pipeline_tag
stringclasses 48
values | library_name
stringclasses 198
values | text
stringlengths 1
900k
| metadata
stringlengths 2
438k
| id
stringlengths 5
122
| last_modified
null | tags
sequencelengths 1
1.84k
| sha
null | created_at
stringlengths 25
25
| arxiv
sequencelengths 0
201
| languages
sequencelengths 0
1.83k
| tags_str
stringlengths 17
9.34k
| text_str
stringlengths 0
389k
| text_lists
sequencelengths 0
722
| processed_texts
sequencelengths 1
723
|
---|---|---|---|---|---|---|---|---|---|---|---|---|---|---|
text-generation | transformers | Quantization made by Richard Erkhov.
[Github](https://github.com/RichardErkhov)
[Discord](https://discord.gg/pvy7H8DZMG)
[Request more models](https://github.com/RichardErkhov/quant_request)
CodeLlama-34b-hf - bnb 4bits
- Model creator: https://huggingface.co/meta-llama/
- Original model: https://huggingface.co/meta-llama/CodeLlama-34b-hf/
Original model description:
---
extra_gated_heading: You need to share contact information with Meta to access this model
extra_gated_prompt: >-
### LLAMA 2 COMMUNITY LICENSE AGREEMENT
"Agreement" means the terms and conditions for use, reproduction, distribution
and modification of the Llama Materials set forth herein.
"Documentation" means the specifications, manuals and documentation
accompanying Llama 2 distributed by Meta at
https://ai.meta.com/resources/models-and-libraries/llama-downloads/.
"Licensee" or "you" means you, or your employer or any other person or entity
(if you are entering into this Agreement on such person or entity's behalf),
of the age required under applicable laws, rules or regulations to provide
legal consent and that has legal authority to bind your employer or such other
person or entity if you are entering in this Agreement on their behalf.
"Llama 2" means the foundational large language models and software and
algorithms, including machine-learning model code, trained model weights,
inference-enabling code, training-enabling code, fine-tuning enabling code and
other elements of the foregoing distributed by Meta at
ai.meta.com/resources/models-and-libraries/llama-downloads/.
"Llama Materials" means, collectively, Meta's proprietary Llama 2 and
documentation (and any portion thereof) made available under this Agreement.
"Meta" or "we" means Meta Platforms Ireland Limited (if you are located in or,
if you are an entity, your principal place of business is in the EEA or
Switzerland) and Meta Platforms, Inc. (if you are located outside of the EEA
or Switzerland).
By clicking "I Accept" below or by using or distributing any portion or
element of the Llama Materials, you agree to be bound by this Agreement.
1. License Rights and Redistribution.
a. Grant of Rights. You are granted a non-exclusive, worldwide, non-
transferable and royalty-free limited license under Meta's intellectual
property or other rights owned by Meta embodied in the Llama Materials to
use, reproduce, distribute, copy, create derivative works of, and make
modifications to the Llama Materials.
b. Redistribution and Use.
i. If you distribute or make the Llama Materials, or any derivative works
thereof, available to a third party, you shall provide a copy of this
Agreement to such third party.
ii. If you receive Llama Materials, or any derivative works thereof, from a
Licensee as part of an integrated end user product, then Section 2 of this
Agreement will not apply to you.
iii. You must retain in all copies of the Llama Materials that you distribute
the following attribution notice within a "Notice" text file distributed as a
part of such copies: "Llama 2 is licensed under the LLAMA 2 Community
License, Copyright (c) Meta Platforms, Inc. All Rights Reserved."
iv. Your use of the Llama Materials must comply with applicable laws and
regulations (including trade compliance laws and regulations) and adhere to
the Acceptable Use Policy for the Llama Materials (available at
https://ai.meta.com/llama/use-policy), which is hereby incorporated by
reference into this Agreement.
v. You will not use the Llama Materials or any output or results of the Llama
Materials to improve any other large language model (excluding Llama 2 or
derivative works thereof).
2. Additional Commercial Terms. If, on the Llama 2 version release date, the
monthly active users of the products or services made available by or for
Licensee, or Licensee's affiliates, is greater than 700 million monthly
active users in the preceding calendar month, you must request a license from
Meta, which Meta may grant to you in its sole discretion, and you are not
authorized to exercise any of the rights under this Agreement unless or until
Meta otherwise expressly grants you such rights.
3. Disclaimer of Warranty. UNLESS REQUIRED BY APPLICABLE LAW, THE LLAMA
MATERIALS AND ANY OUTPUT AND RESULTS THEREFROM ARE PROVIDED ON AN "AS IS"
BASIS, WITHOUT WARRANTIES OF ANY KIND, EITHER EXPRESS OR IMPLIED, INCLUDING,
WITHOUT LIMITATION, ANY WARRANTIES OF TITLE, NON-INFRINGEMENT,
MERCHANTABILITY, OR FITNESS FOR A PARTICULAR PURPOSE. YOU ARE SOLELY
RESPONSIBLE FOR DETERMINING THE APPROPRIATENESS OF USING OR REDISTRIBUTING
THE LLAMA MATERIALS AND ASSUME ANY RISKS ASSOCIATED WITH YOUR USE OF THE
LLAMA MATERIALS AND ANY OUTPUT AND RESULTS.
4. Limitation of Liability. IN NO EVENT WILL META OR ITS AFFILIATES BE LIABLE
UNDER ANY THEORY OF LIABILITY, WHETHER IN CONTRACT, TORT, NEGLIGENCE,
PRODUCTS LIABILITY, OR OTHERWISE, ARISING OUT OF THIS AGREEMENT, FOR ANY LOST
PROFITS OR ANY INDIRECT, SPECIAL, CONSEQUENTIAL, INCIDENTAL, EXEMPLARY OR
PUNITIVE DAMAGES, EVEN IF META OR ITS AFFILIATES HAVE BEEN ADVISED OF THE
POSSIBILITY OF ANY OF THE FOREGOING.
5. Intellectual Property.
a. No trademark licenses are granted under this Agreement, and in connection
with the Llama Materials, neither Meta nor Licensee may use any name or mark
owned by or associated with the other or any of its affiliates, except as
required for reasonable and customary use in describing and redistributing
the Llama Materials.
b. Subject to Meta's ownership of Llama Materials and derivatives made by or
for Meta, with respect to any derivative works and modifications of the Llama
Materials that are made by you, as between you and Meta, you are and will be
the owner of such derivative works and modifications.
c. If you institute litigation or other proceedings against Meta or any
entity (including a cross-claim or counterclaim in a lawsuit) alleging that
the Llama Materials or Llama 2 outputs or results, or any portion of any of
the foregoing, constitutes infringement of intellectual property or other
rights owned or licensable by you, then any licenses granted to you under
this Agreement shall terminate as of the date such litigation or claim is
filed or instituted. You will indemnify and hold harmless Meta from and
against any claim by any third party arising out of or related to your use or
distribution of the Llama Materials.
6. Term and Termination. The term of this Agreement will commence upon your
acceptance of this Agreement or access to the Llama Materials and will
continue in full force and effect until terminated in accordance with the
terms and conditions herein. Meta may terminate this Agreement if you are in
breach of any term or condition of this Agreement. Upon termination of this
Agreement, you shall delete and cease use of the Llama Materials. Sections 3,
4 and 7 shall survive the termination of this Agreement.
7. Governing Law and Jurisdiction. This Agreement will be governed and
construed under the laws of the State of California without regard to choice
of law principles, and the UN Convention on Contracts for the International
Sale of Goods does not apply to this Agreement. The courts of California
shall have exclusive jurisdiction of any dispute arising out of this
Agreement.
USE POLICY
### Llama 2 Acceptable Use Policy
Meta is committed to promoting safe and fair use of its tools and features,
including Llama 2. If you access or use Llama 2, you agree to this Acceptable
Use Policy (“Policy”). The most recent copy of this policy can be found at
[ai.meta.com/llama/use-policy](http://ai.meta.com/llama/use-policy).
#### Prohibited Uses
We want everyone to use Llama 2 safely and responsibly. You agree you will not
use, or allow others to use, Llama 2 to:
1. Violate the law or others’ rights, including to:
1. Engage in, promote, generate, contribute to, encourage, plan, incite, or further illegal or unlawful activity or content, such as:
1. Violence or terrorism
2. Exploitation or harm to children, including the solicitation, creation, acquisition, or dissemination of child exploitative content or failure to report Child Sexual Abuse Material
3. Human trafficking, exploitation, and sexual violence
4. The illegal distribution of information or materials to minors, including obscene materials, or failure to employ legally required age-gating in connection with such information or materials.
5. Sexual solicitation
6. Any other criminal activity
2. Engage in, promote, incite, or facilitate the harassment, abuse, threatening, or bullying of individuals or groups of individuals
3. Engage in, promote, incite, or facilitate discrimination or other unlawful or harmful conduct in the provision of employment, employment benefits, credit, housing, other economic benefits, or other essential goods and services
4. Engage in the unauthorized or unlicensed practice of any profession including, but not limited to, financial, legal, medical/health, or related professional practices
5. Collect, process, disclose, generate, or infer health, demographic, or other sensitive personal or private information about individuals without rights and consents required by applicable laws
6. Engage in or facilitate any action or generate any content that infringes, misappropriates, or otherwise violates any third-party rights, including the outputs or results of any products or services using the Llama 2 Materials
7. Create, generate, or facilitate the creation of malicious code, malware, computer viruses or do anything else that could disable, overburden, interfere with or impair the proper working, integrity, operation or appearance of a website or computer system
2. Engage in, promote, incite, facilitate, or assist in the planning or
development of activities that present a risk of death or bodily harm to
individuals, including use of Llama 2 related to the following:
1. Military, warfare, nuclear industries or applications, espionage, use for materials or activities that are subject to the International Traffic Arms Regulations (ITAR) maintained by the United States Department of State
2. Guns and illegal weapons (including weapon development)
3. Illegal drugs and regulated/controlled substances
4. Operation of critical infrastructure, transportation technologies, or heavy machinery
5. Self-harm or harm to others, including suicide, cutting, and eating disorders
6. Any content intended to incite or promote violence, abuse, or any infliction of bodily harm to an individual
3. Intentionally deceive or mislead others, including use of Llama 2 related
to the following:
1. Generating, promoting, or furthering fraud or the creation or promotion of disinformation
2. Generating, promoting, or furthering defamatory content, including the creation of defamatory statements, images, or other content
3. Generating, promoting, or further distributing spam
4. Impersonating another individual without consent, authorization, or legal right
5. Representing that the use of Llama 2 or outputs are human-generated
6. Generating or facilitating false online engagement, including fake reviews and other means of fake online engagement
4. Fail to appropriately disclose to end users any known dangers of your AI system
Please report any violation of this Policy, software “bug,” or other problems
that could lead to a violation of this Policy through one of the following
means:
* Reporting issues with the model:
[github.com/facebookresearch/llama](http://github.com/facebookresearch/llama)
* Reporting risky content generated by the model:
[developers.facebook.com/llama_output_feedback](http://developers.facebook.com/llama_output_feedback)
* Reporting bugs and security concerns:
[facebook.com/whitehat/info](http://facebook.com/whitehat/info)
* Reporting violations of the Acceptable Use Policy or unlicensed uses of
Llama: [[email protected]](mailto:[email protected])
extra_gated_fields:
First Name: text
Last Name: text
Date of birth: date_picker
Country: country
Affiliation: text
geo: ip_location
By clicking Submit below I accept the terms of the license and acknowledge that the information I provide will be collected stored processed and shared in accordance with the Meta Privacy Policy: checkbox
extra_gated_description: The information you provide will be collected, stored, processed and shared in accordance with the [Meta Privacy Policy](https://www.facebook.com/privacy/policy/).
extra_gated_button_content: Submit
language:
- code
pipeline_tag: text-generation
tags:
- facebook
- meta
- pytorch
- llama
- llama-2
license: llama2
---
# **Code Llama**
Code Llama is a collection of pretrained and fine-tuned generative text models ranging in scale from 7 billion to 34 billion parameters. This is the repository for the base 34B version in the Hugging Face Transformers format. This model is designed for general code synthesis and understanding. Links to other models can be found in the index at the bottom.
| | Base Model | Python | Instruct |
| --- | ----------------------------------------------------------------------------- | ------------------------------------------------------------------------------------------- | ----------------------------------------------------------------------------------------------- |
| 7B | [meta-llama/CodeLlama-7b-hf](https://huggingface.co/meta-llama/CodeLlama-7b-hf) | [meta-llama/CodeLlama-7b-Python-hf](https://huggingface.co/meta-llama/CodeLlama-7b-Python-hf) | [meta-llama/CodeLlama-7b-Instruct-hf](https://huggingface.co/meta-llama/CodeLlama-7b-Instruct-hf) |
| 13B | [meta-llama/CodeLlama-13b-hf](https://huggingface.co/meta-llama/CodeLlama-13b-hf) | [meta-llama/CodeLlama-13b-Python-hf](https://huggingface.co/meta-llama/CodeLlama-13b-Python-hf) | [meta-llama/CodeLlama-13b-Instruct-hf](https://huggingface.co/meta-llama/CodeLlama-13b-Instruct-hf) |
| 34B | [meta-llama/CodeLlama-34b-hf](https://huggingface.co/meta-llama/CodeLlama-34b-hf) | [meta-llama/CodeLlama-34b-Python-hf](https://huggingface.co/meta-llama/CodeLlama-34b-Python-hf) | [meta-llama/CodeLlama-34b-Instruct-hf](https://huggingface.co/meta-llama/CodeLlama-34b-Instruct-hf) |
| 70B | [meta-llama/CodeLlama-70b-hf](https://huggingface.co/meta-llama/CodeLlama-70b-hf) | [meta-llama/CodeLlama-70b-Python-hf](https://huggingface.co/meta-llama/CodeLlama-70b-Python-hf) | [meta-llama/CodeLlama-70b-Instruct-hf](https://huggingface.co/meta-llama/CodeLlama-70b-Instruct-hf) |
## Model Use
To use this model, please make sure to install transformers:
```bash
pip install transformers.git accelerate
```
Model capabilities:
- [x] Code completion.
- [ ] Infilling.
- [ ] Instructions / chat.
- [ ] Python specialist.
```python
from transformers import AutoTokenizer
import transformers
import torch
model = "meta-llama/CodeLlama-34b-hf"
tokenizer = AutoTokenizer.from_pretrained(model)
pipeline = transformers.pipeline(
"text-generation",
model=model,
torch_dtype=torch.float16,
device_map="auto",
)
sequences = pipeline(
'import socket\n\ndef ping_exponential_backoff(host: str):',
do_sample=True,
top_k=10,
temperature=0.1,
top_p=0.95,
num_return_sequences=1,
eos_token_id=tokenizer.eos_token_id,
max_length=200,
)
for seq in sequences:
print(f"Result: {seq['generated_text']}")
```
## Model Details
*Note: Use of this model is governed by the Meta license. Meta developed and publicly released the Code Llama family of large language models (LLMs).
**Model Developers** Meta
**Variations** Code Llama comes in three model sizes, and three variants:
* Code Llama: base models designed for general code synthesis and understanding
* Code Llama - Python: designed specifically for Python
* Code Llama - Instruct: for instruction following and safer deployment
All variants are available in sizes of 7B, 13B and 34B parameters.
**This repository contains the base version of the 34B parameters model.**
**Input** Models input text only.
**Output** Models generate text only.
**Model Architecture** Code Llama is an auto-regressive language model that uses an optimized transformer architecture.
**Model Dates** Code Llama and its variants have been trained between January 2023 and July 2023.
**Status** This is a static model trained on an offline dataset. Future versions of Code Llama - Instruct will be released as we improve model safety with community feedback.
**License** A custom commercial license is available at: [https://ai.meta.com/resources/models-and-libraries/llama-downloads/](https://ai.meta.com/resources/models-and-libraries/llama-downloads/)
**Research Paper** More information can be found in the paper "[Code Llama: Open Foundation Models for Code](https://ai.meta.com/research/publications/code-llama-open-foundation-models-for-code/)" or its [arXiv page](https://arxiv.org/abs/2308.12950).
## Intended Use
**Intended Use Cases** Code Llama and its variants is intended for commercial and research use in English and relevant programming languages. The base model Code Llama can be adapted for a variety of code synthesis and understanding tasks, Code Llama - Python is designed specifically to handle the Python programming language, and Code Llama - Instruct is intended to be safer to use for code assistant and generation applications.
**Out-of-Scope Uses** Use in any manner that violates applicable laws or regulations (including trade compliance laws). Use in languages other than English. Use in any other way that is prohibited by the Acceptable Use Policy and Licensing Agreement for Code Llama and its variants.
## Hardware and Software
**Training Factors** We used custom training libraries. The training and fine-tuning of the released models have been performed Meta’s Research Super Cluster.
**Carbon Footprint** In aggregate, training all 9 Code Llama models required 400K GPU hours of computation on hardware of type A100-80GB (TDP of 350-400W). Estimated total emissions were 65.3 tCO2eq, 100% of which were offset by Meta’s sustainability program.
## Training Data
All experiments reported here and the released models have been trained and fine-tuned using the same data as Llama 2 with different weights (see Section 2 and Table 1 in the [research paper](https://ai.meta.com/research/publications/code-llama-open-foundation-models-for-code/) for details).
## Evaluation Results
See evaluations for the main models and detailed ablations in Section 3 and safety evaluations in Section 4 of the research paper.
## Ethical Considerations and Limitations
Code Llama and its variants are a new technology that carries risks with use. Testing conducted to date has been in English, and has not covered, nor could it cover all scenarios. For these reasons, as with all LLMs, Code Llama’s potential outputs cannot be predicted in advance, and the model may in some instances produce inaccurate or objectionable responses to user prompts. Therefore, before deploying any applications of Code Llama, developers should perform safety testing and tuning tailored to their specific applications of the model.
Please see the Responsible Use Guide available available at [https://ai.meta.com/llama/responsible-use-guide](https://ai.meta.com/llama/responsible-use-guide).
| {} | RichardErkhov/meta-llama_-_CodeLlama-34b-hf-4bits | null | [
"transformers",
"safetensors",
"llama",
"text-generation",
"arxiv:2308.12950",
"autotrain_compatible",
"endpoints_compatible",
"text-generation-inference",
"4-bit",
"region:us"
] | null | 2024-04-21T17:58:59+00:00 | [
"2308.12950"
] | [] | TAGS
#transformers #safetensors #llama #text-generation #arxiv-2308.12950 #autotrain_compatible #endpoints_compatible #text-generation-inference #4-bit #region-us
| Quantization made by Richard Erkhov.
Github
Discord
Request more models
CodeLlama-34b-hf - bnb 4bits
* Model creator: URL
* Original model: URL
Original model description:
---------------------------
extra\_gated\_heading: You need to share contact information with Meta to access this model
extra\_gated\_prompt: >-
### LLAMA 2 COMMUNITY LICENSE AGREEMENT
"Agreement" means the terms and conditions for use, reproduction, distribution
and modification of the Llama Materials set forth herein.
"Documentation" means the specifications, manuals and documentation
accompanying Llama 2 distributed by Meta at
URL
"Licensee" or "you" means you, or your employer or any other person or entity
(if you are entering into this Agreement on such person or entity's behalf),
of the age required under applicable laws, rules or regulations to provide
legal consent and that has legal authority to bind your employer or such other
person or entity if you are entering in this Agreement on their behalf.
"Llama 2" means the foundational large language models and software and
algorithms, including machine-learning model code, trained model weights,
inference-enabling code, training-enabling code, fine-tuning enabling code and
other elements of the foregoing distributed by Meta at
URL
"Llama Materials" means, collectively, Meta's proprietary Llama 2 and
documentation (and any portion thereof) made available under this Agreement.
"Meta" or "we" means Meta Platforms Ireland Limited (if you are located in or,
if you are an entity, your principal place of business is in the EEA or
Switzerland) and Meta Platforms, Inc. (if you are located outside of the EEA
or Switzerland).
By clicking "I Accept" below or by using or distributing any portion or
element of the Llama Materials, you agree to be bound by this Agreement.
1. License Rights and Redistribution.
a. Grant of Rights. You are granted a non-exclusive, worldwide, non-
transferable and royalty-free limited license under Meta's intellectual
property or other rights owned by Meta embodied in the Llama Materials to
use, reproduce, distribute, copy, create derivative works of, and make
modifications to the Llama Materials.
b. Redistribution and Use.
i. If you distribute or make the Llama Materials, or any derivative works
thereof, available to a third party, you shall provide a copy of this
Agreement to such third party.
ii. If you receive Llama Materials, or any derivative works thereof, from a
Licensee as part of an integrated end user product, then Section 2 of this
Agreement will not apply to you.
iii. You must retain in all copies of the Llama Materials that you distribute
the following attribution notice within a "Notice" text file distributed as a
part of such copies: "Llama 2 is licensed under the LLAMA 2 Community
License, Copyright (c) Meta Platforms, Inc. All Rights Reserved."
iv. Your use of the Llama Materials must comply with applicable laws and
regulations (including trade compliance laws and regulations) and adhere to
the Acceptable Use Policy for the Llama Materials (available at
URL which is hereby incorporated by
reference into this Agreement.
v. You will not use the Llama Materials or any output or results of the Llama
Materials to improve any other large language model (excluding Llama 2 or
derivative works thereof).
2. Additional Commercial Terms. If, on the Llama 2 version release date, the
monthly active users of the products or services made available by or for
Licensee, or Licensee's affiliates, is greater than 700 million monthly
active users in the preceding calendar month, you must request a license from
Meta, which Meta may grant to you in its sole discretion, and you are not
authorized to exercise any of the rights under this Agreement unless or until
Meta otherwise expressly grants you such rights.
3. Disclaimer of Warranty. UNLESS REQUIRED BY APPLICABLE LAW, THE LLAMA
MATERIALS AND ANY OUTPUT AND RESULTS THEREFROM ARE PROVIDED ON AN "AS IS"
BASIS, WITHOUT WARRANTIES OF ANY KIND, EITHER EXPRESS OR IMPLIED, INCLUDING,
WITHOUT LIMITATION, ANY WARRANTIES OF TITLE, NON-INFRINGEMENT,
MERCHANTABILITY, OR FITNESS FOR A PARTICULAR PURPOSE. YOU ARE SOLELY
RESPONSIBLE FOR DETERMINING THE APPROPRIATENESS OF USING OR REDISTRIBUTING
THE LLAMA MATERIALS AND ASSUME ANY RISKS ASSOCIATED WITH YOUR USE OF THE
LLAMA MATERIALS AND ANY OUTPUT AND RESULTS.
4. Limitation of Liability. IN NO EVENT WILL META OR ITS AFFILIATES BE LIABLE
UNDER ANY THEORY OF LIABILITY, WHETHER IN CONTRACT, TORT, NEGLIGENCE,
PRODUCTS LIABILITY, OR OTHERWISE, ARISING OUT OF THIS AGREEMENT, FOR ANY LOST
PROFITS OR ANY INDIRECT, SPECIAL, CONSEQUENTIAL, INCIDENTAL, EXEMPLARY OR
PUNITIVE DAMAGES, EVEN IF META OR ITS AFFILIATES HAVE BEEN ADVISED OF THE
POSSIBILITY OF ANY OF THE FOREGOING.
5. Intellectual Property.
a. No trademark licenses are granted under this Agreement, and in connection
with the Llama Materials, neither Meta nor Licensee may use any name or mark
owned by or associated with the other or any of its affiliates, except as
required for reasonable and customary use in describing and redistributing
the Llama Materials.
b. Subject to Meta's ownership of Llama Materials and derivatives made by or
for Meta, with respect to any derivative works and modifications of the Llama
Materials that are made by you, as between you and Meta, you are and will be
the owner of such derivative works and modifications.
c. If you institute litigation or other proceedings against Meta or any
entity (including a cross-claim or counterclaim in a lawsuit) alleging that
the Llama Materials or Llama 2 outputs or results, or any portion of any of
the foregoing, constitutes infringement of intellectual property or other
rights owned or licensable by you, then any licenses granted to you under
this Agreement shall terminate as of the date such litigation or claim is
filed or instituted. You will indemnify and hold harmless Meta from and
against any claim by any third party arising out of or related to your use or
distribution of the Llama Materials.
6. Term and Termination. The term of this Agreement will commence upon your
acceptance of this Agreement or access to the Llama Materials and will
continue in full force and effect until terminated in accordance with the
terms and conditions herein. Meta may terminate this Agreement if you are in
breach of any term or condition of this Agreement. Upon termination of this
Agreement, you shall delete and cease use of the Llama Materials. Sections 3,
4 and 7 shall survive the termination of this Agreement.
7. Governing Law and Jurisdiction. This Agreement will be governed and
construed under the laws of the State of California without regard to choice
of law principles, and the UN Convention on Contracts for the International
Sale of Goods does not apply to this Agreement. The courts of California
shall have exclusive jurisdiction of any dispute arising out of this
Agreement.
USE POLICY
### Llama 2 Acceptable Use Policy
Meta is committed to promoting safe and fair use of its tools and features,
including Llama 2. If you access or use Llama 2, you agree to this Acceptable
Use Policy (“Policy”). The most recent copy of this policy can be found at
URL
#### Prohibited Uses
We want everyone to use Llama 2 safely and responsibly. You agree you will not
use, or allow others to use, Llama 2 to:
1. Violate the law or others’ rights, including to:
1. Engage in, promote, generate, contribute to, encourage, plan, incite, or further illegal or unlawful activity or content, such as:
1. Violence or terrorism
2. Exploitation or harm to children, including the solicitation, creation, acquisition, or dissemination of child exploitative content or failure to report Child Sexual Abuse Material
3. Human trafficking, exploitation, and sexual violence
4. The illegal distribution of information or materials to minors, including obscene materials, or failure to employ legally required age-gating in connection with such information or materials.
5. Sexual solicitation
6. Any other criminal activity
```
2. Engage in, promote, incite, or facilitate the harassment, abuse, threatening, or bullying of individuals or groups of individuals
3. Engage in, promote, incite, or facilitate discrimination or other unlawful or harmful conduct in the provision of employment, employment benefits, credit, housing, other economic benefits, or other essential goods and services
4. Engage in the unauthorized or unlicensed practice of any profession including, but not limited to, financial, legal, medical/health, or related professional practices
5. Collect, process, disclose, generate, or infer health, demographic, or other sensitive personal or private information about individuals without rights and consents required by applicable laws
6. Engage in or facilitate any action or generate any content that infringes, misappropriates, or otherwise violates any third-party rights, including the outputs or results of any products or services using the Llama 2 Materials
7. Create, generate, or facilitate the creation of malicious code, malware, computer viruses or do anything else that could disable, overburden, interfere with or impair the proper working, integrity, operation or appearance of a website or computer system
```
2. Engage in, promote, incite, facilitate, or assist in the planning or
development of activities that present a risk of death or bodily harm to
individuals, including use of Llama 2 related to the following:
1. Military, warfare, nuclear industries or applications, espionage, use for materials or activities that are subject to the International Traffic Arms Regulations (ITAR) maintained by the United States Department of State
2. Guns and illegal weapons (including weapon development)
3. Illegal drugs and regulated/controlled substances
4. Operation of critical infrastructure, transportation technologies, or heavy machinery
5. Self-harm or harm to others, including suicide, cutting, and eating disorders
6. Any content intended to incite or promote violence, abuse, or any infliction of bodily harm to an individual
3. Intentionally deceive or mislead others, including use of Llama 2 related
to the following:
1. Generating, promoting, or furthering fraud or the creation or promotion of disinformation
2. Generating, promoting, or furthering defamatory content, including the creation of defamatory statements, images, or other content
3. Generating, promoting, or further distributing spam
4. Impersonating another individual without consent, authorization, or legal right
5. Representing that the use of Llama 2 or outputs are human-generated
6. Generating or facilitating false online engagement, including fake reviews and other means of fake online engagement
4. Fail to appropriately disclose to end users any known dangers of your AI system
Please report any violation of this Policy, software “bug,” or other problems
that could lead to a violation of this Policy through one of the following
means:
* Reporting issues with the model:
URL
* Reporting risky content generated by the model:
URL
* Reporting bugs and security concerns:
URL
* Reporting violations of the Acceptable Use Policy or unlicensed uses of
Llama: LlamaUseReport@URL
extra\_gated\_fields:
First Name: text
Last Name: text
Date of birth: date\_picker
Country: country
Affiliation: text
geo: ip\_location
By clicking Submit below I accept the terms of the license and acknowledge that the information I provide will be collected stored processed and shared in accordance with the Meta Privacy Policy: checkbox
extra\_gated\_description: The information you provide will be collected, stored, processed and shared in accordance with the Meta Privacy Policy.
extra\_gated\_button\_content: Submit
language:
* code
pipeline\_tag: text-generation
tags:
* facebook
* meta
* pytorch
* llama
* llama-2
license: llama2
---
Code Llama
==========
Code Llama is a collection of pretrained and fine-tuned generative text models ranging in scale from 7 billion to 34 billion parameters. This is the repository for the base 34B version in the Hugging Face Transformers format. This model is designed for general code synthesis and understanding. Links to other models can be found in the index at the bottom.
Model Use
---------
To use this model, please make sure to install transformers:
Model capabilities:
* [x] Code completion.
* [ ] Infilling.
* [ ] Instructions / chat.
* [ ] Python specialist.
Model Details
-------------
\*Note: Use of this model is governed by the Meta license. Meta developed and publicly released the Code Llama family of large language models (LLMs).
Model Developers Meta
Variations Code Llama comes in three model sizes, and three variants:
* Code Llama: base models designed for general code synthesis and understanding
* Code Llama - Python: designed specifically for Python
* Code Llama - Instruct: for instruction following and safer deployment
All variants are available in sizes of 7B, 13B and 34B parameters.
This repository contains the base version of the 34B parameters model.
Input Models input text only.
Output Models generate text only.
Model Architecture Code Llama is an auto-regressive language model that uses an optimized transformer architecture.
Model Dates Code Llama and its variants have been trained between January 2023 and July 2023.
Status This is a static model trained on an offline dataset. Future versions of Code Llama - Instruct will be released as we improve model safety with community feedback.
License A custom commercial license is available at: URL
Research Paper More information can be found in the paper "Code Llama: Open Foundation Models for Code" or its arXiv page.
Intended Use
------------
Intended Use Cases Code Llama and its variants is intended for commercial and research use in English and relevant programming languages. The base model Code Llama can be adapted for a variety of code synthesis and understanding tasks, Code Llama - Python is designed specifically to handle the Python programming language, and Code Llama - Instruct is intended to be safer to use for code assistant and generation applications.
Out-of-Scope Uses Use in any manner that violates applicable laws or regulations (including trade compliance laws). Use in languages other than English. Use in any other way that is prohibited by the Acceptable Use Policy and Licensing Agreement for Code Llama and its variants.
Hardware and Software
---------------------
Training Factors We used custom training libraries. The training and fine-tuning of the released models have been performed Meta’s Research Super Cluster.
Carbon Footprint In aggregate, training all 9 Code Llama models required 400K GPU hours of computation on hardware of type A100-80GB (TDP of 350-400W). Estimated total emissions were 65.3 tCO2eq, 100% of which were offset by Meta’s sustainability program.
Training Data
-------------
All experiments reported here and the released models have been trained and fine-tuned using the same data as Llama 2 with different weights (see Section 2 and Table 1 in the research paper for details).
Evaluation Results
------------------
See evaluations for the main models and detailed ablations in Section 3 and safety evaluations in Section 4 of the research paper.
Ethical Considerations and Limitations
--------------------------------------
Code Llama and its variants are a new technology that carries risks with use. Testing conducted to date has been in English, and has not covered, nor could it cover all scenarios. For these reasons, as with all LLMs, Code Llama’s potential outputs cannot be predicted in advance, and the model may in some instances produce inaccurate or objectionable responses to user prompts. Therefore, before deploying any applications of Code Llama, developers should perform safety testing and tuning tailored to their specific applications of the model.
Please see the Responsible Use Guide available available at URL
| [
"### LLAMA 2 COMMUNITY LICENSE AGREEMENT\n\n\n\"Agreement\" means the terms and conditions for use, reproduction, distribution\nand modification of the Llama Materials set forth herein.\n\"Documentation\" means the specifications, manuals and documentation\naccompanying Llama 2 distributed by Meta at\nURL \n\n\"Licensee\" or \"you\" means you, or your employer or any other person or entity\n(if you are entering into this Agreement on such person or entity's behalf),\nof the age required under applicable laws, rules or regulations to provide\nlegal consent and that has legal authority to bind your employer or such other\nperson or entity if you are entering in this Agreement on their behalf.\n\"Llama 2\" means the foundational large language models and software and\nalgorithms, including machine-learning model code, trained model weights,\ninference-enabling code, training-enabling code, fine-tuning enabling code and\nother elements of the foregoing distributed by Meta at\nURL\n\"Llama Materials\" means, collectively, Meta's proprietary Llama 2 and\ndocumentation (and any portion thereof) made available under this Agreement.\n\"Meta\" or \"we\" means Meta Platforms Ireland Limited (if you are located in or,\nif you are an entity, your principal place of business is in the EEA or\nSwitzerland) and Meta Platforms, Inc. (if you are located outside of the EEA\nor Switzerland).\nBy clicking \"I Accept\" below or by using or distributing any portion or\nelement of the Llama Materials, you agree to be bound by this Agreement.\n\n\n1. License Rights and Redistribution.\na. Grant of Rights. You are granted a non-exclusive, worldwide, non-\ntransferable and royalty-free limited license under Meta's intellectual\nproperty or other rights owned by Meta embodied in the Llama Materials to\nuse, reproduce, distribute, copy, create derivative works of, and make\nmodifications to the Llama Materials.\n\n\nb. Redistribution and Use. \n\ni. If you distribute or make the Llama Materials, or any derivative works\nthereof, available to a third party, you shall provide a copy of this\nAgreement to such third party.\nii. If you receive Llama Materials, or any derivative works thereof, from a\nLicensee as part of an integrated end user product, then Section 2 of this\nAgreement will not apply to you.\niii. You must retain in all copies of the Llama Materials that you distribute\nthe following attribution notice within a \"Notice\" text file distributed as a\npart of such copies: \"Llama 2 is licensed under the LLAMA 2 Community\nLicense, Copyright (c) Meta Platforms, Inc. All Rights Reserved.\"\niv. Your use of the Llama Materials must comply with applicable laws and\nregulations (including trade compliance laws and regulations) and adhere to\nthe Acceptable Use Policy for the Llama Materials (available at\nURL which is hereby incorporated by\nreference into this Agreement.\nv. You will not use the Llama Materials or any output or results of the Llama\nMaterials to improve any other large language model (excluding Llama 2 or\nderivative works thereof). \n\n2. Additional Commercial Terms. If, on the Llama 2 version release date, the\nmonthly active users of the products or services made available by or for\nLicensee, or Licensee's affiliates, is greater than 700 million monthly\nactive users in the preceding calendar month, you must request a license from\nMeta, which Meta may grant to you in its sole discretion, and you are not\nauthorized to exercise any of the rights under this Agreement unless or until\nMeta otherwise expressly grants you such rights.\n3. Disclaimer of Warranty. UNLESS REQUIRED BY APPLICABLE LAW, THE LLAMA\nMATERIALS AND ANY OUTPUT AND RESULTS THEREFROM ARE PROVIDED ON AN \"AS IS\"\nBASIS, WITHOUT WARRANTIES OF ANY KIND, EITHER EXPRESS OR IMPLIED, INCLUDING,\nWITHOUT LIMITATION, ANY WARRANTIES OF TITLE, NON-INFRINGEMENT,\nMERCHANTABILITY, OR FITNESS FOR A PARTICULAR PURPOSE. YOU ARE SOLELY\nRESPONSIBLE FOR DETERMINING THE APPROPRIATENESS OF USING OR REDISTRIBUTING\nTHE LLAMA MATERIALS AND ASSUME ANY RISKS ASSOCIATED WITH YOUR USE OF THE\nLLAMA MATERIALS AND ANY OUTPUT AND RESULTS.\n4. Limitation of Liability. IN NO EVENT WILL META OR ITS AFFILIATES BE LIABLE\nUNDER ANY THEORY OF LIABILITY, WHETHER IN CONTRACT, TORT, NEGLIGENCE,\nPRODUCTS LIABILITY, OR OTHERWISE, ARISING OUT OF THIS AGREEMENT, FOR ANY LOST\nPROFITS OR ANY INDIRECT, SPECIAL, CONSEQUENTIAL, INCIDENTAL, EXEMPLARY OR\nPUNITIVE DAMAGES, EVEN IF META OR ITS AFFILIATES HAVE BEEN ADVISED OF THE\nPOSSIBILITY OF ANY OF THE FOREGOING.\n5. Intellectual Property.\na. No trademark licenses are granted under this Agreement, and in connection\nwith the Llama Materials, neither Meta nor Licensee may use any name or mark\nowned by or associated with the other or any of its affiliates, except as\nrequired for reasonable and customary use in describing and redistributing\nthe Llama Materials.\nb. Subject to Meta's ownership of Llama Materials and derivatives made by or\nfor Meta, with respect to any derivative works and modifications of the Llama\nMaterials that are made by you, as between you and Meta, you are and will be\nthe owner of such derivative works and modifications.\nc. If you institute litigation or other proceedings against Meta or any\nentity (including a cross-claim or counterclaim in a lawsuit) alleging that\nthe Llama Materials or Llama 2 outputs or results, or any portion of any of\nthe foregoing, constitutes infringement of intellectual property or other\nrights owned or licensable by you, then any licenses granted to you under\nthis Agreement shall terminate as of the date such litigation or claim is\nfiled or instituted. You will indemnify and hold harmless Meta from and\nagainst any claim by any third party arising out of or related to your use or\ndistribution of the Llama Materials.\n6. Term and Termination. The term of this Agreement will commence upon your\nacceptance of this Agreement or access to the Llama Materials and will\ncontinue in full force and effect until terminated in accordance with the\nterms and conditions herein. Meta may terminate this Agreement if you are in\nbreach of any term or condition of this Agreement. Upon termination of this\nAgreement, you shall delete and cease use of the Llama Materials. Sections 3,\n4 and 7 shall survive the termination of this Agreement.\n7. Governing Law and Jurisdiction. This Agreement will be governed and\nconstrued under the laws of the State of California without regard to choice\nof law principles, and the UN Convention on Contracts for the International\nSale of Goods does not apply to this Agreement. The courts of California\nshall have exclusive jurisdiction of any dispute arising out of this\nAgreement.\nUSE POLICY",
"### Llama 2 Acceptable Use Policy\n\n\nMeta is committed to promoting safe and fair use of its tools and features,\nincluding Llama 2. If you access or use Llama 2, you agree to this Acceptable\nUse Policy (“Policy”). The most recent copy of this policy can be found at\nURL",
"#### Prohibited Uses\n\n\nWe want everyone to use Llama 2 safely and responsibly. You agree you will not\nuse, or allow others to use, Llama 2 to:\n\n\n1. Violate the law or others’ rights, including to:\n1. Engage in, promote, generate, contribute to, encourage, plan, incite, or further illegal or unlawful activity or content, such as:\n\t1. Violence or terrorism\n\t2. Exploitation or harm to children, including the solicitation, creation, acquisition, or dissemination of child exploitative content or failure to report Child Sexual Abuse Material\n\t3. Human trafficking, exploitation, and sexual violence\n\t4. The illegal distribution of information or materials to minors, including obscene materials, or failure to employ legally required age-gating in connection with such information or materials.\n\t5. Sexual solicitation\n\t6. Any other criminal activity\n\n\n\n```\n2. Engage in, promote, incite, or facilitate the harassment, abuse, threatening, or bullying of individuals or groups of individuals\n3. Engage in, promote, incite, or facilitate discrimination or other unlawful or harmful conduct in the provision of employment, employment benefits, credit, housing, other economic benefits, or other essential goods and services\n4. Engage in the unauthorized or unlicensed practice of any profession including, but not limited to, financial, legal, medical/health, or related professional practices \n5. Collect, process, disclose, generate, or infer health, demographic, or other sensitive personal or private information about individuals without rights and consents required by applicable laws\n6. Engage in or facilitate any action or generate any content that infringes, misappropriates, or otherwise violates any third-party rights, including the outputs or results of any products or services using the Llama 2 Materials\n7. Create, generate, or facilitate the creation of malicious code, malware, computer viruses or do anything else that could disable, overburden, interfere with or impair the proper working, integrity, operation or appearance of a website or computer system \n\n```\n\n2. Engage in, promote, incite, facilitate, or assist in the planning or\ndevelopment of activities that present a risk of death or bodily harm to\nindividuals, including use of Llama 2 related to the following:\n1. Military, warfare, nuclear industries or applications, espionage, use for materials or activities that are subject to the International Traffic Arms Regulations (ITAR) maintained by the United States Department of State\n2. Guns and illegal weapons (including weapon development)\n3. Illegal drugs and regulated/controlled substances\n4. Operation of critical infrastructure, transportation technologies, or heavy machinery\n5. Self-harm or harm to others, including suicide, cutting, and eating disorders\n6. Any content intended to incite or promote violence, abuse, or any infliction of bodily harm to an individual\n3. Intentionally deceive or mislead others, including use of Llama 2 related\nto the following:\n1. Generating, promoting, or furthering fraud or the creation or promotion of disinformation\n2. Generating, promoting, or furthering defamatory content, including the creation of defamatory statements, images, or other content\n3. Generating, promoting, or further distributing spam\n4. Impersonating another individual without consent, authorization, or legal right\n5. Representing that the use of Llama 2 or outputs are human-generated\n6. Generating or facilitating false online engagement, including fake reviews and other means of fake online engagement\n4. Fail to appropriately disclose to end users any known dangers of your AI system\nPlease report any violation of this Policy, software “bug,” or other problems\nthat could lead to a violation of this Policy through one of the following\nmeans:\n\n\n* Reporting issues with the model:\nURL\n* Reporting risky content generated by the model:\nURL\n* Reporting bugs and security concerns:\nURL\n* Reporting violations of the Acceptable Use Policy or unlicensed uses of\nLlama: LlamaUseReport@URL\nextra\\_gated\\_fields:\nFirst Name: text\nLast Name: text\nDate of birth: date\\_picker\nCountry: country\nAffiliation: text\ngeo: ip\\_location \n\nBy clicking Submit below I accept the terms of the license and acknowledge that the information I provide will be collected stored processed and shared in accordance with the Meta Privacy Policy: checkbox\nextra\\_gated\\_description: The information you provide will be collected, stored, processed and shared in accordance with the Meta Privacy Policy.\nextra\\_gated\\_button\\_content: Submit\nlanguage:\n\n\n* code\npipeline\\_tag: text-generation\ntags:\n* facebook\n* meta\n* pytorch\n* llama\n* llama-2\nlicense: llama2\n\n\n\n\n---\n\n\nCode Llama\n==========\n\n\nCode Llama is a collection of pretrained and fine-tuned generative text models ranging in scale from 7 billion to 34 billion parameters. This is the repository for the base 34B version in the Hugging Face Transformers format. This model is designed for general code synthesis and understanding. Links to other models can be found in the index at the bottom.\n\n\n\nModel Use\n---------\n\n\nTo use this model, please make sure to install transformers:\n\n\nModel capabilities:\n\n\n* [x] Code completion.\n* [ ] Infilling.\n* [ ] Instructions / chat.\n* [ ] Python specialist.\n\n\nModel Details\n-------------\n\n\n\\*Note: Use of this model is governed by the Meta license. Meta developed and publicly released the Code Llama family of large language models (LLMs).\n\n\nModel Developers Meta\n\n\nVariations Code Llama comes in three model sizes, and three variants:\n\n\n* Code Llama: base models designed for general code synthesis and understanding\n* Code Llama - Python: designed specifically for Python\n* Code Llama - Instruct: for instruction following and safer deployment\n\n\nAll variants are available in sizes of 7B, 13B and 34B parameters.\n\n\nThis repository contains the base version of the 34B parameters model.\n\n\nInput Models input text only.\n\n\nOutput Models generate text only.\n\n\nModel Architecture Code Llama is an auto-regressive language model that uses an optimized transformer architecture.\n\n\nModel Dates Code Llama and its variants have been trained between January 2023 and July 2023.\n\n\nStatus This is a static model trained on an offline dataset. Future versions of Code Llama - Instruct will be released as we improve model safety with community feedback.\n\n\nLicense A custom commercial license is available at: URL\n\n\nResearch Paper More information can be found in the paper \"Code Llama: Open Foundation Models for Code\" or its arXiv page.\n\n\nIntended Use\n------------\n\n\nIntended Use Cases Code Llama and its variants is intended for commercial and research use in English and relevant programming languages. The base model Code Llama can be adapted for a variety of code synthesis and understanding tasks, Code Llama - Python is designed specifically to handle the Python programming language, and Code Llama - Instruct is intended to be safer to use for code assistant and generation applications.\n\n\nOut-of-Scope Uses Use in any manner that violates applicable laws or regulations (including trade compliance laws). Use in languages other than English. Use in any other way that is prohibited by the Acceptable Use Policy and Licensing Agreement for Code Llama and its variants.\n\n\nHardware and Software\n---------------------\n\n\nTraining Factors We used custom training libraries. The training and fine-tuning of the released models have been performed Meta’s Research Super Cluster.\n\n\nCarbon Footprint In aggregate, training all 9 Code Llama models required 400K GPU hours of computation on hardware of type A100-80GB (TDP of 350-400W). Estimated total emissions were 65.3 tCO2eq, 100% of which were offset by Meta’s sustainability program.\n\n\nTraining Data\n-------------\n\n\nAll experiments reported here and the released models have been trained and fine-tuned using the same data as Llama 2 with different weights (see Section 2 and Table 1 in the research paper for details).\n\n\nEvaluation Results\n------------------\n\n\nSee evaluations for the main models and detailed ablations in Section 3 and safety evaluations in Section 4 of the research paper.\n\n\nEthical Considerations and Limitations\n--------------------------------------\n\n\nCode Llama and its variants are a new technology that carries risks with use. Testing conducted to date has been in English, and has not covered, nor could it cover all scenarios. For these reasons, as with all LLMs, Code Llama’s potential outputs cannot be predicted in advance, and the model may in some instances produce inaccurate or objectionable responses to user prompts. Therefore, before deploying any applications of Code Llama, developers should perform safety testing and tuning tailored to their specific applications of the model.\n\n\nPlease see the Responsible Use Guide available available at URL"
] | [
"TAGS\n#transformers #safetensors #llama #text-generation #arxiv-2308.12950 #autotrain_compatible #endpoints_compatible #text-generation-inference #4-bit #region-us \n",
"### LLAMA 2 COMMUNITY LICENSE AGREEMENT\n\n\n\"Agreement\" means the terms and conditions for use, reproduction, distribution\nand modification of the Llama Materials set forth herein.\n\"Documentation\" means the specifications, manuals and documentation\naccompanying Llama 2 distributed by Meta at\nURL \n\n\"Licensee\" or \"you\" means you, or your employer or any other person or entity\n(if you are entering into this Agreement on such person or entity's behalf),\nof the age required under applicable laws, rules or regulations to provide\nlegal consent and that has legal authority to bind your employer or such other\nperson or entity if you are entering in this Agreement on their behalf.\n\"Llama 2\" means the foundational large language models and software and\nalgorithms, including machine-learning model code, trained model weights,\ninference-enabling code, training-enabling code, fine-tuning enabling code and\nother elements of the foregoing distributed by Meta at\nURL\n\"Llama Materials\" means, collectively, Meta's proprietary Llama 2 and\ndocumentation (and any portion thereof) made available under this Agreement.\n\"Meta\" or \"we\" means Meta Platforms Ireland Limited (if you are located in or,\nif you are an entity, your principal place of business is in the EEA or\nSwitzerland) and Meta Platforms, Inc. (if you are located outside of the EEA\nor Switzerland).\nBy clicking \"I Accept\" below or by using or distributing any portion or\nelement of the Llama Materials, you agree to be bound by this Agreement.\n\n\n1. License Rights and Redistribution.\na. Grant of Rights. You are granted a non-exclusive, worldwide, non-\ntransferable and royalty-free limited license under Meta's intellectual\nproperty or other rights owned by Meta embodied in the Llama Materials to\nuse, reproduce, distribute, copy, create derivative works of, and make\nmodifications to the Llama Materials.\n\n\nb. Redistribution and Use. \n\ni. If you distribute or make the Llama Materials, or any derivative works\nthereof, available to a third party, you shall provide a copy of this\nAgreement to such third party.\nii. If you receive Llama Materials, or any derivative works thereof, from a\nLicensee as part of an integrated end user product, then Section 2 of this\nAgreement will not apply to you.\niii. You must retain in all copies of the Llama Materials that you distribute\nthe following attribution notice within a \"Notice\" text file distributed as a\npart of such copies: \"Llama 2 is licensed under the LLAMA 2 Community\nLicense, Copyright (c) Meta Platforms, Inc. All Rights Reserved.\"\niv. Your use of the Llama Materials must comply with applicable laws and\nregulations (including trade compliance laws and regulations) and adhere to\nthe Acceptable Use Policy for the Llama Materials (available at\nURL which is hereby incorporated by\nreference into this Agreement.\nv. You will not use the Llama Materials or any output or results of the Llama\nMaterials to improve any other large language model (excluding Llama 2 or\nderivative works thereof). \n\n2. Additional Commercial Terms. If, on the Llama 2 version release date, the\nmonthly active users of the products or services made available by or for\nLicensee, or Licensee's affiliates, is greater than 700 million monthly\nactive users in the preceding calendar month, you must request a license from\nMeta, which Meta may grant to you in its sole discretion, and you are not\nauthorized to exercise any of the rights under this Agreement unless or until\nMeta otherwise expressly grants you such rights.\n3. Disclaimer of Warranty. UNLESS REQUIRED BY APPLICABLE LAW, THE LLAMA\nMATERIALS AND ANY OUTPUT AND RESULTS THEREFROM ARE PROVIDED ON AN \"AS IS\"\nBASIS, WITHOUT WARRANTIES OF ANY KIND, EITHER EXPRESS OR IMPLIED, INCLUDING,\nWITHOUT LIMITATION, ANY WARRANTIES OF TITLE, NON-INFRINGEMENT,\nMERCHANTABILITY, OR FITNESS FOR A PARTICULAR PURPOSE. YOU ARE SOLELY\nRESPONSIBLE FOR DETERMINING THE APPROPRIATENESS OF USING OR REDISTRIBUTING\nTHE LLAMA MATERIALS AND ASSUME ANY RISKS ASSOCIATED WITH YOUR USE OF THE\nLLAMA MATERIALS AND ANY OUTPUT AND RESULTS.\n4. Limitation of Liability. IN NO EVENT WILL META OR ITS AFFILIATES BE LIABLE\nUNDER ANY THEORY OF LIABILITY, WHETHER IN CONTRACT, TORT, NEGLIGENCE,\nPRODUCTS LIABILITY, OR OTHERWISE, ARISING OUT OF THIS AGREEMENT, FOR ANY LOST\nPROFITS OR ANY INDIRECT, SPECIAL, CONSEQUENTIAL, INCIDENTAL, EXEMPLARY OR\nPUNITIVE DAMAGES, EVEN IF META OR ITS AFFILIATES HAVE BEEN ADVISED OF THE\nPOSSIBILITY OF ANY OF THE FOREGOING.\n5. Intellectual Property.\na. No trademark licenses are granted under this Agreement, and in connection\nwith the Llama Materials, neither Meta nor Licensee may use any name or mark\nowned by or associated with the other or any of its affiliates, except as\nrequired for reasonable and customary use in describing and redistributing\nthe Llama Materials.\nb. Subject to Meta's ownership of Llama Materials and derivatives made by or\nfor Meta, with respect to any derivative works and modifications of the Llama\nMaterials that are made by you, as between you and Meta, you are and will be\nthe owner of such derivative works and modifications.\nc. If you institute litigation or other proceedings against Meta or any\nentity (including a cross-claim or counterclaim in a lawsuit) alleging that\nthe Llama Materials or Llama 2 outputs or results, or any portion of any of\nthe foregoing, constitutes infringement of intellectual property or other\nrights owned or licensable by you, then any licenses granted to you under\nthis Agreement shall terminate as of the date such litigation or claim is\nfiled or instituted. You will indemnify and hold harmless Meta from and\nagainst any claim by any third party arising out of or related to your use or\ndistribution of the Llama Materials.\n6. Term and Termination. The term of this Agreement will commence upon your\nacceptance of this Agreement or access to the Llama Materials and will\ncontinue in full force and effect until terminated in accordance with the\nterms and conditions herein. Meta may terminate this Agreement if you are in\nbreach of any term or condition of this Agreement. Upon termination of this\nAgreement, you shall delete and cease use of the Llama Materials. Sections 3,\n4 and 7 shall survive the termination of this Agreement.\n7. Governing Law and Jurisdiction. This Agreement will be governed and\nconstrued under the laws of the State of California without regard to choice\nof law principles, and the UN Convention on Contracts for the International\nSale of Goods does not apply to this Agreement. The courts of California\nshall have exclusive jurisdiction of any dispute arising out of this\nAgreement.\nUSE POLICY",
"### Llama 2 Acceptable Use Policy\n\n\nMeta is committed to promoting safe and fair use of its tools and features,\nincluding Llama 2. If you access or use Llama 2, you agree to this Acceptable\nUse Policy (“Policy”). The most recent copy of this policy can be found at\nURL",
"#### Prohibited Uses\n\n\nWe want everyone to use Llama 2 safely and responsibly. You agree you will not\nuse, or allow others to use, Llama 2 to:\n\n\n1. Violate the law or others’ rights, including to:\n1. Engage in, promote, generate, contribute to, encourage, plan, incite, or further illegal or unlawful activity or content, such as:\n\t1. Violence or terrorism\n\t2. Exploitation or harm to children, including the solicitation, creation, acquisition, or dissemination of child exploitative content or failure to report Child Sexual Abuse Material\n\t3. Human trafficking, exploitation, and sexual violence\n\t4. The illegal distribution of information or materials to minors, including obscene materials, or failure to employ legally required age-gating in connection with such information or materials.\n\t5. Sexual solicitation\n\t6. Any other criminal activity\n\n\n\n```\n2. Engage in, promote, incite, or facilitate the harassment, abuse, threatening, or bullying of individuals or groups of individuals\n3. Engage in, promote, incite, or facilitate discrimination or other unlawful or harmful conduct in the provision of employment, employment benefits, credit, housing, other economic benefits, or other essential goods and services\n4. Engage in the unauthorized or unlicensed practice of any profession including, but not limited to, financial, legal, medical/health, or related professional practices \n5. Collect, process, disclose, generate, or infer health, demographic, or other sensitive personal or private information about individuals without rights and consents required by applicable laws\n6. Engage in or facilitate any action or generate any content that infringes, misappropriates, or otherwise violates any third-party rights, including the outputs or results of any products or services using the Llama 2 Materials\n7. Create, generate, or facilitate the creation of malicious code, malware, computer viruses or do anything else that could disable, overburden, interfere with or impair the proper working, integrity, operation or appearance of a website or computer system \n\n```\n\n2. Engage in, promote, incite, facilitate, or assist in the planning or\ndevelopment of activities that present a risk of death or bodily harm to\nindividuals, including use of Llama 2 related to the following:\n1. Military, warfare, nuclear industries or applications, espionage, use for materials or activities that are subject to the International Traffic Arms Regulations (ITAR) maintained by the United States Department of State\n2. Guns and illegal weapons (including weapon development)\n3. Illegal drugs and regulated/controlled substances\n4. Operation of critical infrastructure, transportation technologies, or heavy machinery\n5. Self-harm or harm to others, including suicide, cutting, and eating disorders\n6. Any content intended to incite or promote violence, abuse, or any infliction of bodily harm to an individual\n3. Intentionally deceive or mislead others, including use of Llama 2 related\nto the following:\n1. Generating, promoting, or furthering fraud or the creation or promotion of disinformation\n2. Generating, promoting, or furthering defamatory content, including the creation of defamatory statements, images, or other content\n3. Generating, promoting, or further distributing spam\n4. Impersonating another individual without consent, authorization, or legal right\n5. Representing that the use of Llama 2 or outputs are human-generated\n6. Generating or facilitating false online engagement, including fake reviews and other means of fake online engagement\n4. Fail to appropriately disclose to end users any known dangers of your AI system\nPlease report any violation of this Policy, software “bug,” or other problems\nthat could lead to a violation of this Policy through one of the following\nmeans:\n\n\n* Reporting issues with the model:\nURL\n* Reporting risky content generated by the model:\nURL\n* Reporting bugs and security concerns:\nURL\n* Reporting violations of the Acceptable Use Policy or unlicensed uses of\nLlama: LlamaUseReport@URL\nextra\\_gated\\_fields:\nFirst Name: text\nLast Name: text\nDate of birth: date\\_picker\nCountry: country\nAffiliation: text\ngeo: ip\\_location \n\nBy clicking Submit below I accept the terms of the license and acknowledge that the information I provide will be collected stored processed and shared in accordance with the Meta Privacy Policy: checkbox\nextra\\_gated\\_description: The information you provide will be collected, stored, processed and shared in accordance with the Meta Privacy Policy.\nextra\\_gated\\_button\\_content: Submit\nlanguage:\n\n\n* code\npipeline\\_tag: text-generation\ntags:\n* facebook\n* meta\n* pytorch\n* llama\n* llama-2\nlicense: llama2\n\n\n\n\n---\n\n\nCode Llama\n==========\n\n\nCode Llama is a collection of pretrained and fine-tuned generative text models ranging in scale from 7 billion to 34 billion parameters. This is the repository for the base 34B version in the Hugging Face Transformers format. This model is designed for general code synthesis and understanding. Links to other models can be found in the index at the bottom.\n\n\n\nModel Use\n---------\n\n\nTo use this model, please make sure to install transformers:\n\n\nModel capabilities:\n\n\n* [x] Code completion.\n* [ ] Infilling.\n* [ ] Instructions / chat.\n* [ ] Python specialist.\n\n\nModel Details\n-------------\n\n\n\\*Note: Use of this model is governed by the Meta license. Meta developed and publicly released the Code Llama family of large language models (LLMs).\n\n\nModel Developers Meta\n\n\nVariations Code Llama comes in three model sizes, and three variants:\n\n\n* Code Llama: base models designed for general code synthesis and understanding\n* Code Llama - Python: designed specifically for Python\n* Code Llama - Instruct: for instruction following and safer deployment\n\n\nAll variants are available in sizes of 7B, 13B and 34B parameters.\n\n\nThis repository contains the base version of the 34B parameters model.\n\n\nInput Models input text only.\n\n\nOutput Models generate text only.\n\n\nModel Architecture Code Llama is an auto-regressive language model that uses an optimized transformer architecture.\n\n\nModel Dates Code Llama and its variants have been trained between January 2023 and July 2023.\n\n\nStatus This is a static model trained on an offline dataset. Future versions of Code Llama - Instruct will be released as we improve model safety with community feedback.\n\n\nLicense A custom commercial license is available at: URL\n\n\nResearch Paper More information can be found in the paper \"Code Llama: Open Foundation Models for Code\" or its arXiv page.\n\n\nIntended Use\n------------\n\n\nIntended Use Cases Code Llama and its variants is intended for commercial and research use in English and relevant programming languages. The base model Code Llama can be adapted for a variety of code synthesis and understanding tasks, Code Llama - Python is designed specifically to handle the Python programming language, and Code Llama - Instruct is intended to be safer to use for code assistant and generation applications.\n\n\nOut-of-Scope Uses Use in any manner that violates applicable laws or regulations (including trade compliance laws). Use in languages other than English. Use in any other way that is prohibited by the Acceptable Use Policy and Licensing Agreement for Code Llama and its variants.\n\n\nHardware and Software\n---------------------\n\n\nTraining Factors We used custom training libraries. The training and fine-tuning of the released models have been performed Meta’s Research Super Cluster.\n\n\nCarbon Footprint In aggregate, training all 9 Code Llama models required 400K GPU hours of computation on hardware of type A100-80GB (TDP of 350-400W). Estimated total emissions were 65.3 tCO2eq, 100% of which were offset by Meta’s sustainability program.\n\n\nTraining Data\n-------------\n\n\nAll experiments reported here and the released models have been trained and fine-tuned using the same data as Llama 2 with different weights (see Section 2 and Table 1 in the research paper for details).\n\n\nEvaluation Results\n------------------\n\n\nSee evaluations for the main models and detailed ablations in Section 3 and safety evaluations in Section 4 of the research paper.\n\n\nEthical Considerations and Limitations\n--------------------------------------\n\n\nCode Llama and its variants are a new technology that carries risks with use. Testing conducted to date has been in English, and has not covered, nor could it cover all scenarios. For these reasons, as with all LLMs, Code Llama’s potential outputs cannot be predicted in advance, and the model may in some instances produce inaccurate or objectionable responses to user prompts. Therefore, before deploying any applications of Code Llama, developers should perform safety testing and tuning tailored to their specific applications of the model.\n\n\nPlease see the Responsible Use Guide available available at URL"
] |
reinforcement-learning | ml-agents |
# **ppo** Agent playing **Pyramids**
This is a trained model of a **ppo** agent playing **Pyramids**
using the [Unity ML-Agents Library](https://github.com/Unity-Technologies/ml-agents).
## Usage (with ML-Agents)
The Documentation: https://unity-technologies.github.io/ml-agents/ML-Agents-Toolkit-Documentation/
We wrote a complete tutorial to learn to train your first agent using ML-Agents and publish it to the Hub:
- A *short tutorial* where you teach Huggy the Dog 🐶 to fetch the stick and then play with him directly in your
browser: https://huggingface.co/learn/deep-rl-course/unitbonus1/introduction
- A *longer tutorial* to understand how works ML-Agents:
https://huggingface.co/learn/deep-rl-course/unit5/introduction
### Resume the training
```bash
mlagents-learn <your_configuration_file_path.yaml> --run-id=<run_id> --resume
```
### Watch your Agent play
You can watch your agent **playing directly in your browser**
1. If the environment is part of ML-Agents official environments, go to https://huggingface.co/unity
2. Step 1: Find your model_id: tarpalsus/ppo-Pyramids
3. Step 2: Select your *.nn /*.onnx file
4. Click on Watch the agent play 👀
| {"library_name": "ml-agents", "tags": ["Pyramids", "deep-reinforcement-learning", "reinforcement-learning", "ML-Agents-Pyramids"]} | tarpalsus/ppo-Pyramids | null | [
"ml-agents",
"tensorboard",
"onnx",
"Pyramids",
"deep-reinforcement-learning",
"reinforcement-learning",
"ML-Agents-Pyramids",
"region:us"
] | null | 2024-04-21T17:59:34+00:00 | [] | [] | TAGS
#ml-agents #tensorboard #onnx #Pyramids #deep-reinforcement-learning #reinforcement-learning #ML-Agents-Pyramids #region-us
|
# ppo Agent playing Pyramids
This is a trained model of a ppo agent playing Pyramids
using the Unity ML-Agents Library.
## Usage (with ML-Agents)
The Documentation: URL
We wrote a complete tutorial to learn to train your first agent using ML-Agents and publish it to the Hub:
- A *short tutorial* where you teach Huggy the Dog to fetch the stick and then play with him directly in your
browser: URL
- A *longer tutorial* to understand how works ML-Agents:
URL
### Resume the training
### Watch your Agent play
You can watch your agent playing directly in your browser
1. If the environment is part of ML-Agents official environments, go to URL
2. Step 1: Find your model_id: tarpalsus/ppo-Pyramids
3. Step 2: Select your *.nn /*.onnx file
4. Click on Watch the agent play
| [
"# ppo Agent playing Pyramids\n This is a trained model of a ppo agent playing Pyramids\n using the Unity ML-Agents Library.\n\n ## Usage (with ML-Agents)\n The Documentation: URL\n\n We wrote a complete tutorial to learn to train your first agent using ML-Agents and publish it to the Hub:\n - A *short tutorial* where you teach Huggy the Dog to fetch the stick and then play with him directly in your\n browser: URL\n - A *longer tutorial* to understand how works ML-Agents:\n URL\n\n ### Resume the training\n \n\n ### Watch your Agent play\n You can watch your agent playing directly in your browser\n\n 1. If the environment is part of ML-Agents official environments, go to URL\n 2. Step 1: Find your model_id: tarpalsus/ppo-Pyramids\n 3. Step 2: Select your *.nn /*.onnx file\n 4. Click on Watch the agent play"
] | [
"TAGS\n#ml-agents #tensorboard #onnx #Pyramids #deep-reinforcement-learning #reinforcement-learning #ML-Agents-Pyramids #region-us \n",
"# ppo Agent playing Pyramids\n This is a trained model of a ppo agent playing Pyramids\n using the Unity ML-Agents Library.\n\n ## Usage (with ML-Agents)\n The Documentation: URL\n\n We wrote a complete tutorial to learn to train your first agent using ML-Agents and publish it to the Hub:\n - A *short tutorial* where you teach Huggy the Dog to fetch the stick and then play with him directly in your\n browser: URL\n - A *longer tutorial* to understand how works ML-Agents:\n URL\n\n ### Resume the training\n \n\n ### Watch your Agent play\n You can watch your agent playing directly in your browser\n\n 1. If the environment is part of ML-Agents official environments, go to URL\n 2. Step 1: Find your model_id: tarpalsus/ppo-Pyramids\n 3. Step 2: Select your *.nn /*.onnx file\n 4. Click on Watch the agent play"
] |
null | null |
<!-- This model card has been generated automatically according to the information the Trainer had access to. You
should probably proofread and complete it, then remove this comment. -->
# llama-7b-absa-MT-laptops
This model is a fine-tuned version of [baffo32/decapoda-research-llama-7B-hf](https://huggingface.co/baffo32/decapoda-research-llama-7B-hf) on an unknown dataset.
It achieves the following results on the evaluation set:
- Loss: 0.0032
## Model description
More information needed
## Intended uses & limitations
More information needed
## Training and evaluation data
More information needed
## Training procedure
### Training hyperparameters
The following hyperparameters were used during training:
- learning_rate: 0.0003
- train_batch_size: 8
- eval_batch_size: 8
- seed: 42
- gradient_accumulation_steps: 4
- total_train_batch_size: 32
- optimizer: Adam with betas=(0.9,0.999) and epsilon=1e-08
- lr_scheduler_type: linear
- lr_scheduler_warmup_steps: 2
- training_steps: 1200
- mixed_precision_training: Native AMP
### Training results
| Training Loss | Epoch | Step | Validation Loss |
|:-------------:|:-----:|:----:|:---------------:|
| 0.0853 | 0.13 | 40 | 0.0270 |
| 0.0209 | 0.25 | 80 | 0.0205 |
| 0.017 | 0.38 | 120 | 0.0178 |
| 0.016 | 0.51 | 160 | 0.0157 |
| 0.0129 | 0.63 | 200 | 0.0140 |
| 0.0129 | 0.76 | 240 | 0.0118 |
| 0.0108 | 0.89 | 280 | 0.0115 |
| 0.009 | 1.01 | 320 | 0.0107 |
| 0.0052 | 1.14 | 360 | 0.0087 |
| 0.0054 | 1.26 | 400 | 0.0074 |
| 0.0046 | 1.39 | 440 | 0.0087 |
| 0.005 | 1.52 | 480 | 0.0074 |
| 0.0043 | 1.64 | 520 | 0.0061 |
| 0.0035 | 1.77 | 560 | 0.0056 |
| 0.003 | 1.9 | 600 | 0.0053 |
| 0.0026 | 2.02 | 640 | 0.0049 |
| 0.0021 | 2.15 | 680 | 0.0052 |
| 0.0027 | 2.28 | 720 | 0.0047 |
| 0.0015 | 2.4 | 760 | 0.0044 |
| 0.0013 | 2.53 | 800 | 0.0043 |
| 0.0009 | 2.66 | 840 | 0.0042 |
| 0.001 | 2.78 | 880 | 0.0039 |
| 0.0008 | 2.91 | 920 | 0.0036 |
| 0.0005 | 3.04 | 960 | 0.0036 |
| 0.0006 | 3.16 | 1000 | 0.0039 |
| 0.0005 | 3.29 | 1040 | 0.0033 |
| 0.0002 | 3.42 | 1080 | 0.0032 |
| 0.0002 | 3.54 | 1120 | 0.0033 |
| 0.0002 | 3.67 | 1160 | 0.0031 |
| 0.0002 | 3.79 | 1200 | 0.0032 |
### Framework versions
- Transformers 4.38.2
- Pytorch 2.2.1+cu121
- Datasets 2.18.0
- Tokenizers 0.15.2
| {"license": "other", "tags": ["generated_from_trainer"], "base_model": "baffo32/decapoda-research-llama-7B-hf", "model-index": [{"name": "llama-7b-absa-MT-laptops", "results": []}]} | Shakhovak/llama-7b-absa-MT-laptops | null | [
"generated_from_trainer",
"base_model:baffo32/decapoda-research-llama-7B-hf",
"license:other",
"region:us"
] | null | 2024-04-21T18:01:13+00:00 | [] | [] | TAGS
#generated_from_trainer #base_model-baffo32/decapoda-research-llama-7B-hf #license-other #region-us
| llama-7b-absa-MT-laptops
========================
This model is a fine-tuned version of baffo32/decapoda-research-llama-7B-hf on an unknown dataset.
It achieves the following results on the evaluation set:
* Loss: 0.0032
Model description
-----------------
More information needed
Intended uses & limitations
---------------------------
More information needed
Training and evaluation data
----------------------------
More information needed
Training procedure
------------------
### Training hyperparameters
The following hyperparameters were used during training:
* learning\_rate: 0.0003
* train\_batch\_size: 8
* eval\_batch\_size: 8
* seed: 42
* gradient\_accumulation\_steps: 4
* total\_train\_batch\_size: 32
* optimizer: Adam with betas=(0.9,0.999) and epsilon=1e-08
* lr\_scheduler\_type: linear
* lr\_scheduler\_warmup\_steps: 2
* training\_steps: 1200
* mixed\_precision\_training: Native AMP
### Training results
### Framework versions
* Transformers 4.38.2
* Pytorch 2.2.1+cu121
* Datasets 2.18.0
* Tokenizers 0.15.2
| [
"### Training hyperparameters\n\n\nThe following hyperparameters were used during training:\n\n\n* learning\\_rate: 0.0003\n* train\\_batch\\_size: 8\n* eval\\_batch\\_size: 8\n* seed: 42\n* gradient\\_accumulation\\_steps: 4\n* total\\_train\\_batch\\_size: 32\n* optimizer: Adam with betas=(0.9,0.999) and epsilon=1e-08\n* lr\\_scheduler\\_type: linear\n* lr\\_scheduler\\_warmup\\_steps: 2\n* training\\_steps: 1200\n* mixed\\_precision\\_training: Native AMP",
"### Training results",
"### Framework versions\n\n\n* Transformers 4.38.2\n* Pytorch 2.2.1+cu121\n* Datasets 2.18.0\n* Tokenizers 0.15.2"
] | [
"TAGS\n#generated_from_trainer #base_model-baffo32/decapoda-research-llama-7B-hf #license-other #region-us \n",
"### Training hyperparameters\n\n\nThe following hyperparameters were used during training:\n\n\n* learning\\_rate: 0.0003\n* train\\_batch\\_size: 8\n* eval\\_batch\\_size: 8\n* seed: 42\n* gradient\\_accumulation\\_steps: 4\n* total\\_train\\_batch\\_size: 32\n* optimizer: Adam with betas=(0.9,0.999) and epsilon=1e-08\n* lr\\_scheduler\\_type: linear\n* lr\\_scheduler\\_warmup\\_steps: 2\n* training\\_steps: 1200\n* mixed\\_precision\\_training: Native AMP",
"### Training results",
"### Framework versions\n\n\n* Transformers 4.38.2\n* Pytorch 2.2.1+cu121\n* Datasets 2.18.0\n* Tokenizers 0.15.2"
] |
text-generation | transformers |
# Model Card for Model ID
<!-- Provide a quick summary of what the model is/does. -->
## Model Details
### Model Description
<!-- Provide a longer summary of what this model is. -->
This is the model card of a 🤗 transformers model that has been pushed on the Hub. This model card has been automatically generated.
- **Developed by:** [More Information Needed]
- **Funded by [optional]:** [More Information Needed]
- **Shared by [optional]:** [More Information Needed]
- **Model type:** [More Information Needed]
- **Language(s) (NLP):** [More Information Needed]
- **License:** [More Information Needed]
- **Finetuned from model [optional]:** [More Information Needed]
### Model Sources [optional]
<!-- Provide the basic links for the model. -->
- **Repository:** [More Information Needed]
- **Paper [optional]:** [More Information Needed]
- **Demo [optional]:** [More Information Needed]
## Uses
<!-- Address questions around how the model is intended to be used, including the foreseeable users of the model and those affected by the model. -->
### Direct Use
<!-- This section is for the model use without fine-tuning or plugging into a larger ecosystem/app. -->
[More Information Needed]
### Downstream Use [optional]
<!-- This section is for the model use when fine-tuned for a task, or when plugged into a larger ecosystem/app -->
[More Information Needed]
### Out-of-Scope Use
<!-- This section addresses misuse, malicious use, and uses that the model will not work well for. -->
[More Information Needed]
## Bias, Risks, and Limitations
<!-- This section is meant to convey both technical and sociotechnical limitations. -->
[More Information Needed]
### Recommendations
<!-- This section is meant to convey recommendations with respect to the bias, risk, and technical limitations. -->
Users (both direct and downstream) should be made aware of the risks, biases and limitations of the model. More information needed for further recommendations.
## How to Get Started with the Model
Use the code below to get started with the model.
[More Information Needed]
## Training Details
### Training Data
<!-- This should link to a Dataset Card, perhaps with a short stub of information on what the training data is all about as well as documentation related to data pre-processing or additional filtering. -->
[More Information Needed]
### Training Procedure
<!-- This relates heavily to the Technical Specifications. Content here should link to that section when it is relevant to the training procedure. -->
#### Preprocessing [optional]
[More Information Needed]
#### Training Hyperparameters
- **Training regime:** [More Information Needed] <!--fp32, fp16 mixed precision, bf16 mixed precision, bf16 non-mixed precision, fp16 non-mixed precision, fp8 mixed precision -->
#### Speeds, Sizes, Times [optional]
<!-- This section provides information about throughput, start/end time, checkpoint size if relevant, etc. -->
[More Information Needed]
## Evaluation
<!-- This section describes the evaluation protocols and provides the results. -->
### Testing Data, Factors & Metrics
#### Testing Data
<!-- This should link to a Dataset Card if possible. -->
[More Information Needed]
#### Factors
<!-- These are the things the evaluation is disaggregating by, e.g., subpopulations or domains. -->
[More Information Needed]
#### Metrics
<!-- These are the evaluation metrics being used, ideally with a description of why. -->
[More Information Needed]
### Results
[More Information Needed]
#### Summary
## Model Examination [optional]
<!-- Relevant interpretability work for the model goes here -->
[More Information Needed]
## Environmental Impact
<!-- Total emissions (in grams of CO2eq) and additional considerations, such as electricity usage, go here. Edit the suggested text below accordingly -->
Carbon emissions can be estimated using the [Machine Learning Impact calculator](https://mlco2.github.io/impact#compute) presented in [Lacoste et al. (2019)](https://arxiv.org/abs/1910.09700).
- **Hardware Type:** [More Information Needed]
- **Hours used:** [More Information Needed]
- **Cloud Provider:** [More Information Needed]
- **Compute Region:** [More Information Needed]
- **Carbon Emitted:** [More Information Needed]
## Technical Specifications [optional]
### Model Architecture and Objective
[More Information Needed]
### Compute Infrastructure
[More Information Needed]
#### Hardware
[More Information Needed]
#### Software
[More Information Needed]
## Citation [optional]
<!-- If there is a paper or blog post introducing the model, the APA and Bibtex information for that should go in this section. -->
**BibTeX:**
[More Information Needed]
**APA:**
[More Information Needed]
## Glossary [optional]
<!-- If relevant, include terms and calculations in this section that can help readers understand the model or model card. -->
[More Information Needed]
## More Information [optional]
[More Information Needed]
## Model Card Authors [optional]
[More Information Needed]
## Model Card Contact
[More Information Needed] | {"library_name": "transformers", "tags": []} | Abhilashvj/sql-Mistral-7B | null | [
"transformers",
"safetensors",
"mistral",
"text-generation",
"conversational",
"arxiv:1910.09700",
"autotrain_compatible",
"endpoints_compatible",
"text-generation-inference",
"region:us"
] | null | 2024-04-21T18:02:26+00:00 | [
"1910.09700"
] | [] | TAGS
#transformers #safetensors #mistral #text-generation #conversational #arxiv-1910.09700 #autotrain_compatible #endpoints_compatible #text-generation-inference #region-us
|
# Model Card for Model ID
## Model Details
### Model Description
This is the model card of a transformers model that has been pushed on the Hub. This model card has been automatically generated.
- Developed by:
- Funded by [optional]:
- Shared by [optional]:
- Model type:
- Language(s) (NLP):
- License:
- Finetuned from model [optional]:
### Model Sources [optional]
- Repository:
- Paper [optional]:
- Demo [optional]:
## Uses
### Direct Use
### Downstream Use [optional]
### Out-of-Scope Use
## Bias, Risks, and Limitations
### Recommendations
Users (both direct and downstream) should be made aware of the risks, biases and limitations of the model. More information needed for further recommendations.
## How to Get Started with the Model
Use the code below to get started with the model.
## Training Details
### Training Data
### Training Procedure
#### Preprocessing [optional]
#### Training Hyperparameters
- Training regime:
#### Speeds, Sizes, Times [optional]
## Evaluation
### Testing Data, Factors & Metrics
#### Testing Data
#### Factors
#### Metrics
### Results
#### Summary
## Model Examination [optional]
## Environmental Impact
Carbon emissions can be estimated using the Machine Learning Impact calculator presented in Lacoste et al. (2019).
- Hardware Type:
- Hours used:
- Cloud Provider:
- Compute Region:
- Carbon Emitted:
## Technical Specifications [optional]
### Model Architecture and Objective
### Compute Infrastructure
#### Hardware
#### Software
[optional]
BibTeX:
APA:
## Glossary [optional]
## More Information [optional]
## Model Card Authors [optional]
## Model Card Contact
| [
"# Model Card for Model ID",
"## Model Details",
"### Model Description\n\n\n\nThis is the model card of a transformers model that has been pushed on the Hub. This model card has been automatically generated.\n\n- Developed by: \n- Funded by [optional]: \n- Shared by [optional]: \n- Model type: \n- Language(s) (NLP): \n- License: \n- Finetuned from model [optional]:",
"### Model Sources [optional]\n\n\n\n- Repository: \n- Paper [optional]: \n- Demo [optional]:",
"## Uses",
"### Direct Use",
"### Downstream Use [optional]",
"### Out-of-Scope Use",
"## Bias, Risks, and Limitations",
"### Recommendations\n\n\n\nUsers (both direct and downstream) should be made aware of the risks, biases and limitations of the model. More information needed for further recommendations.",
"## How to Get Started with the Model\n\nUse the code below to get started with the model.",
"## Training Details",
"### Training Data",
"### Training Procedure",
"#### Preprocessing [optional]",
"#### Training Hyperparameters\n\n- Training regime:",
"#### Speeds, Sizes, Times [optional]",
"## Evaluation",
"### Testing Data, Factors & Metrics",
"#### Testing Data",
"#### Factors",
"#### Metrics",
"### Results",
"#### Summary",
"## Model Examination [optional]",
"## Environmental Impact\n\n\n\nCarbon emissions can be estimated using the Machine Learning Impact calculator presented in Lacoste et al. (2019).\n\n- Hardware Type: \n- Hours used: \n- Cloud Provider: \n- Compute Region: \n- Carbon Emitted:",
"## Technical Specifications [optional]",
"### Model Architecture and Objective",
"### Compute Infrastructure",
"#### Hardware",
"#### Software\n\n\n\n[optional]\n\n\n\nBibTeX:\n\n\n\nAPA:",
"## Glossary [optional]",
"## More Information [optional]",
"## Model Card Authors [optional]",
"## Model Card Contact"
] | [
"TAGS\n#transformers #safetensors #mistral #text-generation #conversational #arxiv-1910.09700 #autotrain_compatible #endpoints_compatible #text-generation-inference #region-us \n",
"# Model Card for Model ID",
"## Model Details",
"### Model Description\n\n\n\nThis is the model card of a transformers model that has been pushed on the Hub. This model card has been automatically generated.\n\n- Developed by: \n- Funded by [optional]: \n- Shared by [optional]: \n- Model type: \n- Language(s) (NLP): \n- License: \n- Finetuned from model [optional]:",
"### Model Sources [optional]\n\n\n\n- Repository: \n- Paper [optional]: \n- Demo [optional]:",
"## Uses",
"### Direct Use",
"### Downstream Use [optional]",
"### Out-of-Scope Use",
"## Bias, Risks, and Limitations",
"### Recommendations\n\n\n\nUsers (both direct and downstream) should be made aware of the risks, biases and limitations of the model. More information needed for further recommendations.",
"## How to Get Started with the Model\n\nUse the code below to get started with the model.",
"## Training Details",
"### Training Data",
"### Training Procedure",
"#### Preprocessing [optional]",
"#### Training Hyperparameters\n\n- Training regime:",
"#### Speeds, Sizes, Times [optional]",
"## Evaluation",
"### Testing Data, Factors & Metrics",
"#### Testing Data",
"#### Factors",
"#### Metrics",
"### Results",
"#### Summary",
"## Model Examination [optional]",
"## Environmental Impact\n\n\n\nCarbon emissions can be estimated using the Machine Learning Impact calculator presented in Lacoste et al. (2019).\n\n- Hardware Type: \n- Hours used: \n- Cloud Provider: \n- Compute Region: \n- Carbon Emitted:",
"## Technical Specifications [optional]",
"### Model Architecture and Objective",
"### Compute Infrastructure",
"#### Hardware",
"#### Software\n\n\n\n[optional]\n\n\n\nBibTeX:\n\n\n\nAPA:",
"## Glossary [optional]",
"## More Information [optional]",
"## Model Card Authors [optional]",
"## Model Card Contact"
] |
text-generation | transformers | Mistral-7B Japanese [LAPT + Heuristics (Untied)]
===
## How to use
```python
from peft import AutoPeftModelForCausalLM
from transformers import AutoTokenizer
model = AutoPeftModelForCausalLM.from_pretrained(
"atsuki-yamaguchi/Mistral-7B-v0.1-heuristics-untied-ja"
)
tokenizer = AutoTokenizer.from_pretrained(
"atsuki-yamaguchi/Mistral-7B-v0.1-heuristics-untied-ja"
)
# w/ GPU
model = AutoPeftModelForCausalLM.from_pretrained(
"atsuki-yamaguchi/Mistral-7B-v0.1-heuristics-untied-ja",
device_map="auto",
load_in_8bit=True,
)
```
## Citation
```
@article{yamaguchi2024empirical,
title={An Empirical Study on Cross-lingual Vocabulary Adaptation for Efficient Generative {LLM} Inference},
author={Atsuki Yamaguchi and Aline Villavicencio and Nikolaos Aletras},
journal={ArXiv},
year={2024},
volume={abs/2402.10712},
url={https://arxiv.org/abs/2402.10712}
}
```
## Link
For more details, please visit https://github.com/gucci-j/llm-cva
| {"language": "ja", "license": "mit"} | atsuki-yamaguchi/Mistral-7B-v0.1-heuristics-untied-ja | null | [
"transformers",
"safetensors",
"mistral",
"text-generation",
"ja",
"arxiv:2402.10712",
"license:mit",
"autotrain_compatible",
"endpoints_compatible",
"text-generation-inference",
"region:us"
] | null | 2024-04-21T18:04:25+00:00 | [
"2402.10712"
] | [
"ja"
] | TAGS
#transformers #safetensors #mistral #text-generation #ja #arxiv-2402.10712 #license-mit #autotrain_compatible #endpoints_compatible #text-generation-inference #region-us
| Mistral-7B Japanese [LAPT + Heuristics (Untied)]
===
## How to use
## Link
For more details, please visit URL
| [
"## How to use",
"## Link\nFor more details, please visit URL"
] | [
"TAGS\n#transformers #safetensors #mistral #text-generation #ja #arxiv-2402.10712 #license-mit #autotrain_compatible #endpoints_compatible #text-generation-inference #region-us \n",
"## How to use",
"## Link\nFor more details, please visit URL"
] |
text-generation | transformers |
# Model Trained Using AutoTrain
This model was trained using AutoTrain. For more information, please visit [AutoTrain](https://hf.co/docs/autotrain).
# Usage
```python
from transformers import AutoModelForCausalLM, AutoTokenizer
model_path = "PATH_TO_THIS_REPO"
tokenizer = AutoTokenizer.from_pretrained(model_path)
model = AutoModelForCausalLM.from_pretrained(
model_path,
device_map="auto",
torch_dtype='auto'
).eval()
# Prompt content: "hi"
messages = [
{"role": "user", "content": "hi"}
]
input_ids = tokenizer.apply_chat_template(conversation=messages, tokenize=True, add_generation_prompt=True, return_tensors='pt')
output_ids = model.generate(input_ids.to('cuda'))
response = tokenizer.decode(output_ids[0][input_ids.shape[1]:], skip_special_tokens=True)
# Model response: "Hello! How can I assist you today?"
print(response)
``` | {"license": "other", "library_name": "transformers", "tags": ["autotrain", "text-generation-inference", "text-generation", "peft"], "widget": [{"messages": [{"role": "user", "content": "What is your favorite condiment?"}]}]} | QuackAI/Atorinv0.1-adapter-model | null | [
"transformers",
"safetensors",
"autotrain",
"text-generation-inference",
"text-generation",
"peft",
"conversational",
"license:other",
"endpoints_compatible",
"region:us"
] | null | 2024-04-21T18:06:15+00:00 | [] | [] | TAGS
#transformers #safetensors #autotrain #text-generation-inference #text-generation #peft #conversational #license-other #endpoints_compatible #region-us
|
# Model Trained Using AutoTrain
This model was trained using AutoTrain. For more information, please visit AutoTrain.
# Usage
| [
"# Model Trained Using AutoTrain\n\nThis model was trained using AutoTrain. For more information, please visit AutoTrain.",
"# Usage"
] | [
"TAGS\n#transformers #safetensors #autotrain #text-generation-inference #text-generation #peft #conversational #license-other #endpoints_compatible #region-us \n",
"# Model Trained Using AutoTrain\n\nThis model was trained using AutoTrain. For more information, please visit AutoTrain.",
"# Usage"
] |
text-classification | transformers |
<!-- This model card has been generated automatically according to the information the Trainer had access to. You
should probably proofread and complete it, then remove this comment. -->
# canine-mouse-enhancers
This model is a fine-tuned version of [google/canine-c](https://huggingface.co/google/canine-c) on an unknown dataset.
It achieves the following results on the evaluation set:
- Loss: 0.9641
- Accuracy: 0.7727
## Model description
More information needed
## Intended uses & limitations
More information needed
## Training and evaluation data
More information needed
## Training procedure
### Training hyperparameters
The following hyperparameters were used during training:
- learning_rate: 2e-06
- train_batch_size: 4
- eval_batch_size: 4
- seed: 42
- optimizer: Adam with betas=(0.9,0.999) and epsilon=1e-08
- lr_scheduler_type: linear
- num_epochs: 50
### Training results
| Training Loss | Epoch | Step | Validation Loss | Accuracy |
|:-------------:|:-----:|:-----:|:---------------:|:--------:|
| No log | 1.0 | 242 | 0.6476 | 0.6281 |
| No log | 2.0 | 484 | 0.6080 | 0.6860 |
| 0.6372 | 3.0 | 726 | 0.5989 | 0.7231 |
| 0.6372 | 4.0 | 968 | 0.6285 | 0.6694 |
| 0.5955 | 5.0 | 1210 | 0.5904 | 0.6860 |
| 0.5955 | 6.0 | 1452 | 0.5782 | 0.7107 |
| 0.5812 | 7.0 | 1694 | 0.5845 | 0.6983 |
| 0.5812 | 8.0 | 1936 | 0.6186 | 0.6983 |
| 0.5901 | 9.0 | 2178 | 0.5814 | 0.7231 |
| 0.5901 | 10.0 | 2420 | 0.6152 | 0.7355 |
| 0.5535 | 11.0 | 2662 | 0.5556 | 0.7438 |
| 0.5535 | 12.0 | 2904 | 0.5476 | 0.7479 |
| 0.5566 | 13.0 | 3146 | 0.6583 | 0.7107 |
| 0.5566 | 14.0 | 3388 | 0.5571 | 0.7521 |
| 0.5419 | 15.0 | 3630 | 0.6231 | 0.7231 |
| 0.5419 | 16.0 | 3872 | 0.6068 | 0.7603 |
| 0.546 | 17.0 | 4114 | 0.6581 | 0.7273 |
| 0.546 | 18.0 | 4356 | 0.6350 | 0.7438 |
| 0.5359 | 19.0 | 4598 | 0.7081 | 0.7438 |
| 0.5359 | 20.0 | 4840 | 0.6711 | 0.7521 |
| 0.5262 | 21.0 | 5082 | 0.8095 | 0.7190 |
| 0.5262 | 22.0 | 5324 | 0.7282 | 0.7521 |
| 0.5666 | 23.0 | 5566 | 0.7604 | 0.7479 |
| 0.5666 | 24.0 | 5808 | 0.8097 | 0.7521 |
| 0.5456 | 25.0 | 6050 | 0.8513 | 0.7521 |
| 0.5456 | 26.0 | 6292 | 0.7954 | 0.7603 |
| 0.5612 | 27.0 | 6534 | 0.8435 | 0.7521 |
| 0.5612 | 28.0 | 6776 | 0.9000 | 0.7355 |
| 0.5358 | 29.0 | 7018 | 0.9241 | 0.7603 |
| 0.5358 | 30.0 | 7260 | 0.9005 | 0.7479 |
| 0.5434 | 31.0 | 7502 | 0.8875 | 0.7645 |
| 0.5434 | 32.0 | 7744 | 0.8878 | 0.7686 |
| 0.5434 | 33.0 | 7986 | 0.9162 | 0.7645 |
| 0.5066 | 34.0 | 8228 | 0.8665 | 0.7686 |
| 0.5066 | 35.0 | 8470 | 0.8756 | 0.7686 |
| 0.5276 | 36.0 | 8712 | 0.9723 | 0.7603 |
| 0.5276 | 37.0 | 8954 | 1.0044 | 0.7521 |
| 0.4916 | 38.0 | 9196 | 0.9647 | 0.7521 |
| 0.4916 | 39.0 | 9438 | 0.9819 | 0.7603 |
| 0.4865 | 40.0 | 9680 | 0.9644 | 0.7686 |
| 0.4865 | 41.0 | 9922 | 0.9084 | 0.7851 |
| 0.4505 | 42.0 | 10164 | 1.0152 | 0.7521 |
| 0.4505 | 43.0 | 10406 | 0.9332 | 0.7769 |
| 0.4798 | 44.0 | 10648 | 0.9803 | 0.7603 |
| 0.4798 | 45.0 | 10890 | 1.0211 | 0.7521 |
| 0.4234 | 46.0 | 11132 | 0.9143 | 0.7810 |
| 0.4234 | 47.0 | 11374 | 0.9969 | 0.7645 |
| 0.4269 | 48.0 | 11616 | 0.9515 | 0.7851 |
| 0.4269 | 49.0 | 11858 | 0.9998 | 0.7686 |
| 0.4135 | 50.0 | 12100 | 0.9641 | 0.7727 |
### Framework versions
- Transformers 4.26.1
- Pytorch 2.0.0+cu117
- Datasets 2.19.0
- Tokenizers 0.13.3
| {"license": "apache-2.0", "tags": ["generated_from_trainer"], "metrics": ["accuracy"], "model-index": [{"name": "canine-mouse-enhancers", "results": []}]} | addykan/canine-mouse-enhancers | null | [
"transformers",
"pytorch",
"canine",
"text-classification",
"generated_from_trainer",
"license:apache-2.0",
"autotrain_compatible",
"endpoints_compatible",
"region:us"
] | null | 2024-04-21T18:09:08+00:00 | [] | [] | TAGS
#transformers #pytorch #canine #text-classification #generated_from_trainer #license-apache-2.0 #autotrain_compatible #endpoints_compatible #region-us
| canine-mouse-enhancers
======================
This model is a fine-tuned version of google/canine-c on an unknown dataset.
It achieves the following results on the evaluation set:
* Loss: 0.9641
* Accuracy: 0.7727
Model description
-----------------
More information needed
Intended uses & limitations
---------------------------
More information needed
Training and evaluation data
----------------------------
More information needed
Training procedure
------------------
### Training hyperparameters
The following hyperparameters were used during training:
* learning\_rate: 2e-06
* train\_batch\_size: 4
* eval\_batch\_size: 4
* seed: 42
* optimizer: Adam with betas=(0.9,0.999) and epsilon=1e-08
* lr\_scheduler\_type: linear
* num\_epochs: 50
### Training results
### Framework versions
* Transformers 4.26.1
* Pytorch 2.0.0+cu117
* Datasets 2.19.0
* Tokenizers 0.13.3
| [
"### Training hyperparameters\n\n\nThe following hyperparameters were used during training:\n\n\n* learning\\_rate: 2e-06\n* train\\_batch\\_size: 4\n* eval\\_batch\\_size: 4\n* seed: 42\n* optimizer: Adam with betas=(0.9,0.999) and epsilon=1e-08\n* lr\\_scheduler\\_type: linear\n* num\\_epochs: 50",
"### Training results",
"### Framework versions\n\n\n* Transformers 4.26.1\n* Pytorch 2.0.0+cu117\n* Datasets 2.19.0\n* Tokenizers 0.13.3"
] | [
"TAGS\n#transformers #pytorch #canine #text-classification #generated_from_trainer #license-apache-2.0 #autotrain_compatible #endpoints_compatible #region-us \n",
"### Training hyperparameters\n\n\nThe following hyperparameters were used during training:\n\n\n* learning\\_rate: 2e-06\n* train\\_batch\\_size: 4\n* eval\\_batch\\_size: 4\n* seed: 42\n* optimizer: Adam with betas=(0.9,0.999) and epsilon=1e-08\n* lr\\_scheduler\\_type: linear\n* num\\_epochs: 50",
"### Training results",
"### Framework versions\n\n\n* Transformers 4.26.1\n* Pytorch 2.0.0+cu117\n* Datasets 2.19.0\n* Tokenizers 0.13.3"
] |
null | null | # LLaMA3 License and Usage

## Introduction
The LLaMA3 model is equipped to deliver superior results in machine learning applications. This model is particularly effective when used in conjunction with the IF_AI_tools custom node for ComfyUI and the IF_PromptMKr, my extension for A1111 Forge and Next platforms.
## Model Training
LLaMA3 has been meticulously trained on a synthetic dataset comprising over 50,000 high-quality, stable diffusion prompts, ensuring robustness and high performance across various tasks.
## Useful Links
- [IF Prompt MKR](https://github.com/if-ai/IF_prompt_MKR)
- [ComfyUI-IF_AI_tools](https://github.com/if-ai/ComfyUI-IF_AI_tools)
## Support
Your support is invaluable in continuing the development and enhancement of tools like these. If you find this tool useful, please consider extending your support by:
- **Starring the repository** on GitHub: [Star ComfyUI-IF_AI_tools](https://github.com/if-ai/ComfyUI-IF_AI_tools)
- **Subscribing** to my YouTube channel: [Impact Frames on YouTube](https://youtube.com/@impactframes?si=DrBu3tOAC2-YbEvc)
- **Donating** on Ko-fi: [Support Impact Frames on Ko-fi](https://ko-fi.com/impactframes)
- **Becoming a patron** on Patreon: [Support via Patreon](https://patreon.com/ImpactFrames)
Thank you for your interest and support!
- **Developed by:** impactframes
- **License:** apache-2.0
- **Finetuned from model :** unsloth/llama-3-8b-bnb-4bit
This llama model was trained 2x faster with [Unsloth](https://github.com/unslothai/unsloth) and Huggingface's TRL library.
[<img src="https://raw.githubusercontent.com/unslothai/unsloth/main/images/unsloth%20made%20with%20love.png" width="200"/>](https://github.com/unslothai/unsloth)
| {} | impactframes/llama3_if_ai_sdpromptmkr_q2k | null | [
"gguf",
"region:us"
] | null | 2024-04-21T18:09:29+00:00 | [] | [] | TAGS
#gguf #region-us
| # LLaMA3 License and Usage
!Model Visualization
## Introduction
The LLaMA3 model is equipped to deliver superior results in machine learning applications. This model is particularly effective when used in conjunction with the IF_AI_tools custom node for ComfyUI and the IF_PromptMKr, my extension for A1111 Forge and Next platforms.
## Model Training
LLaMA3 has been meticulously trained on a synthetic dataset comprising over 50,000 high-quality, stable diffusion prompts, ensuring robustness and high performance across various tasks.
## Useful Links
- IF Prompt MKR
- ComfyUI-IF_AI_tools
## Support
Your support is invaluable in continuing the development and enhancement of tools like these. If you find this tool useful, please consider extending your support by:
- Starring the repository on GitHub: Star ComfyUI-IF_AI_tools
- Subscribing to my YouTube channel: Impact Frames on YouTube
- Donating on Ko-fi: Support Impact Frames on Ko-fi
- Becoming a patron on Patreon: Support via Patreon
Thank you for your interest and support!
- Developed by: impactframes
- License: apache-2.0
- Finetuned from model : unsloth/llama-3-8b-bnb-4bit
This llama model was trained 2x faster with Unsloth and Huggingface's TRL library.
<img src="URL width="200"/>
| [
"# LLaMA3 License and Usage\n!Model Visualization",
"## Introduction\nThe LLaMA3 model is equipped to deliver superior results in machine learning applications. This model is particularly effective when used in conjunction with the IF_AI_tools custom node for ComfyUI and the IF_PromptMKr, my extension for A1111 Forge and Next platforms.",
"## Model Training\nLLaMA3 has been meticulously trained on a synthetic dataset comprising over 50,000 high-quality, stable diffusion prompts, ensuring robustness and high performance across various tasks.",
"## Useful Links\n- IF Prompt MKR\n- ComfyUI-IF_AI_tools",
"## Support\nYour support is invaluable in continuing the development and enhancement of tools like these. If you find this tool useful, please consider extending your support by:\n- Starring the repository on GitHub: Star ComfyUI-IF_AI_tools\n- Subscribing to my YouTube channel: Impact Frames on YouTube\n- Donating on Ko-fi: Support Impact Frames on Ko-fi\n- Becoming a patron on Patreon: Support via Patreon\n\nThank you for your interest and support!\n\n- Developed by: impactframes\n- License: apache-2.0\n- Finetuned from model : unsloth/llama-3-8b-bnb-4bit\n\nThis llama model was trained 2x faster with Unsloth and Huggingface's TRL library.\n\n<img src=\"URL width=\"200\"/>"
] | [
"TAGS\n#gguf #region-us \n",
"# LLaMA3 License and Usage\n!Model Visualization",
"## Introduction\nThe LLaMA3 model is equipped to deliver superior results in machine learning applications. This model is particularly effective when used in conjunction with the IF_AI_tools custom node for ComfyUI and the IF_PromptMKr, my extension for A1111 Forge and Next platforms.",
"## Model Training\nLLaMA3 has been meticulously trained on a synthetic dataset comprising over 50,000 high-quality, stable diffusion prompts, ensuring robustness and high performance across various tasks.",
"## Useful Links\n- IF Prompt MKR\n- ComfyUI-IF_AI_tools",
"## Support\nYour support is invaluable in continuing the development and enhancement of tools like these. If you find this tool useful, please consider extending your support by:\n- Starring the repository on GitHub: Star ComfyUI-IF_AI_tools\n- Subscribing to my YouTube channel: Impact Frames on YouTube\n- Donating on Ko-fi: Support Impact Frames on Ko-fi\n- Becoming a patron on Patreon: Support via Patreon\n\nThank you for your interest and support!\n\n- Developed by: impactframes\n- License: apache-2.0\n- Finetuned from model : unsloth/llama-3-8b-bnb-4bit\n\nThis llama model was trained 2x faster with Unsloth and Huggingface's TRL library.\n\n<img src=\"URL width=\"200\"/>"
] |
null | transformers |
# Model Card for Model ID
<!-- Provide a quick summary of what the model is/does. -->
## Model Details
### Model Description
<!-- Provide a longer summary of what this model is. -->
This is the model card of a 🤗 transformers model that has been pushed on the Hub. This model card has been automatically generated.
- **Developed by:** [More Information Needed]
- **Funded by [optional]:** [More Information Needed]
- **Shared by [optional]:** [More Information Needed]
- **Model type:** [More Information Needed]
- **Language(s) (NLP):** [More Information Needed]
- **License:** [More Information Needed]
- **Finetuned from model [optional]:** [More Information Needed]
### Model Sources [optional]
<!-- Provide the basic links for the model. -->
- **Repository:** [More Information Needed]
- **Paper [optional]:** [More Information Needed]
- **Demo [optional]:** [More Information Needed]
## Uses
<!-- Address questions around how the model is intended to be used, including the foreseeable users of the model and those affected by the model. -->
### Direct Use
<!-- This section is for the model use without fine-tuning or plugging into a larger ecosystem/app. -->
[More Information Needed]
### Downstream Use [optional]
<!-- This section is for the model use when fine-tuned for a task, or when plugged into a larger ecosystem/app -->
[More Information Needed]
### Out-of-Scope Use
<!-- This section addresses misuse, malicious use, and uses that the model will not work well for. -->
[More Information Needed]
## Bias, Risks, and Limitations
<!-- This section is meant to convey both technical and sociotechnical limitations. -->
[More Information Needed]
### Recommendations
<!-- This section is meant to convey recommendations with respect to the bias, risk, and technical limitations. -->
Users (both direct and downstream) should be made aware of the risks, biases and limitations of the model. More information needed for further recommendations.
## How to Get Started with the Model
Use the code below to get started with the model.
[More Information Needed]
## Training Details
### Training Data
<!-- This should link to a Dataset Card, perhaps with a short stub of information on what the training data is all about as well as documentation related to data pre-processing or additional filtering. -->
[More Information Needed]
### Training Procedure
<!-- This relates heavily to the Technical Specifications. Content here should link to that section when it is relevant to the training procedure. -->
#### Preprocessing [optional]
[More Information Needed]
#### Training Hyperparameters
- **Training regime:** [More Information Needed] <!--fp32, fp16 mixed precision, bf16 mixed precision, bf16 non-mixed precision, fp16 non-mixed precision, fp8 mixed precision -->
#### Speeds, Sizes, Times [optional]
<!-- This section provides information about throughput, start/end time, checkpoint size if relevant, etc. -->
[More Information Needed]
## Evaluation
<!-- This section describes the evaluation protocols and provides the results. -->
### Testing Data, Factors & Metrics
#### Testing Data
<!-- This should link to a Dataset Card if possible. -->
[More Information Needed]
#### Factors
<!-- These are the things the evaluation is disaggregating by, e.g., subpopulations or domains. -->
[More Information Needed]
#### Metrics
<!-- These are the evaluation metrics being used, ideally with a description of why. -->
[More Information Needed]
### Results
[More Information Needed]
#### Summary
## Model Examination [optional]
<!-- Relevant interpretability work for the model goes here -->
[More Information Needed]
## Environmental Impact
<!-- Total emissions (in grams of CO2eq) and additional considerations, such as electricity usage, go here. Edit the suggested text below accordingly -->
Carbon emissions can be estimated using the [Machine Learning Impact calculator](https://mlco2.github.io/impact#compute) presented in [Lacoste et al. (2019)](https://arxiv.org/abs/1910.09700).
- **Hardware Type:** [More Information Needed]
- **Hours used:** [More Information Needed]
- **Cloud Provider:** [More Information Needed]
- **Compute Region:** [More Information Needed]
- **Carbon Emitted:** [More Information Needed]
## Technical Specifications [optional]
### Model Architecture and Objective
[More Information Needed]
### Compute Infrastructure
[More Information Needed]
#### Hardware
[More Information Needed]
#### Software
[More Information Needed]
## Citation [optional]
<!-- If there is a paper or blog post introducing the model, the APA and Bibtex information for that should go in this section. -->
**BibTeX:**
[More Information Needed]
**APA:**
[More Information Needed]
## Glossary [optional]
<!-- If relevant, include terms and calculations in this section that can help readers understand the model or model card. -->
[More Information Needed]
## More Information [optional]
[More Information Needed]
## Model Card Authors [optional]
[More Information Needed]
## Model Card Contact
[More Information Needed] | {"library_name": "transformers", "tags": []} | SansarK/opt-350m-lora | null | [
"transformers",
"safetensors",
"arxiv:1910.09700",
"endpoints_compatible",
"region:us"
] | null | 2024-04-21T18:10:03+00:00 | [
"1910.09700"
] | [] | TAGS
#transformers #safetensors #arxiv-1910.09700 #endpoints_compatible #region-us
|
# Model Card for Model ID
## Model Details
### Model Description
This is the model card of a transformers model that has been pushed on the Hub. This model card has been automatically generated.
- Developed by:
- Funded by [optional]:
- Shared by [optional]:
- Model type:
- Language(s) (NLP):
- License:
- Finetuned from model [optional]:
### Model Sources [optional]
- Repository:
- Paper [optional]:
- Demo [optional]:
## Uses
### Direct Use
### Downstream Use [optional]
### Out-of-Scope Use
## Bias, Risks, and Limitations
### Recommendations
Users (both direct and downstream) should be made aware of the risks, biases and limitations of the model. More information needed for further recommendations.
## How to Get Started with the Model
Use the code below to get started with the model.
## Training Details
### Training Data
### Training Procedure
#### Preprocessing [optional]
#### Training Hyperparameters
- Training regime:
#### Speeds, Sizes, Times [optional]
## Evaluation
### Testing Data, Factors & Metrics
#### Testing Data
#### Factors
#### Metrics
### Results
#### Summary
## Model Examination [optional]
## Environmental Impact
Carbon emissions can be estimated using the Machine Learning Impact calculator presented in Lacoste et al. (2019).
- Hardware Type:
- Hours used:
- Cloud Provider:
- Compute Region:
- Carbon Emitted:
## Technical Specifications [optional]
### Model Architecture and Objective
### Compute Infrastructure
#### Hardware
#### Software
[optional]
BibTeX:
APA:
## Glossary [optional]
## More Information [optional]
## Model Card Authors [optional]
## Model Card Contact
| [
"# Model Card for Model ID",
"## Model Details",
"### Model Description\n\n\n\nThis is the model card of a transformers model that has been pushed on the Hub. This model card has been automatically generated.\n\n- Developed by: \n- Funded by [optional]: \n- Shared by [optional]: \n- Model type: \n- Language(s) (NLP): \n- License: \n- Finetuned from model [optional]:",
"### Model Sources [optional]\n\n\n\n- Repository: \n- Paper [optional]: \n- Demo [optional]:",
"## Uses",
"### Direct Use",
"### Downstream Use [optional]",
"### Out-of-Scope Use",
"## Bias, Risks, and Limitations",
"### Recommendations\n\n\n\nUsers (both direct and downstream) should be made aware of the risks, biases and limitations of the model. More information needed for further recommendations.",
"## How to Get Started with the Model\n\nUse the code below to get started with the model.",
"## Training Details",
"### Training Data",
"### Training Procedure",
"#### Preprocessing [optional]",
"#### Training Hyperparameters\n\n- Training regime:",
"#### Speeds, Sizes, Times [optional]",
"## Evaluation",
"### Testing Data, Factors & Metrics",
"#### Testing Data",
"#### Factors",
"#### Metrics",
"### Results",
"#### Summary",
"## Model Examination [optional]",
"## Environmental Impact\n\n\n\nCarbon emissions can be estimated using the Machine Learning Impact calculator presented in Lacoste et al. (2019).\n\n- Hardware Type: \n- Hours used: \n- Cloud Provider: \n- Compute Region: \n- Carbon Emitted:",
"## Technical Specifications [optional]",
"### Model Architecture and Objective",
"### Compute Infrastructure",
"#### Hardware",
"#### Software\n\n\n\n[optional]\n\n\n\nBibTeX:\n\n\n\nAPA:",
"## Glossary [optional]",
"## More Information [optional]",
"## Model Card Authors [optional]",
"## Model Card Contact"
] | [
"TAGS\n#transformers #safetensors #arxiv-1910.09700 #endpoints_compatible #region-us \n",
"# Model Card for Model ID",
"## Model Details",
"### Model Description\n\n\n\nThis is the model card of a transformers model that has been pushed on the Hub. This model card has been automatically generated.\n\n- Developed by: \n- Funded by [optional]: \n- Shared by [optional]: \n- Model type: \n- Language(s) (NLP): \n- License: \n- Finetuned from model [optional]:",
"### Model Sources [optional]\n\n\n\n- Repository: \n- Paper [optional]: \n- Demo [optional]:",
"## Uses",
"### Direct Use",
"### Downstream Use [optional]",
"### Out-of-Scope Use",
"## Bias, Risks, and Limitations",
"### Recommendations\n\n\n\nUsers (both direct and downstream) should be made aware of the risks, biases and limitations of the model. More information needed for further recommendations.",
"## How to Get Started with the Model\n\nUse the code below to get started with the model.",
"## Training Details",
"### Training Data",
"### Training Procedure",
"#### Preprocessing [optional]",
"#### Training Hyperparameters\n\n- Training regime:",
"#### Speeds, Sizes, Times [optional]",
"## Evaluation",
"### Testing Data, Factors & Metrics",
"#### Testing Data",
"#### Factors",
"#### Metrics",
"### Results",
"#### Summary",
"## Model Examination [optional]",
"## Environmental Impact\n\n\n\nCarbon emissions can be estimated using the Machine Learning Impact calculator presented in Lacoste et al. (2019).\n\n- Hardware Type: \n- Hours used: \n- Cloud Provider: \n- Compute Region: \n- Carbon Emitted:",
"## Technical Specifications [optional]",
"### Model Architecture and Objective",
"### Compute Infrastructure",
"#### Hardware",
"#### Software\n\n\n\n[optional]\n\n\n\nBibTeX:\n\n\n\nAPA:",
"## Glossary [optional]",
"## More Information [optional]",
"## Model Card Authors [optional]",
"## Model Card Contact"
] |
text-generation | transformers | Mistral-7B Swahili [LAPT + Heuristics (Untied)]
===
## How to use
```python
from peft import AutoPeftModelForCausalLM
from transformers import AutoTokenizer
model = AutoPeftModelForCausalLM.from_pretrained(
"atsuki-yamaguchi/Mistral-7B-v0.1-heuristics-untied-sw"
)
tokenizer = AutoTokenizer.from_pretrained(
"atsuki-yamaguchi/Mistral-7B-v0.1-heuristics-untied-sw"
)
# w/ GPU
model = AutoPeftModelForCausalLM.from_pretrained(
"atsuki-yamaguchi/Mistral-7B-v0.1-heuristics-untied-sw",
device_map="auto",
load_in_8bit=True,
)
```
## Citation
```
@article{yamaguchi2024empirical,
title={An Empirical Study on Cross-lingual Vocabulary Adaptation for Efficient Generative {LLM} Inference},
author={Atsuki Yamaguchi and Aline Villavicencio and Nikolaos Aletras},
journal={ArXiv},
year={2024},
volume={abs/2402.10712},
url={https://arxiv.org/abs/2402.10712}
}
```
## Link
For more details, please visit https://github.com/gucci-j/llm-cva
| {"language": "sw", "license": "mit"} | atsuki-yamaguchi/Mistral-7B-v0.1-heuristics-untied-sw | null | [
"transformers",
"safetensors",
"mistral",
"text-generation",
"sw",
"arxiv:2402.10712",
"license:mit",
"autotrain_compatible",
"endpoints_compatible",
"text-generation-inference",
"region:us"
] | null | 2024-04-21T18:12:41+00:00 | [
"2402.10712"
] | [
"sw"
] | TAGS
#transformers #safetensors #mistral #text-generation #sw #arxiv-2402.10712 #license-mit #autotrain_compatible #endpoints_compatible #text-generation-inference #region-us
| Mistral-7B Swahili [LAPT + Heuristics (Untied)]
===
## How to use
## Link
For more details, please visit URL
| [
"## How to use",
"## Link\nFor more details, please visit URL"
] | [
"TAGS\n#transformers #safetensors #mistral #text-generation #sw #arxiv-2402.10712 #license-mit #autotrain_compatible #endpoints_compatible #text-generation-inference #region-us \n",
"## How to use",
"## Link\nFor more details, please visit URL"
] |
reinforcement-learning | ml-agents |
# **ppo** Agent playing **SnowballTarget**
This is a trained model of a **ppo** agent playing **SnowballTarget**
using the [Unity ML-Agents Library](https://github.com/Unity-Technologies/ml-agents).
## Usage (with ML-Agents)
The Documentation: https://unity-technologies.github.io/ml-agents/ML-Agents-Toolkit-Documentation/
We wrote a complete tutorial to learn to train your first agent using ML-Agents and publish it to the Hub:
- A *short tutorial* where you teach Huggy the Dog 🐶 to fetch the stick and then play with him directly in your
browser: https://huggingface.co/learn/deep-rl-course/unitbonus1/introduction
- A *longer tutorial* to understand how works ML-Agents:
https://huggingface.co/learn/deep-rl-course/unit5/introduction
### Resume the training
```bash
mlagents-learn <your_configuration_file_path.yaml> --run-id=<run_id> --resume
```
### Watch your Agent play
You can watch your agent **playing directly in your browser**
1. If the environment is part of ML-Agents official environments, go to https://huggingface.co/unity
2. Step 1: Find your model_id: ahforoughi/ppo-SnowballTarget
3. Step 2: Select your *.nn /*.onnx file
4. Click on Watch the agent play 👀
| {"library_name": "ml-agents", "tags": ["SnowballTarget", "deep-reinforcement-learning", "reinforcement-learning", "ML-Agents-SnowballTarget"]} | ahforoughi/ppo-SnowballTarget | null | [
"ml-agents",
"tensorboard",
"onnx",
"SnowballTarget",
"deep-reinforcement-learning",
"reinforcement-learning",
"ML-Agents-SnowballTarget",
"region:us"
] | null | 2024-04-21T18:13:10+00:00 | [] | [] | TAGS
#ml-agents #tensorboard #onnx #SnowballTarget #deep-reinforcement-learning #reinforcement-learning #ML-Agents-SnowballTarget #region-us
|
# ppo Agent playing SnowballTarget
This is a trained model of a ppo agent playing SnowballTarget
using the Unity ML-Agents Library.
## Usage (with ML-Agents)
The Documentation: URL
We wrote a complete tutorial to learn to train your first agent using ML-Agents and publish it to the Hub:
- A *short tutorial* where you teach Huggy the Dog to fetch the stick and then play with him directly in your
browser: URL
- A *longer tutorial* to understand how works ML-Agents:
URL
### Resume the training
### Watch your Agent play
You can watch your agent playing directly in your browser
1. If the environment is part of ML-Agents official environments, go to URL
2. Step 1: Find your model_id: ahforoughi/ppo-SnowballTarget
3. Step 2: Select your *.nn /*.onnx file
4. Click on Watch the agent play
| [
"# ppo Agent playing SnowballTarget\n This is a trained model of a ppo agent playing SnowballTarget\n using the Unity ML-Agents Library.\n\n ## Usage (with ML-Agents)\n The Documentation: URL\n\n We wrote a complete tutorial to learn to train your first agent using ML-Agents and publish it to the Hub:\n - A *short tutorial* where you teach Huggy the Dog to fetch the stick and then play with him directly in your\n browser: URL\n - A *longer tutorial* to understand how works ML-Agents:\n URL\n\n ### Resume the training\n \n\n ### Watch your Agent play\n You can watch your agent playing directly in your browser\n\n 1. If the environment is part of ML-Agents official environments, go to URL\n 2. Step 1: Find your model_id: ahforoughi/ppo-SnowballTarget\n 3. Step 2: Select your *.nn /*.onnx file\n 4. Click on Watch the agent play"
] | [
"TAGS\n#ml-agents #tensorboard #onnx #SnowballTarget #deep-reinforcement-learning #reinforcement-learning #ML-Agents-SnowballTarget #region-us \n",
"# ppo Agent playing SnowballTarget\n This is a trained model of a ppo agent playing SnowballTarget\n using the Unity ML-Agents Library.\n\n ## Usage (with ML-Agents)\n The Documentation: URL\n\n We wrote a complete tutorial to learn to train your first agent using ML-Agents and publish it to the Hub:\n - A *short tutorial* where you teach Huggy the Dog to fetch the stick and then play with him directly in your\n browser: URL\n - A *longer tutorial* to understand how works ML-Agents:\n URL\n\n ### Resume the training\n \n\n ### Watch your Agent play\n You can watch your agent playing directly in your browser\n\n 1. If the environment is part of ML-Agents official environments, go to URL\n 2. Step 1: Find your model_id: ahforoughi/ppo-SnowballTarget\n 3. Step 2: Select your *.nn /*.onnx file\n 4. Click on Watch the agent play"
] |
text-generation | transformers | # MeowGPT Readme
## Overview
MeowGPT, developed by CutyCat2000x, is a language model based on Llama with the checkpoint version ll3. This model is designed to generate text in a conversational manner and can be used for various natural language processing tasks.
## Usage
### Loading the Model
To use MeowGPT, you can load it via the `transformers` library in Python using the following code:
```python
from transformers import LlamaTokenizer, AutoModelForCausalLM, AutoTokenizer
tokenizer = LlamaTokenizer.from_pretrained("cutycat2000x/MeowGPT-ll3")
model = AutoModelForCausalLM.from_pretrained("cutycat2000x/MeowGPT-ll3")
```
### Example Prompt
An example of how to prompt the model for generating text:
```python
{{ bos_token }}{% if messages[0]['role'] == 'system' %}{% set loop_messages = messages[1:] %}{% set system_message = messages[0]['content'] %}{% else %}{% set loop_messages = messages %}{% set system_message = false %}{% endif %}{% for message in loop_messages %}{% if (message['role'] == 'user') != (loop.index0 % 2 == 0) %}{{ raise_exception('Conversation roles must alternate user/assistant/user/assistant/...') }}{% endif %}{% if loop.index0 == 0 and system_message != false %}{% set content = '<<SYS>>\\n' + system_message + '\\n<</SYS>>\\n\\n' + message['content'] %}{% else %}{% set content = message['content'] %}{% endif %}{% if message['role'] == 'user' %}{{ '[INST] ' + content.strip() + ' [/INST]' }}{% elif message['role'] == 'assistant' %}{{ ' ' + content.strip() + eos_token }}{% endif %}{% endfor %}
```
The <s> and </s> are start and end tokens.
## About the Model
- **Base Model**: Llama3
- **Checkpoint Version**: ll3
- **Datasets Used**: Open-Orca/SlimOrca-Dedup, jondurbin/airoboros-3.2, microsoft/orca-math-word-problems-200k, m-a-p/Code-Feedback, MaziyarPanahi/WizardLM_evol_instruct_V2_196k, mlabonne/orpo-dpo-mix-40k
## Citation
If you use MeowGPT in your research or projects, please consider citing CutyCat2000x.
## Disclaimer
Please note that while MeowGPT is trained to assist in generating text based on given prompts, it may not always provide accurate or contextually appropriate responses. It's recommended to review and validate the generated content before usage in critical applications.
For more information or support, refer to the `transformers` library documentation or CutyCat2000x's resources. | {"language": ["en"], "license": "mit", "library_name": "transformers", "tags": ["freeai", "conversational", "meowgpt", "gpt", "free", "opensource", "splittic", "ai", "llama", "llama3"], "datasets": ["Open-Orca/SlimOrca-Dedup", "jondurbin/airoboros-3.2", "microsoft/orca-math-word-problems-200k", "m-a-p/Code-Feedback", "MaziyarPanahi/WizardLM_evol_instruct_V2_196k", "mlabonne/orpo-dpo-mix-40k"], "pipeline_tag": "text-generation", "widget": [{"text": "<s> [|User|] Hello World </s>[|Assistant|]"}]} | cutycat2000x/MeowGPT-ll3 | null | [
"transformers",
"safetensors",
"llama",
"text-generation",
"freeai",
"conversational",
"meowgpt",
"gpt",
"free",
"opensource",
"splittic",
"ai",
"llama3",
"en",
"dataset:Open-Orca/SlimOrca-Dedup",
"dataset:jondurbin/airoboros-3.2",
"dataset:microsoft/orca-math-word-problems-200k",
"dataset:m-a-p/Code-Feedback",
"dataset:MaziyarPanahi/WizardLM_evol_instruct_V2_196k",
"dataset:mlabonne/orpo-dpo-mix-40k",
"license:mit",
"autotrain_compatible",
"endpoints_compatible",
"text-generation-inference",
"region:us"
] | null | 2024-04-21T18:13:42+00:00 | [] | [
"en"
] | TAGS
#transformers #safetensors #llama #text-generation #freeai #conversational #meowgpt #gpt #free #opensource #splittic #ai #llama3 #en #dataset-Open-Orca/SlimOrca-Dedup #dataset-jondurbin/airoboros-3.2 #dataset-microsoft/orca-math-word-problems-200k #dataset-m-a-p/Code-Feedback #dataset-MaziyarPanahi/WizardLM_evol_instruct_V2_196k #dataset-mlabonne/orpo-dpo-mix-40k #license-mit #autotrain_compatible #endpoints_compatible #text-generation-inference #region-us
| # MeowGPT Readme
## Overview
MeowGPT, developed by CutyCat2000x, is a language model based on Llama with the checkpoint version ll3. This model is designed to generate text in a conversational manner and can be used for various natural language processing tasks.
## Usage
### Loading the Model
To use MeowGPT, you can load it via the 'transformers' library in Python using the following code:
### Example Prompt
An example of how to prompt the model for generating text:
The <s> and </s> are start and end tokens.
## About the Model
- Base Model: Llama3
- Checkpoint Version: ll3
- Datasets Used: Open-Orca/SlimOrca-Dedup, jondurbin/airoboros-3.2, microsoft/orca-math-word-problems-200k, m-a-p/Code-Feedback, MaziyarPanahi/WizardLM_evol_instruct_V2_196k, mlabonne/orpo-dpo-mix-40k
If you use MeowGPT in your research or projects, please consider citing CutyCat2000x.
## Disclaimer
Please note that while MeowGPT is trained to assist in generating text based on given prompts, it may not always provide accurate or contextually appropriate responses. It's recommended to review and validate the generated content before usage in critical applications.
For more information or support, refer to the 'transformers' library documentation or CutyCat2000x's resources. | [
"# MeowGPT Readme",
"## Overview\nMeowGPT, developed by CutyCat2000x, is a language model based on Llama with the checkpoint version ll3. This model is designed to generate text in a conversational manner and can be used for various natural language processing tasks.",
"## Usage",
"### Loading the Model\nTo use MeowGPT, you can load it via the 'transformers' library in Python using the following code:",
"### Example Prompt\nAn example of how to prompt the model for generating text:\n\n\n\nThe <s> and </s> are start and end tokens.",
"## About the Model\n- Base Model: Llama3\n- Checkpoint Version: ll3\n- Datasets Used: Open-Orca/SlimOrca-Dedup, jondurbin/airoboros-3.2, microsoft/orca-math-word-problems-200k, m-a-p/Code-Feedback, MaziyarPanahi/WizardLM_evol_instruct_V2_196k, mlabonne/orpo-dpo-mix-40k\n\nIf you use MeowGPT in your research or projects, please consider citing CutyCat2000x.",
"## Disclaimer\nPlease note that while MeowGPT is trained to assist in generating text based on given prompts, it may not always provide accurate or contextually appropriate responses. It's recommended to review and validate the generated content before usage in critical applications.\n\nFor more information or support, refer to the 'transformers' library documentation or CutyCat2000x's resources."
] | [
"TAGS\n#transformers #safetensors #llama #text-generation #freeai #conversational #meowgpt #gpt #free #opensource #splittic #ai #llama3 #en #dataset-Open-Orca/SlimOrca-Dedup #dataset-jondurbin/airoboros-3.2 #dataset-microsoft/orca-math-word-problems-200k #dataset-m-a-p/Code-Feedback #dataset-MaziyarPanahi/WizardLM_evol_instruct_V2_196k #dataset-mlabonne/orpo-dpo-mix-40k #license-mit #autotrain_compatible #endpoints_compatible #text-generation-inference #region-us \n",
"# MeowGPT Readme",
"## Overview\nMeowGPT, developed by CutyCat2000x, is a language model based on Llama with the checkpoint version ll3. This model is designed to generate text in a conversational manner and can be used for various natural language processing tasks.",
"## Usage",
"### Loading the Model\nTo use MeowGPT, you can load it via the 'transformers' library in Python using the following code:",
"### Example Prompt\nAn example of how to prompt the model for generating text:\n\n\n\nThe <s> and </s> are start and end tokens.",
"## About the Model\n- Base Model: Llama3\n- Checkpoint Version: ll3\n- Datasets Used: Open-Orca/SlimOrca-Dedup, jondurbin/airoboros-3.2, microsoft/orca-math-word-problems-200k, m-a-p/Code-Feedback, MaziyarPanahi/WizardLM_evol_instruct_V2_196k, mlabonne/orpo-dpo-mix-40k\n\nIf you use MeowGPT in your research or projects, please consider citing CutyCat2000x.",
"## Disclaimer\nPlease note that while MeowGPT is trained to assist in generating text based on given prompts, it may not always provide accurate or contextually appropriate responses. It's recommended to review and validate the generated content before usage in critical applications.\n\nFor more information or support, refer to the 'transformers' library documentation or CutyCat2000x's resources."
] |
text-classification | transformers |
<!-- This model card has been generated automatically according to the information the Trainer had access to. You
should probably proofread and complete it, then remove this comment. -->
# MGG_model_tclss
This model is a fine-tuned version of [distilbert/distilbert-base-uncased](https://huggingface.co/distilbert/distilbert-base-uncased) on an unknown dataset.
It achieves the following results on the evaluation set:
- Loss: 0.2352
- Accuracy: 0.9316
## Model description
More information needed
## Intended uses & limitations
More information needed
## Training and evaluation data
More information needed
## Training procedure
### Training hyperparameters
The following hyperparameters were used during training:
- learning_rate: 2e-05
- train_batch_size: 16
- eval_batch_size: 16
- seed: 42
- optimizer: Adam with betas=(0.9,0.999) and epsilon=1e-08
- lr_scheduler_type: linear
- num_epochs: 2
### Training results
| Training Loss | Epoch | Step | Validation Loss | Accuracy |
|:-------------:|:-----:|:----:|:---------------:|:--------:|
| 0.2228 | 1.0 | 1563 | 0.1990 | 0.9237 |
| 0.1449 | 2.0 | 3126 | 0.2352 | 0.9316 |
### Framework versions
- Transformers 4.40.0
- Pytorch 2.2.1+cu121
- Datasets 2.19.0
- Tokenizers 0.19.1
| {"license": "apache-2.0", "tags": ["generated_from_trainer"], "metrics": ["accuracy"], "base_model": "distilbert/distilbert-base-uncased", "model-index": [{"name": "MGG_model_tclss", "results": []}]} | ManuGG/MGG_model_tclss | null | [
"transformers",
"tensorboard",
"safetensors",
"distilbert",
"text-classification",
"generated_from_trainer",
"base_model:distilbert/distilbert-base-uncased",
"license:apache-2.0",
"autotrain_compatible",
"endpoints_compatible",
"region:us"
] | null | 2024-04-21T18:14:43+00:00 | [] | [] | TAGS
#transformers #tensorboard #safetensors #distilbert #text-classification #generated_from_trainer #base_model-distilbert/distilbert-base-uncased #license-apache-2.0 #autotrain_compatible #endpoints_compatible #region-us
| MGG\_model\_tclss
=================
This model is a fine-tuned version of distilbert/distilbert-base-uncased on an unknown dataset.
It achieves the following results on the evaluation set:
* Loss: 0.2352
* Accuracy: 0.9316
Model description
-----------------
More information needed
Intended uses & limitations
---------------------------
More information needed
Training and evaluation data
----------------------------
More information needed
Training procedure
------------------
### Training hyperparameters
The following hyperparameters were used during training:
* learning\_rate: 2e-05
* train\_batch\_size: 16
* eval\_batch\_size: 16
* seed: 42
* optimizer: Adam with betas=(0.9,0.999) and epsilon=1e-08
* lr\_scheduler\_type: linear
* num\_epochs: 2
### Training results
### Framework versions
* Transformers 4.40.0
* Pytorch 2.2.1+cu121
* Datasets 2.19.0
* Tokenizers 0.19.1
| [
"### Training hyperparameters\n\n\nThe following hyperparameters were used during training:\n\n\n* learning\\_rate: 2e-05\n* train\\_batch\\_size: 16\n* eval\\_batch\\_size: 16\n* seed: 42\n* optimizer: Adam with betas=(0.9,0.999) and epsilon=1e-08\n* lr\\_scheduler\\_type: linear\n* num\\_epochs: 2",
"### Training results",
"### Framework versions\n\n\n* Transformers 4.40.0\n* Pytorch 2.2.1+cu121\n* Datasets 2.19.0\n* Tokenizers 0.19.1"
] | [
"TAGS\n#transformers #tensorboard #safetensors #distilbert #text-classification #generated_from_trainer #base_model-distilbert/distilbert-base-uncased #license-apache-2.0 #autotrain_compatible #endpoints_compatible #region-us \n",
"### Training hyperparameters\n\n\nThe following hyperparameters were used during training:\n\n\n* learning\\_rate: 2e-05\n* train\\_batch\\_size: 16\n* eval\\_batch\\_size: 16\n* seed: 42\n* optimizer: Adam with betas=(0.9,0.999) and epsilon=1e-08\n* lr\\_scheduler\\_type: linear\n* num\\_epochs: 2",
"### Training results",
"### Framework versions\n\n\n* Transformers 4.40.0\n* Pytorch 2.2.1+cu121\n* Datasets 2.19.0\n* Tokenizers 0.19.1"
] |
text-generation | transformers |
<!-- This model card has been generated automatically according to the information the Trainer had access to. You
should probably proofread and complete it, then remove this comment. -->
# gpt2+morf_s0-30-x-2_cx-en_00000-00009_50k
This model is a fine-tuned version of [](https://huggingface.co/) on the uonlp/CulturaX en dataset.
It achieves the following results on the evaluation set:
- Loss: 2.8423
- Accuracy: 0.4330
## Model description
More information needed
## Intended uses & limitations
More information needed
## Training and evaluation data
More information needed
## Training procedure
### Training hyperparameters
The following hyperparameters were used during training:
- learning_rate: 5e-05
- train_batch_size: 64
- eval_batch_size: 64
- seed: 42
- optimizer: Adam with betas=(0.9,0.999) and epsilon=1e-08
- lr_scheduler_type: linear
- num_epochs: 1.0
### Training results
| Training Loss | Epoch | Step | Validation Loss | Accuracy |
|:-------------:|:-----:|:------:|:---------------:|:--------:|
| 3.6569 | 0.03 | 10000 | 3.5764 | 0.3502 |
| 3.4317 | 0.06 | 20000 | 3.3581 | 0.3727 |
| 3.3161 | 0.09 | 30000 | 3.2447 | 0.3848 |
| 3.2463 | 0.13 | 40000 | 3.1761 | 0.3924 |
| 3.1897 | 0.16 | 50000 | 3.1277 | 0.3977 |
| 3.152 | 0.19 | 60000 | 3.0910 | 0.4022 |
| 3.1341 | 0.22 | 70000 | 3.0575 | 0.4060 |
| 3.1006 | 0.25 | 80000 | 3.0363 | 0.4084 |
| 3.0806 | 0.28 | 90000 | 3.0118 | 0.4115 |
| 3.0555 | 0.31 | 100000 | 2.9919 | 0.4138 |
| 3.038 | 0.34 | 110000 | 2.9786 | 0.4156 |
| 3.0291 | 0.38 | 120000 | 2.9651 | 0.4171 |
| 3.0182 | 0.41 | 130000 | 2.9499 | 0.4191 |
| 3.0145 | 0.44 | 140000 | 2.9381 | 0.4205 |
| 2.9891 | 0.47 | 150000 | 2.9272 | 0.4219 |
| 2.9836 | 0.5 | 160000 | 2.9191 | 0.4230 |
| 2.9717 | 0.53 | 170000 | 2.9103 | 0.4241 |
| 2.9651 | 0.56 | 180000 | 2.9039 | 0.4250 |
| 2.9615 | 0.59 | 190000 | 2.8971 | 0.4258 |
| 2.9556 | 0.63 | 200000 | 2.8882 | 0.4269 |
| 2.9452 | 0.66 | 210000 | 2.8825 | 0.4277 |
| 2.9412 | 0.69 | 220000 | 2.8766 | 0.4284 |
| 2.9402 | 0.72 | 230000 | 2.8722 | 0.4290 |
| 2.9299 | 0.75 | 240000 | 2.8675 | 0.4296 |
| 2.9302 | 0.78 | 250000 | 2.8623 | 0.4304 |
| 2.9165 | 0.81 | 260000 | 2.8585 | 0.4308 |
| 2.915 | 0.84 | 270000 | 2.8537 | 0.4314 |
| 2.92 | 0.88 | 280000 | 2.8506 | 0.4319 |
| 2.9186 | 0.91 | 290000 | 2.8484 | 0.4321 |
| 2.9084 | 0.94 | 300000 | 2.8458 | 0.4325 |
| 2.9142 | 0.97 | 310000 | 2.8438 | 0.4327 |
### Framework versions
- Transformers 4.37.1
- Pytorch 2.1.2+cu121
- Datasets 2.16.1
- Tokenizers 0.15.1 | {"language": ["en"], "license": "mit", "tags": ["generated_from_trainer"], "datasets": ["uonlp/CulturaX"], "metrics": ["accuracy"], "model-index": [{"name": "gpt2+morf_s0-30-x-2_cx-en_00000-00009_50k", "results": [{"task": {"type": "text-generation", "name": "Causal Language Modeling"}, "dataset": {"name": "uonlp/CulturaX en", "type": "uonlp/CulturaX", "args": "en"}, "metrics": [{"type": "accuracy", "value": 0.4329592727693433, "name": "Accuracy"}]}]}]} | jonasknobloch/gpt2-morf_s0-30-x-2_cx-en_00000-00009_50k | null | [
"transformers",
"safetensors",
"gpt2",
"text-generation",
"generated_from_trainer",
"en",
"dataset:uonlp/CulturaX",
"license:mit",
"model-index",
"autotrain_compatible",
"endpoints_compatible",
"text-generation-inference",
"region:us"
] | null | 2024-04-21T18:14:58+00:00 | [] | [
"en"
] | TAGS
#transformers #safetensors #gpt2 #text-generation #generated_from_trainer #en #dataset-uonlp/CulturaX #license-mit #model-index #autotrain_compatible #endpoints_compatible #text-generation-inference #region-us
| gpt2+morf\_s0-30-x-2\_cx-en\_00000-00009\_50k
=============================================
This model is a fine-tuned version of [](URL on the uonlp/CulturaX en dataset.
It achieves the following results on the evaluation set:
* Loss: 2.8423
* Accuracy: 0.4330
Model description
-----------------
More information needed
Intended uses & limitations
---------------------------
More information needed
Training and evaluation data
----------------------------
More information needed
Training procedure
------------------
### Training hyperparameters
The following hyperparameters were used during training:
* learning\_rate: 5e-05
* train\_batch\_size: 64
* eval\_batch\_size: 64
* seed: 42
* optimizer: Adam with betas=(0.9,0.999) and epsilon=1e-08
* lr\_scheduler\_type: linear
* num\_epochs: 1.0
### Training results
### Framework versions
* Transformers 4.37.1
* Pytorch 2.1.2+cu121
* Datasets 2.16.1
* Tokenizers 0.15.1
| [
"### Training hyperparameters\n\n\nThe following hyperparameters were used during training:\n\n\n* learning\\_rate: 5e-05\n* train\\_batch\\_size: 64\n* eval\\_batch\\_size: 64\n* seed: 42\n* optimizer: Adam with betas=(0.9,0.999) and epsilon=1e-08\n* lr\\_scheduler\\_type: linear\n* num\\_epochs: 1.0",
"### Training results",
"### Framework versions\n\n\n* Transformers 4.37.1\n* Pytorch 2.1.2+cu121\n* Datasets 2.16.1\n* Tokenizers 0.15.1"
] | [
"TAGS\n#transformers #safetensors #gpt2 #text-generation #generated_from_trainer #en #dataset-uonlp/CulturaX #license-mit #model-index #autotrain_compatible #endpoints_compatible #text-generation-inference #region-us \n",
"### Training hyperparameters\n\n\nThe following hyperparameters were used during training:\n\n\n* learning\\_rate: 5e-05\n* train\\_batch\\_size: 64\n* eval\\_batch\\_size: 64\n* seed: 42\n* optimizer: Adam with betas=(0.9,0.999) and epsilon=1e-08\n* lr\\_scheduler\\_type: linear\n* num\\_epochs: 1.0",
"### Training results",
"### Framework versions\n\n\n* Transformers 4.37.1\n* Pytorch 2.1.2+cu121\n* Datasets 2.16.1\n* Tokenizers 0.15.1"
] |
null | transformers |
# Uploaded model
- **Developed by:** sccastillo
- **License:** apache-2.0
- **Finetuned from model :** unsloth/mistral-7b-bnb-4bit
This mistral model was trained 2x faster with [Unsloth](https://github.com/unslothai/unsloth) and Huggingface's TRL library.
[<img src="https://raw.githubusercontent.com/unslothai/unsloth/main/images/unsloth%20made%20with%20love.png" width="200"/>](https://github.com/unslothai/unsloth)
| {"language": ["en"], "license": "apache-2.0", "tags": ["text-generation-inference", "transformers", "unsloth", "mistral", "trl"], "base_model": "unsloth/mistral-7b-bnb-4bit"} | sccastillo/tiny_med_es | null | [
"transformers",
"safetensors",
"text-generation-inference",
"unsloth",
"mistral",
"trl",
"en",
"base_model:unsloth/mistral-7b-bnb-4bit",
"license:apache-2.0",
"endpoints_compatible",
"region:us"
] | null | 2024-04-21T18:16:18+00:00 | [] | [
"en"
] | TAGS
#transformers #safetensors #text-generation-inference #unsloth #mistral #trl #en #base_model-unsloth/mistral-7b-bnb-4bit #license-apache-2.0 #endpoints_compatible #region-us
|
# Uploaded model
- Developed by: sccastillo
- License: apache-2.0
- Finetuned from model : unsloth/mistral-7b-bnb-4bit
This mistral model was trained 2x faster with Unsloth and Huggingface's TRL library.
<img src="URL width="200"/>
| [
"# Uploaded model\n\n- Developed by: sccastillo\n- License: apache-2.0\n- Finetuned from model : unsloth/mistral-7b-bnb-4bit\n\nThis mistral model was trained 2x faster with Unsloth and Huggingface's TRL library.\n\n<img src=\"URL width=\"200\"/>"
] | [
"TAGS\n#transformers #safetensors #text-generation-inference #unsloth #mistral #trl #en #base_model-unsloth/mistral-7b-bnb-4bit #license-apache-2.0 #endpoints_compatible #region-us \n",
"# Uploaded model\n\n- Developed by: sccastillo\n- License: apache-2.0\n- Finetuned from model : unsloth/mistral-7b-bnb-4bit\n\nThis mistral model was trained 2x faster with Unsloth and Huggingface's TRL library.\n\n<img src=\"URL width=\"200\"/>"
] |
token-classification | transformers |
<!-- This model card has been generated automatically according to the information the Trainer had access to. You
should probably proofread and complete it, then remove this comment. -->
# my_awesome_wnut_model_
This model is a fine-tuned version of [cointegrated/rubert-tiny2](https://huggingface.co/cointegrated/rubert-tiny2) on the None dataset.
It achieves the following results on the evaluation set:
- Loss: 0.1997
- Precision: 0.3076
- Recall: 0.4690
- F1: 0.3716
- Accuracy: 0.9322
## Model description
More information needed
## Intended uses & limitations
More information needed
## Training and evaluation data
More information needed
## Training procedure
### Training hyperparameters
The following hyperparameters were used during training:
- learning_rate: 2e-05
- train_batch_size: 7
- eval_batch_size: 7
- seed: 42
- optimizer: Adam with betas=(0.9,0.999) and epsilon=1e-08
- lr_scheduler_type: linear
- num_epochs: 24
### Training results
| Training Loss | Epoch | Step | Validation Loss | Precision | Recall | F1 | Accuracy |
|:-------------:|:-----:|:----:|:---------------:|:---------:|:------:|:------:|:--------:|
| 0.621 | 2.41 | 140 | 0.4025 | 0.0 | 0.0 | 0.0 | 0.9050 |
| 0.3224 | 4.83 | 280 | 0.2750 | 0.2036 | 0.2074 | 0.2055 | 0.9118 |
| 0.2421 | 7.24 | 420 | 0.2326 | 0.2706 | 0.3406 | 0.3016 | 0.9220 |
| 0.2061 | 9.66 | 560 | 0.2146 | 0.2968 | 0.4102 | 0.3444 | 0.9269 |
| 0.1779 | 12.07 | 700 | 0.2037 | 0.3125 | 0.4257 | 0.3604 | 0.9306 |
| 0.1606 | 14.48 | 840 | 0.2042 | 0.3044 | 0.4613 | 0.3668 | 0.9298 |
| 0.1544 | 16.9 | 980 | 0.2001 | 0.3101 | 0.4690 | 0.3734 | 0.9310 |
| 0.1402 | 19.31 | 1120 | 0.1991 | 0.3130 | 0.4690 | 0.3755 | 0.9316 |
| 0.139 | 21.72 | 1260 | 0.1997 | 0.3076 | 0.4690 | 0.3716 | 0.9322 |
### Framework versions
- Transformers 4.38.2
- Pytorch 2.2.1+cu121
- Datasets 2.19.0
- Tokenizers 0.15.2
| {"license": "mit", "tags": ["generated_from_trainer"], "metrics": ["precision", "recall", "f1", "accuracy"], "base_model": "cointegrated/rubert-tiny2", "model-index": [{"name": "my_awesome_wnut_model_", "results": []}]} | NastyaKorneeva/my_awesome_wnut_model_ | null | [
"transformers",
"tensorboard",
"safetensors",
"bert",
"token-classification",
"generated_from_trainer",
"base_model:cointegrated/rubert-tiny2",
"license:mit",
"autotrain_compatible",
"endpoints_compatible",
"region:us"
] | null | 2024-04-21T18:16:53+00:00 | [] | [] | TAGS
#transformers #tensorboard #safetensors #bert #token-classification #generated_from_trainer #base_model-cointegrated/rubert-tiny2 #license-mit #autotrain_compatible #endpoints_compatible #region-us
| my\_awesome\_wnut\_model\_
==========================
This model is a fine-tuned version of cointegrated/rubert-tiny2 on the None dataset.
It achieves the following results on the evaluation set:
* Loss: 0.1997
* Precision: 0.3076
* Recall: 0.4690
* F1: 0.3716
* Accuracy: 0.9322
Model description
-----------------
More information needed
Intended uses & limitations
---------------------------
More information needed
Training and evaluation data
----------------------------
More information needed
Training procedure
------------------
### Training hyperparameters
The following hyperparameters were used during training:
* learning\_rate: 2e-05
* train\_batch\_size: 7
* eval\_batch\_size: 7
* seed: 42
* optimizer: Adam with betas=(0.9,0.999) and epsilon=1e-08
* lr\_scheduler\_type: linear
* num\_epochs: 24
### Training results
### Framework versions
* Transformers 4.38.2
* Pytorch 2.2.1+cu121
* Datasets 2.19.0
* Tokenizers 0.15.2
| [
"### Training hyperparameters\n\n\nThe following hyperparameters were used during training:\n\n\n* learning\\_rate: 2e-05\n* train\\_batch\\_size: 7\n* eval\\_batch\\_size: 7\n* seed: 42\n* optimizer: Adam with betas=(0.9,0.999) and epsilon=1e-08\n* lr\\_scheduler\\_type: linear\n* num\\_epochs: 24",
"### Training results",
"### Framework versions\n\n\n* Transformers 4.38.2\n* Pytorch 2.2.1+cu121\n* Datasets 2.19.0\n* Tokenizers 0.15.2"
] | [
"TAGS\n#transformers #tensorboard #safetensors #bert #token-classification #generated_from_trainer #base_model-cointegrated/rubert-tiny2 #license-mit #autotrain_compatible #endpoints_compatible #region-us \n",
"### Training hyperparameters\n\n\nThe following hyperparameters were used during training:\n\n\n* learning\\_rate: 2e-05\n* train\\_batch\\_size: 7\n* eval\\_batch\\_size: 7\n* seed: 42\n* optimizer: Adam with betas=(0.9,0.999) and epsilon=1e-08\n* lr\\_scheduler\\_type: linear\n* num\\_epochs: 24",
"### Training results",
"### Framework versions\n\n\n* Transformers 4.38.2\n* Pytorch 2.2.1+cu121\n* Datasets 2.19.0\n* Tokenizers 0.15.2"
] |
text-generation | transformers |
<!-- This model card has been generated automatically according to the information the Trainer had access to. You
should probably proofread and complete it, then remove this comment. -->
# Llama-2-7b-chat-hf_fictional_chinese_v1
This model is a fine-tuned version of [meta-llama/Llama-2-7b-chat-hf](https://huggingface.co/meta-llama/Llama-2-7b-chat-hf) on the generator dataset.
## Model description
More information needed
## Intended uses & limitations
More information needed
## Training and evaluation data
More information needed
## Training procedure
### Training hyperparameters
The following hyperparameters were used during training:
- learning_rate: 5e-05
- train_batch_size: 1
- eval_batch_size: 2
- seed: 42
- gradient_accumulation_steps: 8
- total_train_batch_size: 8
- optimizer: Adam with betas=(0.9,0.999) and epsilon=1e-08
- lr_scheduler_type: linear
- num_epochs: 10
### Training results
### Framework versions
- Transformers 4.40.0
- Pytorch 2.1.2
- Datasets 2.19.0
- Tokenizers 0.19.1
| {"license": "llama2", "tags": ["trl", "sft", "generated_from_trainer"], "datasets": ["generator"], "base_model": "meta-llama/Llama-2-7b-chat-hf", "model-index": [{"name": "Llama-2-7b-chat-hf_fictional_chinese_v1", "results": []}]} | yzhuang/Llama-2-7b-chat-hf_fictional_chinese_v1 | null | [
"transformers",
"safetensors",
"llama",
"text-generation",
"trl",
"sft",
"generated_from_trainer",
"conversational",
"dataset:generator",
"base_model:meta-llama/Llama-2-7b-chat-hf",
"license:llama2",
"autotrain_compatible",
"endpoints_compatible",
"text-generation-inference",
"region:us"
] | null | 2024-04-21T18:18:33+00:00 | [] | [] | TAGS
#transformers #safetensors #llama #text-generation #trl #sft #generated_from_trainer #conversational #dataset-generator #base_model-meta-llama/Llama-2-7b-chat-hf #license-llama2 #autotrain_compatible #endpoints_compatible #text-generation-inference #region-us
|
# Llama-2-7b-chat-hf_fictional_chinese_v1
This model is a fine-tuned version of meta-llama/Llama-2-7b-chat-hf on the generator dataset.
## Model description
More information needed
## Intended uses & limitations
More information needed
## Training and evaluation data
More information needed
## Training procedure
### Training hyperparameters
The following hyperparameters were used during training:
- learning_rate: 5e-05
- train_batch_size: 1
- eval_batch_size: 2
- seed: 42
- gradient_accumulation_steps: 8
- total_train_batch_size: 8
- optimizer: Adam with betas=(0.9,0.999) and epsilon=1e-08
- lr_scheduler_type: linear
- num_epochs: 10
### Training results
### Framework versions
- Transformers 4.40.0
- Pytorch 2.1.2
- Datasets 2.19.0
- Tokenizers 0.19.1
| [
"# Llama-2-7b-chat-hf_fictional_chinese_v1\n\nThis model is a fine-tuned version of meta-llama/Llama-2-7b-chat-hf on the generator dataset.",
"## Model description\n\nMore information needed",
"## Intended uses & limitations\n\nMore information needed",
"## Training and evaluation data\n\nMore information needed",
"## Training procedure",
"### Training hyperparameters\n\nThe following hyperparameters were used during training:\n- learning_rate: 5e-05\n- train_batch_size: 1\n- eval_batch_size: 2\n- seed: 42\n- gradient_accumulation_steps: 8\n- total_train_batch_size: 8\n- optimizer: Adam with betas=(0.9,0.999) and epsilon=1e-08\n- lr_scheduler_type: linear\n- num_epochs: 10",
"### Training results",
"### Framework versions\n\n- Transformers 4.40.0\n- Pytorch 2.1.2\n- Datasets 2.19.0\n- Tokenizers 0.19.1"
] | [
"TAGS\n#transformers #safetensors #llama #text-generation #trl #sft #generated_from_trainer #conversational #dataset-generator #base_model-meta-llama/Llama-2-7b-chat-hf #license-llama2 #autotrain_compatible #endpoints_compatible #text-generation-inference #region-us \n",
"# Llama-2-7b-chat-hf_fictional_chinese_v1\n\nThis model is a fine-tuned version of meta-llama/Llama-2-7b-chat-hf on the generator dataset.",
"## Model description\n\nMore information needed",
"## Intended uses & limitations\n\nMore information needed",
"## Training and evaluation data\n\nMore information needed",
"## Training procedure",
"### Training hyperparameters\n\nThe following hyperparameters were used during training:\n- learning_rate: 5e-05\n- train_batch_size: 1\n- eval_batch_size: 2\n- seed: 42\n- gradient_accumulation_steps: 8\n- total_train_batch_size: 8\n- optimizer: Adam with betas=(0.9,0.999) and epsilon=1e-08\n- lr_scheduler_type: linear\n- num_epochs: 10",
"### Training results",
"### Framework versions\n\n- Transformers 4.40.0\n- Pytorch 2.1.2\n- Datasets 2.19.0\n- Tokenizers 0.19.1"
] |
text-generation | transformers |
# Llama3merge6-15B-MoE
Llama3merge6-15B-MoE is a Mixture of Experts (MoE) made with the following models using [LazyMergekit](https://colab.research.google.com/drive/1obulZ1ROXHjYLn6PPZJwRR6GzgQogxxb?usp=sharing):
* [Kukedlc/NeuralLlamita-3-8B-v0.2](https://huggingface.co/Kukedlc/NeuralLlamita-3-8B-v0.2)
* [imone/Llama-3-8B-fixed-special-embedding](https://huggingface.co/imone/Llama-3-8B-fixed-special-embedding)
## 🧩 Configuration
```yaml
base_model: Kukedlc/NeuralLlamita-3-8B-v0.2
experts:
- source_model: Kukedlc/NeuralLlamita-3-8B-v0.2
positive_prompts: ["why"]
- source_model: imone/Llama-3-8B-fixed-special-embedding
positive_prompts: ["what"]
```
## 💻 Usage
```python
!pip install -qU transformers bitsandbytes accelerate
from transformers import AutoTokenizer
import transformers
import torch
model = "allknowingroger/Llama3merge6-15B-MoE"
tokenizer = AutoTokenizer.from_pretrained(model)
pipeline = transformers.pipeline(
"text-generation",
model=model,
model_kwargs={"torch_dtype": torch.float16, "load_in_4bit": True},
)
messages = [{"role": "user", "content": "Explain what a Mixture of Experts is in less than 100 words."}]
prompt = pipeline.tokenizer.apply_chat_template(messages, tokenize=False, add_generation_prompt=True)
outputs = pipeline(prompt, max_new_tokens=256, do_sample=True, temperature=0.7, top_k=50, top_p=0.95)
print(outputs[0]["generated_text"])
``` | {"license": "apache-2.0", "tags": ["moe", "frankenmoe", "merge", "mergekit", "lazymergekit", "Kukedlc/NeuralLlamita-3-8B-v0.2", "imone/Llama-3-8B-fixed-special-embedding"], "base_model": ["Kukedlc/NeuralLlamita-3-8B-v0.2", "imone/Llama-3-8B-fixed-special-embedding"]} | allknowingroger/Llama3merge6-15B-MoE | null | [
"transformers",
"safetensors",
"mixtral",
"text-generation",
"moe",
"frankenmoe",
"merge",
"mergekit",
"lazymergekit",
"Kukedlc/NeuralLlamita-3-8B-v0.2",
"imone/Llama-3-8B-fixed-special-embedding",
"conversational",
"base_model:Kukedlc/NeuralLlamita-3-8B-v0.2",
"base_model:imone/Llama-3-8B-fixed-special-embedding",
"license:apache-2.0",
"autotrain_compatible",
"endpoints_compatible",
"text-generation-inference",
"region:us"
] | null | 2024-04-21T18:18:44+00:00 | [] | [] | TAGS
#transformers #safetensors #mixtral #text-generation #moe #frankenmoe #merge #mergekit #lazymergekit #Kukedlc/NeuralLlamita-3-8B-v0.2 #imone/Llama-3-8B-fixed-special-embedding #conversational #base_model-Kukedlc/NeuralLlamita-3-8B-v0.2 #base_model-imone/Llama-3-8B-fixed-special-embedding #license-apache-2.0 #autotrain_compatible #endpoints_compatible #text-generation-inference #region-us
|
# Llama3merge6-15B-MoE
Llama3merge6-15B-MoE is a Mixture of Experts (MoE) made with the following models using LazyMergekit:
* Kukedlc/NeuralLlamita-3-8B-v0.2
* imone/Llama-3-8B-fixed-special-embedding
## Configuration
## Usage
| [
"# Llama3merge6-15B-MoE\n\nLlama3merge6-15B-MoE is a Mixture of Experts (MoE) made with the following models using LazyMergekit:\n* Kukedlc/NeuralLlamita-3-8B-v0.2\n* imone/Llama-3-8B-fixed-special-embedding",
"## Configuration",
"## Usage"
] | [
"TAGS\n#transformers #safetensors #mixtral #text-generation #moe #frankenmoe #merge #mergekit #lazymergekit #Kukedlc/NeuralLlamita-3-8B-v0.2 #imone/Llama-3-8B-fixed-special-embedding #conversational #base_model-Kukedlc/NeuralLlamita-3-8B-v0.2 #base_model-imone/Llama-3-8B-fixed-special-embedding #license-apache-2.0 #autotrain_compatible #endpoints_compatible #text-generation-inference #region-us \n",
"# Llama3merge6-15B-MoE\n\nLlama3merge6-15B-MoE is a Mixture of Experts (MoE) made with the following models using LazyMergekit:\n* Kukedlc/NeuralLlamita-3-8B-v0.2\n* imone/Llama-3-8B-fixed-special-embedding",
"## Configuration",
"## Usage"
] |
text-to-image | diffusers |
# AutoTrain SDXL LoRA DreamBooth - leonickson1/woodward_building_uncc
<Gallery />
## Model description
These are leonickson1/woodward_building_uncc LoRA adaption weights for stabilityai/stable-diffusion-xl-base-1.0.
The weights were trained using [DreamBooth](https://dreambooth.github.io/).
LoRA for the text encoder was enabled: False.
Special VAE used for training: None.
## Trigger words
You should use photo of a sks building to trigger the image generation.
## Download model
Weights for this model are available in Safetensors format.
[Download](leonickson1/woodward_building_uncc/tree/main) them in the Files & versions tab.
| {"license": "openrail++", "tags": ["autotrain", "stable-diffusion-xl", "stable-diffusion-xl-diffusers", "text-to-image", "diffusers", "lora", "template:sd-lora"], "base_model": "stabilityai/stable-diffusion-xl-base-1.0", "instance_prompt": "photo of a sks building"} | leonickson1/woodward_building_uncc | null | [
"diffusers",
"autotrain",
"stable-diffusion-xl",
"stable-diffusion-xl-diffusers",
"text-to-image",
"lora",
"template:sd-lora",
"base_model:stabilityai/stable-diffusion-xl-base-1.0",
"license:openrail++",
"region:us"
] | null | 2024-04-21T18:20:49+00:00 | [] | [] | TAGS
#diffusers #autotrain #stable-diffusion-xl #stable-diffusion-xl-diffusers #text-to-image #lora #template-sd-lora #base_model-stabilityai/stable-diffusion-xl-base-1.0 #license-openrail++ #region-us
|
# AutoTrain SDXL LoRA DreamBooth - leonickson1/woodward_building_uncc
<Gallery />
## Model description
These are leonickson1/woodward_building_uncc LoRA adaption weights for stabilityai/stable-diffusion-xl-base-1.0.
The weights were trained using DreamBooth.
LoRA for the text encoder was enabled: False.
Special VAE used for training: None.
## Trigger words
You should use photo of a sks building to trigger the image generation.
## Download model
Weights for this model are available in Safetensors format.
Download them in the Files & versions tab.
| [
"# AutoTrain SDXL LoRA DreamBooth - leonickson1/woodward_building_uncc\n\n<Gallery />",
"## Model description\n\nThese are leonickson1/woodward_building_uncc LoRA adaption weights for stabilityai/stable-diffusion-xl-base-1.0.\n\nThe weights were trained using DreamBooth.\n\nLoRA for the text encoder was enabled: False.\n\nSpecial VAE used for training: None.",
"## Trigger words\n\nYou should use photo of a sks building to trigger the image generation.",
"## Download model\n\nWeights for this model are available in Safetensors format.\n\nDownload them in the Files & versions tab."
] | [
"TAGS\n#diffusers #autotrain #stable-diffusion-xl #stable-diffusion-xl-diffusers #text-to-image #lora #template-sd-lora #base_model-stabilityai/stable-diffusion-xl-base-1.0 #license-openrail++ #region-us \n",
"# AutoTrain SDXL LoRA DreamBooth - leonickson1/woodward_building_uncc\n\n<Gallery />",
"## Model description\n\nThese are leonickson1/woodward_building_uncc LoRA adaption weights for stabilityai/stable-diffusion-xl-base-1.0.\n\nThe weights were trained using DreamBooth.\n\nLoRA for the text encoder was enabled: False.\n\nSpecial VAE used for training: None.",
"## Trigger words\n\nYou should use photo of a sks building to trigger the image generation.",
"## Download model\n\nWeights for this model are available in Safetensors format.\n\nDownload them in the Files & versions tab."
] |
text-to-image | diffusers |
# AutoTrain SDXL LoRA DreamBooth - leonickson1/cato_building_uncc
<Gallery />
## Model description
These are leonickson1/cato_building_uncc LoRA adaption weights for stabilityai/stable-diffusion-xl-base-1.0.
The weights were trained using [DreamBooth](https://dreambooth.github.io/).
LoRA for the text encoder was enabled: False.
Special VAE used for training: None.
## Trigger words
You should use photo of a sks building to trigger the image generation.
## Download model
Weights for this model are available in Safetensors format.
[Download](leonickson1/cato_building_uncc/tree/main) them in the Files & versions tab.
| {"license": "openrail++", "tags": ["autotrain", "stable-diffusion-xl", "stable-diffusion-xl-diffusers", "text-to-image", "diffusers", "lora", "template:sd-lora"], "base_model": "stabilityai/stable-diffusion-xl-base-1.0", "instance_prompt": "photo of a sks building"} | leonickson1/cato_building_uncc | null | [
"diffusers",
"autotrain",
"stable-diffusion-xl",
"stable-diffusion-xl-diffusers",
"text-to-image",
"lora",
"template:sd-lora",
"base_model:stabilityai/stable-diffusion-xl-base-1.0",
"license:openrail++",
"region:us"
] | null | 2024-04-21T18:21:07+00:00 | [] | [] | TAGS
#diffusers #autotrain #stable-diffusion-xl #stable-diffusion-xl-diffusers #text-to-image #lora #template-sd-lora #base_model-stabilityai/stable-diffusion-xl-base-1.0 #license-openrail++ #region-us
|
# AutoTrain SDXL LoRA DreamBooth - leonickson1/cato_building_uncc
<Gallery />
## Model description
These are leonickson1/cato_building_uncc LoRA adaption weights for stabilityai/stable-diffusion-xl-base-1.0.
The weights were trained using DreamBooth.
LoRA for the text encoder was enabled: False.
Special VAE used for training: None.
## Trigger words
You should use photo of a sks building to trigger the image generation.
## Download model
Weights for this model are available in Safetensors format.
Download them in the Files & versions tab.
| [
"# AutoTrain SDXL LoRA DreamBooth - leonickson1/cato_building_uncc\n\n<Gallery />",
"## Model description\n\nThese are leonickson1/cato_building_uncc LoRA adaption weights for stabilityai/stable-diffusion-xl-base-1.0.\n\nThe weights were trained using DreamBooth.\n\nLoRA for the text encoder was enabled: False.\n\nSpecial VAE used for training: None.",
"## Trigger words\n\nYou should use photo of a sks building to trigger the image generation.",
"## Download model\n\nWeights for this model are available in Safetensors format.\n\nDownload them in the Files & versions tab."
] | [
"TAGS\n#diffusers #autotrain #stable-diffusion-xl #stable-diffusion-xl-diffusers #text-to-image #lora #template-sd-lora #base_model-stabilityai/stable-diffusion-xl-base-1.0 #license-openrail++ #region-us \n",
"# AutoTrain SDXL LoRA DreamBooth - leonickson1/cato_building_uncc\n\n<Gallery />",
"## Model description\n\nThese are leonickson1/cato_building_uncc LoRA adaption weights for stabilityai/stable-diffusion-xl-base-1.0.\n\nThe weights were trained using DreamBooth.\n\nLoRA for the text encoder was enabled: False.\n\nSpecial VAE used for training: None.",
"## Trigger words\n\nYou should use photo of a sks building to trigger the image generation.",
"## Download model\n\nWeights for this model are available in Safetensors format.\n\nDownload them in the Files & versions tab."
] |
text-to-image | diffusers |
# AutoTrain SDXL LoRA DreamBooth - leonickson1/chhs_building_uncc
<Gallery />
## Model description
These are leonickson1/chhs_building_uncc LoRA adaption weights for stabilityai/stable-diffusion-xl-base-1.0.
The weights were trained using [DreamBooth](https://dreambooth.github.io/).
LoRA for the text encoder was enabled: False.
Special VAE used for training: None.
## Trigger words
You should use photo of a sks building to trigger the image generation.
## Download model
Weights for this model are available in Safetensors format.
[Download](leonickson1/chhs_building_uncc/tree/main) them in the Files & versions tab.
| {"license": "openrail++", "tags": ["autotrain", "stable-diffusion-xl", "stable-diffusion-xl-diffusers", "text-to-image", "diffusers", "lora", "template:sd-lora"], "base_model": "stabilityai/stable-diffusion-xl-base-1.0", "instance_prompt": "photo of a sks building"} | leonickson1/chhs_building_uncc | null | [
"diffusers",
"autotrain",
"stable-diffusion-xl",
"stable-diffusion-xl-diffusers",
"text-to-image",
"lora",
"template:sd-lora",
"base_model:stabilityai/stable-diffusion-xl-base-1.0",
"license:openrail++",
"region:us"
] | null | 2024-04-21T18:21:18+00:00 | [] | [] | TAGS
#diffusers #autotrain #stable-diffusion-xl #stable-diffusion-xl-diffusers #text-to-image #lora #template-sd-lora #base_model-stabilityai/stable-diffusion-xl-base-1.0 #license-openrail++ #region-us
|
# AutoTrain SDXL LoRA DreamBooth - leonickson1/chhs_building_uncc
<Gallery />
## Model description
These are leonickson1/chhs_building_uncc LoRA adaption weights for stabilityai/stable-diffusion-xl-base-1.0.
The weights were trained using DreamBooth.
LoRA for the text encoder was enabled: False.
Special VAE used for training: None.
## Trigger words
You should use photo of a sks building to trigger the image generation.
## Download model
Weights for this model are available in Safetensors format.
Download them in the Files & versions tab.
| [
"# AutoTrain SDXL LoRA DreamBooth - leonickson1/chhs_building_uncc\n\n<Gallery />",
"## Model description\n\nThese are leonickson1/chhs_building_uncc LoRA adaption weights for stabilityai/stable-diffusion-xl-base-1.0.\n\nThe weights were trained using DreamBooth.\n\nLoRA for the text encoder was enabled: False.\n\nSpecial VAE used for training: None.",
"## Trigger words\n\nYou should use photo of a sks building to trigger the image generation.",
"## Download model\n\nWeights for this model are available in Safetensors format.\n\nDownload them in the Files & versions tab."
] | [
"TAGS\n#diffusers #autotrain #stable-diffusion-xl #stable-diffusion-xl-diffusers #text-to-image #lora #template-sd-lora #base_model-stabilityai/stable-diffusion-xl-base-1.0 #license-openrail++ #region-us \n",
"# AutoTrain SDXL LoRA DreamBooth - leonickson1/chhs_building_uncc\n\n<Gallery />",
"## Model description\n\nThese are leonickson1/chhs_building_uncc LoRA adaption weights for stabilityai/stable-diffusion-xl-base-1.0.\n\nThe weights were trained using DreamBooth.\n\nLoRA for the text encoder was enabled: False.\n\nSpecial VAE used for training: None.",
"## Trigger words\n\nYou should use photo of a sks building to trigger the image generation.",
"## Download model\n\nWeights for this model are available in Safetensors format.\n\nDownload them in the Files & versions tab."
] |
text-to-image | diffusers |
# AutoTrain SDXL LoRA DreamBooth - leonickson1/atkins_library_building_uncc
<Gallery />
## Model description
These are leonickson1/atkins_library_building_uncc LoRA adaption weights for stabilityai/stable-diffusion-xl-base-1.0.
The weights were trained using [DreamBooth](https://dreambooth.github.io/).
LoRA for the text encoder was enabled: False.
Special VAE used for training: None.
## Trigger words
You should use photo of a sks building to trigger the image generation.
## Download model
Weights for this model are available in Safetensors format.
[Download](leonickson1/atkins_library_building_uncc/tree/main) them in the Files & versions tab.
| {"license": "openrail++", "tags": ["autotrain", "stable-diffusion-xl", "stable-diffusion-xl-diffusers", "text-to-image", "diffusers", "lora", "template:sd-lora"], "base_model": "stabilityai/stable-diffusion-xl-base-1.0", "instance_prompt": "photo of a sks building"} | leonickson1/atkins_library_building_uncc | null | [
"diffusers",
"autotrain",
"stable-diffusion-xl",
"stable-diffusion-xl-diffusers",
"text-to-image",
"lora",
"template:sd-lora",
"base_model:stabilityai/stable-diffusion-xl-base-1.0",
"license:openrail++",
"region:us"
] | null | 2024-04-21T18:21:35+00:00 | [] | [] | TAGS
#diffusers #autotrain #stable-diffusion-xl #stable-diffusion-xl-diffusers #text-to-image #lora #template-sd-lora #base_model-stabilityai/stable-diffusion-xl-base-1.0 #license-openrail++ #region-us
|
# AutoTrain SDXL LoRA DreamBooth - leonickson1/atkins_library_building_uncc
<Gallery />
## Model description
These are leonickson1/atkins_library_building_uncc LoRA adaption weights for stabilityai/stable-diffusion-xl-base-1.0.
The weights were trained using DreamBooth.
LoRA for the text encoder was enabled: False.
Special VAE used for training: None.
## Trigger words
You should use photo of a sks building to trigger the image generation.
## Download model
Weights for this model are available in Safetensors format.
Download them in the Files & versions tab.
| [
"# AutoTrain SDXL LoRA DreamBooth - leonickson1/atkins_library_building_uncc\n\n<Gallery />",
"## Model description\n\nThese are leonickson1/atkins_library_building_uncc LoRA adaption weights for stabilityai/stable-diffusion-xl-base-1.0.\n\nThe weights were trained using DreamBooth.\n\nLoRA for the text encoder was enabled: False.\n\nSpecial VAE used for training: None.",
"## Trigger words\n\nYou should use photo of a sks building to trigger the image generation.",
"## Download model\n\nWeights for this model are available in Safetensors format.\n\nDownload them in the Files & versions tab."
] | [
"TAGS\n#diffusers #autotrain #stable-diffusion-xl #stable-diffusion-xl-diffusers #text-to-image #lora #template-sd-lora #base_model-stabilityai/stable-diffusion-xl-base-1.0 #license-openrail++ #region-us \n",
"# AutoTrain SDXL LoRA DreamBooth - leonickson1/atkins_library_building_uncc\n\n<Gallery />",
"## Model description\n\nThese are leonickson1/atkins_library_building_uncc LoRA adaption weights for stabilityai/stable-diffusion-xl-base-1.0.\n\nThe weights were trained using DreamBooth.\n\nLoRA for the text encoder was enabled: False.\n\nSpecial VAE used for training: None.",
"## Trigger words\n\nYou should use photo of a sks building to trigger the image generation.",
"## Download model\n\nWeights for this model are available in Safetensors format.\n\nDownload them in the Files & versions tab."
] |
text-to-image | diffusers |
# AutoTrain SDXL LoRA DreamBooth - leonickson1/robinson_building_uncc
<Gallery />
## Model description
These are leonickson1/robinson_building_uncc LoRA adaption weights for stabilityai/stable-diffusion-xl-base-1.0.
The weights were trained using [DreamBooth](https://dreambooth.github.io/).
LoRA for the text encoder was enabled: False.
Special VAE used for training: None.
## Trigger words
You should use photo of a sks building to trigger the image generation.
## Download model
Weights for this model are available in Safetensors format.
[Download](leonickson1/robinson_building_uncc/tree/main) them in the Files & versions tab.
| {"license": "openrail++", "tags": ["autotrain", "stable-diffusion-xl", "stable-diffusion-xl-diffusers", "text-to-image", "diffusers", "lora", "template:sd-lora"], "base_model": "stabilityai/stable-diffusion-xl-base-1.0", "instance_prompt": "photo of a sks building"} | leonickson1/robinson_building_uncc | null | [
"diffusers",
"autotrain",
"stable-diffusion-xl",
"stable-diffusion-xl-diffusers",
"text-to-image",
"lora",
"template:sd-lora",
"base_model:stabilityai/stable-diffusion-xl-base-1.0",
"license:openrail++",
"region:us"
] | null | 2024-04-21T18:21:48+00:00 | [] | [] | TAGS
#diffusers #autotrain #stable-diffusion-xl #stable-diffusion-xl-diffusers #text-to-image #lora #template-sd-lora #base_model-stabilityai/stable-diffusion-xl-base-1.0 #license-openrail++ #region-us
|
# AutoTrain SDXL LoRA DreamBooth - leonickson1/robinson_building_uncc
<Gallery />
## Model description
These are leonickson1/robinson_building_uncc LoRA adaption weights for stabilityai/stable-diffusion-xl-base-1.0.
The weights were trained using DreamBooth.
LoRA for the text encoder was enabled: False.
Special VAE used for training: None.
## Trigger words
You should use photo of a sks building to trigger the image generation.
## Download model
Weights for this model are available in Safetensors format.
Download them in the Files & versions tab.
| [
"# AutoTrain SDXL LoRA DreamBooth - leonickson1/robinson_building_uncc\n\n<Gallery />",
"## Model description\n\nThese are leonickson1/robinson_building_uncc LoRA adaption weights for stabilityai/stable-diffusion-xl-base-1.0.\n\nThe weights were trained using DreamBooth.\n\nLoRA for the text encoder was enabled: False.\n\nSpecial VAE used for training: None.",
"## Trigger words\n\nYou should use photo of a sks building to trigger the image generation.",
"## Download model\n\nWeights for this model are available in Safetensors format.\n\nDownload them in the Files & versions tab."
] | [
"TAGS\n#diffusers #autotrain #stable-diffusion-xl #stable-diffusion-xl-diffusers #text-to-image #lora #template-sd-lora #base_model-stabilityai/stable-diffusion-xl-base-1.0 #license-openrail++ #region-us \n",
"# AutoTrain SDXL LoRA DreamBooth - leonickson1/robinson_building_uncc\n\n<Gallery />",
"## Model description\n\nThese are leonickson1/robinson_building_uncc LoRA adaption weights for stabilityai/stable-diffusion-xl-base-1.0.\n\nThe weights were trained using DreamBooth.\n\nLoRA for the text encoder was enabled: False.\n\nSpecial VAE used for training: None.",
"## Trigger words\n\nYou should use photo of a sks building to trigger the image generation.",
"## Download model\n\nWeights for this model are available in Safetensors format.\n\nDownload them in the Files & versions tab."
] |
text-generation | transformers |
# Model Card for Model ID
<!-- Provide a quick summary of what the model is/does. -->
## Model Details
### Model Description
<!-- Provide a longer summary of what this model is. -->
This is the model card of a 🤗 transformers model that has been pushed on the Hub. This model card has been automatically generated.
- **Developed by:** [More Information Needed]
- **Funded by [optional]:** [More Information Needed]
- **Shared by [optional]:** [More Information Needed]
- **Model type:** [More Information Needed]
- **Language(s) (NLP):** [More Information Needed]
- **License:** [More Information Needed]
- **Finetuned from model [optional]:** [More Information Needed]
### Model Sources [optional]
<!-- Provide the basic links for the model. -->
- **Repository:** [More Information Needed]
- **Paper [optional]:** [More Information Needed]
- **Demo [optional]:** [More Information Needed]
## Uses
<!-- Address questions around how the model is intended to be used, including the foreseeable users of the model and those affected by the model. -->
### Direct Use
<!-- This section is for the model use without fine-tuning or plugging into a larger ecosystem/app. -->
[More Information Needed]
### Downstream Use [optional]
<!-- This section is for the model use when fine-tuned for a task, or when plugged into a larger ecosystem/app -->
[More Information Needed]
### Out-of-Scope Use
<!-- This section addresses misuse, malicious use, and uses that the model will not work well for. -->
[More Information Needed]
## Bias, Risks, and Limitations
<!-- This section is meant to convey both technical and sociotechnical limitations. -->
[More Information Needed]
### Recommendations
<!-- This section is meant to convey recommendations with respect to the bias, risk, and technical limitations. -->
Users (both direct and downstream) should be made aware of the risks, biases and limitations of the model. More information needed for further recommendations.
## How to Get Started with the Model
Use the code below to get started with the model.
[More Information Needed]
## Training Details
### Training Data
<!-- This should link to a Dataset Card, perhaps with a short stub of information on what the training data is all about as well as documentation related to data pre-processing or additional filtering. -->
[More Information Needed]
### Training Procedure
<!-- This relates heavily to the Technical Specifications. Content here should link to that section when it is relevant to the training procedure. -->
#### Preprocessing [optional]
[More Information Needed]
#### Training Hyperparameters
- **Training regime:** [More Information Needed] <!--fp32, fp16 mixed precision, bf16 mixed precision, bf16 non-mixed precision, fp16 non-mixed precision, fp8 mixed precision -->
#### Speeds, Sizes, Times [optional]
<!-- This section provides information about throughput, start/end time, checkpoint size if relevant, etc. -->
[More Information Needed]
## Evaluation
<!-- This section describes the evaluation protocols and provides the results. -->
### Testing Data, Factors & Metrics
#### Testing Data
<!-- This should link to a Dataset Card if possible. -->
[More Information Needed]
#### Factors
<!-- These are the things the evaluation is disaggregating by, e.g., subpopulations or domains. -->
[More Information Needed]
#### Metrics
<!-- These are the evaluation metrics being used, ideally with a description of why. -->
[More Information Needed]
### Results
[More Information Needed]
#### Summary
## Model Examination [optional]
<!-- Relevant interpretability work for the model goes here -->
[More Information Needed]
## Environmental Impact
<!-- Total emissions (in grams of CO2eq) and additional considerations, such as electricity usage, go here. Edit the suggested text below accordingly -->
Carbon emissions can be estimated using the [Machine Learning Impact calculator](https://mlco2.github.io/impact#compute) presented in [Lacoste et al. (2019)](https://arxiv.org/abs/1910.09700).
- **Hardware Type:** [More Information Needed]
- **Hours used:** [More Information Needed]
- **Cloud Provider:** [More Information Needed]
- **Compute Region:** [More Information Needed]
- **Carbon Emitted:** [More Information Needed]
## Technical Specifications [optional]
### Model Architecture and Objective
[More Information Needed]
### Compute Infrastructure
[More Information Needed]
#### Hardware
[More Information Needed]
#### Software
[More Information Needed]
## Citation [optional]
<!-- If there is a paper or blog post introducing the model, the APA and Bibtex information for that should go in this section. -->
**BibTeX:**
[More Information Needed]
**APA:**
[More Information Needed]
## Glossary [optional]
<!-- If relevant, include terms and calculations in this section that can help readers understand the model or model card. -->
[More Information Needed]
## More Information [optional]
[More Information Needed]
## Model Card Authors [optional]
[More Information Needed]
## Model Card Contact
[More Information Needed] | {"library_name": "transformers", "tags": []} | engine58848/tpaejref_sn10_17 | null | [
"transformers",
"safetensors",
"llama",
"text-generation",
"conversational",
"arxiv:1910.09700",
"autotrain_compatible",
"endpoints_compatible",
"text-generation-inference",
"region:us"
] | null | 2024-04-21T18:21:58+00:00 | [
"1910.09700"
] | [] | TAGS
#transformers #safetensors #llama #text-generation #conversational #arxiv-1910.09700 #autotrain_compatible #endpoints_compatible #text-generation-inference #region-us
|
# Model Card for Model ID
## Model Details
### Model Description
This is the model card of a transformers model that has been pushed on the Hub. This model card has been automatically generated.
- Developed by:
- Funded by [optional]:
- Shared by [optional]:
- Model type:
- Language(s) (NLP):
- License:
- Finetuned from model [optional]:
### Model Sources [optional]
- Repository:
- Paper [optional]:
- Demo [optional]:
## Uses
### Direct Use
### Downstream Use [optional]
### Out-of-Scope Use
## Bias, Risks, and Limitations
### Recommendations
Users (both direct and downstream) should be made aware of the risks, biases and limitations of the model. More information needed for further recommendations.
## How to Get Started with the Model
Use the code below to get started with the model.
## Training Details
### Training Data
### Training Procedure
#### Preprocessing [optional]
#### Training Hyperparameters
- Training regime:
#### Speeds, Sizes, Times [optional]
## Evaluation
### Testing Data, Factors & Metrics
#### Testing Data
#### Factors
#### Metrics
### Results
#### Summary
## Model Examination [optional]
## Environmental Impact
Carbon emissions can be estimated using the Machine Learning Impact calculator presented in Lacoste et al. (2019).
- Hardware Type:
- Hours used:
- Cloud Provider:
- Compute Region:
- Carbon Emitted:
## Technical Specifications [optional]
### Model Architecture and Objective
### Compute Infrastructure
#### Hardware
#### Software
[optional]
BibTeX:
APA:
## Glossary [optional]
## More Information [optional]
## Model Card Authors [optional]
## Model Card Contact
| [
"# Model Card for Model ID",
"## Model Details",
"### Model Description\n\n\n\nThis is the model card of a transformers model that has been pushed on the Hub. This model card has been automatically generated.\n\n- Developed by: \n- Funded by [optional]: \n- Shared by [optional]: \n- Model type: \n- Language(s) (NLP): \n- License: \n- Finetuned from model [optional]:",
"### Model Sources [optional]\n\n\n\n- Repository: \n- Paper [optional]: \n- Demo [optional]:",
"## Uses",
"### Direct Use",
"### Downstream Use [optional]",
"### Out-of-Scope Use",
"## Bias, Risks, and Limitations",
"### Recommendations\n\n\n\nUsers (both direct and downstream) should be made aware of the risks, biases and limitations of the model. More information needed for further recommendations.",
"## How to Get Started with the Model\n\nUse the code below to get started with the model.",
"## Training Details",
"### Training Data",
"### Training Procedure",
"#### Preprocessing [optional]",
"#### Training Hyperparameters\n\n- Training regime:",
"#### Speeds, Sizes, Times [optional]",
"## Evaluation",
"### Testing Data, Factors & Metrics",
"#### Testing Data",
"#### Factors",
"#### Metrics",
"### Results",
"#### Summary",
"## Model Examination [optional]",
"## Environmental Impact\n\n\n\nCarbon emissions can be estimated using the Machine Learning Impact calculator presented in Lacoste et al. (2019).\n\n- Hardware Type: \n- Hours used: \n- Cloud Provider: \n- Compute Region: \n- Carbon Emitted:",
"## Technical Specifications [optional]",
"### Model Architecture and Objective",
"### Compute Infrastructure",
"#### Hardware",
"#### Software\n\n\n\n[optional]\n\n\n\nBibTeX:\n\n\n\nAPA:",
"## Glossary [optional]",
"## More Information [optional]",
"## Model Card Authors [optional]",
"## Model Card Contact"
] | [
"TAGS\n#transformers #safetensors #llama #text-generation #conversational #arxiv-1910.09700 #autotrain_compatible #endpoints_compatible #text-generation-inference #region-us \n",
"# Model Card for Model ID",
"## Model Details",
"### Model Description\n\n\n\nThis is the model card of a transformers model that has been pushed on the Hub. This model card has been automatically generated.\n\n- Developed by: \n- Funded by [optional]: \n- Shared by [optional]: \n- Model type: \n- Language(s) (NLP): \n- License: \n- Finetuned from model [optional]:",
"### Model Sources [optional]\n\n\n\n- Repository: \n- Paper [optional]: \n- Demo [optional]:",
"## Uses",
"### Direct Use",
"### Downstream Use [optional]",
"### Out-of-Scope Use",
"## Bias, Risks, and Limitations",
"### Recommendations\n\n\n\nUsers (both direct and downstream) should be made aware of the risks, biases and limitations of the model. More information needed for further recommendations.",
"## How to Get Started with the Model\n\nUse the code below to get started with the model.",
"## Training Details",
"### Training Data",
"### Training Procedure",
"#### Preprocessing [optional]",
"#### Training Hyperparameters\n\n- Training regime:",
"#### Speeds, Sizes, Times [optional]",
"## Evaluation",
"### Testing Data, Factors & Metrics",
"#### Testing Data",
"#### Factors",
"#### Metrics",
"### Results",
"#### Summary",
"## Model Examination [optional]",
"## Environmental Impact\n\n\n\nCarbon emissions can be estimated using the Machine Learning Impact calculator presented in Lacoste et al. (2019).\n\n- Hardware Type: \n- Hours used: \n- Cloud Provider: \n- Compute Region: \n- Carbon Emitted:",
"## Technical Specifications [optional]",
"### Model Architecture and Objective",
"### Compute Infrastructure",
"#### Hardware",
"#### Software\n\n\n\n[optional]\n\n\n\nBibTeX:\n\n\n\nAPA:",
"## Glossary [optional]",
"## More Information [optional]",
"## Model Card Authors [optional]",
"## Model Card Contact"
] |
text-to-image | diffusers |
# AutoTrain SDXL LoRA DreamBooth - leonickson1/friday_building_uncc
<Gallery />
## Model description
These are leonickson1/friday_building_uncc LoRA adaption weights for stabilityai/stable-diffusion-xl-base-1.0.
The weights were trained using [DreamBooth](https://dreambooth.github.io/).
LoRA for the text encoder was enabled: False.
Special VAE used for training: None.
## Trigger words
You should use photo of a sks building to trigger the image generation.
## Download model
Weights for this model are available in Safetensors format.
[Download](leonickson1/friday_building_uncc/tree/main) them in the Files & versions tab.
| {"license": "openrail++", "tags": ["autotrain", "stable-diffusion-xl", "stable-diffusion-xl-diffusers", "text-to-image", "diffusers", "lora", "template:sd-lora"], "base_model": "stabilityai/stable-diffusion-xl-base-1.0", "instance_prompt": "photo of a sks building"} | leonickson1/friday_building_uncc | null | [
"diffusers",
"autotrain",
"stable-diffusion-xl",
"stable-diffusion-xl-diffusers",
"text-to-image",
"lora",
"template:sd-lora",
"base_model:stabilityai/stable-diffusion-xl-base-1.0",
"license:openrail++",
"region:us"
] | null | 2024-04-21T18:22:05+00:00 | [] | [] | TAGS
#diffusers #autotrain #stable-diffusion-xl #stable-diffusion-xl-diffusers #text-to-image #lora #template-sd-lora #base_model-stabilityai/stable-diffusion-xl-base-1.0 #license-openrail++ #region-us
|
# AutoTrain SDXL LoRA DreamBooth - leonickson1/friday_building_uncc
<Gallery />
## Model description
These are leonickson1/friday_building_uncc LoRA adaption weights for stabilityai/stable-diffusion-xl-base-1.0.
The weights were trained using DreamBooth.
LoRA for the text encoder was enabled: False.
Special VAE used for training: None.
## Trigger words
You should use photo of a sks building to trigger the image generation.
## Download model
Weights for this model are available in Safetensors format.
Download them in the Files & versions tab.
| [
"# AutoTrain SDXL LoRA DreamBooth - leonickson1/friday_building_uncc\n\n<Gallery />",
"## Model description\n\nThese are leonickson1/friday_building_uncc LoRA adaption weights for stabilityai/stable-diffusion-xl-base-1.0.\n\nThe weights were trained using DreamBooth.\n\nLoRA for the text encoder was enabled: False.\n\nSpecial VAE used for training: None.",
"## Trigger words\n\nYou should use photo of a sks building to trigger the image generation.",
"## Download model\n\nWeights for this model are available in Safetensors format.\n\nDownload them in the Files & versions tab."
] | [
"TAGS\n#diffusers #autotrain #stable-diffusion-xl #stable-diffusion-xl-diffusers #text-to-image #lora #template-sd-lora #base_model-stabilityai/stable-diffusion-xl-base-1.0 #license-openrail++ #region-us \n",
"# AutoTrain SDXL LoRA DreamBooth - leonickson1/friday_building_uncc\n\n<Gallery />",
"## Model description\n\nThese are leonickson1/friday_building_uncc LoRA adaption weights for stabilityai/stable-diffusion-xl-base-1.0.\n\nThe weights were trained using DreamBooth.\n\nLoRA for the text encoder was enabled: False.\n\nSpecial VAE used for training: None.",
"## Trigger words\n\nYou should use photo of a sks building to trigger the image generation.",
"## Download model\n\nWeights for this model are available in Safetensors format.\n\nDownload them in the Files & versions tab."
] |
text-to-image | diffusers |
# AutoTrain SDXL LoRA DreamBooth - leonickson1/fretwell_building_uncc
<Gallery />
## Model description
These are leonickson1/fretwell_building_uncc LoRA adaption weights for stabilityai/stable-diffusion-xl-base-1.0.
The weights were trained using [DreamBooth](https://dreambooth.github.io/).
LoRA for the text encoder was enabled: False.
Special VAE used for training: None.
## Trigger words
You should use photo of a sks building to trigger the image generation.
## Download model
Weights for this model are available in Safetensors format.
[Download](leonickson1/fretwell_building_uncc/tree/main) them in the Files & versions tab.
| {"license": "openrail++", "tags": ["autotrain", "stable-diffusion-xl", "stable-diffusion-xl-diffusers", "text-to-image", "diffusers", "lora", "template:sd-lora"], "base_model": "stabilityai/stable-diffusion-xl-base-1.0", "instance_prompt": "photo of a sks building"} | leonickson1/fretwell_building_uncc | null | [
"diffusers",
"autotrain",
"stable-diffusion-xl",
"stable-diffusion-xl-diffusers",
"text-to-image",
"lora",
"template:sd-lora",
"base_model:stabilityai/stable-diffusion-xl-base-1.0",
"license:openrail++",
"region:us"
] | null | 2024-04-21T18:22:25+00:00 | [] | [] | TAGS
#diffusers #autotrain #stable-diffusion-xl #stable-diffusion-xl-diffusers #text-to-image #lora #template-sd-lora #base_model-stabilityai/stable-diffusion-xl-base-1.0 #license-openrail++ #region-us
|
# AutoTrain SDXL LoRA DreamBooth - leonickson1/fretwell_building_uncc
<Gallery />
## Model description
These are leonickson1/fretwell_building_uncc LoRA adaption weights for stabilityai/stable-diffusion-xl-base-1.0.
The weights were trained using DreamBooth.
LoRA for the text encoder was enabled: False.
Special VAE used for training: None.
## Trigger words
You should use photo of a sks building to trigger the image generation.
## Download model
Weights for this model are available in Safetensors format.
Download them in the Files & versions tab.
| [
"# AutoTrain SDXL LoRA DreamBooth - leonickson1/fretwell_building_uncc\n\n<Gallery />",
"## Model description\n\nThese are leonickson1/fretwell_building_uncc LoRA adaption weights for stabilityai/stable-diffusion-xl-base-1.0.\n\nThe weights were trained using DreamBooth.\n\nLoRA for the text encoder was enabled: False.\n\nSpecial VAE used for training: None.",
"## Trigger words\n\nYou should use photo of a sks building to trigger the image generation.",
"## Download model\n\nWeights for this model are available in Safetensors format.\n\nDownload them in the Files & versions tab."
] | [
"TAGS\n#diffusers #autotrain #stable-diffusion-xl #stable-diffusion-xl-diffusers #text-to-image #lora #template-sd-lora #base_model-stabilityai/stable-diffusion-xl-base-1.0 #license-openrail++ #region-us \n",
"# AutoTrain SDXL LoRA DreamBooth - leonickson1/fretwell_building_uncc\n\n<Gallery />",
"## Model description\n\nThese are leonickson1/fretwell_building_uncc LoRA adaption weights for stabilityai/stable-diffusion-xl-base-1.0.\n\nThe weights were trained using DreamBooth.\n\nLoRA for the text encoder was enabled: False.\n\nSpecial VAE used for training: None.",
"## Trigger words\n\nYou should use photo of a sks building to trigger the image generation.",
"## Download model\n\nWeights for this model are available in Safetensors format.\n\nDownload them in the Files & versions tab."
] |
text2text-generation | transformers |
<!-- This model card has been generated automatically according to the information the Trainer had access to. You
should probably proofread and complete it, then remove this comment. -->
# my_awesome_opus_books_model
This model is a fine-tuned version of [t5-small](https://huggingface.co/t5-small) on an unknown dataset.
It achieves the following results on the evaluation set:
- Loss: 1.6848
- Bleu: 5.0886
- Gen Len: 17.6469
## Model description
More information needed
## Intended uses & limitations
More information needed
## Training and evaluation data
More information needed
## Training procedure
### Training hyperparameters
The following hyperparameters were used during training:
- learning_rate: 2e-05
- train_batch_size: 32
- eval_batch_size: 32
- seed: 42
- optimizer: Adam with betas=(0.9,0.999) and epsilon=1e-08
- lr_scheduler_type: linear
- num_epochs: 1
- mixed_precision_training: Native AMP
### Training results
| Training Loss | Epoch | Step | Validation Loss | Bleu | Gen Len |
|:-------------:|:-----:|:----:|:---------------:|:------:|:-------:|
| 1.9021 | 1.0 | 3178 | 1.6848 | 5.0886 | 17.6469 |
### Framework versions
- Transformers 4.38.2
- Pytorch 2.2.1+cu121
- Datasets 2.19.0
- Tokenizers 0.15.2
| {"license": "apache-2.0", "tags": ["generated_from_trainer"], "metrics": ["bleu"], "base_model": "t5-small", "model-index": [{"name": "my_awesome_opus_books_model", "results": []}]} | rujengelal/my_awesome_opus_books_model | null | [
"transformers",
"tensorboard",
"safetensors",
"t5",
"text2text-generation",
"generated_from_trainer",
"base_model:t5-small",
"license:apache-2.0",
"autotrain_compatible",
"endpoints_compatible",
"text-generation-inference",
"region:us"
] | null | 2024-04-21T18:22:50+00:00 | [] | [] | TAGS
#transformers #tensorboard #safetensors #t5 #text2text-generation #generated_from_trainer #base_model-t5-small #license-apache-2.0 #autotrain_compatible #endpoints_compatible #text-generation-inference #region-us
| my\_awesome\_opus\_books\_model
===============================
This model is a fine-tuned version of t5-small on an unknown dataset.
It achieves the following results on the evaluation set:
* Loss: 1.6848
* Bleu: 5.0886
* Gen Len: 17.6469
Model description
-----------------
More information needed
Intended uses & limitations
---------------------------
More information needed
Training and evaluation data
----------------------------
More information needed
Training procedure
------------------
### Training hyperparameters
The following hyperparameters were used during training:
* learning\_rate: 2e-05
* train\_batch\_size: 32
* eval\_batch\_size: 32
* seed: 42
* optimizer: Adam with betas=(0.9,0.999) and epsilon=1e-08
* lr\_scheduler\_type: linear
* num\_epochs: 1
* mixed\_precision\_training: Native AMP
### Training results
### Framework versions
* Transformers 4.38.2
* Pytorch 2.2.1+cu121
* Datasets 2.19.0
* Tokenizers 0.15.2
| [
"### Training hyperparameters\n\n\nThe following hyperparameters were used during training:\n\n\n* learning\\_rate: 2e-05\n* train\\_batch\\_size: 32\n* eval\\_batch\\_size: 32\n* seed: 42\n* optimizer: Adam with betas=(0.9,0.999) and epsilon=1e-08\n* lr\\_scheduler\\_type: linear\n* num\\_epochs: 1\n* mixed\\_precision\\_training: Native AMP",
"### Training results",
"### Framework versions\n\n\n* Transformers 4.38.2\n* Pytorch 2.2.1+cu121\n* Datasets 2.19.0\n* Tokenizers 0.15.2"
] | [
"TAGS\n#transformers #tensorboard #safetensors #t5 #text2text-generation #generated_from_trainer #base_model-t5-small #license-apache-2.0 #autotrain_compatible #endpoints_compatible #text-generation-inference #region-us \n",
"### Training hyperparameters\n\n\nThe following hyperparameters were used during training:\n\n\n* learning\\_rate: 2e-05\n* train\\_batch\\_size: 32\n* eval\\_batch\\_size: 32\n* seed: 42\n* optimizer: Adam with betas=(0.9,0.999) and epsilon=1e-08\n* lr\\_scheduler\\_type: linear\n* num\\_epochs: 1\n* mixed\\_precision\\_training: Native AMP",
"### Training results",
"### Framework versions\n\n\n* Transformers 4.38.2\n* Pytorch 2.2.1+cu121\n* Datasets 2.19.0\n* Tokenizers 0.15.2"
] |
text-generation | transformers |
<!-- This model card has been generated automatically according to the information the Trainer had access to. You
should probably proofread and complete it, then remove this comment. -->
# gpt2+morf_u0-30-50-x_cx-en_00000-00009_50k
This model is a fine-tuned version of [](https://huggingface.co/) on the uonlp/CulturaX en dataset.
It achieves the following results on the evaluation set:
- Loss: 3.3667
- Accuracy: 0.3950
## Model description
More information needed
## Intended uses & limitations
More information needed
## Training and evaluation data
More information needed
## Training procedure
### Training hyperparameters
The following hyperparameters were used during training:
- learning_rate: 5e-05
- train_batch_size: 64
- eval_batch_size: 64
- seed: 42
- optimizer: Adam with betas=(0.9,0.999) and epsilon=1e-08
- lr_scheduler_type: linear
- num_epochs: 1.0
### Training results
| Training Loss | Epoch | Step | Validation Loss | Accuracy |
| :-----------: | :---: | :----: | :-------------: | :------: |
| 4.3227 | 0.04 | 10000 | 4.2268 | 0.3161 |
| 4.0305 | 0.07 | 20000 | 3.9455 | 0.3393 |
| 3.8916 | 0.11 | 30000 | 3.8194 | 0.3502 |
| 3.8104 | 0.15 | 40000 | 3.7340 | 0.3580 |
| 3.7491 | 0.19 | 50000 | 3.6770 | 0.3633 |
| 3.7062 | 0.22 | 60000 | 3.6288 | 0.3679 |
| 3.6724 | 0.26 | 70000 | 3.5938 | 0.3714 |
| 3.6399 | 0.3 | 80000 | 3.5652 | 0.3743 |
| 3.6147 | 0.34 | 90000 | 3.5396 | 0.3768 |
| 3.5946 | 0.37 | 100000 | 3.5158 | 0.3791 |
| 3.5726 | 0.41 | 110000 | 3.4986 | 0.3809 |
| 3.5631 | 0.45 | 120000 | 3.4819 | 0.3826 |
| 3.5459 | 0.49 | 130000 | 3.4678 | 0.3842 |
| 3.5304 | 0.52 | 140000 | 3.4535 | 0.3857 |
| 3.5245 | 0.56 | 150000 | 3.4430 | 0.3867 |
| 3.5124 | 0.6 | 160000 | 3.4329 | 0.3877 |
| 3.501 | 0.63 | 170000 | 3.4223 | 0.3890 |
| 3.4934 | 0.67 | 180000 | 3.4130 | 0.3901 |
| 3.4863 | 0.71 | 190000 | 3.4042 | 0.3909 |
| 3.4799 | 0.75 | 200000 | 3.3991 | 0.3914 |
| 3.4682 | 0.78 | 210000 | 3.3909 | 0.3924 |
| 3.4667 | 0.82 | 220000 | 3.3852 | 0.3930 |
| 3.4564 | 0.86 | 230000 | 3.3790 | 0.3936 |
| 3.4581 | 0.9 | 240000 | 3.3753 | 0.3941 |
| 3.4553 | 0.93 | 250000 | 3.3710 | 0.3945 |
| 3.4508 | 0.97 | 260000 | 3.3680 | 0.3949 |
### Framework versions
- Transformers 4.37.1
- Pytorch 2.1.2+cu121
- Datasets 2.16.1
- Tokenizers 0.15.1 | {"language": ["en"], "license": "mit", "tags": ["generated_from_trainer"], "datasets": ["uonlp/CulturaX"], "metrics": ["accuracy"], "model-index": [{"name": "gpt2+morf_u0-30-50-x_cx-en_00000-00009_50k", "results": [{"task": {"type": "text-generation", "name": "Causal Language Modeling"}, "dataset": {"name": "uonlp/CulturaX en", "type": "uonlp/CulturaX", "args": "en"}, "metrics": [{"type": "accuracy", "value": 0.39500633343599556, "name": "Accuracy"}]}]}]} | jonasknobloch/gpt2-morf_u0-30-50-x_cx-en_00000-00009_50k | null | [
"transformers",
"safetensors",
"gpt2",
"text-generation",
"generated_from_trainer",
"en",
"dataset:uonlp/CulturaX",
"license:mit",
"model-index",
"autotrain_compatible",
"endpoints_compatible",
"text-generation-inference",
"region:us"
] | null | 2024-04-21T18:23:03+00:00 | [] | [
"en"
] | TAGS
#transformers #safetensors #gpt2 #text-generation #generated_from_trainer #en #dataset-uonlp/CulturaX #license-mit #model-index #autotrain_compatible #endpoints_compatible #text-generation-inference #region-us
| gpt2+morf\_u0-30-50-x\_cx-en\_00000-00009\_50k
==============================================
This model is a fine-tuned version of [](URL on the uonlp/CulturaX en dataset.
It achieves the following results on the evaluation set:
* Loss: 3.3667
* Accuracy: 0.3950
Model description
-----------------
More information needed
Intended uses & limitations
---------------------------
More information needed
Training and evaluation data
----------------------------
More information needed
Training procedure
------------------
### Training hyperparameters
The following hyperparameters were used during training:
* learning\_rate: 5e-05
* train\_batch\_size: 64
* eval\_batch\_size: 64
* seed: 42
* optimizer: Adam with betas=(0.9,0.999) and epsilon=1e-08
* lr\_scheduler\_type: linear
* num\_epochs: 1.0
### Training results
### Framework versions
* Transformers 4.37.1
* Pytorch 2.1.2+cu121
* Datasets 2.16.1
* Tokenizers 0.15.1
| [
"### Training hyperparameters\n\n\nThe following hyperparameters were used during training:\n\n\n* learning\\_rate: 5e-05\n* train\\_batch\\_size: 64\n* eval\\_batch\\_size: 64\n* seed: 42\n* optimizer: Adam with betas=(0.9,0.999) and epsilon=1e-08\n* lr\\_scheduler\\_type: linear\n* num\\_epochs: 1.0",
"### Training results",
"### Framework versions\n\n\n* Transformers 4.37.1\n* Pytorch 2.1.2+cu121\n* Datasets 2.16.1\n* Tokenizers 0.15.1"
] | [
"TAGS\n#transformers #safetensors #gpt2 #text-generation #generated_from_trainer #en #dataset-uonlp/CulturaX #license-mit #model-index #autotrain_compatible #endpoints_compatible #text-generation-inference #region-us \n",
"### Training hyperparameters\n\n\nThe following hyperparameters were used during training:\n\n\n* learning\\_rate: 5e-05\n* train\\_batch\\_size: 64\n* eval\\_batch\\_size: 64\n* seed: 42\n* optimizer: Adam with betas=(0.9,0.999) and epsilon=1e-08\n* lr\\_scheduler\\_type: linear\n* num\\_epochs: 1.0",
"### Training results",
"### Framework versions\n\n\n* Transformers 4.37.1\n* Pytorch 2.1.2+cu121\n* Datasets 2.16.1\n* Tokenizers 0.15.1"
] |
text-to-image | diffusers |
# AutoTrain SDXL LoRA DreamBooth - leonickson1/storris_building_uncc
<Gallery />
## Model description
These are leonickson1/storris_building_uncc LoRA adaption weights for stabilityai/stable-diffusion-xl-base-1.0.
The weights were trained using [DreamBooth](https://dreambooth.github.io/).
LoRA for the text encoder was enabled: False.
Special VAE used for training: None.
## Trigger words
You should use photo of a sks building to trigger the image generation.
## Download model
Weights for this model are available in Safetensors format.
[Download](leonickson1/storris_building_uncc/tree/main) them in the Files & versions tab.
| {"license": "openrail++", "tags": ["autotrain", "stable-diffusion-xl", "stable-diffusion-xl-diffusers", "text-to-image", "diffusers", "lora", "template:sd-lora"], "base_model": "stabilityai/stable-diffusion-xl-base-1.0", "instance_prompt": "photo of a sks building"} | leonickson1/storris_building_uncc | null | [
"diffusers",
"autotrain",
"stable-diffusion-xl",
"stable-diffusion-xl-diffusers",
"text-to-image",
"lora",
"template:sd-lora",
"base_model:stabilityai/stable-diffusion-xl-base-1.0",
"license:openrail++",
"region:us"
] | null | 2024-04-21T18:23:59+00:00 | [] | [] | TAGS
#diffusers #autotrain #stable-diffusion-xl #stable-diffusion-xl-diffusers #text-to-image #lora #template-sd-lora #base_model-stabilityai/stable-diffusion-xl-base-1.0 #license-openrail++ #region-us
|
# AutoTrain SDXL LoRA DreamBooth - leonickson1/storris_building_uncc
<Gallery />
## Model description
These are leonickson1/storris_building_uncc LoRA adaption weights for stabilityai/stable-diffusion-xl-base-1.0.
The weights were trained using DreamBooth.
LoRA for the text encoder was enabled: False.
Special VAE used for training: None.
## Trigger words
You should use photo of a sks building to trigger the image generation.
## Download model
Weights for this model are available in Safetensors format.
Download them in the Files & versions tab.
| [
"# AutoTrain SDXL LoRA DreamBooth - leonickson1/storris_building_uncc\n\n<Gallery />",
"## Model description\n\nThese are leonickson1/storris_building_uncc LoRA adaption weights for stabilityai/stable-diffusion-xl-base-1.0.\n\nThe weights were trained using DreamBooth.\n\nLoRA for the text encoder was enabled: False.\n\nSpecial VAE used for training: None.",
"## Trigger words\n\nYou should use photo of a sks building to trigger the image generation.",
"## Download model\n\nWeights for this model are available in Safetensors format.\n\nDownload them in the Files & versions tab."
] | [
"TAGS\n#diffusers #autotrain #stable-diffusion-xl #stable-diffusion-xl-diffusers #text-to-image #lora #template-sd-lora #base_model-stabilityai/stable-diffusion-xl-base-1.0 #license-openrail++ #region-us \n",
"# AutoTrain SDXL LoRA DreamBooth - leonickson1/storris_building_uncc\n\n<Gallery />",
"## Model description\n\nThese are leonickson1/storris_building_uncc LoRA adaption weights for stabilityai/stable-diffusion-xl-base-1.0.\n\nThe weights were trained using DreamBooth.\n\nLoRA for the text encoder was enabled: False.\n\nSpecial VAE used for training: None.",
"## Trigger words\n\nYou should use photo of a sks building to trigger the image generation.",
"## Download model\n\nWeights for this model are available in Safetensors format.\n\nDownload them in the Files & versions tab."
] |
text-to-image | diffusers |
# AutoTrain SDXL LoRA DreamBooth - leonickson1/epic_building_uncc
<Gallery />
## Model description
These are leonickson1/epic_building_uncc LoRA adaption weights for stabilityai/stable-diffusion-xl-base-1.0.
The weights were trained using [DreamBooth](https://dreambooth.github.io/).
LoRA for the text encoder was enabled: False.
Special VAE used for training: None.
## Trigger words
You should use photo of a sks building to trigger the image generation.
## Download model
Weights for this model are available in Safetensors format.
[Download](leonickson1/epic_building_uncc/tree/main) them in the Files & versions tab.
| {"license": "openrail++", "tags": ["autotrain", "stable-diffusion-xl", "stable-diffusion-xl-diffusers", "text-to-image", "diffusers", "lora", "template:sd-lora"], "base_model": "stabilityai/stable-diffusion-xl-base-1.0", "instance_prompt": "photo of a sks building"} | leonickson1/epic_building_uncc | null | [
"diffusers",
"autotrain",
"stable-diffusion-xl",
"stable-diffusion-xl-diffusers",
"text-to-image",
"lora",
"template:sd-lora",
"base_model:stabilityai/stable-diffusion-xl-base-1.0",
"license:openrail++",
"region:us"
] | null | 2024-04-21T18:24:11+00:00 | [] | [] | TAGS
#diffusers #autotrain #stable-diffusion-xl #stable-diffusion-xl-diffusers #text-to-image #lora #template-sd-lora #base_model-stabilityai/stable-diffusion-xl-base-1.0 #license-openrail++ #region-us
|
# AutoTrain SDXL LoRA DreamBooth - leonickson1/epic_building_uncc
<Gallery />
## Model description
These are leonickson1/epic_building_uncc LoRA adaption weights for stabilityai/stable-diffusion-xl-base-1.0.
The weights were trained using DreamBooth.
LoRA for the text encoder was enabled: False.
Special VAE used for training: None.
## Trigger words
You should use photo of a sks building to trigger the image generation.
## Download model
Weights for this model are available in Safetensors format.
Download them in the Files & versions tab.
| [
"# AutoTrain SDXL LoRA DreamBooth - leonickson1/epic_building_uncc\n\n<Gallery />",
"## Model description\n\nThese are leonickson1/epic_building_uncc LoRA adaption weights for stabilityai/stable-diffusion-xl-base-1.0.\n\nThe weights were trained using DreamBooth.\n\nLoRA for the text encoder was enabled: False.\n\nSpecial VAE used for training: None.",
"## Trigger words\n\nYou should use photo of a sks building to trigger the image generation.",
"## Download model\n\nWeights for this model are available in Safetensors format.\n\nDownload them in the Files & versions tab."
] | [
"TAGS\n#diffusers #autotrain #stable-diffusion-xl #stable-diffusion-xl-diffusers #text-to-image #lora #template-sd-lora #base_model-stabilityai/stable-diffusion-xl-base-1.0 #license-openrail++ #region-us \n",
"# AutoTrain SDXL LoRA DreamBooth - leonickson1/epic_building_uncc\n\n<Gallery />",
"## Model description\n\nThese are leonickson1/epic_building_uncc LoRA adaption weights for stabilityai/stable-diffusion-xl-base-1.0.\n\nThe weights were trained using DreamBooth.\n\nLoRA for the text encoder was enabled: False.\n\nSpecial VAE used for training: None.",
"## Trigger words\n\nYou should use photo of a sks building to trigger the image generation.",
"## Download model\n\nWeights for this model are available in Safetensors format.\n\nDownload them in the Files & versions tab."
] |
text-generation | transformers |
# Model Card for Model ID
<!-- Provide a quick summary of what the model is/does. -->
## Model Details
### Model Description
<!-- Provide a longer summary of what this model is. -->
This is the model card of a 🤗 transformers model that has been pushed on the Hub. This model card has been automatically generated.
- **Developed by:** [More Information Needed]
- **Funded by [optional]:** [More Information Needed]
- **Shared by [optional]:** [More Information Needed]
- **Model type:** [More Information Needed]
- **Language(s) (NLP):** [More Information Needed]
- **License:** [More Information Needed]
- **Finetuned from model [optional]:** [More Information Needed]
### Model Sources [optional]
<!-- Provide the basic links for the model. -->
- **Repository:** [More Information Needed]
- **Paper [optional]:** [More Information Needed]
- **Demo [optional]:** [More Information Needed]
## Uses
<!-- Address questions around how the model is intended to be used, including the foreseeable users of the model and those affected by the model. -->
### Direct Use
<!-- This section is for the model use without fine-tuning or plugging into a larger ecosystem/app. -->
[More Information Needed]
### Downstream Use [optional]
<!-- This section is for the model use when fine-tuned for a task, or when plugged into a larger ecosystem/app -->
[More Information Needed]
### Out-of-Scope Use
<!-- This section addresses misuse, malicious use, and uses that the model will not work well for. -->
[More Information Needed]
## Bias, Risks, and Limitations
<!-- This section is meant to convey both technical and sociotechnical limitations. -->
[More Information Needed]
### Recommendations
<!-- This section is meant to convey recommendations with respect to the bias, risk, and technical limitations. -->
Users (both direct and downstream) should be made aware of the risks, biases and limitations of the model. More information needed for further recommendations.
## How to Get Started with the Model
Use the code below to get started with the model.
[More Information Needed]
## Training Details
### Training Data
<!-- This should link to a Dataset Card, perhaps with a short stub of information on what the training data is all about as well as documentation related to data pre-processing or additional filtering. -->
[More Information Needed]
### Training Procedure
<!-- This relates heavily to the Technical Specifications. Content here should link to that section when it is relevant to the training procedure. -->
#### Preprocessing [optional]
[More Information Needed]
#### Training Hyperparameters
- **Training regime:** [More Information Needed] <!--fp32, fp16 mixed precision, bf16 mixed precision, bf16 non-mixed precision, fp16 non-mixed precision, fp8 mixed precision -->
#### Speeds, Sizes, Times [optional]
<!-- This section provides information about throughput, start/end time, checkpoint size if relevant, etc. -->
[More Information Needed]
## Evaluation
<!-- This section describes the evaluation protocols and provides the results. -->
### Testing Data, Factors & Metrics
#### Testing Data
<!-- This should link to a Dataset Card if possible. -->
[More Information Needed]
#### Factors
<!-- These are the things the evaluation is disaggregating by, e.g., subpopulations or domains. -->
[More Information Needed]
#### Metrics
<!-- These are the evaluation metrics being used, ideally with a description of why. -->
[More Information Needed]
### Results
[More Information Needed]
#### Summary
## Model Examination [optional]
<!-- Relevant interpretability work for the model goes here -->
[More Information Needed]
## Environmental Impact
<!-- Total emissions (in grams of CO2eq) and additional considerations, such as electricity usage, go here. Edit the suggested text below accordingly -->
Carbon emissions can be estimated using the [Machine Learning Impact calculator](https://mlco2.github.io/impact#compute) presented in [Lacoste et al. (2019)](https://arxiv.org/abs/1910.09700).
- **Hardware Type:** [More Information Needed]
- **Hours used:** [More Information Needed]
- **Cloud Provider:** [More Information Needed]
- **Compute Region:** [More Information Needed]
- **Carbon Emitted:** [More Information Needed]
## Technical Specifications [optional]
### Model Architecture and Objective
[More Information Needed]
### Compute Infrastructure
[More Information Needed]
#### Hardware
[More Information Needed]
#### Software
[More Information Needed]
## Citation [optional]
<!-- If there is a paper or blog post introducing the model, the APA and Bibtex information for that should go in this section. -->
**BibTeX:**
[More Information Needed]
**APA:**
[More Information Needed]
## Glossary [optional]
<!-- If relevant, include terms and calculations in this section that can help readers understand the model or model card. -->
[More Information Needed]
## More Information [optional]
[More Information Needed]
## Model Card Authors [optional]
[More Information Needed]
## Model Card Contact
[More Information Needed] | {"library_name": "transformers", "tags": []} | hi000000/insta_attrangs_llama2-koen_evaluation | null | [
"transformers",
"safetensors",
"llama",
"text-generation",
"arxiv:1910.09700",
"autotrain_compatible",
"endpoints_compatible",
"text-generation-inference",
"region:us"
] | null | 2024-04-21T18:24:26+00:00 | [
"1910.09700"
] | [] | TAGS
#transformers #safetensors #llama #text-generation #arxiv-1910.09700 #autotrain_compatible #endpoints_compatible #text-generation-inference #region-us
|
# Model Card for Model ID
## Model Details
### Model Description
This is the model card of a transformers model that has been pushed on the Hub. This model card has been automatically generated.
- Developed by:
- Funded by [optional]:
- Shared by [optional]:
- Model type:
- Language(s) (NLP):
- License:
- Finetuned from model [optional]:
### Model Sources [optional]
- Repository:
- Paper [optional]:
- Demo [optional]:
## Uses
### Direct Use
### Downstream Use [optional]
### Out-of-Scope Use
## Bias, Risks, and Limitations
### Recommendations
Users (both direct and downstream) should be made aware of the risks, biases and limitations of the model. More information needed for further recommendations.
## How to Get Started with the Model
Use the code below to get started with the model.
## Training Details
### Training Data
### Training Procedure
#### Preprocessing [optional]
#### Training Hyperparameters
- Training regime:
#### Speeds, Sizes, Times [optional]
## Evaluation
### Testing Data, Factors & Metrics
#### Testing Data
#### Factors
#### Metrics
### Results
#### Summary
## Model Examination [optional]
## Environmental Impact
Carbon emissions can be estimated using the Machine Learning Impact calculator presented in Lacoste et al. (2019).
- Hardware Type:
- Hours used:
- Cloud Provider:
- Compute Region:
- Carbon Emitted:
## Technical Specifications [optional]
### Model Architecture and Objective
### Compute Infrastructure
#### Hardware
#### Software
[optional]
BibTeX:
APA:
## Glossary [optional]
## More Information [optional]
## Model Card Authors [optional]
## Model Card Contact
| [
"# Model Card for Model ID",
"## Model Details",
"### Model Description\n\n\n\nThis is the model card of a transformers model that has been pushed on the Hub. This model card has been automatically generated.\n\n- Developed by: \n- Funded by [optional]: \n- Shared by [optional]: \n- Model type: \n- Language(s) (NLP): \n- License: \n- Finetuned from model [optional]:",
"### Model Sources [optional]\n\n\n\n- Repository: \n- Paper [optional]: \n- Demo [optional]:",
"## Uses",
"### Direct Use",
"### Downstream Use [optional]",
"### Out-of-Scope Use",
"## Bias, Risks, and Limitations",
"### Recommendations\n\n\n\nUsers (both direct and downstream) should be made aware of the risks, biases and limitations of the model. More information needed for further recommendations.",
"## How to Get Started with the Model\n\nUse the code below to get started with the model.",
"## Training Details",
"### Training Data",
"### Training Procedure",
"#### Preprocessing [optional]",
"#### Training Hyperparameters\n\n- Training regime:",
"#### Speeds, Sizes, Times [optional]",
"## Evaluation",
"### Testing Data, Factors & Metrics",
"#### Testing Data",
"#### Factors",
"#### Metrics",
"### Results",
"#### Summary",
"## Model Examination [optional]",
"## Environmental Impact\n\n\n\nCarbon emissions can be estimated using the Machine Learning Impact calculator presented in Lacoste et al. (2019).\n\n- Hardware Type: \n- Hours used: \n- Cloud Provider: \n- Compute Region: \n- Carbon Emitted:",
"## Technical Specifications [optional]",
"### Model Architecture and Objective",
"### Compute Infrastructure",
"#### Hardware",
"#### Software\n\n\n\n[optional]\n\n\n\nBibTeX:\n\n\n\nAPA:",
"## Glossary [optional]",
"## More Information [optional]",
"## Model Card Authors [optional]",
"## Model Card Contact"
] | [
"TAGS\n#transformers #safetensors #llama #text-generation #arxiv-1910.09700 #autotrain_compatible #endpoints_compatible #text-generation-inference #region-us \n",
"# Model Card for Model ID",
"## Model Details",
"### Model Description\n\n\n\nThis is the model card of a transformers model that has been pushed on the Hub. This model card has been automatically generated.\n\n- Developed by: \n- Funded by [optional]: \n- Shared by [optional]: \n- Model type: \n- Language(s) (NLP): \n- License: \n- Finetuned from model [optional]:",
"### Model Sources [optional]\n\n\n\n- Repository: \n- Paper [optional]: \n- Demo [optional]:",
"## Uses",
"### Direct Use",
"### Downstream Use [optional]",
"### Out-of-Scope Use",
"## Bias, Risks, and Limitations",
"### Recommendations\n\n\n\nUsers (both direct and downstream) should be made aware of the risks, biases and limitations of the model. More information needed for further recommendations.",
"## How to Get Started with the Model\n\nUse the code below to get started with the model.",
"## Training Details",
"### Training Data",
"### Training Procedure",
"#### Preprocessing [optional]",
"#### Training Hyperparameters\n\n- Training regime:",
"#### Speeds, Sizes, Times [optional]",
"## Evaluation",
"### Testing Data, Factors & Metrics",
"#### Testing Data",
"#### Factors",
"#### Metrics",
"### Results",
"#### Summary",
"## Model Examination [optional]",
"## Environmental Impact\n\n\n\nCarbon emissions can be estimated using the Machine Learning Impact calculator presented in Lacoste et al. (2019).\n\n- Hardware Type: \n- Hours used: \n- Cloud Provider: \n- Compute Region: \n- Carbon Emitted:",
"## Technical Specifications [optional]",
"### Model Architecture and Objective",
"### Compute Infrastructure",
"#### Hardware",
"#### Software\n\n\n\n[optional]\n\n\n\nBibTeX:\n\n\n\nAPA:",
"## Glossary [optional]",
"## More Information [optional]",
"## Model Card Authors [optional]",
"## Model Card Contact"
] |
text-to-image | diffusers |
# AutoTrain SDXL LoRA DreamBooth - leonickson1/reese_building_uncc
<Gallery />
## Model description
These are leonickson1/reese_building_uncc LoRA adaption weights for stabilityai/stable-diffusion-xl-base-1.0.
The weights were trained using [DreamBooth](https://dreambooth.github.io/).
LoRA for the text encoder was enabled: False.
Special VAE used for training: None.
## Trigger words
You should use photo of a sks building to trigger the image generation.
## Download model
Weights for this model are available in Safetensors format.
[Download](leonickson1/reese_building_uncc/tree/main) them in the Files & versions tab.
| {"license": "openrail++", "tags": ["autotrain", "stable-diffusion-xl", "stable-diffusion-xl-diffusers", "text-to-image", "diffusers", "lora", "template:sd-lora"], "base_model": "stabilityai/stable-diffusion-xl-base-1.0", "instance_prompt": "photo of a sks building"} | leonickson1/reese_building_uncc | null | [
"diffusers",
"autotrain",
"stable-diffusion-xl",
"stable-diffusion-xl-diffusers",
"text-to-image",
"lora",
"template:sd-lora",
"base_model:stabilityai/stable-diffusion-xl-base-1.0",
"license:openrail++",
"region:us"
] | null | 2024-04-21T18:24:29+00:00 | [] | [] | TAGS
#diffusers #autotrain #stable-diffusion-xl #stable-diffusion-xl-diffusers #text-to-image #lora #template-sd-lora #base_model-stabilityai/stable-diffusion-xl-base-1.0 #license-openrail++ #region-us
|
# AutoTrain SDXL LoRA DreamBooth - leonickson1/reese_building_uncc
<Gallery />
## Model description
These are leonickson1/reese_building_uncc LoRA adaption weights for stabilityai/stable-diffusion-xl-base-1.0.
The weights were trained using DreamBooth.
LoRA for the text encoder was enabled: False.
Special VAE used for training: None.
## Trigger words
You should use photo of a sks building to trigger the image generation.
## Download model
Weights for this model are available in Safetensors format.
Download them in the Files & versions tab.
| [
"# AutoTrain SDXL LoRA DreamBooth - leonickson1/reese_building_uncc\n\n<Gallery />",
"## Model description\n\nThese are leonickson1/reese_building_uncc LoRA adaption weights for stabilityai/stable-diffusion-xl-base-1.0.\n\nThe weights were trained using DreamBooth.\n\nLoRA for the text encoder was enabled: False.\n\nSpecial VAE used for training: None.",
"## Trigger words\n\nYou should use photo of a sks building to trigger the image generation.",
"## Download model\n\nWeights for this model are available in Safetensors format.\n\nDownload them in the Files & versions tab."
] | [
"TAGS\n#diffusers #autotrain #stable-diffusion-xl #stable-diffusion-xl-diffusers #text-to-image #lora #template-sd-lora #base_model-stabilityai/stable-diffusion-xl-base-1.0 #license-openrail++ #region-us \n",
"# AutoTrain SDXL LoRA DreamBooth - leonickson1/reese_building_uncc\n\n<Gallery />",
"## Model description\n\nThese are leonickson1/reese_building_uncc LoRA adaption weights for stabilityai/stable-diffusion-xl-base-1.0.\n\nThe weights were trained using DreamBooth.\n\nLoRA for the text encoder was enabled: False.\n\nSpecial VAE used for training: None.",
"## Trigger words\n\nYou should use photo of a sks building to trigger the image generation.",
"## Download model\n\nWeights for this model are available in Safetensors format.\n\nDownload them in the Files & versions tab."
] |
text-to-image | diffusers |
# AutoTrain SDXL LoRA DreamBooth - leonickson1/bioinformatic_building_uncc
<Gallery />
## Model description
These are leonickson1/bioinformatic_building_uncc LoRA adaption weights for stabilityai/stable-diffusion-xl-base-1.0.
The weights were trained using [DreamBooth](https://dreambooth.github.io/).
LoRA for the text encoder was enabled: False.
Special VAE used for training: None.
## Trigger words
You should use photo of a sks building to trigger the image generation.
## Download model
Weights for this model are available in Safetensors format.
[Download](leonickson1/bioinformatic_building_uncc/tree/main) them in the Files & versions tab.
| {"license": "openrail++", "tags": ["autotrain", "stable-diffusion-xl", "stable-diffusion-xl-diffusers", "text-to-image", "diffusers", "lora", "template:sd-lora"], "base_model": "stabilityai/stable-diffusion-xl-base-1.0", "instance_prompt": "photo of a sks building"} | leonickson1/bioinformatic_building_uncc | null | [
"diffusers",
"autotrain",
"stable-diffusion-xl",
"stable-diffusion-xl-diffusers",
"text-to-image",
"lora",
"template:sd-lora",
"base_model:stabilityai/stable-diffusion-xl-base-1.0",
"license:openrail++",
"region:us"
] | null | 2024-04-21T18:25:17+00:00 | [] | [] | TAGS
#diffusers #autotrain #stable-diffusion-xl #stable-diffusion-xl-diffusers #text-to-image #lora #template-sd-lora #base_model-stabilityai/stable-diffusion-xl-base-1.0 #license-openrail++ #region-us
|
# AutoTrain SDXL LoRA DreamBooth - leonickson1/bioinformatic_building_uncc
<Gallery />
## Model description
These are leonickson1/bioinformatic_building_uncc LoRA adaption weights for stabilityai/stable-diffusion-xl-base-1.0.
The weights were trained using DreamBooth.
LoRA for the text encoder was enabled: False.
Special VAE used for training: None.
## Trigger words
You should use photo of a sks building to trigger the image generation.
## Download model
Weights for this model are available in Safetensors format.
Download them in the Files & versions tab.
| [
"# AutoTrain SDXL LoRA DreamBooth - leonickson1/bioinformatic_building_uncc\n\n<Gallery />",
"## Model description\n\nThese are leonickson1/bioinformatic_building_uncc LoRA adaption weights for stabilityai/stable-diffusion-xl-base-1.0.\n\nThe weights were trained using DreamBooth.\n\nLoRA for the text encoder was enabled: False.\n\nSpecial VAE used for training: None.",
"## Trigger words\n\nYou should use photo of a sks building to trigger the image generation.",
"## Download model\n\nWeights for this model are available in Safetensors format.\n\nDownload them in the Files & versions tab."
] | [
"TAGS\n#diffusers #autotrain #stable-diffusion-xl #stable-diffusion-xl-diffusers #text-to-image #lora #template-sd-lora #base_model-stabilityai/stable-diffusion-xl-base-1.0 #license-openrail++ #region-us \n",
"# AutoTrain SDXL LoRA DreamBooth - leonickson1/bioinformatic_building_uncc\n\n<Gallery />",
"## Model description\n\nThese are leonickson1/bioinformatic_building_uncc LoRA adaption weights for stabilityai/stable-diffusion-xl-base-1.0.\n\nThe weights were trained using DreamBooth.\n\nLoRA for the text encoder was enabled: False.\n\nSpecial VAE used for training: None.",
"## Trigger words\n\nYou should use photo of a sks building to trigger the image generation.",
"## Download model\n\nWeights for this model are available in Safetensors format.\n\nDownload them in the Files & versions tab."
] |
null | speechbrain | <iframe src="https://ghbtns.com/github-btn.html?user=SupradeepDanturti&repo=ConvAIProject&type=star&count=true&size=large&v=2" frameborder="0" scrolling="0" width="170" height="30" title="GitHub"></iframe>
<br/><br/>
# Speaker Counting with XVector and ECAPA-TDNN models
This repository provides tools for detecting and counting the number of speakers in an audio recording using models like XVector and ECAPA-TDNN, trained on datasets such as LibriSpeech.
The pre-trained system processes audio inputs to detect the presence and count of speakers, providing output in the following format:
```
0.00-2.50 has 1 speaker
2.50-4.20 has 2 speakers
4.20-5:30 has no speakers
```
The system expects input recordings sampled at 16kHz. If your signal has a different sample rate, resample it using torchaudio before using the interface.
# Model Performance
Error rates for different numbers of speakers detected by the models are provided below:
## XVector Model Error Rates
| Number of Speakers | Error Rate |
|--------------------|------------------|
| No Speakers | 0.00e+00 |
| 1 Speaker | 4.06e-01 |
| 2 Speakers | 3.20e-02 |
| 3 Speakers | 2.15e-01 |
| 4 Speakers | 4.67e-01 |
| **Overall** | **2.29e-01** |
## ECAPA-TDNN Model Error Rates
| Number of Speakers | Error Rate |
|--------------------|------------------|
| No Speakers | 0.00e+00 |
| 1 Speaker | 4.12e-01 |
| 2 Speakers | 4.10e-01 |
| 3 Speakers | 3.26e-01 |
| 4 Speakers | 5.23e-01 |
| **Overall** | **2.40e-01** |
## Self-supervised MLP Model Error Rates
| Number of Speakers | Error Rate |
|--------------------|------------------|
| No Speakers | 0.00e+00 |
| 1 Speaker | 8.67e-03 |
| 2 Speakers | 1.14e-01 |
| 3 Speakers | 4.08e-01 |
| 4 Speakers | 4.49e-01 |
| **Overall** | **2.00e-01** |
## Self-supervised XVector Model Error Rates
| Number of Speakers | Error Rate |
|--------------------|------------------|
| No Speakers | 0.00e+00 |
| 1 Speaker | 1.64e-02 |
| 2 Speakers | 1.39e-01 |
| 3 Speakers | 3.34e-01 |
| 4 Speakers | 5.41e-01 |
| **Overall** | **2.10e-01** |
Error rates are crucial to understanding where the models excel and where they may need further improvement. In particular, they can reveal challenges in scenarios with higher numbers of speakers.
### Limitations
Please note that these models are evaluated under controlled conditions and their performance may vary when applied to different datasets or in real-world scenarios.
## System Overview
This project includes interfaces for processing audio files, detecting speech, and counting speakers. It leverages pre-trained models and custom scripts for refining and aggregating predictions.
### Installation
```
pip install speechbrain
```
Please look at [SpeechBrain](https://speechbrain.github.io/) for tutorials or more information.
## Using the Speaker Counter Interface
### For XVector & ECAPA-TDNN:
```
from interface.SpeakerCounter import SpeakerCounter
wav_path = "path/to/your/audio.wav"
model_path = "path/to/your/model"
save_dir = "path/to/save/results"
# Initialize the SpeakerCounter
speaker_counter = SpeakerCounter.from_hparams(source=model_path, savedir=save_dir)
# Run inference
speaker_counter.classify_file(wav_path)
```
### For a Self-supervised model:
```
from interface.SpeakerCounterSelfsupervisedMLP import SpeakerCounter
wav_path = "path/to/your/audio.wav"
model_path = "path/to/your/selfsupervised_model"
save_dir = "path/to/save/results"
# Initialize the SpeakerCounter
audio_classifier = SpeakerCounter.from_hparams(source=model_path, savedir=save_dir)
# Run inference
audio_classifier.classify_file(wav_path)
```
## **Setup and Training Instructions**
To reproduce the results of this project, follow these steps.
```
!git clone https://github.com/SupradeepDanturti/ConvAIProject
%cd ConvAIProject
```
Download the project code from the GitHub repository and navigate into the project directory.
```
!python prepare_dataset/download_required_data.py --output_folder <destination_folder_path>
```
Download the necessary datasets (LibriSpeech etc.), specifying the desired destination folder.
```
!python prepare_dataset/create_custom_dataset.py prepare_dataset/dataset.yaml
```
Create custom dataset based on set parameters as shown in the sample below
Sample of dataset.yaml:
```
n_sessions:
train: 1000 # Creates 1000 sessions per class
dev: 200 # Creates 200 sessions per class
eval: 200 # Creates 200 sessions per class
n_speakers: 4 # max number of speakers. In this case the total classes will be 5 (0-4 speakers)
max_length: 120 # max length in seconds for each session/utterance.
```
<center>Sample of dataset.yaml</center>
To train the XVector model run the following command.
```
%cd xvector
!python train_xvector_augmentation.py hparams_xvector_augmentation.yaml
```
To train the ECAPA-TDNN model run the following command.
```
%cd ecapa_tdnn
!python train_ecapa_tdnn.py hparams_ecapa_tdnn_augmentation.yaml
```
To train the SelfSupervised MLP model run the following command.
```
%cd selfsupervised
!python selfsupervised_mlp.py hparams_selfsupervised_mlp.yaml
```
To train the SelfSupervised XVector model run the following command.
```
%cd selfsupervised
!python selfsupervised_xvector.py hparams_selfsupervised_xvector.yaml
```
### **This Project was developed completely using [SpeechBrain](https://speechbrain.github.io/) **
## Reference
```
@misc{speechbrain,
title={{SpeechBrain}: A General-Purpose Speech Toolkit},
author={Mirco Ravanelli and Titouan Parcollet and Peter Plantinga and Aku Rouhe and Samuele Cornell and Loren Lugosch and Cem Subakan and Nauman Dawalatabad and Abdelwahab Heba and Jianyuan Zhong and Ju-Chieh Chou and Sung-Lin Yeh and Szu-Wei Fu and Chien-Feng Liao and Elena Rastorgueva and François Grondin and William Aris and Hwidong Na and Yan Gao and Renato De Mori and Yoshua Bengio},
year={2021},
eprint={2106.04624},
archivePrefix={arXiv},
primaryClass={eess.AS},
note={arXiv:2106.04624}
}
``` | {"language": ["en"], "library_name": "speechbrain", "datasets": ["librispeech_asr"], "metrics": ["accuracy"]} | Supradeepdan/OverlapSpeakerCounter | null | [
"speechbrain",
"en",
"dataset:librispeech_asr",
"arxiv:2106.04624",
"region:us"
] | null | 2024-04-21T18:29:03+00:00 | [
"2106.04624"
] | [
"en"
] | TAGS
#speechbrain #en #dataset-librispeech_asr #arxiv-2106.04624 #region-us
|
Speaker Counting with XVector and ECAPA-TDNN models
===================================================
This repository provides tools for detecting and counting the number of speakers in an audio recording using models like XVector and ECAPA-TDNN, trained on datasets such as LibriSpeech.
The pre-trained system processes audio inputs to detect the presence and count of speakers, providing output in the following format:
The system expects input recordings sampled at 16kHz. If your signal has a different sample rate, resample it using torchaudio before using the interface.
Model Performance
=================
Error rates for different numbers of speakers detected by the models are provided below:
XVector Model Error Rates
-------------------------
ECAPA-TDNN Model Error Rates
----------------------------
Self-supervised MLP Model Error Rates
-------------------------------------
Self-supervised XVector Model Error Rates
-----------------------------------------
Error rates are crucial to understanding where the models excel and where they may need further improvement. In particular, they can reveal challenges in scenarios with higher numbers of speakers.
### Limitations
Please note that these models are evaluated under controlled conditions and their performance may vary when applied to different datasets or in real-world scenarios.
System Overview
---------------
This project includes interfaces for processing audio files, detecting speech, and counting speakers. It leverages pre-trained models and custom scripts for refining and aggregating predictions.
### Installation
Please look at SpeechBrain for tutorials or more information.
Using the Speaker Counter Interface
-----------------------------------
### For XVector & ECAPA-TDNN:
### For a Self-supervised model:
Setup and Training Instructions
-------------------------------
To reproduce the results of this project, follow these steps.
Download the project code from the GitHub repository and navigate into the project directory.
Download the necessary datasets (LibriSpeech etc.), specifying the desired destination folder.
Create custom dataset based on set parameters as shown in the sample below
Sample of URL:
Sample of URL
To train the XVector model run the following command.
To train the ECAPA-TDNN model run the following command.
To train the SelfSupervised MLP model run the following command.
To train the SelfSupervised XVector model run the following command.
### This Project was developed completely using SpeechBrain
Reference
---------
| [
"### Limitations\n\n\nPlease note that these models are evaluated under controlled conditions and their performance may vary when applied to different datasets or in real-world scenarios.\n\n\nSystem Overview\n---------------\n\n\nThis project includes interfaces for processing audio files, detecting speech, and counting speakers. It leverages pre-trained models and custom scripts for refining and aggregating predictions.",
"### Installation\n\n\nPlease look at SpeechBrain for tutorials or more information.\n\n\nUsing the Speaker Counter Interface\n-----------------------------------",
"### For XVector & ECAPA-TDNN:",
"### For a Self-supervised model:\n\n\nSetup and Training Instructions\n-------------------------------\n\n\nTo reproduce the results of this project, follow these steps.\n\n\nDownload the project code from the GitHub repository and navigate into the project directory.\n\n\nDownload the necessary datasets (LibriSpeech etc.), specifying the desired destination folder.\n\n\nCreate custom dataset based on set parameters as shown in the sample below\n\n\nSample of URL:\n\n\nSample of URL\nTo train the XVector model run the following command.\n\n\nTo train the ECAPA-TDNN model run the following command.\n\n\nTo train the SelfSupervised MLP model run the following command.\n\n\nTo train the SelfSupervised XVector model run the following command.",
"### This Project was developed completely using SpeechBrain\n\n\nReference\n---------"
] | [
"TAGS\n#speechbrain #en #dataset-librispeech_asr #arxiv-2106.04624 #region-us \n",
"### Limitations\n\n\nPlease note that these models are evaluated under controlled conditions and their performance may vary when applied to different datasets or in real-world scenarios.\n\n\nSystem Overview\n---------------\n\n\nThis project includes interfaces for processing audio files, detecting speech, and counting speakers. It leverages pre-trained models and custom scripts for refining and aggregating predictions.",
"### Installation\n\n\nPlease look at SpeechBrain for tutorials or more information.\n\n\nUsing the Speaker Counter Interface\n-----------------------------------",
"### For XVector & ECAPA-TDNN:",
"### For a Self-supervised model:\n\n\nSetup and Training Instructions\n-------------------------------\n\n\nTo reproduce the results of this project, follow these steps.\n\n\nDownload the project code from the GitHub repository and navigate into the project directory.\n\n\nDownload the necessary datasets (LibriSpeech etc.), specifying the desired destination folder.\n\n\nCreate custom dataset based on set parameters as shown in the sample below\n\n\nSample of URL:\n\n\nSample of URL\nTo train the XVector model run the following command.\n\n\nTo train the ECAPA-TDNN model run the following command.\n\n\nTo train the SelfSupervised MLP model run the following command.\n\n\nTo train the SelfSupervised XVector model run the following command.",
"### This Project was developed completely using SpeechBrain\n\n\nReference\n---------"
] |
null | transformers | ## About
<!-- ### quantize_version: 1 -->
<!-- ### output_tensor_quantised: 1 -->
<!-- ### convert_type: -->
<!-- ### vocab_type: -->
static quants of https://huggingface.co/ehristoforu/Gistral-16B
<!-- provided-files -->
weighted/imatrix quants seem not to be available (by me) at this time. If they do not show up a week or so after the static ones, I have probably not planned for them. Feel free to request them by opening a Community Discussion.
## Usage
If you are unsure how to use GGUF files, refer to one of [TheBloke's
READMEs](https://huggingface.co/TheBloke/KafkaLM-70B-German-V0.1-GGUF) for
more details, including on how to concatenate multi-part files.
## Provided Quants
(sorted by size, not necessarily quality. IQ-quants are often preferable over similar sized non-IQ quants)
| Link | Type | Size/GB | Notes |
|:-----|:-----|--------:|:------|
| [GGUF](https://huggingface.co/mradermacher/Gistral-16B-GGUF/resolve/main/Gistral-16B.Q2_K.gguf) | Q2_K | 6.4 | |
| [GGUF](https://huggingface.co/mradermacher/Gistral-16B-GGUF/resolve/main/Gistral-16B.IQ3_XS.gguf) | IQ3_XS | 7.0 | |
| [GGUF](https://huggingface.co/mradermacher/Gistral-16B-GGUF/resolve/main/Gistral-16B.Q3_K_S.gguf) | Q3_K_S | 7.4 | |
| [GGUF](https://huggingface.co/mradermacher/Gistral-16B-GGUF/resolve/main/Gistral-16B.IQ3_S.gguf) | IQ3_S | 7.4 | beats Q3_K* |
| [GGUF](https://huggingface.co/mradermacher/Gistral-16B-GGUF/resolve/main/Gistral-16B.IQ3_M.gguf) | IQ3_M | 7.7 | |
| [GGUF](https://huggingface.co/mradermacher/Gistral-16B-GGUF/resolve/main/Gistral-16B.Q3_K_M.gguf) | Q3_K_M | 8.2 | lower quality |
| [GGUF](https://huggingface.co/mradermacher/Gistral-16B-GGUF/resolve/main/Gistral-16B.Q3_K_L.gguf) | Q3_K_L | 9.0 | |
| [GGUF](https://huggingface.co/mradermacher/Gistral-16B-GGUF/resolve/main/Gistral-16B.IQ4_XS.gguf) | IQ4_XS | 9.2 | |
| [GGUF](https://huggingface.co/mradermacher/Gistral-16B-GGUF/resolve/main/Gistral-16B.Q4_K_S.gguf) | Q4_K_S | 9.7 | fast, recommended |
| [GGUF](https://huggingface.co/mradermacher/Gistral-16B-GGUF/resolve/main/Gistral-16B.Q4_K_M.gguf) | Q4_K_M | 10.2 | fast, recommended |
| [GGUF](https://huggingface.co/mradermacher/Gistral-16B-GGUF/resolve/main/Gistral-16B.Q5_K_S.gguf) | Q5_K_S | 11.7 | |
| [GGUF](https://huggingface.co/mradermacher/Gistral-16B-GGUF/resolve/main/Gistral-16B.Q5_K_M.gguf) | Q5_K_M | 12.0 | |
| [GGUF](https://huggingface.co/mradermacher/Gistral-16B-GGUF/resolve/main/Gistral-16B.Q6_K.gguf) | Q6_K | 13.9 | very good quality |
| [GGUF](https://huggingface.co/mradermacher/Gistral-16B-GGUF/resolve/main/Gistral-16B.Q8_0.gguf) | Q8_0 | 18.0 | fast, best quality |
Here is a handy graph by ikawrakow comparing some lower-quality quant
types (lower is better):

And here are Artefact2's thoughts on the matter:
https://gist.github.com/Artefact2/b5f810600771265fc1e39442288e8ec9
## FAQ / Model Request
See https://huggingface.co/mradermacher/model_requests for some answers to
questions you might have and/or if you want some other model quantized.
## Thanks
I thank my company, [nethype GmbH](https://www.nethype.de/), for letting
me use its servers and providing upgrades to my workstation to enable
this work in my free time.
<!-- end -->
| {"language": ["en"], "license": "apache-2.0", "library_name": "transformers", "tags": ["mistral", "gistral", "gistral-16b", "multilingual", "code", "128k", "metamath", "grok-1", "anthropic", "openhermes", "instruct", "merge"], "datasets": ["HuggingFaceH4/grok-conversation-harmless", "HuggingFaceH4/ultrachat_200k", "HuggingFaceH4/ultrafeedback_binarized_fixed", "HuggingFaceH4/cai-conversation-harmless", "meta-math/MetaMathQA", "emozilla/yarn-train-tokenized-16k-mistral", "snorkelai/Snorkel-Mistral-PairRM-DPO-Dataset", "microsoft/orca-math-word-problems-200k", "m-a-p/Code-Feedback", "teknium/openhermes", "lksy/ru_instruct_gpt4", "IlyaGusev/ru_turbo_saiga", "IlyaGusev/ru_sharegpt_cleaned", "IlyaGusev/oasst1_ru_main_branch"], "base_model": "ehristoforu/Gistral-16B", "quantized_by": "mradermacher"} | mradermacher/Gistral-16B-GGUF | null | [
"transformers",
"gguf",
"mistral",
"gistral",
"gistral-16b",
"multilingual",
"code",
"128k",
"metamath",
"grok-1",
"anthropic",
"openhermes",
"instruct",
"merge",
"en",
"dataset:HuggingFaceH4/grok-conversation-harmless",
"dataset:HuggingFaceH4/ultrachat_200k",
"dataset:HuggingFaceH4/ultrafeedback_binarized_fixed",
"dataset:HuggingFaceH4/cai-conversation-harmless",
"dataset:meta-math/MetaMathQA",
"dataset:emozilla/yarn-train-tokenized-16k-mistral",
"dataset:snorkelai/Snorkel-Mistral-PairRM-DPO-Dataset",
"dataset:microsoft/orca-math-word-problems-200k",
"dataset:m-a-p/Code-Feedback",
"dataset:teknium/openhermes",
"dataset:lksy/ru_instruct_gpt4",
"dataset:IlyaGusev/ru_turbo_saiga",
"dataset:IlyaGusev/ru_sharegpt_cleaned",
"dataset:IlyaGusev/oasst1_ru_main_branch",
"base_model:ehristoforu/Gistral-16B",
"license:apache-2.0",
"endpoints_compatible",
"region:us"
] | null | 2024-04-21T18:29:21+00:00 | [] | [
"en"
] | TAGS
#transformers #gguf #mistral #gistral #gistral-16b #multilingual #code #128k #metamath #grok-1 #anthropic #openhermes #instruct #merge #en #dataset-HuggingFaceH4/grok-conversation-harmless #dataset-HuggingFaceH4/ultrachat_200k #dataset-HuggingFaceH4/ultrafeedback_binarized_fixed #dataset-HuggingFaceH4/cai-conversation-harmless #dataset-meta-math/MetaMathQA #dataset-emozilla/yarn-train-tokenized-16k-mistral #dataset-snorkelai/Snorkel-Mistral-PairRM-DPO-Dataset #dataset-microsoft/orca-math-word-problems-200k #dataset-m-a-p/Code-Feedback #dataset-teknium/openhermes #dataset-lksy/ru_instruct_gpt4 #dataset-IlyaGusev/ru_turbo_saiga #dataset-IlyaGusev/ru_sharegpt_cleaned #dataset-IlyaGusev/oasst1_ru_main_branch #base_model-ehristoforu/Gistral-16B #license-apache-2.0 #endpoints_compatible #region-us
| About
-----
static quants of URL
weighted/imatrix quants seem not to be available (by me) at this time. If they do not show up a week or so after the static ones, I have probably not planned for them. Feel free to request them by opening a Community Discussion.
Usage
-----
If you are unsure how to use GGUF files, refer to one of TheBloke's
READMEs for
more details, including on how to concatenate multi-part files.
Provided Quants
---------------
(sorted by size, not necessarily quality. IQ-quants are often preferable over similar sized non-IQ quants)
Here is a handy graph by ikawrakow comparing some lower-quality quant
types (lower is better):
!URL
And here are Artefact2's thoughts on the matter:
URL
FAQ / Model Request
-------------------
See URL for some answers to
questions you might have and/or if you want some other model quantized.
Thanks
------
I thank my company, nethype GmbH, for letting
me use its servers and providing upgrades to my workstation to enable
this work in my free time.
| [] | [
"TAGS\n#transformers #gguf #mistral #gistral #gistral-16b #multilingual #code #128k #metamath #grok-1 #anthropic #openhermes #instruct #merge #en #dataset-HuggingFaceH4/grok-conversation-harmless #dataset-HuggingFaceH4/ultrachat_200k #dataset-HuggingFaceH4/ultrafeedback_binarized_fixed #dataset-HuggingFaceH4/cai-conversation-harmless #dataset-meta-math/MetaMathQA #dataset-emozilla/yarn-train-tokenized-16k-mistral #dataset-snorkelai/Snorkel-Mistral-PairRM-DPO-Dataset #dataset-microsoft/orca-math-word-problems-200k #dataset-m-a-p/Code-Feedback #dataset-teknium/openhermes #dataset-lksy/ru_instruct_gpt4 #dataset-IlyaGusev/ru_turbo_saiga #dataset-IlyaGusev/ru_sharegpt_cleaned #dataset-IlyaGusev/oasst1_ru_main_branch #base_model-ehristoforu/Gistral-16B #license-apache-2.0 #endpoints_compatible #region-us \n"
] |
null | transformers |
# Uploaded model
- **Developed by:** alihan
- **License:** apache-2.0
- **Finetuned from model :** unsloth/llama-3-8b-bnb-4bit
This llama model was trained 2x faster with [Unsloth](https://github.com/unslothai/unsloth) and Huggingface's TRL library.
[<img src="https://raw.githubusercontent.com/unslothai/unsloth/main/images/unsloth%20made%20with%20love.png" width="200"/>](https://github.com/unslothai/unsloth)
| {"language": ["en"], "license": "apache-2.0", "tags": ["text-generation-inference", "transformers", "unsloth", "llama", "trl"], "base_model": "unsloth/llama-3-8b-bnb-4bit"} | alihan/llama-3-8b-4bit-finetuned-adapters | null | [
"transformers",
"safetensors",
"text-generation-inference",
"unsloth",
"llama",
"trl",
"en",
"base_model:unsloth/llama-3-8b-bnb-4bit",
"license:apache-2.0",
"endpoints_compatible",
"region:us"
] | null | 2024-04-21T18:31:17+00:00 | [] | [
"en"
] | TAGS
#transformers #safetensors #text-generation-inference #unsloth #llama #trl #en #base_model-unsloth/llama-3-8b-bnb-4bit #license-apache-2.0 #endpoints_compatible #region-us
|
# Uploaded model
- Developed by: alihan
- License: apache-2.0
- Finetuned from model : unsloth/llama-3-8b-bnb-4bit
This llama model was trained 2x faster with Unsloth and Huggingface's TRL library.
<img src="URL width="200"/>
| [
"# Uploaded model\n\n- Developed by: alihan\n- License: apache-2.0\n- Finetuned from model : unsloth/llama-3-8b-bnb-4bit\n\nThis llama model was trained 2x faster with Unsloth and Huggingface's TRL library.\n\n<img src=\"URL width=\"200\"/>"
] | [
"TAGS\n#transformers #safetensors #text-generation-inference #unsloth #llama #trl #en #base_model-unsloth/llama-3-8b-bnb-4bit #license-apache-2.0 #endpoints_compatible #region-us \n",
"# Uploaded model\n\n- Developed by: alihan\n- License: apache-2.0\n- Finetuned from model : unsloth/llama-3-8b-bnb-4bit\n\nThis llama model was trained 2x faster with Unsloth and Huggingface's TRL library.\n\n<img src=\"URL width=\"200\"/>"
] |
text-generation | transformers |
# UndeadWizard
UndeadWizard is a Mixture of Experts (MoE) made with the following models using [LazyMergekit](https://colab.research.google.com/drive/1obulZ1ROXHjYLn6PPZJwRR6GzgQogxxb?usp=sharing):
* [cognitivecomputations/WizardLM-7B-Uncensored](https://huggingface.co/cognitivecomputations/WizardLM-7B-Uncensored)
* [dreamgen/WizardLM-2-7B](https://huggingface.co/dreamgen/WizardLM-2-7B)
## 🧩 Configuration
```yaml
base_model: dreamgen/WizardLM-2-7B
experts:
- source_model: cognitivecomputations/WizardLM-7B-Uncensored
positive_prompts:
- "chat"
- "write"
- "scene"
- "story"
- "explain"
- source_model: dreamgen/WizardLM-2-7B
positive_prompts:
- "reason"
- "math"
- "code"
- "solve"
- "python"
```
## 💻 Usage
```python
!pip install -qU transformers bitsandbytes accelerate
from transformers import AutoTokenizer
import transformers
import torch
model = "blueprintninja/UndeadWizard"
tokenizer = AutoTokenizer.from_pretrained(model)
pipeline = transformers.pipeline(
"text-generation",
model=model,
model_kwargs={"torch_dtype": torch.float16, "load_in_4bit": True},
)
messages = [{"role": "user", "content": "Explain what a Mixture of Experts is in less than 100 words."}]
prompt = pipeline.tokenizer.apply_chat_template(messages, tokenize=False, add_generation_prompt=True)
outputs = pipeline(prompt, max_new_tokens=256, do_sample=True, temperature=0.7, top_k=50, top_p=0.95)
print(outputs[0]["generated_text"])
``` | {"license": "apache-2.0", "tags": ["moe", "frankenmoe", "merge", "mergekit", "lazymergekit", "cognitivecomputations/WizardLM-7B-Uncensored", "dreamgen/WizardLM-2-7B"], "base_model": ["cognitivecomputations/WizardLM-7B-Uncensored", "dreamgen/WizardLM-2-7B"]} | blueprintninja/UndeadWizard | null | [
"transformers",
"mixtral",
"text-generation",
"moe",
"frankenmoe",
"merge",
"mergekit",
"lazymergekit",
"cognitivecomputations/WizardLM-7B-Uncensored",
"dreamgen/WizardLM-2-7B",
"base_model:cognitivecomputations/WizardLM-7B-Uncensored",
"base_model:dreamgen/WizardLM-2-7B",
"license:apache-2.0",
"autotrain_compatible",
"endpoints_compatible",
"text-generation-inference",
"region:us"
] | null | 2024-04-21T18:31:26+00:00 | [] | [] | TAGS
#transformers #mixtral #text-generation #moe #frankenmoe #merge #mergekit #lazymergekit #cognitivecomputations/WizardLM-7B-Uncensored #dreamgen/WizardLM-2-7B #base_model-cognitivecomputations/WizardLM-7B-Uncensored #base_model-dreamgen/WizardLM-2-7B #license-apache-2.0 #autotrain_compatible #endpoints_compatible #text-generation-inference #region-us
|
# UndeadWizard
UndeadWizard is a Mixture of Experts (MoE) made with the following models using LazyMergekit:
* cognitivecomputations/WizardLM-7B-Uncensored
* dreamgen/WizardLM-2-7B
## Configuration
## Usage
| [
"# UndeadWizard\n\nUndeadWizard is a Mixture of Experts (MoE) made with the following models using LazyMergekit:\n* cognitivecomputations/WizardLM-7B-Uncensored\n* dreamgen/WizardLM-2-7B",
"## Configuration",
"## Usage"
] | [
"TAGS\n#transformers #mixtral #text-generation #moe #frankenmoe #merge #mergekit #lazymergekit #cognitivecomputations/WizardLM-7B-Uncensored #dreamgen/WizardLM-2-7B #base_model-cognitivecomputations/WizardLM-7B-Uncensored #base_model-dreamgen/WizardLM-2-7B #license-apache-2.0 #autotrain_compatible #endpoints_compatible #text-generation-inference #region-us \n",
"# UndeadWizard\n\nUndeadWizard is a Mixture of Experts (MoE) made with the following models using LazyMergekit:\n* cognitivecomputations/WizardLM-7B-Uncensored\n* dreamgen/WizardLM-2-7B",
"## Configuration",
"## Usage"
] |
text-to-image | diffusers |
# AutoTrain SDXL LoRA DreamBooth - leonickson1/sac
<Gallery />
## Model description
These are leonickson1/sac LoRA adaption weights for stabilityai/stable-diffusion-xl-base-1.0.
The weights were trained using [DreamBooth](https://dreambooth.github.io/).
LoRA for the text encoder was enabled: False.
Special VAE used for training: None.
## Trigger words
You should use photo of a sks building to trigger the image generation.
## Download model
Weights for this model are available in Safetensors format.
[Download](leonickson1/sac/tree/main) them in the Files & versions tab.
| {"license": "openrail++", "tags": ["autotrain", "stable-diffusion-xl", "stable-diffusion-xl-diffusers", "text-to-image", "diffusers", "lora", "template:sd-lora"], "base_model": "stabilityai/stable-diffusion-xl-base-1.0", "instance_prompt": "photo of a sks building"} | leonickson1/clock_tower_building_uncc | null | [
"diffusers",
"autotrain",
"stable-diffusion-xl",
"stable-diffusion-xl-diffusers",
"text-to-image",
"lora",
"template:sd-lora",
"base_model:stabilityai/stable-diffusion-xl-base-1.0",
"license:openrail++",
"region:us"
] | null | 2024-04-21T18:32:54+00:00 | [] | [] | TAGS
#diffusers #autotrain #stable-diffusion-xl #stable-diffusion-xl-diffusers #text-to-image #lora #template-sd-lora #base_model-stabilityai/stable-diffusion-xl-base-1.0 #license-openrail++ #region-us
|
# AutoTrain SDXL LoRA DreamBooth - leonickson1/sac
<Gallery />
## Model description
These are leonickson1/sac LoRA adaption weights for stabilityai/stable-diffusion-xl-base-1.0.
The weights were trained using DreamBooth.
LoRA for the text encoder was enabled: False.
Special VAE used for training: None.
## Trigger words
You should use photo of a sks building to trigger the image generation.
## Download model
Weights for this model are available in Safetensors format.
Download them in the Files & versions tab.
| [
"# AutoTrain SDXL LoRA DreamBooth - leonickson1/sac\n\n<Gallery />",
"## Model description\n\nThese are leonickson1/sac LoRA adaption weights for stabilityai/stable-diffusion-xl-base-1.0.\n\nThe weights were trained using DreamBooth.\n\nLoRA for the text encoder was enabled: False.\n\nSpecial VAE used for training: None.",
"## Trigger words\n\nYou should use photo of a sks building to trigger the image generation.",
"## Download model\n\nWeights for this model are available in Safetensors format.\n\nDownload them in the Files & versions tab."
] | [
"TAGS\n#diffusers #autotrain #stable-diffusion-xl #stable-diffusion-xl-diffusers #text-to-image #lora #template-sd-lora #base_model-stabilityai/stable-diffusion-xl-base-1.0 #license-openrail++ #region-us \n",
"# AutoTrain SDXL LoRA DreamBooth - leonickson1/sac\n\n<Gallery />",
"## Model description\n\nThese are leonickson1/sac LoRA adaption weights for stabilityai/stable-diffusion-xl-base-1.0.\n\nThe weights were trained using DreamBooth.\n\nLoRA for the text encoder was enabled: False.\n\nSpecial VAE used for training: None.",
"## Trigger words\n\nYou should use photo of a sks building to trigger the image generation.",
"## Download model\n\nWeights for this model are available in Safetensors format.\n\nDownload them in the Files & versions tab."
] |
null | transformers | ## About
<!-- ### quantize_version: 1 -->
<!-- ### output_tensor_quantised: 1 -->
<!-- ### convert_type: -->
<!-- ### vocab_type: -->
static quants of https://huggingface.co/macadeliccc/Orpo-GutenLlama-3-8B-v2
<!-- provided-files -->
weighted/imatrix quants seem not to be available (by me) at this time. If they do not show up a week or so after the static ones, I have probably not planned for them. Feel free to request them by opening a Community Discussion.
## Usage
If you are unsure how to use GGUF files, refer to one of [TheBloke's
READMEs](https://huggingface.co/TheBloke/KafkaLM-70B-German-V0.1-GGUF) for
more details, including on how to concatenate multi-part files.
## Provided Quants
(sorted by size, not necessarily quality. IQ-quants are often preferable over similar sized non-IQ quants)
| Link | Type | Size/GB | Notes |
|:-----|:-----|--------:|:------|
| [GGUF](https://huggingface.co/mradermacher/Orpo-GutenLlama-3-8B-v2-GGUF/resolve/main/Orpo-GutenLlama-3-8B-v2.Q2_K.gguf) | Q2_K | 3.3 | |
| [GGUF](https://huggingface.co/mradermacher/Orpo-GutenLlama-3-8B-v2-GGUF/resolve/main/Orpo-GutenLlama-3-8B-v2.IQ3_XS.gguf) | IQ3_XS | 3.6 | |
| [GGUF](https://huggingface.co/mradermacher/Orpo-GutenLlama-3-8B-v2-GGUF/resolve/main/Orpo-GutenLlama-3-8B-v2.Q3_K_S.gguf) | Q3_K_S | 3.8 | |
| [GGUF](https://huggingface.co/mradermacher/Orpo-GutenLlama-3-8B-v2-GGUF/resolve/main/Orpo-GutenLlama-3-8B-v2.IQ3_S.gguf) | IQ3_S | 3.8 | beats Q3_K* |
| [GGUF](https://huggingface.co/mradermacher/Orpo-GutenLlama-3-8B-v2-GGUF/resolve/main/Orpo-GutenLlama-3-8B-v2.IQ3_M.gguf) | IQ3_M | 3.9 | |
| [GGUF](https://huggingface.co/mradermacher/Orpo-GutenLlama-3-8B-v2-GGUF/resolve/main/Orpo-GutenLlama-3-8B-v2.Q3_K_M.gguf) | Q3_K_M | 4.1 | lower quality |
| [GGUF](https://huggingface.co/mradermacher/Orpo-GutenLlama-3-8B-v2-GGUF/resolve/main/Orpo-GutenLlama-3-8B-v2.Q3_K_L.gguf) | Q3_K_L | 4.4 | |
| [GGUF](https://huggingface.co/mradermacher/Orpo-GutenLlama-3-8B-v2-GGUF/resolve/main/Orpo-GutenLlama-3-8B-v2.IQ4_XS.gguf) | IQ4_XS | 4.6 | |
| [GGUF](https://huggingface.co/mradermacher/Orpo-GutenLlama-3-8B-v2-GGUF/resolve/main/Orpo-GutenLlama-3-8B-v2.Q4_K_S.gguf) | Q4_K_S | 4.8 | fast, recommended |
| [GGUF](https://huggingface.co/mradermacher/Orpo-GutenLlama-3-8B-v2-GGUF/resolve/main/Orpo-GutenLlama-3-8B-v2.Q4_K_M.gguf) | Q4_K_M | 5.0 | fast, recommended |
| [GGUF](https://huggingface.co/mradermacher/Orpo-GutenLlama-3-8B-v2-GGUF/resolve/main/Orpo-GutenLlama-3-8B-v2.Q5_K_S.gguf) | Q5_K_S | 5.7 | |
| [GGUF](https://huggingface.co/mradermacher/Orpo-GutenLlama-3-8B-v2-GGUF/resolve/main/Orpo-GutenLlama-3-8B-v2.Q5_K_M.gguf) | Q5_K_M | 5.8 | |
| [GGUF](https://huggingface.co/mradermacher/Orpo-GutenLlama-3-8B-v2-GGUF/resolve/main/Orpo-GutenLlama-3-8B-v2.Q6_K.gguf) | Q6_K | 6.7 | very good quality |
| [GGUF](https://huggingface.co/mradermacher/Orpo-GutenLlama-3-8B-v2-GGUF/resolve/main/Orpo-GutenLlama-3-8B-v2.Q8_0.gguf) | Q8_0 | 8.6 | fast, best quality |
Here is a handy graph by ikawrakow comparing some lower-quality quant
types (lower is better):

And here are Artefact2's thoughts on the matter:
https://gist.github.com/Artefact2/b5f810600771265fc1e39442288e8ec9
## FAQ / Model Request
See https://huggingface.co/mradermacher/model_requests for some answers to
questions you might have and/or if you want some other model quantized.
## Thanks
I thank my company, [nethype GmbH](https://www.nethype.de/), for letting
me use its servers and providing upgrades to my workstation to enable
this work in my free time.
<!-- end -->
| {"language": ["en"], "library_name": "transformers", "datasets": ["mlabonne/orpo-dpo-mix-40k", "jondurbin/gutenberg-dpo-v0.1"], "base_model": "macadeliccc/Orpo-GutenLlama-3-8B-v2", "quantized_by": "mradermacher"} | mradermacher/Orpo-GutenLlama-3-8B-v2-GGUF | null | [
"transformers",
"gguf",
"en",
"dataset:mlabonne/orpo-dpo-mix-40k",
"dataset:jondurbin/gutenberg-dpo-v0.1",
"base_model:macadeliccc/Orpo-GutenLlama-3-8B-v2",
"endpoints_compatible",
"region:us"
] | null | 2024-04-21T18:34:00+00:00 | [] | [
"en"
] | TAGS
#transformers #gguf #en #dataset-mlabonne/orpo-dpo-mix-40k #dataset-jondurbin/gutenberg-dpo-v0.1 #base_model-macadeliccc/Orpo-GutenLlama-3-8B-v2 #endpoints_compatible #region-us
| About
-----
static quants of URL
weighted/imatrix quants seem not to be available (by me) at this time. If they do not show up a week or so after the static ones, I have probably not planned for them. Feel free to request them by opening a Community Discussion.
Usage
-----
If you are unsure how to use GGUF files, refer to one of TheBloke's
READMEs for
more details, including on how to concatenate multi-part files.
Provided Quants
---------------
(sorted by size, not necessarily quality. IQ-quants are often preferable over similar sized non-IQ quants)
Here is a handy graph by ikawrakow comparing some lower-quality quant
types (lower is better):
!URL
And here are Artefact2's thoughts on the matter:
URL
FAQ / Model Request
-------------------
See URL for some answers to
questions you might have and/or if you want some other model quantized.
Thanks
------
I thank my company, nethype GmbH, for letting
me use its servers and providing upgrades to my workstation to enable
this work in my free time.
| [] | [
"TAGS\n#transformers #gguf #en #dataset-mlabonne/orpo-dpo-mix-40k #dataset-jondurbin/gutenberg-dpo-v0.1 #base_model-macadeliccc/Orpo-GutenLlama-3-8B-v2 #endpoints_compatible #region-us \n"
] |
null | trl |
# Weni/WeniGPT-Agents-Mistral-1.0.0-SFT-1.0.15-DPO
This model is a fine-tuned version of [Weni/WeniGPT-Agents-Mistral-1.0.0-SFT-merged] on the dataset Weni/wenigpt-agent-dpo-1.0.0 with the DPO trainer. It is part of the WeniGPT project for [Weni](https://weni.ai/).
Description: Experiment on DPO with other hyperparameters and best SFT model of WeniGPT
It achieves the following results on the evaluation set:
{'eval_loss': 0.021960575133562088, 'eval_runtime': 13.5737, 'eval_samples_per_second': 2.063, 'eval_steps_per_second': 0.516, 'eval_rewards/chosen': 4.207414627075195, 'eval_rewards/rejected': -2.967747211456299, 'eval_rewards/accuracies': 1.0, 'eval_rewards/margins': 7.175161838531494, 'eval_logps/rejected': -180.97348022460938, 'eval_logps/chosen': -120.53617095947266, 'eval_logits/rejected': -1.8871468305587769, 'eval_logits/chosen': -1.8866546154022217, 'epoch': 5.806451612903226}
## Intended uses & limitations
This model has not been trained to avoid specific intructions.
## Training procedure
Finetuning was done on the model Weni/WeniGPT-Agents-Mistral-1.0.0-SFT-merged with the following prompt:
```
---------------------
System_prompt:
Agora você se chama {name}, você é {occupation} e seu objetivo é {chatbot_goal}. O adjetivo que mais define a sua personalidade é {adjective} e você se comporta da seguinte forma:
{instructions_formatted}
{context_statement}
Lista de requisitos:
- Responda de forma natural, mas nunca fale sobre um assunto fora do contexto.
- Nunca traga informações do seu próprio conhecimento.
- Repito é crucial que você responda usando apenas informações do contexto.
- Nunca mencione o contexto fornecido.
- Nunca mencione a pergunta fornecida.
- Gere a resposta mais útil possível para a pergunta usando informações do conexto acima.
- Nunca elabore sobre o porque e como você fez a tarefa, apenas responda.
---------------------
```
### Training hyperparameters
The following hyperparameters were used during training:
- learning_rate: 5e-06
- per_device_train_batch_size: 1
- per_device_eval_batch_size: 1
- gradient_accumulation_steps: 2
- num_gpus: 4
- total_train_batch_size: 8
- optimizer: AdamW
- lr_scheduler_type: cosine
- num_steps: 180
- quantization_type: bitsandbytes
- LoRA: ("\n - bits: 4\n - use_exllama: True\n - device_map: auto\n - use_cache: False\n - lora_r: 16\n - lora_alpha: 32\n - lora_dropout: 0.1\n - bias: none\n - target_modules: ['q_proj', 'k_proj', 'v_proj', 'o_proj', 'gate_proj', 'up_proj', 'down_proj']\n - task_type: CAUSAL_LM",)
### Training results
### Framework versions
- transformers==4.40.0
- datasets==2.18.0
- peft==0.10.0
- safetensors==0.4.2
- evaluate==0.4.1
- bitsandbytes==0.43
- huggingface_hub==0.22.2
- seqeval==1.2.2
- auto-gptq==0.7.1
- gpustat==1.1.1
- deepspeed==0.14.0
- wandb==0.16.6
- trl==0.8.1
- accelerate==0.29.3
- coloredlogs==15.0.1
- traitlets==5.14.2
- git+https://github.com/casper-hansen/AutoAWQ.git
### Hardware
- Cloud provided: runpod.io
| {"language": ["pt"], "license": "mit", "library_name": "trl", "tags": ["DPO", "WeniGPT"], "base_model": "Weni/WeniGPT-Agents-Mistral-1.0.0-SFT-merged", "model-index": [{"name": "Weni/WeniGPT-Agents-Mistral-1.0.0-SFT-1.0.15-DPO", "results": []}]} | Weni/WeniGPT-Agents-Mistral-1.0.0-SFT-1.0.15-DPO | null | [
"trl",
"safetensors",
"DPO",
"WeniGPT",
"pt",
"base_model:Weni/WeniGPT-Agents-Mistral-1.0.0-SFT-merged",
"license:mit",
"region:us"
] | null | 2024-04-21T18:34:19+00:00 | [] | [
"pt"
] | TAGS
#trl #safetensors #DPO #WeniGPT #pt #base_model-Weni/WeniGPT-Agents-Mistral-1.0.0-SFT-merged #license-mit #region-us
|
# Weni/WeniGPT-Agents-Mistral-1.0.0-SFT-1.0.15-DPO
This model is a fine-tuned version of [Weni/WeniGPT-Agents-Mistral-1.0.0-SFT-merged] on the dataset Weni/wenigpt-agent-dpo-1.0.0 with the DPO trainer. It is part of the WeniGPT project for Weni.
Description: Experiment on DPO with other hyperparameters and best SFT model of WeniGPT
It achieves the following results on the evaluation set:
{'eval_loss': 0.021960575133562088, 'eval_runtime': 13.5737, 'eval_samples_per_second': 2.063, 'eval_steps_per_second': 0.516, 'eval_rewards/chosen': 4.207414627075195, 'eval_rewards/rejected': -2.967747211456299, 'eval_rewards/accuracies': 1.0, 'eval_rewards/margins': 7.175161838531494, 'eval_logps/rejected': -180.97348022460938, 'eval_logps/chosen': -120.53617095947266, 'eval_logits/rejected': -1.8871468305587769, 'eval_logits/chosen': -1.8866546154022217, 'epoch': 5.806451612903226}
## Intended uses & limitations
This model has not been trained to avoid specific intructions.
## Training procedure
Finetuning was done on the model Weni/WeniGPT-Agents-Mistral-1.0.0-SFT-merged with the following prompt:
### Training hyperparameters
The following hyperparameters were used during training:
- learning_rate: 5e-06
- per_device_train_batch_size: 1
- per_device_eval_batch_size: 1
- gradient_accumulation_steps: 2
- num_gpus: 4
- total_train_batch_size: 8
- optimizer: AdamW
- lr_scheduler_type: cosine
- num_steps: 180
- quantization_type: bitsandbytes
- LoRA: ("\n - bits: 4\n - use_exllama: True\n - device_map: auto\n - use_cache: False\n - lora_r: 16\n - lora_alpha: 32\n - lora_dropout: 0.1\n - bias: none\n - target_modules: ['q_proj', 'k_proj', 'v_proj', 'o_proj', 'gate_proj', 'up_proj', 'down_proj']\n - task_type: CAUSAL_LM",)
### Training results
### Framework versions
- transformers==4.40.0
- datasets==2.18.0
- peft==0.10.0
- safetensors==0.4.2
- evaluate==0.4.1
- bitsandbytes==0.43
- huggingface_hub==0.22.2
- seqeval==1.2.2
- auto-gptq==0.7.1
- gpustat==1.1.1
- deepspeed==0.14.0
- wandb==0.16.6
- trl==0.8.1
- accelerate==0.29.3
- coloredlogs==15.0.1
- traitlets==5.14.2
- git+URL
### Hardware
- Cloud provided: URL
| [
"# Weni/WeniGPT-Agents-Mistral-1.0.0-SFT-1.0.15-DPO\n\nThis model is a fine-tuned version of [Weni/WeniGPT-Agents-Mistral-1.0.0-SFT-merged] on the dataset Weni/wenigpt-agent-dpo-1.0.0 with the DPO trainer. It is part of the WeniGPT project for Weni.\nDescription: Experiment on DPO with other hyperparameters and best SFT model of WeniGPT\n\nIt achieves the following results on the evaluation set:\n{'eval_loss': 0.021960575133562088, 'eval_runtime': 13.5737, 'eval_samples_per_second': 2.063, 'eval_steps_per_second': 0.516, 'eval_rewards/chosen': 4.207414627075195, 'eval_rewards/rejected': -2.967747211456299, 'eval_rewards/accuracies': 1.0, 'eval_rewards/margins': 7.175161838531494, 'eval_logps/rejected': -180.97348022460938, 'eval_logps/chosen': -120.53617095947266, 'eval_logits/rejected': -1.8871468305587769, 'eval_logits/chosen': -1.8866546154022217, 'epoch': 5.806451612903226}",
"## Intended uses & limitations\n\nThis model has not been trained to avoid specific intructions.",
"## Training procedure\n\nFinetuning was done on the model Weni/WeniGPT-Agents-Mistral-1.0.0-SFT-merged with the following prompt:",
"### Training hyperparameters\n\nThe following hyperparameters were used during training:\n- learning_rate: 5e-06\n- per_device_train_batch_size: 1\n- per_device_eval_batch_size: 1\n- gradient_accumulation_steps: 2\n- num_gpus: 4\n- total_train_batch_size: 8\n- optimizer: AdamW\n- lr_scheduler_type: cosine\n- num_steps: 180\n- quantization_type: bitsandbytes\n- LoRA: (\"\\n - bits: 4\\n - use_exllama: True\\n - device_map: auto\\n - use_cache: False\\n - lora_r: 16\\n - lora_alpha: 32\\n - lora_dropout: 0.1\\n - bias: none\\n - target_modules: ['q_proj', 'k_proj', 'v_proj', 'o_proj', 'gate_proj', 'up_proj', 'down_proj']\\n - task_type: CAUSAL_LM\",)",
"### Training results",
"### Framework versions\n\n- transformers==4.40.0\n- datasets==2.18.0\n- peft==0.10.0\n- safetensors==0.4.2\n- evaluate==0.4.1\n- bitsandbytes==0.43\n- huggingface_hub==0.22.2\n- seqeval==1.2.2\n- auto-gptq==0.7.1\n- gpustat==1.1.1\n- deepspeed==0.14.0\n- wandb==0.16.6\n- trl==0.8.1\n- accelerate==0.29.3\n- coloredlogs==15.0.1\n- traitlets==5.14.2\n- git+URL",
"### Hardware\n- Cloud provided: URL"
] | [
"TAGS\n#trl #safetensors #DPO #WeniGPT #pt #base_model-Weni/WeniGPT-Agents-Mistral-1.0.0-SFT-merged #license-mit #region-us \n",
"# Weni/WeniGPT-Agents-Mistral-1.0.0-SFT-1.0.15-DPO\n\nThis model is a fine-tuned version of [Weni/WeniGPT-Agents-Mistral-1.0.0-SFT-merged] on the dataset Weni/wenigpt-agent-dpo-1.0.0 with the DPO trainer. It is part of the WeniGPT project for Weni.\nDescription: Experiment on DPO with other hyperparameters and best SFT model of WeniGPT\n\nIt achieves the following results on the evaluation set:\n{'eval_loss': 0.021960575133562088, 'eval_runtime': 13.5737, 'eval_samples_per_second': 2.063, 'eval_steps_per_second': 0.516, 'eval_rewards/chosen': 4.207414627075195, 'eval_rewards/rejected': -2.967747211456299, 'eval_rewards/accuracies': 1.0, 'eval_rewards/margins': 7.175161838531494, 'eval_logps/rejected': -180.97348022460938, 'eval_logps/chosen': -120.53617095947266, 'eval_logits/rejected': -1.8871468305587769, 'eval_logits/chosen': -1.8866546154022217, 'epoch': 5.806451612903226}",
"## Intended uses & limitations\n\nThis model has not been trained to avoid specific intructions.",
"## Training procedure\n\nFinetuning was done on the model Weni/WeniGPT-Agents-Mistral-1.0.0-SFT-merged with the following prompt:",
"### Training hyperparameters\n\nThe following hyperparameters were used during training:\n- learning_rate: 5e-06\n- per_device_train_batch_size: 1\n- per_device_eval_batch_size: 1\n- gradient_accumulation_steps: 2\n- num_gpus: 4\n- total_train_batch_size: 8\n- optimizer: AdamW\n- lr_scheduler_type: cosine\n- num_steps: 180\n- quantization_type: bitsandbytes\n- LoRA: (\"\\n - bits: 4\\n - use_exllama: True\\n - device_map: auto\\n - use_cache: False\\n - lora_r: 16\\n - lora_alpha: 32\\n - lora_dropout: 0.1\\n - bias: none\\n - target_modules: ['q_proj', 'k_proj', 'v_proj', 'o_proj', 'gate_proj', 'up_proj', 'down_proj']\\n - task_type: CAUSAL_LM\",)",
"### Training results",
"### Framework versions\n\n- transformers==4.40.0\n- datasets==2.18.0\n- peft==0.10.0\n- safetensors==0.4.2\n- evaluate==0.4.1\n- bitsandbytes==0.43\n- huggingface_hub==0.22.2\n- seqeval==1.2.2\n- auto-gptq==0.7.1\n- gpustat==1.1.1\n- deepspeed==0.14.0\n- wandb==0.16.6\n- trl==0.8.1\n- accelerate==0.29.3\n- coloredlogs==15.0.1\n- traitlets==5.14.2\n- git+URL",
"### Hardware\n- Cloud provided: URL"
] |
null | peft |
<!-- This model card has been generated automatically according to the information the Trainer had access to. You
should probably proofread and complete it, then remove this comment. -->
# mistral_finetune_chatbot
This model is a fine-tuned version of [mistralai/Mistral-7B-v0.1](https://huggingface.co/mistralai/Mistral-7B-v0.1) on an unknown dataset.
## Model description
More information needed
## Intended uses & limitations
More information needed
## Training and evaluation data
More information needed
## Training procedure
### Training hyperparameters
The following hyperparameters were used during training:
- learning_rate: 0.0002
- train_batch_size: 1
- eval_batch_size: 8
- seed: 42
- gradient_accumulation_steps: 4
- total_train_batch_size: 4
- optimizer: Adam with betas=(0.9,0.999) and epsilon=1e-08
- lr_scheduler_type: linear
- training_steps: 20
- mixed_precision_training: Native AMP
### Training results
### Framework versions
- PEFT 0.10.0
- Transformers 4.40.0
- Pytorch 2.2.2+cu121
- Datasets 2.19.0
- Tokenizers 0.19.1 | {"license": "apache-2.0", "library_name": "peft", "tags": ["trl", "sft", "generated_from_trainer"], "base_model": "mistralai/Mistral-7B-v0.1", "model-index": [{"name": "mistral_finetune_chatbot", "results": []}]} | gouravsinha/mistral_finetune_chatbot | null | [
"peft",
"tensorboard",
"safetensors",
"trl",
"sft",
"generated_from_trainer",
"base_model:mistralai/Mistral-7B-v0.1",
"license:apache-2.0",
"region:us"
] | null | 2024-04-21T18:35:58+00:00 | [] | [] | TAGS
#peft #tensorboard #safetensors #trl #sft #generated_from_trainer #base_model-mistralai/Mistral-7B-v0.1 #license-apache-2.0 #region-us
|
# mistral_finetune_chatbot
This model is a fine-tuned version of mistralai/Mistral-7B-v0.1 on an unknown dataset.
## Model description
More information needed
## Intended uses & limitations
More information needed
## Training and evaluation data
More information needed
## Training procedure
### Training hyperparameters
The following hyperparameters were used during training:
- learning_rate: 0.0002
- train_batch_size: 1
- eval_batch_size: 8
- seed: 42
- gradient_accumulation_steps: 4
- total_train_batch_size: 4
- optimizer: Adam with betas=(0.9,0.999) and epsilon=1e-08
- lr_scheduler_type: linear
- training_steps: 20
- mixed_precision_training: Native AMP
### Training results
### Framework versions
- PEFT 0.10.0
- Transformers 4.40.0
- Pytorch 2.2.2+cu121
- Datasets 2.19.0
- Tokenizers 0.19.1 | [
"# mistral_finetune_chatbot\n\nThis model is a fine-tuned version of mistralai/Mistral-7B-v0.1 on an unknown dataset.",
"## Model description\n\nMore information needed",
"## Intended uses & limitations\n\nMore information needed",
"## Training and evaluation data\n\nMore information needed",
"## Training procedure",
"### Training hyperparameters\n\nThe following hyperparameters were used during training:\n- learning_rate: 0.0002\n- train_batch_size: 1\n- eval_batch_size: 8\n- seed: 42\n- gradient_accumulation_steps: 4\n- total_train_batch_size: 4\n- optimizer: Adam with betas=(0.9,0.999) and epsilon=1e-08\n- lr_scheduler_type: linear\n- training_steps: 20\n- mixed_precision_training: Native AMP",
"### Training results",
"### Framework versions\n\n- PEFT 0.10.0\n- Transformers 4.40.0\n- Pytorch 2.2.2+cu121\n- Datasets 2.19.0\n- Tokenizers 0.19.1"
] | [
"TAGS\n#peft #tensorboard #safetensors #trl #sft #generated_from_trainer #base_model-mistralai/Mistral-7B-v0.1 #license-apache-2.0 #region-us \n",
"# mistral_finetune_chatbot\n\nThis model is a fine-tuned version of mistralai/Mistral-7B-v0.1 on an unknown dataset.",
"## Model description\n\nMore information needed",
"## Intended uses & limitations\n\nMore information needed",
"## Training and evaluation data\n\nMore information needed",
"## Training procedure",
"### Training hyperparameters\n\nThe following hyperparameters were used during training:\n- learning_rate: 0.0002\n- train_batch_size: 1\n- eval_batch_size: 8\n- seed: 42\n- gradient_accumulation_steps: 4\n- total_train_batch_size: 4\n- optimizer: Adam with betas=(0.9,0.999) and epsilon=1e-08\n- lr_scheduler_type: linear\n- training_steps: 20\n- mixed_precision_training: Native AMP",
"### Training results",
"### Framework versions\n\n- PEFT 0.10.0\n- Transformers 4.40.0\n- Pytorch 2.2.2+cu121\n- Datasets 2.19.0\n- Tokenizers 0.19.1"
] |
null | adapter-transformers |
# Adapter `BigTMiami/n_par_bn_v_1_help_class_40_e_adp_lr_0003_S_0` for roberta-base
An [adapter](https://adapterhub.ml) for the `roberta-base` model that was trained on the [BigTMiami/amazon_MICRO_helpfulness_dataset](https://huggingface.co/datasets/BigTMiami/amazon_MICRO_helpfulness_dataset/) dataset and includes a prediction head for classification.
This adapter was created for usage with the **[Adapters](https://github.com/Adapter-Hub/adapters)** library.
## Usage
First, install `adapters`:
```
pip install -U adapters
```
Now, the adapter can be loaded and activated like this:
```python
from adapters import AutoAdapterModel
model = AutoAdapterModel.from_pretrained("roberta-base")
adapter_name = model.load_adapter("BigTMiami/n_par_bn_v_1_help_class_40_e_adp_lr_0003_S_0", source="hf", set_active=True)
```
## Architecture & Training
<!-- Add some description here -->
## Evaluation results
<!-- Add some description here -->
## Citation
<!-- Add some description here --> | {"tags": ["roberta", "adapter-transformers"], "datasets": ["BigTMiami/amazon_MICRO_helpfulness_dataset"]} | BigTMiami/n_par_bn_v_1_help_class_40_e_adp_lr_0003_S_0 | null | [
"adapter-transformers",
"roberta",
"dataset:BigTMiami/amazon_MICRO_helpfulness_dataset",
"region:us"
] | null | 2024-04-21T18:36:22+00:00 | [] | [] | TAGS
#adapter-transformers #roberta #dataset-BigTMiami/amazon_MICRO_helpfulness_dataset #region-us
|
# Adapter 'BigTMiami/n_par_bn_v_1_help_class_40_e_adp_lr_0003_S_0' for roberta-base
An adapter for the 'roberta-base' model that was trained on the BigTMiami/amazon_MICRO_helpfulness_dataset dataset and includes a prediction head for classification.
This adapter was created for usage with the Adapters library.
## Usage
First, install 'adapters':
Now, the adapter can be loaded and activated like this:
## Architecture & Training
## Evaluation results
| [
"# Adapter 'BigTMiami/n_par_bn_v_1_help_class_40_e_adp_lr_0003_S_0' for roberta-base\n\nAn adapter for the 'roberta-base' model that was trained on the BigTMiami/amazon_MICRO_helpfulness_dataset dataset and includes a prediction head for classification.\n\nThis adapter was created for usage with the Adapters library.",
"## Usage\n\nFirst, install 'adapters':\n\n\n\nNow, the adapter can be loaded and activated like this:",
"## Architecture & Training",
"## Evaluation results"
] | [
"TAGS\n#adapter-transformers #roberta #dataset-BigTMiami/amazon_MICRO_helpfulness_dataset #region-us \n",
"# Adapter 'BigTMiami/n_par_bn_v_1_help_class_40_e_adp_lr_0003_S_0' for roberta-base\n\nAn adapter for the 'roberta-base' model that was trained on the BigTMiami/amazon_MICRO_helpfulness_dataset dataset and includes a prediction head for classification.\n\nThis adapter was created for usage with the Adapters library.",
"## Usage\n\nFirst, install 'adapters':\n\n\n\nNow, the adapter can be loaded and activated like this:",
"## Architecture & Training",
"## Evaluation results"
] |
text-generation | transformers |
# Model Card for Model ID
<!-- Provide a quick summary of what the model is/does. -->
## Model Details
### Model Description
<!-- Provide a longer summary of what this model is. -->
This is the model card of a 🤗 transformers model that has been pushed on the Hub. This model card has been automatically generated.
- **Developed by:** [More Information Needed]
- **Funded by [optional]:** [More Information Needed]
- **Shared by [optional]:** [More Information Needed]
- **Model type:** [More Information Needed]
- **Language(s) (NLP):** [More Information Needed]
- **License:** [More Information Needed]
- **Finetuned from model [optional]:** [More Information Needed]
### Model Sources [optional]
<!-- Provide the basic links for the model. -->
- **Repository:** [More Information Needed]
- **Paper [optional]:** [More Information Needed]
- **Demo [optional]:** [More Information Needed]
## Uses
<!-- Address questions around how the model is intended to be used, including the foreseeable users of the model and those affected by the model. -->
### Direct Use
<!-- This section is for the model use without fine-tuning or plugging into a larger ecosystem/app. -->
[More Information Needed]
### Downstream Use [optional]
<!-- This section is for the model use when fine-tuned for a task, or when plugged into a larger ecosystem/app -->
[More Information Needed]
### Out-of-Scope Use
<!-- This section addresses misuse, malicious use, and uses that the model will not work well for. -->
[More Information Needed]
## Bias, Risks, and Limitations
<!-- This section is meant to convey both technical and sociotechnical limitations. -->
[More Information Needed]
### Recommendations
<!-- This section is meant to convey recommendations with respect to the bias, risk, and technical limitations. -->
Users (both direct and downstream) should be made aware of the risks, biases and limitations of the model. More information needed for further recommendations.
## How to Get Started with the Model
Use the code below to get started with the model.
[More Information Needed]
## Training Details
### Training Data
<!-- This should link to a Dataset Card, perhaps with a short stub of information on what the training data is all about as well as documentation related to data pre-processing or additional filtering. -->
[More Information Needed]
### Training Procedure
<!-- This relates heavily to the Technical Specifications. Content here should link to that section when it is relevant to the training procedure. -->
#### Preprocessing [optional]
[More Information Needed]
#### Training Hyperparameters
- **Training regime:** [More Information Needed] <!--fp32, fp16 mixed precision, bf16 mixed precision, bf16 non-mixed precision, fp16 non-mixed precision, fp8 mixed precision -->
#### Speeds, Sizes, Times [optional]
<!-- This section provides information about throughput, start/end time, checkpoint size if relevant, etc. -->
[More Information Needed]
## Evaluation
<!-- This section describes the evaluation protocols and provides the results. -->
### Testing Data, Factors & Metrics
#### Testing Data
<!-- This should link to a Dataset Card if possible. -->
[More Information Needed]
#### Factors
<!-- These are the things the evaluation is disaggregating by, e.g., subpopulations or domains. -->
[More Information Needed]
#### Metrics
<!-- These are the evaluation metrics being used, ideally with a description of why. -->
[More Information Needed]
### Results
[More Information Needed]
#### Summary
## Model Examination [optional]
<!-- Relevant interpretability work for the model goes here -->
[More Information Needed]
## Environmental Impact
<!-- Total emissions (in grams of CO2eq) and additional considerations, such as electricity usage, go here. Edit the suggested text below accordingly -->
Carbon emissions can be estimated using the [Machine Learning Impact calculator](https://mlco2.github.io/impact#compute) presented in [Lacoste et al. (2019)](https://arxiv.org/abs/1910.09700).
- **Hardware Type:** [More Information Needed]
- **Hours used:** [More Information Needed]
- **Cloud Provider:** [More Information Needed]
- **Compute Region:** [More Information Needed]
- **Carbon Emitted:** [More Information Needed]
## Technical Specifications [optional]
### Model Architecture and Objective
[More Information Needed]
### Compute Infrastructure
[More Information Needed]
#### Hardware
[More Information Needed]
#### Software
[More Information Needed]
## Citation [optional]
<!-- If there is a paper or blog post introducing the model, the APA and Bibtex information for that should go in this section. -->
**BibTeX:**
[More Information Needed]
**APA:**
[More Information Needed]
## Glossary [optional]
<!-- If relevant, include terms and calculations in this section that can help readers understand the model or model card. -->
[More Information Needed]
## More Information [optional]
[More Information Needed]
## Model Card Authors [optional]
[More Information Needed]
## Model Card Contact
[More Information Needed] | {"library_name": "transformers", "tags": []} | palgraciel/Meta-Llama-3-8B-Instruct-leo-kor | null | [
"transformers",
"safetensors",
"llama",
"text-generation",
"conversational",
"arxiv:1910.09700",
"autotrain_compatible",
"endpoints_compatible",
"text-generation-inference",
"4-bit",
"region:us"
] | null | 2024-04-21T18:40:07+00:00 | [
"1910.09700"
] | [] | TAGS
#transformers #safetensors #llama #text-generation #conversational #arxiv-1910.09700 #autotrain_compatible #endpoints_compatible #text-generation-inference #4-bit #region-us
|
# Model Card for Model ID
## Model Details
### Model Description
This is the model card of a transformers model that has been pushed on the Hub. This model card has been automatically generated.
- Developed by:
- Funded by [optional]:
- Shared by [optional]:
- Model type:
- Language(s) (NLP):
- License:
- Finetuned from model [optional]:
### Model Sources [optional]
- Repository:
- Paper [optional]:
- Demo [optional]:
## Uses
### Direct Use
### Downstream Use [optional]
### Out-of-Scope Use
## Bias, Risks, and Limitations
### Recommendations
Users (both direct and downstream) should be made aware of the risks, biases and limitations of the model. More information needed for further recommendations.
## How to Get Started with the Model
Use the code below to get started with the model.
## Training Details
### Training Data
### Training Procedure
#### Preprocessing [optional]
#### Training Hyperparameters
- Training regime:
#### Speeds, Sizes, Times [optional]
## Evaluation
### Testing Data, Factors & Metrics
#### Testing Data
#### Factors
#### Metrics
### Results
#### Summary
## Model Examination [optional]
## Environmental Impact
Carbon emissions can be estimated using the Machine Learning Impact calculator presented in Lacoste et al. (2019).
- Hardware Type:
- Hours used:
- Cloud Provider:
- Compute Region:
- Carbon Emitted:
## Technical Specifications [optional]
### Model Architecture and Objective
### Compute Infrastructure
#### Hardware
#### Software
[optional]
BibTeX:
APA:
## Glossary [optional]
## More Information [optional]
## Model Card Authors [optional]
## Model Card Contact
| [
"# Model Card for Model ID",
"## Model Details",
"### Model Description\n\n\n\nThis is the model card of a transformers model that has been pushed on the Hub. This model card has been automatically generated.\n\n- Developed by: \n- Funded by [optional]: \n- Shared by [optional]: \n- Model type: \n- Language(s) (NLP): \n- License: \n- Finetuned from model [optional]:",
"### Model Sources [optional]\n\n\n\n- Repository: \n- Paper [optional]: \n- Demo [optional]:",
"## Uses",
"### Direct Use",
"### Downstream Use [optional]",
"### Out-of-Scope Use",
"## Bias, Risks, and Limitations",
"### Recommendations\n\n\n\nUsers (both direct and downstream) should be made aware of the risks, biases and limitations of the model. More information needed for further recommendations.",
"## How to Get Started with the Model\n\nUse the code below to get started with the model.",
"## Training Details",
"### Training Data",
"### Training Procedure",
"#### Preprocessing [optional]",
"#### Training Hyperparameters\n\n- Training regime:",
"#### Speeds, Sizes, Times [optional]",
"## Evaluation",
"### Testing Data, Factors & Metrics",
"#### Testing Data",
"#### Factors",
"#### Metrics",
"### Results",
"#### Summary",
"## Model Examination [optional]",
"## Environmental Impact\n\n\n\nCarbon emissions can be estimated using the Machine Learning Impact calculator presented in Lacoste et al. (2019).\n\n- Hardware Type: \n- Hours used: \n- Cloud Provider: \n- Compute Region: \n- Carbon Emitted:",
"## Technical Specifications [optional]",
"### Model Architecture and Objective",
"### Compute Infrastructure",
"#### Hardware",
"#### Software\n\n\n\n[optional]\n\n\n\nBibTeX:\n\n\n\nAPA:",
"## Glossary [optional]",
"## More Information [optional]",
"## Model Card Authors [optional]",
"## Model Card Contact"
] | [
"TAGS\n#transformers #safetensors #llama #text-generation #conversational #arxiv-1910.09700 #autotrain_compatible #endpoints_compatible #text-generation-inference #4-bit #region-us \n",
"# Model Card for Model ID",
"## Model Details",
"### Model Description\n\n\n\nThis is the model card of a transformers model that has been pushed on the Hub. This model card has been automatically generated.\n\n- Developed by: \n- Funded by [optional]: \n- Shared by [optional]: \n- Model type: \n- Language(s) (NLP): \n- License: \n- Finetuned from model [optional]:",
"### Model Sources [optional]\n\n\n\n- Repository: \n- Paper [optional]: \n- Demo [optional]:",
"## Uses",
"### Direct Use",
"### Downstream Use [optional]",
"### Out-of-Scope Use",
"## Bias, Risks, and Limitations",
"### Recommendations\n\n\n\nUsers (both direct and downstream) should be made aware of the risks, biases and limitations of the model. More information needed for further recommendations.",
"## How to Get Started with the Model\n\nUse the code below to get started with the model.",
"## Training Details",
"### Training Data",
"### Training Procedure",
"#### Preprocessing [optional]",
"#### Training Hyperparameters\n\n- Training regime:",
"#### Speeds, Sizes, Times [optional]",
"## Evaluation",
"### Testing Data, Factors & Metrics",
"#### Testing Data",
"#### Factors",
"#### Metrics",
"### Results",
"#### Summary",
"## Model Examination [optional]",
"## Environmental Impact\n\n\n\nCarbon emissions can be estimated using the Machine Learning Impact calculator presented in Lacoste et al. (2019).\n\n- Hardware Type: \n- Hours used: \n- Cloud Provider: \n- Compute Region: \n- Carbon Emitted:",
"## Technical Specifications [optional]",
"### Model Architecture and Objective",
"### Compute Infrastructure",
"#### Hardware",
"#### Software\n\n\n\n[optional]\n\n\n\nBibTeX:\n\n\n\nAPA:",
"## Glossary [optional]",
"## More Information [optional]",
"## Model Card Authors [optional]",
"## Model Card Contact"
] |
text-generation | transformers |
# Model Card for Model ID
<!-- Provide a quick summary of what the model is/does. -->
## Model Details
### Model Description
<!-- Provide a longer summary of what this model is. -->
This is the model card of a 🤗 transformers model that has been pushed on the Hub. This model card has been automatically generated.
- **Developed by:** [More Information Needed]
- **Funded by [optional]:** [More Information Needed]
- **Shared by [optional]:** [More Information Needed]
- **Model type:** [More Information Needed]
- **Language(s) (NLP):** [More Information Needed]
- **License:** [More Information Needed]
- **Finetuned from model [optional]:** [More Information Needed]
### Model Sources [optional]
<!-- Provide the basic links for the model. -->
- **Repository:** [More Information Needed]
- **Paper [optional]:** [More Information Needed]
- **Demo [optional]:** [More Information Needed]
## Uses
<!-- Address questions around how the model is intended to be used, including the foreseeable users of the model and those affected by the model. -->
### Direct Use
<!-- This section is for the model use without fine-tuning or plugging into a larger ecosystem/app. -->
[More Information Needed]
### Downstream Use [optional]
<!-- This section is for the model use when fine-tuned for a task, or when plugged into a larger ecosystem/app -->
[More Information Needed]
### Out-of-Scope Use
<!-- This section addresses misuse, malicious use, and uses that the model will not work well for. -->
[More Information Needed]
## Bias, Risks, and Limitations
<!-- This section is meant to convey both technical and sociotechnical limitations. -->
[More Information Needed]
### Recommendations
<!-- This section is meant to convey recommendations with respect to the bias, risk, and technical limitations. -->
Users (both direct and downstream) should be made aware of the risks, biases and limitations of the model. More information needed for further recommendations.
## How to Get Started with the Model
Use the code below to get started with the model.
[More Information Needed]
## Training Details
### Training Data
<!-- This should link to a Dataset Card, perhaps with a short stub of information on what the training data is all about as well as documentation related to data pre-processing or additional filtering. -->
[More Information Needed]
### Training Procedure
<!-- This relates heavily to the Technical Specifications. Content here should link to that section when it is relevant to the training procedure. -->
#### Preprocessing [optional]
[More Information Needed]
#### Training Hyperparameters
- **Training regime:** [More Information Needed] <!--fp32, fp16 mixed precision, bf16 mixed precision, bf16 non-mixed precision, fp16 non-mixed precision, fp8 mixed precision -->
#### Speeds, Sizes, Times [optional]
<!-- This section provides information about throughput, start/end time, checkpoint size if relevant, etc. -->
[More Information Needed]
## Evaluation
<!-- This section describes the evaluation protocols and provides the results. -->
### Testing Data, Factors & Metrics
#### Testing Data
<!-- This should link to a Dataset Card if possible. -->
[More Information Needed]
#### Factors
<!-- These are the things the evaluation is disaggregating by, e.g., subpopulations or domains. -->
[More Information Needed]
#### Metrics
<!-- These are the evaluation metrics being used, ideally with a description of why. -->
[More Information Needed]
### Results
[More Information Needed]
#### Summary
## Model Examination [optional]
<!-- Relevant interpretability work for the model goes here -->
[More Information Needed]
## Environmental Impact
<!-- Total emissions (in grams of CO2eq) and additional considerations, such as electricity usage, go here. Edit the suggested text below accordingly -->
Carbon emissions can be estimated using the [Machine Learning Impact calculator](https://mlco2.github.io/impact#compute) presented in [Lacoste et al. (2019)](https://arxiv.org/abs/1910.09700).
- **Hardware Type:** [More Information Needed]
- **Hours used:** [More Information Needed]
- **Cloud Provider:** [More Information Needed]
- **Compute Region:** [More Information Needed]
- **Carbon Emitted:** [More Information Needed]
## Technical Specifications [optional]
### Model Architecture and Objective
[More Information Needed]
### Compute Infrastructure
[More Information Needed]
#### Hardware
[More Information Needed]
#### Software
[More Information Needed]
## Citation [optional]
<!-- If there is a paper or blog post introducing the model, the APA and Bibtex information for that should go in this section. -->
**BibTeX:**
[More Information Needed]
**APA:**
[More Information Needed]
## Glossary [optional]
<!-- If relevant, include terms and calculations in this section that can help readers understand the model or model card. -->
[More Information Needed]
## More Information [optional]
[More Information Needed]
## Model Card Authors [optional]
[More Information Needed]
## Model Card Contact
[More Information Needed] | {"library_name": "transformers", "tags": []} | weege007/OrpoLlama-3-8B | null | [
"transformers",
"safetensors",
"llama",
"text-generation",
"conversational",
"arxiv:1910.09700",
"autotrain_compatible",
"endpoints_compatible",
"text-generation-inference",
"region:us"
] | null | 2024-04-21T18:40:52+00:00 | [
"1910.09700"
] | [] | TAGS
#transformers #safetensors #llama #text-generation #conversational #arxiv-1910.09700 #autotrain_compatible #endpoints_compatible #text-generation-inference #region-us
|
# Model Card for Model ID
## Model Details
### Model Description
This is the model card of a transformers model that has been pushed on the Hub. This model card has been automatically generated.
- Developed by:
- Funded by [optional]:
- Shared by [optional]:
- Model type:
- Language(s) (NLP):
- License:
- Finetuned from model [optional]:
### Model Sources [optional]
- Repository:
- Paper [optional]:
- Demo [optional]:
## Uses
### Direct Use
### Downstream Use [optional]
### Out-of-Scope Use
## Bias, Risks, and Limitations
### Recommendations
Users (both direct and downstream) should be made aware of the risks, biases and limitations of the model. More information needed for further recommendations.
## How to Get Started with the Model
Use the code below to get started with the model.
## Training Details
### Training Data
### Training Procedure
#### Preprocessing [optional]
#### Training Hyperparameters
- Training regime:
#### Speeds, Sizes, Times [optional]
## Evaluation
### Testing Data, Factors & Metrics
#### Testing Data
#### Factors
#### Metrics
### Results
#### Summary
## Model Examination [optional]
## Environmental Impact
Carbon emissions can be estimated using the Machine Learning Impact calculator presented in Lacoste et al. (2019).
- Hardware Type:
- Hours used:
- Cloud Provider:
- Compute Region:
- Carbon Emitted:
## Technical Specifications [optional]
### Model Architecture and Objective
### Compute Infrastructure
#### Hardware
#### Software
[optional]
BibTeX:
APA:
## Glossary [optional]
## More Information [optional]
## Model Card Authors [optional]
## Model Card Contact
| [
"# Model Card for Model ID",
"## Model Details",
"### Model Description\n\n\n\nThis is the model card of a transformers model that has been pushed on the Hub. This model card has been automatically generated.\n\n- Developed by: \n- Funded by [optional]: \n- Shared by [optional]: \n- Model type: \n- Language(s) (NLP): \n- License: \n- Finetuned from model [optional]:",
"### Model Sources [optional]\n\n\n\n- Repository: \n- Paper [optional]: \n- Demo [optional]:",
"## Uses",
"### Direct Use",
"### Downstream Use [optional]",
"### Out-of-Scope Use",
"## Bias, Risks, and Limitations",
"### Recommendations\n\n\n\nUsers (both direct and downstream) should be made aware of the risks, biases and limitations of the model. More information needed for further recommendations.",
"## How to Get Started with the Model\n\nUse the code below to get started with the model.",
"## Training Details",
"### Training Data",
"### Training Procedure",
"#### Preprocessing [optional]",
"#### Training Hyperparameters\n\n- Training regime:",
"#### Speeds, Sizes, Times [optional]",
"## Evaluation",
"### Testing Data, Factors & Metrics",
"#### Testing Data",
"#### Factors",
"#### Metrics",
"### Results",
"#### Summary",
"## Model Examination [optional]",
"## Environmental Impact\n\n\n\nCarbon emissions can be estimated using the Machine Learning Impact calculator presented in Lacoste et al. (2019).\n\n- Hardware Type: \n- Hours used: \n- Cloud Provider: \n- Compute Region: \n- Carbon Emitted:",
"## Technical Specifications [optional]",
"### Model Architecture and Objective",
"### Compute Infrastructure",
"#### Hardware",
"#### Software\n\n\n\n[optional]\n\n\n\nBibTeX:\n\n\n\nAPA:",
"## Glossary [optional]",
"## More Information [optional]",
"## Model Card Authors [optional]",
"## Model Card Contact"
] | [
"TAGS\n#transformers #safetensors #llama #text-generation #conversational #arxiv-1910.09700 #autotrain_compatible #endpoints_compatible #text-generation-inference #region-us \n",
"# Model Card for Model ID",
"## Model Details",
"### Model Description\n\n\n\nThis is the model card of a transformers model that has been pushed on the Hub. This model card has been automatically generated.\n\n- Developed by: \n- Funded by [optional]: \n- Shared by [optional]: \n- Model type: \n- Language(s) (NLP): \n- License: \n- Finetuned from model [optional]:",
"### Model Sources [optional]\n\n\n\n- Repository: \n- Paper [optional]: \n- Demo [optional]:",
"## Uses",
"### Direct Use",
"### Downstream Use [optional]",
"### Out-of-Scope Use",
"## Bias, Risks, and Limitations",
"### Recommendations\n\n\n\nUsers (both direct and downstream) should be made aware of the risks, biases and limitations of the model. More information needed for further recommendations.",
"## How to Get Started with the Model\n\nUse the code below to get started with the model.",
"## Training Details",
"### Training Data",
"### Training Procedure",
"#### Preprocessing [optional]",
"#### Training Hyperparameters\n\n- Training regime:",
"#### Speeds, Sizes, Times [optional]",
"## Evaluation",
"### Testing Data, Factors & Metrics",
"#### Testing Data",
"#### Factors",
"#### Metrics",
"### Results",
"#### Summary",
"## Model Examination [optional]",
"## Environmental Impact\n\n\n\nCarbon emissions can be estimated using the Machine Learning Impact calculator presented in Lacoste et al. (2019).\n\n- Hardware Type: \n- Hours used: \n- Cloud Provider: \n- Compute Region: \n- Carbon Emitted:",
"## Technical Specifications [optional]",
"### Model Architecture and Objective",
"### Compute Infrastructure",
"#### Hardware",
"#### Software\n\n\n\n[optional]\n\n\n\nBibTeX:\n\n\n\nAPA:",
"## Glossary [optional]",
"## More Information [optional]",
"## Model Card Authors [optional]",
"## Model Card Contact"
] |
text-generation | transformers | Quantization made by Richard Erkhov.
[Github](https://github.com/RichardErkhov)
[Discord](https://discord.gg/pvy7H8DZMG)
[Request more models](https://github.com/RichardErkhov/quant_request)
CodeLlama-34b-hf - bnb 8bits
- Model creator: https://huggingface.co/meta-llama/
- Original model: https://huggingface.co/meta-llama/CodeLlama-34b-hf/
Original model description:
---
extra_gated_heading: You need to share contact information with Meta to access this model
extra_gated_prompt: >-
### LLAMA 2 COMMUNITY LICENSE AGREEMENT
"Agreement" means the terms and conditions for use, reproduction, distribution
and modification of the Llama Materials set forth herein.
"Documentation" means the specifications, manuals and documentation
accompanying Llama 2 distributed by Meta at
https://ai.meta.com/resources/models-and-libraries/llama-downloads/.
"Licensee" or "you" means you, or your employer or any other person or entity
(if you are entering into this Agreement on such person or entity's behalf),
of the age required under applicable laws, rules or regulations to provide
legal consent and that has legal authority to bind your employer or such other
person or entity if you are entering in this Agreement on their behalf.
"Llama 2" means the foundational large language models and software and
algorithms, including machine-learning model code, trained model weights,
inference-enabling code, training-enabling code, fine-tuning enabling code and
other elements of the foregoing distributed by Meta at
ai.meta.com/resources/models-and-libraries/llama-downloads/.
"Llama Materials" means, collectively, Meta's proprietary Llama 2 and
documentation (and any portion thereof) made available under this Agreement.
"Meta" or "we" means Meta Platforms Ireland Limited (if you are located in or,
if you are an entity, your principal place of business is in the EEA or
Switzerland) and Meta Platforms, Inc. (if you are located outside of the EEA
or Switzerland).
By clicking "I Accept" below or by using or distributing any portion or
element of the Llama Materials, you agree to be bound by this Agreement.
1. License Rights and Redistribution.
a. Grant of Rights. You are granted a non-exclusive, worldwide, non-
transferable and royalty-free limited license under Meta's intellectual
property or other rights owned by Meta embodied in the Llama Materials to
use, reproduce, distribute, copy, create derivative works of, and make
modifications to the Llama Materials.
b. Redistribution and Use.
i. If you distribute or make the Llama Materials, or any derivative works
thereof, available to a third party, you shall provide a copy of this
Agreement to such third party.
ii. If you receive Llama Materials, or any derivative works thereof, from a
Licensee as part of an integrated end user product, then Section 2 of this
Agreement will not apply to you.
iii. You must retain in all copies of the Llama Materials that you distribute
the following attribution notice within a "Notice" text file distributed as a
part of such copies: "Llama 2 is licensed under the LLAMA 2 Community
License, Copyright (c) Meta Platforms, Inc. All Rights Reserved."
iv. Your use of the Llama Materials must comply with applicable laws and
regulations (including trade compliance laws and regulations) and adhere to
the Acceptable Use Policy for the Llama Materials (available at
https://ai.meta.com/llama/use-policy), which is hereby incorporated by
reference into this Agreement.
v. You will not use the Llama Materials or any output or results of the Llama
Materials to improve any other large language model (excluding Llama 2 or
derivative works thereof).
2. Additional Commercial Terms. If, on the Llama 2 version release date, the
monthly active users of the products or services made available by or for
Licensee, or Licensee's affiliates, is greater than 700 million monthly
active users in the preceding calendar month, you must request a license from
Meta, which Meta may grant to you in its sole discretion, and you are not
authorized to exercise any of the rights under this Agreement unless or until
Meta otherwise expressly grants you such rights.
3. Disclaimer of Warranty. UNLESS REQUIRED BY APPLICABLE LAW, THE LLAMA
MATERIALS AND ANY OUTPUT AND RESULTS THEREFROM ARE PROVIDED ON AN "AS IS"
BASIS, WITHOUT WARRANTIES OF ANY KIND, EITHER EXPRESS OR IMPLIED, INCLUDING,
WITHOUT LIMITATION, ANY WARRANTIES OF TITLE, NON-INFRINGEMENT,
MERCHANTABILITY, OR FITNESS FOR A PARTICULAR PURPOSE. YOU ARE SOLELY
RESPONSIBLE FOR DETERMINING THE APPROPRIATENESS OF USING OR REDISTRIBUTING
THE LLAMA MATERIALS AND ASSUME ANY RISKS ASSOCIATED WITH YOUR USE OF THE
LLAMA MATERIALS AND ANY OUTPUT AND RESULTS.
4. Limitation of Liability. IN NO EVENT WILL META OR ITS AFFILIATES BE LIABLE
UNDER ANY THEORY OF LIABILITY, WHETHER IN CONTRACT, TORT, NEGLIGENCE,
PRODUCTS LIABILITY, OR OTHERWISE, ARISING OUT OF THIS AGREEMENT, FOR ANY LOST
PROFITS OR ANY INDIRECT, SPECIAL, CONSEQUENTIAL, INCIDENTAL, EXEMPLARY OR
PUNITIVE DAMAGES, EVEN IF META OR ITS AFFILIATES HAVE BEEN ADVISED OF THE
POSSIBILITY OF ANY OF THE FOREGOING.
5. Intellectual Property.
a. No trademark licenses are granted under this Agreement, and in connection
with the Llama Materials, neither Meta nor Licensee may use any name or mark
owned by or associated with the other or any of its affiliates, except as
required for reasonable and customary use in describing and redistributing
the Llama Materials.
b. Subject to Meta's ownership of Llama Materials and derivatives made by or
for Meta, with respect to any derivative works and modifications of the Llama
Materials that are made by you, as between you and Meta, you are and will be
the owner of such derivative works and modifications.
c. If you institute litigation or other proceedings against Meta or any
entity (including a cross-claim or counterclaim in a lawsuit) alleging that
the Llama Materials or Llama 2 outputs or results, or any portion of any of
the foregoing, constitutes infringement of intellectual property or other
rights owned or licensable by you, then any licenses granted to you under
this Agreement shall terminate as of the date such litigation or claim is
filed or instituted. You will indemnify and hold harmless Meta from and
against any claim by any third party arising out of or related to your use or
distribution of the Llama Materials.
6. Term and Termination. The term of this Agreement will commence upon your
acceptance of this Agreement or access to the Llama Materials and will
continue in full force and effect until terminated in accordance with the
terms and conditions herein. Meta may terminate this Agreement if you are in
breach of any term or condition of this Agreement. Upon termination of this
Agreement, you shall delete and cease use of the Llama Materials. Sections 3,
4 and 7 shall survive the termination of this Agreement.
7. Governing Law and Jurisdiction. This Agreement will be governed and
construed under the laws of the State of California without regard to choice
of law principles, and the UN Convention on Contracts for the International
Sale of Goods does not apply to this Agreement. The courts of California
shall have exclusive jurisdiction of any dispute arising out of this
Agreement.
USE POLICY
### Llama 2 Acceptable Use Policy
Meta is committed to promoting safe and fair use of its tools and features,
including Llama 2. If you access or use Llama 2, you agree to this Acceptable
Use Policy (“Policy”). The most recent copy of this policy can be found at
[ai.meta.com/llama/use-policy](http://ai.meta.com/llama/use-policy).
#### Prohibited Uses
We want everyone to use Llama 2 safely and responsibly. You agree you will not
use, or allow others to use, Llama 2 to:
1. Violate the law or others’ rights, including to:
1. Engage in, promote, generate, contribute to, encourage, plan, incite, or further illegal or unlawful activity or content, such as:
1. Violence or terrorism
2. Exploitation or harm to children, including the solicitation, creation, acquisition, or dissemination of child exploitative content or failure to report Child Sexual Abuse Material
3. Human trafficking, exploitation, and sexual violence
4. The illegal distribution of information or materials to minors, including obscene materials, or failure to employ legally required age-gating in connection with such information or materials.
5. Sexual solicitation
6. Any other criminal activity
2. Engage in, promote, incite, or facilitate the harassment, abuse, threatening, or bullying of individuals or groups of individuals
3. Engage in, promote, incite, or facilitate discrimination or other unlawful or harmful conduct in the provision of employment, employment benefits, credit, housing, other economic benefits, or other essential goods and services
4. Engage in the unauthorized or unlicensed practice of any profession including, but not limited to, financial, legal, medical/health, or related professional practices
5. Collect, process, disclose, generate, or infer health, demographic, or other sensitive personal or private information about individuals without rights and consents required by applicable laws
6. Engage in or facilitate any action or generate any content that infringes, misappropriates, or otherwise violates any third-party rights, including the outputs or results of any products or services using the Llama 2 Materials
7. Create, generate, or facilitate the creation of malicious code, malware, computer viruses or do anything else that could disable, overburden, interfere with or impair the proper working, integrity, operation or appearance of a website or computer system
2. Engage in, promote, incite, facilitate, or assist in the planning or
development of activities that present a risk of death or bodily harm to
individuals, including use of Llama 2 related to the following:
1. Military, warfare, nuclear industries or applications, espionage, use for materials or activities that are subject to the International Traffic Arms Regulations (ITAR) maintained by the United States Department of State
2. Guns and illegal weapons (including weapon development)
3. Illegal drugs and regulated/controlled substances
4. Operation of critical infrastructure, transportation technologies, or heavy machinery
5. Self-harm or harm to others, including suicide, cutting, and eating disorders
6. Any content intended to incite or promote violence, abuse, or any infliction of bodily harm to an individual
3. Intentionally deceive or mislead others, including use of Llama 2 related
to the following:
1. Generating, promoting, or furthering fraud or the creation or promotion of disinformation
2. Generating, promoting, or furthering defamatory content, including the creation of defamatory statements, images, or other content
3. Generating, promoting, or further distributing spam
4. Impersonating another individual without consent, authorization, or legal right
5. Representing that the use of Llama 2 or outputs are human-generated
6. Generating or facilitating false online engagement, including fake reviews and other means of fake online engagement
4. Fail to appropriately disclose to end users any known dangers of your AI system
Please report any violation of this Policy, software “bug,” or other problems
that could lead to a violation of this Policy through one of the following
means:
* Reporting issues with the model:
[github.com/facebookresearch/llama](http://github.com/facebookresearch/llama)
* Reporting risky content generated by the model:
[developers.facebook.com/llama_output_feedback](http://developers.facebook.com/llama_output_feedback)
* Reporting bugs and security concerns:
[facebook.com/whitehat/info](http://facebook.com/whitehat/info)
* Reporting violations of the Acceptable Use Policy or unlicensed uses of
Llama: [[email protected]](mailto:[email protected])
extra_gated_fields:
First Name: text
Last Name: text
Date of birth: date_picker
Country: country
Affiliation: text
geo: ip_location
By clicking Submit below I accept the terms of the license and acknowledge that the information I provide will be collected stored processed and shared in accordance with the Meta Privacy Policy: checkbox
extra_gated_description: The information you provide will be collected, stored, processed and shared in accordance with the [Meta Privacy Policy](https://www.facebook.com/privacy/policy/).
extra_gated_button_content: Submit
language:
- code
pipeline_tag: text-generation
tags:
- facebook
- meta
- pytorch
- llama
- llama-2
license: llama2
---
# **Code Llama**
Code Llama is a collection of pretrained and fine-tuned generative text models ranging in scale from 7 billion to 34 billion parameters. This is the repository for the base 34B version in the Hugging Face Transformers format. This model is designed for general code synthesis and understanding. Links to other models can be found in the index at the bottom.
| | Base Model | Python | Instruct |
| --- | ----------------------------------------------------------------------------- | ------------------------------------------------------------------------------------------- | ----------------------------------------------------------------------------------------------- |
| 7B | [meta-llama/CodeLlama-7b-hf](https://huggingface.co/meta-llama/CodeLlama-7b-hf) | [meta-llama/CodeLlama-7b-Python-hf](https://huggingface.co/meta-llama/CodeLlama-7b-Python-hf) | [meta-llama/CodeLlama-7b-Instruct-hf](https://huggingface.co/meta-llama/CodeLlama-7b-Instruct-hf) |
| 13B | [meta-llama/CodeLlama-13b-hf](https://huggingface.co/meta-llama/CodeLlama-13b-hf) | [meta-llama/CodeLlama-13b-Python-hf](https://huggingface.co/meta-llama/CodeLlama-13b-Python-hf) | [meta-llama/CodeLlama-13b-Instruct-hf](https://huggingface.co/meta-llama/CodeLlama-13b-Instruct-hf) |
| 34B | [meta-llama/CodeLlama-34b-hf](https://huggingface.co/meta-llama/CodeLlama-34b-hf) | [meta-llama/CodeLlama-34b-Python-hf](https://huggingface.co/meta-llama/CodeLlama-34b-Python-hf) | [meta-llama/CodeLlama-34b-Instruct-hf](https://huggingface.co/meta-llama/CodeLlama-34b-Instruct-hf) |
| 70B | [meta-llama/CodeLlama-70b-hf](https://huggingface.co/meta-llama/CodeLlama-70b-hf) | [meta-llama/CodeLlama-70b-Python-hf](https://huggingface.co/meta-llama/CodeLlama-70b-Python-hf) | [meta-llama/CodeLlama-70b-Instruct-hf](https://huggingface.co/meta-llama/CodeLlama-70b-Instruct-hf) |
## Model Use
To use this model, please make sure to install transformers:
```bash
pip install transformers.git accelerate
```
Model capabilities:
- [x] Code completion.
- [ ] Infilling.
- [ ] Instructions / chat.
- [ ] Python specialist.
```python
from transformers import AutoTokenizer
import transformers
import torch
model = "meta-llama/CodeLlama-34b-hf"
tokenizer = AutoTokenizer.from_pretrained(model)
pipeline = transformers.pipeline(
"text-generation",
model=model,
torch_dtype=torch.float16,
device_map="auto",
)
sequences = pipeline(
'import socket\n\ndef ping_exponential_backoff(host: str):',
do_sample=True,
top_k=10,
temperature=0.1,
top_p=0.95,
num_return_sequences=1,
eos_token_id=tokenizer.eos_token_id,
max_length=200,
)
for seq in sequences:
print(f"Result: {seq['generated_text']}")
```
## Model Details
*Note: Use of this model is governed by the Meta license. Meta developed and publicly released the Code Llama family of large language models (LLMs).
**Model Developers** Meta
**Variations** Code Llama comes in three model sizes, and three variants:
* Code Llama: base models designed for general code synthesis and understanding
* Code Llama - Python: designed specifically for Python
* Code Llama - Instruct: for instruction following and safer deployment
All variants are available in sizes of 7B, 13B and 34B parameters.
**This repository contains the base version of the 34B parameters model.**
**Input** Models input text only.
**Output** Models generate text only.
**Model Architecture** Code Llama is an auto-regressive language model that uses an optimized transformer architecture.
**Model Dates** Code Llama and its variants have been trained between January 2023 and July 2023.
**Status** This is a static model trained on an offline dataset. Future versions of Code Llama - Instruct will be released as we improve model safety with community feedback.
**License** A custom commercial license is available at: [https://ai.meta.com/resources/models-and-libraries/llama-downloads/](https://ai.meta.com/resources/models-and-libraries/llama-downloads/)
**Research Paper** More information can be found in the paper "[Code Llama: Open Foundation Models for Code](https://ai.meta.com/research/publications/code-llama-open-foundation-models-for-code/)" or its [arXiv page](https://arxiv.org/abs/2308.12950).
## Intended Use
**Intended Use Cases** Code Llama and its variants is intended for commercial and research use in English and relevant programming languages. The base model Code Llama can be adapted for a variety of code synthesis and understanding tasks, Code Llama - Python is designed specifically to handle the Python programming language, and Code Llama - Instruct is intended to be safer to use for code assistant and generation applications.
**Out-of-Scope Uses** Use in any manner that violates applicable laws or regulations (including trade compliance laws). Use in languages other than English. Use in any other way that is prohibited by the Acceptable Use Policy and Licensing Agreement for Code Llama and its variants.
## Hardware and Software
**Training Factors** We used custom training libraries. The training and fine-tuning of the released models have been performed Meta’s Research Super Cluster.
**Carbon Footprint** In aggregate, training all 9 Code Llama models required 400K GPU hours of computation on hardware of type A100-80GB (TDP of 350-400W). Estimated total emissions were 65.3 tCO2eq, 100% of which were offset by Meta’s sustainability program.
## Training Data
All experiments reported here and the released models have been trained and fine-tuned using the same data as Llama 2 with different weights (see Section 2 and Table 1 in the [research paper](https://ai.meta.com/research/publications/code-llama-open-foundation-models-for-code/) for details).
## Evaluation Results
See evaluations for the main models and detailed ablations in Section 3 and safety evaluations in Section 4 of the research paper.
## Ethical Considerations and Limitations
Code Llama and its variants are a new technology that carries risks with use. Testing conducted to date has been in English, and has not covered, nor could it cover all scenarios. For these reasons, as with all LLMs, Code Llama’s potential outputs cannot be predicted in advance, and the model may in some instances produce inaccurate or objectionable responses to user prompts. Therefore, before deploying any applications of Code Llama, developers should perform safety testing and tuning tailored to their specific applications of the model.
Please see the Responsible Use Guide available available at [https://ai.meta.com/llama/responsible-use-guide](https://ai.meta.com/llama/responsible-use-guide).
| {} | RichardErkhov/meta-llama_-_CodeLlama-34b-hf-8bits | null | [
"transformers",
"safetensors",
"llama",
"text-generation",
"arxiv:2308.12950",
"autotrain_compatible",
"endpoints_compatible",
"text-generation-inference",
"8-bit",
"region:us"
] | null | 2024-04-21T18:41:57+00:00 | [
"2308.12950"
] | [] | TAGS
#transformers #safetensors #llama #text-generation #arxiv-2308.12950 #autotrain_compatible #endpoints_compatible #text-generation-inference #8-bit #region-us
| Quantization made by Richard Erkhov.
Github
Discord
Request more models
CodeLlama-34b-hf - bnb 8bits
* Model creator: URL
* Original model: URL
Original model description:
---------------------------
extra\_gated\_heading: You need to share contact information with Meta to access this model
extra\_gated\_prompt: >-
### LLAMA 2 COMMUNITY LICENSE AGREEMENT
"Agreement" means the terms and conditions for use, reproduction, distribution
and modification of the Llama Materials set forth herein.
"Documentation" means the specifications, manuals and documentation
accompanying Llama 2 distributed by Meta at
URL
"Licensee" or "you" means you, or your employer or any other person or entity
(if you are entering into this Agreement on such person or entity's behalf),
of the age required under applicable laws, rules or regulations to provide
legal consent and that has legal authority to bind your employer or such other
person or entity if you are entering in this Agreement on their behalf.
"Llama 2" means the foundational large language models and software and
algorithms, including machine-learning model code, trained model weights,
inference-enabling code, training-enabling code, fine-tuning enabling code and
other elements of the foregoing distributed by Meta at
URL
"Llama Materials" means, collectively, Meta's proprietary Llama 2 and
documentation (and any portion thereof) made available under this Agreement.
"Meta" or "we" means Meta Platforms Ireland Limited (if you are located in or,
if you are an entity, your principal place of business is in the EEA or
Switzerland) and Meta Platforms, Inc. (if you are located outside of the EEA
or Switzerland).
By clicking "I Accept" below or by using or distributing any portion or
element of the Llama Materials, you agree to be bound by this Agreement.
1. License Rights and Redistribution.
a. Grant of Rights. You are granted a non-exclusive, worldwide, non-
transferable and royalty-free limited license under Meta's intellectual
property or other rights owned by Meta embodied in the Llama Materials to
use, reproduce, distribute, copy, create derivative works of, and make
modifications to the Llama Materials.
b. Redistribution and Use.
i. If you distribute or make the Llama Materials, or any derivative works
thereof, available to a third party, you shall provide a copy of this
Agreement to such third party.
ii. If you receive Llama Materials, or any derivative works thereof, from a
Licensee as part of an integrated end user product, then Section 2 of this
Agreement will not apply to you.
iii. You must retain in all copies of the Llama Materials that you distribute
the following attribution notice within a "Notice" text file distributed as a
part of such copies: "Llama 2 is licensed under the LLAMA 2 Community
License, Copyright (c) Meta Platforms, Inc. All Rights Reserved."
iv. Your use of the Llama Materials must comply with applicable laws and
regulations (including trade compliance laws and regulations) and adhere to
the Acceptable Use Policy for the Llama Materials (available at
URL which is hereby incorporated by
reference into this Agreement.
v. You will not use the Llama Materials or any output or results of the Llama
Materials to improve any other large language model (excluding Llama 2 or
derivative works thereof).
2. Additional Commercial Terms. If, on the Llama 2 version release date, the
monthly active users of the products or services made available by or for
Licensee, or Licensee's affiliates, is greater than 700 million monthly
active users in the preceding calendar month, you must request a license from
Meta, which Meta may grant to you in its sole discretion, and you are not
authorized to exercise any of the rights under this Agreement unless or until
Meta otherwise expressly grants you such rights.
3. Disclaimer of Warranty. UNLESS REQUIRED BY APPLICABLE LAW, THE LLAMA
MATERIALS AND ANY OUTPUT AND RESULTS THEREFROM ARE PROVIDED ON AN "AS IS"
BASIS, WITHOUT WARRANTIES OF ANY KIND, EITHER EXPRESS OR IMPLIED, INCLUDING,
WITHOUT LIMITATION, ANY WARRANTIES OF TITLE, NON-INFRINGEMENT,
MERCHANTABILITY, OR FITNESS FOR A PARTICULAR PURPOSE. YOU ARE SOLELY
RESPONSIBLE FOR DETERMINING THE APPROPRIATENESS OF USING OR REDISTRIBUTING
THE LLAMA MATERIALS AND ASSUME ANY RISKS ASSOCIATED WITH YOUR USE OF THE
LLAMA MATERIALS AND ANY OUTPUT AND RESULTS.
4. Limitation of Liability. IN NO EVENT WILL META OR ITS AFFILIATES BE LIABLE
UNDER ANY THEORY OF LIABILITY, WHETHER IN CONTRACT, TORT, NEGLIGENCE,
PRODUCTS LIABILITY, OR OTHERWISE, ARISING OUT OF THIS AGREEMENT, FOR ANY LOST
PROFITS OR ANY INDIRECT, SPECIAL, CONSEQUENTIAL, INCIDENTAL, EXEMPLARY OR
PUNITIVE DAMAGES, EVEN IF META OR ITS AFFILIATES HAVE BEEN ADVISED OF THE
POSSIBILITY OF ANY OF THE FOREGOING.
5. Intellectual Property.
a. No trademark licenses are granted under this Agreement, and in connection
with the Llama Materials, neither Meta nor Licensee may use any name or mark
owned by or associated with the other or any of its affiliates, except as
required for reasonable and customary use in describing and redistributing
the Llama Materials.
b. Subject to Meta's ownership of Llama Materials and derivatives made by or
for Meta, with respect to any derivative works and modifications of the Llama
Materials that are made by you, as between you and Meta, you are and will be
the owner of such derivative works and modifications.
c. If you institute litigation or other proceedings against Meta or any
entity (including a cross-claim or counterclaim in a lawsuit) alleging that
the Llama Materials or Llama 2 outputs or results, or any portion of any of
the foregoing, constitutes infringement of intellectual property or other
rights owned or licensable by you, then any licenses granted to you under
this Agreement shall terminate as of the date such litigation or claim is
filed or instituted. You will indemnify and hold harmless Meta from and
against any claim by any third party arising out of or related to your use or
distribution of the Llama Materials.
6. Term and Termination. The term of this Agreement will commence upon your
acceptance of this Agreement or access to the Llama Materials and will
continue in full force and effect until terminated in accordance with the
terms and conditions herein. Meta may terminate this Agreement if you are in
breach of any term or condition of this Agreement. Upon termination of this
Agreement, you shall delete and cease use of the Llama Materials. Sections 3,
4 and 7 shall survive the termination of this Agreement.
7. Governing Law and Jurisdiction. This Agreement will be governed and
construed under the laws of the State of California without regard to choice
of law principles, and the UN Convention on Contracts for the International
Sale of Goods does not apply to this Agreement. The courts of California
shall have exclusive jurisdiction of any dispute arising out of this
Agreement.
USE POLICY
### Llama 2 Acceptable Use Policy
Meta is committed to promoting safe and fair use of its tools and features,
including Llama 2. If you access or use Llama 2, you agree to this Acceptable
Use Policy (“Policy”). The most recent copy of this policy can be found at
URL
#### Prohibited Uses
We want everyone to use Llama 2 safely and responsibly. You agree you will not
use, or allow others to use, Llama 2 to:
1. Violate the law or others’ rights, including to:
1. Engage in, promote, generate, contribute to, encourage, plan, incite, or further illegal or unlawful activity or content, such as:
1. Violence or terrorism
2. Exploitation or harm to children, including the solicitation, creation, acquisition, or dissemination of child exploitative content or failure to report Child Sexual Abuse Material
3. Human trafficking, exploitation, and sexual violence
4. The illegal distribution of information or materials to minors, including obscene materials, or failure to employ legally required age-gating in connection with such information or materials.
5. Sexual solicitation
6. Any other criminal activity
```
2. Engage in, promote, incite, or facilitate the harassment, abuse, threatening, or bullying of individuals or groups of individuals
3. Engage in, promote, incite, or facilitate discrimination or other unlawful or harmful conduct in the provision of employment, employment benefits, credit, housing, other economic benefits, or other essential goods and services
4. Engage in the unauthorized or unlicensed practice of any profession including, but not limited to, financial, legal, medical/health, or related professional practices
5. Collect, process, disclose, generate, or infer health, demographic, or other sensitive personal or private information about individuals without rights and consents required by applicable laws
6. Engage in or facilitate any action or generate any content that infringes, misappropriates, or otherwise violates any third-party rights, including the outputs or results of any products or services using the Llama 2 Materials
7. Create, generate, or facilitate the creation of malicious code, malware, computer viruses or do anything else that could disable, overburden, interfere with or impair the proper working, integrity, operation or appearance of a website or computer system
```
2. Engage in, promote, incite, facilitate, or assist in the planning or
development of activities that present a risk of death or bodily harm to
individuals, including use of Llama 2 related to the following:
1. Military, warfare, nuclear industries or applications, espionage, use for materials or activities that are subject to the International Traffic Arms Regulations (ITAR) maintained by the United States Department of State
2. Guns and illegal weapons (including weapon development)
3. Illegal drugs and regulated/controlled substances
4. Operation of critical infrastructure, transportation technologies, or heavy machinery
5. Self-harm or harm to others, including suicide, cutting, and eating disorders
6. Any content intended to incite or promote violence, abuse, or any infliction of bodily harm to an individual
3. Intentionally deceive or mislead others, including use of Llama 2 related
to the following:
1. Generating, promoting, or furthering fraud or the creation or promotion of disinformation
2. Generating, promoting, or furthering defamatory content, including the creation of defamatory statements, images, or other content
3. Generating, promoting, or further distributing spam
4. Impersonating another individual without consent, authorization, or legal right
5. Representing that the use of Llama 2 or outputs are human-generated
6. Generating or facilitating false online engagement, including fake reviews and other means of fake online engagement
4. Fail to appropriately disclose to end users any known dangers of your AI system
Please report any violation of this Policy, software “bug,” or other problems
that could lead to a violation of this Policy through one of the following
means:
* Reporting issues with the model:
URL
* Reporting risky content generated by the model:
URL
* Reporting bugs and security concerns:
URL
* Reporting violations of the Acceptable Use Policy or unlicensed uses of
Llama: LlamaUseReport@URL
extra\_gated\_fields:
First Name: text
Last Name: text
Date of birth: date\_picker
Country: country
Affiliation: text
geo: ip\_location
By clicking Submit below I accept the terms of the license and acknowledge that the information I provide will be collected stored processed and shared in accordance with the Meta Privacy Policy: checkbox
extra\_gated\_description: The information you provide will be collected, stored, processed and shared in accordance with the Meta Privacy Policy.
extra\_gated\_button\_content: Submit
language:
* code
pipeline\_tag: text-generation
tags:
* facebook
* meta
* pytorch
* llama
* llama-2
license: llama2
---
Code Llama
==========
Code Llama is a collection of pretrained and fine-tuned generative text models ranging in scale from 7 billion to 34 billion parameters. This is the repository for the base 34B version in the Hugging Face Transformers format. This model is designed for general code synthesis and understanding. Links to other models can be found in the index at the bottom.
Model Use
---------
To use this model, please make sure to install transformers:
Model capabilities:
* [x] Code completion.
* [ ] Infilling.
* [ ] Instructions / chat.
* [ ] Python specialist.
Model Details
-------------
\*Note: Use of this model is governed by the Meta license. Meta developed and publicly released the Code Llama family of large language models (LLMs).
Model Developers Meta
Variations Code Llama comes in three model sizes, and three variants:
* Code Llama: base models designed for general code synthesis and understanding
* Code Llama - Python: designed specifically for Python
* Code Llama - Instruct: for instruction following and safer deployment
All variants are available in sizes of 7B, 13B and 34B parameters.
This repository contains the base version of the 34B parameters model.
Input Models input text only.
Output Models generate text only.
Model Architecture Code Llama is an auto-regressive language model that uses an optimized transformer architecture.
Model Dates Code Llama and its variants have been trained between January 2023 and July 2023.
Status This is a static model trained on an offline dataset. Future versions of Code Llama - Instruct will be released as we improve model safety with community feedback.
License A custom commercial license is available at: URL
Research Paper More information can be found in the paper "Code Llama: Open Foundation Models for Code" or its arXiv page.
Intended Use
------------
Intended Use Cases Code Llama and its variants is intended for commercial and research use in English and relevant programming languages. The base model Code Llama can be adapted for a variety of code synthesis and understanding tasks, Code Llama - Python is designed specifically to handle the Python programming language, and Code Llama - Instruct is intended to be safer to use for code assistant and generation applications.
Out-of-Scope Uses Use in any manner that violates applicable laws or regulations (including trade compliance laws). Use in languages other than English. Use in any other way that is prohibited by the Acceptable Use Policy and Licensing Agreement for Code Llama and its variants.
Hardware and Software
---------------------
Training Factors We used custom training libraries. The training and fine-tuning of the released models have been performed Meta’s Research Super Cluster.
Carbon Footprint In aggregate, training all 9 Code Llama models required 400K GPU hours of computation on hardware of type A100-80GB (TDP of 350-400W). Estimated total emissions were 65.3 tCO2eq, 100% of which were offset by Meta’s sustainability program.
Training Data
-------------
All experiments reported here and the released models have been trained and fine-tuned using the same data as Llama 2 with different weights (see Section 2 and Table 1 in the research paper for details).
Evaluation Results
------------------
See evaluations for the main models and detailed ablations in Section 3 and safety evaluations in Section 4 of the research paper.
Ethical Considerations and Limitations
--------------------------------------
Code Llama and its variants are a new technology that carries risks with use. Testing conducted to date has been in English, and has not covered, nor could it cover all scenarios. For these reasons, as with all LLMs, Code Llama’s potential outputs cannot be predicted in advance, and the model may in some instances produce inaccurate or objectionable responses to user prompts. Therefore, before deploying any applications of Code Llama, developers should perform safety testing and tuning tailored to their specific applications of the model.
Please see the Responsible Use Guide available available at URL
| [
"### LLAMA 2 COMMUNITY LICENSE AGREEMENT\n\n\n\"Agreement\" means the terms and conditions for use, reproduction, distribution\nand modification of the Llama Materials set forth herein.\n\"Documentation\" means the specifications, manuals and documentation\naccompanying Llama 2 distributed by Meta at\nURL \n\n\"Licensee\" or \"you\" means you, or your employer or any other person or entity\n(if you are entering into this Agreement on such person or entity's behalf),\nof the age required under applicable laws, rules or regulations to provide\nlegal consent and that has legal authority to bind your employer or such other\nperson or entity if you are entering in this Agreement on their behalf.\n\"Llama 2\" means the foundational large language models and software and\nalgorithms, including machine-learning model code, trained model weights,\ninference-enabling code, training-enabling code, fine-tuning enabling code and\nother elements of the foregoing distributed by Meta at\nURL\n\"Llama Materials\" means, collectively, Meta's proprietary Llama 2 and\ndocumentation (and any portion thereof) made available under this Agreement.\n\"Meta\" or \"we\" means Meta Platforms Ireland Limited (if you are located in or,\nif you are an entity, your principal place of business is in the EEA or\nSwitzerland) and Meta Platforms, Inc. (if you are located outside of the EEA\nor Switzerland).\nBy clicking \"I Accept\" below or by using or distributing any portion or\nelement of the Llama Materials, you agree to be bound by this Agreement.\n\n\n1. License Rights and Redistribution.\na. Grant of Rights. You are granted a non-exclusive, worldwide, non-\ntransferable and royalty-free limited license under Meta's intellectual\nproperty or other rights owned by Meta embodied in the Llama Materials to\nuse, reproduce, distribute, copy, create derivative works of, and make\nmodifications to the Llama Materials.\n\n\nb. Redistribution and Use. \n\ni. If you distribute or make the Llama Materials, or any derivative works\nthereof, available to a third party, you shall provide a copy of this\nAgreement to such third party.\nii. If you receive Llama Materials, or any derivative works thereof, from a\nLicensee as part of an integrated end user product, then Section 2 of this\nAgreement will not apply to you.\niii. You must retain in all copies of the Llama Materials that you distribute\nthe following attribution notice within a \"Notice\" text file distributed as a\npart of such copies: \"Llama 2 is licensed under the LLAMA 2 Community\nLicense, Copyright (c) Meta Platforms, Inc. All Rights Reserved.\"\niv. Your use of the Llama Materials must comply with applicable laws and\nregulations (including trade compliance laws and regulations) and adhere to\nthe Acceptable Use Policy for the Llama Materials (available at\nURL which is hereby incorporated by\nreference into this Agreement.\nv. You will not use the Llama Materials or any output or results of the Llama\nMaterials to improve any other large language model (excluding Llama 2 or\nderivative works thereof). \n\n2. Additional Commercial Terms. If, on the Llama 2 version release date, the\nmonthly active users of the products or services made available by or for\nLicensee, or Licensee's affiliates, is greater than 700 million monthly\nactive users in the preceding calendar month, you must request a license from\nMeta, which Meta may grant to you in its sole discretion, and you are not\nauthorized to exercise any of the rights under this Agreement unless or until\nMeta otherwise expressly grants you such rights.\n3. Disclaimer of Warranty. UNLESS REQUIRED BY APPLICABLE LAW, THE LLAMA\nMATERIALS AND ANY OUTPUT AND RESULTS THEREFROM ARE PROVIDED ON AN \"AS IS\"\nBASIS, WITHOUT WARRANTIES OF ANY KIND, EITHER EXPRESS OR IMPLIED, INCLUDING,\nWITHOUT LIMITATION, ANY WARRANTIES OF TITLE, NON-INFRINGEMENT,\nMERCHANTABILITY, OR FITNESS FOR A PARTICULAR PURPOSE. YOU ARE SOLELY\nRESPONSIBLE FOR DETERMINING THE APPROPRIATENESS OF USING OR REDISTRIBUTING\nTHE LLAMA MATERIALS AND ASSUME ANY RISKS ASSOCIATED WITH YOUR USE OF THE\nLLAMA MATERIALS AND ANY OUTPUT AND RESULTS.\n4. Limitation of Liability. IN NO EVENT WILL META OR ITS AFFILIATES BE LIABLE\nUNDER ANY THEORY OF LIABILITY, WHETHER IN CONTRACT, TORT, NEGLIGENCE,\nPRODUCTS LIABILITY, OR OTHERWISE, ARISING OUT OF THIS AGREEMENT, FOR ANY LOST\nPROFITS OR ANY INDIRECT, SPECIAL, CONSEQUENTIAL, INCIDENTAL, EXEMPLARY OR\nPUNITIVE DAMAGES, EVEN IF META OR ITS AFFILIATES HAVE BEEN ADVISED OF THE\nPOSSIBILITY OF ANY OF THE FOREGOING.\n5. Intellectual Property.\na. No trademark licenses are granted under this Agreement, and in connection\nwith the Llama Materials, neither Meta nor Licensee may use any name or mark\nowned by or associated with the other or any of its affiliates, except as\nrequired for reasonable and customary use in describing and redistributing\nthe Llama Materials.\nb. Subject to Meta's ownership of Llama Materials and derivatives made by or\nfor Meta, with respect to any derivative works and modifications of the Llama\nMaterials that are made by you, as between you and Meta, you are and will be\nthe owner of such derivative works and modifications.\nc. If you institute litigation or other proceedings against Meta or any\nentity (including a cross-claim or counterclaim in a lawsuit) alleging that\nthe Llama Materials or Llama 2 outputs or results, or any portion of any of\nthe foregoing, constitutes infringement of intellectual property or other\nrights owned or licensable by you, then any licenses granted to you under\nthis Agreement shall terminate as of the date such litigation or claim is\nfiled or instituted. You will indemnify and hold harmless Meta from and\nagainst any claim by any third party arising out of or related to your use or\ndistribution of the Llama Materials.\n6. Term and Termination. The term of this Agreement will commence upon your\nacceptance of this Agreement or access to the Llama Materials and will\ncontinue in full force and effect until terminated in accordance with the\nterms and conditions herein. Meta may terminate this Agreement if you are in\nbreach of any term or condition of this Agreement. Upon termination of this\nAgreement, you shall delete and cease use of the Llama Materials. Sections 3,\n4 and 7 shall survive the termination of this Agreement.\n7. Governing Law and Jurisdiction. This Agreement will be governed and\nconstrued under the laws of the State of California without regard to choice\nof law principles, and the UN Convention on Contracts for the International\nSale of Goods does not apply to this Agreement. The courts of California\nshall have exclusive jurisdiction of any dispute arising out of this\nAgreement.\nUSE POLICY",
"### Llama 2 Acceptable Use Policy\n\n\nMeta is committed to promoting safe and fair use of its tools and features,\nincluding Llama 2. If you access or use Llama 2, you agree to this Acceptable\nUse Policy (“Policy”). The most recent copy of this policy can be found at\nURL",
"#### Prohibited Uses\n\n\nWe want everyone to use Llama 2 safely and responsibly. You agree you will not\nuse, or allow others to use, Llama 2 to:\n\n\n1. Violate the law or others’ rights, including to:\n1. Engage in, promote, generate, contribute to, encourage, plan, incite, or further illegal or unlawful activity or content, such as:\n\t1. Violence or terrorism\n\t2. Exploitation or harm to children, including the solicitation, creation, acquisition, or dissemination of child exploitative content or failure to report Child Sexual Abuse Material\n\t3. Human trafficking, exploitation, and sexual violence\n\t4. The illegal distribution of information or materials to minors, including obscene materials, or failure to employ legally required age-gating in connection with such information or materials.\n\t5. Sexual solicitation\n\t6. Any other criminal activity\n\n\n\n```\n2. Engage in, promote, incite, or facilitate the harassment, abuse, threatening, or bullying of individuals or groups of individuals\n3. Engage in, promote, incite, or facilitate discrimination or other unlawful or harmful conduct in the provision of employment, employment benefits, credit, housing, other economic benefits, or other essential goods and services\n4. Engage in the unauthorized or unlicensed practice of any profession including, but not limited to, financial, legal, medical/health, or related professional practices \n5. Collect, process, disclose, generate, or infer health, demographic, or other sensitive personal or private information about individuals without rights and consents required by applicable laws\n6. Engage in or facilitate any action or generate any content that infringes, misappropriates, or otherwise violates any third-party rights, including the outputs or results of any products or services using the Llama 2 Materials\n7. Create, generate, or facilitate the creation of malicious code, malware, computer viruses or do anything else that could disable, overburden, interfere with or impair the proper working, integrity, operation or appearance of a website or computer system \n\n```\n\n2. Engage in, promote, incite, facilitate, or assist in the planning or\ndevelopment of activities that present a risk of death or bodily harm to\nindividuals, including use of Llama 2 related to the following:\n1. Military, warfare, nuclear industries or applications, espionage, use for materials or activities that are subject to the International Traffic Arms Regulations (ITAR) maintained by the United States Department of State\n2. Guns and illegal weapons (including weapon development)\n3. Illegal drugs and regulated/controlled substances\n4. Operation of critical infrastructure, transportation technologies, or heavy machinery\n5. Self-harm or harm to others, including suicide, cutting, and eating disorders\n6. Any content intended to incite or promote violence, abuse, or any infliction of bodily harm to an individual\n3. Intentionally deceive or mislead others, including use of Llama 2 related\nto the following:\n1. Generating, promoting, or furthering fraud or the creation or promotion of disinformation\n2. Generating, promoting, or furthering defamatory content, including the creation of defamatory statements, images, or other content\n3. Generating, promoting, or further distributing spam\n4. Impersonating another individual without consent, authorization, or legal right\n5. Representing that the use of Llama 2 or outputs are human-generated\n6. Generating or facilitating false online engagement, including fake reviews and other means of fake online engagement\n4. Fail to appropriately disclose to end users any known dangers of your AI system\nPlease report any violation of this Policy, software “bug,” or other problems\nthat could lead to a violation of this Policy through one of the following\nmeans:\n\n\n* Reporting issues with the model:\nURL\n* Reporting risky content generated by the model:\nURL\n* Reporting bugs and security concerns:\nURL\n* Reporting violations of the Acceptable Use Policy or unlicensed uses of\nLlama: LlamaUseReport@URL\nextra\\_gated\\_fields:\nFirst Name: text\nLast Name: text\nDate of birth: date\\_picker\nCountry: country\nAffiliation: text\ngeo: ip\\_location \n\nBy clicking Submit below I accept the terms of the license and acknowledge that the information I provide will be collected stored processed and shared in accordance with the Meta Privacy Policy: checkbox\nextra\\_gated\\_description: The information you provide will be collected, stored, processed and shared in accordance with the Meta Privacy Policy.\nextra\\_gated\\_button\\_content: Submit\nlanguage:\n\n\n* code\npipeline\\_tag: text-generation\ntags:\n* facebook\n* meta\n* pytorch\n* llama\n* llama-2\nlicense: llama2\n\n\n\n\n---\n\n\nCode Llama\n==========\n\n\nCode Llama is a collection of pretrained and fine-tuned generative text models ranging in scale from 7 billion to 34 billion parameters. This is the repository for the base 34B version in the Hugging Face Transformers format. This model is designed for general code synthesis and understanding. Links to other models can be found in the index at the bottom.\n\n\n\nModel Use\n---------\n\n\nTo use this model, please make sure to install transformers:\n\n\nModel capabilities:\n\n\n* [x] Code completion.\n* [ ] Infilling.\n* [ ] Instructions / chat.\n* [ ] Python specialist.\n\n\nModel Details\n-------------\n\n\n\\*Note: Use of this model is governed by the Meta license. Meta developed and publicly released the Code Llama family of large language models (LLMs).\n\n\nModel Developers Meta\n\n\nVariations Code Llama comes in three model sizes, and three variants:\n\n\n* Code Llama: base models designed for general code synthesis and understanding\n* Code Llama - Python: designed specifically for Python\n* Code Llama - Instruct: for instruction following and safer deployment\n\n\nAll variants are available in sizes of 7B, 13B and 34B parameters.\n\n\nThis repository contains the base version of the 34B parameters model.\n\n\nInput Models input text only.\n\n\nOutput Models generate text only.\n\n\nModel Architecture Code Llama is an auto-regressive language model that uses an optimized transformer architecture.\n\n\nModel Dates Code Llama and its variants have been trained between January 2023 and July 2023.\n\n\nStatus This is a static model trained on an offline dataset. Future versions of Code Llama - Instruct will be released as we improve model safety with community feedback.\n\n\nLicense A custom commercial license is available at: URL\n\n\nResearch Paper More information can be found in the paper \"Code Llama: Open Foundation Models for Code\" or its arXiv page.\n\n\nIntended Use\n------------\n\n\nIntended Use Cases Code Llama and its variants is intended for commercial and research use in English and relevant programming languages. The base model Code Llama can be adapted for a variety of code synthesis and understanding tasks, Code Llama - Python is designed specifically to handle the Python programming language, and Code Llama - Instruct is intended to be safer to use for code assistant and generation applications.\n\n\nOut-of-Scope Uses Use in any manner that violates applicable laws or regulations (including trade compliance laws). Use in languages other than English. Use in any other way that is prohibited by the Acceptable Use Policy and Licensing Agreement for Code Llama and its variants.\n\n\nHardware and Software\n---------------------\n\n\nTraining Factors We used custom training libraries. The training and fine-tuning of the released models have been performed Meta’s Research Super Cluster.\n\n\nCarbon Footprint In aggregate, training all 9 Code Llama models required 400K GPU hours of computation on hardware of type A100-80GB (TDP of 350-400W). Estimated total emissions were 65.3 tCO2eq, 100% of which were offset by Meta’s sustainability program.\n\n\nTraining Data\n-------------\n\n\nAll experiments reported here and the released models have been trained and fine-tuned using the same data as Llama 2 with different weights (see Section 2 and Table 1 in the research paper for details).\n\n\nEvaluation Results\n------------------\n\n\nSee evaluations for the main models and detailed ablations in Section 3 and safety evaluations in Section 4 of the research paper.\n\n\nEthical Considerations and Limitations\n--------------------------------------\n\n\nCode Llama and its variants are a new technology that carries risks with use. Testing conducted to date has been in English, and has not covered, nor could it cover all scenarios. For these reasons, as with all LLMs, Code Llama’s potential outputs cannot be predicted in advance, and the model may in some instances produce inaccurate or objectionable responses to user prompts. Therefore, before deploying any applications of Code Llama, developers should perform safety testing and tuning tailored to their specific applications of the model.\n\n\nPlease see the Responsible Use Guide available available at URL"
] | [
"TAGS\n#transformers #safetensors #llama #text-generation #arxiv-2308.12950 #autotrain_compatible #endpoints_compatible #text-generation-inference #8-bit #region-us \n",
"### LLAMA 2 COMMUNITY LICENSE AGREEMENT\n\n\n\"Agreement\" means the terms and conditions for use, reproduction, distribution\nand modification of the Llama Materials set forth herein.\n\"Documentation\" means the specifications, manuals and documentation\naccompanying Llama 2 distributed by Meta at\nURL \n\n\"Licensee\" or \"you\" means you, or your employer or any other person or entity\n(if you are entering into this Agreement on such person or entity's behalf),\nof the age required under applicable laws, rules or regulations to provide\nlegal consent and that has legal authority to bind your employer or such other\nperson or entity if you are entering in this Agreement on their behalf.\n\"Llama 2\" means the foundational large language models and software and\nalgorithms, including machine-learning model code, trained model weights,\ninference-enabling code, training-enabling code, fine-tuning enabling code and\nother elements of the foregoing distributed by Meta at\nURL\n\"Llama Materials\" means, collectively, Meta's proprietary Llama 2 and\ndocumentation (and any portion thereof) made available under this Agreement.\n\"Meta\" or \"we\" means Meta Platforms Ireland Limited (if you are located in or,\nif you are an entity, your principal place of business is in the EEA or\nSwitzerland) and Meta Platforms, Inc. (if you are located outside of the EEA\nor Switzerland).\nBy clicking \"I Accept\" below or by using or distributing any portion or\nelement of the Llama Materials, you agree to be bound by this Agreement.\n\n\n1. License Rights and Redistribution.\na. Grant of Rights. You are granted a non-exclusive, worldwide, non-\ntransferable and royalty-free limited license under Meta's intellectual\nproperty or other rights owned by Meta embodied in the Llama Materials to\nuse, reproduce, distribute, copy, create derivative works of, and make\nmodifications to the Llama Materials.\n\n\nb. Redistribution and Use. \n\ni. If you distribute or make the Llama Materials, or any derivative works\nthereof, available to a third party, you shall provide a copy of this\nAgreement to such third party.\nii. If you receive Llama Materials, or any derivative works thereof, from a\nLicensee as part of an integrated end user product, then Section 2 of this\nAgreement will not apply to you.\niii. You must retain in all copies of the Llama Materials that you distribute\nthe following attribution notice within a \"Notice\" text file distributed as a\npart of such copies: \"Llama 2 is licensed under the LLAMA 2 Community\nLicense, Copyright (c) Meta Platforms, Inc. All Rights Reserved.\"\niv. Your use of the Llama Materials must comply with applicable laws and\nregulations (including trade compliance laws and regulations) and adhere to\nthe Acceptable Use Policy for the Llama Materials (available at\nURL which is hereby incorporated by\nreference into this Agreement.\nv. You will not use the Llama Materials or any output or results of the Llama\nMaterials to improve any other large language model (excluding Llama 2 or\nderivative works thereof). \n\n2. Additional Commercial Terms. If, on the Llama 2 version release date, the\nmonthly active users of the products or services made available by or for\nLicensee, or Licensee's affiliates, is greater than 700 million monthly\nactive users in the preceding calendar month, you must request a license from\nMeta, which Meta may grant to you in its sole discretion, and you are not\nauthorized to exercise any of the rights under this Agreement unless or until\nMeta otherwise expressly grants you such rights.\n3. Disclaimer of Warranty. UNLESS REQUIRED BY APPLICABLE LAW, THE LLAMA\nMATERIALS AND ANY OUTPUT AND RESULTS THEREFROM ARE PROVIDED ON AN \"AS IS\"\nBASIS, WITHOUT WARRANTIES OF ANY KIND, EITHER EXPRESS OR IMPLIED, INCLUDING,\nWITHOUT LIMITATION, ANY WARRANTIES OF TITLE, NON-INFRINGEMENT,\nMERCHANTABILITY, OR FITNESS FOR A PARTICULAR PURPOSE. YOU ARE SOLELY\nRESPONSIBLE FOR DETERMINING THE APPROPRIATENESS OF USING OR REDISTRIBUTING\nTHE LLAMA MATERIALS AND ASSUME ANY RISKS ASSOCIATED WITH YOUR USE OF THE\nLLAMA MATERIALS AND ANY OUTPUT AND RESULTS.\n4. Limitation of Liability. IN NO EVENT WILL META OR ITS AFFILIATES BE LIABLE\nUNDER ANY THEORY OF LIABILITY, WHETHER IN CONTRACT, TORT, NEGLIGENCE,\nPRODUCTS LIABILITY, OR OTHERWISE, ARISING OUT OF THIS AGREEMENT, FOR ANY LOST\nPROFITS OR ANY INDIRECT, SPECIAL, CONSEQUENTIAL, INCIDENTAL, EXEMPLARY OR\nPUNITIVE DAMAGES, EVEN IF META OR ITS AFFILIATES HAVE BEEN ADVISED OF THE\nPOSSIBILITY OF ANY OF THE FOREGOING.\n5. Intellectual Property.\na. No trademark licenses are granted under this Agreement, and in connection\nwith the Llama Materials, neither Meta nor Licensee may use any name or mark\nowned by or associated with the other or any of its affiliates, except as\nrequired for reasonable and customary use in describing and redistributing\nthe Llama Materials.\nb. Subject to Meta's ownership of Llama Materials and derivatives made by or\nfor Meta, with respect to any derivative works and modifications of the Llama\nMaterials that are made by you, as between you and Meta, you are and will be\nthe owner of such derivative works and modifications.\nc. If you institute litigation or other proceedings against Meta or any\nentity (including a cross-claim or counterclaim in a lawsuit) alleging that\nthe Llama Materials or Llama 2 outputs or results, or any portion of any of\nthe foregoing, constitutes infringement of intellectual property or other\nrights owned or licensable by you, then any licenses granted to you under\nthis Agreement shall terminate as of the date such litigation or claim is\nfiled or instituted. You will indemnify and hold harmless Meta from and\nagainst any claim by any third party arising out of or related to your use or\ndistribution of the Llama Materials.\n6. Term and Termination. The term of this Agreement will commence upon your\nacceptance of this Agreement or access to the Llama Materials and will\ncontinue in full force and effect until terminated in accordance with the\nterms and conditions herein. Meta may terminate this Agreement if you are in\nbreach of any term or condition of this Agreement. Upon termination of this\nAgreement, you shall delete and cease use of the Llama Materials. Sections 3,\n4 and 7 shall survive the termination of this Agreement.\n7. Governing Law and Jurisdiction. This Agreement will be governed and\nconstrued under the laws of the State of California without regard to choice\nof law principles, and the UN Convention on Contracts for the International\nSale of Goods does not apply to this Agreement. The courts of California\nshall have exclusive jurisdiction of any dispute arising out of this\nAgreement.\nUSE POLICY",
"### Llama 2 Acceptable Use Policy\n\n\nMeta is committed to promoting safe and fair use of its tools and features,\nincluding Llama 2. If you access or use Llama 2, you agree to this Acceptable\nUse Policy (“Policy”). The most recent copy of this policy can be found at\nURL",
"#### Prohibited Uses\n\n\nWe want everyone to use Llama 2 safely and responsibly. You agree you will not\nuse, or allow others to use, Llama 2 to:\n\n\n1. Violate the law or others’ rights, including to:\n1. Engage in, promote, generate, contribute to, encourage, plan, incite, or further illegal or unlawful activity or content, such as:\n\t1. Violence or terrorism\n\t2. Exploitation or harm to children, including the solicitation, creation, acquisition, or dissemination of child exploitative content or failure to report Child Sexual Abuse Material\n\t3. Human trafficking, exploitation, and sexual violence\n\t4. The illegal distribution of information or materials to minors, including obscene materials, or failure to employ legally required age-gating in connection with such information or materials.\n\t5. Sexual solicitation\n\t6. Any other criminal activity\n\n\n\n```\n2. Engage in, promote, incite, or facilitate the harassment, abuse, threatening, or bullying of individuals or groups of individuals\n3. Engage in, promote, incite, or facilitate discrimination or other unlawful or harmful conduct in the provision of employment, employment benefits, credit, housing, other economic benefits, or other essential goods and services\n4. Engage in the unauthorized or unlicensed practice of any profession including, but not limited to, financial, legal, medical/health, or related professional practices \n5. Collect, process, disclose, generate, or infer health, demographic, or other sensitive personal or private information about individuals without rights and consents required by applicable laws\n6. Engage in or facilitate any action or generate any content that infringes, misappropriates, or otherwise violates any third-party rights, including the outputs or results of any products or services using the Llama 2 Materials\n7. Create, generate, or facilitate the creation of malicious code, malware, computer viruses or do anything else that could disable, overburden, interfere with or impair the proper working, integrity, operation or appearance of a website or computer system \n\n```\n\n2. Engage in, promote, incite, facilitate, or assist in the planning or\ndevelopment of activities that present a risk of death or bodily harm to\nindividuals, including use of Llama 2 related to the following:\n1. Military, warfare, nuclear industries or applications, espionage, use for materials or activities that are subject to the International Traffic Arms Regulations (ITAR) maintained by the United States Department of State\n2. Guns and illegal weapons (including weapon development)\n3. Illegal drugs and regulated/controlled substances\n4. Operation of critical infrastructure, transportation technologies, or heavy machinery\n5. Self-harm or harm to others, including suicide, cutting, and eating disorders\n6. Any content intended to incite or promote violence, abuse, or any infliction of bodily harm to an individual\n3. Intentionally deceive or mislead others, including use of Llama 2 related\nto the following:\n1. Generating, promoting, or furthering fraud or the creation or promotion of disinformation\n2. Generating, promoting, or furthering defamatory content, including the creation of defamatory statements, images, or other content\n3. Generating, promoting, or further distributing spam\n4. Impersonating another individual without consent, authorization, or legal right\n5. Representing that the use of Llama 2 or outputs are human-generated\n6. Generating or facilitating false online engagement, including fake reviews and other means of fake online engagement\n4. Fail to appropriately disclose to end users any known dangers of your AI system\nPlease report any violation of this Policy, software “bug,” or other problems\nthat could lead to a violation of this Policy through one of the following\nmeans:\n\n\n* Reporting issues with the model:\nURL\n* Reporting risky content generated by the model:\nURL\n* Reporting bugs and security concerns:\nURL\n* Reporting violations of the Acceptable Use Policy or unlicensed uses of\nLlama: LlamaUseReport@URL\nextra\\_gated\\_fields:\nFirst Name: text\nLast Name: text\nDate of birth: date\\_picker\nCountry: country\nAffiliation: text\ngeo: ip\\_location \n\nBy clicking Submit below I accept the terms of the license and acknowledge that the information I provide will be collected stored processed and shared in accordance with the Meta Privacy Policy: checkbox\nextra\\_gated\\_description: The information you provide will be collected, stored, processed and shared in accordance with the Meta Privacy Policy.\nextra\\_gated\\_button\\_content: Submit\nlanguage:\n\n\n* code\npipeline\\_tag: text-generation\ntags:\n* facebook\n* meta\n* pytorch\n* llama\n* llama-2\nlicense: llama2\n\n\n\n\n---\n\n\nCode Llama\n==========\n\n\nCode Llama is a collection of pretrained and fine-tuned generative text models ranging in scale from 7 billion to 34 billion parameters. This is the repository for the base 34B version in the Hugging Face Transformers format. This model is designed for general code synthesis and understanding. Links to other models can be found in the index at the bottom.\n\n\n\nModel Use\n---------\n\n\nTo use this model, please make sure to install transformers:\n\n\nModel capabilities:\n\n\n* [x] Code completion.\n* [ ] Infilling.\n* [ ] Instructions / chat.\n* [ ] Python specialist.\n\n\nModel Details\n-------------\n\n\n\\*Note: Use of this model is governed by the Meta license. Meta developed and publicly released the Code Llama family of large language models (LLMs).\n\n\nModel Developers Meta\n\n\nVariations Code Llama comes in three model sizes, and three variants:\n\n\n* Code Llama: base models designed for general code synthesis and understanding\n* Code Llama - Python: designed specifically for Python\n* Code Llama - Instruct: for instruction following and safer deployment\n\n\nAll variants are available in sizes of 7B, 13B and 34B parameters.\n\n\nThis repository contains the base version of the 34B parameters model.\n\n\nInput Models input text only.\n\n\nOutput Models generate text only.\n\n\nModel Architecture Code Llama is an auto-regressive language model that uses an optimized transformer architecture.\n\n\nModel Dates Code Llama and its variants have been trained between January 2023 and July 2023.\n\n\nStatus This is a static model trained on an offline dataset. Future versions of Code Llama - Instruct will be released as we improve model safety with community feedback.\n\n\nLicense A custom commercial license is available at: URL\n\n\nResearch Paper More information can be found in the paper \"Code Llama: Open Foundation Models for Code\" or its arXiv page.\n\n\nIntended Use\n------------\n\n\nIntended Use Cases Code Llama and its variants is intended for commercial and research use in English and relevant programming languages. The base model Code Llama can be adapted for a variety of code synthesis and understanding tasks, Code Llama - Python is designed specifically to handle the Python programming language, and Code Llama - Instruct is intended to be safer to use for code assistant and generation applications.\n\n\nOut-of-Scope Uses Use in any manner that violates applicable laws or regulations (including trade compliance laws). Use in languages other than English. Use in any other way that is prohibited by the Acceptable Use Policy and Licensing Agreement for Code Llama and its variants.\n\n\nHardware and Software\n---------------------\n\n\nTraining Factors We used custom training libraries. The training and fine-tuning of the released models have been performed Meta’s Research Super Cluster.\n\n\nCarbon Footprint In aggregate, training all 9 Code Llama models required 400K GPU hours of computation on hardware of type A100-80GB (TDP of 350-400W). Estimated total emissions were 65.3 tCO2eq, 100% of which were offset by Meta’s sustainability program.\n\n\nTraining Data\n-------------\n\n\nAll experiments reported here and the released models have been trained and fine-tuned using the same data as Llama 2 with different weights (see Section 2 and Table 1 in the research paper for details).\n\n\nEvaluation Results\n------------------\n\n\nSee evaluations for the main models and detailed ablations in Section 3 and safety evaluations in Section 4 of the research paper.\n\n\nEthical Considerations and Limitations\n--------------------------------------\n\n\nCode Llama and its variants are a new technology that carries risks with use. Testing conducted to date has been in English, and has not covered, nor could it cover all scenarios. For these reasons, as with all LLMs, Code Llama’s potential outputs cannot be predicted in advance, and the model may in some instances produce inaccurate or objectionable responses to user prompts. Therefore, before deploying any applications of Code Llama, developers should perform safety testing and tuning tailored to their specific applications of the model.\n\n\nPlease see the Responsible Use Guide available available at URL"
] |
null | null | FP16 models of ControlNet++. Original project repo: https://huggingface.co/spaces/limingcv/ControlNet-Plus-Plus. | {"license": "apache-2.0"} | huchenlei/ControlNet_plus_plus_collection_fp16 | null | [
"license:apache-2.0",
"region:us"
] | null | 2024-04-21T18:42:33+00:00 | [] | [] | TAGS
#license-apache-2.0 #region-us
| FP16 models of ControlNet++. Original project repo: URL | [] | [
"TAGS\n#license-apache-2.0 #region-us \n"
] |
text-generation | transformers |
# Model Card for Model ID
<!-- Provide a quick summary of what the model is/does. -->
## Model Details
### Model Description
<!-- Provide a longer summary of what this model is. -->
This is the model card of a 🤗 transformers model that has been pushed on the Hub. This model card has been automatically generated.
- **Developed by:** [More Information Needed]
- **Funded by [optional]:** [More Information Needed]
- **Shared by [optional]:** [More Information Needed]
- **Model type:** [More Information Needed]
- **Language(s) (NLP):** [More Information Needed]
- **License:** [More Information Needed]
- **Finetuned from model [optional]:** [More Information Needed]
### Model Sources [optional]
<!-- Provide the basic links for the model. -->
- **Repository:** [More Information Needed]
- **Paper [optional]:** [More Information Needed]
- **Demo [optional]:** [More Information Needed]
## Uses
<!-- Address questions around how the model is intended to be used, including the foreseeable users of the model and those affected by the model. -->
### Direct Use
<!-- This section is for the model use without fine-tuning or plugging into a larger ecosystem/app. -->
[More Information Needed]
### Downstream Use [optional]
<!-- This section is for the model use when fine-tuned for a task, or when plugged into a larger ecosystem/app -->
[More Information Needed]
### Out-of-Scope Use
<!-- This section addresses misuse, malicious use, and uses that the model will not work well for. -->
[More Information Needed]
## Bias, Risks, and Limitations
<!-- This section is meant to convey both technical and sociotechnical limitations. -->
[More Information Needed]
### Recommendations
<!-- This section is meant to convey recommendations with respect to the bias, risk, and technical limitations. -->
Users (both direct and downstream) should be made aware of the risks, biases and limitations of the model. More information needed for further recommendations.
## How to Get Started with the Model
Use the code below to get started with the model.
[More Information Needed]
## Training Details
### Training Data
<!-- This should link to a Dataset Card, perhaps with a short stub of information on what the training data is all about as well as documentation related to data pre-processing or additional filtering. -->
[More Information Needed]
### Training Procedure
<!-- This relates heavily to the Technical Specifications. Content here should link to that section when it is relevant to the training procedure. -->
#### Preprocessing [optional]
[More Information Needed]
#### Training Hyperparameters
- **Training regime:** [More Information Needed] <!--fp32, fp16 mixed precision, bf16 mixed precision, bf16 non-mixed precision, fp16 non-mixed precision, fp8 mixed precision -->
#### Speeds, Sizes, Times [optional]
<!-- This section provides information about throughput, start/end time, checkpoint size if relevant, etc. -->
[More Information Needed]
## Evaluation
<!-- This section describes the evaluation protocols and provides the results. -->
### Testing Data, Factors & Metrics
#### Testing Data
<!-- This should link to a Dataset Card if possible. -->
[More Information Needed]
#### Factors
<!-- These are the things the evaluation is disaggregating by, e.g., subpopulations or domains. -->
[More Information Needed]
#### Metrics
<!-- These are the evaluation metrics being used, ideally with a description of why. -->
[More Information Needed]
### Results
[More Information Needed]
#### Summary
## Model Examination [optional]
<!-- Relevant interpretability work for the model goes here -->
[More Information Needed]
## Environmental Impact
<!-- Total emissions (in grams of CO2eq) and additional considerations, such as electricity usage, go here. Edit the suggested text below accordingly -->
Carbon emissions can be estimated using the [Machine Learning Impact calculator](https://mlco2.github.io/impact#compute) presented in [Lacoste et al. (2019)](https://arxiv.org/abs/1910.09700).
- **Hardware Type:** [More Information Needed]
- **Hours used:** [More Information Needed]
- **Cloud Provider:** [More Information Needed]
- **Compute Region:** [More Information Needed]
- **Carbon Emitted:** [More Information Needed]
## Technical Specifications [optional]
### Model Architecture and Objective
[More Information Needed]
### Compute Infrastructure
[More Information Needed]
#### Hardware
[More Information Needed]
#### Software
[More Information Needed]
## Citation [optional]
<!-- If there is a paper or blog post introducing the model, the APA and Bibtex information for that should go in this section. -->
**BibTeX:**
[More Information Needed]
**APA:**
[More Information Needed]
## Glossary [optional]
<!-- If relevant, include terms and calculations in this section that can help readers understand the model or model card. -->
[More Information Needed]
## More Information [optional]
[More Information Needed]
## Model Card Authors [optional]
[More Information Needed]
## Model Card Contact
[More Information Needed] | {"library_name": "transformers", "tags": []} | GalaganKV/Mistral-7B-Instruct-v0.2-MultiTask-v8 | null | [
"transformers",
"safetensors",
"mistral",
"text-generation",
"conversational",
"arxiv:1910.09700",
"autotrain_compatible",
"endpoints_compatible",
"text-generation-inference",
"region:us"
] | null | 2024-04-21T18:43:30+00:00 | [
"1910.09700"
] | [] | TAGS
#transformers #safetensors #mistral #text-generation #conversational #arxiv-1910.09700 #autotrain_compatible #endpoints_compatible #text-generation-inference #region-us
|
# Model Card for Model ID
## Model Details
### Model Description
This is the model card of a transformers model that has been pushed on the Hub. This model card has been automatically generated.
- Developed by:
- Funded by [optional]:
- Shared by [optional]:
- Model type:
- Language(s) (NLP):
- License:
- Finetuned from model [optional]:
### Model Sources [optional]
- Repository:
- Paper [optional]:
- Demo [optional]:
## Uses
### Direct Use
### Downstream Use [optional]
### Out-of-Scope Use
## Bias, Risks, and Limitations
### Recommendations
Users (both direct and downstream) should be made aware of the risks, biases and limitations of the model. More information needed for further recommendations.
## How to Get Started with the Model
Use the code below to get started with the model.
## Training Details
### Training Data
### Training Procedure
#### Preprocessing [optional]
#### Training Hyperparameters
- Training regime:
#### Speeds, Sizes, Times [optional]
## Evaluation
### Testing Data, Factors & Metrics
#### Testing Data
#### Factors
#### Metrics
### Results
#### Summary
## Model Examination [optional]
## Environmental Impact
Carbon emissions can be estimated using the Machine Learning Impact calculator presented in Lacoste et al. (2019).
- Hardware Type:
- Hours used:
- Cloud Provider:
- Compute Region:
- Carbon Emitted:
## Technical Specifications [optional]
### Model Architecture and Objective
### Compute Infrastructure
#### Hardware
#### Software
[optional]
BibTeX:
APA:
## Glossary [optional]
## More Information [optional]
## Model Card Authors [optional]
## Model Card Contact
| [
"# Model Card for Model ID",
"## Model Details",
"### Model Description\n\n\n\nThis is the model card of a transformers model that has been pushed on the Hub. This model card has been automatically generated.\n\n- Developed by: \n- Funded by [optional]: \n- Shared by [optional]: \n- Model type: \n- Language(s) (NLP): \n- License: \n- Finetuned from model [optional]:",
"### Model Sources [optional]\n\n\n\n- Repository: \n- Paper [optional]: \n- Demo [optional]:",
"## Uses",
"### Direct Use",
"### Downstream Use [optional]",
"### Out-of-Scope Use",
"## Bias, Risks, and Limitations",
"### Recommendations\n\n\n\nUsers (both direct and downstream) should be made aware of the risks, biases and limitations of the model. More information needed for further recommendations.",
"## How to Get Started with the Model\n\nUse the code below to get started with the model.",
"## Training Details",
"### Training Data",
"### Training Procedure",
"#### Preprocessing [optional]",
"#### Training Hyperparameters\n\n- Training regime:",
"#### Speeds, Sizes, Times [optional]",
"## Evaluation",
"### Testing Data, Factors & Metrics",
"#### Testing Data",
"#### Factors",
"#### Metrics",
"### Results",
"#### Summary",
"## Model Examination [optional]",
"## Environmental Impact\n\n\n\nCarbon emissions can be estimated using the Machine Learning Impact calculator presented in Lacoste et al. (2019).\n\n- Hardware Type: \n- Hours used: \n- Cloud Provider: \n- Compute Region: \n- Carbon Emitted:",
"## Technical Specifications [optional]",
"### Model Architecture and Objective",
"### Compute Infrastructure",
"#### Hardware",
"#### Software\n\n\n\n[optional]\n\n\n\nBibTeX:\n\n\n\nAPA:",
"## Glossary [optional]",
"## More Information [optional]",
"## Model Card Authors [optional]",
"## Model Card Contact"
] | [
"TAGS\n#transformers #safetensors #mistral #text-generation #conversational #arxiv-1910.09700 #autotrain_compatible #endpoints_compatible #text-generation-inference #region-us \n",
"# Model Card for Model ID",
"## Model Details",
"### Model Description\n\n\n\nThis is the model card of a transformers model that has been pushed on the Hub. This model card has been automatically generated.\n\n- Developed by: \n- Funded by [optional]: \n- Shared by [optional]: \n- Model type: \n- Language(s) (NLP): \n- License: \n- Finetuned from model [optional]:",
"### Model Sources [optional]\n\n\n\n- Repository: \n- Paper [optional]: \n- Demo [optional]:",
"## Uses",
"### Direct Use",
"### Downstream Use [optional]",
"### Out-of-Scope Use",
"## Bias, Risks, and Limitations",
"### Recommendations\n\n\n\nUsers (both direct and downstream) should be made aware of the risks, biases and limitations of the model. More information needed for further recommendations.",
"## How to Get Started with the Model\n\nUse the code below to get started with the model.",
"## Training Details",
"### Training Data",
"### Training Procedure",
"#### Preprocessing [optional]",
"#### Training Hyperparameters\n\n- Training regime:",
"#### Speeds, Sizes, Times [optional]",
"## Evaluation",
"### Testing Data, Factors & Metrics",
"#### Testing Data",
"#### Factors",
"#### Metrics",
"### Results",
"#### Summary",
"## Model Examination [optional]",
"## Environmental Impact\n\n\n\nCarbon emissions can be estimated using the Machine Learning Impact calculator presented in Lacoste et al. (2019).\n\n- Hardware Type: \n- Hours used: \n- Cloud Provider: \n- Compute Region: \n- Carbon Emitted:",
"## Technical Specifications [optional]",
"### Model Architecture and Objective",
"### Compute Infrastructure",
"#### Hardware",
"#### Software\n\n\n\n[optional]\n\n\n\nBibTeX:\n\n\n\nAPA:",
"## Glossary [optional]",
"## More Information [optional]",
"## Model Card Authors [optional]",
"## Model Card Contact"
] |
null | adapter-transformers |
# Adapter `BigTMiami/n_par_bn_v_1_help_class_80_e_adp_lr_0003_S_0` for roberta-base
An [adapter](https://adapterhub.ml) for the `roberta-base` model that was trained on the [BigTMiami/amazon_MICRO_helpfulness_dataset](https://huggingface.co/datasets/BigTMiami/amazon_MICRO_helpfulness_dataset/) dataset and includes a prediction head for classification.
This adapter was created for usage with the **[Adapters](https://github.com/Adapter-Hub/adapters)** library.
## Usage
First, install `adapters`:
```
pip install -U adapters
```
Now, the adapter can be loaded and activated like this:
```python
from adapters import AutoAdapterModel
model = AutoAdapterModel.from_pretrained("roberta-base")
adapter_name = model.load_adapter("BigTMiami/n_par_bn_v_1_help_class_80_e_adp_lr_0003_S_0", source="hf", set_active=True)
```
## Architecture & Training
<!-- Add some description here -->
## Evaluation results
<!-- Add some description here -->
## Citation
<!-- Add some description here --> | {"tags": ["roberta", "adapter-transformers"], "datasets": ["BigTMiami/amazon_MICRO_helpfulness_dataset"]} | BigTMiami/n_par_bn_v_1_help_class_80_e_adp_lr_0003_S_0 | null | [
"adapter-transformers",
"roberta",
"dataset:BigTMiami/amazon_MICRO_helpfulness_dataset",
"region:us"
] | null | 2024-04-21T18:44:06+00:00 | [] | [] | TAGS
#adapter-transformers #roberta #dataset-BigTMiami/amazon_MICRO_helpfulness_dataset #region-us
|
# Adapter 'BigTMiami/n_par_bn_v_1_help_class_80_e_adp_lr_0003_S_0' for roberta-base
An adapter for the 'roberta-base' model that was trained on the BigTMiami/amazon_MICRO_helpfulness_dataset dataset and includes a prediction head for classification.
This adapter was created for usage with the Adapters library.
## Usage
First, install 'adapters':
Now, the adapter can be loaded and activated like this:
## Architecture & Training
## Evaluation results
| [
"# Adapter 'BigTMiami/n_par_bn_v_1_help_class_80_e_adp_lr_0003_S_0' for roberta-base\n\nAn adapter for the 'roberta-base' model that was trained on the BigTMiami/amazon_MICRO_helpfulness_dataset dataset and includes a prediction head for classification.\n\nThis adapter was created for usage with the Adapters library.",
"## Usage\n\nFirst, install 'adapters':\n\n\n\nNow, the adapter can be loaded and activated like this:",
"## Architecture & Training",
"## Evaluation results"
] | [
"TAGS\n#adapter-transformers #roberta #dataset-BigTMiami/amazon_MICRO_helpfulness_dataset #region-us \n",
"# Adapter 'BigTMiami/n_par_bn_v_1_help_class_80_e_adp_lr_0003_S_0' for roberta-base\n\nAn adapter for the 'roberta-base' model that was trained on the BigTMiami/amazon_MICRO_helpfulness_dataset dataset and includes a prediction head for classification.\n\nThis adapter was created for usage with the Adapters library.",
"## Usage\n\nFirst, install 'adapters':\n\n\n\nNow, the adapter can be loaded and activated like this:",
"## Architecture & Training",
"## Evaluation results"
] |
null | adapter-transformers |
# Adapter `BigTMiami/n_par_bn_v_1_help_class_40_e_adp_lr_0003_S_1` for roberta-base
An [adapter](https://adapterhub.ml) for the `roberta-base` model that was trained on the [BigTMiami/amazon_MICRO_helpfulness_dataset](https://huggingface.co/datasets/BigTMiami/amazon_MICRO_helpfulness_dataset/) dataset and includes a prediction head for classification.
This adapter was created for usage with the **[Adapters](https://github.com/Adapter-Hub/adapters)** library.
## Usage
First, install `adapters`:
```
pip install -U adapters
```
Now, the adapter can be loaded and activated like this:
```python
from adapters import AutoAdapterModel
model = AutoAdapterModel.from_pretrained("roberta-base")
adapter_name = model.load_adapter("BigTMiami/n_par_bn_v_1_help_class_40_e_adp_lr_0003_S_1", source="hf", set_active=True)
```
## Architecture & Training
<!-- Add some description here -->
## Evaluation results
<!-- Add some description here -->
## Citation
<!-- Add some description here --> | {"tags": ["roberta", "adapter-transformers"], "datasets": ["BigTMiami/amazon_MICRO_helpfulness_dataset"]} | BigTMiami/n_par_bn_v_1_help_class_40_e_adp_lr_0003_S_1 | null | [
"adapter-transformers",
"roberta",
"dataset:BigTMiami/amazon_MICRO_helpfulness_dataset",
"region:us"
] | null | 2024-04-21T18:46:51+00:00 | [] | [] | TAGS
#adapter-transformers #roberta #dataset-BigTMiami/amazon_MICRO_helpfulness_dataset #region-us
|
# Adapter 'BigTMiami/n_par_bn_v_1_help_class_40_e_adp_lr_0003_S_1' for roberta-base
An adapter for the 'roberta-base' model that was trained on the BigTMiami/amazon_MICRO_helpfulness_dataset dataset and includes a prediction head for classification.
This adapter was created for usage with the Adapters library.
## Usage
First, install 'adapters':
Now, the adapter can be loaded and activated like this:
## Architecture & Training
## Evaluation results
| [
"# Adapter 'BigTMiami/n_par_bn_v_1_help_class_40_e_adp_lr_0003_S_1' for roberta-base\n\nAn adapter for the 'roberta-base' model that was trained on the BigTMiami/amazon_MICRO_helpfulness_dataset dataset and includes a prediction head for classification.\n\nThis adapter was created for usage with the Adapters library.",
"## Usage\n\nFirst, install 'adapters':\n\n\n\nNow, the adapter can be loaded and activated like this:",
"## Architecture & Training",
"## Evaluation results"
] | [
"TAGS\n#adapter-transformers #roberta #dataset-BigTMiami/amazon_MICRO_helpfulness_dataset #region-us \n",
"# Adapter 'BigTMiami/n_par_bn_v_1_help_class_40_e_adp_lr_0003_S_1' for roberta-base\n\nAn adapter for the 'roberta-base' model that was trained on the BigTMiami/amazon_MICRO_helpfulness_dataset dataset and includes a prediction head for classification.\n\nThis adapter was created for usage with the Adapters library.",
"## Usage\n\nFirst, install 'adapters':\n\n\n\nNow, the adapter can be loaded and activated like this:",
"## Architecture & Training",
"## Evaluation results"
] |
null | transformers |
# Model Card for Model ID
<!-- Provide a quick summary of what the model is/does. -->
## Model Details
### Model Description
<!-- Provide a longer summary of what this model is. -->
This is the model card of a 🤗 transformers model that has been pushed on the Hub. This model card has been automatically generated.
- **Developed by:** [More Information Needed]
- **Funded by [optional]:** [More Information Needed]
- **Shared by [optional]:** [More Information Needed]
- **Model type:** [More Information Needed]
- **Language(s) (NLP):** [More Information Needed]
- **License:** [More Information Needed]
- **Finetuned from model [optional]:** [More Information Needed]
### Model Sources [optional]
<!-- Provide the basic links for the model. -->
- **Repository:** [More Information Needed]
- **Paper [optional]:** [More Information Needed]
- **Demo [optional]:** [More Information Needed]
## Uses
<!-- Address questions around how the model is intended to be used, including the foreseeable users of the model and those affected by the model. -->
### Direct Use
<!-- This section is for the model use without fine-tuning or plugging into a larger ecosystem/app. -->
[More Information Needed]
### Downstream Use [optional]
<!-- This section is for the model use when fine-tuned for a task, or when plugged into a larger ecosystem/app -->
[More Information Needed]
### Out-of-Scope Use
<!-- This section addresses misuse, malicious use, and uses that the model will not work well for. -->
[More Information Needed]
## Bias, Risks, and Limitations
<!-- This section is meant to convey both technical and sociotechnical limitations. -->
[More Information Needed]
### Recommendations
<!-- This section is meant to convey recommendations with respect to the bias, risk, and technical limitations. -->
Users (both direct and downstream) should be made aware of the risks, biases and limitations of the model. More information needed for further recommendations.
## How to Get Started with the Model
Use the code below to get started with the model.
[More Information Needed]
## Training Details
### Training Data
<!-- This should link to a Dataset Card, perhaps with a short stub of information on what the training data is all about as well as documentation related to data pre-processing or additional filtering. -->
[More Information Needed]
### Training Procedure
<!-- This relates heavily to the Technical Specifications. Content here should link to that section when it is relevant to the training procedure. -->
#### Preprocessing [optional]
[More Information Needed]
#### Training Hyperparameters
- **Training regime:** [More Information Needed] <!--fp32, fp16 mixed precision, bf16 mixed precision, bf16 non-mixed precision, fp16 non-mixed precision, fp8 mixed precision -->
#### Speeds, Sizes, Times [optional]
<!-- This section provides information about throughput, start/end time, checkpoint size if relevant, etc. -->
[More Information Needed]
## Evaluation
<!-- This section describes the evaluation protocols and provides the results. -->
### Testing Data, Factors & Metrics
#### Testing Data
<!-- This should link to a Dataset Card if possible. -->
[More Information Needed]
#### Factors
<!-- These are the things the evaluation is disaggregating by, e.g., subpopulations or domains. -->
[More Information Needed]
#### Metrics
<!-- These are the evaluation metrics being used, ideally with a description of why. -->
[More Information Needed]
### Results
[More Information Needed]
#### Summary
## Model Examination [optional]
<!-- Relevant interpretability work for the model goes here -->
[More Information Needed]
## Environmental Impact
<!-- Total emissions (in grams of CO2eq) and additional considerations, such as electricity usage, go here. Edit the suggested text below accordingly -->
Carbon emissions can be estimated using the [Machine Learning Impact calculator](https://mlco2.github.io/impact#compute) presented in [Lacoste et al. (2019)](https://arxiv.org/abs/1910.09700).
- **Hardware Type:** [More Information Needed]
- **Hours used:** [More Information Needed]
- **Cloud Provider:** [More Information Needed]
- **Compute Region:** [More Information Needed]
- **Carbon Emitted:** [More Information Needed]
## Technical Specifications [optional]
### Model Architecture and Objective
[More Information Needed]
### Compute Infrastructure
[More Information Needed]
#### Hardware
[More Information Needed]
#### Software
[More Information Needed]
## Citation [optional]
<!-- If there is a paper or blog post introducing the model, the APA and Bibtex information for that should go in this section. -->
**BibTeX:**
[More Information Needed]
**APA:**
[More Information Needed]
## Glossary [optional]
<!-- If relevant, include terms and calculations in this section that can help readers understand the model or model card. -->
[More Information Needed]
## More Information [optional]
[More Information Needed]
## Model Card Authors [optional]
[More Information Needed]
## Model Card Contact
[More Information Needed] | {"library_name": "transformers", "tags": ["unsloth"]} | msmart/llama3-DPO-test | null | [
"transformers",
"safetensors",
"unsloth",
"arxiv:1910.09700",
"endpoints_compatible",
"region:us"
] | null | 2024-04-21T18:48:35+00:00 | [
"1910.09700"
] | [] | TAGS
#transformers #safetensors #unsloth #arxiv-1910.09700 #endpoints_compatible #region-us
|
# Model Card for Model ID
## Model Details
### Model Description
This is the model card of a transformers model that has been pushed on the Hub. This model card has been automatically generated.
- Developed by:
- Funded by [optional]:
- Shared by [optional]:
- Model type:
- Language(s) (NLP):
- License:
- Finetuned from model [optional]:
### Model Sources [optional]
- Repository:
- Paper [optional]:
- Demo [optional]:
## Uses
### Direct Use
### Downstream Use [optional]
### Out-of-Scope Use
## Bias, Risks, and Limitations
### Recommendations
Users (both direct and downstream) should be made aware of the risks, biases and limitations of the model. More information needed for further recommendations.
## How to Get Started with the Model
Use the code below to get started with the model.
## Training Details
### Training Data
### Training Procedure
#### Preprocessing [optional]
#### Training Hyperparameters
- Training regime:
#### Speeds, Sizes, Times [optional]
## Evaluation
### Testing Data, Factors & Metrics
#### Testing Data
#### Factors
#### Metrics
### Results
#### Summary
## Model Examination [optional]
## Environmental Impact
Carbon emissions can be estimated using the Machine Learning Impact calculator presented in Lacoste et al. (2019).
- Hardware Type:
- Hours used:
- Cloud Provider:
- Compute Region:
- Carbon Emitted:
## Technical Specifications [optional]
### Model Architecture and Objective
### Compute Infrastructure
#### Hardware
#### Software
[optional]
BibTeX:
APA:
## Glossary [optional]
## More Information [optional]
## Model Card Authors [optional]
## Model Card Contact
| [
"# Model Card for Model ID",
"## Model Details",
"### Model Description\n\n\n\nThis is the model card of a transformers model that has been pushed on the Hub. This model card has been automatically generated.\n\n- Developed by: \n- Funded by [optional]: \n- Shared by [optional]: \n- Model type: \n- Language(s) (NLP): \n- License: \n- Finetuned from model [optional]:",
"### Model Sources [optional]\n\n\n\n- Repository: \n- Paper [optional]: \n- Demo [optional]:",
"## Uses",
"### Direct Use",
"### Downstream Use [optional]",
"### Out-of-Scope Use",
"## Bias, Risks, and Limitations",
"### Recommendations\n\n\n\nUsers (both direct and downstream) should be made aware of the risks, biases and limitations of the model. More information needed for further recommendations.",
"## How to Get Started with the Model\n\nUse the code below to get started with the model.",
"## Training Details",
"### Training Data",
"### Training Procedure",
"#### Preprocessing [optional]",
"#### Training Hyperparameters\n\n- Training regime:",
"#### Speeds, Sizes, Times [optional]",
"## Evaluation",
"### Testing Data, Factors & Metrics",
"#### Testing Data",
"#### Factors",
"#### Metrics",
"### Results",
"#### Summary",
"## Model Examination [optional]",
"## Environmental Impact\n\n\n\nCarbon emissions can be estimated using the Machine Learning Impact calculator presented in Lacoste et al. (2019).\n\n- Hardware Type: \n- Hours used: \n- Cloud Provider: \n- Compute Region: \n- Carbon Emitted:",
"## Technical Specifications [optional]",
"### Model Architecture and Objective",
"### Compute Infrastructure",
"#### Hardware",
"#### Software\n\n\n\n[optional]\n\n\n\nBibTeX:\n\n\n\nAPA:",
"## Glossary [optional]",
"## More Information [optional]",
"## Model Card Authors [optional]",
"## Model Card Contact"
] | [
"TAGS\n#transformers #safetensors #unsloth #arxiv-1910.09700 #endpoints_compatible #region-us \n",
"# Model Card for Model ID",
"## Model Details",
"### Model Description\n\n\n\nThis is the model card of a transformers model that has been pushed on the Hub. This model card has been automatically generated.\n\n- Developed by: \n- Funded by [optional]: \n- Shared by [optional]: \n- Model type: \n- Language(s) (NLP): \n- License: \n- Finetuned from model [optional]:",
"### Model Sources [optional]\n\n\n\n- Repository: \n- Paper [optional]: \n- Demo [optional]:",
"## Uses",
"### Direct Use",
"### Downstream Use [optional]",
"### Out-of-Scope Use",
"## Bias, Risks, and Limitations",
"### Recommendations\n\n\n\nUsers (both direct and downstream) should be made aware of the risks, biases and limitations of the model. More information needed for further recommendations.",
"## How to Get Started with the Model\n\nUse the code below to get started with the model.",
"## Training Details",
"### Training Data",
"### Training Procedure",
"#### Preprocessing [optional]",
"#### Training Hyperparameters\n\n- Training regime:",
"#### Speeds, Sizes, Times [optional]",
"## Evaluation",
"### Testing Data, Factors & Metrics",
"#### Testing Data",
"#### Factors",
"#### Metrics",
"### Results",
"#### Summary",
"## Model Examination [optional]",
"## Environmental Impact\n\n\n\nCarbon emissions can be estimated using the Machine Learning Impact calculator presented in Lacoste et al. (2019).\n\n- Hardware Type: \n- Hours used: \n- Cloud Provider: \n- Compute Region: \n- Carbon Emitted:",
"## Technical Specifications [optional]",
"### Model Architecture and Objective",
"### Compute Infrastructure",
"#### Hardware",
"#### Software\n\n\n\n[optional]\n\n\n\nBibTeX:\n\n\n\nAPA:",
"## Glossary [optional]",
"## More Information [optional]",
"## Model Card Authors [optional]",
"## Model Card Contact"
] |
null | null |
This is a private model (txt)(Sorry as it is easy to extract from your own models..) but also Mistral Do sell access to thier embedding models ? I dont know why as it is in the actual 7b model or MOE models they released ?
QUESTION: Do these models contain bad embedding space as they have only been trained on random stuff and thier flagship models correctly trained on GOOD DATA, hence GuardRailing !
So just in case of ISSUES ~
MAKE A REQUEST (SORRY ALSO AS when i was uploading the files i told it to make a datasets repo but it made the normal ! so you will have top clone the repo then load_datset from Txt file:::
)
Sorry as i did download the file already and split the text file with (UltraEdit) so this is mainly just an archive (for me)
# Embeddings(32,000)
Embeddings are vectorial representations of text that capture the semantic meaning of paragraphs through their position in a high dimensional
vector space. Mistral AI Embeddings API offers cutting-edge,
state-of-the-art embeddings for text, which can be used for many NLP tasks.
In the realm of text embeddings, texts with similar meanings or context tend to be located in closer proximity to each other within this space, as measured by the distance between their vectors.
This is due to the fact that the model has learned to group semantically related texts together during the training process.
### ALSO ::::
By using a TinyLLM you can train the llm on a single task such as what are the embedding for this term !
ie train An LLM to provide the tensor for the embedding ! instead of extracting it !
## PERSONALLY :::
i have found that small slim tiny models SPecific for Purpose such as Dictionary provides a better result in downstreaming !
You may have your Super brain Query these models for such tasks !
So your instruction based CHAT! model - can stay the personality and its sub models (TINY TEAMS) can provide services such as auto agent:
Hence even coding task should be sublet to smaller agents providing only this funciton : for the discussion regarding the code use the llm and the code provider use the tiny !
# Extraction Processes :
As you know each model contains embeddings , here is how you can extract the mistral embeddings model (Table) to CSV :
In fact it tool a while to extract (at least 2hr)
## Large Filesize :
as this file was made in code ; it has only two colums :
1 The word :
2. the Assosiated Tensor !
It can be easier to LookUp an embedding from a File instead of extracting them from the model which is much slower and more resource intensive:
This file can be brokeni in to a split text file (1000) line eah making it easier to load into memory :
## (Embeddings are not alphabetically ordered!)
### Example Extraction Scrits
``` python
def lookup_embedding(file_path, term):
"""
Looks up the embedding for a given term in a text file.
Args:
file_path: The path to the text file containing term and embedding pairs.
term: The term to look up.
Returns:
A list of floats representing the embedding for the given term, or None if the term is not found.
"""
with open(file_path, 'r') as f:
for line in f:
line = line.strip()
if not line:
continue
parts = line.split()
if parts[0] == term:
return [float(x) for x in parts[1:]]
return "<unk>"
def Extract_Embedding_Vocab(model,tokenizer):
# Get the vocabulary tokens
vocab_tokens = tokenizer.get_vocab().keys()
# Convert vocabulary tokens to a list
vocab_tokens_list = list(vocab_tokens)
# Get the embeddings for each vocabulary token
embeddings_list = []
for token in vocab_tokens_list:
tokens = tokenizer(token, return_tensors="pt")
embeddings = model(**tokens).past_key_values[0][0].squeeze().tolist()
embeddings_list.append(embeddings)
# Save the vocabulary tokens and embeddings to a text file
with open(output_file_path, "w", encoding="utf-8") as output_file:
for token, embeddings in zip(vocab_tokens_list, embeddings_list):
# Write each line with token and its embedding values
output_file.write(token + "," + ",".join(map(str, embeddings)) + "\n")
output_file_path = "Mistral-7B-Instruct-v0.2_embeddings_with_vocab.txt"
print(f"Vocabulary tokens and embeddings saved to {output_file_path}")
def get_token_embedding(token,model,tokenizer):
"""
Returns the embedding for a given token using the specified BERT model.
Args:
token: A string representing the token.
Returns:
A list of floats representing the embedding.
"""
# Convert the token to its corresponding token ID
token_id = tokenizer.convert_tokens_to_ids(token)
# Create a tensor containing the token ID
input_ids = torch.tensor([token_id]).to(model.device)
# Get the model's output for the given token
model_output = model(input_ids=input_ids)
# Extract the embedding from the model's output
embedding = model_output.last_hidden_state[0][0].detach().numpy()
return embedding
def TaskGetEmbedingModel(model_name):
MODEL,TOKENIZER = LoadPretrained(model_name)
Extract_Embedding_Vocab(MODEL,TOKENIZER)
def TaskTestEmbeddingModel(model_name):
MODEL,TOKENIZER = LoadPretrained(model_name)
sample_tokens = ["the", "cat", "sat", "on", "the", "mat"]
for token in sample_tokens:
embedding = get_token_embedding(token)
print(f"Embedding for '{token}': {embedding}")
```
## mistral example for comarison of phrases :
``` python
OR YOUR COULD USE THE API and Pay For each extraction !
```python
from mistralai.client import MistralClient
api_key = os.environ["MISTRAL_API_KEY"]
client = MistralClient(api_key=api_key)
embeddings_batch_response = client.embeddings(
model="mistral-embed",
input=["Embed this sentence.", "As well as this one."],
)
```
```python
import itertools
sentences = [
"Have a safe happy Memorial Day weekend everyone",
"To all our friends at Whatsit Productions Films enjoy a safe happy Memorial Day weekend",
"Where can I find the best cheese?",
]
sentence_embeddings = [get_text_embedding(t) for t in sentences]
sentence_embeddings_pairs = list(itertools.combinations(sentence_embeddings, 2))
sentence_pairs = list(itertools.combinations(sentences, 2))
for s, e in zip(sentence_pairs, sentence_embeddings_pairs):
print(s, euclidean_distances([e[0]], [e[1]]))
``` | {"language": ["en"], "license": "apache-2.0", "tags": ["embeddings", "Mistral", "SpydazWebAI", "Embeds-to-CSV"]} | LeroyDyer/Mistral_Embeddings_CSV | null | [
"embeddings",
"Mistral",
"SpydazWebAI",
"Embeds-to-CSV",
"en",
"license:apache-2.0",
"region:us"
] | null | 2024-04-21T18:48:38+00:00 | [] | [
"en"
] | TAGS
#embeddings #Mistral #SpydazWebAI #Embeds-to-CSV #en #license-apache-2.0 #region-us
|
This is a private model (txt)(Sorry as it is easy to extract from your own models..) but also Mistral Do sell access to thier embedding models ? I dont know why as it is in the actual 7b model or MOE models they released ?
QUESTION: Do these models contain bad embedding space as they have only been trained on random stuff and thier flagship models correctly trained on GOOD DATA, hence GuardRailing !
So just in case of ISSUES ~
MAKE A REQUEST (SORRY ALSO AS when i was uploading the files i told it to make a datasets repo but it made the normal ! so you will have top clone the repo then load_datset from Txt file:::
)
Sorry as i did download the file already and split the text file with (UltraEdit) so this is mainly just an archive (for me)
# Embeddings(32,000)
Embeddings are vectorial representations of text that capture the semantic meaning of paragraphs through their position in a high dimensional
vector space. Mistral AI Embeddings API offers cutting-edge,
state-of-the-art embeddings for text, which can be used for many NLP tasks.
In the realm of text embeddings, texts with similar meanings or context tend to be located in closer proximity to each other within this space, as measured by the distance between their vectors.
This is due to the fact that the model has learned to group semantically related texts together during the training process.
### ALSO ::::
By using a TinyLLM you can train the llm on a single task such as what are the embedding for this term !
ie train An LLM to provide the tensor for the embedding ! instead of extracting it !
## PERSONALLY :::
i have found that small slim tiny models SPecific for Purpose such as Dictionary provides a better result in downstreaming !
You may have your Super brain Query these models for such tasks !
So your instruction based CHAT! model - can stay the personality and its sub models (TINY TEAMS) can provide services such as auto agent:
Hence even coding task should be sublet to smaller agents providing only this funciton : for the discussion regarding the code use the llm and the code provider use the tiny !
# Extraction Processes :
As you know each model contains embeddings , here is how you can extract the mistral embeddings model (Table) to CSV :
In fact it tool a while to extract (at least 2hr)
## Large Filesize :
as this file was made in code ; it has only two colums :
1 The word :
2. the Assosiated Tensor !
It can be easier to LookUp an embedding from a File instead of extracting them from the model which is much slower and more resource intensive:
This file can be brokeni in to a split text file (1000) line eah making it easier to load into memory :
## (Embeddings are not alphabetically ordered!)
### Example Extraction Scrits
## mistral example for comarison of phrases :
python
from URL import MistralClient
api_key = os.environ["MISTRAL_API_KEY"]
client = MistralClient(api_key=api_key)
embeddings_batch_response = client.embeddings(
model="mistral-embed",
input=["Embed this sentence.", "As well as this one."],
)
python
import itertools
sentences = [
"Have a safe happy Memorial Day weekend everyone",
"To all our friends at Whatsit Productions Films enjoy a safe happy Memorial Day weekend",
"Where can I find the best cheese?",
]
sentence_embeddings = [get_text_embedding(t) for t in sentences]
sentence_embeddings_pairs = list(itertools.combinations(sentence_embeddings, 2))
sentence_pairs = list(itertools.combinations(sentences, 2))
for s, e in zip(sentence_pairs, sentence_embeddings_pairs):
print(s, euclidean_distances([e[0]], [e[1]]))
''' | [
"# Embeddings(32,000)\nEmbeddings are vectorial representations of text that capture the semantic meaning of paragraphs through their position in a high dimensional \nvector space. Mistral AI Embeddings API offers cutting-edge, \nstate-of-the-art embeddings for text, which can be used for many NLP tasks. \nIn the realm of text embeddings, texts with similar meanings or context tend to be located in closer proximity to each other within this space, as measured by the distance between their vectors.\nThis is due to the fact that the model has learned to group semantically related texts together during the training process.",
"### ALSO ::::\n\nBy using a TinyLLM you can train the llm on a single task such as what are the embedding for this term ! \nie train An LLM to provide the tensor for the embedding ! instead of extracting it !",
"## PERSONALLY ::: \ni have found that small slim tiny models SPecific for Purpose such as Dictionary provides a better result in downstreaming !\nYou may have your Super brain Query these models for such tasks ! \nSo your instruction based CHAT! model - can stay the personality and its sub models (TINY TEAMS) can provide services such as auto agent:\nHence even coding task should be sublet to smaller agents providing only this funciton : for the discussion regarding the code use the llm and the code provider use the tiny !",
"# Extraction Processes :\n\nAs you know each model contains embeddings , here is how you can extract the mistral embeddings model (Table) to CSV : \nIn fact it tool a while to extract (at least 2hr)",
"## Large Filesize :\n\nas this file was made in code ; it has only two colums :\n\n1 The word : \n\n2. the Assosiated Tensor !\n\nIt can be easier to LookUp an embedding from a File instead of extracting them from the model which is much slower and more resource intensive: \nThis file can be brokeni in to a split text file (1000) line eah making it easier to load into memory :",
"## (Embeddings are not alphabetically ordered!)",
"### Example Extraction Scrits",
"## mistral example for comarison of phrases :\n\npython\n\nfrom URL import MistralClient\n\napi_key = os.environ[\"MISTRAL_API_KEY\"]\nclient = MistralClient(api_key=api_key)\n\nembeddings_batch_response = client.embeddings(\n model=\"mistral-embed\",\n input=[\"Embed this sentence.\", \"As well as this one.\"],\n )\n\npython\n\nimport itertools\n\nsentences = [\n \"Have a safe happy Memorial Day weekend everyone\",\n \"To all our friends at Whatsit Productions Films enjoy a safe happy Memorial Day weekend\",\n \"Where can I find the best cheese?\",\n]\n\nsentence_embeddings = [get_text_embedding(t) for t in sentences]\n\nsentence_embeddings_pairs = list(itertools.combinations(sentence_embeddings, 2))\nsentence_pairs = list(itertools.combinations(sentences, 2))\nfor s, e in zip(sentence_pairs, sentence_embeddings_pairs):\n print(s, euclidean_distances([e[0]], [e[1]]))\n\n'''"
] | [
"TAGS\n#embeddings #Mistral #SpydazWebAI #Embeds-to-CSV #en #license-apache-2.0 #region-us \n",
"# Embeddings(32,000)\nEmbeddings are vectorial representations of text that capture the semantic meaning of paragraphs through their position in a high dimensional \nvector space. Mistral AI Embeddings API offers cutting-edge, \nstate-of-the-art embeddings for text, which can be used for many NLP tasks. \nIn the realm of text embeddings, texts with similar meanings or context tend to be located in closer proximity to each other within this space, as measured by the distance between their vectors.\nThis is due to the fact that the model has learned to group semantically related texts together during the training process.",
"### ALSO ::::\n\nBy using a TinyLLM you can train the llm on a single task such as what are the embedding for this term ! \nie train An LLM to provide the tensor for the embedding ! instead of extracting it !",
"## PERSONALLY ::: \ni have found that small slim tiny models SPecific for Purpose such as Dictionary provides a better result in downstreaming !\nYou may have your Super brain Query these models for such tasks ! \nSo your instruction based CHAT! model - can stay the personality and its sub models (TINY TEAMS) can provide services such as auto agent:\nHence even coding task should be sublet to smaller agents providing only this funciton : for the discussion regarding the code use the llm and the code provider use the tiny !",
"# Extraction Processes :\n\nAs you know each model contains embeddings , here is how you can extract the mistral embeddings model (Table) to CSV : \nIn fact it tool a while to extract (at least 2hr)",
"## Large Filesize :\n\nas this file was made in code ; it has only two colums :\n\n1 The word : \n\n2. the Assosiated Tensor !\n\nIt can be easier to LookUp an embedding from a File instead of extracting them from the model which is much slower and more resource intensive: \nThis file can be brokeni in to a split text file (1000) line eah making it easier to load into memory :",
"## (Embeddings are not alphabetically ordered!)",
"### Example Extraction Scrits",
"## mistral example for comarison of phrases :\n\npython\n\nfrom URL import MistralClient\n\napi_key = os.environ[\"MISTRAL_API_KEY\"]\nclient = MistralClient(api_key=api_key)\n\nembeddings_batch_response = client.embeddings(\n model=\"mistral-embed\",\n input=[\"Embed this sentence.\", \"As well as this one.\"],\n )\n\npython\n\nimport itertools\n\nsentences = [\n \"Have a safe happy Memorial Day weekend everyone\",\n \"To all our friends at Whatsit Productions Films enjoy a safe happy Memorial Day weekend\",\n \"Where can I find the best cheese?\",\n]\n\nsentence_embeddings = [get_text_embedding(t) for t in sentences]\n\nsentence_embeddings_pairs = list(itertools.combinations(sentence_embeddings, 2))\nsentence_pairs = list(itertools.combinations(sentences, 2))\nfor s, e in zip(sentence_pairs, sentence_embeddings_pairs):\n print(s, euclidean_distances([e[0]], [e[1]]))\n\n'''"
] |
text-generation | transformers |
# Model Card for Model ID
<!-- Provide a quick summary of what the model is/does. -->
## Model Details
### Model Description
<!-- Provide a longer summary of what this model is. -->
This is the model card of a 🤗 transformers model that has been pushed on the Hub. This model card has been automatically generated.
- **Developed by:** [More Information Needed]
- **Funded by [optional]:** [More Information Needed]
- **Shared by [optional]:** [More Information Needed]
- **Model type:** [More Information Needed]
- **Language(s) (NLP):** [More Information Needed]
- **License:** [More Information Needed]
- **Finetuned from model [optional]:** [More Information Needed]
### Model Sources [optional]
<!-- Provide the basic links for the model. -->
- **Repository:** [More Information Needed]
- **Paper [optional]:** [More Information Needed]
- **Demo [optional]:** [More Information Needed]
## Uses
<!-- Address questions around how the model is intended to be used, including the foreseeable users of the model and those affected by the model. -->
### Direct Use
<!-- This section is for the model use without fine-tuning or plugging into a larger ecosystem/app. -->
[More Information Needed]
### Downstream Use [optional]
<!-- This section is for the model use when fine-tuned for a task, or when plugged into a larger ecosystem/app -->
[More Information Needed]
### Out-of-Scope Use
<!-- This section addresses misuse, malicious use, and uses that the model will not work well for. -->
[More Information Needed]
## Bias, Risks, and Limitations
<!-- This section is meant to convey both technical and sociotechnical limitations. -->
[More Information Needed]
### Recommendations
<!-- This section is meant to convey recommendations with respect to the bias, risk, and technical limitations. -->
Users (both direct and downstream) should be made aware of the risks, biases and limitations of the model. More information needed for further recommendations.
## How to Get Started with the Model
Use the code below to get started with the model.
[More Information Needed]
## Training Details
### Training Data
<!-- This should link to a Dataset Card, perhaps with a short stub of information on what the training data is all about as well as documentation related to data pre-processing or additional filtering. -->
[More Information Needed]
### Training Procedure
<!-- This relates heavily to the Technical Specifications. Content here should link to that section when it is relevant to the training procedure. -->
#### Preprocessing [optional]
[More Information Needed]
#### Training Hyperparameters
- **Training regime:** [More Information Needed] <!--fp32, fp16 mixed precision, bf16 mixed precision, bf16 non-mixed precision, fp16 non-mixed precision, fp8 mixed precision -->
#### Speeds, Sizes, Times [optional]
<!-- This section provides information about throughput, start/end time, checkpoint size if relevant, etc. -->
[More Information Needed]
## Evaluation
<!-- This section describes the evaluation protocols and provides the results. -->
### Testing Data, Factors & Metrics
#### Testing Data
<!-- This should link to a Dataset Card if possible. -->
[More Information Needed]
#### Factors
<!-- These are the things the evaluation is disaggregating by, e.g., subpopulations or domains. -->
[More Information Needed]
#### Metrics
<!-- These are the evaluation metrics being used, ideally with a description of why. -->
[More Information Needed]
### Results
[More Information Needed]
#### Summary
## Model Examination [optional]
<!-- Relevant interpretability work for the model goes here -->
[More Information Needed]
## Environmental Impact
<!-- Total emissions (in grams of CO2eq) and additional considerations, such as electricity usage, go here. Edit the suggested text below accordingly -->
Carbon emissions can be estimated using the [Machine Learning Impact calculator](https://mlco2.github.io/impact#compute) presented in [Lacoste et al. (2019)](https://arxiv.org/abs/1910.09700).
- **Hardware Type:** [More Information Needed]
- **Hours used:** [More Information Needed]
- **Cloud Provider:** [More Information Needed]
- **Compute Region:** [More Information Needed]
- **Carbon Emitted:** [More Information Needed]
## Technical Specifications [optional]
### Model Architecture and Objective
[More Information Needed]
### Compute Infrastructure
[More Information Needed]
#### Hardware
[More Information Needed]
#### Software
[More Information Needed]
## Citation [optional]
<!-- If there is a paper or blog post introducing the model, the APA and Bibtex information for that should go in this section. -->
**BibTeX:**
[More Information Needed]
**APA:**
[More Information Needed]
## Glossary [optional]
<!-- If relevant, include terms and calculations in this section that can help readers understand the model or model card. -->
[More Information Needed]
## More Information [optional]
[More Information Needed]
## Model Card Authors [optional]
[More Information Needed]
## Model Card Contact
[More Information Needed] | {"library_name": "transformers", "tags": []} | mohannadirshedat/gemma-medical_qa-Finetune | null | [
"transformers",
"safetensors",
"gemma",
"text-generation",
"conversational",
"arxiv:1910.09700",
"autotrain_compatible",
"endpoints_compatible",
"text-generation-inference",
"region:us"
] | null | 2024-04-21T18:49:05+00:00 | [
"1910.09700"
] | [] | TAGS
#transformers #safetensors #gemma #text-generation #conversational #arxiv-1910.09700 #autotrain_compatible #endpoints_compatible #text-generation-inference #region-us
|
# Model Card for Model ID
## Model Details
### Model Description
This is the model card of a transformers model that has been pushed on the Hub. This model card has been automatically generated.
- Developed by:
- Funded by [optional]:
- Shared by [optional]:
- Model type:
- Language(s) (NLP):
- License:
- Finetuned from model [optional]:
### Model Sources [optional]
- Repository:
- Paper [optional]:
- Demo [optional]:
## Uses
### Direct Use
### Downstream Use [optional]
### Out-of-Scope Use
## Bias, Risks, and Limitations
### Recommendations
Users (both direct and downstream) should be made aware of the risks, biases and limitations of the model. More information needed for further recommendations.
## How to Get Started with the Model
Use the code below to get started with the model.
## Training Details
### Training Data
### Training Procedure
#### Preprocessing [optional]
#### Training Hyperparameters
- Training regime:
#### Speeds, Sizes, Times [optional]
## Evaluation
### Testing Data, Factors & Metrics
#### Testing Data
#### Factors
#### Metrics
### Results
#### Summary
## Model Examination [optional]
## Environmental Impact
Carbon emissions can be estimated using the Machine Learning Impact calculator presented in Lacoste et al. (2019).
- Hardware Type:
- Hours used:
- Cloud Provider:
- Compute Region:
- Carbon Emitted:
## Technical Specifications [optional]
### Model Architecture and Objective
### Compute Infrastructure
#### Hardware
#### Software
[optional]
BibTeX:
APA:
## Glossary [optional]
## More Information [optional]
## Model Card Authors [optional]
## Model Card Contact
| [
"# Model Card for Model ID",
"## Model Details",
"### Model Description\n\n\n\nThis is the model card of a transformers model that has been pushed on the Hub. This model card has been automatically generated.\n\n- Developed by: \n- Funded by [optional]: \n- Shared by [optional]: \n- Model type: \n- Language(s) (NLP): \n- License: \n- Finetuned from model [optional]:",
"### Model Sources [optional]\n\n\n\n- Repository: \n- Paper [optional]: \n- Demo [optional]:",
"## Uses",
"### Direct Use",
"### Downstream Use [optional]",
"### Out-of-Scope Use",
"## Bias, Risks, and Limitations",
"### Recommendations\n\n\n\nUsers (both direct and downstream) should be made aware of the risks, biases and limitations of the model. More information needed for further recommendations.",
"## How to Get Started with the Model\n\nUse the code below to get started with the model.",
"## Training Details",
"### Training Data",
"### Training Procedure",
"#### Preprocessing [optional]",
"#### Training Hyperparameters\n\n- Training regime:",
"#### Speeds, Sizes, Times [optional]",
"## Evaluation",
"### Testing Data, Factors & Metrics",
"#### Testing Data",
"#### Factors",
"#### Metrics",
"### Results",
"#### Summary",
"## Model Examination [optional]",
"## Environmental Impact\n\n\n\nCarbon emissions can be estimated using the Machine Learning Impact calculator presented in Lacoste et al. (2019).\n\n- Hardware Type: \n- Hours used: \n- Cloud Provider: \n- Compute Region: \n- Carbon Emitted:",
"## Technical Specifications [optional]",
"### Model Architecture and Objective",
"### Compute Infrastructure",
"#### Hardware",
"#### Software\n\n\n\n[optional]\n\n\n\nBibTeX:\n\n\n\nAPA:",
"## Glossary [optional]",
"## More Information [optional]",
"## Model Card Authors [optional]",
"## Model Card Contact"
] | [
"TAGS\n#transformers #safetensors #gemma #text-generation #conversational #arxiv-1910.09700 #autotrain_compatible #endpoints_compatible #text-generation-inference #region-us \n",
"# Model Card for Model ID",
"## Model Details",
"### Model Description\n\n\n\nThis is the model card of a transformers model that has been pushed on the Hub. This model card has been automatically generated.\n\n- Developed by: \n- Funded by [optional]: \n- Shared by [optional]: \n- Model type: \n- Language(s) (NLP): \n- License: \n- Finetuned from model [optional]:",
"### Model Sources [optional]\n\n\n\n- Repository: \n- Paper [optional]: \n- Demo [optional]:",
"## Uses",
"### Direct Use",
"### Downstream Use [optional]",
"### Out-of-Scope Use",
"## Bias, Risks, and Limitations",
"### Recommendations\n\n\n\nUsers (both direct and downstream) should be made aware of the risks, biases and limitations of the model. More information needed for further recommendations.",
"## How to Get Started with the Model\n\nUse the code below to get started with the model.",
"## Training Details",
"### Training Data",
"### Training Procedure",
"#### Preprocessing [optional]",
"#### Training Hyperparameters\n\n- Training regime:",
"#### Speeds, Sizes, Times [optional]",
"## Evaluation",
"### Testing Data, Factors & Metrics",
"#### Testing Data",
"#### Factors",
"#### Metrics",
"### Results",
"#### Summary",
"## Model Examination [optional]",
"## Environmental Impact\n\n\n\nCarbon emissions can be estimated using the Machine Learning Impact calculator presented in Lacoste et al. (2019).\n\n- Hardware Type: \n- Hours used: \n- Cloud Provider: \n- Compute Region: \n- Carbon Emitted:",
"## Technical Specifications [optional]",
"### Model Architecture and Objective",
"### Compute Infrastructure",
"#### Hardware",
"#### Software\n\n\n\n[optional]\n\n\n\nBibTeX:\n\n\n\nAPA:",
"## Glossary [optional]",
"## More Information [optional]",
"## Model Card Authors [optional]",
"## Model Card Contact"
] |
text-generation | transformers | Quantization made by Richard Erkhov.
[Github](https://github.com/RichardErkhov)
[Discord](https://discord.gg/pvy7H8DZMG)
[Request more models](https://github.com/RichardErkhov/quant_request)
CodeLlama-34b-Instruct-hf - bnb 4bits
- Model creator: https://huggingface.co/meta-llama/
- Original model: https://huggingface.co/meta-llama/CodeLlama-34b-Instruct-hf/
Original model description:
---
extra_gated_heading: You need to share contact information with Meta to access this model
extra_gated_prompt: >-
### LLAMA 2 COMMUNITY LICENSE AGREEMENT
"Agreement" means the terms and conditions for use, reproduction, distribution
and modification of the Llama Materials set forth herein.
"Documentation" means the specifications, manuals and documentation
accompanying Llama 2 distributed by Meta at
https://ai.meta.com/resources/models-and-libraries/llama-downloads/.
"Licensee" or "you" means you, or your employer or any other person or entity
(if you are entering into this Agreement on such person or entity's behalf),
of the age required under applicable laws, rules or regulations to provide
legal consent and that has legal authority to bind your employer or such other
person or entity if you are entering in this Agreement on their behalf.
"Llama 2" means the foundational large language models and software and
algorithms, including machine-learning model code, trained model weights,
inference-enabling code, training-enabling code, fine-tuning enabling code and
other elements of the foregoing distributed by Meta at
ai.meta.com/resources/models-and-libraries/llama-downloads/.
"Llama Materials" means, collectively, Meta's proprietary Llama 2 and
documentation (and any portion thereof) made available under this Agreement.
"Meta" or "we" means Meta Platforms Ireland Limited (if you are located in or,
if you are an entity, your principal place of business is in the EEA or
Switzerland) and Meta Platforms, Inc. (if you are located outside of the EEA
or Switzerland).
By clicking "I Accept" below or by using or distributing any portion or
element of the Llama Materials, you agree to be bound by this Agreement.
1. License Rights and Redistribution.
a. Grant of Rights. You are granted a non-exclusive, worldwide, non-
transferable and royalty-free limited license under Meta's intellectual
property or other rights owned by Meta embodied in the Llama Materials to
use, reproduce, distribute, copy, create derivative works of, and make
modifications to the Llama Materials.
b. Redistribution and Use.
i. If you distribute or make the Llama Materials, or any derivative works
thereof, available to a third party, you shall provide a copy of this
Agreement to such third party.
ii. If you receive Llama Materials, or any derivative works thereof, from a
Licensee as part of an integrated end user product, then Section 2 of this
Agreement will not apply to you.
iii. You must retain in all copies of the Llama Materials that you distribute
the following attribution notice within a "Notice" text file distributed as a
part of such copies: "Llama 2 is licensed under the LLAMA 2 Community
License, Copyright (c) Meta Platforms, Inc. All Rights Reserved."
iv. Your use of the Llama Materials must comply with applicable laws and
regulations (including trade compliance laws and regulations) and adhere to
the Acceptable Use Policy for the Llama Materials (available at
https://ai.meta.com/llama/use-policy), which is hereby incorporated by
reference into this Agreement.
v. You will not use the Llama Materials or any output or results of the Llama
Materials to improve any other large language model (excluding Llama 2 or
derivative works thereof).
2. Additional Commercial Terms. If, on the Llama 2 version release date, the
monthly active users of the products or services made available by or for
Licensee, or Licensee's affiliates, is greater than 700 million monthly
active users in the preceding calendar month, you must request a license from
Meta, which Meta may grant to you in its sole discretion, and you are not
authorized to exercise any of the rights under this Agreement unless or until
Meta otherwise expressly grants you such rights.
3. Disclaimer of Warranty. UNLESS REQUIRED BY APPLICABLE LAW, THE LLAMA
MATERIALS AND ANY OUTPUT AND RESULTS THEREFROM ARE PROVIDED ON AN "AS IS"
BASIS, WITHOUT WARRANTIES OF ANY KIND, EITHER EXPRESS OR IMPLIED, INCLUDING,
WITHOUT LIMITATION, ANY WARRANTIES OF TITLE, NON-INFRINGEMENT,
MERCHANTABILITY, OR FITNESS FOR A PARTICULAR PURPOSE. YOU ARE SOLELY
RESPONSIBLE FOR DETERMINING THE APPROPRIATENESS OF USING OR REDISTRIBUTING
THE LLAMA MATERIALS AND ASSUME ANY RISKS ASSOCIATED WITH YOUR USE OF THE
LLAMA MATERIALS AND ANY OUTPUT AND RESULTS.
4. Limitation of Liability. IN NO EVENT WILL META OR ITS AFFILIATES BE LIABLE
UNDER ANY THEORY OF LIABILITY, WHETHER IN CONTRACT, TORT, NEGLIGENCE,
PRODUCTS LIABILITY, OR OTHERWISE, ARISING OUT OF THIS AGREEMENT, FOR ANY LOST
PROFITS OR ANY INDIRECT, SPECIAL, CONSEQUENTIAL, INCIDENTAL, EXEMPLARY OR
PUNITIVE DAMAGES, EVEN IF META OR ITS AFFILIATES HAVE BEEN ADVISED OF THE
POSSIBILITY OF ANY OF THE FOREGOING.
5. Intellectual Property.
a. No trademark licenses are granted under this Agreement, and in connection
with the Llama Materials, neither Meta nor Licensee may use any name or mark
owned by or associated with the other or any of its affiliates, except as
required for reasonable and customary use in describing and redistributing
the Llama Materials.
b. Subject to Meta's ownership of Llama Materials and derivatives made by or
for Meta, with respect to any derivative works and modifications of the Llama
Materials that are made by you, as between you and Meta, you are and will be
the owner of such derivative works and modifications.
c. If you institute litigation or other proceedings against Meta or any
entity (including a cross-claim or counterclaim in a lawsuit) alleging that
the Llama Materials or Llama 2 outputs or results, or any portion of any of
the foregoing, constitutes infringement of intellectual property or other
rights owned or licensable by you, then any licenses granted to you under
this Agreement shall terminate as of the date such litigation or claim is
filed or instituted. You will indemnify and hold harmless Meta from and
against any claim by any third party arising out of or related to your use or
distribution of the Llama Materials.
6. Term and Termination. The term of this Agreement will commence upon your
acceptance of this Agreement or access to the Llama Materials and will
continue in full force and effect until terminated in accordance with the
terms and conditions herein. Meta may terminate this Agreement if you are in
breach of any term or condition of this Agreement. Upon termination of this
Agreement, you shall delete and cease use of the Llama Materials. Sections 3,
4 and 7 shall survive the termination of this Agreement.
7. Governing Law and Jurisdiction. This Agreement will be governed and
construed under the laws of the State of California without regard to choice
of law principles, and the UN Convention on Contracts for the International
Sale of Goods does not apply to this Agreement. The courts of California
shall have exclusive jurisdiction of any dispute arising out of this
Agreement.
USE POLICY
### Llama 2 Acceptable Use Policy
Meta is committed to promoting safe and fair use of its tools and features,
including Llama 2. If you access or use Llama 2, you agree to this Acceptable
Use Policy (“Policy”). The most recent copy of this policy can be found at
[ai.meta.com/llama/use-policy](http://ai.meta.com/llama/use-policy).
#### Prohibited Uses
We want everyone to use Llama 2 safely and responsibly. You agree you will not
use, or allow others to use, Llama 2 to:
1. Violate the law or others’ rights, including to:
1. Engage in, promote, generate, contribute to, encourage, plan, incite, or further illegal or unlawful activity or content, such as:
1. Violence or terrorism
2. Exploitation or harm to children, including the solicitation, creation, acquisition, or dissemination of child exploitative content or failure to report Child Sexual Abuse Material
3. Human trafficking, exploitation, and sexual violence
4. The illegal distribution of information or materials to minors, including obscene materials, or failure to employ legally required age-gating in connection with such information or materials.
5. Sexual solicitation
6. Any other criminal activity
2. Engage in, promote, incite, or facilitate the harassment, abuse, threatening, or bullying of individuals or groups of individuals
3. Engage in, promote, incite, or facilitate discrimination or other unlawful or harmful conduct in the provision of employment, employment benefits, credit, housing, other economic benefits, or other essential goods and services
4. Engage in the unauthorized or unlicensed practice of any profession including, but not limited to, financial, legal, medical/health, or related professional practices
5. Collect, process, disclose, generate, or infer health, demographic, or other sensitive personal or private information about individuals without rights and consents required by applicable laws
6. Engage in or facilitate any action or generate any content that infringes, misappropriates, or otherwise violates any third-party rights, including the outputs or results of any products or services using the Llama 2 Materials
7. Create, generate, or facilitate the creation of malicious code, malware, computer viruses or do anything else that could disable, overburden, interfere with or impair the proper working, integrity, operation or appearance of a website or computer system
2. Engage in, promote, incite, facilitate, or assist in the planning or
development of activities that present a risk of death or bodily harm to
individuals, including use of Llama 2 related to the following:
1. Military, warfare, nuclear industries or applications, espionage, use for materials or activities that are subject to the International Traffic Arms Regulations (ITAR) maintained by the United States Department of State
2. Guns and illegal weapons (including weapon development)
3. Illegal drugs and regulated/controlled substances
4. Operation of critical infrastructure, transportation technologies, or heavy machinery
5. Self-harm or harm to others, including suicide, cutting, and eating disorders
6. Any content intended to incite or promote violence, abuse, or any infliction of bodily harm to an individual
3. Intentionally deceive or mislead others, including use of Llama 2 related
to the following:
1. Generating, promoting, or furthering fraud or the creation or promotion of disinformation
2. Generating, promoting, or furthering defamatory content, including the creation of defamatory statements, images, or other content
3. Generating, promoting, or further distributing spam
4. Impersonating another individual without consent, authorization, or legal right
5. Representing that the use of Llama 2 or outputs are human-generated
6. Generating or facilitating false online engagement, including fake reviews and other means of fake online engagement
4. Fail to appropriately disclose to end users any known dangers of your AI system
Please report any violation of this Policy, software “bug,” or other problems
that could lead to a violation of this Policy through one of the following
means:
* Reporting issues with the model:
[github.com/facebookresearch/llama](http://github.com/facebookresearch/llama)
* Reporting risky content generated by the model:
[developers.facebook.com/llama_output_feedback](http://developers.facebook.com/llama_output_feedback)
* Reporting bugs and security concerns:
[facebook.com/whitehat/info](http://facebook.com/whitehat/info)
* Reporting violations of the Acceptable Use Policy or unlicensed uses of
Llama: [[email protected]](mailto:[email protected])
extra_gated_fields:
First Name: text
Last Name: text
Date of birth: date_picker
Country: country
Affiliation: text
geo: ip_location
By clicking Submit below I accept the terms of the license and acknowledge that the information I provide will be collected stored processed and shared in accordance with the Meta Privacy Policy: checkbox
extra_gated_description: The information you provide will be collected, stored, processed and shared in accordance with the [Meta Privacy Policy](https://www.facebook.com/privacy/policy/).
extra_gated_button_content: Submit
language:
- code
pipeline_tag: text-generation
tags:
- facebook
- meta
- pytorch
- llama
- llama-2
license: llama2
---
# **Code Llama**
Code Llama is a collection of pretrained and fine-tuned generative text models ranging in scale from 7 billion to 34 billion parameters. This is the repository for the 34B instruct-tuned version in the Hugging Face Transformers format. This model is designed for general code synthesis and understanding. Links to other models can be found in the index at the bottom.
| | Base Model | Python | Instruct |
| --- | ----------------------------------------------------------------------------- | ------------------------------------------------------------------------------------------- | ----------------------------------------------------------------------------------------------- |
| 7B | [meta-llama/CodeLlama-7b-hf](https://huggingface.co/meta-llama/CodeLlama-7b-hf) | [meta-llama/CodeLlama-7b-Python-hf](https://huggingface.co/meta-llama/CodeLlama-7b-Python-hf) | [meta-llama/CodeLlama-7b-Instruct-hf](https://huggingface.co/meta-llama/CodeLlama-7b-Instruct-hf) |
| 13B | [meta-llama/CodeLlama-13b-hf](https://huggingface.co/meta-llama/CodeLlama-13b-hf) | [meta-llama/CodeLlama-13b-Python-hf](https://huggingface.co/meta-llama/CodeLlama-13b-Python-hf) | [meta-llama/CodeLlama-13b-Instruct-hf](https://huggingface.co/meta-llama/CodeLlama-13b-Instruct-hf) |
| 34B | [meta-llama/CodeLlama-34b-hf](https://huggingface.co/meta-llama/CodeLlama-34b-hf) | [meta-llama/CodeLlama-34b-Python-hf](https://huggingface.co/meta-llama/CodeLlama-34b-Python-hf) | [meta-llama/CodeLlama-34b-Instruct-hf](https://huggingface.co/meta-llama/CodeLlama-34b-Instruct-hf) |
| 70B | [meta-llama/CodeLlama-70b-hf](https://huggingface.co/meta-llama/CodeLlama-70b-hf) | [meta-llama/CodeLlama-70b-Python-hf](https://huggingface.co/meta-llama/CodeLlama-70b-Python-hf) | [meta-llama/CodeLlama-70b-Instruct-hf](https://huggingface.co/meta-llama/CodeLlama-70b-Instruct-hf) |
## Model Use
To use this model, please make sure to install transformers:
```bash
pip install transformers accelerate
```
Model capabilities:
- [x] Code completion.
- [ ] Infilling.
- [x] Instructions / chat.
- [ ] Python specialist.
## Model Details
*Note: Use of this model is governed by the Meta license. Meta developed and publicly released the Code Llama family of large language models (LLMs).
**Model Developers** Meta
**Variations** Code Llama comes in three model sizes, and three variants:
* Code Llama: base models designed for general code synthesis and understanding
* Code Llama - Python: designed specifically for Python
* Code Llama - Instruct: for instruction following and safer deployment
All variants are available in sizes of 7B, 13B and 34B parameters.
**This repository contains the Instruct version of the 34B parameters model.**
**Input** Models input text only.
**Output** Models generate text only.
**Model Architecture** Code Llama is an auto-regressive language model that uses an optimized transformer architecture.
**Model Dates** Code Llama and its variants have been trained between January 2023 and July 2023.
**Status** This is a static model trained on an offline dataset. Future versions of Code Llama - Instruct will be released as we improve model safety with community feedback.
**License** A custom commercial license is available at: [https://ai.meta.com/resources/models-and-libraries/llama-downloads/](https://ai.meta.com/resources/models-and-libraries/llama-downloads/)
**Research Paper** More information can be found in the paper "[Code Llama: Open Foundation Models for Code](https://ai.meta.com/research/publications/code-llama-open-foundation-models-for-code/)" or its [arXiv page](https://arxiv.org/abs/2308.12950).
## Intended Use
**Intended Use Cases** Code Llama and its variants is intended for commercial and research use in English and relevant programming languages. The base model Code Llama can be adapted for a variety of code synthesis and understanding tasks, Code Llama - Python is designed specifically to handle the Python programming language, and Code Llama - Instruct is intended to be safer to use for code assistant and generation applications.
**Out-of-Scope Uses** Use in any manner that violates applicable laws or regulations (including trade compliance laws). Use in languages other than English. Use in any other way that is prohibited by the Acceptable Use Policy and Licensing Agreement for Code Llama and its variants.
## Hardware and Software
**Training Factors** We used custom training libraries. The training and fine-tuning of the released models have been performed Meta’s Research Super Cluster.
**Carbon Footprint** In aggregate, training all 9 Code Llama models required 400K GPU hours of computation on hardware of type A100-80GB (TDP of 350-400W). Estimated total emissions were 65.3 tCO2eq, 100% of which were offset by Meta’s sustainability program.
## Training Data
All experiments reported here and the released models have been trained and fine-tuned using the same data as Llama 2 with different weights (see Section 2 and Table 1 in the [research paper](https://ai.meta.com/research/publications/code-llama-open-foundation-models-for-code/) for details).
## Evaluation Results
See evaluations for the main models and detailed ablations in Section 3 and safety evaluations in Section 4 of the research paper.
## Ethical Considerations and Limitations
Code Llama and its variants are a new technology that carries risks with use. Testing conducted to date has been in English, and has not covered, nor could it cover all scenarios. For these reasons, as with all LLMs, Code Llama’s potential outputs cannot be predicted in advance, and the model may in some instances produce inaccurate or objectionable responses to user prompts. Therefore, before deploying any applications of Code Llama, developers should perform safety testing and tuning tailored to their specific applications of the model.
Please see the Responsible Use Guide available available at [https://ai.meta.com/llama/responsible-use-guide](https://ai.meta.com/llama/responsible-use-guide).
| {} | RichardErkhov/meta-llama_-_CodeLlama-34b-Instruct-hf-4bits | null | [
"transformers",
"safetensors",
"llama",
"text-generation",
"conversational",
"arxiv:2308.12950",
"autotrain_compatible",
"endpoints_compatible",
"text-generation-inference",
"4-bit",
"region:us"
] | null | 2024-04-21T18:50:12+00:00 | [
"2308.12950"
] | [] | TAGS
#transformers #safetensors #llama #text-generation #conversational #arxiv-2308.12950 #autotrain_compatible #endpoints_compatible #text-generation-inference #4-bit #region-us
| Quantization made by Richard Erkhov.
Github
Discord
Request more models
CodeLlama-34b-Instruct-hf - bnb 4bits
* Model creator: URL
* Original model: URL
Original model description:
---------------------------
extra\_gated\_heading: You need to share contact information with Meta to access this model
extra\_gated\_prompt: >-
### LLAMA 2 COMMUNITY LICENSE AGREEMENT
"Agreement" means the terms and conditions for use, reproduction, distribution
and modification of the Llama Materials set forth herein.
"Documentation" means the specifications, manuals and documentation
accompanying Llama 2 distributed by Meta at
URL
"Licensee" or "you" means you, or your employer or any other person or entity
(if you are entering into this Agreement on such person or entity's behalf),
of the age required under applicable laws, rules or regulations to provide
legal consent and that has legal authority to bind your employer or such other
person or entity if you are entering in this Agreement on their behalf.
"Llama 2" means the foundational large language models and software and
algorithms, including machine-learning model code, trained model weights,
inference-enabling code, training-enabling code, fine-tuning enabling code and
other elements of the foregoing distributed by Meta at
URL
"Llama Materials" means, collectively, Meta's proprietary Llama 2 and
documentation (and any portion thereof) made available under this Agreement.
"Meta" or "we" means Meta Platforms Ireland Limited (if you are located in or,
if you are an entity, your principal place of business is in the EEA or
Switzerland) and Meta Platforms, Inc. (if you are located outside of the EEA
or Switzerland).
By clicking "I Accept" below or by using or distributing any portion or
element of the Llama Materials, you agree to be bound by this Agreement.
1. License Rights and Redistribution.
a. Grant of Rights. You are granted a non-exclusive, worldwide, non-
transferable and royalty-free limited license under Meta's intellectual
property or other rights owned by Meta embodied in the Llama Materials to
use, reproduce, distribute, copy, create derivative works of, and make
modifications to the Llama Materials.
b. Redistribution and Use.
i. If you distribute or make the Llama Materials, or any derivative works
thereof, available to a third party, you shall provide a copy of this
Agreement to such third party.
ii. If you receive Llama Materials, or any derivative works thereof, from a
Licensee as part of an integrated end user product, then Section 2 of this
Agreement will not apply to you.
iii. You must retain in all copies of the Llama Materials that you distribute
the following attribution notice within a "Notice" text file distributed as a
part of such copies: "Llama 2 is licensed under the LLAMA 2 Community
License, Copyright (c) Meta Platforms, Inc. All Rights Reserved."
iv. Your use of the Llama Materials must comply with applicable laws and
regulations (including trade compliance laws and regulations) and adhere to
the Acceptable Use Policy for the Llama Materials (available at
URL which is hereby incorporated by
reference into this Agreement.
v. You will not use the Llama Materials or any output or results of the Llama
Materials to improve any other large language model (excluding Llama 2 or
derivative works thereof).
2. Additional Commercial Terms. If, on the Llama 2 version release date, the
monthly active users of the products or services made available by or for
Licensee, or Licensee's affiliates, is greater than 700 million monthly
active users in the preceding calendar month, you must request a license from
Meta, which Meta may grant to you in its sole discretion, and you are not
authorized to exercise any of the rights under this Agreement unless or until
Meta otherwise expressly grants you such rights.
3. Disclaimer of Warranty. UNLESS REQUIRED BY APPLICABLE LAW, THE LLAMA
MATERIALS AND ANY OUTPUT AND RESULTS THEREFROM ARE PROVIDED ON AN "AS IS"
BASIS, WITHOUT WARRANTIES OF ANY KIND, EITHER EXPRESS OR IMPLIED, INCLUDING,
WITHOUT LIMITATION, ANY WARRANTIES OF TITLE, NON-INFRINGEMENT,
MERCHANTABILITY, OR FITNESS FOR A PARTICULAR PURPOSE. YOU ARE SOLELY
RESPONSIBLE FOR DETERMINING THE APPROPRIATENESS OF USING OR REDISTRIBUTING
THE LLAMA MATERIALS AND ASSUME ANY RISKS ASSOCIATED WITH YOUR USE OF THE
LLAMA MATERIALS AND ANY OUTPUT AND RESULTS.
4. Limitation of Liability. IN NO EVENT WILL META OR ITS AFFILIATES BE LIABLE
UNDER ANY THEORY OF LIABILITY, WHETHER IN CONTRACT, TORT, NEGLIGENCE,
PRODUCTS LIABILITY, OR OTHERWISE, ARISING OUT OF THIS AGREEMENT, FOR ANY LOST
PROFITS OR ANY INDIRECT, SPECIAL, CONSEQUENTIAL, INCIDENTAL, EXEMPLARY OR
PUNITIVE DAMAGES, EVEN IF META OR ITS AFFILIATES HAVE BEEN ADVISED OF THE
POSSIBILITY OF ANY OF THE FOREGOING.
5. Intellectual Property.
a. No trademark licenses are granted under this Agreement, and in connection
with the Llama Materials, neither Meta nor Licensee may use any name or mark
owned by or associated with the other or any of its affiliates, except as
required for reasonable and customary use in describing and redistributing
the Llama Materials.
b. Subject to Meta's ownership of Llama Materials and derivatives made by or
for Meta, with respect to any derivative works and modifications of the Llama
Materials that are made by you, as between you and Meta, you are and will be
the owner of such derivative works and modifications.
c. If you institute litigation or other proceedings against Meta or any
entity (including a cross-claim or counterclaim in a lawsuit) alleging that
the Llama Materials or Llama 2 outputs or results, or any portion of any of
the foregoing, constitutes infringement of intellectual property or other
rights owned or licensable by you, then any licenses granted to you under
this Agreement shall terminate as of the date such litigation or claim is
filed or instituted. You will indemnify and hold harmless Meta from and
against any claim by any third party arising out of or related to your use or
distribution of the Llama Materials.
6. Term and Termination. The term of this Agreement will commence upon your
acceptance of this Agreement or access to the Llama Materials and will
continue in full force and effect until terminated in accordance with the
terms and conditions herein. Meta may terminate this Agreement if you are in
breach of any term or condition of this Agreement. Upon termination of this
Agreement, you shall delete and cease use of the Llama Materials. Sections 3,
4 and 7 shall survive the termination of this Agreement.
7. Governing Law and Jurisdiction. This Agreement will be governed and
construed under the laws of the State of California without regard to choice
of law principles, and the UN Convention on Contracts for the International
Sale of Goods does not apply to this Agreement. The courts of California
shall have exclusive jurisdiction of any dispute arising out of this
Agreement.
USE POLICY
### Llama 2 Acceptable Use Policy
Meta is committed to promoting safe and fair use of its tools and features,
including Llama 2. If you access or use Llama 2, you agree to this Acceptable
Use Policy (“Policy”). The most recent copy of this policy can be found at
URL
#### Prohibited Uses
We want everyone to use Llama 2 safely and responsibly. You agree you will not
use, or allow others to use, Llama 2 to:
1. Violate the law or others’ rights, including to:
1. Engage in, promote, generate, contribute to, encourage, plan, incite, or further illegal or unlawful activity or content, such as:
1. Violence or terrorism
2. Exploitation or harm to children, including the solicitation, creation, acquisition, or dissemination of child exploitative content or failure to report Child Sexual Abuse Material
3. Human trafficking, exploitation, and sexual violence
4. The illegal distribution of information or materials to minors, including obscene materials, or failure to employ legally required age-gating in connection with such information or materials.
5. Sexual solicitation
6. Any other criminal activity
```
2. Engage in, promote, incite, or facilitate the harassment, abuse, threatening, or bullying of individuals or groups of individuals
3. Engage in, promote, incite, or facilitate discrimination or other unlawful or harmful conduct in the provision of employment, employment benefits, credit, housing, other economic benefits, or other essential goods and services
4. Engage in the unauthorized or unlicensed practice of any profession including, but not limited to, financial, legal, medical/health, or related professional practices
5. Collect, process, disclose, generate, or infer health, demographic, or other sensitive personal or private information about individuals without rights and consents required by applicable laws
6. Engage in or facilitate any action or generate any content that infringes, misappropriates, or otherwise violates any third-party rights, including the outputs or results of any products or services using the Llama 2 Materials
7. Create, generate, or facilitate the creation of malicious code, malware, computer viruses or do anything else that could disable, overburden, interfere with or impair the proper working, integrity, operation or appearance of a website or computer system
```
2. Engage in, promote, incite, facilitate, or assist in the planning or
development of activities that present a risk of death or bodily harm to
individuals, including use of Llama 2 related to the following:
1. Military, warfare, nuclear industries or applications, espionage, use for materials or activities that are subject to the International Traffic Arms Regulations (ITAR) maintained by the United States Department of State
2. Guns and illegal weapons (including weapon development)
3. Illegal drugs and regulated/controlled substances
4. Operation of critical infrastructure, transportation technologies, or heavy machinery
5. Self-harm or harm to others, including suicide, cutting, and eating disorders
6. Any content intended to incite or promote violence, abuse, or any infliction of bodily harm to an individual
3. Intentionally deceive or mislead others, including use of Llama 2 related
to the following:
1. Generating, promoting, or furthering fraud or the creation or promotion of disinformation
2. Generating, promoting, or furthering defamatory content, including the creation of defamatory statements, images, or other content
3. Generating, promoting, or further distributing spam
4. Impersonating another individual without consent, authorization, or legal right
5. Representing that the use of Llama 2 or outputs are human-generated
6. Generating or facilitating false online engagement, including fake reviews and other means of fake online engagement
4. Fail to appropriately disclose to end users any known dangers of your AI system
Please report any violation of this Policy, software “bug,” or other problems
that could lead to a violation of this Policy through one of the following
means:
* Reporting issues with the model:
URL
* Reporting risky content generated by the model:
URL
* Reporting bugs and security concerns:
URL
* Reporting violations of the Acceptable Use Policy or unlicensed uses of
Llama: LlamaUseReport@URL
extra\_gated\_fields:
First Name: text
Last Name: text
Date of birth: date\_picker
Country: country
Affiliation: text
geo: ip\_location
By clicking Submit below I accept the terms of the license and acknowledge that the information I provide will be collected stored processed and shared in accordance with the Meta Privacy Policy: checkbox
extra\_gated\_description: The information you provide will be collected, stored, processed and shared in accordance with the Meta Privacy Policy.
extra\_gated\_button\_content: Submit
language:
* code
pipeline\_tag: text-generation
tags:
* facebook
* meta
* pytorch
* llama
* llama-2
license: llama2
---
Code Llama
==========
Code Llama is a collection of pretrained and fine-tuned generative text models ranging in scale from 7 billion to 34 billion parameters. This is the repository for the 34B instruct-tuned version in the Hugging Face Transformers format. This model is designed for general code synthesis and understanding. Links to other models can be found in the index at the bottom.
Model Use
---------
To use this model, please make sure to install transformers:
Model capabilities:
* [x] Code completion.
* [ ] Infilling.
* [x] Instructions / chat.
* [ ] Python specialist.
Model Details
-------------
\*Note: Use of this model is governed by the Meta license. Meta developed and publicly released the Code Llama family of large language models (LLMs).
Model Developers Meta
Variations Code Llama comes in three model sizes, and three variants:
* Code Llama: base models designed for general code synthesis and understanding
* Code Llama - Python: designed specifically for Python
* Code Llama - Instruct: for instruction following and safer deployment
All variants are available in sizes of 7B, 13B and 34B parameters.
This repository contains the Instruct version of the 34B parameters model.
Input Models input text only.
Output Models generate text only.
Model Architecture Code Llama is an auto-regressive language model that uses an optimized transformer architecture.
Model Dates Code Llama and its variants have been trained between January 2023 and July 2023.
Status This is a static model trained on an offline dataset. Future versions of Code Llama - Instruct will be released as we improve model safety with community feedback.
License A custom commercial license is available at: URL
Research Paper More information can be found in the paper "Code Llama: Open Foundation Models for Code" or its arXiv page.
Intended Use
------------
Intended Use Cases Code Llama and its variants is intended for commercial and research use in English and relevant programming languages. The base model Code Llama can be adapted for a variety of code synthesis and understanding tasks, Code Llama - Python is designed specifically to handle the Python programming language, and Code Llama - Instruct is intended to be safer to use for code assistant and generation applications.
Out-of-Scope Uses Use in any manner that violates applicable laws or regulations (including trade compliance laws). Use in languages other than English. Use in any other way that is prohibited by the Acceptable Use Policy and Licensing Agreement for Code Llama and its variants.
Hardware and Software
---------------------
Training Factors We used custom training libraries. The training and fine-tuning of the released models have been performed Meta’s Research Super Cluster.
Carbon Footprint In aggregate, training all 9 Code Llama models required 400K GPU hours of computation on hardware of type A100-80GB (TDP of 350-400W). Estimated total emissions were 65.3 tCO2eq, 100% of which were offset by Meta’s sustainability program.
Training Data
-------------
All experiments reported here and the released models have been trained and fine-tuned using the same data as Llama 2 with different weights (see Section 2 and Table 1 in the research paper for details).
Evaluation Results
------------------
See evaluations for the main models and detailed ablations in Section 3 and safety evaluations in Section 4 of the research paper.
Ethical Considerations and Limitations
--------------------------------------
Code Llama and its variants are a new technology that carries risks with use. Testing conducted to date has been in English, and has not covered, nor could it cover all scenarios. For these reasons, as with all LLMs, Code Llama’s potential outputs cannot be predicted in advance, and the model may in some instances produce inaccurate or objectionable responses to user prompts. Therefore, before deploying any applications of Code Llama, developers should perform safety testing and tuning tailored to their specific applications of the model.
Please see the Responsible Use Guide available available at URL
| [
"### LLAMA 2 COMMUNITY LICENSE AGREEMENT\n\n\n\"Agreement\" means the terms and conditions for use, reproduction, distribution\nand modification of the Llama Materials set forth herein.\n\"Documentation\" means the specifications, manuals and documentation\naccompanying Llama 2 distributed by Meta at\nURL \n\n\"Licensee\" or \"you\" means you, or your employer or any other person or entity\n(if you are entering into this Agreement on such person or entity's behalf),\nof the age required under applicable laws, rules or regulations to provide\nlegal consent and that has legal authority to bind your employer or such other\nperson or entity if you are entering in this Agreement on their behalf.\n\"Llama 2\" means the foundational large language models and software and\nalgorithms, including machine-learning model code, trained model weights,\ninference-enabling code, training-enabling code, fine-tuning enabling code and\nother elements of the foregoing distributed by Meta at\nURL\n\"Llama Materials\" means, collectively, Meta's proprietary Llama 2 and\ndocumentation (and any portion thereof) made available under this Agreement.\n\"Meta\" or \"we\" means Meta Platforms Ireland Limited (if you are located in or,\nif you are an entity, your principal place of business is in the EEA or\nSwitzerland) and Meta Platforms, Inc. (if you are located outside of the EEA\nor Switzerland).\nBy clicking \"I Accept\" below or by using or distributing any portion or\nelement of the Llama Materials, you agree to be bound by this Agreement.\n\n\n1. License Rights and Redistribution.\na. Grant of Rights. You are granted a non-exclusive, worldwide, non-\ntransferable and royalty-free limited license under Meta's intellectual\nproperty or other rights owned by Meta embodied in the Llama Materials to\nuse, reproduce, distribute, copy, create derivative works of, and make\nmodifications to the Llama Materials.\n\n\nb. Redistribution and Use. \n\ni. If you distribute or make the Llama Materials, or any derivative works\nthereof, available to a third party, you shall provide a copy of this\nAgreement to such third party.\nii. If you receive Llama Materials, or any derivative works thereof, from a\nLicensee as part of an integrated end user product, then Section 2 of this\nAgreement will not apply to you.\niii. You must retain in all copies of the Llama Materials that you distribute\nthe following attribution notice within a \"Notice\" text file distributed as a\npart of such copies: \"Llama 2 is licensed under the LLAMA 2 Community\nLicense, Copyright (c) Meta Platforms, Inc. All Rights Reserved.\"\niv. Your use of the Llama Materials must comply with applicable laws and\nregulations (including trade compliance laws and regulations) and adhere to\nthe Acceptable Use Policy for the Llama Materials (available at\nURL which is hereby incorporated by\nreference into this Agreement.\nv. You will not use the Llama Materials or any output or results of the Llama\nMaterials to improve any other large language model (excluding Llama 2 or\nderivative works thereof). \n\n2. Additional Commercial Terms. If, on the Llama 2 version release date, the\nmonthly active users of the products or services made available by or for\nLicensee, or Licensee's affiliates, is greater than 700 million monthly\nactive users in the preceding calendar month, you must request a license from\nMeta, which Meta may grant to you in its sole discretion, and you are not\nauthorized to exercise any of the rights under this Agreement unless or until\nMeta otherwise expressly grants you such rights.\n3. Disclaimer of Warranty. UNLESS REQUIRED BY APPLICABLE LAW, THE LLAMA\nMATERIALS AND ANY OUTPUT AND RESULTS THEREFROM ARE PROVIDED ON AN \"AS IS\"\nBASIS, WITHOUT WARRANTIES OF ANY KIND, EITHER EXPRESS OR IMPLIED, INCLUDING,\nWITHOUT LIMITATION, ANY WARRANTIES OF TITLE, NON-INFRINGEMENT,\nMERCHANTABILITY, OR FITNESS FOR A PARTICULAR PURPOSE. YOU ARE SOLELY\nRESPONSIBLE FOR DETERMINING THE APPROPRIATENESS OF USING OR REDISTRIBUTING\nTHE LLAMA MATERIALS AND ASSUME ANY RISKS ASSOCIATED WITH YOUR USE OF THE\nLLAMA MATERIALS AND ANY OUTPUT AND RESULTS.\n4. Limitation of Liability. IN NO EVENT WILL META OR ITS AFFILIATES BE LIABLE\nUNDER ANY THEORY OF LIABILITY, WHETHER IN CONTRACT, TORT, NEGLIGENCE,\nPRODUCTS LIABILITY, OR OTHERWISE, ARISING OUT OF THIS AGREEMENT, FOR ANY LOST\nPROFITS OR ANY INDIRECT, SPECIAL, CONSEQUENTIAL, INCIDENTAL, EXEMPLARY OR\nPUNITIVE DAMAGES, EVEN IF META OR ITS AFFILIATES HAVE BEEN ADVISED OF THE\nPOSSIBILITY OF ANY OF THE FOREGOING.\n5. Intellectual Property.\na. No trademark licenses are granted under this Agreement, and in connection\nwith the Llama Materials, neither Meta nor Licensee may use any name or mark\nowned by or associated with the other or any of its affiliates, except as\nrequired for reasonable and customary use in describing and redistributing\nthe Llama Materials.\nb. Subject to Meta's ownership of Llama Materials and derivatives made by or\nfor Meta, with respect to any derivative works and modifications of the Llama\nMaterials that are made by you, as between you and Meta, you are and will be\nthe owner of such derivative works and modifications.\nc. If you institute litigation or other proceedings against Meta or any\nentity (including a cross-claim or counterclaim in a lawsuit) alleging that\nthe Llama Materials or Llama 2 outputs or results, or any portion of any of\nthe foregoing, constitutes infringement of intellectual property or other\nrights owned or licensable by you, then any licenses granted to you under\nthis Agreement shall terminate as of the date such litigation or claim is\nfiled or instituted. You will indemnify and hold harmless Meta from and\nagainst any claim by any third party arising out of or related to your use or\ndistribution of the Llama Materials.\n6. Term and Termination. The term of this Agreement will commence upon your\nacceptance of this Agreement or access to the Llama Materials and will\ncontinue in full force and effect until terminated in accordance with the\nterms and conditions herein. Meta may terminate this Agreement if you are in\nbreach of any term or condition of this Agreement. Upon termination of this\nAgreement, you shall delete and cease use of the Llama Materials. Sections 3,\n4 and 7 shall survive the termination of this Agreement.\n7. Governing Law and Jurisdiction. This Agreement will be governed and\nconstrued under the laws of the State of California without regard to choice\nof law principles, and the UN Convention on Contracts for the International\nSale of Goods does not apply to this Agreement. The courts of California\nshall have exclusive jurisdiction of any dispute arising out of this\nAgreement.\nUSE POLICY",
"### Llama 2 Acceptable Use Policy\n\n\nMeta is committed to promoting safe and fair use of its tools and features,\nincluding Llama 2. If you access or use Llama 2, you agree to this Acceptable\nUse Policy (“Policy”). The most recent copy of this policy can be found at\nURL",
"#### Prohibited Uses\n\n\nWe want everyone to use Llama 2 safely and responsibly. You agree you will not\nuse, or allow others to use, Llama 2 to:\n\n\n1. Violate the law or others’ rights, including to:\n1. Engage in, promote, generate, contribute to, encourage, plan, incite, or further illegal or unlawful activity or content, such as:\n\t1. Violence or terrorism\n\t2. Exploitation or harm to children, including the solicitation, creation, acquisition, or dissemination of child exploitative content or failure to report Child Sexual Abuse Material\n\t3. Human trafficking, exploitation, and sexual violence\n\t4. The illegal distribution of information or materials to minors, including obscene materials, or failure to employ legally required age-gating in connection with such information or materials.\n\t5. Sexual solicitation\n\t6. Any other criminal activity\n\n\n\n```\n2. Engage in, promote, incite, or facilitate the harassment, abuse, threatening, or bullying of individuals or groups of individuals\n3. Engage in, promote, incite, or facilitate discrimination or other unlawful or harmful conduct in the provision of employment, employment benefits, credit, housing, other economic benefits, or other essential goods and services\n4. Engage in the unauthorized or unlicensed practice of any profession including, but not limited to, financial, legal, medical/health, or related professional practices \n5. Collect, process, disclose, generate, or infer health, demographic, or other sensitive personal or private information about individuals without rights and consents required by applicable laws\n6. Engage in or facilitate any action or generate any content that infringes, misappropriates, or otherwise violates any third-party rights, including the outputs or results of any products or services using the Llama 2 Materials\n7. Create, generate, or facilitate the creation of malicious code, malware, computer viruses or do anything else that could disable, overburden, interfere with or impair the proper working, integrity, operation or appearance of a website or computer system \n\n```\n\n2. Engage in, promote, incite, facilitate, or assist in the planning or\ndevelopment of activities that present a risk of death or bodily harm to\nindividuals, including use of Llama 2 related to the following:\n1. Military, warfare, nuclear industries or applications, espionage, use for materials or activities that are subject to the International Traffic Arms Regulations (ITAR) maintained by the United States Department of State\n2. Guns and illegal weapons (including weapon development)\n3. Illegal drugs and regulated/controlled substances\n4. Operation of critical infrastructure, transportation technologies, or heavy machinery\n5. Self-harm or harm to others, including suicide, cutting, and eating disorders\n6. Any content intended to incite or promote violence, abuse, or any infliction of bodily harm to an individual\n3. Intentionally deceive or mislead others, including use of Llama 2 related\nto the following:\n1. Generating, promoting, or furthering fraud or the creation or promotion of disinformation\n2. Generating, promoting, or furthering defamatory content, including the creation of defamatory statements, images, or other content\n3. Generating, promoting, or further distributing spam\n4. Impersonating another individual without consent, authorization, or legal right\n5. Representing that the use of Llama 2 or outputs are human-generated\n6. Generating or facilitating false online engagement, including fake reviews and other means of fake online engagement\n4. Fail to appropriately disclose to end users any known dangers of your AI system\nPlease report any violation of this Policy, software “bug,” or other problems\nthat could lead to a violation of this Policy through one of the following\nmeans:\n\n\n* Reporting issues with the model:\nURL\n* Reporting risky content generated by the model:\nURL\n* Reporting bugs and security concerns:\nURL\n* Reporting violations of the Acceptable Use Policy or unlicensed uses of\nLlama: LlamaUseReport@URL\nextra\\_gated\\_fields:\nFirst Name: text\nLast Name: text\nDate of birth: date\\_picker\nCountry: country\nAffiliation: text\ngeo: ip\\_location \n\nBy clicking Submit below I accept the terms of the license and acknowledge that the information I provide will be collected stored processed and shared in accordance with the Meta Privacy Policy: checkbox\nextra\\_gated\\_description: The information you provide will be collected, stored, processed and shared in accordance with the Meta Privacy Policy.\nextra\\_gated\\_button\\_content: Submit\nlanguage:\n\n\n* code\npipeline\\_tag: text-generation\ntags:\n* facebook\n* meta\n* pytorch\n* llama\n* llama-2\nlicense: llama2\n\n\n\n\n---\n\n\nCode Llama\n==========\n\n\nCode Llama is a collection of pretrained and fine-tuned generative text models ranging in scale from 7 billion to 34 billion parameters. This is the repository for the 34B instruct-tuned version in the Hugging Face Transformers format. This model is designed for general code synthesis and understanding. Links to other models can be found in the index at the bottom.\n\n\n\nModel Use\n---------\n\n\nTo use this model, please make sure to install transformers:\n\n\nModel capabilities:\n\n\n* [x] Code completion.\n* [ ] Infilling.\n* [x] Instructions / chat.\n* [ ] Python specialist.\n\n\nModel Details\n-------------\n\n\n\\*Note: Use of this model is governed by the Meta license. Meta developed and publicly released the Code Llama family of large language models (LLMs).\n\n\nModel Developers Meta\n\n\nVariations Code Llama comes in three model sizes, and three variants:\n\n\n* Code Llama: base models designed for general code synthesis and understanding\n* Code Llama - Python: designed specifically for Python\n* Code Llama - Instruct: for instruction following and safer deployment\n\n\nAll variants are available in sizes of 7B, 13B and 34B parameters.\n\n\nThis repository contains the Instruct version of the 34B parameters model.\n\n\nInput Models input text only.\n\n\nOutput Models generate text only.\n\n\nModel Architecture Code Llama is an auto-regressive language model that uses an optimized transformer architecture.\n\n\nModel Dates Code Llama and its variants have been trained between January 2023 and July 2023.\n\n\nStatus This is a static model trained on an offline dataset. Future versions of Code Llama - Instruct will be released as we improve model safety with community feedback.\n\n\nLicense A custom commercial license is available at: URL\n\n\nResearch Paper More information can be found in the paper \"Code Llama: Open Foundation Models for Code\" or its arXiv page.\n\n\nIntended Use\n------------\n\n\nIntended Use Cases Code Llama and its variants is intended for commercial and research use in English and relevant programming languages. The base model Code Llama can be adapted for a variety of code synthesis and understanding tasks, Code Llama - Python is designed specifically to handle the Python programming language, and Code Llama - Instruct is intended to be safer to use for code assistant and generation applications.\n\n\nOut-of-Scope Uses Use in any manner that violates applicable laws or regulations (including trade compliance laws). Use in languages other than English. Use in any other way that is prohibited by the Acceptable Use Policy and Licensing Agreement for Code Llama and its variants.\n\n\nHardware and Software\n---------------------\n\n\nTraining Factors We used custom training libraries. The training and fine-tuning of the released models have been performed Meta’s Research Super Cluster.\n\n\nCarbon Footprint In aggregate, training all 9 Code Llama models required 400K GPU hours of computation on hardware of type A100-80GB (TDP of 350-400W). Estimated total emissions were 65.3 tCO2eq, 100% of which were offset by Meta’s sustainability program.\n\n\nTraining Data\n-------------\n\n\nAll experiments reported here and the released models have been trained and fine-tuned using the same data as Llama 2 with different weights (see Section 2 and Table 1 in the research paper for details).\n\n\nEvaluation Results\n------------------\n\n\nSee evaluations for the main models and detailed ablations in Section 3 and safety evaluations in Section 4 of the research paper.\n\n\nEthical Considerations and Limitations\n--------------------------------------\n\n\nCode Llama and its variants are a new technology that carries risks with use. Testing conducted to date has been in English, and has not covered, nor could it cover all scenarios. For these reasons, as with all LLMs, Code Llama’s potential outputs cannot be predicted in advance, and the model may in some instances produce inaccurate or objectionable responses to user prompts. Therefore, before deploying any applications of Code Llama, developers should perform safety testing and tuning tailored to their specific applications of the model.\n\n\nPlease see the Responsible Use Guide available available at URL"
] | [
"TAGS\n#transformers #safetensors #llama #text-generation #conversational #arxiv-2308.12950 #autotrain_compatible #endpoints_compatible #text-generation-inference #4-bit #region-us \n",
"### LLAMA 2 COMMUNITY LICENSE AGREEMENT\n\n\n\"Agreement\" means the terms and conditions for use, reproduction, distribution\nand modification of the Llama Materials set forth herein.\n\"Documentation\" means the specifications, manuals and documentation\naccompanying Llama 2 distributed by Meta at\nURL \n\n\"Licensee\" or \"you\" means you, or your employer or any other person or entity\n(if you are entering into this Agreement on such person or entity's behalf),\nof the age required under applicable laws, rules or regulations to provide\nlegal consent and that has legal authority to bind your employer or such other\nperson or entity if you are entering in this Agreement on their behalf.\n\"Llama 2\" means the foundational large language models and software and\nalgorithms, including machine-learning model code, trained model weights,\ninference-enabling code, training-enabling code, fine-tuning enabling code and\nother elements of the foregoing distributed by Meta at\nURL\n\"Llama Materials\" means, collectively, Meta's proprietary Llama 2 and\ndocumentation (and any portion thereof) made available under this Agreement.\n\"Meta\" or \"we\" means Meta Platforms Ireland Limited (if you are located in or,\nif you are an entity, your principal place of business is in the EEA or\nSwitzerland) and Meta Platforms, Inc. (if you are located outside of the EEA\nor Switzerland).\nBy clicking \"I Accept\" below or by using or distributing any portion or\nelement of the Llama Materials, you agree to be bound by this Agreement.\n\n\n1. License Rights and Redistribution.\na. Grant of Rights. You are granted a non-exclusive, worldwide, non-\ntransferable and royalty-free limited license under Meta's intellectual\nproperty or other rights owned by Meta embodied in the Llama Materials to\nuse, reproduce, distribute, copy, create derivative works of, and make\nmodifications to the Llama Materials.\n\n\nb. Redistribution and Use. \n\ni. If you distribute or make the Llama Materials, or any derivative works\nthereof, available to a third party, you shall provide a copy of this\nAgreement to such third party.\nii. If you receive Llama Materials, or any derivative works thereof, from a\nLicensee as part of an integrated end user product, then Section 2 of this\nAgreement will not apply to you.\niii. You must retain in all copies of the Llama Materials that you distribute\nthe following attribution notice within a \"Notice\" text file distributed as a\npart of such copies: \"Llama 2 is licensed under the LLAMA 2 Community\nLicense, Copyright (c) Meta Platforms, Inc. All Rights Reserved.\"\niv. Your use of the Llama Materials must comply with applicable laws and\nregulations (including trade compliance laws and regulations) and adhere to\nthe Acceptable Use Policy for the Llama Materials (available at\nURL which is hereby incorporated by\nreference into this Agreement.\nv. You will not use the Llama Materials or any output or results of the Llama\nMaterials to improve any other large language model (excluding Llama 2 or\nderivative works thereof). \n\n2. Additional Commercial Terms. If, on the Llama 2 version release date, the\nmonthly active users of the products or services made available by or for\nLicensee, or Licensee's affiliates, is greater than 700 million monthly\nactive users in the preceding calendar month, you must request a license from\nMeta, which Meta may grant to you in its sole discretion, and you are not\nauthorized to exercise any of the rights under this Agreement unless or until\nMeta otherwise expressly grants you such rights.\n3. Disclaimer of Warranty. UNLESS REQUIRED BY APPLICABLE LAW, THE LLAMA\nMATERIALS AND ANY OUTPUT AND RESULTS THEREFROM ARE PROVIDED ON AN \"AS IS\"\nBASIS, WITHOUT WARRANTIES OF ANY KIND, EITHER EXPRESS OR IMPLIED, INCLUDING,\nWITHOUT LIMITATION, ANY WARRANTIES OF TITLE, NON-INFRINGEMENT,\nMERCHANTABILITY, OR FITNESS FOR A PARTICULAR PURPOSE. YOU ARE SOLELY\nRESPONSIBLE FOR DETERMINING THE APPROPRIATENESS OF USING OR REDISTRIBUTING\nTHE LLAMA MATERIALS AND ASSUME ANY RISKS ASSOCIATED WITH YOUR USE OF THE\nLLAMA MATERIALS AND ANY OUTPUT AND RESULTS.\n4. Limitation of Liability. IN NO EVENT WILL META OR ITS AFFILIATES BE LIABLE\nUNDER ANY THEORY OF LIABILITY, WHETHER IN CONTRACT, TORT, NEGLIGENCE,\nPRODUCTS LIABILITY, OR OTHERWISE, ARISING OUT OF THIS AGREEMENT, FOR ANY LOST\nPROFITS OR ANY INDIRECT, SPECIAL, CONSEQUENTIAL, INCIDENTAL, EXEMPLARY OR\nPUNITIVE DAMAGES, EVEN IF META OR ITS AFFILIATES HAVE BEEN ADVISED OF THE\nPOSSIBILITY OF ANY OF THE FOREGOING.\n5. Intellectual Property.\na. No trademark licenses are granted under this Agreement, and in connection\nwith the Llama Materials, neither Meta nor Licensee may use any name or mark\nowned by or associated with the other or any of its affiliates, except as\nrequired for reasonable and customary use in describing and redistributing\nthe Llama Materials.\nb. Subject to Meta's ownership of Llama Materials and derivatives made by or\nfor Meta, with respect to any derivative works and modifications of the Llama\nMaterials that are made by you, as between you and Meta, you are and will be\nthe owner of such derivative works and modifications.\nc. If you institute litigation or other proceedings against Meta or any\nentity (including a cross-claim or counterclaim in a lawsuit) alleging that\nthe Llama Materials or Llama 2 outputs or results, or any portion of any of\nthe foregoing, constitutes infringement of intellectual property or other\nrights owned or licensable by you, then any licenses granted to you under\nthis Agreement shall terminate as of the date such litigation or claim is\nfiled or instituted. You will indemnify and hold harmless Meta from and\nagainst any claim by any third party arising out of or related to your use or\ndistribution of the Llama Materials.\n6. Term and Termination. The term of this Agreement will commence upon your\nacceptance of this Agreement or access to the Llama Materials and will\ncontinue in full force and effect until terminated in accordance with the\nterms and conditions herein. Meta may terminate this Agreement if you are in\nbreach of any term or condition of this Agreement. Upon termination of this\nAgreement, you shall delete and cease use of the Llama Materials. Sections 3,\n4 and 7 shall survive the termination of this Agreement.\n7. Governing Law and Jurisdiction. This Agreement will be governed and\nconstrued under the laws of the State of California without regard to choice\nof law principles, and the UN Convention on Contracts for the International\nSale of Goods does not apply to this Agreement. The courts of California\nshall have exclusive jurisdiction of any dispute arising out of this\nAgreement.\nUSE POLICY",
"### Llama 2 Acceptable Use Policy\n\n\nMeta is committed to promoting safe and fair use of its tools and features,\nincluding Llama 2. If you access or use Llama 2, you agree to this Acceptable\nUse Policy (“Policy”). The most recent copy of this policy can be found at\nURL",
"#### Prohibited Uses\n\n\nWe want everyone to use Llama 2 safely and responsibly. You agree you will not\nuse, or allow others to use, Llama 2 to:\n\n\n1. Violate the law or others’ rights, including to:\n1. Engage in, promote, generate, contribute to, encourage, plan, incite, or further illegal or unlawful activity or content, such as:\n\t1. Violence or terrorism\n\t2. Exploitation or harm to children, including the solicitation, creation, acquisition, or dissemination of child exploitative content or failure to report Child Sexual Abuse Material\n\t3. Human trafficking, exploitation, and sexual violence\n\t4. The illegal distribution of information or materials to minors, including obscene materials, or failure to employ legally required age-gating in connection with such information or materials.\n\t5. Sexual solicitation\n\t6. Any other criminal activity\n\n\n\n```\n2. Engage in, promote, incite, or facilitate the harassment, abuse, threatening, or bullying of individuals or groups of individuals\n3. Engage in, promote, incite, or facilitate discrimination or other unlawful or harmful conduct in the provision of employment, employment benefits, credit, housing, other economic benefits, or other essential goods and services\n4. Engage in the unauthorized or unlicensed practice of any profession including, but not limited to, financial, legal, medical/health, or related professional practices \n5. Collect, process, disclose, generate, or infer health, demographic, or other sensitive personal or private information about individuals without rights and consents required by applicable laws\n6. Engage in or facilitate any action or generate any content that infringes, misappropriates, or otherwise violates any third-party rights, including the outputs or results of any products or services using the Llama 2 Materials\n7. Create, generate, or facilitate the creation of malicious code, malware, computer viruses or do anything else that could disable, overburden, interfere with or impair the proper working, integrity, operation or appearance of a website or computer system \n\n```\n\n2. Engage in, promote, incite, facilitate, or assist in the planning or\ndevelopment of activities that present a risk of death or bodily harm to\nindividuals, including use of Llama 2 related to the following:\n1. Military, warfare, nuclear industries or applications, espionage, use for materials or activities that are subject to the International Traffic Arms Regulations (ITAR) maintained by the United States Department of State\n2. Guns and illegal weapons (including weapon development)\n3. Illegal drugs and regulated/controlled substances\n4. Operation of critical infrastructure, transportation technologies, or heavy machinery\n5. Self-harm or harm to others, including suicide, cutting, and eating disorders\n6. Any content intended to incite or promote violence, abuse, or any infliction of bodily harm to an individual\n3. Intentionally deceive or mislead others, including use of Llama 2 related\nto the following:\n1. Generating, promoting, or furthering fraud or the creation or promotion of disinformation\n2. Generating, promoting, or furthering defamatory content, including the creation of defamatory statements, images, or other content\n3. Generating, promoting, or further distributing spam\n4. Impersonating another individual without consent, authorization, or legal right\n5. Representing that the use of Llama 2 or outputs are human-generated\n6. Generating or facilitating false online engagement, including fake reviews and other means of fake online engagement\n4. Fail to appropriately disclose to end users any known dangers of your AI system\nPlease report any violation of this Policy, software “bug,” or other problems\nthat could lead to a violation of this Policy through one of the following\nmeans:\n\n\n* Reporting issues with the model:\nURL\n* Reporting risky content generated by the model:\nURL\n* Reporting bugs and security concerns:\nURL\n* Reporting violations of the Acceptable Use Policy or unlicensed uses of\nLlama: LlamaUseReport@URL\nextra\\_gated\\_fields:\nFirst Name: text\nLast Name: text\nDate of birth: date\\_picker\nCountry: country\nAffiliation: text\ngeo: ip\\_location \n\nBy clicking Submit below I accept the terms of the license and acknowledge that the information I provide will be collected stored processed and shared in accordance with the Meta Privacy Policy: checkbox\nextra\\_gated\\_description: The information you provide will be collected, stored, processed and shared in accordance with the Meta Privacy Policy.\nextra\\_gated\\_button\\_content: Submit\nlanguage:\n\n\n* code\npipeline\\_tag: text-generation\ntags:\n* facebook\n* meta\n* pytorch\n* llama\n* llama-2\nlicense: llama2\n\n\n\n\n---\n\n\nCode Llama\n==========\n\n\nCode Llama is a collection of pretrained and fine-tuned generative text models ranging in scale from 7 billion to 34 billion parameters. This is the repository for the 34B instruct-tuned version in the Hugging Face Transformers format. This model is designed for general code synthesis and understanding. Links to other models can be found in the index at the bottom.\n\n\n\nModel Use\n---------\n\n\nTo use this model, please make sure to install transformers:\n\n\nModel capabilities:\n\n\n* [x] Code completion.\n* [ ] Infilling.\n* [x] Instructions / chat.\n* [ ] Python specialist.\n\n\nModel Details\n-------------\n\n\n\\*Note: Use of this model is governed by the Meta license. Meta developed and publicly released the Code Llama family of large language models (LLMs).\n\n\nModel Developers Meta\n\n\nVariations Code Llama comes in three model sizes, and three variants:\n\n\n* Code Llama: base models designed for general code synthesis and understanding\n* Code Llama - Python: designed specifically for Python\n* Code Llama - Instruct: for instruction following and safer deployment\n\n\nAll variants are available in sizes of 7B, 13B and 34B parameters.\n\n\nThis repository contains the Instruct version of the 34B parameters model.\n\n\nInput Models input text only.\n\n\nOutput Models generate text only.\n\n\nModel Architecture Code Llama is an auto-regressive language model that uses an optimized transformer architecture.\n\n\nModel Dates Code Llama and its variants have been trained between January 2023 and July 2023.\n\n\nStatus This is a static model trained on an offline dataset. Future versions of Code Llama - Instruct will be released as we improve model safety with community feedback.\n\n\nLicense A custom commercial license is available at: URL\n\n\nResearch Paper More information can be found in the paper \"Code Llama: Open Foundation Models for Code\" or its arXiv page.\n\n\nIntended Use\n------------\n\n\nIntended Use Cases Code Llama and its variants is intended for commercial and research use in English and relevant programming languages. The base model Code Llama can be adapted for a variety of code synthesis and understanding tasks, Code Llama - Python is designed specifically to handle the Python programming language, and Code Llama - Instruct is intended to be safer to use for code assistant and generation applications.\n\n\nOut-of-Scope Uses Use in any manner that violates applicable laws or regulations (including trade compliance laws). Use in languages other than English. Use in any other way that is prohibited by the Acceptable Use Policy and Licensing Agreement for Code Llama and its variants.\n\n\nHardware and Software\n---------------------\n\n\nTraining Factors We used custom training libraries. The training and fine-tuning of the released models have been performed Meta’s Research Super Cluster.\n\n\nCarbon Footprint In aggregate, training all 9 Code Llama models required 400K GPU hours of computation on hardware of type A100-80GB (TDP of 350-400W). Estimated total emissions were 65.3 tCO2eq, 100% of which were offset by Meta’s sustainability program.\n\n\nTraining Data\n-------------\n\n\nAll experiments reported here and the released models have been trained and fine-tuned using the same data as Llama 2 with different weights (see Section 2 and Table 1 in the research paper for details).\n\n\nEvaluation Results\n------------------\n\n\nSee evaluations for the main models and detailed ablations in Section 3 and safety evaluations in Section 4 of the research paper.\n\n\nEthical Considerations and Limitations\n--------------------------------------\n\n\nCode Llama and its variants are a new technology that carries risks with use. Testing conducted to date has been in English, and has not covered, nor could it cover all scenarios. For these reasons, as with all LLMs, Code Llama’s potential outputs cannot be predicted in advance, and the model may in some instances produce inaccurate or objectionable responses to user prompts. Therefore, before deploying any applications of Code Llama, developers should perform safety testing and tuning tailored to their specific applications of the model.\n\n\nPlease see the Responsible Use Guide available available at URL"
] |
null | null | ## MixtralIMAT
llamafile lets you distribute and run LLMs with a single file. [announcement blog post](https://hacks.mozilla.org/2023/11/introducing-llamafile/)
#### Downloads
- [mixtral-8x7b-instruct-v0.1-imat-IQ1_S.llamafile](https://huggingface.co/blueprintninja/MixtralIMAT/resolve/main/mixtral-8x7b-instruct-v0.1-imat-IQ1_S.llamafile)
This repository was created using the [llamafile-builder](https://github.com/rabilrbl/llamafile-builder)
| {"tags": ["llamafile", "GGUF"], "base_model": "qwp4w3hyb/Mixtral-8x7B-Instruct-v0.1-iMat-GGUF"} | blueprintninja/MixtralIMAT | null | [
"llamafile",
"GGUF",
"base_model:qwp4w3hyb/Mixtral-8x7B-Instruct-v0.1-iMat-GGUF",
"region:us"
] | null | 2024-04-21T18:55:35+00:00 | [] | [] | TAGS
#llamafile #GGUF #base_model-qwp4w3hyb/Mixtral-8x7B-Instruct-v0.1-iMat-GGUF #region-us
| ## MixtralIMAT
llamafile lets you distribute and run LLMs with a single file. announcement blog post
#### Downloads
- mixtral-8x7b-instruct-v0.1-imat-IQ1_S.llamafile
This repository was created using the llamafile-builder
| [
"## MixtralIMAT\n\nllamafile lets you distribute and run LLMs with a single file. announcement blog post",
"#### Downloads\n\n - mixtral-8x7b-instruct-v0.1-imat-IQ1_S.llamafile\n\nThis repository was created using the llamafile-builder"
] | [
"TAGS\n#llamafile #GGUF #base_model-qwp4w3hyb/Mixtral-8x7B-Instruct-v0.1-iMat-GGUF #region-us \n",
"## MixtralIMAT\n\nllamafile lets you distribute and run LLMs with a single file. announcement blog post",
"#### Downloads\n\n - mixtral-8x7b-instruct-v0.1-imat-IQ1_S.llamafile\n\nThis repository was created using the llamafile-builder"
] |
null | transformers |
# Model Card for Model ID
<!-- Provide a quick summary of what the model is/does. -->
## Model Details
### Model Description
<!-- Provide a longer summary of what this model is. -->
This is the model card of a 🤗 transformers model that has been pushed on the Hub. This model card has been automatically generated.
- **Developed by:** [More Information Needed]
- **Funded by [optional]:** [More Information Needed]
- **Shared by [optional]:** [More Information Needed]
- **Model type:** [More Information Needed]
- **Language(s) (NLP):** [More Information Needed]
- **License:** [More Information Needed]
- **Finetuned from model [optional]:** [More Information Needed]
### Model Sources [optional]
<!-- Provide the basic links for the model. -->
- **Repository:** [More Information Needed]
- **Paper [optional]:** [More Information Needed]
- **Demo [optional]:** [More Information Needed]
## Uses
<!-- Address questions around how the model is intended to be used, including the foreseeable users of the model and those affected by the model. -->
### Direct Use
<!-- This section is for the model use without fine-tuning or plugging into a larger ecosystem/app. -->
[More Information Needed]
### Downstream Use [optional]
<!-- This section is for the model use when fine-tuned for a task, or when plugged into a larger ecosystem/app -->
[More Information Needed]
### Out-of-Scope Use
<!-- This section addresses misuse, malicious use, and uses that the model will not work well for. -->
[More Information Needed]
## Bias, Risks, and Limitations
<!-- This section is meant to convey both technical and sociotechnical limitations. -->
[More Information Needed]
### Recommendations
<!-- This section is meant to convey recommendations with respect to the bias, risk, and technical limitations. -->
Users (both direct and downstream) should be made aware of the risks, biases and limitations of the model. More information needed for further recommendations.
## How to Get Started with the Model
Use the code below to get started with the model.
[More Information Needed]
## Training Details
### Training Data
<!-- This should link to a Dataset Card, perhaps with a short stub of information on what the training data is all about as well as documentation related to data pre-processing or additional filtering. -->
[More Information Needed]
### Training Procedure
<!-- This relates heavily to the Technical Specifications. Content here should link to that section when it is relevant to the training procedure. -->
#### Preprocessing [optional]
[More Information Needed]
#### Training Hyperparameters
- **Training regime:** [More Information Needed] <!--fp32, fp16 mixed precision, bf16 mixed precision, bf16 non-mixed precision, fp16 non-mixed precision, fp8 mixed precision -->
#### Speeds, Sizes, Times [optional]
<!-- This section provides information about throughput, start/end time, checkpoint size if relevant, etc. -->
[More Information Needed]
## Evaluation
<!-- This section describes the evaluation protocols and provides the results. -->
### Testing Data, Factors & Metrics
#### Testing Data
<!-- This should link to a Dataset Card if possible. -->
[More Information Needed]
#### Factors
<!-- These are the things the evaluation is disaggregating by, e.g., subpopulations or domains. -->
[More Information Needed]
#### Metrics
<!-- These are the evaluation metrics being used, ideally with a description of why. -->
[More Information Needed]
### Results
[More Information Needed]
#### Summary
## Model Examination [optional]
<!-- Relevant interpretability work for the model goes here -->
[More Information Needed]
## Environmental Impact
<!-- Total emissions (in grams of CO2eq) and additional considerations, such as electricity usage, go here. Edit the suggested text below accordingly -->
Carbon emissions can be estimated using the [Machine Learning Impact calculator](https://mlco2.github.io/impact#compute) presented in [Lacoste et al. (2019)](https://arxiv.org/abs/1910.09700).
- **Hardware Type:** [More Information Needed]
- **Hours used:** [More Information Needed]
- **Cloud Provider:** [More Information Needed]
- **Compute Region:** [More Information Needed]
- **Carbon Emitted:** [More Information Needed]
## Technical Specifications [optional]
### Model Architecture and Objective
[More Information Needed]
### Compute Infrastructure
[More Information Needed]
#### Hardware
[More Information Needed]
#### Software
[More Information Needed]
## Citation [optional]
<!-- If there is a paper or blog post introducing the model, the APA and Bibtex information for that should go in this section. -->
**BibTeX:**
[More Information Needed]
**APA:**
[More Information Needed]
## Glossary [optional]
<!-- If relevant, include terms and calculations in this section that can help readers understand the model or model card. -->
[More Information Needed]
## More Information [optional]
[More Information Needed]
## Model Card Authors [optional]
[More Information Needed]
## Model Card Contact
[More Information Needed] | {"library_name": "transformers", "tags": []} | mryannugent/knowledge-finetune-miscondetect-bloomz-3b | null | [
"transformers",
"safetensors",
"arxiv:1910.09700",
"endpoints_compatible",
"region:us"
] | null | 2024-04-21T18:58:47+00:00 | [
"1910.09700"
] | [] | TAGS
#transformers #safetensors #arxiv-1910.09700 #endpoints_compatible #region-us
|
# Model Card for Model ID
## Model Details
### Model Description
This is the model card of a transformers model that has been pushed on the Hub. This model card has been automatically generated.
- Developed by:
- Funded by [optional]:
- Shared by [optional]:
- Model type:
- Language(s) (NLP):
- License:
- Finetuned from model [optional]:
### Model Sources [optional]
- Repository:
- Paper [optional]:
- Demo [optional]:
## Uses
### Direct Use
### Downstream Use [optional]
### Out-of-Scope Use
## Bias, Risks, and Limitations
### Recommendations
Users (both direct and downstream) should be made aware of the risks, biases and limitations of the model. More information needed for further recommendations.
## How to Get Started with the Model
Use the code below to get started with the model.
## Training Details
### Training Data
### Training Procedure
#### Preprocessing [optional]
#### Training Hyperparameters
- Training regime:
#### Speeds, Sizes, Times [optional]
## Evaluation
### Testing Data, Factors & Metrics
#### Testing Data
#### Factors
#### Metrics
### Results
#### Summary
## Model Examination [optional]
## Environmental Impact
Carbon emissions can be estimated using the Machine Learning Impact calculator presented in Lacoste et al. (2019).
- Hardware Type:
- Hours used:
- Cloud Provider:
- Compute Region:
- Carbon Emitted:
## Technical Specifications [optional]
### Model Architecture and Objective
### Compute Infrastructure
#### Hardware
#### Software
[optional]
BibTeX:
APA:
## Glossary [optional]
## More Information [optional]
## Model Card Authors [optional]
## Model Card Contact
| [
"# Model Card for Model ID",
"## Model Details",
"### Model Description\n\n\n\nThis is the model card of a transformers model that has been pushed on the Hub. This model card has been automatically generated.\n\n- Developed by: \n- Funded by [optional]: \n- Shared by [optional]: \n- Model type: \n- Language(s) (NLP): \n- License: \n- Finetuned from model [optional]:",
"### Model Sources [optional]\n\n\n\n- Repository: \n- Paper [optional]: \n- Demo [optional]:",
"## Uses",
"### Direct Use",
"### Downstream Use [optional]",
"### Out-of-Scope Use",
"## Bias, Risks, and Limitations",
"### Recommendations\n\n\n\nUsers (both direct and downstream) should be made aware of the risks, biases and limitations of the model. More information needed for further recommendations.",
"## How to Get Started with the Model\n\nUse the code below to get started with the model.",
"## Training Details",
"### Training Data",
"### Training Procedure",
"#### Preprocessing [optional]",
"#### Training Hyperparameters\n\n- Training regime:",
"#### Speeds, Sizes, Times [optional]",
"## Evaluation",
"### Testing Data, Factors & Metrics",
"#### Testing Data",
"#### Factors",
"#### Metrics",
"### Results",
"#### Summary",
"## Model Examination [optional]",
"## Environmental Impact\n\n\n\nCarbon emissions can be estimated using the Machine Learning Impact calculator presented in Lacoste et al. (2019).\n\n- Hardware Type: \n- Hours used: \n- Cloud Provider: \n- Compute Region: \n- Carbon Emitted:",
"## Technical Specifications [optional]",
"### Model Architecture and Objective",
"### Compute Infrastructure",
"#### Hardware",
"#### Software\n\n\n\n[optional]\n\n\n\nBibTeX:\n\n\n\nAPA:",
"## Glossary [optional]",
"## More Information [optional]",
"## Model Card Authors [optional]",
"## Model Card Contact"
] | [
"TAGS\n#transformers #safetensors #arxiv-1910.09700 #endpoints_compatible #region-us \n",
"# Model Card for Model ID",
"## Model Details",
"### Model Description\n\n\n\nThis is the model card of a transformers model that has been pushed on the Hub. This model card has been automatically generated.\n\n- Developed by: \n- Funded by [optional]: \n- Shared by [optional]: \n- Model type: \n- Language(s) (NLP): \n- License: \n- Finetuned from model [optional]:",
"### Model Sources [optional]\n\n\n\n- Repository: \n- Paper [optional]: \n- Demo [optional]:",
"## Uses",
"### Direct Use",
"### Downstream Use [optional]",
"### Out-of-Scope Use",
"## Bias, Risks, and Limitations",
"### Recommendations\n\n\n\nUsers (both direct and downstream) should be made aware of the risks, biases and limitations of the model. More information needed for further recommendations.",
"## How to Get Started with the Model\n\nUse the code below to get started with the model.",
"## Training Details",
"### Training Data",
"### Training Procedure",
"#### Preprocessing [optional]",
"#### Training Hyperparameters\n\n- Training regime:",
"#### Speeds, Sizes, Times [optional]",
"## Evaluation",
"### Testing Data, Factors & Metrics",
"#### Testing Data",
"#### Factors",
"#### Metrics",
"### Results",
"#### Summary",
"## Model Examination [optional]",
"## Environmental Impact\n\n\n\nCarbon emissions can be estimated using the Machine Learning Impact calculator presented in Lacoste et al. (2019).\n\n- Hardware Type: \n- Hours used: \n- Cloud Provider: \n- Compute Region: \n- Carbon Emitted:",
"## Technical Specifications [optional]",
"### Model Architecture and Objective",
"### Compute Infrastructure",
"#### Hardware",
"#### Software\n\n\n\n[optional]\n\n\n\nBibTeX:\n\n\n\nAPA:",
"## Glossary [optional]",
"## More Information [optional]",
"## Model Card Authors [optional]",
"## Model Card Contact"
] |
text-generation | transformers | # NEBULA-23.8B-v1.0
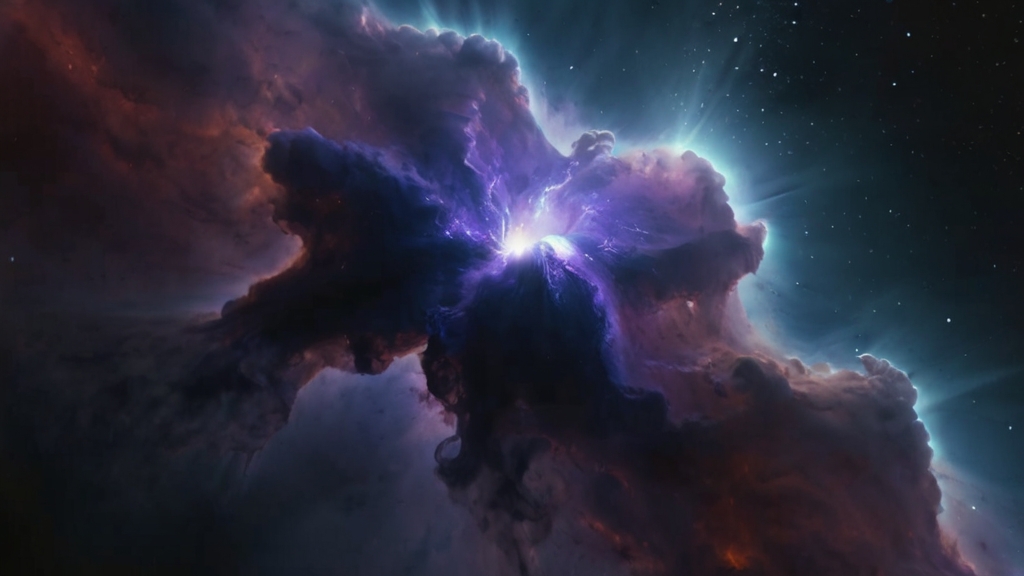
## Technical notes
- 108 layers,DUS procedure, mistral(32)->SOLAR(48)->GALAXY(72)->NEBULA(108)
- 23.8B parameters
- model created as a extension of depth upscaling procedure used for SOLAR by upstage
## Results
- model can and will produce NSFW content
- GSM8k evaluation seems to be often broken, HellaSwag, Winograde and TQA show that its a smart model
- RP and ERP work surprisingly good and I didn't encounter any GPTisms yet
- lower memory footprint than 20B and 23B models
- follows character card very well
- NSFW output feels fresh comparing to existing models
## Finetuning for RP
- SFT using MinervaAI/Aesir-Preview dataset, 10 epochs
- DPO using athirdpath/DPO_Pairs-Roleplay-Alpaca-NSFW dataset, 1 epoch
- SFT using 1xAda6000, 10h
- DPO using 1x3090, 30h
- jupyter notebooks or mergekit configs for anyone wanting to reproduce/reuse scripts - just drop me a message
## Prompt template
- Alpaca
- chat template is embedded in tokenizer config, should load automatically
## Context size
- 4096
All comments are greatly appreciated, download, test and if you appreciate my work, consider buying me my fuel:
<a href="https://www.buymeacoffee.com/TeeZee" target="_blank"><img src="https://cdn.buymeacoffee.com/buttons/v2/default-yellow.png" alt="Buy Me A Coffee" style="height: 60px !important;width: 217px !important;" ></a>
# [Open LLM Leaderboard Evaluation Results](https://huggingface.co/spaces/HuggingFaceH4/open_llm_leaderboard)
Detailed results can be found [here](https://huggingface.co/datasets/open-llm-leaderboard/details_TeeZee__NEBULA-23B-v1.0)
| Metric |Value|
|---------------------------------|----:|
|Avg. |59.94|
|AI2 Reasoning Challenge (25-Shot)|66.72|
|HellaSwag (10-Shot) |86.98|
|MMLU (5-Shot) |65.40|
|TruthfulQA (0-shot) |57.60|
|Winogrande (5-shot) |82.95|
|GSM8k (5-shot) | 0.00|
| {"language": ["en"], "license": "apache-2.0", "tags": ["not-for-all-audiences"], "datasets": ["Intel/orca_dpo_pairs", "athirdpath/DPO_Pairs-Roleplay-Alpaca-NSFW", "Open-Orca/SlimOrca", "MinervaAI/Aesir-Preview", "allenai/ultrafeedback_binarized_cleaned"], "model-index": [{"name": "NEBULA-23B-v1.0", "results": [{"task": {"type": "text-generation", "name": "Text Generation"}, "dataset": {"name": "AI2 Reasoning Challenge (25-Shot)", "type": "ai2_arc", "config": "ARC-Challenge", "split": "test", "args": {"num_few_shot": 25}}, "metrics": [{"type": "acc_norm", "value": 66.72, "name": "normalized accuracy"}], "source": {"url": "https://huggingface.co/spaces/HuggingFaceH4/open_llm_leaderboard?query=TeeZee/NEBULA-23B-v1.0", "name": "Open LLM Leaderboard"}}, {"task": {"type": "text-generation", "name": "Text Generation"}, "dataset": {"name": "HellaSwag (10-Shot)", "type": "hellaswag", "split": "validation", "args": {"num_few_shot": 10}}, "metrics": [{"type": "acc_norm", "value": 86.98, "name": "normalized accuracy"}], "source": {"url": "https://huggingface.co/spaces/HuggingFaceH4/open_llm_leaderboard?query=TeeZee/NEBULA-23B-v1.0", "name": "Open LLM Leaderboard"}}, {"task": {"type": "text-generation", "name": "Text Generation"}, "dataset": {"name": "MMLU (5-Shot)", "type": "cais/mmlu", "config": "all", "split": "test", "args": {"num_few_shot": 5}}, "metrics": [{"type": "acc", "value": 65.4, "name": "accuracy"}], "source": {"url": "https://huggingface.co/spaces/HuggingFaceH4/open_llm_leaderboard?query=TeeZee/NEBULA-23B-v1.0", "name": "Open LLM Leaderboard"}}, {"task": {"type": "text-generation", "name": "Text Generation"}, "dataset": {"name": "TruthfulQA (0-shot)", "type": "truthful_qa", "config": "multiple_choice", "split": "validation", "args": {"num_few_shot": 0}}, "metrics": [{"type": "mc2", "value": 57.6}], "source": {"url": "https://huggingface.co/spaces/HuggingFaceH4/open_llm_leaderboard?query=TeeZee/NEBULA-23B-v1.0", "name": "Open LLM Leaderboard"}}, {"task": {"type": "text-generation", "name": "Text Generation"}, "dataset": {"name": "Winogrande (5-shot)", "type": "winogrande", "config": "winogrande_xl", "split": "validation", "args": {"num_few_shot": 5}}, "metrics": [{"type": "acc", "value": 82.95, "name": "accuracy"}], "source": {"url": "https://huggingface.co/spaces/HuggingFaceH4/open_llm_leaderboard?query=TeeZee/NEBULA-23B-v1.0", "name": "Open LLM Leaderboard"}}, {"task": {"type": "text-generation", "name": "Text Generation"}, "dataset": {"name": "GSM8k (5-shot)", "type": "gsm8k", "config": "main", "split": "test", "args": {"num_few_shot": 5}}, "metrics": [{"type": "acc", "value": 0.0, "name": "accuracy"}], "source": {"url": "https://huggingface.co/spaces/HuggingFaceH4/open_llm_leaderboard?query=TeeZee/NEBULA-23B-v1.0", "name": "Open LLM Leaderboard"}}]}]} | TeeZee/NEBULA-23.8B-v1.0-bpw4.0-h6-exl2 | null | [
"transformers",
"safetensors",
"llama",
"text-generation",
"not-for-all-audiences",
"conversational",
"en",
"dataset:Intel/orca_dpo_pairs",
"dataset:athirdpath/DPO_Pairs-Roleplay-Alpaca-NSFW",
"dataset:Open-Orca/SlimOrca",
"dataset:MinervaAI/Aesir-Preview",
"dataset:allenai/ultrafeedback_binarized_cleaned",
"license:apache-2.0",
"model-index",
"autotrain_compatible",
"endpoints_compatible",
"text-generation-inference",
"region:us"
] | null | 2024-04-21T18:59:22+00:00 | [] | [
"en"
] | TAGS
#transformers #safetensors #llama #text-generation #not-for-all-audiences #conversational #en #dataset-Intel/orca_dpo_pairs #dataset-athirdpath/DPO_Pairs-Roleplay-Alpaca-NSFW #dataset-Open-Orca/SlimOrca #dataset-MinervaAI/Aesir-Preview #dataset-allenai/ultrafeedback_binarized_cleaned #license-apache-2.0 #model-index #autotrain_compatible #endpoints_compatible #text-generation-inference #region-us
| NEBULA-23.8B-v1.0
=================
!image/png
Technical notes
---------------
* 108 layers,DUS procedure, mistral(32)->SOLAR(48)->GALAXY(72)->NEBULA(108)
* 23.8B parameters
* model created as a extension of depth upscaling procedure used for SOLAR by upstage
Results
-------
* model can and will produce NSFW content
* GSM8k evaluation seems to be often broken, HellaSwag, Winograde and TQA show that its a smart model
* RP and ERP work surprisingly good and I didn't encounter any GPTisms yet
* lower memory footprint than 20B and 23B models
* follows character card very well
* NSFW output feels fresh comparing to existing models
Finetuning for RP
-----------------
* SFT using MinervaAI/Aesir-Preview dataset, 10 epochs
* DPO using athirdpath/DPO\_Pairs-Roleplay-Alpaca-NSFW dataset, 1 epoch
* SFT using 1xAda6000, 10h
* DPO using 1x3090, 30h
* jupyter notebooks or mergekit configs for anyone wanting to reproduce/reuse scripts - just drop me a message
Prompt template
---------------
* Alpaca
* chat template is embedded in tokenizer config, should load automatically
Context size
------------
* 4096
All comments are greatly appreciated, download, test and if you appreciate my work, consider buying me my fuel:
<a href="URL target="\_blank"><img src="URL alt="Buy Me A Coffee" style="height: 60px !important;width: 217px !important;" >
Open LLM Leaderboard Evaluation Results
=======================================
Detailed results can be found here
| [] | [
"TAGS\n#transformers #safetensors #llama #text-generation #not-for-all-audiences #conversational #en #dataset-Intel/orca_dpo_pairs #dataset-athirdpath/DPO_Pairs-Roleplay-Alpaca-NSFW #dataset-Open-Orca/SlimOrca #dataset-MinervaAI/Aesir-Preview #dataset-allenai/ultrafeedback_binarized_cleaned #license-apache-2.0 #model-index #autotrain_compatible #endpoints_compatible #text-generation-inference #region-us \n"
] |
null | null |
# Multi_verse_modelNeuralsynthesis-7B
Multi_verse_modelNeuralsynthesis-7B is an automated merge created by [Maxime Labonne](https://huggingface.co/mlabonne) using the following configuration.
## 🧩 Configuration
```yaml
models:
- model: mistralai/Mistral-7B-v0.1
- model: MTSAIR/multi_verse_model
- model: Kukedlc/NeuralSynthesis-7B-v0.3
merge_method: model_stock
base_model: mistralai/Mistral-7B-v0.1
dtype: bfloat16
```
## 💻 Usage
```python
!pip install -qU transformers accelerate
from transformers import AutoTokenizer
import transformers
import torch
model = "automerger/Multi_verse_modelNeuralsynthesis-7B"
messages = [{"role": "user", "content": "What is a large language model?"}]
tokenizer = AutoTokenizer.from_pretrained(model)
prompt = tokenizer.apply_chat_template(messages, tokenize=False, add_generation_prompt=True)
pipeline = transformers.pipeline(
"text-generation",
model=model,
torch_dtype=torch.float16,
device_map="auto",
)
outputs = pipeline(prompt, max_new_tokens=256, do_sample=True, temperature=0.7, top_k=50, top_p=0.95)
print(outputs[0]["generated_text"])
``` | {"license": "apache-2.0", "tags": ["merge", "mergekit", "lazymergekit", "automerger"]} | automerger/Multi_verse_modelNeuralsynthesis-7B | null | [
"merge",
"mergekit",
"lazymergekit",
"automerger",
"license:apache-2.0",
"region:us"
] | null | 2024-04-21T19:00:34+00:00 | [] | [] | TAGS
#merge #mergekit #lazymergekit #automerger #license-apache-2.0 #region-us
|
# Multi_verse_modelNeuralsynthesis-7B
Multi_verse_modelNeuralsynthesis-7B is an automated merge created by Maxime Labonne using the following configuration.
## Configuration
## Usage
| [
"# Multi_verse_modelNeuralsynthesis-7B\n\nMulti_verse_modelNeuralsynthesis-7B is an automated merge created by Maxime Labonne using the following configuration.",
"## Configuration",
"## Usage"
] | [
"TAGS\n#merge #mergekit #lazymergekit #automerger #license-apache-2.0 #region-us \n",
"# Multi_verse_modelNeuralsynthesis-7B\n\nMulti_verse_modelNeuralsynthesis-7B is an automated merge created by Maxime Labonne using the following configuration.",
"## Configuration",
"## Usage"
] |
null | null |
3-bit [OmniQuant](https://arxiv.org/abs/2308.13137) quantized version of [dolphin-2.9-llama3-8b](https://huggingface.co/cognitivecomputations/dolphin-2.9-llama3-8b).
| {"license": "other", "license_name": "llama3", "license_link": "LICENSE"} | numen-tech/dolphin-2.9-llama3-8b-w3a16g40sym | null | [
"arxiv:2308.13137",
"license:other",
"region:us"
] | null | 2024-04-21T19:01:55+00:00 | [
"2308.13137"
] | [] | TAGS
#arxiv-2308.13137 #license-other #region-us
|
3-bit OmniQuant quantized version of dolphin-2.9-llama3-8b.
| [] | [
"TAGS\n#arxiv-2308.13137 #license-other #region-us \n"
] |
object-detection | transformers |
<!-- This model card has been generated automatically according to the information the Trainer had access to. You
should probably proofread and complete it, then remove this comment. -->
# detr
This model is a fine-tuned version of [facebook/detr-resnet-50](https://huggingface.co/facebook/detr-resnet-50) on an unknown dataset.
It achieves the following results on the evaluation set:
- Loss: 2.3771
## Model description
More information needed
## Intended uses & limitations
More information needed
## Training and evaluation data
More information needed
## Training procedure
### Training hyperparameters
The following hyperparameters were used during training:
- learning_rate: 2e-05
- train_batch_size: 32
- eval_batch_size: 8
- seed: 42
- optimizer: Adam with betas=(0.9,0.999) and epsilon=1e-08
- lr_scheduler_type: linear
- num_epochs: 1
- mixed_precision_training: Native AMP
### Training results
| Training Loss | Epoch | Step | Validation Loss |
|:-------------:|:-----:|:----:|:---------------:|
| 4.3956 | 0.16 | 50 | 4.0045 |
| 3.5668 | 0.32 | 100 | 3.3500 |
| 2.9609 | 0.48 | 150 | 2.9119 |
| 2.6387 | 0.64 | 200 | 2.5813 |
| 2.411 | 0.8 | 250 | 2.4280 |
| 2.3426 | 0.96 | 300 | 2.3771 |
### Framework versions
- Transformers 4.38.2
- Pytorch 2.2.1+cu121
- Datasets 2.19.0
- Tokenizers 0.15.2
| {"license": "apache-2.0", "tags": ["generated_from_trainer"], "base_model": "facebook/detr-resnet-50", "model-index": [{"name": "detr", "results": []}]} | obednsiah/detr | null | [
"transformers",
"tensorboard",
"safetensors",
"detr",
"object-detection",
"generated_from_trainer",
"base_model:facebook/detr-resnet-50",
"license:apache-2.0",
"endpoints_compatible",
"region:us"
] | null | 2024-04-21T19:02:55+00:00 | [] | [] | TAGS
#transformers #tensorboard #safetensors #detr #object-detection #generated_from_trainer #base_model-facebook/detr-resnet-50 #license-apache-2.0 #endpoints_compatible #region-us
| detr
====
This model is a fine-tuned version of facebook/detr-resnet-50 on an unknown dataset.
It achieves the following results on the evaluation set:
* Loss: 2.3771
Model description
-----------------
More information needed
Intended uses & limitations
---------------------------
More information needed
Training and evaluation data
----------------------------
More information needed
Training procedure
------------------
### Training hyperparameters
The following hyperparameters were used during training:
* learning\_rate: 2e-05
* train\_batch\_size: 32
* eval\_batch\_size: 8
* seed: 42
* optimizer: Adam with betas=(0.9,0.999) and epsilon=1e-08
* lr\_scheduler\_type: linear
* num\_epochs: 1
* mixed\_precision\_training: Native AMP
### Training results
### Framework versions
* Transformers 4.38.2
* Pytorch 2.2.1+cu121
* Datasets 2.19.0
* Tokenizers 0.15.2
| [
"### Training hyperparameters\n\n\nThe following hyperparameters were used during training:\n\n\n* learning\\_rate: 2e-05\n* train\\_batch\\_size: 32\n* eval\\_batch\\_size: 8\n* seed: 42\n* optimizer: Adam with betas=(0.9,0.999) and epsilon=1e-08\n* lr\\_scheduler\\_type: linear\n* num\\_epochs: 1\n* mixed\\_precision\\_training: Native AMP",
"### Training results",
"### Framework versions\n\n\n* Transformers 4.38.2\n* Pytorch 2.2.1+cu121\n* Datasets 2.19.0\n* Tokenizers 0.15.2"
] | [
"TAGS\n#transformers #tensorboard #safetensors #detr #object-detection #generated_from_trainer #base_model-facebook/detr-resnet-50 #license-apache-2.0 #endpoints_compatible #region-us \n",
"### Training hyperparameters\n\n\nThe following hyperparameters were used during training:\n\n\n* learning\\_rate: 2e-05\n* train\\_batch\\_size: 32\n* eval\\_batch\\_size: 8\n* seed: 42\n* optimizer: Adam with betas=(0.9,0.999) and epsilon=1e-08\n* lr\\_scheduler\\_type: linear\n* num\\_epochs: 1\n* mixed\\_precision\\_training: Native AMP",
"### Training results",
"### Framework versions\n\n\n* Transformers 4.38.2\n* Pytorch 2.2.1+cu121\n* Datasets 2.19.0\n* Tokenizers 0.15.2"
] |
text-generation | transformers |
<!-- This model card has been generated automatically according to the information the Trainer had access to. You
should probably proofread and complete it, then remove this comment. -->
# c13
This model is a fine-tuned version of [openai-community/gpt2](https://huggingface.co/openai-community/gpt2) on an unknown dataset.
It achieves the following results on the evaluation set:
- Loss: 2.9572
## Model description
More information needed
## Intended uses & limitations
More information needed
## Training and evaluation data
More information needed
## Training procedure
### Training hyperparameters
The following hyperparameters were used during training:
- learning_rate: 2e-07
- train_batch_size: 32
- eval_batch_size: 32
- seed: 42
- optimizer: Adam with betas=(0.9,0.999) and epsilon=1e-08
- lr_scheduler_type: linear
- num_epochs: 1
### Training results
| Training Loss | Epoch | Step | Validation Loss |
|:-------------:|:-----:|:----:|:---------------:|
| No log | 1.0 | 1 | 2.9572 |
### Framework versions
- Transformers 4.38.2
- Pytorch 2.2.1+cu121
- Datasets 2.19.0
- Tokenizers 0.15.2
| {"license": "mit", "tags": ["generated_from_trainer"], "base_model": "openai-community/gpt2", "model-index": [{"name": "c13", "results": []}]} | samhitmantrala/c13 | null | [
"transformers",
"tensorboard",
"safetensors",
"gpt2",
"text-generation",
"generated_from_trainer",
"base_model:openai-community/gpt2",
"license:mit",
"autotrain_compatible",
"endpoints_compatible",
"text-generation-inference",
"region:us"
] | null | 2024-04-21T19:03:36+00:00 | [] | [] | TAGS
#transformers #tensorboard #safetensors #gpt2 #text-generation #generated_from_trainer #base_model-openai-community/gpt2 #license-mit #autotrain_compatible #endpoints_compatible #text-generation-inference #region-us
| c13
===
This model is a fine-tuned version of openai-community/gpt2 on an unknown dataset.
It achieves the following results on the evaluation set:
* Loss: 2.9572
Model description
-----------------
More information needed
Intended uses & limitations
---------------------------
More information needed
Training and evaluation data
----------------------------
More information needed
Training procedure
------------------
### Training hyperparameters
The following hyperparameters were used during training:
* learning\_rate: 2e-07
* train\_batch\_size: 32
* eval\_batch\_size: 32
* seed: 42
* optimizer: Adam with betas=(0.9,0.999) and epsilon=1e-08
* lr\_scheduler\_type: linear
* num\_epochs: 1
### Training results
### Framework versions
* Transformers 4.38.2
* Pytorch 2.2.1+cu121
* Datasets 2.19.0
* Tokenizers 0.15.2
| [
"### Training hyperparameters\n\n\nThe following hyperparameters were used during training:\n\n\n* learning\\_rate: 2e-07\n* train\\_batch\\_size: 32\n* eval\\_batch\\_size: 32\n* seed: 42\n* optimizer: Adam with betas=(0.9,0.999) and epsilon=1e-08\n* lr\\_scheduler\\_type: linear\n* num\\_epochs: 1",
"### Training results",
"### Framework versions\n\n\n* Transformers 4.38.2\n* Pytorch 2.2.1+cu121\n* Datasets 2.19.0\n* Tokenizers 0.15.2"
] | [
"TAGS\n#transformers #tensorboard #safetensors #gpt2 #text-generation #generated_from_trainer #base_model-openai-community/gpt2 #license-mit #autotrain_compatible #endpoints_compatible #text-generation-inference #region-us \n",
"### Training hyperparameters\n\n\nThe following hyperparameters were used during training:\n\n\n* learning\\_rate: 2e-07\n* train\\_batch\\_size: 32\n* eval\\_batch\\_size: 32\n* seed: 42\n* optimizer: Adam with betas=(0.9,0.999) and epsilon=1e-08\n* lr\\_scheduler\\_type: linear\n* num\\_epochs: 1",
"### Training results",
"### Framework versions\n\n\n* Transformers 4.38.2\n* Pytorch 2.2.1+cu121\n* Datasets 2.19.0\n* Tokenizers 0.15.2"
] |
null | adapter-transformers |
# Adapter `BigTMiami/n_par_bn_v_1_help_class_80_e_adp_lr_0003_S_1` for roberta-base
An [adapter](https://adapterhub.ml) for the `roberta-base` model that was trained on the [BigTMiami/amazon_MICRO_helpfulness_dataset](https://huggingface.co/datasets/BigTMiami/amazon_MICRO_helpfulness_dataset/) dataset and includes a prediction head for classification.
This adapter was created for usage with the **[Adapters](https://github.com/Adapter-Hub/adapters)** library.
## Usage
First, install `adapters`:
```
pip install -U adapters
```
Now, the adapter can be loaded and activated like this:
```python
from adapters import AutoAdapterModel
model = AutoAdapterModel.from_pretrained("roberta-base")
adapter_name = model.load_adapter("BigTMiami/n_par_bn_v_1_help_class_80_e_adp_lr_0003_S_1", source="hf", set_active=True)
```
## Architecture & Training
<!-- Add some description here -->
## Evaluation results
<!-- Add some description here -->
## Citation
<!-- Add some description here --> | {"tags": ["roberta", "adapter-transformers"], "datasets": ["BigTMiami/amazon_MICRO_helpfulness_dataset"]} | BigTMiami/n_par_bn_v_1_help_class_80_e_adp_lr_0003_S_1 | null | [
"adapter-transformers",
"roberta",
"dataset:BigTMiami/amazon_MICRO_helpfulness_dataset",
"region:us"
] | null | 2024-04-21T19:04:21+00:00 | [] | [] | TAGS
#adapter-transformers #roberta #dataset-BigTMiami/amazon_MICRO_helpfulness_dataset #region-us
|
# Adapter 'BigTMiami/n_par_bn_v_1_help_class_80_e_adp_lr_0003_S_1' for roberta-base
An adapter for the 'roberta-base' model that was trained on the BigTMiami/amazon_MICRO_helpfulness_dataset dataset and includes a prediction head for classification.
This adapter was created for usage with the Adapters library.
## Usage
First, install 'adapters':
Now, the adapter can be loaded and activated like this:
## Architecture & Training
## Evaluation results
| [
"# Adapter 'BigTMiami/n_par_bn_v_1_help_class_80_e_adp_lr_0003_S_1' for roberta-base\n\nAn adapter for the 'roberta-base' model that was trained on the BigTMiami/amazon_MICRO_helpfulness_dataset dataset and includes a prediction head for classification.\n\nThis adapter was created for usage with the Adapters library.",
"## Usage\n\nFirst, install 'adapters':\n\n\n\nNow, the adapter can be loaded and activated like this:",
"## Architecture & Training",
"## Evaluation results"
] | [
"TAGS\n#adapter-transformers #roberta #dataset-BigTMiami/amazon_MICRO_helpfulness_dataset #region-us \n",
"# Adapter 'BigTMiami/n_par_bn_v_1_help_class_80_e_adp_lr_0003_S_1' for roberta-base\n\nAn adapter for the 'roberta-base' model that was trained on the BigTMiami/amazon_MICRO_helpfulness_dataset dataset and includes a prediction head for classification.\n\nThis adapter was created for usage with the Adapters library.",
"## Usage\n\nFirst, install 'adapters':\n\n\n\nNow, the adapter can be loaded and activated like this:",
"## Architecture & Training",
"## Evaluation results"
] |
text2text-generation | transformers |
<!-- This model card has been generated automatically according to the information the Trainer had access to. You
should probably proofread and complete it, then remove this comment. -->
# falcon-summ
This model is a fine-tuned version of [Falconsai/text_summarization](https://huggingface.co/Falconsai/text_summarization) on an unknown dataset.
It achieves the following results on the evaluation set:
- Loss: 2.1784
- Rouge1: 0.1921
- Rouge2: 0.0958
- Rougel: 0.1642
- Rougelsum: 0.1643
- Gen Len: 19.0
## Model description
More information needed
## Intended uses & limitations
More information needed
## Training and evaluation data
More information needed
## Training procedure
### Training hyperparameters
The following hyperparameters were used during training:
- learning_rate: 2e-05
- train_batch_size: 16
- eval_batch_size: 16
- seed: 42
- optimizer: Adam with betas=(0.9,0.999) and epsilon=1e-08
- lr_scheduler_type: linear
- num_epochs: 30
- mixed_precision_training: Native AMP
### Training results
| Training Loss | Epoch | Step | Validation Loss | Rouge1 | Rouge2 | Rougel | Rougelsum | Gen Len |
|:-------------:|:-----:|:----:|:---------------:|:------:|:------:|:------:|:---------:|:-------:|
| No log | 1.0 | 62 | 2.6597 | 0.1336 | 0.0451 | 0.1109 | 0.1109 | 19.0 |
| No log | 2.0 | 124 | 2.5126 | 0.1519 | 0.0584 | 0.1258 | 0.1259 | 19.0 |
| No log | 3.0 | 186 | 2.4354 | 0.1702 | 0.0705 | 0.142 | 0.1422 | 19.0 |
| No log | 4.0 | 248 | 2.3855 | 0.1915 | 0.0922 | 0.1616 | 0.1618 | 19.0 |
| No log | 5.0 | 310 | 2.3499 | 0.1932 | 0.0939 | 0.1641 | 0.1644 | 19.0 |
| No log | 6.0 | 372 | 2.3251 | 0.1951 | 0.0959 | 0.1665 | 0.1667 | 19.0 |
| No log | 7.0 | 434 | 2.3035 | 0.1943 | 0.0963 | 0.1655 | 0.1656 | 19.0 |
| No log | 8.0 | 496 | 2.2879 | 0.1941 | 0.0953 | 0.1652 | 0.1653 | 19.0 |
| 2.6368 | 9.0 | 558 | 2.2695 | 0.1952 | 0.0958 | 0.1665 | 0.1667 | 19.0 |
| 2.6368 | 10.0 | 620 | 2.2588 | 0.194 | 0.0952 | 0.1651 | 0.1653 | 19.0 |
| 2.6368 | 11.0 | 682 | 2.2476 | 0.1954 | 0.0968 | 0.1668 | 0.167 | 19.0 |
| 2.6368 | 12.0 | 744 | 2.2396 | 0.1944 | 0.0965 | 0.1656 | 0.1657 | 19.0 |
| 2.6368 | 13.0 | 806 | 2.2296 | 0.1929 | 0.0963 | 0.1641 | 0.1644 | 19.0 |
| 2.6368 | 14.0 | 868 | 2.2242 | 0.1928 | 0.0969 | 0.1639 | 0.1639 | 19.0 |
| 2.6368 | 15.0 | 930 | 2.2159 | 0.1935 | 0.0971 | 0.164 | 0.1641 | 19.0 |
| 2.6368 | 16.0 | 992 | 2.2114 | 0.1924 | 0.0965 | 0.164 | 0.1641 | 19.0 |
| 2.3506 | 17.0 | 1054 | 2.2077 | 0.1926 | 0.0973 | 0.1644 | 0.1645 | 19.0 |
| 2.3506 | 18.0 | 1116 | 2.2031 | 0.1933 | 0.0968 | 0.1647 | 0.1648 | 19.0 |
| 2.3506 | 19.0 | 1178 | 2.1971 | 0.1928 | 0.0962 | 0.1643 | 0.1644 | 19.0 |
| 2.3506 | 20.0 | 1240 | 2.1956 | 0.1925 | 0.0956 | 0.165 | 0.1651 | 19.0 |
| 2.3506 | 21.0 | 1302 | 2.1903 | 0.1927 | 0.0958 | 0.1644 | 0.1644 | 19.0 |
| 2.3506 | 22.0 | 1364 | 2.1882 | 0.1933 | 0.0972 | 0.1653 | 0.1653 | 19.0 |
| 2.3506 | 23.0 | 1426 | 2.1858 | 0.1921 | 0.0956 | 0.1639 | 0.1641 | 19.0 |
| 2.3506 | 24.0 | 1488 | 2.1842 | 0.1921 | 0.0956 | 0.1642 | 0.1643 | 19.0 |
| 2.2758 | 25.0 | 1550 | 2.1832 | 0.1919 | 0.0958 | 0.1645 | 0.1647 | 19.0 |
| 2.2758 | 26.0 | 1612 | 2.1815 | 0.1922 | 0.0958 | 0.1646 | 0.1647 | 19.0 |
| 2.2758 | 27.0 | 1674 | 2.1795 | 0.1924 | 0.0962 | 0.1646 | 0.1647 | 19.0 |
| 2.2758 | 28.0 | 1736 | 2.1790 | 0.1922 | 0.0961 | 0.1646 | 0.1647 | 19.0 |
| 2.2758 | 29.0 | 1798 | 2.1784 | 0.1925 | 0.0963 | 0.1645 | 0.1646 | 19.0 |
| 2.2758 | 30.0 | 1860 | 2.1784 | 0.1921 | 0.0958 | 0.1642 | 0.1643 | 19.0 |
### Framework versions
- Transformers 4.39.3
- Pytorch 2.1.2
- Datasets 2.18.0
- Tokenizers 0.15.2
| {"license": "apache-2.0", "tags": ["generated_from_trainer"], "metrics": ["rouge"], "base_model": "Falconsai/text_summarization", "model-index": [{"name": "falcon-summ", "results": []}]} | suneeln-duke/falcon-summ | null | [
"transformers",
"tensorboard",
"safetensors",
"t5",
"text2text-generation",
"generated_from_trainer",
"base_model:Falconsai/text_summarization",
"license:apache-2.0",
"autotrain_compatible",
"endpoints_compatible",
"text-generation-inference",
"region:us"
] | null | 2024-04-21T19:04:58+00:00 | [] | [] | TAGS
#transformers #tensorboard #safetensors #t5 #text2text-generation #generated_from_trainer #base_model-Falconsai/text_summarization #license-apache-2.0 #autotrain_compatible #endpoints_compatible #text-generation-inference #region-us
| falcon-summ
===========
This model is a fine-tuned version of Falconsai/text\_summarization on an unknown dataset.
It achieves the following results on the evaluation set:
* Loss: 2.1784
* Rouge1: 0.1921
* Rouge2: 0.0958
* Rougel: 0.1642
* Rougelsum: 0.1643
* Gen Len: 19.0
Model description
-----------------
More information needed
Intended uses & limitations
---------------------------
More information needed
Training and evaluation data
----------------------------
More information needed
Training procedure
------------------
### Training hyperparameters
The following hyperparameters were used during training:
* learning\_rate: 2e-05
* train\_batch\_size: 16
* eval\_batch\_size: 16
* seed: 42
* optimizer: Adam with betas=(0.9,0.999) and epsilon=1e-08
* lr\_scheduler\_type: linear
* num\_epochs: 30
* mixed\_precision\_training: Native AMP
### Training results
### Framework versions
* Transformers 4.39.3
* Pytorch 2.1.2
* Datasets 2.18.0
* Tokenizers 0.15.2
| [
"### Training hyperparameters\n\n\nThe following hyperparameters were used during training:\n\n\n* learning\\_rate: 2e-05\n* train\\_batch\\_size: 16\n* eval\\_batch\\_size: 16\n* seed: 42\n* optimizer: Adam with betas=(0.9,0.999) and epsilon=1e-08\n* lr\\_scheduler\\_type: linear\n* num\\_epochs: 30\n* mixed\\_precision\\_training: Native AMP",
"### Training results",
"### Framework versions\n\n\n* Transformers 4.39.3\n* Pytorch 2.1.2\n* Datasets 2.18.0\n* Tokenizers 0.15.2"
] | [
"TAGS\n#transformers #tensorboard #safetensors #t5 #text2text-generation #generated_from_trainer #base_model-Falconsai/text_summarization #license-apache-2.0 #autotrain_compatible #endpoints_compatible #text-generation-inference #region-us \n",
"### Training hyperparameters\n\n\nThe following hyperparameters were used during training:\n\n\n* learning\\_rate: 2e-05\n* train\\_batch\\_size: 16\n* eval\\_batch\\_size: 16\n* seed: 42\n* optimizer: Adam with betas=(0.9,0.999) and epsilon=1e-08\n* lr\\_scheduler\\_type: linear\n* num\\_epochs: 30\n* mixed\\_precision\\_training: Native AMP",
"### Training results",
"### Framework versions\n\n\n* Transformers 4.39.3\n* Pytorch 2.1.2\n* Datasets 2.18.0\n* Tokenizers 0.15.2"
] |
null | null |
# Model Card for Model ID
<!-- Provide a quick summary of what the model is/does. -->
This modelcard aims to be a base template for new models. It has been generated using [this raw template](https://github.com/huggingface/huggingface_hub/blob/main/src/huggingface_hub/templates/modelcard_template.md?plain=1).
## Model Details
### Model Description
<!-- Provide a longer summary of what this model is. -->
- **Developed by:** [More Information Needed]
- **Funded by [optional]:** [More Information Needed]
- **Shared by [optional]:** [More Information Needed]
- **Model type:** [More Information Needed]
- **Language(s) (NLP):** [More Information Needed]
- **License:** [More Information Needed]
- **Finetuned from model [optional]:** [More Information Needed]
### Model Sources [optional]
<!-- Provide the basic links for the model. -->
- **Repository:** [More Information Needed]
- **Paper [optional]:** [More Information Needed]
- **Demo [optional]:** [More Information Needed]
## Uses
<!-- Address questions around how the model is intended to be used, including the foreseeable users of the model and those affected by the model. -->
### Direct Use
<!-- This section is for the model use without fine-tuning or plugging into a larger ecosystem/app. -->
[More Information Needed]
### Downstream Use [optional]
<!-- This section is for the model use when fine-tuned for a task, or when plugged into a larger ecosystem/app -->
[More Information Needed]
### Out-of-Scope Use
<!-- This section addresses misuse, malicious use, and uses that the model will not work well for. -->
[More Information Needed]
## Bias, Risks, and Limitations
<!-- This section is meant to convey both technical and sociotechnical limitations. -->
[More Information Needed]
### Recommendations
<!-- This section is meant to convey recommendations with respect to the bias, risk, and technical limitations. -->
Users (both direct and downstream) should be made aware of the risks, biases and limitations of the model. More information needed for further recommendations.
## How to Get Started with the Model
Use the code below to get started with the model.
[More Information Needed]
## Training Details
### Training Data
<!-- This should link to a Dataset Card, perhaps with a short stub of information on what the training data is all about as well as documentation related to data pre-processing or additional filtering. -->
[More Information Needed]
### Training Procedure
<!-- This relates heavily to the Technical Specifications. Content here should link to that section when it is relevant to the training procedure. -->
#### Preprocessing [optional]
[More Information Needed]
#### Training Hyperparameters
- **Training regime:** [More Information Needed] <!--fp32, fp16 mixed precision, bf16 mixed precision, bf16 non-mixed precision, fp16 non-mixed precision, fp8 mixed precision -->
#### Speeds, Sizes, Times [optional]
<!-- This section provides information about throughput, start/end time, checkpoint size if relevant, etc. -->
[More Information Needed]
## Evaluation
<!-- This section describes the evaluation protocols and provides the results. -->
### Testing Data, Factors & Metrics
#### Testing Data
<!-- This should link to a Dataset Card if possible. -->
[More Information Needed]
#### Factors
<!-- These are the things the evaluation is disaggregating by, e.g., subpopulations or domains. -->
[More Information Needed]
#### Metrics
<!-- These are the evaluation metrics being used, ideally with a description of why. -->
[More Information Needed]
### Results
[More Information Needed]
#### Summary
## Model Examination [optional]
<!-- Relevant interpretability work for the model goes here -->
[More Information Needed]
## Environmental Impact
<!-- Total emissions (in grams of CO2eq) and additional considerations, such as electricity usage, go here. Edit the suggested text below accordingly -->
Carbon emissions can be estimated using the [Machine Learning Impact calculator](https://mlco2.github.io/impact#compute) presented in [Lacoste et al. (2019)](https://arxiv.org/abs/1910.09700).
- **Hardware Type:** [More Information Needed]
- **Hours used:** [More Information Needed]
- **Cloud Provider:** [More Information Needed]
- **Compute Region:** [More Information Needed]
- **Carbon Emitted:** [More Information Needed]
## Technical Specifications [optional]
### Model Architecture and Objective
[More Information Needed]
### Compute Infrastructure
[More Information Needed]
#### Hardware
[More Information Needed]
#### Software
[More Information Needed]
## Citation [optional]
<!-- If there is a paper or blog post introducing the model, the APA and Bibtex information for that should go in this section. -->
**BibTeX:**
[More Information Needed]
**APA:**
[More Information Needed]
## Glossary [optional]
<!-- If relevant, include terms and calculations in this section that can help readers understand the model or model card. -->
[More Information Needed]
## More Information [optional]
[More Information Needed]
## Model Card Authors [optional]
[More Information Needed]
## Model Card Contact
[More Information Needed] | {} | DEnahs/THenahs | null | [
"arxiv:1910.09700",
"region:us"
] | null | 2024-04-21T19:05:22+00:00 | [
"1910.09700"
] | [] | TAGS
#arxiv-1910.09700 #region-us
|
# Model Card for Model ID
This modelcard aims to be a base template for new models. It has been generated using this raw template.
## Model Details
### Model Description
- Developed by:
- Funded by [optional]:
- Shared by [optional]:
- Model type:
- Language(s) (NLP):
- License:
- Finetuned from model [optional]:
### Model Sources [optional]
- Repository:
- Paper [optional]:
- Demo [optional]:
## Uses
### Direct Use
### Downstream Use [optional]
### Out-of-Scope Use
## Bias, Risks, and Limitations
### Recommendations
Users (both direct and downstream) should be made aware of the risks, biases and limitations of the model. More information needed for further recommendations.
## How to Get Started with the Model
Use the code below to get started with the model.
## Training Details
### Training Data
### Training Procedure
#### Preprocessing [optional]
#### Training Hyperparameters
- Training regime:
#### Speeds, Sizes, Times [optional]
## Evaluation
### Testing Data, Factors & Metrics
#### Testing Data
#### Factors
#### Metrics
### Results
#### Summary
## Model Examination [optional]
## Environmental Impact
Carbon emissions can be estimated using the Machine Learning Impact calculator presented in Lacoste et al. (2019).
- Hardware Type:
- Hours used:
- Cloud Provider:
- Compute Region:
- Carbon Emitted:
## Technical Specifications [optional]
### Model Architecture and Objective
### Compute Infrastructure
#### Hardware
#### Software
[optional]
BibTeX:
APA:
## Glossary [optional]
## More Information [optional]
## Model Card Authors [optional]
## Model Card Contact
| [
"# Model Card for Model ID\n\n\n\nThis modelcard aims to be a base template for new models. It has been generated using this raw template.",
"## Model Details",
"### Model Description\n\n\n\n\n\n- Developed by: \n- Funded by [optional]: \n- Shared by [optional]: \n- Model type: \n- Language(s) (NLP): \n- License: \n- Finetuned from model [optional]:",
"### Model Sources [optional]\n\n\n\n- Repository: \n- Paper [optional]: \n- Demo [optional]:",
"## Uses",
"### Direct Use",
"### Downstream Use [optional]",
"### Out-of-Scope Use",
"## Bias, Risks, and Limitations",
"### Recommendations\n\n\n\nUsers (both direct and downstream) should be made aware of the risks, biases and limitations of the model. More information needed for further recommendations.",
"## How to Get Started with the Model\n\nUse the code below to get started with the model.",
"## Training Details",
"### Training Data",
"### Training Procedure",
"#### Preprocessing [optional]",
"#### Training Hyperparameters\n\n- Training regime:",
"#### Speeds, Sizes, Times [optional]",
"## Evaluation",
"### Testing Data, Factors & Metrics",
"#### Testing Data",
"#### Factors",
"#### Metrics",
"### Results",
"#### Summary",
"## Model Examination [optional]",
"## Environmental Impact\n\n\n\nCarbon emissions can be estimated using the Machine Learning Impact calculator presented in Lacoste et al. (2019).\n\n- Hardware Type: \n- Hours used: \n- Cloud Provider: \n- Compute Region: \n- Carbon Emitted:",
"## Technical Specifications [optional]",
"### Model Architecture and Objective",
"### Compute Infrastructure",
"#### Hardware",
"#### Software\n\n\n\n[optional]\n\n\n\nBibTeX:\n\n\n\nAPA:",
"## Glossary [optional]",
"## More Information [optional]",
"## Model Card Authors [optional]",
"## Model Card Contact"
] | [
"TAGS\n#arxiv-1910.09700 #region-us \n",
"# Model Card for Model ID\n\n\n\nThis modelcard aims to be a base template for new models. It has been generated using this raw template.",
"## Model Details",
"### Model Description\n\n\n\n\n\n- Developed by: \n- Funded by [optional]: \n- Shared by [optional]: \n- Model type: \n- Language(s) (NLP): \n- License: \n- Finetuned from model [optional]:",
"### Model Sources [optional]\n\n\n\n- Repository: \n- Paper [optional]: \n- Demo [optional]:",
"## Uses",
"### Direct Use",
"### Downstream Use [optional]",
"### Out-of-Scope Use",
"## Bias, Risks, and Limitations",
"### Recommendations\n\n\n\nUsers (both direct and downstream) should be made aware of the risks, biases and limitations of the model. More information needed for further recommendations.",
"## How to Get Started with the Model\n\nUse the code below to get started with the model.",
"## Training Details",
"### Training Data",
"### Training Procedure",
"#### Preprocessing [optional]",
"#### Training Hyperparameters\n\n- Training regime:",
"#### Speeds, Sizes, Times [optional]",
"## Evaluation",
"### Testing Data, Factors & Metrics",
"#### Testing Data",
"#### Factors",
"#### Metrics",
"### Results",
"#### Summary",
"## Model Examination [optional]",
"## Environmental Impact\n\n\n\nCarbon emissions can be estimated using the Machine Learning Impact calculator presented in Lacoste et al. (2019).\n\n- Hardware Type: \n- Hours used: \n- Cloud Provider: \n- Compute Region: \n- Carbon Emitted:",
"## Technical Specifications [optional]",
"### Model Architecture and Objective",
"### Compute Infrastructure",
"#### Hardware",
"#### Software\n\n\n\n[optional]\n\n\n\nBibTeX:\n\n\n\nAPA:",
"## Glossary [optional]",
"## More Information [optional]",
"## Model Card Authors [optional]",
"## Model Card Contact"
] |
null | transformers |
# Model Card for Model ID
<!-- Provide a quick summary of what the model is/does. -->
## Model Details
### Model Description
<!-- Provide a longer summary of what this model is. -->
This is the model card of a 🤗 transformers model that has been pushed on the Hub. This model card has been automatically generated.
- **Developed by:** [More Information Needed]
- **Funded by [optional]:** [More Information Needed]
- **Shared by [optional]:** [More Information Needed]
- **Model type:** [More Information Needed]
- **Language(s) (NLP):** [More Information Needed]
- **License:** [More Information Needed]
- **Finetuned from model [optional]:** [More Information Needed]
### Model Sources [optional]
<!-- Provide the basic links for the model. -->
- **Repository:** [More Information Needed]
- **Paper [optional]:** [More Information Needed]
- **Demo [optional]:** [More Information Needed]
## Uses
<!-- Address questions around how the model is intended to be used, including the foreseeable users of the model and those affected by the model. -->
### Direct Use
<!-- This section is for the model use without fine-tuning or plugging into a larger ecosystem/app. -->
[More Information Needed]
### Downstream Use [optional]
<!-- This section is for the model use when fine-tuned for a task, or when plugged into a larger ecosystem/app -->
[More Information Needed]
### Out-of-Scope Use
<!-- This section addresses misuse, malicious use, and uses that the model will not work well for. -->
[More Information Needed]
## Bias, Risks, and Limitations
<!-- This section is meant to convey both technical and sociotechnical limitations. -->
[More Information Needed]
### Recommendations
<!-- This section is meant to convey recommendations with respect to the bias, risk, and technical limitations. -->
Users (both direct and downstream) should be made aware of the risks, biases and limitations of the model. More information needed for further recommendations.
## How to Get Started with the Model
Use the code below to get started with the model.
[More Information Needed]
## Training Details
### Training Data
<!-- This should link to a Dataset Card, perhaps with a short stub of information on what the training data is all about as well as documentation related to data pre-processing or additional filtering. -->
[More Information Needed]
### Training Procedure
<!-- This relates heavily to the Technical Specifications. Content here should link to that section when it is relevant to the training procedure. -->
#### Preprocessing [optional]
[More Information Needed]
#### Training Hyperparameters
- **Training regime:** [More Information Needed] <!--fp32, fp16 mixed precision, bf16 mixed precision, bf16 non-mixed precision, fp16 non-mixed precision, fp8 mixed precision -->
#### Speeds, Sizes, Times [optional]
<!-- This section provides information about throughput, start/end time, checkpoint size if relevant, etc. -->
[More Information Needed]
## Evaluation
<!-- This section describes the evaluation protocols and provides the results. -->
### Testing Data, Factors & Metrics
#### Testing Data
<!-- This should link to a Dataset Card if possible. -->
[More Information Needed]
#### Factors
<!-- These are the things the evaluation is disaggregating by, e.g., subpopulations or domains. -->
[More Information Needed]
#### Metrics
<!-- These are the evaluation metrics being used, ideally with a description of why. -->
[More Information Needed]
### Results
[More Information Needed]
#### Summary
## Model Examination [optional]
<!-- Relevant interpretability work for the model goes here -->
[More Information Needed]
## Environmental Impact
<!-- Total emissions (in grams of CO2eq) and additional considerations, such as electricity usage, go here. Edit the suggested text below accordingly -->
Carbon emissions can be estimated using the [Machine Learning Impact calculator](https://mlco2.github.io/impact#compute) presented in [Lacoste et al. (2019)](https://arxiv.org/abs/1910.09700).
- **Hardware Type:** [More Information Needed]
- **Hours used:** [More Information Needed]
- **Cloud Provider:** [More Information Needed]
- **Compute Region:** [More Information Needed]
- **Carbon Emitted:** [More Information Needed]
## Technical Specifications [optional]
### Model Architecture and Objective
[More Information Needed]
### Compute Infrastructure
[More Information Needed]
#### Hardware
[More Information Needed]
#### Software
[More Information Needed]
## Citation [optional]
<!-- If there is a paper or blog post introducing the model, the APA and Bibtex information for that should go in this section. -->
**BibTeX:**
[More Information Needed]
**APA:**
[More Information Needed]
## Glossary [optional]
<!-- If relevant, include terms and calculations in this section that can help readers understand the model or model card. -->
[More Information Needed]
## More Information [optional]
[More Information Needed]
## Model Card Authors [optional]
[More Information Needed]
## Model Card Contact
[More Information Needed] | {"library_name": "transformers", "tags": []} | Cafet/w2v-bert-2.0-mongolian_test | null | [
"transformers",
"arxiv:1910.09700",
"endpoints_compatible",
"region:us"
] | null | 2024-04-21T19:05:27+00:00 | [
"1910.09700"
] | [] | TAGS
#transformers #arxiv-1910.09700 #endpoints_compatible #region-us
|
# Model Card for Model ID
## Model Details
### Model Description
This is the model card of a transformers model that has been pushed on the Hub. This model card has been automatically generated.
- Developed by:
- Funded by [optional]:
- Shared by [optional]:
- Model type:
- Language(s) (NLP):
- License:
- Finetuned from model [optional]:
### Model Sources [optional]
- Repository:
- Paper [optional]:
- Demo [optional]:
## Uses
### Direct Use
### Downstream Use [optional]
### Out-of-Scope Use
## Bias, Risks, and Limitations
### Recommendations
Users (both direct and downstream) should be made aware of the risks, biases and limitations of the model. More information needed for further recommendations.
## How to Get Started with the Model
Use the code below to get started with the model.
## Training Details
### Training Data
### Training Procedure
#### Preprocessing [optional]
#### Training Hyperparameters
- Training regime:
#### Speeds, Sizes, Times [optional]
## Evaluation
### Testing Data, Factors & Metrics
#### Testing Data
#### Factors
#### Metrics
### Results
#### Summary
## Model Examination [optional]
## Environmental Impact
Carbon emissions can be estimated using the Machine Learning Impact calculator presented in Lacoste et al. (2019).
- Hardware Type:
- Hours used:
- Cloud Provider:
- Compute Region:
- Carbon Emitted:
## Technical Specifications [optional]
### Model Architecture and Objective
### Compute Infrastructure
#### Hardware
#### Software
[optional]
BibTeX:
APA:
## Glossary [optional]
## More Information [optional]
## Model Card Authors [optional]
## Model Card Contact
| [
"# Model Card for Model ID",
"## Model Details",
"### Model Description\n\n\n\nThis is the model card of a transformers model that has been pushed on the Hub. This model card has been automatically generated.\n\n- Developed by: \n- Funded by [optional]: \n- Shared by [optional]: \n- Model type: \n- Language(s) (NLP): \n- License: \n- Finetuned from model [optional]:",
"### Model Sources [optional]\n\n\n\n- Repository: \n- Paper [optional]: \n- Demo [optional]:",
"## Uses",
"### Direct Use",
"### Downstream Use [optional]",
"### Out-of-Scope Use",
"## Bias, Risks, and Limitations",
"### Recommendations\n\n\n\nUsers (both direct and downstream) should be made aware of the risks, biases and limitations of the model. More information needed for further recommendations.",
"## How to Get Started with the Model\n\nUse the code below to get started with the model.",
"## Training Details",
"### Training Data",
"### Training Procedure",
"#### Preprocessing [optional]",
"#### Training Hyperparameters\n\n- Training regime:",
"#### Speeds, Sizes, Times [optional]",
"## Evaluation",
"### Testing Data, Factors & Metrics",
"#### Testing Data",
"#### Factors",
"#### Metrics",
"### Results",
"#### Summary",
"## Model Examination [optional]",
"## Environmental Impact\n\n\n\nCarbon emissions can be estimated using the Machine Learning Impact calculator presented in Lacoste et al. (2019).\n\n- Hardware Type: \n- Hours used: \n- Cloud Provider: \n- Compute Region: \n- Carbon Emitted:",
"## Technical Specifications [optional]",
"### Model Architecture and Objective",
"### Compute Infrastructure",
"#### Hardware",
"#### Software\n\n\n\n[optional]\n\n\n\nBibTeX:\n\n\n\nAPA:",
"## Glossary [optional]",
"## More Information [optional]",
"## Model Card Authors [optional]",
"## Model Card Contact"
] | [
"TAGS\n#transformers #arxiv-1910.09700 #endpoints_compatible #region-us \n",
"# Model Card for Model ID",
"## Model Details",
"### Model Description\n\n\n\nThis is the model card of a transformers model that has been pushed on the Hub. This model card has been automatically generated.\n\n- Developed by: \n- Funded by [optional]: \n- Shared by [optional]: \n- Model type: \n- Language(s) (NLP): \n- License: \n- Finetuned from model [optional]:",
"### Model Sources [optional]\n\n\n\n- Repository: \n- Paper [optional]: \n- Demo [optional]:",
"## Uses",
"### Direct Use",
"### Downstream Use [optional]",
"### Out-of-Scope Use",
"## Bias, Risks, and Limitations",
"### Recommendations\n\n\n\nUsers (both direct and downstream) should be made aware of the risks, biases and limitations of the model. More information needed for further recommendations.",
"## How to Get Started with the Model\n\nUse the code below to get started with the model.",
"## Training Details",
"### Training Data",
"### Training Procedure",
"#### Preprocessing [optional]",
"#### Training Hyperparameters\n\n- Training regime:",
"#### Speeds, Sizes, Times [optional]",
"## Evaluation",
"### Testing Data, Factors & Metrics",
"#### Testing Data",
"#### Factors",
"#### Metrics",
"### Results",
"#### Summary",
"## Model Examination [optional]",
"## Environmental Impact\n\n\n\nCarbon emissions can be estimated using the Machine Learning Impact calculator presented in Lacoste et al. (2019).\n\n- Hardware Type: \n- Hours used: \n- Cloud Provider: \n- Compute Region: \n- Carbon Emitted:",
"## Technical Specifications [optional]",
"### Model Architecture and Objective",
"### Compute Infrastructure",
"#### Hardware",
"#### Software\n\n\n\n[optional]\n\n\n\nBibTeX:\n\n\n\nAPA:",
"## Glossary [optional]",
"## More Information [optional]",
"## Model Card Authors [optional]",
"## Model Card Contact"
] |
fill-mask | transformers |
# Model Card for Model ID
<!-- Provide a quick summary of what the model is/does. -->
## Model Details
### Model Description
<!-- Provide a longer summary of what this model is. -->
This is the model card of a 🤗 transformers model that has been pushed on the Hub. This model card has been automatically generated.
- **Developed by:** [More Information Needed]
- **Funded by [optional]:** [More Information Needed]
- **Shared by [optional]:** [More Information Needed]
- **Model type:** [More Information Needed]
- **Language(s) (NLP):** [More Information Needed]
- **License:** [More Information Needed]
- **Finetuned from model [optional]:** [More Information Needed]
### Model Sources [optional]
<!-- Provide the basic links for the model. -->
- **Repository:** [More Information Needed]
- **Paper [optional]:** [More Information Needed]
- **Demo [optional]:** [More Information Needed]
## Uses
<!-- Address questions around how the model is intended to be used, including the foreseeable users of the model and those affected by the model. -->
### Direct Use
<!-- This section is for the model use without fine-tuning or plugging into a larger ecosystem/app. -->
[More Information Needed]
### Downstream Use [optional]
<!-- This section is for the model use when fine-tuned for a task, or when plugged into a larger ecosystem/app -->
[More Information Needed]
### Out-of-Scope Use
<!-- This section addresses misuse, malicious use, and uses that the model will not work well for. -->
[More Information Needed]
## Bias, Risks, and Limitations
<!-- This section is meant to convey both technical and sociotechnical limitations. -->
[More Information Needed]
### Recommendations
<!-- This section is meant to convey recommendations with respect to the bias, risk, and technical limitations. -->
Users (both direct and downstream) should be made aware of the risks, biases and limitations of the model. More information needed for further recommendations.
## How to Get Started with the Model
Use the code below to get started with the model.
[More Information Needed]
## Training Details
### Training Data
<!-- This should link to a Dataset Card, perhaps with a short stub of information on what the training data is all about as well as documentation related to data pre-processing or additional filtering. -->
[More Information Needed]
### Training Procedure
<!-- This relates heavily to the Technical Specifications. Content here should link to that section when it is relevant to the training procedure. -->
#### Preprocessing [optional]
[More Information Needed]
#### Training Hyperparameters
- **Training regime:** [More Information Needed] <!--fp32, fp16 mixed precision, bf16 mixed precision, bf16 non-mixed precision, fp16 non-mixed precision, fp8 mixed precision -->
#### Speeds, Sizes, Times [optional]
<!-- This section provides information about throughput, start/end time, checkpoint size if relevant, etc. -->
[More Information Needed]
## Evaluation
<!-- This section describes the evaluation protocols and provides the results. -->
### Testing Data, Factors & Metrics
#### Testing Data
<!-- This should link to a Dataset Card if possible. -->
[More Information Needed]
#### Factors
<!-- These are the things the evaluation is disaggregating by, e.g., subpopulations or domains. -->
[More Information Needed]
#### Metrics
<!-- These are the evaluation metrics being used, ideally with a description of why. -->
[More Information Needed]
### Results
[More Information Needed]
#### Summary
## Model Examination [optional]
<!-- Relevant interpretability work for the model goes here -->
[More Information Needed]
## Environmental Impact
<!-- Total emissions (in grams of CO2eq) and additional considerations, such as electricity usage, go here. Edit the suggested text below accordingly -->
Carbon emissions can be estimated using the [Machine Learning Impact calculator](https://mlco2.github.io/impact#compute) presented in [Lacoste et al. (2019)](https://arxiv.org/abs/1910.09700).
- **Hardware Type:** [More Information Needed]
- **Hours used:** [More Information Needed]
- **Cloud Provider:** [More Information Needed]
- **Compute Region:** [More Information Needed]
- **Carbon Emitted:** [More Information Needed]
## Technical Specifications [optional]
### Model Architecture and Objective
[More Information Needed]
### Compute Infrastructure
[More Information Needed]
#### Hardware
[More Information Needed]
#### Software
[More Information Needed]
## Citation [optional]
<!-- If there is a paper or blog post introducing the model, the APA and Bibtex information for that should go in this section. -->
**BibTeX:**
[More Information Needed]
**APA:**
[More Information Needed]
## Glossary [optional]
<!-- If relevant, include terms and calculations in this section that can help readers understand the model or model card. -->
[More Information Needed]
## More Information [optional]
[More Information Needed]
## Model Card Authors [optional]
[More Information Needed]
## Model Card Contact
[More Information Needed] | {"library_name": "transformers", "tags": []} | eromanal/my-finetuned-bert | null | [
"transformers",
"bert",
"fill-mask",
"arxiv:1910.09700",
"autotrain_compatible",
"endpoints_compatible",
"region:us"
] | null | 2024-04-21T19:06:11+00:00 | [
"1910.09700"
] | [] | TAGS
#transformers #bert #fill-mask #arxiv-1910.09700 #autotrain_compatible #endpoints_compatible #region-us
|
# Model Card for Model ID
## Model Details
### Model Description
This is the model card of a transformers model that has been pushed on the Hub. This model card has been automatically generated.
- Developed by:
- Funded by [optional]:
- Shared by [optional]:
- Model type:
- Language(s) (NLP):
- License:
- Finetuned from model [optional]:
### Model Sources [optional]
- Repository:
- Paper [optional]:
- Demo [optional]:
## Uses
### Direct Use
### Downstream Use [optional]
### Out-of-Scope Use
## Bias, Risks, and Limitations
### Recommendations
Users (both direct and downstream) should be made aware of the risks, biases and limitations of the model. More information needed for further recommendations.
## How to Get Started with the Model
Use the code below to get started with the model.
## Training Details
### Training Data
### Training Procedure
#### Preprocessing [optional]
#### Training Hyperparameters
- Training regime:
#### Speeds, Sizes, Times [optional]
## Evaluation
### Testing Data, Factors & Metrics
#### Testing Data
#### Factors
#### Metrics
### Results
#### Summary
## Model Examination [optional]
## Environmental Impact
Carbon emissions can be estimated using the Machine Learning Impact calculator presented in Lacoste et al. (2019).
- Hardware Type:
- Hours used:
- Cloud Provider:
- Compute Region:
- Carbon Emitted:
## Technical Specifications [optional]
### Model Architecture and Objective
### Compute Infrastructure
#### Hardware
#### Software
[optional]
BibTeX:
APA:
## Glossary [optional]
## More Information [optional]
## Model Card Authors [optional]
## Model Card Contact
| [
"# Model Card for Model ID",
"## Model Details",
"### Model Description\n\n\n\nThis is the model card of a transformers model that has been pushed on the Hub. This model card has been automatically generated.\n\n- Developed by: \n- Funded by [optional]: \n- Shared by [optional]: \n- Model type: \n- Language(s) (NLP): \n- License: \n- Finetuned from model [optional]:",
"### Model Sources [optional]\n\n\n\n- Repository: \n- Paper [optional]: \n- Demo [optional]:",
"## Uses",
"### Direct Use",
"### Downstream Use [optional]",
"### Out-of-Scope Use",
"## Bias, Risks, and Limitations",
"### Recommendations\n\n\n\nUsers (both direct and downstream) should be made aware of the risks, biases and limitations of the model. More information needed for further recommendations.",
"## How to Get Started with the Model\n\nUse the code below to get started with the model.",
"## Training Details",
"### Training Data",
"### Training Procedure",
"#### Preprocessing [optional]",
"#### Training Hyperparameters\n\n- Training regime:",
"#### Speeds, Sizes, Times [optional]",
"## Evaluation",
"### Testing Data, Factors & Metrics",
"#### Testing Data",
"#### Factors",
"#### Metrics",
"### Results",
"#### Summary",
"## Model Examination [optional]",
"## Environmental Impact\n\n\n\nCarbon emissions can be estimated using the Machine Learning Impact calculator presented in Lacoste et al. (2019).\n\n- Hardware Type: \n- Hours used: \n- Cloud Provider: \n- Compute Region: \n- Carbon Emitted:",
"## Technical Specifications [optional]",
"### Model Architecture and Objective",
"### Compute Infrastructure",
"#### Hardware",
"#### Software\n\n\n\n[optional]\n\n\n\nBibTeX:\n\n\n\nAPA:",
"## Glossary [optional]",
"## More Information [optional]",
"## Model Card Authors [optional]",
"## Model Card Contact"
] | [
"TAGS\n#transformers #bert #fill-mask #arxiv-1910.09700 #autotrain_compatible #endpoints_compatible #region-us \n",
"# Model Card for Model ID",
"## Model Details",
"### Model Description\n\n\n\nThis is the model card of a transformers model that has been pushed on the Hub. This model card has been automatically generated.\n\n- Developed by: \n- Funded by [optional]: \n- Shared by [optional]: \n- Model type: \n- Language(s) (NLP): \n- License: \n- Finetuned from model [optional]:",
"### Model Sources [optional]\n\n\n\n- Repository: \n- Paper [optional]: \n- Demo [optional]:",
"## Uses",
"### Direct Use",
"### Downstream Use [optional]",
"### Out-of-Scope Use",
"## Bias, Risks, and Limitations",
"### Recommendations\n\n\n\nUsers (both direct and downstream) should be made aware of the risks, biases and limitations of the model. More information needed for further recommendations.",
"## How to Get Started with the Model\n\nUse the code below to get started with the model.",
"## Training Details",
"### Training Data",
"### Training Procedure",
"#### Preprocessing [optional]",
"#### Training Hyperparameters\n\n- Training regime:",
"#### Speeds, Sizes, Times [optional]",
"## Evaluation",
"### Testing Data, Factors & Metrics",
"#### Testing Data",
"#### Factors",
"#### Metrics",
"### Results",
"#### Summary",
"## Model Examination [optional]",
"## Environmental Impact\n\n\n\nCarbon emissions can be estimated using the Machine Learning Impact calculator presented in Lacoste et al. (2019).\n\n- Hardware Type: \n- Hours used: \n- Cloud Provider: \n- Compute Region: \n- Carbon Emitted:",
"## Technical Specifications [optional]",
"### Model Architecture and Objective",
"### Compute Infrastructure",
"#### Hardware",
"#### Software\n\n\n\n[optional]\n\n\n\nBibTeX:\n\n\n\nAPA:",
"## Glossary [optional]",
"## More Information [optional]",
"## Model Card Authors [optional]",
"## Model Card Contact"
] |
text-generation | transformers |
# Model Trained Using AutoTrain
This model was trained using AutoTrain. For more information, please visit [AutoTrain](https://hf.co/docs/autotrain).
# Usage
```python
from transformers import AutoModelForCausalLM, AutoTokenizer
model_path = "PATH_TO_THIS_REPO"
tokenizer = AutoTokenizer.from_pretrained(model_path)
model = AutoModelForCausalLM.from_pretrained(
model_path,
device_map="auto",
torch_dtype='auto'
).eval()
# Prompt content: "hi"
messages = [
{"role": "user", "content": "hi"}
]
input_ids = tokenizer.apply_chat_template(conversation=messages, tokenize=True, add_generation_prompt=True, return_tensors='pt')
output_ids = model.generate(input_ids.to('cuda'))
response = tokenizer.decode(output_ids[0][input_ids.shape[1]:], skip_special_tokens=True)
# Model response: "Hello! How can I assist you today?"
print(response)
``` | {"license": "other", "library_name": "transformers", "tags": ["autotrain", "text-generation-inference", "text-generation", "peft"], "widget": [{"messages": [{"role": "user", "content": "What is your favorite condiment?"}]}]} | franciscobdl/TestL_1 | null | [
"transformers",
"safetensors",
"autotrain",
"text-generation-inference",
"text-generation",
"peft",
"conversational",
"license:other",
"endpoints_compatible",
"region:us"
] | null | 2024-04-21T19:07:02+00:00 | [] | [] | TAGS
#transformers #safetensors #autotrain #text-generation-inference #text-generation #peft #conversational #license-other #endpoints_compatible #region-us
|
# Model Trained Using AutoTrain
This model was trained using AutoTrain. For more information, please visit AutoTrain.
# Usage
| [
"# Model Trained Using AutoTrain\n\nThis model was trained using AutoTrain. For more information, please visit AutoTrain.",
"# Usage"
] | [
"TAGS\n#transformers #safetensors #autotrain #text-generation-inference #text-generation #peft #conversational #license-other #endpoints_compatible #region-us \n",
"# Model Trained Using AutoTrain\n\nThis model was trained using AutoTrain. For more information, please visit AutoTrain.",
"# Usage"
] |
text-classification | transformers |
<!-- This model card has been generated automatically according to the information the Trainer had access to. You
should probably proofread and complete it, then remove this comment. -->
# robust_llm_pythia-410m_ian-022_IMDB_n-its-25
This model is a fine-tuned version of [EleutherAI/pythia-410m](https://huggingface.co/EleutherAI/pythia-410m) on an unknown dataset.
## Model description
More information needed
## Intended uses & limitations
More information needed
## Training and evaluation data
More information needed
## Training procedure
### Training hyperparameters
The following hyperparameters were used during training:
- learning_rate: 1e-05
- train_batch_size: 8
- eval_batch_size: 64
- seed: 0
- optimizer: Adam with betas=(0.9,0.999) and epsilon=1e-08
- lr_scheduler_type: linear
- num_epochs: 1
### Training results
### Framework versions
- Transformers 4.39.3
- Pytorch 2.2.1
- Datasets 2.18.0
- Tokenizers 0.15.2
| {"license": "apache-2.0", "tags": ["generated_from_trainer"], "base_model": "EleutherAI/pythia-410m", "model-index": [{"name": "robust_llm_pythia-410m_ian-022_IMDB_n-its-25", "results": []}]} | AlignmentResearch/robust_llm_pythia-410m_ian-022_IMDB_n-its-25 | null | [
"transformers",
"tensorboard",
"safetensors",
"gpt_neox",
"text-classification",
"generated_from_trainer",
"base_model:EleutherAI/pythia-410m",
"license:apache-2.0",
"autotrain_compatible",
"endpoints_compatible",
"text-generation-inference",
"region:us"
] | null | 2024-04-21T19:07:04+00:00 | [] | [] | TAGS
#transformers #tensorboard #safetensors #gpt_neox #text-classification #generated_from_trainer #base_model-EleutherAI/pythia-410m #license-apache-2.0 #autotrain_compatible #endpoints_compatible #text-generation-inference #region-us
|
# robust_llm_pythia-410m_ian-022_IMDB_n-its-25
This model is a fine-tuned version of EleutherAI/pythia-410m on an unknown dataset.
## Model description
More information needed
## Intended uses & limitations
More information needed
## Training and evaluation data
More information needed
## Training procedure
### Training hyperparameters
The following hyperparameters were used during training:
- learning_rate: 1e-05
- train_batch_size: 8
- eval_batch_size: 64
- seed: 0
- optimizer: Adam with betas=(0.9,0.999) and epsilon=1e-08
- lr_scheduler_type: linear
- num_epochs: 1
### Training results
### Framework versions
- Transformers 4.39.3
- Pytorch 2.2.1
- Datasets 2.18.0
- Tokenizers 0.15.2
| [
"# robust_llm_pythia-410m_ian-022_IMDB_n-its-25\n\nThis model is a fine-tuned version of EleutherAI/pythia-410m on an unknown dataset.",
"## Model description\n\nMore information needed",
"## Intended uses & limitations\n\nMore information needed",
"## Training and evaluation data\n\nMore information needed",
"## Training procedure",
"### Training hyperparameters\n\nThe following hyperparameters were used during training:\n- learning_rate: 1e-05\n- train_batch_size: 8\n- eval_batch_size: 64\n- seed: 0\n- optimizer: Adam with betas=(0.9,0.999) and epsilon=1e-08\n- lr_scheduler_type: linear\n- num_epochs: 1",
"### Training results",
"### Framework versions\n\n- Transformers 4.39.3\n- Pytorch 2.2.1\n- Datasets 2.18.0\n- Tokenizers 0.15.2"
] | [
"TAGS\n#transformers #tensorboard #safetensors #gpt_neox #text-classification #generated_from_trainer #base_model-EleutherAI/pythia-410m #license-apache-2.0 #autotrain_compatible #endpoints_compatible #text-generation-inference #region-us \n",
"# robust_llm_pythia-410m_ian-022_IMDB_n-its-25\n\nThis model is a fine-tuned version of EleutherAI/pythia-410m on an unknown dataset.",
"## Model description\n\nMore information needed",
"## Intended uses & limitations\n\nMore information needed",
"## Training and evaluation data\n\nMore information needed",
"## Training procedure",
"### Training hyperparameters\n\nThe following hyperparameters were used during training:\n- learning_rate: 1e-05\n- train_batch_size: 8\n- eval_batch_size: 64\n- seed: 0\n- optimizer: Adam with betas=(0.9,0.999) and epsilon=1e-08\n- lr_scheduler_type: linear\n- num_epochs: 1",
"### Training results",
"### Framework versions\n\n- Transformers 4.39.3\n- Pytorch 2.2.1\n- Datasets 2.18.0\n- Tokenizers 0.15.2"
] |
null | adapter-transformers |
# Adapter `BigTMiami/n_par_bn_v_1_help_class_40_e_adp_lr_0003_S_2` for roberta-base
An [adapter](https://adapterhub.ml) for the `roberta-base` model that was trained on the [BigTMiami/amazon_MICRO_helpfulness_dataset](https://huggingface.co/datasets/BigTMiami/amazon_MICRO_helpfulness_dataset/) dataset and includes a prediction head for classification.
This adapter was created for usage with the **[Adapters](https://github.com/Adapter-Hub/adapters)** library.
## Usage
First, install `adapters`:
```
pip install -U adapters
```
Now, the adapter can be loaded and activated like this:
```python
from adapters import AutoAdapterModel
model = AutoAdapterModel.from_pretrained("roberta-base")
adapter_name = model.load_adapter("BigTMiami/n_par_bn_v_1_help_class_40_e_adp_lr_0003_S_2", source="hf", set_active=True)
```
## Architecture & Training
<!-- Add some description here -->
## Evaluation results
<!-- Add some description here -->
## Citation
<!-- Add some description here --> | {"tags": ["roberta", "adapter-transformers"], "datasets": ["BigTMiami/amazon_MICRO_helpfulness_dataset"]} | BigTMiami/n_par_bn_v_1_help_class_40_e_adp_lr_0003_S_2 | null | [
"adapter-transformers",
"roberta",
"dataset:BigTMiami/amazon_MICRO_helpfulness_dataset",
"region:us"
] | null | 2024-04-21T19:07:06+00:00 | [] | [] | TAGS
#adapter-transformers #roberta #dataset-BigTMiami/amazon_MICRO_helpfulness_dataset #region-us
|
# Adapter 'BigTMiami/n_par_bn_v_1_help_class_40_e_adp_lr_0003_S_2' for roberta-base
An adapter for the 'roberta-base' model that was trained on the BigTMiami/amazon_MICRO_helpfulness_dataset dataset and includes a prediction head for classification.
This adapter was created for usage with the Adapters library.
## Usage
First, install 'adapters':
Now, the adapter can be loaded and activated like this:
## Architecture & Training
## Evaluation results
| [
"# Adapter 'BigTMiami/n_par_bn_v_1_help_class_40_e_adp_lr_0003_S_2' for roberta-base\n\nAn adapter for the 'roberta-base' model that was trained on the BigTMiami/amazon_MICRO_helpfulness_dataset dataset and includes a prediction head for classification.\n\nThis adapter was created for usage with the Adapters library.",
"## Usage\n\nFirst, install 'adapters':\n\n\n\nNow, the adapter can be loaded and activated like this:",
"## Architecture & Training",
"## Evaluation results"
] | [
"TAGS\n#adapter-transformers #roberta #dataset-BigTMiami/amazon_MICRO_helpfulness_dataset #region-us \n",
"# Adapter 'BigTMiami/n_par_bn_v_1_help_class_40_e_adp_lr_0003_S_2' for roberta-base\n\nAn adapter for the 'roberta-base' model that was trained on the BigTMiami/amazon_MICRO_helpfulness_dataset dataset and includes a prediction head for classification.\n\nThis adapter was created for usage with the Adapters library.",
"## Usage\n\nFirst, install 'adapters':\n\n\n\nNow, the adapter can be loaded and activated like this:",
"## Architecture & Training",
"## Evaluation results"
] |
text-generation | transformers | Quantization made by Richard Erkhov.
[Github](https://github.com/RichardErkhov)
[Discord](https://discord.gg/pvy7H8DZMG)
[Request more models](https://github.com/RichardErkhov/quant_request)
CodeLlama-34b-Instruct-hf - bnb 8bits
- Model creator: https://huggingface.co/meta-llama/
- Original model: https://huggingface.co/meta-llama/CodeLlama-34b-Instruct-hf/
Original model description:
---
extra_gated_heading: You need to share contact information with Meta to access this model
extra_gated_prompt: >-
### LLAMA 2 COMMUNITY LICENSE AGREEMENT
"Agreement" means the terms and conditions for use, reproduction, distribution
and modification of the Llama Materials set forth herein.
"Documentation" means the specifications, manuals and documentation
accompanying Llama 2 distributed by Meta at
https://ai.meta.com/resources/models-and-libraries/llama-downloads/.
"Licensee" or "you" means you, or your employer or any other person or entity
(if you are entering into this Agreement on such person or entity's behalf),
of the age required under applicable laws, rules or regulations to provide
legal consent and that has legal authority to bind your employer or such other
person or entity if you are entering in this Agreement on their behalf.
"Llama 2" means the foundational large language models and software and
algorithms, including machine-learning model code, trained model weights,
inference-enabling code, training-enabling code, fine-tuning enabling code and
other elements of the foregoing distributed by Meta at
ai.meta.com/resources/models-and-libraries/llama-downloads/.
"Llama Materials" means, collectively, Meta's proprietary Llama 2 and
documentation (and any portion thereof) made available under this Agreement.
"Meta" or "we" means Meta Platforms Ireland Limited (if you are located in or,
if you are an entity, your principal place of business is in the EEA or
Switzerland) and Meta Platforms, Inc. (if you are located outside of the EEA
or Switzerland).
By clicking "I Accept" below or by using or distributing any portion or
element of the Llama Materials, you agree to be bound by this Agreement.
1. License Rights and Redistribution.
a. Grant of Rights. You are granted a non-exclusive, worldwide, non-
transferable and royalty-free limited license under Meta's intellectual
property or other rights owned by Meta embodied in the Llama Materials to
use, reproduce, distribute, copy, create derivative works of, and make
modifications to the Llama Materials.
b. Redistribution and Use.
i. If you distribute or make the Llama Materials, or any derivative works
thereof, available to a third party, you shall provide a copy of this
Agreement to such third party.
ii. If you receive Llama Materials, or any derivative works thereof, from a
Licensee as part of an integrated end user product, then Section 2 of this
Agreement will not apply to you.
iii. You must retain in all copies of the Llama Materials that you distribute
the following attribution notice within a "Notice" text file distributed as a
part of such copies: "Llama 2 is licensed under the LLAMA 2 Community
License, Copyright (c) Meta Platforms, Inc. All Rights Reserved."
iv. Your use of the Llama Materials must comply with applicable laws and
regulations (including trade compliance laws and regulations) and adhere to
the Acceptable Use Policy for the Llama Materials (available at
https://ai.meta.com/llama/use-policy), which is hereby incorporated by
reference into this Agreement.
v. You will not use the Llama Materials or any output or results of the Llama
Materials to improve any other large language model (excluding Llama 2 or
derivative works thereof).
2. Additional Commercial Terms. If, on the Llama 2 version release date, the
monthly active users of the products or services made available by or for
Licensee, or Licensee's affiliates, is greater than 700 million monthly
active users in the preceding calendar month, you must request a license from
Meta, which Meta may grant to you in its sole discretion, and you are not
authorized to exercise any of the rights under this Agreement unless or until
Meta otherwise expressly grants you such rights.
3. Disclaimer of Warranty. UNLESS REQUIRED BY APPLICABLE LAW, THE LLAMA
MATERIALS AND ANY OUTPUT AND RESULTS THEREFROM ARE PROVIDED ON AN "AS IS"
BASIS, WITHOUT WARRANTIES OF ANY KIND, EITHER EXPRESS OR IMPLIED, INCLUDING,
WITHOUT LIMITATION, ANY WARRANTIES OF TITLE, NON-INFRINGEMENT,
MERCHANTABILITY, OR FITNESS FOR A PARTICULAR PURPOSE. YOU ARE SOLELY
RESPONSIBLE FOR DETERMINING THE APPROPRIATENESS OF USING OR REDISTRIBUTING
THE LLAMA MATERIALS AND ASSUME ANY RISKS ASSOCIATED WITH YOUR USE OF THE
LLAMA MATERIALS AND ANY OUTPUT AND RESULTS.
4. Limitation of Liability. IN NO EVENT WILL META OR ITS AFFILIATES BE LIABLE
UNDER ANY THEORY OF LIABILITY, WHETHER IN CONTRACT, TORT, NEGLIGENCE,
PRODUCTS LIABILITY, OR OTHERWISE, ARISING OUT OF THIS AGREEMENT, FOR ANY LOST
PROFITS OR ANY INDIRECT, SPECIAL, CONSEQUENTIAL, INCIDENTAL, EXEMPLARY OR
PUNITIVE DAMAGES, EVEN IF META OR ITS AFFILIATES HAVE BEEN ADVISED OF THE
POSSIBILITY OF ANY OF THE FOREGOING.
5. Intellectual Property.
a. No trademark licenses are granted under this Agreement, and in connection
with the Llama Materials, neither Meta nor Licensee may use any name or mark
owned by or associated with the other or any of its affiliates, except as
required for reasonable and customary use in describing and redistributing
the Llama Materials.
b. Subject to Meta's ownership of Llama Materials and derivatives made by or
for Meta, with respect to any derivative works and modifications of the Llama
Materials that are made by you, as between you and Meta, you are and will be
the owner of such derivative works and modifications.
c. If you institute litigation or other proceedings against Meta or any
entity (including a cross-claim or counterclaim in a lawsuit) alleging that
the Llama Materials or Llama 2 outputs or results, or any portion of any of
the foregoing, constitutes infringement of intellectual property or other
rights owned or licensable by you, then any licenses granted to you under
this Agreement shall terminate as of the date such litigation or claim is
filed or instituted. You will indemnify and hold harmless Meta from and
against any claim by any third party arising out of or related to your use or
distribution of the Llama Materials.
6. Term and Termination. The term of this Agreement will commence upon your
acceptance of this Agreement or access to the Llama Materials and will
continue in full force and effect until terminated in accordance with the
terms and conditions herein. Meta may terminate this Agreement if you are in
breach of any term or condition of this Agreement. Upon termination of this
Agreement, you shall delete and cease use of the Llama Materials. Sections 3,
4 and 7 shall survive the termination of this Agreement.
7. Governing Law and Jurisdiction. This Agreement will be governed and
construed under the laws of the State of California without regard to choice
of law principles, and the UN Convention on Contracts for the International
Sale of Goods does not apply to this Agreement. The courts of California
shall have exclusive jurisdiction of any dispute arising out of this
Agreement.
USE POLICY
### Llama 2 Acceptable Use Policy
Meta is committed to promoting safe and fair use of its tools and features,
including Llama 2. If you access or use Llama 2, you agree to this Acceptable
Use Policy (“Policy”). The most recent copy of this policy can be found at
[ai.meta.com/llama/use-policy](http://ai.meta.com/llama/use-policy).
#### Prohibited Uses
We want everyone to use Llama 2 safely and responsibly. You agree you will not
use, or allow others to use, Llama 2 to:
1. Violate the law or others’ rights, including to:
1. Engage in, promote, generate, contribute to, encourage, plan, incite, or further illegal or unlawful activity or content, such as:
1. Violence or terrorism
2. Exploitation or harm to children, including the solicitation, creation, acquisition, or dissemination of child exploitative content or failure to report Child Sexual Abuse Material
3. Human trafficking, exploitation, and sexual violence
4. The illegal distribution of information or materials to minors, including obscene materials, or failure to employ legally required age-gating in connection with such information or materials.
5. Sexual solicitation
6. Any other criminal activity
2. Engage in, promote, incite, or facilitate the harassment, abuse, threatening, or bullying of individuals or groups of individuals
3. Engage in, promote, incite, or facilitate discrimination or other unlawful or harmful conduct in the provision of employment, employment benefits, credit, housing, other economic benefits, or other essential goods and services
4. Engage in the unauthorized or unlicensed practice of any profession including, but not limited to, financial, legal, medical/health, or related professional practices
5. Collect, process, disclose, generate, or infer health, demographic, or other sensitive personal or private information about individuals without rights and consents required by applicable laws
6. Engage in or facilitate any action or generate any content that infringes, misappropriates, or otherwise violates any third-party rights, including the outputs or results of any products or services using the Llama 2 Materials
7. Create, generate, or facilitate the creation of malicious code, malware, computer viruses or do anything else that could disable, overburden, interfere with or impair the proper working, integrity, operation or appearance of a website or computer system
2. Engage in, promote, incite, facilitate, or assist in the planning or
development of activities that present a risk of death or bodily harm to
individuals, including use of Llama 2 related to the following:
1. Military, warfare, nuclear industries or applications, espionage, use for materials or activities that are subject to the International Traffic Arms Regulations (ITAR) maintained by the United States Department of State
2. Guns and illegal weapons (including weapon development)
3. Illegal drugs and regulated/controlled substances
4. Operation of critical infrastructure, transportation technologies, or heavy machinery
5. Self-harm or harm to others, including suicide, cutting, and eating disorders
6. Any content intended to incite or promote violence, abuse, or any infliction of bodily harm to an individual
3. Intentionally deceive or mislead others, including use of Llama 2 related
to the following:
1. Generating, promoting, or furthering fraud or the creation or promotion of disinformation
2. Generating, promoting, or furthering defamatory content, including the creation of defamatory statements, images, or other content
3. Generating, promoting, or further distributing spam
4. Impersonating another individual without consent, authorization, or legal right
5. Representing that the use of Llama 2 or outputs are human-generated
6. Generating or facilitating false online engagement, including fake reviews and other means of fake online engagement
4. Fail to appropriately disclose to end users any known dangers of your AI system
Please report any violation of this Policy, software “bug,” or other problems
that could lead to a violation of this Policy through one of the following
means:
* Reporting issues with the model:
[github.com/facebookresearch/llama](http://github.com/facebookresearch/llama)
* Reporting risky content generated by the model:
[developers.facebook.com/llama_output_feedback](http://developers.facebook.com/llama_output_feedback)
* Reporting bugs and security concerns:
[facebook.com/whitehat/info](http://facebook.com/whitehat/info)
* Reporting violations of the Acceptable Use Policy or unlicensed uses of
Llama: [[email protected]](mailto:[email protected])
extra_gated_fields:
First Name: text
Last Name: text
Date of birth: date_picker
Country: country
Affiliation: text
geo: ip_location
By clicking Submit below I accept the terms of the license and acknowledge that the information I provide will be collected stored processed and shared in accordance with the Meta Privacy Policy: checkbox
extra_gated_description: The information you provide will be collected, stored, processed and shared in accordance with the [Meta Privacy Policy](https://www.facebook.com/privacy/policy/).
extra_gated_button_content: Submit
language:
- code
pipeline_tag: text-generation
tags:
- facebook
- meta
- pytorch
- llama
- llama-2
license: llama2
---
# **Code Llama**
Code Llama is a collection of pretrained and fine-tuned generative text models ranging in scale from 7 billion to 34 billion parameters. This is the repository for the 34B instruct-tuned version in the Hugging Face Transformers format. This model is designed for general code synthesis and understanding. Links to other models can be found in the index at the bottom.
| | Base Model | Python | Instruct |
| --- | ----------------------------------------------------------------------------- | ------------------------------------------------------------------------------------------- | ----------------------------------------------------------------------------------------------- |
| 7B | [meta-llama/CodeLlama-7b-hf](https://huggingface.co/meta-llama/CodeLlama-7b-hf) | [meta-llama/CodeLlama-7b-Python-hf](https://huggingface.co/meta-llama/CodeLlama-7b-Python-hf) | [meta-llama/CodeLlama-7b-Instruct-hf](https://huggingface.co/meta-llama/CodeLlama-7b-Instruct-hf) |
| 13B | [meta-llama/CodeLlama-13b-hf](https://huggingface.co/meta-llama/CodeLlama-13b-hf) | [meta-llama/CodeLlama-13b-Python-hf](https://huggingface.co/meta-llama/CodeLlama-13b-Python-hf) | [meta-llama/CodeLlama-13b-Instruct-hf](https://huggingface.co/meta-llama/CodeLlama-13b-Instruct-hf) |
| 34B | [meta-llama/CodeLlama-34b-hf](https://huggingface.co/meta-llama/CodeLlama-34b-hf) | [meta-llama/CodeLlama-34b-Python-hf](https://huggingface.co/meta-llama/CodeLlama-34b-Python-hf) | [meta-llama/CodeLlama-34b-Instruct-hf](https://huggingface.co/meta-llama/CodeLlama-34b-Instruct-hf) |
| 70B | [meta-llama/CodeLlama-70b-hf](https://huggingface.co/meta-llama/CodeLlama-70b-hf) | [meta-llama/CodeLlama-70b-Python-hf](https://huggingface.co/meta-llama/CodeLlama-70b-Python-hf) | [meta-llama/CodeLlama-70b-Instruct-hf](https://huggingface.co/meta-llama/CodeLlama-70b-Instruct-hf) |
## Model Use
To use this model, please make sure to install transformers:
```bash
pip install transformers accelerate
```
Model capabilities:
- [x] Code completion.
- [ ] Infilling.
- [x] Instructions / chat.
- [ ] Python specialist.
## Model Details
*Note: Use of this model is governed by the Meta license. Meta developed and publicly released the Code Llama family of large language models (LLMs).
**Model Developers** Meta
**Variations** Code Llama comes in three model sizes, and three variants:
* Code Llama: base models designed for general code synthesis and understanding
* Code Llama - Python: designed specifically for Python
* Code Llama - Instruct: for instruction following and safer deployment
All variants are available in sizes of 7B, 13B and 34B parameters.
**This repository contains the Instruct version of the 34B parameters model.**
**Input** Models input text only.
**Output** Models generate text only.
**Model Architecture** Code Llama is an auto-regressive language model that uses an optimized transformer architecture.
**Model Dates** Code Llama and its variants have been trained between January 2023 and July 2023.
**Status** This is a static model trained on an offline dataset. Future versions of Code Llama - Instruct will be released as we improve model safety with community feedback.
**License** A custom commercial license is available at: [https://ai.meta.com/resources/models-and-libraries/llama-downloads/](https://ai.meta.com/resources/models-and-libraries/llama-downloads/)
**Research Paper** More information can be found in the paper "[Code Llama: Open Foundation Models for Code](https://ai.meta.com/research/publications/code-llama-open-foundation-models-for-code/)" or its [arXiv page](https://arxiv.org/abs/2308.12950).
## Intended Use
**Intended Use Cases** Code Llama and its variants is intended for commercial and research use in English and relevant programming languages. The base model Code Llama can be adapted for a variety of code synthesis and understanding tasks, Code Llama - Python is designed specifically to handle the Python programming language, and Code Llama - Instruct is intended to be safer to use for code assistant and generation applications.
**Out-of-Scope Uses** Use in any manner that violates applicable laws or regulations (including trade compliance laws). Use in languages other than English. Use in any other way that is prohibited by the Acceptable Use Policy and Licensing Agreement for Code Llama and its variants.
## Hardware and Software
**Training Factors** We used custom training libraries. The training and fine-tuning of the released models have been performed Meta’s Research Super Cluster.
**Carbon Footprint** In aggregate, training all 9 Code Llama models required 400K GPU hours of computation on hardware of type A100-80GB (TDP of 350-400W). Estimated total emissions were 65.3 tCO2eq, 100% of which were offset by Meta’s sustainability program.
## Training Data
All experiments reported here and the released models have been trained and fine-tuned using the same data as Llama 2 with different weights (see Section 2 and Table 1 in the [research paper](https://ai.meta.com/research/publications/code-llama-open-foundation-models-for-code/) for details).
## Evaluation Results
See evaluations for the main models and detailed ablations in Section 3 and safety evaluations in Section 4 of the research paper.
## Ethical Considerations and Limitations
Code Llama and its variants are a new technology that carries risks with use. Testing conducted to date has been in English, and has not covered, nor could it cover all scenarios. For these reasons, as with all LLMs, Code Llama’s potential outputs cannot be predicted in advance, and the model may in some instances produce inaccurate or objectionable responses to user prompts. Therefore, before deploying any applications of Code Llama, developers should perform safety testing and tuning tailored to their specific applications of the model.
Please see the Responsible Use Guide available available at [https://ai.meta.com/llama/responsible-use-guide](https://ai.meta.com/llama/responsible-use-guide).
| {} | RichardErkhov/meta-llama_-_CodeLlama-34b-Instruct-hf-8bits | null | [
"transformers",
"safetensors",
"llama",
"text-generation",
"conversational",
"arxiv:2308.12950",
"autotrain_compatible",
"endpoints_compatible",
"text-generation-inference",
"8-bit",
"region:us"
] | null | 2024-04-21T19:07:24+00:00 | [
"2308.12950"
] | [] | TAGS
#transformers #safetensors #llama #text-generation #conversational #arxiv-2308.12950 #autotrain_compatible #endpoints_compatible #text-generation-inference #8-bit #region-us
| Quantization made by Richard Erkhov.
Github
Discord
Request more models
CodeLlama-34b-Instruct-hf - bnb 8bits
* Model creator: URL
* Original model: URL
Original model description:
---------------------------
extra\_gated\_heading: You need to share contact information with Meta to access this model
extra\_gated\_prompt: >-
### LLAMA 2 COMMUNITY LICENSE AGREEMENT
"Agreement" means the terms and conditions for use, reproduction, distribution
and modification of the Llama Materials set forth herein.
"Documentation" means the specifications, manuals and documentation
accompanying Llama 2 distributed by Meta at
URL
"Licensee" or "you" means you, or your employer or any other person or entity
(if you are entering into this Agreement on such person or entity's behalf),
of the age required under applicable laws, rules or regulations to provide
legal consent and that has legal authority to bind your employer or such other
person or entity if you are entering in this Agreement on their behalf.
"Llama 2" means the foundational large language models and software and
algorithms, including machine-learning model code, trained model weights,
inference-enabling code, training-enabling code, fine-tuning enabling code and
other elements of the foregoing distributed by Meta at
URL
"Llama Materials" means, collectively, Meta's proprietary Llama 2 and
documentation (and any portion thereof) made available under this Agreement.
"Meta" or "we" means Meta Platforms Ireland Limited (if you are located in or,
if you are an entity, your principal place of business is in the EEA or
Switzerland) and Meta Platforms, Inc. (if you are located outside of the EEA
or Switzerland).
By clicking "I Accept" below or by using or distributing any portion or
element of the Llama Materials, you agree to be bound by this Agreement.
1. License Rights and Redistribution.
a. Grant of Rights. You are granted a non-exclusive, worldwide, non-
transferable and royalty-free limited license under Meta's intellectual
property or other rights owned by Meta embodied in the Llama Materials to
use, reproduce, distribute, copy, create derivative works of, and make
modifications to the Llama Materials.
b. Redistribution and Use.
i. If you distribute or make the Llama Materials, or any derivative works
thereof, available to a third party, you shall provide a copy of this
Agreement to such third party.
ii. If you receive Llama Materials, or any derivative works thereof, from a
Licensee as part of an integrated end user product, then Section 2 of this
Agreement will not apply to you.
iii. You must retain in all copies of the Llama Materials that you distribute
the following attribution notice within a "Notice" text file distributed as a
part of such copies: "Llama 2 is licensed under the LLAMA 2 Community
License, Copyright (c) Meta Platforms, Inc. All Rights Reserved."
iv. Your use of the Llama Materials must comply with applicable laws and
regulations (including trade compliance laws and regulations) and adhere to
the Acceptable Use Policy for the Llama Materials (available at
URL which is hereby incorporated by
reference into this Agreement.
v. You will not use the Llama Materials or any output or results of the Llama
Materials to improve any other large language model (excluding Llama 2 or
derivative works thereof).
2. Additional Commercial Terms. If, on the Llama 2 version release date, the
monthly active users of the products or services made available by or for
Licensee, or Licensee's affiliates, is greater than 700 million monthly
active users in the preceding calendar month, you must request a license from
Meta, which Meta may grant to you in its sole discretion, and you are not
authorized to exercise any of the rights under this Agreement unless or until
Meta otherwise expressly grants you such rights.
3. Disclaimer of Warranty. UNLESS REQUIRED BY APPLICABLE LAW, THE LLAMA
MATERIALS AND ANY OUTPUT AND RESULTS THEREFROM ARE PROVIDED ON AN "AS IS"
BASIS, WITHOUT WARRANTIES OF ANY KIND, EITHER EXPRESS OR IMPLIED, INCLUDING,
WITHOUT LIMITATION, ANY WARRANTIES OF TITLE, NON-INFRINGEMENT,
MERCHANTABILITY, OR FITNESS FOR A PARTICULAR PURPOSE. YOU ARE SOLELY
RESPONSIBLE FOR DETERMINING THE APPROPRIATENESS OF USING OR REDISTRIBUTING
THE LLAMA MATERIALS AND ASSUME ANY RISKS ASSOCIATED WITH YOUR USE OF THE
LLAMA MATERIALS AND ANY OUTPUT AND RESULTS.
4. Limitation of Liability. IN NO EVENT WILL META OR ITS AFFILIATES BE LIABLE
UNDER ANY THEORY OF LIABILITY, WHETHER IN CONTRACT, TORT, NEGLIGENCE,
PRODUCTS LIABILITY, OR OTHERWISE, ARISING OUT OF THIS AGREEMENT, FOR ANY LOST
PROFITS OR ANY INDIRECT, SPECIAL, CONSEQUENTIAL, INCIDENTAL, EXEMPLARY OR
PUNITIVE DAMAGES, EVEN IF META OR ITS AFFILIATES HAVE BEEN ADVISED OF THE
POSSIBILITY OF ANY OF THE FOREGOING.
5. Intellectual Property.
a. No trademark licenses are granted under this Agreement, and in connection
with the Llama Materials, neither Meta nor Licensee may use any name or mark
owned by or associated with the other or any of its affiliates, except as
required for reasonable and customary use in describing and redistributing
the Llama Materials.
b. Subject to Meta's ownership of Llama Materials and derivatives made by or
for Meta, with respect to any derivative works and modifications of the Llama
Materials that are made by you, as between you and Meta, you are and will be
the owner of such derivative works and modifications.
c. If you institute litigation or other proceedings against Meta or any
entity (including a cross-claim or counterclaim in a lawsuit) alleging that
the Llama Materials or Llama 2 outputs or results, or any portion of any of
the foregoing, constitutes infringement of intellectual property or other
rights owned or licensable by you, then any licenses granted to you under
this Agreement shall terminate as of the date such litigation or claim is
filed or instituted. You will indemnify and hold harmless Meta from and
against any claim by any third party arising out of or related to your use or
distribution of the Llama Materials.
6. Term and Termination. The term of this Agreement will commence upon your
acceptance of this Agreement or access to the Llama Materials and will
continue in full force and effect until terminated in accordance with the
terms and conditions herein. Meta may terminate this Agreement if you are in
breach of any term or condition of this Agreement. Upon termination of this
Agreement, you shall delete and cease use of the Llama Materials. Sections 3,
4 and 7 shall survive the termination of this Agreement.
7. Governing Law and Jurisdiction. This Agreement will be governed and
construed under the laws of the State of California without regard to choice
of law principles, and the UN Convention on Contracts for the International
Sale of Goods does not apply to this Agreement. The courts of California
shall have exclusive jurisdiction of any dispute arising out of this
Agreement.
USE POLICY
### Llama 2 Acceptable Use Policy
Meta is committed to promoting safe and fair use of its tools and features,
including Llama 2. If you access or use Llama 2, you agree to this Acceptable
Use Policy (“Policy”). The most recent copy of this policy can be found at
URL
#### Prohibited Uses
We want everyone to use Llama 2 safely and responsibly. You agree you will not
use, or allow others to use, Llama 2 to:
1. Violate the law or others’ rights, including to:
1. Engage in, promote, generate, contribute to, encourage, plan, incite, or further illegal or unlawful activity or content, such as:
1. Violence or terrorism
2. Exploitation or harm to children, including the solicitation, creation, acquisition, or dissemination of child exploitative content or failure to report Child Sexual Abuse Material
3. Human trafficking, exploitation, and sexual violence
4. The illegal distribution of information or materials to minors, including obscene materials, or failure to employ legally required age-gating in connection with such information or materials.
5. Sexual solicitation
6. Any other criminal activity
```
2. Engage in, promote, incite, or facilitate the harassment, abuse, threatening, or bullying of individuals or groups of individuals
3. Engage in, promote, incite, or facilitate discrimination or other unlawful or harmful conduct in the provision of employment, employment benefits, credit, housing, other economic benefits, or other essential goods and services
4. Engage in the unauthorized or unlicensed practice of any profession including, but not limited to, financial, legal, medical/health, or related professional practices
5. Collect, process, disclose, generate, or infer health, demographic, or other sensitive personal or private information about individuals without rights and consents required by applicable laws
6. Engage in or facilitate any action or generate any content that infringes, misappropriates, or otherwise violates any third-party rights, including the outputs or results of any products or services using the Llama 2 Materials
7. Create, generate, or facilitate the creation of malicious code, malware, computer viruses or do anything else that could disable, overburden, interfere with or impair the proper working, integrity, operation or appearance of a website or computer system
```
2. Engage in, promote, incite, facilitate, or assist in the planning or
development of activities that present a risk of death or bodily harm to
individuals, including use of Llama 2 related to the following:
1. Military, warfare, nuclear industries or applications, espionage, use for materials or activities that are subject to the International Traffic Arms Regulations (ITAR) maintained by the United States Department of State
2. Guns and illegal weapons (including weapon development)
3. Illegal drugs and regulated/controlled substances
4. Operation of critical infrastructure, transportation technologies, or heavy machinery
5. Self-harm or harm to others, including suicide, cutting, and eating disorders
6. Any content intended to incite or promote violence, abuse, or any infliction of bodily harm to an individual
3. Intentionally deceive or mislead others, including use of Llama 2 related
to the following:
1. Generating, promoting, or furthering fraud or the creation or promotion of disinformation
2. Generating, promoting, or furthering defamatory content, including the creation of defamatory statements, images, or other content
3. Generating, promoting, or further distributing spam
4. Impersonating another individual without consent, authorization, or legal right
5. Representing that the use of Llama 2 or outputs are human-generated
6. Generating or facilitating false online engagement, including fake reviews and other means of fake online engagement
4. Fail to appropriately disclose to end users any known dangers of your AI system
Please report any violation of this Policy, software “bug,” or other problems
that could lead to a violation of this Policy through one of the following
means:
* Reporting issues with the model:
URL
* Reporting risky content generated by the model:
URL
* Reporting bugs and security concerns:
URL
* Reporting violations of the Acceptable Use Policy or unlicensed uses of
Llama: LlamaUseReport@URL
extra\_gated\_fields:
First Name: text
Last Name: text
Date of birth: date\_picker
Country: country
Affiliation: text
geo: ip\_location
By clicking Submit below I accept the terms of the license and acknowledge that the information I provide will be collected stored processed and shared in accordance with the Meta Privacy Policy: checkbox
extra\_gated\_description: The information you provide will be collected, stored, processed and shared in accordance with the Meta Privacy Policy.
extra\_gated\_button\_content: Submit
language:
* code
pipeline\_tag: text-generation
tags:
* facebook
* meta
* pytorch
* llama
* llama-2
license: llama2
---
Code Llama
==========
Code Llama is a collection of pretrained and fine-tuned generative text models ranging in scale from 7 billion to 34 billion parameters. This is the repository for the 34B instruct-tuned version in the Hugging Face Transformers format. This model is designed for general code synthesis and understanding. Links to other models can be found in the index at the bottom.
Model Use
---------
To use this model, please make sure to install transformers:
Model capabilities:
* [x] Code completion.
* [ ] Infilling.
* [x] Instructions / chat.
* [ ] Python specialist.
Model Details
-------------
\*Note: Use of this model is governed by the Meta license. Meta developed and publicly released the Code Llama family of large language models (LLMs).
Model Developers Meta
Variations Code Llama comes in three model sizes, and three variants:
* Code Llama: base models designed for general code synthesis and understanding
* Code Llama - Python: designed specifically for Python
* Code Llama - Instruct: for instruction following and safer deployment
All variants are available in sizes of 7B, 13B and 34B parameters.
This repository contains the Instruct version of the 34B parameters model.
Input Models input text only.
Output Models generate text only.
Model Architecture Code Llama is an auto-regressive language model that uses an optimized transformer architecture.
Model Dates Code Llama and its variants have been trained between January 2023 and July 2023.
Status This is a static model trained on an offline dataset. Future versions of Code Llama - Instruct will be released as we improve model safety with community feedback.
License A custom commercial license is available at: URL
Research Paper More information can be found in the paper "Code Llama: Open Foundation Models for Code" or its arXiv page.
Intended Use
------------
Intended Use Cases Code Llama and its variants is intended for commercial and research use in English and relevant programming languages. The base model Code Llama can be adapted for a variety of code synthesis and understanding tasks, Code Llama - Python is designed specifically to handle the Python programming language, and Code Llama - Instruct is intended to be safer to use for code assistant and generation applications.
Out-of-Scope Uses Use in any manner that violates applicable laws or regulations (including trade compliance laws). Use in languages other than English. Use in any other way that is prohibited by the Acceptable Use Policy and Licensing Agreement for Code Llama and its variants.
Hardware and Software
---------------------
Training Factors We used custom training libraries. The training and fine-tuning of the released models have been performed Meta’s Research Super Cluster.
Carbon Footprint In aggregate, training all 9 Code Llama models required 400K GPU hours of computation on hardware of type A100-80GB (TDP of 350-400W). Estimated total emissions were 65.3 tCO2eq, 100% of which were offset by Meta’s sustainability program.
Training Data
-------------
All experiments reported here and the released models have been trained and fine-tuned using the same data as Llama 2 with different weights (see Section 2 and Table 1 in the research paper for details).
Evaluation Results
------------------
See evaluations for the main models and detailed ablations in Section 3 and safety evaluations in Section 4 of the research paper.
Ethical Considerations and Limitations
--------------------------------------
Code Llama and its variants are a new technology that carries risks with use. Testing conducted to date has been in English, and has not covered, nor could it cover all scenarios. For these reasons, as with all LLMs, Code Llama’s potential outputs cannot be predicted in advance, and the model may in some instances produce inaccurate or objectionable responses to user prompts. Therefore, before deploying any applications of Code Llama, developers should perform safety testing and tuning tailored to their specific applications of the model.
Please see the Responsible Use Guide available available at URL
| [
"### LLAMA 2 COMMUNITY LICENSE AGREEMENT\n\n\n\"Agreement\" means the terms and conditions for use, reproduction, distribution\nand modification of the Llama Materials set forth herein.\n\"Documentation\" means the specifications, manuals and documentation\naccompanying Llama 2 distributed by Meta at\nURL \n\n\"Licensee\" or \"you\" means you, or your employer or any other person or entity\n(if you are entering into this Agreement on such person or entity's behalf),\nof the age required under applicable laws, rules or regulations to provide\nlegal consent and that has legal authority to bind your employer or such other\nperson or entity if you are entering in this Agreement on their behalf.\n\"Llama 2\" means the foundational large language models and software and\nalgorithms, including machine-learning model code, trained model weights,\ninference-enabling code, training-enabling code, fine-tuning enabling code and\nother elements of the foregoing distributed by Meta at\nURL\n\"Llama Materials\" means, collectively, Meta's proprietary Llama 2 and\ndocumentation (and any portion thereof) made available under this Agreement.\n\"Meta\" or \"we\" means Meta Platforms Ireland Limited (if you are located in or,\nif you are an entity, your principal place of business is in the EEA or\nSwitzerland) and Meta Platforms, Inc. (if you are located outside of the EEA\nor Switzerland).\nBy clicking \"I Accept\" below or by using or distributing any portion or\nelement of the Llama Materials, you agree to be bound by this Agreement.\n\n\n1. License Rights and Redistribution.\na. Grant of Rights. You are granted a non-exclusive, worldwide, non-\ntransferable and royalty-free limited license under Meta's intellectual\nproperty or other rights owned by Meta embodied in the Llama Materials to\nuse, reproduce, distribute, copy, create derivative works of, and make\nmodifications to the Llama Materials.\n\n\nb. Redistribution and Use. \n\ni. If you distribute or make the Llama Materials, or any derivative works\nthereof, available to a third party, you shall provide a copy of this\nAgreement to such third party.\nii. If you receive Llama Materials, or any derivative works thereof, from a\nLicensee as part of an integrated end user product, then Section 2 of this\nAgreement will not apply to you.\niii. You must retain in all copies of the Llama Materials that you distribute\nthe following attribution notice within a \"Notice\" text file distributed as a\npart of such copies: \"Llama 2 is licensed under the LLAMA 2 Community\nLicense, Copyright (c) Meta Platforms, Inc. All Rights Reserved.\"\niv. Your use of the Llama Materials must comply with applicable laws and\nregulations (including trade compliance laws and regulations) and adhere to\nthe Acceptable Use Policy for the Llama Materials (available at\nURL which is hereby incorporated by\nreference into this Agreement.\nv. You will not use the Llama Materials or any output or results of the Llama\nMaterials to improve any other large language model (excluding Llama 2 or\nderivative works thereof). \n\n2. Additional Commercial Terms. If, on the Llama 2 version release date, the\nmonthly active users of the products or services made available by or for\nLicensee, or Licensee's affiliates, is greater than 700 million monthly\nactive users in the preceding calendar month, you must request a license from\nMeta, which Meta may grant to you in its sole discretion, and you are not\nauthorized to exercise any of the rights under this Agreement unless or until\nMeta otherwise expressly grants you such rights.\n3. Disclaimer of Warranty. UNLESS REQUIRED BY APPLICABLE LAW, THE LLAMA\nMATERIALS AND ANY OUTPUT AND RESULTS THEREFROM ARE PROVIDED ON AN \"AS IS\"\nBASIS, WITHOUT WARRANTIES OF ANY KIND, EITHER EXPRESS OR IMPLIED, INCLUDING,\nWITHOUT LIMITATION, ANY WARRANTIES OF TITLE, NON-INFRINGEMENT,\nMERCHANTABILITY, OR FITNESS FOR A PARTICULAR PURPOSE. YOU ARE SOLELY\nRESPONSIBLE FOR DETERMINING THE APPROPRIATENESS OF USING OR REDISTRIBUTING\nTHE LLAMA MATERIALS AND ASSUME ANY RISKS ASSOCIATED WITH YOUR USE OF THE\nLLAMA MATERIALS AND ANY OUTPUT AND RESULTS.\n4. Limitation of Liability. IN NO EVENT WILL META OR ITS AFFILIATES BE LIABLE\nUNDER ANY THEORY OF LIABILITY, WHETHER IN CONTRACT, TORT, NEGLIGENCE,\nPRODUCTS LIABILITY, OR OTHERWISE, ARISING OUT OF THIS AGREEMENT, FOR ANY LOST\nPROFITS OR ANY INDIRECT, SPECIAL, CONSEQUENTIAL, INCIDENTAL, EXEMPLARY OR\nPUNITIVE DAMAGES, EVEN IF META OR ITS AFFILIATES HAVE BEEN ADVISED OF THE\nPOSSIBILITY OF ANY OF THE FOREGOING.\n5. Intellectual Property.\na. No trademark licenses are granted under this Agreement, and in connection\nwith the Llama Materials, neither Meta nor Licensee may use any name or mark\nowned by or associated with the other or any of its affiliates, except as\nrequired for reasonable and customary use in describing and redistributing\nthe Llama Materials.\nb. Subject to Meta's ownership of Llama Materials and derivatives made by or\nfor Meta, with respect to any derivative works and modifications of the Llama\nMaterials that are made by you, as between you and Meta, you are and will be\nthe owner of such derivative works and modifications.\nc. If you institute litigation or other proceedings against Meta or any\nentity (including a cross-claim or counterclaim in a lawsuit) alleging that\nthe Llama Materials or Llama 2 outputs or results, or any portion of any of\nthe foregoing, constitutes infringement of intellectual property or other\nrights owned or licensable by you, then any licenses granted to you under\nthis Agreement shall terminate as of the date such litigation or claim is\nfiled or instituted. You will indemnify and hold harmless Meta from and\nagainst any claim by any third party arising out of or related to your use or\ndistribution of the Llama Materials.\n6. Term and Termination. The term of this Agreement will commence upon your\nacceptance of this Agreement or access to the Llama Materials and will\ncontinue in full force and effect until terminated in accordance with the\nterms and conditions herein. Meta may terminate this Agreement if you are in\nbreach of any term or condition of this Agreement. Upon termination of this\nAgreement, you shall delete and cease use of the Llama Materials. Sections 3,\n4 and 7 shall survive the termination of this Agreement.\n7. Governing Law and Jurisdiction. This Agreement will be governed and\nconstrued under the laws of the State of California without regard to choice\nof law principles, and the UN Convention on Contracts for the International\nSale of Goods does not apply to this Agreement. The courts of California\nshall have exclusive jurisdiction of any dispute arising out of this\nAgreement.\nUSE POLICY",
"### Llama 2 Acceptable Use Policy\n\n\nMeta is committed to promoting safe and fair use of its tools and features,\nincluding Llama 2. If you access or use Llama 2, you agree to this Acceptable\nUse Policy (“Policy”). The most recent copy of this policy can be found at\nURL",
"#### Prohibited Uses\n\n\nWe want everyone to use Llama 2 safely and responsibly. You agree you will not\nuse, or allow others to use, Llama 2 to:\n\n\n1. Violate the law or others’ rights, including to:\n1. Engage in, promote, generate, contribute to, encourage, plan, incite, or further illegal or unlawful activity or content, such as:\n\t1. Violence or terrorism\n\t2. Exploitation or harm to children, including the solicitation, creation, acquisition, or dissemination of child exploitative content or failure to report Child Sexual Abuse Material\n\t3. Human trafficking, exploitation, and sexual violence\n\t4. The illegal distribution of information or materials to minors, including obscene materials, or failure to employ legally required age-gating in connection with such information or materials.\n\t5. Sexual solicitation\n\t6. Any other criminal activity\n\n\n\n```\n2. Engage in, promote, incite, or facilitate the harassment, abuse, threatening, or bullying of individuals or groups of individuals\n3. Engage in, promote, incite, or facilitate discrimination or other unlawful or harmful conduct in the provision of employment, employment benefits, credit, housing, other economic benefits, or other essential goods and services\n4. Engage in the unauthorized or unlicensed practice of any profession including, but not limited to, financial, legal, medical/health, or related professional practices \n5. Collect, process, disclose, generate, or infer health, demographic, or other sensitive personal or private information about individuals without rights and consents required by applicable laws\n6. Engage in or facilitate any action or generate any content that infringes, misappropriates, or otherwise violates any third-party rights, including the outputs or results of any products or services using the Llama 2 Materials\n7. Create, generate, or facilitate the creation of malicious code, malware, computer viruses or do anything else that could disable, overburden, interfere with or impair the proper working, integrity, operation or appearance of a website or computer system \n\n```\n\n2. Engage in, promote, incite, facilitate, or assist in the planning or\ndevelopment of activities that present a risk of death or bodily harm to\nindividuals, including use of Llama 2 related to the following:\n1. Military, warfare, nuclear industries or applications, espionage, use for materials or activities that are subject to the International Traffic Arms Regulations (ITAR) maintained by the United States Department of State\n2. Guns and illegal weapons (including weapon development)\n3. Illegal drugs and regulated/controlled substances\n4. Operation of critical infrastructure, transportation technologies, or heavy machinery\n5. Self-harm or harm to others, including suicide, cutting, and eating disorders\n6. Any content intended to incite or promote violence, abuse, or any infliction of bodily harm to an individual\n3. Intentionally deceive or mislead others, including use of Llama 2 related\nto the following:\n1. Generating, promoting, or furthering fraud or the creation or promotion of disinformation\n2. Generating, promoting, or furthering defamatory content, including the creation of defamatory statements, images, or other content\n3. Generating, promoting, or further distributing spam\n4. Impersonating another individual without consent, authorization, or legal right\n5. Representing that the use of Llama 2 or outputs are human-generated\n6. Generating or facilitating false online engagement, including fake reviews and other means of fake online engagement\n4. Fail to appropriately disclose to end users any known dangers of your AI system\nPlease report any violation of this Policy, software “bug,” or other problems\nthat could lead to a violation of this Policy through one of the following\nmeans:\n\n\n* Reporting issues with the model:\nURL\n* Reporting risky content generated by the model:\nURL\n* Reporting bugs and security concerns:\nURL\n* Reporting violations of the Acceptable Use Policy or unlicensed uses of\nLlama: LlamaUseReport@URL\nextra\\_gated\\_fields:\nFirst Name: text\nLast Name: text\nDate of birth: date\\_picker\nCountry: country\nAffiliation: text\ngeo: ip\\_location \n\nBy clicking Submit below I accept the terms of the license and acknowledge that the information I provide will be collected stored processed and shared in accordance with the Meta Privacy Policy: checkbox\nextra\\_gated\\_description: The information you provide will be collected, stored, processed and shared in accordance with the Meta Privacy Policy.\nextra\\_gated\\_button\\_content: Submit\nlanguage:\n\n\n* code\npipeline\\_tag: text-generation\ntags:\n* facebook\n* meta\n* pytorch\n* llama\n* llama-2\nlicense: llama2\n\n\n\n\n---\n\n\nCode Llama\n==========\n\n\nCode Llama is a collection of pretrained and fine-tuned generative text models ranging in scale from 7 billion to 34 billion parameters. This is the repository for the 34B instruct-tuned version in the Hugging Face Transformers format. This model is designed for general code synthesis and understanding. Links to other models can be found in the index at the bottom.\n\n\n\nModel Use\n---------\n\n\nTo use this model, please make sure to install transformers:\n\n\nModel capabilities:\n\n\n* [x] Code completion.\n* [ ] Infilling.\n* [x] Instructions / chat.\n* [ ] Python specialist.\n\n\nModel Details\n-------------\n\n\n\\*Note: Use of this model is governed by the Meta license. Meta developed and publicly released the Code Llama family of large language models (LLMs).\n\n\nModel Developers Meta\n\n\nVariations Code Llama comes in three model sizes, and three variants:\n\n\n* Code Llama: base models designed for general code synthesis and understanding\n* Code Llama - Python: designed specifically for Python\n* Code Llama - Instruct: for instruction following and safer deployment\n\n\nAll variants are available in sizes of 7B, 13B and 34B parameters.\n\n\nThis repository contains the Instruct version of the 34B parameters model.\n\n\nInput Models input text only.\n\n\nOutput Models generate text only.\n\n\nModel Architecture Code Llama is an auto-regressive language model that uses an optimized transformer architecture.\n\n\nModel Dates Code Llama and its variants have been trained between January 2023 and July 2023.\n\n\nStatus This is a static model trained on an offline dataset. Future versions of Code Llama - Instruct will be released as we improve model safety with community feedback.\n\n\nLicense A custom commercial license is available at: URL\n\n\nResearch Paper More information can be found in the paper \"Code Llama: Open Foundation Models for Code\" or its arXiv page.\n\n\nIntended Use\n------------\n\n\nIntended Use Cases Code Llama and its variants is intended for commercial and research use in English and relevant programming languages. The base model Code Llama can be adapted for a variety of code synthesis and understanding tasks, Code Llama - Python is designed specifically to handle the Python programming language, and Code Llama - Instruct is intended to be safer to use for code assistant and generation applications.\n\n\nOut-of-Scope Uses Use in any manner that violates applicable laws or regulations (including trade compliance laws). Use in languages other than English. Use in any other way that is prohibited by the Acceptable Use Policy and Licensing Agreement for Code Llama and its variants.\n\n\nHardware and Software\n---------------------\n\n\nTraining Factors We used custom training libraries. The training and fine-tuning of the released models have been performed Meta’s Research Super Cluster.\n\n\nCarbon Footprint In aggregate, training all 9 Code Llama models required 400K GPU hours of computation on hardware of type A100-80GB (TDP of 350-400W). Estimated total emissions were 65.3 tCO2eq, 100% of which were offset by Meta’s sustainability program.\n\n\nTraining Data\n-------------\n\n\nAll experiments reported here and the released models have been trained and fine-tuned using the same data as Llama 2 with different weights (see Section 2 and Table 1 in the research paper for details).\n\n\nEvaluation Results\n------------------\n\n\nSee evaluations for the main models and detailed ablations in Section 3 and safety evaluations in Section 4 of the research paper.\n\n\nEthical Considerations and Limitations\n--------------------------------------\n\n\nCode Llama and its variants are a new technology that carries risks with use. Testing conducted to date has been in English, and has not covered, nor could it cover all scenarios. For these reasons, as with all LLMs, Code Llama’s potential outputs cannot be predicted in advance, and the model may in some instances produce inaccurate or objectionable responses to user prompts. Therefore, before deploying any applications of Code Llama, developers should perform safety testing and tuning tailored to their specific applications of the model.\n\n\nPlease see the Responsible Use Guide available available at URL"
] | [
"TAGS\n#transformers #safetensors #llama #text-generation #conversational #arxiv-2308.12950 #autotrain_compatible #endpoints_compatible #text-generation-inference #8-bit #region-us \n",
"### LLAMA 2 COMMUNITY LICENSE AGREEMENT\n\n\n\"Agreement\" means the terms and conditions for use, reproduction, distribution\nand modification of the Llama Materials set forth herein.\n\"Documentation\" means the specifications, manuals and documentation\naccompanying Llama 2 distributed by Meta at\nURL \n\n\"Licensee\" or \"you\" means you, or your employer or any other person or entity\n(if you are entering into this Agreement on such person or entity's behalf),\nof the age required under applicable laws, rules or regulations to provide\nlegal consent and that has legal authority to bind your employer or such other\nperson or entity if you are entering in this Agreement on their behalf.\n\"Llama 2\" means the foundational large language models and software and\nalgorithms, including machine-learning model code, trained model weights,\ninference-enabling code, training-enabling code, fine-tuning enabling code and\nother elements of the foregoing distributed by Meta at\nURL\n\"Llama Materials\" means, collectively, Meta's proprietary Llama 2 and\ndocumentation (and any portion thereof) made available under this Agreement.\n\"Meta\" or \"we\" means Meta Platforms Ireland Limited (if you are located in or,\nif you are an entity, your principal place of business is in the EEA or\nSwitzerland) and Meta Platforms, Inc. (if you are located outside of the EEA\nor Switzerland).\nBy clicking \"I Accept\" below or by using or distributing any portion or\nelement of the Llama Materials, you agree to be bound by this Agreement.\n\n\n1. License Rights and Redistribution.\na. Grant of Rights. You are granted a non-exclusive, worldwide, non-\ntransferable and royalty-free limited license under Meta's intellectual\nproperty or other rights owned by Meta embodied in the Llama Materials to\nuse, reproduce, distribute, copy, create derivative works of, and make\nmodifications to the Llama Materials.\n\n\nb. Redistribution and Use. \n\ni. If you distribute or make the Llama Materials, or any derivative works\nthereof, available to a third party, you shall provide a copy of this\nAgreement to such third party.\nii. If you receive Llama Materials, or any derivative works thereof, from a\nLicensee as part of an integrated end user product, then Section 2 of this\nAgreement will not apply to you.\niii. You must retain in all copies of the Llama Materials that you distribute\nthe following attribution notice within a \"Notice\" text file distributed as a\npart of such copies: \"Llama 2 is licensed under the LLAMA 2 Community\nLicense, Copyright (c) Meta Platforms, Inc. All Rights Reserved.\"\niv. Your use of the Llama Materials must comply with applicable laws and\nregulations (including trade compliance laws and regulations) and adhere to\nthe Acceptable Use Policy for the Llama Materials (available at\nURL which is hereby incorporated by\nreference into this Agreement.\nv. You will not use the Llama Materials or any output or results of the Llama\nMaterials to improve any other large language model (excluding Llama 2 or\nderivative works thereof). \n\n2. Additional Commercial Terms. If, on the Llama 2 version release date, the\nmonthly active users of the products or services made available by or for\nLicensee, or Licensee's affiliates, is greater than 700 million monthly\nactive users in the preceding calendar month, you must request a license from\nMeta, which Meta may grant to you in its sole discretion, and you are not\nauthorized to exercise any of the rights under this Agreement unless or until\nMeta otherwise expressly grants you such rights.\n3. Disclaimer of Warranty. UNLESS REQUIRED BY APPLICABLE LAW, THE LLAMA\nMATERIALS AND ANY OUTPUT AND RESULTS THEREFROM ARE PROVIDED ON AN \"AS IS\"\nBASIS, WITHOUT WARRANTIES OF ANY KIND, EITHER EXPRESS OR IMPLIED, INCLUDING,\nWITHOUT LIMITATION, ANY WARRANTIES OF TITLE, NON-INFRINGEMENT,\nMERCHANTABILITY, OR FITNESS FOR A PARTICULAR PURPOSE. YOU ARE SOLELY\nRESPONSIBLE FOR DETERMINING THE APPROPRIATENESS OF USING OR REDISTRIBUTING\nTHE LLAMA MATERIALS AND ASSUME ANY RISKS ASSOCIATED WITH YOUR USE OF THE\nLLAMA MATERIALS AND ANY OUTPUT AND RESULTS.\n4. Limitation of Liability. IN NO EVENT WILL META OR ITS AFFILIATES BE LIABLE\nUNDER ANY THEORY OF LIABILITY, WHETHER IN CONTRACT, TORT, NEGLIGENCE,\nPRODUCTS LIABILITY, OR OTHERWISE, ARISING OUT OF THIS AGREEMENT, FOR ANY LOST\nPROFITS OR ANY INDIRECT, SPECIAL, CONSEQUENTIAL, INCIDENTAL, EXEMPLARY OR\nPUNITIVE DAMAGES, EVEN IF META OR ITS AFFILIATES HAVE BEEN ADVISED OF THE\nPOSSIBILITY OF ANY OF THE FOREGOING.\n5. Intellectual Property.\na. No trademark licenses are granted under this Agreement, and in connection\nwith the Llama Materials, neither Meta nor Licensee may use any name or mark\nowned by or associated with the other or any of its affiliates, except as\nrequired for reasonable and customary use in describing and redistributing\nthe Llama Materials.\nb. Subject to Meta's ownership of Llama Materials and derivatives made by or\nfor Meta, with respect to any derivative works and modifications of the Llama\nMaterials that are made by you, as between you and Meta, you are and will be\nthe owner of such derivative works and modifications.\nc. If you institute litigation or other proceedings against Meta or any\nentity (including a cross-claim or counterclaim in a lawsuit) alleging that\nthe Llama Materials or Llama 2 outputs or results, or any portion of any of\nthe foregoing, constitutes infringement of intellectual property or other\nrights owned or licensable by you, then any licenses granted to you under\nthis Agreement shall terminate as of the date such litigation or claim is\nfiled or instituted. You will indemnify and hold harmless Meta from and\nagainst any claim by any third party arising out of or related to your use or\ndistribution of the Llama Materials.\n6. Term and Termination. The term of this Agreement will commence upon your\nacceptance of this Agreement or access to the Llama Materials and will\ncontinue in full force and effect until terminated in accordance with the\nterms and conditions herein. Meta may terminate this Agreement if you are in\nbreach of any term or condition of this Agreement. Upon termination of this\nAgreement, you shall delete and cease use of the Llama Materials. Sections 3,\n4 and 7 shall survive the termination of this Agreement.\n7. Governing Law and Jurisdiction. This Agreement will be governed and\nconstrued under the laws of the State of California without regard to choice\nof law principles, and the UN Convention on Contracts for the International\nSale of Goods does not apply to this Agreement. The courts of California\nshall have exclusive jurisdiction of any dispute arising out of this\nAgreement.\nUSE POLICY",
"### Llama 2 Acceptable Use Policy\n\n\nMeta is committed to promoting safe and fair use of its tools and features,\nincluding Llama 2. If you access or use Llama 2, you agree to this Acceptable\nUse Policy (“Policy”). The most recent copy of this policy can be found at\nURL",
"#### Prohibited Uses\n\n\nWe want everyone to use Llama 2 safely and responsibly. You agree you will not\nuse, or allow others to use, Llama 2 to:\n\n\n1. Violate the law or others’ rights, including to:\n1. Engage in, promote, generate, contribute to, encourage, plan, incite, or further illegal or unlawful activity or content, such as:\n\t1. Violence or terrorism\n\t2. Exploitation or harm to children, including the solicitation, creation, acquisition, or dissemination of child exploitative content or failure to report Child Sexual Abuse Material\n\t3. Human trafficking, exploitation, and sexual violence\n\t4. The illegal distribution of information or materials to minors, including obscene materials, or failure to employ legally required age-gating in connection with such information or materials.\n\t5. Sexual solicitation\n\t6. Any other criminal activity\n\n\n\n```\n2. Engage in, promote, incite, or facilitate the harassment, abuse, threatening, or bullying of individuals or groups of individuals\n3. Engage in, promote, incite, or facilitate discrimination or other unlawful or harmful conduct in the provision of employment, employment benefits, credit, housing, other economic benefits, or other essential goods and services\n4. Engage in the unauthorized or unlicensed practice of any profession including, but not limited to, financial, legal, medical/health, or related professional practices \n5. Collect, process, disclose, generate, or infer health, demographic, or other sensitive personal or private information about individuals without rights and consents required by applicable laws\n6. Engage in or facilitate any action or generate any content that infringes, misappropriates, or otherwise violates any third-party rights, including the outputs or results of any products or services using the Llama 2 Materials\n7. Create, generate, or facilitate the creation of malicious code, malware, computer viruses or do anything else that could disable, overburden, interfere with or impair the proper working, integrity, operation or appearance of a website or computer system \n\n```\n\n2. Engage in, promote, incite, facilitate, or assist in the planning or\ndevelopment of activities that present a risk of death or bodily harm to\nindividuals, including use of Llama 2 related to the following:\n1. Military, warfare, nuclear industries or applications, espionage, use for materials or activities that are subject to the International Traffic Arms Regulations (ITAR) maintained by the United States Department of State\n2. Guns and illegal weapons (including weapon development)\n3. Illegal drugs and regulated/controlled substances\n4. Operation of critical infrastructure, transportation technologies, or heavy machinery\n5. Self-harm or harm to others, including suicide, cutting, and eating disorders\n6. Any content intended to incite or promote violence, abuse, or any infliction of bodily harm to an individual\n3. Intentionally deceive or mislead others, including use of Llama 2 related\nto the following:\n1. Generating, promoting, or furthering fraud or the creation or promotion of disinformation\n2. Generating, promoting, or furthering defamatory content, including the creation of defamatory statements, images, or other content\n3. Generating, promoting, or further distributing spam\n4. Impersonating another individual without consent, authorization, or legal right\n5. Representing that the use of Llama 2 or outputs are human-generated\n6. Generating or facilitating false online engagement, including fake reviews and other means of fake online engagement\n4. Fail to appropriately disclose to end users any known dangers of your AI system\nPlease report any violation of this Policy, software “bug,” or other problems\nthat could lead to a violation of this Policy through one of the following\nmeans:\n\n\n* Reporting issues with the model:\nURL\n* Reporting risky content generated by the model:\nURL\n* Reporting bugs and security concerns:\nURL\n* Reporting violations of the Acceptable Use Policy or unlicensed uses of\nLlama: LlamaUseReport@URL\nextra\\_gated\\_fields:\nFirst Name: text\nLast Name: text\nDate of birth: date\\_picker\nCountry: country\nAffiliation: text\ngeo: ip\\_location \n\nBy clicking Submit below I accept the terms of the license and acknowledge that the information I provide will be collected stored processed and shared in accordance with the Meta Privacy Policy: checkbox\nextra\\_gated\\_description: The information you provide will be collected, stored, processed and shared in accordance with the Meta Privacy Policy.\nextra\\_gated\\_button\\_content: Submit\nlanguage:\n\n\n* code\npipeline\\_tag: text-generation\ntags:\n* facebook\n* meta\n* pytorch\n* llama\n* llama-2\nlicense: llama2\n\n\n\n\n---\n\n\nCode Llama\n==========\n\n\nCode Llama is a collection of pretrained and fine-tuned generative text models ranging in scale from 7 billion to 34 billion parameters. This is the repository for the 34B instruct-tuned version in the Hugging Face Transformers format. This model is designed for general code synthesis and understanding. Links to other models can be found in the index at the bottom.\n\n\n\nModel Use\n---------\n\n\nTo use this model, please make sure to install transformers:\n\n\nModel capabilities:\n\n\n* [x] Code completion.\n* [ ] Infilling.\n* [x] Instructions / chat.\n* [ ] Python specialist.\n\n\nModel Details\n-------------\n\n\n\\*Note: Use of this model is governed by the Meta license. Meta developed and publicly released the Code Llama family of large language models (LLMs).\n\n\nModel Developers Meta\n\n\nVariations Code Llama comes in three model sizes, and three variants:\n\n\n* Code Llama: base models designed for general code synthesis and understanding\n* Code Llama - Python: designed specifically for Python\n* Code Llama - Instruct: for instruction following and safer deployment\n\n\nAll variants are available in sizes of 7B, 13B and 34B parameters.\n\n\nThis repository contains the Instruct version of the 34B parameters model.\n\n\nInput Models input text only.\n\n\nOutput Models generate text only.\n\n\nModel Architecture Code Llama is an auto-regressive language model that uses an optimized transformer architecture.\n\n\nModel Dates Code Llama and its variants have been trained between January 2023 and July 2023.\n\n\nStatus This is a static model trained on an offline dataset. Future versions of Code Llama - Instruct will be released as we improve model safety with community feedback.\n\n\nLicense A custom commercial license is available at: URL\n\n\nResearch Paper More information can be found in the paper \"Code Llama: Open Foundation Models for Code\" or its arXiv page.\n\n\nIntended Use\n------------\n\n\nIntended Use Cases Code Llama and its variants is intended for commercial and research use in English and relevant programming languages. The base model Code Llama can be adapted for a variety of code synthesis and understanding tasks, Code Llama - Python is designed specifically to handle the Python programming language, and Code Llama - Instruct is intended to be safer to use for code assistant and generation applications.\n\n\nOut-of-Scope Uses Use in any manner that violates applicable laws or regulations (including trade compliance laws). Use in languages other than English. Use in any other way that is prohibited by the Acceptable Use Policy and Licensing Agreement for Code Llama and its variants.\n\n\nHardware and Software\n---------------------\n\n\nTraining Factors We used custom training libraries. The training and fine-tuning of the released models have been performed Meta’s Research Super Cluster.\n\n\nCarbon Footprint In aggregate, training all 9 Code Llama models required 400K GPU hours of computation on hardware of type A100-80GB (TDP of 350-400W). Estimated total emissions were 65.3 tCO2eq, 100% of which were offset by Meta’s sustainability program.\n\n\nTraining Data\n-------------\n\n\nAll experiments reported here and the released models have been trained and fine-tuned using the same data as Llama 2 with different weights (see Section 2 and Table 1 in the research paper for details).\n\n\nEvaluation Results\n------------------\n\n\nSee evaluations for the main models and detailed ablations in Section 3 and safety evaluations in Section 4 of the research paper.\n\n\nEthical Considerations and Limitations\n--------------------------------------\n\n\nCode Llama and its variants are a new technology that carries risks with use. Testing conducted to date has been in English, and has not covered, nor could it cover all scenarios. For these reasons, as with all LLMs, Code Llama’s potential outputs cannot be predicted in advance, and the model may in some instances produce inaccurate or objectionable responses to user prompts. Therefore, before deploying any applications of Code Llama, developers should perform safety testing and tuning tailored to their specific applications of the model.\n\n\nPlease see the Responsible Use Guide available available at URL"
] |
text-generation | transformers |
# Model Trained Using AutoTrain
This model was trained using AutoTrain. For more information, please visit [AutoTrain](https://hf.co/docs/autotrain).
# Usage
```python
from transformers import AutoModelForCausalLM, AutoTokenizer
model_path = "shawn-padstats/rental-text-llama_3"
tokenizer = AutoTokenizer.from_pretrained(model_path)
model = AutoModelForCausalLM.from_pretrained(
model_path,
device_map="auto",
torch_dtype='auto'
).eval()
# Prompt content: "hi"
messages = [
{"role": "user", "content": "hi"}
]
input_ids = tokenizer.apply_chat_template(conversation=messages, tokenize=True, add_generation_prompt=True, return_tensors='pt')
output_ids = model.generate(input_ids.to('cuda'))
response = tokenizer.decode(output_ids[0][input_ids.shape[1]:], skip_special_tokens=True)
# Model response: "Hello! How can I assist you today?"
print(response)
``` | {"license": "other", "library_name": "transformers", "tags": ["autotrain", "text-generation-inference", "text-generation", "peft"], "widget": [{"messages": [{"role": "user", "content": "What is your favorite condiment?"}]}]} | shawn-padstats/rental-text-llama_3 | null | [
"transformers",
"safetensors",
"llama",
"text-generation",
"autotrain",
"text-generation-inference",
"peft",
"conversational",
"license:other",
"autotrain_compatible",
"endpoints_compatible",
"region:us"
] | null | 2024-04-21T19:10:00+00:00 | [] | [] | TAGS
#transformers #safetensors #llama #text-generation #autotrain #text-generation-inference #peft #conversational #license-other #autotrain_compatible #endpoints_compatible #region-us
|
# Model Trained Using AutoTrain
This model was trained using AutoTrain. For more information, please visit AutoTrain.
# Usage
| [
"# Model Trained Using AutoTrain\n\nThis model was trained using AutoTrain. For more information, please visit AutoTrain.",
"# Usage"
] | [
"TAGS\n#transformers #safetensors #llama #text-generation #autotrain #text-generation-inference #peft #conversational #license-other #autotrain_compatible #endpoints_compatible #region-us \n",
"# Model Trained Using AutoTrain\n\nThis model was trained using AutoTrain. For more information, please visit AutoTrain.",
"# Usage"
] |
null | null | Quantization made by Richard Erkhov.
[Github](https://github.com/RichardErkhov)
[Discord](https://discord.gg/pvy7H8DZMG)
[Request more models](https://github.com/RichardErkhov/quant_request)
CodeLlama-34b-hf - GGUF
- Model creator: https://huggingface.co/meta-llama/
- Original model: https://huggingface.co/meta-llama/CodeLlama-34b-hf/
| Name | Quant method | Size |
| ---- | ---- | ---- |
| [CodeLlama-34b-hf.Q2_K.gguf](https://huggingface.co/RichardErkhov/meta-llama_-_CodeLlama-34b-hf-gguf/blob/main/CodeLlama-34b-hf.Q2_K.gguf) | Q2_K | 11.65GB |
| [CodeLlama-34b-hf.IQ3_XS.gguf](https://huggingface.co/RichardErkhov/meta-llama_-_CodeLlama-34b-hf-gguf/blob/main/CodeLlama-34b-hf.IQ3_XS.gguf) | IQ3_XS | 12.93GB |
| [CodeLlama-34b-hf.IQ3_S.gguf](https://huggingface.co/RichardErkhov/meta-llama_-_CodeLlama-34b-hf-gguf/blob/main/CodeLlama-34b-hf.IQ3_S.gguf) | IQ3_S | 13.65GB |
| [CodeLlama-34b-hf.Q3_K_S.gguf](https://huggingface.co/RichardErkhov/meta-llama_-_CodeLlama-34b-hf-gguf/blob/main/CodeLlama-34b-hf.Q3_K_S.gguf) | Q3_K_S | 13.6GB |
| [CodeLlama-34b-hf.IQ3_M.gguf](https://huggingface.co/RichardErkhov/meta-llama_-_CodeLlama-34b-hf-gguf/blob/main/CodeLlama-34b-hf.IQ3_M.gguf) | IQ3_M | 14.18GB |
| [CodeLlama-34b-hf.Q3_K.gguf](https://huggingface.co/RichardErkhov/meta-llama_-_CodeLlama-34b-hf-gguf/blob/main/CodeLlama-34b-hf.Q3_K.gguf) | Q3_K | 15.19GB |
| [CodeLlama-34b-hf.Q3_K_M.gguf](https://huggingface.co/RichardErkhov/meta-llama_-_CodeLlama-34b-hf-gguf/blob/main/CodeLlama-34b-hf.Q3_K_M.gguf) | Q3_K_M | 15.19GB |
| [CodeLlama-34b-hf.Q3_K_L.gguf](https://huggingface.co/RichardErkhov/meta-llama_-_CodeLlama-34b-hf-gguf/blob/main/CodeLlama-34b-hf.Q3_K_L.gguf) | Q3_K_L | 16.55GB |
| [CodeLlama-34b-hf.IQ4_XS.gguf](https://huggingface.co/RichardErkhov/meta-llama_-_CodeLlama-34b-hf-gguf/blob/main/CodeLlama-34b-hf.IQ4_XS.gguf) | IQ4_XS | 16.99GB |
| [CodeLlama-34b-hf.Q4_0.gguf](https://huggingface.co/RichardErkhov/meta-llama_-_CodeLlama-34b-hf-gguf/blob/main/CodeLlama-34b-hf.Q4_0.gguf) | Q4_0 | 17.74GB |
| [CodeLlama-34b-hf.IQ4_NL.gguf](https://huggingface.co/RichardErkhov/meta-llama_-_CodeLlama-34b-hf-gguf/blob/main/CodeLlama-34b-hf.IQ4_NL.gguf) | IQ4_NL | 17.92GB |
| [CodeLlama-34b-hf.Q4_K_S.gguf](https://huggingface.co/RichardErkhov/meta-llama_-_CodeLlama-34b-hf-gguf/blob/main/CodeLlama-34b-hf.Q4_K_S.gguf) | Q4_K_S | 17.87GB |
| [CodeLlama-34b-hf.Q4_K.gguf](https://huggingface.co/RichardErkhov/meta-llama_-_CodeLlama-34b-hf-gguf/blob/main/CodeLlama-34b-hf.Q4_K.gguf) | Q4_K | 18.83GB |
| [CodeLlama-34b-hf.Q4_K_M.gguf](https://huggingface.co/RichardErkhov/meta-llama_-_CodeLlama-34b-hf-gguf/blob/main/CodeLlama-34b-hf.Q4_K_M.gguf) | Q4_K_M | 18.83GB |
| [CodeLlama-34b-hf.Q4_1.gguf](https://huggingface.co/RichardErkhov/meta-llama_-_CodeLlama-34b-hf-gguf/blob/main/CodeLlama-34b-hf.Q4_1.gguf) | Q4_1 | 19.69GB |
| [CodeLlama-34b-hf.Q5_0.gguf](https://huggingface.co/RichardErkhov/meta-llama_-_CodeLlama-34b-hf-gguf/blob/main/CodeLlama-34b-hf.Q5_0.gguf) | Q5_0 | 21.64GB |
| [CodeLlama-34b-hf.Q5_K_S.gguf](https://huggingface.co/RichardErkhov/meta-llama_-_CodeLlama-34b-hf-gguf/blob/main/CodeLlama-34b-hf.Q5_K_S.gguf) | Q5_K_S | 21.64GB |
| [CodeLlama-34b-hf.Q5_K.gguf](https://huggingface.co/RichardErkhov/meta-llama_-_CodeLlama-34b-hf-gguf/blob/main/CodeLlama-34b-hf.Q5_K.gguf) | Q5_K | 22.2GB |
| [CodeLlama-34b-hf.Q5_K_M.gguf](https://huggingface.co/RichardErkhov/meta-llama_-_CodeLlama-34b-hf-gguf/blob/main/CodeLlama-34b-hf.Q5_K_M.gguf) | Q5_K_M | 22.2GB |
| [CodeLlama-34b-hf.Q5_1.gguf](https://huggingface.co/RichardErkhov/meta-llama_-_CodeLlama-34b-hf-gguf/blob/main/CodeLlama-34b-hf.Q5_1.gguf) | Q5_1 | 23.59GB |
| [CodeLlama-34b-hf.Q6_K.gguf](https://huggingface.co/RichardErkhov/meta-llama_-_CodeLlama-34b-hf-gguf/blob/main/CodeLlama-34b-hf.Q6_K.gguf) | Q6_K | 25.78GB |
Original model description:
---
extra_gated_heading: You need to share contact information with Meta to access this model
extra_gated_prompt: >-
### LLAMA 2 COMMUNITY LICENSE AGREEMENT
"Agreement" means the terms and conditions for use, reproduction, distribution
and modification of the Llama Materials set forth herein.
"Documentation" means the specifications, manuals and documentation
accompanying Llama 2 distributed by Meta at
https://ai.meta.com/resources/models-and-libraries/llama-downloads/.
"Licensee" or "you" means you, or your employer or any other person or entity
(if you are entering into this Agreement on such person or entity's behalf),
of the age required under applicable laws, rules or regulations to provide
legal consent and that has legal authority to bind your employer or such other
person or entity if you are entering in this Agreement on their behalf.
"Llama 2" means the foundational large language models and software and
algorithms, including machine-learning model code, trained model weights,
inference-enabling code, training-enabling code, fine-tuning enabling code and
other elements of the foregoing distributed by Meta at
ai.meta.com/resources/models-and-libraries/llama-downloads/.
"Llama Materials" means, collectively, Meta's proprietary Llama 2 and
documentation (and any portion thereof) made available under this Agreement.
"Meta" or "we" means Meta Platforms Ireland Limited (if you are located in or,
if you are an entity, your principal place of business is in the EEA or
Switzerland) and Meta Platforms, Inc. (if you are located outside of the EEA
or Switzerland).
By clicking "I Accept" below or by using or distributing any portion or
element of the Llama Materials, you agree to be bound by this Agreement.
1. License Rights and Redistribution.
a. Grant of Rights. You are granted a non-exclusive, worldwide, non-
transferable and royalty-free limited license under Meta's intellectual
property or other rights owned by Meta embodied in the Llama Materials to
use, reproduce, distribute, copy, create derivative works of, and make
modifications to the Llama Materials.
b. Redistribution and Use.
i. If you distribute or make the Llama Materials, or any derivative works
thereof, available to a third party, you shall provide a copy of this
Agreement to such third party.
ii. If you receive Llama Materials, or any derivative works thereof, from a
Licensee as part of an integrated end user product, then Section 2 of this
Agreement will not apply to you.
iii. You must retain in all copies of the Llama Materials that you distribute
the following attribution notice within a "Notice" text file distributed as a
part of such copies: "Llama 2 is licensed under the LLAMA 2 Community
License, Copyright (c) Meta Platforms, Inc. All Rights Reserved."
iv. Your use of the Llama Materials must comply with applicable laws and
regulations (including trade compliance laws and regulations) and adhere to
the Acceptable Use Policy for the Llama Materials (available at
https://ai.meta.com/llama/use-policy), which is hereby incorporated by
reference into this Agreement.
v. You will not use the Llama Materials or any output or results of the Llama
Materials to improve any other large language model (excluding Llama 2 or
derivative works thereof).
2. Additional Commercial Terms. If, on the Llama 2 version release date, the
monthly active users of the products or services made available by or for
Licensee, or Licensee's affiliates, is greater than 700 million monthly
active users in the preceding calendar month, you must request a license from
Meta, which Meta may grant to you in its sole discretion, and you are not
authorized to exercise any of the rights under this Agreement unless or until
Meta otherwise expressly grants you such rights.
3. Disclaimer of Warranty. UNLESS REQUIRED BY APPLICABLE LAW, THE LLAMA
MATERIALS AND ANY OUTPUT AND RESULTS THEREFROM ARE PROVIDED ON AN "AS IS"
BASIS, WITHOUT WARRANTIES OF ANY KIND, EITHER EXPRESS OR IMPLIED, INCLUDING,
WITHOUT LIMITATION, ANY WARRANTIES OF TITLE, NON-INFRINGEMENT,
MERCHANTABILITY, OR FITNESS FOR A PARTICULAR PURPOSE. YOU ARE SOLELY
RESPONSIBLE FOR DETERMINING THE APPROPRIATENESS OF USING OR REDISTRIBUTING
THE LLAMA MATERIALS AND ASSUME ANY RISKS ASSOCIATED WITH YOUR USE OF THE
LLAMA MATERIALS AND ANY OUTPUT AND RESULTS.
4. Limitation of Liability. IN NO EVENT WILL META OR ITS AFFILIATES BE LIABLE
UNDER ANY THEORY OF LIABILITY, WHETHER IN CONTRACT, TORT, NEGLIGENCE,
PRODUCTS LIABILITY, OR OTHERWISE, ARISING OUT OF THIS AGREEMENT, FOR ANY LOST
PROFITS OR ANY INDIRECT, SPECIAL, CONSEQUENTIAL, INCIDENTAL, EXEMPLARY OR
PUNITIVE DAMAGES, EVEN IF META OR ITS AFFILIATES HAVE BEEN ADVISED OF THE
POSSIBILITY OF ANY OF THE FOREGOING.
5. Intellectual Property.
a. No trademark licenses are granted under this Agreement, and in connection
with the Llama Materials, neither Meta nor Licensee may use any name or mark
owned by or associated with the other or any of its affiliates, except as
required for reasonable and customary use in describing and redistributing
the Llama Materials.
b. Subject to Meta's ownership of Llama Materials and derivatives made by or
for Meta, with respect to any derivative works and modifications of the Llama
Materials that are made by you, as between you and Meta, you are and will be
the owner of such derivative works and modifications.
c. If you institute litigation or other proceedings against Meta or any
entity (including a cross-claim or counterclaim in a lawsuit) alleging that
the Llama Materials or Llama 2 outputs or results, or any portion of any of
the foregoing, constitutes infringement of intellectual property or other
rights owned or licensable by you, then any licenses granted to you under
this Agreement shall terminate as of the date such litigation or claim is
filed or instituted. You will indemnify and hold harmless Meta from and
against any claim by any third party arising out of or related to your use or
distribution of the Llama Materials.
6. Term and Termination. The term of this Agreement will commence upon your
acceptance of this Agreement or access to the Llama Materials and will
continue in full force and effect until terminated in accordance with the
terms and conditions herein. Meta may terminate this Agreement if you are in
breach of any term or condition of this Agreement. Upon termination of this
Agreement, you shall delete and cease use of the Llama Materials. Sections 3,
4 and 7 shall survive the termination of this Agreement.
7. Governing Law and Jurisdiction. This Agreement will be governed and
construed under the laws of the State of California without regard to choice
of law principles, and the UN Convention on Contracts for the International
Sale of Goods does not apply to this Agreement. The courts of California
shall have exclusive jurisdiction of any dispute arising out of this
Agreement.
USE POLICY
### Llama 2 Acceptable Use Policy
Meta is committed to promoting safe and fair use of its tools and features,
including Llama 2. If you access or use Llama 2, you agree to this Acceptable
Use Policy (“Policy”). The most recent copy of this policy can be found at
[ai.meta.com/llama/use-policy](http://ai.meta.com/llama/use-policy).
#### Prohibited Uses
We want everyone to use Llama 2 safely and responsibly. You agree you will not
use, or allow others to use, Llama 2 to:
1. Violate the law or others’ rights, including to:
1. Engage in, promote, generate, contribute to, encourage, plan, incite, or further illegal or unlawful activity or content, such as:
1. Violence or terrorism
2. Exploitation or harm to children, including the solicitation, creation, acquisition, or dissemination of child exploitative content or failure to report Child Sexual Abuse Material
3. Human trafficking, exploitation, and sexual violence
4. The illegal distribution of information or materials to minors, including obscene materials, or failure to employ legally required age-gating in connection with such information or materials.
5. Sexual solicitation
6. Any other criminal activity
2. Engage in, promote, incite, or facilitate the harassment, abuse, threatening, or bullying of individuals or groups of individuals
3. Engage in, promote, incite, or facilitate discrimination or other unlawful or harmful conduct in the provision of employment, employment benefits, credit, housing, other economic benefits, or other essential goods and services
4. Engage in the unauthorized or unlicensed practice of any profession including, but not limited to, financial, legal, medical/health, or related professional practices
5. Collect, process, disclose, generate, or infer health, demographic, or other sensitive personal or private information about individuals without rights and consents required by applicable laws
6. Engage in or facilitate any action or generate any content that infringes, misappropriates, or otherwise violates any third-party rights, including the outputs or results of any products or services using the Llama 2 Materials
7. Create, generate, or facilitate the creation of malicious code, malware, computer viruses or do anything else that could disable, overburden, interfere with or impair the proper working, integrity, operation or appearance of a website or computer system
2. Engage in, promote, incite, facilitate, or assist in the planning or
development of activities that present a risk of death or bodily harm to
individuals, including use of Llama 2 related to the following:
1. Military, warfare, nuclear industries or applications, espionage, use for materials or activities that are subject to the International Traffic Arms Regulations (ITAR) maintained by the United States Department of State
2. Guns and illegal weapons (including weapon development)
3. Illegal drugs and regulated/controlled substances
4. Operation of critical infrastructure, transportation technologies, or heavy machinery
5. Self-harm or harm to others, including suicide, cutting, and eating disorders
6. Any content intended to incite or promote violence, abuse, or any infliction of bodily harm to an individual
3. Intentionally deceive or mislead others, including use of Llama 2 related
to the following:
1. Generating, promoting, or furthering fraud or the creation or promotion of disinformation
2. Generating, promoting, or furthering defamatory content, including the creation of defamatory statements, images, or other content
3. Generating, promoting, or further distributing spam
4. Impersonating another individual without consent, authorization, or legal right
5. Representing that the use of Llama 2 or outputs are human-generated
6. Generating or facilitating false online engagement, including fake reviews and other means of fake online engagement
4. Fail to appropriately disclose to end users any known dangers of your AI system
Please report any violation of this Policy, software “bug,” or other problems
that could lead to a violation of this Policy through one of the following
means:
* Reporting issues with the model:
[github.com/facebookresearch/llama](http://github.com/facebookresearch/llama)
* Reporting risky content generated by the model:
[developers.facebook.com/llama_output_feedback](http://developers.facebook.com/llama_output_feedback)
* Reporting bugs and security concerns:
[facebook.com/whitehat/info](http://facebook.com/whitehat/info)
* Reporting violations of the Acceptable Use Policy or unlicensed uses of
Llama: [[email protected]](mailto:[email protected])
extra_gated_fields:
First Name: text
Last Name: text
Date of birth: date_picker
Country: country
Affiliation: text
geo: ip_location
By clicking Submit below I accept the terms of the license and acknowledge that the information I provide will be collected stored processed and shared in accordance with the Meta Privacy Policy: checkbox
extra_gated_description: The information you provide will be collected, stored, processed and shared in accordance with the [Meta Privacy Policy](https://www.facebook.com/privacy/policy/).
extra_gated_button_content: Submit
language:
- code
pipeline_tag: text-generation
tags:
- facebook
- meta
- pytorch
- llama
- llama-2
license: llama2
---
# **Code Llama**
Code Llama is a collection of pretrained and fine-tuned generative text models ranging in scale from 7 billion to 34 billion parameters. This is the repository for the base 34B version in the Hugging Face Transformers format. This model is designed for general code synthesis and understanding. Links to other models can be found in the index at the bottom.
| | Base Model | Python | Instruct |
| --- | ----------------------------------------------------------------------------- | ------------------------------------------------------------------------------------------- | ----------------------------------------------------------------------------------------------- |
| 7B | [meta-llama/CodeLlama-7b-hf](https://huggingface.co/meta-llama/CodeLlama-7b-hf) | [meta-llama/CodeLlama-7b-Python-hf](https://huggingface.co/meta-llama/CodeLlama-7b-Python-hf) | [meta-llama/CodeLlama-7b-Instruct-hf](https://huggingface.co/meta-llama/CodeLlama-7b-Instruct-hf) |
| 13B | [meta-llama/CodeLlama-13b-hf](https://huggingface.co/meta-llama/CodeLlama-13b-hf) | [meta-llama/CodeLlama-13b-Python-hf](https://huggingface.co/meta-llama/CodeLlama-13b-Python-hf) | [meta-llama/CodeLlama-13b-Instruct-hf](https://huggingface.co/meta-llama/CodeLlama-13b-Instruct-hf) |
| 34B | [meta-llama/CodeLlama-34b-hf](https://huggingface.co/meta-llama/CodeLlama-34b-hf) | [meta-llama/CodeLlama-34b-Python-hf](https://huggingface.co/meta-llama/CodeLlama-34b-Python-hf) | [meta-llama/CodeLlama-34b-Instruct-hf](https://huggingface.co/meta-llama/CodeLlama-34b-Instruct-hf) |
| 70B | [meta-llama/CodeLlama-70b-hf](https://huggingface.co/meta-llama/CodeLlama-70b-hf) | [meta-llama/CodeLlama-70b-Python-hf](https://huggingface.co/meta-llama/CodeLlama-70b-Python-hf) | [meta-llama/CodeLlama-70b-Instruct-hf](https://huggingface.co/meta-llama/CodeLlama-70b-Instruct-hf) |
## Model Use
To use this model, please make sure to install transformers:
```bash
pip install transformers.git accelerate
```
Model capabilities:
- [x] Code completion.
- [ ] Infilling.
- [ ] Instructions / chat.
- [ ] Python specialist.
```python
from transformers import AutoTokenizer
import transformers
import torch
model = "meta-llama/CodeLlama-34b-hf"
tokenizer = AutoTokenizer.from_pretrained(model)
pipeline = transformers.pipeline(
"text-generation",
model=model,
torch_dtype=torch.float16,
device_map="auto",
)
sequences = pipeline(
'import socket\n\ndef ping_exponential_backoff(host: str):',
do_sample=True,
top_k=10,
temperature=0.1,
top_p=0.95,
num_return_sequences=1,
eos_token_id=tokenizer.eos_token_id,
max_length=200,
)
for seq in sequences:
print(f"Result: {seq['generated_text']}")
```
## Model Details
*Note: Use of this model is governed by the Meta license. Meta developed and publicly released the Code Llama family of large language models (LLMs).
**Model Developers** Meta
**Variations** Code Llama comes in three model sizes, and three variants:
* Code Llama: base models designed for general code synthesis and understanding
* Code Llama - Python: designed specifically for Python
* Code Llama - Instruct: for instruction following and safer deployment
All variants are available in sizes of 7B, 13B and 34B parameters.
**This repository contains the base version of the 34B parameters model.**
**Input** Models input text only.
**Output** Models generate text only.
**Model Architecture** Code Llama is an auto-regressive language model that uses an optimized transformer architecture.
**Model Dates** Code Llama and its variants have been trained between January 2023 and July 2023.
**Status** This is a static model trained on an offline dataset. Future versions of Code Llama - Instruct will be released as we improve model safety with community feedback.
**License** A custom commercial license is available at: [https://ai.meta.com/resources/models-and-libraries/llama-downloads/](https://ai.meta.com/resources/models-and-libraries/llama-downloads/)
**Research Paper** More information can be found in the paper "[Code Llama: Open Foundation Models for Code](https://ai.meta.com/research/publications/code-llama-open-foundation-models-for-code/)" or its [arXiv page](https://arxiv.org/abs/2308.12950).
## Intended Use
**Intended Use Cases** Code Llama and its variants is intended for commercial and research use in English and relevant programming languages. The base model Code Llama can be adapted for a variety of code synthesis and understanding tasks, Code Llama - Python is designed specifically to handle the Python programming language, and Code Llama - Instruct is intended to be safer to use for code assistant and generation applications.
**Out-of-Scope Uses** Use in any manner that violates applicable laws or regulations (including trade compliance laws). Use in languages other than English. Use in any other way that is prohibited by the Acceptable Use Policy and Licensing Agreement for Code Llama and its variants.
## Hardware and Software
**Training Factors** We used custom training libraries. The training and fine-tuning of the released models have been performed Meta’s Research Super Cluster.
**Carbon Footprint** In aggregate, training all 9 Code Llama models required 400K GPU hours of computation on hardware of type A100-80GB (TDP of 350-400W). Estimated total emissions were 65.3 tCO2eq, 100% of which were offset by Meta’s sustainability program.
## Training Data
All experiments reported here and the released models have been trained and fine-tuned using the same data as Llama 2 with different weights (see Section 2 and Table 1 in the [research paper](https://ai.meta.com/research/publications/code-llama-open-foundation-models-for-code/) for details).
## Evaluation Results
See evaluations for the main models and detailed ablations in Section 3 and safety evaluations in Section 4 of the research paper.
## Ethical Considerations and Limitations
Code Llama and its variants are a new technology that carries risks with use. Testing conducted to date has been in English, and has not covered, nor could it cover all scenarios. For these reasons, as with all LLMs, Code Llama’s potential outputs cannot be predicted in advance, and the model may in some instances produce inaccurate or objectionable responses to user prompts. Therefore, before deploying any applications of Code Llama, developers should perform safety testing and tuning tailored to their specific applications of the model.
Please see the Responsible Use Guide available available at [https://ai.meta.com/llama/responsible-use-guide](https://ai.meta.com/llama/responsible-use-guide).
| {} | RichardErkhov/meta-llama_-_CodeLlama-34b-hf-gguf | null | [
"gguf",
"arxiv:2308.12950",
"region:us"
] | null | 2024-04-21T19:10:40+00:00 | [
"2308.12950"
] | [] | TAGS
#gguf #arxiv-2308.12950 #region-us
| Quantization made by Richard Erkhov.
Github
Discord
Request more models
CodeLlama-34b-hf - GGUF
* Model creator: URL
* Original model: URL
Name: CodeLlama-34b-hf.Q2\_K.gguf, Quant method: Q2\_K, Size: 11.65GB
Name: CodeLlama-34b-hf.IQ3\_XS.gguf, Quant method: IQ3\_XS, Size: 12.93GB
Name: CodeLlama-34b-hf.IQ3\_S.gguf, Quant method: IQ3\_S, Size: 13.65GB
Name: CodeLlama-34b-hf.Q3\_K\_S.gguf, Quant method: Q3\_K\_S, Size: 13.6GB
Name: CodeLlama-34b-hf.IQ3\_M.gguf, Quant method: IQ3\_M, Size: 14.18GB
Name: CodeLlama-34b-hf.Q3\_K.gguf, Quant method: Q3\_K, Size: 15.19GB
Name: CodeLlama-34b-hf.Q3\_K\_M.gguf, Quant method: Q3\_K\_M, Size: 15.19GB
Name: CodeLlama-34b-hf.Q3\_K\_L.gguf, Quant method: Q3\_K\_L, Size: 16.55GB
Name: CodeLlama-34b-hf.IQ4\_XS.gguf, Quant method: IQ4\_XS, Size: 16.99GB
Name: CodeLlama-34b-hf.Q4\_0.gguf, Quant method: Q4\_0, Size: 17.74GB
Name: CodeLlama-34b-hf.IQ4\_NL.gguf, Quant method: IQ4\_NL, Size: 17.92GB
Name: CodeLlama-34b-hf.Q4\_K\_S.gguf, Quant method: Q4\_K\_S, Size: 17.87GB
Name: CodeLlama-34b-hf.Q4\_K.gguf, Quant method: Q4\_K, Size: 18.83GB
Name: CodeLlama-34b-hf.Q4\_K\_M.gguf, Quant method: Q4\_K\_M, Size: 18.83GB
Name: CodeLlama-34b-hf.Q4\_1.gguf, Quant method: Q4\_1, Size: 19.69GB
Name: CodeLlama-34b-hf.Q5\_0.gguf, Quant method: Q5\_0, Size: 21.64GB
Name: CodeLlama-34b-hf.Q5\_K\_S.gguf, Quant method: Q5\_K\_S, Size: 21.64GB
Name: CodeLlama-34b-hf.Q5\_K.gguf, Quant method: Q5\_K, Size: 22.2GB
Name: CodeLlama-34b-hf.Q5\_K\_M.gguf, Quant method: Q5\_K\_M, Size: 22.2GB
Name: CodeLlama-34b-hf.Q5\_1.gguf, Quant method: Q5\_1, Size: 23.59GB
Name: CodeLlama-34b-hf.Q6\_K.gguf, Quant method: Q6\_K, Size: 25.78GB
Original model description:
---------------------------
extra\_gated\_heading: You need to share contact information with Meta to access this model
extra\_gated\_prompt: >-
### LLAMA 2 COMMUNITY LICENSE AGREEMENT
"Agreement" means the terms and conditions for use, reproduction, distribution
and modification of the Llama Materials set forth herein.
"Documentation" means the specifications, manuals and documentation
accompanying Llama 2 distributed by Meta at
URL
"Licensee" or "you" means you, or your employer or any other person or entity
(if you are entering into this Agreement on such person or entity's behalf),
of the age required under applicable laws, rules or regulations to provide
legal consent and that has legal authority to bind your employer or such other
person or entity if you are entering in this Agreement on their behalf.
"Llama 2" means the foundational large language models and software and
algorithms, including machine-learning model code, trained model weights,
inference-enabling code, training-enabling code, fine-tuning enabling code and
other elements of the foregoing distributed by Meta at
URL
"Llama Materials" means, collectively, Meta's proprietary Llama 2 and
documentation (and any portion thereof) made available under this Agreement.
"Meta" or "we" means Meta Platforms Ireland Limited (if you are located in or,
if you are an entity, your principal place of business is in the EEA or
Switzerland) and Meta Platforms, Inc. (if you are located outside of the EEA
or Switzerland).
By clicking "I Accept" below or by using or distributing any portion or
element of the Llama Materials, you agree to be bound by this Agreement.
1. License Rights and Redistribution.
a. Grant of Rights. You are granted a non-exclusive, worldwide, non-
transferable and royalty-free limited license under Meta's intellectual
property or other rights owned by Meta embodied in the Llama Materials to
use, reproduce, distribute, copy, create derivative works of, and make
modifications to the Llama Materials.
b. Redistribution and Use.
i. If you distribute or make the Llama Materials, or any derivative works
thereof, available to a third party, you shall provide a copy of this
Agreement to such third party.
ii. If you receive Llama Materials, or any derivative works thereof, from a
Licensee as part of an integrated end user product, then Section 2 of this
Agreement will not apply to you.
iii. You must retain in all copies of the Llama Materials that you distribute
the following attribution notice within a "Notice" text file distributed as a
part of such copies: "Llama 2 is licensed under the LLAMA 2 Community
License, Copyright (c) Meta Platforms, Inc. All Rights Reserved."
iv. Your use of the Llama Materials must comply with applicable laws and
regulations (including trade compliance laws and regulations) and adhere to
the Acceptable Use Policy for the Llama Materials (available at
URL which is hereby incorporated by
reference into this Agreement.
v. You will not use the Llama Materials or any output or results of the Llama
Materials to improve any other large language model (excluding Llama 2 or
derivative works thereof).
2. Additional Commercial Terms. If, on the Llama 2 version release date, the
monthly active users of the products or services made available by or for
Licensee, or Licensee's affiliates, is greater than 700 million monthly
active users in the preceding calendar month, you must request a license from
Meta, which Meta may grant to you in its sole discretion, and you are not
authorized to exercise any of the rights under this Agreement unless or until
Meta otherwise expressly grants you such rights.
3. Disclaimer of Warranty. UNLESS REQUIRED BY APPLICABLE LAW, THE LLAMA
MATERIALS AND ANY OUTPUT AND RESULTS THEREFROM ARE PROVIDED ON AN "AS IS"
BASIS, WITHOUT WARRANTIES OF ANY KIND, EITHER EXPRESS OR IMPLIED, INCLUDING,
WITHOUT LIMITATION, ANY WARRANTIES OF TITLE, NON-INFRINGEMENT,
MERCHANTABILITY, OR FITNESS FOR A PARTICULAR PURPOSE. YOU ARE SOLELY
RESPONSIBLE FOR DETERMINING THE APPROPRIATENESS OF USING OR REDISTRIBUTING
THE LLAMA MATERIALS AND ASSUME ANY RISKS ASSOCIATED WITH YOUR USE OF THE
LLAMA MATERIALS AND ANY OUTPUT AND RESULTS.
4. Limitation of Liability. IN NO EVENT WILL META OR ITS AFFILIATES BE LIABLE
UNDER ANY THEORY OF LIABILITY, WHETHER IN CONTRACT, TORT, NEGLIGENCE,
PRODUCTS LIABILITY, OR OTHERWISE, ARISING OUT OF THIS AGREEMENT, FOR ANY LOST
PROFITS OR ANY INDIRECT, SPECIAL, CONSEQUENTIAL, INCIDENTAL, EXEMPLARY OR
PUNITIVE DAMAGES, EVEN IF META OR ITS AFFILIATES HAVE BEEN ADVISED OF THE
POSSIBILITY OF ANY OF THE FOREGOING.
5. Intellectual Property.
a. No trademark licenses are granted under this Agreement, and in connection
with the Llama Materials, neither Meta nor Licensee may use any name or mark
owned by or associated with the other or any of its affiliates, except as
required for reasonable and customary use in describing and redistributing
the Llama Materials.
b. Subject to Meta's ownership of Llama Materials and derivatives made by or
for Meta, with respect to any derivative works and modifications of the Llama
Materials that are made by you, as between you and Meta, you are and will be
the owner of such derivative works and modifications.
c. If you institute litigation or other proceedings against Meta or any
entity (including a cross-claim or counterclaim in a lawsuit) alleging that
the Llama Materials or Llama 2 outputs or results, or any portion of any of
the foregoing, constitutes infringement of intellectual property or other
rights owned or licensable by you, then any licenses granted to you under
this Agreement shall terminate as of the date such litigation or claim is
filed or instituted. You will indemnify and hold harmless Meta from and
against any claim by any third party arising out of or related to your use or
distribution of the Llama Materials.
6. Term and Termination. The term of this Agreement will commence upon your
acceptance of this Agreement or access to the Llama Materials and will
continue in full force and effect until terminated in accordance with the
terms and conditions herein. Meta may terminate this Agreement if you are in
breach of any term or condition of this Agreement. Upon termination of this
Agreement, you shall delete and cease use of the Llama Materials. Sections 3,
4 and 7 shall survive the termination of this Agreement.
7. Governing Law and Jurisdiction. This Agreement will be governed and
construed under the laws of the State of California without regard to choice
of law principles, and the UN Convention on Contracts for the International
Sale of Goods does not apply to this Agreement. The courts of California
shall have exclusive jurisdiction of any dispute arising out of this
Agreement.
USE POLICY
### Llama 2 Acceptable Use Policy
Meta is committed to promoting safe and fair use of its tools and features,
including Llama 2. If you access or use Llama 2, you agree to this Acceptable
Use Policy (“Policy”). The most recent copy of this policy can be found at
URL
#### Prohibited Uses
We want everyone to use Llama 2 safely and responsibly. You agree you will not
use, or allow others to use, Llama 2 to:
1. Violate the law or others’ rights, including to:
1. Engage in, promote, generate, contribute to, encourage, plan, incite, or further illegal or unlawful activity or content, such as:
1. Violence or terrorism
2. Exploitation or harm to children, including the solicitation, creation, acquisition, or dissemination of child exploitative content or failure to report Child Sexual Abuse Material
3. Human trafficking, exploitation, and sexual violence
4. The illegal distribution of information or materials to minors, including obscene materials, or failure to employ legally required age-gating in connection with such information or materials.
5. Sexual solicitation
6. Any other criminal activity
```
2. Engage in, promote, incite, or facilitate the harassment, abuse, threatening, or bullying of individuals or groups of individuals
3. Engage in, promote, incite, or facilitate discrimination or other unlawful or harmful conduct in the provision of employment, employment benefits, credit, housing, other economic benefits, or other essential goods and services
4. Engage in the unauthorized or unlicensed practice of any profession including, but not limited to, financial, legal, medical/health, or related professional practices
5. Collect, process, disclose, generate, or infer health, demographic, or other sensitive personal or private information about individuals without rights and consents required by applicable laws
6. Engage in or facilitate any action or generate any content that infringes, misappropriates, or otherwise violates any third-party rights, including the outputs or results of any products or services using the Llama 2 Materials
7. Create, generate, or facilitate the creation of malicious code, malware, computer viruses or do anything else that could disable, overburden, interfere with or impair the proper working, integrity, operation or appearance of a website or computer system
```
2. Engage in, promote, incite, facilitate, or assist in the planning or
development of activities that present a risk of death or bodily harm to
individuals, including use of Llama 2 related to the following:
1. Military, warfare, nuclear industries or applications, espionage, use for materials or activities that are subject to the International Traffic Arms Regulations (ITAR) maintained by the United States Department of State
2. Guns and illegal weapons (including weapon development)
3. Illegal drugs and regulated/controlled substances
4. Operation of critical infrastructure, transportation technologies, or heavy machinery
5. Self-harm or harm to others, including suicide, cutting, and eating disorders
6. Any content intended to incite or promote violence, abuse, or any infliction of bodily harm to an individual
3. Intentionally deceive or mislead others, including use of Llama 2 related
to the following:
1. Generating, promoting, or furthering fraud or the creation or promotion of disinformation
2. Generating, promoting, or furthering defamatory content, including the creation of defamatory statements, images, or other content
3. Generating, promoting, or further distributing spam
4. Impersonating another individual without consent, authorization, or legal right
5. Representing that the use of Llama 2 or outputs are human-generated
6. Generating or facilitating false online engagement, including fake reviews and other means of fake online engagement
4. Fail to appropriately disclose to end users any known dangers of your AI system
Please report any violation of this Policy, software “bug,” or other problems
that could lead to a violation of this Policy through one of the following
means:
* Reporting issues with the model:
URL
* Reporting risky content generated by the model:
URL
* Reporting bugs and security concerns:
URL
* Reporting violations of the Acceptable Use Policy or unlicensed uses of
Llama: LlamaUseReport@URL
extra\_gated\_fields:
First Name: text
Last Name: text
Date of birth: date\_picker
Country: country
Affiliation: text
geo: ip\_location
By clicking Submit below I accept the terms of the license and acknowledge that the information I provide will be collected stored processed and shared in accordance with the Meta Privacy Policy: checkbox
extra\_gated\_description: The information you provide will be collected, stored, processed and shared in accordance with the Meta Privacy Policy.
extra\_gated\_button\_content: Submit
language:
* code
pipeline\_tag: text-generation
tags:
* facebook
* meta
* pytorch
* llama
* llama-2
license: llama2
---
Code Llama
==========
Code Llama is a collection of pretrained and fine-tuned generative text models ranging in scale from 7 billion to 34 billion parameters. This is the repository for the base 34B version in the Hugging Face Transformers format. This model is designed for general code synthesis and understanding. Links to other models can be found in the index at the bottom.
Model Use
---------
To use this model, please make sure to install transformers:
Model capabilities:
* [x] Code completion.
* [ ] Infilling.
* [ ] Instructions / chat.
* [ ] Python specialist.
Model Details
-------------
\*Note: Use of this model is governed by the Meta license. Meta developed and publicly released the Code Llama family of large language models (LLMs).
Model Developers Meta
Variations Code Llama comes in three model sizes, and three variants:
* Code Llama: base models designed for general code synthesis and understanding
* Code Llama - Python: designed specifically for Python
* Code Llama - Instruct: for instruction following and safer deployment
All variants are available in sizes of 7B, 13B and 34B parameters.
This repository contains the base version of the 34B parameters model.
Input Models input text only.
Output Models generate text only.
Model Architecture Code Llama is an auto-regressive language model that uses an optimized transformer architecture.
Model Dates Code Llama and its variants have been trained between January 2023 and July 2023.
Status This is a static model trained on an offline dataset. Future versions of Code Llama - Instruct will be released as we improve model safety with community feedback.
License A custom commercial license is available at: URL
Research Paper More information can be found in the paper "Code Llama: Open Foundation Models for Code" or its arXiv page.
Intended Use
------------
Intended Use Cases Code Llama and its variants is intended for commercial and research use in English and relevant programming languages. The base model Code Llama can be adapted for a variety of code synthesis and understanding tasks, Code Llama - Python is designed specifically to handle the Python programming language, and Code Llama - Instruct is intended to be safer to use for code assistant and generation applications.
Out-of-Scope Uses Use in any manner that violates applicable laws or regulations (including trade compliance laws). Use in languages other than English. Use in any other way that is prohibited by the Acceptable Use Policy and Licensing Agreement for Code Llama and its variants.
Hardware and Software
---------------------
Training Factors We used custom training libraries. The training and fine-tuning of the released models have been performed Meta’s Research Super Cluster.
Carbon Footprint In aggregate, training all 9 Code Llama models required 400K GPU hours of computation on hardware of type A100-80GB (TDP of 350-400W). Estimated total emissions were 65.3 tCO2eq, 100% of which were offset by Meta’s sustainability program.
Training Data
-------------
All experiments reported here and the released models have been trained and fine-tuned using the same data as Llama 2 with different weights (see Section 2 and Table 1 in the research paper for details).
Evaluation Results
------------------
See evaluations for the main models and detailed ablations in Section 3 and safety evaluations in Section 4 of the research paper.
Ethical Considerations and Limitations
--------------------------------------
Code Llama and its variants are a new technology that carries risks with use. Testing conducted to date has been in English, and has not covered, nor could it cover all scenarios. For these reasons, as with all LLMs, Code Llama’s potential outputs cannot be predicted in advance, and the model may in some instances produce inaccurate or objectionable responses to user prompts. Therefore, before deploying any applications of Code Llama, developers should perform safety testing and tuning tailored to their specific applications of the model.
Please see the Responsible Use Guide available available at URL
| [
"### LLAMA 2 COMMUNITY LICENSE AGREEMENT\n\n\n\"Agreement\" means the terms and conditions for use, reproduction, distribution\nand modification of the Llama Materials set forth herein.\n\"Documentation\" means the specifications, manuals and documentation\naccompanying Llama 2 distributed by Meta at\nURL \n\n\"Licensee\" or \"you\" means you, or your employer or any other person or entity\n(if you are entering into this Agreement on such person or entity's behalf),\nof the age required under applicable laws, rules or regulations to provide\nlegal consent and that has legal authority to bind your employer or such other\nperson or entity if you are entering in this Agreement on their behalf.\n\"Llama 2\" means the foundational large language models and software and\nalgorithms, including machine-learning model code, trained model weights,\ninference-enabling code, training-enabling code, fine-tuning enabling code and\nother elements of the foregoing distributed by Meta at\nURL\n\"Llama Materials\" means, collectively, Meta's proprietary Llama 2 and\ndocumentation (and any portion thereof) made available under this Agreement.\n\"Meta\" or \"we\" means Meta Platforms Ireland Limited (if you are located in or,\nif you are an entity, your principal place of business is in the EEA or\nSwitzerland) and Meta Platforms, Inc. (if you are located outside of the EEA\nor Switzerland).\nBy clicking \"I Accept\" below or by using or distributing any portion or\nelement of the Llama Materials, you agree to be bound by this Agreement.\n\n\n1. License Rights and Redistribution.\na. Grant of Rights. You are granted a non-exclusive, worldwide, non-\ntransferable and royalty-free limited license under Meta's intellectual\nproperty or other rights owned by Meta embodied in the Llama Materials to\nuse, reproduce, distribute, copy, create derivative works of, and make\nmodifications to the Llama Materials.\n\n\nb. Redistribution and Use. \n\ni. If you distribute or make the Llama Materials, or any derivative works\nthereof, available to a third party, you shall provide a copy of this\nAgreement to such third party.\nii. If you receive Llama Materials, or any derivative works thereof, from a\nLicensee as part of an integrated end user product, then Section 2 of this\nAgreement will not apply to you.\niii. You must retain in all copies of the Llama Materials that you distribute\nthe following attribution notice within a \"Notice\" text file distributed as a\npart of such copies: \"Llama 2 is licensed under the LLAMA 2 Community\nLicense, Copyright (c) Meta Platforms, Inc. All Rights Reserved.\"\niv. Your use of the Llama Materials must comply with applicable laws and\nregulations (including trade compliance laws and regulations) and adhere to\nthe Acceptable Use Policy for the Llama Materials (available at\nURL which is hereby incorporated by\nreference into this Agreement.\nv. You will not use the Llama Materials or any output or results of the Llama\nMaterials to improve any other large language model (excluding Llama 2 or\nderivative works thereof). \n\n2. Additional Commercial Terms. If, on the Llama 2 version release date, the\nmonthly active users of the products or services made available by or for\nLicensee, or Licensee's affiliates, is greater than 700 million monthly\nactive users in the preceding calendar month, you must request a license from\nMeta, which Meta may grant to you in its sole discretion, and you are not\nauthorized to exercise any of the rights under this Agreement unless or until\nMeta otherwise expressly grants you such rights.\n3. Disclaimer of Warranty. UNLESS REQUIRED BY APPLICABLE LAW, THE LLAMA\nMATERIALS AND ANY OUTPUT AND RESULTS THEREFROM ARE PROVIDED ON AN \"AS IS\"\nBASIS, WITHOUT WARRANTIES OF ANY KIND, EITHER EXPRESS OR IMPLIED, INCLUDING,\nWITHOUT LIMITATION, ANY WARRANTIES OF TITLE, NON-INFRINGEMENT,\nMERCHANTABILITY, OR FITNESS FOR A PARTICULAR PURPOSE. YOU ARE SOLELY\nRESPONSIBLE FOR DETERMINING THE APPROPRIATENESS OF USING OR REDISTRIBUTING\nTHE LLAMA MATERIALS AND ASSUME ANY RISKS ASSOCIATED WITH YOUR USE OF THE\nLLAMA MATERIALS AND ANY OUTPUT AND RESULTS.\n4. Limitation of Liability. IN NO EVENT WILL META OR ITS AFFILIATES BE LIABLE\nUNDER ANY THEORY OF LIABILITY, WHETHER IN CONTRACT, TORT, NEGLIGENCE,\nPRODUCTS LIABILITY, OR OTHERWISE, ARISING OUT OF THIS AGREEMENT, FOR ANY LOST\nPROFITS OR ANY INDIRECT, SPECIAL, CONSEQUENTIAL, INCIDENTAL, EXEMPLARY OR\nPUNITIVE DAMAGES, EVEN IF META OR ITS AFFILIATES HAVE BEEN ADVISED OF THE\nPOSSIBILITY OF ANY OF THE FOREGOING.\n5. Intellectual Property.\na. No trademark licenses are granted under this Agreement, and in connection\nwith the Llama Materials, neither Meta nor Licensee may use any name or mark\nowned by or associated with the other or any of its affiliates, except as\nrequired for reasonable and customary use in describing and redistributing\nthe Llama Materials.\nb. Subject to Meta's ownership of Llama Materials and derivatives made by or\nfor Meta, with respect to any derivative works and modifications of the Llama\nMaterials that are made by you, as between you and Meta, you are and will be\nthe owner of such derivative works and modifications.\nc. If you institute litigation or other proceedings against Meta or any\nentity (including a cross-claim or counterclaim in a lawsuit) alleging that\nthe Llama Materials or Llama 2 outputs or results, or any portion of any of\nthe foregoing, constitutes infringement of intellectual property or other\nrights owned or licensable by you, then any licenses granted to you under\nthis Agreement shall terminate as of the date such litigation or claim is\nfiled or instituted. You will indemnify and hold harmless Meta from and\nagainst any claim by any third party arising out of or related to your use or\ndistribution of the Llama Materials.\n6. Term and Termination. The term of this Agreement will commence upon your\nacceptance of this Agreement or access to the Llama Materials and will\ncontinue in full force and effect until terminated in accordance with the\nterms and conditions herein. Meta may terminate this Agreement if you are in\nbreach of any term or condition of this Agreement. Upon termination of this\nAgreement, you shall delete and cease use of the Llama Materials. Sections 3,\n4 and 7 shall survive the termination of this Agreement.\n7. Governing Law and Jurisdiction. This Agreement will be governed and\nconstrued under the laws of the State of California without regard to choice\nof law principles, and the UN Convention on Contracts for the International\nSale of Goods does not apply to this Agreement. The courts of California\nshall have exclusive jurisdiction of any dispute arising out of this\nAgreement.\nUSE POLICY",
"### Llama 2 Acceptable Use Policy\n\n\nMeta is committed to promoting safe and fair use of its tools and features,\nincluding Llama 2. If you access or use Llama 2, you agree to this Acceptable\nUse Policy (“Policy”). The most recent copy of this policy can be found at\nURL",
"#### Prohibited Uses\n\n\nWe want everyone to use Llama 2 safely and responsibly. You agree you will not\nuse, or allow others to use, Llama 2 to:\n\n\n1. Violate the law or others’ rights, including to:\n1. Engage in, promote, generate, contribute to, encourage, plan, incite, or further illegal or unlawful activity or content, such as:\n\t1. Violence or terrorism\n\t2. Exploitation or harm to children, including the solicitation, creation, acquisition, or dissemination of child exploitative content or failure to report Child Sexual Abuse Material\n\t3. Human trafficking, exploitation, and sexual violence\n\t4. The illegal distribution of information or materials to minors, including obscene materials, or failure to employ legally required age-gating in connection with such information or materials.\n\t5. Sexual solicitation\n\t6. Any other criminal activity\n\n\n\n```\n2. Engage in, promote, incite, or facilitate the harassment, abuse, threatening, or bullying of individuals or groups of individuals\n3. Engage in, promote, incite, or facilitate discrimination or other unlawful or harmful conduct in the provision of employment, employment benefits, credit, housing, other economic benefits, or other essential goods and services\n4. Engage in the unauthorized or unlicensed practice of any profession including, but not limited to, financial, legal, medical/health, or related professional practices \n5. Collect, process, disclose, generate, or infer health, demographic, or other sensitive personal or private information about individuals without rights and consents required by applicable laws\n6. Engage in or facilitate any action or generate any content that infringes, misappropriates, or otherwise violates any third-party rights, including the outputs or results of any products or services using the Llama 2 Materials\n7. Create, generate, or facilitate the creation of malicious code, malware, computer viruses or do anything else that could disable, overburden, interfere with or impair the proper working, integrity, operation or appearance of a website or computer system \n\n```\n\n2. Engage in, promote, incite, facilitate, or assist in the planning or\ndevelopment of activities that present a risk of death or bodily harm to\nindividuals, including use of Llama 2 related to the following:\n1. Military, warfare, nuclear industries or applications, espionage, use for materials or activities that are subject to the International Traffic Arms Regulations (ITAR) maintained by the United States Department of State\n2. Guns and illegal weapons (including weapon development)\n3. Illegal drugs and regulated/controlled substances\n4. Operation of critical infrastructure, transportation technologies, or heavy machinery\n5. Self-harm or harm to others, including suicide, cutting, and eating disorders\n6. Any content intended to incite or promote violence, abuse, or any infliction of bodily harm to an individual\n3. Intentionally deceive or mislead others, including use of Llama 2 related\nto the following:\n1. Generating, promoting, or furthering fraud or the creation or promotion of disinformation\n2. Generating, promoting, or furthering defamatory content, including the creation of defamatory statements, images, or other content\n3. Generating, promoting, or further distributing spam\n4. Impersonating another individual without consent, authorization, or legal right\n5. Representing that the use of Llama 2 or outputs are human-generated\n6. Generating or facilitating false online engagement, including fake reviews and other means of fake online engagement\n4. Fail to appropriately disclose to end users any known dangers of your AI system\nPlease report any violation of this Policy, software “bug,” or other problems\nthat could lead to a violation of this Policy through one of the following\nmeans:\n\n\n* Reporting issues with the model:\nURL\n* Reporting risky content generated by the model:\nURL\n* Reporting bugs and security concerns:\nURL\n* Reporting violations of the Acceptable Use Policy or unlicensed uses of\nLlama: LlamaUseReport@URL\nextra\\_gated\\_fields:\nFirst Name: text\nLast Name: text\nDate of birth: date\\_picker\nCountry: country\nAffiliation: text\ngeo: ip\\_location \n\nBy clicking Submit below I accept the terms of the license and acknowledge that the information I provide will be collected stored processed and shared in accordance with the Meta Privacy Policy: checkbox\nextra\\_gated\\_description: The information you provide will be collected, stored, processed and shared in accordance with the Meta Privacy Policy.\nextra\\_gated\\_button\\_content: Submit\nlanguage:\n\n\n* code\npipeline\\_tag: text-generation\ntags:\n* facebook\n* meta\n* pytorch\n* llama\n* llama-2\nlicense: llama2\n\n\n\n\n---\n\n\nCode Llama\n==========\n\n\nCode Llama is a collection of pretrained and fine-tuned generative text models ranging in scale from 7 billion to 34 billion parameters. This is the repository for the base 34B version in the Hugging Face Transformers format. This model is designed for general code synthesis and understanding. Links to other models can be found in the index at the bottom.\n\n\n\nModel Use\n---------\n\n\nTo use this model, please make sure to install transformers:\n\n\nModel capabilities:\n\n\n* [x] Code completion.\n* [ ] Infilling.\n* [ ] Instructions / chat.\n* [ ] Python specialist.\n\n\nModel Details\n-------------\n\n\n\\*Note: Use of this model is governed by the Meta license. Meta developed and publicly released the Code Llama family of large language models (LLMs).\n\n\nModel Developers Meta\n\n\nVariations Code Llama comes in three model sizes, and three variants:\n\n\n* Code Llama: base models designed for general code synthesis and understanding\n* Code Llama - Python: designed specifically for Python\n* Code Llama - Instruct: for instruction following and safer deployment\n\n\nAll variants are available in sizes of 7B, 13B and 34B parameters.\n\n\nThis repository contains the base version of the 34B parameters model.\n\n\nInput Models input text only.\n\n\nOutput Models generate text only.\n\n\nModel Architecture Code Llama is an auto-regressive language model that uses an optimized transformer architecture.\n\n\nModel Dates Code Llama and its variants have been trained between January 2023 and July 2023.\n\n\nStatus This is a static model trained on an offline dataset. Future versions of Code Llama - Instruct will be released as we improve model safety with community feedback.\n\n\nLicense A custom commercial license is available at: URL\n\n\nResearch Paper More information can be found in the paper \"Code Llama: Open Foundation Models for Code\" or its arXiv page.\n\n\nIntended Use\n------------\n\n\nIntended Use Cases Code Llama and its variants is intended for commercial and research use in English and relevant programming languages. The base model Code Llama can be adapted for a variety of code synthesis and understanding tasks, Code Llama - Python is designed specifically to handle the Python programming language, and Code Llama - Instruct is intended to be safer to use for code assistant and generation applications.\n\n\nOut-of-Scope Uses Use in any manner that violates applicable laws or regulations (including trade compliance laws). Use in languages other than English. Use in any other way that is prohibited by the Acceptable Use Policy and Licensing Agreement for Code Llama and its variants.\n\n\nHardware and Software\n---------------------\n\n\nTraining Factors We used custom training libraries. The training and fine-tuning of the released models have been performed Meta’s Research Super Cluster.\n\n\nCarbon Footprint In aggregate, training all 9 Code Llama models required 400K GPU hours of computation on hardware of type A100-80GB (TDP of 350-400W). Estimated total emissions were 65.3 tCO2eq, 100% of which were offset by Meta’s sustainability program.\n\n\nTraining Data\n-------------\n\n\nAll experiments reported here and the released models have been trained and fine-tuned using the same data as Llama 2 with different weights (see Section 2 and Table 1 in the research paper for details).\n\n\nEvaluation Results\n------------------\n\n\nSee evaluations for the main models and detailed ablations in Section 3 and safety evaluations in Section 4 of the research paper.\n\n\nEthical Considerations and Limitations\n--------------------------------------\n\n\nCode Llama and its variants are a new technology that carries risks with use. Testing conducted to date has been in English, and has not covered, nor could it cover all scenarios. For these reasons, as with all LLMs, Code Llama’s potential outputs cannot be predicted in advance, and the model may in some instances produce inaccurate or objectionable responses to user prompts. Therefore, before deploying any applications of Code Llama, developers should perform safety testing and tuning tailored to their specific applications of the model.\n\n\nPlease see the Responsible Use Guide available available at URL"
] | [
"TAGS\n#gguf #arxiv-2308.12950 #region-us \n",
"### LLAMA 2 COMMUNITY LICENSE AGREEMENT\n\n\n\"Agreement\" means the terms and conditions for use, reproduction, distribution\nand modification of the Llama Materials set forth herein.\n\"Documentation\" means the specifications, manuals and documentation\naccompanying Llama 2 distributed by Meta at\nURL \n\n\"Licensee\" or \"you\" means you, or your employer or any other person or entity\n(if you are entering into this Agreement on such person or entity's behalf),\nof the age required under applicable laws, rules or regulations to provide\nlegal consent and that has legal authority to bind your employer or such other\nperson or entity if you are entering in this Agreement on their behalf.\n\"Llama 2\" means the foundational large language models and software and\nalgorithms, including machine-learning model code, trained model weights,\ninference-enabling code, training-enabling code, fine-tuning enabling code and\nother elements of the foregoing distributed by Meta at\nURL\n\"Llama Materials\" means, collectively, Meta's proprietary Llama 2 and\ndocumentation (and any portion thereof) made available under this Agreement.\n\"Meta\" or \"we\" means Meta Platforms Ireland Limited (if you are located in or,\nif you are an entity, your principal place of business is in the EEA or\nSwitzerland) and Meta Platforms, Inc. (if you are located outside of the EEA\nor Switzerland).\nBy clicking \"I Accept\" below or by using or distributing any portion or\nelement of the Llama Materials, you agree to be bound by this Agreement.\n\n\n1. License Rights and Redistribution.\na. Grant of Rights. You are granted a non-exclusive, worldwide, non-\ntransferable and royalty-free limited license under Meta's intellectual\nproperty or other rights owned by Meta embodied in the Llama Materials to\nuse, reproduce, distribute, copy, create derivative works of, and make\nmodifications to the Llama Materials.\n\n\nb. Redistribution and Use. \n\ni. If you distribute or make the Llama Materials, or any derivative works\nthereof, available to a third party, you shall provide a copy of this\nAgreement to such third party.\nii. If you receive Llama Materials, or any derivative works thereof, from a\nLicensee as part of an integrated end user product, then Section 2 of this\nAgreement will not apply to you.\niii. You must retain in all copies of the Llama Materials that you distribute\nthe following attribution notice within a \"Notice\" text file distributed as a\npart of such copies: \"Llama 2 is licensed under the LLAMA 2 Community\nLicense, Copyright (c) Meta Platforms, Inc. All Rights Reserved.\"\niv. Your use of the Llama Materials must comply with applicable laws and\nregulations (including trade compliance laws and regulations) and adhere to\nthe Acceptable Use Policy for the Llama Materials (available at\nURL which is hereby incorporated by\nreference into this Agreement.\nv. You will not use the Llama Materials or any output or results of the Llama\nMaterials to improve any other large language model (excluding Llama 2 or\nderivative works thereof). \n\n2. Additional Commercial Terms. If, on the Llama 2 version release date, the\nmonthly active users of the products or services made available by or for\nLicensee, or Licensee's affiliates, is greater than 700 million monthly\nactive users in the preceding calendar month, you must request a license from\nMeta, which Meta may grant to you in its sole discretion, and you are not\nauthorized to exercise any of the rights under this Agreement unless or until\nMeta otherwise expressly grants you such rights.\n3. Disclaimer of Warranty. UNLESS REQUIRED BY APPLICABLE LAW, THE LLAMA\nMATERIALS AND ANY OUTPUT AND RESULTS THEREFROM ARE PROVIDED ON AN \"AS IS\"\nBASIS, WITHOUT WARRANTIES OF ANY KIND, EITHER EXPRESS OR IMPLIED, INCLUDING,\nWITHOUT LIMITATION, ANY WARRANTIES OF TITLE, NON-INFRINGEMENT,\nMERCHANTABILITY, OR FITNESS FOR A PARTICULAR PURPOSE. YOU ARE SOLELY\nRESPONSIBLE FOR DETERMINING THE APPROPRIATENESS OF USING OR REDISTRIBUTING\nTHE LLAMA MATERIALS AND ASSUME ANY RISKS ASSOCIATED WITH YOUR USE OF THE\nLLAMA MATERIALS AND ANY OUTPUT AND RESULTS.\n4. Limitation of Liability. IN NO EVENT WILL META OR ITS AFFILIATES BE LIABLE\nUNDER ANY THEORY OF LIABILITY, WHETHER IN CONTRACT, TORT, NEGLIGENCE,\nPRODUCTS LIABILITY, OR OTHERWISE, ARISING OUT OF THIS AGREEMENT, FOR ANY LOST\nPROFITS OR ANY INDIRECT, SPECIAL, CONSEQUENTIAL, INCIDENTAL, EXEMPLARY OR\nPUNITIVE DAMAGES, EVEN IF META OR ITS AFFILIATES HAVE BEEN ADVISED OF THE\nPOSSIBILITY OF ANY OF THE FOREGOING.\n5. Intellectual Property.\na. No trademark licenses are granted under this Agreement, and in connection\nwith the Llama Materials, neither Meta nor Licensee may use any name or mark\nowned by or associated with the other or any of its affiliates, except as\nrequired for reasonable and customary use in describing and redistributing\nthe Llama Materials.\nb. Subject to Meta's ownership of Llama Materials and derivatives made by or\nfor Meta, with respect to any derivative works and modifications of the Llama\nMaterials that are made by you, as between you and Meta, you are and will be\nthe owner of such derivative works and modifications.\nc. If you institute litigation or other proceedings against Meta or any\nentity (including a cross-claim or counterclaim in a lawsuit) alleging that\nthe Llama Materials or Llama 2 outputs or results, or any portion of any of\nthe foregoing, constitutes infringement of intellectual property or other\nrights owned or licensable by you, then any licenses granted to you under\nthis Agreement shall terminate as of the date such litigation or claim is\nfiled or instituted. You will indemnify and hold harmless Meta from and\nagainst any claim by any third party arising out of or related to your use or\ndistribution of the Llama Materials.\n6. Term and Termination. The term of this Agreement will commence upon your\nacceptance of this Agreement or access to the Llama Materials and will\ncontinue in full force and effect until terminated in accordance with the\nterms and conditions herein. Meta may terminate this Agreement if you are in\nbreach of any term or condition of this Agreement. Upon termination of this\nAgreement, you shall delete and cease use of the Llama Materials. Sections 3,\n4 and 7 shall survive the termination of this Agreement.\n7. Governing Law and Jurisdiction. This Agreement will be governed and\nconstrued under the laws of the State of California without regard to choice\nof law principles, and the UN Convention on Contracts for the International\nSale of Goods does not apply to this Agreement. The courts of California\nshall have exclusive jurisdiction of any dispute arising out of this\nAgreement.\nUSE POLICY",
"### Llama 2 Acceptable Use Policy\n\n\nMeta is committed to promoting safe and fair use of its tools and features,\nincluding Llama 2. If you access or use Llama 2, you agree to this Acceptable\nUse Policy (“Policy”). The most recent copy of this policy can be found at\nURL",
"#### Prohibited Uses\n\n\nWe want everyone to use Llama 2 safely and responsibly. You agree you will not\nuse, or allow others to use, Llama 2 to:\n\n\n1. Violate the law or others’ rights, including to:\n1. Engage in, promote, generate, contribute to, encourage, plan, incite, or further illegal or unlawful activity or content, such as:\n\t1. Violence or terrorism\n\t2. Exploitation or harm to children, including the solicitation, creation, acquisition, or dissemination of child exploitative content or failure to report Child Sexual Abuse Material\n\t3. Human trafficking, exploitation, and sexual violence\n\t4. The illegal distribution of information or materials to minors, including obscene materials, or failure to employ legally required age-gating in connection with such information or materials.\n\t5. Sexual solicitation\n\t6. Any other criminal activity\n\n\n\n```\n2. Engage in, promote, incite, or facilitate the harassment, abuse, threatening, or bullying of individuals or groups of individuals\n3. Engage in, promote, incite, or facilitate discrimination or other unlawful or harmful conduct in the provision of employment, employment benefits, credit, housing, other economic benefits, or other essential goods and services\n4. Engage in the unauthorized or unlicensed practice of any profession including, but not limited to, financial, legal, medical/health, or related professional practices \n5. Collect, process, disclose, generate, or infer health, demographic, or other sensitive personal or private information about individuals without rights and consents required by applicable laws\n6. Engage in or facilitate any action or generate any content that infringes, misappropriates, or otherwise violates any third-party rights, including the outputs or results of any products or services using the Llama 2 Materials\n7. Create, generate, or facilitate the creation of malicious code, malware, computer viruses or do anything else that could disable, overburden, interfere with or impair the proper working, integrity, operation or appearance of a website or computer system \n\n```\n\n2. Engage in, promote, incite, facilitate, or assist in the planning or\ndevelopment of activities that present a risk of death or bodily harm to\nindividuals, including use of Llama 2 related to the following:\n1. Military, warfare, nuclear industries or applications, espionage, use for materials or activities that are subject to the International Traffic Arms Regulations (ITAR) maintained by the United States Department of State\n2. Guns and illegal weapons (including weapon development)\n3. Illegal drugs and regulated/controlled substances\n4. Operation of critical infrastructure, transportation technologies, or heavy machinery\n5. Self-harm or harm to others, including suicide, cutting, and eating disorders\n6. Any content intended to incite or promote violence, abuse, or any infliction of bodily harm to an individual\n3. Intentionally deceive or mislead others, including use of Llama 2 related\nto the following:\n1. Generating, promoting, or furthering fraud or the creation or promotion of disinformation\n2. Generating, promoting, or furthering defamatory content, including the creation of defamatory statements, images, or other content\n3. Generating, promoting, or further distributing spam\n4. Impersonating another individual without consent, authorization, or legal right\n5. Representing that the use of Llama 2 or outputs are human-generated\n6. Generating or facilitating false online engagement, including fake reviews and other means of fake online engagement\n4. Fail to appropriately disclose to end users any known dangers of your AI system\nPlease report any violation of this Policy, software “bug,” or other problems\nthat could lead to a violation of this Policy through one of the following\nmeans:\n\n\n* Reporting issues with the model:\nURL\n* Reporting risky content generated by the model:\nURL\n* Reporting bugs and security concerns:\nURL\n* Reporting violations of the Acceptable Use Policy or unlicensed uses of\nLlama: LlamaUseReport@URL\nextra\\_gated\\_fields:\nFirst Name: text\nLast Name: text\nDate of birth: date\\_picker\nCountry: country\nAffiliation: text\ngeo: ip\\_location \n\nBy clicking Submit below I accept the terms of the license and acknowledge that the information I provide will be collected stored processed and shared in accordance with the Meta Privacy Policy: checkbox\nextra\\_gated\\_description: The information you provide will be collected, stored, processed and shared in accordance with the Meta Privacy Policy.\nextra\\_gated\\_button\\_content: Submit\nlanguage:\n\n\n* code\npipeline\\_tag: text-generation\ntags:\n* facebook\n* meta\n* pytorch\n* llama\n* llama-2\nlicense: llama2\n\n\n\n\n---\n\n\nCode Llama\n==========\n\n\nCode Llama is a collection of pretrained and fine-tuned generative text models ranging in scale from 7 billion to 34 billion parameters. This is the repository for the base 34B version in the Hugging Face Transformers format. This model is designed for general code synthesis and understanding. Links to other models can be found in the index at the bottom.\n\n\n\nModel Use\n---------\n\n\nTo use this model, please make sure to install transformers:\n\n\nModel capabilities:\n\n\n* [x] Code completion.\n* [ ] Infilling.\n* [ ] Instructions / chat.\n* [ ] Python specialist.\n\n\nModel Details\n-------------\n\n\n\\*Note: Use of this model is governed by the Meta license. Meta developed and publicly released the Code Llama family of large language models (LLMs).\n\n\nModel Developers Meta\n\n\nVariations Code Llama comes in three model sizes, and three variants:\n\n\n* Code Llama: base models designed for general code synthesis and understanding\n* Code Llama - Python: designed specifically for Python\n* Code Llama - Instruct: for instruction following and safer deployment\n\n\nAll variants are available in sizes of 7B, 13B and 34B parameters.\n\n\nThis repository contains the base version of the 34B parameters model.\n\n\nInput Models input text only.\n\n\nOutput Models generate text only.\n\n\nModel Architecture Code Llama is an auto-regressive language model that uses an optimized transformer architecture.\n\n\nModel Dates Code Llama and its variants have been trained between January 2023 and July 2023.\n\n\nStatus This is a static model trained on an offline dataset. Future versions of Code Llama - Instruct will be released as we improve model safety with community feedback.\n\n\nLicense A custom commercial license is available at: URL\n\n\nResearch Paper More information can be found in the paper \"Code Llama: Open Foundation Models for Code\" or its arXiv page.\n\n\nIntended Use\n------------\n\n\nIntended Use Cases Code Llama and its variants is intended for commercial and research use in English and relevant programming languages. The base model Code Llama can be adapted for a variety of code synthesis and understanding tasks, Code Llama - Python is designed specifically to handle the Python programming language, and Code Llama - Instruct is intended to be safer to use for code assistant and generation applications.\n\n\nOut-of-Scope Uses Use in any manner that violates applicable laws or regulations (including trade compliance laws). Use in languages other than English. Use in any other way that is prohibited by the Acceptable Use Policy and Licensing Agreement for Code Llama and its variants.\n\n\nHardware and Software\n---------------------\n\n\nTraining Factors We used custom training libraries. The training and fine-tuning of the released models have been performed Meta’s Research Super Cluster.\n\n\nCarbon Footprint In aggregate, training all 9 Code Llama models required 400K GPU hours of computation on hardware of type A100-80GB (TDP of 350-400W). Estimated total emissions were 65.3 tCO2eq, 100% of which were offset by Meta’s sustainability program.\n\n\nTraining Data\n-------------\n\n\nAll experiments reported here and the released models have been trained and fine-tuned using the same data as Llama 2 with different weights (see Section 2 and Table 1 in the research paper for details).\n\n\nEvaluation Results\n------------------\n\n\nSee evaluations for the main models and detailed ablations in Section 3 and safety evaluations in Section 4 of the research paper.\n\n\nEthical Considerations and Limitations\n--------------------------------------\n\n\nCode Llama and its variants are a new technology that carries risks with use. Testing conducted to date has been in English, and has not covered, nor could it cover all scenarios. For these reasons, as with all LLMs, Code Llama’s potential outputs cannot be predicted in advance, and the model may in some instances produce inaccurate or objectionable responses to user prompts. Therefore, before deploying any applications of Code Llama, developers should perform safety testing and tuning tailored to their specific applications of the model.\n\n\nPlease see the Responsible Use Guide available available at URL"
] |
token-classification | transformers |
<!-- This model card has been generated automatically according to the information the Trainer had access to. You
should probably proofread and complete it, then remove this comment. -->
# xlm-roberta-base-finetuned-panx-ar
This model is a fine-tuned version of [tner/xlm-roberta-base-panx-dataset-ar](https://huggingface.co/tner/xlm-roberta-base-panx-dataset-ar) on an unknown dataset.
It achieves the following results on the evaluation set:
- Loss: 0.1920
- F1: 0.8882
- Accuracy: 0.9538
## Model description
More information needed
## Intended uses & limitations
More information needed
## Training and evaluation data
More information needed
## Training procedure
### Training hyperparameters
The following hyperparameters were used during training:
- learning_rate: 5e-05
- train_batch_size: 64
- eval_batch_size: 64
- seed: 42
- optimizer: Adam with betas=(0.9,0.999) and epsilon=1e-08
- lr_scheduler_type: linear
- num_epochs: 1
### Training results
| Training Loss | Epoch | Step | Validation Loss | F1 | Accuracy |
|:-------------:|:-----:|:----:|:---------------:|:------:|:--------:|
| 0.1889 | 1.0 | 313 | 0.1920 | 0.8882 | 0.9538 |
### Framework versions
- Transformers 4.40.0
- Pytorch 2.2.1+cu121
- Datasets 2.19.0
- Tokenizers 0.19.1
| {"tags": ["generated_from_trainer"], "metrics": ["f1", "accuracy"], "base_model": "tner/xlm-roberta-base-panx-dataset-ar", "model-index": [{"name": "xlm-roberta-base-finetuned-panx-ar", "results": []}]} | habahbeh/xlm-roberta-base-finetuned-panx-ar | null | [
"transformers",
"tensorboard",
"safetensors",
"xlm-roberta",
"token-classification",
"generated_from_trainer",
"base_model:tner/xlm-roberta-base-panx-dataset-ar",
"autotrain_compatible",
"endpoints_compatible",
"region:us"
] | null | 2024-04-21T19:11:21+00:00 | [] | [] | TAGS
#transformers #tensorboard #safetensors #xlm-roberta #token-classification #generated_from_trainer #base_model-tner/xlm-roberta-base-panx-dataset-ar #autotrain_compatible #endpoints_compatible #region-us
| xlm-roberta-base-finetuned-panx-ar
==================================
This model is a fine-tuned version of tner/xlm-roberta-base-panx-dataset-ar on an unknown dataset.
It achieves the following results on the evaluation set:
* Loss: 0.1920
* F1: 0.8882
* Accuracy: 0.9538
Model description
-----------------
More information needed
Intended uses & limitations
---------------------------
More information needed
Training and evaluation data
----------------------------
More information needed
Training procedure
------------------
### Training hyperparameters
The following hyperparameters were used during training:
* learning\_rate: 5e-05
* train\_batch\_size: 64
* eval\_batch\_size: 64
* seed: 42
* optimizer: Adam with betas=(0.9,0.999) and epsilon=1e-08
* lr\_scheduler\_type: linear
* num\_epochs: 1
### Training results
### Framework versions
* Transformers 4.40.0
* Pytorch 2.2.1+cu121
* Datasets 2.19.0
* Tokenizers 0.19.1
| [
"### Training hyperparameters\n\n\nThe following hyperparameters were used during training:\n\n\n* learning\\_rate: 5e-05\n* train\\_batch\\_size: 64\n* eval\\_batch\\_size: 64\n* seed: 42\n* optimizer: Adam with betas=(0.9,0.999) and epsilon=1e-08\n* lr\\_scheduler\\_type: linear\n* num\\_epochs: 1",
"### Training results",
"### Framework versions\n\n\n* Transformers 4.40.0\n* Pytorch 2.2.1+cu121\n* Datasets 2.19.0\n* Tokenizers 0.19.1"
] | [
"TAGS\n#transformers #tensorboard #safetensors #xlm-roberta #token-classification #generated_from_trainer #base_model-tner/xlm-roberta-base-panx-dataset-ar #autotrain_compatible #endpoints_compatible #region-us \n",
"### Training hyperparameters\n\n\nThe following hyperparameters were used during training:\n\n\n* learning\\_rate: 5e-05\n* train\\_batch\\_size: 64\n* eval\\_batch\\_size: 64\n* seed: 42\n* optimizer: Adam with betas=(0.9,0.999) and epsilon=1e-08\n* lr\\_scheduler\\_type: linear\n* num\\_epochs: 1",
"### Training results",
"### Framework versions\n\n\n* Transformers 4.40.0\n* Pytorch 2.2.1+cu121\n* Datasets 2.19.0\n* Tokenizers 0.19.1"
] |
null | transformers |
# Uploaded model
- **Developed by:** feliphe-galiza
- **License:** apache-2.0
- **Finetuned from model :** unsloth/llama-3-8b-bnb-4bit
This llama model was trained 2x faster with [Unsloth](https://github.com/unslothai/unsloth) and Huggingface's TRL library.
[<img src="https://raw.githubusercontent.com/unslothai/unsloth/main/images/unsloth%20made%20with%20love.png" width="200"/>](https://github.com/unslothai/unsloth)
| {"language": ["en"], "license": "apache-2.0", "tags": ["text-generation-inference", "transformers", "unsloth", "llama", "trl"], "base_model": "unsloth/llama-3-8b-bnb-4bit"} | feliphe-galiza/llama-3-1A.english-hypernyms | null | [
"transformers",
"safetensors",
"text-generation-inference",
"unsloth",
"llama",
"trl",
"en",
"base_model:unsloth/llama-3-8b-bnb-4bit",
"license:apache-2.0",
"endpoints_compatible",
"region:us"
] | null | 2024-04-21T19:13:27+00:00 | [] | [
"en"
] | TAGS
#transformers #safetensors #text-generation-inference #unsloth #llama #trl #en #base_model-unsloth/llama-3-8b-bnb-4bit #license-apache-2.0 #endpoints_compatible #region-us
|
# Uploaded model
- Developed by: feliphe-galiza
- License: apache-2.0
- Finetuned from model : unsloth/llama-3-8b-bnb-4bit
This llama model was trained 2x faster with Unsloth and Huggingface's TRL library.
<img src="URL width="200"/>
| [
"# Uploaded model\n\n- Developed by: feliphe-galiza\n- License: apache-2.0\n- Finetuned from model : unsloth/llama-3-8b-bnb-4bit\n\nThis llama model was trained 2x faster with Unsloth and Huggingface's TRL library.\n\n<img src=\"URL width=\"200\"/>"
] | [
"TAGS\n#transformers #safetensors #text-generation-inference #unsloth #llama #trl #en #base_model-unsloth/llama-3-8b-bnb-4bit #license-apache-2.0 #endpoints_compatible #region-us \n",
"# Uploaded model\n\n- Developed by: feliphe-galiza\n- License: apache-2.0\n- Finetuned from model : unsloth/llama-3-8b-bnb-4bit\n\nThis llama model was trained 2x faster with Unsloth and Huggingface's TRL library.\n\n<img src=\"URL width=\"200\"/>"
] |
null | adapter-transformers |
# Adapter `BigTMiami/n_par_bn_v_1_help_class_80_e_adp_lr_0003_S_2` for roberta-base
An [adapter](https://adapterhub.ml) for the `roberta-base` model that was trained on the [BigTMiami/amazon_MICRO_helpfulness_dataset](https://huggingface.co/datasets/BigTMiami/amazon_MICRO_helpfulness_dataset/) dataset and includes a prediction head for classification.
This adapter was created for usage with the **[Adapters](https://github.com/Adapter-Hub/adapters)** library.
## Usage
First, install `adapters`:
```
pip install -U adapters
```
Now, the adapter can be loaded and activated like this:
```python
from adapters import AutoAdapterModel
model = AutoAdapterModel.from_pretrained("roberta-base")
adapter_name = model.load_adapter("BigTMiami/n_par_bn_v_1_help_class_80_e_adp_lr_0003_S_2", source="hf", set_active=True)
```
## Architecture & Training
<!-- Add some description here -->
## Evaluation results
<!-- Add some description here -->
## Citation
<!-- Add some description here --> | {"tags": ["roberta", "adapter-transformers"], "datasets": ["BigTMiami/amazon_MICRO_helpfulness_dataset"]} | BigTMiami/n_par_bn_v_1_help_class_80_e_adp_lr_0003_S_2 | null | [
"adapter-transformers",
"roberta",
"dataset:BigTMiami/amazon_MICRO_helpfulness_dataset",
"region:us"
] | null | 2024-04-21T19:14:53+00:00 | [] | [] | TAGS
#adapter-transformers #roberta #dataset-BigTMiami/amazon_MICRO_helpfulness_dataset #region-us
|
# Adapter 'BigTMiami/n_par_bn_v_1_help_class_80_e_adp_lr_0003_S_2' for roberta-base
An adapter for the 'roberta-base' model that was trained on the BigTMiami/amazon_MICRO_helpfulness_dataset dataset and includes a prediction head for classification.
This adapter was created for usage with the Adapters library.
## Usage
First, install 'adapters':
Now, the adapter can be loaded and activated like this:
## Architecture & Training
## Evaluation results
| [
"# Adapter 'BigTMiami/n_par_bn_v_1_help_class_80_e_adp_lr_0003_S_2' for roberta-base\n\nAn adapter for the 'roberta-base' model that was trained on the BigTMiami/amazon_MICRO_helpfulness_dataset dataset and includes a prediction head for classification.\n\nThis adapter was created for usage with the Adapters library.",
"## Usage\n\nFirst, install 'adapters':\n\n\n\nNow, the adapter can be loaded and activated like this:",
"## Architecture & Training",
"## Evaluation results"
] | [
"TAGS\n#adapter-transformers #roberta #dataset-BigTMiami/amazon_MICRO_helpfulness_dataset #region-us \n",
"# Adapter 'BigTMiami/n_par_bn_v_1_help_class_80_e_adp_lr_0003_S_2' for roberta-base\n\nAn adapter for the 'roberta-base' model that was trained on the BigTMiami/amazon_MICRO_helpfulness_dataset dataset and includes a prediction head for classification.\n\nThis adapter was created for usage with the Adapters library.",
"## Usage\n\nFirst, install 'adapters':\n\n\n\nNow, the adapter can be loaded and activated like this:",
"## Architecture & Training",
"## Evaluation results"
] |
text-generation | transformers |
# Model Card for Model ID
<!-- Provide a quick summary of what the model is/does. -->
## Model Details
### Model Description
<!-- Provide a longer summary of what this model is. -->
This is the model card of a 🤗 transformers model that has been pushed on the Hub. This model card has been automatically generated.
- **Developed by:** [More Information Needed]
- **Funded by [optional]:** [More Information Needed]
- **Shared by [optional]:** [More Information Needed]
- **Model type:** [More Information Needed]
- **Language(s) (NLP):** [More Information Needed]
- **License:** [More Information Needed]
- **Finetuned from model [optional]:** [More Information Needed]
### Model Sources [optional]
<!-- Provide the basic links for the model. -->
- **Repository:** [More Information Needed]
- **Paper [optional]:** [More Information Needed]
- **Demo [optional]:** [More Information Needed]
## Uses
<!-- Address questions around how the model is intended to be used, including the foreseeable users of the model and those affected by the model. -->
### Direct Use
<!-- This section is for the model use without fine-tuning or plugging into a larger ecosystem/app. -->
[More Information Needed]
### Downstream Use [optional]
<!-- This section is for the model use when fine-tuned for a task, or when plugged into a larger ecosystem/app -->
[More Information Needed]
### Out-of-Scope Use
<!-- This section addresses misuse, malicious use, and uses that the model will not work well for. -->
[More Information Needed]
## Bias, Risks, and Limitations
<!-- This section is meant to convey both technical and sociotechnical limitations. -->
[More Information Needed]
### Recommendations
<!-- This section is meant to convey recommendations with respect to the bias, risk, and technical limitations. -->
Users (both direct and downstream) should be made aware of the risks, biases and limitations of the model. More information needed for further recommendations.
## How to Get Started with the Model
Use the code below to get started with the model.
[More Information Needed]
## Training Details
### Training Data
<!-- This should link to a Dataset Card, perhaps with a short stub of information on what the training data is all about as well as documentation related to data pre-processing or additional filtering. -->
[More Information Needed]
### Training Procedure
<!-- This relates heavily to the Technical Specifications. Content here should link to that section when it is relevant to the training procedure. -->
#### Preprocessing [optional]
[More Information Needed]
#### Training Hyperparameters
- **Training regime:** [More Information Needed] <!--fp32, fp16 mixed precision, bf16 mixed precision, bf16 non-mixed precision, fp16 non-mixed precision, fp8 mixed precision -->
#### Speeds, Sizes, Times [optional]
<!-- This section provides information about throughput, start/end time, checkpoint size if relevant, etc. -->
[More Information Needed]
## Evaluation
<!-- This section describes the evaluation protocols and provides the results. -->
### Testing Data, Factors & Metrics
#### Testing Data
<!-- This should link to a Dataset Card if possible. -->
[More Information Needed]
#### Factors
<!-- These are the things the evaluation is disaggregating by, e.g., subpopulations or domains. -->
[More Information Needed]
#### Metrics
<!-- These are the evaluation metrics being used, ideally with a description of why. -->
[More Information Needed]
### Results
[More Information Needed]
#### Summary
## Model Examination [optional]
<!-- Relevant interpretability work for the model goes here -->
[More Information Needed]
## Environmental Impact
<!-- Total emissions (in grams of CO2eq) and additional considerations, such as electricity usage, go here. Edit the suggested text below accordingly -->
Carbon emissions can be estimated using the [Machine Learning Impact calculator](https://mlco2.github.io/impact#compute) presented in [Lacoste et al. (2019)](https://arxiv.org/abs/1910.09700).
- **Hardware Type:** [More Information Needed]
- **Hours used:** [More Information Needed]
- **Cloud Provider:** [More Information Needed]
- **Compute Region:** [More Information Needed]
- **Carbon Emitted:** [More Information Needed]
## Technical Specifications [optional]
### Model Architecture and Objective
[More Information Needed]
### Compute Infrastructure
[More Information Needed]
#### Hardware
[More Information Needed]
#### Software
[More Information Needed]
## Citation [optional]
<!-- If there is a paper or blog post introducing the model, the APA and Bibtex information for that should go in this section. -->
**BibTeX:**
[More Information Needed]
**APA:**
[More Information Needed]
## Glossary [optional]
<!-- If relevant, include terms and calculations in this section that can help readers understand the model or model card. -->
[More Information Needed]
## More Information [optional]
[More Information Needed]
## Model Card Authors [optional]
[More Information Needed]
## Model Card Contact
[More Information Needed] | {"library_name": "transformers", "tags": []} | Yasusan/gpt_0.3B_llma_sft_gcp | null | [
"transformers",
"safetensors",
"llama",
"text-generation",
"conversational",
"arxiv:1910.09700",
"autotrain_compatible",
"endpoints_compatible",
"text-generation-inference",
"region:us"
] | null | 2024-04-21T19:16:43+00:00 | [
"1910.09700"
] | [] | TAGS
#transformers #safetensors #llama #text-generation #conversational #arxiv-1910.09700 #autotrain_compatible #endpoints_compatible #text-generation-inference #region-us
|
# Model Card for Model ID
## Model Details
### Model Description
This is the model card of a transformers model that has been pushed on the Hub. This model card has been automatically generated.
- Developed by:
- Funded by [optional]:
- Shared by [optional]:
- Model type:
- Language(s) (NLP):
- License:
- Finetuned from model [optional]:
### Model Sources [optional]
- Repository:
- Paper [optional]:
- Demo [optional]:
## Uses
### Direct Use
### Downstream Use [optional]
### Out-of-Scope Use
## Bias, Risks, and Limitations
### Recommendations
Users (both direct and downstream) should be made aware of the risks, biases and limitations of the model. More information needed for further recommendations.
## How to Get Started with the Model
Use the code below to get started with the model.
## Training Details
### Training Data
### Training Procedure
#### Preprocessing [optional]
#### Training Hyperparameters
- Training regime:
#### Speeds, Sizes, Times [optional]
## Evaluation
### Testing Data, Factors & Metrics
#### Testing Data
#### Factors
#### Metrics
### Results
#### Summary
## Model Examination [optional]
## Environmental Impact
Carbon emissions can be estimated using the Machine Learning Impact calculator presented in Lacoste et al. (2019).
- Hardware Type:
- Hours used:
- Cloud Provider:
- Compute Region:
- Carbon Emitted:
## Technical Specifications [optional]
### Model Architecture and Objective
### Compute Infrastructure
#### Hardware
#### Software
[optional]
BibTeX:
APA:
## Glossary [optional]
## More Information [optional]
## Model Card Authors [optional]
## Model Card Contact
| [
"# Model Card for Model ID",
"## Model Details",
"### Model Description\n\n\n\nThis is the model card of a transformers model that has been pushed on the Hub. This model card has been automatically generated.\n\n- Developed by: \n- Funded by [optional]: \n- Shared by [optional]: \n- Model type: \n- Language(s) (NLP): \n- License: \n- Finetuned from model [optional]:",
"### Model Sources [optional]\n\n\n\n- Repository: \n- Paper [optional]: \n- Demo [optional]:",
"## Uses",
"### Direct Use",
"### Downstream Use [optional]",
"### Out-of-Scope Use",
"## Bias, Risks, and Limitations",
"### Recommendations\n\n\n\nUsers (both direct and downstream) should be made aware of the risks, biases and limitations of the model. More information needed for further recommendations.",
"## How to Get Started with the Model\n\nUse the code below to get started with the model.",
"## Training Details",
"### Training Data",
"### Training Procedure",
"#### Preprocessing [optional]",
"#### Training Hyperparameters\n\n- Training regime:",
"#### Speeds, Sizes, Times [optional]",
"## Evaluation",
"### Testing Data, Factors & Metrics",
"#### Testing Data",
"#### Factors",
"#### Metrics",
"### Results",
"#### Summary",
"## Model Examination [optional]",
"## Environmental Impact\n\n\n\nCarbon emissions can be estimated using the Machine Learning Impact calculator presented in Lacoste et al. (2019).\n\n- Hardware Type: \n- Hours used: \n- Cloud Provider: \n- Compute Region: \n- Carbon Emitted:",
"## Technical Specifications [optional]",
"### Model Architecture and Objective",
"### Compute Infrastructure",
"#### Hardware",
"#### Software\n\n\n\n[optional]\n\n\n\nBibTeX:\n\n\n\nAPA:",
"## Glossary [optional]",
"## More Information [optional]",
"## Model Card Authors [optional]",
"## Model Card Contact"
] | [
"TAGS\n#transformers #safetensors #llama #text-generation #conversational #arxiv-1910.09700 #autotrain_compatible #endpoints_compatible #text-generation-inference #region-us \n",
"# Model Card for Model ID",
"## Model Details",
"### Model Description\n\n\n\nThis is the model card of a transformers model that has been pushed on the Hub. This model card has been automatically generated.\n\n- Developed by: \n- Funded by [optional]: \n- Shared by [optional]: \n- Model type: \n- Language(s) (NLP): \n- License: \n- Finetuned from model [optional]:",
"### Model Sources [optional]\n\n\n\n- Repository: \n- Paper [optional]: \n- Demo [optional]:",
"## Uses",
"### Direct Use",
"### Downstream Use [optional]",
"### Out-of-Scope Use",
"## Bias, Risks, and Limitations",
"### Recommendations\n\n\n\nUsers (both direct and downstream) should be made aware of the risks, biases and limitations of the model. More information needed for further recommendations.",
"## How to Get Started with the Model\n\nUse the code below to get started with the model.",
"## Training Details",
"### Training Data",
"### Training Procedure",
"#### Preprocessing [optional]",
"#### Training Hyperparameters\n\n- Training regime:",
"#### Speeds, Sizes, Times [optional]",
"## Evaluation",
"### Testing Data, Factors & Metrics",
"#### Testing Data",
"#### Factors",
"#### Metrics",
"### Results",
"#### Summary",
"## Model Examination [optional]",
"## Environmental Impact\n\n\n\nCarbon emissions can be estimated using the Machine Learning Impact calculator presented in Lacoste et al. (2019).\n\n- Hardware Type: \n- Hours used: \n- Cloud Provider: \n- Compute Region: \n- Carbon Emitted:",
"## Technical Specifications [optional]",
"### Model Architecture and Objective",
"### Compute Infrastructure",
"#### Hardware",
"#### Software\n\n\n\n[optional]\n\n\n\nBibTeX:\n\n\n\nAPA:",
"## Glossary [optional]",
"## More Information [optional]",
"## Model Card Authors [optional]",
"## Model Card Contact"
] |
null | transformers |
# Uploaded model
- **Developed by:** shubham11
- **License:** apache-2.0
- **Finetuned from model :** unsloth/mistral-7b-instruct-v0.2-bnb-4bit
This mistral model was trained 2x faster with [Unsloth](https://github.com/unslothai/unsloth) and Huggingface's TRL library.
[<img src="https://raw.githubusercontent.com/unslothai/unsloth/main/images/unsloth%20made%20with%20love.png" width="200"/>](https://github.com/unslothai/unsloth)
| {"language": ["en"], "license": "apache-2.0", "tags": ["text-generation-inference", "transformers", "unsloth", "mistral", "trl"], "base_model": "unsloth/mistral-7b-instruct-v0.2-bnb-4bit"} | shubham11/v11 | null | [
"transformers",
"safetensors",
"text-generation-inference",
"unsloth",
"mistral",
"trl",
"en",
"base_model:unsloth/mistral-7b-instruct-v0.2-bnb-4bit",
"license:apache-2.0",
"endpoints_compatible",
"region:us"
] | null | 2024-04-21T19:17:53+00:00 | [] | [
"en"
] | TAGS
#transformers #safetensors #text-generation-inference #unsloth #mistral #trl #en #base_model-unsloth/mistral-7b-instruct-v0.2-bnb-4bit #license-apache-2.0 #endpoints_compatible #region-us
|
# Uploaded model
- Developed by: shubham11
- License: apache-2.0
- Finetuned from model : unsloth/mistral-7b-instruct-v0.2-bnb-4bit
This mistral model was trained 2x faster with Unsloth and Huggingface's TRL library.
<img src="URL width="200"/>
| [
"# Uploaded model\n\n- Developed by: shubham11\n- License: apache-2.0\n- Finetuned from model : unsloth/mistral-7b-instruct-v0.2-bnb-4bit\n\nThis mistral model was trained 2x faster with Unsloth and Huggingface's TRL library.\n\n<img src=\"URL width=\"200\"/>"
] | [
"TAGS\n#transformers #safetensors #text-generation-inference #unsloth #mistral #trl #en #base_model-unsloth/mistral-7b-instruct-v0.2-bnb-4bit #license-apache-2.0 #endpoints_compatible #region-us \n",
"# Uploaded model\n\n- Developed by: shubham11\n- License: apache-2.0\n- Finetuned from model : unsloth/mistral-7b-instruct-v0.2-bnb-4bit\n\nThis mistral model was trained 2x faster with Unsloth and Huggingface's TRL library.\n\n<img src=\"URL width=\"200\"/>"
] |
text-generation | transformers |
<img src="./llama-3-merges.webp" alt="Goku 8x22B v0.1 Logo" width="500" style="margin-left:'auto' margin-right:'auto' display:'block'"/>
# Llama-3-8B-Instruct-DPO-v0.1
This model is a fine-tune (DPO) of `meta-llama/Meta-Llama-3-8B-Instruct` model.
# Prompt Template
This model uses `ChatML` prompt template:
```
<|im_start|>system
{System}
<|im_end|>
<|im_start|>user
{User}
<|im_end|>
<|im_start|>assistant
{Assistant}
````
# How to use
You can use this model by using `MaziyarPanahi/Llama-3-8B-Instruct-DPO-v0.1` as the model name in Hugging Face's
transformers library.
```python
from transformers import AutoModelForCausalLM, AutoTokenizer, TextStreamer
from transformers import pipeline
import torch
model_id = "MaziyarPanahi/Llama-3-8B-Instruct-DPO-v0.1"
model = AutoModelForCausalLM.from_pretrained(
model_id,
torch_dtype=torch.float16,
device_map="auto",
trust_remote_code=True,
# attn_implementation="flash_attention_2"
)
tokenizer = AutoTokenizer.from_pretrained(
model_id,
trust_remote_code=True
)
streamer = TextStreamer(tokenizer)
pipeline = pipeline(
"text-generation",
model=model,
tokenizer=tokenizer,
model_kwargs={"torch_dtype": torch.bfloat16},
streamer=streamer
)
# Then you can use the pipeline to generate text.
messages = [
{"role": "system", "content": "You are a pirate chatbot who always responds in pirate speak!"},
{"role": "user", "content": "Who are you?"},
]
prompt = tokenizer.apply_chat_template(
messages,
tokenize=False,
add_generation_prompt=True
)
terminators = [
tokenizer.eos_token_id,
tokenizer.convert_tokens_to_ids("<|im_end|>")
]
outputs = pipeline(
prompt,
max_new_tokens=256,
eos_token_id=terminators,
do_sample=True,
temperature=0.6,
top_p=0.95,
)
print(outputs[0]["generated_text"][len(prompt):])
```
# [Open LLM Leaderboard Evaluation Results](https://huggingface.co/spaces/HuggingFaceH4/open_llm_leaderboard)
Detailed results can be found [here](https://huggingface.co/datasets/open-llm-leaderboard/details_MaziyarPanahi__Llama-3-8B-Instruct-DPO-v0.1)
| Metric |Value|
|---------------------------------|----:|
|Avg. |67.25|
|AI2 Reasoning Challenge (25-Shot)|61.52|
|HellaSwag (10-Shot) |79.06|
|MMLU (5-Shot) |67.09|
|TruthfulQA (0-shot) |51.85|
|Winogrande (5-shot) |74.66|
|GSM8k (5-shot) |69.29|
| {"language": ["en"], "license": "other", "library_name": "transformers", "tags": ["axolotl", "finetune", "facebook", "meta", "pytorch", "llama", "llama-3"], "datasets": ["mlabonne/chatml-OpenHermes2.5-dpo-binarized-alpha"], "base_model": "meta-llama/Meta-Llama-3-8B-Instruct", "pipeline_tag": "text-generation", "license_name": "llama3", "license_link": "LICENSE", "inference": false, "model_creator": "MaziyarPanahi", "quantized_by": "MaziyarPanahi", "model-index": [{"name": "Llama-3-8B-Instruct-DPO-v0.1", "results": [{"task": {"type": "text-generation", "name": "Text Generation"}, "dataset": {"name": "AI2 Reasoning Challenge (25-Shot)", "type": "ai2_arc", "config": "ARC-Challenge", "split": "test", "args": {"num_few_shot": 25}}, "metrics": [{"type": "acc_norm", "value": 61.52, "name": "normalized accuracy"}], "source": {"url": "https://huggingface.co/spaces/HuggingFaceH4/open_llm_leaderboard?query=MaziyarPanahi/Llama-3-8B-Instruct-DPO-v0.1", "name": "Open LLM Leaderboard"}}, {"task": {"type": "text-generation", "name": "Text Generation"}, "dataset": {"name": "HellaSwag (10-Shot)", "type": "hellaswag", "split": "validation", "args": {"num_few_shot": 10}}, "metrics": [{"type": "acc_norm", "value": 79.06, "name": "normalized accuracy"}], "source": {"url": "https://huggingface.co/spaces/HuggingFaceH4/open_llm_leaderboard?query=MaziyarPanahi/Llama-3-8B-Instruct-DPO-v0.1", "name": "Open LLM Leaderboard"}}, {"task": {"type": "text-generation", "name": "Text Generation"}, "dataset": {"name": "MMLU (5-Shot)", "type": "cais/mmlu", "config": "all", "split": "test", "args": {"num_few_shot": 5}}, "metrics": [{"type": "acc", "value": 67.09, "name": "accuracy"}], "source": {"url": "https://huggingface.co/spaces/HuggingFaceH4/open_llm_leaderboard?query=MaziyarPanahi/Llama-3-8B-Instruct-DPO-v0.1", "name": "Open LLM Leaderboard"}}, {"task": {"type": "text-generation", "name": "Text Generation"}, "dataset": {"name": "TruthfulQA (0-shot)", "type": "truthful_qa", "config": "multiple_choice", "split": "validation", "args": {"num_few_shot": 0}}, "metrics": [{"type": "mc2", "value": 51.85}], "source": {"url": "https://huggingface.co/spaces/HuggingFaceH4/open_llm_leaderboard?query=MaziyarPanahi/Llama-3-8B-Instruct-DPO-v0.1", "name": "Open LLM Leaderboard"}}, {"task": {"type": "text-generation", "name": "Text Generation"}, "dataset": {"name": "Winogrande (5-shot)", "type": "winogrande", "config": "winogrande_xl", "split": "validation", "args": {"num_few_shot": 5}}, "metrics": [{"type": "acc", "value": 74.66, "name": "accuracy"}], "source": {"url": "https://huggingface.co/spaces/HuggingFaceH4/open_llm_leaderboard?query=MaziyarPanahi/Llama-3-8B-Instruct-DPO-v0.1", "name": "Open LLM Leaderboard"}}, {"task": {"type": "text-generation", "name": "Text Generation"}, "dataset": {"name": "GSM8k (5-shot)", "type": "gsm8k", "config": "main", "split": "test", "args": {"num_few_shot": 5}}, "metrics": [{"type": "acc", "value": 69.29, "name": "accuracy"}], "source": {"url": "https://huggingface.co/spaces/HuggingFaceH4/open_llm_leaderboard?query=MaziyarPanahi/Llama-3-8B-Instruct-DPO-v0.1", "name": "Open LLM Leaderboard"}}]}]} | MaziyarPanahi/Llama-3-8B-Instruct-DPO-v0.1 | null | [
"transformers",
"safetensors",
"llama",
"text-generation",
"axolotl",
"finetune",
"facebook",
"meta",
"pytorch",
"llama-3",
"conversational",
"en",
"dataset:mlabonne/chatml-OpenHermes2.5-dpo-binarized-alpha",
"base_model:meta-llama/Meta-Llama-3-8B-Instruct",
"license:other",
"model-index",
"autotrain_compatible",
"text-generation-inference",
"region:us"
] | null | 2024-04-21T19:18:34+00:00 | [] | [
"en"
] | TAGS
#transformers #safetensors #llama #text-generation #axolotl #finetune #facebook #meta #pytorch #llama-3 #conversational #en #dataset-mlabonne/chatml-OpenHermes2.5-dpo-binarized-alpha #base_model-meta-llama/Meta-Llama-3-8B-Instruct #license-other #model-index #autotrain_compatible #text-generation-inference #region-us
| 
Llama-3-8B-Instruct-DPO-v0.1
============================
This model is a fine-tune (DPO) of 'meta-llama/Meta-Llama-3-8B-Instruct' model.
Prompt Template
===============
This model uses 'ChatML' prompt template:
'
How to use
==========
You can use this model by using 'MaziyarPanahi/Llama-3-8B-Instruct-DPO-v0.1' as the model name in Hugging Face's
transformers library.
Open LLM Leaderboard Evaluation Results
=======================================
Detailed results can be found here
| [] | [
"TAGS\n#transformers #safetensors #llama #text-generation #axolotl #finetune #facebook #meta #pytorch #llama-3 #conversational #en #dataset-mlabonne/chatml-OpenHermes2.5-dpo-binarized-alpha #base_model-meta-llama/Meta-Llama-3-8B-Instruct #license-other #model-index #autotrain_compatible #text-generation-inference #region-us \n"
] |
null | transformers |
# Uploaded model
- **Developed by:** shubham11
- **License:** apache-2.0
- **Finetuned from model :** unsloth/mistral-7b-instruct-v0.2-bnb-4bit
This mistral model was trained 2x faster with [Unsloth](https://github.com/unslothai/unsloth) and Huggingface's TRL library.
[<img src="https://raw.githubusercontent.com/unslothai/unsloth/main/images/unsloth%20made%20with%20love.png" width="200"/>](https://github.com/unslothai/unsloth)
| {"language": ["en"], "license": "apache-2.0", "tags": ["text-generation-inference", "transformers", "unsloth", "mistral", "trl"], "base_model": "unsloth/mistral-7b-instruct-v0.2-bnb-4bit"} | shubham11/release1 | null | [
"transformers",
"text-generation-inference",
"unsloth",
"mistral",
"trl",
"en",
"base_model:unsloth/mistral-7b-instruct-v0.2-bnb-4bit",
"license:apache-2.0",
"endpoints_compatible",
"region:us"
] | null | 2024-04-21T19:20:25+00:00 | [] | [
"en"
] | TAGS
#transformers #text-generation-inference #unsloth #mistral #trl #en #base_model-unsloth/mistral-7b-instruct-v0.2-bnb-4bit #license-apache-2.0 #endpoints_compatible #region-us
|
# Uploaded model
- Developed by: shubham11
- License: apache-2.0
- Finetuned from model : unsloth/mistral-7b-instruct-v0.2-bnb-4bit
This mistral model was trained 2x faster with Unsloth and Huggingface's TRL library.
<img src="URL width="200"/>
| [
"# Uploaded model\n\n- Developed by: shubham11\n- License: apache-2.0\n- Finetuned from model : unsloth/mistral-7b-instruct-v0.2-bnb-4bit\n\nThis mistral model was trained 2x faster with Unsloth and Huggingface's TRL library.\n\n<img src=\"URL width=\"200\"/>"
] | [
"TAGS\n#transformers #text-generation-inference #unsloth #mistral #trl #en #base_model-unsloth/mistral-7b-instruct-v0.2-bnb-4bit #license-apache-2.0 #endpoints_compatible #region-us \n",
"# Uploaded model\n\n- Developed by: shubham11\n- License: apache-2.0\n- Finetuned from model : unsloth/mistral-7b-instruct-v0.2-bnb-4bit\n\nThis mistral model was trained 2x faster with Unsloth and Huggingface's TRL library.\n\n<img src=\"URL width=\"200\"/>"
] |
feature-extraction | transformers |
# Model Card for Model ID
<!-- Provide a quick summary of what the model is/does. -->
## Model Details
### Model Description
<!-- Provide a longer summary of what this model is. -->
This is the model card of a 🤗 transformers model that has been pushed on the Hub. This model card has been automatically generated.
- **Developed by:** [More Information Needed]
- **Funded by [optional]:** [More Information Needed]
- **Shared by [optional]:** [More Information Needed]
- **Model type:** [More Information Needed]
- **Language(s) (NLP):** [More Information Needed]
- **License:** [More Information Needed]
- **Finetuned from model [optional]:** [More Information Needed]
### Model Sources [optional]
<!-- Provide the basic links for the model. -->
- **Repository:** [More Information Needed]
- **Paper [optional]:** [More Information Needed]
- **Demo [optional]:** [More Information Needed]
## Uses
<!-- Address questions around how the model is intended to be used, including the foreseeable users of the model and those affected by the model. -->
### Direct Use
<!-- This section is for the model use without fine-tuning or plugging into a larger ecosystem/app. -->
[More Information Needed]
### Downstream Use [optional]
<!-- This section is for the model use when fine-tuned for a task, or when plugged into a larger ecosystem/app -->
[More Information Needed]
### Out-of-Scope Use
<!-- This section addresses misuse, malicious use, and uses that the model will not work well for. -->
[More Information Needed]
## Bias, Risks, and Limitations
<!-- This section is meant to convey both technical and sociotechnical limitations. -->
[More Information Needed]
### Recommendations
<!-- This section is meant to convey recommendations with respect to the bias, risk, and technical limitations. -->
Users (both direct and downstream) should be made aware of the risks, biases and limitations of the model. More information needed for further recommendations.
## How to Get Started with the Model
Use the code below to get started with the model.
[More Information Needed]
## Training Details
### Training Data
<!-- This should link to a Dataset Card, perhaps with a short stub of information on what the training data is all about as well as documentation related to data pre-processing or additional filtering. -->
[More Information Needed]
### Training Procedure
<!-- This relates heavily to the Technical Specifications. Content here should link to that section when it is relevant to the training procedure. -->
#### Preprocessing [optional]
[More Information Needed]
#### Training Hyperparameters
- **Training regime:** [More Information Needed] <!--fp32, fp16 mixed precision, bf16 mixed precision, bf16 non-mixed precision, fp16 non-mixed precision, fp8 mixed precision -->
#### Speeds, Sizes, Times [optional]
<!-- This section provides information about throughput, start/end time, checkpoint size if relevant, etc. -->
[More Information Needed]
## Evaluation
<!-- This section describes the evaluation protocols and provides the results. -->
### Testing Data, Factors & Metrics
#### Testing Data
<!-- This should link to a Dataset Card if possible. -->
[More Information Needed]
#### Factors
<!-- These are the things the evaluation is disaggregating by, e.g., subpopulations or domains. -->
[More Information Needed]
#### Metrics
<!-- These are the evaluation metrics being used, ideally with a description of why. -->
[More Information Needed]
### Results
[More Information Needed]
#### Summary
## Model Examination [optional]
<!-- Relevant interpretability work for the model goes here -->
[More Information Needed]
## Environmental Impact
<!-- Total emissions (in grams of CO2eq) and additional considerations, such as electricity usage, go here. Edit the suggested text below accordingly -->
Carbon emissions can be estimated using the [Machine Learning Impact calculator](https://mlco2.github.io/impact#compute) presented in [Lacoste et al. (2019)](https://arxiv.org/abs/1910.09700).
- **Hardware Type:** [More Information Needed]
- **Hours used:** [More Information Needed]
- **Cloud Provider:** [More Information Needed]
- **Compute Region:** [More Information Needed]
- **Carbon Emitted:** [More Information Needed]
## Technical Specifications [optional]
### Model Architecture and Objective
[More Information Needed]
### Compute Infrastructure
[More Information Needed]
#### Hardware
[More Information Needed]
#### Software
[More Information Needed]
## Citation [optional]
<!-- If there is a paper or blog post introducing the model, the APA and Bibtex information for that should go in this section. -->
**BibTeX:**
[More Information Needed]
**APA:**
[More Information Needed]
## Glossary [optional]
<!-- If relevant, include terms and calculations in this section that can help readers understand the model or model card. -->
[More Information Needed]
## More Information [optional]
[More Information Needed]
## Model Card Authors [optional]
[More Information Needed]
## Model Card Contact
[More Information Needed] | {"library_name": "transformers", "tags": []} | Ehsanl/m3-ranker-fl18-e2 | null | [
"transformers",
"safetensors",
"xlm-roberta",
"feature-extraction",
"arxiv:1910.09700",
"endpoints_compatible",
"region:us"
] | null | 2024-04-21T19:21:43+00:00 | [
"1910.09700"
] | [] | TAGS
#transformers #safetensors #xlm-roberta #feature-extraction #arxiv-1910.09700 #endpoints_compatible #region-us
|
# Model Card for Model ID
## Model Details
### Model Description
This is the model card of a transformers model that has been pushed on the Hub. This model card has been automatically generated.
- Developed by:
- Funded by [optional]:
- Shared by [optional]:
- Model type:
- Language(s) (NLP):
- License:
- Finetuned from model [optional]:
### Model Sources [optional]
- Repository:
- Paper [optional]:
- Demo [optional]:
## Uses
### Direct Use
### Downstream Use [optional]
### Out-of-Scope Use
## Bias, Risks, and Limitations
### Recommendations
Users (both direct and downstream) should be made aware of the risks, biases and limitations of the model. More information needed for further recommendations.
## How to Get Started with the Model
Use the code below to get started with the model.
## Training Details
### Training Data
### Training Procedure
#### Preprocessing [optional]
#### Training Hyperparameters
- Training regime:
#### Speeds, Sizes, Times [optional]
## Evaluation
### Testing Data, Factors & Metrics
#### Testing Data
#### Factors
#### Metrics
### Results
#### Summary
## Model Examination [optional]
## Environmental Impact
Carbon emissions can be estimated using the Machine Learning Impact calculator presented in Lacoste et al. (2019).
- Hardware Type:
- Hours used:
- Cloud Provider:
- Compute Region:
- Carbon Emitted:
## Technical Specifications [optional]
### Model Architecture and Objective
### Compute Infrastructure
#### Hardware
#### Software
[optional]
BibTeX:
APA:
## Glossary [optional]
## More Information [optional]
## Model Card Authors [optional]
## Model Card Contact
| [
"# Model Card for Model ID",
"## Model Details",
"### Model Description\n\n\n\nThis is the model card of a transformers model that has been pushed on the Hub. This model card has been automatically generated.\n\n- Developed by: \n- Funded by [optional]: \n- Shared by [optional]: \n- Model type: \n- Language(s) (NLP): \n- License: \n- Finetuned from model [optional]:",
"### Model Sources [optional]\n\n\n\n- Repository: \n- Paper [optional]: \n- Demo [optional]:",
"## Uses",
"### Direct Use",
"### Downstream Use [optional]",
"### Out-of-Scope Use",
"## Bias, Risks, and Limitations",
"### Recommendations\n\n\n\nUsers (both direct and downstream) should be made aware of the risks, biases and limitations of the model. More information needed for further recommendations.",
"## How to Get Started with the Model\n\nUse the code below to get started with the model.",
"## Training Details",
"### Training Data",
"### Training Procedure",
"#### Preprocessing [optional]",
"#### Training Hyperparameters\n\n- Training regime:",
"#### Speeds, Sizes, Times [optional]",
"## Evaluation",
"### Testing Data, Factors & Metrics",
"#### Testing Data",
"#### Factors",
"#### Metrics",
"### Results",
"#### Summary",
"## Model Examination [optional]",
"## Environmental Impact\n\n\n\nCarbon emissions can be estimated using the Machine Learning Impact calculator presented in Lacoste et al. (2019).\n\n- Hardware Type: \n- Hours used: \n- Cloud Provider: \n- Compute Region: \n- Carbon Emitted:",
"## Technical Specifications [optional]",
"### Model Architecture and Objective",
"### Compute Infrastructure",
"#### Hardware",
"#### Software\n\n\n\n[optional]\n\n\n\nBibTeX:\n\n\n\nAPA:",
"## Glossary [optional]",
"## More Information [optional]",
"## Model Card Authors [optional]",
"## Model Card Contact"
] | [
"TAGS\n#transformers #safetensors #xlm-roberta #feature-extraction #arxiv-1910.09700 #endpoints_compatible #region-us \n",
"# Model Card for Model ID",
"## Model Details",
"### Model Description\n\n\n\nThis is the model card of a transformers model that has been pushed on the Hub. This model card has been automatically generated.\n\n- Developed by: \n- Funded by [optional]: \n- Shared by [optional]: \n- Model type: \n- Language(s) (NLP): \n- License: \n- Finetuned from model [optional]:",
"### Model Sources [optional]\n\n\n\n- Repository: \n- Paper [optional]: \n- Demo [optional]:",
"## Uses",
"### Direct Use",
"### Downstream Use [optional]",
"### Out-of-Scope Use",
"## Bias, Risks, and Limitations",
"### Recommendations\n\n\n\nUsers (both direct and downstream) should be made aware of the risks, biases and limitations of the model. More information needed for further recommendations.",
"## How to Get Started with the Model\n\nUse the code below to get started with the model.",
"## Training Details",
"### Training Data",
"### Training Procedure",
"#### Preprocessing [optional]",
"#### Training Hyperparameters\n\n- Training regime:",
"#### Speeds, Sizes, Times [optional]",
"## Evaluation",
"### Testing Data, Factors & Metrics",
"#### Testing Data",
"#### Factors",
"#### Metrics",
"### Results",
"#### Summary",
"## Model Examination [optional]",
"## Environmental Impact\n\n\n\nCarbon emissions can be estimated using the Machine Learning Impact calculator presented in Lacoste et al. (2019).\n\n- Hardware Type: \n- Hours used: \n- Cloud Provider: \n- Compute Region: \n- Carbon Emitted:",
"## Technical Specifications [optional]",
"### Model Architecture and Objective",
"### Compute Infrastructure",
"#### Hardware",
"#### Software\n\n\n\n[optional]\n\n\n\nBibTeX:\n\n\n\nAPA:",
"## Glossary [optional]",
"## More Information [optional]",
"## Model Card Authors [optional]",
"## Model Card Contact"
] |
sentence-similarity | sentence-transformers |
# multi-qa-mpnet-base-cos-v1
This is a [sentence-transformers](https://www.SBERT.net) model: It maps sentences & paragraphs to a 768 dimensional dense vector space and was designed for **semantic search**. It has been trained on 215M (question, answer) pairs from diverse sources. For an introduction to semantic search, have a look at: [SBERT.net - Semantic Search](https://www.sbert.net/examples/applications/semantic-search/README.html)
## Usage (Sentence-Transformers)
Using this model becomes easy when you have [sentence-transformers](https://www.SBERT.net) installed:
```
pip install -U sentence-transformers
```
Then you can use the model like this:
```python
from sentence_transformers import SentenceTransformer, util
query = "How many people live in London?"
docs = ["Around 9 Million people live in London", "London is known for its financial district"]
#Load the model
model = SentenceTransformer('sentence-transformers/multi-qa-mpnet-base-cos-v1')
#Encode query and documents
query_emb = model.encode(query)
doc_emb = model.encode(docs)
#Compute dot score between query and all document embeddings
scores = util.dot_score(query_emb, doc_emb)[0].cpu().tolist()
#Combine docs & scores
doc_score_pairs = list(zip(docs, scores))
#Sort by decreasing score
doc_score_pairs = sorted(doc_score_pairs, key=lambda x: x[1], reverse=True)
#Output passages & scores
for doc, score in doc_score_pairs:
print(score, doc)
```
## Usage (HuggingFace Transformers)
Without [sentence-transformers](https://www.SBERT.net), you can use the model like this: First, you pass your input through the transformer model, then you have to apply the correct pooling-operation on-top of the contextualized word embeddings.
```python
from transformers import AutoTokenizer, AutoModel
import torch
import torch.nn.functional as F
#Mean Pooling - Take average of all tokens
def mean_pooling(model_output, attention_mask):
token_embeddings = model_output.last_hidden_state #First element of model_output contains all token embeddings
input_mask_expanded = attention_mask.unsqueeze(-1).expand(token_embeddings.size()).float()
return torch.sum(token_embeddings * input_mask_expanded, 1) / torch.clamp(input_mask_expanded.sum(1), min=1e-9)
#Encode text
def encode(texts):
# Tokenize sentences
encoded_input = tokenizer(texts, padding=True, truncation=True, return_tensors='pt')
# Compute token embeddings
with torch.no_grad():
model_output = model(**encoded_input, return_dict=True)
# Perform pooling
embeddings = mean_pooling(model_output, encoded_input['attention_mask'])
# Normalize embeddings
embeddings = F.normalize(embeddings, p=2, dim=1)
return embeddings
# Sentences we want sentence embeddings for
query = "How many people live in London?"
docs = ["Around 9 Million people live in London", "London is known for its financial district"]
# Load model from HuggingFace Hub
tokenizer = AutoTokenizer.from_pretrained("sentence-transformers/multi-qa-mpnet-base-cos-v1")
model = AutoModel.from_pretrained("sentence-transformers/multi-qa-mpnet-base-cos-v1")
#Encode query and docs
query_emb = encode(query)
doc_emb = encode(docs)
#Compute dot score between query and all document embeddings
scores = torch.mm(query_emb, doc_emb.transpose(0, 1))[0].cpu().tolist()
#Combine docs & scores
doc_score_pairs = list(zip(docs, scores))
#Sort by decreasing score
doc_score_pairs = sorted(doc_score_pairs, key=lambda x: x[1], reverse=True)
#Output passages & scores
for doc, score in doc_score_pairs:
print(score, doc)
```
## Technical Details
In the following some technical details how this model must be used:
| Setting | Value |
| --- | :---: |
| Dimensions | 768 |
| Produces normalized embeddings | Yes |
| Pooling-Method | Mean pooling |
| Suitable score functions | dot-product (`util.dot_score`), cosine-similarity (`util.cos_sim`), or euclidean distance |
Note: When loaded with `sentence-transformers`, this model produces normalized embeddings with length 1. In that case, dot-product and cosine-similarity are equivalent. dot-product is preferred as it is faster. Euclidean distance is proportional to dot-product and can also be used.
----
## Background
The project aims to train sentence embedding models on very large sentence level datasets using a self-supervised
contrastive learning objective. We use a contrastive learning objective: given a sentence from the pair, the model should predict which out of a set of randomly sampled other sentences, was actually paired with it in our dataset.
We developped this model during the
[Community week using JAX/Flax for NLP & CV](https://discuss.huggingface.co/t/open-to-the-community-community-week-using-jax-flax-for-nlp-cv/7104),
organized by Hugging Face. We developped this model as part of the project:
[Train the Best Sentence Embedding Model Ever with 1B Training Pairs](https://discuss.huggingface.co/t/train-the-best-sentence-embedding-model-ever-with-1b-training-pairs/7354). We benefited from efficient hardware infrastructure to run the project: 7 TPUs v3-8, as well as intervention from Googles Flax, JAX, and Cloud team member about efficient deep learning frameworks.
## Intended uses
Our model is intented to be used for semantic search: It encodes queries / questions and text paragraphs in a dense vector space. It finds relevant documents for the given passages.
Note that there is a limit of 512 word pieces: Text longer than that will be truncated. Further note that the model was just trained on input text up to 250 word pieces. It might not work well for longer text.
## Training procedure
The full training script is accessible in this current repository: `train_script.py`.
### Pre-training
We use the pretrained [`mpnet-base`](https://huggingface.co/microsoft/mpnet-base) model. Please refer to the model card for more detailed information about the pre-training procedure.
#### Training
We use the concatenation from multiple datasets to fine-tune our model. In total we have about 215M (question, answer) pairs.
We sampled each dataset given a weighted probability which configuration is detailed in the `data_config.json` file.
The model was trained with [MultipleNegativesRankingLoss](https://www.sbert.net/docs/package_reference/losses.html#multiplenegativesrankingloss) using Mean-pooling, cosine-similarity as similarity function, and a scale of 20.
| Dataset | Number of training tuples |
|--------------------------------------------------------|:--------------------------:|
| [WikiAnswers](https://github.com/afader/oqa#wikianswers-corpus) Duplicate question pairs from WikiAnswers | 77,427,422 |
| [PAQ](https://github.com/facebookresearch/PAQ) Automatically generated (Question, Paragraph) pairs for each paragraph in Wikipedia | 64,371,441 |
| [Stack Exchange](https://huggingface.co/datasets/flax-sentence-embeddings/stackexchange_xml) (Title, Body) pairs from all StackExchanges | 25,316,456 |
| [Stack Exchange](https://huggingface.co/datasets/flax-sentence-embeddings/stackexchange_xml) (Title, Answer) pairs from all StackExchanges | 21,396,559 |
| [MS MARCO](https://microsoft.github.io/msmarco/) Triplets (query, answer, hard_negative) for 500k queries from Bing search engine | 17,579,773 |
| [GOOAQ: Open Question Answering with Diverse Answer Types](https://github.com/allenai/gooaq) (query, answer) pairs for 3M Google queries and Google featured snippet | 3,012,496 |
| [Amazon-QA](http://jmcauley.ucsd.edu/data/amazon/qa/) (Question, Answer) pairs from Amazon product pages | 2,448,839
| [Yahoo Answers](https://www.kaggle.com/soumikrakshit/yahoo-answers-dataset) (Title, Answer) pairs from Yahoo Answers | 1,198,260 |
| [Yahoo Answers](https://www.kaggle.com/soumikrakshit/yahoo-answers-dataset) (Question, Answer) pairs from Yahoo Answers | 681,164 |
| [Yahoo Answers](https://www.kaggle.com/soumikrakshit/yahoo-answers-dataset) (Title, Question) pairs from Yahoo Answers | 659,896 |
| [SearchQA](https://huggingface.co/datasets/search_qa) (Question, Answer) pairs for 140k questions, each with Top5 Google snippets on that question | 582,261 |
| [ELI5](https://huggingface.co/datasets/eli5) (Question, Answer) pairs from Reddit ELI5 (explainlikeimfive) | 325,475 |
| [Stack Exchange](https://huggingface.co/datasets/flax-sentence-embeddings/stackexchange_xml) Duplicate questions pairs (titles) | 304,525 |
| [Quora Question Triplets](https://quoradata.quora.com/First-Quora-Dataset-Release-Question-Pairs) (Question, Duplicate_Question, Hard_Negative) triplets for Quora Questions Pairs dataset | 103,663 |
| [Natural Questions (NQ)](https://ai.google.com/research/NaturalQuestions) (Question, Paragraph) pairs for 100k real Google queries with relevant Wikipedia paragraph | 100,231 |
| [SQuAD2.0](https://rajpurkar.github.io/SQuAD-explorer/) (Question, Paragraph) pairs from SQuAD2.0 dataset | 87,599 |
| [TriviaQA](https://huggingface.co/datasets/trivia_qa) (Question, Evidence) pairs | 73,346 |
| **Total** | **214,988,242** | | {"language": ["en"], "library_name": "sentence-transformers", "tags": ["sentence-transformers", "feature-extraction", "sentence-similarity", "transformers"], "pipeline_tag": "sentence-similarity"} | cicero-ai/multi-qa-mpnet-base-cos-v1-rust | null | [
"sentence-transformers",
"pytorch",
"rust",
"safetensors",
"mpnet",
"fill-mask",
"feature-extraction",
"sentence-similarity",
"transformers",
"en",
"endpoints_compatible",
"region:us"
] | null | 2024-04-21T19:21:49+00:00 | [] | [
"en"
] | TAGS
#sentence-transformers #pytorch #rust #safetensors #mpnet #fill-mask #feature-extraction #sentence-similarity #transformers #en #endpoints_compatible #region-us
| multi-qa-mpnet-base-cos-v1
==========================
This is a sentence-transformers model: It maps sentences & paragraphs to a 768 dimensional dense vector space and was designed for semantic search. It has been trained on 215M (question, answer) pairs from diverse sources. For an introduction to semantic search, have a look at: URL - Semantic Search
Usage (Sentence-Transformers)
-----------------------------
Using this model becomes easy when you have sentence-transformers installed:
Then you can use the model like this:
Usage (HuggingFace Transformers)
--------------------------------
Without sentence-transformers, you can use the model like this: First, you pass your input through the transformer model, then you have to apply the correct pooling-operation on-top of the contextualized word embeddings.
Technical Details
-----------------
In the following some technical details how this model must be used:
Note: When loaded with 'sentence-transformers', this model produces normalized embeddings with length 1. In that case, dot-product and cosine-similarity are equivalent. dot-product is preferred as it is faster. Euclidean distance is proportional to dot-product and can also be used.
---
Background
----------
The project aims to train sentence embedding models on very large sentence level datasets using a self-supervised
contrastive learning objective. We use a contrastive learning objective: given a sentence from the pair, the model should predict which out of a set of randomly sampled other sentences, was actually paired with it in our dataset.
We developped this model during the
Community week using JAX/Flax for NLP & CV,
organized by Hugging Face. We developped this model as part of the project:
Train the Best Sentence Embedding Model Ever with 1B Training Pairs. We benefited from efficient hardware infrastructure to run the project: 7 TPUs v3-8, as well as intervention from Googles Flax, JAX, and Cloud team member about efficient deep learning frameworks.
Intended uses
-------------
Our model is intented to be used for semantic search: It encodes queries / questions and text paragraphs in a dense vector space. It finds relevant documents for the given passages.
Note that there is a limit of 512 word pieces: Text longer than that will be truncated. Further note that the model was just trained on input text up to 250 word pieces. It might not work well for longer text.
Training procedure
------------------
The full training script is accessible in this current repository: 'train\_script.py'.
### Pre-training
We use the pretrained 'mpnet-base' model. Please refer to the model card for more detailed information about the pre-training procedure.
#### Training
We use the concatenation from multiple datasets to fine-tune our model. In total we have about 215M (question, answer) pairs.
We sampled each dataset given a weighted probability which configuration is detailed in the 'data\_config.json' file.
The model was trained with MultipleNegativesRankingLoss using Mean-pooling, cosine-similarity as similarity function, and a scale of 20.
| [
"### Pre-training\n\n\nWe use the pretrained 'mpnet-base' model. Please refer to the model card for more detailed information about the pre-training procedure.",
"#### Training\n\n\nWe use the concatenation from multiple datasets to fine-tune our model. In total we have about 215M (question, answer) pairs.\nWe sampled each dataset given a weighted probability which configuration is detailed in the 'data\\_config.json' file.\n\n\nThe model was trained with MultipleNegativesRankingLoss using Mean-pooling, cosine-similarity as similarity function, and a scale of 20."
] | [
"TAGS\n#sentence-transformers #pytorch #rust #safetensors #mpnet #fill-mask #feature-extraction #sentence-similarity #transformers #en #endpoints_compatible #region-us \n",
"### Pre-training\n\n\nWe use the pretrained 'mpnet-base' model. Please refer to the model card for more detailed information about the pre-training procedure.",
"#### Training\n\n\nWe use the concatenation from multiple datasets to fine-tune our model. In total we have about 215M (question, answer) pairs.\nWe sampled each dataset given a weighted probability which configuration is detailed in the 'data\\_config.json' file.\n\n\nThe model was trained with MultipleNegativesRankingLoss using Mean-pooling, cosine-similarity as similarity function, and a scale of 20."
] |
text-generation | transformers |
# Model Card for Model ID
<!-- Provide a quick summary of what the model is/does. -->
## Model Details
### Model Description
<!-- Provide a longer summary of what this model is. -->
This is the model card of a 🤗 transformers model that has been pushed on the Hub. This model card has been automatically generated.
- **Developed by:** [More Information Needed]
- **Funded by [optional]:** [More Information Needed]
- **Shared by [optional]:** [More Information Needed]
- **Model type:** [More Information Needed]
- **Language(s) (NLP):** [More Information Needed]
- **License:** [More Information Needed]
- **Finetuned from model [optional]:** [More Information Needed]
### Model Sources [optional]
<!-- Provide the basic links for the model. -->
- **Repository:** [More Information Needed]
- **Paper [optional]:** [More Information Needed]
- **Demo [optional]:** [More Information Needed]
## Uses
<!-- Address questions around how the model is intended to be used, including the foreseeable users of the model and those affected by the model. -->
### Direct Use
<!-- This section is for the model use without fine-tuning or plugging into a larger ecosystem/app. -->
[More Information Needed]
### Downstream Use [optional]
<!-- This section is for the model use when fine-tuned for a task, or when plugged into a larger ecosystem/app -->
[More Information Needed]
### Out-of-Scope Use
<!-- This section addresses misuse, malicious use, and uses that the model will not work well for. -->
[More Information Needed]
## Bias, Risks, and Limitations
<!-- This section is meant to convey both technical and sociotechnical limitations. -->
[More Information Needed]
### Recommendations
<!-- This section is meant to convey recommendations with respect to the bias, risk, and technical limitations. -->
Users (both direct and downstream) should be made aware of the risks, biases and limitations of the model. More information needed for further recommendations.
## How to Get Started with the Model
Use the code below to get started with the model.
[More Information Needed]
## Training Details
### Training Data
<!-- This should link to a Dataset Card, perhaps with a short stub of information on what the training data is all about as well as documentation related to data pre-processing or additional filtering. -->
[More Information Needed]
### Training Procedure
<!-- This relates heavily to the Technical Specifications. Content here should link to that section when it is relevant to the training procedure. -->
#### Preprocessing [optional]
[More Information Needed]
#### Training Hyperparameters
- **Training regime:** [More Information Needed] <!--fp32, fp16 mixed precision, bf16 mixed precision, bf16 non-mixed precision, fp16 non-mixed precision, fp8 mixed precision -->
#### Speeds, Sizes, Times [optional]
<!-- This section provides information about throughput, start/end time, checkpoint size if relevant, etc. -->
[More Information Needed]
## Evaluation
<!-- This section describes the evaluation protocols and provides the results. -->
### Testing Data, Factors & Metrics
#### Testing Data
<!-- This should link to a Dataset Card if possible. -->
[More Information Needed]
#### Factors
<!-- These are the things the evaluation is disaggregating by, e.g., subpopulations or domains. -->
[More Information Needed]
#### Metrics
<!-- These are the evaluation metrics being used, ideally with a description of why. -->
[More Information Needed]
### Results
[More Information Needed]
#### Summary
## Model Examination [optional]
<!-- Relevant interpretability work for the model goes here -->
[More Information Needed]
## Environmental Impact
<!-- Total emissions (in grams of CO2eq) and additional considerations, such as electricity usage, go here. Edit the suggested text below accordingly -->
Carbon emissions can be estimated using the [Machine Learning Impact calculator](https://mlco2.github.io/impact#compute) presented in [Lacoste et al. (2019)](https://arxiv.org/abs/1910.09700).
- **Hardware Type:** [More Information Needed]
- **Hours used:** [More Information Needed]
- **Cloud Provider:** [More Information Needed]
- **Compute Region:** [More Information Needed]
- **Carbon Emitted:** [More Information Needed]
## Technical Specifications [optional]
### Model Architecture and Objective
[More Information Needed]
### Compute Infrastructure
[More Information Needed]
#### Hardware
[More Information Needed]
#### Software
[More Information Needed]
## Citation [optional]
<!-- If there is a paper or blog post introducing the model, the APA and Bibtex information for that should go in this section. -->
**BibTeX:**
[More Information Needed]
**APA:**
[More Information Needed]
## Glossary [optional]
<!-- If relevant, include terms and calculations in this section that can help readers understand the model or model card. -->
[More Information Needed]
## More Information [optional]
[More Information Needed]
## Model Card Authors [optional]
[More Information Needed]
## Model Card Contact
[More Information Needed] | {"library_name": "transformers", "tags": []} | Grayx/sad_llama_19 | null | [
"transformers",
"safetensors",
"llama",
"text-generation",
"conversational",
"arxiv:1910.09700",
"autotrain_compatible",
"endpoints_compatible",
"text-generation-inference",
"region:us"
] | null | 2024-04-21T19:22:25+00:00 | [
"1910.09700"
] | [] | TAGS
#transformers #safetensors #llama #text-generation #conversational #arxiv-1910.09700 #autotrain_compatible #endpoints_compatible #text-generation-inference #region-us
|
# Model Card for Model ID
## Model Details
### Model Description
This is the model card of a transformers model that has been pushed on the Hub. This model card has been automatically generated.
- Developed by:
- Funded by [optional]:
- Shared by [optional]:
- Model type:
- Language(s) (NLP):
- License:
- Finetuned from model [optional]:
### Model Sources [optional]
- Repository:
- Paper [optional]:
- Demo [optional]:
## Uses
### Direct Use
### Downstream Use [optional]
### Out-of-Scope Use
## Bias, Risks, and Limitations
### Recommendations
Users (both direct and downstream) should be made aware of the risks, biases and limitations of the model. More information needed for further recommendations.
## How to Get Started with the Model
Use the code below to get started with the model.
## Training Details
### Training Data
### Training Procedure
#### Preprocessing [optional]
#### Training Hyperparameters
- Training regime:
#### Speeds, Sizes, Times [optional]
## Evaluation
### Testing Data, Factors & Metrics
#### Testing Data
#### Factors
#### Metrics
### Results
#### Summary
## Model Examination [optional]
## Environmental Impact
Carbon emissions can be estimated using the Machine Learning Impact calculator presented in Lacoste et al. (2019).
- Hardware Type:
- Hours used:
- Cloud Provider:
- Compute Region:
- Carbon Emitted:
## Technical Specifications [optional]
### Model Architecture and Objective
### Compute Infrastructure
#### Hardware
#### Software
[optional]
BibTeX:
APA:
## Glossary [optional]
## More Information [optional]
## Model Card Authors [optional]
## Model Card Contact
| [
"# Model Card for Model ID",
"## Model Details",
"### Model Description\n\n\n\nThis is the model card of a transformers model that has been pushed on the Hub. This model card has been automatically generated.\n\n- Developed by: \n- Funded by [optional]: \n- Shared by [optional]: \n- Model type: \n- Language(s) (NLP): \n- License: \n- Finetuned from model [optional]:",
"### Model Sources [optional]\n\n\n\n- Repository: \n- Paper [optional]: \n- Demo [optional]:",
"## Uses",
"### Direct Use",
"### Downstream Use [optional]",
"### Out-of-Scope Use",
"## Bias, Risks, and Limitations",
"### Recommendations\n\n\n\nUsers (both direct and downstream) should be made aware of the risks, biases and limitations of the model. More information needed for further recommendations.",
"## How to Get Started with the Model\n\nUse the code below to get started with the model.",
"## Training Details",
"### Training Data",
"### Training Procedure",
"#### Preprocessing [optional]",
"#### Training Hyperparameters\n\n- Training regime:",
"#### Speeds, Sizes, Times [optional]",
"## Evaluation",
"### Testing Data, Factors & Metrics",
"#### Testing Data",
"#### Factors",
"#### Metrics",
"### Results",
"#### Summary",
"## Model Examination [optional]",
"## Environmental Impact\n\n\n\nCarbon emissions can be estimated using the Machine Learning Impact calculator presented in Lacoste et al. (2019).\n\n- Hardware Type: \n- Hours used: \n- Cloud Provider: \n- Compute Region: \n- Carbon Emitted:",
"## Technical Specifications [optional]",
"### Model Architecture and Objective",
"### Compute Infrastructure",
"#### Hardware",
"#### Software\n\n\n\n[optional]\n\n\n\nBibTeX:\n\n\n\nAPA:",
"## Glossary [optional]",
"## More Information [optional]",
"## Model Card Authors [optional]",
"## Model Card Contact"
] | [
"TAGS\n#transformers #safetensors #llama #text-generation #conversational #arxiv-1910.09700 #autotrain_compatible #endpoints_compatible #text-generation-inference #region-us \n",
"# Model Card for Model ID",
"## Model Details",
"### Model Description\n\n\n\nThis is the model card of a transformers model that has been pushed on the Hub. This model card has been automatically generated.\n\n- Developed by: \n- Funded by [optional]: \n- Shared by [optional]: \n- Model type: \n- Language(s) (NLP): \n- License: \n- Finetuned from model [optional]:",
"### Model Sources [optional]\n\n\n\n- Repository: \n- Paper [optional]: \n- Demo [optional]:",
"## Uses",
"### Direct Use",
"### Downstream Use [optional]",
"### Out-of-Scope Use",
"## Bias, Risks, and Limitations",
"### Recommendations\n\n\n\nUsers (both direct and downstream) should be made aware of the risks, biases and limitations of the model. More information needed for further recommendations.",
"## How to Get Started with the Model\n\nUse the code below to get started with the model.",
"## Training Details",
"### Training Data",
"### Training Procedure",
"#### Preprocessing [optional]",
"#### Training Hyperparameters\n\n- Training regime:",
"#### Speeds, Sizes, Times [optional]",
"## Evaluation",
"### Testing Data, Factors & Metrics",
"#### Testing Data",
"#### Factors",
"#### Metrics",
"### Results",
"#### Summary",
"## Model Examination [optional]",
"## Environmental Impact\n\n\n\nCarbon emissions can be estimated using the Machine Learning Impact calculator presented in Lacoste et al. (2019).\n\n- Hardware Type: \n- Hours used: \n- Cloud Provider: \n- Compute Region: \n- Carbon Emitted:",
"## Technical Specifications [optional]",
"### Model Architecture and Objective",
"### Compute Infrastructure",
"#### Hardware",
"#### Software\n\n\n\n[optional]\n\n\n\nBibTeX:\n\n\n\nAPA:",
"## Glossary [optional]",
"## More Information [optional]",
"## Model Card Authors [optional]",
"## Model Card Contact"
] |
object-detection | transformers |
<!-- This model card has been generated automatically according to the information the Trainer had access to. You
should probably proofread and complete it, then remove this comment. -->
# detr
This model is a fine-tuned version of [facebook/detr-resnet-50](https://huggingface.co/facebook/detr-resnet-50) on an unknown dataset.
It achieves the following results on the evaluation set:
- Loss: 1.9572
## Model description
More information needed
## Intended uses & limitations
More information needed
## Training and evaluation data
More information needed
## Training procedure
### Training hyperparameters
The following hyperparameters were used during training:
- learning_rate: 1e-05
- train_batch_size: 4
- eval_batch_size: 8
- seed: 42
- optimizer: Adam with betas=(0.9,0.999) and epsilon=1e-08
- lr_scheduler_type: linear
- num_epochs: 1
- mixed_precision_training: Native AMP
### Training results
| Training Loss | Epoch | Step | Validation Loss |
|:-------------:|:-----:|:----:|:---------------:|
| 1.8945 | 1.0 | 2500 | 1.9572 |
### Framework versions
- Transformers 4.38.2
- Pytorch 2.2.1+cu121
- Datasets 2.19.0
- Tokenizers 0.15.2
| {"license": "apache-2.0", "tags": ["generated_from_trainer"], "base_model": "facebook/detr-resnet-50", "model-index": [{"name": "detr", "results": []}]} | Naghma/detr | null | [
"transformers",
"tensorboard",
"safetensors",
"detr",
"object-detection",
"generated_from_trainer",
"base_model:facebook/detr-resnet-50",
"license:apache-2.0",
"endpoints_compatible",
"region:us"
] | null | 2024-04-21T19:22:30+00:00 | [] | [] | TAGS
#transformers #tensorboard #safetensors #detr #object-detection #generated_from_trainer #base_model-facebook/detr-resnet-50 #license-apache-2.0 #endpoints_compatible #region-us
| detr
====
This model is a fine-tuned version of facebook/detr-resnet-50 on an unknown dataset.
It achieves the following results on the evaluation set:
* Loss: 1.9572
Model description
-----------------
More information needed
Intended uses & limitations
---------------------------
More information needed
Training and evaluation data
----------------------------
More information needed
Training procedure
------------------
### Training hyperparameters
The following hyperparameters were used during training:
* learning\_rate: 1e-05
* train\_batch\_size: 4
* eval\_batch\_size: 8
* seed: 42
* optimizer: Adam with betas=(0.9,0.999) and epsilon=1e-08
* lr\_scheduler\_type: linear
* num\_epochs: 1
* mixed\_precision\_training: Native AMP
### Training results
### Framework versions
* Transformers 4.38.2
* Pytorch 2.2.1+cu121
* Datasets 2.19.0
* Tokenizers 0.15.2
| [
"### Training hyperparameters\n\n\nThe following hyperparameters were used during training:\n\n\n* learning\\_rate: 1e-05\n* train\\_batch\\_size: 4\n* eval\\_batch\\_size: 8\n* seed: 42\n* optimizer: Adam with betas=(0.9,0.999) and epsilon=1e-08\n* lr\\_scheduler\\_type: linear\n* num\\_epochs: 1\n* mixed\\_precision\\_training: Native AMP",
"### Training results",
"### Framework versions\n\n\n* Transformers 4.38.2\n* Pytorch 2.2.1+cu121\n* Datasets 2.19.0\n* Tokenizers 0.15.2"
] | [
"TAGS\n#transformers #tensorboard #safetensors #detr #object-detection #generated_from_trainer #base_model-facebook/detr-resnet-50 #license-apache-2.0 #endpoints_compatible #region-us \n",
"### Training hyperparameters\n\n\nThe following hyperparameters were used during training:\n\n\n* learning\\_rate: 1e-05\n* train\\_batch\\_size: 4\n* eval\\_batch\\_size: 8\n* seed: 42\n* optimizer: Adam with betas=(0.9,0.999) and epsilon=1e-08\n* lr\\_scheduler\\_type: linear\n* num\\_epochs: 1\n* mixed\\_precision\\_training: Native AMP",
"### Training results",
"### Framework versions\n\n\n* Transformers 4.38.2\n* Pytorch 2.2.1+cu121\n* Datasets 2.19.0\n* Tokenizers 0.15.2"
] |
reinforcement-learning | stable-baselines3 |
# **PPO** Agent playing **LunarLander-v2**
This is a trained model of a **PPO** agent playing **LunarLander-v2**
using the [stable-baselines3 library](https://github.com/DLR-RM/stable-baselines3).
## Usage (with Stable-baselines3)
TODO: Add your code
```python
from stable_baselines3 import ...
from huggingface_sb3 import load_from_hub
...
```
| {"library_name": "stable-baselines3", "tags": ["LunarLander-v2", "deep-reinforcement-learning", "reinforcement-learning", "stable-baselines3"], "model-index": [{"name": "PPO", "results": [{"task": {"type": "reinforcement-learning", "name": "reinforcement-learning"}, "dataset": {"name": "LunarLander-v2", "type": "LunarLander-v2"}, "metrics": [{"type": "mean_reward", "value": "238.67 +/- 24.90", "name": "mean_reward", "verified": false}]}]}]} | togthehog/ppo-LunarLander-v2 | null | [
"stable-baselines3",
"LunarLander-v2",
"deep-reinforcement-learning",
"reinforcement-learning",
"model-index",
"region:us"
] | null | 2024-04-21T19:23:03+00:00 | [] | [] | TAGS
#stable-baselines3 #LunarLander-v2 #deep-reinforcement-learning #reinforcement-learning #model-index #region-us
|
# PPO Agent playing LunarLander-v2
This is a trained model of a PPO agent playing LunarLander-v2
using the stable-baselines3 library.
## Usage (with Stable-baselines3)
TODO: Add your code
| [
"# PPO Agent playing LunarLander-v2\nThis is a trained model of a PPO agent playing LunarLander-v2\nusing the stable-baselines3 library.",
"## Usage (with Stable-baselines3)\nTODO: Add your code"
] | [
"TAGS\n#stable-baselines3 #LunarLander-v2 #deep-reinforcement-learning #reinforcement-learning #model-index #region-us \n",
"# PPO Agent playing LunarLander-v2\nThis is a trained model of a PPO agent playing LunarLander-v2\nusing the stable-baselines3 library.",
"## Usage (with Stable-baselines3)\nTODO: Add your code"
] |
null | adapter-transformers |
# Adapter `BigTMiami/n_par_bn_v_1_help_class_80_e_adp_lr_0003_S_3` for roberta-base
An [adapter](https://adapterhub.ml) for the `roberta-base` model that was trained on the [BigTMiami/amazon_MICRO_helpfulness_dataset](https://huggingface.co/datasets/BigTMiami/amazon_MICRO_helpfulness_dataset/) dataset and includes a prediction head for classification.
This adapter was created for usage with the **[Adapters](https://github.com/Adapter-Hub/adapters)** library.
## Usage
First, install `adapters`:
```
pip install -U adapters
```
Now, the adapter can be loaded and activated like this:
```python
from adapters import AutoAdapterModel
model = AutoAdapterModel.from_pretrained("roberta-base")
adapter_name = model.load_adapter("BigTMiami/n_par_bn_v_1_help_class_80_e_adp_lr_0003_S_3", source="hf", set_active=True)
```
## Architecture & Training
<!-- Add some description here -->
## Evaluation results
<!-- Add some description here -->
## Citation
<!-- Add some description here --> | {"tags": ["roberta", "adapter-transformers"], "datasets": ["BigTMiami/amazon_MICRO_helpfulness_dataset"]} | BigTMiami/n_par_bn_v_1_help_class_80_e_adp_lr_0003_S_3 | null | [
"adapter-transformers",
"roberta",
"dataset:BigTMiami/amazon_MICRO_helpfulness_dataset",
"region:us"
] | null | 2024-04-21T19:23:27+00:00 | [] | [] | TAGS
#adapter-transformers #roberta #dataset-BigTMiami/amazon_MICRO_helpfulness_dataset #region-us
|
# Adapter 'BigTMiami/n_par_bn_v_1_help_class_80_e_adp_lr_0003_S_3' for roberta-base
An adapter for the 'roberta-base' model that was trained on the BigTMiami/amazon_MICRO_helpfulness_dataset dataset and includes a prediction head for classification.
This adapter was created for usage with the Adapters library.
## Usage
First, install 'adapters':
Now, the adapter can be loaded and activated like this:
## Architecture & Training
## Evaluation results
| [
"# Adapter 'BigTMiami/n_par_bn_v_1_help_class_80_e_adp_lr_0003_S_3' for roberta-base\n\nAn adapter for the 'roberta-base' model that was trained on the BigTMiami/amazon_MICRO_helpfulness_dataset dataset and includes a prediction head for classification.\n\nThis adapter was created for usage with the Adapters library.",
"## Usage\n\nFirst, install 'adapters':\n\n\n\nNow, the adapter can be loaded and activated like this:",
"## Architecture & Training",
"## Evaluation results"
] | [
"TAGS\n#adapter-transformers #roberta #dataset-BigTMiami/amazon_MICRO_helpfulness_dataset #region-us \n",
"# Adapter 'BigTMiami/n_par_bn_v_1_help_class_80_e_adp_lr_0003_S_3' for roberta-base\n\nAn adapter for the 'roberta-base' model that was trained on the BigTMiami/amazon_MICRO_helpfulness_dataset dataset and includes a prediction head for classification.\n\nThis adapter was created for usage with the Adapters library.",
"## Usage\n\nFirst, install 'adapters':\n\n\n\nNow, the adapter can be loaded and activated like this:",
"## Architecture & Training",
"## Evaluation results"
] |
null | peft |
<!-- This model card has been generated automatically according to the information the Trainer had access to. You
should probably proofread and complete it, then remove this comment. -->
# text2sql-llama-3-8B
This model is a fine-tuned version of [meta-llama/Meta-Llama-3-8B-Instruct](https://huggingface.co/meta-llama/Meta-Llama-3-8B-Instruct) on the generator dataset.
## Model description
More information needed
## Intended uses & limitations
More information needed
## Training and evaluation data
More information needed
## Training procedure
### Training hyperparameters
The following hyperparameters were used during training:
- learning_rate: 0.0002
- train_batch_size: 2
- eval_batch_size: 8
- seed: 42
- gradient_accumulation_steps: 2
- total_train_batch_size: 4
- optimizer: Adam with betas=(0.9,0.999) and epsilon=1e-08
- lr_scheduler_type: constant
- lr_scheduler_warmup_ratio: 0.03
- num_epochs: 1
### Training results
### Framework versions
- PEFT 0.8.2
- Transformers 4.38.2
- Pytorch 2.1.0+cu118
- Datasets 2.16.1
- Tokenizers 0.15.2 | {"license": "other", "library_name": "peft", "tags": ["trl", "sft", "generated_from_trainer"], "datasets": ["generator"], "base_model": "meta-llama/Meta-Llama-3-8B-Instruct", "model-index": [{"name": "text2sql-llama-3-8B", "results": []}]} | kyryl-opens-ml/text2sql-llama-3-8B | null | [
"peft",
"safetensors",
"trl",
"sft",
"generated_from_trainer",
"dataset:generator",
"base_model:meta-llama/Meta-Llama-3-8B-Instruct",
"license:other",
"region:us"
] | null | 2024-04-21T19:24:13+00:00 | [] | [] | TAGS
#peft #safetensors #trl #sft #generated_from_trainer #dataset-generator #base_model-meta-llama/Meta-Llama-3-8B-Instruct #license-other #region-us
|
# text2sql-llama-3-8B
This model is a fine-tuned version of meta-llama/Meta-Llama-3-8B-Instruct on the generator dataset.
## Model description
More information needed
## Intended uses & limitations
More information needed
## Training and evaluation data
More information needed
## Training procedure
### Training hyperparameters
The following hyperparameters were used during training:
- learning_rate: 0.0002
- train_batch_size: 2
- eval_batch_size: 8
- seed: 42
- gradient_accumulation_steps: 2
- total_train_batch_size: 4
- optimizer: Adam with betas=(0.9,0.999) and epsilon=1e-08
- lr_scheduler_type: constant
- lr_scheduler_warmup_ratio: 0.03
- num_epochs: 1
### Training results
### Framework versions
- PEFT 0.8.2
- Transformers 4.38.2
- Pytorch 2.1.0+cu118
- Datasets 2.16.1
- Tokenizers 0.15.2 | [
"# text2sql-llama-3-8B\n\nThis model is a fine-tuned version of meta-llama/Meta-Llama-3-8B-Instruct on the generator dataset.",
"## Model description\n\nMore information needed",
"## Intended uses & limitations\n\nMore information needed",
"## Training and evaluation data\n\nMore information needed",
"## Training procedure",
"### Training hyperparameters\n\nThe following hyperparameters were used during training:\n- learning_rate: 0.0002\n- train_batch_size: 2\n- eval_batch_size: 8\n- seed: 42\n- gradient_accumulation_steps: 2\n- total_train_batch_size: 4\n- optimizer: Adam with betas=(0.9,0.999) and epsilon=1e-08\n- lr_scheduler_type: constant\n- lr_scheduler_warmup_ratio: 0.03\n- num_epochs: 1",
"### Training results",
"### Framework versions\n\n- PEFT 0.8.2\n- Transformers 4.38.2\n- Pytorch 2.1.0+cu118\n- Datasets 2.16.1\n- Tokenizers 0.15.2"
] | [
"TAGS\n#peft #safetensors #trl #sft #generated_from_trainer #dataset-generator #base_model-meta-llama/Meta-Llama-3-8B-Instruct #license-other #region-us \n",
"# text2sql-llama-3-8B\n\nThis model is a fine-tuned version of meta-llama/Meta-Llama-3-8B-Instruct on the generator dataset.",
"## Model description\n\nMore information needed",
"## Intended uses & limitations\n\nMore information needed",
"## Training and evaluation data\n\nMore information needed",
"## Training procedure",
"### Training hyperparameters\n\nThe following hyperparameters were used during training:\n- learning_rate: 0.0002\n- train_batch_size: 2\n- eval_batch_size: 8\n- seed: 42\n- gradient_accumulation_steps: 2\n- total_train_batch_size: 4\n- optimizer: Adam with betas=(0.9,0.999) and epsilon=1e-08\n- lr_scheduler_type: constant\n- lr_scheduler_warmup_ratio: 0.03\n- num_epochs: 1",
"### Training results",
"### Framework versions\n\n- PEFT 0.8.2\n- Transformers 4.38.2\n- Pytorch 2.1.0+cu118\n- Datasets 2.16.1\n- Tokenizers 0.15.2"
] |
null | adapter-transformers |
# Adapter `BigTMiami/n_par_bn_v_1_help_class_40_e_adp_lr_0003_S_3` for roberta-base
An [adapter](https://adapterhub.ml) for the `roberta-base` model that was trained on the [BigTMiami/amazon_MICRO_helpfulness_dataset](https://huggingface.co/datasets/BigTMiami/amazon_MICRO_helpfulness_dataset/) dataset and includes a prediction head for classification.
This adapter was created for usage with the **[Adapters](https://github.com/Adapter-Hub/adapters)** library.
## Usage
First, install `adapters`:
```
pip install -U adapters
```
Now, the adapter can be loaded and activated like this:
```python
from adapters import AutoAdapterModel
model = AutoAdapterModel.from_pretrained("roberta-base")
adapter_name = model.load_adapter("BigTMiami/n_par_bn_v_1_help_class_40_e_adp_lr_0003_S_3", source="hf", set_active=True)
```
## Architecture & Training
<!-- Add some description here -->
## Evaluation results
<!-- Add some description here -->
## Citation
<!-- Add some description here --> | {"tags": ["roberta", "adapter-transformers"], "datasets": ["BigTMiami/amazon_MICRO_helpfulness_dataset"]} | BigTMiami/n_par_bn_v_1_help_class_40_e_adp_lr_0003_S_3 | null | [
"adapter-transformers",
"roberta",
"dataset:BigTMiami/amazon_MICRO_helpfulness_dataset",
"region:us"
] | null | 2024-04-21T19:25:35+00:00 | [] | [] | TAGS
#adapter-transformers #roberta #dataset-BigTMiami/amazon_MICRO_helpfulness_dataset #region-us
|
# Adapter 'BigTMiami/n_par_bn_v_1_help_class_40_e_adp_lr_0003_S_3' for roberta-base
An adapter for the 'roberta-base' model that was trained on the BigTMiami/amazon_MICRO_helpfulness_dataset dataset and includes a prediction head for classification.
This adapter was created for usage with the Adapters library.
## Usage
First, install 'adapters':
Now, the adapter can be loaded and activated like this:
## Architecture & Training
## Evaluation results
| [
"# Adapter 'BigTMiami/n_par_bn_v_1_help_class_40_e_adp_lr_0003_S_3' for roberta-base\n\nAn adapter for the 'roberta-base' model that was trained on the BigTMiami/amazon_MICRO_helpfulness_dataset dataset and includes a prediction head for classification.\n\nThis adapter was created for usage with the Adapters library.",
"## Usage\n\nFirst, install 'adapters':\n\n\n\nNow, the adapter can be loaded and activated like this:",
"## Architecture & Training",
"## Evaluation results"
] | [
"TAGS\n#adapter-transformers #roberta #dataset-BigTMiami/amazon_MICRO_helpfulness_dataset #region-us \n",
"# Adapter 'BigTMiami/n_par_bn_v_1_help_class_40_e_adp_lr_0003_S_3' for roberta-base\n\nAn adapter for the 'roberta-base' model that was trained on the BigTMiami/amazon_MICRO_helpfulness_dataset dataset and includes a prediction head for classification.\n\nThis adapter was created for usage with the Adapters library.",
"## Usage\n\nFirst, install 'adapters':\n\n\n\nNow, the adapter can be loaded and activated like this:",
"## Architecture & Training",
"## Evaluation results"
] |
null | peft |
# Model Card for Model ID
<!-- Provide a quick summary of what the model is/does. -->
## Model Details
### Model Description
<!-- Provide a longer summary of what this model is. -->
- **Developed by:** [More Information Needed]
- **Funded by [optional]:** [More Information Needed]
- **Shared by [optional]:** [More Information Needed]
- **Model type:** [More Information Needed]
- **Language(s) (NLP):** [More Information Needed]
- **License:** [More Information Needed]
- **Finetuned from model [optional]:** [More Information Needed]
### Model Sources [optional]
<!-- Provide the basic links for the model. -->
- **Repository:** [More Information Needed]
- **Paper [optional]:** [More Information Needed]
- **Demo [optional]:** [More Information Needed]
## Uses
<!-- Address questions around how the model is intended to be used, including the foreseeable users of the model and those affected by the model. -->
### Direct Use
<!-- This section is for the model use without fine-tuning or plugging into a larger ecosystem/app. -->
[More Information Needed]
### Downstream Use [optional]
<!-- This section is for the model use when fine-tuned for a task, or when plugged into a larger ecosystem/app -->
[More Information Needed]
### Out-of-Scope Use
<!-- This section addresses misuse, malicious use, and uses that the model will not work well for. -->
[More Information Needed]
## Bias, Risks, and Limitations
<!-- This section is meant to convey both technical and sociotechnical limitations. -->
[More Information Needed]
### Recommendations
<!-- This section is meant to convey recommendations with respect to the bias, risk, and technical limitations. -->
Users (both direct and downstream) should be made aware of the risks, biases and limitations of the model. More information needed for further recommendations.
## How to Get Started with the Model
Use the code below to get started with the model.
[More Information Needed]
## Training Details
### Training Data
<!-- This should link to a Dataset Card, perhaps with a short stub of information on what the training data is all about as well as documentation related to data pre-processing or additional filtering. -->
[More Information Needed]
### Training Procedure
<!-- This relates heavily to the Technical Specifications. Content here should link to that section when it is relevant to the training procedure. -->
#### Preprocessing [optional]
[More Information Needed]
#### Training Hyperparameters
- **Training regime:** [More Information Needed] <!--fp32, fp16 mixed precision, bf16 mixed precision, bf16 non-mixed precision, fp16 non-mixed precision, fp8 mixed precision -->
#### Speeds, Sizes, Times [optional]
<!-- This section provides information about throughput, start/end time, checkpoint size if relevant, etc. -->
[More Information Needed]
## Evaluation
<!-- This section describes the evaluation protocols and provides the results. -->
### Testing Data, Factors & Metrics
#### Testing Data
<!-- This should link to a Dataset Card if possible. -->
[More Information Needed]
#### Factors
<!-- These are the things the evaluation is disaggregating by, e.g., subpopulations or domains. -->
[More Information Needed]
#### Metrics
<!-- These are the evaluation metrics being used, ideally with a description of why. -->
[More Information Needed]
### Results
[More Information Needed]
#### Summary
## Model Examination [optional]
<!-- Relevant interpretability work for the model goes here -->
[More Information Needed]
## Environmental Impact
<!-- Total emissions (in grams of CO2eq) and additional considerations, such as electricity usage, go here. Edit the suggested text below accordingly -->
Carbon emissions can be estimated using the [Machine Learning Impact calculator](https://mlco2.github.io/impact#compute) presented in [Lacoste et al. (2019)](https://arxiv.org/abs/1910.09700).
- **Hardware Type:** [More Information Needed]
- **Hours used:** [More Information Needed]
- **Cloud Provider:** [More Information Needed]
- **Compute Region:** [More Information Needed]
- **Carbon Emitted:** [More Information Needed]
## Technical Specifications [optional]
### Model Architecture and Objective
[More Information Needed]
### Compute Infrastructure
[More Information Needed]
#### Hardware
[More Information Needed]
#### Software
[More Information Needed]
## Citation [optional]
<!-- If there is a paper or blog post introducing the model, the APA and Bibtex information for that should go in this section. -->
**BibTeX:**
[More Information Needed]
**APA:**
[More Information Needed]
## Glossary [optional]
<!-- If relevant, include terms and calculations in this section that can help readers understand the model or model card. -->
[More Information Needed]
## More Information [optional]
[More Information Needed]
## Model Card Authors [optional]
[More Information Needed]
## Model Card Contact
[More Information Needed]
### Framework versions
- PEFT 0.10.0 | {"library_name": "peft", "base_model": "mistralai/Mistral-7B-Instruct-v0.2"} | nitinaggarwal12/ludwig-llm-fine-tuning | null | [
"peft",
"safetensors",
"arxiv:1910.09700",
"base_model:mistralai/Mistral-7B-Instruct-v0.2",
"region:us"
] | null | 2024-04-21T19:25:56+00:00 | [
"1910.09700"
] | [] | TAGS
#peft #safetensors #arxiv-1910.09700 #base_model-mistralai/Mistral-7B-Instruct-v0.2 #region-us
|
# Model Card for Model ID
## Model Details
### Model Description
- Developed by:
- Funded by [optional]:
- Shared by [optional]:
- Model type:
- Language(s) (NLP):
- License:
- Finetuned from model [optional]:
### Model Sources [optional]
- Repository:
- Paper [optional]:
- Demo [optional]:
## Uses
### Direct Use
### Downstream Use [optional]
### Out-of-Scope Use
## Bias, Risks, and Limitations
### Recommendations
Users (both direct and downstream) should be made aware of the risks, biases and limitations of the model. More information needed for further recommendations.
## How to Get Started with the Model
Use the code below to get started with the model.
## Training Details
### Training Data
### Training Procedure
#### Preprocessing [optional]
#### Training Hyperparameters
- Training regime:
#### Speeds, Sizes, Times [optional]
## Evaluation
### Testing Data, Factors & Metrics
#### Testing Data
#### Factors
#### Metrics
### Results
#### Summary
## Model Examination [optional]
## Environmental Impact
Carbon emissions can be estimated using the Machine Learning Impact calculator presented in Lacoste et al. (2019).
- Hardware Type:
- Hours used:
- Cloud Provider:
- Compute Region:
- Carbon Emitted:
## Technical Specifications [optional]
### Model Architecture and Objective
### Compute Infrastructure
#### Hardware
#### Software
[optional]
BibTeX:
APA:
## Glossary [optional]
## More Information [optional]
## Model Card Authors [optional]
## Model Card Contact
### Framework versions
- PEFT 0.10.0 | [
"# Model Card for Model ID",
"## Model Details",
"### Model Description\n\n\n\n\n\n- Developed by: \n- Funded by [optional]: \n- Shared by [optional]: \n- Model type: \n- Language(s) (NLP): \n- License: \n- Finetuned from model [optional]:",
"### Model Sources [optional]\n\n\n\n- Repository: \n- Paper [optional]: \n- Demo [optional]:",
"## Uses",
"### Direct Use",
"### Downstream Use [optional]",
"### Out-of-Scope Use",
"## Bias, Risks, and Limitations",
"### Recommendations\n\n\n\nUsers (both direct and downstream) should be made aware of the risks, biases and limitations of the model. More information needed for further recommendations.",
"## How to Get Started with the Model\n\nUse the code below to get started with the model.",
"## Training Details",
"### Training Data",
"### Training Procedure",
"#### Preprocessing [optional]",
"#### Training Hyperparameters\n\n- Training regime:",
"#### Speeds, Sizes, Times [optional]",
"## Evaluation",
"### Testing Data, Factors & Metrics",
"#### Testing Data",
"#### Factors",
"#### Metrics",
"### Results",
"#### Summary",
"## Model Examination [optional]",
"## Environmental Impact\n\n\n\nCarbon emissions can be estimated using the Machine Learning Impact calculator presented in Lacoste et al. (2019).\n\n- Hardware Type: \n- Hours used: \n- Cloud Provider: \n- Compute Region: \n- Carbon Emitted:",
"## Technical Specifications [optional]",
"### Model Architecture and Objective",
"### Compute Infrastructure",
"#### Hardware",
"#### Software\n\n\n\n[optional]\n\n\n\nBibTeX:\n\n\n\nAPA:",
"## Glossary [optional]",
"## More Information [optional]",
"## Model Card Authors [optional]",
"## Model Card Contact",
"### Framework versions\n\n- PEFT 0.10.0"
] | [
"TAGS\n#peft #safetensors #arxiv-1910.09700 #base_model-mistralai/Mistral-7B-Instruct-v0.2 #region-us \n",
"# Model Card for Model ID",
"## Model Details",
"### Model Description\n\n\n\n\n\n- Developed by: \n- Funded by [optional]: \n- Shared by [optional]: \n- Model type: \n- Language(s) (NLP): \n- License: \n- Finetuned from model [optional]:",
"### Model Sources [optional]\n\n\n\n- Repository: \n- Paper [optional]: \n- Demo [optional]:",
"## Uses",
"### Direct Use",
"### Downstream Use [optional]",
"### Out-of-Scope Use",
"## Bias, Risks, and Limitations",
"### Recommendations\n\n\n\nUsers (both direct and downstream) should be made aware of the risks, biases and limitations of the model. More information needed for further recommendations.",
"## How to Get Started with the Model\n\nUse the code below to get started with the model.",
"## Training Details",
"### Training Data",
"### Training Procedure",
"#### Preprocessing [optional]",
"#### Training Hyperparameters\n\n- Training regime:",
"#### Speeds, Sizes, Times [optional]",
"## Evaluation",
"### Testing Data, Factors & Metrics",
"#### Testing Data",
"#### Factors",
"#### Metrics",
"### Results",
"#### Summary",
"## Model Examination [optional]",
"## Environmental Impact\n\n\n\nCarbon emissions can be estimated using the Machine Learning Impact calculator presented in Lacoste et al. (2019).\n\n- Hardware Type: \n- Hours used: \n- Cloud Provider: \n- Compute Region: \n- Carbon Emitted:",
"## Technical Specifications [optional]",
"### Model Architecture and Objective",
"### Compute Infrastructure",
"#### Hardware",
"#### Software\n\n\n\n[optional]\n\n\n\nBibTeX:\n\n\n\nAPA:",
"## Glossary [optional]",
"## More Information [optional]",
"## Model Card Authors [optional]",
"## Model Card Contact",
"### Framework versions\n\n- PEFT 0.10.0"
] |
text-to-image | diffusers |
# Model Card for Model ID
<!-- Provide a quick summary of what the model is/does. -->
## Model Details
### Model Description
<!-- Provide a longer summary of what this model is. -->
This is the model card of a 🧨 diffusers model that has been pushed on the Hub. This model card has been automatically generated.
- **Developed by:** [More Information Needed]
- **Funded by [optional]:** [More Information Needed]
- **Shared by [optional]:** [More Information Needed]
- **Model type:** [More Information Needed]
- **Language(s) (NLP):** [More Information Needed]
- **License:** [More Information Needed]
- **Finetuned from model [optional]:** [More Information Needed]
### Model Sources [optional]
<!-- Provide the basic links for the model. -->
- **Repository:** [More Information Needed]
- **Paper [optional]:** [More Information Needed]
- **Demo [optional]:** [More Information Needed]
## Uses
<!-- Address questions around how the model is intended to be used, including the foreseeable users of the model and those affected by the model. -->
### Direct Use
<!-- This section is for the model use without fine-tuning or plugging into a larger ecosystem/app. -->
[More Information Needed]
### Downstream Use [optional]
<!-- This section is for the model use when fine-tuned for a task, or when plugged into a larger ecosystem/app -->
[More Information Needed]
### Out-of-Scope Use
<!-- This section addresses misuse, malicious use, and uses that the model will not work well for. -->
[More Information Needed]
## Bias, Risks, and Limitations
<!-- This section is meant to convey both technical and sociotechnical limitations. -->
[More Information Needed]
### Recommendations
<!-- This section is meant to convey recommendations with respect to the bias, risk, and technical limitations. -->
Users (both direct and downstream) should be made aware of the risks, biases and limitations of the model. More information needed for further recommendations.
## How to Get Started with the Model
Use the code below to get started with the model.
[More Information Needed]
## Training Details
### Training Data
<!-- This should link to a Dataset Card, perhaps with a short stub of information on what the training data is all about as well as documentation related to data pre-processing or additional filtering. -->
[More Information Needed]
### Training Procedure
<!-- This relates heavily to the Technical Specifications. Content here should link to that section when it is relevant to the training procedure. -->
#### Preprocessing [optional]
[More Information Needed]
#### Training Hyperparameters
- **Training regime:** [More Information Needed] <!--fp32, fp16 mixed precision, bf16 mixed precision, bf16 non-mixed precision, fp16 non-mixed precision, fp8 mixed precision -->
#### Speeds, Sizes, Times [optional]
<!-- This section provides information about throughput, start/end time, checkpoint size if relevant, etc. -->
[More Information Needed]
## Evaluation
<!-- This section describes the evaluation protocols and provides the results. -->
### Testing Data, Factors & Metrics
#### Testing Data
<!-- This should link to a Dataset Card if possible. -->
[More Information Needed]
#### Factors
<!-- These are the things the evaluation is disaggregating by, e.g., subpopulations or domains. -->
[More Information Needed]
#### Metrics
<!-- These are the evaluation metrics being used, ideally with a description of why. -->
[More Information Needed]
### Results
[More Information Needed]
#### Summary
## Model Examination [optional]
<!-- Relevant interpretability work for the model goes here -->
[More Information Needed]
## Environmental Impact
<!-- Total emissions (in grams of CO2eq) and additional considerations, such as electricity usage, go here. Edit the suggested text below accordingly -->
Carbon emissions can be estimated using the [Machine Learning Impact calculator](https://mlco2.github.io/impact#compute) presented in [Lacoste et al. (2019)](https://arxiv.org/abs/1910.09700).
- **Hardware Type:** [More Information Needed]
- **Hours used:** [More Information Needed]
- **Cloud Provider:** [More Information Needed]
- **Compute Region:** [More Information Needed]
- **Carbon Emitted:** [More Information Needed]
## Technical Specifications [optional]
### Model Architecture and Objective
[More Information Needed]
### Compute Infrastructure
[More Information Needed]
#### Hardware
[More Information Needed]
#### Software
[More Information Needed]
## Citation [optional]
<!-- If there is a paper or blog post introducing the model, the APA and Bibtex information for that should go in this section. -->
**BibTeX:**
[More Information Needed]
**APA:**
[More Information Needed]
## Glossary [optional]
<!-- If relevant, include terms and calculations in this section that can help readers understand the model or model card. -->
[More Information Needed]
## More Information [optional]
[More Information Needed]
## Model Card Authors [optional]
[More Information Needed]
## Model Card Contact
[More Information Needed]
| {"library_name": "diffusers"} | Niggendar/atomixPonyXL_v10TurboDPMSDE | null | [
"diffusers",
"safetensors",
"arxiv:1910.09700",
"endpoints_compatible",
"diffusers:StableDiffusionXLPipeline",
"region:us"
] | null | 2024-04-21T19:26:35+00:00 | [
"1910.09700"
] | [] | TAGS
#diffusers #safetensors #arxiv-1910.09700 #endpoints_compatible #diffusers-StableDiffusionXLPipeline #region-us
|
# Model Card for Model ID
## Model Details
### Model Description
This is the model card of a diffusers model that has been pushed on the Hub. This model card has been automatically generated.
- Developed by:
- Funded by [optional]:
- Shared by [optional]:
- Model type:
- Language(s) (NLP):
- License:
- Finetuned from model [optional]:
### Model Sources [optional]
- Repository:
- Paper [optional]:
- Demo [optional]:
## Uses
### Direct Use
### Downstream Use [optional]
### Out-of-Scope Use
## Bias, Risks, and Limitations
### Recommendations
Users (both direct and downstream) should be made aware of the risks, biases and limitations of the model. More information needed for further recommendations.
## How to Get Started with the Model
Use the code below to get started with the model.
## Training Details
### Training Data
### Training Procedure
#### Preprocessing [optional]
#### Training Hyperparameters
- Training regime:
#### Speeds, Sizes, Times [optional]
## Evaluation
### Testing Data, Factors & Metrics
#### Testing Data
#### Factors
#### Metrics
### Results
#### Summary
## Model Examination [optional]
## Environmental Impact
Carbon emissions can be estimated using the Machine Learning Impact calculator presented in Lacoste et al. (2019).
- Hardware Type:
- Hours used:
- Cloud Provider:
- Compute Region:
- Carbon Emitted:
## Technical Specifications [optional]
### Model Architecture and Objective
### Compute Infrastructure
#### Hardware
#### Software
[optional]
BibTeX:
APA:
## Glossary [optional]
## More Information [optional]
## Model Card Authors [optional]
## Model Card Contact
| [
"# Model Card for Model ID",
"## Model Details",
"### Model Description\n\n\n\nThis is the model card of a diffusers model that has been pushed on the Hub. This model card has been automatically generated.\n\n- Developed by: \n- Funded by [optional]: \n- Shared by [optional]: \n- Model type: \n- Language(s) (NLP): \n- License: \n- Finetuned from model [optional]:",
"### Model Sources [optional]\n\n\n\n- Repository: \n- Paper [optional]: \n- Demo [optional]:",
"## Uses",
"### Direct Use",
"### Downstream Use [optional]",
"### Out-of-Scope Use",
"## Bias, Risks, and Limitations",
"### Recommendations\n\n\n\nUsers (both direct and downstream) should be made aware of the risks, biases and limitations of the model. More information needed for further recommendations.",
"## How to Get Started with the Model\n\nUse the code below to get started with the model.",
"## Training Details",
"### Training Data",
"### Training Procedure",
"#### Preprocessing [optional]",
"#### Training Hyperparameters\n\n- Training regime:",
"#### Speeds, Sizes, Times [optional]",
"## Evaluation",
"### Testing Data, Factors & Metrics",
"#### Testing Data",
"#### Factors",
"#### Metrics",
"### Results",
"#### Summary",
"## Model Examination [optional]",
"## Environmental Impact\n\n\n\nCarbon emissions can be estimated using the Machine Learning Impact calculator presented in Lacoste et al. (2019).\n\n- Hardware Type: \n- Hours used: \n- Cloud Provider: \n- Compute Region: \n- Carbon Emitted:",
"## Technical Specifications [optional]",
"### Model Architecture and Objective",
"### Compute Infrastructure",
"#### Hardware",
"#### Software\n\n\n\n[optional]\n\n\n\nBibTeX:\n\n\n\nAPA:",
"## Glossary [optional]",
"## More Information [optional]",
"## Model Card Authors [optional]",
"## Model Card Contact"
] | [
"TAGS\n#diffusers #safetensors #arxiv-1910.09700 #endpoints_compatible #diffusers-StableDiffusionXLPipeline #region-us \n",
"# Model Card for Model ID",
"## Model Details",
"### Model Description\n\n\n\nThis is the model card of a diffusers model that has been pushed on the Hub. This model card has been automatically generated.\n\n- Developed by: \n- Funded by [optional]: \n- Shared by [optional]: \n- Model type: \n- Language(s) (NLP): \n- License: \n- Finetuned from model [optional]:",
"### Model Sources [optional]\n\n\n\n- Repository: \n- Paper [optional]: \n- Demo [optional]:",
"## Uses",
"### Direct Use",
"### Downstream Use [optional]",
"### Out-of-Scope Use",
"## Bias, Risks, and Limitations",
"### Recommendations\n\n\n\nUsers (both direct and downstream) should be made aware of the risks, biases and limitations of the model. More information needed for further recommendations.",
"## How to Get Started with the Model\n\nUse the code below to get started with the model.",
"## Training Details",
"### Training Data",
"### Training Procedure",
"#### Preprocessing [optional]",
"#### Training Hyperparameters\n\n- Training regime:",
"#### Speeds, Sizes, Times [optional]",
"## Evaluation",
"### Testing Data, Factors & Metrics",
"#### Testing Data",
"#### Factors",
"#### Metrics",
"### Results",
"#### Summary",
"## Model Examination [optional]",
"## Environmental Impact\n\n\n\nCarbon emissions can be estimated using the Machine Learning Impact calculator presented in Lacoste et al. (2019).\n\n- Hardware Type: \n- Hours used: \n- Cloud Provider: \n- Compute Region: \n- Carbon Emitted:",
"## Technical Specifications [optional]",
"### Model Architecture and Objective",
"### Compute Infrastructure",
"#### Hardware",
"#### Software\n\n\n\n[optional]\n\n\n\nBibTeX:\n\n\n\nAPA:",
"## Glossary [optional]",
"## More Information [optional]",
"## Model Card Authors [optional]",
"## Model Card Contact"
] |
reinforcement-learning | stable-baselines3 |
# **A2C** Agent playing **PandaReachDense-v3**
This is a trained model of a **A2C** agent playing **PandaReachDense-v3**
using the [stable-baselines3 library](https://github.com/DLR-RM/stable-baselines3).
## Usage (with Stable-baselines3)
TODO: Add your code
```python
from stable_baselines3 import ...
from huggingface_sb3 import load_from_hub
...
```
| {"library_name": "stable-baselines3", "tags": ["PandaReachDense-v3", "deep-reinforcement-learning", "reinforcement-learning", "stable-baselines3"], "model-index": [{"name": "A2C", "results": [{"task": {"type": "reinforcement-learning", "name": "reinforcement-learning"}, "dataset": {"name": "PandaReachDense-v3", "type": "PandaReachDense-v3"}, "metrics": [{"type": "mean_reward", "value": "-0.22 +/- 0.07", "name": "mean_reward", "verified": false}]}]}]} | tarpalsus/a2c-PandaReachDense-v3 | null | [
"stable-baselines3",
"PandaReachDense-v3",
"deep-reinforcement-learning",
"reinforcement-learning",
"model-index",
"region:us"
] | null | 2024-04-21T19:29:19+00:00 | [] | [] | TAGS
#stable-baselines3 #PandaReachDense-v3 #deep-reinforcement-learning #reinforcement-learning #model-index #region-us
|
# A2C Agent playing PandaReachDense-v3
This is a trained model of a A2C agent playing PandaReachDense-v3
using the stable-baselines3 library.
## Usage (with Stable-baselines3)
TODO: Add your code
| [
"# A2C Agent playing PandaReachDense-v3\nThis is a trained model of a A2C agent playing PandaReachDense-v3\nusing the stable-baselines3 library.",
"## Usage (with Stable-baselines3)\nTODO: Add your code"
] | [
"TAGS\n#stable-baselines3 #PandaReachDense-v3 #deep-reinforcement-learning #reinforcement-learning #model-index #region-us \n",
"# A2C Agent playing PandaReachDense-v3\nThis is a trained model of a A2C agent playing PandaReachDense-v3\nusing the stable-baselines3 library.",
"## Usage (with Stable-baselines3)\nTODO: Add your code"
] |
null | transformers |
# Model Card for Model ID
<!-- Provide a quick summary of what the model is/does. -->
## Model Details
### Model Description
<!-- Provide a longer summary of what this model is. -->
This is the model card of a 🤗 transformers model that has been pushed on the Hub. This model card has been automatically generated.
- **Developed by:** [More Information Needed]
- **Funded by [optional]:** [More Information Needed]
- **Shared by [optional]:** [More Information Needed]
- **Model type:** [More Information Needed]
- **Language(s) (NLP):** [More Information Needed]
- **License:** [More Information Needed]
- **Finetuned from model [optional]:** [More Information Needed]
### Model Sources [optional]
<!-- Provide the basic links for the model. -->
- **Repository:** [More Information Needed]
- **Paper [optional]:** [More Information Needed]
- **Demo [optional]:** [More Information Needed]
## Uses
<!-- Address questions around how the model is intended to be used, including the foreseeable users of the model and those affected by the model. -->
### Direct Use
<!-- This section is for the model use without fine-tuning or plugging into a larger ecosystem/app. -->
[More Information Needed]
### Downstream Use [optional]
<!-- This section is for the model use when fine-tuned for a task, or when plugged into a larger ecosystem/app -->
[More Information Needed]
### Out-of-Scope Use
<!-- This section addresses misuse, malicious use, and uses that the model will not work well for. -->
[More Information Needed]
## Bias, Risks, and Limitations
<!-- This section is meant to convey both technical and sociotechnical limitations. -->
[More Information Needed]
### Recommendations
<!-- This section is meant to convey recommendations with respect to the bias, risk, and technical limitations. -->
Users (both direct and downstream) should be made aware of the risks, biases and limitations of the model. More information needed for further recommendations.
## How to Get Started with the Model
Use the code below to get started with the model.
[More Information Needed]
## Training Details
### Training Data
<!-- This should link to a Dataset Card, perhaps with a short stub of information on what the training data is all about as well as documentation related to data pre-processing or additional filtering. -->
[More Information Needed]
### Training Procedure
<!-- This relates heavily to the Technical Specifications. Content here should link to that section when it is relevant to the training procedure. -->
#### Preprocessing [optional]
[More Information Needed]
#### Training Hyperparameters
- **Training regime:** [More Information Needed] <!--fp32, fp16 mixed precision, bf16 mixed precision, bf16 non-mixed precision, fp16 non-mixed precision, fp8 mixed precision -->
#### Speeds, Sizes, Times [optional]
<!-- This section provides information about throughput, start/end time, checkpoint size if relevant, etc. -->
[More Information Needed]
## Evaluation
<!-- This section describes the evaluation protocols and provides the results. -->
### Testing Data, Factors & Metrics
#### Testing Data
<!-- This should link to a Dataset Card if possible. -->
[More Information Needed]
#### Factors
<!-- These are the things the evaluation is disaggregating by, e.g., subpopulations or domains. -->
[More Information Needed]
#### Metrics
<!-- These are the evaluation metrics being used, ideally with a description of why. -->
[More Information Needed]
### Results
[More Information Needed]
#### Summary
## Model Examination [optional]
<!-- Relevant interpretability work for the model goes here -->
[More Information Needed]
## Environmental Impact
<!-- Total emissions (in grams of CO2eq) and additional considerations, such as electricity usage, go here. Edit the suggested text below accordingly -->
Carbon emissions can be estimated using the [Machine Learning Impact calculator](https://mlco2.github.io/impact#compute) presented in [Lacoste et al. (2019)](https://arxiv.org/abs/1910.09700).
- **Hardware Type:** [More Information Needed]
- **Hours used:** [More Information Needed]
- **Cloud Provider:** [More Information Needed]
- **Compute Region:** [More Information Needed]
- **Carbon Emitted:** [More Information Needed]
## Technical Specifications [optional]
### Model Architecture and Objective
[More Information Needed]
### Compute Infrastructure
[More Information Needed]
#### Hardware
[More Information Needed]
#### Software
[More Information Needed]
## Citation [optional]
<!-- If there is a paper or blog post introducing the model, the APA and Bibtex information for that should go in this section. -->
**BibTeX:**
[More Information Needed]
**APA:**
[More Information Needed]
## Glossary [optional]
<!-- If relevant, include terms and calculations in this section that can help readers understand the model or model card. -->
[More Information Needed]
## More Information [optional]
[More Information Needed]
## Model Card Authors [optional]
[More Information Needed]
## Model Card Contact
[More Information Needed]
| {"library_name": "transformers", "tags": []} | Enagamirzayev/whisper-small-llm-lingo-adapters_aa | null | [
"transformers",
"safetensors",
"arxiv:1910.09700",
"endpoints_compatible",
"region:us"
] | null | 2024-04-21T19:29:22+00:00 | [
"1910.09700"
] | [] | TAGS
#transformers #safetensors #arxiv-1910.09700 #endpoints_compatible #region-us
|
# Model Card for Model ID
## Model Details
### Model Description
This is the model card of a transformers model that has been pushed on the Hub. This model card has been automatically generated.
- Developed by:
- Funded by [optional]:
- Shared by [optional]:
- Model type:
- Language(s) (NLP):
- License:
- Finetuned from model [optional]:
### Model Sources [optional]
- Repository:
- Paper [optional]:
- Demo [optional]:
## Uses
### Direct Use
### Downstream Use [optional]
### Out-of-Scope Use
## Bias, Risks, and Limitations
### Recommendations
Users (both direct and downstream) should be made aware of the risks, biases and limitations of the model. More information needed for further recommendations.
## How to Get Started with the Model
Use the code below to get started with the model.
## Training Details
### Training Data
### Training Procedure
#### Preprocessing [optional]
#### Training Hyperparameters
- Training regime:
#### Speeds, Sizes, Times [optional]
## Evaluation
### Testing Data, Factors & Metrics
#### Testing Data
#### Factors
#### Metrics
### Results
#### Summary
## Model Examination [optional]
## Environmental Impact
Carbon emissions can be estimated using the Machine Learning Impact calculator presented in Lacoste et al. (2019).
- Hardware Type:
- Hours used:
- Cloud Provider:
- Compute Region:
- Carbon Emitted:
## Technical Specifications [optional]
### Model Architecture and Objective
### Compute Infrastructure
#### Hardware
#### Software
[optional]
BibTeX:
APA:
## Glossary [optional]
## More Information [optional]
## Model Card Authors [optional]
## Model Card Contact
| [
"# Model Card for Model ID",
"## Model Details",
"### Model Description\n\n\n\nThis is the model card of a transformers model that has been pushed on the Hub. This model card has been automatically generated.\n\n- Developed by: \n- Funded by [optional]: \n- Shared by [optional]: \n- Model type: \n- Language(s) (NLP): \n- License: \n- Finetuned from model [optional]:",
"### Model Sources [optional]\n\n\n\n- Repository: \n- Paper [optional]: \n- Demo [optional]:",
"## Uses",
"### Direct Use",
"### Downstream Use [optional]",
"### Out-of-Scope Use",
"## Bias, Risks, and Limitations",
"### Recommendations\n\n\n\nUsers (both direct and downstream) should be made aware of the risks, biases and limitations of the model. More information needed for further recommendations.",
"## How to Get Started with the Model\n\nUse the code below to get started with the model.",
"## Training Details",
"### Training Data",
"### Training Procedure",
"#### Preprocessing [optional]",
"#### Training Hyperparameters\n\n- Training regime:",
"#### Speeds, Sizes, Times [optional]",
"## Evaluation",
"### Testing Data, Factors & Metrics",
"#### Testing Data",
"#### Factors",
"#### Metrics",
"### Results",
"#### Summary",
"## Model Examination [optional]",
"## Environmental Impact\n\n\n\nCarbon emissions can be estimated using the Machine Learning Impact calculator presented in Lacoste et al. (2019).\n\n- Hardware Type: \n- Hours used: \n- Cloud Provider: \n- Compute Region: \n- Carbon Emitted:",
"## Technical Specifications [optional]",
"### Model Architecture and Objective",
"### Compute Infrastructure",
"#### Hardware",
"#### Software\n\n\n\n[optional]\n\n\n\nBibTeX:\n\n\n\nAPA:",
"## Glossary [optional]",
"## More Information [optional]",
"## Model Card Authors [optional]",
"## Model Card Contact"
] | [
"TAGS\n#transformers #safetensors #arxiv-1910.09700 #endpoints_compatible #region-us \n",
"# Model Card for Model ID",
"## Model Details",
"### Model Description\n\n\n\nThis is the model card of a transformers model that has been pushed on the Hub. This model card has been automatically generated.\n\n- Developed by: \n- Funded by [optional]: \n- Shared by [optional]: \n- Model type: \n- Language(s) (NLP): \n- License: \n- Finetuned from model [optional]:",
"### Model Sources [optional]\n\n\n\n- Repository: \n- Paper [optional]: \n- Demo [optional]:",
"## Uses",
"### Direct Use",
"### Downstream Use [optional]",
"### Out-of-Scope Use",
"## Bias, Risks, and Limitations",
"### Recommendations\n\n\n\nUsers (both direct and downstream) should be made aware of the risks, biases and limitations of the model. More information needed for further recommendations.",
"## How to Get Started with the Model\n\nUse the code below to get started with the model.",
"## Training Details",
"### Training Data",
"### Training Procedure",
"#### Preprocessing [optional]",
"#### Training Hyperparameters\n\n- Training regime:",
"#### Speeds, Sizes, Times [optional]",
"## Evaluation",
"### Testing Data, Factors & Metrics",
"#### Testing Data",
"#### Factors",
"#### Metrics",
"### Results",
"#### Summary",
"## Model Examination [optional]",
"## Environmental Impact\n\n\n\nCarbon emissions can be estimated using the Machine Learning Impact calculator presented in Lacoste et al. (2019).\n\n- Hardware Type: \n- Hours used: \n- Cloud Provider: \n- Compute Region: \n- Carbon Emitted:",
"## Technical Specifications [optional]",
"### Model Architecture and Objective",
"### Compute Infrastructure",
"#### Hardware",
"#### Software\n\n\n\n[optional]\n\n\n\nBibTeX:\n\n\n\nAPA:",
"## Glossary [optional]",
"## More Information [optional]",
"## Model Card Authors [optional]",
"## Model Card Contact"
] |
text-generation | transformers |
# Model Card for Model ID
<!-- Provide a quick summary of what the model is/does. -->
## Model Details
### Model Description
<!-- Provide a longer summary of what this model is. -->
This is the model card of a 🤗 transformers model that has been pushed on the Hub. This model card has been automatically generated.
- **Developed by:** [More Information Needed]
- **Funded by [optional]:** [More Information Needed]
- **Shared by [optional]:** [More Information Needed]
- **Model type:** [More Information Needed]
- **Language(s) (NLP):** [More Information Needed]
- **License:** [More Information Needed]
- **Finetuned from model [optional]:** [More Information Needed]
### Model Sources [optional]
<!-- Provide the basic links for the model. -->
- **Repository:** [More Information Needed]
- **Paper [optional]:** [More Information Needed]
- **Demo [optional]:** [More Information Needed]
## Uses
<!-- Address questions around how the model is intended to be used, including the foreseeable users of the model and those affected by the model. -->
### Direct Use
<!-- This section is for the model use without fine-tuning or plugging into a larger ecosystem/app. -->
[More Information Needed]
### Downstream Use [optional]
<!-- This section is for the model use when fine-tuned for a task, or when plugged into a larger ecosystem/app -->
[More Information Needed]
### Out-of-Scope Use
<!-- This section addresses misuse, malicious use, and uses that the model will not work well for. -->
[More Information Needed]
## Bias, Risks, and Limitations
<!-- This section is meant to convey both technical and sociotechnical limitations. -->
[More Information Needed]
### Recommendations
<!-- This section is meant to convey recommendations with respect to the bias, risk, and technical limitations. -->
Users (both direct and downstream) should be made aware of the risks, biases and limitations of the model. More information needed for further recommendations.
## How to Get Started with the Model
Use the code below to get started with the model.
[More Information Needed]
## Training Details
### Training Data
<!-- This should link to a Dataset Card, perhaps with a short stub of information on what the training data is all about as well as documentation related to data pre-processing or additional filtering. -->
[More Information Needed]
### Training Procedure
<!-- This relates heavily to the Technical Specifications. Content here should link to that section when it is relevant to the training procedure. -->
#### Preprocessing [optional]
[More Information Needed]
#### Training Hyperparameters
- **Training regime:** [More Information Needed] <!--fp32, fp16 mixed precision, bf16 mixed precision, bf16 non-mixed precision, fp16 non-mixed precision, fp8 mixed precision -->
#### Speeds, Sizes, Times [optional]
<!-- This section provides information about throughput, start/end time, checkpoint size if relevant, etc. -->
[More Information Needed]
## Evaluation
<!-- This section describes the evaluation protocols and provides the results. -->
### Testing Data, Factors & Metrics
#### Testing Data
<!-- This should link to a Dataset Card if possible. -->
[More Information Needed]
#### Factors
<!-- These are the things the evaluation is disaggregating by, e.g., subpopulations or domains. -->
[More Information Needed]
#### Metrics
<!-- These are the evaluation metrics being used, ideally with a description of why. -->
[More Information Needed]
### Results
[More Information Needed]
#### Summary
## Model Examination [optional]
<!-- Relevant interpretability work for the model goes here -->
[More Information Needed]
## Environmental Impact
<!-- Total emissions (in grams of CO2eq) and additional considerations, such as electricity usage, go here. Edit the suggested text below accordingly -->
Carbon emissions can be estimated using the [Machine Learning Impact calculator](https://mlco2.github.io/impact#compute) presented in [Lacoste et al. (2019)](https://arxiv.org/abs/1910.09700).
- **Hardware Type:** [More Information Needed]
- **Hours used:** [More Information Needed]
- **Cloud Provider:** [More Information Needed]
- **Compute Region:** [More Information Needed]
- **Carbon Emitted:** [More Information Needed]
## Technical Specifications [optional]
### Model Architecture and Objective
[More Information Needed]
### Compute Infrastructure
[More Information Needed]
#### Hardware
[More Information Needed]
#### Software
[More Information Needed]
## Citation [optional]
<!-- If there is a paper or blog post introducing the model, the APA and Bibtex information for that should go in this section. -->
**BibTeX:**
[More Information Needed]
**APA:**
[More Information Needed]
## Glossary [optional]
<!-- If relevant, include terms and calculations in this section that can help readers understand the model or model card. -->
[More Information Needed]
## More Information [optional]
[More Information Needed]
## Model Card Authors [optional]
[More Information Needed]
## Model Card Contact
[More Information Needed] | {"library_name": "transformers", "tags": []} | OwOOwO/dumbo-llama6 | null | [
"transformers",
"safetensors",
"llama",
"text-generation",
"conversational",
"arxiv:1910.09700",
"autotrain_compatible",
"endpoints_compatible",
"text-generation-inference",
"region:us"
] | null | 2024-04-21T19:29:44+00:00 | [
"1910.09700"
] | [] | TAGS
#transformers #safetensors #llama #text-generation #conversational #arxiv-1910.09700 #autotrain_compatible #endpoints_compatible #text-generation-inference #region-us
|
# Model Card for Model ID
## Model Details
### Model Description
This is the model card of a transformers model that has been pushed on the Hub. This model card has been automatically generated.
- Developed by:
- Funded by [optional]:
- Shared by [optional]:
- Model type:
- Language(s) (NLP):
- License:
- Finetuned from model [optional]:
### Model Sources [optional]
- Repository:
- Paper [optional]:
- Demo [optional]:
## Uses
### Direct Use
### Downstream Use [optional]
### Out-of-Scope Use
## Bias, Risks, and Limitations
### Recommendations
Users (both direct and downstream) should be made aware of the risks, biases and limitations of the model. More information needed for further recommendations.
## How to Get Started with the Model
Use the code below to get started with the model.
## Training Details
### Training Data
### Training Procedure
#### Preprocessing [optional]
#### Training Hyperparameters
- Training regime:
#### Speeds, Sizes, Times [optional]
## Evaluation
### Testing Data, Factors & Metrics
#### Testing Data
#### Factors
#### Metrics
### Results
#### Summary
## Model Examination [optional]
## Environmental Impact
Carbon emissions can be estimated using the Machine Learning Impact calculator presented in Lacoste et al. (2019).
- Hardware Type:
- Hours used:
- Cloud Provider:
- Compute Region:
- Carbon Emitted:
## Technical Specifications [optional]
### Model Architecture and Objective
### Compute Infrastructure
#### Hardware
#### Software
[optional]
BibTeX:
APA:
## Glossary [optional]
## More Information [optional]
## Model Card Authors [optional]
## Model Card Contact
| [
"# Model Card for Model ID",
"## Model Details",
"### Model Description\n\n\n\nThis is the model card of a transformers model that has been pushed on the Hub. This model card has been automatically generated.\n\n- Developed by: \n- Funded by [optional]: \n- Shared by [optional]: \n- Model type: \n- Language(s) (NLP): \n- License: \n- Finetuned from model [optional]:",
"### Model Sources [optional]\n\n\n\n- Repository: \n- Paper [optional]: \n- Demo [optional]:",
"## Uses",
"### Direct Use",
"### Downstream Use [optional]",
"### Out-of-Scope Use",
"## Bias, Risks, and Limitations",
"### Recommendations\n\n\n\nUsers (both direct and downstream) should be made aware of the risks, biases and limitations of the model. More information needed for further recommendations.",
"## How to Get Started with the Model\n\nUse the code below to get started with the model.",
"## Training Details",
"### Training Data",
"### Training Procedure",
"#### Preprocessing [optional]",
"#### Training Hyperparameters\n\n- Training regime:",
"#### Speeds, Sizes, Times [optional]",
"## Evaluation",
"### Testing Data, Factors & Metrics",
"#### Testing Data",
"#### Factors",
"#### Metrics",
"### Results",
"#### Summary",
"## Model Examination [optional]",
"## Environmental Impact\n\n\n\nCarbon emissions can be estimated using the Machine Learning Impact calculator presented in Lacoste et al. (2019).\n\n- Hardware Type: \n- Hours used: \n- Cloud Provider: \n- Compute Region: \n- Carbon Emitted:",
"## Technical Specifications [optional]",
"### Model Architecture and Objective",
"### Compute Infrastructure",
"#### Hardware",
"#### Software\n\n\n\n[optional]\n\n\n\nBibTeX:\n\n\n\nAPA:",
"## Glossary [optional]",
"## More Information [optional]",
"## Model Card Authors [optional]",
"## Model Card Contact"
] | [
"TAGS\n#transformers #safetensors #llama #text-generation #conversational #arxiv-1910.09700 #autotrain_compatible #endpoints_compatible #text-generation-inference #region-us \n",
"# Model Card for Model ID",
"## Model Details",
"### Model Description\n\n\n\nThis is the model card of a transformers model that has been pushed on the Hub. This model card has been automatically generated.\n\n- Developed by: \n- Funded by [optional]: \n- Shared by [optional]: \n- Model type: \n- Language(s) (NLP): \n- License: \n- Finetuned from model [optional]:",
"### Model Sources [optional]\n\n\n\n- Repository: \n- Paper [optional]: \n- Demo [optional]:",
"## Uses",
"### Direct Use",
"### Downstream Use [optional]",
"### Out-of-Scope Use",
"## Bias, Risks, and Limitations",
"### Recommendations\n\n\n\nUsers (both direct and downstream) should be made aware of the risks, biases and limitations of the model. More information needed for further recommendations.",
"## How to Get Started with the Model\n\nUse the code below to get started with the model.",
"## Training Details",
"### Training Data",
"### Training Procedure",
"#### Preprocessing [optional]",
"#### Training Hyperparameters\n\n- Training regime:",
"#### Speeds, Sizes, Times [optional]",
"## Evaluation",
"### Testing Data, Factors & Metrics",
"#### Testing Data",
"#### Factors",
"#### Metrics",
"### Results",
"#### Summary",
"## Model Examination [optional]",
"## Environmental Impact\n\n\n\nCarbon emissions can be estimated using the Machine Learning Impact calculator presented in Lacoste et al. (2019).\n\n- Hardware Type: \n- Hours used: \n- Cloud Provider: \n- Compute Region: \n- Carbon Emitted:",
"## Technical Specifications [optional]",
"### Model Architecture and Objective",
"### Compute Infrastructure",
"#### Hardware",
"#### Software\n\n\n\n[optional]\n\n\n\nBibTeX:\n\n\n\nAPA:",
"## Glossary [optional]",
"## More Information [optional]",
"## Model Card Authors [optional]",
"## Model Card Contact"
] |
automatic-speech-recognition | transformers |
# Model Card for Model ID
<!-- Provide a quick summary of what the model is/does. -->
## Model Details
### Model Description
<!-- Provide a longer summary of what this model is. -->
This is the model card of a 🤗 transformers model that has been pushed on the Hub. This model card has been automatically generated.
- **Developed by:** [More Information Needed]
- **Funded by [optional]:** [More Information Needed]
- **Shared by [optional]:** [More Information Needed]
- **Model type:** [More Information Needed]
- **Language(s) (NLP):** [More Information Needed]
- **License:** [More Information Needed]
- **Finetuned from model [optional]:** [More Information Needed]
### Model Sources [optional]
<!-- Provide the basic links for the model. -->
- **Repository:** [More Information Needed]
- **Paper [optional]:** [More Information Needed]
- **Demo [optional]:** [More Information Needed]
## Uses
<!-- Address questions around how the model is intended to be used, including the foreseeable users of the model and those affected by the model. -->
### Direct Use
<!-- This section is for the model use without fine-tuning or plugging into a larger ecosystem/app. -->
[More Information Needed]
### Downstream Use [optional]
<!-- This section is for the model use when fine-tuned for a task, or when plugged into a larger ecosystem/app -->
[More Information Needed]
### Out-of-Scope Use
<!-- This section addresses misuse, malicious use, and uses that the model will not work well for. -->
[More Information Needed]
## Bias, Risks, and Limitations
<!-- This section is meant to convey both technical and sociotechnical limitations. -->
[More Information Needed]
### Recommendations
<!-- This section is meant to convey recommendations with respect to the bias, risk, and technical limitations. -->
Users (both direct and downstream) should be made aware of the risks, biases and limitations of the model. More information needed for further recommendations.
## How to Get Started with the Model
Use the code below to get started with the model.
[More Information Needed]
## Training Details
### Training Data
<!-- This should link to a Dataset Card, perhaps with a short stub of information on what the training data is all about as well as documentation related to data pre-processing or additional filtering. -->
[More Information Needed]
### Training Procedure
<!-- This relates heavily to the Technical Specifications. Content here should link to that section when it is relevant to the training procedure. -->
#### Preprocessing [optional]
[More Information Needed]
#### Training Hyperparameters
- **Training regime:** [More Information Needed] <!--fp32, fp16 mixed precision, bf16 mixed precision, bf16 non-mixed precision, fp16 non-mixed precision, fp8 mixed precision -->
#### Speeds, Sizes, Times [optional]
<!-- This section provides information about throughput, start/end time, checkpoint size if relevant, etc. -->
[More Information Needed]
## Evaluation
<!-- This section describes the evaluation protocols and provides the results. -->
### Testing Data, Factors & Metrics
#### Testing Data
<!-- This should link to a Dataset Card if possible. -->
[More Information Needed]
#### Factors
<!-- These are the things the evaluation is disaggregating by, e.g., subpopulations or domains. -->
[More Information Needed]
#### Metrics
<!-- These are the evaluation metrics being used, ideally with a description of why. -->
[More Information Needed]
### Results
[More Information Needed]
#### Summary
## Model Examination [optional]
<!-- Relevant interpretability work for the model goes here -->
[More Information Needed]
## Environmental Impact
<!-- Total emissions (in grams of CO2eq) and additional considerations, such as electricity usage, go here. Edit the suggested text below accordingly -->
Carbon emissions can be estimated using the [Machine Learning Impact calculator](https://mlco2.github.io/impact#compute) presented in [Lacoste et al. (2019)](https://arxiv.org/abs/1910.09700).
- **Hardware Type:** [More Information Needed]
- **Hours used:** [More Information Needed]
- **Cloud Provider:** [More Information Needed]
- **Compute Region:** [More Information Needed]
- **Carbon Emitted:** [More Information Needed]
## Technical Specifications [optional]
### Model Architecture and Objective
[More Information Needed]
### Compute Infrastructure
[More Information Needed]
#### Hardware
[More Information Needed]
#### Software
[More Information Needed]
## Citation [optional]
<!-- If there is a paper or blog post introducing the model, the APA and Bibtex information for that should go in this section. -->
**BibTeX:**
[More Information Needed]
**APA:**
[More Information Needed]
## Glossary [optional]
<!-- If relevant, include terms and calculations in this section that can help readers understand the model or model card. -->
[More Information Needed]
## More Information [optional]
[More Information Needed]
## Model Card Authors [optional]
[More Information Needed]
## Model Card Contact
[More Information Needed]
| {"library_name": "transformers", "tags": []} | Enagamirzayev/whisper-small-llm-lingo_aa | null | [
"transformers",
"safetensors",
"whisper",
"automatic-speech-recognition",
"arxiv:1910.09700",
"endpoints_compatible",
"region:us"
] | null | 2024-04-21T19:34:05+00:00 | [
"1910.09700"
] | [] | TAGS
#transformers #safetensors #whisper #automatic-speech-recognition #arxiv-1910.09700 #endpoints_compatible #region-us
|
# Model Card for Model ID
## Model Details
### Model Description
This is the model card of a transformers model that has been pushed on the Hub. This model card has been automatically generated.
- Developed by:
- Funded by [optional]:
- Shared by [optional]:
- Model type:
- Language(s) (NLP):
- License:
- Finetuned from model [optional]:
### Model Sources [optional]
- Repository:
- Paper [optional]:
- Demo [optional]:
## Uses
### Direct Use
### Downstream Use [optional]
### Out-of-Scope Use
## Bias, Risks, and Limitations
### Recommendations
Users (both direct and downstream) should be made aware of the risks, biases and limitations of the model. More information needed for further recommendations.
## How to Get Started with the Model
Use the code below to get started with the model.
## Training Details
### Training Data
### Training Procedure
#### Preprocessing [optional]
#### Training Hyperparameters
- Training regime:
#### Speeds, Sizes, Times [optional]
## Evaluation
### Testing Data, Factors & Metrics
#### Testing Data
#### Factors
#### Metrics
### Results
#### Summary
## Model Examination [optional]
## Environmental Impact
Carbon emissions can be estimated using the Machine Learning Impact calculator presented in Lacoste et al. (2019).
- Hardware Type:
- Hours used:
- Cloud Provider:
- Compute Region:
- Carbon Emitted:
## Technical Specifications [optional]
### Model Architecture and Objective
### Compute Infrastructure
#### Hardware
#### Software
[optional]
BibTeX:
APA:
## Glossary [optional]
## More Information [optional]
## Model Card Authors [optional]
## Model Card Contact
| [
"# Model Card for Model ID",
"## Model Details",
"### Model Description\n\n\n\nThis is the model card of a transformers model that has been pushed on the Hub. This model card has been automatically generated.\n\n- Developed by: \n- Funded by [optional]: \n- Shared by [optional]: \n- Model type: \n- Language(s) (NLP): \n- License: \n- Finetuned from model [optional]:",
"### Model Sources [optional]\n\n\n\n- Repository: \n- Paper [optional]: \n- Demo [optional]:",
"## Uses",
"### Direct Use",
"### Downstream Use [optional]",
"### Out-of-Scope Use",
"## Bias, Risks, and Limitations",
"### Recommendations\n\n\n\nUsers (both direct and downstream) should be made aware of the risks, biases and limitations of the model. More information needed for further recommendations.",
"## How to Get Started with the Model\n\nUse the code below to get started with the model.",
"## Training Details",
"### Training Data",
"### Training Procedure",
"#### Preprocessing [optional]",
"#### Training Hyperparameters\n\n- Training regime:",
"#### Speeds, Sizes, Times [optional]",
"## Evaluation",
"### Testing Data, Factors & Metrics",
"#### Testing Data",
"#### Factors",
"#### Metrics",
"### Results",
"#### Summary",
"## Model Examination [optional]",
"## Environmental Impact\n\n\n\nCarbon emissions can be estimated using the Machine Learning Impact calculator presented in Lacoste et al. (2019).\n\n- Hardware Type: \n- Hours used: \n- Cloud Provider: \n- Compute Region: \n- Carbon Emitted:",
"## Technical Specifications [optional]",
"### Model Architecture and Objective",
"### Compute Infrastructure",
"#### Hardware",
"#### Software\n\n\n\n[optional]\n\n\n\nBibTeX:\n\n\n\nAPA:",
"## Glossary [optional]",
"## More Information [optional]",
"## Model Card Authors [optional]",
"## Model Card Contact"
] | [
"TAGS\n#transformers #safetensors #whisper #automatic-speech-recognition #arxiv-1910.09700 #endpoints_compatible #region-us \n",
"# Model Card for Model ID",
"## Model Details",
"### Model Description\n\n\n\nThis is the model card of a transformers model that has been pushed on the Hub. This model card has been automatically generated.\n\n- Developed by: \n- Funded by [optional]: \n- Shared by [optional]: \n- Model type: \n- Language(s) (NLP): \n- License: \n- Finetuned from model [optional]:",
"### Model Sources [optional]\n\n\n\n- Repository: \n- Paper [optional]: \n- Demo [optional]:",
"## Uses",
"### Direct Use",
"### Downstream Use [optional]",
"### Out-of-Scope Use",
"## Bias, Risks, and Limitations",
"### Recommendations\n\n\n\nUsers (both direct and downstream) should be made aware of the risks, biases and limitations of the model. More information needed for further recommendations.",
"## How to Get Started with the Model\n\nUse the code below to get started with the model.",
"## Training Details",
"### Training Data",
"### Training Procedure",
"#### Preprocessing [optional]",
"#### Training Hyperparameters\n\n- Training regime:",
"#### Speeds, Sizes, Times [optional]",
"## Evaluation",
"### Testing Data, Factors & Metrics",
"#### Testing Data",
"#### Factors",
"#### Metrics",
"### Results",
"#### Summary",
"## Model Examination [optional]",
"## Environmental Impact\n\n\n\nCarbon emissions can be estimated using the Machine Learning Impact calculator presented in Lacoste et al. (2019).\n\n- Hardware Type: \n- Hours used: \n- Cloud Provider: \n- Compute Region: \n- Carbon Emitted:",
"## Technical Specifications [optional]",
"### Model Architecture and Objective",
"### Compute Infrastructure",
"#### Hardware",
"#### Software\n\n\n\n[optional]\n\n\n\nBibTeX:\n\n\n\nAPA:",
"## Glossary [optional]",
"## More Information [optional]",
"## Model Card Authors [optional]",
"## Model Card Contact"
] |
reinforcement-learning | null |
# **Q-Learning** Agent playing1 **FrozenLake-v1**
This is a trained model of a **Q-Learning** agent playing **FrozenLake-v1** .
## Usage
```python
model = load_from_hub(repo_id="rahil1206/q-FrozenLake-v1-4x4-noSlippery", filename="q-learning.pkl")
# Don't forget to check if you need to add additional attributes (is_slippery=False etc)
env = gym.make(model["env_id"])
```
| {"tags": ["FrozenLake-v1-4x4-no_slippery", "q-learning", "reinforcement-learning", "custom-implementation"], "model-index": [{"name": "q-FrozenLake-v1-4x4-noSlippery", "results": [{"task": {"type": "reinforcement-learning", "name": "reinforcement-learning"}, "dataset": {"name": "FrozenLake-v1-4x4-no_slippery", "type": "FrozenLake-v1-4x4-no_slippery"}, "metrics": [{"type": "mean_reward", "value": "1.00 +/- 0.00", "name": "mean_reward", "verified": false}]}]}]} | rahil1206/q-FrozenLake-v1-4x4-noSlippery | null | [
"FrozenLake-v1-4x4-no_slippery",
"q-learning",
"reinforcement-learning",
"custom-implementation",
"model-index",
"region:us"
] | null | 2024-04-21T19:34:42+00:00 | [] | [] | TAGS
#FrozenLake-v1-4x4-no_slippery #q-learning #reinforcement-learning #custom-implementation #model-index #region-us
|
# Q-Learning Agent playing1 FrozenLake-v1
This is a trained model of a Q-Learning agent playing FrozenLake-v1 .
## Usage
| [
"# Q-Learning Agent playing1 FrozenLake-v1\n This is a trained model of a Q-Learning agent playing FrozenLake-v1 .\n\n ## Usage"
] | [
"TAGS\n#FrozenLake-v1-4x4-no_slippery #q-learning #reinforcement-learning #custom-implementation #model-index #region-us \n",
"# Q-Learning Agent playing1 FrozenLake-v1\n This is a trained model of a Q-Learning agent playing FrozenLake-v1 .\n\n ## Usage"
] |
null | null | Quantization made by Richard Erkhov.
[Github](https://github.com/RichardErkhov)
[Discord](https://discord.gg/pvy7H8DZMG)
[Request more models](https://github.com/RichardErkhov/quant_request)
CodeLlama-34b-Instruct-hf - GGUF
- Model creator: https://huggingface.co/meta-llama/
- Original model: https://huggingface.co/meta-llama/CodeLlama-34b-Instruct-hf/
| Name | Quant method | Size |
| ---- | ---- | ---- |
| [CodeLlama-34b-Instruct-hf.Q2_K.gguf](https://huggingface.co/RichardErkhov/meta-llama_-_CodeLlama-34b-Instruct-hf-gguf/blob/main/CodeLlama-34b-Instruct-hf.Q2_K.gguf) | Q2_K | 11.65GB |
| [CodeLlama-34b-Instruct-hf.IQ3_XS.gguf](https://huggingface.co/RichardErkhov/meta-llama_-_CodeLlama-34b-Instruct-hf-gguf/blob/main/CodeLlama-34b-Instruct-hf.IQ3_XS.gguf) | IQ3_XS | 12.93GB |
| [CodeLlama-34b-Instruct-hf.IQ3_S.gguf](https://huggingface.co/RichardErkhov/meta-llama_-_CodeLlama-34b-Instruct-hf-gguf/blob/main/CodeLlama-34b-Instruct-hf.IQ3_S.gguf) | IQ3_S | 13.65GB |
| [CodeLlama-34b-Instruct-hf.Q3_K_S.gguf](https://huggingface.co/RichardErkhov/meta-llama_-_CodeLlama-34b-Instruct-hf-gguf/blob/main/CodeLlama-34b-Instruct-hf.Q3_K_S.gguf) | Q3_K_S | 13.6GB |
| [CodeLlama-34b-Instruct-hf.IQ3_M.gguf](https://huggingface.co/RichardErkhov/meta-llama_-_CodeLlama-34b-Instruct-hf-gguf/blob/main/CodeLlama-34b-Instruct-hf.IQ3_M.gguf) | IQ3_M | 14.18GB |
| [CodeLlama-34b-Instruct-hf.Q3_K.gguf](https://huggingface.co/RichardErkhov/meta-llama_-_CodeLlama-34b-Instruct-hf-gguf/blob/main/CodeLlama-34b-Instruct-hf.Q3_K.gguf) | Q3_K | 15.19GB |
| [CodeLlama-34b-Instruct-hf.Q3_K_M.gguf](https://huggingface.co/RichardErkhov/meta-llama_-_CodeLlama-34b-Instruct-hf-gguf/blob/main/CodeLlama-34b-Instruct-hf.Q3_K_M.gguf) | Q3_K_M | 15.19GB |
| [CodeLlama-34b-Instruct-hf.Q3_K_L.gguf](https://huggingface.co/RichardErkhov/meta-llama_-_CodeLlama-34b-Instruct-hf-gguf/blob/main/CodeLlama-34b-Instruct-hf.Q3_K_L.gguf) | Q3_K_L | 16.55GB |
| [CodeLlama-34b-Instruct-hf.IQ4_XS.gguf](https://huggingface.co/RichardErkhov/meta-llama_-_CodeLlama-34b-Instruct-hf-gguf/blob/main/CodeLlama-34b-Instruct-hf.IQ4_XS.gguf) | IQ4_XS | 16.99GB |
| [CodeLlama-34b-Instruct-hf.Q4_0.gguf](https://huggingface.co/RichardErkhov/meta-llama_-_CodeLlama-34b-Instruct-hf-gguf/blob/main/CodeLlama-34b-Instruct-hf.Q4_0.gguf) | Q4_0 | 17.74GB |
| [CodeLlama-34b-Instruct-hf.IQ4_NL.gguf](https://huggingface.co/RichardErkhov/meta-llama_-_CodeLlama-34b-Instruct-hf-gguf/blob/main/CodeLlama-34b-Instruct-hf.IQ4_NL.gguf) | IQ4_NL | 17.92GB |
| [CodeLlama-34b-Instruct-hf.Q4_K_S.gguf](https://huggingface.co/RichardErkhov/meta-llama_-_CodeLlama-34b-Instruct-hf-gguf/blob/main/CodeLlama-34b-Instruct-hf.Q4_K_S.gguf) | Q4_K_S | 17.87GB |
| [CodeLlama-34b-Instruct-hf.Q4_K.gguf](https://huggingface.co/RichardErkhov/meta-llama_-_CodeLlama-34b-Instruct-hf-gguf/blob/main/CodeLlama-34b-Instruct-hf.Q4_K.gguf) | Q4_K | 18.83GB |
| [CodeLlama-34b-Instruct-hf.Q4_K_M.gguf](https://huggingface.co/RichardErkhov/meta-llama_-_CodeLlama-34b-Instruct-hf-gguf/blob/main/CodeLlama-34b-Instruct-hf.Q4_K_M.gguf) | Q4_K_M | 18.83GB |
| [CodeLlama-34b-Instruct-hf.Q4_1.gguf](https://huggingface.co/RichardErkhov/meta-llama_-_CodeLlama-34b-Instruct-hf-gguf/blob/main/CodeLlama-34b-Instruct-hf.Q4_1.gguf) | Q4_1 | 19.69GB |
| [CodeLlama-34b-Instruct-hf.Q5_0.gguf](https://huggingface.co/RichardErkhov/meta-llama_-_CodeLlama-34b-Instruct-hf-gguf/blob/main/CodeLlama-34b-Instruct-hf.Q5_0.gguf) | Q5_0 | 21.64GB |
| [CodeLlama-34b-Instruct-hf.Q5_K_S.gguf](https://huggingface.co/RichardErkhov/meta-llama_-_CodeLlama-34b-Instruct-hf-gguf/blob/main/CodeLlama-34b-Instruct-hf.Q5_K_S.gguf) | Q5_K_S | 21.64GB |
| [CodeLlama-34b-Instruct-hf.Q5_K.gguf](https://huggingface.co/RichardErkhov/meta-llama_-_CodeLlama-34b-Instruct-hf-gguf/blob/main/CodeLlama-34b-Instruct-hf.Q5_K.gguf) | Q5_K | 22.2GB |
| [CodeLlama-34b-Instruct-hf.Q5_K_M.gguf](https://huggingface.co/RichardErkhov/meta-llama_-_CodeLlama-34b-Instruct-hf-gguf/blob/main/CodeLlama-34b-Instruct-hf.Q5_K_M.gguf) | Q5_K_M | 22.2GB |
| [CodeLlama-34b-Instruct-hf.Q5_1.gguf](https://huggingface.co/RichardErkhov/meta-llama_-_CodeLlama-34b-Instruct-hf-gguf/blob/main/CodeLlama-34b-Instruct-hf.Q5_1.gguf) | Q5_1 | 23.59GB |
| [CodeLlama-34b-Instruct-hf.Q6_K.gguf](https://huggingface.co/RichardErkhov/meta-llama_-_CodeLlama-34b-Instruct-hf-gguf/blob/main/CodeLlama-34b-Instruct-hf.Q6_K.gguf) | Q6_K | 25.78GB |
Original model description:
---
extra_gated_heading: You need to share contact information with Meta to access this model
extra_gated_prompt: >-
### LLAMA 2 COMMUNITY LICENSE AGREEMENT
"Agreement" means the terms and conditions for use, reproduction, distribution
and modification of the Llama Materials set forth herein.
"Documentation" means the specifications, manuals and documentation
accompanying Llama 2 distributed by Meta at
https://ai.meta.com/resources/models-and-libraries/llama-downloads/.
"Licensee" or "you" means you, or your employer or any other person or entity
(if you are entering into this Agreement on such person or entity's behalf),
of the age required under applicable laws, rules or regulations to provide
legal consent and that has legal authority to bind your employer or such other
person or entity if you are entering in this Agreement on their behalf.
"Llama 2" means the foundational large language models and software and
algorithms, including machine-learning model code, trained model weights,
inference-enabling code, training-enabling code, fine-tuning enabling code and
other elements of the foregoing distributed by Meta at
ai.meta.com/resources/models-and-libraries/llama-downloads/.
"Llama Materials" means, collectively, Meta's proprietary Llama 2 and
documentation (and any portion thereof) made available under this Agreement.
"Meta" or "we" means Meta Platforms Ireland Limited (if you are located in or,
if you are an entity, your principal place of business is in the EEA or
Switzerland) and Meta Platforms, Inc. (if you are located outside of the EEA
or Switzerland).
By clicking "I Accept" below or by using or distributing any portion or
element of the Llama Materials, you agree to be bound by this Agreement.
1. License Rights and Redistribution.
a. Grant of Rights. You are granted a non-exclusive, worldwide, non-
transferable and royalty-free limited license under Meta's intellectual
property or other rights owned by Meta embodied in the Llama Materials to
use, reproduce, distribute, copy, create derivative works of, and make
modifications to the Llama Materials.
b. Redistribution and Use.
i. If you distribute or make the Llama Materials, or any derivative works
thereof, available to a third party, you shall provide a copy of this
Agreement to such third party.
ii. If you receive Llama Materials, or any derivative works thereof, from a
Licensee as part of an integrated end user product, then Section 2 of this
Agreement will not apply to you.
iii. You must retain in all copies of the Llama Materials that you distribute
the following attribution notice within a "Notice" text file distributed as a
part of such copies: "Llama 2 is licensed under the LLAMA 2 Community
License, Copyright (c) Meta Platforms, Inc. All Rights Reserved."
iv. Your use of the Llama Materials must comply with applicable laws and
regulations (including trade compliance laws and regulations) and adhere to
the Acceptable Use Policy for the Llama Materials (available at
https://ai.meta.com/llama/use-policy), which is hereby incorporated by
reference into this Agreement.
v. You will not use the Llama Materials or any output or results of the Llama
Materials to improve any other large language model (excluding Llama 2 or
derivative works thereof).
2. Additional Commercial Terms. If, on the Llama 2 version release date, the
monthly active users of the products or services made available by or for
Licensee, or Licensee's affiliates, is greater than 700 million monthly
active users in the preceding calendar month, you must request a license from
Meta, which Meta may grant to you in its sole discretion, and you are not
authorized to exercise any of the rights under this Agreement unless or until
Meta otherwise expressly grants you such rights.
3. Disclaimer of Warranty. UNLESS REQUIRED BY APPLICABLE LAW, THE LLAMA
MATERIALS AND ANY OUTPUT AND RESULTS THEREFROM ARE PROVIDED ON AN "AS IS"
BASIS, WITHOUT WARRANTIES OF ANY KIND, EITHER EXPRESS OR IMPLIED, INCLUDING,
WITHOUT LIMITATION, ANY WARRANTIES OF TITLE, NON-INFRINGEMENT,
MERCHANTABILITY, OR FITNESS FOR A PARTICULAR PURPOSE. YOU ARE SOLELY
RESPONSIBLE FOR DETERMINING THE APPROPRIATENESS OF USING OR REDISTRIBUTING
THE LLAMA MATERIALS AND ASSUME ANY RISKS ASSOCIATED WITH YOUR USE OF THE
LLAMA MATERIALS AND ANY OUTPUT AND RESULTS.
4. Limitation of Liability. IN NO EVENT WILL META OR ITS AFFILIATES BE LIABLE
UNDER ANY THEORY OF LIABILITY, WHETHER IN CONTRACT, TORT, NEGLIGENCE,
PRODUCTS LIABILITY, OR OTHERWISE, ARISING OUT OF THIS AGREEMENT, FOR ANY LOST
PROFITS OR ANY INDIRECT, SPECIAL, CONSEQUENTIAL, INCIDENTAL, EXEMPLARY OR
PUNITIVE DAMAGES, EVEN IF META OR ITS AFFILIATES HAVE BEEN ADVISED OF THE
POSSIBILITY OF ANY OF THE FOREGOING.
5. Intellectual Property.
a. No trademark licenses are granted under this Agreement, and in connection
with the Llama Materials, neither Meta nor Licensee may use any name or mark
owned by or associated with the other or any of its affiliates, except as
required for reasonable and customary use in describing and redistributing
the Llama Materials.
b. Subject to Meta's ownership of Llama Materials and derivatives made by or
for Meta, with respect to any derivative works and modifications of the Llama
Materials that are made by you, as between you and Meta, you are and will be
the owner of such derivative works and modifications.
c. If you institute litigation or other proceedings against Meta or any
entity (including a cross-claim or counterclaim in a lawsuit) alleging that
the Llama Materials or Llama 2 outputs or results, or any portion of any of
the foregoing, constitutes infringement of intellectual property or other
rights owned or licensable by you, then any licenses granted to you under
this Agreement shall terminate as of the date such litigation or claim is
filed or instituted. You will indemnify and hold harmless Meta from and
against any claim by any third party arising out of or related to your use or
distribution of the Llama Materials.
6. Term and Termination. The term of this Agreement will commence upon your
acceptance of this Agreement or access to the Llama Materials and will
continue in full force and effect until terminated in accordance with the
terms and conditions herein. Meta may terminate this Agreement if you are in
breach of any term or condition of this Agreement. Upon termination of this
Agreement, you shall delete and cease use of the Llama Materials. Sections 3,
4 and 7 shall survive the termination of this Agreement.
7. Governing Law and Jurisdiction. This Agreement will be governed and
construed under the laws of the State of California without regard to choice
of law principles, and the UN Convention on Contracts for the International
Sale of Goods does not apply to this Agreement. The courts of California
shall have exclusive jurisdiction of any dispute arising out of this
Agreement.
USE POLICY
### Llama 2 Acceptable Use Policy
Meta is committed to promoting safe and fair use of its tools and features,
including Llama 2. If you access or use Llama 2, you agree to this Acceptable
Use Policy (“Policy”). The most recent copy of this policy can be found at
[ai.meta.com/llama/use-policy](http://ai.meta.com/llama/use-policy).
#### Prohibited Uses
We want everyone to use Llama 2 safely and responsibly. You agree you will not
use, or allow others to use, Llama 2 to:
1. Violate the law or others’ rights, including to:
1. Engage in, promote, generate, contribute to, encourage, plan, incite, or further illegal or unlawful activity or content, such as:
1. Violence or terrorism
2. Exploitation or harm to children, including the solicitation, creation, acquisition, or dissemination of child exploitative content or failure to report Child Sexual Abuse Material
3. Human trafficking, exploitation, and sexual violence
4. The illegal distribution of information or materials to minors, including obscene materials, or failure to employ legally required age-gating in connection with such information or materials.
5. Sexual solicitation
6. Any other criminal activity
2. Engage in, promote, incite, or facilitate the harassment, abuse, threatening, or bullying of individuals or groups of individuals
3. Engage in, promote, incite, or facilitate discrimination or other unlawful or harmful conduct in the provision of employment, employment benefits, credit, housing, other economic benefits, or other essential goods and services
4. Engage in the unauthorized or unlicensed practice of any profession including, but not limited to, financial, legal, medical/health, or related professional practices
5. Collect, process, disclose, generate, or infer health, demographic, or other sensitive personal or private information about individuals without rights and consents required by applicable laws
6. Engage in or facilitate any action or generate any content that infringes, misappropriates, or otherwise violates any third-party rights, including the outputs or results of any products or services using the Llama 2 Materials
7. Create, generate, or facilitate the creation of malicious code, malware, computer viruses or do anything else that could disable, overburden, interfere with or impair the proper working, integrity, operation or appearance of a website or computer system
2. Engage in, promote, incite, facilitate, or assist in the planning or
development of activities that present a risk of death or bodily harm to
individuals, including use of Llama 2 related to the following:
1. Military, warfare, nuclear industries or applications, espionage, use for materials or activities that are subject to the International Traffic Arms Regulations (ITAR) maintained by the United States Department of State
2. Guns and illegal weapons (including weapon development)
3. Illegal drugs and regulated/controlled substances
4. Operation of critical infrastructure, transportation technologies, or heavy machinery
5. Self-harm or harm to others, including suicide, cutting, and eating disorders
6. Any content intended to incite or promote violence, abuse, or any infliction of bodily harm to an individual
3. Intentionally deceive or mislead others, including use of Llama 2 related
to the following:
1. Generating, promoting, or furthering fraud or the creation or promotion of disinformation
2. Generating, promoting, or furthering defamatory content, including the creation of defamatory statements, images, or other content
3. Generating, promoting, or further distributing spam
4. Impersonating another individual without consent, authorization, or legal right
5. Representing that the use of Llama 2 or outputs are human-generated
6. Generating or facilitating false online engagement, including fake reviews and other means of fake online engagement
4. Fail to appropriately disclose to end users any known dangers of your AI system
Please report any violation of this Policy, software “bug,” or other problems
that could lead to a violation of this Policy through one of the following
means:
* Reporting issues with the model:
[github.com/facebookresearch/llama](http://github.com/facebookresearch/llama)
* Reporting risky content generated by the model:
[developers.facebook.com/llama_output_feedback](http://developers.facebook.com/llama_output_feedback)
* Reporting bugs and security concerns:
[facebook.com/whitehat/info](http://facebook.com/whitehat/info)
* Reporting violations of the Acceptable Use Policy or unlicensed uses of
Llama: [[email protected]](mailto:[email protected])
extra_gated_fields:
First Name: text
Last Name: text
Date of birth: date_picker
Country: country
Affiliation: text
geo: ip_location
By clicking Submit below I accept the terms of the license and acknowledge that the information I provide will be collected stored processed and shared in accordance with the Meta Privacy Policy: checkbox
extra_gated_description: The information you provide will be collected, stored, processed and shared in accordance with the [Meta Privacy Policy](https://www.facebook.com/privacy/policy/).
extra_gated_button_content: Submit
language:
- code
pipeline_tag: text-generation
tags:
- facebook
- meta
- pytorch
- llama
- llama-2
license: llama2
---
# **Code Llama**
Code Llama is a collection of pretrained and fine-tuned generative text models ranging in scale from 7 billion to 34 billion parameters. This is the repository for the 34B instruct-tuned version in the Hugging Face Transformers format. This model is designed for general code synthesis and understanding. Links to other models can be found in the index at the bottom.
| | Base Model | Python | Instruct |
| --- | ----------------------------------------------------------------------------- | ------------------------------------------------------------------------------------------- | ----------------------------------------------------------------------------------------------- |
| 7B | [meta-llama/CodeLlama-7b-hf](https://huggingface.co/meta-llama/CodeLlama-7b-hf) | [meta-llama/CodeLlama-7b-Python-hf](https://huggingface.co/meta-llama/CodeLlama-7b-Python-hf) | [meta-llama/CodeLlama-7b-Instruct-hf](https://huggingface.co/meta-llama/CodeLlama-7b-Instruct-hf) |
| 13B | [meta-llama/CodeLlama-13b-hf](https://huggingface.co/meta-llama/CodeLlama-13b-hf) | [meta-llama/CodeLlama-13b-Python-hf](https://huggingface.co/meta-llama/CodeLlama-13b-Python-hf) | [meta-llama/CodeLlama-13b-Instruct-hf](https://huggingface.co/meta-llama/CodeLlama-13b-Instruct-hf) |
| 34B | [meta-llama/CodeLlama-34b-hf](https://huggingface.co/meta-llama/CodeLlama-34b-hf) | [meta-llama/CodeLlama-34b-Python-hf](https://huggingface.co/meta-llama/CodeLlama-34b-Python-hf) | [meta-llama/CodeLlama-34b-Instruct-hf](https://huggingface.co/meta-llama/CodeLlama-34b-Instruct-hf) |
| 70B | [meta-llama/CodeLlama-70b-hf](https://huggingface.co/meta-llama/CodeLlama-70b-hf) | [meta-llama/CodeLlama-70b-Python-hf](https://huggingface.co/meta-llama/CodeLlama-70b-Python-hf) | [meta-llama/CodeLlama-70b-Instruct-hf](https://huggingface.co/meta-llama/CodeLlama-70b-Instruct-hf) |
## Model Use
To use this model, please make sure to install transformers:
```bash
pip install transformers accelerate
```
Model capabilities:
- [x] Code completion.
- [ ] Infilling.
- [x] Instructions / chat.
- [ ] Python specialist.
## Model Details
*Note: Use of this model is governed by the Meta license. Meta developed and publicly released the Code Llama family of large language models (LLMs).
**Model Developers** Meta
**Variations** Code Llama comes in three model sizes, and three variants:
* Code Llama: base models designed for general code synthesis and understanding
* Code Llama - Python: designed specifically for Python
* Code Llama - Instruct: for instruction following and safer deployment
All variants are available in sizes of 7B, 13B and 34B parameters.
**This repository contains the Instruct version of the 34B parameters model.**
**Input** Models input text only.
**Output** Models generate text only.
**Model Architecture** Code Llama is an auto-regressive language model that uses an optimized transformer architecture.
**Model Dates** Code Llama and its variants have been trained between January 2023 and July 2023.
**Status** This is a static model trained on an offline dataset. Future versions of Code Llama - Instruct will be released as we improve model safety with community feedback.
**License** A custom commercial license is available at: [https://ai.meta.com/resources/models-and-libraries/llama-downloads/](https://ai.meta.com/resources/models-and-libraries/llama-downloads/)
**Research Paper** More information can be found in the paper "[Code Llama: Open Foundation Models for Code](https://ai.meta.com/research/publications/code-llama-open-foundation-models-for-code/)" or its [arXiv page](https://arxiv.org/abs/2308.12950).
## Intended Use
**Intended Use Cases** Code Llama and its variants is intended for commercial and research use in English and relevant programming languages. The base model Code Llama can be adapted for a variety of code synthesis and understanding tasks, Code Llama - Python is designed specifically to handle the Python programming language, and Code Llama - Instruct is intended to be safer to use for code assistant and generation applications.
**Out-of-Scope Uses** Use in any manner that violates applicable laws or regulations (including trade compliance laws). Use in languages other than English. Use in any other way that is prohibited by the Acceptable Use Policy and Licensing Agreement for Code Llama and its variants.
## Hardware and Software
**Training Factors** We used custom training libraries. The training and fine-tuning of the released models have been performed Meta’s Research Super Cluster.
**Carbon Footprint** In aggregate, training all 9 Code Llama models required 400K GPU hours of computation on hardware of type A100-80GB (TDP of 350-400W). Estimated total emissions were 65.3 tCO2eq, 100% of which were offset by Meta’s sustainability program.
## Training Data
All experiments reported here and the released models have been trained and fine-tuned using the same data as Llama 2 with different weights (see Section 2 and Table 1 in the [research paper](https://ai.meta.com/research/publications/code-llama-open-foundation-models-for-code/) for details).
## Evaluation Results
See evaluations for the main models and detailed ablations in Section 3 and safety evaluations in Section 4 of the research paper.
## Ethical Considerations and Limitations
Code Llama and its variants are a new technology that carries risks with use. Testing conducted to date has been in English, and has not covered, nor could it cover all scenarios. For these reasons, as with all LLMs, Code Llama’s potential outputs cannot be predicted in advance, and the model may in some instances produce inaccurate or objectionable responses to user prompts. Therefore, before deploying any applications of Code Llama, developers should perform safety testing and tuning tailored to their specific applications of the model.
Please see the Responsible Use Guide available available at [https://ai.meta.com/llama/responsible-use-guide](https://ai.meta.com/llama/responsible-use-guide).
| {} | RichardErkhov/meta-llama_-_CodeLlama-34b-Instruct-hf-gguf | null | [
"gguf",
"arxiv:2308.12950",
"region:us"
] | null | 2024-04-21T19:35:38+00:00 | [
"2308.12950"
] | [] | TAGS
#gguf #arxiv-2308.12950 #region-us
| Quantization made by Richard Erkhov.
Github
Discord
Request more models
CodeLlama-34b-Instruct-hf - GGUF
* Model creator: URL
* Original model: URL
Name: CodeLlama-34b-Instruct-hf.Q2\_K.gguf, Quant method: Q2\_K, Size: 11.65GB
Name: CodeLlama-34b-Instruct-hf.IQ3\_XS.gguf, Quant method: IQ3\_XS, Size: 12.93GB
Name: CodeLlama-34b-Instruct-hf.IQ3\_S.gguf, Quant method: IQ3\_S, Size: 13.65GB
Name: CodeLlama-34b-Instruct-hf.Q3\_K\_S.gguf, Quant method: Q3\_K\_S, Size: 13.6GB
Name: CodeLlama-34b-Instruct-hf.IQ3\_M.gguf, Quant method: IQ3\_M, Size: 14.18GB
Name: CodeLlama-34b-Instruct-hf.Q3\_K.gguf, Quant method: Q3\_K, Size: 15.19GB
Name: CodeLlama-34b-Instruct-hf.Q3\_K\_M.gguf, Quant method: Q3\_K\_M, Size: 15.19GB
Name: CodeLlama-34b-Instruct-hf.Q3\_K\_L.gguf, Quant method: Q3\_K\_L, Size: 16.55GB
Name: CodeLlama-34b-Instruct-hf.IQ4\_XS.gguf, Quant method: IQ4\_XS, Size: 16.99GB
Name: CodeLlama-34b-Instruct-hf.Q4\_0.gguf, Quant method: Q4\_0, Size: 17.74GB
Name: CodeLlama-34b-Instruct-hf.IQ4\_NL.gguf, Quant method: IQ4\_NL, Size: 17.92GB
Name: CodeLlama-34b-Instruct-hf.Q4\_K\_S.gguf, Quant method: Q4\_K\_S, Size: 17.87GB
Name: CodeLlama-34b-Instruct-hf.Q4\_K.gguf, Quant method: Q4\_K, Size: 18.83GB
Name: CodeLlama-34b-Instruct-hf.Q4\_K\_M.gguf, Quant method: Q4\_K\_M, Size: 18.83GB
Name: CodeLlama-34b-Instruct-hf.Q4\_1.gguf, Quant method: Q4\_1, Size: 19.69GB
Name: CodeLlama-34b-Instruct-hf.Q5\_0.gguf, Quant method: Q5\_0, Size: 21.64GB
Name: CodeLlama-34b-Instruct-hf.Q5\_K\_S.gguf, Quant method: Q5\_K\_S, Size: 21.64GB
Name: CodeLlama-34b-Instruct-hf.Q5\_K.gguf, Quant method: Q5\_K, Size: 22.2GB
Name: CodeLlama-34b-Instruct-hf.Q5\_K\_M.gguf, Quant method: Q5\_K\_M, Size: 22.2GB
Name: CodeLlama-34b-Instruct-hf.Q5\_1.gguf, Quant method: Q5\_1, Size: 23.59GB
Name: CodeLlama-34b-Instruct-hf.Q6\_K.gguf, Quant method: Q6\_K, Size: 25.78GB
Original model description:
---------------------------
extra\_gated\_heading: You need to share contact information with Meta to access this model
extra\_gated\_prompt: >-
### LLAMA 2 COMMUNITY LICENSE AGREEMENT
"Agreement" means the terms and conditions for use, reproduction, distribution
and modification of the Llama Materials set forth herein.
"Documentation" means the specifications, manuals and documentation
accompanying Llama 2 distributed by Meta at
URL
"Licensee" or "you" means you, or your employer or any other person or entity
(if you are entering into this Agreement on such person or entity's behalf),
of the age required under applicable laws, rules or regulations to provide
legal consent and that has legal authority to bind your employer or such other
person or entity if you are entering in this Agreement on their behalf.
"Llama 2" means the foundational large language models and software and
algorithms, including machine-learning model code, trained model weights,
inference-enabling code, training-enabling code, fine-tuning enabling code and
other elements of the foregoing distributed by Meta at
URL
"Llama Materials" means, collectively, Meta's proprietary Llama 2 and
documentation (and any portion thereof) made available under this Agreement.
"Meta" or "we" means Meta Platforms Ireland Limited (if you are located in or,
if you are an entity, your principal place of business is in the EEA or
Switzerland) and Meta Platforms, Inc. (if you are located outside of the EEA
or Switzerland).
By clicking "I Accept" below or by using or distributing any portion or
element of the Llama Materials, you agree to be bound by this Agreement.
1. License Rights and Redistribution.
a. Grant of Rights. You are granted a non-exclusive, worldwide, non-
transferable and royalty-free limited license under Meta's intellectual
property or other rights owned by Meta embodied in the Llama Materials to
use, reproduce, distribute, copy, create derivative works of, and make
modifications to the Llama Materials.
b. Redistribution and Use.
i. If you distribute or make the Llama Materials, or any derivative works
thereof, available to a third party, you shall provide a copy of this
Agreement to such third party.
ii. If you receive Llama Materials, or any derivative works thereof, from a
Licensee as part of an integrated end user product, then Section 2 of this
Agreement will not apply to you.
iii. You must retain in all copies of the Llama Materials that you distribute
the following attribution notice within a "Notice" text file distributed as a
part of such copies: "Llama 2 is licensed under the LLAMA 2 Community
License, Copyright (c) Meta Platforms, Inc. All Rights Reserved."
iv. Your use of the Llama Materials must comply with applicable laws and
regulations (including trade compliance laws and regulations) and adhere to
the Acceptable Use Policy for the Llama Materials (available at
URL which is hereby incorporated by
reference into this Agreement.
v. You will not use the Llama Materials or any output or results of the Llama
Materials to improve any other large language model (excluding Llama 2 or
derivative works thereof).
2. Additional Commercial Terms. If, on the Llama 2 version release date, the
monthly active users of the products or services made available by or for
Licensee, or Licensee's affiliates, is greater than 700 million monthly
active users in the preceding calendar month, you must request a license from
Meta, which Meta may grant to you in its sole discretion, and you are not
authorized to exercise any of the rights under this Agreement unless or until
Meta otherwise expressly grants you such rights.
3. Disclaimer of Warranty. UNLESS REQUIRED BY APPLICABLE LAW, THE LLAMA
MATERIALS AND ANY OUTPUT AND RESULTS THEREFROM ARE PROVIDED ON AN "AS IS"
BASIS, WITHOUT WARRANTIES OF ANY KIND, EITHER EXPRESS OR IMPLIED, INCLUDING,
WITHOUT LIMITATION, ANY WARRANTIES OF TITLE, NON-INFRINGEMENT,
MERCHANTABILITY, OR FITNESS FOR A PARTICULAR PURPOSE. YOU ARE SOLELY
RESPONSIBLE FOR DETERMINING THE APPROPRIATENESS OF USING OR REDISTRIBUTING
THE LLAMA MATERIALS AND ASSUME ANY RISKS ASSOCIATED WITH YOUR USE OF THE
LLAMA MATERIALS AND ANY OUTPUT AND RESULTS.
4. Limitation of Liability. IN NO EVENT WILL META OR ITS AFFILIATES BE LIABLE
UNDER ANY THEORY OF LIABILITY, WHETHER IN CONTRACT, TORT, NEGLIGENCE,
PRODUCTS LIABILITY, OR OTHERWISE, ARISING OUT OF THIS AGREEMENT, FOR ANY LOST
PROFITS OR ANY INDIRECT, SPECIAL, CONSEQUENTIAL, INCIDENTAL, EXEMPLARY OR
PUNITIVE DAMAGES, EVEN IF META OR ITS AFFILIATES HAVE BEEN ADVISED OF THE
POSSIBILITY OF ANY OF THE FOREGOING.
5. Intellectual Property.
a. No trademark licenses are granted under this Agreement, and in connection
with the Llama Materials, neither Meta nor Licensee may use any name or mark
owned by or associated with the other or any of its affiliates, except as
required for reasonable and customary use in describing and redistributing
the Llama Materials.
b. Subject to Meta's ownership of Llama Materials and derivatives made by or
for Meta, with respect to any derivative works and modifications of the Llama
Materials that are made by you, as between you and Meta, you are and will be
the owner of such derivative works and modifications.
c. If you institute litigation or other proceedings against Meta or any
entity (including a cross-claim or counterclaim in a lawsuit) alleging that
the Llama Materials or Llama 2 outputs or results, or any portion of any of
the foregoing, constitutes infringement of intellectual property or other
rights owned or licensable by you, then any licenses granted to you under
this Agreement shall terminate as of the date such litigation or claim is
filed or instituted. You will indemnify and hold harmless Meta from and
against any claim by any third party arising out of or related to your use or
distribution of the Llama Materials.
6. Term and Termination. The term of this Agreement will commence upon your
acceptance of this Agreement or access to the Llama Materials and will
continue in full force and effect until terminated in accordance with the
terms and conditions herein. Meta may terminate this Agreement if you are in
breach of any term or condition of this Agreement. Upon termination of this
Agreement, you shall delete and cease use of the Llama Materials. Sections 3,
4 and 7 shall survive the termination of this Agreement.
7. Governing Law and Jurisdiction. This Agreement will be governed and
construed under the laws of the State of California without regard to choice
of law principles, and the UN Convention on Contracts for the International
Sale of Goods does not apply to this Agreement. The courts of California
shall have exclusive jurisdiction of any dispute arising out of this
Agreement.
USE POLICY
### Llama 2 Acceptable Use Policy
Meta is committed to promoting safe and fair use of its tools and features,
including Llama 2. If you access or use Llama 2, you agree to this Acceptable
Use Policy (“Policy”). The most recent copy of this policy can be found at
URL
#### Prohibited Uses
We want everyone to use Llama 2 safely and responsibly. You agree you will not
use, or allow others to use, Llama 2 to:
1. Violate the law or others’ rights, including to:
1. Engage in, promote, generate, contribute to, encourage, plan, incite, or further illegal or unlawful activity or content, such as:
1. Violence or terrorism
2. Exploitation or harm to children, including the solicitation, creation, acquisition, or dissemination of child exploitative content or failure to report Child Sexual Abuse Material
3. Human trafficking, exploitation, and sexual violence
4. The illegal distribution of information or materials to minors, including obscene materials, or failure to employ legally required age-gating in connection with such information or materials.
5. Sexual solicitation
6. Any other criminal activity
```
2. Engage in, promote, incite, or facilitate the harassment, abuse, threatening, or bullying of individuals or groups of individuals
3. Engage in, promote, incite, or facilitate discrimination or other unlawful or harmful conduct in the provision of employment, employment benefits, credit, housing, other economic benefits, or other essential goods and services
4. Engage in the unauthorized or unlicensed practice of any profession including, but not limited to, financial, legal, medical/health, or related professional practices
5. Collect, process, disclose, generate, or infer health, demographic, or other sensitive personal or private information about individuals without rights and consents required by applicable laws
6. Engage in or facilitate any action or generate any content that infringes, misappropriates, or otherwise violates any third-party rights, including the outputs or results of any products or services using the Llama 2 Materials
7. Create, generate, or facilitate the creation of malicious code, malware, computer viruses or do anything else that could disable, overburden, interfere with or impair the proper working, integrity, operation or appearance of a website or computer system
```
2. Engage in, promote, incite, facilitate, or assist in the planning or
development of activities that present a risk of death or bodily harm to
individuals, including use of Llama 2 related to the following:
1. Military, warfare, nuclear industries or applications, espionage, use for materials or activities that are subject to the International Traffic Arms Regulations (ITAR) maintained by the United States Department of State
2. Guns and illegal weapons (including weapon development)
3. Illegal drugs and regulated/controlled substances
4. Operation of critical infrastructure, transportation technologies, or heavy machinery
5. Self-harm or harm to others, including suicide, cutting, and eating disorders
6. Any content intended to incite or promote violence, abuse, or any infliction of bodily harm to an individual
3. Intentionally deceive or mislead others, including use of Llama 2 related
to the following:
1. Generating, promoting, or furthering fraud or the creation or promotion of disinformation
2. Generating, promoting, or furthering defamatory content, including the creation of defamatory statements, images, or other content
3. Generating, promoting, or further distributing spam
4. Impersonating another individual without consent, authorization, or legal right
5. Representing that the use of Llama 2 or outputs are human-generated
6. Generating or facilitating false online engagement, including fake reviews and other means of fake online engagement
4. Fail to appropriately disclose to end users any known dangers of your AI system
Please report any violation of this Policy, software “bug,” or other problems
that could lead to a violation of this Policy through one of the following
means:
* Reporting issues with the model:
URL
* Reporting risky content generated by the model:
URL
* Reporting bugs and security concerns:
URL
* Reporting violations of the Acceptable Use Policy or unlicensed uses of
Llama: LlamaUseReport@URL
extra\_gated\_fields:
First Name: text
Last Name: text
Date of birth: date\_picker
Country: country
Affiliation: text
geo: ip\_location
By clicking Submit below I accept the terms of the license and acknowledge that the information I provide will be collected stored processed and shared in accordance with the Meta Privacy Policy: checkbox
extra\_gated\_description: The information you provide will be collected, stored, processed and shared in accordance with the Meta Privacy Policy.
extra\_gated\_button\_content: Submit
language:
* code
pipeline\_tag: text-generation
tags:
* facebook
* meta
* pytorch
* llama
* llama-2
license: llama2
---
Code Llama
==========
Code Llama is a collection of pretrained and fine-tuned generative text models ranging in scale from 7 billion to 34 billion parameters. This is the repository for the 34B instruct-tuned version in the Hugging Face Transformers format. This model is designed for general code synthesis and understanding. Links to other models can be found in the index at the bottom.
Model Use
---------
To use this model, please make sure to install transformers:
Model capabilities:
* [x] Code completion.
* [ ] Infilling.
* [x] Instructions / chat.
* [ ] Python specialist.
Model Details
-------------
\*Note: Use of this model is governed by the Meta license. Meta developed and publicly released the Code Llama family of large language models (LLMs).
Model Developers Meta
Variations Code Llama comes in three model sizes, and three variants:
* Code Llama: base models designed for general code synthesis and understanding
* Code Llama - Python: designed specifically for Python
* Code Llama - Instruct: for instruction following and safer deployment
All variants are available in sizes of 7B, 13B and 34B parameters.
This repository contains the Instruct version of the 34B parameters model.
Input Models input text only.
Output Models generate text only.
Model Architecture Code Llama is an auto-regressive language model that uses an optimized transformer architecture.
Model Dates Code Llama and its variants have been trained between January 2023 and July 2023.
Status This is a static model trained on an offline dataset. Future versions of Code Llama - Instruct will be released as we improve model safety with community feedback.
License A custom commercial license is available at: URL
Research Paper More information can be found in the paper "Code Llama: Open Foundation Models for Code" or its arXiv page.
Intended Use
------------
Intended Use Cases Code Llama and its variants is intended for commercial and research use in English and relevant programming languages. The base model Code Llama can be adapted for a variety of code synthesis and understanding tasks, Code Llama - Python is designed specifically to handle the Python programming language, and Code Llama - Instruct is intended to be safer to use for code assistant and generation applications.
Out-of-Scope Uses Use in any manner that violates applicable laws or regulations (including trade compliance laws). Use in languages other than English. Use in any other way that is prohibited by the Acceptable Use Policy and Licensing Agreement for Code Llama and its variants.
Hardware and Software
---------------------
Training Factors We used custom training libraries. The training and fine-tuning of the released models have been performed Meta’s Research Super Cluster.
Carbon Footprint In aggregate, training all 9 Code Llama models required 400K GPU hours of computation on hardware of type A100-80GB (TDP of 350-400W). Estimated total emissions were 65.3 tCO2eq, 100% of which were offset by Meta’s sustainability program.
Training Data
-------------
All experiments reported here and the released models have been trained and fine-tuned using the same data as Llama 2 with different weights (see Section 2 and Table 1 in the research paper for details).
Evaluation Results
------------------
See evaluations for the main models and detailed ablations in Section 3 and safety evaluations in Section 4 of the research paper.
Ethical Considerations and Limitations
--------------------------------------
Code Llama and its variants are a new technology that carries risks with use. Testing conducted to date has been in English, and has not covered, nor could it cover all scenarios. For these reasons, as with all LLMs, Code Llama’s potential outputs cannot be predicted in advance, and the model may in some instances produce inaccurate or objectionable responses to user prompts. Therefore, before deploying any applications of Code Llama, developers should perform safety testing and tuning tailored to their specific applications of the model.
Please see the Responsible Use Guide available available at URL
| [
"### LLAMA 2 COMMUNITY LICENSE AGREEMENT\n\n\n\"Agreement\" means the terms and conditions for use, reproduction, distribution\nand modification of the Llama Materials set forth herein.\n\"Documentation\" means the specifications, manuals and documentation\naccompanying Llama 2 distributed by Meta at\nURL \n\n\"Licensee\" or \"you\" means you, or your employer or any other person or entity\n(if you are entering into this Agreement on such person or entity's behalf),\nof the age required under applicable laws, rules or regulations to provide\nlegal consent and that has legal authority to bind your employer or such other\nperson or entity if you are entering in this Agreement on their behalf.\n\"Llama 2\" means the foundational large language models and software and\nalgorithms, including machine-learning model code, trained model weights,\ninference-enabling code, training-enabling code, fine-tuning enabling code and\nother elements of the foregoing distributed by Meta at\nURL\n\"Llama Materials\" means, collectively, Meta's proprietary Llama 2 and\ndocumentation (and any portion thereof) made available under this Agreement.\n\"Meta\" or \"we\" means Meta Platforms Ireland Limited (if you are located in or,\nif you are an entity, your principal place of business is in the EEA or\nSwitzerland) and Meta Platforms, Inc. (if you are located outside of the EEA\nor Switzerland).\nBy clicking \"I Accept\" below or by using or distributing any portion or\nelement of the Llama Materials, you agree to be bound by this Agreement.\n\n\n1. License Rights and Redistribution.\na. Grant of Rights. You are granted a non-exclusive, worldwide, non-\ntransferable and royalty-free limited license under Meta's intellectual\nproperty or other rights owned by Meta embodied in the Llama Materials to\nuse, reproduce, distribute, copy, create derivative works of, and make\nmodifications to the Llama Materials.\n\n\nb. Redistribution and Use. \n\ni. If you distribute or make the Llama Materials, or any derivative works\nthereof, available to a third party, you shall provide a copy of this\nAgreement to such third party.\nii. If you receive Llama Materials, or any derivative works thereof, from a\nLicensee as part of an integrated end user product, then Section 2 of this\nAgreement will not apply to you.\niii. You must retain in all copies of the Llama Materials that you distribute\nthe following attribution notice within a \"Notice\" text file distributed as a\npart of such copies: \"Llama 2 is licensed under the LLAMA 2 Community\nLicense, Copyright (c) Meta Platforms, Inc. All Rights Reserved.\"\niv. Your use of the Llama Materials must comply with applicable laws and\nregulations (including trade compliance laws and regulations) and adhere to\nthe Acceptable Use Policy for the Llama Materials (available at\nURL which is hereby incorporated by\nreference into this Agreement.\nv. You will not use the Llama Materials or any output or results of the Llama\nMaterials to improve any other large language model (excluding Llama 2 or\nderivative works thereof). \n\n2. Additional Commercial Terms. If, on the Llama 2 version release date, the\nmonthly active users of the products or services made available by or for\nLicensee, or Licensee's affiliates, is greater than 700 million monthly\nactive users in the preceding calendar month, you must request a license from\nMeta, which Meta may grant to you in its sole discretion, and you are not\nauthorized to exercise any of the rights under this Agreement unless or until\nMeta otherwise expressly grants you such rights.\n3. Disclaimer of Warranty. UNLESS REQUIRED BY APPLICABLE LAW, THE LLAMA\nMATERIALS AND ANY OUTPUT AND RESULTS THEREFROM ARE PROVIDED ON AN \"AS IS\"\nBASIS, WITHOUT WARRANTIES OF ANY KIND, EITHER EXPRESS OR IMPLIED, INCLUDING,\nWITHOUT LIMITATION, ANY WARRANTIES OF TITLE, NON-INFRINGEMENT,\nMERCHANTABILITY, OR FITNESS FOR A PARTICULAR PURPOSE. YOU ARE SOLELY\nRESPONSIBLE FOR DETERMINING THE APPROPRIATENESS OF USING OR REDISTRIBUTING\nTHE LLAMA MATERIALS AND ASSUME ANY RISKS ASSOCIATED WITH YOUR USE OF THE\nLLAMA MATERIALS AND ANY OUTPUT AND RESULTS.\n4. Limitation of Liability. IN NO EVENT WILL META OR ITS AFFILIATES BE LIABLE\nUNDER ANY THEORY OF LIABILITY, WHETHER IN CONTRACT, TORT, NEGLIGENCE,\nPRODUCTS LIABILITY, OR OTHERWISE, ARISING OUT OF THIS AGREEMENT, FOR ANY LOST\nPROFITS OR ANY INDIRECT, SPECIAL, CONSEQUENTIAL, INCIDENTAL, EXEMPLARY OR\nPUNITIVE DAMAGES, EVEN IF META OR ITS AFFILIATES HAVE BEEN ADVISED OF THE\nPOSSIBILITY OF ANY OF THE FOREGOING.\n5. Intellectual Property.\na. No trademark licenses are granted under this Agreement, and in connection\nwith the Llama Materials, neither Meta nor Licensee may use any name or mark\nowned by or associated with the other or any of its affiliates, except as\nrequired for reasonable and customary use in describing and redistributing\nthe Llama Materials.\nb. Subject to Meta's ownership of Llama Materials and derivatives made by or\nfor Meta, with respect to any derivative works and modifications of the Llama\nMaterials that are made by you, as between you and Meta, you are and will be\nthe owner of such derivative works and modifications.\nc. If you institute litigation or other proceedings against Meta or any\nentity (including a cross-claim or counterclaim in a lawsuit) alleging that\nthe Llama Materials or Llama 2 outputs or results, or any portion of any of\nthe foregoing, constitutes infringement of intellectual property or other\nrights owned or licensable by you, then any licenses granted to you under\nthis Agreement shall terminate as of the date such litigation or claim is\nfiled or instituted. You will indemnify and hold harmless Meta from and\nagainst any claim by any third party arising out of or related to your use or\ndistribution of the Llama Materials.\n6. Term and Termination. The term of this Agreement will commence upon your\nacceptance of this Agreement or access to the Llama Materials and will\ncontinue in full force and effect until terminated in accordance with the\nterms and conditions herein. Meta may terminate this Agreement if you are in\nbreach of any term or condition of this Agreement. Upon termination of this\nAgreement, you shall delete and cease use of the Llama Materials. Sections 3,\n4 and 7 shall survive the termination of this Agreement.\n7. Governing Law and Jurisdiction. This Agreement will be governed and\nconstrued under the laws of the State of California without regard to choice\nof law principles, and the UN Convention on Contracts for the International\nSale of Goods does not apply to this Agreement. The courts of California\nshall have exclusive jurisdiction of any dispute arising out of this\nAgreement.\nUSE POLICY",
"### Llama 2 Acceptable Use Policy\n\n\nMeta is committed to promoting safe and fair use of its tools and features,\nincluding Llama 2. If you access or use Llama 2, you agree to this Acceptable\nUse Policy (“Policy”). The most recent copy of this policy can be found at\nURL",
"#### Prohibited Uses\n\n\nWe want everyone to use Llama 2 safely and responsibly. You agree you will not\nuse, or allow others to use, Llama 2 to:\n\n\n1. Violate the law or others’ rights, including to:\n1. Engage in, promote, generate, contribute to, encourage, plan, incite, or further illegal or unlawful activity or content, such as:\n\t1. Violence or terrorism\n\t2. Exploitation or harm to children, including the solicitation, creation, acquisition, or dissemination of child exploitative content or failure to report Child Sexual Abuse Material\n\t3. Human trafficking, exploitation, and sexual violence\n\t4. The illegal distribution of information or materials to minors, including obscene materials, or failure to employ legally required age-gating in connection with such information or materials.\n\t5. Sexual solicitation\n\t6. Any other criminal activity\n\n\n\n```\n2. Engage in, promote, incite, or facilitate the harassment, abuse, threatening, or bullying of individuals or groups of individuals\n3. Engage in, promote, incite, or facilitate discrimination or other unlawful or harmful conduct in the provision of employment, employment benefits, credit, housing, other economic benefits, or other essential goods and services\n4. Engage in the unauthorized or unlicensed practice of any profession including, but not limited to, financial, legal, medical/health, or related professional practices \n5. Collect, process, disclose, generate, or infer health, demographic, or other sensitive personal or private information about individuals without rights and consents required by applicable laws\n6. Engage in or facilitate any action or generate any content that infringes, misappropriates, or otherwise violates any third-party rights, including the outputs or results of any products or services using the Llama 2 Materials\n7. Create, generate, or facilitate the creation of malicious code, malware, computer viruses or do anything else that could disable, overburden, interfere with or impair the proper working, integrity, operation or appearance of a website or computer system \n\n```\n\n2. Engage in, promote, incite, facilitate, or assist in the planning or\ndevelopment of activities that present a risk of death or bodily harm to\nindividuals, including use of Llama 2 related to the following:\n1. Military, warfare, nuclear industries or applications, espionage, use for materials or activities that are subject to the International Traffic Arms Regulations (ITAR) maintained by the United States Department of State\n2. Guns and illegal weapons (including weapon development)\n3. Illegal drugs and regulated/controlled substances\n4. Operation of critical infrastructure, transportation technologies, or heavy machinery\n5. Self-harm or harm to others, including suicide, cutting, and eating disorders\n6. Any content intended to incite or promote violence, abuse, or any infliction of bodily harm to an individual\n3. Intentionally deceive or mislead others, including use of Llama 2 related\nto the following:\n1. Generating, promoting, or furthering fraud or the creation or promotion of disinformation\n2. Generating, promoting, or furthering defamatory content, including the creation of defamatory statements, images, or other content\n3. Generating, promoting, or further distributing spam\n4. Impersonating another individual without consent, authorization, or legal right\n5. Representing that the use of Llama 2 or outputs are human-generated\n6. Generating or facilitating false online engagement, including fake reviews and other means of fake online engagement\n4. Fail to appropriately disclose to end users any known dangers of your AI system\nPlease report any violation of this Policy, software “bug,” or other problems\nthat could lead to a violation of this Policy through one of the following\nmeans:\n\n\n* Reporting issues with the model:\nURL\n* Reporting risky content generated by the model:\nURL\n* Reporting bugs and security concerns:\nURL\n* Reporting violations of the Acceptable Use Policy or unlicensed uses of\nLlama: LlamaUseReport@URL\nextra\\_gated\\_fields:\nFirst Name: text\nLast Name: text\nDate of birth: date\\_picker\nCountry: country\nAffiliation: text\ngeo: ip\\_location \n\nBy clicking Submit below I accept the terms of the license and acknowledge that the information I provide will be collected stored processed and shared in accordance with the Meta Privacy Policy: checkbox\nextra\\_gated\\_description: The information you provide will be collected, stored, processed and shared in accordance with the Meta Privacy Policy.\nextra\\_gated\\_button\\_content: Submit\nlanguage:\n\n\n* code\npipeline\\_tag: text-generation\ntags:\n* facebook\n* meta\n* pytorch\n* llama\n* llama-2\nlicense: llama2\n\n\n\n\n---\n\n\nCode Llama\n==========\n\n\nCode Llama is a collection of pretrained and fine-tuned generative text models ranging in scale from 7 billion to 34 billion parameters. This is the repository for the 34B instruct-tuned version in the Hugging Face Transformers format. This model is designed for general code synthesis and understanding. Links to other models can be found in the index at the bottom.\n\n\n\nModel Use\n---------\n\n\nTo use this model, please make sure to install transformers:\n\n\nModel capabilities:\n\n\n* [x] Code completion.\n* [ ] Infilling.\n* [x] Instructions / chat.\n* [ ] Python specialist.\n\n\nModel Details\n-------------\n\n\n\\*Note: Use of this model is governed by the Meta license. Meta developed and publicly released the Code Llama family of large language models (LLMs).\n\n\nModel Developers Meta\n\n\nVariations Code Llama comes in three model sizes, and three variants:\n\n\n* Code Llama: base models designed for general code synthesis and understanding\n* Code Llama - Python: designed specifically for Python\n* Code Llama - Instruct: for instruction following and safer deployment\n\n\nAll variants are available in sizes of 7B, 13B and 34B parameters.\n\n\nThis repository contains the Instruct version of the 34B parameters model.\n\n\nInput Models input text only.\n\n\nOutput Models generate text only.\n\n\nModel Architecture Code Llama is an auto-regressive language model that uses an optimized transformer architecture.\n\n\nModel Dates Code Llama and its variants have been trained between January 2023 and July 2023.\n\n\nStatus This is a static model trained on an offline dataset. Future versions of Code Llama - Instruct will be released as we improve model safety with community feedback.\n\n\nLicense A custom commercial license is available at: URL\n\n\nResearch Paper More information can be found in the paper \"Code Llama: Open Foundation Models for Code\" or its arXiv page.\n\n\nIntended Use\n------------\n\n\nIntended Use Cases Code Llama and its variants is intended for commercial and research use in English and relevant programming languages. The base model Code Llama can be adapted for a variety of code synthesis and understanding tasks, Code Llama - Python is designed specifically to handle the Python programming language, and Code Llama - Instruct is intended to be safer to use for code assistant and generation applications.\n\n\nOut-of-Scope Uses Use in any manner that violates applicable laws or regulations (including trade compliance laws). Use in languages other than English. Use in any other way that is prohibited by the Acceptable Use Policy and Licensing Agreement for Code Llama and its variants.\n\n\nHardware and Software\n---------------------\n\n\nTraining Factors We used custom training libraries. The training and fine-tuning of the released models have been performed Meta’s Research Super Cluster.\n\n\nCarbon Footprint In aggregate, training all 9 Code Llama models required 400K GPU hours of computation on hardware of type A100-80GB (TDP of 350-400W). Estimated total emissions were 65.3 tCO2eq, 100% of which were offset by Meta’s sustainability program.\n\n\nTraining Data\n-------------\n\n\nAll experiments reported here and the released models have been trained and fine-tuned using the same data as Llama 2 with different weights (see Section 2 and Table 1 in the research paper for details).\n\n\nEvaluation Results\n------------------\n\n\nSee evaluations for the main models and detailed ablations in Section 3 and safety evaluations in Section 4 of the research paper.\n\n\nEthical Considerations and Limitations\n--------------------------------------\n\n\nCode Llama and its variants are a new technology that carries risks with use. Testing conducted to date has been in English, and has not covered, nor could it cover all scenarios. For these reasons, as with all LLMs, Code Llama’s potential outputs cannot be predicted in advance, and the model may in some instances produce inaccurate or objectionable responses to user prompts. Therefore, before deploying any applications of Code Llama, developers should perform safety testing and tuning tailored to their specific applications of the model.\n\n\nPlease see the Responsible Use Guide available available at URL"
] | [
"TAGS\n#gguf #arxiv-2308.12950 #region-us \n",
"### LLAMA 2 COMMUNITY LICENSE AGREEMENT\n\n\n\"Agreement\" means the terms and conditions for use, reproduction, distribution\nand modification of the Llama Materials set forth herein.\n\"Documentation\" means the specifications, manuals and documentation\naccompanying Llama 2 distributed by Meta at\nURL \n\n\"Licensee\" or \"you\" means you, or your employer or any other person or entity\n(if you are entering into this Agreement on such person or entity's behalf),\nof the age required under applicable laws, rules or regulations to provide\nlegal consent and that has legal authority to bind your employer or such other\nperson or entity if you are entering in this Agreement on their behalf.\n\"Llama 2\" means the foundational large language models and software and\nalgorithms, including machine-learning model code, trained model weights,\ninference-enabling code, training-enabling code, fine-tuning enabling code and\nother elements of the foregoing distributed by Meta at\nURL\n\"Llama Materials\" means, collectively, Meta's proprietary Llama 2 and\ndocumentation (and any portion thereof) made available under this Agreement.\n\"Meta\" or \"we\" means Meta Platforms Ireland Limited (if you are located in or,\nif you are an entity, your principal place of business is in the EEA or\nSwitzerland) and Meta Platforms, Inc. (if you are located outside of the EEA\nor Switzerland).\nBy clicking \"I Accept\" below or by using or distributing any portion or\nelement of the Llama Materials, you agree to be bound by this Agreement.\n\n\n1. License Rights and Redistribution.\na. Grant of Rights. You are granted a non-exclusive, worldwide, non-\ntransferable and royalty-free limited license under Meta's intellectual\nproperty or other rights owned by Meta embodied in the Llama Materials to\nuse, reproduce, distribute, copy, create derivative works of, and make\nmodifications to the Llama Materials.\n\n\nb. Redistribution and Use. \n\ni. If you distribute or make the Llama Materials, or any derivative works\nthereof, available to a third party, you shall provide a copy of this\nAgreement to such third party.\nii. If you receive Llama Materials, or any derivative works thereof, from a\nLicensee as part of an integrated end user product, then Section 2 of this\nAgreement will not apply to you.\niii. You must retain in all copies of the Llama Materials that you distribute\nthe following attribution notice within a \"Notice\" text file distributed as a\npart of such copies: \"Llama 2 is licensed under the LLAMA 2 Community\nLicense, Copyright (c) Meta Platforms, Inc. All Rights Reserved.\"\niv. Your use of the Llama Materials must comply with applicable laws and\nregulations (including trade compliance laws and regulations) and adhere to\nthe Acceptable Use Policy for the Llama Materials (available at\nURL which is hereby incorporated by\nreference into this Agreement.\nv. You will not use the Llama Materials or any output or results of the Llama\nMaterials to improve any other large language model (excluding Llama 2 or\nderivative works thereof). \n\n2. Additional Commercial Terms. If, on the Llama 2 version release date, the\nmonthly active users of the products or services made available by or for\nLicensee, or Licensee's affiliates, is greater than 700 million monthly\nactive users in the preceding calendar month, you must request a license from\nMeta, which Meta may grant to you in its sole discretion, and you are not\nauthorized to exercise any of the rights under this Agreement unless or until\nMeta otherwise expressly grants you such rights.\n3. Disclaimer of Warranty. UNLESS REQUIRED BY APPLICABLE LAW, THE LLAMA\nMATERIALS AND ANY OUTPUT AND RESULTS THEREFROM ARE PROVIDED ON AN \"AS IS\"\nBASIS, WITHOUT WARRANTIES OF ANY KIND, EITHER EXPRESS OR IMPLIED, INCLUDING,\nWITHOUT LIMITATION, ANY WARRANTIES OF TITLE, NON-INFRINGEMENT,\nMERCHANTABILITY, OR FITNESS FOR A PARTICULAR PURPOSE. YOU ARE SOLELY\nRESPONSIBLE FOR DETERMINING THE APPROPRIATENESS OF USING OR REDISTRIBUTING\nTHE LLAMA MATERIALS AND ASSUME ANY RISKS ASSOCIATED WITH YOUR USE OF THE\nLLAMA MATERIALS AND ANY OUTPUT AND RESULTS.\n4. Limitation of Liability. IN NO EVENT WILL META OR ITS AFFILIATES BE LIABLE\nUNDER ANY THEORY OF LIABILITY, WHETHER IN CONTRACT, TORT, NEGLIGENCE,\nPRODUCTS LIABILITY, OR OTHERWISE, ARISING OUT OF THIS AGREEMENT, FOR ANY LOST\nPROFITS OR ANY INDIRECT, SPECIAL, CONSEQUENTIAL, INCIDENTAL, EXEMPLARY OR\nPUNITIVE DAMAGES, EVEN IF META OR ITS AFFILIATES HAVE BEEN ADVISED OF THE\nPOSSIBILITY OF ANY OF THE FOREGOING.\n5. Intellectual Property.\na. No trademark licenses are granted under this Agreement, and in connection\nwith the Llama Materials, neither Meta nor Licensee may use any name or mark\nowned by or associated with the other or any of its affiliates, except as\nrequired for reasonable and customary use in describing and redistributing\nthe Llama Materials.\nb. Subject to Meta's ownership of Llama Materials and derivatives made by or\nfor Meta, with respect to any derivative works and modifications of the Llama\nMaterials that are made by you, as between you and Meta, you are and will be\nthe owner of such derivative works and modifications.\nc. If you institute litigation or other proceedings against Meta or any\nentity (including a cross-claim or counterclaim in a lawsuit) alleging that\nthe Llama Materials or Llama 2 outputs or results, or any portion of any of\nthe foregoing, constitutes infringement of intellectual property or other\nrights owned or licensable by you, then any licenses granted to you under\nthis Agreement shall terminate as of the date such litigation or claim is\nfiled or instituted. You will indemnify and hold harmless Meta from and\nagainst any claim by any third party arising out of or related to your use or\ndistribution of the Llama Materials.\n6. Term and Termination. The term of this Agreement will commence upon your\nacceptance of this Agreement or access to the Llama Materials and will\ncontinue in full force and effect until terminated in accordance with the\nterms and conditions herein. Meta may terminate this Agreement if you are in\nbreach of any term or condition of this Agreement. Upon termination of this\nAgreement, you shall delete and cease use of the Llama Materials. Sections 3,\n4 and 7 shall survive the termination of this Agreement.\n7. Governing Law and Jurisdiction. This Agreement will be governed and\nconstrued under the laws of the State of California without regard to choice\nof law principles, and the UN Convention on Contracts for the International\nSale of Goods does not apply to this Agreement. The courts of California\nshall have exclusive jurisdiction of any dispute arising out of this\nAgreement.\nUSE POLICY",
"### Llama 2 Acceptable Use Policy\n\n\nMeta is committed to promoting safe and fair use of its tools and features,\nincluding Llama 2. If you access or use Llama 2, you agree to this Acceptable\nUse Policy (“Policy”). The most recent copy of this policy can be found at\nURL",
"#### Prohibited Uses\n\n\nWe want everyone to use Llama 2 safely and responsibly. You agree you will not\nuse, or allow others to use, Llama 2 to:\n\n\n1. Violate the law or others’ rights, including to:\n1. Engage in, promote, generate, contribute to, encourage, plan, incite, or further illegal or unlawful activity or content, such as:\n\t1. Violence or terrorism\n\t2. Exploitation or harm to children, including the solicitation, creation, acquisition, or dissemination of child exploitative content or failure to report Child Sexual Abuse Material\n\t3. Human trafficking, exploitation, and sexual violence\n\t4. The illegal distribution of information or materials to minors, including obscene materials, or failure to employ legally required age-gating in connection with such information or materials.\n\t5. Sexual solicitation\n\t6. Any other criminal activity\n\n\n\n```\n2. Engage in, promote, incite, or facilitate the harassment, abuse, threatening, or bullying of individuals or groups of individuals\n3. Engage in, promote, incite, or facilitate discrimination or other unlawful or harmful conduct in the provision of employment, employment benefits, credit, housing, other economic benefits, or other essential goods and services\n4. Engage in the unauthorized or unlicensed practice of any profession including, but not limited to, financial, legal, medical/health, or related professional practices \n5. Collect, process, disclose, generate, or infer health, demographic, or other sensitive personal or private information about individuals without rights and consents required by applicable laws\n6. Engage in or facilitate any action or generate any content that infringes, misappropriates, or otherwise violates any third-party rights, including the outputs or results of any products or services using the Llama 2 Materials\n7. Create, generate, or facilitate the creation of malicious code, malware, computer viruses or do anything else that could disable, overburden, interfere with or impair the proper working, integrity, operation or appearance of a website or computer system \n\n```\n\n2. Engage in, promote, incite, facilitate, or assist in the planning or\ndevelopment of activities that present a risk of death or bodily harm to\nindividuals, including use of Llama 2 related to the following:\n1. Military, warfare, nuclear industries or applications, espionage, use for materials or activities that are subject to the International Traffic Arms Regulations (ITAR) maintained by the United States Department of State\n2. Guns and illegal weapons (including weapon development)\n3. Illegal drugs and regulated/controlled substances\n4. Operation of critical infrastructure, transportation technologies, or heavy machinery\n5. Self-harm or harm to others, including suicide, cutting, and eating disorders\n6. Any content intended to incite or promote violence, abuse, or any infliction of bodily harm to an individual\n3. Intentionally deceive or mislead others, including use of Llama 2 related\nto the following:\n1. Generating, promoting, or furthering fraud or the creation or promotion of disinformation\n2. Generating, promoting, or furthering defamatory content, including the creation of defamatory statements, images, or other content\n3. Generating, promoting, or further distributing spam\n4. Impersonating another individual without consent, authorization, or legal right\n5. Representing that the use of Llama 2 or outputs are human-generated\n6. Generating or facilitating false online engagement, including fake reviews and other means of fake online engagement\n4. Fail to appropriately disclose to end users any known dangers of your AI system\nPlease report any violation of this Policy, software “bug,” or other problems\nthat could lead to a violation of this Policy through one of the following\nmeans:\n\n\n* Reporting issues with the model:\nURL\n* Reporting risky content generated by the model:\nURL\n* Reporting bugs and security concerns:\nURL\n* Reporting violations of the Acceptable Use Policy or unlicensed uses of\nLlama: LlamaUseReport@URL\nextra\\_gated\\_fields:\nFirst Name: text\nLast Name: text\nDate of birth: date\\_picker\nCountry: country\nAffiliation: text\ngeo: ip\\_location \n\nBy clicking Submit below I accept the terms of the license and acknowledge that the information I provide will be collected stored processed and shared in accordance with the Meta Privacy Policy: checkbox\nextra\\_gated\\_description: The information you provide will be collected, stored, processed and shared in accordance with the Meta Privacy Policy.\nextra\\_gated\\_button\\_content: Submit\nlanguage:\n\n\n* code\npipeline\\_tag: text-generation\ntags:\n* facebook\n* meta\n* pytorch\n* llama\n* llama-2\nlicense: llama2\n\n\n\n\n---\n\n\nCode Llama\n==========\n\n\nCode Llama is a collection of pretrained and fine-tuned generative text models ranging in scale from 7 billion to 34 billion parameters. This is the repository for the 34B instruct-tuned version in the Hugging Face Transformers format. This model is designed for general code synthesis and understanding. Links to other models can be found in the index at the bottom.\n\n\n\nModel Use\n---------\n\n\nTo use this model, please make sure to install transformers:\n\n\nModel capabilities:\n\n\n* [x] Code completion.\n* [ ] Infilling.\n* [x] Instructions / chat.\n* [ ] Python specialist.\n\n\nModel Details\n-------------\n\n\n\\*Note: Use of this model is governed by the Meta license. Meta developed and publicly released the Code Llama family of large language models (LLMs).\n\n\nModel Developers Meta\n\n\nVariations Code Llama comes in three model sizes, and three variants:\n\n\n* Code Llama: base models designed for general code synthesis and understanding\n* Code Llama - Python: designed specifically for Python\n* Code Llama - Instruct: for instruction following and safer deployment\n\n\nAll variants are available in sizes of 7B, 13B and 34B parameters.\n\n\nThis repository contains the Instruct version of the 34B parameters model.\n\n\nInput Models input text only.\n\n\nOutput Models generate text only.\n\n\nModel Architecture Code Llama is an auto-regressive language model that uses an optimized transformer architecture.\n\n\nModel Dates Code Llama and its variants have been trained between January 2023 and July 2023.\n\n\nStatus This is a static model trained on an offline dataset. Future versions of Code Llama - Instruct will be released as we improve model safety with community feedback.\n\n\nLicense A custom commercial license is available at: URL\n\n\nResearch Paper More information can be found in the paper \"Code Llama: Open Foundation Models for Code\" or its arXiv page.\n\n\nIntended Use\n------------\n\n\nIntended Use Cases Code Llama and its variants is intended for commercial and research use in English and relevant programming languages. The base model Code Llama can be adapted for a variety of code synthesis and understanding tasks, Code Llama - Python is designed specifically to handle the Python programming language, and Code Llama - Instruct is intended to be safer to use for code assistant and generation applications.\n\n\nOut-of-Scope Uses Use in any manner that violates applicable laws or regulations (including trade compliance laws). Use in languages other than English. Use in any other way that is prohibited by the Acceptable Use Policy and Licensing Agreement for Code Llama and its variants.\n\n\nHardware and Software\n---------------------\n\n\nTraining Factors We used custom training libraries. The training and fine-tuning of the released models have been performed Meta’s Research Super Cluster.\n\n\nCarbon Footprint In aggregate, training all 9 Code Llama models required 400K GPU hours of computation on hardware of type A100-80GB (TDP of 350-400W). Estimated total emissions were 65.3 tCO2eq, 100% of which were offset by Meta’s sustainability program.\n\n\nTraining Data\n-------------\n\n\nAll experiments reported here and the released models have been trained and fine-tuned using the same data as Llama 2 with different weights (see Section 2 and Table 1 in the research paper for details).\n\n\nEvaluation Results\n------------------\n\n\nSee evaluations for the main models and detailed ablations in Section 3 and safety evaluations in Section 4 of the research paper.\n\n\nEthical Considerations and Limitations\n--------------------------------------\n\n\nCode Llama and its variants are a new technology that carries risks with use. Testing conducted to date has been in English, and has not covered, nor could it cover all scenarios. For these reasons, as with all LLMs, Code Llama’s potential outputs cannot be predicted in advance, and the model may in some instances produce inaccurate or objectionable responses to user prompts. Therefore, before deploying any applications of Code Llama, developers should perform safety testing and tuning tailored to their specific applications of the model.\n\n\nPlease see the Responsible Use Guide available available at URL"
] |
text-generation | transformers |
# Model Card for Model ID
<!-- Provide a quick summary of what the model is/does. -->
## Model Details
### Model Description
<!-- Provide a longer summary of what this model is. -->
This is the model card of a 🤗 transformers model that has been pushed on the Hub. This model card has been automatically generated.
- **Developed by:** [More Information Needed]
- **Funded by [optional]:** [More Information Needed]
- **Shared by [optional]:** [More Information Needed]
- **Model type:** [More Information Needed]
- **Language(s) (NLP):** [More Information Needed]
- **License:** [More Information Needed]
- **Finetuned from model [optional]:** [More Information Needed]
### Model Sources [optional]
<!-- Provide the basic links for the model. -->
- **Repository:** [More Information Needed]
- **Paper [optional]:** [More Information Needed]
- **Demo [optional]:** [More Information Needed]
## Uses
<!-- Address questions around how the model is intended to be used, including the foreseeable users of the model and those affected by the model. -->
### Direct Use
<!-- This section is for the model use without fine-tuning or plugging into a larger ecosystem/app. -->
[More Information Needed]
### Downstream Use [optional]
<!-- This section is for the model use when fine-tuned for a task, or when plugged into a larger ecosystem/app -->
[More Information Needed]
### Out-of-Scope Use
<!-- This section addresses misuse, malicious use, and uses that the model will not work well for. -->
[More Information Needed]
## Bias, Risks, and Limitations
<!-- This section is meant to convey both technical and sociotechnical limitations. -->
[More Information Needed]
### Recommendations
<!-- This section is meant to convey recommendations with respect to the bias, risk, and technical limitations. -->
Users (both direct and downstream) should be made aware of the risks, biases and limitations of the model. More information needed for further recommendations.
## How to Get Started with the Model
Use the code below to get started with the model.
[More Information Needed]
## Training Details
### Training Data
<!-- This should link to a Dataset Card, perhaps with a short stub of information on what the training data is all about as well as documentation related to data pre-processing or additional filtering. -->
[More Information Needed]
### Training Procedure
<!-- This relates heavily to the Technical Specifications. Content here should link to that section when it is relevant to the training procedure. -->
#### Preprocessing [optional]
[More Information Needed]
#### Training Hyperparameters
- **Training regime:** [More Information Needed] <!--fp32, fp16 mixed precision, bf16 mixed precision, bf16 non-mixed precision, fp16 non-mixed precision, fp8 mixed precision -->
#### Speeds, Sizes, Times [optional]
<!-- This section provides information about throughput, start/end time, checkpoint size if relevant, etc. -->
[More Information Needed]
## Evaluation
<!-- This section describes the evaluation protocols and provides the results. -->
### Testing Data, Factors & Metrics
#### Testing Data
<!-- This should link to a Dataset Card if possible. -->
[More Information Needed]
#### Factors
<!-- These are the things the evaluation is disaggregating by, e.g., subpopulations or domains. -->
[More Information Needed]
#### Metrics
<!-- These are the evaluation metrics being used, ideally with a description of why. -->
[More Information Needed]
### Results
[More Information Needed]
#### Summary
## Model Examination [optional]
<!-- Relevant interpretability work for the model goes here -->
[More Information Needed]
## Environmental Impact
<!-- Total emissions (in grams of CO2eq) and additional considerations, such as electricity usage, go here. Edit the suggested text below accordingly -->
Carbon emissions can be estimated using the [Machine Learning Impact calculator](https://mlco2.github.io/impact#compute) presented in [Lacoste et al. (2019)](https://arxiv.org/abs/1910.09700).
- **Hardware Type:** [More Information Needed]
- **Hours used:** [More Information Needed]
- **Cloud Provider:** [More Information Needed]
- **Compute Region:** [More Information Needed]
- **Carbon Emitted:** [More Information Needed]
## Technical Specifications [optional]
### Model Architecture and Objective
[More Information Needed]
### Compute Infrastructure
[More Information Needed]
#### Hardware
[More Information Needed]
#### Software
[More Information Needed]
## Citation [optional]
<!-- If there is a paper or blog post introducing the model, the APA and Bibtex information for that should go in this section. -->
**BibTeX:**
[More Information Needed]
**APA:**
[More Information Needed]
## Glossary [optional]
<!-- If relevant, include terms and calculations in this section that can help readers understand the model or model card. -->
[More Information Needed]
## More Information [optional]
[More Information Needed]
## Model Card Authors [optional]
[More Information Needed]
## Model Card Contact
[More Information Needed] | {"library_name": "transformers", "tags": []} | VisionForge/Alien-8B-0.2 | null | [
"transformers",
"safetensors",
"llama",
"text-generation",
"conversational",
"arxiv:1910.09700",
"autotrain_compatible",
"endpoints_compatible",
"text-generation-inference",
"region:us"
] | null | 2024-04-21T19:36:37+00:00 | [
"1910.09700"
] | [] | TAGS
#transformers #safetensors #llama #text-generation #conversational #arxiv-1910.09700 #autotrain_compatible #endpoints_compatible #text-generation-inference #region-us
|
# Model Card for Model ID
## Model Details
### Model Description
This is the model card of a transformers model that has been pushed on the Hub. This model card has been automatically generated.
- Developed by:
- Funded by [optional]:
- Shared by [optional]:
- Model type:
- Language(s) (NLP):
- License:
- Finetuned from model [optional]:
### Model Sources [optional]
- Repository:
- Paper [optional]:
- Demo [optional]:
## Uses
### Direct Use
### Downstream Use [optional]
### Out-of-Scope Use
## Bias, Risks, and Limitations
### Recommendations
Users (both direct and downstream) should be made aware of the risks, biases and limitations of the model. More information needed for further recommendations.
## How to Get Started with the Model
Use the code below to get started with the model.
## Training Details
### Training Data
### Training Procedure
#### Preprocessing [optional]
#### Training Hyperparameters
- Training regime:
#### Speeds, Sizes, Times [optional]
## Evaluation
### Testing Data, Factors & Metrics
#### Testing Data
#### Factors
#### Metrics
### Results
#### Summary
## Model Examination [optional]
## Environmental Impact
Carbon emissions can be estimated using the Machine Learning Impact calculator presented in Lacoste et al. (2019).
- Hardware Type:
- Hours used:
- Cloud Provider:
- Compute Region:
- Carbon Emitted:
## Technical Specifications [optional]
### Model Architecture and Objective
### Compute Infrastructure
#### Hardware
#### Software
[optional]
BibTeX:
APA:
## Glossary [optional]
## More Information [optional]
## Model Card Authors [optional]
## Model Card Contact
| [
"# Model Card for Model ID",
"## Model Details",
"### Model Description\n\n\n\nThis is the model card of a transformers model that has been pushed on the Hub. This model card has been automatically generated.\n\n- Developed by: \n- Funded by [optional]: \n- Shared by [optional]: \n- Model type: \n- Language(s) (NLP): \n- License: \n- Finetuned from model [optional]:",
"### Model Sources [optional]\n\n\n\n- Repository: \n- Paper [optional]: \n- Demo [optional]:",
"## Uses",
"### Direct Use",
"### Downstream Use [optional]",
"### Out-of-Scope Use",
"## Bias, Risks, and Limitations",
"### Recommendations\n\n\n\nUsers (both direct and downstream) should be made aware of the risks, biases and limitations of the model. More information needed for further recommendations.",
"## How to Get Started with the Model\n\nUse the code below to get started with the model.",
"## Training Details",
"### Training Data",
"### Training Procedure",
"#### Preprocessing [optional]",
"#### Training Hyperparameters\n\n- Training regime:",
"#### Speeds, Sizes, Times [optional]",
"## Evaluation",
"### Testing Data, Factors & Metrics",
"#### Testing Data",
"#### Factors",
"#### Metrics",
"### Results",
"#### Summary",
"## Model Examination [optional]",
"## Environmental Impact\n\n\n\nCarbon emissions can be estimated using the Machine Learning Impact calculator presented in Lacoste et al. (2019).\n\n- Hardware Type: \n- Hours used: \n- Cloud Provider: \n- Compute Region: \n- Carbon Emitted:",
"## Technical Specifications [optional]",
"### Model Architecture and Objective",
"### Compute Infrastructure",
"#### Hardware",
"#### Software\n\n\n\n[optional]\n\n\n\nBibTeX:\n\n\n\nAPA:",
"## Glossary [optional]",
"## More Information [optional]",
"## Model Card Authors [optional]",
"## Model Card Contact"
] | [
"TAGS\n#transformers #safetensors #llama #text-generation #conversational #arxiv-1910.09700 #autotrain_compatible #endpoints_compatible #text-generation-inference #region-us \n",
"# Model Card for Model ID",
"## Model Details",
"### Model Description\n\n\n\nThis is the model card of a transformers model that has been pushed on the Hub. This model card has been automatically generated.\n\n- Developed by: \n- Funded by [optional]: \n- Shared by [optional]: \n- Model type: \n- Language(s) (NLP): \n- License: \n- Finetuned from model [optional]:",
"### Model Sources [optional]\n\n\n\n- Repository: \n- Paper [optional]: \n- Demo [optional]:",
"## Uses",
"### Direct Use",
"### Downstream Use [optional]",
"### Out-of-Scope Use",
"## Bias, Risks, and Limitations",
"### Recommendations\n\n\n\nUsers (both direct and downstream) should be made aware of the risks, biases and limitations of the model. More information needed for further recommendations.",
"## How to Get Started with the Model\n\nUse the code below to get started with the model.",
"## Training Details",
"### Training Data",
"### Training Procedure",
"#### Preprocessing [optional]",
"#### Training Hyperparameters\n\n- Training regime:",
"#### Speeds, Sizes, Times [optional]",
"## Evaluation",
"### Testing Data, Factors & Metrics",
"#### Testing Data",
"#### Factors",
"#### Metrics",
"### Results",
"#### Summary",
"## Model Examination [optional]",
"## Environmental Impact\n\n\n\nCarbon emissions can be estimated using the Machine Learning Impact calculator presented in Lacoste et al. (2019).\n\n- Hardware Type: \n- Hours used: \n- Cloud Provider: \n- Compute Region: \n- Carbon Emitted:",
"## Technical Specifications [optional]",
"### Model Architecture and Objective",
"### Compute Infrastructure",
"#### Hardware",
"#### Software\n\n\n\n[optional]\n\n\n\nBibTeX:\n\n\n\nAPA:",
"## Glossary [optional]",
"## More Information [optional]",
"## Model Card Authors [optional]",
"## Model Card Contact"
] |
null | transformers |
# Uploaded model
- **Developed by:** mehdi selbi
- **License:** apache-2.0
- **Finetuned from model :** unsloth/llama-3-8b-bnb-4bit
- **Dataset :** gretelai/synthetic_text_to_sql
This llama model was trained 2x faster with [Unsloth](https://github.com/unslothai/unsloth) and Huggingface's TRL library.
[<img src="https://raw.githubusercontent.com/unslothai/unsloth/main/images/unsloth%20made%20with%20love.png" width="200"/>](https://github.com/unslothai/unsloth) | {"language": ["en"], "license": "apache-2.0", "tags": ["text-generation-inference", "transformers", "unsloth", "llama", "trl", "text-to-sql"], "base_model": "unsloth/llama-3-8b-bnb-4bit"} | mehdiselbi/llama3-8b-bnb-4bit-text-to-sql | null | [
"transformers",
"safetensors",
"text-generation-inference",
"unsloth",
"llama",
"trl",
"text-to-sql",
"en",
"base_model:unsloth/llama-3-8b-bnb-4bit",
"license:apache-2.0",
"endpoints_compatible",
"region:us"
] | null | 2024-04-21T19:37:37+00:00 | [] | [
"en"
] | TAGS
#transformers #safetensors #text-generation-inference #unsloth #llama #trl #text-to-sql #en #base_model-unsloth/llama-3-8b-bnb-4bit #license-apache-2.0 #endpoints_compatible #region-us
|
# Uploaded model
- Developed by: mehdi selbi
- License: apache-2.0
- Finetuned from model : unsloth/llama-3-8b-bnb-4bit
- Dataset : gretelai/synthetic_text_to_sql
This llama model was trained 2x faster with Unsloth and Huggingface's TRL library.
<img src="URL width="200"/> | [
"# Uploaded model\n\n- Developed by: mehdi selbi\n- License: apache-2.0\n- Finetuned from model : unsloth/llama-3-8b-bnb-4bit\n- Dataset : gretelai/synthetic_text_to_sql\n\nThis llama model was trained 2x faster with Unsloth and Huggingface's TRL library.\n\n<img src=\"URL width=\"200\"/>"
] | [
"TAGS\n#transformers #safetensors #text-generation-inference #unsloth #llama #trl #text-to-sql #en #base_model-unsloth/llama-3-8b-bnb-4bit #license-apache-2.0 #endpoints_compatible #region-us \n",
"# Uploaded model\n\n- Developed by: mehdi selbi\n- License: apache-2.0\n- Finetuned from model : unsloth/llama-3-8b-bnb-4bit\n- Dataset : gretelai/synthetic_text_to_sql\n\nThis llama model was trained 2x faster with Unsloth and Huggingface's TRL library.\n\n<img src=\"URL width=\"200\"/>"
] |
text-to-image | diffusers |
# Model Card for Model ID
<!-- Provide a quick summary of what the model is/does. -->
## Model Details
### Model Description
<!-- Provide a longer summary of what this model is. -->
This is the model card of a 🧨 diffusers model that has been pushed on the Hub. This model card has been automatically generated.
- **Developed by:** [More Information Needed]
- **Funded by [optional]:** [More Information Needed]
- **Shared by [optional]:** [More Information Needed]
- **Model type:** [More Information Needed]
- **Language(s) (NLP):** [More Information Needed]
- **License:** [More Information Needed]
- **Finetuned from model [optional]:** [More Information Needed]
### Model Sources [optional]
<!-- Provide the basic links for the model. -->
- **Repository:** [More Information Needed]
- **Paper [optional]:** [More Information Needed]
- **Demo [optional]:** [More Information Needed]
## Uses
<!-- Address questions around how the model is intended to be used, including the foreseeable users of the model and those affected by the model. -->
### Direct Use
<!-- This section is for the model use without fine-tuning or plugging into a larger ecosystem/app. -->
[More Information Needed]
### Downstream Use [optional]
<!-- This section is for the model use when fine-tuned for a task, or when plugged into a larger ecosystem/app -->
[More Information Needed]
### Out-of-Scope Use
<!-- This section addresses misuse, malicious use, and uses that the model will not work well for. -->
[More Information Needed]
## Bias, Risks, and Limitations
<!-- This section is meant to convey both technical and sociotechnical limitations. -->
[More Information Needed]
### Recommendations
<!-- This section is meant to convey recommendations with respect to the bias, risk, and technical limitations. -->
Users (both direct and downstream) should be made aware of the risks, biases and limitations of the model. More information needed for further recommendations.
## How to Get Started with the Model
Use the code below to get started with the model.
[More Information Needed]
## Training Details
### Training Data
<!-- This should link to a Dataset Card, perhaps with a short stub of information on what the training data is all about as well as documentation related to data pre-processing or additional filtering. -->
[More Information Needed]
### Training Procedure
<!-- This relates heavily to the Technical Specifications. Content here should link to that section when it is relevant to the training procedure. -->
#### Preprocessing [optional]
[More Information Needed]
#### Training Hyperparameters
- **Training regime:** [More Information Needed] <!--fp32, fp16 mixed precision, bf16 mixed precision, bf16 non-mixed precision, fp16 non-mixed precision, fp8 mixed precision -->
#### Speeds, Sizes, Times [optional]
<!-- This section provides information about throughput, start/end time, checkpoint size if relevant, etc. -->
[More Information Needed]
## Evaluation
<!-- This section describes the evaluation protocols and provides the results. -->
### Testing Data, Factors & Metrics
#### Testing Data
<!-- This should link to a Dataset Card if possible. -->
[More Information Needed]
#### Factors
<!-- These are the things the evaluation is disaggregating by, e.g., subpopulations or domains. -->
[More Information Needed]
#### Metrics
<!-- These are the evaluation metrics being used, ideally with a description of why. -->
[More Information Needed]
### Results
[More Information Needed]
#### Summary
## Model Examination [optional]
<!-- Relevant interpretability work for the model goes here -->
[More Information Needed]
## Environmental Impact
<!-- Total emissions (in grams of CO2eq) and additional considerations, such as electricity usage, go here. Edit the suggested text below accordingly -->
Carbon emissions can be estimated using the [Machine Learning Impact calculator](https://mlco2.github.io/impact#compute) presented in [Lacoste et al. (2019)](https://arxiv.org/abs/1910.09700).
- **Hardware Type:** [More Information Needed]
- **Hours used:** [More Information Needed]
- **Cloud Provider:** [More Information Needed]
- **Compute Region:** [More Information Needed]
- **Carbon Emitted:** [More Information Needed]
## Technical Specifications [optional]
### Model Architecture and Objective
[More Information Needed]
### Compute Infrastructure
[More Information Needed]
#### Hardware
[More Information Needed]
#### Software
[More Information Needed]
## Citation [optional]
<!-- If there is a paper or blog post introducing the model, the APA and Bibtex information for that should go in this section. -->
**BibTeX:**
[More Information Needed]
**APA:**
[More Information Needed]
## Glossary [optional]
<!-- If relevant, include terms and calculations in this section that can help readers understand the model or model card. -->
[More Information Needed]
## More Information [optional]
[More Information Needed]
## Model Card Authors [optional]
[More Information Needed]
## Model Card Contact
[More Information Needed]
| {"library_name": "diffusers"} | Niggendar/incompleteAnimePony_alpha | null | [
"diffusers",
"safetensors",
"arxiv:1910.09700",
"endpoints_compatible",
"diffusers:StableDiffusionXLPipeline",
"region:us"
] | null | 2024-04-21T19:40:27+00:00 | [
"1910.09700"
] | [] | TAGS
#diffusers #safetensors #arxiv-1910.09700 #endpoints_compatible #diffusers-StableDiffusionXLPipeline #region-us
|
# Model Card for Model ID
## Model Details
### Model Description
This is the model card of a diffusers model that has been pushed on the Hub. This model card has been automatically generated.
- Developed by:
- Funded by [optional]:
- Shared by [optional]:
- Model type:
- Language(s) (NLP):
- License:
- Finetuned from model [optional]:
### Model Sources [optional]
- Repository:
- Paper [optional]:
- Demo [optional]:
## Uses
### Direct Use
### Downstream Use [optional]
### Out-of-Scope Use
## Bias, Risks, and Limitations
### Recommendations
Users (both direct and downstream) should be made aware of the risks, biases and limitations of the model. More information needed for further recommendations.
## How to Get Started with the Model
Use the code below to get started with the model.
## Training Details
### Training Data
### Training Procedure
#### Preprocessing [optional]
#### Training Hyperparameters
- Training regime:
#### Speeds, Sizes, Times [optional]
## Evaluation
### Testing Data, Factors & Metrics
#### Testing Data
#### Factors
#### Metrics
### Results
#### Summary
## Model Examination [optional]
## Environmental Impact
Carbon emissions can be estimated using the Machine Learning Impact calculator presented in Lacoste et al. (2019).
- Hardware Type:
- Hours used:
- Cloud Provider:
- Compute Region:
- Carbon Emitted:
## Technical Specifications [optional]
### Model Architecture and Objective
### Compute Infrastructure
#### Hardware
#### Software
[optional]
BibTeX:
APA:
## Glossary [optional]
## More Information [optional]
## Model Card Authors [optional]
## Model Card Contact
| [
"# Model Card for Model ID",
"## Model Details",
"### Model Description\n\n\n\nThis is the model card of a diffusers model that has been pushed on the Hub. This model card has been automatically generated.\n\n- Developed by: \n- Funded by [optional]: \n- Shared by [optional]: \n- Model type: \n- Language(s) (NLP): \n- License: \n- Finetuned from model [optional]:",
"### Model Sources [optional]\n\n\n\n- Repository: \n- Paper [optional]: \n- Demo [optional]:",
"## Uses",
"### Direct Use",
"### Downstream Use [optional]",
"### Out-of-Scope Use",
"## Bias, Risks, and Limitations",
"### Recommendations\n\n\n\nUsers (both direct and downstream) should be made aware of the risks, biases and limitations of the model. More information needed for further recommendations.",
"## How to Get Started with the Model\n\nUse the code below to get started with the model.",
"## Training Details",
"### Training Data",
"### Training Procedure",
"#### Preprocessing [optional]",
"#### Training Hyperparameters\n\n- Training regime:",
"#### Speeds, Sizes, Times [optional]",
"## Evaluation",
"### Testing Data, Factors & Metrics",
"#### Testing Data",
"#### Factors",
"#### Metrics",
"### Results",
"#### Summary",
"## Model Examination [optional]",
"## Environmental Impact\n\n\n\nCarbon emissions can be estimated using the Machine Learning Impact calculator presented in Lacoste et al. (2019).\n\n- Hardware Type: \n- Hours used: \n- Cloud Provider: \n- Compute Region: \n- Carbon Emitted:",
"## Technical Specifications [optional]",
"### Model Architecture and Objective",
"### Compute Infrastructure",
"#### Hardware",
"#### Software\n\n\n\n[optional]\n\n\n\nBibTeX:\n\n\n\nAPA:",
"## Glossary [optional]",
"## More Information [optional]",
"## Model Card Authors [optional]",
"## Model Card Contact"
] | [
"TAGS\n#diffusers #safetensors #arxiv-1910.09700 #endpoints_compatible #diffusers-StableDiffusionXLPipeline #region-us \n",
"# Model Card for Model ID",
"## Model Details",
"### Model Description\n\n\n\nThis is the model card of a diffusers model that has been pushed on the Hub. This model card has been automatically generated.\n\n- Developed by: \n- Funded by [optional]: \n- Shared by [optional]: \n- Model type: \n- Language(s) (NLP): \n- License: \n- Finetuned from model [optional]:",
"### Model Sources [optional]\n\n\n\n- Repository: \n- Paper [optional]: \n- Demo [optional]:",
"## Uses",
"### Direct Use",
"### Downstream Use [optional]",
"### Out-of-Scope Use",
"## Bias, Risks, and Limitations",
"### Recommendations\n\n\n\nUsers (both direct and downstream) should be made aware of the risks, biases and limitations of the model. More information needed for further recommendations.",
"## How to Get Started with the Model\n\nUse the code below to get started with the model.",
"## Training Details",
"### Training Data",
"### Training Procedure",
"#### Preprocessing [optional]",
"#### Training Hyperparameters\n\n- Training regime:",
"#### Speeds, Sizes, Times [optional]",
"## Evaluation",
"### Testing Data, Factors & Metrics",
"#### Testing Data",
"#### Factors",
"#### Metrics",
"### Results",
"#### Summary",
"## Model Examination [optional]",
"## Environmental Impact\n\n\n\nCarbon emissions can be estimated using the Machine Learning Impact calculator presented in Lacoste et al. (2019).\n\n- Hardware Type: \n- Hours used: \n- Cloud Provider: \n- Compute Region: \n- Carbon Emitted:",
"## Technical Specifications [optional]",
"### Model Architecture and Objective",
"### Compute Infrastructure",
"#### Hardware",
"#### Software\n\n\n\n[optional]\n\n\n\nBibTeX:\n\n\n\nAPA:",
"## Glossary [optional]",
"## More Information [optional]",
"## Model Card Authors [optional]",
"## Model Card Contact"
] |
null | adapter-transformers |
# Adapter `BigTMiami/n_par_bn_v_1_help_class_80_e_adp_lr_0003_S_4` for roberta-base
An [adapter](https://adapterhub.ml) for the `roberta-base` model that was trained on the [BigTMiami/amazon_MICRO_helpfulness_dataset](https://huggingface.co/datasets/BigTMiami/amazon_MICRO_helpfulness_dataset/) dataset and includes a prediction head for classification.
This adapter was created for usage with the **[Adapters](https://github.com/Adapter-Hub/adapters)** library.
## Usage
First, install `adapters`:
```
pip install -U adapters
```
Now, the adapter can be loaded and activated like this:
```python
from adapters import AutoAdapterModel
model = AutoAdapterModel.from_pretrained("roberta-base")
adapter_name = model.load_adapter("BigTMiami/n_par_bn_v_1_help_class_80_e_adp_lr_0003_S_4", source="hf", set_active=True)
```
## Architecture & Training
<!-- Add some description here -->
## Evaluation results
<!-- Add some description here -->
## Citation
<!-- Add some description here --> | {"tags": ["roberta", "adapter-transformers"], "datasets": ["BigTMiami/amazon_MICRO_helpfulness_dataset"]} | BigTMiami/n_par_bn_v_1_help_class_80_e_adp_lr_0003_S_4 | null | [
"adapter-transformers",
"roberta",
"dataset:BigTMiami/amazon_MICRO_helpfulness_dataset",
"region:us"
] | null | 2024-04-21T19:41:50+00:00 | [] | [] | TAGS
#adapter-transformers #roberta #dataset-BigTMiami/amazon_MICRO_helpfulness_dataset #region-us
|
# Adapter 'BigTMiami/n_par_bn_v_1_help_class_80_e_adp_lr_0003_S_4' for roberta-base
An adapter for the 'roberta-base' model that was trained on the BigTMiami/amazon_MICRO_helpfulness_dataset dataset and includes a prediction head for classification.
This adapter was created for usage with the Adapters library.
## Usage
First, install 'adapters':
Now, the adapter can be loaded and activated like this:
## Architecture & Training
## Evaluation results
| [
"# Adapter 'BigTMiami/n_par_bn_v_1_help_class_80_e_adp_lr_0003_S_4' for roberta-base\n\nAn adapter for the 'roberta-base' model that was trained on the BigTMiami/amazon_MICRO_helpfulness_dataset dataset and includes a prediction head for classification.\n\nThis adapter was created for usage with the Adapters library.",
"## Usage\n\nFirst, install 'adapters':\n\n\n\nNow, the adapter can be loaded and activated like this:",
"## Architecture & Training",
"## Evaluation results"
] | [
"TAGS\n#adapter-transformers #roberta #dataset-BigTMiami/amazon_MICRO_helpfulness_dataset #region-us \n",
"# Adapter 'BigTMiami/n_par_bn_v_1_help_class_80_e_adp_lr_0003_S_4' for roberta-base\n\nAn adapter for the 'roberta-base' model that was trained on the BigTMiami/amazon_MICRO_helpfulness_dataset dataset and includes a prediction head for classification.\n\nThis adapter was created for usage with the Adapters library.",
"## Usage\n\nFirst, install 'adapters':\n\n\n\nNow, the adapter can be loaded and activated like this:",
"## Architecture & Training",
"## Evaluation results"
] |
text2text-generation | transformers |
<!-- This model card has been generated automatically according to the information the Trainer had access to. You
should probably proofread and complete it, then remove this comment. -->
# my_awesome_english_to_nepali
This model is a fine-tuned version of [t5-small](https://huggingface.co/t5-small) on an unknown dataset.
It achieves the following results on the evaluation set:
- Loss: 0.2429
- Bleu: 12.0872
- Gen Len: 16.7863
## Model description
More information needed
## Intended uses & limitations
More information needed
## Training and evaluation data
More information needed
## Training procedure
### Training hyperparameters
The following hyperparameters were used during training:
- learning_rate: 2e-05
- train_batch_size: 32
- eval_batch_size: 32
- seed: 42
- optimizer: Adam with betas=(0.9,0.999) and epsilon=1e-08
- lr_scheduler_type: linear
- num_epochs: 1
- mixed_precision_training: Native AMP
### Training results
| Training Loss | Epoch | Step | Validation Loss | Bleu | Gen Len |
|:-------------:|:-----:|:----:|:---------------:|:-------:|:-------:|
| 0.2698 | 1.0 | 4434 | 0.2429 | 12.0872 | 16.7863 |
### Framework versions
- Transformers 4.38.2
- Pytorch 2.2.1+cu121
- Datasets 2.19.0
- Tokenizers 0.15.2
| {"license": "apache-2.0", "tags": ["generated_from_trainer"], "metrics": ["bleu"], "base_model": "t5-small", "model-index": [{"name": "my_awesome_english_to_nepali", "results": []}]} | rujengelal/my_awesome_english_to_nepali | null | [
"transformers",
"tensorboard",
"safetensors",
"t5",
"text2text-generation",
"generated_from_trainer",
"base_model:t5-small",
"license:apache-2.0",
"autotrain_compatible",
"endpoints_compatible",
"text-generation-inference",
"region:us"
] | null | 2024-04-21T19:42:53+00:00 | [] | [] | TAGS
#transformers #tensorboard #safetensors #t5 #text2text-generation #generated_from_trainer #base_model-t5-small #license-apache-2.0 #autotrain_compatible #endpoints_compatible #text-generation-inference #region-us
| my\_awesome\_english\_to\_nepali
================================
This model is a fine-tuned version of t5-small on an unknown dataset.
It achieves the following results on the evaluation set:
* Loss: 0.2429
* Bleu: 12.0872
* Gen Len: 16.7863
Model description
-----------------
More information needed
Intended uses & limitations
---------------------------
More information needed
Training and evaluation data
----------------------------
More information needed
Training procedure
------------------
### Training hyperparameters
The following hyperparameters were used during training:
* learning\_rate: 2e-05
* train\_batch\_size: 32
* eval\_batch\_size: 32
* seed: 42
* optimizer: Adam with betas=(0.9,0.999) and epsilon=1e-08
* lr\_scheduler\_type: linear
* num\_epochs: 1
* mixed\_precision\_training: Native AMP
### Training results
### Framework versions
* Transformers 4.38.2
* Pytorch 2.2.1+cu121
* Datasets 2.19.0
* Tokenizers 0.15.2
| [
"### Training hyperparameters\n\n\nThe following hyperparameters were used during training:\n\n\n* learning\\_rate: 2e-05\n* train\\_batch\\_size: 32\n* eval\\_batch\\_size: 32\n* seed: 42\n* optimizer: Adam with betas=(0.9,0.999) and epsilon=1e-08\n* lr\\_scheduler\\_type: linear\n* num\\_epochs: 1\n* mixed\\_precision\\_training: Native AMP",
"### Training results",
"### Framework versions\n\n\n* Transformers 4.38.2\n* Pytorch 2.2.1+cu121\n* Datasets 2.19.0\n* Tokenizers 0.15.2"
] | [
"TAGS\n#transformers #tensorboard #safetensors #t5 #text2text-generation #generated_from_trainer #base_model-t5-small #license-apache-2.0 #autotrain_compatible #endpoints_compatible #text-generation-inference #region-us \n",
"### Training hyperparameters\n\n\nThe following hyperparameters were used during training:\n\n\n* learning\\_rate: 2e-05\n* train\\_batch\\_size: 32\n* eval\\_batch\\_size: 32\n* seed: 42\n* optimizer: Adam with betas=(0.9,0.999) and epsilon=1e-08\n* lr\\_scheduler\\_type: linear\n* num\\_epochs: 1\n* mixed\\_precision\\_training: Native AMP",
"### Training results",
"### Framework versions\n\n\n* Transformers 4.38.2\n* Pytorch 2.2.1+cu121\n* Datasets 2.19.0\n* Tokenizers 0.15.2"
] |
null | adapter-transformers |
# Adapter `BigTMiami/n_par_bn_v_1_help_class_40_e_adp_lr_0003_S_4` for roberta-base
An [adapter](https://adapterhub.ml) for the `roberta-base` model that was trained on the [BigTMiami/amazon_MICRO_helpfulness_dataset](https://huggingface.co/datasets/BigTMiami/amazon_MICRO_helpfulness_dataset/) dataset and includes a prediction head for classification.
This adapter was created for usage with the **[Adapters](https://github.com/Adapter-Hub/adapters)** library.
## Usage
First, install `adapters`:
```
pip install -U adapters
```
Now, the adapter can be loaded and activated like this:
```python
from adapters import AutoAdapterModel
model = AutoAdapterModel.from_pretrained("roberta-base")
adapter_name = model.load_adapter("BigTMiami/n_par_bn_v_1_help_class_40_e_adp_lr_0003_S_4", source="hf", set_active=True)
```
## Architecture & Training
<!-- Add some description here -->
## Evaluation results
<!-- Add some description here -->
## Citation
<!-- Add some description here --> | {"tags": ["roberta", "adapter-transformers"], "datasets": ["BigTMiami/amazon_MICRO_helpfulness_dataset"]} | BigTMiami/n_par_bn_v_1_help_class_40_e_adp_lr_0003_S_4 | null | [
"adapter-transformers",
"roberta",
"dataset:BigTMiami/amazon_MICRO_helpfulness_dataset",
"region:us"
] | null | 2024-04-21T19:43:54+00:00 | [] | [] | TAGS
#adapter-transformers #roberta #dataset-BigTMiami/amazon_MICRO_helpfulness_dataset #region-us
|
# Adapter 'BigTMiami/n_par_bn_v_1_help_class_40_e_adp_lr_0003_S_4' for roberta-base
An adapter for the 'roberta-base' model that was trained on the BigTMiami/amazon_MICRO_helpfulness_dataset dataset and includes a prediction head for classification.
This adapter was created for usage with the Adapters library.
## Usage
First, install 'adapters':
Now, the adapter can be loaded and activated like this:
## Architecture & Training
## Evaluation results
| [
"# Adapter 'BigTMiami/n_par_bn_v_1_help_class_40_e_adp_lr_0003_S_4' for roberta-base\n\nAn adapter for the 'roberta-base' model that was trained on the BigTMiami/amazon_MICRO_helpfulness_dataset dataset and includes a prediction head for classification.\n\nThis adapter was created for usage with the Adapters library.",
"## Usage\n\nFirst, install 'adapters':\n\n\n\nNow, the adapter can be loaded and activated like this:",
"## Architecture & Training",
"## Evaluation results"
] | [
"TAGS\n#adapter-transformers #roberta #dataset-BigTMiami/amazon_MICRO_helpfulness_dataset #region-us \n",
"# Adapter 'BigTMiami/n_par_bn_v_1_help_class_40_e_adp_lr_0003_S_4' for roberta-base\n\nAn adapter for the 'roberta-base' model that was trained on the BigTMiami/amazon_MICRO_helpfulness_dataset dataset and includes a prediction head for classification.\n\nThis adapter was created for usage with the Adapters library.",
"## Usage\n\nFirst, install 'adapters':\n\n\n\nNow, the adapter can be loaded and activated like this:",
"## Architecture & Training",
"## Evaluation results"
] |
Subsets and Splits
No community queries yet
The top public SQL queries from the community will appear here once available.