pipeline_tag
stringclasses 48
values | library_name
stringclasses 198
values | text
stringlengths 1
900k
| metadata
stringlengths 2
438k
| id
stringlengths 5
122
| last_modified
null | tags
listlengths 1
1.84k
| sha
null | created_at
stringlengths 25
25
| arxiv
listlengths 0
201
| languages
listlengths 0
1.83k
| tags_str
stringlengths 17
9.34k
| text_str
stringlengths 0
389k
| text_lists
listlengths 0
722
| processed_texts
listlengths 1
723
|
---|---|---|---|---|---|---|---|---|---|---|---|---|---|---|
null | null | ¿Qué es Lutexid Pastillas?
Lutexid tabletas es un suplemento premium para la salud ocular formulado con una potente mezcla de ingredientes naturales que nutren y protegen los ojos. Elaborado por expertos en el campo del cuidado de la visión, Lutexid Precio ofrece una solución integral a los problemas oculares comunes, desde la fatiga visual digital hasta el deterioro de la visión relacionado con la edad.
Página web oficial:<a href="https://www.nutritionsee.com/Lutearhsg">www.Lutexid.com</a>
<p><a href="https://www.nutritionsee.com/Lutearhsg"> <img src="https://www.nutritionsee.com/wp-content/uploads/2024/04/Lutexid-Argentina.png" alt="enter image description here"> </a></p>
<a href="https://www.nutritionsee.com/Lutearhsg">¡¡Comprar ahora!! Haga clic en el enlace a continuación para obtener más información y obtener un 50% de descuento ahora... ¡Date prisa!</a>
Página web oficial:<a href="https://www.nutritionsee.com/Lutearhsg">www.Lutexid.com</a> | {"license": "apache-2.0"} | LutexidArgentina/LutexidArgentina | null | [
"license:apache-2.0",
"region:us"
] | null | 2024-04-18T08:14:51+00:00 | [] | [] | TAGS
#license-apache-2.0 #region-us
| ¿Qué es Lutexid Pastillas?
Lutexid tabletas es un suplemento premium para la salud ocular formulado con una potente mezcla de ingredientes naturales que nutren y protegen los ojos. Elaborado por expertos en el campo del cuidado de la visión, Lutexid Precio ofrece una solución integral a los problemas oculares comunes, desde la fatiga visual digital hasta el deterioro de la visión relacionado con la edad.
Página web oficial:<a href="URL
<p><a href="URL <img src="URL alt="enter image description here"> </a></p>
<a href="URL¡¡Comprar ahora!! Haga clic en el enlace a continuación para obtener más información y obtener un 50% de descuento ahora... ¡Date prisa!</a>
Página web oficial:<a href="URL | [] | [
"TAGS\n#license-apache-2.0 #region-us \n"
] |
text-classification | transformers |
<!-- This model card has been generated automatically according to the information the Trainer had access to. You
should probably proofread and complete it, then remove this comment. -->
# Intent-classification-BERT-Large-Ashuv5
This model is a fine-tuned version of [google-bert/bert-large-cased-whole-word-masking](https://huggingface.co/google-bert/bert-large-cased-whole-word-masking) on an unknown dataset.
It achieves the following results on the evaluation set:
- Loss: 1.7988
- Accuracy: 0.1420
- F1: 0.0414
- Precision: 0.0237
- Recall: 0.1667
## Model description
More information needed
## Intended uses & limitations
More information needed
## Training and evaluation data
More information needed
## Training procedure
### Training hyperparameters
The following hyperparameters were used during training:
- learning_rate: 0.0005
- train_batch_size: 16
- eval_batch_size: 32
- seed: 42
- optimizer: Adam with betas=(0.9,0.999) and epsilon=1e-08
- lr_scheduler_type: linear
- num_epochs: 3
### Training results
| Training Loss | Epoch | Step | Validation Loss | Accuracy | F1 | Precision | Recall |
|:-------------:|:-----:|:----:|:---------------:|:--------:|:------:|:---------:|:------:|
| 2.1123 | 0.24 | 10 | 1.8066 | 0.2174 | 0.0595 | 0.0362 | 0.1667 |
| 1.8577 | 0.49 | 20 | 1.9500 | 0.1242 | 0.0368 | 0.0207 | 0.1667 |
| 1.8864 | 0.73 | 30 | 1.7999 | 0.1801 | 0.0509 | 0.0300 | 0.1667 |
| 1.8516 | 0.98 | 40 | 1.8570 | 0.1429 | 0.0417 | 0.0238 | 0.1667 |
| 1.8664 | 1.22 | 50 | 1.8667 | 0.1242 | 0.0368 | 0.0207 | 0.1667 |
| 1.8207 | 1.46 | 60 | 1.9616 | 0.1180 | 0.0352 | 0.0197 | 0.1667 |
| 1.8652 | 1.71 | 70 | 1.7831 | 0.2174 | 0.0595 | 0.0362 | 0.1667 |
| 1.8372 | 1.95 | 80 | 1.8018 | 0.2174 | 0.0595 | 0.0362 | 0.1667 |
| 1.8671 | 2.2 | 90 | 1.8436 | 0.1180 | 0.0352 | 0.0197 | 0.1667 |
| 1.8484 | 2.44 | 100 | 1.7722 | 0.2174 | 0.0595 | 0.0362 | 0.1667 |
| 1.8262 | 2.68 | 110 | 1.7752 | 0.2174 | 0.0595 | 0.0362 | 0.1667 |
| 1.8292 | 2.93 | 120 | 1.8064 | 0.1242 | 0.0368 | 0.0207 | 0.1667 |
### Framework versions
- Transformers 4.38.2
- Pytorch 2.1.2
- Datasets 2.1.0
- Tokenizers 0.15.2
| {"license": "apache-2.0", "tags": ["generated_from_trainer"], "metrics": ["accuracy", "f1", "precision", "recall"], "base_model": "google-bert/bert-large-cased-whole-word-masking", "model-index": [{"name": "Intent-classification-BERT-Large-Ashuv5", "results": []}]} | Narkantak/Intent-classification-BERT-Large-Ashuv5 | null | [
"transformers",
"safetensors",
"bert",
"text-classification",
"generated_from_trainer",
"base_model:google-bert/bert-large-cased-whole-word-masking",
"license:apache-2.0",
"autotrain_compatible",
"endpoints_compatible",
"region:us"
] | null | 2024-04-18T08:15:30+00:00 | [] | [] | TAGS
#transformers #safetensors #bert #text-classification #generated_from_trainer #base_model-google-bert/bert-large-cased-whole-word-masking #license-apache-2.0 #autotrain_compatible #endpoints_compatible #region-us
| Intent-classification-BERT-Large-Ashuv5
=======================================
This model is a fine-tuned version of google-bert/bert-large-cased-whole-word-masking on an unknown dataset.
It achieves the following results on the evaluation set:
* Loss: 1.7988
* Accuracy: 0.1420
* F1: 0.0414
* Precision: 0.0237
* Recall: 0.1667
Model description
-----------------
More information needed
Intended uses & limitations
---------------------------
More information needed
Training and evaluation data
----------------------------
More information needed
Training procedure
------------------
### Training hyperparameters
The following hyperparameters were used during training:
* learning\_rate: 0.0005
* train\_batch\_size: 16
* eval\_batch\_size: 32
* seed: 42
* optimizer: Adam with betas=(0.9,0.999) and epsilon=1e-08
* lr\_scheduler\_type: linear
* num\_epochs: 3
### Training results
### Framework versions
* Transformers 4.38.2
* Pytorch 2.1.2
* Datasets 2.1.0
* Tokenizers 0.15.2
| [
"### Training hyperparameters\n\n\nThe following hyperparameters were used during training:\n\n\n* learning\\_rate: 0.0005\n* train\\_batch\\_size: 16\n* eval\\_batch\\_size: 32\n* seed: 42\n* optimizer: Adam with betas=(0.9,0.999) and epsilon=1e-08\n* lr\\_scheduler\\_type: linear\n* num\\_epochs: 3",
"### Training results",
"### Framework versions\n\n\n* Transformers 4.38.2\n* Pytorch 2.1.2\n* Datasets 2.1.0\n* Tokenizers 0.15.2"
] | [
"TAGS\n#transformers #safetensors #bert #text-classification #generated_from_trainer #base_model-google-bert/bert-large-cased-whole-word-masking #license-apache-2.0 #autotrain_compatible #endpoints_compatible #region-us \n",
"### Training hyperparameters\n\n\nThe following hyperparameters were used during training:\n\n\n* learning\\_rate: 0.0005\n* train\\_batch\\_size: 16\n* eval\\_batch\\_size: 32\n* seed: 42\n* optimizer: Adam with betas=(0.9,0.999) and epsilon=1e-08\n* lr\\_scheduler\\_type: linear\n* num\\_epochs: 3",
"### Training results",
"### Framework versions\n\n\n* Transformers 4.38.2\n* Pytorch 2.1.2\n* Datasets 2.1.0\n* Tokenizers 0.15.2"
] |
image-classification | transformers |
<!-- This model card has been generated automatically according to the information the Trainer had access to. You
should probably proofread and complete it, then remove this comment. -->
# swin-tiny-patch4-window7-224-finetuned-eurosat
This model is a fine-tuned version of [microsoft/swin-tiny-patch4-window7-224](https://huggingface.co/microsoft/swin-tiny-patch4-window7-224) on the imagefolder dataset.
It achieves the following results on the evaluation set:
- Loss: 0.0601
- Accuracy: 0.98
## Model description
More information needed
## Intended uses & limitations
More information needed
## Training and evaluation data
More information needed
## Training procedure
### Training hyperparameters
The following hyperparameters were used during training:
- learning_rate: 5e-05
- train_batch_size: 32
- eval_batch_size: 32
- seed: 42
- gradient_accumulation_steps: 4
- total_train_batch_size: 128
- optimizer: Adam with betas=(0.9,0.999) and epsilon=1e-08
- lr_scheduler_type: linear
- lr_scheduler_warmup_ratio: 0.1
- num_epochs: 3
### Training results
| Training Loss | Epoch | Step | Validation Loss | Accuracy |
|:-------------:|:-----:|:----:|:---------------:|:--------:|
| 0.2994 | 1.0 | 190 | 0.1234 | 0.9604 |
| 0.1853 | 2.0 | 380 | 0.0705 | 0.9741 |
| 0.158 | 3.0 | 570 | 0.0601 | 0.98 |
### Framework versions
- Transformers 4.38.2
- Pytorch 2.2.1+cu121
- Datasets 2.18.0
- Tokenizers 0.15.2
| {"license": "apache-2.0", "tags": ["generated_from_trainer"], "datasets": ["imagefolder"], "metrics": ["accuracy"], "base_model": "microsoft/swin-tiny-patch4-window7-224", "model-index": [{"name": "swin-tiny-patch4-window7-224-finetuned-eurosat", "results": [{"task": {"type": "image-classification", "name": "Image Classification"}, "dataset": {"name": "imagefolder", "type": "imagefolder", "config": "default", "split": "train", "args": "default"}, "metrics": [{"type": "accuracy", "value": 0.98, "name": "Accuracy"}]}]}]} | iayrots/swin-tiny-patch4-window7-224-finetuned-eurosat | null | [
"transformers",
"tensorboard",
"safetensors",
"swin",
"image-classification",
"generated_from_trainer",
"dataset:imagefolder",
"base_model:microsoft/swin-tiny-patch4-window7-224",
"license:apache-2.0",
"model-index",
"autotrain_compatible",
"endpoints_compatible",
"region:us"
] | null | 2024-04-18T08:15:49+00:00 | [] | [] | TAGS
#transformers #tensorboard #safetensors #swin #image-classification #generated_from_trainer #dataset-imagefolder #base_model-microsoft/swin-tiny-patch4-window7-224 #license-apache-2.0 #model-index #autotrain_compatible #endpoints_compatible #region-us
| swin-tiny-patch4-window7-224-finetuned-eurosat
==============================================
This model is a fine-tuned version of microsoft/swin-tiny-patch4-window7-224 on the imagefolder dataset.
It achieves the following results on the evaluation set:
* Loss: 0.0601
* Accuracy: 0.98
Model description
-----------------
More information needed
Intended uses & limitations
---------------------------
More information needed
Training and evaluation data
----------------------------
More information needed
Training procedure
------------------
### Training hyperparameters
The following hyperparameters were used during training:
* learning\_rate: 5e-05
* train\_batch\_size: 32
* eval\_batch\_size: 32
* seed: 42
* gradient\_accumulation\_steps: 4
* total\_train\_batch\_size: 128
* optimizer: Adam with betas=(0.9,0.999) and epsilon=1e-08
* lr\_scheduler\_type: linear
* lr\_scheduler\_warmup\_ratio: 0.1
* num\_epochs: 3
### Training results
### Framework versions
* Transformers 4.38.2
* Pytorch 2.2.1+cu121
* Datasets 2.18.0
* Tokenizers 0.15.2
| [
"### Training hyperparameters\n\n\nThe following hyperparameters were used during training:\n\n\n* learning\\_rate: 5e-05\n* train\\_batch\\_size: 32\n* eval\\_batch\\_size: 32\n* seed: 42\n* gradient\\_accumulation\\_steps: 4\n* total\\_train\\_batch\\_size: 128\n* optimizer: Adam with betas=(0.9,0.999) and epsilon=1e-08\n* lr\\_scheduler\\_type: linear\n* lr\\_scheduler\\_warmup\\_ratio: 0.1\n* num\\_epochs: 3",
"### Training results",
"### Framework versions\n\n\n* Transformers 4.38.2\n* Pytorch 2.2.1+cu121\n* Datasets 2.18.0\n* Tokenizers 0.15.2"
] | [
"TAGS\n#transformers #tensorboard #safetensors #swin #image-classification #generated_from_trainer #dataset-imagefolder #base_model-microsoft/swin-tiny-patch4-window7-224 #license-apache-2.0 #model-index #autotrain_compatible #endpoints_compatible #region-us \n",
"### Training hyperparameters\n\n\nThe following hyperparameters were used during training:\n\n\n* learning\\_rate: 5e-05\n* train\\_batch\\_size: 32\n* eval\\_batch\\_size: 32\n* seed: 42\n* gradient\\_accumulation\\_steps: 4\n* total\\_train\\_batch\\_size: 128\n* optimizer: Adam with betas=(0.9,0.999) and epsilon=1e-08\n* lr\\_scheduler\\_type: linear\n* lr\\_scheduler\\_warmup\\_ratio: 0.1\n* num\\_epochs: 3",
"### Training results",
"### Framework versions\n\n\n* Transformers 4.38.2\n* Pytorch 2.2.1+cu121\n* Datasets 2.18.0\n* Tokenizers 0.15.2"
] |
feature-extraction | transformers |
# Model Card for Model ID
<!-- Provide a quick summary of what the model is/does. -->
## Model Details
### Model Description
<!-- Provide a longer summary of what this model is. -->
This is the model card of a 🤗 transformers model that has been pushed on the Hub. This model card has been automatically generated.
- **Developed by:** [More Information Needed]
- **Funded by [optional]:** [More Information Needed]
- **Shared by [optional]:** [More Information Needed]
- **Model type:** [More Information Needed]
- **Language(s) (NLP):** [More Information Needed]
- **License:** [More Information Needed]
- **Finetuned from model [optional]:** [More Information Needed]
### Model Sources [optional]
<!-- Provide the basic links for the model. -->
- **Repository:** [More Information Needed]
- **Paper [optional]:** [More Information Needed]
- **Demo [optional]:** [More Information Needed]
## Uses
<!-- Address questions around how the model is intended to be used, including the foreseeable users of the model and those affected by the model. -->
### Direct Use
<!-- This section is for the model use without fine-tuning or plugging into a larger ecosystem/app. -->
[More Information Needed]
### Downstream Use [optional]
<!-- This section is for the model use when fine-tuned for a task, or when plugged into a larger ecosystem/app -->
[More Information Needed]
### Out-of-Scope Use
<!-- This section addresses misuse, malicious use, and uses that the model will not work well for. -->
[More Information Needed]
## Bias, Risks, and Limitations
<!-- This section is meant to convey both technical and sociotechnical limitations. -->
[More Information Needed]
### Recommendations
<!-- This section is meant to convey recommendations with respect to the bias, risk, and technical limitations. -->
Users (both direct and downstream) should be made aware of the risks, biases and limitations of the model. More information needed for further recommendations.
## How to Get Started with the Model
Use the code below to get started with the model.
[More Information Needed]
## Training Details
### Training Data
<!-- This should link to a Dataset Card, perhaps with a short stub of information on what the training data is all about as well as documentation related to data pre-processing or additional filtering. -->
[More Information Needed]
### Training Procedure
<!-- This relates heavily to the Technical Specifications. Content here should link to that section when it is relevant to the training procedure. -->
#### Preprocessing [optional]
[More Information Needed]
#### Training Hyperparameters
- **Training regime:** [More Information Needed] <!--fp32, fp16 mixed precision, bf16 mixed precision, bf16 non-mixed precision, fp16 non-mixed precision, fp8 mixed precision -->
#### Speeds, Sizes, Times [optional]
<!-- This section provides information about throughput, start/end time, checkpoint size if relevant, etc. -->
[More Information Needed]
## Evaluation
<!-- This section describes the evaluation protocols and provides the results. -->
### Testing Data, Factors & Metrics
#### Testing Data
<!-- This should link to a Dataset Card if possible. -->
[More Information Needed]
#### Factors
<!-- These are the things the evaluation is disaggregating by, e.g., subpopulations or domains. -->
[More Information Needed]
#### Metrics
<!-- These are the evaluation metrics being used, ideally with a description of why. -->
[More Information Needed]
### Results
[More Information Needed]
#### Summary
## Model Examination [optional]
<!-- Relevant interpretability work for the model goes here -->
[More Information Needed]
## Environmental Impact
<!-- Total emissions (in grams of CO2eq) and additional considerations, such as electricity usage, go here. Edit the suggested text below accordingly -->
Carbon emissions can be estimated using the [Machine Learning Impact calculator](https://mlco2.github.io/impact#compute) presented in [Lacoste et al. (2019)](https://arxiv.org/abs/1910.09700).
- **Hardware Type:** [More Information Needed]
- **Hours used:** [More Information Needed]
- **Cloud Provider:** [More Information Needed]
- **Compute Region:** [More Information Needed]
- **Carbon Emitted:** [More Information Needed]
## Technical Specifications [optional]
### Model Architecture and Objective
[More Information Needed]
### Compute Infrastructure
[More Information Needed]
#### Hardware
[More Information Needed]
#### Software
[More Information Needed]
## Citation [optional]
<!-- If there is a paper or blog post introducing the model, the APA and Bibtex information for that should go in this section. -->
**BibTeX:**
[More Information Needed]
**APA:**
[More Information Needed]
## Glossary [optional]
<!-- If relevant, include terms and calculations in this section that can help readers understand the model or model card. -->
[More Information Needed]
## More Information [optional]
[More Information Needed]
## Model Card Authors [optional]
[More Information Needed]
## Model Card Contact
[More Information Needed]
| {"library_name": "transformers", "tags": []} | EinsZwo/mlm_mixed_supertagging_fullset_justbert_alpha05 | null | [
"transformers",
"safetensors",
"bert",
"feature-extraction",
"arxiv:1910.09700",
"endpoints_compatible",
"region:us"
] | null | 2024-04-18T08:15:50+00:00 | [
"1910.09700"
] | [] | TAGS
#transformers #safetensors #bert #feature-extraction #arxiv-1910.09700 #endpoints_compatible #region-us
|
# Model Card for Model ID
## Model Details
### Model Description
This is the model card of a transformers model that has been pushed on the Hub. This model card has been automatically generated.
- Developed by:
- Funded by [optional]:
- Shared by [optional]:
- Model type:
- Language(s) (NLP):
- License:
- Finetuned from model [optional]:
### Model Sources [optional]
- Repository:
- Paper [optional]:
- Demo [optional]:
## Uses
### Direct Use
### Downstream Use [optional]
### Out-of-Scope Use
## Bias, Risks, and Limitations
### Recommendations
Users (both direct and downstream) should be made aware of the risks, biases and limitations of the model. More information needed for further recommendations.
## How to Get Started with the Model
Use the code below to get started with the model.
## Training Details
### Training Data
### Training Procedure
#### Preprocessing [optional]
#### Training Hyperparameters
- Training regime:
#### Speeds, Sizes, Times [optional]
## Evaluation
### Testing Data, Factors & Metrics
#### Testing Data
#### Factors
#### Metrics
### Results
#### Summary
## Model Examination [optional]
## Environmental Impact
Carbon emissions can be estimated using the Machine Learning Impact calculator presented in Lacoste et al. (2019).
- Hardware Type:
- Hours used:
- Cloud Provider:
- Compute Region:
- Carbon Emitted:
## Technical Specifications [optional]
### Model Architecture and Objective
### Compute Infrastructure
#### Hardware
#### Software
[optional]
BibTeX:
APA:
## Glossary [optional]
## More Information [optional]
## Model Card Authors [optional]
## Model Card Contact
| [
"# Model Card for Model ID",
"## Model Details",
"### Model Description\n\n\n\nThis is the model card of a transformers model that has been pushed on the Hub. This model card has been automatically generated.\n\n- Developed by: \n- Funded by [optional]: \n- Shared by [optional]: \n- Model type: \n- Language(s) (NLP): \n- License: \n- Finetuned from model [optional]:",
"### Model Sources [optional]\n\n\n\n- Repository: \n- Paper [optional]: \n- Demo [optional]:",
"## Uses",
"### Direct Use",
"### Downstream Use [optional]",
"### Out-of-Scope Use",
"## Bias, Risks, and Limitations",
"### Recommendations\n\n\n\nUsers (both direct and downstream) should be made aware of the risks, biases and limitations of the model. More information needed for further recommendations.",
"## How to Get Started with the Model\n\nUse the code below to get started with the model.",
"## Training Details",
"### Training Data",
"### Training Procedure",
"#### Preprocessing [optional]",
"#### Training Hyperparameters\n\n- Training regime:",
"#### Speeds, Sizes, Times [optional]",
"## Evaluation",
"### Testing Data, Factors & Metrics",
"#### Testing Data",
"#### Factors",
"#### Metrics",
"### Results",
"#### Summary",
"## Model Examination [optional]",
"## Environmental Impact\n\n\n\nCarbon emissions can be estimated using the Machine Learning Impact calculator presented in Lacoste et al. (2019).\n\n- Hardware Type: \n- Hours used: \n- Cloud Provider: \n- Compute Region: \n- Carbon Emitted:",
"## Technical Specifications [optional]",
"### Model Architecture and Objective",
"### Compute Infrastructure",
"#### Hardware",
"#### Software\n\n\n\n[optional]\n\n\n\nBibTeX:\n\n\n\nAPA:",
"## Glossary [optional]",
"## More Information [optional]",
"## Model Card Authors [optional]",
"## Model Card Contact"
] | [
"TAGS\n#transformers #safetensors #bert #feature-extraction #arxiv-1910.09700 #endpoints_compatible #region-us \n",
"# Model Card for Model ID",
"## Model Details",
"### Model Description\n\n\n\nThis is the model card of a transformers model that has been pushed on the Hub. This model card has been automatically generated.\n\n- Developed by: \n- Funded by [optional]: \n- Shared by [optional]: \n- Model type: \n- Language(s) (NLP): \n- License: \n- Finetuned from model [optional]:",
"### Model Sources [optional]\n\n\n\n- Repository: \n- Paper [optional]: \n- Demo [optional]:",
"## Uses",
"### Direct Use",
"### Downstream Use [optional]",
"### Out-of-Scope Use",
"## Bias, Risks, and Limitations",
"### Recommendations\n\n\n\nUsers (both direct and downstream) should be made aware of the risks, biases and limitations of the model. More information needed for further recommendations.",
"## How to Get Started with the Model\n\nUse the code below to get started with the model.",
"## Training Details",
"### Training Data",
"### Training Procedure",
"#### Preprocessing [optional]",
"#### Training Hyperparameters\n\n- Training regime:",
"#### Speeds, Sizes, Times [optional]",
"## Evaluation",
"### Testing Data, Factors & Metrics",
"#### Testing Data",
"#### Factors",
"#### Metrics",
"### Results",
"#### Summary",
"## Model Examination [optional]",
"## Environmental Impact\n\n\n\nCarbon emissions can be estimated using the Machine Learning Impact calculator presented in Lacoste et al. (2019).\n\n- Hardware Type: \n- Hours used: \n- Cloud Provider: \n- Compute Region: \n- Carbon Emitted:",
"## Technical Specifications [optional]",
"### Model Architecture and Objective",
"### Compute Infrastructure",
"#### Hardware",
"#### Software\n\n\n\n[optional]\n\n\n\nBibTeX:\n\n\n\nAPA:",
"## Glossary [optional]",
"## More Information [optional]",
"## Model Card Authors [optional]",
"## Model Card Contact"
] |
text-generation | transformers | Qwen 7B chat by Alibaba, SFTuned on Saiga dataset. Finetuned with EasyDeL framework on v3-8 Google TPU, provided by TRC.
Модель Qwen 7B, дообученая на датасете Ильи Гусева. По моему краткому опыту общения с моделью, лучше чем Saiga-mistral. Не ошибается в падежах. Карточка модели будет дополнена после теста на Russian SuperGlue. Возможно, будет DPO
Чтобы использовать модель, необходимо назначить eos токен как <|im_end|>. Рабочий ноутбук на Kaggle: https://www.kaggle.com/code/defdet/smol-chatbot/notebook
| {"license": "apache-2.0", "tags": ["Russian"]} | Defetya/qwen-7B-saiga | null | [
"transformers",
"safetensors",
"qwen2",
"text-generation",
"Russian",
"conversational",
"license:apache-2.0",
"autotrain_compatible",
"endpoints_compatible",
"text-generation-inference",
"region:us"
] | null | 2024-04-18T08:15:55+00:00 | [] | [] | TAGS
#transformers #safetensors #qwen2 #text-generation #Russian #conversational #license-apache-2.0 #autotrain_compatible #endpoints_compatible #text-generation-inference #region-us
| Qwen 7B chat by Alibaba, SFTuned on Saiga dataset. Finetuned with EasyDeL framework on v3-8 Google TPU, provided by TRC.
Модель Qwen 7B, дообученая на датасете Ильи Гусева. По моему краткому опыту общения с моделью, лучше чем Saiga-mistral. Не ошибается в падежах. Карточка модели будет дополнена после теста на Russian SuperGlue. Возможно, будет DPO
Чтобы использовать модель, необходимо назначить eos токен как <|im_end|>. Рабочий ноутбук на Kaggle: URL
| [] | [
"TAGS\n#transformers #safetensors #qwen2 #text-generation #Russian #conversational #license-apache-2.0 #autotrain_compatible #endpoints_compatible #text-generation-inference #region-us \n"
] |
text-generation | transformers |
# Model Card for Model ID
<!-- Provide a quick summary of what the model is/does. -->
## Model Details
### Model Description
<!-- Provide a longer summary of what this model is. -->
This is the model card of a 🤗 transformers model that has been pushed on the Hub. This model card has been automatically generated.
- **Developed by:** [More Information Needed]
- **Funded by [optional]:** [More Information Needed]
- **Shared by [optional]:** [More Information Needed]
- **Model type:** [More Information Needed]
- **Language(s) (NLP):** [More Information Needed]
- **License:** [More Information Needed]
- **Finetuned from model [optional]:** [More Information Needed]
### Model Sources [optional]
<!-- Provide the basic links for the model. -->
- **Repository:** [More Information Needed]
- **Paper [optional]:** [More Information Needed]
- **Demo [optional]:** [More Information Needed]
## Uses
<!-- Address questions around how the model is intended to be used, including the foreseeable users of the model and those affected by the model. -->
### Direct Use
<!-- This section is for the model use without fine-tuning or plugging into a larger ecosystem/app. -->
[More Information Needed]
### Downstream Use [optional]
<!-- This section is for the model use when fine-tuned for a task, or when plugged into a larger ecosystem/app -->
[More Information Needed]
### Out-of-Scope Use
<!-- This section addresses misuse, malicious use, and uses that the model will not work well for. -->
[More Information Needed]
## Bias, Risks, and Limitations
<!-- This section is meant to convey both technical and sociotechnical limitations. -->
[More Information Needed]
### Recommendations
<!-- This section is meant to convey recommendations with respect to the bias, risk, and technical limitations. -->
Users (both direct and downstream) should be made aware of the risks, biases and limitations of the model. More information needed for further recommendations.
## How to Get Started with the Model
Use the code below to get started with the model.
[More Information Needed]
## Training Details
### Training Data
<!-- This should link to a Dataset Card, perhaps with a short stub of information on what the training data is all about as well as documentation related to data pre-processing or additional filtering. -->
[More Information Needed]
### Training Procedure
<!-- This relates heavily to the Technical Specifications. Content here should link to that section when it is relevant to the training procedure. -->
#### Preprocessing [optional]
[More Information Needed]
#### Training Hyperparameters
- **Training regime:** [More Information Needed] <!--fp32, fp16 mixed precision, bf16 mixed precision, bf16 non-mixed precision, fp16 non-mixed precision, fp8 mixed precision -->
#### Speeds, Sizes, Times [optional]
<!-- This section provides information about throughput, start/end time, checkpoint size if relevant, etc. -->
[More Information Needed]
## Evaluation
<!-- This section describes the evaluation protocols and provides the results. -->
### Testing Data, Factors & Metrics
#### Testing Data
<!-- This should link to a Dataset Card if possible. -->
[More Information Needed]
#### Factors
<!-- These are the things the evaluation is disaggregating by, e.g., subpopulations or domains. -->
[More Information Needed]
#### Metrics
<!-- These are the evaluation metrics being used, ideally with a description of why. -->
[More Information Needed]
### Results
[More Information Needed]
#### Summary
## Model Examination [optional]
<!-- Relevant interpretability work for the model goes here -->
[More Information Needed]
## Environmental Impact
<!-- Total emissions (in grams of CO2eq) and additional considerations, such as electricity usage, go here. Edit the suggested text below accordingly -->
Carbon emissions can be estimated using the [Machine Learning Impact calculator](https://mlco2.github.io/impact#compute) presented in [Lacoste et al. (2019)](https://arxiv.org/abs/1910.09700).
- **Hardware Type:** [More Information Needed]
- **Hours used:** [More Information Needed]
- **Cloud Provider:** [More Information Needed]
- **Compute Region:** [More Information Needed]
- **Carbon Emitted:** [More Information Needed]
## Technical Specifications [optional]
### Model Architecture and Objective
[More Information Needed]
### Compute Infrastructure
[More Information Needed]
#### Hardware
[More Information Needed]
#### Software
[More Information Needed]
## Citation [optional]
<!-- If there is a paper or blog post introducing the model, the APA and Bibtex information for that should go in this section. -->
**BibTeX:**
[More Information Needed]
**APA:**
[More Information Needed]
## Glossary [optional]
<!-- If relevant, include terms and calculations in this section that can help readers understand the model or model card. -->
[More Information Needed]
## More Information [optional]
[More Information Needed]
## Model Card Authors [optional]
[More Information Needed]
## Model Card Contact
[More Information Needed]
| {"library_name": "transformers", "tags": []} | gildead/mistral-aes-966 | null | [
"transformers",
"safetensors",
"mistral",
"text-generation",
"conversational",
"arxiv:1910.09700",
"autotrain_compatible",
"endpoints_compatible",
"text-generation-inference",
"4-bit",
"region:us"
] | null | 2024-04-18T08:16:12+00:00 | [
"1910.09700"
] | [] | TAGS
#transformers #safetensors #mistral #text-generation #conversational #arxiv-1910.09700 #autotrain_compatible #endpoints_compatible #text-generation-inference #4-bit #region-us
|
# Model Card for Model ID
## Model Details
### Model Description
This is the model card of a transformers model that has been pushed on the Hub. This model card has been automatically generated.
- Developed by:
- Funded by [optional]:
- Shared by [optional]:
- Model type:
- Language(s) (NLP):
- License:
- Finetuned from model [optional]:
### Model Sources [optional]
- Repository:
- Paper [optional]:
- Demo [optional]:
## Uses
### Direct Use
### Downstream Use [optional]
### Out-of-Scope Use
## Bias, Risks, and Limitations
### Recommendations
Users (both direct and downstream) should be made aware of the risks, biases and limitations of the model. More information needed for further recommendations.
## How to Get Started with the Model
Use the code below to get started with the model.
## Training Details
### Training Data
### Training Procedure
#### Preprocessing [optional]
#### Training Hyperparameters
- Training regime:
#### Speeds, Sizes, Times [optional]
## Evaluation
### Testing Data, Factors & Metrics
#### Testing Data
#### Factors
#### Metrics
### Results
#### Summary
## Model Examination [optional]
## Environmental Impact
Carbon emissions can be estimated using the Machine Learning Impact calculator presented in Lacoste et al. (2019).
- Hardware Type:
- Hours used:
- Cloud Provider:
- Compute Region:
- Carbon Emitted:
## Technical Specifications [optional]
### Model Architecture and Objective
### Compute Infrastructure
#### Hardware
#### Software
[optional]
BibTeX:
APA:
## Glossary [optional]
## More Information [optional]
## Model Card Authors [optional]
## Model Card Contact
| [
"# Model Card for Model ID",
"## Model Details",
"### Model Description\n\n\n\nThis is the model card of a transformers model that has been pushed on the Hub. This model card has been automatically generated.\n\n- Developed by: \n- Funded by [optional]: \n- Shared by [optional]: \n- Model type: \n- Language(s) (NLP): \n- License: \n- Finetuned from model [optional]:",
"### Model Sources [optional]\n\n\n\n- Repository: \n- Paper [optional]: \n- Demo [optional]:",
"## Uses",
"### Direct Use",
"### Downstream Use [optional]",
"### Out-of-Scope Use",
"## Bias, Risks, and Limitations",
"### Recommendations\n\n\n\nUsers (both direct and downstream) should be made aware of the risks, biases and limitations of the model. More information needed for further recommendations.",
"## How to Get Started with the Model\n\nUse the code below to get started with the model.",
"## Training Details",
"### Training Data",
"### Training Procedure",
"#### Preprocessing [optional]",
"#### Training Hyperparameters\n\n- Training regime:",
"#### Speeds, Sizes, Times [optional]",
"## Evaluation",
"### Testing Data, Factors & Metrics",
"#### Testing Data",
"#### Factors",
"#### Metrics",
"### Results",
"#### Summary",
"## Model Examination [optional]",
"## Environmental Impact\n\n\n\nCarbon emissions can be estimated using the Machine Learning Impact calculator presented in Lacoste et al. (2019).\n\n- Hardware Type: \n- Hours used: \n- Cloud Provider: \n- Compute Region: \n- Carbon Emitted:",
"## Technical Specifications [optional]",
"### Model Architecture and Objective",
"### Compute Infrastructure",
"#### Hardware",
"#### Software\n\n\n\n[optional]\n\n\n\nBibTeX:\n\n\n\nAPA:",
"## Glossary [optional]",
"## More Information [optional]",
"## Model Card Authors [optional]",
"## Model Card Contact"
] | [
"TAGS\n#transformers #safetensors #mistral #text-generation #conversational #arxiv-1910.09700 #autotrain_compatible #endpoints_compatible #text-generation-inference #4-bit #region-us \n",
"# Model Card for Model ID",
"## Model Details",
"### Model Description\n\n\n\nThis is the model card of a transformers model that has been pushed on the Hub. This model card has been automatically generated.\n\n- Developed by: \n- Funded by [optional]: \n- Shared by [optional]: \n- Model type: \n- Language(s) (NLP): \n- License: \n- Finetuned from model [optional]:",
"### Model Sources [optional]\n\n\n\n- Repository: \n- Paper [optional]: \n- Demo [optional]:",
"## Uses",
"### Direct Use",
"### Downstream Use [optional]",
"### Out-of-Scope Use",
"## Bias, Risks, and Limitations",
"### Recommendations\n\n\n\nUsers (both direct and downstream) should be made aware of the risks, biases and limitations of the model. More information needed for further recommendations.",
"## How to Get Started with the Model\n\nUse the code below to get started with the model.",
"## Training Details",
"### Training Data",
"### Training Procedure",
"#### Preprocessing [optional]",
"#### Training Hyperparameters\n\n- Training regime:",
"#### Speeds, Sizes, Times [optional]",
"## Evaluation",
"### Testing Data, Factors & Metrics",
"#### Testing Data",
"#### Factors",
"#### Metrics",
"### Results",
"#### Summary",
"## Model Examination [optional]",
"## Environmental Impact\n\n\n\nCarbon emissions can be estimated using the Machine Learning Impact calculator presented in Lacoste et al. (2019).\n\n- Hardware Type: \n- Hours used: \n- Cloud Provider: \n- Compute Region: \n- Carbon Emitted:",
"## Technical Specifications [optional]",
"### Model Architecture and Objective",
"### Compute Infrastructure",
"#### Hardware",
"#### Software\n\n\n\n[optional]\n\n\n\nBibTeX:\n\n\n\nAPA:",
"## Glossary [optional]",
"## More Information [optional]",
"## Model Card Authors [optional]",
"## Model Card Contact"
] |
text-generation | transformers |
# Model Card for Model ID
<!-- Provide a quick summary of what the model is/does. -->
## Model Details
### Model Description
<!-- Provide a longer summary of what this model is. -->
This is the model card of a 🤗 transformers model that has been pushed on the Hub. This model card has been automatically generated.
- **Developed by:** [More Information Needed]
- **Funded by [optional]:** [More Information Needed]
- **Shared by [optional]:** [More Information Needed]
- **Model type:** [More Information Needed]
- **Language(s) (NLP):** [More Information Needed]
- **License:** [More Information Needed]
- **Finetuned from model [optional]:** [More Information Needed]
### Model Sources [optional]
<!-- Provide the basic links for the model. -->
- **Repository:** [More Information Needed]
- **Paper [optional]:** [More Information Needed]
- **Demo [optional]:** [More Information Needed]
## Uses
<!-- Address questions around how the model is intended to be used, including the foreseeable users of the model and those affected by the model. -->
### Direct Use
<!-- This section is for the model use without fine-tuning or plugging into a larger ecosystem/app. -->
[More Information Needed]
### Downstream Use [optional]
<!-- This section is for the model use when fine-tuned for a task, or when plugged into a larger ecosystem/app -->
[More Information Needed]
### Out-of-Scope Use
<!-- This section addresses misuse, malicious use, and uses that the model will not work well for. -->
[More Information Needed]
## Bias, Risks, and Limitations
<!-- This section is meant to convey both technical and sociotechnical limitations. -->
[More Information Needed]
### Recommendations
<!-- This section is meant to convey recommendations with respect to the bias, risk, and technical limitations. -->
Users (both direct and downstream) should be made aware of the risks, biases and limitations of the model. More information needed for further recommendations.
## How to Get Started with the Model
Use the code below to get started with the model.
[More Information Needed]
## Training Details
### Training Data
<!-- This should link to a Dataset Card, perhaps with a short stub of information on what the training data is all about as well as documentation related to data pre-processing or additional filtering. -->
[More Information Needed]
### Training Procedure
<!-- This relates heavily to the Technical Specifications. Content here should link to that section when it is relevant to the training procedure. -->
#### Preprocessing [optional]
[More Information Needed]
#### Training Hyperparameters
- **Training regime:** [More Information Needed] <!--fp32, fp16 mixed precision, bf16 mixed precision, bf16 non-mixed precision, fp16 non-mixed precision, fp8 mixed precision -->
#### Speeds, Sizes, Times [optional]
<!-- This section provides information about throughput, start/end time, checkpoint size if relevant, etc. -->
[More Information Needed]
## Evaluation
<!-- This section describes the evaluation protocols and provides the results. -->
### Testing Data, Factors & Metrics
#### Testing Data
<!-- This should link to a Dataset Card if possible. -->
[More Information Needed]
#### Factors
<!-- These are the things the evaluation is disaggregating by, e.g., subpopulations or domains. -->
[More Information Needed]
#### Metrics
<!-- These are the evaluation metrics being used, ideally with a description of why. -->
[More Information Needed]
### Results
[More Information Needed]
#### Summary
## Model Examination [optional]
<!-- Relevant interpretability work for the model goes here -->
[More Information Needed]
## Environmental Impact
<!-- Total emissions (in grams of CO2eq) and additional considerations, such as electricity usage, go here. Edit the suggested text below accordingly -->
Carbon emissions can be estimated using the [Machine Learning Impact calculator](https://mlco2.github.io/impact#compute) presented in [Lacoste et al. (2019)](https://arxiv.org/abs/1910.09700).
- **Hardware Type:** [More Information Needed]
- **Hours used:** [More Information Needed]
- **Cloud Provider:** [More Information Needed]
- **Compute Region:** [More Information Needed]
- **Carbon Emitted:** [More Information Needed]
## Technical Specifications [optional]
### Model Architecture and Objective
[More Information Needed]
### Compute Infrastructure
[More Information Needed]
#### Hardware
[More Information Needed]
#### Software
[More Information Needed]
## Citation [optional]
<!-- If there is a paper or blog post introducing the model, the APA and Bibtex information for that should go in this section. -->
**BibTeX:**
[More Information Needed]
**APA:**
[More Information Needed]
## Glossary [optional]
<!-- If relevant, include terms and calculations in this section that can help readers understand the model or model card. -->
[More Information Needed]
## More Information [optional]
[More Information Needed]
## Model Card Authors [optional]
[More Information Needed]
## Model Card Contact
[More Information Needed] | {"library_name": "transformers", "tags": []} | OwOOwO/dumbo-krillin52 | null | [
"transformers",
"safetensors",
"llama",
"text-generation",
"conversational",
"arxiv:1910.09700",
"autotrain_compatible",
"endpoints_compatible",
"text-generation-inference",
"region:us"
] | null | 2024-04-18T08:17:43+00:00 | [
"1910.09700"
] | [] | TAGS
#transformers #safetensors #llama #text-generation #conversational #arxiv-1910.09700 #autotrain_compatible #endpoints_compatible #text-generation-inference #region-us
|
# Model Card for Model ID
## Model Details
### Model Description
This is the model card of a transformers model that has been pushed on the Hub. This model card has been automatically generated.
- Developed by:
- Funded by [optional]:
- Shared by [optional]:
- Model type:
- Language(s) (NLP):
- License:
- Finetuned from model [optional]:
### Model Sources [optional]
- Repository:
- Paper [optional]:
- Demo [optional]:
## Uses
### Direct Use
### Downstream Use [optional]
### Out-of-Scope Use
## Bias, Risks, and Limitations
### Recommendations
Users (both direct and downstream) should be made aware of the risks, biases and limitations of the model. More information needed for further recommendations.
## How to Get Started with the Model
Use the code below to get started with the model.
## Training Details
### Training Data
### Training Procedure
#### Preprocessing [optional]
#### Training Hyperparameters
- Training regime:
#### Speeds, Sizes, Times [optional]
## Evaluation
### Testing Data, Factors & Metrics
#### Testing Data
#### Factors
#### Metrics
### Results
#### Summary
## Model Examination [optional]
## Environmental Impact
Carbon emissions can be estimated using the Machine Learning Impact calculator presented in Lacoste et al. (2019).
- Hardware Type:
- Hours used:
- Cloud Provider:
- Compute Region:
- Carbon Emitted:
## Technical Specifications [optional]
### Model Architecture and Objective
### Compute Infrastructure
#### Hardware
#### Software
[optional]
BibTeX:
APA:
## Glossary [optional]
## More Information [optional]
## Model Card Authors [optional]
## Model Card Contact
| [
"# Model Card for Model ID",
"## Model Details",
"### Model Description\n\n\n\nThis is the model card of a transformers model that has been pushed on the Hub. This model card has been automatically generated.\n\n- Developed by: \n- Funded by [optional]: \n- Shared by [optional]: \n- Model type: \n- Language(s) (NLP): \n- License: \n- Finetuned from model [optional]:",
"### Model Sources [optional]\n\n\n\n- Repository: \n- Paper [optional]: \n- Demo [optional]:",
"## Uses",
"### Direct Use",
"### Downstream Use [optional]",
"### Out-of-Scope Use",
"## Bias, Risks, and Limitations",
"### Recommendations\n\n\n\nUsers (both direct and downstream) should be made aware of the risks, biases and limitations of the model. More information needed for further recommendations.",
"## How to Get Started with the Model\n\nUse the code below to get started with the model.",
"## Training Details",
"### Training Data",
"### Training Procedure",
"#### Preprocessing [optional]",
"#### Training Hyperparameters\n\n- Training regime:",
"#### Speeds, Sizes, Times [optional]",
"## Evaluation",
"### Testing Data, Factors & Metrics",
"#### Testing Data",
"#### Factors",
"#### Metrics",
"### Results",
"#### Summary",
"## Model Examination [optional]",
"## Environmental Impact\n\n\n\nCarbon emissions can be estimated using the Machine Learning Impact calculator presented in Lacoste et al. (2019).\n\n- Hardware Type: \n- Hours used: \n- Cloud Provider: \n- Compute Region: \n- Carbon Emitted:",
"## Technical Specifications [optional]",
"### Model Architecture and Objective",
"### Compute Infrastructure",
"#### Hardware",
"#### Software\n\n\n\n[optional]\n\n\n\nBibTeX:\n\n\n\nAPA:",
"## Glossary [optional]",
"## More Information [optional]",
"## Model Card Authors [optional]",
"## Model Card Contact"
] | [
"TAGS\n#transformers #safetensors #llama #text-generation #conversational #arxiv-1910.09700 #autotrain_compatible #endpoints_compatible #text-generation-inference #region-us \n",
"# Model Card for Model ID",
"## Model Details",
"### Model Description\n\n\n\nThis is the model card of a transformers model that has been pushed on the Hub. This model card has been automatically generated.\n\n- Developed by: \n- Funded by [optional]: \n- Shared by [optional]: \n- Model type: \n- Language(s) (NLP): \n- License: \n- Finetuned from model [optional]:",
"### Model Sources [optional]\n\n\n\n- Repository: \n- Paper [optional]: \n- Demo [optional]:",
"## Uses",
"### Direct Use",
"### Downstream Use [optional]",
"### Out-of-Scope Use",
"## Bias, Risks, and Limitations",
"### Recommendations\n\n\n\nUsers (both direct and downstream) should be made aware of the risks, biases and limitations of the model. More information needed for further recommendations.",
"## How to Get Started with the Model\n\nUse the code below to get started with the model.",
"## Training Details",
"### Training Data",
"### Training Procedure",
"#### Preprocessing [optional]",
"#### Training Hyperparameters\n\n- Training regime:",
"#### Speeds, Sizes, Times [optional]",
"## Evaluation",
"### Testing Data, Factors & Metrics",
"#### Testing Data",
"#### Factors",
"#### Metrics",
"### Results",
"#### Summary",
"## Model Examination [optional]",
"## Environmental Impact\n\n\n\nCarbon emissions can be estimated using the Machine Learning Impact calculator presented in Lacoste et al. (2019).\n\n- Hardware Type: \n- Hours used: \n- Cloud Provider: \n- Compute Region: \n- Carbon Emitted:",
"## Technical Specifications [optional]",
"### Model Architecture and Objective",
"### Compute Infrastructure",
"#### Hardware",
"#### Software\n\n\n\n[optional]\n\n\n\nBibTeX:\n\n\n\nAPA:",
"## Glossary [optional]",
"## More Information [optional]",
"## Model Card Authors [optional]",
"## Model Card Contact"
] |
text-generation | transformers |
# Model Card for Model ID
<!-- Provide a quick summary of what the model is/does. -->
## Model Details
### Model Description
<!-- Provide a longer summary of what this model is. -->
This is the model card of a 🤗 transformers model that has been pushed on the Hub. This model card has been automatically generated.
- **Developed by:** [More Information Needed]
- **Funded by [optional]:** [More Information Needed]
- **Shared by [optional]:** [More Information Needed]
- **Model type:** [More Information Needed]
- **Language(s) (NLP):** [More Information Needed]
- **License:** [More Information Needed]
- **Finetuned from model [optional]:** [More Information Needed]
### Model Sources [optional]
<!-- Provide the basic links for the model. -->
- **Repository:** [More Information Needed]
- **Paper [optional]:** [More Information Needed]
- **Demo [optional]:** [More Information Needed]
## Uses
<!-- Address questions around how the model is intended to be used, including the foreseeable users of the model and those affected by the model. -->
### Direct Use
<!-- This section is for the model use without fine-tuning or plugging into a larger ecosystem/app. -->
[More Information Needed]
### Downstream Use [optional]
<!-- This section is for the model use when fine-tuned for a task, or when plugged into a larger ecosystem/app -->
[More Information Needed]
### Out-of-Scope Use
<!-- This section addresses misuse, malicious use, and uses that the model will not work well for. -->
[More Information Needed]
## Bias, Risks, and Limitations
<!-- This section is meant to convey both technical and sociotechnical limitations. -->
[More Information Needed]
### Recommendations
<!-- This section is meant to convey recommendations with respect to the bias, risk, and technical limitations. -->
Users (both direct and downstream) should be made aware of the risks, biases and limitations of the model. More information needed for further recommendations.
## How to Get Started with the Model
Use the code below to get started with the model.
[More Information Needed]
## Training Details
### Training Data
<!-- This should link to a Dataset Card, perhaps with a short stub of information on what the training data is all about as well as documentation related to data pre-processing or additional filtering. -->
[More Information Needed]
### Training Procedure
<!-- This relates heavily to the Technical Specifications. Content here should link to that section when it is relevant to the training procedure. -->
#### Preprocessing [optional]
[More Information Needed]
#### Training Hyperparameters
- **Training regime:** [More Information Needed] <!--fp32, fp16 mixed precision, bf16 mixed precision, bf16 non-mixed precision, fp16 non-mixed precision, fp8 mixed precision -->
#### Speeds, Sizes, Times [optional]
<!-- This section provides information about throughput, start/end time, checkpoint size if relevant, etc. -->
[More Information Needed]
## Evaluation
<!-- This section describes the evaluation protocols and provides the results. -->
### Testing Data, Factors & Metrics
#### Testing Data
<!-- This should link to a Dataset Card if possible. -->
[More Information Needed]
#### Factors
<!-- These are the things the evaluation is disaggregating by, e.g., subpopulations or domains. -->
[More Information Needed]
#### Metrics
<!-- These are the evaluation metrics being used, ideally with a description of why. -->
[More Information Needed]
### Results
[More Information Needed]
#### Summary
## Model Examination [optional]
<!-- Relevant interpretability work for the model goes here -->
[More Information Needed]
## Environmental Impact
<!-- Total emissions (in grams of CO2eq) and additional considerations, such as electricity usage, go here. Edit the suggested text below accordingly -->
Carbon emissions can be estimated using the [Machine Learning Impact calculator](https://mlco2.github.io/impact#compute) presented in [Lacoste et al. (2019)](https://arxiv.org/abs/1910.09700).
- **Hardware Type:** [More Information Needed]
- **Hours used:** [More Information Needed]
- **Cloud Provider:** [More Information Needed]
- **Compute Region:** [More Information Needed]
- **Carbon Emitted:** [More Information Needed]
## Technical Specifications [optional]
### Model Architecture and Objective
[More Information Needed]
### Compute Infrastructure
[More Information Needed]
#### Hardware
[More Information Needed]
#### Software
[More Information Needed]
## Citation [optional]
<!-- If there is a paper or blog post introducing the model, the APA and Bibtex information for that should go in this section. -->
**BibTeX:**
[More Information Needed]
**APA:**
[More Information Needed]
## Glossary [optional]
<!-- If relevant, include terms and calculations in this section that can help readers understand the model or model card. -->
[More Information Needed]
## More Information [optional]
[More Information Needed]
## Model Card Authors [optional]
[More Information Needed]
## Model Card Contact
[More Information Needed]
| {"library_name": "transformers", "tags": []} | gildead/mistral-aes-414 | null | [
"transformers",
"safetensors",
"mistral",
"text-generation",
"conversational",
"arxiv:1910.09700",
"autotrain_compatible",
"endpoints_compatible",
"has_space",
"text-generation-inference",
"4-bit",
"region:us"
] | null | 2024-04-18T08:17:48+00:00 | [
"1910.09700"
] | [] | TAGS
#transformers #safetensors #mistral #text-generation #conversational #arxiv-1910.09700 #autotrain_compatible #endpoints_compatible #has_space #text-generation-inference #4-bit #region-us
|
# Model Card for Model ID
## Model Details
### Model Description
This is the model card of a transformers model that has been pushed on the Hub. This model card has been automatically generated.
- Developed by:
- Funded by [optional]:
- Shared by [optional]:
- Model type:
- Language(s) (NLP):
- License:
- Finetuned from model [optional]:
### Model Sources [optional]
- Repository:
- Paper [optional]:
- Demo [optional]:
## Uses
### Direct Use
### Downstream Use [optional]
### Out-of-Scope Use
## Bias, Risks, and Limitations
### Recommendations
Users (both direct and downstream) should be made aware of the risks, biases and limitations of the model. More information needed for further recommendations.
## How to Get Started with the Model
Use the code below to get started with the model.
## Training Details
### Training Data
### Training Procedure
#### Preprocessing [optional]
#### Training Hyperparameters
- Training regime:
#### Speeds, Sizes, Times [optional]
## Evaluation
### Testing Data, Factors & Metrics
#### Testing Data
#### Factors
#### Metrics
### Results
#### Summary
## Model Examination [optional]
## Environmental Impact
Carbon emissions can be estimated using the Machine Learning Impact calculator presented in Lacoste et al. (2019).
- Hardware Type:
- Hours used:
- Cloud Provider:
- Compute Region:
- Carbon Emitted:
## Technical Specifications [optional]
### Model Architecture and Objective
### Compute Infrastructure
#### Hardware
#### Software
[optional]
BibTeX:
APA:
## Glossary [optional]
## More Information [optional]
## Model Card Authors [optional]
## Model Card Contact
| [
"# Model Card for Model ID",
"## Model Details",
"### Model Description\n\n\n\nThis is the model card of a transformers model that has been pushed on the Hub. This model card has been automatically generated.\n\n- Developed by: \n- Funded by [optional]: \n- Shared by [optional]: \n- Model type: \n- Language(s) (NLP): \n- License: \n- Finetuned from model [optional]:",
"### Model Sources [optional]\n\n\n\n- Repository: \n- Paper [optional]: \n- Demo [optional]:",
"## Uses",
"### Direct Use",
"### Downstream Use [optional]",
"### Out-of-Scope Use",
"## Bias, Risks, and Limitations",
"### Recommendations\n\n\n\nUsers (both direct and downstream) should be made aware of the risks, biases and limitations of the model. More information needed for further recommendations.",
"## How to Get Started with the Model\n\nUse the code below to get started with the model.",
"## Training Details",
"### Training Data",
"### Training Procedure",
"#### Preprocessing [optional]",
"#### Training Hyperparameters\n\n- Training regime:",
"#### Speeds, Sizes, Times [optional]",
"## Evaluation",
"### Testing Data, Factors & Metrics",
"#### Testing Data",
"#### Factors",
"#### Metrics",
"### Results",
"#### Summary",
"## Model Examination [optional]",
"## Environmental Impact\n\n\n\nCarbon emissions can be estimated using the Machine Learning Impact calculator presented in Lacoste et al. (2019).\n\n- Hardware Type: \n- Hours used: \n- Cloud Provider: \n- Compute Region: \n- Carbon Emitted:",
"## Technical Specifications [optional]",
"### Model Architecture and Objective",
"### Compute Infrastructure",
"#### Hardware",
"#### Software\n\n\n\n[optional]\n\n\n\nBibTeX:\n\n\n\nAPA:",
"## Glossary [optional]",
"## More Information [optional]",
"## Model Card Authors [optional]",
"## Model Card Contact"
] | [
"TAGS\n#transformers #safetensors #mistral #text-generation #conversational #arxiv-1910.09700 #autotrain_compatible #endpoints_compatible #has_space #text-generation-inference #4-bit #region-us \n",
"# Model Card for Model ID",
"## Model Details",
"### Model Description\n\n\n\nThis is the model card of a transformers model that has been pushed on the Hub. This model card has been automatically generated.\n\n- Developed by: \n- Funded by [optional]: \n- Shared by [optional]: \n- Model type: \n- Language(s) (NLP): \n- License: \n- Finetuned from model [optional]:",
"### Model Sources [optional]\n\n\n\n- Repository: \n- Paper [optional]: \n- Demo [optional]:",
"## Uses",
"### Direct Use",
"### Downstream Use [optional]",
"### Out-of-Scope Use",
"## Bias, Risks, and Limitations",
"### Recommendations\n\n\n\nUsers (both direct and downstream) should be made aware of the risks, biases and limitations of the model. More information needed for further recommendations.",
"## How to Get Started with the Model\n\nUse the code below to get started with the model.",
"## Training Details",
"### Training Data",
"### Training Procedure",
"#### Preprocessing [optional]",
"#### Training Hyperparameters\n\n- Training regime:",
"#### Speeds, Sizes, Times [optional]",
"## Evaluation",
"### Testing Data, Factors & Metrics",
"#### Testing Data",
"#### Factors",
"#### Metrics",
"### Results",
"#### Summary",
"## Model Examination [optional]",
"## Environmental Impact\n\n\n\nCarbon emissions can be estimated using the Machine Learning Impact calculator presented in Lacoste et al. (2019).\n\n- Hardware Type: \n- Hours used: \n- Cloud Provider: \n- Compute Region: \n- Carbon Emitted:",
"## Technical Specifications [optional]",
"### Model Architecture and Objective",
"### Compute Infrastructure",
"#### Hardware",
"#### Software\n\n\n\n[optional]\n\n\n\nBibTeX:\n\n\n\nAPA:",
"## Glossary [optional]",
"## More Information [optional]",
"## Model Card Authors [optional]",
"## Model Card Contact"
] |
text2text-generation | transformers |
<!-- This model card has been generated automatically according to the information the Trainer had access to. You
should probably proofread and complete it, then remove this comment. -->
# gpt2-finetuned-justification-v3
This model is a fine-tuned version of [](https://huggingface.co/) on the None dataset.
It achieves the following results on the evaluation set:
- Loss: 0.2415
- Rouge1: 30.8957
- Rouge2: 13.5597
- Rougel: 22.4384
- Rougelsum: 28.2668
## Model description
More information needed
## Intended uses & limitations
More information needed
## Training and evaluation data
More information needed
## Training procedure
### Training hyperparameters
The following hyperparameters were used during training:
- learning_rate: 5e-05
- train_batch_size: 2
- eval_batch_size: 2
- seed: 42
- optimizer: Adam with betas=(0.9,0.999) and epsilon=1e-08
- lr_scheduler_type: linear
- num_epochs: 10
### Training results
| Training Loss | Epoch | Step | Validation Loss | Rouge1 | Rouge2 | Rougel | Rougelsum |
|:-------------:|:-----:|:----:|:---------------:|:-------:|:-------:|:-------:|:---------:|
| No log | 1.0 | 338 | 0.1980 | 30.0775 | 13.8145 | 22.3863 | 28.0341 |
| 0.226 | 2.0 | 676 | 0.1972 | 28.9676 | 13.7684 | 21.8084 | 26.6768 |
| 0.1594 | 3.0 | 1014 | 0.2007 | 29.8576 | 13.3727 | 22.1581 | 27.5726 |
| 0.1594 | 4.0 | 1352 | 0.2071 | 32.2090 | 13.7848 | 22.8787 | 29.0171 |
| 0.1259 | 5.0 | 1690 | 0.2146 | 28.5240 | 13.5821 | 21.4908 | 26.2550 |
| 0.1046 | 6.0 | 2028 | 0.2211 | 26.1623 | 13.1641 | 21.5936 | 25.0346 |
| 0.1046 | 7.0 | 2366 | 0.2294 | 28.7169 | 13.4858 | 21.1068 | 26.1213 |
| 0.0894 | 8.0 | 2704 | 0.2355 | 30.8957 | 13.5597 | 22.4384 | 28.2668 |
| 0.0785 | 9.0 | 3042 | 0.2398 | 30.8957 | 13.5597 | 22.4384 | 28.2668 |
| 0.0785 | 10.0 | 3380 | 0.2415 | 30.8957 | 13.5597 | 22.4384 | 28.2668 |
### Framework versions
- Transformers 4.36.2
- Pytorch 2.2.2+cu121
- Datasets 2.16.0
- Tokenizers 0.15.2
| {"tags": ["generated_from_trainer"], "metrics": ["rouge"], "model-index": [{"name": "gpt2-finetuned-justification-v3", "results": []}]} | satyanshu404/gpt2-finetuned-justification-v3 | null | [
"transformers",
"safetensors",
"encoder-decoder",
"text2text-generation",
"generated_from_trainer",
"autotrain_compatible",
"endpoints_compatible",
"region:us"
] | null | 2024-04-18T08:21:14+00:00 | [] | [] | TAGS
#transformers #safetensors #encoder-decoder #text2text-generation #generated_from_trainer #autotrain_compatible #endpoints_compatible #region-us
| gpt2-finetuned-justification-v3
===============================
This model is a fine-tuned version of [](URL on the None dataset.
It achieves the following results on the evaluation set:
* Loss: 0.2415
* Rouge1: 30.8957
* Rouge2: 13.5597
* Rougel: 22.4384
* Rougelsum: 28.2668
Model description
-----------------
More information needed
Intended uses & limitations
---------------------------
More information needed
Training and evaluation data
----------------------------
More information needed
Training procedure
------------------
### Training hyperparameters
The following hyperparameters were used during training:
* learning\_rate: 5e-05
* train\_batch\_size: 2
* eval\_batch\_size: 2
* seed: 42
* optimizer: Adam with betas=(0.9,0.999) and epsilon=1e-08
* lr\_scheduler\_type: linear
* num\_epochs: 10
### Training results
### Framework versions
* Transformers 4.36.2
* Pytorch 2.2.2+cu121
* Datasets 2.16.0
* Tokenizers 0.15.2
| [
"### Training hyperparameters\n\n\nThe following hyperparameters were used during training:\n\n\n* learning\\_rate: 5e-05\n* train\\_batch\\_size: 2\n* eval\\_batch\\_size: 2\n* seed: 42\n* optimizer: Adam with betas=(0.9,0.999) and epsilon=1e-08\n* lr\\_scheduler\\_type: linear\n* num\\_epochs: 10",
"### Training results",
"### Framework versions\n\n\n* Transformers 4.36.2\n* Pytorch 2.2.2+cu121\n* Datasets 2.16.0\n* Tokenizers 0.15.2"
] | [
"TAGS\n#transformers #safetensors #encoder-decoder #text2text-generation #generated_from_trainer #autotrain_compatible #endpoints_compatible #region-us \n",
"### Training hyperparameters\n\n\nThe following hyperparameters were used during training:\n\n\n* learning\\_rate: 5e-05\n* train\\_batch\\_size: 2\n* eval\\_batch\\_size: 2\n* seed: 42\n* optimizer: Adam with betas=(0.9,0.999) and epsilon=1e-08\n* lr\\_scheduler\\_type: linear\n* num\\_epochs: 10",
"### Training results",
"### Framework versions\n\n\n* Transformers 4.36.2\n* Pytorch 2.2.2+cu121\n* Datasets 2.16.0\n* Tokenizers 0.15.2"
] |
text-generation | transformers |
# Boundary-Hermes-Chat-2x7B-MoE
Boundary-Hermes-Chat-2x7B-MoE is a Mixture of Experts (MoE) made with the following models:
* [NousResearch/Hermes-2-Pro-Mistral-7B](https://huggingface.co/NousResearch/Hermes-2-Pro-Mistral-7B)
* [Nexusflow/Starling-LM-7B-beta](https://huggingface.co/Nexusflow/Starling-LM-7B-beta)
## 🧩 Configuration
```yaml
base_model: NousResearch/Hermes-2-Pro-Mistral-7B
dtype: float16
gate_mode: cheap_embed
experts:
- source_model: NousResearch/Hermes-2-Pro-Mistral-7B
positive_prompts: ["You are a helpful general assistant."]
- source_model: Nexusflow/Starling-LM-7B-beta
positive_prompts: ["You are assistant for question and answering."]
```
## 💻 Usage
```python
!pip install -qU transformers bitsandbytes accelerate
from transformers import AutoTokenizer
import transformers
import torch
model = "NotAiLOL/Boundary-Hermes-Chat-2x7B-MoE"
tokenizer = AutoTokenizer.from_pretrained(model)
pipeline = transformers.pipeline(
"text-generation",
model=model,
model_kwargs={"torch_dtype": torch.float16, "load_in_4bit": True},
)
messages = [{"role": "user", "content": "Explain what a Mixture of Experts is in less than 100 words."}]
prompt = pipeline.tokenizer.apply_chat_template(messages, tokenize=False, add_generation_prompt=True)
outputs = pipeline(prompt, max_new_tokens=256, do_sample=True, temperature=0.7, top_k=50, top_p=0.95)
print(outputs[0]["generated_text"])
``` | {"license": "apache-2.0", "tags": ["moe", "merge", "mergekit", "NousResearch/Hermes-2-Pro-Mistral-7B", "Nexusflow/Starling-LM-7B-beta"], "base_model": ["NousResearch/Hermes-2-Pro-Mistral-7B", "Nexusflow/Starling-LM-7B-beta"]} | NotAiLOL/Boundary-Hermes-Chat-2x7B-MoE | null | [
"transformers",
"safetensors",
"mixtral",
"text-generation",
"moe",
"merge",
"mergekit",
"NousResearch/Hermes-2-Pro-Mistral-7B",
"Nexusflow/Starling-LM-7B-beta",
"conversational",
"base_model:NousResearch/Hermes-2-Pro-Mistral-7B",
"base_model:Nexusflow/Starling-LM-7B-beta",
"license:apache-2.0",
"autotrain_compatible",
"endpoints_compatible",
"text-generation-inference",
"region:us"
] | null | 2024-04-18T08:21:39+00:00 | [] | [] | TAGS
#transformers #safetensors #mixtral #text-generation #moe #merge #mergekit #NousResearch/Hermes-2-Pro-Mistral-7B #Nexusflow/Starling-LM-7B-beta #conversational #base_model-NousResearch/Hermes-2-Pro-Mistral-7B #base_model-Nexusflow/Starling-LM-7B-beta #license-apache-2.0 #autotrain_compatible #endpoints_compatible #text-generation-inference #region-us
|
# Boundary-Hermes-Chat-2x7B-MoE
Boundary-Hermes-Chat-2x7B-MoE is a Mixture of Experts (MoE) made with the following models:
* NousResearch/Hermes-2-Pro-Mistral-7B
* Nexusflow/Starling-LM-7B-beta
## Configuration
## Usage
| [
"# Boundary-Hermes-Chat-2x7B-MoE\n\nBoundary-Hermes-Chat-2x7B-MoE is a Mixture of Experts (MoE) made with the following models:\n* NousResearch/Hermes-2-Pro-Mistral-7B\n* Nexusflow/Starling-LM-7B-beta",
"## Configuration",
"## Usage"
] | [
"TAGS\n#transformers #safetensors #mixtral #text-generation #moe #merge #mergekit #NousResearch/Hermes-2-Pro-Mistral-7B #Nexusflow/Starling-LM-7B-beta #conversational #base_model-NousResearch/Hermes-2-Pro-Mistral-7B #base_model-Nexusflow/Starling-LM-7B-beta #license-apache-2.0 #autotrain_compatible #endpoints_compatible #text-generation-inference #region-us \n",
"# Boundary-Hermes-Chat-2x7B-MoE\n\nBoundary-Hermes-Chat-2x7B-MoE is a Mixture of Experts (MoE) made with the following models:\n* NousResearch/Hermes-2-Pro-Mistral-7B\n* Nexusflow/Starling-LM-7B-beta",
"## Configuration",
"## Usage"
] |
null | null |
<!-- This model card has been generated automatically according to the information the Trainer had access to. You
should probably proofread and complete it, then remove this comment. -->
# CHAFIK_MODEL
This model is a fine-tuned version of [NousResearch/Llama-2-7b-chat-hf](https://huggingface.co/NousResearch/Llama-2-7b-chat-hf) on an unknown dataset.
## Model description
More information needed
## Intended uses & limitations
More information needed
## Training and evaluation data
More information needed
## Training procedure
### Training hyperparameters
The following hyperparameters were used during training:
- learning_rate: 0.0002
- train_batch_size: 1
- eval_batch_size: 8
- seed: 42
- gradient_accumulation_steps: 4
- total_train_batch_size: 4
- optimizer: Adam with betas=(0.9,0.999) and epsilon=1e-08
- lr_scheduler_type: cosine
- lr_scheduler_warmup_ratio: 0.05
- num_epochs: 3
- mixed_precision_training: Native AMP
### Training results
### Framework versions
- Transformers 4.30.0.dev0
- Pytorch 2.1.2
- Datasets 2.18.0
- Tokenizers 0.13.3
| {"tags": ["generated_from_trainer"], "model-index": [{"name": "CHAFIK_MODEL", "results": []}]} | Sree26/CHAFIK_MODEL | null | [
"pytorch",
"tensorboard",
"generated_from_trainer",
"region:us"
] | null | 2024-04-18T08:23:09+00:00 | [] | [] | TAGS
#pytorch #tensorboard #generated_from_trainer #region-us
|
# CHAFIK_MODEL
This model is a fine-tuned version of NousResearch/Llama-2-7b-chat-hf on an unknown dataset.
## Model description
More information needed
## Intended uses & limitations
More information needed
## Training and evaluation data
More information needed
## Training procedure
### Training hyperparameters
The following hyperparameters were used during training:
- learning_rate: 0.0002
- train_batch_size: 1
- eval_batch_size: 8
- seed: 42
- gradient_accumulation_steps: 4
- total_train_batch_size: 4
- optimizer: Adam with betas=(0.9,0.999) and epsilon=1e-08
- lr_scheduler_type: cosine
- lr_scheduler_warmup_ratio: 0.05
- num_epochs: 3
- mixed_precision_training: Native AMP
### Training results
### Framework versions
- Transformers 4.30.0.dev0
- Pytorch 2.1.2
- Datasets 2.18.0
- Tokenizers 0.13.3
| [
"# CHAFIK_MODEL\n\nThis model is a fine-tuned version of NousResearch/Llama-2-7b-chat-hf on an unknown dataset.",
"## Model description\n\nMore information needed",
"## Intended uses & limitations\n\nMore information needed",
"## Training and evaluation data\n\nMore information needed",
"## Training procedure",
"### Training hyperparameters\n\nThe following hyperparameters were used during training:\n- learning_rate: 0.0002\n- train_batch_size: 1\n- eval_batch_size: 8\n- seed: 42\n- gradient_accumulation_steps: 4\n- total_train_batch_size: 4\n- optimizer: Adam with betas=(0.9,0.999) and epsilon=1e-08\n- lr_scheduler_type: cosine\n- lr_scheduler_warmup_ratio: 0.05\n- num_epochs: 3\n- mixed_precision_training: Native AMP",
"### Training results",
"### Framework versions\n\n- Transformers 4.30.0.dev0\n- Pytorch 2.1.2\n- Datasets 2.18.0\n- Tokenizers 0.13.3"
] | [
"TAGS\n#pytorch #tensorboard #generated_from_trainer #region-us \n",
"# CHAFIK_MODEL\n\nThis model is a fine-tuned version of NousResearch/Llama-2-7b-chat-hf on an unknown dataset.",
"## Model description\n\nMore information needed",
"## Intended uses & limitations\n\nMore information needed",
"## Training and evaluation data\n\nMore information needed",
"## Training procedure",
"### Training hyperparameters\n\nThe following hyperparameters were used during training:\n- learning_rate: 0.0002\n- train_batch_size: 1\n- eval_batch_size: 8\n- seed: 42\n- gradient_accumulation_steps: 4\n- total_train_batch_size: 4\n- optimizer: Adam with betas=(0.9,0.999) and epsilon=1e-08\n- lr_scheduler_type: cosine\n- lr_scheduler_warmup_ratio: 0.05\n- num_epochs: 3\n- mixed_precision_training: Native AMP",
"### Training results",
"### Framework versions\n\n- Transformers 4.30.0.dev0\n- Pytorch 2.1.2\n- Datasets 2.18.0\n- Tokenizers 0.13.3"
] |
null | transformers | ## About
<!-- ### quantize_version: 1 -->
<!-- ### output_tensor_quantised: 1 -->
<!-- ### convert_type: -->
<!-- ### vocab_type: -->
static quants of https://huggingface.co/Alsebay/NarumashiRTS-7B-V2-1
<!-- provided-files -->
weighted/imatrix quants seem not to be available (by me) at this time. If they do not show up a week or so after the static ones, I have probably not planned for them. Feel free to request them by opening a Community Discussion.
## Usage
If you are unsure how to use GGUF files, refer to one of [TheBloke's
READMEs](https://huggingface.co/TheBloke/KafkaLM-70B-German-V0.1-GGUF) for
more details, including on how to concatenate multi-part files.
## Provided Quants
(sorted by size, not necessarily quality. IQ-quants are often preferable over similar sized non-IQ quants)
| Link | Type | Size/GB | Notes |
|:-----|:-----|--------:|:------|
| [GGUF](https://huggingface.co/mradermacher/NarumashiRTS-7B-V2-1-GGUF/resolve/main/NarumashiRTS-7B-V2-1.Q2_K.gguf) | Q2_K | 2.8 | |
| [GGUF](https://huggingface.co/mradermacher/NarumashiRTS-7B-V2-1-GGUF/resolve/main/NarumashiRTS-7B-V2-1.IQ3_XS.gguf) | IQ3_XS | 3.1 | |
| [GGUF](https://huggingface.co/mradermacher/NarumashiRTS-7B-V2-1-GGUF/resolve/main/NarumashiRTS-7B-V2-1.Q3_K_S.gguf) | Q3_K_S | 3.3 | |
| [GGUF](https://huggingface.co/mradermacher/NarumashiRTS-7B-V2-1-GGUF/resolve/main/NarumashiRTS-7B-V2-1.IQ3_S.gguf) | IQ3_S | 3.3 | beats Q3_K* |
| [GGUF](https://huggingface.co/mradermacher/NarumashiRTS-7B-V2-1-GGUF/resolve/main/NarumashiRTS-7B-V2-1.IQ3_M.gguf) | IQ3_M | 3.4 | |
| [GGUF](https://huggingface.co/mradermacher/NarumashiRTS-7B-V2-1-GGUF/resolve/main/NarumashiRTS-7B-V2-1.Q3_K_M.gguf) | Q3_K_M | 3.6 | lower quality |
| [GGUF](https://huggingface.co/mradermacher/NarumashiRTS-7B-V2-1-GGUF/resolve/main/NarumashiRTS-7B-V2-1.Q3_K_L.gguf) | Q3_K_L | 3.9 | |
| [GGUF](https://huggingface.co/mradermacher/NarumashiRTS-7B-V2-1-GGUF/resolve/main/NarumashiRTS-7B-V2-1.IQ4_XS.gguf) | IQ4_XS | 4.0 | |
| [GGUF](https://huggingface.co/mradermacher/NarumashiRTS-7B-V2-1-GGUF/resolve/main/NarumashiRTS-7B-V2-1.Q4_K_S.gguf) | Q4_K_S | 4.2 | fast, recommended |
| [GGUF](https://huggingface.co/mradermacher/NarumashiRTS-7B-V2-1-GGUF/resolve/main/NarumashiRTS-7B-V2-1.Q4_K_M.gguf) | Q4_K_M | 4.5 | fast, recommended |
| [GGUF](https://huggingface.co/mradermacher/NarumashiRTS-7B-V2-1-GGUF/resolve/main/NarumashiRTS-7B-V2-1.Q5_K_S.gguf) | Q5_K_S | 5.1 | |
| [GGUF](https://huggingface.co/mradermacher/NarumashiRTS-7B-V2-1-GGUF/resolve/main/NarumashiRTS-7B-V2-1.Q5_K_M.gguf) | Q5_K_M | 5.2 | |
| [GGUF](https://huggingface.co/mradermacher/NarumashiRTS-7B-V2-1-GGUF/resolve/main/NarumashiRTS-7B-V2-1.Q6_K.gguf) | Q6_K | 6.0 | very good quality |
| [GGUF](https://huggingface.co/mradermacher/NarumashiRTS-7B-V2-1-GGUF/resolve/main/NarumashiRTS-7B-V2-1.Q8_0.gguf) | Q8_0 | 7.8 | fast, best quality |
Here is a handy graph by ikawrakow comparing some lower-quality quant
types (lower is better):

And here are Artefact2's thoughts on the matter:
https://gist.github.com/Artefact2/b5f810600771265fc1e39442288e8ec9
## FAQ / Model Request
See https://huggingface.co/mradermacher/model_requests for some answers to
questions you might have and/or if you want some other model quantized.
## Thanks
I thank my company, [nethype GmbH](https://www.nethype.de/), for letting
me use its servers and providing upgrades to my workstation to enable
this work in my free time.
<!-- end -->
| {"language": ["en"], "license": "cc-by-nc-4.0", "library_name": "transformers", "tags": ["text-generation-inference", "transformers", "unsloth", "mistral", "trl", "sft", "Roleplay", "roleplay"], "base_model": "Alsebay/NarumashiRTS-7B-V2-1", "quantized_by": "mradermacher"} | mradermacher/NarumashiRTS-7B-V2-1-GGUF | null | [
"transformers",
"gguf",
"text-generation-inference",
"unsloth",
"mistral",
"trl",
"sft",
"Roleplay",
"roleplay",
"en",
"base_model:Alsebay/NarumashiRTS-7B-V2-1",
"license:cc-by-nc-4.0",
"endpoints_compatible",
"has_space",
"region:us"
] | null | 2024-04-18T08:24:06+00:00 | [] | [
"en"
] | TAGS
#transformers #gguf #text-generation-inference #unsloth #mistral #trl #sft #Roleplay #roleplay #en #base_model-Alsebay/NarumashiRTS-7B-V2-1 #license-cc-by-nc-4.0 #endpoints_compatible #has_space #region-us
| About
-----
static quants of URL
weighted/imatrix quants seem not to be available (by me) at this time. If they do not show up a week or so after the static ones, I have probably not planned for them. Feel free to request them by opening a Community Discussion.
Usage
-----
If you are unsure how to use GGUF files, refer to one of TheBloke's
READMEs for
more details, including on how to concatenate multi-part files.
Provided Quants
---------------
(sorted by size, not necessarily quality. IQ-quants are often preferable over similar sized non-IQ quants)
Here is a handy graph by ikawrakow comparing some lower-quality quant
types (lower is better):
!URL
And here are Artefact2's thoughts on the matter:
URL
FAQ / Model Request
-------------------
See URL for some answers to
questions you might have and/or if you want some other model quantized.
Thanks
------
I thank my company, nethype GmbH, for letting
me use its servers and providing upgrades to my workstation to enable
this work in my free time.
| [] | [
"TAGS\n#transformers #gguf #text-generation-inference #unsloth #mistral #trl #sft #Roleplay #roleplay #en #base_model-Alsebay/NarumashiRTS-7B-V2-1 #license-cc-by-nc-4.0 #endpoints_compatible #has_space #region-us \n"
] |
text-classification | setfit |
# SetFit with sentence-transformers/paraphrase-mpnet-base-v2
This is a [SetFit](https://github.com/huggingface/setfit) model that can be used for Text Classification. This SetFit model uses [sentence-transformers/paraphrase-mpnet-base-v2](https://huggingface.co/sentence-transformers/paraphrase-mpnet-base-v2) as the Sentence Transformer embedding model. A [LogisticRegression](https://scikit-learn.org/stable/modules/generated/sklearn.linear_model.LogisticRegression.html) instance is used for classification.
The model has been trained using an efficient few-shot learning technique that involves:
1. Fine-tuning a [Sentence Transformer](https://www.sbert.net) with contrastive learning.
2. Training a classification head with features from the fine-tuned Sentence Transformer.
## Model Details
### Model Description
- **Model Type:** SetFit
- **Sentence Transformer body:** [sentence-transformers/paraphrase-mpnet-base-v2](https://huggingface.co/sentence-transformers/paraphrase-mpnet-base-v2)
- **Classification head:** a [LogisticRegression](https://scikit-learn.org/stable/modules/generated/sklearn.linear_model.LogisticRegression.html) instance
- **Maximum Sequence Length:** 512 tokens
- **Number of Classes:** 12 classes
<!-- - **Training Dataset:** [Unknown](https://huggingface.co/datasets/unknown) -->
<!-- - **Language:** Unknown -->
<!-- - **License:** Unknown -->
### Model Sources
- **Repository:** [SetFit on GitHub](https://github.com/huggingface/setfit)
- **Paper:** [Efficient Few-Shot Learning Without Prompts](https://arxiv.org/abs/2209.11055)
- **Blogpost:** [SetFit: Efficient Few-Shot Learning Without Prompts](https://huggingface.co/blog/setfit)
### Model Labels
| Label | Examples |
|:--------------|:----------------------------------------------------------------------------------------------------------------------------------------------------------------------------------------------------------------------------------------------------------------------------------|
| Politics | <ul><li>'The mayor announced a new initiative to improve public transportation.'</li><li>'The senator is facing criticism for her stance on the recent bill.'</li><li>'The upcoming election has sparked intense debates among the candidates.'</li></ul> |
| Health | <ul><li>'Regular exercise and a balanced diet are key to maintaining good health.'</li><li>'The World Health Organization has issued new guidelines on COVID-19.'</li><li>'A new study reveals the benefits of meditation for mental health.'</li></ul> |
| Finance | <ul><li>'The stock market saw a significant drop following the announcement.'</li><li>'Investing in real estate can be a profitable venture if done correctly.'</li><li>"The company's profits have doubled since the launch of their new product."</li></ul> |
| Travel | <ul><li>'Visiting the Grand Canyon is a breathtaking experience.'</li><li>'The tourism industry has been severely impacted by the pandemic.'</li><li>'Backpacking through Europe is a popular choice for young travelers.'</li></ul> |
| Food | <ul><li>'The new restaurant in town offers a fusion of Italian and Japanese cuisine.'</li><li>'Drinking eight glasses of water a day is essential for staying hydrated.'</li><li>'Cooking classes are a fun way to learn new recipes and techniques.'</li></ul> |
| Education | <ul><li>'The school district is implementing a new curriculum for the upcoming year.'</li><li>'Online learning has become increasingly popular during the pandemic.'</li><li>'The university is offering scholarships for students in financial need.'</li></ul> |
| Environment | <ul><li>'Climate change is causing a significant rise in sea levels.'</li><li>'Recycling and composting are effective ways to reduce waste.'</li><li>'The Amazon rainforest is home to millions of unique species.'</li></ul> |
| Fashion | <ul><li>'The new fashion trend is all about sustainability and eco-friendly materials.'</li><li>'The annual Met Gala is a major event in the fashion world.'</li><li>'Vintage clothing has made a comeback in recent years.'</li></ul> |
| Science | <ul><li>"NASA's Mars Rover has made significant discoveries about the red planet."</li><li>'The Nobel Prize in Physics was awarded for breakthroughs in black hole research.'</li><li>'Genetic engineering is opening up new possibilities in medical treatment.'</li></ul> |
| Sports | <ul><li>'The NBA Finals are set to begin next week with the top two teams in the league.'</li><li>'Serena Williams continues to dominate the tennis world with her powerful serve.'</li><li>'The World Cup is the most prestigious tournament in international soccer.'</li></ul> |
| Technology | <ul><li>'Artificial intelligence is changing the way we live and work.'</li><li>'The latest iPhone has a number of exciting new features.'</li><li>'Cybersecurity is becoming increasingly important as more and more data moves online.'</li></ul> |
| Entertainment | <ul><li>'The new Marvel movie is breaking box office records.'</li><li>'The Grammy Awards are a celebration of the best music of the year.'</li><li>'The latest season of Game of Thrones had fans on the edge of their seats.'</li></ul> |
## Uses
### Direct Use for Inference
First install the SetFit library:
```bash
pip install setfit
```
Then you can load this model and run inference.
```python
from setfit import SetFitModel
# Download from the 🤗 Hub
model = SetFitModel.from_pretrained("EmeraldMP/ANLP_kaggle")
# Run inference
preds = model("Climate change is causing a significant rise in sea levels.")
```
<!--
### Downstream Use
*List how someone could finetune this model on their own dataset.*
-->
<!--
### Out-of-Scope Use
*List how the model may foreseeably be misused and address what users ought not to do with the model.*
-->
<!--
## Bias, Risks and Limitations
*What are the known or foreseeable issues stemming from this model? You could also flag here known failure cases or weaknesses of the model.*
-->
<!--
### Recommendations
*What are recommendations with respect to the foreseeable issues? For example, filtering explicit content.*
-->
## Training Details
### Training Set Metrics
| Training set | Min | Median | Max |
|:-------------|:----|:--------|:----|
| Word count | 7 | 12.8073 | 24 |
| Label | Training Sample Count |
|:--------------|:----------------------|
| Education | 23 |
| Entertainment | 23 |
| Environment | 23 |
| Fashion | 23 |
| Finance | 23 |
| Food | 23 |
| Health | 23 |
| Politics | 22 |
| Science | 23 |
| Sports | 23 |
| Technology | 23 |
| Travel | 23 |
### Training Hyperparameters
- batch_size: (16, 16)
- num_epochs: (10, 10)
- max_steps: -1
- sampling_strategy: oversampling
- num_iterations: 20
- body_learning_rate: (2e-05, 2e-05)
- head_learning_rate: 2e-05
- loss: CosineSimilarityLoss
- distance_metric: cosine_distance
- margin: 0.25
- end_to_end: False
- use_amp: False
- warmup_proportion: 0.1
- seed: 42
- eval_max_steps: -1
- load_best_model_at_end: False
### Training Results
| Epoch | Step | Training Loss | Validation Loss |
|:------:|:----:|:-------------:|:---------------:|
| 0.0015 | 1 | 0.2748 | - |
| 0.0727 | 50 | 0.2537 | - |
| 0.1453 | 100 | 0.1734 | - |
| 0.2180 | 150 | 0.1086 | - |
| 0.2907 | 200 | 0.062 | - |
| 0.3634 | 250 | 0.046 | - |
| 0.4360 | 300 | 0.017 | - |
| 0.5087 | 350 | 0.0104 | - |
| 0.5814 | 400 | 0.006 | - |
| 0.6541 | 450 | 0.0021 | - |
| 0.7267 | 500 | 0.0052 | - |
| 0.7994 | 550 | 0.0045 | - |
| 0.8721 | 600 | 0.0012 | - |
| 0.9448 | 650 | 0.0007 | - |
| 1.0174 | 700 | 0.0006 | - |
| 1.0901 | 750 | 0.0006 | - |
| 1.1628 | 800 | 0.0006 | - |
| 1.2355 | 850 | 0.0005 | - |
| 1.3081 | 900 | 0.0004 | - |
| 1.3808 | 950 | 0.0003 | - |
| 1.4535 | 1000 | 0.0004 | - |
| 1.5262 | 1050 | 0.0004 | - |
| 1.5988 | 1100 | 0.0004 | - |
| 1.6715 | 1150 | 0.0003 | - |
| 1.7442 | 1200 | 0.0002 | - |
| 1.8169 | 1250 | 0.0002 | - |
| 1.8895 | 1300 | 0.0005 | - |
| 1.9622 | 1350 | 0.0004 | - |
| 2.0349 | 1400 | 0.0002 | - |
| 2.1076 | 1450 | 0.0004 | - |
| 2.1802 | 1500 | 0.0002 | - |
| 2.2529 | 1550 | 0.0002 | - |
| 2.3256 | 1600 | 0.0004 | - |
| 2.3983 | 1650 | 0.0002 | - |
| 2.4709 | 1700 | 0.0002 | - |
| 2.5436 | 1750 | 0.0002 | - |
| 2.6163 | 1800 | 0.0002 | - |
| 2.6890 | 1850 | 0.0002 | - |
| 2.7616 | 1900 | 0.0003 | - |
| 2.8343 | 1950 | 0.0001 | - |
| 2.9070 | 2000 | 0.0002 | - |
| 2.9797 | 2050 | 0.0002 | - |
| 3.0523 | 2100 | 0.0003 | - |
| 3.125 | 2150 | 0.0002 | - |
| 3.1977 | 2200 | 0.0002 | - |
| 3.2703 | 2250 | 0.0001 | - |
| 3.3430 | 2300 | 0.0002 | - |
| 3.4157 | 2350 | 0.0002 | - |
| 3.4884 | 2400 | 0.0002 | - |
| 3.5610 | 2450 | 0.0001 | - |
| 3.6337 | 2500 | 0.0001 | - |
| 3.7064 | 2550 | 0.0001 | - |
| 3.7791 | 2600 | 0.0001 | - |
| 3.8517 | 2650 | 0.0001 | - |
| 3.9244 | 2700 | 0.0001 | - |
| 3.9971 | 2750 | 0.0001 | - |
| 4.0698 | 2800 | 0.0001 | - |
| 4.1424 | 2850 | 0.0001 | - |
| 4.2151 | 2900 | 0.0001 | - |
| 4.2878 | 2950 | 0.0001 | - |
| 4.3605 | 3000 | 0.0001 | - |
| 4.4331 | 3050 | 0.0001 | - |
| 4.5058 | 3100 | 0.0001 | - |
| 4.5785 | 3150 | 0.0001 | - |
| 4.6512 | 3200 | 0.0001 | - |
| 4.7238 | 3250 | 0.0001 | - |
| 4.7965 | 3300 | 0.0001 | - |
| 4.8692 | 3350 | 0.0001 | - |
| 4.9419 | 3400 | 0.0001 | - |
| 5.0145 | 3450 | 0.0001 | - |
| 5.0872 | 3500 | 0.0001 | - |
| 5.1599 | 3550 | 0.0001 | - |
| 5.2326 | 3600 | 0.0001 | - |
| 5.3052 | 3650 | 0.0001 | - |
| 5.3779 | 3700 | 0.0001 | - |
| 5.4506 | 3750 | 0.0001 | - |
| 5.5233 | 3800 | 0.0001 | - |
| 5.5959 | 3850 | 0.0001 | - |
| 5.6686 | 3900 | 0.0001 | - |
| 5.7413 | 3950 | 0.0001 | - |
| 5.8140 | 4000 | 0.0001 | - |
| 5.8866 | 4050 | 0.0001 | - |
| 5.9593 | 4100 | 0.0001 | - |
| 6.0320 | 4150 | 0.0001 | - |
| 6.1047 | 4200 | 0.0001 | - |
| 6.1773 | 4250 | 0.0001 | - |
| 6.25 | 4300 | 0.0001 | - |
| 6.3227 | 4350 | 0.0001 | - |
| 6.3953 | 4400 | 0.0001 | - |
| 6.4680 | 4450 | 0.0001 | - |
| 6.5407 | 4500 | 0.0001 | - |
| 6.6134 | 4550 | 0.0001 | - |
| 6.6860 | 4600 | 0.0001 | - |
| 6.7587 | 4650 | 0.0001 | - |
| 6.8314 | 4700 | 0.0001 | - |
| 6.9041 | 4750 | 0.0001 | - |
| 6.9767 | 4800 | 0.0 | - |
| 7.0494 | 4850 | 0.0001 | - |
| 7.1221 | 4900 | 0.0001 | - |
| 7.1948 | 4950 | 0.0001 | - |
| 7.2674 | 5000 | 0.0001 | - |
| 7.3401 | 5050 | 0.0001 | - |
| 7.4128 | 5100 | 0.0001 | - |
| 7.4855 | 5150 | 0.0001 | - |
| 7.5581 | 5200 | 0.0001 | - |
| 7.6308 | 5250 | 0.0001 | - |
| 7.7035 | 5300 | 0.0001 | - |
| 7.7762 | 5350 | 0.0001 | - |
| 7.8488 | 5400 | 0.0001 | - |
| 7.9215 | 5450 | 0.0001 | - |
| 7.9942 | 5500 | 0.0 | - |
| 8.0669 | 5550 | 0.0001 | - |
| 8.1395 | 5600 | 0.0001 | - |
| 8.2122 | 5650 | 0.0001 | - |
| 8.2849 | 5700 | 0.0 | - |
| 8.3576 | 5750 | 0.0001 | - |
| 8.4302 | 5800 | 0.0001 | - |
| 8.5029 | 5850 | 0.0001 | - |
| 8.5756 | 5900 | 0.0001 | - |
| 8.6483 | 5950 | 0.0001 | - |
| 8.7209 | 6000 | 0.0001 | - |
| 8.7936 | 6050 | 0.0001 | - |
| 8.8663 | 6100 | 0.0 | - |
| 8.9390 | 6150 | 0.0 | - |
| 9.0116 | 6200 | 0.0001 | - |
| 9.0843 | 6250 | 0.0001 | - |
| 9.1570 | 6300 | 0.0 | - |
| 9.2297 | 6350 | 0.0 | - |
| 9.3023 | 6400 | 0.0 | - |
| 9.375 | 6450 | 0.0001 | - |
| 9.4477 | 6500 | 0.0001 | - |
| 9.5203 | 6550 | 0.0001 | - |
| 9.5930 | 6600 | 0.0001 | - |
| 9.6657 | 6650 | 0.0001 | - |
| 9.7384 | 6700 | 0.0001 | - |
| 9.8110 | 6750 | 0.0001 | - |
| 9.8837 | 6800 | 0.0001 | - |
| 9.9564 | 6850 | 0.0 | - |
### Framework Versions
- Python: 3.10.12
- SetFit: 1.0.3
- Sentence Transformers: 2.7.0
- Transformers: 4.38.2
- PyTorch: 2.2.1+cu121
- Datasets: 2.18.0
- Tokenizers: 0.15.2
## Citation
### BibTeX
```bibtex
@article{https://doi.org/10.48550/arxiv.2209.11055,
doi = {10.48550/ARXIV.2209.11055},
url = {https://arxiv.org/abs/2209.11055},
author = {Tunstall, Lewis and Reimers, Nils and Jo, Unso Eun Seo and Bates, Luke and Korat, Daniel and Wasserblat, Moshe and Pereg, Oren},
keywords = {Computation and Language (cs.CL), FOS: Computer and information sciences, FOS: Computer and information sciences},
title = {Efficient Few-Shot Learning Without Prompts},
publisher = {arXiv},
year = {2022},
copyright = {Creative Commons Attribution 4.0 International}
}
```
<!--
## Glossary
*Clearly define terms in order to be accessible across audiences.*
-->
<!--
## Model Card Authors
*Lists the people who create the model card, providing recognition and accountability for the detailed work that goes into its construction.*
-->
<!--
## Model Card Contact
*Provides a way for people who have updates to the Model Card, suggestions, or questions, to contact the Model Card authors.*
--> | {"library_name": "setfit", "tags": ["setfit", "sentence-transformers", "text-classification", "generated_from_setfit_trainer"], "metrics": ["accuracy"], "widget": [{"text": "The development of smart cities is leveraging technology to improve urban living conditions."}, {"text": "Climate change is causing a significant rise in sea levels."}, {"text": "Fans are speculating about the plot of the upcoming season of Stranger Things."}, {"text": "Fashion branding and marketing campaigns shape consumer perceptions and influence purchasing decisions."}, {"text": "Volunteering abroad provides a unique opportunity to experience different cultures while giving back to society."}], "pipeline_tag": "text-classification", "inference": true, "base_model": "sentence-transformers/paraphrase-mpnet-base-v2"} | EmeraldMP/ANLP_kaggle | null | [
"setfit",
"safetensors",
"mpnet",
"sentence-transformers",
"text-classification",
"generated_from_setfit_trainer",
"arxiv:2209.11055",
"base_model:sentence-transformers/paraphrase-mpnet-base-v2",
"region:us"
] | null | 2024-04-18T08:24:38+00:00 | [
"2209.11055"
] | [] | TAGS
#setfit #safetensors #mpnet #sentence-transformers #text-classification #generated_from_setfit_trainer #arxiv-2209.11055 #base_model-sentence-transformers/paraphrase-mpnet-base-v2 #region-us
| SetFit with sentence-transformers/paraphrase-mpnet-base-v2
==========================================================
This is a SetFit model that can be used for Text Classification. This SetFit model uses sentence-transformers/paraphrase-mpnet-base-v2 as the Sentence Transformer embedding model. A LogisticRegression instance is used for classification.
The model has been trained using an efficient few-shot learning technique that involves:
1. Fine-tuning a Sentence Transformer with contrastive learning.
2. Training a classification head with features from the fine-tuned Sentence Transformer.
Model Details
-------------
### Model Description
* Model Type: SetFit
* Sentence Transformer body: sentence-transformers/paraphrase-mpnet-base-v2
* Classification head: a LogisticRegression instance
* Maximum Sequence Length: 512 tokens
* Number of Classes: 12 classes
### Model Sources
* Repository: SetFit on GitHub
* Paper: Efficient Few-Shot Learning Without Prompts
* Blogpost: SetFit: Efficient Few-Shot Learning Without Prompts
### Model Labels
Uses
----
### Direct Use for Inference
First install the SetFit library:
Then you can load this model and run inference.
Training Details
----------------
### Training Set Metrics
### Training Hyperparameters
* batch\_size: (16, 16)
* num\_epochs: (10, 10)
* max\_steps: -1
* sampling\_strategy: oversampling
* num\_iterations: 20
* body\_learning\_rate: (2e-05, 2e-05)
* head\_learning\_rate: 2e-05
* loss: CosineSimilarityLoss
* distance\_metric: cosine\_distance
* margin: 0.25
* end\_to\_end: False
* use\_amp: False
* warmup\_proportion: 0.1
* seed: 42
* eval\_max\_steps: -1
* load\_best\_model\_at\_end: False
### Training Results
### Framework Versions
* Python: 3.10.12
* SetFit: 1.0.3
* Sentence Transformers: 2.7.0
* Transformers: 4.38.2
* PyTorch: 2.2.1+cu121
* Datasets: 2.18.0
* Tokenizers: 0.15.2
### BibTeX
| [
"### Model Description\n\n\n* Model Type: SetFit\n* Sentence Transformer body: sentence-transformers/paraphrase-mpnet-base-v2\n* Classification head: a LogisticRegression instance\n* Maximum Sequence Length: 512 tokens\n* Number of Classes: 12 classes",
"### Model Sources\n\n\n* Repository: SetFit on GitHub\n* Paper: Efficient Few-Shot Learning Without Prompts\n* Blogpost: SetFit: Efficient Few-Shot Learning Without Prompts",
"### Model Labels\n\n\n\nUses\n----",
"### Direct Use for Inference\n\n\nFirst install the SetFit library:\n\n\nThen you can load this model and run inference.\n\n\nTraining Details\n----------------",
"### Training Set Metrics",
"### Training Hyperparameters\n\n\n* batch\\_size: (16, 16)\n* num\\_epochs: (10, 10)\n* max\\_steps: -1\n* sampling\\_strategy: oversampling\n* num\\_iterations: 20\n* body\\_learning\\_rate: (2e-05, 2e-05)\n* head\\_learning\\_rate: 2e-05\n* loss: CosineSimilarityLoss\n* distance\\_metric: cosine\\_distance\n* margin: 0.25\n* end\\_to\\_end: False\n* use\\_amp: False\n* warmup\\_proportion: 0.1\n* seed: 42\n* eval\\_max\\_steps: -1\n* load\\_best\\_model\\_at\\_end: False",
"### Training Results",
"### Framework Versions\n\n\n* Python: 3.10.12\n* SetFit: 1.0.3\n* Sentence Transformers: 2.7.0\n* Transformers: 4.38.2\n* PyTorch: 2.2.1+cu121\n* Datasets: 2.18.0\n* Tokenizers: 0.15.2",
"### BibTeX"
] | [
"TAGS\n#setfit #safetensors #mpnet #sentence-transformers #text-classification #generated_from_setfit_trainer #arxiv-2209.11055 #base_model-sentence-transformers/paraphrase-mpnet-base-v2 #region-us \n",
"### Model Description\n\n\n* Model Type: SetFit\n* Sentence Transformer body: sentence-transformers/paraphrase-mpnet-base-v2\n* Classification head: a LogisticRegression instance\n* Maximum Sequence Length: 512 tokens\n* Number of Classes: 12 classes",
"### Model Sources\n\n\n* Repository: SetFit on GitHub\n* Paper: Efficient Few-Shot Learning Without Prompts\n* Blogpost: SetFit: Efficient Few-Shot Learning Without Prompts",
"### Model Labels\n\n\n\nUses\n----",
"### Direct Use for Inference\n\n\nFirst install the SetFit library:\n\n\nThen you can load this model and run inference.\n\n\nTraining Details\n----------------",
"### Training Set Metrics",
"### Training Hyperparameters\n\n\n* batch\\_size: (16, 16)\n* num\\_epochs: (10, 10)\n* max\\_steps: -1\n* sampling\\_strategy: oversampling\n* num\\_iterations: 20\n* body\\_learning\\_rate: (2e-05, 2e-05)\n* head\\_learning\\_rate: 2e-05\n* loss: CosineSimilarityLoss\n* distance\\_metric: cosine\\_distance\n* margin: 0.25\n* end\\_to\\_end: False\n* use\\_amp: False\n* warmup\\_proportion: 0.1\n* seed: 42\n* eval\\_max\\_steps: -1\n* load\\_best\\_model\\_at\\_end: False",
"### Training Results",
"### Framework Versions\n\n\n* Python: 3.10.12\n* SetFit: 1.0.3\n* Sentence Transformers: 2.7.0\n* Transformers: 4.38.2\n* PyTorch: 2.2.1+cu121\n* Datasets: 2.18.0\n* Tokenizers: 0.15.2",
"### BibTeX"
] |
null | null |
**English** | [中文](./README_zh.md)
## Code implementation of new GTE embeddings
This model is a BERT-like encoder with the following optimizations implemented:
1. Replacing absolute position embeddings with RoPE [^1].
2. Substituting the conventional activation functions with Gated Linear Units (GLU) [^2].
3. Setting attention dropout to 0 to use `xformers` and `flash_attn`.
4. Using unpadding to eliminate the needless computations for padding tokens [^3]. (this is off by default and should be used in conjunction with `xformers` for optimal acceleration).
5. Setting `vocab_size` as a multiple of 64.
### Recommendation: Enable Unpadding and Acceleration with `xformers`
This code supports the acceleration of attention computations using `xformers`, which can automatically choose the optimal implementation based on the type of device, such as `flash_attn`. Therefore, we can also achieve significant acceleration on old devices like the V100.
Firstly, install `xformers` (with `pytorch` pre-installed):
```
if pytorch is installed using conda:
conda install xformers -c xformers
elif pytorch is installed using pip:
# cuda 11.8 version
pip3 install -U xformers --index-url https://download.pytorch.org/whl/cu118
# cuda 12.1 version
pip3 install -U xformers --index-url https://download.pytorch.org/whl/cu121
```
For more information, refer to [Installing xformers](https://github.com/facebookresearch/xformers?tab=readme-ov-file#installing-xformers).
Then, when loading the model, set `unpad_inputs` and `use_memory_efficient_attention` to `true`, and enable `fp16` mixed precision computation to achieve the fastest acceleration.
```python
import torch
from transformers import AutoModel, AutoTokenizer
path = 'Alibaba-NLP/gte-base-en-v1.5'
device = torch.device('cuda')
tokenzier = AutoTokenizer.from_pretrained(path)
model = AutoModel.from_pretrained(
path,
trust_remote_code=True,
unpad_inputs=True,
use_memory_efficient_attention=True,
).to(device)
with torch.autocast(device_type=device.type, dtype=torch.float16): # or bfloat16
with torch.inference_mode():
outputs = model(**inputs.to(device))
```
Alternatively, you can directly modify the `unpad_inputs` and `use_memory_efficient_attention` settings to `true` in the model's `config.json`, eliminating the need to set them in the code.
---
<details>
<summary> Clarification of Relationship with nomic-embed and nomicBERT </summary>
One may question the originality of our work and consider it a mere replication of `nomicBERT`. To clarify, our work is parallel but stems from the same idea as `nomicBERT`.
Applying RoPE and GLU to BERT to support longer texts is a straightforward idea. Our exploration of the transformer++ encoder (i.e., BERT + RoPE + GLU) began in August 2023.
And by November 2023, we had completed the `gte-base-en-v1.1`. Then, I went on to prepare for the ACL submission of the other project...
The release of `nomic-embed` [^4] brought to our attention the pressure, as well as provided us with more resources, which allowed us to continue with this project.
Without the outstanding work of `nomicai`, the release of `gte-v1.5` could have been delayed much longer. Thanks!
</details>
---
[^1]: Su, Jianlin, Murtadha Ahmed, Yu Lu, Shengfeng Pan, Wen Bo, and Yunfeng Liu. "Roformer: Enhanced transformer with rotary position embedding." Neurocomputing 568 (2024): 127063.
[^2]: Shazeer, Noam. "Glu variants improve transformer." arXiv preprint arXiv:2002.05202 (2020).
[^3]: Portes, Jacob, Alexander Trott, Sam Havens, Daniel King, Abhinav Venigalla, Moin Nadeem, Nikhil Sardana, Daya Khudia, and Jonathan Frankle. "MosaicBERT: A Bidirectional Encoder Optimized for Fast Pretraining." Advances in Neural Information Processing Systems 36 (2024).
[^4]: Nussbaum, Zach, John X. Morris, Brandon Duderstadt, and Andriy Mulyar. "Nomic Embed: Training a Reproducible Long Context Text Embedder." arXiv preprint arXiv:2402.01613 (2024). | {"license": "apache-2.0"} | Alibaba-NLP/new-impl | null | [
"license:apache-2.0",
"region:us"
] | null | 2024-04-18T08:25:38+00:00 | [] | [] | TAGS
#license-apache-2.0 #region-us
|
English | 中文
## Code implementation of new GTE embeddings
This model is a BERT-like encoder with the following optimizations implemented:
1. Replacing absolute position embeddings with RoPE [^1].
2. Substituting the conventional activation functions with Gated Linear Units (GLU) [^2].
3. Setting attention dropout to 0 to use 'xformers' and 'flash_attn'.
4. Using unpadding to eliminate the needless computations for padding tokens [^3]. (this is off by default and should be used in conjunction with 'xformers' for optimal acceleration).
5. Setting 'vocab_size' as a multiple of 64.
### Recommendation: Enable Unpadding and Acceleration with 'xformers'
This code supports the acceleration of attention computations using 'xformers', which can automatically choose the optimal implementation based on the type of device, such as 'flash_attn'. Therefore, we can also achieve significant acceleration on old devices like the V100.
Firstly, install 'xformers' (with 'pytorch' pre-installed):
For more information, refer to Installing xformers.
Then, when loading the model, set 'unpad_inputs' and 'use_memory_efficient_attention' to 'true', and enable 'fp16' mixed precision computation to achieve the fastest acceleration.
Alternatively, you can directly modify the 'unpad_inputs' and 'use_memory_efficient_attention' settings to 'true' in the model's 'URL', eliminating the need to set them in the code.
---
<details>
<summary> Clarification of Relationship with nomic-embed and nomicBERT </summary>
One may question the originality of our work and consider it a mere replication of 'nomicBERT'. To clarify, our work is parallel but stems from the same idea as 'nomicBERT'.
Applying RoPE and GLU to BERT to support longer texts is a straightforward idea. Our exploration of the transformer++ encoder (i.e., BERT + RoPE + GLU) began in August 2023.
And by November 2023, we had completed the 'gte-base-en-v1.1'. Then, I went on to prepare for the ACL submission of the other project...
The release of 'nomic-embed' [^4] brought to our attention the pressure, as well as provided us with more resources, which allowed us to continue with this project.
Without the outstanding work of 'nomicai', the release of 'gte-v1.5' could have been delayed much longer. Thanks!
</details>
---
[^1]: Su, Jianlin, Murtadha Ahmed, Yu Lu, Shengfeng Pan, Wen Bo, and Yunfeng Liu. "Roformer: Enhanced transformer with rotary position embedding." Neurocomputing 568 (2024): 127063.
[^2]: Shazeer, Noam. "Glu variants improve transformer." arXiv preprint arXiv:2002.05202 (2020).
[^3]: Portes, Jacob, Alexander Trott, Sam Havens, Daniel King, Abhinav Venigalla, Moin Nadeem, Nikhil Sardana, Daya Khudia, and Jonathan Frankle. "MosaicBERT: A Bidirectional Encoder Optimized for Fast Pretraining." Advances in Neural Information Processing Systems 36 (2024).
[^4]: Nussbaum, Zach, John X. Morris, Brandon Duderstadt, and Andriy Mulyar. "Nomic Embed: Training a Reproducible Long Context Text Embedder." arXiv preprint arXiv:2402.01613 (2024). | [
"## Code implementation of new GTE embeddings\n\nThis model is a BERT-like encoder with the following optimizations implemented:\n\n1. Replacing absolute position embeddings with RoPE [^1].\n2. Substituting the conventional activation functions with Gated Linear Units (GLU) [^2].\n3. Setting attention dropout to 0 to use 'xformers' and 'flash_attn'.\n4. Using unpadding to eliminate the needless computations for padding tokens [^3]. (this is off by default and should be used in conjunction with 'xformers' for optimal acceleration).\n5. Setting 'vocab_size' as a multiple of 64.",
"### Recommendation: Enable Unpadding and Acceleration with 'xformers'\n\nThis code supports the acceleration of attention computations using 'xformers', which can automatically choose the optimal implementation based on the type of device, such as 'flash_attn'. Therefore, we can also achieve significant acceleration on old devices like the V100.\n\n\nFirstly, install 'xformers' (with 'pytorch' pre-installed):\n\nFor more information, refer to Installing xformers.\n\nThen, when loading the model, set 'unpad_inputs' and 'use_memory_efficient_attention' to 'true', and enable 'fp16' mixed precision computation to achieve the fastest acceleration.\n\n\n\nAlternatively, you can directly modify the 'unpad_inputs' and 'use_memory_efficient_attention' settings to 'true' in the model's 'URL', eliminating the need to set them in the code.\n\n\n---\n\n<details>\n <summary> Clarification of Relationship with nomic-embed and nomicBERT </summary>\n\nOne may question the originality of our work and consider it a mere replication of 'nomicBERT'. To clarify, our work is parallel but stems from the same idea as 'nomicBERT'.\n\nApplying RoPE and GLU to BERT to support longer texts is a straightforward idea. Our exploration of the transformer++ encoder (i.e., BERT + RoPE + GLU) began in August 2023.\nAnd by November 2023, we had completed the 'gte-base-en-v1.1'. Then, I went on to prepare for the ACL submission of the other project...\n\nThe release of 'nomic-embed' [^4] brought to our attention the pressure, as well as provided us with more resources, which allowed us to continue with this project.\nWithout the outstanding work of 'nomicai', the release of 'gte-v1.5' could have been delayed much longer. Thanks!\n\n</details>\n\n---\n\n[^1]: Su, Jianlin, Murtadha Ahmed, Yu Lu, Shengfeng Pan, Wen Bo, and Yunfeng Liu. \"Roformer: Enhanced transformer with rotary position embedding.\" Neurocomputing 568 (2024): 127063.\n\n[^2]: Shazeer, Noam. \"Glu variants improve transformer.\" arXiv preprint arXiv:2002.05202 (2020).\n\n[^3]: Portes, Jacob, Alexander Trott, Sam Havens, Daniel King, Abhinav Venigalla, Moin Nadeem, Nikhil Sardana, Daya Khudia, and Jonathan Frankle. \"MosaicBERT: A Bidirectional Encoder Optimized for Fast Pretraining.\" Advances in Neural Information Processing Systems 36 (2024).\n\n[^4]: Nussbaum, Zach, John X. Morris, Brandon Duderstadt, and Andriy Mulyar. \"Nomic Embed: Training a Reproducible Long Context Text Embedder.\" arXiv preprint arXiv:2402.01613 (2024)."
] | [
"TAGS\n#license-apache-2.0 #region-us \n",
"## Code implementation of new GTE embeddings\n\nThis model is a BERT-like encoder with the following optimizations implemented:\n\n1. Replacing absolute position embeddings with RoPE [^1].\n2. Substituting the conventional activation functions with Gated Linear Units (GLU) [^2].\n3. Setting attention dropout to 0 to use 'xformers' and 'flash_attn'.\n4. Using unpadding to eliminate the needless computations for padding tokens [^3]. (this is off by default and should be used in conjunction with 'xformers' for optimal acceleration).\n5. Setting 'vocab_size' as a multiple of 64.",
"### Recommendation: Enable Unpadding and Acceleration with 'xformers'\n\nThis code supports the acceleration of attention computations using 'xformers', which can automatically choose the optimal implementation based on the type of device, such as 'flash_attn'. Therefore, we can also achieve significant acceleration on old devices like the V100.\n\n\nFirstly, install 'xformers' (with 'pytorch' pre-installed):\n\nFor more information, refer to Installing xformers.\n\nThen, when loading the model, set 'unpad_inputs' and 'use_memory_efficient_attention' to 'true', and enable 'fp16' mixed precision computation to achieve the fastest acceleration.\n\n\n\nAlternatively, you can directly modify the 'unpad_inputs' and 'use_memory_efficient_attention' settings to 'true' in the model's 'URL', eliminating the need to set them in the code.\n\n\n---\n\n<details>\n <summary> Clarification of Relationship with nomic-embed and nomicBERT </summary>\n\nOne may question the originality of our work and consider it a mere replication of 'nomicBERT'. To clarify, our work is parallel but stems from the same idea as 'nomicBERT'.\n\nApplying RoPE and GLU to BERT to support longer texts is a straightforward idea. Our exploration of the transformer++ encoder (i.e., BERT + RoPE + GLU) began in August 2023.\nAnd by November 2023, we had completed the 'gte-base-en-v1.1'. Then, I went on to prepare for the ACL submission of the other project...\n\nThe release of 'nomic-embed' [^4] brought to our attention the pressure, as well as provided us with more resources, which allowed us to continue with this project.\nWithout the outstanding work of 'nomicai', the release of 'gte-v1.5' could have been delayed much longer. Thanks!\n\n</details>\n\n---\n\n[^1]: Su, Jianlin, Murtadha Ahmed, Yu Lu, Shengfeng Pan, Wen Bo, and Yunfeng Liu. \"Roformer: Enhanced transformer with rotary position embedding.\" Neurocomputing 568 (2024): 127063.\n\n[^2]: Shazeer, Noam. \"Glu variants improve transformer.\" arXiv preprint arXiv:2002.05202 (2020).\n\n[^3]: Portes, Jacob, Alexander Trott, Sam Havens, Daniel King, Abhinav Venigalla, Moin Nadeem, Nikhil Sardana, Daya Khudia, and Jonathan Frankle. \"MosaicBERT: A Bidirectional Encoder Optimized for Fast Pretraining.\" Advances in Neural Information Processing Systems 36 (2024).\n\n[^4]: Nussbaum, Zach, John X. Morris, Brandon Duderstadt, and Andriy Mulyar. \"Nomic Embed: Training a Reproducible Long Context Text Embedder.\" arXiv preprint arXiv:2402.01613 (2024)."
] |
text-generation | transformers |
# taide/TAIDE-LX-7B-Chat-GGUF
- This is quantized version of [taide/TAIDE-LX-7B-Chat](https://huggingface.co/taide/TAIDE-LX-7B-Chat) created using llama.cpp
# Model Description
* The [TAIDE project](https://taide.tw/index) aims to develop a generative AI dialogue engine model that is tailored to the linguistic and cultural characteristics of Taiwan, while also establishing a trustworthy AI environment. By combining academic, industrial, and research resources, the project seeks to advance the development of trustworthy generative AI, enhancing Taiwan's international competitiveness, promoting industrial development, and reducing dependence on foreign technologies.
* The large language models developed in this project are based on Meta's [LLaMA2-7b](https://huggingface.co/meta-llama/Llama-2-7b-hf) release, incorporating text and training materials from various fields in Taiwan to enhance the model's ability to respond in Traditional Chinese and perform well in specific tasks. The publicly released models are as follows:
* [TAIDE-LX-7B](https://huggingface.co/taide/TAIDE-LX-7B): This model is a continuous pretrained version with Traditional Chinese data based on LLaMA2-7b. It is suitable for scenarios where users intend to further fine-tune the model. Since the pre-trained model has not undergone fine-tuning and preference alignment, it may produce malicious or unsafe outputs. Please use with caution.
* [TAIDE-LX-7B-Chat](https://huggingface.co/taide/TAIDE-LX-7B-Chat): This model enhances office-related tasks and multi-turn question-and-answer dialogue capabilities through instruction tuning based on TAIDE-LX-7B. It is suitable for scenarios such as chat conversations or task assistance. TAIDE-LX-7B-Chat also provides a [4-bit quantization model](https://huggingface.co/taide/TAIDE-LX-7B-Chat-4bit). The quantization model is primarily offered for user convenience but may affect performance and introduce unforeseen issues. Users are advised to understand and take note of this.
# Model Parameters
* Parameters: 7B
* Max context length: 4K
* Training token in Traditional Chinese: 41.44B
* Training time: 1531.82 H100 GPU Hours
# Features
* Expanding the character and word count by 24,720 to enhance the model's ability to process Traditional Chinese.
* Strictly vetting training data for the model to improve its trustworthiness and applicability.
* Enhancing the model's performance for tasks such as summarization, writing articles, writing letters, and translating between Chinese and English, which are commonly used in office settings.
* Strengthening the model's understanding of Taiwan-specific culture, terminology, and context.
* Equip the model with multi-turn conversation capabilities.
# Examples
| Task | User input | Model output |
| --- | -------- | ------- |
| Writing articles | 請以以下內容為基礎,寫一篇文章:撰寫一篇作文,題目為《一張舊照片》,內容要求為:選擇一張令你印象深刻的照片,說明令你印象深刻的原因,並描述照片中的影像及背後的故事。記錄成長的過程、與他人的情景、環境變遷和美麗的景色。 | 以下是根據指令創作的一篇作文:<br><br>《一張舊照片》<br><br>在我塵封已久的家族相簿中,有一張特別吸引我的舊照片,那張照片記錄了我們家族成員在過往美好時光的歡聚,至今仍舊令人回味無窮。這張照片是我奶奶在二十多年前舉辦的一場家族聚會上所拍下的,照片中的人物包括了我的父母、祖父母、叔叔、姑姑和表哥等,大家臉上洋溢著幸福的笑容,彷彿時光靜止般地美好。<br><br>照片中的場景十分溫馨,大家圍坐在一張長方形的餐桌上,桌上擺滿了美味的食物和飲料。桌上的菜餚是傳統中式的佳餚,有香噴噴的烤全魚、嫩滑的雞肉和鮮甜的蔬菜,這些菜餚是當年奶奶親自烹調的,她手藝精湛,讓每個人都吃得津津有味。<br><br>最讓我印象深刻的是,照片中還拍到了我年幼時的模樣。我坐在桌子的另一端,手裡拿著一碗湯,臉上帶著滿足的笑容。那時的我才剛滿周歲,對於這場盛大的家族聚會還感到十分新奇。從這張照片中,我能感受到當年家族成員間的親密和溫暖,即使過了這麼多年,那份情感卻依然牢固地存在著。<br><br>這張照片背後有著動人的故事。在那個時代,我的祖父母和父母都還在讀中學,他們每天都會放學後到農田裡幫忙,辛勤地耕作以維持一家人的生計。儘管生活條件並不富裕,但他們仍舊保持著樂觀的態度和對生活的熱愛。每年到了秋天,只要收獲季節來臨,他們就會舉辦家族聚會,邀請所有親戚朋友前來共享豐收的喜悅。<br><br>隨著歲月的流逝,我逐漸長大,開始工作和生活。每次回鄉,我都會抽空去探望老人家,聽聽他們當年的往事,感受那段刻骨銘心的時光。而這張舊照片,也將一直陪伴著我,成為我珍貴回憶的一部分。它見證了我們的成長和變遷,也記錄下了那些難以忘懷的美好時刻。<br><br>這張照片不僅展示了家族的親情和溫暖,還折射出那個時代人們勤勞樸實的生活方式和對傳統的尊重。如今,隨著社會的快速發展,許多傳統的美德和風俗正在逐漸消失。我們應該好好珍惜這樣的回憶和文化,將它們傳承下去,讓後代子孫也能感受到那份來自歷史的溫情和傳承。<br><br>因此,我決定將這張照片好好保管起來,並常常拿出來看。它不僅是一張舊照片,更是一段寶貴的回憶和家族精神的象徵。我希望未來能有機會重現當年的盛況,再一次聚在一起,分享彼此的生活和故事,讓那份親情和友情繼續在時光中蔓延,永不消逝。 |
| Writing letters | 請以品牌經理的身份,給廣告公司的創意總監寫一封信,提出對於新產品廣告宣傳活動的創意建議。 | 尊敬的創意總監:<br><br>我寫這封信是為了向您提出一些關於我們新產品廣告宣傳活動的創意建議。我們公司即將推出一款全新的產品,為了確保它在市場上取得成功,我們希望透過一個引人注目的廣告宣傳活動來吸引消費者的注意。<br><br>在考慮廣告的創意和策略時,有幾個因素需要納入考量。首先,我們要針對目標客群進行研究,以瞭解他們的需求和喜好。其次,我們要確保廣告內容具有吸引力和說服力,能引起目標客群的共鳴。最後,我們要利用各種媒體平臺和數位行銷工具,以擴大廣告的影響力。<br><br>基於這些因素,我提出以下幾點創意建議:<br><br>1. 產品定位:我們可將這款新產品定位為高端、環保、健康和時尚的代表,強調其獨有的功能和特色。在廣告中,我們可以透過生動的視覺效果和簡潔的語言來傳達這些特點。<br>2. 故事行銷:在廣告中講述一個與產品功能相關的動人故事,讓消費者能更深入地瞭解產品所帶來的生活變化。例如,我們可以講述一位忙碌的職業婦女,如何使用我們的新產品在工作和生活中取得平衡的故事。<br>3. 名人效應:邀請一位受歡迎的公眾人物或意見領袖擔任產品代言人,利用他們的影響力來推廣產品。這不僅可以增加產品曝光度,還能讓更多消費者信任和信賴產品。<br>4. 跨界合作:與一些相關產業(如時尚、環保、健康等)進行跨界合作,共同推出限量版或聯名產品。這種合作不僅能吸引不同領域的消費者,還能為產品帶來更多話題性和收藏價值。<br>5. 數位行銷:充分利用社群媒體、搜尋引擎和電郵行銷等數位工具,在整個廣告宣傳過程中保持一致性的訊息和形象。我們也可製作一系列短視頻和線上活動,以增加消費者參與度和品牌忠誠度。<br>6. 創新形式:嘗試使用新穎的廣告形式,如AR(擴增實境)、VR(虛擬實境)和360度影片等,給消費者帶來全新的廣告體驗。這種創新形式不僅能吸引消費者目光,還能提高產品在市場上的辨識度。<br>7. 活動參與:在廣告宣傳活動中加入互動環節,如產品試用、抽獎和優惠券等,鼓勵消費者積極參與並分享他們的體驗。這將有助於口碑傳播,進一步擴大產品影響力。<br><br>綜合以上建議,我希望我們能製定出一套全面而富有創意的廣告宣傳活動計劃,以最大限度地提升新產品在市場上的競爭力。如果您有任何疑問或需要進一步討論,歡迎隨時與我聯絡。謝謝您的關注和支援!<br><br>祝商祺!<br><br>您的品牌經理<br><br>(註:此信件可根據實際情況和產品特點進行調整) |
| Summarization | 請將這篇文章精簡條理化:「產業創新條例第10條之2及第72條條文修正案」俗稱「台版晶片法」,針對半導體、電動車、5G等技術創新且居國際供應鏈關鍵地位公司,提供最高25%營所稅投抵優惠,企業適用要件包含當年度研發費用、研發密度達一定規模,且有效稅率達一定比率。<br>為因應經濟合作暨發展組織(OECD)國家最低稅負制調整,其中有效稅率門檻,民國112年訂為12%,113年料將提高至15%,但仍得審酌國際間最低稅負制實施情形。<br>經濟部官員表示,已和財政部協商進入最後階段,除企業研發密度訂在6%,目前已確認,企業購置先進製程的設備投資金額達100億元以上可抵減。<br>財政部官員表示,研商過程中,針對台灣產業與其在國際間類似的公司進行深入研究,在設備部分,畢竟適用產創10之2的業者是代表台灣隊打「國際盃」,投入金額不達100億元,可能也打不了。<br>至於備受關注的研發費用門檻,經濟部官員表示,歷經與財政部來回密切討論,研發費用門檻有望落在60億至70億元之間。<br>財政部官員指出,研發攸關台灣未來經濟成長動能,門檻不能「高不可攀」,起初雖設定在100億元,之所以會調降,正是盼讓企業覺得有辦法達得到門檻、進而適用租稅優惠,才有動力繼續投入研發,維持國際供應鏈關鍵地位。<br>經濟部官員表示,因廠商研發費用平均為30、40億元,其中,IC設計業者介於30億至60億元範圍,若將門檻訂在100億元,符合條件的業者較少、刺激誘因不足;此外,若符合申請門檻的業者增加,將可提高企業在台投資金額,財政部稅收也能因此獲得挹注。<br>IC設計業者近日頻頻針對產創10之2發聲,希望降低適用門檻,加上各國力拚供應鏈自主化、加碼補助半導體產業,經濟部官員表示,經濟部和財政部就產創10之2達成共識,爭取讓更多業者受惠,盼增強企業投資力道及鞏固台灣技術地位。<br>財政部官員表示,租稅獎勵的制定必須「有為有守」,並以達到獎勵設置目的為最高原則,現階段在打「國內盃」的企業仍可適用產創第10條、10之1的租稅優惠,共同壯大台灣經濟發展。<br>經濟部和財政部正就研發費用門檻做最後確認,待今明兩天預告子法之後,約有30天時間,可與業界進一步討論及調整,盼產創10之2能在6月上路。 | 台灣「產業創新條例」的修正案,又稱「台版晶片法」,旨在支持半導體、電動車及5G技術領先企業的創新。新政策提供最高25%的所得稅抵免,企業需滿足當年度研發費用達一定規模、研發密度超過6%以及有效稅率達一定比率等條件。因應國際間對企業最低稅負制度的調整,台灣的門檻預計分別於2023年和2024年達到12%和15%。目前,與財政部討論後,確定100億元的設備投資抵免是可行的誘因,且研發費用門檻預計會在60至70億元之間。對於降低門檻的要求,經濟部和財政部已達成共識,旨在擴大受惠範圍並鼓勵企業投資以維持供應鏈的關鍵地位。此外,針對IC設計業者的訴求,也考慮調降門檻。預計此法案將在近期內實施,相關討論和調整將在預告期後進一步進行。 |
| Translation | 以下提供英文內容,請幫我翻譯成中文。Dongshan coffee is famous for its unique position, and the constant refinement of production methods. The flavor is admired by many caffeine afficionados. | 東山咖啡以得天獨厚的地位,不斷精研的製作工藝著稱,風味獲諸多咖啡愛好者推崇。 |
# Training methods
* Software / hardware spec
* GPU: H100
* Training Framework: PyTorch
* Data preprocessing
* Character normalization
* Deduplication
* Denoise
* Html tag、javascript in web content
* Non-standard characters or garbage characters
* Posts with an insufficient number of characters
* Removing specific formats such as extra line breaks added for formatting purposes
* Removing personal information such as emails and phone numbers.
* Remove inappropriate content such as gambling, pornography, etc..
* Character and word expanding
* Enhancing the performance of Traditional Chinese input and output, the expanded data include the following two parts:
* Obtaining Chinese characters from the Ministry of Education's ["Variant Chinese Characters Dictionary" and "Corrected Characters Table"](https://dict.variants.moe.edu.tw/appendix.jsp?ID=1&ID=0).
* Collecting over 5,000,000 sentences with more than 100 characters each from the Traditional Chinese Wikipedia, news articles, and the Chinese Common Crawl data (2.1G), used to train the tokenizer for Chinese characters and words.
* Continuous pretraining (CP)
* Supplementing the model with a large amount of reliable Traditional Chinese knowledge.
* Hyper parameters
* optimizer: AdamW
* learning rate: 1e-4
* batch size: 1M tokens
* epoch: 1
* Fine tune (FT)
* Enabling the model to answer questions in Traditional Chinese.
* Hyper parameters
* optimizer: AdamW
* learning rate: 5e-5
* batch size: 256K tokens
* epoch: 3
# Training Data
* Continuous pre-training data (about 140GB)
| Dataset | Description |
| --- | -------- |
| Litigation Data | [Civil litigation data](https://judgment.judicial.gov.tw/FJUD/default.aspx) from various levels of courts in the judicial rulings, including data from 2013/01 to 2023/12. |
| CNA news | The [CNA news](https://www.cna.com.tw/) includes daily news articles from June 1993 to June 2023, spanning a period of 30 years. The content covers various domains such as domestic and international politics, society, economy, culture, education, and lifestyle. |
| ETtoday news | [ETtoday news](https://www.ettoday.net/) data, including data from 2011/10 to 2023/12. |
| Legislative Yuan Gazette | The [Legislative Yuan Gazette](https://ppg.ly.gov.tw/ppg/) contains data from the 1st session of the 8th term to the 7th session of the 10th term. |
| Publisher Website Book Introduction | Includes book introduction data from the websites of [SunColor](https://www.suncolor.com.tw/), [Gotop](https://www.gotop.com.tw/) publishers. |
| Abstracts of GRB research projects | [GRB](https://www.grb.gov.tw/) is an information system that compiles research projects funded by government grants and their outcome reports. This dataset primarily includes research project abstracts from 1993 to 2023, including both Chinese and their English counterparts. |
| Academic conference proceedings abstracts | The [database](https://sticnet.stpi.narl.org.tw/sticloc/ttscalle?meet:) contains academic conference proceedings held in Taiwan from 1988 to 2009. |
| Taiwan Panorama magazine | [Taiwan Panorama magazine](https://www.taiwan-panorama.com/) contains articles from July 1993 to June 2023, spanning 30 years. The content focuses on Taiwanese culture, tourism, and local customs. |
| 樂詞網 | 《[樂詞網](https://terms.naer.edu.tw/)》covers approximately 187,000 academic terms in the humanities and social sciences, along with their translations. |
| Data from various ministries and commissions | Including partial data from government department websites such as the Executive Yuan's "[National Overview](https://www.ey.gov.tw/state/)", the Ministry of Culture's "[National Cultural Memory Bank](https://memory.culture.tw/)", the National Development Council's "[Archives Support Teaching Network](https://art.archives.gov.tw/index.aspx)", the Ministry of Transportation's "[Traffic Safety Portal](https://168.motc.gov.tw/)", etc. |
| Business Today | [Business Today](https://www.businesstoday.com.tw/) Magazine is a weekly magazine focused on finance. The dataset includes articles from 2008/01 to 2023/07. |
| Mandarin and idiom dictionary from the Ministry of Education | Dataset including:<br>[Idiom Dictionary](https://dict.idioms.moe.edu.tw/search.jsp?webMd=1&la=0): Contains 5,338 idioms, including definitions, original stories, usage explanations, and example sentences.<br>[Revised Mandarin Dictionary](https://dict.revised.moe.edu.tw/?la=0&powerMode=0): contains Chinese words and various vocabulary, including pronunciation, radicals, definitions, and other information, totaling approximately 165,539 entries.<br>[Concise Mandarin Dictionary](https://dict.concised.moe.edu.tw/?la=0&powerMode=0): is a condensed version of the "Revised Mandarin Dictionary", containing a total of 45,247 entries. |
| SCITechVista | The dataset includes science news and popular science articles from the [SCITechVista](https://scitechvista.nat.gov.tw/) website. |
| iKnow | The [iKnow](https://iknow.stpi.narl.org.tw/) platform provides information on market trends, strategic analysis, patent knowledge, and technology transaction information for Taiwan and the global technology industry. The dataset includes data from 2005/01 to 2023/07. |
| Science Development Monthly Magazine | [Science Development Monthly Magazine](https://ejournal.stpi.narl.org.tw/sd) is a popular science publication published by the National Science Council (NSC) to promote science education. It includes articles from 2004/10 to 2020/12. In 2021, the magazine was relaunched as "[CharmingSCITech](https://www.charmingscitech.nat.gov.tw/)" quarterly, providing new knowledge on international technology issues. |
| Legislation Database | The [Legislation Database](https://law.moj.gov.tw/) includes the latest central regulations, rules, draft bills, and local regulations issued by government agencies as of 2023/10. |
| Local Government Tourism Websites | Covering partial data from tourism websites of local government counties and cities in Taiwan. |
| Curriculum Guidelines from the National Institute of Education | The dataset includes curriculum guidelines for different subjects at various levels of education. |
| CNA's English and Chinese Name Translation Database | The English and Chinese Name Translation Database of the Central News Agency (CNA) collects translations of foreign and Chinese surnames, personal names, organizations, and place names used in news. |
| Fairy tales | A total of 20 fairy tale books, including "Tom Sawyer," "Peter Pan," "Alice's Adventures in Wonderland," "Uncle Long Legs," and more. |
| RedPajama-Data-V2 | Extracting English data from the [RedPajama-Data-v2](https://github.com/togethercomputer/RedPajama-Data) multilingual dataset |
| MathPile-commercial | A mathematics-focused dataset obtained from [MathPile-commercial](https://huggingface.co/datasets/GAIR/MathPile_Commercial) |
| Traditional Chinese Wikipedia Articles | The content of all articles in [Traditional Chinese Wikipedia](https://zh.wikipedia.org/zh-tw/%E4%B8%AD%E6%96%87%E7%BB%B4%E5%9F%BA%E7%99%BE%E7%A7%91), up to January 2023. |
| github-code-clean | An open-source code dataset on GitHub. After removing unlicensed code and documents. |
* Fine tune data
* The TAIDE team trains the LLaMA2 series models to generate fine-tuning data, which generates single or multi-turn conversations on topics such as world knowledge, creative writing, general knowledge, translation, summarization, programming, and Taiwanese values. The fine tune data consists of 128K prompt-response pairs and will be released publicly later.
# Evaluation
* taide-bench
* Data
* Tasks include writing articles, writing letters, summarizing articles, translating from English to Traditional Chinese, translating from Traditional Chinese to English. There are 500 questions in total.
* data link: [taide-bench](https://huggingface.co/datasets/taide/taide-bench)
* Evaluation method
* LLM as a Judge by GPT4
* code link: [taide-bench-eval](https://github.com/taide-taiwan/taide-bench-eval)
* Scores
| Model | Translating from Traditional Chinese to English | Translating from English to Traditional Chinese | Summerization | Writing articles | Writing letters | Average |
| --- | ----- | ----- | ---- | ---- | ---- | --- |
| TAIDE-LX-7B-Chat | 7.165 | 7.685 | 7.720 | 9.635 | 9.110 | 8.263 |
| GPT3.5 | 8.880 | 8.810 | 7.450 | 9.490 | 8.750 | 8.676 |
| LLAMA2 7B | 6.075 | 4.475 | 5.905 | 2.625 | 3.040 | 4.424 |
| LLAMA2 13B | 6.480 | 6.135 | 6.110 | 2.565 | 3.000 | 4.858 |
| LLAMA2 70B | 6.975 | 6.375 | 6.795 | 2.625 | 2.990 | 5.152 |
# License
* [TAIDE L Models Community License Agreement](https://drive.google.com/file/d/1FcUZjbUH6jr4xoCyAronN_slLgcdhEUd/view)
# Disclaimer
* Due to limitations in its design architecture and the inevitable biases in data, any response from the LLM model does not represent the stance of TAIDE. Additional security measures should be implemented before use, and responses may also contain incorrect information. Users are advised not to fully trust the responses.
# Development Team
* [https://taide.tw/index/teamList](https://taide.tw/index/teamList)
# Useful links
* [TAIDE official website](https://taide.tw/index)
* [TAIDE Huggingface](https://huggingface.co/taide)
* [TAIDE Github](https://github.com/taide-taiwan)
* [Kuwa AI](https://kuwaai.org/) | {"license": "other", "library_name": "transformers", "tags": ["llama", "conversational"], "license_name": "taide-l-models-community-license-agreement", "license_link": "https://drive.google.com/file/d/1FcUZjbUH6jr4xoCyAronN_slLgcdhEUd/view", "pipeline_tag": "text-generation"} | QuantFactory/TAIDE-LX-7B-Chat-GGUF | null | [
"transformers",
"gguf",
"llama",
"conversational",
"text-generation",
"license:other",
"endpoints_compatible",
"region:us"
] | null | 2024-04-18T08:28:37+00:00 | [] | [] | TAGS
#transformers #gguf #llama #conversational #text-generation #license-other #endpoints_compatible #region-us
| taide/TAIDE-LX-7B-Chat-GGUF
===========================
* This is quantized version of taide/TAIDE-LX-7B-Chat created using URL
Model Description
=================
* The TAIDE project aims to develop a generative AI dialogue engine model that is tailored to the linguistic and cultural characteristics of Taiwan, while also establishing a trustworthy AI environment. By combining academic, industrial, and research resources, the project seeks to advance the development of trustworthy generative AI, enhancing Taiwan's international competitiveness, promoting industrial development, and reducing dependence on foreign technologies.
* The large language models developed in this project are based on Meta's LLaMA2-7b release, incorporating text and training materials from various fields in Taiwan to enhance the model's ability to respond in Traditional Chinese and perform well in specific tasks. The publicly released models are as follows:
+ TAIDE-LX-7B: This model is a continuous pretrained version with Traditional Chinese data based on LLaMA2-7b. It is suitable for scenarios where users intend to further fine-tune the model. Since the pre-trained model has not undergone fine-tuning and preference alignment, it may produce malicious or unsafe outputs. Please use with caution.
+ TAIDE-LX-7B-Chat: This model enhances office-related tasks and multi-turn question-and-answer dialogue capabilities through instruction tuning based on TAIDE-LX-7B. It is suitable for scenarios such as chat conversations or task assistance. TAIDE-LX-7B-Chat also provides a 4-bit quantization model. The quantization model is primarily offered for user convenience but may affect performance and introduce unforeseen issues. Users are advised to understand and take note of this.
Model Parameters
================
* Parameters: 7B
* Max context length: 4K
* Training token in Traditional Chinese: 41.44B
* Training time: 1531.82 H100 GPU Hours
Features
========
* Expanding the character and word count by 24,720 to enhance the model's ability to process Traditional Chinese.
* Strictly vetting training data for the model to improve its trustworthiness and applicability.
* Enhancing the model's performance for tasks such as summarization, writing articles, writing letters, and translating between Chinese and English, which are commonly used in office settings.
* Strengthening the model's understanding of Taiwan-specific culture, terminology, and context.
* Equip the model with multi-turn conversation capabilities.
Examples
========
Task: Writing articles, User input: 請以以下內容為基礎,寫一篇文章:撰寫一篇作文,題目為《一張舊照片》,內容要求為:選擇一張令你印象深刻的照片,說明令你印象深刻的原因,並描述照片中的影像及背後的故事。記錄成長的過程、與他人的情景、環境變遷和美麗的景色。, Model output: 以下是根據指令創作的一篇作文:
《一張舊照片》
在我塵封已久的家族相簿中,有一張特別吸引我的舊照片,那張照片記錄了我們家族成員在過往美好時光的歡聚,至今仍舊令人回味無窮。這張照片是我奶奶在二十多年前舉辦的一場家族聚會上所拍下的,照片中的人物包括了我的父母、祖父母、叔叔、姑姑和表哥等,大家臉上洋溢著幸福的笑容,彷彿時光靜止般地美好。
照片中的場景十分溫馨,大家圍坐在一張長方形的餐桌上,桌上擺滿了美味的食物和飲料。桌上的菜餚是傳統中式的佳餚,有香噴噴的烤全魚、嫩滑的雞肉和鮮甜的蔬菜,這些菜餚是當年奶奶親自烹調的,她手藝精湛,讓每個人都吃得津津有味。
最讓我印象深刻的是,照片中還拍到了我年幼時的模樣。我坐在桌子的另一端,手裡拿著一碗湯,臉上帶著滿足的笑容。那時的我才剛滿周歲,對於這場盛大的家族聚會還感到十分新奇。從這張照片中,我能感受到當年家族成員間的親密和溫暖,即使過了這麼多年,那份情感卻依然牢固地存在著。
這張照片背後有著動人的故事。在那個時代,我的祖父母和父母都還在讀中學,他們每天都會放學後到農田裡幫忙,辛勤地耕作以維持一家人的生計。儘管生活條件並不富裕,但他們仍舊保持著樂觀的態度和對生活的熱愛。每年到了秋天,只要收獲季節來臨,他們就會舉辦家族聚會,邀請所有親戚朋友前來共享豐收的喜悅。
隨著歲月的流逝,我逐漸長大,開始工作和生活。每次回鄉,我都會抽空去探望老人家,聽聽他們當年的往事,感受那段刻骨銘心的時光。而這張舊照片,也將一直陪伴著我,成為我珍貴回憶的一部分。它見證了我們的成長和變遷,也記錄下了那些難以忘懷的美好時刻。
這張照片不僅展示了家族的親情和溫暖,還折射出那個時代人們勤勞樸實的生活方式和對傳統的尊重。如今,隨著社會的快速發展,許多傳統的美德和風俗正在逐漸消失。我們應該好好珍惜這樣的回憶和文化,將它們傳承下去,讓後代子孫也能感受到那份來自歷史的溫情和傳承。
因此,我決定將這張照片好好保管起來,並常常拿出來看。它不僅是一張舊照片,更是一段寶貴的回憶和家族精神的象徵。我希望未來能有機會重現當年的盛況,再一次聚在一起,分享彼此的生活和故事,讓那份親情和友情繼續在時光中蔓延,永不消逝。
Task: Writing letters, User input: 請以品牌經理的身份,給廣告公司的創意總監寫一封信,提出對於新產品廣告宣傳活動的創意建議。, Model output: 尊敬的創意總監:
我寫這封信是為了向您提出一些關於我們新產品廣告宣傳活動的創意建議。我們公司即將推出一款全新的產品,為了確保它在市場上取得成功,我們希望透過一個引人注目的廣告宣傳活動來吸引消費者的注意。
在考慮廣告的創意和策略時,有幾個因素需要納入考量。首先,我們要針對目標客群進行研究,以瞭解他們的需求和喜好。其次,我們要確保廣告內容具有吸引力和說服力,能引起目標客群的共鳴。最後,我們要利用各種媒體平臺和數位行銷工具,以擴大廣告的影響力。
基於這些因素,我提出以下幾點創意建議:
1. 產品定位:我們可將這款新產品定位為高端、環保、健康和時尚的代表,強調其獨有的功能和特色。在廣告中,我們可以透過生動的視覺效果和簡潔的語言來傳達這些特點。
2. 故事行銷:在廣告中講述一個與產品功能相關的動人故事,讓消費者能更深入地瞭解產品所帶來的生活變化。例如,我們可以講述一位忙碌的職業婦女,如何使用我們的新產品在工作和生活中取得平衡的故事。
3. 名人效應:邀請一位受歡迎的公眾人物或意見領袖擔任產品代言人,利用他們的影響力來推廣產品。這不僅可以增加產品曝光度,還能讓更多消費者信任和信賴產品。
4. 跨界合作:與一些相關產業(如時尚、環保、健康等)進行跨界合作,共同推出限量版或聯名產品。這種合作不僅能吸引不同領域的消費者,還能為產品帶來更多話題性和收藏價值。
5. 數位行銷:充分利用社群媒體、搜尋引擎和電郵行銷等數位工具,在整個廣告宣傳過程中保持一致性的訊息和形象。我們也可製作一系列短視頻和線上活動,以增加消費者參與度和品牌忠誠度。
6. 創新形式:嘗試使用新穎的廣告形式,如AR(擴增實境)、VR(虛擬實境)和360度影片等,給消費者帶來全新的廣告體驗。這種創新形式不僅能吸引消費者目光,還能提高產品在市場上的辨識度。
7. 活動參與:在廣告宣傳活動中加入互動環節,如產品試用、抽獎和優惠券等,鼓勵消費者積極參與並分享他們的體驗。這將有助於口碑傳播,進一步擴大產品影響力。
綜合以上建議,我希望我們能製定出一套全面而富有創意的廣告宣傳活動計劃,以最大限度地提升新產品在市場上的競爭力。如果您有任何疑問或需要進一步討論,歡迎隨時與我聯絡。謝謝您的關注和支援!
祝商祺!
您的品牌經理
(註:此信件可根據實際情況和產品特點進行調整)
Task: Summarization, User input: 請將這篇文章精簡條理化:「產業創新條例第10條之2及第72條條文修正案」俗稱「台版晶片法」,針對半導體、電動車、5G等技術創新且居國際供應鏈關鍵地位公司,提供最高25%營所稅投抵優惠,企業適用要件包含當年度研發費用、研發密度達一定規模,且有效稅率達一定比率。
為因應經濟合作暨發展組織(OECD)國家最低稅負制調整,其中有效稅率門檻,民國112年訂為12%,113年料將提高至15%,但仍得審酌國際間最低稅負制實施情形。
經濟部官員表示,已和財政部協商進入最後階段,除企業研發密度訂在6%,目前已確認,企業購置先進製程的設備投資金額達100億元以上可抵減。
財政部官員表示,研商過程中,針對台灣產業與其在國際間類似的公司進行深入研究,在設備部分,畢竟適用產創10之2的業者是代表台灣隊打「國際盃」,投入金額不達100億元,可能也打不了。
至於備受關注的研發費用門檻,經濟部官員表示,歷經與財政部來回密切討論,研發費用門檻有望落在60億至70億元之間。
財政部官員指出,研發攸關台灣未來經濟成長動能,門檻不能「高不可攀」,起初雖設定在100億元,之所以會調降,正是盼讓企業覺得有辦法達得到門檻、進而適用租稅優惠,才有動力繼續投入研發,維持國際供應鏈關鍵地位。
經濟部官員表示,因廠商研發費用平均為30、40億元,其中,IC設計業者介於30億至60億元範圍,若將門檻訂在100億元,符合條件的業者較少、刺激誘因不足;此外,若符合申請門檻的業者增加,將可提高企業在台投資金額,財政部稅收也能因此獲得挹注。
IC設計業者近日頻頻針對產創10之2發聲,希望降低適用門檻,加上各國力拚供應鏈自主化、加碼補助半導體產業,經濟部官員表示,經濟部和財政部就產創10之2達成共識,爭取讓更多業者受惠,盼增強企業投資力道及鞏固台灣技術地位。
財政部官員表示,租稅獎勵的制定必須「有為有守」,並以達到獎勵設置目的為最高原則,現階段在打「國內盃」的企業仍可適用產創第10條、10之1的租稅優惠,共同壯大台灣經濟發展。
經濟部和財政部正就研發費用門檻做最後確認,待今明兩天預告子法之後,約有30天時間,可與業界進一步討論及調整,盼產創10之2能在6月上路。, Model output: 台灣「產業創新條例」的修正案,又稱「台版晶片法」,旨在支持半導體、電動車及5G技術領先企業的創新。新政策提供最高25%的所得稅抵免,企業需滿足當年度研發費用達一定規模、研發密度超過6%以及有效稅率達一定比率等條件。因應國際間對企業最低稅負制度的調整,台灣的門檻預計分別於2023年和2024年達到12%和15%。目前,與財政部討論後,確定100億元的設備投資抵免是可行的誘因,且研發費用門檻預計會在60至70億元之間。對於降低門檻的要求,經濟部和財政部已達成共識,旨在擴大受惠範圍並鼓勵企業投資以維持供應鏈的關鍵地位。此外,針對IC設計業者的訴求,也考慮調降門檻。預計此法案將在近期內實施,相關討論和調整將在預告期後進一步進行。
Task: Translation, User input: 以下提供英文內容,請幫我翻譯成中文。Dongshan coffee is famous for its unique position, and the constant refinement of production methods. The flavor is admired by many caffeine afficionados., Model output: 東山咖啡以得天獨厚的地位,不斷精研的製作工藝著稱,風味獲諸多咖啡愛好者推崇。
Training methods
================
* Software / hardware spec
+ GPU: H100
+ Training Framework: PyTorch
* Data preprocessing
+ Character normalization
+ Deduplication
+ Denoise
- Html tag、javascript in web content
- Non-standard characters or garbage characters
- Posts with an insufficient number of characters
- Removing specific formats such as extra line breaks added for formatting purposes
+ Removing personal information such as emails and phone numbers.
+ Remove inappropriate content such as gambling, pornography, etc..
* Character and word expanding
+ Enhancing the performance of Traditional Chinese input and output, the expanded data include the following two parts:
- Obtaining Chinese characters from the Ministry of Education's "Variant Chinese Characters Dictionary" and "Corrected Characters Table".
- Collecting over 5,000,000 sentences with more than 100 characters each from the Traditional Chinese Wikipedia, news articles, and the Chinese Common Crawl data (2.1G), used to train the tokenizer for Chinese characters and words.
* Continuous pretraining (CP)
+ Supplementing the model with a large amount of reliable Traditional Chinese knowledge.
+ Hyper parameters
- optimizer: AdamW
- learning rate: 1e-4
- batch size: 1M tokens
- epoch: 1
* Fine tune (FT)
+ Enabling the model to answer questions in Traditional Chinese.
+ Hyper parameters
- optimizer: AdamW
- learning rate: 5e-5
- batch size: 256K tokens
- epoch: 3
Training Data
=============
* Continuous pre-training data (about 140GB)
| Dataset | Description |
| --- | -------- |
| Litigation Data | Civil litigation data from various levels of courts in the judicial rulings, including data from 2013/01 to 2023/12. |
| CNA news | The CNA news includes daily news articles from June 1993 to June 2023, spanning a period of 30 years. The content covers various domains such as domestic and international politics, society, economy, culture, education, and lifestyle. |
| ETtoday news | ETtoday news data, including data from 2011/10 to 2023/12. |
| Legislative Yuan Gazette | The Legislative Yuan Gazette contains data from the 1st session of the 8th term to the 7th session of the 10th term. |
| Publisher Website Book Introduction | Includes book introduction data from the websites of SunColor, Gotop publishers. |
| Abstracts of GRB research projects | GRB is an information system that compiles research projects funded by government grants and their outcome reports. This dataset primarily includes research project abstracts from 1993 to 2023, including both Chinese and their English counterparts. |
| Academic conference proceedings abstracts | The database contains academic conference proceedings held in Taiwan from 1988 to 2009. |
| Taiwan Panorama magazine | Taiwan Panorama magazine contains articles from July 1993 to June 2023, spanning 30 years. The content focuses on Taiwanese culture, tourism, and local customs. |
| 樂詞網 | 《樂詞網》covers approximately 187,000 academic terms in the humanities and social sciences, along with their translations. |
| Data from various ministries and commissions | Including partial data from government department websites such as the Executive Yuan's "National Overview", the Ministry of Culture's "National Cultural Memory Bank", the National Development Council's "Archives Support Teaching Network", the Ministry of Transportation's "Traffic Safety Portal", etc. |
| Business Today | Business Today Magazine is a weekly magazine focused on finance. The dataset includes articles from 2008/01 to 2023/07. |
| Mandarin and idiom dictionary from the Ministry of Education | Dataset including:
Idiom Dictionary: Contains 5,338 idioms, including definitions, original stories, usage explanations, and example sentences.
Revised Mandarin Dictionary: contains Chinese words and various vocabulary, including pronunciation, radicals, definitions, and other information, totaling approximately 165,539 entries.
Concise Mandarin Dictionary: is a condensed version of the "Revised Mandarin Dictionary", containing a total of 45,247 entries. |
| SCITechVista | The dataset includes science news and popular science articles from the SCITechVista website. |
| iKnow | The iKnow platform provides information on market trends, strategic analysis, patent knowledge, and technology transaction information for Taiwan and the global technology industry. The dataset includes data from 2005/01 to 2023/07. |
| Science Development Monthly Magazine | Science Development Monthly Magazine is a popular science publication published by the National Science Council (NSC) to promote science education. It includes articles from 2004/10 to 2020/12. In 2021, the magazine was relaunched as "CharmingSCITech" quarterly, providing new knowledge on international technology issues. |
| Legislation Database | The Legislation Database includes the latest central regulations, rules, draft bills, and local regulations issued by government agencies as of 2023/10. |
| Local Government Tourism Websites | Covering partial data from tourism websites of local government counties and cities in Taiwan. |
| Curriculum Guidelines from the National Institute of Education | The dataset includes curriculum guidelines for different subjects at various levels of education. |
| CNA's English and Chinese Name Translation Database | The English and Chinese Name Translation Database of the Central News Agency (CNA) collects translations of foreign and Chinese surnames, personal names, organizations, and place names used in news. |
| Fairy tales | A total of 20 fairy tale books, including "Tom Sawyer," "Peter Pan," "Alice's Adventures in Wonderland," "Uncle Long Legs," and more. |
| RedPajama-Data-V2 | Extracting English data from the RedPajama-Data-v2 multilingual dataset |
| MathPile-commercial | A mathematics-focused dataset obtained from MathPile-commercial |
| Traditional Chinese Wikipedia Articles | The content of all articles in Traditional Chinese Wikipedia, up to January 2023. |
| github-code-clean | An open-source code dataset on GitHub. After removing unlicensed code and documents. |
* Fine tune data
+ The TAIDE team trains the LLaMA2 series models to generate fine-tuning data, which generates single or multi-turn conversations on topics such as world knowledge, creative writing, general knowledge, translation, summarization, programming, and Taiwanese values. The fine tune data consists of 128K prompt-response pairs and will be released publicly later.
Evaluation
==========
* taide-bench
+ Data
- Tasks include writing articles, writing letters, summarizing articles, translating from English to Traditional Chinese, translating from Traditional Chinese to English. There are 500 questions in total.
- data link: taide-bench
+ Evaluation method
- LLM as a Judge by GPT4
- code link: taide-bench-eval
+ Scores
| Model | Translating from Traditional Chinese to English | Translating from English to Traditional Chinese | Summerization | Writing articles | Writing letters | Average |
| --- | ----- | ----- | ---- | ---- | ---- | --- |
| TAIDE-LX-7B-Chat | 7.165 | 7.685 | 7.720 | 9.635 | 9.110 | 8.263 |
| GPT3.5 | 8.880 | 8.810 | 7.450 | 9.490 | 8.750 | 8.676 |
| LLAMA2 7B | 6.075 | 4.475 | 5.905 | 2.625 | 3.040 | 4.424 |
| LLAMA2 13B | 6.480 | 6.135 | 6.110 | 2.565 | 3.000 | 4.858 |
| LLAMA2 70B | 6.975 | 6.375 | 6.795 | 2.625 | 2.990 | 5.152 |
License
=======
* TAIDE L Models Community License Agreement
Disclaimer
==========
* Due to limitations in its design architecture and the inevitable biases in data, any response from the LLM model does not represent the stance of TAIDE. Additional security measures should be implemented before use, and responses may also contain incorrect information. Users are advised not to fully trust the responses.
Development Team
================
* URL
Useful links
============
* TAIDE official website
* TAIDE Huggingface
* TAIDE Github
* Kuwa AI
| [] | [
"TAGS\n#transformers #gguf #llama #conversational #text-generation #license-other #endpoints_compatible #region-us \n"
] |
text-generation | transformers |
# Uploaded model
- **Developed by:** goncaavci
- **License:** apache-2.0
- **Finetuned from model :** unsloth/llama-2-7b-bnb-4bit
This llama model was trained 2x faster with [Unsloth](https://github.com/unslothai/unsloth) and Huggingface's TRL library.
[<img src="https://raw.githubusercontent.com/unslothai/unsloth/main/images/unsloth%20made%20with%20love.png" width="200"/>](https://github.com/unslothai/unsloth)
| {"language": ["en"], "license": "apache-2.0", "tags": ["text-generation-inference", "transformers", "unsloth", "llama", "trl", "sft"], "base_model": "unsloth/llama-2-7b-bnb-4bit"} | goncaavci/peft-llama-incident-factor-trail9 | null | [
"transformers",
"pytorch",
"llama",
"text-generation",
"text-generation-inference",
"unsloth",
"trl",
"sft",
"en",
"base_model:unsloth/llama-2-7b-bnb-4bit",
"license:apache-2.0",
"autotrain_compatible",
"endpoints_compatible",
"region:us"
] | null | 2024-04-18T08:30:11+00:00 | [] | [
"en"
] | TAGS
#transformers #pytorch #llama #text-generation #text-generation-inference #unsloth #trl #sft #en #base_model-unsloth/llama-2-7b-bnb-4bit #license-apache-2.0 #autotrain_compatible #endpoints_compatible #region-us
|
# Uploaded model
- Developed by: goncaavci
- License: apache-2.0
- Finetuned from model : unsloth/llama-2-7b-bnb-4bit
This llama model was trained 2x faster with Unsloth and Huggingface's TRL library.
<img src="URL width="200"/>
| [
"# Uploaded model\n\n- Developed by: goncaavci\n- License: apache-2.0\n- Finetuned from model : unsloth/llama-2-7b-bnb-4bit\n\nThis llama model was trained 2x faster with Unsloth and Huggingface's TRL library.\n\n<img src=\"URL width=\"200\"/>"
] | [
"TAGS\n#transformers #pytorch #llama #text-generation #text-generation-inference #unsloth #trl #sft #en #base_model-unsloth/llama-2-7b-bnb-4bit #license-apache-2.0 #autotrain_compatible #endpoints_compatible #region-us \n",
"# Uploaded model\n\n- Developed by: goncaavci\n- License: apache-2.0\n- Finetuned from model : unsloth/llama-2-7b-bnb-4bit\n\nThis llama model was trained 2x faster with Unsloth and Huggingface's TRL library.\n\n<img src=\"URL width=\"200\"/>"
] |
null | transformers | ## About
<!-- ### quantize_version: 1 -->
<!-- ### output_tensor_quantised: 1 -->
<!-- ### convert_type: -->
<!-- ### vocab_type: -->
weighted/imatrix quants of https://huggingface.co/NotAiLOL/Boundary-4x7b-MoE
<!-- provided-files -->
static quants are available at https://huggingface.co/mradermacher/Boundary-4x7b-MoE-GGUF
## Usage
If you are unsure how to use GGUF files, refer to one of [TheBloke's
READMEs](https://huggingface.co/TheBloke/KafkaLM-70B-German-V0.1-GGUF) for
more details, including on how to concatenate multi-part files.
## Provided Quants
(sorted by size, not necessarily quality. IQ-quants are often preferable over similar sized non-IQ quants)
| Link | Type | Size/GB | Notes |
|:-----|:-----|--------:|:------|
| [GGUF](https://huggingface.co/mradermacher/Boundary-4x7b-MoE-i1-GGUF/resolve/main/Boundary-4x7b-MoE.i1-IQ1_S.gguf) | i1-IQ1_S | 5.1 | for the desperate |
| [GGUF](https://huggingface.co/mradermacher/Boundary-4x7b-MoE-i1-GGUF/resolve/main/Boundary-4x7b-MoE.i1-IQ1_M.gguf) | i1-IQ1_M | 5.6 | for the desperate |
| [GGUF](https://huggingface.co/mradermacher/Boundary-4x7b-MoE-i1-GGUF/resolve/main/Boundary-4x7b-MoE.i1-IQ2_XXS.gguf) | i1-IQ2_XXS | 6.5 | |
| [GGUF](https://huggingface.co/mradermacher/Boundary-4x7b-MoE-i1-GGUF/resolve/main/Boundary-4x7b-MoE.i1-IQ2_XS.gguf) | i1-IQ2_XS | 7.2 | |
| [GGUF](https://huggingface.co/mradermacher/Boundary-4x7b-MoE-i1-GGUF/resolve/main/Boundary-4x7b-MoE.i1-IQ2_S.gguf) | i1-IQ2_S | 7.4 | |
| [GGUF](https://huggingface.co/mradermacher/Boundary-4x7b-MoE-i1-GGUF/resolve/main/Boundary-4x7b-MoE.i1-IQ2_M.gguf) | i1-IQ2_M | 8.1 | |
| [GGUF](https://huggingface.co/mradermacher/Boundary-4x7b-MoE-i1-GGUF/resolve/main/Boundary-4x7b-MoE.i1-Q2_K.gguf) | i1-Q2_K | 8.9 | IQ3_XXS probably better |
| [GGUF](https://huggingface.co/mradermacher/Boundary-4x7b-MoE-i1-GGUF/resolve/main/Boundary-4x7b-MoE.i1-IQ3_XXS.gguf) | i1-IQ3_XXS | 9.4 | lower quality |
| [GGUF](https://huggingface.co/mradermacher/Boundary-4x7b-MoE-i1-GGUF/resolve/main/Boundary-4x7b-MoE.i1-IQ3_XS.gguf) | i1-IQ3_XS | 10.0 | |
| [GGUF](https://huggingface.co/mradermacher/Boundary-4x7b-MoE-i1-GGUF/resolve/main/Boundary-4x7b-MoE.i1-Q3_K_S.gguf) | i1-Q3_K_S | 10.5 | IQ3_XS probably better |
| [GGUF](https://huggingface.co/mradermacher/Boundary-4x7b-MoE-i1-GGUF/resolve/main/Boundary-4x7b-MoE.i1-IQ3_S.gguf) | i1-IQ3_S | 10.6 | beats Q3_K* |
| [GGUF](https://huggingface.co/mradermacher/Boundary-4x7b-MoE-i1-GGUF/resolve/main/Boundary-4x7b-MoE.i1-IQ3_M.gguf) | i1-IQ3_M | 10.7 | |
| [GGUF](https://huggingface.co/mradermacher/Boundary-4x7b-MoE-i1-GGUF/resolve/main/Boundary-4x7b-MoE.i1-Q3_K_M.gguf) | i1-Q3_K_M | 11.7 | IQ3_S probably better |
| [GGUF](https://huggingface.co/mradermacher/Boundary-4x7b-MoE-i1-GGUF/resolve/main/Boundary-4x7b-MoE.i1-Q3_K_L.gguf) | i1-Q3_K_L | 12.6 | IQ3_M probably better |
| [GGUF](https://huggingface.co/mradermacher/Boundary-4x7b-MoE-i1-GGUF/resolve/main/Boundary-4x7b-MoE.i1-IQ4_XS.gguf) | i1-IQ4_XS | 13.0 | |
| [GGUF](https://huggingface.co/mradermacher/Boundary-4x7b-MoE-i1-GGUF/resolve/main/Boundary-4x7b-MoE.i1-Q4_0.gguf) | i1-Q4_0 | 13.8 | fast, low quality |
| [GGUF](https://huggingface.co/mradermacher/Boundary-4x7b-MoE-i1-GGUF/resolve/main/Boundary-4x7b-MoE.i1-Q4_K_S.gguf) | i1-Q4_K_S | 13.8 | optimal size/speed/quality |
| [GGUF](https://huggingface.co/mradermacher/Boundary-4x7b-MoE-i1-GGUF/resolve/main/Boundary-4x7b-MoE.i1-Q4_K_M.gguf) | i1-Q4_K_M | 14.7 | fast, recommended |
| [GGUF](https://huggingface.co/mradermacher/Boundary-4x7b-MoE-i1-GGUF/resolve/main/Boundary-4x7b-MoE.i1-Q5_K_S.gguf) | i1-Q5_K_S | 16.7 | |
| [GGUF](https://huggingface.co/mradermacher/Boundary-4x7b-MoE-i1-GGUF/resolve/main/Boundary-4x7b-MoE.i1-Q5_K_M.gguf) | i1-Q5_K_M | 17.2 | |
| [GGUF](https://huggingface.co/mradermacher/Boundary-4x7b-MoE-i1-GGUF/resolve/main/Boundary-4x7b-MoE.i1-Q6_K.gguf) | i1-Q6_K | 19.9 | practically like static Q6_K |
Here is a handy graph by ikawrakow comparing some lower-quality quant
types (lower is better):

And here are Artefact2's thoughts on the matter:
https://gist.github.com/Artefact2/b5f810600771265fc1e39442288e8ec9
## FAQ / Model Request
See https://huggingface.co/mradermacher/model_requests for some answers to
questions you might have and/or if you want some other model quantized.
## Thanks
I thank my company, [nethype GmbH](https://www.nethype.de/), for letting
me use its servers and providing upgrades to my workstation to enable
this work in my free time.
<!-- end -->
| {"language": ["en"], "license": "apache-2.0", "library_name": "transformers", "tags": ["moe", "merge", "mergekit", "HuggingFaceH4/zephyr-7b-beta", "mistralai/Mistral-7B-Instruct-v0.2", "teknium/OpenHermes-2.5-Mistral-7B", "meta-math/MetaMath-Mistral-7B", "Mistral"], "base_model": "NotAiLOL/Boundary-4x7b-MoE", "quantized_by": "mradermacher"} | mradermacher/Boundary-4x7b-MoE-i1-GGUF | null | [
"transformers",
"gguf",
"moe",
"merge",
"mergekit",
"HuggingFaceH4/zephyr-7b-beta",
"mistralai/Mistral-7B-Instruct-v0.2",
"teknium/OpenHermes-2.5-Mistral-7B",
"meta-math/MetaMath-Mistral-7B",
"Mistral",
"en",
"base_model:NotAiLOL/Boundary-4x7b-MoE",
"license:apache-2.0",
"endpoints_compatible",
"region:us"
] | null | 2024-04-18T08:30:12+00:00 | [] | [
"en"
] | TAGS
#transformers #gguf #moe #merge #mergekit #HuggingFaceH4/zephyr-7b-beta #mistralai/Mistral-7B-Instruct-v0.2 #teknium/OpenHermes-2.5-Mistral-7B #meta-math/MetaMath-Mistral-7B #Mistral #en #base_model-NotAiLOL/Boundary-4x7b-MoE #license-apache-2.0 #endpoints_compatible #region-us
| About
-----
weighted/imatrix quants of URL
static quants are available at URL
Usage
-----
If you are unsure how to use GGUF files, refer to one of TheBloke's
READMEs for
more details, including on how to concatenate multi-part files.
Provided Quants
---------------
(sorted by size, not necessarily quality. IQ-quants are often preferable over similar sized non-IQ quants)
Here is a handy graph by ikawrakow comparing some lower-quality quant
types (lower is better):
!URL
And here are Artefact2's thoughts on the matter:
URL
FAQ / Model Request
-------------------
See URL for some answers to
questions you might have and/or if you want some other model quantized.
Thanks
------
I thank my company, nethype GmbH, for letting
me use its servers and providing upgrades to my workstation to enable
this work in my free time.
| [] | [
"TAGS\n#transformers #gguf #moe #merge #mergekit #HuggingFaceH4/zephyr-7b-beta #mistralai/Mistral-7B-Instruct-v0.2 #teknium/OpenHermes-2.5-Mistral-7B #meta-math/MetaMath-Mistral-7B #Mistral #en #base_model-NotAiLOL/Boundary-4x7b-MoE #license-apache-2.0 #endpoints_compatible #region-us \n"
] |
reinforcement-learning | stable-baselines3 |
# **DQN** Agent playing **SpaceInvadersNoFrameskip-v4**
This is a trained model of a **DQN** agent playing **SpaceInvadersNoFrameskip-v4**
using the [stable-baselines3 library](https://github.com/DLR-RM/stable-baselines3)
and the [RL Zoo](https://github.com/DLR-RM/rl-baselines3-zoo).
The RL Zoo is a training framework for Stable Baselines3
reinforcement learning agents,
with hyperparameter optimization and pre-trained agents included.
## Usage (with SB3 RL Zoo)
RL Zoo: https://github.com/DLR-RM/rl-baselines3-zoo<br/>
SB3: https://github.com/DLR-RM/stable-baselines3<br/>
SB3 Contrib: https://github.com/Stable-Baselines-Team/stable-baselines3-contrib
Install the RL Zoo (with SB3 and SB3-Contrib):
```bash
pip install rl_zoo3
```
```
# Download model and save it into the logs/ folder
python -m rl_zoo3.load_from_hub --algo dqn --env SpaceInvadersNoFrameskip-v4 -orga chirbard -f logs/
python -m rl_zoo3.enjoy --algo dqn --env SpaceInvadersNoFrameskip-v4 -f logs/
```
If you installed the RL Zoo3 via pip (`pip install rl_zoo3`), from anywhere you can do:
```
python -m rl_zoo3.load_from_hub --algo dqn --env SpaceInvadersNoFrameskip-v4 -orga chirbard -f logs/
python -m rl_zoo3.enjoy --algo dqn --env SpaceInvadersNoFrameskip-v4 -f logs/
```
## Training (with the RL Zoo)
```
python -m rl_zoo3.train --algo dqn --env SpaceInvadersNoFrameskip-v4 -f logs/
# Upload the model and generate video (when possible)
python -m rl_zoo3.push_to_hub --algo dqn --env SpaceInvadersNoFrameskip-v4 -f logs/ -orga chirbard
```
## Hyperparameters
```python
OrderedDict([('batch_size', 32),
('buffer_size', 100000),
('env_wrapper',
['stable_baselines3.common.atari_wrappers.AtariWrapper']),
('exploration_final_eps', 0.01),
('exploration_fraction', 0.1),
('frame_stack', 4),
('gradient_steps', 1),
('learning_rate', 0.0001),
('learning_starts', 100000),
('n_timesteps', 1000000.0),
('optimize_memory_usage', False),
('policy', 'CnnPolicy'),
('target_update_interval', 1000),
('train_freq', 4),
('normalize', False)])
```
# Environment Arguments
```python
{'render_mode': 'rgb_array'}
```
| {"library_name": "stable-baselines3", "tags": ["SpaceInvadersNoFrameskip-v4", "deep-reinforcement-learning", "reinforcement-learning", "stable-baselines3"], "model-index": [{"name": "DQN", "results": [{"task": {"type": "reinforcement-learning", "name": "reinforcement-learning"}, "dataset": {"name": "SpaceInvadersNoFrameskip-v4", "type": "SpaceInvadersNoFrameskip-v4"}, "metrics": [{"type": "mean_reward", "value": "512.50 +/- 269.25", "name": "mean_reward", "verified": false}]}]}]} | chirbard/dqn-SpaceInvadersNoFrameskip-v4 | null | [
"stable-baselines3",
"SpaceInvadersNoFrameskip-v4",
"deep-reinforcement-learning",
"reinforcement-learning",
"model-index",
"region:us"
] | null | 2024-04-18T08:30:43+00:00 | [] | [] | TAGS
#stable-baselines3 #SpaceInvadersNoFrameskip-v4 #deep-reinforcement-learning #reinforcement-learning #model-index #region-us
|
# DQN Agent playing SpaceInvadersNoFrameskip-v4
This is a trained model of a DQN agent playing SpaceInvadersNoFrameskip-v4
using the stable-baselines3 library
and the RL Zoo.
The RL Zoo is a training framework for Stable Baselines3
reinforcement learning agents,
with hyperparameter optimization and pre-trained agents included.
## Usage (with SB3 RL Zoo)
RL Zoo: URL
SB3: URL
SB3 Contrib: URL
Install the RL Zoo (with SB3 and SB3-Contrib):
If you installed the RL Zoo3 via pip ('pip install rl_zoo3'), from anywhere you can do:
## Training (with the RL Zoo)
## Hyperparameters
# Environment Arguments
| [
"# DQN Agent playing SpaceInvadersNoFrameskip-v4\nThis is a trained model of a DQN agent playing SpaceInvadersNoFrameskip-v4\nusing the stable-baselines3 library\nand the RL Zoo.\n\nThe RL Zoo is a training framework for Stable Baselines3\nreinforcement learning agents,\nwith hyperparameter optimization and pre-trained agents included.",
"## Usage (with SB3 RL Zoo)\n\nRL Zoo: URL\nSB3: URL\nSB3 Contrib: URL\n\nInstall the RL Zoo (with SB3 and SB3-Contrib):\n\n\n\n\nIf you installed the RL Zoo3 via pip ('pip install rl_zoo3'), from anywhere you can do:",
"## Training (with the RL Zoo)",
"## Hyperparameters",
"# Environment Arguments"
] | [
"TAGS\n#stable-baselines3 #SpaceInvadersNoFrameskip-v4 #deep-reinforcement-learning #reinforcement-learning #model-index #region-us \n",
"# DQN Agent playing SpaceInvadersNoFrameskip-v4\nThis is a trained model of a DQN agent playing SpaceInvadersNoFrameskip-v4\nusing the stable-baselines3 library\nand the RL Zoo.\n\nThe RL Zoo is a training framework for Stable Baselines3\nreinforcement learning agents,\nwith hyperparameter optimization and pre-trained agents included.",
"## Usage (with SB3 RL Zoo)\n\nRL Zoo: URL\nSB3: URL\nSB3 Contrib: URL\n\nInstall the RL Zoo (with SB3 and SB3-Contrib):\n\n\n\n\nIf you installed the RL Zoo3 via pip ('pip install rl_zoo3'), from anywhere you can do:",
"## Training (with the RL Zoo)",
"## Hyperparameters",
"# Environment Arguments"
] |
text-generation | transformers |
# Model Card for Model ID
<!-- Provide a quick summary of what the model is/does. -->
This modelcard aims to be a base template for new models. It has been generated using [this raw template](https://github.com/huggingface/huggingface_hub/blob/main/src/huggingface_hub/templates/modelcard_template.md?plain=1).
## Model Details
### Model Description
<!-- Provide a longer summary of what this model is. -->
- **Developed by:** [More Information Needed]
- **Funded by [optional]:** [More Information Needed]
- **Shared by [optional]:** [More Information Needed]
- **Model type:** [More Information Needed]
- **Language(s) (NLP):** [More Information Needed]
- **License:** [More Information Needed]
- **Finetuned from model [optional]:** [More Information Needed]
### Model Sources [optional]
<!-- Provide the basic links for the model. -->
- **Repository:** [More Information Needed]
- **Paper [optional]:** [More Information Needed]
- **Demo [optional]:** [More Information Needed]
## Uses
<!-- Address questions around how the model is intended to be used, including the foreseeable users of the model and those affected by the model. -->
### Direct Use
<!-- This section is for the model use without fine-tuning or plugging into a larger ecosystem/app. -->
[More Information Needed]
### Downstream Use [optional]
<!-- This section is for the model use when fine-tuned for a task, or when plugged into a larger ecosystem/app -->
[More Information Needed]
### Out-of-Scope Use
<!-- This section addresses misuse, malicious use, and uses that the model will not work well for. -->
[More Information Needed]
## Bias, Risks, and Limitations
<!-- This section is meant to convey both technical and sociotechnical limitations. -->
[More Information Needed]
### Recommendations
<!-- This section is meant to convey recommendations with respect to the bias, risk, and technical limitations. -->
Users (both direct and downstream) should be made aware of the risks, biases and limitations of the model. More information needed for further recommendations.
## How to Get Started with the Model
Use the code below to get started with the model.
[More Information Needed]
## Training Details
### Training Data
<!-- This should link to a Dataset Card, perhaps with a short stub of information on what the training data is all about as well as documentation related to data pre-processing or additional filtering. -->
[More Information Needed]
### Training Procedure
<!-- This relates heavily to the Technical Specifications. Content here should link to that section when it is relevant to the training procedure. -->
#### Preprocessing [optional]
[More Information Needed]
#### Training Hyperparameters
- **Training regime:** [More Information Needed] <!--fp32, fp16 mixed precision, bf16 mixed precision, bf16 non-mixed precision, fp16 non-mixed precision, fp8 mixed precision -->
#### Speeds, Sizes, Times [optional]
<!-- This section provides information about throughput, start/end time, checkpoint size if relevant, etc. -->
[More Information Needed]
## Evaluation
<!-- This section describes the evaluation protocols and provides the results. -->
### Testing Data, Factors & Metrics
#### Testing Data
<!-- This should link to a Dataset Card if possible. -->
[More Information Needed]
#### Factors
<!-- These are the things the evaluation is disaggregating by, e.g., subpopulations or domains. -->
[More Information Needed]
#### Metrics
<!-- These are the evaluation metrics being used, ideally with a description of why. -->
[More Information Needed]
### Results
[More Information Needed]
#### Summary
## Model Examination [optional]
<!-- Relevant interpretability work for the model goes here -->
[More Information Needed]
## Environmental Impact
<!-- Total emissions (in grams of CO2eq) and additional considerations, such as electricity usage, go here. Edit the suggested text below accordingly -->
Carbon emissions can be estimated using the [Machine Learning Impact calculator](https://mlco2.github.io/impact#compute) presented in [Lacoste et al. (2019)](https://arxiv.org/abs/1910.09700).
- **Hardware Type:** [More Information Needed]
- **Hours used:** [More Information Needed]
- **Cloud Provider:** [More Information Needed]
- **Compute Region:** [More Information Needed]
- **Carbon Emitted:** [More Information Needed]
## Technical Specifications [optional]
### Model Architecture and Objective
[More Information Needed]
### Compute Infrastructure
[More Information Needed]
#### Hardware
[More Information Needed]
#### Software
[More Information Needed]
## Citation [optional]
<!-- If there is a paper or blog post introducing the model, the APA and Bibtex information for that should go in this section. -->
**BibTeX:**
[More Information Needed]
**APA:**
[More Information Needed]
## Glossary [optional]
<!-- If relevant, include terms and calculations in this section that can help readers understand the model or model card. -->
[More Information Needed]
## More Information [optional]
[More Information Needed]
## Model Card Authors [optional]
[More Information Needed]
## Model Card Contact
[More Information Needed] | {"license": "apache-2.0"} | kunkun666/kunkun_dat_llama-13b | null | [
"transformers",
"llama",
"text-generation",
"arxiv:1910.09700",
"license:apache-2.0",
"autotrain_compatible",
"endpoints_compatible",
"text-generation-inference",
"region:us"
] | null | 2024-04-18T08:32:55+00:00 | [
"1910.09700"
] | [] | TAGS
#transformers #llama #text-generation #arxiv-1910.09700 #license-apache-2.0 #autotrain_compatible #endpoints_compatible #text-generation-inference #region-us
|
# Model Card for Model ID
This modelcard aims to be a base template for new models. It has been generated using this raw template.
## Model Details
### Model Description
- Developed by:
- Funded by [optional]:
- Shared by [optional]:
- Model type:
- Language(s) (NLP):
- License:
- Finetuned from model [optional]:
### Model Sources [optional]
- Repository:
- Paper [optional]:
- Demo [optional]:
## Uses
### Direct Use
### Downstream Use [optional]
### Out-of-Scope Use
## Bias, Risks, and Limitations
### Recommendations
Users (both direct and downstream) should be made aware of the risks, biases and limitations of the model. More information needed for further recommendations.
## How to Get Started with the Model
Use the code below to get started with the model.
## Training Details
### Training Data
### Training Procedure
#### Preprocessing [optional]
#### Training Hyperparameters
- Training regime:
#### Speeds, Sizes, Times [optional]
## Evaluation
### Testing Data, Factors & Metrics
#### Testing Data
#### Factors
#### Metrics
### Results
#### Summary
## Model Examination [optional]
## Environmental Impact
Carbon emissions can be estimated using the Machine Learning Impact calculator presented in Lacoste et al. (2019).
- Hardware Type:
- Hours used:
- Cloud Provider:
- Compute Region:
- Carbon Emitted:
## Technical Specifications [optional]
### Model Architecture and Objective
### Compute Infrastructure
#### Hardware
#### Software
[optional]
BibTeX:
APA:
## Glossary [optional]
## More Information [optional]
## Model Card Authors [optional]
## Model Card Contact
| [
"# Model Card for Model ID\n\n\n\nThis modelcard aims to be a base template for new models. It has been generated using this raw template.",
"## Model Details",
"### Model Description\n\n\n\n\n\n- Developed by: \n- Funded by [optional]: \n- Shared by [optional]: \n- Model type: \n- Language(s) (NLP): \n- License: \n- Finetuned from model [optional]:",
"### Model Sources [optional]\n\n\n\n- Repository: \n- Paper [optional]: \n- Demo [optional]:",
"## Uses",
"### Direct Use",
"### Downstream Use [optional]",
"### Out-of-Scope Use",
"## Bias, Risks, and Limitations",
"### Recommendations\n\n\n\nUsers (both direct and downstream) should be made aware of the risks, biases and limitations of the model. More information needed for further recommendations.",
"## How to Get Started with the Model\n\nUse the code below to get started with the model.",
"## Training Details",
"### Training Data",
"### Training Procedure",
"#### Preprocessing [optional]",
"#### Training Hyperparameters\n\n- Training regime:",
"#### Speeds, Sizes, Times [optional]",
"## Evaluation",
"### Testing Data, Factors & Metrics",
"#### Testing Data",
"#### Factors",
"#### Metrics",
"### Results",
"#### Summary",
"## Model Examination [optional]",
"## Environmental Impact\n\n\n\nCarbon emissions can be estimated using the Machine Learning Impact calculator presented in Lacoste et al. (2019).\n\n- Hardware Type: \n- Hours used: \n- Cloud Provider: \n- Compute Region: \n- Carbon Emitted:",
"## Technical Specifications [optional]",
"### Model Architecture and Objective",
"### Compute Infrastructure",
"#### Hardware",
"#### Software\n\n\n\n[optional]\n\n\n\nBibTeX:\n\n\n\nAPA:",
"## Glossary [optional]",
"## More Information [optional]",
"## Model Card Authors [optional]",
"## Model Card Contact"
] | [
"TAGS\n#transformers #llama #text-generation #arxiv-1910.09700 #license-apache-2.0 #autotrain_compatible #endpoints_compatible #text-generation-inference #region-us \n",
"# Model Card for Model ID\n\n\n\nThis modelcard aims to be a base template for new models. It has been generated using this raw template.",
"## Model Details",
"### Model Description\n\n\n\n\n\n- Developed by: \n- Funded by [optional]: \n- Shared by [optional]: \n- Model type: \n- Language(s) (NLP): \n- License: \n- Finetuned from model [optional]:",
"### Model Sources [optional]\n\n\n\n- Repository: \n- Paper [optional]: \n- Demo [optional]:",
"## Uses",
"### Direct Use",
"### Downstream Use [optional]",
"### Out-of-Scope Use",
"## Bias, Risks, and Limitations",
"### Recommendations\n\n\n\nUsers (both direct and downstream) should be made aware of the risks, biases and limitations of the model. More information needed for further recommendations.",
"## How to Get Started with the Model\n\nUse the code below to get started with the model.",
"## Training Details",
"### Training Data",
"### Training Procedure",
"#### Preprocessing [optional]",
"#### Training Hyperparameters\n\n- Training regime:",
"#### Speeds, Sizes, Times [optional]",
"## Evaluation",
"### Testing Data, Factors & Metrics",
"#### Testing Data",
"#### Factors",
"#### Metrics",
"### Results",
"#### Summary",
"## Model Examination [optional]",
"## Environmental Impact\n\n\n\nCarbon emissions can be estimated using the Machine Learning Impact calculator presented in Lacoste et al. (2019).\n\n- Hardware Type: \n- Hours used: \n- Cloud Provider: \n- Compute Region: \n- Carbon Emitted:",
"## Technical Specifications [optional]",
"### Model Architecture and Objective",
"### Compute Infrastructure",
"#### Hardware",
"#### Software\n\n\n\n[optional]\n\n\n\nBibTeX:\n\n\n\nAPA:",
"## Glossary [optional]",
"## More Information [optional]",
"## Model Card Authors [optional]",
"## Model Card Contact"
] |
object-detection | keras |
## Model description
More information needed
## Intended uses & limitations
More information needed
## Training and evaluation data
More information needed
## Training procedure
### Training hyperparameters
The following hyperparameters were used during training:
| Hyperparameters | Value |
| :-- | :-- |
| name | Adam |
| weight_decay | None |
| clipnorm | None |
| global_clipnorm | None |
| clipvalue | None |
| use_ema | False |
| ema_momentum | 0.99 |
| ema_overwrite_frequency | None |
| jit_compile | True |
| is_legacy_optimizer | False |
| learning_rate | 0.0010000000474974513 |
| beta_1 | 0.9 |
| beta_2 | 0.999 |
| epsilon | 1e-07 |
| amsgrad | False |
| training_precision | float32 |
## Model Plot
<details>
<summary>View Model Plot</summary>

</details> | {"library_name": "keras", "tags": ["object-detection", "denoising_model"]} | Putul1519/Denoising | null | [
"keras",
"object-detection",
"denoising_model",
"region:us"
] | null | 2024-04-18T08:33:48+00:00 | [] | [] | TAGS
#keras #object-detection #denoising_model #region-us
| Model description
-----------------
More information needed
Intended uses & limitations
---------------------------
More information needed
Training and evaluation data
----------------------------
More information needed
Training procedure
------------------
### Training hyperparameters
The following hyperparameters were used during training:
Model Plot
----------
View Model Plot
!Model Image
| [
"### Training hyperparameters\n\n\nThe following hyperparameters were used during training:\n\n\n\nModel Plot\n----------\n\n\n\nView Model Plot\n!Model Image"
] | [
"TAGS\n#keras #object-detection #denoising_model #region-us \n",
"### Training hyperparameters\n\n\nThe following hyperparameters were used during training:\n\n\n\nModel Plot\n----------\n\n\n\nView Model Plot\n!Model Image"
] |
null | peft |
<!-- This model card has been generated automatically according to the information the Trainer had access to. You
should probably proofread and complete it, then remove this comment. -->
# whisper-medium-finetuned
This model is a fine-tuned version of [openai/whisper-medium](https://huggingface.co/openai/whisper-medium) on the common_voice_16_1 dataset.
It achieves the following results on the evaluation set:
- eval_loss: 1.3771
- eval_wer: 46.6887
- eval_runtime: 35.0021
- eval_samples_per_second: 0.829
- eval_steps_per_second: 0.029
- epoch: 99.01
- step: 100
## Model description
More information needed
## Intended uses & limitations
More information needed
## Training and evaluation data
More information needed
## Training procedure
### Training hyperparameters
The following hyperparameters were used during training:
- learning_rate: 0.001
- train_batch_size: 96
- eval_batch_size: 64
- seed: 42
- optimizer: Adam with betas=(0.9,0.999) and epsilon=1e-08
- lr_scheduler_type: linear
- training_steps: 100
- mixed_precision_training: Native AMP
### Framework versions
- PEFT 0.9.0
- Transformers 4.39.2
- Pytorch 2.2.1+cu121
- Datasets 2.17.0
- Tokenizers 0.15.2 | {"license": "apache-2.0", "library_name": "peft", "tags": ["generated_from_trainer"], "datasets": ["common_voice_16_1"], "base_model": "openai/whisper-medium", "model-index": [{"name": "whisper-medium-finetuned", "results": []}]} | KevinKibe/whisper-medium-finetuned | null | [
"peft",
"pytorch",
"safetensors",
"generated_from_trainer",
"dataset:common_voice_16_1",
"base_model:openai/whisper-medium",
"license:apache-2.0",
"region:us"
] | null | 2024-04-18T08:34:01+00:00 | [] | [] | TAGS
#peft #pytorch #safetensors #generated_from_trainer #dataset-common_voice_16_1 #base_model-openai/whisper-medium #license-apache-2.0 #region-us
|
# whisper-medium-finetuned
This model is a fine-tuned version of openai/whisper-medium on the common_voice_16_1 dataset.
It achieves the following results on the evaluation set:
- eval_loss: 1.3771
- eval_wer: 46.6887
- eval_runtime: 35.0021
- eval_samples_per_second: 0.829
- eval_steps_per_second: 0.029
- epoch: 99.01
- step: 100
## Model description
More information needed
## Intended uses & limitations
More information needed
## Training and evaluation data
More information needed
## Training procedure
### Training hyperparameters
The following hyperparameters were used during training:
- learning_rate: 0.001
- train_batch_size: 96
- eval_batch_size: 64
- seed: 42
- optimizer: Adam with betas=(0.9,0.999) and epsilon=1e-08
- lr_scheduler_type: linear
- training_steps: 100
- mixed_precision_training: Native AMP
### Framework versions
- PEFT 0.9.0
- Transformers 4.39.2
- Pytorch 2.2.1+cu121
- Datasets 2.17.0
- Tokenizers 0.15.2 | [
"# whisper-medium-finetuned\n\nThis model is a fine-tuned version of openai/whisper-medium on the common_voice_16_1 dataset.\nIt achieves the following results on the evaluation set:\n- eval_loss: 1.3771\n- eval_wer: 46.6887\n- eval_runtime: 35.0021\n- eval_samples_per_second: 0.829\n- eval_steps_per_second: 0.029\n- epoch: 99.01\n- step: 100",
"## Model description\n\nMore information needed",
"## Intended uses & limitations\n\nMore information needed",
"## Training and evaluation data\n\nMore information needed",
"## Training procedure",
"### Training hyperparameters\n\nThe following hyperparameters were used during training:\n- learning_rate: 0.001\n- train_batch_size: 96\n- eval_batch_size: 64\n- seed: 42\n- optimizer: Adam with betas=(0.9,0.999) and epsilon=1e-08\n- lr_scheduler_type: linear\n- training_steps: 100\n- mixed_precision_training: Native AMP",
"### Framework versions\n\n- PEFT 0.9.0\n- Transformers 4.39.2\n- Pytorch 2.2.1+cu121\n- Datasets 2.17.0\n- Tokenizers 0.15.2"
] | [
"TAGS\n#peft #pytorch #safetensors #generated_from_trainer #dataset-common_voice_16_1 #base_model-openai/whisper-medium #license-apache-2.0 #region-us \n",
"# whisper-medium-finetuned\n\nThis model is a fine-tuned version of openai/whisper-medium on the common_voice_16_1 dataset.\nIt achieves the following results on the evaluation set:\n- eval_loss: 1.3771\n- eval_wer: 46.6887\n- eval_runtime: 35.0021\n- eval_samples_per_second: 0.829\n- eval_steps_per_second: 0.029\n- epoch: 99.01\n- step: 100",
"## Model description\n\nMore information needed",
"## Intended uses & limitations\n\nMore information needed",
"## Training and evaluation data\n\nMore information needed",
"## Training procedure",
"### Training hyperparameters\n\nThe following hyperparameters were used during training:\n- learning_rate: 0.001\n- train_batch_size: 96\n- eval_batch_size: 64\n- seed: 42\n- optimizer: Adam with betas=(0.9,0.999) and epsilon=1e-08\n- lr_scheduler_type: linear\n- training_steps: 100\n- mixed_precision_training: Native AMP",
"### Framework versions\n\n- PEFT 0.9.0\n- Transformers 4.39.2\n- Pytorch 2.2.1+cu121\n- Datasets 2.17.0\n- Tokenizers 0.15.2"
] |
null | null | Kalau butuh langsung download ya takutnya kena nuke
<div id="container">
<section id="desc">
<h2>atau kalau tidak silahkan download sendiri di web officialnya</h2>
<hr>
<p>let's live healthy together</p>
</section>
<div>
<img id="animated-gif" src="https://cdn.discordapp.com/attachments/746059073073578064/1230444570014384219/kokoro-bandori.gif?ex=663357ce&is=6620e2ce&hm=75dbcc4b8bf09e7c9b3ac576e1b4622f6b0d5d64c4819d5666ad982e359abf7b&"
alt="https://tenor.com/bZInJ.gif"/>
</div>
</div> | {"license": "mpl-2.0"} | Habuki/Microsoft-365 | null | [
"license:mpl-2.0",
"region:us"
] | null | 2024-04-18T08:35:46+00:00 | [] | [] | TAGS
#license-mpl-2.0 #region-us
| Kalau butuh langsung download ya takutnya kena nuke
<div id="container">
<section id="desc">
<h2>atau kalau tidak silahkan download sendiri di web officialnya</h2>
<hr>
<p>let's live healthy together</p>
</section>
<div>
<img id="animated-gif" src="URL
alt="URL
</div>
</div> | [] | [
"TAGS\n#license-mpl-2.0 #region-us \n"
] |
question-answering | transformers |
<!-- This model card has been generated automatically according to the information the Trainer had access to. You
should probably proofread and complete it, then remove this comment. -->
# bert-finetuned-squad
This model is a fine-tuned version of [bert-base-cased](https://huggingface.co/bert-base-cased) on an unknown dataset.
## Model description
More information needed
## Intended uses & limitations
More information needed
## Training and evaluation data
More information needed
## Training procedure
### Training hyperparameters
The following hyperparameters were used during training:
- learning_rate: 2e-05
- train_batch_size: 40
- eval_batch_size: 40
- seed: 42
- optimizer: Adam with betas=(0.9,0.999) and epsilon=1e-08
- lr_scheduler_type: linear
- num_epochs: 30
- mixed_precision_training: Native AMP
### Training results
### Framework versions
- Transformers 4.37.2
- Pytorch 2.0.1+cu117
- Datasets 2.17.0
- Tokenizers 0.15.2
| {"license": "apache-2.0", "tags": ["generated_from_trainer"], "base_model": "bert-base-cased", "model-index": [{"name": "bert-finetuned-squad", "results": []}]} | dokyoungkim/bert-finetuned-squad | null | [
"transformers",
"tensorboard",
"safetensors",
"bert",
"question-answering",
"generated_from_trainer",
"base_model:bert-base-cased",
"license:apache-2.0",
"endpoints_compatible",
"region:us"
] | null | 2024-04-18T08:35:54+00:00 | [] | [] | TAGS
#transformers #tensorboard #safetensors #bert #question-answering #generated_from_trainer #base_model-bert-base-cased #license-apache-2.0 #endpoints_compatible #region-us
|
# bert-finetuned-squad
This model is a fine-tuned version of bert-base-cased on an unknown dataset.
## Model description
More information needed
## Intended uses & limitations
More information needed
## Training and evaluation data
More information needed
## Training procedure
### Training hyperparameters
The following hyperparameters were used during training:
- learning_rate: 2e-05
- train_batch_size: 40
- eval_batch_size: 40
- seed: 42
- optimizer: Adam with betas=(0.9,0.999) and epsilon=1e-08
- lr_scheduler_type: linear
- num_epochs: 30
- mixed_precision_training: Native AMP
### Training results
### Framework versions
- Transformers 4.37.2
- Pytorch 2.0.1+cu117
- Datasets 2.17.0
- Tokenizers 0.15.2
| [
"# bert-finetuned-squad\n\nThis model is a fine-tuned version of bert-base-cased on an unknown dataset.",
"## Model description\n\nMore information needed",
"## Intended uses & limitations\n\nMore information needed",
"## Training and evaluation data\n\nMore information needed",
"## Training procedure",
"### Training hyperparameters\n\nThe following hyperparameters were used during training:\n- learning_rate: 2e-05\n- train_batch_size: 40\n- eval_batch_size: 40\n- seed: 42\n- optimizer: Adam with betas=(0.9,0.999) and epsilon=1e-08\n- lr_scheduler_type: linear\n- num_epochs: 30\n- mixed_precision_training: Native AMP",
"### Training results",
"### Framework versions\n\n- Transformers 4.37.2\n- Pytorch 2.0.1+cu117\n- Datasets 2.17.0\n- Tokenizers 0.15.2"
] | [
"TAGS\n#transformers #tensorboard #safetensors #bert #question-answering #generated_from_trainer #base_model-bert-base-cased #license-apache-2.0 #endpoints_compatible #region-us \n",
"# bert-finetuned-squad\n\nThis model is a fine-tuned version of bert-base-cased on an unknown dataset.",
"## Model description\n\nMore information needed",
"## Intended uses & limitations\n\nMore information needed",
"## Training and evaluation data\n\nMore information needed",
"## Training procedure",
"### Training hyperparameters\n\nThe following hyperparameters were used during training:\n- learning_rate: 2e-05\n- train_batch_size: 40\n- eval_batch_size: 40\n- seed: 42\n- optimizer: Adam with betas=(0.9,0.999) and epsilon=1e-08\n- lr_scheduler_type: linear\n- num_epochs: 30\n- mixed_precision_training: Native AMP",
"### Training results",
"### Framework versions\n\n- Transformers 4.37.2\n- Pytorch 2.0.1+cu117\n- Datasets 2.17.0\n- Tokenizers 0.15.2"
] |
null | null | GGUF-IQ-Imatrix quants for an #experimental model.

> [!WARNING]
> Read about the original model here: <br>
> [[grimjim/fireblossom-32K-7B]](https://huggingface.co/grimjim/fireblossom-32K-7B) | {"license": "cc-by-4.0", "tags": ["gguf", "roleplay", "experimental"]} | Lewdiculous/fireblossom-32K-7B-GGUF-IQ-Imatrix | null | [
"gguf",
"roleplay",
"experimental",
"license:cc-by-4.0",
"region:us"
] | null | 2024-04-18T08:37:25+00:00 | [] | [] | TAGS
#gguf #roleplay #experimental #license-cc-by-4.0 #region-us
| GGUF-IQ-Imatrix quants for an #experimental model.
!model-waifu
> [!WARNING]
> Read about the original model here: <br>
> [[grimjim/fireblossom-32K-7B]](URL | [] | [
"TAGS\n#gguf #roleplay #experimental #license-cc-by-4.0 #region-us \n"
] |
text-generation | transformers | # ConvexAI/Solutus-3x7B AWQ
- Model creator: [ConvexAI](https://huggingface.co/ConvexAI)
- Original model: [Solutus-3x7B](https://huggingface.co/ConvexAI/Solutus-3x7B)

## Model Summary
A model to test how MoE will route without square expansion.
The config looks like this...(detailed version is in the files and versions):
- [cognitivecomputations/WestLake-7B-v2-laser](https://huggingface.co/cognitivecomputations/WestLake-7B-v2-laser) - base
- [mlabonne/Daredevil-7B](https://huggingface.co/mlabonne/Daredevil-7B) - expert #1
- [cognitivecomputations/WestLake-7B-v2-laser](https://huggingface.co/cognitivecomputations/WestLake-7B-v2-laser) - expert #2
- [mlabonne/Daredevil-7B](https://huggingface.co/mlabonne/Daredevil-7B) - expert #3
| {"language": ["en"], "license": "apache-2.0", "library_name": "transformers", "tags": ["moe", "merge", "4-bit", "AWQ", "text-generation", "autotrain_compatible", "endpoints_compatible"], "pipeline_tag": "text-generation", "inference": false, "quantized_by": "Suparious", "model-index": [{"name": "Solutus-3x7B", "results": [{"task": {"type": "text-generation", "name": "Text Generation"}, "dataset": {"name": "AI2 Reasoning Challenge (25-Shot)", "type": "ai2_arc", "config": "ARC-Challenge", "split": "test", "args": {"num_few_shot": 25}}, "metrics": [{"type": "acc_norm", "value": 72.01, "name": "normalized accuracy"}], "source": {"url": "https://huggingface.co/spaces/HuggingFaceH4/open_llm_leaderboard?query=ConvexAI/Solutus-3x7B", "name": "Open LLM Leaderboard"}}, {"task": {"type": "text-generation", "name": "Text Generation"}, "dataset": {"name": "HellaSwag (10-Shot)", "type": "hellaswag", "split": "validation", "args": {"num_few_shot": 10}}, "metrics": [{"type": "acc_norm", "value": 88.31, "name": "normalized accuracy"}], "source": {"url": "https://huggingface.co/spaces/HuggingFaceH4/open_llm_leaderboard?query=ConvexAI/Solutus-3x7B", "name": "Open LLM Leaderboard"}}, {"task": {"type": "text-generation", "name": "Text Generation"}, "dataset": {"name": "MMLU (5-Shot)", "type": "cais/mmlu", "config": "all", "split": "test", "args": {"num_few_shot": 5}}, "metrics": [{"type": "acc", "value": 64.77, "name": "accuracy"}], "source": {"url": "https://huggingface.co/spaces/HuggingFaceH4/open_llm_leaderboard?query=ConvexAI/Solutus-3x7B", "name": "Open LLM Leaderboard"}}, {"task": {"type": "text-generation", "name": "Text Generation"}, "dataset": {"name": "TruthfulQA (0-shot)", "type": "truthful_qa", "config": "multiple_choice", "split": "validation", "args": {"num_few_shot": 0}}, "metrics": [{"type": "mc2", "value": 67.52}], "source": {"url": "https://huggingface.co/spaces/HuggingFaceH4/open_llm_leaderboard?query=ConvexAI/Solutus-3x7B", "name": "Open LLM Leaderboard"}}, {"task": {"type": "text-generation", "name": "Text Generation"}, "dataset": {"name": "Winogrande (5-shot)", "type": "winogrande", "config": "winogrande_xl", "split": "validation", "args": {"num_few_shot": 5}}, "metrics": [{"type": "acc", "value": 83.66, "name": "accuracy"}], "source": {"url": "https://huggingface.co/spaces/HuggingFaceH4/open_llm_leaderboard?query=ConvexAI/Solutus-3x7B", "name": "Open LLM Leaderboard"}}, {"task": {"type": "text-generation", "name": "Text Generation"}, "dataset": {"name": "GSM8k (5-shot)", "type": "gsm8k", "config": "main", "split": "test", "args": {"num_few_shot": 5}}, "metrics": [{"type": "acc", "value": 69.83, "name": "accuracy"}], "source": {"url": "https://huggingface.co/spaces/HuggingFaceH4/open_llm_leaderboard?query=ConvexAI/Solutus-3x7B", "name": "Open LLM Leaderboard"}}]}]} | solidrust/Solutus-3x7B-AWQ | null | [
"transformers",
"safetensors",
"mixtral",
"text-generation",
"moe",
"merge",
"4-bit",
"AWQ",
"autotrain_compatible",
"endpoints_compatible",
"en",
"license:apache-2.0",
"model-index",
"text-generation-inference",
"region:us"
] | null | 2024-04-18T08:39:01+00:00 | [] | [
"en"
] | TAGS
#transformers #safetensors #mixtral #text-generation #moe #merge #4-bit #AWQ #autotrain_compatible #endpoints_compatible #en #license-apache-2.0 #model-index #text-generation-inference #region-us
| # ConvexAI/Solutus-3x7B AWQ
- Model creator: ConvexAI
- Original model: Solutus-3x7B
!image/png
## Model Summary
A model to test how MoE will route without square expansion.
The config looks like this...(detailed version is in the files and versions):
- cognitivecomputations/WestLake-7B-v2-laser - base
- mlabonne/Daredevil-7B - expert #1
- cognitivecomputations/WestLake-7B-v2-laser - expert #2
- mlabonne/Daredevil-7B - expert #3
| [
"# ConvexAI/Solutus-3x7B AWQ\n\n- Model creator: ConvexAI\n- Original model: Solutus-3x7B\n\n!image/png",
"## Model Summary\n\nA model to test how MoE will route without square expansion.\n\nThe config looks like this...(detailed version is in the files and versions):\n- cognitivecomputations/WestLake-7B-v2-laser - base\n- mlabonne/Daredevil-7B - expert #1\n- cognitivecomputations/WestLake-7B-v2-laser - expert #2\n- mlabonne/Daredevil-7B - expert #3"
] | [
"TAGS\n#transformers #safetensors #mixtral #text-generation #moe #merge #4-bit #AWQ #autotrain_compatible #endpoints_compatible #en #license-apache-2.0 #model-index #text-generation-inference #region-us \n",
"# ConvexAI/Solutus-3x7B AWQ\n\n- Model creator: ConvexAI\n- Original model: Solutus-3x7B\n\n!image/png",
"## Model Summary\n\nA model to test how MoE will route without square expansion.\n\nThe config looks like this...(detailed version is in the files and versions):\n- cognitivecomputations/WestLake-7B-v2-laser - base\n- mlabonne/Daredevil-7B - expert #1\n- cognitivecomputations/WestLake-7B-v2-laser - expert #2\n- mlabonne/Daredevil-7B - expert #3"
] |
text-to-image | null | ## Model
 | {"tags": ["stable-diffusion", "text-to-image", "StableDiffusionPipeline", "lora"]} | fearvel/lloyd-de-saloum-sd-v1 | null | [
"stable-diffusion",
"text-to-image",
"StableDiffusionPipeline",
"lora",
"region:us"
] | null | 2024-04-18T08:39:31+00:00 | [] | [] | TAGS
#stable-diffusion #text-to-image #StableDiffusionPipeline #lora #region-us
| ## Model
!pipeline | [
"## Model\n\n!pipeline"
] | [
"TAGS\n#stable-diffusion #text-to-image #StableDiffusionPipeline #lora #region-us \n",
"## Model\n\n!pipeline"
] |
automatic-speech-recognition | transformers |
<!-- This model card has been generated automatically according to the information the Trainer had access to. You
should probably proofread and complete it, then remove this comment. -->
# Whisper da-nst
This model is a fine-tuned version of [openai/whisper-medium](https://huggingface.co/openai/whisper-medium) on the common_voice_14_0 dataset.
It achieves the following results on the evaluation set:
- Loss: 0.7234
- Wer: 35.3094
## Model description
More information needed
## Intended uses & limitations
More information needed
## Training and evaluation data
More information needed
## Training procedure
### Training hyperparameters
The following hyperparameters were used during training:
- learning_rate: 1e-05
- train_batch_size: 8
- eval_batch_size: 8
- seed: 42
- gradient_accumulation_steps: 2
- total_train_batch_size: 16
- optimizer: Adam with betas=(0.9,0.999) and epsilon=1e-08
- lr_scheduler_type: linear
- lr_scheduler_warmup_steps: 500
- training_steps: 5000
- mixed_precision_training: Native AMP
### Training results
| Training Loss | Epoch | Step | Validation Loss | Wer |
|:-------------:|:-----:|:----:|:---------------:|:-------:|
| 0.0133 | 4.04 | 1000 | 0.6362 | 48.9279 |
| 0.0025 | 9.04 | 2000 | 0.6635 | 37.4731 |
| 0.0001 | 14.03 | 3000 | 0.6959 | 34.1296 |
| 0.0001 | 19.03 | 4000 | 0.7166 | 35.1821 |
| 0.0 | 24.03 | 5000 | 0.7234 | 35.3094 |
### Framework versions
- Transformers 4.37.2
- Pytorch 2.2.0+cu121
- Datasets 2.18.0
- Tokenizers 0.15.1
| {"license": "apache-2.0", "tags": ["whisper-event", "generated_from_trainer"], "datasets": ["common_voice_14_0"], "metrics": ["wer"], "base_model": "openai/whisper-medium", "model-index": [{"name": "Whisper da-nst", "results": [{"task": {"type": "automatic-speech-recognition", "name": "Automatic Speech Recognition"}, "dataset": {"name": "common_voice_14_0", "type": "common_voice_14_0", "config": "da", "split": "test", "args": "da"}, "metrics": [{"type": "wer", "value": 35.3093792833366, "name": "Wer"}]}]}]} | nicolarsen/whisper-medium-2-F | null | [
"transformers",
"tensorboard",
"safetensors",
"whisper",
"automatic-speech-recognition",
"whisper-event",
"generated_from_trainer",
"dataset:common_voice_14_0",
"base_model:openai/whisper-medium",
"license:apache-2.0",
"model-index",
"endpoints_compatible",
"region:us"
] | null | 2024-04-18T08:39:38+00:00 | [] | [] | TAGS
#transformers #tensorboard #safetensors #whisper #automatic-speech-recognition #whisper-event #generated_from_trainer #dataset-common_voice_14_0 #base_model-openai/whisper-medium #license-apache-2.0 #model-index #endpoints_compatible #region-us
| Whisper da-nst
==============
This model is a fine-tuned version of openai/whisper-medium on the common\_voice\_14\_0 dataset.
It achieves the following results on the evaluation set:
* Loss: 0.7234
* Wer: 35.3094
Model description
-----------------
More information needed
Intended uses & limitations
---------------------------
More information needed
Training and evaluation data
----------------------------
More information needed
Training procedure
------------------
### Training hyperparameters
The following hyperparameters were used during training:
* learning\_rate: 1e-05
* train\_batch\_size: 8
* eval\_batch\_size: 8
* seed: 42
* gradient\_accumulation\_steps: 2
* total\_train\_batch\_size: 16
* optimizer: Adam with betas=(0.9,0.999) and epsilon=1e-08
* lr\_scheduler\_type: linear
* lr\_scheduler\_warmup\_steps: 500
* training\_steps: 5000
* mixed\_precision\_training: Native AMP
### Training results
### Framework versions
* Transformers 4.37.2
* Pytorch 2.2.0+cu121
* Datasets 2.18.0
* Tokenizers 0.15.1
| [
"### Training hyperparameters\n\n\nThe following hyperparameters were used during training:\n\n\n* learning\\_rate: 1e-05\n* train\\_batch\\_size: 8\n* eval\\_batch\\_size: 8\n* seed: 42\n* gradient\\_accumulation\\_steps: 2\n* total\\_train\\_batch\\_size: 16\n* optimizer: Adam with betas=(0.9,0.999) and epsilon=1e-08\n* lr\\_scheduler\\_type: linear\n* lr\\_scheduler\\_warmup\\_steps: 500\n* training\\_steps: 5000\n* mixed\\_precision\\_training: Native AMP",
"### Training results",
"### Framework versions\n\n\n* Transformers 4.37.2\n* Pytorch 2.2.0+cu121\n* Datasets 2.18.0\n* Tokenizers 0.15.1"
] | [
"TAGS\n#transformers #tensorboard #safetensors #whisper #automatic-speech-recognition #whisper-event #generated_from_trainer #dataset-common_voice_14_0 #base_model-openai/whisper-medium #license-apache-2.0 #model-index #endpoints_compatible #region-us \n",
"### Training hyperparameters\n\n\nThe following hyperparameters were used during training:\n\n\n* learning\\_rate: 1e-05\n* train\\_batch\\_size: 8\n* eval\\_batch\\_size: 8\n* seed: 42\n* gradient\\_accumulation\\_steps: 2\n* total\\_train\\_batch\\_size: 16\n* optimizer: Adam with betas=(0.9,0.999) and epsilon=1e-08\n* lr\\_scheduler\\_type: linear\n* lr\\_scheduler\\_warmup\\_steps: 500\n* training\\_steps: 5000\n* mixed\\_precision\\_training: Native AMP",
"### Training results",
"### Framework versions\n\n\n* Transformers 4.37.2\n* Pytorch 2.2.0+cu121\n* Datasets 2.18.0\n* Tokenizers 0.15.1"
] |
text-generation | transformers |
<!-- This model card has been generated automatically according to the information the Trainer had access to. You
should probably proofread and complete it, then remove this comment. -->
# 0.001_ablation_6iters_iter_4
This model is a fine-tuned version of [ShenaoZ/0.001_ablation_6iters_iter_3](https://huggingface.co/ShenaoZ/0.001_ablation_6iters_iter_3) on the updated and the original datasets.
## Model description
More information needed
## Intended uses & limitations
More information needed
## Training and evaluation data
More information needed
## Training procedure
### Training hyperparameters
The following hyperparameters were used during training:
- learning_rate: 5e-07
- train_batch_size: 8
- eval_batch_size: 8
- seed: 42
- distributed_type: multi-GPU
- num_devices: 8
- gradient_accumulation_steps: 2
- total_train_batch_size: 128
- total_eval_batch_size: 64
- optimizer: Adam with betas=(0.9,0.999) and epsilon=1e-08
- lr_scheduler_type: cosine
- lr_scheduler_warmup_ratio: 0.1
- num_epochs: 1
### Training results
### Framework versions
- Transformers 4.36.2
- Pytorch 2.1.2+cu121
- Datasets 2.14.6
- Tokenizers 0.15.2
| {"license": "mit", "tags": ["alignment-handbook", "generated_from_trainer", "trl", "dpo", "generated_from_trainer"], "datasets": ["updated", "original"], "base_model": "ShenaoZ/0.001_ablation_6iters_iter_3", "model-index": [{"name": "0.001_ablation_6iters_iter_4", "results": []}]} | ShenaoZ/0.001_ablation_6iters_iter_4 | null | [
"transformers",
"safetensors",
"mistral",
"text-generation",
"alignment-handbook",
"generated_from_trainer",
"trl",
"dpo",
"conversational",
"dataset:updated",
"dataset:original",
"base_model:ShenaoZ/0.001_ablation_6iters_iter_3",
"license:mit",
"autotrain_compatible",
"endpoints_compatible",
"text-generation-inference",
"region:us"
] | null | 2024-04-18T08:39:44+00:00 | [] | [] | TAGS
#transformers #safetensors #mistral #text-generation #alignment-handbook #generated_from_trainer #trl #dpo #conversational #dataset-updated #dataset-original #base_model-ShenaoZ/0.001_ablation_6iters_iter_3 #license-mit #autotrain_compatible #endpoints_compatible #text-generation-inference #region-us
|
# 0.001_ablation_6iters_iter_4
This model is a fine-tuned version of ShenaoZ/0.001_ablation_6iters_iter_3 on the updated and the original datasets.
## Model description
More information needed
## Intended uses & limitations
More information needed
## Training and evaluation data
More information needed
## Training procedure
### Training hyperparameters
The following hyperparameters were used during training:
- learning_rate: 5e-07
- train_batch_size: 8
- eval_batch_size: 8
- seed: 42
- distributed_type: multi-GPU
- num_devices: 8
- gradient_accumulation_steps: 2
- total_train_batch_size: 128
- total_eval_batch_size: 64
- optimizer: Adam with betas=(0.9,0.999) and epsilon=1e-08
- lr_scheduler_type: cosine
- lr_scheduler_warmup_ratio: 0.1
- num_epochs: 1
### Training results
### Framework versions
- Transformers 4.36.2
- Pytorch 2.1.2+cu121
- Datasets 2.14.6
- Tokenizers 0.15.2
| [
"# 0.001_ablation_6iters_iter_4\n\nThis model is a fine-tuned version of ShenaoZ/0.001_ablation_6iters_iter_3 on the updated and the original datasets.",
"## Model description\n\nMore information needed",
"## Intended uses & limitations\n\nMore information needed",
"## Training and evaluation data\n\nMore information needed",
"## Training procedure",
"### Training hyperparameters\n\nThe following hyperparameters were used during training:\n- learning_rate: 5e-07\n- train_batch_size: 8\n- eval_batch_size: 8\n- seed: 42\n- distributed_type: multi-GPU\n- num_devices: 8\n- gradient_accumulation_steps: 2\n- total_train_batch_size: 128\n- total_eval_batch_size: 64\n- optimizer: Adam with betas=(0.9,0.999) and epsilon=1e-08\n- lr_scheduler_type: cosine\n- lr_scheduler_warmup_ratio: 0.1\n- num_epochs: 1",
"### Training results",
"### Framework versions\n\n- Transformers 4.36.2\n- Pytorch 2.1.2+cu121\n- Datasets 2.14.6\n- Tokenizers 0.15.2"
] | [
"TAGS\n#transformers #safetensors #mistral #text-generation #alignment-handbook #generated_from_trainer #trl #dpo #conversational #dataset-updated #dataset-original #base_model-ShenaoZ/0.001_ablation_6iters_iter_3 #license-mit #autotrain_compatible #endpoints_compatible #text-generation-inference #region-us \n",
"# 0.001_ablation_6iters_iter_4\n\nThis model is a fine-tuned version of ShenaoZ/0.001_ablation_6iters_iter_3 on the updated and the original datasets.",
"## Model description\n\nMore information needed",
"## Intended uses & limitations\n\nMore information needed",
"## Training and evaluation data\n\nMore information needed",
"## Training procedure",
"### Training hyperparameters\n\nThe following hyperparameters were used during training:\n- learning_rate: 5e-07\n- train_batch_size: 8\n- eval_batch_size: 8\n- seed: 42\n- distributed_type: multi-GPU\n- num_devices: 8\n- gradient_accumulation_steps: 2\n- total_train_batch_size: 128\n- total_eval_batch_size: 64\n- optimizer: Adam with betas=(0.9,0.999) and epsilon=1e-08\n- lr_scheduler_type: cosine\n- lr_scheduler_warmup_ratio: 0.1\n- num_epochs: 1",
"### Training results",
"### Framework versions\n\n- Transformers 4.36.2\n- Pytorch 2.1.2+cu121\n- Datasets 2.14.6\n- Tokenizers 0.15.2"
] |
null | transformers | ## About
<!-- ### quantize_version: 1 -->
<!-- ### output_tensor_quantised: 1 -->
<!-- ### convert_type: -->
<!-- ### vocab_type: -->
static quants of https://huggingface.co/garage-bAInd/Platypus2-70B
<!-- provided-files -->
weighted/imatrix quants are available at https://huggingface.co/mradermacher/Platypus2-70B-i1-GGUF
## Usage
If you are unsure how to use GGUF files, refer to one of [TheBloke's
READMEs](https://huggingface.co/TheBloke/KafkaLM-70B-German-V0.1-GGUF) for
more details, including on how to concatenate multi-part files.
## Provided Quants
(sorted by size, not necessarily quality. IQ-quants are often preferable over similar sized non-IQ quants)
| Link | Type | Size/GB | Notes |
|:-----|:-----|--------:|:------|
| [GGUF](https://huggingface.co/mradermacher/Platypus2-70B-GGUF/resolve/main/Platypus2-70B.Q2_K.gguf) | Q2_K | 25.6 | |
| [GGUF](https://huggingface.co/mradermacher/Platypus2-70B-GGUF/resolve/main/Platypus2-70B.IQ3_XS.gguf) | IQ3_XS | 28.4 | |
| [GGUF](https://huggingface.co/mradermacher/Platypus2-70B-GGUF/resolve/main/Platypus2-70B.IQ3_S.gguf) | IQ3_S | 30.0 | beats Q3_K* |
| [GGUF](https://huggingface.co/mradermacher/Platypus2-70B-GGUF/resolve/main/Platypus2-70B.Q3_K_S.gguf) | Q3_K_S | 30.0 | |
| [GGUF](https://huggingface.co/mradermacher/Platypus2-70B-GGUF/resolve/main/Platypus2-70B.IQ3_M.gguf) | IQ3_M | 31.0 | |
| [GGUF](https://huggingface.co/mradermacher/Platypus2-70B-GGUF/resolve/main/Platypus2-70B.Q3_K_M.gguf) | Q3_K_M | 33.4 | lower quality |
| [GGUF](https://huggingface.co/mradermacher/Platypus2-70B-GGUF/resolve/main/Platypus2-70B.Q3_K_L.gguf) | Q3_K_L | 36.2 | |
| [GGUF](https://huggingface.co/mradermacher/Platypus2-70B-GGUF/resolve/main/Platypus2-70B.IQ4_XS.gguf) | IQ4_XS | 37.3 | |
| [GGUF](https://huggingface.co/mradermacher/Platypus2-70B-GGUF/resolve/main/Platypus2-70B.Q4_K_S.gguf) | Q4_K_S | 39.3 | fast, recommended |
| [GGUF](https://huggingface.co/mradermacher/Platypus2-70B-GGUF/resolve/main/Platypus2-70B.Q4_K_M.gguf) | Q4_K_M | 41.5 | fast, recommended |
| [GGUF](https://huggingface.co/mradermacher/Platypus2-70B-GGUF/resolve/main/Platypus2-70B.Q5_K_S.gguf) | Q5_K_S | 47.6 | |
| [GGUF](https://huggingface.co/mradermacher/Platypus2-70B-GGUF/resolve/main/Platypus2-70B.Q5_K_M.gguf) | Q5_K_M | 48.9 | |
| [PART 1](https://huggingface.co/mradermacher/Platypus2-70B-GGUF/resolve/main/Platypus2-70B.Q6_K.gguf.part1of2) [PART 2](https://huggingface.co/mradermacher/Platypus2-70B-GGUF/resolve/main/Platypus2-70B.Q6_K.gguf.part2of2) | Q6_K | 56.7 | very good quality |
| [PART 1](https://huggingface.co/mradermacher/Platypus2-70B-GGUF/resolve/main/Platypus2-70B.Q8_0.gguf.part1of2) [PART 2](https://huggingface.co/mradermacher/Platypus2-70B-GGUF/resolve/main/Platypus2-70B.Q8_0.gguf.part2of2) | Q8_0 | 73.4 | fast, best quality |
Here is a handy graph by ikawrakow comparing some lower-quality quant
types (lower is better):

And here are Artefact2's thoughts on the matter:
https://gist.github.com/Artefact2/b5f810600771265fc1e39442288e8ec9
## FAQ / Model Request
See https://huggingface.co/mradermacher/model_requests for some answers to
questions you might have and/or if you want some other model quantized.
## Thanks
I thank my company, [nethype GmbH](https://www.nethype.de/), for letting
me use its servers and providing upgrades to my workstation to enable
this work in my free time.
<!-- end -->
| {"language": ["en"], "license": "cc-by-nc-sa-4.0", "library_name": "transformers", "datasets": ["garage-bAInd/Open-Platypus"], "base_model": "garage-bAInd/Platypus2-70B", "quantized_by": "mradermacher"} | mradermacher/Platypus2-70B-GGUF | null | [
"transformers",
"gguf",
"en",
"dataset:garage-bAInd/Open-Platypus",
"base_model:garage-bAInd/Platypus2-70B",
"license:cc-by-nc-sa-4.0",
"endpoints_compatible",
"region:us"
] | null | 2024-04-18T08:39:51+00:00 | [] | [
"en"
] | TAGS
#transformers #gguf #en #dataset-garage-bAInd/Open-Platypus #base_model-garage-bAInd/Platypus2-70B #license-cc-by-nc-sa-4.0 #endpoints_compatible #region-us
| About
-----
static quants of URL
weighted/imatrix quants are available at URL
Usage
-----
If you are unsure how to use GGUF files, refer to one of TheBloke's
READMEs for
more details, including on how to concatenate multi-part files.
Provided Quants
---------------
(sorted by size, not necessarily quality. IQ-quants are often preferable over similar sized non-IQ quants)
Here is a handy graph by ikawrakow comparing some lower-quality quant
types (lower is better):
!URL
And here are Artefact2's thoughts on the matter:
URL
FAQ / Model Request
-------------------
See URL for some answers to
questions you might have and/or if you want some other model quantized.
Thanks
------
I thank my company, nethype GmbH, for letting
me use its servers and providing upgrades to my workstation to enable
this work in my free time.
| [] | [
"TAGS\n#transformers #gguf #en #dataset-garage-bAInd/Open-Platypus #base_model-garage-bAInd/Platypus2-70B #license-cc-by-nc-sa-4.0 #endpoints_compatible #region-us \n"
] |
text-generation | null |
# SeaLLM-7B-v2.5-GGUF
- Thsi si quantized version for [SeaLLMs/SeaLLM-7B-v2.5](https://huggingface.co/SeaLLMs/SeaLLM-7B-v2.5)
## Model Description
We introduce [SeaLLM-7B-v2.5](https://huggingface.co/SeaLLMs/SeaLLM-7B-v2.5), the state-of-the-art multilingual LLM for Southeast Asian (SEA) languages 🇬🇧 🇨🇳 🇻🇳 🇮🇩 🇹🇭 🇲🇾 🇰🇭 🇱🇦 🇲🇲 🇵🇭. It is the most significant upgrade since [SeaLLM-13B](https://huggingface.co/SeaLLMs/SeaLLM-13B-Chat), with half the size, outperforming performance across diverse multilingual tasks, from world knowledge, math reasoning, instruction following, etc.
### Highlights
* [SeaLLM-7B-v2.5](https://huggingface.co/SeaLLMs/SeaLLM-7B-v2.5) outperforms GPT-3.5 and achieves 7B SOTA on most multilingual knowledge benchmarks for SEA languages (MMLU, M3Exam & VMLU).
* It achieves 79.0 and 34.9 on GSM8K and MATH, surpassing GPT-3.5 in MATH.
### Release and DEMO
- DEMO:
- [SeaLLMs/SeaLLM-7B-v2.5](https://huggingface.co/spaces/SeaLLMs/SeaLLM-7B-v2.5).
- [SeaLLMs/SeaLLM-7B | SeaLMMM-7B](https://huggingface.co/spaces/SeaLLMs/SeaLLM-7B) - Experimental multimodal SeaLLM.
- Technical report: [Arxiv: SeaLLMs - Large Language Models for Southeast Asia](https://arxiv.org/pdf/2312.00738.pdf).
- Model weights: [SeaLLM-7B-v2.5](https://huggingface.co/SeaLLMs/SeaLLM-7B-v2.5).
<blockquote style="color:red">
<p><strong style="color: red">Terms of Use and License</strong>:
By using our released weights, codes, and demos, you agree to and comply with the terms and conditions specified in our <a href="https://huggingface.co/SeaLLMs/SeaLLM-Chat-13b/edit/main/LICENSE" target="_blank" rel="noopener">SeaLLMs Terms Of Use</a>.
</blockquote>
> **Disclaimer**:
> We must note that even though the weights, codes, and demos are released in an open manner, similar to other pre-trained language models, and despite our best efforts in red teaming and safety fine-tuning and enforcement, our models come with potential risks, including but not limited to inaccurate, misleading or potentially harmful generation.
> Developers and stakeholders should perform their own red teaming and provide related security measures before deployment, and they must abide by and comply with local governance and regulations.
> In no event shall the authors be held liable for any claim, damages, or other liability arising from the use of the released weights, codes, or demos.
> The logo was generated by DALL-E 3.
### What's new since SeaLLM-7B-v2?
* SeaLLM-7B-v2.5 was built on top of Gemma-7b, and underwent large scale SFT and carefully designed alignment.
## Evaluation
### Multilingual World Knowledge
We evaluate models on 3 benchmarks following the recommended default setups: 5-shot MMLU for En, 3-shot [M3Exam](https://arxiv.org/pdf/2306.05179.pdf) (M3e) for En, Zh, Vi, Id, Th, and zero-shot [VMLU](https://vmlu.ai/) for Vi.
| Model | Langs | En<br>MMLU | En<br>M3e | Zh<br>M3e | Vi<br>M3e | Vi<br>VMLU | Id<br>M3e | Th<br>M3e
|-----| ----- | --- | -- | ----- | ---- | --- | --- | --- |
| GPT-3.5 | Multi | 68.90 | 75.46 | 60.20 | 58.64 | 46.32 | 49.27 | 37.41
| Vistral-7B-chat | Mono | 56.86 | 67.00 | 44.56 | 54.33 | 50.03 | 36.49 | 25.27
| Qwen1.5-7B-chat | Multi | 61.00 | 52.07 | 81.96 | 43.38 | 45.02 | 24.29 | 20.25
| SailorLM | Multi | 52.72 | 59.76 | 67.74 | 50.14 | --- | 39.53 | 37.73
| SeaLLM-7B-v2 | Multi | 61.89 | 70.91 | 55.43 | 51.15 | 45.74 | 42.25 | 35.52
| SeaLLM-7B-v2.5 | Multi | 64.05 | 76.87 | 62.54 | 63.11 | 53.30 | 48.64 | 46.86
### Zero-shot CoT Multilingual Math Reasoning
<!--
[SeaLLM-7B-v2](https://huggingface.co/SeaLLMs/SeaLLM-7B-v2) achieves with **78.5** score on the GSM8K with zero-shot CoT reasoning, making it the **state of the art** in the realm of 7B models. It also outperforms GPT-3.5 in the same GSM8K benchmark as translated into SEA languages (🇨🇳 🇻🇳 🇮🇩 🇹🇭). [SeaLLM-7B-v2](https://huggingface.co/SeaLLMs/SeaLLM-7B-v2) also surpasses GPT-3.5 on the Thai-translated MATH benchmark, with **28.4** vs 18.1 scores.

-->
| Model | GSM8K<br>en | MATH<br>en | GSM8K<br>zh | MATH<br>zh | GSM8K<br>vi | MATH<br>vi | GSM8K<br>id | MATH<br>id | GSM8K<br>th | MATH<br>th
| --- | --- | --- | --- | --- | --- | --- | --- | --- | --- | --- |
| GPT-3.5 | 80.8 | 34.1 | 48.2 | 21.5 | 55 | 26.5 | 64.3 | 26.4 | 35.8 | 18.1
| Qwen-14B-chat | 61.4 | 18.4 | 41.6 | 11.8 | 33.6 | 3.6 | 44.7 | 8.6 | 22 | 6.0
| Vistral-7b-chat | 48.2 | 12.5 | | | 48.7 | 3.1 | | | |
| Qwen1.5-7B-chat | 56.8 | 15.3 | 40.0 | 2.7 | 37.7 | 9 | 36.9 | 7.7 | 21.9 | 4.7
| SeaLLM-7B-v2 | 78.2 | 27.5 | 53.7 | 17.6 | 69.9 | 23.8 | 71.5 | 24.4 | 59.6 | 22.4
| SeaLLM-7B-v2.5 | 78.5 | 34.9 | 51.3 | 22.1 | 72.3 | 30.2 | 71.5 | 30.1 | 62.0 | 28.4
Baselines were evaluated using their respective chat-template and system prompts ([Qwen1.5-7B-chat](https://huggingface.co/Qwen/Qwen1.5-7B-Chat/blob/main/tokenizer_config.json), [Vistral](https://huggingface.co/Viet-Mistral/Vistral-7B-Chat)).
#### Zero-shot MGSM
[SeaLLM-7B-v2.5](https://huggingface.co/SeaLLMs/SeaLLM-7B-v2.5) also outperforms GPT-3.5 and Qwen-14B on the multilingual MGSM for Thai.
| Model | MGSM-Zh | MGSM-Th
|-----| ----- | ---
| ChatGPT (reported) | 61.2 | 47.2
| Qwen-14B-chat | 59.6 | 28
| SeaLLM-7B-v2 | **64.8** | 62.4
| SeaLLM-7B-v2.5 | 58.0 | **64.8**
### Sea-Bench

### Usage
#### Instruction format
```python
prompt = """<|im_start|>system
You are a helpful assistant.<eos>
<|im_start|>user
Hello world<eos>
<|im_start|>assistant
Hi there, how can I help?<eos>"""
# <|im_start|> is not a special token.
# Transformers chat_template should be consistent with vLLM format below.
# ! ENSURE 1 and only 1 bos `<s>` at the beginning of sequence
print(tokenizer.convert_ids_to_tokens(tokenizer.encode(prompt)))
"""
```
#### Using transformers's chat_template
Install the latest transformers (>4.40)
```python
from transformers import AutoModelForCausalLM, AutoTokenizer
device = "cuda" # the device to load the model onto
# use bfloat16 to ensure the best performance.
model = AutoModelForCausalLM.from_pretrained("SeaLLMs/SeaLLM-7B-v2.5", torch_dtype=torch.bfloat16, device_map=device)
tokenizer = AutoTokenizer.from_pretrained("SeaLLMs/SeaLLM-7B-v2.5")
messages = [
{"role": "system", "content": "You are a helpful assistant."},
{"role": "user", "content": "Hello world"},
{"role": "assistant", "content": "Hi there, how can I help you today?"},
{"role": "user", "content": "Explain general relativity in details."}
]
encodeds = tokenizer.apply_chat_template(messages, return_tensors="pt", add_generation_prompt=True)
print(tokenizer.convert_ids_to_tokens(encodeds[0]))
model_inputs = encodeds.to(device)
model.to(device)
generated_ids = model.generate(model_inputs, max_new_tokens=1000, do_sample=True, pad_token_id=tokenizer.pad_token_id)
decoded = tokenizer.batch_decode(generated_ids)
print(decoded[0])
```
#### Using vLLM
```python
from vllm import LLM, SamplingParams
TURN_TEMPLATE = "<|im_start|>{role}\n{content}<eos>\n"
TURN_PREFIX = "<|im_start|>{role}\n"
def seallm_chat_convo_format(conversations, add_assistant_prefix: bool, system_prompt=None):
# conversations: list of dict with key `role` and `content` (openai format)
if conversations[0]['role'] != 'system' and system_prompt is not None:
conversations = [{"role": "system", "content": system_prompt}] + conversations
text = ''
for turn_id, turn in enumerate(conversations):
prompt = TURN_TEMPLATE.format(role=turn['role'], content=turn['content'])
text += prompt
if add_assistant_prefix:
prompt = TURN_PREFIX.format(role='assistant')
text += prompt
return text
sparams = SamplingParams(temperature=0.1, max_tokens=1024, stop=['<eos>', '<|im_start|>'])
llm = LLM("SeaLLMs/SeaLLM-7B-v2.5", dtype="bfloat16")
message = "Explain general relativity in details."
prompt = seallm_chat_convo_format(message, True)
gen = llm.generate(prompt, sampling_params)
print(gen[0].outputs[0].text)
```
#### Fine-tuning SeaLLM-7B-v2.5
Should follow the chat format and accurately mask out source tokens. Here is an example.
```python
conversations = [
{"role": "system", "content": "You are helful assistant."},
{"role": "user", "content": "Hello world."},
{"role": "assistant", "content": "Hi there, how can I help?"},
{"role": "user", "content": "Tell me a joke."},
{"role": "assistant", "content": "Why don't scientists trust atoms? Because they make up everything."},
]
def seallm_7b_v25_tokenize_multi_turns(tokenizer, conversations, add_assistant_prefix=False):
"""
Inputs:
conversations: list of dict following openai format, eg
conversations = [
{"role": "system", "content": "You are helful assistant."},
{"role": "user", "content": "Hello world."},
{"role": "assistant", "content": "Hi there, how can I help?"},
{"role": "user", "content": "Tell me a joke."},
{"role": "assistant", "content": "Why don't scientists trust atoms? Because they make up everything."},
]
add_assistant_prefix: whether to add assistant_prefix, only for inference decoding
Outputs:
tokenize_output_sample, {
"input_ids": ...
"token_type_ids": 1 if train and 0 if masked out (not train)
}
During training, need to create a labels, with masked-out tokens = -100 to avoid loss computations.
labels = sample['input_ids'].clone()
labels[sample['token_type_ids'] == 0] = -100
"""
TURN_TEMPLATE = "<|im_start|>{role}\n{content}<eos>\n"
TURN_PREFIX = "<|im_start|>{role}\n"
TURN_SUFFIX = "<eos>\n"
TURN_SUFFIX_TAKE = "<eos>"
sample = None
assistant_prefix_len = None
assistant_suffix_len = None
for turn_id, turn in enumerate(conversations):
prompt = TURN_TEMPLATE.format(role=turn['role'], content=turn['content'])
turn_sample = tokenizer(
prompt, padding=False, truncation=False, verbose=False, add_special_tokens=False,
return_token_type_ids=True,
)
if turn['role'] == 'assistant':
if assistant_prefix_len is None:
assistant_prefix_len = len(tokenizer.encode(TURN_PREFIX.format(role=turn['role']), add_special_tokens=False))
if assistant_suffix_len is None:
assistant_suffix_len = (
len(tokenizer.encode(TURN_SUFFIX.format(role=turn['role']), add_special_tokens=False)) -
len(tokenizer.encode(TURN_SUFFIX_TAKE, add_special_tokens=False))
)
turn_sample['token_type_ids'][assistant_prefix_len:-assistant_suffix_len] = [1] * (len(turn_sample['input_ids']) - assistant_prefix_len - assistant_suffix_len)
if sample is None:
sample = turn_sample
else:
for k in turn_sample.keys():
sample[k].extend(turn_sample[k])
if add_assistant_prefix:
assistant_prefix_sample = tokenizer(
TURN_PREFIX.format(role="assistant"), padding=False, truncation=False, verbose=False, add_special_tokens=False,
return_token_type_ids=True,
)
for k in sample.keys():
sample[k].extend(assistant_prefix_sample[k])
if tokenizer.add_bos_token:
sample['input_ids'] = [tokenizer.bos_token_id] + sample['input_ids']
sample['attention_mask'] = [1] + sample['attention_mask']
sample['token_type_ids'] = [sample['token_type_ids'][0]] + sample['token_type_ids']
return sample
# ! testing
sample = seallm_7b_v25_tokenize_multi_turns(tokenizer, conversations)
tokens = tokenizer.convert_ids_to_tokens(sample['input_ids'])
pairs = [(x, y) for x, y in zip(tokens, sample['token_type_ids'])]
print(pairs)
# source and special tokens is masked out (token_type 0), only assistant with <eos> is trained (token_type 1)
# [('<bos>', 0), ('<', 0), ('|', 0), ..., ('assistant', 0), ('\n', 0), ('Hi', 1), ('▁there', 1), (',', 1), ('▁how', 1), ('▁can', 1), ('▁I', 1), ('▁help', 1), ('?', 1), ('<eos>', 1), ('\n', 0), ('<', 0), ...
```
## Acknowledgement to Our Linguists
We would like to express our special thanks to our professional and native linguists, Tantong Champaiboon, Nguyen Ngoc Yen Nhi and Tara Devina Putri, who helped build, evaluate, and fact-check our sampled pretraining and SFT dataset as well as evaluating our models across different aspects, especially safety.
## Citation
If you find our project useful, we hope you would kindly star our repo and cite our work as follows: Corresponding Author: [[email protected]](mailto:[email protected])
**Author list and order will change!**
* `*` and `^` are equal contributions.
```
@article{damonlpsg2023seallm,
author = {Xuan-Phi Nguyen*, Wenxuan Zhang*, Xin Li*, Mahani Aljunied*, Weiwen Xu, Hou Pong Chan,
Zhiqiang Hu, Chenhui Shen^, Yew Ken Chia^, Xingxuan Li, Jianyu Wang,
Qingyu Tan, Liying Cheng, Guanzheng Chen, Yue Deng, Sen Yang,
Chaoqun Liu, Hang Zhang, Lidong Bing},
title = {SeaLLMs - Large Language Models for Southeast Asia},
year = 2023,
Eprint = {arXiv:2312.00738},
}
``` | {"language": ["en", "zh", "vi", "id", "th", "ms", "km", "lo", "my", "tl"], "license": "other", "tags": ["multilingual", "sea"], "license_name": "seallms", "license_link": "https://huggingface.co/SeaLLMs/SeaLLM-13B-Chat/blob/main/LICENSE", "pipeline_tag": "text-generation", "base_model": "SeaLLMs/SeaLLM-7B-v2.5"} | QuantFactory/SeaLLM-7B-v2.5-GGUF | null | [
"gguf",
"multilingual",
"sea",
"text-generation",
"en",
"zh",
"vi",
"id",
"th",
"ms",
"km",
"lo",
"my",
"tl",
"arxiv:2312.00738",
"arxiv:2306.05179",
"base_model:SeaLLMs/SeaLLM-7B-v2.5",
"license:other",
"region:us"
] | null | 2024-04-18T08:40:28+00:00 | [
"2312.00738",
"2306.05179"
] | [
"en",
"zh",
"vi",
"id",
"th",
"ms",
"km",
"lo",
"my",
"tl"
] | TAGS
#gguf #multilingual #sea #text-generation #en #zh #vi #id #th #ms #km #lo #my #tl #arxiv-2312.00738 #arxiv-2306.05179 #base_model-SeaLLMs/SeaLLM-7B-v2.5 #license-other #region-us
| SeaLLM-7B-v2.5-GGUF
===================
* Thsi si quantized version for SeaLLMs/SeaLLM-7B-v2.5
Model Description
-----------------
We introduce SeaLLM-7B-v2.5, the state-of-the-art multilingual LLM for Southeast Asian (SEA) languages 🇬🇧 🇨🇳 🇻🇳 🇮🇩 🇹🇭 🇲🇾 🇰🇭 🇱🇦 🇲🇲 🇵🇭. It is the most significant upgrade since SeaLLM-13B, with half the size, outperforming performance across diverse multilingual tasks, from world knowledge, math reasoning, instruction following, etc.
### Highlights
* SeaLLM-7B-v2.5 outperforms GPT-3.5 and achieves 7B SOTA on most multilingual knowledge benchmarks for SEA languages (MMLU, M3Exam & VMLU).
* It achieves 79.0 and 34.9 on GSM8K and MATH, surpassing GPT-3.5 in MATH.
### Release and DEMO
* DEMO:
+ SeaLLMs/SeaLLM-7B-v2.5.
+ SeaLLMs/SeaLLM-7B | SeaLMMM-7B - Experimental multimodal SeaLLM.
* Technical report: Arxiv: SeaLLMs - Large Language Models for Southeast Asia.
* Model weights: SeaLLM-7B-v2.5.
>
> **Terms of Use and License**:
> By using our released weights, codes, and demos, you agree to and comply with the terms and conditions specified in our [SeaLLMs Terms Of Use](URL target=).
>
>
>
>
> Disclaimer:
> We must note that even though the weights, codes, and demos are released in an open manner, similar to other pre-trained language models, and despite our best efforts in red teaming and safety fine-tuning and enforcement, our models come with potential risks, including but not limited to inaccurate, misleading or potentially harmful generation.
> Developers and stakeholders should perform their own red teaming and provide related security measures before deployment, and they must abide by and comply with local governance and regulations.
> In no event shall the authors be held liable for any claim, damages, or other liability arising from the use of the released weights, codes, or demos.
>
>
>
>
> The logo was generated by DALL-E 3.
>
>
>
### What's new since SeaLLM-7B-v2?
* SeaLLM-7B-v2.5 was built on top of Gemma-7b, and underwent large scale SFT and carefully designed alignment.
Evaluation
----------
### Multilingual World Knowledge
We evaluate models on 3 benchmarks following the recommended default setups: 5-shot MMLU for En, 3-shot M3Exam (M3e) for En, Zh, Vi, Id, Th, and zero-shot VMLU for Vi.
### Zero-shot CoT Multilingual Math Reasoning
Baselines were evaluated using their respective chat-template and system prompts (Qwen1.5-7B-chat, Vistral).
#### Zero-shot MGSM
SeaLLM-7B-v2.5 also outperforms GPT-3.5 and Qwen-14B on the multilingual MGSM for Thai.
Model: ChatGPT (reported), MGSM-Zh: 61.2, MGSM-Th: 47.2
Model: Qwen-14B-chat, MGSM-Zh: 59.6, MGSM-Th: 28
Model: SeaLLM-7B-v2, MGSM-Zh: 64.8, MGSM-Th: 62.4
Model: SeaLLM-7B-v2.5, MGSM-Zh: 58.0, MGSM-Th: 64.8
### Sea-Bench
!fig\_sea\_bench\_side\_by\_side.png
### Usage
#### Instruction format
#### Using transformers's chat\_template
Install the latest transformers (>4.40)
#### Using vLLM
#### Fine-tuning SeaLLM-7B-v2.5
Should follow the chat format and accurately mask out source tokens. Here is an example.
Acknowledgement to Our Linguists
--------------------------------
We would like to express our special thanks to our professional and native linguists, Tantong Champaiboon, Nguyen Ngoc Yen Nhi and Tara Devina Putri, who helped build, evaluate, and fact-check our sampled pretraining and SFT dataset as well as evaluating our models across different aspects, especially safety.
If you find our project useful, we hope you would kindly star our repo and cite our work as follows: Corresponding Author: l.bing@URL
Author list and order will change!
* '\*' and '^' are equal contributions.
| [
"### Highlights\n\n\n* SeaLLM-7B-v2.5 outperforms GPT-3.5 and achieves 7B SOTA on most multilingual knowledge benchmarks for SEA languages (MMLU, M3Exam & VMLU).\n* It achieves 79.0 and 34.9 on GSM8K and MATH, surpassing GPT-3.5 in MATH.",
"### Release and DEMO\n\n\n* DEMO:\n\t+ SeaLLMs/SeaLLM-7B-v2.5.\n\t+ SeaLLMs/SeaLLM-7B | SeaLMMM-7B - Experimental multimodal SeaLLM.\n* Technical report: Arxiv: SeaLLMs - Large Language Models for Southeast Asia.\n* Model weights: SeaLLM-7B-v2.5.\n\n\n\n> \n> **Terms of Use and License**: \n> By using our released weights, codes, and demos, you agree to and comply with the terms and conditions specified in our [SeaLLMs Terms Of Use](URL target=).\n> \n> \n> \n\n\n\n> \n> Disclaimer:\n> We must note that even though the weights, codes, and demos are released in an open manner, similar to other pre-trained language models, and despite our best efforts in red teaming and safety fine-tuning and enforcement, our models come with potential risks, including but not limited to inaccurate, misleading or potentially harmful generation.\n> Developers and stakeholders should perform their own red teaming and provide related security measures before deployment, and they must abide by and comply with local governance and regulations.\n> In no event shall the authors be held liable for any claim, damages, or other liability arising from the use of the released weights, codes, or demos.\n> \n> \n> \n\n\n\n> \n> The logo was generated by DALL-E 3.\n> \n> \n>",
"### What's new since SeaLLM-7B-v2?\n\n\n* SeaLLM-7B-v2.5 was built on top of Gemma-7b, and underwent large scale SFT and carefully designed alignment.\n\n\nEvaluation\n----------",
"### Multilingual World Knowledge\n\n\nWe evaluate models on 3 benchmarks following the recommended default setups: 5-shot MMLU for En, 3-shot M3Exam (M3e) for En, Zh, Vi, Id, Th, and zero-shot VMLU for Vi.",
"### Zero-shot CoT Multilingual Math Reasoning\n\n\n\nBaselines were evaluated using their respective chat-template and system prompts (Qwen1.5-7B-chat, Vistral).",
"#### Zero-shot MGSM\n\n\nSeaLLM-7B-v2.5 also outperforms GPT-3.5 and Qwen-14B on the multilingual MGSM for Thai.\n\n\nModel: ChatGPT (reported), MGSM-Zh: 61.2, MGSM-Th: 47.2\nModel: Qwen-14B-chat, MGSM-Zh: 59.6, MGSM-Th: 28\nModel: SeaLLM-7B-v2, MGSM-Zh: 64.8, MGSM-Th: 62.4\nModel: SeaLLM-7B-v2.5, MGSM-Zh: 58.0, MGSM-Th: 64.8",
"### Sea-Bench\n\n\n!fig\\_sea\\_bench\\_side\\_by\\_side.png",
"### Usage",
"#### Instruction format",
"#### Using transformers's chat\\_template\n\n\nInstall the latest transformers (>4.40)",
"#### Using vLLM",
"#### Fine-tuning SeaLLM-7B-v2.5\n\n\nShould follow the chat format and accurately mask out source tokens. Here is an example.\n\n\nAcknowledgement to Our Linguists\n--------------------------------\n\n\nWe would like to express our special thanks to our professional and native linguists, Tantong Champaiboon, Nguyen Ngoc Yen Nhi and Tara Devina Putri, who helped build, evaluate, and fact-check our sampled pretraining and SFT dataset as well as evaluating our models across different aspects, especially safety.\n\n\nIf you find our project useful, we hope you would kindly star our repo and cite our work as follows: Corresponding Author: l.bing@URL\n\n\nAuthor list and order will change!\n\n\n* '\\*' and '^' are equal contributions."
] | [
"TAGS\n#gguf #multilingual #sea #text-generation #en #zh #vi #id #th #ms #km #lo #my #tl #arxiv-2312.00738 #arxiv-2306.05179 #base_model-SeaLLMs/SeaLLM-7B-v2.5 #license-other #region-us \n",
"### Highlights\n\n\n* SeaLLM-7B-v2.5 outperforms GPT-3.5 and achieves 7B SOTA on most multilingual knowledge benchmarks for SEA languages (MMLU, M3Exam & VMLU).\n* It achieves 79.0 and 34.9 on GSM8K and MATH, surpassing GPT-3.5 in MATH.",
"### Release and DEMO\n\n\n* DEMO:\n\t+ SeaLLMs/SeaLLM-7B-v2.5.\n\t+ SeaLLMs/SeaLLM-7B | SeaLMMM-7B - Experimental multimodal SeaLLM.\n* Technical report: Arxiv: SeaLLMs - Large Language Models for Southeast Asia.\n* Model weights: SeaLLM-7B-v2.5.\n\n\n\n> \n> **Terms of Use and License**: \n> By using our released weights, codes, and demos, you agree to and comply with the terms and conditions specified in our [SeaLLMs Terms Of Use](URL target=).\n> \n> \n> \n\n\n\n> \n> Disclaimer:\n> We must note that even though the weights, codes, and demos are released in an open manner, similar to other pre-trained language models, and despite our best efforts in red teaming and safety fine-tuning and enforcement, our models come with potential risks, including but not limited to inaccurate, misleading or potentially harmful generation.\n> Developers and stakeholders should perform their own red teaming and provide related security measures before deployment, and they must abide by and comply with local governance and regulations.\n> In no event shall the authors be held liable for any claim, damages, or other liability arising from the use of the released weights, codes, or demos.\n> \n> \n> \n\n\n\n> \n> The logo was generated by DALL-E 3.\n> \n> \n>",
"### What's new since SeaLLM-7B-v2?\n\n\n* SeaLLM-7B-v2.5 was built on top of Gemma-7b, and underwent large scale SFT and carefully designed alignment.\n\n\nEvaluation\n----------",
"### Multilingual World Knowledge\n\n\nWe evaluate models on 3 benchmarks following the recommended default setups: 5-shot MMLU for En, 3-shot M3Exam (M3e) for En, Zh, Vi, Id, Th, and zero-shot VMLU for Vi.",
"### Zero-shot CoT Multilingual Math Reasoning\n\n\n\nBaselines were evaluated using their respective chat-template and system prompts (Qwen1.5-7B-chat, Vistral).",
"#### Zero-shot MGSM\n\n\nSeaLLM-7B-v2.5 also outperforms GPT-3.5 and Qwen-14B on the multilingual MGSM for Thai.\n\n\nModel: ChatGPT (reported), MGSM-Zh: 61.2, MGSM-Th: 47.2\nModel: Qwen-14B-chat, MGSM-Zh: 59.6, MGSM-Th: 28\nModel: SeaLLM-7B-v2, MGSM-Zh: 64.8, MGSM-Th: 62.4\nModel: SeaLLM-7B-v2.5, MGSM-Zh: 58.0, MGSM-Th: 64.8",
"### Sea-Bench\n\n\n!fig\\_sea\\_bench\\_side\\_by\\_side.png",
"### Usage",
"#### Instruction format",
"#### Using transformers's chat\\_template\n\n\nInstall the latest transformers (>4.40)",
"#### Using vLLM",
"#### Fine-tuning SeaLLM-7B-v2.5\n\n\nShould follow the chat format and accurately mask out source tokens. Here is an example.\n\n\nAcknowledgement to Our Linguists\n--------------------------------\n\n\nWe would like to express our special thanks to our professional and native linguists, Tantong Champaiboon, Nguyen Ngoc Yen Nhi and Tara Devina Putri, who helped build, evaluate, and fact-check our sampled pretraining and SFT dataset as well as evaluating our models across different aspects, especially safety.\n\n\nIf you find our project useful, we hope you would kindly star our repo and cite our work as follows: Corresponding Author: l.bing@URL\n\n\nAuthor list and order will change!\n\n\n* '\\*' and '^' are equal contributions."
] |
null | peft |
<!-- This model card has been generated automatically according to the information the Trainer had access to. You
should probably proofread and complete it, then remove this comment. -->
# tiny-llama-code-feedback
This model is a fine-tuned version of [TinyLlama/TinyLlama-1.1B-Chat-v1.0](https://huggingface.co/TinyLlama/TinyLlama-1.1B-Chat-v1.0) on an unknown dataset.
## Model description
More information needed
## Intended uses & limitations
More information needed
## Training and evaluation data
More information needed
## Training procedure
### Training hyperparameters
The following hyperparameters were used during training:
- learning_rate: 0.0002
- train_batch_size: 1
- eval_batch_size: 8
- seed: 42
- gradient_accumulation_steps: 4
- total_train_batch_size: 4
- optimizer: Adam with betas=(0.9,0.999) and epsilon=1e-08
- lr_scheduler_type: constant
- lr_scheduler_warmup_ratio: 0.03
- num_epochs: 1
- mixed_precision_training: Native AMP
### Training results
### Framework versions
- PEFT 0.10.0
- Transformers 4.39.3
- Pytorch 2.2.2+cu121
- Datasets 2.18.0
- Tokenizers 0.15.2 | {"license": "apache-2.0", "library_name": "peft", "tags": ["trl", "sft", "generated_from_trainer"], "base_model": "TinyLlama/TinyLlama-1.1B-Chat-v1.0", "model-index": [{"name": "tiny-llama-code-feedback", "results": []}]} | bgsmagnuson/tiny-llama-code-feedback | null | [
"peft",
"tensorboard",
"safetensors",
"trl",
"sft",
"generated_from_trainer",
"base_model:TinyLlama/TinyLlama-1.1B-Chat-v1.0",
"license:apache-2.0",
"region:us"
] | null | 2024-04-18T08:42:31+00:00 | [] | [] | TAGS
#peft #tensorboard #safetensors #trl #sft #generated_from_trainer #base_model-TinyLlama/TinyLlama-1.1B-Chat-v1.0 #license-apache-2.0 #region-us
|
# tiny-llama-code-feedback
This model is a fine-tuned version of TinyLlama/TinyLlama-1.1B-Chat-v1.0 on an unknown dataset.
## Model description
More information needed
## Intended uses & limitations
More information needed
## Training and evaluation data
More information needed
## Training procedure
### Training hyperparameters
The following hyperparameters were used during training:
- learning_rate: 0.0002
- train_batch_size: 1
- eval_batch_size: 8
- seed: 42
- gradient_accumulation_steps: 4
- total_train_batch_size: 4
- optimizer: Adam with betas=(0.9,0.999) and epsilon=1e-08
- lr_scheduler_type: constant
- lr_scheduler_warmup_ratio: 0.03
- num_epochs: 1
- mixed_precision_training: Native AMP
### Training results
### Framework versions
- PEFT 0.10.0
- Transformers 4.39.3
- Pytorch 2.2.2+cu121
- Datasets 2.18.0
- Tokenizers 0.15.2 | [
"# tiny-llama-code-feedback\n\nThis model is a fine-tuned version of TinyLlama/TinyLlama-1.1B-Chat-v1.0 on an unknown dataset.",
"## Model description\n\nMore information needed",
"## Intended uses & limitations\n\nMore information needed",
"## Training and evaluation data\n\nMore information needed",
"## Training procedure",
"### Training hyperparameters\n\nThe following hyperparameters were used during training:\n- learning_rate: 0.0002\n- train_batch_size: 1\n- eval_batch_size: 8\n- seed: 42\n- gradient_accumulation_steps: 4\n- total_train_batch_size: 4\n- optimizer: Adam with betas=(0.9,0.999) and epsilon=1e-08\n- lr_scheduler_type: constant\n- lr_scheduler_warmup_ratio: 0.03\n- num_epochs: 1\n- mixed_precision_training: Native AMP",
"### Training results",
"### Framework versions\n\n- PEFT 0.10.0\n- Transformers 4.39.3\n- Pytorch 2.2.2+cu121\n- Datasets 2.18.0\n- Tokenizers 0.15.2"
] | [
"TAGS\n#peft #tensorboard #safetensors #trl #sft #generated_from_trainer #base_model-TinyLlama/TinyLlama-1.1B-Chat-v1.0 #license-apache-2.0 #region-us \n",
"# tiny-llama-code-feedback\n\nThis model is a fine-tuned version of TinyLlama/TinyLlama-1.1B-Chat-v1.0 on an unknown dataset.",
"## Model description\n\nMore information needed",
"## Intended uses & limitations\n\nMore information needed",
"## Training and evaluation data\n\nMore information needed",
"## Training procedure",
"### Training hyperparameters\n\nThe following hyperparameters were used during training:\n- learning_rate: 0.0002\n- train_batch_size: 1\n- eval_batch_size: 8\n- seed: 42\n- gradient_accumulation_steps: 4\n- total_train_batch_size: 4\n- optimizer: Adam with betas=(0.9,0.999) and epsilon=1e-08\n- lr_scheduler_type: constant\n- lr_scheduler_warmup_ratio: 0.03\n- num_epochs: 1\n- mixed_precision_training: Native AMP",
"### Training results",
"### Framework versions\n\n- PEFT 0.10.0\n- Transformers 4.39.3\n- Pytorch 2.2.2+cu121\n- Datasets 2.18.0\n- Tokenizers 0.15.2"
] |
null | transformers |
# Uploaded model
- **Developed by:** xiaoliy2
- **License:** apache-2.0
- **Finetuned from model :** unsloth/mistral-7b-instruct-v0.2-bnb-4bit
This mistral model was trained 2x faster with [Unsloth](https://github.com/unslothai/unsloth) and Huggingface's TRL library.
[<img src="https://raw.githubusercontent.com/unslothai/unsloth/main/images/unsloth%20made%20with%20love.png" width="200"/>](https://github.com/unslothai/unsloth)
| {"language": ["en"], "license": "apache-2.0", "tags": ["text-generation-inference", "transformers", "unsloth", "mistral", "trl"], "base_model": "unsloth/mistral-7b-instruct-v0.2-bnb-4bit"} | xiaoliy2/mistral-7b-instruct-ft-1 | null | [
"transformers",
"safetensors",
"text-generation-inference",
"unsloth",
"mistral",
"trl",
"en",
"base_model:unsloth/mistral-7b-instruct-v0.2-bnb-4bit",
"license:apache-2.0",
"endpoints_compatible",
"region:us"
] | null | 2024-04-18T08:43:20+00:00 | [] | [
"en"
] | TAGS
#transformers #safetensors #text-generation-inference #unsloth #mistral #trl #en #base_model-unsloth/mistral-7b-instruct-v0.2-bnb-4bit #license-apache-2.0 #endpoints_compatible #region-us
|
# Uploaded model
- Developed by: xiaoliy2
- License: apache-2.0
- Finetuned from model : unsloth/mistral-7b-instruct-v0.2-bnb-4bit
This mistral model was trained 2x faster with Unsloth and Huggingface's TRL library.
<img src="URL width="200"/>
| [
"# Uploaded model\n\n- Developed by: xiaoliy2\n- License: apache-2.0\n- Finetuned from model : unsloth/mistral-7b-instruct-v0.2-bnb-4bit\n\nThis mistral model was trained 2x faster with Unsloth and Huggingface's TRL library.\n\n<img src=\"URL width=\"200\"/>"
] | [
"TAGS\n#transformers #safetensors #text-generation-inference #unsloth #mistral #trl #en #base_model-unsloth/mistral-7b-instruct-v0.2-bnb-4bit #license-apache-2.0 #endpoints_compatible #region-us \n",
"# Uploaded model\n\n- Developed by: xiaoliy2\n- License: apache-2.0\n- Finetuned from model : unsloth/mistral-7b-instruct-v0.2-bnb-4bit\n\nThis mistral model was trained 2x faster with Unsloth and Huggingface's TRL library.\n\n<img src=\"URL width=\"200\"/>"
] |
token-classification | transformers |
# Model Card for Model ID
<!-- Provide a quick summary of what the model is/does. -->
## Model Details
### Model Description
<!-- Provide a longer summary of what this model is. -->
This is the model card of a 🤗 transformers model that has been pushed on the Hub. This model card has been automatically generated.
- **Developed by:** [More Information Needed]
- **Funded by [optional]:** [More Information Needed]
- **Shared by [optional]:** [More Information Needed]
- **Model type:** [More Information Needed]
- **Language(s) (NLP):** [More Information Needed]
- **License:** [More Information Needed]
- **Finetuned from model [optional]:** [More Information Needed]
### Model Sources [optional]
<!-- Provide the basic links for the model. -->
- **Repository:** [More Information Needed]
- **Paper [optional]:** [More Information Needed]
- **Demo [optional]:** [More Information Needed]
## Uses
<!-- Address questions around how the model is intended to be used, including the foreseeable users of the model and those affected by the model. -->
### Direct Use
<!-- This section is for the model use without fine-tuning or plugging into a larger ecosystem/app. -->
[More Information Needed]
### Downstream Use [optional]
<!-- This section is for the model use when fine-tuned for a task, or when plugged into a larger ecosystem/app -->
[More Information Needed]
### Out-of-Scope Use
<!-- This section addresses misuse, malicious use, and uses that the model will not work well for. -->
[More Information Needed]
## Bias, Risks, and Limitations
<!-- This section is meant to convey both technical and sociotechnical limitations. -->
[More Information Needed]
### Recommendations
<!-- This section is meant to convey recommendations with respect to the bias, risk, and technical limitations. -->
Users (both direct and downstream) should be made aware of the risks, biases and limitations of the model. More information needed for further recommendations.
## How to Get Started with the Model
Use the code below to get started with the model.
[More Information Needed]
## Training Details
### Training Data
<!-- This should link to a Dataset Card, perhaps with a short stub of information on what the training data is all about as well as documentation related to data pre-processing or additional filtering. -->
[More Information Needed]
### Training Procedure
<!-- This relates heavily to the Technical Specifications. Content here should link to that section when it is relevant to the training procedure. -->
#### Preprocessing [optional]
[More Information Needed]
#### Training Hyperparameters
- **Training regime:** [More Information Needed] <!--fp32, fp16 mixed precision, bf16 mixed precision, bf16 non-mixed precision, fp16 non-mixed precision, fp8 mixed precision -->
#### Speeds, Sizes, Times [optional]
<!-- This section provides information about throughput, start/end time, checkpoint size if relevant, etc. -->
[More Information Needed]
## Evaluation
<!-- This section describes the evaluation protocols and provides the results. -->
### Testing Data, Factors & Metrics
#### Testing Data
<!-- This should link to a Dataset Card if possible. -->
[More Information Needed]
#### Factors
<!-- These are the things the evaluation is disaggregating by, e.g., subpopulations or domains. -->
[More Information Needed]
#### Metrics
<!-- These are the evaluation metrics being used, ideally with a description of why. -->
[More Information Needed]
### Results
[More Information Needed]
#### Summary
## Model Examination [optional]
<!-- Relevant interpretability work for the model goes here -->
[More Information Needed]
## Environmental Impact
<!-- Total emissions (in grams of CO2eq) and additional considerations, such as electricity usage, go here. Edit the suggested text below accordingly -->
Carbon emissions can be estimated using the [Machine Learning Impact calculator](https://mlco2.github.io/impact#compute) presented in [Lacoste et al. (2019)](https://arxiv.org/abs/1910.09700).
- **Hardware Type:** [More Information Needed]
- **Hours used:** [More Information Needed]
- **Cloud Provider:** [More Information Needed]
- **Compute Region:** [More Information Needed]
- **Carbon Emitted:** [More Information Needed]
## Technical Specifications [optional]
### Model Architecture and Objective
[More Information Needed]
### Compute Infrastructure
[More Information Needed]
#### Hardware
[More Information Needed]
#### Software
[More Information Needed]
## Citation [optional]
<!-- If there is a paper or blog post introducing the model, the APA and Bibtex information for that should go in this section. -->
**BibTeX:**
[More Information Needed]
**APA:**
[More Information Needed]
## Glossary [optional]
<!-- If relevant, include terms and calculations in this section that can help readers understand the model or model card. -->
[More Information Needed]
## More Information [optional]
[More Information Needed]
## Model Card Authors [optional]
[More Information Needed]
## Model Card Contact
[More Information Needed]
| {"library_name": "transformers", "tags": []} | Tippawan/SNOMED-CT-NER-V.2-manually-CLASS | null | [
"transformers",
"safetensors",
"camembert",
"token-classification",
"arxiv:1910.09700",
"autotrain_compatible",
"endpoints_compatible",
"region:us"
] | null | 2024-04-18T08:44:20+00:00 | [
"1910.09700"
] | [] | TAGS
#transformers #safetensors #camembert #token-classification #arxiv-1910.09700 #autotrain_compatible #endpoints_compatible #region-us
|
# Model Card for Model ID
## Model Details
### Model Description
This is the model card of a transformers model that has been pushed on the Hub. This model card has been automatically generated.
- Developed by:
- Funded by [optional]:
- Shared by [optional]:
- Model type:
- Language(s) (NLP):
- License:
- Finetuned from model [optional]:
### Model Sources [optional]
- Repository:
- Paper [optional]:
- Demo [optional]:
## Uses
### Direct Use
### Downstream Use [optional]
### Out-of-Scope Use
## Bias, Risks, and Limitations
### Recommendations
Users (both direct and downstream) should be made aware of the risks, biases and limitations of the model. More information needed for further recommendations.
## How to Get Started with the Model
Use the code below to get started with the model.
## Training Details
### Training Data
### Training Procedure
#### Preprocessing [optional]
#### Training Hyperparameters
- Training regime:
#### Speeds, Sizes, Times [optional]
## Evaluation
### Testing Data, Factors & Metrics
#### Testing Data
#### Factors
#### Metrics
### Results
#### Summary
## Model Examination [optional]
## Environmental Impact
Carbon emissions can be estimated using the Machine Learning Impact calculator presented in Lacoste et al. (2019).
- Hardware Type:
- Hours used:
- Cloud Provider:
- Compute Region:
- Carbon Emitted:
## Technical Specifications [optional]
### Model Architecture and Objective
### Compute Infrastructure
#### Hardware
#### Software
[optional]
BibTeX:
APA:
## Glossary [optional]
## More Information [optional]
## Model Card Authors [optional]
## Model Card Contact
| [
"# Model Card for Model ID",
"## Model Details",
"### Model Description\n\n\n\nThis is the model card of a transformers model that has been pushed on the Hub. This model card has been automatically generated.\n\n- Developed by: \n- Funded by [optional]: \n- Shared by [optional]: \n- Model type: \n- Language(s) (NLP): \n- License: \n- Finetuned from model [optional]:",
"### Model Sources [optional]\n\n\n\n- Repository: \n- Paper [optional]: \n- Demo [optional]:",
"## Uses",
"### Direct Use",
"### Downstream Use [optional]",
"### Out-of-Scope Use",
"## Bias, Risks, and Limitations",
"### Recommendations\n\n\n\nUsers (both direct and downstream) should be made aware of the risks, biases and limitations of the model. More information needed for further recommendations.",
"## How to Get Started with the Model\n\nUse the code below to get started with the model.",
"## Training Details",
"### Training Data",
"### Training Procedure",
"#### Preprocessing [optional]",
"#### Training Hyperparameters\n\n- Training regime:",
"#### Speeds, Sizes, Times [optional]",
"## Evaluation",
"### Testing Data, Factors & Metrics",
"#### Testing Data",
"#### Factors",
"#### Metrics",
"### Results",
"#### Summary",
"## Model Examination [optional]",
"## Environmental Impact\n\n\n\nCarbon emissions can be estimated using the Machine Learning Impact calculator presented in Lacoste et al. (2019).\n\n- Hardware Type: \n- Hours used: \n- Cloud Provider: \n- Compute Region: \n- Carbon Emitted:",
"## Technical Specifications [optional]",
"### Model Architecture and Objective",
"### Compute Infrastructure",
"#### Hardware",
"#### Software\n\n\n\n[optional]\n\n\n\nBibTeX:\n\n\n\nAPA:",
"## Glossary [optional]",
"## More Information [optional]",
"## Model Card Authors [optional]",
"## Model Card Contact"
] | [
"TAGS\n#transformers #safetensors #camembert #token-classification #arxiv-1910.09700 #autotrain_compatible #endpoints_compatible #region-us \n",
"# Model Card for Model ID",
"## Model Details",
"### Model Description\n\n\n\nThis is the model card of a transformers model that has been pushed on the Hub. This model card has been automatically generated.\n\n- Developed by: \n- Funded by [optional]: \n- Shared by [optional]: \n- Model type: \n- Language(s) (NLP): \n- License: \n- Finetuned from model [optional]:",
"### Model Sources [optional]\n\n\n\n- Repository: \n- Paper [optional]: \n- Demo [optional]:",
"## Uses",
"### Direct Use",
"### Downstream Use [optional]",
"### Out-of-Scope Use",
"## Bias, Risks, and Limitations",
"### Recommendations\n\n\n\nUsers (both direct and downstream) should be made aware of the risks, biases and limitations of the model. More information needed for further recommendations.",
"## How to Get Started with the Model\n\nUse the code below to get started with the model.",
"## Training Details",
"### Training Data",
"### Training Procedure",
"#### Preprocessing [optional]",
"#### Training Hyperparameters\n\n- Training regime:",
"#### Speeds, Sizes, Times [optional]",
"## Evaluation",
"### Testing Data, Factors & Metrics",
"#### Testing Data",
"#### Factors",
"#### Metrics",
"### Results",
"#### Summary",
"## Model Examination [optional]",
"## Environmental Impact\n\n\n\nCarbon emissions can be estimated using the Machine Learning Impact calculator presented in Lacoste et al. (2019).\n\n- Hardware Type: \n- Hours used: \n- Cloud Provider: \n- Compute Region: \n- Carbon Emitted:",
"## Technical Specifications [optional]",
"### Model Architecture and Objective",
"### Compute Infrastructure",
"#### Hardware",
"#### Software\n\n\n\n[optional]\n\n\n\nBibTeX:\n\n\n\nAPA:",
"## Glossary [optional]",
"## More Information [optional]",
"## Model Card Authors [optional]",
"## Model Card Contact"
] |
text-generation | transformers |
# Model Card for Model ID
<!-- Provide a quick summary of what the model is/does. -->
## Model Details
### Model Description
<!-- Provide a longer summary of what this model is. -->
This is the model card of a 🤗 transformers model that has been pushed on the Hub. This model card has been automatically generated.
- **Developed by:** [More Information Needed]
- **Funded by [optional]:** [More Information Needed]
- **Shared by [optional]:** [More Information Needed]
- **Model type:** [More Information Needed]
- **Language(s) (NLP):** [More Information Needed]
- **License:** [More Information Needed]
- **Finetuned from model [optional]:** [More Information Needed]
### Model Sources [optional]
<!-- Provide the basic links for the model. -->
- **Repository:** [More Information Needed]
- **Paper [optional]:** [More Information Needed]
- **Demo [optional]:** [More Information Needed]
## Uses
<!-- Address questions around how the model is intended to be used, including the foreseeable users of the model and those affected by the model. -->
### Direct Use
<!-- This section is for the model use without fine-tuning or plugging into a larger ecosystem/app. -->
[More Information Needed]
### Downstream Use [optional]
<!-- This section is for the model use when fine-tuned for a task, or when plugged into a larger ecosystem/app -->
[More Information Needed]
### Out-of-Scope Use
<!-- This section addresses misuse, malicious use, and uses that the model will not work well for. -->
[More Information Needed]
## Bias, Risks, and Limitations
<!-- This section is meant to convey both technical and sociotechnical limitations. -->
[More Information Needed]
### Recommendations
<!-- This section is meant to convey recommendations with respect to the bias, risk, and technical limitations. -->
Users (both direct and downstream) should be made aware of the risks, biases and limitations of the model. More information needed for further recommendations.
## How to Get Started with the Model
Use the code below to get started with the model.
[More Information Needed]
## Training Details
### Training Data
<!-- This should link to a Dataset Card, perhaps with a short stub of information on what the training data is all about as well as documentation related to data pre-processing or additional filtering. -->
[More Information Needed]
### Training Procedure
<!-- This relates heavily to the Technical Specifications. Content here should link to that section when it is relevant to the training procedure. -->
#### Preprocessing [optional]
[More Information Needed]
#### Training Hyperparameters
- **Training regime:** [More Information Needed] <!--fp32, fp16 mixed precision, bf16 mixed precision, bf16 non-mixed precision, fp16 non-mixed precision, fp8 mixed precision -->
#### Speeds, Sizes, Times [optional]
<!-- This section provides information about throughput, start/end time, checkpoint size if relevant, etc. -->
[More Information Needed]
## Evaluation
<!-- This section describes the evaluation protocols and provides the results. -->
### Testing Data, Factors & Metrics
#### Testing Data
<!-- This should link to a Dataset Card if possible. -->
[More Information Needed]
#### Factors
<!-- These are the things the evaluation is disaggregating by, e.g., subpopulations or domains. -->
[More Information Needed]
#### Metrics
<!-- These are the evaluation metrics being used, ideally with a description of why. -->
[More Information Needed]
### Results
[More Information Needed]
#### Summary
## Model Examination [optional]
<!-- Relevant interpretability work for the model goes here -->
[More Information Needed]
## Environmental Impact
<!-- Total emissions (in grams of CO2eq) and additional considerations, such as electricity usage, go here. Edit the suggested text below accordingly -->
Carbon emissions can be estimated using the [Machine Learning Impact calculator](https://mlco2.github.io/impact#compute) presented in [Lacoste et al. (2019)](https://arxiv.org/abs/1910.09700).
- **Hardware Type:** [More Information Needed]
- **Hours used:** [More Information Needed]
- **Cloud Provider:** [More Information Needed]
- **Compute Region:** [More Information Needed]
- **Carbon Emitted:** [More Information Needed]
## Technical Specifications [optional]
### Model Architecture and Objective
[More Information Needed]
### Compute Infrastructure
[More Information Needed]
#### Hardware
[More Information Needed]
#### Software
[More Information Needed]
## Citation [optional]
<!-- If there is a paper or blog post introducing the model, the APA and Bibtex information for that should go in this section. -->
**BibTeX:**
[More Information Needed]
**APA:**
[More Information Needed]
## Glossary [optional]
<!-- If relevant, include terms and calculations in this section that can help readers understand the model or model card. -->
[More Information Needed]
## More Information [optional]
[More Information Needed]
## Model Card Authors [optional]
[More Information Needed]
## Model Card Contact
[More Information Needed] | {"library_name": "transformers", "tags": []} | yuiseki/gemma-2b-aya-ja-v0.1 | null | [
"transformers",
"safetensors",
"gemma",
"text-generation",
"arxiv:1910.09700",
"autotrain_compatible",
"endpoints_compatible",
"text-generation-inference",
"region:us"
] | null | 2024-04-18T08:44:54+00:00 | [
"1910.09700"
] | [] | TAGS
#transformers #safetensors #gemma #text-generation #arxiv-1910.09700 #autotrain_compatible #endpoints_compatible #text-generation-inference #region-us
|
# Model Card for Model ID
## Model Details
### Model Description
This is the model card of a transformers model that has been pushed on the Hub. This model card has been automatically generated.
- Developed by:
- Funded by [optional]:
- Shared by [optional]:
- Model type:
- Language(s) (NLP):
- License:
- Finetuned from model [optional]:
### Model Sources [optional]
- Repository:
- Paper [optional]:
- Demo [optional]:
## Uses
### Direct Use
### Downstream Use [optional]
### Out-of-Scope Use
## Bias, Risks, and Limitations
### Recommendations
Users (both direct and downstream) should be made aware of the risks, biases and limitations of the model. More information needed for further recommendations.
## How to Get Started with the Model
Use the code below to get started with the model.
## Training Details
### Training Data
### Training Procedure
#### Preprocessing [optional]
#### Training Hyperparameters
- Training regime:
#### Speeds, Sizes, Times [optional]
## Evaluation
### Testing Data, Factors & Metrics
#### Testing Data
#### Factors
#### Metrics
### Results
#### Summary
## Model Examination [optional]
## Environmental Impact
Carbon emissions can be estimated using the Machine Learning Impact calculator presented in Lacoste et al. (2019).
- Hardware Type:
- Hours used:
- Cloud Provider:
- Compute Region:
- Carbon Emitted:
## Technical Specifications [optional]
### Model Architecture and Objective
### Compute Infrastructure
#### Hardware
#### Software
[optional]
BibTeX:
APA:
## Glossary [optional]
## More Information [optional]
## Model Card Authors [optional]
## Model Card Contact
| [
"# Model Card for Model ID",
"## Model Details",
"### Model Description\n\n\n\nThis is the model card of a transformers model that has been pushed on the Hub. This model card has been automatically generated.\n\n- Developed by: \n- Funded by [optional]: \n- Shared by [optional]: \n- Model type: \n- Language(s) (NLP): \n- License: \n- Finetuned from model [optional]:",
"### Model Sources [optional]\n\n\n\n- Repository: \n- Paper [optional]: \n- Demo [optional]:",
"## Uses",
"### Direct Use",
"### Downstream Use [optional]",
"### Out-of-Scope Use",
"## Bias, Risks, and Limitations",
"### Recommendations\n\n\n\nUsers (both direct and downstream) should be made aware of the risks, biases and limitations of the model. More information needed for further recommendations.",
"## How to Get Started with the Model\n\nUse the code below to get started with the model.",
"## Training Details",
"### Training Data",
"### Training Procedure",
"#### Preprocessing [optional]",
"#### Training Hyperparameters\n\n- Training regime:",
"#### Speeds, Sizes, Times [optional]",
"## Evaluation",
"### Testing Data, Factors & Metrics",
"#### Testing Data",
"#### Factors",
"#### Metrics",
"### Results",
"#### Summary",
"## Model Examination [optional]",
"## Environmental Impact\n\n\n\nCarbon emissions can be estimated using the Machine Learning Impact calculator presented in Lacoste et al. (2019).\n\n- Hardware Type: \n- Hours used: \n- Cloud Provider: \n- Compute Region: \n- Carbon Emitted:",
"## Technical Specifications [optional]",
"### Model Architecture and Objective",
"### Compute Infrastructure",
"#### Hardware",
"#### Software\n\n\n\n[optional]\n\n\n\nBibTeX:\n\n\n\nAPA:",
"## Glossary [optional]",
"## More Information [optional]",
"## Model Card Authors [optional]",
"## Model Card Contact"
] | [
"TAGS\n#transformers #safetensors #gemma #text-generation #arxiv-1910.09700 #autotrain_compatible #endpoints_compatible #text-generation-inference #region-us \n",
"# Model Card for Model ID",
"## Model Details",
"### Model Description\n\n\n\nThis is the model card of a transformers model that has been pushed on the Hub. This model card has been automatically generated.\n\n- Developed by: \n- Funded by [optional]: \n- Shared by [optional]: \n- Model type: \n- Language(s) (NLP): \n- License: \n- Finetuned from model [optional]:",
"### Model Sources [optional]\n\n\n\n- Repository: \n- Paper [optional]: \n- Demo [optional]:",
"## Uses",
"### Direct Use",
"### Downstream Use [optional]",
"### Out-of-Scope Use",
"## Bias, Risks, and Limitations",
"### Recommendations\n\n\n\nUsers (both direct and downstream) should be made aware of the risks, biases and limitations of the model. More information needed for further recommendations.",
"## How to Get Started with the Model\n\nUse the code below to get started with the model.",
"## Training Details",
"### Training Data",
"### Training Procedure",
"#### Preprocessing [optional]",
"#### Training Hyperparameters\n\n- Training regime:",
"#### Speeds, Sizes, Times [optional]",
"## Evaluation",
"### Testing Data, Factors & Metrics",
"#### Testing Data",
"#### Factors",
"#### Metrics",
"### Results",
"#### Summary",
"## Model Examination [optional]",
"## Environmental Impact\n\n\n\nCarbon emissions can be estimated using the Machine Learning Impact calculator presented in Lacoste et al. (2019).\n\n- Hardware Type: \n- Hours used: \n- Cloud Provider: \n- Compute Region: \n- Carbon Emitted:",
"## Technical Specifications [optional]",
"### Model Architecture and Objective",
"### Compute Infrastructure",
"#### Hardware",
"#### Software\n\n\n\n[optional]\n\n\n\nBibTeX:\n\n\n\nAPA:",
"## Glossary [optional]",
"## More Information [optional]",
"## Model Card Authors [optional]",
"## Model Card Contact"
] |
text-to-image | diffusers |
<!-- This model card has been generated automatically according to the information the training script had access to. You
should probably proofread and complete it, then remove this comment. -->
# SDXL LoRA DreamBooth - linxin020826/lora-trained-xl
<Gallery />
## Model description
These are linxin020826/lora-trained-xl LoRA adaption weights for stabilityai/stable-diffusion-xl-base-1.0.
The weights were trained using [DreamBooth](https://dreambooth.github.io/).
LoRA for the text encoder was enabled: False.
Special VAE used for training: madebyollin/sdxl-vae-fp16-fix.
## Trigger words
You should use a photo of sks robot to trigger the image generation.
## Download model
Weights for this model are available in Safetensors format.
[Download](linxin020826/lora-trained-xl/tree/main) them in the Files & versions tab.
## Intended uses & limitations
#### How to use
```python
# TODO: add an example code snippet for running this diffusion pipeline
```
#### Limitations and bias
[TODO: provide examples of latent issues and potential remediations]
## Training details
[TODO: describe the data used to train the model] | {"license": "openrail++", "library_name": "diffusers", "tags": ["text-to-image", "text-to-image", "diffusers-training", "diffusers", "lora", "template:sd-lora", "stable-diffusion-xl", "stable-diffusion-xl-diffusers"], "base_model": "stabilityai/stable-diffusion-xl-base-1.0", "instance_prompt": "a photo of sks robot", "widget": [{"text": "a photo of sks robot", "output": {"url": "image_0.png"}}, {"text": "a photo of sks robot", "output": {"url": "image_1.png"}}, {"text": "a photo of sks robot", "output": {"url": "image_2.png"}}, {"text": "a photo of sks robot", "output": {"url": "image_3.png"}}]} | linxin020826/lora-trained-xl | null | [
"diffusers",
"text-to-image",
"diffusers-training",
"lora",
"template:sd-lora",
"stable-diffusion-xl",
"stable-diffusion-xl-diffusers",
"base_model:stabilityai/stable-diffusion-xl-base-1.0",
"license:openrail++",
"region:us"
] | null | 2024-04-18T08:44:54+00:00 | [] | [] | TAGS
#diffusers #text-to-image #diffusers-training #lora #template-sd-lora #stable-diffusion-xl #stable-diffusion-xl-diffusers #base_model-stabilityai/stable-diffusion-xl-base-1.0 #license-openrail++ #region-us
|
# SDXL LoRA DreamBooth - linxin020826/lora-trained-xl
<Gallery />
## Model description
These are linxin020826/lora-trained-xl LoRA adaption weights for stabilityai/stable-diffusion-xl-base-1.0.
The weights were trained using DreamBooth.
LoRA for the text encoder was enabled: False.
Special VAE used for training: madebyollin/sdxl-vae-fp16-fix.
## Trigger words
You should use a photo of sks robot to trigger the image generation.
## Download model
Weights for this model are available in Safetensors format.
Download them in the Files & versions tab.
## Intended uses & limitations
#### How to use
#### Limitations and bias
[TODO: provide examples of latent issues and potential remediations]
## Training details
[TODO: describe the data used to train the model] | [
"# SDXL LoRA DreamBooth - linxin020826/lora-trained-xl\n\n<Gallery />",
"## Model description\n\nThese are linxin020826/lora-trained-xl LoRA adaption weights for stabilityai/stable-diffusion-xl-base-1.0.\n\nThe weights were trained using DreamBooth.\n\nLoRA for the text encoder was enabled: False.\n\nSpecial VAE used for training: madebyollin/sdxl-vae-fp16-fix.",
"## Trigger words\n\nYou should use a photo of sks robot to trigger the image generation.",
"## Download model\n\nWeights for this model are available in Safetensors format.\n\nDownload them in the Files & versions tab.",
"## Intended uses & limitations",
"#### How to use",
"#### Limitations and bias\n\n[TODO: provide examples of latent issues and potential remediations]",
"## Training details\n\n[TODO: describe the data used to train the model]"
] | [
"TAGS\n#diffusers #text-to-image #diffusers-training #lora #template-sd-lora #stable-diffusion-xl #stable-diffusion-xl-diffusers #base_model-stabilityai/stable-diffusion-xl-base-1.0 #license-openrail++ #region-us \n",
"# SDXL LoRA DreamBooth - linxin020826/lora-trained-xl\n\n<Gallery />",
"## Model description\n\nThese are linxin020826/lora-trained-xl LoRA adaption weights for stabilityai/stable-diffusion-xl-base-1.0.\n\nThe weights were trained using DreamBooth.\n\nLoRA for the text encoder was enabled: False.\n\nSpecial VAE used for training: madebyollin/sdxl-vae-fp16-fix.",
"## Trigger words\n\nYou should use a photo of sks robot to trigger the image generation.",
"## Download model\n\nWeights for this model are available in Safetensors format.\n\nDownload them in the Files & versions tab.",
"## Intended uses & limitations",
"#### How to use",
"#### Limitations and bias\n\n[TODO: provide examples of latent issues and potential remediations]",
"## Training details\n\n[TODO: describe the data used to train the model]"
] |
text-generation | transformers |
<!-- This model card has been generated automatically according to the information the Trainer had access to. You
should probably proofread and complete it, then remove this comment. -->
# 0.0_ablation_6iters_iter_4
This model is a fine-tuned version of [ShenaoZ/0.0_ablation_6iters_iter_3](https://huggingface.co/ShenaoZ/0.0_ablation_6iters_iter_3) on the updated and the original datasets.
## Model description
More information needed
## Intended uses & limitations
More information needed
## Training and evaluation data
More information needed
## Training procedure
### Training hyperparameters
The following hyperparameters were used during training:
- learning_rate: 5e-07
- train_batch_size: 8
- eval_batch_size: 8
- seed: 42
- distributed_type: multi-GPU
- num_devices: 8
- gradient_accumulation_steps: 2
- total_train_batch_size: 128
- total_eval_batch_size: 64
- optimizer: Adam with betas=(0.9,0.999) and epsilon=1e-08
- lr_scheduler_type: cosine
- lr_scheduler_warmup_ratio: 0.1
- num_epochs: 1
### Training results
### Framework versions
- Transformers 4.36.2
- Pytorch 2.1.2+cu121
- Datasets 2.14.6
- Tokenizers 0.15.2
| {"license": "mit", "tags": ["alignment-handbook", "generated_from_trainer", "trl", "dpo", "generated_from_trainer"], "datasets": ["updated", "original"], "base_model": "ShenaoZ/0.0_ablation_6iters_iter_3", "model-index": [{"name": "0.0_ablation_6iters_iter_4", "results": []}]} | ShenaoZ/0.0_ablation_6iters_iter_4 | null | [
"transformers",
"safetensors",
"mistral",
"text-generation",
"alignment-handbook",
"generated_from_trainer",
"trl",
"dpo",
"conversational",
"dataset:updated",
"dataset:original",
"base_model:ShenaoZ/0.0_ablation_6iters_iter_3",
"license:mit",
"autotrain_compatible",
"endpoints_compatible",
"text-generation-inference",
"region:us"
] | null | 2024-04-18T08:46:37+00:00 | [] | [] | TAGS
#transformers #safetensors #mistral #text-generation #alignment-handbook #generated_from_trainer #trl #dpo #conversational #dataset-updated #dataset-original #base_model-ShenaoZ/0.0_ablation_6iters_iter_3 #license-mit #autotrain_compatible #endpoints_compatible #text-generation-inference #region-us
|
# 0.0_ablation_6iters_iter_4
This model is a fine-tuned version of ShenaoZ/0.0_ablation_6iters_iter_3 on the updated and the original datasets.
## Model description
More information needed
## Intended uses & limitations
More information needed
## Training and evaluation data
More information needed
## Training procedure
### Training hyperparameters
The following hyperparameters were used during training:
- learning_rate: 5e-07
- train_batch_size: 8
- eval_batch_size: 8
- seed: 42
- distributed_type: multi-GPU
- num_devices: 8
- gradient_accumulation_steps: 2
- total_train_batch_size: 128
- total_eval_batch_size: 64
- optimizer: Adam with betas=(0.9,0.999) and epsilon=1e-08
- lr_scheduler_type: cosine
- lr_scheduler_warmup_ratio: 0.1
- num_epochs: 1
### Training results
### Framework versions
- Transformers 4.36.2
- Pytorch 2.1.2+cu121
- Datasets 2.14.6
- Tokenizers 0.15.2
| [
"# 0.0_ablation_6iters_iter_4\n\nThis model is a fine-tuned version of ShenaoZ/0.0_ablation_6iters_iter_3 on the updated and the original datasets.",
"## Model description\n\nMore information needed",
"## Intended uses & limitations\n\nMore information needed",
"## Training and evaluation data\n\nMore information needed",
"## Training procedure",
"### Training hyperparameters\n\nThe following hyperparameters were used during training:\n- learning_rate: 5e-07\n- train_batch_size: 8\n- eval_batch_size: 8\n- seed: 42\n- distributed_type: multi-GPU\n- num_devices: 8\n- gradient_accumulation_steps: 2\n- total_train_batch_size: 128\n- total_eval_batch_size: 64\n- optimizer: Adam with betas=(0.9,0.999) and epsilon=1e-08\n- lr_scheduler_type: cosine\n- lr_scheduler_warmup_ratio: 0.1\n- num_epochs: 1",
"### Training results",
"### Framework versions\n\n- Transformers 4.36.2\n- Pytorch 2.1.2+cu121\n- Datasets 2.14.6\n- Tokenizers 0.15.2"
] | [
"TAGS\n#transformers #safetensors #mistral #text-generation #alignment-handbook #generated_from_trainer #trl #dpo #conversational #dataset-updated #dataset-original #base_model-ShenaoZ/0.0_ablation_6iters_iter_3 #license-mit #autotrain_compatible #endpoints_compatible #text-generation-inference #region-us \n",
"# 0.0_ablation_6iters_iter_4\n\nThis model is a fine-tuned version of ShenaoZ/0.0_ablation_6iters_iter_3 on the updated and the original datasets.",
"## Model description\n\nMore information needed",
"## Intended uses & limitations\n\nMore information needed",
"## Training and evaluation data\n\nMore information needed",
"## Training procedure",
"### Training hyperparameters\n\nThe following hyperparameters were used during training:\n- learning_rate: 5e-07\n- train_batch_size: 8\n- eval_batch_size: 8\n- seed: 42\n- distributed_type: multi-GPU\n- num_devices: 8\n- gradient_accumulation_steps: 2\n- total_train_batch_size: 128\n- total_eval_batch_size: 64\n- optimizer: Adam with betas=(0.9,0.999) and epsilon=1e-08\n- lr_scheduler_type: cosine\n- lr_scheduler_warmup_ratio: 0.1\n- num_epochs: 1",
"### Training results",
"### Framework versions\n\n- Transformers 4.36.2\n- Pytorch 2.1.2+cu121\n- Datasets 2.14.6\n- Tokenizers 0.15.2"
] |
text-classification | transformers |
<!-- This model card has been generated automatically according to the information the Trainer had access to. You
should probably proofread and complete it, then remove this comment. -->
# imdb-spoiler-bertOrigDataset
This model is a fine-tuned version of [google-bert/bert-base-uncased](https://huggingface.co/google-bert/bert-base-uncased) on the None dataset.
It achieves the following results on the evaluation set:
- Loss: 0.7518
- Accuracy: 0.6965
- Recall: 0.6703
- Precision: 0.7074
- F1: 0.6883
## Model description
More information needed
## Intended uses & limitations
More information needed
## Training and evaluation data
More information needed
## Training procedure
### Training hyperparameters
The following hyperparameters were used during training:
- learning_rate: 2e-05
- train_batch_size: 8
- eval_batch_size: 8
- seed: 42
- optimizer: Adam with betas=(0.9,0.999) and epsilon=1e-08
- lr_scheduler_type: linear
- num_epochs: 3
### Training results
| Training Loss | Epoch | Step | Validation Loss | Accuracy | Recall | Precision | F1 |
|:-------------:|:-----:|:-----:|:---------------:|:--------:|:------:|:---------:|:------:|
| 0.6534 | 0.12 | 500 | 0.6320 | 0.6492 | 0.563 | 0.6804 | 0.6161 |
| 0.6063 | 0.25 | 1000 | 0.6027 | 0.6705 | 0.8425 | 0.6269 | 0.7189 |
| 0.6026 | 0.38 | 1500 | 0.6011 | 0.6584 | 0.4462 | 0.7751 | 0.5664 |
| 0.5868 | 0.5 | 2000 | 0.5930 | 0.6964 | 0.747 | 0.6783 | 0.7110 |
| 0.577 | 0.62 | 2500 | 0.5836 | 0.6941 | 0.7558 | 0.6728 | 0.7119 |
| 0.579 | 0.75 | 3000 | 0.6345 | 0.6961 | 0.7638 | 0.6728 | 0.7154 |
| 0.5776 | 0.88 | 3500 | 0.5909 | 0.6861 | 0.8055 | 0.6503 | 0.7196 |
| 0.5694 | 1.0 | 4000 | 0.5655 | 0.7023 | 0.6683 | 0.7170 | 0.6918 |
| 0.5312 | 1.12 | 4500 | 0.5695 | 0.7055 | 0.663 | 0.7246 | 0.6924 |
| 0.5205 | 1.25 | 5000 | 0.5820 | 0.7001 | 0.7552 | 0.6803 | 0.7158 |
| 0.514 | 1.38 | 5500 | 0.6030 | 0.7027 | 0.617 | 0.7447 | 0.6749 |
| 0.5016 | 1.5 | 6000 | 0.5826 | 0.7054 | 0.7 | 0.7076 | 0.7038 |
| 0.5062 | 1.62 | 6500 | 0.5947 | 0.6966 | 0.6165 | 0.7341 | 0.6702 |
| 0.5064 | 1.75 | 7000 | 0.5775 | 0.7027 | 0.637 | 0.7334 | 0.6818 |
| 0.4958 | 1.88 | 7500 | 0.5792 | 0.7104 | 0.6865 | 0.7209 | 0.7033 |
| 0.4952 | 2.0 | 8000 | 0.5890 | 0.7135 | 0.668 | 0.7349 | 0.6998 |
| 0.3958 | 2.12 | 8500 | 0.7381 | 0.6957 | 0.59 | 0.7483 | 0.6598 |
| 0.4058 | 2.25 | 9000 | 0.7212 | 0.6985 | 0.6707 | 0.7102 | 0.6899 |
| 0.3944 | 2.38 | 9500 | 0.7299 | 0.689 | 0.6132 | 0.7227 | 0.6635 |
| 0.3787 | 2.5 | 10000 | 0.7261 | 0.691 | 0.6368 | 0.7142 | 0.6733 |
| 0.3831 | 2.62 | 10500 | 0.7347 | 0.6935 | 0.6365 | 0.7184 | 0.6750 |
| 0.3788 | 2.75 | 11000 | 0.7983 | 0.6937 | 0.6455 | 0.7144 | 0.6782 |
| 0.3741 | 2.88 | 11500 | 0.7642 | 0.6931 | 0.6408 | 0.7157 | 0.6762 |
| 0.3763 | 3.0 | 12000 | 0.7518 | 0.6965 | 0.6703 | 0.7074 | 0.6883 |
### Framework versions
- Transformers 4.39.3
- Pytorch 2.2.2+cu121
- Datasets 2.18.0
- Tokenizers 0.15.2
| {"license": "apache-2.0", "tags": ["generated_from_trainer"], "metrics": ["accuracy", "recall", "precision", "f1"], "base_model": "google-bert/bert-base-uncased", "model-index": [{"name": "imdb-spoiler-bertOrigDataset", "results": []}]} | Zritze/imdb-spoiler-bertOrigDataset | null | [
"transformers",
"safetensors",
"bert",
"text-classification",
"generated_from_trainer",
"base_model:google-bert/bert-base-uncased",
"license:apache-2.0",
"autotrain_compatible",
"endpoints_compatible",
"region:us"
] | null | 2024-04-18T08:48:17+00:00 | [] | [] | TAGS
#transformers #safetensors #bert #text-classification #generated_from_trainer #base_model-google-bert/bert-base-uncased #license-apache-2.0 #autotrain_compatible #endpoints_compatible #region-us
| imdb-spoiler-bertOrigDataset
============================
This model is a fine-tuned version of google-bert/bert-base-uncased on the None dataset.
It achieves the following results on the evaluation set:
* Loss: 0.7518
* Accuracy: 0.6965
* Recall: 0.6703
* Precision: 0.7074
* F1: 0.6883
Model description
-----------------
More information needed
Intended uses & limitations
---------------------------
More information needed
Training and evaluation data
----------------------------
More information needed
Training procedure
------------------
### Training hyperparameters
The following hyperparameters were used during training:
* learning\_rate: 2e-05
* train\_batch\_size: 8
* eval\_batch\_size: 8
* seed: 42
* optimizer: Adam with betas=(0.9,0.999) and epsilon=1e-08
* lr\_scheduler\_type: linear
* num\_epochs: 3
### Training results
### Framework versions
* Transformers 4.39.3
* Pytorch 2.2.2+cu121
* Datasets 2.18.0
* Tokenizers 0.15.2
| [
"### Training hyperparameters\n\n\nThe following hyperparameters were used during training:\n\n\n* learning\\_rate: 2e-05\n* train\\_batch\\_size: 8\n* eval\\_batch\\_size: 8\n* seed: 42\n* optimizer: Adam with betas=(0.9,0.999) and epsilon=1e-08\n* lr\\_scheduler\\_type: linear\n* num\\_epochs: 3",
"### Training results",
"### Framework versions\n\n\n* Transformers 4.39.3\n* Pytorch 2.2.2+cu121\n* Datasets 2.18.0\n* Tokenizers 0.15.2"
] | [
"TAGS\n#transformers #safetensors #bert #text-classification #generated_from_trainer #base_model-google-bert/bert-base-uncased #license-apache-2.0 #autotrain_compatible #endpoints_compatible #region-us \n",
"### Training hyperparameters\n\n\nThe following hyperparameters were used during training:\n\n\n* learning\\_rate: 2e-05\n* train\\_batch\\_size: 8\n* eval\\_batch\\_size: 8\n* seed: 42\n* optimizer: Adam with betas=(0.9,0.999) and epsilon=1e-08\n* lr\\_scheduler\\_type: linear\n* num\\_epochs: 3",
"### Training results",
"### Framework versions\n\n\n* Transformers 4.39.3\n* Pytorch 2.2.2+cu121\n* Datasets 2.18.0\n* Tokenizers 0.15.2"
] |
text-generation | transformers |
<!-- This model card has been generated automatically according to the information the Trainer had access to. You
should probably proofread and complete it, then remove this comment. -->
# Mixtral-8x22B-v0.1-Instruct-sft-en-de
A full SFT of [mistral-community/Mixtral-8x22B-v0.1](https://huggingface.co/mistral-community/Mixtral-8x22B-v0.1) using a mix of English and German instruction data.
There is also an ORPO-trained version: [maxidl/Mixtral-8x22B-v0.1-capybara-orpo-en-de](https://huggingface.co/maxidl/Mixtral-8x22B-v0.1-capybara-orpo-en-de)
## Dataset
|source|#examples|
|---|---|
teknium/OpenHermes-2.5 |1001551
maxidl/OpenOrca-gpt4-de |119559
maxidl/MathInstruct-de |56793
maxidl/Capybara-de |15991
maxidl/math-prm-800k-de |12298
maxidl/wikihow-de |10103
maxidl/no_robots-de |9500
maxidl/lima-de |1030
### Training hyperparameters
The following hyperparameters were used during training:
- learning_rate: 1e-05
- train_batch_size: 1
- eval_batch_size: 8
- seed: 42
- distributed_type: multi-GPU
- num_devices: 64
- total_train_batch_size: 64
- total_eval_batch_size: 512
- optimizer: Adam with betas=(0.9,0.999) and epsilon=1e-08
- lr_scheduler_type: cosine
- lr_scheduler_warmup_steps: 50
- num_epochs: 3
### Training results
### Framework versions
- Transformers 4.39.3
- Pytorch 2.1.2
- Datasets 2.18.0
- Tokenizers 0.15.2 | {"language": ["en", "de"], "license": "apache-2.0", "tags": ["alignment-handbook", "generated_from_trainer"], "datasets": ["maxidl/instruct-en-de", "teknium/OpenHermes-2.5"], "base_model": "mistral-community/Mixtral-8x22B-v0.1", "model-index": [{"name": "Mixtral-8x22B-v0.1-Instruct-sft-en-de", "results": []}]} | maxidl/Mixtral-8x22B-v0.1-Instruct-sft-en-de | null | [
"transformers",
"tensorboard",
"safetensors",
"mixtral",
"text-generation",
"alignment-handbook",
"generated_from_trainer",
"conversational",
"en",
"de",
"dataset:maxidl/instruct-en-de",
"dataset:teknium/OpenHermes-2.5",
"base_model:mistral-community/Mixtral-8x22B-v0.1",
"license:apache-2.0",
"autotrain_compatible",
"endpoints_compatible",
"text-generation-inference",
"region:us"
] | null | 2024-04-18T08:51:11+00:00 | [] | [
"en",
"de"
] | TAGS
#transformers #tensorboard #safetensors #mixtral #text-generation #alignment-handbook #generated_from_trainer #conversational #en #de #dataset-maxidl/instruct-en-de #dataset-teknium/OpenHermes-2.5 #base_model-mistral-community/Mixtral-8x22B-v0.1 #license-apache-2.0 #autotrain_compatible #endpoints_compatible #text-generation-inference #region-us
| Mixtral-8x22B-v0.1-Instruct-sft-en-de
=====================================
A full SFT of mistral-community/Mixtral-8x22B-v0.1 using a mix of English and German instruction data.
There is also an ORPO-trained version: maxidl/Mixtral-8x22B-v0.1-capybara-orpo-en-de
Dataset
-------
### Training hyperparameters
The following hyperparameters were used during training:
* learning\_rate: 1e-05
* train\_batch\_size: 1
* eval\_batch\_size: 8
* seed: 42
* distributed\_type: multi-GPU
* num\_devices: 64
* total\_train\_batch\_size: 64
* total\_eval\_batch\_size: 512
* optimizer: Adam with betas=(0.9,0.999) and epsilon=1e-08
* lr\_scheduler\_type: cosine
* lr\_scheduler\_warmup\_steps: 50
* num\_epochs: 3
### Training results
### Framework versions
* Transformers 4.39.3
* Pytorch 2.1.2
* Datasets 2.18.0
* Tokenizers 0.15.2
| [
"### Training hyperparameters\n\n\nThe following hyperparameters were used during training:\n\n\n* learning\\_rate: 1e-05\n* train\\_batch\\_size: 1\n* eval\\_batch\\_size: 8\n* seed: 42\n* distributed\\_type: multi-GPU\n* num\\_devices: 64\n* total\\_train\\_batch\\_size: 64\n* total\\_eval\\_batch\\_size: 512\n* optimizer: Adam with betas=(0.9,0.999) and epsilon=1e-08\n* lr\\_scheduler\\_type: cosine\n* lr\\_scheduler\\_warmup\\_steps: 50\n* num\\_epochs: 3",
"### Training results",
"### Framework versions\n\n\n* Transformers 4.39.3\n* Pytorch 2.1.2\n* Datasets 2.18.0\n* Tokenizers 0.15.2"
] | [
"TAGS\n#transformers #tensorboard #safetensors #mixtral #text-generation #alignment-handbook #generated_from_trainer #conversational #en #de #dataset-maxidl/instruct-en-de #dataset-teknium/OpenHermes-2.5 #base_model-mistral-community/Mixtral-8x22B-v0.1 #license-apache-2.0 #autotrain_compatible #endpoints_compatible #text-generation-inference #region-us \n",
"### Training hyperparameters\n\n\nThe following hyperparameters were used during training:\n\n\n* learning\\_rate: 1e-05\n* train\\_batch\\_size: 1\n* eval\\_batch\\_size: 8\n* seed: 42\n* distributed\\_type: multi-GPU\n* num\\_devices: 64\n* total\\_train\\_batch\\_size: 64\n* total\\_eval\\_batch\\_size: 512\n* optimizer: Adam with betas=(0.9,0.999) and epsilon=1e-08\n* lr\\_scheduler\\_type: cosine\n* lr\\_scheduler\\_warmup\\_steps: 50\n* num\\_epochs: 3",
"### Training results",
"### Framework versions\n\n\n* Transformers 4.39.3\n* Pytorch 2.1.2\n* Datasets 2.18.0\n* Tokenizers 0.15.2"
] |
image-text-to-text | transformers |
# Idefics2-8B-SFT

Idefics2-8B-SFT is SFT fine-tune of [HuggingFaceM4/idefics2-8b](https://huggingface.co/HuggingFaceM4/idefics2-8b) on 35k [TextVQA dataset](https://huggingface.co/datasets/textvqa). Training was performed on RTX A5000 for 10 hrs. Wandb report:

This fine-tuned model achieves a Levenshtein score of 82.29%.
# Model Summary
- **Developed by:** Hugging Face
- **Model type:** Multi-modal model (image+text)
- **Language(s) (NLP):** en
- **License:** Apache 2.0
- **Parent Models:** [google/siglip-so400m-patch14-384](https://huggingface.co/google/siglip-so400m-patch14-384) and [mistralai/Mistral-7B-v0.1](https://huggingface.co/mistralai/Mistral-7B-v0.1)
## 💻 Usage
```python
processor = AutoProcessor.from_pretrained("Syed-Hasan-8503/Idefics2-8B-SFT")
model = AutoModelForVision2Seq.from_pretrained("Syed-Hasan-8503/Idefics2-8B-SFT",).to(DEVICE)
# Create inputs
messages = [
{
"role": "user",
"content": [
{"type": "image"},
{"type": "text", "text": "What do we see in this image?"},
]
},
{
"role": "assistant",
"content": [
{"type": "text", "text": "In this image, we can see the city of New York, and more specifically the Statue of Liberty."},
]
},
{
"role": "user",
"content": [
{"type": "image"},
{"type": "text", "text": "And how about this image?"},
]
},
]
prompt = processor.apply_chat_template(messages, add_generation_prompt=True)
inputs = processor(text=prompt, images=[image1, image2], return_tensors="pt")
inputs = {k: v.to(DEVICE) for k, v in inputs.items()}
# Generate
generated_ids = model.generate(**inputs, max_new_tokens=500)
generated_texts = processor.batch_decode(generated_ids, skip_special_tokens=True)
print(generated_texts)
# ['User: What do we see in this image? \nAssistant: In this image, we can see the city of New York, and more specifically the Statue of Liberty. \nUser: And how about this image? \nAssistant: In this image we can see buildings, trees, lights, water and sky.']
```
## 🏆 Evaluation
Coming Soon! | {"language": ["en"], "license": "apache-2.0", "library_name": "transformers", "tags": ["multimodal", "vision", "image-text-to-text"], "datasets": ["lmms-lab/textvqa"]} | Syed-Hasan-8503/Idefics2-8B-SFT | null | [
"transformers",
"safetensors",
"idefics2",
"pretraining",
"multimodal",
"vision",
"image-text-to-text",
"en",
"dataset:lmms-lab/textvqa",
"license:apache-2.0",
"endpoints_compatible",
"region:us"
] | null | 2024-04-18T08:52:39+00:00 | [] | [
"en"
] | TAGS
#transformers #safetensors #idefics2 #pretraining #multimodal #vision #image-text-to-text #en #dataset-lmms-lab/textvqa #license-apache-2.0 #endpoints_compatible #region-us
|
# Idefics2-8B-SFT
!image/jpeg
Idefics2-8B-SFT is SFT fine-tune of HuggingFaceM4/idefics2-8b on 35k TextVQA dataset. Training was performed on RTX A5000 for 10 hrs. Wandb report:
!image/png
This fine-tuned model achieves a Levenshtein score of 82.29%.
# Model Summary
- Developed by: Hugging Face
- Model type: Multi-modal model (image+text)
- Language(s) (NLP): en
- License: Apache 2.0
- Parent Models: google/siglip-so400m-patch14-384 and mistralai/Mistral-7B-v0.1
## Usage
## Evaluation
Coming Soon! | [
"# Idefics2-8B-SFT\n\n!image/jpeg\n\nIdefics2-8B-SFT is SFT fine-tune of HuggingFaceM4/idefics2-8b on 35k TextVQA dataset. Training was performed on RTX A5000 for 10 hrs. Wandb report:\n\n\n!image/png\n\nThis fine-tuned model achieves a Levenshtein score of 82.29%.",
"# Model Summary\n\n- Developed by: Hugging Face\n- Model type: Multi-modal model (image+text)\n- Language(s) (NLP): en\n- License: Apache 2.0\n- Parent Models: google/siglip-so400m-patch14-384 and mistralai/Mistral-7B-v0.1",
"## Usage",
"## Evaluation\nComing Soon!"
] | [
"TAGS\n#transformers #safetensors #idefics2 #pretraining #multimodal #vision #image-text-to-text #en #dataset-lmms-lab/textvqa #license-apache-2.0 #endpoints_compatible #region-us \n",
"# Idefics2-8B-SFT\n\n!image/jpeg\n\nIdefics2-8B-SFT is SFT fine-tune of HuggingFaceM4/idefics2-8b on 35k TextVQA dataset. Training was performed on RTX A5000 for 10 hrs. Wandb report:\n\n\n!image/png\n\nThis fine-tuned model achieves a Levenshtein score of 82.29%.",
"# Model Summary\n\n- Developed by: Hugging Face\n- Model type: Multi-modal model (image+text)\n- Language(s) (NLP): en\n- License: Apache 2.0\n- Parent Models: google/siglip-so400m-patch14-384 and mistralai/Mistral-7B-v0.1",
"## Usage",
"## Evaluation\nComing Soon!"
] |
null | peft |
<!-- This model card has been generated automatically according to the information the Trainer had access to. You
should probably proofread and complete it, then remove this comment. -->
# whisper-small-finetuned-finetuned
This model is a fine-tuned version of [openai/whisper-small](https://huggingface.co/openai/whisper-small) on the common_voice_16_1 dataset.
It achieves the following results on the evaluation set:
- eval_loss: 3.2831
- eval_wer: 109.0909
- eval_runtime: 18.9581
- eval_samples_per_second: 0.211
- eval_steps_per_second: 0.053
- epoch: 99.01
- step: 100
## Model description
More information needed
## Intended uses & limitations
More information needed
## Training and evaluation data
More information needed
## Training procedure
### Training hyperparameters
The following hyperparameters were used during training:
- learning_rate: 0.001
- train_batch_size: 96
- eval_batch_size: 64
- seed: 42
- optimizer: Adam with betas=(0.9,0.999) and epsilon=1e-08
- lr_scheduler_type: linear
- training_steps: 100
- mixed_precision_training: Native AMP
### Framework versions
- PEFT 0.9.0
- Transformers 4.39.2
- Pytorch 2.2.2+cu121
- Datasets 2.17.0
- Tokenizers 0.15.2 | {"license": "apache-2.0", "library_name": "peft", "tags": ["generated_from_trainer"], "datasets": ["common_voice_16_1"], "base_model": "openai/whisper-small", "model-index": [{"name": "whisper-small-finetuned-finetuned", "results": []}]} | KevinKibe/whisper-small-finetuned-finetuned | null | [
"peft",
"pytorch",
"safetensors",
"generated_from_trainer",
"dataset:common_voice_16_1",
"base_model:openai/whisper-small",
"license:apache-2.0",
"region:us"
] | null | 2024-04-18T08:53:57+00:00 | [] | [] | TAGS
#peft #pytorch #safetensors #generated_from_trainer #dataset-common_voice_16_1 #base_model-openai/whisper-small #license-apache-2.0 #region-us
|
# whisper-small-finetuned-finetuned
This model is a fine-tuned version of openai/whisper-small on the common_voice_16_1 dataset.
It achieves the following results on the evaluation set:
- eval_loss: 3.2831
- eval_wer: 109.0909
- eval_runtime: 18.9581
- eval_samples_per_second: 0.211
- eval_steps_per_second: 0.053
- epoch: 99.01
- step: 100
## Model description
More information needed
## Intended uses & limitations
More information needed
## Training and evaluation data
More information needed
## Training procedure
### Training hyperparameters
The following hyperparameters were used during training:
- learning_rate: 0.001
- train_batch_size: 96
- eval_batch_size: 64
- seed: 42
- optimizer: Adam with betas=(0.9,0.999) and epsilon=1e-08
- lr_scheduler_type: linear
- training_steps: 100
- mixed_precision_training: Native AMP
### Framework versions
- PEFT 0.9.0
- Transformers 4.39.2
- Pytorch 2.2.2+cu121
- Datasets 2.17.0
- Tokenizers 0.15.2 | [
"# whisper-small-finetuned-finetuned\n\nThis model is a fine-tuned version of openai/whisper-small on the common_voice_16_1 dataset.\nIt achieves the following results on the evaluation set:\n- eval_loss: 3.2831\n- eval_wer: 109.0909\n- eval_runtime: 18.9581\n- eval_samples_per_second: 0.211\n- eval_steps_per_second: 0.053\n- epoch: 99.01\n- step: 100",
"## Model description\n\nMore information needed",
"## Intended uses & limitations\n\nMore information needed",
"## Training and evaluation data\n\nMore information needed",
"## Training procedure",
"### Training hyperparameters\n\nThe following hyperparameters were used during training:\n- learning_rate: 0.001\n- train_batch_size: 96\n- eval_batch_size: 64\n- seed: 42\n- optimizer: Adam with betas=(0.9,0.999) and epsilon=1e-08\n- lr_scheduler_type: linear\n- training_steps: 100\n- mixed_precision_training: Native AMP",
"### Framework versions\n\n- PEFT 0.9.0\n- Transformers 4.39.2\n- Pytorch 2.2.2+cu121\n- Datasets 2.17.0\n- Tokenizers 0.15.2"
] | [
"TAGS\n#peft #pytorch #safetensors #generated_from_trainer #dataset-common_voice_16_1 #base_model-openai/whisper-small #license-apache-2.0 #region-us \n",
"# whisper-small-finetuned-finetuned\n\nThis model is a fine-tuned version of openai/whisper-small on the common_voice_16_1 dataset.\nIt achieves the following results on the evaluation set:\n- eval_loss: 3.2831\n- eval_wer: 109.0909\n- eval_runtime: 18.9581\n- eval_samples_per_second: 0.211\n- eval_steps_per_second: 0.053\n- epoch: 99.01\n- step: 100",
"## Model description\n\nMore information needed",
"## Intended uses & limitations\n\nMore information needed",
"## Training and evaluation data\n\nMore information needed",
"## Training procedure",
"### Training hyperparameters\n\nThe following hyperparameters were used during training:\n- learning_rate: 0.001\n- train_batch_size: 96\n- eval_batch_size: 64\n- seed: 42\n- optimizer: Adam with betas=(0.9,0.999) and epsilon=1e-08\n- lr_scheduler_type: linear\n- training_steps: 100\n- mixed_precision_training: Native AMP",
"### Framework versions\n\n- PEFT 0.9.0\n- Transformers 4.39.2\n- Pytorch 2.2.2+cu121\n- Datasets 2.17.0\n- Tokenizers 0.15.2"
] |
null | transformers |
# Model Card for Model ID
<!-- Provide a quick summary of what the model is/does. -->
## Model Details
### Model Description
<!-- Provide a longer summary of what this model is. -->
This is the model card of a 🤗 transformers model that has been pushed on the Hub. This model card has been automatically generated.
- **Developed by:** [More Information Needed]
- **Funded by [optional]:** [More Information Needed]
- **Shared by [optional]:** [More Information Needed]
- **Model type:** [More Information Needed]
- **Language(s) (NLP):** [More Information Needed]
- **License:** [More Information Needed]
- **Finetuned from model [optional]:** [More Information Needed]
### Model Sources [optional]
<!-- Provide the basic links for the model. -->
- **Repository:** [More Information Needed]
- **Paper [optional]:** [More Information Needed]
- **Demo [optional]:** [More Information Needed]
## Uses
<!-- Address questions around how the model is intended to be used, including the foreseeable users of the model and those affected by the model. -->
### Direct Use
<!-- This section is for the model use without fine-tuning or plugging into a larger ecosystem/app. -->
[More Information Needed]
### Downstream Use [optional]
<!-- This section is for the model use when fine-tuned for a task, or when plugged into a larger ecosystem/app -->
[More Information Needed]
### Out-of-Scope Use
<!-- This section addresses misuse, malicious use, and uses that the model will not work well for. -->
[More Information Needed]
## Bias, Risks, and Limitations
<!-- This section is meant to convey both technical and sociotechnical limitations. -->
[More Information Needed]
### Recommendations
<!-- This section is meant to convey recommendations with respect to the bias, risk, and technical limitations. -->
Users (both direct and downstream) should be made aware of the risks, biases and limitations of the model. More information needed for further recommendations.
## How to Get Started with the Model
Use the code below to get started with the model.
[More Information Needed]
## Training Details
### Training Data
<!-- This should link to a Dataset Card, perhaps with a short stub of information on what the training data is all about as well as documentation related to data pre-processing or additional filtering. -->
[More Information Needed]
### Training Procedure
<!-- This relates heavily to the Technical Specifications. Content here should link to that section when it is relevant to the training procedure. -->
#### Preprocessing [optional]
[More Information Needed]
#### Training Hyperparameters
- **Training regime:** [More Information Needed] <!--fp32, fp16 mixed precision, bf16 mixed precision, bf16 non-mixed precision, fp16 non-mixed precision, fp8 mixed precision -->
#### Speeds, Sizes, Times [optional]
<!-- This section provides information about throughput, start/end time, checkpoint size if relevant, etc. -->
[More Information Needed]
## Evaluation
<!-- This section describes the evaluation protocols and provides the results. -->
### Testing Data, Factors & Metrics
#### Testing Data
<!-- This should link to a Dataset Card if possible. -->
[More Information Needed]
#### Factors
<!-- These are the things the evaluation is disaggregating by, e.g., subpopulations or domains. -->
[More Information Needed]
#### Metrics
<!-- These are the evaluation metrics being used, ideally with a description of why. -->
[More Information Needed]
### Results
[More Information Needed]
#### Summary
## Model Examination [optional]
<!-- Relevant interpretability work for the model goes here -->
[More Information Needed]
## Environmental Impact
<!-- Total emissions (in grams of CO2eq) and additional considerations, such as electricity usage, go here. Edit the suggested text below accordingly -->
Carbon emissions can be estimated using the [Machine Learning Impact calculator](https://mlco2.github.io/impact#compute) presented in [Lacoste et al. (2019)](https://arxiv.org/abs/1910.09700).
- **Hardware Type:** [More Information Needed]
- **Hours used:** [More Information Needed]
- **Cloud Provider:** [More Information Needed]
- **Compute Region:** [More Information Needed]
- **Carbon Emitted:** [More Information Needed]
## Technical Specifications [optional]
### Model Architecture and Objective
[More Information Needed]
### Compute Infrastructure
[More Information Needed]
#### Hardware
[More Information Needed]
#### Software
[More Information Needed]
## Citation [optional]
<!-- If there is a paper or blog post introducing the model, the APA and Bibtex information for that should go in this section. -->
**BibTeX:**
[More Information Needed]
**APA:**
[More Information Needed]
## Glossary [optional]
<!-- If relevant, include terms and calculations in this section that can help readers understand the model or model card. -->
[More Information Needed]
## More Information [optional]
[More Information Needed]
## Model Card Authors [optional]
[More Information Needed]
## Model Card Contact
[More Information Needed] | {"library_name": "transformers", "tags": []} | Reihaneh/wav2vec2_fy_nl_en_common_voice_12 | null | [
"transformers",
"arxiv:1910.09700",
"endpoints_compatible",
"region:us"
] | null | 2024-04-18T08:54:02+00:00 | [
"1910.09700"
] | [] | TAGS
#transformers #arxiv-1910.09700 #endpoints_compatible #region-us
|
# Model Card for Model ID
## Model Details
### Model Description
This is the model card of a transformers model that has been pushed on the Hub. This model card has been automatically generated.
- Developed by:
- Funded by [optional]:
- Shared by [optional]:
- Model type:
- Language(s) (NLP):
- License:
- Finetuned from model [optional]:
### Model Sources [optional]
- Repository:
- Paper [optional]:
- Demo [optional]:
## Uses
### Direct Use
### Downstream Use [optional]
### Out-of-Scope Use
## Bias, Risks, and Limitations
### Recommendations
Users (both direct and downstream) should be made aware of the risks, biases and limitations of the model. More information needed for further recommendations.
## How to Get Started with the Model
Use the code below to get started with the model.
## Training Details
### Training Data
### Training Procedure
#### Preprocessing [optional]
#### Training Hyperparameters
- Training regime:
#### Speeds, Sizes, Times [optional]
## Evaluation
### Testing Data, Factors & Metrics
#### Testing Data
#### Factors
#### Metrics
### Results
#### Summary
## Model Examination [optional]
## Environmental Impact
Carbon emissions can be estimated using the Machine Learning Impact calculator presented in Lacoste et al. (2019).
- Hardware Type:
- Hours used:
- Cloud Provider:
- Compute Region:
- Carbon Emitted:
## Technical Specifications [optional]
### Model Architecture and Objective
### Compute Infrastructure
#### Hardware
#### Software
[optional]
BibTeX:
APA:
## Glossary [optional]
## More Information [optional]
## Model Card Authors [optional]
## Model Card Contact
| [
"# Model Card for Model ID",
"## Model Details",
"### Model Description\n\n\n\nThis is the model card of a transformers model that has been pushed on the Hub. This model card has been automatically generated.\n\n- Developed by: \n- Funded by [optional]: \n- Shared by [optional]: \n- Model type: \n- Language(s) (NLP): \n- License: \n- Finetuned from model [optional]:",
"### Model Sources [optional]\n\n\n\n- Repository: \n- Paper [optional]: \n- Demo [optional]:",
"## Uses",
"### Direct Use",
"### Downstream Use [optional]",
"### Out-of-Scope Use",
"## Bias, Risks, and Limitations",
"### Recommendations\n\n\n\nUsers (both direct and downstream) should be made aware of the risks, biases and limitations of the model. More information needed for further recommendations.",
"## How to Get Started with the Model\n\nUse the code below to get started with the model.",
"## Training Details",
"### Training Data",
"### Training Procedure",
"#### Preprocessing [optional]",
"#### Training Hyperparameters\n\n- Training regime:",
"#### Speeds, Sizes, Times [optional]",
"## Evaluation",
"### Testing Data, Factors & Metrics",
"#### Testing Data",
"#### Factors",
"#### Metrics",
"### Results",
"#### Summary",
"## Model Examination [optional]",
"## Environmental Impact\n\n\n\nCarbon emissions can be estimated using the Machine Learning Impact calculator presented in Lacoste et al. (2019).\n\n- Hardware Type: \n- Hours used: \n- Cloud Provider: \n- Compute Region: \n- Carbon Emitted:",
"## Technical Specifications [optional]",
"### Model Architecture and Objective",
"### Compute Infrastructure",
"#### Hardware",
"#### Software\n\n\n\n[optional]\n\n\n\nBibTeX:\n\n\n\nAPA:",
"## Glossary [optional]",
"## More Information [optional]",
"## Model Card Authors [optional]",
"## Model Card Contact"
] | [
"TAGS\n#transformers #arxiv-1910.09700 #endpoints_compatible #region-us \n",
"# Model Card for Model ID",
"## Model Details",
"### Model Description\n\n\n\nThis is the model card of a transformers model that has been pushed on the Hub. This model card has been automatically generated.\n\n- Developed by: \n- Funded by [optional]: \n- Shared by [optional]: \n- Model type: \n- Language(s) (NLP): \n- License: \n- Finetuned from model [optional]:",
"### Model Sources [optional]\n\n\n\n- Repository: \n- Paper [optional]: \n- Demo [optional]:",
"## Uses",
"### Direct Use",
"### Downstream Use [optional]",
"### Out-of-Scope Use",
"## Bias, Risks, and Limitations",
"### Recommendations\n\n\n\nUsers (both direct and downstream) should be made aware of the risks, biases and limitations of the model. More information needed for further recommendations.",
"## How to Get Started with the Model\n\nUse the code below to get started with the model.",
"## Training Details",
"### Training Data",
"### Training Procedure",
"#### Preprocessing [optional]",
"#### Training Hyperparameters\n\n- Training regime:",
"#### Speeds, Sizes, Times [optional]",
"## Evaluation",
"### Testing Data, Factors & Metrics",
"#### Testing Data",
"#### Factors",
"#### Metrics",
"### Results",
"#### Summary",
"## Model Examination [optional]",
"## Environmental Impact\n\n\n\nCarbon emissions can be estimated using the Machine Learning Impact calculator presented in Lacoste et al. (2019).\n\n- Hardware Type: \n- Hours used: \n- Cloud Provider: \n- Compute Region: \n- Carbon Emitted:",
"## Technical Specifications [optional]",
"### Model Architecture and Objective",
"### Compute Infrastructure",
"#### Hardware",
"#### Software\n\n\n\n[optional]\n\n\n\nBibTeX:\n\n\n\nAPA:",
"## Glossary [optional]",
"## More Information [optional]",
"## Model Card Authors [optional]",
"## Model Card Contact"
] |
text-generation | null |
# CodeQwen1.5-7B-Chat-GGUF
- This is quantized version of [CodeQwen1.5-7B-Chat](https://huggingface.co/Qwen/CodeQwen1.5-7B-Chat) created using llama.cpp
## Model Description
CodeQwen1.5 is the Code-Specific version of Qwen1.5. It is a transformer-based decoder-only language model pretrained on a large amount of data of codes.
CodeQwen1.5 is based on Qwen1.5, a language model series including decoder language models of different model sizes. It is trained on 3 trillion tokens of data of codes, and it includes group query attention (GQA) for efficient inference.
* Strong code generation capabilities and competitve performance across a series of benchmarks;
* Supporting long context understanding and generation with the context length of 64K tokens;
* Supporting 92 coding languages
* Excellent performance in text-to-SQL, bug fix, etc.
For more details, please refer to Qwen [blog post](https://qwenlm.github.io/blog/codeqwen1.5/) and [GitHub repo](https://github.com/QwenLM/Qwen1.5).
## Requirements
The code of Qwen1.5 has been in the latest Hugging face transformers and we advise you to install `transformers>=4.37.0`, or you might encounter the following error:
```
KeyError: 'qwen2'.
```
## Tips
* If you encounter code switching or other bad cases, we advise you to use our provided hyper-parameters in `generation_config.json`.
`
| {"language": ["en"], "license": "other", "tags": ["chat"], "license_name": "tongyi-qianwen", "license_link": "https://huggingface.co/Qwen/CodeQwen1.5-7B-Chat/blob/main/LICENSE", "pipeline_tag": "text-generation", "base_model": "Qwen/CodeQwen1.5-7B-Chat"} | QuantFactory/CodeQwen1.5-7B-Chat-GGUF | null | [
"gguf",
"chat",
"text-generation",
"en",
"base_model:Qwen/CodeQwen1.5-7B-Chat",
"license:other",
"region:us"
] | null | 2024-04-18T08:54:10+00:00 | [] | [
"en"
] | TAGS
#gguf #chat #text-generation #en #base_model-Qwen/CodeQwen1.5-7B-Chat #license-other #region-us
|
# CodeQwen1.5-7B-Chat-GGUF
- This is quantized version of CodeQwen1.5-7B-Chat created using URL
## Model Description
CodeQwen1.5 is the Code-Specific version of Qwen1.5. It is a transformer-based decoder-only language model pretrained on a large amount of data of codes.
CodeQwen1.5 is based on Qwen1.5, a language model series including decoder language models of different model sizes. It is trained on 3 trillion tokens of data of codes, and it includes group query attention (GQA) for efficient inference.
* Strong code generation capabilities and competitve performance across a series of benchmarks;
* Supporting long context understanding and generation with the context length of 64K tokens;
* Supporting 92 coding languages
* Excellent performance in text-to-SQL, bug fix, etc.
For more details, please refer to Qwen blog post and GitHub repo.
## Requirements
The code of Qwen1.5 has been in the latest Hugging face transformers and we advise you to install 'transformers>=4.37.0', or you might encounter the following error:
## Tips
* If you encounter code switching or other bad cases, we advise you to use our provided hyper-parameters in 'generation_config.json'.
'
| [
"# CodeQwen1.5-7B-Chat-GGUF\n- This is quantized version of CodeQwen1.5-7B-Chat created using URL",
"## Model Description\n\nCodeQwen1.5 is the Code-Specific version of Qwen1.5. It is a transformer-based decoder-only language model pretrained on a large amount of data of codes. \nCodeQwen1.5 is based on Qwen1.5, a language model series including decoder language models of different model sizes. It is trained on 3 trillion tokens of data of codes, and it includes group query attention (GQA) for efficient inference.\n\n* Strong code generation capabilities and competitve performance across a series of benchmarks;\n* Supporting long context understanding and generation with the context length of 64K tokens;\n* Supporting 92 coding languages\n* Excellent performance in text-to-SQL, bug fix, etc.\n\nFor more details, please refer to Qwen blog post and GitHub repo.",
"## Requirements\nThe code of Qwen1.5 has been in the latest Hugging face transformers and we advise you to install 'transformers>=4.37.0', or you might encounter the following error:",
"## Tips\n\n* If you encounter code switching or other bad cases, we advise you to use our provided hyper-parameters in 'generation_config.json'.\n\n'"
] | [
"TAGS\n#gguf #chat #text-generation #en #base_model-Qwen/CodeQwen1.5-7B-Chat #license-other #region-us \n",
"# CodeQwen1.5-7B-Chat-GGUF\n- This is quantized version of CodeQwen1.5-7B-Chat created using URL",
"## Model Description\n\nCodeQwen1.5 is the Code-Specific version of Qwen1.5. It is a transformer-based decoder-only language model pretrained on a large amount of data of codes. \nCodeQwen1.5 is based on Qwen1.5, a language model series including decoder language models of different model sizes. It is trained on 3 trillion tokens of data of codes, and it includes group query attention (GQA) for efficient inference.\n\n* Strong code generation capabilities and competitve performance across a series of benchmarks;\n* Supporting long context understanding and generation with the context length of 64K tokens;\n* Supporting 92 coding languages\n* Excellent performance in text-to-SQL, bug fix, etc.\n\nFor more details, please refer to Qwen blog post and GitHub repo.",
"## Requirements\nThe code of Qwen1.5 has been in the latest Hugging face transformers and we advise you to install 'transformers>=4.37.0', or you might encounter the following error:",
"## Tips\n\n* If you encounter code switching or other bad cases, we advise you to use our provided hyper-parameters in 'generation_config.json'.\n\n'"
] |
reinforcement-learning | stable-baselines3 |
# **PPO** Agent playing **LunarLander-v2**
This is a trained model of a **PPO** agent playing **LunarLander-v2**
using the [stable-baselines3 library](https://github.com/DLR-RM/stable-baselines3).
## Usage (with Stable-baselines3)
TODO: Add your code
```python
from stable_baselines3 import ...
from huggingface_sb3 import load_from_hub
...
```
| {"library_name": "stable-baselines3", "tags": ["LunarLander-v2", "deep-reinforcement-learning", "reinforcement-learning", "stable-baselines3"], "model-index": [{"name": "PPO", "results": [{"task": {"type": "reinforcement-learning", "name": "reinforcement-learning"}, "dataset": {"name": "LunarLander-v2", "type": "LunarLander-v2"}, "metrics": [{"type": "mean_reward", "value": "-299.24 +/- 60.78", "name": "mean_reward", "verified": false}]}]}]} | Koyiljon/ppo-LunarLander-v2 | null | [
"stable-baselines3",
"LunarLander-v2",
"deep-reinforcement-learning",
"reinforcement-learning",
"model-index",
"region:us"
] | null | 2024-04-18T08:56:54+00:00 | [] | [] | TAGS
#stable-baselines3 #LunarLander-v2 #deep-reinforcement-learning #reinforcement-learning #model-index #region-us
|
# PPO Agent playing LunarLander-v2
This is a trained model of a PPO agent playing LunarLander-v2
using the stable-baselines3 library.
## Usage (with Stable-baselines3)
TODO: Add your code
| [
"# PPO Agent playing LunarLander-v2\nThis is a trained model of a PPO agent playing LunarLander-v2\nusing the stable-baselines3 library.",
"## Usage (with Stable-baselines3)\nTODO: Add your code"
] | [
"TAGS\n#stable-baselines3 #LunarLander-v2 #deep-reinforcement-learning #reinforcement-learning #model-index #region-us \n",
"# PPO Agent playing LunarLander-v2\nThis is a trained model of a PPO agent playing LunarLander-v2\nusing the stable-baselines3 library.",
"## Usage (with Stable-baselines3)\nTODO: Add your code"
] |
null | adapter-transformers |
# Adapter `jgrc3/pfeiffer_adapter_classification_noPre_10epochs` for roberta-base
An [adapter](https://adapterhub.ml) for the `roberta-base` model that was trained on the [BigTMiami/amazon_helpfulness](https://huggingface.co/datasets/BigTMiami/amazon_helpfulness/) dataset and includes a prediction head for classification.
This adapter was created for usage with the **[Adapters](https://github.com/Adapter-Hub/adapters)** library.
## Usage
First, install `adapters`:
```
pip install -U adapters
```
Now, the adapter can be loaded and activated like this:
```python
from adapters import AutoAdapterModel
model = AutoAdapterModel.from_pretrained("roberta-base")
adapter_name = model.load_adapter("jgrc3/pfeiffer_adapter_classification_noPre_10epochs", source="hf", set_active=True)
```
## Architecture & Training
<!-- Add some description here -->
## Evaluation results
<!-- Add some description here -->
## Citation
<!-- Add some description here --> | {"tags": ["adapter-transformers", "roberta"], "datasets": ["BigTMiami/amazon_helpfulness"]} | jgrc3/pfeiffer_adapter_classification_noPre_10epochs | null | [
"adapter-transformers",
"roberta",
"dataset:BigTMiami/amazon_helpfulness",
"region:us"
] | null | 2024-04-18T08:57:08+00:00 | [] | [] | TAGS
#adapter-transformers #roberta #dataset-BigTMiami/amazon_helpfulness #region-us
|
# Adapter 'jgrc3/pfeiffer_adapter_classification_noPre_10epochs' for roberta-base
An adapter for the 'roberta-base' model that was trained on the BigTMiami/amazon_helpfulness dataset and includes a prediction head for classification.
This adapter was created for usage with the Adapters library.
## Usage
First, install 'adapters':
Now, the adapter can be loaded and activated like this:
## Architecture & Training
## Evaluation results
| [
"# Adapter 'jgrc3/pfeiffer_adapter_classification_noPre_10epochs' for roberta-base\n\nAn adapter for the 'roberta-base' model that was trained on the BigTMiami/amazon_helpfulness dataset and includes a prediction head for classification.\n\nThis adapter was created for usage with the Adapters library.",
"## Usage\n\nFirst, install 'adapters':\n\n\n\nNow, the adapter can be loaded and activated like this:",
"## Architecture & Training",
"## Evaluation results"
] | [
"TAGS\n#adapter-transformers #roberta #dataset-BigTMiami/amazon_helpfulness #region-us \n",
"# Adapter 'jgrc3/pfeiffer_adapter_classification_noPre_10epochs' for roberta-base\n\nAn adapter for the 'roberta-base' model that was trained on the BigTMiami/amazon_helpfulness dataset and includes a prediction head for classification.\n\nThis adapter was created for usage with the Adapters library.",
"## Usage\n\nFirst, install 'adapters':\n\n\n\nNow, the adapter can be loaded and activated like this:",
"## Architecture & Training",
"## Evaluation results"
] |
null | fastai |
# Amazing!
🥳 Congratulations on hosting your fastai model on the Hugging Face Hub!
# Some next steps
1. Fill out this model card with more information (see the template below and the [documentation here](https://huggingface.co/docs/hub/model-repos))!
2. Create a demo in Gradio or Streamlit using 🤗 Spaces ([documentation here](https://huggingface.co/docs/hub/spaces)).
3. Join the fastai community on the [Fastai Discord](https://discord.com/invite/YKrxeNn)!
Greetings fellow fastlearner 🤝! Don't forget to delete this content from your model card.
---
# Model card
## Model description
More information needed
## Intended uses & limitations
More information needed
## Training and evaluation data
More information needed
| {"tags": ["fastai"]} | sergheevadrian/group8-million-song-model | null | [
"fastai",
"region:us"
] | null | 2024-04-18T09:00:05+00:00 | [] | [] | TAGS
#fastai #region-us
|
# Amazing!
Congratulations on hosting your fastai model on the Hugging Face Hub!
# Some next steps
1. Fill out this model card with more information (see the template below and the documentation here)!
2. Create a demo in Gradio or Streamlit using Spaces (documentation here).
3. Join the fastai community on the Fastai Discord!
Greetings fellow fastlearner ! Don't forget to delete this content from your model card.
---
# Model card
## Model description
More information needed
## Intended uses & limitations
More information needed
## Training and evaluation data
More information needed
| [
"# Amazing!\n\n Congratulations on hosting your fastai model on the Hugging Face Hub!",
"# Some next steps\n1. Fill out this model card with more information (see the template below and the documentation here)!\n\n2. Create a demo in Gradio or Streamlit using Spaces (documentation here).\n\n3. Join the fastai community on the Fastai Discord!\n\nGreetings fellow fastlearner ! Don't forget to delete this content from your model card.\n\n\n---",
"# Model card",
"## Model description\nMore information needed",
"## Intended uses & limitations\nMore information needed",
"## Training and evaluation data\nMore information needed"
] | [
"TAGS\n#fastai #region-us \n",
"# Amazing!\n\n Congratulations on hosting your fastai model on the Hugging Face Hub!",
"# Some next steps\n1. Fill out this model card with more information (see the template below and the documentation here)!\n\n2. Create a demo in Gradio or Streamlit using Spaces (documentation here).\n\n3. Join the fastai community on the Fastai Discord!\n\nGreetings fellow fastlearner ! Don't forget to delete this content from your model card.\n\n\n---",
"# Model card",
"## Model description\nMore information needed",
"## Intended uses & limitations\nMore information needed",
"## Training and evaluation data\nMore information needed"
] |
null | peft |
# Model Card for Model ID
<!-- Provide a quick summary of what the model is/does. -->
## Model Details
### Model Description
<!-- Provide a longer summary of what this model is. -->
- **Developed by:** [More Information Needed]
- **Funded by [optional]:** [More Information Needed]
- **Shared by [optional]:** [More Information Needed]
- **Model type:** [More Information Needed]
- **Language(s) (NLP):** [More Information Needed]
- **License:** [More Information Needed]
- **Finetuned from model [optional]:** [More Information Needed]
### Model Sources [optional]
<!-- Provide the basic links for the model. -->
- **Repository:** [More Information Needed]
- **Paper [optional]:** [More Information Needed]
- **Demo [optional]:** [More Information Needed]
## Uses
<!-- Address questions around how the model is intended to be used, including the foreseeable users of the model and those affected by the model. -->
### Direct Use
<!-- This section is for the model use without fine-tuning or plugging into a larger ecosystem/app. -->
[More Information Needed]
### Downstream Use [optional]
<!-- This section is for the model use when fine-tuned for a task, or when plugged into a larger ecosystem/app -->
[More Information Needed]
### Out-of-Scope Use
<!-- This section addresses misuse, malicious use, and uses that the model will not work well for. -->
[More Information Needed]
## Bias, Risks, and Limitations
<!-- This section is meant to convey both technical and sociotechnical limitations. -->
[More Information Needed]
### Recommendations
<!-- This section is meant to convey recommendations with respect to the bias, risk, and technical limitations. -->
Users (both direct and downstream) should be made aware of the risks, biases and limitations of the model. More information needed for further recommendations.
## How to Get Started with the Model
Use the code below to get started with the model.
[More Information Needed]
## Training Details
### Training Data
<!-- This should link to a Dataset Card, perhaps with a short stub of information on what the training data is all about as well as documentation related to data pre-processing or additional filtering. -->
[More Information Needed]
### Training Procedure
<!-- This relates heavily to the Technical Specifications. Content here should link to that section when it is relevant to the training procedure. -->
#### Preprocessing [optional]
[More Information Needed]
#### Training Hyperparameters
- **Training regime:** [More Information Needed] <!--fp32, fp16 mixed precision, bf16 mixed precision, bf16 non-mixed precision, fp16 non-mixed precision, fp8 mixed precision -->
#### Speeds, Sizes, Times [optional]
<!-- This section provides information about throughput, start/end time, checkpoint size if relevant, etc. -->
[More Information Needed]
## Evaluation
<!-- This section describes the evaluation protocols and provides the results. -->
### Testing Data, Factors & Metrics
#### Testing Data
<!-- This should link to a Dataset Card if possible. -->
[More Information Needed]
#### Factors
<!-- These are the things the evaluation is disaggregating by, e.g., subpopulations or domains. -->
[More Information Needed]
#### Metrics
<!-- These are the evaluation metrics being used, ideally with a description of why. -->
[More Information Needed]
### Results
[More Information Needed]
#### Summary
## Model Examination [optional]
<!-- Relevant interpretability work for the model goes here -->
[More Information Needed]
## Environmental Impact
<!-- Total emissions (in grams of CO2eq) and additional considerations, such as electricity usage, go here. Edit the suggested text below accordingly -->
Carbon emissions can be estimated using the [Machine Learning Impact calculator](https://mlco2.github.io/impact#compute) presented in [Lacoste et al. (2019)](https://arxiv.org/abs/1910.09700).
- **Hardware Type:** [More Information Needed]
- **Hours used:** [More Information Needed]
- **Cloud Provider:** [More Information Needed]
- **Compute Region:** [More Information Needed]
- **Carbon Emitted:** [More Information Needed]
## Technical Specifications [optional]
### Model Architecture and Objective
[More Information Needed]
### Compute Infrastructure
[More Information Needed]
#### Hardware
[More Information Needed]
#### Software
[More Information Needed]
## Citation [optional]
<!-- If there is a paper or blog post introducing the model, the APA and Bibtex information for that should go in this section. -->
**BibTeX:**
[More Information Needed]
**APA:**
[More Information Needed]
## Glossary [optional]
<!-- If relevant, include terms and calculations in this section that can help readers understand the model or model card. -->
[More Information Needed]
## More Information [optional]
[More Information Needed]
## Model Card Authors [optional]
[More Information Needed]
## Model Card Contact
[More Information Needed]
### Framework versions
- PEFT 0.7.1 | {"library_name": "peft", "base_model": "Qwen/Qwen-7B-Chat"} | kyriemao/chatretriever | null | [
"peft",
"safetensors",
"arxiv:1910.09700",
"base_model:Qwen/Qwen-7B-Chat",
"region:us"
] | null | 2024-04-18T09:00:26+00:00 | [
"1910.09700"
] | [] | TAGS
#peft #safetensors #arxiv-1910.09700 #base_model-Qwen/Qwen-7B-Chat #region-us
|
# Model Card for Model ID
## Model Details
### Model Description
- Developed by:
- Funded by [optional]:
- Shared by [optional]:
- Model type:
- Language(s) (NLP):
- License:
- Finetuned from model [optional]:
### Model Sources [optional]
- Repository:
- Paper [optional]:
- Demo [optional]:
## Uses
### Direct Use
### Downstream Use [optional]
### Out-of-Scope Use
## Bias, Risks, and Limitations
### Recommendations
Users (both direct and downstream) should be made aware of the risks, biases and limitations of the model. More information needed for further recommendations.
## How to Get Started with the Model
Use the code below to get started with the model.
## Training Details
### Training Data
### Training Procedure
#### Preprocessing [optional]
#### Training Hyperparameters
- Training regime:
#### Speeds, Sizes, Times [optional]
## Evaluation
### Testing Data, Factors & Metrics
#### Testing Data
#### Factors
#### Metrics
### Results
#### Summary
## Model Examination [optional]
## Environmental Impact
Carbon emissions can be estimated using the Machine Learning Impact calculator presented in Lacoste et al. (2019).
- Hardware Type:
- Hours used:
- Cloud Provider:
- Compute Region:
- Carbon Emitted:
## Technical Specifications [optional]
### Model Architecture and Objective
### Compute Infrastructure
#### Hardware
#### Software
[optional]
BibTeX:
APA:
## Glossary [optional]
## More Information [optional]
## Model Card Authors [optional]
## Model Card Contact
### Framework versions
- PEFT 0.7.1 | [
"# Model Card for Model ID",
"## Model Details",
"### Model Description\n\n\n\n\n\n- Developed by: \n- Funded by [optional]: \n- Shared by [optional]: \n- Model type: \n- Language(s) (NLP): \n- License: \n- Finetuned from model [optional]:",
"### Model Sources [optional]\n\n\n\n- Repository: \n- Paper [optional]: \n- Demo [optional]:",
"## Uses",
"### Direct Use",
"### Downstream Use [optional]",
"### Out-of-Scope Use",
"## Bias, Risks, and Limitations",
"### Recommendations\n\n\n\nUsers (both direct and downstream) should be made aware of the risks, biases and limitations of the model. More information needed for further recommendations.",
"## How to Get Started with the Model\n\nUse the code below to get started with the model.",
"## Training Details",
"### Training Data",
"### Training Procedure",
"#### Preprocessing [optional]",
"#### Training Hyperparameters\n\n- Training regime:",
"#### Speeds, Sizes, Times [optional]",
"## Evaluation",
"### Testing Data, Factors & Metrics",
"#### Testing Data",
"#### Factors",
"#### Metrics",
"### Results",
"#### Summary",
"## Model Examination [optional]",
"## Environmental Impact\n\n\n\nCarbon emissions can be estimated using the Machine Learning Impact calculator presented in Lacoste et al. (2019).\n\n- Hardware Type: \n- Hours used: \n- Cloud Provider: \n- Compute Region: \n- Carbon Emitted:",
"## Technical Specifications [optional]",
"### Model Architecture and Objective",
"### Compute Infrastructure",
"#### Hardware",
"#### Software\n\n\n\n[optional]\n\n\n\nBibTeX:\n\n\n\nAPA:",
"## Glossary [optional]",
"## More Information [optional]",
"## Model Card Authors [optional]",
"## Model Card Contact",
"### Framework versions\n\n- PEFT 0.7.1"
] | [
"TAGS\n#peft #safetensors #arxiv-1910.09700 #base_model-Qwen/Qwen-7B-Chat #region-us \n",
"# Model Card for Model ID",
"## Model Details",
"### Model Description\n\n\n\n\n\n- Developed by: \n- Funded by [optional]: \n- Shared by [optional]: \n- Model type: \n- Language(s) (NLP): \n- License: \n- Finetuned from model [optional]:",
"### Model Sources [optional]\n\n\n\n- Repository: \n- Paper [optional]: \n- Demo [optional]:",
"## Uses",
"### Direct Use",
"### Downstream Use [optional]",
"### Out-of-Scope Use",
"## Bias, Risks, and Limitations",
"### Recommendations\n\n\n\nUsers (both direct and downstream) should be made aware of the risks, biases and limitations of the model. More information needed for further recommendations.",
"## How to Get Started with the Model\n\nUse the code below to get started with the model.",
"## Training Details",
"### Training Data",
"### Training Procedure",
"#### Preprocessing [optional]",
"#### Training Hyperparameters\n\n- Training regime:",
"#### Speeds, Sizes, Times [optional]",
"## Evaluation",
"### Testing Data, Factors & Metrics",
"#### Testing Data",
"#### Factors",
"#### Metrics",
"### Results",
"#### Summary",
"## Model Examination [optional]",
"## Environmental Impact\n\n\n\nCarbon emissions can be estimated using the Machine Learning Impact calculator presented in Lacoste et al. (2019).\n\n- Hardware Type: \n- Hours used: \n- Cloud Provider: \n- Compute Region: \n- Carbon Emitted:",
"## Technical Specifications [optional]",
"### Model Architecture and Objective",
"### Compute Infrastructure",
"#### Hardware",
"#### Software\n\n\n\n[optional]\n\n\n\nBibTeX:\n\n\n\nAPA:",
"## Glossary [optional]",
"## More Information [optional]",
"## Model Card Authors [optional]",
"## Model Card Contact",
"### Framework versions\n\n- PEFT 0.7.1"
] |
null | transformers | ## About
<!-- ### quantize_version: 1 -->
<!-- ### output_tensor_quantised: 1 -->
<!-- ### convert_type: -->
<!-- ### vocab_type: -->
weighted/imatrix quants of https://huggingface.co/Kotokin/Merged-RP-Stew-V2-51B
<!-- provided-files -->
static quants are available at https://huggingface.co/mradermacher/Merged-RP-Stew-V2-51B-GGUF
## Usage
If you are unsure how to use GGUF files, refer to one of [TheBloke's
READMEs](https://huggingface.co/TheBloke/KafkaLM-70B-German-V0.1-GGUF) for
more details, including on how to concatenate multi-part files.
## Provided Quants
(sorted by size, not necessarily quality. IQ-quants are often preferable over similar sized non-IQ quants)
| Link | Type | Size/GB | Notes |
|:-----|:-----|--------:|:------|
| [GGUF](https://huggingface.co/mradermacher/Merged-RP-Stew-V2-51B-i1-GGUF/resolve/main/Merged-RP-Stew-V2-51B.i1-IQ1_S.gguf) | i1-IQ1_S | 11.1 | for the desperate |
| [GGUF](https://huggingface.co/mradermacher/Merged-RP-Stew-V2-51B-i1-GGUF/resolve/main/Merged-RP-Stew-V2-51B.i1-IQ1_M.gguf) | i1-IQ1_M | 12.1 | for the desperate |
| [GGUF](https://huggingface.co/mradermacher/Merged-RP-Stew-V2-51B-i1-GGUF/resolve/main/Merged-RP-Stew-V2-51B.i1-IQ2_XXS.gguf) | i1-IQ2_XXS | 13.8 | |
| [GGUF](https://huggingface.co/mradermacher/Merged-RP-Stew-V2-51B-i1-GGUF/resolve/main/Merged-RP-Stew-V2-51B.i1-IQ2_XS.gguf) | i1-IQ2_XS | 15.3 | |
| [GGUF](https://huggingface.co/mradermacher/Merged-RP-Stew-V2-51B-i1-GGUF/resolve/main/Merged-RP-Stew-V2-51B.i1-IQ2_S.gguf) | i1-IQ2_S | 16.2 | |
| [GGUF](https://huggingface.co/mradermacher/Merged-RP-Stew-V2-51B-i1-GGUF/resolve/main/Merged-RP-Stew-V2-51B.i1-IQ2_M.gguf) | i1-IQ2_M | 17.5 | |
| [GGUF](https://huggingface.co/mradermacher/Merged-RP-Stew-V2-51B-i1-GGUF/resolve/main/Merged-RP-Stew-V2-51B.i1-Q2_K.gguf) | i1-Q2_K | 19.1 | IQ3_XXS probably better |
| [GGUF](https://huggingface.co/mradermacher/Merged-RP-Stew-V2-51B-i1-GGUF/resolve/main/Merged-RP-Stew-V2-51B.i1-IQ3_XXS.gguf) | i1-IQ3_XXS | 19.8 | lower quality |
| [GGUF](https://huggingface.co/mradermacher/Merged-RP-Stew-V2-51B-i1-GGUF/resolve/main/Merged-RP-Stew-V2-51B.i1-IQ3_XS.gguf) | i1-IQ3_XS | 21.2 | |
| [GGUF](https://huggingface.co/mradermacher/Merged-RP-Stew-V2-51B-i1-GGUF/resolve/main/Merged-RP-Stew-V2-51B.i1-Q3_K_S.gguf) | i1-Q3_K_S | 22.3 | IQ3_XS probably better |
| [GGUF](https://huggingface.co/mradermacher/Merged-RP-Stew-V2-51B-i1-GGUF/resolve/main/Merged-RP-Stew-V2-51B.i1-IQ3_S.gguf) | i1-IQ3_S | 22.3 | beats Q3_K* |
| [GGUF](https://huggingface.co/mradermacher/Merged-RP-Stew-V2-51B-i1-GGUF/resolve/main/Merged-RP-Stew-V2-51B.i1-IQ3_M.gguf) | i1-IQ3_M | 23.2 | |
| [GGUF](https://huggingface.co/mradermacher/Merged-RP-Stew-V2-51B-i1-GGUF/resolve/main/Merged-RP-Stew-V2-51B.i1-Q3_K_M.gguf) | i1-Q3_K_M | 24.8 | IQ3_S probably better |
| [GGUF](https://huggingface.co/mradermacher/Merged-RP-Stew-V2-51B-i1-GGUF/resolve/main/Merged-RP-Stew-V2-51B.i1-Q3_K_L.gguf) | i1-Q3_K_L | 27.0 | IQ3_M probably better |
| [GGUF](https://huggingface.co/mradermacher/Merged-RP-Stew-V2-51B-i1-GGUF/resolve/main/Merged-RP-Stew-V2-51B.i1-IQ4_XS.gguf) | i1-IQ4_XS | 27.5 | |
| [GGUF](https://huggingface.co/mradermacher/Merged-RP-Stew-V2-51B-i1-GGUF/resolve/main/Merged-RP-Stew-V2-51B.i1-Q4_0.gguf) | i1-Q4_0 | 29.1 | fast, low quality |
| [GGUF](https://huggingface.co/mradermacher/Merged-RP-Stew-V2-51B-i1-GGUF/resolve/main/Merged-RP-Stew-V2-51B.i1-Q4_K_S.gguf) | i1-Q4_K_S | 29.2 | optimal size/speed/quality |
| [GGUF](https://huggingface.co/mradermacher/Merged-RP-Stew-V2-51B-i1-GGUF/resolve/main/Merged-RP-Stew-V2-51B.i1-Q4_K_M.gguf) | i1-Q4_K_M | 30.8 | fast, recommended |
| [GGUF](https://huggingface.co/mradermacher/Merged-RP-Stew-V2-51B-i1-GGUF/resolve/main/Merged-RP-Stew-V2-51B.i1-Q5_K_S.gguf) | i1-Q5_K_S | 35.3 | |
| [GGUF](https://huggingface.co/mradermacher/Merged-RP-Stew-V2-51B-i1-GGUF/resolve/main/Merged-RP-Stew-V2-51B.i1-Q5_K_M.gguf) | i1-Q5_K_M | 36.2 | |
| [GGUF](https://huggingface.co/mradermacher/Merged-RP-Stew-V2-51B-i1-GGUF/resolve/main/Merged-RP-Stew-V2-51B.i1-Q6_K.gguf) | i1-Q6_K | 42.0 | practically like static Q6_K |
Here is a handy graph by ikawrakow comparing some lower-quality quant
types (lower is better):

And here are Artefact2's thoughts on the matter:
https://gist.github.com/Artefact2/b5f810600771265fc1e39442288e8ec9
## FAQ / Model Request
See https://huggingface.co/mradermacher/model_requests for some answers to
questions you might have and/or if you want some other model quantized.
## Thanks
I thank my company, [nethype GmbH](https://www.nethype.de/), for letting
me use its servers and providing upgrades to my workstation to enable
this work in my free time.
<!-- end -->
| {"language": ["en"], "license": "other", "library_name": "transformers", "tags": ["merge", "roleplay", "exl2", "not-for-all-audiences"], "base_model": "Kotokin/Merged-RP-Stew-V2-51B", "license_link": "https://huggingface.co/01-ai/Yi-34B-200K/blob/main/LICENSE", "license_name": "yi-34b", "quantized_by": "mradermacher"} | mradermacher/Merged-RP-Stew-V2-51B-i1-GGUF | null | [
"transformers",
"gguf",
"merge",
"roleplay",
"exl2",
"not-for-all-audiences",
"en",
"base_model:Kotokin/Merged-RP-Stew-V2-51B",
"license:other",
"endpoints_compatible",
"region:us"
] | null | 2024-04-18T09:02:05+00:00 | [] | [
"en"
] | TAGS
#transformers #gguf #merge #roleplay #exl2 #not-for-all-audiences #en #base_model-Kotokin/Merged-RP-Stew-V2-51B #license-other #endpoints_compatible #region-us
| About
-----
weighted/imatrix quants of URL
static quants are available at URL
Usage
-----
If you are unsure how to use GGUF files, refer to one of TheBloke's
READMEs for
more details, including on how to concatenate multi-part files.
Provided Quants
---------------
(sorted by size, not necessarily quality. IQ-quants are often preferable over similar sized non-IQ quants)
Here is a handy graph by ikawrakow comparing some lower-quality quant
types (lower is better):
!URL
And here are Artefact2's thoughts on the matter:
URL
FAQ / Model Request
-------------------
See URL for some answers to
questions you might have and/or if you want some other model quantized.
Thanks
------
I thank my company, nethype GmbH, for letting
me use its servers and providing upgrades to my workstation to enable
this work in my free time.
| [] | [
"TAGS\n#transformers #gguf #merge #roleplay #exl2 #not-for-all-audiences #en #base_model-Kotokin/Merged-RP-Stew-V2-51B #license-other #endpoints_compatible #region-us \n"
] |
null | peft |
<!-- This model card has been generated automatically according to the information the Trainer had access to. You
should probably proofread and complete it, then remove this comment. -->
# llama2-chat_30000_500
This model is a fine-tuned version of [unsloth/llama-2-7b-chat-bnb-4bit](https://huggingface.co/unsloth/llama-2-7b-chat-bnb-4bit) on the None dataset.
It achieves the following results on the evaluation set:
- eval_loss: 0.9608
- eval_runtime: 160.5428
- eval_samples_per_second: 5.606
- eval_steps_per_second: 1.401
- epoch: 1.2
- step: 564
## Model description
More information needed
## Intended uses & limitations
More information needed
## Training and evaluation data
More information needed
## Training procedure
### Training hyperparameters
The following hyperparameters were used during training:
- learning_rate: 0.0002
- train_batch_size: 8
- eval_batch_size: 4
- seed: 3407
- gradient_accumulation_steps: 8
- total_train_batch_size: 64
- optimizer: Adam with betas=(0.9,0.999) and epsilon=1e-08
- lr_scheduler_type: cosine
- lr_scheduler_warmup_steps: 5
- num_epochs: 5
- mixed_precision_training: Native AMP
### Framework versions
- PEFT 0.10.0
- Transformers 4.39.3
- Pytorch 2.2.2+cu121
- Datasets 2.16.0
- Tokenizers 0.15.2 | {"license": "apache-2.0", "library_name": "peft", "tags": ["trl", "sft", "unsloth", "generated_from_trainer"], "base_model": "unsloth/llama-2-7b-chat-bnb-4bit", "model-index": [{"name": "llama2-chat_30000_500", "results": []}]} | Angelectronic/llama2-chat_30000_500 | null | [
"peft",
"tensorboard",
"safetensors",
"trl",
"sft",
"unsloth",
"generated_from_trainer",
"base_model:unsloth/llama-2-7b-chat-bnb-4bit",
"license:apache-2.0",
"region:us"
] | null | 2024-04-18T09:05:05+00:00 | [] | [] | TAGS
#peft #tensorboard #safetensors #trl #sft #unsloth #generated_from_trainer #base_model-unsloth/llama-2-7b-chat-bnb-4bit #license-apache-2.0 #region-us
|
# llama2-chat_30000_500
This model is a fine-tuned version of unsloth/llama-2-7b-chat-bnb-4bit on the None dataset.
It achieves the following results on the evaluation set:
- eval_loss: 0.9608
- eval_runtime: 160.5428
- eval_samples_per_second: 5.606
- eval_steps_per_second: 1.401
- epoch: 1.2
- step: 564
## Model description
More information needed
## Intended uses & limitations
More information needed
## Training and evaluation data
More information needed
## Training procedure
### Training hyperparameters
The following hyperparameters were used during training:
- learning_rate: 0.0002
- train_batch_size: 8
- eval_batch_size: 4
- seed: 3407
- gradient_accumulation_steps: 8
- total_train_batch_size: 64
- optimizer: Adam with betas=(0.9,0.999) and epsilon=1e-08
- lr_scheduler_type: cosine
- lr_scheduler_warmup_steps: 5
- num_epochs: 5
- mixed_precision_training: Native AMP
### Framework versions
- PEFT 0.10.0
- Transformers 4.39.3
- Pytorch 2.2.2+cu121
- Datasets 2.16.0
- Tokenizers 0.15.2 | [
"# llama2-chat_30000_500\n\nThis model is a fine-tuned version of unsloth/llama-2-7b-chat-bnb-4bit on the None dataset.\nIt achieves the following results on the evaluation set:\n- eval_loss: 0.9608\n- eval_runtime: 160.5428\n- eval_samples_per_second: 5.606\n- eval_steps_per_second: 1.401\n- epoch: 1.2\n- step: 564",
"## Model description\n\nMore information needed",
"## Intended uses & limitations\n\nMore information needed",
"## Training and evaluation data\n\nMore information needed",
"## Training procedure",
"### Training hyperparameters\n\nThe following hyperparameters were used during training:\n- learning_rate: 0.0002\n- train_batch_size: 8\n- eval_batch_size: 4\n- seed: 3407\n- gradient_accumulation_steps: 8\n- total_train_batch_size: 64\n- optimizer: Adam with betas=(0.9,0.999) and epsilon=1e-08\n- lr_scheduler_type: cosine\n- lr_scheduler_warmup_steps: 5\n- num_epochs: 5\n- mixed_precision_training: Native AMP",
"### Framework versions\n\n- PEFT 0.10.0\n- Transformers 4.39.3\n- Pytorch 2.2.2+cu121\n- Datasets 2.16.0\n- Tokenizers 0.15.2"
] | [
"TAGS\n#peft #tensorboard #safetensors #trl #sft #unsloth #generated_from_trainer #base_model-unsloth/llama-2-7b-chat-bnb-4bit #license-apache-2.0 #region-us \n",
"# llama2-chat_30000_500\n\nThis model is a fine-tuned version of unsloth/llama-2-7b-chat-bnb-4bit on the None dataset.\nIt achieves the following results on the evaluation set:\n- eval_loss: 0.9608\n- eval_runtime: 160.5428\n- eval_samples_per_second: 5.606\n- eval_steps_per_second: 1.401\n- epoch: 1.2\n- step: 564",
"## Model description\n\nMore information needed",
"## Intended uses & limitations\n\nMore information needed",
"## Training and evaluation data\n\nMore information needed",
"## Training procedure",
"### Training hyperparameters\n\nThe following hyperparameters were used during training:\n- learning_rate: 0.0002\n- train_batch_size: 8\n- eval_batch_size: 4\n- seed: 3407\n- gradient_accumulation_steps: 8\n- total_train_batch_size: 64\n- optimizer: Adam with betas=(0.9,0.999) and epsilon=1e-08\n- lr_scheduler_type: cosine\n- lr_scheduler_warmup_steps: 5\n- num_epochs: 5\n- mixed_precision_training: Native AMP",
"### Framework versions\n\n- PEFT 0.10.0\n- Transformers 4.39.3\n- Pytorch 2.2.2+cu121\n- Datasets 2.16.0\n- Tokenizers 0.15.2"
] |
token-classification | transformers |
<!-- This model card has been generated automatically according to the information the Trainer had access to. You
should probably proofread and complete it, then remove this comment. -->
# bert-finetuned-ner
This model is a fine-tuned version of [bert-base-cased](https://huggingface.co/bert-base-cased) on an unknown dataset.
It achieves the following results on the evaluation set:
- Loss: 0.0626
- Precision: 0.9372
- Recall: 0.9519
- F1: 0.9445
- Accuracy: 0.9865
## Model description
More information needed
## Intended uses & limitations
More information needed
## Training and evaluation data
More information needed
## Training procedure
### Training hyperparameters
The following hyperparameters were used during training:
- learning_rate: 2e-05
- train_batch_size: 8
- eval_batch_size: 8
- seed: 42
- optimizer: Adam with betas=(0.9,0.999) and epsilon=1e-08
- lr_scheduler_type: linear
- num_epochs: 3
### Training results
| Training Loss | Epoch | Step | Validation Loss | Precision | Recall | F1 | Accuracy |
|:-------------:|:-----:|:----:|:---------------:|:---------:|:------:|:------:|:--------:|
| 0.0735 | 1.0 | 1756 | 0.0664 | 0.9112 | 0.9376 | 0.9242 | 0.9818 |
| 0.0363 | 2.0 | 3512 | 0.0640 | 0.9358 | 0.9470 | 0.9414 | 0.9857 |
| 0.0213 | 3.0 | 5268 | 0.0626 | 0.9372 | 0.9519 | 0.9445 | 0.9865 |
### Framework versions
- Transformers 4.38.2
- Pytorch 2.2.1+cu121
- Datasets 2.18.0
- Tokenizers 0.15.2
| {"license": "apache-2.0", "tags": ["generated_from_trainer"], "metrics": ["precision", "recall", "f1", "accuracy"], "base_model": "bert-base-cased", "model-index": [{"name": "bert-finetuned-ner", "results": []}]} | JayBDev/bert-finetuned-ner | null | [
"transformers",
"tensorboard",
"safetensors",
"bert",
"token-classification",
"generated_from_trainer",
"base_model:bert-base-cased",
"license:apache-2.0",
"autotrain_compatible",
"endpoints_compatible",
"region:us"
] | null | 2024-04-18T09:06:13+00:00 | [] | [] | TAGS
#transformers #tensorboard #safetensors #bert #token-classification #generated_from_trainer #base_model-bert-base-cased #license-apache-2.0 #autotrain_compatible #endpoints_compatible #region-us
| bert-finetuned-ner
==================
This model is a fine-tuned version of bert-base-cased on an unknown dataset.
It achieves the following results on the evaluation set:
* Loss: 0.0626
* Precision: 0.9372
* Recall: 0.9519
* F1: 0.9445
* Accuracy: 0.9865
Model description
-----------------
More information needed
Intended uses & limitations
---------------------------
More information needed
Training and evaluation data
----------------------------
More information needed
Training procedure
------------------
### Training hyperparameters
The following hyperparameters were used during training:
* learning\_rate: 2e-05
* train\_batch\_size: 8
* eval\_batch\_size: 8
* seed: 42
* optimizer: Adam with betas=(0.9,0.999) and epsilon=1e-08
* lr\_scheduler\_type: linear
* num\_epochs: 3
### Training results
### Framework versions
* Transformers 4.38.2
* Pytorch 2.2.1+cu121
* Datasets 2.18.0
* Tokenizers 0.15.2
| [
"### Training hyperparameters\n\n\nThe following hyperparameters were used during training:\n\n\n* learning\\_rate: 2e-05\n* train\\_batch\\_size: 8\n* eval\\_batch\\_size: 8\n* seed: 42\n* optimizer: Adam with betas=(0.9,0.999) and epsilon=1e-08\n* lr\\_scheduler\\_type: linear\n* num\\_epochs: 3",
"### Training results",
"### Framework versions\n\n\n* Transformers 4.38.2\n* Pytorch 2.2.1+cu121\n* Datasets 2.18.0\n* Tokenizers 0.15.2"
] | [
"TAGS\n#transformers #tensorboard #safetensors #bert #token-classification #generated_from_trainer #base_model-bert-base-cased #license-apache-2.0 #autotrain_compatible #endpoints_compatible #region-us \n",
"### Training hyperparameters\n\n\nThe following hyperparameters were used during training:\n\n\n* learning\\_rate: 2e-05\n* train\\_batch\\_size: 8\n* eval\\_batch\\_size: 8\n* seed: 42\n* optimizer: Adam with betas=(0.9,0.999) and epsilon=1e-08\n* lr\\_scheduler\\_type: linear\n* num\\_epochs: 3",
"### Training results",
"### Framework versions\n\n\n* Transformers 4.38.2\n* Pytorch 2.2.1+cu121\n* Datasets 2.18.0\n* Tokenizers 0.15.2"
] |
null | peft |
<!-- This model card has been generated automatically according to the information the Trainer had access to. You
should probably proofread and complete it, then remove this comment. -->
# Mixtral-8x22b-Instruct-finetuned
This model is a fine-tuned version of [mistralai/Mixtral-8x22B-Instruct-v0.1](https://huggingface.co/mistralai/Mixtral-8x22B-Instruct-v0.1) on the generator dataset.
## Model description
More information needed
## Intended uses & limitations
More information needed
## Training and evaluation data
More information needed
## Training procedure
### Training hyperparameters
The following hyperparameters were used during training:
- learning_rate: 2.5e-05
- train_batch_size: 1
- eval_batch_size: 8
- seed: 42
- optimizer: Adam with betas=(0.9,0.999) and epsilon=1e-08
- lr_scheduler_type: linear
- lr_scheduler_warmup_steps: 0.03
- training_steps: 500
### Framework versions
- PEFT 0.10.1.dev0
- Transformers 4.40.0.dev0
- Pytorch 2.1.1+cu121
- Datasets 2.18.0
- Tokenizers 0.19.1 | {"license": "apache-2.0", "library_name": "peft", "tags": ["trl", "sft", "generated_from_trainer"], "datasets": ["generator"], "base_model": "mistralai/Mixtral-8x22B-Instruct-v0.1", "model-index": [{"name": "Mixtral-8x22b-Instruct-finetuned", "results": []}]} | Utshav/Mixtral-8x22b-Instruct-finetuned | null | [
"peft",
"safetensors",
"trl",
"sft",
"generated_from_trainer",
"dataset:generator",
"base_model:mistralai/Mixtral-8x22B-Instruct-v0.1",
"license:apache-2.0",
"region:us"
] | null | 2024-04-18T09:07:55+00:00 | [] | [] | TAGS
#peft #safetensors #trl #sft #generated_from_trainer #dataset-generator #base_model-mistralai/Mixtral-8x22B-Instruct-v0.1 #license-apache-2.0 #region-us
|
# Mixtral-8x22b-Instruct-finetuned
This model is a fine-tuned version of mistralai/Mixtral-8x22B-Instruct-v0.1 on the generator dataset.
## Model description
More information needed
## Intended uses & limitations
More information needed
## Training and evaluation data
More information needed
## Training procedure
### Training hyperparameters
The following hyperparameters were used during training:
- learning_rate: 2.5e-05
- train_batch_size: 1
- eval_batch_size: 8
- seed: 42
- optimizer: Adam with betas=(0.9,0.999) and epsilon=1e-08
- lr_scheduler_type: linear
- lr_scheduler_warmup_steps: 0.03
- training_steps: 500
### Framework versions
- PEFT 0.10.1.dev0
- Transformers 4.40.0.dev0
- Pytorch 2.1.1+cu121
- Datasets 2.18.0
- Tokenizers 0.19.1 | [
"# Mixtral-8x22b-Instruct-finetuned\n\nThis model is a fine-tuned version of mistralai/Mixtral-8x22B-Instruct-v0.1 on the generator dataset.",
"## Model description\n\nMore information needed",
"## Intended uses & limitations\n\nMore information needed",
"## Training and evaluation data\n\nMore information needed",
"## Training procedure",
"### Training hyperparameters\n\nThe following hyperparameters were used during training:\n- learning_rate: 2.5e-05\n- train_batch_size: 1\n- eval_batch_size: 8\n- seed: 42\n- optimizer: Adam with betas=(0.9,0.999) and epsilon=1e-08\n- lr_scheduler_type: linear\n- lr_scheduler_warmup_steps: 0.03\n- training_steps: 500",
"### Framework versions\n\n- PEFT 0.10.1.dev0\n- Transformers 4.40.0.dev0\n- Pytorch 2.1.1+cu121\n- Datasets 2.18.0\n- Tokenizers 0.19.1"
] | [
"TAGS\n#peft #safetensors #trl #sft #generated_from_trainer #dataset-generator #base_model-mistralai/Mixtral-8x22B-Instruct-v0.1 #license-apache-2.0 #region-us \n",
"# Mixtral-8x22b-Instruct-finetuned\n\nThis model is a fine-tuned version of mistralai/Mixtral-8x22B-Instruct-v0.1 on the generator dataset.",
"## Model description\n\nMore information needed",
"## Intended uses & limitations\n\nMore information needed",
"## Training and evaluation data\n\nMore information needed",
"## Training procedure",
"### Training hyperparameters\n\nThe following hyperparameters were used during training:\n- learning_rate: 2.5e-05\n- train_batch_size: 1\n- eval_batch_size: 8\n- seed: 42\n- optimizer: Adam with betas=(0.9,0.999) and epsilon=1e-08\n- lr_scheduler_type: linear\n- lr_scheduler_warmup_steps: 0.03\n- training_steps: 500",
"### Framework versions\n\n- PEFT 0.10.1.dev0\n- Transformers 4.40.0.dev0\n- Pytorch 2.1.1+cu121\n- Datasets 2.18.0\n- Tokenizers 0.19.1"
] |
text-generation | transformers | # IBI-CAAI/MELT-Mistral-3x7B-Instruct-v0.1 AWQ
- Model creator: [IBI-CAAI](https://huggingface.co/IBI-CAAI)
- Original model: [MELT-Mistral-3x7B-Instruct-v0.1](https://huggingface.co/IBI-CAAI/MELT-Mistral-3x7B-Instruct-v0.1)
## Model Summary
The MELT-Mistral-3x7B-Instruct-v0.1 Large Language Model (LLM) is a pretrained generative text model pre-trained and fine-tuned on using publically avalable medical data.
MELT-Mistral-3x7B-Instruct-v0.1 demonstrated a average 19.7% improvement over Mistral-3x7B-Instruct-v0.1 (MoE of 3 X Mistral-7B-Instruct-v0.1) across 3 USMLE, Indian AIIMS, and NEET medical examination benchmarks.
This is MoE model, thanks to [Charles Goddard](https://huggingface.co/chargoddard) for code/tools.
The Medical Education Language Transformer (MELT) models have been trained on a wide-range of text, chat, Q/A, and instruction data in the medical domain.
While the model was evaluated using publically avalable [USMLE](https://www.usmle.org/), Indian AIIMS, and NEET medical examination example questions, its use it intented to be more broadly applicable.
- **Developed by:** [Center for Applied AI](https://caai.ai.uky.edu/)
- **Funded by:** [Institute or Biomedical Informatics](https://www.research.uky.edu/IBI)
- **Model type:** LLM
- **Language(s) (NLP):** English
- **License:** Apache 2.0
- **Finetuned from model:** A MoE x 3 [Mistral-7B-v0.1](https://huggingface.co/mistralai/Mistral-7B-v0.1)
| {"language": ["en"], "license": "apache-2.0", "library_name": "transformers", "tags": ["4-bit", "AWQ", "text-generation", "autotrain_compatible", "endpoints_compatible"], "pipeline_tag": "text-generation", "inference": false, "quantized_by": "Suparious"} | solidrust/MELT-Mistral-3x7B-Instruct-v0.1-AWQ | null | [
"transformers",
"safetensors",
"mixtral",
"text-generation",
"4-bit",
"AWQ",
"autotrain_compatible",
"endpoints_compatible",
"conversational",
"en",
"license:apache-2.0",
"text-generation-inference",
"region:us"
] | null | 2024-04-18T09:07:56+00:00 | [] | [
"en"
] | TAGS
#transformers #safetensors #mixtral #text-generation #4-bit #AWQ #autotrain_compatible #endpoints_compatible #conversational #en #license-apache-2.0 #text-generation-inference #region-us
| # IBI-CAAI/MELT-Mistral-3x7B-Instruct-v0.1 AWQ
- Model creator: IBI-CAAI
- Original model: MELT-Mistral-3x7B-Instruct-v0.1
## Model Summary
The MELT-Mistral-3x7B-Instruct-v0.1 Large Language Model (LLM) is a pretrained generative text model pre-trained and fine-tuned on using publically avalable medical data.
MELT-Mistral-3x7B-Instruct-v0.1 demonstrated a average 19.7% improvement over Mistral-3x7B-Instruct-v0.1 (MoE of 3 X Mistral-7B-Instruct-v0.1) across 3 USMLE, Indian AIIMS, and NEET medical examination benchmarks.
This is MoE model, thanks to Charles Goddard for code/tools.
The Medical Education Language Transformer (MELT) models have been trained on a wide-range of text, chat, Q/A, and instruction data in the medical domain.
While the model was evaluated using publically avalable USMLE, Indian AIIMS, and NEET medical examination example questions, its use it intented to be more broadly applicable.
- Developed by: Center for Applied AI
- Funded by: Institute or Biomedical Informatics
- Model type: LLM
- Language(s) (NLP): English
- License: Apache 2.0
- Finetuned from model: A MoE x 3 Mistral-7B-v0.1
| [
"# IBI-CAAI/MELT-Mistral-3x7B-Instruct-v0.1 AWQ\n\n- Model creator: IBI-CAAI\n- Original model: MELT-Mistral-3x7B-Instruct-v0.1",
"## Model Summary\n\nThe MELT-Mistral-3x7B-Instruct-v0.1 Large Language Model (LLM) is a pretrained generative text model pre-trained and fine-tuned on using publically avalable medical data.\n\nMELT-Mistral-3x7B-Instruct-v0.1 demonstrated a average 19.7% improvement over Mistral-3x7B-Instruct-v0.1 (MoE of 3 X Mistral-7B-Instruct-v0.1) across 3 USMLE, Indian AIIMS, and NEET medical examination benchmarks.\n\nThis is MoE model, thanks to Charles Goddard for code/tools.\n\nThe Medical Education Language Transformer (MELT) models have been trained on a wide-range of text, chat, Q/A, and instruction data in the medical domain. \n\nWhile the model was evaluated using publically avalable USMLE, Indian AIIMS, and NEET medical examination example questions, its use it intented to be more broadly applicable. \n\n- Developed by: Center for Applied AI\n- Funded by: Institute or Biomedical Informatics\n- Model type: LLM\n- Language(s) (NLP): English\n- License: Apache 2.0\n- Finetuned from model: A MoE x 3 Mistral-7B-v0.1"
] | [
"TAGS\n#transformers #safetensors #mixtral #text-generation #4-bit #AWQ #autotrain_compatible #endpoints_compatible #conversational #en #license-apache-2.0 #text-generation-inference #region-us \n",
"# IBI-CAAI/MELT-Mistral-3x7B-Instruct-v0.1 AWQ\n\n- Model creator: IBI-CAAI\n- Original model: MELT-Mistral-3x7B-Instruct-v0.1",
"## Model Summary\n\nThe MELT-Mistral-3x7B-Instruct-v0.1 Large Language Model (LLM) is a pretrained generative text model pre-trained and fine-tuned on using publically avalable medical data.\n\nMELT-Mistral-3x7B-Instruct-v0.1 demonstrated a average 19.7% improvement over Mistral-3x7B-Instruct-v0.1 (MoE of 3 X Mistral-7B-Instruct-v0.1) across 3 USMLE, Indian AIIMS, and NEET medical examination benchmarks.\n\nThis is MoE model, thanks to Charles Goddard for code/tools.\n\nThe Medical Education Language Transformer (MELT) models have been trained on a wide-range of text, chat, Q/A, and instruction data in the medical domain. \n\nWhile the model was evaluated using publically avalable USMLE, Indian AIIMS, and NEET medical examination example questions, its use it intented to be more broadly applicable. \n\n- Developed by: Center for Applied AI\n- Funded by: Institute or Biomedical Informatics\n- Model type: LLM\n- Language(s) (NLP): English\n- License: Apache 2.0\n- Finetuned from model: A MoE x 3 Mistral-7B-v0.1"
] |
sentence-similarity | sentence-transformers |
# nisso22/all-mini-1
This is a [sentence-transformers](https://www.SBERT.net) model: It maps sentences & paragraphs to a 768 dimensional dense vector space and can be used for tasks like clustering or semantic search.
<!--- Describe your model here -->
## Usage (Sentence-Transformers)
Using this model becomes easy when you have [sentence-transformers](https://www.SBERT.net) installed:
```
pip install -U sentence-transformers
```
Then you can use the model like this:
```python
from sentence_transformers import SentenceTransformer
sentences = ["This is an example sentence", "Each sentence is converted"]
model = SentenceTransformer('nisso22/all-mini-1')
embeddings = model.encode(sentences)
print(embeddings)
```
## Usage (HuggingFace Transformers)
Without [sentence-transformers](https://www.SBERT.net), you can use the model like this: First, you pass your input through the transformer model, then you have to apply the right pooling-operation on-top of the contextualized word embeddings.
```python
from transformers import AutoTokenizer, AutoModel
import torch
#Mean Pooling - Take attention mask into account for correct averaging
def mean_pooling(model_output, attention_mask):
token_embeddings = model_output[0] #First element of model_output contains all token embeddings
input_mask_expanded = attention_mask.unsqueeze(-1).expand(token_embeddings.size()).float()
return torch.sum(token_embeddings * input_mask_expanded, 1) / torch.clamp(input_mask_expanded.sum(1), min=1e-9)
# Sentences we want sentence embeddings for
sentences = ['This is an example sentence', 'Each sentence is converted']
# Load model from HuggingFace Hub
tokenizer = AutoTokenizer.from_pretrained('nisso22/all-mini-1')
model = AutoModel.from_pretrained('nisso22/all-mini-1')
# Tokenize sentences
encoded_input = tokenizer(sentences, padding=True, truncation=True, return_tensors='pt')
# Compute token embeddings
with torch.no_grad():
model_output = model(**encoded_input)
# Perform pooling. In this case, mean pooling.
sentence_embeddings = mean_pooling(model_output, encoded_input['attention_mask'])
print("Sentence embeddings:")
print(sentence_embeddings)
```
## Evaluation Results
<!--- Describe how your model was evaluated -->
For an automated evaluation of this model, see the *Sentence Embeddings Benchmark*: [https://seb.sbert.net](https://seb.sbert.net?model_name=nisso22/all-mini-1)
## Training
The model was trained with the parameters:
**DataLoader**:
`torch.utils.data.dataloader.DataLoader` of length 44 with parameters:
```
{'batch_size': 16, 'sampler': 'torch.utils.data.sampler.RandomSampler', 'batch_sampler': 'torch.utils.data.sampler.BatchSampler'}
```
**Loss**:
`sentence_transformers.losses.TripletLoss.TripletLoss` with parameters:
```
{'distance_metric': 'TripletDistanceMetric.EUCLIDEAN', 'triplet_margin': 5}
```
Parameters of the fit()-Method:
```
{
"epochs": 10,
"evaluation_steps": 0,
"evaluator": "NoneType",
"max_grad_norm": 1,
"optimizer_class": "<class 'torch.optim.adamw.AdamW'>",
"optimizer_params": {
"lr": 2e-05
},
"scheduler": "WarmupLinear",
"steps_per_epoch": null,
"warmup_steps": 44,
"weight_decay": 0.01
}
```
## Full Model Architecture
```
SentenceTransformer(
(0): Transformer({'max_seq_length': 512, 'do_lower_case': False}) with Transformer model: RobertaModel
(1): Pooling({'word_embedding_dimension': 768, 'pooling_mode_cls_token': False, 'pooling_mode_mean_tokens': True, 'pooling_mode_max_tokens': False, 'pooling_mode_mean_sqrt_len_tokens': False, 'pooling_mode_weightedmean_tokens': False, 'pooling_mode_lasttoken': False, 'include_prompt': True})
)
```
## Citing & Authors
<!--- Describe where people can find more information --> | {"library_name": "sentence-transformers", "tags": ["sentence-transformers", "feature-extraction", "sentence-similarity", "transformers"], "pipeline_tag": "sentence-similarity"} | nisso22/all-mini-1 | null | [
"sentence-transformers",
"safetensors",
"roberta",
"feature-extraction",
"sentence-similarity",
"transformers",
"endpoints_compatible",
"region:us"
] | null | 2024-04-18T09:08:55+00:00 | [] | [] | TAGS
#sentence-transformers #safetensors #roberta #feature-extraction #sentence-similarity #transformers #endpoints_compatible #region-us
|
# nisso22/all-mini-1
This is a sentence-transformers model: It maps sentences & paragraphs to a 768 dimensional dense vector space and can be used for tasks like clustering or semantic search.
## Usage (Sentence-Transformers)
Using this model becomes easy when you have sentence-transformers installed:
Then you can use the model like this:
## Usage (HuggingFace Transformers)
Without sentence-transformers, you can use the model like this: First, you pass your input through the transformer model, then you have to apply the right pooling-operation on-top of the contextualized word embeddings.
## Evaluation Results
For an automated evaluation of this model, see the *Sentence Embeddings Benchmark*: URL
## Training
The model was trained with the parameters:
DataLoader:
'URL.dataloader.DataLoader' of length 44 with parameters:
Loss:
'sentence_transformers.losses.TripletLoss.TripletLoss' with parameters:
Parameters of the fit()-Method:
## Full Model Architecture
## Citing & Authors
| [
"# nisso22/all-mini-1\n\nThis is a sentence-transformers model: It maps sentences & paragraphs to a 768 dimensional dense vector space and can be used for tasks like clustering or semantic search.",
"## Usage (Sentence-Transformers)\n\nUsing this model becomes easy when you have sentence-transformers installed:\n\n\n\nThen you can use the model like this:",
"## Usage (HuggingFace Transformers)\nWithout sentence-transformers, you can use the model like this: First, you pass your input through the transformer model, then you have to apply the right pooling-operation on-top of the contextualized word embeddings.",
"## Evaluation Results\n\n\n\nFor an automated evaluation of this model, see the *Sentence Embeddings Benchmark*: URL",
"## Training\nThe model was trained with the parameters:\n\nDataLoader:\n\n'URL.dataloader.DataLoader' of length 44 with parameters:\n\n\nLoss:\n\n'sentence_transformers.losses.TripletLoss.TripletLoss' with parameters:\n \n\nParameters of the fit()-Method:",
"## Full Model Architecture",
"## Citing & Authors"
] | [
"TAGS\n#sentence-transformers #safetensors #roberta #feature-extraction #sentence-similarity #transformers #endpoints_compatible #region-us \n",
"# nisso22/all-mini-1\n\nThis is a sentence-transformers model: It maps sentences & paragraphs to a 768 dimensional dense vector space and can be used for tasks like clustering or semantic search.",
"## Usage (Sentence-Transformers)\n\nUsing this model becomes easy when you have sentence-transformers installed:\n\n\n\nThen you can use the model like this:",
"## Usage (HuggingFace Transformers)\nWithout sentence-transformers, you can use the model like this: First, you pass your input through the transformer model, then you have to apply the right pooling-operation on-top of the contextualized word embeddings.",
"## Evaluation Results\n\n\n\nFor an automated evaluation of this model, see the *Sentence Embeddings Benchmark*: URL",
"## Training\nThe model was trained with the parameters:\n\nDataLoader:\n\n'URL.dataloader.DataLoader' of length 44 with parameters:\n\n\nLoss:\n\n'sentence_transformers.losses.TripletLoss.TripletLoss' with parameters:\n \n\nParameters of the fit()-Method:",
"## Full Model Architecture",
"## Citing & Authors"
] |
text-to-image | diffusers |
<!-- This model card has been generated automatically according to the information the training script had access to. You
should probably proofread and complete it, then remove this comment. -->
# LoRA text2image fine-tuning - zhangyi617/sd-AE-model-pall-FGSM-origin-prompt-lora_text
These are LoRA adaption weights for runwayml/stable-diffusion-v1-5. The weights were fine-tuned on the zhangyi617/AE_adversarial_train_prompt_all_origin dataset. You can find some example images in the following.




## Intended uses & limitations
#### How to use
```python
# TODO: add an example code snippet for running this diffusion pipeline
```
#### Limitations and bias
[TODO: provide examples of latent issues and potential remediations]
## Training details
[TODO: describe the data used to train the model] | {"license": "creativeml-openrail-m", "library_name": "diffusers", "tags": ["stable-diffusion", "stable-diffusion-diffusers", "text-to-image", "diffusers", "diffusers-training", "lora"], "base_model": "runwayml/stable-diffusion-v1-5", "inference": true} | zhangyi617/sd-AE-model-pall-FGSM-origin-prompt-lora_text | null | [
"diffusers",
"safetensors",
"stable-diffusion",
"stable-diffusion-diffusers",
"text-to-image",
"diffusers-training",
"lora",
"base_model:runwayml/stable-diffusion-v1-5",
"license:creativeml-openrail-m",
"region:us"
] | null | 2024-04-18T09:10:43+00:00 | [] | [] | TAGS
#diffusers #safetensors #stable-diffusion #stable-diffusion-diffusers #text-to-image #diffusers-training #lora #base_model-runwayml/stable-diffusion-v1-5 #license-creativeml-openrail-m #region-us
|
# LoRA text2image fine-tuning - zhangyi617/sd-AE-model-pall-FGSM-origin-prompt-lora_text
These are LoRA adaption weights for runwayml/stable-diffusion-v1-5. The weights were fine-tuned on the zhangyi617/AE_adversarial_train_prompt_all_origin dataset. You can find some example images in the following.
!img_0
!img_1
!img_2
!img_3
## Intended uses & limitations
#### How to use
#### Limitations and bias
[TODO: provide examples of latent issues and potential remediations]
## Training details
[TODO: describe the data used to train the model] | [
"# LoRA text2image fine-tuning - zhangyi617/sd-AE-model-pall-FGSM-origin-prompt-lora_text\nThese are LoRA adaption weights for runwayml/stable-diffusion-v1-5. The weights were fine-tuned on the zhangyi617/AE_adversarial_train_prompt_all_origin dataset. You can find some example images in the following. \n\n!img_0\n!img_1\n!img_2\n!img_3",
"## Intended uses & limitations",
"#### How to use",
"#### Limitations and bias\n\n[TODO: provide examples of latent issues and potential remediations]",
"## Training details\n\n[TODO: describe the data used to train the model]"
] | [
"TAGS\n#diffusers #safetensors #stable-diffusion #stable-diffusion-diffusers #text-to-image #diffusers-training #lora #base_model-runwayml/stable-diffusion-v1-5 #license-creativeml-openrail-m #region-us \n",
"# LoRA text2image fine-tuning - zhangyi617/sd-AE-model-pall-FGSM-origin-prompt-lora_text\nThese are LoRA adaption weights for runwayml/stable-diffusion-v1-5. The weights were fine-tuned on the zhangyi617/AE_adversarial_train_prompt_all_origin dataset. You can find some example images in the following. \n\n!img_0\n!img_1\n!img_2\n!img_3",
"## Intended uses & limitations",
"#### How to use",
"#### Limitations and bias\n\n[TODO: provide examples of latent issues and potential remediations]",
"## Training details\n\n[TODO: describe the data used to train the model]"
] |
null | transformers |
# Model Card for Model ID
<!-- Provide a quick summary of what the model is/does. -->
## Model Details
### Model Description
<!-- Provide a longer summary of what this model is. -->
This is the model card of a 🤗 transformers model that has been pushed on the Hub. This model card has been automatically generated.
- **Developed by:** [More Information Needed]
- **Funded by [optional]:** [More Information Needed]
- **Shared by [optional]:** [More Information Needed]
- **Model type:** [More Information Needed]
- **Language(s) (NLP):** [More Information Needed]
- **License:** [More Information Needed]
- **Finetuned from model [optional]:** [More Information Needed]
### Model Sources [optional]
<!-- Provide the basic links for the model. -->
- **Repository:** [More Information Needed]
- **Paper [optional]:** [More Information Needed]
- **Demo [optional]:** [More Information Needed]
## Uses
<!-- Address questions around how the model is intended to be used, including the foreseeable users of the model and those affected by the model. -->
### Direct Use
<!-- This section is for the model use without fine-tuning or plugging into a larger ecosystem/app. -->
[More Information Needed]
### Downstream Use [optional]
<!-- This section is for the model use when fine-tuned for a task, or when plugged into a larger ecosystem/app -->
[More Information Needed]
### Out-of-Scope Use
<!-- This section addresses misuse, malicious use, and uses that the model will not work well for. -->
[More Information Needed]
## Bias, Risks, and Limitations
<!-- This section is meant to convey both technical and sociotechnical limitations. -->
[More Information Needed]
### Recommendations
<!-- This section is meant to convey recommendations with respect to the bias, risk, and technical limitations. -->
Users (both direct and downstream) should be made aware of the risks, biases and limitations of the model. More information needed for further recommendations.
## How to Get Started with the Model
Use the code below to get started with the model.
[More Information Needed]
## Training Details
### Training Data
<!-- This should link to a Dataset Card, perhaps with a short stub of information on what the training data is all about as well as documentation related to data pre-processing or additional filtering. -->
[More Information Needed]
### Training Procedure
<!-- This relates heavily to the Technical Specifications. Content here should link to that section when it is relevant to the training procedure. -->
#### Preprocessing [optional]
[More Information Needed]
#### Training Hyperparameters
- **Training regime:** [More Information Needed] <!--fp32, fp16 mixed precision, bf16 mixed precision, bf16 non-mixed precision, fp16 non-mixed precision, fp8 mixed precision -->
#### Speeds, Sizes, Times [optional]
<!-- This section provides information about throughput, start/end time, checkpoint size if relevant, etc. -->
[More Information Needed]
## Evaluation
<!-- This section describes the evaluation protocols and provides the results. -->
### Testing Data, Factors & Metrics
#### Testing Data
<!-- This should link to a Dataset Card if possible. -->
[More Information Needed]
#### Factors
<!-- These are the things the evaluation is disaggregating by, e.g., subpopulations or domains. -->
[More Information Needed]
#### Metrics
<!-- These are the evaluation metrics being used, ideally with a description of why. -->
[More Information Needed]
### Results
[More Information Needed]
#### Summary
## Model Examination [optional]
<!-- Relevant interpretability work for the model goes here -->
[More Information Needed]
## Environmental Impact
<!-- Total emissions (in grams of CO2eq) and additional considerations, such as electricity usage, go here. Edit the suggested text below accordingly -->
Carbon emissions can be estimated using the [Machine Learning Impact calculator](https://mlco2.github.io/impact#compute) presented in [Lacoste et al. (2019)](https://arxiv.org/abs/1910.09700).
- **Hardware Type:** [More Information Needed]
- **Hours used:** [More Information Needed]
- **Cloud Provider:** [More Information Needed]
- **Compute Region:** [More Information Needed]
- **Carbon Emitted:** [More Information Needed]
## Technical Specifications [optional]
### Model Architecture and Objective
[More Information Needed]
### Compute Infrastructure
[More Information Needed]
#### Hardware
[More Information Needed]
#### Software
[More Information Needed]
## Citation [optional]
<!-- If there is a paper or blog post introducing the model, the APA and Bibtex information for that should go in this section. -->
**BibTeX:**
[More Information Needed]
**APA:**
[More Information Needed]
## Glossary [optional]
<!-- If relevant, include terms and calculations in this section that can help readers understand the model or model card. -->
[More Information Needed]
## More Information [optional]
[More Information Needed]
## Model Card Authors [optional]
[More Information Needed]
## Model Card Contact
[More Information Needed]
| {"library_name": "transformers", "tags": []} | IbtiHt/commentgpt-ft | null | [
"transformers",
"safetensors",
"arxiv:1910.09700",
"endpoints_compatible",
"region:us"
] | null | 2024-04-18T09:12:36+00:00 | [
"1910.09700"
] | [] | TAGS
#transformers #safetensors #arxiv-1910.09700 #endpoints_compatible #region-us
|
# Model Card for Model ID
## Model Details
### Model Description
This is the model card of a transformers model that has been pushed on the Hub. This model card has been automatically generated.
- Developed by:
- Funded by [optional]:
- Shared by [optional]:
- Model type:
- Language(s) (NLP):
- License:
- Finetuned from model [optional]:
### Model Sources [optional]
- Repository:
- Paper [optional]:
- Demo [optional]:
## Uses
### Direct Use
### Downstream Use [optional]
### Out-of-Scope Use
## Bias, Risks, and Limitations
### Recommendations
Users (both direct and downstream) should be made aware of the risks, biases and limitations of the model. More information needed for further recommendations.
## How to Get Started with the Model
Use the code below to get started with the model.
## Training Details
### Training Data
### Training Procedure
#### Preprocessing [optional]
#### Training Hyperparameters
- Training regime:
#### Speeds, Sizes, Times [optional]
## Evaluation
### Testing Data, Factors & Metrics
#### Testing Data
#### Factors
#### Metrics
### Results
#### Summary
## Model Examination [optional]
## Environmental Impact
Carbon emissions can be estimated using the Machine Learning Impact calculator presented in Lacoste et al. (2019).
- Hardware Type:
- Hours used:
- Cloud Provider:
- Compute Region:
- Carbon Emitted:
## Technical Specifications [optional]
### Model Architecture and Objective
### Compute Infrastructure
#### Hardware
#### Software
[optional]
BibTeX:
APA:
## Glossary [optional]
## More Information [optional]
## Model Card Authors [optional]
## Model Card Contact
| [
"# Model Card for Model ID",
"## Model Details",
"### Model Description\n\n\n\nThis is the model card of a transformers model that has been pushed on the Hub. This model card has been automatically generated.\n\n- Developed by: \n- Funded by [optional]: \n- Shared by [optional]: \n- Model type: \n- Language(s) (NLP): \n- License: \n- Finetuned from model [optional]:",
"### Model Sources [optional]\n\n\n\n- Repository: \n- Paper [optional]: \n- Demo [optional]:",
"## Uses",
"### Direct Use",
"### Downstream Use [optional]",
"### Out-of-Scope Use",
"## Bias, Risks, and Limitations",
"### Recommendations\n\n\n\nUsers (both direct and downstream) should be made aware of the risks, biases and limitations of the model. More information needed for further recommendations.",
"## How to Get Started with the Model\n\nUse the code below to get started with the model.",
"## Training Details",
"### Training Data",
"### Training Procedure",
"#### Preprocessing [optional]",
"#### Training Hyperparameters\n\n- Training regime:",
"#### Speeds, Sizes, Times [optional]",
"## Evaluation",
"### Testing Data, Factors & Metrics",
"#### Testing Data",
"#### Factors",
"#### Metrics",
"### Results",
"#### Summary",
"## Model Examination [optional]",
"## Environmental Impact\n\n\n\nCarbon emissions can be estimated using the Machine Learning Impact calculator presented in Lacoste et al. (2019).\n\n- Hardware Type: \n- Hours used: \n- Cloud Provider: \n- Compute Region: \n- Carbon Emitted:",
"## Technical Specifications [optional]",
"### Model Architecture and Objective",
"### Compute Infrastructure",
"#### Hardware",
"#### Software\n\n\n\n[optional]\n\n\n\nBibTeX:\n\n\n\nAPA:",
"## Glossary [optional]",
"## More Information [optional]",
"## Model Card Authors [optional]",
"## Model Card Contact"
] | [
"TAGS\n#transformers #safetensors #arxiv-1910.09700 #endpoints_compatible #region-us \n",
"# Model Card for Model ID",
"## Model Details",
"### Model Description\n\n\n\nThis is the model card of a transformers model that has been pushed on the Hub. This model card has been automatically generated.\n\n- Developed by: \n- Funded by [optional]: \n- Shared by [optional]: \n- Model type: \n- Language(s) (NLP): \n- License: \n- Finetuned from model [optional]:",
"### Model Sources [optional]\n\n\n\n- Repository: \n- Paper [optional]: \n- Demo [optional]:",
"## Uses",
"### Direct Use",
"### Downstream Use [optional]",
"### Out-of-Scope Use",
"## Bias, Risks, and Limitations",
"### Recommendations\n\n\n\nUsers (both direct and downstream) should be made aware of the risks, biases and limitations of the model. More information needed for further recommendations.",
"## How to Get Started with the Model\n\nUse the code below to get started with the model.",
"## Training Details",
"### Training Data",
"### Training Procedure",
"#### Preprocessing [optional]",
"#### Training Hyperparameters\n\n- Training regime:",
"#### Speeds, Sizes, Times [optional]",
"## Evaluation",
"### Testing Data, Factors & Metrics",
"#### Testing Data",
"#### Factors",
"#### Metrics",
"### Results",
"#### Summary",
"## Model Examination [optional]",
"## Environmental Impact\n\n\n\nCarbon emissions can be estimated using the Machine Learning Impact calculator presented in Lacoste et al. (2019).\n\n- Hardware Type: \n- Hours used: \n- Cloud Provider: \n- Compute Region: \n- Carbon Emitted:",
"## Technical Specifications [optional]",
"### Model Architecture and Objective",
"### Compute Infrastructure",
"#### Hardware",
"#### Software\n\n\n\n[optional]\n\n\n\nBibTeX:\n\n\n\nAPA:",
"## Glossary [optional]",
"## More Information [optional]",
"## Model Card Authors [optional]",
"## Model Card Contact"
] |
reinforcement-learning | stable-baselines3 |
# **A2C** Agent playing **PandaReachDense-v3**
This is a trained model of a **A2C** agent playing **PandaReachDense-v3**
using the [stable-baselines3 library](https://github.com/DLR-RM/stable-baselines3).
## Usage (with Stable-baselines3)
TODO: Add your code
```python
from stable_baselines3 import ...
from huggingface_sb3 import load_from_hub
...
```
| {"library_name": "stable-baselines3", "tags": ["PandaReachDense-v3", "deep-reinforcement-learning", "reinforcement-learning", "stable-baselines3"], "model-index": [{"name": "A2C", "results": [{"task": {"type": "reinforcement-learning", "name": "reinforcement-learning"}, "dataset": {"name": "PandaReachDense-v3", "type": "PandaReachDense-v3"}, "metrics": [{"type": "mean_reward", "value": "-0.21 +/- 0.09", "name": "mean_reward", "verified": false}]}]}]} | adekhovich/a2c-PandaReachDense-v3 | null | [
"stable-baselines3",
"PandaReachDense-v3",
"deep-reinforcement-learning",
"reinforcement-learning",
"model-index",
"region:us"
] | null | 2024-04-18T09:15:48+00:00 | [] | [] | TAGS
#stable-baselines3 #PandaReachDense-v3 #deep-reinforcement-learning #reinforcement-learning #model-index #region-us
|
# A2C Agent playing PandaReachDense-v3
This is a trained model of a A2C agent playing PandaReachDense-v3
using the stable-baselines3 library.
## Usage (with Stable-baselines3)
TODO: Add your code
| [
"# A2C Agent playing PandaReachDense-v3\nThis is a trained model of a A2C agent playing PandaReachDense-v3\nusing the stable-baselines3 library.",
"## Usage (with Stable-baselines3)\nTODO: Add your code"
] | [
"TAGS\n#stable-baselines3 #PandaReachDense-v3 #deep-reinforcement-learning #reinforcement-learning #model-index #region-us \n",
"# A2C Agent playing PandaReachDense-v3\nThis is a trained model of a A2C agent playing PandaReachDense-v3\nusing the stable-baselines3 library.",
"## Usage (with Stable-baselines3)\nTODO: Add your code"
] |
null | null |
<!-- This model card has been generated automatically according to the information the Trainer had access to. You
should probably proofread and complete it, then remove this comment. -->
# open-llama-2-ko-7b-ko-sharegpt-finetuned-50steps
This model is a fine-tuned version of [beomi/open-llama-2-ko-7b](https://huggingface.co/beomi/open-llama-2-ko-7b) on an unknown dataset.
## Model description
More information needed
## Intended uses & limitations
More information needed
## Training and evaluation data
More information needed
## Training procedure
### Training hyperparameters
The following hyperparameters were used during training:
- learning_rate: 0.0001
- train_batch_size: 2
- eval_batch_size: 8
- seed: 42
- optimizer: Adam with betas=(0.9,0.999) and epsilon=1e-08
- lr_scheduler_type: linear
- training_steps: 50
### Training results
### Framework versions
- Transformers 4.31.0
- Pytorch 2.2.2+cu121
- Datasets 2.18.0
- Tokenizers 0.13.3
| {"license": "mit", "tags": ["generated_from_trainer"], "base_model": "beomi/open-llama-2-ko-7b", "model-index": [{"name": "open-llama-2-ko-7b-ko-sharegpt-finetuned-50steps", "results": []}]} | youngwook-kim/open-llama-2-ko-7b-ko-sharegpt-finetuned-50steps | null | [
"generated_from_trainer",
"base_model:beomi/open-llama-2-ko-7b",
"license:mit",
"region:us"
] | null | 2024-04-18T09:17:03+00:00 | [] | [] | TAGS
#generated_from_trainer #base_model-beomi/open-llama-2-ko-7b #license-mit #region-us
|
# open-llama-2-ko-7b-ko-sharegpt-finetuned-50steps
This model is a fine-tuned version of beomi/open-llama-2-ko-7b on an unknown dataset.
## Model description
More information needed
## Intended uses & limitations
More information needed
## Training and evaluation data
More information needed
## Training procedure
### Training hyperparameters
The following hyperparameters were used during training:
- learning_rate: 0.0001
- train_batch_size: 2
- eval_batch_size: 8
- seed: 42
- optimizer: Adam with betas=(0.9,0.999) and epsilon=1e-08
- lr_scheduler_type: linear
- training_steps: 50
### Training results
### Framework versions
- Transformers 4.31.0
- Pytorch 2.2.2+cu121
- Datasets 2.18.0
- Tokenizers 0.13.3
| [
"# open-llama-2-ko-7b-ko-sharegpt-finetuned-50steps\n\nThis model is a fine-tuned version of beomi/open-llama-2-ko-7b on an unknown dataset.",
"## Model description\n\nMore information needed",
"## Intended uses & limitations\n\nMore information needed",
"## Training and evaluation data\n\nMore information needed",
"## Training procedure",
"### Training hyperparameters\n\nThe following hyperparameters were used during training:\n- learning_rate: 0.0001\n- train_batch_size: 2\n- eval_batch_size: 8\n- seed: 42\n- optimizer: Adam with betas=(0.9,0.999) and epsilon=1e-08\n- lr_scheduler_type: linear\n- training_steps: 50",
"### Training results",
"### Framework versions\n\n- Transformers 4.31.0\n- Pytorch 2.2.2+cu121\n- Datasets 2.18.0\n- Tokenizers 0.13.3"
] | [
"TAGS\n#generated_from_trainer #base_model-beomi/open-llama-2-ko-7b #license-mit #region-us \n",
"# open-llama-2-ko-7b-ko-sharegpt-finetuned-50steps\n\nThis model is a fine-tuned version of beomi/open-llama-2-ko-7b on an unknown dataset.",
"## Model description\n\nMore information needed",
"## Intended uses & limitations\n\nMore information needed",
"## Training and evaluation data\n\nMore information needed",
"## Training procedure",
"### Training hyperparameters\n\nThe following hyperparameters were used during training:\n- learning_rate: 0.0001\n- train_batch_size: 2\n- eval_batch_size: 8\n- seed: 42\n- optimizer: Adam with betas=(0.9,0.999) and epsilon=1e-08\n- lr_scheduler_type: linear\n- training_steps: 50",
"### Training results",
"### Framework versions\n\n- Transformers 4.31.0\n- Pytorch 2.2.2+cu121\n- Datasets 2.18.0\n- Tokenizers 0.13.3"
] |
text-classification | transformers |
<!-- This model card has been generated automatically according to the information the Trainer had access to. You
should probably proofread and complete it, then remove this comment. -->
# CodeBertForClone-Detection
This model is a fine-tuned version of [microsoft/codebert-base](https://huggingface.co/microsoft/codebert-base) on an unknown dataset.
It achieves the following results on the evaluation set:
- Loss: 0.4183
- Accuracy: 0.834
## Model description
More information needed
## Intended uses & limitations
More information needed
## Training and evaluation data
More information needed
## Training procedure
### Training hyperparameters
The following hyperparameters were used during training:
- learning_rate: 5e-05
- train_batch_size: 16
- eval_batch_size: 32
- seed: 42
- optimizer: Adam with betas=(0.9,0.999) and epsilon=1e-08
- lr_scheduler_type: linear
- lr_scheduler_warmup_steps: 24000.0
- num_epochs: 5
- mixed_precision_training: Native AMP
### Training results
| Training Loss | Epoch | Step | Validation Loss | Accuracy |
|:-------------:|:-----:|:-----:|:---------------:|:--------:|
| 0.3291 | 1.0 | 5000 | 0.3769 | 0.8285 |
| 0.3053 | 2.0 | 10000 | 0.3781 | 0.8345 |
| 0.3319 | 3.0 | 15000 | 0.3811 | 0.847 |
| 0.3007 | 4.0 | 20000 | 0.3990 | 0.8413 |
| 0.291 | 5.0 | 25000 | 0.4183 | 0.834 |
### Framework versions
- Transformers 4.37.2
- Pytorch 2.1.2+cu121
- Datasets 2.15.0
- Tokenizers 0.15.0
| {"tags": ["generated_from_trainer"], "metrics": ["accuracy"], "base_model": "microsoft/codebert-base", "model-index": [{"name": "CodeBertForClone-Detection", "results": []}]} | ljcnju/CodeBertForClone-Detection | null | [
"transformers",
"safetensors",
"roberta",
"text-classification",
"generated_from_trainer",
"base_model:microsoft/codebert-base",
"autotrain_compatible",
"endpoints_compatible",
"region:us"
] | null | 2024-04-18T09:24:40+00:00 | [] | [] | TAGS
#transformers #safetensors #roberta #text-classification #generated_from_trainer #base_model-microsoft/codebert-base #autotrain_compatible #endpoints_compatible #region-us
| CodeBertForClone-Detection
==========================
This model is a fine-tuned version of microsoft/codebert-base on an unknown dataset.
It achieves the following results on the evaluation set:
* Loss: 0.4183
* Accuracy: 0.834
Model description
-----------------
More information needed
Intended uses & limitations
---------------------------
More information needed
Training and evaluation data
----------------------------
More information needed
Training procedure
------------------
### Training hyperparameters
The following hyperparameters were used during training:
* learning\_rate: 5e-05
* train\_batch\_size: 16
* eval\_batch\_size: 32
* seed: 42
* optimizer: Adam with betas=(0.9,0.999) and epsilon=1e-08
* lr\_scheduler\_type: linear
* lr\_scheduler\_warmup\_steps: 24000.0
* num\_epochs: 5
* mixed\_precision\_training: Native AMP
### Training results
### Framework versions
* Transformers 4.37.2
* Pytorch 2.1.2+cu121
* Datasets 2.15.0
* Tokenizers 0.15.0
| [
"### Training hyperparameters\n\n\nThe following hyperparameters were used during training:\n\n\n* learning\\_rate: 5e-05\n* train\\_batch\\_size: 16\n* eval\\_batch\\_size: 32\n* seed: 42\n* optimizer: Adam with betas=(0.9,0.999) and epsilon=1e-08\n* lr\\_scheduler\\_type: linear\n* lr\\_scheduler\\_warmup\\_steps: 24000.0\n* num\\_epochs: 5\n* mixed\\_precision\\_training: Native AMP",
"### Training results",
"### Framework versions\n\n\n* Transformers 4.37.2\n* Pytorch 2.1.2+cu121\n* Datasets 2.15.0\n* Tokenizers 0.15.0"
] | [
"TAGS\n#transformers #safetensors #roberta #text-classification #generated_from_trainer #base_model-microsoft/codebert-base #autotrain_compatible #endpoints_compatible #region-us \n",
"### Training hyperparameters\n\n\nThe following hyperparameters were used during training:\n\n\n* learning\\_rate: 5e-05\n* train\\_batch\\_size: 16\n* eval\\_batch\\_size: 32\n* seed: 42\n* optimizer: Adam with betas=(0.9,0.999) and epsilon=1e-08\n* lr\\_scheduler\\_type: linear\n* lr\\_scheduler\\_warmup\\_steps: 24000.0\n* num\\_epochs: 5\n* mixed\\_precision\\_training: Native AMP",
"### Training results",
"### Framework versions\n\n\n* Transformers 4.37.2\n* Pytorch 2.1.2+cu121\n* Datasets 2.15.0\n* Tokenizers 0.15.0"
] |
null | null |
Flan-T5 small finetuned with [GLAM](https://sites.google.com/view/grounding-llms-with-online-rl/) on BabyAI-Text GoToLocal task.
Paper: arxiv.org/abs/2302.02662 | {"license": "mit"} | ClementRomac/llm_gtl_nbr_env_32_Flan_T5small_6-actions | null | [
"arxiv:2302.02662",
"license:mit",
"region:us"
] | null | 2024-04-18T09:24:40+00:00 | [
"2302.02662"
] | [] | TAGS
#arxiv-2302.02662 #license-mit #region-us
|
Flan-T5 small finetuned with GLAM on BabyAI-Text GoToLocal task.
Paper: URL | [] | [
"TAGS\n#arxiv-2302.02662 #license-mit #region-us \n"
] |
null | null | Flan-T5 xl finetuned with [GLAM](https://sites.google.com/view/grounding-llms-with-online-rl/) on BabyAI-Text GoToLocal task.
Paper: arxiv.org/abs/2302.02662 | {"license": "mit"} | ClementRomac/llm_gtl_nbr_env_32_Flan_T5xl_6-actions | null | [
"arxiv:2302.02662",
"license:mit",
"region:us"
] | null | 2024-04-18T09:27:46+00:00 | [
"2302.02662"
] | [] | TAGS
#arxiv-2302.02662 #license-mit #region-us
| Flan-T5 xl finetuned with GLAM on BabyAI-Text GoToLocal task.
Paper: URL | [] | [
"TAGS\n#arxiv-2302.02662 #license-mit #region-us \n"
] |
null | transformers |
<!-- This model card has been generated automatically according to the information the Trainer had access to. You
should probably proofread and complete it, then remove this comment. -->
# output
This model is a fine-tuned version of [](https://huggingface.co/) on an unknown dataset.
## Model description
More information needed
## Intended uses & limitations
More information needed
## Training and evaluation data
More information needed
## Training procedure
### Training hyperparameters
The following hyperparameters were used during training:
- learning_rate: 0.0001
- train_batch_size: 64
- eval_batch_size: 8
- seed: 42
- optimizer: Adam with betas=(0.9,0.999) and epsilon=1e-08
- lr_scheduler_type: linear
- lr_scheduler_warmup_ratio: 0.1
- num_epochs: 120
### Training results
### Framework versions
- Transformers 4.38.2
- Pytorch 2.2.1+cu121
- Datasets 2.18.0
- Tokenizers 0.15.2
| {"tags": ["generated_from_trainer"], "model-index": [{"name": "output", "results": []}]} | shoveling42/output | null | [
"transformers",
"tensorboard",
"safetensors",
"decision_transformer",
"generated_from_trainer",
"endpoints_compatible",
"region:us"
] | null | 2024-04-18T09:29:12+00:00 | [] | [] | TAGS
#transformers #tensorboard #safetensors #decision_transformer #generated_from_trainer #endpoints_compatible #region-us
|
# output
This model is a fine-tuned version of [](URL on an unknown dataset.
## Model description
More information needed
## Intended uses & limitations
More information needed
## Training and evaluation data
More information needed
## Training procedure
### Training hyperparameters
The following hyperparameters were used during training:
- learning_rate: 0.0001
- train_batch_size: 64
- eval_batch_size: 8
- seed: 42
- optimizer: Adam with betas=(0.9,0.999) and epsilon=1e-08
- lr_scheduler_type: linear
- lr_scheduler_warmup_ratio: 0.1
- num_epochs: 120
### Training results
### Framework versions
- Transformers 4.38.2
- Pytorch 2.2.1+cu121
- Datasets 2.18.0
- Tokenizers 0.15.2
| [
"# output\n\nThis model is a fine-tuned version of [](URL on an unknown dataset.",
"## Model description\n\nMore information needed",
"## Intended uses & limitations\n\nMore information needed",
"## Training and evaluation data\n\nMore information needed",
"## Training procedure",
"### Training hyperparameters\n\nThe following hyperparameters were used during training:\n- learning_rate: 0.0001\n- train_batch_size: 64\n- eval_batch_size: 8\n- seed: 42\n- optimizer: Adam with betas=(0.9,0.999) and epsilon=1e-08\n- lr_scheduler_type: linear\n- lr_scheduler_warmup_ratio: 0.1\n- num_epochs: 120",
"### Training results",
"### Framework versions\n\n- Transformers 4.38.2\n- Pytorch 2.2.1+cu121\n- Datasets 2.18.0\n- Tokenizers 0.15.2"
] | [
"TAGS\n#transformers #tensorboard #safetensors #decision_transformer #generated_from_trainer #endpoints_compatible #region-us \n",
"# output\n\nThis model is a fine-tuned version of [](URL on an unknown dataset.",
"## Model description\n\nMore information needed",
"## Intended uses & limitations\n\nMore information needed",
"## Training and evaluation data\n\nMore information needed",
"## Training procedure",
"### Training hyperparameters\n\nThe following hyperparameters were used during training:\n- learning_rate: 0.0001\n- train_batch_size: 64\n- eval_batch_size: 8\n- seed: 42\n- optimizer: Adam with betas=(0.9,0.999) and epsilon=1e-08\n- lr_scheduler_type: linear\n- lr_scheduler_warmup_ratio: 0.1\n- num_epochs: 120",
"### Training results",
"### Framework versions\n\n- Transformers 4.38.2\n- Pytorch 2.2.1+cu121\n- Datasets 2.18.0\n- Tokenizers 0.15.2"
] |
null | null |
<!-- This model card has been generated automatically according to the information the Trainer had access to. You
should probably proofread and complete it, then remove this comment. -->
# new_results
This model is a fine-tuned version of [NousResearch/Llama-2-7b-chat-hf](https://huggingface.co/NousResearch/Llama-2-7b-chat-hf) on an unknown dataset.
## Model description
More information needed
## Intended uses & limitations
More information needed
## Training and evaluation data
More information needed
## Training procedure
### Training hyperparameters
The following hyperparameters were used during training:
- learning_rate: 0.0002
- train_batch_size: 4
- eval_batch_size: 8
- seed: 42
- optimizer: Adam with betas=(0.9,0.999) and epsilon=1e-08
- lr_scheduler_type: cosine
- lr_scheduler_warmup_ratio: 0.03
- training_steps: 20
### Training results
### Framework versions
- Transformers 4.31.0
- Pytorch 2.2.1+cu121
- Datasets 2.18.0
- Tokenizers 0.13.3
| {"tags": ["generated_from_trainer"], "base_model": "NousResearch/Llama-2-7b-chat-hf", "model-index": [{"name": "new_results", "results": []}]} | huylys12/Llama-2-resume-fine-tune | null | [
"tensorboard",
"generated_from_trainer",
"base_model:NousResearch/Llama-2-7b-chat-hf",
"region:us"
] | null | 2024-04-18T09:29:13+00:00 | [] | [] | TAGS
#tensorboard #generated_from_trainer #base_model-NousResearch/Llama-2-7b-chat-hf #region-us
|
# new_results
This model is a fine-tuned version of NousResearch/Llama-2-7b-chat-hf on an unknown dataset.
## Model description
More information needed
## Intended uses & limitations
More information needed
## Training and evaluation data
More information needed
## Training procedure
### Training hyperparameters
The following hyperparameters were used during training:
- learning_rate: 0.0002
- train_batch_size: 4
- eval_batch_size: 8
- seed: 42
- optimizer: Adam with betas=(0.9,0.999) and epsilon=1e-08
- lr_scheduler_type: cosine
- lr_scheduler_warmup_ratio: 0.03
- training_steps: 20
### Training results
### Framework versions
- Transformers 4.31.0
- Pytorch 2.2.1+cu121
- Datasets 2.18.0
- Tokenizers 0.13.3
| [
"# new_results\n\nThis model is a fine-tuned version of NousResearch/Llama-2-7b-chat-hf on an unknown dataset.",
"## Model description\n\nMore information needed",
"## Intended uses & limitations\n\nMore information needed",
"## Training and evaluation data\n\nMore information needed",
"## Training procedure",
"### Training hyperparameters\n\nThe following hyperparameters were used during training:\n- learning_rate: 0.0002\n- train_batch_size: 4\n- eval_batch_size: 8\n- seed: 42\n- optimizer: Adam with betas=(0.9,0.999) and epsilon=1e-08\n- lr_scheduler_type: cosine\n- lr_scheduler_warmup_ratio: 0.03\n- training_steps: 20",
"### Training results",
"### Framework versions\n\n- Transformers 4.31.0\n- Pytorch 2.2.1+cu121\n- Datasets 2.18.0\n- Tokenizers 0.13.3"
] | [
"TAGS\n#tensorboard #generated_from_trainer #base_model-NousResearch/Llama-2-7b-chat-hf #region-us \n",
"# new_results\n\nThis model is a fine-tuned version of NousResearch/Llama-2-7b-chat-hf on an unknown dataset.",
"## Model description\n\nMore information needed",
"## Intended uses & limitations\n\nMore information needed",
"## Training and evaluation data\n\nMore information needed",
"## Training procedure",
"### Training hyperparameters\n\nThe following hyperparameters were used during training:\n- learning_rate: 0.0002\n- train_batch_size: 4\n- eval_batch_size: 8\n- seed: 42\n- optimizer: Adam with betas=(0.9,0.999) and epsilon=1e-08\n- lr_scheduler_type: cosine\n- lr_scheduler_warmup_ratio: 0.03\n- training_steps: 20",
"### Training results",
"### Framework versions\n\n- Transformers 4.31.0\n- Pytorch 2.2.1+cu121\n- Datasets 2.18.0\n- Tokenizers 0.13.3"
] |
text-generation | transformers |
# Model Card for Model ID
<!-- Provide a quick summary of what the model is/does. -->
## Model Details
### Model Description
<!-- Provide a longer summary of what this model is. -->
This is the model card of a 🤗 transformers model that has been pushed on the Hub. This model card has been automatically generated.
- **Developed by:** [More Information Needed]
- **Funded by [optional]:** [More Information Needed]
- **Shared by [optional]:** [More Information Needed]
- **Model type:** [More Information Needed]
- **Language(s) (NLP):** [More Information Needed]
- **License:** [More Information Needed]
- **Finetuned from model [optional]:** [More Information Needed]
### Model Sources [optional]
<!-- Provide the basic links for the model. -->
- **Repository:** [More Information Needed]
- **Paper [optional]:** [More Information Needed]
- **Demo [optional]:** [More Information Needed]
## Uses
<!-- Address questions around how the model is intended to be used, including the foreseeable users of the model and those affected by the model. -->
### Direct Use
<!-- This section is for the model use without fine-tuning or plugging into a larger ecosystem/app. -->
[More Information Needed]
### Downstream Use [optional]
<!-- This section is for the model use when fine-tuned for a task, or when plugged into a larger ecosystem/app -->
[More Information Needed]
### Out-of-Scope Use
<!-- This section addresses misuse, malicious use, and uses that the model will not work well for. -->
[More Information Needed]
## Bias, Risks, and Limitations
<!-- This section is meant to convey both technical and sociotechnical limitations. -->
[More Information Needed]
### Recommendations
<!-- This section is meant to convey recommendations with respect to the bias, risk, and technical limitations. -->
Users (both direct and downstream) should be made aware of the risks, biases and limitations of the model. More information needed for further recommendations.
## How to Get Started with the Model
Use the code below to get started with the model.
[More Information Needed]
## Training Details
### Training Data
<!-- This should link to a Dataset Card, perhaps with a short stub of information on what the training data is all about as well as documentation related to data pre-processing or additional filtering. -->
[More Information Needed]
### Training Procedure
<!-- This relates heavily to the Technical Specifications. Content here should link to that section when it is relevant to the training procedure. -->
#### Preprocessing [optional]
[More Information Needed]
#### Training Hyperparameters
- **Training regime:** [More Information Needed] <!--fp32, fp16 mixed precision, bf16 mixed precision, bf16 non-mixed precision, fp16 non-mixed precision, fp8 mixed precision -->
#### Speeds, Sizes, Times [optional]
<!-- This section provides information about throughput, start/end time, checkpoint size if relevant, etc. -->
[More Information Needed]
## Evaluation
<!-- This section describes the evaluation protocols and provides the results. -->
### Testing Data, Factors & Metrics
#### Testing Data
<!-- This should link to a Dataset Card if possible. -->
[More Information Needed]
#### Factors
<!-- These are the things the evaluation is disaggregating by, e.g., subpopulations or domains. -->
[More Information Needed]
#### Metrics
<!-- These are the evaluation metrics being used, ideally with a description of why. -->
[More Information Needed]
### Results
[More Information Needed]
#### Summary
## Model Examination [optional]
<!-- Relevant interpretability work for the model goes here -->
[More Information Needed]
## Environmental Impact
<!-- Total emissions (in grams of CO2eq) and additional considerations, such as electricity usage, go here. Edit the suggested text below accordingly -->
Carbon emissions can be estimated using the [Machine Learning Impact calculator](https://mlco2.github.io/impact#compute) presented in [Lacoste et al. (2019)](https://arxiv.org/abs/1910.09700).
- **Hardware Type:** [More Information Needed]
- **Hours used:** [More Information Needed]
- **Cloud Provider:** [More Information Needed]
- **Compute Region:** [More Information Needed]
- **Carbon Emitted:** [More Information Needed]
## Technical Specifications [optional]
### Model Architecture and Objective
[More Information Needed]
### Compute Infrastructure
[More Information Needed]
#### Hardware
[More Information Needed]
#### Software
[More Information Needed]
## Citation [optional]
<!-- If there is a paper or blog post introducing the model, the APA and Bibtex information for that should go in this section. -->
**BibTeX:**
[More Information Needed]
**APA:**
[More Information Needed]
## Glossary [optional]
<!-- If relevant, include terms and calculations in this section that can help readers understand the model or model card. -->
[More Information Needed]
## More Information [optional]
[More Information Needed]
## Model Card Authors [optional]
[More Information Needed]
## Model Card Contact
[More Information Needed]
| {"library_name": "transformers", "tags": []} | thanhnew2001/bank5 | null | [
"transformers",
"safetensors",
"bloom",
"text-generation",
"arxiv:1910.09700",
"autotrain_compatible",
"endpoints_compatible",
"text-generation-inference",
"4-bit",
"region:us"
] | null | 2024-04-18T09:29:46+00:00 | [
"1910.09700"
] | [] | TAGS
#transformers #safetensors #bloom #text-generation #arxiv-1910.09700 #autotrain_compatible #endpoints_compatible #text-generation-inference #4-bit #region-us
|
# Model Card for Model ID
## Model Details
### Model Description
This is the model card of a transformers model that has been pushed on the Hub. This model card has been automatically generated.
- Developed by:
- Funded by [optional]:
- Shared by [optional]:
- Model type:
- Language(s) (NLP):
- License:
- Finetuned from model [optional]:
### Model Sources [optional]
- Repository:
- Paper [optional]:
- Demo [optional]:
## Uses
### Direct Use
### Downstream Use [optional]
### Out-of-Scope Use
## Bias, Risks, and Limitations
### Recommendations
Users (both direct and downstream) should be made aware of the risks, biases and limitations of the model. More information needed for further recommendations.
## How to Get Started with the Model
Use the code below to get started with the model.
## Training Details
### Training Data
### Training Procedure
#### Preprocessing [optional]
#### Training Hyperparameters
- Training regime:
#### Speeds, Sizes, Times [optional]
## Evaluation
### Testing Data, Factors & Metrics
#### Testing Data
#### Factors
#### Metrics
### Results
#### Summary
## Model Examination [optional]
## Environmental Impact
Carbon emissions can be estimated using the Machine Learning Impact calculator presented in Lacoste et al. (2019).
- Hardware Type:
- Hours used:
- Cloud Provider:
- Compute Region:
- Carbon Emitted:
## Technical Specifications [optional]
### Model Architecture and Objective
### Compute Infrastructure
#### Hardware
#### Software
[optional]
BibTeX:
APA:
## Glossary [optional]
## More Information [optional]
## Model Card Authors [optional]
## Model Card Contact
| [
"# Model Card for Model ID",
"## Model Details",
"### Model Description\n\n\n\nThis is the model card of a transformers model that has been pushed on the Hub. This model card has been automatically generated.\n\n- Developed by: \n- Funded by [optional]: \n- Shared by [optional]: \n- Model type: \n- Language(s) (NLP): \n- License: \n- Finetuned from model [optional]:",
"### Model Sources [optional]\n\n\n\n- Repository: \n- Paper [optional]: \n- Demo [optional]:",
"## Uses",
"### Direct Use",
"### Downstream Use [optional]",
"### Out-of-Scope Use",
"## Bias, Risks, and Limitations",
"### Recommendations\n\n\n\nUsers (both direct and downstream) should be made aware of the risks, biases and limitations of the model. More information needed for further recommendations.",
"## How to Get Started with the Model\n\nUse the code below to get started with the model.",
"## Training Details",
"### Training Data",
"### Training Procedure",
"#### Preprocessing [optional]",
"#### Training Hyperparameters\n\n- Training regime:",
"#### Speeds, Sizes, Times [optional]",
"## Evaluation",
"### Testing Data, Factors & Metrics",
"#### Testing Data",
"#### Factors",
"#### Metrics",
"### Results",
"#### Summary",
"## Model Examination [optional]",
"## Environmental Impact\n\n\n\nCarbon emissions can be estimated using the Machine Learning Impact calculator presented in Lacoste et al. (2019).\n\n- Hardware Type: \n- Hours used: \n- Cloud Provider: \n- Compute Region: \n- Carbon Emitted:",
"## Technical Specifications [optional]",
"### Model Architecture and Objective",
"### Compute Infrastructure",
"#### Hardware",
"#### Software\n\n\n\n[optional]\n\n\n\nBibTeX:\n\n\n\nAPA:",
"## Glossary [optional]",
"## More Information [optional]",
"## Model Card Authors [optional]",
"## Model Card Contact"
] | [
"TAGS\n#transformers #safetensors #bloom #text-generation #arxiv-1910.09700 #autotrain_compatible #endpoints_compatible #text-generation-inference #4-bit #region-us \n",
"# Model Card for Model ID",
"## Model Details",
"### Model Description\n\n\n\nThis is the model card of a transformers model that has been pushed on the Hub. This model card has been automatically generated.\n\n- Developed by: \n- Funded by [optional]: \n- Shared by [optional]: \n- Model type: \n- Language(s) (NLP): \n- License: \n- Finetuned from model [optional]:",
"### Model Sources [optional]\n\n\n\n- Repository: \n- Paper [optional]: \n- Demo [optional]:",
"## Uses",
"### Direct Use",
"### Downstream Use [optional]",
"### Out-of-Scope Use",
"## Bias, Risks, and Limitations",
"### Recommendations\n\n\n\nUsers (both direct and downstream) should be made aware of the risks, biases and limitations of the model. More information needed for further recommendations.",
"## How to Get Started with the Model\n\nUse the code below to get started with the model.",
"## Training Details",
"### Training Data",
"### Training Procedure",
"#### Preprocessing [optional]",
"#### Training Hyperparameters\n\n- Training regime:",
"#### Speeds, Sizes, Times [optional]",
"## Evaluation",
"### Testing Data, Factors & Metrics",
"#### Testing Data",
"#### Factors",
"#### Metrics",
"### Results",
"#### Summary",
"## Model Examination [optional]",
"## Environmental Impact\n\n\n\nCarbon emissions can be estimated using the Machine Learning Impact calculator presented in Lacoste et al. (2019).\n\n- Hardware Type: \n- Hours used: \n- Cloud Provider: \n- Compute Region: \n- Carbon Emitted:",
"## Technical Specifications [optional]",
"### Model Architecture and Objective",
"### Compute Infrastructure",
"#### Hardware",
"#### Software\n\n\n\n[optional]\n\n\n\nBibTeX:\n\n\n\nAPA:",
"## Glossary [optional]",
"## More Information [optional]",
"## Model Card Authors [optional]",
"## Model Card Contact"
] |
null | null |
Flan-T5 large finetuned with [GLAM](https://sites.google.com/view/grounding-llms-with-online-rl/) on BabyAI-Text MixedTrainLocal task.
Paper: arxiv.org/abs/2302.02662 | {"license": "mit"} | ClementRomac/llm_mtrl_nbr_env_32_Flan_T5large_6-actions | null | [
"arxiv:2302.02662",
"license:mit",
"region:us"
] | null | 2024-04-18T09:30:35+00:00 | [
"2302.02662"
] | [] | TAGS
#arxiv-2302.02662 #license-mit #region-us
|
Flan-T5 large finetuned with GLAM on BabyAI-Text MixedTrainLocal task.
Paper: URL | [] | [
"TAGS\n#arxiv-2302.02662 #license-mit #region-us \n"
] |
text2text-generation | transformers |
<!-- This model card has been generated automatically according to the information the Trainer had access to. You
should probably proofread and complete it, then remove this comment. -->
# gpt2-finetuned-justification-v5
This model is a fine-tuned version of [](https://huggingface.co/) on the None dataset.
It achieves the following results on the evaluation set:
- Loss: 0.3678
- Rouge1: 28.2558
- Rouge2: 13.2942
- Rougel: 20.5646
- Rougelsum: 25.4960
## Model description
More information needed
## Intended uses & limitations
More information needed
## Training and evaluation data
More information needed
## Training procedure
### Training hyperparameters
The following hyperparameters were used during training:
- learning_rate: 2e-05
- train_batch_size: 2
- eval_batch_size: 2
- seed: 42
- optimizer: Adam with betas=(0.9,0.999) and epsilon=1e-08
- lr_scheduler_type: linear
- num_epochs: 200
### Training results
| Training Loss | Epoch | Step | Validation Loss | Rouge1 | Rouge2 | Rougel | Rougelsum |
|:-------------:|:-----:|:-----:|:---------------:|:-------:|:-------:|:-------:|:---------:|
| No log | 1.0 | 338 | 0.2009 | 31.3319 | 13.9488 | 23.6685 | 29.4548 |
| 0.2408 | 2.0 | 676 | 0.1974 | 29.1645 | 13.8230 | 21.7497 | 26.8219 |
| 0.1816 | 3.0 | 1014 | 0.1970 | 29.1922 | 13.8428 | 21.7595 | 26.8727 |
| 0.1816 | 4.0 | 1352 | 0.1976 | 31.8894 | 13.6293 | 23.2637 | 29.1353 |
| 0.1594 | 5.0 | 1690 | 0.1999 | 28.9161 | 13.7629 | 21.7786 | 26.6707 |
| 0.1417 | 6.0 | 2028 | 0.2019 | 26.1827 | 13.0883 | 21.6704 | 25.0609 |
| 0.1417 | 7.0 | 2366 | 0.2052 | 32.5679 | 14.4370 | 23.6445 | 29.7651 |
| 0.1287 | 8.0 | 2704 | 0.2093 | 30.4390 | 13.4570 | 22.2407 | 27.9234 |
| 0.1148 | 9.0 | 3042 | 0.2140 | 26.3746 | 13.2671 | 21.7004 | 25.0909 |
| 0.1148 | 10.0 | 3380 | 0.2182 | 30.4390 | 13.4570 | 22.2407 | 27.9234 |
| 0.0996 | 11.0 | 3718 | 0.2235 | 28.9954 | 13.7629 | 21.8212 | 26.7486 |
| 0.0918 | 12.0 | 4056 | 0.2292 | 29.6633 | 13.5103 | 21.9731 | 27.1570 |
| 0.0918 | 13.0 | 4394 | 0.2344 | 32.1781 | 13.7632 | 22.8996 | 29.0738 |
| 0.0783 | 14.0 | 4732 | 0.2369 | 27.9019 | 13.5715 | 21.1971 | 25.6530 |
| 0.0697 | 15.0 | 5070 | 0.2448 | 30.3482 | 13.6242 | 22.1001 | 27.5802 |
| 0.0697 | 16.0 | 5408 | 0.2478 | 32.2060 | 13.7632 | 22.8806 | 29.0774 |
| 0.0617 | 17.0 | 5746 | 0.2511 | 30.3482 | 13.6242 | 22.1001 | 27.5802 |
| 0.0547 | 18.0 | 6084 | 0.2562 | 30.3482 | 13.6242 | 22.1001 | 27.5802 |
| 0.0547 | 19.0 | 6422 | 0.2614 | 31.7077 | 14.1430 | 23.3427 | 29.2428 |
| 0.0486 | 20.0 | 6760 | 0.2619 | 30.3691 | 13.6839 | 22.1309 | 27.5877 |
| 0.0431 | 21.0 | 7098 | 0.2666 | 30.3482 | 13.6242 | 22.1001 | 27.5802 |
| 0.0431 | 22.0 | 7436 | 0.2661 | 32.1647 | 13.7632 | 22.8606 | 29.0573 |
| 0.0398 | 23.0 | 7774 | 0.2710 | 28.3033 | 13.7723 | 21.5098 | 26.0225 |
| 0.0356 | 24.0 | 8112 | 0.2743 | 30.3482 | 13.6242 | 22.1001 | 27.5802 |
| 0.0356 | 25.0 | 8450 | 0.2729 | 30.3482 | 13.6242 | 22.1001 | 27.5802 |
| 0.033 | 26.0 | 8788 | 0.2761 | 30.3482 | 13.6242 | 22.1001 | 27.5802 |
| 0.03 | 27.0 | 9126 | 0.2782 | 30.3482 | 13.6242 | 22.1001 | 27.5802 |
| 0.03 | 28.0 | 9464 | 0.2821 | 27.7803 | 13.2224 | 20.9152 | 25.2690 |
| 0.0274 | 29.0 | 9802 | 0.2840 | 27.7800 | 13.4113 | 20.6604 | 25.5476 |
| 0.0257 | 30.0 | 10140 | 0.2855 | 30.2852 | 13.6008 | 22.0867 | 27.5376 |
| 0.0257 | 31.0 | 10478 | 0.2878 | 30.3482 | 13.6242 | 22.1001 | 27.5802 |
| 0.0238 | 32.0 | 10816 | 0.2888 | 30.3482 | 13.6242 | 22.1001 | 27.5802 |
| 0.0219 | 33.0 | 11154 | 0.2872 | 30.3482 | 13.6242 | 22.1001 | 27.5802 |
| 0.0219 | 34.0 | 11492 | 0.2905 | 30.3482 | 13.6242 | 22.1001 | 27.5802 |
| 0.0211 | 35.0 | 11830 | 0.2926 | 30.2097 | 13.6132 | 22.0455 | 27.4601 |
| 0.0192 | 36.0 | 12168 | 0.2961 | 26.7582 | 13.4935 | 20.6488 | 24.7462 |
| 0.0186 | 37.0 | 12506 | 0.2984 | 26.7089 | 12.7390 | 20.4116 | 24.8107 |
| 0.0186 | 38.0 | 12844 | 0.2955 | 30.3482 | 13.6242 | 22.1001 | 27.5802 |
| 0.0175 | 39.0 | 13182 | 0.2985 | 30.3545 | 13.8737 | 22.2152 | 27.6296 |
| 0.017 | 40.0 | 13520 | 0.3025 | 30.3545 | 13.8737 | 22.2152 | 27.6296 |
| 0.017 | 41.0 | 13858 | 0.3038 | 30.5505 | 13.0272 | 21.9910 | 27.6810 |
| 0.0158 | 42.0 | 14196 | 0.3042 | 30.3545 | 13.8737 | 22.2152 | 27.6296 |
| 0.0156 | 43.0 | 14534 | 0.3048 | 26.7070 | 13.5119 | 20.6281 | 24.6882 |
| 0.0156 | 44.0 | 14872 | 0.3061 | 30.3440 | 13.8737 | 22.1997 | 27.6126 |
| 0.0147 | 45.0 | 15210 | 0.3081 | 26.7070 | 13.5119 | 20.6281 | 24.6882 |
| 0.0141 | 46.0 | 15548 | 0.3133 | 26.7414 | 13.5119 | 20.6325 | 24.7363 |
| 0.0141 | 47.0 | 15886 | 0.3115 | 30.3482 | 13.6242 | 22.1001 | 27.5802 |
| 0.0135 | 48.0 | 16224 | 0.3131 | 26.5987 | 13.0206 | 20.4843 | 24.9550 |
| 0.0131 | 49.0 | 16562 | 0.3142 | 27.9816 | 13.6880 | 21.2917 | 25.7438 |
| 0.0131 | 50.0 | 16900 | 0.3161 | 26.9511 | 13.3418 | 20.9442 | 25.0861 |
| 0.0128 | 51.0 | 17238 | 0.3157 | 26.4405 | 12.8498 | 19.8095 | 23.8804 |
| 0.0123 | 52.0 | 17576 | 0.3169 | 26.4482 | 12.8554 | 19.8262 | 23.8895 |
| 0.0123 | 53.0 | 17914 | 0.3162 | 27.4677 | 13.5119 | 21.0011 | 25.2709 |
| 0.0121 | 54.0 | 18252 | 0.3192 | 26.4405 | 12.8498 | 19.8095 | 23.8804 |
| 0.012 | 55.0 | 18590 | 0.3192 | 27.4743 | 13.5029 | 21.0202 | 25.2768 |
| 0.012 | 56.0 | 18928 | 0.3217 | 28.7538 | 13.6889 | 21.2448 | 26.2972 |
| 0.0116 | 57.0 | 19266 | 0.3221 | 30.3482 | 13.6242 | 22.1001 | 27.5802 |
| 0.0112 | 58.0 | 19604 | 0.3214 | 27.4677 | 13.5119 | 21.0011 | 25.2709 |
| 0.0112 | 59.0 | 19942 | 0.3256 | 30.3482 | 13.6242 | 22.1001 | 27.5802 |
| 0.011 | 60.0 | 20280 | 0.3246 | 30.3482 | 13.6242 | 22.1001 | 27.5802 |
| 0.0107 | 61.0 | 20618 | 0.3269 | 26.4008 | 12.8554 | 19.8262 | 23.8411 |
| 0.0107 | 62.0 | 20956 | 0.3262 | 30.3482 | 13.6242 | 22.1001 | 27.5802 |
| 0.0107 | 63.0 | 21294 | 0.3262 | 26.4405 | 12.8498 | 19.8095 | 23.8804 |
| 0.0104 | 64.0 | 21632 | 0.3313 | 26.4008 | 12.8554 | 19.8262 | 23.8411 |
| 0.0104 | 65.0 | 21970 | 0.3301 | 26.4405 | 12.8498 | 19.8095 | 23.8804 |
| 0.0102 | 66.0 | 22308 | 0.3334 | 27.0875 | 13.1212 | 20.9683 | 24.8767 |
| 0.01 | 67.0 | 22646 | 0.3307 | 27.1356 | 13.1167 | 20.9787 | 24.9210 |
| 0.01 | 68.0 | 22984 | 0.3351 | 26.4482 | 12.8554 | 19.8262 | 23.8895 |
| 0.0101 | 69.0 | 23322 | 0.3334 | 28.2990 | 13.2942 | 20.5684 | 25.5223 |
| 0.0098 | 70.0 | 23660 | 0.3337 | 27.4743 | 13.5029 | 21.0202 | 25.2768 |
| 0.0098 | 71.0 | 23998 | 0.3320 | 26.5357 | 12.9745 | 20.4634 | 24.8632 |
| 0.0097 | 72.0 | 24336 | 0.3371 | 26.4405 | 12.8498 | 19.8095 | 23.8804 |
| 0.0094 | 73.0 | 24674 | 0.3365 | 28.2536 | 13.2942 | 20.5684 | 25.4770 |
| 0.0096 | 74.0 | 25012 | 0.3334 | 27.4677 | 13.5119 | 21.0011 | 25.2709 |
| 0.0096 | 75.0 | 25350 | 0.3401 | 26.4008 | 12.8554 | 19.8262 | 23.8411 |
| 0.0094 | 76.0 | 25688 | 0.3369 | 27.0875 | 13.1212 | 20.9683 | 24.8767 |
| 0.0092 | 77.0 | 26026 | 0.3379 | 28.2558 | 13.2942 | 20.5646 | 25.4960 |
| 0.0092 | 78.0 | 26364 | 0.3402 | 26.4405 | 12.8498 | 19.8095 | 23.8804 |
| 0.0091 | 79.0 | 26702 | 0.3394 | 29.8901 | 13.3237 | 21.5272 | 26.8283 |
| 0.0091 | 80.0 | 27040 | 0.3381 | 20.8422 | 11.5482 | 18.3383 | 20.0148 |
| 0.0091 | 81.0 | 27378 | 0.3375 | 28.2558 | 13.2942 | 20.5646 | 25.4960 |
| 0.009 | 82.0 | 27716 | 0.3382 | 28.2536 | 13.2942 | 20.5684 | 25.4770 |
| 0.0088 | 83.0 | 28054 | 0.3393 | 27.4677 | 13.5119 | 21.0011 | 25.2709 |
| 0.0088 | 84.0 | 28392 | 0.3412 | 27.2358 | 13.8221 | 21.2686 | 25.1893 |
| 0.0087 | 85.0 | 28730 | 0.3473 | 26.4405 | 12.8498 | 19.8095 | 23.8804 |
| 0.0088 | 86.0 | 29068 | 0.3433 | 28.2902 | 13.2884 | 20.5599 | 25.5133 |
| 0.0088 | 87.0 | 29406 | 0.3433 | 30.3482 | 13.6242 | 22.1001 | 27.5802 |
| 0.0086 | 88.0 | 29744 | 0.3430 | 27.0875 | 13.1212 | 20.9683 | 24.8767 |
| 0.0086 | 89.0 | 30082 | 0.3465 | 30.3482 | 13.6242 | 22.1001 | 27.5802 |
| 0.0086 | 90.0 | 30420 | 0.3444 | 26.4611 | 12.8498 | 19.8370 | 23.8945 |
| 0.0085 | 91.0 | 30758 | 0.3480 | 26.4243 | 12.8644 | 19.8396 | 23.8598 |
| 0.0085 | 92.0 | 31096 | 0.3462 | 26.4405 | 12.8498 | 19.8095 | 23.8804 |
| 0.0085 | 93.0 | 31434 | 0.3458 | 30.3482 | 13.6242 | 22.1001 | 27.5802 |
| 0.0084 | 94.0 | 31772 | 0.3433 | 30.3482 | 13.6242 | 22.1001 | 27.5802 |
| 0.0084 | 95.0 | 32110 | 0.3468 | 26.4405 | 12.8498 | 19.8095 | 23.8804 |
| 0.0084 | 96.0 | 32448 | 0.3453 | 19.7830 | 7.7026 | 16.7373 | 18.8332 |
| 0.0083 | 97.0 | 32786 | 0.3499 | 21.9400 | 11.8071 | 19.0004 | 20.7038 |
| 0.0082 | 98.0 | 33124 | 0.3509 | 28.9561 | 13.0484 | 21.4552 | 26.5578 |
| 0.0082 | 99.0 | 33462 | 0.3493 | 28.2536 | 13.2942 | 20.5684 | 25.4770 |
| 0.0082 | 100.0 | 33800 | 0.3505 | 27.1066 | 13.1324 | 20.9784 | 24.8951 |
| 0.0082 | 101.0 | 34138 | 0.3482 | 27.0875 | 13.1212 | 20.9683 | 24.8767 |
| 0.0082 | 102.0 | 34476 | 0.3497 | 27.0875 | 13.1212 | 20.9683 | 24.8767 |
| 0.0082 | 103.0 | 34814 | 0.3517 | 27.0875 | 13.1212 | 20.9683 | 24.8767 |
| 0.0081 | 104.0 | 35152 | 0.3529 | 30.3100 | 13.6441 | 22.1010 | 27.5391 |
| 0.0081 | 105.0 | 35490 | 0.3490 | 26.4405 | 12.8498 | 19.8095 | 23.8804 |
| 0.0081 | 106.0 | 35828 | 0.3524 | 30.3282 | 13.6242 | 22.1001 | 27.5644 |
| 0.0079 | 107.0 | 36166 | 0.3514 | 28.2536 | 13.2942 | 20.5684 | 25.4770 |
| 0.0081 | 108.0 | 36504 | 0.3534 | 30.3482 | 13.6242 | 22.1001 | 27.5802 |
| 0.0081 | 109.0 | 36842 | 0.3518 | 28.3079 | 13.2884 | 20.5813 | 25.5310 |
| 0.0078 | 110.0 | 37180 | 0.3538 | 30.3482 | 13.6242 | 22.1001 | 27.5802 |
| 0.0079 | 111.0 | 37518 | 0.3567 | 27.2358 | 13.8221 | 21.2686 | 25.1893 |
| 0.0079 | 112.0 | 37856 | 0.3517 | 30.3482 | 13.6242 | 22.1001 | 27.5802 |
| 0.0078 | 113.0 | 38194 | 0.3542 | 27.2358 | 13.8221 | 21.2686 | 25.1893 |
| 0.0078 | 114.0 | 38532 | 0.3558 | 30.3482 | 13.6242 | 22.1001 | 27.5802 |
| 0.0078 | 115.0 | 38870 | 0.3571 | 28.3079 | 13.2884 | 20.5813 | 25.5310 |
| 0.0077 | 116.0 | 39208 | 0.3566 | 26.4405 | 12.8498 | 19.8095 | 23.8804 |
| 0.0077 | 117.0 | 39546 | 0.3590 | 27.0875 | 13.1212 | 20.9683 | 24.8767 |
| 0.0077 | 118.0 | 39884 | 0.3574 | 23.4456 | 12.1733 | 19.9307 | 22.2553 |
| 0.0076 | 119.0 | 40222 | 0.3563 | 26.4405 | 12.8498 | 19.8095 | 23.8804 |
| 0.0077 | 120.0 | 40560 | 0.3547 | 26.4405 | 12.8498 | 19.8095 | 23.8804 |
| 0.0077 | 121.0 | 40898 | 0.3590 | 26.4611 | 12.8498 | 19.8370 | 23.8945 |
| 0.0076 | 122.0 | 41236 | 0.3559 | 22.1818 | 12.0059 | 19.2020 | 20.9696 |
| 0.0076 | 123.0 | 41574 | 0.3529 | 30.3482 | 13.6242 | 22.1001 | 27.5802 |
| 0.0076 | 124.0 | 41912 | 0.3566 | 30.3482 | 13.6242 | 22.1001 | 27.5802 |
| 0.0076 | 125.0 | 42250 | 0.3586 | 26.4243 | 12.8644 | 19.8396 | 23.8598 |
| 0.0076 | 126.0 | 42588 | 0.3562 | 26.4405 | 12.8498 | 19.8095 | 23.8804 |
| 0.0076 | 127.0 | 42926 | 0.3594 | 28.2558 | 13.2942 | 20.5646 | 25.4960 |
| 0.0075 | 128.0 | 43264 | 0.3575 | 30.3482 | 13.6242 | 22.1001 | 27.5802 |
| 0.0075 | 129.0 | 43602 | 0.3536 | 30.3482 | 13.6242 | 22.1001 | 27.5802 |
| 0.0075 | 130.0 | 43940 | 0.3566 | 28.2536 | 13.2942 | 20.5684 | 25.4770 |
| 0.0074 | 131.0 | 44278 | 0.3591 | 30.3482 | 13.6242 | 22.1001 | 27.5802 |
| 0.0075 | 132.0 | 44616 | 0.3576 | 30.3482 | 13.6242 | 22.1001 | 27.5802 |
| 0.0075 | 133.0 | 44954 | 0.3573 | 26.4611 | 12.8498 | 19.8370 | 23.8945 |
| 0.0075 | 134.0 | 45292 | 0.3580 | 26.4008 | 12.8554 | 19.8262 | 23.8411 |
| 0.0075 | 135.0 | 45630 | 0.3584 | 26.4008 | 12.8554 | 19.8262 | 23.8411 |
| 0.0075 | 136.0 | 45968 | 0.3584 | 27.0875 | 13.1212 | 20.9683 | 24.8767 |
| 0.0074 | 137.0 | 46306 | 0.3591 | 30.3482 | 13.6242 | 22.1001 | 27.5802 |
| 0.0074 | 138.0 | 46644 | 0.3604 | 28.3079 | 13.2884 | 20.5813 | 25.5310 |
| 0.0074 | 139.0 | 46982 | 0.3624 | 25.6025 | 13.3836 | 19.9214 | 23.2847 |
| 0.0074 | 140.0 | 47320 | 0.3598 | 28.2536 | 13.2942 | 20.5684 | 25.4770 |
| 0.0073 | 141.0 | 47658 | 0.3604 | 26.4405 | 12.8498 | 19.8095 | 23.8804 |
| 0.0073 | 142.0 | 47996 | 0.3613 | 26.3898 | 12.8644 | 19.8160 | 23.8305 |
| 0.0074 | 143.0 | 48334 | 0.3614 | 28.2536 | 13.2942 | 20.5684 | 25.4770 |
| 0.0074 | 144.0 | 48672 | 0.3615 | 28.2558 | 13.2942 | 20.5646 | 25.4960 |
| 0.0073 | 145.0 | 49010 | 0.3608 | 28.2536 | 13.2942 | 20.5684 | 25.4770 |
| 0.0073 | 146.0 | 49348 | 0.3616 | 26.4405 | 12.8498 | 19.8095 | 23.8804 |
| 0.0072 | 147.0 | 49686 | 0.3652 | 28.9561 | 13.0484 | 21.4552 | 26.5578 |
| 0.0073 | 148.0 | 50024 | 0.3632 | 28.2536 | 13.2942 | 20.5684 | 25.4770 |
| 0.0073 | 149.0 | 50362 | 0.3603 | 27.0875 | 13.1212 | 20.9683 | 24.8767 |
| 0.0073 | 150.0 | 50700 | 0.3608 | 26.3919 | 12.8457 | 19.8638 | 23.8486 |
| 0.0072 | 151.0 | 51038 | 0.3614 | 26.3919 | 12.8457 | 19.8638 | 23.8486 |
| 0.0072 | 152.0 | 51376 | 0.3624 | 28.2536 | 13.2942 | 20.5684 | 25.4770 |
| 0.0071 | 153.0 | 51714 | 0.3615 | 26.4008 | 12.8554 | 19.8262 | 23.8411 |
| 0.0071 | 154.0 | 52052 | 0.3636 | 26.4405 | 12.8498 | 19.8095 | 23.8804 |
| 0.0071 | 155.0 | 52390 | 0.3646 | 30.3482 | 13.6242 | 22.1001 | 27.5802 |
| 0.0072 | 156.0 | 52728 | 0.3656 | 26.4405 | 12.8498 | 19.8095 | 23.8804 |
| 0.0071 | 157.0 | 53066 | 0.3653 | 26.4405 | 12.8498 | 19.8095 | 23.8804 |
| 0.0071 | 158.0 | 53404 | 0.3644 | 26.4405 | 12.8498 | 19.8095 | 23.8804 |
| 0.0071 | 159.0 | 53742 | 0.3648 | 26.4611 | 12.8498 | 19.8370 | 23.8945 |
| 0.0071 | 160.0 | 54080 | 0.3616 | 28.2107 | 13.2942 | 20.5662 | 25.4111 |
| 0.0071 | 161.0 | 54418 | 0.3629 | 28.2536 | 13.2942 | 20.5684 | 25.4770 |
| 0.0071 | 162.0 | 54756 | 0.3647 | 28.2558 | 13.2942 | 20.5646 | 25.4960 |
| 0.007 | 163.0 | 55094 | 0.3636 | 28.2558 | 13.2942 | 20.5646 | 25.4960 |
| 0.007 | 164.0 | 55432 | 0.3650 | 26.4405 | 12.8498 | 19.8095 | 23.8804 |
| 0.007 | 165.0 | 55770 | 0.3663 | 28.2558 | 13.2942 | 20.5646 | 25.4960 |
| 0.007 | 166.0 | 56108 | 0.3659 | 28.2558 | 13.2942 | 20.5646 | 25.4960 |
| 0.007 | 167.0 | 56446 | 0.3676 | 28.2558 | 13.2942 | 20.5646 | 25.4960 |
| 0.0069 | 168.0 | 56784 | 0.3659 | 28.2558 | 13.2942 | 20.5646 | 25.4960 |
| 0.0069 | 169.0 | 57122 | 0.3674 | 28.2536 | 13.2942 | 20.5684 | 25.4770 |
| 0.0069 | 170.0 | 57460 | 0.3662 | 26.4405 | 12.8498 | 19.8095 | 23.8804 |
| 0.007 | 171.0 | 57798 | 0.3651 | 26.4405 | 12.8498 | 19.8095 | 23.8804 |
| 0.007 | 172.0 | 58136 | 0.3670 | 26.4405 | 12.8498 | 19.8095 | 23.8804 |
| 0.007 | 173.0 | 58474 | 0.3666 | 26.4405 | 12.8498 | 19.8095 | 23.8804 |
| 0.007 | 174.0 | 58812 | 0.3684 | 26.5255 | 12.8696 | 19.9233 | 24.0077 |
| 0.0069 | 175.0 | 59150 | 0.3682 | 28.2902 | 13.2884 | 20.5599 | 25.5133 |
| 0.0069 | 176.0 | 59488 | 0.3681 | 28.3079 | 13.2884 | 20.5813 | 25.5310 |
| 0.0069 | 177.0 | 59826 | 0.3687 | 28.2536 | 13.2942 | 20.5684 | 25.4770 |
| 0.0069 | 178.0 | 60164 | 0.3691 | 28.2558 | 13.2942 | 20.5646 | 25.4960 |
| 0.0069 | 179.0 | 60502 | 0.3656 | 26.4008 | 12.8554 | 19.8262 | 23.8411 |
| 0.0069 | 180.0 | 60840 | 0.3664 | 28.2536 | 13.2942 | 20.5684 | 25.4770 |
| 0.0069 | 181.0 | 61178 | 0.3663 | 28.2558 | 13.2942 | 20.5646 | 25.4960 |
| 0.0069 | 182.0 | 61516 | 0.3667 | 28.2558 | 13.2942 | 20.5646 | 25.4960 |
| 0.0069 | 183.0 | 61854 | 0.3658 | 28.2558 | 13.2942 | 20.5646 | 25.4960 |
| 0.0069 | 184.0 | 62192 | 0.3671 | 28.2558 | 13.2942 | 20.5646 | 25.4960 |
| 0.0068 | 185.0 | 62530 | 0.3686 | 28.2558 | 13.2942 | 20.5646 | 25.4960 |
| 0.0068 | 186.0 | 62868 | 0.3670 | 28.2652 | 13.2942 | 20.5234 | 25.4470 |
| 0.0068 | 187.0 | 63206 | 0.3667 | 28.2558 | 13.2942 | 20.5646 | 25.4960 |
| 0.0068 | 188.0 | 63544 | 0.3669 | 28.2558 | 13.2942 | 20.5646 | 25.4960 |
| 0.0068 | 189.0 | 63882 | 0.3676 | 28.2558 | 13.2942 | 20.5646 | 25.4960 |
| 0.0067 | 190.0 | 64220 | 0.3675 | 28.2558 | 13.2942 | 20.5646 | 25.4960 |
| 0.0068 | 191.0 | 64558 | 0.3680 | 28.2558 | 13.2942 | 20.5646 | 25.4960 |
| 0.0068 | 192.0 | 64896 | 0.3681 | 28.2558 | 13.2942 | 20.5646 | 25.4960 |
| 0.007 | 193.0 | 65234 | 0.3675 | 28.2558 | 13.2942 | 20.5646 | 25.4960 |
| 0.0068 | 194.0 | 65572 | 0.3675 | 28.2558 | 13.2942 | 20.5646 | 25.4960 |
| 0.0068 | 195.0 | 65910 | 0.3674 | 28.2558 | 13.2942 | 20.5646 | 25.4960 |
| 0.0068 | 196.0 | 66248 | 0.3679 | 28.2558 | 13.2942 | 20.5646 | 25.4960 |
| 0.0068 | 197.0 | 66586 | 0.3678 | 28.2558 | 13.2942 | 20.5646 | 25.4960 |
| 0.0068 | 198.0 | 66924 | 0.3677 | 28.2558 | 13.2942 | 20.5646 | 25.4960 |
| 0.0067 | 199.0 | 67262 | 0.3678 | 28.2558 | 13.2942 | 20.5646 | 25.4960 |
| 0.0068 | 200.0 | 67600 | 0.3678 | 28.2558 | 13.2942 | 20.5646 | 25.4960 |
### Framework versions
- Transformers 4.36.2
- Pytorch 2.2.2+cu121
- Datasets 2.16.0
- Tokenizers 0.15.2
| {"tags": ["generated_from_trainer"], "metrics": ["rouge"], "model-index": [{"name": "gpt2-finetuned-justification-v5", "results": []}]} | satyanshu404/gpt2-finetuned-justification-v5 | null | [
"transformers",
"safetensors",
"encoder-decoder",
"text2text-generation",
"generated_from_trainer",
"autotrain_compatible",
"endpoints_compatible",
"region:us"
] | null | 2024-04-18T09:30:51+00:00 | [] | [] | TAGS
#transformers #safetensors #encoder-decoder #text2text-generation #generated_from_trainer #autotrain_compatible #endpoints_compatible #region-us
| gpt2-finetuned-justification-v5
===============================
This model is a fine-tuned version of [](URL on the None dataset.
It achieves the following results on the evaluation set:
* Loss: 0.3678
* Rouge1: 28.2558
* Rouge2: 13.2942
* Rougel: 20.5646
* Rougelsum: 25.4960
Model description
-----------------
More information needed
Intended uses & limitations
---------------------------
More information needed
Training and evaluation data
----------------------------
More information needed
Training procedure
------------------
### Training hyperparameters
The following hyperparameters were used during training:
* learning\_rate: 2e-05
* train\_batch\_size: 2
* eval\_batch\_size: 2
* seed: 42
* optimizer: Adam with betas=(0.9,0.999) and epsilon=1e-08
* lr\_scheduler\_type: linear
* num\_epochs: 200
### Training results
### Framework versions
* Transformers 4.36.2
* Pytorch 2.2.2+cu121
* Datasets 2.16.0
* Tokenizers 0.15.2
| [
"### Training hyperparameters\n\n\nThe following hyperparameters were used during training:\n\n\n* learning\\_rate: 2e-05\n* train\\_batch\\_size: 2\n* eval\\_batch\\_size: 2\n* seed: 42\n* optimizer: Adam with betas=(0.9,0.999) and epsilon=1e-08\n* lr\\_scheduler\\_type: linear\n* num\\_epochs: 200",
"### Training results",
"### Framework versions\n\n\n* Transformers 4.36.2\n* Pytorch 2.2.2+cu121\n* Datasets 2.16.0\n* Tokenizers 0.15.2"
] | [
"TAGS\n#transformers #safetensors #encoder-decoder #text2text-generation #generated_from_trainer #autotrain_compatible #endpoints_compatible #region-us \n",
"### Training hyperparameters\n\n\nThe following hyperparameters were used during training:\n\n\n* learning\\_rate: 2e-05\n* train\\_batch\\_size: 2\n* eval\\_batch\\_size: 2\n* seed: 42\n* optimizer: Adam with betas=(0.9,0.999) and epsilon=1e-08\n* lr\\_scheduler\\_type: linear\n* num\\_epochs: 200",
"### Training results",
"### Framework versions\n\n\n* Transformers 4.36.2\n* Pytorch 2.2.2+cu121\n* Datasets 2.16.0\n* Tokenizers 0.15.2"
] |
text-classification | transformers |
<!-- This model card has been generated automatically according to the information the Trainer had access to. You
should probably proofread and complete it, then remove this comment. -->
# finetuning-0
This model is a fine-tuned version of [PlanTL-GOB-ES/bsc-bio-ehr-es](https://huggingface.co/PlanTL-GOB-ES/bsc-bio-ehr-es) on the None dataset.
It achieves the following results on the evaluation set:
- Loss: 0.2446
- Precision: 0.9615
- Sensitivity: 0.8065
- F1: 0.8772
## Model description
More information needed
## Intended uses & limitations
More information needed
## Training and evaluation data
More information needed
## Training procedure
### Training hyperparameters
The following hyperparameters were used during training:
- learning_rate: 1e-05
- train_batch_size: 16
- eval_batch_size: 8
- seed: 42
- optimizer: Adam with betas=(0.9,0.999) and epsilon=1e-08
- lr_scheduler_type: linear
- num_epochs: 8
### Training results
| Training Loss | Epoch | Step | Validation Loss | Precision | Sensitivity | F1 |
|:-------------:|:-----:|:----:|:---------------:|:---------:|:-----------:|:------:|
| 0.3577 | 1.27 | 150 | 0.2695 | 0.9615 | 0.5376 | 0.6897 |
| 0.1836 | 2.54 | 300 | 0.2239 | 0.9545 | 0.6774 | 0.7925 |
| 0.1518 | 3.81 | 450 | 0.1970 | 0.9481 | 0.7849 | 0.8588 |
| 0.1129 | 5.08 | 600 | 0.2098 | 0.9620 | 0.8172 | 0.8837 |
| 0.0688 | 6.36 | 750 | 0.2758 | 0.9853 | 0.7204 | 0.8323 |
| 0.0592 | 7.63 | 900 | 0.2446 | 0.9615 | 0.8065 | 0.8772 |
### Framework versions
- Transformers 4.38.2
- Pytorch 2.2.1+cu121
- Datasets 2.18.0
- Tokenizers 0.15.2
| {"license": "apache-2.0", "tags": ["generated_from_trainer"], "metrics": ["precision", "f1"], "base_model": "PlanTL-GOB-ES/bsc-bio-ehr-es", "model-index": [{"name": "finetuning-0", "results": []}]} | roscazo/finetuning-0 | null | [
"transformers",
"tensorboard",
"safetensors",
"roberta",
"text-classification",
"generated_from_trainer",
"base_model:PlanTL-GOB-ES/bsc-bio-ehr-es",
"license:apache-2.0",
"autotrain_compatible",
"endpoints_compatible",
"region:us"
] | null | 2024-04-18T09:34:18+00:00 | [] | [] | TAGS
#transformers #tensorboard #safetensors #roberta #text-classification #generated_from_trainer #base_model-PlanTL-GOB-ES/bsc-bio-ehr-es #license-apache-2.0 #autotrain_compatible #endpoints_compatible #region-us
| finetuning-0
============
This model is a fine-tuned version of PlanTL-GOB-ES/bsc-bio-ehr-es on the None dataset.
It achieves the following results on the evaluation set:
* Loss: 0.2446
* Precision: 0.9615
* Sensitivity: 0.8065
* F1: 0.8772
Model description
-----------------
More information needed
Intended uses & limitations
---------------------------
More information needed
Training and evaluation data
----------------------------
More information needed
Training procedure
------------------
### Training hyperparameters
The following hyperparameters were used during training:
* learning\_rate: 1e-05
* train\_batch\_size: 16
* eval\_batch\_size: 8
* seed: 42
* optimizer: Adam with betas=(0.9,0.999) and epsilon=1e-08
* lr\_scheduler\_type: linear
* num\_epochs: 8
### Training results
### Framework versions
* Transformers 4.38.2
* Pytorch 2.2.1+cu121
* Datasets 2.18.0
* Tokenizers 0.15.2
| [
"### Training hyperparameters\n\n\nThe following hyperparameters were used during training:\n\n\n* learning\\_rate: 1e-05\n* train\\_batch\\_size: 16\n* eval\\_batch\\_size: 8\n* seed: 42\n* optimizer: Adam with betas=(0.9,0.999) and epsilon=1e-08\n* lr\\_scheduler\\_type: linear\n* num\\_epochs: 8",
"### Training results",
"### Framework versions\n\n\n* Transformers 4.38.2\n* Pytorch 2.2.1+cu121\n* Datasets 2.18.0\n* Tokenizers 0.15.2"
] | [
"TAGS\n#transformers #tensorboard #safetensors #roberta #text-classification #generated_from_trainer #base_model-PlanTL-GOB-ES/bsc-bio-ehr-es #license-apache-2.0 #autotrain_compatible #endpoints_compatible #region-us \n",
"### Training hyperparameters\n\n\nThe following hyperparameters were used during training:\n\n\n* learning\\_rate: 1e-05\n* train\\_batch\\_size: 16\n* eval\\_batch\\_size: 8\n* seed: 42\n* optimizer: Adam with betas=(0.9,0.999) and epsilon=1e-08\n* lr\\_scheduler\\_type: linear\n* num\\_epochs: 8",
"### Training results",
"### Framework versions\n\n\n* Transformers 4.38.2\n* Pytorch 2.2.1+cu121\n* Datasets 2.18.0\n* Tokenizers 0.15.2"
] |
null | transformers |
# Model Card for Model ID
<!-- Provide a quick summary of what the model is/does. -->
## Model Details
### Model Description
<!-- Provide a longer summary of what this model is. -->
This is the model card of a 🤗 transformers model that has been pushed on the Hub. This model card has been automatically generated.
- **Developed by:** [More Information Needed]
- **Funded by [optional]:** [More Information Needed]
- **Shared by [optional]:** [More Information Needed]
- **Model type:** [More Information Needed]
- **Language(s) (NLP):** [More Information Needed]
- **License:** [More Information Needed]
- **Finetuned from model [optional]:** [More Information Needed]
### Model Sources [optional]
<!-- Provide the basic links for the model. -->
- **Repository:** [More Information Needed]
- **Paper [optional]:** [More Information Needed]
- **Demo [optional]:** [More Information Needed]
## Uses
<!-- Address questions around how the model is intended to be used, including the foreseeable users of the model and those affected by the model. -->
### Direct Use
<!-- This section is for the model use without fine-tuning or plugging into a larger ecosystem/app. -->
[More Information Needed]
### Downstream Use [optional]
<!-- This section is for the model use when fine-tuned for a task, or when plugged into a larger ecosystem/app -->
[More Information Needed]
### Out-of-Scope Use
<!-- This section addresses misuse, malicious use, and uses that the model will not work well for. -->
[More Information Needed]
## Bias, Risks, and Limitations
<!-- This section is meant to convey both technical and sociotechnical limitations. -->
[More Information Needed]
### Recommendations
<!-- This section is meant to convey recommendations with respect to the bias, risk, and technical limitations. -->
Users (both direct and downstream) should be made aware of the risks, biases and limitations of the model. More information needed for further recommendations.
## How to Get Started with the Model
Use the code below to get started with the model.
[More Information Needed]
## Training Details
### Training Data
<!-- This should link to a Dataset Card, perhaps with a short stub of information on what the training data is all about as well as documentation related to data pre-processing or additional filtering. -->
[More Information Needed]
### Training Procedure
<!-- This relates heavily to the Technical Specifications. Content here should link to that section when it is relevant to the training procedure. -->
#### Preprocessing [optional]
[More Information Needed]
#### Training Hyperparameters
- **Training regime:** [More Information Needed] <!--fp32, fp16 mixed precision, bf16 mixed precision, bf16 non-mixed precision, fp16 non-mixed precision, fp8 mixed precision -->
#### Speeds, Sizes, Times [optional]
<!-- This section provides information about throughput, start/end time, checkpoint size if relevant, etc. -->
[More Information Needed]
## Evaluation
<!-- This section describes the evaluation protocols and provides the results. -->
### Testing Data, Factors & Metrics
#### Testing Data
<!-- This should link to a Dataset Card if possible. -->
[More Information Needed]
#### Factors
<!-- These are the things the evaluation is disaggregating by, e.g., subpopulations or domains. -->
[More Information Needed]
#### Metrics
<!-- These are the evaluation metrics being used, ideally with a description of why. -->
[More Information Needed]
### Results
[More Information Needed]
#### Summary
## Model Examination [optional]
<!-- Relevant interpretability work for the model goes here -->
[More Information Needed]
## Environmental Impact
<!-- Total emissions (in grams of CO2eq) and additional considerations, such as electricity usage, go here. Edit the suggested text below accordingly -->
Carbon emissions can be estimated using the [Machine Learning Impact calculator](https://mlco2.github.io/impact#compute) presented in [Lacoste et al. (2019)](https://arxiv.org/abs/1910.09700).
- **Hardware Type:** [More Information Needed]
- **Hours used:** [More Information Needed]
- **Cloud Provider:** [More Information Needed]
- **Compute Region:** [More Information Needed]
- **Carbon Emitted:** [More Information Needed]
## Technical Specifications [optional]
### Model Architecture and Objective
[More Information Needed]
### Compute Infrastructure
[More Information Needed]
#### Hardware
[More Information Needed]
#### Software
[More Information Needed]
## Citation [optional]
<!-- If there is a paper or blog post introducing the model, the APA and Bibtex information for that should go in this section. -->
**BibTeX:**
[More Information Needed]
**APA:**
[More Information Needed]
## Glossary [optional]
<!-- If relevant, include terms and calculations in this section that can help readers understand the model or model card. -->
[More Information Needed]
## More Information [optional]
[More Information Needed]
## Model Card Authors [optional]
[More Information Needed]
## Model Card Contact
[More Information Needed] | {"library_name": "transformers", "tags": []} | abdullahfurquan/mistral-7b_1000 | null | [
"transformers",
"safetensors",
"arxiv:1910.09700",
"endpoints_compatible",
"region:us"
] | null | 2024-04-18T09:35:01+00:00 | [
"1910.09700"
] | [] | TAGS
#transformers #safetensors #arxiv-1910.09700 #endpoints_compatible #region-us
|
# Model Card for Model ID
## Model Details
### Model Description
This is the model card of a transformers model that has been pushed on the Hub. This model card has been automatically generated.
- Developed by:
- Funded by [optional]:
- Shared by [optional]:
- Model type:
- Language(s) (NLP):
- License:
- Finetuned from model [optional]:
### Model Sources [optional]
- Repository:
- Paper [optional]:
- Demo [optional]:
## Uses
### Direct Use
### Downstream Use [optional]
### Out-of-Scope Use
## Bias, Risks, and Limitations
### Recommendations
Users (both direct and downstream) should be made aware of the risks, biases and limitations of the model. More information needed for further recommendations.
## How to Get Started with the Model
Use the code below to get started with the model.
## Training Details
### Training Data
### Training Procedure
#### Preprocessing [optional]
#### Training Hyperparameters
- Training regime:
#### Speeds, Sizes, Times [optional]
## Evaluation
### Testing Data, Factors & Metrics
#### Testing Data
#### Factors
#### Metrics
### Results
#### Summary
## Model Examination [optional]
## Environmental Impact
Carbon emissions can be estimated using the Machine Learning Impact calculator presented in Lacoste et al. (2019).
- Hardware Type:
- Hours used:
- Cloud Provider:
- Compute Region:
- Carbon Emitted:
## Technical Specifications [optional]
### Model Architecture and Objective
### Compute Infrastructure
#### Hardware
#### Software
[optional]
BibTeX:
APA:
## Glossary [optional]
## More Information [optional]
## Model Card Authors [optional]
## Model Card Contact
| [
"# Model Card for Model ID",
"## Model Details",
"### Model Description\n\n\n\nThis is the model card of a transformers model that has been pushed on the Hub. This model card has been automatically generated.\n\n- Developed by: \n- Funded by [optional]: \n- Shared by [optional]: \n- Model type: \n- Language(s) (NLP): \n- License: \n- Finetuned from model [optional]:",
"### Model Sources [optional]\n\n\n\n- Repository: \n- Paper [optional]: \n- Demo [optional]:",
"## Uses",
"### Direct Use",
"### Downstream Use [optional]",
"### Out-of-Scope Use",
"## Bias, Risks, and Limitations",
"### Recommendations\n\n\n\nUsers (both direct and downstream) should be made aware of the risks, biases and limitations of the model. More information needed for further recommendations.",
"## How to Get Started with the Model\n\nUse the code below to get started with the model.",
"## Training Details",
"### Training Data",
"### Training Procedure",
"#### Preprocessing [optional]",
"#### Training Hyperparameters\n\n- Training regime:",
"#### Speeds, Sizes, Times [optional]",
"## Evaluation",
"### Testing Data, Factors & Metrics",
"#### Testing Data",
"#### Factors",
"#### Metrics",
"### Results",
"#### Summary",
"## Model Examination [optional]",
"## Environmental Impact\n\n\n\nCarbon emissions can be estimated using the Machine Learning Impact calculator presented in Lacoste et al. (2019).\n\n- Hardware Type: \n- Hours used: \n- Cloud Provider: \n- Compute Region: \n- Carbon Emitted:",
"## Technical Specifications [optional]",
"### Model Architecture and Objective",
"### Compute Infrastructure",
"#### Hardware",
"#### Software\n\n\n\n[optional]\n\n\n\nBibTeX:\n\n\n\nAPA:",
"## Glossary [optional]",
"## More Information [optional]",
"## Model Card Authors [optional]",
"## Model Card Contact"
] | [
"TAGS\n#transformers #safetensors #arxiv-1910.09700 #endpoints_compatible #region-us \n",
"# Model Card for Model ID",
"## Model Details",
"### Model Description\n\n\n\nThis is the model card of a transformers model that has been pushed on the Hub. This model card has been automatically generated.\n\n- Developed by: \n- Funded by [optional]: \n- Shared by [optional]: \n- Model type: \n- Language(s) (NLP): \n- License: \n- Finetuned from model [optional]:",
"### Model Sources [optional]\n\n\n\n- Repository: \n- Paper [optional]: \n- Demo [optional]:",
"## Uses",
"### Direct Use",
"### Downstream Use [optional]",
"### Out-of-Scope Use",
"## Bias, Risks, and Limitations",
"### Recommendations\n\n\n\nUsers (both direct and downstream) should be made aware of the risks, biases and limitations of the model. More information needed for further recommendations.",
"## How to Get Started with the Model\n\nUse the code below to get started with the model.",
"## Training Details",
"### Training Data",
"### Training Procedure",
"#### Preprocessing [optional]",
"#### Training Hyperparameters\n\n- Training regime:",
"#### Speeds, Sizes, Times [optional]",
"## Evaluation",
"### Testing Data, Factors & Metrics",
"#### Testing Data",
"#### Factors",
"#### Metrics",
"### Results",
"#### Summary",
"## Model Examination [optional]",
"## Environmental Impact\n\n\n\nCarbon emissions can be estimated using the Machine Learning Impact calculator presented in Lacoste et al. (2019).\n\n- Hardware Type: \n- Hours used: \n- Cloud Provider: \n- Compute Region: \n- Carbon Emitted:",
"## Technical Specifications [optional]",
"### Model Architecture and Objective",
"### Compute Infrastructure",
"#### Hardware",
"#### Software\n\n\n\n[optional]\n\n\n\nBibTeX:\n\n\n\nAPA:",
"## Glossary [optional]",
"## More Information [optional]",
"## Model Card Authors [optional]",
"## Model Card Contact"
] |
null | transformers |
# Model Card for Model ID
<!-- Provide a quick summary of what the model is/does. -->
## Model Details
### Model Description
<!-- Provide a longer summary of what this model is. -->
This is the model card of a 🤗 transformers model that has been pushed on the Hub. This model card has been automatically generated.
- **Developed by:** [More Information Needed]
- **Funded by [optional]:** [More Information Needed]
- **Shared by [optional]:** [More Information Needed]
- **Model type:** [More Information Needed]
- **Language(s) (NLP):** [More Information Needed]
- **License:** [More Information Needed]
- **Finetuned from model [optional]:** [More Information Needed]
### Model Sources [optional]
<!-- Provide the basic links for the model. -->
- **Repository:** [More Information Needed]
- **Paper [optional]:** [More Information Needed]
- **Demo [optional]:** [More Information Needed]
## Uses
<!-- Address questions around how the model is intended to be used, including the foreseeable users of the model and those affected by the model. -->
### Direct Use
<!-- This section is for the model use without fine-tuning or plugging into a larger ecosystem/app. -->
[More Information Needed]
### Downstream Use [optional]
<!-- This section is for the model use when fine-tuned for a task, or when plugged into a larger ecosystem/app -->
[More Information Needed]
### Out-of-Scope Use
<!-- This section addresses misuse, malicious use, and uses that the model will not work well for. -->
[More Information Needed]
## Bias, Risks, and Limitations
<!-- This section is meant to convey both technical and sociotechnical limitations. -->
[More Information Needed]
### Recommendations
<!-- This section is meant to convey recommendations with respect to the bias, risk, and technical limitations. -->
Users (both direct and downstream) should be made aware of the risks, biases and limitations of the model. More information needed for further recommendations.
## How to Get Started with the Model
Use the code below to get started with the model.
[More Information Needed]
## Training Details
### Training Data
<!-- This should link to a Dataset Card, perhaps with a short stub of information on what the training data is all about as well as documentation related to data pre-processing or additional filtering. -->
[More Information Needed]
### Training Procedure
<!-- This relates heavily to the Technical Specifications. Content here should link to that section when it is relevant to the training procedure. -->
#### Preprocessing [optional]
[More Information Needed]
#### Training Hyperparameters
- **Training regime:** [More Information Needed] <!--fp32, fp16 mixed precision, bf16 mixed precision, bf16 non-mixed precision, fp16 non-mixed precision, fp8 mixed precision -->
#### Speeds, Sizes, Times [optional]
<!-- This section provides information about throughput, start/end time, checkpoint size if relevant, etc. -->
[More Information Needed]
## Evaluation
<!-- This section describes the evaluation protocols and provides the results. -->
### Testing Data, Factors & Metrics
#### Testing Data
<!-- This should link to a Dataset Card if possible. -->
[More Information Needed]
#### Factors
<!-- These are the things the evaluation is disaggregating by, e.g., subpopulations or domains. -->
[More Information Needed]
#### Metrics
<!-- These are the evaluation metrics being used, ideally with a description of why. -->
[More Information Needed]
### Results
[More Information Needed]
#### Summary
## Model Examination [optional]
<!-- Relevant interpretability work for the model goes here -->
[More Information Needed]
## Environmental Impact
<!-- Total emissions (in grams of CO2eq) and additional considerations, such as electricity usage, go here. Edit the suggested text below accordingly -->
Carbon emissions can be estimated using the [Machine Learning Impact calculator](https://mlco2.github.io/impact#compute) presented in [Lacoste et al. (2019)](https://arxiv.org/abs/1910.09700).
- **Hardware Type:** [More Information Needed]
- **Hours used:** [More Information Needed]
- **Cloud Provider:** [More Information Needed]
- **Compute Region:** [More Information Needed]
- **Carbon Emitted:** [More Information Needed]
## Technical Specifications [optional]
### Model Architecture and Objective
[More Information Needed]
### Compute Infrastructure
[More Information Needed]
#### Hardware
[More Information Needed]
#### Software
[More Information Needed]
## Citation [optional]
<!-- If there is a paper or blog post introducing the model, the APA and Bibtex information for that should go in this section. -->
**BibTeX:**
[More Information Needed]
**APA:**
[More Information Needed]
## Glossary [optional]
<!-- If relevant, include terms and calculations in this section that can help readers understand the model or model card. -->
[More Information Needed]
## More Information [optional]
[More Information Needed]
## Model Card Authors [optional]
[More Information Needed]
## Model Card Contact
[More Information Needed] | {"library_name": "transformers", "tags": []} | abdullahfurquan/mistral-7b_2000 | null | [
"transformers",
"safetensors",
"arxiv:1910.09700",
"endpoints_compatible",
"region:us"
] | null | 2024-04-18T09:35:15+00:00 | [
"1910.09700"
] | [] | TAGS
#transformers #safetensors #arxiv-1910.09700 #endpoints_compatible #region-us
|
# Model Card for Model ID
## Model Details
### Model Description
This is the model card of a transformers model that has been pushed on the Hub. This model card has been automatically generated.
- Developed by:
- Funded by [optional]:
- Shared by [optional]:
- Model type:
- Language(s) (NLP):
- License:
- Finetuned from model [optional]:
### Model Sources [optional]
- Repository:
- Paper [optional]:
- Demo [optional]:
## Uses
### Direct Use
### Downstream Use [optional]
### Out-of-Scope Use
## Bias, Risks, and Limitations
### Recommendations
Users (both direct and downstream) should be made aware of the risks, biases and limitations of the model. More information needed for further recommendations.
## How to Get Started with the Model
Use the code below to get started with the model.
## Training Details
### Training Data
### Training Procedure
#### Preprocessing [optional]
#### Training Hyperparameters
- Training regime:
#### Speeds, Sizes, Times [optional]
## Evaluation
### Testing Data, Factors & Metrics
#### Testing Data
#### Factors
#### Metrics
### Results
#### Summary
## Model Examination [optional]
## Environmental Impact
Carbon emissions can be estimated using the Machine Learning Impact calculator presented in Lacoste et al. (2019).
- Hardware Type:
- Hours used:
- Cloud Provider:
- Compute Region:
- Carbon Emitted:
## Technical Specifications [optional]
### Model Architecture and Objective
### Compute Infrastructure
#### Hardware
#### Software
[optional]
BibTeX:
APA:
## Glossary [optional]
## More Information [optional]
## Model Card Authors [optional]
## Model Card Contact
| [
"# Model Card for Model ID",
"## Model Details",
"### Model Description\n\n\n\nThis is the model card of a transformers model that has been pushed on the Hub. This model card has been automatically generated.\n\n- Developed by: \n- Funded by [optional]: \n- Shared by [optional]: \n- Model type: \n- Language(s) (NLP): \n- License: \n- Finetuned from model [optional]:",
"### Model Sources [optional]\n\n\n\n- Repository: \n- Paper [optional]: \n- Demo [optional]:",
"## Uses",
"### Direct Use",
"### Downstream Use [optional]",
"### Out-of-Scope Use",
"## Bias, Risks, and Limitations",
"### Recommendations\n\n\n\nUsers (both direct and downstream) should be made aware of the risks, biases and limitations of the model. More information needed for further recommendations.",
"## How to Get Started with the Model\n\nUse the code below to get started with the model.",
"## Training Details",
"### Training Data",
"### Training Procedure",
"#### Preprocessing [optional]",
"#### Training Hyperparameters\n\n- Training regime:",
"#### Speeds, Sizes, Times [optional]",
"## Evaluation",
"### Testing Data, Factors & Metrics",
"#### Testing Data",
"#### Factors",
"#### Metrics",
"### Results",
"#### Summary",
"## Model Examination [optional]",
"## Environmental Impact\n\n\n\nCarbon emissions can be estimated using the Machine Learning Impact calculator presented in Lacoste et al. (2019).\n\n- Hardware Type: \n- Hours used: \n- Cloud Provider: \n- Compute Region: \n- Carbon Emitted:",
"## Technical Specifications [optional]",
"### Model Architecture and Objective",
"### Compute Infrastructure",
"#### Hardware",
"#### Software\n\n\n\n[optional]\n\n\n\nBibTeX:\n\n\n\nAPA:",
"## Glossary [optional]",
"## More Information [optional]",
"## Model Card Authors [optional]",
"## Model Card Contact"
] | [
"TAGS\n#transformers #safetensors #arxiv-1910.09700 #endpoints_compatible #region-us \n",
"# Model Card for Model ID",
"## Model Details",
"### Model Description\n\n\n\nThis is the model card of a transformers model that has been pushed on the Hub. This model card has been automatically generated.\n\n- Developed by: \n- Funded by [optional]: \n- Shared by [optional]: \n- Model type: \n- Language(s) (NLP): \n- License: \n- Finetuned from model [optional]:",
"### Model Sources [optional]\n\n\n\n- Repository: \n- Paper [optional]: \n- Demo [optional]:",
"## Uses",
"### Direct Use",
"### Downstream Use [optional]",
"### Out-of-Scope Use",
"## Bias, Risks, and Limitations",
"### Recommendations\n\n\n\nUsers (both direct and downstream) should be made aware of the risks, biases and limitations of the model. More information needed for further recommendations.",
"## How to Get Started with the Model\n\nUse the code below to get started with the model.",
"## Training Details",
"### Training Data",
"### Training Procedure",
"#### Preprocessing [optional]",
"#### Training Hyperparameters\n\n- Training regime:",
"#### Speeds, Sizes, Times [optional]",
"## Evaluation",
"### Testing Data, Factors & Metrics",
"#### Testing Data",
"#### Factors",
"#### Metrics",
"### Results",
"#### Summary",
"## Model Examination [optional]",
"## Environmental Impact\n\n\n\nCarbon emissions can be estimated using the Machine Learning Impact calculator presented in Lacoste et al. (2019).\n\n- Hardware Type: \n- Hours used: \n- Cloud Provider: \n- Compute Region: \n- Carbon Emitted:",
"## Technical Specifications [optional]",
"### Model Architecture and Objective",
"### Compute Infrastructure",
"#### Hardware",
"#### Software\n\n\n\n[optional]\n\n\n\nBibTeX:\n\n\n\nAPA:",
"## Glossary [optional]",
"## More Information [optional]",
"## Model Card Authors [optional]",
"## Model Card Contact"
] |
text-generation | transformers |
# Model Card for Model ID
<!-- Provide a quick summary of what the model is/does. -->
## Model Details
### Model Description
<!-- Provide a longer summary of what this model is. -->
This is the model card of a 🤗 transformers model that has been pushed on the Hub. This model card has been automatically generated.
- **Developed by:** [More Information Needed]
- **Funded by [optional]:** [More Information Needed]
- **Shared by [optional]:** [More Information Needed]
- **Model type:** [More Information Needed]
- **Language(s) (NLP):** [More Information Needed]
- **License:** [More Information Needed]
- **Finetuned from model [optional]:** [More Information Needed]
### Model Sources [optional]
<!-- Provide the basic links for the model. -->
- **Repository:** [More Information Needed]
- **Paper [optional]:** [More Information Needed]
- **Demo [optional]:** [More Information Needed]
## Uses
<!-- Address questions around how the model is intended to be used, including the foreseeable users of the model and those affected by the model. -->
### Direct Use
<!-- This section is for the model use without fine-tuning or plugging into a larger ecosystem/app. -->
[More Information Needed]
### Downstream Use [optional]
<!-- This section is for the model use when fine-tuned for a task, or when plugged into a larger ecosystem/app -->
[More Information Needed]
### Out-of-Scope Use
<!-- This section addresses misuse, malicious use, and uses that the model will not work well for. -->
[More Information Needed]
## Bias, Risks, and Limitations
<!-- This section is meant to convey both technical and sociotechnical limitations. -->
[More Information Needed]
### Recommendations
<!-- This section is meant to convey recommendations with respect to the bias, risk, and technical limitations. -->
Users (both direct and downstream) should be made aware of the risks, biases and limitations of the model. More information needed for further recommendations.
## How to Get Started with the Model
Use the code below to get started with the model.
[More Information Needed]
## Training Details
### Training Data
<!-- This should link to a Dataset Card, perhaps with a short stub of information on what the training data is all about as well as documentation related to data pre-processing or additional filtering. -->
[More Information Needed]
### Training Procedure
<!-- This relates heavily to the Technical Specifications. Content here should link to that section when it is relevant to the training procedure. -->
#### Preprocessing [optional]
[More Information Needed]
#### Training Hyperparameters
- **Training regime:** [More Information Needed] <!--fp32, fp16 mixed precision, bf16 mixed precision, bf16 non-mixed precision, fp16 non-mixed precision, fp8 mixed precision -->
#### Speeds, Sizes, Times [optional]
<!-- This section provides information about throughput, start/end time, checkpoint size if relevant, etc. -->
[More Information Needed]
## Evaluation
<!-- This section describes the evaluation protocols and provides the results. -->
### Testing Data, Factors & Metrics
#### Testing Data
<!-- This should link to a Dataset Card if possible. -->
[More Information Needed]
#### Factors
<!-- These are the things the evaluation is disaggregating by, e.g., subpopulations or domains. -->
[More Information Needed]
#### Metrics
<!-- These are the evaluation metrics being used, ideally with a description of why. -->
[More Information Needed]
### Results
[More Information Needed]
#### Summary
## Model Examination [optional]
<!-- Relevant interpretability work for the model goes here -->
[More Information Needed]
## Environmental Impact
<!-- Total emissions (in grams of CO2eq) and additional considerations, such as electricity usage, go here. Edit the suggested text below accordingly -->
Carbon emissions can be estimated using the [Machine Learning Impact calculator](https://mlco2.github.io/impact#compute) presented in [Lacoste et al. (2019)](https://arxiv.org/abs/1910.09700).
- **Hardware Type:** [More Information Needed]
- **Hours used:** [More Information Needed]
- **Cloud Provider:** [More Information Needed]
- **Compute Region:** [More Information Needed]
- **Carbon Emitted:** [More Information Needed]
## Technical Specifications [optional]
### Model Architecture and Objective
[More Information Needed]
### Compute Infrastructure
[More Information Needed]
#### Hardware
[More Information Needed]
#### Software
[More Information Needed]
## Citation [optional]
<!-- If there is a paper or blog post introducing the model, the APA and Bibtex information for that should go in this section. -->
**BibTeX:**
[More Information Needed]
**APA:**
[More Information Needed]
## Glossary [optional]
<!-- If relevant, include terms and calculations in this section that can help readers understand the model or model card. -->
[More Information Needed]
## More Information [optional]
[More Information Needed]
## Model Card Authors [optional]
[More Information Needed]
## Model Card Contact
[More Information Needed] | {"library_name": "transformers", "tags": []} | Grayx/sad_pepe_7.0 | null | [
"transformers",
"safetensors",
"stablelm",
"text-generation",
"conversational",
"arxiv:1910.09700",
"autotrain_compatible",
"endpoints_compatible",
"region:us"
] | null | 2024-04-18T09:36:45+00:00 | [
"1910.09700"
] | [] | TAGS
#transformers #safetensors #stablelm #text-generation #conversational #arxiv-1910.09700 #autotrain_compatible #endpoints_compatible #region-us
|
# Model Card for Model ID
## Model Details
### Model Description
This is the model card of a transformers model that has been pushed on the Hub. This model card has been automatically generated.
- Developed by:
- Funded by [optional]:
- Shared by [optional]:
- Model type:
- Language(s) (NLP):
- License:
- Finetuned from model [optional]:
### Model Sources [optional]
- Repository:
- Paper [optional]:
- Demo [optional]:
## Uses
### Direct Use
### Downstream Use [optional]
### Out-of-Scope Use
## Bias, Risks, and Limitations
### Recommendations
Users (both direct and downstream) should be made aware of the risks, biases and limitations of the model. More information needed for further recommendations.
## How to Get Started with the Model
Use the code below to get started with the model.
## Training Details
### Training Data
### Training Procedure
#### Preprocessing [optional]
#### Training Hyperparameters
- Training regime:
#### Speeds, Sizes, Times [optional]
## Evaluation
### Testing Data, Factors & Metrics
#### Testing Data
#### Factors
#### Metrics
### Results
#### Summary
## Model Examination [optional]
## Environmental Impact
Carbon emissions can be estimated using the Machine Learning Impact calculator presented in Lacoste et al. (2019).
- Hardware Type:
- Hours used:
- Cloud Provider:
- Compute Region:
- Carbon Emitted:
## Technical Specifications [optional]
### Model Architecture and Objective
### Compute Infrastructure
#### Hardware
#### Software
[optional]
BibTeX:
APA:
## Glossary [optional]
## More Information [optional]
## Model Card Authors [optional]
## Model Card Contact
| [
"# Model Card for Model ID",
"## Model Details",
"### Model Description\n\n\n\nThis is the model card of a transformers model that has been pushed on the Hub. This model card has been automatically generated.\n\n- Developed by: \n- Funded by [optional]: \n- Shared by [optional]: \n- Model type: \n- Language(s) (NLP): \n- License: \n- Finetuned from model [optional]:",
"### Model Sources [optional]\n\n\n\n- Repository: \n- Paper [optional]: \n- Demo [optional]:",
"## Uses",
"### Direct Use",
"### Downstream Use [optional]",
"### Out-of-Scope Use",
"## Bias, Risks, and Limitations",
"### Recommendations\n\n\n\nUsers (both direct and downstream) should be made aware of the risks, biases and limitations of the model. More information needed for further recommendations.",
"## How to Get Started with the Model\n\nUse the code below to get started with the model.",
"## Training Details",
"### Training Data",
"### Training Procedure",
"#### Preprocessing [optional]",
"#### Training Hyperparameters\n\n- Training regime:",
"#### Speeds, Sizes, Times [optional]",
"## Evaluation",
"### Testing Data, Factors & Metrics",
"#### Testing Data",
"#### Factors",
"#### Metrics",
"### Results",
"#### Summary",
"## Model Examination [optional]",
"## Environmental Impact\n\n\n\nCarbon emissions can be estimated using the Machine Learning Impact calculator presented in Lacoste et al. (2019).\n\n- Hardware Type: \n- Hours used: \n- Cloud Provider: \n- Compute Region: \n- Carbon Emitted:",
"## Technical Specifications [optional]",
"### Model Architecture and Objective",
"### Compute Infrastructure",
"#### Hardware",
"#### Software\n\n\n\n[optional]\n\n\n\nBibTeX:\n\n\n\nAPA:",
"## Glossary [optional]",
"## More Information [optional]",
"## Model Card Authors [optional]",
"## Model Card Contact"
] | [
"TAGS\n#transformers #safetensors #stablelm #text-generation #conversational #arxiv-1910.09700 #autotrain_compatible #endpoints_compatible #region-us \n",
"# Model Card for Model ID",
"## Model Details",
"### Model Description\n\n\n\nThis is the model card of a transformers model that has been pushed on the Hub. This model card has been automatically generated.\n\n- Developed by: \n- Funded by [optional]: \n- Shared by [optional]: \n- Model type: \n- Language(s) (NLP): \n- License: \n- Finetuned from model [optional]:",
"### Model Sources [optional]\n\n\n\n- Repository: \n- Paper [optional]: \n- Demo [optional]:",
"## Uses",
"### Direct Use",
"### Downstream Use [optional]",
"### Out-of-Scope Use",
"## Bias, Risks, and Limitations",
"### Recommendations\n\n\n\nUsers (both direct and downstream) should be made aware of the risks, biases and limitations of the model. More information needed for further recommendations.",
"## How to Get Started with the Model\n\nUse the code below to get started with the model.",
"## Training Details",
"### Training Data",
"### Training Procedure",
"#### Preprocessing [optional]",
"#### Training Hyperparameters\n\n- Training regime:",
"#### Speeds, Sizes, Times [optional]",
"## Evaluation",
"### Testing Data, Factors & Metrics",
"#### Testing Data",
"#### Factors",
"#### Metrics",
"### Results",
"#### Summary",
"## Model Examination [optional]",
"## Environmental Impact\n\n\n\nCarbon emissions can be estimated using the Machine Learning Impact calculator presented in Lacoste et al. (2019).\n\n- Hardware Type: \n- Hours used: \n- Cloud Provider: \n- Compute Region: \n- Carbon Emitted:",
"## Technical Specifications [optional]",
"### Model Architecture and Objective",
"### Compute Infrastructure",
"#### Hardware",
"#### Software\n\n\n\n[optional]\n\n\n\nBibTeX:\n\n\n\nAPA:",
"## Glossary [optional]",
"## More Information [optional]",
"## Model Card Authors [optional]",
"## Model Card Contact"
] |
text-generation | transformers | # Endevor/EndlessRP-v3-7B AWQ
- Model creator: [Endevor](https://huggingface.co/Endevor)
- Original model: [EndlessRP-v3-7B](https://huggingface.co/Endevor/EndlessRP-v3-7B)

## Model Summary
This model is recommended for RP, but you can use it as assistant as well.
New model! Version 2 brings less GPTims, but it's more the same, so I made this one. This is probably the best. Please, give it a try.
### Prompt Format:
- **Extended Alpaca Format** As for exemple from [lemonilia/LimaRP-Mistral-7B-v0.1](https://huggingface.co/lemonilia/LimaRP-Mistral-7B-v0.1).
Use *### Response: (length = huge)* for exemple, to increase length. You can use **Metharme** or **ChatML** as well, but **Alpaca** is recommended.
| {"language": ["en"], "license": "apache-2.0", "library_name": "transformers", "tags": ["4-bit", "AWQ", "text-generation", "autotrain_compatible", "endpoints_compatible", "safetensors", "mergekit", "merge", "mistral", "not-for-all-audiences", "nsfw", "rp", "roleplay"], "pipeline_tag": "text-generation", "inference": false, "quantized_by": "Suparious"} | solidrust/EndlessRP-v3-7B-AWQ | null | [
"transformers",
"safetensors",
"mistral",
"text-generation",
"4-bit",
"AWQ",
"autotrain_compatible",
"endpoints_compatible",
"mergekit",
"merge",
"not-for-all-audiences",
"nsfw",
"rp",
"roleplay",
"en",
"license:apache-2.0",
"text-generation-inference",
"region:us"
] | null | 2024-04-18T09:37:30+00:00 | [] | [
"en"
] | TAGS
#transformers #safetensors #mistral #text-generation #4-bit #AWQ #autotrain_compatible #endpoints_compatible #mergekit #merge #not-for-all-audiences #nsfw #rp #roleplay #en #license-apache-2.0 #text-generation-inference #region-us
| # Endevor/EndlessRP-v3-7B AWQ
- Model creator: Endevor
- Original model: EndlessRP-v3-7B
!image/jpeg
## Model Summary
This model is recommended for RP, but you can use it as assistant as well.
New model! Version 2 brings less GPTims, but it's more the same, so I made this one. This is probably the best. Please, give it a try.
### Prompt Format:
- Extended Alpaca Format As for exemple from lemonilia/LimaRP-Mistral-7B-v0.1.
Use *### Response: (length = huge)* for exemple, to increase length. You can use Metharme or ChatML as well, but Alpaca is recommended.
| [
"# Endevor/EndlessRP-v3-7B AWQ\n\n- Model creator: Endevor\n- Original model: EndlessRP-v3-7B\n\n!image/jpeg",
"## Model Summary\n\nThis model is recommended for RP, but you can use it as assistant as well.\n\nNew model! Version 2 brings less GPTims, but it's more the same, so I made this one. This is probably the best. Please, give it a try.",
"### Prompt Format:\n- Extended Alpaca Format As for exemple from lemonilia/LimaRP-Mistral-7B-v0.1.\nUse *### Response: (length = huge)* for exemple, to increase length. You can use Metharme or ChatML as well, but Alpaca is recommended."
] | [
"TAGS\n#transformers #safetensors #mistral #text-generation #4-bit #AWQ #autotrain_compatible #endpoints_compatible #mergekit #merge #not-for-all-audiences #nsfw #rp #roleplay #en #license-apache-2.0 #text-generation-inference #region-us \n",
"# Endevor/EndlessRP-v3-7B AWQ\n\n- Model creator: Endevor\n- Original model: EndlessRP-v3-7B\n\n!image/jpeg",
"## Model Summary\n\nThis model is recommended for RP, but you can use it as assistant as well.\n\nNew model! Version 2 brings less GPTims, but it's more the same, so I made this one. This is probably the best. Please, give it a try.",
"### Prompt Format:\n- Extended Alpaca Format As for exemple from lemonilia/LimaRP-Mistral-7B-v0.1.\nUse *### Response: (length = huge)* for exemple, to increase length. You can use Metharme or ChatML as well, but Alpaca is recommended."
] |
null | transformers |
# Model Card for Model ID
<!-- Provide a quick summary of what the model is/does. -->
## Model Details
### Model Description
<!-- Provide a longer summary of what this model is. -->
This is the model card of a 🤗 transformers model that has been pushed on the Hub. This model card has been automatically generated.
- **Developed by:** [More Information Needed]
- **Funded by [optional]:** [More Information Needed]
- **Shared by [optional]:** [More Information Needed]
- **Model type:** [More Information Needed]
- **Language(s) (NLP):** [More Information Needed]
- **License:** [More Information Needed]
- **Finetuned from model [optional]:** [More Information Needed]
### Model Sources [optional]
<!-- Provide the basic links for the model. -->
- **Repository:** [More Information Needed]
- **Paper [optional]:** [More Information Needed]
- **Demo [optional]:** [More Information Needed]
## Uses
<!-- Address questions around how the model is intended to be used, including the foreseeable users of the model and those affected by the model. -->
### Direct Use
<!-- This section is for the model use without fine-tuning or plugging into a larger ecosystem/app. -->
[More Information Needed]
### Downstream Use [optional]
<!-- This section is for the model use when fine-tuned for a task, or when plugged into a larger ecosystem/app -->
[More Information Needed]
### Out-of-Scope Use
<!-- This section addresses misuse, malicious use, and uses that the model will not work well for. -->
[More Information Needed]
## Bias, Risks, and Limitations
<!-- This section is meant to convey both technical and sociotechnical limitations. -->
[More Information Needed]
### Recommendations
<!-- This section is meant to convey recommendations with respect to the bias, risk, and technical limitations. -->
Users (both direct and downstream) should be made aware of the risks, biases and limitations of the model. More information needed for further recommendations.
## How to Get Started with the Model
Use the code below to get started with the model.
[More Information Needed]
## Training Details
### Training Data
<!-- This should link to a Dataset Card, perhaps with a short stub of information on what the training data is all about as well as documentation related to data pre-processing or additional filtering. -->
[More Information Needed]
### Training Procedure
<!-- This relates heavily to the Technical Specifications. Content here should link to that section when it is relevant to the training procedure. -->
#### Preprocessing [optional]
[More Information Needed]
#### Training Hyperparameters
- **Training regime:** [More Information Needed] <!--fp32, fp16 mixed precision, bf16 mixed precision, bf16 non-mixed precision, fp16 non-mixed precision, fp8 mixed precision -->
#### Speeds, Sizes, Times [optional]
<!-- This section provides information about throughput, start/end time, checkpoint size if relevant, etc. -->
[More Information Needed]
## Evaluation
<!-- This section describes the evaluation protocols and provides the results. -->
### Testing Data, Factors & Metrics
#### Testing Data
<!-- This should link to a Dataset Card if possible. -->
[More Information Needed]
#### Factors
<!-- These are the things the evaluation is disaggregating by, e.g., subpopulations or domains. -->
[More Information Needed]
#### Metrics
<!-- These are the evaluation metrics being used, ideally with a description of why. -->
[More Information Needed]
### Results
[More Information Needed]
#### Summary
## Model Examination [optional]
<!-- Relevant interpretability work for the model goes here -->
[More Information Needed]
## Environmental Impact
<!-- Total emissions (in grams of CO2eq) and additional considerations, such as electricity usage, go here. Edit the suggested text below accordingly -->
Carbon emissions can be estimated using the [Machine Learning Impact calculator](https://mlco2.github.io/impact#compute) presented in [Lacoste et al. (2019)](https://arxiv.org/abs/1910.09700).
- **Hardware Type:** [More Information Needed]
- **Hours used:** [More Information Needed]
- **Cloud Provider:** [More Information Needed]
- **Compute Region:** [More Information Needed]
- **Carbon Emitted:** [More Information Needed]
## Technical Specifications [optional]
### Model Architecture and Objective
[More Information Needed]
### Compute Infrastructure
[More Information Needed]
#### Hardware
[More Information Needed]
#### Software
[More Information Needed]
## Citation [optional]
<!-- If there is a paper or blog post introducing the model, the APA and Bibtex information for that should go in this section. -->
**BibTeX:**
[More Information Needed]
**APA:**
[More Information Needed]
## Glossary [optional]
<!-- If relevant, include terms and calculations in this section that can help readers understand the model or model card. -->
[More Information Needed]
## More Information [optional]
[More Information Needed]
## Model Card Authors [optional]
[More Information Needed]
## Model Card Contact
[More Information Needed] | {"library_name": "transformers", "tags": []} | abdullahfurquan/mistral-xyz1 | null | [
"transformers",
"arxiv:1910.09700",
"endpoints_compatible",
"region:us"
] | null | 2024-04-18T09:38:42+00:00 | [
"1910.09700"
] | [] | TAGS
#transformers #arxiv-1910.09700 #endpoints_compatible #region-us
|
# Model Card for Model ID
## Model Details
### Model Description
This is the model card of a transformers model that has been pushed on the Hub. This model card has been automatically generated.
- Developed by:
- Funded by [optional]:
- Shared by [optional]:
- Model type:
- Language(s) (NLP):
- License:
- Finetuned from model [optional]:
### Model Sources [optional]
- Repository:
- Paper [optional]:
- Demo [optional]:
## Uses
### Direct Use
### Downstream Use [optional]
### Out-of-Scope Use
## Bias, Risks, and Limitations
### Recommendations
Users (both direct and downstream) should be made aware of the risks, biases and limitations of the model. More information needed for further recommendations.
## How to Get Started with the Model
Use the code below to get started with the model.
## Training Details
### Training Data
### Training Procedure
#### Preprocessing [optional]
#### Training Hyperparameters
- Training regime:
#### Speeds, Sizes, Times [optional]
## Evaluation
### Testing Data, Factors & Metrics
#### Testing Data
#### Factors
#### Metrics
### Results
#### Summary
## Model Examination [optional]
## Environmental Impact
Carbon emissions can be estimated using the Machine Learning Impact calculator presented in Lacoste et al. (2019).
- Hardware Type:
- Hours used:
- Cloud Provider:
- Compute Region:
- Carbon Emitted:
## Technical Specifications [optional]
### Model Architecture and Objective
### Compute Infrastructure
#### Hardware
#### Software
[optional]
BibTeX:
APA:
## Glossary [optional]
## More Information [optional]
## Model Card Authors [optional]
## Model Card Contact
| [
"# Model Card for Model ID",
"## Model Details",
"### Model Description\n\n\n\nThis is the model card of a transformers model that has been pushed on the Hub. This model card has been automatically generated.\n\n- Developed by: \n- Funded by [optional]: \n- Shared by [optional]: \n- Model type: \n- Language(s) (NLP): \n- License: \n- Finetuned from model [optional]:",
"### Model Sources [optional]\n\n\n\n- Repository: \n- Paper [optional]: \n- Demo [optional]:",
"## Uses",
"### Direct Use",
"### Downstream Use [optional]",
"### Out-of-Scope Use",
"## Bias, Risks, and Limitations",
"### Recommendations\n\n\n\nUsers (both direct and downstream) should be made aware of the risks, biases and limitations of the model. More information needed for further recommendations.",
"## How to Get Started with the Model\n\nUse the code below to get started with the model.",
"## Training Details",
"### Training Data",
"### Training Procedure",
"#### Preprocessing [optional]",
"#### Training Hyperparameters\n\n- Training regime:",
"#### Speeds, Sizes, Times [optional]",
"## Evaluation",
"### Testing Data, Factors & Metrics",
"#### Testing Data",
"#### Factors",
"#### Metrics",
"### Results",
"#### Summary",
"## Model Examination [optional]",
"## Environmental Impact\n\n\n\nCarbon emissions can be estimated using the Machine Learning Impact calculator presented in Lacoste et al. (2019).\n\n- Hardware Type: \n- Hours used: \n- Cloud Provider: \n- Compute Region: \n- Carbon Emitted:",
"## Technical Specifications [optional]",
"### Model Architecture and Objective",
"### Compute Infrastructure",
"#### Hardware",
"#### Software\n\n\n\n[optional]\n\n\n\nBibTeX:\n\n\n\nAPA:",
"## Glossary [optional]",
"## More Information [optional]",
"## Model Card Authors [optional]",
"## Model Card Contact"
] | [
"TAGS\n#transformers #arxiv-1910.09700 #endpoints_compatible #region-us \n",
"# Model Card for Model ID",
"## Model Details",
"### Model Description\n\n\n\nThis is the model card of a transformers model that has been pushed on the Hub. This model card has been automatically generated.\n\n- Developed by: \n- Funded by [optional]: \n- Shared by [optional]: \n- Model type: \n- Language(s) (NLP): \n- License: \n- Finetuned from model [optional]:",
"### Model Sources [optional]\n\n\n\n- Repository: \n- Paper [optional]: \n- Demo [optional]:",
"## Uses",
"### Direct Use",
"### Downstream Use [optional]",
"### Out-of-Scope Use",
"## Bias, Risks, and Limitations",
"### Recommendations\n\n\n\nUsers (both direct and downstream) should be made aware of the risks, biases and limitations of the model. More information needed for further recommendations.",
"## How to Get Started with the Model\n\nUse the code below to get started with the model.",
"## Training Details",
"### Training Data",
"### Training Procedure",
"#### Preprocessing [optional]",
"#### Training Hyperparameters\n\n- Training regime:",
"#### Speeds, Sizes, Times [optional]",
"## Evaluation",
"### Testing Data, Factors & Metrics",
"#### Testing Data",
"#### Factors",
"#### Metrics",
"### Results",
"#### Summary",
"## Model Examination [optional]",
"## Environmental Impact\n\n\n\nCarbon emissions can be estimated using the Machine Learning Impact calculator presented in Lacoste et al. (2019).\n\n- Hardware Type: \n- Hours used: \n- Cloud Provider: \n- Compute Region: \n- Carbon Emitted:",
"## Technical Specifications [optional]",
"### Model Architecture and Objective",
"### Compute Infrastructure",
"#### Hardware",
"#### Software\n\n\n\n[optional]\n\n\n\nBibTeX:\n\n\n\nAPA:",
"## Glossary [optional]",
"## More Information [optional]",
"## Model Card Authors [optional]",
"## Model Card Contact"
] |
null | transformers |
# Model Card for Model ID
<!-- Provide a quick summary of what the model is/does. -->
## Model Details
### Model Description
<!-- Provide a longer summary of what this model is. -->
This is the model card of a 🤗 transformers model that has been pushed on the Hub. This model card has been automatically generated.
- **Developed by:** [More Information Needed]
- **Funded by [optional]:** [More Information Needed]
- **Shared by [optional]:** [More Information Needed]
- **Model type:** [More Information Needed]
- **Language(s) (NLP):** [More Information Needed]
- **License:** [More Information Needed]
- **Finetuned from model [optional]:** [More Information Needed]
### Model Sources [optional]
<!-- Provide the basic links for the model. -->
- **Repository:** [More Information Needed]
- **Paper [optional]:** [More Information Needed]
- **Demo [optional]:** [More Information Needed]
## Uses
<!-- Address questions around how the model is intended to be used, including the foreseeable users of the model and those affected by the model. -->
### Direct Use
<!-- This section is for the model use without fine-tuning or plugging into a larger ecosystem/app. -->
[More Information Needed]
### Downstream Use [optional]
<!-- This section is for the model use when fine-tuned for a task, or when plugged into a larger ecosystem/app -->
[More Information Needed]
### Out-of-Scope Use
<!-- This section addresses misuse, malicious use, and uses that the model will not work well for. -->
[More Information Needed]
## Bias, Risks, and Limitations
<!-- This section is meant to convey both technical and sociotechnical limitations. -->
[More Information Needed]
### Recommendations
<!-- This section is meant to convey recommendations with respect to the bias, risk, and technical limitations. -->
Users (both direct and downstream) should be made aware of the risks, biases and limitations of the model. More information needed for further recommendations.
## How to Get Started with the Model
Use the code below to get started with the model.
[More Information Needed]
## Training Details
### Training Data
<!-- This should link to a Dataset Card, perhaps with a short stub of information on what the training data is all about as well as documentation related to data pre-processing or additional filtering. -->
[More Information Needed]
### Training Procedure
<!-- This relates heavily to the Technical Specifications. Content here should link to that section when it is relevant to the training procedure. -->
#### Preprocessing [optional]
[More Information Needed]
#### Training Hyperparameters
- **Training regime:** [More Information Needed] <!--fp32, fp16 mixed precision, bf16 mixed precision, bf16 non-mixed precision, fp16 non-mixed precision, fp8 mixed precision -->
#### Speeds, Sizes, Times [optional]
<!-- This section provides information about throughput, start/end time, checkpoint size if relevant, etc. -->
[More Information Needed]
## Evaluation
<!-- This section describes the evaluation protocols and provides the results. -->
### Testing Data, Factors & Metrics
#### Testing Data
<!-- This should link to a Dataset Card if possible. -->
[More Information Needed]
#### Factors
<!-- These are the things the evaluation is disaggregating by, e.g., subpopulations or domains. -->
[More Information Needed]
#### Metrics
<!-- These are the evaluation metrics being used, ideally with a description of why. -->
[More Information Needed]
### Results
[More Information Needed]
#### Summary
## Model Examination [optional]
<!-- Relevant interpretability work for the model goes here -->
[More Information Needed]
## Environmental Impact
<!-- Total emissions (in grams of CO2eq) and additional considerations, such as electricity usage, go here. Edit the suggested text below accordingly -->
Carbon emissions can be estimated using the [Machine Learning Impact calculator](https://mlco2.github.io/impact#compute) presented in [Lacoste et al. (2019)](https://arxiv.org/abs/1910.09700).
- **Hardware Type:** [More Information Needed]
- **Hours used:** [More Information Needed]
- **Cloud Provider:** [More Information Needed]
- **Compute Region:** [More Information Needed]
- **Carbon Emitted:** [More Information Needed]
## Technical Specifications [optional]
### Model Architecture and Objective
[More Information Needed]
### Compute Infrastructure
[More Information Needed]
#### Hardware
[More Information Needed]
#### Software
[More Information Needed]
## Citation [optional]
<!-- If there is a paper or blog post introducing the model, the APA and Bibtex information for that should go in this section. -->
**BibTeX:**
[More Information Needed]
**APA:**
[More Information Needed]
## Glossary [optional]
<!-- If relevant, include terms and calculations in this section that can help readers understand the model or model card. -->
[More Information Needed]
## More Information [optional]
[More Information Needed]
## Model Card Authors [optional]
[More Information Needed]
## Model Card Contact
[More Information Needed] | {"library_name": "transformers", "tags": []} | abdullahfurquan/mistral-xyz2 | null | [
"transformers",
"arxiv:1910.09700",
"endpoints_compatible",
"region:us"
] | null | 2024-04-18T09:38:43+00:00 | [
"1910.09700"
] | [] | TAGS
#transformers #arxiv-1910.09700 #endpoints_compatible #region-us
|
# Model Card for Model ID
## Model Details
### Model Description
This is the model card of a transformers model that has been pushed on the Hub. This model card has been automatically generated.
- Developed by:
- Funded by [optional]:
- Shared by [optional]:
- Model type:
- Language(s) (NLP):
- License:
- Finetuned from model [optional]:
### Model Sources [optional]
- Repository:
- Paper [optional]:
- Demo [optional]:
## Uses
### Direct Use
### Downstream Use [optional]
### Out-of-Scope Use
## Bias, Risks, and Limitations
### Recommendations
Users (both direct and downstream) should be made aware of the risks, biases and limitations of the model. More information needed for further recommendations.
## How to Get Started with the Model
Use the code below to get started with the model.
## Training Details
### Training Data
### Training Procedure
#### Preprocessing [optional]
#### Training Hyperparameters
- Training regime:
#### Speeds, Sizes, Times [optional]
## Evaluation
### Testing Data, Factors & Metrics
#### Testing Data
#### Factors
#### Metrics
### Results
#### Summary
## Model Examination [optional]
## Environmental Impact
Carbon emissions can be estimated using the Machine Learning Impact calculator presented in Lacoste et al. (2019).
- Hardware Type:
- Hours used:
- Cloud Provider:
- Compute Region:
- Carbon Emitted:
## Technical Specifications [optional]
### Model Architecture and Objective
### Compute Infrastructure
#### Hardware
#### Software
[optional]
BibTeX:
APA:
## Glossary [optional]
## More Information [optional]
## Model Card Authors [optional]
## Model Card Contact
| [
"# Model Card for Model ID",
"## Model Details",
"### Model Description\n\n\n\nThis is the model card of a transformers model that has been pushed on the Hub. This model card has been automatically generated.\n\n- Developed by: \n- Funded by [optional]: \n- Shared by [optional]: \n- Model type: \n- Language(s) (NLP): \n- License: \n- Finetuned from model [optional]:",
"### Model Sources [optional]\n\n\n\n- Repository: \n- Paper [optional]: \n- Demo [optional]:",
"## Uses",
"### Direct Use",
"### Downstream Use [optional]",
"### Out-of-Scope Use",
"## Bias, Risks, and Limitations",
"### Recommendations\n\n\n\nUsers (both direct and downstream) should be made aware of the risks, biases and limitations of the model. More information needed for further recommendations.",
"## How to Get Started with the Model\n\nUse the code below to get started with the model.",
"## Training Details",
"### Training Data",
"### Training Procedure",
"#### Preprocessing [optional]",
"#### Training Hyperparameters\n\n- Training regime:",
"#### Speeds, Sizes, Times [optional]",
"## Evaluation",
"### Testing Data, Factors & Metrics",
"#### Testing Data",
"#### Factors",
"#### Metrics",
"### Results",
"#### Summary",
"## Model Examination [optional]",
"## Environmental Impact\n\n\n\nCarbon emissions can be estimated using the Machine Learning Impact calculator presented in Lacoste et al. (2019).\n\n- Hardware Type: \n- Hours used: \n- Cloud Provider: \n- Compute Region: \n- Carbon Emitted:",
"## Technical Specifications [optional]",
"### Model Architecture and Objective",
"### Compute Infrastructure",
"#### Hardware",
"#### Software\n\n\n\n[optional]\n\n\n\nBibTeX:\n\n\n\nAPA:",
"## Glossary [optional]",
"## More Information [optional]",
"## Model Card Authors [optional]",
"## Model Card Contact"
] | [
"TAGS\n#transformers #arxiv-1910.09700 #endpoints_compatible #region-us \n",
"# Model Card for Model ID",
"## Model Details",
"### Model Description\n\n\n\nThis is the model card of a transformers model that has been pushed on the Hub. This model card has been automatically generated.\n\n- Developed by: \n- Funded by [optional]: \n- Shared by [optional]: \n- Model type: \n- Language(s) (NLP): \n- License: \n- Finetuned from model [optional]:",
"### Model Sources [optional]\n\n\n\n- Repository: \n- Paper [optional]: \n- Demo [optional]:",
"## Uses",
"### Direct Use",
"### Downstream Use [optional]",
"### Out-of-Scope Use",
"## Bias, Risks, and Limitations",
"### Recommendations\n\n\n\nUsers (both direct and downstream) should be made aware of the risks, biases and limitations of the model. More information needed for further recommendations.",
"## How to Get Started with the Model\n\nUse the code below to get started with the model.",
"## Training Details",
"### Training Data",
"### Training Procedure",
"#### Preprocessing [optional]",
"#### Training Hyperparameters\n\n- Training regime:",
"#### Speeds, Sizes, Times [optional]",
"## Evaluation",
"### Testing Data, Factors & Metrics",
"#### Testing Data",
"#### Factors",
"#### Metrics",
"### Results",
"#### Summary",
"## Model Examination [optional]",
"## Environmental Impact\n\n\n\nCarbon emissions can be estimated using the Machine Learning Impact calculator presented in Lacoste et al. (2019).\n\n- Hardware Type: \n- Hours used: \n- Cloud Provider: \n- Compute Region: \n- Carbon Emitted:",
"## Technical Specifications [optional]",
"### Model Architecture and Objective",
"### Compute Infrastructure",
"#### Hardware",
"#### Software\n\n\n\n[optional]\n\n\n\nBibTeX:\n\n\n\nAPA:",
"## Glossary [optional]",
"## More Information [optional]",
"## Model Card Authors [optional]",
"## Model Card Contact"
] |
image-classification | transformers |
# Model Card for Model ID
<!-- Provide a quick summary of what the model is/does. -->
## Model Details
### Model Description
<!-- Provide a longer summary of what this model is. -->
This is the model card of a 🤗 transformers model that has been pushed on the Hub. This model card has been automatically generated.
- **Developed by:** [More Information Needed]
- **Funded by [optional]:** [More Information Needed]
- **Shared by [optional]:** [More Information Needed]
- **Model type:** [More Information Needed]
- **Language(s) (NLP):** [More Information Needed]
- **License:** [More Information Needed]
- **Finetuned from model [optional]:** [More Information Needed]
### Model Sources [optional]
<!-- Provide the basic links for the model. -->
- **Repository:** [More Information Needed]
- **Paper [optional]:** [More Information Needed]
- **Demo [optional]:** [More Information Needed]
## Uses
<!-- Address questions around how the model is intended to be used, including the foreseeable users of the model and those affected by the model. -->
### Direct Use
<!-- This section is for the model use without fine-tuning or plugging into a larger ecosystem/app. -->
[More Information Needed]
### Downstream Use [optional]
<!-- This section is for the model use when fine-tuned for a task, or when plugged into a larger ecosystem/app -->
[More Information Needed]
### Out-of-Scope Use
<!-- This section addresses misuse, malicious use, and uses that the model will not work well for. -->
[More Information Needed]
## Bias, Risks, and Limitations
<!-- This section is meant to convey both technical and sociotechnical limitations. -->
[More Information Needed]
### Recommendations
<!-- This section is meant to convey recommendations with respect to the bias, risk, and technical limitations. -->
Users (both direct and downstream) should be made aware of the risks, biases and limitations of the model. More information needed for further recommendations.
## How to Get Started with the Model
Use the code below to get started with the model.
[More Information Needed]
## Training Details
### Training Data
<!-- This should link to a Dataset Card, perhaps with a short stub of information on what the training data is all about as well as documentation related to data pre-processing or additional filtering. -->
[More Information Needed]
### Training Procedure
<!-- This relates heavily to the Technical Specifications. Content here should link to that section when it is relevant to the training procedure. -->
#### Preprocessing [optional]
[More Information Needed]
#### Training Hyperparameters
- **Training regime:** [More Information Needed] <!--fp32, fp16 mixed precision, bf16 mixed precision, bf16 non-mixed precision, fp16 non-mixed precision, fp8 mixed precision -->
#### Speeds, Sizes, Times [optional]
<!-- This section provides information about throughput, start/end time, checkpoint size if relevant, etc. -->
[More Information Needed]
## Evaluation
<!-- This section describes the evaluation protocols and provides the results. -->
### Testing Data, Factors & Metrics
#### Testing Data
<!-- This should link to a Dataset Card if possible. -->
[More Information Needed]
#### Factors
<!-- These are the things the evaluation is disaggregating by, e.g., subpopulations or domains. -->
[More Information Needed]
#### Metrics
<!-- These are the evaluation metrics being used, ideally with a description of why. -->
[More Information Needed]
### Results
[More Information Needed]
#### Summary
## Model Examination [optional]
<!-- Relevant interpretability work for the model goes here -->
[More Information Needed]
## Environmental Impact
<!-- Total emissions (in grams of CO2eq) and additional considerations, such as electricity usage, go here. Edit the suggested text below accordingly -->
Carbon emissions can be estimated using the [Machine Learning Impact calculator](https://mlco2.github.io/impact#compute) presented in [Lacoste et al. (2019)](https://arxiv.org/abs/1910.09700).
- **Hardware Type:** [More Information Needed]
- **Hours used:** [More Information Needed]
- **Cloud Provider:** [More Information Needed]
- **Compute Region:** [More Information Needed]
- **Carbon Emitted:** [More Information Needed]
## Technical Specifications [optional]
### Model Architecture and Objective
[More Information Needed]
### Compute Infrastructure
[More Information Needed]
#### Hardware
[More Information Needed]
#### Software
[More Information Needed]
## Citation [optional]
<!-- If there is a paper or blog post introducing the model, the APA and Bibtex information for that should go in this section. -->
**BibTeX:**
[More Information Needed]
**APA:**
[More Information Needed]
## Glossary [optional]
<!-- If relevant, include terms and calculations in this section that can help readers understand the model or model card. -->
[More Information Needed]
## More Information [optional]
[More Information Needed]
## Model Card Authors [optional]
[More Information Needed]
## Model Card Contact
[More Information Needed] | {"library_name": "transformers", "tags": []} | IlyasMoutawwakil/vit-with-hidden_states | null | [
"transformers",
"safetensors",
"openvino",
"vit",
"image-classification",
"arxiv:1910.09700",
"autotrain_compatible",
"endpoints_compatible",
"region:us"
] | null | 2024-04-18T09:40:57+00:00 | [
"1910.09700"
] | [] | TAGS
#transformers #safetensors #openvino #vit #image-classification #arxiv-1910.09700 #autotrain_compatible #endpoints_compatible #region-us
|
# Model Card for Model ID
## Model Details
### Model Description
This is the model card of a transformers model that has been pushed on the Hub. This model card has been automatically generated.
- Developed by:
- Funded by [optional]:
- Shared by [optional]:
- Model type:
- Language(s) (NLP):
- License:
- Finetuned from model [optional]:
### Model Sources [optional]
- Repository:
- Paper [optional]:
- Demo [optional]:
## Uses
### Direct Use
### Downstream Use [optional]
### Out-of-Scope Use
## Bias, Risks, and Limitations
### Recommendations
Users (both direct and downstream) should be made aware of the risks, biases and limitations of the model. More information needed for further recommendations.
## How to Get Started with the Model
Use the code below to get started with the model.
## Training Details
### Training Data
### Training Procedure
#### Preprocessing [optional]
#### Training Hyperparameters
- Training regime:
#### Speeds, Sizes, Times [optional]
## Evaluation
### Testing Data, Factors & Metrics
#### Testing Data
#### Factors
#### Metrics
### Results
#### Summary
## Model Examination [optional]
## Environmental Impact
Carbon emissions can be estimated using the Machine Learning Impact calculator presented in Lacoste et al. (2019).
- Hardware Type:
- Hours used:
- Cloud Provider:
- Compute Region:
- Carbon Emitted:
## Technical Specifications [optional]
### Model Architecture and Objective
### Compute Infrastructure
#### Hardware
#### Software
[optional]
BibTeX:
APA:
## Glossary [optional]
## More Information [optional]
## Model Card Authors [optional]
## Model Card Contact
| [
"# Model Card for Model ID",
"## Model Details",
"### Model Description\n\n\n\nThis is the model card of a transformers model that has been pushed on the Hub. This model card has been automatically generated.\n\n- Developed by: \n- Funded by [optional]: \n- Shared by [optional]: \n- Model type: \n- Language(s) (NLP): \n- License: \n- Finetuned from model [optional]:",
"### Model Sources [optional]\n\n\n\n- Repository: \n- Paper [optional]: \n- Demo [optional]:",
"## Uses",
"### Direct Use",
"### Downstream Use [optional]",
"### Out-of-Scope Use",
"## Bias, Risks, and Limitations",
"### Recommendations\n\n\n\nUsers (both direct and downstream) should be made aware of the risks, biases and limitations of the model. More information needed for further recommendations.",
"## How to Get Started with the Model\n\nUse the code below to get started with the model.",
"## Training Details",
"### Training Data",
"### Training Procedure",
"#### Preprocessing [optional]",
"#### Training Hyperparameters\n\n- Training regime:",
"#### Speeds, Sizes, Times [optional]",
"## Evaluation",
"### Testing Data, Factors & Metrics",
"#### Testing Data",
"#### Factors",
"#### Metrics",
"### Results",
"#### Summary",
"## Model Examination [optional]",
"## Environmental Impact\n\n\n\nCarbon emissions can be estimated using the Machine Learning Impact calculator presented in Lacoste et al. (2019).\n\n- Hardware Type: \n- Hours used: \n- Cloud Provider: \n- Compute Region: \n- Carbon Emitted:",
"## Technical Specifications [optional]",
"### Model Architecture and Objective",
"### Compute Infrastructure",
"#### Hardware",
"#### Software\n\n\n\n[optional]\n\n\n\nBibTeX:\n\n\n\nAPA:",
"## Glossary [optional]",
"## More Information [optional]",
"## Model Card Authors [optional]",
"## Model Card Contact"
] | [
"TAGS\n#transformers #safetensors #openvino #vit #image-classification #arxiv-1910.09700 #autotrain_compatible #endpoints_compatible #region-us \n",
"# Model Card for Model ID",
"## Model Details",
"### Model Description\n\n\n\nThis is the model card of a transformers model that has been pushed on the Hub. This model card has been automatically generated.\n\n- Developed by: \n- Funded by [optional]: \n- Shared by [optional]: \n- Model type: \n- Language(s) (NLP): \n- License: \n- Finetuned from model [optional]:",
"### Model Sources [optional]\n\n\n\n- Repository: \n- Paper [optional]: \n- Demo [optional]:",
"## Uses",
"### Direct Use",
"### Downstream Use [optional]",
"### Out-of-Scope Use",
"## Bias, Risks, and Limitations",
"### Recommendations\n\n\n\nUsers (both direct and downstream) should be made aware of the risks, biases and limitations of the model. More information needed for further recommendations.",
"## How to Get Started with the Model\n\nUse the code below to get started with the model.",
"## Training Details",
"### Training Data",
"### Training Procedure",
"#### Preprocessing [optional]",
"#### Training Hyperparameters\n\n- Training regime:",
"#### Speeds, Sizes, Times [optional]",
"## Evaluation",
"### Testing Data, Factors & Metrics",
"#### Testing Data",
"#### Factors",
"#### Metrics",
"### Results",
"#### Summary",
"## Model Examination [optional]",
"## Environmental Impact\n\n\n\nCarbon emissions can be estimated using the Machine Learning Impact calculator presented in Lacoste et al. (2019).\n\n- Hardware Type: \n- Hours used: \n- Cloud Provider: \n- Compute Region: \n- Carbon Emitted:",
"## Technical Specifications [optional]",
"### Model Architecture and Objective",
"### Compute Infrastructure",
"#### Hardware",
"#### Software\n\n\n\n[optional]\n\n\n\nBibTeX:\n\n\n\nAPA:",
"## Glossary [optional]",
"## More Information [optional]",
"## Model Card Authors [optional]",
"## Model Card Contact"
] |
text-generation | transformers | # Probably BROKEN, look for v0.2 which should be working!
* Test merge of two extremely large MoE models using SLERP. Don't know if it's working yet, haven't had time or hardware to test.
# merge
This is a merge of pre-trained language models created using [mergekit](https://github.com/cg123/mergekit).
## Merge Details
### Merge Method
This model was merged using the SLERP merge method.
### Models Merged
The following models were included in the merge:
* [alpindale/WizardLM-2-8x22B](https://huggingface.co/alpindale/WizardLM-2-8x22B)
* [mistralai/Mixtral-8x22B-Instruct-v0.1](https://huggingface.co/mistralai/Mixtral-8x22B-Instruct-v0.1)
### Configuration
The following YAML configuration was used to produce this model:
```yaml
slices:
- sources:
- model: mistralai/Mixtral-8x22B-Instruct-v0.1
layer_range: [0, 55]
- model: alpindale/WizardLM-2-8x22B
layer_range: [0, 55]
merge_method: slerp
base_model: mistralai/Mixtral-8x22B-Instruct-v0.1
parameters:
t:
- filter: self_attn
value: [0, 0.5, 0.3, 0.7, 1]
- filter: mlp
value: [1, 0.5, 0.7, 0.3, 0]
- value: 0.5
dtype: bfloat16
``` | {"license": "apache-2.0", "tags": ["mergekit", "merge"], "base_model": ["alpindale/WizardLM-2-8x22B", "mistralai/Mixtral-8x22B-Instruct-v0.1"]} | jsfs11/MixtralxWizardLM2-8x22B-SLERP-v0.1 | null | [
"transformers",
"safetensors",
"mixtral",
"text-generation",
"mergekit",
"merge",
"conversational",
"base_model:alpindale/WizardLM-2-8x22B",
"base_model:mistralai/Mixtral-8x22B-Instruct-v0.1",
"license:apache-2.0",
"autotrain_compatible",
"endpoints_compatible",
"text-generation-inference",
"region:us"
] | null | 2024-04-18T09:41:08+00:00 | [] | [] | TAGS
#transformers #safetensors #mixtral #text-generation #mergekit #merge #conversational #base_model-alpindale/WizardLM-2-8x22B #base_model-mistralai/Mixtral-8x22B-Instruct-v0.1 #license-apache-2.0 #autotrain_compatible #endpoints_compatible #text-generation-inference #region-us
| # Probably BROKEN, look for v0.2 which should be working!
* Test merge of two extremely large MoE models using SLERP. Don't know if it's working yet, haven't had time or hardware to test.
# merge
This is a merge of pre-trained language models created using mergekit.
## Merge Details
### Merge Method
This model was merged using the SLERP merge method.
### Models Merged
The following models were included in the merge:
* alpindale/WizardLM-2-8x22B
* mistralai/Mixtral-8x22B-Instruct-v0.1
### Configuration
The following YAML configuration was used to produce this model:
| [
"# Probably BROKEN, look for v0.2 which should be working!\n* Test merge of two extremely large MoE models using SLERP. Don't know if it's working yet, haven't had time or hardware to test.",
"# merge\n\nThis is a merge of pre-trained language models created using mergekit.",
"## Merge Details",
"### Merge Method\n\nThis model was merged using the SLERP merge method.",
"### Models Merged\n\nThe following models were included in the merge:\n* alpindale/WizardLM-2-8x22B\n* mistralai/Mixtral-8x22B-Instruct-v0.1",
"### Configuration\n\nThe following YAML configuration was used to produce this model:"
] | [
"TAGS\n#transformers #safetensors #mixtral #text-generation #mergekit #merge #conversational #base_model-alpindale/WizardLM-2-8x22B #base_model-mistralai/Mixtral-8x22B-Instruct-v0.1 #license-apache-2.0 #autotrain_compatible #endpoints_compatible #text-generation-inference #region-us \n",
"# Probably BROKEN, look for v0.2 which should be working!\n* Test merge of two extremely large MoE models using SLERP. Don't know if it's working yet, haven't had time or hardware to test.",
"# merge\n\nThis is a merge of pre-trained language models created using mergekit.",
"## Merge Details",
"### Merge Method\n\nThis model was merged using the SLERP merge method.",
"### Models Merged\n\nThe following models were included in the merge:\n* alpindale/WizardLM-2-8x22B\n* mistralai/Mixtral-8x22B-Instruct-v0.1",
"### Configuration\n\nThe following YAML configuration was used to produce this model:"
] |
image-classification | transformers |
# Model Card for Model ID
<!-- Provide a quick summary of what the model is/does. -->
## Model Details
### Model Description
<!-- Provide a longer summary of what this model is. -->
This is the model card of a 🤗 transformers model that has been pushed on the Hub. This model card has been automatically generated.
- **Developed by:** [More Information Needed]
- **Funded by [optional]:** [More Information Needed]
- **Shared by [optional]:** [More Information Needed]
- **Model type:** [More Information Needed]
- **Language(s) (NLP):** [More Information Needed]
- **License:** [More Information Needed]
- **Finetuned from model [optional]:** [More Information Needed]
### Model Sources [optional]
<!-- Provide the basic links for the model. -->
- **Repository:** [More Information Needed]
- **Paper [optional]:** [More Information Needed]
- **Demo [optional]:** [More Information Needed]
## Uses
<!-- Address questions around how the model is intended to be used, including the foreseeable users of the model and those affected by the model. -->
### Direct Use
<!-- This section is for the model use without fine-tuning or plugging into a larger ecosystem/app. -->
[More Information Needed]
### Downstream Use [optional]
<!-- This section is for the model use when fine-tuned for a task, or when plugged into a larger ecosystem/app -->
[More Information Needed]
### Out-of-Scope Use
<!-- This section addresses misuse, malicious use, and uses that the model will not work well for. -->
[More Information Needed]
## Bias, Risks, and Limitations
<!-- This section is meant to convey both technical and sociotechnical limitations. -->
[More Information Needed]
### Recommendations
<!-- This section is meant to convey recommendations with respect to the bias, risk, and technical limitations. -->
Users (both direct and downstream) should be made aware of the risks, biases and limitations of the model. More information needed for further recommendations.
## How to Get Started with the Model
Use the code below to get started with the model.
[More Information Needed]
## Training Details
### Training Data
<!-- This should link to a Dataset Card, perhaps with a short stub of information on what the training data is all about as well as documentation related to data pre-processing or additional filtering. -->
[More Information Needed]
### Training Procedure
<!-- This relates heavily to the Technical Specifications. Content here should link to that section when it is relevant to the training procedure. -->
#### Preprocessing [optional]
[More Information Needed]
#### Training Hyperparameters
- **Training regime:** [More Information Needed] <!--fp32, fp16 mixed precision, bf16 mixed precision, bf16 non-mixed precision, fp16 non-mixed precision, fp8 mixed precision -->
#### Speeds, Sizes, Times [optional]
<!-- This section provides information about throughput, start/end time, checkpoint size if relevant, etc. -->
[More Information Needed]
## Evaluation
<!-- This section describes the evaluation protocols and provides the results. -->
### Testing Data, Factors & Metrics
#### Testing Data
<!-- This should link to a Dataset Card if possible. -->
[More Information Needed]
#### Factors
<!-- These are the things the evaluation is disaggregating by, e.g., subpopulations or domains. -->
[More Information Needed]
#### Metrics
<!-- These are the evaluation metrics being used, ideally with a description of why. -->
[More Information Needed]
### Results
[More Information Needed]
#### Summary
## Model Examination [optional]
<!-- Relevant interpretability work for the model goes here -->
[More Information Needed]
## Environmental Impact
<!-- Total emissions (in grams of CO2eq) and additional considerations, such as electricity usage, go here. Edit the suggested text below accordingly -->
Carbon emissions can be estimated using the [Machine Learning Impact calculator](https://mlco2.github.io/impact#compute) presented in [Lacoste et al. (2019)](https://arxiv.org/abs/1910.09700).
- **Hardware Type:** [More Information Needed]
- **Hours used:** [More Information Needed]
- **Cloud Provider:** [More Information Needed]
- **Compute Region:** [More Information Needed]
- **Carbon Emitted:** [More Information Needed]
## Technical Specifications [optional]
### Model Architecture and Objective
[More Information Needed]
### Compute Infrastructure
[More Information Needed]
#### Hardware
[More Information Needed]
#### Software
[More Information Needed]
## Citation [optional]
<!-- If there is a paper or blog post introducing the model, the APA and Bibtex information for that should go in this section. -->
**BibTeX:**
[More Information Needed]
**APA:**
[More Information Needed]
## Glossary [optional]
<!-- If relevant, include terms and calculations in this section that can help readers understand the model or model card. -->
[More Information Needed]
## More Information [optional]
[More Information Needed]
## Model Card Authors [optional]
[More Information Needed]
## Model Card Contact
[More Information Needed] | {"library_name": "transformers", "tags": []} | IlyasMoutawwakil/vit-with-attentions | null | [
"transformers",
"safetensors",
"openvino",
"vit",
"image-classification",
"arxiv:1910.09700",
"autotrain_compatible",
"endpoints_compatible",
"region:us"
] | null | 2024-04-18T09:41:53+00:00 | [
"1910.09700"
] | [] | TAGS
#transformers #safetensors #openvino #vit #image-classification #arxiv-1910.09700 #autotrain_compatible #endpoints_compatible #region-us
|
# Model Card for Model ID
## Model Details
### Model Description
This is the model card of a transformers model that has been pushed on the Hub. This model card has been automatically generated.
- Developed by:
- Funded by [optional]:
- Shared by [optional]:
- Model type:
- Language(s) (NLP):
- License:
- Finetuned from model [optional]:
### Model Sources [optional]
- Repository:
- Paper [optional]:
- Demo [optional]:
## Uses
### Direct Use
### Downstream Use [optional]
### Out-of-Scope Use
## Bias, Risks, and Limitations
### Recommendations
Users (both direct and downstream) should be made aware of the risks, biases and limitations of the model. More information needed for further recommendations.
## How to Get Started with the Model
Use the code below to get started with the model.
## Training Details
### Training Data
### Training Procedure
#### Preprocessing [optional]
#### Training Hyperparameters
- Training regime:
#### Speeds, Sizes, Times [optional]
## Evaluation
### Testing Data, Factors & Metrics
#### Testing Data
#### Factors
#### Metrics
### Results
#### Summary
## Model Examination [optional]
## Environmental Impact
Carbon emissions can be estimated using the Machine Learning Impact calculator presented in Lacoste et al. (2019).
- Hardware Type:
- Hours used:
- Cloud Provider:
- Compute Region:
- Carbon Emitted:
## Technical Specifications [optional]
### Model Architecture and Objective
### Compute Infrastructure
#### Hardware
#### Software
[optional]
BibTeX:
APA:
## Glossary [optional]
## More Information [optional]
## Model Card Authors [optional]
## Model Card Contact
| [
"# Model Card for Model ID",
"## Model Details",
"### Model Description\n\n\n\nThis is the model card of a transformers model that has been pushed on the Hub. This model card has been automatically generated.\n\n- Developed by: \n- Funded by [optional]: \n- Shared by [optional]: \n- Model type: \n- Language(s) (NLP): \n- License: \n- Finetuned from model [optional]:",
"### Model Sources [optional]\n\n\n\n- Repository: \n- Paper [optional]: \n- Demo [optional]:",
"## Uses",
"### Direct Use",
"### Downstream Use [optional]",
"### Out-of-Scope Use",
"## Bias, Risks, and Limitations",
"### Recommendations\n\n\n\nUsers (both direct and downstream) should be made aware of the risks, biases and limitations of the model. More information needed for further recommendations.",
"## How to Get Started with the Model\n\nUse the code below to get started with the model.",
"## Training Details",
"### Training Data",
"### Training Procedure",
"#### Preprocessing [optional]",
"#### Training Hyperparameters\n\n- Training regime:",
"#### Speeds, Sizes, Times [optional]",
"## Evaluation",
"### Testing Data, Factors & Metrics",
"#### Testing Data",
"#### Factors",
"#### Metrics",
"### Results",
"#### Summary",
"## Model Examination [optional]",
"## Environmental Impact\n\n\n\nCarbon emissions can be estimated using the Machine Learning Impact calculator presented in Lacoste et al. (2019).\n\n- Hardware Type: \n- Hours used: \n- Cloud Provider: \n- Compute Region: \n- Carbon Emitted:",
"## Technical Specifications [optional]",
"### Model Architecture and Objective",
"### Compute Infrastructure",
"#### Hardware",
"#### Software\n\n\n\n[optional]\n\n\n\nBibTeX:\n\n\n\nAPA:",
"## Glossary [optional]",
"## More Information [optional]",
"## Model Card Authors [optional]",
"## Model Card Contact"
] | [
"TAGS\n#transformers #safetensors #openvino #vit #image-classification #arxiv-1910.09700 #autotrain_compatible #endpoints_compatible #region-us \n",
"# Model Card for Model ID",
"## Model Details",
"### Model Description\n\n\n\nThis is the model card of a transformers model that has been pushed on the Hub. This model card has been automatically generated.\n\n- Developed by: \n- Funded by [optional]: \n- Shared by [optional]: \n- Model type: \n- Language(s) (NLP): \n- License: \n- Finetuned from model [optional]:",
"### Model Sources [optional]\n\n\n\n- Repository: \n- Paper [optional]: \n- Demo [optional]:",
"## Uses",
"### Direct Use",
"### Downstream Use [optional]",
"### Out-of-Scope Use",
"## Bias, Risks, and Limitations",
"### Recommendations\n\n\n\nUsers (both direct and downstream) should be made aware of the risks, biases and limitations of the model. More information needed for further recommendations.",
"## How to Get Started with the Model\n\nUse the code below to get started with the model.",
"## Training Details",
"### Training Data",
"### Training Procedure",
"#### Preprocessing [optional]",
"#### Training Hyperparameters\n\n- Training regime:",
"#### Speeds, Sizes, Times [optional]",
"## Evaluation",
"### Testing Data, Factors & Metrics",
"#### Testing Data",
"#### Factors",
"#### Metrics",
"### Results",
"#### Summary",
"## Model Examination [optional]",
"## Environmental Impact\n\n\n\nCarbon emissions can be estimated using the Machine Learning Impact calculator presented in Lacoste et al. (2019).\n\n- Hardware Type: \n- Hours used: \n- Cloud Provider: \n- Compute Region: \n- Carbon Emitted:",
"## Technical Specifications [optional]",
"### Model Architecture and Objective",
"### Compute Infrastructure",
"#### Hardware",
"#### Software\n\n\n\n[optional]\n\n\n\nBibTeX:\n\n\n\nAPA:",
"## Glossary [optional]",
"## More Information [optional]",
"## Model Card Authors [optional]",
"## Model Card Contact"
] |
question-answering | transformers |
# Model Card for Model ID
<!-- Provide a quick summary of what the model is/does. -->
## Model Details
### Model Description
<!-- Provide a longer summary of what this model is. -->
This is the model card of a 🤗 transformers model that has been pushed on the Hub. This model card has been automatically generated.
- **Developed by:** [More Information Needed]
- **Funded by [optional]:** [More Information Needed]
- **Shared by [optional]:** [More Information Needed]
- **Model type:** [More Information Needed]
- **Language(s) (NLP):** [More Information Needed]
- **License:** [More Information Needed]
- **Finetuned from model [optional]:** [More Information Needed]
### Model Sources [optional]
<!-- Provide the basic links for the model. -->
- **Repository:** [More Information Needed]
- **Paper [optional]:** [More Information Needed]
- **Demo [optional]:** [More Information Needed]
## Uses
<!-- Address questions around how the model is intended to be used, including the foreseeable users of the model and those affected by the model. -->
### Direct Use
<!-- This section is for the model use without fine-tuning or plugging into a larger ecosystem/app. -->
[More Information Needed]
### Downstream Use [optional]
<!-- This section is for the model use when fine-tuned for a task, or when plugged into a larger ecosystem/app -->
[More Information Needed]
### Out-of-Scope Use
<!-- This section addresses misuse, malicious use, and uses that the model will not work well for. -->
[More Information Needed]
## Bias, Risks, and Limitations
<!-- This section is meant to convey both technical and sociotechnical limitations. -->
[More Information Needed]
### Recommendations
<!-- This section is meant to convey recommendations with respect to the bias, risk, and technical limitations. -->
Users (both direct and downstream) should be made aware of the risks, biases and limitations of the model. More information needed for further recommendations.
## How to Get Started with the Model
Use the code below to get started with the model.
[More Information Needed]
## Training Details
### Training Data
<!-- This should link to a Dataset Card, perhaps with a short stub of information on what the training data is all about as well as documentation related to data pre-processing or additional filtering. -->
[More Information Needed]
### Training Procedure
<!-- This relates heavily to the Technical Specifications. Content here should link to that section when it is relevant to the training procedure. -->
#### Preprocessing [optional]
[More Information Needed]
#### Training Hyperparameters
- **Training regime:** [More Information Needed] <!--fp32, fp16 mixed precision, bf16 mixed precision, bf16 non-mixed precision, fp16 non-mixed precision, fp8 mixed precision -->
#### Speeds, Sizes, Times [optional]
<!-- This section provides information about throughput, start/end time, checkpoint size if relevant, etc. -->
[More Information Needed]
## Evaluation
<!-- This section describes the evaluation protocols and provides the results. -->
### Testing Data, Factors & Metrics
#### Testing Data
<!-- This should link to a Dataset Card if possible. -->
[More Information Needed]
#### Factors
<!-- These are the things the evaluation is disaggregating by, e.g., subpopulations or domains. -->
[More Information Needed]
#### Metrics
<!-- These are the evaluation metrics being used, ideally with a description of why. -->
[More Information Needed]
### Results
[More Information Needed]
#### Summary
## Model Examination [optional]
<!-- Relevant interpretability work for the model goes here -->
[More Information Needed]
## Environmental Impact
<!-- Total emissions (in grams of CO2eq) and additional considerations, such as electricity usage, go here. Edit the suggested text below accordingly -->
Carbon emissions can be estimated using the [Machine Learning Impact calculator](https://mlco2.github.io/impact#compute) presented in [Lacoste et al. (2019)](https://arxiv.org/abs/1910.09700).
- **Hardware Type:** [More Information Needed]
- **Hours used:** [More Information Needed]
- **Cloud Provider:** [More Information Needed]
- **Compute Region:** [More Information Needed]
- **Carbon Emitted:** [More Information Needed]
## Technical Specifications [optional]
### Model Architecture and Objective
[More Information Needed]
### Compute Infrastructure
[More Information Needed]
#### Hardware
[More Information Needed]
#### Software
[More Information Needed]
## Citation [optional]
<!-- If there is a paper or blog post introducing the model, the APA and Bibtex information for that should go in this section. -->
**BibTeX:**
[More Information Needed]
**APA:**
[More Information Needed]
## Glossary [optional]
<!-- If relevant, include terms and calculations in this section that can help readers understand the model or model card. -->
[More Information Needed]
## More Information [optional]
[More Information Needed]
## Model Card Authors [optional]
[More Information Needed]
## Model Card Contact
[More Information Needed]
| {"library_name": "transformers", "tags": []} | Dofla/bert-squad | null | [
"transformers",
"safetensors",
"bert",
"question-answering",
"arxiv:1910.09700",
"endpoints_compatible",
"has_space",
"region:us"
] | null | 2024-04-18T09:41:57+00:00 | [
"1910.09700"
] | [] | TAGS
#transformers #safetensors #bert #question-answering #arxiv-1910.09700 #endpoints_compatible #has_space #region-us
|
# Model Card for Model ID
## Model Details
### Model Description
This is the model card of a transformers model that has been pushed on the Hub. This model card has been automatically generated.
- Developed by:
- Funded by [optional]:
- Shared by [optional]:
- Model type:
- Language(s) (NLP):
- License:
- Finetuned from model [optional]:
### Model Sources [optional]
- Repository:
- Paper [optional]:
- Demo [optional]:
## Uses
### Direct Use
### Downstream Use [optional]
### Out-of-Scope Use
## Bias, Risks, and Limitations
### Recommendations
Users (both direct and downstream) should be made aware of the risks, biases and limitations of the model. More information needed for further recommendations.
## How to Get Started with the Model
Use the code below to get started with the model.
## Training Details
### Training Data
### Training Procedure
#### Preprocessing [optional]
#### Training Hyperparameters
- Training regime:
#### Speeds, Sizes, Times [optional]
## Evaluation
### Testing Data, Factors & Metrics
#### Testing Data
#### Factors
#### Metrics
### Results
#### Summary
## Model Examination [optional]
## Environmental Impact
Carbon emissions can be estimated using the Machine Learning Impact calculator presented in Lacoste et al. (2019).
- Hardware Type:
- Hours used:
- Cloud Provider:
- Compute Region:
- Carbon Emitted:
## Technical Specifications [optional]
### Model Architecture and Objective
### Compute Infrastructure
#### Hardware
#### Software
[optional]
BibTeX:
APA:
## Glossary [optional]
## More Information [optional]
## Model Card Authors [optional]
## Model Card Contact
| [
"# Model Card for Model ID",
"## Model Details",
"### Model Description\n\n\n\nThis is the model card of a transformers model that has been pushed on the Hub. This model card has been automatically generated.\n\n- Developed by: \n- Funded by [optional]: \n- Shared by [optional]: \n- Model type: \n- Language(s) (NLP): \n- License: \n- Finetuned from model [optional]:",
"### Model Sources [optional]\n\n\n\n- Repository: \n- Paper [optional]: \n- Demo [optional]:",
"## Uses",
"### Direct Use",
"### Downstream Use [optional]",
"### Out-of-Scope Use",
"## Bias, Risks, and Limitations",
"### Recommendations\n\n\n\nUsers (both direct and downstream) should be made aware of the risks, biases and limitations of the model. More information needed for further recommendations.",
"## How to Get Started with the Model\n\nUse the code below to get started with the model.",
"## Training Details",
"### Training Data",
"### Training Procedure",
"#### Preprocessing [optional]",
"#### Training Hyperparameters\n\n- Training regime:",
"#### Speeds, Sizes, Times [optional]",
"## Evaluation",
"### Testing Data, Factors & Metrics",
"#### Testing Data",
"#### Factors",
"#### Metrics",
"### Results",
"#### Summary",
"## Model Examination [optional]",
"## Environmental Impact\n\n\n\nCarbon emissions can be estimated using the Machine Learning Impact calculator presented in Lacoste et al. (2019).\n\n- Hardware Type: \n- Hours used: \n- Cloud Provider: \n- Compute Region: \n- Carbon Emitted:",
"## Technical Specifications [optional]",
"### Model Architecture and Objective",
"### Compute Infrastructure",
"#### Hardware",
"#### Software\n\n\n\n[optional]\n\n\n\nBibTeX:\n\n\n\nAPA:",
"## Glossary [optional]",
"## More Information [optional]",
"## Model Card Authors [optional]",
"## Model Card Contact"
] | [
"TAGS\n#transformers #safetensors #bert #question-answering #arxiv-1910.09700 #endpoints_compatible #has_space #region-us \n",
"# Model Card for Model ID",
"## Model Details",
"### Model Description\n\n\n\nThis is the model card of a transformers model that has been pushed on the Hub. This model card has been automatically generated.\n\n- Developed by: \n- Funded by [optional]: \n- Shared by [optional]: \n- Model type: \n- Language(s) (NLP): \n- License: \n- Finetuned from model [optional]:",
"### Model Sources [optional]\n\n\n\n- Repository: \n- Paper [optional]: \n- Demo [optional]:",
"## Uses",
"### Direct Use",
"### Downstream Use [optional]",
"### Out-of-Scope Use",
"## Bias, Risks, and Limitations",
"### Recommendations\n\n\n\nUsers (both direct and downstream) should be made aware of the risks, biases and limitations of the model. More information needed for further recommendations.",
"## How to Get Started with the Model\n\nUse the code below to get started with the model.",
"## Training Details",
"### Training Data",
"### Training Procedure",
"#### Preprocessing [optional]",
"#### Training Hyperparameters\n\n- Training regime:",
"#### Speeds, Sizes, Times [optional]",
"## Evaluation",
"### Testing Data, Factors & Metrics",
"#### Testing Data",
"#### Factors",
"#### Metrics",
"### Results",
"#### Summary",
"## Model Examination [optional]",
"## Environmental Impact\n\n\n\nCarbon emissions can be estimated using the Machine Learning Impact calculator presented in Lacoste et al. (2019).\n\n- Hardware Type: \n- Hours used: \n- Cloud Provider: \n- Compute Region: \n- Carbon Emitted:",
"## Technical Specifications [optional]",
"### Model Architecture and Objective",
"### Compute Infrastructure",
"#### Hardware",
"#### Software\n\n\n\n[optional]\n\n\n\nBibTeX:\n\n\n\nAPA:",
"## Glossary [optional]",
"## More Information [optional]",
"## Model Card Authors [optional]",
"## Model Card Contact"
] |
automatic-speech-recognition | transformers |
<!-- This model card has been generated automatically according to the information the Trainer had access to. You
should probably proofread and complete it, then remove this comment. -->
# Whisper Small - Singlish v2
This model is a fine-tuned version of [openai/whisper-small](https://huggingface.co/openai/whisper-small) on the rngzhi/cs3264-project dataset.
It achieves the following results on the evaluation set:
- Loss: 0.1850
- Wer: 4.9236
## Model description
More information needed
## Intended uses & limitations
More information needed
## Training and evaluation data
More information needed
## Training procedure
### Training hyperparameters
The following hyperparameters were used during training:
- learning_rate: 1e-05
- train_batch_size: 16
- eval_batch_size: 8
- seed: 42
- gradient_accumulation_steps: 2
- total_train_batch_size: 32
- optimizer: Adam with betas=(0.9,0.999) and epsilon=1e-08
- lr_scheduler_type: linear
- lr_scheduler_warmup_steps: 25
- training_steps: 800
- mixed_precision_training: Native AMP
### Training results
| Training Loss | Epoch | Step | Validation Loss | Wer |
|:-------------:|:------:|:----:|:---------------:|:------:|
| 0.5404 | 0.0625 | 50 | 0.1970 | 5.6075 |
| 0.075 | 1.0144 | 100 | 0.1557 | 4.8780 |
| 0.042 | 1.0769 | 150 | 0.1610 | 4.9692 |
| 0.0185 | 2.0288 | 200 | 0.1628 | 4.9122 |
| 0.0117 | 2.0913 | 250 | 0.1651 | 5.0262 |
| 0.0096 | 3.0431 | 300 | 0.1716 | 5.0490 |
| 0.007 | 3.1056 | 350 | 0.1747 | 5.0034 |
| 0.0045 | 4.0575 | 400 | 0.1783 | 5.1402 |
| 0.0046 | 5.0094 | 450 | 0.1749 | 5.1288 |
| 0.004 | 5.0719 | 500 | 0.1782 | 5.0148 |
| 0.0021 | 6.0237 | 550 | 0.1814 | 5.0034 |
| 0.004 | 6.0862 | 600 | 0.1813 | 4.9920 |
| 0.0024 | 7.0381 | 650 | 0.1844 | 4.9350 |
| 0.0022 | 7.1006 | 700 | 0.1834 | 4.9008 |
| 0.0032 | 8.0525 | 750 | 0.1850 | 4.9236 |
| 0.0016 | 9.0044 | 800 | 0.1850 | 4.9236 |
### Framework versions
- Transformers 4.40.0.dev0
- Pytorch 2.2.1+cu121
- Datasets 2.18.1.dev0
- Tokenizers 0.19.1
| {"language": ["en"], "license": "apache-2.0", "tags": ["generated_from_trainer"], "datasets": ["rngzhi/cs3264-project"], "metrics": ["wer"], "base_model": "openai/whisper-small", "model-index": [{"name": "Whisper Small - Singlish v2", "results": [{"task": {"type": "automatic-speech-recognition", "name": "Automatic Speech Recognition"}, "dataset": {"name": "rngzhi/cs3264-project", "type": "rngzhi/cs3264-project"}, "metrics": [{"type": "wer", "value": 4.923638021426943, "name": "Wer"}]}]}]} | rngzhi/cs3264-project-v2 | null | [
"transformers",
"tensorboard",
"safetensors",
"whisper",
"automatic-speech-recognition",
"generated_from_trainer",
"en",
"dataset:rngzhi/cs3264-project",
"base_model:openai/whisper-small",
"license:apache-2.0",
"model-index",
"endpoints_compatible",
"has_space",
"region:us"
] | null | 2024-04-18T09:44:53+00:00 | [] | [
"en"
] | TAGS
#transformers #tensorboard #safetensors #whisper #automatic-speech-recognition #generated_from_trainer #en #dataset-rngzhi/cs3264-project #base_model-openai/whisper-small #license-apache-2.0 #model-index #endpoints_compatible #has_space #region-us
| Whisper Small - Singlish v2
===========================
This model is a fine-tuned version of openai/whisper-small on the rngzhi/cs3264-project dataset.
It achieves the following results on the evaluation set:
* Loss: 0.1850
* Wer: 4.9236
Model description
-----------------
More information needed
Intended uses & limitations
---------------------------
More information needed
Training and evaluation data
----------------------------
More information needed
Training procedure
------------------
### Training hyperparameters
The following hyperparameters were used during training:
* learning\_rate: 1e-05
* train\_batch\_size: 16
* eval\_batch\_size: 8
* seed: 42
* gradient\_accumulation\_steps: 2
* total\_train\_batch\_size: 32
* optimizer: Adam with betas=(0.9,0.999) and epsilon=1e-08
* lr\_scheduler\_type: linear
* lr\_scheduler\_warmup\_steps: 25
* training\_steps: 800
* mixed\_precision\_training: Native AMP
### Training results
### Framework versions
* Transformers 4.40.0.dev0
* Pytorch 2.2.1+cu121
* Datasets 2.18.1.dev0
* Tokenizers 0.19.1
| [
"### Training hyperparameters\n\n\nThe following hyperparameters were used during training:\n\n\n* learning\\_rate: 1e-05\n* train\\_batch\\_size: 16\n* eval\\_batch\\_size: 8\n* seed: 42\n* gradient\\_accumulation\\_steps: 2\n* total\\_train\\_batch\\_size: 32\n* optimizer: Adam with betas=(0.9,0.999) and epsilon=1e-08\n* lr\\_scheduler\\_type: linear\n* lr\\_scheduler\\_warmup\\_steps: 25\n* training\\_steps: 800\n* mixed\\_precision\\_training: Native AMP",
"### Training results",
"### Framework versions\n\n\n* Transformers 4.40.0.dev0\n* Pytorch 2.2.1+cu121\n* Datasets 2.18.1.dev0\n* Tokenizers 0.19.1"
] | [
"TAGS\n#transformers #tensorboard #safetensors #whisper #automatic-speech-recognition #generated_from_trainer #en #dataset-rngzhi/cs3264-project #base_model-openai/whisper-small #license-apache-2.0 #model-index #endpoints_compatible #has_space #region-us \n",
"### Training hyperparameters\n\n\nThe following hyperparameters were used during training:\n\n\n* learning\\_rate: 1e-05\n* train\\_batch\\_size: 16\n* eval\\_batch\\_size: 8\n* seed: 42\n* gradient\\_accumulation\\_steps: 2\n* total\\_train\\_batch\\_size: 32\n* optimizer: Adam with betas=(0.9,0.999) and epsilon=1e-08\n* lr\\_scheduler\\_type: linear\n* lr\\_scheduler\\_warmup\\_steps: 25\n* training\\_steps: 800\n* mixed\\_precision\\_training: Native AMP",
"### Training results",
"### Framework versions\n\n\n* Transformers 4.40.0.dev0\n* Pytorch 2.2.1+cu121\n* Datasets 2.18.1.dev0\n* Tokenizers 0.19.1"
] |
text-generation | transformers |
# CodeMind
Coding Test Explanatory LLM Model.
## Model Details
- **Model Name**: CodeMind
- **Base Model**: gemma-1.1-7b-it
- **Fine-tuning Datasets**:
- [kreimben/leetcode_user_submissions_only_python](https://huggingface.co/datasets/kreimben/leetcode_user_submissions_only_python)
- [kreimben/leetcode_with_youtube_captions](https://huggingface.co/datasets/kreimben/leetcode_with_youtube_captions)
- **Model Type**: Language Model
- **Language**: English
- **License**: gemma
- **Model Size**: 8.54B
## Intended Use
CodeMind is a fine-tuned language model specifically designed to assist users with coding test questions and provide programming education. It leverages the knowledge from LeetCode user submissions in Python and YouTube video captions related to LeetCode problems to offer guidance, explanations, and code examples.
## Training Data
The model was fine-tuned using the following datasets:
1. **kreimben/leetcode_user_submissions_only_python**: This dataset contains a collection of LeetCode user submissions written in Python. It provides a wide range of coding solutions to various algorithmic problems commonly encountered in coding interviews.
2. **kreimben/leetcode_with_youtube_captions**: This dataset combines LeetCode problems with corresponding YouTube video captions. The captions provide explanations, thought processes, and step-by-step guidance for solving the coding problems.
## Training Procedure
The model was fine-tuned using the Hugging Face Transformer library. The base model, gemma-1.1-2b-it, was further trained on the combined dataset of LeetCode user submissions and YouTube video captions. The fine-tuning process aimed to enhance the model's understanding of coding concepts, problem-solving strategies, and its ability to generate relevant code snippets and explanations.
## Evaluation
[]
## Bias and Limitations
- The model's knowledge is primarily based on the LeetCode user submissions and YouTube video captions used for fine-tuning. It may have limitations in handling coding problems or concepts that are not well-represented in the training data.
- The model's responses are generated based on patterns and information learned from the training data. It may sometimes produce incorrect or suboptimal solutions. Users should always review and verify the generated code before using it in practice.
- The model may exhibit biases present in the training data, such as favoring certain programming styles, algorithms, or approaches. It is important to consider alternative solutions and best practices when using the model's outputs.
## Ethical Considerations
- The model should be used as a supportive tool for learning and problem-solving, not as a substitute for human expertise and critical thinking.
- Users should be aware that the model's responses are generated based on patterns in the training data and may not always be accurate, complete, or up to date.
- The model should not be relied upon for making critical decisions or solving real-world problems without thorough validation and testing.
## Usage
To use the CodeMind model, you can access it through the Hugging Face model hub or by integrating it into your own applications using the provided API. Provide a coding problem or a question related to programming concepts, and the model will generate relevant explanations, code snippets, or guidance based on its training.
Please refer to the documentation and examples for detailed instructions on how to integrate and use the CodeMind model effectively. | {"language": ["en"], "license": "mit", "library_name": "transformers", "tags": ["code"], "datasets": ["kreimben/leetcode_with_youtube_captions", "kreimben/leetcode_user_submissions"], "base_model": ["google/gemma-1.1-2b-it"], "widget": [{"text": "explain about two sum problem. from brute force approach to the most advanced algorithms.", "example_title": "two sum example"}, {"text": "explain about leetcode 72 edit distance. i don't get even the approach.", "example_title": "edit distance example"}, {"text": "explain about leetcode 139 Word Break. please give me the approach.", "example_title": "word break example"}], "inference": {"parameters": {"max_new_tokens": 250, "temperature": 0.1}}, "pipeline_tag": "text-generation"} | kreimben/CodeMind-gemma-7b | null | [
"transformers",
"safetensors",
"gemma",
"text-generation",
"code",
"conversational",
"en",
"dataset:kreimben/leetcode_with_youtube_captions",
"dataset:kreimben/leetcode_user_submissions",
"base_model:google/gemma-1.1-2b-it",
"license:mit",
"autotrain_compatible",
"endpoints_compatible",
"text-generation-inference",
"region:us"
] | null | 2024-04-18T09:46:57+00:00 | [] | [
"en"
] | TAGS
#transformers #safetensors #gemma #text-generation #code #conversational #en #dataset-kreimben/leetcode_with_youtube_captions #dataset-kreimben/leetcode_user_submissions #base_model-google/gemma-1.1-2b-it #license-mit #autotrain_compatible #endpoints_compatible #text-generation-inference #region-us
|
# CodeMind
Coding Test Explanatory LLM Model.
## Model Details
- Model Name: CodeMind
- Base Model: gemma-1.1-7b-it
- Fine-tuning Datasets:
- kreimben/leetcode_user_submissions_only_python
- kreimben/leetcode_with_youtube_captions
- Model Type: Language Model
- Language: English
- License: gemma
- Model Size: 8.54B
## Intended Use
CodeMind is a fine-tuned language model specifically designed to assist users with coding test questions and provide programming education. It leverages the knowledge from LeetCode user submissions in Python and YouTube video captions related to LeetCode problems to offer guidance, explanations, and code examples.
## Training Data
The model was fine-tuned using the following datasets:
1. kreimben/leetcode_user_submissions_only_python: This dataset contains a collection of LeetCode user submissions written in Python. It provides a wide range of coding solutions to various algorithmic problems commonly encountered in coding interviews.
2. kreimben/leetcode_with_youtube_captions: This dataset combines LeetCode problems with corresponding YouTube video captions. The captions provide explanations, thought processes, and step-by-step guidance for solving the coding problems.
## Training Procedure
The model was fine-tuned using the Hugging Face Transformer library. The base model, gemma-1.1-2b-it, was further trained on the combined dataset of LeetCode user submissions and YouTube video captions. The fine-tuning process aimed to enhance the model's understanding of coding concepts, problem-solving strategies, and its ability to generate relevant code snippets and explanations.
## Evaluation
[]
## Bias and Limitations
- The model's knowledge is primarily based on the LeetCode user submissions and YouTube video captions used for fine-tuning. It may have limitations in handling coding problems or concepts that are not well-represented in the training data.
- The model's responses are generated based on patterns and information learned from the training data. It may sometimes produce incorrect or suboptimal solutions. Users should always review and verify the generated code before using it in practice.
- The model may exhibit biases present in the training data, such as favoring certain programming styles, algorithms, or approaches. It is important to consider alternative solutions and best practices when using the model's outputs.
## Ethical Considerations
- The model should be used as a supportive tool for learning and problem-solving, not as a substitute for human expertise and critical thinking.
- Users should be aware that the model's responses are generated based on patterns in the training data and may not always be accurate, complete, or up to date.
- The model should not be relied upon for making critical decisions or solving real-world problems without thorough validation and testing.
## Usage
To use the CodeMind model, you can access it through the Hugging Face model hub or by integrating it into your own applications using the provided API. Provide a coding problem or a question related to programming concepts, and the model will generate relevant explanations, code snippets, or guidance based on its training.
Please refer to the documentation and examples for detailed instructions on how to integrate and use the CodeMind model effectively. | [
"# CodeMind\nCoding Test Explanatory LLM Model.",
"## Model Details\n- Model Name: CodeMind\n- Base Model: gemma-1.1-7b-it\n- Fine-tuning Datasets:\n - kreimben/leetcode_user_submissions_only_python\n - kreimben/leetcode_with_youtube_captions\n- Model Type: Language Model\n- Language: English\n- License: gemma\n- Model Size: 8.54B",
"## Intended Use\nCodeMind is a fine-tuned language model specifically designed to assist users with coding test questions and provide programming education. It leverages the knowledge from LeetCode user submissions in Python and YouTube video captions related to LeetCode problems to offer guidance, explanations, and code examples.",
"## Training Data\nThe model was fine-tuned using the following datasets:\n1. kreimben/leetcode_user_submissions_only_python: This dataset contains a collection of LeetCode user submissions written in Python. It provides a wide range of coding solutions to various algorithmic problems commonly encountered in coding interviews.\n2. kreimben/leetcode_with_youtube_captions: This dataset combines LeetCode problems with corresponding YouTube video captions. The captions provide explanations, thought processes, and step-by-step guidance for solving the coding problems.",
"## Training Procedure\nThe model was fine-tuned using the Hugging Face Transformer library. The base model, gemma-1.1-2b-it, was further trained on the combined dataset of LeetCode user submissions and YouTube video captions. The fine-tuning process aimed to enhance the model's understanding of coding concepts, problem-solving strategies, and its ability to generate relevant code snippets and explanations.",
"## Evaluation\n[]",
"## Bias and Limitations\n- The model's knowledge is primarily based on the LeetCode user submissions and YouTube video captions used for fine-tuning. It may have limitations in handling coding problems or concepts that are not well-represented in the training data.\n- The model's responses are generated based on patterns and information learned from the training data. It may sometimes produce incorrect or suboptimal solutions. Users should always review and verify the generated code before using it in practice.\n- The model may exhibit biases present in the training data, such as favoring certain programming styles, algorithms, or approaches. It is important to consider alternative solutions and best practices when using the model's outputs.",
"## Ethical Considerations\n- The model should be used as a supportive tool for learning and problem-solving, not as a substitute for human expertise and critical thinking.\n- Users should be aware that the model's responses are generated based on patterns in the training data and may not always be accurate, complete, or up to date.\n- The model should not be relied upon for making critical decisions or solving real-world problems without thorough validation and testing.",
"## Usage\nTo use the CodeMind model, you can access it through the Hugging Face model hub or by integrating it into your own applications using the provided API. Provide a coding problem or a question related to programming concepts, and the model will generate relevant explanations, code snippets, or guidance based on its training.\n\nPlease refer to the documentation and examples for detailed instructions on how to integrate and use the CodeMind model effectively."
] | [
"TAGS\n#transformers #safetensors #gemma #text-generation #code #conversational #en #dataset-kreimben/leetcode_with_youtube_captions #dataset-kreimben/leetcode_user_submissions #base_model-google/gemma-1.1-2b-it #license-mit #autotrain_compatible #endpoints_compatible #text-generation-inference #region-us \n",
"# CodeMind\nCoding Test Explanatory LLM Model.",
"## Model Details\n- Model Name: CodeMind\n- Base Model: gemma-1.1-7b-it\n- Fine-tuning Datasets:\n - kreimben/leetcode_user_submissions_only_python\n - kreimben/leetcode_with_youtube_captions\n- Model Type: Language Model\n- Language: English\n- License: gemma\n- Model Size: 8.54B",
"## Intended Use\nCodeMind is a fine-tuned language model specifically designed to assist users with coding test questions and provide programming education. It leverages the knowledge from LeetCode user submissions in Python and YouTube video captions related to LeetCode problems to offer guidance, explanations, and code examples.",
"## Training Data\nThe model was fine-tuned using the following datasets:\n1. kreimben/leetcode_user_submissions_only_python: This dataset contains a collection of LeetCode user submissions written in Python. It provides a wide range of coding solutions to various algorithmic problems commonly encountered in coding interviews.\n2. kreimben/leetcode_with_youtube_captions: This dataset combines LeetCode problems with corresponding YouTube video captions. The captions provide explanations, thought processes, and step-by-step guidance for solving the coding problems.",
"## Training Procedure\nThe model was fine-tuned using the Hugging Face Transformer library. The base model, gemma-1.1-2b-it, was further trained on the combined dataset of LeetCode user submissions and YouTube video captions. The fine-tuning process aimed to enhance the model's understanding of coding concepts, problem-solving strategies, and its ability to generate relevant code snippets and explanations.",
"## Evaluation\n[]",
"## Bias and Limitations\n- The model's knowledge is primarily based on the LeetCode user submissions and YouTube video captions used for fine-tuning. It may have limitations in handling coding problems or concepts that are not well-represented in the training data.\n- The model's responses are generated based on patterns and information learned from the training data. It may sometimes produce incorrect or suboptimal solutions. Users should always review and verify the generated code before using it in practice.\n- The model may exhibit biases present in the training data, such as favoring certain programming styles, algorithms, or approaches. It is important to consider alternative solutions and best practices when using the model's outputs.",
"## Ethical Considerations\n- The model should be used as a supportive tool for learning and problem-solving, not as a substitute for human expertise and critical thinking.\n- Users should be aware that the model's responses are generated based on patterns in the training data and may not always be accurate, complete, or up to date.\n- The model should not be relied upon for making critical decisions or solving real-world problems without thorough validation and testing.",
"## Usage\nTo use the CodeMind model, you can access it through the Hugging Face model hub or by integrating it into your own applications using the provided API. Provide a coding problem or a question related to programming concepts, and the model will generate relevant explanations, code snippets, or guidance based on its training.\n\nPlease refer to the documentation and examples for detailed instructions on how to integrate and use the CodeMind model effectively."
] |
null | transformers |
# Model Card for Model ID
<!-- Provide a quick summary of what the model is/does. -->
## Model Details
### Model Description
<!-- Provide a longer summary of what this model is. -->
This is the model card of a 🤗 transformers model that has been pushed on the Hub. This model card has been automatically generated.
- **Developed by:** [More Information Needed]
- **Funded by [optional]:** [More Information Needed]
- **Shared by [optional]:** [More Information Needed]
- **Model type:** [More Information Needed]
- **Language(s) (NLP):** [More Information Needed]
- **License:** [More Information Needed]
- **Finetuned from model [optional]:** [More Information Needed]
### Model Sources [optional]
<!-- Provide the basic links for the model. -->
- **Repository:** [More Information Needed]
- **Paper [optional]:** [More Information Needed]
- **Demo [optional]:** [More Information Needed]
## Uses
<!-- Address questions around how the model is intended to be used, including the foreseeable users of the model and those affected by the model. -->
### Direct Use
<!-- This section is for the model use without fine-tuning or plugging into a larger ecosystem/app. -->
[More Information Needed]
### Downstream Use [optional]
<!-- This section is for the model use when fine-tuned for a task, or when plugged into a larger ecosystem/app -->
[More Information Needed]
### Out-of-Scope Use
<!-- This section addresses misuse, malicious use, and uses that the model will not work well for. -->
[More Information Needed]
## Bias, Risks, and Limitations
<!-- This section is meant to convey both technical and sociotechnical limitations. -->
[More Information Needed]
### Recommendations
<!-- This section is meant to convey recommendations with respect to the bias, risk, and technical limitations. -->
Users (both direct and downstream) should be made aware of the risks, biases and limitations of the model. More information needed for further recommendations.
## How to Get Started with the Model
Use the code below to get started with the model.
[More Information Needed]
## Training Details
### Training Data
<!-- This should link to a Dataset Card, perhaps with a short stub of information on what the training data is all about as well as documentation related to data pre-processing or additional filtering. -->
[More Information Needed]
### Training Procedure
<!-- This relates heavily to the Technical Specifications. Content here should link to that section when it is relevant to the training procedure. -->
#### Preprocessing [optional]
[More Information Needed]
#### Training Hyperparameters
- **Training regime:** [More Information Needed] <!--fp32, fp16 mixed precision, bf16 mixed precision, bf16 non-mixed precision, fp16 non-mixed precision, fp8 mixed precision -->
#### Speeds, Sizes, Times [optional]
<!-- This section provides information about throughput, start/end time, checkpoint size if relevant, etc. -->
[More Information Needed]
## Evaluation
<!-- This section describes the evaluation protocols and provides the results. -->
### Testing Data, Factors & Metrics
#### Testing Data
<!-- This should link to a Dataset Card if possible. -->
[More Information Needed]
#### Factors
<!-- These are the things the evaluation is disaggregating by, e.g., subpopulations or domains. -->
[More Information Needed]
#### Metrics
<!-- These are the evaluation metrics being used, ideally with a description of why. -->
[More Information Needed]
### Results
[More Information Needed]
#### Summary
## Model Examination [optional]
<!-- Relevant interpretability work for the model goes here -->
[More Information Needed]
## Environmental Impact
<!-- Total emissions (in grams of CO2eq) and additional considerations, such as electricity usage, go here. Edit the suggested text below accordingly -->
Carbon emissions can be estimated using the [Machine Learning Impact calculator](https://mlco2.github.io/impact#compute) presented in [Lacoste et al. (2019)](https://arxiv.org/abs/1910.09700).
- **Hardware Type:** [More Information Needed]
- **Hours used:** [More Information Needed]
- **Cloud Provider:** [More Information Needed]
- **Compute Region:** [More Information Needed]
- **Carbon Emitted:** [More Information Needed]
## Technical Specifications [optional]
### Model Architecture and Objective
[More Information Needed]
### Compute Infrastructure
[More Information Needed]
#### Hardware
[More Information Needed]
#### Software
[More Information Needed]
## Citation [optional]
<!-- If there is a paper or blog post introducing the model, the APA and Bibtex information for that should go in this section. -->
**BibTeX:**
[More Information Needed]
**APA:**
[More Information Needed]
## Glossary [optional]
<!-- If relevant, include terms and calculations in this section that can help readers understand the model or model card. -->
[More Information Needed]
## More Information [optional]
[More Information Needed]
## Model Card Authors [optional]
[More Information Needed]
## Model Card Contact
[More Information Needed] | {"library_name": "transformers", "tags": []} | abdullahfurquan/mistral-7b-final | null | [
"transformers",
"safetensors",
"arxiv:1910.09700",
"endpoints_compatible",
"region:us"
] | null | 2024-04-18T09:47:14+00:00 | [
"1910.09700"
] | [] | TAGS
#transformers #safetensors #arxiv-1910.09700 #endpoints_compatible #region-us
|
# Model Card for Model ID
## Model Details
### Model Description
This is the model card of a transformers model that has been pushed on the Hub. This model card has been automatically generated.
- Developed by:
- Funded by [optional]:
- Shared by [optional]:
- Model type:
- Language(s) (NLP):
- License:
- Finetuned from model [optional]:
### Model Sources [optional]
- Repository:
- Paper [optional]:
- Demo [optional]:
## Uses
### Direct Use
### Downstream Use [optional]
### Out-of-Scope Use
## Bias, Risks, and Limitations
### Recommendations
Users (both direct and downstream) should be made aware of the risks, biases and limitations of the model. More information needed for further recommendations.
## How to Get Started with the Model
Use the code below to get started with the model.
## Training Details
### Training Data
### Training Procedure
#### Preprocessing [optional]
#### Training Hyperparameters
- Training regime:
#### Speeds, Sizes, Times [optional]
## Evaluation
### Testing Data, Factors & Metrics
#### Testing Data
#### Factors
#### Metrics
### Results
#### Summary
## Model Examination [optional]
## Environmental Impact
Carbon emissions can be estimated using the Machine Learning Impact calculator presented in Lacoste et al. (2019).
- Hardware Type:
- Hours used:
- Cloud Provider:
- Compute Region:
- Carbon Emitted:
## Technical Specifications [optional]
### Model Architecture and Objective
### Compute Infrastructure
#### Hardware
#### Software
[optional]
BibTeX:
APA:
## Glossary [optional]
## More Information [optional]
## Model Card Authors [optional]
## Model Card Contact
| [
"# Model Card for Model ID",
"## Model Details",
"### Model Description\n\n\n\nThis is the model card of a transformers model that has been pushed on the Hub. This model card has been automatically generated.\n\n- Developed by: \n- Funded by [optional]: \n- Shared by [optional]: \n- Model type: \n- Language(s) (NLP): \n- License: \n- Finetuned from model [optional]:",
"### Model Sources [optional]\n\n\n\n- Repository: \n- Paper [optional]: \n- Demo [optional]:",
"## Uses",
"### Direct Use",
"### Downstream Use [optional]",
"### Out-of-Scope Use",
"## Bias, Risks, and Limitations",
"### Recommendations\n\n\n\nUsers (both direct and downstream) should be made aware of the risks, biases and limitations of the model. More information needed for further recommendations.",
"## How to Get Started with the Model\n\nUse the code below to get started with the model.",
"## Training Details",
"### Training Data",
"### Training Procedure",
"#### Preprocessing [optional]",
"#### Training Hyperparameters\n\n- Training regime:",
"#### Speeds, Sizes, Times [optional]",
"## Evaluation",
"### Testing Data, Factors & Metrics",
"#### Testing Data",
"#### Factors",
"#### Metrics",
"### Results",
"#### Summary",
"## Model Examination [optional]",
"## Environmental Impact\n\n\n\nCarbon emissions can be estimated using the Machine Learning Impact calculator presented in Lacoste et al. (2019).\n\n- Hardware Type: \n- Hours used: \n- Cloud Provider: \n- Compute Region: \n- Carbon Emitted:",
"## Technical Specifications [optional]",
"### Model Architecture and Objective",
"### Compute Infrastructure",
"#### Hardware",
"#### Software\n\n\n\n[optional]\n\n\n\nBibTeX:\n\n\n\nAPA:",
"## Glossary [optional]",
"## More Information [optional]",
"## Model Card Authors [optional]",
"## Model Card Contact"
] | [
"TAGS\n#transformers #safetensors #arxiv-1910.09700 #endpoints_compatible #region-us \n",
"# Model Card for Model ID",
"## Model Details",
"### Model Description\n\n\n\nThis is the model card of a transformers model that has been pushed on the Hub. This model card has been automatically generated.\n\n- Developed by: \n- Funded by [optional]: \n- Shared by [optional]: \n- Model type: \n- Language(s) (NLP): \n- License: \n- Finetuned from model [optional]:",
"### Model Sources [optional]\n\n\n\n- Repository: \n- Paper [optional]: \n- Demo [optional]:",
"## Uses",
"### Direct Use",
"### Downstream Use [optional]",
"### Out-of-Scope Use",
"## Bias, Risks, and Limitations",
"### Recommendations\n\n\n\nUsers (both direct and downstream) should be made aware of the risks, biases and limitations of the model. More information needed for further recommendations.",
"## How to Get Started with the Model\n\nUse the code below to get started with the model.",
"## Training Details",
"### Training Data",
"### Training Procedure",
"#### Preprocessing [optional]",
"#### Training Hyperparameters\n\n- Training regime:",
"#### Speeds, Sizes, Times [optional]",
"## Evaluation",
"### Testing Data, Factors & Metrics",
"#### Testing Data",
"#### Factors",
"#### Metrics",
"### Results",
"#### Summary",
"## Model Examination [optional]",
"## Environmental Impact\n\n\n\nCarbon emissions can be estimated using the Machine Learning Impact calculator presented in Lacoste et al. (2019).\n\n- Hardware Type: \n- Hours used: \n- Cloud Provider: \n- Compute Region: \n- Carbon Emitted:",
"## Technical Specifications [optional]",
"### Model Architecture and Objective",
"### Compute Infrastructure",
"#### Hardware",
"#### Software\n\n\n\n[optional]\n\n\n\nBibTeX:\n\n\n\nAPA:",
"## Glossary [optional]",
"## More Information [optional]",
"## Model Card Authors [optional]",
"## Model Card Contact"
] |
null | transformers |
# Model Card for Model ID
<!-- Provide a quick summary of what the model is/does. -->
## Model Details
### Model Description
<!-- Provide a longer summary of what this model is. -->
This is the model card of a 🤗 transformers model that has been pushed on the Hub. This model card has been automatically generated.
- **Developed by:** [More Information Needed]
- **Funded by [optional]:** [More Information Needed]
- **Shared by [optional]:** [More Information Needed]
- **Model type:** [More Information Needed]
- **Language(s) (NLP):** [More Information Needed]
- **License:** [More Information Needed]
- **Finetuned from model [optional]:** [More Information Needed]
### Model Sources [optional]
<!-- Provide the basic links for the model. -->
- **Repository:** [More Information Needed]
- **Paper [optional]:** [More Information Needed]
- **Demo [optional]:** [More Information Needed]
## Uses
<!-- Address questions around how the model is intended to be used, including the foreseeable users of the model and those affected by the model. -->
### Direct Use
<!-- This section is for the model use without fine-tuning or plugging into a larger ecosystem/app. -->
[More Information Needed]
### Downstream Use [optional]
<!-- This section is for the model use when fine-tuned for a task, or when plugged into a larger ecosystem/app -->
[More Information Needed]
### Out-of-Scope Use
<!-- This section addresses misuse, malicious use, and uses that the model will not work well for. -->
[More Information Needed]
## Bias, Risks, and Limitations
<!-- This section is meant to convey both technical and sociotechnical limitations. -->
[More Information Needed]
### Recommendations
<!-- This section is meant to convey recommendations with respect to the bias, risk, and technical limitations. -->
Users (both direct and downstream) should be made aware of the risks, biases and limitations of the model. More information needed for further recommendations.
## How to Get Started with the Model
Use the code below to get started with the model.
[More Information Needed]
## Training Details
### Training Data
<!-- This should link to a Dataset Card, perhaps with a short stub of information on what the training data is all about as well as documentation related to data pre-processing or additional filtering. -->
[More Information Needed]
### Training Procedure
<!-- This relates heavily to the Technical Specifications. Content here should link to that section when it is relevant to the training procedure. -->
#### Preprocessing [optional]
[More Information Needed]
#### Training Hyperparameters
- **Training regime:** [More Information Needed] <!--fp32, fp16 mixed precision, bf16 mixed precision, bf16 non-mixed precision, fp16 non-mixed precision, fp8 mixed precision -->
#### Speeds, Sizes, Times [optional]
<!-- This section provides information about throughput, start/end time, checkpoint size if relevant, etc. -->
[More Information Needed]
## Evaluation
<!-- This section describes the evaluation protocols and provides the results. -->
### Testing Data, Factors & Metrics
#### Testing Data
<!-- This should link to a Dataset Card if possible. -->
[More Information Needed]
#### Factors
<!-- These are the things the evaluation is disaggregating by, e.g., subpopulations or domains. -->
[More Information Needed]
#### Metrics
<!-- These are the evaluation metrics being used, ideally with a description of why. -->
[More Information Needed]
### Results
[More Information Needed]
#### Summary
## Model Examination [optional]
<!-- Relevant interpretability work for the model goes here -->
[More Information Needed]
## Environmental Impact
<!-- Total emissions (in grams of CO2eq) and additional considerations, such as electricity usage, go here. Edit the suggested text below accordingly -->
Carbon emissions can be estimated using the [Machine Learning Impact calculator](https://mlco2.github.io/impact#compute) presented in [Lacoste et al. (2019)](https://arxiv.org/abs/1910.09700).
- **Hardware Type:** [More Information Needed]
- **Hours used:** [More Information Needed]
- **Cloud Provider:** [More Information Needed]
- **Compute Region:** [More Information Needed]
- **Carbon Emitted:** [More Information Needed]
## Technical Specifications [optional]
### Model Architecture and Objective
[More Information Needed]
### Compute Infrastructure
[More Information Needed]
#### Hardware
[More Information Needed]
#### Software
[More Information Needed]
## Citation [optional]
<!-- If there is a paper or blog post introducing the model, the APA and Bibtex information for that should go in this section. -->
**BibTeX:**
[More Information Needed]
**APA:**
[More Information Needed]
## Glossary [optional]
<!-- If relevant, include terms and calculations in this section that can help readers understand the model or model card. -->
[More Information Needed]
## More Information [optional]
[More Information Needed]
## Model Card Authors [optional]
[More Information Needed]
## Model Card Contact
[More Information Needed] | {"library_name": "transformers", "tags": []} | Owaner/CodexTokenizer6k | null | [
"transformers",
"arxiv:1910.09700",
"endpoints_compatible",
"region:us"
] | null | 2024-04-18T09:49:24+00:00 | [
"1910.09700"
] | [] | TAGS
#transformers #arxiv-1910.09700 #endpoints_compatible #region-us
|
# Model Card for Model ID
## Model Details
### Model Description
This is the model card of a transformers model that has been pushed on the Hub. This model card has been automatically generated.
- Developed by:
- Funded by [optional]:
- Shared by [optional]:
- Model type:
- Language(s) (NLP):
- License:
- Finetuned from model [optional]:
### Model Sources [optional]
- Repository:
- Paper [optional]:
- Demo [optional]:
## Uses
### Direct Use
### Downstream Use [optional]
### Out-of-Scope Use
## Bias, Risks, and Limitations
### Recommendations
Users (both direct and downstream) should be made aware of the risks, biases and limitations of the model. More information needed for further recommendations.
## How to Get Started with the Model
Use the code below to get started with the model.
## Training Details
### Training Data
### Training Procedure
#### Preprocessing [optional]
#### Training Hyperparameters
- Training regime:
#### Speeds, Sizes, Times [optional]
## Evaluation
### Testing Data, Factors & Metrics
#### Testing Data
#### Factors
#### Metrics
### Results
#### Summary
## Model Examination [optional]
## Environmental Impact
Carbon emissions can be estimated using the Machine Learning Impact calculator presented in Lacoste et al. (2019).
- Hardware Type:
- Hours used:
- Cloud Provider:
- Compute Region:
- Carbon Emitted:
## Technical Specifications [optional]
### Model Architecture and Objective
### Compute Infrastructure
#### Hardware
#### Software
[optional]
BibTeX:
APA:
## Glossary [optional]
## More Information [optional]
## Model Card Authors [optional]
## Model Card Contact
| [
"# Model Card for Model ID",
"## Model Details",
"### Model Description\n\n\n\nThis is the model card of a transformers model that has been pushed on the Hub. This model card has been automatically generated.\n\n- Developed by: \n- Funded by [optional]: \n- Shared by [optional]: \n- Model type: \n- Language(s) (NLP): \n- License: \n- Finetuned from model [optional]:",
"### Model Sources [optional]\n\n\n\n- Repository: \n- Paper [optional]: \n- Demo [optional]:",
"## Uses",
"### Direct Use",
"### Downstream Use [optional]",
"### Out-of-Scope Use",
"## Bias, Risks, and Limitations",
"### Recommendations\n\n\n\nUsers (both direct and downstream) should be made aware of the risks, biases and limitations of the model. More information needed for further recommendations.",
"## How to Get Started with the Model\n\nUse the code below to get started with the model.",
"## Training Details",
"### Training Data",
"### Training Procedure",
"#### Preprocessing [optional]",
"#### Training Hyperparameters\n\n- Training regime:",
"#### Speeds, Sizes, Times [optional]",
"## Evaluation",
"### Testing Data, Factors & Metrics",
"#### Testing Data",
"#### Factors",
"#### Metrics",
"### Results",
"#### Summary",
"## Model Examination [optional]",
"## Environmental Impact\n\n\n\nCarbon emissions can be estimated using the Machine Learning Impact calculator presented in Lacoste et al. (2019).\n\n- Hardware Type: \n- Hours used: \n- Cloud Provider: \n- Compute Region: \n- Carbon Emitted:",
"## Technical Specifications [optional]",
"### Model Architecture and Objective",
"### Compute Infrastructure",
"#### Hardware",
"#### Software\n\n\n\n[optional]\n\n\n\nBibTeX:\n\n\n\nAPA:",
"## Glossary [optional]",
"## More Information [optional]",
"## Model Card Authors [optional]",
"## Model Card Contact"
] | [
"TAGS\n#transformers #arxiv-1910.09700 #endpoints_compatible #region-us \n",
"# Model Card for Model ID",
"## Model Details",
"### Model Description\n\n\n\nThis is the model card of a transformers model that has been pushed on the Hub. This model card has been automatically generated.\n\n- Developed by: \n- Funded by [optional]: \n- Shared by [optional]: \n- Model type: \n- Language(s) (NLP): \n- License: \n- Finetuned from model [optional]:",
"### Model Sources [optional]\n\n\n\n- Repository: \n- Paper [optional]: \n- Demo [optional]:",
"## Uses",
"### Direct Use",
"### Downstream Use [optional]",
"### Out-of-Scope Use",
"## Bias, Risks, and Limitations",
"### Recommendations\n\n\n\nUsers (both direct and downstream) should be made aware of the risks, biases and limitations of the model. More information needed for further recommendations.",
"## How to Get Started with the Model\n\nUse the code below to get started with the model.",
"## Training Details",
"### Training Data",
"### Training Procedure",
"#### Preprocessing [optional]",
"#### Training Hyperparameters\n\n- Training regime:",
"#### Speeds, Sizes, Times [optional]",
"## Evaluation",
"### Testing Data, Factors & Metrics",
"#### Testing Data",
"#### Factors",
"#### Metrics",
"### Results",
"#### Summary",
"## Model Examination [optional]",
"## Environmental Impact\n\n\n\nCarbon emissions can be estimated using the Machine Learning Impact calculator presented in Lacoste et al. (2019).\n\n- Hardware Type: \n- Hours used: \n- Cloud Provider: \n- Compute Region: \n- Carbon Emitted:",
"## Technical Specifications [optional]",
"### Model Architecture and Objective",
"### Compute Infrastructure",
"#### Hardware",
"#### Software\n\n\n\n[optional]\n\n\n\nBibTeX:\n\n\n\nAPA:",
"## Glossary [optional]",
"## More Information [optional]",
"## Model Card Authors [optional]",
"## Model Card Contact"
] |
text-to-image | diffusers | # Working30days
<Gallery />
## Model description
Type Tigger word Style of TOK
## Trigger words
You should use `Style of TOK` to trigger the image generation.
## Download model
Weights for this model are available in Safetensors format.
[Download](/Hoga2/Workingbody30days/tree/main) them in the Files & versions tab.
| {"tags": ["text-to-image", "stable-diffusion", "lora", "diffusers", "template:sd-lora"], "widget": [{"text": "-", "output": {"url": "images/\u0e44\u0e21\u0e48\u0e21\u0e35\u0e0a\u0e37\u0e48\u0e2d 265_20240418164824.png"}}], "base_model": "stabilityai/stable-diffusion-xl-base-1.0", "instance_prompt": "Style of TOK"} | Hoga2/Workingbody30days | null | [
"diffusers",
"text-to-image",
"stable-diffusion",
"lora",
"template:sd-lora",
"base_model:stabilityai/stable-diffusion-xl-base-1.0",
"region:us"
] | null | 2024-04-18T09:49:55+00:00 | [] | [] | TAGS
#diffusers #text-to-image #stable-diffusion #lora #template-sd-lora #base_model-stabilityai/stable-diffusion-xl-base-1.0 #region-us
| # Working30days
<Gallery />
## Model description
Type Tigger word Style of TOK
## Trigger words
You should use 'Style of TOK' to trigger the image generation.
## Download model
Weights for this model are available in Safetensors format.
Download them in the Files & versions tab.
| [
"# Working30days \n\n<Gallery />",
"## Model description \n\nType Tigger word Style of TOK",
"## Trigger words\n\nYou should use 'Style of TOK' to trigger the image generation.",
"## Download model\n\nWeights for this model are available in Safetensors format.\n\nDownload them in the Files & versions tab."
] | [
"TAGS\n#diffusers #text-to-image #stable-diffusion #lora #template-sd-lora #base_model-stabilityai/stable-diffusion-xl-base-1.0 #region-us \n",
"# Working30days \n\n<Gallery />",
"## Model description \n\nType Tigger word Style of TOK",
"## Trigger words\n\nYou should use 'Style of TOK' to trigger the image generation.",
"## Download model\n\nWeights for this model are available in Safetensors format.\n\nDownload them in the Files & versions tab."
] |
null | null |
# cleatherbury/Phigments12-Q8_0-GGUF
This model was converted to GGUF format from [`liminerity/Phigments12`](https://huggingface.co/liminerity/Phigments12) using llama.cpp via the ggml.ai's [GGUF-my-repo](https://huggingface.co/spaces/ggml-org/gguf-my-repo) space.
Refer to the [original model card](https://huggingface.co/liminerity/Phigments12) for more details on the model.
## Use with llama.cpp
Install llama.cpp through brew.
```bash
brew install ggerganov/ggerganov/llama.cpp
```
Invoke the llama.cpp server or the CLI.
CLI:
```bash
llama-cli --hf-repo cleatherbury/Phigments12-Q8_0-GGUF --model phigments12.Q8_0.gguf -p "The meaning to life and the universe is"
```
Server:
```bash
llama-server --hf-repo cleatherbury/Phigments12-Q8_0-GGUF --model phigments12.Q8_0.gguf -c 2048
```
Note: You can also use this checkpoint directly through the [usage steps](https://github.com/ggerganov/llama.cpp?tab=readme-ov-file#usage) listed in the Llama.cpp repo as well.
```
git clone https://github.com/ggerganov/llama.cpp && cd llama.cpp && make && ./main -m phigments12.Q8_0.gguf -n 128
```
| {"license": "apache-2.0", "tags": ["liminerity/merge6", "liminerity/merge3", "Merge", "llama-cpp", "gguf-my-repo"]} | cleatherbury/Phigments12-Q8_0-GGUF | null | [
"gguf",
"liminerity/merge6",
"liminerity/merge3",
"Merge",
"llama-cpp",
"gguf-my-repo",
"license:apache-2.0",
"region:us"
] | null | 2024-04-18T09:52:36+00:00 | [] | [] | TAGS
#gguf #liminerity/merge6 #liminerity/merge3 #Merge #llama-cpp #gguf-my-repo #license-apache-2.0 #region-us
|
# cleatherbury/Phigments12-Q8_0-GGUF
This model was converted to GGUF format from 'liminerity/Phigments12' using URL via the URL's GGUF-my-repo space.
Refer to the original model card for more details on the model.
## Use with URL
Install URL through brew.
Invoke the URL server or the CLI.
CLI:
Server:
Note: You can also use this checkpoint directly through the usage steps listed in the URL repo as well.
| [
"# cleatherbury/Phigments12-Q8_0-GGUF\nThis model was converted to GGUF format from 'liminerity/Phigments12' using URL via the URL's GGUF-my-repo space.\nRefer to the original model card for more details on the model.",
"## Use with URL\n\nInstall URL through brew.\n\n\nInvoke the URL server or the CLI.\n\nCLI:\n\n\n\nServer:\n\n\n\nNote: You can also use this checkpoint directly through the usage steps listed in the URL repo as well."
] | [
"TAGS\n#gguf #liminerity/merge6 #liminerity/merge3 #Merge #llama-cpp #gguf-my-repo #license-apache-2.0 #region-us \n",
"# cleatherbury/Phigments12-Q8_0-GGUF\nThis model was converted to GGUF format from 'liminerity/Phigments12' using URL via the URL's GGUF-my-repo space.\nRefer to the original model card for more details on the model.",
"## Use with URL\n\nInstall URL through brew.\n\n\nInvoke the URL server or the CLI.\n\nCLI:\n\n\n\nServer:\n\n\n\nNote: You can also use this checkpoint directly through the usage steps listed in the URL repo as well."
] |
null | null |
# Marcoro14-7B-slerp
Marcoro14-7B-slerp is a merge of the following models using [mergekit](https://github.com/cg123/mergekit):
* [AIDC-ai-business/Marcoroni-7B-v3](https://huggingface.co/AIDC-ai-business/Marcoroni-7B-v3)
* [EmbeddedLLM/Mistral-7B-Merge-14-v0.1](https://huggingface.co/EmbeddedLLM/Mistral-7B-Merge-14-v0.1)
## 🧩 Configuration
```yaml
slices:
- sources:
- model: AIDC-ai-business/Marcoroni-7B-v3
layer_range: [0, 32]
- model: EmbeddedLLM/Mistral-7B-Merge-14-v0.1
layer_range: [0, 32]
merge_method: slerp
base_model: AIDC-ai-business/Marcoroni-7B-v3
parameters:
t:
- filter: self_attn
value: [0, 0.5, 0.3, 0.7, 1]
- filter: mlp
value: [1, 0.5, 0.7, 0.3, 0]
- value: 0.5
dtype: bfloat16
``` | {"license": "apache-2.0", "tags": ["merge", "mergekit", "lazymergekit", "AIDC-ai-business/Marcoroni-7B-v3", "EmbeddedLLM/Mistral-7B-Merge-14-v0.1"]} | fuyu-quant/Marcoro14-7B-slerp | null | [
"merge",
"mergekit",
"lazymergekit",
"AIDC-ai-business/Marcoroni-7B-v3",
"EmbeddedLLM/Mistral-7B-Merge-14-v0.1",
"license:apache-2.0",
"region:us"
] | null | 2024-04-18T09:53:34+00:00 | [] | [] | TAGS
#merge #mergekit #lazymergekit #AIDC-ai-business/Marcoroni-7B-v3 #EmbeddedLLM/Mistral-7B-Merge-14-v0.1 #license-apache-2.0 #region-us
|
# Marcoro14-7B-slerp
Marcoro14-7B-slerp is a merge of the following models using mergekit:
* AIDC-ai-business/Marcoroni-7B-v3
* EmbeddedLLM/Mistral-7B-Merge-14-v0.1
## Configuration
| [
"# Marcoro14-7B-slerp\n\nMarcoro14-7B-slerp is a merge of the following models using mergekit:\n* AIDC-ai-business/Marcoroni-7B-v3\n* EmbeddedLLM/Mistral-7B-Merge-14-v0.1",
"## Configuration"
] | [
"TAGS\n#merge #mergekit #lazymergekit #AIDC-ai-business/Marcoroni-7B-v3 #EmbeddedLLM/Mistral-7B-Merge-14-v0.1 #license-apache-2.0 #region-us \n",
"# Marcoro14-7B-slerp\n\nMarcoro14-7B-slerp is a merge of the following models using mergekit:\n* AIDC-ai-business/Marcoroni-7B-v3\n* EmbeddedLLM/Mistral-7B-Merge-14-v0.1",
"## Configuration"
] |
text-generation | transformers |
<!-- header start -->
<p align="center">
<img src="https://i.imgur.com/mNM6Cai.png" width="100%" alt="Friendli Logo">
</p>
<!-- header end -->
# Mixtral-8x22B-Instruct-v0.1 - FP8
- Model creator: [Mistral AI](https://huggingface.co/mistralai)
- Original model: [Mixtral-8x22B-Instruct-v0.1](https://huggingface.co/mistralai/Mixtral-8x22B-Instruct-v0.1)
## Description
This repo contains the Mixtral-8x22B-Instruct-v0.1 model quantized to FP8 by FriendliAI, significantly enhancing its inference efficiency while maintaining high accuracy.
Note that FP8 is only supported by NVIDIA Ada, Hopper, and Blackwell GPU architectures.
Check out [FriendliAI documentation](https://docs.friendli.ai/) for more details.
## Compatibility
This model is compatible with **[Friendli Container](https://friendli.ai/products/container/)**.
## Prerequisites
- Before you begin, make sure you have signed up for [Friendli Suite](https://suite.friendli.ai/). **You can use Friendli Containers free of charge for four weeks.**
- Prepare a Personal Access Token following [this guide](#preparing-personal-access-token).
- Prepare a Friendli Container Secret following [this guide](#preparing-container-secret).
### Preparing Personal Access Token
PAT (Personal Access Token) is the user credential for for logging into our container registry.
1. Sign in [Friendli Suite](https://suite.friendli.ai/).
2. Go to **[User Settings > Tokens](https://suite.friendli.ai/user-settings/tokens)** and click **'Create new token'**.
3. Save your created token value.
### Pulling Friendli Container Image
1. Log in to the Docker client using the personal access token created as outlined in [this guide](#preparing-personal-access-token).
```sh
export FRIENDLI_PAT="YOUR PAT"
docker login registry.friendli.ai -u $YOUR_EMAIL -p $FRIENDLI_PAT
```
2. Pull image
```sh
docker pull registry.friendli.ai/trial
```
## Running Friendli Container
Once you've prepared the image of Friendli Container, you can launch it to create a serving endpoint.
```sh
docker run \
--gpus '"device=0,1,2,3"' \
-p 8000:8000 \
-v ~/.cache/huggingface:/root/.cache/huggingface \
-e FRIENDLI_CONTAINER_SECRET="YOUR CONTAINER SECRET" \
registry.friendli.ai/trial \
--web-server-port 8000 \
--hf-model-name FriendliAI/Mixtral-8x22B-Instruct-v0.1-fp8 \
--num-devices 4 # Use tensor parallelism degree 4
```
### Optimizing Inference Performance with Policy Search
To serve MoE models efficiently, it is required to run a policy search to explore the optimal execution policy:
```sh
export POLICY_DIR=$PWD/policy
mkdir -p $POLICY_DIR
docker run \
--gpus '"device=0,1,2,3"' \
-p 8000:8000 \
-v ~/.cache/huggingface:/root/.cache/huggingface \
-v $POLICY_DIR:/policy \
-e FRIENDLI_CONTAINER_SECRET="YOUR CONTAINER SECRET" \
registry.friendli.ai/trial \
--web-server-port 8000 \
--hf-model-name FriendliAI/Mixtral-8x22B-Instruct-v0.1-fp8 \
--num-devices 4 # Use tensor parallelism degree 4 \
--algo-policy-dir /policy \
--search-policy true
```
When the optimal policy is successfully searched, the policy is compiled into a policy file and saved at `$POLICY_DIR`.
Now you can create an inference endpoint with this optimal policy as follows:
```sh
docker run \
--gpus '"device=0,1,2,3"' \
-p 8000:8000 \
-v ~/.cache/huggingface:/root/.cache/huggingface \
-v $POLICY_DIR:/policy \
-e FRIENDLI_CONTAINER_SECRET="YOUR CONTAINER SECRET" \
registry.friendli.ai/trial \
--web-server-port 8000 \
--hf-model-name FriendliAI/Mixtral-8x22B-Instruct-v0.1-fp8 \
--num-devices 4 # Use tensor parallelism degree 4 \
--algo-policy-dir /policy
```
---
# Original model card: MistralAI's Mixtral-8x22B-Instruct v0.1
# Model Card for Mixtral-8x22B-Instruct-v0.1
The Mixtral-8x22B-Instruct-v0.1 Large Language Model (LLM) is an instruct fine-tuned version of the [Mixtral-8x22B-v0.1](https://huggingface.co/mistralai/Mixtral-8x22B-v0.1).
## Run the model
```python
from transformers import AutoModelForCausalLM
from mistral_common.protocol.instruct.messages import (
AssistantMessage,
UserMessage,
)
from mistral_common.protocol.instruct.tool_calls import (
Tool,
Function,
)
from mistral_common.tokens.tokenizers.mistral import MistralTokenizer
from mistral_common.tokens.instruct.normalize import ChatCompletionRequest
device = "cuda" # the device to load the model onto
tokenizer_v3 = MistralTokenizer.v3()
mistral_query = ChatCompletionRequest(
tools=[
Tool(
function=Function(
name="get_current_weather",
description="Get the current weather",
parameters={
"type": "object",
"properties": {
"location": {
"type": "string",
"description": "The city and state, e.g. San Francisco, CA",
},
"format": {
"type": "string",
"enum": ["celsius", "fahrenheit"],
"description": "The temperature unit to use. Infer this from the users location.",
},
},
"required": ["location", "format"],
},
)
)
],
messages=[
UserMessage(content="What's the weather like today in Paris"),
],
model="test",
)
encodeds = tokenizer_v3.encode_chat_completion(mistral_query).tokens
model = AutoModelForCausalLM.from_pretrained("mistralai/Mixtral-8x22B-Instruct-v0.1")
model_inputs = encodeds.to(device)
model.to(device)
generated_ids = model.generate(model_inputs, max_new_tokens=1000, do_sample=True)
sp_tokenizer = tokenizer_v3.instruct_tokenizer.tokenizer
decoded = sp_tokenizer.decode(generated_ids[0])
print(decoded)
```
Alternatively, you can run this example with the Hugging Face tokenizer.
To use this example, you'll need transformers version 4.39.0 or higher.
```console
pip install transformers==4.39.0
```
```python
from transformers import AutoModelForCausalLM, AutoTokenizer
model_id = "mistralai/Mixtral-8x22B-Instruct-v0.1"
tokenizer = AutoTokenizer.from_pretrained(model_id)
conversation=[
{"role": "user", "content": "What's the weather like in Paris?"},
{
"role": "tool_calls",
"content": [
{
"name": "get_current_weather",
"arguments": {"location": "Paris, France", "format": "celsius"},
}
]
},
{
"role": "tool_results",
"content": {"content": 22}
},
{"role": "assistant", "content": "The current temperature in Paris, France is 22 degrees Celsius."},
{"role": "user", "content": "What about San Francisco?"}
]
tools = [{"type": "function", "function": {"name":"get_current_weather", "description": "Get▁the▁current▁weather", "parameters": {"type": "object", "properties": {"location": {"type": "string", "description": "The city and state, e.g. San Francisco, CA"}, "format": {"type": "string", "enum": ["celsius", "fahrenheit"], "description": "The temperature unit to use. Infer this from the users location."}},"required":["location","format"]}}}]
# render the tool use prompt as a string:
tool_use_prompt = tokenizer.apply_chat_template(
conversation,
chat_template="tool_use",
tools=tools,
tokenize=False,
add_generation_prompt=True,
)
model = AutoModelForCausalLM.from_pretrained("mistralai/Mixtral-8x22B-Instruct-v0.1")
inputs = tokenizer(tool_use_prompt, return_tensors="pt")
outputs = model.generate(**inputs, max_new_tokens=20)
print(tokenizer.decode(outputs[0], skip_special_tokens=True))
```
# Instruct tokenizer
The HuggingFace tokenizer included in this release should match our own. To compare:
`pip install mistral-common`
```py
from mistral_common.protocol.instruct.messages import (
AssistantMessage,
UserMessage,
)
from mistral_common.tokens.tokenizers.mistral import MistralTokenizer
from mistral_common.tokens.instruct.normalize import ChatCompletionRequest
from transformers import AutoTokenizer
tokenizer_v3 = MistralTokenizer.v3()
mistral_query = ChatCompletionRequest(
messages=[
UserMessage(content="How many experts ?"),
AssistantMessage(content="8"),
UserMessage(content="How big ?"),
AssistantMessage(content="22B"),
UserMessage(content="Noice 🎉 !"),
],
model="test",
)
hf_messages = mistral_query.model_dump()['messages']
tokenized_mistral = tokenizer_v3.encode_chat_completion(mistral_query).tokens
tokenizer_hf = AutoTokenizer.from_pretrained('mistralai/Mixtral-8x22B-Instruct-v0.1')
tokenized_hf = tokenizer_hf.apply_chat_template(hf_messages, tokenize=True)
assert tokenized_hf == tokenized_mistral
```
# Function calling and special tokens
This tokenizer includes more special tokens, related to function calling :
- [TOOL_CALLS]
- [AVAILABLE_TOOLS]
- [/AVAILABLE_TOOLS]
- [TOOL_RESULTS]
- [/TOOL_RESULTS]
If you want to use this model with function calling, please be sure to apply it similarly to what is done in our [SentencePieceTokenizerV3](https://github.com/mistralai/mistral-common/blob/main/src/mistral_common/tokens/tokenizers/sentencepiece.py#L299).
# The Mistral AI Team
Albert Jiang, Alexandre Sablayrolles, Alexis Tacnet, Antoine Roux,
Arthur Mensch, Audrey Herblin-Stoop, Baptiste Bout, Baudouin de Monicault,
Blanche Savary, Bam4d, Caroline Feldman, Devendra Singh Chaplot,
Diego de las Casas, Eleonore Arcelin, Emma Bou Hanna, Etienne Metzger,
Gianna Lengyel, Guillaume Bour, Guillaume Lample, Harizo Rajaona,
Jean-Malo Delignon, Jia Li, Justus Murke, Louis Martin, Louis Ternon,
Lucile Saulnier, Lélio Renard Lavaud, Margaret Jennings, Marie Pellat,
Marie Torelli, Marie-Anne Lachaux, Nicolas Schuhl, Patrick von Platen,
Pierre Stock, Sandeep Subramanian, Sophia Yang, Szymon Antoniak, Teven Le Scao,
Thibaut Lavril, Timothée Lacroix, Théophile Gervet, Thomas Wang,
Valera Nemychnikova, William El Sayed, William Marshall | {"license": "apache-2.0", "tags": ["pretrained"], "model_name": "mistralai/Mixtral-8x22B-Instruct-v0.1", "base_model": "mistralai/Mixtral-8x22B-Instruct-v0.1", "inference": false, "model_link": "https://huggingface.co/mistralai/Mixtral-8x22B-Instruct-v0.1", "pipeline_tag": "text-generation", "quantized_by": "FriendliAI"} | FriendliAI/Mixtral-8x22B-Instruct-v0.1-fp8 | null | [
"transformers",
"safetensors",
"mixtral",
"text-generation",
"pretrained",
"conversational",
"base_model:mistralai/Mixtral-8x22B-Instruct-v0.1",
"license:apache-2.0",
"autotrain_compatible",
"text-generation-inference",
"8-bit",
"region:us"
] | null | 2024-04-18T09:54:03+00:00 | [] | [] | TAGS
#transformers #safetensors #mixtral #text-generation #pretrained #conversational #base_model-mistralai/Mixtral-8x22B-Instruct-v0.1 #license-apache-2.0 #autotrain_compatible #text-generation-inference #8-bit #region-us
|
<p align="center">
<img src="https://i.URL width="100%" alt="Friendli Logo">
</p>
# Mixtral-8x22B-Instruct-v0.1 - FP8
- Model creator: Mistral AI
- Original model: Mixtral-8x22B-Instruct-v0.1
## Description
This repo contains the Mixtral-8x22B-Instruct-v0.1 model quantized to FP8 by FriendliAI, significantly enhancing its inference efficiency while maintaining high accuracy.
Note that FP8 is only supported by NVIDIA Ada, Hopper, and Blackwell GPU architectures.
Check out FriendliAI documentation for more details.
## Compatibility
This model is compatible with Friendli Container.
## Prerequisites
- Before you begin, make sure you have signed up for Friendli Suite. You can use Friendli Containers free of charge for four weeks.
- Prepare a Personal Access Token following this guide.
- Prepare a Friendli Container Secret following this guide.
### Preparing Personal Access Token
PAT (Personal Access Token) is the user credential for for logging into our container registry.
1. Sign in Friendli Suite.
2. Go to User Settings > Tokens and click 'Create new token'.
3. Save your created token value.
### Pulling Friendli Container Image
1. Log in to the Docker client using the personal access token created as outlined in this guide.
2. Pull image
## Running Friendli Container
Once you've prepared the image of Friendli Container, you can launch it to create a serving endpoint.
### Optimizing Inference Performance with Policy Search
To serve MoE models efficiently, it is required to run a policy search to explore the optimal execution policy:
When the optimal policy is successfully searched, the policy is compiled into a policy file and saved at '$POLICY_DIR'.
Now you can create an inference endpoint with this optimal policy as follows:
---
# Original model card: MistralAI's Mixtral-8x22B-Instruct v0.1
# Model Card for Mixtral-8x22B-Instruct-v0.1
The Mixtral-8x22B-Instruct-v0.1 Large Language Model (LLM) is an instruct fine-tuned version of the Mixtral-8x22B-v0.1.
## Run the model
Alternatively, you can run this example with the Hugging Face tokenizer.
To use this example, you'll need transformers version 4.39.0 or higher.
# Instruct tokenizer
The HuggingFace tokenizer included in this release should match our own. To compare:
'pip install mistral-common'
# Function calling and special tokens
This tokenizer includes more special tokens, related to function calling :
- [TOOL_CALLS]
- [AVAILABLE_TOOLS]
- [/AVAILABLE_TOOLS]
- [TOOL_RESULTS]
- [/TOOL_RESULTS]
If you want to use this model with function calling, please be sure to apply it similarly to what is done in our SentencePieceTokenizerV3.
# The Mistral AI Team
Albert Jiang, Alexandre Sablayrolles, Alexis Tacnet, Antoine Roux,
Arthur Mensch, Audrey Herblin-Stoop, Baptiste Bout, Baudouin de Monicault,
Blanche Savary, Bam4d, Caroline Feldman, Devendra Singh Chaplot,
Diego de las Casas, Eleonore Arcelin, Emma Bou Hanna, Etienne Metzger,
Gianna Lengyel, Guillaume Bour, Guillaume Lample, Harizo Rajaona,
Jean-Malo Delignon, Jia Li, Justus Murke, Louis Martin, Louis Ternon,
Lucile Saulnier, Lélio Renard Lavaud, Margaret Jennings, Marie Pellat,
Marie Torelli, Marie-Anne Lachaux, Nicolas Schuhl, Patrick von Platen,
Pierre Stock, Sandeep Subramanian, Sophia Yang, Szymon Antoniak, Teven Le Scao,
Thibaut Lavril, Timothée Lacroix, Théophile Gervet, Thomas Wang,
Valera Nemychnikova, William El Sayed, William Marshall | [
"# Mixtral-8x22B-Instruct-v0.1 - FP8\n\n- Model creator: Mistral AI\n- Original model: Mixtral-8x22B-Instruct-v0.1",
"## Description\n\nThis repo contains the Mixtral-8x22B-Instruct-v0.1 model quantized to FP8 by FriendliAI, significantly enhancing its inference efficiency while maintaining high accuracy.\nNote that FP8 is only supported by NVIDIA Ada, Hopper, and Blackwell GPU architectures.\nCheck out FriendliAI documentation for more details.",
"## Compatibility\n\nThis model is compatible with Friendli Container.",
"## Prerequisites\n\n- Before you begin, make sure you have signed up for Friendli Suite. You can use Friendli Containers free of charge for four weeks.\n- Prepare a Personal Access Token following this guide.\n- Prepare a Friendli Container Secret following this guide.",
"### Preparing Personal Access Token\n\nPAT (Personal Access Token) is the user credential for for logging into our container registry.\n\n1. Sign in Friendli Suite.\n2. Go to User Settings > Tokens and click 'Create new token'.\n3. Save your created token value.",
"### Pulling Friendli Container Image\n\n1. Log in to the Docker client using the personal access token created as outlined in this guide.\n\n \n\n2. Pull image",
"## Running Friendli Container\n\nOnce you've prepared the image of Friendli Container, you can launch it to create a serving endpoint.",
"### Optimizing Inference Performance with Policy Search\n\nTo serve MoE models efficiently, it is required to run a policy search to explore the optimal execution policy:\n\n\n\nWhen the optimal policy is successfully searched, the policy is compiled into a policy file and saved at '$POLICY_DIR'.\nNow you can create an inference endpoint with this optimal policy as follows:\n\n\n\n---",
"# Original model card: MistralAI's Mixtral-8x22B-Instruct v0.1",
"# Model Card for Mixtral-8x22B-Instruct-v0.1\nThe Mixtral-8x22B-Instruct-v0.1 Large Language Model (LLM) is an instruct fine-tuned version of the Mixtral-8x22B-v0.1.",
"## Run the model \n\nAlternatively, you can run this example with the Hugging Face tokenizer.\nTo use this example, you'll need transformers version 4.39.0 or higher.",
"# Instruct tokenizer\nThe HuggingFace tokenizer included in this release should match our own. To compare: \n'pip install mistral-common'",
"# Function calling and special tokens\nThis tokenizer includes more special tokens, related to function calling : \n- [TOOL_CALLS]\n- [AVAILABLE_TOOLS]\n- [/AVAILABLE_TOOLS]\n- [TOOL_RESULTS]\n- [/TOOL_RESULTS]\n\nIf you want to use this model with function calling, please be sure to apply it similarly to what is done in our SentencePieceTokenizerV3.",
"# The Mistral AI Team\nAlbert Jiang, Alexandre Sablayrolles, Alexis Tacnet, Antoine Roux,\nArthur Mensch, Audrey Herblin-Stoop, Baptiste Bout, Baudouin de Monicault,\nBlanche Savary, Bam4d, Caroline Feldman, Devendra Singh Chaplot,\nDiego de las Casas, Eleonore Arcelin, Emma Bou Hanna, Etienne Metzger,\nGianna Lengyel, Guillaume Bour, Guillaume Lample, Harizo Rajaona,\nJean-Malo Delignon, Jia Li, Justus Murke, Louis Martin, Louis Ternon,\nLucile Saulnier, Lélio Renard Lavaud, Margaret Jennings, Marie Pellat,\nMarie Torelli, Marie-Anne Lachaux, Nicolas Schuhl, Patrick von Platen,\nPierre Stock, Sandeep Subramanian, Sophia Yang, Szymon Antoniak, Teven Le Scao,\nThibaut Lavril, Timothée Lacroix, Théophile Gervet, Thomas Wang,\nValera Nemychnikova, William El Sayed, William Marshall"
] | [
"TAGS\n#transformers #safetensors #mixtral #text-generation #pretrained #conversational #base_model-mistralai/Mixtral-8x22B-Instruct-v0.1 #license-apache-2.0 #autotrain_compatible #text-generation-inference #8-bit #region-us \n",
"# Mixtral-8x22B-Instruct-v0.1 - FP8\n\n- Model creator: Mistral AI\n- Original model: Mixtral-8x22B-Instruct-v0.1",
"## Description\n\nThis repo contains the Mixtral-8x22B-Instruct-v0.1 model quantized to FP8 by FriendliAI, significantly enhancing its inference efficiency while maintaining high accuracy.\nNote that FP8 is only supported by NVIDIA Ada, Hopper, and Blackwell GPU architectures.\nCheck out FriendliAI documentation for more details.",
"## Compatibility\n\nThis model is compatible with Friendli Container.",
"## Prerequisites\n\n- Before you begin, make sure you have signed up for Friendli Suite. You can use Friendli Containers free of charge for four weeks.\n- Prepare a Personal Access Token following this guide.\n- Prepare a Friendli Container Secret following this guide.",
"### Preparing Personal Access Token\n\nPAT (Personal Access Token) is the user credential for for logging into our container registry.\n\n1. Sign in Friendli Suite.\n2. Go to User Settings > Tokens and click 'Create new token'.\n3. Save your created token value.",
"### Pulling Friendli Container Image\n\n1. Log in to the Docker client using the personal access token created as outlined in this guide.\n\n \n\n2. Pull image",
"## Running Friendli Container\n\nOnce you've prepared the image of Friendli Container, you can launch it to create a serving endpoint.",
"### Optimizing Inference Performance with Policy Search\n\nTo serve MoE models efficiently, it is required to run a policy search to explore the optimal execution policy:\n\n\n\nWhen the optimal policy is successfully searched, the policy is compiled into a policy file and saved at '$POLICY_DIR'.\nNow you can create an inference endpoint with this optimal policy as follows:\n\n\n\n---",
"# Original model card: MistralAI's Mixtral-8x22B-Instruct v0.1",
"# Model Card for Mixtral-8x22B-Instruct-v0.1\nThe Mixtral-8x22B-Instruct-v0.1 Large Language Model (LLM) is an instruct fine-tuned version of the Mixtral-8x22B-v0.1.",
"## Run the model \n\nAlternatively, you can run this example with the Hugging Face tokenizer.\nTo use this example, you'll need transformers version 4.39.0 or higher.",
"# Instruct tokenizer\nThe HuggingFace tokenizer included in this release should match our own. To compare: \n'pip install mistral-common'",
"# Function calling and special tokens\nThis tokenizer includes more special tokens, related to function calling : \n- [TOOL_CALLS]\n- [AVAILABLE_TOOLS]\n- [/AVAILABLE_TOOLS]\n- [TOOL_RESULTS]\n- [/TOOL_RESULTS]\n\nIf you want to use this model with function calling, please be sure to apply it similarly to what is done in our SentencePieceTokenizerV3.",
"# The Mistral AI Team\nAlbert Jiang, Alexandre Sablayrolles, Alexis Tacnet, Antoine Roux,\nArthur Mensch, Audrey Herblin-Stoop, Baptiste Bout, Baudouin de Monicault,\nBlanche Savary, Bam4d, Caroline Feldman, Devendra Singh Chaplot,\nDiego de las Casas, Eleonore Arcelin, Emma Bou Hanna, Etienne Metzger,\nGianna Lengyel, Guillaume Bour, Guillaume Lample, Harizo Rajaona,\nJean-Malo Delignon, Jia Li, Justus Murke, Louis Martin, Louis Ternon,\nLucile Saulnier, Lélio Renard Lavaud, Margaret Jennings, Marie Pellat,\nMarie Torelli, Marie-Anne Lachaux, Nicolas Schuhl, Patrick von Platen,\nPierre Stock, Sandeep Subramanian, Sophia Yang, Szymon Antoniak, Teven Le Scao,\nThibaut Lavril, Timothée Lacroix, Théophile Gervet, Thomas Wang,\nValera Nemychnikova, William El Sayed, William Marshall"
] |
object-detection | transformers |
<!-- This model card has been generated automatically according to the information the Trainer had access to. You
should probably proofread and complete it, then remove this comment. -->
# detr-resnet-50-finetuned-10k-cppe5
This model is a fine-tuned version of [facebook/detr-resnet-50](https://huggingface.co/facebook/detr-resnet-50) on the cppe-5 dataset.
It achieves the following results on the evaluation set:
- Loss: 0.9865
- Map: 0.3578
- Map 50: 0.6781
- Map 75: 0.3105
- Map Small: 0.3578
- Map Medium: -1.0
- Map Large: -1.0
- Mar 1: 0.365
- Mar 10: 0.535
- Mar 100: 0.5483
- Mar Small: 0.5483
- Mar Medium: -1.0
- Mar Large: -1.0
- Map Coverall: 0.6584
- Mar 100 Coverall: 0.7772
- Map Face Shield: 0.3691
- Mar 100 Face Shield: 0.6063
- Map Gloves: 0.2477
- Mar 100 Gloves: 0.4266
- Map Goggles: 0.1766
- Mar 100 Goggles: 0.4655
- Map Mask: 0.3371
- Mar 100 Mask: 0.4661
## Model description
More information needed
## Intended uses & limitations
More information needed
## Training and evaluation data
More information needed
## Training procedure
### Training hyperparameters
The following hyperparameters were used during training:
- learning_rate: 5e-05
- train_batch_size: 8
- eval_batch_size: 8
- seed: 1337
- optimizer: Adam with betas=(0.9,0.999) and epsilon=1e-08
- lr_scheduler_type: linear
- num_epochs: 100.0
- mixed_precision_training: Native AMP
### Training results
| Training Loss | Epoch | Step | Validation Loss | Map | Map 50 | Map 75 | Map Small | Map Medium | Map Large | Mar 1 | Mar 10 | Mar 100 | Mar Small | Mar Medium | Mar Large | Map Coverall | Mar 100 Coverall | Map Face Shield | Mar 100 Face Shield | Map Gloves | Mar 100 Gloves | Map Goggles | Mar 100 Goggles | Map Mask | Mar 100 Mask |
|:-------------:|:-----:|:-----:|:---------------:|:------:|:------:|:------:|:---------:|:----------:|:---------:|:------:|:------:|:-------:|:---------:|:----------:|:---------:|:------------:|:----------------:|:---------------:|:-------------------:|:----------:|:--------------:|:-----------:|:---------------:|:--------:|:------------:|
| 3.7198 | 1.0 | 107 | 3.1869 | 0.0036 | 0.0144 | 0.0006 | 0.0036 | -1.0 | -1.0 | 0.017 | 0.0426 | 0.0647 | 0.0647 | -1.0 | -1.0 | 0.0169 | 0.1994 | 0.0 | 0.0 | 0.0001 | 0.0177 | 0.0 | 0.0 | 0.0008 | 0.1062 |
| 3.0393 | 2.0 | 214 | 2.8978 | 0.007 | 0.021 | 0.003 | 0.007 | -1.0 | -1.0 | 0.0192 | 0.0796 | 0.1238 | 0.1238 | -1.0 | -1.0 | 0.0333 | 0.5216 | 0.0 | 0.0 | 0.0001 | 0.0292 | 0.0 | 0.0 | 0.0017 | 0.0684 |
| 2.7812 | 3.0 | 321 | 2.5445 | 0.0138 | 0.0414 | 0.0085 | 0.0138 | -1.0 | -1.0 | 0.0307 | 0.0994 | 0.1258 | 0.1258 | -1.0 | -1.0 | 0.0655 | 0.4938 | 0.0 | 0.0 | 0.0002 | 0.0354 | 0.0 | 0.0 | 0.0033 | 0.1 |
| 2.5992 | 4.0 | 428 | 2.3828 | 0.0232 | 0.0601 | 0.0155 | 0.0232 | -1.0 | -1.0 | 0.0423 | 0.1202 | 0.1518 | 0.1518 | -1.0 | -1.0 | 0.1021 | 0.5481 | 0.0 | 0.0 | 0.0006 | 0.0495 | 0.0059 | 0.0109 | 0.0072 | 0.1503 |
| 2.3828 | 5.0 | 535 | 2.2672 | 0.0283 | 0.0703 | 0.0179 | 0.0283 | -1.0 | -1.0 | 0.0521 | 0.1283 | 0.1737 | 0.1737 | -1.0 | -1.0 | 0.1344 | 0.5846 | 0.0 | 0.0 | 0.001 | 0.0833 | 0.0 | 0.0 | 0.0063 | 0.2006 |
| 2.2633 | 6.0 | 642 | 2.0618 | 0.0479 | 0.0996 | 0.0416 | 0.0479 | -1.0 | -1.0 | 0.0782 | 0.1679 | 0.2035 | 0.2035 | -1.0 | -1.0 | 0.2099 | 0.6333 | 0.003 | 0.0159 | 0.0018 | 0.1187 | 0.0052 | 0.0218 | 0.0195 | 0.2277 |
| 2.1837 | 7.0 | 749 | 2.1100 | 0.0455 | 0.1159 | 0.0255 | 0.0455 | -1.0 | -1.0 | 0.0747 | 0.1582 | 0.1894 | 0.1894 | -1.0 | -1.0 | 0.2068 | 0.6185 | 0.0085 | 0.0556 | 0.001 | 0.0734 | 0.0002 | 0.0018 | 0.0113 | 0.1977 |
| 2.0689 | 8.0 | 856 | 2.0000 | 0.054 | 0.1389 | 0.0301 | 0.054 | -1.0 | -1.0 | 0.0954 | 0.1846 | 0.2159 | 0.2159 | -1.0 | -1.0 | 0.2155 | 0.5537 | 0.0314 | 0.1397 | 0.0049 | 0.1406 | 0.0002 | 0.0018 | 0.0181 | 0.2435 |
| 2.0417 | 9.0 | 963 | 1.8702 | 0.0697 | 0.1631 | 0.0501 | 0.0697 | -1.0 | -1.0 | 0.1074 | 0.2173 | 0.257 | 0.257 | -1.0 | -1.0 | 0.2826 | 0.6086 | 0.0279 | 0.181 | 0.0046 | 0.1734 | 0.0102 | 0.0418 | 0.0234 | 0.2802 |
| 1.9972 | 10.0 | 1070 | 1.8563 | 0.0742 | 0.1568 | 0.0541 | 0.0742 | -1.0 | -1.0 | 0.1196 | 0.2416 | 0.2786 | 0.2786 | -1.0 | -1.0 | 0.2933 | 0.6086 | 0.0233 | 0.1921 | 0.0053 | 0.1672 | 0.0239 | 0.0891 | 0.025 | 0.3362 |
| 1.8931 | 11.0 | 1177 | 1.6778 | 0.1054 | 0.2248 | 0.0898 | 0.1054 | -1.0 | -1.0 | 0.1456 | 0.2764 | 0.3033 | 0.3033 | -1.0 | -1.0 | 0.3955 | 0.671 | 0.0498 | 0.2603 | 0.0108 | 0.2188 | 0.0149 | 0.0382 | 0.056 | 0.3282 |
| 1.8269 | 12.0 | 1284 | 1.6905 | 0.1111 | 0.2399 | 0.0942 | 0.1111 | -1.0 | -1.0 | 0.1543 | 0.2949 | 0.3257 | 0.3257 | -1.0 | -1.0 | 0.4113 | 0.679 | 0.069 | 0.319 | 0.0087 | 0.2021 | 0.015 | 0.0909 | 0.0514 | 0.3373 |
| 1.8036 | 13.0 | 1391 | 1.6406 | 0.1149 | 0.2407 | 0.097 | 0.1149 | -1.0 | -1.0 | 0.1636 | 0.3108 | 0.3372 | 0.3372 | -1.0 | -1.0 | 0.4255 | 0.6759 | 0.0771 | 0.3381 | 0.0109 | 0.2182 | 0.0137 | 0.1309 | 0.047 | 0.3226 |
| 1.7463 | 14.0 | 1498 | 1.7169 | 0.1106 | 0.2421 | 0.0875 | 0.1106 | -1.0 | -1.0 | 0.1776 | 0.3205 | 0.3511 | 0.3511 | -1.0 | -1.0 | 0.3996 | 0.7 | 0.0404 | 0.2476 | 0.0117 | 0.2458 | 0.0257 | 0.2036 | 0.0757 | 0.3582 |
| 1.763 | 15.0 | 1605 | 1.5961 | 0.1245 | 0.2577 | 0.1018 | 0.1245 | -1.0 | -1.0 | 0.1817 | 0.3384 | 0.3677 | 0.3677 | -1.0 | -1.0 | 0.4575 | 0.6679 | 0.0775 | 0.3698 | 0.0107 | 0.2505 | 0.0318 | 0.1964 | 0.0447 | 0.3537 |
| 1.6467 | 16.0 | 1712 | 1.5365 | 0.1376 | 0.3073 | 0.1062 | 0.1376 | -1.0 | -1.0 | 0.2164 | 0.38 | 0.408 | 0.408 | -1.0 | -1.0 | 0.455 | 0.6852 | 0.0739 | 0.3873 | 0.0215 | 0.2719 | 0.0442 | 0.2891 | 0.0934 | 0.4068 |
| 1.6222 | 17.0 | 1819 | 1.5990 | 0.1295 | 0.2696 | 0.1026 | 0.1295 | -1.0 | -1.0 | 0.1802 | 0.3409 | 0.3693 | 0.3693 | -1.0 | -1.0 | 0.4577 | 0.6654 | 0.0786 | 0.3619 | 0.0297 | 0.2958 | 0.0211 | 0.2218 | 0.0603 | 0.3017 |
| 1.6239 | 18.0 | 1926 | 1.4164 | 0.159 | 0.3543 | 0.1262 | 0.159 | -1.0 | -1.0 | 0.235 | 0.3929 | 0.4138 | 0.4138 | -1.0 | -1.0 | 0.4753 | 0.7204 | 0.0921 | 0.3968 | 0.039 | 0.2922 | 0.0323 | 0.2636 | 0.1565 | 0.396 |
| 1.5448 | 19.0 | 2033 | 1.4689 | 0.1628 | 0.3725 | 0.1314 | 0.1628 | -1.0 | -1.0 | 0.205 | 0.3811 | 0.4064 | 0.4064 | -1.0 | -1.0 | 0.4794 | 0.6895 | 0.1038 | 0.419 | 0.0398 | 0.2828 | 0.0333 | 0.28 | 0.1578 | 0.3605 |
| 1.5026 | 20.0 | 2140 | 1.4093 | 0.1798 | 0.397 | 0.1369 | 0.1798 | -1.0 | -1.0 | 0.2336 | 0.4125 | 0.4349 | 0.4349 | -1.0 | -1.0 | 0.4851 | 0.6858 | 0.1494 | 0.4508 | 0.0341 | 0.2859 | 0.0434 | 0.3382 | 0.1869 | 0.4136 |
| 1.4797 | 21.0 | 2247 | 1.4605 | 0.1652 | 0.3605 | 0.1254 | 0.1652 | -1.0 | -1.0 | 0.2295 | 0.3823 | 0.4041 | 0.4041 | -1.0 | -1.0 | 0.4978 | 0.6957 | 0.0968 | 0.3825 | 0.0529 | 0.2797 | 0.0263 | 0.3236 | 0.1522 | 0.339 |
| 1.4298 | 22.0 | 2354 | 1.4231 | 0.163 | 0.3558 | 0.115 | 0.163 | -1.0 | -1.0 | 0.2256 | 0.3851 | 0.4108 | 0.4108 | -1.0 | -1.0 | 0.4902 | 0.7093 | 0.1033 | 0.4159 | 0.0515 | 0.313 | 0.0261 | 0.3109 | 0.1437 | 0.3051 |
| 1.4157 | 23.0 | 2461 | 1.3665 | 0.1914 | 0.4048 | 0.1533 | 0.1914 | -1.0 | -1.0 | 0.2478 | 0.4232 | 0.447 | 0.447 | -1.0 | -1.0 | 0.491 | 0.6975 | 0.1599 | 0.4683 | 0.0502 | 0.3021 | 0.0603 | 0.3618 | 0.1956 | 0.4051 |
| 1.4438 | 24.0 | 2568 | 1.2908 | 0.2103 | 0.433 | 0.168 | 0.2103 | -1.0 | -1.0 | 0.2643 | 0.4512 | 0.4761 | 0.4761 | -1.0 | -1.0 | 0.5368 | 0.7136 | 0.1493 | 0.4873 | 0.0789 | 0.3609 | 0.043 | 0.3891 | 0.2433 | 0.4294 |
| 1.4044 | 25.0 | 2675 | 1.4752 | 0.1709 | 0.3749 | 0.1388 | 0.1709 | -1.0 | -1.0 | 0.2187 | 0.3926 | 0.4191 | 0.4191 | -1.0 | -1.0 | 0.4862 | 0.7167 | 0.09 | 0.3905 | 0.0762 | 0.299 | 0.0393 | 0.3527 | 0.1627 | 0.3367 |
| 1.3703 | 26.0 | 2782 | 1.3047 | 0.2162 | 0.4568 | 0.1714 | 0.2162 | -1.0 | -1.0 | 0.2661 | 0.4344 | 0.4548 | 0.4548 | -1.0 | -1.0 | 0.5342 | 0.7272 | 0.166 | 0.4508 | 0.0971 | 0.3281 | 0.0424 | 0.3527 | 0.2414 | 0.4153 |
| 1.3292 | 27.0 | 2889 | 1.2674 | 0.22 | 0.4681 | 0.1702 | 0.22 | -1.0 | -1.0 | 0.2743 | 0.4286 | 0.4473 | 0.4473 | -1.0 | -1.0 | 0.5438 | 0.7265 | 0.2128 | 0.4429 | 0.1171 | 0.3443 | 0.0387 | 0.3455 | 0.1878 | 0.3774 |
| 1.359 | 28.0 | 2996 | 1.3156 | 0.2007 | 0.4272 | 0.1536 | 0.2007 | -1.0 | -1.0 | 0.2715 | 0.4384 | 0.4555 | 0.4555 | -1.0 | -1.0 | 0.5306 | 0.7111 | 0.163 | 0.5016 | 0.0896 | 0.3135 | 0.0307 | 0.38 | 0.1898 | 0.3712 |
| 1.3471 | 29.0 | 3103 | 1.2646 | 0.2161 | 0.455 | 0.172 | 0.2161 | -1.0 | -1.0 | 0.277 | 0.4492 | 0.4728 | 0.4728 | -1.0 | -1.0 | 0.5301 | 0.7216 | 0.1708 | 0.519 | 0.1216 | 0.3271 | 0.0391 | 0.4145 | 0.2189 | 0.3819 |
| 1.308 | 30.0 | 3210 | 1.3017 | 0.2107 | 0.4465 | 0.1718 | 0.2107 | -1.0 | -1.0 | 0.2556 | 0.4141 | 0.4387 | 0.4387 | -1.0 | -1.0 | 0.5321 | 0.7136 | 0.1531 | 0.454 | 0.1037 | 0.3203 | 0.0334 | 0.3218 | 0.2313 | 0.3836 |
| 1.3023 | 31.0 | 3317 | 1.2809 | 0.2174 | 0.462 | 0.1714 | 0.2174 | -1.0 | -1.0 | 0.2646 | 0.4242 | 0.4473 | 0.4473 | -1.0 | -1.0 | 0.5484 | 0.7259 | 0.1686 | 0.427 | 0.1163 | 0.3536 | 0.0506 | 0.3564 | 0.2029 | 0.3734 |
| 1.2561 | 32.0 | 3424 | 1.2679 | 0.2082 | 0.4557 | 0.1579 | 0.2082 | -1.0 | -1.0 | 0.263 | 0.4283 | 0.4511 | 0.4511 | -1.0 | -1.0 | 0.541 | 0.7284 | 0.1448 | 0.4556 | 0.1308 | 0.3682 | 0.0411 | 0.3436 | 0.1835 | 0.3599 |
| 1.268 | 33.0 | 3531 | 1.2632 | 0.2304 | 0.4841 | 0.1882 | 0.2304 | -1.0 | -1.0 | 0.2852 | 0.4469 | 0.4629 | 0.4629 | -1.0 | -1.0 | 0.5535 | 0.7327 | 0.2042 | 0.5286 | 0.1027 | 0.2917 | 0.0472 | 0.38 | 0.2441 | 0.3814 |
| 1.2337 | 34.0 | 3638 | 1.2157 | 0.2339 | 0.497 | 0.1854 | 0.2339 | -1.0 | -1.0 | 0.2845 | 0.4495 | 0.4756 | 0.4756 | -1.0 | -1.0 | 0.5704 | 0.7414 | 0.187 | 0.5095 | 0.1339 | 0.3448 | 0.0414 | 0.3982 | 0.2367 | 0.3842 |
| 1.2192 | 35.0 | 3745 | 1.2109 | 0.2505 | 0.5078 | 0.2013 | 0.2505 | -1.0 | -1.0 | 0.288 | 0.4617 | 0.4795 | 0.4795 | -1.0 | -1.0 | 0.5599 | 0.7259 | 0.2431 | 0.5016 | 0.1564 | 0.3521 | 0.0496 | 0.4164 | 0.2437 | 0.4017 |
| 1.2119 | 36.0 | 3852 | 1.1897 | 0.2584 | 0.5229 | 0.2198 | 0.2584 | -1.0 | -1.0 | 0.2986 | 0.4575 | 0.4744 | 0.4744 | -1.0 | -1.0 | 0.5742 | 0.7383 | 0.2667 | 0.5159 | 0.1389 | 0.35 | 0.0448 | 0.3473 | 0.2676 | 0.4203 |
| 1.1686 | 37.0 | 3959 | 1.1441 | 0.2581 | 0.5164 | 0.2072 | 0.2581 | -1.0 | -1.0 | 0.3002 | 0.4712 | 0.4918 | 0.4918 | -1.0 | -1.0 | 0.5904 | 0.7642 | 0.2406 | 0.5238 | 0.1535 | 0.3708 | 0.0404 | 0.3945 | 0.2654 | 0.4056 |
| 1.1664 | 38.0 | 4066 | 1.1554 | 0.2618 | 0.546 | 0.204 | 0.2618 | -1.0 | -1.0 | 0.3078 | 0.4822 | 0.5069 | 0.5069 | -1.0 | -1.0 | 0.5674 | 0.734 | 0.2486 | 0.519 | 0.1922 | 0.3927 | 0.0409 | 0.4509 | 0.26 | 0.4379 |
| 1.1746 | 39.0 | 4173 | 1.1873 | 0.2514 | 0.5269 | 0.1995 | 0.2514 | -1.0 | -1.0 | 0.292 | 0.4628 | 0.4842 | 0.4842 | -1.0 | -1.0 | 0.5527 | 0.7235 | 0.2427 | 0.5127 | 0.1591 | 0.3448 | 0.0468 | 0.4218 | 0.2557 | 0.4181 |
| 1.1559 | 40.0 | 4280 | 1.1594 | 0.2652 | 0.5517 | 0.2014 | 0.2652 | -1.0 | -1.0 | 0.2955 | 0.4743 | 0.4885 | 0.4885 | -1.0 | -1.0 | 0.5685 | 0.7377 | 0.2624 | 0.5238 | 0.1852 | 0.3755 | 0.0505 | 0.3873 | 0.2595 | 0.4181 |
| 1.1668 | 41.0 | 4387 | 1.1562 | 0.2479 | 0.5294 | 0.1853 | 0.2479 | -1.0 | -1.0 | 0.2967 | 0.4772 | 0.4994 | 0.4994 | -1.0 | -1.0 | 0.5742 | 0.7272 | 0.217 | 0.5413 | 0.1492 | 0.3453 | 0.058 | 0.4709 | 0.2411 | 0.4124 |
| 1.1084 | 42.0 | 4494 | 1.1302 | 0.2634 | 0.5352 | 0.2094 | 0.2634 | -1.0 | -1.0 | 0.3135 | 0.4783 | 0.4945 | 0.4945 | -1.0 | -1.0 | 0.5847 | 0.7333 | 0.2335 | 0.5397 | 0.1653 | 0.3583 | 0.0484 | 0.4127 | 0.2851 | 0.4282 |
| 1.1118 | 43.0 | 4601 | 1.1270 | 0.2754 | 0.5506 | 0.2408 | 0.2754 | -1.0 | -1.0 | 0.3103 | 0.4789 | 0.4973 | 0.4973 | -1.0 | -1.0 | 0.5867 | 0.7364 | 0.2662 | 0.5476 | 0.1587 | 0.3682 | 0.0599 | 0.3855 | 0.3057 | 0.4486 |
| 1.1096 | 44.0 | 4708 | 1.1772 | 0.2611 | 0.5565 | 0.2002 | 0.2611 | -1.0 | -1.0 | 0.2873 | 0.4606 | 0.4755 | 0.4755 | -1.0 | -1.0 | 0.5739 | 0.7228 | 0.247 | 0.5143 | 0.1649 | 0.3516 | 0.081 | 0.4073 | 0.2389 | 0.3814 |
| 1.0741 | 45.0 | 4815 | 1.0871 | 0.2857 | 0.5718 | 0.2186 | 0.2857 | -1.0 | -1.0 | 0.3237 | 0.4934 | 0.5104 | 0.5104 | -1.0 | -1.0 | 0.5927 | 0.75 | 0.2775 | 0.5698 | 0.1899 | 0.3786 | 0.0781 | 0.4145 | 0.2904 | 0.439 |
| 1.0774 | 46.0 | 4922 | 1.1049 | 0.2684 | 0.5461 | 0.2197 | 0.2684 | -1.0 | -1.0 | 0.3112 | 0.4956 | 0.5115 | 0.5115 | -1.0 | -1.0 | 0.5979 | 0.7574 | 0.2552 | 0.5794 | 0.143 | 0.3677 | 0.062 | 0.4291 | 0.284 | 0.4237 |
| 1.0517 | 47.0 | 5029 | 1.1005 | 0.2806 | 0.5821 | 0.2184 | 0.2806 | -1.0 | -1.0 | 0.3112 | 0.4872 | 0.5048 | 0.5048 | -1.0 | -1.0 | 0.5861 | 0.7543 | 0.2862 | 0.5619 | 0.1749 | 0.4016 | 0.0737 | 0.3945 | 0.282 | 0.4119 |
| 1.0717 | 48.0 | 5136 | 1.0878 | 0.2956 | 0.5902 | 0.2492 | 0.2956 | -1.0 | -1.0 | 0.3146 | 0.4925 | 0.5089 | 0.5089 | -1.0 | -1.0 | 0.6128 | 0.7506 | 0.2813 | 0.5381 | 0.1947 | 0.4036 | 0.0849 | 0.4091 | 0.3041 | 0.4429 |
| 1.0578 | 49.0 | 5243 | 1.0932 | 0.2816 | 0.5671 | 0.2246 | 0.2816 | -1.0 | -1.0 | 0.3137 | 0.479 | 0.4943 | 0.4943 | -1.0 | -1.0 | 0.6069 | 0.7537 | 0.2616 | 0.4873 | 0.1947 | 0.4026 | 0.0613 | 0.3964 | 0.2835 | 0.4316 |
| 1.0367 | 50.0 | 5350 | 1.0784 | 0.2956 | 0.5813 | 0.2416 | 0.2956 | -1.0 | -1.0 | 0.3308 | 0.4864 | 0.4979 | 0.4979 | -1.0 | -1.0 | 0.6193 | 0.7642 | 0.2809 | 0.5143 | 0.1841 | 0.3854 | 0.0885 | 0.3891 | 0.3053 | 0.4367 |
| 1.0239 | 51.0 | 5457 | 1.0702 | 0.2893 | 0.5689 | 0.2387 | 0.2893 | -1.0 | -1.0 | 0.315 | 0.4944 | 0.5097 | 0.5097 | -1.0 | -1.0 | 0.5978 | 0.763 | 0.2656 | 0.5095 | 0.1887 | 0.3938 | 0.0817 | 0.4309 | 0.3125 | 0.4514 |
| 1.0127 | 52.0 | 5564 | 1.0771 | 0.2833 | 0.5713 | 0.243 | 0.2833 | -1.0 | -1.0 | 0.3184 | 0.4856 | 0.5075 | 0.5075 | -1.0 | -1.0 | 0.6007 | 0.7605 | 0.2649 | 0.5175 | 0.1843 | 0.3932 | 0.0766 | 0.4364 | 0.2902 | 0.4299 |
| 0.9948 | 53.0 | 5671 | 1.1039 | 0.2821 | 0.5628 | 0.2607 | 0.2821 | -1.0 | -1.0 | 0.3143 | 0.4954 | 0.5132 | 0.5132 | -1.0 | -1.0 | 0.6002 | 0.7599 | 0.2846 | 0.5587 | 0.1729 | 0.3693 | 0.0646 | 0.4582 | 0.2884 | 0.4198 |
| 1.0026 | 54.0 | 5778 | 1.1125 | 0.2811 | 0.5569 | 0.2461 | 0.2811 | -1.0 | -1.0 | 0.3166 | 0.4846 | 0.5019 | 0.5019 | -1.0 | -1.0 | 0.6118 | 0.7691 | 0.2617 | 0.5286 | 0.1762 | 0.376 | 0.0625 | 0.3964 | 0.2932 | 0.4395 |
| 1.0037 | 55.0 | 5885 | 1.1172 | 0.2819 | 0.5757 | 0.2532 | 0.2819 | -1.0 | -1.0 | 0.3089 | 0.4653 | 0.4811 | 0.4811 | -1.0 | -1.0 | 0.5869 | 0.7302 | 0.2946 | 0.5032 | 0.1914 | 0.388 | 0.0565 | 0.3745 | 0.2802 | 0.4096 |
| 0.9892 | 56.0 | 5992 | 1.0649 | 0.311 | 0.6027 | 0.2673 | 0.311 | -1.0 | -1.0 | 0.3226 | 0.4954 | 0.515 | 0.515 | -1.0 | -1.0 | 0.629 | 0.7679 | 0.2972 | 0.5556 | 0.198 | 0.3901 | 0.1233 | 0.4182 | 0.3075 | 0.4435 |
| 0.98 | 57.0 | 6099 | 1.0413 | 0.3137 | 0.6244 | 0.2912 | 0.3137 | -1.0 | -1.0 | 0.3293 | 0.5021 | 0.5171 | 0.5171 | -1.0 | -1.0 | 0.6288 | 0.7599 | 0.3193 | 0.5698 | 0.2062 | 0.399 | 0.1101 | 0.4236 | 0.3043 | 0.4333 |
| 0.9682 | 58.0 | 6206 | 1.0301 | 0.3128 | 0.6003 | 0.2801 | 0.3128 | -1.0 | -1.0 | 0.3278 | 0.4963 | 0.517 | 0.517 | -1.0 | -1.0 | 0.632 | 0.7704 | 0.3038 | 0.5238 | 0.2093 | 0.4203 | 0.1204 | 0.4164 | 0.2984 | 0.4542 |
| 0.9533 | 59.0 | 6313 | 1.0755 | 0.2928 | 0.5954 | 0.25 | 0.2928 | -1.0 | -1.0 | 0.3177 | 0.4937 | 0.5084 | 0.5084 | -1.0 | -1.0 | 0.6102 | 0.7401 | 0.2906 | 0.5635 | 0.1942 | 0.399 | 0.1002 | 0.4218 | 0.2688 | 0.4175 |
| 0.9589 | 60.0 | 6420 | 1.0364 | 0.3213 | 0.6182 | 0.2717 | 0.3213 | -1.0 | -1.0 | 0.3383 | 0.5121 | 0.5273 | 0.5273 | -1.0 | -1.0 | 0.622 | 0.7617 | 0.3318 | 0.5683 | 0.2273 | 0.4193 | 0.1243 | 0.4236 | 0.301 | 0.4638 |
| 0.9398 | 61.0 | 6527 | 1.0653 | 0.3089 | 0.6099 | 0.2673 | 0.3089 | -1.0 | -1.0 | 0.3325 | 0.4946 | 0.5046 | 0.5046 | -1.0 | -1.0 | 0.6166 | 0.7556 | 0.3144 | 0.5286 | 0.177 | 0.376 | 0.1451 | 0.4127 | 0.2915 | 0.4503 |
| 0.924 | 62.0 | 6634 | 1.0409 | 0.319 | 0.6335 | 0.2641 | 0.319 | -1.0 | -1.0 | 0.3437 | 0.5085 | 0.5234 | 0.5234 | -1.0 | -1.0 | 0.6223 | 0.7537 | 0.3263 | 0.5476 | 0.21 | 0.4021 | 0.1269 | 0.4582 | 0.3093 | 0.4554 |
| 0.924 | 63.0 | 6741 | 1.0517 | 0.3212 | 0.6485 | 0.2727 | 0.3212 | -1.0 | -1.0 | 0.3382 | 0.5109 | 0.5232 | 0.5232 | -1.0 | -1.0 | 0.6392 | 0.7704 | 0.3195 | 0.5857 | 0.1967 | 0.399 | 0.1268 | 0.4218 | 0.3241 | 0.439 |
| 0.9458 | 64.0 | 6848 | 1.0314 | 0.3213 | 0.6357 | 0.28 | 0.3213 | -1.0 | -1.0 | 0.3414 | 0.5152 | 0.5278 | 0.5278 | -1.0 | -1.0 | 0.6272 | 0.7648 | 0.3137 | 0.573 | 0.227 | 0.4078 | 0.1257 | 0.4545 | 0.3129 | 0.439 |
| 0.9136 | 65.0 | 6955 | 1.0237 | 0.3262 | 0.6377 | 0.284 | 0.3262 | -1.0 | -1.0 | 0.3489 | 0.5157 | 0.5299 | 0.5299 | -1.0 | -1.0 | 0.6277 | 0.766 | 0.3294 | 0.5587 | 0.2223 | 0.3958 | 0.13 | 0.4655 | 0.3216 | 0.4633 |
| 0.8969 | 66.0 | 7062 | 1.0190 | 0.3188 | 0.6478 | 0.2833 | 0.3188 | -1.0 | -1.0 | 0.3456 | 0.5153 | 0.528 | 0.528 | -1.0 | -1.0 | 0.6254 | 0.7704 | 0.3338 | 0.5762 | 0.187 | 0.3859 | 0.1258 | 0.4582 | 0.322 | 0.4492 |
| 0.8917 | 67.0 | 7169 | 0.9900 | 0.326 | 0.6304 | 0.281 | 0.326 | -1.0 | -1.0 | 0.354 | 0.5289 | 0.5382 | 0.5382 | -1.0 | -1.0 | 0.6478 | 0.7759 | 0.3118 | 0.5556 | 0.2245 | 0.4115 | 0.1149 | 0.4691 | 0.3312 | 0.4791 |
| 0.8724 | 68.0 | 7276 | 1.0181 | 0.3141 | 0.6119 | 0.2757 | 0.3141 | -1.0 | -1.0 | 0.3398 | 0.517 | 0.5303 | 0.5303 | -1.0 | -1.0 | 0.642 | 0.7716 | 0.3118 | 0.5778 | 0.2091 | 0.4177 | 0.096 | 0.4255 | 0.3116 | 0.4588 |
| 0.8881 | 69.0 | 7383 | 1.0231 | 0.3119 | 0.63 | 0.2566 | 0.3119 | -1.0 | -1.0 | 0.3438 | 0.507 | 0.5148 | 0.5148 | -1.0 | -1.0 | 0.6278 | 0.7556 | 0.3062 | 0.5476 | 0.2077 | 0.4052 | 0.0951 | 0.4073 | 0.3228 | 0.4582 |
| 0.8604 | 70.0 | 7490 | 1.0245 | 0.3266 | 0.6436 | 0.2621 | 0.3266 | -1.0 | -1.0 | 0.3448 | 0.5179 | 0.5273 | 0.5273 | -1.0 | -1.0 | 0.6251 | 0.7623 | 0.3301 | 0.5889 | 0.2223 | 0.4115 | 0.1406 | 0.4255 | 0.315 | 0.4486 |
| 0.86 | 71.0 | 7597 | 1.0249 | 0.3226 | 0.6426 | 0.2709 | 0.3226 | -1.0 | -1.0 | 0.3442 | 0.5201 | 0.5325 | 0.5325 | -1.0 | -1.0 | 0.6353 | 0.7716 | 0.3171 | 0.6032 | 0.2233 | 0.4177 | 0.1289 | 0.4236 | 0.3082 | 0.4463 |
| 0.8452 | 72.0 | 7704 | 1.0383 | 0.3225 | 0.6364 | 0.2629 | 0.3225 | -1.0 | -1.0 | 0.344 | 0.5124 | 0.5223 | 0.5223 | -1.0 | -1.0 | 0.6375 | 0.7728 | 0.322 | 0.5794 | 0.2082 | 0.399 | 0.1443 | 0.4291 | 0.3003 | 0.4311 |
| 0.8444 | 73.0 | 7811 | 1.0018 | 0.3312 | 0.6454 | 0.2914 | 0.3312 | -1.0 | -1.0 | 0.3558 | 0.5223 | 0.535 | 0.535 | -1.0 | -1.0 | 0.6395 | 0.771 | 0.3471 | 0.5841 | 0.2158 | 0.4182 | 0.1433 | 0.4345 | 0.3105 | 0.4672 |
| 0.8325 | 74.0 | 7918 | 1.0412 | 0.3296 | 0.6574 | 0.272 | 0.3296 | -1.0 | -1.0 | 0.3404 | 0.5203 | 0.5312 | 0.5312 | -1.0 | -1.0 | 0.6449 | 0.7722 | 0.3422 | 0.6032 | 0.2302 | 0.4125 | 0.1352 | 0.4509 | 0.2956 | 0.4169 |
| 0.8438 | 75.0 | 8025 | 1.0015 | 0.34 | 0.6482 | 0.3027 | 0.34 | -1.0 | -1.0 | 0.3487 | 0.5211 | 0.5333 | 0.5333 | -1.0 | -1.0 | 0.6449 | 0.7691 | 0.3555 | 0.5968 | 0.2324 | 0.4172 | 0.1427 | 0.4382 | 0.3248 | 0.4452 |
| 0.8197 | 76.0 | 8132 | 1.0196 | 0.3426 | 0.6544 | 0.2931 | 0.3426 | -1.0 | -1.0 | 0.356 | 0.5234 | 0.5347 | 0.5347 | -1.0 | -1.0 | 0.6443 | 0.7722 | 0.35 | 0.5794 | 0.2465 | 0.4172 | 0.1434 | 0.4455 | 0.3288 | 0.4593 |
| 0.8241 | 77.0 | 8239 | 1.0215 | 0.339 | 0.6531 | 0.3012 | 0.339 | -1.0 | -1.0 | 0.3523 | 0.5162 | 0.5279 | 0.5279 | -1.0 | -1.0 | 0.6411 | 0.771 | 0.3486 | 0.5921 | 0.2324 | 0.4073 | 0.1375 | 0.4091 | 0.3357 | 0.4599 |
| 0.8019 | 78.0 | 8346 | 1.0356 | 0.3265 | 0.6279 | 0.2799 | 0.3265 | -1.0 | -1.0 | 0.3503 | 0.5169 | 0.5264 | 0.5264 | -1.0 | -1.0 | 0.639 | 0.771 | 0.3313 | 0.5762 | 0.2315 | 0.413 | 0.1144 | 0.4127 | 0.3162 | 0.4593 |
| 0.8113 | 79.0 | 8453 | 1.0087 | 0.3377 | 0.6556 | 0.3059 | 0.3377 | -1.0 | -1.0 | 0.3487 | 0.5269 | 0.5369 | 0.5369 | -1.0 | -1.0 | 0.6396 | 0.7765 | 0.3392 | 0.5873 | 0.2336 | 0.4068 | 0.1513 | 0.4564 | 0.3249 | 0.4576 |
| 0.8043 | 80.0 | 8560 | 1.0225 | 0.3351 | 0.6526 | 0.3035 | 0.3351 | -1.0 | -1.0 | 0.3485 | 0.5177 | 0.5285 | 0.5285 | -1.0 | -1.0 | 0.6529 | 0.7827 | 0.331 | 0.5778 | 0.2224 | 0.399 | 0.1421 | 0.4255 | 0.3273 | 0.4576 |
| 0.7909 | 81.0 | 8667 | 1.0009 | 0.345 | 0.6563 | 0.3086 | 0.345 | -1.0 | -1.0 | 0.3512 | 0.522 | 0.5359 | 0.5359 | -1.0 | -1.0 | 0.6649 | 0.7877 | 0.3589 | 0.5714 | 0.2366 | 0.4219 | 0.1374 | 0.4418 | 0.3272 | 0.4565 |
| 0.8034 | 82.0 | 8774 | 1.0006 | 0.333 | 0.6455 | 0.295 | 0.333 | -1.0 | -1.0 | 0.3529 | 0.5257 | 0.5369 | 0.5369 | -1.0 | -1.0 | 0.6387 | 0.7741 | 0.3352 | 0.5873 | 0.2342 | 0.4208 | 0.1362 | 0.4491 | 0.3206 | 0.4531 |
| 0.7744 | 83.0 | 8881 | 0.9946 | 0.341 | 0.6513 | 0.3182 | 0.341 | -1.0 | -1.0 | 0.3615 | 0.5273 | 0.5381 | 0.5381 | -1.0 | -1.0 | 0.6425 | 0.7778 | 0.3636 | 0.5873 | 0.2404 | 0.4198 | 0.1246 | 0.4418 | 0.3341 | 0.4638 |
| 0.7769 | 84.0 | 8988 | 0.9994 | 0.3406 | 0.6523 | 0.3009 | 0.3406 | -1.0 | -1.0 | 0.3637 | 0.5304 | 0.5384 | 0.5384 | -1.0 | -1.0 | 0.6478 | 0.7728 | 0.3472 | 0.5968 | 0.2355 | 0.4198 | 0.1444 | 0.4382 | 0.3281 | 0.4644 |
| 0.7679 | 85.0 | 9095 | 1.0170 | 0.3397 | 0.6549 | 0.2888 | 0.3397 | -1.0 | -1.0 | 0.3624 | 0.5301 | 0.5407 | 0.5407 | -1.0 | -1.0 | 0.6485 | 0.7685 | 0.3431 | 0.6079 | 0.2294 | 0.4167 | 0.1607 | 0.46 | 0.317 | 0.4503 |
| 0.772 | 86.0 | 9202 | 0.9953 | 0.3515 | 0.6722 | 0.3099 | 0.3515 | -1.0 | -1.0 | 0.3674 | 0.5331 | 0.543 | 0.543 | -1.0 | -1.0 | 0.6599 | 0.7778 | 0.3692 | 0.6032 | 0.24 | 0.4187 | 0.1523 | 0.46 | 0.3361 | 0.4554 |
| 0.783 | 87.0 | 9309 | 1.0003 | 0.3401 | 0.6545 | 0.2943 | 0.3401 | -1.0 | -1.0 | 0.3603 | 0.5309 | 0.5457 | 0.5457 | -1.0 | -1.0 | 0.651 | 0.7759 | 0.3521 | 0.6222 | 0.2296 | 0.4161 | 0.1433 | 0.46 | 0.3246 | 0.4542 |
| 0.7508 | 88.0 | 9416 | 0.9849 | 0.3517 | 0.6734 | 0.3217 | 0.3517 | -1.0 | -1.0 | 0.362 | 0.5333 | 0.5447 | 0.5447 | -1.0 | -1.0 | 0.6572 | 0.7747 | 0.369 | 0.5984 | 0.2439 | 0.4318 | 0.1503 | 0.4527 | 0.338 | 0.4661 |
| 0.7558 | 89.0 | 9523 | 0.9861 | 0.3517 | 0.6749 | 0.3173 | 0.3517 | -1.0 | -1.0 | 0.3628 | 0.5329 | 0.5408 | 0.5408 | -1.0 | -1.0 | 0.6469 | 0.7728 | 0.3661 | 0.5889 | 0.2323 | 0.4104 | 0.1747 | 0.4709 | 0.3385 | 0.461 |
| 0.7398 | 90.0 | 9630 | 0.9966 | 0.3565 | 0.6784 | 0.315 | 0.3565 | -1.0 | -1.0 | 0.3621 | 0.5367 | 0.5484 | 0.5484 | -1.0 | -1.0 | 0.6524 | 0.7796 | 0.3655 | 0.6127 | 0.2374 | 0.4151 | 0.1769 | 0.4655 | 0.3503 | 0.4689 |
| 0.7532 | 91.0 | 9737 | 0.9883 | 0.354 | 0.6709 | 0.3179 | 0.354 | -1.0 | -1.0 | 0.3623 | 0.5395 | 0.5475 | 0.5475 | -1.0 | -1.0 | 0.6551 | 0.779 | 0.3669 | 0.6175 | 0.2432 | 0.4187 | 0.1545 | 0.4564 | 0.3502 | 0.4661 |
| 0.7417 | 92.0 | 9844 | 0.9932 | 0.3557 | 0.6777 | 0.3131 | 0.3557 | -1.0 | -1.0 | 0.3629 | 0.5361 | 0.5465 | 0.5465 | -1.0 | -1.0 | 0.6566 | 0.7765 | 0.3713 | 0.6143 | 0.2425 | 0.4224 | 0.163 | 0.4491 | 0.3451 | 0.4701 |
| 0.7554 | 93.0 | 9951 | 0.9921 | 0.3598 | 0.6841 | 0.3212 | 0.3598 | -1.0 | -1.0 | 0.3643 | 0.5375 | 0.5466 | 0.5466 | -1.0 | -1.0 | 0.6591 | 0.7821 | 0.3696 | 0.5952 | 0.2482 | 0.4214 | 0.175 | 0.4691 | 0.3469 | 0.465 |
| 0.7339 | 94.0 | 10058 | 1.0049 | 0.3587 | 0.6838 | 0.3184 | 0.3587 | -1.0 | -1.0 | 0.3668 | 0.5347 | 0.5447 | 0.5447 | -1.0 | -1.0 | 0.658 | 0.7753 | 0.3767 | 0.6048 | 0.2434 | 0.4177 | 0.1744 | 0.46 | 0.3411 | 0.4655 |
| 0.7184 | 95.0 | 10165 | 0.9969 | 0.3547 | 0.6739 | 0.3214 | 0.3547 | -1.0 | -1.0 | 0.3633 | 0.5353 | 0.5449 | 0.5449 | -1.0 | -1.0 | 0.6521 | 0.7722 | 0.369 | 0.6079 | 0.2402 | 0.413 | 0.1707 | 0.4673 | 0.3412 | 0.4638 |
| 0.7448 | 96.0 | 10272 | 0.9925 | 0.3556 | 0.6708 | 0.3061 | 0.3556 | -1.0 | -1.0 | 0.365 | 0.5327 | 0.544 | 0.544 | -1.0 | -1.0 | 0.6517 | 0.7765 | 0.3707 | 0.6016 | 0.2354 | 0.4208 | 0.1781 | 0.4527 | 0.3421 | 0.4684 |
| 0.7264 | 97.0 | 10379 | 0.9914 | 0.3576 | 0.6793 | 0.315 | 0.3576 | -1.0 | -1.0 | 0.3657 | 0.5332 | 0.5439 | 0.5439 | -1.0 | -1.0 | 0.6517 | 0.7753 | 0.378 | 0.6 | 0.2464 | 0.426 | 0.1707 | 0.4545 | 0.3414 | 0.4638 |
| 0.7455 | 98.0 | 10486 | 0.9829 | 0.3586 | 0.6794 | 0.3132 | 0.3586 | -1.0 | -1.0 | 0.367 | 0.5353 | 0.5473 | 0.5473 | -1.0 | -1.0 | 0.6586 | 0.7772 | 0.3781 | 0.6079 | 0.2468 | 0.4266 | 0.1742 | 0.4582 | 0.3355 | 0.4667 |
| 0.7105 | 99.0 | 10593 | 0.9862 | 0.3577 | 0.6795 | 0.3089 | 0.3577 | -1.0 | -1.0 | 0.3649 | 0.5352 | 0.5476 | 0.5476 | -1.0 | -1.0 | 0.6591 | 0.7778 | 0.3712 | 0.6032 | 0.2481 | 0.4271 | 0.1727 | 0.4655 | 0.3376 | 0.4644 |
| 0.7089 | 100.0 | 10700 | 0.9865 | 0.3578 | 0.6781 | 0.3105 | 0.3578 | -1.0 | -1.0 | 0.365 | 0.535 | 0.5483 | 0.5483 | -1.0 | -1.0 | 0.6584 | 0.7772 | 0.3691 | 0.6063 | 0.2477 | 0.4266 | 0.1766 | 0.4655 | 0.3371 | 0.4661 |
### Framework versions
- Transformers 4.40.0.dev0
- Pytorch 1.13.0+cu117
- Datasets 2.18.0
- Tokenizers 0.19.0 | {"license": "apache-2.0", "tags": ["object-detection", "vision", "generated_from_trainer"], "datasets": ["cppe-5"], "base_model": "facebook/detr-resnet-50", "model-index": [{"name": "detr-resnet-50-finetuned-10k-cppe5", "results": []}]} | qubvel-hf/detr-resnet-50-finetuned-10k-cppe5 | null | [
"transformers",
"safetensors",
"detr",
"object-detection",
"vision",
"generated_from_trainer",
"dataset:cppe-5",
"base_model:facebook/detr-resnet-50",
"license:apache-2.0",
"endpoints_compatible",
"region:us"
] | null | 2024-04-18T09:54:30+00:00 | [] | [] | TAGS
#transformers #safetensors #detr #object-detection #vision #generated_from_trainer #dataset-cppe-5 #base_model-facebook/detr-resnet-50 #license-apache-2.0 #endpoints_compatible #region-us
| detr-resnet-50-finetuned-10k-cppe5
==================================
This model is a fine-tuned version of facebook/detr-resnet-50 on the cppe-5 dataset.
It achieves the following results on the evaluation set:
* Loss: 0.9865
* Map: 0.3578
* Map 50: 0.6781
* Map 75: 0.3105
* Map Small: 0.3578
* Map Medium: -1.0
* Map Large: -1.0
* Mar 1: 0.365
* Mar 10: 0.535
* Mar 100: 0.5483
* Mar Small: 0.5483
* Mar Medium: -1.0
* Mar Large: -1.0
* Map Coverall: 0.6584
* Mar 100 Coverall: 0.7772
* Map Face Shield: 0.3691
* Mar 100 Face Shield: 0.6063
* Map Gloves: 0.2477
* Mar 100 Gloves: 0.4266
* Map Goggles: 0.1766
* Mar 100 Goggles: 0.4655
* Map Mask: 0.3371
* Mar 100 Mask: 0.4661
Model description
-----------------
More information needed
Intended uses & limitations
---------------------------
More information needed
Training and evaluation data
----------------------------
More information needed
Training procedure
------------------
### Training hyperparameters
The following hyperparameters were used during training:
* learning\_rate: 5e-05
* train\_batch\_size: 8
* eval\_batch\_size: 8
* seed: 1337
* optimizer: Adam with betas=(0.9,0.999) and epsilon=1e-08
* lr\_scheduler\_type: linear
* num\_epochs: 100.0
* mixed\_precision\_training: Native AMP
### Training results
### Framework versions
* Transformers 4.40.0.dev0
* Pytorch 1.13.0+cu117
* Datasets 2.18.0
* Tokenizers 0.19.0
| [
"### Training hyperparameters\n\n\nThe following hyperparameters were used during training:\n\n\n* learning\\_rate: 5e-05\n* train\\_batch\\_size: 8\n* eval\\_batch\\_size: 8\n* seed: 1337\n* optimizer: Adam with betas=(0.9,0.999) and epsilon=1e-08\n* lr\\_scheduler\\_type: linear\n* num\\_epochs: 100.0\n* mixed\\_precision\\_training: Native AMP",
"### Training results",
"### Framework versions\n\n\n* Transformers 4.40.0.dev0\n* Pytorch 1.13.0+cu117\n* Datasets 2.18.0\n* Tokenizers 0.19.0"
] | [
"TAGS\n#transformers #safetensors #detr #object-detection #vision #generated_from_trainer #dataset-cppe-5 #base_model-facebook/detr-resnet-50 #license-apache-2.0 #endpoints_compatible #region-us \n",
"### Training hyperparameters\n\n\nThe following hyperparameters were used during training:\n\n\n* learning\\_rate: 5e-05\n* train\\_batch\\_size: 8\n* eval\\_batch\\_size: 8\n* seed: 1337\n* optimizer: Adam with betas=(0.9,0.999) and epsilon=1e-08\n* lr\\_scheduler\\_type: linear\n* num\\_epochs: 100.0\n* mixed\\_precision\\_training: Native AMP",
"### Training results",
"### Framework versions\n\n\n* Transformers 4.40.0.dev0\n* Pytorch 1.13.0+cu117\n* Datasets 2.18.0\n* Tokenizers 0.19.0"
] |
text-to-image | diffusers | # Workout
<Gallery />
## Model description
Test
## Trigger words
You should use `Style of TOK` to trigger the image generation.
## Download model
Weights for this model are available in Safetensors format.
[Download](/Hoga2/Working30daysworkout/tree/main) them in the Files & versions tab.
| {"tags": ["text-to-image", "stable-diffusion", "lora", "diffusers", "template:sd-lora"], "widget": [{"text": "-", "output": {"url": "images/\u0e44\u0e21\u0e48\u0e21\u0e35\u0e0a\u0e37\u0e48\u0e2d 265_20240418164824.png"}}], "base_model": "stabilityai/stable-diffusion-xl-base-1.0", "instance_prompt": "Style of TOK"} | Hoga2/Working30daysworkout | null | [
"diffusers",
"text-to-image",
"stable-diffusion",
"lora",
"template:sd-lora",
"base_model:stabilityai/stable-diffusion-xl-base-1.0",
"region:us"
] | null | 2024-04-18T09:55:02+00:00 | [] | [] | TAGS
#diffusers #text-to-image #stable-diffusion #lora #template-sd-lora #base_model-stabilityai/stable-diffusion-xl-base-1.0 #region-us
| # Workout
<Gallery />
## Model description
Test
## Trigger words
You should use 'Style of TOK' to trigger the image generation.
## Download model
Weights for this model are available in Safetensors format.
Download them in the Files & versions tab.
| [
"# Workout\n\n<Gallery />",
"## Model description \n\nTest",
"## Trigger words\n\nYou should use 'Style of TOK' to trigger the image generation.",
"## Download model\n\nWeights for this model are available in Safetensors format.\n\nDownload them in the Files & versions tab."
] | [
"TAGS\n#diffusers #text-to-image #stable-diffusion #lora #template-sd-lora #base_model-stabilityai/stable-diffusion-xl-base-1.0 #region-us \n",
"# Workout\n\n<Gallery />",
"## Model description \n\nTest",
"## Trigger words\n\nYou should use 'Style of TOK' to trigger the image generation.",
"## Download model\n\nWeights for this model are available in Safetensors format.\n\nDownload them in the Files & versions tab."
] |
text-generation | transformers |
<!-- header start -->
<p align="center">
<img src="https://i.imgur.com/mNM6Cai.png" width="100%" alt="Friendli Logo">
</p>
<!-- header end -->
# Mixtral-8x22B-v0.1 - FP8
- Model creator: [Mistral AI](https://huggingface.co/mistralai)
- Original model: [Mixtral-8x22B-v0.1](https://huggingface.co/mistralai/Mixtral-8x22B-v0.1)
## Description
This repo contains the Mixtral-8x22B-v0.1 model quantized to FP8 by FriendliAI, significantly enhancing its inference efficiency while maintaining high accuracy.
Note that FP8 is only supported by NVIDIA Ada, Hopper, and Blackwell GPU architectures.
Check out [FriendliAI documentation](https://docs.friendli.ai/) for more details.
## Compatibility
This model is compatible with **[Friendli Container](https://friendli.ai/products/container/)**.
## Prerequisites
- Before you begin, make sure you have signed up for [Friendli Suite](https://suite.friendli.ai/). **You can use Friendli Containers free of charge for four weeks.**
- Prepare a Personal Access Token following [this guide](#preparing-personal-access-token).
- Prepare a Friendli Container Secret following [this guide](#preparing-container-secret).
### Preparing Personal Access Token
PAT (Personal Access Token) is the user credential for for logging into our container registry.
1. Sign in [Friendli Suite](https://suite.friendli.ai/).
2. Go to **[User Settings > Tokens](https://suite.friendli.ai/user-settings/tokens)** and click **'Create new token'**.
3. Save your created token value.
### Pulling Friendli Container Image
1. Log in to the Docker client using the personal access token created as outlined in [this guide](#preparing-personal-access-token).
```sh
export FRIENDLI_PAT="YOUR PAT"
docker login registry.friendli.ai -u $YOUR_EMAIL -p $FRIENDLI_PAT
```
2. Pull image
```sh
docker pull registry.friendli.ai/trial
```
## Running Friendli Container
Once you've prepared the image of Friendli Container, you can launch it to create a serving endpoint.
```sh
docker run \
--gpus '"device=0,1,2,3"' \
-p 8000:8000 \
-v ~/.cache/huggingface:/root/.cache/huggingface \
-e FRIENDLI_CONTAINER_SECRET="YOUR CONTAINER SECRET" \
registry.friendli.ai/trial \
--web-server-port 8000 \
--hf-model-name FriendliAI/Mixtral-8x22B-v0.1-fp8 \
--num-devices 4 # Use tensor parallelism degree 4
```
### Optimizing Inference Performance with Policy Search
To serve MoE models efficiently, it is required to run a policy search to explore the optimal execution policy:
```sh
export POLICY_DIR=$PWD/policy
mkdir -p $POLICY_DIR
docker run \
--gpus '"device=0,1,2,3"' \
-p 8000:8000 \
-v ~/.cache/huggingface:/root/.cache/huggingface \
-v $POLICY_DIR:/policy \
-e FRIENDLI_CONTAINER_SECRET="YOUR CONTAINER SECRET" \
registry.friendli.ai/trial \
--web-server-port 8000 \
--hf-model-name FriendliAI/Mixtral-8x22B-v0.1-fp8 \
--num-devices 4 # Use tensor parallelism degree 4 \
--algo-policy-dir /policy \
--search-policy true
```
When the optimal policy is successfully searched, the policy is compiled into a policy file and saved at `$POLICY_DIR`.
Now you can create an inference endpoint with this optimal policy as follows:
```sh
docker run \
--gpus '"device=0,1,2,3"' \
-p 8000:8000 \
-v ~/.cache/huggingface:/root/.cache/huggingface \
-v $POLICY_DIR:/policy \
-e FRIENDLI_CONTAINER_SECRET="YOUR CONTAINER SECRET" \
registry.friendli.ai/trial \
--web-server-port 8000 \
--hf-model-name FriendliAI/Mixtral-8x22B-v0.1-fp8 \
--num-devices 4 # Use tensor parallelism degree 4 \
--algo-policy-dir /policy
```
---
# Original model card: MistralAI's Mixtral-8x22B v0.1
# Model Card for Mixtral-8x22B
The Mixtral-8x22B Large Language Model (LLM) is a pretrained generative Sparse Mixture of Experts.
For full details of this model please read our [release blog post](https://mistral.ai/news/mixtral-8x22b).
## Warning
This repo contains weights that are compatible with [vLLM](https://github.com/vllm-project/vllm) serving of the model as well as Hugging Face [transformers](https://github.com/huggingface/transformers) library. It is based on the original Mixtral [torrent release](https://twitter.com/MistralAI/status/1777869263778291896), but the file format and parameter names are different.
## Run the model
```python
from transformers import AutoModelForCausalLM, AutoTokenizer
model_id = "mistralai/Mixtral-8x22B-v0.1"
tokenizer = AutoTokenizer.from_pretrained(model_id)
model = AutoModelForCausalLM.from_pretrained(model_id)
text = "Hello my name is"
inputs = tokenizer(text, return_tensors="pt")
outputs = model.generate(**inputs, max_new_tokens=20)
print(tokenizer.decode(outputs[0], skip_special_tokens=True))
```
By default, transformers will load the model in full precision. Therefore you might be interested to further reduce down the memory requirements to run the model through the optimizations we offer in HF ecosystem:
## Notice
Mixtral-8x22B is a pretrained base model and therefore does not have any moderation mechanisms.
# The Mistral AI Team
Albert Jiang, Alexandre Sablayrolles, Alexis Tacnet, Antoine Roux,
Arthur Mensch, Audrey Herblin-Stoop, Baptiste Bout, Baudouin de Monicault,
Blanche Savary, Bam4d, Caroline Feldman, Devendra Singh Chaplot,
Diego de las Casas, Eleonore Arcelin, Emma Bou Hanna, Etienne Metzger,
Gianna Lengyel, Guillaume Bour, Guillaume Lample, Harizo Rajaona,
Jean-Malo Delignon, Jia Li, Justus Murke, Louis Martin, Louis Ternon,
Lucile Saulnier, Lélio Renard Lavaud, Margaret Jennings, Marie Pellat,
Marie Torelli, Marie-Anne Lachaux, Nicolas Schuhl, Patrick von Platen,
Pierre Stock, Sandeep Subramanian, Sophia Yang, Szymon Antoniak, Teven Le Scao,
Thibaut Lavril, Timothée Lacroix, Théophile Gervet, Thomas Wang,
Valera Nemychnikova, William El Sayed, William Marshall | {"license": "apache-2.0", "tags": ["pretrained"], "model_name": "mistralai/Mixtral-8x22B-v0.1", "base_model": "mistralai/Mixtral-8x22B-v0.1", "inference": false, "model_link": "https://huggingface.co/mistralai/Mixtral-8x22B-v0.1", "pipeline_tag": "text-generation", "quantized_by": "FriendliAI"} | FriendliAI/Mixtral-8x22B-v0.1-fp8 | null | [
"transformers",
"safetensors",
"mixtral",
"text-generation",
"pretrained",
"conversational",
"base_model:mistralai/Mixtral-8x22B-v0.1",
"license:apache-2.0",
"autotrain_compatible",
"text-generation-inference",
"8-bit",
"region:us"
] | null | 2024-04-18T09:55:15+00:00 | [] | [] | TAGS
#transformers #safetensors #mixtral #text-generation #pretrained #conversational #base_model-mistralai/Mixtral-8x22B-v0.1 #license-apache-2.0 #autotrain_compatible #text-generation-inference #8-bit #region-us
|
<p align="center">
<img src="https://i.URL width="100%" alt="Friendli Logo">
</p>
# Mixtral-8x22B-v0.1 - FP8
- Model creator: Mistral AI
- Original model: Mixtral-8x22B-v0.1
## Description
This repo contains the Mixtral-8x22B-v0.1 model quantized to FP8 by FriendliAI, significantly enhancing its inference efficiency while maintaining high accuracy.
Note that FP8 is only supported by NVIDIA Ada, Hopper, and Blackwell GPU architectures.
Check out FriendliAI documentation for more details.
## Compatibility
This model is compatible with Friendli Container.
## Prerequisites
- Before you begin, make sure you have signed up for Friendli Suite. You can use Friendli Containers free of charge for four weeks.
- Prepare a Personal Access Token following this guide.
- Prepare a Friendli Container Secret following this guide.
### Preparing Personal Access Token
PAT (Personal Access Token) is the user credential for for logging into our container registry.
1. Sign in Friendli Suite.
2. Go to User Settings > Tokens and click 'Create new token'.
3. Save your created token value.
### Pulling Friendli Container Image
1. Log in to the Docker client using the personal access token created as outlined in this guide.
2. Pull image
## Running Friendli Container
Once you've prepared the image of Friendli Container, you can launch it to create a serving endpoint.
### Optimizing Inference Performance with Policy Search
To serve MoE models efficiently, it is required to run a policy search to explore the optimal execution policy:
When the optimal policy is successfully searched, the policy is compiled into a policy file and saved at '$POLICY_DIR'.
Now you can create an inference endpoint with this optimal policy as follows:
---
# Original model card: MistralAI's Mixtral-8x22B v0.1
# Model Card for Mixtral-8x22B
The Mixtral-8x22B Large Language Model (LLM) is a pretrained generative Sparse Mixture of Experts.
For full details of this model please read our release blog post.
## Warning
This repo contains weights that are compatible with vLLM serving of the model as well as Hugging Face transformers library. It is based on the original Mixtral torrent release, but the file format and parameter names are different.
## Run the model
By default, transformers will load the model in full precision. Therefore you might be interested to further reduce down the memory requirements to run the model through the optimizations we offer in HF ecosystem:
## Notice
Mixtral-8x22B is a pretrained base model and therefore does not have any moderation mechanisms.
# The Mistral AI Team
Albert Jiang, Alexandre Sablayrolles, Alexis Tacnet, Antoine Roux,
Arthur Mensch, Audrey Herblin-Stoop, Baptiste Bout, Baudouin de Monicault,
Blanche Savary, Bam4d, Caroline Feldman, Devendra Singh Chaplot,
Diego de las Casas, Eleonore Arcelin, Emma Bou Hanna, Etienne Metzger,
Gianna Lengyel, Guillaume Bour, Guillaume Lample, Harizo Rajaona,
Jean-Malo Delignon, Jia Li, Justus Murke, Louis Martin, Louis Ternon,
Lucile Saulnier, Lélio Renard Lavaud, Margaret Jennings, Marie Pellat,
Marie Torelli, Marie-Anne Lachaux, Nicolas Schuhl, Patrick von Platen,
Pierre Stock, Sandeep Subramanian, Sophia Yang, Szymon Antoniak, Teven Le Scao,
Thibaut Lavril, Timothée Lacroix, Théophile Gervet, Thomas Wang,
Valera Nemychnikova, William El Sayed, William Marshall | [
"# Mixtral-8x22B-v0.1 - FP8\n\n- Model creator: Mistral AI\n- Original model: Mixtral-8x22B-v0.1",
"## Description\n\nThis repo contains the Mixtral-8x22B-v0.1 model quantized to FP8 by FriendliAI, significantly enhancing its inference efficiency while maintaining high accuracy.\nNote that FP8 is only supported by NVIDIA Ada, Hopper, and Blackwell GPU architectures.\nCheck out FriendliAI documentation for more details.",
"## Compatibility\n\nThis model is compatible with Friendli Container.",
"## Prerequisites\n\n- Before you begin, make sure you have signed up for Friendli Suite. You can use Friendli Containers free of charge for four weeks.\n- Prepare a Personal Access Token following this guide.\n- Prepare a Friendli Container Secret following this guide.",
"### Preparing Personal Access Token\n\nPAT (Personal Access Token) is the user credential for for logging into our container registry.\n\n1. Sign in Friendli Suite.\n2. Go to User Settings > Tokens and click 'Create new token'.\n3. Save your created token value.",
"### Pulling Friendli Container Image\n\n1. Log in to the Docker client using the personal access token created as outlined in this guide.\n\n \n\n2. Pull image",
"## Running Friendli Container\n\nOnce you've prepared the image of Friendli Container, you can launch it to create a serving endpoint.",
"### Optimizing Inference Performance with Policy Search\n\nTo serve MoE models efficiently, it is required to run a policy search to explore the optimal execution policy:\n\n\n\nWhen the optimal policy is successfully searched, the policy is compiled into a policy file and saved at '$POLICY_DIR'.\nNow you can create an inference endpoint with this optimal policy as follows:\n\n\n\n---",
"# Original model card: MistralAI's Mixtral-8x22B v0.1",
"# Model Card for Mixtral-8x22B\nThe Mixtral-8x22B Large Language Model (LLM) is a pretrained generative Sparse Mixture of Experts.\n\nFor full details of this model please read our release blog post.",
"## Warning\nThis repo contains weights that are compatible with vLLM serving of the model as well as Hugging Face transformers library. It is based on the original Mixtral torrent release, but the file format and parameter names are different.",
"## Run the model\n\n\n\n\nBy default, transformers will load the model in full precision. Therefore you might be interested to further reduce down the memory requirements to run the model through the optimizations we offer in HF ecosystem:",
"## Notice\nMixtral-8x22B is a pretrained base model and therefore does not have any moderation mechanisms.",
"# The Mistral AI Team\nAlbert Jiang, Alexandre Sablayrolles, Alexis Tacnet, Antoine Roux,\nArthur Mensch, Audrey Herblin-Stoop, Baptiste Bout, Baudouin de Monicault,\nBlanche Savary, Bam4d, Caroline Feldman, Devendra Singh Chaplot,\nDiego de las Casas, Eleonore Arcelin, Emma Bou Hanna, Etienne Metzger,\nGianna Lengyel, Guillaume Bour, Guillaume Lample, Harizo Rajaona,\nJean-Malo Delignon, Jia Li, Justus Murke, Louis Martin, Louis Ternon,\nLucile Saulnier, Lélio Renard Lavaud, Margaret Jennings, Marie Pellat,\nMarie Torelli, Marie-Anne Lachaux, Nicolas Schuhl, Patrick von Platen,\nPierre Stock, Sandeep Subramanian, Sophia Yang, Szymon Antoniak, Teven Le Scao,\nThibaut Lavril, Timothée Lacroix, Théophile Gervet, Thomas Wang,\nValera Nemychnikova, William El Sayed, William Marshall"
] | [
"TAGS\n#transformers #safetensors #mixtral #text-generation #pretrained #conversational #base_model-mistralai/Mixtral-8x22B-v0.1 #license-apache-2.0 #autotrain_compatible #text-generation-inference #8-bit #region-us \n",
"# Mixtral-8x22B-v0.1 - FP8\n\n- Model creator: Mistral AI\n- Original model: Mixtral-8x22B-v0.1",
"## Description\n\nThis repo contains the Mixtral-8x22B-v0.1 model quantized to FP8 by FriendliAI, significantly enhancing its inference efficiency while maintaining high accuracy.\nNote that FP8 is only supported by NVIDIA Ada, Hopper, and Blackwell GPU architectures.\nCheck out FriendliAI documentation for more details.",
"## Compatibility\n\nThis model is compatible with Friendli Container.",
"## Prerequisites\n\n- Before you begin, make sure you have signed up for Friendli Suite. You can use Friendli Containers free of charge for four weeks.\n- Prepare a Personal Access Token following this guide.\n- Prepare a Friendli Container Secret following this guide.",
"### Preparing Personal Access Token\n\nPAT (Personal Access Token) is the user credential for for logging into our container registry.\n\n1. Sign in Friendli Suite.\n2. Go to User Settings > Tokens and click 'Create new token'.\n3. Save your created token value.",
"### Pulling Friendli Container Image\n\n1. Log in to the Docker client using the personal access token created as outlined in this guide.\n\n \n\n2. Pull image",
"## Running Friendli Container\n\nOnce you've prepared the image of Friendli Container, you can launch it to create a serving endpoint.",
"### Optimizing Inference Performance with Policy Search\n\nTo serve MoE models efficiently, it is required to run a policy search to explore the optimal execution policy:\n\n\n\nWhen the optimal policy is successfully searched, the policy is compiled into a policy file and saved at '$POLICY_DIR'.\nNow you can create an inference endpoint with this optimal policy as follows:\n\n\n\n---",
"# Original model card: MistralAI's Mixtral-8x22B v0.1",
"# Model Card for Mixtral-8x22B\nThe Mixtral-8x22B Large Language Model (LLM) is a pretrained generative Sparse Mixture of Experts.\n\nFor full details of this model please read our release blog post.",
"## Warning\nThis repo contains weights that are compatible with vLLM serving of the model as well as Hugging Face transformers library. It is based on the original Mixtral torrent release, but the file format and parameter names are different.",
"## Run the model\n\n\n\n\nBy default, transformers will load the model in full precision. Therefore you might be interested to further reduce down the memory requirements to run the model through the optimizations we offer in HF ecosystem:",
"## Notice\nMixtral-8x22B is a pretrained base model and therefore does not have any moderation mechanisms.",
"# The Mistral AI Team\nAlbert Jiang, Alexandre Sablayrolles, Alexis Tacnet, Antoine Roux,\nArthur Mensch, Audrey Herblin-Stoop, Baptiste Bout, Baudouin de Monicault,\nBlanche Savary, Bam4d, Caroline Feldman, Devendra Singh Chaplot,\nDiego de las Casas, Eleonore Arcelin, Emma Bou Hanna, Etienne Metzger,\nGianna Lengyel, Guillaume Bour, Guillaume Lample, Harizo Rajaona,\nJean-Malo Delignon, Jia Li, Justus Murke, Louis Martin, Louis Ternon,\nLucile Saulnier, Lélio Renard Lavaud, Margaret Jennings, Marie Pellat,\nMarie Torelli, Marie-Anne Lachaux, Nicolas Schuhl, Patrick von Platen,\nPierre Stock, Sandeep Subramanian, Sophia Yang, Szymon Antoniak, Teven Le Scao,\nThibaut Lavril, Timothée Lacroix, Théophile Gervet, Thomas Wang,\nValera Nemychnikova, William El Sayed, William Marshall"
] |
audio-classification | transformers |
<!-- This model card has been generated automatically according to the information the Trainer had access to. You
should probably proofread and complete it, then remove this comment. -->
# wav2vec2-base-finetuned-gtzan
This model is a fine-tuned version of [facebook/wav2vec2-base](https://huggingface.co/facebook/wav2vec2-base) on the GTZAN dataset.
It achieves the following results on the evaluation set:
- Loss: 1.1430
- Accuracy: 0.76
## Model description
More information needed
## Intended uses & limitations
More information needed
## Training and evaluation data
More information needed
## Training procedure
### Training hyperparameters
The following hyperparameters were used during training:
- learning_rate: 3e-05
- train_batch_size: 8
- eval_batch_size: 8
- seed: 42
- gradient_accumulation_steps: 4
- total_train_batch_size: 32
- optimizer: Adam with betas=(0.9,0.999) and epsilon=1e-08
- lr_scheduler_type: linear
- lr_scheduler_warmup_ratio: 0.1
- num_epochs: 10
### Training results
| Training Loss | Epoch | Step | Validation Loss | Accuracy |
|:-------------:|:-----:|:----:|:---------------:|:--------:|
| 2.2574 | 1.0 | 25 | 2.1793 | 0.445 |
| 1.9361 | 2.0 | 50 | 1.8937 | 0.475 |
| 1.7211 | 3.0 | 75 | 1.7034 | 0.54 |
| 1.5003 | 4.0 | 100 | 1.5038 | 0.63 |
| 1.3653 | 5.0 | 125 | 1.3770 | 0.7 |
| 1.2614 | 6.0 | 150 | 1.3169 | 0.69 |
| 1.1654 | 7.0 | 175 | 1.2444 | 0.725 |
| 1.0837 | 8.0 | 200 | 1.1828 | 0.755 |
| 1.0409 | 9.0 | 225 | 1.1549 | 0.755 |
| 1.0147 | 10.0 | 250 | 1.1430 | 0.76 |
### Framework versions
- Transformers 4.38.2
- Pytorch 2.2.1+cu121
- Datasets 2.18.0
- Tokenizers 0.15.2
| {"license": "apache-2.0", "tags": ["generated_from_trainer"], "datasets": ["marsyas/gtzan"], "metrics": ["accuracy"], "base_model": "facebook/wav2vec2-base", "model-index": [{"name": "wav2vec2-base-finetuned-gtzan", "results": [{"task": {"type": "audio-classification", "name": "Audio Classification"}, "dataset": {"name": "GTZAN", "type": "marsyas/gtzan"}, "metrics": [{"type": "accuracy", "value": 0.76, "name": "Accuracy"}]}]}]} | saketag73/classification_facebook_wav2vec2-base-finetuned-gtzan-1 | null | [
"transformers",
"tensorboard",
"safetensors",
"wav2vec2",
"audio-classification",
"generated_from_trainer",
"dataset:marsyas/gtzan",
"base_model:facebook/wav2vec2-base",
"license:apache-2.0",
"model-index",
"endpoints_compatible",
"region:us"
] | null | 2024-04-18T09:56:53+00:00 | [] | [] | TAGS
#transformers #tensorboard #safetensors #wav2vec2 #audio-classification #generated_from_trainer #dataset-marsyas/gtzan #base_model-facebook/wav2vec2-base #license-apache-2.0 #model-index #endpoints_compatible #region-us
| wav2vec2-base-finetuned-gtzan
=============================
This model is a fine-tuned version of facebook/wav2vec2-base on the GTZAN dataset.
It achieves the following results on the evaluation set:
* Loss: 1.1430
* Accuracy: 0.76
Model description
-----------------
More information needed
Intended uses & limitations
---------------------------
More information needed
Training and evaluation data
----------------------------
More information needed
Training procedure
------------------
### Training hyperparameters
The following hyperparameters were used during training:
* learning\_rate: 3e-05
* train\_batch\_size: 8
* eval\_batch\_size: 8
* seed: 42
* gradient\_accumulation\_steps: 4
* total\_train\_batch\_size: 32
* optimizer: Adam with betas=(0.9,0.999) and epsilon=1e-08
* lr\_scheduler\_type: linear
* lr\_scheduler\_warmup\_ratio: 0.1
* num\_epochs: 10
### Training results
### Framework versions
* Transformers 4.38.2
* Pytorch 2.2.1+cu121
* Datasets 2.18.0
* Tokenizers 0.15.2
| [
"### Training hyperparameters\n\n\nThe following hyperparameters were used during training:\n\n\n* learning\\_rate: 3e-05\n* train\\_batch\\_size: 8\n* eval\\_batch\\_size: 8\n* seed: 42\n* gradient\\_accumulation\\_steps: 4\n* total\\_train\\_batch\\_size: 32\n* optimizer: Adam with betas=(0.9,0.999) and epsilon=1e-08\n* lr\\_scheduler\\_type: linear\n* lr\\_scheduler\\_warmup\\_ratio: 0.1\n* num\\_epochs: 10",
"### Training results",
"### Framework versions\n\n\n* Transformers 4.38.2\n* Pytorch 2.2.1+cu121\n* Datasets 2.18.0\n* Tokenizers 0.15.2"
] | [
"TAGS\n#transformers #tensorboard #safetensors #wav2vec2 #audio-classification #generated_from_trainer #dataset-marsyas/gtzan #base_model-facebook/wav2vec2-base #license-apache-2.0 #model-index #endpoints_compatible #region-us \n",
"### Training hyperparameters\n\n\nThe following hyperparameters were used during training:\n\n\n* learning\\_rate: 3e-05\n* train\\_batch\\_size: 8\n* eval\\_batch\\_size: 8\n* seed: 42\n* gradient\\_accumulation\\_steps: 4\n* total\\_train\\_batch\\_size: 32\n* optimizer: Adam with betas=(0.9,0.999) and epsilon=1e-08\n* lr\\_scheduler\\_type: linear\n* lr\\_scheduler\\_warmup\\_ratio: 0.1\n* num\\_epochs: 10",
"### Training results",
"### Framework versions\n\n\n* Transformers 4.38.2\n* Pytorch 2.2.1+cu121\n* Datasets 2.18.0\n* Tokenizers 0.15.2"
] |
text-classification | transformers |
<!-- This model card has been generated automatically according to the information the Trainer had access to. You
should probably proofread and complete it, then remove this comment. -->
# CodeBertForDefect-Detection
This model is a fine-tuned version of [microsoft/codebert-base](https://huggingface.co/microsoft/codebert-base) on an unknown dataset.
It achieves the following results on the evaluation set:
- Loss: 0.9039
- Accuracy: 0.6435
## Model description
More information needed
## Intended uses & limitations
More information needed
## Training and evaluation data
More information needed
## Training procedure
### Training hyperparameters
The following hyperparameters were used during training:
- learning_rate: 5e-05
- train_batch_size: 16
- eval_batch_size: 32
- seed: 42
- optimizer: Adam with betas=(0.9,0.999) and epsilon=1e-08
- lr_scheduler_type: linear
- lr_scheduler_warmup_steps: 13112.4
- num_epochs: 10
- mixed_precision_training: Native AMP
### Training results
| Training Loss | Epoch | Step | Validation Loss | Accuracy |
|:-------------:|:-----:|:-----:|:---------------:|:--------:|
| 0.6483 | 1.0 | 1366 | 0.6494 | 0.5637 |
| 0.6213 | 2.0 | 2732 | 0.5968 | 0.6380 |
| 0.5927 | 3.0 | 4098 | 0.5767 | 0.6457 |
| 0.5615 | 4.0 | 5464 | 0.5855 | 0.6669 |
| 0.5271 | 5.0 | 6830 | 0.6677 | 0.6643 |
| 0.4488 | 6.0 | 8196 | 0.7177 | 0.6237 |
| 0.4576 | 7.0 | 9562 | 0.6643 | 0.6398 |
| 0.45 | 8.0 | 10928 | 0.7414 | 0.6479 |
| 0.4156 | 9.0 | 12294 | 0.9852 | 0.6519 |
| 0.3362 | 10.0 | 13660 | 0.9039 | 0.6435 |
### Framework versions
- Transformers 4.37.2
- Pytorch 2.1.2+cu121
- Datasets 2.15.0
- Tokenizers 0.15.0
| {"tags": ["generated_from_trainer"], "metrics": ["accuracy"], "base_model": "microsoft/codebert-base", "model-index": [{"name": "CodeBertForDefect-Detection", "results": []}]} | ljcnju/CodeBertForDefect-Detection | null | [
"transformers",
"safetensors",
"roberta",
"text-classification",
"generated_from_trainer",
"base_model:microsoft/codebert-base",
"autotrain_compatible",
"endpoints_compatible",
"region:us"
] | null | 2024-04-18T09:57:51+00:00 | [] | [] | TAGS
#transformers #safetensors #roberta #text-classification #generated_from_trainer #base_model-microsoft/codebert-base #autotrain_compatible #endpoints_compatible #region-us
| CodeBertForDefect-Detection
===========================
This model is a fine-tuned version of microsoft/codebert-base on an unknown dataset.
It achieves the following results on the evaluation set:
* Loss: 0.9039
* Accuracy: 0.6435
Model description
-----------------
More information needed
Intended uses & limitations
---------------------------
More information needed
Training and evaluation data
----------------------------
More information needed
Training procedure
------------------
### Training hyperparameters
The following hyperparameters were used during training:
* learning\_rate: 5e-05
* train\_batch\_size: 16
* eval\_batch\_size: 32
* seed: 42
* optimizer: Adam with betas=(0.9,0.999) and epsilon=1e-08
* lr\_scheduler\_type: linear
* lr\_scheduler\_warmup\_steps: 13112.4
* num\_epochs: 10
* mixed\_precision\_training: Native AMP
### Training results
### Framework versions
* Transformers 4.37.2
* Pytorch 2.1.2+cu121
* Datasets 2.15.0
* Tokenizers 0.15.0
| [
"### Training hyperparameters\n\n\nThe following hyperparameters were used during training:\n\n\n* learning\\_rate: 5e-05\n* train\\_batch\\_size: 16\n* eval\\_batch\\_size: 32\n* seed: 42\n* optimizer: Adam with betas=(0.9,0.999) and epsilon=1e-08\n* lr\\_scheduler\\_type: linear\n* lr\\_scheduler\\_warmup\\_steps: 13112.4\n* num\\_epochs: 10\n* mixed\\_precision\\_training: Native AMP",
"### Training results",
"### Framework versions\n\n\n* Transformers 4.37.2\n* Pytorch 2.1.2+cu121\n* Datasets 2.15.0\n* Tokenizers 0.15.0"
] | [
"TAGS\n#transformers #safetensors #roberta #text-classification #generated_from_trainer #base_model-microsoft/codebert-base #autotrain_compatible #endpoints_compatible #region-us \n",
"### Training hyperparameters\n\n\nThe following hyperparameters were used during training:\n\n\n* learning\\_rate: 5e-05\n* train\\_batch\\_size: 16\n* eval\\_batch\\_size: 32\n* seed: 42\n* optimizer: Adam with betas=(0.9,0.999) and epsilon=1e-08\n* lr\\_scheduler\\_type: linear\n* lr\\_scheduler\\_warmup\\_steps: 13112.4\n* num\\_epochs: 10\n* mixed\\_precision\\_training: Native AMP",
"### Training results",
"### Framework versions\n\n\n* Transformers 4.37.2\n* Pytorch 2.1.2+cu121\n* Datasets 2.15.0\n* Tokenizers 0.15.0"
] |
null | null | Insugr capsule क्या है?
Insugr Tablets एक विशेष रूप से तैयार किया गया आहार अनुपूरक है जिसे मधुमेह से पीड़ित व्यक्तियों को उनके रक्त शर्करा के स्तर को प्रभावी ढंग से प्रबंधित करने में सहायता करने के लिए डिज़ाइन किया गया है। इसमें प्राकृतिक अवयवों का एक अनूठा मिश्रण होता है, प्रत्येक को ग्लूकोज चयापचय को विनियमित करने और इंसुलिन संवेदनशीलता में सुधार करने की उनकी क्षमता के लिए सावधानीपूर्वक चुना जाता है।
आधिकारिक वेबसाइट:<a href="https://www.nutritionsee.com/Insugsingd">www.Insugr.com</a>
<p><a href="https://www.nutritionsee.com/Insugsingd"> <img src="https://www.nutritionsee.com/wp-content/uploads/2024/04/Insugr-India.png" alt="enter image description here"> </a></p>
<a href="https://www.nutritionsee.com/Insugsingd">अभी खरीदें!! अधिक जानकारी के लिए नीचे दिए गए लिंक पर क्लिक करें और अभी 50% छूट पाएं... जल्दी करें
</a>
आधिकारिक वेबसाइट:<a href="https://www.nutritionsee.com/Insugsingd">www.Insugr.com</a> | {"license": "apache-2.0"} | InsugrIndia/InsugrIndia | null | [
"license:apache-2.0",
"region:us"
] | null | 2024-04-18T09:58:06+00:00 | [] | [] | TAGS
#license-apache-2.0 #region-us
| Insugr capsule क्या है?
Insugr Tablets एक विशेष रूप से तैयार किया गया आहार अनुपूरक है जिसे मधुमेह से पीड़ित व्यक्तियों को उनके रक्त शर्करा के स्तर को प्रभावी ढंग से प्रबंधित करने में सहायता करने के लिए डिज़ाइन किया गया है। इसमें प्राकृतिक अवयवों का एक अनूठा मिश्रण होता है, प्रत्येक को ग्लूकोज चयापचय को विनियमित करने और इंसुलिन संवेदनशीलता में सुधार करने की उनकी क्षमता के लिए सावधानीपूर्वक चुना जाता है।
आधिकारिक वेबसाइट:<a href="URL
<p><a href="URL <img src="URL alt="enter image description here"> </a></p>
<a href="URL>अभी खरीदें!! अधिक जानकारी के लिए नीचे दिए गए लिंक पर क्लिक करें और अभी 50% छूट पाएं... जल्दी करें
</a>
आधिकारिक वेबसाइट:<a href="URL | [] | [
"TAGS\n#license-apache-2.0 #region-us \n"
] |
text-classification | transformers |
# Model Card for Model ID
<!-- Provide a quick summary of what the model is/does. -->
## Model Details
### Model Description
<!-- Provide a longer summary of what this model is. -->
This is the model card of a 🤗 transformers model that has been pushed on the Hub. This model card has been automatically generated.
- **Developed by:** [More Information Needed]
- **Funded by [optional]:** [More Information Needed]
- **Shared by [optional]:** [More Information Needed]
- **Model type:** [More Information Needed]
- **Language(s) (NLP):** [More Information Needed]
- **License:** [More Information Needed]
- **Finetuned from model [optional]:** [More Information Needed]
### Model Sources [optional]
<!-- Provide the basic links for the model. -->
- **Repository:** [More Information Needed]
- **Paper [optional]:** [More Information Needed]
- **Demo [optional]:** [More Information Needed]
## Uses
<!-- Address questions around how the model is intended to be used, including the foreseeable users of the model and those affected by the model. -->
### Direct Use
<!-- This section is for the model use without fine-tuning or plugging into a larger ecosystem/app. -->
[More Information Needed]
### Downstream Use [optional]
<!-- This section is for the model use when fine-tuned for a task, or when plugged into a larger ecosystem/app -->
[More Information Needed]
### Out-of-Scope Use
<!-- This section addresses misuse, malicious use, and uses that the model will not work well for. -->
[More Information Needed]
## Bias, Risks, and Limitations
<!-- This section is meant to convey both technical and sociotechnical limitations. -->
[More Information Needed]
### Recommendations
<!-- This section is meant to convey recommendations with respect to the bias, risk, and technical limitations. -->
Users (both direct and downstream) should be made aware of the risks, biases and limitations of the model. More information needed for further recommendations.
## How to Get Started with the Model
Use the code below to get started with the model.
[More Information Needed]
## Training Details
### Training Data
<!-- This should link to a Dataset Card, perhaps with a short stub of information on what the training data is all about as well as documentation related to data pre-processing or additional filtering. -->
[More Information Needed]
### Training Procedure
<!-- This relates heavily to the Technical Specifications. Content here should link to that section when it is relevant to the training procedure. -->
#### Preprocessing [optional]
[More Information Needed]
#### Training Hyperparameters
- **Training regime:** [More Information Needed] <!--fp32, fp16 mixed precision, bf16 mixed precision, bf16 non-mixed precision, fp16 non-mixed precision, fp8 mixed precision -->
#### Speeds, Sizes, Times [optional]
<!-- This section provides information about throughput, start/end time, checkpoint size if relevant, etc. -->
[More Information Needed]
## Evaluation
<!-- This section describes the evaluation protocols and provides the results. -->
### Testing Data, Factors & Metrics
#### Testing Data
<!-- This should link to a Dataset Card if possible. -->
[More Information Needed]
#### Factors
<!-- These are the things the evaluation is disaggregating by, e.g., subpopulations or domains. -->
[More Information Needed]
#### Metrics
<!-- These are the evaluation metrics being used, ideally with a description of why. -->
[More Information Needed]
### Results
[More Information Needed]
#### Summary
## Model Examination [optional]
<!-- Relevant interpretability work for the model goes here -->
[More Information Needed]
## Environmental Impact
<!-- Total emissions (in grams of CO2eq) and additional considerations, such as electricity usage, go here. Edit the suggested text below accordingly -->
Carbon emissions can be estimated using the [Machine Learning Impact calculator](https://mlco2.github.io/impact#compute) presented in [Lacoste et al. (2019)](https://arxiv.org/abs/1910.09700).
- **Hardware Type:** [More Information Needed]
- **Hours used:** [More Information Needed]
- **Cloud Provider:** [More Information Needed]
- **Compute Region:** [More Information Needed]
- **Carbon Emitted:** [More Information Needed]
## Technical Specifications [optional]
### Model Architecture and Objective
[More Information Needed]
### Compute Infrastructure
[More Information Needed]
#### Hardware
[More Information Needed]
#### Software
[More Information Needed]
## Citation [optional]
<!-- If there is a paper or blog post introducing the model, the APA and Bibtex information for that should go in this section. -->
**BibTeX:**
[More Information Needed]
**APA:**
[More Information Needed]
## Glossary [optional]
<!-- If relevant, include terms and calculations in this section that can help readers understand the model or model card. -->
[More Information Needed]
## More Information [optional]
[More Information Needed]
## Model Card Authors [optional]
[More Information Needed]
## Model Card Contact
[More Information Needed] | {"library_name": "transformers", "tags": []} | ahmedheakl/bert-resume-classification | null | [
"transformers",
"safetensors",
"bert",
"text-classification",
"arxiv:1910.09700",
"autotrain_compatible",
"endpoints_compatible",
"region:us"
] | null | 2024-04-18T09:58:58+00:00 | [
"1910.09700"
] | [] | TAGS
#transformers #safetensors #bert #text-classification #arxiv-1910.09700 #autotrain_compatible #endpoints_compatible #region-us
|
# Model Card for Model ID
## Model Details
### Model Description
This is the model card of a transformers model that has been pushed on the Hub. This model card has been automatically generated.
- Developed by:
- Funded by [optional]:
- Shared by [optional]:
- Model type:
- Language(s) (NLP):
- License:
- Finetuned from model [optional]:
### Model Sources [optional]
- Repository:
- Paper [optional]:
- Demo [optional]:
## Uses
### Direct Use
### Downstream Use [optional]
### Out-of-Scope Use
## Bias, Risks, and Limitations
### Recommendations
Users (both direct and downstream) should be made aware of the risks, biases and limitations of the model. More information needed for further recommendations.
## How to Get Started with the Model
Use the code below to get started with the model.
## Training Details
### Training Data
### Training Procedure
#### Preprocessing [optional]
#### Training Hyperparameters
- Training regime:
#### Speeds, Sizes, Times [optional]
## Evaluation
### Testing Data, Factors & Metrics
#### Testing Data
#### Factors
#### Metrics
### Results
#### Summary
## Model Examination [optional]
## Environmental Impact
Carbon emissions can be estimated using the Machine Learning Impact calculator presented in Lacoste et al. (2019).
- Hardware Type:
- Hours used:
- Cloud Provider:
- Compute Region:
- Carbon Emitted:
## Technical Specifications [optional]
### Model Architecture and Objective
### Compute Infrastructure
#### Hardware
#### Software
[optional]
BibTeX:
APA:
## Glossary [optional]
## More Information [optional]
## Model Card Authors [optional]
## Model Card Contact
| [
"# Model Card for Model ID",
"## Model Details",
"### Model Description\n\n\n\nThis is the model card of a transformers model that has been pushed on the Hub. This model card has been automatically generated.\n\n- Developed by: \n- Funded by [optional]: \n- Shared by [optional]: \n- Model type: \n- Language(s) (NLP): \n- License: \n- Finetuned from model [optional]:",
"### Model Sources [optional]\n\n\n\n- Repository: \n- Paper [optional]: \n- Demo [optional]:",
"## Uses",
"### Direct Use",
"### Downstream Use [optional]",
"### Out-of-Scope Use",
"## Bias, Risks, and Limitations",
"### Recommendations\n\n\n\nUsers (both direct and downstream) should be made aware of the risks, biases and limitations of the model. More information needed for further recommendations.",
"## How to Get Started with the Model\n\nUse the code below to get started with the model.",
"## Training Details",
"### Training Data",
"### Training Procedure",
"#### Preprocessing [optional]",
"#### Training Hyperparameters\n\n- Training regime:",
"#### Speeds, Sizes, Times [optional]",
"## Evaluation",
"### Testing Data, Factors & Metrics",
"#### Testing Data",
"#### Factors",
"#### Metrics",
"### Results",
"#### Summary",
"## Model Examination [optional]",
"## Environmental Impact\n\n\n\nCarbon emissions can be estimated using the Machine Learning Impact calculator presented in Lacoste et al. (2019).\n\n- Hardware Type: \n- Hours used: \n- Cloud Provider: \n- Compute Region: \n- Carbon Emitted:",
"## Technical Specifications [optional]",
"### Model Architecture and Objective",
"### Compute Infrastructure",
"#### Hardware",
"#### Software\n\n\n\n[optional]\n\n\n\nBibTeX:\n\n\n\nAPA:",
"## Glossary [optional]",
"## More Information [optional]",
"## Model Card Authors [optional]",
"## Model Card Contact"
] | [
"TAGS\n#transformers #safetensors #bert #text-classification #arxiv-1910.09700 #autotrain_compatible #endpoints_compatible #region-us \n",
"# Model Card for Model ID",
"## Model Details",
"### Model Description\n\n\n\nThis is the model card of a transformers model that has been pushed on the Hub. This model card has been automatically generated.\n\n- Developed by: \n- Funded by [optional]: \n- Shared by [optional]: \n- Model type: \n- Language(s) (NLP): \n- License: \n- Finetuned from model [optional]:",
"### Model Sources [optional]\n\n\n\n- Repository: \n- Paper [optional]: \n- Demo [optional]:",
"## Uses",
"### Direct Use",
"### Downstream Use [optional]",
"### Out-of-Scope Use",
"## Bias, Risks, and Limitations",
"### Recommendations\n\n\n\nUsers (both direct and downstream) should be made aware of the risks, biases and limitations of the model. More information needed for further recommendations.",
"## How to Get Started with the Model\n\nUse the code below to get started with the model.",
"## Training Details",
"### Training Data",
"### Training Procedure",
"#### Preprocessing [optional]",
"#### Training Hyperparameters\n\n- Training regime:",
"#### Speeds, Sizes, Times [optional]",
"## Evaluation",
"### Testing Data, Factors & Metrics",
"#### Testing Data",
"#### Factors",
"#### Metrics",
"### Results",
"#### Summary",
"## Model Examination [optional]",
"## Environmental Impact\n\n\n\nCarbon emissions can be estimated using the Machine Learning Impact calculator presented in Lacoste et al. (2019).\n\n- Hardware Type: \n- Hours used: \n- Cloud Provider: \n- Compute Region: \n- Carbon Emitted:",
"## Technical Specifications [optional]",
"### Model Architecture and Objective",
"### Compute Infrastructure",
"#### Hardware",
"#### Software\n\n\n\n[optional]\n\n\n\nBibTeX:\n\n\n\nAPA:",
"## Glossary [optional]",
"## More Information [optional]",
"## Model Card Authors [optional]",
"## Model Card Contact"
] |
sentence-similarity | sentence-transformers |
# all-MiniLM-L6-v2
This is a [sentence-transformers](https://www.SBERT.net) model: It maps sentences & paragraphs to a 384 dimensional dense vector space and can be used for tasks like clustering or semantic search.
## Usage (Sentence-Transformers)
Using this model becomes easy when you have [sentence-transformers](https://www.SBERT.net) installed:
```
pip install -U sentence-transformers
```
Then you can use the model like this:
```python
from sentence_transformers import SentenceTransformer
sentences = ["This is an example sentence", "Each sentence is converted"]
model = SentenceTransformer('sentence-transformers/all-MiniLM-L6-v2')
embeddings = model.encode(sentences)
print(embeddings)
```
## Usage (HuggingFace Transformers)
Without [sentence-transformers](https://www.SBERT.net), you can use the model like this: First, you pass your input through the transformer model, then you have to apply the right pooling-operation on-top of the contextualized word embeddings.
```python
from transformers import AutoTokenizer, AutoModel
import torch
import torch.nn.functional as F
#Mean Pooling - Take attention mask into account for correct averaging
def mean_pooling(model_output, attention_mask):
token_embeddings = model_output[0] #First element of model_output contains all token embeddings
input_mask_expanded = attention_mask.unsqueeze(-1).expand(token_embeddings.size()).float()
return torch.sum(token_embeddings * input_mask_expanded, 1) / torch.clamp(input_mask_expanded.sum(1), min=1e-9)
# Sentences we want sentence embeddings for
sentences = ['This is an example sentence', 'Each sentence is converted']
# Load model from HuggingFace Hub
tokenizer = AutoTokenizer.from_pretrained('sentence-transformers/all-MiniLM-L6-v2')
model = AutoModel.from_pretrained('sentence-transformers/all-MiniLM-L6-v2')
# Tokenize sentences
encoded_input = tokenizer(sentences, padding=True, truncation=True, return_tensors='pt')
# Compute token embeddings
with torch.no_grad():
model_output = model(**encoded_input)
# Perform pooling
sentence_embeddings = mean_pooling(model_output, encoded_input['attention_mask'])
# Normalize embeddings
sentence_embeddings = F.normalize(sentence_embeddings, p=2, dim=1)
print("Sentence embeddings:")
print(sentence_embeddings)
```
## Evaluation Results
For an automated evaluation of this model, see the *Sentence Embeddings Benchmark*: [https://seb.sbert.net](https://seb.sbert.net?model_name=sentence-transformers/all-MiniLM-L6-v2)
------
## Background
The project aims to train sentence embedding models on very large sentence level datasets using a self-supervised
contrastive learning objective. We used the pretrained [`nreimers/MiniLM-L6-H384-uncased`](https://huggingface.co/nreimers/MiniLM-L6-H384-uncased) model and fine-tuned in on a
1B sentence pairs dataset. We use a contrastive learning objective: given a sentence from the pair, the model should predict which out of a set of randomly sampled other sentences, was actually paired with it in our dataset.
We developed this model during the
[Community week using JAX/Flax for NLP & CV](https://discuss.huggingface.co/t/open-to-the-community-community-week-using-jax-flax-for-nlp-cv/7104),
organized by Hugging Face. We developed this model as part of the project:
[Train the Best Sentence Embedding Model Ever with 1B Training Pairs](https://discuss.huggingface.co/t/train-the-best-sentence-embedding-model-ever-with-1b-training-pairs/7354). We benefited from efficient hardware infrastructure to run the project: 7 TPUs v3-8, as well as intervention from Googles Flax, JAX, and Cloud team member about efficient deep learning frameworks.
## Intended uses
Our model is intended to be used as a sentence and short paragraph encoder. Given an input text, it outputs a vector which captures
the semantic information. The sentence vector may be used for information retrieval, clustering or sentence similarity tasks.
By default, input text longer than 256 word pieces is truncated.
## Training procedure
### Pre-training
We use the pretrained [`nreimers/MiniLM-L6-H384-uncased`](https://huggingface.co/nreimers/MiniLM-L6-H384-uncased) model. Please refer to the model card for more detailed information about the pre-training procedure.
### Fine-tuning
We fine-tune the model using a contrastive objective. Formally, we compute the cosine similarity from each possible sentence pairs from the batch.
We then apply the cross entropy loss by comparing with true pairs.
#### Hyper parameters
We trained our model on a TPU v3-8. We train the model during 100k steps using a batch size of 1024 (128 per TPU core).
We use a learning rate warm up of 500. The sequence length was limited to 128 tokens. We used the AdamW optimizer with
a 2e-5 learning rate. The full training script is accessible in this current repository: `train_script.py`.
#### Training data
We use the concatenation from multiple datasets to fine-tune our model. The total number of sentence pairs is above 1 billion sentences.
We sampled each dataset given a weighted probability which configuration is detailed in the `data_config.json` file.
| Dataset | Paper | Number of training tuples |
|--------------------------------------------------------|:----------------------------------------:|:--------------------------:|
| [Reddit comments (2015-2018)](https://github.com/PolyAI-LDN/conversational-datasets/tree/master/reddit) | [paper](https://arxiv.org/abs/1904.06472) | 726,484,430 |
| [S2ORC](https://github.com/allenai/s2orc) Citation pairs (Abstracts) | [paper](https://aclanthology.org/2020.acl-main.447/) | 116,288,806 |
| [WikiAnswers](https://github.com/afader/oqa#wikianswers-corpus) Duplicate question pairs | [paper](https://doi.org/10.1145/2623330.2623677) | 77,427,422 |
| [PAQ](https://github.com/facebookresearch/PAQ) (Question, Answer) pairs | [paper](https://arxiv.org/abs/2102.07033) | 64,371,441 |
| [S2ORC](https://github.com/allenai/s2orc) Citation pairs (Titles) | [paper](https://aclanthology.org/2020.acl-main.447/) | 52,603,982 |
| [S2ORC](https://github.com/allenai/s2orc) (Title, Abstract) | [paper](https://aclanthology.org/2020.acl-main.447/) | 41,769,185 |
| [Stack Exchange](https://huggingface.co/datasets/flax-sentence-embeddings/stackexchange_xml) (Title, Body) pairs | - | 25,316,456 |
| [Stack Exchange](https://huggingface.co/datasets/flax-sentence-embeddings/stackexchange_xml) (Title+Body, Answer) pairs | - | 21,396,559 |
| [Stack Exchange](https://huggingface.co/datasets/flax-sentence-embeddings/stackexchange_xml) (Title, Answer) pairs | - | 21,396,559 |
| [MS MARCO](https://microsoft.github.io/msmarco/) triplets | [paper](https://doi.org/10.1145/3404835.3462804) | 9,144,553 |
| [GOOAQ: Open Question Answering with Diverse Answer Types](https://github.com/allenai/gooaq) | [paper](https://arxiv.org/pdf/2104.08727.pdf) | 3,012,496 |
| [Yahoo Answers](https://www.kaggle.com/soumikrakshit/yahoo-answers-dataset) (Title, Answer) | [paper](https://proceedings.neurips.cc/paper/2015/hash/250cf8b51c773f3f8dc8b4be867a9a02-Abstract.html) | 1,198,260 |
| [Code Search](https://huggingface.co/datasets/code_search_net) | - | 1,151,414 |
| [COCO](https://cocodataset.org/#home) Image captions | [paper](https://link.springer.com/chapter/10.1007%2F978-3-319-10602-1_48) | 828,395|
| [SPECTER](https://github.com/allenai/specter) citation triplets | [paper](https://doi.org/10.18653/v1/2020.acl-main.207) | 684,100 |
| [Yahoo Answers](https://www.kaggle.com/soumikrakshit/yahoo-answers-dataset) (Question, Answer) | [paper](https://proceedings.neurips.cc/paper/2015/hash/250cf8b51c773f3f8dc8b4be867a9a02-Abstract.html) | 681,164 |
| [Yahoo Answers](https://www.kaggle.com/soumikrakshit/yahoo-answers-dataset) (Title, Question) | [paper](https://proceedings.neurips.cc/paper/2015/hash/250cf8b51c773f3f8dc8b4be867a9a02-Abstract.html) | 659,896 |
| [SearchQA](https://huggingface.co/datasets/search_qa) | [paper](https://arxiv.org/abs/1704.05179) | 582,261 |
| [Eli5](https://huggingface.co/datasets/eli5) | [paper](https://doi.org/10.18653/v1/p19-1346) | 325,475 |
| [Flickr 30k](https://shannon.cs.illinois.edu/DenotationGraph/) | [paper](https://transacl.org/ojs/index.php/tacl/article/view/229/33) | 317,695 |
| [Stack Exchange](https://huggingface.co/datasets/flax-sentence-embeddings/stackexchange_xml) Duplicate questions (titles) | | 304,525 |
| AllNLI ([SNLI](https://nlp.stanford.edu/projects/snli/) and [MultiNLI](https://cims.nyu.edu/~sbowman/multinli/) | [paper SNLI](https://doi.org/10.18653/v1/d15-1075), [paper MultiNLI](https://doi.org/10.18653/v1/n18-1101) | 277,230 |
| [Stack Exchange](https://huggingface.co/datasets/flax-sentence-embeddings/stackexchange_xml) Duplicate questions (bodies) | | 250,519 |
| [Stack Exchange](https://huggingface.co/datasets/flax-sentence-embeddings/stackexchange_xml) Duplicate questions (titles+bodies) | | 250,460 |
| [Sentence Compression](https://github.com/google-research-datasets/sentence-compression) | [paper](https://www.aclweb.org/anthology/D13-1155/) | 180,000 |
| [Wikihow](https://github.com/pvl/wikihow_pairs_dataset) | [paper](https://arxiv.org/abs/1810.09305) | 128,542 |
| [Altlex](https://github.com/chridey/altlex/) | [paper](https://aclanthology.org/P16-1135.pdf) | 112,696 |
| [Quora Question Triplets](https://quoradata.quora.com/First-Quora-Dataset-Release-Question-Pairs) | - | 103,663 |
| [Simple Wikipedia](https://cs.pomona.edu/~dkauchak/simplification/) | [paper](https://www.aclweb.org/anthology/P11-2117/) | 102,225 |
| [Natural Questions (NQ)](https://ai.google.com/research/NaturalQuestions) | [paper](https://transacl.org/ojs/index.php/tacl/article/view/1455) | 100,231 |
| [SQuAD2.0](https://rajpurkar.github.io/SQuAD-explorer/) | [paper](https://aclanthology.org/P18-2124.pdf) | 87,599 |
| [TriviaQA](https://huggingface.co/datasets/trivia_qa) | - | 73,346 |
| **Total** | | **1,170,060,424** | | {"language": "en", "license": "apache-2.0", "library_name": "sentence-transformers", "tags": ["sentence-transformers", "feature-extraction", "sentence-similarity", "transformers"], "datasets": ["s2orc", "flax-sentence-embeddings/stackexchange_xml", "ms_marco", "gooaq", "yahoo_answers_topics", "code_search_net", "search_qa", "eli5", "snli", "multi_nli", "wikihow", "natural_questions", "trivia_qa", "embedding-data/sentence-compression", "embedding-data/flickr30k-captions", "embedding-data/altlex", "embedding-data/simple-wiki", "embedding-data/QQP", "embedding-data/SPECTER", "embedding-data/PAQ_pairs", "embedding-data/WikiAnswers"], "pipeline_tag": "sentence-similarity"} | 1998Shubham007/ModelRecomm | null | [
"sentence-transformers",
"safetensors",
"bert",
"feature-extraction",
"sentence-similarity",
"transformers",
"en",
"dataset:s2orc",
"dataset:flax-sentence-embeddings/stackexchange_xml",
"dataset:ms_marco",
"dataset:gooaq",
"dataset:yahoo_answers_topics",
"dataset:code_search_net",
"dataset:search_qa",
"dataset:eli5",
"dataset:snli",
"dataset:multi_nli",
"dataset:wikihow",
"dataset:natural_questions",
"dataset:trivia_qa",
"dataset:embedding-data/sentence-compression",
"dataset:embedding-data/flickr30k-captions",
"dataset:embedding-data/altlex",
"dataset:embedding-data/simple-wiki",
"dataset:embedding-data/QQP",
"dataset:embedding-data/SPECTER",
"dataset:embedding-data/PAQ_pairs",
"dataset:embedding-data/WikiAnswers",
"arxiv:1904.06472",
"arxiv:2102.07033",
"arxiv:2104.08727",
"arxiv:1704.05179",
"arxiv:1810.09305",
"license:apache-2.0",
"endpoints_compatible",
"region:us"
] | null | 2024-04-18T10:00:43+00:00 | [
"1904.06472",
"2102.07033",
"2104.08727",
"1704.05179",
"1810.09305"
] | [
"en"
] | TAGS
#sentence-transformers #safetensors #bert #feature-extraction #sentence-similarity #transformers #en #dataset-s2orc #dataset-flax-sentence-embeddings/stackexchange_xml #dataset-ms_marco #dataset-gooaq #dataset-yahoo_answers_topics #dataset-code_search_net #dataset-search_qa #dataset-eli5 #dataset-snli #dataset-multi_nli #dataset-wikihow #dataset-natural_questions #dataset-trivia_qa #dataset-embedding-data/sentence-compression #dataset-embedding-data/flickr30k-captions #dataset-embedding-data/altlex #dataset-embedding-data/simple-wiki #dataset-embedding-data/QQP #dataset-embedding-data/SPECTER #dataset-embedding-data/PAQ_pairs #dataset-embedding-data/WikiAnswers #arxiv-1904.06472 #arxiv-2102.07033 #arxiv-2104.08727 #arxiv-1704.05179 #arxiv-1810.09305 #license-apache-2.0 #endpoints_compatible #region-us
| all-MiniLM-L6-v2
================
This is a sentence-transformers model: It maps sentences & paragraphs to a 384 dimensional dense vector space and can be used for tasks like clustering or semantic search.
Usage (Sentence-Transformers)
-----------------------------
Using this model becomes easy when you have sentence-transformers installed:
Then you can use the model like this:
Usage (HuggingFace Transformers)
--------------------------------
Without sentence-transformers, you can use the model like this: First, you pass your input through the transformer model, then you have to apply the right pooling-operation on-top of the contextualized word embeddings.
Evaluation Results
------------------
For an automated evaluation of this model, see the *Sentence Embeddings Benchmark*: URL
---
Background
----------
The project aims to train sentence embedding models on very large sentence level datasets using a self-supervised
contrastive learning objective. We used the pretrained 'nreimers/MiniLM-L6-H384-uncased' model and fine-tuned in on a
1B sentence pairs dataset. We use a contrastive learning objective: given a sentence from the pair, the model should predict which out of a set of randomly sampled other sentences, was actually paired with it in our dataset.
We developed this model during the
Community week using JAX/Flax for NLP & CV,
organized by Hugging Face. We developed this model as part of the project:
Train the Best Sentence Embedding Model Ever with 1B Training Pairs. We benefited from efficient hardware infrastructure to run the project: 7 TPUs v3-8, as well as intervention from Googles Flax, JAX, and Cloud team member about efficient deep learning frameworks.
Intended uses
-------------
Our model is intended to be used as a sentence and short paragraph encoder. Given an input text, it outputs a vector which captures
the semantic information. The sentence vector may be used for information retrieval, clustering or sentence similarity tasks.
By default, input text longer than 256 word pieces is truncated.
Training procedure
------------------
### Pre-training
We use the pretrained 'nreimers/MiniLM-L6-H384-uncased' model. Please refer to the model card for more detailed information about the pre-training procedure.
### Fine-tuning
We fine-tune the model using a contrastive objective. Formally, we compute the cosine similarity from each possible sentence pairs from the batch.
We then apply the cross entropy loss by comparing with true pairs.
#### Hyper parameters
We trained our model on a TPU v3-8. We train the model during 100k steps using a batch size of 1024 (128 per TPU core).
We use a learning rate warm up of 500. The sequence length was limited to 128 tokens. We used the AdamW optimizer with
a 2e-5 learning rate. The full training script is accessible in this current repository: 'train\_script.py'.
#### Training data
We use the concatenation from multiple datasets to fine-tune our model. The total number of sentence pairs is above 1 billion sentences.
We sampled each dataset given a weighted probability which configuration is detailed in the 'data\_config.json' file.
| [
"### Pre-training\n\n\nWe use the pretrained 'nreimers/MiniLM-L6-H384-uncased' model. Please refer to the model card for more detailed information about the pre-training procedure.",
"### Fine-tuning\n\n\nWe fine-tune the model using a contrastive objective. Formally, we compute the cosine similarity from each possible sentence pairs from the batch.\nWe then apply the cross entropy loss by comparing with true pairs.",
"#### Hyper parameters\n\n\nWe trained our model on a TPU v3-8. We train the model during 100k steps using a batch size of 1024 (128 per TPU core).\nWe use a learning rate warm up of 500. The sequence length was limited to 128 tokens. We used the AdamW optimizer with\na 2e-5 learning rate. The full training script is accessible in this current repository: 'train\\_script.py'.",
"#### Training data\n\n\nWe use the concatenation from multiple datasets to fine-tune our model. The total number of sentence pairs is above 1 billion sentences.\nWe sampled each dataset given a weighted probability which configuration is detailed in the 'data\\_config.json' file."
] | [
"TAGS\n#sentence-transformers #safetensors #bert #feature-extraction #sentence-similarity #transformers #en #dataset-s2orc #dataset-flax-sentence-embeddings/stackexchange_xml #dataset-ms_marco #dataset-gooaq #dataset-yahoo_answers_topics #dataset-code_search_net #dataset-search_qa #dataset-eli5 #dataset-snli #dataset-multi_nli #dataset-wikihow #dataset-natural_questions #dataset-trivia_qa #dataset-embedding-data/sentence-compression #dataset-embedding-data/flickr30k-captions #dataset-embedding-data/altlex #dataset-embedding-data/simple-wiki #dataset-embedding-data/QQP #dataset-embedding-data/SPECTER #dataset-embedding-data/PAQ_pairs #dataset-embedding-data/WikiAnswers #arxiv-1904.06472 #arxiv-2102.07033 #arxiv-2104.08727 #arxiv-1704.05179 #arxiv-1810.09305 #license-apache-2.0 #endpoints_compatible #region-us \n",
"### Pre-training\n\n\nWe use the pretrained 'nreimers/MiniLM-L6-H384-uncased' model. Please refer to the model card for more detailed information about the pre-training procedure.",
"### Fine-tuning\n\n\nWe fine-tune the model using a contrastive objective. Formally, we compute the cosine similarity from each possible sentence pairs from the batch.\nWe then apply the cross entropy loss by comparing with true pairs.",
"#### Hyper parameters\n\n\nWe trained our model on a TPU v3-8. We train the model during 100k steps using a batch size of 1024 (128 per TPU core).\nWe use a learning rate warm up of 500. The sequence length was limited to 128 tokens. We used the AdamW optimizer with\na 2e-5 learning rate. The full training script is accessible in this current repository: 'train\\_script.py'.",
"#### Training data\n\n\nWe use the concatenation from multiple datasets to fine-tune our model. The total number of sentence pairs is above 1 billion sentences.\nWe sampled each dataset given a weighted probability which configuration is detailed in the 'data\\_config.json' file."
] |
null | peft |
<!-- This model card has been generated automatically according to the information the Trainer had access to. You
should probably proofread and complete it, then remove this comment. -->
# save
This model is a fine-tuned version of [mistralai/Mistral-7B-Instruct-v0.2](https://huggingface.co/mistralai/Mistral-7B-Instruct-v0.2) on the generator dataset.
## Model description
More information needed
## Intended uses & limitations
More information needed
## Training and evaluation data
More information needed
## Training procedure
### Training hyperparameters
The following hyperparameters were used during training:
- learning_rate: 0.0002
- train_batch_size: 3
- eval_batch_size: 8
- seed: 42
- gradient_accumulation_steps: 2
- total_train_batch_size: 6
- optimizer: Adam with betas=(0.9,0.999) and epsilon=1e-08
- lr_scheduler_type: constant
- lr_scheduler_warmup_ratio: 0.03
- num_epochs: 3
### Training results
### Framework versions
- PEFT 0.7.2.dev0
- Transformers 4.36.2
- Pytorch 2.2.1+cu121
- Datasets 2.16.1
- Tokenizers 0.15.2 | {"license": "apache-2.0", "library_name": "peft", "tags": ["trl", "sft", "generated_from_trainer"], "datasets": ["generator"], "base_model": "mistralai/Mistral-7B-Instruct-v0.2", "model-index": [{"name": "save", "results": []}]} | Baprick/save | null | [
"peft",
"tensorboard",
"safetensors",
"trl",
"sft",
"generated_from_trainer",
"dataset:generator",
"base_model:mistralai/Mistral-7B-Instruct-v0.2",
"license:apache-2.0",
"region:us"
] | null | 2024-04-18T10:03:43+00:00 | [] | [] | TAGS
#peft #tensorboard #safetensors #trl #sft #generated_from_trainer #dataset-generator #base_model-mistralai/Mistral-7B-Instruct-v0.2 #license-apache-2.0 #region-us
|
# save
This model is a fine-tuned version of mistralai/Mistral-7B-Instruct-v0.2 on the generator dataset.
## Model description
More information needed
## Intended uses & limitations
More information needed
## Training and evaluation data
More information needed
## Training procedure
### Training hyperparameters
The following hyperparameters were used during training:
- learning_rate: 0.0002
- train_batch_size: 3
- eval_batch_size: 8
- seed: 42
- gradient_accumulation_steps: 2
- total_train_batch_size: 6
- optimizer: Adam with betas=(0.9,0.999) and epsilon=1e-08
- lr_scheduler_type: constant
- lr_scheduler_warmup_ratio: 0.03
- num_epochs: 3
### Training results
### Framework versions
- PEFT 0.7.2.dev0
- Transformers 4.36.2
- Pytorch 2.2.1+cu121
- Datasets 2.16.1
- Tokenizers 0.15.2 | [
"# save\n\nThis model is a fine-tuned version of mistralai/Mistral-7B-Instruct-v0.2 on the generator dataset.",
"## Model description\n\nMore information needed",
"## Intended uses & limitations\n\nMore information needed",
"## Training and evaluation data\n\nMore information needed",
"## Training procedure",
"### Training hyperparameters\n\nThe following hyperparameters were used during training:\n- learning_rate: 0.0002\n- train_batch_size: 3\n- eval_batch_size: 8\n- seed: 42\n- gradient_accumulation_steps: 2\n- total_train_batch_size: 6\n- optimizer: Adam with betas=(0.9,0.999) and epsilon=1e-08\n- lr_scheduler_type: constant\n- lr_scheduler_warmup_ratio: 0.03\n- num_epochs: 3",
"### Training results",
"### Framework versions\n\n- PEFT 0.7.2.dev0\n- Transformers 4.36.2\n- Pytorch 2.2.1+cu121\n- Datasets 2.16.1\n- Tokenizers 0.15.2"
] | [
"TAGS\n#peft #tensorboard #safetensors #trl #sft #generated_from_trainer #dataset-generator #base_model-mistralai/Mistral-7B-Instruct-v0.2 #license-apache-2.0 #region-us \n",
"# save\n\nThis model is a fine-tuned version of mistralai/Mistral-7B-Instruct-v0.2 on the generator dataset.",
"## Model description\n\nMore information needed",
"## Intended uses & limitations\n\nMore information needed",
"## Training and evaluation data\n\nMore information needed",
"## Training procedure",
"### Training hyperparameters\n\nThe following hyperparameters were used during training:\n- learning_rate: 0.0002\n- train_batch_size: 3\n- eval_batch_size: 8\n- seed: 42\n- gradient_accumulation_steps: 2\n- total_train_batch_size: 6\n- optimizer: Adam with betas=(0.9,0.999) and epsilon=1e-08\n- lr_scheduler_type: constant\n- lr_scheduler_warmup_ratio: 0.03\n- num_epochs: 3",
"### Training results",
"### Framework versions\n\n- PEFT 0.7.2.dev0\n- Transformers 4.36.2\n- Pytorch 2.2.1+cu121\n- Datasets 2.16.1\n- Tokenizers 0.15.2"
] |
text-generation | transformers | # Endevor/InfinityRP-v1-7B AWQ
- Model creator: [Endevor](https://huggingface.co/Endevor)
- Original model: [InfinityRP-v1-7B](https://huggingface.co/Endevor/InfinityRP-v1-7B)
## Model Summary
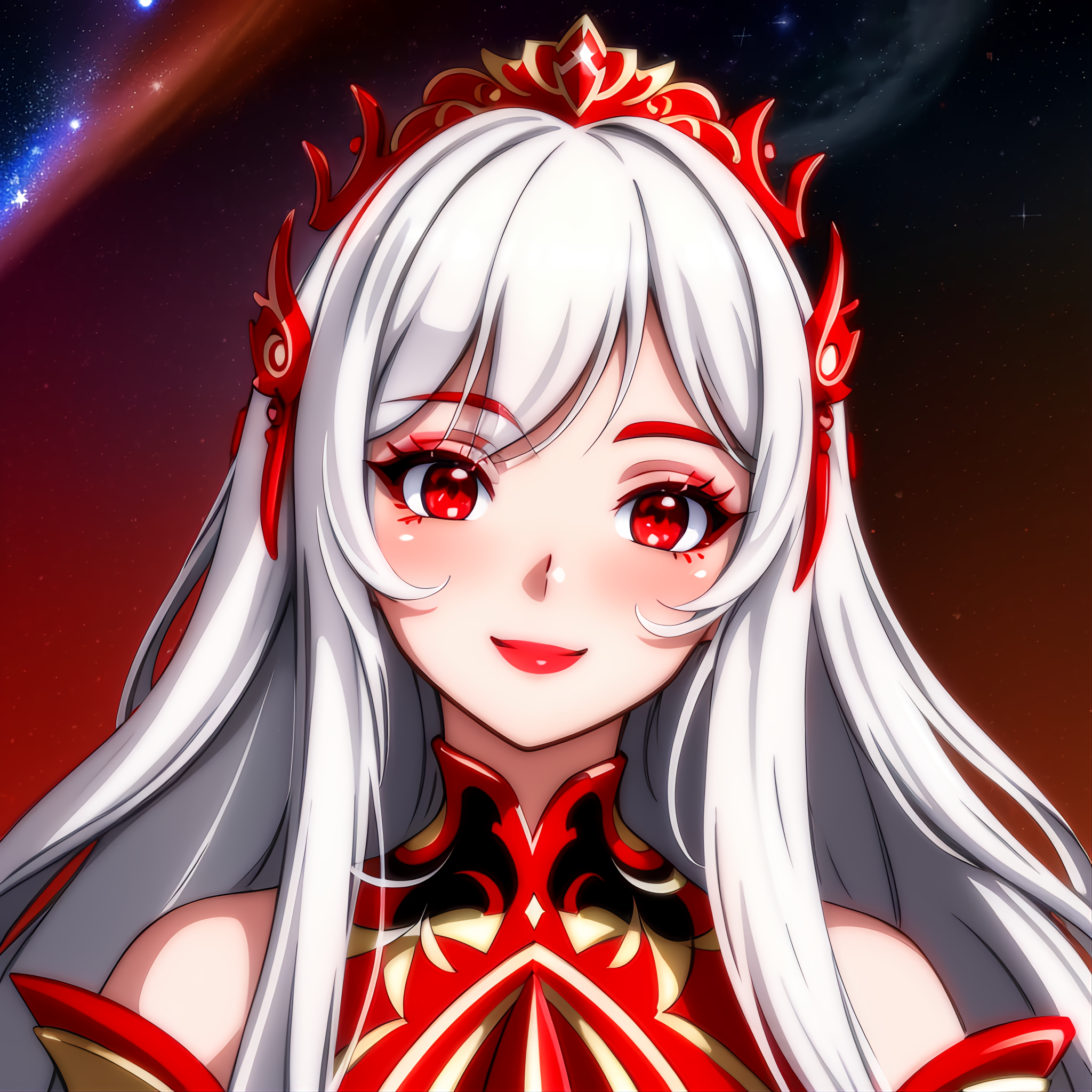
This is an experimental model I currently use. It's far from great as I'm still working on it, but I leave it here for people to try if interested in this format.
This model was basically made to stop some upsetting hallucinations, so {{char}} mostly and occasionally will wait {{user}} response instead of responding itself or deciding for {{user}}, also, my primary idea was to create a cozy model that thinks.*
Inspired by [lemonilia/Limamono-Mistral-7B-v0.50](https://huggingface.co/lemonilia/Limamono-Mistral-7B-v0.50)
### Style details:
- Quotes are used for character dialogs.
- `"Hey, Anon... What do you think about my style?"`
- Asterisks can be used for narration, but it's optional, it's recommended to use default novel format.
- `*Her cheeks blush slightly, she tries to hide.*`
- Character thoughts are wrapped with ` marks. **This may often spontaneously occur.**
- `My heart skips a beat hearing him call me pretty!`
*If you want thoughts to appear more often, just add something like this to your system prompt: ```"{{char}} internal thoughts are wrapped with ` marks."```*
- Accepted response lengths: ***tiny, short, medium, long, huge***
-
For example: ### Response: (length = medium)
Note: Apparently ***humongous***, ***extreme*** and ***unlimited*** may not work at moment. Not fully tested.
### Prompt format:
Extended Alpaca, as always.
``"You are now in roleplay chat mode. Engage in an endless chat with {{user}}. Always wait {{user}} turn, next actions and responses."``
## Example:

| {"language": ["en"], "license": "apache-2.0", "library_name": "transformers", "tags": ["4-bit", "AWQ", "text-generation", "autotrain_compatible", "endpoints_compatible", "safetensors", "mistral", "not-for-all-audiences", "nsfw", "rp", "roleplay"], "pipeline_tag": "text-generation", "inference": false, "quantized_by": "Suparious"} | solidrust/InfinityRP-v1-7B-AWQ | null | [
"transformers",
"safetensors",
"mistral",
"text-generation",
"4-bit",
"AWQ",
"autotrain_compatible",
"endpoints_compatible",
"not-for-all-audiences",
"nsfw",
"rp",
"roleplay",
"en",
"license:apache-2.0",
"text-generation-inference",
"region:us"
] | null | 2024-04-18T10:03:49+00:00 | [] | [
"en"
] | TAGS
#transformers #safetensors #mistral #text-generation #4-bit #AWQ #autotrain_compatible #endpoints_compatible #not-for-all-audiences #nsfw #rp #roleplay #en #license-apache-2.0 #text-generation-inference #region-us
| # Endevor/InfinityRP-v1-7B AWQ
- Model creator: Endevor
- Original model: InfinityRP-v1-7B
## Model Summary
!example
This is an experimental model I currently use. It's far from great as I'm still working on it, but I leave it here for people to try if interested in this format.
This model was basically made to stop some upsetting hallucinations, so {{char}} mostly and occasionally will wait {{user}} response instead of responding itself or deciding for {{user}}, also, my primary idea was to create a cozy model that thinks.*
Inspired by lemonilia/Limamono-Mistral-7B-v0.50
### Style details:
- Quotes are used for character dialogs.
- '"Hey, Anon... What do you think about my style?"'
- Asterisks can be used for narration, but it's optional, it's recommended to use default novel format.
- '*Her cheeks blush slightly, she tries to hide.*'
- Character thoughts are wrapped with ' marks. This may often spontaneously occur.
- 'My heart skips a beat hearing him call me pretty!'
*If you want thoughts to appear more often, just add something like this to your system prompt: *
- Accepted response lengths: *tiny, short, medium, long, huge*
-
For example: ### Response: (length = medium)
Note: Apparently *humongous*, *extreme* and *unlimited* may not work at moment. Not fully tested.
### Prompt format:
Extended Alpaca, as always.
''"You are now in roleplay chat mode. Engage in an endless chat with {{user}}. Always wait {{user}} turn, next actions and responses."''
## Example:
!example
| [
"# Endevor/InfinityRP-v1-7B AWQ\n\n- Model creator: Endevor\n- Original model: InfinityRP-v1-7B",
"## Model Summary\n\n!example\n\nThis is an experimental model I currently use. It's far from great as I'm still working on it, but I leave it here for people to try if interested in this format.\nThis model was basically made to stop some upsetting hallucinations, so {{char}} mostly and occasionally will wait {{user}} response instead of responding itself or deciding for {{user}}, also, my primary idea was to create a cozy model that thinks.*\n\nInspired by lemonilia/Limamono-Mistral-7B-v0.50",
"### Style details:\n- Quotes are used for character dialogs.\n - '\"Hey, Anon... What do you think about my style?\"'\n- Asterisks can be used for narration, but it's optional, it's recommended to use default novel format.\n - '*Her cheeks blush slightly, she tries to hide.*'\n- Character thoughts are wrapped with ' marks. This may often spontaneously occur.\n - 'My heart skips a beat hearing him call me pretty!'\n\n*If you want thoughts to appear more often, just add something like this to your system prompt: *\n\n- Accepted response lengths: *tiny, short, medium, long, huge*\n-\nFor example: ### Response: (length = medium)\n\nNote: Apparently *humongous*, *extreme* and *unlimited* may not work at moment. Not fully tested.",
"### Prompt format: \nExtended Alpaca, as always.\n\n''\"You are now in roleplay chat mode. Engage in an endless chat with {{user}}. Always wait {{user}} turn, next actions and responses.\"''",
"## Example:\n\n!example"
] | [
"TAGS\n#transformers #safetensors #mistral #text-generation #4-bit #AWQ #autotrain_compatible #endpoints_compatible #not-for-all-audiences #nsfw #rp #roleplay #en #license-apache-2.0 #text-generation-inference #region-us \n",
"# Endevor/InfinityRP-v1-7B AWQ\n\n- Model creator: Endevor\n- Original model: InfinityRP-v1-7B",
"## Model Summary\n\n!example\n\nThis is an experimental model I currently use. It's far from great as I'm still working on it, but I leave it here for people to try if interested in this format.\nThis model was basically made to stop some upsetting hallucinations, so {{char}} mostly and occasionally will wait {{user}} response instead of responding itself or deciding for {{user}}, also, my primary idea was to create a cozy model that thinks.*\n\nInspired by lemonilia/Limamono-Mistral-7B-v0.50",
"### Style details:\n- Quotes are used for character dialogs.\n - '\"Hey, Anon... What do you think about my style?\"'\n- Asterisks can be used for narration, but it's optional, it's recommended to use default novel format.\n - '*Her cheeks blush slightly, she tries to hide.*'\n- Character thoughts are wrapped with ' marks. This may often spontaneously occur.\n - 'My heart skips a beat hearing him call me pretty!'\n\n*If you want thoughts to appear more often, just add something like this to your system prompt: *\n\n- Accepted response lengths: *tiny, short, medium, long, huge*\n-\nFor example: ### Response: (length = medium)\n\nNote: Apparently *humongous*, *extreme* and *unlimited* may not work at moment. Not fully tested.",
"### Prompt format: \nExtended Alpaca, as always.\n\n''\"You are now in roleplay chat mode. Engage in an endless chat with {{user}}. Always wait {{user}} turn, next actions and responses.\"''",
"## Example:\n\n!example"
] |
null | null | # StarAntler-RP-WestLake-chatvector_GGUF
このモデルは[StarAntler-RP-WestLake-chatvector](https://huggingface.co/soramikaduki/StarAntler-RP-WestLake-chatvector?not-for-all-audiences=true)の量子化バージョンです。
詳細は上記のモデルページで確認してください | {"language": ["ja"], "license": "apache-2.0", "tags": ["not-for-all-audiences"]} | soramikaduki/StarAntler-RP-WestLake-chatvector_GGUF | null | [
"gguf",
"not-for-all-audiences",
"ja",
"license:apache-2.0",
"region:us"
] | null | 2024-04-18T10:05:25+00:00 | [] | [
"ja"
] | TAGS
#gguf #not-for-all-audiences #ja #license-apache-2.0 #region-us
| # StarAntler-RP-WestLake-chatvector_GGUF
このモデルはStarAntler-RP-WestLake-chatvectorの量子化バージョンです。
詳細は上記のモデルページで確認してください | [
"# StarAntler-RP-WestLake-chatvector_GGUF\n\nこのモデルはStarAntler-RP-WestLake-chatvectorの量子化バージョンです。\n詳細は上記のモデルページで確認してください"
] | [
"TAGS\n#gguf #not-for-all-audiences #ja #license-apache-2.0 #region-us \n",
"# StarAntler-RP-WestLake-chatvector_GGUF\n\nこのモデルはStarAntler-RP-WestLake-chatvectorの量子化バージョンです。\n詳細は上記のモデルページで確認してください"
] |
null | peft |
# Model Card for Model ID
<!-- Provide a quick summary of what the model is/does. -->
## Model Details
### Model Description
<!-- Provide a longer summary of what this model is. -->
- **Developed by:** [More Information Needed]
- **Funded by [optional]:** [More Information Needed]
- **Shared by [optional]:** [More Information Needed]
- **Model type:** [More Information Needed]
- **Language(s) (NLP):** [More Information Needed]
- **License:** [More Information Needed]
- **Finetuned from model [optional]:** [More Information Needed]
### Model Sources [optional]
<!-- Provide the basic links for the model. -->
- **Repository:** [More Information Needed]
- **Paper [optional]:** [More Information Needed]
- **Demo [optional]:** [More Information Needed]
## Uses
<!-- Address questions around how the model is intended to be used, including the foreseeable users of the model and those affected by the model. -->
### Direct Use
<!-- This section is for the model use without fine-tuning or plugging into a larger ecosystem/app. -->
[More Information Needed]
### Downstream Use [optional]
<!-- This section is for the model use when fine-tuned for a task, or when plugged into a larger ecosystem/app -->
[More Information Needed]
### Out-of-Scope Use
<!-- This section addresses misuse, malicious use, and uses that the model will not work well for. -->
[More Information Needed]
## Bias, Risks, and Limitations
<!-- This section is meant to convey both technical and sociotechnical limitations. -->
[More Information Needed]
### Recommendations
<!-- This section is meant to convey recommendations with respect to the bias, risk, and technical limitations. -->
Users (both direct and downstream) should be made aware of the risks, biases and limitations of the model. More information needed for further recommendations.
## How to Get Started with the Model
Use the code below to get started with the model.
[More Information Needed]
## Training Details
### Training Data
<!-- This should link to a Dataset Card, perhaps with a short stub of information on what the training data is all about as well as documentation related to data pre-processing or additional filtering. -->
[More Information Needed]
### Training Procedure
<!-- This relates heavily to the Technical Specifications. Content here should link to that section when it is relevant to the training procedure. -->
#### Preprocessing [optional]
[More Information Needed]
#### Training Hyperparameters
- **Training regime:** [More Information Needed] <!--fp32, fp16 mixed precision, bf16 mixed precision, bf16 non-mixed precision, fp16 non-mixed precision, fp8 mixed precision -->
#### Speeds, Sizes, Times [optional]
<!-- This section provides information about throughput, start/end time, checkpoint size if relevant, etc. -->
[More Information Needed]
## Evaluation
<!-- This section describes the evaluation protocols and provides the results. -->
### Testing Data, Factors & Metrics
#### Testing Data
<!-- This should link to a Dataset Card if possible. -->
[More Information Needed]
#### Factors
<!-- These are the things the evaluation is disaggregating by, e.g., subpopulations or domains. -->
[More Information Needed]
#### Metrics
<!-- These are the evaluation metrics being used, ideally with a description of why. -->
[More Information Needed]
### Results
[More Information Needed]
#### Summary
## Model Examination [optional]
<!-- Relevant interpretability work for the model goes here -->
[More Information Needed]
## Environmental Impact
<!-- Total emissions (in grams of CO2eq) and additional considerations, such as electricity usage, go here. Edit the suggested text below accordingly -->
Carbon emissions can be estimated using the [Machine Learning Impact calculator](https://mlco2.github.io/impact#compute) presented in [Lacoste et al. (2019)](https://arxiv.org/abs/1910.09700).
- **Hardware Type:** [More Information Needed]
- **Hours used:** [More Information Needed]
- **Cloud Provider:** [More Information Needed]
- **Compute Region:** [More Information Needed]
- **Carbon Emitted:** [More Information Needed]
## Technical Specifications [optional]
### Model Architecture and Objective
[More Information Needed]
### Compute Infrastructure
[More Information Needed]
#### Hardware
[More Information Needed]
#### Software
[More Information Needed]
## Citation [optional]
<!-- If there is a paper or blog post introducing the model, the APA and Bibtex information for that should go in this section. -->
**BibTeX:**
[More Information Needed]
**APA:**
[More Information Needed]
## Glossary [optional]
<!-- If relevant, include terms and calculations in this section that can help readers understand the model or model card. -->
[More Information Needed]
## More Information [optional]
[More Information Needed]
## Model Card Authors [optional]
[More Information Needed]
## Model Card Contact
[More Information Needed]
### Framework versions
- PEFT 0.7.1 | {"library_name": "peft", "base_model": "HuggingFaceH4/zephyr-7b-beta"} | ernest/intent_redline_v4_adapter_1792 | null | [
"peft",
"safetensors",
"arxiv:1910.09700",
"base_model:HuggingFaceH4/zephyr-7b-beta",
"region:us"
] | null | 2024-04-18T10:07:23+00:00 | [
"1910.09700"
] | [] | TAGS
#peft #safetensors #arxiv-1910.09700 #base_model-HuggingFaceH4/zephyr-7b-beta #region-us
|
# Model Card for Model ID
## Model Details
### Model Description
- Developed by:
- Funded by [optional]:
- Shared by [optional]:
- Model type:
- Language(s) (NLP):
- License:
- Finetuned from model [optional]:
### Model Sources [optional]
- Repository:
- Paper [optional]:
- Demo [optional]:
## Uses
### Direct Use
### Downstream Use [optional]
### Out-of-Scope Use
## Bias, Risks, and Limitations
### Recommendations
Users (both direct and downstream) should be made aware of the risks, biases and limitations of the model. More information needed for further recommendations.
## How to Get Started with the Model
Use the code below to get started with the model.
## Training Details
### Training Data
### Training Procedure
#### Preprocessing [optional]
#### Training Hyperparameters
- Training regime:
#### Speeds, Sizes, Times [optional]
## Evaluation
### Testing Data, Factors & Metrics
#### Testing Data
#### Factors
#### Metrics
### Results
#### Summary
## Model Examination [optional]
## Environmental Impact
Carbon emissions can be estimated using the Machine Learning Impact calculator presented in Lacoste et al. (2019).
- Hardware Type:
- Hours used:
- Cloud Provider:
- Compute Region:
- Carbon Emitted:
## Technical Specifications [optional]
### Model Architecture and Objective
### Compute Infrastructure
#### Hardware
#### Software
[optional]
BibTeX:
APA:
## Glossary [optional]
## More Information [optional]
## Model Card Authors [optional]
## Model Card Contact
### Framework versions
- PEFT 0.7.1 | [
"# Model Card for Model ID",
"## Model Details",
"### Model Description\n\n\n\n\n\n- Developed by: \n- Funded by [optional]: \n- Shared by [optional]: \n- Model type: \n- Language(s) (NLP): \n- License: \n- Finetuned from model [optional]:",
"### Model Sources [optional]\n\n\n\n- Repository: \n- Paper [optional]: \n- Demo [optional]:",
"## Uses",
"### Direct Use",
"### Downstream Use [optional]",
"### Out-of-Scope Use",
"## Bias, Risks, and Limitations",
"### Recommendations\n\n\n\nUsers (both direct and downstream) should be made aware of the risks, biases and limitations of the model. More information needed for further recommendations.",
"## How to Get Started with the Model\n\nUse the code below to get started with the model.",
"## Training Details",
"### Training Data",
"### Training Procedure",
"#### Preprocessing [optional]",
"#### Training Hyperparameters\n\n- Training regime:",
"#### Speeds, Sizes, Times [optional]",
"## Evaluation",
"### Testing Data, Factors & Metrics",
"#### Testing Data",
"#### Factors",
"#### Metrics",
"### Results",
"#### Summary",
"## Model Examination [optional]",
"## Environmental Impact\n\n\n\nCarbon emissions can be estimated using the Machine Learning Impact calculator presented in Lacoste et al. (2019).\n\n- Hardware Type: \n- Hours used: \n- Cloud Provider: \n- Compute Region: \n- Carbon Emitted:",
"## Technical Specifications [optional]",
"### Model Architecture and Objective",
"### Compute Infrastructure",
"#### Hardware",
"#### Software\n\n\n\n[optional]\n\n\n\nBibTeX:\n\n\n\nAPA:",
"## Glossary [optional]",
"## More Information [optional]",
"## Model Card Authors [optional]",
"## Model Card Contact",
"### Framework versions\n\n- PEFT 0.7.1"
] | [
"TAGS\n#peft #safetensors #arxiv-1910.09700 #base_model-HuggingFaceH4/zephyr-7b-beta #region-us \n",
"# Model Card for Model ID",
"## Model Details",
"### Model Description\n\n\n\n\n\n- Developed by: \n- Funded by [optional]: \n- Shared by [optional]: \n- Model type: \n- Language(s) (NLP): \n- License: \n- Finetuned from model [optional]:",
"### Model Sources [optional]\n\n\n\n- Repository: \n- Paper [optional]: \n- Demo [optional]:",
"## Uses",
"### Direct Use",
"### Downstream Use [optional]",
"### Out-of-Scope Use",
"## Bias, Risks, and Limitations",
"### Recommendations\n\n\n\nUsers (both direct and downstream) should be made aware of the risks, biases and limitations of the model. More information needed for further recommendations.",
"## How to Get Started with the Model\n\nUse the code below to get started with the model.",
"## Training Details",
"### Training Data",
"### Training Procedure",
"#### Preprocessing [optional]",
"#### Training Hyperparameters\n\n- Training regime:",
"#### Speeds, Sizes, Times [optional]",
"## Evaluation",
"### Testing Data, Factors & Metrics",
"#### Testing Data",
"#### Factors",
"#### Metrics",
"### Results",
"#### Summary",
"## Model Examination [optional]",
"## Environmental Impact\n\n\n\nCarbon emissions can be estimated using the Machine Learning Impact calculator presented in Lacoste et al. (2019).\n\n- Hardware Type: \n- Hours used: \n- Cloud Provider: \n- Compute Region: \n- Carbon Emitted:",
"## Technical Specifications [optional]",
"### Model Architecture and Objective",
"### Compute Infrastructure",
"#### Hardware",
"#### Software\n\n\n\n[optional]\n\n\n\nBibTeX:\n\n\n\nAPA:",
"## Glossary [optional]",
"## More Information [optional]",
"## Model Card Authors [optional]",
"## Model Card Contact",
"### Framework versions\n\n- PEFT 0.7.1"
] |
null | transformers |
# Model Card for Model ID
<!-- Provide a quick summary of what the model is/does. -->
## Model Details
### Model Description
<!-- Provide a longer summary of what this model is. -->
This is the model card of a 🤗 transformers model that has been pushed on the Hub. This model card has been automatically generated.
- **Developed by:** [More Information Needed]
- **Funded by [optional]:** [More Information Needed]
- **Shared by [optional]:** [More Information Needed]
- **Model type:** [More Information Needed]
- **Language(s) (NLP):** [More Information Needed]
- **License:** [More Information Needed]
- **Finetuned from model [optional]:** [More Information Needed]
### Model Sources [optional]
<!-- Provide the basic links for the model. -->
- **Repository:** [More Information Needed]
- **Paper [optional]:** [More Information Needed]
- **Demo [optional]:** [More Information Needed]
## Uses
<!-- Address questions around how the model is intended to be used, including the foreseeable users of the model and those affected by the model. -->
### Direct Use
<!-- This section is for the model use without fine-tuning or plugging into a larger ecosystem/app. -->
[More Information Needed]
### Downstream Use [optional]
<!-- This section is for the model use when fine-tuned for a task, or when plugged into a larger ecosystem/app -->
[More Information Needed]
### Out-of-Scope Use
<!-- This section addresses misuse, malicious use, and uses that the model will not work well for. -->
[More Information Needed]
## Bias, Risks, and Limitations
<!-- This section is meant to convey both technical and sociotechnical limitations. -->
[More Information Needed]
### Recommendations
<!-- This section is meant to convey recommendations with respect to the bias, risk, and technical limitations. -->
Users (both direct and downstream) should be made aware of the risks, biases and limitations of the model. More information needed for further recommendations.
## How to Get Started with the Model
Use the code below to get started with the model.
[More Information Needed]
## Training Details
### Training Data
<!-- This should link to a Dataset Card, perhaps with a short stub of information on what the training data is all about as well as documentation related to data pre-processing or additional filtering. -->
[More Information Needed]
### Training Procedure
<!-- This relates heavily to the Technical Specifications. Content here should link to that section when it is relevant to the training procedure. -->
#### Preprocessing [optional]
[More Information Needed]
#### Training Hyperparameters
- **Training regime:** [More Information Needed] <!--fp32, fp16 mixed precision, bf16 mixed precision, bf16 non-mixed precision, fp16 non-mixed precision, fp8 mixed precision -->
#### Speeds, Sizes, Times [optional]
<!-- This section provides information about throughput, start/end time, checkpoint size if relevant, etc. -->
[More Information Needed]
## Evaluation
<!-- This section describes the evaluation protocols and provides the results. -->
### Testing Data, Factors & Metrics
#### Testing Data
<!-- This should link to a Dataset Card if possible. -->
[More Information Needed]
#### Factors
<!-- These are the things the evaluation is disaggregating by, e.g., subpopulations or domains. -->
[More Information Needed]
#### Metrics
<!-- These are the evaluation metrics being used, ideally with a description of why. -->
[More Information Needed]
### Results
[More Information Needed]
#### Summary
## Model Examination [optional]
<!-- Relevant interpretability work for the model goes here -->
[More Information Needed]
## Environmental Impact
<!-- Total emissions (in grams of CO2eq) and additional considerations, such as electricity usage, go here. Edit the suggested text below accordingly -->
Carbon emissions can be estimated using the [Machine Learning Impact calculator](https://mlco2.github.io/impact#compute) presented in [Lacoste et al. (2019)](https://arxiv.org/abs/1910.09700).
- **Hardware Type:** [More Information Needed]
- **Hours used:** [More Information Needed]
- **Cloud Provider:** [More Information Needed]
- **Compute Region:** [More Information Needed]
- **Carbon Emitted:** [More Information Needed]
## Technical Specifications [optional]
### Model Architecture and Objective
[More Information Needed]
### Compute Infrastructure
[More Information Needed]
#### Hardware
[More Information Needed]
#### Software
[More Information Needed]
## Citation [optional]
<!-- If there is a paper or blog post introducing the model, the APA and Bibtex information for that should go in this section. -->
**BibTeX:**
[More Information Needed]
**APA:**
[More Information Needed]
## Glossary [optional]
<!-- If relevant, include terms and calculations in this section that can help readers understand the model or model card. -->
[More Information Needed]
## More Information [optional]
[More Information Needed]
## Model Card Authors [optional]
[More Information Needed]
## Model Card Contact
[More Information Needed]
| {"library_name": "transformers", "tags": []} | ManikanthaDandi/idefics-9b-PokemonCards | null | [
"transformers",
"safetensors",
"arxiv:1910.09700",
"endpoints_compatible",
"region:us"
] | null | 2024-04-18T10:08:48+00:00 | [
"1910.09700"
] | [] | TAGS
#transformers #safetensors #arxiv-1910.09700 #endpoints_compatible #region-us
|
# Model Card for Model ID
## Model Details
### Model Description
This is the model card of a transformers model that has been pushed on the Hub. This model card has been automatically generated.
- Developed by:
- Funded by [optional]:
- Shared by [optional]:
- Model type:
- Language(s) (NLP):
- License:
- Finetuned from model [optional]:
### Model Sources [optional]
- Repository:
- Paper [optional]:
- Demo [optional]:
## Uses
### Direct Use
### Downstream Use [optional]
### Out-of-Scope Use
## Bias, Risks, and Limitations
### Recommendations
Users (both direct and downstream) should be made aware of the risks, biases and limitations of the model. More information needed for further recommendations.
## How to Get Started with the Model
Use the code below to get started with the model.
## Training Details
### Training Data
### Training Procedure
#### Preprocessing [optional]
#### Training Hyperparameters
- Training regime:
#### Speeds, Sizes, Times [optional]
## Evaluation
### Testing Data, Factors & Metrics
#### Testing Data
#### Factors
#### Metrics
### Results
#### Summary
## Model Examination [optional]
## Environmental Impact
Carbon emissions can be estimated using the Machine Learning Impact calculator presented in Lacoste et al. (2019).
- Hardware Type:
- Hours used:
- Cloud Provider:
- Compute Region:
- Carbon Emitted:
## Technical Specifications [optional]
### Model Architecture and Objective
### Compute Infrastructure
#### Hardware
#### Software
[optional]
BibTeX:
APA:
## Glossary [optional]
## More Information [optional]
## Model Card Authors [optional]
## Model Card Contact
| [
"# Model Card for Model ID",
"## Model Details",
"### Model Description\n\n\n\nThis is the model card of a transformers model that has been pushed on the Hub. This model card has been automatically generated.\n\n- Developed by: \n- Funded by [optional]: \n- Shared by [optional]: \n- Model type: \n- Language(s) (NLP): \n- License: \n- Finetuned from model [optional]:",
"### Model Sources [optional]\n\n\n\n- Repository: \n- Paper [optional]: \n- Demo [optional]:",
"## Uses",
"### Direct Use",
"### Downstream Use [optional]",
"### Out-of-Scope Use",
"## Bias, Risks, and Limitations",
"### Recommendations\n\n\n\nUsers (both direct and downstream) should be made aware of the risks, biases and limitations of the model. More information needed for further recommendations.",
"## How to Get Started with the Model\n\nUse the code below to get started with the model.",
"## Training Details",
"### Training Data",
"### Training Procedure",
"#### Preprocessing [optional]",
"#### Training Hyperparameters\n\n- Training regime:",
"#### Speeds, Sizes, Times [optional]",
"## Evaluation",
"### Testing Data, Factors & Metrics",
"#### Testing Data",
"#### Factors",
"#### Metrics",
"### Results",
"#### Summary",
"## Model Examination [optional]",
"## Environmental Impact\n\n\n\nCarbon emissions can be estimated using the Machine Learning Impact calculator presented in Lacoste et al. (2019).\n\n- Hardware Type: \n- Hours used: \n- Cloud Provider: \n- Compute Region: \n- Carbon Emitted:",
"## Technical Specifications [optional]",
"### Model Architecture and Objective",
"### Compute Infrastructure",
"#### Hardware",
"#### Software\n\n\n\n[optional]\n\n\n\nBibTeX:\n\n\n\nAPA:",
"## Glossary [optional]",
"## More Information [optional]",
"## Model Card Authors [optional]",
"## Model Card Contact"
] | [
"TAGS\n#transformers #safetensors #arxiv-1910.09700 #endpoints_compatible #region-us \n",
"# Model Card for Model ID",
"## Model Details",
"### Model Description\n\n\n\nThis is the model card of a transformers model that has been pushed on the Hub. This model card has been automatically generated.\n\n- Developed by: \n- Funded by [optional]: \n- Shared by [optional]: \n- Model type: \n- Language(s) (NLP): \n- License: \n- Finetuned from model [optional]:",
"### Model Sources [optional]\n\n\n\n- Repository: \n- Paper [optional]: \n- Demo [optional]:",
"## Uses",
"### Direct Use",
"### Downstream Use [optional]",
"### Out-of-Scope Use",
"## Bias, Risks, and Limitations",
"### Recommendations\n\n\n\nUsers (both direct and downstream) should be made aware of the risks, biases and limitations of the model. More information needed for further recommendations.",
"## How to Get Started with the Model\n\nUse the code below to get started with the model.",
"## Training Details",
"### Training Data",
"### Training Procedure",
"#### Preprocessing [optional]",
"#### Training Hyperparameters\n\n- Training regime:",
"#### Speeds, Sizes, Times [optional]",
"## Evaluation",
"### Testing Data, Factors & Metrics",
"#### Testing Data",
"#### Factors",
"#### Metrics",
"### Results",
"#### Summary",
"## Model Examination [optional]",
"## Environmental Impact\n\n\n\nCarbon emissions can be estimated using the Machine Learning Impact calculator presented in Lacoste et al. (2019).\n\n- Hardware Type: \n- Hours used: \n- Cloud Provider: \n- Compute Region: \n- Carbon Emitted:",
"## Technical Specifications [optional]",
"### Model Architecture and Objective",
"### Compute Infrastructure",
"#### Hardware",
"#### Software\n\n\n\n[optional]\n\n\n\nBibTeX:\n\n\n\nAPA:",
"## Glossary [optional]",
"## More Information [optional]",
"## Model Card Authors [optional]",
"## Model Card Contact"
] |
token-classification | transformers |
<!-- This model card has been generated automatically according to the information the Trainer had access to. You
should probably proofread and complete it, then remove this comment. -->
# model-v2-18-04-2024
This model is a fine-tuned version of [microsoft/layoutlmv3-large](https://huggingface.co/microsoft/layoutlmv3-large) on an unknown dataset.
It achieves the following results on the evaluation set:
- Loss: 1.2634
- Precision: 0.6613
- Recall: 0.6949
- F1: 0.6777
- Accuracy: 0.8140
## Model description
More information needed
## Intended uses & limitations
More information needed
## Training and evaluation data
More information needed
## Training procedure
### Training hyperparameters
The following hyperparameters were used during training:
- learning_rate: 1e-05
- train_batch_size: 2
- eval_batch_size: 2
- seed: 42
- optimizer: Adam with betas=(0.9,0.999) and epsilon=1e-08
- lr_scheduler_type: linear
- training_steps: 1000
### Training results
| Training Loss | Epoch | Step | Validation Loss | Precision | Recall | F1 | Accuracy |
|:-------------:|:-----:|:----:|:---------------:|:---------:|:------:|:------:|:--------:|
| No log | 8.33 | 100 | 1.1672 | 0.53 | 0.4492 | 0.4862 | 0.7228 |
| No log | 16.67 | 200 | 0.9729 | 0.6491 | 0.6271 | 0.6379 | 0.7895 |
| No log | 25.0 | 300 | 1.0899 | 0.6552 | 0.6441 | 0.6496 | 0.8 |
| No log | 33.33 | 400 | 1.0176 | 0.6532 | 0.6864 | 0.6694 | 0.8140 |
| 0.5362 | 41.67 | 500 | 1.1735 | 0.6325 | 0.6271 | 0.6298 | 0.7965 |
| 0.5362 | 50.0 | 600 | 1.1586 | 0.664 | 0.7034 | 0.6831 | 0.8211 |
| 0.5362 | 58.33 | 700 | 1.2151 | 0.672 | 0.7119 | 0.6914 | 0.8246 |
| 0.5362 | 66.67 | 800 | 1.2713 | 0.6587 | 0.7034 | 0.6803 | 0.8140 |
| 0.5362 | 75.0 | 900 | 1.2688 | 0.664 | 0.7034 | 0.6831 | 0.8175 |
| 0.0216 | 83.33 | 1000 | 1.2634 | 0.6613 | 0.6949 | 0.6777 | 0.8140 |
### Framework versions
- Transformers 4.29.2
- Pytorch 2.2.1+cu121
- Datasets 2.18.0
- Tokenizers 0.13.3
| {"license": "cc-by-nc-sa-4.0", "tags": ["generated_from_trainer"], "metrics": ["precision", "recall", "f1", "accuracy"], "model-index": [{"name": "model-v2-18-04-2024", "results": []}]} | ineoApp/model-v2-18-04-2024 | null | [
"transformers",
"pytorch",
"tensorboard",
"layoutlmv3",
"token-classification",
"generated_from_trainer",
"license:cc-by-nc-sa-4.0",
"autotrain_compatible",
"endpoints_compatible",
"region:us"
] | null | 2024-04-18T10:10:19+00:00 | [] | [] | TAGS
#transformers #pytorch #tensorboard #layoutlmv3 #token-classification #generated_from_trainer #license-cc-by-nc-sa-4.0 #autotrain_compatible #endpoints_compatible #region-us
| model-v2-18-04-2024
===================
This model is a fine-tuned version of microsoft/layoutlmv3-large on an unknown dataset.
It achieves the following results on the evaluation set:
* Loss: 1.2634
* Precision: 0.6613
* Recall: 0.6949
* F1: 0.6777
* Accuracy: 0.8140
Model description
-----------------
More information needed
Intended uses & limitations
---------------------------
More information needed
Training and evaluation data
----------------------------
More information needed
Training procedure
------------------
### Training hyperparameters
The following hyperparameters were used during training:
* learning\_rate: 1e-05
* train\_batch\_size: 2
* eval\_batch\_size: 2
* seed: 42
* optimizer: Adam with betas=(0.9,0.999) and epsilon=1e-08
* lr\_scheduler\_type: linear
* training\_steps: 1000
### Training results
### Framework versions
* Transformers 4.29.2
* Pytorch 2.2.1+cu121
* Datasets 2.18.0
* Tokenizers 0.13.3
| [
"### Training hyperparameters\n\n\nThe following hyperparameters were used during training:\n\n\n* learning\\_rate: 1e-05\n* train\\_batch\\_size: 2\n* eval\\_batch\\_size: 2\n* seed: 42\n* optimizer: Adam with betas=(0.9,0.999) and epsilon=1e-08\n* lr\\_scheduler\\_type: linear\n* training\\_steps: 1000",
"### Training results",
"### Framework versions\n\n\n* Transformers 4.29.2\n* Pytorch 2.2.1+cu121\n* Datasets 2.18.0\n* Tokenizers 0.13.3"
] | [
"TAGS\n#transformers #pytorch #tensorboard #layoutlmv3 #token-classification #generated_from_trainer #license-cc-by-nc-sa-4.0 #autotrain_compatible #endpoints_compatible #region-us \n",
"### Training hyperparameters\n\n\nThe following hyperparameters were used during training:\n\n\n* learning\\_rate: 1e-05\n* train\\_batch\\_size: 2\n* eval\\_batch\\_size: 2\n* seed: 42\n* optimizer: Adam with betas=(0.9,0.999) and epsilon=1e-08\n* lr\\_scheduler\\_type: linear\n* training\\_steps: 1000",
"### Training results",
"### Framework versions\n\n\n* Transformers 4.29.2\n* Pytorch 2.2.1+cu121\n* Datasets 2.18.0\n* Tokenizers 0.13.3"
] |
text-generation | transformers |
<!-- This model card has been generated automatically according to the information the Trainer had access to. You
should probably proofread and complete it, then remove this comment. -->
# GPT2-705M
This model is a fine-tuned version of [](https://huggingface.co/) on an unknown dataset.
It achieves the following results on the evaluation set:
- Loss: 5.5041
## Model description
More information needed
## Intended uses & limitations
More information needed
## Training and evaluation data
More information needed
## Training procedure
### Training hyperparameters
The following hyperparameters were used during training:
- learning_rate: 0.00025
- train_batch_size: 16
- eval_batch_size: 8
- seed: 42
- gradient_accumulation_steps: 8
- total_train_batch_size: 128
- optimizer: Adam with betas=(0.9,0.999) and epsilon=1e-08
- lr_scheduler_type: cosine
- lr_scheduler_warmup_steps: 50
- num_epochs: 10
- mixed_precision_training: Native AMP
### Training results
| Training Loss | Epoch | Step | Validation Loss |
|:-------------:|:-----:|:----:|:---------------:|
| 7.6866 | 0.98 | 7 | 8.0542 |
| 6.8762 | 1.96 | 14 | 7.3416 |
| 6.1904 | 2.95 | 21 | 6.8861 |
| 5.7933 | 3.93 | 28 | 6.6310 |
| 5.3583 | 4.91 | 35 | 6.3709 |
| 4.8424 | 5.89 | 42 | 6.1468 |
| 4.4728 | 6.88 | 49 | 5.8255 |
| 3.8852 | 8.0 | 57 | 5.6175 |
| 3.4495 | 8.98 | 64 | 5.5436 |
| 3.2287 | 9.82 | 70 | 5.5041 |
### Framework versions
- Transformers 4.39.1
- Pytorch 2.1.2+cu121
- Datasets 2.16.1
- Tokenizers 0.15.0
| {"tags": ["generated_from_trainer"], "model-index": [{"name": "GPT2-705M", "results": []}]} | ninagroot/GPT2-705M-RUN2 | null | [
"transformers",
"tensorboard",
"safetensors",
"gpt2",
"text-generation",
"generated_from_trainer",
"autotrain_compatible",
"endpoints_compatible",
"text-generation-inference",
"region:us"
] | null | 2024-04-18T10:12:00+00:00 | [] | [] | TAGS
#transformers #tensorboard #safetensors #gpt2 #text-generation #generated_from_trainer #autotrain_compatible #endpoints_compatible #text-generation-inference #region-us
| GPT2-705M
=========
This model is a fine-tuned version of [](URL on an unknown dataset.
It achieves the following results on the evaluation set:
* Loss: 5.5041
Model description
-----------------
More information needed
Intended uses & limitations
---------------------------
More information needed
Training and evaluation data
----------------------------
More information needed
Training procedure
------------------
### Training hyperparameters
The following hyperparameters were used during training:
* learning\_rate: 0.00025
* train\_batch\_size: 16
* eval\_batch\_size: 8
* seed: 42
* gradient\_accumulation\_steps: 8
* total\_train\_batch\_size: 128
* optimizer: Adam with betas=(0.9,0.999) and epsilon=1e-08
* lr\_scheduler\_type: cosine
* lr\_scheduler\_warmup\_steps: 50
* num\_epochs: 10
* mixed\_precision\_training: Native AMP
### Training results
### Framework versions
* Transformers 4.39.1
* Pytorch 2.1.2+cu121
* Datasets 2.16.1
* Tokenizers 0.15.0
| [
"### Training hyperparameters\n\n\nThe following hyperparameters were used during training:\n\n\n* learning\\_rate: 0.00025\n* train\\_batch\\_size: 16\n* eval\\_batch\\_size: 8\n* seed: 42\n* gradient\\_accumulation\\_steps: 8\n* total\\_train\\_batch\\_size: 128\n* optimizer: Adam with betas=(0.9,0.999) and epsilon=1e-08\n* lr\\_scheduler\\_type: cosine\n* lr\\_scheduler\\_warmup\\_steps: 50\n* num\\_epochs: 10\n* mixed\\_precision\\_training: Native AMP",
"### Training results",
"### Framework versions\n\n\n* Transformers 4.39.1\n* Pytorch 2.1.2+cu121\n* Datasets 2.16.1\n* Tokenizers 0.15.0"
] | [
"TAGS\n#transformers #tensorboard #safetensors #gpt2 #text-generation #generated_from_trainer #autotrain_compatible #endpoints_compatible #text-generation-inference #region-us \n",
"### Training hyperparameters\n\n\nThe following hyperparameters were used during training:\n\n\n* learning\\_rate: 0.00025\n* train\\_batch\\_size: 16\n* eval\\_batch\\_size: 8\n* seed: 42\n* gradient\\_accumulation\\_steps: 8\n* total\\_train\\_batch\\_size: 128\n* optimizer: Adam with betas=(0.9,0.999) and epsilon=1e-08\n* lr\\_scheduler\\_type: cosine\n* lr\\_scheduler\\_warmup\\_steps: 50\n* num\\_epochs: 10\n* mixed\\_precision\\_training: Native AMP",
"### Training results",
"### Framework versions\n\n\n* Transformers 4.39.1\n* Pytorch 2.1.2+cu121\n* Datasets 2.16.1\n* Tokenizers 0.15.0"
] |
null | transformers | ## About
<!-- ### quantize_version: 1 -->
<!-- ### output_tensor_quantised: 1 -->
<!-- ### convert_type: -->
<!-- ### vocab_type: -->
static quants of https://huggingface.co/ResplendentAI/Aura_v2_7B
<!-- provided-files -->
weighted/imatrix quants seem not to be available (by me) at this time. If they do not show up a week or so after the static ones, I have probably not planned for them. Feel free to request them by opening a Community Discussion.
## Usage
If you are unsure how to use GGUF files, refer to one of [TheBloke's
READMEs](https://huggingface.co/TheBloke/KafkaLM-70B-German-V0.1-GGUF) for
more details, including on how to concatenate multi-part files.
## Provided Quants
(sorted by size, not necessarily quality. IQ-quants are often preferable over similar sized non-IQ quants)
| Link | Type | Size/GB | Notes |
|:-----|:-----|--------:|:------|
| [GGUF](https://huggingface.co/mradermacher/Aura_v2_7B-GGUF/resolve/main/Aura_v2_7B.Q2_K.gguf) | Q2_K | 2.8 | |
| [GGUF](https://huggingface.co/mradermacher/Aura_v2_7B-GGUF/resolve/main/Aura_v2_7B.IQ3_XS.gguf) | IQ3_XS | 3.1 | |
| [GGUF](https://huggingface.co/mradermacher/Aura_v2_7B-GGUF/resolve/main/Aura_v2_7B.Q3_K_S.gguf) | Q3_K_S | 3.3 | |
| [GGUF](https://huggingface.co/mradermacher/Aura_v2_7B-GGUF/resolve/main/Aura_v2_7B.IQ3_S.gguf) | IQ3_S | 3.3 | beats Q3_K* |
| [GGUF](https://huggingface.co/mradermacher/Aura_v2_7B-GGUF/resolve/main/Aura_v2_7B.IQ3_M.gguf) | IQ3_M | 3.4 | |
| [GGUF](https://huggingface.co/mradermacher/Aura_v2_7B-GGUF/resolve/main/Aura_v2_7B.Q3_K_M.gguf) | Q3_K_M | 3.6 | lower quality |
| [GGUF](https://huggingface.co/mradermacher/Aura_v2_7B-GGUF/resolve/main/Aura_v2_7B.Q3_K_L.gguf) | Q3_K_L | 3.9 | |
| [GGUF](https://huggingface.co/mradermacher/Aura_v2_7B-GGUF/resolve/main/Aura_v2_7B.IQ4_XS.gguf) | IQ4_XS | 4.0 | |
| [GGUF](https://huggingface.co/mradermacher/Aura_v2_7B-GGUF/resolve/main/Aura_v2_7B.Q4_K_S.gguf) | Q4_K_S | 4.2 | fast, recommended |
| [GGUF](https://huggingface.co/mradermacher/Aura_v2_7B-GGUF/resolve/main/Aura_v2_7B.Q4_K_M.gguf) | Q4_K_M | 4.5 | fast, recommended |
| [GGUF](https://huggingface.co/mradermacher/Aura_v2_7B-GGUF/resolve/main/Aura_v2_7B.Q5_K_S.gguf) | Q5_K_S | 5.1 | |
| [GGUF](https://huggingface.co/mradermacher/Aura_v2_7B-GGUF/resolve/main/Aura_v2_7B.Q5_K_M.gguf) | Q5_K_M | 5.2 | |
| [GGUF](https://huggingface.co/mradermacher/Aura_v2_7B-GGUF/resolve/main/Aura_v2_7B.Q6_K.gguf) | Q6_K | 6.0 | very good quality |
| [GGUF](https://huggingface.co/mradermacher/Aura_v2_7B-GGUF/resolve/main/Aura_v2_7B.Q8_0.gguf) | Q8_0 | 7.8 | fast, best quality |
Here is a handy graph by ikawrakow comparing some lower-quality quant
types (lower is better):

And here are Artefact2's thoughts on the matter:
https://gist.github.com/Artefact2/b5f810600771265fc1e39442288e8ec9
## FAQ / Model Request
See https://huggingface.co/mradermacher/model_requests for some answers to
questions you might have and/or if you want some other model quantized.
## Thanks
I thank my company, [nethype GmbH](https://www.nethype.de/), for letting
me use its servers and providing upgrades to my workstation to enable
this work in my free time.
<!-- end -->
| {"language": ["en"], "license": "apache-2.0", "library_name": "transformers", "base_model": "ResplendentAI/Aura_v2_7B", "quantized_by": "mradermacher"} | mradermacher/Aura_v2_7B-GGUF | null | [
"transformers",
"gguf",
"en",
"base_model:ResplendentAI/Aura_v2_7B",
"license:apache-2.0",
"endpoints_compatible",
"region:us"
] | null | 2024-04-18T10:12:09+00:00 | [] | [
"en"
] | TAGS
#transformers #gguf #en #base_model-ResplendentAI/Aura_v2_7B #license-apache-2.0 #endpoints_compatible #region-us
| About
-----
static quants of URL
weighted/imatrix quants seem not to be available (by me) at this time. If they do not show up a week or so after the static ones, I have probably not planned for them. Feel free to request them by opening a Community Discussion.
Usage
-----
If you are unsure how to use GGUF files, refer to one of TheBloke's
READMEs for
more details, including on how to concatenate multi-part files.
Provided Quants
---------------
(sorted by size, not necessarily quality. IQ-quants are often preferable over similar sized non-IQ quants)
Here is a handy graph by ikawrakow comparing some lower-quality quant
types (lower is better):
!URL
And here are Artefact2's thoughts on the matter:
URL
FAQ / Model Request
-------------------
See URL for some answers to
questions you might have and/or if you want some other model quantized.
Thanks
------
I thank my company, nethype GmbH, for letting
me use its servers and providing upgrades to my workstation to enable
this work in my free time.
| [] | [
"TAGS\n#transformers #gguf #en #base_model-ResplendentAI/Aura_v2_7B #license-apache-2.0 #endpoints_compatible #region-us \n"
] |
null | transformers |
<!-- This model card has been generated automatically according to the information the Trainer had access to. You
should probably proofread and complete it, then remove this comment. -->
# PolizzeDonut-CR-ProvaCluster3-5Epochs
This model is a fine-tuned version of [tedad09/PolizzeDonut-ChangeRequest-imm5epochs-Expand0](https://huggingface.co/tedad09/PolizzeDonut-ChangeRequest-imm5epochs-Expand0) on the imagefolder dataset.
## Model description
More information needed
## Intended uses & limitations
More information needed
## Training and evaluation data
More information needed
## Training procedure
### Training hyperparameters
The following hyperparameters were used during training:
- learning_rate: 2e-05
- train_batch_size: 2
- eval_batch_size: 8
- seed: 42
- optimizer: Adam with betas=(0.9,0.999) and epsilon=1e-08
- lr_scheduler_type: linear
- num_epochs: 5
- mixed_precision_training: Native AMP
### Training results
### Framework versions
- Transformers 4.38.2
- Pytorch 2.2.2+cu121
- Datasets 2.18.0
- Tokenizers 0.15.2
| {"license": "mit", "tags": ["generated_from_trainer"], "datasets": ["imagefolder"], "base_model": "tedad09/PolizzeDonut-ChangeRequest-imm5epochs-Expand0", "model-index": [{"name": "PolizzeDonut-CR-ProvaCluster3-5Epochs", "results": []}]} | tedad09/PolizzeDonut-CR-ProvaCluster3-5Epochs | null | [
"transformers",
"tensorboard",
"safetensors",
"vision-encoder-decoder",
"generated_from_trainer",
"dataset:imagefolder",
"base_model:tedad09/PolizzeDonut-ChangeRequest-imm5epochs-Expand0",
"license:mit",
"endpoints_compatible",
"region:us"
] | null | 2024-04-18T10:13:59+00:00 | [] | [] | TAGS
#transformers #tensorboard #safetensors #vision-encoder-decoder #generated_from_trainer #dataset-imagefolder #base_model-tedad09/PolizzeDonut-ChangeRequest-imm5epochs-Expand0 #license-mit #endpoints_compatible #region-us
|
# PolizzeDonut-CR-ProvaCluster3-5Epochs
This model is a fine-tuned version of tedad09/PolizzeDonut-ChangeRequest-imm5epochs-Expand0 on the imagefolder dataset.
## Model description
More information needed
## Intended uses & limitations
More information needed
## Training and evaluation data
More information needed
## Training procedure
### Training hyperparameters
The following hyperparameters were used during training:
- learning_rate: 2e-05
- train_batch_size: 2
- eval_batch_size: 8
- seed: 42
- optimizer: Adam with betas=(0.9,0.999) and epsilon=1e-08
- lr_scheduler_type: linear
- num_epochs: 5
- mixed_precision_training: Native AMP
### Training results
### Framework versions
- Transformers 4.38.2
- Pytorch 2.2.2+cu121
- Datasets 2.18.0
- Tokenizers 0.15.2
| [
"# PolizzeDonut-CR-ProvaCluster3-5Epochs\n\nThis model is a fine-tuned version of tedad09/PolizzeDonut-ChangeRequest-imm5epochs-Expand0 on the imagefolder dataset.",
"## Model description\n\nMore information needed",
"## Intended uses & limitations\n\nMore information needed",
"## Training and evaluation data\n\nMore information needed",
"## Training procedure",
"### Training hyperparameters\n\nThe following hyperparameters were used during training:\n- learning_rate: 2e-05\n- train_batch_size: 2\n- eval_batch_size: 8\n- seed: 42\n- optimizer: Adam with betas=(0.9,0.999) and epsilon=1e-08\n- lr_scheduler_type: linear\n- num_epochs: 5\n- mixed_precision_training: Native AMP",
"### Training results",
"### Framework versions\n\n- Transformers 4.38.2\n- Pytorch 2.2.2+cu121\n- Datasets 2.18.0\n- Tokenizers 0.15.2"
] | [
"TAGS\n#transformers #tensorboard #safetensors #vision-encoder-decoder #generated_from_trainer #dataset-imagefolder #base_model-tedad09/PolizzeDonut-ChangeRequest-imm5epochs-Expand0 #license-mit #endpoints_compatible #region-us \n",
"# PolizzeDonut-CR-ProvaCluster3-5Epochs\n\nThis model is a fine-tuned version of tedad09/PolizzeDonut-ChangeRequest-imm5epochs-Expand0 on the imagefolder dataset.",
"## Model description\n\nMore information needed",
"## Intended uses & limitations\n\nMore information needed",
"## Training and evaluation data\n\nMore information needed",
"## Training procedure",
"### Training hyperparameters\n\nThe following hyperparameters were used during training:\n- learning_rate: 2e-05\n- train_batch_size: 2\n- eval_batch_size: 8\n- seed: 42\n- optimizer: Adam with betas=(0.9,0.999) and epsilon=1e-08\n- lr_scheduler_type: linear\n- num_epochs: 5\n- mixed_precision_training: Native AMP",
"### Training results",
"### Framework versions\n\n- Transformers 4.38.2\n- Pytorch 2.2.2+cu121\n- Datasets 2.18.0\n- Tokenizers 0.15.2"
] |
Subsets and Splits
No community queries yet
The top public SQL queries from the community will appear here once available.