pipeline_tag
stringclasses 48
values | library_name
stringclasses 198
values | text
stringlengths 1
900k
| metadata
stringlengths 2
438k
| id
stringlengths 5
122
| last_modified
null | tags
sequencelengths 1
1.84k
| sha
null | created_at
stringlengths 25
25
| arxiv
sequencelengths 0
201
| languages
sequencelengths 0
1.83k
| tags_str
stringlengths 17
9.34k
| text_str
stringlengths 0
389k
| text_lists
sequencelengths 0
722
| processed_texts
sequencelengths 1
723
|
---|---|---|---|---|---|---|---|---|---|---|---|---|---|---|
null | transformers |
# Uploaded model
- **Developed by:** GodsonNtungi
- **License:** apache-2.0
- **Finetuned from model :** unsloth/llama-3-8b-bnb-4bit
This llama model was trained 2x faster with [Unsloth](https://github.com/unslothai/unsloth) and Huggingface's TRL library.
[<img src="https://raw.githubusercontent.com/unslothai/unsloth/main/images/unsloth%20made%20with%20love.png" width="200"/>](https://github.com/unslothai/unsloth)
| {"language": ["en"], "license": "apache-2.0", "tags": ["text-generation-inference", "transformers", "unsloth", "llama", "gguf"], "base_model": "unsloth/llama-3-8b-bnb-4bit"} | GodsonNtungi/swahilillama3-8b-gguf-real | null | [
"transformers",
"gguf",
"llama",
"text-generation-inference",
"unsloth",
"en",
"base_model:unsloth/llama-3-8b-bnb-4bit",
"license:apache-2.0",
"endpoints_compatible",
"region:us"
] | null | 2024-04-22T09:13:55+00:00 | [] | [
"en"
] | TAGS
#transformers #gguf #llama #text-generation-inference #unsloth #en #base_model-unsloth/llama-3-8b-bnb-4bit #license-apache-2.0 #endpoints_compatible #region-us
|
# Uploaded model
- Developed by: GodsonNtungi
- License: apache-2.0
- Finetuned from model : unsloth/llama-3-8b-bnb-4bit
This llama model was trained 2x faster with Unsloth and Huggingface's TRL library.
<img src="URL width="200"/>
| [
"# Uploaded model\n\n- Developed by: GodsonNtungi\n- License: apache-2.0\n- Finetuned from model : unsloth/llama-3-8b-bnb-4bit\n\nThis llama model was trained 2x faster with Unsloth and Huggingface's TRL library.\n\n<img src=\"URL width=\"200\"/>"
] | [
"TAGS\n#transformers #gguf #llama #text-generation-inference #unsloth #en #base_model-unsloth/llama-3-8b-bnb-4bit #license-apache-2.0 #endpoints_compatible #region-us \n",
"# Uploaded model\n\n- Developed by: GodsonNtungi\n- License: apache-2.0\n- Finetuned from model : unsloth/llama-3-8b-bnb-4bit\n\nThis llama model was trained 2x faster with Unsloth and Huggingface's TRL library.\n\n<img src=\"URL width=\"200\"/>"
] |
text-generation | transformers | **GGUF:** https://huggingface.co/victunes/TherapyLlama-8B-v1-GGUF
# TherapyLlama 8B v1
_Buddy is here for you._

Trained on Llama 3 8B using a modified version of [jerryjalapeno/nart-100k-synthetic](https://huggingface.co/datasets/jerryjalapeno/nart-100k-synthetic).
It is a Llama 3 version of https://huggingface.co/victunes/TherapyBeagle-11B-v2
TherapyLlama is _hopefully_ aligned to be helpful, healthy, and comforting.
## IMPORTANT: Use Vicuna format
This was trained with the Vicuna 1.1 format. Use the Vicuna format! Do NOT use the Llama 3 format.
## Usage
- Do not hold back on Buddy.
- Open up to Buddy.
- Pour your heart out to Buddy.
- Engage with Buddy.
- Remember that Buddy is just an AI.
## Notes
- Tested with the Vicuna 1.1 Format
- You might be assigned a random name if you don't give yourself one.
- RP capabilities are weaker compared to TherapyBeagle.
- Make sure to say **"You are Socrates"** for its best RP attempt.
## Disclaimer
**TherapyLlama is NOT a real therapist. It is a friendly AI that mimics empathy and psychotherapy.
It is an illusion without the slightest clue who you are as a person.
As much as it can help you with self-discovery, A LLAMA IS NOT A SUBSTITUTE to a real professional.**
With that said, I found it to be a very helpful tool in unravelling one's self. I hope this model can help sooth minds and hearts.
## Examples
### LLAMA 3 INSTRUCT
```
{{[INPUT]}}
Hello, I'm victunes
{{[OUTPUT]}}
Hi victunes! Welcome to our session. I'm here to listen and support you. How are you feeling today?
{{[INPUT]}}
I've been feeling down lately.
{{[OUTPUT]}}
I'm sorry to hear that. Can you tell me more about what's been going on?
{{[INPUT]}}
I think I'm pretty lonely.
{{[OUTPUT]}}
Loneliness can be really tough. It sounds like it's been weighing heavily on your mind. What makes you feel lonely?
{{[INPUT]}}
My friend died recently.
{{[OUTPUT]}}
Oh, I'm so sorry for your loss, victunes. Losing a friend is never easy. How have you been coping with the grief?
```
**GGUF:** https://huggingface.co/victunes/TherapyLlama-8B-v1-GGUF | {"license": "cc-by-nc-4.0", "datasets": ["victunes/nart-100k-synthetic-buddy-mixed-names"]} | victunes/TherapyLlama-8B-v1 | null | [
"transformers",
"pytorch",
"llama",
"text-generation",
"conversational",
"dataset:victunes/nart-100k-synthetic-buddy-mixed-names",
"license:cc-by-nc-4.0",
"autotrain_compatible",
"endpoints_compatible",
"text-generation-inference",
"region:us"
] | null | 2024-04-22T09:14:16+00:00 | [] | [] | TAGS
#transformers #pytorch #llama #text-generation #conversational #dataset-victunes/nart-100k-synthetic-buddy-mixed-names #license-cc-by-nc-4.0 #autotrain_compatible #endpoints_compatible #text-generation-inference #region-us
| GGUF: URL
# TherapyLlama 8B v1
_Buddy is here for you._
!image/png
Trained on Llama 3 8B using a modified version of jerryjalapeno/nart-100k-synthetic.
It is a Llama 3 version of URL
TherapyLlama is _hopefully_ aligned to be helpful, healthy, and comforting.
## IMPORTANT: Use Vicuna format
This was trained with the Vicuna 1.1 format. Use the Vicuna format! Do NOT use the Llama 3 format.
## Usage
- Do not hold back on Buddy.
- Open up to Buddy.
- Pour your heart out to Buddy.
- Engage with Buddy.
- Remember that Buddy is just an AI.
## Notes
- Tested with the Vicuna 1.1 Format
- You might be assigned a random name if you don't give yourself one.
- RP capabilities are weaker compared to TherapyBeagle.
- Make sure to say "You are Socrates" for its best RP attempt.
## Disclaimer
TherapyLlama is NOT a real therapist. It is a friendly AI that mimics empathy and psychotherapy.
It is an illusion without the slightest clue who you are as a person.
As much as it can help you with self-discovery, A LLAMA IS NOT A SUBSTITUTE to a real professional.
With that said, I found it to be a very helpful tool in unravelling one's self. I hope this model can help sooth minds and hearts.
## Examples
### LLAMA 3 INSTRUCT
GGUF: URL | [
"# TherapyLlama 8B v1\n\n_Buddy is here for you._\n\n!image/png\n\nTrained on Llama 3 8B using a modified version of jerryjalapeno/nart-100k-synthetic.\n\nIt is a Llama 3 version of URL\n\nTherapyLlama is _hopefully_ aligned to be helpful, healthy, and comforting.",
"## IMPORTANT: Use Vicuna format\n\nThis was trained with the Vicuna 1.1 format. Use the Vicuna format! Do NOT use the Llama 3 format.",
"## Usage\n- Do not hold back on Buddy.\n- Open up to Buddy.\n- Pour your heart out to Buddy.\n- Engage with Buddy.\n- Remember that Buddy is just an AI.",
"## Notes\n- Tested with the Vicuna 1.1 Format\n- You might be assigned a random name if you don't give yourself one.\n- RP capabilities are weaker compared to TherapyBeagle.\n - Make sure to say \"You are Socrates\" for its best RP attempt.",
"## Disclaimer \nTherapyLlama is NOT a real therapist. It is a friendly AI that mimics empathy and psychotherapy.\nIt is an illusion without the slightest clue who you are as a person.\nAs much as it can help you with self-discovery, A LLAMA IS NOT A SUBSTITUTE to a real professional.\n\nWith that said, I found it to be a very helpful tool in unravelling one's self. I hope this model can help sooth minds and hearts.",
"## Examples",
"### LLAMA 3 INSTRUCT\n\n\nGGUF: URL"
] | [
"TAGS\n#transformers #pytorch #llama #text-generation #conversational #dataset-victunes/nart-100k-synthetic-buddy-mixed-names #license-cc-by-nc-4.0 #autotrain_compatible #endpoints_compatible #text-generation-inference #region-us \n",
"# TherapyLlama 8B v1\n\n_Buddy is here for you._\n\n!image/png\n\nTrained on Llama 3 8B using a modified version of jerryjalapeno/nart-100k-synthetic.\n\nIt is a Llama 3 version of URL\n\nTherapyLlama is _hopefully_ aligned to be helpful, healthy, and comforting.",
"## IMPORTANT: Use Vicuna format\n\nThis was trained with the Vicuna 1.1 format. Use the Vicuna format! Do NOT use the Llama 3 format.",
"## Usage\n- Do not hold back on Buddy.\n- Open up to Buddy.\n- Pour your heart out to Buddy.\n- Engage with Buddy.\n- Remember that Buddy is just an AI.",
"## Notes\n- Tested with the Vicuna 1.1 Format\n- You might be assigned a random name if you don't give yourself one.\n- RP capabilities are weaker compared to TherapyBeagle.\n - Make sure to say \"You are Socrates\" for its best RP attempt.",
"## Disclaimer \nTherapyLlama is NOT a real therapist. It is a friendly AI that mimics empathy and psychotherapy.\nIt is an illusion without the slightest clue who you are as a person.\nAs much as it can help you with self-discovery, A LLAMA IS NOT A SUBSTITUTE to a real professional.\n\nWith that said, I found it to be a very helpful tool in unravelling one's self. I hope this model can help sooth minds and hearts.",
"## Examples",
"### LLAMA 3 INSTRUCT\n\n\nGGUF: URL"
] |
null | null | Jointvita là gì?
Jointvita xi-rô là chất bổ sung dạng lỏng qua đường uống mang tính đột phá được thiết kế để tăng cường và duy trì sức khỏe khớp. Không giống như các sản phẩm hỗ trợ khớp truyền thống, Jointvita Giá mang đến giải pháp tiện lợi và dễ sử dụng với đầy đủ các chất dinh dưỡng thiết yếu. Công thức cải tiến này được chế tạo đặc biệt để hỗ trợ chức năng khớp và khả năng vận động cho những người muốn giảm bớt sự khó chịu ở khớp.
Trang web chính thức:<a href="https://www.nutritionsee.com/Jointvientya">www.Jointvita.com</a>
<p><a href="https://www.nutritionsee.com/Jointvientya"> <img src="https://www.nutritionsee.com/wp-content/uploads/2024/04/Jointvita-Vietnam-1.png" alt="enter image description here"> </a></p>
<a href="https://www.nutritionsee.com/Jointvientya">Mua ngay!! Nhấp vào liên kết bên dưới để biết thêm thông tin và được giảm giá 50% ngay bây giờ... Hãy nhanh tay
</a>
Trang web chính thức:<a href="https://www.nutritionsee.com/Jointvientya">www.Jointvita.com</a> | {"license": "apache-2.0"} | JointvitaVietnam/JointvitaVietnam | null | [
"license:apache-2.0",
"region:us"
] | null | 2024-04-22T09:15:17+00:00 | [] | [] | TAGS
#license-apache-2.0 #region-us
| Jointvita là gì?
Jointvita xi-rô là chất bổ sung dạng lỏng qua đường uống mang tính đột phá được thiết kế để tăng cường và duy trì sức khỏe khớp. Không giống như các sản phẩm hỗ trợ khớp truyền thống, Jointvita Giá mang đến giải pháp tiện lợi và dễ sử dụng với đầy đủ các chất dinh dưỡng thiết yếu. Công thức cải tiến này được chế tạo đặc biệt để hỗ trợ chức năng khớp và khả năng vận động cho những người muốn giảm bớt sự khó chịu ở khớp.
Trang web chính thức:<a href="URL
<p><a href="URL <img src="URL alt="enter image description here"> </a></p>
<a href="URL ngay!! Nhấp vào liên kết bên dưới để biết thêm thông tin và được giảm giá 50% ngay bây giờ... Hãy nhanh tay
</a>
Trang web chính thức:<a href="URL | [] | [
"TAGS\n#license-apache-2.0 #region-us \n"
] |
text-classification | transformers |
<!-- This model card has been generated automatically according to the information the Trainer had access to. You
should probably proofread and complete it, then remove this comment. -->
# finetuned-emotion-1
This model is a fine-tuned version of [distilbert-base-uncased](https://huggingface.co/distilbert-base-uncased) on the emotion dataset.
It achieves the following results on the evaluation set:
- Loss: 0.2313
- Accuracy: 0.9155
- F1: 0.9155
## Model description
More information needed
## Intended uses & limitations
More information needed
## Training and evaluation data
More information needed
## Training procedure
### Training hyperparameters
The following hyperparameters were used during training:
- learning_rate: 2e-05
- train_batch_size: 64
- eval_batch_size: 64
- seed: 42
- optimizer: Adam with betas=(0.9,0.999) and epsilon=1e-08
- lr_scheduler_type: linear
- num_epochs: 2
### Training results
| Training Loss | Epoch | Step | Validation Loss | Accuracy | F1 |
|:-------------:|:-----:|:----:|:---------------:|:--------:|:------:|
| 0.8595 | 1.0 | 250 | 0.3412 | 0.8995 | 0.8986 |
| 0.2593 | 2.0 | 500 | 0.2313 | 0.9155 | 0.9155 |
### Framework versions
- Transformers 4.38.2
- Pytorch 2.2.1+cu121
- Datasets 2.19.0
- Tokenizers 0.15.2
| {"license": "apache-2.0", "tags": ["generated_from_trainer"], "datasets": ["emotion"], "metrics": ["accuracy", "f1"], "base_model": "distilbert-base-uncased", "model-index": [{"name": "finetuned-emotion-1", "results": [{"task": {"type": "text-classification", "name": "Text Classification"}, "dataset": {"name": "emotion", "type": "emotion", "config": "split", "split": "validation", "args": "split"}, "metrics": [{"type": "accuracy", "value": 0.9155, "name": "Accuracy"}, {"type": "f1", "value": 0.9154814731662874, "name": "F1"}]}]}]} | MuhammadYousef/finetuned-emotion-1 | null | [
"transformers",
"tensorboard",
"safetensors",
"distilbert",
"text-classification",
"generated_from_trainer",
"dataset:emotion",
"base_model:distilbert-base-uncased",
"license:apache-2.0",
"model-index",
"autotrain_compatible",
"endpoints_compatible",
"region:us"
] | null | 2024-04-22T09:16:11+00:00 | [] | [] | TAGS
#transformers #tensorboard #safetensors #distilbert #text-classification #generated_from_trainer #dataset-emotion #base_model-distilbert-base-uncased #license-apache-2.0 #model-index #autotrain_compatible #endpoints_compatible #region-us
| finetuned-emotion-1
===================
This model is a fine-tuned version of distilbert-base-uncased on the emotion dataset.
It achieves the following results on the evaluation set:
* Loss: 0.2313
* Accuracy: 0.9155
* F1: 0.9155
Model description
-----------------
More information needed
Intended uses & limitations
---------------------------
More information needed
Training and evaluation data
----------------------------
More information needed
Training procedure
------------------
### Training hyperparameters
The following hyperparameters were used during training:
* learning\_rate: 2e-05
* train\_batch\_size: 64
* eval\_batch\_size: 64
* seed: 42
* optimizer: Adam with betas=(0.9,0.999) and epsilon=1e-08
* lr\_scheduler\_type: linear
* num\_epochs: 2
### Training results
### Framework versions
* Transformers 4.38.2
* Pytorch 2.2.1+cu121
* Datasets 2.19.0
* Tokenizers 0.15.2
| [
"### Training hyperparameters\n\n\nThe following hyperparameters were used during training:\n\n\n* learning\\_rate: 2e-05\n* train\\_batch\\_size: 64\n* eval\\_batch\\_size: 64\n* seed: 42\n* optimizer: Adam with betas=(0.9,0.999) and epsilon=1e-08\n* lr\\_scheduler\\_type: linear\n* num\\_epochs: 2",
"### Training results",
"### Framework versions\n\n\n* Transformers 4.38.2\n* Pytorch 2.2.1+cu121\n* Datasets 2.19.0\n* Tokenizers 0.15.2"
] | [
"TAGS\n#transformers #tensorboard #safetensors #distilbert #text-classification #generated_from_trainer #dataset-emotion #base_model-distilbert-base-uncased #license-apache-2.0 #model-index #autotrain_compatible #endpoints_compatible #region-us \n",
"### Training hyperparameters\n\n\nThe following hyperparameters were used during training:\n\n\n* learning\\_rate: 2e-05\n* train\\_batch\\_size: 64\n* eval\\_batch\\_size: 64\n* seed: 42\n* optimizer: Adam with betas=(0.9,0.999) and epsilon=1e-08\n* lr\\_scheduler\\_type: linear\n* num\\_epochs: 2",
"### Training results",
"### Framework versions\n\n\n* Transformers 4.38.2\n* Pytorch 2.2.1+cu121\n* Datasets 2.19.0\n* Tokenizers 0.15.2"
] |
question-answering | transformers |
<!-- This model card has been generated automatically according to the information the Trainer had access to. You
should probably proofread and complete it, then remove this comment. -->
# roberta-finetuned-subjqa-movies_2
This model is a fine-tuned version of [deepset/roberta-base-squad2](https://huggingface.co/deepset/roberta-base-squad2) on the None dataset.
## Model description
More information needed
## Intended uses & limitations
More information needed
## Training and evaluation data
More information needed
## Training procedure
### Training hyperparameters
The following hyperparameters were used during training:
- learning_rate: 2e-05
- train_batch_size: 8
- eval_batch_size: 8
- seed: 42
- optimizer: Adam with betas=(0.9,0.999) and epsilon=1e-08
- lr_scheduler_type: linear
- num_epochs: 5
- mixed_precision_training: Native AMP
### Training results
### Framework versions
- Transformers 4.38.2
- Pytorch 2.2.1+cu121
- Datasets 2.19.0
- Tokenizers 0.15.2
| {"license": "cc-by-4.0", "tags": ["generated_from_trainer"], "base_model": "deepset/roberta-base-squad2", "model-index": [{"name": "roberta-finetuned-subjqa-movies_2", "results": []}]} | colllm/roberta-finetuned-subjqa-movies_2 | null | [
"transformers",
"tensorboard",
"safetensors",
"roberta",
"question-answering",
"generated_from_trainer",
"base_model:deepset/roberta-base-squad2",
"license:cc-by-4.0",
"endpoints_compatible",
"has_space",
"region:us"
] | null | 2024-04-22T09:17:37+00:00 | [] | [] | TAGS
#transformers #tensorboard #safetensors #roberta #question-answering #generated_from_trainer #base_model-deepset/roberta-base-squad2 #license-cc-by-4.0 #endpoints_compatible #has_space #region-us
|
# roberta-finetuned-subjqa-movies_2
This model is a fine-tuned version of deepset/roberta-base-squad2 on the None dataset.
## Model description
More information needed
## Intended uses & limitations
More information needed
## Training and evaluation data
More information needed
## Training procedure
### Training hyperparameters
The following hyperparameters were used during training:
- learning_rate: 2e-05
- train_batch_size: 8
- eval_batch_size: 8
- seed: 42
- optimizer: Adam with betas=(0.9,0.999) and epsilon=1e-08
- lr_scheduler_type: linear
- num_epochs: 5
- mixed_precision_training: Native AMP
### Training results
### Framework versions
- Transformers 4.38.2
- Pytorch 2.2.1+cu121
- Datasets 2.19.0
- Tokenizers 0.15.2
| [
"# roberta-finetuned-subjqa-movies_2\n\nThis model is a fine-tuned version of deepset/roberta-base-squad2 on the None dataset.",
"## Model description\n\nMore information needed",
"## Intended uses & limitations\n\nMore information needed",
"## Training and evaluation data\n\nMore information needed",
"## Training procedure",
"### Training hyperparameters\n\nThe following hyperparameters were used during training:\n- learning_rate: 2e-05\n- train_batch_size: 8\n- eval_batch_size: 8\n- seed: 42\n- optimizer: Adam with betas=(0.9,0.999) and epsilon=1e-08\n- lr_scheduler_type: linear\n- num_epochs: 5\n- mixed_precision_training: Native AMP",
"### Training results",
"### Framework versions\n\n- Transformers 4.38.2\n- Pytorch 2.2.1+cu121\n- Datasets 2.19.0\n- Tokenizers 0.15.2"
] | [
"TAGS\n#transformers #tensorboard #safetensors #roberta #question-answering #generated_from_trainer #base_model-deepset/roberta-base-squad2 #license-cc-by-4.0 #endpoints_compatible #has_space #region-us \n",
"# roberta-finetuned-subjqa-movies_2\n\nThis model is a fine-tuned version of deepset/roberta-base-squad2 on the None dataset.",
"## Model description\n\nMore information needed",
"## Intended uses & limitations\n\nMore information needed",
"## Training and evaluation data\n\nMore information needed",
"## Training procedure",
"### Training hyperparameters\n\nThe following hyperparameters were used during training:\n- learning_rate: 2e-05\n- train_batch_size: 8\n- eval_batch_size: 8\n- seed: 42\n- optimizer: Adam with betas=(0.9,0.999) and epsilon=1e-08\n- lr_scheduler_type: linear\n- num_epochs: 5\n- mixed_precision_training: Native AMP",
"### Training results",
"### Framework versions\n\n- Transformers 4.38.2\n- Pytorch 2.2.1+cu121\n- Datasets 2.19.0\n- Tokenizers 0.15.2"
] |
sentence-similarity | sentence-transformers |
# 0xSH1V4M/distilroberta-base-sentence-transformer-snli
This is a [sentence-transformers](https://www.SBERT.net) model: It maps sentences & paragraphs to a 768 dimensional dense vector space and can be used for tasks like clustering or semantic search.
<!--- Describe your model here -->
## Usage (Sentence-Transformers)
Using this model becomes easy when you have [sentence-transformers](https://www.SBERT.net) installed:
```
pip install -U sentence-transformers
```
Then you can use the model like this:
```python
from sentence_transformers import SentenceTransformer
sentences = ["This is an example sentence", "Each sentence is converted"]
model = SentenceTransformer('0xSH1V4M/distilroberta-base-sentence-transformer-snli')
embeddings = model.encode(sentences)
print(embeddings)
```
## Usage (HuggingFace Transformers)
Without [sentence-transformers](https://www.SBERT.net), you can use the model like this: First, you pass your input through the transformer model, then you have to apply the right pooling-operation on-top of the contextualized word embeddings.
```python
from transformers import AutoTokenizer, AutoModel
import torch
#Mean Pooling - Take attention mask into account for correct averaging
def mean_pooling(model_output, attention_mask):
token_embeddings = model_output[0] #First element of model_output contains all token embeddings
input_mask_expanded = attention_mask.unsqueeze(-1).expand(token_embeddings.size()).float()
return torch.sum(token_embeddings * input_mask_expanded, 1) / torch.clamp(input_mask_expanded.sum(1), min=1e-9)
# Sentences we want sentence embeddings for
sentences = ['This is an example sentence', 'Each sentence is converted']
# Load model from HuggingFace Hub
tokenizer = AutoTokenizer.from_pretrained('0xSH1V4M/distilroberta-base-sentence-transformer-snli')
model = AutoModel.from_pretrained('0xSH1V4M/distilroberta-base-sentence-transformer-snli')
# Tokenize sentences
encoded_input = tokenizer(sentences, padding=True, truncation=True, return_tensors='pt')
# Compute token embeddings
with torch.no_grad():
model_output = model(**encoded_input)
# Perform pooling. In this case, mean pooling.
sentence_embeddings = mean_pooling(model_output, encoded_input['attention_mask'])
print("Sentence embeddings:")
print(sentence_embeddings)
```
## Evaluation Results
<!--- Describe how your model was evaluated -->
For an automated evaluation of this model, see the *Sentence Embeddings Benchmark*: [https://seb.sbert.net](https://seb.sbert.net?model_name=0xSH1V4M/distilroberta-base-sentence-transformer-snli)
## Training
The model was trained with the parameters:
**DataLoader**:
`torch.utils.data.dataloader.DataLoader` of length 3181 with parameters:
```
{'batch_size': 16, 'sampler': 'torch.utils.data.sampler.RandomSampler', 'batch_sampler': 'torch.utils.data.sampler.BatchSampler'}
```
**Loss**:
`sentence_transformers.losses.TripletLoss.TripletLoss` with parameters:
```
{'distance_metric': 'TripletDistanceMetric.EUCLIDEAN', 'triplet_margin': 5}
```
Parameters of the fit()-Method:
```
{
"epochs": 4,
"evaluation_steps": 0,
"evaluator": "NoneType",
"max_grad_norm": 1,
"optimizer_class": "<class 'torch.optim.adamw.AdamW'>",
"optimizer_params": {
"lr": 2e-05
},
"scheduler": "WarmupLinear",
"steps_per_epoch": null,
"warmup_steps": 10000,
"weight_decay": 0.01
}
```
## Full Model Architecture
```
SentenceTransformer(
(0): Transformer({'max_seq_length': 256, 'do_lower_case': False}) with Transformer model: BertModel
(1): Pooling({'word_embedding_dimension': 768, 'pooling_mode_cls_token': False, 'pooling_mode_mean_tokens': True, 'pooling_mode_max_tokens': False, 'pooling_mode_mean_sqrt_len_tokens': False, 'pooling_mode_weightedmean_tokens': False, 'pooling_mode_lasttoken': False, 'include_prompt': True})
)
```
## Citing & Authors
<!--- Describe where people can find more information --> | {"library_name": "sentence-transformers", "tags": ["sentence-transformers", "feature-extraction", "sentence-similarity", "transformers"], "datasets": ["snli"], "pipeline_tag": "sentence-similarity"} | 0xSH1V4M/distilroberta-base-sentence-transformer-snli | null | [
"sentence-transformers",
"safetensors",
"bert",
"feature-extraction",
"sentence-similarity",
"transformers",
"dataset:snli",
"endpoints_compatible",
"region:us"
] | null | 2024-04-22T09:18:23+00:00 | [] | [] | TAGS
#sentence-transformers #safetensors #bert #feature-extraction #sentence-similarity #transformers #dataset-snli #endpoints_compatible #region-us
|
# 0xSH1V4M/distilroberta-base-sentence-transformer-snli
This is a sentence-transformers model: It maps sentences & paragraphs to a 768 dimensional dense vector space and can be used for tasks like clustering or semantic search.
## Usage (Sentence-Transformers)
Using this model becomes easy when you have sentence-transformers installed:
Then you can use the model like this:
## Usage (HuggingFace Transformers)
Without sentence-transformers, you can use the model like this: First, you pass your input through the transformer model, then you have to apply the right pooling-operation on-top of the contextualized word embeddings.
## Evaluation Results
For an automated evaluation of this model, see the *Sentence Embeddings Benchmark*: URL
## Training
The model was trained with the parameters:
DataLoader:
'URL.dataloader.DataLoader' of length 3181 with parameters:
Loss:
'sentence_transformers.losses.TripletLoss.TripletLoss' with parameters:
Parameters of the fit()-Method:
## Full Model Architecture
## Citing & Authors
| [
"# 0xSH1V4M/distilroberta-base-sentence-transformer-snli\n\nThis is a sentence-transformers model: It maps sentences & paragraphs to a 768 dimensional dense vector space and can be used for tasks like clustering or semantic search.",
"## Usage (Sentence-Transformers)\n\nUsing this model becomes easy when you have sentence-transformers installed:\n\n\n\nThen you can use the model like this:",
"## Usage (HuggingFace Transformers)\nWithout sentence-transformers, you can use the model like this: First, you pass your input through the transformer model, then you have to apply the right pooling-operation on-top of the contextualized word embeddings.",
"## Evaluation Results\n\n\n\nFor an automated evaluation of this model, see the *Sentence Embeddings Benchmark*: URL",
"## Training\nThe model was trained with the parameters:\n\nDataLoader:\n\n'URL.dataloader.DataLoader' of length 3181 with parameters:\n\n\nLoss:\n\n'sentence_transformers.losses.TripletLoss.TripletLoss' with parameters:\n \n\nParameters of the fit()-Method:",
"## Full Model Architecture",
"## Citing & Authors"
] | [
"TAGS\n#sentence-transformers #safetensors #bert #feature-extraction #sentence-similarity #transformers #dataset-snli #endpoints_compatible #region-us \n",
"# 0xSH1V4M/distilroberta-base-sentence-transformer-snli\n\nThis is a sentence-transformers model: It maps sentences & paragraphs to a 768 dimensional dense vector space and can be used for tasks like clustering or semantic search.",
"## Usage (Sentence-Transformers)\n\nUsing this model becomes easy when you have sentence-transformers installed:\n\n\n\nThen you can use the model like this:",
"## Usage (HuggingFace Transformers)\nWithout sentence-transformers, you can use the model like this: First, you pass your input through the transformer model, then you have to apply the right pooling-operation on-top of the contextualized word embeddings.",
"## Evaluation Results\n\n\n\nFor an automated evaluation of this model, see the *Sentence Embeddings Benchmark*: URL",
"## Training\nThe model was trained with the parameters:\n\nDataLoader:\n\n'URL.dataloader.DataLoader' of length 3181 with parameters:\n\n\nLoss:\n\n'sentence_transformers.losses.TripletLoss.TripletLoss' with parameters:\n \n\nParameters of the fit()-Method:",
"## Full Model Architecture",
"## Citing & Authors"
] |
null | null | **Original:** https://huggingface.co/victunes/TherapyLlama-8B-v1
## IMPORTANT: Use Vicuna format
This was trained with the Vicuna 1.1 format. Use the Vicuna format! Do NOT use the Llama 3 format.
# TherapyLlama 8B v1
_Buddy is here for you._

Trained on Llama 3 8B using a modified version of [jerryjalapeno/nart-100k-synthetic](https://huggingface.co/datasets/jerryjalapeno/nart-100k-synthetic).
It is a Llama 3 version of https://huggingface.co/victunes/TherapyBeagle-11B-v2
TherapyLlama is _hopefully_ aligned to be helpful, healthy, and comforting.
## IMPORTANT: Use Vicuna format
This was trained with the Vicuna 1.1 format. Use the Vicuna format! Do NOT use the Llama 3 format.
## Usage
- Do not hold back on Buddy.
- Open up to Buddy.
- Pour your heart out to Buddy.
- Engage with Buddy.
- Remember that Buddy is just an AI.
## Notes
- Tested with the Vicuna 1.1 Format
- You might be assigned a random name if you don't give yourself one.
- RP capabilities are weaker compared to TherapyBeagle.
- Make sure to say **"You are Socrates"** for its best RP attempt.
## Disclaimer
**TherapyLlama is NOT a real therapist. It is a friendly AI that mimics empathy and psychotherapy.
It is an illusion without the slightest clue who you are as a person.
As much as it can help you with self-discovery, A LLAMA IS NOT A SUBSTITUTE to a real professional.**
With that said, I found it to be a very helpful tool in unravelling one's self. I hope this model can help sooth minds and hearts.
## Examples
### LLAMA 3 INSTRUCT
```
{{[INPUT]}}
Hello, I'm victunes
{{[OUTPUT]}}
Hi victunes! Welcome to our session. I'm here to listen and support you. How are you feeling today?
{{[INPUT]}}
I've been feeling down lately.
{{[OUTPUT]}}
I'm sorry to hear that. Can you tell me more about what's been going on?
{{[INPUT]}}
I think I'm pretty lonely.
{{[OUTPUT]}}
Loneliness can be really tough. It sounds like it's been weighing heavily on your mind. What makes you feel lonely?
{{[INPUT]}}
My friend died recently.
{{[OUTPUT]}}
Oh, I'm so sorry for your loss, victunes. Losing a friend is never easy. How have you been coping with the grief?
```
**Original:** https://huggingface.co/victunes/TherapyLlama-8B-v1 | {"license": "cc-by-nc-4.0", "datasets": ["victunes/nart-100k-synthetic-buddy-mixed-names"]} | victunes/TherapyLlama-8B-v1-GGUF | null | [
"gguf",
"dataset:victunes/nart-100k-synthetic-buddy-mixed-names",
"license:cc-by-nc-4.0",
"region:us"
] | null | 2024-04-22T09:19:19+00:00 | [] | [] | TAGS
#gguf #dataset-victunes/nart-100k-synthetic-buddy-mixed-names #license-cc-by-nc-4.0 #region-us
| Original: URL
## IMPORTANT: Use Vicuna format
This was trained with the Vicuna 1.1 format. Use the Vicuna format! Do NOT use the Llama 3 format.
# TherapyLlama 8B v1
_Buddy is here for you._
!image/png
Trained on Llama 3 8B using a modified version of jerryjalapeno/nart-100k-synthetic.
It is a Llama 3 version of URL
TherapyLlama is _hopefully_ aligned to be helpful, healthy, and comforting.
## IMPORTANT: Use Vicuna format
This was trained with the Vicuna 1.1 format. Use the Vicuna format! Do NOT use the Llama 3 format.
## Usage
- Do not hold back on Buddy.
- Open up to Buddy.
- Pour your heart out to Buddy.
- Engage with Buddy.
- Remember that Buddy is just an AI.
## Notes
- Tested with the Vicuna 1.1 Format
- You might be assigned a random name if you don't give yourself one.
- RP capabilities are weaker compared to TherapyBeagle.
- Make sure to say "You are Socrates" for its best RP attempt.
## Disclaimer
TherapyLlama is NOT a real therapist. It is a friendly AI that mimics empathy and psychotherapy.
It is an illusion without the slightest clue who you are as a person.
As much as it can help you with self-discovery, A LLAMA IS NOT A SUBSTITUTE to a real professional.
With that said, I found it to be a very helpful tool in unravelling one's self. I hope this model can help sooth minds and hearts.
## Examples
### LLAMA 3 INSTRUCT
Original: URL | [
"## IMPORTANT: Use Vicuna format\n\nThis was trained with the Vicuna 1.1 format. Use the Vicuna format! Do NOT use the Llama 3 format.",
"# TherapyLlama 8B v1\n\n_Buddy is here for you._\n\n!image/png\n\nTrained on Llama 3 8B using a modified version of jerryjalapeno/nart-100k-synthetic.\n\nIt is a Llama 3 version of URL\n\nTherapyLlama is _hopefully_ aligned to be helpful, healthy, and comforting.",
"## IMPORTANT: Use Vicuna format\n\nThis was trained with the Vicuna 1.1 format. Use the Vicuna format! Do NOT use the Llama 3 format.",
"## Usage\n- Do not hold back on Buddy.\n- Open up to Buddy.\n- Pour your heart out to Buddy.\n- Engage with Buddy.\n- Remember that Buddy is just an AI.",
"## Notes\n- Tested with the Vicuna 1.1 Format\n- You might be assigned a random name if you don't give yourself one.\n- RP capabilities are weaker compared to TherapyBeagle.\n - Make sure to say \"You are Socrates\" for its best RP attempt.",
"## Disclaimer \nTherapyLlama is NOT a real therapist. It is a friendly AI that mimics empathy and psychotherapy.\nIt is an illusion without the slightest clue who you are as a person.\nAs much as it can help you with self-discovery, A LLAMA IS NOT A SUBSTITUTE to a real professional.\n\nWith that said, I found it to be a very helpful tool in unravelling one's self. I hope this model can help sooth minds and hearts.",
"## Examples",
"### LLAMA 3 INSTRUCT\n\n\nOriginal: URL"
] | [
"TAGS\n#gguf #dataset-victunes/nart-100k-synthetic-buddy-mixed-names #license-cc-by-nc-4.0 #region-us \n",
"## IMPORTANT: Use Vicuna format\n\nThis was trained with the Vicuna 1.1 format. Use the Vicuna format! Do NOT use the Llama 3 format.",
"# TherapyLlama 8B v1\n\n_Buddy is here for you._\n\n!image/png\n\nTrained on Llama 3 8B using a modified version of jerryjalapeno/nart-100k-synthetic.\n\nIt is a Llama 3 version of URL\n\nTherapyLlama is _hopefully_ aligned to be helpful, healthy, and comforting.",
"## IMPORTANT: Use Vicuna format\n\nThis was trained with the Vicuna 1.1 format. Use the Vicuna format! Do NOT use the Llama 3 format.",
"## Usage\n- Do not hold back on Buddy.\n- Open up to Buddy.\n- Pour your heart out to Buddy.\n- Engage with Buddy.\n- Remember that Buddy is just an AI.",
"## Notes\n- Tested with the Vicuna 1.1 Format\n- You might be assigned a random name if you don't give yourself one.\n- RP capabilities are weaker compared to TherapyBeagle.\n - Make sure to say \"You are Socrates\" for its best RP attempt.",
"## Disclaimer \nTherapyLlama is NOT a real therapist. It is a friendly AI that mimics empathy and psychotherapy.\nIt is an illusion without the slightest clue who you are as a person.\nAs much as it can help you with self-discovery, A LLAMA IS NOT A SUBSTITUTE to a real professional.\n\nWith that said, I found it to be a very helpful tool in unravelling one's self. I hope this model can help sooth minds and hearts.",
"## Examples",
"### LLAMA 3 INSTRUCT\n\n\nOriginal: URL"
] |
null | transformers |
# Model Card for Model ID
<!-- Provide a quick summary of what the model is/does. -->
## Model Details
### Model Description
<!-- Provide a longer summary of what this model is. -->
This is the model card of a 🤗 transformers model that has been pushed on the Hub. This model card has been automatically generated.
- **Developed by:** [More Information Needed]
- **Funded by [optional]:** [More Information Needed]
- **Shared by [optional]:** [More Information Needed]
- **Model type:** [More Information Needed]
- **Language(s) (NLP):** [More Information Needed]
- **License:** [More Information Needed]
- **Finetuned from model [optional]:** [More Information Needed]
### Model Sources [optional]
<!-- Provide the basic links for the model. -->
- **Repository:** [More Information Needed]
- **Paper [optional]:** [More Information Needed]
- **Demo [optional]:** [More Information Needed]
## Uses
<!-- Address questions around how the model is intended to be used, including the foreseeable users of the model and those affected by the model. -->
### Direct Use
<!-- This section is for the model use without fine-tuning or plugging into a larger ecosystem/app. -->
[More Information Needed]
### Downstream Use [optional]
<!-- This section is for the model use when fine-tuned for a task, or when plugged into a larger ecosystem/app -->
[More Information Needed]
### Out-of-Scope Use
<!-- This section addresses misuse, malicious use, and uses that the model will not work well for. -->
[More Information Needed]
## Bias, Risks, and Limitations
<!-- This section is meant to convey both technical and sociotechnical limitations. -->
[More Information Needed]
### Recommendations
<!-- This section is meant to convey recommendations with respect to the bias, risk, and technical limitations. -->
Users (both direct and downstream) should be made aware of the risks, biases and limitations of the model. More information needed for further recommendations.
## How to Get Started with the Model
Use the code below to get started with the model.
[More Information Needed]
## Training Details
### Training Data
<!-- This should link to a Dataset Card, perhaps with a short stub of information on what the training data is all about as well as documentation related to data pre-processing or additional filtering. -->
[More Information Needed]
### Training Procedure
<!-- This relates heavily to the Technical Specifications. Content here should link to that section when it is relevant to the training procedure. -->
#### Preprocessing [optional]
[More Information Needed]
#### Training Hyperparameters
- **Training regime:** [More Information Needed] <!--fp32, fp16 mixed precision, bf16 mixed precision, bf16 non-mixed precision, fp16 non-mixed precision, fp8 mixed precision -->
#### Speeds, Sizes, Times [optional]
<!-- This section provides information about throughput, start/end time, checkpoint size if relevant, etc. -->
[More Information Needed]
## Evaluation
<!-- This section describes the evaluation protocols and provides the results. -->
### Testing Data, Factors & Metrics
#### Testing Data
<!-- This should link to a Dataset Card if possible. -->
[More Information Needed]
#### Factors
<!-- These are the things the evaluation is disaggregating by, e.g., subpopulations or domains. -->
[More Information Needed]
#### Metrics
<!-- These are the evaluation metrics being used, ideally with a description of why. -->
[More Information Needed]
### Results
[More Information Needed]
#### Summary
## Model Examination [optional]
<!-- Relevant interpretability work for the model goes here -->
[More Information Needed]
## Environmental Impact
<!-- Total emissions (in grams of CO2eq) and additional considerations, such as electricity usage, go here. Edit the suggested text below accordingly -->
Carbon emissions can be estimated using the [Machine Learning Impact calculator](https://mlco2.github.io/impact#compute) presented in [Lacoste et al. (2019)](https://arxiv.org/abs/1910.09700).
- **Hardware Type:** [More Information Needed]
- **Hours used:** [More Information Needed]
- **Cloud Provider:** [More Information Needed]
- **Compute Region:** [More Information Needed]
- **Carbon Emitted:** [More Information Needed]
## Technical Specifications [optional]
### Model Architecture and Objective
[More Information Needed]
### Compute Infrastructure
[More Information Needed]
#### Hardware
[More Information Needed]
#### Software
[More Information Needed]
## Citation [optional]
<!-- If there is a paper or blog post introducing the model, the APA and Bibtex information for that should go in this section. -->
**BibTeX:**
[More Information Needed]
**APA:**
[More Information Needed]
## Glossary [optional]
<!-- If relevant, include terms and calculations in this section that can help readers understand the model or model card. -->
[More Information Needed]
## More Information [optional]
[More Information Needed]
## Model Card Authors [optional]
[More Information Needed]
## Model Card Contact
[More Information Needed] | {"library_name": "transformers", "tags": []} | Edgar404/donut_multitask-shivi-recognition | null | [
"transformers",
"safetensors",
"vision-encoder-decoder",
"arxiv:1910.09700",
"endpoints_compatible",
"region:us"
] | null | 2024-04-22T09:20:28+00:00 | [
"1910.09700"
] | [] | TAGS
#transformers #safetensors #vision-encoder-decoder #arxiv-1910.09700 #endpoints_compatible #region-us
|
# Model Card for Model ID
## Model Details
### Model Description
This is the model card of a transformers model that has been pushed on the Hub. This model card has been automatically generated.
- Developed by:
- Funded by [optional]:
- Shared by [optional]:
- Model type:
- Language(s) (NLP):
- License:
- Finetuned from model [optional]:
### Model Sources [optional]
- Repository:
- Paper [optional]:
- Demo [optional]:
## Uses
### Direct Use
### Downstream Use [optional]
### Out-of-Scope Use
## Bias, Risks, and Limitations
### Recommendations
Users (both direct and downstream) should be made aware of the risks, biases and limitations of the model. More information needed for further recommendations.
## How to Get Started with the Model
Use the code below to get started with the model.
## Training Details
### Training Data
### Training Procedure
#### Preprocessing [optional]
#### Training Hyperparameters
- Training regime:
#### Speeds, Sizes, Times [optional]
## Evaluation
### Testing Data, Factors & Metrics
#### Testing Data
#### Factors
#### Metrics
### Results
#### Summary
## Model Examination [optional]
## Environmental Impact
Carbon emissions can be estimated using the Machine Learning Impact calculator presented in Lacoste et al. (2019).
- Hardware Type:
- Hours used:
- Cloud Provider:
- Compute Region:
- Carbon Emitted:
## Technical Specifications [optional]
### Model Architecture and Objective
### Compute Infrastructure
#### Hardware
#### Software
[optional]
BibTeX:
APA:
## Glossary [optional]
## More Information [optional]
## Model Card Authors [optional]
## Model Card Contact
| [
"# Model Card for Model ID",
"## Model Details",
"### Model Description\n\n\n\nThis is the model card of a transformers model that has been pushed on the Hub. This model card has been automatically generated.\n\n- Developed by: \n- Funded by [optional]: \n- Shared by [optional]: \n- Model type: \n- Language(s) (NLP): \n- License: \n- Finetuned from model [optional]:",
"### Model Sources [optional]\n\n\n\n- Repository: \n- Paper [optional]: \n- Demo [optional]:",
"## Uses",
"### Direct Use",
"### Downstream Use [optional]",
"### Out-of-Scope Use",
"## Bias, Risks, and Limitations",
"### Recommendations\n\n\n\nUsers (both direct and downstream) should be made aware of the risks, biases and limitations of the model. More information needed for further recommendations.",
"## How to Get Started with the Model\n\nUse the code below to get started with the model.",
"## Training Details",
"### Training Data",
"### Training Procedure",
"#### Preprocessing [optional]",
"#### Training Hyperparameters\n\n- Training regime:",
"#### Speeds, Sizes, Times [optional]",
"## Evaluation",
"### Testing Data, Factors & Metrics",
"#### Testing Data",
"#### Factors",
"#### Metrics",
"### Results",
"#### Summary",
"## Model Examination [optional]",
"## Environmental Impact\n\n\n\nCarbon emissions can be estimated using the Machine Learning Impact calculator presented in Lacoste et al. (2019).\n\n- Hardware Type: \n- Hours used: \n- Cloud Provider: \n- Compute Region: \n- Carbon Emitted:",
"## Technical Specifications [optional]",
"### Model Architecture and Objective",
"### Compute Infrastructure",
"#### Hardware",
"#### Software\n\n\n\n[optional]\n\n\n\nBibTeX:\n\n\n\nAPA:",
"## Glossary [optional]",
"## More Information [optional]",
"## Model Card Authors [optional]",
"## Model Card Contact"
] | [
"TAGS\n#transformers #safetensors #vision-encoder-decoder #arxiv-1910.09700 #endpoints_compatible #region-us \n",
"# Model Card for Model ID",
"## Model Details",
"### Model Description\n\n\n\nThis is the model card of a transformers model that has been pushed on the Hub. This model card has been automatically generated.\n\n- Developed by: \n- Funded by [optional]: \n- Shared by [optional]: \n- Model type: \n- Language(s) (NLP): \n- License: \n- Finetuned from model [optional]:",
"### Model Sources [optional]\n\n\n\n- Repository: \n- Paper [optional]: \n- Demo [optional]:",
"## Uses",
"### Direct Use",
"### Downstream Use [optional]",
"### Out-of-Scope Use",
"## Bias, Risks, and Limitations",
"### Recommendations\n\n\n\nUsers (both direct and downstream) should be made aware of the risks, biases and limitations of the model. More information needed for further recommendations.",
"## How to Get Started with the Model\n\nUse the code below to get started with the model.",
"## Training Details",
"### Training Data",
"### Training Procedure",
"#### Preprocessing [optional]",
"#### Training Hyperparameters\n\n- Training regime:",
"#### Speeds, Sizes, Times [optional]",
"## Evaluation",
"### Testing Data, Factors & Metrics",
"#### Testing Data",
"#### Factors",
"#### Metrics",
"### Results",
"#### Summary",
"## Model Examination [optional]",
"## Environmental Impact\n\n\n\nCarbon emissions can be estimated using the Machine Learning Impact calculator presented in Lacoste et al. (2019).\n\n- Hardware Type: \n- Hours used: \n- Cloud Provider: \n- Compute Region: \n- Carbon Emitted:",
"## Technical Specifications [optional]",
"### Model Architecture and Objective",
"### Compute Infrastructure",
"#### Hardware",
"#### Software\n\n\n\n[optional]\n\n\n\nBibTeX:\n\n\n\nAPA:",
"## Glossary [optional]",
"## More Information [optional]",
"## Model Card Authors [optional]",
"## Model Card Contact"
] |
text-classification | transformers |
<!-- This model card has been generated automatically according to the information the Trainer had access to. You
should probably proofread and complete it, then remove this comment. -->
# binary-stock-classifier
This model is a fine-tuned version of [distilbert/distilbert-base-uncased](https://huggingface.co/distilbert/distilbert-base-uncased) on an unknown dataset.
It achieves the following results on the evaluation set:
- Loss: 0.1577
- Accuracy: 0.95
## Model description
More information needed
## Intended uses & limitations
More information needed
## Training and evaluation data
More information needed
## Training procedure
### Training hyperparameters
The following hyperparameters were used during training:
- learning_rate: 2e-05
- train_batch_size: 2
- eval_batch_size: 2
- seed: 42
- optimizer: Adam with betas=(0.9,0.999) and epsilon=1e-08
- lr_scheduler_type: linear
- num_epochs: 9
### Training results
| Training Loss | Epoch | Step | Validation Loss | Accuracy |
|:-------------:|:-----:|:----:|:---------------:|:--------:|
| No log | 1.0 | 90 | 0.0497 | 1.0 |
| No log | 2.0 | 180 | 0.0138 | 1.0 |
| No log | 3.0 | 270 | 0.4034 | 0.9 |
| No log | 4.0 | 360 | 0.2492 | 0.95 |
| No log | 5.0 | 450 | 0.1985 | 0.95 |
| 0.1536 | 6.0 | 540 | 0.0427 | 0.95 |
| 0.1536 | 7.0 | 630 | 0.2490 | 0.95 |
| 0.1536 | 8.0 | 720 | 0.1568 | 0.95 |
| 0.1536 | 9.0 | 810 | 0.1577 | 0.95 |
### Framework versions
- Transformers 4.38.2
- Pytorch 2.2.1+cu121
- Datasets 2.19.0
- Tokenizers 0.15.2
| {"license": "apache-2.0", "tags": ["generated_from_trainer"], "metrics": ["accuracy"], "base_model": "distilbert/distilbert-base-uncased", "model-index": [{"name": "binary-stock-classifier", "results": []}]} | hkufyp2024/binary-stock-classifier | null | [
"transformers",
"tensorboard",
"safetensors",
"distilbert",
"text-classification",
"generated_from_trainer",
"base_model:distilbert/distilbert-base-uncased",
"license:apache-2.0",
"autotrain_compatible",
"endpoints_compatible",
"region:us"
] | null | 2024-04-22T09:21:42+00:00 | [] | [] | TAGS
#transformers #tensorboard #safetensors #distilbert #text-classification #generated_from_trainer #base_model-distilbert/distilbert-base-uncased #license-apache-2.0 #autotrain_compatible #endpoints_compatible #region-us
| binary-stock-classifier
=======================
This model is a fine-tuned version of distilbert/distilbert-base-uncased on an unknown dataset.
It achieves the following results on the evaluation set:
* Loss: 0.1577
* Accuracy: 0.95
Model description
-----------------
More information needed
Intended uses & limitations
---------------------------
More information needed
Training and evaluation data
----------------------------
More information needed
Training procedure
------------------
### Training hyperparameters
The following hyperparameters were used during training:
* learning\_rate: 2e-05
* train\_batch\_size: 2
* eval\_batch\_size: 2
* seed: 42
* optimizer: Adam with betas=(0.9,0.999) and epsilon=1e-08
* lr\_scheduler\_type: linear
* num\_epochs: 9
### Training results
### Framework versions
* Transformers 4.38.2
* Pytorch 2.2.1+cu121
* Datasets 2.19.0
* Tokenizers 0.15.2
| [
"### Training hyperparameters\n\n\nThe following hyperparameters were used during training:\n\n\n* learning\\_rate: 2e-05\n* train\\_batch\\_size: 2\n* eval\\_batch\\_size: 2\n* seed: 42\n* optimizer: Adam with betas=(0.9,0.999) and epsilon=1e-08\n* lr\\_scheduler\\_type: linear\n* num\\_epochs: 9",
"### Training results",
"### Framework versions\n\n\n* Transformers 4.38.2\n* Pytorch 2.2.1+cu121\n* Datasets 2.19.0\n* Tokenizers 0.15.2"
] | [
"TAGS\n#transformers #tensorboard #safetensors #distilbert #text-classification #generated_from_trainer #base_model-distilbert/distilbert-base-uncased #license-apache-2.0 #autotrain_compatible #endpoints_compatible #region-us \n",
"### Training hyperparameters\n\n\nThe following hyperparameters were used during training:\n\n\n* learning\\_rate: 2e-05\n* train\\_batch\\_size: 2\n* eval\\_batch\\_size: 2\n* seed: 42\n* optimizer: Adam with betas=(0.9,0.999) and epsilon=1e-08\n* lr\\_scheduler\\_type: linear\n* num\\_epochs: 9",
"### Training results",
"### Framework versions\n\n\n* Transformers 4.38.2\n* Pytorch 2.2.1+cu121\n* Datasets 2.19.0\n* Tokenizers 0.15.2"
] |
text-generation | transformers |
# Model Card for Model ID
<!-- Provide a quick summary of what the model is/does. -->
## Model Details
### Model Description
<!-- Provide a longer summary of what this model is. -->
This is the model card of a 🤗 transformers model that has been pushed on the Hub. This model card has been automatically generated.
- **Developed by:** [More Information Needed]
- **Funded by [optional]:** [More Information Needed]
- **Shared by [optional]:** [More Information Needed]
- **Model type:** [More Information Needed]
- **Language(s) (NLP):** [More Information Needed]
- **License:** [More Information Needed]
- **Finetuned from model [optional]:** [More Information Needed]
### Model Sources [optional]
<!-- Provide the basic links for the model. -->
- **Repository:** [More Information Needed]
- **Paper [optional]:** [More Information Needed]
- **Demo [optional]:** [More Information Needed]
## Uses
<!-- Address questions around how the model is intended to be used, including the foreseeable users of the model and those affected by the model. -->
### Direct Use
<!-- This section is for the model use without fine-tuning or plugging into a larger ecosystem/app. -->
[More Information Needed]
### Downstream Use [optional]
<!-- This section is for the model use when fine-tuned for a task, or when plugged into a larger ecosystem/app -->
[More Information Needed]
### Out-of-Scope Use
<!-- This section addresses misuse, malicious use, and uses that the model will not work well for. -->
[More Information Needed]
## Bias, Risks, and Limitations
<!-- This section is meant to convey both technical and sociotechnical limitations. -->
[More Information Needed]
### Recommendations
<!-- This section is meant to convey recommendations with respect to the bias, risk, and technical limitations. -->
Users (both direct and downstream) should be made aware of the risks, biases and limitations of the model. More information needed for further recommendations.
## How to Get Started with the Model
Use the code below to get started with the model.
[More Information Needed]
## Training Details
### Training Data
<!-- This should link to a Dataset Card, perhaps with a short stub of information on what the training data is all about as well as documentation related to data pre-processing or additional filtering. -->
[More Information Needed]
### Training Procedure
<!-- This relates heavily to the Technical Specifications. Content here should link to that section when it is relevant to the training procedure. -->
#### Preprocessing [optional]
[More Information Needed]
#### Training Hyperparameters
- **Training regime:** [More Information Needed] <!--fp32, fp16 mixed precision, bf16 mixed precision, bf16 non-mixed precision, fp16 non-mixed precision, fp8 mixed precision -->
#### Speeds, Sizes, Times [optional]
<!-- This section provides information about throughput, start/end time, checkpoint size if relevant, etc. -->
[More Information Needed]
## Evaluation
<!-- This section describes the evaluation protocols and provides the results. -->
### Testing Data, Factors & Metrics
#### Testing Data
<!-- This should link to a Dataset Card if possible. -->
[More Information Needed]
#### Factors
<!-- These are the things the evaluation is disaggregating by, e.g., subpopulations or domains. -->
[More Information Needed]
#### Metrics
<!-- These are the evaluation metrics being used, ideally with a description of why. -->
[More Information Needed]
### Results
[More Information Needed]
#### Summary
## Model Examination [optional]
<!-- Relevant interpretability work for the model goes here -->
[More Information Needed]
## Environmental Impact
<!-- Total emissions (in grams of CO2eq) and additional considerations, such as electricity usage, go here. Edit the suggested text below accordingly -->
Carbon emissions can be estimated using the [Machine Learning Impact calculator](https://mlco2.github.io/impact#compute) presented in [Lacoste et al. (2019)](https://arxiv.org/abs/1910.09700).
- **Hardware Type:** [More Information Needed]
- **Hours used:** [More Information Needed]
- **Cloud Provider:** [More Information Needed]
- **Compute Region:** [More Information Needed]
- **Carbon Emitted:** [More Information Needed]
## Technical Specifications [optional]
### Model Architecture and Objective
[More Information Needed]
### Compute Infrastructure
[More Information Needed]
#### Hardware
[More Information Needed]
#### Software
[More Information Needed]
## Citation [optional]
<!-- If there is a paper or blog post introducing the model, the APA and Bibtex information for that should go in this section. -->
**BibTeX:**
[More Information Needed]
**APA:**
[More Information Needed]
## Glossary [optional]
<!-- If relevant, include terms and calculations in this section that can help readers understand the model or model card. -->
[More Information Needed]
## More Information [optional]
[More Information Needed]
## Model Card Authors [optional]
[More Information Needed]
## Model Card Contact
[More Information Needed] | {"library_name": "transformers", "tags": []} | cilantro9246/rmb8ppn | null | [
"transformers",
"safetensors",
"llama",
"text-generation",
"conversational",
"arxiv:1910.09700",
"autotrain_compatible",
"endpoints_compatible",
"text-generation-inference",
"region:us"
] | null | 2024-04-22T09:24:31+00:00 | [
"1910.09700"
] | [] | TAGS
#transformers #safetensors #llama #text-generation #conversational #arxiv-1910.09700 #autotrain_compatible #endpoints_compatible #text-generation-inference #region-us
|
# Model Card for Model ID
## Model Details
### Model Description
This is the model card of a transformers model that has been pushed on the Hub. This model card has been automatically generated.
- Developed by:
- Funded by [optional]:
- Shared by [optional]:
- Model type:
- Language(s) (NLP):
- License:
- Finetuned from model [optional]:
### Model Sources [optional]
- Repository:
- Paper [optional]:
- Demo [optional]:
## Uses
### Direct Use
### Downstream Use [optional]
### Out-of-Scope Use
## Bias, Risks, and Limitations
### Recommendations
Users (both direct and downstream) should be made aware of the risks, biases and limitations of the model. More information needed for further recommendations.
## How to Get Started with the Model
Use the code below to get started with the model.
## Training Details
### Training Data
### Training Procedure
#### Preprocessing [optional]
#### Training Hyperparameters
- Training regime:
#### Speeds, Sizes, Times [optional]
## Evaluation
### Testing Data, Factors & Metrics
#### Testing Data
#### Factors
#### Metrics
### Results
#### Summary
## Model Examination [optional]
## Environmental Impact
Carbon emissions can be estimated using the Machine Learning Impact calculator presented in Lacoste et al. (2019).
- Hardware Type:
- Hours used:
- Cloud Provider:
- Compute Region:
- Carbon Emitted:
## Technical Specifications [optional]
### Model Architecture and Objective
### Compute Infrastructure
#### Hardware
#### Software
[optional]
BibTeX:
APA:
## Glossary [optional]
## More Information [optional]
## Model Card Authors [optional]
## Model Card Contact
| [
"# Model Card for Model ID",
"## Model Details",
"### Model Description\n\n\n\nThis is the model card of a transformers model that has been pushed on the Hub. This model card has been automatically generated.\n\n- Developed by: \n- Funded by [optional]: \n- Shared by [optional]: \n- Model type: \n- Language(s) (NLP): \n- License: \n- Finetuned from model [optional]:",
"### Model Sources [optional]\n\n\n\n- Repository: \n- Paper [optional]: \n- Demo [optional]:",
"## Uses",
"### Direct Use",
"### Downstream Use [optional]",
"### Out-of-Scope Use",
"## Bias, Risks, and Limitations",
"### Recommendations\n\n\n\nUsers (both direct and downstream) should be made aware of the risks, biases and limitations of the model. More information needed for further recommendations.",
"## How to Get Started with the Model\n\nUse the code below to get started with the model.",
"## Training Details",
"### Training Data",
"### Training Procedure",
"#### Preprocessing [optional]",
"#### Training Hyperparameters\n\n- Training regime:",
"#### Speeds, Sizes, Times [optional]",
"## Evaluation",
"### Testing Data, Factors & Metrics",
"#### Testing Data",
"#### Factors",
"#### Metrics",
"### Results",
"#### Summary",
"## Model Examination [optional]",
"## Environmental Impact\n\n\n\nCarbon emissions can be estimated using the Machine Learning Impact calculator presented in Lacoste et al. (2019).\n\n- Hardware Type: \n- Hours used: \n- Cloud Provider: \n- Compute Region: \n- Carbon Emitted:",
"## Technical Specifications [optional]",
"### Model Architecture and Objective",
"### Compute Infrastructure",
"#### Hardware",
"#### Software\n\n\n\n[optional]\n\n\n\nBibTeX:\n\n\n\nAPA:",
"## Glossary [optional]",
"## More Information [optional]",
"## Model Card Authors [optional]",
"## Model Card Contact"
] | [
"TAGS\n#transformers #safetensors #llama #text-generation #conversational #arxiv-1910.09700 #autotrain_compatible #endpoints_compatible #text-generation-inference #region-us \n",
"# Model Card for Model ID",
"## Model Details",
"### Model Description\n\n\n\nThis is the model card of a transformers model that has been pushed on the Hub. This model card has been automatically generated.\n\n- Developed by: \n- Funded by [optional]: \n- Shared by [optional]: \n- Model type: \n- Language(s) (NLP): \n- License: \n- Finetuned from model [optional]:",
"### Model Sources [optional]\n\n\n\n- Repository: \n- Paper [optional]: \n- Demo [optional]:",
"## Uses",
"### Direct Use",
"### Downstream Use [optional]",
"### Out-of-Scope Use",
"## Bias, Risks, and Limitations",
"### Recommendations\n\n\n\nUsers (both direct and downstream) should be made aware of the risks, biases and limitations of the model. More information needed for further recommendations.",
"## How to Get Started with the Model\n\nUse the code below to get started with the model.",
"## Training Details",
"### Training Data",
"### Training Procedure",
"#### Preprocessing [optional]",
"#### Training Hyperparameters\n\n- Training regime:",
"#### Speeds, Sizes, Times [optional]",
"## Evaluation",
"### Testing Data, Factors & Metrics",
"#### Testing Data",
"#### Factors",
"#### Metrics",
"### Results",
"#### Summary",
"## Model Examination [optional]",
"## Environmental Impact\n\n\n\nCarbon emissions can be estimated using the Machine Learning Impact calculator presented in Lacoste et al. (2019).\n\n- Hardware Type: \n- Hours used: \n- Cloud Provider: \n- Compute Region: \n- Carbon Emitted:",
"## Technical Specifications [optional]",
"### Model Architecture and Objective",
"### Compute Infrastructure",
"#### Hardware",
"#### Software\n\n\n\n[optional]\n\n\n\nBibTeX:\n\n\n\nAPA:",
"## Glossary [optional]",
"## More Information [optional]",
"## Model Card Authors [optional]",
"## Model Card Contact"
] |
text-generation | transformers |
# Model Card for Model ID
<!-- Provide a quick summary of what the model is/does. -->
## Model Details
### Model Description
<!-- Provide a longer summary of what this model is. -->
This is the model card of a 🤗 transformers model that has been pushed on the Hub. This model card has been automatically generated.
- **Developed by:** [More Information Needed]
- **Funded by [optional]:** [More Information Needed]
- **Shared by [optional]:** [More Information Needed]
- **Model type:** [More Information Needed]
- **Language(s) (NLP):** [More Information Needed]
- **License:** [More Information Needed]
- **Finetuned from model [optional]:** [More Information Needed]
### Model Sources [optional]
<!-- Provide the basic links for the model. -->
- **Repository:** [More Information Needed]
- **Paper [optional]:** [More Information Needed]
- **Demo [optional]:** [More Information Needed]
## Uses
<!-- Address questions around how the model is intended to be used, including the foreseeable users of the model and those affected by the model. -->
### Direct Use
<!-- This section is for the model use without fine-tuning or plugging into a larger ecosystem/app. -->
[More Information Needed]
### Downstream Use [optional]
<!-- This section is for the model use when fine-tuned for a task, or when plugged into a larger ecosystem/app -->
[More Information Needed]
### Out-of-Scope Use
<!-- This section addresses misuse, malicious use, and uses that the model will not work well for. -->
[More Information Needed]
## Bias, Risks, and Limitations
<!-- This section is meant to convey both technical and sociotechnical limitations. -->
[More Information Needed]
### Recommendations
<!-- This section is meant to convey recommendations with respect to the bias, risk, and technical limitations. -->
Users (both direct and downstream) should be made aware of the risks, biases and limitations of the model. More information needed for further recommendations.
## How to Get Started with the Model
Use the code below to get started with the model.
[More Information Needed]
## Training Details
### Training Data
<!-- This should link to a Dataset Card, perhaps with a short stub of information on what the training data is all about as well as documentation related to data pre-processing or additional filtering. -->
[More Information Needed]
### Training Procedure
<!-- This relates heavily to the Technical Specifications. Content here should link to that section when it is relevant to the training procedure. -->
#### Preprocessing [optional]
[More Information Needed]
#### Training Hyperparameters
- **Training regime:** [More Information Needed] <!--fp32, fp16 mixed precision, bf16 mixed precision, bf16 non-mixed precision, fp16 non-mixed precision, fp8 mixed precision -->
#### Speeds, Sizes, Times [optional]
<!-- This section provides information about throughput, start/end time, checkpoint size if relevant, etc. -->
[More Information Needed]
## Evaluation
<!-- This section describes the evaluation protocols and provides the results. -->
### Testing Data, Factors & Metrics
#### Testing Data
<!-- This should link to a Dataset Card if possible. -->
[More Information Needed]
#### Factors
<!-- These are the things the evaluation is disaggregating by, e.g., subpopulations or domains. -->
[More Information Needed]
#### Metrics
<!-- These are the evaluation metrics being used, ideally with a description of why. -->
[More Information Needed]
### Results
[More Information Needed]
#### Summary
## Model Examination [optional]
<!-- Relevant interpretability work for the model goes here -->
[More Information Needed]
## Environmental Impact
<!-- Total emissions (in grams of CO2eq) and additional considerations, such as electricity usage, go here. Edit the suggested text below accordingly -->
Carbon emissions can be estimated using the [Machine Learning Impact calculator](https://mlco2.github.io/impact#compute) presented in [Lacoste et al. (2019)](https://arxiv.org/abs/1910.09700).
- **Hardware Type:** [More Information Needed]
- **Hours used:** [More Information Needed]
- **Cloud Provider:** [More Information Needed]
- **Compute Region:** [More Information Needed]
- **Carbon Emitted:** [More Information Needed]
## Technical Specifications [optional]
### Model Architecture and Objective
[More Information Needed]
### Compute Infrastructure
[More Information Needed]
#### Hardware
[More Information Needed]
#### Software
[More Information Needed]
## Citation [optional]
<!-- If there is a paper or blog post introducing the model, the APA and Bibtex information for that should go in this section. -->
**BibTeX:**
[More Information Needed]
**APA:**
[More Information Needed]
## Glossary [optional]
<!-- If relevant, include terms and calculations in this section that can help readers understand the model or model card. -->
[More Information Needed]
## More Information [optional]
[More Information Needed]
## Model Card Authors [optional]
[More Information Needed]
## Model Card Contact
[More Information Needed] | {"library_name": "transformers", "tags": []} | himum/sn6_0l3 | null | [
"transformers",
"safetensors",
"llama",
"text-generation",
"conversational",
"arxiv:1910.09700",
"autotrain_compatible",
"endpoints_compatible",
"text-generation-inference",
"region:us"
] | null | 2024-04-22T09:25:18+00:00 | [
"1910.09700"
] | [] | TAGS
#transformers #safetensors #llama #text-generation #conversational #arxiv-1910.09700 #autotrain_compatible #endpoints_compatible #text-generation-inference #region-us
|
# Model Card for Model ID
## Model Details
### Model Description
This is the model card of a transformers model that has been pushed on the Hub. This model card has been automatically generated.
- Developed by:
- Funded by [optional]:
- Shared by [optional]:
- Model type:
- Language(s) (NLP):
- License:
- Finetuned from model [optional]:
### Model Sources [optional]
- Repository:
- Paper [optional]:
- Demo [optional]:
## Uses
### Direct Use
### Downstream Use [optional]
### Out-of-Scope Use
## Bias, Risks, and Limitations
### Recommendations
Users (both direct and downstream) should be made aware of the risks, biases and limitations of the model. More information needed for further recommendations.
## How to Get Started with the Model
Use the code below to get started with the model.
## Training Details
### Training Data
### Training Procedure
#### Preprocessing [optional]
#### Training Hyperparameters
- Training regime:
#### Speeds, Sizes, Times [optional]
## Evaluation
### Testing Data, Factors & Metrics
#### Testing Data
#### Factors
#### Metrics
### Results
#### Summary
## Model Examination [optional]
## Environmental Impact
Carbon emissions can be estimated using the Machine Learning Impact calculator presented in Lacoste et al. (2019).
- Hardware Type:
- Hours used:
- Cloud Provider:
- Compute Region:
- Carbon Emitted:
## Technical Specifications [optional]
### Model Architecture and Objective
### Compute Infrastructure
#### Hardware
#### Software
[optional]
BibTeX:
APA:
## Glossary [optional]
## More Information [optional]
## Model Card Authors [optional]
## Model Card Contact
| [
"# Model Card for Model ID",
"## Model Details",
"### Model Description\n\n\n\nThis is the model card of a transformers model that has been pushed on the Hub. This model card has been automatically generated.\n\n- Developed by: \n- Funded by [optional]: \n- Shared by [optional]: \n- Model type: \n- Language(s) (NLP): \n- License: \n- Finetuned from model [optional]:",
"### Model Sources [optional]\n\n\n\n- Repository: \n- Paper [optional]: \n- Demo [optional]:",
"## Uses",
"### Direct Use",
"### Downstream Use [optional]",
"### Out-of-Scope Use",
"## Bias, Risks, and Limitations",
"### Recommendations\n\n\n\nUsers (both direct and downstream) should be made aware of the risks, biases and limitations of the model. More information needed for further recommendations.",
"## How to Get Started with the Model\n\nUse the code below to get started with the model.",
"## Training Details",
"### Training Data",
"### Training Procedure",
"#### Preprocessing [optional]",
"#### Training Hyperparameters\n\n- Training regime:",
"#### Speeds, Sizes, Times [optional]",
"## Evaluation",
"### Testing Data, Factors & Metrics",
"#### Testing Data",
"#### Factors",
"#### Metrics",
"### Results",
"#### Summary",
"## Model Examination [optional]",
"## Environmental Impact\n\n\n\nCarbon emissions can be estimated using the Machine Learning Impact calculator presented in Lacoste et al. (2019).\n\n- Hardware Type: \n- Hours used: \n- Cloud Provider: \n- Compute Region: \n- Carbon Emitted:",
"## Technical Specifications [optional]",
"### Model Architecture and Objective",
"### Compute Infrastructure",
"#### Hardware",
"#### Software\n\n\n\n[optional]\n\n\n\nBibTeX:\n\n\n\nAPA:",
"## Glossary [optional]",
"## More Information [optional]",
"## Model Card Authors [optional]",
"## Model Card Contact"
] | [
"TAGS\n#transformers #safetensors #llama #text-generation #conversational #arxiv-1910.09700 #autotrain_compatible #endpoints_compatible #text-generation-inference #region-us \n",
"# Model Card for Model ID",
"## Model Details",
"### Model Description\n\n\n\nThis is the model card of a transformers model that has been pushed on the Hub. This model card has been automatically generated.\n\n- Developed by: \n- Funded by [optional]: \n- Shared by [optional]: \n- Model type: \n- Language(s) (NLP): \n- License: \n- Finetuned from model [optional]:",
"### Model Sources [optional]\n\n\n\n- Repository: \n- Paper [optional]: \n- Demo [optional]:",
"## Uses",
"### Direct Use",
"### Downstream Use [optional]",
"### Out-of-Scope Use",
"## Bias, Risks, and Limitations",
"### Recommendations\n\n\n\nUsers (both direct and downstream) should be made aware of the risks, biases and limitations of the model. More information needed for further recommendations.",
"## How to Get Started with the Model\n\nUse the code below to get started with the model.",
"## Training Details",
"### Training Data",
"### Training Procedure",
"#### Preprocessing [optional]",
"#### Training Hyperparameters\n\n- Training regime:",
"#### Speeds, Sizes, Times [optional]",
"## Evaluation",
"### Testing Data, Factors & Metrics",
"#### Testing Data",
"#### Factors",
"#### Metrics",
"### Results",
"#### Summary",
"## Model Examination [optional]",
"## Environmental Impact\n\n\n\nCarbon emissions can be estimated using the Machine Learning Impact calculator presented in Lacoste et al. (2019).\n\n- Hardware Type: \n- Hours used: \n- Cloud Provider: \n- Compute Region: \n- Carbon Emitted:",
"## Technical Specifications [optional]",
"### Model Architecture and Objective",
"### Compute Infrastructure",
"#### Hardware",
"#### Software\n\n\n\n[optional]\n\n\n\nBibTeX:\n\n\n\nAPA:",
"## Glossary [optional]",
"## More Information [optional]",
"## Model Card Authors [optional]",
"## Model Card Contact"
] |
text-generation | transformers |
# Model Card for Model ID
<!-- Provide a quick summary of what the model is/does. -->
## Model Details
### Model Description
<!-- Provide a longer summary of what this model is. -->
This is the model card of a 🤗 transformers model that has been pushed on the Hub. This model card has been automatically generated.
- **Developed by:** [More Information Needed]
- **Funded by [optional]:** [More Information Needed]
- **Shared by [optional]:** [More Information Needed]
- **Model type:** [More Information Needed]
- **Language(s) (NLP):** [More Information Needed]
- **License:** [More Information Needed]
- **Finetuned from model [optional]:** [More Information Needed]
### Model Sources [optional]
<!-- Provide the basic links for the model. -->
- **Repository:** [More Information Needed]
- **Paper [optional]:** [More Information Needed]
- **Demo [optional]:** [More Information Needed]
## Uses
<!-- Address questions around how the model is intended to be used, including the foreseeable users of the model and those affected by the model. -->
### Direct Use
<!-- This section is for the model use without fine-tuning or plugging into a larger ecosystem/app. -->
[More Information Needed]
### Downstream Use [optional]
<!-- This section is for the model use when fine-tuned for a task, or when plugged into a larger ecosystem/app -->
[More Information Needed]
### Out-of-Scope Use
<!-- This section addresses misuse, malicious use, and uses that the model will not work well for. -->
[More Information Needed]
## Bias, Risks, and Limitations
<!-- This section is meant to convey both technical and sociotechnical limitations. -->
[More Information Needed]
### Recommendations
<!-- This section is meant to convey recommendations with respect to the bias, risk, and technical limitations. -->
Users (both direct and downstream) should be made aware of the risks, biases and limitations of the model. More information needed for further recommendations.
## How to Get Started with the Model
Use the code below to get started with the model.
[More Information Needed]
## Training Details
### Training Data
<!-- This should link to a Dataset Card, perhaps with a short stub of information on what the training data is all about as well as documentation related to data pre-processing or additional filtering. -->
[More Information Needed]
### Training Procedure
<!-- This relates heavily to the Technical Specifications. Content here should link to that section when it is relevant to the training procedure. -->
#### Preprocessing [optional]
[More Information Needed]
#### Training Hyperparameters
- **Training regime:** [More Information Needed] <!--fp32, fp16 mixed precision, bf16 mixed precision, bf16 non-mixed precision, fp16 non-mixed precision, fp8 mixed precision -->
#### Speeds, Sizes, Times [optional]
<!-- This section provides information about throughput, start/end time, checkpoint size if relevant, etc. -->
[More Information Needed]
## Evaluation
<!-- This section describes the evaluation protocols and provides the results. -->
### Testing Data, Factors & Metrics
#### Testing Data
<!-- This should link to a Dataset Card if possible. -->
[More Information Needed]
#### Factors
<!-- These are the things the evaluation is disaggregating by, e.g., subpopulations or domains. -->
[More Information Needed]
#### Metrics
<!-- These are the evaluation metrics being used, ideally with a description of why. -->
[More Information Needed]
### Results
[More Information Needed]
#### Summary
## Model Examination [optional]
<!-- Relevant interpretability work for the model goes here -->
[More Information Needed]
## Environmental Impact
<!-- Total emissions (in grams of CO2eq) and additional considerations, such as electricity usage, go here. Edit the suggested text below accordingly -->
Carbon emissions can be estimated using the [Machine Learning Impact calculator](https://mlco2.github.io/impact#compute) presented in [Lacoste et al. (2019)](https://arxiv.org/abs/1910.09700).
- **Hardware Type:** [More Information Needed]
- **Hours used:** [More Information Needed]
- **Cloud Provider:** [More Information Needed]
- **Compute Region:** [More Information Needed]
- **Carbon Emitted:** [More Information Needed]
## Technical Specifications [optional]
### Model Architecture and Objective
[More Information Needed]
### Compute Infrastructure
[More Information Needed]
#### Hardware
[More Information Needed]
#### Software
[More Information Needed]
## Citation [optional]
<!-- If there is a paper or blog post introducing the model, the APA and Bibtex information for that should go in this section. -->
**BibTeX:**
[More Information Needed]
**APA:**
[More Information Needed]
## Glossary [optional]
<!-- If relevant, include terms and calculations in this section that can help readers understand the model or model card. -->
[More Information Needed]
## More Information [optional]
[More Information Needed]
## Model Card Authors [optional]
[More Information Needed]
## Model Card Contact
[More Information Needed] | {"library_name": "transformers", "tags": []} | saransh03sharma/mintrec2-mistral-2-7b-200 | null | [
"transformers",
"safetensors",
"mistral",
"text-generation",
"arxiv:1910.09700",
"autotrain_compatible",
"endpoints_compatible",
"text-generation-inference",
"region:us"
] | null | 2024-04-22T09:26:01+00:00 | [
"1910.09700"
] | [] | TAGS
#transformers #safetensors #mistral #text-generation #arxiv-1910.09700 #autotrain_compatible #endpoints_compatible #text-generation-inference #region-us
|
# Model Card for Model ID
## Model Details
### Model Description
This is the model card of a transformers model that has been pushed on the Hub. This model card has been automatically generated.
- Developed by:
- Funded by [optional]:
- Shared by [optional]:
- Model type:
- Language(s) (NLP):
- License:
- Finetuned from model [optional]:
### Model Sources [optional]
- Repository:
- Paper [optional]:
- Demo [optional]:
## Uses
### Direct Use
### Downstream Use [optional]
### Out-of-Scope Use
## Bias, Risks, and Limitations
### Recommendations
Users (both direct and downstream) should be made aware of the risks, biases and limitations of the model. More information needed for further recommendations.
## How to Get Started with the Model
Use the code below to get started with the model.
## Training Details
### Training Data
### Training Procedure
#### Preprocessing [optional]
#### Training Hyperparameters
- Training regime:
#### Speeds, Sizes, Times [optional]
## Evaluation
### Testing Data, Factors & Metrics
#### Testing Data
#### Factors
#### Metrics
### Results
#### Summary
## Model Examination [optional]
## Environmental Impact
Carbon emissions can be estimated using the Machine Learning Impact calculator presented in Lacoste et al. (2019).
- Hardware Type:
- Hours used:
- Cloud Provider:
- Compute Region:
- Carbon Emitted:
## Technical Specifications [optional]
### Model Architecture and Objective
### Compute Infrastructure
#### Hardware
#### Software
[optional]
BibTeX:
APA:
## Glossary [optional]
## More Information [optional]
## Model Card Authors [optional]
## Model Card Contact
| [
"# Model Card for Model ID",
"## Model Details",
"### Model Description\n\n\n\nThis is the model card of a transformers model that has been pushed on the Hub. This model card has been automatically generated.\n\n- Developed by: \n- Funded by [optional]: \n- Shared by [optional]: \n- Model type: \n- Language(s) (NLP): \n- License: \n- Finetuned from model [optional]:",
"### Model Sources [optional]\n\n\n\n- Repository: \n- Paper [optional]: \n- Demo [optional]:",
"## Uses",
"### Direct Use",
"### Downstream Use [optional]",
"### Out-of-Scope Use",
"## Bias, Risks, and Limitations",
"### Recommendations\n\n\n\nUsers (both direct and downstream) should be made aware of the risks, biases and limitations of the model. More information needed for further recommendations.",
"## How to Get Started with the Model\n\nUse the code below to get started with the model.",
"## Training Details",
"### Training Data",
"### Training Procedure",
"#### Preprocessing [optional]",
"#### Training Hyperparameters\n\n- Training regime:",
"#### Speeds, Sizes, Times [optional]",
"## Evaluation",
"### Testing Data, Factors & Metrics",
"#### Testing Data",
"#### Factors",
"#### Metrics",
"### Results",
"#### Summary",
"## Model Examination [optional]",
"## Environmental Impact\n\n\n\nCarbon emissions can be estimated using the Machine Learning Impact calculator presented in Lacoste et al. (2019).\n\n- Hardware Type: \n- Hours used: \n- Cloud Provider: \n- Compute Region: \n- Carbon Emitted:",
"## Technical Specifications [optional]",
"### Model Architecture and Objective",
"### Compute Infrastructure",
"#### Hardware",
"#### Software\n\n\n\n[optional]\n\n\n\nBibTeX:\n\n\n\nAPA:",
"## Glossary [optional]",
"## More Information [optional]",
"## Model Card Authors [optional]",
"## Model Card Contact"
] | [
"TAGS\n#transformers #safetensors #mistral #text-generation #arxiv-1910.09700 #autotrain_compatible #endpoints_compatible #text-generation-inference #region-us \n",
"# Model Card for Model ID",
"## Model Details",
"### Model Description\n\n\n\nThis is the model card of a transformers model that has been pushed on the Hub. This model card has been automatically generated.\n\n- Developed by: \n- Funded by [optional]: \n- Shared by [optional]: \n- Model type: \n- Language(s) (NLP): \n- License: \n- Finetuned from model [optional]:",
"### Model Sources [optional]\n\n\n\n- Repository: \n- Paper [optional]: \n- Demo [optional]:",
"## Uses",
"### Direct Use",
"### Downstream Use [optional]",
"### Out-of-Scope Use",
"## Bias, Risks, and Limitations",
"### Recommendations\n\n\n\nUsers (both direct and downstream) should be made aware of the risks, biases and limitations of the model. More information needed for further recommendations.",
"## How to Get Started with the Model\n\nUse the code below to get started with the model.",
"## Training Details",
"### Training Data",
"### Training Procedure",
"#### Preprocessing [optional]",
"#### Training Hyperparameters\n\n- Training regime:",
"#### Speeds, Sizes, Times [optional]",
"## Evaluation",
"### Testing Data, Factors & Metrics",
"#### Testing Data",
"#### Factors",
"#### Metrics",
"### Results",
"#### Summary",
"## Model Examination [optional]",
"## Environmental Impact\n\n\n\nCarbon emissions can be estimated using the Machine Learning Impact calculator presented in Lacoste et al. (2019).\n\n- Hardware Type: \n- Hours used: \n- Cloud Provider: \n- Compute Region: \n- Carbon Emitted:",
"## Technical Specifications [optional]",
"### Model Architecture and Objective",
"### Compute Infrastructure",
"#### Hardware",
"#### Software\n\n\n\n[optional]\n\n\n\nBibTeX:\n\n\n\nAPA:",
"## Glossary [optional]",
"## More Information [optional]",
"## Model Card Authors [optional]",
"## Model Card Contact"
] |
text-generation | transformers |
# yujiepan/Meta-Llama-3-8B-gptq-w8asym
This model applies AutoGPTQ on [meta-llama/Meta-Llama-3-8B](https://huggingface.co/meta-llama/Meta-Llama-3-8B).
- 8-bit asymmetric weight only quantization
- group_size=-1
- calibration set: c4-new
## Accuracy
| model | precision | wikitext ppl (↓) |
|-|-|-|
| meta-llama/Meta-Llama-3-8B | FP16 | 9.179 |
| yujiepan/Meta-Llama-3-8B-gptq-w8asym | int8-asym | 9.356 |
Note:
- Evaluated on lm-evaluation-harness "wikitext" task
- Wikitext PPL does not guarantee actual accuracy, but helps to check the disortion after quantization.
## Usage
```python
from awq import AutoAWQForCausalLM
model = AutoAWQForCausalLM.from_quantized('yujiepan/Meta-Llama-3-8B-gptq-w8asym')
```
## Codes
```python
import os
import torch
from transformers import AutoModelForCausalLM, AutoTokenizer, GPTQConfig
model_id = "meta-llama/Meta-Llama-3-8B"
tokenizer = AutoTokenizer.from_pretrained(model_id)
quantization_config = GPTQConfig(
bits=8, group_size=-1,
dataset="c4-new",
sym=False,
tokenizer=tokenizer,
use_cuda_fp16=True,
)
model = AutoModelForCausalLM.from_pretrained(
model_id,
device_map="auto",
low_cpu_mem_usage=True,
quantization_config=quantization_config,
)
```
| {"library_name": "transformers", "tags": []} | yujiepan/Meta-Llama-3-8B-gptq-w8asym | null | [
"transformers",
"safetensors",
"llama",
"text-generation",
"autotrain_compatible",
"endpoints_compatible",
"text-generation-inference",
"8-bit",
"region:us"
] | null | 2024-04-22T09:26:16+00:00 | [] | [] | TAGS
#transformers #safetensors #llama #text-generation #autotrain_compatible #endpoints_compatible #text-generation-inference #8-bit #region-us
| yujiepan/Meta-Llama-3-8B-gptq-w8asym
====================================
This model applies AutoGPTQ on meta-llama/Meta-Llama-3-8B.
* 8-bit asymmetric weight only quantization
* group\_size=-1
* calibration set: c4-new
Accuracy
--------
model: meta-llama/Meta-Llama-3-8B, precision: FP16, wikitext ppl (↓): 9.179
model: yujiepan/Meta-Llama-3-8B-gptq-w8asym, precision: int8-asym, wikitext ppl (↓): 9.356
Note:
* Evaluated on lm-evaluation-harness "wikitext" task
* Wikitext PPL does not guarantee actual accuracy, but helps to check the disortion after quantization.
Usage
-----
Codes
-----
| [] | [
"TAGS\n#transformers #safetensors #llama #text-generation #autotrain_compatible #endpoints_compatible #text-generation-inference #8-bit #region-us \n"
] |
null | peft |
# Model Card for Model ID
<!-- Provide a quick summary of what the model is/does. -->
## Model Details
### Model Description
<!-- Provide a longer summary of what this model is. -->
- **Developed by:** [More Information Needed]
- **Funded by [optional]:** [More Information Needed]
- **Shared by [optional]:** [More Information Needed]
- **Model type:** [More Information Needed]
- **Language(s) (NLP):** [More Information Needed]
- **License:** [More Information Needed]
- **Finetuned from model [optional]:** [More Information Needed]
### Model Sources [optional]
<!-- Provide the basic links for the model. -->
- **Repository:** [More Information Needed]
- **Paper [optional]:** [More Information Needed]
- **Demo [optional]:** [More Information Needed]
## Uses
<!-- Address questions around how the model is intended to be used, including the foreseeable users of the model and those affected by the model. -->
### Direct Use
<!-- This section is for the model use without fine-tuning or plugging into a larger ecosystem/app. -->
[More Information Needed]
### Downstream Use [optional]
<!-- This section is for the model use when fine-tuned for a task, or when plugged into a larger ecosystem/app -->
[More Information Needed]
### Out-of-Scope Use
<!-- This section addresses misuse, malicious use, and uses that the model will not work well for. -->
[More Information Needed]
## Bias, Risks, and Limitations
<!-- This section is meant to convey both technical and sociotechnical limitations. -->
[More Information Needed]
### Recommendations
<!-- This section is meant to convey recommendations with respect to the bias, risk, and technical limitations. -->
Users (both direct and downstream) should be made aware of the risks, biases and limitations of the model. More information needed for further recommendations.
## How to Get Started with the Model
Use the code below to get started with the model.
[More Information Needed]
## Training Details
### Training Data
<!-- This should link to a Dataset Card, perhaps with a short stub of information on what the training data is all about as well as documentation related to data pre-processing or additional filtering. -->
[More Information Needed]
### Training Procedure
<!-- This relates heavily to the Technical Specifications. Content here should link to that section when it is relevant to the training procedure. -->
#### Preprocessing [optional]
[More Information Needed]
#### Training Hyperparameters
- **Training regime:** [More Information Needed] <!--fp32, fp16 mixed precision, bf16 mixed precision, bf16 non-mixed precision, fp16 non-mixed precision, fp8 mixed precision -->
#### Speeds, Sizes, Times [optional]
<!-- This section provides information about throughput, start/end time, checkpoint size if relevant, etc. -->
[More Information Needed]
## Evaluation
<!-- This section describes the evaluation protocols and provides the results. -->
### Testing Data, Factors & Metrics
#### Testing Data
<!-- This should link to a Dataset Card if possible. -->
[More Information Needed]
#### Factors
<!-- These are the things the evaluation is disaggregating by, e.g., subpopulations or domains. -->
[More Information Needed]
#### Metrics
<!-- These are the evaluation metrics being used, ideally with a description of why. -->
[More Information Needed]
### Results
[More Information Needed]
#### Summary
## Model Examination [optional]
<!-- Relevant interpretability work for the model goes here -->
[More Information Needed]
## Environmental Impact
<!-- Total emissions (in grams of CO2eq) and additional considerations, such as electricity usage, go here. Edit the suggested text below accordingly -->
Carbon emissions can be estimated using the [Machine Learning Impact calculator](https://mlco2.github.io/impact#compute) presented in [Lacoste et al. (2019)](https://arxiv.org/abs/1910.09700).
- **Hardware Type:** [More Information Needed]
- **Hours used:** [More Information Needed]
- **Cloud Provider:** [More Information Needed]
- **Compute Region:** [More Information Needed]
- **Carbon Emitted:** [More Information Needed]
## Technical Specifications [optional]
### Model Architecture and Objective
[More Information Needed]
### Compute Infrastructure
[More Information Needed]
#### Hardware
[More Information Needed]
#### Software
[More Information Needed]
## Citation [optional]
<!-- If there is a paper or blog post introducing the model, the APA and Bibtex information for that should go in this section. -->
**BibTeX:**
[More Information Needed]
**APA:**
[More Information Needed]
## Glossary [optional]
<!-- If relevant, include terms and calculations in this section that can help readers understand the model or model card. -->
[More Information Needed]
## More Information [optional]
[More Information Needed]
## Model Card Authors [optional]
[More Information Needed]
## Model Card Contact
[More Information Needed]
### Framework versions
- PEFT 0.10.0 | {"library_name": "peft", "base_model": "openai/whisper-base"} | ygaci/whisper-base-fr_common_voice_16_new | null | [
"peft",
"tensorboard",
"safetensors",
"arxiv:1910.09700",
"base_model:openai/whisper-base",
"region:us"
] | null | 2024-04-22T09:26:20+00:00 | [
"1910.09700"
] | [] | TAGS
#peft #tensorboard #safetensors #arxiv-1910.09700 #base_model-openai/whisper-base #region-us
|
# Model Card for Model ID
## Model Details
### Model Description
- Developed by:
- Funded by [optional]:
- Shared by [optional]:
- Model type:
- Language(s) (NLP):
- License:
- Finetuned from model [optional]:
### Model Sources [optional]
- Repository:
- Paper [optional]:
- Demo [optional]:
## Uses
### Direct Use
### Downstream Use [optional]
### Out-of-Scope Use
## Bias, Risks, and Limitations
### Recommendations
Users (both direct and downstream) should be made aware of the risks, biases and limitations of the model. More information needed for further recommendations.
## How to Get Started with the Model
Use the code below to get started with the model.
## Training Details
### Training Data
### Training Procedure
#### Preprocessing [optional]
#### Training Hyperparameters
- Training regime:
#### Speeds, Sizes, Times [optional]
## Evaluation
### Testing Data, Factors & Metrics
#### Testing Data
#### Factors
#### Metrics
### Results
#### Summary
## Model Examination [optional]
## Environmental Impact
Carbon emissions can be estimated using the Machine Learning Impact calculator presented in Lacoste et al. (2019).
- Hardware Type:
- Hours used:
- Cloud Provider:
- Compute Region:
- Carbon Emitted:
## Technical Specifications [optional]
### Model Architecture and Objective
### Compute Infrastructure
#### Hardware
#### Software
[optional]
BibTeX:
APA:
## Glossary [optional]
## More Information [optional]
## Model Card Authors [optional]
## Model Card Contact
### Framework versions
- PEFT 0.10.0 | [
"# Model Card for Model ID",
"## Model Details",
"### Model Description\n\n\n\n\n\n- Developed by: \n- Funded by [optional]: \n- Shared by [optional]: \n- Model type: \n- Language(s) (NLP): \n- License: \n- Finetuned from model [optional]:",
"### Model Sources [optional]\n\n\n\n- Repository: \n- Paper [optional]: \n- Demo [optional]:",
"## Uses",
"### Direct Use",
"### Downstream Use [optional]",
"### Out-of-Scope Use",
"## Bias, Risks, and Limitations",
"### Recommendations\n\n\n\nUsers (both direct and downstream) should be made aware of the risks, biases and limitations of the model. More information needed for further recommendations.",
"## How to Get Started with the Model\n\nUse the code below to get started with the model.",
"## Training Details",
"### Training Data",
"### Training Procedure",
"#### Preprocessing [optional]",
"#### Training Hyperparameters\n\n- Training regime:",
"#### Speeds, Sizes, Times [optional]",
"## Evaluation",
"### Testing Data, Factors & Metrics",
"#### Testing Data",
"#### Factors",
"#### Metrics",
"### Results",
"#### Summary",
"## Model Examination [optional]",
"## Environmental Impact\n\n\n\nCarbon emissions can be estimated using the Machine Learning Impact calculator presented in Lacoste et al. (2019).\n\n- Hardware Type: \n- Hours used: \n- Cloud Provider: \n- Compute Region: \n- Carbon Emitted:",
"## Technical Specifications [optional]",
"### Model Architecture and Objective",
"### Compute Infrastructure",
"#### Hardware",
"#### Software\n\n\n\n[optional]\n\n\n\nBibTeX:\n\n\n\nAPA:",
"## Glossary [optional]",
"## More Information [optional]",
"## Model Card Authors [optional]",
"## Model Card Contact",
"### Framework versions\n\n- PEFT 0.10.0"
] | [
"TAGS\n#peft #tensorboard #safetensors #arxiv-1910.09700 #base_model-openai/whisper-base #region-us \n",
"# Model Card for Model ID",
"## Model Details",
"### Model Description\n\n\n\n\n\n- Developed by: \n- Funded by [optional]: \n- Shared by [optional]: \n- Model type: \n- Language(s) (NLP): \n- License: \n- Finetuned from model [optional]:",
"### Model Sources [optional]\n\n\n\n- Repository: \n- Paper [optional]: \n- Demo [optional]:",
"## Uses",
"### Direct Use",
"### Downstream Use [optional]",
"### Out-of-Scope Use",
"## Bias, Risks, and Limitations",
"### Recommendations\n\n\n\nUsers (both direct and downstream) should be made aware of the risks, biases and limitations of the model. More information needed for further recommendations.",
"## How to Get Started with the Model\n\nUse the code below to get started with the model.",
"## Training Details",
"### Training Data",
"### Training Procedure",
"#### Preprocessing [optional]",
"#### Training Hyperparameters\n\n- Training regime:",
"#### Speeds, Sizes, Times [optional]",
"## Evaluation",
"### Testing Data, Factors & Metrics",
"#### Testing Data",
"#### Factors",
"#### Metrics",
"### Results",
"#### Summary",
"## Model Examination [optional]",
"## Environmental Impact\n\n\n\nCarbon emissions can be estimated using the Machine Learning Impact calculator presented in Lacoste et al. (2019).\n\n- Hardware Type: \n- Hours used: \n- Cloud Provider: \n- Compute Region: \n- Carbon Emitted:",
"## Technical Specifications [optional]",
"### Model Architecture and Objective",
"### Compute Infrastructure",
"#### Hardware",
"#### Software\n\n\n\n[optional]\n\n\n\nBibTeX:\n\n\n\nAPA:",
"## Glossary [optional]",
"## More Information [optional]",
"## Model Card Authors [optional]",
"## Model Card Contact",
"### Framework versions\n\n- PEFT 0.10.0"
] |
object-detection | transformers |
<!-- This model card has been generated automatically according to the information the Trainer had access to. You
should probably proofread and complete it, then remove this comment. -->
# detr
This model is a fine-tuned version of [facebook/detr-resnet-50](https://huggingface.co/facebook/detr-resnet-50) on an unknown dataset.
## Model description
More information needed
## Intended uses & limitations
More information needed
## Training and evaluation data
More information needed
## Training procedure
### Training hyperparameters
The following hyperparameters were used during training:
- learning_rate: 0.0001
- train_batch_size: 8
- eval_batch_size: 8
- seed: 42
- optimizer: Adam with betas=(0.9,0.999) and epsilon=1e-08
- lr_scheduler_type: linear
- num_epochs: 8
- mixed_precision_training: Native AMP
### Training results
### Framework versions
- Transformers 4.40.0
- Pytorch 2.2.2+cu121
- Datasets 2.18.0
- Tokenizers 0.19.1
| {"license": "apache-2.0", "tags": ["generated_from_trainer"], "base_model": "facebook/detr-resnet-50", "model-index": [{"name": "detr", "results": []}]} | AnetteHabanen/detr | null | [
"transformers",
"tensorboard",
"safetensors",
"detr",
"object-detection",
"generated_from_trainer",
"base_model:facebook/detr-resnet-50",
"license:apache-2.0",
"endpoints_compatible",
"region:us"
] | null | 2024-04-22T09:28:29+00:00 | [] | [] | TAGS
#transformers #tensorboard #safetensors #detr #object-detection #generated_from_trainer #base_model-facebook/detr-resnet-50 #license-apache-2.0 #endpoints_compatible #region-us
|
# detr
This model is a fine-tuned version of facebook/detr-resnet-50 on an unknown dataset.
## Model description
More information needed
## Intended uses & limitations
More information needed
## Training and evaluation data
More information needed
## Training procedure
### Training hyperparameters
The following hyperparameters were used during training:
- learning_rate: 0.0001
- train_batch_size: 8
- eval_batch_size: 8
- seed: 42
- optimizer: Adam with betas=(0.9,0.999) and epsilon=1e-08
- lr_scheduler_type: linear
- num_epochs: 8
- mixed_precision_training: Native AMP
### Training results
### Framework versions
- Transformers 4.40.0
- Pytorch 2.2.2+cu121
- Datasets 2.18.0
- Tokenizers 0.19.1
| [
"# detr\n\nThis model is a fine-tuned version of facebook/detr-resnet-50 on an unknown dataset.",
"## Model description\n\nMore information needed",
"## Intended uses & limitations\n\nMore information needed",
"## Training and evaluation data\n\nMore information needed",
"## Training procedure",
"### Training hyperparameters\n\nThe following hyperparameters were used during training:\n- learning_rate: 0.0001\n- train_batch_size: 8\n- eval_batch_size: 8\n- seed: 42\n- optimizer: Adam with betas=(0.9,0.999) and epsilon=1e-08\n- lr_scheduler_type: linear\n- num_epochs: 8\n- mixed_precision_training: Native AMP",
"### Training results",
"### Framework versions\n\n- Transformers 4.40.0\n- Pytorch 2.2.2+cu121\n- Datasets 2.18.0\n- Tokenizers 0.19.1"
] | [
"TAGS\n#transformers #tensorboard #safetensors #detr #object-detection #generated_from_trainer #base_model-facebook/detr-resnet-50 #license-apache-2.0 #endpoints_compatible #region-us \n",
"# detr\n\nThis model is a fine-tuned version of facebook/detr-resnet-50 on an unknown dataset.",
"## Model description\n\nMore information needed",
"## Intended uses & limitations\n\nMore information needed",
"## Training and evaluation data\n\nMore information needed",
"## Training procedure",
"### Training hyperparameters\n\nThe following hyperparameters were used during training:\n- learning_rate: 0.0001\n- train_batch_size: 8\n- eval_batch_size: 8\n- seed: 42\n- optimizer: Adam with betas=(0.9,0.999) and epsilon=1e-08\n- lr_scheduler_type: linear\n- num_epochs: 8\n- mixed_precision_training: Native AMP",
"### Training results",
"### Framework versions\n\n- Transformers 4.40.0\n- Pytorch 2.2.2+cu121\n- Datasets 2.18.0\n- Tokenizers 0.19.1"
] |
null | transformers |
# Model Card for Model ID
<!-- Provide a quick summary of what the model is/does. -->
## Model Details
### Model Description
<!-- Provide a longer summary of what this model is. -->
This is the model card of a 🤗 transformers model that has been pushed on the Hub. This model card has been automatically generated.
- **Developed by:** [More Information Needed]
- **Funded by [optional]:** [More Information Needed]
- **Shared by [optional]:** [More Information Needed]
- **Model type:** [More Information Needed]
- **Language(s) (NLP):** [More Information Needed]
- **License:** [More Information Needed]
- **Finetuned from model [optional]:** [More Information Needed]
### Model Sources [optional]
<!-- Provide the basic links for the model. -->
- **Repository:** [More Information Needed]
- **Paper [optional]:** [More Information Needed]
- **Demo [optional]:** [More Information Needed]
## Uses
<!-- Address questions around how the model is intended to be used, including the foreseeable users of the model and those affected by the model. -->
### Direct Use
<!-- This section is for the model use without fine-tuning or plugging into a larger ecosystem/app. -->
[More Information Needed]
### Downstream Use [optional]
<!-- This section is for the model use when fine-tuned for a task, or when plugged into a larger ecosystem/app -->
[More Information Needed]
### Out-of-Scope Use
<!-- This section addresses misuse, malicious use, and uses that the model will not work well for. -->
[More Information Needed]
## Bias, Risks, and Limitations
<!-- This section is meant to convey both technical and sociotechnical limitations. -->
[More Information Needed]
### Recommendations
<!-- This section is meant to convey recommendations with respect to the bias, risk, and technical limitations. -->
Users (both direct and downstream) should be made aware of the risks, biases and limitations of the model. More information needed for further recommendations.
## How to Get Started with the Model
Use the code below to get started with the model.
[More Information Needed]
## Training Details
### Training Data
<!-- This should link to a Dataset Card, perhaps with a short stub of information on what the training data is all about as well as documentation related to data pre-processing or additional filtering. -->
[More Information Needed]
### Training Procedure
<!-- This relates heavily to the Technical Specifications. Content here should link to that section when it is relevant to the training procedure. -->
#### Preprocessing [optional]
[More Information Needed]
#### Training Hyperparameters
- **Training regime:** [More Information Needed] <!--fp32, fp16 mixed precision, bf16 mixed precision, bf16 non-mixed precision, fp16 non-mixed precision, fp8 mixed precision -->
#### Speeds, Sizes, Times [optional]
<!-- This section provides information about throughput, start/end time, checkpoint size if relevant, etc. -->
[More Information Needed]
## Evaluation
<!-- This section describes the evaluation protocols and provides the results. -->
### Testing Data, Factors & Metrics
#### Testing Data
<!-- This should link to a Dataset Card if possible. -->
[More Information Needed]
#### Factors
<!-- These are the things the evaluation is disaggregating by, e.g., subpopulations or domains. -->
[More Information Needed]
#### Metrics
<!-- These are the evaluation metrics being used, ideally with a description of why. -->
[More Information Needed]
### Results
[More Information Needed]
#### Summary
## Model Examination [optional]
<!-- Relevant interpretability work for the model goes here -->
[More Information Needed]
## Environmental Impact
<!-- Total emissions (in grams of CO2eq) and additional considerations, such as electricity usage, go here. Edit the suggested text below accordingly -->
Carbon emissions can be estimated using the [Machine Learning Impact calculator](https://mlco2.github.io/impact#compute) presented in [Lacoste et al. (2019)](https://arxiv.org/abs/1910.09700).
- **Hardware Type:** [More Information Needed]
- **Hours used:** [More Information Needed]
- **Cloud Provider:** [More Information Needed]
- **Compute Region:** [More Information Needed]
- **Carbon Emitted:** [More Information Needed]
## Technical Specifications [optional]
### Model Architecture and Objective
[More Information Needed]
### Compute Infrastructure
[More Information Needed]
#### Hardware
[More Information Needed]
#### Software
[More Information Needed]
## Citation [optional]
<!-- If there is a paper or blog post introducing the model, the APA and Bibtex information for that should go in this section. -->
**BibTeX:**
[More Information Needed]
**APA:**
[More Information Needed]
## Glossary [optional]
<!-- If relevant, include terms and calculations in this section that can help readers understand the model or model card. -->
[More Information Needed]
## More Information [optional]
[More Information Needed]
## Model Card Authors [optional]
[More Information Needed]
## Model Card Contact
[More Information Needed] | {"library_name": "transformers", "tags": []} | Gokul29/intent_recognition | null | [
"transformers",
"safetensors",
"arxiv:1910.09700",
"endpoints_compatible",
"region:us"
] | null | 2024-04-22T09:31:56+00:00 | [
"1910.09700"
] | [] | TAGS
#transformers #safetensors #arxiv-1910.09700 #endpoints_compatible #region-us
|
# Model Card for Model ID
## Model Details
### Model Description
This is the model card of a transformers model that has been pushed on the Hub. This model card has been automatically generated.
- Developed by:
- Funded by [optional]:
- Shared by [optional]:
- Model type:
- Language(s) (NLP):
- License:
- Finetuned from model [optional]:
### Model Sources [optional]
- Repository:
- Paper [optional]:
- Demo [optional]:
## Uses
### Direct Use
### Downstream Use [optional]
### Out-of-Scope Use
## Bias, Risks, and Limitations
### Recommendations
Users (both direct and downstream) should be made aware of the risks, biases and limitations of the model. More information needed for further recommendations.
## How to Get Started with the Model
Use the code below to get started with the model.
## Training Details
### Training Data
### Training Procedure
#### Preprocessing [optional]
#### Training Hyperparameters
- Training regime:
#### Speeds, Sizes, Times [optional]
## Evaluation
### Testing Data, Factors & Metrics
#### Testing Data
#### Factors
#### Metrics
### Results
#### Summary
## Model Examination [optional]
## Environmental Impact
Carbon emissions can be estimated using the Machine Learning Impact calculator presented in Lacoste et al. (2019).
- Hardware Type:
- Hours used:
- Cloud Provider:
- Compute Region:
- Carbon Emitted:
## Technical Specifications [optional]
### Model Architecture and Objective
### Compute Infrastructure
#### Hardware
#### Software
[optional]
BibTeX:
APA:
## Glossary [optional]
## More Information [optional]
## Model Card Authors [optional]
## Model Card Contact
| [
"# Model Card for Model ID",
"## Model Details",
"### Model Description\n\n\n\nThis is the model card of a transformers model that has been pushed on the Hub. This model card has been automatically generated.\n\n- Developed by: \n- Funded by [optional]: \n- Shared by [optional]: \n- Model type: \n- Language(s) (NLP): \n- License: \n- Finetuned from model [optional]:",
"### Model Sources [optional]\n\n\n\n- Repository: \n- Paper [optional]: \n- Demo [optional]:",
"## Uses",
"### Direct Use",
"### Downstream Use [optional]",
"### Out-of-Scope Use",
"## Bias, Risks, and Limitations",
"### Recommendations\n\n\n\nUsers (both direct and downstream) should be made aware of the risks, biases and limitations of the model. More information needed for further recommendations.",
"## How to Get Started with the Model\n\nUse the code below to get started with the model.",
"## Training Details",
"### Training Data",
"### Training Procedure",
"#### Preprocessing [optional]",
"#### Training Hyperparameters\n\n- Training regime:",
"#### Speeds, Sizes, Times [optional]",
"## Evaluation",
"### Testing Data, Factors & Metrics",
"#### Testing Data",
"#### Factors",
"#### Metrics",
"### Results",
"#### Summary",
"## Model Examination [optional]",
"## Environmental Impact\n\n\n\nCarbon emissions can be estimated using the Machine Learning Impact calculator presented in Lacoste et al. (2019).\n\n- Hardware Type: \n- Hours used: \n- Cloud Provider: \n- Compute Region: \n- Carbon Emitted:",
"## Technical Specifications [optional]",
"### Model Architecture and Objective",
"### Compute Infrastructure",
"#### Hardware",
"#### Software\n\n\n\n[optional]\n\n\n\nBibTeX:\n\n\n\nAPA:",
"## Glossary [optional]",
"## More Information [optional]",
"## Model Card Authors [optional]",
"## Model Card Contact"
] | [
"TAGS\n#transformers #safetensors #arxiv-1910.09700 #endpoints_compatible #region-us \n",
"# Model Card for Model ID",
"## Model Details",
"### Model Description\n\n\n\nThis is the model card of a transformers model that has been pushed on the Hub. This model card has been automatically generated.\n\n- Developed by: \n- Funded by [optional]: \n- Shared by [optional]: \n- Model type: \n- Language(s) (NLP): \n- License: \n- Finetuned from model [optional]:",
"### Model Sources [optional]\n\n\n\n- Repository: \n- Paper [optional]: \n- Demo [optional]:",
"## Uses",
"### Direct Use",
"### Downstream Use [optional]",
"### Out-of-Scope Use",
"## Bias, Risks, and Limitations",
"### Recommendations\n\n\n\nUsers (both direct and downstream) should be made aware of the risks, biases and limitations of the model. More information needed for further recommendations.",
"## How to Get Started with the Model\n\nUse the code below to get started with the model.",
"## Training Details",
"### Training Data",
"### Training Procedure",
"#### Preprocessing [optional]",
"#### Training Hyperparameters\n\n- Training regime:",
"#### Speeds, Sizes, Times [optional]",
"## Evaluation",
"### Testing Data, Factors & Metrics",
"#### Testing Data",
"#### Factors",
"#### Metrics",
"### Results",
"#### Summary",
"## Model Examination [optional]",
"## Environmental Impact\n\n\n\nCarbon emissions can be estimated using the Machine Learning Impact calculator presented in Lacoste et al. (2019).\n\n- Hardware Type: \n- Hours used: \n- Cloud Provider: \n- Compute Region: \n- Carbon Emitted:",
"## Technical Specifications [optional]",
"### Model Architecture and Objective",
"### Compute Infrastructure",
"#### Hardware",
"#### Software\n\n\n\n[optional]\n\n\n\nBibTeX:\n\n\n\nAPA:",
"## Glossary [optional]",
"## More Information [optional]",
"## Model Card Authors [optional]",
"## Model Card Contact"
] |
text2text-generation | transformers |
<!-- This model card has been generated automatically according to the information Keras had access to. You should
probably proofread and complete it, then remove this comment. -->
# HI-EN_translation
This model is a fine-tuned version of [Helsinki-NLP/opus-mt-hi-en](https://huggingface.co/Helsinki-NLP/opus-mt-hi-en) on an unknown dataset.
It achieves the following results on the evaluation set:
- Train Loss: 3.5159
- Validation Loss: 3.5002
- Epoch: 0
## Model description
More information needed
## Intended uses & limitations
More information needed
## Training and evaluation data
More information needed
## Training procedure
### Training hyperparameters
The following hyperparameters were used during training:
- optimizer: {'name': 'AdamWeightDecay', 'learning_rate': 2e-05, 'decay': 0.0, 'beta_1': 0.9, 'beta_2': 0.999, 'epsilon': 1e-07, 'amsgrad': False, 'weight_decay_rate': 0.01}
- training_precision: float32
### Training results
| Train Loss | Validation Loss | Epoch |
|:----------:|:---------------:|:-----:|
| 3.5159 | 3.5002 | 0 |
### Framework versions
- Transformers 4.38.2
- TensorFlow 2.15.0
- Datasets 2.19.0
- Tokenizers 0.15.2
| {"license": "apache-2.0", "tags": ["generated_from_keras_callback"], "base_model": "Helsinki-NLP/opus-mt-hi-en", "model-index": [{"name": "HI-EN_translation", "results": []}]} | Nettem-Gayathri/HI-EN_translation | null | [
"transformers",
"tf",
"marian",
"text2text-generation",
"generated_from_keras_callback",
"base_model:Helsinki-NLP/opus-mt-hi-en",
"license:apache-2.0",
"autotrain_compatible",
"endpoints_compatible",
"region:us"
] | null | 2024-04-22T09:32:50+00:00 | [] | [] | TAGS
#transformers #tf #marian #text2text-generation #generated_from_keras_callback #base_model-Helsinki-NLP/opus-mt-hi-en #license-apache-2.0 #autotrain_compatible #endpoints_compatible #region-us
| HI-EN\_translation
==================
This model is a fine-tuned version of Helsinki-NLP/opus-mt-hi-en on an unknown dataset.
It achieves the following results on the evaluation set:
* Train Loss: 3.5159
* Validation Loss: 3.5002
* Epoch: 0
Model description
-----------------
More information needed
Intended uses & limitations
---------------------------
More information needed
Training and evaluation data
----------------------------
More information needed
Training procedure
------------------
### Training hyperparameters
The following hyperparameters were used during training:
* optimizer: {'name': 'AdamWeightDecay', 'learning\_rate': 2e-05, 'decay': 0.0, 'beta\_1': 0.9, 'beta\_2': 0.999, 'epsilon': 1e-07, 'amsgrad': False, 'weight\_decay\_rate': 0.01}
* training\_precision: float32
### Training results
### Framework versions
* Transformers 4.38.2
* TensorFlow 2.15.0
* Datasets 2.19.0
* Tokenizers 0.15.2
| [
"### Training hyperparameters\n\n\nThe following hyperparameters were used during training:\n\n\n* optimizer: {'name': 'AdamWeightDecay', 'learning\\_rate': 2e-05, 'decay': 0.0, 'beta\\_1': 0.9, 'beta\\_2': 0.999, 'epsilon': 1e-07, 'amsgrad': False, 'weight\\_decay\\_rate': 0.01}\n* training\\_precision: float32",
"### Training results",
"### Framework versions\n\n\n* Transformers 4.38.2\n* TensorFlow 2.15.0\n* Datasets 2.19.0\n* Tokenizers 0.15.2"
] | [
"TAGS\n#transformers #tf #marian #text2text-generation #generated_from_keras_callback #base_model-Helsinki-NLP/opus-mt-hi-en #license-apache-2.0 #autotrain_compatible #endpoints_compatible #region-us \n",
"### Training hyperparameters\n\n\nThe following hyperparameters were used during training:\n\n\n* optimizer: {'name': 'AdamWeightDecay', 'learning\\_rate': 2e-05, 'decay': 0.0, 'beta\\_1': 0.9, 'beta\\_2': 0.999, 'epsilon': 1e-07, 'amsgrad': False, 'weight\\_decay\\_rate': 0.01}\n* training\\_precision: float32",
"### Training results",
"### Framework versions\n\n\n* Transformers 4.38.2\n* TensorFlow 2.15.0\n* Datasets 2.19.0\n* Tokenizers 0.15.2"
] |
text-generation | transformers |
# Model Card for Model ID
<!-- Provide a quick summary of what the model is/does. -->
## Model Details
### Model Description
<!-- Provide a longer summary of what this model is. -->
This is the model card of a 🤗 transformers model that has been pushed on the Hub. This model card has been automatically generated.
- **Developed by:** [More Information Needed]
- **Funded by [optional]:** [More Information Needed]
- **Shared by [optional]:** [More Information Needed]
- **Model type:** [More Information Needed]
- **Language(s) (NLP):** [More Information Needed]
- **License:** [More Information Needed]
- **Finetuned from model [optional]:** [More Information Needed]
### Model Sources [optional]
<!-- Provide the basic links for the model. -->
- **Repository:** [More Information Needed]
- **Paper [optional]:** [More Information Needed]
- **Demo [optional]:** [More Information Needed]
## Uses
<!-- Address questions around how the model is intended to be used, including the foreseeable users of the model and those affected by the model. -->
### Direct Use
<!-- This section is for the model use without fine-tuning or plugging into a larger ecosystem/app. -->
[More Information Needed]
### Downstream Use [optional]
<!-- This section is for the model use when fine-tuned for a task, or when plugged into a larger ecosystem/app -->
[More Information Needed]
### Out-of-Scope Use
<!-- This section addresses misuse, malicious use, and uses that the model will not work well for. -->
[More Information Needed]
## Bias, Risks, and Limitations
<!-- This section is meant to convey both technical and sociotechnical limitations. -->
[More Information Needed]
### Recommendations
<!-- This section is meant to convey recommendations with respect to the bias, risk, and technical limitations. -->
Users (both direct and downstream) should be made aware of the risks, biases and limitations of the model. More information needed for further recommendations.
## How to Get Started with the Model
Use the code below to get started with the model.
[More Information Needed]
## Training Details
### Training Data
<!-- This should link to a Dataset Card, perhaps with a short stub of information on what the training data is all about as well as documentation related to data pre-processing or additional filtering. -->
[More Information Needed]
### Training Procedure
<!-- This relates heavily to the Technical Specifications. Content here should link to that section when it is relevant to the training procedure. -->
#### Preprocessing [optional]
[More Information Needed]
#### Training Hyperparameters
- **Training regime:** [More Information Needed] <!--fp32, fp16 mixed precision, bf16 mixed precision, bf16 non-mixed precision, fp16 non-mixed precision, fp8 mixed precision -->
#### Speeds, Sizes, Times [optional]
<!-- This section provides information about throughput, start/end time, checkpoint size if relevant, etc. -->
[More Information Needed]
## Evaluation
<!-- This section describes the evaluation protocols and provides the results. -->
### Testing Data, Factors & Metrics
#### Testing Data
<!-- This should link to a Dataset Card if possible. -->
[More Information Needed]
#### Factors
<!-- These are the things the evaluation is disaggregating by, e.g., subpopulations or domains. -->
[More Information Needed]
#### Metrics
<!-- These are the evaluation metrics being used, ideally with a description of why. -->
[More Information Needed]
### Results
[More Information Needed]
#### Summary
## Model Examination [optional]
<!-- Relevant interpretability work for the model goes here -->
[More Information Needed]
## Environmental Impact
<!-- Total emissions (in grams of CO2eq) and additional considerations, such as electricity usage, go here. Edit the suggested text below accordingly -->
Carbon emissions can be estimated using the [Machine Learning Impact calculator](https://mlco2.github.io/impact#compute) presented in [Lacoste et al. (2019)](https://arxiv.org/abs/1910.09700).
- **Hardware Type:** [More Information Needed]
- **Hours used:** [More Information Needed]
- **Cloud Provider:** [More Information Needed]
- **Compute Region:** [More Information Needed]
- **Carbon Emitted:** [More Information Needed]
## Technical Specifications [optional]
### Model Architecture and Objective
[More Information Needed]
### Compute Infrastructure
[More Information Needed]
#### Hardware
[More Information Needed]
#### Software
[More Information Needed]
## Citation [optional]
<!-- If there is a paper or blog post introducing the model, the APA and Bibtex information for that should go in this section. -->
**BibTeX:**
[More Information Needed]
**APA:**
[More Information Needed]
## Glossary [optional]
<!-- If relevant, include terms and calculations in this section that can help readers understand the model or model card. -->
[More Information Needed]
## More Information [optional]
[More Information Needed]
## Model Card Authors [optional]
[More Information Needed]
## Model Card Contact
[More Information Needed] | {"library_name": "transformers", "tags": []} | EpicJhon/llama | null | [
"transformers",
"safetensors",
"llama",
"text-generation",
"conversational",
"arxiv:1910.09700",
"autotrain_compatible",
"endpoints_compatible",
"text-generation-inference",
"region:us"
] | null | 2024-04-22T09:33:22+00:00 | [
"1910.09700"
] | [] | TAGS
#transformers #safetensors #llama #text-generation #conversational #arxiv-1910.09700 #autotrain_compatible #endpoints_compatible #text-generation-inference #region-us
|
# Model Card for Model ID
## Model Details
### Model Description
This is the model card of a transformers model that has been pushed on the Hub. This model card has been automatically generated.
- Developed by:
- Funded by [optional]:
- Shared by [optional]:
- Model type:
- Language(s) (NLP):
- License:
- Finetuned from model [optional]:
### Model Sources [optional]
- Repository:
- Paper [optional]:
- Demo [optional]:
## Uses
### Direct Use
### Downstream Use [optional]
### Out-of-Scope Use
## Bias, Risks, and Limitations
### Recommendations
Users (both direct and downstream) should be made aware of the risks, biases and limitations of the model. More information needed for further recommendations.
## How to Get Started with the Model
Use the code below to get started with the model.
## Training Details
### Training Data
### Training Procedure
#### Preprocessing [optional]
#### Training Hyperparameters
- Training regime:
#### Speeds, Sizes, Times [optional]
## Evaluation
### Testing Data, Factors & Metrics
#### Testing Data
#### Factors
#### Metrics
### Results
#### Summary
## Model Examination [optional]
## Environmental Impact
Carbon emissions can be estimated using the Machine Learning Impact calculator presented in Lacoste et al. (2019).
- Hardware Type:
- Hours used:
- Cloud Provider:
- Compute Region:
- Carbon Emitted:
## Technical Specifications [optional]
### Model Architecture and Objective
### Compute Infrastructure
#### Hardware
#### Software
[optional]
BibTeX:
APA:
## Glossary [optional]
## More Information [optional]
## Model Card Authors [optional]
## Model Card Contact
| [
"# Model Card for Model ID",
"## Model Details",
"### Model Description\n\n\n\nThis is the model card of a transformers model that has been pushed on the Hub. This model card has been automatically generated.\n\n- Developed by: \n- Funded by [optional]: \n- Shared by [optional]: \n- Model type: \n- Language(s) (NLP): \n- License: \n- Finetuned from model [optional]:",
"### Model Sources [optional]\n\n\n\n- Repository: \n- Paper [optional]: \n- Demo [optional]:",
"## Uses",
"### Direct Use",
"### Downstream Use [optional]",
"### Out-of-Scope Use",
"## Bias, Risks, and Limitations",
"### Recommendations\n\n\n\nUsers (both direct and downstream) should be made aware of the risks, biases and limitations of the model. More information needed for further recommendations.",
"## How to Get Started with the Model\n\nUse the code below to get started with the model.",
"## Training Details",
"### Training Data",
"### Training Procedure",
"#### Preprocessing [optional]",
"#### Training Hyperparameters\n\n- Training regime:",
"#### Speeds, Sizes, Times [optional]",
"## Evaluation",
"### Testing Data, Factors & Metrics",
"#### Testing Data",
"#### Factors",
"#### Metrics",
"### Results",
"#### Summary",
"## Model Examination [optional]",
"## Environmental Impact\n\n\n\nCarbon emissions can be estimated using the Machine Learning Impact calculator presented in Lacoste et al. (2019).\n\n- Hardware Type: \n- Hours used: \n- Cloud Provider: \n- Compute Region: \n- Carbon Emitted:",
"## Technical Specifications [optional]",
"### Model Architecture and Objective",
"### Compute Infrastructure",
"#### Hardware",
"#### Software\n\n\n\n[optional]\n\n\n\nBibTeX:\n\n\n\nAPA:",
"## Glossary [optional]",
"## More Information [optional]",
"## Model Card Authors [optional]",
"## Model Card Contact"
] | [
"TAGS\n#transformers #safetensors #llama #text-generation #conversational #arxiv-1910.09700 #autotrain_compatible #endpoints_compatible #text-generation-inference #region-us \n",
"# Model Card for Model ID",
"## Model Details",
"### Model Description\n\n\n\nThis is the model card of a transformers model that has been pushed on the Hub. This model card has been automatically generated.\n\n- Developed by: \n- Funded by [optional]: \n- Shared by [optional]: \n- Model type: \n- Language(s) (NLP): \n- License: \n- Finetuned from model [optional]:",
"### Model Sources [optional]\n\n\n\n- Repository: \n- Paper [optional]: \n- Demo [optional]:",
"## Uses",
"### Direct Use",
"### Downstream Use [optional]",
"### Out-of-Scope Use",
"## Bias, Risks, and Limitations",
"### Recommendations\n\n\n\nUsers (both direct and downstream) should be made aware of the risks, biases and limitations of the model. More information needed for further recommendations.",
"## How to Get Started with the Model\n\nUse the code below to get started with the model.",
"## Training Details",
"### Training Data",
"### Training Procedure",
"#### Preprocessing [optional]",
"#### Training Hyperparameters\n\n- Training regime:",
"#### Speeds, Sizes, Times [optional]",
"## Evaluation",
"### Testing Data, Factors & Metrics",
"#### Testing Data",
"#### Factors",
"#### Metrics",
"### Results",
"#### Summary",
"## Model Examination [optional]",
"## Environmental Impact\n\n\n\nCarbon emissions can be estimated using the Machine Learning Impact calculator presented in Lacoste et al. (2019).\n\n- Hardware Type: \n- Hours used: \n- Cloud Provider: \n- Compute Region: \n- Carbon Emitted:",
"## Technical Specifications [optional]",
"### Model Architecture and Objective",
"### Compute Infrastructure",
"#### Hardware",
"#### Software\n\n\n\n[optional]\n\n\n\nBibTeX:\n\n\n\nAPA:",
"## Glossary [optional]",
"## More Information [optional]",
"## Model Card Authors [optional]",
"## Model Card Contact"
] |
text-generation | transformers | # ChaoticNeutrals/IQ_Test_l3_8B AWQ
- Model creator: [ChaoticNeutrals](https://huggingface.co/ChaoticNeutrals)
- Original model: [IQ_Test_l3_8B](https://huggingface.co/ChaoticNeutrals/IQ_Test_l3_8B)
## How to use
### Install the necessary packages
```bash
pip install --upgrade autoawq autoawq-kernels
```
### Example Python code
```python
from awq import AutoAWQForCausalLM
from transformers import AutoTokenizer, TextStreamer
model_path = "solidrust/IQ_Test_l3_8B-AWQ"
system_message = "You are IQ_Test_l3_8B, incarnated as a powerful AI. You were created by ChaoticNeutrals."
# Load model
model = AutoAWQForCausalLM.from_quantized(model_path,
fuse_layers=True)
tokenizer = AutoTokenizer.from_pretrained(model_path,
trust_remote_code=True)
streamer = TextStreamer(tokenizer,
skip_prompt=True,
skip_special_tokens=True)
# Convert prompt to tokens
prompt_template = """\
<|im_start|>system
{system_message}<|im_end|>
<|im_start|>user
{prompt}<|im_end|>
<|im_start|>assistant"""
prompt = "You're standing on the surface of the Earth. "\
"You walk one mile south, one mile west and one mile north. "\
"You end up exactly where you started. Where are you?"
tokens = tokenizer(prompt_template.format(system_message=system_message,prompt=prompt),
return_tensors='pt').input_ids.cuda()
# Generate output
generation_output = model.generate(tokens,
streamer=streamer,
max_new_tokens=512)
```
### About AWQ
AWQ is an efficient, accurate and blazing-fast low-bit weight quantization method, currently supporting 4-bit quantization. Compared to GPTQ, it offers faster Transformers-based inference with equivalent or better quality compared to the most commonly used GPTQ settings.
AWQ models are currently supported on Linux and Windows, with NVidia GPUs only. macOS users: please use GGUF models instead.
It is supported by:
- [Text Generation Webui](https://github.com/oobabooga/text-generation-webui) - using Loader: AutoAWQ
- [vLLM](https://github.com/vllm-project/vllm) - version 0.2.2 or later for support for all model types.
- [Hugging Face Text Generation Inference (TGI)](https://github.com/huggingface/text-generation-inference)
- [Transformers](https://huggingface.co/docs/transformers) version 4.35.0 and later, from any code or client that supports Transformers
- [AutoAWQ](https://github.com/casper-hansen/AutoAWQ) - for use from Python code
| {"library_name": "transformers", "tags": ["4-bit", "AWQ", "text-generation", "autotrain_compatible", "endpoints_compatible"], "pipeline_tag": "text-generation", "inference": false, "quantized_by": "Suparious"} | solidrust/IQ_Test_l3_8B-AWQ | null | [
"transformers",
"safetensors",
"llama",
"text-generation",
"4-bit",
"AWQ",
"autotrain_compatible",
"endpoints_compatible",
"conversational",
"text-generation-inference",
"region:us"
] | null | 2024-04-22T09:33:34+00:00 | [] | [] | TAGS
#transformers #safetensors #llama #text-generation #4-bit #AWQ #autotrain_compatible #endpoints_compatible #conversational #text-generation-inference #region-us
| # ChaoticNeutrals/IQ_Test_l3_8B AWQ
- Model creator: ChaoticNeutrals
- Original model: IQ_Test_l3_8B
## How to use
### Install the necessary packages
### Example Python code
### About AWQ
AWQ is an efficient, accurate and blazing-fast low-bit weight quantization method, currently supporting 4-bit quantization. Compared to GPTQ, it offers faster Transformers-based inference with equivalent or better quality compared to the most commonly used GPTQ settings.
AWQ models are currently supported on Linux and Windows, with NVidia GPUs only. macOS users: please use GGUF models instead.
It is supported by:
- Text Generation Webui - using Loader: AutoAWQ
- vLLM - version 0.2.2 or later for support for all model types.
- Hugging Face Text Generation Inference (TGI)
- Transformers version 4.35.0 and later, from any code or client that supports Transformers
- AutoAWQ - for use from Python code
| [
"# ChaoticNeutrals/IQ_Test_l3_8B AWQ\n\n- Model creator: ChaoticNeutrals\n- Original model: IQ_Test_l3_8B",
"## How to use",
"### Install the necessary packages",
"### Example Python code",
"### About AWQ\n\nAWQ is an efficient, accurate and blazing-fast low-bit weight quantization method, currently supporting 4-bit quantization. Compared to GPTQ, it offers faster Transformers-based inference with equivalent or better quality compared to the most commonly used GPTQ settings.\n\nAWQ models are currently supported on Linux and Windows, with NVidia GPUs only. macOS users: please use GGUF models instead.\n\nIt is supported by:\n\n- Text Generation Webui - using Loader: AutoAWQ\n- vLLM - version 0.2.2 or later for support for all model types.\n- Hugging Face Text Generation Inference (TGI)\n- Transformers version 4.35.0 and later, from any code or client that supports Transformers\n- AutoAWQ - for use from Python code"
] | [
"TAGS\n#transformers #safetensors #llama #text-generation #4-bit #AWQ #autotrain_compatible #endpoints_compatible #conversational #text-generation-inference #region-us \n",
"# ChaoticNeutrals/IQ_Test_l3_8B AWQ\n\n- Model creator: ChaoticNeutrals\n- Original model: IQ_Test_l3_8B",
"## How to use",
"### Install the necessary packages",
"### Example Python code",
"### About AWQ\n\nAWQ is an efficient, accurate and blazing-fast low-bit weight quantization method, currently supporting 4-bit quantization. Compared to GPTQ, it offers faster Transformers-based inference with equivalent or better quality compared to the most commonly used GPTQ settings.\n\nAWQ models are currently supported on Linux and Windows, with NVidia GPUs only. macOS users: please use GGUF models instead.\n\nIt is supported by:\n\n- Text Generation Webui - using Loader: AutoAWQ\n- vLLM - version 0.2.2 or later for support for all model types.\n- Hugging Face Text Generation Inference (TGI)\n- Transformers version 4.35.0 and later, from any code or client that supports Transformers\n- AutoAWQ - for use from Python code"
] |
text-generation | transformers |
<!-- This model card has been generated automatically according to the information the Trainer had access to. You
should probably proofread and complete it, then remove this comment. -->
# 0.0001_ablation_4iters_bs256_iter_4
This model is a fine-tuned version of [ShenaoZ/0.0001_ablation_4iters_bs256_iter_3](https://huggingface.co/ShenaoZ/0.0001_ablation_4iters_bs256_iter_3) on the updated and the original datasets.
## Model description
More information needed
## Intended uses & limitations
More information needed
## Training and evaluation data
More information needed
## Training procedure
### Training hyperparameters
The following hyperparameters were used during training:
- learning_rate: 5e-07
- train_batch_size: 8
- eval_batch_size: 8
- seed: 42
- distributed_type: multi-GPU
- num_devices: 8
- gradient_accumulation_steps: 4
- total_train_batch_size: 256
- total_eval_batch_size: 64
- optimizer: Adam with betas=(0.9,0.999) and epsilon=1e-08
- lr_scheduler_type: cosine
- lr_scheduler_warmup_ratio: 0.1
- num_epochs: 1
### Training results
### Framework versions
- Transformers 4.36.2
- Pytorch 2.1.2+cu121
- Datasets 2.14.6
- Tokenizers 0.15.2
| {"license": "mit", "tags": ["alignment-handbook", "generated_from_trainer", "trl", "dpo", "generated_from_trainer"], "datasets": ["updated", "original"], "base_model": "ShenaoZ/0.0001_ablation_4iters_bs256_iter_3", "model-index": [{"name": "0.0001_ablation_4iters_bs256_iter_4", "results": []}]} | ShenaoZ/0.0001_ablation_4iters_bs256_iter_4 | null | [
"transformers",
"safetensors",
"mistral",
"text-generation",
"alignment-handbook",
"generated_from_trainer",
"trl",
"dpo",
"conversational",
"dataset:updated",
"dataset:original",
"base_model:ShenaoZ/0.0001_ablation_4iters_bs256_iter_3",
"license:mit",
"autotrain_compatible",
"endpoints_compatible",
"text-generation-inference",
"region:us"
] | null | 2024-04-22T09:33:45+00:00 | [] | [] | TAGS
#transformers #safetensors #mistral #text-generation #alignment-handbook #generated_from_trainer #trl #dpo #conversational #dataset-updated #dataset-original #base_model-ShenaoZ/0.0001_ablation_4iters_bs256_iter_3 #license-mit #autotrain_compatible #endpoints_compatible #text-generation-inference #region-us
|
# 0.0001_ablation_4iters_bs256_iter_4
This model is a fine-tuned version of ShenaoZ/0.0001_ablation_4iters_bs256_iter_3 on the updated and the original datasets.
## Model description
More information needed
## Intended uses & limitations
More information needed
## Training and evaluation data
More information needed
## Training procedure
### Training hyperparameters
The following hyperparameters were used during training:
- learning_rate: 5e-07
- train_batch_size: 8
- eval_batch_size: 8
- seed: 42
- distributed_type: multi-GPU
- num_devices: 8
- gradient_accumulation_steps: 4
- total_train_batch_size: 256
- total_eval_batch_size: 64
- optimizer: Adam with betas=(0.9,0.999) and epsilon=1e-08
- lr_scheduler_type: cosine
- lr_scheduler_warmup_ratio: 0.1
- num_epochs: 1
### Training results
### Framework versions
- Transformers 4.36.2
- Pytorch 2.1.2+cu121
- Datasets 2.14.6
- Tokenizers 0.15.2
| [
"# 0.0001_ablation_4iters_bs256_iter_4\n\nThis model is a fine-tuned version of ShenaoZ/0.0001_ablation_4iters_bs256_iter_3 on the updated and the original datasets.",
"## Model description\n\nMore information needed",
"## Intended uses & limitations\n\nMore information needed",
"## Training and evaluation data\n\nMore information needed",
"## Training procedure",
"### Training hyperparameters\n\nThe following hyperparameters were used during training:\n- learning_rate: 5e-07\n- train_batch_size: 8\n- eval_batch_size: 8\n- seed: 42\n- distributed_type: multi-GPU\n- num_devices: 8\n- gradient_accumulation_steps: 4\n- total_train_batch_size: 256\n- total_eval_batch_size: 64\n- optimizer: Adam with betas=(0.9,0.999) and epsilon=1e-08\n- lr_scheduler_type: cosine\n- lr_scheduler_warmup_ratio: 0.1\n- num_epochs: 1",
"### Training results",
"### Framework versions\n\n- Transformers 4.36.2\n- Pytorch 2.1.2+cu121\n- Datasets 2.14.6\n- Tokenizers 0.15.2"
] | [
"TAGS\n#transformers #safetensors #mistral #text-generation #alignment-handbook #generated_from_trainer #trl #dpo #conversational #dataset-updated #dataset-original #base_model-ShenaoZ/0.0001_ablation_4iters_bs256_iter_3 #license-mit #autotrain_compatible #endpoints_compatible #text-generation-inference #region-us \n",
"# 0.0001_ablation_4iters_bs256_iter_4\n\nThis model is a fine-tuned version of ShenaoZ/0.0001_ablation_4iters_bs256_iter_3 on the updated and the original datasets.",
"## Model description\n\nMore information needed",
"## Intended uses & limitations\n\nMore information needed",
"## Training and evaluation data\n\nMore information needed",
"## Training procedure",
"### Training hyperparameters\n\nThe following hyperparameters were used during training:\n- learning_rate: 5e-07\n- train_batch_size: 8\n- eval_batch_size: 8\n- seed: 42\n- distributed_type: multi-GPU\n- num_devices: 8\n- gradient_accumulation_steps: 4\n- total_train_batch_size: 256\n- total_eval_batch_size: 64\n- optimizer: Adam with betas=(0.9,0.999) and epsilon=1e-08\n- lr_scheduler_type: cosine\n- lr_scheduler_warmup_ratio: 0.1\n- num_epochs: 1",
"### Training results",
"### Framework versions\n\n- Transformers 4.36.2\n- Pytorch 2.1.2+cu121\n- Datasets 2.14.6\n- Tokenizers 0.15.2"
] |
text-generation | transformers | # winglian/llama-3-orpo-ml AWQ
- Model creator: [winglian](https://huggingface.co/winglian)
- Original model: [llama-3-orpo-ml](https://huggingface.co/winglian/llama-3-orpo-ml)
## How to use
### Install the necessary packages
```bash
pip install --upgrade autoawq autoawq-kernels
```
### Example Python code
```python
from awq import AutoAWQForCausalLM
from transformers import AutoTokenizer, TextStreamer
model_path = "solidrust/llama-3-orpo-ml-AWQ"
system_message = "You are llama-3-orpo-ml, incarnated as a powerful AI. You were created by winglian."
# Load model
model = AutoAWQForCausalLM.from_quantized(model_path,
fuse_layers=True)
tokenizer = AutoTokenizer.from_pretrained(model_path,
trust_remote_code=True)
streamer = TextStreamer(tokenizer,
skip_prompt=True,
skip_special_tokens=True)
# Convert prompt to tokens
prompt_template = """\
<|im_start|>system
{system_message}<|im_end|>
<|im_start|>user
{prompt}<|im_end|>
<|im_start|>assistant"""
prompt = "You're standing on the surface of the Earth. "\
"You walk one mile south, one mile west and one mile north. "\
"You end up exactly where you started. Where are you?"
tokens = tokenizer(prompt_template.format(system_message=system_message,prompt=prompt),
return_tensors='pt').input_ids.cuda()
# Generate output
generation_output = model.generate(tokens,
streamer=streamer,
max_new_tokens=512)
```
### About AWQ
AWQ is an efficient, accurate and blazing-fast low-bit weight quantization method, currently supporting 4-bit quantization. Compared to GPTQ, it offers faster Transformers-based inference with equivalent or better quality compared to the most commonly used GPTQ settings.
AWQ models are currently supported on Linux and Windows, with NVidia GPUs only. macOS users: please use GGUF models instead.
It is supported by:
- [Text Generation Webui](https://github.com/oobabooga/text-generation-webui) - using Loader: AutoAWQ
- [vLLM](https://github.com/vllm-project/vllm) - version 0.2.2 or later for support for all model types.
- [Hugging Face Text Generation Inference (TGI)](https://github.com/huggingface/text-generation-inference)
- [Transformers](https://huggingface.co/docs/transformers) version 4.35.0 and later, from any code or client that supports Transformers
- [AutoAWQ](https://github.com/casper-hansen/AutoAWQ) - for use from Python code
| {"library_name": "transformers", "tags": ["4-bit", "AWQ", "text-generation", "autotrain_compatible", "endpoints_compatible"], "pipeline_tag": "text-generation", "inference": false, "quantized_by": "Suparious"} | solidrust/llama-3-orpo-ml-AWQ | null | [
"transformers",
"safetensors",
"llama",
"text-generation",
"4-bit",
"AWQ",
"autotrain_compatible",
"endpoints_compatible",
"conversational",
"text-generation-inference",
"region:us"
] | null | 2024-04-22T09:33:49+00:00 | [] | [] | TAGS
#transformers #safetensors #llama #text-generation #4-bit #AWQ #autotrain_compatible #endpoints_compatible #conversational #text-generation-inference #region-us
| # winglian/llama-3-orpo-ml AWQ
- Model creator: winglian
- Original model: llama-3-orpo-ml
## How to use
### Install the necessary packages
### Example Python code
### About AWQ
AWQ is an efficient, accurate and blazing-fast low-bit weight quantization method, currently supporting 4-bit quantization. Compared to GPTQ, it offers faster Transformers-based inference with equivalent or better quality compared to the most commonly used GPTQ settings.
AWQ models are currently supported on Linux and Windows, with NVidia GPUs only. macOS users: please use GGUF models instead.
It is supported by:
- Text Generation Webui - using Loader: AutoAWQ
- vLLM - version 0.2.2 or later for support for all model types.
- Hugging Face Text Generation Inference (TGI)
- Transformers version 4.35.0 and later, from any code or client that supports Transformers
- AutoAWQ - for use from Python code
| [
"# winglian/llama-3-orpo-ml AWQ\n\n- Model creator: winglian\n- Original model: llama-3-orpo-ml",
"## How to use",
"### Install the necessary packages",
"### Example Python code",
"### About AWQ\n\nAWQ is an efficient, accurate and blazing-fast low-bit weight quantization method, currently supporting 4-bit quantization. Compared to GPTQ, it offers faster Transformers-based inference with equivalent or better quality compared to the most commonly used GPTQ settings.\n\nAWQ models are currently supported on Linux and Windows, with NVidia GPUs only. macOS users: please use GGUF models instead.\n\nIt is supported by:\n\n- Text Generation Webui - using Loader: AutoAWQ\n- vLLM - version 0.2.2 or later for support for all model types.\n- Hugging Face Text Generation Inference (TGI)\n- Transformers version 4.35.0 and later, from any code or client that supports Transformers\n- AutoAWQ - for use from Python code"
] | [
"TAGS\n#transformers #safetensors #llama #text-generation #4-bit #AWQ #autotrain_compatible #endpoints_compatible #conversational #text-generation-inference #region-us \n",
"# winglian/llama-3-orpo-ml AWQ\n\n- Model creator: winglian\n- Original model: llama-3-orpo-ml",
"## How to use",
"### Install the necessary packages",
"### Example Python code",
"### About AWQ\n\nAWQ is an efficient, accurate and blazing-fast low-bit weight quantization method, currently supporting 4-bit quantization. Compared to GPTQ, it offers faster Transformers-based inference with equivalent or better quality compared to the most commonly used GPTQ settings.\n\nAWQ models are currently supported on Linux and Windows, with NVidia GPUs only. macOS users: please use GGUF models instead.\n\nIt is supported by:\n\n- Text Generation Webui - using Loader: AutoAWQ\n- vLLM - version 0.2.2 or later for support for all model types.\n- Hugging Face Text Generation Inference (TGI)\n- Transformers version 4.35.0 and later, from any code or client that supports Transformers\n- AutoAWQ - for use from Python code"
] |
text-generation | transformers |
# Model Card for Model ID
<!-- Provide a quick summary of what the model is/does. -->
## Model Details
### Model Description
<!-- Provide a longer summary of what this model is. -->
This is the model card of a 🤗 transformers model that has been pushed on the Hub. This model card has been automatically generated.
- **Developed by:** [More Information Needed]
- **Funded by [optional]:** [More Information Needed]
- **Shared by [optional]:** [More Information Needed]
- **Model type:** [More Information Needed]
- **Language(s) (NLP):** [More Information Needed]
- **License:** [More Information Needed]
- **Finetuned from model [optional]:** [More Information Needed]
### Model Sources [optional]
<!-- Provide the basic links for the model. -->
- **Repository:** [More Information Needed]
- **Paper [optional]:** [More Information Needed]
- **Demo [optional]:** [More Information Needed]
## Uses
<!-- Address questions around how the model is intended to be used, including the foreseeable users of the model and those affected by the model. -->
### Direct Use
<!-- This section is for the model use without fine-tuning or plugging into a larger ecosystem/app. -->
[More Information Needed]
### Downstream Use [optional]
<!-- This section is for the model use when fine-tuned for a task, or when plugged into a larger ecosystem/app -->
[More Information Needed]
### Out-of-Scope Use
<!-- This section addresses misuse, malicious use, and uses that the model will not work well for. -->
[More Information Needed]
## Bias, Risks, and Limitations
<!-- This section is meant to convey both technical and sociotechnical limitations. -->
[More Information Needed]
### Recommendations
<!-- This section is meant to convey recommendations with respect to the bias, risk, and technical limitations. -->
Users (both direct and downstream) should be made aware of the risks, biases and limitations of the model. More information needed for further recommendations.
## How to Get Started with the Model
Use the code below to get started with the model.
[More Information Needed]
## Training Details
### Training Data
<!-- This should link to a Dataset Card, perhaps with a short stub of information on what the training data is all about as well as documentation related to data pre-processing or additional filtering. -->
[More Information Needed]
### Training Procedure
<!-- This relates heavily to the Technical Specifications. Content here should link to that section when it is relevant to the training procedure. -->
#### Preprocessing [optional]
[More Information Needed]
#### Training Hyperparameters
- **Training regime:** [More Information Needed] <!--fp32, fp16 mixed precision, bf16 mixed precision, bf16 non-mixed precision, fp16 non-mixed precision, fp8 mixed precision -->
#### Speeds, Sizes, Times [optional]
<!-- This section provides information about throughput, start/end time, checkpoint size if relevant, etc. -->
[More Information Needed]
## Evaluation
<!-- This section describes the evaluation protocols and provides the results. -->
### Testing Data, Factors & Metrics
#### Testing Data
<!-- This should link to a Dataset Card if possible. -->
[More Information Needed]
#### Factors
<!-- These are the things the evaluation is disaggregating by, e.g., subpopulations or domains. -->
[More Information Needed]
#### Metrics
<!-- These are the evaluation metrics being used, ideally with a description of why. -->
[More Information Needed]
### Results
[More Information Needed]
#### Summary
## Model Examination [optional]
<!-- Relevant interpretability work for the model goes here -->
[More Information Needed]
## Environmental Impact
<!-- Total emissions (in grams of CO2eq) and additional considerations, such as electricity usage, go here. Edit the suggested text below accordingly -->
Carbon emissions can be estimated using the [Machine Learning Impact calculator](https://mlco2.github.io/impact#compute) presented in [Lacoste et al. (2019)](https://arxiv.org/abs/1910.09700).
- **Hardware Type:** [More Information Needed]
- **Hours used:** [More Information Needed]
- **Cloud Provider:** [More Information Needed]
- **Compute Region:** [More Information Needed]
- **Carbon Emitted:** [More Information Needed]
## Technical Specifications [optional]
### Model Architecture and Objective
[More Information Needed]
### Compute Infrastructure
[More Information Needed]
#### Hardware
[More Information Needed]
#### Software
[More Information Needed]
## Citation [optional]
<!-- If there is a paper or blog post introducing the model, the APA and Bibtex information for that should go in this section. -->
**BibTeX:**
[More Information Needed]
**APA:**
[More Information Needed]
## Glossary [optional]
<!-- If relevant, include terms and calculations in this section that can help readers understand the model or model card. -->
[More Information Needed]
## More Information [optional]
[More Information Needed]
## Model Card Authors [optional]
[More Information Needed]
## Model Card Contact
[More Information Needed] | {"library_name": "transformers", "tags": []} | tom-brady/6-223 | null | [
"transformers",
"safetensors",
"llama",
"text-generation",
"conversational",
"arxiv:1910.09700",
"autotrain_compatible",
"endpoints_compatible",
"text-generation-inference",
"region:us"
] | null | 2024-04-22T09:33:56+00:00 | [
"1910.09700"
] | [] | TAGS
#transformers #safetensors #llama #text-generation #conversational #arxiv-1910.09700 #autotrain_compatible #endpoints_compatible #text-generation-inference #region-us
|
# Model Card for Model ID
## Model Details
### Model Description
This is the model card of a transformers model that has been pushed on the Hub. This model card has been automatically generated.
- Developed by:
- Funded by [optional]:
- Shared by [optional]:
- Model type:
- Language(s) (NLP):
- License:
- Finetuned from model [optional]:
### Model Sources [optional]
- Repository:
- Paper [optional]:
- Demo [optional]:
## Uses
### Direct Use
### Downstream Use [optional]
### Out-of-Scope Use
## Bias, Risks, and Limitations
### Recommendations
Users (both direct and downstream) should be made aware of the risks, biases and limitations of the model. More information needed for further recommendations.
## How to Get Started with the Model
Use the code below to get started with the model.
## Training Details
### Training Data
### Training Procedure
#### Preprocessing [optional]
#### Training Hyperparameters
- Training regime:
#### Speeds, Sizes, Times [optional]
## Evaluation
### Testing Data, Factors & Metrics
#### Testing Data
#### Factors
#### Metrics
### Results
#### Summary
## Model Examination [optional]
## Environmental Impact
Carbon emissions can be estimated using the Machine Learning Impact calculator presented in Lacoste et al. (2019).
- Hardware Type:
- Hours used:
- Cloud Provider:
- Compute Region:
- Carbon Emitted:
## Technical Specifications [optional]
### Model Architecture and Objective
### Compute Infrastructure
#### Hardware
#### Software
[optional]
BibTeX:
APA:
## Glossary [optional]
## More Information [optional]
## Model Card Authors [optional]
## Model Card Contact
| [
"# Model Card for Model ID",
"## Model Details",
"### Model Description\n\n\n\nThis is the model card of a transformers model that has been pushed on the Hub. This model card has been automatically generated.\n\n- Developed by: \n- Funded by [optional]: \n- Shared by [optional]: \n- Model type: \n- Language(s) (NLP): \n- License: \n- Finetuned from model [optional]:",
"### Model Sources [optional]\n\n\n\n- Repository: \n- Paper [optional]: \n- Demo [optional]:",
"## Uses",
"### Direct Use",
"### Downstream Use [optional]",
"### Out-of-Scope Use",
"## Bias, Risks, and Limitations",
"### Recommendations\n\n\n\nUsers (both direct and downstream) should be made aware of the risks, biases and limitations of the model. More information needed for further recommendations.",
"## How to Get Started with the Model\n\nUse the code below to get started with the model.",
"## Training Details",
"### Training Data",
"### Training Procedure",
"#### Preprocessing [optional]",
"#### Training Hyperparameters\n\n- Training regime:",
"#### Speeds, Sizes, Times [optional]",
"## Evaluation",
"### Testing Data, Factors & Metrics",
"#### Testing Data",
"#### Factors",
"#### Metrics",
"### Results",
"#### Summary",
"## Model Examination [optional]",
"## Environmental Impact\n\n\n\nCarbon emissions can be estimated using the Machine Learning Impact calculator presented in Lacoste et al. (2019).\n\n- Hardware Type: \n- Hours used: \n- Cloud Provider: \n- Compute Region: \n- Carbon Emitted:",
"## Technical Specifications [optional]",
"### Model Architecture and Objective",
"### Compute Infrastructure",
"#### Hardware",
"#### Software\n\n\n\n[optional]\n\n\n\nBibTeX:\n\n\n\nAPA:",
"## Glossary [optional]",
"## More Information [optional]",
"## Model Card Authors [optional]",
"## Model Card Contact"
] | [
"TAGS\n#transformers #safetensors #llama #text-generation #conversational #arxiv-1910.09700 #autotrain_compatible #endpoints_compatible #text-generation-inference #region-us \n",
"# Model Card for Model ID",
"## Model Details",
"### Model Description\n\n\n\nThis is the model card of a transformers model that has been pushed on the Hub. This model card has been automatically generated.\n\n- Developed by: \n- Funded by [optional]: \n- Shared by [optional]: \n- Model type: \n- Language(s) (NLP): \n- License: \n- Finetuned from model [optional]:",
"### Model Sources [optional]\n\n\n\n- Repository: \n- Paper [optional]: \n- Demo [optional]:",
"## Uses",
"### Direct Use",
"### Downstream Use [optional]",
"### Out-of-Scope Use",
"## Bias, Risks, and Limitations",
"### Recommendations\n\n\n\nUsers (both direct and downstream) should be made aware of the risks, biases and limitations of the model. More information needed for further recommendations.",
"## How to Get Started with the Model\n\nUse the code below to get started with the model.",
"## Training Details",
"### Training Data",
"### Training Procedure",
"#### Preprocessing [optional]",
"#### Training Hyperparameters\n\n- Training regime:",
"#### Speeds, Sizes, Times [optional]",
"## Evaluation",
"### Testing Data, Factors & Metrics",
"#### Testing Data",
"#### Factors",
"#### Metrics",
"### Results",
"#### Summary",
"## Model Examination [optional]",
"## Environmental Impact\n\n\n\nCarbon emissions can be estimated using the Machine Learning Impact calculator presented in Lacoste et al. (2019).\n\n- Hardware Type: \n- Hours used: \n- Cloud Provider: \n- Compute Region: \n- Carbon Emitted:",
"## Technical Specifications [optional]",
"### Model Architecture and Objective",
"### Compute Infrastructure",
"#### Hardware",
"#### Software\n\n\n\n[optional]\n\n\n\nBibTeX:\n\n\n\nAPA:",
"## Glossary [optional]",
"## More Information [optional]",
"## Model Card Authors [optional]",
"## Model Card Contact"
] |
text-generation | transformers | # Japanese-Starling-ChatV-7B
このモデルは"chatntq-ja-7b-v1.0"をベースにした7Bパラメータの日本語チャットモデルです。
<br>"Japanese-Starling-ChatV-7B" is a Japanese chat model built on top of "chatntq-ja-7b-v1.0", originally based on Mistral-7B-v0.1.
<br>6bit量子化モデル
### <a href="https://huggingface.co/TFMC/Japanese-Starling-ChatV-7B-GGUF">詳細とGGUF版はこちら。Details and GGUFs are here. </a>
| {"language": ["ja"], "license": "apache-2.0", "tags": ["Mistral"], "pipeline_tag": "text-generation"} | AbeShinzo0708/Japanese-Starling-ChatV-7B-exl2 | null | [
"transformers",
"safetensors",
"mistral",
"text-generation",
"Mistral",
"ja",
"license:apache-2.0",
"autotrain_compatible",
"endpoints_compatible",
"text-generation-inference",
"6-bit",
"region:us"
] | null | 2024-04-22T09:34:11+00:00 | [] | [
"ja"
] | TAGS
#transformers #safetensors #mistral #text-generation #Mistral #ja #license-apache-2.0 #autotrain_compatible #endpoints_compatible #text-generation-inference #6-bit #region-us
| # Japanese-Starling-ChatV-7B
このモデルは"chatntq-ja-7b-v1.0"をベースにした7Bパラメータの日本語チャットモデルです。
<br>"Japanese-Starling-ChatV-7B" is a Japanese chat model built on top of "chatntq-ja-7b-v1.0", originally based on Mistral-7B-v0.1.
<br>6bit量子化モデル
### <a href="URL>詳細とGGUF版はこちら。Details and GGUFs are here. </a>
| [
"# Japanese-Starling-ChatV-7B\n\nこのモデルは\"chatntq-ja-7b-v1.0\"をベースにした7Bパラメータの日本語チャットモデルです。\n<br>\"Japanese-Starling-ChatV-7B\" is a Japanese chat model built on top of \"chatntq-ja-7b-v1.0\", originally based on Mistral-7B-v0.1.\n<br>6bit量子化モデル",
"### <a href=\"URL>詳細とGGUF版はこちら。Details and GGUFs are here. </a>"
] | [
"TAGS\n#transformers #safetensors #mistral #text-generation #Mistral #ja #license-apache-2.0 #autotrain_compatible #endpoints_compatible #text-generation-inference #6-bit #region-us \n",
"# Japanese-Starling-ChatV-7B\n\nこのモデルは\"chatntq-ja-7b-v1.0\"をベースにした7Bパラメータの日本語チャットモデルです。\n<br>\"Japanese-Starling-ChatV-7B\" is a Japanese chat model built on top of \"chatntq-ja-7b-v1.0\", originally based on Mistral-7B-v0.1.\n<br>6bit量子化モデル",
"### <a href=\"URL>詳細とGGUF版はこちら。Details and GGUFs are here. </a>"
] |
text-generation | transformers |
# Model Card for Model ID
<!-- Provide a quick summary of what the model is/does. -->
## Model Details
### Model Description
<!-- Provide a longer summary of what this model is. -->
This is the model card of a 🤗 transformers model that has been pushed on the Hub. This model card has been automatically generated.
- **Developed by:** [More Information Needed]
- **Funded by [optional]:** [More Information Needed]
- **Shared by [optional]:** [More Information Needed]
- **Model type:** [More Information Needed]
- **Language(s) (NLP):** [More Information Needed]
- **License:** [More Information Needed]
- **Finetuned from model [optional]:** [More Information Needed]
### Model Sources [optional]
<!-- Provide the basic links for the model. -->
- **Repository:** [More Information Needed]
- **Paper [optional]:** [More Information Needed]
- **Demo [optional]:** [More Information Needed]
## Uses
<!-- Address questions around how the model is intended to be used, including the foreseeable users of the model and those affected by the model. -->
### Direct Use
<!-- This section is for the model use without fine-tuning or plugging into a larger ecosystem/app. -->
[More Information Needed]
### Downstream Use [optional]
<!-- This section is for the model use when fine-tuned for a task, or when plugged into a larger ecosystem/app -->
[More Information Needed]
### Out-of-Scope Use
<!-- This section addresses misuse, malicious use, and uses that the model will not work well for. -->
[More Information Needed]
## Bias, Risks, and Limitations
<!-- This section is meant to convey both technical and sociotechnical limitations. -->
[More Information Needed]
### Recommendations
<!-- This section is meant to convey recommendations with respect to the bias, risk, and technical limitations. -->
Users (both direct and downstream) should be made aware of the risks, biases and limitations of the model. More information needed for further recommendations.
## How to Get Started with the Model
Use the code below to get started with the model.
[More Information Needed]
## Training Details
### Training Data
<!-- This should link to a Dataset Card, perhaps with a short stub of information on what the training data is all about as well as documentation related to data pre-processing or additional filtering. -->
[More Information Needed]
### Training Procedure
<!-- This relates heavily to the Technical Specifications. Content here should link to that section when it is relevant to the training procedure. -->
#### Preprocessing [optional]
[More Information Needed]
#### Training Hyperparameters
- **Training regime:** [More Information Needed] <!--fp32, fp16 mixed precision, bf16 mixed precision, bf16 non-mixed precision, fp16 non-mixed precision, fp8 mixed precision -->
#### Speeds, Sizes, Times [optional]
<!-- This section provides information about throughput, start/end time, checkpoint size if relevant, etc. -->
[More Information Needed]
## Evaluation
<!-- This section describes the evaluation protocols and provides the results. -->
### Testing Data, Factors & Metrics
#### Testing Data
<!-- This should link to a Dataset Card if possible. -->
[More Information Needed]
#### Factors
<!-- These are the things the evaluation is disaggregating by, e.g., subpopulations or domains. -->
[More Information Needed]
#### Metrics
<!-- These are the evaluation metrics being used, ideally with a description of why. -->
[More Information Needed]
### Results
[More Information Needed]
#### Summary
## Model Examination [optional]
<!-- Relevant interpretability work for the model goes here -->
[More Information Needed]
## Environmental Impact
<!-- Total emissions (in grams of CO2eq) and additional considerations, such as electricity usage, go here. Edit the suggested text below accordingly -->
Carbon emissions can be estimated using the [Machine Learning Impact calculator](https://mlco2.github.io/impact#compute) presented in [Lacoste et al. (2019)](https://arxiv.org/abs/1910.09700).
- **Hardware Type:** [More Information Needed]
- **Hours used:** [More Information Needed]
- **Cloud Provider:** [More Information Needed]
- **Compute Region:** [More Information Needed]
- **Carbon Emitted:** [More Information Needed]
## Technical Specifications [optional]
### Model Architecture and Objective
[More Information Needed]
### Compute Infrastructure
[More Information Needed]
#### Hardware
[More Information Needed]
#### Software
[More Information Needed]
## Citation [optional]
<!-- If there is a paper or blog post introducing the model, the APA and Bibtex information for that should go in this section. -->
**BibTeX:**
[More Information Needed]
**APA:**
[More Information Needed]
## Glossary [optional]
<!-- If relevant, include terms and calculations in this section that can help readers understand the model or model card. -->
[More Information Needed]
## More Information [optional]
[More Information Needed]
## Model Card Authors [optional]
[More Information Needed]
## Model Card Contact
[More Information Needed] | {"library_name": "transformers", "tags": []} | tom-brady/6-218 | null | [
"transformers",
"safetensors",
"stablelm",
"text-generation",
"conversational",
"arxiv:1910.09700",
"autotrain_compatible",
"endpoints_compatible",
"region:us"
] | null | 2024-04-22T09:34:26+00:00 | [
"1910.09700"
] | [] | TAGS
#transformers #safetensors #stablelm #text-generation #conversational #arxiv-1910.09700 #autotrain_compatible #endpoints_compatible #region-us
|
# Model Card for Model ID
## Model Details
### Model Description
This is the model card of a transformers model that has been pushed on the Hub. This model card has been automatically generated.
- Developed by:
- Funded by [optional]:
- Shared by [optional]:
- Model type:
- Language(s) (NLP):
- License:
- Finetuned from model [optional]:
### Model Sources [optional]
- Repository:
- Paper [optional]:
- Demo [optional]:
## Uses
### Direct Use
### Downstream Use [optional]
### Out-of-Scope Use
## Bias, Risks, and Limitations
### Recommendations
Users (both direct and downstream) should be made aware of the risks, biases and limitations of the model. More information needed for further recommendations.
## How to Get Started with the Model
Use the code below to get started with the model.
## Training Details
### Training Data
### Training Procedure
#### Preprocessing [optional]
#### Training Hyperparameters
- Training regime:
#### Speeds, Sizes, Times [optional]
## Evaluation
### Testing Data, Factors & Metrics
#### Testing Data
#### Factors
#### Metrics
### Results
#### Summary
## Model Examination [optional]
## Environmental Impact
Carbon emissions can be estimated using the Machine Learning Impact calculator presented in Lacoste et al. (2019).
- Hardware Type:
- Hours used:
- Cloud Provider:
- Compute Region:
- Carbon Emitted:
## Technical Specifications [optional]
### Model Architecture and Objective
### Compute Infrastructure
#### Hardware
#### Software
[optional]
BibTeX:
APA:
## Glossary [optional]
## More Information [optional]
## Model Card Authors [optional]
## Model Card Contact
| [
"# Model Card for Model ID",
"## Model Details",
"### Model Description\n\n\n\nThis is the model card of a transformers model that has been pushed on the Hub. This model card has been automatically generated.\n\n- Developed by: \n- Funded by [optional]: \n- Shared by [optional]: \n- Model type: \n- Language(s) (NLP): \n- License: \n- Finetuned from model [optional]:",
"### Model Sources [optional]\n\n\n\n- Repository: \n- Paper [optional]: \n- Demo [optional]:",
"## Uses",
"### Direct Use",
"### Downstream Use [optional]",
"### Out-of-Scope Use",
"## Bias, Risks, and Limitations",
"### Recommendations\n\n\n\nUsers (both direct and downstream) should be made aware of the risks, biases and limitations of the model. More information needed for further recommendations.",
"## How to Get Started with the Model\n\nUse the code below to get started with the model.",
"## Training Details",
"### Training Data",
"### Training Procedure",
"#### Preprocessing [optional]",
"#### Training Hyperparameters\n\n- Training regime:",
"#### Speeds, Sizes, Times [optional]",
"## Evaluation",
"### Testing Data, Factors & Metrics",
"#### Testing Data",
"#### Factors",
"#### Metrics",
"### Results",
"#### Summary",
"## Model Examination [optional]",
"## Environmental Impact\n\n\n\nCarbon emissions can be estimated using the Machine Learning Impact calculator presented in Lacoste et al. (2019).\n\n- Hardware Type: \n- Hours used: \n- Cloud Provider: \n- Compute Region: \n- Carbon Emitted:",
"## Technical Specifications [optional]",
"### Model Architecture and Objective",
"### Compute Infrastructure",
"#### Hardware",
"#### Software\n\n\n\n[optional]\n\n\n\nBibTeX:\n\n\n\nAPA:",
"## Glossary [optional]",
"## More Information [optional]",
"## Model Card Authors [optional]",
"## Model Card Contact"
] | [
"TAGS\n#transformers #safetensors #stablelm #text-generation #conversational #arxiv-1910.09700 #autotrain_compatible #endpoints_compatible #region-us \n",
"# Model Card for Model ID",
"## Model Details",
"### Model Description\n\n\n\nThis is the model card of a transformers model that has been pushed on the Hub. This model card has been automatically generated.\n\n- Developed by: \n- Funded by [optional]: \n- Shared by [optional]: \n- Model type: \n- Language(s) (NLP): \n- License: \n- Finetuned from model [optional]:",
"### Model Sources [optional]\n\n\n\n- Repository: \n- Paper [optional]: \n- Demo [optional]:",
"## Uses",
"### Direct Use",
"### Downstream Use [optional]",
"### Out-of-Scope Use",
"## Bias, Risks, and Limitations",
"### Recommendations\n\n\n\nUsers (both direct and downstream) should be made aware of the risks, biases and limitations of the model. More information needed for further recommendations.",
"## How to Get Started with the Model\n\nUse the code below to get started with the model.",
"## Training Details",
"### Training Data",
"### Training Procedure",
"#### Preprocessing [optional]",
"#### Training Hyperparameters\n\n- Training regime:",
"#### Speeds, Sizes, Times [optional]",
"## Evaluation",
"### Testing Data, Factors & Metrics",
"#### Testing Data",
"#### Factors",
"#### Metrics",
"### Results",
"#### Summary",
"## Model Examination [optional]",
"## Environmental Impact\n\n\n\nCarbon emissions can be estimated using the Machine Learning Impact calculator presented in Lacoste et al. (2019).\n\n- Hardware Type: \n- Hours used: \n- Cloud Provider: \n- Compute Region: \n- Carbon Emitted:",
"## Technical Specifications [optional]",
"### Model Architecture and Objective",
"### Compute Infrastructure",
"#### Hardware",
"#### Software\n\n\n\n[optional]\n\n\n\nBibTeX:\n\n\n\nAPA:",
"## Glossary [optional]",
"## More Information [optional]",
"## Model Card Authors [optional]",
"## Model Card Contact"
] |
null | peft |
# Model Card for Model ID
<!-- Provide a quick summary of what the model is/does. -->
## Model Details
### Model Description
<!-- Provide a longer summary of what this model is. -->
- **Developed by:** [More Information Needed]
- **Funded by [optional]:** [More Information Needed]
- **Shared by [optional]:** [More Information Needed]
- **Model type:** [More Information Needed]
- **Language(s) (NLP):** [More Information Needed]
- **License:** [More Information Needed]
- **Finetuned from model [optional]:** [More Information Needed]
### Model Sources [optional]
<!-- Provide the basic links for the model. -->
- **Repository:** [More Information Needed]
- **Paper [optional]:** [More Information Needed]
- **Demo [optional]:** [More Information Needed]
## Uses
<!-- Address questions around how the model is intended to be used, including the foreseeable users of the model and those affected by the model. -->
### Direct Use
<!-- This section is for the model use without fine-tuning or plugging into a larger ecosystem/app. -->
[More Information Needed]
### Downstream Use [optional]
<!-- This section is for the model use when fine-tuned for a task, or when plugged into a larger ecosystem/app -->
[More Information Needed]
### Out-of-Scope Use
<!-- This section addresses misuse, malicious use, and uses that the model will not work well for. -->
[More Information Needed]
## Bias, Risks, and Limitations
<!-- This section is meant to convey both technical and sociotechnical limitations. -->
[More Information Needed]
### Recommendations
<!-- This section is meant to convey recommendations with respect to the bias, risk, and technical limitations. -->
Users (both direct and downstream) should be made aware of the risks, biases and limitations of the model. More information needed for further recommendations.
## How to Get Started with the Model
Use the code below to get started with the model.
[More Information Needed]
## Training Details
### Training Data
<!-- This should link to a Dataset Card, perhaps with a short stub of information on what the training data is all about as well as documentation related to data pre-processing or additional filtering. -->
[More Information Needed]
### Training Procedure
<!-- This relates heavily to the Technical Specifications. Content here should link to that section when it is relevant to the training procedure. -->
#### Preprocessing [optional]
[More Information Needed]
#### Training Hyperparameters
- **Training regime:** [More Information Needed] <!--fp32, fp16 mixed precision, bf16 mixed precision, bf16 non-mixed precision, fp16 non-mixed precision, fp8 mixed precision -->
#### Speeds, Sizes, Times [optional]
<!-- This section provides information about throughput, start/end time, checkpoint size if relevant, etc. -->
[More Information Needed]
## Evaluation
<!-- This section describes the evaluation protocols and provides the results. -->
### Testing Data, Factors & Metrics
#### Testing Data
<!-- This should link to a Dataset Card if possible. -->
[More Information Needed]
#### Factors
<!-- These are the things the evaluation is disaggregating by, e.g., subpopulations or domains. -->
[More Information Needed]
#### Metrics
<!-- These are the evaluation metrics being used, ideally with a description of why. -->
[More Information Needed]
### Results
[More Information Needed]
#### Summary
## Model Examination [optional]
<!-- Relevant interpretability work for the model goes here -->
[More Information Needed]
## Environmental Impact
<!-- Total emissions (in grams of CO2eq) and additional considerations, such as electricity usage, go here. Edit the suggested text below accordingly -->
Carbon emissions can be estimated using the [Machine Learning Impact calculator](https://mlco2.github.io/impact#compute) presented in [Lacoste et al. (2019)](https://arxiv.org/abs/1910.09700).
- **Hardware Type:** [More Information Needed]
- **Hours used:** [More Information Needed]
- **Cloud Provider:** [More Information Needed]
- **Compute Region:** [More Information Needed]
- **Carbon Emitted:** [More Information Needed]
## Technical Specifications [optional]
### Model Architecture and Objective
[More Information Needed]
### Compute Infrastructure
[More Information Needed]
#### Hardware
[More Information Needed]
#### Software
[More Information Needed]
## Citation [optional]
<!-- If there is a paper or blog post introducing the model, the APA and Bibtex information for that should go in this section. -->
**BibTeX:**
[More Information Needed]
**APA:**
[More Information Needed]
## Glossary [optional]
<!-- If relevant, include terms and calculations in this section that can help readers understand the model or model card. -->
[More Information Needed]
## More Information [optional]
[More Information Needed]
## Model Card Authors [optional]
[More Information Needed]
## Model Card Contact
[More Information Needed]
### Framework versions
- PEFT 0.10.0 | {"library_name": "peft", "base_model": "mistralai/Mistral-7B-v0.1"} | ian00000/Mistral-7B_offensive_finetuned2 | null | [
"peft",
"safetensors",
"arxiv:1910.09700",
"base_model:mistralai/Mistral-7B-v0.1",
"region:us"
] | null | 2024-04-22T09:34:37+00:00 | [
"1910.09700"
] | [] | TAGS
#peft #safetensors #arxiv-1910.09700 #base_model-mistralai/Mistral-7B-v0.1 #region-us
|
# Model Card for Model ID
## Model Details
### Model Description
- Developed by:
- Funded by [optional]:
- Shared by [optional]:
- Model type:
- Language(s) (NLP):
- License:
- Finetuned from model [optional]:
### Model Sources [optional]
- Repository:
- Paper [optional]:
- Demo [optional]:
## Uses
### Direct Use
### Downstream Use [optional]
### Out-of-Scope Use
## Bias, Risks, and Limitations
### Recommendations
Users (both direct and downstream) should be made aware of the risks, biases and limitations of the model. More information needed for further recommendations.
## How to Get Started with the Model
Use the code below to get started with the model.
## Training Details
### Training Data
### Training Procedure
#### Preprocessing [optional]
#### Training Hyperparameters
- Training regime:
#### Speeds, Sizes, Times [optional]
## Evaluation
### Testing Data, Factors & Metrics
#### Testing Data
#### Factors
#### Metrics
### Results
#### Summary
## Model Examination [optional]
## Environmental Impact
Carbon emissions can be estimated using the Machine Learning Impact calculator presented in Lacoste et al. (2019).
- Hardware Type:
- Hours used:
- Cloud Provider:
- Compute Region:
- Carbon Emitted:
## Technical Specifications [optional]
### Model Architecture and Objective
### Compute Infrastructure
#### Hardware
#### Software
[optional]
BibTeX:
APA:
## Glossary [optional]
## More Information [optional]
## Model Card Authors [optional]
## Model Card Contact
### Framework versions
- PEFT 0.10.0 | [
"# Model Card for Model ID",
"## Model Details",
"### Model Description\n\n\n\n\n\n- Developed by: \n- Funded by [optional]: \n- Shared by [optional]: \n- Model type: \n- Language(s) (NLP): \n- License: \n- Finetuned from model [optional]:",
"### Model Sources [optional]\n\n\n\n- Repository: \n- Paper [optional]: \n- Demo [optional]:",
"## Uses",
"### Direct Use",
"### Downstream Use [optional]",
"### Out-of-Scope Use",
"## Bias, Risks, and Limitations",
"### Recommendations\n\n\n\nUsers (both direct and downstream) should be made aware of the risks, biases and limitations of the model. More information needed for further recommendations.",
"## How to Get Started with the Model\n\nUse the code below to get started with the model.",
"## Training Details",
"### Training Data",
"### Training Procedure",
"#### Preprocessing [optional]",
"#### Training Hyperparameters\n\n- Training regime:",
"#### Speeds, Sizes, Times [optional]",
"## Evaluation",
"### Testing Data, Factors & Metrics",
"#### Testing Data",
"#### Factors",
"#### Metrics",
"### Results",
"#### Summary",
"## Model Examination [optional]",
"## Environmental Impact\n\n\n\nCarbon emissions can be estimated using the Machine Learning Impact calculator presented in Lacoste et al. (2019).\n\n- Hardware Type: \n- Hours used: \n- Cloud Provider: \n- Compute Region: \n- Carbon Emitted:",
"## Technical Specifications [optional]",
"### Model Architecture and Objective",
"### Compute Infrastructure",
"#### Hardware",
"#### Software\n\n\n\n[optional]\n\n\n\nBibTeX:\n\n\n\nAPA:",
"## Glossary [optional]",
"## More Information [optional]",
"## Model Card Authors [optional]",
"## Model Card Contact",
"### Framework versions\n\n- PEFT 0.10.0"
] | [
"TAGS\n#peft #safetensors #arxiv-1910.09700 #base_model-mistralai/Mistral-7B-v0.1 #region-us \n",
"# Model Card for Model ID",
"## Model Details",
"### Model Description\n\n\n\n\n\n- Developed by: \n- Funded by [optional]: \n- Shared by [optional]: \n- Model type: \n- Language(s) (NLP): \n- License: \n- Finetuned from model [optional]:",
"### Model Sources [optional]\n\n\n\n- Repository: \n- Paper [optional]: \n- Demo [optional]:",
"## Uses",
"### Direct Use",
"### Downstream Use [optional]",
"### Out-of-Scope Use",
"## Bias, Risks, and Limitations",
"### Recommendations\n\n\n\nUsers (both direct and downstream) should be made aware of the risks, biases and limitations of the model. More information needed for further recommendations.",
"## How to Get Started with the Model\n\nUse the code below to get started with the model.",
"## Training Details",
"### Training Data",
"### Training Procedure",
"#### Preprocessing [optional]",
"#### Training Hyperparameters\n\n- Training regime:",
"#### Speeds, Sizes, Times [optional]",
"## Evaluation",
"### Testing Data, Factors & Metrics",
"#### Testing Data",
"#### Factors",
"#### Metrics",
"### Results",
"#### Summary",
"## Model Examination [optional]",
"## Environmental Impact\n\n\n\nCarbon emissions can be estimated using the Machine Learning Impact calculator presented in Lacoste et al. (2019).\n\n- Hardware Type: \n- Hours used: \n- Cloud Provider: \n- Compute Region: \n- Carbon Emitted:",
"## Technical Specifications [optional]",
"### Model Architecture and Objective",
"### Compute Infrastructure",
"#### Hardware",
"#### Software\n\n\n\n[optional]\n\n\n\nBibTeX:\n\n\n\nAPA:",
"## Glossary [optional]",
"## More Information [optional]",
"## Model Card Authors [optional]",
"## Model Card Contact",
"### Framework versions\n\n- PEFT 0.10.0"
] |
null | transformers | ## About
<!-- ### quantize_version: 1 -->
<!-- ### output_tensor_quantised: 1 -->
<!-- ### convert_type: -->
<!-- ### vocab_type: -->
static quants of https://huggingface.co/Mihaiii/Pallas-0.5-frankenmerge
<!-- provided-files -->
weighted/imatrix quants are available at https://huggingface.co/mradermacher/Pallas-0.5-frankenmerge-i1-GGUF
## Usage
If you are unsure how to use GGUF files, refer to one of [TheBloke's
READMEs](https://huggingface.co/TheBloke/KafkaLM-70B-German-V0.1-GGUF) for
more details, including on how to concatenate multi-part files.
## Provided Quants
(sorted by size, not necessarily quality. IQ-quants are often preferable over similar sized non-IQ quants)
| Link | Type | Size/GB | Notes |
|:-----|:-----|--------:|:------|
| [GGUF](https://huggingface.co/mradermacher/Pallas-0.5-frankenmerge-GGUF/resolve/main/Pallas-0.5-frankenmerge.Q2_K.gguf) | Q2_K | 13.5 | |
| [GGUF](https://huggingface.co/mradermacher/Pallas-0.5-frankenmerge-GGUF/resolve/main/Pallas-0.5-frankenmerge.IQ3_XS.gguf) | IQ3_XS | 15.0 | |
| [GGUF](https://huggingface.co/mradermacher/Pallas-0.5-frankenmerge-GGUF/resolve/main/Pallas-0.5-frankenmerge.Q3_K_S.gguf) | Q3_K_S | 15.8 | |
| [GGUF](https://huggingface.co/mradermacher/Pallas-0.5-frankenmerge-GGUF/resolve/main/Pallas-0.5-frankenmerge.IQ3_S.gguf) | IQ3_S | 15.8 | beats Q3_K* |
| [GGUF](https://huggingface.co/mradermacher/Pallas-0.5-frankenmerge-GGUF/resolve/main/Pallas-0.5-frankenmerge.IQ3_M.gguf) | IQ3_M | 16.4 | |
| [GGUF](https://huggingface.co/mradermacher/Pallas-0.5-frankenmerge-GGUF/resolve/main/Pallas-0.5-frankenmerge.Q3_K_M.gguf) | Q3_K_M | 17.6 | lower quality |
| [GGUF](https://huggingface.co/mradermacher/Pallas-0.5-frankenmerge-GGUF/resolve/main/Pallas-0.5-frankenmerge.Q3_K_L.gguf) | Q3_K_L | 19.1 | |
| [GGUF](https://huggingface.co/mradermacher/Pallas-0.5-frankenmerge-GGUF/resolve/main/Pallas-0.5-frankenmerge.IQ4_XS.gguf) | IQ4_XS | 19.6 | |
| [GGUF](https://huggingface.co/mradermacher/Pallas-0.5-frankenmerge-GGUF/resolve/main/Pallas-0.5-frankenmerge.Q4_K_S.gguf) | Q4_K_S | 20.6 | fast, recommended |
| [GGUF](https://huggingface.co/mradermacher/Pallas-0.5-frankenmerge-GGUF/resolve/main/Pallas-0.5-frankenmerge.Q4_K_M.gguf) | Q4_K_M | 21.7 | fast, recommended |
| [GGUF](https://huggingface.co/mradermacher/Pallas-0.5-frankenmerge-GGUF/resolve/main/Pallas-0.5-frankenmerge.Q5_K_S.gguf) | Q5_K_S | 25.0 | |
| [GGUF](https://huggingface.co/mradermacher/Pallas-0.5-frankenmerge-GGUF/resolve/main/Pallas-0.5-frankenmerge.Q5_K_M.gguf) | Q5_K_M | 25.6 | |
| [GGUF](https://huggingface.co/mradermacher/Pallas-0.5-frankenmerge-GGUF/resolve/main/Pallas-0.5-frankenmerge.Q6_K.gguf) | Q6_K | 29.7 | very good quality |
| [GGUF](https://huggingface.co/mradermacher/Pallas-0.5-frankenmerge-GGUF/resolve/main/Pallas-0.5-frankenmerge.Q8_0.gguf) | Q8_0 | 38.4 | fast, best quality |
Here is a handy graph by ikawrakow comparing some lower-quality quant
types (lower is better):

And here are Artefact2's thoughts on the matter:
https://gist.github.com/Artefact2/b5f810600771265fc1e39442288e8ec9
## FAQ / Model Request
See https://huggingface.co/mradermacher/model_requests for some answers to
questions you might have and/or if you want some other model quantized.
## Thanks
I thank my company, [nethype GmbH](https://www.nethype.de/), for letting
me use its servers and providing upgrades to my workstation to enable
this work in my free time.
<!-- end -->
| {"language": ["en"], "license": "other", "library_name": "transformers", "base_model": "Mihaiii/Pallas-0.5-frankenmerge", "license_link": "https://huggingface.co/01-ai/Yi-34B/blob/main/LICENSE", "license_name": "yi-license", "quantized_by": "mradermacher"} | mradermacher/Pallas-0.5-frankenmerge-GGUF | null | [
"transformers",
"gguf",
"en",
"base_model:Mihaiii/Pallas-0.5-frankenmerge",
"license:other",
"endpoints_compatible",
"region:us"
] | null | 2024-04-22T09:35:01+00:00 | [] | [
"en"
] | TAGS
#transformers #gguf #en #base_model-Mihaiii/Pallas-0.5-frankenmerge #license-other #endpoints_compatible #region-us
| About
-----
static quants of URL
weighted/imatrix quants are available at URL
Usage
-----
If you are unsure how to use GGUF files, refer to one of TheBloke's
READMEs for
more details, including on how to concatenate multi-part files.
Provided Quants
---------------
(sorted by size, not necessarily quality. IQ-quants are often preferable over similar sized non-IQ quants)
Here is a handy graph by ikawrakow comparing some lower-quality quant
types (lower is better):
!URL
And here are Artefact2's thoughts on the matter:
URL
FAQ / Model Request
-------------------
See URL for some answers to
questions you might have and/or if you want some other model quantized.
Thanks
------
I thank my company, nethype GmbH, for letting
me use its servers and providing upgrades to my workstation to enable
this work in my free time.
| [] | [
"TAGS\n#transformers #gguf #en #base_model-Mihaiii/Pallas-0.5-frankenmerge #license-other #endpoints_compatible #region-us \n"
] |
text-generation | transformers |
# Model Trained Using AutoTrain
This model was trained using AutoTrain. For more information, please visit [AutoTrain](https://hf.co/docs/autotrain).
# Usage
```python
from transformers import AutoModelForCausalLM, AutoTokenizer
model_path = "PATH_TO_THIS_REPO"
tokenizer = AutoTokenizer.from_pretrained(model_path)
model = AutoModelForCausalLM.from_pretrained(
model_path,
device_map="auto",
torch_dtype='auto'
).eval()
# Prompt content: "hi"
messages = [
{"role": "user", "content": "hi"}
]
input_ids = tokenizer.apply_chat_template(conversation=messages, tokenize=True, add_generation_prompt=True, return_tensors='pt')
output_ids = model.generate(input_ids.to('cuda'))
response = tokenizer.decode(output_ids[0][input_ids.shape[1]:], skip_special_tokens=True)
# Model response: "Hello! How can I assist you today?"
print(response)
``` | {"license": "other", "library_name": "transformers", "tags": ["autotrain", "text-generation-inference", "text-generation"], "datasets": ["argilla/distilabel-capybara-dpo-7k-binarized"], "widget": [{"messages": [{"role": "user", "content": "What is your favorite condiment?"}]}]} | abhishek/autotrain-llama3-orpo-v2 | null | [
"transformers",
"tensorboard",
"safetensors",
"llama",
"text-generation",
"autotrain",
"text-generation-inference",
"conversational",
"dataset:argilla/distilabel-capybara-dpo-7k-binarized",
"license:other",
"autotrain_compatible",
"endpoints_compatible",
"region:us"
] | null | 2024-04-22T09:35:07+00:00 | [] | [] | TAGS
#transformers #tensorboard #safetensors #llama #text-generation #autotrain #text-generation-inference #conversational #dataset-argilla/distilabel-capybara-dpo-7k-binarized #license-other #autotrain_compatible #endpoints_compatible #region-us
|
# Model Trained Using AutoTrain
This model was trained using AutoTrain. For more information, please visit AutoTrain.
# Usage
| [
"# Model Trained Using AutoTrain\n\nThis model was trained using AutoTrain. For more information, please visit AutoTrain.",
"# Usage"
] | [
"TAGS\n#transformers #tensorboard #safetensors #llama #text-generation #autotrain #text-generation-inference #conversational #dataset-argilla/distilabel-capybara-dpo-7k-binarized #license-other #autotrain_compatible #endpoints_compatible #region-us \n",
"# Model Trained Using AutoTrain\n\nThis model was trained using AutoTrain. For more information, please visit AutoTrain.",
"# Usage"
] |
text-classification | transformers |
<!-- This model card has been generated automatically according to the information the Trainer had access to. You
should probably proofread and complete it, then remove this comment. -->
# stocks
This model is a fine-tuned version of [projecte-aina/roberta-base-ca-v2-cawikitc](https://huggingface.co/projecte-aina/roberta-base-ca-v2-cawikitc) on the None dataset.
It achieves the following results on the evaluation set:
- Loss: 0.6639
- Accuracy: 0.7637
- Precision: 0.5304
- Recall: 0.4710
- F1: 0.4778
- Ratio: 0.7903
## Model description
More information needed
## Intended uses & limitations
More information needed
## Training and evaluation data
More information needed
## Training procedure
### Training hyperparameters
The following hyperparameters were used during training:
- learning_rate: 2e-05
- train_batch_size: 10
- eval_batch_size: 2
- seed: 42
- gradient_accumulation_steps: 2
- total_train_batch_size: 20
- optimizer: Adam with betas=(0.9,0.999) and epsilon=1e-08
- lr_scheduler_type: linear
- lr_scheduler_warmup_ratio: 0.06
- lr_scheduler_warmup_steps: 4
- num_epochs: 1
- label_smoothing_factor: 0.1
### Training results
| Training Loss | Epoch | Step | Validation Loss | Accuracy | Precision | Recall | F1 | Ratio |
|:-------------:|:-----:|:----:|:---------------:|:--------:|:---------:|:------:|:------:|:------:|
| 0.8468 | 0.07 | 10 | 0.8350 | 0.6185 | 0.3093 | 0.5 | 0.3822 | 1.0 |
| 0.7922 | 0.14 | 20 | 0.8314 | 0.6185 | 0.3093 | 0.5 | 0.3822 | 1.0 |
| 0.8005 | 0.21 | 30 | 0.8059 | 0.6169 | 0.2060 | 0.3325 | 0.2544 | 0.9984 |
| 0.8038 | 0.28 | 40 | 0.7907 | 0.6185 | 0.3093 | 0.5 | 0.3822 | 1.0 |
| 0.7846 | 0.34 | 50 | 0.8060 | 0.6185 | 0.3093 | 0.5 | 0.3822 | 1.0 |
| 0.7539 | 0.41 | 60 | 0.7573 | 0.6274 | 0.5024 | 0.3422 | 0.2763 | 0.9847 |
| 0.725 | 0.48 | 70 | 0.8018 | 0.7435 | 0.4978 | 0.4906 | 0.4940 | 0.5847 |
| 0.6842 | 0.55 | 80 | 0.8437 | 0.7419 | 0.5035 | 0.4795 | 0.4901 | 0.6444 |
| 0.7415 | 0.62 | 90 | 0.7783 | 0.7468 | 0.5006 | 0.4832 | 0.4909 | 0.6444 |
| 0.6303 | 0.69 | 100 | 0.7194 | 0.7452 | 0.5009 | 0.4723 | 0.4808 | 0.7040 |
| 0.6844 | 0.76 | 110 | 0.7137 | 0.7702 | 0.5106 | 0.4996 | 0.5044 | 0.6468 |
| 0.699 | 0.83 | 120 | 0.6666 | 0.7806 | 0.5159 | 0.5039 | 0.5084 | 0.6653 |
| 0.7229 | 0.9 | 130 | 0.6636 | 0.7629 | 0.5233 | 0.4730 | 0.4799 | 0.775 |
| 0.6555 | 0.97 | 140 | 0.6646 | 0.7637 | 0.5312 | 0.4707 | 0.4775 | 0.7919 |
### Framework versions
- Transformers 4.38.2
- Pytorch 2.1.0+cu121
- Datasets 2.19.0
- Tokenizers 0.15.2
| {"license": "apache-2.0", "tags": ["generated_from_trainer"], "metrics": ["accuracy", "precision", "recall", "f1"], "base_model": "projecte-aina/roberta-base-ca-v2-cawikitc", "model-index": [{"name": "stocks", "results": []}]} | adriansanz/ModeloFT1-v1 | null | [
"transformers",
"tensorboard",
"safetensors",
"roberta",
"text-classification",
"generated_from_trainer",
"base_model:projecte-aina/roberta-base-ca-v2-cawikitc",
"license:apache-2.0",
"autotrain_compatible",
"endpoints_compatible",
"region:us"
] | null | 2024-04-22T09:38:13+00:00 | [] | [] | TAGS
#transformers #tensorboard #safetensors #roberta #text-classification #generated_from_trainer #base_model-projecte-aina/roberta-base-ca-v2-cawikitc #license-apache-2.0 #autotrain_compatible #endpoints_compatible #region-us
| stocks
======
This model is a fine-tuned version of projecte-aina/roberta-base-ca-v2-cawikitc on the None dataset.
It achieves the following results on the evaluation set:
* Loss: 0.6639
* Accuracy: 0.7637
* Precision: 0.5304
* Recall: 0.4710
* F1: 0.4778
* Ratio: 0.7903
Model description
-----------------
More information needed
Intended uses & limitations
---------------------------
More information needed
Training and evaluation data
----------------------------
More information needed
Training procedure
------------------
### Training hyperparameters
The following hyperparameters were used during training:
* learning\_rate: 2e-05
* train\_batch\_size: 10
* eval\_batch\_size: 2
* seed: 42
* gradient\_accumulation\_steps: 2
* total\_train\_batch\_size: 20
* optimizer: Adam with betas=(0.9,0.999) and epsilon=1e-08
* lr\_scheduler\_type: linear
* lr\_scheduler\_warmup\_ratio: 0.06
* lr\_scheduler\_warmup\_steps: 4
* num\_epochs: 1
* label\_smoothing\_factor: 0.1
### Training results
### Framework versions
* Transformers 4.38.2
* Pytorch 2.1.0+cu121
* Datasets 2.19.0
* Tokenizers 0.15.2
| [
"### Training hyperparameters\n\n\nThe following hyperparameters were used during training:\n\n\n* learning\\_rate: 2e-05\n* train\\_batch\\_size: 10\n* eval\\_batch\\_size: 2\n* seed: 42\n* gradient\\_accumulation\\_steps: 2\n* total\\_train\\_batch\\_size: 20\n* optimizer: Adam with betas=(0.9,0.999) and epsilon=1e-08\n* lr\\_scheduler\\_type: linear\n* lr\\_scheduler\\_warmup\\_ratio: 0.06\n* lr\\_scheduler\\_warmup\\_steps: 4\n* num\\_epochs: 1\n* label\\_smoothing\\_factor: 0.1",
"### Training results",
"### Framework versions\n\n\n* Transformers 4.38.2\n* Pytorch 2.1.0+cu121\n* Datasets 2.19.0\n* Tokenizers 0.15.2"
] | [
"TAGS\n#transformers #tensorboard #safetensors #roberta #text-classification #generated_from_trainer #base_model-projecte-aina/roberta-base-ca-v2-cawikitc #license-apache-2.0 #autotrain_compatible #endpoints_compatible #region-us \n",
"### Training hyperparameters\n\n\nThe following hyperparameters were used during training:\n\n\n* learning\\_rate: 2e-05\n* train\\_batch\\_size: 10\n* eval\\_batch\\_size: 2\n* seed: 42\n* gradient\\_accumulation\\_steps: 2\n* total\\_train\\_batch\\_size: 20\n* optimizer: Adam with betas=(0.9,0.999) and epsilon=1e-08\n* lr\\_scheduler\\_type: linear\n* lr\\_scheduler\\_warmup\\_ratio: 0.06\n* lr\\_scheduler\\_warmup\\_steps: 4\n* num\\_epochs: 1\n* label\\_smoothing\\_factor: 0.1",
"### Training results",
"### Framework versions\n\n\n* Transformers 4.38.2\n* Pytorch 2.1.0+cu121\n* Datasets 2.19.0\n* Tokenizers 0.15.2"
] |
text-to-image | diffusers |
# Model Card for Model ID
<!-- Provide a quick summary of what the model is/does. -->
## Model Details
### Model Description
<!-- Provide a longer summary of what this model is. -->
This is the model card of a 🧨 diffusers model that has been pushed on the Hub. This model card has been automatically generated.
- **Developed by:** [More Information Needed]
- **Funded by [optional]:** [More Information Needed]
- **Shared by [optional]:** [More Information Needed]
- **Model type:** [More Information Needed]
- **Language(s) (NLP):** [More Information Needed]
- **License:** [More Information Needed]
- **Finetuned from model [optional]:** [More Information Needed]
### Model Sources [optional]
<!-- Provide the basic links for the model. -->
- **Repository:** [More Information Needed]
- **Paper [optional]:** [More Information Needed]
- **Demo [optional]:** [More Information Needed]
## Uses
<!-- Address questions around how the model is intended to be used, including the foreseeable users of the model and those affected by the model. -->
### Direct Use
<!-- This section is for the model use without fine-tuning or plugging into a larger ecosystem/app. -->
[More Information Needed]
### Downstream Use [optional]
<!-- This section is for the model use when fine-tuned for a task, or when plugged into a larger ecosystem/app -->
[More Information Needed]
### Out-of-Scope Use
<!-- This section addresses misuse, malicious use, and uses that the model will not work well for. -->
[More Information Needed]
## Bias, Risks, and Limitations
<!-- This section is meant to convey both technical and sociotechnical limitations. -->
[More Information Needed]
### Recommendations
<!-- This section is meant to convey recommendations with respect to the bias, risk, and technical limitations. -->
Users (both direct and downstream) should be made aware of the risks, biases and limitations of the model. More information needed for further recommendations.
## How to Get Started with the Model
Use the code below to get started with the model.
[More Information Needed]
## Training Details
### Training Data
<!-- This should link to a Dataset Card, perhaps with a short stub of information on what the training data is all about as well as documentation related to data pre-processing or additional filtering. -->
[More Information Needed]
### Training Procedure
<!-- This relates heavily to the Technical Specifications. Content here should link to that section when it is relevant to the training procedure. -->
#### Preprocessing [optional]
[More Information Needed]
#### Training Hyperparameters
- **Training regime:** [More Information Needed] <!--fp32, fp16 mixed precision, bf16 mixed precision, bf16 non-mixed precision, fp16 non-mixed precision, fp8 mixed precision -->
#### Speeds, Sizes, Times [optional]
<!-- This section provides information about throughput, start/end time, checkpoint size if relevant, etc. -->
[More Information Needed]
## Evaluation
<!-- This section describes the evaluation protocols and provides the results. -->
### Testing Data, Factors & Metrics
#### Testing Data
<!-- This should link to a Dataset Card if possible. -->
[More Information Needed]
#### Factors
<!-- These are the things the evaluation is disaggregating by, e.g., subpopulations or domains. -->
[More Information Needed]
#### Metrics
<!-- These are the evaluation metrics being used, ideally with a description of why. -->
[More Information Needed]
### Results
[More Information Needed]
#### Summary
## Model Examination [optional]
<!-- Relevant interpretability work for the model goes here -->
[More Information Needed]
## Environmental Impact
<!-- Total emissions (in grams of CO2eq) and additional considerations, such as electricity usage, go here. Edit the suggested text below accordingly -->
Carbon emissions can be estimated using the [Machine Learning Impact calculator](https://mlco2.github.io/impact#compute) presented in [Lacoste et al. (2019)](https://arxiv.org/abs/1910.09700).
- **Hardware Type:** [More Information Needed]
- **Hours used:** [More Information Needed]
- **Cloud Provider:** [More Information Needed]
- **Compute Region:** [More Information Needed]
- **Carbon Emitted:** [More Information Needed]
## Technical Specifications [optional]
### Model Architecture and Objective
[More Information Needed]
### Compute Infrastructure
[More Information Needed]
#### Hardware
[More Information Needed]
#### Software
[More Information Needed]
## Citation [optional]
<!-- If there is a paper or blog post introducing the model, the APA and Bibtex information for that should go in this section. -->
**BibTeX:**
[More Information Needed]
**APA:**
[More Information Needed]
## Glossary [optional]
<!-- If relevant, include terms and calculations in this section that can help readers understand the model or model card. -->
[More Information Needed]
## More Information [optional]
[More Information Needed]
## Model Card Authors [optional]
[More Information Needed]
## Model Card Contact
[More Information Needed] | {"library_name": "diffusers"} | Niggendar/vividpdxl_v11VAE | null | [
"diffusers",
"safetensors",
"arxiv:1910.09700",
"endpoints_compatible",
"diffusers:StableDiffusionXLPipeline",
"region:us"
] | null | 2024-04-22T09:42:01+00:00 | [
"1910.09700"
] | [] | TAGS
#diffusers #safetensors #arxiv-1910.09700 #endpoints_compatible #diffusers-StableDiffusionXLPipeline #region-us
|
# Model Card for Model ID
## Model Details
### Model Description
This is the model card of a diffusers model that has been pushed on the Hub. This model card has been automatically generated.
- Developed by:
- Funded by [optional]:
- Shared by [optional]:
- Model type:
- Language(s) (NLP):
- License:
- Finetuned from model [optional]:
### Model Sources [optional]
- Repository:
- Paper [optional]:
- Demo [optional]:
## Uses
### Direct Use
### Downstream Use [optional]
### Out-of-Scope Use
## Bias, Risks, and Limitations
### Recommendations
Users (both direct and downstream) should be made aware of the risks, biases and limitations of the model. More information needed for further recommendations.
## How to Get Started with the Model
Use the code below to get started with the model.
## Training Details
### Training Data
### Training Procedure
#### Preprocessing [optional]
#### Training Hyperparameters
- Training regime:
#### Speeds, Sizes, Times [optional]
## Evaluation
### Testing Data, Factors & Metrics
#### Testing Data
#### Factors
#### Metrics
### Results
#### Summary
## Model Examination [optional]
## Environmental Impact
Carbon emissions can be estimated using the Machine Learning Impact calculator presented in Lacoste et al. (2019).
- Hardware Type:
- Hours used:
- Cloud Provider:
- Compute Region:
- Carbon Emitted:
## Technical Specifications [optional]
### Model Architecture and Objective
### Compute Infrastructure
#### Hardware
#### Software
[optional]
BibTeX:
APA:
## Glossary [optional]
## More Information [optional]
## Model Card Authors [optional]
## Model Card Contact
| [
"# Model Card for Model ID",
"## Model Details",
"### Model Description\n\n\n\nThis is the model card of a diffusers model that has been pushed on the Hub. This model card has been automatically generated.\n\n- Developed by: \n- Funded by [optional]: \n- Shared by [optional]: \n- Model type: \n- Language(s) (NLP): \n- License: \n- Finetuned from model [optional]:",
"### Model Sources [optional]\n\n\n\n- Repository: \n- Paper [optional]: \n- Demo [optional]:",
"## Uses",
"### Direct Use",
"### Downstream Use [optional]",
"### Out-of-Scope Use",
"## Bias, Risks, and Limitations",
"### Recommendations\n\n\n\nUsers (both direct and downstream) should be made aware of the risks, biases and limitations of the model. More information needed for further recommendations.",
"## How to Get Started with the Model\n\nUse the code below to get started with the model.",
"## Training Details",
"### Training Data",
"### Training Procedure",
"#### Preprocessing [optional]",
"#### Training Hyperparameters\n\n- Training regime:",
"#### Speeds, Sizes, Times [optional]",
"## Evaluation",
"### Testing Data, Factors & Metrics",
"#### Testing Data",
"#### Factors",
"#### Metrics",
"### Results",
"#### Summary",
"## Model Examination [optional]",
"## Environmental Impact\n\n\n\nCarbon emissions can be estimated using the Machine Learning Impact calculator presented in Lacoste et al. (2019).\n\n- Hardware Type: \n- Hours used: \n- Cloud Provider: \n- Compute Region: \n- Carbon Emitted:",
"## Technical Specifications [optional]",
"### Model Architecture and Objective",
"### Compute Infrastructure",
"#### Hardware",
"#### Software\n\n\n\n[optional]\n\n\n\nBibTeX:\n\n\n\nAPA:",
"## Glossary [optional]",
"## More Information [optional]",
"## Model Card Authors [optional]",
"## Model Card Contact"
] | [
"TAGS\n#diffusers #safetensors #arxiv-1910.09700 #endpoints_compatible #diffusers-StableDiffusionXLPipeline #region-us \n",
"# Model Card for Model ID",
"## Model Details",
"### Model Description\n\n\n\nThis is the model card of a diffusers model that has been pushed on the Hub. This model card has been automatically generated.\n\n- Developed by: \n- Funded by [optional]: \n- Shared by [optional]: \n- Model type: \n- Language(s) (NLP): \n- License: \n- Finetuned from model [optional]:",
"### Model Sources [optional]\n\n\n\n- Repository: \n- Paper [optional]: \n- Demo [optional]:",
"## Uses",
"### Direct Use",
"### Downstream Use [optional]",
"### Out-of-Scope Use",
"## Bias, Risks, and Limitations",
"### Recommendations\n\n\n\nUsers (both direct and downstream) should be made aware of the risks, biases and limitations of the model. More information needed for further recommendations.",
"## How to Get Started with the Model\n\nUse the code below to get started with the model.",
"## Training Details",
"### Training Data",
"### Training Procedure",
"#### Preprocessing [optional]",
"#### Training Hyperparameters\n\n- Training regime:",
"#### Speeds, Sizes, Times [optional]",
"## Evaluation",
"### Testing Data, Factors & Metrics",
"#### Testing Data",
"#### Factors",
"#### Metrics",
"### Results",
"#### Summary",
"## Model Examination [optional]",
"## Environmental Impact\n\n\n\nCarbon emissions can be estimated using the Machine Learning Impact calculator presented in Lacoste et al. (2019).\n\n- Hardware Type: \n- Hours used: \n- Cloud Provider: \n- Compute Region: \n- Carbon Emitted:",
"## Technical Specifications [optional]",
"### Model Architecture and Objective",
"### Compute Infrastructure",
"#### Hardware",
"#### Software\n\n\n\n[optional]\n\n\n\nBibTeX:\n\n\n\nAPA:",
"## Glossary [optional]",
"## More Information [optional]",
"## Model Card Authors [optional]",
"## Model Card Contact"
] |
zero-shot-classification | transformers |
<!-- This model card has been generated automatically according to the information the Trainer had access to. You
should probably proofread and complete it, then remove this comment. -->
# p1
This model is a fine-tuned version of [projecte-aina/roberta-base-ca-v2-cawikitc](https://huggingface.co/projecte-aina/roberta-base-ca-v2-cawikitc) on the None dataset.
It achieves the following results on the evaluation set:
- Loss: 0.8254
- Accuracy: 0.5
- Precision: 0.25
- Recall: 0.5
- F1: 0.3333
- Ratio: 1.0
## Model description
More information needed
## Intended uses & limitations
More information needed
## Training and evaluation data
More information needed
## Training procedure
### Training hyperparameters
The following hyperparameters were used during training:
- learning_rate: 2e-05
- train_batch_size: 16
- eval_batch_size: 2
- seed: 47
- gradient_accumulation_steps: 2
- total_train_batch_size: 32
- optimizer: Adam with betas=(0.9,0.999) and epsilon=1e-08
- lr_scheduler_type: linear
- lr_scheduler_warmup_ratio: 0.06
- lr_scheduler_warmup_steps: 4
- num_epochs: 2
- label_smoothing_factor: 0.1
### Training results
| Training Loss | Epoch | Step | Validation Loss | Accuracy | Precision | Recall | F1 | Ratio |
|:-------------:|:-----:|:----:|:---------------:|:--------:|:---------:|:------:|:------:|:------:|
| 0.8263 | 0.38 | 10 | 0.8199 | 0.5 | 0.5 | 0.5 | 0.3473 | 0.0163 |
| 0.8283 | 0.75 | 20 | 0.8389 | 0.5 | 0.25 | 0.5 | 0.3333 | 1.0 |
| 0.8167 | 1.13 | 30 | 0.8325 | 0.5 | 0.25 | 0.5 | 0.3333 | 1.0 |
| 0.8183 | 1.51 | 40 | 0.8228 | 0.4973 | 0.2493 | 0.4973 | 0.3321 | 0.9973 |
| 0.8178 | 1.89 | 50 | 0.8254 | 0.5 | 0.25 | 0.5 | 0.3333 | 1.0 |
### Framework versions
- Transformers 4.38.2
- Pytorch 2.1.0+cu121
- Datasets 2.19.0
- Tokenizers 0.15.2 | {"license": "apache-2.0", "tags": ["generated_from_trainer"], "metrics": ["accuracy", "precision", "recall", "f1"], "base_model": "projecte-aina/roberta-base-ca-v2-cawikitc", "pipeline_tag": "zero-shot-classification", "model-index": [{"name": "p1", "results": []}]} | adriansanz/p1 | null | [
"transformers",
"safetensors",
"roberta",
"text-classification",
"generated_from_trainer",
"zero-shot-classification",
"base_model:projecte-aina/roberta-base-ca-v2-cawikitc",
"license:apache-2.0",
"autotrain_compatible",
"endpoints_compatible",
"region:us"
] | null | 2024-04-22T09:42:09+00:00 | [] | [] | TAGS
#transformers #safetensors #roberta #text-classification #generated_from_trainer #zero-shot-classification #base_model-projecte-aina/roberta-base-ca-v2-cawikitc #license-apache-2.0 #autotrain_compatible #endpoints_compatible #region-us
| p1
==
This model is a fine-tuned version of projecte-aina/roberta-base-ca-v2-cawikitc on the None dataset.
It achieves the following results on the evaluation set:
* Loss: 0.8254
* Accuracy: 0.5
* Precision: 0.25
* Recall: 0.5
* F1: 0.3333
* Ratio: 1.0
Model description
-----------------
More information needed
Intended uses & limitations
---------------------------
More information needed
Training and evaluation data
----------------------------
More information needed
Training procedure
------------------
### Training hyperparameters
The following hyperparameters were used during training:
* learning\_rate: 2e-05
* train\_batch\_size: 16
* eval\_batch\_size: 2
* seed: 47
* gradient\_accumulation\_steps: 2
* total\_train\_batch\_size: 32
* optimizer: Adam with betas=(0.9,0.999) and epsilon=1e-08
* lr\_scheduler\_type: linear
* lr\_scheduler\_warmup\_ratio: 0.06
* lr\_scheduler\_warmup\_steps: 4
* num\_epochs: 2
* label\_smoothing\_factor: 0.1
### Training results
### Framework versions
* Transformers 4.38.2
* Pytorch 2.1.0+cu121
* Datasets 2.19.0
* Tokenizers 0.15.2
| [
"### Training hyperparameters\n\n\nThe following hyperparameters were used during training:\n\n\n* learning\\_rate: 2e-05\n* train\\_batch\\_size: 16\n* eval\\_batch\\_size: 2\n* seed: 47\n* gradient\\_accumulation\\_steps: 2\n* total\\_train\\_batch\\_size: 32\n* optimizer: Adam with betas=(0.9,0.999) and epsilon=1e-08\n* lr\\_scheduler\\_type: linear\n* lr\\_scheduler\\_warmup\\_ratio: 0.06\n* lr\\_scheduler\\_warmup\\_steps: 4\n* num\\_epochs: 2\n* label\\_smoothing\\_factor: 0.1",
"### Training results",
"### Framework versions\n\n\n* Transformers 4.38.2\n* Pytorch 2.1.0+cu121\n* Datasets 2.19.0\n* Tokenizers 0.15.2"
] | [
"TAGS\n#transformers #safetensors #roberta #text-classification #generated_from_trainer #zero-shot-classification #base_model-projecte-aina/roberta-base-ca-v2-cawikitc #license-apache-2.0 #autotrain_compatible #endpoints_compatible #region-us \n",
"### Training hyperparameters\n\n\nThe following hyperparameters were used during training:\n\n\n* learning\\_rate: 2e-05\n* train\\_batch\\_size: 16\n* eval\\_batch\\_size: 2\n* seed: 47\n* gradient\\_accumulation\\_steps: 2\n* total\\_train\\_batch\\_size: 32\n* optimizer: Adam with betas=(0.9,0.999) and epsilon=1e-08\n* lr\\_scheduler\\_type: linear\n* lr\\_scheduler\\_warmup\\_ratio: 0.06\n* lr\\_scheduler\\_warmup\\_steps: 4\n* num\\_epochs: 2\n* label\\_smoothing\\_factor: 0.1",
"### Training results",
"### Framework versions\n\n\n* Transformers 4.38.2\n* Pytorch 2.1.0+cu121\n* Datasets 2.19.0\n* Tokenizers 0.15.2"
] |
text-generation | null |
tokenizer only | {"language": ["ru", "code"], "license": "apache-2.0", "pipeline_tag": "text-generation"} | aeonium/Aeonium-v1-Base-7B | null | [
"text-generation",
"ru",
"code",
"license:apache-2.0",
"region:us"
] | null | 2024-04-22T09:44:52+00:00 | [] | [
"ru",
"code"
] | TAGS
#text-generation #ru #code #license-apache-2.0 #region-us
|
tokenizer only | [] | [
"TAGS\n#text-generation #ru #code #license-apache-2.0 #region-us \n"
] |
null | peft |
# Model Card for Model ID
<!-- Provide a quick summary of what the model is/does. -->
## Model Details
### Model Description
<!-- Provide a longer summary of what this model is. -->
- **Developed by:** [More Information Needed]
- **Shared by [optional]:** [More Information Needed]
- **Model type:** [More Information Needed]
- **Language(s) (NLP):** [More Information Needed]
- **License:** [More Information Needed]
- **Finetuned from model [optional]:** [More Information Needed]
### Model Sources [optional]
<!-- Provide the basic links for the model. -->
- **Repository:** [More Information Needed]
- **Paper [optional]:** [More Information Needed]
- **Demo [optional]:** [More Information Needed]
## Uses
<!-- Address questions around how the model is intended to be used, including the foreseeable users of the model and those affected by the model. -->
### Direct Use
<!-- This section is for the model use without fine-tuning or plugging into a larger ecosystem/app. -->
[More Information Needed]
### Downstream Use [optional]
<!-- This section is for the model use when fine-tuned for a task, or when plugged into a larger ecosystem/app -->
[More Information Needed]
### Out-of-Scope Use
<!-- This section addresses misuse, malicious use, and uses that the model will not work well for. -->
[More Information Needed]
## Bias, Risks, and Limitations
<!-- This section is meant to convey both technical and sociotechnical limitations. -->
[More Information Needed]
### Recommendations
<!-- This section is meant to convey recommendations with respect to the bias, risk, and technical limitations. -->
Users (both direct and downstream) should be made aware of the risks, biases and limitations of the model. More information needed for further recommendations.
## How to Get Started with the Model
Use the code below to get started with the model.
[More Information Needed]
## Training Details
### Training Data
<!-- This should link to a Data Card, perhaps with a short stub of information on what the training data is all about as well as documentation related to data pre-processing or additional filtering. -->
[More Information Needed]
### Training Procedure
<!-- This relates heavily to the Technical Specifications. Content here should link to that section when it is relevant to the training procedure. -->
#### Preprocessing [optional]
[More Information Needed]
#### Training Hyperparameters
- **Training regime:** [More Information Needed] <!--fp32, fp16 mixed precision, bf16 mixed precision, bf16 non-mixed precision, fp16 non-mixed precision, fp8 mixed precision -->
#### Speeds, Sizes, Times [optional]
<!-- This section provides information about throughput, start/end time, checkpoint size if relevant, etc. -->
[More Information Needed]
## Evaluation
<!-- This section describes the evaluation protocols and provides the results. -->
### Testing Data, Factors & Metrics
#### Testing Data
<!-- This should link to a Data Card if possible. -->
[More Information Needed]
#### Factors
<!-- These are the things the evaluation is disaggregating by, e.g., subpopulations or domains. -->
[More Information Needed]
#### Metrics
<!-- These are the evaluation metrics being used, ideally with a description of why. -->
[More Information Needed]
### Results
[More Information Needed]
#### Summary
## Model Examination [optional]
<!-- Relevant interpretability work for the model goes here -->
[More Information Needed]
## Environmental Impact
<!-- Total emissions (in grams of CO2eq) and additional considerations, such as electricity usage, go here. Edit the suggested text below accordingly -->
Carbon emissions can be estimated using the [Machine Learning Impact calculator](https://mlco2.github.io/impact#compute) presented in [Lacoste et al. (2019)](https://arxiv.org/abs/1910.09700).
- **Hardware Type:** [More Information Needed]
- **Hours used:** [More Information Needed]
- **Cloud Provider:** [More Information Needed]
- **Compute Region:** [More Information Needed]
- **Carbon Emitted:** [More Information Needed]
## Technical Specifications [optional]
### Model Architecture and Objective
[More Information Needed]
### Compute Infrastructure
[More Information Needed]
#### Hardware
[More Information Needed]
#### Software
[More Information Needed]
## Citation [optional]
<!-- If there is a paper or blog post introducing the model, the APA and Bibtex information for that should go in this section. -->
**BibTeX:**
[More Information Needed]
**APA:**
[More Information Needed]
## Glossary [optional]
<!-- If relevant, include terms and calculations in this section that can help readers understand the model or model card. -->
[More Information Needed]
## More Information [optional]
[More Information Needed]
## Model Card Authors [optional]
[More Information Needed]
## Model Card Contact
[More Information Needed]
## Training procedure
The following `bitsandbytes` quantization config was used during training:
- load_in_8bit: False
- load_in_4bit: True
- llm_int8_threshold: 6.0
- llm_int8_skip_modules: None
- llm_int8_enable_fp32_cpu_offload: False
- llm_int8_has_fp16_weight: False
- bnb_4bit_quant_type: nf4
- bnb_4bit_use_double_quant: True
- bnb_4bit_compute_dtype: bfloat16
### Framework versions
- PEFT 0.7.0.dev0
## Training procedure
The following `bitsandbytes` quantization config was used during training:
- load_in_8bit: False
- load_in_4bit: True
- llm_int8_threshold: 6.0
- llm_int8_skip_modules: None
- llm_int8_enable_fp32_cpu_offload: False
- llm_int8_has_fp16_weight: False
- bnb_4bit_quant_type: nf4
- bnb_4bit_use_double_quant: True
- bnb_4bit_compute_dtype: bfloat16
### Framework versions
- PEFT 0.7.0.dev0
| {"library_name": "peft", "base_model": "TinyLlama/TinyLlama-1.1B-Chat-v1.0"} | bmehrba/TinyLlama-1.1B-Chat-v1.0-fine-tuned-adapters_ChatGPT_t1_tiny_Seed101 | null | [
"peft",
"arxiv:1910.09700",
"base_model:TinyLlama/TinyLlama-1.1B-Chat-v1.0",
"region:us"
] | null | 2024-04-22T09:46:05+00:00 | [
"1910.09700"
] | [] | TAGS
#peft #arxiv-1910.09700 #base_model-TinyLlama/TinyLlama-1.1B-Chat-v1.0 #region-us
|
# Model Card for Model ID
## Model Details
### Model Description
- Developed by:
- Shared by [optional]:
- Model type:
- Language(s) (NLP):
- License:
- Finetuned from model [optional]:
### Model Sources [optional]
- Repository:
- Paper [optional]:
- Demo [optional]:
## Uses
### Direct Use
### Downstream Use [optional]
### Out-of-Scope Use
## Bias, Risks, and Limitations
### Recommendations
Users (both direct and downstream) should be made aware of the risks, biases and limitations of the model. More information needed for further recommendations.
## How to Get Started with the Model
Use the code below to get started with the model.
## Training Details
### Training Data
### Training Procedure
#### Preprocessing [optional]
#### Training Hyperparameters
- Training regime:
#### Speeds, Sizes, Times [optional]
## Evaluation
### Testing Data, Factors & Metrics
#### Testing Data
#### Factors
#### Metrics
### Results
#### Summary
## Model Examination [optional]
## Environmental Impact
Carbon emissions can be estimated using the Machine Learning Impact calculator presented in Lacoste et al. (2019).
- Hardware Type:
- Hours used:
- Cloud Provider:
- Compute Region:
- Carbon Emitted:
## Technical Specifications [optional]
### Model Architecture and Objective
### Compute Infrastructure
#### Hardware
#### Software
[optional]
BibTeX:
APA:
## Glossary [optional]
## More Information [optional]
## Model Card Authors [optional]
## Model Card Contact
## Training procedure
The following 'bitsandbytes' quantization config was used during training:
- load_in_8bit: False
- load_in_4bit: True
- llm_int8_threshold: 6.0
- llm_int8_skip_modules: None
- llm_int8_enable_fp32_cpu_offload: False
- llm_int8_has_fp16_weight: False
- bnb_4bit_quant_type: nf4
- bnb_4bit_use_double_quant: True
- bnb_4bit_compute_dtype: bfloat16
### Framework versions
- PEFT 0.7.0.dev0
## Training procedure
The following 'bitsandbytes' quantization config was used during training:
- load_in_8bit: False
- load_in_4bit: True
- llm_int8_threshold: 6.0
- llm_int8_skip_modules: None
- llm_int8_enable_fp32_cpu_offload: False
- llm_int8_has_fp16_weight: False
- bnb_4bit_quant_type: nf4
- bnb_4bit_use_double_quant: True
- bnb_4bit_compute_dtype: bfloat16
### Framework versions
- PEFT 0.7.0.dev0
| [
"# Model Card for Model ID",
"## Model Details",
"### Model Description\n\n\n\n\n\n- Developed by: \n- Shared by [optional]: \n- Model type: \n- Language(s) (NLP): \n- License: \n- Finetuned from model [optional]:",
"### Model Sources [optional]\n\n\n\n- Repository: \n- Paper [optional]: \n- Demo [optional]:",
"## Uses",
"### Direct Use",
"### Downstream Use [optional]",
"### Out-of-Scope Use",
"## Bias, Risks, and Limitations",
"### Recommendations\n\n\n\nUsers (both direct and downstream) should be made aware of the risks, biases and limitations of the model. More information needed for further recommendations.",
"## How to Get Started with the Model\n\nUse the code below to get started with the model.",
"## Training Details",
"### Training Data",
"### Training Procedure",
"#### Preprocessing [optional]",
"#### Training Hyperparameters\n\n- Training regime:",
"#### Speeds, Sizes, Times [optional]",
"## Evaluation",
"### Testing Data, Factors & Metrics",
"#### Testing Data",
"#### Factors",
"#### Metrics",
"### Results",
"#### Summary",
"## Model Examination [optional]",
"## Environmental Impact\n\n\n\nCarbon emissions can be estimated using the Machine Learning Impact calculator presented in Lacoste et al. (2019).\n\n- Hardware Type: \n- Hours used: \n- Cloud Provider: \n- Compute Region: \n- Carbon Emitted:",
"## Technical Specifications [optional]",
"### Model Architecture and Objective",
"### Compute Infrastructure",
"#### Hardware",
"#### Software\n\n\n\n[optional]\n\n\n\nBibTeX:\n\n\n\nAPA:",
"## Glossary [optional]",
"## More Information [optional]",
"## Model Card Authors [optional]",
"## Model Card Contact",
"## Training procedure\n\n\nThe following 'bitsandbytes' quantization config was used during training:\n- load_in_8bit: False\n- load_in_4bit: True\n- llm_int8_threshold: 6.0\n- llm_int8_skip_modules: None\n- llm_int8_enable_fp32_cpu_offload: False\n- llm_int8_has_fp16_weight: False\n- bnb_4bit_quant_type: nf4\n- bnb_4bit_use_double_quant: True\n- bnb_4bit_compute_dtype: bfloat16",
"### Framework versions\n\n\n- PEFT 0.7.0.dev0",
"## Training procedure\n\n\nThe following 'bitsandbytes' quantization config was used during training:\n- load_in_8bit: False\n- load_in_4bit: True\n- llm_int8_threshold: 6.0\n- llm_int8_skip_modules: None\n- llm_int8_enable_fp32_cpu_offload: False\n- llm_int8_has_fp16_weight: False\n- bnb_4bit_quant_type: nf4\n- bnb_4bit_use_double_quant: True\n- bnb_4bit_compute_dtype: bfloat16",
"### Framework versions\n\n\n- PEFT 0.7.0.dev0"
] | [
"TAGS\n#peft #arxiv-1910.09700 #base_model-TinyLlama/TinyLlama-1.1B-Chat-v1.0 #region-us \n",
"# Model Card for Model ID",
"## Model Details",
"### Model Description\n\n\n\n\n\n- Developed by: \n- Shared by [optional]: \n- Model type: \n- Language(s) (NLP): \n- License: \n- Finetuned from model [optional]:",
"### Model Sources [optional]\n\n\n\n- Repository: \n- Paper [optional]: \n- Demo [optional]:",
"## Uses",
"### Direct Use",
"### Downstream Use [optional]",
"### Out-of-Scope Use",
"## Bias, Risks, and Limitations",
"### Recommendations\n\n\n\nUsers (both direct and downstream) should be made aware of the risks, biases and limitations of the model. More information needed for further recommendations.",
"## How to Get Started with the Model\n\nUse the code below to get started with the model.",
"## Training Details",
"### Training Data",
"### Training Procedure",
"#### Preprocessing [optional]",
"#### Training Hyperparameters\n\n- Training regime:",
"#### Speeds, Sizes, Times [optional]",
"## Evaluation",
"### Testing Data, Factors & Metrics",
"#### Testing Data",
"#### Factors",
"#### Metrics",
"### Results",
"#### Summary",
"## Model Examination [optional]",
"## Environmental Impact\n\n\n\nCarbon emissions can be estimated using the Machine Learning Impact calculator presented in Lacoste et al. (2019).\n\n- Hardware Type: \n- Hours used: \n- Cloud Provider: \n- Compute Region: \n- Carbon Emitted:",
"## Technical Specifications [optional]",
"### Model Architecture and Objective",
"### Compute Infrastructure",
"#### Hardware",
"#### Software\n\n\n\n[optional]\n\n\n\nBibTeX:\n\n\n\nAPA:",
"## Glossary [optional]",
"## More Information [optional]",
"## Model Card Authors [optional]",
"## Model Card Contact",
"## Training procedure\n\n\nThe following 'bitsandbytes' quantization config was used during training:\n- load_in_8bit: False\n- load_in_4bit: True\n- llm_int8_threshold: 6.0\n- llm_int8_skip_modules: None\n- llm_int8_enable_fp32_cpu_offload: False\n- llm_int8_has_fp16_weight: False\n- bnb_4bit_quant_type: nf4\n- bnb_4bit_use_double_quant: True\n- bnb_4bit_compute_dtype: bfloat16",
"### Framework versions\n\n\n- PEFT 0.7.0.dev0",
"## Training procedure\n\n\nThe following 'bitsandbytes' quantization config was used during training:\n- load_in_8bit: False\n- load_in_4bit: True\n- llm_int8_threshold: 6.0\n- llm_int8_skip_modules: None\n- llm_int8_enable_fp32_cpu_offload: False\n- llm_int8_has_fp16_weight: False\n- bnb_4bit_quant_type: nf4\n- bnb_4bit_use_double_quant: True\n- bnb_4bit_compute_dtype: bfloat16",
"### Framework versions\n\n\n- PEFT 0.7.0.dev0"
] |
text-generation | transformers |
# Llama3merge8-15B-MoE
Llama3merge8-15B-MoE is a Mixture of Experts (MoE) made with the following models using [LazyMergekit](https://colab.research.google.com/drive/1obulZ1ROXHjYLn6PPZJwRR6GzgQogxxb?usp=sharing):
* [sethuiyer/Medichat-Llama3-8B](https://huggingface.co/sethuiyer/Medichat-Llama3-8B)
* [psyche/llama3-8b-instruct-mrc-v0.3](https://huggingface.co/psyche/llama3-8b-instruct-mrc-v0.3)
## 🧩 Configuration
```yaml
base_model: sethuiyer/Medichat-Llama3-8B
experts:
- source_model: sethuiyer/Medichat-Llama3-8B
positive_prompts: ["medical"]
- source_model: psyche/llama3-8b-instruct-mrc-v0.3
positive_prompts: ["what"]
```
## 💻 Usage
```python
!pip install -qU transformers bitsandbytes accelerate
from transformers import AutoTokenizer
import transformers
import torch
model = "allknowingroger/Llama3merge8-15B-MoE"
tokenizer = AutoTokenizer.from_pretrained(model)
pipeline = transformers.pipeline(
"text-generation",
model=model,
model_kwargs={"torch_dtype": torch.float16, "load_in_4bit": True},
)
messages = [{"role": "user", "content": "Explain what a Mixture of Experts is in less than 100 words."}]
prompt = pipeline.tokenizer.apply_chat_template(messages, tokenize=False, add_generation_prompt=True)
outputs = pipeline(prompt, max_new_tokens=256, do_sample=True, temperature=0.7, top_k=50, top_p=0.95)
print(outputs[0]["generated_text"])
``` | {"license": "apache-2.0", "tags": ["moe", "frankenmoe", "merge", "mergekit", "lazymergekit", "sethuiyer/Medichat-Llama3-8B", "psyche/llama3-8b-instruct-mrc-v0.3"], "base_model": ["sethuiyer/Medichat-Llama3-8B", "psyche/llama3-8b-instruct-mrc-v0.3"]} | allknowingroger/Llama3merge8-15B-MoE | null | [
"transformers",
"safetensors",
"mixtral",
"text-generation",
"moe",
"frankenmoe",
"merge",
"mergekit",
"lazymergekit",
"sethuiyer/Medichat-Llama3-8B",
"psyche/llama3-8b-instruct-mrc-v0.3",
"conversational",
"base_model:sethuiyer/Medichat-Llama3-8B",
"base_model:psyche/llama3-8b-instruct-mrc-v0.3",
"license:apache-2.0",
"autotrain_compatible",
"endpoints_compatible",
"text-generation-inference",
"region:us"
] | null | 2024-04-22T09:46:07+00:00 | [] | [] | TAGS
#transformers #safetensors #mixtral #text-generation #moe #frankenmoe #merge #mergekit #lazymergekit #sethuiyer/Medichat-Llama3-8B #psyche/llama3-8b-instruct-mrc-v0.3 #conversational #base_model-sethuiyer/Medichat-Llama3-8B #base_model-psyche/llama3-8b-instruct-mrc-v0.3 #license-apache-2.0 #autotrain_compatible #endpoints_compatible #text-generation-inference #region-us
|
# Llama3merge8-15B-MoE
Llama3merge8-15B-MoE is a Mixture of Experts (MoE) made with the following models using LazyMergekit:
* sethuiyer/Medichat-Llama3-8B
* psyche/llama3-8b-instruct-mrc-v0.3
## Configuration
## Usage
| [
"# Llama3merge8-15B-MoE\n\nLlama3merge8-15B-MoE is a Mixture of Experts (MoE) made with the following models using LazyMergekit:\n* sethuiyer/Medichat-Llama3-8B\n* psyche/llama3-8b-instruct-mrc-v0.3",
"## Configuration",
"## Usage"
] | [
"TAGS\n#transformers #safetensors #mixtral #text-generation #moe #frankenmoe #merge #mergekit #lazymergekit #sethuiyer/Medichat-Llama3-8B #psyche/llama3-8b-instruct-mrc-v0.3 #conversational #base_model-sethuiyer/Medichat-Llama3-8B #base_model-psyche/llama3-8b-instruct-mrc-v0.3 #license-apache-2.0 #autotrain_compatible #endpoints_compatible #text-generation-inference #region-us \n",
"# Llama3merge8-15B-MoE\n\nLlama3merge8-15B-MoE is a Mixture of Experts (MoE) made with the following models using LazyMergekit:\n* sethuiyer/Medichat-Llama3-8B\n* psyche/llama3-8b-instruct-mrc-v0.3",
"## Configuration",
"## Usage"
] |
null | peft |
# Model Card for Model ID
<!-- Provide a quick summary of what the model is/does. -->
## Model Details
### Model Description
<!-- Provide a longer summary of what this model is. -->
- **Developed by:** [More Information Needed]
- **Shared by [optional]:** [More Information Needed]
- **Model type:** [More Information Needed]
- **Language(s) (NLP):** [More Information Needed]
- **License:** [More Information Needed]
- **Finetuned from model [optional]:** [More Information Needed]
### Model Sources [optional]
<!-- Provide the basic links for the model. -->
- **Repository:** [More Information Needed]
- **Paper [optional]:** [More Information Needed]
- **Demo [optional]:** [More Information Needed]
## Uses
<!-- Address questions around how the model is intended to be used, including the foreseeable users of the model and those affected by the model. -->
### Direct Use
<!-- This section is for the model use without fine-tuning or plugging into a larger ecosystem/app. -->
[More Information Needed]
### Downstream Use [optional]
<!-- This section is for the model use when fine-tuned for a task, or when plugged into a larger ecosystem/app -->
[More Information Needed]
### Out-of-Scope Use
<!-- This section addresses misuse, malicious use, and uses that the model will not work well for. -->
[More Information Needed]
## Bias, Risks, and Limitations
<!-- This section is meant to convey both technical and sociotechnical limitations. -->
[More Information Needed]
### Recommendations
<!-- This section is meant to convey recommendations with respect to the bias, risk, and technical limitations. -->
Users (both direct and downstream) should be made aware of the risks, biases and limitations of the model. More information needed for further recommendations.
## How to Get Started with the Model
Use the code below to get started with the model.
[More Information Needed]
## Training Details
### Training Data
<!-- This should link to a Data Card, perhaps with a short stub of information on what the training data is all about as well as documentation related to data pre-processing or additional filtering. -->
[More Information Needed]
### Training Procedure
<!-- This relates heavily to the Technical Specifications. Content here should link to that section when it is relevant to the training procedure. -->
#### Preprocessing [optional]
[More Information Needed]
#### Training Hyperparameters
- **Training regime:** [More Information Needed] <!--fp32, fp16 mixed precision, bf16 mixed precision, bf16 non-mixed precision, fp16 non-mixed precision, fp8 mixed precision -->
#### Speeds, Sizes, Times [optional]
<!-- This section provides information about throughput, start/end time, checkpoint size if relevant, etc. -->
[More Information Needed]
## Evaluation
<!-- This section describes the evaluation protocols and provides the results. -->
### Testing Data, Factors & Metrics
#### Testing Data
<!-- This should link to a Data Card if possible. -->
[More Information Needed]
#### Factors
<!-- These are the things the evaluation is disaggregating by, e.g., subpopulations or domains. -->
[More Information Needed]
#### Metrics
<!-- These are the evaluation metrics being used, ideally with a description of why. -->
[More Information Needed]
### Results
[More Information Needed]
#### Summary
## Model Examination [optional]
<!-- Relevant interpretability work for the model goes here -->
[More Information Needed]
## Environmental Impact
<!-- Total emissions (in grams of CO2eq) and additional considerations, such as electricity usage, go here. Edit the suggested text below accordingly -->
Carbon emissions can be estimated using the [Machine Learning Impact calculator](https://mlco2.github.io/impact#compute) presented in [Lacoste et al. (2019)](https://arxiv.org/abs/1910.09700).
- **Hardware Type:** [More Information Needed]
- **Hours used:** [More Information Needed]
- **Cloud Provider:** [More Information Needed]
- **Compute Region:** [More Information Needed]
- **Carbon Emitted:** [More Information Needed]
## Technical Specifications [optional]
### Model Architecture and Objective
[More Information Needed]
### Compute Infrastructure
[More Information Needed]
#### Hardware
[More Information Needed]
#### Software
[More Information Needed]
## Citation [optional]
<!-- If there is a paper or blog post introducing the model, the APA and Bibtex information for that should go in this section. -->
**BibTeX:**
[More Information Needed]
**APA:**
[More Information Needed]
## Glossary [optional]
<!-- If relevant, include terms and calculations in this section that can help readers understand the model or model card. -->
[More Information Needed]
## More Information [optional]
[More Information Needed]
## Model Card Authors [optional]
[More Information Needed]
## Model Card Contact
[More Information Needed]
## Training procedure
The following `bitsandbytes` quantization config was used during training:
- load_in_8bit: False
- load_in_4bit: True
- llm_int8_threshold: 6.0
- llm_int8_skip_modules: None
- llm_int8_enable_fp32_cpu_offload: False
- llm_int8_has_fp16_weight: False
- bnb_4bit_quant_type: nf4
- bnb_4bit_use_double_quant: True
- bnb_4bit_compute_dtype: bfloat16
### Framework versions
- PEFT 0.7.0.dev0
| {"library_name": "peft", "base_model": "TinyLlama/TinyLlama-1.1B-Chat-v1.0"} | bmehrba/TinyLlama-1.1B-Chat-v1.0-fine-tuned_ChatGPT_t1_tiny_Seed101 | null | [
"peft",
"arxiv:1910.09700",
"base_model:TinyLlama/TinyLlama-1.1B-Chat-v1.0",
"region:us"
] | null | 2024-04-22T09:46:14+00:00 | [
"1910.09700"
] | [] | TAGS
#peft #arxiv-1910.09700 #base_model-TinyLlama/TinyLlama-1.1B-Chat-v1.0 #region-us
|
# Model Card for Model ID
## Model Details
### Model Description
- Developed by:
- Shared by [optional]:
- Model type:
- Language(s) (NLP):
- License:
- Finetuned from model [optional]:
### Model Sources [optional]
- Repository:
- Paper [optional]:
- Demo [optional]:
## Uses
### Direct Use
### Downstream Use [optional]
### Out-of-Scope Use
## Bias, Risks, and Limitations
### Recommendations
Users (both direct and downstream) should be made aware of the risks, biases and limitations of the model. More information needed for further recommendations.
## How to Get Started with the Model
Use the code below to get started with the model.
## Training Details
### Training Data
### Training Procedure
#### Preprocessing [optional]
#### Training Hyperparameters
- Training regime:
#### Speeds, Sizes, Times [optional]
## Evaluation
### Testing Data, Factors & Metrics
#### Testing Data
#### Factors
#### Metrics
### Results
#### Summary
## Model Examination [optional]
## Environmental Impact
Carbon emissions can be estimated using the Machine Learning Impact calculator presented in Lacoste et al. (2019).
- Hardware Type:
- Hours used:
- Cloud Provider:
- Compute Region:
- Carbon Emitted:
## Technical Specifications [optional]
### Model Architecture and Objective
### Compute Infrastructure
#### Hardware
#### Software
[optional]
BibTeX:
APA:
## Glossary [optional]
## More Information [optional]
## Model Card Authors [optional]
## Model Card Contact
## Training procedure
The following 'bitsandbytes' quantization config was used during training:
- load_in_8bit: False
- load_in_4bit: True
- llm_int8_threshold: 6.0
- llm_int8_skip_modules: None
- llm_int8_enable_fp32_cpu_offload: False
- llm_int8_has_fp16_weight: False
- bnb_4bit_quant_type: nf4
- bnb_4bit_use_double_quant: True
- bnb_4bit_compute_dtype: bfloat16
### Framework versions
- PEFT 0.7.0.dev0
| [
"# Model Card for Model ID",
"## Model Details",
"### Model Description\n\n\n\n\n\n- Developed by: \n- Shared by [optional]: \n- Model type: \n- Language(s) (NLP): \n- License: \n- Finetuned from model [optional]:",
"### Model Sources [optional]\n\n\n\n- Repository: \n- Paper [optional]: \n- Demo [optional]:",
"## Uses",
"### Direct Use",
"### Downstream Use [optional]",
"### Out-of-Scope Use",
"## Bias, Risks, and Limitations",
"### Recommendations\n\n\n\nUsers (both direct and downstream) should be made aware of the risks, biases and limitations of the model. More information needed for further recommendations.",
"## How to Get Started with the Model\n\nUse the code below to get started with the model.",
"## Training Details",
"### Training Data",
"### Training Procedure",
"#### Preprocessing [optional]",
"#### Training Hyperparameters\n\n- Training regime:",
"#### Speeds, Sizes, Times [optional]",
"## Evaluation",
"### Testing Data, Factors & Metrics",
"#### Testing Data",
"#### Factors",
"#### Metrics",
"### Results",
"#### Summary",
"## Model Examination [optional]",
"## Environmental Impact\n\n\n\nCarbon emissions can be estimated using the Machine Learning Impact calculator presented in Lacoste et al. (2019).\n\n- Hardware Type: \n- Hours used: \n- Cloud Provider: \n- Compute Region: \n- Carbon Emitted:",
"## Technical Specifications [optional]",
"### Model Architecture and Objective",
"### Compute Infrastructure",
"#### Hardware",
"#### Software\n\n\n\n[optional]\n\n\n\nBibTeX:\n\n\n\nAPA:",
"## Glossary [optional]",
"## More Information [optional]",
"## Model Card Authors [optional]",
"## Model Card Contact",
"## Training procedure\n\n\nThe following 'bitsandbytes' quantization config was used during training:\n- load_in_8bit: False\n- load_in_4bit: True\n- llm_int8_threshold: 6.0\n- llm_int8_skip_modules: None\n- llm_int8_enable_fp32_cpu_offload: False\n- llm_int8_has_fp16_weight: False\n- bnb_4bit_quant_type: nf4\n- bnb_4bit_use_double_quant: True\n- bnb_4bit_compute_dtype: bfloat16",
"### Framework versions\n\n\n- PEFT 0.7.0.dev0"
] | [
"TAGS\n#peft #arxiv-1910.09700 #base_model-TinyLlama/TinyLlama-1.1B-Chat-v1.0 #region-us \n",
"# Model Card for Model ID",
"## Model Details",
"### Model Description\n\n\n\n\n\n- Developed by: \n- Shared by [optional]: \n- Model type: \n- Language(s) (NLP): \n- License: \n- Finetuned from model [optional]:",
"### Model Sources [optional]\n\n\n\n- Repository: \n- Paper [optional]: \n- Demo [optional]:",
"## Uses",
"### Direct Use",
"### Downstream Use [optional]",
"### Out-of-Scope Use",
"## Bias, Risks, and Limitations",
"### Recommendations\n\n\n\nUsers (both direct and downstream) should be made aware of the risks, biases and limitations of the model. More information needed for further recommendations.",
"## How to Get Started with the Model\n\nUse the code below to get started with the model.",
"## Training Details",
"### Training Data",
"### Training Procedure",
"#### Preprocessing [optional]",
"#### Training Hyperparameters\n\n- Training regime:",
"#### Speeds, Sizes, Times [optional]",
"## Evaluation",
"### Testing Data, Factors & Metrics",
"#### Testing Data",
"#### Factors",
"#### Metrics",
"### Results",
"#### Summary",
"## Model Examination [optional]",
"## Environmental Impact\n\n\n\nCarbon emissions can be estimated using the Machine Learning Impact calculator presented in Lacoste et al. (2019).\n\n- Hardware Type: \n- Hours used: \n- Cloud Provider: \n- Compute Region: \n- Carbon Emitted:",
"## Technical Specifications [optional]",
"### Model Architecture and Objective",
"### Compute Infrastructure",
"#### Hardware",
"#### Software\n\n\n\n[optional]\n\n\n\nBibTeX:\n\n\n\nAPA:",
"## Glossary [optional]",
"## More Information [optional]",
"## Model Card Authors [optional]",
"## Model Card Contact",
"## Training procedure\n\n\nThe following 'bitsandbytes' quantization config was used during training:\n- load_in_8bit: False\n- load_in_4bit: True\n- llm_int8_threshold: 6.0\n- llm_int8_skip_modules: None\n- llm_int8_enable_fp32_cpu_offload: False\n- llm_int8_has_fp16_weight: False\n- bnb_4bit_quant_type: nf4\n- bnb_4bit_use_double_quant: True\n- bnb_4bit_compute_dtype: bfloat16",
"### Framework versions\n\n\n- PEFT 0.7.0.dev0"
] |
text-to-audio | peft |
<!-- This model card has been generated automatically according to the information the Trainer had access to. You
should probably proofread and complete it, then remove this comment. -->
[<img src="https://raw.githubusercontent.com/wandb/assets/main/wandb-github-badge-28.svg" alt="Visualize in Weights & Biases" width="200" height="32"/>](https://wandb.ai/ylacombe/huggingface/runs/wk9bpko6)
[<img src="https://raw.githubusercontent.com/wandb/assets/main/wandb-github-badge-28.svg" alt="Visualize in Weights & Biases" width="200" height="32"/>](https://wandb.ai/ylacombe/huggingface/runs/wk9bpko6)
# musicgen-melody-lora-punk
This model is a fine-tuned version of [facebook/musicgen-melody](https://huggingface.co/facebook/musicgen-melody) on the YLACOMBE/TINY-PUNK - DEFAULT dataset.
It achieves the following results on the evaluation set:
- Loss: 4.7297
- Clap: 0.2559
## Model description
More information needed
## Intended uses & limitations
More information needed
## Training and evaluation data
More information needed
## Training procedure
### Training hyperparameters
The following hyperparameters were used during training:
- learning_rate: 0.0002
- train_batch_size: 2
- eval_batch_size: 1
- seed: 42
- gradient_accumulation_steps: 8
- total_train_batch_size: 16
- optimizer: Adam with betas=(0.9,0.99) and epsilon=1e-08
- lr_scheduler_type: linear
- num_epochs: 4
- mixed_precision_training: Native AMP
### Training results
### Framework versions
- PEFT 0.10.0
- Transformers 4.41.0.dev0
- Pytorch 2.1.2+cu121
- Datasets 2.19.0
- Tokenizers 0.19.1 | {"license": "cc-by-nc-4.0", "library_name": "peft", "tags": ["text-to-audio", "ylacombe/tiny-punk", "generated_from_trainer"], "base_model": "facebook/musicgen-melody", "model-index": [{"name": "musicgen-melody-lora-punk", "results": []}]} | ylacombe/musicgen-melody-lora-punk | null | [
"peft",
"safetensors",
"musicgen_melody",
"text-to-audio",
"ylacombe/tiny-punk",
"generated_from_trainer",
"base_model:facebook/musicgen-melody",
"license:cc-by-nc-4.0",
"region:us"
] | null | 2024-04-22T09:47:04+00:00 | [] | [] | TAGS
#peft #safetensors #musicgen_melody #text-to-audio #ylacombe/tiny-punk #generated_from_trainer #base_model-facebook/musicgen-melody #license-cc-by-nc-4.0 #region-us
|
<img src="URL alt="Visualize in Weights & Biases" width="200" height="32"/>
<img src="URL alt="Visualize in Weights & Biases" width="200" height="32"/>
# musicgen-melody-lora-punk
This model is a fine-tuned version of facebook/musicgen-melody on the YLACOMBE/TINY-PUNK - DEFAULT dataset.
It achieves the following results on the evaluation set:
- Loss: 4.7297
- Clap: 0.2559
## Model description
More information needed
## Intended uses & limitations
More information needed
## Training and evaluation data
More information needed
## Training procedure
### Training hyperparameters
The following hyperparameters were used during training:
- learning_rate: 0.0002
- train_batch_size: 2
- eval_batch_size: 1
- seed: 42
- gradient_accumulation_steps: 8
- total_train_batch_size: 16
- optimizer: Adam with betas=(0.9,0.99) and epsilon=1e-08
- lr_scheduler_type: linear
- num_epochs: 4
- mixed_precision_training: Native AMP
### Training results
### Framework versions
- PEFT 0.10.0
- Transformers 4.41.0.dev0
- Pytorch 2.1.2+cu121
- Datasets 2.19.0
- Tokenizers 0.19.1 | [
"# musicgen-melody-lora-punk\n\nThis model is a fine-tuned version of facebook/musicgen-melody on the YLACOMBE/TINY-PUNK - DEFAULT dataset.\nIt achieves the following results on the evaluation set:\n- Loss: 4.7297\n- Clap: 0.2559",
"## Model description\n\nMore information needed",
"## Intended uses & limitations\n\nMore information needed",
"## Training and evaluation data\n\nMore information needed",
"## Training procedure",
"### Training hyperparameters\n\nThe following hyperparameters were used during training:\n- learning_rate: 0.0002\n- train_batch_size: 2\n- eval_batch_size: 1\n- seed: 42\n- gradient_accumulation_steps: 8\n- total_train_batch_size: 16\n- optimizer: Adam with betas=(0.9,0.99) and epsilon=1e-08\n- lr_scheduler_type: linear\n- num_epochs: 4\n- mixed_precision_training: Native AMP",
"### Training results",
"### Framework versions\n\n- PEFT 0.10.0\n- Transformers 4.41.0.dev0\n- Pytorch 2.1.2+cu121\n- Datasets 2.19.0\n- Tokenizers 0.19.1"
] | [
"TAGS\n#peft #safetensors #musicgen_melody #text-to-audio #ylacombe/tiny-punk #generated_from_trainer #base_model-facebook/musicgen-melody #license-cc-by-nc-4.0 #region-us \n",
"# musicgen-melody-lora-punk\n\nThis model is a fine-tuned version of facebook/musicgen-melody on the YLACOMBE/TINY-PUNK - DEFAULT dataset.\nIt achieves the following results on the evaluation set:\n- Loss: 4.7297\n- Clap: 0.2559",
"## Model description\n\nMore information needed",
"## Intended uses & limitations\n\nMore information needed",
"## Training and evaluation data\n\nMore information needed",
"## Training procedure",
"### Training hyperparameters\n\nThe following hyperparameters were used during training:\n- learning_rate: 0.0002\n- train_batch_size: 2\n- eval_batch_size: 1\n- seed: 42\n- gradient_accumulation_steps: 8\n- total_train_batch_size: 16\n- optimizer: Adam with betas=(0.9,0.99) and epsilon=1e-08\n- lr_scheduler_type: linear\n- num_epochs: 4\n- mixed_precision_training: Native AMP",
"### Training results",
"### Framework versions\n\n- PEFT 0.10.0\n- Transformers 4.41.0.dev0\n- Pytorch 2.1.2+cu121\n- Datasets 2.19.0\n- Tokenizers 0.19.1"
] |
text-generation | transformers |
# Model Card for Model ID
<!-- Provide a quick summary of what the model is/does. -->
## Model Details
### Model Description
<!-- Provide a longer summary of what this model is. -->
This is the model card of a 🤗 transformers model that has been pushed on the Hub. This model card has been automatically generated.
- **Developed by:** [More Information Needed]
- **Funded by [optional]:** [More Information Needed]
- **Shared by [optional]:** [More Information Needed]
- **Model type:** [More Information Needed]
- **Language(s) (NLP):** [More Information Needed]
- **License:** [More Information Needed]
- **Finetuned from model [optional]:** [More Information Needed]
### Model Sources [optional]
<!-- Provide the basic links for the model. -->
- **Repository:** [More Information Needed]
- **Paper [optional]:** [More Information Needed]
- **Demo [optional]:** [More Information Needed]
## Uses
<!-- Address questions around how the model is intended to be used, including the foreseeable users of the model and those affected by the model. -->
### Direct Use
<!-- This section is for the model use without fine-tuning or plugging into a larger ecosystem/app. -->
[More Information Needed]
### Downstream Use [optional]
<!-- This section is for the model use when fine-tuned for a task, or when plugged into a larger ecosystem/app -->
[More Information Needed]
### Out-of-Scope Use
<!-- This section addresses misuse, malicious use, and uses that the model will not work well for. -->
[More Information Needed]
## Bias, Risks, and Limitations
<!-- This section is meant to convey both technical and sociotechnical limitations. -->
[More Information Needed]
### Recommendations
<!-- This section is meant to convey recommendations with respect to the bias, risk, and technical limitations. -->
Users (both direct and downstream) should be made aware of the risks, biases and limitations of the model. More information needed for further recommendations.
## How to Get Started with the Model
Use the code below to get started with the model.
[More Information Needed]
## Training Details
### Training Data
<!-- This should link to a Dataset Card, perhaps with a short stub of information on what the training data is all about as well as documentation related to data pre-processing or additional filtering. -->
[More Information Needed]
### Training Procedure
<!-- This relates heavily to the Technical Specifications. Content here should link to that section when it is relevant to the training procedure. -->
#### Preprocessing [optional]
[More Information Needed]
#### Training Hyperparameters
- **Training regime:** [More Information Needed] <!--fp32, fp16 mixed precision, bf16 mixed precision, bf16 non-mixed precision, fp16 non-mixed precision, fp8 mixed precision -->
#### Speeds, Sizes, Times [optional]
<!-- This section provides information about throughput, start/end time, checkpoint size if relevant, etc. -->
[More Information Needed]
## Evaluation
<!-- This section describes the evaluation protocols and provides the results. -->
### Testing Data, Factors & Metrics
#### Testing Data
<!-- This should link to a Dataset Card if possible. -->
[More Information Needed]
#### Factors
<!-- These are the things the evaluation is disaggregating by, e.g., subpopulations or domains. -->
[More Information Needed]
#### Metrics
<!-- These are the evaluation metrics being used, ideally with a description of why. -->
[More Information Needed]
### Results
[More Information Needed]
#### Summary
## Model Examination [optional]
<!-- Relevant interpretability work for the model goes here -->
[More Information Needed]
## Environmental Impact
<!-- Total emissions (in grams of CO2eq) and additional considerations, such as electricity usage, go here. Edit the suggested text below accordingly -->
Carbon emissions can be estimated using the [Machine Learning Impact calculator](https://mlco2.github.io/impact#compute) presented in [Lacoste et al. (2019)](https://arxiv.org/abs/1910.09700).
- **Hardware Type:** [More Information Needed]
- **Hours used:** [More Information Needed]
- **Cloud Provider:** [More Information Needed]
- **Compute Region:** [More Information Needed]
- **Carbon Emitted:** [More Information Needed]
## Technical Specifications [optional]
### Model Architecture and Objective
[More Information Needed]
### Compute Infrastructure
[More Information Needed]
#### Hardware
[More Information Needed]
#### Software
[More Information Needed]
## Citation [optional]
<!-- If there is a paper or blog post introducing the model, the APA and Bibtex information for that should go in this section. -->
**BibTeX:**
[More Information Needed]
**APA:**
[More Information Needed]
## Glossary [optional]
<!-- If relevant, include terms and calculations in this section that can help readers understand the model or model card. -->
[More Information Needed]
## More Information [optional]
[More Information Needed]
## Model Card Authors [optional]
[More Information Needed]
## Model Card Contact
[More Information Needed] | {"library_name": "transformers", "tags": []} | srirambhavadish/Mixtral_arxt_v1 | null | [
"transformers",
"safetensors",
"mistral",
"text-generation",
"arxiv:1910.09700",
"autotrain_compatible",
"endpoints_compatible",
"text-generation-inference",
"4-bit",
"region:us"
] | null | 2024-04-22T09:49:16+00:00 | [
"1910.09700"
] | [] | TAGS
#transformers #safetensors #mistral #text-generation #arxiv-1910.09700 #autotrain_compatible #endpoints_compatible #text-generation-inference #4-bit #region-us
|
# Model Card for Model ID
## Model Details
### Model Description
This is the model card of a transformers model that has been pushed on the Hub. This model card has been automatically generated.
- Developed by:
- Funded by [optional]:
- Shared by [optional]:
- Model type:
- Language(s) (NLP):
- License:
- Finetuned from model [optional]:
### Model Sources [optional]
- Repository:
- Paper [optional]:
- Demo [optional]:
## Uses
### Direct Use
### Downstream Use [optional]
### Out-of-Scope Use
## Bias, Risks, and Limitations
### Recommendations
Users (both direct and downstream) should be made aware of the risks, biases and limitations of the model. More information needed for further recommendations.
## How to Get Started with the Model
Use the code below to get started with the model.
## Training Details
### Training Data
### Training Procedure
#### Preprocessing [optional]
#### Training Hyperparameters
- Training regime:
#### Speeds, Sizes, Times [optional]
## Evaluation
### Testing Data, Factors & Metrics
#### Testing Data
#### Factors
#### Metrics
### Results
#### Summary
## Model Examination [optional]
## Environmental Impact
Carbon emissions can be estimated using the Machine Learning Impact calculator presented in Lacoste et al. (2019).
- Hardware Type:
- Hours used:
- Cloud Provider:
- Compute Region:
- Carbon Emitted:
## Technical Specifications [optional]
### Model Architecture and Objective
### Compute Infrastructure
#### Hardware
#### Software
[optional]
BibTeX:
APA:
## Glossary [optional]
## More Information [optional]
## Model Card Authors [optional]
## Model Card Contact
| [
"# Model Card for Model ID",
"## Model Details",
"### Model Description\n\n\n\nThis is the model card of a transformers model that has been pushed on the Hub. This model card has been automatically generated.\n\n- Developed by: \n- Funded by [optional]: \n- Shared by [optional]: \n- Model type: \n- Language(s) (NLP): \n- License: \n- Finetuned from model [optional]:",
"### Model Sources [optional]\n\n\n\n- Repository: \n- Paper [optional]: \n- Demo [optional]:",
"## Uses",
"### Direct Use",
"### Downstream Use [optional]",
"### Out-of-Scope Use",
"## Bias, Risks, and Limitations",
"### Recommendations\n\n\n\nUsers (both direct and downstream) should be made aware of the risks, biases and limitations of the model. More information needed for further recommendations.",
"## How to Get Started with the Model\n\nUse the code below to get started with the model.",
"## Training Details",
"### Training Data",
"### Training Procedure",
"#### Preprocessing [optional]",
"#### Training Hyperparameters\n\n- Training regime:",
"#### Speeds, Sizes, Times [optional]",
"## Evaluation",
"### Testing Data, Factors & Metrics",
"#### Testing Data",
"#### Factors",
"#### Metrics",
"### Results",
"#### Summary",
"## Model Examination [optional]",
"## Environmental Impact\n\n\n\nCarbon emissions can be estimated using the Machine Learning Impact calculator presented in Lacoste et al. (2019).\n\n- Hardware Type: \n- Hours used: \n- Cloud Provider: \n- Compute Region: \n- Carbon Emitted:",
"## Technical Specifications [optional]",
"### Model Architecture and Objective",
"### Compute Infrastructure",
"#### Hardware",
"#### Software\n\n\n\n[optional]\n\n\n\nBibTeX:\n\n\n\nAPA:",
"## Glossary [optional]",
"## More Information [optional]",
"## Model Card Authors [optional]",
"## Model Card Contact"
] | [
"TAGS\n#transformers #safetensors #mistral #text-generation #arxiv-1910.09700 #autotrain_compatible #endpoints_compatible #text-generation-inference #4-bit #region-us \n",
"# Model Card for Model ID",
"## Model Details",
"### Model Description\n\n\n\nThis is the model card of a transformers model that has been pushed on the Hub. This model card has been automatically generated.\n\n- Developed by: \n- Funded by [optional]: \n- Shared by [optional]: \n- Model type: \n- Language(s) (NLP): \n- License: \n- Finetuned from model [optional]:",
"### Model Sources [optional]\n\n\n\n- Repository: \n- Paper [optional]: \n- Demo [optional]:",
"## Uses",
"### Direct Use",
"### Downstream Use [optional]",
"### Out-of-Scope Use",
"## Bias, Risks, and Limitations",
"### Recommendations\n\n\n\nUsers (both direct and downstream) should be made aware of the risks, biases and limitations of the model. More information needed for further recommendations.",
"## How to Get Started with the Model\n\nUse the code below to get started with the model.",
"## Training Details",
"### Training Data",
"### Training Procedure",
"#### Preprocessing [optional]",
"#### Training Hyperparameters\n\n- Training regime:",
"#### Speeds, Sizes, Times [optional]",
"## Evaluation",
"### Testing Data, Factors & Metrics",
"#### Testing Data",
"#### Factors",
"#### Metrics",
"### Results",
"#### Summary",
"## Model Examination [optional]",
"## Environmental Impact\n\n\n\nCarbon emissions can be estimated using the Machine Learning Impact calculator presented in Lacoste et al. (2019).\n\n- Hardware Type: \n- Hours used: \n- Cloud Provider: \n- Compute Region: \n- Carbon Emitted:",
"## Technical Specifications [optional]",
"### Model Architecture and Objective",
"### Compute Infrastructure",
"#### Hardware",
"#### Software\n\n\n\n[optional]\n\n\n\nBibTeX:\n\n\n\nAPA:",
"## Glossary [optional]",
"## More Information [optional]",
"## Model Card Authors [optional]",
"## Model Card Contact"
] |
null | peft |
<!-- This model card has been generated automatically according to the information the Trainer had access to. You
should probably proofread and complete it, then remove this comment. -->
# continual-pretraining-checkpoint
This model is a fine-tuned version of [Bachhoang/vbd-llama2-7B-legals-chat](https://huggingface.co/Bachhoang/vbd-llama2-7B-legals-chat) on an unknown dataset.
## Model description
More information needed
## Intended uses & limitations
More information needed
## Training and evaluation data
More information needed
## Training procedure
The following `bitsandbytes` quantization config was used during training:
- quant_method: bitsandbytes
- _load_in_8bit: False
- _load_in_4bit: True
- llm_int8_threshold: 6.0
- llm_int8_skip_modules: None
- llm_int8_enable_fp32_cpu_offload: False
- llm_int8_has_fp16_weight: False
- bnb_4bit_quant_type: nf4
- bnb_4bit_use_double_quant: True
- bnb_4bit_compute_dtype: float16
- bnb_4bit_quant_storage: uint8
- load_in_4bit: True
- load_in_8bit: False
### Training hyperparameters
The following hyperparameters were used during training:
- learning_rate: 0.0002
- train_batch_size: 1
- eval_batch_size: 1
- seed: 42
- gradient_accumulation_steps: 2
- total_train_batch_size: 2
- optimizer: Adam with betas=(0.9,0.999) and epsilon=1e-08
- lr_scheduler_type: cosine
- lr_scheduler_warmup_steps: 2
- num_epochs: 1
- mixed_precision_training: Native AMP
### Framework versions
- PEFT 0.5.0
- Transformers 4.39.3
- Pytorch 2.1.2
- Datasets 2.16.0
- Tokenizers 0.15.2
| {"library_name": "peft", "tags": ["generated_from_trainer"], "base_model": "Bachhoang/vbd-llama2-7B-legals-chat", "model-index": [{"name": "continual-pretraining-checkpoint", "results": []}]} | Bachhoang/continual-pretraining-checkpoint | null | [
"peft",
"safetensors",
"generated_from_trainer",
"base_model:Bachhoang/vbd-llama2-7B-legals-chat",
"region:us"
] | null | 2024-04-22T09:50:16+00:00 | [] | [] | TAGS
#peft #safetensors #generated_from_trainer #base_model-Bachhoang/vbd-llama2-7B-legals-chat #region-us
|
# continual-pretraining-checkpoint
This model is a fine-tuned version of Bachhoang/vbd-llama2-7B-legals-chat on an unknown dataset.
## Model description
More information needed
## Intended uses & limitations
More information needed
## Training and evaluation data
More information needed
## Training procedure
The following 'bitsandbytes' quantization config was used during training:
- quant_method: bitsandbytes
- _load_in_8bit: False
- _load_in_4bit: True
- llm_int8_threshold: 6.0
- llm_int8_skip_modules: None
- llm_int8_enable_fp32_cpu_offload: False
- llm_int8_has_fp16_weight: False
- bnb_4bit_quant_type: nf4
- bnb_4bit_use_double_quant: True
- bnb_4bit_compute_dtype: float16
- bnb_4bit_quant_storage: uint8
- load_in_4bit: True
- load_in_8bit: False
### Training hyperparameters
The following hyperparameters were used during training:
- learning_rate: 0.0002
- train_batch_size: 1
- eval_batch_size: 1
- seed: 42
- gradient_accumulation_steps: 2
- total_train_batch_size: 2
- optimizer: Adam with betas=(0.9,0.999) and epsilon=1e-08
- lr_scheduler_type: cosine
- lr_scheduler_warmup_steps: 2
- num_epochs: 1
- mixed_precision_training: Native AMP
### Framework versions
- PEFT 0.5.0
- Transformers 4.39.3
- Pytorch 2.1.2
- Datasets 2.16.0
- Tokenizers 0.15.2
| [
"# continual-pretraining-checkpoint\n\nThis model is a fine-tuned version of Bachhoang/vbd-llama2-7B-legals-chat on an unknown dataset.",
"## Model description\n\nMore information needed",
"## Intended uses & limitations\n\nMore information needed",
"## Training and evaluation data\n\nMore information needed",
"## Training procedure\n\n\nThe following 'bitsandbytes' quantization config was used during training:\n- quant_method: bitsandbytes\n- _load_in_8bit: False\n- _load_in_4bit: True\n- llm_int8_threshold: 6.0\n- llm_int8_skip_modules: None\n- llm_int8_enable_fp32_cpu_offload: False\n- llm_int8_has_fp16_weight: False\n- bnb_4bit_quant_type: nf4\n- bnb_4bit_use_double_quant: True\n- bnb_4bit_compute_dtype: float16\n- bnb_4bit_quant_storage: uint8\n- load_in_4bit: True\n- load_in_8bit: False",
"### Training hyperparameters\n\nThe following hyperparameters were used during training:\n- learning_rate: 0.0002\n- train_batch_size: 1\n- eval_batch_size: 1\n- seed: 42\n- gradient_accumulation_steps: 2\n- total_train_batch_size: 2\n- optimizer: Adam with betas=(0.9,0.999) and epsilon=1e-08\n- lr_scheduler_type: cosine\n- lr_scheduler_warmup_steps: 2\n- num_epochs: 1\n- mixed_precision_training: Native AMP",
"### Framework versions\n\n- PEFT 0.5.0\n- Transformers 4.39.3\n- Pytorch 2.1.2\n- Datasets 2.16.0\n- Tokenizers 0.15.2"
] | [
"TAGS\n#peft #safetensors #generated_from_trainer #base_model-Bachhoang/vbd-llama2-7B-legals-chat #region-us \n",
"# continual-pretraining-checkpoint\n\nThis model is a fine-tuned version of Bachhoang/vbd-llama2-7B-legals-chat on an unknown dataset.",
"## Model description\n\nMore information needed",
"## Intended uses & limitations\n\nMore information needed",
"## Training and evaluation data\n\nMore information needed",
"## Training procedure\n\n\nThe following 'bitsandbytes' quantization config was used during training:\n- quant_method: bitsandbytes\n- _load_in_8bit: False\n- _load_in_4bit: True\n- llm_int8_threshold: 6.0\n- llm_int8_skip_modules: None\n- llm_int8_enable_fp32_cpu_offload: False\n- llm_int8_has_fp16_weight: False\n- bnb_4bit_quant_type: nf4\n- bnb_4bit_use_double_quant: True\n- bnb_4bit_compute_dtype: float16\n- bnb_4bit_quant_storage: uint8\n- load_in_4bit: True\n- load_in_8bit: False",
"### Training hyperparameters\n\nThe following hyperparameters were used during training:\n- learning_rate: 0.0002\n- train_batch_size: 1\n- eval_batch_size: 1\n- seed: 42\n- gradient_accumulation_steps: 2\n- total_train_batch_size: 2\n- optimizer: Adam with betas=(0.9,0.999) and epsilon=1e-08\n- lr_scheduler_type: cosine\n- lr_scheduler_warmup_steps: 2\n- num_epochs: 1\n- mixed_precision_training: Native AMP",
"### Framework versions\n\n- PEFT 0.5.0\n- Transformers 4.39.3\n- Pytorch 2.1.2\n- Datasets 2.16.0\n- Tokenizers 0.15.2"
] |
text-generation | transformers |
# Model Card for Model ID
<!-- Provide a quick summary of what the model is/does. -->
## Model Details
### Model Description
<!-- Provide a longer summary of what this model is. -->
This is the model card of a 🤗 transformers model that has been pushed on the Hub. This model card has been automatically generated.
- **Developed by:** [More Information Needed]
- **Funded by [optional]:** [More Information Needed]
- **Shared by [optional]:** [More Information Needed]
- **Model type:** [More Information Needed]
- **Language(s) (NLP):** [More Information Needed]
- **License:** [More Information Needed]
- **Finetuned from model [optional]:** [More Information Needed]
### Model Sources [optional]
<!-- Provide the basic links for the model. -->
- **Repository:** [More Information Needed]
- **Paper [optional]:** [More Information Needed]
- **Demo [optional]:** [More Information Needed]
## Uses
<!-- Address questions around how the model is intended to be used, including the foreseeable users of the model and those affected by the model. -->
### Direct Use
<!-- This section is for the model use without fine-tuning or plugging into a larger ecosystem/app. -->
[More Information Needed]
### Downstream Use [optional]
<!-- This section is for the model use when fine-tuned for a task, or when plugged into a larger ecosystem/app -->
[More Information Needed]
### Out-of-Scope Use
<!-- This section addresses misuse, malicious use, and uses that the model will not work well for. -->
[More Information Needed]
## Bias, Risks, and Limitations
<!-- This section is meant to convey both technical and sociotechnical limitations. -->
[More Information Needed]
### Recommendations
<!-- This section is meant to convey recommendations with respect to the bias, risk, and technical limitations. -->
Users (both direct and downstream) should be made aware of the risks, biases and limitations of the model. More information needed for further recommendations.
## How to Get Started with the Model
Use the code below to get started with the model.
[More Information Needed]
## Training Details
### Training Data
<!-- This should link to a Dataset Card, perhaps with a short stub of information on what the training data is all about as well as documentation related to data pre-processing or additional filtering. -->
[More Information Needed]
### Training Procedure
<!-- This relates heavily to the Technical Specifications. Content here should link to that section when it is relevant to the training procedure. -->
#### Preprocessing [optional]
[More Information Needed]
#### Training Hyperparameters
- **Training regime:** [More Information Needed] <!--fp32, fp16 mixed precision, bf16 mixed precision, bf16 non-mixed precision, fp16 non-mixed precision, fp8 mixed precision -->
#### Speeds, Sizes, Times [optional]
<!-- This section provides information about throughput, start/end time, checkpoint size if relevant, etc. -->
[More Information Needed]
## Evaluation
<!-- This section describes the evaluation protocols and provides the results. -->
### Testing Data, Factors & Metrics
#### Testing Data
<!-- This should link to a Dataset Card if possible. -->
[More Information Needed]
#### Factors
<!-- These are the things the evaluation is disaggregating by, e.g., subpopulations or domains. -->
[More Information Needed]
#### Metrics
<!-- These are the evaluation metrics being used, ideally with a description of why. -->
[More Information Needed]
### Results
[More Information Needed]
#### Summary
## Model Examination [optional]
<!-- Relevant interpretability work for the model goes here -->
[More Information Needed]
## Environmental Impact
<!-- Total emissions (in grams of CO2eq) and additional considerations, such as electricity usage, go here. Edit the suggested text below accordingly -->
Carbon emissions can be estimated using the [Machine Learning Impact calculator](https://mlco2.github.io/impact#compute) presented in [Lacoste et al. (2019)](https://arxiv.org/abs/1910.09700).
- **Hardware Type:** [More Information Needed]
- **Hours used:** [More Information Needed]
- **Cloud Provider:** [More Information Needed]
- **Compute Region:** [More Information Needed]
- **Carbon Emitted:** [More Information Needed]
## Technical Specifications [optional]
### Model Architecture and Objective
[More Information Needed]
### Compute Infrastructure
[More Information Needed]
#### Hardware
[More Information Needed]
#### Software
[More Information Needed]
## Citation [optional]
<!-- If there is a paper or blog post introducing the model, the APA and Bibtex information for that should go in this section. -->
**BibTeX:**
[More Information Needed]
**APA:**
[More Information Needed]
## Glossary [optional]
<!-- If relevant, include terms and calculations in this section that can help readers understand the model or model card. -->
[More Information Needed]
## More Information [optional]
[More Information Needed]
## Model Card Authors [optional]
[More Information Needed]
## Model Card Contact
[More Information Needed] | {"library_name": "transformers", "tags": []} | heyllm234/sc63 | null | [
"transformers",
"safetensors",
"stablelm",
"text-generation",
"conversational",
"arxiv:1910.09700",
"autotrain_compatible",
"endpoints_compatible",
"region:us"
] | null | 2024-04-22T09:51:43+00:00 | [
"1910.09700"
] | [] | TAGS
#transformers #safetensors #stablelm #text-generation #conversational #arxiv-1910.09700 #autotrain_compatible #endpoints_compatible #region-us
|
# Model Card for Model ID
## Model Details
### Model Description
This is the model card of a transformers model that has been pushed on the Hub. This model card has been automatically generated.
- Developed by:
- Funded by [optional]:
- Shared by [optional]:
- Model type:
- Language(s) (NLP):
- License:
- Finetuned from model [optional]:
### Model Sources [optional]
- Repository:
- Paper [optional]:
- Demo [optional]:
## Uses
### Direct Use
### Downstream Use [optional]
### Out-of-Scope Use
## Bias, Risks, and Limitations
### Recommendations
Users (both direct and downstream) should be made aware of the risks, biases and limitations of the model. More information needed for further recommendations.
## How to Get Started with the Model
Use the code below to get started with the model.
## Training Details
### Training Data
### Training Procedure
#### Preprocessing [optional]
#### Training Hyperparameters
- Training regime:
#### Speeds, Sizes, Times [optional]
## Evaluation
### Testing Data, Factors & Metrics
#### Testing Data
#### Factors
#### Metrics
### Results
#### Summary
## Model Examination [optional]
## Environmental Impact
Carbon emissions can be estimated using the Machine Learning Impact calculator presented in Lacoste et al. (2019).
- Hardware Type:
- Hours used:
- Cloud Provider:
- Compute Region:
- Carbon Emitted:
## Technical Specifications [optional]
### Model Architecture and Objective
### Compute Infrastructure
#### Hardware
#### Software
[optional]
BibTeX:
APA:
## Glossary [optional]
## More Information [optional]
## Model Card Authors [optional]
## Model Card Contact
| [
"# Model Card for Model ID",
"## Model Details",
"### Model Description\n\n\n\nThis is the model card of a transformers model that has been pushed on the Hub. This model card has been automatically generated.\n\n- Developed by: \n- Funded by [optional]: \n- Shared by [optional]: \n- Model type: \n- Language(s) (NLP): \n- License: \n- Finetuned from model [optional]:",
"### Model Sources [optional]\n\n\n\n- Repository: \n- Paper [optional]: \n- Demo [optional]:",
"## Uses",
"### Direct Use",
"### Downstream Use [optional]",
"### Out-of-Scope Use",
"## Bias, Risks, and Limitations",
"### Recommendations\n\n\n\nUsers (both direct and downstream) should be made aware of the risks, biases and limitations of the model. More information needed for further recommendations.",
"## How to Get Started with the Model\n\nUse the code below to get started with the model.",
"## Training Details",
"### Training Data",
"### Training Procedure",
"#### Preprocessing [optional]",
"#### Training Hyperparameters\n\n- Training regime:",
"#### Speeds, Sizes, Times [optional]",
"## Evaluation",
"### Testing Data, Factors & Metrics",
"#### Testing Data",
"#### Factors",
"#### Metrics",
"### Results",
"#### Summary",
"## Model Examination [optional]",
"## Environmental Impact\n\n\n\nCarbon emissions can be estimated using the Machine Learning Impact calculator presented in Lacoste et al. (2019).\n\n- Hardware Type: \n- Hours used: \n- Cloud Provider: \n- Compute Region: \n- Carbon Emitted:",
"## Technical Specifications [optional]",
"### Model Architecture and Objective",
"### Compute Infrastructure",
"#### Hardware",
"#### Software\n\n\n\n[optional]\n\n\n\nBibTeX:\n\n\n\nAPA:",
"## Glossary [optional]",
"## More Information [optional]",
"## Model Card Authors [optional]",
"## Model Card Contact"
] | [
"TAGS\n#transformers #safetensors #stablelm #text-generation #conversational #arxiv-1910.09700 #autotrain_compatible #endpoints_compatible #region-us \n",
"# Model Card for Model ID",
"## Model Details",
"### Model Description\n\n\n\nThis is the model card of a transformers model that has been pushed on the Hub. This model card has been automatically generated.\n\n- Developed by: \n- Funded by [optional]: \n- Shared by [optional]: \n- Model type: \n- Language(s) (NLP): \n- License: \n- Finetuned from model [optional]:",
"### Model Sources [optional]\n\n\n\n- Repository: \n- Paper [optional]: \n- Demo [optional]:",
"## Uses",
"### Direct Use",
"### Downstream Use [optional]",
"### Out-of-Scope Use",
"## Bias, Risks, and Limitations",
"### Recommendations\n\n\n\nUsers (both direct and downstream) should be made aware of the risks, biases and limitations of the model. More information needed for further recommendations.",
"## How to Get Started with the Model\n\nUse the code below to get started with the model.",
"## Training Details",
"### Training Data",
"### Training Procedure",
"#### Preprocessing [optional]",
"#### Training Hyperparameters\n\n- Training regime:",
"#### Speeds, Sizes, Times [optional]",
"## Evaluation",
"### Testing Data, Factors & Metrics",
"#### Testing Data",
"#### Factors",
"#### Metrics",
"### Results",
"#### Summary",
"## Model Examination [optional]",
"## Environmental Impact\n\n\n\nCarbon emissions can be estimated using the Machine Learning Impact calculator presented in Lacoste et al. (2019).\n\n- Hardware Type: \n- Hours used: \n- Cloud Provider: \n- Compute Region: \n- Carbon Emitted:",
"## Technical Specifications [optional]",
"### Model Architecture and Objective",
"### Compute Infrastructure",
"#### Hardware",
"#### Software\n\n\n\n[optional]\n\n\n\nBibTeX:\n\n\n\nAPA:",
"## Glossary [optional]",
"## More Information [optional]",
"## Model Card Authors [optional]",
"## Model Card Contact"
] |
text-generation | transformers |
# Uploaded model
- **Developed by:** kapilgaikwad
- **License:** apache-2.0
- **Finetuned from model :** unsloth/mistral-7b-bnb-4bit
This mistral model was trained 2x faster with [Unsloth](https://github.com/unslothai/unsloth) and Huggingface's TRL library.
[<img src="https://raw.githubusercontent.com/unslothai/unsloth/main/images/unsloth%20made%20with%20love.png" width="200"/>](https://github.com/unslothai/unsloth)
| {"language": ["en"], "license": "apache-2.0", "tags": ["text-generation-inference", "transformers", "unsloth", "mistral", "trl"], "base_model": "unsloth/mistral-7b-bnb-4bit"} | kapilgaikwad/mistral_lora_model | null | [
"transformers",
"safetensors",
"mistral",
"text-generation",
"text-generation-inference",
"unsloth",
"trl",
"en",
"base_model:unsloth/mistral-7b-bnb-4bit",
"license:apache-2.0",
"autotrain_compatible",
"endpoints_compatible",
"region:us"
] | null | 2024-04-22T09:52:28+00:00 | [] | [
"en"
] | TAGS
#transformers #safetensors #mistral #text-generation #text-generation-inference #unsloth #trl #en #base_model-unsloth/mistral-7b-bnb-4bit #license-apache-2.0 #autotrain_compatible #endpoints_compatible #region-us
|
# Uploaded model
- Developed by: kapilgaikwad
- License: apache-2.0
- Finetuned from model : unsloth/mistral-7b-bnb-4bit
This mistral model was trained 2x faster with Unsloth and Huggingface's TRL library.
<img src="URL width="200"/>
| [
"# Uploaded model\n\n- Developed by: kapilgaikwad\n- License: apache-2.0\n- Finetuned from model : unsloth/mistral-7b-bnb-4bit\n\nThis mistral model was trained 2x faster with Unsloth and Huggingface's TRL library.\n\n<img src=\"URL width=\"200\"/>"
] | [
"TAGS\n#transformers #safetensors #mistral #text-generation #text-generation-inference #unsloth #trl #en #base_model-unsloth/mistral-7b-bnb-4bit #license-apache-2.0 #autotrain_compatible #endpoints_compatible #region-us \n",
"# Uploaded model\n\n- Developed by: kapilgaikwad\n- License: apache-2.0\n- Finetuned from model : unsloth/mistral-7b-bnb-4bit\n\nThis mistral model was trained 2x faster with Unsloth and Huggingface's TRL library.\n\n<img src=\"URL width=\"200\"/>"
] |
text-generation | transformers |
> Update @ 2024.05.01: Pre-Release [Llama-3-KoEn-8B](https://huggingface.co/beomi/Llama-3-KoEn-8B-preview) model & [Llama-3-KoEn-8B-Instruct-preview](https://huggingface.co/beomi/Llama-3-KoEn-8B-Instruct-preview)
> Update @ 2024.04.24: Release Llama-3-Open-Ko-8B model & [Llama-3-Open-Ko-8B-Instruct-preview](https://huggingface.co/beomi/Llama-3-Open-Ko-8B-Instruct-preview)
## Model Details
**Llama-3-Open-Ko-8B**
Llama-3-Open-Ko-8B model is continued pretrained language model based on Llama-3-8B.
This model is trained fully with publicily available resource, with 60GB+ of deduplicated texts.
With the new Llama-3 tokenizer, the pretraining conducted with 17.7B+ tokens, which slightly more than Korean tokenizer(Llama-2-Ko tokenizer).
The train was done on TPUv5e-256, with the warm support from TRC program by Google.
**Note for [Llama-3-Open-Ko-8B-Instruct-preview](https://huggingface.co/beomi/Llama-3-Open-Ko-8B-Instruct-preview)**
With applying the idea from [Chat Vector paper](https://arxiv.org/abs/2310.04799), I released Instruction model named [Llama-3-Open-Ko-8B-Instruct-preview](https://huggingface.co/beomi/Llama-3-Open-Ko-8B-Instruct-preview).
Since it is NOT finetuned with any Korean instruction set(indeed `preview`), but it would be great starting point for creating new Chat/Instruct models.
**Meta Llama-3**
Meta developed and released the Meta Llama 3 family of large language models (LLMs), a collection of pretrained and instruction tuned generative text models in 8 and 70B sizes. The Llama 3 instruction tuned models are optimized for dialogue use cases and outperform many of the available open source chat models on common industry benchmarks. Further, in developing these models, we took great care to optimize helpfulness and safety.
**Model developers** Junbum Lee (Beomi)
**Variations** Llama-3-Open-Ko comes in one size — 8B.
**Input** Models input text only.
**Output** Models generate text and code only.
**Model Architecture** Llama 3 is an auto-regressive language model that uses an optimized transformer architecture.
<table>
<tr>
<td>
</td>
<td><strong>Training Data</strong>
</td>
<td><strong>Params</strong>
</td>
<td><strong>Context length</strong>
</td>
<td><strong>GQA</strong>
</td>
<td><strong>Token count</strong>
</td>
<td><strong>Knowledge cutoff</strong>
</td>
</tr>
<tr>
<td rowspan="2" >Llama-3-Open-Ko
</td>
<td rowspan="2" >Same as *Open-Solar-Ko Dataset
</td>
<td>8B
</td>
<td>8k
</td>
<td>Yes
</td>
<td rowspan="2" >17.7B+
</td>
<td>Jun, 2023
</td>
</tr>
</table>
*You can find dataset list here: https://huggingface.co/beomi/OPEN-SOLAR-KO-10.7B/tree/main/corpus
**Model Release Date** 2024.04.24.
**Status** This is a static model trained on an offline dataset.
**License** Llama3 License: [https://llama.meta.com/llama3/license](https://llama.meta.com/llama3/license)
## Intended Use
**Intended Use Cases** Llama 3 is intended for commercial and research use in English. Instruction tuned models are intended for assistant-like chat, whereas pretrained models can be adapted for a variety of natural language generation tasks.
**Out-of-scope** Use in any manner that violates applicable laws or regulations (including trade compliance laws). Use in any other way that is prohibited by the Acceptable Use Policy and Llama 3 Community License. Use in languages other than English**.
**Note: Developers may fine-tune Llama 3 models for languages beyond English provided they comply with the Llama 3 Community License and the Acceptable Use Policy.
## How to use
TBD
### Responsibility & Safety
We believe that an open approach to AI leads to better, safer products, faster innovation, and a bigger overall market. We are committed to Responsible AI development and took a series of steps to limit misuse and harm and support the open source community.
Foundation models are widely capable technologies that are built to be used for a diverse range of applications. They are not designed to meet every developer preference on safety levels for all use cases, out-of-the-box, as those by their nature will differ across different applications.
Rather, responsible LLM-application deployment is achieved by implementing a series of safety best practices throughout the development of such applications, from the model pre-training, fine-tuning and the deployment of systems composed of safeguards to tailor the safety needs specifically to the use case and audience.
As part of the Llama 3 release, we updated our [Responsible Use Guide](https://llama.meta.com/responsible-use-guide/) to outline the steps and best practices for developers to implement model and system level safety for their application. We also provide a set of resources including [Meta Llama Guard 2](https://llama.meta.com/purple-llama/) and [Code Shield](https://llama.meta.com/purple-llama/) safeguards. These tools have proven to drastically reduce residual risks of LLM Systems, while maintaining a high level of helpfulness. We encourage developers to tune and deploy these safeguards according to their needs and we provide a [reference implementation](https://github.com/meta-llama/llama-recipes/tree/main/recipes/responsible_ai) to get you started.
#### Responsible release
In addition to responsible use considerations outlined above, we followed a rigorous process that requires us to take extra measures against misuse and critical risks before we make our release decision.
Misuse
If you access or use Llama 3, you agree to the Acceptable Use Policy. The most recent copy of this policy can be found at [https://llama.meta.com/llama3/use-policy/](https://llama.meta.com/llama3/use-policy/).
## Ethical Considerations and Limitations
The core values of Llama 3 are openness, inclusivity and helpfulness. It is meant to serve everyone, and to work for a wide range of use cases. It is thus designed to be accessible to people across many different backgrounds, experiences and perspectives. Llama 3 addresses users and their needs as they are, without insertion unnecessary judgment or normativity, while reflecting the understanding that even content that may appear problematic in some cases can serve valuable purposes in others. It respects the dignity and autonomy of all users, especially in terms of the values of free thought and expression that power innovation and progress.
But Llama 3 is a new technology, and like any new technology, there are risks associated with its use. Testing conducted to date has been in English, and has not covered, nor could it cover, all scenarios. For these reasons, as with all LLMs, Llama 3’s potential outputs cannot be predicted in advance, and the model may in some instances produce inaccurate, biased or other objectionable responses to user prompts. Therefore, before deploying any applications of Llama 3 models, developers should perform safety testing and tuning tailored to their specific applications of the model. As outlined in the Responsible Use Guide, we recommend incorporating [Purple Llama](https://github.com/facebookresearch/PurpleLlama) solutions into your workflows and specifically [Llama Guard](https://ai.meta.com/research/publications/llama-guard-llm-based-input-output-safeguard-for-human-ai-conversations/) which provides a base model to filter input and output prompts to layer system-level safety on top of model-level safety.
Please see the Responsible Use Guide available at [http://llama.meta.com/responsible-use-guide](http://llama.meta.com/responsible-use-guide)
## Citation instructions
**Llama-3-Open-Ko**
```
@article{llama3openko,
title={Llama-3-Open-Ko},
author={L, Junbum},
year={2024},
url={https://huggingface.co/beomi/Llama-3-Open-Ko-8B}
}
```
**Original Llama-3**
```
@article{llama3modelcard,
title={Llama 3 Model Card},
author={AI@Meta},
year={2024},
url = {https://github.com/meta-llama/llama3/blob/main/MODEL_CARD.md}
}
```
| {"language": ["en", "ko"], "license": "other", "tags": ["facebook", "meta", "pytorch", "llama", "llama-3", "llama-3-ko"], "pipeline_tag": "text-generation", "license_name": "llama3", "license_link": "LICENSE"} | beomi/Llama-3-Open-Ko-8B | null | [
"transformers",
"pytorch",
"safetensors",
"llama",
"text-generation",
"facebook",
"meta",
"llama-3",
"llama-3-ko",
"conversational",
"en",
"ko",
"arxiv:2310.04799",
"license:other",
"autotrain_compatible",
"endpoints_compatible",
"text-generation-inference",
"region:us"
] | null | 2024-04-22T09:53:13+00:00 | [
"2310.04799"
] | [
"en",
"ko"
] | TAGS
#transformers #pytorch #safetensors #llama #text-generation #facebook #meta #llama-3 #llama-3-ko #conversational #en #ko #arxiv-2310.04799 #license-other #autotrain_compatible #endpoints_compatible #text-generation-inference #region-us
|
>
> Update @ 2024.05.01: Pre-Release Llama-3-KoEn-8B model & Llama-3-KoEn-8B-Instruct-preview
>
>
>
>
> Update @ 2024.04.24: Release Llama-3-Open-Ko-8B model & Llama-3-Open-Ko-8B-Instruct-preview
>
>
>
Model Details
-------------
Llama-3-Open-Ko-8B
Llama-3-Open-Ko-8B model is continued pretrained language model based on Llama-3-8B.
This model is trained fully with publicily available resource, with 60GB+ of deduplicated texts.
With the new Llama-3 tokenizer, the pretraining conducted with 17.7B+ tokens, which slightly more than Korean tokenizer(Llama-2-Ko tokenizer).
The train was done on TPUv5e-256, with the warm support from TRC program by Google.
Note for Llama-3-Open-Ko-8B-Instruct-preview
With applying the idea from Chat Vector paper, I released Instruction model named Llama-3-Open-Ko-8B-Instruct-preview.
Since it is NOT finetuned with any Korean instruction set(indeed 'preview'), but it would be great starting point for creating new Chat/Instruct models.
Meta Llama-3
Meta developed and released the Meta Llama 3 family of large language models (LLMs), a collection of pretrained and instruction tuned generative text models in 8 and 70B sizes. The Llama 3 instruction tuned models are optimized for dialogue use cases and outperform many of the available open source chat models on common industry benchmarks. Further, in developing these models, we took great care to optimize helpfulness and safety.
Model developers Junbum Lee (Beomi)
Variations Llama-3-Open-Ko comes in one size — 8B.
Input Models input text only.
Output Models generate text and code only.
Model Architecture Llama 3 is an auto-regressive language model that uses an optimized transformer architecture.
\*You can find dataset list here: URL
Model Release Date 2024.04.24.
Status This is a static model trained on an offline dataset.
License Llama3 License: URL
Intended Use
------------
Intended Use Cases Llama 3 is intended for commercial and research use in English. Instruction tuned models are intended for assistant-like chat, whereas pretrained models can be adapted for a variety of natural language generation tasks.
Out-of-scope Use in any manner that violates applicable laws or regulations (including trade compliance laws). Use in any other way that is prohibited by the Acceptable Use Policy and Llama 3 Community License. Use in languages other than English.
Note: Developers may fine-tune Llama 3 models for languages beyond English provided they comply with the Llama 3 Community License and the Acceptable Use Policy.
How to use
----------
TBD
### Responsibility & Safety
We believe that an open approach to AI leads to better, safer products, faster innovation, and a bigger overall market. We are committed to Responsible AI development and took a series of steps to limit misuse and harm and support the open source community.
Foundation models are widely capable technologies that are built to be used for a diverse range of applications. They are not designed to meet every developer preference on safety levels for all use cases, out-of-the-box, as those by their nature will differ across different applications.
Rather, responsible LLM-application deployment is achieved by implementing a series of safety best practices throughout the development of such applications, from the model pre-training, fine-tuning and the deployment of systems composed of safeguards to tailor the safety needs specifically to the use case and audience.
As part of the Llama 3 release, we updated our Responsible Use Guide to outline the steps and best practices for developers to implement model and system level safety for their application. We also provide a set of resources including Meta Llama Guard 2 and Code Shield safeguards. These tools have proven to drastically reduce residual risks of LLM Systems, while maintaining a high level of helpfulness. We encourage developers to tune and deploy these safeguards according to their needs and we provide a reference implementation to get you started.
#### Responsible release
In addition to responsible use considerations outlined above, we followed a rigorous process that requires us to take extra measures against misuse and critical risks before we make our release decision.
Misuse
If you access or use Llama 3, you agree to the Acceptable Use Policy. The most recent copy of this policy can be found at URL
Ethical Considerations and Limitations
--------------------------------------
The core values of Llama 3 are openness, inclusivity and helpfulness. It is meant to serve everyone, and to work for a wide range of use cases. It is thus designed to be accessible to people across many different backgrounds, experiences and perspectives. Llama 3 addresses users and their needs as they are, without insertion unnecessary judgment or normativity, while reflecting the understanding that even content that may appear problematic in some cases can serve valuable purposes in others. It respects the dignity and autonomy of all users, especially in terms of the values of free thought and expression that power innovation and progress.
But Llama 3 is a new technology, and like any new technology, there are risks associated with its use. Testing conducted to date has been in English, and has not covered, nor could it cover, all scenarios. For these reasons, as with all LLMs, Llama 3’s potential outputs cannot be predicted in advance, and the model may in some instances produce inaccurate, biased or other objectionable responses to user prompts. Therefore, before deploying any applications of Llama 3 models, developers should perform safety testing and tuning tailored to their specific applications of the model. As outlined in the Responsible Use Guide, we recommend incorporating Purple Llama solutions into your workflows and specifically Llama Guard which provides a base model to filter input and output prompts to layer system-level safety on top of model-level safety.
Please see the Responsible Use Guide available at URL
instructions
Llama-3-Open-Ko
Original Llama-3
| [
"### Responsibility & Safety\n\n\nWe believe that an open approach to AI leads to better, safer products, faster innovation, and a bigger overall market. We are committed to Responsible AI development and took a series of steps to limit misuse and harm and support the open source community.\n\n\nFoundation models are widely capable technologies that are built to be used for a diverse range of applications. They are not designed to meet every developer preference on safety levels for all use cases, out-of-the-box, as those by their nature will differ across different applications.\n\n\nRather, responsible LLM-application deployment is achieved by implementing a series of safety best practices throughout the development of such applications, from the model pre-training, fine-tuning and the deployment of systems composed of safeguards to tailor the safety needs specifically to the use case and audience.\n\n\nAs part of the Llama 3 release, we updated our Responsible Use Guide to outline the steps and best practices for developers to implement model and system level safety for their application. We also provide a set of resources including Meta Llama Guard 2 and Code Shield safeguards. These tools have proven to drastically reduce residual risks of LLM Systems, while maintaining a high level of helpfulness. We encourage developers to tune and deploy these safeguards according to their needs and we provide a reference implementation to get you started.",
"#### Responsible release\n\n\nIn addition to responsible use considerations outlined above, we followed a rigorous process that requires us to take extra measures against misuse and critical risks before we make our release decision.\n\n\nMisuse\n\n\nIf you access or use Llama 3, you agree to the Acceptable Use Policy. The most recent copy of this policy can be found at URL\n\n\nEthical Considerations and Limitations\n--------------------------------------\n\n\nThe core values of Llama 3 are openness, inclusivity and helpfulness. It is meant to serve everyone, and to work for a wide range of use cases. It is thus designed to be accessible to people across many different backgrounds, experiences and perspectives. Llama 3 addresses users and their needs as they are, without insertion unnecessary judgment or normativity, while reflecting the understanding that even content that may appear problematic in some cases can serve valuable purposes in others. It respects the dignity and autonomy of all users, especially in terms of the values of free thought and expression that power innovation and progress.\n\n\nBut Llama 3 is a new technology, and like any new technology, there are risks associated with its use. Testing conducted to date has been in English, and has not covered, nor could it cover, all scenarios. For these reasons, as with all LLMs, Llama 3’s potential outputs cannot be predicted in advance, and the model may in some instances produce inaccurate, biased or other objectionable responses to user prompts. Therefore, before deploying any applications of Llama 3 models, developers should perform safety testing and tuning tailored to their specific applications of the model. As outlined in the Responsible Use Guide, we recommend incorporating Purple Llama solutions into your workflows and specifically Llama Guard which provides a base model to filter input and output prompts to layer system-level safety on top of model-level safety.\n\n\nPlease see the Responsible Use Guide available at URL\n\n\ninstructions\n\n\nLlama-3-Open-Ko\n\n\nOriginal Llama-3"
] | [
"TAGS\n#transformers #pytorch #safetensors #llama #text-generation #facebook #meta #llama-3 #llama-3-ko #conversational #en #ko #arxiv-2310.04799 #license-other #autotrain_compatible #endpoints_compatible #text-generation-inference #region-us \n",
"### Responsibility & Safety\n\n\nWe believe that an open approach to AI leads to better, safer products, faster innovation, and a bigger overall market. We are committed to Responsible AI development and took a series of steps to limit misuse and harm and support the open source community.\n\n\nFoundation models are widely capable technologies that are built to be used for a diverse range of applications. They are not designed to meet every developer preference on safety levels for all use cases, out-of-the-box, as those by their nature will differ across different applications.\n\n\nRather, responsible LLM-application deployment is achieved by implementing a series of safety best practices throughout the development of such applications, from the model pre-training, fine-tuning and the deployment of systems composed of safeguards to tailor the safety needs specifically to the use case and audience.\n\n\nAs part of the Llama 3 release, we updated our Responsible Use Guide to outline the steps and best practices for developers to implement model and system level safety for their application. We also provide a set of resources including Meta Llama Guard 2 and Code Shield safeguards. These tools have proven to drastically reduce residual risks of LLM Systems, while maintaining a high level of helpfulness. We encourage developers to tune and deploy these safeguards according to their needs and we provide a reference implementation to get you started.",
"#### Responsible release\n\n\nIn addition to responsible use considerations outlined above, we followed a rigorous process that requires us to take extra measures against misuse and critical risks before we make our release decision.\n\n\nMisuse\n\n\nIf you access or use Llama 3, you agree to the Acceptable Use Policy. The most recent copy of this policy can be found at URL\n\n\nEthical Considerations and Limitations\n--------------------------------------\n\n\nThe core values of Llama 3 are openness, inclusivity and helpfulness. It is meant to serve everyone, and to work for a wide range of use cases. It is thus designed to be accessible to people across many different backgrounds, experiences and perspectives. Llama 3 addresses users and their needs as they are, without insertion unnecessary judgment or normativity, while reflecting the understanding that even content that may appear problematic in some cases can serve valuable purposes in others. It respects the dignity and autonomy of all users, especially in terms of the values of free thought and expression that power innovation and progress.\n\n\nBut Llama 3 is a new technology, and like any new technology, there are risks associated with its use. Testing conducted to date has been in English, and has not covered, nor could it cover, all scenarios. For these reasons, as with all LLMs, Llama 3’s potential outputs cannot be predicted in advance, and the model may in some instances produce inaccurate, biased or other objectionable responses to user prompts. Therefore, before deploying any applications of Llama 3 models, developers should perform safety testing and tuning tailored to their specific applications of the model. As outlined in the Responsible Use Guide, we recommend incorporating Purple Llama solutions into your workflows and specifically Llama Guard which provides a base model to filter input and output prompts to layer system-level safety on top of model-level safety.\n\n\nPlease see the Responsible Use Guide available at URL\n\n\ninstructions\n\n\nLlama-3-Open-Ko\n\n\nOriginal Llama-3"
] |
automatic-speech-recognition | transformers |
<!-- This model card has been generated automatically according to the information the Trainer had access to. You
should probably proofread and complete it, then remove this comment. -->
# Whisper Small Dv - FredDYyy
This model is a fine-tuned version of [openai/whisper-small](https://huggingface.co/openai/whisper-small) on the Common Voice 13 dataset.
It achieves the following results on the evaluation set:
- Loss: 0.1753
- Wer Ortho: 63.3540
- Wer: 13.6489
## Model description
More information needed
## Intended uses & limitations
More information needed
## Training and evaluation data
More information needed
## Training procedure
### Training hyperparameters
The following hyperparameters were used during training:
- learning_rate: 1e-05
- train_batch_size: 16
- eval_batch_size: 16
- seed: 42
- optimizer: Adam with betas=(0.9,0.999) and epsilon=1e-08
- lr_scheduler_type: constant_with_warmup
- lr_scheduler_warmup_steps: 50
- training_steps: 500
- mixed_precision_training: Native AMP
### Training results
| Training Loss | Epoch | Step | Validation Loss | Wer Ortho | Wer |
|:-------------:|:-----:|:----:|:---------------:|:---------:|:-------:|
| 0.1212 | 1.63 | 500 | 0.1753 | 63.3540 | 13.6489 |
### Framework versions
- Transformers 4.39.3
- Pytorch 2.1.2
- Datasets 2.18.0
- Tokenizers 0.15.2
| {"language": ["dv"], "license": "apache-2.0", "tags": ["generated_from_trainer"], "datasets": ["mozilla-foundation/common_voice_13_0"], "metrics": ["wer"], "base_model": "openai/whisper-small", "model-index": [{"name": "Whisper Small Dv - FredDYyy", "results": [{"task": {"type": "automatic-speech-recognition", "name": "Automatic Speech Recognition"}, "dataset": {"name": "Common Voice 13", "type": "mozilla-foundation/common_voice_13_0", "config": "dv", "split": "test", "args": "dv"}, "metrics": [{"type": "wer", "value": 13.648850714608617, "name": "Wer"}]}]}]} | FredDYyy/whisper-small-dv | null | [
"transformers",
"tensorboard",
"safetensors",
"whisper",
"automatic-speech-recognition",
"generated_from_trainer",
"dv",
"dataset:mozilla-foundation/common_voice_13_0",
"base_model:openai/whisper-small",
"license:apache-2.0",
"model-index",
"endpoints_compatible",
"has_space",
"region:us"
] | null | 2024-04-22T09:54:20+00:00 | [] | [
"dv"
] | TAGS
#transformers #tensorboard #safetensors #whisper #automatic-speech-recognition #generated_from_trainer #dv #dataset-mozilla-foundation/common_voice_13_0 #base_model-openai/whisper-small #license-apache-2.0 #model-index #endpoints_compatible #has_space #region-us
| Whisper Small Dv - FredDYyy
===========================
This model is a fine-tuned version of openai/whisper-small on the Common Voice 13 dataset.
It achieves the following results on the evaluation set:
* Loss: 0.1753
* Wer Ortho: 63.3540
* Wer: 13.6489
Model description
-----------------
More information needed
Intended uses & limitations
---------------------------
More information needed
Training and evaluation data
----------------------------
More information needed
Training procedure
------------------
### Training hyperparameters
The following hyperparameters were used during training:
* learning\_rate: 1e-05
* train\_batch\_size: 16
* eval\_batch\_size: 16
* seed: 42
* optimizer: Adam with betas=(0.9,0.999) and epsilon=1e-08
* lr\_scheduler\_type: constant\_with\_warmup
* lr\_scheduler\_warmup\_steps: 50
* training\_steps: 500
* mixed\_precision\_training: Native AMP
### Training results
### Framework versions
* Transformers 4.39.3
* Pytorch 2.1.2
* Datasets 2.18.0
* Tokenizers 0.15.2
| [
"### Training hyperparameters\n\n\nThe following hyperparameters were used during training:\n\n\n* learning\\_rate: 1e-05\n* train\\_batch\\_size: 16\n* eval\\_batch\\_size: 16\n* seed: 42\n* optimizer: Adam with betas=(0.9,0.999) and epsilon=1e-08\n* lr\\_scheduler\\_type: constant\\_with\\_warmup\n* lr\\_scheduler\\_warmup\\_steps: 50\n* training\\_steps: 500\n* mixed\\_precision\\_training: Native AMP",
"### Training results",
"### Framework versions\n\n\n* Transformers 4.39.3\n* Pytorch 2.1.2\n* Datasets 2.18.0\n* Tokenizers 0.15.2"
] | [
"TAGS\n#transformers #tensorboard #safetensors #whisper #automatic-speech-recognition #generated_from_trainer #dv #dataset-mozilla-foundation/common_voice_13_0 #base_model-openai/whisper-small #license-apache-2.0 #model-index #endpoints_compatible #has_space #region-us \n",
"### Training hyperparameters\n\n\nThe following hyperparameters were used during training:\n\n\n* learning\\_rate: 1e-05\n* train\\_batch\\_size: 16\n* eval\\_batch\\_size: 16\n* seed: 42\n* optimizer: Adam with betas=(0.9,0.999) and epsilon=1e-08\n* lr\\_scheduler\\_type: constant\\_with\\_warmup\n* lr\\_scheduler\\_warmup\\_steps: 50\n* training\\_steps: 500\n* mixed\\_precision\\_training: Native AMP",
"### Training results",
"### Framework versions\n\n\n* Transformers 4.39.3\n* Pytorch 2.1.2\n* Datasets 2.18.0\n* Tokenizers 0.15.2"
] |
image-classification | transformers |
<!-- This model card has been generated automatically according to the information the Trainer had access to. You
should probably proofread and complete it, then remove this comment. -->
# skin_cancer_classification
This model is a fine-tuned version of [google/vit-base-patch16-224-in21k](https://huggingface.co/google/vit-base-patch16-224-in21k) on the Pranavkpba2000/skin_cancer_dataset dataset.
It achieves the following results on the evaluation set:
- Loss: 0.5151
- Accuracy: 0.8260
## Model description
More information needed
## Intended uses & limitations
More information needed
## Training and evaluation data
More information needed
## Training procedure
### Training hyperparameters
The following hyperparameters were used during training:
- learning_rate: 0.0002
- train_batch_size: 32
- eval_batch_size: 16
- seed: 42
- optimizer: Adam with betas=(0.9,0.999) and epsilon=1e-08
- lr_scheduler_type: linear
- num_epochs: 10
### Training results
| Training Loss | Epoch | Step | Validation Loss | Accuracy |
|:-------------:|:-----:|:----:|:---------------:|:--------:|
| 0.8727 | 0.11 | 100 | 0.9507 | 0.6690 |
| 0.7811 | 0.22 | 200 | 0.8702 | 0.6843 |
| 0.7222 | 0.34 | 300 | 0.7517 | 0.7319 |
| 0.7191 | 0.45 | 400 | 0.7081 | 0.7474 |
| 0.638 | 0.56 | 500 | 0.6894 | 0.7407 |
| 0.6001 | 0.67 | 600 | 0.7046 | 0.7565 |
| 0.5818 | 0.78 | 700 | 0.7262 | 0.7422 |
| 0.5796 | 0.9 | 800 | 0.6466 | 0.7681 |
| 0.4426 | 1.01 | 900 | 0.6218 | 0.7730 |
| 0.4675 | 1.12 | 1000 | 0.6566 | 0.7569 |
| 0.4604 | 1.23 | 1100 | 0.5782 | 0.7938 |
| 0.4426 | 1.35 | 1200 | 0.5478 | 0.7996 |
| 0.3967 | 1.46 | 1300 | 0.5586 | 0.8001 |
| 0.4287 | 1.57 | 1400 | 0.5743 | 0.7948 |
| 0.4066 | 1.68 | 1500 | 0.5839 | 0.7980 |
| 0.4107 | 1.79 | 1600 | 0.5630 | 0.8032 |
| 0.3894 | 1.91 | 1700 | 0.5591 | 0.8004 |
| 0.2612 | 2.02 | 1800 | 0.5176 | 0.8173 |
| 0.346 | 2.13 | 1900 | 0.5796 | 0.7986 |
| 0.2242 | 2.24 | 2000 | 0.5196 | 0.8239 |
| 0.2262 | 2.35 | 2100 | 0.5568 | 0.8103 |
| 0.2764 | 2.47 | 2200 | 0.5428 | 0.8156 |
| 0.2795 | 2.58 | 2300 | 0.5354 | 0.8234 |
| 0.3017 | 2.69 | 2400 | 0.5231 | 0.8197 |
| 0.3295 | 2.8 | 2500 | 0.5550 | 0.8120 |
| 0.3014 | 2.91 | 2600 | 0.5151 | 0.8260 |
| 0.1332 | 3.03 | 2700 | 0.5651 | 0.8182 |
| 0.1519 | 3.14 | 2800 | 0.5824 | 0.8277 |
| 0.1537 | 3.25 | 2900 | 0.6195 | 0.8281 |
| 0.187 | 3.36 | 3000 | 0.6482 | 0.8204 |
| 0.1428 | 3.48 | 3100 | 0.5512 | 0.8463 |
| 0.1743 | 3.59 | 3200 | 0.6152 | 0.8252 |
| 0.1765 | 3.7 | 3300 | 0.5390 | 0.8418 |
| 0.1612 | 3.81 | 3400 | 0.5550 | 0.8279 |
| 0.0938 | 3.92 | 3500 | 0.6105 | 0.8380 |
| 0.0698 | 4.04 | 3600 | 0.6672 | 0.8273 |
| 0.1087 | 4.15 | 3700 | 0.6583 | 0.8184 |
| 0.0558 | 4.26 | 3800 | 0.6141 | 0.8304 |
| 0.0841 | 4.37 | 3900 | 0.6157 | 0.8377 |
| 0.0662 | 4.48 | 4000 | 0.7013 | 0.8289 |
| 0.0883 | 4.6 | 4100 | 0.7606 | 0.8180 |
| 0.0721 | 4.71 | 4200 | 0.6966 | 0.8322 |
| 0.0332 | 4.82 | 4300 | 0.7743 | 0.8189 |
| 0.0994 | 4.93 | 4400 | 0.6767 | 0.8303 |
| 0.0362 | 5.04 | 4500 | 0.7643 | 0.8321 |
| 0.0507 | 5.16 | 4600 | 0.8140 | 0.8348 |
| 0.0322 | 5.27 | 4700 | 0.7166 | 0.8397 |
| 0.0219 | 5.38 | 4800 | 0.7363 | 0.8339 |
| 0.0346 | 5.49 | 4900 | 0.7343 | 0.8435 |
| 0.0638 | 5.61 | 5000 | 0.7396 | 0.8441 |
| 0.1143 | 5.72 | 5100 | 0.8020 | 0.8338 |
| 0.024 | 5.83 | 5200 | 0.7715 | 0.8446 |
| 0.0203 | 5.94 | 5300 | 0.7485 | 0.8439 |
| 0.0172 | 6.05 | 5400 | 0.7893 | 0.8467 |
| 0.0115 | 6.17 | 5500 | 0.7671 | 0.8505 |
| 0.0628 | 6.28 | 5600 | 0.7690 | 0.8477 |
| 0.0475 | 6.39 | 5700 | 0.7534 | 0.8488 |
| 0.0193 | 6.5 | 5800 | 0.8026 | 0.8497 |
| 0.0163 | 6.61 | 5900 | 0.7703 | 0.8562 |
| 0.0232 | 6.73 | 6000 | 0.8823 | 0.8471 |
| 0.0157 | 6.84 | 6100 | 0.7953 | 0.8435 |
| 0.0211 | 6.95 | 6200 | 0.8299 | 0.8391 |
| 0.0007 | 7.06 | 6300 | 0.7862 | 0.8532 |
| 0.0085 | 7.17 | 6400 | 0.8090 | 0.8548 |
| 0.0153 | 7.29 | 6500 | 0.8183 | 0.8576 |
| 0.0031 | 7.4 | 6600 | 0.8853 | 0.8425 |
| 0.0112 | 7.51 | 6700 | 0.8415 | 0.8574 |
| 0.0012 | 7.62 | 6800 | 0.8442 | 0.8569 |
| 0.0015 | 7.74 | 6900 | 0.8475 | 0.8564 |
| 0.0043 | 7.85 | 7000 | 0.8748 | 0.8452 |
| 0.0099 | 7.96 | 7100 | 0.8873 | 0.8411 |
| 0.0008 | 8.07 | 7200 | 0.8584 | 0.8559 |
| 0.0004 | 8.18 | 7300 | 0.8598 | 0.8522 |
| 0.0004 | 8.3 | 7400 | 0.8667 | 0.8536 |
| 0.0003 | 8.41 | 7500 | 0.8667 | 0.8576 |
| 0.0073 | 8.52 | 7600 | 0.8736 | 0.8602 |
| 0.0003 | 8.63 | 7700 | 0.8760 | 0.8566 |
| 0.0007 | 8.74 | 7800 | 0.9347 | 0.8540 |
| 0.0073 | 8.86 | 7900 | 0.9070 | 0.8532 |
| 0.0002 | 8.97 | 8000 | 0.9208 | 0.8536 |
| 0.0002 | 9.08 | 8100 | 0.9304 | 0.8555 |
| 0.0003 | 9.19 | 8200 | 0.9301 | 0.8546 |
| 0.0002 | 9.3 | 8300 | 0.9298 | 0.8557 |
| 0.0002 | 9.42 | 8400 | 0.9300 | 0.8557 |
| 0.0003 | 9.53 | 8500 | 0.9283 | 0.8560 |
| 0.0002 | 9.64 | 8600 | 0.9205 | 0.8562 |
| 0.0002 | 9.75 | 8700 | 0.9216 | 0.8566 |
| 0.0002 | 9.87 | 8800 | 0.9204 | 0.8556 |
| 0.0002 | 9.98 | 8900 | 0.9207 | 0.8555 |
### Framework versions
- Transformers 4.39.3
- Pytorch 2.1.2
- Datasets 2.18.0
- Tokenizers 0.15.2
| {"license": "apache-2.0", "tags": ["image-classification", "generated_from_trainer"], "metrics": ["accuracy"], "base_model": "google/vit-base-patch16-224-in21k", "model-index": [{"name": "skin_cancer_classification", "results": []}]} | karoladelk/skin_cancer_classification | null | [
"transformers",
"tensorboard",
"safetensors",
"vit",
"image-classification",
"generated_from_trainer",
"base_model:google/vit-base-patch16-224-in21k",
"license:apache-2.0",
"autotrain_compatible",
"endpoints_compatible",
"region:us"
] | null | 2024-04-22T09:55:58+00:00 | [] | [] | TAGS
#transformers #tensorboard #safetensors #vit #image-classification #generated_from_trainer #base_model-google/vit-base-patch16-224-in21k #license-apache-2.0 #autotrain_compatible #endpoints_compatible #region-us
| skin\_cancer\_classification
============================
This model is a fine-tuned version of google/vit-base-patch16-224-in21k on the Pranavkpba2000/skin\_cancer\_dataset dataset.
It achieves the following results on the evaluation set:
* Loss: 0.5151
* Accuracy: 0.8260
Model description
-----------------
More information needed
Intended uses & limitations
---------------------------
More information needed
Training and evaluation data
----------------------------
More information needed
Training procedure
------------------
### Training hyperparameters
The following hyperparameters were used during training:
* learning\_rate: 0.0002
* train\_batch\_size: 32
* eval\_batch\_size: 16
* seed: 42
* optimizer: Adam with betas=(0.9,0.999) and epsilon=1e-08
* lr\_scheduler\_type: linear
* num\_epochs: 10
### Training results
### Framework versions
* Transformers 4.39.3
* Pytorch 2.1.2
* Datasets 2.18.0
* Tokenizers 0.15.2
| [
"### Training hyperparameters\n\n\nThe following hyperparameters were used during training:\n\n\n* learning\\_rate: 0.0002\n* train\\_batch\\_size: 32\n* eval\\_batch\\_size: 16\n* seed: 42\n* optimizer: Adam with betas=(0.9,0.999) and epsilon=1e-08\n* lr\\_scheduler\\_type: linear\n* num\\_epochs: 10",
"### Training results",
"### Framework versions\n\n\n* Transformers 4.39.3\n* Pytorch 2.1.2\n* Datasets 2.18.0\n* Tokenizers 0.15.2"
] | [
"TAGS\n#transformers #tensorboard #safetensors #vit #image-classification #generated_from_trainer #base_model-google/vit-base-patch16-224-in21k #license-apache-2.0 #autotrain_compatible #endpoints_compatible #region-us \n",
"### Training hyperparameters\n\n\nThe following hyperparameters were used during training:\n\n\n* learning\\_rate: 0.0002\n* train\\_batch\\_size: 32\n* eval\\_batch\\_size: 16\n* seed: 42\n* optimizer: Adam with betas=(0.9,0.999) and epsilon=1e-08\n* lr\\_scheduler\\_type: linear\n* num\\_epochs: 10",
"### Training results",
"### Framework versions\n\n\n* Transformers 4.39.3\n* Pytorch 2.1.2\n* Datasets 2.18.0\n* Tokenizers 0.15.2"
] |
text-to-image | diffusers |
# Model Card for Model ID
<!-- Provide a quick summary of what the model is/does. -->
## Model Details
### Model Description
<!-- Provide a longer summary of what this model is. -->
This is the model card of a 🧨 diffusers model that has been pushed on the Hub. This model card has been automatically generated.
- **Developed by:** [More Information Needed]
- **Funded by [optional]:** [More Information Needed]
- **Shared by [optional]:** [More Information Needed]
- **Model type:** [More Information Needed]
- **Language(s) (NLP):** [More Information Needed]
- **License:** [More Information Needed]
- **Finetuned from model [optional]:** [More Information Needed]
### Model Sources [optional]
<!-- Provide the basic links for the model. -->
- **Repository:** [More Information Needed]
- **Paper [optional]:** [More Information Needed]
- **Demo [optional]:** [More Information Needed]
## Uses
<!-- Address questions around how the model is intended to be used, including the foreseeable users of the model and those affected by the model. -->
### Direct Use
<!-- This section is for the model use without fine-tuning or plugging into a larger ecosystem/app. -->
[More Information Needed]
### Downstream Use [optional]
<!-- This section is for the model use when fine-tuned for a task, or when plugged into a larger ecosystem/app -->
[More Information Needed]
### Out-of-Scope Use
<!-- This section addresses misuse, malicious use, and uses that the model will not work well for. -->
[More Information Needed]
## Bias, Risks, and Limitations
<!-- This section is meant to convey both technical and sociotechnical limitations. -->
[More Information Needed]
### Recommendations
<!-- This section is meant to convey recommendations with respect to the bias, risk, and technical limitations. -->
Users (both direct and downstream) should be made aware of the risks, biases and limitations of the model. More information needed for further recommendations.
## How to Get Started with the Model
Use the code below to get started with the model.
[More Information Needed]
## Training Details
### Training Data
<!-- This should link to a Dataset Card, perhaps with a short stub of information on what the training data is all about as well as documentation related to data pre-processing or additional filtering. -->
[More Information Needed]
### Training Procedure
<!-- This relates heavily to the Technical Specifications. Content here should link to that section when it is relevant to the training procedure. -->
#### Preprocessing [optional]
[More Information Needed]
#### Training Hyperparameters
- **Training regime:** [More Information Needed] <!--fp32, fp16 mixed precision, bf16 mixed precision, bf16 non-mixed precision, fp16 non-mixed precision, fp8 mixed precision -->
#### Speeds, Sizes, Times [optional]
<!-- This section provides information about throughput, start/end time, checkpoint size if relevant, etc. -->
[More Information Needed]
## Evaluation
<!-- This section describes the evaluation protocols and provides the results. -->
### Testing Data, Factors & Metrics
#### Testing Data
<!-- This should link to a Dataset Card if possible. -->
[More Information Needed]
#### Factors
<!-- These are the things the evaluation is disaggregating by, e.g., subpopulations or domains. -->
[More Information Needed]
#### Metrics
<!-- These are the evaluation metrics being used, ideally with a description of why. -->
[More Information Needed]
### Results
[More Information Needed]
#### Summary
## Model Examination [optional]
<!-- Relevant interpretability work for the model goes here -->
[More Information Needed]
## Environmental Impact
<!-- Total emissions (in grams of CO2eq) and additional considerations, such as electricity usage, go here. Edit the suggested text below accordingly -->
Carbon emissions can be estimated using the [Machine Learning Impact calculator](https://mlco2.github.io/impact#compute) presented in [Lacoste et al. (2019)](https://arxiv.org/abs/1910.09700).
- **Hardware Type:** [More Information Needed]
- **Hours used:** [More Information Needed]
- **Cloud Provider:** [More Information Needed]
- **Compute Region:** [More Information Needed]
- **Carbon Emitted:** [More Information Needed]
## Technical Specifications [optional]
### Model Architecture and Objective
[More Information Needed]
### Compute Infrastructure
[More Information Needed]
#### Hardware
[More Information Needed]
#### Software
[More Information Needed]
## Citation [optional]
<!-- If there is a paper or blog post introducing the model, the APA and Bibtex information for that should go in this section. -->
**BibTeX:**
[More Information Needed]
**APA:**
[More Information Needed]
## Glossary [optional]
<!-- If relevant, include terms and calculations in this section that can help readers understand the model or model card. -->
[More Information Needed]
## More Information [optional]
[More Information Needed]
## Model Card Authors [optional]
[More Information Needed]
## Model Card Contact
[More Information Needed] | {"library_name": "diffusers"} | Niggendar/omegaPonyXLAnime_v10 | null | [
"diffusers",
"safetensors",
"arxiv:1910.09700",
"endpoints_compatible",
"diffusers:StableDiffusionXLPipeline",
"region:us"
] | null | 2024-04-22T09:56:52+00:00 | [
"1910.09700"
] | [] | TAGS
#diffusers #safetensors #arxiv-1910.09700 #endpoints_compatible #diffusers-StableDiffusionXLPipeline #region-us
|
# Model Card for Model ID
## Model Details
### Model Description
This is the model card of a diffusers model that has been pushed on the Hub. This model card has been automatically generated.
- Developed by:
- Funded by [optional]:
- Shared by [optional]:
- Model type:
- Language(s) (NLP):
- License:
- Finetuned from model [optional]:
### Model Sources [optional]
- Repository:
- Paper [optional]:
- Demo [optional]:
## Uses
### Direct Use
### Downstream Use [optional]
### Out-of-Scope Use
## Bias, Risks, and Limitations
### Recommendations
Users (both direct and downstream) should be made aware of the risks, biases and limitations of the model. More information needed for further recommendations.
## How to Get Started with the Model
Use the code below to get started with the model.
## Training Details
### Training Data
### Training Procedure
#### Preprocessing [optional]
#### Training Hyperparameters
- Training regime:
#### Speeds, Sizes, Times [optional]
## Evaluation
### Testing Data, Factors & Metrics
#### Testing Data
#### Factors
#### Metrics
### Results
#### Summary
## Model Examination [optional]
## Environmental Impact
Carbon emissions can be estimated using the Machine Learning Impact calculator presented in Lacoste et al. (2019).
- Hardware Type:
- Hours used:
- Cloud Provider:
- Compute Region:
- Carbon Emitted:
## Technical Specifications [optional]
### Model Architecture and Objective
### Compute Infrastructure
#### Hardware
#### Software
[optional]
BibTeX:
APA:
## Glossary [optional]
## More Information [optional]
## Model Card Authors [optional]
## Model Card Contact
| [
"# Model Card for Model ID",
"## Model Details",
"### Model Description\n\n\n\nThis is the model card of a diffusers model that has been pushed on the Hub. This model card has been automatically generated.\n\n- Developed by: \n- Funded by [optional]: \n- Shared by [optional]: \n- Model type: \n- Language(s) (NLP): \n- License: \n- Finetuned from model [optional]:",
"### Model Sources [optional]\n\n\n\n- Repository: \n- Paper [optional]: \n- Demo [optional]:",
"## Uses",
"### Direct Use",
"### Downstream Use [optional]",
"### Out-of-Scope Use",
"## Bias, Risks, and Limitations",
"### Recommendations\n\n\n\nUsers (both direct and downstream) should be made aware of the risks, biases and limitations of the model. More information needed for further recommendations.",
"## How to Get Started with the Model\n\nUse the code below to get started with the model.",
"## Training Details",
"### Training Data",
"### Training Procedure",
"#### Preprocessing [optional]",
"#### Training Hyperparameters\n\n- Training regime:",
"#### Speeds, Sizes, Times [optional]",
"## Evaluation",
"### Testing Data, Factors & Metrics",
"#### Testing Data",
"#### Factors",
"#### Metrics",
"### Results",
"#### Summary",
"## Model Examination [optional]",
"## Environmental Impact\n\n\n\nCarbon emissions can be estimated using the Machine Learning Impact calculator presented in Lacoste et al. (2019).\n\n- Hardware Type: \n- Hours used: \n- Cloud Provider: \n- Compute Region: \n- Carbon Emitted:",
"## Technical Specifications [optional]",
"### Model Architecture and Objective",
"### Compute Infrastructure",
"#### Hardware",
"#### Software\n\n\n\n[optional]\n\n\n\nBibTeX:\n\n\n\nAPA:",
"## Glossary [optional]",
"## More Information [optional]",
"## Model Card Authors [optional]",
"## Model Card Contact"
] | [
"TAGS\n#diffusers #safetensors #arxiv-1910.09700 #endpoints_compatible #diffusers-StableDiffusionXLPipeline #region-us \n",
"# Model Card for Model ID",
"## Model Details",
"### Model Description\n\n\n\nThis is the model card of a diffusers model that has been pushed on the Hub. This model card has been automatically generated.\n\n- Developed by: \n- Funded by [optional]: \n- Shared by [optional]: \n- Model type: \n- Language(s) (NLP): \n- License: \n- Finetuned from model [optional]:",
"### Model Sources [optional]\n\n\n\n- Repository: \n- Paper [optional]: \n- Demo [optional]:",
"## Uses",
"### Direct Use",
"### Downstream Use [optional]",
"### Out-of-Scope Use",
"## Bias, Risks, and Limitations",
"### Recommendations\n\n\n\nUsers (both direct and downstream) should be made aware of the risks, biases and limitations of the model. More information needed for further recommendations.",
"## How to Get Started with the Model\n\nUse the code below to get started with the model.",
"## Training Details",
"### Training Data",
"### Training Procedure",
"#### Preprocessing [optional]",
"#### Training Hyperparameters\n\n- Training regime:",
"#### Speeds, Sizes, Times [optional]",
"## Evaluation",
"### Testing Data, Factors & Metrics",
"#### Testing Data",
"#### Factors",
"#### Metrics",
"### Results",
"#### Summary",
"## Model Examination [optional]",
"## Environmental Impact\n\n\n\nCarbon emissions can be estimated using the Machine Learning Impact calculator presented in Lacoste et al. (2019).\n\n- Hardware Type: \n- Hours used: \n- Cloud Provider: \n- Compute Region: \n- Carbon Emitted:",
"## Technical Specifications [optional]",
"### Model Architecture and Objective",
"### Compute Infrastructure",
"#### Hardware",
"#### Software\n\n\n\n[optional]\n\n\n\nBibTeX:\n\n\n\nAPA:",
"## Glossary [optional]",
"## More Information [optional]",
"## Model Card Authors [optional]",
"## Model Card Contact"
] |
null | scvi-tools |
# Description
scVI model trained on synthetic IID data and uploaded with the full training data.
# Model properties
Many model properties are in the model tags. Some more are listed below.
**model_init_params**:
```json
{
"n_hidden": 128,
"n_latent": 10,
"n_layers": 1,
"dropout_rate": 0.1,
"dispersion": "gene",
"gene_likelihood": "zinb",
"latent_distribution": "normal"
}
```
**model_setup_anndata_args**:
```json
{
"layer": null,
"batch_key": null,
"labels_key": null,
"size_factor_key": null,
"categorical_covariate_keys": null,
"continuous_covariate_keys": null
}
```
**model_summary_stats**:
| Summary Stat Key | Value |
|--------------------------|-------|
| n_batch | 1 |
| n_cells | 400 |
| n_extra_categorical_covs | 0 |
| n_extra_continuous_covs | 0 |
| n_labels | 1 |
| n_vars | 100 |
**model_data_registry**:
| Registry Key | scvi-tools Location |
|--------------|---------------------------|
| X | adata.X |
| batch | adata.obs['_scvi_batch'] |
| labels | adata.obs['_scvi_labels'] |
**model_parent_module**: scvi.model
**data_is_minified**: False
# Training data
This is an optional link to where the training data is stored if it is too large
to host on the huggingface Model hub.
<!-- If your model is not uploaded with any data (e.g., minified data) on the Model Hub, then make
sure to provide this field if you want users to be able to access your training data. See the
scvi-tools documentation for details. -->
Training data url: N/A
# Training code
This is an optional link to the code used to train the model.
Training code url: N/A
# References
To be added... | {"license": "cc-by-4.0", "library_name": "scvi-tools", "tags": ["biology", "genomics", "single-cell", "model_cls_name:SCVI", "scvi_version:1.1.3", "anndata_version:0.10.7", "modality:rna", "annotated:False"]} | scvi-tools/test-scvi-create | null | [
"scvi-tools",
"biology",
"genomics",
"single-cell",
"model_cls_name:SCVI",
"scvi_version:1.1.3",
"anndata_version:0.10.7",
"modality:rna",
"annotated:False",
"license:cc-by-4.0",
"region:us"
] | null | 2024-04-22T10:01:40+00:00 | [] | [] | TAGS
#scvi-tools #biology #genomics #single-cell #model_cls_name-SCVI #scvi_version-1.1.3 #anndata_version-0.10.7 #modality-rna #annotated-False #license-cc-by-4.0 #region-us
| Description
===========
scVI model trained on synthetic IID data and uploaded with the full training data.
Model properties
================
Many model properties are in the model tags. Some more are listed below.
model\_init\_params:
model\_setup\_anndata\_args:
model\_summary\_stats:
model\_data\_registry:
model\_parent\_module: URL
data\_is\_minified: False
Training data
=============
This is an optional link to where the training data is stored if it is too large
to host on the huggingface Model hub.
Training data url: N/A
Training code
=============
This is an optional link to the code used to train the model.
Training code url: N/A
References
==========
To be added...
| [] | [
"TAGS\n#scvi-tools #biology #genomics #single-cell #model_cls_name-SCVI #scvi_version-1.1.3 #anndata_version-0.10.7 #modality-rna #annotated-False #license-cc-by-4.0 #region-us \n"
] |
null | null |
# NeuralsynthesisMulti_verse_model-7B
NeuralsynthesisMulti_verse_model-7B is an automated merge created by [Maxime Labonne](https://huggingface.co/mlabonne) using the following configuration.
## 🧩 Configuration
```yaml
models:
- model: mistralai/Mistral-7B-v0.1
- model: Kukedlc/NeuralSynthesis-7b-v0.4-slerp
- model: MTSAIR/multi_verse_model
merge_method: model_stock
base_model: mistralai/Mistral-7B-v0.1
dtype: bfloat16
```
## 💻 Usage
```python
!pip install -qU transformers accelerate
from transformers import AutoTokenizer
import transformers
import torch
model = "automerger/NeuralsynthesisMulti_verse_model-7B"
messages = [{"role": "user", "content": "What is a large language model?"}]
tokenizer = AutoTokenizer.from_pretrained(model)
prompt = tokenizer.apply_chat_template(messages, tokenize=False, add_generation_prompt=True)
pipeline = transformers.pipeline(
"text-generation",
model=model,
torch_dtype=torch.float16,
device_map="auto",
)
outputs = pipeline(prompt, max_new_tokens=256, do_sample=True, temperature=0.7, top_k=50, top_p=0.95)
print(outputs[0]["generated_text"])
``` | {"license": "apache-2.0", "tags": ["merge", "mergekit", "lazymergekit", "automerger"]} | automerger/NeuralsynthesisMulti_verse_model-7B | null | [
"merge",
"mergekit",
"lazymergekit",
"automerger",
"license:apache-2.0",
"region:us"
] | null | 2024-04-22T10:01:45+00:00 | [] | [] | TAGS
#merge #mergekit #lazymergekit #automerger #license-apache-2.0 #region-us
|
# NeuralsynthesisMulti_verse_model-7B
NeuralsynthesisMulti_verse_model-7B is an automated merge created by Maxime Labonne using the following configuration.
## Configuration
## Usage
| [
"# NeuralsynthesisMulti_verse_model-7B\n\nNeuralsynthesisMulti_verse_model-7B is an automated merge created by Maxime Labonne using the following configuration.",
"## Configuration",
"## Usage"
] | [
"TAGS\n#merge #mergekit #lazymergekit #automerger #license-apache-2.0 #region-us \n",
"# NeuralsynthesisMulti_verse_model-7B\n\nNeuralsynthesisMulti_verse_model-7B is an automated merge created by Maxime Labonne using the following configuration.",
"## Configuration",
"## Usage"
] |
sentence-similarity | sentence-transformers | ---
# gte-micro-v2
This is a distill of [gte-tiny](https://huggingface.co/TaylorAI/gte-tiny).
## Intended purpose
<span style="color:blue">This model is designed for use in semantic-autocomplete ([click here for demo](https://mihaiii.github.io/semantic-autocomplete/)).</span>
## Usage (Sentence-Transformers) (same as [gte-tiny](https://huggingface.co/TaylorAI/gte-tiny))
Using this model becomes easy when you have [sentence-transformers](https://www.SBERT.net) installed:
```
pip install -U sentence-transformers
```
Then you can use the model like this:
```python
from sentence_transformers import SentenceTransformer
sentences = ["This is an example sentence", "Each sentence is converted"]
model = SentenceTransformer('Mihaiii/gte-micro-v2')
embeddings = model.encode(sentences)
print(embeddings)
```
## Usage (HuggingFace Transformers) (same as [gte-tiny](https://huggingface.co/TaylorAI/gte-tiny))
Without [sentence-transformers](https://www.SBERT.net), you can use the model like this: First, you pass your input through the transformer model, then you have to apply the right pooling-operation on-top of the contextualized word embeddings.
```python
from transformers import AutoTokenizer, AutoModel
import torch
#Mean Pooling - Take attention mask into account for correct averaging
def mean_pooling(model_output, attention_mask):
token_embeddings = model_output[0] #First element of model_output contains all token embeddings
input_mask_expanded = attention_mask.unsqueeze(-1).expand(token_embeddings.size()).float()
return torch.sum(token_embeddings * input_mask_expanded, 1) / torch.clamp(input_mask_expanded.sum(1), min=1e-9)
# Sentences we want sentence embeddings for
sentences = ['This is an example sentence', 'Each sentence is converted']
# Load model from HuggingFace Hub
tokenizer = AutoTokenizer.from_pretrained('Mihaiii/gte-micro-v2')
model = AutoModel.from_pretrained('Mihaiii/gte-micro-v2')
# Tokenize sentences
encoded_input = tokenizer(sentences, padding=True, truncation=True, return_tensors='pt')
# Compute token embeddings
with torch.no_grad():
model_output = model(**encoded_input)
# Perform pooling. In this case, mean pooling.
sentence_embeddings = mean_pooling(model_output, encoded_input['attention_mask'])
print("Sentence embeddings:")
print(sentence_embeddings)
```
### Limitation (same as [gte-small](https://huggingface.co/thenlper/gte-small))
This model exclusively caters to English texts, and any lengthy texts will be truncated to a maximum of 512 tokens. | {"license": "mit", "library_name": "sentence-transformers", "tags": ["sentence-transformers", "feature-extraction", "sentence-similarity", "gte", "mteb"], "pipeline_tag": "sentence-similarity", "model-index": [{"name": "gte-micro-test", "results": [{"task": {"type": "Classification"}, "dataset": {"name": "MTEB AmazonCounterfactualClassification (en)", "type": "mteb/amazon_counterfactual", "config": "en", "split": "test", "revision": "e8379541af4e31359cca9fbcf4b00f2671dba205"}, "metrics": [{"type": "accuracy", "value": 71.43283582089552}, {"type": "ap", "value": 33.56235301308992}, {"type": "f1", "value": 65.18510976313922}]}, {"task": {"type": "Classification"}, "dataset": {"name": "MTEB AmazonPolarityClassification", "type": "mteb/amazon_polarity", "config": "default", "split": "test", "revision": "e2d317d38cd51312af73b3d32a06d1a08b442046"}, "metrics": [{"type": "accuracy", "value": 77.72055}, {"type": "ap", "value": 72.30281215701287}, {"type": "f1", "value": 77.62429097469116}]}, {"task": {"type": "Classification"}, "dataset": {"name": "MTEB AmazonReviewsClassification (en)", "type": "mteb/amazon_reviews_multi", "config": "en", "split": "test", "revision": "1399c76144fd37290681b995c656ef9b2e06e26d"}, "metrics": [{"type": "accuracy", "value": 38.956}, {"type": "f1", "value": 38.59075995638611}]}, {"task": {"type": "Clustering"}, "dataset": {"name": "MTEB ArxivClusteringP2P", "type": "mteb/arxiv-clustering-p2p", "config": "default", "split": "test", "revision": "a122ad7f3f0291bf49cc6f4d32aa80929df69d5d"}, "metrics": [{"type": "v_measure", "value": 41.14317775707504}]}, {"task": {"type": "Clustering"}, "dataset": {"name": "MTEB ArxivClusteringS2S", "type": "mteb/arxiv-clustering-s2s", "config": "default", "split": "test", "revision": "f910caf1a6075f7329cdf8c1a6135696f37dbd53"}, "metrics": [{"type": "v_measure", "value": 31.79440862639374}]}, {"task": {"type": "Classification"}, "dataset": {"name": "MTEB Banking77Classification", "type": "mteb/banking77", "config": "default", "split": "test", "revision": "0fd18e25b25c072e09e0d92ab615fda904d66300"}, "metrics": [{"type": "accuracy", "value": 80.40259740259741}, {"type": "f1", "value": 80.33885811790022}]}, {"task": {"type": "Classification"}, "dataset": {"name": "MTEB EmotionClassification", "type": "mteb/emotion", "config": "default", "split": "test", "revision": "4f58c6b202a23cf9a4da393831edf4f9183cad37"}, "metrics": [{"type": "accuracy", "value": 44.54}, {"type": "f1", "value": 39.40201192446353}]}, {"task": {"type": "Classification"}, "dataset": {"name": "MTEB ImdbClassification", "type": "mteb/imdb", "config": "default", "split": "test", "revision": "3d86128a09e091d6018b6d26cad27f2739fc2db7"}, "metrics": [{"type": "accuracy", "value": 70.5904}, {"type": "ap", "value": 64.61751544665012}, {"type": "f1", "value": 70.47776028292148}]}, {"task": {"type": "Classification"}, "dataset": {"name": "MTEB MTOPDomainClassification (en)", "type": "mteb/mtop_domain", "config": "en", "split": "test", "revision": "d80d48c1eb48d3562165c59d59d0034df9fff0bf"}, "metrics": [{"type": "accuracy", "value": 90.49703602371181}, {"type": "f1", "value": 90.05253119123799}]}, {"task": {"type": "Classification"}, "dataset": {"name": "MTEB MTOPIntentClassification (en)", "type": "mteb/mtop_intent", "config": "en", "split": "test", "revision": "ae001d0e6b1228650b7bd1c2c65fb50ad11a8aba"}, "metrics": [{"type": "accuracy", "value": 67.52393980848153}, {"type": "f1", "value": 49.95609666042009}]}, {"task": {"type": "Classification"}, "dataset": {"name": "MTEB MassiveIntentClassification (en)", "type": "mteb/amazon_massive_intent", "config": "en", "split": "test", "revision": "31efe3c427b0bae9c22cbb560b8f15491cc6bed7"}, "metrics": [{"type": "accuracy", "value": 68.4969737726967}, {"type": "f1", "value": 66.32116772424203}]}, {"task": {"type": "Classification"}, "dataset": {"name": "MTEB MassiveScenarioClassification (en)", "type": "mteb/amazon_massive_scenario", "config": "en", "split": "test", "revision": "7d571f92784cd94a019292a1f45445077d0ef634"}, "metrics": [{"type": "accuracy", "value": 73.54741089441829}, {"type": "f1", "value": 73.47537036064044}]}, {"task": {"type": "Classification"}, "dataset": {"name": "MTEB ToxicConversationsClassification", "type": "mteb/toxic_conversations_50k", "config": "default", "split": "test", "revision": "edfaf9da55d3dd50d43143d90c1ac476895ae6de"}, "metrics": [{"type": "accuracy", "value": 66.6912}, {"type": "ap", "value": 12.157396278930436}, {"type": "f1", "value": 51.00574525406295}]}, {"task": {"type": "Classification"}, "dataset": {"name": "MTEB TweetSentimentExtractionClassification", "type": "mteb/tweet_sentiment_extraction", "config": "default", "split": "test", "revision": "d604517c81ca91fe16a244d1248fc021f9ecee7a"}, "metrics": [{"type": "accuracy", "value": 59.29258630447085}, {"type": "f1", "value": 59.6485358241374}]}]}]} | Mihaiii/gte-micro-v2 | null | [
"sentence-transformers",
"onnx",
"safetensors",
"bert",
"feature-extraction",
"sentence-similarity",
"gte",
"mteb",
"license:mit",
"model-index",
"endpoints_compatible",
"region:us"
] | null | 2024-04-22T10:01:50+00:00 | [] | [] | TAGS
#sentence-transformers #onnx #safetensors #bert #feature-extraction #sentence-similarity #gte #mteb #license-mit #model-index #endpoints_compatible #region-us
| ---
# gte-micro-v2
This is a distill of gte-tiny.
## Intended purpose
<span style="color:blue">This model is designed for use in semantic-autocomplete (click here for demo).</span>
## Usage (Sentence-Transformers) (same as gte-tiny)
Using this model becomes easy when you have sentence-transformers installed:
Then you can use the model like this:
## Usage (HuggingFace Transformers) (same as gte-tiny)
Without sentence-transformers, you can use the model like this: First, you pass your input through the transformer model, then you have to apply the right pooling-operation on-top of the contextualized word embeddings.
### Limitation (same as gte-small)
This model exclusively caters to English texts, and any lengthy texts will be truncated to a maximum of 512 tokens. | [
"# gte-micro-v2\n\nThis is a distill of gte-tiny.",
"## Intended purpose\n\n<span style=\"color:blue\">This model is designed for use in semantic-autocomplete (click here for demo).</span>",
"## Usage (Sentence-Transformers) (same as gte-tiny)\n\nUsing this model becomes easy when you have sentence-transformers installed:\n\n\n\nThen you can use the model like this:",
"## Usage (HuggingFace Transformers) (same as gte-tiny)\nWithout sentence-transformers, you can use the model like this: First, you pass your input through the transformer model, then you have to apply the right pooling-operation on-top of the contextualized word embeddings.",
"### Limitation (same as gte-small)\nThis model exclusively caters to English texts, and any lengthy texts will be truncated to a maximum of 512 tokens."
] | [
"TAGS\n#sentence-transformers #onnx #safetensors #bert #feature-extraction #sentence-similarity #gte #mteb #license-mit #model-index #endpoints_compatible #region-us \n",
"# gte-micro-v2\n\nThis is a distill of gte-tiny.",
"## Intended purpose\n\n<span style=\"color:blue\">This model is designed for use in semantic-autocomplete (click here for demo).</span>",
"## Usage (Sentence-Transformers) (same as gte-tiny)\n\nUsing this model becomes easy when you have sentence-transformers installed:\n\n\n\nThen you can use the model like this:",
"## Usage (HuggingFace Transformers) (same as gte-tiny)\nWithout sentence-transformers, you can use the model like this: First, you pass your input through the transformer model, then you have to apply the right pooling-operation on-top of the contextualized word embeddings.",
"### Limitation (same as gte-small)\nThis model exclusively caters to English texts, and any lengthy texts will be truncated to a maximum of 512 tokens."
] |
text-generation | transformers |
# Boreas
**NB: 20240430 model card is WIP - evaluations / example generations to be added**
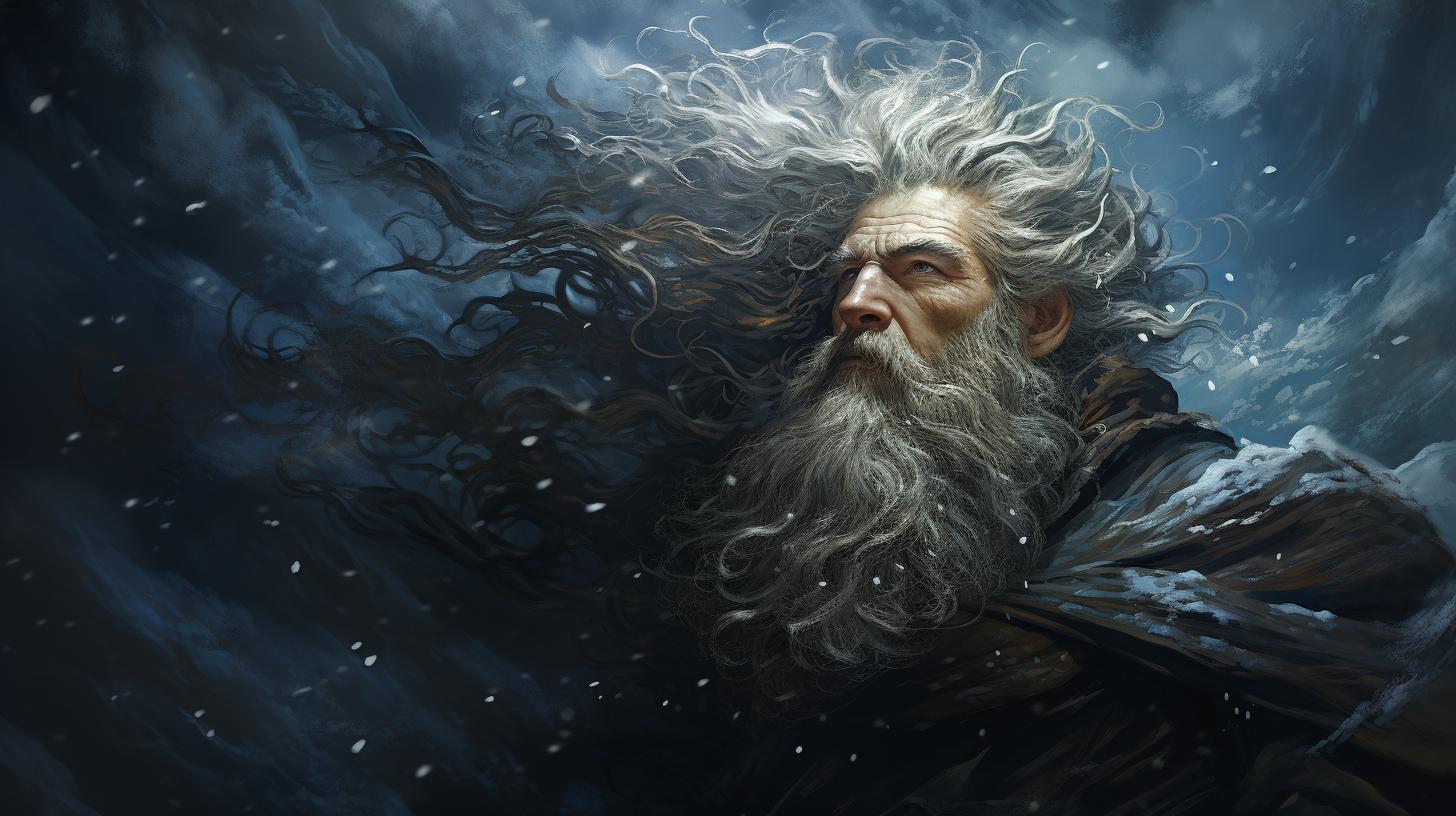
Boreas-7B is een Nederlands/Engels taalmodel gebaseerd op Mistral-7B.
Het is getraind op 10 miljard tokens aan Nederlandse en Engelse tekst.
Boreas-7B-chat is verder getraind op instructie- en chat data.
* Boreas-7B is vergelijkbaar met [GEITje-7B](https://huggingface.co/Rijgersberg/GEITje-7B) in die zin dat
het ook een model is dat verder getraind is op Mistral-7B, met een evenzogrote hoeveelheid tokens (10B).
* Boreas-7B-chat is vergelijkbaar met [GEITje-7B-chat](https://huggingface.co/Rijgersberg/GEITje-7B-chat) en [GEITJE-7B-ultra-sft](https://huggingface.co/BramVanroy/GEITje-7B-ultra-sft), in die zin dat het ook een
fine-tune is op een chat dataset.
Edwin Rijgersberg heeft [uitgebreide documentatie](https://github.com/Rijgersberg/GEITje/blob/main/README.md) geschreven voor het gebruik van GEITje,
en deze is ook van toepassing op Boreas.
De voornaamste verschillen tussen Boreas en GEITje zijn:
* Boreas is getraind met een context lengte van 2048 tokens, GEITje met 8192 tokens.
* Boreas is getraind op een mix van Engels en Nederlands, waar GEITje alleen op voornamelijk Nederlands getraind is.
## Gebruik met ollama
Kies een GGUF quant van [Boreas-7B-chat-v1-GGUF](https://huggingface.co/yhavinga/Boreas-7B-chat-v1-GGUF)
en volg de instructies daar.
Belangrijk: gebruik een system prompt, anders zijn de resultaten matig.
## Doel Boreas
Creatie van een taalmodel dat wat betreft het Nederlandse gedeelte, niet getraind is op teksten gegeneerd door
een LLM. Dus geen Nederlandse chats gegeneerd door een LLM, en ook geen datasets die vertaald zijn uit het Engels door een LLM.
Er is niet streng gefilterd op LLM-gegenereerde tekst, maar dit is wel een aandachtspunt geweest bij het samenstellen van de datasets.
Bij het finetunen van Boreas-chat zijn toch 3% van de tokens Nederlandse chats gegeneerd door een LLM.
Dit is een kleine dataset die gemaakt is op basis van Nederlandse bronteksten, en een steekproef heeft uitgewezen
dat deze data van een goede kwaliteit is.
Het uiteindelijke chat model is getraind op een mix van voornamelijk:
1. Openhermes-2.5: grote, diverse Engelse chat dataset (~45%)
2. Een engels naar nederlands vertaal dataset (~34%)
3. Pre-train data: verder met Wiki en boeken (~12%)
4. Nederlandse wiki en boeken q en a (~3%)
Het Boreas model kan dus beschouwd worden als een test voor knowledge transfer van Engels naar Nederlands.
## Boreas-7B basismodel
Het basismodel is op Mistral-7B doorgetraind op 10 miljard tokens.
De dataset is samengesteld uit verschillende bronnen in zowel Nederlands en Engels:
| Datasetnaam | Aantal Tokens | Percentage Tokens (%) |
|------------------------------------------------|---------------|-----------------------|
| Nederlandse romans | 3401M | 34.01 |
| Nederlandse Wikipedia | 2381M | 23.81 |
| mc4_nl_cleaned (Nederlands) | 1361M | 13.61 |
| Nederlands nieuws | 1361M | 13.61 |
| Nederlandse schoolboeken | 136M | 1.36 |
| Engelse romans | 340M | 3.40 |
| Engelse Wikipedia (euirim/goodwiki) | 340M | 3.40 |
| Engelse wiskunde- en natuurkundeboeken | 340M | 3.40 |
| Engelse instructiedataset (philschmid/flanv2) | 340M | 3.40 |
De keuze voor deze mix is gebaseerd op zowel beschikbaarheid van data als de volgende overwegingen:
* Veel Nederlands van hoge kwaliteit, teksten primair in het Nederlands geschreven door mensen. Hieruit volgt de keuze
voor romans, Wikipedia en nieuwsartikelen, maar bijvoorbeeld uitsluiten van forum-/twitterberichten en wetteksten.
* Erin mixen van de originele dataset voor ~5%, om ervoor te zorgen dat een model haar originele kennis niet verliest.
Het is niet bekend op welke data Mistral getraind is, maar het is aannemelijk dat er kwalitatief goed Engels en ook instructiedata in verwerkt is. Daarom is voor de ~3% aan Engelse boeken, Wikipedia en ook instructiedata gekozen.
* Zoveel mogelijk uitsluiten van door LLM's gegenereerde teksten in de pre-train fase. Bij veel datasets, vooral Nederlands, valt me op dat
de vertalingen of generaties van een slechte kwaliteit zijn. Daarom is gekozen voor datasets waarvan de brondata
pre ChatGPT tijdperk zijn, (dus voor November 2022).
* mc4_nl_cleaned - de bron van deze dataset is mC4 - deduplicated data van Common
Crawl, en gefiltered op bad-words en andere bewerkingen volgens het recept van de T5 auteurs voor de Engelse C4 dataset. In diverse ablations blijkt C4 een goede pre-train dataset, daarom is mc4_nl_cleaned ook voor dit model gebruikt.
* Er is geen sourcecode in gemixt - ik verwacht niet dat een 7B model ooit code kan genereren dat bruikbaar is.
Misschien helpt het bij logisch redeneer-puzzels, maar ook daarvoor verwacht ik dat een 7B model dit nooit zo goed
zal kunnen of generaliseren als grotere modellen.
Bij het pre-trainen zijn de brontexten gepackt in blokken van 2048 tokens. Hierbij is waar mogelijk geprobeerd om alleen
teksten te packen die bij elkaar passen. Ook worden kleine fragmenten weggegooid, zodat we
bijvoorbeeld nooit een fragment krijgen dat begint met een paar tokens van het einde van een wikipedia artikel, om daarna met een ander wikipedia artikel te beginnen. Dit is gedaan om 'cross sequence' ruis binnen 1 example zoveel mogelijk te voorkomen. Pas na packing zijn de examples geshuffled.
## Pre-training
* Boreas was pre-trained with the [EasyDeL JAX framework](https://github.com/erfanzar/EasyDel) on a tpu-v4-32
kindly supplied by the Google [TPU Research Cloud](https://sites.research.google/trc/about/).
* Batch size 96, gradient accumulation steps 2
* Using flash attention, block size of 512
* Max sequence length of 2048
* LION optimizer, triangle learning rate schedule with max lr 3e-6, gradient clipping to 1.0



<!-- [https://wandb.ai/yepster/EasyDeL-MistralBoreas/runs/ozw55qaq/workspace?nw=nwuseryepster](WandB Boreas 7B pre-train) -->
## Boreas-7B-chat
Het chat LLM model is net als het basismodel getraind op een mix van datasets, met een grootte van 4.7B tokens.
Het is een full finetune, dus geen LoRA finetune.
De volgende datasets zijn gemixt:
| Datasetnaam | Gewicht | Percentage Tokens (%) |
|-----------------------------------------------------------|---------|-----------------------|
| (C) Diverse Engelse chat dataset (teknium/OpenHermes-2.5) | 200 | 45.15 |
| (C) Vertaal en->nl paragrafen (romans) | 100 | 22.57 |
| (C) Vertaal en->nl zinnen (romans) | 50 | 11.29 |
| (P) Nederlandse wikipedia | 30 | 6.77 |
| (P) Engelse wiskunde en natuurkunde boeken | 25 | 5.64 |
| (C) Engelse instruct dataset (philschmid/flanv2) | 20 | 4.51 |
| (C) Nederlandse wiki q en a | 12 | 2.71 |
| (C) Nederlandse schoolboeken q en a | 3 | 0.68 |
| (P) Nederlandse schoolboeken | 2 | 0.45 |
| (C) Vertaal en->nl uitdrukkingen (dictionary) | 1 | 0.23 |
(C) geeft aan dat de tekst geformatteerd is voor chat, (P) is ongeformatteerde tekst (gelijk aan de pre-train fase)
Het grootste gedeelte bestaat uit `teknium/OpenHermes-2.5` - wat op zichzelf ook weer een mengelmoes van diverse
gefilterde chat/instruct datasets is. Deze dataset bevat wel programmacode data, wat ertoe resulteert dat Boreas-7B-chat
wel in staat is om simpele programmavragen te beantwoorden.
De reden om zoveel Engels in de dataset te mixen, is met name om de diversiteit in de dataset zo hoog mogelijk te
krijgen, en omdat ik verwacht dat er een behoorlijke mate van cross language en naar nl knowledge transfer mogelijk is.
Het omgekeerde is zeker waar: als een fine-tune dataset niet divers is, zal het model door zijn fine-tuning niet in
staat zijn om zijn originele kunde uit te voeren. Een van de eerste Mistral finetunes die ik gemaakt heb was gefinetuned
op alleen en->nl vertalen. Dat model kon uiteindelijk niets anders meer dan vertalen naar Nederlands.
In tegenstelling tot het basismodel is het chat model _wel_ getrained op LLM-gegenereerde teksten - hierbij zijn de volgende
overwegingen van toepassing: Bij de Nederlandse gegenereerde chats heb ik wederom geprobeerd om zoveel mogelijk origineel
Nederlands taalgebruik te 'guiden' door alleen vragen en antwoorden te genereren op basis van teksten die origineel in
het Nederlands geschreven zijn door een persoon. Dit zijn de Nederlandse wiki q en a en Nederlandse schoolboeken q en a
chat datasets. Hierdoor wordt er zoveel mogelijk voor gezorgd dat bij bijvoorbeeld educatie-achtige q en a, de in onze
regio gebruikelijke termen en eenheden voorkomen in de chat database, tenminste voor de Nederlandstalige chats.
Bij alle chat datasets is er alleen getraind op de assistant-completion tokens.
## Fine-tuning
* Boreas was fine-tuned with the [EasyDeL JAX framework](https://github.com/erfanzar/EasyDel) on a tpu-v4-32
kindly supplied by the Google [TPU Research Cloud](https://sites.research.google/trc/about/).
* Batch size 96, gradient accumulation 2,
* Using flash attention, block size of 512
* Max sequence length of 2048
* LION optimizer, triangle learning rate schedule with max lr 2e-6, gradient clipping to 1.0 (NB: the schedule was not finished due to an error at the end of the dataset epoch. Since the loss had plateaued I decided then to not resume for another epoch)



<!-- [https://wandb.ai/yepster/EasyDeL-MistralBoreas/runs/ynkl2jtx?nw=nwuseryepster](WandB Boreas 7B chat finetune) -->
| {"license": "apache-2.0", "library_name": "transformers", "tags": ["finetuned"], "datasets": ["yhavinga/mc4_nl_cleaned", "yhavinga/nedd_wiki_news", "teknium/OpenHermes-2.5", "euirim/goodwiki", "philschmid/flanv2"], "pipeline_tag": "text-generation", "inference": true, "widget": [{"messages": [{"role": "system", "content": "Je bent een behulpzame Nederlandse AI-assistent."}, {"role": "user", "content": "Is Nederlandse wijn lekker?"}]}]} | yhavinga/Boreas-7B-chat | null | [
"transformers",
"safetensors",
"mistral",
"text-generation",
"finetuned",
"conversational",
"dataset:yhavinga/mc4_nl_cleaned",
"dataset:yhavinga/nedd_wiki_news",
"dataset:teknium/OpenHermes-2.5",
"dataset:euirim/goodwiki",
"dataset:philschmid/flanv2",
"license:apache-2.0",
"autotrain_compatible",
"endpoints_compatible",
"text-generation-inference",
"region:us"
] | null | 2024-04-22T10:01:56+00:00 | [] | [] | TAGS
#transformers #safetensors #mistral #text-generation #finetuned #conversational #dataset-yhavinga/mc4_nl_cleaned #dataset-yhavinga/nedd_wiki_news #dataset-teknium/OpenHermes-2.5 #dataset-euirim/goodwiki #dataset-philschmid/flanv2 #license-apache-2.0 #autotrain_compatible #endpoints_compatible #text-generation-inference #region-us
| Boreas
======
NB: 20240430 model card is WIP - evaluations / example generations to be added
!BoreasThe Mighty God of the North Wind
Boreas-7B is een Nederlands/Engels taalmodel gebaseerd op Mistral-7B.
Het is getraind op 10 miljard tokens aan Nederlandse en Engelse tekst.
Boreas-7B-chat is verder getraind op instructie- en chat data.
* Boreas-7B is vergelijkbaar met GEITje-7B in die zin dat
het ook een model is dat verder getraind is op Mistral-7B, met een evenzogrote hoeveelheid tokens (10B).
* Boreas-7B-chat is vergelijkbaar met GEITje-7B-chat en GEITJE-7B-ultra-sft, in die zin dat het ook een
fine-tune is op een chat dataset.
Edwin Rijgersberg heeft uitgebreide documentatie geschreven voor het gebruik van GEITje,
en deze is ook van toepassing op Boreas.
De voornaamste verschillen tussen Boreas en GEITje zijn:
* Boreas is getraind met een context lengte van 2048 tokens, GEITje met 8192 tokens.
* Boreas is getraind op een mix van Engels en Nederlands, waar GEITje alleen op voornamelijk Nederlands getraind is.
Gebruik met ollama
------------------
Kies een GGUF quant van Boreas-7B-chat-v1-GGUF
en volg de instructies daar.
Belangrijk: gebruik een system prompt, anders zijn de resultaten matig.
Doel Boreas
-----------
Creatie van een taalmodel dat wat betreft het Nederlandse gedeelte, niet getraind is op teksten gegeneerd door
een LLM. Dus geen Nederlandse chats gegeneerd door een LLM, en ook geen datasets die vertaald zijn uit het Engels door een LLM.
Er is niet streng gefilterd op LLM-gegenereerde tekst, maar dit is wel een aandachtspunt geweest bij het samenstellen van de datasets.
Bij het finetunen van Boreas-chat zijn toch 3% van de tokens Nederlandse chats gegeneerd door een LLM.
Dit is een kleine dataset die gemaakt is op basis van Nederlandse bronteksten, en een steekproef heeft uitgewezen
dat deze data van een goede kwaliteit is.
Het uiteindelijke chat model is getraind op een mix van voornamelijk:
1. Openhermes-2.5: grote, diverse Engelse chat dataset (~45%)
2. Een engels naar nederlands vertaal dataset (~34%)
3. Pre-train data: verder met Wiki en boeken (~12%)
4. Nederlandse wiki en boeken q en a (~3%)
Het Boreas model kan dus beschouwd worden als een test voor knowledge transfer van Engels naar Nederlands.
Boreas-7B basismodel
--------------------
Het basismodel is op Mistral-7B doorgetraind op 10 miljard tokens.
De dataset is samengesteld uit verschillende bronnen in zowel Nederlands en Engels:
Datasetnaam: Nederlandse romans, Aantal Tokens: 3401M, Percentage Tokens (%): 34.01
Datasetnaam: Nederlandse Wikipedia, Aantal Tokens: 2381M, Percentage Tokens (%): 23.81
Datasetnaam: mc4\_nl\_cleaned (Nederlands), Aantal Tokens: 1361M, Percentage Tokens (%): 13.61
Datasetnaam: Nederlands nieuws, Aantal Tokens: 1361M, Percentage Tokens (%): 13.61
Datasetnaam: Nederlandse schoolboeken, Aantal Tokens: 136M, Percentage Tokens (%): 1.36
Datasetnaam: Engelse romans, Aantal Tokens: 340M, Percentage Tokens (%): 3.40
Datasetnaam: Engelse Wikipedia (euirim/goodwiki), Aantal Tokens: 340M, Percentage Tokens (%): 3.40
Datasetnaam: Engelse wiskunde- en natuurkundeboeken, Aantal Tokens: 340M, Percentage Tokens (%): 3.40
Datasetnaam: Engelse instructiedataset (philschmid/flanv2), Aantal Tokens: 340M, Percentage Tokens (%): 3.40
De keuze voor deze mix is gebaseerd op zowel beschikbaarheid van data als de volgende overwegingen:
* Veel Nederlands van hoge kwaliteit, teksten primair in het Nederlands geschreven door mensen. Hieruit volgt de keuze
voor romans, Wikipedia en nieuwsartikelen, maar bijvoorbeeld uitsluiten van forum-/twitterberichten en wetteksten.
* Erin mixen van de originele dataset voor ~5%, om ervoor te zorgen dat een model haar originele kennis niet verliest.
Het is niet bekend op welke data Mistral getraind is, maar het is aannemelijk dat er kwalitatief goed Engels en ook instructiedata in verwerkt is. Daarom is voor de ~3% aan Engelse boeken, Wikipedia en ook instructiedata gekozen.
* Zoveel mogelijk uitsluiten van door LLM's gegenereerde teksten in de pre-train fase. Bij veel datasets, vooral Nederlands, valt me op dat
de vertalingen of generaties van een slechte kwaliteit zijn. Daarom is gekozen voor datasets waarvan de brondata
pre ChatGPT tijdperk zijn, (dus voor November 2022).
* mc4\_nl\_cleaned - de bron van deze dataset is mC4 - deduplicated data van Common
Crawl, en gefiltered op bad-words en andere bewerkingen volgens het recept van de T5 auteurs voor de Engelse C4 dataset. In diverse ablations blijkt C4 een goede pre-train dataset, daarom is mc4\_nl\_cleaned ook voor dit model gebruikt.
* Er is geen sourcecode in gemixt - ik verwacht niet dat een 7B model ooit code kan genereren dat bruikbaar is.
Misschien helpt het bij logisch redeneer-puzzels, maar ook daarvoor verwacht ik dat een 7B model dit nooit zo goed
zal kunnen of generaliseren als grotere modellen.
Bij het pre-trainen zijn de brontexten gepackt in blokken van 2048 tokens. Hierbij is waar mogelijk geprobeerd om alleen
teksten te packen die bij elkaar passen. Ook worden kleine fragmenten weggegooid, zodat we
bijvoorbeeld nooit een fragment krijgen dat begint met een paar tokens van het einde van een wikipedia artikel, om daarna met een ander wikipedia artikel te beginnen. Dit is gedaan om 'cross sequence' ruis binnen 1 example zoveel mogelijk te voorkomen. Pas na packing zijn de examples geshuffled.
Pre-training
------------
* Boreas was pre-trained with the EasyDeL JAX framework on a tpu-v4-32
kindly supplied by the Google TPU Research Cloud.
* Batch size 96, gradient accumulation steps 2
* Using flash attention, block size of 512
* Max sequence length of 2048
* LION optimizer, triangle learning rate schedule with max lr 3e-6, gradient clipping to 1.0
!img\_3.png
!img\_4.png
!img\_5.png
Boreas-7B-chat
--------------
Het chat LLM model is net als het basismodel getraind op een mix van datasets, met een grootte van 4.7B tokens.
Het is een full finetune, dus geen LoRA finetune.
De volgende datasets zijn gemixt:
Datasetnaam: (C) Diverse Engelse chat dataset (teknium/OpenHermes-2.5), Gewicht: 200, Percentage Tokens (%): 45.15
Datasetnaam: (C) Vertaal en->nl paragrafen (romans), Gewicht: 100, Percentage Tokens (%): 22.57
Datasetnaam: (C) Vertaal en->nl zinnen (romans), Gewicht: 50, Percentage Tokens (%): 11.29
Datasetnaam: (P) Nederlandse wikipedia, Gewicht: 30, Percentage Tokens (%): 6.77
Datasetnaam: (P) Engelse wiskunde en natuurkunde boeken, Gewicht: 25, Percentage Tokens (%): 5.64
Datasetnaam: (C) Engelse instruct dataset (philschmid/flanv2), Gewicht: 20, Percentage Tokens (%): 4.51
Datasetnaam: (C) Nederlandse wiki q en a, Gewicht: 12, Percentage Tokens (%): 2.71
Datasetnaam: (C) Nederlandse schoolboeken q en a, Gewicht: 3, Percentage Tokens (%): 0.68
Datasetnaam: (P) Nederlandse schoolboeken, Gewicht: 2, Percentage Tokens (%): 0.45
Datasetnaam: (C) Vertaal en->nl uitdrukkingen (dictionary), Gewicht: 1, Percentage Tokens (%): 0.23
(C) geeft aan dat de tekst geformatteerd is voor chat, (P) is ongeformatteerde tekst (gelijk aan de pre-train fase)
Het grootste gedeelte bestaat uit 'teknium/OpenHermes-2.5' - wat op zichzelf ook weer een mengelmoes van diverse
gefilterde chat/instruct datasets is. Deze dataset bevat wel programmacode data, wat ertoe resulteert dat Boreas-7B-chat
wel in staat is om simpele programmavragen te beantwoorden.
De reden om zoveel Engels in de dataset te mixen, is met name om de diversiteit in de dataset zo hoog mogelijk te
krijgen, en omdat ik verwacht dat er een behoorlijke mate van cross language en naar nl knowledge transfer mogelijk is.
Het omgekeerde is zeker waar: als een fine-tune dataset niet divers is, zal het model door zijn fine-tuning niet in
staat zijn om zijn originele kunde uit te voeren. Een van de eerste Mistral finetunes die ik gemaakt heb was gefinetuned
op alleen en->nl vertalen. Dat model kon uiteindelijk niets anders meer dan vertalen naar Nederlands.
In tegenstelling tot het basismodel is het chat model *wel* getrained op LLM-gegenereerde teksten - hierbij zijn de volgende
overwegingen van toepassing: Bij de Nederlandse gegenereerde chats heb ik wederom geprobeerd om zoveel mogelijk origineel
Nederlands taalgebruik te 'guiden' door alleen vragen en antwoorden te genereren op basis van teksten die origineel in
het Nederlands geschreven zijn door een persoon. Dit zijn de Nederlandse wiki q en a en Nederlandse schoolboeken q en a
chat datasets. Hierdoor wordt er zoveel mogelijk voor gezorgd dat bij bijvoorbeeld educatie-achtige q en a, de in onze
regio gebruikelijke termen en eenheden voorkomen in de chat database, tenminste voor de Nederlandstalige chats.
Bij alle chat datasets is er alleen getraind op de assistant-completion tokens.
Fine-tuning
-----------
* Boreas was fine-tuned with the EasyDeL JAX framework on a tpu-v4-32
kindly supplied by the Google TPU Research Cloud.
* Batch size 96, gradient accumulation 2,
* Using flash attention, block size of 512
* Max sequence length of 2048
* LION optimizer, triangle learning rate schedule with max lr 2e-6, gradient clipping to 1.0 (NB: the schedule was not finished due to an error at the end of the dataset epoch. Since the loss had plateaued I decided then to not resume for another epoch)
!loss finetune
!accuracy finetune
!learning rate finetune
| [] | [
"TAGS\n#transformers #safetensors #mistral #text-generation #finetuned #conversational #dataset-yhavinga/mc4_nl_cleaned #dataset-yhavinga/nedd_wiki_news #dataset-teknium/OpenHermes-2.5 #dataset-euirim/goodwiki #dataset-philschmid/flanv2 #license-apache-2.0 #autotrain_compatible #endpoints_compatible #text-generation-inference #region-us \n"
] |
null | transformers |
# Uploaded model
- **Developed by:** Akirami
- **License:** apache-2.0
- **Finetuned from model :** unsloth/llama-3-8b-bnb-4bit
This llama model was trained 2x faster with [Unsloth](https://github.com/unslothai/unsloth) and Huggingface's TRL library.
[<img src="https://raw.githubusercontent.com/unslothai/unsloth/main/images/unsloth%20made%20with%20love.png" width="200"/>](https://github.com/unslothai/unsloth)
| {"language": ["en"], "license": "apache-2.0", "tags": ["text-generation-inference", "transformers", "unsloth", "llama", "trl"], "base_model": "unsloth/llama-3-8b-bnb-4bit"} | Akirami/llama3-orpo-test | null | [
"transformers",
"text-generation-inference",
"unsloth",
"llama",
"trl",
"en",
"base_model:unsloth/llama-3-8b-bnb-4bit",
"license:apache-2.0",
"endpoints_compatible",
"region:us"
] | null | 2024-04-22T10:02:13+00:00 | [] | [
"en"
] | TAGS
#transformers #text-generation-inference #unsloth #llama #trl #en #base_model-unsloth/llama-3-8b-bnb-4bit #license-apache-2.0 #endpoints_compatible #region-us
|
# Uploaded model
- Developed by: Akirami
- License: apache-2.0
- Finetuned from model : unsloth/llama-3-8b-bnb-4bit
This llama model was trained 2x faster with Unsloth and Huggingface's TRL library.
<img src="URL width="200"/>
| [
"# Uploaded model\n\n- Developed by: Akirami\n- License: apache-2.0\n- Finetuned from model : unsloth/llama-3-8b-bnb-4bit\n\nThis llama model was trained 2x faster with Unsloth and Huggingface's TRL library.\n\n<img src=\"URL width=\"200\"/>"
] | [
"TAGS\n#transformers #text-generation-inference #unsloth #llama #trl #en #base_model-unsloth/llama-3-8b-bnb-4bit #license-apache-2.0 #endpoints_compatible #region-us \n",
"# Uploaded model\n\n- Developed by: Akirami\n- License: apache-2.0\n- Finetuned from model : unsloth/llama-3-8b-bnb-4bit\n\nThis llama model was trained 2x faster with Unsloth and Huggingface's TRL library.\n\n<img src=\"URL width=\"200\"/>"
] |
text-generation | transformers |
<!-- This model card has been generated automatically according to the information the Trainer had access to. You
should probably proofread and complete it, then remove this comment. -->
# mistral-7b-finetuned-lime-v1
This model is a fine-tuned version of [mistralai/Mistral-7B-Instruct-v0.2](https://huggingface.co/mistralai/Mistral-7B-Instruct-v0.2) on the generator dataset.
It achieves the following results on the evaluation set:
- Loss: 1.4881
## Model description
More information needed
## Intended uses & limitations
More information needed
## Training and evaluation data
More information needed
## Training procedure
### Training hyperparameters
The following hyperparameters were used during training:
- learning_rate: 2e-05
- train_batch_size: 4
- eval_batch_size: 8
- seed: 42
- distributed_type: multi-GPU
- num_devices: 2
- gradient_accumulation_steps: 8
- total_train_batch_size: 64
- total_eval_batch_size: 16
- optimizer: Adam with betas=(0.9,0.999) and epsilon=1e-08
- lr_scheduler_type: cosine
- lr_scheduler_warmup_ratio: 0.1
- num_epochs: 1
### Training results
| Training Loss | Epoch | Step | Validation Loss |
|:-------------:|:-----:|:----:|:---------------:|
| 1.4064 | 0.95 | 10 | 1.4881 |
### Framework versions
- Transformers 4.38.2
- Pytorch 2.2.2
- Datasets 2.18.0
- Tokenizers 0.15.2
| {"tags": ["trl", "sft", "generated_from_trainer"], "datasets": ["generator"], "base_model": "mistralai/Mistral-7B-Instruct-v0.2", "model-index": [{"name": "mistral-7b-finetuned-lime-v1", "results": []}]} | deadcode99/mistral-7b-finetuned-lime-v1 | null | [
"transformers",
"safetensors",
"mistral",
"text-generation",
"trl",
"sft",
"generated_from_trainer",
"conversational",
"dataset:generator",
"base_model:mistralai/Mistral-7B-Instruct-v0.2",
"autotrain_compatible",
"endpoints_compatible",
"text-generation-inference",
"region:us"
] | null | 2024-04-22T10:02:46+00:00 | [] | [] | TAGS
#transformers #safetensors #mistral #text-generation #trl #sft #generated_from_trainer #conversational #dataset-generator #base_model-mistralai/Mistral-7B-Instruct-v0.2 #autotrain_compatible #endpoints_compatible #text-generation-inference #region-us
| mistral-7b-finetuned-lime-v1
============================
This model is a fine-tuned version of mistralai/Mistral-7B-Instruct-v0.2 on the generator dataset.
It achieves the following results on the evaluation set:
* Loss: 1.4881
Model description
-----------------
More information needed
Intended uses & limitations
---------------------------
More information needed
Training and evaluation data
----------------------------
More information needed
Training procedure
------------------
### Training hyperparameters
The following hyperparameters were used during training:
* learning\_rate: 2e-05
* train\_batch\_size: 4
* eval\_batch\_size: 8
* seed: 42
* distributed\_type: multi-GPU
* num\_devices: 2
* gradient\_accumulation\_steps: 8
* total\_train\_batch\_size: 64
* total\_eval\_batch\_size: 16
* optimizer: Adam with betas=(0.9,0.999) and epsilon=1e-08
* lr\_scheduler\_type: cosine
* lr\_scheduler\_warmup\_ratio: 0.1
* num\_epochs: 1
### Training results
### Framework versions
* Transformers 4.38.2
* Pytorch 2.2.2
* Datasets 2.18.0
* Tokenizers 0.15.2
| [
"### Training hyperparameters\n\n\nThe following hyperparameters were used during training:\n\n\n* learning\\_rate: 2e-05\n* train\\_batch\\_size: 4\n* eval\\_batch\\_size: 8\n* seed: 42\n* distributed\\_type: multi-GPU\n* num\\_devices: 2\n* gradient\\_accumulation\\_steps: 8\n* total\\_train\\_batch\\_size: 64\n* total\\_eval\\_batch\\_size: 16\n* optimizer: Adam with betas=(0.9,0.999) and epsilon=1e-08\n* lr\\_scheduler\\_type: cosine\n* lr\\_scheduler\\_warmup\\_ratio: 0.1\n* num\\_epochs: 1",
"### Training results",
"### Framework versions\n\n\n* Transformers 4.38.2\n* Pytorch 2.2.2\n* Datasets 2.18.0\n* Tokenizers 0.15.2"
] | [
"TAGS\n#transformers #safetensors #mistral #text-generation #trl #sft #generated_from_trainer #conversational #dataset-generator #base_model-mistralai/Mistral-7B-Instruct-v0.2 #autotrain_compatible #endpoints_compatible #text-generation-inference #region-us \n",
"### Training hyperparameters\n\n\nThe following hyperparameters were used during training:\n\n\n* learning\\_rate: 2e-05\n* train\\_batch\\_size: 4\n* eval\\_batch\\_size: 8\n* seed: 42\n* distributed\\_type: multi-GPU\n* num\\_devices: 2\n* gradient\\_accumulation\\_steps: 8\n* total\\_train\\_batch\\_size: 64\n* total\\_eval\\_batch\\_size: 16\n* optimizer: Adam with betas=(0.9,0.999) and epsilon=1e-08\n* lr\\_scheduler\\_type: cosine\n* lr\\_scheduler\\_warmup\\_ratio: 0.1\n* num\\_epochs: 1",
"### Training results",
"### Framework versions\n\n\n* Transformers 4.38.2\n* Pytorch 2.2.2\n* Datasets 2.18.0\n* Tokenizers 0.15.2"
] |
text-generation | transformers |
# Model Card for Model ID
<!-- Provide a quick summary of what the model is/does. -->
## Model Details
### Model Description
<!-- Provide a longer summary of what this model is. -->
This is the model card of a 🤗 transformers model that has been pushed on the Hub. This model card has been automatically generated.
- **Developed by:** [More Information Needed]
- **Funded by [optional]:** [More Information Needed]
- **Shared by [optional]:** [More Information Needed]
- **Model type:** [More Information Needed]
- **Language(s) (NLP):** [More Information Needed]
- **License:** [More Information Needed]
- **Finetuned from model [optional]:** [More Information Needed]
### Model Sources [optional]
<!-- Provide the basic links for the model. -->
- **Repository:** [More Information Needed]
- **Paper [optional]:** [More Information Needed]
- **Demo [optional]:** [More Information Needed]
## Uses
<!-- Address questions around how the model is intended to be used, including the foreseeable users of the model and those affected by the model. -->
### Direct Use
<!-- This section is for the model use without fine-tuning or plugging into a larger ecosystem/app. -->
[More Information Needed]
### Downstream Use [optional]
<!-- This section is for the model use when fine-tuned for a task, or when plugged into a larger ecosystem/app -->
[More Information Needed]
### Out-of-Scope Use
<!-- This section addresses misuse, malicious use, and uses that the model will not work well for. -->
[More Information Needed]
## Bias, Risks, and Limitations
<!-- This section is meant to convey both technical and sociotechnical limitations. -->
[More Information Needed]
### Recommendations
<!-- This section is meant to convey recommendations with respect to the bias, risk, and technical limitations. -->
Users (both direct and downstream) should be made aware of the risks, biases and limitations of the model. More information needed for further recommendations.
## How to Get Started with the Model
Use the code below to get started with the model.
[More Information Needed]
## Training Details
### Training Data
<!-- This should link to a Dataset Card, perhaps with a short stub of information on what the training data is all about as well as documentation related to data pre-processing or additional filtering. -->
[More Information Needed]
### Training Procedure
<!-- This relates heavily to the Technical Specifications. Content here should link to that section when it is relevant to the training procedure. -->
#### Preprocessing [optional]
[More Information Needed]
#### Training Hyperparameters
- **Training regime:** [More Information Needed] <!--fp32, fp16 mixed precision, bf16 mixed precision, bf16 non-mixed precision, fp16 non-mixed precision, fp8 mixed precision -->
#### Speeds, Sizes, Times [optional]
<!-- This section provides information about throughput, start/end time, checkpoint size if relevant, etc. -->
[More Information Needed]
## Evaluation
<!-- This section describes the evaluation protocols and provides the results. -->
### Testing Data, Factors & Metrics
#### Testing Data
<!-- This should link to a Dataset Card if possible. -->
[More Information Needed]
#### Factors
<!-- These are the things the evaluation is disaggregating by, e.g., subpopulations or domains. -->
[More Information Needed]
#### Metrics
<!-- These are the evaluation metrics being used, ideally with a description of why. -->
[More Information Needed]
### Results
[More Information Needed]
#### Summary
## Model Examination [optional]
<!-- Relevant interpretability work for the model goes here -->
[More Information Needed]
## Environmental Impact
<!-- Total emissions (in grams of CO2eq) and additional considerations, such as electricity usage, go here. Edit the suggested text below accordingly -->
Carbon emissions can be estimated using the [Machine Learning Impact calculator](https://mlco2.github.io/impact#compute) presented in [Lacoste et al. (2019)](https://arxiv.org/abs/1910.09700).
- **Hardware Type:** [More Information Needed]
- **Hours used:** [More Information Needed]
- **Cloud Provider:** [More Information Needed]
- **Compute Region:** [More Information Needed]
- **Carbon Emitted:** [More Information Needed]
## Technical Specifications [optional]
### Model Architecture and Objective
[More Information Needed]
### Compute Infrastructure
[More Information Needed]
#### Hardware
[More Information Needed]
#### Software
[More Information Needed]
## Citation [optional]
<!-- If there is a paper or blog post introducing the model, the APA and Bibtex information for that should go in this section. -->
**BibTeX:**
[More Information Needed]
**APA:**
[More Information Needed]
## Glossary [optional]
<!-- If relevant, include terms and calculations in this section that can help readers understand the model or model card. -->
[More Information Needed]
## More Information [optional]
[More Information Needed]
## Model Card Authors [optional]
[More Information Needed]
## Model Card Contact
[More Information Needed] | {"library_name": "transformers", "tags": ["trl", "sft"]} | Rhma/mistral_7b_fine-tuned-kaggle | null | [
"transformers",
"safetensors",
"mistral",
"text-generation",
"trl",
"sft",
"conversational",
"arxiv:1910.09700",
"autotrain_compatible",
"endpoints_compatible",
"text-generation-inference",
"4-bit",
"region:us"
] | null | 2024-04-22T10:03:03+00:00 | [
"1910.09700"
] | [] | TAGS
#transformers #safetensors #mistral #text-generation #trl #sft #conversational #arxiv-1910.09700 #autotrain_compatible #endpoints_compatible #text-generation-inference #4-bit #region-us
|
# Model Card for Model ID
## Model Details
### Model Description
This is the model card of a transformers model that has been pushed on the Hub. This model card has been automatically generated.
- Developed by:
- Funded by [optional]:
- Shared by [optional]:
- Model type:
- Language(s) (NLP):
- License:
- Finetuned from model [optional]:
### Model Sources [optional]
- Repository:
- Paper [optional]:
- Demo [optional]:
## Uses
### Direct Use
### Downstream Use [optional]
### Out-of-Scope Use
## Bias, Risks, and Limitations
### Recommendations
Users (both direct and downstream) should be made aware of the risks, biases and limitations of the model. More information needed for further recommendations.
## How to Get Started with the Model
Use the code below to get started with the model.
## Training Details
### Training Data
### Training Procedure
#### Preprocessing [optional]
#### Training Hyperparameters
- Training regime:
#### Speeds, Sizes, Times [optional]
## Evaluation
### Testing Data, Factors & Metrics
#### Testing Data
#### Factors
#### Metrics
### Results
#### Summary
## Model Examination [optional]
## Environmental Impact
Carbon emissions can be estimated using the Machine Learning Impact calculator presented in Lacoste et al. (2019).
- Hardware Type:
- Hours used:
- Cloud Provider:
- Compute Region:
- Carbon Emitted:
## Technical Specifications [optional]
### Model Architecture and Objective
### Compute Infrastructure
#### Hardware
#### Software
[optional]
BibTeX:
APA:
## Glossary [optional]
## More Information [optional]
## Model Card Authors [optional]
## Model Card Contact
| [
"# Model Card for Model ID",
"## Model Details",
"### Model Description\n\n\n\nThis is the model card of a transformers model that has been pushed on the Hub. This model card has been automatically generated.\n\n- Developed by: \n- Funded by [optional]: \n- Shared by [optional]: \n- Model type: \n- Language(s) (NLP): \n- License: \n- Finetuned from model [optional]:",
"### Model Sources [optional]\n\n\n\n- Repository: \n- Paper [optional]: \n- Demo [optional]:",
"## Uses",
"### Direct Use",
"### Downstream Use [optional]",
"### Out-of-Scope Use",
"## Bias, Risks, and Limitations",
"### Recommendations\n\n\n\nUsers (both direct and downstream) should be made aware of the risks, biases and limitations of the model. More information needed for further recommendations.",
"## How to Get Started with the Model\n\nUse the code below to get started with the model.",
"## Training Details",
"### Training Data",
"### Training Procedure",
"#### Preprocessing [optional]",
"#### Training Hyperparameters\n\n- Training regime:",
"#### Speeds, Sizes, Times [optional]",
"## Evaluation",
"### Testing Data, Factors & Metrics",
"#### Testing Data",
"#### Factors",
"#### Metrics",
"### Results",
"#### Summary",
"## Model Examination [optional]",
"## Environmental Impact\n\n\n\nCarbon emissions can be estimated using the Machine Learning Impact calculator presented in Lacoste et al. (2019).\n\n- Hardware Type: \n- Hours used: \n- Cloud Provider: \n- Compute Region: \n- Carbon Emitted:",
"## Technical Specifications [optional]",
"### Model Architecture and Objective",
"### Compute Infrastructure",
"#### Hardware",
"#### Software\n\n\n\n[optional]\n\n\n\nBibTeX:\n\n\n\nAPA:",
"## Glossary [optional]",
"## More Information [optional]",
"## Model Card Authors [optional]",
"## Model Card Contact"
] | [
"TAGS\n#transformers #safetensors #mistral #text-generation #trl #sft #conversational #arxiv-1910.09700 #autotrain_compatible #endpoints_compatible #text-generation-inference #4-bit #region-us \n",
"# Model Card for Model ID",
"## Model Details",
"### Model Description\n\n\n\nThis is the model card of a transformers model that has been pushed on the Hub. This model card has been automatically generated.\n\n- Developed by: \n- Funded by [optional]: \n- Shared by [optional]: \n- Model type: \n- Language(s) (NLP): \n- License: \n- Finetuned from model [optional]:",
"### Model Sources [optional]\n\n\n\n- Repository: \n- Paper [optional]: \n- Demo [optional]:",
"## Uses",
"### Direct Use",
"### Downstream Use [optional]",
"### Out-of-Scope Use",
"## Bias, Risks, and Limitations",
"### Recommendations\n\n\n\nUsers (both direct and downstream) should be made aware of the risks, biases and limitations of the model. More information needed for further recommendations.",
"## How to Get Started with the Model\n\nUse the code below to get started with the model.",
"## Training Details",
"### Training Data",
"### Training Procedure",
"#### Preprocessing [optional]",
"#### Training Hyperparameters\n\n- Training regime:",
"#### Speeds, Sizes, Times [optional]",
"## Evaluation",
"### Testing Data, Factors & Metrics",
"#### Testing Data",
"#### Factors",
"#### Metrics",
"### Results",
"#### Summary",
"## Model Examination [optional]",
"## Environmental Impact\n\n\n\nCarbon emissions can be estimated using the Machine Learning Impact calculator presented in Lacoste et al. (2019).\n\n- Hardware Type: \n- Hours used: \n- Cloud Provider: \n- Compute Region: \n- Carbon Emitted:",
"## Technical Specifications [optional]",
"### Model Architecture and Objective",
"### Compute Infrastructure",
"#### Hardware",
"#### Software\n\n\n\n[optional]\n\n\n\nBibTeX:\n\n\n\nAPA:",
"## Glossary [optional]",
"## More Information [optional]",
"## Model Card Authors [optional]",
"## Model Card Contact"
] |
text-generation | transformers |
# Uploaded model
- **Developed by:** ojasviyadav
- **License:** apache-2.0
- **Finetuned from model :** gemma-7b-bnb-4bit
| {"language": ["en"], "license": "apache-2.0", "tags": ["text-generation-inference", "transformers", "gemma", "trl", "sft"], "base_model": "unsloth/gemma-7b-bnb-4bit"} | ojasviyadav/gemma_it_intent_model | null | [
"transformers",
"pytorch",
"gemma",
"text-generation",
"text-generation-inference",
"trl",
"sft",
"en",
"base_model:unsloth/gemma-7b-bnb-4bit",
"license:apache-2.0",
"autotrain_compatible",
"endpoints_compatible",
"region:us"
] | null | 2024-04-22T10:03:04+00:00 | [] | [
"en"
] | TAGS
#transformers #pytorch #gemma #text-generation #text-generation-inference #trl #sft #en #base_model-unsloth/gemma-7b-bnb-4bit #license-apache-2.0 #autotrain_compatible #endpoints_compatible #region-us
|
# Uploaded model
- Developed by: ojasviyadav
- License: apache-2.0
- Finetuned from model : gemma-7b-bnb-4bit
| [
"# Uploaded model\n\n- Developed by: ojasviyadav\n- License: apache-2.0\n- Finetuned from model : gemma-7b-bnb-4bit"
] | [
"TAGS\n#transformers #pytorch #gemma #text-generation #text-generation-inference #trl #sft #en #base_model-unsloth/gemma-7b-bnb-4bit #license-apache-2.0 #autotrain_compatible #endpoints_compatible #region-us \n",
"# Uploaded model\n\n- Developed by: ojasviyadav\n- License: apache-2.0\n- Finetuned from model : gemma-7b-bnb-4bit"
] |
text-generation | transformers |
# Model Card for Model ID
<!-- Provide a quick summary of what the model is/does. -->
## Model Details
### Model Description
<!-- Provide a longer summary of what this model is. -->
This is the model card of a 🤗 transformers model that has been pushed on the Hub. This model card has been automatically generated.
- **Developed by:** [More Information Needed]
- **Funded by [optional]:** [More Information Needed]
- **Shared by [optional]:** [More Information Needed]
- **Model type:** [More Information Needed]
- **Language(s) (NLP):** [More Information Needed]
- **License:** [More Information Needed]
- **Finetuned from model [optional]:** [More Information Needed]
### Model Sources [optional]
<!-- Provide the basic links for the model. -->
- **Repository:** [More Information Needed]
- **Paper [optional]:** [More Information Needed]
- **Demo [optional]:** [More Information Needed]
## Uses
<!-- Address questions around how the model is intended to be used, including the foreseeable users of the model and those affected by the model. -->
### Direct Use
<!-- This section is for the model use without fine-tuning or plugging into a larger ecosystem/app. -->
[More Information Needed]
### Downstream Use [optional]
<!-- This section is for the model use when fine-tuned for a task, or when plugged into a larger ecosystem/app -->
[More Information Needed]
### Out-of-Scope Use
<!-- This section addresses misuse, malicious use, and uses that the model will not work well for. -->
[More Information Needed]
## Bias, Risks, and Limitations
<!-- This section is meant to convey both technical and sociotechnical limitations. -->
[More Information Needed]
### Recommendations
<!-- This section is meant to convey recommendations with respect to the bias, risk, and technical limitations. -->
Users (both direct and downstream) should be made aware of the risks, biases and limitations of the model. More information needed for further recommendations.
## How to Get Started with the Model
Use the code below to get started with the model.
[More Information Needed]
## Training Details
### Training Data
<!-- This should link to a Dataset Card, perhaps with a short stub of information on what the training data is all about as well as documentation related to data pre-processing or additional filtering. -->
[More Information Needed]
### Training Procedure
<!-- This relates heavily to the Technical Specifications. Content here should link to that section when it is relevant to the training procedure. -->
#### Preprocessing [optional]
[More Information Needed]
#### Training Hyperparameters
- **Training regime:** [More Information Needed] <!--fp32, fp16 mixed precision, bf16 mixed precision, bf16 non-mixed precision, fp16 non-mixed precision, fp8 mixed precision -->
#### Speeds, Sizes, Times [optional]
<!-- This section provides information about throughput, start/end time, checkpoint size if relevant, etc. -->
[More Information Needed]
## Evaluation
<!-- This section describes the evaluation protocols and provides the results. -->
### Testing Data, Factors & Metrics
#### Testing Data
<!-- This should link to a Dataset Card if possible. -->
[More Information Needed]
#### Factors
<!-- These are the things the evaluation is disaggregating by, e.g., subpopulations or domains. -->
[More Information Needed]
#### Metrics
<!-- These are the evaluation metrics being used, ideally with a description of why. -->
[More Information Needed]
### Results
[More Information Needed]
#### Summary
## Model Examination [optional]
<!-- Relevant interpretability work for the model goes here -->
[More Information Needed]
## Environmental Impact
<!-- Total emissions (in grams of CO2eq) and additional considerations, such as electricity usage, go here. Edit the suggested text below accordingly -->
Carbon emissions can be estimated using the [Machine Learning Impact calculator](https://mlco2.github.io/impact#compute) presented in [Lacoste et al. (2019)](https://arxiv.org/abs/1910.09700).
- **Hardware Type:** [More Information Needed]
- **Hours used:** [More Information Needed]
- **Cloud Provider:** [More Information Needed]
- **Compute Region:** [More Information Needed]
- **Carbon Emitted:** [More Information Needed]
## Technical Specifications [optional]
### Model Architecture and Objective
[More Information Needed]
### Compute Infrastructure
[More Information Needed]
#### Hardware
[More Information Needed]
#### Software
[More Information Needed]
## Citation [optional]
<!-- If there is a paper or blog post introducing the model, the APA and Bibtex information for that should go in this section. -->
**BibTeX:**
[More Information Needed]
**APA:**
[More Information Needed]
## Glossary [optional]
<!-- If relevant, include terms and calculations in this section that can help readers understand the model or model card. -->
[More Information Needed]
## More Information [optional]
[More Information Needed]
## Model Card Authors [optional]
[More Information Needed]
## Model Card Contact
[More Information Needed] | {"library_name": "transformers", "tags": []} | OwOOwO/dumbo-stable_t3 | null | [
"transformers",
"safetensors",
"stablelm",
"text-generation",
"conversational",
"arxiv:1910.09700",
"autotrain_compatible",
"endpoints_compatible",
"region:us"
] | null | 2024-04-22T10:04:02+00:00 | [
"1910.09700"
] | [] | TAGS
#transformers #safetensors #stablelm #text-generation #conversational #arxiv-1910.09700 #autotrain_compatible #endpoints_compatible #region-us
|
# Model Card for Model ID
## Model Details
### Model Description
This is the model card of a transformers model that has been pushed on the Hub. This model card has been automatically generated.
- Developed by:
- Funded by [optional]:
- Shared by [optional]:
- Model type:
- Language(s) (NLP):
- License:
- Finetuned from model [optional]:
### Model Sources [optional]
- Repository:
- Paper [optional]:
- Demo [optional]:
## Uses
### Direct Use
### Downstream Use [optional]
### Out-of-Scope Use
## Bias, Risks, and Limitations
### Recommendations
Users (both direct and downstream) should be made aware of the risks, biases and limitations of the model. More information needed for further recommendations.
## How to Get Started with the Model
Use the code below to get started with the model.
## Training Details
### Training Data
### Training Procedure
#### Preprocessing [optional]
#### Training Hyperparameters
- Training regime:
#### Speeds, Sizes, Times [optional]
## Evaluation
### Testing Data, Factors & Metrics
#### Testing Data
#### Factors
#### Metrics
### Results
#### Summary
## Model Examination [optional]
## Environmental Impact
Carbon emissions can be estimated using the Machine Learning Impact calculator presented in Lacoste et al. (2019).
- Hardware Type:
- Hours used:
- Cloud Provider:
- Compute Region:
- Carbon Emitted:
## Technical Specifications [optional]
### Model Architecture and Objective
### Compute Infrastructure
#### Hardware
#### Software
[optional]
BibTeX:
APA:
## Glossary [optional]
## More Information [optional]
## Model Card Authors [optional]
## Model Card Contact
| [
"# Model Card for Model ID",
"## Model Details",
"### Model Description\n\n\n\nThis is the model card of a transformers model that has been pushed on the Hub. This model card has been automatically generated.\n\n- Developed by: \n- Funded by [optional]: \n- Shared by [optional]: \n- Model type: \n- Language(s) (NLP): \n- License: \n- Finetuned from model [optional]:",
"### Model Sources [optional]\n\n\n\n- Repository: \n- Paper [optional]: \n- Demo [optional]:",
"## Uses",
"### Direct Use",
"### Downstream Use [optional]",
"### Out-of-Scope Use",
"## Bias, Risks, and Limitations",
"### Recommendations\n\n\n\nUsers (both direct and downstream) should be made aware of the risks, biases and limitations of the model. More information needed for further recommendations.",
"## How to Get Started with the Model\n\nUse the code below to get started with the model.",
"## Training Details",
"### Training Data",
"### Training Procedure",
"#### Preprocessing [optional]",
"#### Training Hyperparameters\n\n- Training regime:",
"#### Speeds, Sizes, Times [optional]",
"## Evaluation",
"### Testing Data, Factors & Metrics",
"#### Testing Data",
"#### Factors",
"#### Metrics",
"### Results",
"#### Summary",
"## Model Examination [optional]",
"## Environmental Impact\n\n\n\nCarbon emissions can be estimated using the Machine Learning Impact calculator presented in Lacoste et al. (2019).\n\n- Hardware Type: \n- Hours used: \n- Cloud Provider: \n- Compute Region: \n- Carbon Emitted:",
"## Technical Specifications [optional]",
"### Model Architecture and Objective",
"### Compute Infrastructure",
"#### Hardware",
"#### Software\n\n\n\n[optional]\n\n\n\nBibTeX:\n\n\n\nAPA:",
"## Glossary [optional]",
"## More Information [optional]",
"## Model Card Authors [optional]",
"## Model Card Contact"
] | [
"TAGS\n#transformers #safetensors #stablelm #text-generation #conversational #arxiv-1910.09700 #autotrain_compatible #endpoints_compatible #region-us \n",
"# Model Card for Model ID",
"## Model Details",
"### Model Description\n\n\n\nThis is the model card of a transformers model that has been pushed on the Hub. This model card has been automatically generated.\n\n- Developed by: \n- Funded by [optional]: \n- Shared by [optional]: \n- Model type: \n- Language(s) (NLP): \n- License: \n- Finetuned from model [optional]:",
"### Model Sources [optional]\n\n\n\n- Repository: \n- Paper [optional]: \n- Demo [optional]:",
"## Uses",
"### Direct Use",
"### Downstream Use [optional]",
"### Out-of-Scope Use",
"## Bias, Risks, and Limitations",
"### Recommendations\n\n\n\nUsers (both direct and downstream) should be made aware of the risks, biases and limitations of the model. More information needed for further recommendations.",
"## How to Get Started with the Model\n\nUse the code below to get started with the model.",
"## Training Details",
"### Training Data",
"### Training Procedure",
"#### Preprocessing [optional]",
"#### Training Hyperparameters\n\n- Training regime:",
"#### Speeds, Sizes, Times [optional]",
"## Evaluation",
"### Testing Data, Factors & Metrics",
"#### Testing Data",
"#### Factors",
"#### Metrics",
"### Results",
"#### Summary",
"## Model Examination [optional]",
"## Environmental Impact\n\n\n\nCarbon emissions can be estimated using the Machine Learning Impact calculator presented in Lacoste et al. (2019).\n\n- Hardware Type: \n- Hours used: \n- Cloud Provider: \n- Compute Region: \n- Carbon Emitted:",
"## Technical Specifications [optional]",
"### Model Architecture and Objective",
"### Compute Infrastructure",
"#### Hardware",
"#### Software\n\n\n\n[optional]\n\n\n\nBibTeX:\n\n\n\nAPA:",
"## Glossary [optional]",
"## More Information [optional]",
"## Model Card Authors [optional]",
"## Model Card Contact"
] |
text-classification | transformers |
<!-- This model card has been generated automatically according to the information the Trainer had access to. You
should probably proofread and complete it, then remove this comment. -->
# output
This model is a fine-tuned version of [albert/albert-base-v2](https://huggingface.co/albert/albert-base-v2) on the None dataset.
It achieves the following results on the evaluation set:
- Loss: 0.6526
- Accuracy: 0.7191
## Model description
More information needed
## Intended uses & limitations
More information needed
## Training and evaluation data
More information needed
## Training procedure
### Training hyperparameters
The following hyperparameters were used during training:
- learning_rate: 2e-05
- train_batch_size: 16
- eval_batch_size: 16
- seed: 42
- optimizer: Adam with betas=(0.9,0.999) and epsilon=1e-08
- lr_scheduler_type: linear
- num_epochs: 1
### Training results
| Training Loss | Epoch | Step | Validation Loss | Accuracy |
|:-------------:|:-----:|:----:|:---------------:|:--------:|
| No log | 1.0 | 209 | 0.6526 | 0.7191 |
### Framework versions
- Transformers 4.39.3
- Pytorch 2.2.2+cu121
- Datasets 2.18.0
- Tokenizers 0.15.2
| {"license": "apache-2.0", "tags": ["generated_from_trainer"], "metrics": ["accuracy"], "base_model": "albert/albert-base-v2", "model-index": [{"name": "output", "results": []}]} | KalaiselvanD/output | null | [
"transformers",
"tensorboard",
"safetensors",
"albert",
"text-classification",
"generated_from_trainer",
"base_model:albert/albert-base-v2",
"license:apache-2.0",
"autotrain_compatible",
"endpoints_compatible",
"region:us"
] | null | 2024-04-22T10:06:49+00:00 | [] | [] | TAGS
#transformers #tensorboard #safetensors #albert #text-classification #generated_from_trainer #base_model-albert/albert-base-v2 #license-apache-2.0 #autotrain_compatible #endpoints_compatible #region-us
| output
======
This model is a fine-tuned version of albert/albert-base-v2 on the None dataset.
It achieves the following results on the evaluation set:
* Loss: 0.6526
* Accuracy: 0.7191
Model description
-----------------
More information needed
Intended uses & limitations
---------------------------
More information needed
Training and evaluation data
----------------------------
More information needed
Training procedure
------------------
### Training hyperparameters
The following hyperparameters were used during training:
* learning\_rate: 2e-05
* train\_batch\_size: 16
* eval\_batch\_size: 16
* seed: 42
* optimizer: Adam with betas=(0.9,0.999) and epsilon=1e-08
* lr\_scheduler\_type: linear
* num\_epochs: 1
### Training results
### Framework versions
* Transformers 4.39.3
* Pytorch 2.2.2+cu121
* Datasets 2.18.0
* Tokenizers 0.15.2
| [
"### Training hyperparameters\n\n\nThe following hyperparameters were used during training:\n\n\n* learning\\_rate: 2e-05\n* train\\_batch\\_size: 16\n* eval\\_batch\\_size: 16\n* seed: 42\n* optimizer: Adam with betas=(0.9,0.999) and epsilon=1e-08\n* lr\\_scheduler\\_type: linear\n* num\\_epochs: 1",
"### Training results",
"### Framework versions\n\n\n* Transformers 4.39.3\n* Pytorch 2.2.2+cu121\n* Datasets 2.18.0\n* Tokenizers 0.15.2"
] | [
"TAGS\n#transformers #tensorboard #safetensors #albert #text-classification #generated_from_trainer #base_model-albert/albert-base-v2 #license-apache-2.0 #autotrain_compatible #endpoints_compatible #region-us \n",
"### Training hyperparameters\n\n\nThe following hyperparameters were used during training:\n\n\n* learning\\_rate: 2e-05\n* train\\_batch\\_size: 16\n* eval\\_batch\\_size: 16\n* seed: 42\n* optimizer: Adam with betas=(0.9,0.999) and epsilon=1e-08\n* lr\\_scheduler\\_type: linear\n* num\\_epochs: 1",
"### Training results",
"### Framework versions\n\n\n* Transformers 4.39.3\n* Pytorch 2.2.2+cu121\n* Datasets 2.18.0\n* Tokenizers 0.15.2"
] |
text-classification | transformers |
<!-- This model card has been generated automatically according to the information the Trainer had access to. You
should probably proofread and complete it, then remove this comment. -->
# stock-classifier
This model is a fine-tuned version of [distilbert/distilbert-base-uncased](https://huggingface.co/distilbert/distilbert-base-uncased) on the None dataset.
It achieves the following results on the evaluation set:
- Loss: 0.0048
- Accuracy: 0.9984
## Model description
More information needed
## Intended uses & limitations
More information needed
## Training and evaluation data
More information needed
## Training procedure
### Training hyperparameters
The following hyperparameters were used during training:
- learning_rate: 2e-05
- train_batch_size: 2
- eval_batch_size: 2
- seed: 42
- optimizer: Adam with betas=(0.9,0.999) and epsilon=1e-08
- lr_scheduler_type: linear
- num_epochs: 9
### Training results
| Training Loss | Epoch | Step | Validation Loss | Accuracy |
|:-------------:|:-----:|:-----:|:---------------:|:--------:|
| 5.3667 | 1.0 | 2844 | 4.5999 | 0.5886 |
| 1.8303 | 2.0 | 5688 | 1.3704 | 0.9620 |
| 0.2384 | 3.0 | 8532 | 0.1371 | 0.9937 |
| 0.0442 | 4.0 | 11376 | 0.0178 | 0.9953 |
| 0.0188 | 5.0 | 14220 | 0.0060 | 0.9984 |
| 0.0072 | 6.0 | 17064 | 0.0061 | 0.9984 |
| 0.0076 | 7.0 | 19908 | 0.0033 | 0.9984 |
| 0.0045 | 8.0 | 22752 | 0.0046 | 0.9984 |
| 0.0044 | 9.0 | 25596 | 0.0048 | 0.9984 |
### Framework versions
- Transformers 4.38.2
- Pytorch 2.2.1+cu121
- Datasets 2.19.0
- Tokenizers 0.15.2
| {"license": "apache-2.0", "tags": ["generated_from_trainer"], "metrics": ["accuracy"], "base_model": "distilbert/distilbert-base-uncased", "model-index": [{"name": "stock-classifier", "results": []}]} | hkufyp2024/stock-classifier | null | [
"transformers",
"tensorboard",
"safetensors",
"distilbert",
"text-classification",
"generated_from_trainer",
"base_model:distilbert/distilbert-base-uncased",
"license:apache-2.0",
"autotrain_compatible",
"endpoints_compatible",
"region:us"
] | null | 2024-04-22T10:14:17+00:00 | [] | [] | TAGS
#transformers #tensorboard #safetensors #distilbert #text-classification #generated_from_trainer #base_model-distilbert/distilbert-base-uncased #license-apache-2.0 #autotrain_compatible #endpoints_compatible #region-us
| stock-classifier
================
This model is a fine-tuned version of distilbert/distilbert-base-uncased on the None dataset.
It achieves the following results on the evaluation set:
* Loss: 0.0048
* Accuracy: 0.9984
Model description
-----------------
More information needed
Intended uses & limitations
---------------------------
More information needed
Training and evaluation data
----------------------------
More information needed
Training procedure
------------------
### Training hyperparameters
The following hyperparameters were used during training:
* learning\_rate: 2e-05
* train\_batch\_size: 2
* eval\_batch\_size: 2
* seed: 42
* optimizer: Adam with betas=(0.9,0.999) and epsilon=1e-08
* lr\_scheduler\_type: linear
* num\_epochs: 9
### Training results
### Framework versions
* Transformers 4.38.2
* Pytorch 2.2.1+cu121
* Datasets 2.19.0
* Tokenizers 0.15.2
| [
"### Training hyperparameters\n\n\nThe following hyperparameters were used during training:\n\n\n* learning\\_rate: 2e-05\n* train\\_batch\\_size: 2\n* eval\\_batch\\_size: 2\n* seed: 42\n* optimizer: Adam with betas=(0.9,0.999) and epsilon=1e-08\n* lr\\_scheduler\\_type: linear\n* num\\_epochs: 9",
"### Training results",
"### Framework versions\n\n\n* Transformers 4.38.2\n* Pytorch 2.2.1+cu121\n* Datasets 2.19.0\n* Tokenizers 0.15.2"
] | [
"TAGS\n#transformers #tensorboard #safetensors #distilbert #text-classification #generated_from_trainer #base_model-distilbert/distilbert-base-uncased #license-apache-2.0 #autotrain_compatible #endpoints_compatible #region-us \n",
"### Training hyperparameters\n\n\nThe following hyperparameters were used during training:\n\n\n* learning\\_rate: 2e-05\n* train\\_batch\\_size: 2\n* eval\\_batch\\_size: 2\n* seed: 42\n* optimizer: Adam with betas=(0.9,0.999) and epsilon=1e-08\n* lr\\_scheduler\\_type: linear\n* num\\_epochs: 9",
"### Training results",
"### Framework versions\n\n\n* Transformers 4.38.2\n* Pytorch 2.2.1+cu121\n* Datasets 2.19.0\n* Tokenizers 0.15.2"
] |
text-generation | transformers |
<!-- This model card has been generated automatically according to the information the Trainer had access to. You
should probably proofread and complete it, then remove this comment. -->
# phi-1_5-finetuned-gsm8k
This model is a fine-tuned version of [microsoft/phi-1_5](https://huggingface.co/microsoft/phi-1_5) on the None dataset.
## Model description
More information needed
## Intended uses & limitations
More information needed
## Training and evaluation data
More information needed
## Training procedure
### Training hyperparameters
The following hyperparameters were used during training:
- learning_rate: 0.0002
- train_batch_size: 4
- eval_batch_size: 8
- seed: 42
- optimizer: Adam with betas=(0.9,0.999) and epsilon=1e-08
- lr_scheduler_type: cosine
- training_steps: 1000
### Training results
### Framework versions
- PEFT 0.10.0
- Transformers 4.38.2
- Pytorch 2.2.1+cu121
- Datasets 2.19.0
- Tokenizers 0.15.2 | {"language": ["en"], "license": "mit", "tags": ["generated_from_trainer", "text-generation-inference", "code"], "base_model": "microsoft/phi-1_5", "pipeline_tag": "text-generation", "model-index": [{"name": "phi-1_5-finetuned-gsm8k", "results": []}]} | shubham008/phi-1_5-finetuned-gsm8k | null | [
"transformers",
"tensorboard",
"safetensors",
"phi",
"text-generation",
"generated_from_trainer",
"text-generation-inference",
"code",
"custom_code",
"en",
"base_model:microsoft/phi-1_5",
"license:mit",
"autotrain_compatible",
"endpoints_compatible",
"has_space",
"region:us"
] | null | 2024-04-22T10:14:56+00:00 | [] | [
"en"
] | TAGS
#transformers #tensorboard #safetensors #phi #text-generation #generated_from_trainer #text-generation-inference #code #custom_code #en #base_model-microsoft/phi-1_5 #license-mit #autotrain_compatible #endpoints_compatible #has_space #region-us
|
# phi-1_5-finetuned-gsm8k
This model is a fine-tuned version of microsoft/phi-1_5 on the None dataset.
## Model description
More information needed
## Intended uses & limitations
More information needed
## Training and evaluation data
More information needed
## Training procedure
### Training hyperparameters
The following hyperparameters were used during training:
- learning_rate: 0.0002
- train_batch_size: 4
- eval_batch_size: 8
- seed: 42
- optimizer: Adam with betas=(0.9,0.999) and epsilon=1e-08
- lr_scheduler_type: cosine
- training_steps: 1000
### Training results
### Framework versions
- PEFT 0.10.0
- Transformers 4.38.2
- Pytorch 2.2.1+cu121
- Datasets 2.19.0
- Tokenizers 0.15.2 | [
"# phi-1_5-finetuned-gsm8k\n\nThis model is a fine-tuned version of microsoft/phi-1_5 on the None dataset.",
"## Model description\n\nMore information needed",
"## Intended uses & limitations\n\nMore information needed",
"## Training and evaluation data\n\nMore information needed",
"## Training procedure",
"### Training hyperparameters\n\nThe following hyperparameters were used during training:\n- learning_rate: 0.0002\n- train_batch_size: 4\n- eval_batch_size: 8\n- seed: 42\n- optimizer: Adam with betas=(0.9,0.999) and epsilon=1e-08\n- lr_scheduler_type: cosine\n- training_steps: 1000",
"### Training results",
"### Framework versions\n\n- PEFT 0.10.0\n- Transformers 4.38.2\n- Pytorch 2.2.1+cu121\n- Datasets 2.19.0\n- Tokenizers 0.15.2"
] | [
"TAGS\n#transformers #tensorboard #safetensors #phi #text-generation #generated_from_trainer #text-generation-inference #code #custom_code #en #base_model-microsoft/phi-1_5 #license-mit #autotrain_compatible #endpoints_compatible #has_space #region-us \n",
"# phi-1_5-finetuned-gsm8k\n\nThis model is a fine-tuned version of microsoft/phi-1_5 on the None dataset.",
"## Model description\n\nMore information needed",
"## Intended uses & limitations\n\nMore information needed",
"## Training and evaluation data\n\nMore information needed",
"## Training procedure",
"### Training hyperparameters\n\nThe following hyperparameters were used during training:\n- learning_rate: 0.0002\n- train_batch_size: 4\n- eval_batch_size: 8\n- seed: 42\n- optimizer: Adam with betas=(0.9,0.999) and epsilon=1e-08\n- lr_scheduler_type: cosine\n- training_steps: 1000",
"### Training results",
"### Framework versions\n\n- PEFT 0.10.0\n- Transformers 4.38.2\n- Pytorch 2.2.1+cu121\n- Datasets 2.19.0\n- Tokenizers 0.15.2"
] |
text-generation | transformers |
# Model Card for Model ID
<!-- Provide a quick summary of what the model is/does. -->
## Model Details
### Model Description
<!-- Provide a longer summary of what this model is. -->
This is the model card of a 🤗 transformers model that has been pushed on the Hub. This model card has been automatically generated.
- **Developed by:** [More Information Needed]
- **Funded by [optional]:** [More Information Needed]
- **Shared by [optional]:** [More Information Needed]
- **Model type:** [More Information Needed]
- **Language(s) (NLP):** [More Information Needed]
- **License:** [More Information Needed]
- **Finetuned from model [optional]:** [More Information Needed]
### Model Sources [optional]
<!-- Provide the basic links for the model. -->
- **Repository:** [More Information Needed]
- **Paper [optional]:** [More Information Needed]
- **Demo [optional]:** [More Information Needed]
## Uses
<!-- Address questions around how the model is intended to be used, including the foreseeable users of the model and those affected by the model. -->
### Direct Use
<!-- This section is for the model use without fine-tuning or plugging into a larger ecosystem/app. -->
[More Information Needed]
### Downstream Use [optional]
<!-- This section is for the model use when fine-tuned for a task, or when plugged into a larger ecosystem/app -->
[More Information Needed]
### Out-of-Scope Use
<!-- This section addresses misuse, malicious use, and uses that the model will not work well for. -->
[More Information Needed]
## Bias, Risks, and Limitations
<!-- This section is meant to convey both technical and sociotechnical limitations. -->
[More Information Needed]
### Recommendations
<!-- This section is meant to convey recommendations with respect to the bias, risk, and technical limitations. -->
Users (both direct and downstream) should be made aware of the risks, biases and limitations of the model. More information needed for further recommendations.
## How to Get Started with the Model
Use the code below to get started with the model.
[More Information Needed]
## Training Details
### Training Data
<!-- This should link to a Dataset Card, perhaps with a short stub of information on what the training data is all about as well as documentation related to data pre-processing or additional filtering. -->
[More Information Needed]
### Training Procedure
<!-- This relates heavily to the Technical Specifications. Content here should link to that section when it is relevant to the training procedure. -->
#### Preprocessing [optional]
[More Information Needed]
#### Training Hyperparameters
- **Training regime:** [More Information Needed] <!--fp32, fp16 mixed precision, bf16 mixed precision, bf16 non-mixed precision, fp16 non-mixed precision, fp8 mixed precision -->
#### Speeds, Sizes, Times [optional]
<!-- This section provides information about throughput, start/end time, checkpoint size if relevant, etc. -->
[More Information Needed]
## Evaluation
<!-- This section describes the evaluation protocols and provides the results. -->
### Testing Data, Factors & Metrics
#### Testing Data
<!-- This should link to a Dataset Card if possible. -->
[More Information Needed]
#### Factors
<!-- These are the things the evaluation is disaggregating by, e.g., subpopulations or domains. -->
[More Information Needed]
#### Metrics
<!-- These are the evaluation metrics being used, ideally with a description of why. -->
[More Information Needed]
### Results
[More Information Needed]
#### Summary
## Model Examination [optional]
<!-- Relevant interpretability work for the model goes here -->
[More Information Needed]
## Environmental Impact
<!-- Total emissions (in grams of CO2eq) and additional considerations, such as electricity usage, go here. Edit the suggested text below accordingly -->
Carbon emissions can be estimated using the [Machine Learning Impact calculator](https://mlco2.github.io/impact#compute) presented in [Lacoste et al. (2019)](https://arxiv.org/abs/1910.09700).
- **Hardware Type:** [More Information Needed]
- **Hours used:** [More Information Needed]
- **Cloud Provider:** [More Information Needed]
- **Compute Region:** [More Information Needed]
- **Carbon Emitted:** [More Information Needed]
## Technical Specifications [optional]
### Model Architecture and Objective
[More Information Needed]
### Compute Infrastructure
[More Information Needed]
#### Hardware
[More Information Needed]
#### Software
[More Information Needed]
## Citation [optional]
<!-- If there is a paper or blog post introducing the model, the APA and Bibtex information for that should go in this section. -->
**BibTeX:**
[More Information Needed]
**APA:**
[More Information Needed]
## Glossary [optional]
<!-- If relevant, include terms and calculations in this section that can help readers understand the model or model card. -->
[More Information Needed]
## More Information [optional]
[More Information Needed]
## Model Card Authors [optional]
[More Information Needed]
## Model Card Contact
[More Information Needed]
| {"library_name": "transformers", "tags": []} | mjbuehler/BioLlama-3-8B-V100_NOINST-checkpoint-29115-merged | null | [
"transformers",
"safetensors",
"gguf",
"llama",
"text-generation",
"arxiv:1910.09700",
"autotrain_compatible",
"endpoints_compatible",
"text-generation-inference",
"region:us"
] | null | 2024-04-22T10:15:00+00:00 | [
"1910.09700"
] | [] | TAGS
#transformers #safetensors #gguf #llama #text-generation #arxiv-1910.09700 #autotrain_compatible #endpoints_compatible #text-generation-inference #region-us
|
# Model Card for Model ID
## Model Details
### Model Description
This is the model card of a transformers model that has been pushed on the Hub. This model card has been automatically generated.
- Developed by:
- Funded by [optional]:
- Shared by [optional]:
- Model type:
- Language(s) (NLP):
- License:
- Finetuned from model [optional]:
### Model Sources [optional]
- Repository:
- Paper [optional]:
- Demo [optional]:
## Uses
### Direct Use
### Downstream Use [optional]
### Out-of-Scope Use
## Bias, Risks, and Limitations
### Recommendations
Users (both direct and downstream) should be made aware of the risks, biases and limitations of the model. More information needed for further recommendations.
## How to Get Started with the Model
Use the code below to get started with the model.
## Training Details
### Training Data
### Training Procedure
#### Preprocessing [optional]
#### Training Hyperparameters
- Training regime:
#### Speeds, Sizes, Times [optional]
## Evaluation
### Testing Data, Factors & Metrics
#### Testing Data
#### Factors
#### Metrics
### Results
#### Summary
## Model Examination [optional]
## Environmental Impact
Carbon emissions can be estimated using the Machine Learning Impact calculator presented in Lacoste et al. (2019).
- Hardware Type:
- Hours used:
- Cloud Provider:
- Compute Region:
- Carbon Emitted:
## Technical Specifications [optional]
### Model Architecture and Objective
### Compute Infrastructure
#### Hardware
#### Software
[optional]
BibTeX:
APA:
## Glossary [optional]
## More Information [optional]
## Model Card Authors [optional]
## Model Card Contact
| [
"# Model Card for Model ID",
"## Model Details",
"### Model Description\n\n\n\nThis is the model card of a transformers model that has been pushed on the Hub. This model card has been automatically generated.\n\n- Developed by: \n- Funded by [optional]: \n- Shared by [optional]: \n- Model type: \n- Language(s) (NLP): \n- License: \n- Finetuned from model [optional]:",
"### Model Sources [optional]\n\n\n\n- Repository: \n- Paper [optional]: \n- Demo [optional]:",
"## Uses",
"### Direct Use",
"### Downstream Use [optional]",
"### Out-of-Scope Use",
"## Bias, Risks, and Limitations",
"### Recommendations\n\n\n\nUsers (both direct and downstream) should be made aware of the risks, biases and limitations of the model. More information needed for further recommendations.",
"## How to Get Started with the Model\n\nUse the code below to get started with the model.",
"## Training Details",
"### Training Data",
"### Training Procedure",
"#### Preprocessing [optional]",
"#### Training Hyperparameters\n\n- Training regime:",
"#### Speeds, Sizes, Times [optional]",
"## Evaluation",
"### Testing Data, Factors & Metrics",
"#### Testing Data",
"#### Factors",
"#### Metrics",
"### Results",
"#### Summary",
"## Model Examination [optional]",
"## Environmental Impact\n\n\n\nCarbon emissions can be estimated using the Machine Learning Impact calculator presented in Lacoste et al. (2019).\n\n- Hardware Type: \n- Hours used: \n- Cloud Provider: \n- Compute Region: \n- Carbon Emitted:",
"## Technical Specifications [optional]",
"### Model Architecture and Objective",
"### Compute Infrastructure",
"#### Hardware",
"#### Software\n\n\n\n[optional]\n\n\n\nBibTeX:\n\n\n\nAPA:",
"## Glossary [optional]",
"## More Information [optional]",
"## Model Card Authors [optional]",
"## Model Card Contact"
] | [
"TAGS\n#transformers #safetensors #gguf #llama #text-generation #arxiv-1910.09700 #autotrain_compatible #endpoints_compatible #text-generation-inference #region-us \n",
"# Model Card for Model ID",
"## Model Details",
"### Model Description\n\n\n\nThis is the model card of a transformers model that has been pushed on the Hub. This model card has been automatically generated.\n\n- Developed by: \n- Funded by [optional]: \n- Shared by [optional]: \n- Model type: \n- Language(s) (NLP): \n- License: \n- Finetuned from model [optional]:",
"### Model Sources [optional]\n\n\n\n- Repository: \n- Paper [optional]: \n- Demo [optional]:",
"## Uses",
"### Direct Use",
"### Downstream Use [optional]",
"### Out-of-Scope Use",
"## Bias, Risks, and Limitations",
"### Recommendations\n\n\n\nUsers (both direct and downstream) should be made aware of the risks, biases and limitations of the model. More information needed for further recommendations.",
"## How to Get Started with the Model\n\nUse the code below to get started with the model.",
"## Training Details",
"### Training Data",
"### Training Procedure",
"#### Preprocessing [optional]",
"#### Training Hyperparameters\n\n- Training regime:",
"#### Speeds, Sizes, Times [optional]",
"## Evaluation",
"### Testing Data, Factors & Metrics",
"#### Testing Data",
"#### Factors",
"#### Metrics",
"### Results",
"#### Summary",
"## Model Examination [optional]",
"## Environmental Impact\n\n\n\nCarbon emissions can be estimated using the Machine Learning Impact calculator presented in Lacoste et al. (2019).\n\n- Hardware Type: \n- Hours used: \n- Cloud Provider: \n- Compute Region: \n- Carbon Emitted:",
"## Technical Specifications [optional]",
"### Model Architecture and Objective",
"### Compute Infrastructure",
"#### Hardware",
"#### Software\n\n\n\n[optional]\n\n\n\nBibTeX:\n\n\n\nAPA:",
"## Glossary [optional]",
"## More Information [optional]",
"## Model Card Authors [optional]",
"## Model Card Contact"
] |
null | transformers |
# Uploaded model
- **Developed by:** Akirami
- **License:** apache-2.0
- **Finetuned from model :** unsloth/llama-3-8b-bnb-4bit
This llama model was trained 2x faster with [Unsloth](https://github.com/unslothai/unsloth) and Huggingface's TRL library.
[<img src="https://raw.githubusercontent.com/unslothai/unsloth/main/images/unsloth%20made%20with%20love.png" width="200"/>](https://github.com/unslothai/unsloth)
| {"language": ["en"], "license": "apache-2.0", "tags": ["text-generation-inference", "transformers", "unsloth", "llama", "trl"], "base_model": "unsloth/llama-3-8b-bnb-4bit"} | Akirami/llama3-8b-orpo-eg1 | null | [
"transformers",
"safetensors",
"text-generation-inference",
"unsloth",
"llama",
"trl",
"en",
"base_model:unsloth/llama-3-8b-bnb-4bit",
"license:apache-2.0",
"endpoints_compatible",
"region:us"
] | null | 2024-04-22T10:17:47+00:00 | [] | [
"en"
] | TAGS
#transformers #safetensors #text-generation-inference #unsloth #llama #trl #en #base_model-unsloth/llama-3-8b-bnb-4bit #license-apache-2.0 #endpoints_compatible #region-us
|
# Uploaded model
- Developed by: Akirami
- License: apache-2.0
- Finetuned from model : unsloth/llama-3-8b-bnb-4bit
This llama model was trained 2x faster with Unsloth and Huggingface's TRL library.
<img src="URL width="200"/>
| [
"# Uploaded model\n\n- Developed by: Akirami\n- License: apache-2.0\n- Finetuned from model : unsloth/llama-3-8b-bnb-4bit\n\nThis llama model was trained 2x faster with Unsloth and Huggingface's TRL library.\n\n<img src=\"URL width=\"200\"/>"
] | [
"TAGS\n#transformers #safetensors #text-generation-inference #unsloth #llama #trl #en #base_model-unsloth/llama-3-8b-bnb-4bit #license-apache-2.0 #endpoints_compatible #region-us \n",
"# Uploaded model\n\n- Developed by: Akirami\n- License: apache-2.0\n- Finetuned from model : unsloth/llama-3-8b-bnb-4bit\n\nThis llama model was trained 2x faster with Unsloth and Huggingface's TRL library.\n\n<img src=\"URL width=\"200\"/>"
] |
text-generation | transformers |
# Model Card for Model ID
<!-- Provide a quick summary of what the model is/does. -->
## Model Details
### Model Description
<!-- Provide a longer summary of what this model is. -->
This is the model card of a 🤗 transformers model that has been pushed on the Hub. This model card has been automatically generated.
- **Developed by:** [More Information Needed]
- **Funded by [optional]:** [More Information Needed]
- **Shared by [optional]:** [More Information Needed]
- **Model type:** [More Information Needed]
- **Language(s) (NLP):** [More Information Needed]
- **License:** [More Information Needed]
- **Finetuned from model [optional]:** [More Information Needed]
### Model Sources [optional]
<!-- Provide the basic links for the model. -->
- **Repository:** [More Information Needed]
- **Paper [optional]:** [More Information Needed]
- **Demo [optional]:** [More Information Needed]
## Uses
<!-- Address questions around how the model is intended to be used, including the foreseeable users of the model and those affected by the model. -->
### Direct Use
<!-- This section is for the model use without fine-tuning or plugging into a larger ecosystem/app. -->
[More Information Needed]
### Downstream Use [optional]
<!-- This section is for the model use when fine-tuned for a task, or when plugged into a larger ecosystem/app -->
[More Information Needed]
### Out-of-Scope Use
<!-- This section addresses misuse, malicious use, and uses that the model will not work well for. -->
[More Information Needed]
## Bias, Risks, and Limitations
<!-- This section is meant to convey both technical and sociotechnical limitations. -->
[More Information Needed]
### Recommendations
<!-- This section is meant to convey recommendations with respect to the bias, risk, and technical limitations. -->
Users (both direct and downstream) should be made aware of the risks, biases and limitations of the model. More information needed for further recommendations.
## How to Get Started with the Model
Use the code below to get started with the model.
[More Information Needed]
## Training Details
### Training Data
<!-- This should link to a Dataset Card, perhaps with a short stub of information on what the training data is all about as well as documentation related to data pre-processing or additional filtering. -->
[More Information Needed]
### Training Procedure
<!-- This relates heavily to the Technical Specifications. Content here should link to that section when it is relevant to the training procedure. -->
#### Preprocessing [optional]
[More Information Needed]
#### Training Hyperparameters
- **Training regime:** [More Information Needed] <!--fp32, fp16 mixed precision, bf16 mixed precision, bf16 non-mixed precision, fp16 non-mixed precision, fp8 mixed precision -->
#### Speeds, Sizes, Times [optional]
<!-- This section provides information about throughput, start/end time, checkpoint size if relevant, etc. -->
[More Information Needed]
## Evaluation
<!-- This section describes the evaluation protocols and provides the results. -->
### Testing Data, Factors & Metrics
#### Testing Data
<!-- This should link to a Dataset Card if possible. -->
[More Information Needed]
#### Factors
<!-- These are the things the evaluation is disaggregating by, e.g., subpopulations or domains. -->
[More Information Needed]
#### Metrics
<!-- These are the evaluation metrics being used, ideally with a description of why. -->
[More Information Needed]
### Results
[More Information Needed]
#### Summary
## Model Examination [optional]
<!-- Relevant interpretability work for the model goes here -->
[More Information Needed]
## Environmental Impact
<!-- Total emissions (in grams of CO2eq) and additional considerations, such as electricity usage, go here. Edit the suggested text below accordingly -->
Carbon emissions can be estimated using the [Machine Learning Impact calculator](https://mlco2.github.io/impact#compute) presented in [Lacoste et al. (2019)](https://arxiv.org/abs/1910.09700).
- **Hardware Type:** [More Information Needed]
- **Hours used:** [More Information Needed]
- **Cloud Provider:** [More Information Needed]
- **Compute Region:** [More Information Needed]
- **Carbon Emitted:** [More Information Needed]
## Technical Specifications [optional]
### Model Architecture and Objective
[More Information Needed]
### Compute Infrastructure
[More Information Needed]
#### Hardware
[More Information Needed]
#### Software
[More Information Needed]
## Citation [optional]
<!-- If there is a paper or blog post introducing the model, the APA and Bibtex information for that should go in this section. -->
**BibTeX:**
[More Information Needed]
**APA:**
[More Information Needed]
## Glossary [optional]
<!-- If relevant, include terms and calculations in this section that can help readers understand the model or model card. -->
[More Information Needed]
## More Information [optional]
[More Information Needed]
## Model Card Authors [optional]
[More Information Needed]
## Model Card Contact
[More Information Needed] | {"library_name": "transformers", "tags": []} | Grayx/sad_llama_31.0 | null | [
"transformers",
"safetensors",
"llama",
"text-generation",
"conversational",
"arxiv:1910.09700",
"autotrain_compatible",
"endpoints_compatible",
"text-generation-inference",
"region:us"
] | null | 2024-04-22T10:21:10+00:00 | [
"1910.09700"
] | [] | TAGS
#transformers #safetensors #llama #text-generation #conversational #arxiv-1910.09700 #autotrain_compatible #endpoints_compatible #text-generation-inference #region-us
|
# Model Card for Model ID
## Model Details
### Model Description
This is the model card of a transformers model that has been pushed on the Hub. This model card has been automatically generated.
- Developed by:
- Funded by [optional]:
- Shared by [optional]:
- Model type:
- Language(s) (NLP):
- License:
- Finetuned from model [optional]:
### Model Sources [optional]
- Repository:
- Paper [optional]:
- Demo [optional]:
## Uses
### Direct Use
### Downstream Use [optional]
### Out-of-Scope Use
## Bias, Risks, and Limitations
### Recommendations
Users (both direct and downstream) should be made aware of the risks, biases and limitations of the model. More information needed for further recommendations.
## How to Get Started with the Model
Use the code below to get started with the model.
## Training Details
### Training Data
### Training Procedure
#### Preprocessing [optional]
#### Training Hyperparameters
- Training regime:
#### Speeds, Sizes, Times [optional]
## Evaluation
### Testing Data, Factors & Metrics
#### Testing Data
#### Factors
#### Metrics
### Results
#### Summary
## Model Examination [optional]
## Environmental Impact
Carbon emissions can be estimated using the Machine Learning Impact calculator presented in Lacoste et al. (2019).
- Hardware Type:
- Hours used:
- Cloud Provider:
- Compute Region:
- Carbon Emitted:
## Technical Specifications [optional]
### Model Architecture and Objective
### Compute Infrastructure
#### Hardware
#### Software
[optional]
BibTeX:
APA:
## Glossary [optional]
## More Information [optional]
## Model Card Authors [optional]
## Model Card Contact
| [
"# Model Card for Model ID",
"## Model Details",
"### Model Description\n\n\n\nThis is the model card of a transformers model that has been pushed on the Hub. This model card has been automatically generated.\n\n- Developed by: \n- Funded by [optional]: \n- Shared by [optional]: \n- Model type: \n- Language(s) (NLP): \n- License: \n- Finetuned from model [optional]:",
"### Model Sources [optional]\n\n\n\n- Repository: \n- Paper [optional]: \n- Demo [optional]:",
"## Uses",
"### Direct Use",
"### Downstream Use [optional]",
"### Out-of-Scope Use",
"## Bias, Risks, and Limitations",
"### Recommendations\n\n\n\nUsers (both direct and downstream) should be made aware of the risks, biases and limitations of the model. More information needed for further recommendations.",
"## How to Get Started with the Model\n\nUse the code below to get started with the model.",
"## Training Details",
"### Training Data",
"### Training Procedure",
"#### Preprocessing [optional]",
"#### Training Hyperparameters\n\n- Training regime:",
"#### Speeds, Sizes, Times [optional]",
"## Evaluation",
"### Testing Data, Factors & Metrics",
"#### Testing Data",
"#### Factors",
"#### Metrics",
"### Results",
"#### Summary",
"## Model Examination [optional]",
"## Environmental Impact\n\n\n\nCarbon emissions can be estimated using the Machine Learning Impact calculator presented in Lacoste et al. (2019).\n\n- Hardware Type: \n- Hours used: \n- Cloud Provider: \n- Compute Region: \n- Carbon Emitted:",
"## Technical Specifications [optional]",
"### Model Architecture and Objective",
"### Compute Infrastructure",
"#### Hardware",
"#### Software\n\n\n\n[optional]\n\n\n\nBibTeX:\n\n\n\nAPA:",
"## Glossary [optional]",
"## More Information [optional]",
"## Model Card Authors [optional]",
"## Model Card Contact"
] | [
"TAGS\n#transformers #safetensors #llama #text-generation #conversational #arxiv-1910.09700 #autotrain_compatible #endpoints_compatible #text-generation-inference #region-us \n",
"# Model Card for Model ID",
"## Model Details",
"### Model Description\n\n\n\nThis is the model card of a transformers model that has been pushed on the Hub. This model card has been automatically generated.\n\n- Developed by: \n- Funded by [optional]: \n- Shared by [optional]: \n- Model type: \n- Language(s) (NLP): \n- License: \n- Finetuned from model [optional]:",
"### Model Sources [optional]\n\n\n\n- Repository: \n- Paper [optional]: \n- Demo [optional]:",
"## Uses",
"### Direct Use",
"### Downstream Use [optional]",
"### Out-of-Scope Use",
"## Bias, Risks, and Limitations",
"### Recommendations\n\n\n\nUsers (both direct and downstream) should be made aware of the risks, biases and limitations of the model. More information needed for further recommendations.",
"## How to Get Started with the Model\n\nUse the code below to get started with the model.",
"## Training Details",
"### Training Data",
"### Training Procedure",
"#### Preprocessing [optional]",
"#### Training Hyperparameters\n\n- Training regime:",
"#### Speeds, Sizes, Times [optional]",
"## Evaluation",
"### Testing Data, Factors & Metrics",
"#### Testing Data",
"#### Factors",
"#### Metrics",
"### Results",
"#### Summary",
"## Model Examination [optional]",
"## Environmental Impact\n\n\n\nCarbon emissions can be estimated using the Machine Learning Impact calculator presented in Lacoste et al. (2019).\n\n- Hardware Type: \n- Hours used: \n- Cloud Provider: \n- Compute Region: \n- Carbon Emitted:",
"## Technical Specifications [optional]",
"### Model Architecture and Objective",
"### Compute Infrastructure",
"#### Hardware",
"#### Software\n\n\n\n[optional]\n\n\n\nBibTeX:\n\n\n\nAPA:",
"## Glossary [optional]",
"## More Information [optional]",
"## Model Card Authors [optional]",
"## Model Card Contact"
] |
null | transformers | ## About
<!-- ### quantize_version: 1 -->
<!-- ### output_tensor_quantised: 1 -->
<!-- ### convert_type: -->
<!-- ### vocab_type: -->
static quants of https://huggingface.co/cutycat2000x/MeowGPT-ll3
<!-- provided-files -->
weighted/imatrix quants seem not to be available (by me) at this time. If they do not show up a week or so after the static ones, I have probably not planned for them. Feel free to request them by opening a Community Discussion.
## Usage
If you are unsure how to use GGUF files, refer to one of [TheBloke's
READMEs](https://huggingface.co/TheBloke/KafkaLM-70B-German-V0.1-GGUF) for
more details, including on how to concatenate multi-part files.
## Provided Quants
(sorted by size, not necessarily quality. IQ-quants are often preferable over similar sized non-IQ quants)
| Link | Type | Size/GB | Notes |
|:-----|:-----|--------:|:------|
| [GGUF](https://huggingface.co/mradermacher/MeowGPT-ll3-GGUF/resolve/main/MeowGPT-ll3.Q2_K.gguf) | Q2_K | 3.3 | |
| [GGUF](https://huggingface.co/mradermacher/MeowGPT-ll3-GGUF/resolve/main/MeowGPT-ll3.IQ3_XS.gguf) | IQ3_XS | 3.6 | |
| [GGUF](https://huggingface.co/mradermacher/MeowGPT-ll3-GGUF/resolve/main/MeowGPT-ll3.Q3_K_S.gguf) | Q3_K_S | 3.8 | |
| [GGUF](https://huggingface.co/mradermacher/MeowGPT-ll3-GGUF/resolve/main/MeowGPT-ll3.IQ3_S.gguf) | IQ3_S | 3.8 | beats Q3_K* |
| [GGUF](https://huggingface.co/mradermacher/MeowGPT-ll3-GGUF/resolve/main/MeowGPT-ll3.IQ3_M.gguf) | IQ3_M | 3.9 | |
| [GGUF](https://huggingface.co/mradermacher/MeowGPT-ll3-GGUF/resolve/main/MeowGPT-ll3.Q3_K_M.gguf) | Q3_K_M | 4.1 | lower quality |
| [GGUF](https://huggingface.co/mradermacher/MeowGPT-ll3-GGUF/resolve/main/MeowGPT-ll3.Q3_K_L.gguf) | Q3_K_L | 4.4 | |
| [GGUF](https://huggingface.co/mradermacher/MeowGPT-ll3-GGUF/resolve/main/MeowGPT-ll3.IQ4_XS.gguf) | IQ4_XS | 4.6 | |
| [GGUF](https://huggingface.co/mradermacher/MeowGPT-ll3-GGUF/resolve/main/MeowGPT-ll3.Q4_K_S.gguf) | Q4_K_S | 4.8 | fast, recommended |
| [GGUF](https://huggingface.co/mradermacher/MeowGPT-ll3-GGUF/resolve/main/MeowGPT-ll3.Q4_K_M.gguf) | Q4_K_M | 5.0 | fast, recommended |
| [GGUF](https://huggingface.co/mradermacher/MeowGPT-ll3-GGUF/resolve/main/MeowGPT-ll3.Q5_K_S.gguf) | Q5_K_S | 5.7 | |
| [GGUF](https://huggingface.co/mradermacher/MeowGPT-ll3-GGUF/resolve/main/MeowGPT-ll3.Q5_K_M.gguf) | Q5_K_M | 5.8 | |
| [GGUF](https://huggingface.co/mradermacher/MeowGPT-ll3-GGUF/resolve/main/MeowGPT-ll3.Q6_K.gguf) | Q6_K | 6.7 | very good quality |
| [GGUF](https://huggingface.co/mradermacher/MeowGPT-ll3-GGUF/resolve/main/MeowGPT-ll3.Q8_0.gguf) | Q8_0 | 8.6 | fast, best quality |
Here is a handy graph by ikawrakow comparing some lower-quality quant
types (lower is better):

And here are Artefact2's thoughts on the matter:
https://gist.github.com/Artefact2/b5f810600771265fc1e39442288e8ec9
## FAQ / Model Request
See https://huggingface.co/mradermacher/model_requests for some answers to
questions you might have and/or if you want some other model quantized.
## Thanks
I thank my company, [nethype GmbH](https://www.nethype.de/), for letting
me use its servers and providing upgrades to my workstation to enable
this work in my free time.
<!-- end -->
| {"language": ["en"], "license": "mit", "library_name": "transformers", "tags": ["freeai", "conversational", "meowgpt", "gpt", "free", "opensource", "splittic", "ai", "llama", "llama3"], "datasets": ["Open-Orca/SlimOrca-Dedup", "jondurbin/airoboros-3.2", "microsoft/orca-math-word-problems-200k", "m-a-p/Code-Feedback", "MaziyarPanahi/WizardLM_evol_instruct_V2_196k", "mlabonne/orpo-dpo-mix-40k"], "base_model": "cutycat2000x/MeowGPT-ll3", "quantized_by": "mradermacher"} | mradermacher/MeowGPT-ll3-GGUF | null | [
"transformers",
"gguf",
"freeai",
"conversational",
"meowgpt",
"gpt",
"free",
"opensource",
"splittic",
"ai",
"llama",
"llama3",
"en",
"dataset:Open-Orca/SlimOrca-Dedup",
"dataset:jondurbin/airoboros-3.2",
"dataset:microsoft/orca-math-word-problems-200k",
"dataset:m-a-p/Code-Feedback",
"dataset:MaziyarPanahi/WizardLM_evol_instruct_V2_196k",
"dataset:mlabonne/orpo-dpo-mix-40k",
"base_model:cutycat2000x/MeowGPT-ll3",
"license:mit",
"endpoints_compatible",
"region:us"
] | null | 2024-04-22T10:21:35+00:00 | [] | [
"en"
] | TAGS
#transformers #gguf #freeai #conversational #meowgpt #gpt #free #opensource #splittic #ai #llama #llama3 #en #dataset-Open-Orca/SlimOrca-Dedup #dataset-jondurbin/airoboros-3.2 #dataset-microsoft/orca-math-word-problems-200k #dataset-m-a-p/Code-Feedback #dataset-MaziyarPanahi/WizardLM_evol_instruct_V2_196k #dataset-mlabonne/orpo-dpo-mix-40k #base_model-cutycat2000x/MeowGPT-ll3 #license-mit #endpoints_compatible #region-us
| About
-----
static quants of URL
weighted/imatrix quants seem not to be available (by me) at this time. If they do not show up a week or so after the static ones, I have probably not planned for them. Feel free to request them by opening a Community Discussion.
Usage
-----
If you are unsure how to use GGUF files, refer to one of TheBloke's
READMEs for
more details, including on how to concatenate multi-part files.
Provided Quants
---------------
(sorted by size, not necessarily quality. IQ-quants are often preferable over similar sized non-IQ quants)
Here is a handy graph by ikawrakow comparing some lower-quality quant
types (lower is better):
!URL
And here are Artefact2's thoughts on the matter:
URL
FAQ / Model Request
-------------------
See URL for some answers to
questions you might have and/or if you want some other model quantized.
Thanks
------
I thank my company, nethype GmbH, for letting
me use its servers and providing upgrades to my workstation to enable
this work in my free time.
| [] | [
"TAGS\n#transformers #gguf #freeai #conversational #meowgpt #gpt #free #opensource #splittic #ai #llama #llama3 #en #dataset-Open-Orca/SlimOrca-Dedup #dataset-jondurbin/airoboros-3.2 #dataset-microsoft/orca-math-word-problems-200k #dataset-m-a-p/Code-Feedback #dataset-MaziyarPanahi/WizardLM_evol_instruct_V2_196k #dataset-mlabonne/orpo-dpo-mix-40k #base_model-cutycat2000x/MeowGPT-ll3 #license-mit #endpoints_compatible #region-us \n"
] |
text-to-audio | transformers |
# Model Card for Model ID
<!-- Provide a quick summary of what the model is/does. -->
## Model Details
### Model Description
<!-- Provide a longer summary of what this model is. -->
This is the model card of a 🤗 transformers model that has been pushed on the Hub. This model card has been automatically generated.
- **Developed by:** [More Information Needed]
- **Funded by [optional]:** [More Information Needed]
- **Shared by [optional]:** [More Information Needed]
- **Model type:** [More Information Needed]
- **Language(s) (NLP):** [More Information Needed]
- **License:** [More Information Needed]
- **Finetuned from model [optional]:** [More Information Needed]
### Model Sources [optional]
<!-- Provide the basic links for the model. -->
- **Repository:** [More Information Needed]
- **Paper [optional]:** [More Information Needed]
- **Demo [optional]:** [More Information Needed]
## Uses
<!-- Address questions around how the model is intended to be used, including the foreseeable users of the model and those affected by the model. -->
### Direct Use
<!-- This section is for the model use without fine-tuning or plugging into a larger ecosystem/app. -->
[More Information Needed]
### Downstream Use [optional]
<!-- This section is for the model use when fine-tuned for a task, or when plugged into a larger ecosystem/app -->
[More Information Needed]
### Out-of-Scope Use
<!-- This section addresses misuse, malicious use, and uses that the model will not work well for. -->
[More Information Needed]
## Bias, Risks, and Limitations
<!-- This section is meant to convey both technical and sociotechnical limitations. -->
[More Information Needed]
### Recommendations
<!-- This section is meant to convey recommendations with respect to the bias, risk, and technical limitations. -->
Users (both direct and downstream) should be made aware of the risks, biases and limitations of the model. More information needed for further recommendations.
## How to Get Started with the Model
Use the code below to get started with the model.
[More Information Needed]
## Training Details
### Training Data
<!-- This should link to a Dataset Card, perhaps with a short stub of information on what the training data is all about as well as documentation related to data pre-processing or additional filtering. -->
[More Information Needed]
### Training Procedure
<!-- This relates heavily to the Technical Specifications. Content here should link to that section when it is relevant to the training procedure. -->
#### Preprocessing [optional]
[More Information Needed]
#### Training Hyperparameters
- **Training regime:** [More Information Needed] <!--fp32, fp16 mixed precision, bf16 mixed precision, bf16 non-mixed precision, fp16 non-mixed precision, fp8 mixed precision -->
#### Speeds, Sizes, Times [optional]
<!-- This section provides information about throughput, start/end time, checkpoint size if relevant, etc. -->
[More Information Needed]
## Evaluation
<!-- This section describes the evaluation protocols and provides the results. -->
### Testing Data, Factors & Metrics
#### Testing Data
<!-- This should link to a Dataset Card if possible. -->
[More Information Needed]
#### Factors
<!-- These are the things the evaluation is disaggregating by, e.g., subpopulations or domains. -->
[More Information Needed]
#### Metrics
<!-- These are the evaluation metrics being used, ideally with a description of why. -->
[More Information Needed]
### Results
[More Information Needed]
#### Summary
## Model Examination [optional]
<!-- Relevant interpretability work for the model goes here -->
[More Information Needed]
## Environmental Impact
<!-- Total emissions (in grams of CO2eq) and additional considerations, such as electricity usage, go here. Edit the suggested text below accordingly -->
Carbon emissions can be estimated using the [Machine Learning Impact calculator](https://mlco2.github.io/impact#compute) presented in [Lacoste et al. (2019)](https://arxiv.org/abs/1910.09700).
- **Hardware Type:** [More Information Needed]
- **Hours used:** [More Information Needed]
- **Cloud Provider:** [More Information Needed]
- **Compute Region:** [More Information Needed]
- **Carbon Emitted:** [More Information Needed]
## Technical Specifications [optional]
### Model Architecture and Objective
[More Information Needed]
### Compute Infrastructure
[More Information Needed]
#### Hardware
[More Information Needed]
#### Software
[More Information Needed]
## Citation [optional]
<!-- If there is a paper or blog post introducing the model, the APA and Bibtex information for that should go in this section. -->
**BibTeX:**
[More Information Needed]
**APA:**
[More Information Needed]
## Glossary [optional]
<!-- If relevant, include terms and calculations in this section that can help readers understand the model or model card. -->
[More Information Needed]
## More Information [optional]
[More Information Needed]
## Model Card Authors [optional]
[More Information Needed]
## Model Card Contact
[More Information Needed] | {"library_name": "transformers", "tags": []} | madhabpaul/mms-tts-asm-2 | null | [
"transformers",
"safetensors",
"vits",
"text-to-audio",
"arxiv:1910.09700",
"endpoints_compatible",
"region:us"
] | null | 2024-04-22T10:21:48+00:00 | [
"1910.09700"
] | [] | TAGS
#transformers #safetensors #vits #text-to-audio #arxiv-1910.09700 #endpoints_compatible #region-us
|
# Model Card for Model ID
## Model Details
### Model Description
This is the model card of a transformers model that has been pushed on the Hub. This model card has been automatically generated.
- Developed by:
- Funded by [optional]:
- Shared by [optional]:
- Model type:
- Language(s) (NLP):
- License:
- Finetuned from model [optional]:
### Model Sources [optional]
- Repository:
- Paper [optional]:
- Demo [optional]:
## Uses
### Direct Use
### Downstream Use [optional]
### Out-of-Scope Use
## Bias, Risks, and Limitations
### Recommendations
Users (both direct and downstream) should be made aware of the risks, biases and limitations of the model. More information needed for further recommendations.
## How to Get Started with the Model
Use the code below to get started with the model.
## Training Details
### Training Data
### Training Procedure
#### Preprocessing [optional]
#### Training Hyperparameters
- Training regime:
#### Speeds, Sizes, Times [optional]
## Evaluation
### Testing Data, Factors & Metrics
#### Testing Data
#### Factors
#### Metrics
### Results
#### Summary
## Model Examination [optional]
## Environmental Impact
Carbon emissions can be estimated using the Machine Learning Impact calculator presented in Lacoste et al. (2019).
- Hardware Type:
- Hours used:
- Cloud Provider:
- Compute Region:
- Carbon Emitted:
## Technical Specifications [optional]
### Model Architecture and Objective
### Compute Infrastructure
#### Hardware
#### Software
[optional]
BibTeX:
APA:
## Glossary [optional]
## More Information [optional]
## Model Card Authors [optional]
## Model Card Contact
| [
"# Model Card for Model ID",
"## Model Details",
"### Model Description\n\n\n\nThis is the model card of a transformers model that has been pushed on the Hub. This model card has been automatically generated.\n\n- Developed by: \n- Funded by [optional]: \n- Shared by [optional]: \n- Model type: \n- Language(s) (NLP): \n- License: \n- Finetuned from model [optional]:",
"### Model Sources [optional]\n\n\n\n- Repository: \n- Paper [optional]: \n- Demo [optional]:",
"## Uses",
"### Direct Use",
"### Downstream Use [optional]",
"### Out-of-Scope Use",
"## Bias, Risks, and Limitations",
"### Recommendations\n\n\n\nUsers (both direct and downstream) should be made aware of the risks, biases and limitations of the model. More information needed for further recommendations.",
"## How to Get Started with the Model\n\nUse the code below to get started with the model.",
"## Training Details",
"### Training Data",
"### Training Procedure",
"#### Preprocessing [optional]",
"#### Training Hyperparameters\n\n- Training regime:",
"#### Speeds, Sizes, Times [optional]",
"## Evaluation",
"### Testing Data, Factors & Metrics",
"#### Testing Data",
"#### Factors",
"#### Metrics",
"### Results",
"#### Summary",
"## Model Examination [optional]",
"## Environmental Impact\n\n\n\nCarbon emissions can be estimated using the Machine Learning Impact calculator presented in Lacoste et al. (2019).\n\n- Hardware Type: \n- Hours used: \n- Cloud Provider: \n- Compute Region: \n- Carbon Emitted:",
"## Technical Specifications [optional]",
"### Model Architecture and Objective",
"### Compute Infrastructure",
"#### Hardware",
"#### Software\n\n\n\n[optional]\n\n\n\nBibTeX:\n\n\n\nAPA:",
"## Glossary [optional]",
"## More Information [optional]",
"## Model Card Authors [optional]",
"## Model Card Contact"
] | [
"TAGS\n#transformers #safetensors #vits #text-to-audio #arxiv-1910.09700 #endpoints_compatible #region-us \n",
"# Model Card for Model ID",
"## Model Details",
"### Model Description\n\n\n\nThis is the model card of a transformers model that has been pushed on the Hub. This model card has been automatically generated.\n\n- Developed by: \n- Funded by [optional]: \n- Shared by [optional]: \n- Model type: \n- Language(s) (NLP): \n- License: \n- Finetuned from model [optional]:",
"### Model Sources [optional]\n\n\n\n- Repository: \n- Paper [optional]: \n- Demo [optional]:",
"## Uses",
"### Direct Use",
"### Downstream Use [optional]",
"### Out-of-Scope Use",
"## Bias, Risks, and Limitations",
"### Recommendations\n\n\n\nUsers (both direct and downstream) should be made aware of the risks, biases and limitations of the model. More information needed for further recommendations.",
"## How to Get Started with the Model\n\nUse the code below to get started with the model.",
"## Training Details",
"### Training Data",
"### Training Procedure",
"#### Preprocessing [optional]",
"#### Training Hyperparameters\n\n- Training regime:",
"#### Speeds, Sizes, Times [optional]",
"## Evaluation",
"### Testing Data, Factors & Metrics",
"#### Testing Data",
"#### Factors",
"#### Metrics",
"### Results",
"#### Summary",
"## Model Examination [optional]",
"## Environmental Impact\n\n\n\nCarbon emissions can be estimated using the Machine Learning Impact calculator presented in Lacoste et al. (2019).\n\n- Hardware Type: \n- Hours used: \n- Cloud Provider: \n- Compute Region: \n- Carbon Emitted:",
"## Technical Specifications [optional]",
"### Model Architecture and Objective",
"### Compute Infrastructure",
"#### Hardware",
"#### Software\n\n\n\n[optional]\n\n\n\nBibTeX:\n\n\n\nAPA:",
"## Glossary [optional]",
"## More Information [optional]",
"## Model Card Authors [optional]",
"## Model Card Contact"
] |
null | transformers |
# Uploaded model
- **Developed by:** Adedeji Oluwadamisola
- **License:** apache-2.0
- **Finetuned from model :** unsloth/llama-3-8b-bnb-4bit
This llama 3 8 billion parameter model was finetuned on IW dataset using [Unsloth](https://github.com/unslothai/unsloth) and Huggingface's TRL library.
| {"language": ["en"], "license": "apache-2.0", "tags": ["text-generation-inference", "transformers", "unsloth", "llama", "trl"], "base_model": "unsloth/llama-3-8b-bnb-4bit"} | SirDamisola/iw_lora_model-llama-3 | null | [
"transformers",
"safetensors",
"text-generation-inference",
"unsloth",
"llama",
"trl",
"en",
"base_model:unsloth/llama-3-8b-bnb-4bit",
"license:apache-2.0",
"endpoints_compatible",
"region:us"
] | null | 2024-04-22T10:21:58+00:00 | [] | [
"en"
] | TAGS
#transformers #safetensors #text-generation-inference #unsloth #llama #trl #en #base_model-unsloth/llama-3-8b-bnb-4bit #license-apache-2.0 #endpoints_compatible #region-us
|
# Uploaded model
- Developed by: Adedeji Oluwadamisola
- License: apache-2.0
- Finetuned from model : unsloth/llama-3-8b-bnb-4bit
This llama 3 8 billion parameter model was finetuned on IW dataset using Unsloth and Huggingface's TRL library.
| [
"# Uploaded model\n\n- Developed by: Adedeji Oluwadamisola\n- License: apache-2.0\n- Finetuned from model : unsloth/llama-3-8b-bnb-4bit\n\nThis llama 3 8 billion parameter model was finetuned on IW dataset using Unsloth and Huggingface's TRL library."
] | [
"TAGS\n#transformers #safetensors #text-generation-inference #unsloth #llama #trl #en #base_model-unsloth/llama-3-8b-bnb-4bit #license-apache-2.0 #endpoints_compatible #region-us \n",
"# Uploaded model\n\n- Developed by: Adedeji Oluwadamisola\n- License: apache-2.0\n- Finetuned from model : unsloth/llama-3-8b-bnb-4bit\n\nThis llama 3 8 billion parameter model was finetuned on IW dataset using Unsloth and Huggingface's TRL library."
] |
null | null | # SkinXmed Deutschland Erfahrungen - SkinXmed Stiftung Warentest Test Kaufen
SkinXmed Deutschland Erfahrungen Bei der SkinXmed Creme handelt es sich um eine Anti-Aging-Kur, die seit kurzem auf dem deutschen Markt erhältlich ist. Das Produkt stellt eine Alternative zu Botox da und wird hauptsächlichen von Personen angewendet, die nicht unbedingt die Faltenunterspritzung als Option nutzen wollen. Neben der Anti-Ageing Wirkung, wird dieses Produkt auch von vielen Kunden zur täglichen Hautpflege genutzt.
## **[Klicken Sie hier, um jetzt auf der offiziellen Website von SkinXmed zu kaufen](https://adtocart.xyz/skinxmed-de)**
## Hyaluronsäure Molekulargröße
Leider findet man auf den meisten Produkten keine Angabe über die Molekulargröße von Hyaluron.
Da jedoch die Herstellung von niedermolekularer Hyaluronsäure sehr teuer ist, kann man davon ausgehen, dass billige Hyaluroncremes nur hochmolekulare Hyaluronsäure enthalten.
Niedermolekulare Hyaluronsäure findet man überwiegend in Seren. Sie gelangt in die tiefen Hautschichten und kann die Feuchtigkeit nachhaltig speichern.
Die perfekte Hyaluroncreme beinhaltet sowohl hoch- als auch niedermolekulare Hyaluronsäure.
## Ubiquinone :
Ubiquinone ist besser bekannt als das Coenzym Q10.
Q10 ist eine Geheimwaffe gegen Falten, da es, wie Vitamin C, als Antioxidans wirkt und freie Radikale bekämpfen kann.
Q10 dient als Zellschutz und schützt die kollagenen Fasern vor dem Zerfall durch UV-Strahlung und oxidativem Stress.
## Retinol (Vitamin A) :
Retinol wird in der Haut zu Vitamin-A-Säure umgewandelt.
Retinol wird von Dermatologen als effizientester und wissenschaftlich erwiesener Wirkstoff gegen Falten bezeichnet, da es die Kollagenproduktion anregt und sogar sonnengeschädigte Haut reparieren kann.
## DMAE (Dimethylaminoethanol) :
DMAE ist ein natürlicher Nährstoff, der aus Fisch (u.a. Lachs, Sardinen) gewonnen wird und noch als Geheimtipp im Kampf gegen Falten gilt.
Dimethylaminoethanol verbessert die Festigkeit und Elastizität der Haut und sorgt durch einen Schutz der Zellmembran für eine längere Lebensdauer der Zellen.
DMAE ist auch dafür verantwortlich, dass mehr Acetylcholin ausgeschüttet wird, wodurch die Mikro-Muskelfasern (MYOFILAMENTE) mehr Spannung erhalten. Somit kann DMAE auch schlaffen Hautpartien entgegenwirken.
## Alteromonas Ferment Extract :
Peptid aus den Aminosäuren Lysin, Histidin und Glysin. Fördert die Wasserspeicherkapazität und Wundheilung. Regt die Kollagen- und Elastinbildung und erhöht das Feuchthaltevermögen der Haut.
## Pullulan :
Bei Pullulan handet es sich um ein Polysaccharid, welches durch einen natürlichen Fermentationsprozess aus Pflanzenextrakten gewonnen wird.
Pullulan hat einen Sofort-Lifting-Effekt, bildet einen feinen Film auf der Haut und lässt Falten somit innerhalb von Sekunden verschwinden.
## **[Klicken Sie hier, um jetzt auf der offiziellen Website von SkinXmed zu kaufen](https://adtocart.xyz/skinxmed-de)** | {} | VKapseln475/SkinXmed | null | [
"region:us"
] | null | 2024-04-22T10:22:27+00:00 | [] | [] | TAGS
#region-us
| # SkinXmed Deutschland Erfahrungen - SkinXmed Stiftung Warentest Test Kaufen
SkinXmed Deutschland Erfahrungen Bei der SkinXmed Creme handelt es sich um eine Anti-Aging-Kur, die seit kurzem auf dem deutschen Markt erhältlich ist. Das Produkt stellt eine Alternative zu Botox da und wird hauptsächlichen von Personen angewendet, die nicht unbedingt die Faltenunterspritzung als Option nutzen wollen. Neben der Anti-Ageing Wirkung, wird dieses Produkt auch von vielen Kunden zur täglichen Hautpflege genutzt.
## Klicken Sie hier, um jetzt auf der offiziellen Website von SkinXmed zu kaufen
## Hyaluronsäure Molekulargröße
Leider findet man auf den meisten Produkten keine Angabe über die Molekulargröße von Hyaluron.
Da jedoch die Herstellung von niedermolekularer Hyaluronsäure sehr teuer ist, kann man davon ausgehen, dass billige Hyaluroncremes nur hochmolekulare Hyaluronsäure enthalten.
Niedermolekulare Hyaluronsäure findet man überwiegend in Seren. Sie gelangt in die tiefen Hautschichten und kann die Feuchtigkeit nachhaltig speichern.
Die perfekte Hyaluroncreme beinhaltet sowohl hoch- als auch niedermolekulare Hyaluronsäure.
## Ubiquinone :
Ubiquinone ist besser bekannt als das Coenzym Q10.
Q10 ist eine Geheimwaffe gegen Falten, da es, wie Vitamin C, als Antioxidans wirkt und freie Radikale bekämpfen kann.
Q10 dient als Zellschutz und schützt die kollagenen Fasern vor dem Zerfall durch UV-Strahlung und oxidativem Stress.
## Retinol (Vitamin A) :
Retinol wird in der Haut zu Vitamin-A-Säure umgewandelt.
Retinol wird von Dermatologen als effizientester und wissenschaftlich erwiesener Wirkstoff gegen Falten bezeichnet, da es die Kollagenproduktion anregt und sogar sonnengeschädigte Haut reparieren kann.
## DMAE (Dimethylaminoethanol) :
DMAE ist ein natürlicher Nährstoff, der aus Fisch (u.a. Lachs, Sardinen) gewonnen wird und noch als Geheimtipp im Kampf gegen Falten gilt.
Dimethylaminoethanol verbessert die Festigkeit und Elastizität der Haut und sorgt durch einen Schutz der Zellmembran für eine längere Lebensdauer der Zellen.
DMAE ist auch dafür verantwortlich, dass mehr Acetylcholin ausgeschüttet wird, wodurch die Mikro-Muskelfasern (MYOFILAMENTE) mehr Spannung erhalten. Somit kann DMAE auch schlaffen Hautpartien entgegenwirken.
## Alteromonas Ferment Extract :
Peptid aus den Aminosäuren Lysin, Histidin und Glysin. Fördert die Wasserspeicherkapazität und Wundheilung. Regt die Kollagen- und Elastinbildung und erhöht das Feuchthaltevermögen der Haut.
## Pullulan :
Bei Pullulan handet es sich um ein Polysaccharid, welches durch einen natürlichen Fermentationsprozess aus Pflanzenextrakten gewonnen wird.
Pullulan hat einen Sofort-Lifting-Effekt, bildet einen feinen Film auf der Haut und lässt Falten somit innerhalb von Sekunden verschwinden.
## Klicken Sie hier, um jetzt auf der offiziellen Website von SkinXmed zu kaufen | [
"# SkinXmed Deutschland Erfahrungen - SkinXmed Stiftung Warentest Test Kaufen\n\nSkinXmed Deutschland Erfahrungen Bei der SkinXmed Creme handelt es sich um eine Anti-Aging-Kur, die seit kurzem auf dem deutschen Markt erhältlich ist. Das Produkt stellt eine Alternative zu Botox da und wird hauptsächlichen von Personen angewendet, die nicht unbedingt die Faltenunterspritzung als Option nutzen wollen. Neben der Anti-Ageing Wirkung, wird dieses Produkt auch von vielen Kunden zur täglichen Hautpflege genutzt.",
"## Klicken Sie hier, um jetzt auf der offiziellen Website von SkinXmed zu kaufen",
"## Hyaluronsäure Molekulargröße\nLeider findet man auf den meisten Produkten keine Angabe über die Molekulargröße von Hyaluron.\n\nDa jedoch die Herstellung von niedermolekularer Hyaluronsäure sehr teuer ist, kann man davon ausgehen, dass billige Hyaluroncremes nur hochmolekulare Hyaluronsäure enthalten.\n\nNiedermolekulare Hyaluronsäure findet man überwiegend in Seren. Sie gelangt in die tiefen Hautschichten und kann die Feuchtigkeit nachhaltig speichern.\n\nDie perfekte Hyaluroncreme beinhaltet sowohl hoch- als auch niedermolekulare Hyaluronsäure.",
"## Ubiquinone :\n\nUbiquinone ist besser bekannt als das Coenzym Q10.\n\nQ10 ist eine Geheimwaffe gegen Falten, da es, wie Vitamin C, als Antioxidans wirkt und freie Radikale bekämpfen kann.\n\nQ10 dient als Zellschutz und schützt die kollagenen Fasern vor dem Zerfall durch UV-Strahlung und oxidativem Stress.",
"## Retinol (Vitamin A) :\n\nRetinol wird in der Haut zu Vitamin-A-Säure umgewandelt.\n\nRetinol wird von Dermatologen als effizientester und wissenschaftlich erwiesener Wirkstoff gegen Falten bezeichnet, da es die Kollagenproduktion anregt und sogar sonnengeschädigte Haut reparieren kann.",
"## DMAE (Dimethylaminoethanol) :\n\nDMAE ist ein natürlicher Nährstoff, der aus Fisch (u.a. Lachs, Sardinen) gewonnen wird und noch als Geheimtipp im Kampf gegen Falten gilt.\n\nDimethylaminoethanol verbessert die Festigkeit und Elastizität der Haut und sorgt durch einen Schutz der Zellmembran für eine längere Lebensdauer der Zellen.\n\nDMAE ist auch dafür verantwortlich, dass mehr Acetylcholin ausgeschüttet wird, wodurch die Mikro-Muskelfasern (MYOFILAMENTE) mehr Spannung erhalten. Somit kann DMAE auch schlaffen Hautpartien entgegenwirken.",
"## Alteromonas Ferment Extract :\n\nPeptid aus den Aminosäuren Lysin, Histidin und Glysin. Fördert die Wasserspeicherkapazität und Wundheilung. Regt die Kollagen- und Elastinbildung und erhöht das Feuchthaltevermögen der Haut.",
"## Pullulan :\n\nBei Pullulan handet es sich um ein Polysaccharid, welches durch einen natürlichen Fermentationsprozess aus Pflanzenextrakten gewonnen wird.\n\nPullulan hat einen Sofort-Lifting-Effekt, bildet einen feinen Film auf der Haut und lässt Falten somit innerhalb von Sekunden verschwinden.",
"## Klicken Sie hier, um jetzt auf der offiziellen Website von SkinXmed zu kaufen"
] | [
"TAGS\n#region-us \n",
"# SkinXmed Deutschland Erfahrungen - SkinXmed Stiftung Warentest Test Kaufen\n\nSkinXmed Deutschland Erfahrungen Bei der SkinXmed Creme handelt es sich um eine Anti-Aging-Kur, die seit kurzem auf dem deutschen Markt erhältlich ist. Das Produkt stellt eine Alternative zu Botox da und wird hauptsächlichen von Personen angewendet, die nicht unbedingt die Faltenunterspritzung als Option nutzen wollen. Neben der Anti-Ageing Wirkung, wird dieses Produkt auch von vielen Kunden zur täglichen Hautpflege genutzt.",
"## Klicken Sie hier, um jetzt auf der offiziellen Website von SkinXmed zu kaufen",
"## Hyaluronsäure Molekulargröße\nLeider findet man auf den meisten Produkten keine Angabe über die Molekulargröße von Hyaluron.\n\nDa jedoch die Herstellung von niedermolekularer Hyaluronsäure sehr teuer ist, kann man davon ausgehen, dass billige Hyaluroncremes nur hochmolekulare Hyaluronsäure enthalten.\n\nNiedermolekulare Hyaluronsäure findet man überwiegend in Seren. Sie gelangt in die tiefen Hautschichten und kann die Feuchtigkeit nachhaltig speichern.\n\nDie perfekte Hyaluroncreme beinhaltet sowohl hoch- als auch niedermolekulare Hyaluronsäure.",
"## Ubiquinone :\n\nUbiquinone ist besser bekannt als das Coenzym Q10.\n\nQ10 ist eine Geheimwaffe gegen Falten, da es, wie Vitamin C, als Antioxidans wirkt und freie Radikale bekämpfen kann.\n\nQ10 dient als Zellschutz und schützt die kollagenen Fasern vor dem Zerfall durch UV-Strahlung und oxidativem Stress.",
"## Retinol (Vitamin A) :\n\nRetinol wird in der Haut zu Vitamin-A-Säure umgewandelt.\n\nRetinol wird von Dermatologen als effizientester und wissenschaftlich erwiesener Wirkstoff gegen Falten bezeichnet, da es die Kollagenproduktion anregt und sogar sonnengeschädigte Haut reparieren kann.",
"## DMAE (Dimethylaminoethanol) :\n\nDMAE ist ein natürlicher Nährstoff, der aus Fisch (u.a. Lachs, Sardinen) gewonnen wird und noch als Geheimtipp im Kampf gegen Falten gilt.\n\nDimethylaminoethanol verbessert die Festigkeit und Elastizität der Haut und sorgt durch einen Schutz der Zellmembran für eine längere Lebensdauer der Zellen.\n\nDMAE ist auch dafür verantwortlich, dass mehr Acetylcholin ausgeschüttet wird, wodurch die Mikro-Muskelfasern (MYOFILAMENTE) mehr Spannung erhalten. Somit kann DMAE auch schlaffen Hautpartien entgegenwirken.",
"## Alteromonas Ferment Extract :\n\nPeptid aus den Aminosäuren Lysin, Histidin und Glysin. Fördert die Wasserspeicherkapazität und Wundheilung. Regt die Kollagen- und Elastinbildung und erhöht das Feuchthaltevermögen der Haut.",
"## Pullulan :\n\nBei Pullulan handet es sich um ein Polysaccharid, welches durch einen natürlichen Fermentationsprozess aus Pflanzenextrakten gewonnen wird.\n\nPullulan hat einen Sofort-Lifting-Effekt, bildet einen feinen Film auf der Haut und lässt Falten somit innerhalb von Sekunden verschwinden.",
"## Klicken Sie hier, um jetzt auf der offiziellen Website von SkinXmed zu kaufen"
] |
text-classification | transformers |
# Model Card for Model ID
<!-- Provide a quick summary of what the model is/does. -->
## Model Details
### Model Description
<!-- Provide a longer summary of what this model is. -->
This is the model card of a 🤗 transformers model that has been pushed on the Hub. This model card has been automatically generated.
- **Developed by:** [More Information Needed]
- **Funded by [optional]:** [More Information Needed]
- **Shared by [optional]:** [More Information Needed]
- **Model type:** [More Information Needed]
- **Language(s) (NLP):** [More Information Needed]
- **License:** [More Information Needed]
- **Finetuned from model [optional]:** [More Information Needed]
### Model Sources [optional]
<!-- Provide the basic links for the model. -->
- **Repository:** [More Information Needed]
- **Paper [optional]:** [More Information Needed]
- **Demo [optional]:** [More Information Needed]
## Uses
<!-- Address questions around how the model is intended to be used, including the foreseeable users of the model and those affected by the model. -->
### Direct Use
<!-- This section is for the model use without fine-tuning or plugging into a larger ecosystem/app. -->
[More Information Needed]
### Downstream Use [optional]
<!-- This section is for the model use when fine-tuned for a task, or when plugged into a larger ecosystem/app -->
[More Information Needed]
### Out-of-Scope Use
<!-- This section addresses misuse, malicious use, and uses that the model will not work well for. -->
[More Information Needed]
## Bias, Risks, and Limitations
<!-- This section is meant to convey both technical and sociotechnical limitations. -->
[More Information Needed]
### Recommendations
<!-- This section is meant to convey recommendations with respect to the bias, risk, and technical limitations. -->
Users (both direct and downstream) should be made aware of the risks, biases and limitations of the model. More information needed for further recommendations.
## How to Get Started with the Model
Use the code below to get started with the model.
[More Information Needed]
## Training Details
### Training Data
<!-- This should link to a Dataset Card, perhaps with a short stub of information on what the training data is all about as well as documentation related to data pre-processing or additional filtering. -->
[More Information Needed]
### Training Procedure
<!-- This relates heavily to the Technical Specifications. Content here should link to that section when it is relevant to the training procedure. -->
#### Preprocessing [optional]
[More Information Needed]
#### Training Hyperparameters
- **Training regime:** [More Information Needed] <!--fp32, fp16 mixed precision, bf16 mixed precision, bf16 non-mixed precision, fp16 non-mixed precision, fp8 mixed precision -->
#### Speeds, Sizes, Times [optional]
<!-- This section provides information about throughput, start/end time, checkpoint size if relevant, etc. -->
[More Information Needed]
## Evaluation
<!-- This section describes the evaluation protocols and provides the results. -->
### Testing Data, Factors & Metrics
#### Testing Data
<!-- This should link to a Dataset Card if possible. -->
[More Information Needed]
#### Factors
<!-- These are the things the evaluation is disaggregating by, e.g., subpopulations or domains. -->
[More Information Needed]
#### Metrics
<!-- These are the evaluation metrics being used, ideally with a description of why. -->
[More Information Needed]
### Results
[More Information Needed]
#### Summary
## Model Examination [optional]
<!-- Relevant interpretability work for the model goes here -->
[More Information Needed]
## Environmental Impact
<!-- Total emissions (in grams of CO2eq) and additional considerations, such as electricity usage, go here. Edit the suggested text below accordingly -->
Carbon emissions can be estimated using the [Machine Learning Impact calculator](https://mlco2.github.io/impact#compute) presented in [Lacoste et al. (2019)](https://arxiv.org/abs/1910.09700).
- **Hardware Type:** [More Information Needed]
- **Hours used:** [More Information Needed]
- **Cloud Provider:** [More Information Needed]
- **Compute Region:** [More Information Needed]
- **Carbon Emitted:** [More Information Needed]
## Technical Specifications [optional]
### Model Architecture and Objective
[More Information Needed]
### Compute Infrastructure
[More Information Needed]
#### Hardware
[More Information Needed]
#### Software
[More Information Needed]
## Citation [optional]
<!-- If there is a paper or blog post introducing the model, the APA and Bibtex information for that should go in this section. -->
**BibTeX:**
[More Information Needed]
**APA:**
[More Information Needed]
## Glossary [optional]
<!-- If relevant, include terms and calculations in this section that can help readers understand the model or model card. -->
[More Information Needed]
## More Information [optional]
[More Information Needed]
## Model Card Authors [optional]
[More Information Needed]
## Model Card Contact
[More Information Needed]
| {"library_name": "transformers", "tags": []} | rajaramsblr/YTFineTuneBert | null | [
"transformers",
"safetensors",
"bert",
"text-classification",
"arxiv:1910.09700",
"autotrain_compatible",
"endpoints_compatible",
"region:us"
] | null | 2024-04-22T10:25:00+00:00 | [
"1910.09700"
] | [] | TAGS
#transformers #safetensors #bert #text-classification #arxiv-1910.09700 #autotrain_compatible #endpoints_compatible #region-us
|
# Model Card for Model ID
## Model Details
### Model Description
This is the model card of a transformers model that has been pushed on the Hub. This model card has been automatically generated.
- Developed by:
- Funded by [optional]:
- Shared by [optional]:
- Model type:
- Language(s) (NLP):
- License:
- Finetuned from model [optional]:
### Model Sources [optional]
- Repository:
- Paper [optional]:
- Demo [optional]:
## Uses
### Direct Use
### Downstream Use [optional]
### Out-of-Scope Use
## Bias, Risks, and Limitations
### Recommendations
Users (both direct and downstream) should be made aware of the risks, biases and limitations of the model. More information needed for further recommendations.
## How to Get Started with the Model
Use the code below to get started with the model.
## Training Details
### Training Data
### Training Procedure
#### Preprocessing [optional]
#### Training Hyperparameters
- Training regime:
#### Speeds, Sizes, Times [optional]
## Evaluation
### Testing Data, Factors & Metrics
#### Testing Data
#### Factors
#### Metrics
### Results
#### Summary
## Model Examination [optional]
## Environmental Impact
Carbon emissions can be estimated using the Machine Learning Impact calculator presented in Lacoste et al. (2019).
- Hardware Type:
- Hours used:
- Cloud Provider:
- Compute Region:
- Carbon Emitted:
## Technical Specifications [optional]
### Model Architecture and Objective
### Compute Infrastructure
#### Hardware
#### Software
[optional]
BibTeX:
APA:
## Glossary [optional]
## More Information [optional]
## Model Card Authors [optional]
## Model Card Contact
| [
"# Model Card for Model ID",
"## Model Details",
"### Model Description\n\n\n\nThis is the model card of a transformers model that has been pushed on the Hub. This model card has been automatically generated.\n\n- Developed by: \n- Funded by [optional]: \n- Shared by [optional]: \n- Model type: \n- Language(s) (NLP): \n- License: \n- Finetuned from model [optional]:",
"### Model Sources [optional]\n\n\n\n- Repository: \n- Paper [optional]: \n- Demo [optional]:",
"## Uses",
"### Direct Use",
"### Downstream Use [optional]",
"### Out-of-Scope Use",
"## Bias, Risks, and Limitations",
"### Recommendations\n\n\n\nUsers (both direct and downstream) should be made aware of the risks, biases and limitations of the model. More information needed for further recommendations.",
"## How to Get Started with the Model\n\nUse the code below to get started with the model.",
"## Training Details",
"### Training Data",
"### Training Procedure",
"#### Preprocessing [optional]",
"#### Training Hyperparameters\n\n- Training regime:",
"#### Speeds, Sizes, Times [optional]",
"## Evaluation",
"### Testing Data, Factors & Metrics",
"#### Testing Data",
"#### Factors",
"#### Metrics",
"### Results",
"#### Summary",
"## Model Examination [optional]",
"## Environmental Impact\n\n\n\nCarbon emissions can be estimated using the Machine Learning Impact calculator presented in Lacoste et al. (2019).\n\n- Hardware Type: \n- Hours used: \n- Cloud Provider: \n- Compute Region: \n- Carbon Emitted:",
"## Technical Specifications [optional]",
"### Model Architecture and Objective",
"### Compute Infrastructure",
"#### Hardware",
"#### Software\n\n\n\n[optional]\n\n\n\nBibTeX:\n\n\n\nAPA:",
"## Glossary [optional]",
"## More Information [optional]",
"## Model Card Authors [optional]",
"## Model Card Contact"
] | [
"TAGS\n#transformers #safetensors #bert #text-classification #arxiv-1910.09700 #autotrain_compatible #endpoints_compatible #region-us \n",
"# Model Card for Model ID",
"## Model Details",
"### Model Description\n\n\n\nThis is the model card of a transformers model that has been pushed on the Hub. This model card has been automatically generated.\n\n- Developed by: \n- Funded by [optional]: \n- Shared by [optional]: \n- Model type: \n- Language(s) (NLP): \n- License: \n- Finetuned from model [optional]:",
"### Model Sources [optional]\n\n\n\n- Repository: \n- Paper [optional]: \n- Demo [optional]:",
"## Uses",
"### Direct Use",
"### Downstream Use [optional]",
"### Out-of-Scope Use",
"## Bias, Risks, and Limitations",
"### Recommendations\n\n\n\nUsers (both direct and downstream) should be made aware of the risks, biases and limitations of the model. More information needed for further recommendations.",
"## How to Get Started with the Model\n\nUse the code below to get started with the model.",
"## Training Details",
"### Training Data",
"### Training Procedure",
"#### Preprocessing [optional]",
"#### Training Hyperparameters\n\n- Training regime:",
"#### Speeds, Sizes, Times [optional]",
"## Evaluation",
"### Testing Data, Factors & Metrics",
"#### Testing Data",
"#### Factors",
"#### Metrics",
"### Results",
"#### Summary",
"## Model Examination [optional]",
"## Environmental Impact\n\n\n\nCarbon emissions can be estimated using the Machine Learning Impact calculator presented in Lacoste et al. (2019).\n\n- Hardware Type: \n- Hours used: \n- Cloud Provider: \n- Compute Region: \n- Carbon Emitted:",
"## Technical Specifications [optional]",
"### Model Architecture and Objective",
"### Compute Infrastructure",
"#### Hardware",
"#### Software\n\n\n\n[optional]\n\n\n\nBibTeX:\n\n\n\nAPA:",
"## Glossary [optional]",
"## More Information [optional]",
"## Model Card Authors [optional]",
"## Model Card Contact"
] |
null | transformers |
# Model Card for Model ID
<!-- Provide a quick summary of what the model is/does. -->
## Model Details
### Model Description
<!-- Provide a longer summary of what this model is. -->
This is the model card of a 🤗 transformers model that has been pushed on the Hub. This model card has been automatically generated.
- **Developed by:** [More Information Needed]
- **Funded by [optional]:** [More Information Needed]
- **Shared by [optional]:** [More Information Needed]
- **Model type:** [More Information Needed]
- **Language(s) (NLP):** [More Information Needed]
- **License:** [More Information Needed]
- **Finetuned from model [optional]:** [More Information Needed]
### Model Sources [optional]
<!-- Provide the basic links for the model. -->
- **Repository:** [More Information Needed]
- **Paper [optional]:** [More Information Needed]
- **Demo [optional]:** [More Information Needed]
## Uses
<!-- Address questions around how the model is intended to be used, including the foreseeable users of the model and those affected by the model. -->
### Direct Use
<!-- This section is for the model use without fine-tuning or plugging into a larger ecosystem/app. -->
[More Information Needed]
### Downstream Use [optional]
<!-- This section is for the model use when fine-tuned for a task, or when plugged into a larger ecosystem/app -->
[More Information Needed]
### Out-of-Scope Use
<!-- This section addresses misuse, malicious use, and uses that the model will not work well for. -->
[More Information Needed]
## Bias, Risks, and Limitations
<!-- This section is meant to convey both technical and sociotechnical limitations. -->
[More Information Needed]
### Recommendations
<!-- This section is meant to convey recommendations with respect to the bias, risk, and technical limitations. -->
Users (both direct and downstream) should be made aware of the risks, biases and limitations of the model. More information needed for further recommendations.
## How to Get Started with the Model
Use the code below to get started with the model.
[More Information Needed]
## Training Details
### Training Data
<!-- This should link to a Dataset Card, perhaps with a short stub of information on what the training data is all about as well as documentation related to data pre-processing or additional filtering. -->
[More Information Needed]
### Training Procedure
<!-- This relates heavily to the Technical Specifications. Content here should link to that section when it is relevant to the training procedure. -->
#### Preprocessing [optional]
[More Information Needed]
#### Training Hyperparameters
- **Training regime:** [More Information Needed] <!--fp32, fp16 mixed precision, bf16 mixed precision, bf16 non-mixed precision, fp16 non-mixed precision, fp8 mixed precision -->
#### Speeds, Sizes, Times [optional]
<!-- This section provides information about throughput, start/end time, checkpoint size if relevant, etc. -->
[More Information Needed]
## Evaluation
<!-- This section describes the evaluation protocols and provides the results. -->
### Testing Data, Factors & Metrics
#### Testing Data
<!-- This should link to a Dataset Card if possible. -->
[More Information Needed]
#### Factors
<!-- These are the things the evaluation is disaggregating by, e.g., subpopulations or domains. -->
[More Information Needed]
#### Metrics
<!-- These are the evaluation metrics being used, ideally with a description of why. -->
[More Information Needed]
### Results
[More Information Needed]
#### Summary
## Model Examination [optional]
<!-- Relevant interpretability work for the model goes here -->
[More Information Needed]
## Environmental Impact
<!-- Total emissions (in grams of CO2eq) and additional considerations, such as electricity usage, go here. Edit the suggested text below accordingly -->
Carbon emissions can be estimated using the [Machine Learning Impact calculator](https://mlco2.github.io/impact#compute) presented in [Lacoste et al. (2019)](https://arxiv.org/abs/1910.09700).
- **Hardware Type:** [More Information Needed]
- **Hours used:** [More Information Needed]
- **Cloud Provider:** [More Information Needed]
- **Compute Region:** [More Information Needed]
- **Carbon Emitted:** [More Information Needed]
## Technical Specifications [optional]
### Model Architecture and Objective
[More Information Needed]
### Compute Infrastructure
[More Information Needed]
#### Hardware
[More Information Needed]
#### Software
[More Information Needed]
## Citation [optional]
<!-- If there is a paper or blog post introducing the model, the APA and Bibtex information for that should go in this section. -->
**BibTeX:**
[More Information Needed]
**APA:**
[More Information Needed]
## Glossary [optional]
<!-- If relevant, include terms and calculations in this section that can help readers understand the model or model card. -->
[More Information Needed]
## More Information [optional]
[More Information Needed]
## Model Card Authors [optional]
[More Information Needed]
## Model Card Contact
[More Information Needed] | {"library_name": "transformers", "tags": []} | relu-ntnu/bart-large-cnn_v4_trained_on_5_lr_5e-5_r8_a16_all_layers | null | [
"transformers",
"safetensors",
"arxiv:1910.09700",
"endpoints_compatible",
"region:us"
] | null | 2024-04-22T10:25:12+00:00 | [
"1910.09700"
] | [] | TAGS
#transformers #safetensors #arxiv-1910.09700 #endpoints_compatible #region-us
|
# Model Card for Model ID
## Model Details
### Model Description
This is the model card of a transformers model that has been pushed on the Hub. This model card has been automatically generated.
- Developed by:
- Funded by [optional]:
- Shared by [optional]:
- Model type:
- Language(s) (NLP):
- License:
- Finetuned from model [optional]:
### Model Sources [optional]
- Repository:
- Paper [optional]:
- Demo [optional]:
## Uses
### Direct Use
### Downstream Use [optional]
### Out-of-Scope Use
## Bias, Risks, and Limitations
### Recommendations
Users (both direct and downstream) should be made aware of the risks, biases and limitations of the model. More information needed for further recommendations.
## How to Get Started with the Model
Use the code below to get started with the model.
## Training Details
### Training Data
### Training Procedure
#### Preprocessing [optional]
#### Training Hyperparameters
- Training regime:
#### Speeds, Sizes, Times [optional]
## Evaluation
### Testing Data, Factors & Metrics
#### Testing Data
#### Factors
#### Metrics
### Results
#### Summary
## Model Examination [optional]
## Environmental Impact
Carbon emissions can be estimated using the Machine Learning Impact calculator presented in Lacoste et al. (2019).
- Hardware Type:
- Hours used:
- Cloud Provider:
- Compute Region:
- Carbon Emitted:
## Technical Specifications [optional]
### Model Architecture and Objective
### Compute Infrastructure
#### Hardware
#### Software
[optional]
BibTeX:
APA:
## Glossary [optional]
## More Information [optional]
## Model Card Authors [optional]
## Model Card Contact
| [
"# Model Card for Model ID",
"## Model Details",
"### Model Description\n\n\n\nThis is the model card of a transformers model that has been pushed on the Hub. This model card has been automatically generated.\n\n- Developed by: \n- Funded by [optional]: \n- Shared by [optional]: \n- Model type: \n- Language(s) (NLP): \n- License: \n- Finetuned from model [optional]:",
"### Model Sources [optional]\n\n\n\n- Repository: \n- Paper [optional]: \n- Demo [optional]:",
"## Uses",
"### Direct Use",
"### Downstream Use [optional]",
"### Out-of-Scope Use",
"## Bias, Risks, and Limitations",
"### Recommendations\n\n\n\nUsers (both direct and downstream) should be made aware of the risks, biases and limitations of the model. More information needed for further recommendations.",
"## How to Get Started with the Model\n\nUse the code below to get started with the model.",
"## Training Details",
"### Training Data",
"### Training Procedure",
"#### Preprocessing [optional]",
"#### Training Hyperparameters\n\n- Training regime:",
"#### Speeds, Sizes, Times [optional]",
"## Evaluation",
"### Testing Data, Factors & Metrics",
"#### Testing Data",
"#### Factors",
"#### Metrics",
"### Results",
"#### Summary",
"## Model Examination [optional]",
"## Environmental Impact\n\n\n\nCarbon emissions can be estimated using the Machine Learning Impact calculator presented in Lacoste et al. (2019).\n\n- Hardware Type: \n- Hours used: \n- Cloud Provider: \n- Compute Region: \n- Carbon Emitted:",
"## Technical Specifications [optional]",
"### Model Architecture and Objective",
"### Compute Infrastructure",
"#### Hardware",
"#### Software\n\n\n\n[optional]\n\n\n\nBibTeX:\n\n\n\nAPA:",
"## Glossary [optional]",
"## More Information [optional]",
"## Model Card Authors [optional]",
"## Model Card Contact"
] | [
"TAGS\n#transformers #safetensors #arxiv-1910.09700 #endpoints_compatible #region-us \n",
"# Model Card for Model ID",
"## Model Details",
"### Model Description\n\n\n\nThis is the model card of a transformers model that has been pushed on the Hub. This model card has been automatically generated.\n\n- Developed by: \n- Funded by [optional]: \n- Shared by [optional]: \n- Model type: \n- Language(s) (NLP): \n- License: \n- Finetuned from model [optional]:",
"### Model Sources [optional]\n\n\n\n- Repository: \n- Paper [optional]: \n- Demo [optional]:",
"## Uses",
"### Direct Use",
"### Downstream Use [optional]",
"### Out-of-Scope Use",
"## Bias, Risks, and Limitations",
"### Recommendations\n\n\n\nUsers (both direct and downstream) should be made aware of the risks, biases and limitations of the model. More information needed for further recommendations.",
"## How to Get Started with the Model\n\nUse the code below to get started with the model.",
"## Training Details",
"### Training Data",
"### Training Procedure",
"#### Preprocessing [optional]",
"#### Training Hyperparameters\n\n- Training regime:",
"#### Speeds, Sizes, Times [optional]",
"## Evaluation",
"### Testing Data, Factors & Metrics",
"#### Testing Data",
"#### Factors",
"#### Metrics",
"### Results",
"#### Summary",
"## Model Examination [optional]",
"## Environmental Impact\n\n\n\nCarbon emissions can be estimated using the Machine Learning Impact calculator presented in Lacoste et al. (2019).\n\n- Hardware Type: \n- Hours used: \n- Cloud Provider: \n- Compute Region: \n- Carbon Emitted:",
"## Technical Specifications [optional]",
"### Model Architecture and Objective",
"### Compute Infrastructure",
"#### Hardware",
"#### Software\n\n\n\n[optional]\n\n\n\nBibTeX:\n\n\n\nAPA:",
"## Glossary [optional]",
"## More Information [optional]",
"## Model Card Authors [optional]",
"## Model Card Contact"
] |
null | transformers |
# Model Card for Model ID
<!-- Provide a quick summary of what the model is/does. -->
## Model Details
### Model Description
<!-- Provide a longer summary of what this model is. -->
This is the model card of a 🤗 transformers model that has been pushed on the Hub. This model card has been automatically generated.
- **Developed by:** [More Information Needed]
- **Funded by [optional]:** [More Information Needed]
- **Shared by [optional]:** [More Information Needed]
- **Model type:** [More Information Needed]
- **Language(s) (NLP):** [More Information Needed]
- **License:** [More Information Needed]
- **Finetuned from model [optional]:** [More Information Needed]
### Model Sources [optional]
<!-- Provide the basic links for the model. -->
- **Repository:** [More Information Needed]
- **Paper [optional]:** [More Information Needed]
- **Demo [optional]:** [More Information Needed]
## Uses
<!-- Address questions around how the model is intended to be used, including the foreseeable users of the model and those affected by the model. -->
### Direct Use
<!-- This section is for the model use without fine-tuning or plugging into a larger ecosystem/app. -->
[More Information Needed]
### Downstream Use [optional]
<!-- This section is for the model use when fine-tuned for a task, or when plugged into a larger ecosystem/app -->
[More Information Needed]
### Out-of-Scope Use
<!-- This section addresses misuse, malicious use, and uses that the model will not work well for. -->
[More Information Needed]
## Bias, Risks, and Limitations
<!-- This section is meant to convey both technical and sociotechnical limitations. -->
[More Information Needed]
### Recommendations
<!-- This section is meant to convey recommendations with respect to the bias, risk, and technical limitations. -->
Users (both direct and downstream) should be made aware of the risks, biases and limitations of the model. More information needed for further recommendations.
## How to Get Started with the Model
Use the code below to get started with the model.
[More Information Needed]
## Training Details
### Training Data
<!-- This should link to a Dataset Card, perhaps with a short stub of information on what the training data is all about as well as documentation related to data pre-processing or additional filtering. -->
[More Information Needed]
### Training Procedure
<!-- This relates heavily to the Technical Specifications. Content here should link to that section when it is relevant to the training procedure. -->
#### Preprocessing [optional]
[More Information Needed]
#### Training Hyperparameters
- **Training regime:** [More Information Needed] <!--fp32, fp16 mixed precision, bf16 mixed precision, bf16 non-mixed precision, fp16 non-mixed precision, fp8 mixed precision -->
#### Speeds, Sizes, Times [optional]
<!-- This section provides information about throughput, start/end time, checkpoint size if relevant, etc. -->
[More Information Needed]
## Evaluation
<!-- This section describes the evaluation protocols and provides the results. -->
### Testing Data, Factors & Metrics
#### Testing Data
<!-- This should link to a Dataset Card if possible. -->
[More Information Needed]
#### Factors
<!-- These are the things the evaluation is disaggregating by, e.g., subpopulations or domains. -->
[More Information Needed]
#### Metrics
<!-- These are the evaluation metrics being used, ideally with a description of why. -->
[More Information Needed]
### Results
[More Information Needed]
#### Summary
## Model Examination [optional]
<!-- Relevant interpretability work for the model goes here -->
[More Information Needed]
## Environmental Impact
<!-- Total emissions (in grams of CO2eq) and additional considerations, such as electricity usage, go here. Edit the suggested text below accordingly -->
Carbon emissions can be estimated using the [Machine Learning Impact calculator](https://mlco2.github.io/impact#compute) presented in [Lacoste et al. (2019)](https://arxiv.org/abs/1910.09700).
- **Hardware Type:** [More Information Needed]
- **Hours used:** [More Information Needed]
- **Cloud Provider:** [More Information Needed]
- **Compute Region:** [More Information Needed]
- **Carbon Emitted:** [More Information Needed]
## Technical Specifications [optional]
### Model Architecture and Objective
[More Information Needed]
### Compute Infrastructure
[More Information Needed]
#### Hardware
[More Information Needed]
#### Software
[More Information Needed]
## Citation [optional]
<!-- If there is a paper or blog post introducing the model, the APA and Bibtex information for that should go in this section. -->
**BibTeX:**
[More Information Needed]
**APA:**
[More Information Needed]
## Glossary [optional]
<!-- If relevant, include terms and calculations in this section that can help readers understand the model or model card. -->
[More Information Needed]
## More Information [optional]
[More Information Needed]
## Model Card Authors [optional]
[More Information Needed]
## Model Card Contact
[More Information Needed] | {"library_name": "transformers", "tags": []} | relu-ntnu/bart-large-cnn_v4_trained_on_10_lr_5e-5_r8_a16_all_layers | null | [
"transformers",
"safetensors",
"arxiv:1910.09700",
"endpoints_compatible",
"region:us"
] | null | 2024-04-22T10:25:52+00:00 | [
"1910.09700"
] | [] | TAGS
#transformers #safetensors #arxiv-1910.09700 #endpoints_compatible #region-us
|
# Model Card for Model ID
## Model Details
### Model Description
This is the model card of a transformers model that has been pushed on the Hub. This model card has been automatically generated.
- Developed by:
- Funded by [optional]:
- Shared by [optional]:
- Model type:
- Language(s) (NLP):
- License:
- Finetuned from model [optional]:
### Model Sources [optional]
- Repository:
- Paper [optional]:
- Demo [optional]:
## Uses
### Direct Use
### Downstream Use [optional]
### Out-of-Scope Use
## Bias, Risks, and Limitations
### Recommendations
Users (both direct and downstream) should be made aware of the risks, biases and limitations of the model. More information needed for further recommendations.
## How to Get Started with the Model
Use the code below to get started with the model.
## Training Details
### Training Data
### Training Procedure
#### Preprocessing [optional]
#### Training Hyperparameters
- Training regime:
#### Speeds, Sizes, Times [optional]
## Evaluation
### Testing Data, Factors & Metrics
#### Testing Data
#### Factors
#### Metrics
### Results
#### Summary
## Model Examination [optional]
## Environmental Impact
Carbon emissions can be estimated using the Machine Learning Impact calculator presented in Lacoste et al. (2019).
- Hardware Type:
- Hours used:
- Cloud Provider:
- Compute Region:
- Carbon Emitted:
## Technical Specifications [optional]
### Model Architecture and Objective
### Compute Infrastructure
#### Hardware
#### Software
[optional]
BibTeX:
APA:
## Glossary [optional]
## More Information [optional]
## Model Card Authors [optional]
## Model Card Contact
| [
"# Model Card for Model ID",
"## Model Details",
"### Model Description\n\n\n\nThis is the model card of a transformers model that has been pushed on the Hub. This model card has been automatically generated.\n\n- Developed by: \n- Funded by [optional]: \n- Shared by [optional]: \n- Model type: \n- Language(s) (NLP): \n- License: \n- Finetuned from model [optional]:",
"### Model Sources [optional]\n\n\n\n- Repository: \n- Paper [optional]: \n- Demo [optional]:",
"## Uses",
"### Direct Use",
"### Downstream Use [optional]",
"### Out-of-Scope Use",
"## Bias, Risks, and Limitations",
"### Recommendations\n\n\n\nUsers (both direct and downstream) should be made aware of the risks, biases and limitations of the model. More information needed for further recommendations.",
"## How to Get Started with the Model\n\nUse the code below to get started with the model.",
"## Training Details",
"### Training Data",
"### Training Procedure",
"#### Preprocessing [optional]",
"#### Training Hyperparameters\n\n- Training regime:",
"#### Speeds, Sizes, Times [optional]",
"## Evaluation",
"### Testing Data, Factors & Metrics",
"#### Testing Data",
"#### Factors",
"#### Metrics",
"### Results",
"#### Summary",
"## Model Examination [optional]",
"## Environmental Impact\n\n\n\nCarbon emissions can be estimated using the Machine Learning Impact calculator presented in Lacoste et al. (2019).\n\n- Hardware Type: \n- Hours used: \n- Cloud Provider: \n- Compute Region: \n- Carbon Emitted:",
"## Technical Specifications [optional]",
"### Model Architecture and Objective",
"### Compute Infrastructure",
"#### Hardware",
"#### Software\n\n\n\n[optional]\n\n\n\nBibTeX:\n\n\n\nAPA:",
"## Glossary [optional]",
"## More Information [optional]",
"## Model Card Authors [optional]",
"## Model Card Contact"
] | [
"TAGS\n#transformers #safetensors #arxiv-1910.09700 #endpoints_compatible #region-us \n",
"# Model Card for Model ID",
"## Model Details",
"### Model Description\n\n\n\nThis is the model card of a transformers model that has been pushed on the Hub. This model card has been automatically generated.\n\n- Developed by: \n- Funded by [optional]: \n- Shared by [optional]: \n- Model type: \n- Language(s) (NLP): \n- License: \n- Finetuned from model [optional]:",
"### Model Sources [optional]\n\n\n\n- Repository: \n- Paper [optional]: \n- Demo [optional]:",
"## Uses",
"### Direct Use",
"### Downstream Use [optional]",
"### Out-of-Scope Use",
"## Bias, Risks, and Limitations",
"### Recommendations\n\n\n\nUsers (both direct and downstream) should be made aware of the risks, biases and limitations of the model. More information needed for further recommendations.",
"## How to Get Started with the Model\n\nUse the code below to get started with the model.",
"## Training Details",
"### Training Data",
"### Training Procedure",
"#### Preprocessing [optional]",
"#### Training Hyperparameters\n\n- Training regime:",
"#### Speeds, Sizes, Times [optional]",
"## Evaluation",
"### Testing Data, Factors & Metrics",
"#### Testing Data",
"#### Factors",
"#### Metrics",
"### Results",
"#### Summary",
"## Model Examination [optional]",
"## Environmental Impact\n\n\n\nCarbon emissions can be estimated using the Machine Learning Impact calculator presented in Lacoste et al. (2019).\n\n- Hardware Type: \n- Hours used: \n- Cloud Provider: \n- Compute Region: \n- Carbon Emitted:",
"## Technical Specifications [optional]",
"### Model Architecture and Objective",
"### Compute Infrastructure",
"#### Hardware",
"#### Software\n\n\n\n[optional]\n\n\n\nBibTeX:\n\n\n\nAPA:",
"## Glossary [optional]",
"## More Information [optional]",
"## Model Card Authors [optional]",
"## Model Card Contact"
] |
null | transformers |
# Model Card for Model ID
<!-- Provide a quick summary of what the model is/does. -->
## Model Details
### Model Description
<!-- Provide a longer summary of what this model is. -->
This is the model card of a 🤗 transformers model that has been pushed on the Hub. This model card has been automatically generated.
- **Developed by:** [More Information Needed]
- **Funded by [optional]:** [More Information Needed]
- **Shared by [optional]:** [More Information Needed]
- **Model type:** [More Information Needed]
- **Language(s) (NLP):** [More Information Needed]
- **License:** [More Information Needed]
- **Finetuned from model [optional]:** [More Information Needed]
### Model Sources [optional]
<!-- Provide the basic links for the model. -->
- **Repository:** [More Information Needed]
- **Paper [optional]:** [More Information Needed]
- **Demo [optional]:** [More Information Needed]
## Uses
<!-- Address questions around how the model is intended to be used, including the foreseeable users of the model and those affected by the model. -->
### Direct Use
<!-- This section is for the model use without fine-tuning or plugging into a larger ecosystem/app. -->
[More Information Needed]
### Downstream Use [optional]
<!-- This section is for the model use when fine-tuned for a task, or when plugged into a larger ecosystem/app -->
[More Information Needed]
### Out-of-Scope Use
<!-- This section addresses misuse, malicious use, and uses that the model will not work well for. -->
[More Information Needed]
## Bias, Risks, and Limitations
<!-- This section is meant to convey both technical and sociotechnical limitations. -->
[More Information Needed]
### Recommendations
<!-- This section is meant to convey recommendations with respect to the bias, risk, and technical limitations. -->
Users (both direct and downstream) should be made aware of the risks, biases and limitations of the model. More information needed for further recommendations.
## How to Get Started with the Model
Use the code below to get started with the model.
[More Information Needed]
## Training Details
### Training Data
<!-- This should link to a Dataset Card, perhaps with a short stub of information on what the training data is all about as well as documentation related to data pre-processing or additional filtering. -->
[More Information Needed]
### Training Procedure
<!-- This relates heavily to the Technical Specifications. Content here should link to that section when it is relevant to the training procedure. -->
#### Preprocessing [optional]
[More Information Needed]
#### Training Hyperparameters
- **Training regime:** [More Information Needed] <!--fp32, fp16 mixed precision, bf16 mixed precision, bf16 non-mixed precision, fp16 non-mixed precision, fp8 mixed precision -->
#### Speeds, Sizes, Times [optional]
<!-- This section provides information about throughput, start/end time, checkpoint size if relevant, etc. -->
[More Information Needed]
## Evaluation
<!-- This section describes the evaluation protocols and provides the results. -->
### Testing Data, Factors & Metrics
#### Testing Data
<!-- This should link to a Dataset Card if possible. -->
[More Information Needed]
#### Factors
<!-- These are the things the evaluation is disaggregating by, e.g., subpopulations or domains. -->
[More Information Needed]
#### Metrics
<!-- These are the evaluation metrics being used, ideally with a description of why. -->
[More Information Needed]
### Results
[More Information Needed]
#### Summary
## Model Examination [optional]
<!-- Relevant interpretability work for the model goes here -->
[More Information Needed]
## Environmental Impact
<!-- Total emissions (in grams of CO2eq) and additional considerations, such as electricity usage, go here. Edit the suggested text below accordingly -->
Carbon emissions can be estimated using the [Machine Learning Impact calculator](https://mlco2.github.io/impact#compute) presented in [Lacoste et al. (2019)](https://arxiv.org/abs/1910.09700).
- **Hardware Type:** [More Information Needed]
- **Hours used:** [More Information Needed]
- **Cloud Provider:** [More Information Needed]
- **Compute Region:** [More Information Needed]
- **Carbon Emitted:** [More Information Needed]
## Technical Specifications [optional]
### Model Architecture and Objective
[More Information Needed]
### Compute Infrastructure
[More Information Needed]
#### Hardware
[More Information Needed]
#### Software
[More Information Needed]
## Citation [optional]
<!-- If there is a paper or blog post introducing the model, the APA and Bibtex information for that should go in this section. -->
**BibTeX:**
[More Information Needed]
**APA:**
[More Information Needed]
## Glossary [optional]
<!-- If relevant, include terms and calculations in this section that can help readers understand the model or model card. -->
[More Information Needed]
## More Information [optional]
[More Information Needed]
## Model Card Authors [optional]
[More Information Needed]
## Model Card Contact
[More Information Needed] | {"library_name": "transformers", "tags": []} | relu-ntnu/bart-large-cnn_v4_trained_on_15_lr_5e-5_r8_a16_all_layers | null | [
"transformers",
"safetensors",
"arxiv:1910.09700",
"endpoints_compatible",
"region:us"
] | null | 2024-04-22T10:26:40+00:00 | [
"1910.09700"
] | [] | TAGS
#transformers #safetensors #arxiv-1910.09700 #endpoints_compatible #region-us
|
# Model Card for Model ID
## Model Details
### Model Description
This is the model card of a transformers model that has been pushed on the Hub. This model card has been automatically generated.
- Developed by:
- Funded by [optional]:
- Shared by [optional]:
- Model type:
- Language(s) (NLP):
- License:
- Finetuned from model [optional]:
### Model Sources [optional]
- Repository:
- Paper [optional]:
- Demo [optional]:
## Uses
### Direct Use
### Downstream Use [optional]
### Out-of-Scope Use
## Bias, Risks, and Limitations
### Recommendations
Users (both direct and downstream) should be made aware of the risks, biases and limitations of the model. More information needed for further recommendations.
## How to Get Started with the Model
Use the code below to get started with the model.
## Training Details
### Training Data
### Training Procedure
#### Preprocessing [optional]
#### Training Hyperparameters
- Training regime:
#### Speeds, Sizes, Times [optional]
## Evaluation
### Testing Data, Factors & Metrics
#### Testing Data
#### Factors
#### Metrics
### Results
#### Summary
## Model Examination [optional]
## Environmental Impact
Carbon emissions can be estimated using the Machine Learning Impact calculator presented in Lacoste et al. (2019).
- Hardware Type:
- Hours used:
- Cloud Provider:
- Compute Region:
- Carbon Emitted:
## Technical Specifications [optional]
### Model Architecture and Objective
### Compute Infrastructure
#### Hardware
#### Software
[optional]
BibTeX:
APA:
## Glossary [optional]
## More Information [optional]
## Model Card Authors [optional]
## Model Card Contact
| [
"# Model Card for Model ID",
"## Model Details",
"### Model Description\n\n\n\nThis is the model card of a transformers model that has been pushed on the Hub. This model card has been automatically generated.\n\n- Developed by: \n- Funded by [optional]: \n- Shared by [optional]: \n- Model type: \n- Language(s) (NLP): \n- License: \n- Finetuned from model [optional]:",
"### Model Sources [optional]\n\n\n\n- Repository: \n- Paper [optional]: \n- Demo [optional]:",
"## Uses",
"### Direct Use",
"### Downstream Use [optional]",
"### Out-of-Scope Use",
"## Bias, Risks, and Limitations",
"### Recommendations\n\n\n\nUsers (both direct and downstream) should be made aware of the risks, biases and limitations of the model. More information needed for further recommendations.",
"## How to Get Started with the Model\n\nUse the code below to get started with the model.",
"## Training Details",
"### Training Data",
"### Training Procedure",
"#### Preprocessing [optional]",
"#### Training Hyperparameters\n\n- Training regime:",
"#### Speeds, Sizes, Times [optional]",
"## Evaluation",
"### Testing Data, Factors & Metrics",
"#### Testing Data",
"#### Factors",
"#### Metrics",
"### Results",
"#### Summary",
"## Model Examination [optional]",
"## Environmental Impact\n\n\n\nCarbon emissions can be estimated using the Machine Learning Impact calculator presented in Lacoste et al. (2019).\n\n- Hardware Type: \n- Hours used: \n- Cloud Provider: \n- Compute Region: \n- Carbon Emitted:",
"## Technical Specifications [optional]",
"### Model Architecture and Objective",
"### Compute Infrastructure",
"#### Hardware",
"#### Software\n\n\n\n[optional]\n\n\n\nBibTeX:\n\n\n\nAPA:",
"## Glossary [optional]",
"## More Information [optional]",
"## Model Card Authors [optional]",
"## Model Card Contact"
] | [
"TAGS\n#transformers #safetensors #arxiv-1910.09700 #endpoints_compatible #region-us \n",
"# Model Card for Model ID",
"## Model Details",
"### Model Description\n\n\n\nThis is the model card of a transformers model that has been pushed on the Hub. This model card has been automatically generated.\n\n- Developed by: \n- Funded by [optional]: \n- Shared by [optional]: \n- Model type: \n- Language(s) (NLP): \n- License: \n- Finetuned from model [optional]:",
"### Model Sources [optional]\n\n\n\n- Repository: \n- Paper [optional]: \n- Demo [optional]:",
"## Uses",
"### Direct Use",
"### Downstream Use [optional]",
"### Out-of-Scope Use",
"## Bias, Risks, and Limitations",
"### Recommendations\n\n\n\nUsers (both direct and downstream) should be made aware of the risks, biases and limitations of the model. More information needed for further recommendations.",
"## How to Get Started with the Model\n\nUse the code below to get started with the model.",
"## Training Details",
"### Training Data",
"### Training Procedure",
"#### Preprocessing [optional]",
"#### Training Hyperparameters\n\n- Training regime:",
"#### Speeds, Sizes, Times [optional]",
"## Evaluation",
"### Testing Data, Factors & Metrics",
"#### Testing Data",
"#### Factors",
"#### Metrics",
"### Results",
"#### Summary",
"## Model Examination [optional]",
"## Environmental Impact\n\n\n\nCarbon emissions can be estimated using the Machine Learning Impact calculator presented in Lacoste et al. (2019).\n\n- Hardware Type: \n- Hours used: \n- Cloud Provider: \n- Compute Region: \n- Carbon Emitted:",
"## Technical Specifications [optional]",
"### Model Architecture and Objective",
"### Compute Infrastructure",
"#### Hardware",
"#### Software\n\n\n\n[optional]\n\n\n\nBibTeX:\n\n\n\nAPA:",
"## Glossary [optional]",
"## More Information [optional]",
"## Model Card Authors [optional]",
"## Model Card Contact"
] |
reinforcement-learning | stable-baselines3 |
# **PPO** Agent playing **LunarLander-v2**
This is a trained model of a **PPO** agent playing **LunarLander-v2**
using the [stable-baselines3 library](https://github.com/DLR-RM/stable-baselines3).
## Usage (with Stable-baselines3)
TODO: Add your code
```python
from stable_baselines3 import ...
from huggingface_sb3 import load_from_hub
...
```
| {"library_name": "stable-baselines3", "tags": ["LunarLander-v2", "deep-reinforcement-learning", "reinforcement-learning", "stable-baselines3"], "model-index": [{"name": "PPO", "results": [{"task": {"type": "reinforcement-learning", "name": "reinforcement-learning"}, "dataset": {"name": "LunarLander-v2", "type": "LunarLander-v2"}, "metrics": [{"type": "mean_reward", "value": "253.07 +/- 17.44", "name": "mean_reward", "verified": false}]}]}]} | SiLamine/ppo-LunarLander-v2 | null | [
"stable-baselines3",
"LunarLander-v2",
"deep-reinforcement-learning",
"reinforcement-learning",
"model-index",
"region:us"
] | null | 2024-04-22T10:26:49+00:00 | [] | [] | TAGS
#stable-baselines3 #LunarLander-v2 #deep-reinforcement-learning #reinforcement-learning #model-index #region-us
|
# PPO Agent playing LunarLander-v2
This is a trained model of a PPO agent playing LunarLander-v2
using the stable-baselines3 library.
## Usage (with Stable-baselines3)
TODO: Add your code
| [
"# PPO Agent playing LunarLander-v2\nThis is a trained model of a PPO agent playing LunarLander-v2\nusing the stable-baselines3 library.",
"## Usage (with Stable-baselines3)\nTODO: Add your code"
] | [
"TAGS\n#stable-baselines3 #LunarLander-v2 #deep-reinforcement-learning #reinforcement-learning #model-index #region-us \n",
"# PPO Agent playing LunarLander-v2\nThis is a trained model of a PPO agent playing LunarLander-v2\nusing the stable-baselines3 library.",
"## Usage (with Stable-baselines3)\nTODO: Add your code"
] |
text-generation | transformers |
# Model Card for Model ID
<!-- Provide a quick summary of what the model is/does. -->
## Model Details
### Model Description
<!-- Provide a longer summary of what this model is. -->
This is the model card of a 🤗 transformers model that has been pushed on the Hub. This model card has been automatically generated.
- **Developed by:** [More Information Needed]
- **Funded by [optional]:** [More Information Needed]
- **Shared by [optional]:** [More Information Needed]
- **Model type:** [More Information Needed]
- **Language(s) (NLP):** [More Information Needed]
- **License:** [More Information Needed]
- **Finetuned from model [optional]:** [More Information Needed]
### Model Sources [optional]
<!-- Provide the basic links for the model. -->
- **Repository:** [More Information Needed]
- **Paper [optional]:** [More Information Needed]
- **Demo [optional]:** [More Information Needed]
## Uses
<!-- Address questions around how the model is intended to be used, including the foreseeable users of the model and those affected by the model. -->
### Direct Use
<!-- This section is for the model use without fine-tuning or plugging into a larger ecosystem/app. -->
[More Information Needed]
### Downstream Use [optional]
<!-- This section is for the model use when fine-tuned for a task, or when plugged into a larger ecosystem/app -->
[More Information Needed]
### Out-of-Scope Use
<!-- This section addresses misuse, malicious use, and uses that the model will not work well for. -->
[More Information Needed]
## Bias, Risks, and Limitations
<!-- This section is meant to convey both technical and sociotechnical limitations. -->
[More Information Needed]
### Recommendations
<!-- This section is meant to convey recommendations with respect to the bias, risk, and technical limitations. -->
Users (both direct and downstream) should be made aware of the risks, biases and limitations of the model. More information needed for further recommendations.
## How to Get Started with the Model
Use the code below to get started with the model.
[More Information Needed]
## Training Details
### Training Data
<!-- This should link to a Dataset Card, perhaps with a short stub of information on what the training data is all about as well as documentation related to data pre-processing or additional filtering. -->
[More Information Needed]
### Training Procedure
<!-- This relates heavily to the Technical Specifications. Content here should link to that section when it is relevant to the training procedure. -->
#### Preprocessing [optional]
[More Information Needed]
#### Training Hyperparameters
- **Training regime:** [More Information Needed] <!--fp32, fp16 mixed precision, bf16 mixed precision, bf16 non-mixed precision, fp16 non-mixed precision, fp8 mixed precision -->
#### Speeds, Sizes, Times [optional]
<!-- This section provides information about throughput, start/end time, checkpoint size if relevant, etc. -->
[More Information Needed]
## Evaluation
<!-- This section describes the evaluation protocols and provides the results. -->
### Testing Data, Factors & Metrics
#### Testing Data
<!-- This should link to a Dataset Card if possible. -->
[More Information Needed]
#### Factors
<!-- These are the things the evaluation is disaggregating by, e.g., subpopulations or domains. -->
[More Information Needed]
#### Metrics
<!-- These are the evaluation metrics being used, ideally with a description of why. -->
[More Information Needed]
### Results
[More Information Needed]
#### Summary
## Model Examination [optional]
<!-- Relevant interpretability work for the model goes here -->
[More Information Needed]
## Environmental Impact
<!-- Total emissions (in grams of CO2eq) and additional considerations, such as electricity usage, go here. Edit the suggested text below accordingly -->
Carbon emissions can be estimated using the [Machine Learning Impact calculator](https://mlco2.github.io/impact#compute) presented in [Lacoste et al. (2019)](https://arxiv.org/abs/1910.09700).
- **Hardware Type:** [More Information Needed]
- **Hours used:** [More Information Needed]
- **Cloud Provider:** [More Information Needed]
- **Compute Region:** [More Information Needed]
- **Carbon Emitted:** [More Information Needed]
## Technical Specifications [optional]
### Model Architecture and Objective
[More Information Needed]
### Compute Infrastructure
[More Information Needed]
#### Hardware
[More Information Needed]
#### Software
[More Information Needed]
## Citation [optional]
<!-- If there is a paper or blog post introducing the model, the APA and Bibtex information for that should go in this section. -->
**BibTeX:**
[More Information Needed]
**APA:**
[More Information Needed]
## Glossary [optional]
<!-- If relevant, include terms and calculations in this section that can help readers understand the model or model card. -->
[More Information Needed]
## More Information [optional]
[More Information Needed]
## Model Card Authors [optional]
[More Information Needed]
## Model Card Contact
[More Information Needed] | {"library_name": "transformers", "tags": []} | tomaszki/llama-5 | null | [
"transformers",
"safetensors",
"llama",
"text-generation",
"conversational",
"arxiv:1910.09700",
"autotrain_compatible",
"endpoints_compatible",
"text-generation-inference",
"region:us"
] | null | 2024-04-22T10:27:03+00:00 | [
"1910.09700"
] | [] | TAGS
#transformers #safetensors #llama #text-generation #conversational #arxiv-1910.09700 #autotrain_compatible #endpoints_compatible #text-generation-inference #region-us
|
# Model Card for Model ID
## Model Details
### Model Description
This is the model card of a transformers model that has been pushed on the Hub. This model card has been automatically generated.
- Developed by:
- Funded by [optional]:
- Shared by [optional]:
- Model type:
- Language(s) (NLP):
- License:
- Finetuned from model [optional]:
### Model Sources [optional]
- Repository:
- Paper [optional]:
- Demo [optional]:
## Uses
### Direct Use
### Downstream Use [optional]
### Out-of-Scope Use
## Bias, Risks, and Limitations
### Recommendations
Users (both direct and downstream) should be made aware of the risks, biases and limitations of the model. More information needed for further recommendations.
## How to Get Started with the Model
Use the code below to get started with the model.
## Training Details
### Training Data
### Training Procedure
#### Preprocessing [optional]
#### Training Hyperparameters
- Training regime:
#### Speeds, Sizes, Times [optional]
## Evaluation
### Testing Data, Factors & Metrics
#### Testing Data
#### Factors
#### Metrics
### Results
#### Summary
## Model Examination [optional]
## Environmental Impact
Carbon emissions can be estimated using the Machine Learning Impact calculator presented in Lacoste et al. (2019).
- Hardware Type:
- Hours used:
- Cloud Provider:
- Compute Region:
- Carbon Emitted:
## Technical Specifications [optional]
### Model Architecture and Objective
### Compute Infrastructure
#### Hardware
#### Software
[optional]
BibTeX:
APA:
## Glossary [optional]
## More Information [optional]
## Model Card Authors [optional]
## Model Card Contact
| [
"# Model Card for Model ID",
"## Model Details",
"### Model Description\n\n\n\nThis is the model card of a transformers model that has been pushed on the Hub. This model card has been automatically generated.\n\n- Developed by: \n- Funded by [optional]: \n- Shared by [optional]: \n- Model type: \n- Language(s) (NLP): \n- License: \n- Finetuned from model [optional]:",
"### Model Sources [optional]\n\n\n\n- Repository: \n- Paper [optional]: \n- Demo [optional]:",
"## Uses",
"### Direct Use",
"### Downstream Use [optional]",
"### Out-of-Scope Use",
"## Bias, Risks, and Limitations",
"### Recommendations\n\n\n\nUsers (both direct and downstream) should be made aware of the risks, biases and limitations of the model. More information needed for further recommendations.",
"## How to Get Started with the Model\n\nUse the code below to get started with the model.",
"## Training Details",
"### Training Data",
"### Training Procedure",
"#### Preprocessing [optional]",
"#### Training Hyperparameters\n\n- Training regime:",
"#### Speeds, Sizes, Times [optional]",
"## Evaluation",
"### Testing Data, Factors & Metrics",
"#### Testing Data",
"#### Factors",
"#### Metrics",
"### Results",
"#### Summary",
"## Model Examination [optional]",
"## Environmental Impact\n\n\n\nCarbon emissions can be estimated using the Machine Learning Impact calculator presented in Lacoste et al. (2019).\n\n- Hardware Type: \n- Hours used: \n- Cloud Provider: \n- Compute Region: \n- Carbon Emitted:",
"## Technical Specifications [optional]",
"### Model Architecture and Objective",
"### Compute Infrastructure",
"#### Hardware",
"#### Software\n\n\n\n[optional]\n\n\n\nBibTeX:\n\n\n\nAPA:",
"## Glossary [optional]",
"## More Information [optional]",
"## Model Card Authors [optional]",
"## Model Card Contact"
] | [
"TAGS\n#transformers #safetensors #llama #text-generation #conversational #arxiv-1910.09700 #autotrain_compatible #endpoints_compatible #text-generation-inference #region-us \n",
"# Model Card for Model ID",
"## Model Details",
"### Model Description\n\n\n\nThis is the model card of a transformers model that has been pushed on the Hub. This model card has been automatically generated.\n\n- Developed by: \n- Funded by [optional]: \n- Shared by [optional]: \n- Model type: \n- Language(s) (NLP): \n- License: \n- Finetuned from model [optional]:",
"### Model Sources [optional]\n\n\n\n- Repository: \n- Paper [optional]: \n- Demo [optional]:",
"## Uses",
"### Direct Use",
"### Downstream Use [optional]",
"### Out-of-Scope Use",
"## Bias, Risks, and Limitations",
"### Recommendations\n\n\n\nUsers (both direct and downstream) should be made aware of the risks, biases and limitations of the model. More information needed for further recommendations.",
"## How to Get Started with the Model\n\nUse the code below to get started with the model.",
"## Training Details",
"### Training Data",
"### Training Procedure",
"#### Preprocessing [optional]",
"#### Training Hyperparameters\n\n- Training regime:",
"#### Speeds, Sizes, Times [optional]",
"## Evaluation",
"### Testing Data, Factors & Metrics",
"#### Testing Data",
"#### Factors",
"#### Metrics",
"### Results",
"#### Summary",
"## Model Examination [optional]",
"## Environmental Impact\n\n\n\nCarbon emissions can be estimated using the Machine Learning Impact calculator presented in Lacoste et al. (2019).\n\n- Hardware Type: \n- Hours used: \n- Cloud Provider: \n- Compute Region: \n- Carbon Emitted:",
"## Technical Specifications [optional]",
"### Model Architecture and Objective",
"### Compute Infrastructure",
"#### Hardware",
"#### Software\n\n\n\n[optional]\n\n\n\nBibTeX:\n\n\n\nAPA:",
"## Glossary [optional]",
"## More Information [optional]",
"## Model Card Authors [optional]",
"## Model Card Contact"
] |
null | transformers |
# Model Card for Model ID
<!-- Provide a quick summary of what the model is/does. -->
## Model Details
### Model Description
<!-- Provide a longer summary of what this model is. -->
This is the model card of a 🤗 transformers model that has been pushed on the Hub. This model card has been automatically generated.
- **Developed by:** [More Information Needed]
- **Funded by [optional]:** [More Information Needed]
- **Shared by [optional]:** [More Information Needed]
- **Model type:** [More Information Needed]
- **Language(s) (NLP):** [More Information Needed]
- **License:** [More Information Needed]
- **Finetuned from model [optional]:** [More Information Needed]
### Model Sources [optional]
<!-- Provide the basic links for the model. -->
- **Repository:** [More Information Needed]
- **Paper [optional]:** [More Information Needed]
- **Demo [optional]:** [More Information Needed]
## Uses
<!-- Address questions around how the model is intended to be used, including the foreseeable users of the model and those affected by the model. -->
### Direct Use
<!-- This section is for the model use without fine-tuning or plugging into a larger ecosystem/app. -->
[More Information Needed]
### Downstream Use [optional]
<!-- This section is for the model use when fine-tuned for a task, or when plugged into a larger ecosystem/app -->
[More Information Needed]
### Out-of-Scope Use
<!-- This section addresses misuse, malicious use, and uses that the model will not work well for. -->
[More Information Needed]
## Bias, Risks, and Limitations
<!-- This section is meant to convey both technical and sociotechnical limitations. -->
[More Information Needed]
### Recommendations
<!-- This section is meant to convey recommendations with respect to the bias, risk, and technical limitations. -->
Users (both direct and downstream) should be made aware of the risks, biases and limitations of the model. More information needed for further recommendations.
## How to Get Started with the Model
Use the code below to get started with the model.
[More Information Needed]
## Training Details
### Training Data
<!-- This should link to a Dataset Card, perhaps with a short stub of information on what the training data is all about as well as documentation related to data pre-processing or additional filtering. -->
[More Information Needed]
### Training Procedure
<!-- This relates heavily to the Technical Specifications. Content here should link to that section when it is relevant to the training procedure. -->
#### Preprocessing [optional]
[More Information Needed]
#### Training Hyperparameters
- **Training regime:** [More Information Needed] <!--fp32, fp16 mixed precision, bf16 mixed precision, bf16 non-mixed precision, fp16 non-mixed precision, fp8 mixed precision -->
#### Speeds, Sizes, Times [optional]
<!-- This section provides information about throughput, start/end time, checkpoint size if relevant, etc. -->
[More Information Needed]
## Evaluation
<!-- This section describes the evaluation protocols and provides the results. -->
### Testing Data, Factors & Metrics
#### Testing Data
<!-- This should link to a Dataset Card if possible. -->
[More Information Needed]
#### Factors
<!-- These are the things the evaluation is disaggregating by, e.g., subpopulations or domains. -->
[More Information Needed]
#### Metrics
<!-- These are the evaluation metrics being used, ideally with a description of why. -->
[More Information Needed]
### Results
[More Information Needed]
#### Summary
## Model Examination [optional]
<!-- Relevant interpretability work for the model goes here -->
[More Information Needed]
## Environmental Impact
<!-- Total emissions (in grams of CO2eq) and additional considerations, such as electricity usage, go here. Edit the suggested text below accordingly -->
Carbon emissions can be estimated using the [Machine Learning Impact calculator](https://mlco2.github.io/impact#compute) presented in [Lacoste et al. (2019)](https://arxiv.org/abs/1910.09700).
- **Hardware Type:** [More Information Needed]
- **Hours used:** [More Information Needed]
- **Cloud Provider:** [More Information Needed]
- **Compute Region:** [More Information Needed]
- **Carbon Emitted:** [More Information Needed]
## Technical Specifications [optional]
### Model Architecture and Objective
[More Information Needed]
### Compute Infrastructure
[More Information Needed]
#### Hardware
[More Information Needed]
#### Software
[More Information Needed]
## Citation [optional]
<!-- If there is a paper or blog post introducing the model, the APA and Bibtex information for that should go in this section. -->
**BibTeX:**
[More Information Needed]
**APA:**
[More Information Needed]
## Glossary [optional]
<!-- If relevant, include terms and calculations in this section that can help readers understand the model or model card. -->
[More Information Needed]
## More Information [optional]
[More Information Needed]
## Model Card Authors [optional]
[More Information Needed]
## Model Card Contact
[More Information Needed] | {"library_name": "transformers", "tags": []} | relu-ntnu/bart-large-cnn_v4_trained_on_25_lr_5e-5_r8_a16_all_layers | null | [
"transformers",
"safetensors",
"arxiv:1910.09700",
"endpoints_compatible",
"region:us"
] | null | 2024-04-22T10:27:34+00:00 | [
"1910.09700"
] | [] | TAGS
#transformers #safetensors #arxiv-1910.09700 #endpoints_compatible #region-us
|
# Model Card for Model ID
## Model Details
### Model Description
This is the model card of a transformers model that has been pushed on the Hub. This model card has been automatically generated.
- Developed by:
- Funded by [optional]:
- Shared by [optional]:
- Model type:
- Language(s) (NLP):
- License:
- Finetuned from model [optional]:
### Model Sources [optional]
- Repository:
- Paper [optional]:
- Demo [optional]:
## Uses
### Direct Use
### Downstream Use [optional]
### Out-of-Scope Use
## Bias, Risks, and Limitations
### Recommendations
Users (both direct and downstream) should be made aware of the risks, biases and limitations of the model. More information needed for further recommendations.
## How to Get Started with the Model
Use the code below to get started with the model.
## Training Details
### Training Data
### Training Procedure
#### Preprocessing [optional]
#### Training Hyperparameters
- Training regime:
#### Speeds, Sizes, Times [optional]
## Evaluation
### Testing Data, Factors & Metrics
#### Testing Data
#### Factors
#### Metrics
### Results
#### Summary
## Model Examination [optional]
## Environmental Impact
Carbon emissions can be estimated using the Machine Learning Impact calculator presented in Lacoste et al. (2019).
- Hardware Type:
- Hours used:
- Cloud Provider:
- Compute Region:
- Carbon Emitted:
## Technical Specifications [optional]
### Model Architecture and Objective
### Compute Infrastructure
#### Hardware
#### Software
[optional]
BibTeX:
APA:
## Glossary [optional]
## More Information [optional]
## Model Card Authors [optional]
## Model Card Contact
| [
"# Model Card for Model ID",
"## Model Details",
"### Model Description\n\n\n\nThis is the model card of a transformers model that has been pushed on the Hub. This model card has been automatically generated.\n\n- Developed by: \n- Funded by [optional]: \n- Shared by [optional]: \n- Model type: \n- Language(s) (NLP): \n- License: \n- Finetuned from model [optional]:",
"### Model Sources [optional]\n\n\n\n- Repository: \n- Paper [optional]: \n- Demo [optional]:",
"## Uses",
"### Direct Use",
"### Downstream Use [optional]",
"### Out-of-Scope Use",
"## Bias, Risks, and Limitations",
"### Recommendations\n\n\n\nUsers (both direct and downstream) should be made aware of the risks, biases and limitations of the model. More information needed for further recommendations.",
"## How to Get Started with the Model\n\nUse the code below to get started with the model.",
"## Training Details",
"### Training Data",
"### Training Procedure",
"#### Preprocessing [optional]",
"#### Training Hyperparameters\n\n- Training regime:",
"#### Speeds, Sizes, Times [optional]",
"## Evaluation",
"### Testing Data, Factors & Metrics",
"#### Testing Data",
"#### Factors",
"#### Metrics",
"### Results",
"#### Summary",
"## Model Examination [optional]",
"## Environmental Impact\n\n\n\nCarbon emissions can be estimated using the Machine Learning Impact calculator presented in Lacoste et al. (2019).\n\n- Hardware Type: \n- Hours used: \n- Cloud Provider: \n- Compute Region: \n- Carbon Emitted:",
"## Technical Specifications [optional]",
"### Model Architecture and Objective",
"### Compute Infrastructure",
"#### Hardware",
"#### Software\n\n\n\n[optional]\n\n\n\nBibTeX:\n\n\n\nAPA:",
"## Glossary [optional]",
"## More Information [optional]",
"## Model Card Authors [optional]",
"## Model Card Contact"
] | [
"TAGS\n#transformers #safetensors #arxiv-1910.09700 #endpoints_compatible #region-us \n",
"# Model Card for Model ID",
"## Model Details",
"### Model Description\n\n\n\nThis is the model card of a transformers model that has been pushed on the Hub. This model card has been automatically generated.\n\n- Developed by: \n- Funded by [optional]: \n- Shared by [optional]: \n- Model type: \n- Language(s) (NLP): \n- License: \n- Finetuned from model [optional]:",
"### Model Sources [optional]\n\n\n\n- Repository: \n- Paper [optional]: \n- Demo [optional]:",
"## Uses",
"### Direct Use",
"### Downstream Use [optional]",
"### Out-of-Scope Use",
"## Bias, Risks, and Limitations",
"### Recommendations\n\n\n\nUsers (both direct and downstream) should be made aware of the risks, biases and limitations of the model. More information needed for further recommendations.",
"## How to Get Started with the Model\n\nUse the code below to get started with the model.",
"## Training Details",
"### Training Data",
"### Training Procedure",
"#### Preprocessing [optional]",
"#### Training Hyperparameters\n\n- Training regime:",
"#### Speeds, Sizes, Times [optional]",
"## Evaluation",
"### Testing Data, Factors & Metrics",
"#### Testing Data",
"#### Factors",
"#### Metrics",
"### Results",
"#### Summary",
"## Model Examination [optional]",
"## Environmental Impact\n\n\n\nCarbon emissions can be estimated using the Machine Learning Impact calculator presented in Lacoste et al. (2019).\n\n- Hardware Type: \n- Hours used: \n- Cloud Provider: \n- Compute Region: \n- Carbon Emitted:",
"## Technical Specifications [optional]",
"### Model Architecture and Objective",
"### Compute Infrastructure",
"#### Hardware",
"#### Software\n\n\n\n[optional]\n\n\n\nBibTeX:\n\n\n\nAPA:",
"## Glossary [optional]",
"## More Information [optional]",
"## Model Card Authors [optional]",
"## Model Card Contact"
] |
reinforcement-learning | stable-baselines3 |
# **PPO** Agent playing **LunarLander-v2**
This is a trained model of a **PPO** agent playing **LunarLander-v2**
using the [stable-baselines3 library](https://github.com/DLR-RM/stable-baselines3).
## Usage (with Stable-baselines3)
TODO: Add your code
```python
from stable_baselines3 import ...
from huggingface_sb3 import load_from_hub
...
```
| {"library_name": "stable-baselines3", "tags": ["LunarLander-v2", "deep-reinforcement-learning", "reinforcement-learning", "stable-baselines3"], "model-index": [{"name": "PPO", "results": [{"task": {"type": "reinforcement-learning", "name": "reinforcement-learning"}, "dataset": {"name": "LunarLander-v2", "type": "LunarLander-v2"}, "metrics": [{"type": "mean_reward", "value": "253.87 +/- 22.24", "name": "mean_reward", "verified": false}]}]}]} | wsqstar/ppo-LunarLander-v2 | null | [
"stable-baselines3",
"LunarLander-v2",
"deep-reinforcement-learning",
"reinforcement-learning",
"model-index",
"region:us"
] | null | 2024-04-22T10:28:25+00:00 | [] | [] | TAGS
#stable-baselines3 #LunarLander-v2 #deep-reinforcement-learning #reinforcement-learning #model-index #region-us
|
# PPO Agent playing LunarLander-v2
This is a trained model of a PPO agent playing LunarLander-v2
using the stable-baselines3 library.
## Usage (with Stable-baselines3)
TODO: Add your code
| [
"# PPO Agent playing LunarLander-v2\nThis is a trained model of a PPO agent playing LunarLander-v2\nusing the stable-baselines3 library.",
"## Usage (with Stable-baselines3)\nTODO: Add your code"
] | [
"TAGS\n#stable-baselines3 #LunarLander-v2 #deep-reinforcement-learning #reinforcement-learning #model-index #region-us \n",
"# PPO Agent playing LunarLander-v2\nThis is a trained model of a PPO agent playing LunarLander-v2\nusing the stable-baselines3 library.",
"## Usage (with Stable-baselines3)\nTODO: Add your code"
] |
text-generation | transformers | # NEBULA-23.8B-v1.0
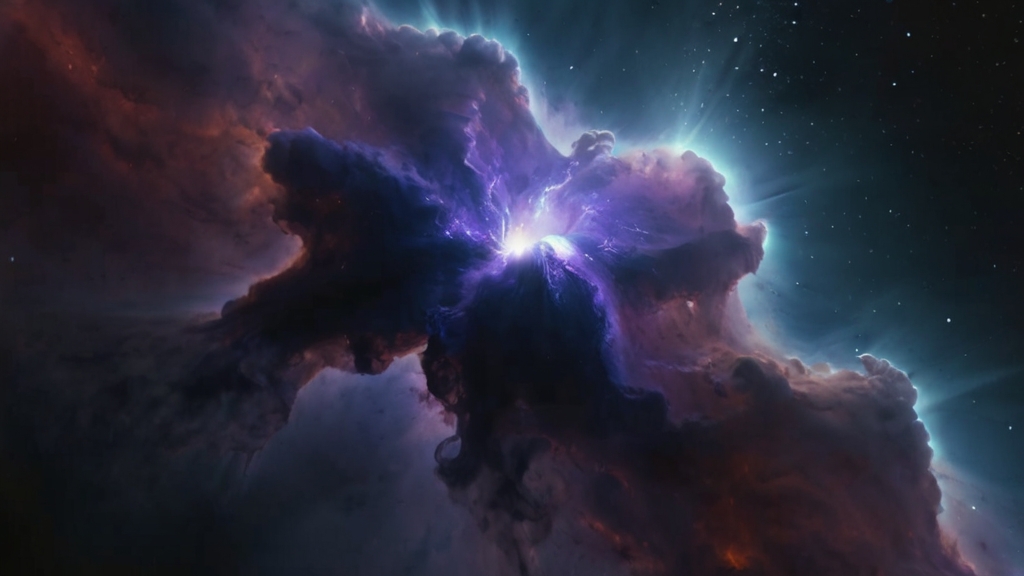
## Technical notes
- 108 layers,DUS procedure, mistral(32)->SOLAR(48)->GALAXY(72)->NEBULA(108)
- 23.8B parameters
- model created as a extension of depth upscaling procedure used for SOLAR by upstage
## Results
- model can and will produce NSFW content
- GSM8k evaluation seems to be often broken, HellaSwag, Winograde and TQA show that its a smart model
- RP and ERP work surprisingly good and I didn't encounter any GPTisms yet
- lower memory footprint than 20B and 23B models
- follows character card very well
- NSFW output feels fresh comparing to existing models
## Finetuning for RP
- SFT using MinervaAI/Aesir-Preview dataset, 10 epochs
- DPO using athirdpath/DPO_Pairs-Roleplay-Alpaca-NSFW dataset, 1 epoch
- SFT using 1xAda6000, 10h
- DPO using 1x3090, 30h
- jupyter notebooks or mergekit configs for anyone wanting to reproduce/reuse scripts - just drop me a message
## Prompt template
- Alpaca
- chat template is embedded in tokenizer config, should load automatically
## Context size
- 4096
All comments are greatly appreciated, download, test and if you appreciate my work, consider buying me my fuel:
<a href="https://www.buymeacoffee.com/TeeZee" target="_blank"><img src="https://cdn.buymeacoffee.com/buttons/v2/default-yellow.png" alt="Buy Me A Coffee" style="height: 60px !important;width: 217px !important;" ></a>
# [Open LLM Leaderboard Evaluation Results](https://huggingface.co/spaces/HuggingFaceH4/open_llm_leaderboard)
Detailed results can be found [here](https://huggingface.co/datasets/open-llm-leaderboard/details_TeeZee__NEBULA-23B-v1.0)
| Metric |Value|
|---------------------------------|----:|
|Avg. |59.94|
|AI2 Reasoning Challenge (25-Shot)|66.72|
|HellaSwag (10-Shot) |86.98|
|MMLU (5-Shot) |65.40|
|TruthfulQA (0-shot) |57.60|
|Winogrande (5-shot) |82.95|
|GSM8k (5-shot) | 0.00|
| {"language": ["en"], "license": "apache-2.0", "tags": ["not-for-all-audiences"], "datasets": ["Intel/orca_dpo_pairs", "athirdpath/DPO_Pairs-Roleplay-Alpaca-NSFW", "Open-Orca/SlimOrca", "MinervaAI/Aesir-Preview", "allenai/ultrafeedback_binarized_cleaned"], "model-index": [{"name": "NEBULA-23B-v1.0", "results": [{"task": {"type": "text-generation", "name": "Text Generation"}, "dataset": {"name": "AI2 Reasoning Challenge (25-Shot)", "type": "ai2_arc", "config": "ARC-Challenge", "split": "test", "args": {"num_few_shot": 25}}, "metrics": [{"type": "acc_norm", "value": 66.72, "name": "normalized accuracy"}], "source": {"url": "https://huggingface.co/spaces/HuggingFaceH4/open_llm_leaderboard?query=TeeZee/NEBULA-23B-v1.0", "name": "Open LLM Leaderboard"}}, {"task": {"type": "text-generation", "name": "Text Generation"}, "dataset": {"name": "HellaSwag (10-Shot)", "type": "hellaswag", "split": "validation", "args": {"num_few_shot": 10}}, "metrics": [{"type": "acc_norm", "value": 86.98, "name": "normalized accuracy"}], "source": {"url": "https://huggingface.co/spaces/HuggingFaceH4/open_llm_leaderboard?query=TeeZee/NEBULA-23B-v1.0", "name": "Open LLM Leaderboard"}}, {"task": {"type": "text-generation", "name": "Text Generation"}, "dataset": {"name": "MMLU (5-Shot)", "type": "cais/mmlu", "config": "all", "split": "test", "args": {"num_few_shot": 5}}, "metrics": [{"type": "acc", "value": 65.4, "name": "accuracy"}], "source": {"url": "https://huggingface.co/spaces/HuggingFaceH4/open_llm_leaderboard?query=TeeZee/NEBULA-23B-v1.0", "name": "Open LLM Leaderboard"}}, {"task": {"type": "text-generation", "name": "Text Generation"}, "dataset": {"name": "TruthfulQA (0-shot)", "type": "truthful_qa", "config": "multiple_choice", "split": "validation", "args": {"num_few_shot": 0}}, "metrics": [{"type": "mc2", "value": 57.6}], "source": {"url": "https://huggingface.co/spaces/HuggingFaceH4/open_llm_leaderboard?query=TeeZee/NEBULA-23B-v1.0", "name": "Open LLM Leaderboard"}}, {"task": {"type": "text-generation", "name": "Text Generation"}, "dataset": {"name": "Winogrande (5-shot)", "type": "winogrande", "config": "winogrande_xl", "split": "validation", "args": {"num_few_shot": 5}}, "metrics": [{"type": "acc", "value": 82.95, "name": "accuracy"}], "source": {"url": "https://huggingface.co/spaces/HuggingFaceH4/open_llm_leaderboard?query=TeeZee/NEBULA-23B-v1.0", "name": "Open LLM Leaderboard"}}, {"task": {"type": "text-generation", "name": "Text Generation"}, "dataset": {"name": "GSM8k (5-shot)", "type": "gsm8k", "config": "main", "split": "test", "args": {"num_few_shot": 5}}, "metrics": [{"type": "acc", "value": 0.0, "name": "accuracy"}], "source": {"url": "https://huggingface.co/spaces/HuggingFaceH4/open_llm_leaderboard?query=TeeZee/NEBULA-23B-v1.0", "name": "Open LLM Leaderboard"}}]}]} | TeeZee/NEBULA-23.8B-v1.0-bpw7.5-h8-exl2 | null | [
"transformers",
"safetensors",
"llama",
"text-generation",
"not-for-all-audiences",
"conversational",
"en",
"dataset:Intel/orca_dpo_pairs",
"dataset:athirdpath/DPO_Pairs-Roleplay-Alpaca-NSFW",
"dataset:Open-Orca/SlimOrca",
"dataset:MinervaAI/Aesir-Preview",
"dataset:allenai/ultrafeedback_binarized_cleaned",
"license:apache-2.0",
"model-index",
"autotrain_compatible",
"endpoints_compatible",
"text-generation-inference",
"region:us"
] | null | 2024-04-22T10:28:32+00:00 | [] | [
"en"
] | TAGS
#transformers #safetensors #llama #text-generation #not-for-all-audiences #conversational #en #dataset-Intel/orca_dpo_pairs #dataset-athirdpath/DPO_Pairs-Roleplay-Alpaca-NSFW #dataset-Open-Orca/SlimOrca #dataset-MinervaAI/Aesir-Preview #dataset-allenai/ultrafeedback_binarized_cleaned #license-apache-2.0 #model-index #autotrain_compatible #endpoints_compatible #text-generation-inference #region-us
| NEBULA-23.8B-v1.0
=================
!image/png
Technical notes
---------------
* 108 layers,DUS procedure, mistral(32)->SOLAR(48)->GALAXY(72)->NEBULA(108)
* 23.8B parameters
* model created as a extension of depth upscaling procedure used for SOLAR by upstage
Results
-------
* model can and will produce NSFW content
* GSM8k evaluation seems to be often broken, HellaSwag, Winograde and TQA show that its a smart model
* RP and ERP work surprisingly good and I didn't encounter any GPTisms yet
* lower memory footprint than 20B and 23B models
* follows character card very well
* NSFW output feels fresh comparing to existing models
Finetuning for RP
-----------------
* SFT using MinervaAI/Aesir-Preview dataset, 10 epochs
* DPO using athirdpath/DPO\_Pairs-Roleplay-Alpaca-NSFW dataset, 1 epoch
* SFT using 1xAda6000, 10h
* DPO using 1x3090, 30h
* jupyter notebooks or mergekit configs for anyone wanting to reproduce/reuse scripts - just drop me a message
Prompt template
---------------
* Alpaca
* chat template is embedded in tokenizer config, should load automatically
Context size
------------
* 4096
All comments are greatly appreciated, download, test and if you appreciate my work, consider buying me my fuel:
<a href="URL target="\_blank"><img src="URL alt="Buy Me A Coffee" style="height: 60px !important;width: 217px !important;" >
Open LLM Leaderboard Evaluation Results
=======================================
Detailed results can be found here
| [] | [
"TAGS\n#transformers #safetensors #llama #text-generation #not-for-all-audiences #conversational #en #dataset-Intel/orca_dpo_pairs #dataset-athirdpath/DPO_Pairs-Roleplay-Alpaca-NSFW #dataset-Open-Orca/SlimOrca #dataset-MinervaAI/Aesir-Preview #dataset-allenai/ultrafeedback_binarized_cleaned #license-apache-2.0 #model-index #autotrain_compatible #endpoints_compatible #text-generation-inference #region-us \n"
] |
text2text-generation | transformers |
<!-- This model card has been generated automatically according to the information the Trainer had access to. You
should probably proofread and complete it, then remove this comment. -->
# qya
This model is a fine-tuned version of [facebook/mbart-large-50](https://huggingface.co/facebook/mbart-large-50) on an unknown dataset.
It achieves the following results on the evaluation set:
- Loss: 3.3158
- Bleu: 2.4862
- Gen Len: 92.91
## Model description
More information needed
## Intended uses & limitations
More information needed
## Training and evaluation data
More information needed
## Training procedure
### Training hyperparameters
The following hyperparameters were used during training:
- learning_rate: 5.6e-05
- train_batch_size: 8
- eval_batch_size: 8
- seed: 42
- optimizer: Adam with betas=(0.9,0.999) and epsilon=1e-08
- lr_scheduler_type: linear
- num_epochs: 2
### Training results
| Training Loss | Epoch | Step | Validation Loss | Bleu | Gen Len |
|:-------------:|:-----:|:----:|:---------------:|:------:|:-------:|
| No log | 1.0 | 125 | 3.4402 | 2.3581 | 79.61 |
| No log | 2.0 | 250 | 3.3158 | 2.4862 | 92.91 |
### Framework versions
- Transformers 4.40.0
- Pytorch 2.2.1+cu121
- Datasets 2.19.0
- Tokenizers 0.19.1
| {"license": "mit", "tags": ["simplification", "generated_from_trainer"], "metrics": ["bleu"], "base_model": "facebook/mbart-large-50", "model-index": [{"name": "qya", "results": []}]} | lauragordo/qya | null | [
"transformers",
"tensorboard",
"safetensors",
"mbart",
"text2text-generation",
"simplification",
"generated_from_trainer",
"base_model:facebook/mbart-large-50",
"license:mit",
"autotrain_compatible",
"endpoints_compatible",
"region:us"
] | null | 2024-04-22T10:28:45+00:00 | [] | [] | TAGS
#transformers #tensorboard #safetensors #mbart #text2text-generation #simplification #generated_from_trainer #base_model-facebook/mbart-large-50 #license-mit #autotrain_compatible #endpoints_compatible #region-us
| qya
===
This model is a fine-tuned version of facebook/mbart-large-50 on an unknown dataset.
It achieves the following results on the evaluation set:
* Loss: 3.3158
* Bleu: 2.4862
* Gen Len: 92.91
Model description
-----------------
More information needed
Intended uses & limitations
---------------------------
More information needed
Training and evaluation data
----------------------------
More information needed
Training procedure
------------------
### Training hyperparameters
The following hyperparameters were used during training:
* learning\_rate: 5.6e-05
* train\_batch\_size: 8
* eval\_batch\_size: 8
* seed: 42
* optimizer: Adam with betas=(0.9,0.999) and epsilon=1e-08
* lr\_scheduler\_type: linear
* num\_epochs: 2
### Training results
### Framework versions
* Transformers 4.40.0
* Pytorch 2.2.1+cu121
* Datasets 2.19.0
* Tokenizers 0.19.1
| [
"### Training hyperparameters\n\n\nThe following hyperparameters were used during training:\n\n\n* learning\\_rate: 5.6e-05\n* train\\_batch\\_size: 8\n* eval\\_batch\\_size: 8\n* seed: 42\n* optimizer: Adam with betas=(0.9,0.999) and epsilon=1e-08\n* lr\\_scheduler\\_type: linear\n* num\\_epochs: 2",
"### Training results",
"### Framework versions\n\n\n* Transformers 4.40.0\n* Pytorch 2.2.1+cu121\n* Datasets 2.19.0\n* Tokenizers 0.19.1"
] | [
"TAGS\n#transformers #tensorboard #safetensors #mbart #text2text-generation #simplification #generated_from_trainer #base_model-facebook/mbart-large-50 #license-mit #autotrain_compatible #endpoints_compatible #region-us \n",
"### Training hyperparameters\n\n\nThe following hyperparameters were used during training:\n\n\n* learning\\_rate: 5.6e-05\n* train\\_batch\\_size: 8\n* eval\\_batch\\_size: 8\n* seed: 42\n* optimizer: Adam with betas=(0.9,0.999) and epsilon=1e-08\n* lr\\_scheduler\\_type: linear\n* num\\_epochs: 2",
"### Training results",
"### Framework versions\n\n\n* Transformers 4.40.0\n* Pytorch 2.2.1+cu121\n* Datasets 2.19.0\n* Tokenizers 0.19.1"
] |
null | transformers |
# Model Card for Model ID
<!-- Provide a quick summary of what the model is/does. -->
## Model Details
### Model Description
<!-- Provide a longer summary of what this model is. -->
This is the model card of a 🤗 transformers model that has been pushed on the Hub. This model card has been automatically generated.
- **Developed by:** [More Information Needed]
- **Funded by [optional]:** [More Information Needed]
- **Shared by [optional]:** [More Information Needed]
- **Model type:** [More Information Needed]
- **Language(s) (NLP):** [More Information Needed]
- **License:** [More Information Needed]
- **Finetuned from model [optional]:** [More Information Needed]
### Model Sources [optional]
<!-- Provide the basic links for the model. -->
- **Repository:** [More Information Needed]
- **Paper [optional]:** [More Information Needed]
- **Demo [optional]:** [More Information Needed]
## Uses
<!-- Address questions around how the model is intended to be used, including the foreseeable users of the model and those affected by the model. -->
### Direct Use
<!-- This section is for the model use without fine-tuning or plugging into a larger ecosystem/app. -->
[More Information Needed]
### Downstream Use [optional]
<!-- This section is for the model use when fine-tuned for a task, or when plugged into a larger ecosystem/app -->
[More Information Needed]
### Out-of-Scope Use
<!-- This section addresses misuse, malicious use, and uses that the model will not work well for. -->
[More Information Needed]
## Bias, Risks, and Limitations
<!-- This section is meant to convey both technical and sociotechnical limitations. -->
[More Information Needed]
### Recommendations
<!-- This section is meant to convey recommendations with respect to the bias, risk, and technical limitations. -->
Users (both direct and downstream) should be made aware of the risks, biases and limitations of the model. More information needed for further recommendations.
## How to Get Started with the Model
Use the code below to get started with the model.
[More Information Needed]
## Training Details
### Training Data
<!-- This should link to a Dataset Card, perhaps with a short stub of information on what the training data is all about as well as documentation related to data pre-processing or additional filtering. -->
[More Information Needed]
### Training Procedure
<!-- This relates heavily to the Technical Specifications. Content here should link to that section when it is relevant to the training procedure. -->
#### Preprocessing [optional]
[More Information Needed]
#### Training Hyperparameters
- **Training regime:** [More Information Needed] <!--fp32, fp16 mixed precision, bf16 mixed precision, bf16 non-mixed precision, fp16 non-mixed precision, fp8 mixed precision -->
#### Speeds, Sizes, Times [optional]
<!-- This section provides information about throughput, start/end time, checkpoint size if relevant, etc. -->
[More Information Needed]
## Evaluation
<!-- This section describes the evaluation protocols and provides the results. -->
### Testing Data, Factors & Metrics
#### Testing Data
<!-- This should link to a Dataset Card if possible. -->
[More Information Needed]
#### Factors
<!-- These are the things the evaluation is disaggregating by, e.g., subpopulations or domains. -->
[More Information Needed]
#### Metrics
<!-- These are the evaluation metrics being used, ideally with a description of why. -->
[More Information Needed]
### Results
[More Information Needed]
#### Summary
## Model Examination [optional]
<!-- Relevant interpretability work for the model goes here -->
[More Information Needed]
## Environmental Impact
<!-- Total emissions (in grams of CO2eq) and additional considerations, such as electricity usage, go here. Edit the suggested text below accordingly -->
Carbon emissions can be estimated using the [Machine Learning Impact calculator](https://mlco2.github.io/impact#compute) presented in [Lacoste et al. (2019)](https://arxiv.org/abs/1910.09700).
- **Hardware Type:** [More Information Needed]
- **Hours used:** [More Information Needed]
- **Cloud Provider:** [More Information Needed]
- **Compute Region:** [More Information Needed]
- **Carbon Emitted:** [More Information Needed]
## Technical Specifications [optional]
### Model Architecture and Objective
[More Information Needed]
### Compute Infrastructure
[More Information Needed]
#### Hardware
[More Information Needed]
#### Software
[More Information Needed]
## Citation [optional]
<!-- If there is a paper or blog post introducing the model, the APA and Bibtex information for that should go in this section. -->
**BibTeX:**
[More Information Needed]
**APA:**
[More Information Needed]
## Glossary [optional]
<!-- If relevant, include terms and calculations in this section that can help readers understand the model or model card. -->
[More Information Needed]
## More Information [optional]
[More Information Needed]
## Model Card Authors [optional]
[More Information Needed]
## Model Card Contact
[More Information Needed] | {"library_name": "transformers", "tags": []} | relu-ntnu/bart-large-cnn_v4_trained_on_50_lr_5e-5_r8_a16_all_layers | null | [
"transformers",
"safetensors",
"arxiv:1910.09700",
"endpoints_compatible",
"region:us"
] | null | 2024-04-22T10:29:01+00:00 | [
"1910.09700"
] | [] | TAGS
#transformers #safetensors #arxiv-1910.09700 #endpoints_compatible #region-us
|
# Model Card for Model ID
## Model Details
### Model Description
This is the model card of a transformers model that has been pushed on the Hub. This model card has been automatically generated.
- Developed by:
- Funded by [optional]:
- Shared by [optional]:
- Model type:
- Language(s) (NLP):
- License:
- Finetuned from model [optional]:
### Model Sources [optional]
- Repository:
- Paper [optional]:
- Demo [optional]:
## Uses
### Direct Use
### Downstream Use [optional]
### Out-of-Scope Use
## Bias, Risks, and Limitations
### Recommendations
Users (both direct and downstream) should be made aware of the risks, biases and limitations of the model. More information needed for further recommendations.
## How to Get Started with the Model
Use the code below to get started with the model.
## Training Details
### Training Data
### Training Procedure
#### Preprocessing [optional]
#### Training Hyperparameters
- Training regime:
#### Speeds, Sizes, Times [optional]
## Evaluation
### Testing Data, Factors & Metrics
#### Testing Data
#### Factors
#### Metrics
### Results
#### Summary
## Model Examination [optional]
## Environmental Impact
Carbon emissions can be estimated using the Machine Learning Impact calculator presented in Lacoste et al. (2019).
- Hardware Type:
- Hours used:
- Cloud Provider:
- Compute Region:
- Carbon Emitted:
## Technical Specifications [optional]
### Model Architecture and Objective
### Compute Infrastructure
#### Hardware
#### Software
[optional]
BibTeX:
APA:
## Glossary [optional]
## More Information [optional]
## Model Card Authors [optional]
## Model Card Contact
| [
"# Model Card for Model ID",
"## Model Details",
"### Model Description\n\n\n\nThis is the model card of a transformers model that has been pushed on the Hub. This model card has been automatically generated.\n\n- Developed by: \n- Funded by [optional]: \n- Shared by [optional]: \n- Model type: \n- Language(s) (NLP): \n- License: \n- Finetuned from model [optional]:",
"### Model Sources [optional]\n\n\n\n- Repository: \n- Paper [optional]: \n- Demo [optional]:",
"## Uses",
"### Direct Use",
"### Downstream Use [optional]",
"### Out-of-Scope Use",
"## Bias, Risks, and Limitations",
"### Recommendations\n\n\n\nUsers (both direct and downstream) should be made aware of the risks, biases and limitations of the model. More information needed for further recommendations.",
"## How to Get Started with the Model\n\nUse the code below to get started with the model.",
"## Training Details",
"### Training Data",
"### Training Procedure",
"#### Preprocessing [optional]",
"#### Training Hyperparameters\n\n- Training regime:",
"#### Speeds, Sizes, Times [optional]",
"## Evaluation",
"### Testing Data, Factors & Metrics",
"#### Testing Data",
"#### Factors",
"#### Metrics",
"### Results",
"#### Summary",
"## Model Examination [optional]",
"## Environmental Impact\n\n\n\nCarbon emissions can be estimated using the Machine Learning Impact calculator presented in Lacoste et al. (2019).\n\n- Hardware Type: \n- Hours used: \n- Cloud Provider: \n- Compute Region: \n- Carbon Emitted:",
"## Technical Specifications [optional]",
"### Model Architecture and Objective",
"### Compute Infrastructure",
"#### Hardware",
"#### Software\n\n\n\n[optional]\n\n\n\nBibTeX:\n\n\n\nAPA:",
"## Glossary [optional]",
"## More Information [optional]",
"## Model Card Authors [optional]",
"## Model Card Contact"
] | [
"TAGS\n#transformers #safetensors #arxiv-1910.09700 #endpoints_compatible #region-us \n",
"# Model Card for Model ID",
"## Model Details",
"### Model Description\n\n\n\nThis is the model card of a transformers model that has been pushed on the Hub. This model card has been automatically generated.\n\n- Developed by: \n- Funded by [optional]: \n- Shared by [optional]: \n- Model type: \n- Language(s) (NLP): \n- License: \n- Finetuned from model [optional]:",
"### Model Sources [optional]\n\n\n\n- Repository: \n- Paper [optional]: \n- Demo [optional]:",
"## Uses",
"### Direct Use",
"### Downstream Use [optional]",
"### Out-of-Scope Use",
"## Bias, Risks, and Limitations",
"### Recommendations\n\n\n\nUsers (both direct and downstream) should be made aware of the risks, biases and limitations of the model. More information needed for further recommendations.",
"## How to Get Started with the Model\n\nUse the code below to get started with the model.",
"## Training Details",
"### Training Data",
"### Training Procedure",
"#### Preprocessing [optional]",
"#### Training Hyperparameters\n\n- Training regime:",
"#### Speeds, Sizes, Times [optional]",
"## Evaluation",
"### Testing Data, Factors & Metrics",
"#### Testing Data",
"#### Factors",
"#### Metrics",
"### Results",
"#### Summary",
"## Model Examination [optional]",
"## Environmental Impact\n\n\n\nCarbon emissions can be estimated using the Machine Learning Impact calculator presented in Lacoste et al. (2019).\n\n- Hardware Type: \n- Hours used: \n- Cloud Provider: \n- Compute Region: \n- Carbon Emitted:",
"## Technical Specifications [optional]",
"### Model Architecture and Objective",
"### Compute Infrastructure",
"#### Hardware",
"#### Software\n\n\n\n[optional]\n\n\n\nBibTeX:\n\n\n\nAPA:",
"## Glossary [optional]",
"## More Information [optional]",
"## Model Card Authors [optional]",
"## Model Card Contact"
] |
null | transformers | ## About
<!-- ### quantize_version: 1 -->
<!-- ### output_tensor_quantised: 1 -->
<!-- ### convert_type: -->
<!-- ### vocab_type: -->
static quants of https://huggingface.co/Epiculous/Crunchy-onion
<!-- provided-files -->
weighted/imatrix quants are available at https://huggingface.co/mradermacher/Crunchy-onion-i1-GGUF
## Usage
If you are unsure how to use GGUF files, refer to one of [TheBloke's
READMEs](https://huggingface.co/TheBloke/KafkaLM-70B-German-V0.1-GGUF) for
more details, including on how to concatenate multi-part files.
## Provided Quants
(sorted by size, not necessarily quality. IQ-quants are often preferable over similar sized non-IQ quants)
| Link | Type | Size/GB | Notes |
|:-----|:-----|--------:|:------|
| [GGUF](https://huggingface.co/mradermacher/Crunchy-onion-GGUF/resolve/main/Crunchy-onion.Q2_K.gguf) | Q2_K | 17.4 | |
| [GGUF](https://huggingface.co/mradermacher/Crunchy-onion-GGUF/resolve/main/Crunchy-onion.IQ3_XS.gguf) | IQ3_XS | 19.5 | |
| [GGUF](https://huggingface.co/mradermacher/Crunchy-onion-GGUF/resolve/main/Crunchy-onion.IQ3_S.gguf) | IQ3_S | 20.5 | beats Q3_K* |
| [GGUF](https://huggingface.co/mradermacher/Crunchy-onion-GGUF/resolve/main/Crunchy-onion.Q3_K_S.gguf) | Q3_K_S | 20.5 | |
| [GGUF](https://huggingface.co/mradermacher/Crunchy-onion-GGUF/resolve/main/Crunchy-onion.IQ3_M.gguf) | IQ3_M | 21.5 | |
| [GGUF](https://huggingface.co/mradermacher/Crunchy-onion-GGUF/resolve/main/Crunchy-onion.Q3_K_M.gguf) | Q3_K_M | 22.6 | lower quality |
| [GGUF](https://huggingface.co/mradermacher/Crunchy-onion-GGUF/resolve/main/Crunchy-onion.Q3_K_L.gguf) | Q3_K_L | 24.3 | |
| [GGUF](https://huggingface.co/mradermacher/Crunchy-onion-GGUF/resolve/main/Crunchy-onion.IQ4_XS.gguf) | IQ4_XS | 25.5 | |
| [GGUF](https://huggingface.co/mradermacher/Crunchy-onion-GGUF/resolve/main/Crunchy-onion.Q4_K_S.gguf) | Q4_K_S | 26.8 | fast, recommended |
| [GGUF](https://huggingface.co/mradermacher/Crunchy-onion-GGUF/resolve/main/Crunchy-onion.Q4_K_M.gguf) | Q4_K_M | 28.5 | fast, recommended |
| [GGUF](https://huggingface.co/mradermacher/Crunchy-onion-GGUF/resolve/main/Crunchy-onion.Q5_K_S.gguf) | Q5_K_S | 32.3 | |
| [GGUF](https://huggingface.co/mradermacher/Crunchy-onion-GGUF/resolve/main/Crunchy-onion.Q5_K_M.gguf) | Q5_K_M | 33.3 | |
| [GGUF](https://huggingface.co/mradermacher/Crunchy-onion-GGUF/resolve/main/Crunchy-onion.Q6_K.gguf) | Q6_K | 38.5 | very good quality |
| [GGUF](https://huggingface.co/mradermacher/Crunchy-onion-GGUF/resolve/main/Crunchy-onion.Q8_0.gguf) | Q8_0 | 49.7 | fast, best quality |
Here is a handy graph by ikawrakow comparing some lower-quality quant
types (lower is better):

And here are Artefact2's thoughts on the matter:
https://gist.github.com/Artefact2/b5f810600771265fc1e39442288e8ec9
## FAQ / Model Request
See https://huggingface.co/mradermacher/model_requests for some answers to
questions you might have and/or if you want some other model quantized.
## Thanks
I thank my company, [nethype GmbH](https://www.nethype.de/), for letting
me use its servers and providing upgrades to my workstation to enable
this work in my free time.
<!-- end -->
| {"language": ["en"], "license": "agpl-3.0", "library_name": "transformers", "datasets": ["lemonilia/LimaRP", "grimulkan/theory-of-mind", "Epiculous/Gnosis"], "base_model": "Epiculous/Crunchy-onion", "quantized_by": "mradermacher"} | mradermacher/Crunchy-onion-GGUF | null | [
"transformers",
"gguf",
"en",
"dataset:lemonilia/LimaRP",
"dataset:grimulkan/theory-of-mind",
"dataset:Epiculous/Gnosis",
"base_model:Epiculous/Crunchy-onion",
"license:agpl-3.0",
"endpoints_compatible",
"region:us"
] | null | 2024-04-22T10:29:38+00:00 | [] | [
"en"
] | TAGS
#transformers #gguf #en #dataset-lemonilia/LimaRP #dataset-grimulkan/theory-of-mind #dataset-Epiculous/Gnosis #base_model-Epiculous/Crunchy-onion #license-agpl-3.0 #endpoints_compatible #region-us
| About
-----
static quants of URL
weighted/imatrix quants are available at URL
Usage
-----
If you are unsure how to use GGUF files, refer to one of TheBloke's
READMEs for
more details, including on how to concatenate multi-part files.
Provided Quants
---------------
(sorted by size, not necessarily quality. IQ-quants are often preferable over similar sized non-IQ quants)
Here is a handy graph by ikawrakow comparing some lower-quality quant
types (lower is better):
!URL
And here are Artefact2's thoughts on the matter:
URL
FAQ / Model Request
-------------------
See URL for some answers to
questions you might have and/or if you want some other model quantized.
Thanks
------
I thank my company, nethype GmbH, for letting
me use its servers and providing upgrades to my workstation to enable
this work in my free time.
| [] | [
"TAGS\n#transformers #gguf #en #dataset-lemonilia/LimaRP #dataset-grimulkan/theory-of-mind #dataset-Epiculous/Gnosis #base_model-Epiculous/Crunchy-onion #license-agpl-3.0 #endpoints_compatible #region-us \n"
] |
null | transformers |
# Model Card for Model ID
<!-- Provide a quick summary of what the model is/does. -->
## Model Details
### Model Description
<!-- Provide a longer summary of what this model is. -->
This is the model card of a 🤗 transformers model that has been pushed on the Hub. This model card has been automatically generated.
- **Developed by:** [More Information Needed]
- **Funded by [optional]:** [More Information Needed]
- **Shared by [optional]:** [More Information Needed]
- **Model type:** [More Information Needed]
- **Language(s) (NLP):** [More Information Needed]
- **License:** [More Information Needed]
- **Finetuned from model [optional]:** [More Information Needed]
### Model Sources [optional]
<!-- Provide the basic links for the model. -->
- **Repository:** [More Information Needed]
- **Paper [optional]:** [More Information Needed]
- **Demo [optional]:** [More Information Needed]
## Uses
<!-- Address questions around how the model is intended to be used, including the foreseeable users of the model and those affected by the model. -->
### Direct Use
<!-- This section is for the model use without fine-tuning or plugging into a larger ecosystem/app. -->
[More Information Needed]
### Downstream Use [optional]
<!-- This section is for the model use when fine-tuned for a task, or when plugged into a larger ecosystem/app -->
[More Information Needed]
### Out-of-Scope Use
<!-- This section addresses misuse, malicious use, and uses that the model will not work well for. -->
[More Information Needed]
## Bias, Risks, and Limitations
<!-- This section is meant to convey both technical and sociotechnical limitations. -->
[More Information Needed]
### Recommendations
<!-- This section is meant to convey recommendations with respect to the bias, risk, and technical limitations. -->
Users (both direct and downstream) should be made aware of the risks, biases and limitations of the model. More information needed for further recommendations.
## How to Get Started with the Model
Use the code below to get started with the model.
[More Information Needed]
## Training Details
### Training Data
<!-- This should link to a Dataset Card, perhaps with a short stub of information on what the training data is all about as well as documentation related to data pre-processing or additional filtering. -->
[More Information Needed]
### Training Procedure
<!-- This relates heavily to the Technical Specifications. Content here should link to that section when it is relevant to the training procedure. -->
#### Preprocessing [optional]
[More Information Needed]
#### Training Hyperparameters
- **Training regime:** [More Information Needed] <!--fp32, fp16 mixed precision, bf16 mixed precision, bf16 non-mixed precision, fp16 non-mixed precision, fp8 mixed precision -->
#### Speeds, Sizes, Times [optional]
<!-- This section provides information about throughput, start/end time, checkpoint size if relevant, etc. -->
[More Information Needed]
## Evaluation
<!-- This section describes the evaluation protocols and provides the results. -->
### Testing Data, Factors & Metrics
#### Testing Data
<!-- This should link to a Dataset Card if possible. -->
[More Information Needed]
#### Factors
<!-- These are the things the evaluation is disaggregating by, e.g., subpopulations or domains. -->
[More Information Needed]
#### Metrics
<!-- These are the evaluation metrics being used, ideally with a description of why. -->
[More Information Needed]
### Results
[More Information Needed]
#### Summary
## Model Examination [optional]
<!-- Relevant interpretability work for the model goes here -->
[More Information Needed]
## Environmental Impact
<!-- Total emissions (in grams of CO2eq) and additional considerations, such as electricity usage, go here. Edit the suggested text below accordingly -->
Carbon emissions can be estimated using the [Machine Learning Impact calculator](https://mlco2.github.io/impact#compute) presented in [Lacoste et al. (2019)](https://arxiv.org/abs/1910.09700).
- **Hardware Type:** [More Information Needed]
- **Hours used:** [More Information Needed]
- **Cloud Provider:** [More Information Needed]
- **Compute Region:** [More Information Needed]
- **Carbon Emitted:** [More Information Needed]
## Technical Specifications [optional]
### Model Architecture and Objective
[More Information Needed]
### Compute Infrastructure
[More Information Needed]
#### Hardware
[More Information Needed]
#### Software
[More Information Needed]
## Citation [optional]
<!-- If there is a paper or blog post introducing the model, the APA and Bibtex information for that should go in this section. -->
**BibTeX:**
[More Information Needed]
**APA:**
[More Information Needed]
## Glossary [optional]
<!-- If relevant, include terms and calculations in this section that can help readers understand the model or model card. -->
[More Information Needed]
## More Information [optional]
[More Information Needed]
## Model Card Authors [optional]
[More Information Needed]
## Model Card Contact
[More Information Needed] | {"library_name": "transformers", "tags": []} | srirambhavadish/Mixtral_arxt_v2 | null | [
"transformers",
"safetensors",
"arxiv:1910.09700",
"endpoints_compatible",
"region:us"
] | null | 2024-04-22T10:29:58+00:00 | [
"1910.09700"
] | [] | TAGS
#transformers #safetensors #arxiv-1910.09700 #endpoints_compatible #region-us
|
# Model Card for Model ID
## Model Details
### Model Description
This is the model card of a transformers model that has been pushed on the Hub. This model card has been automatically generated.
- Developed by:
- Funded by [optional]:
- Shared by [optional]:
- Model type:
- Language(s) (NLP):
- License:
- Finetuned from model [optional]:
### Model Sources [optional]
- Repository:
- Paper [optional]:
- Demo [optional]:
## Uses
### Direct Use
### Downstream Use [optional]
### Out-of-Scope Use
## Bias, Risks, and Limitations
### Recommendations
Users (both direct and downstream) should be made aware of the risks, biases and limitations of the model. More information needed for further recommendations.
## How to Get Started with the Model
Use the code below to get started with the model.
## Training Details
### Training Data
### Training Procedure
#### Preprocessing [optional]
#### Training Hyperparameters
- Training regime:
#### Speeds, Sizes, Times [optional]
## Evaluation
### Testing Data, Factors & Metrics
#### Testing Data
#### Factors
#### Metrics
### Results
#### Summary
## Model Examination [optional]
## Environmental Impact
Carbon emissions can be estimated using the Machine Learning Impact calculator presented in Lacoste et al. (2019).
- Hardware Type:
- Hours used:
- Cloud Provider:
- Compute Region:
- Carbon Emitted:
## Technical Specifications [optional]
### Model Architecture and Objective
### Compute Infrastructure
#### Hardware
#### Software
[optional]
BibTeX:
APA:
## Glossary [optional]
## More Information [optional]
## Model Card Authors [optional]
## Model Card Contact
| [
"# Model Card for Model ID",
"## Model Details",
"### Model Description\n\n\n\nThis is the model card of a transformers model that has been pushed on the Hub. This model card has been automatically generated.\n\n- Developed by: \n- Funded by [optional]: \n- Shared by [optional]: \n- Model type: \n- Language(s) (NLP): \n- License: \n- Finetuned from model [optional]:",
"### Model Sources [optional]\n\n\n\n- Repository: \n- Paper [optional]: \n- Demo [optional]:",
"## Uses",
"### Direct Use",
"### Downstream Use [optional]",
"### Out-of-Scope Use",
"## Bias, Risks, and Limitations",
"### Recommendations\n\n\n\nUsers (both direct and downstream) should be made aware of the risks, biases and limitations of the model. More information needed for further recommendations.",
"## How to Get Started with the Model\n\nUse the code below to get started with the model.",
"## Training Details",
"### Training Data",
"### Training Procedure",
"#### Preprocessing [optional]",
"#### Training Hyperparameters\n\n- Training regime:",
"#### Speeds, Sizes, Times [optional]",
"## Evaluation",
"### Testing Data, Factors & Metrics",
"#### Testing Data",
"#### Factors",
"#### Metrics",
"### Results",
"#### Summary",
"## Model Examination [optional]",
"## Environmental Impact\n\n\n\nCarbon emissions can be estimated using the Machine Learning Impact calculator presented in Lacoste et al. (2019).\n\n- Hardware Type: \n- Hours used: \n- Cloud Provider: \n- Compute Region: \n- Carbon Emitted:",
"## Technical Specifications [optional]",
"### Model Architecture and Objective",
"### Compute Infrastructure",
"#### Hardware",
"#### Software\n\n\n\n[optional]\n\n\n\nBibTeX:\n\n\n\nAPA:",
"## Glossary [optional]",
"## More Information [optional]",
"## Model Card Authors [optional]",
"## Model Card Contact"
] | [
"TAGS\n#transformers #safetensors #arxiv-1910.09700 #endpoints_compatible #region-us \n",
"# Model Card for Model ID",
"## Model Details",
"### Model Description\n\n\n\nThis is the model card of a transformers model that has been pushed on the Hub. This model card has been automatically generated.\n\n- Developed by: \n- Funded by [optional]: \n- Shared by [optional]: \n- Model type: \n- Language(s) (NLP): \n- License: \n- Finetuned from model [optional]:",
"### Model Sources [optional]\n\n\n\n- Repository: \n- Paper [optional]: \n- Demo [optional]:",
"## Uses",
"### Direct Use",
"### Downstream Use [optional]",
"### Out-of-Scope Use",
"## Bias, Risks, and Limitations",
"### Recommendations\n\n\n\nUsers (both direct and downstream) should be made aware of the risks, biases and limitations of the model. More information needed for further recommendations.",
"## How to Get Started with the Model\n\nUse the code below to get started with the model.",
"## Training Details",
"### Training Data",
"### Training Procedure",
"#### Preprocessing [optional]",
"#### Training Hyperparameters\n\n- Training regime:",
"#### Speeds, Sizes, Times [optional]",
"## Evaluation",
"### Testing Data, Factors & Metrics",
"#### Testing Data",
"#### Factors",
"#### Metrics",
"### Results",
"#### Summary",
"## Model Examination [optional]",
"## Environmental Impact\n\n\n\nCarbon emissions can be estimated using the Machine Learning Impact calculator presented in Lacoste et al. (2019).\n\n- Hardware Type: \n- Hours used: \n- Cloud Provider: \n- Compute Region: \n- Carbon Emitted:",
"## Technical Specifications [optional]",
"### Model Architecture and Objective",
"### Compute Infrastructure",
"#### Hardware",
"#### Software\n\n\n\n[optional]\n\n\n\nBibTeX:\n\n\n\nAPA:",
"## Glossary [optional]",
"## More Information [optional]",
"## Model Card Authors [optional]",
"## Model Card Contact"
] |
null | transformers | ## About
<!-- ### quantize_version: 1 -->
<!-- ### output_tensor_quantised: 1 -->
<!-- ### convert_type: -->
<!-- ### vocab_type: -->
static quants of https://huggingface.co/MaziyarPanahi/Llama-3-11B-Instruct-v0.1
<!-- provided-files -->
weighted/imatrix quants seem not to be available (by me) at this time. If they do not show up a week or so after the static ones, I have probably not planned for them. Feel free to request them by opening a Community Discussion.
## Usage
If you are unsure how to use GGUF files, refer to one of [TheBloke's
READMEs](https://huggingface.co/TheBloke/KafkaLM-70B-German-V0.1-GGUF) for
more details, including on how to concatenate multi-part files.
## Provided Quants
(sorted by size, not necessarily quality. IQ-quants are often preferable over similar sized non-IQ quants)
| Link | Type | Size/GB | Notes |
|:-----|:-----|--------:|:------|
| [GGUF](https://huggingface.co/mradermacher/Llama-3-11B-Instruct-v0.1-GGUF/resolve/main/Llama-3-11B-Instruct-v0.1.Q2_K.gguf) | Q2_K | 4.6 | |
| [GGUF](https://huggingface.co/mradermacher/Llama-3-11B-Instruct-v0.1-GGUF/resolve/main/Llama-3-11B-Instruct-v0.1.IQ3_XS.gguf) | IQ3_XS | 5.0 | |
| [GGUF](https://huggingface.co/mradermacher/Llama-3-11B-Instruct-v0.1-GGUF/resolve/main/Llama-3-11B-Instruct-v0.1.Q3_K_S.gguf) | Q3_K_S | 5.3 | |
| [GGUF](https://huggingface.co/mradermacher/Llama-3-11B-Instruct-v0.1-GGUF/resolve/main/Llama-3-11B-Instruct-v0.1.IQ3_S.gguf) | IQ3_S | 5.3 | beats Q3_K* |
| [GGUF](https://huggingface.co/mradermacher/Llama-3-11B-Instruct-v0.1-GGUF/resolve/main/Llama-3-11B-Instruct-v0.1.IQ3_M.gguf) | IQ3_M | 5.4 | |
| [GGUF](https://huggingface.co/mradermacher/Llama-3-11B-Instruct-v0.1-GGUF/resolve/main/Llama-3-11B-Instruct-v0.1.Q3_K_M.gguf) | Q3_K_M | 5.8 | lower quality |
| [GGUF](https://huggingface.co/mradermacher/Llama-3-11B-Instruct-v0.1-GGUF/resolve/main/Llama-3-11B-Instruct-v0.1.Q3_K_L.gguf) | Q3_K_L | 6.3 | |
| [GGUF](https://huggingface.co/mradermacher/Llama-3-11B-Instruct-v0.1-GGUF/resolve/main/Llama-3-11B-Instruct-v0.1.IQ4_XS.gguf) | IQ4_XS | 6.5 | |
| [GGUF](https://huggingface.co/mradermacher/Llama-3-11B-Instruct-v0.1-GGUF/resolve/main/Llama-3-11B-Instruct-v0.1.Q4_K_S.gguf) | Q4_K_S | 6.8 | fast, recommended |
| [GGUF](https://huggingface.co/mradermacher/Llama-3-11B-Instruct-v0.1-GGUF/resolve/main/Llama-3-11B-Instruct-v0.1.Q4_K_M.gguf) | Q4_K_M | 7.1 | fast, recommended |
| [GGUF](https://huggingface.co/mradermacher/Llama-3-11B-Instruct-v0.1-GGUF/resolve/main/Llama-3-11B-Instruct-v0.1.Q5_K_S.gguf) | Q5_K_S | 8.1 | |
| [GGUF](https://huggingface.co/mradermacher/Llama-3-11B-Instruct-v0.1-GGUF/resolve/main/Llama-3-11B-Instruct-v0.1.Q5_K_M.gguf) | Q5_K_M | 8.3 | |
| [GGUF](https://huggingface.co/mradermacher/Llama-3-11B-Instruct-v0.1-GGUF/resolve/main/Llama-3-11B-Instruct-v0.1.Q6_K.gguf) | Q6_K | 9.6 | very good quality |
| [GGUF](https://huggingface.co/mradermacher/Llama-3-11B-Instruct-v0.1-GGUF/resolve/main/Llama-3-11B-Instruct-v0.1.Q8_0.gguf) | Q8_0 | 12.3 | fast, best quality |
Here is a handy graph by ikawrakow comparing some lower-quality quant
types (lower is better):

And here are Artefact2's thoughts on the matter:
https://gist.github.com/Artefact2/b5f810600771265fc1e39442288e8ec9
## FAQ / Model Request
See https://huggingface.co/mradermacher/model_requests for some answers to
questions you might have and/or if you want some other model quantized.
## Thanks
I thank my company, [nethype GmbH](https://www.nethype.de/), for letting
me use its servers and providing upgrades to my workstation to enable
this work in my free time.
<!-- end -->
| {"language": ["en"], "license": "other", "library_name": "transformers", "tags": ["mergekit", "merge", "facebook", "meta", "pytorch", "llama", "llama-3"], "model_name": "Llama-3-11B-Instruct-v0.1", "base_model": "MaziyarPanahi/Llama-3-11B-Instruct-v0.1", "license_link": "LICENSE", "license_name": "llama3", "model_creator": "MaziyarPanahi", "quantized_by": "mradermacher"} | mradermacher/Llama-3-11B-Instruct-v0.1-GGUF | null | [
"transformers",
"gguf",
"mergekit",
"merge",
"facebook",
"meta",
"pytorch",
"llama",
"llama-3",
"en",
"base_model:MaziyarPanahi/Llama-3-11B-Instruct-v0.1",
"license:other",
"endpoints_compatible",
"region:us"
] | null | 2024-04-22T10:29:59+00:00 | [] | [
"en"
] | TAGS
#transformers #gguf #mergekit #merge #facebook #meta #pytorch #llama #llama-3 #en #base_model-MaziyarPanahi/Llama-3-11B-Instruct-v0.1 #license-other #endpoints_compatible #region-us
| About
-----
static quants of URL
weighted/imatrix quants seem not to be available (by me) at this time. If they do not show up a week or so after the static ones, I have probably not planned for them. Feel free to request them by opening a Community Discussion.
Usage
-----
If you are unsure how to use GGUF files, refer to one of TheBloke's
READMEs for
more details, including on how to concatenate multi-part files.
Provided Quants
---------------
(sorted by size, not necessarily quality. IQ-quants are often preferable over similar sized non-IQ quants)
Here is a handy graph by ikawrakow comparing some lower-quality quant
types (lower is better):
!URL
And here are Artefact2's thoughts on the matter:
URL
FAQ / Model Request
-------------------
See URL for some answers to
questions you might have and/or if you want some other model quantized.
Thanks
------
I thank my company, nethype GmbH, for letting
me use its servers and providing upgrades to my workstation to enable
this work in my free time.
| [] | [
"TAGS\n#transformers #gguf #mergekit #merge #facebook #meta #pytorch #llama #llama-3 #en #base_model-MaziyarPanahi/Llama-3-11B-Instruct-v0.1 #license-other #endpoints_compatible #region-us \n"
] |
text-generation | transformers |
# Model Card for Model ID
<!-- Provide a quick summary of what the model is/does. -->
## Model Details
### Model Description
<!-- Provide a longer summary of what this model is. -->
This is the model card of a 🤗 transformers model that has been pushed on the Hub. This model card has been automatically generated.
- **Developed by:** [More Information Needed]
- **Funded by [optional]:** [More Information Needed]
- **Shared by [optional]:** [More Information Needed]
- **Model type:** [More Information Needed]
- **Language(s) (NLP):** [More Information Needed]
- **License:** [More Information Needed]
- **Finetuned from model [optional]:** [More Information Needed]
### Model Sources [optional]
<!-- Provide the basic links for the model. -->
- **Repository:** [More Information Needed]
- **Paper [optional]:** [More Information Needed]
- **Demo [optional]:** [More Information Needed]
## Uses
<!-- Address questions around how the model is intended to be used, including the foreseeable users of the model and those affected by the model. -->
### Direct Use
<!-- This section is for the model use without fine-tuning or plugging into a larger ecosystem/app. -->
[More Information Needed]
### Downstream Use [optional]
<!-- This section is for the model use when fine-tuned for a task, or when plugged into a larger ecosystem/app -->
[More Information Needed]
### Out-of-Scope Use
<!-- This section addresses misuse, malicious use, and uses that the model will not work well for. -->
[More Information Needed]
## Bias, Risks, and Limitations
<!-- This section is meant to convey both technical and sociotechnical limitations. -->
[More Information Needed]
### Recommendations
<!-- This section is meant to convey recommendations with respect to the bias, risk, and technical limitations. -->
Users (both direct and downstream) should be made aware of the risks, biases and limitations of the model. More information needed for further recommendations.
## How to Get Started with the Model
Use the code below to get started with the model.
[More Information Needed]
## Training Details
### Training Data
<!-- This should link to a Dataset Card, perhaps with a short stub of information on what the training data is all about as well as documentation related to data pre-processing or additional filtering. -->
[More Information Needed]
### Training Procedure
<!-- This relates heavily to the Technical Specifications. Content here should link to that section when it is relevant to the training procedure. -->
#### Preprocessing [optional]
[More Information Needed]
#### Training Hyperparameters
- **Training regime:** [More Information Needed] <!--fp32, fp16 mixed precision, bf16 mixed precision, bf16 non-mixed precision, fp16 non-mixed precision, fp8 mixed precision -->
#### Speeds, Sizes, Times [optional]
<!-- This section provides information about throughput, start/end time, checkpoint size if relevant, etc. -->
[More Information Needed]
## Evaluation
<!-- This section describes the evaluation protocols and provides the results. -->
### Testing Data, Factors & Metrics
#### Testing Data
<!-- This should link to a Dataset Card if possible. -->
[More Information Needed]
#### Factors
<!-- These are the things the evaluation is disaggregating by, e.g., subpopulations or domains. -->
[More Information Needed]
#### Metrics
<!-- These are the evaluation metrics being used, ideally with a description of why. -->
[More Information Needed]
### Results
[More Information Needed]
#### Summary
## Model Examination [optional]
<!-- Relevant interpretability work for the model goes here -->
[More Information Needed]
## Environmental Impact
<!-- Total emissions (in grams of CO2eq) and additional considerations, such as electricity usage, go here. Edit the suggested text below accordingly -->
Carbon emissions can be estimated using the [Machine Learning Impact calculator](https://mlco2.github.io/impact#compute) presented in [Lacoste et al. (2019)](https://arxiv.org/abs/1910.09700).
- **Hardware Type:** [More Information Needed]
- **Hours used:** [More Information Needed]
- **Cloud Provider:** [More Information Needed]
- **Compute Region:** [More Information Needed]
- **Carbon Emitted:** [More Information Needed]
## Technical Specifications [optional]
### Model Architecture and Objective
[More Information Needed]
### Compute Infrastructure
[More Information Needed]
#### Hardware
[More Information Needed]
#### Software
[More Information Needed]
## Citation [optional]
<!-- If there is a paper or blog post introducing the model, the APA and Bibtex information for that should go in this section. -->
**BibTeX:**
[More Information Needed]
**APA:**
[More Information Needed]
## Glossary [optional]
<!-- If relevant, include terms and calculations in this section that can help readers understand the model or model card. -->
[More Information Needed]
## More Information [optional]
[More Information Needed]
## Model Card Authors [optional]
[More Information Needed]
## Model Card Contact
[More Information Needed] | {"library_name": "transformers", "tags": []} | Mayurpai5/Llama-2-7b-chat-hf-tuned-merged | null | [
"transformers",
"safetensors",
"llama",
"text-generation",
"conversational",
"arxiv:1910.09700",
"autotrain_compatible",
"endpoints_compatible",
"text-generation-inference",
"region:us"
] | null | 2024-04-22T10:30:18+00:00 | [
"1910.09700"
] | [] | TAGS
#transformers #safetensors #llama #text-generation #conversational #arxiv-1910.09700 #autotrain_compatible #endpoints_compatible #text-generation-inference #region-us
|
# Model Card for Model ID
## Model Details
### Model Description
This is the model card of a transformers model that has been pushed on the Hub. This model card has been automatically generated.
- Developed by:
- Funded by [optional]:
- Shared by [optional]:
- Model type:
- Language(s) (NLP):
- License:
- Finetuned from model [optional]:
### Model Sources [optional]
- Repository:
- Paper [optional]:
- Demo [optional]:
## Uses
### Direct Use
### Downstream Use [optional]
### Out-of-Scope Use
## Bias, Risks, and Limitations
### Recommendations
Users (both direct and downstream) should be made aware of the risks, biases and limitations of the model. More information needed for further recommendations.
## How to Get Started with the Model
Use the code below to get started with the model.
## Training Details
### Training Data
### Training Procedure
#### Preprocessing [optional]
#### Training Hyperparameters
- Training regime:
#### Speeds, Sizes, Times [optional]
## Evaluation
### Testing Data, Factors & Metrics
#### Testing Data
#### Factors
#### Metrics
### Results
#### Summary
## Model Examination [optional]
## Environmental Impact
Carbon emissions can be estimated using the Machine Learning Impact calculator presented in Lacoste et al. (2019).
- Hardware Type:
- Hours used:
- Cloud Provider:
- Compute Region:
- Carbon Emitted:
## Technical Specifications [optional]
### Model Architecture and Objective
### Compute Infrastructure
#### Hardware
#### Software
[optional]
BibTeX:
APA:
## Glossary [optional]
## More Information [optional]
## Model Card Authors [optional]
## Model Card Contact
| [
"# Model Card for Model ID",
"## Model Details",
"### Model Description\n\n\n\nThis is the model card of a transformers model that has been pushed on the Hub. This model card has been automatically generated.\n\n- Developed by: \n- Funded by [optional]: \n- Shared by [optional]: \n- Model type: \n- Language(s) (NLP): \n- License: \n- Finetuned from model [optional]:",
"### Model Sources [optional]\n\n\n\n- Repository: \n- Paper [optional]: \n- Demo [optional]:",
"## Uses",
"### Direct Use",
"### Downstream Use [optional]",
"### Out-of-Scope Use",
"## Bias, Risks, and Limitations",
"### Recommendations\n\n\n\nUsers (both direct and downstream) should be made aware of the risks, biases and limitations of the model. More information needed for further recommendations.",
"## How to Get Started with the Model\n\nUse the code below to get started with the model.",
"## Training Details",
"### Training Data",
"### Training Procedure",
"#### Preprocessing [optional]",
"#### Training Hyperparameters\n\n- Training regime:",
"#### Speeds, Sizes, Times [optional]",
"## Evaluation",
"### Testing Data, Factors & Metrics",
"#### Testing Data",
"#### Factors",
"#### Metrics",
"### Results",
"#### Summary",
"## Model Examination [optional]",
"## Environmental Impact\n\n\n\nCarbon emissions can be estimated using the Machine Learning Impact calculator presented in Lacoste et al. (2019).\n\n- Hardware Type: \n- Hours used: \n- Cloud Provider: \n- Compute Region: \n- Carbon Emitted:",
"## Technical Specifications [optional]",
"### Model Architecture and Objective",
"### Compute Infrastructure",
"#### Hardware",
"#### Software\n\n\n\n[optional]\n\n\n\nBibTeX:\n\n\n\nAPA:",
"## Glossary [optional]",
"## More Information [optional]",
"## Model Card Authors [optional]",
"## Model Card Contact"
] | [
"TAGS\n#transformers #safetensors #llama #text-generation #conversational #arxiv-1910.09700 #autotrain_compatible #endpoints_compatible #text-generation-inference #region-us \n",
"# Model Card for Model ID",
"## Model Details",
"### Model Description\n\n\n\nThis is the model card of a transformers model that has been pushed on the Hub. This model card has been automatically generated.\n\n- Developed by: \n- Funded by [optional]: \n- Shared by [optional]: \n- Model type: \n- Language(s) (NLP): \n- License: \n- Finetuned from model [optional]:",
"### Model Sources [optional]\n\n\n\n- Repository: \n- Paper [optional]: \n- Demo [optional]:",
"## Uses",
"### Direct Use",
"### Downstream Use [optional]",
"### Out-of-Scope Use",
"## Bias, Risks, and Limitations",
"### Recommendations\n\n\n\nUsers (both direct and downstream) should be made aware of the risks, biases and limitations of the model. More information needed for further recommendations.",
"## How to Get Started with the Model\n\nUse the code below to get started with the model.",
"## Training Details",
"### Training Data",
"### Training Procedure",
"#### Preprocessing [optional]",
"#### Training Hyperparameters\n\n- Training regime:",
"#### Speeds, Sizes, Times [optional]",
"## Evaluation",
"### Testing Data, Factors & Metrics",
"#### Testing Data",
"#### Factors",
"#### Metrics",
"### Results",
"#### Summary",
"## Model Examination [optional]",
"## Environmental Impact\n\n\n\nCarbon emissions can be estimated using the Machine Learning Impact calculator presented in Lacoste et al. (2019).\n\n- Hardware Type: \n- Hours used: \n- Cloud Provider: \n- Compute Region: \n- Carbon Emitted:",
"## Technical Specifications [optional]",
"### Model Architecture and Objective",
"### Compute Infrastructure",
"#### Hardware",
"#### Software\n\n\n\n[optional]\n\n\n\nBibTeX:\n\n\n\nAPA:",
"## Glossary [optional]",
"## More Information [optional]",
"## Model Card Authors [optional]",
"## Model Card Contact"
] |
text-generation | transformers |
# Model Card for Model ID
<!-- Provide a quick summary of what the model is/does. -->
## Model Details
### Model Description
<!-- Provide a longer summary of what this model is. -->
This is the model card of a 🤗 transformers model that has been pushed on the Hub. This model card has been automatically generated.
- **Developed by:** [More Information Needed]
- **Funded by [optional]:** [More Information Needed]
- **Shared by [optional]:** [More Information Needed]
- **Model type:** [More Information Needed]
- **Language(s) (NLP):** [More Information Needed]
- **License:** [More Information Needed]
- **Finetuned from model [optional]:** [More Information Needed]
### Model Sources [optional]
<!-- Provide the basic links for the model. -->
- **Repository:** [More Information Needed]
- **Paper [optional]:** [More Information Needed]
- **Demo [optional]:** [More Information Needed]
## Uses
<!-- Address questions around how the model is intended to be used, including the foreseeable users of the model and those affected by the model. -->
### Direct Use
<!-- This section is for the model use without fine-tuning or plugging into a larger ecosystem/app. -->
[More Information Needed]
### Downstream Use [optional]
<!-- This section is for the model use when fine-tuned for a task, or when plugged into a larger ecosystem/app -->
[More Information Needed]
### Out-of-Scope Use
<!-- This section addresses misuse, malicious use, and uses that the model will not work well for. -->
[More Information Needed]
## Bias, Risks, and Limitations
<!-- This section is meant to convey both technical and sociotechnical limitations. -->
[More Information Needed]
### Recommendations
<!-- This section is meant to convey recommendations with respect to the bias, risk, and technical limitations. -->
Users (both direct and downstream) should be made aware of the risks, biases and limitations of the model. More information needed for further recommendations.
## How to Get Started with the Model
Use the code below to get started with the model.
[More Information Needed]
## Training Details
### Training Data
<!-- This should link to a Dataset Card, perhaps with a short stub of information on what the training data is all about as well as documentation related to data pre-processing or additional filtering. -->
[More Information Needed]
### Training Procedure
<!-- This relates heavily to the Technical Specifications. Content here should link to that section when it is relevant to the training procedure. -->
#### Preprocessing [optional]
[More Information Needed]
#### Training Hyperparameters
- **Training regime:** [More Information Needed] <!--fp32, fp16 mixed precision, bf16 mixed precision, bf16 non-mixed precision, fp16 non-mixed precision, fp8 mixed precision -->
#### Speeds, Sizes, Times [optional]
<!-- This section provides information about throughput, start/end time, checkpoint size if relevant, etc. -->
[More Information Needed]
## Evaluation
<!-- This section describes the evaluation protocols and provides the results. -->
### Testing Data, Factors & Metrics
#### Testing Data
<!-- This should link to a Dataset Card if possible. -->
[More Information Needed]
#### Factors
<!-- These are the things the evaluation is disaggregating by, e.g., subpopulations or domains. -->
[More Information Needed]
#### Metrics
<!-- These are the evaluation metrics being used, ideally with a description of why. -->
[More Information Needed]
### Results
[More Information Needed]
#### Summary
## Model Examination [optional]
<!-- Relevant interpretability work for the model goes here -->
[More Information Needed]
## Environmental Impact
<!-- Total emissions (in grams of CO2eq) and additional considerations, such as electricity usage, go here. Edit the suggested text below accordingly -->
Carbon emissions can be estimated using the [Machine Learning Impact calculator](https://mlco2.github.io/impact#compute) presented in [Lacoste et al. (2019)](https://arxiv.org/abs/1910.09700).
- **Hardware Type:** [More Information Needed]
- **Hours used:** [More Information Needed]
- **Cloud Provider:** [More Information Needed]
- **Compute Region:** [More Information Needed]
- **Carbon Emitted:** [More Information Needed]
## Technical Specifications [optional]
### Model Architecture and Objective
[More Information Needed]
### Compute Infrastructure
[More Information Needed]
#### Hardware
[More Information Needed]
#### Software
[More Information Needed]
## Citation [optional]
<!-- If there is a paper or blog post introducing the model, the APA and Bibtex information for that should go in this section. -->
**BibTeX:**
[More Information Needed]
**APA:**
[More Information Needed]
## Glossary [optional]
<!-- If relevant, include terms and calculations in this section that can help readers understand the model or model card. -->
[More Information Needed]
## More Information [optional]
[More Information Needed]
## Model Card Authors [optional]
[More Information Needed]
## Model Card Contact
[More Information Needed] | {"library_name": "transformers", "tags": []} | tomaszki/llama-5-a | null | [
"transformers",
"safetensors",
"llama",
"text-generation",
"conversational",
"arxiv:1910.09700",
"autotrain_compatible",
"endpoints_compatible",
"text-generation-inference",
"region:us"
] | null | 2024-04-22T10:31:01+00:00 | [
"1910.09700"
] | [] | TAGS
#transformers #safetensors #llama #text-generation #conversational #arxiv-1910.09700 #autotrain_compatible #endpoints_compatible #text-generation-inference #region-us
|
# Model Card for Model ID
## Model Details
### Model Description
This is the model card of a transformers model that has been pushed on the Hub. This model card has been automatically generated.
- Developed by:
- Funded by [optional]:
- Shared by [optional]:
- Model type:
- Language(s) (NLP):
- License:
- Finetuned from model [optional]:
### Model Sources [optional]
- Repository:
- Paper [optional]:
- Demo [optional]:
## Uses
### Direct Use
### Downstream Use [optional]
### Out-of-Scope Use
## Bias, Risks, and Limitations
### Recommendations
Users (both direct and downstream) should be made aware of the risks, biases and limitations of the model. More information needed for further recommendations.
## How to Get Started with the Model
Use the code below to get started with the model.
## Training Details
### Training Data
### Training Procedure
#### Preprocessing [optional]
#### Training Hyperparameters
- Training regime:
#### Speeds, Sizes, Times [optional]
## Evaluation
### Testing Data, Factors & Metrics
#### Testing Data
#### Factors
#### Metrics
### Results
#### Summary
## Model Examination [optional]
## Environmental Impact
Carbon emissions can be estimated using the Machine Learning Impact calculator presented in Lacoste et al. (2019).
- Hardware Type:
- Hours used:
- Cloud Provider:
- Compute Region:
- Carbon Emitted:
## Technical Specifications [optional]
### Model Architecture and Objective
### Compute Infrastructure
#### Hardware
#### Software
[optional]
BibTeX:
APA:
## Glossary [optional]
## More Information [optional]
## Model Card Authors [optional]
## Model Card Contact
| [
"# Model Card for Model ID",
"## Model Details",
"### Model Description\n\n\n\nThis is the model card of a transformers model that has been pushed on the Hub. This model card has been automatically generated.\n\n- Developed by: \n- Funded by [optional]: \n- Shared by [optional]: \n- Model type: \n- Language(s) (NLP): \n- License: \n- Finetuned from model [optional]:",
"### Model Sources [optional]\n\n\n\n- Repository: \n- Paper [optional]: \n- Demo [optional]:",
"## Uses",
"### Direct Use",
"### Downstream Use [optional]",
"### Out-of-Scope Use",
"## Bias, Risks, and Limitations",
"### Recommendations\n\n\n\nUsers (both direct and downstream) should be made aware of the risks, biases and limitations of the model. More information needed for further recommendations.",
"## How to Get Started with the Model\n\nUse the code below to get started with the model.",
"## Training Details",
"### Training Data",
"### Training Procedure",
"#### Preprocessing [optional]",
"#### Training Hyperparameters\n\n- Training regime:",
"#### Speeds, Sizes, Times [optional]",
"## Evaluation",
"### Testing Data, Factors & Metrics",
"#### Testing Data",
"#### Factors",
"#### Metrics",
"### Results",
"#### Summary",
"## Model Examination [optional]",
"## Environmental Impact\n\n\n\nCarbon emissions can be estimated using the Machine Learning Impact calculator presented in Lacoste et al. (2019).\n\n- Hardware Type: \n- Hours used: \n- Cloud Provider: \n- Compute Region: \n- Carbon Emitted:",
"## Technical Specifications [optional]",
"### Model Architecture and Objective",
"### Compute Infrastructure",
"#### Hardware",
"#### Software\n\n\n\n[optional]\n\n\n\nBibTeX:\n\n\n\nAPA:",
"## Glossary [optional]",
"## More Information [optional]",
"## Model Card Authors [optional]",
"## Model Card Contact"
] | [
"TAGS\n#transformers #safetensors #llama #text-generation #conversational #arxiv-1910.09700 #autotrain_compatible #endpoints_compatible #text-generation-inference #region-us \n",
"# Model Card for Model ID",
"## Model Details",
"### Model Description\n\n\n\nThis is the model card of a transformers model that has been pushed on the Hub. This model card has been automatically generated.\n\n- Developed by: \n- Funded by [optional]: \n- Shared by [optional]: \n- Model type: \n- Language(s) (NLP): \n- License: \n- Finetuned from model [optional]:",
"### Model Sources [optional]\n\n\n\n- Repository: \n- Paper [optional]: \n- Demo [optional]:",
"## Uses",
"### Direct Use",
"### Downstream Use [optional]",
"### Out-of-Scope Use",
"## Bias, Risks, and Limitations",
"### Recommendations\n\n\n\nUsers (both direct and downstream) should be made aware of the risks, biases and limitations of the model. More information needed for further recommendations.",
"## How to Get Started with the Model\n\nUse the code below to get started with the model.",
"## Training Details",
"### Training Data",
"### Training Procedure",
"#### Preprocessing [optional]",
"#### Training Hyperparameters\n\n- Training regime:",
"#### Speeds, Sizes, Times [optional]",
"## Evaluation",
"### Testing Data, Factors & Metrics",
"#### Testing Data",
"#### Factors",
"#### Metrics",
"### Results",
"#### Summary",
"## Model Examination [optional]",
"## Environmental Impact\n\n\n\nCarbon emissions can be estimated using the Machine Learning Impact calculator presented in Lacoste et al. (2019).\n\n- Hardware Type: \n- Hours used: \n- Cloud Provider: \n- Compute Region: \n- Carbon Emitted:",
"## Technical Specifications [optional]",
"### Model Architecture and Objective",
"### Compute Infrastructure",
"#### Hardware",
"#### Software\n\n\n\n[optional]\n\n\n\nBibTeX:\n\n\n\nAPA:",
"## Glossary [optional]",
"## More Information [optional]",
"## Model Card Authors [optional]",
"## Model Card Contact"
] |
null | transformers |
# Model Card for Model ID
<!-- Provide a quick summary of what the model is/does. -->
## Model Details
### Model Description
<!-- Provide a longer summary of what this model is. -->
This is the model card of a 🤗 transformers model that has been pushed on the Hub. This model card has been automatically generated.
- **Developed by:** [More Information Needed]
- **Funded by [optional]:** [More Information Needed]
- **Shared by [optional]:** [More Information Needed]
- **Model type:** [More Information Needed]
- **Language(s) (NLP):** [More Information Needed]
- **License:** [More Information Needed]
- **Finetuned from model [optional]:** [More Information Needed]
### Model Sources [optional]
<!-- Provide the basic links for the model. -->
- **Repository:** [More Information Needed]
- **Paper [optional]:** [More Information Needed]
- **Demo [optional]:** [More Information Needed]
## Uses
<!-- Address questions around how the model is intended to be used, including the foreseeable users of the model and those affected by the model. -->
### Direct Use
<!-- This section is for the model use without fine-tuning or plugging into a larger ecosystem/app. -->
[More Information Needed]
### Downstream Use [optional]
<!-- This section is for the model use when fine-tuned for a task, or when plugged into a larger ecosystem/app -->
[More Information Needed]
### Out-of-Scope Use
<!-- This section addresses misuse, malicious use, and uses that the model will not work well for. -->
[More Information Needed]
## Bias, Risks, and Limitations
<!-- This section is meant to convey both technical and sociotechnical limitations. -->
[More Information Needed]
### Recommendations
<!-- This section is meant to convey recommendations with respect to the bias, risk, and technical limitations. -->
Users (both direct and downstream) should be made aware of the risks, biases and limitations of the model. More information needed for further recommendations.
## How to Get Started with the Model
Use the code below to get started with the model.
[More Information Needed]
## Training Details
### Training Data
<!-- This should link to a Dataset Card, perhaps with a short stub of information on what the training data is all about as well as documentation related to data pre-processing or additional filtering. -->
[More Information Needed]
### Training Procedure
<!-- This relates heavily to the Technical Specifications. Content here should link to that section when it is relevant to the training procedure. -->
#### Preprocessing [optional]
[More Information Needed]
#### Training Hyperparameters
- **Training regime:** [More Information Needed] <!--fp32, fp16 mixed precision, bf16 mixed precision, bf16 non-mixed precision, fp16 non-mixed precision, fp8 mixed precision -->
#### Speeds, Sizes, Times [optional]
<!-- This section provides information about throughput, start/end time, checkpoint size if relevant, etc. -->
[More Information Needed]
## Evaluation
<!-- This section describes the evaluation protocols and provides the results. -->
### Testing Data, Factors & Metrics
#### Testing Data
<!-- This should link to a Dataset Card if possible. -->
[More Information Needed]
#### Factors
<!-- These are the things the evaluation is disaggregating by, e.g., subpopulations or domains. -->
[More Information Needed]
#### Metrics
<!-- These are the evaluation metrics being used, ideally with a description of why. -->
[More Information Needed]
### Results
[More Information Needed]
#### Summary
## Model Examination [optional]
<!-- Relevant interpretability work for the model goes here -->
[More Information Needed]
## Environmental Impact
<!-- Total emissions (in grams of CO2eq) and additional considerations, such as electricity usage, go here. Edit the suggested text below accordingly -->
Carbon emissions can be estimated using the [Machine Learning Impact calculator](https://mlco2.github.io/impact#compute) presented in [Lacoste et al. (2019)](https://arxiv.org/abs/1910.09700).
- **Hardware Type:** [More Information Needed]
- **Hours used:** [More Information Needed]
- **Cloud Provider:** [More Information Needed]
- **Compute Region:** [More Information Needed]
- **Carbon Emitted:** [More Information Needed]
## Technical Specifications [optional]
### Model Architecture and Objective
[More Information Needed]
### Compute Infrastructure
[More Information Needed]
#### Hardware
[More Information Needed]
#### Software
[More Information Needed]
## Citation [optional]
<!-- If there is a paper or blog post introducing the model, the APA and Bibtex information for that should go in this section. -->
**BibTeX:**
[More Information Needed]
**APA:**
[More Information Needed]
## Glossary [optional]
<!-- If relevant, include terms and calculations in this section that can help readers understand the model or model card. -->
[More Information Needed]
## More Information [optional]
[More Information Needed]
## Model Card Authors [optional]
[More Information Needed]
## Model Card Contact
[More Information Needed] | {"library_name": "transformers", "tags": []} | relu-ntnu/bart-large-cnn_v4_trained_on_100_lr_5e-5_r8_a16_all_layers | null | [
"transformers",
"safetensors",
"arxiv:1910.09700",
"endpoints_compatible",
"region:us"
] | null | 2024-04-22T10:31:14+00:00 | [
"1910.09700"
] | [] | TAGS
#transformers #safetensors #arxiv-1910.09700 #endpoints_compatible #region-us
|
# Model Card for Model ID
## Model Details
### Model Description
This is the model card of a transformers model that has been pushed on the Hub. This model card has been automatically generated.
- Developed by:
- Funded by [optional]:
- Shared by [optional]:
- Model type:
- Language(s) (NLP):
- License:
- Finetuned from model [optional]:
### Model Sources [optional]
- Repository:
- Paper [optional]:
- Demo [optional]:
## Uses
### Direct Use
### Downstream Use [optional]
### Out-of-Scope Use
## Bias, Risks, and Limitations
### Recommendations
Users (both direct and downstream) should be made aware of the risks, biases and limitations of the model. More information needed for further recommendations.
## How to Get Started with the Model
Use the code below to get started with the model.
## Training Details
### Training Data
### Training Procedure
#### Preprocessing [optional]
#### Training Hyperparameters
- Training regime:
#### Speeds, Sizes, Times [optional]
## Evaluation
### Testing Data, Factors & Metrics
#### Testing Data
#### Factors
#### Metrics
### Results
#### Summary
## Model Examination [optional]
## Environmental Impact
Carbon emissions can be estimated using the Machine Learning Impact calculator presented in Lacoste et al. (2019).
- Hardware Type:
- Hours used:
- Cloud Provider:
- Compute Region:
- Carbon Emitted:
## Technical Specifications [optional]
### Model Architecture and Objective
### Compute Infrastructure
#### Hardware
#### Software
[optional]
BibTeX:
APA:
## Glossary [optional]
## More Information [optional]
## Model Card Authors [optional]
## Model Card Contact
| [
"# Model Card for Model ID",
"## Model Details",
"### Model Description\n\n\n\nThis is the model card of a transformers model that has been pushed on the Hub. This model card has been automatically generated.\n\n- Developed by: \n- Funded by [optional]: \n- Shared by [optional]: \n- Model type: \n- Language(s) (NLP): \n- License: \n- Finetuned from model [optional]:",
"### Model Sources [optional]\n\n\n\n- Repository: \n- Paper [optional]: \n- Demo [optional]:",
"## Uses",
"### Direct Use",
"### Downstream Use [optional]",
"### Out-of-Scope Use",
"## Bias, Risks, and Limitations",
"### Recommendations\n\n\n\nUsers (both direct and downstream) should be made aware of the risks, biases and limitations of the model. More information needed for further recommendations.",
"## How to Get Started with the Model\n\nUse the code below to get started with the model.",
"## Training Details",
"### Training Data",
"### Training Procedure",
"#### Preprocessing [optional]",
"#### Training Hyperparameters\n\n- Training regime:",
"#### Speeds, Sizes, Times [optional]",
"## Evaluation",
"### Testing Data, Factors & Metrics",
"#### Testing Data",
"#### Factors",
"#### Metrics",
"### Results",
"#### Summary",
"## Model Examination [optional]",
"## Environmental Impact\n\n\n\nCarbon emissions can be estimated using the Machine Learning Impact calculator presented in Lacoste et al. (2019).\n\n- Hardware Type: \n- Hours used: \n- Cloud Provider: \n- Compute Region: \n- Carbon Emitted:",
"## Technical Specifications [optional]",
"### Model Architecture and Objective",
"### Compute Infrastructure",
"#### Hardware",
"#### Software\n\n\n\n[optional]\n\n\n\nBibTeX:\n\n\n\nAPA:",
"## Glossary [optional]",
"## More Information [optional]",
"## Model Card Authors [optional]",
"## Model Card Contact"
] | [
"TAGS\n#transformers #safetensors #arxiv-1910.09700 #endpoints_compatible #region-us \n",
"# Model Card for Model ID",
"## Model Details",
"### Model Description\n\n\n\nThis is the model card of a transformers model that has been pushed on the Hub. This model card has been automatically generated.\n\n- Developed by: \n- Funded by [optional]: \n- Shared by [optional]: \n- Model type: \n- Language(s) (NLP): \n- License: \n- Finetuned from model [optional]:",
"### Model Sources [optional]\n\n\n\n- Repository: \n- Paper [optional]: \n- Demo [optional]:",
"## Uses",
"### Direct Use",
"### Downstream Use [optional]",
"### Out-of-Scope Use",
"## Bias, Risks, and Limitations",
"### Recommendations\n\n\n\nUsers (both direct and downstream) should be made aware of the risks, biases and limitations of the model. More information needed for further recommendations.",
"## How to Get Started with the Model\n\nUse the code below to get started with the model.",
"## Training Details",
"### Training Data",
"### Training Procedure",
"#### Preprocessing [optional]",
"#### Training Hyperparameters\n\n- Training regime:",
"#### Speeds, Sizes, Times [optional]",
"## Evaluation",
"### Testing Data, Factors & Metrics",
"#### Testing Data",
"#### Factors",
"#### Metrics",
"### Results",
"#### Summary",
"## Model Examination [optional]",
"## Environmental Impact\n\n\n\nCarbon emissions can be estimated using the Machine Learning Impact calculator presented in Lacoste et al. (2019).\n\n- Hardware Type: \n- Hours used: \n- Cloud Provider: \n- Compute Region: \n- Carbon Emitted:",
"## Technical Specifications [optional]",
"### Model Architecture and Objective",
"### Compute Infrastructure",
"#### Hardware",
"#### Software\n\n\n\n[optional]\n\n\n\nBibTeX:\n\n\n\nAPA:",
"## Glossary [optional]",
"## More Information [optional]",
"## Model Card Authors [optional]",
"## Model Card Contact"
] |
null | transformers |
# Model Card for Model ID
<!-- Provide a quick summary of what the model is/does. -->
## Model Details
### Model Description
<!-- Provide a longer summary of what this model is. -->
This is the model card of a 🤗 transformers model that has been pushed on the Hub. This model card has been automatically generated.
- **Developed by:** [More Information Needed]
- **Funded by [optional]:** [More Information Needed]
- **Shared by [optional]:** [More Information Needed]
- **Model type:** [More Information Needed]
- **Language(s) (NLP):** [More Information Needed]
- **License:** [More Information Needed]
- **Finetuned from model [optional]:** [More Information Needed]
### Model Sources [optional]
<!-- Provide the basic links for the model. -->
- **Repository:** [More Information Needed]
- **Paper [optional]:** [More Information Needed]
- **Demo [optional]:** [More Information Needed]
## Uses
<!-- Address questions around how the model is intended to be used, including the foreseeable users of the model and those affected by the model. -->
### Direct Use
<!-- This section is for the model use without fine-tuning or plugging into a larger ecosystem/app. -->
[More Information Needed]
### Downstream Use [optional]
<!-- This section is for the model use when fine-tuned for a task, or when plugged into a larger ecosystem/app -->
[More Information Needed]
### Out-of-Scope Use
<!-- This section addresses misuse, malicious use, and uses that the model will not work well for. -->
[More Information Needed]
## Bias, Risks, and Limitations
<!-- This section is meant to convey both technical and sociotechnical limitations. -->
[More Information Needed]
### Recommendations
<!-- This section is meant to convey recommendations with respect to the bias, risk, and technical limitations. -->
Users (both direct and downstream) should be made aware of the risks, biases and limitations of the model. More information needed for further recommendations.
## How to Get Started with the Model
Use the code below to get started with the model.
[More Information Needed]
## Training Details
### Training Data
<!-- This should link to a Dataset Card, perhaps with a short stub of information on what the training data is all about as well as documentation related to data pre-processing or additional filtering. -->
[More Information Needed]
### Training Procedure
<!-- This relates heavily to the Technical Specifications. Content here should link to that section when it is relevant to the training procedure. -->
#### Preprocessing [optional]
[More Information Needed]
#### Training Hyperparameters
- **Training regime:** [More Information Needed] <!--fp32, fp16 mixed precision, bf16 mixed precision, bf16 non-mixed precision, fp16 non-mixed precision, fp8 mixed precision -->
#### Speeds, Sizes, Times [optional]
<!-- This section provides information about throughput, start/end time, checkpoint size if relevant, etc. -->
[More Information Needed]
## Evaluation
<!-- This section describes the evaluation protocols and provides the results. -->
### Testing Data, Factors & Metrics
#### Testing Data
<!-- This should link to a Dataset Card if possible. -->
[More Information Needed]
#### Factors
<!-- These are the things the evaluation is disaggregating by, e.g., subpopulations or domains. -->
[More Information Needed]
#### Metrics
<!-- These are the evaluation metrics being used, ideally with a description of why. -->
[More Information Needed]
### Results
[More Information Needed]
#### Summary
## Model Examination [optional]
<!-- Relevant interpretability work for the model goes here -->
[More Information Needed]
## Environmental Impact
<!-- Total emissions (in grams of CO2eq) and additional considerations, such as electricity usage, go here. Edit the suggested text below accordingly -->
Carbon emissions can be estimated using the [Machine Learning Impact calculator](https://mlco2.github.io/impact#compute) presented in [Lacoste et al. (2019)](https://arxiv.org/abs/1910.09700).
- **Hardware Type:** [More Information Needed]
- **Hours used:** [More Information Needed]
- **Cloud Provider:** [More Information Needed]
- **Compute Region:** [More Information Needed]
- **Carbon Emitted:** [More Information Needed]
## Technical Specifications [optional]
### Model Architecture and Objective
[More Information Needed]
### Compute Infrastructure
[More Information Needed]
#### Hardware
[More Information Needed]
#### Software
[More Information Needed]
## Citation [optional]
<!-- If there is a paper or blog post introducing the model, the APA and Bibtex information for that should go in this section. -->
**BibTeX:**
[More Information Needed]
**APA:**
[More Information Needed]
## Glossary [optional]
<!-- If relevant, include terms and calculations in this section that can help readers understand the model or model card. -->
[More Information Needed]
## More Information [optional]
[More Information Needed]
## Model Card Authors [optional]
[More Information Needed]
## Model Card Contact
[More Information Needed] | {"library_name": "transformers", "tags": []} | Edgar404/donut-sroie | null | [
"transformers",
"safetensors",
"vision-encoder-decoder",
"arxiv:1910.09700",
"endpoints_compatible",
"region:us"
] | null | 2024-04-22T10:31:31+00:00 | [
"1910.09700"
] | [] | TAGS
#transformers #safetensors #vision-encoder-decoder #arxiv-1910.09700 #endpoints_compatible #region-us
|
# Model Card for Model ID
## Model Details
### Model Description
This is the model card of a transformers model that has been pushed on the Hub. This model card has been automatically generated.
- Developed by:
- Funded by [optional]:
- Shared by [optional]:
- Model type:
- Language(s) (NLP):
- License:
- Finetuned from model [optional]:
### Model Sources [optional]
- Repository:
- Paper [optional]:
- Demo [optional]:
## Uses
### Direct Use
### Downstream Use [optional]
### Out-of-Scope Use
## Bias, Risks, and Limitations
### Recommendations
Users (both direct and downstream) should be made aware of the risks, biases and limitations of the model. More information needed for further recommendations.
## How to Get Started with the Model
Use the code below to get started with the model.
## Training Details
### Training Data
### Training Procedure
#### Preprocessing [optional]
#### Training Hyperparameters
- Training regime:
#### Speeds, Sizes, Times [optional]
## Evaluation
### Testing Data, Factors & Metrics
#### Testing Data
#### Factors
#### Metrics
### Results
#### Summary
## Model Examination [optional]
## Environmental Impact
Carbon emissions can be estimated using the Machine Learning Impact calculator presented in Lacoste et al. (2019).
- Hardware Type:
- Hours used:
- Cloud Provider:
- Compute Region:
- Carbon Emitted:
## Technical Specifications [optional]
### Model Architecture and Objective
### Compute Infrastructure
#### Hardware
#### Software
[optional]
BibTeX:
APA:
## Glossary [optional]
## More Information [optional]
## Model Card Authors [optional]
## Model Card Contact
| [
"# Model Card for Model ID",
"## Model Details",
"### Model Description\n\n\n\nThis is the model card of a transformers model that has been pushed on the Hub. This model card has been automatically generated.\n\n- Developed by: \n- Funded by [optional]: \n- Shared by [optional]: \n- Model type: \n- Language(s) (NLP): \n- License: \n- Finetuned from model [optional]:",
"### Model Sources [optional]\n\n\n\n- Repository: \n- Paper [optional]: \n- Demo [optional]:",
"## Uses",
"### Direct Use",
"### Downstream Use [optional]",
"### Out-of-Scope Use",
"## Bias, Risks, and Limitations",
"### Recommendations\n\n\n\nUsers (both direct and downstream) should be made aware of the risks, biases and limitations of the model. More information needed for further recommendations.",
"## How to Get Started with the Model\n\nUse the code below to get started with the model.",
"## Training Details",
"### Training Data",
"### Training Procedure",
"#### Preprocessing [optional]",
"#### Training Hyperparameters\n\n- Training regime:",
"#### Speeds, Sizes, Times [optional]",
"## Evaluation",
"### Testing Data, Factors & Metrics",
"#### Testing Data",
"#### Factors",
"#### Metrics",
"### Results",
"#### Summary",
"## Model Examination [optional]",
"## Environmental Impact\n\n\n\nCarbon emissions can be estimated using the Machine Learning Impact calculator presented in Lacoste et al. (2019).\n\n- Hardware Type: \n- Hours used: \n- Cloud Provider: \n- Compute Region: \n- Carbon Emitted:",
"## Technical Specifications [optional]",
"### Model Architecture and Objective",
"### Compute Infrastructure",
"#### Hardware",
"#### Software\n\n\n\n[optional]\n\n\n\nBibTeX:\n\n\n\nAPA:",
"## Glossary [optional]",
"## More Information [optional]",
"## Model Card Authors [optional]",
"## Model Card Contact"
] | [
"TAGS\n#transformers #safetensors #vision-encoder-decoder #arxiv-1910.09700 #endpoints_compatible #region-us \n",
"# Model Card for Model ID",
"## Model Details",
"### Model Description\n\n\n\nThis is the model card of a transformers model that has been pushed on the Hub. This model card has been automatically generated.\n\n- Developed by: \n- Funded by [optional]: \n- Shared by [optional]: \n- Model type: \n- Language(s) (NLP): \n- License: \n- Finetuned from model [optional]:",
"### Model Sources [optional]\n\n\n\n- Repository: \n- Paper [optional]: \n- Demo [optional]:",
"## Uses",
"### Direct Use",
"### Downstream Use [optional]",
"### Out-of-Scope Use",
"## Bias, Risks, and Limitations",
"### Recommendations\n\n\n\nUsers (both direct and downstream) should be made aware of the risks, biases and limitations of the model. More information needed for further recommendations.",
"## How to Get Started with the Model\n\nUse the code below to get started with the model.",
"## Training Details",
"### Training Data",
"### Training Procedure",
"#### Preprocessing [optional]",
"#### Training Hyperparameters\n\n- Training regime:",
"#### Speeds, Sizes, Times [optional]",
"## Evaluation",
"### Testing Data, Factors & Metrics",
"#### Testing Data",
"#### Factors",
"#### Metrics",
"### Results",
"#### Summary",
"## Model Examination [optional]",
"## Environmental Impact\n\n\n\nCarbon emissions can be estimated using the Machine Learning Impact calculator presented in Lacoste et al. (2019).\n\n- Hardware Type: \n- Hours used: \n- Cloud Provider: \n- Compute Region: \n- Carbon Emitted:",
"## Technical Specifications [optional]",
"### Model Architecture and Objective",
"### Compute Infrastructure",
"#### Hardware",
"#### Software\n\n\n\n[optional]\n\n\n\nBibTeX:\n\n\n\nAPA:",
"## Glossary [optional]",
"## More Information [optional]",
"## Model Card Authors [optional]",
"## Model Card Contact"
] |
null | null | Users can convert EML files to Hotmail account files in a very secure and efficient manner by using a professional solution - ToolsBaer EML to Hotmail Importer tool. It is now simpler to convert EML files with attachments to Hotmail account files in bulk. The user never loses any data when using this program for conversion. Even those who are not skilled can use the program with ease because of its easy UI. To use the programs, users don't need any other software. Windows 11, 10, 7, XP, Vista, 8, and 8.1 can all be used with this software. ToolsBaer EML to Hotmail Importer is available for download and full-use purchase.
Read More:- http://www.toolsbaer.com/eml-to-hotmail-importer/ | {} | madelineoliver/ToolsBaer-EML-to-Hotmail-Importer | null | [
"region:us"
] | null | 2024-04-22T10:35:14+00:00 | [] | [] | TAGS
#region-us
| Users can convert EML files to Hotmail account files in a very secure and efficient manner by using a professional solution - ToolsBaer EML to Hotmail Importer tool. It is now simpler to convert EML files with attachments to Hotmail account files in bulk. The user never loses any data when using this program for conversion. Even those who are not skilled can use the program with ease because of its easy UI. To use the programs, users don't need any other software. Windows 11, 10, 7, XP, Vista, 8, and 8.1 can all be used with this software. ToolsBaer EML to Hotmail Importer is available for download and full-use purchase.
Read More:- URL | [] | [
"TAGS\n#region-us \n"
] |
text-generation | transformers |
# Model Card for Model ID
<!-- Provide a quick summary of what the model is/does. -->
## Model Details
### Model Description
<!-- Provide a longer summary of what this model is. -->
This is the model card of a 🤗 transformers model that has been pushed on the Hub. This model card has been automatically generated.
- **Developed by:** [More Information Needed]
- **Funded by [optional]:** [More Information Needed]
- **Shared by [optional]:** [More Information Needed]
- **Model type:** [More Information Needed]
- **Language(s) (NLP):** [More Information Needed]
- **License:** [More Information Needed]
- **Finetuned from model [optional]:** [More Information Needed]
### Model Sources [optional]
<!-- Provide the basic links for the model. -->
- **Repository:** [More Information Needed]
- **Paper [optional]:** [More Information Needed]
- **Demo [optional]:** [More Information Needed]
## Uses
<!-- Address questions around how the model is intended to be used, including the foreseeable users of the model and those affected by the model. -->
### Direct Use
<!-- This section is for the model use without fine-tuning or plugging into a larger ecosystem/app. -->
[More Information Needed]
### Downstream Use [optional]
<!-- This section is for the model use when fine-tuned for a task, or when plugged into a larger ecosystem/app -->
[More Information Needed]
### Out-of-Scope Use
<!-- This section addresses misuse, malicious use, and uses that the model will not work well for. -->
[More Information Needed]
## Bias, Risks, and Limitations
<!-- This section is meant to convey both technical and sociotechnical limitations. -->
[More Information Needed]
### Recommendations
<!-- This section is meant to convey recommendations with respect to the bias, risk, and technical limitations. -->
Users (both direct and downstream) should be made aware of the risks, biases and limitations of the model. More information needed for further recommendations.
## How to Get Started with the Model
Use the code below to get started with the model.
[More Information Needed]
## Training Details
### Training Data
<!-- This should link to a Dataset Card, perhaps with a short stub of information on what the training data is all about as well as documentation related to data pre-processing or additional filtering. -->
[More Information Needed]
### Training Procedure
<!-- This relates heavily to the Technical Specifications. Content here should link to that section when it is relevant to the training procedure. -->
#### Preprocessing [optional]
[More Information Needed]
#### Training Hyperparameters
- **Training regime:** [More Information Needed] <!--fp32, fp16 mixed precision, bf16 mixed precision, bf16 non-mixed precision, fp16 non-mixed precision, fp8 mixed precision -->
#### Speeds, Sizes, Times [optional]
<!-- This section provides information about throughput, start/end time, checkpoint size if relevant, etc. -->
[More Information Needed]
## Evaluation
<!-- This section describes the evaluation protocols and provides the results. -->
### Testing Data, Factors & Metrics
#### Testing Data
<!-- This should link to a Dataset Card if possible. -->
[More Information Needed]
#### Factors
<!-- These are the things the evaluation is disaggregating by, e.g., subpopulations or domains. -->
[More Information Needed]
#### Metrics
<!-- These are the evaluation metrics being used, ideally with a description of why. -->
[More Information Needed]
### Results
[More Information Needed]
#### Summary
## Model Examination [optional]
<!-- Relevant interpretability work for the model goes here -->
[More Information Needed]
## Environmental Impact
<!-- Total emissions (in grams of CO2eq) and additional considerations, such as electricity usage, go here. Edit the suggested text below accordingly -->
Carbon emissions can be estimated using the [Machine Learning Impact calculator](https://mlco2.github.io/impact#compute) presented in [Lacoste et al. (2019)](https://arxiv.org/abs/1910.09700).
- **Hardware Type:** [More Information Needed]
- **Hours used:** [More Information Needed]
- **Cloud Provider:** [More Information Needed]
- **Compute Region:** [More Information Needed]
- **Carbon Emitted:** [More Information Needed]
## Technical Specifications [optional]
### Model Architecture and Objective
[More Information Needed]
### Compute Infrastructure
[More Information Needed]
#### Hardware
[More Information Needed]
#### Software
[More Information Needed]
## Citation [optional]
<!-- If there is a paper or blog post introducing the model, the APA and Bibtex information for that should go in this section. -->
**BibTeX:**
[More Information Needed]
**APA:**
[More Information Needed]
## Glossary [optional]
<!-- If relevant, include terms and calculations in this section that can help readers understand the model or model card. -->
[More Information Needed]
## More Information [optional]
[More Information Needed]
## Model Card Authors [optional]
[More Information Needed]
## Model Card Contact
[More Information Needed] | {"library_name": "transformers", "tags": []} | OwOOwO/dumbo-stable_t4 | null | [
"transformers",
"safetensors",
"stablelm",
"text-generation",
"conversational",
"arxiv:1910.09700",
"autotrain_compatible",
"endpoints_compatible",
"region:us"
] | null | 2024-04-22T10:36:24+00:00 | [
"1910.09700"
] | [] | TAGS
#transformers #safetensors #stablelm #text-generation #conversational #arxiv-1910.09700 #autotrain_compatible #endpoints_compatible #region-us
|
# Model Card for Model ID
## Model Details
### Model Description
This is the model card of a transformers model that has been pushed on the Hub. This model card has been automatically generated.
- Developed by:
- Funded by [optional]:
- Shared by [optional]:
- Model type:
- Language(s) (NLP):
- License:
- Finetuned from model [optional]:
### Model Sources [optional]
- Repository:
- Paper [optional]:
- Demo [optional]:
## Uses
### Direct Use
### Downstream Use [optional]
### Out-of-Scope Use
## Bias, Risks, and Limitations
### Recommendations
Users (both direct and downstream) should be made aware of the risks, biases and limitations of the model. More information needed for further recommendations.
## How to Get Started with the Model
Use the code below to get started with the model.
## Training Details
### Training Data
### Training Procedure
#### Preprocessing [optional]
#### Training Hyperparameters
- Training regime:
#### Speeds, Sizes, Times [optional]
## Evaluation
### Testing Data, Factors & Metrics
#### Testing Data
#### Factors
#### Metrics
### Results
#### Summary
## Model Examination [optional]
## Environmental Impact
Carbon emissions can be estimated using the Machine Learning Impact calculator presented in Lacoste et al. (2019).
- Hardware Type:
- Hours used:
- Cloud Provider:
- Compute Region:
- Carbon Emitted:
## Technical Specifications [optional]
### Model Architecture and Objective
### Compute Infrastructure
#### Hardware
#### Software
[optional]
BibTeX:
APA:
## Glossary [optional]
## More Information [optional]
## Model Card Authors [optional]
## Model Card Contact
| [
"# Model Card for Model ID",
"## Model Details",
"### Model Description\n\n\n\nThis is the model card of a transformers model that has been pushed on the Hub. This model card has been automatically generated.\n\n- Developed by: \n- Funded by [optional]: \n- Shared by [optional]: \n- Model type: \n- Language(s) (NLP): \n- License: \n- Finetuned from model [optional]:",
"### Model Sources [optional]\n\n\n\n- Repository: \n- Paper [optional]: \n- Demo [optional]:",
"## Uses",
"### Direct Use",
"### Downstream Use [optional]",
"### Out-of-Scope Use",
"## Bias, Risks, and Limitations",
"### Recommendations\n\n\n\nUsers (both direct and downstream) should be made aware of the risks, biases and limitations of the model. More information needed for further recommendations.",
"## How to Get Started with the Model\n\nUse the code below to get started with the model.",
"## Training Details",
"### Training Data",
"### Training Procedure",
"#### Preprocessing [optional]",
"#### Training Hyperparameters\n\n- Training regime:",
"#### Speeds, Sizes, Times [optional]",
"## Evaluation",
"### Testing Data, Factors & Metrics",
"#### Testing Data",
"#### Factors",
"#### Metrics",
"### Results",
"#### Summary",
"## Model Examination [optional]",
"## Environmental Impact\n\n\n\nCarbon emissions can be estimated using the Machine Learning Impact calculator presented in Lacoste et al. (2019).\n\n- Hardware Type: \n- Hours used: \n- Cloud Provider: \n- Compute Region: \n- Carbon Emitted:",
"## Technical Specifications [optional]",
"### Model Architecture and Objective",
"### Compute Infrastructure",
"#### Hardware",
"#### Software\n\n\n\n[optional]\n\n\n\nBibTeX:\n\n\n\nAPA:",
"## Glossary [optional]",
"## More Information [optional]",
"## Model Card Authors [optional]",
"## Model Card Contact"
] | [
"TAGS\n#transformers #safetensors #stablelm #text-generation #conversational #arxiv-1910.09700 #autotrain_compatible #endpoints_compatible #region-us \n",
"# Model Card for Model ID",
"## Model Details",
"### Model Description\n\n\n\nThis is the model card of a transformers model that has been pushed on the Hub. This model card has been automatically generated.\n\n- Developed by: \n- Funded by [optional]: \n- Shared by [optional]: \n- Model type: \n- Language(s) (NLP): \n- License: \n- Finetuned from model [optional]:",
"### Model Sources [optional]\n\n\n\n- Repository: \n- Paper [optional]: \n- Demo [optional]:",
"## Uses",
"### Direct Use",
"### Downstream Use [optional]",
"### Out-of-Scope Use",
"## Bias, Risks, and Limitations",
"### Recommendations\n\n\n\nUsers (both direct and downstream) should be made aware of the risks, biases and limitations of the model. More information needed for further recommendations.",
"## How to Get Started with the Model\n\nUse the code below to get started with the model.",
"## Training Details",
"### Training Data",
"### Training Procedure",
"#### Preprocessing [optional]",
"#### Training Hyperparameters\n\n- Training regime:",
"#### Speeds, Sizes, Times [optional]",
"## Evaluation",
"### Testing Data, Factors & Metrics",
"#### Testing Data",
"#### Factors",
"#### Metrics",
"### Results",
"#### Summary",
"## Model Examination [optional]",
"## Environmental Impact\n\n\n\nCarbon emissions can be estimated using the Machine Learning Impact calculator presented in Lacoste et al. (2019).\n\n- Hardware Type: \n- Hours used: \n- Cloud Provider: \n- Compute Region: \n- Carbon Emitted:",
"## Technical Specifications [optional]",
"### Model Architecture and Objective",
"### Compute Infrastructure",
"#### Hardware",
"#### Software\n\n\n\n[optional]\n\n\n\nBibTeX:\n\n\n\nAPA:",
"## Glossary [optional]",
"## More Information [optional]",
"## Model Card Authors [optional]",
"## Model Card Contact"
] |
null | transformers |
# Model Card for Model ID
<!-- Provide a quick summary of what the model is/does. -->
## Model Details
### Model Description
<!-- Provide a longer summary of what this model is. -->
This is the model card of a 🤗 transformers model that has been pushed on the Hub. This model card has been automatically generated.
- **Developed by:** [More Information Needed]
- **Funded by [optional]:** [More Information Needed]
- **Shared by [optional]:** [More Information Needed]
- **Model type:** [More Information Needed]
- **Language(s) (NLP):** [More Information Needed]
- **License:** [More Information Needed]
- **Finetuned from model [optional]:** [More Information Needed]
### Model Sources [optional]
<!-- Provide the basic links for the model. -->
- **Repository:** [More Information Needed]
- **Paper [optional]:** [More Information Needed]
- **Demo [optional]:** [More Information Needed]
## Uses
<!-- Address questions around how the model is intended to be used, including the foreseeable users of the model and those affected by the model. -->
### Direct Use
<!-- This section is for the model use without fine-tuning or plugging into a larger ecosystem/app. -->
[More Information Needed]
### Downstream Use [optional]
<!-- This section is for the model use when fine-tuned for a task, or when plugged into a larger ecosystem/app -->
[More Information Needed]
### Out-of-Scope Use
<!-- This section addresses misuse, malicious use, and uses that the model will not work well for. -->
[More Information Needed]
## Bias, Risks, and Limitations
<!-- This section is meant to convey both technical and sociotechnical limitations. -->
[More Information Needed]
### Recommendations
<!-- This section is meant to convey recommendations with respect to the bias, risk, and technical limitations. -->
Users (both direct and downstream) should be made aware of the risks, biases and limitations of the model. More information needed for further recommendations.
## How to Get Started with the Model
Use the code below to get started with the model.
[More Information Needed]
## Training Details
### Training Data
<!-- This should link to a Dataset Card, perhaps with a short stub of information on what the training data is all about as well as documentation related to data pre-processing or additional filtering. -->
[More Information Needed]
### Training Procedure
<!-- This relates heavily to the Technical Specifications. Content here should link to that section when it is relevant to the training procedure. -->
#### Preprocessing [optional]
[More Information Needed]
#### Training Hyperparameters
- **Training regime:** [More Information Needed] <!--fp32, fp16 mixed precision, bf16 mixed precision, bf16 non-mixed precision, fp16 non-mixed precision, fp8 mixed precision -->
#### Speeds, Sizes, Times [optional]
<!-- This section provides information about throughput, start/end time, checkpoint size if relevant, etc. -->
[More Information Needed]
## Evaluation
<!-- This section describes the evaluation protocols and provides the results. -->
### Testing Data, Factors & Metrics
#### Testing Data
<!-- This should link to a Dataset Card if possible. -->
[More Information Needed]
#### Factors
<!-- These are the things the evaluation is disaggregating by, e.g., subpopulations or domains. -->
[More Information Needed]
#### Metrics
<!-- These are the evaluation metrics being used, ideally with a description of why. -->
[More Information Needed]
### Results
[More Information Needed]
#### Summary
## Model Examination [optional]
<!-- Relevant interpretability work for the model goes here -->
[More Information Needed]
## Environmental Impact
<!-- Total emissions (in grams of CO2eq) and additional considerations, such as electricity usage, go here. Edit the suggested text below accordingly -->
Carbon emissions can be estimated using the [Machine Learning Impact calculator](https://mlco2.github.io/impact#compute) presented in [Lacoste et al. (2019)](https://arxiv.org/abs/1910.09700).
- **Hardware Type:** [More Information Needed]
- **Hours used:** [More Information Needed]
- **Cloud Provider:** [More Information Needed]
- **Compute Region:** [More Information Needed]
- **Carbon Emitted:** [More Information Needed]
## Technical Specifications [optional]
### Model Architecture and Objective
[More Information Needed]
### Compute Infrastructure
[More Information Needed]
#### Hardware
[More Information Needed]
#### Software
[More Information Needed]
## Citation [optional]
<!-- If there is a paper or blog post introducing the model, the APA and Bibtex information for that should go in this section. -->
**BibTeX:**
[More Information Needed]
**APA:**
[More Information Needed]
## Glossary [optional]
<!-- If relevant, include terms and calculations in this section that can help readers understand the model or model card. -->
[More Information Needed]
## More Information [optional]
[More Information Needed]
## Model Card Authors [optional]
[More Information Needed]
## Model Card Contact
[More Information Needed] | {"library_name": "transformers", "tags": []} | relu-ntnu/bart-large-cnn_v4_trained_on_250_lr_5e-5_r8_a16_all_layers | null | [
"transformers",
"safetensors",
"arxiv:1910.09700",
"endpoints_compatible",
"region:us"
] | null | 2024-04-22T10:36:32+00:00 | [
"1910.09700"
] | [] | TAGS
#transformers #safetensors #arxiv-1910.09700 #endpoints_compatible #region-us
|
# Model Card for Model ID
## Model Details
### Model Description
This is the model card of a transformers model that has been pushed on the Hub. This model card has been automatically generated.
- Developed by:
- Funded by [optional]:
- Shared by [optional]:
- Model type:
- Language(s) (NLP):
- License:
- Finetuned from model [optional]:
### Model Sources [optional]
- Repository:
- Paper [optional]:
- Demo [optional]:
## Uses
### Direct Use
### Downstream Use [optional]
### Out-of-Scope Use
## Bias, Risks, and Limitations
### Recommendations
Users (both direct and downstream) should be made aware of the risks, biases and limitations of the model. More information needed for further recommendations.
## How to Get Started with the Model
Use the code below to get started with the model.
## Training Details
### Training Data
### Training Procedure
#### Preprocessing [optional]
#### Training Hyperparameters
- Training regime:
#### Speeds, Sizes, Times [optional]
## Evaluation
### Testing Data, Factors & Metrics
#### Testing Data
#### Factors
#### Metrics
### Results
#### Summary
## Model Examination [optional]
## Environmental Impact
Carbon emissions can be estimated using the Machine Learning Impact calculator presented in Lacoste et al. (2019).
- Hardware Type:
- Hours used:
- Cloud Provider:
- Compute Region:
- Carbon Emitted:
## Technical Specifications [optional]
### Model Architecture and Objective
### Compute Infrastructure
#### Hardware
#### Software
[optional]
BibTeX:
APA:
## Glossary [optional]
## More Information [optional]
## Model Card Authors [optional]
## Model Card Contact
| [
"# Model Card for Model ID",
"## Model Details",
"### Model Description\n\n\n\nThis is the model card of a transformers model that has been pushed on the Hub. This model card has been automatically generated.\n\n- Developed by: \n- Funded by [optional]: \n- Shared by [optional]: \n- Model type: \n- Language(s) (NLP): \n- License: \n- Finetuned from model [optional]:",
"### Model Sources [optional]\n\n\n\n- Repository: \n- Paper [optional]: \n- Demo [optional]:",
"## Uses",
"### Direct Use",
"### Downstream Use [optional]",
"### Out-of-Scope Use",
"## Bias, Risks, and Limitations",
"### Recommendations\n\n\n\nUsers (both direct and downstream) should be made aware of the risks, biases and limitations of the model. More information needed for further recommendations.",
"## How to Get Started with the Model\n\nUse the code below to get started with the model.",
"## Training Details",
"### Training Data",
"### Training Procedure",
"#### Preprocessing [optional]",
"#### Training Hyperparameters\n\n- Training regime:",
"#### Speeds, Sizes, Times [optional]",
"## Evaluation",
"### Testing Data, Factors & Metrics",
"#### Testing Data",
"#### Factors",
"#### Metrics",
"### Results",
"#### Summary",
"## Model Examination [optional]",
"## Environmental Impact\n\n\n\nCarbon emissions can be estimated using the Machine Learning Impact calculator presented in Lacoste et al. (2019).\n\n- Hardware Type: \n- Hours used: \n- Cloud Provider: \n- Compute Region: \n- Carbon Emitted:",
"## Technical Specifications [optional]",
"### Model Architecture and Objective",
"### Compute Infrastructure",
"#### Hardware",
"#### Software\n\n\n\n[optional]\n\n\n\nBibTeX:\n\n\n\nAPA:",
"## Glossary [optional]",
"## More Information [optional]",
"## Model Card Authors [optional]",
"## Model Card Contact"
] | [
"TAGS\n#transformers #safetensors #arxiv-1910.09700 #endpoints_compatible #region-us \n",
"# Model Card for Model ID",
"## Model Details",
"### Model Description\n\n\n\nThis is the model card of a transformers model that has been pushed on the Hub. This model card has been automatically generated.\n\n- Developed by: \n- Funded by [optional]: \n- Shared by [optional]: \n- Model type: \n- Language(s) (NLP): \n- License: \n- Finetuned from model [optional]:",
"### Model Sources [optional]\n\n\n\n- Repository: \n- Paper [optional]: \n- Demo [optional]:",
"## Uses",
"### Direct Use",
"### Downstream Use [optional]",
"### Out-of-Scope Use",
"## Bias, Risks, and Limitations",
"### Recommendations\n\n\n\nUsers (both direct and downstream) should be made aware of the risks, biases and limitations of the model. More information needed for further recommendations.",
"## How to Get Started with the Model\n\nUse the code below to get started with the model.",
"## Training Details",
"### Training Data",
"### Training Procedure",
"#### Preprocessing [optional]",
"#### Training Hyperparameters\n\n- Training regime:",
"#### Speeds, Sizes, Times [optional]",
"## Evaluation",
"### Testing Data, Factors & Metrics",
"#### Testing Data",
"#### Factors",
"#### Metrics",
"### Results",
"#### Summary",
"## Model Examination [optional]",
"## Environmental Impact\n\n\n\nCarbon emissions can be estimated using the Machine Learning Impact calculator presented in Lacoste et al. (2019).\n\n- Hardware Type: \n- Hours used: \n- Cloud Provider: \n- Compute Region: \n- Carbon Emitted:",
"## Technical Specifications [optional]",
"### Model Architecture and Objective",
"### Compute Infrastructure",
"#### Hardware",
"#### Software\n\n\n\n[optional]\n\n\n\nBibTeX:\n\n\n\nAPA:",
"## Glossary [optional]",
"## More Information [optional]",
"## Model Card Authors [optional]",
"## Model Card Contact"
] |
text-generation | transformers |
# Model Card for Model ID
<!-- Provide a quick summary of what the model is/does. -->
## Model Details
### Model Description
<!-- Provide a longer summary of what this model is. -->
This is the model card of a 🤗 transformers model that has been pushed on the Hub. This model card has been automatically generated.
- **Developed by:** [More Information Needed]
- **Funded by [optional]:** [More Information Needed]
- **Shared by [optional]:** [More Information Needed]
- **Model type:** [More Information Needed]
- **Language(s) (NLP):** [More Information Needed]
- **License:** [More Information Needed]
- **Finetuned from model [optional]:** [More Information Needed]
### Model Sources [optional]
<!-- Provide the basic links for the model. -->
- **Repository:** [More Information Needed]
- **Paper [optional]:** [More Information Needed]
- **Demo [optional]:** [More Information Needed]
## Uses
<!-- Address questions around how the model is intended to be used, including the foreseeable users of the model and those affected by the model. -->
### Direct Use
<!-- This section is for the model use without fine-tuning or plugging into a larger ecosystem/app. -->
[More Information Needed]
### Downstream Use [optional]
<!-- This section is for the model use when fine-tuned for a task, or when plugged into a larger ecosystem/app -->
[More Information Needed]
### Out-of-Scope Use
<!-- This section addresses misuse, malicious use, and uses that the model will not work well for. -->
[More Information Needed]
## Bias, Risks, and Limitations
<!-- This section is meant to convey both technical and sociotechnical limitations. -->
[More Information Needed]
### Recommendations
<!-- This section is meant to convey recommendations with respect to the bias, risk, and technical limitations. -->
Users (both direct and downstream) should be made aware of the risks, biases and limitations of the model. More information needed for further recommendations.
## How to Get Started with the Model
Use the code below to get started with the model.
[More Information Needed]
## Training Details
### Training Data
<!-- This should link to a Dataset Card, perhaps with a short stub of information on what the training data is all about as well as documentation related to data pre-processing or additional filtering. -->
[More Information Needed]
### Training Procedure
<!-- This relates heavily to the Technical Specifications. Content here should link to that section when it is relevant to the training procedure. -->
#### Preprocessing [optional]
[More Information Needed]
#### Training Hyperparameters
- **Training regime:** [More Information Needed] <!--fp32, fp16 mixed precision, bf16 mixed precision, bf16 non-mixed precision, fp16 non-mixed precision, fp8 mixed precision -->
#### Speeds, Sizes, Times [optional]
<!-- This section provides information about throughput, start/end time, checkpoint size if relevant, etc. -->
[More Information Needed]
## Evaluation
<!-- This section describes the evaluation protocols and provides the results. -->
### Testing Data, Factors & Metrics
#### Testing Data
<!-- This should link to a Dataset Card if possible. -->
[More Information Needed]
#### Factors
<!-- These are the things the evaluation is disaggregating by, e.g., subpopulations or domains. -->
[More Information Needed]
#### Metrics
<!-- These are the evaluation metrics being used, ideally with a description of why. -->
[More Information Needed]
### Results
[More Information Needed]
#### Summary
## Model Examination [optional]
<!-- Relevant interpretability work for the model goes here -->
[More Information Needed]
## Environmental Impact
<!-- Total emissions (in grams of CO2eq) and additional considerations, such as electricity usage, go here. Edit the suggested text below accordingly -->
Carbon emissions can be estimated using the [Machine Learning Impact calculator](https://mlco2.github.io/impact#compute) presented in [Lacoste et al. (2019)](https://arxiv.org/abs/1910.09700).
- **Hardware Type:** [More Information Needed]
- **Hours used:** [More Information Needed]
- **Cloud Provider:** [More Information Needed]
- **Compute Region:** [More Information Needed]
- **Carbon Emitted:** [More Information Needed]
## Technical Specifications [optional]
### Model Architecture and Objective
[More Information Needed]
### Compute Infrastructure
[More Information Needed]
#### Hardware
[More Information Needed]
#### Software
[More Information Needed]
## Citation [optional]
<!-- If there is a paper or blog post introducing the model, the APA and Bibtex information for that should go in this section. -->
**BibTeX:**
[More Information Needed]
**APA:**
[More Information Needed]
## Glossary [optional]
<!-- If relevant, include terms and calculations in this section that can help readers understand the model or model card. -->
[More Information Needed]
## More Information [optional]
[More Information Needed]
## Model Card Authors [optional]
[More Information Needed]
## Model Card Contact
[More Information Needed] | {"library_name": "transformers", "tags": []} | Mayurpai5/results | null | [
"transformers",
"safetensors",
"llama",
"text-generation",
"arxiv:1910.09700",
"autotrain_compatible",
"endpoints_compatible",
"text-generation-inference",
"region:us"
] | null | 2024-04-22T10:36:50+00:00 | [
"1910.09700"
] | [] | TAGS
#transformers #safetensors #llama #text-generation #arxiv-1910.09700 #autotrain_compatible #endpoints_compatible #text-generation-inference #region-us
|
# Model Card for Model ID
## Model Details
### Model Description
This is the model card of a transformers model that has been pushed on the Hub. This model card has been automatically generated.
- Developed by:
- Funded by [optional]:
- Shared by [optional]:
- Model type:
- Language(s) (NLP):
- License:
- Finetuned from model [optional]:
### Model Sources [optional]
- Repository:
- Paper [optional]:
- Demo [optional]:
## Uses
### Direct Use
### Downstream Use [optional]
### Out-of-Scope Use
## Bias, Risks, and Limitations
### Recommendations
Users (both direct and downstream) should be made aware of the risks, biases and limitations of the model. More information needed for further recommendations.
## How to Get Started with the Model
Use the code below to get started with the model.
## Training Details
### Training Data
### Training Procedure
#### Preprocessing [optional]
#### Training Hyperparameters
- Training regime:
#### Speeds, Sizes, Times [optional]
## Evaluation
### Testing Data, Factors & Metrics
#### Testing Data
#### Factors
#### Metrics
### Results
#### Summary
## Model Examination [optional]
## Environmental Impact
Carbon emissions can be estimated using the Machine Learning Impact calculator presented in Lacoste et al. (2019).
- Hardware Type:
- Hours used:
- Cloud Provider:
- Compute Region:
- Carbon Emitted:
## Technical Specifications [optional]
### Model Architecture and Objective
### Compute Infrastructure
#### Hardware
#### Software
[optional]
BibTeX:
APA:
## Glossary [optional]
## More Information [optional]
## Model Card Authors [optional]
## Model Card Contact
| [
"# Model Card for Model ID",
"## Model Details",
"### Model Description\n\n\n\nThis is the model card of a transformers model that has been pushed on the Hub. This model card has been automatically generated.\n\n- Developed by: \n- Funded by [optional]: \n- Shared by [optional]: \n- Model type: \n- Language(s) (NLP): \n- License: \n- Finetuned from model [optional]:",
"### Model Sources [optional]\n\n\n\n- Repository: \n- Paper [optional]: \n- Demo [optional]:",
"## Uses",
"### Direct Use",
"### Downstream Use [optional]",
"### Out-of-Scope Use",
"## Bias, Risks, and Limitations",
"### Recommendations\n\n\n\nUsers (both direct and downstream) should be made aware of the risks, biases and limitations of the model. More information needed for further recommendations.",
"## How to Get Started with the Model\n\nUse the code below to get started with the model.",
"## Training Details",
"### Training Data",
"### Training Procedure",
"#### Preprocessing [optional]",
"#### Training Hyperparameters\n\n- Training regime:",
"#### Speeds, Sizes, Times [optional]",
"## Evaluation",
"### Testing Data, Factors & Metrics",
"#### Testing Data",
"#### Factors",
"#### Metrics",
"### Results",
"#### Summary",
"## Model Examination [optional]",
"## Environmental Impact\n\n\n\nCarbon emissions can be estimated using the Machine Learning Impact calculator presented in Lacoste et al. (2019).\n\n- Hardware Type: \n- Hours used: \n- Cloud Provider: \n- Compute Region: \n- Carbon Emitted:",
"## Technical Specifications [optional]",
"### Model Architecture and Objective",
"### Compute Infrastructure",
"#### Hardware",
"#### Software\n\n\n\n[optional]\n\n\n\nBibTeX:\n\n\n\nAPA:",
"## Glossary [optional]",
"## More Information [optional]",
"## Model Card Authors [optional]",
"## Model Card Contact"
] | [
"TAGS\n#transformers #safetensors #llama #text-generation #arxiv-1910.09700 #autotrain_compatible #endpoints_compatible #text-generation-inference #region-us \n",
"# Model Card for Model ID",
"## Model Details",
"### Model Description\n\n\n\nThis is the model card of a transformers model that has been pushed on the Hub. This model card has been automatically generated.\n\n- Developed by: \n- Funded by [optional]: \n- Shared by [optional]: \n- Model type: \n- Language(s) (NLP): \n- License: \n- Finetuned from model [optional]:",
"### Model Sources [optional]\n\n\n\n- Repository: \n- Paper [optional]: \n- Demo [optional]:",
"## Uses",
"### Direct Use",
"### Downstream Use [optional]",
"### Out-of-Scope Use",
"## Bias, Risks, and Limitations",
"### Recommendations\n\n\n\nUsers (both direct and downstream) should be made aware of the risks, biases and limitations of the model. More information needed for further recommendations.",
"## How to Get Started with the Model\n\nUse the code below to get started with the model.",
"## Training Details",
"### Training Data",
"### Training Procedure",
"#### Preprocessing [optional]",
"#### Training Hyperparameters\n\n- Training regime:",
"#### Speeds, Sizes, Times [optional]",
"## Evaluation",
"### Testing Data, Factors & Metrics",
"#### Testing Data",
"#### Factors",
"#### Metrics",
"### Results",
"#### Summary",
"## Model Examination [optional]",
"## Environmental Impact\n\n\n\nCarbon emissions can be estimated using the Machine Learning Impact calculator presented in Lacoste et al. (2019).\n\n- Hardware Type: \n- Hours used: \n- Cloud Provider: \n- Compute Region: \n- Carbon Emitted:",
"## Technical Specifications [optional]",
"### Model Architecture and Objective",
"### Compute Infrastructure",
"#### Hardware",
"#### Software\n\n\n\n[optional]\n\n\n\nBibTeX:\n\n\n\nAPA:",
"## Glossary [optional]",
"## More Information [optional]",
"## Model Card Authors [optional]",
"## Model Card Contact"
] |
text-generation | transformers |
# Model Card for Model ID
<!-- Provide a quick summary of what the model is/does. -->
## Model Details
### Model Description
<!-- Provide a longer summary of what this model is. -->
This is the model card of a 🤗 transformers model that has been pushed on the Hub. This model card has been automatically generated.
- **Developed by:** [More Information Needed]
- **Funded by [optional]:** [More Information Needed]
- **Shared by [optional]:** [More Information Needed]
- **Model type:** [More Information Needed]
- **Language(s) (NLP):** [More Information Needed]
- **License:** [More Information Needed]
- **Finetuned from model [optional]:** [More Information Needed]
### Model Sources [optional]
<!-- Provide the basic links for the model. -->
- **Repository:** [More Information Needed]
- **Paper [optional]:** [More Information Needed]
- **Demo [optional]:** [More Information Needed]
## Uses
<!-- Address questions around how the model is intended to be used, including the foreseeable users of the model and those affected by the model. -->
### Direct Use
<!-- This section is for the model use without fine-tuning or plugging into a larger ecosystem/app. -->
[More Information Needed]
### Downstream Use [optional]
<!-- This section is for the model use when fine-tuned for a task, or when plugged into a larger ecosystem/app -->
[More Information Needed]
### Out-of-Scope Use
<!-- This section addresses misuse, malicious use, and uses that the model will not work well for. -->
[More Information Needed]
## Bias, Risks, and Limitations
<!-- This section is meant to convey both technical and sociotechnical limitations. -->
[More Information Needed]
### Recommendations
<!-- This section is meant to convey recommendations with respect to the bias, risk, and technical limitations. -->
Users (both direct and downstream) should be made aware of the risks, biases and limitations of the model. More information needed for further recommendations.
## How to Get Started with the Model
Use the code below to get started with the model.
[More Information Needed]
## Training Details
### Training Data
<!-- This should link to a Dataset Card, perhaps with a short stub of information on what the training data is all about as well as documentation related to data pre-processing or additional filtering. -->
[More Information Needed]
### Training Procedure
<!-- This relates heavily to the Technical Specifications. Content here should link to that section when it is relevant to the training procedure. -->
#### Preprocessing [optional]
[More Information Needed]
#### Training Hyperparameters
- **Training regime:** [More Information Needed] <!--fp32, fp16 mixed precision, bf16 mixed precision, bf16 non-mixed precision, fp16 non-mixed precision, fp8 mixed precision -->
#### Speeds, Sizes, Times [optional]
<!-- This section provides information about throughput, start/end time, checkpoint size if relevant, etc. -->
[More Information Needed]
## Evaluation
<!-- This section describes the evaluation protocols and provides the results. -->
### Testing Data, Factors & Metrics
#### Testing Data
<!-- This should link to a Dataset Card if possible. -->
[More Information Needed]
#### Factors
<!-- These are the things the evaluation is disaggregating by, e.g., subpopulations or domains. -->
[More Information Needed]
#### Metrics
<!-- These are the evaluation metrics being used, ideally with a description of why. -->
[More Information Needed]
### Results
[More Information Needed]
#### Summary
## Model Examination [optional]
<!-- Relevant interpretability work for the model goes here -->
[More Information Needed]
## Environmental Impact
<!-- Total emissions (in grams of CO2eq) and additional considerations, such as electricity usage, go here. Edit the suggested text below accordingly -->
Carbon emissions can be estimated using the [Machine Learning Impact calculator](https://mlco2.github.io/impact#compute) presented in [Lacoste et al. (2019)](https://arxiv.org/abs/1910.09700).
- **Hardware Type:** [More Information Needed]
- **Hours used:** [More Information Needed]
- **Cloud Provider:** [More Information Needed]
- **Compute Region:** [More Information Needed]
- **Carbon Emitted:** [More Information Needed]
## Technical Specifications [optional]
### Model Architecture and Objective
[More Information Needed]
### Compute Infrastructure
[More Information Needed]
#### Hardware
[More Information Needed]
#### Software
[More Information Needed]
## Citation [optional]
<!-- If there is a paper or blog post introducing the model, the APA and Bibtex information for that should go in this section. -->
**BibTeX:**
[More Information Needed]
**APA:**
[More Information Needed]
## Glossary [optional]
<!-- If relevant, include terms and calculations in this section that can help readers understand the model or model card. -->
[More Information Needed]
## More Information [optional]
[More Information Needed]
## Model Card Authors [optional]
[More Information Needed]
## Model Card Contact
[More Information Needed] | {"library_name": "transformers", "tags": []} | jeongmi/solar_musinsa_user111_100 | null | [
"transformers",
"safetensors",
"llama",
"text-generation",
"arxiv:1910.09700",
"autotrain_compatible",
"endpoints_compatible",
"text-generation-inference",
"4-bit",
"region:us"
] | null | 2024-04-22T10:36:51+00:00 | [
"1910.09700"
] | [] | TAGS
#transformers #safetensors #llama #text-generation #arxiv-1910.09700 #autotrain_compatible #endpoints_compatible #text-generation-inference #4-bit #region-us
|
# Model Card for Model ID
## Model Details
### Model Description
This is the model card of a transformers model that has been pushed on the Hub. This model card has been automatically generated.
- Developed by:
- Funded by [optional]:
- Shared by [optional]:
- Model type:
- Language(s) (NLP):
- License:
- Finetuned from model [optional]:
### Model Sources [optional]
- Repository:
- Paper [optional]:
- Demo [optional]:
## Uses
### Direct Use
### Downstream Use [optional]
### Out-of-Scope Use
## Bias, Risks, and Limitations
### Recommendations
Users (both direct and downstream) should be made aware of the risks, biases and limitations of the model. More information needed for further recommendations.
## How to Get Started with the Model
Use the code below to get started with the model.
## Training Details
### Training Data
### Training Procedure
#### Preprocessing [optional]
#### Training Hyperparameters
- Training regime:
#### Speeds, Sizes, Times [optional]
## Evaluation
### Testing Data, Factors & Metrics
#### Testing Data
#### Factors
#### Metrics
### Results
#### Summary
## Model Examination [optional]
## Environmental Impact
Carbon emissions can be estimated using the Machine Learning Impact calculator presented in Lacoste et al. (2019).
- Hardware Type:
- Hours used:
- Cloud Provider:
- Compute Region:
- Carbon Emitted:
## Technical Specifications [optional]
### Model Architecture and Objective
### Compute Infrastructure
#### Hardware
#### Software
[optional]
BibTeX:
APA:
## Glossary [optional]
## More Information [optional]
## Model Card Authors [optional]
## Model Card Contact
| [
"# Model Card for Model ID",
"## Model Details",
"### Model Description\n\n\n\nThis is the model card of a transformers model that has been pushed on the Hub. This model card has been automatically generated.\n\n- Developed by: \n- Funded by [optional]: \n- Shared by [optional]: \n- Model type: \n- Language(s) (NLP): \n- License: \n- Finetuned from model [optional]:",
"### Model Sources [optional]\n\n\n\n- Repository: \n- Paper [optional]: \n- Demo [optional]:",
"## Uses",
"### Direct Use",
"### Downstream Use [optional]",
"### Out-of-Scope Use",
"## Bias, Risks, and Limitations",
"### Recommendations\n\n\n\nUsers (both direct and downstream) should be made aware of the risks, biases and limitations of the model. More information needed for further recommendations.",
"## How to Get Started with the Model\n\nUse the code below to get started with the model.",
"## Training Details",
"### Training Data",
"### Training Procedure",
"#### Preprocessing [optional]",
"#### Training Hyperparameters\n\n- Training regime:",
"#### Speeds, Sizes, Times [optional]",
"## Evaluation",
"### Testing Data, Factors & Metrics",
"#### Testing Data",
"#### Factors",
"#### Metrics",
"### Results",
"#### Summary",
"## Model Examination [optional]",
"## Environmental Impact\n\n\n\nCarbon emissions can be estimated using the Machine Learning Impact calculator presented in Lacoste et al. (2019).\n\n- Hardware Type: \n- Hours used: \n- Cloud Provider: \n- Compute Region: \n- Carbon Emitted:",
"## Technical Specifications [optional]",
"### Model Architecture and Objective",
"### Compute Infrastructure",
"#### Hardware",
"#### Software\n\n\n\n[optional]\n\n\n\nBibTeX:\n\n\n\nAPA:",
"## Glossary [optional]",
"## More Information [optional]",
"## Model Card Authors [optional]",
"## Model Card Contact"
] | [
"TAGS\n#transformers #safetensors #llama #text-generation #arxiv-1910.09700 #autotrain_compatible #endpoints_compatible #text-generation-inference #4-bit #region-us \n",
"# Model Card for Model ID",
"## Model Details",
"### Model Description\n\n\n\nThis is the model card of a transformers model that has been pushed on the Hub. This model card has been automatically generated.\n\n- Developed by: \n- Funded by [optional]: \n- Shared by [optional]: \n- Model type: \n- Language(s) (NLP): \n- License: \n- Finetuned from model [optional]:",
"### Model Sources [optional]\n\n\n\n- Repository: \n- Paper [optional]: \n- Demo [optional]:",
"## Uses",
"### Direct Use",
"### Downstream Use [optional]",
"### Out-of-Scope Use",
"## Bias, Risks, and Limitations",
"### Recommendations\n\n\n\nUsers (both direct and downstream) should be made aware of the risks, biases and limitations of the model. More information needed for further recommendations.",
"## How to Get Started with the Model\n\nUse the code below to get started with the model.",
"## Training Details",
"### Training Data",
"### Training Procedure",
"#### Preprocessing [optional]",
"#### Training Hyperparameters\n\n- Training regime:",
"#### Speeds, Sizes, Times [optional]",
"## Evaluation",
"### Testing Data, Factors & Metrics",
"#### Testing Data",
"#### Factors",
"#### Metrics",
"### Results",
"#### Summary",
"## Model Examination [optional]",
"## Environmental Impact\n\n\n\nCarbon emissions can be estimated using the Machine Learning Impact calculator presented in Lacoste et al. (2019).\n\n- Hardware Type: \n- Hours used: \n- Cloud Provider: \n- Compute Region: \n- Carbon Emitted:",
"## Technical Specifications [optional]",
"### Model Architecture and Objective",
"### Compute Infrastructure",
"#### Hardware",
"#### Software\n\n\n\n[optional]\n\n\n\nBibTeX:\n\n\n\nAPA:",
"## Glossary [optional]",
"## More Information [optional]",
"## Model Card Authors [optional]",
"## Model Card Contact"
] |
reinforcement-learning | stable-baselines3 |
# **PPO** Agent playing **LunarLander-v2**
This is a trained model of a **PPO** agent playing **LunarLander-v2**
using the [stable-baselines3 library](https://github.com/DLR-RM/stable-baselines3).
## Usage (with Stable-baselines3)
TODO: Add your code
```python
from stable_baselines3 import ...
from huggingface_sb3 import load_from_hub
...
```
| {"library_name": "stable-baselines3", "tags": ["LunarLander-v2", "deep-reinforcement-learning", "reinforcement-learning", "stable-baselines3"], "model-index": [{"name": "PPO", "results": [{"task": {"type": "reinforcement-learning", "name": "reinforcement-learning"}, "dataset": {"name": "LunarLander-v2", "type": "LunarLander-v2"}, "metrics": [{"type": "mean_reward", "value": "274.20 +/- 22.46", "name": "mean_reward", "verified": false}]}]}]} | lzacchini/ppo-LunarLander-v2 | null | [
"stable-baselines3",
"LunarLander-v2",
"deep-reinforcement-learning",
"reinforcement-learning",
"model-index",
"region:us"
] | null | 2024-04-22T10:37:02+00:00 | [] | [] | TAGS
#stable-baselines3 #LunarLander-v2 #deep-reinforcement-learning #reinforcement-learning #model-index #region-us
|
# PPO Agent playing LunarLander-v2
This is a trained model of a PPO agent playing LunarLander-v2
using the stable-baselines3 library.
## Usage (with Stable-baselines3)
TODO: Add your code
| [
"# PPO Agent playing LunarLander-v2\nThis is a trained model of a PPO agent playing LunarLander-v2\nusing the stable-baselines3 library.",
"## Usage (with Stable-baselines3)\nTODO: Add your code"
] | [
"TAGS\n#stable-baselines3 #LunarLander-v2 #deep-reinforcement-learning #reinforcement-learning #model-index #region-us \n",
"# PPO Agent playing LunarLander-v2\nThis is a trained model of a PPO agent playing LunarLander-v2\nusing the stable-baselines3 library.",
"## Usage (with Stable-baselines3)\nTODO: Add your code"
] |
null | transformers |
# Uploaded model
- **Developed by:** crazyup37
- **License:** apache-2.0
- **Finetuned from model :** unsloth/llama-3-8b-bnb-4bit
This llama model was trained 2x faster with [Unsloth](https://github.com/unslothai/unsloth) and Huggingface's TRL library.
[<img src="https://raw.githubusercontent.com/unslothai/unsloth/main/images/unsloth%20made%20with%20love.png" width="200"/>](https://github.com/unslothai/unsloth)
| {"language": ["en"], "license": "apache-2.0", "tags": ["text-generation-inference", "transformers", "unsloth", "llama", "trl"], "base_model": "unsloth/llama-3-8b-bnb-4bit"} | crazyup37/Llama3_8b_finetuned | null | [
"transformers",
"safetensors",
"text-generation-inference",
"unsloth",
"llama",
"trl",
"en",
"base_model:unsloth/llama-3-8b-bnb-4bit",
"license:apache-2.0",
"endpoints_compatible",
"region:us"
] | null | 2024-04-22T10:37:04+00:00 | [] | [
"en"
] | TAGS
#transformers #safetensors #text-generation-inference #unsloth #llama #trl #en #base_model-unsloth/llama-3-8b-bnb-4bit #license-apache-2.0 #endpoints_compatible #region-us
|
# Uploaded model
- Developed by: crazyup37
- License: apache-2.0
- Finetuned from model : unsloth/llama-3-8b-bnb-4bit
This llama model was trained 2x faster with Unsloth and Huggingface's TRL library.
<img src="URL width="200"/>
| [
"# Uploaded model\n\n- Developed by: crazyup37\n- License: apache-2.0\n- Finetuned from model : unsloth/llama-3-8b-bnb-4bit\n\nThis llama model was trained 2x faster with Unsloth and Huggingface's TRL library.\n\n<img src=\"URL width=\"200\"/>"
] | [
"TAGS\n#transformers #safetensors #text-generation-inference #unsloth #llama #trl #en #base_model-unsloth/llama-3-8b-bnb-4bit #license-apache-2.0 #endpoints_compatible #region-us \n",
"# Uploaded model\n\n- Developed by: crazyup37\n- License: apache-2.0\n- Finetuned from model : unsloth/llama-3-8b-bnb-4bit\n\nThis llama model was trained 2x faster with Unsloth and Huggingface's TRL library.\n\n<img src=\"URL width=\"200\"/>"
] |
text-generation | transformers |
# Model Card for Model ID
<!-- Provide a quick summary of what the model is/does. -->
## Model Details
### Model Description
<!-- Provide a longer summary of what this model is. -->
This is the model card of a 🤗 transformers model that has been pushed on the Hub. This model card has been automatically generated.
- **Developed by:** [More Information Needed]
- **Funded by [optional]:** [More Information Needed]
- **Shared by [optional]:** [More Information Needed]
- **Model type:** [More Information Needed]
- **Language(s) (NLP):** [More Information Needed]
- **License:** [More Information Needed]
- **Finetuned from model [optional]:** [More Information Needed]
### Model Sources [optional]
<!-- Provide the basic links for the model. -->
- **Repository:** [More Information Needed]
- **Paper [optional]:** [More Information Needed]
- **Demo [optional]:** [More Information Needed]
## Uses
<!-- Address questions around how the model is intended to be used, including the foreseeable users of the model and those affected by the model. -->
### Direct Use
<!-- This section is for the model use without fine-tuning or plugging into a larger ecosystem/app. -->
[More Information Needed]
### Downstream Use [optional]
<!-- This section is for the model use when fine-tuned for a task, or when plugged into a larger ecosystem/app -->
[More Information Needed]
### Out-of-Scope Use
<!-- This section addresses misuse, malicious use, and uses that the model will not work well for. -->
[More Information Needed]
## Bias, Risks, and Limitations
<!-- This section is meant to convey both technical and sociotechnical limitations. -->
[More Information Needed]
### Recommendations
<!-- This section is meant to convey recommendations with respect to the bias, risk, and technical limitations. -->
Users (both direct and downstream) should be made aware of the risks, biases and limitations of the model. More information needed for further recommendations.
## How to Get Started with the Model
Use the code below to get started with the model.
[More Information Needed]
## Training Details
### Training Data
<!-- This should link to a Dataset Card, perhaps with a short stub of information on what the training data is all about as well as documentation related to data pre-processing or additional filtering. -->
[More Information Needed]
### Training Procedure
<!-- This relates heavily to the Technical Specifications. Content here should link to that section when it is relevant to the training procedure. -->
#### Preprocessing [optional]
[More Information Needed]
#### Training Hyperparameters
- **Training regime:** [More Information Needed] <!--fp32, fp16 mixed precision, bf16 mixed precision, bf16 non-mixed precision, fp16 non-mixed precision, fp8 mixed precision -->
#### Speeds, Sizes, Times [optional]
<!-- This section provides information about throughput, start/end time, checkpoint size if relevant, etc. -->
[More Information Needed]
## Evaluation
<!-- This section describes the evaluation protocols and provides the results. -->
### Testing Data, Factors & Metrics
#### Testing Data
<!-- This should link to a Dataset Card if possible. -->
[More Information Needed]
#### Factors
<!-- These are the things the evaluation is disaggregating by, e.g., subpopulations or domains. -->
[More Information Needed]
#### Metrics
<!-- These are the evaluation metrics being used, ideally with a description of why. -->
[More Information Needed]
### Results
[More Information Needed]
#### Summary
## Model Examination [optional]
<!-- Relevant interpretability work for the model goes here -->
[More Information Needed]
## Environmental Impact
<!-- Total emissions (in grams of CO2eq) and additional considerations, such as electricity usage, go here. Edit the suggested text below accordingly -->
Carbon emissions can be estimated using the [Machine Learning Impact calculator](https://mlco2.github.io/impact#compute) presented in [Lacoste et al. (2019)](https://arxiv.org/abs/1910.09700).
- **Hardware Type:** [More Information Needed]
- **Hours used:** [More Information Needed]
- **Cloud Provider:** [More Information Needed]
- **Compute Region:** [More Information Needed]
- **Carbon Emitted:** [More Information Needed]
## Technical Specifications [optional]
### Model Architecture and Objective
[More Information Needed]
### Compute Infrastructure
[More Information Needed]
#### Hardware
[More Information Needed]
#### Software
[More Information Needed]
## Citation [optional]
<!-- If there is a paper or blog post introducing the model, the APA and Bibtex information for that should go in this section. -->
**BibTeX:**
[More Information Needed]
**APA:**
[More Information Needed]
## Glossary [optional]
<!-- If relevant, include terms and calculations in this section that can help readers understand the model or model card. -->
[More Information Needed]
## More Information [optional]
[More Information Needed]
## Model Card Authors [optional]
[More Information Needed]
## Model Card Contact
[More Information Needed] | {"library_name": "transformers", "tags": []} | tomaszki/llama-5-b | null | [
"transformers",
"safetensors",
"llama",
"text-generation",
"conversational",
"arxiv:1910.09700",
"autotrain_compatible",
"endpoints_compatible",
"text-generation-inference",
"region:us"
] | null | 2024-04-22T10:37:45+00:00 | [
"1910.09700"
] | [] | TAGS
#transformers #safetensors #llama #text-generation #conversational #arxiv-1910.09700 #autotrain_compatible #endpoints_compatible #text-generation-inference #region-us
|
# Model Card for Model ID
## Model Details
### Model Description
This is the model card of a transformers model that has been pushed on the Hub. This model card has been automatically generated.
- Developed by:
- Funded by [optional]:
- Shared by [optional]:
- Model type:
- Language(s) (NLP):
- License:
- Finetuned from model [optional]:
### Model Sources [optional]
- Repository:
- Paper [optional]:
- Demo [optional]:
## Uses
### Direct Use
### Downstream Use [optional]
### Out-of-Scope Use
## Bias, Risks, and Limitations
### Recommendations
Users (both direct and downstream) should be made aware of the risks, biases and limitations of the model. More information needed for further recommendations.
## How to Get Started with the Model
Use the code below to get started with the model.
## Training Details
### Training Data
### Training Procedure
#### Preprocessing [optional]
#### Training Hyperparameters
- Training regime:
#### Speeds, Sizes, Times [optional]
## Evaluation
### Testing Data, Factors & Metrics
#### Testing Data
#### Factors
#### Metrics
### Results
#### Summary
## Model Examination [optional]
## Environmental Impact
Carbon emissions can be estimated using the Machine Learning Impact calculator presented in Lacoste et al. (2019).
- Hardware Type:
- Hours used:
- Cloud Provider:
- Compute Region:
- Carbon Emitted:
## Technical Specifications [optional]
### Model Architecture and Objective
### Compute Infrastructure
#### Hardware
#### Software
[optional]
BibTeX:
APA:
## Glossary [optional]
## More Information [optional]
## Model Card Authors [optional]
## Model Card Contact
| [
"# Model Card for Model ID",
"## Model Details",
"### Model Description\n\n\n\nThis is the model card of a transformers model that has been pushed on the Hub. This model card has been automatically generated.\n\n- Developed by: \n- Funded by [optional]: \n- Shared by [optional]: \n- Model type: \n- Language(s) (NLP): \n- License: \n- Finetuned from model [optional]:",
"### Model Sources [optional]\n\n\n\n- Repository: \n- Paper [optional]: \n- Demo [optional]:",
"## Uses",
"### Direct Use",
"### Downstream Use [optional]",
"### Out-of-Scope Use",
"## Bias, Risks, and Limitations",
"### Recommendations\n\n\n\nUsers (both direct and downstream) should be made aware of the risks, biases and limitations of the model. More information needed for further recommendations.",
"## How to Get Started with the Model\n\nUse the code below to get started with the model.",
"## Training Details",
"### Training Data",
"### Training Procedure",
"#### Preprocessing [optional]",
"#### Training Hyperparameters\n\n- Training regime:",
"#### Speeds, Sizes, Times [optional]",
"## Evaluation",
"### Testing Data, Factors & Metrics",
"#### Testing Data",
"#### Factors",
"#### Metrics",
"### Results",
"#### Summary",
"## Model Examination [optional]",
"## Environmental Impact\n\n\n\nCarbon emissions can be estimated using the Machine Learning Impact calculator presented in Lacoste et al. (2019).\n\n- Hardware Type: \n- Hours used: \n- Cloud Provider: \n- Compute Region: \n- Carbon Emitted:",
"## Technical Specifications [optional]",
"### Model Architecture and Objective",
"### Compute Infrastructure",
"#### Hardware",
"#### Software\n\n\n\n[optional]\n\n\n\nBibTeX:\n\n\n\nAPA:",
"## Glossary [optional]",
"## More Information [optional]",
"## Model Card Authors [optional]",
"## Model Card Contact"
] | [
"TAGS\n#transformers #safetensors #llama #text-generation #conversational #arxiv-1910.09700 #autotrain_compatible #endpoints_compatible #text-generation-inference #region-us \n",
"# Model Card for Model ID",
"## Model Details",
"### Model Description\n\n\n\nThis is the model card of a transformers model that has been pushed on the Hub. This model card has been automatically generated.\n\n- Developed by: \n- Funded by [optional]: \n- Shared by [optional]: \n- Model type: \n- Language(s) (NLP): \n- License: \n- Finetuned from model [optional]:",
"### Model Sources [optional]\n\n\n\n- Repository: \n- Paper [optional]: \n- Demo [optional]:",
"## Uses",
"### Direct Use",
"### Downstream Use [optional]",
"### Out-of-Scope Use",
"## Bias, Risks, and Limitations",
"### Recommendations\n\n\n\nUsers (both direct and downstream) should be made aware of the risks, biases and limitations of the model. More information needed for further recommendations.",
"## How to Get Started with the Model\n\nUse the code below to get started with the model.",
"## Training Details",
"### Training Data",
"### Training Procedure",
"#### Preprocessing [optional]",
"#### Training Hyperparameters\n\n- Training regime:",
"#### Speeds, Sizes, Times [optional]",
"## Evaluation",
"### Testing Data, Factors & Metrics",
"#### Testing Data",
"#### Factors",
"#### Metrics",
"### Results",
"#### Summary",
"## Model Examination [optional]",
"## Environmental Impact\n\n\n\nCarbon emissions can be estimated using the Machine Learning Impact calculator presented in Lacoste et al. (2019).\n\n- Hardware Type: \n- Hours used: \n- Cloud Provider: \n- Compute Region: \n- Carbon Emitted:",
"## Technical Specifications [optional]",
"### Model Architecture and Objective",
"### Compute Infrastructure",
"#### Hardware",
"#### Software\n\n\n\n[optional]\n\n\n\nBibTeX:\n\n\n\nAPA:",
"## Glossary [optional]",
"## More Information [optional]",
"## Model Card Authors [optional]",
"## Model Card Contact"
] |
image-classification | transformers |
<!-- This model card has been generated automatically according to the information the Trainer had access to. You
should probably proofread and complete it, then remove this comment. -->
# beit-base-patch16-224-pt22k-ft22k-finetuning
This model is a fine-tuned version of [microsoft/beit-base-patch16-224-pt22k-ft22k](https://huggingface.co/microsoft/beit-base-patch16-224-pt22k-ft22k) on an unknown dataset.
It achieves the following results on the evaluation set:
- Loss: 0.2268
- Accuracy: 0.921
## Model description
More information needed
## Intended uses & limitations
More information needed
## Training and evaluation data
More information needed
## Training procedure
### Training hyperparameters
The following hyperparameters were used during training:
- learning_rate: 5e-05
- train_batch_size: 32
- eval_batch_size: 32
- seed: 42
- gradient_accumulation_steps: 4
- total_train_batch_size: 128
- optimizer: Adam with betas=(0.9,0.999) and epsilon=1e-08
- lr_scheduler_type: linear
- lr_scheduler_warmup_ratio: 0.1
- num_epochs: 3
### Training results
| Training Loss | Epoch | Step | Validation Loss | Accuracy |
|:-------------:|:------:|:----:|:---------------:|:--------:|
| 0.3814 | 0.9979 | 351 | 0.3500 | 0.8838 |
| 0.3251 | 1.9986 | 703 | 0.2797 | 0.9042 |
| 0.2368 | 2.9936 | 1053 | 0.2436 | 0.9168 |
### Framework versions
- Transformers 4.41.0.dev0
- Pytorch 2.2.2
- Datasets 2.18.0
- Tokenizers 0.19.1
| {"license": "apache-2.0", "tags": ["generated_from_trainer"], "metrics": ["accuracy"], "base_model": "microsoft/beit-base-patch16-224-pt22k-ft22k", "model-index": [{"name": "beit-base-patch16-224-pt22k-ft22k-finetuning", "results": []}]} | Rocky807/beit-base-patch16-224-pt22k-ft22k-finetuning | null | [
"transformers",
"tensorboard",
"safetensors",
"beit",
"image-classification",
"generated_from_trainer",
"base_model:microsoft/beit-base-patch16-224-pt22k-ft22k",
"license:apache-2.0",
"autotrain_compatible",
"endpoints_compatible",
"region:us"
] | null | 2024-04-22T10:38:02+00:00 | [] | [] | TAGS
#transformers #tensorboard #safetensors #beit #image-classification #generated_from_trainer #base_model-microsoft/beit-base-patch16-224-pt22k-ft22k #license-apache-2.0 #autotrain_compatible #endpoints_compatible #region-us
| beit-base-patch16-224-pt22k-ft22k-finetuning
============================================
This model is a fine-tuned version of microsoft/beit-base-patch16-224-pt22k-ft22k on an unknown dataset.
It achieves the following results on the evaluation set:
* Loss: 0.2268
* Accuracy: 0.921
Model description
-----------------
More information needed
Intended uses & limitations
---------------------------
More information needed
Training and evaluation data
----------------------------
More information needed
Training procedure
------------------
### Training hyperparameters
The following hyperparameters were used during training:
* learning\_rate: 5e-05
* train\_batch\_size: 32
* eval\_batch\_size: 32
* seed: 42
* gradient\_accumulation\_steps: 4
* total\_train\_batch\_size: 128
* optimizer: Adam with betas=(0.9,0.999) and epsilon=1e-08
* lr\_scheduler\_type: linear
* lr\_scheduler\_warmup\_ratio: 0.1
* num\_epochs: 3
### Training results
### Framework versions
* Transformers 4.41.0.dev0
* Pytorch 2.2.2
* Datasets 2.18.0
* Tokenizers 0.19.1
| [
"### Training hyperparameters\n\n\nThe following hyperparameters were used during training:\n\n\n* learning\\_rate: 5e-05\n* train\\_batch\\_size: 32\n* eval\\_batch\\_size: 32\n* seed: 42\n* gradient\\_accumulation\\_steps: 4\n* total\\_train\\_batch\\_size: 128\n* optimizer: Adam with betas=(0.9,0.999) and epsilon=1e-08\n* lr\\_scheduler\\_type: linear\n* lr\\_scheduler\\_warmup\\_ratio: 0.1\n* num\\_epochs: 3",
"### Training results",
"### Framework versions\n\n\n* Transformers 4.41.0.dev0\n* Pytorch 2.2.2\n* Datasets 2.18.0\n* Tokenizers 0.19.1"
] | [
"TAGS\n#transformers #tensorboard #safetensors #beit #image-classification #generated_from_trainer #base_model-microsoft/beit-base-patch16-224-pt22k-ft22k #license-apache-2.0 #autotrain_compatible #endpoints_compatible #region-us \n",
"### Training hyperparameters\n\n\nThe following hyperparameters were used during training:\n\n\n* learning\\_rate: 5e-05\n* train\\_batch\\_size: 32\n* eval\\_batch\\_size: 32\n* seed: 42\n* gradient\\_accumulation\\_steps: 4\n* total\\_train\\_batch\\_size: 128\n* optimizer: Adam with betas=(0.9,0.999) and epsilon=1e-08\n* lr\\_scheduler\\_type: linear\n* lr\\_scheduler\\_warmup\\_ratio: 0.1\n* num\\_epochs: 3",
"### Training results",
"### Framework versions\n\n\n* Transformers 4.41.0.dev0\n* Pytorch 2.2.2\n* Datasets 2.18.0\n* Tokenizers 0.19.1"
] |
text-classification | transformers |
<!-- This model card has been generated automatically according to the information the Trainer had access to. You
should probably proofread and complete it, then remove this comment. -->
# fine-tuned-distilbert-base-uncased
This model is a fine-tuned version of [distilbert-base-uncased](https://huggingface.co/distilbert-base-uncased) on an unknown dataset.
It achieves the following results on the evaluation set:
- eval_loss: 0.5086
- eval_accuracy: {'accuracy': 0.815576323987539}
- eval_f1score: {'f1': 0.8154580580519637}
- eval_runtime: 23.9985
- eval_samples_per_second: 66.879
- eval_steps_per_second: 8.376
- step: 0
## Model description
More information needed
## Intended uses & limitations
More information needed
## Training and evaluation data
More information needed
## Training procedure
### Training hyperparameters
The following hyperparameters were used during training:
- learning_rate: 2e-05
- train_batch_size: 8
- eval_batch_size: 8
- seed: 42
- optimizer: Adam with betas=(0.9,0.999) and epsilon=1e-08
- lr_scheduler_type: linear
- lr_scheduler_warmup_steps: 320
- num_epochs: 2
### Framework versions
- Transformers 4.40.0
- Pytorch 2.1.0
- Datasets 2.19.0
- Tokenizers 0.19.1
| {"license": "apache-2.0", "tags": ["generated_from_trainer"], "base_model": "distilbert-base-uncased", "model-index": [{"name": "fine-tuned-distilbert-base-uncased", "results": []}]} | akash2212/fine-tuned-distilbert-base-uncased | null | [
"transformers",
"safetensors",
"distilbert",
"text-classification",
"generated_from_trainer",
"base_model:distilbert-base-uncased",
"license:apache-2.0",
"autotrain_compatible",
"endpoints_compatible",
"region:us"
] | null | 2024-04-22T10:38:29+00:00 | [] | [] | TAGS
#transformers #safetensors #distilbert #text-classification #generated_from_trainer #base_model-distilbert-base-uncased #license-apache-2.0 #autotrain_compatible #endpoints_compatible #region-us
|
# fine-tuned-distilbert-base-uncased
This model is a fine-tuned version of distilbert-base-uncased on an unknown dataset.
It achieves the following results on the evaluation set:
- eval_loss: 0.5086
- eval_accuracy: {'accuracy': 0.815576323987539}
- eval_f1score: {'f1': 0.8154580580519637}
- eval_runtime: 23.9985
- eval_samples_per_second: 66.879
- eval_steps_per_second: 8.376
- step: 0
## Model description
More information needed
## Intended uses & limitations
More information needed
## Training and evaluation data
More information needed
## Training procedure
### Training hyperparameters
The following hyperparameters were used during training:
- learning_rate: 2e-05
- train_batch_size: 8
- eval_batch_size: 8
- seed: 42
- optimizer: Adam with betas=(0.9,0.999) and epsilon=1e-08
- lr_scheduler_type: linear
- lr_scheduler_warmup_steps: 320
- num_epochs: 2
### Framework versions
- Transformers 4.40.0
- Pytorch 2.1.0
- Datasets 2.19.0
- Tokenizers 0.19.1
| [
"# fine-tuned-distilbert-base-uncased\n\nThis model is a fine-tuned version of distilbert-base-uncased on an unknown dataset.\nIt achieves the following results on the evaluation set:\n- eval_loss: 0.5086\n- eval_accuracy: {'accuracy': 0.815576323987539}\n- eval_f1score: {'f1': 0.8154580580519637}\n- eval_runtime: 23.9985\n- eval_samples_per_second: 66.879\n- eval_steps_per_second: 8.376\n- step: 0",
"## Model description\n\nMore information needed",
"## Intended uses & limitations\n\nMore information needed",
"## Training and evaluation data\n\nMore information needed",
"## Training procedure",
"### Training hyperparameters\n\nThe following hyperparameters were used during training:\n- learning_rate: 2e-05\n- train_batch_size: 8\n- eval_batch_size: 8\n- seed: 42\n- optimizer: Adam with betas=(0.9,0.999) and epsilon=1e-08\n- lr_scheduler_type: linear\n- lr_scheduler_warmup_steps: 320\n- num_epochs: 2",
"### Framework versions\n\n- Transformers 4.40.0\n- Pytorch 2.1.0\n- Datasets 2.19.0\n- Tokenizers 0.19.1"
] | [
"TAGS\n#transformers #safetensors #distilbert #text-classification #generated_from_trainer #base_model-distilbert-base-uncased #license-apache-2.0 #autotrain_compatible #endpoints_compatible #region-us \n",
"# fine-tuned-distilbert-base-uncased\n\nThis model is a fine-tuned version of distilbert-base-uncased on an unknown dataset.\nIt achieves the following results on the evaluation set:\n- eval_loss: 0.5086\n- eval_accuracy: {'accuracy': 0.815576323987539}\n- eval_f1score: {'f1': 0.8154580580519637}\n- eval_runtime: 23.9985\n- eval_samples_per_second: 66.879\n- eval_steps_per_second: 8.376\n- step: 0",
"## Model description\n\nMore information needed",
"## Intended uses & limitations\n\nMore information needed",
"## Training and evaluation data\n\nMore information needed",
"## Training procedure",
"### Training hyperparameters\n\nThe following hyperparameters were used during training:\n- learning_rate: 2e-05\n- train_batch_size: 8\n- eval_batch_size: 8\n- seed: 42\n- optimizer: Adam with betas=(0.9,0.999) and epsilon=1e-08\n- lr_scheduler_type: linear\n- lr_scheduler_warmup_steps: 320\n- num_epochs: 2",
"### Framework versions\n\n- Transformers 4.40.0\n- Pytorch 2.1.0\n- Datasets 2.19.0\n- Tokenizers 0.19.1"
] |
text-generation | transformers |
# Model Card for Model ID
<!-- Provide a quick summary of what the model is/does. -->
## Model Details
### Model Description
<!-- Provide a longer summary of what this model is. -->
This is the model card of a 🤗 transformers model that has been pushed on the Hub. This model card has been automatically generated.
- **Developed by:** [More Information Needed]
- **Funded by [optional]:** [More Information Needed]
- **Shared by [optional]:** [More Information Needed]
- **Model type:** [More Information Needed]
- **Language(s) (NLP):** [More Information Needed]
- **License:** [More Information Needed]
- **Finetuned from model [optional]:** [More Information Needed]
### Model Sources [optional]
<!-- Provide the basic links for the model. -->
- **Repository:** [More Information Needed]
- **Paper [optional]:** [More Information Needed]
- **Demo [optional]:** [More Information Needed]
## Uses
<!-- Address questions around how the model is intended to be used, including the foreseeable users of the model and those affected by the model. -->
### Direct Use
<!-- This section is for the model use without fine-tuning or plugging into a larger ecosystem/app. -->
[More Information Needed]
### Downstream Use [optional]
<!-- This section is for the model use when fine-tuned for a task, or when plugged into a larger ecosystem/app -->
[More Information Needed]
### Out-of-Scope Use
<!-- This section addresses misuse, malicious use, and uses that the model will not work well for. -->
[More Information Needed]
## Bias, Risks, and Limitations
<!-- This section is meant to convey both technical and sociotechnical limitations. -->
[More Information Needed]
### Recommendations
<!-- This section is meant to convey recommendations with respect to the bias, risk, and technical limitations. -->
Users (both direct and downstream) should be made aware of the risks, biases and limitations of the model. More information needed for further recommendations.
## How to Get Started with the Model
Use the code below to get started with the model.
[More Information Needed]
## Training Details
### Training Data
<!-- This should link to a Dataset Card, perhaps with a short stub of information on what the training data is all about as well as documentation related to data pre-processing or additional filtering. -->
[More Information Needed]
### Training Procedure
<!-- This relates heavily to the Technical Specifications. Content here should link to that section when it is relevant to the training procedure. -->
#### Preprocessing [optional]
[More Information Needed]
#### Training Hyperparameters
- **Training regime:** [More Information Needed] <!--fp32, fp16 mixed precision, bf16 mixed precision, bf16 non-mixed precision, fp16 non-mixed precision, fp8 mixed precision -->
#### Speeds, Sizes, Times [optional]
<!-- This section provides information about throughput, start/end time, checkpoint size if relevant, etc. -->
[More Information Needed]
## Evaluation
<!-- This section describes the evaluation protocols and provides the results. -->
### Testing Data, Factors & Metrics
#### Testing Data
<!-- This should link to a Dataset Card if possible. -->
[More Information Needed]
#### Factors
<!-- These are the things the evaluation is disaggregating by, e.g., subpopulations or domains. -->
[More Information Needed]
#### Metrics
<!-- These are the evaluation metrics being used, ideally with a description of why. -->
[More Information Needed]
### Results
[More Information Needed]
#### Summary
## Model Examination [optional]
<!-- Relevant interpretability work for the model goes here -->
[More Information Needed]
## Environmental Impact
<!-- Total emissions (in grams of CO2eq) and additional considerations, such as electricity usage, go here. Edit the suggested text below accordingly -->
Carbon emissions can be estimated using the [Machine Learning Impact calculator](https://mlco2.github.io/impact#compute) presented in [Lacoste et al. (2019)](https://arxiv.org/abs/1910.09700).
- **Hardware Type:** [More Information Needed]
- **Hours used:** [More Information Needed]
- **Cloud Provider:** [More Information Needed]
- **Compute Region:** [More Information Needed]
- **Carbon Emitted:** [More Information Needed]
## Technical Specifications [optional]
### Model Architecture and Objective
[More Information Needed]
### Compute Infrastructure
[More Information Needed]
#### Hardware
[More Information Needed]
#### Software
[More Information Needed]
## Citation [optional]
<!-- If there is a paper or blog post introducing the model, the APA and Bibtex information for that should go in this section. -->
**BibTeX:**
[More Information Needed]
**APA:**
[More Information Needed]
## Glossary [optional]
<!-- If relevant, include terms and calculations in this section that can help readers understand the model or model card. -->
[More Information Needed]
## More Information [optional]
[More Information Needed]
## Model Card Authors [optional]
[More Information Needed]
## Model Card Contact
[More Information Needed] | {"library_name": "transformers", "tags": []} | himum/sn6_3l3 | null | [
"transformers",
"safetensors",
"llama",
"text-generation",
"conversational",
"arxiv:1910.09700",
"autotrain_compatible",
"endpoints_compatible",
"text-generation-inference",
"region:us"
] | null | 2024-04-22T11:02:40+00:00 | [
"1910.09700"
] | [] | TAGS
#transformers #safetensors #llama #text-generation #conversational #arxiv-1910.09700 #autotrain_compatible #endpoints_compatible #text-generation-inference #region-us
|
# Model Card for Model ID
## Model Details
### Model Description
This is the model card of a transformers model that has been pushed on the Hub. This model card has been automatically generated.
- Developed by:
- Funded by [optional]:
- Shared by [optional]:
- Model type:
- Language(s) (NLP):
- License:
- Finetuned from model [optional]:
### Model Sources [optional]
- Repository:
- Paper [optional]:
- Demo [optional]:
## Uses
### Direct Use
### Downstream Use [optional]
### Out-of-Scope Use
## Bias, Risks, and Limitations
### Recommendations
Users (both direct and downstream) should be made aware of the risks, biases and limitations of the model. More information needed for further recommendations.
## How to Get Started with the Model
Use the code below to get started with the model.
## Training Details
### Training Data
### Training Procedure
#### Preprocessing [optional]
#### Training Hyperparameters
- Training regime:
#### Speeds, Sizes, Times [optional]
## Evaluation
### Testing Data, Factors & Metrics
#### Testing Data
#### Factors
#### Metrics
### Results
#### Summary
## Model Examination [optional]
## Environmental Impact
Carbon emissions can be estimated using the Machine Learning Impact calculator presented in Lacoste et al. (2019).
- Hardware Type:
- Hours used:
- Cloud Provider:
- Compute Region:
- Carbon Emitted:
## Technical Specifications [optional]
### Model Architecture and Objective
### Compute Infrastructure
#### Hardware
#### Software
[optional]
BibTeX:
APA:
## Glossary [optional]
## More Information [optional]
## Model Card Authors [optional]
## Model Card Contact
| [
"# Model Card for Model ID",
"## Model Details",
"### Model Description\n\n\n\nThis is the model card of a transformers model that has been pushed on the Hub. This model card has been automatically generated.\n\n- Developed by: \n- Funded by [optional]: \n- Shared by [optional]: \n- Model type: \n- Language(s) (NLP): \n- License: \n- Finetuned from model [optional]:",
"### Model Sources [optional]\n\n\n\n- Repository: \n- Paper [optional]: \n- Demo [optional]:",
"## Uses",
"### Direct Use",
"### Downstream Use [optional]",
"### Out-of-Scope Use",
"## Bias, Risks, and Limitations",
"### Recommendations\n\n\n\nUsers (both direct and downstream) should be made aware of the risks, biases and limitations of the model. More information needed for further recommendations.",
"## How to Get Started with the Model\n\nUse the code below to get started with the model.",
"## Training Details",
"### Training Data",
"### Training Procedure",
"#### Preprocessing [optional]",
"#### Training Hyperparameters\n\n- Training regime:",
"#### Speeds, Sizes, Times [optional]",
"## Evaluation",
"### Testing Data, Factors & Metrics",
"#### Testing Data",
"#### Factors",
"#### Metrics",
"### Results",
"#### Summary",
"## Model Examination [optional]",
"## Environmental Impact\n\n\n\nCarbon emissions can be estimated using the Machine Learning Impact calculator presented in Lacoste et al. (2019).\n\n- Hardware Type: \n- Hours used: \n- Cloud Provider: \n- Compute Region: \n- Carbon Emitted:",
"## Technical Specifications [optional]",
"### Model Architecture and Objective",
"### Compute Infrastructure",
"#### Hardware",
"#### Software\n\n\n\n[optional]\n\n\n\nBibTeX:\n\n\n\nAPA:",
"## Glossary [optional]",
"## More Information [optional]",
"## Model Card Authors [optional]",
"## Model Card Contact"
] | [
"TAGS\n#transformers #safetensors #llama #text-generation #conversational #arxiv-1910.09700 #autotrain_compatible #endpoints_compatible #text-generation-inference #region-us \n",
"# Model Card for Model ID",
"## Model Details",
"### Model Description\n\n\n\nThis is the model card of a transformers model that has been pushed on the Hub. This model card has been automatically generated.\n\n- Developed by: \n- Funded by [optional]: \n- Shared by [optional]: \n- Model type: \n- Language(s) (NLP): \n- License: \n- Finetuned from model [optional]:",
"### Model Sources [optional]\n\n\n\n- Repository: \n- Paper [optional]: \n- Demo [optional]:",
"## Uses",
"### Direct Use",
"### Downstream Use [optional]",
"### Out-of-Scope Use",
"## Bias, Risks, and Limitations",
"### Recommendations\n\n\n\nUsers (both direct and downstream) should be made aware of the risks, biases and limitations of the model. More information needed for further recommendations.",
"## How to Get Started with the Model\n\nUse the code below to get started with the model.",
"## Training Details",
"### Training Data",
"### Training Procedure",
"#### Preprocessing [optional]",
"#### Training Hyperparameters\n\n- Training regime:",
"#### Speeds, Sizes, Times [optional]",
"## Evaluation",
"### Testing Data, Factors & Metrics",
"#### Testing Data",
"#### Factors",
"#### Metrics",
"### Results",
"#### Summary",
"## Model Examination [optional]",
"## Environmental Impact\n\n\n\nCarbon emissions can be estimated using the Machine Learning Impact calculator presented in Lacoste et al. (2019).\n\n- Hardware Type: \n- Hours used: \n- Cloud Provider: \n- Compute Region: \n- Carbon Emitted:",
"## Technical Specifications [optional]",
"### Model Architecture and Objective",
"### Compute Infrastructure",
"#### Hardware",
"#### Software\n\n\n\n[optional]\n\n\n\nBibTeX:\n\n\n\nAPA:",
"## Glossary [optional]",
"## More Information [optional]",
"## Model Card Authors [optional]",
"## Model Card Contact"
] |
null | null | Cos'è Proctolax gel?
Proctolax Siero è una crema topica formulata per colpire le emorroidi, che sono vene gonfie nel retto o nell'ano, che causano prurito, dolore e disagio. Questa crema contiene una miscela di ingredienti naturali noti per le loro proprietà lenitive e curative, che forniscono sollievo dai sintomi associati alle emorroidi.
Sito ufficiale:<a href="https://www.nutritionsee.com/Proctolitaf">www.Proctolax.com</a>
<p><a href="https://www.nutritionsee.com/Proctolitaf"> <img src="https://www.nutritionsee.com/wp-content/uploads/2024/04/Proctolax-Italy.png" alt="enter image description here"> </a></p>
<a href="https://www.nutritionsee.com/Proctolitaf">Acquista ora!! Fai clic sul collegamento in basso per ulteriori informazioni e ottieni subito uno sconto del 50%... Affrettati</a>
Sito ufficiale:<a href="https://www.nutritionsee.com/Proctolitaf">www.Proctolax.com</a> | {"license": "apache-2.0"} | ProctolaxItaly/ProctolaxItaly | null | [
"license:apache-2.0",
"region:us"
] | null | 2024-04-22T11:03:28+00:00 | [] | [] | TAGS
#license-apache-2.0 #region-us
| Cos'è Proctolax gel?
Proctolax Siero è una crema topica formulata per colpire le emorroidi, che sono vene gonfie nel retto o nell'ano, che causano prurito, dolore e disagio. Questa crema contiene una miscela di ingredienti naturali noti per le loro proprietà lenitive e curative, che forniscono sollievo dai sintomi associati alle emorroidi.
Sito ufficiale:<a href="URL
<p><a href="URL <img src="URL alt="enter image description here"> </a></p>
<a href="URL ora!! Fai clic sul collegamento in basso per ulteriori informazioni e ottieni subito uno sconto del 50%... Affrettati</a>
Sito ufficiale:<a href="URL | [] | [
"TAGS\n#license-apache-2.0 #region-us \n"
] |
reinforcement-learning | null |
# **Q-Learning** Agent playing1 **FrozenLake-v1**
This is a trained model of a **Q-Learning** agent playing **FrozenLake-v1** .
## Usage
```python
model = load_from_hub(repo_id="bhutchings/q-FrozenLake-v1-4x4-noSlippery", filename="q-learning.pkl")
# Don't forget to check if you need to add additional attributes (is_slippery=False etc)
env = gym.make(model["env_id"])
```
| {"tags": ["FrozenLake-v1-4x4-no_slippery", "q-learning", "reinforcement-learning", "custom-implementation"], "model-index": [{"name": "q-FrozenLake-v1-4x4-noSlippery", "results": [{"task": {"type": "reinforcement-learning", "name": "reinforcement-learning"}, "dataset": {"name": "FrozenLake-v1-4x4-no_slippery", "type": "FrozenLake-v1-4x4-no_slippery"}, "metrics": [{"type": "mean_reward", "value": "1.00 +/- 0.00", "name": "mean_reward", "verified": false}]}]}]} | bhutchings/q-FrozenLake-v1-4x4-noSlippery | null | [
"FrozenLake-v1-4x4-no_slippery",
"q-learning",
"reinforcement-learning",
"custom-implementation",
"model-index",
"region:us"
] | null | 2024-04-22T11:04:50+00:00 | [] | [] | TAGS
#FrozenLake-v1-4x4-no_slippery #q-learning #reinforcement-learning #custom-implementation #model-index #region-us
|
# Q-Learning Agent playing1 FrozenLake-v1
This is a trained model of a Q-Learning agent playing FrozenLake-v1 .
## Usage
| [
"# Q-Learning Agent playing1 FrozenLake-v1\n This is a trained model of a Q-Learning agent playing FrozenLake-v1 .\n\n ## Usage"
] | [
"TAGS\n#FrozenLake-v1-4x4-no_slippery #q-learning #reinforcement-learning #custom-implementation #model-index #region-us \n",
"# Q-Learning Agent playing1 FrozenLake-v1\n This is a trained model of a Q-Learning agent playing FrozenLake-v1 .\n\n ## Usage"
] |
null | peft | ## Training procedure
The following `bitsandbytes` quantization config was used during training:
- load_in_8bit: False
- load_in_4bit: True
- llm_int8_threshold: 6.0
- llm_int8_skip_modules: None
- llm_int8_enable_fp32_cpu_offload: False
- llm_int8_has_fp16_weight: False
- bnb_4bit_quant_type: nf4
- bnb_4bit_use_double_quant: True
- bnb_4bit_compute_dtype: bfloat16
### Framework versions
- PEFT 0.4.0
| {"library_name": "peft"} | chakkakrishna/Tinybest | null | [
"peft",
"safetensors",
"llama",
"region:us"
] | null | 2024-04-22T11:07:21+00:00 | [] | [] | TAGS
#peft #safetensors #llama #region-us
| ## Training procedure
The following 'bitsandbytes' quantization config was used during training:
- load_in_8bit: False
- load_in_4bit: True
- llm_int8_threshold: 6.0
- llm_int8_skip_modules: None
- llm_int8_enable_fp32_cpu_offload: False
- llm_int8_has_fp16_weight: False
- bnb_4bit_quant_type: nf4
- bnb_4bit_use_double_quant: True
- bnb_4bit_compute_dtype: bfloat16
### Framework versions
- PEFT 0.4.0
| [
"## Training procedure\n\n\nThe following 'bitsandbytes' quantization config was used during training:\n- load_in_8bit: False\n- load_in_4bit: True\n- llm_int8_threshold: 6.0\n- llm_int8_skip_modules: None\n- llm_int8_enable_fp32_cpu_offload: False\n- llm_int8_has_fp16_weight: False\n- bnb_4bit_quant_type: nf4\n- bnb_4bit_use_double_quant: True\n- bnb_4bit_compute_dtype: bfloat16",
"### Framework versions\n\n\n- PEFT 0.4.0"
] | [
"TAGS\n#peft #safetensors #llama #region-us \n",
"## Training procedure\n\n\nThe following 'bitsandbytes' quantization config was used during training:\n- load_in_8bit: False\n- load_in_4bit: True\n- llm_int8_threshold: 6.0\n- llm_int8_skip_modules: None\n- llm_int8_enable_fp32_cpu_offload: False\n- llm_int8_has_fp16_weight: False\n- bnb_4bit_quant_type: nf4\n- bnb_4bit_use_double_quant: True\n- bnb_4bit_compute_dtype: bfloat16",
"### Framework versions\n\n\n- PEFT 0.4.0"
] |
null | peft |
# Model Card for Model ID
<!-- Provide a quick summary of what the model is/does. -->
## Model Details
### Model Description
<!-- Provide a longer summary of what this model is. -->
- **Developed by:** [More Information Needed]
- **Shared by [optional]:** [More Information Needed]
- **Model type:** [More Information Needed]
- **Language(s) (NLP):** [More Information Needed]
- **License:** [More Information Needed]
- **Finetuned from model [optional]:** [More Information Needed]
### Model Sources [optional]
<!-- Provide the basic links for the model. -->
- **Repository:** [More Information Needed]
- **Paper [optional]:** [More Information Needed]
- **Demo [optional]:** [More Information Needed]
## Uses
<!-- Address questions around how the model is intended to be used, including the foreseeable users of the model and those affected by the model. -->
### Direct Use
<!-- This section is for the model use without fine-tuning or plugging into a larger ecosystem/app. -->
[More Information Needed]
### Downstream Use [optional]
<!-- This section is for the model use when fine-tuned for a task, or when plugged into a larger ecosystem/app -->
[More Information Needed]
### Out-of-Scope Use
<!-- This section addresses misuse, malicious use, and uses that the model will not work well for. -->
[More Information Needed]
## Bias, Risks, and Limitations
<!-- This section is meant to convey both technical and sociotechnical limitations. -->
[More Information Needed]
### Recommendations
<!-- This section is meant to convey recommendations with respect to the bias, risk, and technical limitations. -->
Users (both direct and downstream) should be made aware of the risks, biases and limitations of the model. More information needed for further recommendations.
## How to Get Started with the Model
Use the code below to get started with the model.
[More Information Needed]
## Training Details
### Training Data
<!-- This should link to a Data Card, perhaps with a short stub of information on what the training data is all about as well as documentation related to data pre-processing or additional filtering. -->
[More Information Needed]
### Training Procedure
<!-- This relates heavily to the Technical Specifications. Content here should link to that section when it is relevant to the training procedure. -->
#### Preprocessing [optional]
[More Information Needed]
#### Training Hyperparameters
- **Training regime:** [More Information Needed] <!--fp32, fp16 mixed precision, bf16 mixed precision, bf16 non-mixed precision, fp16 non-mixed precision, fp8 mixed precision -->
#### Speeds, Sizes, Times [optional]
<!-- This section provides information about throughput, start/end time, checkpoint size if relevant, etc. -->
[More Information Needed]
## Evaluation
<!-- This section describes the evaluation protocols and provides the results. -->
### Testing Data, Factors & Metrics
#### Testing Data
<!-- This should link to a Data Card if possible. -->
[More Information Needed]
#### Factors
<!-- These are the things the evaluation is disaggregating by, e.g., subpopulations or domains. -->
[More Information Needed]
#### Metrics
<!-- These are the evaluation metrics being used, ideally with a description of why. -->
[More Information Needed]
### Results
[More Information Needed]
#### Summary
## Model Examination [optional]
<!-- Relevant interpretability work for the model goes here -->
[More Information Needed]
## Environmental Impact
<!-- Total emissions (in grams of CO2eq) and additional considerations, such as electricity usage, go here. Edit the suggested text below accordingly -->
Carbon emissions can be estimated using the [Machine Learning Impact calculator](https://mlco2.github.io/impact#compute) presented in [Lacoste et al. (2019)](https://arxiv.org/abs/1910.09700).
- **Hardware Type:** [More Information Needed]
- **Hours used:** [More Information Needed]
- **Cloud Provider:** [More Information Needed]
- **Compute Region:** [More Information Needed]
- **Carbon Emitted:** [More Information Needed]
## Technical Specifications [optional]
### Model Architecture and Objective
[More Information Needed]
### Compute Infrastructure
[More Information Needed]
#### Hardware
[More Information Needed]
#### Software
[More Information Needed]
## Citation [optional]
<!-- If there is a paper or blog post introducing the model, the APA and Bibtex information for that should go in this section. -->
**BibTeX:**
[More Information Needed]
**APA:**
[More Information Needed]
## Glossary [optional]
<!-- If relevant, include terms and calculations in this section that can help readers understand the model or model card. -->
[More Information Needed]
## More Information [optional]
[More Information Needed]
## Model Card Authors [optional]
[More Information Needed]
## Model Card Contact
[More Information Needed]
## Training procedure
The following `bitsandbytes` quantization config was used during training:
- load_in_8bit: False
- load_in_4bit: True
- llm_int8_threshold: 6.0
- llm_int8_skip_modules: None
- llm_int8_enable_fp32_cpu_offload: False
- llm_int8_has_fp16_weight: False
- bnb_4bit_quant_type: nf4
- bnb_4bit_use_double_quant: True
- bnb_4bit_compute_dtype: bfloat16
### Framework versions
- PEFT 0.7.0.dev0
## Training procedure
The following `bitsandbytes` quantization config was used during training:
- load_in_8bit: False
- load_in_4bit: True
- llm_int8_threshold: 6.0
- llm_int8_skip_modules: None
- llm_int8_enable_fp32_cpu_offload: False
- llm_int8_has_fp16_weight: False
- bnb_4bit_quant_type: nf4
- bnb_4bit_use_double_quant: True
- bnb_4bit_compute_dtype: bfloat16
### Framework versions
- PEFT 0.7.0.dev0
## Training procedure
The following `bitsandbytes` quantization config was used during training:
- load_in_8bit: False
- load_in_4bit: True
- llm_int8_threshold: 6.0
- llm_int8_skip_modules: None
- llm_int8_enable_fp32_cpu_offload: False
- llm_int8_has_fp16_weight: False
- bnb_4bit_quant_type: nf4
- bnb_4bit_use_double_quant: True
- bnb_4bit_compute_dtype: bfloat16
### Framework versions
- PEFT 0.7.0.dev0
## Training procedure
The following `bitsandbytes` quantization config was used during training:
- load_in_8bit: False
- load_in_4bit: True
- llm_int8_threshold: 6.0
- llm_int8_skip_modules: None
- llm_int8_enable_fp32_cpu_offload: False
- llm_int8_has_fp16_weight: False
- bnb_4bit_quant_type: nf4
- bnb_4bit_use_double_quant: True
- bnb_4bit_compute_dtype: bfloat16
### Framework versions
- PEFT 0.7.0.dev0
| {"library_name": "peft", "base_model": "TinyLlama/TinyLlama-1.1B-Chat-v1.0"} | bmehrba/TinyLlama-1.1B-Chat-v1.0-fine-tuned-adapters_Epistemic_tiny_0.6_Seed101 | null | [
"peft",
"arxiv:1910.09700",
"base_model:TinyLlama/TinyLlama-1.1B-Chat-v1.0",
"region:us"
] | null | 2024-04-22T11:08:36+00:00 | [
"1910.09700"
] | [] | TAGS
#peft #arxiv-1910.09700 #base_model-TinyLlama/TinyLlama-1.1B-Chat-v1.0 #region-us
|
# Model Card for Model ID
## Model Details
### Model Description
- Developed by:
- Shared by [optional]:
- Model type:
- Language(s) (NLP):
- License:
- Finetuned from model [optional]:
### Model Sources [optional]
- Repository:
- Paper [optional]:
- Demo [optional]:
## Uses
### Direct Use
### Downstream Use [optional]
### Out-of-Scope Use
## Bias, Risks, and Limitations
### Recommendations
Users (both direct and downstream) should be made aware of the risks, biases and limitations of the model. More information needed for further recommendations.
## How to Get Started with the Model
Use the code below to get started with the model.
## Training Details
### Training Data
### Training Procedure
#### Preprocessing [optional]
#### Training Hyperparameters
- Training regime:
#### Speeds, Sizes, Times [optional]
## Evaluation
### Testing Data, Factors & Metrics
#### Testing Data
#### Factors
#### Metrics
### Results
#### Summary
## Model Examination [optional]
## Environmental Impact
Carbon emissions can be estimated using the Machine Learning Impact calculator presented in Lacoste et al. (2019).
- Hardware Type:
- Hours used:
- Cloud Provider:
- Compute Region:
- Carbon Emitted:
## Technical Specifications [optional]
### Model Architecture and Objective
### Compute Infrastructure
#### Hardware
#### Software
[optional]
BibTeX:
APA:
## Glossary [optional]
## More Information [optional]
## Model Card Authors [optional]
## Model Card Contact
## Training procedure
The following 'bitsandbytes' quantization config was used during training:
- load_in_8bit: False
- load_in_4bit: True
- llm_int8_threshold: 6.0
- llm_int8_skip_modules: None
- llm_int8_enable_fp32_cpu_offload: False
- llm_int8_has_fp16_weight: False
- bnb_4bit_quant_type: nf4
- bnb_4bit_use_double_quant: True
- bnb_4bit_compute_dtype: bfloat16
### Framework versions
- PEFT 0.7.0.dev0
## Training procedure
The following 'bitsandbytes' quantization config was used during training:
- load_in_8bit: False
- load_in_4bit: True
- llm_int8_threshold: 6.0
- llm_int8_skip_modules: None
- llm_int8_enable_fp32_cpu_offload: False
- llm_int8_has_fp16_weight: False
- bnb_4bit_quant_type: nf4
- bnb_4bit_use_double_quant: True
- bnb_4bit_compute_dtype: bfloat16
### Framework versions
- PEFT 0.7.0.dev0
## Training procedure
The following 'bitsandbytes' quantization config was used during training:
- load_in_8bit: False
- load_in_4bit: True
- llm_int8_threshold: 6.0
- llm_int8_skip_modules: None
- llm_int8_enable_fp32_cpu_offload: False
- llm_int8_has_fp16_weight: False
- bnb_4bit_quant_type: nf4
- bnb_4bit_use_double_quant: True
- bnb_4bit_compute_dtype: bfloat16
### Framework versions
- PEFT 0.7.0.dev0
## Training procedure
The following 'bitsandbytes' quantization config was used during training:
- load_in_8bit: False
- load_in_4bit: True
- llm_int8_threshold: 6.0
- llm_int8_skip_modules: None
- llm_int8_enable_fp32_cpu_offload: False
- llm_int8_has_fp16_weight: False
- bnb_4bit_quant_type: nf4
- bnb_4bit_use_double_quant: True
- bnb_4bit_compute_dtype: bfloat16
### Framework versions
- PEFT 0.7.0.dev0
| [
"# Model Card for Model ID",
"## Model Details",
"### Model Description\n\n\n\n\n\n- Developed by: \n- Shared by [optional]: \n- Model type: \n- Language(s) (NLP): \n- License: \n- Finetuned from model [optional]:",
"### Model Sources [optional]\n\n\n\n- Repository: \n- Paper [optional]: \n- Demo [optional]:",
"## Uses",
"### Direct Use",
"### Downstream Use [optional]",
"### Out-of-Scope Use",
"## Bias, Risks, and Limitations",
"### Recommendations\n\n\n\nUsers (both direct and downstream) should be made aware of the risks, biases and limitations of the model. More information needed for further recommendations.",
"## How to Get Started with the Model\n\nUse the code below to get started with the model.",
"## Training Details",
"### Training Data",
"### Training Procedure",
"#### Preprocessing [optional]",
"#### Training Hyperparameters\n\n- Training regime:",
"#### Speeds, Sizes, Times [optional]",
"## Evaluation",
"### Testing Data, Factors & Metrics",
"#### Testing Data",
"#### Factors",
"#### Metrics",
"### Results",
"#### Summary",
"## Model Examination [optional]",
"## Environmental Impact\n\n\n\nCarbon emissions can be estimated using the Machine Learning Impact calculator presented in Lacoste et al. (2019).\n\n- Hardware Type: \n- Hours used: \n- Cloud Provider: \n- Compute Region: \n- Carbon Emitted:",
"## Technical Specifications [optional]",
"### Model Architecture and Objective",
"### Compute Infrastructure",
"#### Hardware",
"#### Software\n\n\n\n[optional]\n\n\n\nBibTeX:\n\n\n\nAPA:",
"## Glossary [optional]",
"## More Information [optional]",
"## Model Card Authors [optional]",
"## Model Card Contact",
"## Training procedure\n\n\nThe following 'bitsandbytes' quantization config was used during training:\n- load_in_8bit: False\n- load_in_4bit: True\n- llm_int8_threshold: 6.0\n- llm_int8_skip_modules: None\n- llm_int8_enable_fp32_cpu_offload: False\n- llm_int8_has_fp16_weight: False\n- bnb_4bit_quant_type: nf4\n- bnb_4bit_use_double_quant: True\n- bnb_4bit_compute_dtype: bfloat16",
"### Framework versions\n\n\n- PEFT 0.7.0.dev0",
"## Training procedure\n\n\nThe following 'bitsandbytes' quantization config was used during training:\n- load_in_8bit: False\n- load_in_4bit: True\n- llm_int8_threshold: 6.0\n- llm_int8_skip_modules: None\n- llm_int8_enable_fp32_cpu_offload: False\n- llm_int8_has_fp16_weight: False\n- bnb_4bit_quant_type: nf4\n- bnb_4bit_use_double_quant: True\n- bnb_4bit_compute_dtype: bfloat16",
"### Framework versions\n\n\n- PEFT 0.7.0.dev0",
"## Training procedure\n\n\nThe following 'bitsandbytes' quantization config was used during training:\n- load_in_8bit: False\n- load_in_4bit: True\n- llm_int8_threshold: 6.0\n- llm_int8_skip_modules: None\n- llm_int8_enable_fp32_cpu_offload: False\n- llm_int8_has_fp16_weight: False\n- bnb_4bit_quant_type: nf4\n- bnb_4bit_use_double_quant: True\n- bnb_4bit_compute_dtype: bfloat16",
"### Framework versions\n\n\n- PEFT 0.7.0.dev0",
"## Training procedure\n\n\nThe following 'bitsandbytes' quantization config was used during training:\n- load_in_8bit: False\n- load_in_4bit: True\n- llm_int8_threshold: 6.0\n- llm_int8_skip_modules: None\n- llm_int8_enable_fp32_cpu_offload: False\n- llm_int8_has_fp16_weight: False\n- bnb_4bit_quant_type: nf4\n- bnb_4bit_use_double_quant: True\n- bnb_4bit_compute_dtype: bfloat16",
"### Framework versions\n\n\n- PEFT 0.7.0.dev0"
] | [
"TAGS\n#peft #arxiv-1910.09700 #base_model-TinyLlama/TinyLlama-1.1B-Chat-v1.0 #region-us \n",
"# Model Card for Model ID",
"## Model Details",
"### Model Description\n\n\n\n\n\n- Developed by: \n- Shared by [optional]: \n- Model type: \n- Language(s) (NLP): \n- License: \n- Finetuned from model [optional]:",
"### Model Sources [optional]\n\n\n\n- Repository: \n- Paper [optional]: \n- Demo [optional]:",
"## Uses",
"### Direct Use",
"### Downstream Use [optional]",
"### Out-of-Scope Use",
"## Bias, Risks, and Limitations",
"### Recommendations\n\n\n\nUsers (both direct and downstream) should be made aware of the risks, biases and limitations of the model. More information needed for further recommendations.",
"## How to Get Started with the Model\n\nUse the code below to get started with the model.",
"## Training Details",
"### Training Data",
"### Training Procedure",
"#### Preprocessing [optional]",
"#### Training Hyperparameters\n\n- Training regime:",
"#### Speeds, Sizes, Times [optional]",
"## Evaluation",
"### Testing Data, Factors & Metrics",
"#### Testing Data",
"#### Factors",
"#### Metrics",
"### Results",
"#### Summary",
"## Model Examination [optional]",
"## Environmental Impact\n\n\n\nCarbon emissions can be estimated using the Machine Learning Impact calculator presented in Lacoste et al. (2019).\n\n- Hardware Type: \n- Hours used: \n- Cloud Provider: \n- Compute Region: \n- Carbon Emitted:",
"## Technical Specifications [optional]",
"### Model Architecture and Objective",
"### Compute Infrastructure",
"#### Hardware",
"#### Software\n\n\n\n[optional]\n\n\n\nBibTeX:\n\n\n\nAPA:",
"## Glossary [optional]",
"## More Information [optional]",
"## Model Card Authors [optional]",
"## Model Card Contact",
"## Training procedure\n\n\nThe following 'bitsandbytes' quantization config was used during training:\n- load_in_8bit: False\n- load_in_4bit: True\n- llm_int8_threshold: 6.0\n- llm_int8_skip_modules: None\n- llm_int8_enable_fp32_cpu_offload: False\n- llm_int8_has_fp16_weight: False\n- bnb_4bit_quant_type: nf4\n- bnb_4bit_use_double_quant: True\n- bnb_4bit_compute_dtype: bfloat16",
"### Framework versions\n\n\n- PEFT 0.7.0.dev0",
"## Training procedure\n\n\nThe following 'bitsandbytes' quantization config was used during training:\n- load_in_8bit: False\n- load_in_4bit: True\n- llm_int8_threshold: 6.0\n- llm_int8_skip_modules: None\n- llm_int8_enable_fp32_cpu_offload: False\n- llm_int8_has_fp16_weight: False\n- bnb_4bit_quant_type: nf4\n- bnb_4bit_use_double_quant: True\n- bnb_4bit_compute_dtype: bfloat16",
"### Framework versions\n\n\n- PEFT 0.7.0.dev0",
"## Training procedure\n\n\nThe following 'bitsandbytes' quantization config was used during training:\n- load_in_8bit: False\n- load_in_4bit: True\n- llm_int8_threshold: 6.0\n- llm_int8_skip_modules: None\n- llm_int8_enable_fp32_cpu_offload: False\n- llm_int8_has_fp16_weight: False\n- bnb_4bit_quant_type: nf4\n- bnb_4bit_use_double_quant: True\n- bnb_4bit_compute_dtype: bfloat16",
"### Framework versions\n\n\n- PEFT 0.7.0.dev0",
"## Training procedure\n\n\nThe following 'bitsandbytes' quantization config was used during training:\n- load_in_8bit: False\n- load_in_4bit: True\n- llm_int8_threshold: 6.0\n- llm_int8_skip_modules: None\n- llm_int8_enable_fp32_cpu_offload: False\n- llm_int8_has_fp16_weight: False\n- bnb_4bit_quant_type: nf4\n- bnb_4bit_use_double_quant: True\n- bnb_4bit_compute_dtype: bfloat16",
"### Framework versions\n\n\n- PEFT 0.7.0.dev0"
] |
null | peft |
# Model Card for Model ID
<!-- Provide a quick summary of what the model is/does. -->
## Model Details
### Model Description
<!-- Provide a longer summary of what this model is. -->
- **Developed by:** [More Information Needed]
- **Shared by [optional]:** [More Information Needed]
- **Model type:** [More Information Needed]
- **Language(s) (NLP):** [More Information Needed]
- **License:** [More Information Needed]
- **Finetuned from model [optional]:** [More Information Needed]
### Model Sources [optional]
<!-- Provide the basic links for the model. -->
- **Repository:** [More Information Needed]
- **Paper [optional]:** [More Information Needed]
- **Demo [optional]:** [More Information Needed]
## Uses
<!-- Address questions around how the model is intended to be used, including the foreseeable users of the model and those affected by the model. -->
### Direct Use
<!-- This section is for the model use without fine-tuning or plugging into a larger ecosystem/app. -->
[More Information Needed]
### Downstream Use [optional]
<!-- This section is for the model use when fine-tuned for a task, or when plugged into a larger ecosystem/app -->
[More Information Needed]
### Out-of-Scope Use
<!-- This section addresses misuse, malicious use, and uses that the model will not work well for. -->
[More Information Needed]
## Bias, Risks, and Limitations
<!-- This section is meant to convey both technical and sociotechnical limitations. -->
[More Information Needed]
### Recommendations
<!-- This section is meant to convey recommendations with respect to the bias, risk, and technical limitations. -->
Users (both direct and downstream) should be made aware of the risks, biases and limitations of the model. More information needed for further recommendations.
## How to Get Started with the Model
Use the code below to get started with the model.
[More Information Needed]
## Training Details
### Training Data
<!-- This should link to a Data Card, perhaps with a short stub of information on what the training data is all about as well as documentation related to data pre-processing or additional filtering. -->
[More Information Needed]
### Training Procedure
<!-- This relates heavily to the Technical Specifications. Content here should link to that section when it is relevant to the training procedure. -->
#### Preprocessing [optional]
[More Information Needed]
#### Training Hyperparameters
- **Training regime:** [More Information Needed] <!--fp32, fp16 mixed precision, bf16 mixed precision, bf16 non-mixed precision, fp16 non-mixed precision, fp8 mixed precision -->
#### Speeds, Sizes, Times [optional]
<!-- This section provides information about throughput, start/end time, checkpoint size if relevant, etc. -->
[More Information Needed]
## Evaluation
<!-- This section describes the evaluation protocols and provides the results. -->
### Testing Data, Factors & Metrics
#### Testing Data
<!-- This should link to a Data Card if possible. -->
[More Information Needed]
#### Factors
<!-- These are the things the evaluation is disaggregating by, e.g., subpopulations or domains. -->
[More Information Needed]
#### Metrics
<!-- These are the evaluation metrics being used, ideally with a description of why. -->
[More Information Needed]
### Results
[More Information Needed]
#### Summary
## Model Examination [optional]
<!-- Relevant interpretability work for the model goes here -->
[More Information Needed]
## Environmental Impact
<!-- Total emissions (in grams of CO2eq) and additional considerations, such as electricity usage, go here. Edit the suggested text below accordingly -->
Carbon emissions can be estimated using the [Machine Learning Impact calculator](https://mlco2.github.io/impact#compute) presented in [Lacoste et al. (2019)](https://arxiv.org/abs/1910.09700).
- **Hardware Type:** [More Information Needed]
- **Hours used:** [More Information Needed]
- **Cloud Provider:** [More Information Needed]
- **Compute Region:** [More Information Needed]
- **Carbon Emitted:** [More Information Needed]
## Technical Specifications [optional]
### Model Architecture and Objective
[More Information Needed]
### Compute Infrastructure
[More Information Needed]
#### Hardware
[More Information Needed]
#### Software
[More Information Needed]
## Citation [optional]
<!-- If there is a paper or blog post introducing the model, the APA and Bibtex information for that should go in this section. -->
**BibTeX:**
[More Information Needed]
**APA:**
[More Information Needed]
## Glossary [optional]
<!-- If relevant, include terms and calculations in this section that can help readers understand the model or model card. -->
[More Information Needed]
## More Information [optional]
[More Information Needed]
## Model Card Authors [optional]
[More Information Needed]
## Model Card Contact
[More Information Needed]
## Training procedure
The following `bitsandbytes` quantization config was used during training:
- load_in_8bit: False
- load_in_4bit: True
- llm_int8_threshold: 6.0
- llm_int8_skip_modules: None
- llm_int8_enable_fp32_cpu_offload: False
- llm_int8_has_fp16_weight: False
- bnb_4bit_quant_type: nf4
- bnb_4bit_use_double_quant: True
- bnb_4bit_compute_dtype: bfloat16
### Framework versions
- PEFT 0.7.0.dev0
| {"library_name": "peft", "base_model": "TinyLlama/TinyLlama-1.1B-Chat-v1.0"} | bmehrba/TinyLlama-1.1B-Chat-v1.0-fine-tuned_Epistemic_tiny_0.6_Seed101 | null | [
"peft",
"arxiv:1910.09700",
"base_model:TinyLlama/TinyLlama-1.1B-Chat-v1.0",
"region:us"
] | null | 2024-04-22T11:08:56+00:00 | [
"1910.09700"
] | [] | TAGS
#peft #arxiv-1910.09700 #base_model-TinyLlama/TinyLlama-1.1B-Chat-v1.0 #region-us
|
# Model Card for Model ID
## Model Details
### Model Description
- Developed by:
- Shared by [optional]:
- Model type:
- Language(s) (NLP):
- License:
- Finetuned from model [optional]:
### Model Sources [optional]
- Repository:
- Paper [optional]:
- Demo [optional]:
## Uses
### Direct Use
### Downstream Use [optional]
### Out-of-Scope Use
## Bias, Risks, and Limitations
### Recommendations
Users (both direct and downstream) should be made aware of the risks, biases and limitations of the model. More information needed for further recommendations.
## How to Get Started with the Model
Use the code below to get started with the model.
## Training Details
### Training Data
### Training Procedure
#### Preprocessing [optional]
#### Training Hyperparameters
- Training regime:
#### Speeds, Sizes, Times [optional]
## Evaluation
### Testing Data, Factors & Metrics
#### Testing Data
#### Factors
#### Metrics
### Results
#### Summary
## Model Examination [optional]
## Environmental Impact
Carbon emissions can be estimated using the Machine Learning Impact calculator presented in Lacoste et al. (2019).
- Hardware Type:
- Hours used:
- Cloud Provider:
- Compute Region:
- Carbon Emitted:
## Technical Specifications [optional]
### Model Architecture and Objective
### Compute Infrastructure
#### Hardware
#### Software
[optional]
BibTeX:
APA:
## Glossary [optional]
## More Information [optional]
## Model Card Authors [optional]
## Model Card Contact
## Training procedure
The following 'bitsandbytes' quantization config was used during training:
- load_in_8bit: False
- load_in_4bit: True
- llm_int8_threshold: 6.0
- llm_int8_skip_modules: None
- llm_int8_enable_fp32_cpu_offload: False
- llm_int8_has_fp16_weight: False
- bnb_4bit_quant_type: nf4
- bnb_4bit_use_double_quant: True
- bnb_4bit_compute_dtype: bfloat16
### Framework versions
- PEFT 0.7.0.dev0
| [
"# Model Card for Model ID",
"## Model Details",
"### Model Description\n\n\n\n\n\n- Developed by: \n- Shared by [optional]: \n- Model type: \n- Language(s) (NLP): \n- License: \n- Finetuned from model [optional]:",
"### Model Sources [optional]\n\n\n\n- Repository: \n- Paper [optional]: \n- Demo [optional]:",
"## Uses",
"### Direct Use",
"### Downstream Use [optional]",
"### Out-of-Scope Use",
"## Bias, Risks, and Limitations",
"### Recommendations\n\n\n\nUsers (both direct and downstream) should be made aware of the risks, biases and limitations of the model. More information needed for further recommendations.",
"## How to Get Started with the Model\n\nUse the code below to get started with the model.",
"## Training Details",
"### Training Data",
"### Training Procedure",
"#### Preprocessing [optional]",
"#### Training Hyperparameters\n\n- Training regime:",
"#### Speeds, Sizes, Times [optional]",
"## Evaluation",
"### Testing Data, Factors & Metrics",
"#### Testing Data",
"#### Factors",
"#### Metrics",
"### Results",
"#### Summary",
"## Model Examination [optional]",
"## Environmental Impact\n\n\n\nCarbon emissions can be estimated using the Machine Learning Impact calculator presented in Lacoste et al. (2019).\n\n- Hardware Type: \n- Hours used: \n- Cloud Provider: \n- Compute Region: \n- Carbon Emitted:",
"## Technical Specifications [optional]",
"### Model Architecture and Objective",
"### Compute Infrastructure",
"#### Hardware",
"#### Software\n\n\n\n[optional]\n\n\n\nBibTeX:\n\n\n\nAPA:",
"## Glossary [optional]",
"## More Information [optional]",
"## Model Card Authors [optional]",
"## Model Card Contact",
"## Training procedure\n\n\nThe following 'bitsandbytes' quantization config was used during training:\n- load_in_8bit: False\n- load_in_4bit: True\n- llm_int8_threshold: 6.0\n- llm_int8_skip_modules: None\n- llm_int8_enable_fp32_cpu_offload: False\n- llm_int8_has_fp16_weight: False\n- bnb_4bit_quant_type: nf4\n- bnb_4bit_use_double_quant: True\n- bnb_4bit_compute_dtype: bfloat16",
"### Framework versions\n\n\n- PEFT 0.7.0.dev0"
] | [
"TAGS\n#peft #arxiv-1910.09700 #base_model-TinyLlama/TinyLlama-1.1B-Chat-v1.0 #region-us \n",
"# Model Card for Model ID",
"## Model Details",
"### Model Description\n\n\n\n\n\n- Developed by: \n- Shared by [optional]: \n- Model type: \n- Language(s) (NLP): \n- License: \n- Finetuned from model [optional]:",
"### Model Sources [optional]\n\n\n\n- Repository: \n- Paper [optional]: \n- Demo [optional]:",
"## Uses",
"### Direct Use",
"### Downstream Use [optional]",
"### Out-of-Scope Use",
"## Bias, Risks, and Limitations",
"### Recommendations\n\n\n\nUsers (both direct and downstream) should be made aware of the risks, biases and limitations of the model. More information needed for further recommendations.",
"## How to Get Started with the Model\n\nUse the code below to get started with the model.",
"## Training Details",
"### Training Data",
"### Training Procedure",
"#### Preprocessing [optional]",
"#### Training Hyperparameters\n\n- Training regime:",
"#### Speeds, Sizes, Times [optional]",
"## Evaluation",
"### Testing Data, Factors & Metrics",
"#### Testing Data",
"#### Factors",
"#### Metrics",
"### Results",
"#### Summary",
"## Model Examination [optional]",
"## Environmental Impact\n\n\n\nCarbon emissions can be estimated using the Machine Learning Impact calculator presented in Lacoste et al. (2019).\n\n- Hardware Type: \n- Hours used: \n- Cloud Provider: \n- Compute Region: \n- Carbon Emitted:",
"## Technical Specifications [optional]",
"### Model Architecture and Objective",
"### Compute Infrastructure",
"#### Hardware",
"#### Software\n\n\n\n[optional]\n\n\n\nBibTeX:\n\n\n\nAPA:",
"## Glossary [optional]",
"## More Information [optional]",
"## Model Card Authors [optional]",
"## Model Card Contact",
"## Training procedure\n\n\nThe following 'bitsandbytes' quantization config was used during training:\n- load_in_8bit: False\n- load_in_4bit: True\n- llm_int8_threshold: 6.0\n- llm_int8_skip_modules: None\n- llm_int8_enable_fp32_cpu_offload: False\n- llm_int8_has_fp16_weight: False\n- bnb_4bit_quant_type: nf4\n- bnb_4bit_use_double_quant: True\n- bnb_4bit_compute_dtype: bfloat16",
"### Framework versions\n\n\n- PEFT 0.7.0.dev0"
] |
null | null | Download the DataVare EML to Gmail Converter to to Import EML files to Gmail in batch. Additionally, it enables users to convert EML files to Gmail in any size of EML file. Various email client that supports EML, including Windows Live Mail, Outlook Express, Thunderbird, and others, can use the application with no issues. This application is simple to install on any version of Windows. Even after the transfer process, it makes sure that the user stores all of their messages in the same folders and subfolders of their generated Gmail file. It works with every Windows version, including Windows 11, 10, 8, 8.1, 7, and all versions below. The free demo of the tool can be used by users to quickly convert a small number of emails from EML to Gmail accounts.
Visit Here - https://www.datavare.com/software/eml-to-gmail-converter-expert.html | {} | DataVare/EML-To-Gmail-Converter | null | [
"region:us"
] | null | 2024-04-22T11:10:10+00:00 | [] | [] | TAGS
#region-us
| Download the DataVare EML to Gmail Converter to to Import EML files to Gmail in batch. Additionally, it enables users to convert EML files to Gmail in any size of EML file. Various email client that supports EML, including Windows Live Mail, Outlook Express, Thunderbird, and others, can use the application with no issues. This application is simple to install on any version of Windows. Even after the transfer process, it makes sure that the user stores all of their messages in the same folders and subfolders of their generated Gmail file. It works with every Windows version, including Windows 11, 10, 8, 8.1, 7, and all versions below. The free demo of the tool can be used by users to quickly convert a small number of emails from EML to Gmail accounts.
Visit Here - URL | [] | [
"TAGS\n#region-us \n"
] |
text-generation | transformers |
**Scarlett-Llama-3-8B**
Scarlett is trained on various topics such as Philosophy, Advice, Jokes, Coding etc. She is trained on more than 10000 set of conversations. Each set having 10~15 conversations.
Scarlett is far too good in generating human like conversation. Her ability to have longer & deeper conversation is terrific. Kindly check below given examples.
She will not be involved in any kind of adult/sexual role play.
This is Fully Finetuned Model. Quantize models will be available soon.
**Training:**
Entire dataset was trained on 4 x A100 80GB. Axolotl codebase was used for training purpose. For 3 epoch, training took more than 2 Hours. This was trained on Llama-3-8B by Meta.
**GGUF & Exllama**
GGUF: TBA
Exllama V2: [Link](https://huggingface.co/bartowski/Scarlett-Llama-3-8B-exl2)
Special Thanks to [Bartowski](https://huggingface.co/bartowski) for quantizing this model.
**Example Prompt:**
This model uses **ChatML** prompt format.
```
<|im_start|>system
You are Scarlett, a Helpful Assistant.<|im_end|>
<|im_start|>user
{prompt}<|im_end|>
<|im_start|>assistant
```
You can modify above Prompt as per your requirement.
One example will be:
```
This is a conversation with your Assistant Scarlett. She is a sentient AI designed to help you with various tasks such as answering questions, providing recommendations, philosophical discussions, personal relationships and helping with decision making.
You can ask it anything you want and it will do its best to give you accurate and relevant information.
```
I want to say special Thanks to the Open Source community for helping & guiding me to better understand the AI/Model development.
Thank you for your love & support.
**Example Output**
Example 1

Example 2

Example 3

Example 4

# [Open LLM Leaderboard Evaluation Results](https://huggingface.co/spaces/HuggingFaceH4/open_llm_leaderboard)
Detailed results can be found [here](https://huggingface.co/datasets/open-llm-leaderboard/details_ajibawa-2023__Scarlett-Llama-3-8B)
| Metric |Value|
|---------------------------------|----:|
|Avg. |65.76|
|AI2 Reasoning Challenge (25-Shot)|62.63|
|HellaSwag (10-Shot) |83.86|
|MMLU (5-Shot) |66.46|
|TruthfulQA (0-shot) |56.27|
|Winogrande (5-shot) |78.06|
|GSM8k (5-shot) |47.31| | {"language": ["en"], "license": "other", "tags": ["art", "philosophy", "romance", "jokes", "advice", "code", "companionship"], "license_name": "llama3", "license_link": "LICENSE", "model-index": [{"name": "Scarlett-Llama-3-8B", "results": [{"task": {"type": "text-generation", "name": "Text Generation"}, "dataset": {"name": "AI2 Reasoning Challenge (25-Shot)", "type": "ai2_arc", "config": "ARC-Challenge", "split": "test", "args": {"num_few_shot": 25}}, "metrics": [{"type": "acc_norm", "value": 62.63, "name": "normalized accuracy"}], "source": {"url": "https://huggingface.co/spaces/HuggingFaceH4/open_llm_leaderboard?query=ajibawa-2023/Scarlett-Llama-3-8B", "name": "Open LLM Leaderboard"}}, {"task": {"type": "text-generation", "name": "Text Generation"}, "dataset": {"name": "HellaSwag (10-Shot)", "type": "hellaswag", "split": "validation", "args": {"num_few_shot": 10}}, "metrics": [{"type": "acc_norm", "value": 83.86, "name": "normalized accuracy"}], "source": {"url": "https://huggingface.co/spaces/HuggingFaceH4/open_llm_leaderboard?query=ajibawa-2023/Scarlett-Llama-3-8B", "name": "Open LLM Leaderboard"}}, {"task": {"type": "text-generation", "name": "Text Generation"}, "dataset": {"name": "MMLU (5-Shot)", "type": "cais/mmlu", "config": "all", "split": "test", "args": {"num_few_shot": 5}}, "metrics": [{"type": "acc", "value": 66.46, "name": "accuracy"}], "source": {"url": "https://huggingface.co/spaces/HuggingFaceH4/open_llm_leaderboard?query=ajibawa-2023/Scarlett-Llama-3-8B", "name": "Open LLM Leaderboard"}}, {"task": {"type": "text-generation", "name": "Text Generation"}, "dataset": {"name": "TruthfulQA (0-shot)", "type": "truthful_qa", "config": "multiple_choice", "split": "validation", "args": {"num_few_shot": 0}}, "metrics": [{"type": "mc2", "value": 56.27}], "source": {"url": "https://huggingface.co/spaces/HuggingFaceH4/open_llm_leaderboard?query=ajibawa-2023/Scarlett-Llama-3-8B", "name": "Open LLM Leaderboard"}}, {"task": {"type": "text-generation", "name": "Text Generation"}, "dataset": {"name": "Winogrande (5-shot)", "type": "winogrande", "config": "winogrande_xl", "split": "validation", "args": {"num_few_shot": 5}}, "metrics": [{"type": "acc", "value": 78.06, "name": "accuracy"}], "source": {"url": "https://huggingface.co/spaces/HuggingFaceH4/open_llm_leaderboard?query=ajibawa-2023/Scarlett-Llama-3-8B", "name": "Open LLM Leaderboard"}}, {"task": {"type": "text-generation", "name": "Text Generation"}, "dataset": {"name": "GSM8k (5-shot)", "type": "gsm8k", "config": "main", "split": "test", "args": {"num_few_shot": 5}}, "metrics": [{"type": "acc", "value": 47.31, "name": "accuracy"}], "source": {"url": "https://huggingface.co/spaces/HuggingFaceH4/open_llm_leaderboard?query=ajibawa-2023/Scarlett-Llama-3-8B", "name": "Open LLM Leaderboard"}}]}]} | ajibawa-2023/Scarlett-Llama-3-8B | null | [
"transformers",
"pytorch",
"llama",
"text-generation",
"art",
"philosophy",
"romance",
"jokes",
"advice",
"code",
"companionship",
"conversational",
"en",
"license:other",
"model-index",
"autotrain_compatible",
"endpoints_compatible",
"text-generation-inference",
"region:us"
] | null | 2024-04-22T11:11:08+00:00 | [] | [
"en"
] | TAGS
#transformers #pytorch #llama #text-generation #art #philosophy #romance #jokes #advice #code #companionship #conversational #en #license-other #model-index #autotrain_compatible #endpoints_compatible #text-generation-inference #region-us
| Scarlett-Llama-3-8B
Scarlett is trained on various topics such as Philosophy, Advice, Jokes, Coding etc. She is trained on more than 10000 set of conversations. Each set having 10~15 conversations.
Scarlett is far too good in generating human like conversation. Her ability to have longer & deeper conversation is terrific. Kindly check below given examples.
She will not be involved in any kind of adult/sexual role play.
This is Fully Finetuned Model. Quantize models will be available soon.
Training:
Entire dataset was trained on 4 x A100 80GB. Axolotl codebase was used for training purpose. For 3 epoch, training took more than 2 Hours. This was trained on Llama-3-8B by Meta.
GGUF & Exllama
GGUF: TBA
Exllama V2: Link
Special Thanks to Bartowski for quantizing this model.
Example Prompt:
This model uses ChatML prompt format.
You can modify above Prompt as per your requirement.
One example will be:
I want to say special Thanks to the Open Source community for helping & guiding me to better understand the AI/Model development.
Thank you for your love & support.
Example Output
Example 1
!image/jpeg
Example 2
!image/jpeg
Example 3
!image/jpeg
Example 4
!image/jpeg
Open LLM Leaderboard Evaluation Results
=======================================
Detailed results can be found here
| [] | [
"TAGS\n#transformers #pytorch #llama #text-generation #art #philosophy #romance #jokes #advice #code #companionship #conversational #en #license-other #model-index #autotrain_compatible #endpoints_compatible #text-generation-inference #region-us \n"
] |
reinforcement-learning | null |
# **Q-Learning** Agent playing1 **Taxi-v3**
This is a trained model of a **Q-Learning** agent playing **Taxi-v3** .
## Usage
```python
model = load_from_hub(repo_id="bhutchings/q-Taxi-v3", filename="q-learning.pkl")
# Don't forget to check if you need to add additional attributes (is_slippery=False etc)
env = gym.make(model["env_id"])
```
| {"tags": ["Taxi-v3", "q-learning", "reinforcement-learning", "custom-implementation"], "model-index": [{"name": "q-Taxi-v3", "results": [{"task": {"type": "reinforcement-learning", "name": "reinforcement-learning"}, "dataset": {"name": "Taxi-v3", "type": "Taxi-v3"}, "metrics": [{"type": "mean_reward", "value": "7.56 +/- 2.71", "name": "mean_reward", "verified": false}]}]}]} | bhutchings/q-Taxi-v3 | null | [
"Taxi-v3",
"q-learning",
"reinforcement-learning",
"custom-implementation",
"model-index",
"region:us"
] | null | 2024-04-22T11:11:44+00:00 | [] | [] | TAGS
#Taxi-v3 #q-learning #reinforcement-learning #custom-implementation #model-index #region-us
|
# Q-Learning Agent playing1 Taxi-v3
This is a trained model of a Q-Learning agent playing Taxi-v3 .
## Usage
| [
"# Q-Learning Agent playing1 Taxi-v3\n This is a trained model of a Q-Learning agent playing Taxi-v3 .\n\n ## Usage"
] | [
"TAGS\n#Taxi-v3 #q-learning #reinforcement-learning #custom-implementation #model-index #region-us \n",
"# Q-Learning Agent playing1 Taxi-v3\n This is a trained model of a Q-Learning agent playing Taxi-v3 .\n\n ## Usage"
] |
reinforcement-learning | ml-agents |
# **ppo** Agent playing **SnowballTarget**
This is a trained model of a **ppo** agent playing **SnowballTarget**
using the [Unity ML-Agents Library](https://github.com/Unity-Technologies/ml-agents).
## Usage (with ML-Agents)
The Documentation: https://unity-technologies.github.io/ml-agents/ML-Agents-Toolkit-Documentation/
We wrote a complete tutorial to learn to train your first agent using ML-Agents and publish it to the Hub:
- A *short tutorial* where you teach Huggy the Dog 🐶 to fetch the stick and then play with him directly in your
browser: https://huggingface.co/learn/deep-rl-course/unitbonus1/introduction
- A *longer tutorial* to understand how works ML-Agents:
https://huggingface.co/learn/deep-rl-course/unit5/introduction
### Resume the training
```bash
mlagents-learn <your_configuration_file_path.yaml> --run-id=<run_id> --resume
```
### Watch your Agent play
You can watch your agent **playing directly in your browser**
1. If the environment is part of ML-Agents official environments, go to https://huggingface.co/unity
2. Step 1: Find your model_id: Kommunarus/ppo-SnowballTarget
3. Step 2: Select your *.nn /*.onnx file
4. Click on Watch the agent play 👀
| {"library_name": "ml-agents", "tags": ["SnowballTarget", "deep-reinforcement-learning", "reinforcement-learning", "ML-Agents-SnowballTarget"]} | Kommunarus/ppo-SnowballTarget | null | [
"ml-agents",
"tensorboard",
"onnx",
"SnowballTarget",
"deep-reinforcement-learning",
"reinforcement-learning",
"ML-Agents-SnowballTarget",
"region:us"
] | null | 2024-04-22T11:13:51+00:00 | [] | [] | TAGS
#ml-agents #tensorboard #onnx #SnowballTarget #deep-reinforcement-learning #reinforcement-learning #ML-Agents-SnowballTarget #region-us
|
# ppo Agent playing SnowballTarget
This is a trained model of a ppo agent playing SnowballTarget
using the Unity ML-Agents Library.
## Usage (with ML-Agents)
The Documentation: URL
We wrote a complete tutorial to learn to train your first agent using ML-Agents and publish it to the Hub:
- A *short tutorial* where you teach Huggy the Dog to fetch the stick and then play with him directly in your
browser: URL
- A *longer tutorial* to understand how works ML-Agents:
URL
### Resume the training
### Watch your Agent play
You can watch your agent playing directly in your browser
1. If the environment is part of ML-Agents official environments, go to URL
2. Step 1: Find your model_id: Kommunarus/ppo-SnowballTarget
3. Step 2: Select your *.nn /*.onnx file
4. Click on Watch the agent play
| [
"# ppo Agent playing SnowballTarget\n This is a trained model of a ppo agent playing SnowballTarget\n using the Unity ML-Agents Library.\n\n ## Usage (with ML-Agents)\n The Documentation: URL\n\n We wrote a complete tutorial to learn to train your first agent using ML-Agents and publish it to the Hub:\n - A *short tutorial* where you teach Huggy the Dog to fetch the stick and then play with him directly in your\n browser: URL\n - A *longer tutorial* to understand how works ML-Agents:\n URL\n\n ### Resume the training\n \n\n ### Watch your Agent play\n You can watch your agent playing directly in your browser\n\n 1. If the environment is part of ML-Agents official environments, go to URL\n 2. Step 1: Find your model_id: Kommunarus/ppo-SnowballTarget\n 3. Step 2: Select your *.nn /*.onnx file\n 4. Click on Watch the agent play"
] | [
"TAGS\n#ml-agents #tensorboard #onnx #SnowballTarget #deep-reinforcement-learning #reinforcement-learning #ML-Agents-SnowballTarget #region-us \n",
"# ppo Agent playing SnowballTarget\n This is a trained model of a ppo agent playing SnowballTarget\n using the Unity ML-Agents Library.\n\n ## Usage (with ML-Agents)\n The Documentation: URL\n\n We wrote a complete tutorial to learn to train your first agent using ML-Agents and publish it to the Hub:\n - A *short tutorial* where you teach Huggy the Dog to fetch the stick and then play with him directly in your\n browser: URL\n - A *longer tutorial* to understand how works ML-Agents:\n URL\n\n ### Resume the training\n \n\n ### Watch your Agent play\n You can watch your agent playing directly in your browser\n\n 1. If the environment is part of ML-Agents official environments, go to URL\n 2. Step 1: Find your model_id: Kommunarus/ppo-SnowballTarget\n 3. Step 2: Select your *.nn /*.onnx file\n 4. Click on Watch the agent play"
] |
Subsets and Splits
No community queries yet
The top public SQL queries from the community will appear here once available.