.00018311, -0.00137329, ..., 0.00079346, 0.00091553, 0.00085449], dtype=float32),
'sampling_rate': 48000
},
'sentence': 'Tasub kokku saada inimestega, keda tunned juba ammust ajast saati.',
'up_votes': 2,
'down_votes': 0,
'age': 'twenties',
'gender': 'male',
'accent': '',
'locale': 'et',
'segment': ''
}
```
### Data Fields
`client_id` (`string`): An id for which client (voice) made the recording
`path` (`string`): The path to the audio file
`audio` (`dict`): A dictionary containing the path to the downloaded audio file, the decoded audio array, and the sampling rate. Note that when accessing the audio column: `dataset[0]["audio"]` the audio file is automatically decoded and resampled to `dataset.features["audio"].sampling_rate`. Decoding and resampling of a large number of audio files might take a significant amount of time. Thus it is important to first query the sample index before the `"audio"` column, *i.e.* `dataset[0]["audio"]` should **always** be preferred over `dataset["audio"][0]`.
`sentence` (`string`): The sentence the user was prompted to speak
`up_votes` (`int64`): How many upvotes the audio file has received from reviewers
`down_votes` (`int64`): How many downvotes the audio file has received from reviewers
`age` (`string`): The age of the speaker (e.g. `teens`, `twenties`, `fifties`)
`gender` (`string`): The gender of the speaker
`accent` (`string`): Accent of the speaker
`locale` (`string`): The locale of the speaker
`segment` (`string`): Usually an empty field
### Data Splits
The speech material has been subdivided into portions for dev, train, test, validated, invalidated, reported and other.
The validated data is data that has been validated with reviewers and received upvotes that the data is of high quality.
The invalidated data is data has been invalidated by reviewers
and received downvotes indicating that the data is of low quality.
The reported data is data that has been reported, for different reasons.
The other data is data that has not yet been reviewed.
The dev, test, train are all data that has been reviewed, deemed of high quality and split into dev, test and train.
## Data Preprocessing Recommended by Hugging Face
The following are data preprocessing steps advised by the Hugging Face team. They are accompanied by an example code snippet that shows how to put them to practice.
Many examples in this dataset have trailing quotations marks, e.g _“the cat sat on the mat.“_. These trailing quotation marks do not change the actual meaning of the sentence, and it is near impossible to infer whether a sentence is a quotation or not a quotation from audio data alone. In these cases, it is advised to strip the quotation marks, leaving: _the cat sat on the mat_.
In addition, the majority of training sentences end in punctuation ( . or ? or ! ), whereas just a small proportion do not. In the dev set, **almost all** sentences end in punctuation. Thus, it is recommended to append a full-stop ( . ) to the end of the small number of training examples that do not end in punctuation.
```python
from datasets import load_dataset
ds = load_dataset("mozilla-foundation/common_voice_6_0", "en", use_auth_token=True)
def prepare_dataset(batch):
"""Function to preprocess the dataset with the .map method"""
transcription = batch["sentence"]
if transcription.startswith('"') and transcription.endswith('"'):
# we can remove trailing quotation marks as they do not affect the transcription
transcription = transcription[1:-1]
if transcription[-1] not in [".", "?", "!"]:
# append a full-stop to sentences that do not end in punctuation
transcription = transcription + "."
batch["sentence"] = transcription
return batch
ds = ds.map(prepare_dataset, desc="preprocess dataset")
```
## Dataset Creation
### Curation Rationale
[Needs More Information]
### Source Data
#### Initial Data Collection and Normalization
[Needs More Information]
#### Who are the source language producers?
[Needs More Information]
### Annotations
#### Annotation process
[Needs More Information]
#### Who are the annotators?
[Needs More Information]
### Personal and Sensitive Information
The dataset consists of people who have donated their voice online. You agree to not attempt to determine the identity of speakers in the Common Voice dataset.
## Considerations for Using the Data
### Social Impact of Dataset
The dataset consists of people who have donated their voice online. You agree to not attempt to determine the identity of speakers in the Common Voice dataset.
### Discussion of Biases
[More Information Needed]
### Other Known Limitations
[More Information Needed]
## Additional Information
### Dataset Curators
[More Information Needed]
### Licensing Information
Public Domain, [CC-0](https://creativecommons.org/share-your-work/public-domain/cc0/)
### Citation Information
```
@inproceedings{commonvoice:2020,
author = {Ardila, R. and Branson, M. and Davis, K. and Henretty, M. and Kohler, M. and Meyer, J. and Morais, R. and Saunders, L. and Tyers, F. M. and Weber, G.},
title = {Common Voice: A Massively-Multilingual Speech Corpus},
booktitle = {Proceedings of the 12th Conference on Language Resources and Evaluation (LREC 2020)},
pages = {4211--4215},
year = 2020
}
```
| mozilla-foundation/common_voice_6_0 | [
"task_categories:automatic-speech-recognition",
"annotations_creators:crowdsourced",
"language_creators:crowdsourced",
"multilinguality:multilingual",
"source_datasets:extended|common_voice",
"license:cc0-1.0",
"arxiv:1912.06670",
"region:us"
] | 2022-03-02T23:29:22+00:00 | {"annotations_creators": ["crowdsourced"], "language_creators": ["crowdsourced"], "license": ["cc0-1.0"], "multilinguality": ["multilingual"], "size_categories": {"ab": ["n<1K"], "ar": ["10K<n<100K"], "as": ["n<1K"], "br": ["10K<n<100K"], "ca": ["100K<n<1M"], "cnh": ["1K<n<10K"], "cs": ["10K<n<100K"], "cv": ["10K<n<100K"], "cy": ["10K<n<100K"], "de": ["100K<n<1M"], "dv": ["10K<n<100K"], "el": ["10K<n<100K"], "en": ["1M<n<10M"], "eo": ["10K<n<100K"], "es": ["100K<n<1M"], "et": ["10K<n<100K"], "eu": ["10K<n<100K"], "fa": ["100K<n<1M"], "fi": ["1K<n<10K"], "fr": ["100K<n<1M"], "fy-NL": ["10K<n<100K"], "ga-IE": ["1K<n<10K"], "hi": ["n<1K"], "hsb": ["1K<n<10K"], "hu": ["1K<n<10K"], "ia": ["1K<n<10K"], "id": ["10K<n<100K"], "it": ["100K<n<1M"], "ja": ["1K<n<10K"], "ka": ["1K<n<10K"], "kab": ["100K<n<1M"], "ky": ["10K<n<100K"], "lg": ["1K<n<10K"], "lt": ["1K<n<10K"], "lv": ["1K<n<10K"], "mn": ["10K<n<100K"], "mt": ["10K<n<100K"], "nl": ["10K<n<100K"], "or": ["1K<n<10K"], "pa-IN": ["1K<n<10K"], "pl": ["100K<n<1M"], "pt": ["10K<n<100K"], "rm-sursilv": ["1K<n<10K"], "rm-vallader": ["1K<n<10K"], "ro": ["1K<n<10K"], "ru": ["10K<n<100K"], "rw": ["1M<n<10M"], "sah": ["1K<n<10K"], "sl": ["1K<n<10K"], "sv-SE": ["10K<n<100K"], "ta": ["10K<n<100K"], "th": ["10K<n<100K"], "tr": ["10K<n<100K"], "tt": ["10K<n<100K"], "uk": ["10K<n<100K"], "vi": ["1K<n<10K"], "vot": ["n<1K"], "zh-CN": ["10K<n<100K"], "zh-HK": ["10K<n<100K"], "zh-TW": ["10K<n<100K"]}, "source_datasets": ["extended|common_voice"], "task_categories": ["automatic-speech-recognition"], "paperswithcode_id": "common-voice", "pretty_name": "Common Voice Corpus 6.0", "language_bcp47": ["ab", "ar", "as", "br", "ca", "cnh", "cs", "cv", "cy", "de", "dv", "el", "en", "eo", "es", "et", "eu", "fa", "fi", "fr", "fy-NL", "ga-IE", "hi", "hsb", "hu", "ia", "id", "it", "ja", "ka", "kab", "ky", "lg", "lt", "lv", "mn", "mt", "nl", "or", "pa-IN", "pl", "pt", "rm-sursilv", "rm-vallader", "ro", "ru", "rw", "sah", "sl", "sv-SE", "ta", "th", "tr", "tt", "uk", "vi", "vot", "zh-CN", "zh-HK", "zh-TW"], "extra_gated_prompt": "By clicking on \u201cAccess repository\u201d below, you also agree to not attempt to determine the identity of speakers in the Common Voice dataset."} | 2023-07-29T15:00:06+00:00 | [
"1912.06670"
] | [] | TAGS
#task_categories-automatic-speech-recognition #annotations_creators-crowdsourced #language_creators-crowdsourced #multilinguality-multilingual #source_datasets-extended|common_voice #license-cc0-1.0 #arxiv-1912.06670 #region-us
|
# Dataset Card for Common Voice Corpus 6.0
## Table of Contents
- Dataset Description
- Dataset Summary
- Supported Tasks and Leaderboards
- Languages
- Dataset Structure
- Data Instances
- Data Fields
- Data Splits
- Dataset Creation
- Curation Rationale
- Source Data
- Annotations
- Personal and Sensitive Information
- Considerations for Using the Data
- Social Impact of Dataset
- Discussion of Biases
- Other Known Limitations
- Additional Information
- Dataset Curators
- Licensing Information
- Citation Information
- Contributions
## Dataset Description
- Homepage: URL
- Repository: URL
- Paper: URL
- Leaderboard: URL
- Point of Contact: Anton Lozhkov
### Dataset Summary
The Common Voice dataset consists of a unique MP3 and corresponding text file.
Many of the 9261 recorded hours in the dataset also include demographic metadata like age, sex, and accent
that can help improve the accuracy of speech recognition engines.
The dataset currently consists of 7327 validated hours in 60 languages, but more voices and languages are always added.
Take a look at the Languages page to request a language or start contributing.
### Supported Tasks and Leaderboards
The results for models trained on the Common Voice datasets are available via the
Speech Bench
### Languages
## Dataset Structure
### Data Instances
A typical data point comprises the 'path' to the audio file and its 'sentence'.
Additional fields include 'accent', 'age', 'client_id', 'up_votes', 'down_votes', 'gender', 'locale' and 'segment'.
### Data Fields
'client_id' ('string'): An id for which client (voice) made the recording
'path' ('string'): The path to the audio file
'audio' ('dict'): A dictionary containing the path to the downloaded audio file, the decoded audio array, and the sampling rate. Note that when accessing the audio column: 'dataset[0]["audio"]' the audio file is automatically decoded and resampled to 'dataset.features["audio"].sampling_rate'. Decoding and resampling of a large number of audio files might take a significant amount of time. Thus it is important to first query the sample index before the '"audio"' column, *i.e.* 'dataset[0]["audio"]' should always be preferred over 'dataset["audio"][0]'.
'sentence' ('string'): The sentence the user was prompted to speak
'up_votes' ('int64'): How many upvotes the audio file has received from reviewers
'down_votes' ('int64'): How many downvotes the audio file has received from reviewers
'age' ('string'): The age of the speaker (e.g. 'teens', 'twenties', 'fifties')
'gender' ('string'): The gender of the speaker
'accent' ('string'): Accent of the speaker
'locale' ('string'): The locale of the speaker
'segment' ('string'): Usually an empty field
### Data Splits
The speech material has been subdivided into portions for dev, train, test, validated, invalidated, reported and other.
The validated data is data that has been validated with reviewers and received upvotes that the data is of high quality.
The invalidated data is data has been invalidated by reviewers
and received downvotes indicating that the data is of low quality.
The reported data is data that has been reported, for different reasons.
The other data is data that has not yet been reviewed.
The dev, test, train are all data that has been reviewed, deemed of high quality and split into dev, test and train.
## Data Preprocessing Recommended by Hugging Face
The following are data preprocessing steps advised by the Hugging Face team. They are accompanied by an example code snippet that shows how to put them to practice.
Many examples in this dataset have trailing quotations marks, e.g _“the cat sat on the mat.“_. These trailing quotation marks do not change the actual meaning of the sentence, and it is near impossible to infer whether a sentence is a quotation or not a quotation from audio data alone. In these cases, it is advised to strip the quotation marks, leaving: _the cat sat on the mat_.
In addition, the majority of training sentences end in punctuation ( . or ? or ! ), whereas just a small proportion do not. In the dev set, almost all sentences end in punctuation. Thus, it is recommended to append a full-stop ( . ) to the end of the small number of training examples that do not end in punctuation.
## Dataset Creation
### Curation Rationale
### Source Data
#### Initial Data Collection and Normalization
#### Who are the source language producers?
### Annotations
#### Annotation process
#### Who are the annotators?
### Personal and Sensitive Information
The dataset consists of people who have donated their voice online. You agree to not attempt to determine the identity of speakers in the Common Voice dataset.
## Considerations for Using the Data
### Social Impact of Dataset
The dataset consists of people who have donated their voice online. You agree to not attempt to determine the identity of speakers in the Common Voice dataset.
### Discussion of Biases
### Other Known Limitations
## Additional Information
### Dataset Curators
### Licensing Information
Public Domain, CC-0
| [
"# Dataset Card for Common Voice Corpus 6.0",
"## Table of Contents\n- Dataset Description\n - Dataset Summary\n - Supported Tasks and Leaderboards\n - Languages\n- Dataset Structure\n - Data Instances\n - Data Fields\n - Data Splits\n- Dataset Creation\n - Curation Rationale\n - Source Data\n - Annotations\n - Personal and Sensitive Information\n- Considerations for Using the Data\n - Social Impact of Dataset\n - Discussion of Biases\n - Other Known Limitations\n- Additional Information\n - Dataset Curators\n - Licensing Information\n - Citation Information\n - Contributions",
"## Dataset Description\n\n- Homepage: URL\n- Repository: URL\n- Paper: URL\n- Leaderboard: URL\n- Point of Contact: Anton Lozhkov",
"### Dataset Summary\n\nThe Common Voice dataset consists of a unique MP3 and corresponding text file. \nMany of the 9261 recorded hours in the dataset also include demographic metadata like age, sex, and accent \nthat can help improve the accuracy of speech recognition engines.\n\nThe dataset currently consists of 7327 validated hours in 60 languages, but more voices and languages are always added. \nTake a look at the Languages page to request a language or start contributing.",
"### Supported Tasks and Leaderboards\n\nThe results for models trained on the Common Voice datasets are available via the \n Speech Bench",
"### Languages",
"## Dataset Structure",
"### Data Instances\n\nA typical data point comprises the 'path' to the audio file and its 'sentence'. \nAdditional fields include 'accent', 'age', 'client_id', 'up_votes', 'down_votes', 'gender', 'locale' and 'segment'.",
"### Data Fields\n\n'client_id' ('string'): An id for which client (voice) made the recording\n\n'path' ('string'): The path to the audio file\n\n'audio' ('dict'): A dictionary containing the path to the downloaded audio file, the decoded audio array, and the sampling rate. Note that when accessing the audio column: 'dataset[0][\"audio\"]' the audio file is automatically decoded and resampled to 'dataset.features[\"audio\"].sampling_rate'. Decoding and resampling of a large number of audio files might take a significant amount of time. Thus it is important to first query the sample index before the '\"audio\"' column, *i.e.* 'dataset[0][\"audio\"]' should always be preferred over 'dataset[\"audio\"][0]'.\n\n'sentence' ('string'): The sentence the user was prompted to speak\n\n'up_votes' ('int64'): How many upvotes the audio file has received from reviewers\n\n'down_votes' ('int64'): How many downvotes the audio file has received from reviewers\n\n'age' ('string'): The age of the speaker (e.g. 'teens', 'twenties', 'fifties')\n\n'gender' ('string'): The gender of the speaker\n\n'accent' ('string'): Accent of the speaker\n\n'locale' ('string'): The locale of the speaker\n\n'segment' ('string'): Usually an empty field",
"### Data Splits\n\nThe speech material has been subdivided into portions for dev, train, test, validated, invalidated, reported and other.\n\nThe validated data is data that has been validated with reviewers and received upvotes that the data is of high quality.\n\nThe invalidated data is data has been invalidated by reviewers\nand received downvotes indicating that the data is of low quality.\n\nThe reported data is data that has been reported, for different reasons.\n\nThe other data is data that has not yet been reviewed.\n\nThe dev, test, train are all data that has been reviewed, deemed of high quality and split into dev, test and train.",
"## Data Preprocessing Recommended by Hugging Face\n\nThe following are data preprocessing steps advised by the Hugging Face team. They are accompanied by an example code snippet that shows how to put them to practice. \n\nMany examples in this dataset have trailing quotations marks, e.g _“the cat sat on the mat.“_. These trailing quotation marks do not change the actual meaning of the sentence, and it is near impossible to infer whether a sentence is a quotation or not a quotation from audio data alone. In these cases, it is advised to strip the quotation marks, leaving: _the cat sat on the mat_.\n\nIn addition, the majority of training sentences end in punctuation ( . or ? or ! ), whereas just a small proportion do not. In the dev set, almost all sentences end in punctuation. Thus, it is recommended to append a full-stop ( . ) to the end of the small number of training examples that do not end in punctuation.",
"## Dataset Creation",
"### Curation Rationale",
"### Source Data",
"#### Initial Data Collection and Normalization",
"#### Who are the source language producers?",
"### Annotations",
"#### Annotation process",
"#### Who are the annotators?",
"### Personal and Sensitive Information\n\nThe dataset consists of people who have donated their voice online. You agree to not attempt to determine the identity of speakers in the Common Voice dataset.",
"## Considerations for Using the Data",
"### Social Impact of Dataset\n\nThe dataset consists of people who have donated their voice online. You agree to not attempt to determine the identity of speakers in the Common Voice dataset.",
"### Discussion of Biases",
"### Other Known Limitations",
"## Additional Information",
"### Dataset Curators",
"### Licensing Information\n\nPublic Domain, CC-0"
] | [
"TAGS\n#task_categories-automatic-speech-recognition #annotations_creators-crowdsourced #language_creators-crowdsourced #multilinguality-multilingual #source_datasets-extended|common_voice #license-cc0-1.0 #arxiv-1912.06670 #region-us \n",
"# Dataset Card for Common Voice Corpus 6.0",
"## Table of Contents\n- Dataset Description\n - Dataset Summary\n - Supported Tasks and Leaderboards\n - Languages\n- Dataset Structure\n - Data Instances\n - Data Fields\n - Data Splits\n- Dataset Creation\n - Curation Rationale\n - Source Data\n - Annotations\n - Personal and Sensitive Information\n- Considerations for Using the Data\n - Social Impact of Dataset\n - Discussion of Biases\n - Other Known Limitations\n- Additional Information\n - Dataset Curators\n - Licensing Information\n - Citation Information\n - Contributions",
"## Dataset Description\n\n- Homepage: URL\n- Repository: URL\n- Paper: URL\n- Leaderboard: URL\n- Point of Contact: Anton Lozhkov",
"### Dataset Summary\n\nThe Common Voice dataset consists of a unique MP3 and corresponding text file. \nMany of the 9261 recorded hours in the dataset also include demographic metadata like age, sex, and accent \nthat can help improve the accuracy of speech recognition engines.\n\nThe dataset currently consists of 7327 validated hours in 60 languages, but more voices and languages are always added. \nTake a look at the Languages page to request a language or start contributing.",
"### Supported Tasks and Leaderboards\n\nThe results for models trained on the Common Voice datasets are available via the \n Speech Bench",
"### Languages",
"## Dataset Structure",
"### Data Instances\n\nA typical data point comprises the 'path' to the audio file and its 'sentence'. \nAdditional fields include 'accent', 'age', 'client_id', 'up_votes', 'down_votes', 'gender', 'locale' and 'segment'.",
"### Data Fields\n\n'client_id' ('string'): An id for which client (voice) made the recording\n\n'path' ('string'): The path to the audio file\n\n'audio' ('dict'): A dictionary containing the path to the downloaded audio file, the decoded audio array, and the sampling rate. Note that when accessing the audio column: 'dataset[0][\"audio\"]' the audio file is automatically decoded and resampled to 'dataset.features[\"audio\"].sampling_rate'. Decoding and resampling of a large number of audio files might take a significant amount of time. Thus it is important to first query the sample index before the '\"audio\"' column, *i.e.* 'dataset[0][\"audio\"]' should always be preferred over 'dataset[\"audio\"][0]'.\n\n'sentence' ('string'): The sentence the user was prompted to speak\n\n'up_votes' ('int64'): How many upvotes the audio file has received from reviewers\n\n'down_votes' ('int64'): How many downvotes the audio file has received from reviewers\n\n'age' ('string'): The age of the speaker (e.g. 'teens', 'twenties', 'fifties')\n\n'gender' ('string'): The gender of the speaker\n\n'accent' ('string'): Accent of the speaker\n\n'locale' ('string'): The locale of the speaker\n\n'segment' ('string'): Usually an empty field",
"### Data Splits\n\nThe speech material has been subdivided into portions for dev, train, test, validated, invalidated, reported and other.\n\nThe validated data is data that has been validated with reviewers and received upvotes that the data is of high quality.\n\nThe invalidated data is data has been invalidated by reviewers\nand received downvotes indicating that the data is of low quality.\n\nThe reported data is data that has been reported, for different reasons.\n\nThe other data is data that has not yet been reviewed.\n\nThe dev, test, train are all data that has been reviewed, deemed of high quality and split into dev, test and train.",
"## Data Preprocessing Recommended by Hugging Face\n\nThe following are data preprocessing steps advised by the Hugging Face team. They are accompanied by an example code snippet that shows how to put them to practice. \n\nMany examples in this dataset have trailing quotations marks, e.g _“the cat sat on the mat.“_. These trailing quotation marks do not change the actual meaning of the sentence, and it is near impossible to infer whether a sentence is a quotation or not a quotation from audio data alone. In these cases, it is advised to strip the quotation marks, leaving: _the cat sat on the mat_.\n\nIn addition, the majority of training sentences end in punctuation ( . or ? or ! ), whereas just a small proportion do not. In the dev set, almost all sentences end in punctuation. Thus, it is recommended to append a full-stop ( . ) to the end of the small number of training examples that do not end in punctuation.",
"## Dataset Creation",
"### Curation Rationale",
"### Source Data",
"#### Initial Data Collection and Normalization",
"#### Who are the source language producers?",
"### Annotations",
"#### Annotation process",
"#### Who are the annotators?",
"### Personal and Sensitive Information\n\nThe dataset consists of people who have donated their voice online. You agree to not attempt to determine the identity of speakers in the Common Voice dataset.",
"## Considerations for Using the Data",
"### Social Impact of Dataset\n\nThe dataset consists of people who have donated their voice online. You agree to not attempt to determine the identity of speakers in the Common Voice dataset.",
"### Discussion of Biases",
"### Other Known Limitations",
"## Additional Information",
"### Dataset Curators",
"### Licensing Information\n\nPublic Domain, CC-0"
] |
7b7e1cbaa386bb3f5c4fbdb66b734bf699b51c33 |
# Dataset Card for Common Voice Corpus 6.1
## Table of Contents
- [Dataset Description](#dataset-description)
- [Dataset Summary](#dataset-summary)
- [Supported Tasks and Leaderboards](#supported-tasks-and-leaderboards)
- [Languages](#languages)
- [Dataset Structure](#dataset-structure)
- [Data Instances](#data-instances)
- [Data Fields](#data-fields)
- [Data Splits](#data-splits)
- [Dataset Creation](#dataset-creation)
- [Curation Rationale](#curation-rationale)
- [Source Data](#source-data)
- [Annotations](#annotations)
- [Personal and Sensitive Information](#personal-and-sensitive-information)
- [Considerations for Using the Data](#considerations-for-using-the-data)
- [Social Impact of Dataset](#social-impact-of-dataset)
- [Discussion of Biases](#discussion-of-biases)
- [Other Known Limitations](#other-known-limitations)
- [Additional Information](#additional-information)
- [Dataset Curators](#dataset-curators)
- [Licensing Information](#licensing-information)
- [Citation Information](#citation-information)
- [Contributions](#contributions)
## Dataset Description
- **Homepage:** https://commonvoice.mozilla.org/en/datasets
- **Repository:** https://github.com/common-voice/common-voice
- **Paper:** https://arxiv.org/abs/1912.06670
- **Leaderboard:** https://paperswithcode.com/dataset/common-voice
- **Point of Contact:** [Anton Lozhkov](mailto:[email protected])
### Dataset Summary
The Common Voice dataset consists of a unique MP3 and corresponding text file.
Many of the 9283 recorded hours in the dataset also include demographic metadata like age, sex, and accent
that can help improve the accuracy of speech recognition engines.
The dataset currently consists of 7335 validated hours in 60 languages, but more voices and languages are always added.
Take a look at the [Languages](https://commonvoice.mozilla.org/en/languages) page to request a language or start contributing.
### Supported Tasks and Leaderboards
The results for models trained on the Common Voice datasets are available via the
[🤗 Speech Bench](https://huggingface.co/spaces/huggingface/hf-speech-bench)
### Languages
```
Abkhaz, Arabic, Assamese, Basque, Breton, Catalan, Chinese (China), Chinese (Hong Kong), Chinese (Taiwan), Chuvash, Czech, Dhivehi, Dutch, English, Esperanto, Estonian, Finnish, French, Frisian, Georgian, German, Greek, Hakha Chin, Hindi, Hungarian, Indonesian, Interlingua, Irish, Italian, Japanese, Kabyle, Kinyarwanda, Kyrgyz, Latvian, Lithuanian, Luganda, Maltese, Mongolian, Odia, Persian, Polish, Portuguese, Punjabi, Romanian, Romansh Sursilvan, Romansh Vallader, Russian, Sakha, Slovenian, Sorbian, Upper, Spanish, Swedish, Tamil, Tatar, Thai, Turkish, Ukrainian, Vietnamese, Votic, Welsh
```
## Dataset Structure
### Data Instances
A typical data point comprises the `path` to the audio file and its `sentence`.
Additional fields include `accent`, `age`, `client_id`, `up_votes`, `down_votes`, `gender`, `locale` and `segment`.
```python
{
'client_id': 'd59478fbc1ee646a28a3c652a119379939123784d99131b865a89f8b21c81f69276c48bd574b81267d9d1a77b83b43e6d475a6cfc79c232ddbca946ae9c7afc5',
'path': 'et/clips/common_voice_et_18318995.mp3',
'audio': {
'path': 'et/clips/common_voice_et_18318995.mp3',
'array': array([-0.00048828, -0.00018311, -0.00137329, ..., 0.00079346, 0.00091553, 0.00085449], dtype=float32),
'sampling_rate': 48000
},
'sentence': 'Tasub kokku saada inimestega, keda tunned juba ammust ajast saati.',
'up_votes': 2,
'down_votes': 0,
'age': 'twenties',
'gender': 'male',
'accent': '',
'locale': 'et',
'segment': ''
}
```
### Data Fields
`client_id` (`string`): An id for which client (voice) made the recording
`path` (`string`): The path to the audio file
`audio` (`dict`): A dictionary containing the path to the downloaded audio file, the decoded audio array, and the sampling rate. Note that when accessing the audio column: `dataset[0]["audio"]` the audio file is automatically decoded and resampled to `dataset.features["audio"].sampling_rate`. Decoding and resampling of a large number of audio files might take a significant amount of time. Thus it is important to first query the sample index before the `"audio"` column, *i.e.* `dataset[0]["audio"]` should **always** be preferred over `dataset["audio"][0]`.
`sentence` (`string`): The sentence the user was prompted to speak
`up_votes` (`int64`): How many upvotes the audio file has received from reviewers
`down_votes` (`int64`): How many downvotes the audio file has received from reviewers
`age` (`string`): The age of the speaker (e.g. `teens`, `twenties`, `fifties`)
`gender` (`string`): The gender of the speaker
`accent` (`string`): Accent of the speaker
`locale` (`string`): The locale of the speaker
`segment` (`string`): Usually an empty field
### Data Splits
The speech material has been subdivided into portions for dev, train, test, validated, invalidated, reported and other.
The validated data is data that has been validated with reviewers and received upvotes that the data is of high quality.
The invalidated data is data has been invalidated by reviewers
and received downvotes indicating that the data is of low quality.
The reported data is data that has been reported, for different reasons.
The other data is data that has not yet been reviewed.
The dev, test, train are all data that has been reviewed, deemed of high quality and split into dev, test and train.
## Data Preprocessing Recommended by Hugging Face
The following are data preprocessing steps advised by the Hugging Face team. They are accompanied by an example code snippet that shows how to put them to practice.
Many examples in this dataset have trailing quotations marks, e.g _“the cat sat on the mat.“_. These trailing quotation marks do not change the actual meaning of the sentence, and it is near impossible to infer whether a sentence is a quotation or not a quotation from audio data alone. In these cases, it is advised to strip the quotation marks, leaving: _the cat sat on the mat_.
In addition, the majority of training sentences end in punctuation ( . or ? or ! ), whereas just a small proportion do not. In the dev set, **almost all** sentences end in punctuation. Thus, it is recommended to append a full-stop ( . ) to the end of the small number of training examples that do not end in punctuation.
```python
from datasets import load_dataset
ds = load_dataset("mozilla-foundation/common_voice_6_1", "en", use_auth_token=True)
def prepare_dataset(batch):
"""Function to preprocess the dataset with the .map method"""
transcription = batch["sentence"]
if transcription.startswith('"') and transcription.endswith('"'):
# we can remove trailing quotation marks as they do not affect the transcription
transcription = transcription[1:-1]
if transcription[-1] not in [".", "?", "!"]:
# append a full-stop to sentences that do not end in punctuation
transcription = transcription + "."
batch["sentence"] = transcription
return batch
ds = ds.map(prepare_dataset, desc="preprocess dataset")
```
## Dataset Creation
### Curation Rationale
[Needs More Information]
### Source Data
#### Initial Data Collection and Normalization
[Needs More Information]
#### Who are the source language producers?
[Needs More Information]
### Annotations
#### Annotation process
[Needs More Information]
#### Who are the annotators?
[Needs More Information]
### Personal and Sensitive Information
The dataset consists of people who have donated their voice online. You agree to not attempt to determine the identity of speakers in the Common Voice dataset.
## Considerations for Using the Data
### Social Impact of Dataset
The dataset consists of people who have donated their voice online. You agree to not attempt to determine the identity of speakers in the Common Voice dataset.
### Discussion of Biases
[More Information Needed]
### Other Known Limitations
[More Information Needed]
## Additional Information
### Dataset Curators
[More Information Needed]
### Licensing Information
Public Domain, [CC-0](https://creativecommons.org/share-your-work/public-domain/cc0/)
### Citation Information
```
@inproceedings{commonvoice:2020,
author = {Ardila, R. and Branson, M. and Davis, K. and Henretty, M. and Kohler, M. and Meyer, J. and Morais, R. and Saunders, L. and Tyers, F. M. and Weber, G.},
title = {Common Voice: A Massively-Multilingual Speech Corpus},
booktitle = {Proceedings of the 12th Conference on Language Resources and Evaluation (LREC 2020)},
pages = {4211--4215},
year = 2020
}
```
| mozilla-foundation/common_voice_6_1 | [
"task_categories:automatic-speech-recognition",
"annotations_creators:crowdsourced",
"language_creators:crowdsourced",
"multilinguality:multilingual",
"source_datasets:extended|common_voice",
"license:cc0-1.0",
"arxiv:1912.06670",
"region:us"
] | 2022-03-02T23:29:22+00:00 | {"annotations_creators": ["crowdsourced"], "language_creators": ["crowdsourced"], "license": ["cc0-1.0"], "multilinguality": ["multilingual"], "size_categories": {"ab": ["n<1K"], "ar": ["10K<n<100K"], "as": ["n<1K"], "br": ["10K<n<100K"], "ca": ["100K<n<1M"], "cnh": ["1K<n<10K"], "cs": ["10K<n<100K"], "cv": ["10K<n<100K"], "cy": ["10K<n<100K"], "de": ["100K<n<1M"], "dv": ["10K<n<100K"], "el": ["10K<n<100K"], "en": ["1M<n<10M"], "eo": ["10K<n<100K"], "es": ["100K<n<1M"], "et": ["10K<n<100K"], "eu": ["10K<n<100K"], "fa": ["100K<n<1M"], "fi": ["1K<n<10K"], "fr": ["100K<n<1M"], "fy-NL": ["10K<n<100K"], "ga-IE": ["1K<n<10K"], "hi": ["n<1K"], "hsb": ["1K<n<10K"], "hu": ["1K<n<10K"], "ia": ["1K<n<10K"], "id": ["10K<n<100K"], "it": ["100K<n<1M"], "ja": ["1K<n<10K"], "ka": ["1K<n<10K"], "kab": ["100K<n<1M"], "ky": ["10K<n<100K"], "lg": ["1K<n<10K"], "lt": ["1K<n<10K"], "lv": ["1K<n<10K"], "mn": ["10K<n<100K"], "mt": ["10K<n<100K"], "nl": ["10K<n<100K"], "or": ["1K<n<10K"], "pa-IN": ["1K<n<10K"], "pl": ["100K<n<1M"], "pt": ["10K<n<100K"], "rm-sursilv": ["1K<n<10K"], "rm-vallader": ["1K<n<10K"], "ro": ["1K<n<10K"], "ru": ["10K<n<100K"], "rw": ["1M<n<10M"], "sah": ["1K<n<10K"], "sl": ["1K<n<10K"], "sv-SE": ["10K<n<100K"], "ta": ["10K<n<100K"], "th": ["10K<n<100K"], "tr": ["10K<n<100K"], "tt": ["10K<n<100K"], "uk": ["10K<n<100K"], "vi": ["1K<n<10K"], "vot": ["n<1K"], "zh-CN": ["10K<n<100K"], "zh-HK": ["10K<n<100K"], "zh-TW": ["10K<n<100K"]}, "source_datasets": ["extended|common_voice"], "task_categories": ["automatic-speech-recognition"], "paperswithcode_id": "common-voice", "pretty_name": "Common Voice Corpus 6.1", "language_bcp47": ["ab", "ar", "as", "br", "ca", "cnh", "cs", "cv", "cy", "de", "dv", "el", "en", "eo", "es", "et", "eu", "fa", "fi", "fr", "fy-NL", "ga-IE", "hi", "hsb", "hu", "ia", "id", "it", "ja", "ka", "kab", "ky", "lg", "lt", "lv", "mn", "mt", "nl", "or", "pa-IN", "pl", "pt", "rm-sursilv", "rm-vallader", "ro", "ru", "rw", "sah", "sl", "sv-SE", "ta", "th", "tr", "tt", "uk", "vi", "vot", "zh-CN", "zh-HK", "zh-TW"], "extra_gated_prompt": "By clicking on \u201cAccess repository\u201d below, you also agree to not attempt to determine the identity of speakers in the Common Voice dataset."} | 2023-07-29T15:00:07+00:00 | [
"1912.06670"
] | [] | TAGS
#task_categories-automatic-speech-recognition #annotations_creators-crowdsourced #language_creators-crowdsourced #multilinguality-multilingual #source_datasets-extended|common_voice #license-cc0-1.0 #arxiv-1912.06670 #region-us
|
# Dataset Card for Common Voice Corpus 6.1
## Table of Contents
- Dataset Description
- Dataset Summary
- Supported Tasks and Leaderboards
- Languages
- Dataset Structure
- Data Instances
- Data Fields
- Data Splits
- Dataset Creation
- Curation Rationale
- Source Data
- Annotations
- Personal and Sensitive Information
- Considerations for Using the Data
- Social Impact of Dataset
- Discussion of Biases
- Other Known Limitations
- Additional Information
- Dataset Curators
- Licensing Information
- Citation Information
- Contributions
## Dataset Description
- Homepage: URL
- Repository: URL
- Paper: URL
- Leaderboard: URL
- Point of Contact: Anton Lozhkov
### Dataset Summary
The Common Voice dataset consists of a unique MP3 and corresponding text file.
Many of the 9283 recorded hours in the dataset also include demographic metadata like age, sex, and accent
that can help improve the accuracy of speech recognition engines.
The dataset currently consists of 7335 validated hours in 60 languages, but more voices and languages are always added.
Take a look at the Languages page to request a language or start contributing.
### Supported Tasks and Leaderboards
The results for models trained on the Common Voice datasets are available via the
Speech Bench
### Languages
## Dataset Structure
### Data Instances
A typical data point comprises the 'path' to the audio file and its 'sentence'.
Additional fields include 'accent', 'age', 'client_id', 'up_votes', 'down_votes', 'gender', 'locale' and 'segment'.
### Data Fields
'client_id' ('string'): An id for which client (voice) made the recording
'path' ('string'): The path to the audio file
'audio' ('dict'): A dictionary containing the path to the downloaded audio file, the decoded audio array, and the sampling rate. Note that when accessing the audio column: 'dataset[0]["audio"]' the audio file is automatically decoded and resampled to 'dataset.features["audio"].sampling_rate'. Decoding and resampling of a large number of audio files might take a significant amount of time. Thus it is important to first query the sample index before the '"audio"' column, *i.e.* 'dataset[0]["audio"]' should always be preferred over 'dataset["audio"][0]'.
'sentence' ('string'): The sentence the user was prompted to speak
'up_votes' ('int64'): How many upvotes the audio file has received from reviewers
'down_votes' ('int64'): How many downvotes the audio file has received from reviewers
'age' ('string'): The age of the speaker (e.g. 'teens', 'twenties', 'fifties')
'gender' ('string'): The gender of the speaker
'accent' ('string'): Accent of the speaker
'locale' ('string'): The locale of the speaker
'segment' ('string'): Usually an empty field
### Data Splits
The speech material has been subdivided into portions for dev, train, test, validated, invalidated, reported and other.
The validated data is data that has been validated with reviewers and received upvotes that the data is of high quality.
The invalidated data is data has been invalidated by reviewers
and received downvotes indicating that the data is of low quality.
The reported data is data that has been reported, for different reasons.
The other data is data that has not yet been reviewed.
The dev, test, train are all data that has been reviewed, deemed of high quality and split into dev, test and train.
## Data Preprocessing Recommended by Hugging Face
The following are data preprocessing steps advised by the Hugging Face team. They are accompanied by an example code snippet that shows how to put them to practice.
Many examples in this dataset have trailing quotations marks, e.g _“the cat sat on the mat.“_. These trailing quotation marks do not change the actual meaning of the sentence, and it is near impossible to infer whether a sentence is a quotation or not a quotation from audio data alone. In these cases, it is advised to strip the quotation marks, leaving: _the cat sat on the mat_.
In addition, the majority of training sentences end in punctuation ( . or ? or ! ), whereas just a small proportion do not. In the dev set, almost all sentences end in punctuation. Thus, it is recommended to append a full-stop ( . ) to the end of the small number of training examples that do not end in punctuation.
## Dataset Creation
### Curation Rationale
### Source Data
#### Initial Data Collection and Normalization
#### Who are the source language producers?
### Annotations
#### Annotation process
#### Who are the annotators?
### Personal and Sensitive Information
The dataset consists of people who have donated their voice online. You agree to not attempt to determine the identity of speakers in the Common Voice dataset.
## Considerations for Using the Data
### Social Impact of Dataset
The dataset consists of people who have donated their voice online. You agree to not attempt to determine the identity of speakers in the Common Voice dataset.
### Discussion of Biases
### Other Known Limitations
## Additional Information
### Dataset Curators
### Licensing Information
Public Domain, CC-0
| [
"# Dataset Card for Common Voice Corpus 6.1",
"## Table of Contents\n- Dataset Description\n - Dataset Summary\n - Supported Tasks and Leaderboards\n - Languages\n- Dataset Structure\n - Data Instances\n - Data Fields\n - Data Splits\n- Dataset Creation\n - Curation Rationale\n - Source Data\n - Annotations\n - Personal and Sensitive Information\n- Considerations for Using the Data\n - Social Impact of Dataset\n - Discussion of Biases\n - Other Known Limitations\n- Additional Information\n - Dataset Curators\n - Licensing Information\n - Citation Information\n - Contributions",
"## Dataset Description\n\n- Homepage: URL\n- Repository: URL\n- Paper: URL\n- Leaderboard: URL\n- Point of Contact: Anton Lozhkov",
"### Dataset Summary\n\nThe Common Voice dataset consists of a unique MP3 and corresponding text file. \nMany of the 9283 recorded hours in the dataset also include demographic metadata like age, sex, and accent \nthat can help improve the accuracy of speech recognition engines.\n\nThe dataset currently consists of 7335 validated hours in 60 languages, but more voices and languages are always added. \nTake a look at the Languages page to request a language or start contributing.",
"### Supported Tasks and Leaderboards\n\nThe results for models trained on the Common Voice datasets are available via the \n Speech Bench",
"### Languages",
"## Dataset Structure",
"### Data Instances\n\nA typical data point comprises the 'path' to the audio file and its 'sentence'. \nAdditional fields include 'accent', 'age', 'client_id', 'up_votes', 'down_votes', 'gender', 'locale' and 'segment'.",
"### Data Fields\n\n'client_id' ('string'): An id for which client (voice) made the recording\n\n'path' ('string'): The path to the audio file\n\n'audio' ('dict'): A dictionary containing the path to the downloaded audio file, the decoded audio array, and the sampling rate. Note that when accessing the audio column: 'dataset[0][\"audio\"]' the audio file is automatically decoded and resampled to 'dataset.features[\"audio\"].sampling_rate'. Decoding and resampling of a large number of audio files might take a significant amount of time. Thus it is important to first query the sample index before the '\"audio\"' column, *i.e.* 'dataset[0][\"audio\"]' should always be preferred over 'dataset[\"audio\"][0]'.\n\n'sentence' ('string'): The sentence the user was prompted to speak\n\n'up_votes' ('int64'): How many upvotes the audio file has received from reviewers\n\n'down_votes' ('int64'): How many downvotes the audio file has received from reviewers\n\n'age' ('string'): The age of the speaker (e.g. 'teens', 'twenties', 'fifties')\n\n'gender' ('string'): The gender of the speaker\n\n'accent' ('string'): Accent of the speaker\n\n'locale' ('string'): The locale of the speaker\n\n'segment' ('string'): Usually an empty field",
"### Data Splits\n\nThe speech material has been subdivided into portions for dev, train, test, validated, invalidated, reported and other.\n\nThe validated data is data that has been validated with reviewers and received upvotes that the data is of high quality.\n\nThe invalidated data is data has been invalidated by reviewers\nand received downvotes indicating that the data is of low quality.\n\nThe reported data is data that has been reported, for different reasons.\n\nThe other data is data that has not yet been reviewed.\n\nThe dev, test, train are all data that has been reviewed, deemed of high quality and split into dev, test and train.",
"## Data Preprocessing Recommended by Hugging Face\n\nThe following are data preprocessing steps advised by the Hugging Face team. They are accompanied by an example code snippet that shows how to put them to practice. \n\nMany examples in this dataset have trailing quotations marks, e.g _“the cat sat on the mat.“_. These trailing quotation marks do not change the actual meaning of the sentence, and it is near impossible to infer whether a sentence is a quotation or not a quotation from audio data alone. In these cases, it is advised to strip the quotation marks, leaving: _the cat sat on the mat_.\n\nIn addition, the majority of training sentences end in punctuation ( . or ? or ! ), whereas just a small proportion do not. In the dev set, almost all sentences end in punctuation. Thus, it is recommended to append a full-stop ( . ) to the end of the small number of training examples that do not end in punctuation.",
"## Dataset Creation",
"### Curation Rationale",
"### Source Data",
"#### Initial Data Collection and Normalization",
"#### Who are the source language producers?",
"### Annotations",
"#### Annotation process",
"#### Who are the annotators?",
"### Personal and Sensitive Information\n\nThe dataset consists of people who have donated their voice online. You agree to not attempt to determine the identity of speakers in the Common Voice dataset.",
"## Considerations for Using the Data",
"### Social Impact of Dataset\n\nThe dataset consists of people who have donated their voice online. You agree to not attempt to determine the identity of speakers in the Common Voice dataset.",
"### Discussion of Biases",
"### Other Known Limitations",
"## Additional Information",
"### Dataset Curators",
"### Licensing Information\n\nPublic Domain, CC-0"
] | [
"TAGS\n#task_categories-automatic-speech-recognition #annotations_creators-crowdsourced #language_creators-crowdsourced #multilinguality-multilingual #source_datasets-extended|common_voice #license-cc0-1.0 #arxiv-1912.06670 #region-us \n",
"# Dataset Card for Common Voice Corpus 6.1",
"## Table of Contents\n- Dataset Description\n - Dataset Summary\n - Supported Tasks and Leaderboards\n - Languages\n- Dataset Structure\n - Data Instances\n - Data Fields\n - Data Splits\n- Dataset Creation\n - Curation Rationale\n - Source Data\n - Annotations\n - Personal and Sensitive Information\n- Considerations for Using the Data\n - Social Impact of Dataset\n - Discussion of Biases\n - Other Known Limitations\n- Additional Information\n - Dataset Curators\n - Licensing Information\n - Citation Information\n - Contributions",
"## Dataset Description\n\n- Homepage: URL\n- Repository: URL\n- Paper: URL\n- Leaderboard: URL\n- Point of Contact: Anton Lozhkov",
"### Dataset Summary\n\nThe Common Voice dataset consists of a unique MP3 and corresponding text file. \nMany of the 9283 recorded hours in the dataset also include demographic metadata like age, sex, and accent \nthat can help improve the accuracy of speech recognition engines.\n\nThe dataset currently consists of 7335 validated hours in 60 languages, but more voices and languages are always added. \nTake a look at the Languages page to request a language or start contributing.",
"### Supported Tasks and Leaderboards\n\nThe results for models trained on the Common Voice datasets are available via the \n Speech Bench",
"### Languages",
"## Dataset Structure",
"### Data Instances\n\nA typical data point comprises the 'path' to the audio file and its 'sentence'. \nAdditional fields include 'accent', 'age', 'client_id', 'up_votes', 'down_votes', 'gender', 'locale' and 'segment'.",
"### Data Fields\n\n'client_id' ('string'): An id for which client (voice) made the recording\n\n'path' ('string'): The path to the audio file\n\n'audio' ('dict'): A dictionary containing the path to the downloaded audio file, the decoded audio array, and the sampling rate. Note that when accessing the audio column: 'dataset[0][\"audio\"]' the audio file is automatically decoded and resampled to 'dataset.features[\"audio\"].sampling_rate'. Decoding and resampling of a large number of audio files might take a significant amount of time. Thus it is important to first query the sample index before the '\"audio\"' column, *i.e.* 'dataset[0][\"audio\"]' should always be preferred over 'dataset[\"audio\"][0]'.\n\n'sentence' ('string'): The sentence the user was prompted to speak\n\n'up_votes' ('int64'): How many upvotes the audio file has received from reviewers\n\n'down_votes' ('int64'): How many downvotes the audio file has received from reviewers\n\n'age' ('string'): The age of the speaker (e.g. 'teens', 'twenties', 'fifties')\n\n'gender' ('string'): The gender of the speaker\n\n'accent' ('string'): Accent of the speaker\n\n'locale' ('string'): The locale of the speaker\n\n'segment' ('string'): Usually an empty field",
"### Data Splits\n\nThe speech material has been subdivided into portions for dev, train, test, validated, invalidated, reported and other.\n\nThe validated data is data that has been validated with reviewers and received upvotes that the data is of high quality.\n\nThe invalidated data is data has been invalidated by reviewers\nand received downvotes indicating that the data is of low quality.\n\nThe reported data is data that has been reported, for different reasons.\n\nThe other data is data that has not yet been reviewed.\n\nThe dev, test, train are all data that has been reviewed, deemed of high quality and split into dev, test and train.",
"## Data Preprocessing Recommended by Hugging Face\n\nThe following are data preprocessing steps advised by the Hugging Face team. They are accompanied by an example code snippet that shows how to put them to practice. \n\nMany examples in this dataset have trailing quotations marks, e.g _“the cat sat on the mat.“_. These trailing quotation marks do not change the actual meaning of the sentence, and it is near impossible to infer whether a sentence is a quotation or not a quotation from audio data alone. In these cases, it is advised to strip the quotation marks, leaving: _the cat sat on the mat_.\n\nIn addition, the majority of training sentences end in punctuation ( . or ? or ! ), whereas just a small proportion do not. In the dev set, almost all sentences end in punctuation. Thus, it is recommended to append a full-stop ( . ) to the end of the small number of training examples that do not end in punctuation.",
"## Dataset Creation",
"### Curation Rationale",
"### Source Data",
"#### Initial Data Collection and Normalization",
"#### Who are the source language producers?",
"### Annotations",
"#### Annotation process",
"#### Who are the annotators?",
"### Personal and Sensitive Information\n\nThe dataset consists of people who have donated their voice online. You agree to not attempt to determine the identity of speakers in the Common Voice dataset.",
"## Considerations for Using the Data",
"### Social Impact of Dataset\n\nThe dataset consists of people who have donated their voice online. You agree to not attempt to determine the identity of speakers in the Common Voice dataset.",
"### Discussion of Biases",
"### Other Known Limitations",
"## Additional Information",
"### Dataset Curators",
"### Licensing Information\n\nPublic Domain, CC-0"
] |
73bc0bd17153e7c80a628030bc0d3c604c0112f0 |
# Dataset Card for Common Voice Corpus 7.0
## Table of Contents
- [Dataset Description](#dataset-description)
- [Dataset Summary](#dataset-summary)
- [Supported Tasks and Leaderboards](#supported-tasks-and-leaderboards)
- [Languages](#languages)
- [Dataset Structure](#dataset-structure)
- [Data Instances](#data-instances)
- [Data Fields](#data-fields)
- [Data Splits](#data-splits)
- [Dataset Creation](#dataset-creation)
- [Curation Rationale](#curation-rationale)
- [Source Data](#source-data)
- [Annotations](#annotations)
- [Personal and Sensitive Information](#personal-and-sensitive-information)
- [Considerations for Using the Data](#considerations-for-using-the-data)
- [Social Impact of Dataset](#social-impact-of-dataset)
- [Discussion of Biases](#discussion-of-biases)
- [Other Known Limitations](#other-known-limitations)
- [Additional Information](#additional-information)
- [Dataset Curators](#dataset-curators)
- [Licensing Information](#licensing-information)
- [Citation Information](#citation-information)
- [Contributions](#contributions)
## Dataset Description
- **Homepage:** https://commonvoice.mozilla.org/en/datasets
- **Repository:** https://github.com/common-voice/common-voice
- **Paper:** https://arxiv.org/abs/1912.06670
- **Leaderboard:** https://paperswithcode.com/dataset/common-voice
- **Point of Contact:** [Anton Lozhkov](mailto:[email protected])
### Dataset Summary
The Common Voice dataset consists of a unique MP3 and corresponding text file.
Many of the 13905 recorded hours in the dataset also include demographic metadata like age, sex, and accent
that can help improve the accuracy of speech recognition engines.
The dataset currently consists of 11192 validated hours in 76 languages, but more voices and languages are always added.
Take a look at the [Languages](https://commonvoice.mozilla.org/en/languages) page to request a language or start contributing.
### Supported Tasks and Leaderboards
The results for models trained on the Common Voice datasets are available via the
[🤗 Speech Bench](https://huggingface.co/spaces/huggingface/hf-speech-bench)
### Languages
```
Abkhaz, Arabic, Armenian, Assamese, Azerbaijani, Basaa, Bashkir, Basque, Belarusian, Breton, Bulgarian, Catalan, Chinese (China), Chinese (Hong Kong), Chinese (Taiwan), Chuvash, Czech, Dhivehi, Dutch, English, Esperanto, Estonian, Finnish, French, Frisian, Galician, Georgian, German, Greek, Guarani, Hakha Chin, Hausa, Hindi, Hungarian, Indonesian, Interlingua, Irish, Italian, Japanese, Kabyle, Kazakh, Kinyarwanda, Kurmanji Kurdish, Kyrgyz, Latvian, Lithuanian, Luganda, Maltese, Mongolian, Odia, Persian, Polish, Portuguese, Punjabi, Romanian, Romansh Sursilvan, Romansh Vallader, Russian, Sakha, Serbian, Slovak, Slovenian, Sorbian, Upper, Spanish, Swedish, Tamil, Tatar, Thai, Turkish, Ukrainian, Urdu, Uyghur, Uzbek, Vietnamese, Votic, Welsh
```
## Dataset Structure
### Data Instances
A typical data point comprises the `path` to the audio file and its `sentence`.
Additional fields include `accent`, `age`, `client_id`, `up_votes`, `down_votes`, `gender`, `locale` and `segment`.
```python
{
'client_id': 'd59478fbc1ee646a28a3c652a119379939123784d99131b865a89f8b21c81f69276c48bd574b81267d9d1a77b83b43e6d475a6cfc79c232ddbca946ae9c7afc5',
'path': 'et/clips/common_voice_et_18318995.mp3',
'audio': {
'path': 'et/clips/common_voice_et_18318995.mp3',
'array': array([-0.00048828, -0.00018311, -0.00137329, ..., 0.00079346, 0.00091553, 0.00085449], dtype=float32),
'sampling_rate': 48000
},
'sentence': 'Tasub kokku saada inimestega, keda tunned juba ammust ajast saati.',
'up_votes': 2,
'down_votes': 0,
'age': 'twenties',
'gender': 'male',
'accent': '',
'locale': 'et',
'segment': ''
}
```
### Data Fields
`client_id` (`string`): An id for which client (voice) made the recording
`path` (`string`): The path to the audio file
`audio` (`dict`): A dictionary containing the path to the downloaded audio file, the decoded audio array, and the sampling rate. Note that when accessing the audio column: `dataset[0]["audio"]` the audio file is automatically decoded and resampled to `dataset.features["audio"].sampling_rate`. Decoding and resampling of a large number of audio files might take a significant amount of time. Thus it is important to first query the sample index before the `"audio"` column, *i.e.* `dataset[0]["audio"]` should **always** be preferred over `dataset["audio"][0]`.
`sentence` (`string`): The sentence the user was prompted to speak
`up_votes` (`int64`): How many upvotes the audio file has received from reviewers
`down_votes` (`int64`): How many downvotes the audio file has received from reviewers
`age` (`string`): The age of the speaker (e.g. `teens`, `twenties`, `fifties`)
`gender` (`string`): The gender of the speaker
`accent` (`string`): Accent of the speaker
`locale` (`string`): The locale of the speaker
`segment` (`string`): Usually an empty field
### Data Splits
The speech material has been subdivided into portions for dev, train, test, validated, invalidated, reported and other.
The validated data is data that has been validated with reviewers and received upvotes that the data is of high quality.
The invalidated data is data has been invalidated by reviewers
and received downvotes indicating that the data is of low quality.
The reported data is data that has been reported, for different reasons.
The other data is data that has not yet been reviewed.
The dev, test, train are all data that has been reviewed, deemed of high quality and split into dev, test and train.
## Data Preprocessing Recommended by Hugging Face
The following are data preprocessing steps advised by the Hugging Face team. They are accompanied by an example code snippet that shows how to put them to practice.
Many examples in this dataset have trailing quotations marks, e.g _“the cat sat on the mat.“_. These trailing quotation marks do not change the actual meaning of the sentence, and it is near impossible to infer whether a sentence is a quotation or not a quotation from audio data alone. In these cases, it is advised to strip the quotation marks, leaving: _the cat sat on the mat_.
In addition, the majority of training sentences end in punctuation ( . or ? or ! ), whereas just a small proportion do not. In the dev set, **almost all** sentences end in punctuation. Thus, it is recommended to append a full-stop ( . ) to the end of the small number of training examples that do not end in punctuation.
```python
from datasets import load_dataset
ds = load_dataset("mozilla-foundation/common_voice_7_0", "en", use_auth_token=True)
def prepare_dataset(batch):
"""Function to preprocess the dataset with the .map method"""
transcription = batch["sentence"]
if transcription.startswith('"') and transcription.endswith('"'):
# we can remove trailing quotation marks as they do not affect the transcription
transcription = transcription[1:-1]
if transcription[-1] not in [".", "?", "!"]:
# append a full-stop to sentences that do not end in punctuation
transcription = transcription + "."
batch["sentence"] = transcription
return batch
ds = ds.map(prepare_dataset, desc="preprocess dataset")
```
## Dataset Creation
### Curation Rationale
[Needs More Information]
### Source Data
#### Initial Data Collection and Normalization
[Needs More Information]
#### Who are the source language producers?
[Needs More Information]
### Annotations
#### Annotation process
[Needs More Information]
#### Who are the annotators?
[Needs More Information]
### Personal and Sensitive Information
The dataset consists of people who have donated their voice online. You agree to not attempt to determine the identity of speakers in the Common Voice dataset.
## Considerations for Using the Data
### Social Impact of Dataset
The dataset consists of people who have donated their voice online. You agree to not attempt to determine the identity of speakers in the Common Voice dataset.
### Discussion of Biases
[More Information Needed]
### Other Known Limitations
[More Information Needed]
## Additional Information
### Dataset Curators
[More Information Needed]
### Licensing Information
Public Domain, [CC-0](https://creativecommons.org/share-your-work/public-domain/cc0/)
### Citation Information
```
@inproceedings{commonvoice:2020,
author = {Ardila, R. and Branson, M. and Davis, K. and Henretty, M. and Kohler, M. and Meyer, J. and Morais, R. and Saunders, L. and Tyers, F. M. and Weber, G.},
title = {Common Voice: A Massively-Multilingual Speech Corpus},
booktitle = {Proceedings of the 12th Conference on Language Resources and Evaluation (LREC 2020)},
pages = {4211--4215},
year = 2020
}
```
| mozilla-foundation/common_voice_7_0 | [
"task_categories:automatic-speech-recognition",
"annotations_creators:crowdsourced",
"language_creators:crowdsourced",
"multilinguality:multilingual",
"source_datasets:extended|common_voice",
"license:cc0-1.0",
"arxiv:1912.06670",
"region:us"
] | 2022-03-02T23:29:22+00:00 | {"annotations_creators": ["crowdsourced"], "language_creators": ["crowdsourced"], "license": ["cc0-1.0"], "multilinguality": ["multilingual"], "size_categories": {"ab": ["1K<n<10K"], "ar": ["100K<n<1M"], "as": ["n<1K"], "az": ["n<1K"], "ba": ["100K<n<1M"], "bas": ["1K<n<10K"], "be": ["100K<n<1M"], "bg": ["1K<n<10K"], "br": ["10K<n<100K"], "ca": ["100K<n<1M"], "cnh": ["1K<n<10K"], "cs": ["10K<n<100K"], "cv": ["10K<n<100K"], "cy": ["100K<n<1M"], "de": ["100K<n<1M"], "dv": ["10K<n<100K"], "el": ["10K<n<100K"], "en": ["1M<n<10M"], "eo": ["100K<n<1M"], "es": ["100K<n<1M"], "et": ["10K<n<100K"], "eu": ["10K<n<100K"], "fa": ["100K<n<1M"], "fi": ["1K<n<10K"], "fr": ["100K<n<1M"], "fy-NL": ["10K<n<100K"], "ga-IE": ["1K<n<10K"], "gl": ["1K<n<10K"], "gn": ["1K<n<10K"], "ha": ["1K<n<10K"], "hi": ["1K<n<10K"], "hsb": ["1K<n<10K"], "hu": ["10K<n<100K"], "hy-AM": ["1K<n<10K"], "ia": ["10K<n<100K"], "id": ["10K<n<100K"], "it": ["100K<n<1M"], "ja": ["10K<n<100K"], "ka": ["1K<n<10K"], "kab": ["100K<n<1M"], "kk": ["1K<n<10K"], "kmr": ["10K<n<100K"], "ky": ["10K<n<100K"], "lg": ["10K<n<100K"], "lt": ["10K<n<100K"], "lv": ["1K<n<10K"], "mn": ["10K<n<100K"], "mt": ["10K<n<100K"], "nl": ["10K<n<100K"], "or": ["1K<n<10K"], "pa-IN": ["1K<n<10K"], "pl": ["100K<n<1M"], "pt": ["10K<n<100K"], "rm-sursilv": ["1K<n<10K"], "rm-vallader": ["1K<n<10K"], "ro": ["10K<n<100K"], "ru": ["100K<n<1M"], "rw": ["1M<n<10M"], "sah": ["1K<n<10K"], "sk": ["10K<n<100K"], "sl": ["1K<n<10K"], "sr": ["n<1K"], "sv-SE": ["10K<n<100K"], "ta": ["100K<n<1M"], "th": ["100K<n<1M"], "tr": ["10K<n<100K"], "tt": ["10K<n<100K"], "ug": ["10K<n<100K"], "uk": ["10K<n<100K"], "ur": ["1K<n<10K"], "uz": ["n<1K"], "vi": ["10K<n<100K"], "vot": ["n<1K"], "zh-CN": ["10K<n<100K"], "zh-HK": ["10K<n<100K"], "zh-TW": ["10K<n<100K"]}, "source_datasets": ["extended|common_voice"], "task_categories": ["automatic-speech-recognition"], "paperswithcode_id": "common-voice", "pretty_name": "Common Voice Corpus 7.0", "language_bcp47": ["ab", "ar", "as", "az", "ba", "bas", "be", "bg", "br", "ca", "cnh", "cs", "cv", "cy", "de", "dv", "el", "en", "eo", "es", "et", "eu", "fa", "fi", "fr", "fy-NL", "ga-IE", "gl", "gn", "ha", "hi", "hsb", "hu", "hy-AM", "ia", "id", "it", "ja", "ka", "kab", "kk", "kmr", "ky", "lg", "lt", "lv", "mn", "mt", "nl", "or", "pa-IN", "pl", "pt", "rm-sursilv", "rm-vallader", "ro", "ru", "rw", "sah", "sk", "sl", "sr", "sv-SE", "ta", "th", "tr", "tt", "ug", "uk", "ur", "uz", "vi", "vot", "zh-CN", "zh-HK", "zh-TW"], "extra_gated_prompt": "By clicking on \u201cAccess repository\u201d below, you also agree to not attempt to determine the identity of speakers in the Common Voice dataset."} | 2023-07-29T15:00:09+00:00 | [
"1912.06670"
] | [] | TAGS
#task_categories-automatic-speech-recognition #annotations_creators-crowdsourced #language_creators-crowdsourced #multilinguality-multilingual #source_datasets-extended|common_voice #license-cc0-1.0 #arxiv-1912.06670 #region-us
|
# Dataset Card for Common Voice Corpus 7.0
## Table of Contents
- Dataset Description
- Dataset Summary
- Supported Tasks and Leaderboards
- Languages
- Dataset Structure
- Data Instances
- Data Fields
- Data Splits
- Dataset Creation
- Curation Rationale
- Source Data
- Annotations
- Personal and Sensitive Information
- Considerations for Using the Data
- Social Impact of Dataset
- Discussion of Biases
- Other Known Limitations
- Additional Information
- Dataset Curators
- Licensing Information
- Citation Information
- Contributions
## Dataset Description
- Homepage: URL
- Repository: URL
- Paper: URL
- Leaderboard: URL
- Point of Contact: Anton Lozhkov
### Dataset Summary
The Common Voice dataset consists of a unique MP3 and corresponding text file.
Many of the 13905 recorded hours in the dataset also include demographic metadata like age, sex, and accent
that can help improve the accuracy of speech recognition engines.
The dataset currently consists of 11192 validated hours in 76 languages, but more voices and languages are always added.
Take a look at the Languages page to request a language or start contributing.
### Supported Tasks and Leaderboards
The results for models trained on the Common Voice datasets are available via the
Speech Bench
### Languages
## Dataset Structure
### Data Instances
A typical data point comprises the 'path' to the audio file and its 'sentence'.
Additional fields include 'accent', 'age', 'client_id', 'up_votes', 'down_votes', 'gender', 'locale' and 'segment'.
### Data Fields
'client_id' ('string'): An id for which client (voice) made the recording
'path' ('string'): The path to the audio file
'audio' ('dict'): A dictionary containing the path to the downloaded audio file, the decoded audio array, and the sampling rate. Note that when accessing the audio column: 'dataset[0]["audio"]' the audio file is automatically decoded and resampled to 'dataset.features["audio"].sampling_rate'. Decoding and resampling of a large number of audio files might take a significant amount of time. Thus it is important to first query the sample index before the '"audio"' column, *i.e.* 'dataset[0]["audio"]' should always be preferred over 'dataset["audio"][0]'.
'sentence' ('string'): The sentence the user was prompted to speak
'up_votes' ('int64'): How many upvotes the audio file has received from reviewers
'down_votes' ('int64'): How many downvotes the audio file has received from reviewers
'age' ('string'): The age of the speaker (e.g. 'teens', 'twenties', 'fifties')
'gender' ('string'): The gender of the speaker
'accent' ('string'): Accent of the speaker
'locale' ('string'): The locale of the speaker
'segment' ('string'): Usually an empty field
### Data Splits
The speech material has been subdivided into portions for dev, train, test, validated, invalidated, reported and other.
The validated data is data that has been validated with reviewers and received upvotes that the data is of high quality.
The invalidated data is data has been invalidated by reviewers
and received downvotes indicating that the data is of low quality.
The reported data is data that has been reported, for different reasons.
The other data is data that has not yet been reviewed.
The dev, test, train are all data that has been reviewed, deemed of high quality and split into dev, test and train.
## Data Preprocessing Recommended by Hugging Face
The following are data preprocessing steps advised by the Hugging Face team. They are accompanied by an example code snippet that shows how to put them to practice.
Many examples in this dataset have trailing quotations marks, e.g _“the cat sat on the mat.“_. These trailing quotation marks do not change the actual meaning of the sentence, and it is near impossible to infer whether a sentence is a quotation or not a quotation from audio data alone. In these cases, it is advised to strip the quotation marks, leaving: _the cat sat on the mat_.
In addition, the majority of training sentences end in punctuation ( . or ? or ! ), whereas just a small proportion do not. In the dev set, almost all sentences end in punctuation. Thus, it is recommended to append a full-stop ( . ) to the end of the small number of training examples that do not end in punctuation.
## Dataset Creation
### Curation Rationale
### Source Data
#### Initial Data Collection and Normalization
#### Who are the source language producers?
### Annotations
#### Annotation process
#### Who are the annotators?
### Personal and Sensitive Information
The dataset consists of people who have donated their voice online. You agree to not attempt to determine the identity of speakers in the Common Voice dataset.
## Considerations for Using the Data
### Social Impact of Dataset
The dataset consists of people who have donated their voice online. You agree to not attempt to determine the identity of speakers in the Common Voice dataset.
### Discussion of Biases
### Other Known Limitations
## Additional Information
### Dataset Curators
### Licensing Information
Public Domain, CC-0
| [
"# Dataset Card for Common Voice Corpus 7.0",
"## Table of Contents\n- Dataset Description\n - Dataset Summary\n - Supported Tasks and Leaderboards\n - Languages\n- Dataset Structure\n - Data Instances\n - Data Fields\n - Data Splits\n- Dataset Creation\n - Curation Rationale\n - Source Data\n - Annotations\n - Personal and Sensitive Information\n- Considerations for Using the Data\n - Social Impact of Dataset\n - Discussion of Biases\n - Other Known Limitations\n- Additional Information\n - Dataset Curators\n - Licensing Information\n - Citation Information\n - Contributions",
"## Dataset Description\n\n- Homepage: URL\n- Repository: URL\n- Paper: URL\n- Leaderboard: URL\n- Point of Contact: Anton Lozhkov",
"### Dataset Summary\n\nThe Common Voice dataset consists of a unique MP3 and corresponding text file. \nMany of the 13905 recorded hours in the dataset also include demographic metadata like age, sex, and accent \nthat can help improve the accuracy of speech recognition engines.\n\nThe dataset currently consists of 11192 validated hours in 76 languages, but more voices and languages are always added. \nTake a look at the Languages page to request a language or start contributing.",
"### Supported Tasks and Leaderboards\n\nThe results for models trained on the Common Voice datasets are available via the \n Speech Bench",
"### Languages",
"## Dataset Structure",
"### Data Instances\n\nA typical data point comprises the 'path' to the audio file and its 'sentence'. \nAdditional fields include 'accent', 'age', 'client_id', 'up_votes', 'down_votes', 'gender', 'locale' and 'segment'.",
"### Data Fields\n\n'client_id' ('string'): An id for which client (voice) made the recording\n\n'path' ('string'): The path to the audio file\n\n'audio' ('dict'): A dictionary containing the path to the downloaded audio file, the decoded audio array, and the sampling rate. Note that when accessing the audio column: 'dataset[0][\"audio\"]' the audio file is automatically decoded and resampled to 'dataset.features[\"audio\"].sampling_rate'. Decoding and resampling of a large number of audio files might take a significant amount of time. Thus it is important to first query the sample index before the '\"audio\"' column, *i.e.* 'dataset[0][\"audio\"]' should always be preferred over 'dataset[\"audio\"][0]'.\n\n'sentence' ('string'): The sentence the user was prompted to speak\n\n'up_votes' ('int64'): How many upvotes the audio file has received from reviewers\n\n'down_votes' ('int64'): How many downvotes the audio file has received from reviewers\n\n'age' ('string'): The age of the speaker (e.g. 'teens', 'twenties', 'fifties')\n\n'gender' ('string'): The gender of the speaker\n\n'accent' ('string'): Accent of the speaker\n\n'locale' ('string'): The locale of the speaker\n\n'segment' ('string'): Usually an empty field",
"### Data Splits\n\nThe speech material has been subdivided into portions for dev, train, test, validated, invalidated, reported and other.\n\nThe validated data is data that has been validated with reviewers and received upvotes that the data is of high quality.\n\nThe invalidated data is data has been invalidated by reviewers\nand received downvotes indicating that the data is of low quality.\n\nThe reported data is data that has been reported, for different reasons.\n\nThe other data is data that has not yet been reviewed.\n\nThe dev, test, train are all data that has been reviewed, deemed of high quality and split into dev, test and train.",
"## Data Preprocessing Recommended by Hugging Face\n\nThe following are data preprocessing steps advised by the Hugging Face team. They are accompanied by an example code snippet that shows how to put them to practice. \n\nMany examples in this dataset have trailing quotations marks, e.g _“the cat sat on the mat.“_. These trailing quotation marks do not change the actual meaning of the sentence, and it is near impossible to infer whether a sentence is a quotation or not a quotation from audio data alone. In these cases, it is advised to strip the quotation marks, leaving: _the cat sat on the mat_.\n\nIn addition, the majority of training sentences end in punctuation ( . or ? or ! ), whereas just a small proportion do not. In the dev set, almost all sentences end in punctuation. Thus, it is recommended to append a full-stop ( . ) to the end of the small number of training examples that do not end in punctuation.",
"## Dataset Creation",
"### Curation Rationale",
"### Source Data",
"#### Initial Data Collection and Normalization",
"#### Who are the source language producers?",
"### Annotations",
"#### Annotation process",
"#### Who are the annotators?",
"### Personal and Sensitive Information\n\nThe dataset consists of people who have donated their voice online. You agree to not attempt to determine the identity of speakers in the Common Voice dataset.",
"## Considerations for Using the Data",
"### Social Impact of Dataset\n\nThe dataset consists of people who have donated their voice online. You agree to not attempt to determine the identity of speakers in the Common Voice dataset.",
"### Discussion of Biases",
"### Other Known Limitations",
"## Additional Information",
"### Dataset Curators",
"### Licensing Information\n\nPublic Domain, CC-0"
] | [
"TAGS\n#task_categories-automatic-speech-recognition #annotations_creators-crowdsourced #language_creators-crowdsourced #multilinguality-multilingual #source_datasets-extended|common_voice #license-cc0-1.0 #arxiv-1912.06670 #region-us \n",
"# Dataset Card for Common Voice Corpus 7.0",
"## Table of Contents\n- Dataset Description\n - Dataset Summary\n - Supported Tasks and Leaderboards\n - Languages\n- Dataset Structure\n - Data Instances\n - Data Fields\n - Data Splits\n- Dataset Creation\n - Curation Rationale\n - Source Data\n - Annotations\n - Personal and Sensitive Information\n- Considerations for Using the Data\n - Social Impact of Dataset\n - Discussion of Biases\n - Other Known Limitations\n- Additional Information\n - Dataset Curators\n - Licensing Information\n - Citation Information\n - Contributions",
"## Dataset Description\n\n- Homepage: URL\n- Repository: URL\n- Paper: URL\n- Leaderboard: URL\n- Point of Contact: Anton Lozhkov",
"### Dataset Summary\n\nThe Common Voice dataset consists of a unique MP3 and corresponding text file. \nMany of the 13905 recorded hours in the dataset also include demographic metadata like age, sex, and accent \nthat can help improve the accuracy of speech recognition engines.\n\nThe dataset currently consists of 11192 validated hours in 76 languages, but more voices and languages are always added. \nTake a look at the Languages page to request a language or start contributing.",
"### Supported Tasks and Leaderboards\n\nThe results for models trained on the Common Voice datasets are available via the \n Speech Bench",
"### Languages",
"## Dataset Structure",
"### Data Instances\n\nA typical data point comprises the 'path' to the audio file and its 'sentence'. \nAdditional fields include 'accent', 'age', 'client_id', 'up_votes', 'down_votes', 'gender', 'locale' and 'segment'.",
"### Data Fields\n\n'client_id' ('string'): An id for which client (voice) made the recording\n\n'path' ('string'): The path to the audio file\n\n'audio' ('dict'): A dictionary containing the path to the downloaded audio file, the decoded audio array, and the sampling rate. Note that when accessing the audio column: 'dataset[0][\"audio\"]' the audio file is automatically decoded and resampled to 'dataset.features[\"audio\"].sampling_rate'. Decoding and resampling of a large number of audio files might take a significant amount of time. Thus it is important to first query the sample index before the '\"audio\"' column, *i.e.* 'dataset[0][\"audio\"]' should always be preferred over 'dataset[\"audio\"][0]'.\n\n'sentence' ('string'): The sentence the user was prompted to speak\n\n'up_votes' ('int64'): How many upvotes the audio file has received from reviewers\n\n'down_votes' ('int64'): How many downvotes the audio file has received from reviewers\n\n'age' ('string'): The age of the speaker (e.g. 'teens', 'twenties', 'fifties')\n\n'gender' ('string'): The gender of the speaker\n\n'accent' ('string'): Accent of the speaker\n\n'locale' ('string'): The locale of the speaker\n\n'segment' ('string'): Usually an empty field",
"### Data Splits\n\nThe speech material has been subdivided into portions for dev, train, test, validated, invalidated, reported and other.\n\nThe validated data is data that has been validated with reviewers and received upvotes that the data is of high quality.\n\nThe invalidated data is data has been invalidated by reviewers\nand received downvotes indicating that the data is of low quality.\n\nThe reported data is data that has been reported, for different reasons.\n\nThe other data is data that has not yet been reviewed.\n\nThe dev, test, train are all data that has been reviewed, deemed of high quality and split into dev, test and train.",
"## Data Preprocessing Recommended by Hugging Face\n\nThe following are data preprocessing steps advised by the Hugging Face team. They are accompanied by an example code snippet that shows how to put them to practice. \n\nMany examples in this dataset have trailing quotations marks, e.g _“the cat sat on the mat.“_. These trailing quotation marks do not change the actual meaning of the sentence, and it is near impossible to infer whether a sentence is a quotation or not a quotation from audio data alone. In these cases, it is advised to strip the quotation marks, leaving: _the cat sat on the mat_.\n\nIn addition, the majority of training sentences end in punctuation ( . or ? or ! ), whereas just a small proportion do not. In the dev set, almost all sentences end in punctuation. Thus, it is recommended to append a full-stop ( . ) to the end of the small number of training examples that do not end in punctuation.",
"## Dataset Creation",
"### Curation Rationale",
"### Source Data",
"#### Initial Data Collection and Normalization",
"#### Who are the source language producers?",
"### Annotations",
"#### Annotation process",
"#### Who are the annotators?",
"### Personal and Sensitive Information\n\nThe dataset consists of people who have donated their voice online. You agree to not attempt to determine the identity of speakers in the Common Voice dataset.",
"## Considerations for Using the Data",
"### Social Impact of Dataset\n\nThe dataset consists of people who have donated their voice online. You agree to not attempt to determine the identity of speakers in the Common Voice dataset.",
"### Discussion of Biases",
"### Other Known Limitations",
"## Additional Information",
"### Dataset Curators",
"### Licensing Information\n\nPublic Domain, CC-0"
] |
791a941ef037d187d6cafb8d69da9bede3cce492 |
# Dataset Card for Common Voice Corpus 8.0
## Table of Contents
- [Dataset Description](#dataset-description)
- [Dataset Summary](#dataset-summary)
- [Supported Tasks and Leaderboards](#supported-tasks-and-leaderboards)
- [Languages](#languages)
- [Dataset Structure](#dataset-structure)
- [Data Instances](#data-instances)
- [Data Fields](#data-fields)
- [Data Splits](#data-splits)
- [Dataset Creation](#dataset-creation)
- [Curation Rationale](#curation-rationale)
- [Source Data](#source-data)
- [Annotations](#annotations)
- [Personal and Sensitive Information](#personal-and-sensitive-information)
- [Considerations for Using the Data](#considerations-for-using-the-data)
- [Social Impact of Dataset](#social-impact-of-dataset)
- [Discussion of Biases](#discussion-of-biases)
- [Other Known Limitations](#other-known-limitations)
- [Additional Information](#additional-information)
- [Dataset Curators](#dataset-curators)
- [Licensing Information](#licensing-information)
- [Citation Information](#citation-information)
- [Contributions](#contributions)
## Dataset Description
- **Homepage:** https://commonvoice.mozilla.org/en/datasets
- **Repository:** https://github.com/common-voice/common-voice
- **Paper:** https://arxiv.org/abs/1912.06670
- **Leaderboard:** https://paperswithcode.com/dataset/common-voice
- **Point of Contact:** [Anton Lozhkov](mailto:[email protected])
### Dataset Summary
The Common Voice dataset consists of a unique MP3 and corresponding text file.
Many of the 18243 recorded hours in the dataset also include demographic metadata like age, sex, and accent
that can help improve the accuracy of speech recognition engines.
The dataset currently consists of 14122 validated hours in 87 languages, but more voices and languages are always added.
Take a look at the [Languages](https://commonvoice.mozilla.org/en/languages) page to request a language or start contributing.
### Supported Tasks and Leaderboards
The results for models trained on the Common Voice datasets are available via the
[🤗 Speech Bench](https://huggingface.co/spaces/huggingface/hf-speech-bench)
### Languages
```
Abkhaz, Arabic, Armenian, Assamese, Azerbaijani, Basaa, Bashkir, Basque, Belarusian, Breton, Bulgarian, Catalan, Central Kurdish, Chinese (China), Chinese (Hong Kong), Chinese (Taiwan), Chuvash, Czech, Danish, Dhivehi, Dutch, English, Erzya, Esperanto, Estonian, Finnish, French, Frisian, Galician, Georgian, German, Greek, Guarani, Hakha Chin, Hausa, Hindi, Hungarian, Igbo, Indonesian, Interlingua, Irish, Italian, Japanese, Kabyle, Kazakh, Kinyarwanda, Kurmanji Kurdish, Kyrgyz, Latvian, Lithuanian, Luganda, Macedonian, Malayalam, Maltese, Marathi, Moksha, Mongolian, Norwegian Nynorsk, Odia, Persian, Polish, Portuguese, Punjabi, Romanian, Romansh Sursilvan, Romansh Vallader, Russian, Sakha, Santali (Ol Chiki), Serbian, Slovak, Slovenian, Sorbian, Upper, Spanish, Swahili, Swedish, Tamil, Tatar, Thai, Turkish, Ukrainian, Urdu, Uyghur, Uzbek, Vietnamese, Votic, Welsh
```
## Dataset Structure
### Data Instances
A typical data point comprises the `path` to the audio file and its `sentence`.
Additional fields include `accent`, `age`, `client_id`, `up_votes`, `down_votes`, `gender`, `locale` and `segment`.
```python
{
'client_id': 'd59478fbc1ee646a28a3c652a119379939123784d99131b865a89f8b21c81f69276c48bd574b81267d9d1a77b83b43e6d475a6cfc79c232ddbca946ae9c7afc5',
'path': 'et/clips/common_voice_et_18318995.mp3',
'audio': {
'path': 'et/clips/common_voice_et_18318995.mp3',
'array': array([-0.00048828, -0.00018311, -0.00137329, ..., 0.00079346, 0.00091553, 0.00085449], dtype=float32),
'sampling_rate': 48000
},
'sentence': 'Tasub kokku saada inimestega, keda tunned juba ammust ajast saati.',
'up_votes': 2,
'down_votes': 0,
'age': 'twenties',
'gender': 'male',
'accent': '',
'locale': 'et',
'segment': ''
}
```
### Data Fields
`client_id` (`string`): An id for which client (voice) made the recording
`path` (`string`): The path to the audio file
`audio` (`dict`): A dictionary containing the path to the downloaded audio file, the decoded audio array, and the sampling rate. Note that when accessing the audio column: `dataset[0]["audio"]` the audio file is automatically decoded and resampled to `dataset.features["audio"].sampling_rate`. Decoding and resampling of a large number of audio files might take a significant amount of time. Thus it is important to first query the sample index before the `"audio"` column, *i.e.* `dataset[0]["audio"]` should **always** be preferred over `dataset["audio"][0]`.
`sentence` (`string`): The sentence the user was prompted to speak
`up_votes` (`int64`): How many upvotes the audio file has received from reviewers
`down_votes` (`int64`): How many downvotes the audio file has received from reviewers
`age` (`string`): The age of the speaker (e.g. `teens`, `twenties`, `fifties`)
`gender` (`string`): The gender of the speaker
`accent` (`string`): Accent of the speaker
`locale` (`string`): The locale of the speaker
`segment` (`string`): Usually an empty field
### Data Splits
The speech material has been subdivided into portions for dev, train, test, validated, invalidated, reported and other.
The validated data is data that has been validated with reviewers and received upvotes that the data is of high quality.
The invalidated data is data has been invalidated by reviewers
and received downvotes indicating that the data is of low quality.
The reported data is data that has been reported, for different reasons.
The other data is data that has not yet been reviewed.
The dev, test, train are all data that has been reviewed, deemed of high quality and split into dev, test and train.
## Data Preprocessing Recommended by Hugging Face
The following are data preprocessing steps advised by the Hugging Face team. They are accompanied by an example code snippet that shows how to put them to practice.
Many examples in this dataset have trailing quotations marks, e.g _“the cat sat on the mat.“_. These trailing quotation marks do not change the actual meaning of the sentence, and it is near impossible to infer whether a sentence is a quotation or not a quotation from audio data alone. In these cases, it is advised to strip the quotation marks, leaving: _the cat sat on the mat_.
In addition, the majority of training sentences end in punctuation ( . or ? or ! ), whereas just a small proportion do not. In the dev set, **almost all** sentences end in punctuation. Thus, it is recommended to append a full-stop ( . ) to the end of the small number of training examples that do not end in punctuation.
```python
from datasets import load_dataset
ds = load_dataset("mozilla-foundation/common_voice_8_0", "en", use_auth_token=True)
def prepare_dataset(batch):
"""Function to preprocess the dataset with the .map method"""
transcription = batch["sentence"]
if transcription.startswith('"') and transcription.endswith('"'):
# we can remove trailing quotation marks as they do not affect the transcription
transcription = transcription[1:-1]
if transcription[-1] not in [".", "?", "!"]:
# append a full-stop to sentences that do not end in punctuation
transcription = transcription + "."
batch["sentence"] = transcription
return batch
ds = ds.map(prepare_dataset, desc="preprocess dataset")
```
## Dataset Creation
### Curation Rationale
[Needs More Information]
### Source Data
#### Initial Data Collection and Normalization
[Needs More Information]
#### Who are the source language producers?
[Needs More Information]
### Annotations
#### Annotation process
[Needs More Information]
#### Who are the annotators?
[Needs More Information]
### Personal and Sensitive Information
The dataset consists of people who have donated their voice online. You agree to not attempt to determine the identity of speakers in the Common Voice dataset.
## Considerations for Using the Data
### Social Impact of Dataset
The dataset consists of people who have donated their voice online. You agree to not attempt to determine the identity of speakers in the Common Voice dataset.
### Discussion of Biases
[More Information Needed]
### Other Known Limitations
[More Information Needed]
## Additional Information
### Dataset Curators
[More Information Needed]
### Licensing Information
Public Domain, [CC-0](https://creativecommons.org/share-your-work/public-domain/cc0/)
### Citation Information
```
@inproceedings{commonvoice:2020,
author = {Ardila, R. and Branson, M. and Davis, K. and Henretty, M. and Kohler, M. and Meyer, J. and Morais, R. and Saunders, L. and Tyers, F. M. and Weber, G.},
title = {Common Voice: A Massively-Multilingual Speech Corpus},
booktitle = {Proceedings of the 12th Conference on Language Resources and Evaluation (LREC 2020)},
pages = {4211--4215},
year = 2020
}
```
| mozilla-foundation/common_voice_8_0 | [
"task_categories:automatic-speech-recognition",
"annotations_creators:crowdsourced",
"language_creators:crowdsourced",
"multilinguality:multilingual",
"source_datasets:extended|common_voice",
"license:cc0-1.0",
"arxiv:1912.06670",
"region:us"
] | 2022-03-02T23:29:22+00:00 | {"annotations_creators": ["crowdsourced"], "language_creators": ["crowdsourced"], "license": ["cc0-1.0"], "multilinguality": ["multilingual"], "size_categories": {"ab": ["10K<n<100K"], "ar": ["100K<n<1M"], "as": ["n<1K"], "az": ["n<1K"], "ba": ["100K<n<1M"], "bas": ["1K<n<10K"], "be": ["100K<n<1M"], "bg": ["1K<n<10K"], "br": ["10K<n<100K"], "ca": ["100K<n<1M"], "ckb": ["10K<n<100K"], "cnh": ["1K<n<10K"], "cs": ["10K<n<100K"], "cv": ["10K<n<100K"], "cy": ["100K<n<1M"], "da": ["1K<n<10K"], "de": ["100K<n<1M"], "dv": ["10K<n<100K"], "el": ["10K<n<100K"], "en": ["1M<n<10M"], "eo": ["1M<n<10M"], "es": ["100K<n<1M"], "et": ["10K<n<100K"], "eu": ["100K<n<1M"], "fa": ["100K<n<1M"], "fi": ["10K<n<100K"], "fr": ["100K<n<1M"], "fy-NL": ["10K<n<100K"], "ga-IE": ["1K<n<10K"], "gl": ["10K<n<100K"], "gn": ["1K<n<10K"], "ha": ["1K<n<10K"], "hi": ["10K<n<100K"], "hsb": ["1K<n<10K"], "hu": ["10K<n<100K"], "hy-AM": ["1K<n<10K"], "ia": ["10K<n<100K"], "id": ["10K<n<100K"], "ig": ["n<1K"], "it": ["100K<n<1M"], "ja": ["10K<n<100K"], "ka": ["1K<n<10K"], "kab": ["100K<n<1M"], "kk": ["1K<n<10K"], "kmr": ["10K<n<100K"], "ky": ["10K<n<100K"], "lg": ["100K<n<1M"], "lt": ["10K<n<100K"], "lv": ["1K<n<10K"], "mdf": ["n<1K"], "mk": ["n<1K"], "ml": ["1K<n<10K"], "mn": ["10K<n<100K"], "mr": ["1K<n<10K"], "mt": ["10K<n<100K"], "myv": ["1K<n<10K"], "nl": ["10K<n<100K"], "nn-NO": ["n<1K"], "or": ["1K<n<10K"], "pa-IN": ["1K<n<10K"], "pl": ["100K<n<1M"], "pt": ["100K<n<1M"], "rm-sursilv": ["1K<n<10K"], "rm-vallader": ["1K<n<10K"], "ro": ["10K<n<100K"], "ru": ["100K<n<1M"], "rw": ["1M<n<10M"], "sah": ["1K<n<10K"], "sat": ["n<1K"], "sk": ["10K<n<100K"], "sl": ["10K<n<100K"], "sr": ["1K<n<10K"], "sv-SE": ["10K<n<100K"], "sw": ["100K<n<1M"], "ta": ["100K<n<1M"], "th": ["100K<n<1M"], "tr": ["10K<n<100K"], "tt": ["10K<n<100K"], "ug": ["10K<n<100K"], "uk": ["10K<n<100K"], "ur": ["1K<n<10K"], "uz": ["100K<n<1M"], "vi": ["10K<n<100K"], "vot": ["n<1K"], "zh-CN": ["10K<n<100K"], "zh-HK": ["100K<n<1M"], "zh-TW": ["10K<n<100K"]}, "source_datasets": ["extended|common_voice"], "task_categories": ["automatic-speech-recognition"], "paperswithcode_id": "common-voice", "pretty_name": "Common Voice Corpus 8.0", "language_bcp47": ["ab", "ar", "as", "az", "ba", "bas", "be", "bg", "br", "ca", "ckb", "cnh", "cs", "cv", "cy", "da", "de", "dv", "el", "en", "eo", "es", "et", "eu", "fa", "fi", "fr", "fy-NL", "ga-IE", "gl", "gn", "ha", "hi", "hsb", "hu", "hy-AM", "ia", "id", "ig", "it", "ja", "ka", "kab", "kk", "kmr", "ky", "lg", "lt", "lv", "mdf", "mk", "ml", "mn", "mr", "mt", "myv", "nl", "nn-NO", "or", "pa-IN", "pl", "pt", "rm-sursilv", "rm-vallader", "ro", "ru", "rw", "sah", "sat", "sk", "sl", "sr", "sv-SE", "sw", "ta", "th", "tr", "tt", "ug", "uk", "ur", "uz", "vi", "vot", "zh-CN", "zh-HK", "zh-TW"], "extra_gated_prompt": "By clicking on \u201cAccess repository\u201d below, you also agree to not attempt to determine the identity of speakers in the Common Voice dataset."} | 2023-07-29T15:00:11+00:00 | [
"1912.06670"
] | [] | TAGS
#task_categories-automatic-speech-recognition #annotations_creators-crowdsourced #language_creators-crowdsourced #multilinguality-multilingual #source_datasets-extended|common_voice #license-cc0-1.0 #arxiv-1912.06670 #region-us
|
# Dataset Card for Common Voice Corpus 8.0
## Table of Contents
- Dataset Description
- Dataset Summary
- Supported Tasks and Leaderboards
- Languages
- Dataset Structure
- Data Instances
- Data Fields
- Data Splits
- Dataset Creation
- Curation Rationale
- Source Data
- Annotations
- Personal and Sensitive Information
- Considerations for Using the Data
- Social Impact of Dataset
- Discussion of Biases
- Other Known Limitations
- Additional Information
- Dataset Curators
- Licensing Information
- Citation Information
- Contributions
## Dataset Description
- Homepage: URL
- Repository: URL
- Paper: URL
- Leaderboard: URL
- Point of Contact: Anton Lozhkov
### Dataset Summary
The Common Voice dataset consists of a unique MP3 and corresponding text file.
Many of the 18243 recorded hours in the dataset also include demographic metadata like age, sex, and accent
that can help improve the accuracy of speech recognition engines.
The dataset currently consists of 14122 validated hours in 87 languages, but more voices and languages are always added.
Take a look at the Languages page to request a language or start contributing.
### Supported Tasks and Leaderboards
The results for models trained on the Common Voice datasets are available via the
Speech Bench
### Languages
## Dataset Structure
### Data Instances
A typical data point comprises the 'path' to the audio file and its 'sentence'.
Additional fields include 'accent', 'age', 'client_id', 'up_votes', 'down_votes', 'gender', 'locale' and 'segment'.
### Data Fields
'client_id' ('string'): An id for which client (voice) made the recording
'path' ('string'): The path to the audio file
'audio' ('dict'): A dictionary containing the path to the downloaded audio file, the decoded audio array, and the sampling rate. Note that when accessing the audio column: 'dataset[0]["audio"]' the audio file is automatically decoded and resampled to 'dataset.features["audio"].sampling_rate'. Decoding and resampling of a large number of audio files might take a significant amount of time. Thus it is important to first query the sample index before the '"audio"' column, *i.e.* 'dataset[0]["audio"]' should always be preferred over 'dataset["audio"][0]'.
'sentence' ('string'): The sentence the user was prompted to speak
'up_votes' ('int64'): How many upvotes the audio file has received from reviewers
'down_votes' ('int64'): How many downvotes the audio file has received from reviewers
'age' ('string'): The age of the speaker (e.g. 'teens', 'twenties', 'fifties')
'gender' ('string'): The gender of the speaker
'accent' ('string'): Accent of the speaker
'locale' ('string'): The locale of the speaker
'segment' ('string'): Usually an empty field
### Data Splits
The speech material has been subdivided into portions for dev, train, test, validated, invalidated, reported and other.
The validated data is data that has been validated with reviewers and received upvotes that the data is of high quality.
The invalidated data is data has been invalidated by reviewers
and received downvotes indicating that the data is of low quality.
The reported data is data that has been reported, for different reasons.
The other data is data that has not yet been reviewed.
The dev, test, train are all data that has been reviewed, deemed of high quality and split into dev, test and train.
## Data Preprocessing Recommended by Hugging Face
The following are data preprocessing steps advised by the Hugging Face team. They are accompanied by an example code snippet that shows how to put them to practice.
Many examples in this dataset have trailing quotations marks, e.g _“the cat sat on the mat.“_. These trailing quotation marks do not change the actual meaning of the sentence, and it is near impossible to infer whether a sentence is a quotation or not a quotation from audio data alone. In these cases, it is advised to strip the quotation marks, leaving: _the cat sat on the mat_.
In addition, the majority of training sentences end in punctuation ( . or ? or ! ), whereas just a small proportion do not. In the dev set, almost all sentences end in punctuation. Thus, it is recommended to append a full-stop ( . ) to the end of the small number of training examples that do not end in punctuation.
## Dataset Creation
### Curation Rationale
### Source Data
#### Initial Data Collection and Normalization
#### Who are the source language producers?
### Annotations
#### Annotation process
#### Who are the annotators?
### Personal and Sensitive Information
The dataset consists of people who have donated their voice online. You agree to not attempt to determine the identity of speakers in the Common Voice dataset.
## Considerations for Using the Data
### Social Impact of Dataset
The dataset consists of people who have donated their voice online. You agree to not attempt to determine the identity of speakers in the Common Voice dataset.
### Discussion of Biases
### Other Known Limitations
## Additional Information
### Dataset Curators
### Licensing Information
Public Domain, CC-0
| [
"# Dataset Card for Common Voice Corpus 8.0",
"## Table of Contents\n- Dataset Description\n - Dataset Summary\n - Supported Tasks and Leaderboards\n - Languages\n- Dataset Structure\n - Data Instances\n - Data Fields\n - Data Splits\n- Dataset Creation\n - Curation Rationale\n - Source Data\n - Annotations\n - Personal and Sensitive Information\n- Considerations for Using the Data\n - Social Impact of Dataset\n - Discussion of Biases\n - Other Known Limitations\n- Additional Information\n - Dataset Curators\n - Licensing Information\n - Citation Information\n - Contributions",
"## Dataset Description\n\n- Homepage: URL\n- Repository: URL\n- Paper: URL\n- Leaderboard: URL\n- Point of Contact: Anton Lozhkov",
"### Dataset Summary\n\nThe Common Voice dataset consists of a unique MP3 and corresponding text file. \nMany of the 18243 recorded hours in the dataset also include demographic metadata like age, sex, and accent \nthat can help improve the accuracy of speech recognition engines.\n\nThe dataset currently consists of 14122 validated hours in 87 languages, but more voices and languages are always added. \nTake a look at the Languages page to request a language or start contributing.",
"### Supported Tasks and Leaderboards\n\nThe results for models trained on the Common Voice datasets are available via the \n Speech Bench",
"### Languages",
"## Dataset Structure",
"### Data Instances\n\nA typical data point comprises the 'path' to the audio file and its 'sentence'. \nAdditional fields include 'accent', 'age', 'client_id', 'up_votes', 'down_votes', 'gender', 'locale' and 'segment'.",
"### Data Fields\n\n'client_id' ('string'): An id for which client (voice) made the recording\n\n'path' ('string'): The path to the audio file\n\n'audio' ('dict'): A dictionary containing the path to the downloaded audio file, the decoded audio array, and the sampling rate. Note that when accessing the audio column: 'dataset[0][\"audio\"]' the audio file is automatically decoded and resampled to 'dataset.features[\"audio\"].sampling_rate'. Decoding and resampling of a large number of audio files might take a significant amount of time. Thus it is important to first query the sample index before the '\"audio\"' column, *i.e.* 'dataset[0][\"audio\"]' should always be preferred over 'dataset[\"audio\"][0]'.\n\n'sentence' ('string'): The sentence the user was prompted to speak\n\n'up_votes' ('int64'): How many upvotes the audio file has received from reviewers\n\n'down_votes' ('int64'): How many downvotes the audio file has received from reviewers\n\n'age' ('string'): The age of the speaker (e.g. 'teens', 'twenties', 'fifties')\n\n'gender' ('string'): The gender of the speaker\n\n'accent' ('string'): Accent of the speaker\n\n'locale' ('string'): The locale of the speaker\n\n'segment' ('string'): Usually an empty field",
"### Data Splits\n\nThe speech material has been subdivided into portions for dev, train, test, validated, invalidated, reported and other.\n\nThe validated data is data that has been validated with reviewers and received upvotes that the data is of high quality.\n\nThe invalidated data is data has been invalidated by reviewers\nand received downvotes indicating that the data is of low quality.\n\nThe reported data is data that has been reported, for different reasons.\n\nThe other data is data that has not yet been reviewed.\n\nThe dev, test, train are all data that has been reviewed, deemed of high quality and split into dev, test and train.",
"## Data Preprocessing Recommended by Hugging Face\n\nThe following are data preprocessing steps advised by the Hugging Face team. They are accompanied by an example code snippet that shows how to put them to practice. \n\nMany examples in this dataset have trailing quotations marks, e.g _“the cat sat on the mat.“_. These trailing quotation marks do not change the actual meaning of the sentence, and it is near impossible to infer whether a sentence is a quotation or not a quotation from audio data alone. In these cases, it is advised to strip the quotation marks, leaving: _the cat sat on the mat_.\n\nIn addition, the majority of training sentences end in punctuation ( . or ? or ! ), whereas just a small proportion do not. In the dev set, almost all sentences end in punctuation. Thus, it is recommended to append a full-stop ( . ) to the end of the small number of training examples that do not end in punctuation.",
"## Dataset Creation",
"### Curation Rationale",
"### Source Data",
"#### Initial Data Collection and Normalization",
"#### Who are the source language producers?",
"### Annotations",
"#### Annotation process",
"#### Who are the annotators?",
"### Personal and Sensitive Information\n\nThe dataset consists of people who have donated their voice online. You agree to not attempt to determine the identity of speakers in the Common Voice dataset.",
"## Considerations for Using the Data",
"### Social Impact of Dataset\n\nThe dataset consists of people who have donated their voice online. You agree to not attempt to determine the identity of speakers in the Common Voice dataset.",
"### Discussion of Biases",
"### Other Known Limitations",
"## Additional Information",
"### Dataset Curators",
"### Licensing Information\n\nPublic Domain, CC-0"
] | [
"TAGS\n#task_categories-automatic-speech-recognition #annotations_creators-crowdsourced #language_creators-crowdsourced #multilinguality-multilingual #source_datasets-extended|common_voice #license-cc0-1.0 #arxiv-1912.06670 #region-us \n",
"# Dataset Card for Common Voice Corpus 8.0",
"## Table of Contents\n- Dataset Description\n - Dataset Summary\n - Supported Tasks and Leaderboards\n - Languages\n- Dataset Structure\n - Data Instances\n - Data Fields\n - Data Splits\n- Dataset Creation\n - Curation Rationale\n - Source Data\n - Annotations\n - Personal and Sensitive Information\n- Considerations for Using the Data\n - Social Impact of Dataset\n - Discussion of Biases\n - Other Known Limitations\n- Additional Information\n - Dataset Curators\n - Licensing Information\n - Citation Information\n - Contributions",
"## Dataset Description\n\n- Homepage: URL\n- Repository: URL\n- Paper: URL\n- Leaderboard: URL\n- Point of Contact: Anton Lozhkov",
"### Dataset Summary\n\nThe Common Voice dataset consists of a unique MP3 and corresponding text file. \nMany of the 18243 recorded hours in the dataset also include demographic metadata like age, sex, and accent \nthat can help improve the accuracy of speech recognition engines.\n\nThe dataset currently consists of 14122 validated hours in 87 languages, but more voices and languages are always added. \nTake a look at the Languages page to request a language or start contributing.",
"### Supported Tasks and Leaderboards\n\nThe results for models trained on the Common Voice datasets are available via the \n Speech Bench",
"### Languages",
"## Dataset Structure",
"### Data Instances\n\nA typical data point comprises the 'path' to the audio file and its 'sentence'. \nAdditional fields include 'accent', 'age', 'client_id', 'up_votes', 'down_votes', 'gender', 'locale' and 'segment'.",
"### Data Fields\n\n'client_id' ('string'): An id for which client (voice) made the recording\n\n'path' ('string'): The path to the audio file\n\n'audio' ('dict'): A dictionary containing the path to the downloaded audio file, the decoded audio array, and the sampling rate. Note that when accessing the audio column: 'dataset[0][\"audio\"]' the audio file is automatically decoded and resampled to 'dataset.features[\"audio\"].sampling_rate'. Decoding and resampling of a large number of audio files might take a significant amount of time. Thus it is important to first query the sample index before the '\"audio\"' column, *i.e.* 'dataset[0][\"audio\"]' should always be preferred over 'dataset[\"audio\"][0]'.\n\n'sentence' ('string'): The sentence the user was prompted to speak\n\n'up_votes' ('int64'): How many upvotes the audio file has received from reviewers\n\n'down_votes' ('int64'): How many downvotes the audio file has received from reviewers\n\n'age' ('string'): The age of the speaker (e.g. 'teens', 'twenties', 'fifties')\n\n'gender' ('string'): The gender of the speaker\n\n'accent' ('string'): Accent of the speaker\n\n'locale' ('string'): The locale of the speaker\n\n'segment' ('string'): Usually an empty field",
"### Data Splits\n\nThe speech material has been subdivided into portions for dev, train, test, validated, invalidated, reported and other.\n\nThe validated data is data that has been validated with reviewers and received upvotes that the data is of high quality.\n\nThe invalidated data is data has been invalidated by reviewers\nand received downvotes indicating that the data is of low quality.\n\nThe reported data is data that has been reported, for different reasons.\n\nThe other data is data that has not yet been reviewed.\n\nThe dev, test, train are all data that has been reviewed, deemed of high quality and split into dev, test and train.",
"## Data Preprocessing Recommended by Hugging Face\n\nThe following are data preprocessing steps advised by the Hugging Face team. They are accompanied by an example code snippet that shows how to put them to practice. \n\nMany examples in this dataset have trailing quotations marks, e.g _“the cat sat on the mat.“_. These trailing quotation marks do not change the actual meaning of the sentence, and it is near impossible to infer whether a sentence is a quotation or not a quotation from audio data alone. In these cases, it is advised to strip the quotation marks, leaving: _the cat sat on the mat_.\n\nIn addition, the majority of training sentences end in punctuation ( . or ? or ! ), whereas just a small proportion do not. In the dev set, almost all sentences end in punctuation. Thus, it is recommended to append a full-stop ( . ) to the end of the small number of training examples that do not end in punctuation.",
"## Dataset Creation",
"### Curation Rationale",
"### Source Data",
"#### Initial Data Collection and Normalization",
"#### Who are the source language producers?",
"### Annotations",
"#### Annotation process",
"#### Who are the annotators?",
"### Personal and Sensitive Information\n\nThe dataset consists of people who have donated their voice online. You agree to not attempt to determine the identity of speakers in the Common Voice dataset.",
"## Considerations for Using the Data",
"### Social Impact of Dataset\n\nThe dataset consists of people who have donated their voice online. You agree to not attempt to determine the identity of speakers in the Common Voice dataset.",
"### Discussion of Biases",
"### Other Known Limitations",
"## Additional Information",
"### Dataset Curators",
"### Licensing Information\n\nPublic Domain, CC-0"
] |
649dd8041e8074738c81be5dce2c7ff6b32d7d4b | kmsdkmksm | mr-robot/ec | [
"region:us"
] | 2022-03-02T23:29:22+00:00 | {} | 2021-08-09T19:22:21+00:00 | [] | [] | TAGS
#region-us
| kmsdkmksm | [] | [
"TAGS\n#region-us \n"
] |
bd3ed9a7817b7a9f0742593ac893ab7b2dc2b996 | # GoEmotions
**GoEmotions** is a corpus of 58k carefully curated comments extracted from Reddit,
with human annotations to 27 emotion categories or Neutral.
* Number of examples: 58,009.
* Number of labels: 27 + Neutral.
* Maximum sequence length in training and evaluation datasets: 30.
On top of the raw data, we also include a version filtered based on reter-agreement, which contains a train/test/validation split:
* Size of training dataset: 43,410.
* Size of test dataset: 5,427.
* Size of validation dataset: 5,426.
The emotion categories are: _admiration, amusement, anger, annoyance, approval,
caring, confusion, curiosity, desire, disappointment, disapproval, disgust,
embarrassment, excitement, fear, gratitude, grief, joy, love, nervousness,
optimism, pride, realization, relief, remorse, sadness, surprise_.
For more details on the design and content of the dataset, please see our
[paper](https://arxiv.org/abs/2005.00547).
## Data
Our raw dataset can be retrieved by running:
```
wget -P data/full_dataset/ https://storage.googleapis.com/gresearch/goemotions/data/full_dataset/goemotions_1.csv
wget -P data/full_dataset/ https://storage.googleapis.com/gresearch/goemotions/data/full_dataset/goemotions_2.csv
wget -P data/full_dataset/ https://storage.googleapis.com/gresearch/goemotions/data/full_dataset/goemotions_3.csv
```
See the `data` folder for more detailed data information.
### Data Format
Our raw dataset, split into three csv files, includes all annotations as well as metadata on the comments. Each row represents a single rater's annotation for a single example. This file includes the following columns:
* `text`: The text of the comment (with masked tokens, as described in the paper).
* `id`: The unique id of the comment.
* `author`: The Reddit username of the comment's author.
* `subreddit`: The subreddit that the comment belongs to.
* `link_id`: The link id of the comment.
* `parent_id`: The parent id of the comment.
* `created_utc`: The timestamp of the comment.
* `rater_id`: The unique id of the annotator.
* `example_very_unclear`: Whether the annotator marked the example as being very unclear or difficult to label (in this case they did not choose any emotion labels).
* separate columns representing each of the emotion categories, with binary labels (0 or 1)
The data we used for training the models includes examples where there is agreement between at least 2 raters. Our data includes 43,410 training examples (`train.tsv`), 5426 dev examples (`dev.tsv`) and 5427 test examples (`test.tsv`). These files have _no header row_ and have the following columns:
1. text
2. comma-separated list of emotion ids (the ids are indexed based on the order of emotions in `emotions.txt`)
3. id of the comment
### Visualization
[Here](https://nlp.stanford.edu/~ddemszky/goemotions/tsne.html) you can view a TSNE projection showing a random sample of the data. The plot is generated using PPCA (see scripts below). Each point in the plot represents a single example and the text and the labels are shown on mouse-hover. The color of each point is the weighted average of the RGB values of the those emotions.
## Data Analysis
See each script for more documentation and descriptive command line flags.
* `python3 -m analyze_data`: get high-level statistics of the
data and correlation among emotion ratings.
* `python3 -m extract_words`: get the words that are significantly
associated with each emotion, in contrast to the other emotions, based on
their log odds ratio.
* `python3 -m ppca`: run PPCA
[(Cowen et al., 2019)](https://www.nature.com/articles/s41562-019-0533-6) on
the data and generate plots.
### Tutorial
We released a [detailed tutorial](https://github.com/tensorflow/models/blob/master/research/seq_flow_lite/demo/colab/emotion_colab.ipynb)
for training a neural emotion prediction model. In it, we work through training
a model architecture available on TensorFlow Model Garden using GoEmotions and
applying it for the task of suggesting emojis based on conversational text.
## Citation
If you use this code for your publication, please cite the original paper:
```
@inproceedings{demszky2020goemotions,
author = {Demszky, Dorottya and Movshovitz-Attias, Dana and Ko, Jeongwoo and Cowen, Alan and Nemade, Gaurav and Ravi, Sujith},
booktitle = {58th Annual Meeting of the Association for Computational Linguistics (ACL)},
title = {{GoEmotions: A Dataset of Fine-Grained Emotions}},
year = {2020}
}
```
## Contact
[Dora Demszky](https://nlp.stanford.edu/~ddemszky/index.html)
## Disclaimer
- We are aware that the dataset contains biases and is not representative of global diversity.
- We are aware that the dataset contains potentially problematic content.
- Potential biases in the data include: Inherent biases in Reddit and user base biases, the offensive/vulgar word lists used for data filtering, inherent or unconscious bias in assessment of offensive identity labels, annotators were all native English speakers from India. All these likely affect labelling, precision, and recall for a trained model.
- The emotion pilot model used for sentiment labeling, was trained on examples reviewed by the research team.
- Anyone using this dataset should be aware of these limitations of the dataset.
## Dataset Metadata
The following table is necessary for this dataset to be indexed by search
engines such as <a href="https://g.co/datasetsearch">Google Dataset Search</a>.
<div itemscope itemtype="http://schema.org/Dataset">
<table>
<tr>
<th>property</th>
<th>value</th>
</tr>
<tr>
<td>name</td>
<td><code itemprop="name">GoEmotions</code></td>
</tr>
<tr>
<td>description</td>
<td><code itemprop="description">GoEmotions contains 58k carefully curated Reddit comments labeled for 27 emotion categories or Neutral. The emotion categories are _admiration, amusement, anger, annoyance, approval, caring, confusion, curiosity, desire, disappointment, disapproval, disgust, embarrassment, excitement, fear, gratitude, grief, joy, love, nervousness, optimism, pride, realization, relief, remorse, sadness, surprise_.</code></td>
</tr>
<tr>
<td>sameAs</td>
<td><code itemprop="sameAs">https://github.com/google-research/google-research/tree/master/goemotions</code></td>
</tr>
<tr>
<td>citation</td>
<td><code itemprop="citation">https://identifiers.org/arxiv:2005.00547</code></td>
</tr>
<tr>
<td>provider</td>
<td>
<div itemscope="" itemtype="http://schema.org/Organization" itemprop="provider">
<table>
<tbody><tr>
<th>property</th>
<th>value</th>
</tr>
<tr>
<td>name</td>
<td><code itemprop="name">Google</code></td>
</tr>
<tr>
<td>sameAs</td>
<td><code itemprop="sameAs">https://en.wikipedia.org/wiki/Google</code></td>
</tr>
</tbody></table>
</div>
</td>
</tr>
</table>
</div> | mrm8488/goemotions | [
"arxiv:2005.00547",
"region:us"
] | 2022-03-02T23:29:22+00:00 | {} | 2021-12-28T17:49:54+00:00 | [
"2005.00547"
] | [] | TAGS
#arxiv-2005.00547 #region-us
| GoEmotions
==========
GoEmotions is a corpus of 58k carefully curated comments extracted from Reddit,
with human annotations to 27 emotion categories or Neutral.
* Number of examples: 58,009.
* Number of labels: 27 + Neutral.
* Maximum sequence length in training and evaluation datasets: 30.
On top of the raw data, we also include a version filtered based on reter-agreement, which contains a train/test/validation split:
* Size of training dataset: 43,410.
* Size of test dataset: 5,427.
* Size of validation dataset: 5,426.
The emotion categories are: *admiration, amusement, anger, annoyance, approval,
caring, confusion, curiosity, desire, disappointment, disapproval, disgust,
embarrassment, excitement, fear, gratitude, grief, joy, love, nervousness,
optimism, pride, realization, relief, remorse, sadness, surprise*.
For more details on the design and content of the dataset, please see our
paper.
Data
----
Our raw dataset can be retrieved by running:
See the 'data' folder for more detailed data information.
### Data Format
Our raw dataset, split into three csv files, includes all annotations as well as metadata on the comments. Each row represents a single rater's annotation for a single example. This file includes the following columns:
* 'text': The text of the comment (with masked tokens, as described in the paper).
* 'id': The unique id of the comment.
* 'author': The Reddit username of the comment's author.
* 'subreddit': The subreddit that the comment belongs to.
* 'link\_id': The link id of the comment.
* 'parent\_id': The parent id of the comment.
* 'created\_utc': The timestamp of the comment.
* 'rater\_id': The unique id of the annotator.
* 'example\_very\_unclear': Whether the annotator marked the example as being very unclear or difficult to label (in this case they did not choose any emotion labels).
* separate columns representing each of the emotion categories, with binary labels (0 or 1)
The data we used for training the models includes examples where there is agreement between at least 2 raters. Our data includes 43,410 training examples ('URL'), 5426 dev examples ('URL') and 5427 test examples ('URL'). These files have *no header row* and have the following columns:
1. text
2. comma-separated list of emotion ids (the ids are indexed based on the order of emotions in 'URL')
3. id of the comment
### Visualization
Here you can view a TSNE projection showing a random sample of the data. The plot is generated using PPCA (see scripts below). Each point in the plot represents a single example and the text and the labels are shown on mouse-hover. The color of each point is the weighted average of the RGB values of the those emotions.
Data Analysis
-------------
See each script for more documentation and descriptive command line flags.
* 'python3 -m analyze\_data': get high-level statistics of the
data and correlation among emotion ratings.
* 'python3 -m extract\_words': get the words that are significantly
associated with each emotion, in contrast to the other emotions, based on
their log odds ratio.
* 'python3 -m ppca': run PPCA
(Cowen et al., 2019) on
the data and generate plots.
### Tutorial
We released a detailed tutorial
for training a neural emotion prediction model. In it, we work through training
a model architecture available on TensorFlow Model Garden using GoEmotions and
applying it for the task of suggesting emojis based on conversational text.
If you use this code for your publication, please cite the original paper:
Contact
-------
Dora Demszky
Disclaimer
----------
* We are aware that the dataset contains biases and is not representative of global diversity.
* We are aware that the dataset contains potentially problematic content.
* Potential biases in the data include: Inherent biases in Reddit and user base biases, the offensive/vulgar word lists used for data filtering, inherent or unconscious bias in assessment of offensive identity labels, annotators were all native English speakers from India. All these likely affect labelling, precision, and recall for a trained model.
* The emotion pilot model used for sentiment labeling, was trained on examples reviewed by the research team.
* Anyone using this dataset should be aware of these limitations of the dataset.
Dataset Metadata
----------------
The following table is necessary for this dataset to be indexed by search
engines such as [Google Dataset Search](https://g.co/datasetsearch).
<div itemscope itemtype="URL
<p>
| [
"### Data Format\n\n\nOur raw dataset, split into three csv files, includes all annotations as well as metadata on the comments. Each row represents a single rater's annotation for a single example. This file includes the following columns:\n\n\n* 'text': The text of the comment (with masked tokens, as described in the paper).\n* 'id': The unique id of the comment.\n* 'author': The Reddit username of the comment's author.\n* 'subreddit': The subreddit that the comment belongs to.\n* 'link\\_id': The link id of the comment.\n* 'parent\\_id': The parent id of the comment.\n* 'created\\_utc': The timestamp of the comment.\n* 'rater\\_id': The unique id of the annotator.\n* 'example\\_very\\_unclear': Whether the annotator marked the example as being very unclear or difficult to label (in this case they did not choose any emotion labels).\n* separate columns representing each of the emotion categories, with binary labels (0 or 1)\n\n\nThe data we used for training the models includes examples where there is agreement between at least 2 raters. Our data includes 43,410 training examples ('URL'), 5426 dev examples ('URL') and 5427 test examples ('URL'). These files have *no header row* and have the following columns:\n\n\n1. text\n2. comma-separated list of emotion ids (the ids are indexed based on the order of emotions in 'URL')\n3. id of the comment",
"### Visualization\n\n\nHere you can view a TSNE projection showing a random sample of the data. The plot is generated using PPCA (see scripts below). Each point in the plot represents a single example and the text and the labels are shown on mouse-hover. The color of each point is the weighted average of the RGB values of the those emotions.\n\n\nData Analysis\n-------------\n\n\nSee each script for more documentation and descriptive command line flags.\n\n\n* 'python3 -m analyze\\_data': get high-level statistics of the\ndata and correlation among emotion ratings.\n* 'python3 -m extract\\_words': get the words that are significantly\nassociated with each emotion, in contrast to the other emotions, based on\ntheir log odds ratio.\n* 'python3 -m ppca': run PPCA\n(Cowen et al., 2019) on\nthe data and generate plots.",
"### Tutorial\n\n\nWe released a detailed tutorial\nfor training a neural emotion prediction model. In it, we work through training\na model architecture available on TensorFlow Model Garden using GoEmotions and\napplying it for the task of suggesting emojis based on conversational text.\n\n\nIf you use this code for your publication, please cite the original paper:\n\n\nContact\n-------\n\n\nDora Demszky\n\n\nDisclaimer\n----------\n\n\n* We are aware that the dataset contains biases and is not representative of global diversity.\n* We are aware that the dataset contains potentially problematic content.\n* Potential biases in the data include: Inherent biases in Reddit and user base biases, the offensive/vulgar word lists used for data filtering, inherent or unconscious bias in assessment of offensive identity labels, annotators were all native English speakers from India. All these likely affect labelling, precision, and recall for a trained model.\n* The emotion pilot model used for sentiment labeling, was trained on examples reviewed by the research team.\n* Anyone using this dataset should be aware of these limitations of the dataset.\n\n\nDataset Metadata\n----------------\n\n\nThe following table is necessary for this dataset to be indexed by search\nengines such as [Google Dataset Search](https://g.co/datasetsearch).\n\n\n<div itemscope itemtype=\"URL\n <p>"
] | [
"TAGS\n#arxiv-2005.00547 #region-us \n",
"### Data Format\n\n\nOur raw dataset, split into three csv files, includes all annotations as well as metadata on the comments. Each row represents a single rater's annotation for a single example. This file includes the following columns:\n\n\n* 'text': The text of the comment (with masked tokens, as described in the paper).\n* 'id': The unique id of the comment.\n* 'author': The Reddit username of the comment's author.\n* 'subreddit': The subreddit that the comment belongs to.\n* 'link\\_id': The link id of the comment.\n* 'parent\\_id': The parent id of the comment.\n* 'created\\_utc': The timestamp of the comment.\n* 'rater\\_id': The unique id of the annotator.\n* 'example\\_very\\_unclear': Whether the annotator marked the example as being very unclear or difficult to label (in this case they did not choose any emotion labels).\n* separate columns representing each of the emotion categories, with binary labels (0 or 1)\n\n\nThe data we used for training the models includes examples where there is agreement between at least 2 raters. Our data includes 43,410 training examples ('URL'), 5426 dev examples ('URL') and 5427 test examples ('URL'). These files have *no header row* and have the following columns:\n\n\n1. text\n2. comma-separated list of emotion ids (the ids are indexed based on the order of emotions in 'URL')\n3. id of the comment",
"### Visualization\n\n\nHere you can view a TSNE projection showing a random sample of the data. The plot is generated using PPCA (see scripts below). Each point in the plot represents a single example and the text and the labels are shown on mouse-hover. The color of each point is the weighted average of the RGB values of the those emotions.\n\n\nData Analysis\n-------------\n\n\nSee each script for more documentation and descriptive command line flags.\n\n\n* 'python3 -m analyze\\_data': get high-level statistics of the\ndata and correlation among emotion ratings.\n* 'python3 -m extract\\_words': get the words that are significantly\nassociated with each emotion, in contrast to the other emotions, based on\ntheir log odds ratio.\n* 'python3 -m ppca': run PPCA\n(Cowen et al., 2019) on\nthe data and generate plots.",
"### Tutorial\n\n\nWe released a detailed tutorial\nfor training a neural emotion prediction model. In it, we work through training\na model architecture available on TensorFlow Model Garden using GoEmotions and\napplying it for the task of suggesting emojis based on conversational text.\n\n\nIf you use this code for your publication, please cite the original paper:\n\n\nContact\n-------\n\n\nDora Demszky\n\n\nDisclaimer\n----------\n\n\n* We are aware that the dataset contains biases and is not representative of global diversity.\n* We are aware that the dataset contains potentially problematic content.\n* Potential biases in the data include: Inherent biases in Reddit and user base biases, the offensive/vulgar word lists used for data filtering, inherent or unconscious bias in assessment of offensive identity labels, annotators were all native English speakers from India. All these likely affect labelling, precision, and recall for a trained model.\n* The emotion pilot model used for sentiment labeling, was trained on examples reviewed by the research team.\n* Anyone using this dataset should be aware of these limitations of the dataset.\n\n\nDataset Metadata\n----------------\n\n\nThe following table is necessary for this dataset to be indexed by search\nengines such as [Google Dataset Search](https://g.co/datasetsearch).\n\n\n<div itemscope itemtype=\"URL\n <p>"
] |
f94bfc7e593334581ceb28962278ce43e0afe4af | Sentence representation plays a crucial role in NLP downstream tasks such as NLI, text classification, and STS. Recent sentence representation training techniques require NLI or STS datasets. However, there are no equivalent Thai NLI or STS datasets for sentence representation training.
To address this problem we provide the Thai sentence vector benchmark. We evaluate the Spearman correlation score of the sentence representations’ performance on Thai STS-B (translated version of [STS-B](https://github.com/facebookresearch/SentEval)).
# Thai semantic textual similarity benchmark
- We use [STS-B translated ver.](https://github.com/mrpeerat/Thai-Sentence-Vector-Benchmark/blob/main/sts-test_th.csv) in which we translate STS-B from [SentEval](https://github.com/facebookresearch/SentEval) by using google-translate.
- How to evaluate sentence representation: [SentEval.ipynb](https://github.com/mrpeerat/Thai-Sentence-Vector-Benchmark/blob/main/SentEval.ipynb)
- How to evaluate sentence representation on Google Colab: https://colab.research.google.com/github/mrpeerat/Thai-Sentence-Vector-Benchmark/blob/main/SentEval.ipynb
| Base Model | Spearman's Correlation (*100) | Supervised? |
| ------------- | :-------------: | :-------------: |
| [simcse-model-distil-m-bert](https://huggingface.co/mrp/simcse-model-distil-m-bert) | 38.84 |
| [simcse-model-m-bert-thai-cased](https://huggingface.co/mrp/simcse-model-m-bert-thai-cased) | 39.26 |
| [simcse-model-roberta-base-thai](https://huggingface.co/mrp/simcse-model-roberta-base-thai) | 62.60 |
| [distiluse-base-multilingual-cased-v2](https://huggingface.co/sentence-transformers/distiluse-base-multilingual-cased-v2) | 63.50 | ✓
| [paraphrase-multilingual-mpnet-base-v2](https://huggingface.co/sentence-transformers/paraphrase-multilingual-mpnet-base-v2) | 80.11 | ✓ | mrp/Thai-Semantic-Textual-Similarity-Benchmark | [
"region:us"
] | 2022-03-02T23:29:22+00:00 | {} | 2021-11-29T06:15:34+00:00 | [] | [] | TAGS
#region-us
| Sentence representation plays a crucial role in NLP downstream tasks such as NLI, text classification, and STS. Recent sentence representation training techniques require NLI or STS datasets. However, there are no equivalent Thai NLI or STS datasets for sentence representation training.
To address this problem we provide the Thai sentence vector benchmark. We evaluate the Spearman correlation score of the sentence representations’ performance on Thai STS-B (translated version of STS-B).
Thai semantic textual similarity benchmark
==========================================
* We use STS-B translated ver. in which we translate STS-B from SentEval by using google-translate.
* How to evaluate sentence representation: URL
* How to evaluate sentence representation on Google Colab: URL
| [] | [
"TAGS\n#region-us \n"
] |
d29aba88703a445763c2ff7344b2de28960d3554 |
# Dataset Card for english-korean-multitarget-ted-talks-task
## Table of Contents
- [Table of Contents](#table-of-contents)
- [Dataset Description](#dataset-description)
- [Dataset Summary](#dataset-summary)
- [Supported Tasks and Leaderboards](#supported-tasks-and-leaderboards)
- [Languages](#languages)
- [Dataset Structure](#dataset-structure)
- [Data Instances](#data-instances)
- [Data Fields](#data-fields)
- [Data Splits](#data-splits)
- [Dataset Creation](#dataset-creation)
- [Curation Rationale](#curation-rationale)
- [Source Data](#source-data)
- [Annotations](#annotations)
- [Personal and Sensitive Information](#personal-and-sensitive-information)
- [Considerations for Using the Data](#considerations-for-using-the-data)
- [Social Impact of Dataset](#social-impact-of-dataset)
- [Discussion of Biases](#discussion-of-biases)
- [Other Known Limitations](#other-known-limitations)
- [Additional Information](#additional-information)
- [Dataset Curators](#dataset-curators)
- [Licensing Information](#licensing-information)
- [Citation Information](#citation-information)
- [Contributions](#contributions)
## Dataset Description
- **Homepage:** https://www.cs.jhu.edu/~kevinduh/a/multitarget-tedtalks/
### Dataset Summary
- Parallel English-Korean Text Corpus
- Text was originally transcribed to English from various Ted Talks, then translated to Korean by TED translators
- Approximately 166k train, 2k validation, and 2k test sentence pairs.
### Supported Tasks and Leaderboards
- Machine Translation
### Languages
- English
- Korean
## Additional Information
### Dataset Curators
Kevin Duh, "The Multitarget TED Talks Task", http://www.cs.jhu.edu/~kevinduh/a/multitarget-tedtalks/, 2018
### Licensing Information
TED makes its collection available under the Creative Commons BY-NC-ND license. Please acknowledge TED when using this data. We acknowledge the authorship of TED Talks (BY condition). We are not redistributing the transcripts for commercial purposes (NC condition) nor making derivative works of the original contents (ND condition).
### Citation Information
@misc{duh18multitarget,
author = {Kevin Duh},
title = {The Multitarget TED Talks Task},
howpublished = {\url{http://www.cs.jhu.edu/~kevinduh/a/multitarget-tedtalks/}},
year = {2018},
} | msarmi9/korean-english-multitarget-ted-talks-task | [
"annotations_creators:expert-generated",
"language_creators:other",
"multilinguality:translation",
"multilinguality:multilingual",
"language:en",
"language:ko",
"license:cc-by-nc-nd-4.0",
"region:us"
] | 2022-03-02T23:29:22+00:00 | {"annotations_creators": ["expert-generated"], "language_creators": ["other"], "language": ["en", "ko"], "license": ["cc-by-nc-nd-4.0"], "multilinguality": ["translation", "multilingual"], "task_categories": ["conditional-text-generation"], "task_ids": ["machine-translation"], "pretty_name": "English-Korean Multitarget Ted Talks Task (MTTT)", "language_bcp47": ["en-US", "ko-KR"]} | 2022-10-22T14:05:15+00:00 | [] | [
"en",
"ko"
] | TAGS
#annotations_creators-expert-generated #language_creators-other #multilinguality-translation #multilinguality-multilingual #language-English #language-Korean #license-cc-by-nc-nd-4.0 #region-us
|
# Dataset Card for english-korean-multitarget-ted-talks-task
## Table of Contents
- Table of Contents
- Dataset Description
- Dataset Summary
- Supported Tasks and Leaderboards
- Languages
- Dataset Structure
- Data Instances
- Data Fields
- Data Splits
- Dataset Creation
- Curation Rationale
- Source Data
- Annotations
- Personal and Sensitive Information
- Considerations for Using the Data
- Social Impact of Dataset
- Discussion of Biases
- Other Known Limitations
- Additional Information
- Dataset Curators
- Licensing Information
- Citation Information
- Contributions
## Dataset Description
- Homepage: URL
### Dataset Summary
- Parallel English-Korean Text Corpus
- Text was originally transcribed to English from various Ted Talks, then translated to Korean by TED translators
- Approximately 166k train, 2k validation, and 2k test sentence pairs.
### Supported Tasks and Leaderboards
- Machine Translation
### Languages
- English
- Korean
## Additional Information
### Dataset Curators
Kevin Duh, "The Multitarget TED Talks Task", URL 2018
### Licensing Information
TED makes its collection available under the Creative Commons BY-NC-ND license. Please acknowledge TED when using this data. We acknowledge the authorship of TED Talks (BY condition). We are not redistributing the transcripts for commercial purposes (NC condition) nor making derivative works of the original contents (ND condition).
@misc{duh18multitarget,
author = {Kevin Duh},
title = {The Multitarget TED Talks Task},
howpublished = {\url{URL
year = {2018},
} | [
"# Dataset Card for english-korean-multitarget-ted-talks-task",
"## Table of Contents\n- Table of Contents\n- Dataset Description\n - Dataset Summary\n - Supported Tasks and Leaderboards\n - Languages\n- Dataset Structure\n - Data Instances\n - Data Fields\n - Data Splits\n- Dataset Creation\n - Curation Rationale\n - Source Data\n - Annotations\n - Personal and Sensitive Information\n- Considerations for Using the Data\n - Social Impact of Dataset\n - Discussion of Biases\n - Other Known Limitations\n- Additional Information\n - Dataset Curators\n - Licensing Information\n - Citation Information\n - Contributions",
"## Dataset Description\n\n- Homepage: URL",
"### Dataset Summary\n\n- Parallel English-Korean Text Corpus\n- Text was originally transcribed to English from various Ted Talks, then translated to Korean by TED translators\n- Approximately 166k train, 2k validation, and 2k test sentence pairs.",
"### Supported Tasks and Leaderboards\n\n- Machine Translation",
"### Languages\n\n- English\n- Korean",
"## Additional Information",
"### Dataset Curators\n\nKevin Duh, \"The Multitarget TED Talks Task\", URL 2018",
"### Licensing Information\n\nTED makes its collection available under the Creative Commons BY-NC-ND license. Please acknowledge TED when using this data. We acknowledge the authorship of TED Talks (BY condition). We are not redistributing the transcripts for commercial purposes (NC condition) nor making derivative works of the original contents (ND condition).\n\n\n\n@misc{duh18multitarget,\n author = {Kevin Duh},\n title = {The Multitarget TED Talks Task},\n howpublished = {\\url{URL\n year = {2018},\n}"
] | [
"TAGS\n#annotations_creators-expert-generated #language_creators-other #multilinguality-translation #multilinguality-multilingual #language-English #language-Korean #license-cc-by-nc-nd-4.0 #region-us \n",
"# Dataset Card for english-korean-multitarget-ted-talks-task",
"## Table of Contents\n- Table of Contents\n- Dataset Description\n - Dataset Summary\n - Supported Tasks and Leaderboards\n - Languages\n- Dataset Structure\n - Data Instances\n - Data Fields\n - Data Splits\n- Dataset Creation\n - Curation Rationale\n - Source Data\n - Annotations\n - Personal and Sensitive Information\n- Considerations for Using the Data\n - Social Impact of Dataset\n - Discussion of Biases\n - Other Known Limitations\n- Additional Information\n - Dataset Curators\n - Licensing Information\n - Citation Information\n - Contributions",
"## Dataset Description\n\n- Homepage: URL",
"### Dataset Summary\n\n- Parallel English-Korean Text Corpus\n- Text was originally transcribed to English from various Ted Talks, then translated to Korean by TED translators\n- Approximately 166k train, 2k validation, and 2k test sentence pairs.",
"### Supported Tasks and Leaderboards\n\n- Machine Translation",
"### Languages\n\n- English\n- Korean",
"## Additional Information",
"### Dataset Curators\n\nKevin Duh, \"The Multitarget TED Talks Task\", URL 2018",
"### Licensing Information\n\nTED makes its collection available under the Creative Commons BY-NC-ND license. Please acknowledge TED when using this data. We acknowledge the authorship of TED Talks (BY condition). We are not redistributing the transcripts for commercial purposes (NC condition) nor making derivative works of the original contents (ND condition).\n\n\n\n@misc{duh18multitarget,\n author = {Kevin Duh},\n title = {The Multitarget TED Talks Task},\n howpublished = {\\url{URL\n year = {2018},\n}"
] |
978a45cb5c1c78bb4e8f03d22c17138b38d6a15c | this is my test demo | mtfelix/datasetdemo | [
"region:us"
] | 2022-03-02T23:29:22+00:00 | {} | 2022-02-14T10:09:12+00:00 | [] | [] | TAGS
#region-us
| this is my test demo | [] | [
"TAGS\n#region-us \n"
] |
59a8c8289335ea176c54284b6462746b6dc4e9c3 | # AutoNLP Dataset for project: Doctor_DE
## Table of content
- [Dataset Description](#dataset-description)
- [Languages](#languages)
- [Dataset Structure](#dataset-structure)
- [Data Instances](#data-instances)
- [Data Fields](#data-fields)
- [Data Splits](#data-splits)
## Dataset Descritpion
This dataset has been automatically processed by AutoNLP for project Doctor_DE.
### Languages
The BCP-47 code for the dataset's language is de.
## Dataset Structure
### Data Instances
A sample from this dataset looks as follows:
```json
[
{
"text": "Ich bin nun seit ca 12 Jahren Patientin in dieser Praxis und kann einige der Kommentare hier ehrlich gesagt \u00fcberhaupt nicht nachvollziehen.<br />\nFr. Dr. Gr\u00f6ber Pohl ist in meinen Augen eine unglaublich nette und kompetente \u00c4rztin. Ich kenne in meinem Familien- und Bekanntenkreis viele die bei ihr in Behandlung sind, und alle sind sehr zufrieden!<br />\nSie nimmt sich immer viel Zeit und auch in meiner Schwangerschaft habe ich mich bei ihr immer gut versorgt gef\u00fchlt, und musste daf\u00fcr kein einziges Mal in die Tasche greifen!<br />\nDas einzig negative ist die lange Wartezeit in der Praxis. Daf\u00fcr nimmt sie sich aber auch Zeit und arbeitet nicht wie andere \u00c4rzte wie am Flie\u00dfband.<br />\nIch kann sie nur weiter empfehlen!",
"target": 1.0
},
{
"text": "Ich hatte nie den Eindruck \"Der N\u00e4chste bitte\" Er hatte sofort meine Beschwerden erkannt und Abhilfe geschafft.",
"target": 1.0
}
]
```
### Dataset Fields
The dataset has the following fields (also called "features"):
```json
{
"text": "Value(dtype='string', id=None)",
"target": "Value(dtype='float32', id=None)"
}
```
### Dataset Splits
This dataset is split into a train and validation split. The split sizes are as follow:
| Split name | Num samples |
| ------------ | ------------------- |
| train | 280191 |
| valid | 70050 |
| muhtasham/autonlp-data-Doctor_DE | [
"task_categories:text-classification",
"task_ids:text-scoring",
"language:de",
"region:us"
] | 2022-03-02T23:29:22+00:00 | {"language": ["de"], "task_categories": ["text-classification"], "task_ids": ["text-scoring"]} | 2022-10-27T17:52:42+00:00 | [] | [
"de"
] | TAGS
#task_categories-text-classification #task_ids-text-scoring #language-German #region-us
| AutoNLP Dataset for project: Doctor\_DE
=======================================
Table of content
----------------
* Dataset Description
+ Languages
* Dataset Structure
+ Data Instances
+ Data Fields
+ Data Splits
Dataset Descritpion
-------------------
This dataset has been automatically processed by AutoNLP for project Doctor\_DE.
### Languages
The BCP-47 code for the dataset's language is de.
Dataset Structure
-----------------
### Data Instances
A sample from this dataset looks as follows:
### Dataset Fields
The dataset has the following fields (also called "features"):
### Dataset Splits
This dataset is split into a train and validation split. The split sizes are as follow:
| [
"### Languages\n\n\nThe BCP-47 code for the dataset's language is de.\n\n\nDataset Structure\n-----------------",
"### Data Instances\n\n\nA sample from this dataset looks as follows:",
"### Dataset Fields\n\n\nThe dataset has the following fields (also called \"features\"):",
"### Dataset Splits\n\n\nThis dataset is split into a train and validation split. The split sizes are as follow:"
] | [
"TAGS\n#task_categories-text-classification #task_ids-text-scoring #language-German #region-us \n",
"### Languages\n\n\nThe BCP-47 code for the dataset's language is de.\n\n\nDataset Structure\n-----------------",
"### Data Instances\n\n\nA sample from this dataset looks as follows:",
"### Dataset Fields\n\n\nThe dataset has the following fields (also called \"features\"):",
"### Dataset Splits\n\n\nThis dataset is split into a train and validation split. The split sizes are as follow:"
] |
38479a7a477f2388e20048c6161dc3b122575ea9 |
# Dataset Card for Clean(maybe) Indonesia mC4
## Dataset Description
- **Original Homepage:** [HF Hub](https://huggingface.co/datasets/allenai/c4)
- **Paper:** [ArXiv](https://arxiv.org/abs/1910.10683)
### Dataset Summary
A thoroughly cleaned version of the Indonesia split of the multilingual colossal, cleaned version of Common Crawl's web crawl corpus (mC4). Based on the [Common Crawl dataset](https://commoncrawl.org). The original version was prepared by [AllenAI](https://allenai.org/), hosted at the address [https://huggingface.co/datasets/allenai/c4](https://huggingface.co/datasets/allenai/c4).
### Data Fields
The data contains the following fields:
- `url`: url of the source as a string
- `text`: text content as a string
- `timestamp`: timestamp of extraction as a string
### Data Splits
You can load any subset like this:
```python
from datasets import load_dataset
mc4_id_tiny = load_dataset("munggok/mc4-id", "tiny")
```
Since splits are quite large, you may want to traverse them using the streaming mode available starting from 🤗 Datasets v1.9.0:
```python
from datasets import load_dataset
mc4_id_full_stream = load_dataset("munggok/mc4-id", "full", split='train', streaming=True)
print(next(iter(mc4_id_full_stream))) # Prints the example presented above
```
## Dataset Creation
Refer to the original paper for more considerations regarding the choice of sources and the scraping process for creating `mC4`.
## Considerations for Using the Data
### Discussion of Biases
Despite the cleaning procedure aimed at removing vulgarity and profanity, it must be considered that model trained on this scraped corpus will inevitably reflect biases present in blog articles and comments on the Internet. This makes the corpus especially interesting in the context of studying data biases and how to limit their impacts.
## Additional Information
### Dataset Curators
Authors at AllenAI are the original curators for the `mc4` corpus.
### Licensing Information
AllenAI are releasing this dataset under the terms of ODC-BY. By using this, you are also bound by the Common Crawl terms of use in respect of the content contained in the dataset.
### Citation Information
If you use this dataset in your work, please cite us and the original mC4 authors as:
```
@inproceedings{xue-etal-2021-mt5,
title = "m{T}5: A Massively Multilingual Pre-trained Text-to-Text Transformer",
author = "Xue, Linting and
Constant, Noah and
Roberts, Adam and
Kale, Mihir and
Al-Rfou, Rami and
Siddhant, Aditya and
Barua, Aditya and
Raffel, Colin",
booktitle = "Proceedings of the 2021 Conference of the North American Chapter of the Association for Computational Linguistics: Human Language Technologies",
month = jun,
year = "2021",
address = "Online",
publisher = "Association for Computational Linguistics",
url = "https://aclanthology.org/2021.naacl-main.41",
doi = "10.18653/v1/2021.naacl-main.41",
pages = "483--498",
}
```
### Contributions
Thanks to [@dirkgr](https://github.com/dirkgr) and [@lhoestq](https://github.com/lhoestq) for adding this dataset.
| indonesian-nlp/mc4-id | [
"task_categories:text-generation",
"task_ids:language-modeling",
"annotations_creators:no-annotation",
"language_creators:found",
"multilinguality:monolingual",
"source_datasets:extended",
"language:id",
"license:odc-by",
"arxiv:1910.10683",
"region:us"
] | 2022-03-02T23:29:22+00:00 | {"annotations_creators": ["no-annotation"], "language_creators": ["found"], "language": ["id"], "license": ["odc-by"], "multilinguality": ["monolingual"], "size_categories": {"tiny": ["1M<n<10M"], "small": ["10M<n<100M"], "medium": ["10M<n<100M"], "large": ["10M<n<100M"], "full": ["100M<n<1B"]}, "source_datasets": ["extended"], "task_categories": ["text-generation"], "task_ids": ["language-modeling"], "paperswithcode_id": "mc4", "pretty_name": "mC4-id"} | 2022-10-25T10:52:34+00:00 | [
"1910.10683"
] | [
"id"
] | TAGS
#task_categories-text-generation #task_ids-language-modeling #annotations_creators-no-annotation #language_creators-found #multilinguality-monolingual #source_datasets-extended #language-Indonesian #license-odc-by #arxiv-1910.10683 #region-us
|
# Dataset Card for Clean(maybe) Indonesia mC4
## Dataset Description
- Original Homepage: HF Hub
- Paper: ArXiv
### Dataset Summary
A thoroughly cleaned version of the Indonesia split of the multilingual colossal, cleaned version of Common Crawl's web crawl corpus (mC4). Based on the Common Crawl dataset. The original version was prepared by AllenAI, hosted at the address URL
### Data Fields
The data contains the following fields:
- 'url': url of the source as a string
- 'text': text content as a string
- 'timestamp': timestamp of extraction as a string
### Data Splits
You can load any subset like this:
Since splits are quite large, you may want to traverse them using the streaming mode available starting from Datasets v1.9.0:
## Dataset Creation
Refer to the original paper for more considerations regarding the choice of sources and the scraping process for creating 'mC4'.
## Considerations for Using the Data
### Discussion of Biases
Despite the cleaning procedure aimed at removing vulgarity and profanity, it must be considered that model trained on this scraped corpus will inevitably reflect biases present in blog articles and comments on the Internet. This makes the corpus especially interesting in the context of studying data biases and how to limit their impacts.
## Additional Information
### Dataset Curators
Authors at AllenAI are the original curators for the 'mc4' corpus.
### Licensing Information
AllenAI are releasing this dataset under the terms of ODC-BY. By using this, you are also bound by the Common Crawl terms of use in respect of the content contained in the dataset.
If you use this dataset in your work, please cite us and the original mC4 authors as:
### Contributions
Thanks to @dirkgr and @lhoestq for adding this dataset.
| [
"# Dataset Card for Clean(maybe) Indonesia mC4",
"## Dataset Description\n\n- Original Homepage: HF Hub\n- Paper: ArXiv",
"### Dataset Summary\n\nA thoroughly cleaned version of the Indonesia split of the multilingual colossal, cleaned version of Common Crawl's web crawl corpus (mC4). Based on the Common Crawl dataset. The original version was prepared by AllenAI, hosted at the address URL",
"### Data Fields\n\nThe data contains the following fields:\n\n- 'url': url of the source as a string\n- 'text': text content as a string\n- 'timestamp': timestamp of extraction as a string",
"### Data Splits\n\n\nYou can load any subset like this:\n\n\n\nSince splits are quite large, you may want to traverse them using the streaming mode available starting from Datasets v1.9.0:",
"## Dataset Creation\n\nRefer to the original paper for more considerations regarding the choice of sources and the scraping process for creating 'mC4'.",
"## Considerations for Using the Data",
"### Discussion of Biases\n\nDespite the cleaning procedure aimed at removing vulgarity and profanity, it must be considered that model trained on this scraped corpus will inevitably reflect biases present in blog articles and comments on the Internet. This makes the corpus especially interesting in the context of studying data biases and how to limit their impacts.",
"## Additional Information",
"### Dataset Curators\n\nAuthors at AllenAI are the original curators for the 'mc4' corpus.",
"### Licensing Information\n\nAllenAI are releasing this dataset under the terms of ODC-BY. By using this, you are also bound by the Common Crawl terms of use in respect of the content contained in the dataset.\n\n\n\nIf you use this dataset in your work, please cite us and the original mC4 authors as:",
"### Contributions\n\nThanks to @dirkgr and @lhoestq for adding this dataset."
] | [
"TAGS\n#task_categories-text-generation #task_ids-language-modeling #annotations_creators-no-annotation #language_creators-found #multilinguality-monolingual #source_datasets-extended #language-Indonesian #license-odc-by #arxiv-1910.10683 #region-us \n",
"# Dataset Card for Clean(maybe) Indonesia mC4",
"## Dataset Description\n\n- Original Homepage: HF Hub\n- Paper: ArXiv",
"### Dataset Summary\n\nA thoroughly cleaned version of the Indonesia split of the multilingual colossal, cleaned version of Common Crawl's web crawl corpus (mC4). Based on the Common Crawl dataset. The original version was prepared by AllenAI, hosted at the address URL",
"### Data Fields\n\nThe data contains the following fields:\n\n- 'url': url of the source as a string\n- 'text': text content as a string\n- 'timestamp': timestamp of extraction as a string",
"### Data Splits\n\n\nYou can load any subset like this:\n\n\n\nSince splits are quite large, you may want to traverse them using the streaming mode available starting from Datasets v1.9.0:",
"## Dataset Creation\n\nRefer to the original paper for more considerations regarding the choice of sources and the scraping process for creating 'mC4'.",
"## Considerations for Using the Data",
"### Discussion of Biases\n\nDespite the cleaning procedure aimed at removing vulgarity and profanity, it must be considered that model trained on this scraped corpus will inevitably reflect biases present in blog articles and comments on the Internet. This makes the corpus especially interesting in the context of studying data biases and how to limit their impacts.",
"## Additional Information",
"### Dataset Curators\n\nAuthors at AllenAI are the original curators for the 'mc4' corpus.",
"### Licensing Information\n\nAllenAI are releasing this dataset under the terms of ODC-BY. By using this, you are also bound by the Common Crawl terms of use in respect of the content contained in the dataset.\n\n\n\nIf you use this dataset in your work, please cite us and the original mC4 authors as:",
"### Contributions\n\nThanks to @dirkgr and @lhoestq for adding this dataset."
] |
5a267c00ebafb624847136d25503824f1bf66b4e | https://vouproifsc.com/forums/topic/watch-tom-jerry-2021-full-movie-hd-online-free/
https://vouproifsc.com/forums/topic/watch-boogie-2021-full-movie-hd-online-free/
https://vouproifsc.com/forums/topic/watch-crazy-about-her-2021-full-movie-hd-online-free/
https://vouproifsc.com/forums/topic/watch-coming-2-america-2021-full-movie-hd-online-free/
https://vouproifsc.com/forums/topic/watch-raya-and-the-last-dragon-2021-full-movie-hd-online-free/
https://vouproifsc.com/forums/topic/watch-the-girl-on-the-train-2021-full-movie-hd-online-free/
https://vouproifsc.com/forums/topic/watch-the-world-to-come-2021-full-movie-hd-online-free/
https://vouproifsc.com/forums/topic/watch-godzilla-vs-kong-2021-full-movie-hd-online-free/
https://vouproifsc.com/forums/topic/watch-the-marksman-2021-full-movie-hd-online-free/
https://vouproifsc.com/forums/topic/watch-the-mauritanian-2021-full-movie-hd-online-free/
https://vouproifsc.com/forums/topic/watch-cosmic-sin-2021-full-movie-hd-online-free/
https://vouproifsc.com/forums/topic/watch-nobody-2021-full-movie-hd-online-free/
https://vouproifsc.com/forums/topic/watch-cherry-2021-full-movie-hd-online-free/
https://vouproifsc.com/forums/topic/watch-land-2021-full-movie-hd-online-free/
https://vouproifsc.com/forums/topic/watch-to-all-the-boys-always-and-forever-2021-full-movie-hd-online-free/
https://vouproifsc.com/forums/topic/watch-run-hide-fight-2021-full-movie-hd-online-free/
https://vouproifsc.com/forums/topic/watch-chaos-walking-2021-full-movie-hd-online-free/
https://vouproifsc.com/forums/topic/watch-willys-wonderland-2021-full-movie-hd-online-free/
https://vouproifsc.com/forums/topic/watch-the-mole-agent-2020-full-movie-hd-online-free/
https://vouproifsc.com/forums/topic/watch-soul-2020-full-movie-hd-online-free/
https://vouproifsc.com/forums/topic/watch-demon-slayer-mugen-train-2020-full-movie-hd-online-free/
https://vouproifsc.com/forums/topic/watch-wonder-woman-1984-2020-full-movie-hd-online-free/
https://vouproifsc.com/forums/topic/watch-the-little-things-2021-full-movie-hd-online-free/
https://vouproifsc.com/forums/topic/watch-promising-young-woman-2020-full-movie-hd-online-free/
https://vouproifsc.com/forums/topic/watch-mortal-kombat-2021-full-movie-hd-online-free/ | mustafa12/db_ee | [
"region:us"
] | 2022-03-02T23:29:22+00:00 | {} | 2021-03-07T09:20:06+00:00 | [] | [] | TAGS
#region-us
| URL
URL
URL
URL
URL
URL
URL
URL
URL
URL
URL
URL
URL
URL
URL
URL
URL
URL
URL
URL
URL
URL
URL
URL
URL | [] | [
"TAGS\n#region-us \n"
] |
c3e0a0f06a7d3c167b0017da0bd4ab6f89845348 | https://www.wda.org/advert/watch-tom-jerry-2021-full-hd-movie-online-free-123movies
https://www.wda.org/advert/watch-the-little-things-2021-full-hd-movie-online-free-123movies
https://www.wda.org/advert/watch-raya-and-the-last-dragon-2021-full-hd-movie-online-free-123movies
https://www.wda.org/advert/watch-i-care-a-lot-2021-full-hd-movie-online-free-123movies
https://www.wda.org/advert/watch-the-marksman-2021-full-hd-movie-online-free-123movies
https://www.wda.org/advert/watch-the-mauritanian-2021-full-hd-movie-online-free-123movies
https://www.wda.org/advert/watch-godzilla-vs-kong-2021-full-hd-movie-online-free-123movies
https://www.wda.org/advert/watch-judas-and-the-black-messiah-2021-full-hd-movie-online-free-123movies
https://www.wda.org/advert/watch-mortal-kombat-2021-full-hd-movie-online-free-123movies
https://www.wda.org/advert/watch-willys-wonderland-2021-full-hd-movie-online-free-123movies
https://www.wda.org/advert/watch-chaos-walking-2021-full-hd-movie-online-free-123movies
https://www.wda.org/advert/watch-nobody-2021-full-hd-movie-online-free-123movies
https://www.wda.org/advert/watch-aew-revolution-2021-2021-full-hd-movie-online-free-123movies
https://www.wda.org/advert/watch-cherry-2021-full-hd-movie-online-free-123movies
https://www.wda.org/advert/watch-the-world-to-come-2021-full-hd-movie-online-free-123movies
https://www.wda.org/advert/watch-crazy-about-her-2021-full-hd-movie-online-free-123movies
https://www.wda.org/advert/watch-cosmic-sin-2021-full-hd-movie-online-free-123movies
https://www.wda.org/advert/watch-boogie-2021-full-hd-movie-online-free-123movies
https://www.wda.org/advert/watch-to-all-the-boys-always-and-forever-2021-full-hd-movie-online-free-123movies
https://www.wda.org/advert/watch-soul-2020-full-hd-movie-online-free-123movies
https://www.wda.org/advert/watch-wonder-woman-1984-2020-full-hd-movie-online-free-123movies
https://www.wda.org/advert/watch-the-king-of-staten-island-2020-full-hd-movie-online-free-123movies
https://www.wda.org/advert/watch-the-girl-on-the-train-2021-full-hd-movie-online-free-123movies
https://www.wda.org/advert/watch-coming-2-america-2021-full-hd-movie-online-free-123movies
https://www.wda.org/advert/watch-after-we-fell-2021-full-hd-movie-online-free-123movies
https://www.wda.org/advert/watch-a-writers-odyssey-2021-full-hd-movie-online-free-123movies | mustafa12/edaaaas | [
"region:us"
] | 2022-03-02T23:29:22+00:00 | {} | 2021-03-08T09:46:55+00:00 | [] | [] | TAGS
#region-us
| URL
URL
URL
URL
URL
URL
URL
URL
URL
URL
URL
URL
URL
URL
URL
URL
URL
URL
URL
URL
URL
URL
URL
URL
URL
URL | [] | [
"TAGS\n#region-us \n"
] |
a316e3aea1723cec211ab9576ba5cb26ed1f3650 | https://www.sparkblue.org/content/watch-tom-jerry-2021-full-hd-movie-online-free-123movies
https://www.sparkblue.org/content/watch-little-things-2021-full-hd-movie-online-free-123movies
https://www.sparkblue.org/content/watch-raya-and-last-dragon-2021-full-hd-movie-online-free-123movies
https://www.sparkblue.org/content/watch-i-care-lot-2021-full-hd-movie-online-free-123movies
https://www.sparkblue.org/content/watch-marksman-2021-full-hd-movie-online-free-123movies
https://www.sparkblue.org/content/watch-mauritanian-2021-full-hd-movie-online-free-123movies
https://www.sparkblue.org/content/watch-godzilla-vs-kong-2021-full-hd-movie-online-free-123movies
https://www.sparkblue.org/content/watch-judas-and-black-messiah-2021-full-hd-movie-online-free-123movies
https://www.sparkblue.org/content/watch-mortal-kombat-2021-full-hd-movie-online-free-123movies
https://www.sparkblue.org/content/watch-willys-wonderland-2021-full-hd-movie-online-free-123movies
https://www.sparkblue.org/content/watch-chaos-walking-2021-full-hd-movie-online-free-123movies
https://www.sparkblue.org/content/watch-nobody-2021-full-hd-movie-online-free-123movies
https://www.sparkblue.org/content/watch-aew-revolution-2021-2021-full-hd-movie-online-free-123movies
https://www.sparkblue.org/content/watch-cherry-2021-full-hd-movie-online-free-123movies
https://www.sparkblue.org/content/watch-world-come-2021-full-hd-movie-online-free-123movies
https://www.sparkblue.org/content/watch-crazy-about-her-2021-full-hd-movie-online-free-123movies
https://www.sparkblue.org/content/watch-cosmic-sin-2021-full-hd-movie-online-free-123movies
https://www.sparkblue.org/content/watch-boogie-2021-full-hd-movie-online-free-123movies
https://www.sparkblue.org/content/watch-all-boys-always-and-forever-2021-full-hd-movie-online-free-123movies
https://www.sparkblue.org/content/watch-soul-2020-full-hd-movie-online-free-123movies
https://www.sparkblue.org/content/watch-wonder-woman-1984-2020-full-hd-movie-online-free-123movies
https://www.sparkblue.org/content/watch-king-staten-island-2020-full-hd-movie-online-free-123movies
https://www.sparkblue.org/content/watch-girl-train-2021-full-hd-movie-online-free-123movies
https://www.sparkblue.org/content/watch-coming-2-america-2021-full-hd-movie-online-free-123movies
https://www.sparkblue.org/content/watch-after-we-fell-2021-full-hd-movie-online-free-123movies
https://www.sparkblue.org/content/watch-writers-odyssey-2021-full-hd-movie-online-free-123movies | mustafa12/thors | [
"region:us"
] | 2022-03-02T23:29:22+00:00 | {} | 2021-03-08T08:21:22+00:00 | [] | [] | TAGS
#region-us
| URL
URL
URL
URL
URL
URL
URL
URL
URL
URL
URL
URL
URL
URL
URL
URL
URL
URL
URL
URL
URL
URL
URL
URL
URL
URL | [] | [
"TAGS\n#region-us \n"
] |
7d9f19d0cb4e7dcedfe2dafdda3ac8d6b7c9dbd9 |
# Dataset Card for MedWiki
## Table of Contents
- [Table of Contents](#table-of-contents)
- [Dataset Description](#dataset-description)
- [Dataset Summary](#dataset-summary)
- [Languages](#languages)
- [Dataset Structure](#dataset-structure)
- [Data Instances](#data-instances)
- [Data Fields](#data-fields)
- [Data Splits](#data-splits)
- [Dataset Creation](#dataset-creation)
- [Curation Rationale](#curation-rationale)
- [Source Data](#source-data)
- [Annotations](#annotations)
- [Personal and Sensitive Information](#personal-and-sensitive-information)
- [Considerations for Using the Data](#considerations-for-using-the-data)
- [Social Impact of Dataset](#social-impact-of-dataset)
- [Discussion of Biases](#discussion-of-biases)
- [Other Known Limitations](#other-known-limitations)
- [Additional Information](#additional-information)
- [Dataset Curators](#dataset-curators)
- [Licensing Information](#licensing-information)
- [Citation Information](#citation-information)
- [Contributions](#contributions)
## Dataset Description
- **Repository:** [Github](https://github.com/HazyResearch/medical-ned-integration)
- **Paper:** [Cross-Domain Data Integration for Named Entity Disambiguation in Biomedical Text](https://arxiv.org/abs/2110.08228)
- **Point of Contact:** [Maya Varma](mailto:[email protected])
### Dataset Summary
MedWiki is a large sentence dataset collected from a medically-relevant subset of Wikipedia and annotated with biomedical entities in the Unified Medical Language System (UMLS) knowledge base. For each entity, we include a rich set of types sourced from both UMLS and WikiData. Consisting of over 13 million sentences and 17 million entity annotations, MedWiki can be utilized as a pretraining resource for language models and can improve performance of medical named entity recognition and disambiguation systems, especially on rare entities.
Here, we include two configurations of MedWiki (further details in [Dataset Creation](#dataset-creation)):
- `MedWiki-Full` is a large sentence dataset with UMLS medical entity annotations generated through the following two steps: (1) a weak labeling proecedure to annotate WikiData entities in sentences and (2) a data integration approach that maps WikiData entities to their counterparts in UMLS.
- `MedWiki-HQ` is a subset of MedWiki-Full with higher quality labels designed to limit noise that arises from the annotation procedure listed above.
### Languages
The text in the dataset is in English and was obtained from English Wikipedia.
## Dataset Structure
### Data Instances
A typical data point includes a sentence collected from Wikipedia annotated with UMLS medical entities and associated titles and types.
An example from the MedWiki test set looks as follows:
```
{'sent_idx_unq': 57000409,
'sentence': "The hair , teeth , and skeletal side effects of TDO are lifelong , and treatment is used to manage those effects .",
'mentions': ['tdo'],
'entities': ['C2931236'],
'entity_titles': ['Tricho-dento-osseous syndrome 1'],
'types': [['Disease or Syndrome', 'disease', 'rare disease', 'developmental defect during embryogenesis', 'malformation syndrome with odontal and/or periodontal component', 'primary bone dysplasia with increased bone density', 'syndromic hair shaft abnormality']],
'spans': [[10, 11]]}
```
### Data Fields
- `sent_idx_unq`: a unique integer identifier for the data instance
- `sentence`: a string sentence collected from English Wikipedia. Punctuation is separated from words, and the sentence can be tokenized into word-pieces with the .split() method.
- `mentions`: list of medical mentions in the sentence.
- `entities`: list of UMLS medical entity identifiers corresponding to mentions. There is exactly one entity for each mention, and the length of the `entities` list is equal to the length of the `mentions` list.
- `entity_titles`: List of English titles collected from UMLS that describe each entity. The length of the `entity_titles` list is equal to the length of the `entities` list.
- `types`: List of category types associated with each entity, including types collected from UMLS and WikiData.
- `spans`: List of integer pairs representing the word span of each mention in the sentence.
### Data Splits
MedWiki includes two configurations: MedWiki-Full and MedWiki-HQ (described further in [Dataset Creation](#dataset-creation)). For each configuration, data is split into training, development, and test sets. The split sizes are as follow:
| | Train | Dev | Test |
| ----- | ------ | ----- | ---- |
| MedWiki-Full Sentences |11,784,235 | 649,132 | 648,608 |
| MedWiki-Full Mentions |15,981,347 | 876,586 | 877,090 |
| MedWiki-Full Unique Entities | 230,871 | 55,002 | 54,772 |
| MedWiki-HQ Sentences | 2,962,089 | 165,941 | 164,193 |
| MedWiki-HQ Mentions | 3,366,108 | 188,957 | 186,622 |
| MedWiki-HQ Unique Entities | 118,572 | 19,725 | 19,437 |
## Dataset Creation
### Curation Rationale
Existing medical text datasets are generally limited in scope, often obtaining low coverage over the entities and structural resources in the UMLS medical knowledge base. When language models are trained across such datasets, the lack of adequate examples may prevent models from learning the complex reasoning patterns that are necessary for performing effective entity linking or disambiguation, especially for rare entities as shown in prior work by [Orr et al.](http://cidrdb.org/cidr2021/papers/cidr2021_paper13.pdf). Wikipedia, which is often utilized as a rich knowledge source in general text settings, contains references to medical terms and can help address this issue. Here, we curate the MedWiki dataset, which is a large-scale, weakly-labeled dataset that consists of sentences from Wikipedia annotated with medical entities in the UMLS knowledge base. MedWiki can serve as a pretraining dataset for language models and holds potential for improving performance on medical named entity recognition tasks, especially on rare entities.
### Source Data
#### Initial Data Collection and Normalization
MedWiki consists of sentences obtained from the November 2019 dump of English Wikipedia. We split pages into an 80/10/10 train/dev/test split and then segment each page at the sentence-level. This ensures that all sentences associated with a single Wikipedia page are placed in the same split.
#### Who are the source language producers?
The source language producers are editors on English Wikipedia.
### Annotations
#### Annotation process
We create two configurations of our dataset: MedWiki-Full and MedWiki-HQ. We label MedWiki-Full by first annotating all English Wikipedia articles with textual mentions and corresponding WikiData entities; we do so by obtaining gold entity labels from internal page links as well as generating weak labels based on pronouns and alternative entity names (see [Orr et al. 2020](http://cidrdb.org/cidr2021/papers/cidr2021_paper13.pdf) for additional information). Then, we use the off-the-shelf entity linker [Bootleg](https://github.com/HazyResearch/bootleg) to map entities in WikiData to their counterparts in the 2017AA release of the Unified Medical Language System (UMLS), a standard knowledge base for biomedical entities (additional implementation details in forthcoming publication). Any sentence containing at least one UMLS entity is included in MedWiki-Full. We also include types associated with each entity, which are collected from both WikiData and UMLS using the generated UMLS-Wikidata mapping. It is important to note that types obtained from WikiData are filtered according to methods described in [Orr et al. 2020](http://cidrdb.org/cidr2021/papers/cidr2021_paper13.pdf).
Since our labeling procedure introduces some noise into annotations, we also release the MedWiki-HQ dataset configuration with higher-quality labels. To generate MedWiki-HQ, we filtered the UMLS-Wikidata mappings to only include pairs of UMLS medical entities and WikiData items that share a high textual overlap between titles. MedWiki-HQ is a subset of MedWiki-Full.
To evaluate the quality of our UMLS-Wikidata mappings, we find that WikiData includes a small set of "true" labeled mappings between UMLS entities and WikiData items. (Note that we only include WikiData items associated with linked Wikipedia pages.) This set comprises approximately 9.3k UMLS entities in the original UMLS-Wikidata mapping (used for MedWiki-Full) and 5.6k entities in the filtered UMLS-Wikidata mapping (used for MedWiki-HQ). Using these labeled sets, we find that our mapping accuracy is 80.2% for the original UMLS-Wikidata mapping and 94.5% for the filtered UMLS-Wikidata mapping. We also evaluate integration performance on this segment as the proportion of mapped WikiData entities that share a WikiData type with the true entity, suggesting the predicted mapping adds relevant structural resources. Integration performance is 85.4% for the original UMLS-Wikidata mapping and 95.9% for the filtered UMLS-Wikidata mapping. The remainder of items in UMLS have no “true” mappings to WikiData.
#### Who are the annotators?
The dataset was labeled using weak-labeling techniques as described above.
### Personal and Sensitive Information
No personal or sensitive information is included in MedWiki.
## Considerations for Using the Data
### Social Impact of Dataset
The purpose of this dataset is to enable the creation of better named entity recognition systems for biomedical text. MedWiki encompasses a large set of entities in the UMLS knowledge base and includes a rich set of types associated with each entity, which can enable the creation of models that achieve high performance on named entity recognition tasks, especially on rare or unpopular entities. Such systems hold potential for improving automated parsing and information retrieval from large quantities of biomedical text.
### Discussion of Biases
The data included in MedWiki comes from English Wikipedia. Generally, Wikipedia articles are neutral in point of view and aim to avoid bias. However, some [prior work](https://www.hbs.edu/ris/Publication%20Files/15-023_e044cf50-f621-4759-a827-e9a3bf8920c0.pdf) has shown that ideological biases may exist within some Wikipedia articles, especially those that are focused on political issues or those that are written by fewer authors. We anticipate that such biases are rare for medical articles, which are typically comprised of scientific facts. However, it is important to note that bias encoded in Wikipedia is likely to be reflected by MedWiki.
### Other Known Limitations
Since MedWiki was annotated using weak labeling techniques, there is likely some noise in entity annotations. (Note that to address this, we include the MedWiki-HQ configuration, which is a subset of MedWiki-Full with higher quality labels. Additional details in [Dataset Creation](#dataset-creation)).
## Additional Information
### Dataset Curators
MedWiki was curated by Maya Varma, Laurel Orr, Sen Wu, Megan Leszczynski, Xiao Ling, and Chris Ré.
### Licensing Information
Dataset licensed under CC BY 4.0.
### Citation Information
```
@inproceedings{varma-etal-2021-cross-domain,
title = "Cross-Domain Data Integration for Named Entity Disambiguation in Biomedical Text",
author = "Varma, Maya and
Orr, Laurel and
Wu, Sen and
Leszczynski, Megan and
Ling, Xiao and
R{\'e}, Christopher",
booktitle = "Findings of the Association for Computational Linguistics: EMNLP 2021",
month = nov,
year = "2021",
address = "Punta Cana, Dominican Republic",
publisher = "Association for Computational Linguistics",
url = "https://aclanthology.org/2021.findings-emnlp.388",
pages = "4566--4575",
}
```
### Contributions
Thanks to [@maya124](https://github.com/maya124) for adding this dataset.
| mvarma/medwiki | [
"task_categories:text-retrieval",
"task_ids:entity-linking-retrieval",
"annotations_creators:machine-generated",
"language_creators:crowdsourced",
"multilinguality:monolingual",
"size_categories:unknown",
"source_datasets:extended|wikipedia",
"license:cc-by-4.0",
"arxiv:2110.08228",
"region:us"
] | 2022-03-02T23:29:22+00:00 | {"annotations_creators": ["machine-generated"], "language_creators": ["crowdsourced"], "language": ["en-US", "en"], "license": ["cc-by-4.0"], "multilinguality": ["monolingual"], "size_categories": ["unknown"], "source_datasets": ["extended|wikipedia"], "task_categories": ["text-retrieval"], "task_ids": ["entity-linking-retrieval"], "pretty_name": "medwiki"} | 2022-10-25T08:51:06+00:00 | [
"2110.08228"
] | [
"en-US",
"en"
] | TAGS
#task_categories-text-retrieval #task_ids-entity-linking-retrieval #annotations_creators-machine-generated #language_creators-crowdsourced #multilinguality-monolingual #size_categories-unknown #source_datasets-extended|wikipedia #license-cc-by-4.0 #arxiv-2110.08228 #region-us
| Dataset Card for MedWiki
========================
Table of Contents
-----------------
* Table of Contents
* Dataset Description
+ Dataset Summary
+ Languages
* Dataset Structure
+ Data Instances
+ Data Fields
+ Data Splits
* Dataset Creation
+ Curation Rationale
+ Source Data
+ Annotations
+ Personal and Sensitive Information
* Considerations for Using the Data
+ Social Impact of Dataset
+ Discussion of Biases
+ Other Known Limitations
* Additional Information
+ Dataset Curators
+ Licensing Information
+ Citation Information
+ Contributions
Dataset Description
-------------------
* Repository: Github
* Paper: Cross-Domain Data Integration for Named Entity Disambiguation in Biomedical Text
* Point of Contact: Maya Varma
### Dataset Summary
MedWiki is a large sentence dataset collected from a medically-relevant subset of Wikipedia and annotated with biomedical entities in the Unified Medical Language System (UMLS) knowledge base. For each entity, we include a rich set of types sourced from both UMLS and WikiData. Consisting of over 13 million sentences and 17 million entity annotations, MedWiki can be utilized as a pretraining resource for language models and can improve performance of medical named entity recognition and disambiguation systems, especially on rare entities.
Here, we include two configurations of MedWiki (further details in Dataset Creation):
* 'MedWiki-Full' is a large sentence dataset with UMLS medical entity annotations generated through the following two steps: (1) a weak labeling proecedure to annotate WikiData entities in sentences and (2) a data integration approach that maps WikiData entities to their counterparts in UMLS.
* 'MedWiki-HQ' is a subset of MedWiki-Full with higher quality labels designed to limit noise that arises from the annotation procedure listed above.
### Languages
The text in the dataset is in English and was obtained from English Wikipedia.
Dataset Structure
-----------------
### Data Instances
A typical data point includes a sentence collected from Wikipedia annotated with UMLS medical entities and associated titles and types.
An example from the MedWiki test set looks as follows:
### Data Fields
* 'sent\_idx\_unq': a unique integer identifier for the data instance
* 'sentence': a string sentence collected from English Wikipedia. Punctuation is separated from words, and the sentence can be tokenized into word-pieces with the .split() method.
* 'mentions': list of medical mentions in the sentence.
* 'entities': list of UMLS medical entity identifiers corresponding to mentions. There is exactly one entity for each mention, and the length of the 'entities' list is equal to the length of the 'mentions' list.
* 'entity\_titles': List of English titles collected from UMLS that describe each entity. The length of the 'entity\_titles' list is equal to the length of the 'entities' list.
* 'types': List of category types associated with each entity, including types collected from UMLS and WikiData.
* 'spans': List of integer pairs representing the word span of each mention in the sentence.
### Data Splits
MedWiki includes two configurations: MedWiki-Full and MedWiki-HQ (described further in Dataset Creation). For each configuration, data is split into training, development, and test sets. The split sizes are as follow:
Dataset Creation
----------------
### Curation Rationale
Existing medical text datasets are generally limited in scope, often obtaining low coverage over the entities and structural resources in the UMLS medical knowledge base. When language models are trained across such datasets, the lack of adequate examples may prevent models from learning the complex reasoning patterns that are necessary for performing effective entity linking or disambiguation, especially for rare entities as shown in prior work by Orr et al.. Wikipedia, which is often utilized as a rich knowledge source in general text settings, contains references to medical terms and can help address this issue. Here, we curate the MedWiki dataset, which is a large-scale, weakly-labeled dataset that consists of sentences from Wikipedia annotated with medical entities in the UMLS knowledge base. MedWiki can serve as a pretraining dataset for language models and holds potential for improving performance on medical named entity recognition tasks, especially on rare entities.
### Source Data
#### Initial Data Collection and Normalization
MedWiki consists of sentences obtained from the November 2019 dump of English Wikipedia. We split pages into an 80/10/10 train/dev/test split and then segment each page at the sentence-level. This ensures that all sentences associated with a single Wikipedia page are placed in the same split.
#### Who are the source language producers?
The source language producers are editors on English Wikipedia.
### Annotations
#### Annotation process
We create two configurations of our dataset: MedWiki-Full and MedWiki-HQ. We label MedWiki-Full by first annotating all English Wikipedia articles with textual mentions and corresponding WikiData entities; we do so by obtaining gold entity labels from internal page links as well as generating weak labels based on pronouns and alternative entity names (see Orr et al. 2020 for additional information). Then, we use the off-the-shelf entity linker Bootleg to map entities in WikiData to their counterparts in the 2017AA release of the Unified Medical Language System (UMLS), a standard knowledge base for biomedical entities (additional implementation details in forthcoming publication). Any sentence containing at least one UMLS entity is included in MedWiki-Full. We also include types associated with each entity, which are collected from both WikiData and UMLS using the generated UMLS-Wikidata mapping. It is important to note that types obtained from WikiData are filtered according to methods described in Orr et al. 2020.
Since our labeling procedure introduces some noise into annotations, we also release the MedWiki-HQ dataset configuration with higher-quality labels. To generate MedWiki-HQ, we filtered the UMLS-Wikidata mappings to only include pairs of UMLS medical entities and WikiData items that share a high textual overlap between titles. MedWiki-HQ is a subset of MedWiki-Full.
To evaluate the quality of our UMLS-Wikidata mappings, we find that WikiData includes a small set of "true" labeled mappings between UMLS entities and WikiData items. (Note that we only include WikiData items associated with linked Wikipedia pages.) This set comprises approximately 9.3k UMLS entities in the original UMLS-Wikidata mapping (used for MedWiki-Full) and 5.6k entities in the filtered UMLS-Wikidata mapping (used for MedWiki-HQ). Using these labeled sets, we find that our mapping accuracy is 80.2% for the original UMLS-Wikidata mapping and 94.5% for the filtered UMLS-Wikidata mapping. We also evaluate integration performance on this segment as the proportion of mapped WikiData entities that share a WikiData type with the true entity, suggesting the predicted mapping adds relevant structural resources. Integration performance is 85.4% for the original UMLS-Wikidata mapping and 95.9% for the filtered UMLS-Wikidata mapping. The remainder of items in UMLS have no “true” mappings to WikiData.
#### Who are the annotators?
The dataset was labeled using weak-labeling techniques as described above.
### Personal and Sensitive Information
No personal or sensitive information is included in MedWiki.
Considerations for Using the Data
---------------------------------
### Social Impact of Dataset
The purpose of this dataset is to enable the creation of better named entity recognition systems for biomedical text. MedWiki encompasses a large set of entities in the UMLS knowledge base and includes a rich set of types associated with each entity, which can enable the creation of models that achieve high performance on named entity recognition tasks, especially on rare or unpopular entities. Such systems hold potential for improving automated parsing and information retrieval from large quantities of biomedical text.
### Discussion of Biases
The data included in MedWiki comes from English Wikipedia. Generally, Wikipedia articles are neutral in point of view and aim to avoid bias. However, some prior work has shown that ideological biases may exist within some Wikipedia articles, especially those that are focused on political issues or those that are written by fewer authors. We anticipate that such biases are rare for medical articles, which are typically comprised of scientific facts. However, it is important to note that bias encoded in Wikipedia is likely to be reflected by MedWiki.
### Other Known Limitations
Since MedWiki was annotated using weak labeling techniques, there is likely some noise in entity annotations. (Note that to address this, we include the MedWiki-HQ configuration, which is a subset of MedWiki-Full with higher quality labels. Additional details in Dataset Creation).
Additional Information
----------------------
### Dataset Curators
MedWiki was curated by Maya Varma, Laurel Orr, Sen Wu, Megan Leszczynski, Xiao Ling, and Chris Ré.
### Licensing Information
Dataset licensed under CC BY 4.0.
### Contributions
Thanks to @maya124 for adding this dataset.
| [
"### Dataset Summary\n\n\nMedWiki is a large sentence dataset collected from a medically-relevant subset of Wikipedia and annotated with biomedical entities in the Unified Medical Language System (UMLS) knowledge base. For each entity, we include a rich set of types sourced from both UMLS and WikiData. Consisting of over 13 million sentences and 17 million entity annotations, MedWiki can be utilized as a pretraining resource for language models and can improve performance of medical named entity recognition and disambiguation systems, especially on rare entities.\n\n\nHere, we include two configurations of MedWiki (further details in Dataset Creation):\n\n\n* 'MedWiki-Full' is a large sentence dataset with UMLS medical entity annotations generated through the following two steps: (1) a weak labeling proecedure to annotate WikiData entities in sentences and (2) a data integration approach that maps WikiData entities to their counterparts in UMLS.\n* 'MedWiki-HQ' is a subset of MedWiki-Full with higher quality labels designed to limit noise that arises from the annotation procedure listed above.",
"### Languages\n\n\nThe text in the dataset is in English and was obtained from English Wikipedia.\n\n\nDataset Structure\n-----------------",
"### Data Instances\n\n\nA typical data point includes a sentence collected from Wikipedia annotated with UMLS medical entities and associated titles and types.\n\n\nAn example from the MedWiki test set looks as follows:",
"### Data Fields\n\n\n* 'sent\\_idx\\_unq': a unique integer identifier for the data instance\n* 'sentence': a string sentence collected from English Wikipedia. Punctuation is separated from words, and the sentence can be tokenized into word-pieces with the .split() method.\n* 'mentions': list of medical mentions in the sentence.\n* 'entities': list of UMLS medical entity identifiers corresponding to mentions. There is exactly one entity for each mention, and the length of the 'entities' list is equal to the length of the 'mentions' list.\n* 'entity\\_titles': List of English titles collected from UMLS that describe each entity. The length of the 'entity\\_titles' list is equal to the length of the 'entities' list.\n* 'types': List of category types associated with each entity, including types collected from UMLS and WikiData.\n* 'spans': List of integer pairs representing the word span of each mention in the sentence.",
"### Data Splits\n\n\nMedWiki includes two configurations: MedWiki-Full and MedWiki-HQ (described further in Dataset Creation). For each configuration, data is split into training, development, and test sets. The split sizes are as follow:\n\n\n\nDataset Creation\n----------------",
"### Curation Rationale\n\n\nExisting medical text datasets are generally limited in scope, often obtaining low coverage over the entities and structural resources in the UMLS medical knowledge base. When language models are trained across such datasets, the lack of adequate examples may prevent models from learning the complex reasoning patterns that are necessary for performing effective entity linking or disambiguation, especially for rare entities as shown in prior work by Orr et al.. Wikipedia, which is often utilized as a rich knowledge source in general text settings, contains references to medical terms and can help address this issue. Here, we curate the MedWiki dataset, which is a large-scale, weakly-labeled dataset that consists of sentences from Wikipedia annotated with medical entities in the UMLS knowledge base. MedWiki can serve as a pretraining dataset for language models and holds potential for improving performance on medical named entity recognition tasks, especially on rare entities.",
"### Source Data",
"#### Initial Data Collection and Normalization\n\n\nMedWiki consists of sentences obtained from the November 2019 dump of English Wikipedia. We split pages into an 80/10/10 train/dev/test split and then segment each page at the sentence-level. This ensures that all sentences associated with a single Wikipedia page are placed in the same split.",
"#### Who are the source language producers?\n\n\nThe source language producers are editors on English Wikipedia.",
"### Annotations",
"#### Annotation process\n\n\nWe create two configurations of our dataset: MedWiki-Full and MedWiki-HQ. We label MedWiki-Full by first annotating all English Wikipedia articles with textual mentions and corresponding WikiData entities; we do so by obtaining gold entity labels from internal page links as well as generating weak labels based on pronouns and alternative entity names (see Orr et al. 2020 for additional information). Then, we use the off-the-shelf entity linker Bootleg to map entities in WikiData to their counterparts in the 2017AA release of the Unified Medical Language System (UMLS), a standard knowledge base for biomedical entities (additional implementation details in forthcoming publication). Any sentence containing at least one UMLS entity is included in MedWiki-Full. We also include types associated with each entity, which are collected from both WikiData and UMLS using the generated UMLS-Wikidata mapping. It is important to note that types obtained from WikiData are filtered according to methods described in Orr et al. 2020.\n\n\nSince our labeling procedure introduces some noise into annotations, we also release the MedWiki-HQ dataset configuration with higher-quality labels. To generate MedWiki-HQ, we filtered the UMLS-Wikidata mappings to only include pairs of UMLS medical entities and WikiData items that share a high textual overlap between titles. MedWiki-HQ is a subset of MedWiki-Full.\n\n\nTo evaluate the quality of our UMLS-Wikidata mappings, we find that WikiData includes a small set of \"true\" labeled mappings between UMLS entities and WikiData items. (Note that we only include WikiData items associated with linked Wikipedia pages.) This set comprises approximately 9.3k UMLS entities in the original UMLS-Wikidata mapping (used for MedWiki-Full) and 5.6k entities in the filtered UMLS-Wikidata mapping (used for MedWiki-HQ). Using these labeled sets, we find that our mapping accuracy is 80.2% for the original UMLS-Wikidata mapping and 94.5% for the filtered UMLS-Wikidata mapping. We also evaluate integration performance on this segment as the proportion of mapped WikiData entities that share a WikiData type with the true entity, suggesting the predicted mapping adds relevant structural resources. Integration performance is 85.4% for the original UMLS-Wikidata mapping and 95.9% for the filtered UMLS-Wikidata mapping. The remainder of items in UMLS have no “true” mappings to WikiData.",
"#### Who are the annotators?\n\n\nThe dataset was labeled using weak-labeling techniques as described above.",
"### Personal and Sensitive Information\n\n\nNo personal or sensitive information is included in MedWiki.\n\n\nConsiderations for Using the Data\n---------------------------------",
"### Social Impact of Dataset\n\n\nThe purpose of this dataset is to enable the creation of better named entity recognition systems for biomedical text. MedWiki encompasses a large set of entities in the UMLS knowledge base and includes a rich set of types associated with each entity, which can enable the creation of models that achieve high performance on named entity recognition tasks, especially on rare or unpopular entities. Such systems hold potential for improving automated parsing and information retrieval from large quantities of biomedical text.",
"### Discussion of Biases\n\n\nThe data included in MedWiki comes from English Wikipedia. Generally, Wikipedia articles are neutral in point of view and aim to avoid bias. However, some prior work has shown that ideological biases may exist within some Wikipedia articles, especially those that are focused on political issues or those that are written by fewer authors. We anticipate that such biases are rare for medical articles, which are typically comprised of scientific facts. However, it is important to note that bias encoded in Wikipedia is likely to be reflected by MedWiki.",
"### Other Known Limitations\n\n\nSince MedWiki was annotated using weak labeling techniques, there is likely some noise in entity annotations. (Note that to address this, we include the MedWiki-HQ configuration, which is a subset of MedWiki-Full with higher quality labels. Additional details in Dataset Creation).\n\n\nAdditional Information\n----------------------",
"### Dataset Curators\n\n\nMedWiki was curated by Maya Varma, Laurel Orr, Sen Wu, Megan Leszczynski, Xiao Ling, and Chris Ré.",
"### Licensing Information\n\n\nDataset licensed under CC BY 4.0.",
"### Contributions\n\n\nThanks to @maya124 for adding this dataset."
] | [
"TAGS\n#task_categories-text-retrieval #task_ids-entity-linking-retrieval #annotations_creators-machine-generated #language_creators-crowdsourced #multilinguality-monolingual #size_categories-unknown #source_datasets-extended|wikipedia #license-cc-by-4.0 #arxiv-2110.08228 #region-us \n",
"### Dataset Summary\n\n\nMedWiki is a large sentence dataset collected from a medically-relevant subset of Wikipedia and annotated with biomedical entities in the Unified Medical Language System (UMLS) knowledge base. For each entity, we include a rich set of types sourced from both UMLS and WikiData. Consisting of over 13 million sentences and 17 million entity annotations, MedWiki can be utilized as a pretraining resource for language models and can improve performance of medical named entity recognition and disambiguation systems, especially on rare entities.\n\n\nHere, we include two configurations of MedWiki (further details in Dataset Creation):\n\n\n* 'MedWiki-Full' is a large sentence dataset with UMLS medical entity annotations generated through the following two steps: (1) a weak labeling proecedure to annotate WikiData entities in sentences and (2) a data integration approach that maps WikiData entities to their counterparts in UMLS.\n* 'MedWiki-HQ' is a subset of MedWiki-Full with higher quality labels designed to limit noise that arises from the annotation procedure listed above.",
"### Languages\n\n\nThe text in the dataset is in English and was obtained from English Wikipedia.\n\n\nDataset Structure\n-----------------",
"### Data Instances\n\n\nA typical data point includes a sentence collected from Wikipedia annotated with UMLS medical entities and associated titles and types.\n\n\nAn example from the MedWiki test set looks as follows:",
"### Data Fields\n\n\n* 'sent\\_idx\\_unq': a unique integer identifier for the data instance\n* 'sentence': a string sentence collected from English Wikipedia. Punctuation is separated from words, and the sentence can be tokenized into word-pieces with the .split() method.\n* 'mentions': list of medical mentions in the sentence.\n* 'entities': list of UMLS medical entity identifiers corresponding to mentions. There is exactly one entity for each mention, and the length of the 'entities' list is equal to the length of the 'mentions' list.\n* 'entity\\_titles': List of English titles collected from UMLS that describe each entity. The length of the 'entity\\_titles' list is equal to the length of the 'entities' list.\n* 'types': List of category types associated with each entity, including types collected from UMLS and WikiData.\n* 'spans': List of integer pairs representing the word span of each mention in the sentence.",
"### Data Splits\n\n\nMedWiki includes two configurations: MedWiki-Full and MedWiki-HQ (described further in Dataset Creation). For each configuration, data is split into training, development, and test sets. The split sizes are as follow:\n\n\n\nDataset Creation\n----------------",
"### Curation Rationale\n\n\nExisting medical text datasets are generally limited in scope, often obtaining low coverage over the entities and structural resources in the UMLS medical knowledge base. When language models are trained across such datasets, the lack of adequate examples may prevent models from learning the complex reasoning patterns that are necessary for performing effective entity linking or disambiguation, especially for rare entities as shown in prior work by Orr et al.. Wikipedia, which is often utilized as a rich knowledge source in general text settings, contains references to medical terms and can help address this issue. Here, we curate the MedWiki dataset, which is a large-scale, weakly-labeled dataset that consists of sentences from Wikipedia annotated with medical entities in the UMLS knowledge base. MedWiki can serve as a pretraining dataset for language models and holds potential for improving performance on medical named entity recognition tasks, especially on rare entities.",
"### Source Data",
"#### Initial Data Collection and Normalization\n\n\nMedWiki consists of sentences obtained from the November 2019 dump of English Wikipedia. We split pages into an 80/10/10 train/dev/test split and then segment each page at the sentence-level. This ensures that all sentences associated with a single Wikipedia page are placed in the same split.",
"#### Who are the source language producers?\n\n\nThe source language producers are editors on English Wikipedia.",
"### Annotations",
"#### Annotation process\n\n\nWe create two configurations of our dataset: MedWiki-Full and MedWiki-HQ. We label MedWiki-Full by first annotating all English Wikipedia articles with textual mentions and corresponding WikiData entities; we do so by obtaining gold entity labels from internal page links as well as generating weak labels based on pronouns and alternative entity names (see Orr et al. 2020 for additional information). Then, we use the off-the-shelf entity linker Bootleg to map entities in WikiData to their counterparts in the 2017AA release of the Unified Medical Language System (UMLS), a standard knowledge base for biomedical entities (additional implementation details in forthcoming publication). Any sentence containing at least one UMLS entity is included in MedWiki-Full. We also include types associated with each entity, which are collected from both WikiData and UMLS using the generated UMLS-Wikidata mapping. It is important to note that types obtained from WikiData are filtered according to methods described in Orr et al. 2020.\n\n\nSince our labeling procedure introduces some noise into annotations, we also release the MedWiki-HQ dataset configuration with higher-quality labels. To generate MedWiki-HQ, we filtered the UMLS-Wikidata mappings to only include pairs of UMLS medical entities and WikiData items that share a high textual overlap between titles. MedWiki-HQ is a subset of MedWiki-Full.\n\n\nTo evaluate the quality of our UMLS-Wikidata mappings, we find that WikiData includes a small set of \"true\" labeled mappings between UMLS entities and WikiData items. (Note that we only include WikiData items associated with linked Wikipedia pages.) This set comprises approximately 9.3k UMLS entities in the original UMLS-Wikidata mapping (used for MedWiki-Full) and 5.6k entities in the filtered UMLS-Wikidata mapping (used for MedWiki-HQ). Using these labeled sets, we find that our mapping accuracy is 80.2% for the original UMLS-Wikidata mapping and 94.5% for the filtered UMLS-Wikidata mapping. We also evaluate integration performance on this segment as the proportion of mapped WikiData entities that share a WikiData type with the true entity, suggesting the predicted mapping adds relevant structural resources. Integration performance is 85.4% for the original UMLS-Wikidata mapping and 95.9% for the filtered UMLS-Wikidata mapping. The remainder of items in UMLS have no “true” mappings to WikiData.",
"#### Who are the annotators?\n\n\nThe dataset was labeled using weak-labeling techniques as described above.",
"### Personal and Sensitive Information\n\n\nNo personal or sensitive information is included in MedWiki.\n\n\nConsiderations for Using the Data\n---------------------------------",
"### Social Impact of Dataset\n\n\nThe purpose of this dataset is to enable the creation of better named entity recognition systems for biomedical text. MedWiki encompasses a large set of entities in the UMLS knowledge base and includes a rich set of types associated with each entity, which can enable the creation of models that achieve high performance on named entity recognition tasks, especially on rare or unpopular entities. Such systems hold potential for improving automated parsing and information retrieval from large quantities of biomedical text.",
"### Discussion of Biases\n\n\nThe data included in MedWiki comes from English Wikipedia. Generally, Wikipedia articles are neutral in point of view and aim to avoid bias. However, some prior work has shown that ideological biases may exist within some Wikipedia articles, especially those that are focused on political issues or those that are written by fewer authors. We anticipate that such biases are rare for medical articles, which are typically comprised of scientific facts. However, it is important to note that bias encoded in Wikipedia is likely to be reflected by MedWiki.",
"### Other Known Limitations\n\n\nSince MedWiki was annotated using weak labeling techniques, there is likely some noise in entity annotations. (Note that to address this, we include the MedWiki-HQ configuration, which is a subset of MedWiki-Full with higher quality labels. Additional details in Dataset Creation).\n\n\nAdditional Information\n----------------------",
"### Dataset Curators\n\n\nMedWiki was curated by Maya Varma, Laurel Orr, Sen Wu, Megan Leszczynski, Xiao Ling, and Chris Ré.",
"### Licensing Information\n\n\nDataset licensed under CC BY 4.0.",
"### Contributions\n\n\nThanks to @maya124 for adding this dataset."
] |
Subsets and Splits