pipeline_tag
stringclasses 48
values | library_name
stringclasses 198
values | text
stringlengths 1
900k
| metadata
stringlengths 2
438k
| id
stringlengths 5
122
| last_modified
null | tags
sequencelengths 1
1.84k
| sha
null | created_at
stringlengths 25
25
| arxiv
sequencelengths 0
201
| languages
sequencelengths 0
1.83k
| tags_str
stringlengths 17
9.34k
| text_str
stringlengths 0
389k
| text_lists
sequencelengths 0
722
| processed_texts
sequencelengths 1
723
|
---|---|---|---|---|---|---|---|---|---|---|---|---|---|---|
reinforcement-learning | stable-baselines3 |
# **PPO** Agent playing **LunarLander-v2**
This is a trained model of a **PPO** agent playing **LunarLander-v2**
using the [stable-baselines3 library](https://github.com/DLR-RM/stable-baselines3).
## Usage (with Stable-baselines3)
TODO: Add your code
```python
from stable_baselines3 import ...
from huggingface_sb3 import load_from_hub
...
```
| {"library_name": "stable-baselines3", "tags": ["LunarLander-v2", "deep-reinforcement-learning", "reinforcement-learning", "stable-baselines3"], "model-index": [{"name": "PPO", "results": [{"task": {"type": "reinforcement-learning", "name": "reinforcement-learning"}, "dataset": {"name": "LunarLander-v2", "type": "LunarLander-v2"}, "metrics": [{"type": "mean_reward", "value": "253.33 +/- 11.60", "name": "mean_reward", "verified": false}]}]}]} | Novski/PPO-LunarLander-v2 | null | [
"stable-baselines3",
"LunarLander-v2",
"deep-reinforcement-learning",
"reinforcement-learning",
"model-index",
"region:us"
] | null | 2024-04-26T17:46:00+00:00 | [] | [] | TAGS
#stable-baselines3 #LunarLander-v2 #deep-reinforcement-learning #reinforcement-learning #model-index #region-us
|
# PPO Agent playing LunarLander-v2
This is a trained model of a PPO agent playing LunarLander-v2
using the stable-baselines3 library.
## Usage (with Stable-baselines3)
TODO: Add your code
| [
"# PPO Agent playing LunarLander-v2\nThis is a trained model of a PPO agent playing LunarLander-v2\nusing the stable-baselines3 library.",
"## Usage (with Stable-baselines3)\nTODO: Add your code"
] | [
"TAGS\n#stable-baselines3 #LunarLander-v2 #deep-reinforcement-learning #reinforcement-learning #model-index #region-us \n",
"# PPO Agent playing LunarLander-v2\nThis is a trained model of a PPO agent playing LunarLander-v2\nusing the stable-baselines3 library.",
"## Usage (with Stable-baselines3)\nTODO: Add your code"
] |
reinforcement-learning | stable-baselines3 |
# **PPO** Agent playing **LunarLander-v2**
This is a trained model of a **PPO** agent playing **LunarLander-v2**
using the [stable-baselines3 library](https://github.com/DLR-RM/stable-baselines3).
## Usage (with Stable-baselines3)
TODO: Add your code
```python
from stable_baselines3 import ...
from huggingface_sb3 import load_from_hub
...
```
| {"library_name": "stable-baselines3", "tags": ["LunarLander-v2", "deep-reinforcement-learning", "reinforcement-learning", "stable-baselines3"], "model-index": [{"name": "PPO", "results": [{"task": {"type": "reinforcement-learning", "name": "reinforcement-learning"}, "dataset": {"name": "LunarLander-v2", "type": "LunarLander-v2"}, "metrics": [{"type": "mean_reward", "value": "255.07 +/- 18.00", "name": "mean_reward", "verified": false}]}]}]} | Unclad3610/ppo-LunarLander-v2 | null | [
"stable-baselines3",
"LunarLander-v2",
"deep-reinforcement-learning",
"reinforcement-learning",
"model-index",
"region:us"
] | null | 2024-04-26T17:49:18+00:00 | [] | [] | TAGS
#stable-baselines3 #LunarLander-v2 #deep-reinforcement-learning #reinforcement-learning #model-index #region-us
|
# PPO Agent playing LunarLander-v2
This is a trained model of a PPO agent playing LunarLander-v2
using the stable-baselines3 library.
## Usage (with Stable-baselines3)
TODO: Add your code
| [
"# PPO Agent playing LunarLander-v2\nThis is a trained model of a PPO agent playing LunarLander-v2\nusing the stable-baselines3 library.",
"## Usage (with Stable-baselines3)\nTODO: Add your code"
] | [
"TAGS\n#stable-baselines3 #LunarLander-v2 #deep-reinforcement-learning #reinforcement-learning #model-index #region-us \n",
"# PPO Agent playing LunarLander-v2\nThis is a trained model of a PPO agent playing LunarLander-v2\nusing the stable-baselines3 library.",
"## Usage (with Stable-baselines3)\nTODO: Add your code"
] |
text-classification | transformers |
<!-- This model card has been generated automatically according to the information the Trainer had access to. You
should probably proofread and complete it, then remove this comment. -->
# seq
This model is a fine-tuned version of [autoevaluate/binary-classification](https://huggingface.co/autoevaluate/binary-classification) on an unknown dataset.
It achieves the following results on the evaluation set:
- Loss: 0.2408
- Accuracy: 0.9352
## Model description
More information needed
## Intended uses & limitations
More information needed
## Training and evaluation data
More information needed
## Training procedure
### Training hyperparameters
The following hyperparameters were used during training:
- learning_rate: 2e-05
- train_batch_size: 16
- eval_batch_size: 16
- seed: 42
- optimizer: Adam with betas=(0.9,0.999) and epsilon=1e-08
- lr_scheduler_type: linear
- num_epochs: 2
### Training results
| Training Loss | Epoch | Step | Validation Loss | Accuracy |
|:-------------:|:-----:|:----:|:---------------:|:--------:|
| 0.212 | 1.0 | 1563 | 0.1816 | 0.9304 |
| 0.132 | 2.0 | 3126 | 0.2408 | 0.9352 |
### Framework versions
- Transformers 4.40.1
- Pytorch 2.2.1+cu121
- Datasets 2.19.0
- Tokenizers 0.19.1
| {"license": "apache-2.0", "tags": ["generated_from_trainer"], "metrics": ["accuracy"], "base_model": "autoevaluate/binary-classification", "model-index": [{"name": "seq", "results": []}]} | qianyihuang1203/seq | null | [
"transformers",
"tensorboard",
"safetensors",
"distilbert",
"text-classification",
"generated_from_trainer",
"base_model:autoevaluate/binary-classification",
"license:apache-2.0",
"autotrain_compatible",
"endpoints_compatible",
"region:us"
] | null | 2024-04-26T17:49:40+00:00 | [] | [] | TAGS
#transformers #tensorboard #safetensors #distilbert #text-classification #generated_from_trainer #base_model-autoevaluate/binary-classification #license-apache-2.0 #autotrain_compatible #endpoints_compatible #region-us
| seq
===
This model is a fine-tuned version of autoevaluate/binary-classification on an unknown dataset.
It achieves the following results on the evaluation set:
* Loss: 0.2408
* Accuracy: 0.9352
Model description
-----------------
More information needed
Intended uses & limitations
---------------------------
More information needed
Training and evaluation data
----------------------------
More information needed
Training procedure
------------------
### Training hyperparameters
The following hyperparameters were used during training:
* learning\_rate: 2e-05
* train\_batch\_size: 16
* eval\_batch\_size: 16
* seed: 42
* optimizer: Adam with betas=(0.9,0.999) and epsilon=1e-08
* lr\_scheduler\_type: linear
* num\_epochs: 2
### Training results
### Framework versions
* Transformers 4.40.1
* Pytorch 2.2.1+cu121
* Datasets 2.19.0
* Tokenizers 0.19.1
| [
"### Training hyperparameters\n\n\nThe following hyperparameters were used during training:\n\n\n* learning\\_rate: 2e-05\n* train\\_batch\\_size: 16\n* eval\\_batch\\_size: 16\n* seed: 42\n* optimizer: Adam with betas=(0.9,0.999) and epsilon=1e-08\n* lr\\_scheduler\\_type: linear\n* num\\_epochs: 2",
"### Training results",
"### Framework versions\n\n\n* Transformers 4.40.1\n* Pytorch 2.2.1+cu121\n* Datasets 2.19.0\n* Tokenizers 0.19.1"
] | [
"TAGS\n#transformers #tensorboard #safetensors #distilbert #text-classification #generated_from_trainer #base_model-autoevaluate/binary-classification #license-apache-2.0 #autotrain_compatible #endpoints_compatible #region-us \n",
"### Training hyperparameters\n\n\nThe following hyperparameters were used during training:\n\n\n* learning\\_rate: 2e-05\n* train\\_batch\\_size: 16\n* eval\\_batch\\_size: 16\n* seed: 42\n* optimizer: Adam with betas=(0.9,0.999) and epsilon=1e-08\n* lr\\_scheduler\\_type: linear\n* num\\_epochs: 2",
"### Training results",
"### Framework versions\n\n\n* Transformers 4.40.1\n* Pytorch 2.2.1+cu121\n* Datasets 2.19.0\n* Tokenizers 0.19.1"
] |
null | fastai |
# Amazing!
🥳 Congratulations on hosting your fastai model on the Hugging Face Hub!
# Some next steps
1. Fill out this model card with more information (see the template below and the [documentation here](https://huggingface.co/docs/hub/model-repos))!
2. Create a demo in Gradio or Streamlit using 🤗 Spaces ([documentation here](https://huggingface.co/docs/hub/spaces)).
3. Join the fastai community on the [Fastai Discord](https://discord.com/invite/YKrxeNn)!
Greetings fellow fastlearner 🤝! Don't forget to delete this content from your model card.
---
# Model card
## Model description
More information needed
## Intended uses & limitations
More information needed
## Training and evaluation data
More information needed
| {"tags": ["fastai"]} | cesaenv/hojasVid | null | [
"fastai",
"region:us",
"has_space"
] | null | 2024-04-26T17:50:24+00:00 | [] | [] | TAGS
#fastai #region-us #has_space
|
# Amazing!
Congratulations on hosting your fastai model on the Hugging Face Hub!
# Some next steps
1. Fill out this model card with more information (see the template below and the documentation here)!
2. Create a demo in Gradio or Streamlit using Spaces (documentation here).
3. Join the fastai community on the Fastai Discord!
Greetings fellow fastlearner ! Don't forget to delete this content from your model card.
---
# Model card
## Model description
More information needed
## Intended uses & limitations
More information needed
## Training and evaluation data
More information needed
| [
"# Amazing!\n\n Congratulations on hosting your fastai model on the Hugging Face Hub!",
"# Some next steps\n1. Fill out this model card with more information (see the template below and the documentation here)!\n\n2. Create a demo in Gradio or Streamlit using Spaces (documentation here).\n\n3. Join the fastai community on the Fastai Discord!\n\nGreetings fellow fastlearner ! Don't forget to delete this content from your model card.\n\n\n---",
"# Model card",
"## Model description\nMore information needed",
"## Intended uses & limitations\nMore information needed",
"## Training and evaluation data\nMore information needed"
] | [
"TAGS\n#fastai #region-us #has_space \n",
"# Amazing!\n\n Congratulations on hosting your fastai model on the Hugging Face Hub!",
"# Some next steps\n1. Fill out this model card with more information (see the template below and the documentation here)!\n\n2. Create a demo in Gradio or Streamlit using Spaces (documentation here).\n\n3. Join the fastai community on the Fastai Discord!\n\nGreetings fellow fastlearner ! Don't forget to delete this content from your model card.\n\n\n---",
"# Model card",
"## Model description\nMore information needed",
"## Intended uses & limitations\nMore information needed",
"## Training and evaluation data\nMore information needed"
] |
null | null |
# NotAiLOL/Boundary-Coder-Yi-2x9B-MoE-Q4_K_M-GGUF
This model was converted to GGUF format from [`NotAiLOL/Boundary-Coder-Yi-2x9B-MoE`](https://huggingface.co/NotAiLOL/Boundary-Coder-Yi-2x9B-MoE) using llama.cpp via the ggml.ai's [GGUF-my-repo](https://huggingface.co/spaces/ggml-org/gguf-my-repo) space.
Refer to the [original model card](https://huggingface.co/NotAiLOL/Boundary-Coder-Yi-2x9B-MoE) for more details on the model.
## Use with llama.cpp
Install llama.cpp through brew.
```bash
brew install ggerganov/ggerganov/llama.cpp
```
Invoke the llama.cpp server or the CLI.
CLI:
```bash
llama-cli --hf-repo NotAiLOL/Boundary-Coder-Yi-2x9B-MoE-Q4_K_M-GGUF --model boundary-coder-yi-2x9b-moe.Q4_K_M.gguf -p "The meaning to life and the universe is"
```
Server:
```bash
llama-server --hf-repo NotAiLOL/Boundary-Coder-Yi-2x9B-MoE-Q4_K_M-GGUF --model boundary-coder-yi-2x9b-moe.Q4_K_M.gguf -c 2048
```
Note: You can also use this checkpoint directly through the [usage steps](https://github.com/ggerganov/llama.cpp?tab=readme-ov-file#usage) listed in the Llama.cpp repo as well.
```
git clone https://github.com/ggerganov/llama.cpp && cd llama.cpp && make && ./main -m boundary-coder-yi-2x9b-moe.Q4_K_M.gguf -n 128
```
| {"license": "apache-2.0", "tags": ["moe", "merge", "mergekit", "01-ai/Yi-9B-200K", "TechxGenus/Yi-9B-Coder", "llama-cpp", "gguf-my-repo"], "base_model": ["01-ai/Yi-9B-200K", "TechxGenus/Yi-9B-Coder"]} | NotAiLOL/Boundary-Coder-Yi-2x9B-MoE-Q4_K_M-GGUF | null | [
"gguf",
"moe",
"merge",
"mergekit",
"01-ai/Yi-9B-200K",
"TechxGenus/Yi-9B-Coder",
"llama-cpp",
"gguf-my-repo",
"base_model:01-ai/Yi-9B-200K",
"base_model:TechxGenus/Yi-9B-Coder",
"license:apache-2.0",
"region:us"
] | null | 2024-04-26T17:50:47+00:00 | [] | [] | TAGS
#gguf #moe #merge #mergekit #01-ai/Yi-9B-200K #TechxGenus/Yi-9B-Coder #llama-cpp #gguf-my-repo #base_model-01-ai/Yi-9B-200K #base_model-TechxGenus/Yi-9B-Coder #license-apache-2.0 #region-us
|
# NotAiLOL/Boundary-Coder-Yi-2x9B-MoE-Q4_K_M-GGUF
This model was converted to GGUF format from 'NotAiLOL/Boundary-Coder-Yi-2x9B-MoE' using URL via the URL's GGUF-my-repo space.
Refer to the original model card for more details on the model.
## Use with URL
Install URL through brew.
Invoke the URL server or the CLI.
CLI:
Server:
Note: You can also use this checkpoint directly through the usage steps listed in the URL repo as well.
| [
"# NotAiLOL/Boundary-Coder-Yi-2x9B-MoE-Q4_K_M-GGUF\nThis model was converted to GGUF format from 'NotAiLOL/Boundary-Coder-Yi-2x9B-MoE' using URL via the URL's GGUF-my-repo space.\nRefer to the original model card for more details on the model.",
"## Use with URL\n\nInstall URL through brew.\n\n\nInvoke the URL server or the CLI.\n\nCLI:\n\n\n\nServer:\n\n\n\nNote: You can also use this checkpoint directly through the usage steps listed in the URL repo as well."
] | [
"TAGS\n#gguf #moe #merge #mergekit #01-ai/Yi-9B-200K #TechxGenus/Yi-9B-Coder #llama-cpp #gguf-my-repo #base_model-01-ai/Yi-9B-200K #base_model-TechxGenus/Yi-9B-Coder #license-apache-2.0 #region-us \n",
"# NotAiLOL/Boundary-Coder-Yi-2x9B-MoE-Q4_K_M-GGUF\nThis model was converted to GGUF format from 'NotAiLOL/Boundary-Coder-Yi-2x9B-MoE' using URL via the URL's GGUF-my-repo space.\nRefer to the original model card for more details on the model.",
"## Use with URL\n\nInstall URL through brew.\n\n\nInvoke the URL server or the CLI.\n\nCLI:\n\n\n\nServer:\n\n\n\nNote: You can also use this checkpoint directly through the usage steps listed in the URL repo as well."
] |
text-classification | transformers |
<!-- This model card has been generated automatically according to the information the Trainer had access to. You
should probably proofread and complete it, then remove this comment. -->
# distilbert-base-uncased-finetuned-clinc
This model is a fine-tuned version of [distilbert-base-uncased](https://huggingface.co/distilbert-base-uncased) on an unknown dataset.
It achieves the following results on the evaluation set:
- Loss: 0.8019
- Accuracy: 0.9165
## Model description
More information needed
## Intended uses & limitations
More information needed
## Training and evaluation data
More information needed
## Training procedure
### Training hyperparameters
The following hyperparameters were used during training:
- learning_rate: 2e-05
- train_batch_size: 48
- eval_batch_size: 48
- seed: 42
- optimizer: Adam with betas=(0.9,0.999) and epsilon=1e-08
- lr_scheduler_type: linear
- num_epochs: 5
### Training results
| Training Loss | Epoch | Step | Validation Loss | Accuracy |
|:-------------:|:-----:|:----:|:---------------:|:--------:|
| No log | 1.0 | 318 | 3.3056 | 0.7232 |
| 3.8093 | 2.0 | 636 | 1.9063 | 0.8445 |
| 3.8093 | 3.0 | 954 | 1.1856 | 0.8897 |
| 1.7292 | 4.0 | 1272 | 0.8850 | 0.9106 |
| 0.9328 | 5.0 | 1590 | 0.8019 | 0.9165 |
### Framework versions
- Transformers 4.37.2
- Pytorch 2.2.0+cu121
- Datasets 2.17.0
- Tokenizers 0.15.2
| {"license": "apache-2.0", "tags": ["generated_from_trainer"], "metrics": ["accuracy"], "base_model": "distilbert-base-uncased", "model-index": [{"name": "distilbert-base-uncased-finetuned-clinc", "results": []}]} | saqidr/distilbert-base-uncased-finetuned-clinc | null | [
"transformers",
"safetensors",
"distilbert",
"text-classification",
"generated_from_trainer",
"base_model:distilbert-base-uncased",
"license:apache-2.0",
"autotrain_compatible",
"endpoints_compatible",
"region:us"
] | null | 2024-04-26T17:51:02+00:00 | [] | [] | TAGS
#transformers #safetensors #distilbert #text-classification #generated_from_trainer #base_model-distilbert-base-uncased #license-apache-2.0 #autotrain_compatible #endpoints_compatible #region-us
| distilbert-base-uncased-finetuned-clinc
=======================================
This model is a fine-tuned version of distilbert-base-uncased on an unknown dataset.
It achieves the following results on the evaluation set:
* Loss: 0.8019
* Accuracy: 0.9165
Model description
-----------------
More information needed
Intended uses & limitations
---------------------------
More information needed
Training and evaluation data
----------------------------
More information needed
Training procedure
------------------
### Training hyperparameters
The following hyperparameters were used during training:
* learning\_rate: 2e-05
* train\_batch\_size: 48
* eval\_batch\_size: 48
* seed: 42
* optimizer: Adam with betas=(0.9,0.999) and epsilon=1e-08
* lr\_scheduler\_type: linear
* num\_epochs: 5
### Training results
### Framework versions
* Transformers 4.37.2
* Pytorch 2.2.0+cu121
* Datasets 2.17.0
* Tokenizers 0.15.2
| [
"### Training hyperparameters\n\n\nThe following hyperparameters were used during training:\n\n\n* learning\\_rate: 2e-05\n* train\\_batch\\_size: 48\n* eval\\_batch\\_size: 48\n* seed: 42\n* optimizer: Adam with betas=(0.9,0.999) and epsilon=1e-08\n* lr\\_scheduler\\_type: linear\n* num\\_epochs: 5",
"### Training results",
"### Framework versions\n\n\n* Transformers 4.37.2\n* Pytorch 2.2.0+cu121\n* Datasets 2.17.0\n* Tokenizers 0.15.2"
] | [
"TAGS\n#transformers #safetensors #distilbert #text-classification #generated_from_trainer #base_model-distilbert-base-uncased #license-apache-2.0 #autotrain_compatible #endpoints_compatible #region-us \n",
"### Training hyperparameters\n\n\nThe following hyperparameters were used during training:\n\n\n* learning\\_rate: 2e-05\n* train\\_batch\\_size: 48\n* eval\\_batch\\_size: 48\n* seed: 42\n* optimizer: Adam with betas=(0.9,0.999) and epsilon=1e-08\n* lr\\_scheduler\\_type: linear\n* num\\_epochs: 5",
"### Training results",
"### Framework versions\n\n\n* Transformers 4.37.2\n* Pytorch 2.2.0+cu121\n* Datasets 2.17.0\n* Tokenizers 0.15.2"
] |
image-classification | transformers |
<!-- This model card has been generated automatically according to the information the Trainer had access to. You
should probably proofread and complete it, then remove this comment. -->
# Boya1_RMSProp_1-e5_10Epoch_swin-large-patch4-window7-224-in22k_fold5
This model is a fine-tuned version of [microsoft/swin-large-patch4-window7-224-in22k](https://huggingface.co/microsoft/swin-large-patch4-window7-224-in22k) on the imagefolder dataset.
It achieves the following results on the evaluation set:
- Loss: 1.1157
- Accuracy: 0.6804
## Model description
More information needed
## Intended uses & limitations
More information needed
## Training and evaluation data
More information needed
## Training procedure
### Training hyperparameters
The following hyperparameters were used during training:
- learning_rate: 1e-05
- train_batch_size: 16
- eval_batch_size: 16
- seed: 42
- optimizer: Adam with betas=(0.9,0.999) and epsilon=1e-08
- lr_scheduler_type: linear
- lr_scheduler_warmup_ratio: 0.1
- num_epochs: 10
### Training results
| Training Loss | Epoch | Step | Validation Loss | Accuracy |
|:-------------:|:-----:|:----:|:---------------:|:--------:|
| 1.1701 | 1.0 | 924 | 1.1808 | 0.5937 |
| 0.9552 | 2.0 | 1848 | 0.9904 | 0.6598 |
| 0.7355 | 3.0 | 2772 | 0.9231 | 0.6861 |
| 0.549 | 4.0 | 3696 | 0.9522 | 0.6842 |
| 0.5075 | 5.0 | 4620 | 0.9671 | 0.6796 |
| 0.6648 | 6.0 | 5544 | 0.9868 | 0.6831 |
| 0.4425 | 7.0 | 6468 | 1.0296 | 0.6856 |
| 0.366 | 8.0 | 7392 | 1.0722 | 0.6853 |
| 0.2413 | 9.0 | 8316 | 1.1113 | 0.6812 |
| 0.2526 | 10.0 | 9240 | 1.1157 | 0.6804 |
### Framework versions
- Transformers 4.35.0
- Pytorch 2.1.0
- Datasets 2.14.6
- Tokenizers 0.14.1
| {"license": "apache-2.0", "tags": ["generated_from_trainer"], "datasets": ["imagefolder"], "metrics": ["accuracy"], "base_model": "microsoft/swin-large-patch4-window7-224-in22k", "model-index": [{"name": "Boya1_RMSProp_1-e5_10Epoch_swin-large-patch4-window7-224-in22k_fold5", "results": [{"task": {"type": "image-classification", "name": "Image Classification"}, "dataset": {"name": "imagefolder", "type": "imagefolder", "config": "default", "split": "test", "args": "default"}, "metrics": [{"type": "accuracy", "value": 0.6804011927351585, "name": "Accuracy"}]}]}]} | onizukal/Boya1_RMSProp_1-e5_10Epoch_swin-large-patch4-window7-224-in22k_fold5 | null | [
"transformers",
"safetensors",
"swin",
"image-classification",
"generated_from_trainer",
"dataset:imagefolder",
"base_model:microsoft/swin-large-patch4-window7-224-in22k",
"license:apache-2.0",
"model-index",
"autotrain_compatible",
"endpoints_compatible",
"region:us"
] | null | 2024-04-26T17:51:17+00:00 | [] | [] | TAGS
#transformers #safetensors #swin #image-classification #generated_from_trainer #dataset-imagefolder #base_model-microsoft/swin-large-patch4-window7-224-in22k #license-apache-2.0 #model-index #autotrain_compatible #endpoints_compatible #region-us
| Boya1\_RMSProp\_1-e5\_10Epoch\_swin-large-patch4-window7-224-in22k\_fold5
=========================================================================
This model is a fine-tuned version of microsoft/swin-large-patch4-window7-224-in22k on the imagefolder dataset.
It achieves the following results on the evaluation set:
* Loss: 1.1157
* Accuracy: 0.6804
Model description
-----------------
More information needed
Intended uses & limitations
---------------------------
More information needed
Training and evaluation data
----------------------------
More information needed
Training procedure
------------------
### Training hyperparameters
The following hyperparameters were used during training:
* learning\_rate: 1e-05
* train\_batch\_size: 16
* eval\_batch\_size: 16
* seed: 42
* optimizer: Adam with betas=(0.9,0.999) and epsilon=1e-08
* lr\_scheduler\_type: linear
* lr\_scheduler\_warmup\_ratio: 0.1
* num\_epochs: 10
### Training results
### Framework versions
* Transformers 4.35.0
* Pytorch 2.1.0
* Datasets 2.14.6
* Tokenizers 0.14.1
| [
"### Training hyperparameters\n\n\nThe following hyperparameters were used during training:\n\n\n* learning\\_rate: 1e-05\n* train\\_batch\\_size: 16\n* eval\\_batch\\_size: 16\n* seed: 42\n* optimizer: Adam with betas=(0.9,0.999) and epsilon=1e-08\n* lr\\_scheduler\\_type: linear\n* lr\\_scheduler\\_warmup\\_ratio: 0.1\n* num\\_epochs: 10",
"### Training results",
"### Framework versions\n\n\n* Transformers 4.35.0\n* Pytorch 2.1.0\n* Datasets 2.14.6\n* Tokenizers 0.14.1"
] | [
"TAGS\n#transformers #safetensors #swin #image-classification #generated_from_trainer #dataset-imagefolder #base_model-microsoft/swin-large-patch4-window7-224-in22k #license-apache-2.0 #model-index #autotrain_compatible #endpoints_compatible #region-us \n",
"### Training hyperparameters\n\n\nThe following hyperparameters were used during training:\n\n\n* learning\\_rate: 1e-05\n* train\\_batch\\_size: 16\n* eval\\_batch\\_size: 16\n* seed: 42\n* optimizer: Adam with betas=(0.9,0.999) and epsilon=1e-08\n* lr\\_scheduler\\_type: linear\n* lr\\_scheduler\\_warmup\\_ratio: 0.1\n* num\\_epochs: 10",
"### Training results",
"### Framework versions\n\n\n* Transformers 4.35.0\n* Pytorch 2.1.0\n* Datasets 2.14.6\n* Tokenizers 0.14.1"
] |
null | transformers |
# Uploaded model
- **Developed by:** cchakons
- **License:** apache-2.0
- **Finetuned from model :** unsloth/llama-3-8b-bnb-4bit
This llama model was trained 2x faster with [Unsloth](https://github.com/unslothai/unsloth) and Huggingface's TRL library.
[<img src="https://raw.githubusercontent.com/unslothai/unsloth/main/images/unsloth%20made%20with%20love.png" width="200"/>](https://github.com/unslothai/unsloth)
| {"language": ["en"], "license": "apache-2.0", "tags": ["text-generation-inference", "transformers", "unsloth", "llama", "trl"], "base_model": "unsloth/llama-3-8b-bnb-4bit"} | cchakons/sv_model_try | null | [
"transformers",
"safetensors",
"text-generation-inference",
"unsloth",
"llama",
"trl",
"en",
"base_model:unsloth/llama-3-8b-bnb-4bit",
"license:apache-2.0",
"endpoints_compatible",
"region:us"
] | null | 2024-04-26T17:52:54+00:00 | [] | [
"en"
] | TAGS
#transformers #safetensors #text-generation-inference #unsloth #llama #trl #en #base_model-unsloth/llama-3-8b-bnb-4bit #license-apache-2.0 #endpoints_compatible #region-us
|
# Uploaded model
- Developed by: cchakons
- License: apache-2.0
- Finetuned from model : unsloth/llama-3-8b-bnb-4bit
This llama model was trained 2x faster with Unsloth and Huggingface's TRL library.
<img src="URL width="200"/>
| [
"# Uploaded model\n\n- Developed by: cchakons\n- License: apache-2.0\n- Finetuned from model : unsloth/llama-3-8b-bnb-4bit\n\nThis llama model was trained 2x faster with Unsloth and Huggingface's TRL library.\n\n<img src=\"URL width=\"200\"/>"
] | [
"TAGS\n#transformers #safetensors #text-generation-inference #unsloth #llama #trl #en #base_model-unsloth/llama-3-8b-bnb-4bit #license-apache-2.0 #endpoints_compatible #region-us \n",
"# Uploaded model\n\n- Developed by: cchakons\n- License: apache-2.0\n- Finetuned from model : unsloth/llama-3-8b-bnb-4bit\n\nThis llama model was trained 2x faster with Unsloth and Huggingface's TRL library.\n\n<img src=\"URL width=\"200\"/>"
] |
text2text-generation | transformers |
<!-- This model card has been generated automatically according to the information the Trainer had access to. You
should probably proofread and complete it, then remove this comment. -->
# trans
This model is a fine-tuned version of [t5-small](https://huggingface.co/t5-small) on an unknown dataset.
It achieves the following results on the evaluation set:
- Loss: 3.1920
- Bleu: 0.2223
- Gen Len: 18.1849
## Model description
More information needed
## Intended uses & limitations
More information needed
## Training and evaluation data
More information needed
## Training procedure
### Training hyperparameters
The following hyperparameters were used during training:
- learning_rate: 2e-05
- train_batch_size: 16
- eval_batch_size: 16
- seed: 42
- optimizer: Adam with betas=(0.9,0.999) and epsilon=1e-08
- lr_scheduler_type: linear
- num_epochs: 2
- mixed_precision_training: Native AMP
### Training results
| Training Loss | Epoch | Step | Validation Loss | Bleu | Gen Len |
|:-------------:|:-----:|:----:|:---------------:|:------:|:-------:|
| 3.651 | 1.0 | 1617 | 3.2746 | 0.1854 | 18.197 |
| 3.5127 | 2.0 | 3234 | 3.1920 | 0.2223 | 18.1849 |
### Framework versions
- Transformers 4.40.1
- Pytorch 2.2.1+cu121
- Datasets 2.19.0
- Tokenizers 0.19.1
| {"license": "apache-2.0", "tags": ["generated_from_trainer"], "metrics": ["bleu"], "base_model": "t5-small", "model-index": [{"name": "trans", "results": []}]} | qianyihuang1203/trans | null | [
"transformers",
"tensorboard",
"safetensors",
"t5",
"text2text-generation",
"generated_from_trainer",
"base_model:t5-small",
"license:apache-2.0",
"autotrain_compatible",
"endpoints_compatible",
"text-generation-inference",
"region:us"
] | null | 2024-04-26T17:54:14+00:00 | [] | [] | TAGS
#transformers #tensorboard #safetensors #t5 #text2text-generation #generated_from_trainer #base_model-t5-small #license-apache-2.0 #autotrain_compatible #endpoints_compatible #text-generation-inference #region-us
| trans
=====
This model is a fine-tuned version of t5-small on an unknown dataset.
It achieves the following results on the evaluation set:
* Loss: 3.1920
* Bleu: 0.2223
* Gen Len: 18.1849
Model description
-----------------
More information needed
Intended uses & limitations
---------------------------
More information needed
Training and evaluation data
----------------------------
More information needed
Training procedure
------------------
### Training hyperparameters
The following hyperparameters were used during training:
* learning\_rate: 2e-05
* train\_batch\_size: 16
* eval\_batch\_size: 16
* seed: 42
* optimizer: Adam with betas=(0.9,0.999) and epsilon=1e-08
* lr\_scheduler\_type: linear
* num\_epochs: 2
* mixed\_precision\_training: Native AMP
### Training results
### Framework versions
* Transformers 4.40.1
* Pytorch 2.2.1+cu121
* Datasets 2.19.0
* Tokenizers 0.19.1
| [
"### Training hyperparameters\n\n\nThe following hyperparameters were used during training:\n\n\n* learning\\_rate: 2e-05\n* train\\_batch\\_size: 16\n* eval\\_batch\\_size: 16\n* seed: 42\n* optimizer: Adam with betas=(0.9,0.999) and epsilon=1e-08\n* lr\\_scheduler\\_type: linear\n* num\\_epochs: 2\n* mixed\\_precision\\_training: Native AMP",
"### Training results",
"### Framework versions\n\n\n* Transformers 4.40.1\n* Pytorch 2.2.1+cu121\n* Datasets 2.19.0\n* Tokenizers 0.19.1"
] | [
"TAGS\n#transformers #tensorboard #safetensors #t5 #text2text-generation #generated_from_trainer #base_model-t5-small #license-apache-2.0 #autotrain_compatible #endpoints_compatible #text-generation-inference #region-us \n",
"### Training hyperparameters\n\n\nThe following hyperparameters were used during training:\n\n\n* learning\\_rate: 2e-05\n* train\\_batch\\_size: 16\n* eval\\_batch\\_size: 16\n* seed: 42\n* optimizer: Adam with betas=(0.9,0.999) and epsilon=1e-08\n* lr\\_scheduler\\_type: linear\n* num\\_epochs: 2\n* mixed\\_precision\\_training: Native AMP",
"### Training results",
"### Framework versions\n\n\n* Transformers 4.40.1\n* Pytorch 2.2.1+cu121\n* Datasets 2.19.0\n* Tokenizers 0.19.1"
] |
null | peft |
<!-- This model card has been generated automatically according to the information the Trainer had access to. You
should probably proofread and complete it, then remove this comment. -->
# GUE_mouse_4-seqsight_4096_512_27M-L1_f
This model is a fine-tuned version of [mahdibaghbanzadeh/seqsight_4096_512_27M](https://huggingface.co/mahdibaghbanzadeh/seqsight_4096_512_27M) on the [mahdibaghbanzadeh/GUE_mouse_4](https://huggingface.co/datasets/mahdibaghbanzadeh/GUE_mouse_4) dataset.
It achieves the following results on the evaluation set:
- Loss: 0.5795
- F1 Score: 0.6958
- Accuracy: 0.6968
## Model description
More information needed
## Intended uses & limitations
More information needed
## Training and evaluation data
More information needed
## Training procedure
### Training hyperparameters
The following hyperparameters were used during training:
- learning_rate: 0.0005
- train_batch_size: 128
- eval_batch_size: 128
- seed: 42
- optimizer: Adam with betas=(0.9,0.999) and epsilon=1e-08
- lr_scheduler_type: linear
- training_steps: 10000
### Training results
| Training Loss | Epoch | Step | Validation Loss | F1 Score | Accuracy |
|:-------------:|:-----:|:-----:|:---------------:|:--------:|:--------:|
| 0.6419 | 1.69 | 200 | 0.6112 | 0.6628 | 0.6644 |
| 0.6144 | 3.39 | 400 | 0.5983 | 0.6768 | 0.6782 |
| 0.6007 | 5.08 | 600 | 0.5833 | 0.6898 | 0.6904 |
| 0.5915 | 6.78 | 800 | 0.5721 | 0.7031 | 0.7031 |
| 0.5841 | 8.47 | 1000 | 0.5657 | 0.6978 | 0.6984 |
| 0.5765 | 10.17 | 1200 | 0.5641 | 0.7033 | 0.7047 |
| 0.5678 | 11.86 | 1400 | 0.5533 | 0.7163 | 0.7164 |
| 0.5624 | 13.56 | 1600 | 0.5476 | 0.7162 | 0.7164 |
| 0.5566 | 15.25 | 1800 | 0.5443 | 0.7206 | 0.7207 |
| 0.5486 | 16.95 | 2000 | 0.5561 | 0.6972 | 0.7010 |
| 0.5477 | 18.64 | 2200 | 0.5388 | 0.7249 | 0.7254 |
| 0.5425 | 20.34 | 2400 | 0.5378 | 0.7271 | 0.7270 |
| 0.5429 | 22.03 | 2600 | 0.5655 | 0.6949 | 0.7005 |
| 0.5375 | 23.73 | 2800 | 0.5385 | 0.7287 | 0.7297 |
| 0.5342 | 25.42 | 3000 | 0.5326 | 0.7284 | 0.7286 |
| 0.5303 | 27.12 | 3200 | 0.5541 | 0.7034 | 0.7084 |
| 0.5316 | 28.81 | 3400 | 0.5365 | 0.7255 | 0.7270 |
| 0.5256 | 30.51 | 3600 | 0.5331 | 0.7350 | 0.7361 |
| 0.5259 | 32.2 | 3800 | 0.5372 | 0.7236 | 0.7254 |
| 0.5238 | 33.9 | 4000 | 0.5425 | 0.7121 | 0.7153 |
| 0.5196 | 35.59 | 4200 | 0.5500 | 0.7087 | 0.7132 |
| 0.5167 | 37.29 | 4400 | 0.5352 | 0.7225 | 0.7244 |
| 0.5195 | 38.98 | 4600 | 0.5284 | 0.7276 | 0.7286 |
| 0.5196 | 40.68 | 4800 | 0.5423 | 0.7105 | 0.7143 |
| 0.508 | 42.37 | 5000 | 0.5374 | 0.7269 | 0.7286 |
| 0.5154 | 44.07 | 5200 | 0.5329 | 0.7272 | 0.7286 |
| 0.5111 | 45.76 | 5400 | 0.5363 | 0.7235 | 0.7254 |
| 0.5114 | 47.46 | 5600 | 0.5320 | 0.7324 | 0.7334 |
| 0.5154 | 49.15 | 5800 | 0.5371 | 0.7189 | 0.7217 |
| 0.5123 | 50.85 | 6000 | 0.5333 | 0.7227 | 0.7249 |
| 0.5095 | 52.54 | 6200 | 0.5277 | 0.7341 | 0.7350 |
| 0.5105 | 54.24 | 6400 | 0.5279 | 0.7324 | 0.7334 |
| 0.5087 | 55.93 | 6600 | 0.5286 | 0.7296 | 0.7307 |
| 0.5064 | 57.63 | 6800 | 0.5314 | 0.7279 | 0.7292 |
| 0.507 | 59.32 | 7000 | 0.5451 | 0.7142 | 0.7180 |
| 0.5066 | 61.02 | 7200 | 0.5310 | 0.7277 | 0.7292 |
| 0.5055 | 62.71 | 7400 | 0.5305 | 0.7263 | 0.7276 |
| 0.5025 | 64.41 | 7600 | 0.5361 | 0.7222 | 0.7244 |
| 0.5046 | 66.1 | 7800 | 0.5343 | 0.7231 | 0.7249 |
| 0.5045 | 67.8 | 8000 | 0.5301 | 0.7258 | 0.7270 |
| 0.4996 | 69.49 | 8200 | 0.5279 | 0.7288 | 0.7297 |
| 0.5016 | 71.19 | 8400 | 0.5300 | 0.7291 | 0.7302 |
| 0.5046 | 72.88 | 8600 | 0.5298 | 0.7287 | 0.7297 |
| 0.4966 | 74.58 | 8800 | 0.5351 | 0.7233 | 0.7249 |
| 0.5012 | 76.27 | 9000 | 0.5319 | 0.7258 | 0.7270 |
| 0.5017 | 77.97 | 9200 | 0.5358 | 0.7221 | 0.7238 |
| 0.4974 | 79.66 | 9400 | 0.5318 | 0.7286 | 0.7297 |
| 0.5002 | 81.36 | 9600 | 0.5322 | 0.7264 | 0.7276 |
| 0.4999 | 83.05 | 9800 | 0.5320 | 0.7264 | 0.7276 |
| 0.4989 | 84.75 | 10000 | 0.5335 | 0.7251 | 0.7265 |
### Framework versions
- PEFT 0.9.0
- Transformers 4.38.2
- Pytorch 2.2.0+cu121
- Datasets 2.17.1
- Tokenizers 0.15.2 | {"library_name": "peft", "tags": ["generated_from_trainer"], "metrics": ["accuracy"], "base_model": "mahdibaghbanzadeh/seqsight_4096_512_27M", "model-index": [{"name": "GUE_mouse_4-seqsight_4096_512_27M-L1_f", "results": []}]} | mahdibaghbanzadeh/GUE_mouse_4-seqsight_4096_512_27M-L1_f | null | [
"peft",
"safetensors",
"generated_from_trainer",
"base_model:mahdibaghbanzadeh/seqsight_4096_512_27M",
"region:us"
] | null | 2024-04-26T17:55:46+00:00 | [] | [] | TAGS
#peft #safetensors #generated_from_trainer #base_model-mahdibaghbanzadeh/seqsight_4096_512_27M #region-us
| GUE\_mouse\_4-seqsight\_4096\_512\_27M-L1\_f
============================================
This model is a fine-tuned version of mahdibaghbanzadeh/seqsight\_4096\_512\_27M on the mahdibaghbanzadeh/GUE\_mouse\_4 dataset.
It achieves the following results on the evaluation set:
* Loss: 0.5795
* F1 Score: 0.6958
* Accuracy: 0.6968
Model description
-----------------
More information needed
Intended uses & limitations
---------------------------
More information needed
Training and evaluation data
----------------------------
More information needed
Training procedure
------------------
### Training hyperparameters
The following hyperparameters were used during training:
* learning\_rate: 0.0005
* train\_batch\_size: 128
* eval\_batch\_size: 128
* seed: 42
* optimizer: Adam with betas=(0.9,0.999) and epsilon=1e-08
* lr\_scheduler\_type: linear
* training\_steps: 10000
### Training results
### Framework versions
* PEFT 0.9.0
* Transformers 4.38.2
* Pytorch 2.2.0+cu121
* Datasets 2.17.1
* Tokenizers 0.15.2
| [
"### Training hyperparameters\n\n\nThe following hyperparameters were used during training:\n\n\n* learning\\_rate: 0.0005\n* train\\_batch\\_size: 128\n* eval\\_batch\\_size: 128\n* seed: 42\n* optimizer: Adam with betas=(0.9,0.999) and epsilon=1e-08\n* lr\\_scheduler\\_type: linear\n* training\\_steps: 10000",
"### Training results",
"### Framework versions\n\n\n* PEFT 0.9.0\n* Transformers 4.38.2\n* Pytorch 2.2.0+cu121\n* Datasets 2.17.1\n* Tokenizers 0.15.2"
] | [
"TAGS\n#peft #safetensors #generated_from_trainer #base_model-mahdibaghbanzadeh/seqsight_4096_512_27M #region-us \n",
"### Training hyperparameters\n\n\nThe following hyperparameters were used during training:\n\n\n* learning\\_rate: 0.0005\n* train\\_batch\\_size: 128\n* eval\\_batch\\_size: 128\n* seed: 42\n* optimizer: Adam with betas=(0.9,0.999) and epsilon=1e-08\n* lr\\_scheduler\\_type: linear\n* training\\_steps: 10000",
"### Training results",
"### Framework versions\n\n\n* PEFT 0.9.0\n* Transformers 4.38.2\n* Pytorch 2.2.0+cu121\n* Datasets 2.17.1\n* Tokenizers 0.15.2"
] |
text-generation | transformers | ## KoDolph-2x8b
> **Update @ 2024.04.26:** Linear Merge of [Llama-3-Open-Ko-8B-Instruct-preview](https://huggingface.co/beomi/Llama-3-Open-Ko-8B-Instruct-preview) and [dolphin-2.9-llama3-8b](https://huggingface.co/cognitivecomputations/dolphin-2.9-llama3-8b)
### Model Details
**KoDolph-2x8b:**
I had this idea at night that it would make sense to make a Linear Merge
**Model Merge:**
Linear Merge
### Composition
1. **Base Layers from Llama-3-Open-Ko-8B-Instruct-preview:**
- **Range:** Layers 0 to 20
- **Purpose:** These layers are utilized for their strong foundational language processing capabilities specifically in Korean. They are crucial for processing and understanding Korean text effectively, handling basic linguistic functions and intermediate language understanding.
2. **Advanced Layers from Dolphin-2.9-llama3-8b:**
- **Range:** Layers 15 to 24
- **Purpose:** These layers provide advanced domain-specific capabilities, particularly suited for coding and technical tasks. Beginning integration from layer 15 enhances the model's ability to manage complex scenarios involving technical language and coding tasks.
**Purpose and Utility:**
This "Linear Merge" strategically combines the strengths of both models through weighted averaging, ensuring a balanced influence in the merged output. This approach is designed to provide robust performance in applications requiring a deep understanding and generation of Korean text, along with the capability to handle specialized tasks involving technical descriptions and coding. It is ideal for creating advanced AI assistants, coding bots, or any application where high linguistic and technical precision is needed.
### Configuration
```yaml
models:
- model: beomi/Llama-3-Open-Ko-8B-Instruct-preview
parameters:
weight: 0.5 # Equal weight to maintain balance between foundational language processing and advanced technical tasks
layer_range: [0, 20] # Use foundational and intermediate language processing layers in Korean
- model: cognitivecomputations/dolphin-2.9-llama3-8b
parameters:
weight: 0.5 # Equal weight to complement and balance the capabilities of the Llama model
layer_range: [15, 24] # Utilize advanced coding and domain-specific layers
merge_method: linear # Balanced combination of layers using a weighted average
dtype: float16 # Efficient resource usage for computational performance
```
### Test Result
**Root Cause:**
- **Bad Response:** There were some strange answers, so I think there may have been a problem during the merge process. We are merging and investigating again as the instructions are not in the Korean version.
<a href="https://ibb.co/nLtW1QF"><img src="https://i.ibb.co/ZM7Zgc0/Screenshot-2024-04-27-at-12-25-46-PM.png" alt="Screenshot-2024-04-27-at-12-25-46-PM" border="0" /></a>
| {"language": ["en", "ko"], "license": "other", "tags": ["mergekit", "merge", "facebook", "meta", "pytorch", "llama", "llama-3"], "pipeline_tag": "text-generation", "license_name": "llama3", "license_link": "LICENSE", "extra_gated_prompt": "### META LLAMA 3 COMMUNITY LICENSE AGREEMENT Meta Llama 3 Version Release Date: April 18, 2024\n\"Agreement\" means the terms and conditions for use, reproduction, distribution and modification of the Llama Materials set forth herein.\n\"Documentation\" means the specifications, manuals and documentation accompanying Meta Llama 3 distributed by Meta at https://llama.meta.com/get-started/.\n\"Licensee\" or \"you\" means you, or your employer or any other person or entity (if you are entering into this Agreement on such person or entity\u2019s behalf), of the age required under applicable laws, rules or regulations to provide legal consent and that has legal authority to bind your employer or such other person or entity if you are entering in this Agreement on their behalf.\n\"Meta Llama 3\" means the foundational large language models and software and algorithms, including machine-learning model code, trained model weights, inference-enabling code, training-enabling code, fine-tuning enabling code and other elements of the foregoing distributed by Meta at https://llama.meta.com/llama-downloads.\n\"Llama Materials\" means, collectively, Meta\u2019s proprietary Meta Llama 3 and Documentation (and any portion thereof) made available under this Agreement.\n\"Meta\" or \"we\" means Meta Platforms Ireland Limited (if you are located in or, if you are an entity, your principal place of business is in the EEA or Switzerland) and Meta Platforms, Inc. (if you are located outside of the EEA or Switzerland).\n \n1. License Rights and Redistribution.\na. Grant of Rights. You are granted a non-exclusive, worldwide, non-transferable and royalty-free limited license under Meta\u2019s intellectual property or other rights owned by Meta embodied in the Llama Materials to use, reproduce, distribute, copy, create derivative works of, and make modifications to the Llama Materials.\nb. Redistribution and Use.\ni. If you distribute or make available the Llama Materials (or any derivative works thereof), or a product or service that uses any of them, including another AI model, you shall (A) provide a copy of this Agreement with any such Llama Materials; and (B) prominently display \u201cBuilt with Meta Llama 3\u201d on a related website, user interface, blogpost, about page, or product documentation. If you use the Llama Materials to create, train, fine tune, or otherwise improve an AI model, which is distributed or made available, you shall also include \u201cLlama 3\u201d at the beginning of any such AI model name.\nii. If you receive Llama Materials, or any derivative works thereof, from a Licensee as part of an integrated end user product, then Section 2 of this Agreement will not apply to you.\niii. You must retain in all copies of the Llama Materials that you distribute the following attribution notice within a \u201cNotice\u201d text file distributed as a part of such copies: \u201cMeta Llama 3 is licensed under the Meta Llama 3 Community License, Copyright \u00a9 Meta Platforms, Inc. All Rights Reserved.\u201d\niv. Your use of the Llama Materials must comply with applicable laws and regulations (including trade compliance laws and regulations) and adhere to the Acceptable Use Policy for the Llama Materials (available at https://llama.meta.com/llama3/use-policy), which is hereby incorporated by reference into this Agreement.\nv. You will not use the Llama Materials or any output or results of the Llama Materials to improve any other large language model (excluding Meta Llama 3 or derivative works thereof).\n2. Additional Commercial Terms. If, on the Meta Llama 3 version release date, the monthly active users of the products or services made available by or for Licensee, or Licensee\u2019s affiliates, is greater than 700 million monthly active users in the preceding calendar month, you must request a license from Meta, which Meta may grant to you in its sole discretion, and you are not authorized to exercise any of the rights under this Agreement unless or until Meta otherwise expressly grants you such rights.\n3. Disclaimer of Warranty. UNLESS REQUIRED BY APPLICABLE LAW, THE LLAMA MATERIALS AND ANY OUTPUT AND RESULTS THEREFROM ARE PROVIDED ON AN \u201cAS IS\u201d BASIS, WITHOUT WARRANTIES OF ANY KIND, AND META DISCLAIMS ALL WARRANTIES OF ANY KIND, BOTH EXPRESS AND IMPLIED, INCLUDING, WITHOUT LIMITATION, ANY WARRANTIES OF TITLE, NON-INFRINGEMENT, MERCHANTABILITY, OR FITNESS FOR A PARTICULAR PURPOSE. YOU ARE SOLELY RESPONSIBLE FOR DETERMINING THE APPROPRIATENESS OF USING OR REDISTRIBUTING THE LLAMA MATERIALS AND ASSUME ANY RISKS ASSOCIATED WITH YOUR USE OF THE LLAMA MATERIALS AND ANY OUTPUT AND RESULTS.\n4. Limitation of Liability. IN NO EVENT WILL META OR ITS AFFILIATES BE LIABLE UNDER ANY THEORY OF LIABILITY, WHETHER IN CONTRACT, TORT, NEGLIGENCE, PRODUCTS LIABILITY, OR OTHERWISE, ARISING OUT OF THIS AGREEMENT, FOR ANY LOST PROFITS OR ANY INDIRECT, SPECIAL, CONSEQUENTIAL, INCIDENTAL, EXEMPLARY OR PUNITIVE DAMAGES, EVEN IF META OR ITS AFFILIATES HAVE BEEN ADVISED OF THE POSSIBILITY OF ANY OF THE FOREGOING.\n5. Intellectual Property.\na. No trademark licenses are granted under this Agreement, and in connection with the Llama Materials, neither Meta nor Licensee may use any name or mark owned by or associated with the other or any of its affiliates, except as required for reasonable and customary use in describing and redistributing the Llama Materials or as set forth in this Section 5(a). Meta hereby grants you a license to use \u201cLlama 3\u201d (the \u201cMark\u201d) solely as required to comply with the last sentence of Section 1.b.i. You will comply with Meta\u2019s brand guidelines (currently accessible at https://about.meta.com/brand/resources/meta/company-brand/ ). All goodwill arising out of your use of the Mark will inure to the benefit of Meta.\nb. Subject to Meta\u2019s ownership of Llama Materials and derivatives made by or for Meta, with respect to any derivative works and modifications of the Llama Materials that are made by you, as between you and Meta, you are and will be the owner of such derivative works and modifications.\nc. If you institute litigation or other proceedings against Meta or any entity (including a cross-claim or counterclaim in a lawsuit) alleging that the Llama Materials or Meta Llama 3 outputs or results, or any portion of any of the foregoing, constitutes infringement of intellectual property or other rights owned or licensable by you, then any licenses granted to you under this Agreement shall terminate as of the date such litigation or claim is filed or instituted. You will indemnify and hold harmless Meta from and against any claim by any third party arising out of or related to your use or distribution of the Llama Materials.\n6. Term and Termination. The term of this Agreement will commence upon your acceptance of this Agreement or access to the Llama Materials and will continue in full force and effect until terminated in accordance with the terms and conditions herein. Meta may terminate this Agreement if you are in breach of any term or condition of this Agreement. Upon termination of this Agreement, you shall delete and cease use of the Llama Materials. Sections 3, 4 and 7 shall survive the termination of this Agreement.\n7. Governing Law and Jurisdiction. This Agreement will be governed and construed under the laws of the State of California without regard to choice of law principles, and the UN Convention on Contracts for the International Sale of Goods does not apply to this Agreement. The courts of California shall have exclusive jurisdiction of any dispute arising out of this Agreement.\n### Meta Llama 3 Acceptable Use Policy\nMeta is committed to promoting safe and fair use of its tools and features, including Meta Llama 3. If you access or use Meta Llama 3, you agree to this Acceptable Use Policy (\u201cPolicy\u201d). The most recent copy of this policy can be found at [https://llama.meta.com/llama3/use-policy](https://llama.meta.com/llama3/use-policy)\n#### Prohibited Uses\nWe want everyone to use Meta Llama 3 safely and responsibly. You agree you will not use, or allow others to use, Meta Llama 3 to: 1. Violate the law or others\u2019 rights, including to:\n 1. Engage in, promote, generate, contribute to, encourage, plan, incite, or further illegal or unlawful activity or content, such as:\n 1. Violence or terrorism\n 2. Exploitation or harm to children, including the solicitation, creation, acquisition, or dissemination of child exploitative content or failure to report Child Sexual Abuse Material\n 3. Human trafficking, exploitation, and sexual violence\n 4. The illegal distribution of information or materials to minors, including obscene materials, or failure to employ legally required age-gating in connection with such information or materials.\n 5. Sexual solicitation\n 6. Any other criminal activity\n 2. Engage in, promote, incite, or facilitate the harassment, abuse, threatening, or bullying of individuals or groups of individuals\n 3. Engage in, promote, incite, or facilitate discrimination or other unlawful or harmful conduct in the provision of employment, employment benefits, credit, housing, other economic benefits, or other essential goods and services\n 4. Engage in the unauthorized or unlicensed practice of any profession including, but not limited to, financial, legal, medical/health, or related professional practices\n 5. Collect, process, disclose, generate, or infer health, demographic, or other sensitive personal or private information about individuals without rights and consents required by applicable laws\n 6. Engage in or facilitate any action or generate any content that infringes, misappropriates, or otherwise violates any third-party rights, including the outputs or results of any products or services using the Llama Materials\n 7. Create, generate, or facilitate the creation of malicious code, malware, computer viruses or do anything else that could disable, overburden, interfere with or impair the proper working, integrity, operation or appearance of a website or computer system\n2. Engage in, promote, incite, facilitate, or assist in the planning or development of activities that present a risk of death or bodily harm to individuals, including use of Meta Llama 3 related to the following:\n 1. Military, warfare, nuclear industries or applications, espionage, use for materials or activities that are subject to the International Traffic Arms Regulations (ITAR) maintained by the United States Department of State\n 2. Guns and illegal weapons (including weapon development)\n 3. Illegal drugs and regulated/controlled substances\n 4. Operation of critical infrastructure, transportation technologies, or heavy machinery\n 5. Self-harm or harm to others, including suicide, cutting, and eating disorders\n 6. Any content intended to incite or promote violence, abuse, or any infliction of bodily harm to an individual\n3. Intentionally deceive or mislead others, including use of Meta Llama 3 related to the following:\n 1. Generating, promoting, or furthering fraud or the creation or promotion of disinformation\n 2. Generating, promoting, or furthering defamatory content, including the creation of defamatory statements, images, or other content\n 3. Generating, promoting, or further distributing spam\n 4. Impersonating another individual without consent, authorization, or legal right\n 5. Representing that the use of Meta Llama 3 or outputs are human-generated\n 6. Generating or facilitating false online engagement, including fake reviews and other means of fake online engagement\n4. Fail to appropriately disclose to end users any known dangers of your AI system\nPlease report any violation of this Policy, software \u201cbug,\u201d or other problems that could lead to a violation of this Policy through one of the following means:\n * Reporting issues with the model: [https://github.com/meta-llama/llama3](https://github.com/meta-llama/llama3)\n * Reporting risky content generated by the model:\n developers.facebook.com/llama_output_feedback\n * Reporting bugs and security concerns: facebook.com/whitehat/info\n * Reporting violations of the Acceptable Use Policy or unlicensed uses of Meta Llama 3: [email protected]", "extra_gated_fields": {"First Name": "text", "Last Name": "text", "Date of birth": "date_picker", "Country": "country", "Affiliation": "text", "geo": "ip_location", "By clicking Submit below I accept the terms of the license and acknowledge that the information I provide will be collected stored processed and shared in accordance with the Meta Privacy Policy": "checkbox"}, "extra_gated_description": "The information you provide will be collected, stored, processed and shared in accordance with the [Meta Privacy Policy](https://www.facebook.com/privacy/policy/).", "extra_gated_button_content": "Submit"} | asiansoul/KoDolph-2x8b-Instruct | null | [
"transformers",
"safetensors",
"llama",
"text-generation",
"mergekit",
"merge",
"facebook",
"meta",
"pytorch",
"llama-3",
"conversational",
"en",
"ko",
"license:other",
"autotrain_compatible",
"endpoints_compatible",
"text-generation-inference",
"region:us"
] | null | 2024-04-26T17:56:35+00:00 | [] | [
"en",
"ko"
] | TAGS
#transformers #safetensors #llama #text-generation #mergekit #merge #facebook #meta #pytorch #llama-3 #conversational #en #ko #license-other #autotrain_compatible #endpoints_compatible #text-generation-inference #region-us
| ## KoDolph-2x8b
> Update @ 2024.04.26: Linear Merge of Llama-3-Open-Ko-8B-Instruct-preview and dolphin-2.9-llama3-8b
### Model Details
KoDolph-2x8b:
I had this idea at night that it would make sense to make a Linear Merge
Model Merge:
Linear Merge
### Composition
1. Base Layers from Llama-3-Open-Ko-8B-Instruct-preview:
- Range: Layers 0 to 20
- Purpose: These layers are utilized for their strong foundational language processing capabilities specifically in Korean. They are crucial for processing and understanding Korean text effectively, handling basic linguistic functions and intermediate language understanding.
2. Advanced Layers from Dolphin-2.9-llama3-8b:
- Range: Layers 15 to 24
- Purpose: These layers provide advanced domain-specific capabilities, particularly suited for coding and technical tasks. Beginning integration from layer 15 enhances the model's ability to manage complex scenarios involving technical language and coding tasks.
Purpose and Utility:
This "Linear Merge" strategically combines the strengths of both models through weighted averaging, ensuring a balanced influence in the merged output. This approach is designed to provide robust performance in applications requiring a deep understanding and generation of Korean text, along with the capability to handle specialized tasks involving technical descriptions and coding. It is ideal for creating advanced AI assistants, coding bots, or any application where high linguistic and technical precision is needed.
### Configuration
### Test Result
Root Cause:
- Bad Response: There were some strange answers, so I think there may have been a problem during the merge process. We are merging and investigating again as the instructions are not in the Korean version.
<a href="URL src="https://i.URL alt="Screenshot-2024-04-27-at-12-25-46-PM" border="0" /></a>
| [
"## KoDolph-2x8b\n\n> Update @ 2024.04.26: Linear Merge of Llama-3-Open-Ko-8B-Instruct-preview and dolphin-2.9-llama3-8b",
"### Model Details\n\nKoDolph-2x8b: \nI had this idea at night that it would make sense to make a Linear Merge\n\nModel Merge: \nLinear Merge",
"### Composition\n\n1. Base Layers from Llama-3-Open-Ko-8B-Instruct-preview:\n - Range: Layers 0 to 20\n - Purpose: These layers are utilized for their strong foundational language processing capabilities specifically in Korean. They are crucial for processing and understanding Korean text effectively, handling basic linguistic functions and intermediate language understanding.\n\n2. Advanced Layers from Dolphin-2.9-llama3-8b:\n - Range: Layers 15 to 24\n - Purpose: These layers provide advanced domain-specific capabilities, particularly suited for coding and technical tasks. Beginning integration from layer 15 enhances the model's ability to manage complex scenarios involving technical language and coding tasks.\n\nPurpose and Utility:\n\nThis \"Linear Merge\" strategically combines the strengths of both models through weighted averaging, ensuring a balanced influence in the merged output. This approach is designed to provide robust performance in applications requiring a deep understanding and generation of Korean text, along with the capability to handle specialized tasks involving technical descriptions and coding. It is ideal for creating advanced AI assistants, coding bots, or any application where high linguistic and technical precision is needed.",
"### Configuration",
"### Test Result\n\nRoot Cause:\n- Bad Response: There were some strange answers, so I think there may have been a problem during the merge process. We are merging and investigating again as the instructions are not in the Korean version.\n\n\n<a href=\"URL src=\"https://i.URL alt=\"Screenshot-2024-04-27-at-12-25-46-PM\" border=\"0\" /></a>"
] | [
"TAGS\n#transformers #safetensors #llama #text-generation #mergekit #merge #facebook #meta #pytorch #llama-3 #conversational #en #ko #license-other #autotrain_compatible #endpoints_compatible #text-generation-inference #region-us \n",
"## KoDolph-2x8b\n\n> Update @ 2024.04.26: Linear Merge of Llama-3-Open-Ko-8B-Instruct-preview and dolphin-2.9-llama3-8b",
"### Model Details\n\nKoDolph-2x8b: \nI had this idea at night that it would make sense to make a Linear Merge\n\nModel Merge: \nLinear Merge",
"### Composition\n\n1. Base Layers from Llama-3-Open-Ko-8B-Instruct-preview:\n - Range: Layers 0 to 20\n - Purpose: These layers are utilized for their strong foundational language processing capabilities specifically in Korean. They are crucial for processing and understanding Korean text effectively, handling basic linguistic functions and intermediate language understanding.\n\n2. Advanced Layers from Dolphin-2.9-llama3-8b:\n - Range: Layers 15 to 24\n - Purpose: These layers provide advanced domain-specific capabilities, particularly suited for coding and technical tasks. Beginning integration from layer 15 enhances the model's ability to manage complex scenarios involving technical language and coding tasks.\n\nPurpose and Utility:\n\nThis \"Linear Merge\" strategically combines the strengths of both models through weighted averaging, ensuring a balanced influence in the merged output. This approach is designed to provide robust performance in applications requiring a deep understanding and generation of Korean text, along with the capability to handle specialized tasks involving technical descriptions and coding. It is ideal for creating advanced AI assistants, coding bots, or any application where high linguistic and technical precision is needed.",
"### Configuration",
"### Test Result\n\nRoot Cause:\n- Bad Response: There were some strange answers, so I think there may have been a problem during the merge process. We are merging and investigating again as the instructions are not in the Korean version.\n\n\n<a href=\"URL src=\"https://i.URL alt=\"Screenshot-2024-04-27-at-12-25-46-PM\" border=\"0\" /></a>"
] |
null | peft |
<!-- This model card has been generated automatically according to the information the Trainer had access to. You
should probably proofread and complete it, then remove this comment. -->
# GUE_mouse_4-seqsight_4096_512_27M-L8_f
This model is a fine-tuned version of [mahdibaghbanzadeh/seqsight_4096_512_27M](https://huggingface.co/mahdibaghbanzadeh/seqsight_4096_512_27M) on the [mahdibaghbanzadeh/GUE_mouse_4](https://huggingface.co/datasets/mahdibaghbanzadeh/GUE_mouse_4) dataset.
It achieves the following results on the evaluation set:
- Loss: 0.5900
- F1 Score: 0.7031
- Accuracy: 0.7031
## Model description
More information needed
## Intended uses & limitations
More information needed
## Training and evaluation data
More information needed
## Training procedure
### Training hyperparameters
The following hyperparameters were used during training:
- learning_rate: 0.0005
- train_batch_size: 128
- eval_batch_size: 128
- seed: 42
- optimizer: Adam with betas=(0.9,0.999) and epsilon=1e-08
- lr_scheduler_type: linear
- training_steps: 10000
### Training results
| Training Loss | Epoch | Step | Validation Loss | F1 Score | Accuracy |
|:-------------:|:-----:|:-----:|:---------------:|:--------:|:--------:|
| 0.6363 | 1.69 | 200 | 0.6028 | 0.6692 | 0.6713 |
| 0.6046 | 3.39 | 400 | 0.5806 | 0.6875 | 0.6877 |
| 0.578 | 5.08 | 600 | 0.5600 | 0.7020 | 0.7021 |
| 0.563 | 6.78 | 800 | 0.5518 | 0.7144 | 0.7153 |
| 0.5515 | 8.47 | 1000 | 0.5677 | 0.6998 | 0.7037 |
| 0.5425 | 10.17 | 1200 | 0.5560 | 0.7170 | 0.7191 |
| 0.5324 | 11.86 | 1400 | 0.5374 | 0.7222 | 0.7223 |
| 0.5228 | 13.56 | 1600 | 0.5384 | 0.7270 | 0.7276 |
| 0.5178 | 15.25 | 1800 | 0.5315 | 0.7287 | 0.7286 |
| 0.5061 | 16.95 | 2000 | 0.5386 | 0.7174 | 0.7196 |
| 0.5024 | 18.64 | 2200 | 0.5359 | 0.7272 | 0.7281 |
| 0.4935 | 20.34 | 2400 | 0.5336 | 0.7386 | 0.7387 |
| 0.4902 | 22.03 | 2600 | 0.5667 | 0.7048 | 0.7095 |
| 0.4789 | 23.73 | 2800 | 0.5478 | 0.7295 | 0.7302 |
| 0.4735 | 25.42 | 3000 | 0.5389 | 0.7430 | 0.7430 |
| 0.4662 | 27.12 | 3200 | 0.5511 | 0.7300 | 0.7307 |
| 0.4688 | 28.81 | 3400 | 0.5470 | 0.7275 | 0.7281 |
| 0.4593 | 30.51 | 3600 | 0.5487 | 0.7386 | 0.7387 |
| 0.4533 | 32.2 | 3800 | 0.5518 | 0.7379 | 0.7382 |
| 0.4504 | 33.9 | 4000 | 0.5498 | 0.7342 | 0.7345 |
| 0.4437 | 35.59 | 4200 | 0.5627 | 0.7258 | 0.7270 |
| 0.4365 | 37.29 | 4400 | 0.5641 | 0.7409 | 0.7408 |
| 0.4357 | 38.98 | 4600 | 0.5610 | 0.7372 | 0.7371 |
| 0.4325 | 40.68 | 4800 | 0.5738 | 0.7262 | 0.7281 |
| 0.4191 | 42.37 | 5000 | 0.5743 | 0.7382 | 0.7382 |
| 0.4259 | 44.07 | 5200 | 0.5645 | 0.7377 | 0.7377 |
| 0.4203 | 45.76 | 5400 | 0.5657 | 0.7323 | 0.7323 |
| 0.4148 | 47.46 | 5600 | 0.5784 | 0.7340 | 0.7339 |
| 0.4168 | 49.15 | 5800 | 0.5707 | 0.7344 | 0.7345 |
| 0.4112 | 50.85 | 6000 | 0.5666 | 0.7399 | 0.7398 |
| 0.4077 | 52.54 | 6200 | 0.5729 | 0.7324 | 0.7323 |
| 0.4053 | 54.24 | 6400 | 0.5809 | 0.7328 | 0.7329 |
| 0.4028 | 55.93 | 6600 | 0.5787 | 0.7340 | 0.7339 |
| 0.4001 | 57.63 | 6800 | 0.5831 | 0.7356 | 0.7355 |
| 0.3952 | 59.32 | 7000 | 0.5903 | 0.7293 | 0.7297 |
| 0.3945 | 61.02 | 7200 | 0.5842 | 0.7358 | 0.7361 |
| 0.3911 | 62.71 | 7400 | 0.5891 | 0.7345 | 0.7345 |
| 0.3881 | 64.41 | 7600 | 0.5956 | 0.7344 | 0.7345 |
| 0.3885 | 66.1 | 7800 | 0.5911 | 0.7338 | 0.7339 |
| 0.3858 | 67.8 | 8000 | 0.5961 | 0.7319 | 0.7318 |
| 0.3822 | 69.49 | 8200 | 0.5897 | 0.7345 | 0.7345 |
| 0.3823 | 71.19 | 8400 | 0.5972 | 0.7340 | 0.7339 |
| 0.3827 | 72.88 | 8600 | 0.5985 | 0.7329 | 0.7329 |
| 0.3736 | 74.58 | 8800 | 0.6000 | 0.7313 | 0.7313 |
| 0.3798 | 76.27 | 9000 | 0.5993 | 0.7335 | 0.7334 |
| 0.3768 | 77.97 | 9200 | 0.6022 | 0.7311 | 0.7313 |
| 0.3743 | 79.66 | 9400 | 0.6030 | 0.7324 | 0.7323 |
| 0.3715 | 81.36 | 9600 | 0.6037 | 0.7346 | 0.7345 |
| 0.3748 | 83.05 | 9800 | 0.6037 | 0.7323 | 0.7323 |
| 0.3752 | 84.75 | 10000 | 0.6033 | 0.7324 | 0.7323 |
### Framework versions
- PEFT 0.9.0
- Transformers 4.38.2
- Pytorch 2.2.0+cu121
- Datasets 2.17.1
- Tokenizers 0.15.2 | {"library_name": "peft", "tags": ["generated_from_trainer"], "metrics": ["accuracy"], "base_model": "mahdibaghbanzadeh/seqsight_4096_512_27M", "model-index": [{"name": "GUE_mouse_4-seqsight_4096_512_27M-L8_f", "results": []}]} | mahdibaghbanzadeh/GUE_mouse_4-seqsight_4096_512_27M-L8_f | null | [
"peft",
"safetensors",
"generated_from_trainer",
"base_model:mahdibaghbanzadeh/seqsight_4096_512_27M",
"region:us"
] | null | 2024-04-26T17:57:13+00:00 | [] | [] | TAGS
#peft #safetensors #generated_from_trainer #base_model-mahdibaghbanzadeh/seqsight_4096_512_27M #region-us
| GUE\_mouse\_4-seqsight\_4096\_512\_27M-L8\_f
============================================
This model is a fine-tuned version of mahdibaghbanzadeh/seqsight\_4096\_512\_27M on the mahdibaghbanzadeh/GUE\_mouse\_4 dataset.
It achieves the following results on the evaluation set:
* Loss: 0.5900
* F1 Score: 0.7031
* Accuracy: 0.7031
Model description
-----------------
More information needed
Intended uses & limitations
---------------------------
More information needed
Training and evaluation data
----------------------------
More information needed
Training procedure
------------------
### Training hyperparameters
The following hyperparameters were used during training:
* learning\_rate: 0.0005
* train\_batch\_size: 128
* eval\_batch\_size: 128
* seed: 42
* optimizer: Adam with betas=(0.9,0.999) and epsilon=1e-08
* lr\_scheduler\_type: linear
* training\_steps: 10000
### Training results
### Framework versions
* PEFT 0.9.0
* Transformers 4.38.2
* Pytorch 2.2.0+cu121
* Datasets 2.17.1
* Tokenizers 0.15.2
| [
"### Training hyperparameters\n\n\nThe following hyperparameters were used during training:\n\n\n* learning\\_rate: 0.0005\n* train\\_batch\\_size: 128\n* eval\\_batch\\_size: 128\n* seed: 42\n* optimizer: Adam with betas=(0.9,0.999) and epsilon=1e-08\n* lr\\_scheduler\\_type: linear\n* training\\_steps: 10000",
"### Training results",
"### Framework versions\n\n\n* PEFT 0.9.0\n* Transformers 4.38.2\n* Pytorch 2.2.0+cu121\n* Datasets 2.17.1\n* Tokenizers 0.15.2"
] | [
"TAGS\n#peft #safetensors #generated_from_trainer #base_model-mahdibaghbanzadeh/seqsight_4096_512_27M #region-us \n",
"### Training hyperparameters\n\n\nThe following hyperparameters were used during training:\n\n\n* learning\\_rate: 0.0005\n* train\\_batch\\_size: 128\n* eval\\_batch\\_size: 128\n* seed: 42\n* optimizer: Adam with betas=(0.9,0.999) and epsilon=1e-08\n* lr\\_scheduler\\_type: linear\n* training\\_steps: 10000",
"### Training results",
"### Framework versions\n\n\n* PEFT 0.9.0\n* Transformers 4.38.2\n* Pytorch 2.2.0+cu121\n* Datasets 2.17.1\n* Tokenizers 0.15.2"
] |
null | transformers |
# Uploaded model
- **Developed by:** kchopra04
- **License:** apache-2.0
- **Finetuned from model :** unsloth/llama-3-8b-bnb-4bit
This llama model was trained 2x faster with [Unsloth](https://github.com/unslothai/unsloth) and Huggingface's TRL library.
[<img src="https://raw.githubusercontent.com/unslothai/unsloth/main/images/unsloth%20made%20with%20love.png" width="200"/>](https://github.com/unslothai/unsloth)
| {"language": ["en"], "license": "apache-2.0", "tags": ["text-generation-inference", "transformers", "unsloth", "llama", "gguf"], "base_model": "unsloth/llama-3-8b-bnb-4bit"} | kchopra04/llama3-finetune-saxs-gguf | null | [
"transformers",
"gguf",
"llama",
"text-generation-inference",
"unsloth",
"en",
"base_model:unsloth/llama-3-8b-bnb-4bit",
"license:apache-2.0",
"endpoints_compatible",
"region:us"
] | null | 2024-04-26T17:57:14+00:00 | [] | [
"en"
] | TAGS
#transformers #gguf #llama #text-generation-inference #unsloth #en #base_model-unsloth/llama-3-8b-bnb-4bit #license-apache-2.0 #endpoints_compatible #region-us
|
# Uploaded model
- Developed by: kchopra04
- License: apache-2.0
- Finetuned from model : unsloth/llama-3-8b-bnb-4bit
This llama model was trained 2x faster with Unsloth and Huggingface's TRL library.
<img src="URL width="200"/>
| [
"# Uploaded model\n\n- Developed by: kchopra04\n- License: apache-2.0\n- Finetuned from model : unsloth/llama-3-8b-bnb-4bit\n\nThis llama model was trained 2x faster with Unsloth and Huggingface's TRL library.\n\n<img src=\"URL width=\"200\"/>"
] | [
"TAGS\n#transformers #gguf #llama #text-generation-inference #unsloth #en #base_model-unsloth/llama-3-8b-bnb-4bit #license-apache-2.0 #endpoints_compatible #region-us \n",
"# Uploaded model\n\n- Developed by: kchopra04\n- License: apache-2.0\n- Finetuned from model : unsloth/llama-3-8b-bnb-4bit\n\nThis llama model was trained 2x faster with Unsloth and Huggingface's TRL library.\n\n<img src=\"URL width=\"200\"/>"
] |
null | peft |
<!-- This model card has been generated automatically according to the information the Trainer had access to. You
should probably proofread and complete it, then remove this comment. -->
# GUE_mouse_4-seqsight_4096_512_27M-L32_f
This model is a fine-tuned version of [mahdibaghbanzadeh/seqsight_4096_512_27M](https://huggingface.co/mahdibaghbanzadeh/seqsight_4096_512_27M) on the [mahdibaghbanzadeh/GUE_mouse_4](https://huggingface.co/datasets/mahdibaghbanzadeh/GUE_mouse_4) dataset.
It achieves the following results on the evaluation set:
- Loss: 0.6336
- F1 Score: 0.7125
- Accuracy: 0.7127
## Model description
More information needed
## Intended uses & limitations
More information needed
## Training and evaluation data
More information needed
## Training procedure
### Training hyperparameters
The following hyperparameters were used during training:
- learning_rate: 0.0005
- train_batch_size: 128
- eval_batch_size: 128
- seed: 42
- optimizer: Adam with betas=(0.9,0.999) and epsilon=1e-08
- lr_scheduler_type: linear
- training_steps: 10000
### Training results
| Training Loss | Epoch | Step | Validation Loss | F1 Score | Accuracy |
|:-------------:|:-----:|:-----:|:---------------:|:--------:|:--------:|
| 0.6279 | 1.69 | 200 | 0.5839 | 0.6830 | 0.6845 |
| 0.5853 | 3.39 | 400 | 0.5650 | 0.6931 | 0.6941 |
| 0.5545 | 5.08 | 600 | 0.5485 | 0.7199 | 0.7217 |
| 0.5336 | 6.78 | 800 | 0.5356 | 0.7229 | 0.7228 |
| 0.5168 | 8.47 | 1000 | 0.5650 | 0.7094 | 0.7122 |
| 0.4969 | 10.17 | 1200 | 0.5444 | 0.7244 | 0.7249 |
| 0.4813 | 11.86 | 1400 | 0.5433 | 0.7324 | 0.7323 |
| 0.4647 | 13.56 | 1600 | 0.5394 | 0.7349 | 0.7350 |
| 0.4506 | 15.25 | 1800 | 0.5537 | 0.7298 | 0.7297 |
| 0.4298 | 16.95 | 2000 | 0.5423 | 0.7350 | 0.7350 |
| 0.4194 | 18.64 | 2200 | 0.5853 | 0.7273 | 0.7281 |
| 0.4051 | 20.34 | 2400 | 0.5825 | 0.7365 | 0.7366 |
| 0.3975 | 22.03 | 2600 | 0.5968 | 0.7281 | 0.7281 |
| 0.3785 | 23.73 | 2800 | 0.5912 | 0.7304 | 0.7307 |
| 0.3721 | 25.42 | 3000 | 0.6188 | 0.7243 | 0.7244 |
| 0.3548 | 27.12 | 3200 | 0.6495 | 0.7239 | 0.7244 |
| 0.3496 | 28.81 | 3400 | 0.6275 | 0.7259 | 0.7260 |
| 0.3371 | 30.51 | 3600 | 0.6488 | 0.7249 | 0.7249 |
| 0.3225 | 32.2 | 3800 | 0.6788 | 0.7276 | 0.7276 |
| 0.3177 | 33.9 | 4000 | 0.6711 | 0.7170 | 0.7175 |
| 0.3058 | 35.59 | 4200 | 0.7007 | 0.7230 | 0.7233 |
| 0.2987 | 37.29 | 4400 | 0.7340 | 0.7253 | 0.7254 |
| 0.2881 | 38.98 | 4600 | 0.7403 | 0.7223 | 0.7223 |
| 0.2849 | 40.68 | 4800 | 0.7458 | 0.7205 | 0.7212 |
| 0.2704 | 42.37 | 5000 | 0.7612 | 0.7234 | 0.7233 |
| 0.2664 | 44.07 | 5200 | 0.7594 | 0.7217 | 0.7217 |
| 0.2599 | 45.76 | 5400 | 0.7650 | 0.7186 | 0.7185 |
| 0.2534 | 47.46 | 5600 | 0.8010 | 0.7186 | 0.7185 |
| 0.2531 | 49.15 | 5800 | 0.8018 | 0.7217 | 0.7217 |
| 0.2484 | 50.85 | 6000 | 0.7817 | 0.7195 | 0.7196 |
| 0.2361 | 52.54 | 6200 | 0.8236 | 0.7160 | 0.7159 |
| 0.2337 | 54.24 | 6400 | 0.8249 | 0.7180 | 0.7180 |
| 0.2316 | 55.93 | 6600 | 0.8268 | 0.7185 | 0.7185 |
| 0.2235 | 57.63 | 6800 | 0.8636 | 0.7112 | 0.7111 |
| 0.2172 | 59.32 | 7000 | 0.8511 | 0.7197 | 0.7196 |
| 0.2181 | 61.02 | 7200 | 0.8387 | 0.7202 | 0.7201 |
| 0.2106 | 62.71 | 7400 | 0.8557 | 0.7197 | 0.7196 |
| 0.2095 | 64.41 | 7600 | 0.8786 | 0.7165 | 0.7164 |
| 0.2089 | 66.1 | 7800 | 0.8671 | 0.7136 | 0.7138 |
| 0.1999 | 67.8 | 8000 | 0.9168 | 0.7176 | 0.7175 |
| 0.1967 | 69.49 | 8200 | 0.9169 | 0.7165 | 0.7164 |
| 0.1968 | 71.19 | 8400 | 0.9093 | 0.7138 | 0.7138 |
| 0.1967 | 72.88 | 8600 | 0.9135 | 0.7123 | 0.7122 |
| 0.1863 | 74.58 | 8800 | 0.9310 | 0.7080 | 0.7079 |
| 0.1905 | 76.27 | 9000 | 0.9374 | 0.7128 | 0.7127 |
| 0.1888 | 77.97 | 9200 | 0.9292 | 0.7160 | 0.7159 |
| 0.1878 | 79.66 | 9400 | 0.9351 | 0.7154 | 0.7153 |
| 0.1874 | 81.36 | 9600 | 0.9415 | 0.7139 | 0.7138 |
| 0.1858 | 83.05 | 9800 | 0.9370 | 0.7139 | 0.7138 |
| 0.1836 | 84.75 | 10000 | 0.9407 | 0.7117 | 0.7116 |
### Framework versions
- PEFT 0.9.0
- Transformers 4.38.2
- Pytorch 2.2.0+cu121
- Datasets 2.17.1
- Tokenizers 0.15.2 | {"library_name": "peft", "tags": ["generated_from_trainer"], "metrics": ["accuracy"], "base_model": "mahdibaghbanzadeh/seqsight_4096_512_27M", "model-index": [{"name": "GUE_mouse_4-seqsight_4096_512_27M-L32_f", "results": []}]} | mahdibaghbanzadeh/GUE_mouse_4-seqsight_4096_512_27M-L32_f | null | [
"peft",
"safetensors",
"generated_from_trainer",
"base_model:mahdibaghbanzadeh/seqsight_4096_512_27M",
"region:us"
] | null | 2024-04-26T17:57:20+00:00 | [] | [] | TAGS
#peft #safetensors #generated_from_trainer #base_model-mahdibaghbanzadeh/seqsight_4096_512_27M #region-us
| GUE\_mouse\_4-seqsight\_4096\_512\_27M-L32\_f
=============================================
This model is a fine-tuned version of mahdibaghbanzadeh/seqsight\_4096\_512\_27M on the mahdibaghbanzadeh/GUE\_mouse\_4 dataset.
It achieves the following results on the evaluation set:
* Loss: 0.6336
* F1 Score: 0.7125
* Accuracy: 0.7127
Model description
-----------------
More information needed
Intended uses & limitations
---------------------------
More information needed
Training and evaluation data
----------------------------
More information needed
Training procedure
------------------
### Training hyperparameters
The following hyperparameters were used during training:
* learning\_rate: 0.0005
* train\_batch\_size: 128
* eval\_batch\_size: 128
* seed: 42
* optimizer: Adam with betas=(0.9,0.999) and epsilon=1e-08
* lr\_scheduler\_type: linear
* training\_steps: 10000
### Training results
### Framework versions
* PEFT 0.9.0
* Transformers 4.38.2
* Pytorch 2.2.0+cu121
* Datasets 2.17.1
* Tokenizers 0.15.2
| [
"### Training hyperparameters\n\n\nThe following hyperparameters were used during training:\n\n\n* learning\\_rate: 0.0005\n* train\\_batch\\_size: 128\n* eval\\_batch\\_size: 128\n* seed: 42\n* optimizer: Adam with betas=(0.9,0.999) and epsilon=1e-08\n* lr\\_scheduler\\_type: linear\n* training\\_steps: 10000",
"### Training results",
"### Framework versions\n\n\n* PEFT 0.9.0\n* Transformers 4.38.2\n* Pytorch 2.2.0+cu121\n* Datasets 2.17.1\n* Tokenizers 0.15.2"
] | [
"TAGS\n#peft #safetensors #generated_from_trainer #base_model-mahdibaghbanzadeh/seqsight_4096_512_27M #region-us \n",
"### Training hyperparameters\n\n\nThe following hyperparameters were used during training:\n\n\n* learning\\_rate: 0.0005\n* train\\_batch\\_size: 128\n* eval\\_batch\\_size: 128\n* seed: 42\n* optimizer: Adam with betas=(0.9,0.999) and epsilon=1e-08\n* lr\\_scheduler\\_type: linear\n* training\\_steps: 10000",
"### Training results",
"### Framework versions\n\n\n* PEFT 0.9.0\n* Transformers 4.38.2\n* Pytorch 2.2.0+cu121\n* Datasets 2.17.1\n* Tokenizers 0.15.2"
] |
null | peft |
<!-- This model card has been generated automatically according to the information the Trainer had access to. You
should probably proofread and complete it, then remove this comment. -->
# GUE_mouse_3-seqsight_4096_512_27M-L1_f
This model is a fine-tuned version of [mahdibaghbanzadeh/seqsight_4096_512_27M](https://huggingface.co/mahdibaghbanzadeh/seqsight_4096_512_27M) on the [mahdibaghbanzadeh/GUE_mouse_3](https://huggingface.co/datasets/mahdibaghbanzadeh/GUE_mouse_3) dataset.
It achieves the following results on the evaluation set:
- Loss: 0.6345
- F1 Score: 0.7991
- Accuracy: 0.7992
## Model description
More information needed
## Intended uses & limitations
More information needed
## Training and evaluation data
More information needed
## Training procedure
### Training hyperparameters
The following hyperparameters were used during training:
- learning_rate: 0.0005
- train_batch_size: 128
- eval_batch_size: 128
- seed: 42
- optimizer: Adam with betas=(0.9,0.999) and epsilon=1e-08
- lr_scheduler_type: linear
- training_steps: 10000
### Training results
| Training Loss | Epoch | Step | Validation Loss | F1 Score | Accuracy |
|:-------------:|:------:|:-----:|:---------------:|:--------:|:--------:|
| 0.5903 | 13.33 | 200 | 0.4986 | 0.7528 | 0.7531 |
| 0.4264 | 26.67 | 400 | 0.3442 | 0.8410 | 0.8410 |
| 0.3372 | 40.0 | 600 | 0.3347 | 0.8533 | 0.8536 |
| 0.2919 | 53.33 | 800 | 0.3285 | 0.8493 | 0.8494 |
| 0.2622 | 66.67 | 1000 | 0.3342 | 0.8577 | 0.8577 |
| 0.2369 | 80.0 | 1200 | 0.3757 | 0.8451 | 0.8452 |
| 0.2092 | 93.33 | 1400 | 0.4033 | 0.8536 | 0.8536 |
| 0.1922 | 106.67 | 1600 | 0.4138 | 0.8619 | 0.8619 |
| 0.1724 | 120.0 | 1800 | 0.4177 | 0.8703 | 0.8703 |
| 0.1647 | 133.33 | 2000 | 0.4359 | 0.8619 | 0.8619 |
| 0.1562 | 146.67 | 2200 | 0.4706 | 0.8576 | 0.8577 |
| 0.1457 | 160.0 | 2400 | 0.4755 | 0.8534 | 0.8536 |
| 0.136 | 173.33 | 2600 | 0.5017 | 0.8452 | 0.8452 |
| 0.1266 | 186.67 | 2800 | 0.5199 | 0.8452 | 0.8452 |
| 0.1197 | 200.0 | 3000 | 0.5534 | 0.8409 | 0.8410 |
| 0.1183 | 213.33 | 3200 | 0.5627 | 0.8452 | 0.8452 |
| 0.1147 | 226.67 | 3400 | 0.5535 | 0.8535 | 0.8536 |
| 0.1051 | 240.0 | 3600 | 0.5945 | 0.8410 | 0.8410 |
| 0.1006 | 253.33 | 3800 | 0.5833 | 0.8494 | 0.8494 |
| 0.0976 | 266.67 | 4000 | 0.5997 | 0.8494 | 0.8494 |
| 0.0921 | 280.0 | 4200 | 0.6151 | 0.8535 | 0.8536 |
| 0.0936 | 293.33 | 4400 | 0.6167 | 0.8577 | 0.8577 |
| 0.0913 | 306.67 | 4600 | 0.6181 | 0.8536 | 0.8536 |
| 0.0859 | 320.0 | 4800 | 0.6072 | 0.8493 | 0.8494 |
| 0.0806 | 333.33 | 5000 | 0.6417 | 0.8494 | 0.8494 |
| 0.0831 | 346.67 | 5200 | 0.6390 | 0.8619 | 0.8619 |
| 0.0816 | 360.0 | 5400 | 0.6398 | 0.8410 | 0.8410 |
| 0.0776 | 373.33 | 5600 | 0.6627 | 0.8535 | 0.8536 |
| 0.0775 | 386.67 | 5800 | 0.6513 | 0.8534 | 0.8536 |
| 0.0776 | 400.0 | 6000 | 0.6444 | 0.8577 | 0.8577 |
| 0.0722 | 413.33 | 6200 | 0.6659 | 0.8493 | 0.8494 |
| 0.0709 | 426.67 | 6400 | 0.6696 | 0.8493 | 0.8494 |
| 0.0757 | 440.0 | 6600 | 0.6711 | 0.8452 | 0.8452 |
| 0.0712 | 453.33 | 6800 | 0.6671 | 0.8368 | 0.8368 |
| 0.0691 | 466.67 | 7000 | 0.6419 | 0.8535 | 0.8536 |
| 0.0703 | 480.0 | 7200 | 0.6407 | 0.8494 | 0.8494 |
| 0.0712 | 493.33 | 7400 | 0.6420 | 0.8451 | 0.8452 |
| 0.0685 | 506.67 | 7600 | 0.6664 | 0.8451 | 0.8452 |
| 0.0673 | 520.0 | 7800 | 0.6903 | 0.8534 | 0.8536 |
| 0.0652 | 533.33 | 8000 | 0.6751 | 0.8577 | 0.8577 |
| 0.0688 | 546.67 | 8200 | 0.6641 | 0.8577 | 0.8577 |
| 0.067 | 560.0 | 8400 | 0.6697 | 0.8493 | 0.8494 |
| 0.0613 | 573.33 | 8600 | 0.6724 | 0.8493 | 0.8494 |
| 0.0615 | 586.67 | 8800 | 0.6757 | 0.8493 | 0.8494 |
| 0.0619 | 600.0 | 9000 | 0.6615 | 0.8577 | 0.8577 |
| 0.0615 | 613.33 | 9200 | 0.6634 | 0.8535 | 0.8536 |
| 0.0632 | 626.67 | 9400 | 0.6642 | 0.8493 | 0.8494 |
| 0.0611 | 640.0 | 9600 | 0.6666 | 0.8535 | 0.8536 |
| 0.0629 | 653.33 | 9800 | 0.6698 | 0.8493 | 0.8494 |
| 0.063 | 666.67 | 10000 | 0.6690 | 0.8493 | 0.8494 |
### Framework versions
- PEFT 0.9.0
- Transformers 4.38.2
- Pytorch 2.2.0+cu121
- Datasets 2.17.1
- Tokenizers 0.15.2 | {"library_name": "peft", "tags": ["generated_from_trainer"], "metrics": ["accuracy"], "base_model": "mahdibaghbanzadeh/seqsight_4096_512_27M", "model-index": [{"name": "GUE_mouse_3-seqsight_4096_512_27M-L1_f", "results": []}]} | mahdibaghbanzadeh/GUE_mouse_3-seqsight_4096_512_27M-L1_f | null | [
"peft",
"safetensors",
"generated_from_trainer",
"base_model:mahdibaghbanzadeh/seqsight_4096_512_27M",
"region:us"
] | null | 2024-04-26T17:58:58+00:00 | [] | [] | TAGS
#peft #safetensors #generated_from_trainer #base_model-mahdibaghbanzadeh/seqsight_4096_512_27M #region-us
| GUE\_mouse\_3-seqsight\_4096\_512\_27M-L1\_f
============================================
This model is a fine-tuned version of mahdibaghbanzadeh/seqsight\_4096\_512\_27M on the mahdibaghbanzadeh/GUE\_mouse\_3 dataset.
It achieves the following results on the evaluation set:
* Loss: 0.6345
* F1 Score: 0.7991
* Accuracy: 0.7992
Model description
-----------------
More information needed
Intended uses & limitations
---------------------------
More information needed
Training and evaluation data
----------------------------
More information needed
Training procedure
------------------
### Training hyperparameters
The following hyperparameters were used during training:
* learning\_rate: 0.0005
* train\_batch\_size: 128
* eval\_batch\_size: 128
* seed: 42
* optimizer: Adam with betas=(0.9,0.999) and epsilon=1e-08
* lr\_scheduler\_type: linear
* training\_steps: 10000
### Training results
### Framework versions
* PEFT 0.9.0
* Transformers 4.38.2
* Pytorch 2.2.0+cu121
* Datasets 2.17.1
* Tokenizers 0.15.2
| [
"### Training hyperparameters\n\n\nThe following hyperparameters were used during training:\n\n\n* learning\\_rate: 0.0005\n* train\\_batch\\_size: 128\n* eval\\_batch\\_size: 128\n* seed: 42\n* optimizer: Adam with betas=(0.9,0.999) and epsilon=1e-08\n* lr\\_scheduler\\_type: linear\n* training\\_steps: 10000",
"### Training results",
"### Framework versions\n\n\n* PEFT 0.9.0\n* Transformers 4.38.2\n* Pytorch 2.2.0+cu121\n* Datasets 2.17.1\n* Tokenizers 0.15.2"
] | [
"TAGS\n#peft #safetensors #generated_from_trainer #base_model-mahdibaghbanzadeh/seqsight_4096_512_27M #region-us \n",
"### Training hyperparameters\n\n\nThe following hyperparameters were used during training:\n\n\n* learning\\_rate: 0.0005\n* train\\_batch\\_size: 128\n* eval\\_batch\\_size: 128\n* seed: 42\n* optimizer: Adam with betas=(0.9,0.999) and epsilon=1e-08\n* lr\\_scheduler\\_type: linear\n* training\\_steps: 10000",
"### Training results",
"### Framework versions\n\n\n* PEFT 0.9.0\n* Transformers 4.38.2\n* Pytorch 2.2.0+cu121\n* Datasets 2.17.1\n* Tokenizers 0.15.2"
] |
null | peft |
<!-- This model card has been generated automatically according to the information the Trainer had access to. You
should probably proofread and complete it, then remove this comment. -->
# GUE_mouse_3-seqsight_4096_512_27M-L8_f
This model is a fine-tuned version of [mahdibaghbanzadeh/seqsight_4096_512_27M](https://huggingface.co/mahdibaghbanzadeh/seqsight_4096_512_27M) on the [mahdibaghbanzadeh/GUE_mouse_3](https://huggingface.co/datasets/mahdibaghbanzadeh/GUE_mouse_3) dataset.
It achieves the following results on the evaluation set:
- Loss: 0.4676
- F1 Score: 0.8326
- Accuracy: 0.8326
## Model description
More information needed
## Intended uses & limitations
More information needed
## Training and evaluation data
More information needed
## Training procedure
### Training hyperparameters
The following hyperparameters were used during training:
- learning_rate: 0.0005
- train_batch_size: 128
- eval_batch_size: 128
- seed: 42
- optimizer: Adam with betas=(0.9,0.999) and epsilon=1e-08
- lr_scheduler_type: linear
- training_steps: 10000
### Training results
| Training Loss | Epoch | Step | Validation Loss | F1 Score | Accuracy |
|:-------------:|:------:|:-----:|:---------------:|:--------:|:--------:|
| 0.5177 | 13.33 | 200 | 0.3281 | 0.8410 | 0.8410 |
| 0.2973 | 26.67 | 400 | 0.3334 | 0.8786 | 0.8787 |
| 0.2174 | 40.0 | 600 | 0.3442 | 0.8745 | 0.8745 |
| 0.1643 | 53.33 | 800 | 0.3881 | 0.8577 | 0.8577 |
| 0.1259 | 66.67 | 1000 | 0.4468 | 0.8576 | 0.8577 |
| 0.1017 | 80.0 | 1200 | 0.4680 | 0.8702 | 0.8703 |
| 0.0819 | 93.33 | 1400 | 0.4787 | 0.8619 | 0.8619 |
| 0.0701 | 106.67 | 1600 | 0.4808 | 0.8660 | 0.8661 |
| 0.0669 | 120.0 | 1800 | 0.4591 | 0.8535 | 0.8536 |
| 0.053 | 133.33 | 2000 | 0.5055 | 0.8619 | 0.8619 |
| 0.0519 | 146.67 | 2200 | 0.4725 | 0.8703 | 0.8703 |
| 0.0438 | 160.0 | 2400 | 0.5250 | 0.8702 | 0.8703 |
| 0.039 | 173.33 | 2600 | 0.5993 | 0.8574 | 0.8577 |
| 0.0375 | 186.67 | 2800 | 0.5148 | 0.8660 | 0.8661 |
| 0.0321 | 200.0 | 3000 | 0.5897 | 0.8660 | 0.8661 |
| 0.0333 | 213.33 | 3200 | 0.6052 | 0.8703 | 0.8703 |
| 0.031 | 226.67 | 3400 | 0.6084 | 0.8659 | 0.8661 |
| 0.0268 | 240.0 | 3600 | 0.6494 | 0.8619 | 0.8619 |
| 0.0248 | 253.33 | 3800 | 0.6453 | 0.8492 | 0.8494 |
| 0.0224 | 266.67 | 4000 | 0.7034 | 0.8661 | 0.8661 |
| 0.0204 | 280.0 | 4200 | 0.7270 | 0.8535 | 0.8536 |
| 0.0212 | 293.33 | 4400 | 0.6803 | 0.8702 | 0.8703 |
| 0.0188 | 306.67 | 4600 | 0.6722 | 0.8577 | 0.8577 |
| 0.0205 | 320.0 | 4800 | 0.6428 | 0.8619 | 0.8619 |
| 0.0181 | 333.33 | 5000 | 0.7518 | 0.8703 | 0.8703 |
| 0.0171 | 346.67 | 5200 | 0.7052 | 0.8661 | 0.8661 |
| 0.0163 | 360.0 | 5400 | 0.7272 | 0.8661 | 0.8661 |
| 0.016 | 373.33 | 5600 | 0.6844 | 0.8536 | 0.8536 |
| 0.0127 | 386.67 | 5800 | 0.7118 | 0.8618 | 0.8619 |
| 0.0121 | 400.0 | 6000 | 0.7362 | 0.8703 | 0.8703 |
| 0.0114 | 413.33 | 6200 | 0.7393 | 0.8703 | 0.8703 |
| 0.0135 | 426.67 | 6400 | 0.8458 | 0.8744 | 0.8745 |
| 0.0122 | 440.0 | 6600 | 0.8097 | 0.8577 | 0.8577 |
| 0.0117 | 453.33 | 6800 | 0.8333 | 0.8619 | 0.8619 |
| 0.0096 | 466.67 | 7000 | 0.7518 | 0.8744 | 0.8745 |
| 0.0109 | 480.0 | 7200 | 0.7978 | 0.8661 | 0.8661 |
| 0.0109 | 493.33 | 7400 | 0.8350 | 0.8661 | 0.8661 |
| 0.0103 | 506.67 | 7600 | 0.8452 | 0.8745 | 0.8745 |
| 0.0105 | 520.0 | 7800 | 0.7969 | 0.8745 | 0.8745 |
| 0.01 | 533.33 | 8000 | 0.8034 | 0.8703 | 0.8703 |
| 0.0091 | 546.67 | 8200 | 0.7955 | 0.8661 | 0.8661 |
| 0.0107 | 560.0 | 8400 | 0.7913 | 0.8661 | 0.8661 |
| 0.0082 | 573.33 | 8600 | 0.8030 | 0.8703 | 0.8703 |
| 0.0068 | 586.67 | 8800 | 0.8139 | 0.8703 | 0.8703 |
| 0.0083 | 600.0 | 9000 | 0.7869 | 0.8703 | 0.8703 |
| 0.0068 | 613.33 | 9200 | 0.8095 | 0.8703 | 0.8703 |
| 0.0072 | 626.67 | 9400 | 0.8403 | 0.8745 | 0.8745 |
| 0.0074 | 640.0 | 9600 | 0.8105 | 0.8703 | 0.8703 |
| 0.0068 | 653.33 | 9800 | 0.8260 | 0.8745 | 0.8745 |
| 0.0078 | 666.67 | 10000 | 0.8269 | 0.8745 | 0.8745 |
### Framework versions
- PEFT 0.9.0
- Transformers 4.38.2
- Pytorch 2.2.0+cu121
- Datasets 2.17.1
- Tokenizers 0.15.2 | {"library_name": "peft", "tags": ["generated_from_trainer"], "metrics": ["accuracy"], "base_model": "mahdibaghbanzadeh/seqsight_4096_512_27M", "model-index": [{"name": "GUE_mouse_3-seqsight_4096_512_27M-L8_f", "results": []}]} | mahdibaghbanzadeh/GUE_mouse_3-seqsight_4096_512_27M-L8_f | null | [
"peft",
"safetensors",
"generated_from_trainer",
"base_model:mahdibaghbanzadeh/seqsight_4096_512_27M",
"region:us"
] | null | 2024-04-26T17:59:06+00:00 | [] | [] | TAGS
#peft #safetensors #generated_from_trainer #base_model-mahdibaghbanzadeh/seqsight_4096_512_27M #region-us
| GUE\_mouse\_3-seqsight\_4096\_512\_27M-L8\_f
============================================
This model is a fine-tuned version of mahdibaghbanzadeh/seqsight\_4096\_512\_27M on the mahdibaghbanzadeh/GUE\_mouse\_3 dataset.
It achieves the following results on the evaluation set:
* Loss: 0.4676
* F1 Score: 0.8326
* Accuracy: 0.8326
Model description
-----------------
More information needed
Intended uses & limitations
---------------------------
More information needed
Training and evaluation data
----------------------------
More information needed
Training procedure
------------------
### Training hyperparameters
The following hyperparameters were used during training:
* learning\_rate: 0.0005
* train\_batch\_size: 128
* eval\_batch\_size: 128
* seed: 42
* optimizer: Adam with betas=(0.9,0.999) and epsilon=1e-08
* lr\_scheduler\_type: linear
* training\_steps: 10000
### Training results
### Framework versions
* PEFT 0.9.0
* Transformers 4.38.2
* Pytorch 2.2.0+cu121
* Datasets 2.17.1
* Tokenizers 0.15.2
| [
"### Training hyperparameters\n\n\nThe following hyperparameters were used during training:\n\n\n* learning\\_rate: 0.0005\n* train\\_batch\\_size: 128\n* eval\\_batch\\_size: 128\n* seed: 42\n* optimizer: Adam with betas=(0.9,0.999) and epsilon=1e-08\n* lr\\_scheduler\\_type: linear\n* training\\_steps: 10000",
"### Training results",
"### Framework versions\n\n\n* PEFT 0.9.0\n* Transformers 4.38.2\n* Pytorch 2.2.0+cu121\n* Datasets 2.17.1\n* Tokenizers 0.15.2"
] | [
"TAGS\n#peft #safetensors #generated_from_trainer #base_model-mahdibaghbanzadeh/seqsight_4096_512_27M #region-us \n",
"### Training hyperparameters\n\n\nThe following hyperparameters were used during training:\n\n\n* learning\\_rate: 0.0005\n* train\\_batch\\_size: 128\n* eval\\_batch\\_size: 128\n* seed: 42\n* optimizer: Adam with betas=(0.9,0.999) and epsilon=1e-08\n* lr\\_scheduler\\_type: linear\n* training\\_steps: 10000",
"### Training results",
"### Framework versions\n\n\n* PEFT 0.9.0\n* Transformers 4.38.2\n* Pytorch 2.2.0+cu121\n* Datasets 2.17.1\n* Tokenizers 0.15.2"
] |
null | peft |
<!-- This model card has been generated automatically according to the information the Trainer had access to. You
should probably proofread and complete it, then remove this comment. -->
# GUE_mouse_3-seqsight_4096_512_27M-L32_f
This model is a fine-tuned version of [mahdibaghbanzadeh/seqsight_4096_512_27M](https://huggingface.co/mahdibaghbanzadeh/seqsight_4096_512_27M) on the [mahdibaghbanzadeh/GUE_mouse_3](https://huggingface.co/datasets/mahdibaghbanzadeh/GUE_mouse_3) dataset.
It achieves the following results on the evaluation set:
- Loss: 1.1748
- F1 Score: 0.8451
- Accuracy: 0.8452
## Model description
More information needed
## Intended uses & limitations
More information needed
## Training and evaluation data
More information needed
## Training procedure
### Training hyperparameters
The following hyperparameters were used during training:
- learning_rate: 0.0005
- train_batch_size: 128
- eval_batch_size: 128
- seed: 42
- optimizer: Adam with betas=(0.9,0.999) and epsilon=1e-08
- lr_scheduler_type: linear
- training_steps: 10000
### Training results
| Training Loss | Epoch | Step | Validation Loss | F1 Score | Accuracy |
|:-------------:|:------:|:-----:|:---------------:|:--------:|:--------:|
| 0.4542 | 13.33 | 200 | 0.3389 | 0.8659 | 0.8661 |
| 0.2137 | 26.67 | 400 | 0.4034 | 0.8618 | 0.8619 |
| 0.1324 | 40.0 | 600 | 0.4920 | 0.8535 | 0.8536 |
| 0.078 | 53.33 | 800 | 0.5033 | 0.8744 | 0.8745 |
| 0.056 | 66.67 | 1000 | 0.5309 | 0.8577 | 0.8577 |
| 0.0423 | 80.0 | 1200 | 0.5277 | 0.8786 | 0.8787 |
| 0.0318 | 93.33 | 1400 | 0.6270 | 0.8827 | 0.8828 |
| 0.0283 | 106.67 | 1600 | 0.5893 | 0.8703 | 0.8703 |
| 0.023 | 120.0 | 1800 | 0.5917 | 0.8869 | 0.8870 |
| 0.016 | 133.33 | 2000 | 0.6910 | 0.8702 | 0.8703 |
| 0.0188 | 146.67 | 2200 | 0.6562 | 0.8745 | 0.8745 |
| 0.0145 | 160.0 | 2400 | 0.6896 | 0.8661 | 0.8661 |
| 0.013 | 173.33 | 2600 | 0.7359 | 0.8661 | 0.8661 |
| 0.0125 | 186.67 | 2800 | 0.7159 | 0.8577 | 0.8577 |
| 0.0114 | 200.0 | 3000 | 0.6982 | 0.8745 | 0.8745 |
| 0.0104 | 213.33 | 3200 | 0.7969 | 0.8619 | 0.8619 |
| 0.0116 | 226.67 | 3400 | 0.6981 | 0.8619 | 0.8619 |
| 0.0074 | 240.0 | 3600 | 0.7122 | 0.8661 | 0.8661 |
| 0.0065 | 253.33 | 3800 | 0.8584 | 0.8658 | 0.8661 |
| 0.0075 | 266.67 | 4000 | 0.7644 | 0.8661 | 0.8661 |
| 0.0047 | 280.0 | 4200 | 0.7431 | 0.8828 | 0.8828 |
| 0.007 | 293.33 | 4400 | 0.7701 | 0.8745 | 0.8745 |
| 0.0047 | 306.67 | 4600 | 0.8499 | 0.8535 | 0.8536 |
| 0.0066 | 320.0 | 4800 | 0.8531 | 0.8577 | 0.8577 |
| 0.0045 | 333.33 | 5000 | 0.8765 | 0.8577 | 0.8577 |
| 0.0042 | 346.67 | 5200 | 0.9121 | 0.8660 | 0.8661 |
| 0.0046 | 360.0 | 5400 | 0.8047 | 0.8703 | 0.8703 |
| 0.0045 | 373.33 | 5600 | 0.9306 | 0.8577 | 0.8577 |
| 0.0039 | 386.67 | 5800 | 0.9161 | 0.8619 | 0.8619 |
| 0.0035 | 400.0 | 6000 | 0.9040 | 0.8786 | 0.8787 |
| 0.0026 | 413.33 | 6200 | 0.9097 | 0.8828 | 0.8828 |
| 0.0053 | 426.67 | 6400 | 0.7852 | 0.8703 | 0.8703 |
| 0.0037 | 440.0 | 6600 | 0.8481 | 0.8703 | 0.8703 |
| 0.0025 | 453.33 | 6800 | 0.8393 | 0.8745 | 0.8745 |
| 0.004 | 466.67 | 7000 | 0.8626 | 0.8828 | 0.8828 |
| 0.0038 | 480.0 | 7200 | 0.7929 | 0.8787 | 0.8787 |
| 0.003 | 493.33 | 7400 | 0.8016 | 0.8828 | 0.8828 |
| 0.0035 | 506.67 | 7600 | 0.8058 | 0.8828 | 0.8828 |
| 0.0024 | 520.0 | 7800 | 0.7645 | 0.8786 | 0.8787 |
| 0.0022 | 533.33 | 8000 | 0.7874 | 0.8870 | 0.8870 |
| 0.0027 | 546.67 | 8200 | 0.7565 | 0.8954 | 0.8954 |
| 0.0021 | 560.0 | 8400 | 0.8255 | 0.8745 | 0.8745 |
| 0.0012 | 573.33 | 8600 | 0.8135 | 0.8786 | 0.8787 |
| 0.0015 | 586.67 | 8800 | 0.8468 | 0.8701 | 0.8703 |
| 0.0015 | 600.0 | 9000 | 0.8308 | 0.8912 | 0.8912 |
| 0.0016 | 613.33 | 9200 | 0.8519 | 0.8828 | 0.8828 |
| 0.0011 | 626.67 | 9400 | 0.8783 | 0.8786 | 0.8787 |
| 0.0007 | 640.0 | 9600 | 0.8817 | 0.8828 | 0.8828 |
| 0.0011 | 653.33 | 9800 | 0.8989 | 0.8786 | 0.8787 |
| 0.0014 | 666.67 | 10000 | 0.8990 | 0.8786 | 0.8787 |
### Framework versions
- PEFT 0.9.0
- Transformers 4.38.2
- Pytorch 2.2.0+cu121
- Datasets 2.17.1
- Tokenizers 0.15.2 | {"library_name": "peft", "tags": ["generated_from_trainer"], "metrics": ["accuracy"], "base_model": "mahdibaghbanzadeh/seqsight_4096_512_27M", "model-index": [{"name": "GUE_mouse_3-seqsight_4096_512_27M-L32_f", "results": []}]} | mahdibaghbanzadeh/GUE_mouse_3-seqsight_4096_512_27M-L32_f | null | [
"peft",
"safetensors",
"generated_from_trainer",
"base_model:mahdibaghbanzadeh/seqsight_4096_512_27M",
"region:us"
] | null | 2024-04-26T17:59:23+00:00 | [] | [] | TAGS
#peft #safetensors #generated_from_trainer #base_model-mahdibaghbanzadeh/seqsight_4096_512_27M #region-us
| GUE\_mouse\_3-seqsight\_4096\_512\_27M-L32\_f
=============================================
This model is a fine-tuned version of mahdibaghbanzadeh/seqsight\_4096\_512\_27M on the mahdibaghbanzadeh/GUE\_mouse\_3 dataset.
It achieves the following results on the evaluation set:
* Loss: 1.1748
* F1 Score: 0.8451
* Accuracy: 0.8452
Model description
-----------------
More information needed
Intended uses & limitations
---------------------------
More information needed
Training and evaluation data
----------------------------
More information needed
Training procedure
------------------
### Training hyperparameters
The following hyperparameters were used during training:
* learning\_rate: 0.0005
* train\_batch\_size: 128
* eval\_batch\_size: 128
* seed: 42
* optimizer: Adam with betas=(0.9,0.999) and epsilon=1e-08
* lr\_scheduler\_type: linear
* training\_steps: 10000
### Training results
### Framework versions
* PEFT 0.9.0
* Transformers 4.38.2
* Pytorch 2.2.0+cu121
* Datasets 2.17.1
* Tokenizers 0.15.2
| [
"### Training hyperparameters\n\n\nThe following hyperparameters were used during training:\n\n\n* learning\\_rate: 0.0005\n* train\\_batch\\_size: 128\n* eval\\_batch\\_size: 128\n* seed: 42\n* optimizer: Adam with betas=(0.9,0.999) and epsilon=1e-08\n* lr\\_scheduler\\_type: linear\n* training\\_steps: 10000",
"### Training results",
"### Framework versions\n\n\n* PEFT 0.9.0\n* Transformers 4.38.2\n* Pytorch 2.2.0+cu121\n* Datasets 2.17.1\n* Tokenizers 0.15.2"
] | [
"TAGS\n#peft #safetensors #generated_from_trainer #base_model-mahdibaghbanzadeh/seqsight_4096_512_27M #region-us \n",
"### Training hyperparameters\n\n\nThe following hyperparameters were used during training:\n\n\n* learning\\_rate: 0.0005\n* train\\_batch\\_size: 128\n* eval\\_batch\\_size: 128\n* seed: 42\n* optimizer: Adam with betas=(0.9,0.999) and epsilon=1e-08\n* lr\\_scheduler\\_type: linear\n* training\\_steps: 10000",
"### Training results",
"### Framework versions\n\n\n* PEFT 0.9.0\n* Transformers 4.38.2\n* Pytorch 2.2.0+cu121\n* Datasets 2.17.1\n* Tokenizers 0.15.2"
] |
text-generation | null |
## Llamacpp imatrix Quantizations of OpenBioLLM-Llama3-8B
Using <a href="https://github.com/ggerganov/llama.cpp/">llama.cpp</a> release <a href="https://github.com/ggerganov/llama.cpp/releases/tag/b2717">b2717</a> for quantization.
Original model: https://huggingface.co/aaditya/OpenBioLLM-Llama3-8B
All quants made using imatrix option with dataset provided by Kalomaze [here](https://github.com/ggerganov/llama.cpp/discussions/5263#discussioncomment-8395384)
## Prompt format
No chat template specified so default is used. This may be incorrect, check original model card for details.
```
<|im_start|>system
{system_prompt}<|im_end|>
<|im_start|>user
{prompt}<|im_end|>
<|im_start|>assistant
```
## Download a file (not the whole branch) from below:
| Filename | Quant type | File Size | Description |
| -------- | ---------- | --------- | ----------- |
| [OpenBioLLM-Llama3-8B-Q8_0.gguf](https://huggingface.co/bartowski/OpenBioLLM-Llama3-8B-GGUF/blob/main/OpenBioLLM-Llama3-8B-Q8_0.gguf) | Q8_0 | 8.54GB | Extremely high quality, generally unneeded but max available quant. |
| [OpenBioLLM-Llama3-8B-Q6_K.gguf](https://huggingface.co/bartowski/OpenBioLLM-Llama3-8B-GGUF/blob/main/OpenBioLLM-Llama3-8B-Q6_K.gguf) | Q6_K | 6.59GB | Very high quality, near perfect, *recommended*. |
| [OpenBioLLM-Llama3-8B-Q5_K_M.gguf](https://huggingface.co/bartowski/OpenBioLLM-Llama3-8B-GGUF/blob/main/OpenBioLLM-Llama3-8B-Q5_K_M.gguf) | Q5_K_M | 5.73GB | High quality, *recommended*. |
| [OpenBioLLM-Llama3-8B-Q5_K_S.gguf](https://huggingface.co/bartowski/OpenBioLLM-Llama3-8B-GGUF/blob/main/OpenBioLLM-Llama3-8B-Q5_K_S.gguf) | Q5_K_S | 5.59GB | High quality, *recommended*. |
| [OpenBioLLM-Llama3-8B-Q4_K_M.gguf](https://huggingface.co/bartowski/OpenBioLLM-Llama3-8B-GGUF/blob/main/OpenBioLLM-Llama3-8B-Q4_K_M.gguf) | Q4_K_M | 4.92GB | Good quality, uses about 4.83 bits per weight, *recommended*. |
| [OpenBioLLM-Llama3-8B-Q4_K_S.gguf](https://huggingface.co/bartowski/OpenBioLLM-Llama3-8B-GGUF/blob/main/OpenBioLLM-Llama3-8B-Q4_K_S.gguf) | Q4_K_S | 4.69GB | Slightly lower quality with more space savings, *recommended*. |
| [OpenBioLLM-Llama3-8B-IQ4_NL.gguf](https://huggingface.co/bartowski/OpenBioLLM-Llama3-8B-GGUF/blob/main/OpenBioLLM-Llama3-8B-IQ4_NL.gguf) | IQ4_NL | 4.67GB | Decent quality, slightly smaller than Q4_K_S with similar performance *recommended*. |
| [OpenBioLLM-Llama3-8B-IQ4_XS.gguf](https://huggingface.co/bartowski/OpenBioLLM-Llama3-8B-GGUF/blob/main/OpenBioLLM-Llama3-8B-IQ4_XS.gguf) | IQ4_XS | 4.44GB | Decent quality, smaller than Q4_K_S with similar performance, *recommended*. |
| [OpenBioLLM-Llama3-8B-Q3_K_L.gguf](https://huggingface.co/bartowski/OpenBioLLM-Llama3-8B-GGUF/blob/main/OpenBioLLM-Llama3-8B-Q3_K_L.gguf) | Q3_K_L | 4.32GB | Lower quality but usable, good for low RAM availability. |
| [OpenBioLLM-Llama3-8B-Q3_K_M.gguf](https://huggingface.co/bartowski/OpenBioLLM-Llama3-8B-GGUF/blob/main/OpenBioLLM-Llama3-8B-Q3_K_M.gguf) | Q3_K_M | 4.01GB | Even lower quality. |
| [OpenBioLLM-Llama3-8B-IQ3_M.gguf](https://huggingface.co/bartowski/OpenBioLLM-Llama3-8B-GGUF/blob/main/OpenBioLLM-Llama3-8B-IQ3_M.gguf) | IQ3_M | 3.78GB | Medium-low quality, new method with decent performance comparable to Q3_K_M. |
| [OpenBioLLM-Llama3-8B-IQ3_S.gguf](https://huggingface.co/bartowski/OpenBioLLM-Llama3-8B-GGUF/blob/main/OpenBioLLM-Llama3-8B-IQ3_S.gguf) | IQ3_S | 3.68GB | Lower quality, new method with decent performance, recommended over Q3_K_S quant, same size with better performance. |
| [OpenBioLLM-Llama3-8B-Q3_K_S.gguf](https://huggingface.co/bartowski/OpenBioLLM-Llama3-8B-GGUF/blob/main/OpenBioLLM-Llama3-8B-Q3_K_S.gguf) | Q3_K_S | 3.66GB | Low quality, not recommended. |
| [OpenBioLLM-Llama3-8B-IQ3_XS.gguf](https://huggingface.co/bartowski/OpenBioLLM-Llama3-8B-GGUF/blob/main/OpenBioLLM-Llama3-8B-IQ3_XS.gguf) | IQ3_XS | 3.51GB | Lower quality, new method with decent performance, slightly better than Q3_K_S. |
| [OpenBioLLM-Llama3-8B-IQ3_XXS.gguf](https://huggingface.co/bartowski/OpenBioLLM-Llama3-8B-GGUF/blob/main/OpenBioLLM-Llama3-8B-IQ3_XXS.gguf) | IQ3_XXS | 3.27GB | Lower quality, new method with decent performance, comparable to Q3 quants. |
| [OpenBioLLM-Llama3-8B-Q2_K.gguf](https://huggingface.co/bartowski/OpenBioLLM-Llama3-8B-GGUF/blob/main/OpenBioLLM-Llama3-8B-Q2_K.gguf) | Q2_K | 3.17GB | Very low quality but surprisingly usable. |
| [OpenBioLLM-Llama3-8B-IQ2_M.gguf](https://huggingface.co/bartowski/OpenBioLLM-Llama3-8B-GGUF/blob/main/OpenBioLLM-Llama3-8B-IQ2_M.gguf) | IQ2_M | 2.94GB | Very low quality, uses SOTA techniques to also be surprisingly usable. |
| [OpenBioLLM-Llama3-8B-IQ2_S.gguf](https://huggingface.co/bartowski/OpenBioLLM-Llama3-8B-GGUF/blob/main/OpenBioLLM-Llama3-8B-IQ2_S.gguf) | IQ2_S | 2.75GB | Very low quality, uses SOTA techniques to be usable. |
| [OpenBioLLM-Llama3-8B-IQ2_XS.gguf](https://huggingface.co/bartowski/OpenBioLLM-Llama3-8B-GGUF/blob/main/OpenBioLLM-Llama3-8B-IQ2_XS.gguf) | IQ2_XS | 2.60GB | Very low quality, uses SOTA techniques to be usable. |
| [OpenBioLLM-Llama3-8B-IQ2_XXS.gguf](https://huggingface.co/bartowski/OpenBioLLM-Llama3-8B-GGUF/blob/main/OpenBioLLM-Llama3-8B-IQ2_XXS.gguf) | IQ2_XXS | 2.39GB | Lower quality, uses SOTA techniques to be usable. |
| [OpenBioLLM-Llama3-8B-IQ1_M.gguf](https://huggingface.co/bartowski/OpenBioLLM-Llama3-8B-GGUF/blob/main/OpenBioLLM-Llama3-8B-IQ1_M.gguf) | IQ1_M | 2.16GB | Extremely low quality, *not* recommended. |
| [OpenBioLLM-Llama3-8B-IQ1_S.gguf](https://huggingface.co/bartowski/OpenBioLLM-Llama3-8B-GGUF/blob/main/OpenBioLLM-Llama3-8B-IQ1_S.gguf) | IQ1_S | 2.01GB | Extremely low quality, *not* recommended. |
## Which file should I choose?
A great write up with charts showing various performances is provided by Artefact2 [here](https://gist.github.com/Artefact2/b5f810600771265fc1e39442288e8ec9)
The first thing to figure out is how big a model you can run. To do this, you'll need to figure out how much RAM and/or VRAM you have.
If you want your model running as FAST as possible, you'll want to fit the whole thing on your GPU's VRAM. Aim for a quant with a file size 1-2GB smaller than your GPU's total VRAM.
If you want the absolute maximum quality, add both your system RAM and your GPU's VRAM together, then similarly grab a quant with a file size 1-2GB Smaller than that total.
Next, you'll need to decide if you want to use an 'I-quant' or a 'K-quant'.
If you don't want to think too much, grab one of the K-quants. These are in format 'QX_K_X', like Q5_K_M.
If you want to get more into the weeds, you can check out this extremely useful feature chart:
[llama.cpp feature matrix](https://github.com/ggerganov/llama.cpp/wiki/Feature-matrix)
But basically, if you're aiming for below Q4, and you're running cuBLAS (Nvidia) or rocBLAS (AMD), you should look towards the I-quants. These are in format IQX_X, like IQ3_M. These are newer and offer better performance for their size.
These I-quants can also be used on CPU and Apple Metal, but will be slower than their K-quant equivalent, so speed vs performance is a tradeoff you'll have to decide.
The I-quants are *not* compatible with Vulcan, which is also AMD, so if you have an AMD card double check if you're using the rocBLAS build or the Vulcan build. At the time of writing this, LM Studio has a preview with ROCm support, and other inference engines have specific builds for ROCm.
Want to support my work? Visit my ko-fi page here: https://ko-fi.com/bartowski
| {"language": ["en"], "license": "llama3", "tags": ["llama-3", "llama", "Mixtral", "instruct", "finetune", "chatml", "DPO", "RLHF", "gpt4", "distillation"], "base_model": "meta-llama/Meta-Llama-3-8B", "widget": [{"example_title": "OpenBioLLM-8B", "messages": [{"role": "system", "content": "You are an expert and experienced from the healthcare and biomedical domain with extensive medical knowledge and practical experience."}, {"role": "user", "content": "How long does it take for newborn jaundice to go away?"}], "output": {"text": "Newborn jaundice, also known as neonatal jaundice, is a common condition in newborns where the yellowing of the skin and eyes occurs due to an elevated level of bilirubin in the blood. Bilirubin is a yellow pigment that forms when red blood cells break down. In most cases, newborn jaundice resolves on its own without any specific treatment.\nThe duration of newborn jaundice can vary depending on several factors such as the underlying cause, gestational age at birth, and individual variations in bilirubin metabolism. Here are some general guidelines:\n1. Physiological jaundice: This is the most common type of newborn jaundice and usually appears within 24-72 hours after birth. It tends to peak between the second and fifth day of life and gradually improves over the next week or two. By the time the baby is one week old, the jaundice should have mostly resolved. 2. Breast milk jaundice: This type of jaundice occurs in breastfed babies and may appear later than physiological jaundice, typically between the fifth and fourteenth day of life. It tends to persist for a longer duration but usually resolves within six weeks after birth. 3. Pathological jaundice: This type of jaundice is less common and occurs due to an underlying medical condition that affects bilirubin metabolism or liver function. The duration of pathological jaundice depends on the specific cause and may require treatment.\nIt's important for parents to monitor their newborn's jaundice closely and seek medical advice if the jaundice progresses rapidly, becomes severe, or is accompanied by other symptoms such as poor feeding, lethargy, or excessive sleepiness. In these cases, further evaluation and management may be necessary. Remember that each baby is unique, and the timing of jaundice resolution can vary. If you have concerns about your newborn's jaundice, it's always best to consult with a healthcare professional for personalized advice and guidance."}}], "quantized_by": "bartowski", "pipeline_tag": "text-generation", "model-index": [{"name": "OpenBioLLM-8B", "results": []}]} | nitsuai/OpenBioLLM-Llama3-8B-GGUF | null | [
"gguf",
"llama-3",
"llama",
"Mixtral",
"instruct",
"finetune",
"chatml",
"DPO",
"RLHF",
"gpt4",
"distillation",
"text-generation",
"en",
"base_model:meta-llama/Meta-Llama-3-8B",
"license:llama3",
"region:us"
] | null | 2024-04-26T18:02:16+00:00 | [] | [
"en"
] | TAGS
#gguf #llama-3 #llama #Mixtral #instruct #finetune #chatml #DPO #RLHF #gpt4 #distillation #text-generation #en #base_model-meta-llama/Meta-Llama-3-8B #license-llama3 #region-us
| Llamacpp imatrix Quantizations of OpenBioLLM-Llama3-8B
------------------------------------------------------
Using <a href="URL release <a href="URL for quantization.
Original model: URL
All quants made using imatrix option with dataset provided by Kalomaze here
Prompt format
-------------
No chat template specified so default is used. This may be incorrect, check original model card for details.
Download a file (not the whole branch) from below:
--------------------------------------------------
Which file should I choose?
---------------------------
A great write up with charts showing various performances is provided by Artefact2 here
The first thing to figure out is how big a model you can run. To do this, you'll need to figure out how much RAM and/or VRAM you have.
If you want your model running as FAST as possible, you'll want to fit the whole thing on your GPU's VRAM. Aim for a quant with a file size 1-2GB smaller than your GPU's total VRAM.
If you want the absolute maximum quality, add both your system RAM and your GPU's VRAM together, then similarly grab a quant with a file size 1-2GB Smaller than that total.
Next, you'll need to decide if you want to use an 'I-quant' or a 'K-quant'.
If you don't want to think too much, grab one of the K-quants. These are in format 'QX\_K\_X', like Q5\_K\_M.
If you want to get more into the weeds, you can check out this extremely useful feature chart:
URL feature matrix
But basically, if you're aiming for below Q4, and you're running cuBLAS (Nvidia) or rocBLAS (AMD), you should look towards the I-quants. These are in format IQX\_X, like IQ3\_M. These are newer and offer better performance for their size.
These I-quants can also be used on CPU and Apple Metal, but will be slower than their K-quant equivalent, so speed vs performance is a tradeoff you'll have to decide.
The I-quants are *not* compatible with Vulcan, which is also AMD, so if you have an AMD card double check if you're using the rocBLAS build or the Vulcan build. At the time of writing this, LM Studio has a preview with ROCm support, and other inference engines have specific builds for ROCm.
Want to support my work? Visit my ko-fi page here: URL
| [] | [
"TAGS\n#gguf #llama-3 #llama #Mixtral #instruct #finetune #chatml #DPO #RLHF #gpt4 #distillation #text-generation #en #base_model-meta-llama/Meta-Llama-3-8B #license-llama3 #region-us \n"
] |
token-classification | transformers |
<!-- This model card has been generated automatically according to the information the Trainer had access to. You
should probably proofread and complete it, then remove this comment. -->
# group1_non_all_zero
This model is a fine-tuned version of [microsoft/deberta-v3-base](https://huggingface.co/microsoft/deberta-v3-base) on an unknown dataset.
It achieves the following results on the evaluation set:
- Loss: 0.7437
- Precision: 0.0149
- Recall: 0.1076
- F1: 0.0262
- Accuracy: 0.9260
## Model description
More information needed
## Intended uses & limitations
More information needed
## Training and evaluation data
More information needed
## Training procedure
### Training hyperparameters
The following hyperparameters were used during training:
- learning_rate: 3e-05
- train_batch_size: 32
- eval_batch_size: 32
- seed: 42
- optimizer: Adam with betas=(0.9,0.999) and epsilon=1e-08
- lr_scheduler_type: linear
- num_epochs: 15
### Training results
| Training Loss | Epoch | Step | Validation Loss | Precision | Recall | F1 | Accuracy |
|:-------------:|:-----:|:----:|:---------------:|:---------:|:------:|:------:|:--------:|
| No log | 1.0 | 15 | 1.0746 | 0.0007 | 0.0633 | 0.0013 | 0.4145 |
| No log | 2.0 | 30 | 0.8623 | 0.0023 | 0.1139 | 0.0045 | 0.6250 |
| No log | 3.0 | 45 | 0.7242 | 0.0024 | 0.0696 | 0.0046 | 0.7334 |
| No log | 4.0 | 60 | 0.6181 | 0.0037 | 0.0696 | 0.0070 | 0.8030 |
| No log | 5.0 | 75 | 0.6489 | 0.0090 | 0.1329 | 0.0169 | 0.8282 |
| No log | 6.0 | 90 | 0.6538 | 0.0091 | 0.1266 | 0.0170 | 0.8445 |
| No log | 7.0 | 105 | 0.6189 | 0.0103 | 0.1013 | 0.0188 | 0.8893 |
| No log | 8.0 | 120 | 0.6328 | 0.0101 | 0.1013 | 0.0183 | 0.8917 |
| No log | 9.0 | 135 | 0.6561 | 0.0119 | 0.1076 | 0.0215 | 0.9099 |
| No log | 10.0 | 150 | 0.6537 | 0.0152 | 0.1139 | 0.0267 | 0.9265 |
| No log | 11.0 | 165 | 0.6939 | 0.0182 | 0.1139 | 0.0314 | 0.9385 |
| No log | 12.0 | 180 | 0.7481 | 0.0113 | 0.0949 | 0.0203 | 0.9103 |
| No log | 13.0 | 195 | 0.7242 | 0.0150 | 0.1203 | 0.0267 | 0.9209 |
| No log | 14.0 | 210 | 0.7553 | 0.0140 | 0.1013 | 0.0247 | 0.9229 |
| No log | 15.0 | 225 | 0.7437 | 0.0149 | 0.1076 | 0.0262 | 0.9260 |
### Framework versions
- Transformers 4.30.0
- Pytorch 2.2.2+cu121
- Datasets 2.19.0
- Tokenizers 0.13.3
| {"license": "mit", "tags": ["generated_from_trainer"], "metrics": ["precision", "recall", "f1", "accuracy"], "model-index": [{"name": "group1_non_all_zero", "results": []}]} | anismahmahi/group1_non_all_zero | null | [
"transformers",
"pytorch",
"tensorboard",
"deberta-v2",
"token-classification",
"generated_from_trainer",
"license:mit",
"autotrain_compatible",
"endpoints_compatible",
"region:us"
] | null | 2024-04-26T18:02:17+00:00 | [] | [] | TAGS
#transformers #pytorch #tensorboard #deberta-v2 #token-classification #generated_from_trainer #license-mit #autotrain_compatible #endpoints_compatible #region-us
| group1\_non\_all\_zero
======================
This model is a fine-tuned version of microsoft/deberta-v3-base on an unknown dataset.
It achieves the following results on the evaluation set:
* Loss: 0.7437
* Precision: 0.0149
* Recall: 0.1076
* F1: 0.0262
* Accuracy: 0.9260
Model description
-----------------
More information needed
Intended uses & limitations
---------------------------
More information needed
Training and evaluation data
----------------------------
More information needed
Training procedure
------------------
### Training hyperparameters
The following hyperparameters were used during training:
* learning\_rate: 3e-05
* train\_batch\_size: 32
* eval\_batch\_size: 32
* seed: 42
* optimizer: Adam with betas=(0.9,0.999) and epsilon=1e-08
* lr\_scheduler\_type: linear
* num\_epochs: 15
### Training results
### Framework versions
* Transformers 4.30.0
* Pytorch 2.2.2+cu121
* Datasets 2.19.0
* Tokenizers 0.13.3
| [
"### Training hyperparameters\n\n\nThe following hyperparameters were used during training:\n\n\n* learning\\_rate: 3e-05\n* train\\_batch\\_size: 32\n* eval\\_batch\\_size: 32\n* seed: 42\n* optimizer: Adam with betas=(0.9,0.999) and epsilon=1e-08\n* lr\\_scheduler\\_type: linear\n* num\\_epochs: 15",
"### Training results",
"### Framework versions\n\n\n* Transformers 4.30.0\n* Pytorch 2.2.2+cu121\n* Datasets 2.19.0\n* Tokenizers 0.13.3"
] | [
"TAGS\n#transformers #pytorch #tensorboard #deberta-v2 #token-classification #generated_from_trainer #license-mit #autotrain_compatible #endpoints_compatible #region-us \n",
"### Training hyperparameters\n\n\nThe following hyperparameters were used during training:\n\n\n* learning\\_rate: 3e-05\n* train\\_batch\\_size: 32\n* eval\\_batch\\_size: 32\n* seed: 42\n* optimizer: Adam with betas=(0.9,0.999) and epsilon=1e-08\n* lr\\_scheduler\\_type: linear\n* num\\_epochs: 15",
"### Training results",
"### Framework versions\n\n\n* Transformers 4.30.0\n* Pytorch 2.2.2+cu121\n* Datasets 2.19.0\n* Tokenizers 0.13.3"
] |
text-generation | transformers |
# Keiana-L3-Test5.75-8B-13.5
Keiana-L3-Test5.75-8B-13.5 is a merge of the following models using [LazyMergekit](https://colab.research.google.com/drive/1obulZ1ROXHjYLn6PPZJwRR6GzgQogxxb?usp=sharing):
# Keep in mind that, this merged model isn't usually tested at the moment, which could benefit in vocabulary error.
* [Kaoeiri/Keiana-L3-Test5.4-8B-10](https://huggingface.co/Kaoeiri/Keiana-L3-Test5.4-8B-10)
* [Undi95/Llama-3-LewdPlay-8B](https://huggingface.co/Undi95/Llama-3-LewdPlay-8B)
* [Kaoeiri/Keiana-L3-Test4.7-8B-3](https://huggingface.co/Kaoeiri/Keiana-L3-Test4.7-8B-3)
## 🧩 Configuration
```yaml
merge_method: model_stock
dtype: float16
base_model: Kaoeiri/Keiana-L3-Test5.2-8B-8
models:
- model: Kaoeiri/Keiana-L3-Test5.4-8B-10
parameters:
weight: .23
density: .26
- model: Undi95/Llama-3-LewdPlay-8B
parameters:
weight: .26
density: .38
- model: Kaoeiri/Keiana-L3-Test4.7-8B-3
parameters:
weight: .24
density: .42
parameters:
int8_mask: true
```
## 💻 Usage
```python
!pip install -qU transformers accelerate
from transformers import AutoTokenizer
import transformers
import torch
model = "Kaoeiri/Keiana-L3-Test5.75-8B-13.5"
messages = [{"role": "user", "content": "What is a large language model?"}]
tokenizer = AutoTokenizer.from_pretrained(model)
prompt = tokenizer.apply_chat_template(messages, tokenize=False, add_generation_prompt=True)
pipeline = transformers.pipeline(
"text-generation",
model=model,
torch_dtype=torch.float16,
device_map="auto",
)
outputs = pipeline(prompt, max_new_tokens=256, do_sample=True, temperature=0.7, top_k=50, top_p=0.95)
print(outputs[0]["generated_text"])
``` | {"tags": ["merge", "mergekit", "lazymergekit", "Kaoeiri/Keiana-L3-Test5.4-8B-10", "Undi95/Llama-3-LewdPlay-8B", "Kaoeiri/Keiana-L3-Test4.7-8B-3"], "base_model": ["Kaoeiri/Keiana-L3-Test5.4-8B-10", "Undi95/Llama-3-LewdPlay-8B", "Kaoeiri/Keiana-L3-Test4.7-8B-3"]} | Kaoeiri/Keiana-L3-Test5.75-8B-13.5 | null | [
"transformers",
"safetensors",
"llama",
"text-generation",
"merge",
"mergekit",
"lazymergekit",
"Kaoeiri/Keiana-L3-Test5.4-8B-10",
"Undi95/Llama-3-LewdPlay-8B",
"Kaoeiri/Keiana-L3-Test4.7-8B-3",
"conversational",
"base_model:Kaoeiri/Keiana-L3-Test5.4-8B-10",
"base_model:Undi95/Llama-3-LewdPlay-8B",
"base_model:Kaoeiri/Keiana-L3-Test4.7-8B-3",
"autotrain_compatible",
"endpoints_compatible",
"text-generation-inference",
"region:us"
] | null | 2024-04-26T18:02:28+00:00 | [] | [] | TAGS
#transformers #safetensors #llama #text-generation #merge #mergekit #lazymergekit #Kaoeiri/Keiana-L3-Test5.4-8B-10 #Undi95/Llama-3-LewdPlay-8B #Kaoeiri/Keiana-L3-Test4.7-8B-3 #conversational #base_model-Kaoeiri/Keiana-L3-Test5.4-8B-10 #base_model-Undi95/Llama-3-LewdPlay-8B #base_model-Kaoeiri/Keiana-L3-Test4.7-8B-3 #autotrain_compatible #endpoints_compatible #text-generation-inference #region-us
|
# Keiana-L3-Test5.75-8B-13.5
Keiana-L3-Test5.75-8B-13.5 is a merge of the following models using LazyMergekit:
# Keep in mind that, this merged model isn't usually tested at the moment, which could benefit in vocabulary error.
* Kaoeiri/Keiana-L3-Test5.4-8B-10
* Undi95/Llama-3-LewdPlay-8B
* Kaoeiri/Keiana-L3-Test4.7-8B-3
## Configuration
## Usage
| [
"# Keiana-L3-Test5.75-8B-13.5\n\nKeiana-L3-Test5.75-8B-13.5 is a merge of the following models using LazyMergekit:",
"# Keep in mind that, this merged model isn't usually tested at the moment, which could benefit in vocabulary error.\n* Kaoeiri/Keiana-L3-Test5.4-8B-10\n* Undi95/Llama-3-LewdPlay-8B\n* Kaoeiri/Keiana-L3-Test4.7-8B-3",
"## Configuration",
"## Usage"
] | [
"TAGS\n#transformers #safetensors #llama #text-generation #merge #mergekit #lazymergekit #Kaoeiri/Keiana-L3-Test5.4-8B-10 #Undi95/Llama-3-LewdPlay-8B #Kaoeiri/Keiana-L3-Test4.7-8B-3 #conversational #base_model-Kaoeiri/Keiana-L3-Test5.4-8B-10 #base_model-Undi95/Llama-3-LewdPlay-8B #base_model-Kaoeiri/Keiana-L3-Test4.7-8B-3 #autotrain_compatible #endpoints_compatible #text-generation-inference #region-us \n",
"# Keiana-L3-Test5.75-8B-13.5\n\nKeiana-L3-Test5.75-8B-13.5 is a merge of the following models using LazyMergekit:",
"# Keep in mind that, this merged model isn't usually tested at the moment, which could benefit in vocabulary error.\n* Kaoeiri/Keiana-L3-Test5.4-8B-10\n* Undi95/Llama-3-LewdPlay-8B\n* Kaoeiri/Keiana-L3-Test4.7-8B-3",
"## Configuration",
"## Usage"
] |
text-generation | transformers |
# Model Card for Model ID
<!-- Provide a quick summary of what the model is/does. -->
## Model Details
### Model Description
<!-- Provide a longer summary of what this model is. -->
This is the model card of a 🤗 transformers model that has been pushed on the Hub. This model card has been automatically generated.
- **Developed by:** [More Information Needed]
- **Funded by [optional]:** [More Information Needed]
- **Shared by [optional]:** [More Information Needed]
- **Model type:** [More Information Needed]
- **Language(s) (NLP):** [More Information Needed]
- **License:** [More Information Needed]
- **Finetuned from model [optional]:** [More Information Needed]
### Model Sources [optional]
<!-- Provide the basic links for the model. -->
- **Repository:** [More Information Needed]
- **Paper [optional]:** [More Information Needed]
- **Demo [optional]:** [More Information Needed]
## Uses
<!-- Address questions around how the model is intended to be used, including the foreseeable users of the model and those affected by the model. -->
### Direct Use
<!-- This section is for the model use without fine-tuning or plugging into a larger ecosystem/app. -->
[More Information Needed]
### Downstream Use [optional]
<!-- This section is for the model use when fine-tuned for a task, or when plugged into a larger ecosystem/app -->
[More Information Needed]
### Out-of-Scope Use
<!-- This section addresses misuse, malicious use, and uses that the model will not work well for. -->
[More Information Needed]
## Bias, Risks, and Limitations
<!-- This section is meant to convey both technical and sociotechnical limitations. -->
[More Information Needed]
### Recommendations
<!-- This section is meant to convey recommendations with respect to the bias, risk, and technical limitations. -->
Users (both direct and downstream) should be made aware of the risks, biases and limitations of the model. More information needed for further recommendations.
## How to Get Started with the Model
Use the code below to get started with the model.
[More Information Needed]
## Training Details
### Training Data
<!-- This should link to a Dataset Card, perhaps with a short stub of information on what the training data is all about as well as documentation related to data pre-processing or additional filtering. -->
[More Information Needed]
### Training Procedure
<!-- This relates heavily to the Technical Specifications. Content here should link to that section when it is relevant to the training procedure. -->
#### Preprocessing [optional]
[More Information Needed]
#### Training Hyperparameters
- **Training regime:** [More Information Needed] <!--fp32, fp16 mixed precision, bf16 mixed precision, bf16 non-mixed precision, fp16 non-mixed precision, fp8 mixed precision -->
#### Speeds, Sizes, Times [optional]
<!-- This section provides information about throughput, start/end time, checkpoint size if relevant, etc. -->
[More Information Needed]
## Evaluation
<!-- This section describes the evaluation protocols and provides the results. -->
### Testing Data, Factors & Metrics
#### Testing Data
<!-- This should link to a Dataset Card if possible. -->
[More Information Needed]
#### Factors
<!-- These are the things the evaluation is disaggregating by, e.g., subpopulations or domains. -->
[More Information Needed]
#### Metrics
<!-- These are the evaluation metrics being used, ideally with a description of why. -->
[More Information Needed]
### Results
[More Information Needed]
#### Summary
## Model Examination [optional]
<!-- Relevant interpretability work for the model goes here -->
[More Information Needed]
## Environmental Impact
<!-- Total emissions (in grams of CO2eq) and additional considerations, such as electricity usage, go here. Edit the suggested text below accordingly -->
Carbon emissions can be estimated using the [Machine Learning Impact calculator](https://mlco2.github.io/impact#compute) presented in [Lacoste et al. (2019)](https://arxiv.org/abs/1910.09700).
- **Hardware Type:** [More Information Needed]
- **Hours used:** [More Information Needed]
- **Cloud Provider:** [More Information Needed]
- **Compute Region:** [More Information Needed]
- **Carbon Emitted:** [More Information Needed]
## Technical Specifications [optional]
### Model Architecture and Objective
[More Information Needed]
### Compute Infrastructure
[More Information Needed]
#### Hardware
[More Information Needed]
#### Software
[More Information Needed]
## Citation [optional]
<!-- If there is a paper or blog post introducing the model, the APA and Bibtex information for that should go in this section. -->
**BibTeX:**
[More Information Needed]
**APA:**
[More Information Needed]
## Glossary [optional]
<!-- If relevant, include terms and calculations in this section that can help readers understand the model or model card. -->
[More Information Needed]
## More Information [optional]
[More Information Needed]
## Model Card Authors [optional]
[More Information Needed]
## Model Card Contact
[More Information Needed] | {"library_name": "transformers", "tags": []} | orpo-explorers/kaist-mistral-orpo-capybara-beta-0.05-1epoch | null | [
"transformers",
"safetensors",
"mistral",
"text-generation",
"conversational",
"arxiv:1910.09700",
"autotrain_compatible",
"endpoints_compatible",
"text-generation-inference",
"region:us"
] | null | 2024-04-26T18:03:25+00:00 | [
"1910.09700"
] | [] | TAGS
#transformers #safetensors #mistral #text-generation #conversational #arxiv-1910.09700 #autotrain_compatible #endpoints_compatible #text-generation-inference #region-us
|
# Model Card for Model ID
## Model Details
### Model Description
This is the model card of a transformers model that has been pushed on the Hub. This model card has been automatically generated.
- Developed by:
- Funded by [optional]:
- Shared by [optional]:
- Model type:
- Language(s) (NLP):
- License:
- Finetuned from model [optional]:
### Model Sources [optional]
- Repository:
- Paper [optional]:
- Demo [optional]:
## Uses
### Direct Use
### Downstream Use [optional]
### Out-of-Scope Use
## Bias, Risks, and Limitations
### Recommendations
Users (both direct and downstream) should be made aware of the risks, biases and limitations of the model. More information needed for further recommendations.
## How to Get Started with the Model
Use the code below to get started with the model.
## Training Details
### Training Data
### Training Procedure
#### Preprocessing [optional]
#### Training Hyperparameters
- Training regime:
#### Speeds, Sizes, Times [optional]
## Evaluation
### Testing Data, Factors & Metrics
#### Testing Data
#### Factors
#### Metrics
### Results
#### Summary
## Model Examination [optional]
## Environmental Impact
Carbon emissions can be estimated using the Machine Learning Impact calculator presented in Lacoste et al. (2019).
- Hardware Type:
- Hours used:
- Cloud Provider:
- Compute Region:
- Carbon Emitted:
## Technical Specifications [optional]
### Model Architecture and Objective
### Compute Infrastructure
#### Hardware
#### Software
[optional]
BibTeX:
APA:
## Glossary [optional]
## More Information [optional]
## Model Card Authors [optional]
## Model Card Contact
| [
"# Model Card for Model ID",
"## Model Details",
"### Model Description\n\n\n\nThis is the model card of a transformers model that has been pushed on the Hub. This model card has been automatically generated.\n\n- Developed by: \n- Funded by [optional]: \n- Shared by [optional]: \n- Model type: \n- Language(s) (NLP): \n- License: \n- Finetuned from model [optional]:",
"### Model Sources [optional]\n\n\n\n- Repository: \n- Paper [optional]: \n- Demo [optional]:",
"## Uses",
"### Direct Use",
"### Downstream Use [optional]",
"### Out-of-Scope Use",
"## Bias, Risks, and Limitations",
"### Recommendations\n\n\n\nUsers (both direct and downstream) should be made aware of the risks, biases and limitations of the model. More information needed for further recommendations.",
"## How to Get Started with the Model\n\nUse the code below to get started with the model.",
"## Training Details",
"### Training Data",
"### Training Procedure",
"#### Preprocessing [optional]",
"#### Training Hyperparameters\n\n- Training regime:",
"#### Speeds, Sizes, Times [optional]",
"## Evaluation",
"### Testing Data, Factors & Metrics",
"#### Testing Data",
"#### Factors",
"#### Metrics",
"### Results",
"#### Summary",
"## Model Examination [optional]",
"## Environmental Impact\n\n\n\nCarbon emissions can be estimated using the Machine Learning Impact calculator presented in Lacoste et al. (2019).\n\n- Hardware Type: \n- Hours used: \n- Cloud Provider: \n- Compute Region: \n- Carbon Emitted:",
"## Technical Specifications [optional]",
"### Model Architecture and Objective",
"### Compute Infrastructure",
"#### Hardware",
"#### Software\n\n\n\n[optional]\n\n\n\nBibTeX:\n\n\n\nAPA:",
"## Glossary [optional]",
"## More Information [optional]",
"## Model Card Authors [optional]",
"## Model Card Contact"
] | [
"TAGS\n#transformers #safetensors #mistral #text-generation #conversational #arxiv-1910.09700 #autotrain_compatible #endpoints_compatible #text-generation-inference #region-us \n",
"# Model Card for Model ID",
"## Model Details",
"### Model Description\n\n\n\nThis is the model card of a transformers model that has been pushed on the Hub. This model card has been automatically generated.\n\n- Developed by: \n- Funded by [optional]: \n- Shared by [optional]: \n- Model type: \n- Language(s) (NLP): \n- License: \n- Finetuned from model [optional]:",
"### Model Sources [optional]\n\n\n\n- Repository: \n- Paper [optional]: \n- Demo [optional]:",
"## Uses",
"### Direct Use",
"### Downstream Use [optional]",
"### Out-of-Scope Use",
"## Bias, Risks, and Limitations",
"### Recommendations\n\n\n\nUsers (both direct and downstream) should be made aware of the risks, biases and limitations of the model. More information needed for further recommendations.",
"## How to Get Started with the Model\n\nUse the code below to get started with the model.",
"## Training Details",
"### Training Data",
"### Training Procedure",
"#### Preprocessing [optional]",
"#### Training Hyperparameters\n\n- Training regime:",
"#### Speeds, Sizes, Times [optional]",
"## Evaluation",
"### Testing Data, Factors & Metrics",
"#### Testing Data",
"#### Factors",
"#### Metrics",
"### Results",
"#### Summary",
"## Model Examination [optional]",
"## Environmental Impact\n\n\n\nCarbon emissions can be estimated using the Machine Learning Impact calculator presented in Lacoste et al. (2019).\n\n- Hardware Type: \n- Hours used: \n- Cloud Provider: \n- Compute Region: \n- Carbon Emitted:",
"## Technical Specifications [optional]",
"### Model Architecture and Objective",
"### Compute Infrastructure",
"#### Hardware",
"#### Software\n\n\n\n[optional]\n\n\n\nBibTeX:\n\n\n\nAPA:",
"## Glossary [optional]",
"## More Information [optional]",
"## Model Card Authors [optional]",
"## Model Card Contact"
] |
text-generation | null |
## Llamacpp imatrix Quantizations of Llama-3-8B-LexiFun-Uncensored-V1
Using <a href="https://github.com/ggerganov/llama.cpp/">llama.cpp</a> release <a href="https://github.com/ggerganov/llama.cpp/releases/tag/b2717">b2717</a> for quantization.
Original model: https://huggingface.co/Orenguteng/Llama-3-8B-LexiFun-Uncensored-V1
All quants made using imatrix option with dataset provided by Kalomaze [here](https://github.com/ggerganov/llama.cpp/discussions/5263#discussioncomment-8395384)
## Prompt format
```
<|begin_of_text|><|start_header_id|>system<|end_header_id|>
{system_prompt}<|end_of_text|><|start_header_id|>user<|end_header_id|>
{prompt}<|end_of_text|><|start_header_id|>assistant<|end_header_id|>
```
## Download a file (not the whole branch) from below:
| Filename | Quant type | File Size | Description |
| -------- | ---------- | --------- | ----------- |
| [Llama-3-8B-LexiFun-Uncensored-V1-Q8_0.gguf](https://huggingface.co/bartowski/Llama-3-8B-LexiFun-Uncensored-V1-GGUF/blob/main/Llama-3-8B-LexiFun-Uncensored-V1-Q8_0.gguf) | Q8_0 | 8.54GB | Extremely high quality, generally unneeded but max available quant. |
| [Llama-3-8B-LexiFun-Uncensored-V1-Q6_K.gguf](https://huggingface.co/bartowski/Llama-3-8B-LexiFun-Uncensored-V1-GGUF/blob/main/Llama-3-8B-LexiFun-Uncensored-V1-Q6_K.gguf) | Q6_K | 6.59GB | Very high quality, near perfect, *recommended*. |
| [Llama-3-8B-LexiFun-Uncensored-V1-Q5_K_M.gguf](https://huggingface.co/bartowski/Llama-3-8B-LexiFun-Uncensored-V1-GGUF/blob/main/Llama-3-8B-LexiFun-Uncensored-V1-Q5_K_M.gguf) | Q5_K_M | 5.73GB | High quality, *recommended*. |
| [Llama-3-8B-LexiFun-Uncensored-V1-Q5_K_S.gguf](https://huggingface.co/bartowski/Llama-3-8B-LexiFun-Uncensored-V1-GGUF/blob/main/Llama-3-8B-LexiFun-Uncensored-V1-Q5_K_S.gguf) | Q5_K_S | 5.59GB | High quality, *recommended*. |
| [Llama-3-8B-LexiFun-Uncensored-V1-Q4_K_M.gguf](https://huggingface.co/bartowski/Llama-3-8B-LexiFun-Uncensored-V1-GGUF/blob/main/Llama-3-8B-LexiFun-Uncensored-V1-Q4_K_M.gguf) | Q4_K_M | 4.92GB | Good quality, uses about 4.83 bits per weight, *recommended*. |
| [Llama-3-8B-LexiFun-Uncensored-V1-Q4_K_S.gguf](https://huggingface.co/bartowski/Llama-3-8B-LexiFun-Uncensored-V1-GGUF/blob/main/Llama-3-8B-LexiFun-Uncensored-V1-Q4_K_S.gguf) | Q4_K_S | 4.69GB | Slightly lower quality with more space savings, *recommended*. |
| [Llama-3-8B-LexiFun-Uncensored-V1-IQ4_NL.gguf](https://huggingface.co/bartowski/Llama-3-8B-LexiFun-Uncensored-V1-GGUF/blob/main/Llama-3-8B-LexiFun-Uncensored-V1-IQ4_NL.gguf) | IQ4_NL | 4.67GB | Decent quality, slightly smaller than Q4_K_S with similar performance *recommended*. |
| [Llama-3-8B-LexiFun-Uncensored-V1-IQ4_XS.gguf](https://huggingface.co/bartowski/Llama-3-8B-LexiFun-Uncensored-V1-GGUF/blob/main/Llama-3-8B-LexiFun-Uncensored-V1-IQ4_XS.gguf) | IQ4_XS | 4.44GB | Decent quality, smaller than Q4_K_S with similar performance, *recommended*. |
| [Llama-3-8B-LexiFun-Uncensored-V1-Q3_K_L.gguf](https://huggingface.co/bartowski/Llama-3-8B-LexiFun-Uncensored-V1-GGUF/blob/main/Llama-3-8B-LexiFun-Uncensored-V1-Q3_K_L.gguf) | Q3_K_L | 4.32GB | Lower quality but usable, good for low RAM availability. |
| [Llama-3-8B-LexiFun-Uncensored-V1-Q3_K_M.gguf](https://huggingface.co/bartowski/Llama-3-8B-LexiFun-Uncensored-V1-GGUF/blob/main/Llama-3-8B-LexiFun-Uncensored-V1-Q3_K_M.gguf) | Q3_K_M | 4.01GB | Even lower quality. |
| [Llama-3-8B-LexiFun-Uncensored-V1-IQ3_M.gguf](https://huggingface.co/bartowski/Llama-3-8B-LexiFun-Uncensored-V1-GGUF/blob/main/Llama-3-8B-LexiFun-Uncensored-V1-IQ3_M.gguf) | IQ3_M | 3.78GB | Medium-low quality, new method with decent performance comparable to Q3_K_M. |
| [Llama-3-8B-LexiFun-Uncensored-V1-IQ3_S.gguf](https://huggingface.co/bartowski/Llama-3-8B-LexiFun-Uncensored-V1-GGUF/blob/main/Llama-3-8B-LexiFun-Uncensored-V1-IQ3_S.gguf) | IQ3_S | 3.68GB | Lower quality, new method with decent performance, recommended over Q3_K_S quant, same size with better performance. |
| [Llama-3-8B-LexiFun-Uncensored-V1-Q3_K_S.gguf](https://huggingface.co/bartowski/Llama-3-8B-LexiFun-Uncensored-V1-GGUF/blob/main/Llama-3-8B-LexiFun-Uncensored-V1-Q3_K_S.gguf) | Q3_K_S | 3.66GB | Low quality, not recommended. |
| [Llama-3-8B-LexiFun-Uncensored-V1-IQ3_XS.gguf](https://huggingface.co/bartowski/Llama-3-8B-LexiFun-Uncensored-V1-GGUF/blob/main/Llama-3-8B-LexiFun-Uncensored-V1-IQ3_XS.gguf) | IQ3_XS | 3.51GB | Lower quality, new method with decent performance, slightly better than Q3_K_S. |
| [Llama-3-8B-LexiFun-Uncensored-V1-IQ3_XXS.gguf](https://huggingface.co/bartowski/Llama-3-8B-LexiFun-Uncensored-V1-GGUF/blob/main/Llama-3-8B-LexiFun-Uncensored-V1-IQ3_XXS.gguf) | IQ3_XXS | 3.27GB | Lower quality, new method with decent performance, comparable to Q3 quants. |
| [Llama-3-8B-LexiFun-Uncensored-V1-Q2_K.gguf](https://huggingface.co/bartowski/Llama-3-8B-LexiFun-Uncensored-V1-GGUF/blob/main/Llama-3-8B-LexiFun-Uncensored-V1-Q2_K.gguf) | Q2_K | 3.17GB | Very low quality but surprisingly usable. |
| [Llama-3-8B-LexiFun-Uncensored-V1-IQ2_M.gguf](https://huggingface.co/bartowski/Llama-3-8B-LexiFun-Uncensored-V1-GGUF/blob/main/Llama-3-8B-LexiFun-Uncensored-V1-IQ2_M.gguf) | IQ2_M | 2.94GB | Very low quality, uses SOTA techniques to also be surprisingly usable. |
| [Llama-3-8B-LexiFun-Uncensored-V1-IQ2_S.gguf](https://huggingface.co/bartowski/Llama-3-8B-LexiFun-Uncensored-V1-GGUF/blob/main/Llama-3-8B-LexiFun-Uncensored-V1-IQ2_S.gguf) | IQ2_S | 2.75GB | Very low quality, uses SOTA techniques to be usable. |
| [Llama-3-8B-LexiFun-Uncensored-V1-IQ2_XS.gguf](https://huggingface.co/bartowski/Llama-3-8B-LexiFun-Uncensored-V1-GGUF/blob/main/Llama-3-8B-LexiFun-Uncensored-V1-IQ2_XS.gguf) | IQ2_XS | 2.60GB | Very low quality, uses SOTA techniques to be usable. |
| [Llama-3-8B-LexiFun-Uncensored-V1-IQ2_XXS.gguf](https://huggingface.co/bartowski/Llama-3-8B-LexiFun-Uncensored-V1-GGUF/blob/main/Llama-3-8B-LexiFun-Uncensored-V1-IQ2_XXS.gguf) | IQ2_XXS | 2.39GB | Lower quality, uses SOTA techniques to be usable. |
| [Llama-3-8B-LexiFun-Uncensored-V1-IQ1_M.gguf](https://huggingface.co/bartowski/Llama-3-8B-LexiFun-Uncensored-V1-GGUF/blob/main/Llama-3-8B-LexiFun-Uncensored-V1-IQ1_M.gguf) | IQ1_M | 2.16GB | Extremely low quality, *not* recommended. |
| [Llama-3-8B-LexiFun-Uncensored-V1-IQ1_S.gguf](https://huggingface.co/bartowski/Llama-3-8B-LexiFun-Uncensored-V1-GGUF/blob/main/Llama-3-8B-LexiFun-Uncensored-V1-IQ1_S.gguf) | IQ1_S | 2.01GB | Extremely low quality, *not* recommended. |
## Which file should I choose?
A great write up with charts showing various performances is provided by Artefact2 [here](https://gist.github.com/Artefact2/b5f810600771265fc1e39442288e8ec9)
The first thing to figure out is how big a model you can run. To do this, you'll need to figure out how much RAM and/or VRAM you have.
If you want your model running as FAST as possible, you'll want to fit the whole thing on your GPU's VRAM. Aim for a quant with a file size 1-2GB smaller than your GPU's total VRAM.
If you want the absolute maximum quality, add both your system RAM and your GPU's VRAM together, then similarly grab a quant with a file size 1-2GB Smaller than that total.
Next, you'll need to decide if you want to use an 'I-quant' or a 'K-quant'.
If you don't want to think too much, grab one of the K-quants. These are in format 'QX_K_X', like Q5_K_M.
If you want to get more into the weeds, you can check out this extremely useful feature chart:
[llama.cpp feature matrix](https://github.com/ggerganov/llama.cpp/wiki/Feature-matrix)
But basically, if you're aiming for below Q4, and you're running cuBLAS (Nvidia) or rocBLAS (AMD), you should look towards the I-quants. These are in format IQX_X, like IQ3_M. These are newer and offer better performance for their size.
These I-quants can also be used on CPU and Apple Metal, but will be slower than their K-quant equivalent, so speed vs performance is a tradeoff you'll have to decide.
The I-quants are *not* compatible with Vulcan, which is also AMD, so if you have an AMD card double check if you're using the rocBLAS build or the Vulcan build. At the time of writing this, LM Studio has a preview with ROCm support, and other inference engines have specific builds for ROCm.
Want to support my work? Visit my ko-fi page here: https://ko-fi.com/bartowski
| {"language": ["en"], "license": "other", "tags": ["llama3", "comedy", "comedian", "fun", "funny", "llama38b", "laugh", "sarcasm", "roleplay"], "license_name": "llama3", "license_link": "https://llama.meta.com/llama3/license/", "quantized_by": "bartowski", "pipeline_tag": "text-generation"} | nitsuai/Llama-3-8B-LexiFun-Uncensored-V1-GGUF | null | [
"gguf",
"llama3",
"comedy",
"comedian",
"fun",
"funny",
"llama38b",
"laugh",
"sarcasm",
"roleplay",
"text-generation",
"en",
"license:other",
"region:us"
] | null | 2024-04-26T18:05:51+00:00 | [] | [
"en"
] | TAGS
#gguf #llama3 #comedy #comedian #fun #funny #llama38b #laugh #sarcasm #roleplay #text-generation #en #license-other #region-us
| Llamacpp imatrix Quantizations of Llama-3-8B-LexiFun-Uncensored-V1
------------------------------------------------------------------
Using <a href="URL release <a href="URL for quantization.
Original model: URL
All quants made using imatrix option with dataset provided by Kalomaze here
Prompt format
-------------
Download a file (not the whole branch) from below:
--------------------------------------------------
Which file should I choose?
---------------------------
A great write up with charts showing various performances is provided by Artefact2 here
The first thing to figure out is how big a model you can run. To do this, you'll need to figure out how much RAM and/or VRAM you have.
If you want your model running as FAST as possible, you'll want to fit the whole thing on your GPU's VRAM. Aim for a quant with a file size 1-2GB smaller than your GPU's total VRAM.
If you want the absolute maximum quality, add both your system RAM and your GPU's VRAM together, then similarly grab a quant with a file size 1-2GB Smaller than that total.
Next, you'll need to decide if you want to use an 'I-quant' or a 'K-quant'.
If you don't want to think too much, grab one of the K-quants. These are in format 'QX\_K\_X', like Q5\_K\_M.
If you want to get more into the weeds, you can check out this extremely useful feature chart:
URL feature matrix
But basically, if you're aiming for below Q4, and you're running cuBLAS (Nvidia) or rocBLAS (AMD), you should look towards the I-quants. These are in format IQX\_X, like IQ3\_M. These are newer and offer better performance for their size.
These I-quants can also be used on CPU and Apple Metal, but will be slower than their K-quant equivalent, so speed vs performance is a tradeoff you'll have to decide.
The I-quants are *not* compatible with Vulcan, which is also AMD, so if you have an AMD card double check if you're using the rocBLAS build or the Vulcan build. At the time of writing this, LM Studio has a preview with ROCm support, and other inference engines have specific builds for ROCm.
Want to support my work? Visit my ko-fi page here: URL
| [] | [
"TAGS\n#gguf #llama3 #comedy #comedian #fun #funny #llama38b #laugh #sarcasm #roleplay #text-generation #en #license-other #region-us \n"
] |
null | peft |
<!-- This model card has been generated automatically according to the information the Trainer had access to. You
should probably proofread and complete it, then remove this comment. -->
# GUE_mouse_2-seqsight_4096_512_27M-L1_f
This model is a fine-tuned version of [mahdibaghbanzadeh/seqsight_4096_512_27M](https://huggingface.co/mahdibaghbanzadeh/seqsight_4096_512_27M) on the [mahdibaghbanzadeh/GUE_mouse_2](https://huggingface.co/datasets/mahdibaghbanzadeh/GUE_mouse_2) dataset.
It achieves the following results on the evaluation set:
- Loss: 0.2800
- F1 Score: 0.8689
- Accuracy: 0.8689
## Model description
More information needed
## Intended uses & limitations
More information needed
## Training and evaluation data
More information needed
## Training procedure
### Training hyperparameters
The following hyperparameters were used during training:
- learning_rate: 0.0005
- train_batch_size: 128
- eval_batch_size: 128
- seed: 42
- optimizer: Adam with betas=(0.9,0.999) and epsilon=1e-08
- lr_scheduler_type: linear
- training_steps: 10000
### Training results
| Training Loss | Epoch | Step | Validation Loss | F1 Score | Accuracy |
|:-------------:|:------:|:-----:|:---------------:|:--------:|:--------:|
| 0.4059 | 9.52 | 200 | 0.3052 | 0.8445 | 0.8445 |
| 0.2826 | 19.05 | 400 | 0.2839 | 0.8688 | 0.8689 |
| 0.2512 | 28.57 | 600 | 0.2766 | 0.8932 | 0.8933 |
| 0.2319 | 38.1 | 800 | 0.2613 | 0.8994 | 0.8994 |
| 0.2134 | 47.62 | 1000 | 0.2590 | 0.9085 | 0.9085 |
| 0.1954 | 57.14 | 1200 | 0.2581 | 0.9116 | 0.9116 |
| 0.1728 | 66.67 | 1400 | 0.2820 | 0.8931 | 0.8933 |
| 0.1584 | 76.19 | 1600 | 0.2905 | 0.8899 | 0.8902 |
| 0.1443 | 85.71 | 1800 | 0.2892 | 0.9054 | 0.9055 |
| 0.1333 | 95.24 | 2000 | 0.2959 | 0.8993 | 0.8994 |
| 0.1298 | 104.76 | 2200 | 0.3010 | 0.9054 | 0.9055 |
| 0.1116 | 114.29 | 2400 | 0.3154 | 0.9024 | 0.9024 |
| 0.1103 | 123.81 | 2600 | 0.3135 | 0.8963 | 0.8963 |
| 0.1014 | 133.33 | 2800 | 0.3388 | 0.8993 | 0.8994 |
| 0.0963 | 142.86 | 3000 | 0.3886 | 0.8932 | 0.8933 |
| 0.0924 | 152.38 | 3200 | 0.4090 | 0.8870 | 0.8872 |
| 0.0889 | 161.9 | 3400 | 0.3970 | 0.8902 | 0.8902 |
| 0.0837 | 171.43 | 3600 | 0.4102 | 0.8932 | 0.8933 |
| 0.0795 | 180.95 | 3800 | 0.4117 | 0.8902 | 0.8902 |
| 0.0793 | 190.48 | 4000 | 0.4228 | 0.8872 | 0.8872 |
| 0.0746 | 200.0 | 4200 | 0.4878 | 0.8901 | 0.8902 |
| 0.0725 | 209.52 | 4400 | 0.4712 | 0.8933 | 0.8933 |
| 0.0695 | 219.05 | 4600 | 0.4784 | 0.8963 | 0.8963 |
| 0.063 | 228.57 | 4800 | 0.4905 | 0.8963 | 0.8963 |
| 0.0645 | 238.1 | 5000 | 0.5177 | 0.8933 | 0.8933 |
| 0.0633 | 247.62 | 5200 | 0.5055 | 0.8872 | 0.8872 |
| 0.0631 | 257.14 | 5400 | 0.5056 | 0.8902 | 0.8902 |
| 0.0584 | 266.67 | 5600 | 0.5230 | 0.8872 | 0.8872 |
| 0.0586 | 276.19 | 5800 | 0.5650 | 0.8932 | 0.8933 |
| 0.056 | 285.71 | 6000 | 0.5467 | 0.8872 | 0.8872 |
| 0.0576 | 295.24 | 6200 | 0.5626 | 0.8871 | 0.8872 |
| 0.0553 | 304.76 | 6400 | 0.5777 | 0.8871 | 0.8872 |
| 0.0591 | 314.29 | 6600 | 0.5562 | 0.8902 | 0.8902 |
| 0.0551 | 323.81 | 6800 | 0.5597 | 0.8932 | 0.8933 |
| 0.0517 | 333.33 | 7000 | 0.5533 | 0.8933 | 0.8933 |
| 0.0504 | 342.86 | 7200 | 0.5743 | 0.8963 | 0.8963 |
| 0.0519 | 352.38 | 7400 | 0.5927 | 0.8901 | 0.8902 |
| 0.0514 | 361.9 | 7600 | 0.5765 | 0.8872 | 0.8872 |
| 0.0447 | 371.43 | 7800 | 0.5952 | 0.8932 | 0.8933 |
| 0.0535 | 380.95 | 8000 | 0.5915 | 0.8932 | 0.8933 |
| 0.0494 | 390.48 | 8200 | 0.5995 | 0.8901 | 0.8902 |
| 0.047 | 400.0 | 8400 | 0.6098 | 0.8932 | 0.8933 |
| 0.0467 | 409.52 | 8600 | 0.6046 | 0.8901 | 0.8902 |
| 0.0457 | 419.05 | 8800 | 0.5916 | 0.8963 | 0.8963 |
| 0.0457 | 428.57 | 9000 | 0.5954 | 0.8993 | 0.8994 |
| 0.0461 | 438.1 | 9200 | 0.5841 | 0.8963 | 0.8963 |
| 0.0453 | 447.62 | 9400 | 0.6011 | 0.8963 | 0.8963 |
| 0.0406 | 457.14 | 9600 | 0.5970 | 0.8902 | 0.8902 |
| 0.0428 | 466.67 | 9800 | 0.5993 | 0.8902 | 0.8902 |
| 0.0423 | 476.19 | 10000 | 0.6044 | 0.8963 | 0.8963 |
### Framework versions
- PEFT 0.9.0
- Transformers 4.38.2
- Pytorch 2.2.0+cu121
- Datasets 2.17.1
- Tokenizers 0.15.2 | {"library_name": "peft", "tags": ["generated_from_trainer"], "metrics": ["accuracy"], "base_model": "mahdibaghbanzadeh/seqsight_4096_512_27M", "model-index": [{"name": "GUE_mouse_2-seqsight_4096_512_27M-L1_f", "results": []}]} | mahdibaghbanzadeh/GUE_mouse_2-seqsight_4096_512_27M-L1_f | null | [
"peft",
"safetensors",
"generated_from_trainer",
"base_model:mahdibaghbanzadeh/seqsight_4096_512_27M",
"region:us"
] | null | 2024-04-26T18:07:09+00:00 | [] | [] | TAGS
#peft #safetensors #generated_from_trainer #base_model-mahdibaghbanzadeh/seqsight_4096_512_27M #region-us
| GUE\_mouse\_2-seqsight\_4096\_512\_27M-L1\_f
============================================
This model is a fine-tuned version of mahdibaghbanzadeh/seqsight\_4096\_512\_27M on the mahdibaghbanzadeh/GUE\_mouse\_2 dataset.
It achieves the following results on the evaluation set:
* Loss: 0.2800
* F1 Score: 0.8689
* Accuracy: 0.8689
Model description
-----------------
More information needed
Intended uses & limitations
---------------------------
More information needed
Training and evaluation data
----------------------------
More information needed
Training procedure
------------------
### Training hyperparameters
The following hyperparameters were used during training:
* learning\_rate: 0.0005
* train\_batch\_size: 128
* eval\_batch\_size: 128
* seed: 42
* optimizer: Adam with betas=(0.9,0.999) and epsilon=1e-08
* lr\_scheduler\_type: linear
* training\_steps: 10000
### Training results
### Framework versions
* PEFT 0.9.0
* Transformers 4.38.2
* Pytorch 2.2.0+cu121
* Datasets 2.17.1
* Tokenizers 0.15.2
| [
"### Training hyperparameters\n\n\nThe following hyperparameters were used during training:\n\n\n* learning\\_rate: 0.0005\n* train\\_batch\\_size: 128\n* eval\\_batch\\_size: 128\n* seed: 42\n* optimizer: Adam with betas=(0.9,0.999) and epsilon=1e-08\n* lr\\_scheduler\\_type: linear\n* training\\_steps: 10000",
"### Training results",
"### Framework versions\n\n\n* PEFT 0.9.0\n* Transformers 4.38.2\n* Pytorch 2.2.0+cu121\n* Datasets 2.17.1\n* Tokenizers 0.15.2"
] | [
"TAGS\n#peft #safetensors #generated_from_trainer #base_model-mahdibaghbanzadeh/seqsight_4096_512_27M #region-us \n",
"### Training hyperparameters\n\n\nThe following hyperparameters were used during training:\n\n\n* learning\\_rate: 0.0005\n* train\\_batch\\_size: 128\n* eval\\_batch\\_size: 128\n* seed: 42\n* optimizer: Adam with betas=(0.9,0.999) and epsilon=1e-08\n* lr\\_scheduler\\_type: linear\n* training\\_steps: 10000",
"### Training results",
"### Framework versions\n\n\n* PEFT 0.9.0\n* Transformers 4.38.2\n* Pytorch 2.2.0+cu121\n* Datasets 2.17.1\n* Tokenizers 0.15.2"
] |
null | peft |
<!-- This model card has been generated automatically according to the information the Trainer had access to. You
should probably proofread and complete it, then remove this comment. -->
# GUE_mouse_2-seqsight_4096_512_27M-L8_f
This model is a fine-tuned version of [mahdibaghbanzadeh/seqsight_4096_512_27M](https://huggingface.co/mahdibaghbanzadeh/seqsight_4096_512_27M) on the [mahdibaghbanzadeh/GUE_mouse_2](https://huggingface.co/datasets/mahdibaghbanzadeh/GUE_mouse_2) dataset.
It achieves the following results on the evaluation set:
- Loss: 0.4841
- F1 Score: 0.8841
- Accuracy: 0.8841
## Model description
More information needed
## Intended uses & limitations
More information needed
## Training and evaluation data
More information needed
## Training procedure
### Training hyperparameters
The following hyperparameters were used during training:
- learning_rate: 0.0005
- train_batch_size: 128
- eval_batch_size: 128
- seed: 42
- optimizer: Adam with betas=(0.9,0.999) and epsilon=1e-08
- lr_scheduler_type: linear
- training_steps: 10000
### Training results
| Training Loss | Epoch | Step | Validation Loss | F1 Score | Accuracy |
|:-------------:|:------:|:-----:|:---------------:|:--------:|:--------:|
| 0.3677 | 9.52 | 200 | 0.2949 | 0.8595 | 0.8598 |
| 0.2382 | 19.05 | 400 | 0.2746 | 0.8902 | 0.8902 |
| 0.1951 | 28.57 | 600 | 0.2833 | 0.8872 | 0.8872 |
| 0.1554 | 38.1 | 800 | 0.3011 | 0.8778 | 0.8780 |
| 0.1272 | 47.62 | 1000 | 0.3565 | 0.8749 | 0.875 |
| 0.1002 | 57.14 | 1200 | 0.4124 | 0.8929 | 0.8933 |
| 0.0815 | 66.67 | 1400 | 0.4215 | 0.8841 | 0.8841 |
| 0.0679 | 76.19 | 1600 | 0.4577 | 0.8870 | 0.8872 |
| 0.0562 | 85.71 | 1800 | 0.4996 | 0.8933 | 0.8933 |
| 0.0499 | 95.24 | 2000 | 0.5216 | 0.8932 | 0.8933 |
| 0.0463 | 104.76 | 2200 | 0.5312 | 0.9085 | 0.9085 |
| 0.0351 | 114.29 | 2400 | 0.6140 | 0.8841 | 0.8841 |
| 0.0337 | 123.81 | 2600 | 0.6153 | 0.8811 | 0.8811 |
| 0.0302 | 133.33 | 2800 | 0.5750 | 0.8933 | 0.8933 |
| 0.0288 | 142.86 | 3000 | 0.6441 | 0.8902 | 0.8902 |
| 0.026 | 152.38 | 3200 | 0.6817 | 0.8902 | 0.8902 |
| 0.0225 | 161.9 | 3400 | 0.7127 | 0.8933 | 0.8933 |
| 0.0202 | 171.43 | 3600 | 0.7430 | 0.8932 | 0.8933 |
| 0.0191 | 180.95 | 3800 | 0.7142 | 0.8902 | 0.8902 |
| 0.0217 | 190.48 | 4000 | 0.7186 | 0.8963 | 0.8963 |
| 0.0201 | 200.0 | 4200 | 0.7240 | 0.8901 | 0.8902 |
| 0.0164 | 209.52 | 4400 | 0.7281 | 0.8841 | 0.8841 |
| 0.0184 | 219.05 | 4600 | 0.7269 | 0.8933 | 0.8933 |
| 0.0138 | 228.57 | 4800 | 0.7507 | 0.8872 | 0.8872 |
| 0.0131 | 238.1 | 5000 | 0.8044 | 0.8872 | 0.8872 |
| 0.0138 | 247.62 | 5200 | 0.7881 | 0.8932 | 0.8933 |
| 0.0159 | 257.14 | 5400 | 0.7528 | 0.8901 | 0.8902 |
| 0.0132 | 266.67 | 5600 | 0.7890 | 0.8870 | 0.8872 |
| 0.0135 | 276.19 | 5800 | 0.8025 | 0.8962 | 0.8963 |
| 0.0122 | 285.71 | 6000 | 0.7585 | 0.9055 | 0.9055 |
| 0.0122 | 295.24 | 6200 | 0.7767 | 0.8963 | 0.8963 |
| 0.01 | 304.76 | 6400 | 0.7881 | 0.8871 | 0.8872 |
| 0.0095 | 314.29 | 6600 | 0.8054 | 0.8902 | 0.8902 |
| 0.009 | 323.81 | 6800 | 0.8139 | 0.8902 | 0.8902 |
| 0.0102 | 333.33 | 7000 | 0.8491 | 0.8902 | 0.8902 |
| 0.0101 | 342.86 | 7200 | 0.8116 | 0.8902 | 0.8902 |
| 0.0075 | 352.38 | 7400 | 0.8772 | 0.8811 | 0.8811 |
| 0.0099 | 361.9 | 7600 | 0.8711 | 0.8933 | 0.8933 |
| 0.008 | 371.43 | 7800 | 0.8187 | 0.8902 | 0.8902 |
| 0.0069 | 380.95 | 8000 | 0.8350 | 0.8872 | 0.8872 |
| 0.0056 | 390.48 | 8200 | 0.8756 | 0.8841 | 0.8841 |
| 0.0091 | 400.0 | 8400 | 0.8785 | 0.8872 | 0.8872 |
| 0.0059 | 409.52 | 8600 | 0.8792 | 0.8902 | 0.8902 |
| 0.0068 | 419.05 | 8800 | 0.8813 | 0.8933 | 0.8933 |
| 0.0063 | 428.57 | 9000 | 0.9176 | 0.8902 | 0.8902 |
| 0.0072 | 438.1 | 9200 | 0.9083 | 0.8871 | 0.8872 |
| 0.0064 | 447.62 | 9400 | 0.9086 | 0.8841 | 0.8841 |
| 0.0054 | 457.14 | 9600 | 0.9012 | 0.8872 | 0.8872 |
| 0.006 | 466.67 | 9800 | 0.8947 | 0.8933 | 0.8933 |
| 0.0052 | 476.19 | 10000 | 0.9082 | 0.8872 | 0.8872 |
### Framework versions
- PEFT 0.9.0
- Transformers 4.38.2
- Pytorch 2.2.0+cu121
- Datasets 2.17.1
- Tokenizers 0.15.2 | {"library_name": "peft", "tags": ["generated_from_trainer"], "metrics": ["accuracy"], "base_model": "mahdibaghbanzadeh/seqsight_4096_512_27M", "model-index": [{"name": "GUE_mouse_2-seqsight_4096_512_27M-L8_f", "results": []}]} | mahdibaghbanzadeh/GUE_mouse_2-seqsight_4096_512_27M-L8_f | null | [
"peft",
"safetensors",
"generated_from_trainer",
"base_model:mahdibaghbanzadeh/seqsight_4096_512_27M",
"region:us"
] | null | 2024-04-26T18:08:05+00:00 | [] | [] | TAGS
#peft #safetensors #generated_from_trainer #base_model-mahdibaghbanzadeh/seqsight_4096_512_27M #region-us
| GUE\_mouse\_2-seqsight\_4096\_512\_27M-L8\_f
============================================
This model is a fine-tuned version of mahdibaghbanzadeh/seqsight\_4096\_512\_27M on the mahdibaghbanzadeh/GUE\_mouse\_2 dataset.
It achieves the following results on the evaluation set:
* Loss: 0.4841
* F1 Score: 0.8841
* Accuracy: 0.8841
Model description
-----------------
More information needed
Intended uses & limitations
---------------------------
More information needed
Training and evaluation data
----------------------------
More information needed
Training procedure
------------------
### Training hyperparameters
The following hyperparameters were used during training:
* learning\_rate: 0.0005
* train\_batch\_size: 128
* eval\_batch\_size: 128
* seed: 42
* optimizer: Adam with betas=(0.9,0.999) and epsilon=1e-08
* lr\_scheduler\_type: linear
* training\_steps: 10000
### Training results
### Framework versions
* PEFT 0.9.0
* Transformers 4.38.2
* Pytorch 2.2.0+cu121
* Datasets 2.17.1
* Tokenizers 0.15.2
| [
"### Training hyperparameters\n\n\nThe following hyperparameters were used during training:\n\n\n* learning\\_rate: 0.0005\n* train\\_batch\\_size: 128\n* eval\\_batch\\_size: 128\n* seed: 42\n* optimizer: Adam with betas=(0.9,0.999) and epsilon=1e-08\n* lr\\_scheduler\\_type: linear\n* training\\_steps: 10000",
"### Training results",
"### Framework versions\n\n\n* PEFT 0.9.0\n* Transformers 4.38.2\n* Pytorch 2.2.0+cu121\n* Datasets 2.17.1\n* Tokenizers 0.15.2"
] | [
"TAGS\n#peft #safetensors #generated_from_trainer #base_model-mahdibaghbanzadeh/seqsight_4096_512_27M #region-us \n",
"### Training hyperparameters\n\n\nThe following hyperparameters were used during training:\n\n\n* learning\\_rate: 0.0005\n* train\\_batch\\_size: 128\n* eval\\_batch\\_size: 128\n* seed: 42\n* optimizer: Adam with betas=(0.9,0.999) and epsilon=1e-08\n* lr\\_scheduler\\_type: linear\n* training\\_steps: 10000",
"### Training results",
"### Framework versions\n\n\n* PEFT 0.9.0\n* Transformers 4.38.2\n* Pytorch 2.2.0+cu121\n* Datasets 2.17.1\n* Tokenizers 0.15.2"
] |
null | peft |
<!-- This model card has been generated automatically according to the information the Trainer had access to. You
should probably proofread and complete it, then remove this comment. -->
# GUE_mouse_2-seqsight_4096_512_27M-L32_f
This model is a fine-tuned version of [mahdibaghbanzadeh/seqsight_4096_512_27M](https://huggingface.co/mahdibaghbanzadeh/seqsight_4096_512_27M) on the [mahdibaghbanzadeh/GUE_mouse_2](https://huggingface.co/datasets/mahdibaghbanzadeh/GUE_mouse_2) dataset.
It achieves the following results on the evaluation set:
- Loss: 0.6199
- F1 Score: 0.8993
- Accuracy: 0.8994
## Model description
More information needed
## Intended uses & limitations
More information needed
## Training and evaluation data
More information needed
## Training procedure
### Training hyperparameters
The following hyperparameters were used during training:
- learning_rate: 0.0005
- train_batch_size: 128
- eval_batch_size: 128
- seed: 42
- optimizer: Adam with betas=(0.9,0.999) and epsilon=1e-08
- lr_scheduler_type: linear
- training_steps: 10000
### Training results
| Training Loss | Epoch | Step | Validation Loss | F1 Score | Accuracy |
|:-------------:|:------:|:-----:|:---------------:|:--------:|:--------:|
| 0.3367 | 9.52 | 200 | 0.2672 | 0.8811 | 0.8811 |
| 0.1924 | 19.05 | 400 | 0.2664 | 0.9054 | 0.9055 |
| 0.1281 | 28.57 | 600 | 0.3610 | 0.8839 | 0.8841 |
| 0.0803 | 38.1 | 800 | 0.4049 | 0.8872 | 0.8872 |
| 0.0548 | 47.62 | 1000 | 0.4676 | 0.8899 | 0.8902 |
| 0.0396 | 57.14 | 1200 | 0.4522 | 0.8992 | 0.8994 |
| 0.0264 | 66.67 | 1400 | 0.4723 | 0.8932 | 0.8933 |
| 0.0245 | 76.19 | 1600 | 0.5330 | 0.8900 | 0.8902 |
| 0.0228 | 85.71 | 1800 | 0.6126 | 0.8992 | 0.8994 |
| 0.0181 | 95.24 | 2000 | 0.5333 | 0.8932 | 0.8933 |
| 0.0145 | 104.76 | 2200 | 0.5952 | 0.8993 | 0.8994 |
| 0.0108 | 114.29 | 2400 | 0.6096 | 0.8963 | 0.8963 |
| 0.0127 | 123.81 | 2600 | 0.5955 | 0.9024 | 0.9024 |
| 0.0095 | 133.33 | 2800 | 0.6507 | 0.8902 | 0.8902 |
| 0.0103 | 142.86 | 3000 | 0.5953 | 0.9085 | 0.9085 |
| 0.0094 | 152.38 | 3200 | 0.6162 | 0.9023 | 0.9024 |
| 0.0076 | 161.9 | 3400 | 0.6577 | 0.9083 | 0.9085 |
| 0.0065 | 171.43 | 3600 | 0.6651 | 0.9023 | 0.9024 |
| 0.0086 | 180.95 | 3800 | 0.6336 | 0.9145 | 0.9146 |
| 0.0044 | 190.48 | 4000 | 0.6888 | 0.9054 | 0.9055 |
| 0.0058 | 200.0 | 4200 | 0.6846 | 0.8963 | 0.8963 |
| 0.0045 | 209.52 | 4400 | 0.6625 | 0.9054 | 0.9055 |
| 0.0063 | 219.05 | 4600 | 0.6380 | 0.9085 | 0.9085 |
| 0.004 | 228.57 | 4800 | 0.7722 | 0.8898 | 0.8902 |
| 0.0052 | 238.1 | 5000 | 0.6179 | 0.9085 | 0.9085 |
| 0.0059 | 247.62 | 5200 | 0.5979 | 0.9085 | 0.9085 |
| 0.0041 | 257.14 | 5400 | 0.5972 | 0.9023 | 0.9024 |
| 0.0025 | 266.67 | 5600 | 0.6238 | 0.9085 | 0.9085 |
| 0.0044 | 276.19 | 5800 | 0.6865 | 0.8963 | 0.8963 |
| 0.0035 | 285.71 | 6000 | 0.7340 | 0.8963 | 0.8963 |
| 0.0034 | 295.24 | 6200 | 0.6925 | 0.8932 | 0.8933 |
| 0.0024 | 304.76 | 6400 | 0.7674 | 0.8931 | 0.8933 |
| 0.0017 | 314.29 | 6600 | 0.7933 | 0.8991 | 0.8994 |
| 0.0023 | 323.81 | 6800 | 0.7734 | 0.9055 | 0.9055 |
| 0.0018 | 333.33 | 7000 | 0.8113 | 0.8961 | 0.8963 |
| 0.0019 | 342.86 | 7200 | 0.8432 | 0.9054 | 0.9055 |
| 0.0025 | 352.38 | 7400 | 0.7970 | 0.8994 | 0.8994 |
| 0.0023 | 361.9 | 7600 | 0.7408 | 0.9055 | 0.9055 |
| 0.0029 | 371.43 | 7800 | 0.7113 | 0.9116 | 0.9116 |
| 0.0019 | 380.95 | 8000 | 0.7800 | 0.8992 | 0.8994 |
| 0.0012 | 390.48 | 8200 | 0.7262 | 0.9055 | 0.9055 |
| 0.0017 | 400.0 | 8400 | 0.7920 | 0.8962 | 0.8963 |
| 0.0011 | 409.52 | 8600 | 0.7884 | 0.9054 | 0.9055 |
| 0.0011 | 419.05 | 8800 | 0.7962 | 0.8871 | 0.8872 |
| 0.0015 | 428.57 | 9000 | 0.8056 | 0.8902 | 0.8902 |
| 0.0013 | 438.1 | 9200 | 0.8130 | 0.8932 | 0.8933 |
| 0.0014 | 447.62 | 9400 | 0.8064 | 0.8932 | 0.8933 |
| 0.0013 | 457.14 | 9600 | 0.7994 | 0.8993 | 0.8994 |
| 0.0013 | 466.67 | 9800 | 0.7875 | 0.8963 | 0.8963 |
| 0.001 | 476.19 | 10000 | 0.7926 | 0.8963 | 0.8963 |
### Framework versions
- PEFT 0.9.0
- Transformers 4.38.2
- Pytorch 2.2.0+cu121
- Datasets 2.17.1
- Tokenizers 0.15.2 | {"library_name": "peft", "tags": ["generated_from_trainer"], "metrics": ["accuracy"], "base_model": "mahdibaghbanzadeh/seqsight_4096_512_27M", "model-index": [{"name": "GUE_mouse_2-seqsight_4096_512_27M-L32_f", "results": []}]} | mahdibaghbanzadeh/GUE_mouse_2-seqsight_4096_512_27M-L32_f | null | [
"peft",
"safetensors",
"generated_from_trainer",
"base_model:mahdibaghbanzadeh/seqsight_4096_512_27M",
"region:us"
] | null | 2024-04-26T18:08:13+00:00 | [] | [] | TAGS
#peft #safetensors #generated_from_trainer #base_model-mahdibaghbanzadeh/seqsight_4096_512_27M #region-us
| GUE\_mouse\_2-seqsight\_4096\_512\_27M-L32\_f
=============================================
This model is a fine-tuned version of mahdibaghbanzadeh/seqsight\_4096\_512\_27M on the mahdibaghbanzadeh/GUE\_mouse\_2 dataset.
It achieves the following results on the evaluation set:
* Loss: 0.6199
* F1 Score: 0.8993
* Accuracy: 0.8994
Model description
-----------------
More information needed
Intended uses & limitations
---------------------------
More information needed
Training and evaluation data
----------------------------
More information needed
Training procedure
------------------
### Training hyperparameters
The following hyperparameters were used during training:
* learning\_rate: 0.0005
* train\_batch\_size: 128
* eval\_batch\_size: 128
* seed: 42
* optimizer: Adam with betas=(0.9,0.999) and epsilon=1e-08
* lr\_scheduler\_type: linear
* training\_steps: 10000
### Training results
### Framework versions
* PEFT 0.9.0
* Transformers 4.38.2
* Pytorch 2.2.0+cu121
* Datasets 2.17.1
* Tokenizers 0.15.2
| [
"### Training hyperparameters\n\n\nThe following hyperparameters were used during training:\n\n\n* learning\\_rate: 0.0005\n* train\\_batch\\_size: 128\n* eval\\_batch\\_size: 128\n* seed: 42\n* optimizer: Adam with betas=(0.9,0.999) and epsilon=1e-08\n* lr\\_scheduler\\_type: linear\n* training\\_steps: 10000",
"### Training results",
"### Framework versions\n\n\n* PEFT 0.9.0\n* Transformers 4.38.2\n* Pytorch 2.2.0+cu121\n* Datasets 2.17.1\n* Tokenizers 0.15.2"
] | [
"TAGS\n#peft #safetensors #generated_from_trainer #base_model-mahdibaghbanzadeh/seqsight_4096_512_27M #region-us \n",
"### Training hyperparameters\n\n\nThe following hyperparameters were used during training:\n\n\n* learning\\_rate: 0.0005\n* train\\_batch\\_size: 128\n* eval\\_batch\\_size: 128\n* seed: 42\n* optimizer: Adam with betas=(0.9,0.999) and epsilon=1e-08\n* lr\\_scheduler\\_type: linear\n* training\\_steps: 10000",
"### Training results",
"### Framework versions\n\n\n* PEFT 0.9.0\n* Transformers 4.38.2\n* Pytorch 2.2.0+cu121\n* Datasets 2.17.1\n* Tokenizers 0.15.2"
] |
null | peft |
<!-- This model card has been generated automatically according to the information the Trainer had access to. You
should probably proofread and complete it, then remove this comment. -->
# GUE_splice_reconstructed-seqsight_4096_512_27M-L1_f
This model is a fine-tuned version of [mahdibaghbanzadeh/seqsight_4096_512_27M](https://huggingface.co/mahdibaghbanzadeh/seqsight_4096_512_27M) on the [mahdibaghbanzadeh/GUE_splice_reconstructed](https://huggingface.co/datasets/mahdibaghbanzadeh/GUE_splice_reconstructed) dataset.
It achieves the following results on the evaluation set:
- Loss: 0.3574
- F1 Score: 0.8608
- Accuracy: 0.8601
## Model description
More information needed
## Intended uses & limitations
More information needed
## Training and evaluation data
More information needed
## Training procedure
### Training hyperparameters
The following hyperparameters were used during training:
- learning_rate: 0.0005
- train_batch_size: 128
- eval_batch_size: 128
- seed: 42
- optimizer: Adam with betas=(0.9,0.999) and epsilon=1e-08
- lr_scheduler_type: linear
- training_steps: 10000
### Training results
| Training Loss | Epoch | Step | Validation Loss | F1 Score | Accuracy |
|:-------------:|:-----:|:-----:|:---------------:|:--------:|:--------:|
| 0.9468 | 0.7 | 200 | 0.8792 | 0.6136 | 0.6339 |
| 0.6592 | 1.4 | 400 | 0.5421 | 0.7802 | 0.7790 |
| 0.5071 | 2.1 | 600 | 0.5161 | 0.7921 | 0.7911 |
| 0.4728 | 2.8 | 800 | 0.4809 | 0.8082 | 0.8071 |
| 0.4585 | 3.5 | 1000 | 0.4611 | 0.8164 | 0.8157 |
| 0.4494 | 4.2 | 1200 | 0.4537 | 0.8161 | 0.8152 |
| 0.4334 | 4.9 | 1400 | 0.4327 | 0.8296 | 0.8290 |
| 0.43 | 5.59 | 1600 | 0.4469 | 0.8196 | 0.8187 |
| 0.4188 | 6.29 | 1800 | 0.4392 | 0.8214 | 0.8205 |
| 0.4117 | 6.99 | 2000 | 0.4360 | 0.8252 | 0.8242 |
| 0.4112 | 7.69 | 2200 | 0.4004 | 0.8378 | 0.8376 |
| 0.4032 | 8.39 | 2400 | 0.4192 | 0.8314 | 0.8306 |
| 0.4008 | 9.09 | 2600 | 0.4166 | 0.8336 | 0.8325 |
| 0.3956 | 9.79 | 2800 | 0.3997 | 0.8407 | 0.8400 |
| 0.3974 | 10.49 | 3000 | 0.4094 | 0.8351 | 0.8343 |
| 0.3832 | 11.19 | 3200 | 0.4284 | 0.8295 | 0.8284 |
| 0.3922 | 11.89 | 3400 | 0.3979 | 0.8399 | 0.8391 |
| 0.3776 | 12.59 | 3600 | 0.3968 | 0.8404 | 0.8395 |
| 0.3856 | 13.29 | 3800 | 0.3994 | 0.8373 | 0.8365 |
| 0.3776 | 13.99 | 4000 | 0.3954 | 0.8409 | 0.8400 |
| 0.3739 | 14.69 | 4200 | 0.3957 | 0.8426 | 0.8417 |
| 0.377 | 15.38 | 4400 | 0.3957 | 0.8416 | 0.8406 |
| 0.3746 | 16.08 | 4600 | 0.4019 | 0.8384 | 0.8376 |
| 0.3732 | 16.78 | 4800 | 0.3955 | 0.8395 | 0.8384 |
| 0.3687 | 17.48 | 5000 | 0.4004 | 0.8389 | 0.8378 |
| 0.3672 | 18.18 | 5200 | 0.3752 | 0.8479 | 0.8474 |
| 0.3694 | 18.88 | 5400 | 0.3908 | 0.8423 | 0.8413 |
| 0.3637 | 19.58 | 5600 | 0.3805 | 0.8485 | 0.8479 |
| 0.3696 | 20.28 | 5800 | 0.3856 | 0.8464 | 0.8455 |
| 0.3646 | 20.98 | 6000 | 0.3780 | 0.8476 | 0.8468 |
| 0.3648 | 21.68 | 6200 | 0.3885 | 0.8428 | 0.8420 |
| 0.3569 | 22.38 | 6400 | 0.3840 | 0.8454 | 0.8446 |
| 0.3567 | 23.08 | 6600 | 0.3801 | 0.8477 | 0.8468 |
| 0.3592 | 23.78 | 6800 | 0.3767 | 0.8476 | 0.8468 |
| 0.3566 | 24.48 | 7000 | 0.3875 | 0.8438 | 0.8428 |
| 0.357 | 25.17 | 7200 | 0.3758 | 0.8474 | 0.8466 |
| 0.3573 | 25.87 | 7400 | 0.3861 | 0.8460 | 0.8450 |
| 0.3543 | 26.57 | 7600 | 0.3952 | 0.8402 | 0.8391 |
| 0.3504 | 27.27 | 7800 | 0.3812 | 0.8461 | 0.8452 |
| 0.3557 | 27.97 | 8000 | 0.3781 | 0.8477 | 0.8468 |
| 0.3583 | 28.67 | 8200 | 0.3841 | 0.8426 | 0.8417 |
| 0.3502 | 29.37 | 8400 | 0.3761 | 0.8479 | 0.8470 |
| 0.3481 | 30.07 | 8600 | 0.3709 | 0.8499 | 0.8492 |
| 0.3506 | 30.77 | 8800 | 0.3778 | 0.8474 | 0.8466 |
| 0.3517 | 31.47 | 9000 | 0.3776 | 0.8479 | 0.8470 |
| 0.3556 | 32.17 | 9200 | 0.3750 | 0.8483 | 0.8474 |
| 0.3495 | 32.87 | 9400 | 0.3805 | 0.8457 | 0.8448 |
| 0.3548 | 33.57 | 9600 | 0.3788 | 0.8483 | 0.8474 |
| 0.3463 | 34.27 | 9800 | 0.3761 | 0.8488 | 0.8479 |
| 0.3474 | 34.97 | 10000 | 0.3776 | 0.8481 | 0.8472 |
### Framework versions
- PEFT 0.9.0
- Transformers 4.38.2
- Pytorch 2.2.0+cu121
- Datasets 2.17.1
- Tokenizers 0.15.2 | {"library_name": "peft", "tags": ["generated_from_trainer"], "metrics": ["accuracy"], "base_model": "mahdibaghbanzadeh/seqsight_4096_512_27M", "model-index": [{"name": "GUE_splice_reconstructed-seqsight_4096_512_27M-L1_f", "results": []}]} | mahdibaghbanzadeh/GUE_splice_reconstructed-seqsight_4096_512_27M-L1_f | null | [
"peft",
"safetensors",
"generated_from_trainer",
"base_model:mahdibaghbanzadeh/seqsight_4096_512_27M",
"region:us"
] | null | 2024-04-26T18:09:21+00:00 | [] | [] | TAGS
#peft #safetensors #generated_from_trainer #base_model-mahdibaghbanzadeh/seqsight_4096_512_27M #region-us
| GUE\_splice\_reconstructed-seqsight\_4096\_512\_27M-L1\_f
=========================================================
This model is a fine-tuned version of mahdibaghbanzadeh/seqsight\_4096\_512\_27M on the mahdibaghbanzadeh/GUE\_splice\_reconstructed dataset.
It achieves the following results on the evaluation set:
* Loss: 0.3574
* F1 Score: 0.8608
* Accuracy: 0.8601
Model description
-----------------
More information needed
Intended uses & limitations
---------------------------
More information needed
Training and evaluation data
----------------------------
More information needed
Training procedure
------------------
### Training hyperparameters
The following hyperparameters were used during training:
* learning\_rate: 0.0005
* train\_batch\_size: 128
* eval\_batch\_size: 128
* seed: 42
* optimizer: Adam with betas=(0.9,0.999) and epsilon=1e-08
* lr\_scheduler\_type: linear
* training\_steps: 10000
### Training results
### Framework versions
* PEFT 0.9.0
* Transformers 4.38.2
* Pytorch 2.2.0+cu121
* Datasets 2.17.1
* Tokenizers 0.15.2
| [
"### Training hyperparameters\n\n\nThe following hyperparameters were used during training:\n\n\n* learning\\_rate: 0.0005\n* train\\_batch\\_size: 128\n* eval\\_batch\\_size: 128\n* seed: 42\n* optimizer: Adam with betas=(0.9,0.999) and epsilon=1e-08\n* lr\\_scheduler\\_type: linear\n* training\\_steps: 10000",
"### Training results",
"### Framework versions\n\n\n* PEFT 0.9.0\n* Transformers 4.38.2\n* Pytorch 2.2.0+cu121\n* Datasets 2.17.1\n* Tokenizers 0.15.2"
] | [
"TAGS\n#peft #safetensors #generated_from_trainer #base_model-mahdibaghbanzadeh/seqsight_4096_512_27M #region-us \n",
"### Training hyperparameters\n\n\nThe following hyperparameters were used during training:\n\n\n* learning\\_rate: 0.0005\n* train\\_batch\\_size: 128\n* eval\\_batch\\_size: 128\n* seed: 42\n* optimizer: Adam with betas=(0.9,0.999) and epsilon=1e-08\n* lr\\_scheduler\\_type: linear\n* training\\_steps: 10000",
"### Training results",
"### Framework versions\n\n\n* PEFT 0.9.0\n* Transformers 4.38.2\n* Pytorch 2.2.0+cu121\n* Datasets 2.17.1\n* Tokenizers 0.15.2"
] |
null | peft |
<!-- This model card has been generated automatically according to the information the Trainer had access to. You
should probably proofread and complete it, then remove this comment. -->
# GUE_splice_reconstructed-seqsight_4096_512_27M-L8_f
This model is a fine-tuned version of [mahdibaghbanzadeh/seqsight_4096_512_27M](https://huggingface.co/mahdibaghbanzadeh/seqsight_4096_512_27M) on the [mahdibaghbanzadeh/GUE_splice_reconstructed](https://huggingface.co/datasets/mahdibaghbanzadeh/GUE_splice_reconstructed) dataset.
It achieves the following results on the evaluation set:
- Loss: 0.2943
- F1 Score: 0.8937
- Accuracy: 0.8932
## Model description
More information needed
## Intended uses & limitations
More information needed
## Training and evaluation data
More information needed
## Training procedure
### Training hyperparameters
The following hyperparameters were used during training:
- learning_rate: 0.0005
- train_batch_size: 128
- eval_batch_size: 128
- seed: 42
- optimizer: Adam with betas=(0.9,0.999) and epsilon=1e-08
- lr_scheduler_type: linear
- training_steps: 10000
### Training results
| Training Loss | Epoch | Step | Validation Loss | F1 Score | Accuracy |
|:-------------:|:-----:|:-----:|:---------------:|:--------:|:--------:|
| 0.9118 | 0.7 | 200 | 0.6731 | 0.7270 | 0.7245 |
| 0.5089 | 1.4 | 400 | 0.4856 | 0.8024 | 0.8012 |
| 0.4473 | 2.1 | 600 | 0.4612 | 0.8142 | 0.8132 |
| 0.4169 | 2.8 | 800 | 0.4382 | 0.8249 | 0.8240 |
| 0.4008 | 3.5 | 1000 | 0.4092 | 0.8357 | 0.8349 |
| 0.3902 | 4.2 | 1200 | 0.4061 | 0.8369 | 0.8360 |
| 0.3746 | 4.9 | 1400 | 0.3952 | 0.8438 | 0.8431 |
| 0.3655 | 5.59 | 1600 | 0.3879 | 0.8461 | 0.8455 |
| 0.3549 | 6.29 | 1800 | 0.3853 | 0.8489 | 0.8481 |
| 0.345 | 6.99 | 2000 | 0.3826 | 0.8503 | 0.8494 |
| 0.3426 | 7.69 | 2200 | 0.3463 | 0.8641 | 0.8637 |
| 0.3326 | 8.39 | 2400 | 0.3651 | 0.8549 | 0.8542 |
| 0.3295 | 9.09 | 2600 | 0.3599 | 0.8620 | 0.8612 |
| 0.3185 | 9.79 | 2800 | 0.3449 | 0.8669 | 0.8663 |
| 0.3196 | 10.49 | 3000 | 0.3411 | 0.8700 | 0.8696 |
| 0.3042 | 11.19 | 3200 | 0.3686 | 0.8603 | 0.8595 |
| 0.3156 | 11.89 | 3400 | 0.3473 | 0.8653 | 0.8645 |
| 0.2972 | 12.59 | 3600 | 0.3409 | 0.8698 | 0.8691 |
| 0.2999 | 13.29 | 3800 | 0.3465 | 0.8647 | 0.8641 |
| 0.2932 | 13.99 | 4000 | 0.3441 | 0.8683 | 0.8676 |
| 0.2884 | 14.69 | 4200 | 0.3422 | 0.8702 | 0.8696 |
| 0.2895 | 15.38 | 4400 | 0.3515 | 0.8677 | 0.8669 |
| 0.2842 | 16.08 | 4600 | 0.3592 | 0.8666 | 0.8658 |
| 0.283 | 16.78 | 4800 | 0.3399 | 0.8698 | 0.8691 |
| 0.2764 | 17.48 | 5000 | 0.3582 | 0.8660 | 0.8652 |
| 0.2791 | 18.18 | 5200 | 0.3222 | 0.8788 | 0.8783 |
| 0.2762 | 18.88 | 5400 | 0.3183 | 0.8821 | 0.8816 |
| 0.2731 | 19.58 | 5600 | 0.3289 | 0.8780 | 0.8775 |
| 0.2741 | 20.28 | 5800 | 0.3360 | 0.8773 | 0.8766 |
| 0.2693 | 20.98 | 6000 | 0.3149 | 0.8843 | 0.8838 |
| 0.271 | 21.68 | 6200 | 0.3285 | 0.8767 | 0.8762 |
| 0.2607 | 22.38 | 6400 | 0.3240 | 0.8805 | 0.8799 |
| 0.2574 | 23.08 | 6600 | 0.3228 | 0.8792 | 0.8786 |
| 0.2641 | 23.78 | 6800 | 0.3254 | 0.8780 | 0.8775 |
| 0.2544 | 24.48 | 7000 | 0.3438 | 0.8733 | 0.8726 |
| 0.259 | 25.17 | 7200 | 0.3308 | 0.8779 | 0.8772 |
| 0.2588 | 25.87 | 7400 | 0.3351 | 0.8755 | 0.8748 |
| 0.2523 | 26.57 | 7600 | 0.3550 | 0.8707 | 0.8698 |
| 0.2547 | 27.27 | 7800 | 0.3396 | 0.8748 | 0.8742 |
| 0.2514 | 27.97 | 8000 | 0.3253 | 0.8801 | 0.8794 |
| 0.2587 | 28.67 | 8200 | 0.3315 | 0.8776 | 0.8770 |
| 0.2493 | 29.37 | 8400 | 0.3226 | 0.8819 | 0.8812 |
| 0.2471 | 30.07 | 8600 | 0.3239 | 0.8803 | 0.8797 |
| 0.2489 | 30.77 | 8800 | 0.3322 | 0.8794 | 0.8788 |
| 0.2488 | 31.47 | 9000 | 0.3347 | 0.8777 | 0.8770 |
| 0.2501 | 32.17 | 9200 | 0.3269 | 0.8814 | 0.8808 |
| 0.2465 | 32.87 | 9400 | 0.3340 | 0.8770 | 0.8764 |
| 0.2465 | 33.57 | 9600 | 0.3342 | 0.8782 | 0.8775 |
| 0.246 | 34.27 | 9800 | 0.3280 | 0.8794 | 0.8788 |
| 0.2455 | 34.97 | 10000 | 0.3302 | 0.8786 | 0.8779 |
### Framework versions
- PEFT 0.9.0
- Transformers 4.38.2
- Pytorch 2.2.0+cu121
- Datasets 2.17.1
- Tokenizers 0.15.2 | {"library_name": "peft", "tags": ["generated_from_trainer"], "metrics": ["accuracy"], "base_model": "mahdibaghbanzadeh/seqsight_4096_512_27M", "model-index": [{"name": "GUE_splice_reconstructed-seqsight_4096_512_27M-L8_f", "results": []}]} | mahdibaghbanzadeh/GUE_splice_reconstructed-seqsight_4096_512_27M-L8_f | null | [
"peft",
"safetensors",
"generated_from_trainer",
"base_model:mahdibaghbanzadeh/seqsight_4096_512_27M",
"region:us"
] | null | 2024-04-26T18:09:27+00:00 | [] | [] | TAGS
#peft #safetensors #generated_from_trainer #base_model-mahdibaghbanzadeh/seqsight_4096_512_27M #region-us
| GUE\_splice\_reconstructed-seqsight\_4096\_512\_27M-L8\_f
=========================================================
This model is a fine-tuned version of mahdibaghbanzadeh/seqsight\_4096\_512\_27M on the mahdibaghbanzadeh/GUE\_splice\_reconstructed dataset.
It achieves the following results on the evaluation set:
* Loss: 0.2943
* F1 Score: 0.8937
* Accuracy: 0.8932
Model description
-----------------
More information needed
Intended uses & limitations
---------------------------
More information needed
Training and evaluation data
----------------------------
More information needed
Training procedure
------------------
### Training hyperparameters
The following hyperparameters were used during training:
* learning\_rate: 0.0005
* train\_batch\_size: 128
* eval\_batch\_size: 128
* seed: 42
* optimizer: Adam with betas=(0.9,0.999) and epsilon=1e-08
* lr\_scheduler\_type: linear
* training\_steps: 10000
### Training results
### Framework versions
* PEFT 0.9.0
* Transformers 4.38.2
* Pytorch 2.2.0+cu121
* Datasets 2.17.1
* Tokenizers 0.15.2
| [
"### Training hyperparameters\n\n\nThe following hyperparameters were used during training:\n\n\n* learning\\_rate: 0.0005\n* train\\_batch\\_size: 128\n* eval\\_batch\\_size: 128\n* seed: 42\n* optimizer: Adam with betas=(0.9,0.999) and epsilon=1e-08\n* lr\\_scheduler\\_type: linear\n* training\\_steps: 10000",
"### Training results",
"### Framework versions\n\n\n* PEFT 0.9.0\n* Transformers 4.38.2\n* Pytorch 2.2.0+cu121\n* Datasets 2.17.1\n* Tokenizers 0.15.2"
] | [
"TAGS\n#peft #safetensors #generated_from_trainer #base_model-mahdibaghbanzadeh/seqsight_4096_512_27M #region-us \n",
"### Training hyperparameters\n\n\nThe following hyperparameters were used during training:\n\n\n* learning\\_rate: 0.0005\n* train\\_batch\\_size: 128\n* eval\\_batch\\_size: 128\n* seed: 42\n* optimizer: Adam with betas=(0.9,0.999) and epsilon=1e-08\n* lr\\_scheduler\\_type: linear\n* training\\_steps: 10000",
"### Training results",
"### Framework versions\n\n\n* PEFT 0.9.0\n* Transformers 4.38.2\n* Pytorch 2.2.0+cu121\n* Datasets 2.17.1\n* Tokenizers 0.15.2"
] |
null | peft |
<!-- This model card has been generated automatically according to the information the Trainer had access to. You
should probably proofread and complete it, then remove this comment. -->
# GUE_splice_reconstructed-seqsight_4096_512_27M-L32_f
This model is a fine-tuned version of [mahdibaghbanzadeh/seqsight_4096_512_27M](https://huggingface.co/mahdibaghbanzadeh/seqsight_4096_512_27M) on the [mahdibaghbanzadeh/GUE_splice_reconstructed](https://huggingface.co/datasets/mahdibaghbanzadeh/GUE_splice_reconstructed) dataset.
It achieves the following results on the evaluation set:
- Loss: 0.3111
- F1 Score: 0.8970
- Accuracy: 0.8965
## Model description
More information needed
## Intended uses & limitations
More information needed
## Training and evaluation data
More information needed
## Training procedure
### Training hyperparameters
The following hyperparameters were used during training:
- learning_rate: 0.0005
- train_batch_size: 128
- eval_batch_size: 128
- seed: 42
- optimizer: Adam with betas=(0.9,0.999) and epsilon=1e-08
- lr_scheduler_type: linear
- training_steps: 10000
### Training results
| Training Loss | Epoch | Step | Validation Loss | F1 Score | Accuracy |
|:-------------:|:-----:|:-----:|:---------------:|:--------:|:--------:|
| 0.7991 | 0.7 | 200 | 0.4999 | 0.7953 | 0.7944 |
| 0.4452 | 1.4 | 400 | 0.4695 | 0.8051 | 0.8029 |
| 0.4067 | 2.1 | 600 | 0.4252 | 0.8265 | 0.8253 |
| 0.373 | 2.8 | 800 | 0.4182 | 0.8326 | 0.8317 |
| 0.353 | 3.5 | 1000 | 0.3556 | 0.8592 | 0.8584 |
| 0.3414 | 4.2 | 1200 | 0.3692 | 0.8549 | 0.8538 |
| 0.3219 | 4.9 | 1400 | 0.3385 | 0.8660 | 0.8654 |
| 0.3127 | 5.59 | 1600 | 0.3501 | 0.8613 | 0.8608 |
| 0.3011 | 6.29 | 1800 | 0.3740 | 0.8565 | 0.8558 |
| 0.2879 | 6.99 | 2000 | 0.3557 | 0.8627 | 0.8619 |
| 0.2866 | 7.69 | 2200 | 0.3355 | 0.8676 | 0.8669 |
| 0.2723 | 8.39 | 2400 | 0.3221 | 0.8801 | 0.8797 |
| 0.2706 | 9.09 | 2600 | 0.3381 | 0.8723 | 0.8713 |
| 0.2615 | 9.79 | 2800 | 0.3347 | 0.8740 | 0.8733 |
| 0.2576 | 10.49 | 3000 | 0.3304 | 0.8754 | 0.8748 |
| 0.2474 | 11.19 | 3200 | 0.3436 | 0.8771 | 0.8764 |
| 0.2543 | 11.89 | 3400 | 0.3337 | 0.8727 | 0.8720 |
| 0.238 | 12.59 | 3600 | 0.3263 | 0.8811 | 0.8805 |
| 0.2345 | 13.29 | 3800 | 0.3264 | 0.8817 | 0.8810 |
| 0.2325 | 13.99 | 4000 | 0.3437 | 0.8758 | 0.8751 |
| 0.2224 | 14.69 | 4200 | 0.3280 | 0.8805 | 0.8799 |
| 0.2252 | 15.38 | 4400 | 0.3443 | 0.8760 | 0.8753 |
| 0.2201 | 16.08 | 4600 | 0.3445 | 0.8752 | 0.8744 |
| 0.2178 | 16.78 | 4800 | 0.3368 | 0.8798 | 0.8790 |
| 0.2105 | 17.48 | 5000 | 0.3454 | 0.8800 | 0.8792 |
| 0.2109 | 18.18 | 5200 | 0.3173 | 0.8879 | 0.8873 |
| 0.2071 | 18.88 | 5400 | 0.3111 | 0.8917 | 0.8913 |
| 0.2015 | 19.58 | 5600 | 0.3206 | 0.8870 | 0.8865 |
| 0.2031 | 20.28 | 5800 | 0.3209 | 0.8876 | 0.8869 |
| 0.1984 | 20.98 | 6000 | 0.3164 | 0.8935 | 0.8930 |
| 0.1964 | 21.68 | 6200 | 0.3139 | 0.8922 | 0.8917 |
| 0.1879 | 22.38 | 6400 | 0.3183 | 0.8922 | 0.8917 |
| 0.1846 | 23.08 | 6600 | 0.3172 | 0.8911 | 0.8906 |
| 0.1853 | 23.78 | 6800 | 0.3230 | 0.8912 | 0.8906 |
| 0.1792 | 24.48 | 7000 | 0.3389 | 0.8885 | 0.8880 |
| 0.1768 | 25.17 | 7200 | 0.3415 | 0.8843 | 0.8836 |
| 0.1784 | 25.87 | 7400 | 0.3276 | 0.8884 | 0.8878 |
| 0.1728 | 26.57 | 7600 | 0.3354 | 0.8893 | 0.8886 |
| 0.1752 | 27.27 | 7800 | 0.3378 | 0.8879 | 0.8873 |
| 0.1748 | 27.97 | 8000 | 0.3199 | 0.8959 | 0.8954 |
| 0.1767 | 28.67 | 8200 | 0.3285 | 0.8908 | 0.8902 |
| 0.1678 | 29.37 | 8400 | 0.3282 | 0.8938 | 0.8932 |
| 0.1669 | 30.07 | 8600 | 0.3265 | 0.8949 | 0.8943 |
| 0.1683 | 30.77 | 8800 | 0.3290 | 0.8938 | 0.8932 |
| 0.1672 | 31.47 | 9000 | 0.3345 | 0.8925 | 0.8919 |
| 0.1654 | 32.17 | 9200 | 0.3296 | 0.8927 | 0.8922 |
| 0.1618 | 32.87 | 9400 | 0.3357 | 0.8925 | 0.8919 |
| 0.1608 | 33.57 | 9600 | 0.3390 | 0.8901 | 0.8895 |
| 0.1577 | 34.27 | 9800 | 0.3358 | 0.8923 | 0.8917 |
| 0.1624 | 34.97 | 10000 | 0.3360 | 0.8923 | 0.8917 |
### Framework versions
- PEFT 0.9.0
- Transformers 4.38.2
- Pytorch 2.2.0+cu121
- Datasets 2.17.1
- Tokenizers 0.15.2 | {"library_name": "peft", "tags": ["generated_from_trainer"], "metrics": ["accuracy"], "base_model": "mahdibaghbanzadeh/seqsight_4096_512_27M", "model-index": [{"name": "GUE_splice_reconstructed-seqsight_4096_512_27M-L32_f", "results": []}]} | mahdibaghbanzadeh/GUE_splice_reconstructed-seqsight_4096_512_27M-L32_f | null | [
"peft",
"safetensors",
"generated_from_trainer",
"base_model:mahdibaghbanzadeh/seqsight_4096_512_27M",
"region:us"
] | null | 2024-04-26T18:09:49+00:00 | [] | [] | TAGS
#peft #safetensors #generated_from_trainer #base_model-mahdibaghbanzadeh/seqsight_4096_512_27M #region-us
| GUE\_splice\_reconstructed-seqsight\_4096\_512\_27M-L32\_f
==========================================================
This model is a fine-tuned version of mahdibaghbanzadeh/seqsight\_4096\_512\_27M on the mahdibaghbanzadeh/GUE\_splice\_reconstructed dataset.
It achieves the following results on the evaluation set:
* Loss: 0.3111
* F1 Score: 0.8970
* Accuracy: 0.8965
Model description
-----------------
More information needed
Intended uses & limitations
---------------------------
More information needed
Training and evaluation data
----------------------------
More information needed
Training procedure
------------------
### Training hyperparameters
The following hyperparameters were used during training:
* learning\_rate: 0.0005
* train\_batch\_size: 128
* eval\_batch\_size: 128
* seed: 42
* optimizer: Adam with betas=(0.9,0.999) and epsilon=1e-08
* lr\_scheduler\_type: linear
* training\_steps: 10000
### Training results
### Framework versions
* PEFT 0.9.0
* Transformers 4.38.2
* Pytorch 2.2.0+cu121
* Datasets 2.17.1
* Tokenizers 0.15.2
| [
"### Training hyperparameters\n\n\nThe following hyperparameters were used during training:\n\n\n* learning\\_rate: 0.0005\n* train\\_batch\\_size: 128\n* eval\\_batch\\_size: 128\n* seed: 42\n* optimizer: Adam with betas=(0.9,0.999) and epsilon=1e-08\n* lr\\_scheduler\\_type: linear\n* training\\_steps: 10000",
"### Training results",
"### Framework versions\n\n\n* PEFT 0.9.0\n* Transformers 4.38.2\n* Pytorch 2.2.0+cu121\n* Datasets 2.17.1\n* Tokenizers 0.15.2"
] | [
"TAGS\n#peft #safetensors #generated_from_trainer #base_model-mahdibaghbanzadeh/seqsight_4096_512_27M #region-us \n",
"### Training hyperparameters\n\n\nThe following hyperparameters were used during training:\n\n\n* learning\\_rate: 0.0005\n* train\\_batch\\_size: 128\n* eval\\_batch\\_size: 128\n* seed: 42\n* optimizer: Adam with betas=(0.9,0.999) and epsilon=1e-08\n* lr\\_scheduler\\_type: linear\n* training\\_steps: 10000",
"### Training results",
"### Framework versions\n\n\n* PEFT 0.9.0\n* Transformers 4.38.2\n* Pytorch 2.2.0+cu121\n* Datasets 2.17.1\n* Tokenizers 0.15.2"
] |
text-generation | transformers |
# Model Card for Model ID
<!-- Provide a quick summary of what the model is/does. -->
## Model Details
### Model Description
<!-- Provide a longer summary of what this model is. -->
This is the model card of a 🤗 transformers model that has been pushed on the Hub. This model card has been automatically generated.
- **Developed by:** [More Information Needed]
- **Funded by [optional]:** [More Information Needed]
- **Shared by [optional]:** [More Information Needed]
- **Model type:** [More Information Needed]
- **Language(s) (NLP):** [More Information Needed]
- **License:** [More Information Needed]
- **Finetuned from model [optional]:** [More Information Needed]
### Model Sources [optional]
<!-- Provide the basic links for the model. -->
- **Repository:** [More Information Needed]
- **Paper [optional]:** [More Information Needed]
- **Demo [optional]:** [More Information Needed]
## Uses
<!-- Address questions around how the model is intended to be used, including the foreseeable users of the model and those affected by the model. -->
### Direct Use
<!-- This section is for the model use without fine-tuning or plugging into a larger ecosystem/app. -->
[More Information Needed]
### Downstream Use [optional]
<!-- This section is for the model use when fine-tuned for a task, or when plugged into a larger ecosystem/app -->
[More Information Needed]
### Out-of-Scope Use
<!-- This section addresses misuse, malicious use, and uses that the model will not work well for. -->
[More Information Needed]
## Bias, Risks, and Limitations
<!-- This section is meant to convey both technical and sociotechnical limitations. -->
[More Information Needed]
### Recommendations
<!-- This section is meant to convey recommendations with respect to the bias, risk, and technical limitations. -->
Users (both direct and downstream) should be made aware of the risks, biases and limitations of the model. More information needed for further recommendations.
## How to Get Started with the Model
Use the code below to get started with the model.
[More Information Needed]
## Training Details
### Training Data
<!-- This should link to a Dataset Card, perhaps with a short stub of information on what the training data is all about as well as documentation related to data pre-processing or additional filtering. -->
[More Information Needed]
### Training Procedure
<!-- This relates heavily to the Technical Specifications. Content here should link to that section when it is relevant to the training procedure. -->
#### Preprocessing [optional]
[More Information Needed]
#### Training Hyperparameters
- **Training regime:** [More Information Needed] <!--fp32, fp16 mixed precision, bf16 mixed precision, bf16 non-mixed precision, fp16 non-mixed precision, fp8 mixed precision -->
#### Speeds, Sizes, Times [optional]
<!-- This section provides information about throughput, start/end time, checkpoint size if relevant, etc. -->
[More Information Needed]
## Evaluation
<!-- This section describes the evaluation protocols and provides the results. -->
### Testing Data, Factors & Metrics
#### Testing Data
<!-- This should link to a Dataset Card if possible. -->
[More Information Needed]
#### Factors
<!-- These are the things the evaluation is disaggregating by, e.g., subpopulations or domains. -->
[More Information Needed]
#### Metrics
<!-- These are the evaluation metrics being used, ideally with a description of why. -->
[More Information Needed]
### Results
[More Information Needed]
#### Summary
## Model Examination [optional]
<!-- Relevant interpretability work for the model goes here -->
[More Information Needed]
## Environmental Impact
<!-- Total emissions (in grams of CO2eq) and additional considerations, such as electricity usage, go here. Edit the suggested text below accordingly -->
Carbon emissions can be estimated using the [Machine Learning Impact calculator](https://mlco2.github.io/impact#compute) presented in [Lacoste et al. (2019)](https://arxiv.org/abs/1910.09700).
- **Hardware Type:** [More Information Needed]
- **Hours used:** [More Information Needed]
- **Cloud Provider:** [More Information Needed]
- **Compute Region:** [More Information Needed]
- **Carbon Emitted:** [More Information Needed]
## Technical Specifications [optional]
### Model Architecture and Objective
[More Information Needed]
### Compute Infrastructure
[More Information Needed]
#### Hardware
[More Information Needed]
#### Software
[More Information Needed]
## Citation [optional]
<!-- If there is a paper or blog post introducing the model, the APA and Bibtex information for that should go in this section. -->
**BibTeX:**
[More Information Needed]
**APA:**
[More Information Needed]
## Glossary [optional]
<!-- If relevant, include terms and calculations in this section that can help readers understand the model or model card. -->
[More Information Needed]
## More Information [optional]
[More Information Needed]
## Model Card Authors [optional]
[More Information Needed]
## Model Card Contact
[More Information Needed] | {"library_name": "transformers", "tags": []} | orpo-explorers/kaist-mistral-orpo-capybara-beta-0.05-2epoch | null | [
"transformers",
"safetensors",
"mistral",
"text-generation",
"conversational",
"arxiv:1910.09700",
"autotrain_compatible",
"endpoints_compatible",
"text-generation-inference",
"region:us"
] | null | 2024-04-26T18:10:43+00:00 | [
"1910.09700"
] | [] | TAGS
#transformers #safetensors #mistral #text-generation #conversational #arxiv-1910.09700 #autotrain_compatible #endpoints_compatible #text-generation-inference #region-us
|
# Model Card for Model ID
## Model Details
### Model Description
This is the model card of a transformers model that has been pushed on the Hub. This model card has been automatically generated.
- Developed by:
- Funded by [optional]:
- Shared by [optional]:
- Model type:
- Language(s) (NLP):
- License:
- Finetuned from model [optional]:
### Model Sources [optional]
- Repository:
- Paper [optional]:
- Demo [optional]:
## Uses
### Direct Use
### Downstream Use [optional]
### Out-of-Scope Use
## Bias, Risks, and Limitations
### Recommendations
Users (both direct and downstream) should be made aware of the risks, biases and limitations of the model. More information needed for further recommendations.
## How to Get Started with the Model
Use the code below to get started with the model.
## Training Details
### Training Data
### Training Procedure
#### Preprocessing [optional]
#### Training Hyperparameters
- Training regime:
#### Speeds, Sizes, Times [optional]
## Evaluation
### Testing Data, Factors & Metrics
#### Testing Data
#### Factors
#### Metrics
### Results
#### Summary
## Model Examination [optional]
## Environmental Impact
Carbon emissions can be estimated using the Machine Learning Impact calculator presented in Lacoste et al. (2019).
- Hardware Type:
- Hours used:
- Cloud Provider:
- Compute Region:
- Carbon Emitted:
## Technical Specifications [optional]
### Model Architecture and Objective
### Compute Infrastructure
#### Hardware
#### Software
[optional]
BibTeX:
APA:
## Glossary [optional]
## More Information [optional]
## Model Card Authors [optional]
## Model Card Contact
| [
"# Model Card for Model ID",
"## Model Details",
"### Model Description\n\n\n\nThis is the model card of a transformers model that has been pushed on the Hub. This model card has been automatically generated.\n\n- Developed by: \n- Funded by [optional]: \n- Shared by [optional]: \n- Model type: \n- Language(s) (NLP): \n- License: \n- Finetuned from model [optional]:",
"### Model Sources [optional]\n\n\n\n- Repository: \n- Paper [optional]: \n- Demo [optional]:",
"## Uses",
"### Direct Use",
"### Downstream Use [optional]",
"### Out-of-Scope Use",
"## Bias, Risks, and Limitations",
"### Recommendations\n\n\n\nUsers (both direct and downstream) should be made aware of the risks, biases and limitations of the model. More information needed for further recommendations.",
"## How to Get Started with the Model\n\nUse the code below to get started with the model.",
"## Training Details",
"### Training Data",
"### Training Procedure",
"#### Preprocessing [optional]",
"#### Training Hyperparameters\n\n- Training regime:",
"#### Speeds, Sizes, Times [optional]",
"## Evaluation",
"### Testing Data, Factors & Metrics",
"#### Testing Data",
"#### Factors",
"#### Metrics",
"### Results",
"#### Summary",
"## Model Examination [optional]",
"## Environmental Impact\n\n\n\nCarbon emissions can be estimated using the Machine Learning Impact calculator presented in Lacoste et al. (2019).\n\n- Hardware Type: \n- Hours used: \n- Cloud Provider: \n- Compute Region: \n- Carbon Emitted:",
"## Technical Specifications [optional]",
"### Model Architecture and Objective",
"### Compute Infrastructure",
"#### Hardware",
"#### Software\n\n\n\n[optional]\n\n\n\nBibTeX:\n\n\n\nAPA:",
"## Glossary [optional]",
"## More Information [optional]",
"## Model Card Authors [optional]",
"## Model Card Contact"
] | [
"TAGS\n#transformers #safetensors #mistral #text-generation #conversational #arxiv-1910.09700 #autotrain_compatible #endpoints_compatible #text-generation-inference #region-us \n",
"# Model Card for Model ID",
"## Model Details",
"### Model Description\n\n\n\nThis is the model card of a transformers model that has been pushed on the Hub. This model card has been automatically generated.\n\n- Developed by: \n- Funded by [optional]: \n- Shared by [optional]: \n- Model type: \n- Language(s) (NLP): \n- License: \n- Finetuned from model [optional]:",
"### Model Sources [optional]\n\n\n\n- Repository: \n- Paper [optional]: \n- Demo [optional]:",
"## Uses",
"### Direct Use",
"### Downstream Use [optional]",
"### Out-of-Scope Use",
"## Bias, Risks, and Limitations",
"### Recommendations\n\n\n\nUsers (both direct and downstream) should be made aware of the risks, biases and limitations of the model. More information needed for further recommendations.",
"## How to Get Started with the Model\n\nUse the code below to get started with the model.",
"## Training Details",
"### Training Data",
"### Training Procedure",
"#### Preprocessing [optional]",
"#### Training Hyperparameters\n\n- Training regime:",
"#### Speeds, Sizes, Times [optional]",
"## Evaluation",
"### Testing Data, Factors & Metrics",
"#### Testing Data",
"#### Factors",
"#### Metrics",
"### Results",
"#### Summary",
"## Model Examination [optional]",
"## Environmental Impact\n\n\n\nCarbon emissions can be estimated using the Machine Learning Impact calculator presented in Lacoste et al. (2019).\n\n- Hardware Type: \n- Hours used: \n- Cloud Provider: \n- Compute Region: \n- Carbon Emitted:",
"## Technical Specifications [optional]",
"### Model Architecture and Objective",
"### Compute Infrastructure",
"#### Hardware",
"#### Software\n\n\n\n[optional]\n\n\n\nBibTeX:\n\n\n\nAPA:",
"## Glossary [optional]",
"## More Information [optional]",
"## Model Card Authors [optional]",
"## Model Card Contact"
] |
null | peft |
<!-- This model card has been generated automatically according to the information the Trainer had access to. You
should probably proofread and complete it, then remove this comment. -->
# GUE_tf_0-seqsight_4096_512_27M-L1_f
This model is a fine-tuned version of [mahdibaghbanzadeh/seqsight_4096_512_27M](https://huggingface.co/mahdibaghbanzadeh/seqsight_4096_512_27M) on the [mahdibaghbanzadeh/GUE_tf_0](https://huggingface.co/datasets/mahdibaghbanzadeh/GUE_tf_0) dataset.
It achieves the following results on the evaluation set:
- Loss: 0.3628
- F1 Score: 0.8287
- Accuracy: 0.829
## Model description
More information needed
## Intended uses & limitations
More information needed
## Training and evaluation data
More information needed
## Training procedure
### Training hyperparameters
The following hyperparameters were used during training:
- learning_rate: 0.0005
- train_batch_size: 128
- eval_batch_size: 128
- seed: 42
- optimizer: Adam with betas=(0.9,0.999) and epsilon=1e-08
- lr_scheduler_type: linear
- training_steps: 10000
### Training results
| Training Loss | Epoch | Step | Validation Loss | F1 Score | Accuracy |
|:-------------:|:-----:|:-----:|:---------------:|:--------:|:--------:|
| 0.5368 | 0.79 | 200 | 0.4777 | 0.7615 | 0.762 |
| 0.4791 | 1.58 | 400 | 0.4668 | 0.7706 | 0.771 |
| 0.475 | 2.37 | 600 | 0.4695 | 0.7775 | 0.779 |
| 0.467 | 3.16 | 800 | 0.4623 | 0.7741 | 0.774 |
| 0.4632 | 3.95 | 1000 | 0.4601 | 0.7725 | 0.773 |
| 0.4589 | 4.74 | 1200 | 0.4607 | 0.7710 | 0.771 |
| 0.46 | 5.53 | 1400 | 0.4565 | 0.7751 | 0.775 |
| 0.45 | 6.32 | 1600 | 0.4560 | 0.7810 | 0.781 |
| 0.4547 | 7.11 | 1800 | 0.4560 | 0.7801 | 0.78 |
| 0.4546 | 7.91 | 2000 | 0.4564 | 0.7840 | 0.784 |
| 0.4498 | 8.7 | 2200 | 0.4513 | 0.7849 | 0.785 |
| 0.4482 | 9.49 | 2400 | 0.4492 | 0.7754 | 0.776 |
| 0.4481 | 10.28 | 2600 | 0.4586 | 0.7799 | 0.78 |
| 0.4436 | 11.07 | 2800 | 0.4518 | 0.7840 | 0.784 |
| 0.4431 | 11.86 | 3000 | 0.4520 | 0.7820 | 0.782 |
| 0.4432 | 12.65 | 3200 | 0.4529 | 0.7829 | 0.783 |
| 0.4435 | 13.44 | 3400 | 0.4450 | 0.7858 | 0.786 |
| 0.4418 | 14.23 | 3600 | 0.4439 | 0.7840 | 0.784 |
| 0.4413 | 15.02 | 3800 | 0.4462 | 0.7891 | 0.789 |
| 0.4417 | 15.81 | 4000 | 0.4486 | 0.7870 | 0.787 |
| 0.4358 | 16.6 | 4200 | 0.4429 | 0.7838 | 0.784 |
| 0.4389 | 17.39 | 4400 | 0.4510 | 0.7909 | 0.791 |
| 0.4375 | 18.18 | 4600 | 0.4467 | 0.7910 | 0.791 |
| 0.4372 | 18.97 | 4800 | 0.4495 | 0.7870 | 0.787 |
| 0.4389 | 19.76 | 5000 | 0.4432 | 0.7901 | 0.79 |
| 0.4349 | 20.55 | 5200 | 0.4397 | 0.7965 | 0.797 |
| 0.4314 | 21.34 | 5400 | 0.4421 | 0.7931 | 0.793 |
| 0.4309 | 22.13 | 5600 | 0.4436 | 0.7951 | 0.795 |
| 0.4365 | 22.92 | 5800 | 0.4440 | 0.7941 | 0.794 |
| 0.4319 | 23.72 | 6000 | 0.4426 | 0.7951 | 0.795 |
| 0.43 | 24.51 | 6200 | 0.4386 | 0.7949 | 0.795 |
| 0.4363 | 25.3 | 6400 | 0.4399 | 0.8001 | 0.8 |
| 0.4283 | 26.09 | 6600 | 0.4401 | 0.7918 | 0.792 |
| 0.4298 | 26.88 | 6800 | 0.4428 | 0.7921 | 0.792 |
| 0.4328 | 27.67 | 7000 | 0.4381 | 0.7949 | 0.795 |
| 0.4286 | 28.46 | 7200 | 0.4377 | 0.7965 | 0.797 |
| 0.4325 | 29.25 | 7400 | 0.4383 | 0.7959 | 0.796 |
| 0.4314 | 30.04 | 7600 | 0.4395 | 0.7929 | 0.793 |
| 0.429 | 30.83 | 7800 | 0.4394 | 0.7969 | 0.797 |
| 0.4297 | 31.62 | 8000 | 0.4380 | 0.7956 | 0.796 |
| 0.4302 | 32.41 | 8200 | 0.4424 | 0.7951 | 0.795 |
| 0.4248 | 33.2 | 8400 | 0.4404 | 0.794 | 0.794 |
| 0.4335 | 33.99 | 8600 | 0.4395 | 0.7929 | 0.793 |
| 0.4294 | 34.78 | 8800 | 0.4382 | 0.7938 | 0.794 |
| 0.4247 | 35.57 | 9000 | 0.4382 | 0.7928 | 0.793 |
| 0.4262 | 36.36 | 9200 | 0.4413 | 0.7941 | 0.794 |
| 0.4287 | 37.15 | 9400 | 0.4410 | 0.7951 | 0.795 |
| 0.4282 | 37.94 | 9600 | 0.4392 | 0.7959 | 0.796 |
| 0.4277 | 38.74 | 9800 | 0.4398 | 0.7950 | 0.795 |
| 0.4288 | 39.53 | 10000 | 0.4395 | 0.7930 | 0.793 |
### Framework versions
- PEFT 0.9.0
- Transformers 4.38.2
- Pytorch 2.2.0+cu121
- Datasets 2.17.1
- Tokenizers 0.15.2 | {"library_name": "peft", "tags": ["generated_from_trainer"], "metrics": ["accuracy"], "base_model": "mahdibaghbanzadeh/seqsight_4096_512_27M", "model-index": [{"name": "GUE_tf_0-seqsight_4096_512_27M-L1_f", "results": []}]} | mahdibaghbanzadeh/GUE_tf_0-seqsight_4096_512_27M-L1_f | null | [
"peft",
"safetensors",
"generated_from_trainer",
"base_model:mahdibaghbanzadeh/seqsight_4096_512_27M",
"region:us"
] | null | 2024-04-26T18:11:09+00:00 | [] | [] | TAGS
#peft #safetensors #generated_from_trainer #base_model-mahdibaghbanzadeh/seqsight_4096_512_27M #region-us
| GUE\_tf\_0-seqsight\_4096\_512\_27M-L1\_f
=========================================
This model is a fine-tuned version of mahdibaghbanzadeh/seqsight\_4096\_512\_27M on the mahdibaghbanzadeh/GUE\_tf\_0 dataset.
It achieves the following results on the evaluation set:
* Loss: 0.3628
* F1 Score: 0.8287
* Accuracy: 0.829
Model description
-----------------
More information needed
Intended uses & limitations
---------------------------
More information needed
Training and evaluation data
----------------------------
More information needed
Training procedure
------------------
### Training hyperparameters
The following hyperparameters were used during training:
* learning\_rate: 0.0005
* train\_batch\_size: 128
* eval\_batch\_size: 128
* seed: 42
* optimizer: Adam with betas=(0.9,0.999) and epsilon=1e-08
* lr\_scheduler\_type: linear
* training\_steps: 10000
### Training results
### Framework versions
* PEFT 0.9.0
* Transformers 4.38.2
* Pytorch 2.2.0+cu121
* Datasets 2.17.1
* Tokenizers 0.15.2
| [
"### Training hyperparameters\n\n\nThe following hyperparameters were used during training:\n\n\n* learning\\_rate: 0.0005\n* train\\_batch\\_size: 128\n* eval\\_batch\\_size: 128\n* seed: 42\n* optimizer: Adam with betas=(0.9,0.999) and epsilon=1e-08\n* lr\\_scheduler\\_type: linear\n* training\\_steps: 10000",
"### Training results",
"### Framework versions\n\n\n* PEFT 0.9.0\n* Transformers 4.38.2\n* Pytorch 2.2.0+cu121\n* Datasets 2.17.1\n* Tokenizers 0.15.2"
] | [
"TAGS\n#peft #safetensors #generated_from_trainer #base_model-mahdibaghbanzadeh/seqsight_4096_512_27M #region-us \n",
"### Training hyperparameters\n\n\nThe following hyperparameters were used during training:\n\n\n* learning\\_rate: 0.0005\n* train\\_batch\\_size: 128\n* eval\\_batch\\_size: 128\n* seed: 42\n* optimizer: Adam with betas=(0.9,0.999) and epsilon=1e-08\n* lr\\_scheduler\\_type: linear\n* training\\_steps: 10000",
"### Training results",
"### Framework versions\n\n\n* PEFT 0.9.0\n* Transformers 4.38.2\n* Pytorch 2.2.0+cu121\n* Datasets 2.17.1\n* Tokenizers 0.15.2"
] |
sentence-similarity | sentence-transformers | # # Fast-Inference with Ctranslate2
Speedup inference while reducing memory by 2x-4x using int8 inference in C++ on CPU or GPU.
quantized version of [sentence-transformers/all-MiniLM-L6-v2](https://huggingface.co/sentence-transformers/all-MiniLM-L6-v2)
```bash
pip install hf-hub-ctranslate2>=2.12.0 ctranslate2>=3.17.1
```
```python
# from transformers import AutoTokenizer
model_name = "michaelfeil/ct2fast-all-MiniLM-L6-v2"
model_name_orig="sentence-transformers/all-MiniLM-L6-v2"
from hf_hub_ctranslate2 import EncoderCT2fromHfHub
model = EncoderCT2fromHfHub(
# load in int8 on CUDA
model_name_or_path=model_name,
device="cuda",
compute_type="int8_float16"
)
outputs = model.generate(
text=["I like soccer", "I like tennis", "The eiffel tower is in Paris"],
max_length=64,
) # perform downstream tasks on outputs
outputs["pooler_output"]
outputs["last_hidden_state"]
outputs["attention_mask"]
# alternative, use SentenceTransformer Mix-In
# for end-to-end Sentence embeddings generation
# (not pulling from this CT2fast-HF repo)
from hf_hub_ctranslate2 import CT2SentenceTransformer
model = CT2SentenceTransformer(
model_name_orig, compute_type="int8_float16", device="cuda"
)
embeddings = model.encode(
["I like soccer", "I like tennis", "The eiffel tower is in Paris"],
batch_size=32,
convert_to_numpy=True,
normalize_embeddings=True,
)
print(embeddings.shape, embeddings)
scores = (embeddings @ embeddings.T) * 100
# Hint: you can also host this code via REST API and
# via github.com/michaelfeil/infinity
```
Checkpoint compatible to [ctranslate2>=3.17.1](https://github.com/OpenNMT/CTranslate2)
and [hf-hub-ctranslate2>=2.12.0](https://github.com/michaelfeil/hf-hub-ctranslate2)
- `compute_type=int8_float16` for `device="cuda"`
- `compute_type=int8` for `device="cpu"`
Converted on 2023-10-13 using
```
LLama-2 -> removed <pad> token.
```
# Licence and other remarks:
This is just a quantized version. Licence conditions are intended to be idential to original huggingface repo.
# Original description
# all-MiniLM-L6-v2
This is a [sentence-transformers](https://www.SBERT.net) model: It maps sentences & paragraphs to a 384 dimensional dense vector space and can be used for tasks like clustering or semantic search.
## Usage (Sentence-Transformers)
Using this model becomes easy when you have [sentence-transformers](https://www.SBERT.net) installed:
```
pip install -U sentence-transformers
```
Then you can use the model like this:
```python
from sentence_transformers import SentenceTransformer
sentences = ["This is an example sentence", "Each sentence is converted"]
model = SentenceTransformer('sentence-transformers/all-MiniLM-L6-v2')
embeddings = model.encode(sentences)
print(embeddings)
```
## Usage (HuggingFace Transformers)
Without [sentence-transformers](https://www.SBERT.net), you can use the model like this: First, you pass your input through the transformer model, then you have to apply the right pooling-operation on-top of the contextualized word embeddings.
```python
from transformers import AutoTokenizer, AutoModel
import torch
import torch.nn.functional as F
#Mean Pooling - Take attention mask into account for correct averaging
def mean_pooling(model_output, attention_mask):
token_embeddings = model_output[0] #First element of model_output contains all token embeddings
input_mask_expanded = attention_mask.unsqueeze(-1).expand(token_embeddings.size()).float()
return torch.sum(token_embeddings * input_mask_expanded, 1) / torch.clamp(input_mask_expanded.sum(1), min=1e-9)
# Sentences we want sentence embeddings for
sentences = ['This is an example sentence', 'Each sentence is converted']
# Load model from HuggingFace Hub
tokenizer = AutoTokenizer.from_pretrained('sentence-transformers/all-MiniLM-L6-v2')
model = AutoModel.from_pretrained('sentence-transformers/all-MiniLM-L6-v2')
# Tokenize sentences
encoded_input = tokenizer(sentences, padding=True, truncation=True, return_tensors='pt')
# Compute token embeddings
with torch.no_grad():
model_output = model(**encoded_input)
# Perform pooling
sentence_embeddings = mean_pooling(model_output, encoded_input['attention_mask'])
# Normalize embeddings
sentence_embeddings = F.normalize(sentence_embeddings, p=2, dim=1)
print("Sentence embeddings:")
print(sentence_embeddings)
```
## Evaluation Results
For an automated evaluation of this model, see the *Sentence Embeddings Benchmark*: [https://seb.sbert.net](https://seb.sbert.net?model_name=sentence-transformers/all-MiniLM-L6-v2)
------
## Background
The project aims to train sentence embedding models on very large sentence level datasets using a self-supervised
contrastive learning objective. We used the pretrained [`nreimers/MiniLM-L6-H384-uncased`](https://huggingface.co/nreimers/MiniLM-L6-H384-uncased) model and fine-tuned in on a
1B sentence pairs dataset. We use a contrastive learning objective: given a sentence from the pair, the model should predict which out of a set of randomly sampled other sentences, was actually paired with it in our dataset.
We developped this model during the
[Community week using JAX/Flax for NLP & CV](https://discuss.huggingface.co/t/open-to-the-community-community-week-using-jax-flax-for-nlp-cv/7104),
organized by Hugging Face. We developped this model as part of the project:
[Train the Best Sentence Embedding Model Ever with 1B Training Pairs](https://discuss.huggingface.co/t/train-the-best-sentence-embedding-model-ever-with-1b-training-pairs/7354). We benefited from efficient hardware infrastructure to run the project: 7 TPUs v3-8, as well as intervention from Googles Flax, JAX, and Cloud team member about efficient deep learning frameworks.
## Intended uses
Our model is intented to be used as a sentence and short paragraph encoder. Given an input text, it ouptuts a vector which captures
the semantic information. The sentence vector may be used for information retrieval, clustering or sentence similarity tasks.
By default, input text longer than 256 word pieces is truncated.
## Training procedure
### Pre-training
We use the pretrained [`nreimers/MiniLM-L6-H384-uncased`](https://huggingface.co/nreimers/MiniLM-L6-H384-uncased) model. Please refer to the model card for more detailed information about the pre-training procedure.
### Fine-tuning
We fine-tune the model using a contrastive objective. Formally, we compute the cosine similarity from each possible sentence pairs from the batch.
We then apply the cross entropy loss by comparing with true pairs.
#### Hyper parameters
We trained ou model on a TPU v3-8. We train the model during 100k steps using a batch size of 1024 (128 per TPU core).
We use a learning rate warm up of 500. The sequence length was limited to 128 tokens. We used the AdamW optimizer with
a 2e-5 learning rate. The full training script is accessible in this current repository: `train_script.py`.
#### Training data
We use the concatenation from multiple datasets to fine-tune our model. The total number of sentence pairs is above 1 billion sentences.
We sampled each dataset given a weighted probability which configuration is detailed in the `data_config.json` file.
| Dataset | Paper | Number of training tuples |
|--------------------------------------------------------|:----------------------------------------:|:--------------------------:|
| [Reddit comments (2015-2018)](https://github.com/PolyAI-LDN/conversational-datasets/tree/master/reddit) | [paper](https://arxiv.org/abs/1904.06472) | 726,484,430 |
| [S2ORC](https://github.com/allenai/s2orc) Citation pairs (Abstracts) | [paper](https://aclanthology.org/2020.acl-main.447/) | 116,288,806 |
| [WikiAnswers](https://github.com/afader/oqa#wikianswers-corpus) Duplicate question pairs | [paper](https://doi.org/10.1145/2623330.2623677) | 77,427,422 |
| [PAQ](https://github.com/facebookresearch/PAQ) (Question, Answer) pairs | [paper](https://arxiv.org/abs/2102.07033) | 64,371,441 |
| [S2ORC](https://github.com/allenai/s2orc) Citation pairs (Titles) | [paper](https://aclanthology.org/2020.acl-main.447/) | 52,603,982 |
| [S2ORC](https://github.com/allenai/s2orc) (Title, Abstract) | [paper](https://aclanthology.org/2020.acl-main.447/) | 41,769,185 |
| [Stack Exchange](https://huggingface.co/datasets/flax-sentence-embeddings/stackexchange_xml) (Title, Body) pairs | - | 25,316,456 |
| [Stack Exchange](https://huggingface.co/datasets/flax-sentence-embeddings/stackexchange_xml) (Title+Body, Answer) pairs | - | 21,396,559 |
| [Stack Exchange](https://huggingface.co/datasets/flax-sentence-embeddings/stackexchange_xml) (Title, Answer) pairs | - | 21,396,559 |
| [MS MARCO](https://microsoft.github.io/msmarco/) triplets | [paper](https://doi.org/10.1145/3404835.3462804) | 9,144,553 |
| [GOOAQ: Open Question Answering with Diverse Answer Types](https://github.com/allenai/gooaq) | [paper](https://arxiv.org/pdf/2104.08727.pdf) | 3,012,496 |
| [Yahoo Answers](https://www.kaggle.com/soumikrakshit/yahoo-answers-dataset) (Title, Answer) | [paper](https://proceedings.neurips.cc/paper/2015/hash/250cf8b51c773f3f8dc8b4be867a9a02-Abstract.html) | 1,198,260 |
| [Code Search](https://huggingface.co/datasets/code_search_net) | - | 1,151,414 |
| [COCO](https://cocodataset.org/#home) Image captions | [paper](https://link.springer.com/chapter/10.1007%2F978-3-319-10602-1_48) | 828,395|
| [SPECTER](https://github.com/allenai/specter) citation triplets | [paper](https://doi.org/10.18653/v1/2020.acl-main.207) | 684,100 |
| [Yahoo Answers](https://www.kaggle.com/soumikrakshit/yahoo-answers-dataset) (Question, Answer) | [paper](https://proceedings.neurips.cc/paper/2015/hash/250cf8b51c773f3f8dc8b4be867a9a02-Abstract.html) | 681,164 |
| [Yahoo Answers](https://www.kaggle.com/soumikrakshit/yahoo-answers-dataset) (Title, Question) | [paper](https://proceedings.neurips.cc/paper/2015/hash/250cf8b51c773f3f8dc8b4be867a9a02-Abstract.html) | 659,896 |
| [SearchQA](https://huggingface.co/datasets/search_qa) | [paper](https://arxiv.org/abs/1704.05179) | 582,261 |
| [Eli5](https://huggingface.co/datasets/eli5) | [paper](https://doi.org/10.18653/v1/p19-1346) | 325,475 |
| [Flickr 30k](https://shannon.cs.illinois.edu/DenotationGraph/) | [paper](https://transacl.org/ojs/index.php/tacl/article/view/229/33) | 317,695 |
| [Stack Exchange](https://huggingface.co/datasets/flax-sentence-embeddings/stackexchange_xml) Duplicate questions (titles) | | 304,525 |
| AllNLI ([SNLI](https://nlp.stanford.edu/projects/snli/) and [MultiNLI](https://cims.nyu.edu/~sbowman/multinli/) | [paper SNLI](https://doi.org/10.18653/v1/d15-1075), [paper MultiNLI](https://doi.org/10.18653/v1/n18-1101) | 277,230 |
| [Stack Exchange](https://huggingface.co/datasets/flax-sentence-embeddings/stackexchange_xml) Duplicate questions (bodies) | | 250,519 |
| [Stack Exchange](https://huggingface.co/datasets/flax-sentence-embeddings/stackexchange_xml) Duplicate questions (titles+bodies) | | 250,460 |
| [Sentence Compression](https://github.com/google-research-datasets/sentence-compression) | [paper](https://www.aclweb.org/anthology/D13-1155/) | 180,000 |
| [Wikihow](https://github.com/pvl/wikihow_pairs_dataset) | [paper](https://arxiv.org/abs/1810.09305) | 128,542 |
| [Altlex](https://github.com/chridey/altlex/) | [paper](https://aclanthology.org/P16-1135.pdf) | 112,696 |
| [Quora Question Triplets](https://quoradata.quora.com/First-Quora-Dataset-Release-Question-Pairs) | - | 103,663 |
| [Simple Wikipedia](https://cs.pomona.edu/~dkauchak/simplification/) | [paper](https://www.aclweb.org/anthology/P11-2117/) | 102,225 |
| [Natural Questions (NQ)](https://ai.google.com/research/NaturalQuestions) | [paper](https://transacl.org/ojs/index.php/tacl/article/view/1455) | 100,231 |
| [SQuAD2.0](https://rajpurkar.github.io/SQuAD-explorer/) | [paper](https://aclanthology.org/P18-2124.pdf) | 87,599 |
| [TriviaQA](https://huggingface.co/datasets/trivia_qa) | - | 73,346 |
| **Total** | | **1,170,060,424** | | {"language": "en", "license": "apache-2.0", "tags": ["ctranslate2", "int8", "float16", "sentence-transformers", "feature-extraction", "sentence-similarity"], "datasets": ["s2orc", "flax-sentence-embeddings/stackexchange_xml", "ms_marco", "gooaq", "yahoo_answers_topics", "code_search_net", "search_qa", "eli5", "snli", "multi_nli", "wikihow", "natural_questions", "trivia_qa", "embedding-data/sentence-compression", "embedding-data/flickr30k-captions", "embedding-data/altlex", "embedding-data/simple-wiki", "embedding-data/QQP", "embedding-data/SPECTER", "embedding-data/PAQ_pairs", "embedding-data/WikiAnswers"], "pipeline_tag": "sentence-similarity"} | nitsuai/ct2fast-all-MiniLM-L6-v2 | null | [
"sentence-transformers",
"bert",
"ctranslate2",
"int8",
"float16",
"feature-extraction",
"sentence-similarity",
"en",
"dataset:s2orc",
"dataset:flax-sentence-embeddings/stackexchange_xml",
"dataset:ms_marco",
"dataset:gooaq",
"dataset:yahoo_answers_topics",
"dataset:code_search_net",
"dataset:search_qa",
"dataset:eli5",
"dataset:snli",
"dataset:multi_nli",
"dataset:wikihow",
"dataset:natural_questions",
"dataset:trivia_qa",
"dataset:embedding-data/sentence-compression",
"dataset:embedding-data/flickr30k-captions",
"dataset:embedding-data/altlex",
"dataset:embedding-data/simple-wiki",
"dataset:embedding-data/QQP",
"dataset:embedding-data/SPECTER",
"dataset:embedding-data/PAQ_pairs",
"dataset:embedding-data/WikiAnswers",
"arxiv:1904.06472",
"arxiv:2102.07033",
"arxiv:2104.08727",
"arxiv:1704.05179",
"arxiv:1810.09305",
"license:apache-2.0",
"endpoints_compatible",
"region:us"
] | null | 2024-04-26T18:14:40+00:00 | [
"1904.06472",
"2102.07033",
"2104.08727",
"1704.05179",
"1810.09305"
] | [
"en"
] | TAGS
#sentence-transformers #bert #ctranslate2 #int8 #float16 #feature-extraction #sentence-similarity #en #dataset-s2orc #dataset-flax-sentence-embeddings/stackexchange_xml #dataset-ms_marco #dataset-gooaq #dataset-yahoo_answers_topics #dataset-code_search_net #dataset-search_qa #dataset-eli5 #dataset-snli #dataset-multi_nli #dataset-wikihow #dataset-natural_questions #dataset-trivia_qa #dataset-embedding-data/sentence-compression #dataset-embedding-data/flickr30k-captions #dataset-embedding-data/altlex #dataset-embedding-data/simple-wiki #dataset-embedding-data/QQP #dataset-embedding-data/SPECTER #dataset-embedding-data/PAQ_pairs #dataset-embedding-data/WikiAnswers #arxiv-1904.06472 #arxiv-2102.07033 #arxiv-2104.08727 #arxiv-1704.05179 #arxiv-1810.09305 #license-apache-2.0 #endpoints_compatible #region-us
| # Fast-Inference with Ctranslate2
=================================
Speedup inference while reducing memory by 2x-4x using int8 inference in C++ on CPU or GPU.
quantized version of sentence-transformers/all-MiniLM-L6-v2
Checkpoint compatible to ctranslate2>=3.17.1
and hf-hub-ctranslate2>=2.12.0
* 'compute\_type=int8\_float16' for 'device="cuda"'
* 'compute\_type=int8' for 'device="cpu"'
Converted on 2023-10-13 using
Licence and other remarks:
==========================
This is just a quantized version. Licence conditions are intended to be idential to original huggingface repo.
Original description
====================
all-MiniLM-L6-v2
================
This is a sentence-transformers model: It maps sentences & paragraphs to a 384 dimensional dense vector space and can be used for tasks like clustering or semantic search.
Usage (Sentence-Transformers)
-----------------------------
Using this model becomes easy when you have sentence-transformers installed:
Then you can use the model like this:
Usage (HuggingFace Transformers)
--------------------------------
Without sentence-transformers, you can use the model like this: First, you pass your input through the transformer model, then you have to apply the right pooling-operation on-top of the contextualized word embeddings.
Evaluation Results
------------------
For an automated evaluation of this model, see the *Sentence Embeddings Benchmark*: URL
---
Background
----------
The project aims to train sentence embedding models on very large sentence level datasets using a self-supervised
contrastive learning objective. We used the pretrained 'nreimers/MiniLM-L6-H384-uncased' model and fine-tuned in on a
1B sentence pairs dataset. We use a contrastive learning objective: given a sentence from the pair, the model should predict which out of a set of randomly sampled other sentences, was actually paired with it in our dataset.
We developped this model during the
Community week using JAX/Flax for NLP & CV,
organized by Hugging Face. We developped this model as part of the project:
Train the Best Sentence Embedding Model Ever with 1B Training Pairs. We benefited from efficient hardware infrastructure to run the project: 7 TPUs v3-8, as well as intervention from Googles Flax, JAX, and Cloud team member about efficient deep learning frameworks.
Intended uses
-------------
Our model is intented to be used as a sentence and short paragraph encoder. Given an input text, it ouptuts a vector which captures
the semantic information. The sentence vector may be used for information retrieval, clustering or sentence similarity tasks.
By default, input text longer than 256 word pieces is truncated.
Training procedure
------------------
### Pre-training
We use the pretrained 'nreimers/MiniLM-L6-H384-uncased' model. Please refer to the model card for more detailed information about the pre-training procedure.
### Fine-tuning
We fine-tune the model using a contrastive objective. Formally, we compute the cosine similarity from each possible sentence pairs from the batch.
We then apply the cross entropy loss by comparing with true pairs.
#### Hyper parameters
We trained ou model on a TPU v3-8. We train the model during 100k steps using a batch size of 1024 (128 per TPU core).
We use a learning rate warm up of 500. The sequence length was limited to 128 tokens. We used the AdamW optimizer with
a 2e-5 learning rate. The full training script is accessible in this current repository: 'train\_script.py'.
#### Training data
We use the concatenation from multiple datasets to fine-tune our model. The total number of sentence pairs is above 1 billion sentences.
We sampled each dataset given a weighted probability which configuration is detailed in the 'data\_config.json' file.
| [
"# Fast-Inference with Ctranslate2\n=================================\n\n\nSpeedup inference while reducing memory by 2x-4x using int8 inference in C++ on CPU or GPU.\n\n\nquantized version of sentence-transformers/all-MiniLM-L6-v2\n\n\nCheckpoint compatible to ctranslate2>=3.17.1\nand hf-hub-ctranslate2>=2.12.0\n\n\n* 'compute\\_type=int8\\_float16' for 'device=\"cuda\"'\n* 'compute\\_type=int8' for 'device=\"cpu\"'\n\n\nConverted on 2023-10-13 using\n\n\nLicence and other remarks:\n==========================\n\n\nThis is just a quantized version. Licence conditions are intended to be idential to original huggingface repo.\n\n\nOriginal description\n====================\n\n\nall-MiniLM-L6-v2\n================\n\n\nThis is a sentence-transformers model: It maps sentences & paragraphs to a 384 dimensional dense vector space and can be used for tasks like clustering or semantic search.\n\n\nUsage (Sentence-Transformers)\n-----------------------------\n\n\nUsing this model becomes easy when you have sentence-transformers installed:\n\n\nThen you can use the model like this:\n\n\nUsage (HuggingFace Transformers)\n--------------------------------\n\n\nWithout sentence-transformers, you can use the model like this: First, you pass your input through the transformer model, then you have to apply the right pooling-operation on-top of the contextualized word embeddings.\n\n\nEvaluation Results\n------------------\n\n\nFor an automated evaluation of this model, see the *Sentence Embeddings Benchmark*: URL\n\n\n\n\n---\n\n\nBackground\n----------\n\n\nThe project aims to train sentence embedding models on very large sentence level datasets using a self-supervised\ncontrastive learning objective. We used the pretrained 'nreimers/MiniLM-L6-H384-uncased' model and fine-tuned in on a\n1B sentence pairs dataset. We use a contrastive learning objective: given a sentence from the pair, the model should predict which out of a set of randomly sampled other sentences, was actually paired with it in our dataset.\n\n\nWe developped this model during the\nCommunity week using JAX/Flax for NLP & CV,\norganized by Hugging Face. We developped this model as part of the project:\nTrain the Best Sentence Embedding Model Ever with 1B Training Pairs. We benefited from efficient hardware infrastructure to run the project: 7 TPUs v3-8, as well as intervention from Googles Flax, JAX, and Cloud team member about efficient deep learning frameworks.\n\n\nIntended uses\n-------------\n\n\nOur model is intented to be used as a sentence and short paragraph encoder. Given an input text, it ouptuts a vector which captures\nthe semantic information. The sentence vector may be used for information retrieval, clustering or sentence similarity tasks.\n\n\nBy default, input text longer than 256 word pieces is truncated.\n\n\nTraining procedure\n------------------",
"### Pre-training\n\n\nWe use the pretrained 'nreimers/MiniLM-L6-H384-uncased' model. Please refer to the model card for more detailed information about the pre-training procedure.",
"### Fine-tuning\n\n\nWe fine-tune the model using a contrastive objective. Formally, we compute the cosine similarity from each possible sentence pairs from the batch.\nWe then apply the cross entropy loss by comparing with true pairs.",
"#### Hyper parameters\n\n\nWe trained ou model on a TPU v3-8. We train the model during 100k steps using a batch size of 1024 (128 per TPU core).\nWe use a learning rate warm up of 500. The sequence length was limited to 128 tokens. We used the AdamW optimizer with\na 2e-5 learning rate. The full training script is accessible in this current repository: 'train\\_script.py'.",
"#### Training data\n\n\nWe use the concatenation from multiple datasets to fine-tune our model. The total number of sentence pairs is above 1 billion sentences.\nWe sampled each dataset given a weighted probability which configuration is detailed in the 'data\\_config.json' file."
] | [
"TAGS\n#sentence-transformers #bert #ctranslate2 #int8 #float16 #feature-extraction #sentence-similarity #en #dataset-s2orc #dataset-flax-sentence-embeddings/stackexchange_xml #dataset-ms_marco #dataset-gooaq #dataset-yahoo_answers_topics #dataset-code_search_net #dataset-search_qa #dataset-eli5 #dataset-snli #dataset-multi_nli #dataset-wikihow #dataset-natural_questions #dataset-trivia_qa #dataset-embedding-data/sentence-compression #dataset-embedding-data/flickr30k-captions #dataset-embedding-data/altlex #dataset-embedding-data/simple-wiki #dataset-embedding-data/QQP #dataset-embedding-data/SPECTER #dataset-embedding-data/PAQ_pairs #dataset-embedding-data/WikiAnswers #arxiv-1904.06472 #arxiv-2102.07033 #arxiv-2104.08727 #arxiv-1704.05179 #arxiv-1810.09305 #license-apache-2.0 #endpoints_compatible #region-us \n",
"# Fast-Inference with Ctranslate2\n=================================\n\n\nSpeedup inference while reducing memory by 2x-4x using int8 inference in C++ on CPU or GPU.\n\n\nquantized version of sentence-transformers/all-MiniLM-L6-v2\n\n\nCheckpoint compatible to ctranslate2>=3.17.1\nand hf-hub-ctranslate2>=2.12.0\n\n\n* 'compute\\_type=int8\\_float16' for 'device=\"cuda\"'\n* 'compute\\_type=int8' for 'device=\"cpu\"'\n\n\nConverted on 2023-10-13 using\n\n\nLicence and other remarks:\n==========================\n\n\nThis is just a quantized version. Licence conditions are intended to be idential to original huggingface repo.\n\n\nOriginal description\n====================\n\n\nall-MiniLM-L6-v2\n================\n\n\nThis is a sentence-transformers model: It maps sentences & paragraphs to a 384 dimensional dense vector space and can be used for tasks like clustering or semantic search.\n\n\nUsage (Sentence-Transformers)\n-----------------------------\n\n\nUsing this model becomes easy when you have sentence-transformers installed:\n\n\nThen you can use the model like this:\n\n\nUsage (HuggingFace Transformers)\n--------------------------------\n\n\nWithout sentence-transformers, you can use the model like this: First, you pass your input through the transformer model, then you have to apply the right pooling-operation on-top of the contextualized word embeddings.\n\n\nEvaluation Results\n------------------\n\n\nFor an automated evaluation of this model, see the *Sentence Embeddings Benchmark*: URL\n\n\n\n\n---\n\n\nBackground\n----------\n\n\nThe project aims to train sentence embedding models on very large sentence level datasets using a self-supervised\ncontrastive learning objective. We used the pretrained 'nreimers/MiniLM-L6-H384-uncased' model and fine-tuned in on a\n1B sentence pairs dataset. We use a contrastive learning objective: given a sentence from the pair, the model should predict which out of a set of randomly sampled other sentences, was actually paired with it in our dataset.\n\n\nWe developped this model during the\nCommunity week using JAX/Flax for NLP & CV,\norganized by Hugging Face. We developped this model as part of the project:\nTrain the Best Sentence Embedding Model Ever with 1B Training Pairs. We benefited from efficient hardware infrastructure to run the project: 7 TPUs v3-8, as well as intervention from Googles Flax, JAX, and Cloud team member about efficient deep learning frameworks.\n\n\nIntended uses\n-------------\n\n\nOur model is intented to be used as a sentence and short paragraph encoder. Given an input text, it ouptuts a vector which captures\nthe semantic information. The sentence vector may be used for information retrieval, clustering or sentence similarity tasks.\n\n\nBy default, input text longer than 256 word pieces is truncated.\n\n\nTraining procedure\n------------------",
"### Pre-training\n\n\nWe use the pretrained 'nreimers/MiniLM-L6-H384-uncased' model. Please refer to the model card for more detailed information about the pre-training procedure.",
"### Fine-tuning\n\n\nWe fine-tune the model using a contrastive objective. Formally, we compute the cosine similarity from each possible sentence pairs from the batch.\nWe then apply the cross entropy loss by comparing with true pairs.",
"#### Hyper parameters\n\n\nWe trained ou model on a TPU v3-8. We train the model during 100k steps using a batch size of 1024 (128 per TPU core).\nWe use a learning rate warm up of 500. The sequence length was limited to 128 tokens. We used the AdamW optimizer with\na 2e-5 learning rate. The full training script is accessible in this current repository: 'train\\_script.py'.",
"#### Training data\n\n\nWe use the concatenation from multiple datasets to fine-tune our model. The total number of sentence pairs is above 1 billion sentences.\nWe sampled each dataset given a weighted probability which configuration is detailed in the 'data\\_config.json' file."
] |
null | transformers | '---
pipeline_tag: sentence-similarity
tags:
- ctranslate2
- int8
- float16
- sentence-transformers
- feature-extraction
- sentence-similarity
language: en
license: apache-2.0
datasets:
- s2orc
- flax-sentence-embeddings/stackexchange_xml
- MS Marco
- gooaq
- yahoo_answers_topics
- code_search_net
- search_qa
- eli5
- snli
- multi_nli
- wikihow
- natural_questions
- trivia_qa
- embedding-data/sentence-compression
- embedding-data/flickr30k-captions
- embedding-data/altlex
- embedding-data/simple-wiki
- embedding-data/QQP
- embedding-data/SPECTER
- embedding-data/PAQ_pairs
- embedding-data/WikiAnswers
---
# # Fast-Inference with Ctranslate2
Speedup inference while reducing memory by 2x-4x using int8 inference in C++ on CPU or GPU.
quantized version of [sentence-transformers/all-MiniLM-L12-v2](https://huggingface.co/sentence-transformers/all-MiniLM-L12-v2)
```bash
pip install hf-hub-ctranslate2>=2.12.0 ctranslate2>=3.17.1
```
```python
# from transformers import AutoTokenizer
model_name = "michaelfeil/ct2fast-all-MiniLM-L12-v2"
model_name_orig="sentence-transformers/all-MiniLM-L12-v2"
from hf_hub_ctranslate2 import EncoderCT2fromHfHub
model = EncoderCT2fromHfHub(
# load in int8 on CUDA
model_name_or_path=model_name,
device="cuda",
compute_type="int8_float16"
)
outputs = model.generate(
text=["I like soccer", "I like tennis", "The eiffel tower is in Paris"],
max_length=64,
) # perform downstream tasks on outputs
outputs["pooler_output"]
outputs["last_hidden_state"]
outputs["attention_mask"]
# alternative, use SentenceTransformer Mix-In
# for end-to-end Sentence embeddings generation
# (not pulling from this CT2fast-HF repo)
from hf_hub_ctranslate2 import CT2SentenceTransformer
model = CT2SentenceTransformer(
model_name_orig, compute_type="int8_float16", device="cuda"
)
embeddings = model.encode(
["I like soccer", "I like tennis", "The eiffel tower is in Paris"],
batch_size=32,
convert_to_numpy=True,
normalize_embeddings=True,
)
print(embeddings.shape, embeddings)
scores = (embeddings @ embeddings.T) * 100
# Hint: you can also host this code via REST API and
# via github.com/michaelfeil/infinity
```
Checkpoint compatible to [ctranslate2>=3.17.1](https://github.com/OpenNMT/CTranslate2)
and [hf-hub-ctranslate2>=2.12.0](https://github.com/michaelfeil/hf-hub-ctranslate2)
- `compute_type=int8_float16` for `device="cuda"`
- `compute_type=int8` for `device="cpu"`
Converted on 2023-10-13 using
```
LLama-2 -> removed <pad> token.
```
# Licence and other remarks:
This is just a quantized version. Licence conditions are intended to be idential to original huggingface repo.
# Original description
| {} | nitsuai/ct2fast-all-MiniLM-L12-v2 | null | [
"transformers",
"bert",
"endpoints_compatible",
"region:us"
] | null | 2024-04-26T18:15:05+00:00 | [] | [] | TAGS
#transformers #bert #endpoints_compatible #region-us
| '---
pipeline_tag: sentence-similarity
tags:
- ctranslate2
- int8
- float16
- sentence-transformers
- feature-extraction
- sentence-similarity
language: en
license: apache-2.0
datasets:
- s2orc
- flax-sentence-embeddings/stackexchange_xml
- MS Marco
- gooaq
- yahoo_answers_topics
- code_search_net
- search_qa
- eli5
- snli
- multi_nli
- wikihow
- natural_questions
- trivia_qa
- embedding-data/sentence-compression
- embedding-data/flickr30k-captions
- embedding-data/altlex
- embedding-data/simple-wiki
- embedding-data/QQP
- embedding-data/SPECTER
- embedding-data/PAQ_pairs
- embedding-data/WikiAnswers
---
# # Fast-Inference with Ctranslate2
Speedup inference while reducing memory by 2x-4x using int8 inference in C++ on CPU or GPU.
quantized version of sentence-transformers/all-MiniLM-L12-v2
Checkpoint compatible to ctranslate2>=3.17.1
and hf-hub-ctranslate2>=2.12.0
- 'compute_type=int8_float16' for 'device="cuda"'
- 'compute_type=int8' for 'device="cpu"'
Converted on 2023-10-13 using
# Licence and other remarks:
This is just a quantized version. Licence conditions are intended to be idential to original huggingface repo.
# Original description
| [
"# # Fast-Inference with Ctranslate2\nSpeedup inference while reducing memory by 2x-4x using int8 inference in C++ on CPU or GPU.\n\nquantized version of sentence-transformers/all-MiniLM-L12-v2\n\n\n\n\nCheckpoint compatible to ctranslate2>=3.17.1\nand hf-hub-ctranslate2>=2.12.0\n- 'compute_type=int8_float16' for 'device=\"cuda\"'\n- 'compute_type=int8' for 'device=\"cpu\"'\n\nConverted on 2023-10-13 using",
"# Licence and other remarks:\nThis is just a quantized version. Licence conditions are intended to be idential to original huggingface repo.",
"# Original description"
] | [
"TAGS\n#transformers #bert #endpoints_compatible #region-us \n",
"# # Fast-Inference with Ctranslate2\nSpeedup inference while reducing memory by 2x-4x using int8 inference in C++ on CPU or GPU.\n\nquantized version of sentence-transformers/all-MiniLM-L12-v2\n\n\n\n\nCheckpoint compatible to ctranslate2>=3.17.1\nand hf-hub-ctranslate2>=2.12.0\n- 'compute_type=int8_float16' for 'device=\"cuda\"'\n- 'compute_type=int8' for 'device=\"cpu\"'\n\nConverted on 2023-10-13 using",
"# Licence and other remarks:\nThis is just a quantized version. Licence conditions are intended to be idential to original huggingface repo.",
"# Original description"
] |
sentence-similarity | sentence-transformers | # # Fast-Inference with Ctranslate2
Speedup inference while reducing memory by 2x-4x using int8 inference in C++ on CPU or GPU.
quantized version of [sentence-transformers/paraphrase-multilingual-MiniLM-L12-v2](https://huggingface.co/sentence-transformers/paraphrase-multilingual-MiniLM-L12-v2)
```bash
pip install hf-hub-ctranslate2>=2.12.0 ctranslate2>=3.17.1
```
```python
# from transformers import AutoTokenizer
model_name = "michaelfeil/ct2fast-paraphrase-multilingual-MiniLM-L12-v2"
model_name_orig="sentence-transformers/paraphrase-multilingual-MiniLM-L12-v2"
from hf_hub_ctranslate2 import EncoderCT2fromHfHub
model = EncoderCT2fromHfHub(
# load in int8 on CUDA
model_name_or_path=model_name,
device="cuda",
compute_type="int8_float16"
)
outputs = model.generate(
text=["I like soccer", "I like tennis", "The eiffel tower is in Paris"],
max_length=64,
) # perform downstream tasks on outputs
outputs["pooler_output"]
outputs["last_hidden_state"]
outputs["attention_mask"]
# alternative, use SentenceTransformer Mix-In
# for end-to-end Sentence embeddings generation
# (not pulling from this CT2fast-HF repo)
from hf_hub_ctranslate2 import CT2SentenceTransformer
model = CT2SentenceTransformer(
model_name_orig, compute_type="int8_float16", device="cuda"
)
embeddings = model.encode(
["I like soccer", "I like tennis", "The eiffel tower is in Paris"],
batch_size=32,
convert_to_numpy=True,
normalize_embeddings=True,
)
print(embeddings.shape, embeddings)
scores = (embeddings @ embeddings.T) * 100
# Hint: you can also host this code via REST API and
# via github.com/michaelfeil/infinity
```
Checkpoint compatible to [ctranslate2>=3.17.1](https://github.com/OpenNMT/CTranslate2)
and [hf-hub-ctranslate2>=2.12.0](https://github.com/michaelfeil/hf-hub-ctranslate2)
- `compute_type=int8_float16` for `device="cuda"`
- `compute_type=int8` for `device="cpu"`
Converted on 2023-10-13 using
```
LLama-2 -> removed <pad> token.
```
# Licence and other remarks:
This is just a quantized version. Licence conditions are intended to be idential to original huggingface repo.
# Original description
# sentence-transformers/paraphrase-multilingual-MiniLM-L12-v2
This is a [sentence-transformers](https://www.SBERT.net) model: It maps sentences & paragraphs to a 384 dimensional dense vector space and can be used for tasks like clustering or semantic search.
## Usage (Sentence-Transformers)
Using this model becomes easy when you have [sentence-transformers](https://www.SBERT.net) installed:
```
pip install -U sentence-transformers
```
Then you can use the model like this:
```python
from sentence_transformers import SentenceTransformer
sentences = ["This is an example sentence", "Each sentence is converted"]
model = SentenceTransformer('sentence-transformers/paraphrase-multilingual-MiniLM-L12-v2')
embeddings = model.encode(sentences)
print(embeddings)
```
## Usage (HuggingFace Transformers)
Without [sentence-transformers](https://www.SBERT.net), you can use the model like this: First, you pass your input through the transformer model, then you have to apply the right pooling-operation on-top of the contextualized word embeddings.
```python
from transformers import AutoTokenizer, AutoModel
import torch
#Mean Pooling - Take attention mask into account for correct averaging
def mean_pooling(model_output, attention_mask):
token_embeddings = model_output[0] #First element of model_output contains all token embeddings
input_mask_expanded = attention_mask.unsqueeze(-1).expand(token_embeddings.size()).float()
return torch.sum(token_embeddings * input_mask_expanded, 1) / torch.clamp(input_mask_expanded.sum(1), min=1e-9)
# Sentences we want sentence embeddings for
sentences = ['This is an example sentence', 'Each sentence is converted']
# Load model from HuggingFace Hub
tokenizer = AutoTokenizer.from_pretrained('sentence-transformers/paraphrase-multilingual-MiniLM-L12-v2')
model = AutoModel.from_pretrained('sentence-transformers/paraphrase-multilingual-MiniLM-L12-v2')
# Tokenize sentences
encoded_input = tokenizer(sentences, padding=True, truncation=True, return_tensors='pt')
# Compute token embeddings
with torch.no_grad():
model_output = model(**encoded_input)
# Perform pooling. In this case, max pooling.
sentence_embeddings = mean_pooling(model_output, encoded_input['attention_mask'])
print("Sentence embeddings:")
print(sentence_embeddings)
```
## Evaluation Results
For an automated evaluation of this model, see the *Sentence Embeddings Benchmark*: [https://seb.sbert.net](https://seb.sbert.net?model_name=sentence-transformers/paraphrase-multilingual-MiniLM-L12-v2)
## Full Model Architecture
```
SentenceTransformer(
(0): Transformer({'max_seq_length': 128, 'do_lower_case': False}) with Transformer model: BertModel
(1): Pooling({'word_embedding_dimension': 384, 'pooling_mode_cls_token': False, 'pooling_mode_mean_tokens': True, 'pooling_mode_max_tokens': False, 'pooling_mode_mean_sqrt_len_tokens': False})
)
```
## Citing & Authors
This model was trained by [sentence-transformers](https://www.sbert.net/).
If you find this model helpful, feel free to cite our publication [Sentence-BERT: Sentence Embeddings using Siamese BERT-Networks](https://arxiv.org/abs/1908.10084):
```bibtex
@inproceedings{reimers-2019-sentence-bert,
title = "Sentence-BERT: Sentence Embeddings using Siamese BERT-Networks",
author = "Reimers, Nils and Gurevych, Iryna",
booktitle = "Proceedings of the 2019 Conference on Empirical Methods in Natural Language Processing",
month = "11",
year = "2019",
publisher = "Association for Computational Linguistics",
url = "http://arxiv.org/abs/1908.10084",
}
``` | {"language": "multilingual", "license": "apache-2.0", "tags": ["ctranslate2", "int8", "float16", "sentence-transformers", "feature-extraction", "sentence-similarity", "transformers"], "pipeline_tag": "sentence-similarity"} | nitsuai/ct2fast-paraphrase-multilingual-MiniLM-L12-v2 | null | [
"sentence-transformers",
"bert",
"feature-extraction",
"ctranslate2",
"int8",
"float16",
"sentence-similarity",
"transformers",
"multilingual",
"arxiv:1908.10084",
"license:apache-2.0",
"endpoints_compatible",
"region:us"
] | null | 2024-04-26T18:15:24+00:00 | [
"1908.10084"
] | [
"multilingual"
] | TAGS
#sentence-transformers #bert #feature-extraction #ctranslate2 #int8 #float16 #sentence-similarity #transformers #multilingual #arxiv-1908.10084 #license-apache-2.0 #endpoints_compatible #region-us
| # # Fast-Inference with Ctranslate2
Speedup inference while reducing memory by 2x-4x using int8 inference in C++ on CPU or GPU.
quantized version of sentence-transformers/paraphrase-multilingual-MiniLM-L12-v2
Checkpoint compatible to ctranslate2>=3.17.1
and hf-hub-ctranslate2>=2.12.0
- 'compute_type=int8_float16' for 'device="cuda"'
- 'compute_type=int8' for 'device="cpu"'
Converted on 2023-10-13 using
# Licence and other remarks:
This is just a quantized version. Licence conditions are intended to be idential to original huggingface repo.
# Original description
# sentence-transformers/paraphrase-multilingual-MiniLM-L12-v2
This is a sentence-transformers model: It maps sentences & paragraphs to a 384 dimensional dense vector space and can be used for tasks like clustering or semantic search.
## Usage (Sentence-Transformers)
Using this model becomes easy when you have sentence-transformers installed:
Then you can use the model like this:
## Usage (HuggingFace Transformers)
Without sentence-transformers, you can use the model like this: First, you pass your input through the transformer model, then you have to apply the right pooling-operation on-top of the contextualized word embeddings.
## Evaluation Results
For an automated evaluation of this model, see the *Sentence Embeddings Benchmark*: URL
## Full Model Architecture
## Citing & Authors
This model was trained by sentence-transformers.
If you find this model helpful, feel free to cite our publication Sentence-BERT: Sentence Embeddings using Siamese BERT-Networks:
| [
"# # Fast-Inference with Ctranslate2\nSpeedup inference while reducing memory by 2x-4x using int8 inference in C++ on CPU or GPU.\n\nquantized version of sentence-transformers/paraphrase-multilingual-MiniLM-L12-v2\n\n\n\n\nCheckpoint compatible to ctranslate2>=3.17.1\nand hf-hub-ctranslate2>=2.12.0\n- 'compute_type=int8_float16' for 'device=\"cuda\"'\n- 'compute_type=int8' for 'device=\"cpu\"'\n\nConverted on 2023-10-13 using",
"# Licence and other remarks:\nThis is just a quantized version. Licence conditions are intended to be idential to original huggingface repo.",
"# Original description",
"# sentence-transformers/paraphrase-multilingual-MiniLM-L12-v2\n\nThis is a sentence-transformers model: It maps sentences & paragraphs to a 384 dimensional dense vector space and can be used for tasks like clustering or semantic search.",
"## Usage (Sentence-Transformers)\n\nUsing this model becomes easy when you have sentence-transformers installed:\n\n\n\nThen you can use the model like this:",
"## Usage (HuggingFace Transformers)\nWithout sentence-transformers, you can use the model like this: First, you pass your input through the transformer model, then you have to apply the right pooling-operation on-top of the contextualized word embeddings.",
"## Evaluation Results\n\n\n\nFor an automated evaluation of this model, see the *Sentence Embeddings Benchmark*: URL",
"## Full Model Architecture",
"## Citing & Authors\n\nThis model was trained by sentence-transformers. \n \nIf you find this model helpful, feel free to cite our publication Sentence-BERT: Sentence Embeddings using Siamese BERT-Networks:"
] | [
"TAGS\n#sentence-transformers #bert #feature-extraction #ctranslate2 #int8 #float16 #sentence-similarity #transformers #multilingual #arxiv-1908.10084 #license-apache-2.0 #endpoints_compatible #region-us \n",
"# # Fast-Inference with Ctranslate2\nSpeedup inference while reducing memory by 2x-4x using int8 inference in C++ on CPU or GPU.\n\nquantized version of sentence-transformers/paraphrase-multilingual-MiniLM-L12-v2\n\n\n\n\nCheckpoint compatible to ctranslate2>=3.17.1\nand hf-hub-ctranslate2>=2.12.0\n- 'compute_type=int8_float16' for 'device=\"cuda\"'\n- 'compute_type=int8' for 'device=\"cpu\"'\n\nConverted on 2023-10-13 using",
"# Licence and other remarks:\nThis is just a quantized version. Licence conditions are intended to be idential to original huggingface repo.",
"# Original description",
"# sentence-transformers/paraphrase-multilingual-MiniLM-L12-v2\n\nThis is a sentence-transformers model: It maps sentences & paragraphs to a 384 dimensional dense vector space and can be used for tasks like clustering or semantic search.",
"## Usage (Sentence-Transformers)\n\nUsing this model becomes easy when you have sentence-transformers installed:\n\n\n\nThen you can use the model like this:",
"## Usage (HuggingFace Transformers)\nWithout sentence-transformers, you can use the model like this: First, you pass your input through the transformer model, then you have to apply the right pooling-operation on-top of the contextualized word embeddings.",
"## Evaluation Results\n\n\n\nFor an automated evaluation of this model, see the *Sentence Embeddings Benchmark*: URL",
"## Full Model Architecture",
"## Citing & Authors\n\nThis model was trained by sentence-transformers. \n \nIf you find this model helpful, feel free to cite our publication Sentence-BERT: Sentence Embeddings using Siamese BERT-Networks:"
] |
null | peft |
<!-- This model card has been generated automatically according to the information the Trainer had access to. You
should probably proofread and complete it, then remove this comment. -->
# GUE_tf_0-seqsight_4096_512_27M-L8_f
This model is a fine-tuned version of [mahdibaghbanzadeh/seqsight_4096_512_27M](https://huggingface.co/mahdibaghbanzadeh/seqsight_4096_512_27M) on the [mahdibaghbanzadeh/GUE_tf_0](https://huggingface.co/datasets/mahdibaghbanzadeh/GUE_tf_0) dataset.
It achieves the following results on the evaluation set:
- Loss: 0.3647
- F1 Score: 0.8277
- Accuracy: 0.828
## Model description
More information needed
## Intended uses & limitations
More information needed
## Training and evaluation data
More information needed
## Training procedure
### Training hyperparameters
The following hyperparameters were used during training:
- learning_rate: 0.0005
- train_batch_size: 128
- eval_batch_size: 128
- seed: 42
- optimizer: Adam with betas=(0.9,0.999) and epsilon=1e-08
- lr_scheduler_type: linear
- training_steps: 10000
### Training results
| Training Loss | Epoch | Step | Validation Loss | F1 Score | Accuracy |
|:-------------:|:-----:|:-----:|:---------------:|:--------:|:--------:|
| 0.5227 | 0.79 | 200 | 0.4716 | 0.7619 | 0.762 |
| 0.4731 | 1.58 | 400 | 0.4631 | 0.7735 | 0.774 |
| 0.4657 | 2.37 | 600 | 0.4624 | 0.7763 | 0.778 |
| 0.4577 | 3.16 | 800 | 0.4528 | 0.7860 | 0.786 |
| 0.453 | 3.95 | 1000 | 0.4531 | 0.7761 | 0.777 |
| 0.446 | 4.74 | 1200 | 0.4525 | 0.7860 | 0.786 |
| 0.4477 | 5.53 | 1400 | 0.4549 | 0.7829 | 0.783 |
| 0.4359 | 6.32 | 1600 | 0.4490 | 0.7920 | 0.792 |
| 0.4393 | 7.11 | 1800 | 0.4507 | 0.7830 | 0.783 |
| 0.4382 | 7.91 | 2000 | 0.4492 | 0.7850 | 0.785 |
| 0.4328 | 8.7 | 2200 | 0.4425 | 0.7880 | 0.788 |
| 0.4295 | 9.49 | 2400 | 0.4400 | 0.7875 | 0.788 |
| 0.429 | 10.28 | 2600 | 0.4504 | 0.7879 | 0.788 |
| 0.4242 | 11.07 | 2800 | 0.4516 | 0.7828 | 0.783 |
| 0.4234 | 11.86 | 3000 | 0.4484 | 0.7990 | 0.799 |
| 0.4201 | 12.65 | 3200 | 0.4426 | 0.8050 | 0.805 |
| 0.4197 | 13.44 | 3400 | 0.4388 | 0.7979 | 0.798 |
| 0.4173 | 14.23 | 3600 | 0.4388 | 0.8060 | 0.806 |
| 0.4189 | 15.02 | 3800 | 0.4416 | 0.8010 | 0.801 |
| 0.4158 | 15.81 | 4000 | 0.4381 | 0.7951 | 0.795 |
| 0.4092 | 16.6 | 4200 | 0.4366 | 0.8018 | 0.802 |
| 0.4122 | 17.39 | 4400 | 0.4429 | 0.7980 | 0.798 |
| 0.4106 | 18.18 | 4600 | 0.4403 | 0.7970 | 0.797 |
| 0.4084 | 18.97 | 4800 | 0.4408 | 0.7970 | 0.797 |
| 0.4122 | 19.76 | 5000 | 0.4358 | 0.7970 | 0.797 |
| 0.4068 | 20.55 | 5200 | 0.4329 | 0.8010 | 0.801 |
| 0.401 | 21.34 | 5400 | 0.4385 | 0.7961 | 0.796 |
| 0.4026 | 22.13 | 5600 | 0.4375 | 0.7970 | 0.797 |
| 0.4048 | 22.92 | 5800 | 0.4382 | 0.7931 | 0.793 |
| 0.3982 | 23.72 | 6000 | 0.4368 | 0.7991 | 0.799 |
| 0.3976 | 24.51 | 6200 | 0.4328 | 0.7980 | 0.798 |
| 0.4035 | 25.3 | 6400 | 0.4315 | 0.8070 | 0.807 |
| 0.3963 | 26.09 | 6600 | 0.4324 | 0.7969 | 0.797 |
| 0.3947 | 26.88 | 6800 | 0.4388 | 0.7921 | 0.792 |
| 0.3987 | 27.67 | 7000 | 0.4337 | 0.7990 | 0.799 |
| 0.3944 | 28.46 | 7200 | 0.4327 | 0.7978 | 0.798 |
| 0.3971 | 29.25 | 7400 | 0.4329 | 0.7969 | 0.797 |
| 0.3971 | 30.04 | 7600 | 0.4354 | 0.7991 | 0.799 |
| 0.394 | 30.83 | 7800 | 0.4345 | 0.7969 | 0.797 |
| 0.3925 | 31.62 | 8000 | 0.4331 | 0.7958 | 0.796 |
| 0.3943 | 32.41 | 8200 | 0.4377 | 0.8011 | 0.801 |
| 0.3876 | 33.2 | 8400 | 0.4365 | 0.7960 | 0.796 |
| 0.3939 | 33.99 | 8600 | 0.4372 | 0.798 | 0.798 |
| 0.3924 | 34.78 | 8800 | 0.4340 | 0.7959 | 0.796 |
| 0.3861 | 35.57 | 9000 | 0.4344 | 0.7989 | 0.799 |
| 0.3906 | 36.36 | 9200 | 0.4380 | 0.7971 | 0.797 |
| 0.3887 | 37.15 | 9400 | 0.4390 | 0.7961 | 0.796 |
| 0.3889 | 37.94 | 9600 | 0.4360 | 0.7990 | 0.799 |
| 0.3879 | 38.74 | 9800 | 0.4369 | 0.796 | 0.796 |
| 0.39 | 39.53 | 10000 | 0.4366 | 0.7980 | 0.798 |
### Framework versions
- PEFT 0.9.0
- Transformers 4.38.2
- Pytorch 2.2.0+cu121
- Datasets 2.17.1
- Tokenizers 0.15.2 | {"library_name": "peft", "tags": ["generated_from_trainer"], "metrics": ["accuracy"], "base_model": "mahdibaghbanzadeh/seqsight_4096_512_27M", "model-index": [{"name": "GUE_tf_0-seqsight_4096_512_27M-L8_f", "results": []}]} | mahdibaghbanzadeh/GUE_tf_0-seqsight_4096_512_27M-L8_f | null | [
"peft",
"safetensors",
"generated_from_trainer",
"base_model:mahdibaghbanzadeh/seqsight_4096_512_27M",
"region:us"
] | null | 2024-04-26T18:17:38+00:00 | [] | [] | TAGS
#peft #safetensors #generated_from_trainer #base_model-mahdibaghbanzadeh/seqsight_4096_512_27M #region-us
| GUE\_tf\_0-seqsight\_4096\_512\_27M-L8\_f
=========================================
This model is a fine-tuned version of mahdibaghbanzadeh/seqsight\_4096\_512\_27M on the mahdibaghbanzadeh/GUE\_tf\_0 dataset.
It achieves the following results on the evaluation set:
* Loss: 0.3647
* F1 Score: 0.8277
* Accuracy: 0.828
Model description
-----------------
More information needed
Intended uses & limitations
---------------------------
More information needed
Training and evaluation data
----------------------------
More information needed
Training procedure
------------------
### Training hyperparameters
The following hyperparameters were used during training:
* learning\_rate: 0.0005
* train\_batch\_size: 128
* eval\_batch\_size: 128
* seed: 42
* optimizer: Adam with betas=(0.9,0.999) and epsilon=1e-08
* lr\_scheduler\_type: linear
* training\_steps: 10000
### Training results
### Framework versions
* PEFT 0.9.0
* Transformers 4.38.2
* Pytorch 2.2.0+cu121
* Datasets 2.17.1
* Tokenizers 0.15.2
| [
"### Training hyperparameters\n\n\nThe following hyperparameters were used during training:\n\n\n* learning\\_rate: 0.0005\n* train\\_batch\\_size: 128\n* eval\\_batch\\_size: 128\n* seed: 42\n* optimizer: Adam with betas=(0.9,0.999) and epsilon=1e-08\n* lr\\_scheduler\\_type: linear\n* training\\_steps: 10000",
"### Training results",
"### Framework versions\n\n\n* PEFT 0.9.0\n* Transformers 4.38.2\n* Pytorch 2.2.0+cu121\n* Datasets 2.17.1\n* Tokenizers 0.15.2"
] | [
"TAGS\n#peft #safetensors #generated_from_trainer #base_model-mahdibaghbanzadeh/seqsight_4096_512_27M #region-us \n",
"### Training hyperparameters\n\n\nThe following hyperparameters were used during training:\n\n\n* learning\\_rate: 0.0005\n* train\\_batch\\_size: 128\n* eval\\_batch\\_size: 128\n* seed: 42\n* optimizer: Adam with betas=(0.9,0.999) and epsilon=1e-08\n* lr\\_scheduler\\_type: linear\n* training\\_steps: 10000",
"### Training results",
"### Framework versions\n\n\n* PEFT 0.9.0\n* Transformers 4.38.2\n* Pytorch 2.2.0+cu121\n* Datasets 2.17.1\n* Tokenizers 0.15.2"
] |
image-classification | transformers |
<!-- This model card has been generated automatically according to the information the Trainer had access to. You
should probably proofread and complete it, then remove this comment. -->
# dog-or-food-arturo-guerrero
This model is a fine-tuned version of [google/vit-base-patch16-224-in21k](https://huggingface.co/google/vit-base-patch16-224-in21k) on the lewtun/dog_food dataset.
It achieves the following results on the evaluation set:
- Loss: 0.0048
- Accuracy: 0.9987
## Model description
More information needed
## Intended uses & limitations
More information needed
## Training and evaluation data
More information needed
## Training procedure
### Training hyperparameters
The following hyperparameters were used during training:
- learning_rate: 0.0002
- train_batch_size: 8
- eval_batch_size: 8
- seed: 42
- optimizer: Adam with betas=(0.9,0.999) and epsilon=1e-08
- lr_scheduler_type: linear
- num_epochs: 4
### Training results
| Training Loss | Epoch | Step | Validation Loss | Accuracy |
|:-------------:|:-----:|:----:|:---------------:|:--------:|
| 0.0697 | 1.77 | 500 | 0.0513 | 0.988 |
| 0.0087 | 3.55 | 1000 | 0.0048 | 0.9987 |
### Framework versions
- Transformers 4.30.2
- Pytorch 2.2.1+cu121
- Datasets 2.19.0
- Tokenizers 0.13.3
| {"license": "apache-2.0", "tags": ["image-clasification", "generated_from_trainer"], "metrics": ["accuracy"], "model-index": [{"name": "dog-or-food-arturo-guerrero", "results": []}]} | arturoxdev/dog-or-food-arturo-guerrero | null | [
"transformers",
"pytorch",
"tensorboard",
"vit",
"image-classification",
"image-clasification",
"generated_from_trainer",
"license:apache-2.0",
"autotrain_compatible",
"endpoints_compatible",
"region:us"
] | null | 2024-04-26T18:18:01+00:00 | [] | [] | TAGS
#transformers #pytorch #tensorboard #vit #image-classification #image-clasification #generated_from_trainer #license-apache-2.0 #autotrain_compatible #endpoints_compatible #region-us
| dog-or-food-arturo-guerrero
===========================
This model is a fine-tuned version of google/vit-base-patch16-224-in21k on the lewtun/dog\_food dataset.
It achieves the following results on the evaluation set:
* Loss: 0.0048
* Accuracy: 0.9987
Model description
-----------------
More information needed
Intended uses & limitations
---------------------------
More information needed
Training and evaluation data
----------------------------
More information needed
Training procedure
------------------
### Training hyperparameters
The following hyperparameters were used during training:
* learning\_rate: 0.0002
* train\_batch\_size: 8
* eval\_batch\_size: 8
* seed: 42
* optimizer: Adam with betas=(0.9,0.999) and epsilon=1e-08
* lr\_scheduler\_type: linear
* num\_epochs: 4
### Training results
### Framework versions
* Transformers 4.30.2
* Pytorch 2.2.1+cu121
* Datasets 2.19.0
* Tokenizers 0.13.3
| [
"### Training hyperparameters\n\n\nThe following hyperparameters were used during training:\n\n\n* learning\\_rate: 0.0002\n* train\\_batch\\_size: 8\n* eval\\_batch\\_size: 8\n* seed: 42\n* optimizer: Adam with betas=(0.9,0.999) and epsilon=1e-08\n* lr\\_scheduler\\_type: linear\n* num\\_epochs: 4",
"### Training results",
"### Framework versions\n\n\n* Transformers 4.30.2\n* Pytorch 2.2.1+cu121\n* Datasets 2.19.0\n* Tokenizers 0.13.3"
] | [
"TAGS\n#transformers #pytorch #tensorboard #vit #image-classification #image-clasification #generated_from_trainer #license-apache-2.0 #autotrain_compatible #endpoints_compatible #region-us \n",
"### Training hyperparameters\n\n\nThe following hyperparameters were used during training:\n\n\n* learning\\_rate: 0.0002\n* train\\_batch\\_size: 8\n* eval\\_batch\\_size: 8\n* seed: 42\n* optimizer: Adam with betas=(0.9,0.999) and epsilon=1e-08\n* lr\\_scheduler\\_type: linear\n* num\\_epochs: 4",
"### Training results",
"### Framework versions\n\n\n* Transformers 4.30.2\n* Pytorch 2.2.1+cu121\n* Datasets 2.19.0\n* Tokenizers 0.13.3"
] |
null | peft |
<!-- This model card has been generated automatically according to the information the Trainer had access to. You
should probably proofread and complete it, then remove this comment. -->
# GUE_tf_0-seqsight_4096_512_27M-L32_f
This model is a fine-tuned version of [mahdibaghbanzadeh/seqsight_4096_512_27M](https://huggingface.co/mahdibaghbanzadeh/seqsight_4096_512_27M) on the [mahdibaghbanzadeh/GUE_tf_0](https://huggingface.co/datasets/mahdibaghbanzadeh/GUE_tf_0) dataset.
It achieves the following results on the evaluation set:
- Loss: 0.3656
- F1 Score: 0.8327
- Accuracy: 0.833
## Model description
More information needed
## Intended uses & limitations
More information needed
## Training and evaluation data
More information needed
## Training procedure
### Training hyperparameters
The following hyperparameters were used during training:
- learning_rate: 0.0005
- train_batch_size: 128
- eval_batch_size: 128
- seed: 42
- optimizer: Adam with betas=(0.9,0.999) and epsilon=1e-08
- lr_scheduler_type: linear
- training_steps: 10000
### Training results
| Training Loss | Epoch | Step | Validation Loss | F1 Score | Accuracy |
|:-------------:|:-----:|:-----:|:---------------:|:--------:|:--------:|
| 0.5152 | 0.79 | 200 | 0.4668 | 0.7718 | 0.772 |
| 0.4682 | 1.58 | 400 | 0.4565 | 0.7805 | 0.781 |
| 0.4581 | 2.37 | 600 | 0.4580 | 0.7813 | 0.783 |
| 0.4495 | 3.16 | 800 | 0.4467 | 0.7869 | 0.787 |
| 0.4415 | 3.95 | 1000 | 0.4442 | 0.7906 | 0.791 |
| 0.4324 | 4.74 | 1200 | 0.4511 | 0.7859 | 0.786 |
| 0.4318 | 5.53 | 1400 | 0.4537 | 0.7827 | 0.783 |
| 0.4202 | 6.32 | 1600 | 0.4390 | 0.8009 | 0.801 |
| 0.423 | 7.11 | 1800 | 0.4471 | 0.7850 | 0.785 |
| 0.419 | 7.91 | 2000 | 0.4472 | 0.8000 | 0.8 |
| 0.4109 | 8.7 | 2200 | 0.4454 | 0.7861 | 0.786 |
| 0.4079 | 9.49 | 2400 | 0.4356 | 0.7958 | 0.796 |
| 0.4083 | 10.28 | 2600 | 0.4475 | 0.7909 | 0.791 |
| 0.4009 | 11.07 | 2800 | 0.4439 | 0.7960 | 0.796 |
| 0.399 | 11.86 | 3000 | 0.4379 | 0.8001 | 0.8 |
| 0.3942 | 12.65 | 3200 | 0.4344 | 0.8081 | 0.808 |
| 0.3919 | 13.44 | 3400 | 0.4354 | 0.7970 | 0.797 |
| 0.3857 | 14.23 | 3600 | 0.4343 | 0.8 | 0.8 |
| 0.3904 | 15.02 | 3800 | 0.4448 | 0.794 | 0.794 |
| 0.3829 | 15.81 | 4000 | 0.4396 | 0.7970 | 0.797 |
| 0.3767 | 16.6 | 4200 | 0.4474 | 0.8000 | 0.8 |
| 0.3768 | 17.39 | 4400 | 0.4500 | 0.7900 | 0.79 |
| 0.3743 | 18.18 | 4600 | 0.4564 | 0.7971 | 0.797 |
| 0.3693 | 18.97 | 4800 | 0.4530 | 0.8030 | 0.803 |
| 0.3724 | 19.76 | 5000 | 0.4475 | 0.8041 | 0.804 |
| 0.3642 | 20.55 | 5200 | 0.4460 | 0.7930 | 0.793 |
| 0.3598 | 21.34 | 5400 | 0.4572 | 0.7958 | 0.796 |
| 0.3604 | 22.13 | 5600 | 0.4570 | 0.7989 | 0.799 |
| 0.3584 | 22.92 | 5800 | 0.4659 | 0.7997 | 0.8 |
| 0.3513 | 23.72 | 6000 | 0.4589 | 0.7919 | 0.792 |
| 0.3468 | 24.51 | 6200 | 0.4569 | 0.8070 | 0.807 |
| 0.353 | 25.3 | 6400 | 0.4592 | 0.8040 | 0.804 |
| 0.346 | 26.09 | 6600 | 0.4631 | 0.7990 | 0.799 |
| 0.3435 | 26.88 | 6800 | 0.4623 | 0.8030 | 0.803 |
| 0.3432 | 27.67 | 7000 | 0.4671 | 0.7960 | 0.796 |
| 0.3394 | 28.46 | 7200 | 0.4669 | 0.8030 | 0.803 |
| 0.3398 | 29.25 | 7400 | 0.4704 | 0.8040 | 0.804 |
| 0.3417 | 30.04 | 7600 | 0.4749 | 0.7958 | 0.796 |
| 0.3358 | 30.83 | 7800 | 0.4716 | 0.7950 | 0.795 |
| 0.3315 | 31.62 | 8000 | 0.4776 | 0.7920 | 0.792 |
| 0.3339 | 32.41 | 8200 | 0.4808 | 0.7967 | 0.797 |
| 0.327 | 33.2 | 8400 | 0.4812 | 0.7919 | 0.792 |
| 0.3303 | 33.99 | 8600 | 0.4808 | 0.7969 | 0.797 |
| 0.3287 | 34.78 | 8800 | 0.4768 | 0.7961 | 0.796 |
| 0.3248 | 35.57 | 9000 | 0.4781 | 0.7921 | 0.792 |
| 0.3286 | 36.36 | 9200 | 0.4839 | 0.7889 | 0.789 |
| 0.3237 | 37.15 | 9400 | 0.4869 | 0.7919 | 0.792 |
| 0.3224 | 37.94 | 9600 | 0.4845 | 0.794 | 0.794 |
| 0.3215 | 38.74 | 9800 | 0.4851 | 0.7930 | 0.793 |
| 0.3227 | 39.53 | 10000 | 0.4859 | 0.7940 | 0.794 |
### Framework versions
- PEFT 0.9.0
- Transformers 4.38.2
- Pytorch 2.2.0+cu121
- Datasets 2.17.1
- Tokenizers 0.15.2 | {"library_name": "peft", "tags": ["generated_from_trainer"], "metrics": ["accuracy"], "base_model": "mahdibaghbanzadeh/seqsight_4096_512_27M", "model-index": [{"name": "GUE_tf_0-seqsight_4096_512_27M-L32_f", "results": []}]} | mahdibaghbanzadeh/GUE_tf_0-seqsight_4096_512_27M-L32_f | null | [
"peft",
"safetensors",
"generated_from_trainer",
"base_model:mahdibaghbanzadeh/seqsight_4096_512_27M",
"region:us"
] | null | 2024-04-26T18:18:29+00:00 | [] | [] | TAGS
#peft #safetensors #generated_from_trainer #base_model-mahdibaghbanzadeh/seqsight_4096_512_27M #region-us
| GUE\_tf\_0-seqsight\_4096\_512\_27M-L32\_f
==========================================
This model is a fine-tuned version of mahdibaghbanzadeh/seqsight\_4096\_512\_27M on the mahdibaghbanzadeh/GUE\_tf\_0 dataset.
It achieves the following results on the evaluation set:
* Loss: 0.3656
* F1 Score: 0.8327
* Accuracy: 0.833
Model description
-----------------
More information needed
Intended uses & limitations
---------------------------
More information needed
Training and evaluation data
----------------------------
More information needed
Training procedure
------------------
### Training hyperparameters
The following hyperparameters were used during training:
* learning\_rate: 0.0005
* train\_batch\_size: 128
* eval\_batch\_size: 128
* seed: 42
* optimizer: Adam with betas=(0.9,0.999) and epsilon=1e-08
* lr\_scheduler\_type: linear
* training\_steps: 10000
### Training results
### Framework versions
* PEFT 0.9.0
* Transformers 4.38.2
* Pytorch 2.2.0+cu121
* Datasets 2.17.1
* Tokenizers 0.15.2
| [
"### Training hyperparameters\n\n\nThe following hyperparameters were used during training:\n\n\n* learning\\_rate: 0.0005\n* train\\_batch\\_size: 128\n* eval\\_batch\\_size: 128\n* seed: 42\n* optimizer: Adam with betas=(0.9,0.999) and epsilon=1e-08\n* lr\\_scheduler\\_type: linear\n* training\\_steps: 10000",
"### Training results",
"### Framework versions\n\n\n* PEFT 0.9.0\n* Transformers 4.38.2\n* Pytorch 2.2.0+cu121\n* Datasets 2.17.1\n* Tokenizers 0.15.2"
] | [
"TAGS\n#peft #safetensors #generated_from_trainer #base_model-mahdibaghbanzadeh/seqsight_4096_512_27M #region-us \n",
"### Training hyperparameters\n\n\nThe following hyperparameters were used during training:\n\n\n* learning\\_rate: 0.0005\n* train\\_batch\\_size: 128\n* eval\\_batch\\_size: 128\n* seed: 42\n* optimizer: Adam with betas=(0.9,0.999) and epsilon=1e-08\n* lr\\_scheduler\\_type: linear\n* training\\_steps: 10000",
"### Training results",
"### Framework versions\n\n\n* PEFT 0.9.0\n* Transformers 4.38.2\n* Pytorch 2.2.0+cu121\n* Datasets 2.17.1\n* Tokenizers 0.15.2"
] |
null | peft |
<!-- This model card has been generated automatically according to the information the Trainer had access to. You
should probably proofread and complete it, then remove this comment. -->
# GUE_tf_1-seqsight_4096_512_27M-L1_f
This model is a fine-tuned version of [mahdibaghbanzadeh/seqsight_4096_512_27M](https://huggingface.co/mahdibaghbanzadeh/seqsight_4096_512_27M) on the [mahdibaghbanzadeh/GUE_tf_1](https://huggingface.co/datasets/mahdibaghbanzadeh/GUE_tf_1) dataset.
It achieves the following results on the evaluation set:
- Loss: 0.3351
- F1 Score: 0.8588
- Accuracy: 0.859
## Model description
More information needed
## Intended uses & limitations
More information needed
## Training and evaluation data
More information needed
## Training procedure
### Training hyperparameters
The following hyperparameters were used during training:
- learning_rate: 0.0005
- train_batch_size: 128
- eval_batch_size: 128
- seed: 42
- optimizer: Adam with betas=(0.9,0.999) and epsilon=1e-08
- lr_scheduler_type: linear
- training_steps: 10000
### Training results
| Training Loss | Epoch | Step | Validation Loss | F1 Score | Accuracy |
|:-------------:|:-----:|:-----:|:---------------:|:--------:|:--------:|
| 0.5527 | 0.83 | 200 | 0.5266 | 0.7480 | 0.748 |
| 0.501 | 1.67 | 400 | 0.5182 | 0.7460 | 0.746 |
| 0.4919 | 2.5 | 600 | 0.5166 | 0.7499 | 0.75 |
| 0.4846 | 3.33 | 800 | 0.5136 | 0.7575 | 0.758 |
| 0.4874 | 4.17 | 1000 | 0.5085 | 0.7580 | 0.758 |
| 0.4815 | 5.0 | 1200 | 0.5102 | 0.7550 | 0.755 |
| 0.4773 | 5.83 | 1400 | 0.5036 | 0.7630 | 0.763 |
| 0.4795 | 6.67 | 1600 | 0.5061 | 0.7519 | 0.752 |
| 0.4763 | 7.5 | 1800 | 0.5025 | 0.7480 | 0.748 |
| 0.4704 | 8.33 | 2000 | 0.5010 | 0.7609 | 0.761 |
| 0.4738 | 9.17 | 2200 | 0.5006 | 0.7549 | 0.755 |
| 0.4705 | 10.0 | 2400 | 0.5108 | 0.7531 | 0.754 |
| 0.4703 | 10.83 | 2600 | 0.5067 | 0.7542 | 0.755 |
| 0.4694 | 11.67 | 2800 | 0.5071 | 0.7559 | 0.757 |
| 0.4611 | 12.5 | 3000 | 0.5065 | 0.7593 | 0.76 |
| 0.4712 | 13.33 | 3200 | 0.4969 | 0.7686 | 0.769 |
| 0.4626 | 14.17 | 3400 | 0.4961 | 0.7570 | 0.757 |
| 0.4623 | 15.0 | 3600 | 0.5074 | 0.7598 | 0.761 |
| 0.4607 | 15.83 | 3800 | 0.4962 | 0.7657 | 0.766 |
| 0.4628 | 16.67 | 4000 | 0.5014 | 0.7636 | 0.764 |
| 0.4608 | 17.5 | 4200 | 0.4992 | 0.7653 | 0.766 |
| 0.4566 | 18.33 | 4400 | 0.5019 | 0.7659 | 0.766 |
| 0.4607 | 19.17 | 4600 | 0.5015 | 0.7695 | 0.77 |
| 0.4592 | 20.0 | 4800 | 0.4947 | 0.7698 | 0.77 |
| 0.4604 | 20.83 | 5000 | 0.4966 | 0.7698 | 0.77 |
| 0.4545 | 21.67 | 5200 | 0.4955 | 0.7727 | 0.773 |
| 0.4562 | 22.5 | 5400 | 0.4935 | 0.7650 | 0.765 |
| 0.4566 | 23.33 | 5600 | 0.4947 | 0.7767 | 0.777 |
| 0.4542 | 24.17 | 5800 | 0.4994 | 0.7707 | 0.771 |
| 0.4548 | 25.0 | 6000 | 0.4958 | 0.7690 | 0.769 |
| 0.4503 | 25.83 | 6200 | 0.4945 | 0.7699 | 0.77 |
| 0.4503 | 26.67 | 6400 | 0.4939 | 0.7680 | 0.768 |
| 0.4542 | 27.5 | 6600 | 0.4966 | 0.7720 | 0.772 |
| 0.4521 | 28.33 | 6800 | 0.4945 | 0.7689 | 0.769 |
| 0.4515 | 29.17 | 7000 | 0.4961 | 0.7668 | 0.767 |
| 0.4537 | 30.0 | 7200 | 0.4940 | 0.7718 | 0.772 |
| 0.4512 | 30.83 | 7400 | 0.5001 | 0.7693 | 0.77 |
| 0.45 | 31.67 | 7600 | 0.4930 | 0.7700 | 0.77 |
| 0.4507 | 32.5 | 7800 | 0.4951 | 0.7708 | 0.771 |
| 0.4491 | 33.33 | 8000 | 0.4942 | 0.7708 | 0.771 |
| 0.4535 | 34.17 | 8200 | 0.4935 | 0.7678 | 0.768 |
| 0.4473 | 35.0 | 8400 | 0.4941 | 0.7678 | 0.768 |
| 0.4504 | 35.83 | 8600 | 0.4930 | 0.7688 | 0.769 |
| 0.4489 | 36.67 | 8800 | 0.4940 | 0.7698 | 0.77 |
| 0.4487 | 37.5 | 9000 | 0.4979 | 0.7705 | 0.771 |
| 0.4486 | 38.33 | 9200 | 0.4928 | 0.7709 | 0.771 |
| 0.4466 | 39.17 | 9400 | 0.4936 | 0.7689 | 0.769 |
| 0.4488 | 40.0 | 9600 | 0.4936 | 0.7658 | 0.766 |
| 0.4493 | 40.83 | 9800 | 0.4923 | 0.7699 | 0.77 |
| 0.4489 | 41.67 | 10000 | 0.4929 | 0.7689 | 0.769 |
### Framework versions
- PEFT 0.9.0
- Transformers 4.38.2
- Pytorch 2.2.0+cu121
- Datasets 2.17.1
- Tokenizers 0.15.2 | {"library_name": "peft", "tags": ["generated_from_trainer"], "metrics": ["accuracy"], "base_model": "mahdibaghbanzadeh/seqsight_4096_512_27M", "model-index": [{"name": "GUE_tf_1-seqsight_4096_512_27M-L1_f", "results": []}]} | mahdibaghbanzadeh/GUE_tf_1-seqsight_4096_512_27M-L1_f | null | [
"peft",
"safetensors",
"generated_from_trainer",
"base_model:mahdibaghbanzadeh/seqsight_4096_512_27M",
"region:us"
] | null | 2024-04-26T18:18:42+00:00 | [] | [] | TAGS
#peft #safetensors #generated_from_trainer #base_model-mahdibaghbanzadeh/seqsight_4096_512_27M #region-us
| GUE\_tf\_1-seqsight\_4096\_512\_27M-L1\_f
=========================================
This model is a fine-tuned version of mahdibaghbanzadeh/seqsight\_4096\_512\_27M on the mahdibaghbanzadeh/GUE\_tf\_1 dataset.
It achieves the following results on the evaluation set:
* Loss: 0.3351
* F1 Score: 0.8588
* Accuracy: 0.859
Model description
-----------------
More information needed
Intended uses & limitations
---------------------------
More information needed
Training and evaluation data
----------------------------
More information needed
Training procedure
------------------
### Training hyperparameters
The following hyperparameters were used during training:
* learning\_rate: 0.0005
* train\_batch\_size: 128
* eval\_batch\_size: 128
* seed: 42
* optimizer: Adam with betas=(0.9,0.999) and epsilon=1e-08
* lr\_scheduler\_type: linear
* training\_steps: 10000
### Training results
### Framework versions
* PEFT 0.9.0
* Transformers 4.38.2
* Pytorch 2.2.0+cu121
* Datasets 2.17.1
* Tokenizers 0.15.2
| [
"### Training hyperparameters\n\n\nThe following hyperparameters were used during training:\n\n\n* learning\\_rate: 0.0005\n* train\\_batch\\_size: 128\n* eval\\_batch\\_size: 128\n* seed: 42\n* optimizer: Adam with betas=(0.9,0.999) and epsilon=1e-08\n* lr\\_scheduler\\_type: linear\n* training\\_steps: 10000",
"### Training results",
"### Framework versions\n\n\n* PEFT 0.9.0\n* Transformers 4.38.2\n* Pytorch 2.2.0+cu121\n* Datasets 2.17.1\n* Tokenizers 0.15.2"
] | [
"TAGS\n#peft #safetensors #generated_from_trainer #base_model-mahdibaghbanzadeh/seqsight_4096_512_27M #region-us \n",
"### Training hyperparameters\n\n\nThe following hyperparameters were used during training:\n\n\n* learning\\_rate: 0.0005\n* train\\_batch\\_size: 128\n* eval\\_batch\\_size: 128\n* seed: 42\n* optimizer: Adam with betas=(0.9,0.999) and epsilon=1e-08\n* lr\\_scheduler\\_type: linear\n* training\\_steps: 10000",
"### Training results",
"### Framework versions\n\n\n* PEFT 0.9.0\n* Transformers 4.38.2\n* Pytorch 2.2.0+cu121\n* Datasets 2.17.1\n* Tokenizers 0.15.2"
] |
null | null |
<!-- This model card has been generated automatically according to the information the Trainer had access to. You
should probably proofread and complete it, then remove this comment. -->
# Mistral-7B-Instruct-v0.2-absa-MT-laptops
This model is a fine-tuned version of [mistralai/Mistral-7B-Instruct-v0.2](https://huggingface.co/mistralai/Mistral-7B-Instruct-v0.2) on the None dataset.
It achieves the following results on the evaluation set:
- Loss: 0.0060
## Model description
More information needed
## Intended uses & limitations
More information needed
## Training and evaluation data
More information needed
## Training procedure
### Training hyperparameters
The following hyperparameters were used during training:
- learning_rate: 3e-05
- train_batch_size: 8
- eval_batch_size: 8
- seed: 42
- gradient_accumulation_steps: 4
- total_train_batch_size: 32
- optimizer: Adam with betas=(0.9,0.999) and epsilon=1e-08
- lr_scheduler_type: linear
- lr_scheduler_warmup_steps: 2
- training_steps: 1200
- mixed_precision_training: Native AMP
### Training results
| Training Loss | Epoch | Step | Validation Loss |
|:-------------:|:-----:|:----:|:---------------:|
| 0.8786 | 0.13 | 40 | 0.1392 |
| 0.0627 | 0.25 | 80 | 0.0165 |
| 0.0162 | 0.38 | 120 | 0.0143 |
| 0.0139 | 0.5 | 160 | 0.0125 |
| 0.0131 | 0.63 | 200 | 0.0110 |
| 0.0115 | 0.75 | 240 | 0.0106 |
| 0.0111 | 0.88 | 280 | 0.0105 |
| 0.0091 | 1.0 | 320 | 0.0093 |
| 0.0073 | 1.13 | 360 | 0.0090 |
| 0.0079 | 1.25 | 400 | 0.0090 |
| 0.0068 | 1.38 | 440 | 0.0083 |
| 0.0065 | 1.5 | 480 | 0.0076 |
| 0.0071 | 1.63 | 520 | 0.0076 |
| 0.0062 | 1.75 | 560 | 0.0077 |
| 0.0062 | 1.88 | 600 | 0.0069 |
| 0.0058 | 2.0 | 640 | 0.0069 |
| 0.0034 | 2.13 | 680 | 0.0070 |
| 0.0034 | 2.25 | 720 | 0.0066 |
| 0.0034 | 2.38 | 760 | 0.0071 |
| 0.0038 | 2.5 | 800 | 0.0064 |
| 0.0032 | 2.63 | 840 | 0.0070 |
| 0.0031 | 2.75 | 880 | 0.0062 |
| 0.0032 | 2.88 | 920 | 0.0058 |
| 0.0026 | 3.0 | 960 | 0.0059 |
| 0.0018 | 3.13 | 1000 | 0.0058 |
| 0.0014 | 3.26 | 1040 | 0.0059 |
| 0.0014 | 3.38 | 1080 | 0.0060 |
| 0.0012 | 3.51 | 1120 | 0.0060 |
| 0.0014 | 3.63 | 1160 | 0.0060 |
| 0.001 | 3.76 | 1200 | 0.0060 |
### Framework versions
- Transformers 4.38.2
- Pytorch 2.2.1+cu121
- Datasets 2.18.0
- Tokenizers 0.15.2
| {"license": "apache-2.0", "tags": ["generated_from_trainer"], "base_model": "mistralai/Mistral-7B-Instruct-v0.2", "model-index": [{"name": "Mistral-7B-Instruct-v0.2-absa-MT-laptops", "results": []}]} | Shakhovak/Mistral-7B-Instruct-v0.2-absa-MT-laptops | null | [
"generated_from_trainer",
"base_model:mistralai/Mistral-7B-Instruct-v0.2",
"license:apache-2.0",
"region:us"
] | null | 2024-04-26T18:18:42+00:00 | [] | [] | TAGS
#generated_from_trainer #base_model-mistralai/Mistral-7B-Instruct-v0.2 #license-apache-2.0 #region-us
| Mistral-7B-Instruct-v0.2-absa-MT-laptops
========================================
This model is a fine-tuned version of mistralai/Mistral-7B-Instruct-v0.2 on the None dataset.
It achieves the following results on the evaluation set:
* Loss: 0.0060
Model description
-----------------
More information needed
Intended uses & limitations
---------------------------
More information needed
Training and evaluation data
----------------------------
More information needed
Training procedure
------------------
### Training hyperparameters
The following hyperparameters were used during training:
* learning\_rate: 3e-05
* train\_batch\_size: 8
* eval\_batch\_size: 8
* seed: 42
* gradient\_accumulation\_steps: 4
* total\_train\_batch\_size: 32
* optimizer: Adam with betas=(0.9,0.999) and epsilon=1e-08
* lr\_scheduler\_type: linear
* lr\_scheduler\_warmup\_steps: 2
* training\_steps: 1200
* mixed\_precision\_training: Native AMP
### Training results
### Framework versions
* Transformers 4.38.2
* Pytorch 2.2.1+cu121
* Datasets 2.18.0
* Tokenizers 0.15.2
| [
"### Training hyperparameters\n\n\nThe following hyperparameters were used during training:\n\n\n* learning\\_rate: 3e-05\n* train\\_batch\\_size: 8\n* eval\\_batch\\_size: 8\n* seed: 42\n* gradient\\_accumulation\\_steps: 4\n* total\\_train\\_batch\\_size: 32\n* optimizer: Adam with betas=(0.9,0.999) and epsilon=1e-08\n* lr\\_scheduler\\_type: linear\n* lr\\_scheduler\\_warmup\\_steps: 2\n* training\\_steps: 1200\n* mixed\\_precision\\_training: Native AMP",
"### Training results",
"### Framework versions\n\n\n* Transformers 4.38.2\n* Pytorch 2.2.1+cu121\n* Datasets 2.18.0\n* Tokenizers 0.15.2"
] | [
"TAGS\n#generated_from_trainer #base_model-mistralai/Mistral-7B-Instruct-v0.2 #license-apache-2.0 #region-us \n",
"### Training hyperparameters\n\n\nThe following hyperparameters were used during training:\n\n\n* learning\\_rate: 3e-05\n* train\\_batch\\_size: 8\n* eval\\_batch\\_size: 8\n* seed: 42\n* gradient\\_accumulation\\_steps: 4\n* total\\_train\\_batch\\_size: 32\n* optimizer: Adam with betas=(0.9,0.999) and epsilon=1e-08\n* lr\\_scheduler\\_type: linear\n* lr\\_scheduler\\_warmup\\_steps: 2\n* training\\_steps: 1200\n* mixed\\_precision\\_training: Native AMP",
"### Training results",
"### Framework versions\n\n\n* Transformers 4.38.2\n* Pytorch 2.2.1+cu121\n* Datasets 2.18.0\n* Tokenizers 0.15.2"
] |
text-generation | transformers |
# Keiana-L3-Test5.8-8B-14
Keiana-L3-Test5.8-8B-14 is a merge of the following models using [LazyMergekit](https://colab.research.google.com/drive/1obulZ1ROXHjYLn6PPZJwRR6GzgQogxxb?usp=sharing):
# Keep in mind that, this merged model isn't usually tested at the moment, which could benefit in vocabulary error.
* [Kaoeiri/Keiana-L3-Test5.4-8B-10](https://huggingface.co/Kaoeiri/Keiana-L3-Test5.4-8B-10)
* [Undi95/Llama-3-LewdPlay-8B](https://huggingface.co/Undi95/Llama-3-LewdPlay-8B)
* [Kaoeiri/Keiana-L3-Test4.7-8B-3](https://huggingface.co/Kaoeiri/Keiana-L3-Test4.7-8B-3)
## 🧩 Configuration
```yaml
merge_method: model_stock
dtype: float16
base_model: Kaoeiri/Keiana-L3-Test5.6-8B-12
models:
- model: Kaoeiri/Keiana-L3-Test5.4-8B-10
parameters:
weight: .2
density: .24
- model: Undi95/Llama-3-LewdPlay-8B
parameters:
weight: .26
density: .38
- model: Kaoeiri/Keiana-L3-Test4.7-8B-3
parameters:
weight: .24
density: .42
parameters:
int8_mask: true
```
## 💻 Usage
```python
!pip install -qU transformers accelerate
from transformers import AutoTokenizer
import transformers
import torch
model = "Kaoeiri/Keiana-L3-Test5.8-8B-14"
messages = [{"role": "user", "content": "What is a large language model?"}]
tokenizer = AutoTokenizer.from_pretrained(model)
prompt = tokenizer.apply_chat_template(messages, tokenize=False, add_generation_prompt=True)
pipeline = transformers.pipeline(
"text-generation",
model=model,
torch_dtype=torch.float16,
device_map="auto",
)
outputs = pipeline(prompt, max_new_tokens=256, do_sample=True, temperature=0.7, top_k=50, top_p=0.95)
print(outputs[0]["generated_text"])
``` | {"tags": ["merge", "mergekit", "lazymergekit", "Kaoeiri/Keiana-L3-Test5.4-8B-10", "Undi95/Llama-3-LewdPlay-8B", "Kaoeiri/Keiana-L3-Test4.7-8B-3"], "base_model": ["Kaoeiri/Keiana-L3-Test5.4-8B-10", "Undi95/Llama-3-LewdPlay-8B", "Kaoeiri/Keiana-L3-Test4.7-8B-3"]} | Kaoeiri/Keiana-L3-Test5.8-8B-14 | null | [
"transformers",
"safetensors",
"llama",
"text-generation",
"merge",
"mergekit",
"lazymergekit",
"Kaoeiri/Keiana-L3-Test5.4-8B-10",
"Undi95/Llama-3-LewdPlay-8B",
"Kaoeiri/Keiana-L3-Test4.7-8B-3",
"conversational",
"base_model:Kaoeiri/Keiana-L3-Test5.4-8B-10",
"base_model:Undi95/Llama-3-LewdPlay-8B",
"base_model:Kaoeiri/Keiana-L3-Test4.7-8B-3",
"autotrain_compatible",
"endpoints_compatible",
"text-generation-inference",
"region:us"
] | null | 2024-04-26T18:18:52+00:00 | [] | [] | TAGS
#transformers #safetensors #llama #text-generation #merge #mergekit #lazymergekit #Kaoeiri/Keiana-L3-Test5.4-8B-10 #Undi95/Llama-3-LewdPlay-8B #Kaoeiri/Keiana-L3-Test4.7-8B-3 #conversational #base_model-Kaoeiri/Keiana-L3-Test5.4-8B-10 #base_model-Undi95/Llama-3-LewdPlay-8B #base_model-Kaoeiri/Keiana-L3-Test4.7-8B-3 #autotrain_compatible #endpoints_compatible #text-generation-inference #region-us
|
# Keiana-L3-Test5.8-8B-14
Keiana-L3-Test5.8-8B-14 is a merge of the following models using LazyMergekit:
# Keep in mind that, this merged model isn't usually tested at the moment, which could benefit in vocabulary error.
* Kaoeiri/Keiana-L3-Test5.4-8B-10
* Undi95/Llama-3-LewdPlay-8B
* Kaoeiri/Keiana-L3-Test4.7-8B-3
## Configuration
## Usage
| [
"# Keiana-L3-Test5.8-8B-14\n\nKeiana-L3-Test5.8-8B-14 is a merge of the following models using LazyMergekit:",
"# Keep in mind that, this merged model isn't usually tested at the moment, which could benefit in vocabulary error.\n* Kaoeiri/Keiana-L3-Test5.4-8B-10\n* Undi95/Llama-3-LewdPlay-8B\n* Kaoeiri/Keiana-L3-Test4.7-8B-3",
"## Configuration",
"## Usage"
] | [
"TAGS\n#transformers #safetensors #llama #text-generation #merge #mergekit #lazymergekit #Kaoeiri/Keiana-L3-Test5.4-8B-10 #Undi95/Llama-3-LewdPlay-8B #Kaoeiri/Keiana-L3-Test4.7-8B-3 #conversational #base_model-Kaoeiri/Keiana-L3-Test5.4-8B-10 #base_model-Undi95/Llama-3-LewdPlay-8B #base_model-Kaoeiri/Keiana-L3-Test4.7-8B-3 #autotrain_compatible #endpoints_compatible #text-generation-inference #region-us \n",
"# Keiana-L3-Test5.8-8B-14\n\nKeiana-L3-Test5.8-8B-14 is a merge of the following models using LazyMergekit:",
"# Keep in mind that, this merged model isn't usually tested at the moment, which could benefit in vocabulary error.\n* Kaoeiri/Keiana-L3-Test5.4-8B-10\n* Undi95/Llama-3-LewdPlay-8B\n* Kaoeiri/Keiana-L3-Test4.7-8B-3",
"## Configuration",
"## Usage"
] |
text-generation | transformers |
# Model Card for Model ID
<!-- Provide a quick summary of what the model is/does. -->
## Model Details
### Model Description
<!-- Provide a longer summary of what this model is. -->
This is the model card of a 🤗 transformers model that has been pushed on the Hub. This model card has been automatically generated.
- **Developed by:** [More Information Needed]
- **Funded by [optional]:** [More Information Needed]
- **Shared by [optional]:** [More Information Needed]
- **Model type:** [More Information Needed]
- **Language(s) (NLP):** [More Information Needed]
- **License:** [More Information Needed]
- **Finetuned from model [optional]:** [More Information Needed]
### Model Sources [optional]
<!-- Provide the basic links for the model. -->
- **Repository:** [More Information Needed]
- **Paper [optional]:** [More Information Needed]
- **Demo [optional]:** [More Information Needed]
## Uses
<!-- Address questions around how the model is intended to be used, including the foreseeable users of the model and those affected by the model. -->
### Direct Use
<!-- This section is for the model use without fine-tuning or plugging into a larger ecosystem/app. -->
[More Information Needed]
### Downstream Use [optional]
<!-- This section is for the model use when fine-tuned for a task, or when plugged into a larger ecosystem/app -->
[More Information Needed]
### Out-of-Scope Use
<!-- This section addresses misuse, malicious use, and uses that the model will not work well for. -->
[More Information Needed]
## Bias, Risks, and Limitations
<!-- This section is meant to convey both technical and sociotechnical limitations. -->
[More Information Needed]
### Recommendations
<!-- This section is meant to convey recommendations with respect to the bias, risk, and technical limitations. -->
Users (both direct and downstream) should be made aware of the risks, biases and limitations of the model. More information needed for further recommendations.
## How to Get Started with the Model
Use the code below to get started with the model.
[More Information Needed]
## Training Details
### Training Data
<!-- This should link to a Dataset Card, perhaps with a short stub of information on what the training data is all about as well as documentation related to data pre-processing or additional filtering. -->
[More Information Needed]
### Training Procedure
<!-- This relates heavily to the Technical Specifications. Content here should link to that section when it is relevant to the training procedure. -->
#### Preprocessing [optional]
[More Information Needed]
#### Training Hyperparameters
- **Training regime:** [More Information Needed] <!--fp32, fp16 mixed precision, bf16 mixed precision, bf16 non-mixed precision, fp16 non-mixed precision, fp8 mixed precision -->
#### Speeds, Sizes, Times [optional]
<!-- This section provides information about throughput, start/end time, checkpoint size if relevant, etc. -->
[More Information Needed]
## Evaluation
<!-- This section describes the evaluation protocols and provides the results. -->
### Testing Data, Factors & Metrics
#### Testing Data
<!-- This should link to a Dataset Card if possible. -->
[More Information Needed]
#### Factors
<!-- These are the things the evaluation is disaggregating by, e.g., subpopulations or domains. -->
[More Information Needed]
#### Metrics
<!-- These are the evaluation metrics being used, ideally with a description of why. -->
[More Information Needed]
### Results
[More Information Needed]
#### Summary
## Model Examination [optional]
<!-- Relevant interpretability work for the model goes here -->
[More Information Needed]
## Environmental Impact
<!-- Total emissions (in grams of CO2eq) and additional considerations, such as electricity usage, go here. Edit the suggested text below accordingly -->
Carbon emissions can be estimated using the [Machine Learning Impact calculator](https://mlco2.github.io/impact#compute) presented in [Lacoste et al. (2019)](https://arxiv.org/abs/1910.09700).
- **Hardware Type:** [More Information Needed]
- **Hours used:** [More Information Needed]
- **Cloud Provider:** [More Information Needed]
- **Compute Region:** [More Information Needed]
- **Carbon Emitted:** [More Information Needed]
## Technical Specifications [optional]
### Model Architecture and Objective
[More Information Needed]
### Compute Infrastructure
[More Information Needed]
#### Hardware
[More Information Needed]
#### Software
[More Information Needed]
## Citation [optional]
<!-- If there is a paper or blog post introducing the model, the APA and Bibtex information for that should go in this section. -->
**BibTeX:**
[More Information Needed]
**APA:**
[More Information Needed]
## Glossary [optional]
<!-- If relevant, include terms and calculations in this section that can help readers understand the model or model card. -->
[More Information Needed]
## More Information [optional]
[More Information Needed]
## Model Card Authors [optional]
[More Information Needed]
## Model Card Contact
[More Information Needed] | {"library_name": "transformers", "tags": []} | CMU-AIR2/math-deepseek-FULL-ArithHardC11 | null | [
"transformers",
"safetensors",
"llama",
"text-generation",
"conversational",
"arxiv:1910.09700",
"autotrain_compatible",
"endpoints_compatible",
"text-generation-inference",
"region:us"
] | null | 2024-04-26T18:21:08+00:00 | [
"1910.09700"
] | [] | TAGS
#transformers #safetensors #llama #text-generation #conversational #arxiv-1910.09700 #autotrain_compatible #endpoints_compatible #text-generation-inference #region-us
|
# Model Card for Model ID
## Model Details
### Model Description
This is the model card of a transformers model that has been pushed on the Hub. This model card has been automatically generated.
- Developed by:
- Funded by [optional]:
- Shared by [optional]:
- Model type:
- Language(s) (NLP):
- License:
- Finetuned from model [optional]:
### Model Sources [optional]
- Repository:
- Paper [optional]:
- Demo [optional]:
## Uses
### Direct Use
### Downstream Use [optional]
### Out-of-Scope Use
## Bias, Risks, and Limitations
### Recommendations
Users (both direct and downstream) should be made aware of the risks, biases and limitations of the model. More information needed for further recommendations.
## How to Get Started with the Model
Use the code below to get started with the model.
## Training Details
### Training Data
### Training Procedure
#### Preprocessing [optional]
#### Training Hyperparameters
- Training regime:
#### Speeds, Sizes, Times [optional]
## Evaluation
### Testing Data, Factors & Metrics
#### Testing Data
#### Factors
#### Metrics
### Results
#### Summary
## Model Examination [optional]
## Environmental Impact
Carbon emissions can be estimated using the Machine Learning Impact calculator presented in Lacoste et al. (2019).
- Hardware Type:
- Hours used:
- Cloud Provider:
- Compute Region:
- Carbon Emitted:
## Technical Specifications [optional]
### Model Architecture and Objective
### Compute Infrastructure
#### Hardware
#### Software
[optional]
BibTeX:
APA:
## Glossary [optional]
## More Information [optional]
## Model Card Authors [optional]
## Model Card Contact
| [
"# Model Card for Model ID",
"## Model Details",
"### Model Description\n\n\n\nThis is the model card of a transformers model that has been pushed on the Hub. This model card has been automatically generated.\n\n- Developed by: \n- Funded by [optional]: \n- Shared by [optional]: \n- Model type: \n- Language(s) (NLP): \n- License: \n- Finetuned from model [optional]:",
"### Model Sources [optional]\n\n\n\n- Repository: \n- Paper [optional]: \n- Demo [optional]:",
"## Uses",
"### Direct Use",
"### Downstream Use [optional]",
"### Out-of-Scope Use",
"## Bias, Risks, and Limitations",
"### Recommendations\n\n\n\nUsers (both direct and downstream) should be made aware of the risks, biases and limitations of the model. More information needed for further recommendations.",
"## How to Get Started with the Model\n\nUse the code below to get started with the model.",
"## Training Details",
"### Training Data",
"### Training Procedure",
"#### Preprocessing [optional]",
"#### Training Hyperparameters\n\n- Training regime:",
"#### Speeds, Sizes, Times [optional]",
"## Evaluation",
"### Testing Data, Factors & Metrics",
"#### Testing Data",
"#### Factors",
"#### Metrics",
"### Results",
"#### Summary",
"## Model Examination [optional]",
"## Environmental Impact\n\n\n\nCarbon emissions can be estimated using the Machine Learning Impact calculator presented in Lacoste et al. (2019).\n\n- Hardware Type: \n- Hours used: \n- Cloud Provider: \n- Compute Region: \n- Carbon Emitted:",
"## Technical Specifications [optional]",
"### Model Architecture and Objective",
"### Compute Infrastructure",
"#### Hardware",
"#### Software\n\n\n\n[optional]\n\n\n\nBibTeX:\n\n\n\nAPA:",
"## Glossary [optional]",
"## More Information [optional]",
"## Model Card Authors [optional]",
"## Model Card Contact"
] | [
"TAGS\n#transformers #safetensors #llama #text-generation #conversational #arxiv-1910.09700 #autotrain_compatible #endpoints_compatible #text-generation-inference #region-us \n",
"# Model Card for Model ID",
"## Model Details",
"### Model Description\n\n\n\nThis is the model card of a transformers model that has been pushed on the Hub. This model card has been automatically generated.\n\n- Developed by: \n- Funded by [optional]: \n- Shared by [optional]: \n- Model type: \n- Language(s) (NLP): \n- License: \n- Finetuned from model [optional]:",
"### Model Sources [optional]\n\n\n\n- Repository: \n- Paper [optional]: \n- Demo [optional]:",
"## Uses",
"### Direct Use",
"### Downstream Use [optional]",
"### Out-of-Scope Use",
"## Bias, Risks, and Limitations",
"### Recommendations\n\n\n\nUsers (both direct and downstream) should be made aware of the risks, biases and limitations of the model. More information needed for further recommendations.",
"## How to Get Started with the Model\n\nUse the code below to get started with the model.",
"## Training Details",
"### Training Data",
"### Training Procedure",
"#### Preprocessing [optional]",
"#### Training Hyperparameters\n\n- Training regime:",
"#### Speeds, Sizes, Times [optional]",
"## Evaluation",
"### Testing Data, Factors & Metrics",
"#### Testing Data",
"#### Factors",
"#### Metrics",
"### Results",
"#### Summary",
"## Model Examination [optional]",
"## Environmental Impact\n\n\n\nCarbon emissions can be estimated using the Machine Learning Impact calculator presented in Lacoste et al. (2019).\n\n- Hardware Type: \n- Hours used: \n- Cloud Provider: \n- Compute Region: \n- Carbon Emitted:",
"## Technical Specifications [optional]",
"### Model Architecture and Objective",
"### Compute Infrastructure",
"#### Hardware",
"#### Software\n\n\n\n[optional]\n\n\n\nBibTeX:\n\n\n\nAPA:",
"## Glossary [optional]",
"## More Information [optional]",
"## Model Card Authors [optional]",
"## Model Card Contact"
] |
token-classification | transformers |
<!-- This model card has been generated automatically according to the information the Trainer had access to. You
should probably proofread and complete it, then remove this comment. -->
# group4_non_all_zero
This model is a fine-tuned version of [microsoft/deberta-v3-base](https://huggingface.co/microsoft/deberta-v3-base) on an unknown dataset.
It achieves the following results on the evaluation set:
- Loss: 1.2820
- Precision: 0.0006
- Recall: 0.08
- F1: 0.0012
- Accuracy: 0.4380
## Model description
More information needed
## Intended uses & limitations
More information needed
## Training and evaluation data
More information needed
## Training procedure
### Training hyperparameters
The following hyperparameters were used during training:
- learning_rate: 3e-05
- train_batch_size: 32
- eval_batch_size: 32
- seed: 42
- optimizer: Adam with betas=(0.9,0.999) and epsilon=1e-08
- lr_scheduler_type: linear
- num_epochs: 15
### Training results
| Training Loss | Epoch | Step | Validation Loss | Precision | Recall | F1 | Accuracy |
|:-------------:|:-----:|:----:|:---------------:|:---------:|:------:|:------:|:--------:|
| No log | 1.0 | 5 | 2.1670 | 0.0 | 0.0 | 0.0 | 0.0084 |
| No log | 2.0 | 10 | 2.3289 | 0.0 | 0.0 | 0.0 | 0.0078 |
| No log | 3.0 | 15 | 2.3316 | 0.0 | 0.0 | 0.0 | 0.0078 |
| No log | 4.0 | 20 | 2.0441 | 0.0 | 0.0 | 0.0 | 0.0078 |
| No log | 5.0 | 25 | 2.4322 | 0.0 | 0.0 | 0.0 | 0.0078 |
| No log | 6.0 | 30 | 1.7898 | 0.0 | 0.0 | 0.0 | 0.0106 |
| No log | 7.0 | 35 | 1.8590 | 0.0002 | 0.0133 | 0.0004 | 0.0104 |
| No log | 8.0 | 40 | 1.7022 | 0.0002 | 0.0133 | 0.0004 | 0.0250 |
| No log | 9.0 | 45 | 1.5775 | 0.0004 | 0.04 | 0.0007 | 0.1004 |
| No log | 10.0 | 50 | 1.4837 | 0.0006 | 0.08 | 0.0011 | 0.1939 |
| No log | 11.0 | 55 | 1.3180 | 0.0004 | 0.0533 | 0.0008 | 0.3309 |
| No log | 12.0 | 60 | 1.3418 | 0.0005 | 0.0667 | 0.0011 | 0.3799 |
| No log | 13.0 | 65 | 1.3140 | 0.0005 | 0.0667 | 0.0010 | 0.4117 |
| No log | 14.0 | 70 | 1.3444 | 0.0004 | 0.0533 | 0.0008 | 0.4048 |
| No log | 15.0 | 75 | 1.2820 | 0.0006 | 0.08 | 0.0012 | 0.4380 |
### Framework versions
- Transformers 4.30.0
- Pytorch 2.2.2+cu121
- Datasets 2.19.0
- Tokenizers 0.13.3
| {"license": "mit", "tags": ["generated_from_trainer"], "metrics": ["precision", "recall", "f1", "accuracy"], "model-index": [{"name": "group4_non_all_zero", "results": []}]} | anismahmahi/group4_non_all_zero | null | [
"transformers",
"pytorch",
"tensorboard",
"deberta-v2",
"token-classification",
"generated_from_trainer",
"license:mit",
"autotrain_compatible",
"endpoints_compatible",
"region:us"
] | null | 2024-04-26T18:21:13+00:00 | [] | [] | TAGS
#transformers #pytorch #tensorboard #deberta-v2 #token-classification #generated_from_trainer #license-mit #autotrain_compatible #endpoints_compatible #region-us
| group4\_non\_all\_zero
======================
This model is a fine-tuned version of microsoft/deberta-v3-base on an unknown dataset.
It achieves the following results on the evaluation set:
* Loss: 1.2820
* Precision: 0.0006
* Recall: 0.08
* F1: 0.0012
* Accuracy: 0.4380
Model description
-----------------
More information needed
Intended uses & limitations
---------------------------
More information needed
Training and evaluation data
----------------------------
More information needed
Training procedure
------------------
### Training hyperparameters
The following hyperparameters were used during training:
* learning\_rate: 3e-05
* train\_batch\_size: 32
* eval\_batch\_size: 32
* seed: 42
* optimizer: Adam with betas=(0.9,0.999) and epsilon=1e-08
* lr\_scheduler\_type: linear
* num\_epochs: 15
### Training results
### Framework versions
* Transformers 4.30.0
* Pytorch 2.2.2+cu121
* Datasets 2.19.0
* Tokenizers 0.13.3
| [
"### Training hyperparameters\n\n\nThe following hyperparameters were used during training:\n\n\n* learning\\_rate: 3e-05\n* train\\_batch\\_size: 32\n* eval\\_batch\\_size: 32\n* seed: 42\n* optimizer: Adam with betas=(0.9,0.999) and epsilon=1e-08\n* lr\\_scheduler\\_type: linear\n* num\\_epochs: 15",
"### Training results",
"### Framework versions\n\n\n* Transformers 4.30.0\n* Pytorch 2.2.2+cu121\n* Datasets 2.19.0\n* Tokenizers 0.13.3"
] | [
"TAGS\n#transformers #pytorch #tensorboard #deberta-v2 #token-classification #generated_from_trainer #license-mit #autotrain_compatible #endpoints_compatible #region-us \n",
"### Training hyperparameters\n\n\nThe following hyperparameters were used during training:\n\n\n* learning\\_rate: 3e-05\n* train\\_batch\\_size: 32\n* eval\\_batch\\_size: 32\n* seed: 42\n* optimizer: Adam with betas=(0.9,0.999) and epsilon=1e-08\n* lr\\_scheduler\\_type: linear\n* num\\_epochs: 15",
"### Training results",
"### Framework versions\n\n\n* Transformers 4.30.0\n* Pytorch 2.2.2+cu121\n* Datasets 2.19.0\n* Tokenizers 0.13.3"
] |
null | transformers |
# acidsound/Bllossom-Q4_K_M-GGUF
This model was converted to GGUF format from [`MLP-KTLim/Bllossom`](https://huggingface.co/MLP-KTLim/Bllossom) using llama.cpp via the ggml.ai's [GGUF-my-repo](https://huggingface.co/spaces/ggml-org/gguf-my-repo) space.
Refer to the [original model card](https://huggingface.co/MLP-KTLim/Bllossom) for more details on the model.
## Use with llama.cpp
Install llama.cpp through brew.
```bash
brew install ggerganov/ggerganov/llama.cpp
```
Invoke the llama.cpp server or the CLI.
CLI:
```bash
llama-cli --hf-repo acidsound/Bllossom-Q4_K_M-GGUF --model bllossom.Q4_K_M.gguf -p "The meaning to life and the universe is"
```
Server:
```bash
llama-server --hf-repo acidsound/Bllossom-Q4_K_M-GGUF --model bllossom.Q4_K_M.gguf -c 2048
```
Note: You can also use this checkpoint directly through the [usage steps](https://github.com/ggerganov/llama.cpp?tab=readme-ov-file#usage) listed in the Llama.cpp repo as well.
```
git clone https://github.com/ggerganov/llama.cpp && cd llama.cpp && make && ./main -m bllossom.Q4_K_M.gguf -n 128
```
| {"language": ["en", "ko"], "license": "apache-2.0", "library_name": "transformers", "tags": ["llama-cpp", "gguf-my-repo"], "base_model": ["meta-llama/Meta-Llama-3-8B"]} | acidsound/Bllossom-Q4_K_M-GGUF | null | [
"transformers",
"gguf",
"llama-cpp",
"gguf-my-repo",
"en",
"ko",
"base_model:meta-llama/Meta-Llama-3-8B",
"license:apache-2.0",
"endpoints_compatible",
"region:us"
] | null | 2024-04-26T18:22:26+00:00 | [] | [
"en",
"ko"
] | TAGS
#transformers #gguf #llama-cpp #gguf-my-repo #en #ko #base_model-meta-llama/Meta-Llama-3-8B #license-apache-2.0 #endpoints_compatible #region-us
|
# acidsound/Bllossom-Q4_K_M-GGUF
This model was converted to GGUF format from 'MLP-KTLim/Bllossom' using URL via the URL's GGUF-my-repo space.
Refer to the original model card for more details on the model.
## Use with URL
Install URL through brew.
Invoke the URL server or the CLI.
CLI:
Server:
Note: You can also use this checkpoint directly through the usage steps listed in the URL repo as well.
| [
"# acidsound/Bllossom-Q4_K_M-GGUF\nThis model was converted to GGUF format from 'MLP-KTLim/Bllossom' using URL via the URL's GGUF-my-repo space.\nRefer to the original model card for more details on the model.",
"## Use with URL\n\nInstall URL through brew.\n\n\nInvoke the URL server or the CLI.\n\nCLI:\n\n\n\nServer:\n\n\n\nNote: You can also use this checkpoint directly through the usage steps listed in the URL repo as well."
] | [
"TAGS\n#transformers #gguf #llama-cpp #gguf-my-repo #en #ko #base_model-meta-llama/Meta-Llama-3-8B #license-apache-2.0 #endpoints_compatible #region-us \n",
"# acidsound/Bllossom-Q4_K_M-GGUF\nThis model was converted to GGUF format from 'MLP-KTLim/Bllossom' using URL via the URL's GGUF-my-repo space.\nRefer to the original model card for more details on the model.",
"## Use with URL\n\nInstall URL through brew.\n\n\nInvoke the URL server or the CLI.\n\nCLI:\n\n\n\nServer:\n\n\n\nNote: You can also use this checkpoint directly through the usage steps listed in the URL repo as well."
] |
null | peft |
<!-- This model card has been generated automatically according to the information the Trainer had access to. You
should probably proofread and complete it, then remove this comment. -->
# GUE_tf_1-seqsight_4096_512_27M-L8_f
This model is a fine-tuned version of [mahdibaghbanzadeh/seqsight_4096_512_27M](https://huggingface.co/mahdibaghbanzadeh/seqsight_4096_512_27M) on the [mahdibaghbanzadeh/GUE_tf_1](https://huggingface.co/datasets/mahdibaghbanzadeh/GUE_tf_1) dataset.
It achieves the following results on the evaluation set:
- Loss: 0.3286
- F1 Score: 0.8679
- Accuracy: 0.868
## Model description
More information needed
## Intended uses & limitations
More information needed
## Training and evaluation data
More information needed
## Training procedure
### Training hyperparameters
The following hyperparameters were used during training:
- learning_rate: 0.0005
- train_batch_size: 128
- eval_batch_size: 128
- seed: 42
- optimizer: Adam with betas=(0.9,0.999) and epsilon=1e-08
- lr_scheduler_type: linear
- training_steps: 10000
### Training results
| Training Loss | Epoch | Step | Validation Loss | F1 Score | Accuracy |
|:-------------:|:-----:|:-----:|:---------------:|:--------:|:--------:|
| 0.5402 | 0.83 | 200 | 0.5197 | 0.7560 | 0.756 |
| 0.4919 | 1.67 | 400 | 0.5123 | 0.7478 | 0.748 |
| 0.4824 | 2.5 | 600 | 0.5077 | 0.7520 | 0.752 |
| 0.4747 | 3.33 | 800 | 0.5003 | 0.7560 | 0.756 |
| 0.4756 | 4.17 | 1000 | 0.5011 | 0.7635 | 0.764 |
| 0.4689 | 5.0 | 1200 | 0.4994 | 0.7610 | 0.761 |
| 0.4627 | 5.83 | 1400 | 0.4949 | 0.7648 | 0.765 |
| 0.4651 | 6.67 | 1600 | 0.4980 | 0.7587 | 0.759 |
| 0.461 | 7.5 | 1800 | 0.4923 | 0.7610 | 0.761 |
| 0.4533 | 8.33 | 2000 | 0.4905 | 0.7649 | 0.765 |
| 0.4545 | 9.17 | 2200 | 0.4904 | 0.7609 | 0.761 |
| 0.4521 | 10.0 | 2400 | 0.5044 | 0.7565 | 0.758 |
| 0.4505 | 10.83 | 2600 | 0.4954 | 0.7679 | 0.769 |
| 0.4476 | 11.67 | 2800 | 0.4965 | 0.7620 | 0.763 |
| 0.4388 | 12.5 | 3000 | 0.4945 | 0.7664 | 0.767 |
| 0.448 | 13.33 | 3200 | 0.4893 | 0.7603 | 0.761 |
| 0.4406 | 14.17 | 3400 | 0.4833 | 0.7550 | 0.755 |
| 0.4375 | 15.0 | 3600 | 0.4912 | 0.7610 | 0.762 |
| 0.4346 | 15.83 | 3800 | 0.4847 | 0.7642 | 0.765 |
| 0.4369 | 16.67 | 4000 | 0.4937 | 0.7592 | 0.76 |
| 0.4349 | 17.5 | 4200 | 0.4926 | 0.7580 | 0.759 |
| 0.4274 | 18.33 | 4400 | 0.4937 | 0.7677 | 0.768 |
| 0.431 | 19.17 | 4600 | 0.4918 | 0.7634 | 0.764 |
| 0.4297 | 20.0 | 4800 | 0.4845 | 0.7597 | 0.76 |
| 0.4307 | 20.83 | 5000 | 0.4880 | 0.7598 | 0.76 |
| 0.4258 | 21.67 | 5200 | 0.4854 | 0.7656 | 0.766 |
| 0.424 | 22.5 | 5400 | 0.4860 | 0.7558 | 0.756 |
| 0.4267 | 23.33 | 5600 | 0.4872 | 0.7654 | 0.766 |
| 0.4229 | 24.17 | 5800 | 0.4926 | 0.7693 | 0.77 |
| 0.4232 | 25.0 | 6000 | 0.4894 | 0.7620 | 0.762 |
| 0.4176 | 25.83 | 6200 | 0.4894 | 0.7606 | 0.761 |
| 0.4173 | 26.67 | 6400 | 0.4842 | 0.7640 | 0.764 |
| 0.422 | 27.5 | 6600 | 0.4889 | 0.7618 | 0.762 |
| 0.4188 | 28.33 | 6800 | 0.4862 | 0.7578 | 0.758 |
| 0.4174 | 29.17 | 7000 | 0.4912 | 0.7615 | 0.762 |
| 0.4185 | 30.0 | 7200 | 0.4862 | 0.7596 | 0.76 |
| 0.4167 | 30.83 | 7400 | 0.4959 | 0.7608 | 0.762 |
| 0.4122 | 31.67 | 7600 | 0.4883 | 0.7628 | 0.763 |
| 0.4155 | 32.5 | 7800 | 0.4911 | 0.7615 | 0.762 |
| 0.413 | 33.33 | 8000 | 0.4909 | 0.7626 | 0.763 |
| 0.4161 | 34.17 | 8200 | 0.4889 | 0.7636 | 0.764 |
| 0.411 | 35.0 | 8400 | 0.4886 | 0.7638 | 0.764 |
| 0.4126 | 35.83 | 8600 | 0.4886 | 0.7617 | 0.762 |
| 0.4101 | 36.67 | 8800 | 0.4902 | 0.7605 | 0.761 |
| 0.4089 | 37.5 | 9000 | 0.4951 | 0.7610 | 0.762 |
| 0.4109 | 38.33 | 9200 | 0.4903 | 0.7647 | 0.765 |
| 0.4106 | 39.17 | 9400 | 0.4901 | 0.7626 | 0.763 |
| 0.4109 | 40.0 | 9600 | 0.4900 | 0.7646 | 0.765 |
| 0.409 | 40.83 | 9800 | 0.4887 | 0.7658 | 0.766 |
| 0.4095 | 41.67 | 10000 | 0.4898 | 0.7646 | 0.765 |
### Framework versions
- PEFT 0.9.0
- Transformers 4.38.2
- Pytorch 2.2.0+cu121
- Datasets 2.17.1
- Tokenizers 0.15.2 | {"library_name": "peft", "tags": ["generated_from_trainer"], "metrics": ["accuracy"], "base_model": "mahdibaghbanzadeh/seqsight_4096_512_27M", "model-index": [{"name": "GUE_tf_1-seqsight_4096_512_27M-L8_f", "results": []}]} | mahdibaghbanzadeh/GUE_tf_1-seqsight_4096_512_27M-L8_f | null | [
"peft",
"safetensors",
"generated_from_trainer",
"base_model:mahdibaghbanzadeh/seqsight_4096_512_27M",
"region:us"
] | null | 2024-04-26T18:22:34+00:00 | [] | [] | TAGS
#peft #safetensors #generated_from_trainer #base_model-mahdibaghbanzadeh/seqsight_4096_512_27M #region-us
| GUE\_tf\_1-seqsight\_4096\_512\_27M-L8\_f
=========================================
This model is a fine-tuned version of mahdibaghbanzadeh/seqsight\_4096\_512\_27M on the mahdibaghbanzadeh/GUE\_tf\_1 dataset.
It achieves the following results on the evaluation set:
* Loss: 0.3286
* F1 Score: 0.8679
* Accuracy: 0.868
Model description
-----------------
More information needed
Intended uses & limitations
---------------------------
More information needed
Training and evaluation data
----------------------------
More information needed
Training procedure
------------------
### Training hyperparameters
The following hyperparameters were used during training:
* learning\_rate: 0.0005
* train\_batch\_size: 128
* eval\_batch\_size: 128
* seed: 42
* optimizer: Adam with betas=(0.9,0.999) and epsilon=1e-08
* lr\_scheduler\_type: linear
* training\_steps: 10000
### Training results
### Framework versions
* PEFT 0.9.0
* Transformers 4.38.2
* Pytorch 2.2.0+cu121
* Datasets 2.17.1
* Tokenizers 0.15.2
| [
"### Training hyperparameters\n\n\nThe following hyperparameters were used during training:\n\n\n* learning\\_rate: 0.0005\n* train\\_batch\\_size: 128\n* eval\\_batch\\_size: 128\n* seed: 42\n* optimizer: Adam with betas=(0.9,0.999) and epsilon=1e-08\n* lr\\_scheduler\\_type: linear\n* training\\_steps: 10000",
"### Training results",
"### Framework versions\n\n\n* PEFT 0.9.0\n* Transformers 4.38.2\n* Pytorch 2.2.0+cu121\n* Datasets 2.17.1\n* Tokenizers 0.15.2"
] | [
"TAGS\n#peft #safetensors #generated_from_trainer #base_model-mahdibaghbanzadeh/seqsight_4096_512_27M #region-us \n",
"### Training hyperparameters\n\n\nThe following hyperparameters were used during training:\n\n\n* learning\\_rate: 0.0005\n* train\\_batch\\_size: 128\n* eval\\_batch\\_size: 128\n* seed: 42\n* optimizer: Adam with betas=(0.9,0.999) and epsilon=1e-08\n* lr\\_scheduler\\_type: linear\n* training\\_steps: 10000",
"### Training results",
"### Framework versions\n\n\n* PEFT 0.9.0\n* Transformers 4.38.2\n* Pytorch 2.2.0+cu121\n* Datasets 2.17.1\n* Tokenizers 0.15.2"
] |
text-generation | transformers | # merge
This is a merge of pre-trained language models created using [mergekit](https://github.com/cg123/mergekit).
## Merge Details
### Merge Method
This model was merged using the [Model Stock](https://arxiv.org/abs/2403.19522) merge method using [ResplendentAI/Aura_Uncensored_l3_8B](https://huggingface.co/ResplendentAI/Aura_Uncensored_l3_8B) as a base.
### Models Merged
The following models were included in the merge:
* [vicgalle/Roleplay-Llama-3-8B](https://huggingface.co/vicgalle/Roleplay-Llama-3-8B)
* [Undi95/Llama-3-LewdPlay-8B-evo](https://huggingface.co/Undi95/Llama-3-LewdPlay-8B-evo)
* [abhishek/autotrain-llama3-orpo](https://huggingface.co/abhishek/autotrain-llama3-orpo)
### Configuration
The following YAML configuration was used to produce this model:
```yaml
models:
- model: ResplendentAI/Aura_Uncensored_l3_8B
- model: abhishek/autotrain-llama3-orpo
- model: vicgalle/Roleplay-Llama-3-8B
- model: Undi95/Llama-3-LewdPlay-8B-evo
merge_method: model_stock
base_model: ResplendentAI/Aura_Uncensored_l3_8B
dtype: bfloat16
```
| {"library_name": "transformers", "tags": ["mergekit", "merge"], "base_model": ["ResplendentAI/Aura_Uncensored_l3_8B", "vicgalle/Roleplay-Llama-3-8B", "Undi95/Llama-3-LewdPlay-8B-evo", "abhishek/autotrain-llama3-orpo"]} | Azazelle/Llama-3-8B-contaminated-roleplay | null | [
"transformers",
"safetensors",
"llama",
"text-generation",
"mergekit",
"merge",
"conversational",
"arxiv:2403.19522",
"base_model:ResplendentAI/Aura_Uncensored_l3_8B",
"base_model:vicgalle/Roleplay-Llama-3-8B",
"base_model:Undi95/Llama-3-LewdPlay-8B-evo",
"base_model:abhishek/autotrain-llama3-orpo",
"autotrain_compatible",
"endpoints_compatible",
"text-generation-inference",
"region:us"
] | null | 2024-04-26T18:23:03+00:00 | [
"2403.19522"
] | [] | TAGS
#transformers #safetensors #llama #text-generation #mergekit #merge #conversational #arxiv-2403.19522 #base_model-ResplendentAI/Aura_Uncensored_l3_8B #base_model-vicgalle/Roleplay-Llama-3-8B #base_model-Undi95/Llama-3-LewdPlay-8B-evo #base_model-abhishek/autotrain-llama3-orpo #autotrain_compatible #endpoints_compatible #text-generation-inference #region-us
| # merge
This is a merge of pre-trained language models created using mergekit.
## Merge Details
### Merge Method
This model was merged using the Model Stock merge method using ResplendentAI/Aura_Uncensored_l3_8B as a base.
### Models Merged
The following models were included in the merge:
* vicgalle/Roleplay-Llama-3-8B
* Undi95/Llama-3-LewdPlay-8B-evo
* abhishek/autotrain-llama3-orpo
### Configuration
The following YAML configuration was used to produce this model:
| [
"# merge\n\nThis is a merge of pre-trained language models created using mergekit.",
"## Merge Details",
"### Merge Method\n\nThis model was merged using the Model Stock merge method using ResplendentAI/Aura_Uncensored_l3_8B as a base.",
"### Models Merged\n\nThe following models were included in the merge:\n* vicgalle/Roleplay-Llama-3-8B\n* Undi95/Llama-3-LewdPlay-8B-evo\n* abhishek/autotrain-llama3-orpo",
"### Configuration\n\nThe following YAML configuration was used to produce this model:"
] | [
"TAGS\n#transformers #safetensors #llama #text-generation #mergekit #merge #conversational #arxiv-2403.19522 #base_model-ResplendentAI/Aura_Uncensored_l3_8B #base_model-vicgalle/Roleplay-Llama-3-8B #base_model-Undi95/Llama-3-LewdPlay-8B-evo #base_model-abhishek/autotrain-llama3-orpo #autotrain_compatible #endpoints_compatible #text-generation-inference #region-us \n",
"# merge\n\nThis is a merge of pre-trained language models created using mergekit.",
"## Merge Details",
"### Merge Method\n\nThis model was merged using the Model Stock merge method using ResplendentAI/Aura_Uncensored_l3_8B as a base.",
"### Models Merged\n\nThe following models were included in the merge:\n* vicgalle/Roleplay-Llama-3-8B\n* Undi95/Llama-3-LewdPlay-8B-evo\n* abhishek/autotrain-llama3-orpo",
"### Configuration\n\nThe following YAML configuration was used to produce this model:"
] |
question-answering | transformers |
<!-- This model card has been generated automatically according to the information Keras had access to. You should
probably proofread and complete it, then remove this comment. -->
# AlessandraAbreu/ftllm_loggi
This model is a fine-tuned version of [distilbert/distilbert-base-uncased](https://huggingface.co/distilbert/distilbert-base-uncased) on an unknown dataset.
It achieves the following results on the evaluation set:
- Train Loss: 1.9755
- Validation Loss: 1.9604
- Epoch: 1
## Model description
More information needed
## Intended uses & limitations
More information needed
## Training and evaluation data
More information needed
## Training procedure
### Training hyperparameters
The following hyperparameters were used during training:
- optimizer: {'name': 'Adam', 'weight_decay': None, 'clipnorm': None, 'global_clipnorm': None, 'clipvalue': None, 'use_ema': False, 'ema_momentum': 0.99, 'ema_overwrite_frequency': None, 'jit_compile': True, 'is_legacy_optimizer': False, 'learning_rate': {'module': 'keras.optimizers.schedules', 'class_name': 'PolynomialDecay', 'config': {'initial_learning_rate': 2e-05, 'decay_steps': 436, 'end_learning_rate': 0.0, 'power': 1.0, 'cycle': False, 'name': None}, 'registered_name': None}, 'beta_1': 0.9, 'beta_2': 0.999, 'epsilon': 1e-08, 'amsgrad': False}
- training_precision: float32
### Training results
| Train Loss | Validation Loss | Epoch |
|:----------:|:---------------:|:-----:|
| 3.6195 | 2.4226 | 0 |
| 1.9755 | 1.9604 | 1 |
### Framework versions
- Transformers 4.40.0
- TensorFlow 2.15.0
- Datasets 2.19.0
- Tokenizers 0.19.1
| {"license": "apache-2.0", "tags": ["generated_from_keras_callback"], "base_model": "distilbert/distilbert-base-uncased", "model-index": [{"name": "AlessandraAbreu/ftllm_loggi", "results": []}]} | AlessandraAbreu/ftllm_loggi | null | [
"transformers",
"tf",
"distilbert",
"question-answering",
"generated_from_keras_callback",
"base_model:distilbert/distilbert-base-uncased",
"license:apache-2.0",
"endpoints_compatible",
"region:us"
] | null | 2024-04-26T18:24:09+00:00 | [] | [] | TAGS
#transformers #tf #distilbert #question-answering #generated_from_keras_callback #base_model-distilbert/distilbert-base-uncased #license-apache-2.0 #endpoints_compatible #region-us
| AlessandraAbreu/ftllm\_loggi
============================
This model is a fine-tuned version of distilbert/distilbert-base-uncased on an unknown dataset.
It achieves the following results on the evaluation set:
* Train Loss: 1.9755
* Validation Loss: 1.9604
* Epoch: 1
Model description
-----------------
More information needed
Intended uses & limitations
---------------------------
More information needed
Training and evaluation data
----------------------------
More information needed
Training procedure
------------------
### Training hyperparameters
The following hyperparameters were used during training:
* optimizer: {'name': 'Adam', 'weight\_decay': None, 'clipnorm': None, 'global\_clipnorm': None, 'clipvalue': None, 'use\_ema': False, 'ema\_momentum': 0.99, 'ema\_overwrite\_frequency': None, 'jit\_compile': True, 'is\_legacy\_optimizer': False, 'learning\_rate': {'module': 'keras.optimizers.schedules', 'class\_name': 'PolynomialDecay', 'config': {'initial\_learning\_rate': 2e-05, 'decay\_steps': 436, 'end\_learning\_rate': 0.0, 'power': 1.0, 'cycle': False, 'name': None}, 'registered\_name': None}, 'beta\_1': 0.9, 'beta\_2': 0.999, 'epsilon': 1e-08, 'amsgrad': False}
* training\_precision: float32
### Training results
### Framework versions
* Transformers 4.40.0
* TensorFlow 2.15.0
* Datasets 2.19.0
* Tokenizers 0.19.1
| [
"### Training hyperparameters\n\n\nThe following hyperparameters were used during training:\n\n\n* optimizer: {'name': 'Adam', 'weight\\_decay': None, 'clipnorm': None, 'global\\_clipnorm': None, 'clipvalue': None, 'use\\_ema': False, 'ema\\_momentum': 0.99, 'ema\\_overwrite\\_frequency': None, 'jit\\_compile': True, 'is\\_legacy\\_optimizer': False, 'learning\\_rate': {'module': 'keras.optimizers.schedules', 'class\\_name': 'PolynomialDecay', 'config': {'initial\\_learning\\_rate': 2e-05, 'decay\\_steps': 436, 'end\\_learning\\_rate': 0.0, 'power': 1.0, 'cycle': False, 'name': None}, 'registered\\_name': None}, 'beta\\_1': 0.9, 'beta\\_2': 0.999, 'epsilon': 1e-08, 'amsgrad': False}\n* training\\_precision: float32",
"### Training results",
"### Framework versions\n\n\n* Transformers 4.40.0\n* TensorFlow 2.15.0\n* Datasets 2.19.0\n* Tokenizers 0.19.1"
] | [
"TAGS\n#transformers #tf #distilbert #question-answering #generated_from_keras_callback #base_model-distilbert/distilbert-base-uncased #license-apache-2.0 #endpoints_compatible #region-us \n",
"### Training hyperparameters\n\n\nThe following hyperparameters were used during training:\n\n\n* optimizer: {'name': 'Adam', 'weight\\_decay': None, 'clipnorm': None, 'global\\_clipnorm': None, 'clipvalue': None, 'use\\_ema': False, 'ema\\_momentum': 0.99, 'ema\\_overwrite\\_frequency': None, 'jit\\_compile': True, 'is\\_legacy\\_optimizer': False, 'learning\\_rate': {'module': 'keras.optimizers.schedules', 'class\\_name': 'PolynomialDecay', 'config': {'initial\\_learning\\_rate': 2e-05, 'decay\\_steps': 436, 'end\\_learning\\_rate': 0.0, 'power': 1.0, 'cycle': False, 'name': None}, 'registered\\_name': None}, 'beta\\_1': 0.9, 'beta\\_2': 0.999, 'epsilon': 1e-08, 'amsgrad': False}\n* training\\_precision: float32",
"### Training results",
"### Framework versions\n\n\n* Transformers 4.40.0\n* TensorFlow 2.15.0\n* Datasets 2.19.0\n* Tokenizers 0.19.1"
] |
text2text-generation | transformers |
<!-- This model card has been generated automatically according to the information the Trainer had access to. You
should probably proofread and complete it, then remove this comment. -->
# my_awesome_billsum_model
This model is a fine-tuned version of [t5-small](https://huggingface.co/t5-small) on an unknown dataset.
It achieves the following results on the evaluation set:
- Loss: 2.4539
- Rouge1: 0.1468
- Rouge2: 0.0569
- Rougel: 0.1209
- Rougelsum: 0.1212
- Gen Len: 19.0
## Model description
More information needed
## Intended uses & limitations
More information needed
## Training and evaluation data
More information needed
## Training procedure
### Training hyperparameters
The following hyperparameters were used during training:
- learning_rate: 2e-05
- train_batch_size: 16
- eval_batch_size: 16
- seed: 42
- optimizer: Adam with betas=(0.9,0.999) and epsilon=1e-08
- lr_scheduler_type: linear
- num_epochs: 4
- mixed_precision_training: Native AMP
### Training results
| Training Loss | Epoch | Step | Validation Loss | Rouge1 | Rouge2 | Rougel | Rougelsum | Gen Len |
|:-------------:|:-----:|:----:|:---------------:|:------:|:------:|:------:|:---------:|:-------:|
| No log | 1.0 | 62 | 2.7578 | 0.1326 | 0.0415 | 0.1103 | 0.1105 | 19.0 |
| No log | 2.0 | 124 | 2.5392 | 0.1368 | 0.0491 | 0.1134 | 0.1136 | 19.0 |
| No log | 3.0 | 186 | 2.4711 | 0.1456 | 0.0563 | 0.1193 | 0.1196 | 19.0 |
| No log | 4.0 | 248 | 2.4539 | 0.1468 | 0.0569 | 0.1209 | 0.1212 | 19.0 |
### Framework versions
- Transformers 4.39.3
- Pytorch 2.1.2
- Datasets 2.18.0
- Tokenizers 0.15.2
| {"license": "apache-2.0", "tags": ["generated_from_trainer"], "metrics": ["rouge"], "base_model": "t5-small", "model-index": [{"name": "my_awesome_billsum_model", "results": []}]} | josiahgottfried/my_awesome_billsum_model | null | [
"transformers",
"tensorboard",
"safetensors",
"t5",
"text2text-generation",
"generated_from_trainer",
"base_model:t5-small",
"license:apache-2.0",
"autotrain_compatible",
"endpoints_compatible",
"text-generation-inference",
"region:us"
] | null | 2024-04-26T18:25:30+00:00 | [] | [] | TAGS
#transformers #tensorboard #safetensors #t5 #text2text-generation #generated_from_trainer #base_model-t5-small #license-apache-2.0 #autotrain_compatible #endpoints_compatible #text-generation-inference #region-us
| my\_awesome\_billsum\_model
===========================
This model is a fine-tuned version of t5-small on an unknown dataset.
It achieves the following results on the evaluation set:
* Loss: 2.4539
* Rouge1: 0.1468
* Rouge2: 0.0569
* Rougel: 0.1209
* Rougelsum: 0.1212
* Gen Len: 19.0
Model description
-----------------
More information needed
Intended uses & limitations
---------------------------
More information needed
Training and evaluation data
----------------------------
More information needed
Training procedure
------------------
### Training hyperparameters
The following hyperparameters were used during training:
* learning\_rate: 2e-05
* train\_batch\_size: 16
* eval\_batch\_size: 16
* seed: 42
* optimizer: Adam with betas=(0.9,0.999) and epsilon=1e-08
* lr\_scheduler\_type: linear
* num\_epochs: 4
* mixed\_precision\_training: Native AMP
### Training results
### Framework versions
* Transformers 4.39.3
* Pytorch 2.1.2
* Datasets 2.18.0
* Tokenizers 0.15.2
| [
"### Training hyperparameters\n\n\nThe following hyperparameters were used during training:\n\n\n* learning\\_rate: 2e-05\n* train\\_batch\\_size: 16\n* eval\\_batch\\_size: 16\n* seed: 42\n* optimizer: Adam with betas=(0.9,0.999) and epsilon=1e-08\n* lr\\_scheduler\\_type: linear\n* num\\_epochs: 4\n* mixed\\_precision\\_training: Native AMP",
"### Training results",
"### Framework versions\n\n\n* Transformers 4.39.3\n* Pytorch 2.1.2\n* Datasets 2.18.0\n* Tokenizers 0.15.2"
] | [
"TAGS\n#transformers #tensorboard #safetensors #t5 #text2text-generation #generated_from_trainer #base_model-t5-small #license-apache-2.0 #autotrain_compatible #endpoints_compatible #text-generation-inference #region-us \n",
"### Training hyperparameters\n\n\nThe following hyperparameters were used during training:\n\n\n* learning\\_rate: 2e-05\n* train\\_batch\\_size: 16\n* eval\\_batch\\_size: 16\n* seed: 42\n* optimizer: Adam with betas=(0.9,0.999) and epsilon=1e-08\n* lr\\_scheduler\\_type: linear\n* num\\_epochs: 4\n* mixed\\_precision\\_training: Native AMP",
"### Training results",
"### Framework versions\n\n\n* Transformers 4.39.3\n* Pytorch 2.1.2\n* Datasets 2.18.0\n* Tokenizers 0.15.2"
] |
text-generation | transformers |
# Qwen1.5-110B-Chat
## About Quantization
我们使用modelscope [swift](https://github.com/modelscope/swift/)仓库进行GPTQ量化. 量化文档可以查看[这里](https://github.com/modelscope/swift/blob/main/docs/source/LLM/LLM%E9%87%8F%E5%8C%96%E6%96%87%E6%A1%A3.md). 量化命令如下:
We use the modelscope [swift](https://github.com/modelscope/swift/) repository to perform GPTQ quantization. Quantization documentation can be found [here](https://github.com/modelscope/swift/blob/main/docs/source_en/LLM/LLM-quantization.md). The quantization command is as follows:
```bash
OMP_NUM_THREADS=14 CUDA_VISIBLE_DEVICES=0 swift export \
--model_type qwen1half-110b-chat --quant_bits 8 \
--dataset sharegpt-gpt4-mini alpaca-zh alpaca-en \
--quant_method gptq --quant_seqlen 8192
```
## Introduction
Qwen1.5 is the beta version of Qwen2, a transformer-based decoder-only language model pretrained on a large amount of data. In comparison with the previous released Qwen, the improvements include:
* 9 model sizes, including 0.5B, 1.8B, 4B, 7B, 14B, 32B, 72B, and 110B dense models, and an MoE model of 14B with 2.7B activated;
* Significant performance improvement in human preference for chat models;
* Multilingual support of both base and chat models;
* Stable support of 32K context length for models of all sizes
* No need of `trust_remote_code`.
For more details, please refer to our [blog post](https://qwenlm.github.io/blog/qwen1.5/) and [GitHub repo](https://github.com/QwenLM/Qwen1.5).
<br>
## Model Details
Qwen1.5 is a language model series including decoder language models of different model sizes. For each size, we release the base language model and the aligned chat model. It is based on the Transformer architecture with SwiGLU activation, attention QKV bias, group query attention, mixture of sliding window attention and full attention, etc. Additionally, we have an improved tokenizer adaptive to multiple natural languages and codes. For the beta version, temporarily we did not include GQA (except for 32B and 110B) and the mixture of SWA and full attention.
## Training details
We pretrained the models with a large amount of data, and we post-trained the models with both supervised finetuning and direct preference optimization.
## Requirements
The code of Qwen1.5 has been in the latest Hugging face transformers and we advise you to install `transformers>=4.37.0`, or you might encounter the following error:
```
KeyError: 'qwen2'
```
## Quickstart
Here provides a code snippet with `apply_chat_template` to show you how to load the tokenizer and model and how to generate contents.
```python
from transformers import AutoModelForCausalLM, AutoTokenizer
device = "cuda" # the device to load the model onto
model = AutoModelForCausalLM.from_pretrained(
"study-hjt/Qwen1.5-110B-Chat-GPTQ-Int8",
torch_dtype="auto",
device_map="auto"
)
tokenizer = AutoTokenizer.from_pretrained("study-hjt/Qwen1.5-110B-Chat-GPTQ-Int8")
prompt = "Give me a short introduction to large language model."
messages = [
{"role": "system", "content": "You are a helpful assistant."},
{"role": "user", "content": prompt}
]
text = tokenizer.apply_chat_template(
messages,
tokenize=False,
add_generation_prompt=True
)
model_inputs = tokenizer([text], return_tensors="pt").to(device)
generated_ids = model.generate(
model_inputs.input_ids,
max_new_tokens=512
)
generated_ids = [
output_ids[len(input_ids):] for input_ids, output_ids in zip(model_inputs.input_ids, generated_ids)
]
response = tokenizer.batch_decode(generated_ids, skip_special_tokens=True)[0]
```
## Tips
* If you encounter code switching or other bad cases, we advise you to use our provided hyper-parameters in `generation_config.json`.
## Citation
If you find our work helpful, feel free to give us a cite.
```
@article{qwen,
title={Qwen Technical Report},
author={Jinze Bai and Shuai Bai and Yunfei Chu and Zeyu Cui and Kai Dang and Xiaodong Deng and Yang Fan and Wenbin Ge and Yu Han and Fei Huang and Binyuan Hui and Luo Ji and Mei Li and Junyang Lin and Runji Lin and Dayiheng Liu and Gao Liu and Chengqiang Lu and Keming Lu and Jianxin Ma and Rui Men and Xingzhang Ren and Xuancheng Ren and Chuanqi Tan and Sinan Tan and Jianhong Tu and Peng Wang and Shijie Wang and Wei Wang and Shengguang Wu and Benfeng Xu and Jin Xu and An Yang and Hao Yang and Jian Yang and Shusheng Yang and Yang Yao and Bowen Yu and Hongyi Yuan and Zheng Yuan and Jianwei Zhang and Xingxuan Zhang and Yichang Zhang and Zhenru Zhang and Chang Zhou and Jingren Zhou and Xiaohuan Zhou and Tianhang Zhu},
journal={arXiv preprint arXiv:2309.16609},
year={2023}
}
```
| {"language": ["en"], "license": "other", "tags": ["chat", "qwen", "gptq", "int8", "8bits", "110b"], "license_name": "tongyi-qianwen", "license_link": "https://huggingface.co/Qwen/Qwen1.5-110B-Chat/blob/main/LICENSE", "pipeline_tag": "text-generation"} | study-hjt/Qwen1.5-110B-Chat-GPTQ-Int8 | null | [
"transformers",
"safetensors",
"qwen2",
"text-generation",
"chat",
"qwen",
"gptq",
"int8",
"8bits",
"110b",
"conversational",
"en",
"license:other",
"autotrain_compatible",
"endpoints_compatible",
"text-generation-inference",
"8-bit",
"region:us"
] | null | 2024-04-26T18:25:47+00:00 | [] | [
"en"
] | TAGS
#transformers #safetensors #qwen2 #text-generation #chat #qwen #gptq #int8 #8bits #110b #conversational #en #license-other #autotrain_compatible #endpoints_compatible #text-generation-inference #8-bit #region-us
|
# Qwen1.5-110B-Chat
## About Quantization
我们使用modelscope swift仓库进行GPTQ量化. 量化文档可以查看这里. 量化命令如下:
We use the modelscope swift repository to perform GPTQ quantization. Quantization documentation can be found here. The quantization command is as follows:
## Introduction
Qwen1.5 is the beta version of Qwen2, a transformer-based decoder-only language model pretrained on a large amount of data. In comparison with the previous released Qwen, the improvements include:
* 9 model sizes, including 0.5B, 1.8B, 4B, 7B, 14B, 32B, 72B, and 110B dense models, and an MoE model of 14B with 2.7B activated;
* Significant performance improvement in human preference for chat models;
* Multilingual support of both base and chat models;
* Stable support of 32K context length for models of all sizes
* No need of 'trust_remote_code'.
For more details, please refer to our blog post and GitHub repo.
<br>
## Model Details
Qwen1.5 is a language model series including decoder language models of different model sizes. For each size, we release the base language model and the aligned chat model. It is based on the Transformer architecture with SwiGLU activation, attention QKV bias, group query attention, mixture of sliding window attention and full attention, etc. Additionally, we have an improved tokenizer adaptive to multiple natural languages and codes. For the beta version, temporarily we did not include GQA (except for 32B and 110B) and the mixture of SWA and full attention.
## Training details
We pretrained the models with a large amount of data, and we post-trained the models with both supervised finetuning and direct preference optimization.
## Requirements
The code of Qwen1.5 has been in the latest Hugging face transformers and we advise you to install 'transformers>=4.37.0', or you might encounter the following error:
## Quickstart
Here provides a code snippet with 'apply_chat_template' to show you how to load the tokenizer and model and how to generate contents.
## Tips
* If you encounter code switching or other bad cases, we advise you to use our provided hyper-parameters in 'generation_config.json'.
If you find our work helpful, feel free to give us a cite.
| [
"# Qwen1.5-110B-Chat",
"## About Quantization\n我们使用modelscope swift仓库进行GPTQ量化. 量化文档可以查看这里. 量化命令如下:\n\nWe use the modelscope swift repository to perform GPTQ quantization. Quantization documentation can be found here. The quantization command is as follows:",
"## Introduction\n\nQwen1.5 is the beta version of Qwen2, a transformer-based decoder-only language model pretrained on a large amount of data. In comparison with the previous released Qwen, the improvements include: \n\n* 9 model sizes, including 0.5B, 1.8B, 4B, 7B, 14B, 32B, 72B, and 110B dense models, and an MoE model of 14B with 2.7B activated;\n* Significant performance improvement in human preference for chat models;\n* Multilingual support of both base and chat models;\n* Stable support of 32K context length for models of all sizes\n* No need of 'trust_remote_code'.\n\nFor more details, please refer to our blog post and GitHub repo.\n<br>",
"## Model Details\nQwen1.5 is a language model series including decoder language models of different model sizes. For each size, we release the base language model and the aligned chat model. It is based on the Transformer architecture with SwiGLU activation, attention QKV bias, group query attention, mixture of sliding window attention and full attention, etc. Additionally, we have an improved tokenizer adaptive to multiple natural languages and codes. For the beta version, temporarily we did not include GQA (except for 32B and 110B) and the mixture of SWA and full attention.",
"## Training details\nWe pretrained the models with a large amount of data, and we post-trained the models with both supervised finetuning and direct preference optimization.",
"## Requirements\nThe code of Qwen1.5 has been in the latest Hugging face transformers and we advise you to install 'transformers>=4.37.0', or you might encounter the following error:",
"## Quickstart\n\nHere provides a code snippet with 'apply_chat_template' to show you how to load the tokenizer and model and how to generate contents.",
"## Tips\n\n* If you encounter code switching or other bad cases, we advise you to use our provided hyper-parameters in 'generation_config.json'.\n\n\nIf you find our work helpful, feel free to give us a cite."
] | [
"TAGS\n#transformers #safetensors #qwen2 #text-generation #chat #qwen #gptq #int8 #8bits #110b #conversational #en #license-other #autotrain_compatible #endpoints_compatible #text-generation-inference #8-bit #region-us \n",
"# Qwen1.5-110B-Chat",
"## About Quantization\n我们使用modelscope swift仓库进行GPTQ量化. 量化文档可以查看这里. 量化命令如下:\n\nWe use the modelscope swift repository to perform GPTQ quantization. Quantization documentation can be found here. The quantization command is as follows:",
"## Introduction\n\nQwen1.5 is the beta version of Qwen2, a transformer-based decoder-only language model pretrained on a large amount of data. In comparison with the previous released Qwen, the improvements include: \n\n* 9 model sizes, including 0.5B, 1.8B, 4B, 7B, 14B, 32B, 72B, and 110B dense models, and an MoE model of 14B with 2.7B activated;\n* Significant performance improvement in human preference for chat models;\n* Multilingual support of both base and chat models;\n* Stable support of 32K context length for models of all sizes\n* No need of 'trust_remote_code'.\n\nFor more details, please refer to our blog post and GitHub repo.\n<br>",
"## Model Details\nQwen1.5 is a language model series including decoder language models of different model sizes. For each size, we release the base language model and the aligned chat model. It is based on the Transformer architecture with SwiGLU activation, attention QKV bias, group query attention, mixture of sliding window attention and full attention, etc. Additionally, we have an improved tokenizer adaptive to multiple natural languages and codes. For the beta version, temporarily we did not include GQA (except for 32B and 110B) and the mixture of SWA and full attention.",
"## Training details\nWe pretrained the models with a large amount of data, and we post-trained the models with both supervised finetuning and direct preference optimization.",
"## Requirements\nThe code of Qwen1.5 has been in the latest Hugging face transformers and we advise you to install 'transformers>=4.37.0', or you might encounter the following error:",
"## Quickstart\n\nHere provides a code snippet with 'apply_chat_template' to show you how to load the tokenizer and model and how to generate contents.",
"## Tips\n\n* If you encounter code switching or other bad cases, we advise you to use our provided hyper-parameters in 'generation_config.json'.\n\n\nIf you find our work helpful, feel free to give us a cite."
] |
null | peft |
# Model Card for Model ID
<!-- Provide a quick summary of what the model is/does. -->
## Model Details
### Model Description
<!-- Provide a longer summary of what this model is. -->
- **Developed by:** [More Information Needed]
- **Funded by [optional]:** [More Information Needed]
- **Shared by [optional]:** [More Information Needed]
- **Model type:** [More Information Needed]
- **Language(s) (NLP):** [More Information Needed]
- **License:** [More Information Needed]
- **Finetuned from model [optional]:** [More Information Needed]
### Model Sources [optional]
<!-- Provide the basic links for the model. -->
- **Repository:** [More Information Needed]
- **Paper [optional]:** [More Information Needed]
- **Demo [optional]:** [More Information Needed]
## Uses
<!-- Address questions around how the model is intended to be used, including the foreseeable users of the model and those affected by the model. -->
### Direct Use
<!-- This section is for the model use without fine-tuning or plugging into a larger ecosystem/app. -->
[More Information Needed]
### Downstream Use [optional]
<!-- This section is for the model use when fine-tuned for a task, or when plugged into a larger ecosystem/app -->
[More Information Needed]
### Out-of-Scope Use
<!-- This section addresses misuse, malicious use, and uses that the model will not work well for. -->
[More Information Needed]
## Bias, Risks, and Limitations
<!-- This section is meant to convey both technical and sociotechnical limitations. -->
[More Information Needed]
### Recommendations
<!-- This section is meant to convey recommendations with respect to the bias, risk, and technical limitations. -->
Users (both direct and downstream) should be made aware of the risks, biases and limitations of the model. More information needed for further recommendations.
## How to Get Started with the Model
Use the code below to get started with the model.
[More Information Needed]
## Training Details
### Training Data
<!-- This should link to a Dataset Card, perhaps with a short stub of information on what the training data is all about as well as documentation related to data pre-processing or additional filtering. -->
[More Information Needed]
### Training Procedure
<!-- This relates heavily to the Technical Specifications. Content here should link to that section when it is relevant to the training procedure. -->
#### Preprocessing [optional]
[More Information Needed]
#### Training Hyperparameters
- **Training regime:** [More Information Needed] <!--fp32, fp16 mixed precision, bf16 mixed precision, bf16 non-mixed precision, fp16 non-mixed precision, fp8 mixed precision -->
#### Speeds, Sizes, Times [optional]
<!-- This section provides information about throughput, start/end time, checkpoint size if relevant, etc. -->
[More Information Needed]
## Evaluation
<!-- This section describes the evaluation protocols and provides the results. -->
### Testing Data, Factors & Metrics
#### Testing Data
<!-- This should link to a Dataset Card if possible. -->
[More Information Needed]
#### Factors
<!-- These are the things the evaluation is disaggregating by, e.g., subpopulations or domains. -->
[More Information Needed]
#### Metrics
<!-- These are the evaluation metrics being used, ideally with a description of why. -->
[More Information Needed]
### Results
[More Information Needed]
#### Summary
## Model Examination [optional]
<!-- Relevant interpretability work for the model goes here -->
[More Information Needed]
## Environmental Impact
<!-- Total emissions (in grams of CO2eq) and additional considerations, such as electricity usage, go here. Edit the suggested text below accordingly -->
Carbon emissions can be estimated using the [Machine Learning Impact calculator](https://mlco2.github.io/impact#compute) presented in [Lacoste et al. (2019)](https://arxiv.org/abs/1910.09700).
- **Hardware Type:** [More Information Needed]
- **Hours used:** [More Information Needed]
- **Cloud Provider:** [More Information Needed]
- **Compute Region:** [More Information Needed]
- **Carbon Emitted:** [More Information Needed]
## Technical Specifications [optional]
### Model Architecture and Objective
[More Information Needed]
### Compute Infrastructure
[More Information Needed]
#### Hardware
[More Information Needed]
#### Software
[More Information Needed]
## Citation [optional]
<!-- If there is a paper or blog post introducing the model, the APA and Bibtex information for that should go in this section. -->
**BibTeX:**
[More Information Needed]
**APA:**
[More Information Needed]
## Glossary [optional]
<!-- If relevant, include terms and calculations in this section that can help readers understand the model or model card. -->
[More Information Needed]
## More Information [optional]
[More Information Needed]
## Model Card Authors [optional]
[More Information Needed]
## Model Card Contact
[More Information Needed]
### Framework versions
- PEFT 0.9.0 | {"library_name": "peft", "base_model": "unsloth/mistral-7b-instruct-v0.2-bnb-4bit"} | Hajas0/hun_emotion_modification | null | [
"peft",
"safetensors",
"arxiv:1910.09700",
"base_model:unsloth/mistral-7b-instruct-v0.2-bnb-4bit",
"region:us"
] | null | 2024-04-26T18:25:49+00:00 | [
"1910.09700"
] | [] | TAGS
#peft #safetensors #arxiv-1910.09700 #base_model-unsloth/mistral-7b-instruct-v0.2-bnb-4bit #region-us
|
# Model Card for Model ID
## Model Details
### Model Description
- Developed by:
- Funded by [optional]:
- Shared by [optional]:
- Model type:
- Language(s) (NLP):
- License:
- Finetuned from model [optional]:
### Model Sources [optional]
- Repository:
- Paper [optional]:
- Demo [optional]:
## Uses
### Direct Use
### Downstream Use [optional]
### Out-of-Scope Use
## Bias, Risks, and Limitations
### Recommendations
Users (both direct and downstream) should be made aware of the risks, biases and limitations of the model. More information needed for further recommendations.
## How to Get Started with the Model
Use the code below to get started with the model.
## Training Details
### Training Data
### Training Procedure
#### Preprocessing [optional]
#### Training Hyperparameters
- Training regime:
#### Speeds, Sizes, Times [optional]
## Evaluation
### Testing Data, Factors & Metrics
#### Testing Data
#### Factors
#### Metrics
### Results
#### Summary
## Model Examination [optional]
## Environmental Impact
Carbon emissions can be estimated using the Machine Learning Impact calculator presented in Lacoste et al. (2019).
- Hardware Type:
- Hours used:
- Cloud Provider:
- Compute Region:
- Carbon Emitted:
## Technical Specifications [optional]
### Model Architecture and Objective
### Compute Infrastructure
#### Hardware
#### Software
[optional]
BibTeX:
APA:
## Glossary [optional]
## More Information [optional]
## Model Card Authors [optional]
## Model Card Contact
### Framework versions
- PEFT 0.9.0 | [
"# Model Card for Model ID",
"## Model Details",
"### Model Description\n\n\n\n\n\n- Developed by: \n- Funded by [optional]: \n- Shared by [optional]: \n- Model type: \n- Language(s) (NLP): \n- License: \n- Finetuned from model [optional]:",
"### Model Sources [optional]\n\n\n\n- Repository: \n- Paper [optional]: \n- Demo [optional]:",
"## Uses",
"### Direct Use",
"### Downstream Use [optional]",
"### Out-of-Scope Use",
"## Bias, Risks, and Limitations",
"### Recommendations\n\n\n\nUsers (both direct and downstream) should be made aware of the risks, biases and limitations of the model. More information needed for further recommendations.",
"## How to Get Started with the Model\n\nUse the code below to get started with the model.",
"## Training Details",
"### Training Data",
"### Training Procedure",
"#### Preprocessing [optional]",
"#### Training Hyperparameters\n\n- Training regime:",
"#### Speeds, Sizes, Times [optional]",
"## Evaluation",
"### Testing Data, Factors & Metrics",
"#### Testing Data",
"#### Factors",
"#### Metrics",
"### Results",
"#### Summary",
"## Model Examination [optional]",
"## Environmental Impact\n\n\n\nCarbon emissions can be estimated using the Machine Learning Impact calculator presented in Lacoste et al. (2019).\n\n- Hardware Type: \n- Hours used: \n- Cloud Provider: \n- Compute Region: \n- Carbon Emitted:",
"## Technical Specifications [optional]",
"### Model Architecture and Objective",
"### Compute Infrastructure",
"#### Hardware",
"#### Software\n\n\n\n[optional]\n\n\n\nBibTeX:\n\n\n\nAPA:",
"## Glossary [optional]",
"## More Information [optional]",
"## Model Card Authors [optional]",
"## Model Card Contact",
"### Framework versions\n\n- PEFT 0.9.0"
] | [
"TAGS\n#peft #safetensors #arxiv-1910.09700 #base_model-unsloth/mistral-7b-instruct-v0.2-bnb-4bit #region-us \n",
"# Model Card for Model ID",
"## Model Details",
"### Model Description\n\n\n\n\n\n- Developed by: \n- Funded by [optional]: \n- Shared by [optional]: \n- Model type: \n- Language(s) (NLP): \n- License: \n- Finetuned from model [optional]:",
"### Model Sources [optional]\n\n\n\n- Repository: \n- Paper [optional]: \n- Demo [optional]:",
"## Uses",
"### Direct Use",
"### Downstream Use [optional]",
"### Out-of-Scope Use",
"## Bias, Risks, and Limitations",
"### Recommendations\n\n\n\nUsers (both direct and downstream) should be made aware of the risks, biases and limitations of the model. More information needed for further recommendations.",
"## How to Get Started with the Model\n\nUse the code below to get started with the model.",
"## Training Details",
"### Training Data",
"### Training Procedure",
"#### Preprocessing [optional]",
"#### Training Hyperparameters\n\n- Training regime:",
"#### Speeds, Sizes, Times [optional]",
"## Evaluation",
"### Testing Data, Factors & Metrics",
"#### Testing Data",
"#### Factors",
"#### Metrics",
"### Results",
"#### Summary",
"## Model Examination [optional]",
"## Environmental Impact\n\n\n\nCarbon emissions can be estimated using the Machine Learning Impact calculator presented in Lacoste et al. (2019).\n\n- Hardware Type: \n- Hours used: \n- Cloud Provider: \n- Compute Region: \n- Carbon Emitted:",
"## Technical Specifications [optional]",
"### Model Architecture and Objective",
"### Compute Infrastructure",
"#### Hardware",
"#### Software\n\n\n\n[optional]\n\n\n\nBibTeX:\n\n\n\nAPA:",
"## Glossary [optional]",
"## More Information [optional]",
"## Model Card Authors [optional]",
"## Model Card Contact",
"### Framework versions\n\n- PEFT 0.9.0"
] |
null | peft |
<!-- This model card has been generated automatically according to the information the Trainer had access to. You
should probably proofread and complete it, then remove this comment. -->
# GUE_tf_1-seqsight_4096_512_27M-L32_f
This model is a fine-tuned version of [mahdibaghbanzadeh/seqsight_4096_512_27M](https://huggingface.co/mahdibaghbanzadeh/seqsight_4096_512_27M) on the [mahdibaghbanzadeh/GUE_tf_1](https://huggingface.co/datasets/mahdibaghbanzadeh/GUE_tf_1) dataset.
It achieves the following results on the evaluation set:
- Loss: 0.3607
- F1 Score: 0.8438
- Accuracy: 0.844
## Model description
More information needed
## Intended uses & limitations
More information needed
## Training and evaluation data
More information needed
## Training procedure
### Training hyperparameters
The following hyperparameters were used during training:
- learning_rate: 0.0005
- train_batch_size: 128
- eval_batch_size: 128
- seed: 42
- optimizer: Adam with betas=(0.9,0.999) and epsilon=1e-08
- lr_scheduler_type: linear
- training_steps: 10000
### Training results
| Training Loss | Epoch | Step | Validation Loss | F1 Score | Accuracy |
|:-------------:|:-----:|:-----:|:---------------:|:--------:|:--------:|
| 0.5322 | 0.83 | 200 | 0.5168 | 0.7507 | 0.751 |
| 0.4855 | 1.67 | 400 | 0.5076 | 0.7468 | 0.747 |
| 0.4743 | 2.5 | 600 | 0.5012 | 0.7610 | 0.761 |
| 0.4659 | 3.33 | 800 | 0.4963 | 0.7569 | 0.757 |
| 0.4642 | 4.17 | 1000 | 0.4950 | 0.7635 | 0.764 |
| 0.4551 | 5.0 | 1200 | 0.5011 | 0.7468 | 0.747 |
| 0.4472 | 5.83 | 1400 | 0.4920 | 0.7588 | 0.759 |
| 0.4466 | 6.67 | 1600 | 0.4961 | 0.7530 | 0.753 |
| 0.4421 | 7.5 | 1800 | 0.4901 | 0.7538 | 0.754 |
| 0.432 | 8.33 | 2000 | 0.4895 | 0.7640 | 0.764 |
| 0.4337 | 9.17 | 2200 | 0.4938 | 0.7597 | 0.76 |
| 0.4283 | 10.0 | 2400 | 0.5123 | 0.7516 | 0.753 |
| 0.4268 | 10.83 | 2600 | 0.4997 | 0.7588 | 0.76 |
| 0.4206 | 11.67 | 2800 | 0.5024 | 0.7511 | 0.752 |
| 0.4111 | 12.5 | 3000 | 0.5004 | 0.7592 | 0.76 |
| 0.4173 | 13.33 | 3200 | 0.5004 | 0.7591 | 0.76 |
| 0.4106 | 14.17 | 3400 | 0.4989 | 0.7610 | 0.761 |
| 0.407 | 15.0 | 3600 | 0.5104 | 0.7607 | 0.762 |
| 0.4008 | 15.83 | 3800 | 0.5001 | 0.7562 | 0.757 |
| 0.4007 | 16.67 | 4000 | 0.5059 | 0.7616 | 0.762 |
| 0.4002 | 17.5 | 4200 | 0.5137 | 0.7611 | 0.762 |
| 0.3894 | 18.33 | 4400 | 0.5167 | 0.7637 | 0.764 |
| 0.3899 | 19.17 | 4600 | 0.5273 | 0.7604 | 0.761 |
| 0.3875 | 20.0 | 4800 | 0.5129 | 0.7557 | 0.756 |
| 0.3849 | 20.83 | 5000 | 0.5354 | 0.7564 | 0.757 |
| 0.3795 | 21.67 | 5200 | 0.5259 | 0.7553 | 0.756 |
| 0.3769 | 22.5 | 5400 | 0.5273 | 0.7513 | 0.752 |
| 0.3804 | 23.33 | 5600 | 0.5323 | 0.7483 | 0.75 |
| 0.3712 | 24.17 | 5800 | 0.5403 | 0.7490 | 0.75 |
| 0.3714 | 25.0 | 6000 | 0.5343 | 0.7494 | 0.75 |
| 0.3639 | 25.83 | 6200 | 0.5363 | 0.7492 | 0.75 |
| 0.3609 | 26.67 | 6400 | 0.5273 | 0.7608 | 0.761 |
| 0.3658 | 27.5 | 6600 | 0.5361 | 0.7547 | 0.755 |
| 0.3616 | 28.33 | 6800 | 0.5276 | 0.7668 | 0.767 |
| 0.3575 | 29.17 | 7000 | 0.5445 | 0.7555 | 0.756 |
| 0.3585 | 30.0 | 7200 | 0.5391 | 0.7552 | 0.756 |
| 0.3528 | 30.83 | 7400 | 0.5566 | 0.7463 | 0.748 |
| 0.3449 | 31.67 | 7600 | 0.5522 | 0.7564 | 0.757 |
| 0.351 | 32.5 | 7800 | 0.5517 | 0.7511 | 0.752 |
| 0.3458 | 33.33 | 8000 | 0.5577 | 0.7501 | 0.751 |
| 0.3478 | 34.17 | 8200 | 0.5542 | 0.7410 | 0.742 |
| 0.3427 | 35.0 | 8400 | 0.5523 | 0.7502 | 0.751 |
| 0.3415 | 35.83 | 8600 | 0.5551 | 0.7484 | 0.749 |
| 0.3404 | 36.67 | 8800 | 0.5604 | 0.7492 | 0.75 |
| 0.3384 | 37.5 | 9000 | 0.5706 | 0.7441 | 0.746 |
| 0.3356 | 38.33 | 9200 | 0.5693 | 0.7481 | 0.749 |
| 0.3367 | 39.17 | 9400 | 0.5691 | 0.7541 | 0.755 |
| 0.3367 | 40.0 | 9600 | 0.5704 | 0.7510 | 0.752 |
| 0.3327 | 40.83 | 9800 | 0.5670 | 0.7524 | 0.753 |
| 0.3344 | 41.67 | 10000 | 0.5708 | 0.7491 | 0.75 |
### Framework versions
- PEFT 0.9.0
- Transformers 4.38.2
- Pytorch 2.2.0+cu121
- Datasets 2.17.1
- Tokenizers 0.15.2 | {"library_name": "peft", "tags": ["generated_from_trainer"], "metrics": ["accuracy"], "base_model": "mahdibaghbanzadeh/seqsight_4096_512_27M", "model-index": [{"name": "GUE_tf_1-seqsight_4096_512_27M-L32_f", "results": []}]} | mahdibaghbanzadeh/GUE_tf_1-seqsight_4096_512_27M-L32_f | null | [
"peft",
"safetensors",
"generated_from_trainer",
"base_model:mahdibaghbanzadeh/seqsight_4096_512_27M",
"region:us"
] | null | 2024-04-26T18:28:31+00:00 | [] | [] | TAGS
#peft #safetensors #generated_from_trainer #base_model-mahdibaghbanzadeh/seqsight_4096_512_27M #region-us
| GUE\_tf\_1-seqsight\_4096\_512\_27M-L32\_f
==========================================
This model is a fine-tuned version of mahdibaghbanzadeh/seqsight\_4096\_512\_27M on the mahdibaghbanzadeh/GUE\_tf\_1 dataset.
It achieves the following results on the evaluation set:
* Loss: 0.3607
* F1 Score: 0.8438
* Accuracy: 0.844
Model description
-----------------
More information needed
Intended uses & limitations
---------------------------
More information needed
Training and evaluation data
----------------------------
More information needed
Training procedure
------------------
### Training hyperparameters
The following hyperparameters were used during training:
* learning\_rate: 0.0005
* train\_batch\_size: 128
* eval\_batch\_size: 128
* seed: 42
* optimizer: Adam with betas=(0.9,0.999) and epsilon=1e-08
* lr\_scheduler\_type: linear
* training\_steps: 10000
### Training results
### Framework versions
* PEFT 0.9.0
* Transformers 4.38.2
* Pytorch 2.2.0+cu121
* Datasets 2.17.1
* Tokenizers 0.15.2
| [
"### Training hyperparameters\n\n\nThe following hyperparameters were used during training:\n\n\n* learning\\_rate: 0.0005\n* train\\_batch\\_size: 128\n* eval\\_batch\\_size: 128\n* seed: 42\n* optimizer: Adam with betas=(0.9,0.999) and epsilon=1e-08\n* lr\\_scheduler\\_type: linear\n* training\\_steps: 10000",
"### Training results",
"### Framework versions\n\n\n* PEFT 0.9.0\n* Transformers 4.38.2\n* Pytorch 2.2.0+cu121\n* Datasets 2.17.1\n* Tokenizers 0.15.2"
] | [
"TAGS\n#peft #safetensors #generated_from_trainer #base_model-mahdibaghbanzadeh/seqsight_4096_512_27M #region-us \n",
"### Training hyperparameters\n\n\nThe following hyperparameters were used during training:\n\n\n* learning\\_rate: 0.0005\n* train\\_batch\\_size: 128\n* eval\\_batch\\_size: 128\n* seed: 42\n* optimizer: Adam with betas=(0.9,0.999) and epsilon=1e-08\n* lr\\_scheduler\\_type: linear\n* training\\_steps: 10000",
"### Training results",
"### Framework versions\n\n\n* PEFT 0.9.0\n* Transformers 4.38.2\n* Pytorch 2.2.0+cu121\n* Datasets 2.17.1\n* Tokenizers 0.15.2"
] |
text-generation | transformers |
# Model Card for Model ID
<!-- Provide a quick summary of what the model is/does. -->
## Model Details
### Model Description
<!-- Provide a longer summary of what this model is. -->
This is the model card of a 🤗 transformers model that has been pushed on the Hub. This model card has been automatically generated.
- **Developed by:** [More Information Needed]
- **Funded by [optional]:** [More Information Needed]
- **Shared by [optional]:** [More Information Needed]
- **Model type:** [More Information Needed]
- **Language(s) (NLP):** [More Information Needed]
- **License:** [More Information Needed]
- **Finetuned from model [optional]:** [More Information Needed]
### Model Sources [optional]
<!-- Provide the basic links for the model. -->
- **Repository:** [More Information Needed]
- **Paper [optional]:** [More Information Needed]
- **Demo [optional]:** [More Information Needed]
## Uses
<!-- Address questions around how the model is intended to be used, including the foreseeable users of the model and those affected by the model. -->
### Direct Use
<!-- This section is for the model use without fine-tuning or plugging into a larger ecosystem/app. -->
[More Information Needed]
### Downstream Use [optional]
<!-- This section is for the model use when fine-tuned for a task, or when plugged into a larger ecosystem/app -->
[More Information Needed]
### Out-of-Scope Use
<!-- This section addresses misuse, malicious use, and uses that the model will not work well for. -->
[More Information Needed]
## Bias, Risks, and Limitations
<!-- This section is meant to convey both technical and sociotechnical limitations. -->
[More Information Needed]
### Recommendations
<!-- This section is meant to convey recommendations with respect to the bias, risk, and technical limitations. -->
Users (both direct and downstream) should be made aware of the risks, biases and limitations of the model. More information needed for further recommendations.
## How to Get Started with the Model
Use the code below to get started with the model.
[More Information Needed]
## Training Details
### Training Data
<!-- This should link to a Dataset Card, perhaps with a short stub of information on what the training data is all about as well as documentation related to data pre-processing or additional filtering. -->
[More Information Needed]
### Training Procedure
<!-- This relates heavily to the Technical Specifications. Content here should link to that section when it is relevant to the training procedure. -->
#### Preprocessing [optional]
[More Information Needed]
#### Training Hyperparameters
- **Training regime:** [More Information Needed] <!--fp32, fp16 mixed precision, bf16 mixed precision, bf16 non-mixed precision, fp16 non-mixed precision, fp8 mixed precision -->
#### Speeds, Sizes, Times [optional]
<!-- This section provides information about throughput, start/end time, checkpoint size if relevant, etc. -->
[More Information Needed]
## Evaluation
<!-- This section describes the evaluation protocols and provides the results. -->
### Testing Data, Factors & Metrics
#### Testing Data
<!-- This should link to a Dataset Card if possible. -->
[More Information Needed]
#### Factors
<!-- These are the things the evaluation is disaggregating by, e.g., subpopulations or domains. -->
[More Information Needed]
#### Metrics
<!-- These are the evaluation metrics being used, ideally with a description of why. -->
[More Information Needed]
### Results
[More Information Needed]
#### Summary
## Model Examination [optional]
<!-- Relevant interpretability work for the model goes here -->
[More Information Needed]
## Environmental Impact
<!-- Total emissions (in grams of CO2eq) and additional considerations, such as electricity usage, go here. Edit the suggested text below accordingly -->
Carbon emissions can be estimated using the [Machine Learning Impact calculator](https://mlco2.github.io/impact#compute) presented in [Lacoste et al. (2019)](https://arxiv.org/abs/1910.09700).
- **Hardware Type:** [More Information Needed]
- **Hours used:** [More Information Needed]
- **Cloud Provider:** [More Information Needed]
- **Compute Region:** [More Information Needed]
- **Carbon Emitted:** [More Information Needed]
## Technical Specifications [optional]
### Model Architecture and Objective
[More Information Needed]
### Compute Infrastructure
[More Information Needed]
#### Hardware
[More Information Needed]
#### Software
[More Information Needed]
## Citation [optional]
<!-- If there is a paper or blog post introducing the model, the APA and Bibtex information for that should go in this section. -->
**BibTeX:**
[More Information Needed]
**APA:**
[More Information Needed]
## Glossary [optional]
<!-- If relevant, include terms and calculations in this section that can help readers understand the model or model card. -->
[More Information Needed]
## More Information [optional]
[More Information Needed]
## Model Card Authors [optional]
[More Information Needed]
## Model Card Contact
[More Information Needed] | {"library_name": "transformers", "tags": []} | nem012/gemma2B-r16MHCv2 | null | [
"transformers",
"safetensors",
"gemma",
"text-generation",
"conversational",
"arxiv:1910.09700",
"autotrain_compatible",
"endpoints_compatible",
"text-generation-inference",
"region:us"
] | null | 2024-04-26T18:28:56+00:00 | [
"1910.09700"
] | [] | TAGS
#transformers #safetensors #gemma #text-generation #conversational #arxiv-1910.09700 #autotrain_compatible #endpoints_compatible #text-generation-inference #region-us
|
# Model Card for Model ID
## Model Details
### Model Description
This is the model card of a transformers model that has been pushed on the Hub. This model card has been automatically generated.
- Developed by:
- Funded by [optional]:
- Shared by [optional]:
- Model type:
- Language(s) (NLP):
- License:
- Finetuned from model [optional]:
### Model Sources [optional]
- Repository:
- Paper [optional]:
- Demo [optional]:
## Uses
### Direct Use
### Downstream Use [optional]
### Out-of-Scope Use
## Bias, Risks, and Limitations
### Recommendations
Users (both direct and downstream) should be made aware of the risks, biases and limitations of the model. More information needed for further recommendations.
## How to Get Started with the Model
Use the code below to get started with the model.
## Training Details
### Training Data
### Training Procedure
#### Preprocessing [optional]
#### Training Hyperparameters
- Training regime:
#### Speeds, Sizes, Times [optional]
## Evaluation
### Testing Data, Factors & Metrics
#### Testing Data
#### Factors
#### Metrics
### Results
#### Summary
## Model Examination [optional]
## Environmental Impact
Carbon emissions can be estimated using the Machine Learning Impact calculator presented in Lacoste et al. (2019).
- Hardware Type:
- Hours used:
- Cloud Provider:
- Compute Region:
- Carbon Emitted:
## Technical Specifications [optional]
### Model Architecture and Objective
### Compute Infrastructure
#### Hardware
#### Software
[optional]
BibTeX:
APA:
## Glossary [optional]
## More Information [optional]
## Model Card Authors [optional]
## Model Card Contact
| [
"# Model Card for Model ID",
"## Model Details",
"### Model Description\n\n\n\nThis is the model card of a transformers model that has been pushed on the Hub. This model card has been automatically generated.\n\n- Developed by: \n- Funded by [optional]: \n- Shared by [optional]: \n- Model type: \n- Language(s) (NLP): \n- License: \n- Finetuned from model [optional]:",
"### Model Sources [optional]\n\n\n\n- Repository: \n- Paper [optional]: \n- Demo [optional]:",
"## Uses",
"### Direct Use",
"### Downstream Use [optional]",
"### Out-of-Scope Use",
"## Bias, Risks, and Limitations",
"### Recommendations\n\n\n\nUsers (both direct and downstream) should be made aware of the risks, biases and limitations of the model. More information needed for further recommendations.",
"## How to Get Started with the Model\n\nUse the code below to get started with the model.",
"## Training Details",
"### Training Data",
"### Training Procedure",
"#### Preprocessing [optional]",
"#### Training Hyperparameters\n\n- Training regime:",
"#### Speeds, Sizes, Times [optional]",
"## Evaluation",
"### Testing Data, Factors & Metrics",
"#### Testing Data",
"#### Factors",
"#### Metrics",
"### Results",
"#### Summary",
"## Model Examination [optional]",
"## Environmental Impact\n\n\n\nCarbon emissions can be estimated using the Machine Learning Impact calculator presented in Lacoste et al. (2019).\n\n- Hardware Type: \n- Hours used: \n- Cloud Provider: \n- Compute Region: \n- Carbon Emitted:",
"## Technical Specifications [optional]",
"### Model Architecture and Objective",
"### Compute Infrastructure",
"#### Hardware",
"#### Software\n\n\n\n[optional]\n\n\n\nBibTeX:\n\n\n\nAPA:",
"## Glossary [optional]",
"## More Information [optional]",
"## Model Card Authors [optional]",
"## Model Card Contact"
] | [
"TAGS\n#transformers #safetensors #gemma #text-generation #conversational #arxiv-1910.09700 #autotrain_compatible #endpoints_compatible #text-generation-inference #region-us \n",
"# Model Card for Model ID",
"## Model Details",
"### Model Description\n\n\n\nThis is the model card of a transformers model that has been pushed on the Hub. This model card has been automatically generated.\n\n- Developed by: \n- Funded by [optional]: \n- Shared by [optional]: \n- Model type: \n- Language(s) (NLP): \n- License: \n- Finetuned from model [optional]:",
"### Model Sources [optional]\n\n\n\n- Repository: \n- Paper [optional]: \n- Demo [optional]:",
"## Uses",
"### Direct Use",
"### Downstream Use [optional]",
"### Out-of-Scope Use",
"## Bias, Risks, and Limitations",
"### Recommendations\n\n\n\nUsers (both direct and downstream) should be made aware of the risks, biases and limitations of the model. More information needed for further recommendations.",
"## How to Get Started with the Model\n\nUse the code below to get started with the model.",
"## Training Details",
"### Training Data",
"### Training Procedure",
"#### Preprocessing [optional]",
"#### Training Hyperparameters\n\n- Training regime:",
"#### Speeds, Sizes, Times [optional]",
"## Evaluation",
"### Testing Data, Factors & Metrics",
"#### Testing Data",
"#### Factors",
"#### Metrics",
"### Results",
"#### Summary",
"## Model Examination [optional]",
"## Environmental Impact\n\n\n\nCarbon emissions can be estimated using the Machine Learning Impact calculator presented in Lacoste et al. (2019).\n\n- Hardware Type: \n- Hours used: \n- Cloud Provider: \n- Compute Region: \n- Carbon Emitted:",
"## Technical Specifications [optional]",
"### Model Architecture and Objective",
"### Compute Infrastructure",
"#### Hardware",
"#### Software\n\n\n\n[optional]\n\n\n\nBibTeX:\n\n\n\nAPA:",
"## Glossary [optional]",
"## More Information [optional]",
"## Model Card Authors [optional]",
"## Model Card Contact"
] |
null | peft |
<!-- This model card has been generated automatically according to the information the Trainer had access to. You
should probably proofread and complete it, then remove this comment. -->
# GUE_tf_4-seqsight_4096_512_27M-L1_f
This model is a fine-tuned version of [mahdibaghbanzadeh/seqsight_4096_512_27M](https://huggingface.co/mahdibaghbanzadeh/seqsight_4096_512_27M) on the [mahdibaghbanzadeh/GUE_tf_4](https://huggingface.co/datasets/mahdibaghbanzadeh/GUE_tf_4) dataset.
It achieves the following results on the evaluation set:
- Loss: 0.3500
- F1 Score: 0.8474
- Accuracy: 0.848
## Model description
More information needed
## Intended uses & limitations
More information needed
## Training and evaluation data
More information needed
## Training procedure
### Training hyperparameters
The following hyperparameters were used during training:
- learning_rate: 0.0005
- train_batch_size: 128
- eval_batch_size: 128
- seed: 42
- optimizer: Adam with betas=(0.9,0.999) and epsilon=1e-08
- lr_scheduler_type: linear
- training_steps: 10000
### Training results
| Training Loss | Epoch | Step | Validation Loss | F1 Score | Accuracy |
|:-------------:|:-----:|:-----:|:---------------:|:--------:|:--------:|
| 0.5501 | 1.34 | 200 | 0.5144 | 0.7339 | 0.734 |
| 0.4861 | 2.68 | 400 | 0.5033 | 0.7314 | 0.732 |
| 0.4736 | 4.03 | 600 | 0.4949 | 0.7432 | 0.744 |
| 0.461 | 5.37 | 800 | 0.4889 | 0.7579 | 0.758 |
| 0.4512 | 6.71 | 1000 | 0.4796 | 0.7616 | 0.762 |
| 0.4443 | 8.05 | 1200 | 0.4725 | 0.7678 | 0.768 |
| 0.442 | 9.4 | 1400 | 0.4713 | 0.7670 | 0.767 |
| 0.4365 | 10.74 | 1600 | 0.4703 | 0.7737 | 0.774 |
| 0.4289 | 12.08 | 1800 | 0.4711 | 0.7748 | 0.775 |
| 0.4297 | 13.42 | 2000 | 0.4588 | 0.7780 | 0.778 |
| 0.4205 | 14.77 | 2200 | 0.4674 | 0.7734 | 0.774 |
| 0.4218 | 16.11 | 2400 | 0.4602 | 0.7809 | 0.781 |
| 0.419 | 17.45 | 2600 | 0.4594 | 0.7789 | 0.779 |
| 0.4155 | 18.79 | 2800 | 0.4609 | 0.7799 | 0.78 |
| 0.4191 | 20.13 | 3000 | 0.4531 | 0.7789 | 0.779 |
| 0.41 | 21.48 | 3200 | 0.4549 | 0.7780 | 0.778 |
| 0.4086 | 22.82 | 3400 | 0.4540 | 0.7769 | 0.777 |
| 0.4081 | 24.16 | 3600 | 0.4505 | 0.7820 | 0.782 |
| 0.4055 | 25.5 | 3800 | 0.4513 | 0.7888 | 0.789 |
| 0.4033 | 26.85 | 4000 | 0.4509 | 0.7809 | 0.781 |
| 0.4045 | 28.19 | 4200 | 0.4496 | 0.7799 | 0.78 |
| 0.3972 | 29.53 | 4400 | 0.4562 | 0.7807 | 0.781 |
| 0.3973 | 30.87 | 4600 | 0.4523 | 0.7817 | 0.782 |
| 0.4009 | 32.21 | 4800 | 0.4488 | 0.7808 | 0.781 |
| 0.3938 | 33.56 | 5000 | 0.4506 | 0.7799 | 0.78 |
| 0.3972 | 34.9 | 5200 | 0.4467 | 0.7779 | 0.778 |
| 0.3951 | 36.24 | 5400 | 0.4458 | 0.7819 | 0.782 |
| 0.3906 | 37.58 | 5600 | 0.4470 | 0.7860 | 0.786 |
| 0.3931 | 38.93 | 5800 | 0.4470 | 0.7839 | 0.784 |
| 0.3928 | 40.27 | 6000 | 0.4462 | 0.7870 | 0.787 |
| 0.391 | 41.61 | 6200 | 0.4462 | 0.7850 | 0.785 |
| 0.3938 | 42.95 | 6400 | 0.4442 | 0.7850 | 0.785 |
| 0.3911 | 44.3 | 6600 | 0.4425 | 0.7880 | 0.788 |
| 0.3877 | 45.64 | 6800 | 0.4473 | 0.7796 | 0.78 |
| 0.3908 | 46.98 | 7000 | 0.4427 | 0.7880 | 0.788 |
| 0.3894 | 48.32 | 7200 | 0.4385 | 0.7930 | 0.793 |
| 0.3874 | 49.66 | 7400 | 0.4413 | 0.7879 | 0.788 |
| 0.387 | 51.01 | 7600 | 0.4391 | 0.7900 | 0.79 |
| 0.3884 | 52.35 | 7800 | 0.4398 | 0.7969 | 0.797 |
| 0.3844 | 53.69 | 8000 | 0.4421 | 0.7889 | 0.789 |
| 0.3867 | 55.03 | 8200 | 0.4454 | 0.7817 | 0.782 |
| 0.3857 | 56.38 | 8400 | 0.4407 | 0.7890 | 0.789 |
| 0.3878 | 57.72 | 8600 | 0.4388 | 0.7959 | 0.796 |
| 0.3842 | 59.06 | 8800 | 0.4422 | 0.7859 | 0.786 |
| 0.3824 | 60.4 | 9000 | 0.4408 | 0.7880 | 0.788 |
| 0.3826 | 61.74 | 9200 | 0.4397 | 0.7940 | 0.794 |
| 0.3816 | 63.09 | 9400 | 0.4411 | 0.7900 | 0.79 |
| 0.3874 | 64.43 | 9600 | 0.4395 | 0.7930 | 0.793 |
| 0.3828 | 65.77 | 9800 | 0.4402 | 0.7900 | 0.79 |
| 0.3817 | 67.11 | 10000 | 0.4404 | 0.7900 | 0.79 |
### Framework versions
- PEFT 0.9.0
- Transformers 4.38.2
- Pytorch 2.2.0+cu121
- Datasets 2.17.1
- Tokenizers 0.15.2 | {"library_name": "peft", "tags": ["generated_from_trainer"], "metrics": ["accuracy"], "base_model": "mahdibaghbanzadeh/seqsight_4096_512_27M", "model-index": [{"name": "GUE_tf_4-seqsight_4096_512_27M-L1_f", "results": []}]} | mahdibaghbanzadeh/GUE_tf_4-seqsight_4096_512_27M-L1_f | null | [
"peft",
"safetensors",
"generated_from_trainer",
"base_model:mahdibaghbanzadeh/seqsight_4096_512_27M",
"region:us"
] | null | 2024-04-26T18:29:13+00:00 | [] | [] | TAGS
#peft #safetensors #generated_from_trainer #base_model-mahdibaghbanzadeh/seqsight_4096_512_27M #region-us
| GUE\_tf\_4-seqsight\_4096\_512\_27M-L1\_f
=========================================
This model is a fine-tuned version of mahdibaghbanzadeh/seqsight\_4096\_512\_27M on the mahdibaghbanzadeh/GUE\_tf\_4 dataset.
It achieves the following results on the evaluation set:
* Loss: 0.3500
* F1 Score: 0.8474
* Accuracy: 0.848
Model description
-----------------
More information needed
Intended uses & limitations
---------------------------
More information needed
Training and evaluation data
----------------------------
More information needed
Training procedure
------------------
### Training hyperparameters
The following hyperparameters were used during training:
* learning\_rate: 0.0005
* train\_batch\_size: 128
* eval\_batch\_size: 128
* seed: 42
* optimizer: Adam with betas=(0.9,0.999) and epsilon=1e-08
* lr\_scheduler\_type: linear
* training\_steps: 10000
### Training results
### Framework versions
* PEFT 0.9.0
* Transformers 4.38.2
* Pytorch 2.2.0+cu121
* Datasets 2.17.1
* Tokenizers 0.15.2
| [
"### Training hyperparameters\n\n\nThe following hyperparameters were used during training:\n\n\n* learning\\_rate: 0.0005\n* train\\_batch\\_size: 128\n* eval\\_batch\\_size: 128\n* seed: 42\n* optimizer: Adam with betas=(0.9,0.999) and epsilon=1e-08\n* lr\\_scheduler\\_type: linear\n* training\\_steps: 10000",
"### Training results",
"### Framework versions\n\n\n* PEFT 0.9.0\n* Transformers 4.38.2\n* Pytorch 2.2.0+cu121\n* Datasets 2.17.1\n* Tokenizers 0.15.2"
] | [
"TAGS\n#peft #safetensors #generated_from_trainer #base_model-mahdibaghbanzadeh/seqsight_4096_512_27M #region-us \n",
"### Training hyperparameters\n\n\nThe following hyperparameters were used during training:\n\n\n* learning\\_rate: 0.0005\n* train\\_batch\\_size: 128\n* eval\\_batch\\_size: 128\n* seed: 42\n* optimizer: Adam with betas=(0.9,0.999) and epsilon=1e-08\n* lr\\_scheduler\\_type: linear\n* training\\_steps: 10000",
"### Training results",
"### Framework versions\n\n\n* PEFT 0.9.0\n* Transformers 4.38.2\n* Pytorch 2.2.0+cu121\n* Datasets 2.17.1\n* Tokenizers 0.15.2"
] |
null | peft |
<!-- This model card has been generated automatically according to the information the Trainer had access to. You
should probably proofread and complete it, then remove this comment. -->
# GUE_tf_4-seqsight_4096_512_27M-L8_f
This model is a fine-tuned version of [mahdibaghbanzadeh/seqsight_4096_512_27M](https://huggingface.co/mahdibaghbanzadeh/seqsight_4096_512_27M) on the [mahdibaghbanzadeh/GUE_tf_4](https://huggingface.co/datasets/mahdibaghbanzadeh/GUE_tf_4) dataset.
It achieves the following results on the evaluation set:
- Loss: 0.3638
- F1 Score: 0.8528
- Accuracy: 0.853
## Model description
More information needed
## Intended uses & limitations
More information needed
## Training and evaluation data
More information needed
## Training procedure
### Training hyperparameters
The following hyperparameters were used during training:
- learning_rate: 0.0005
- train_batch_size: 128
- eval_batch_size: 128
- seed: 42
- optimizer: Adam with betas=(0.9,0.999) and epsilon=1e-08
- lr_scheduler_type: linear
- training_steps: 10000
### Training results
| Training Loss | Epoch | Step | Validation Loss | F1 Score | Accuracy |
|:-------------:|:-----:|:-----:|:---------------:|:--------:|:--------:|
| 0.5313 | 1.34 | 200 | 0.5008 | 0.7468 | 0.747 |
| 0.4686 | 2.68 | 400 | 0.4823 | 0.7527 | 0.753 |
| 0.4476 | 4.03 | 600 | 0.4752 | 0.7657 | 0.766 |
| 0.4317 | 5.37 | 800 | 0.4797 | 0.7818 | 0.782 |
| 0.4219 | 6.71 | 1000 | 0.4683 | 0.7674 | 0.768 |
| 0.4125 | 8.05 | 1200 | 0.4625 | 0.7838 | 0.784 |
| 0.407 | 9.4 | 1400 | 0.4541 | 0.7850 | 0.785 |
| 0.4018 | 10.74 | 1600 | 0.4510 | 0.782 | 0.782 |
| 0.3926 | 12.08 | 1800 | 0.4601 | 0.7779 | 0.778 |
| 0.3909 | 13.42 | 2000 | 0.4530 | 0.7836 | 0.784 |
| 0.3818 | 14.77 | 2200 | 0.4502 | 0.7857 | 0.786 |
| 0.3789 | 16.11 | 2400 | 0.4478 | 0.7965 | 0.797 |
| 0.3761 | 17.45 | 2600 | 0.4495 | 0.7897 | 0.79 |
| 0.371 | 18.79 | 2800 | 0.4445 | 0.8010 | 0.801 |
| 0.3705 | 20.13 | 3000 | 0.4337 | 0.8000 | 0.8 |
| 0.3599 | 21.48 | 3200 | 0.4375 | 0.7990 | 0.799 |
| 0.3563 | 22.82 | 3400 | 0.4411 | 0.7976 | 0.798 |
| 0.3539 | 24.16 | 3600 | 0.4323 | 0.8050 | 0.805 |
| 0.3527 | 25.5 | 3800 | 0.4368 | 0.8021 | 0.803 |
| 0.3425 | 26.85 | 4000 | 0.4337 | 0.7990 | 0.799 |
| 0.3432 | 28.19 | 4200 | 0.4360 | 0.7949 | 0.795 |
| 0.3355 | 29.53 | 4400 | 0.4297 | 0.8070 | 0.807 |
| 0.3332 | 30.87 | 4600 | 0.4427 | 0.7924 | 0.793 |
| 0.3347 | 32.21 | 4800 | 0.4296 | 0.8080 | 0.808 |
| 0.3237 | 33.56 | 5000 | 0.4420 | 0.8029 | 0.803 |
| 0.3285 | 34.9 | 5200 | 0.4285 | 0.8120 | 0.812 |
| 0.3225 | 36.24 | 5400 | 0.4245 | 0.8079 | 0.808 |
| 0.3157 | 37.58 | 5600 | 0.4291 | 0.8160 | 0.816 |
| 0.3182 | 38.93 | 5800 | 0.4305 | 0.8090 | 0.809 |
| 0.3149 | 40.27 | 6000 | 0.4315 | 0.8179 | 0.818 |
| 0.3104 | 41.61 | 6200 | 0.4353 | 0.8089 | 0.809 |
| 0.3152 | 42.95 | 6400 | 0.4278 | 0.8150 | 0.815 |
| 0.3131 | 44.3 | 6600 | 0.4228 | 0.8089 | 0.809 |
| 0.3032 | 45.64 | 6800 | 0.4346 | 0.8169 | 0.817 |
| 0.3059 | 46.98 | 7000 | 0.4223 | 0.8170 | 0.817 |
| 0.3012 | 48.32 | 7200 | 0.4269 | 0.8168 | 0.817 |
| 0.2998 | 49.66 | 7400 | 0.4292 | 0.8160 | 0.816 |
| 0.2988 | 51.01 | 7600 | 0.4291 | 0.8170 | 0.817 |
| 0.2952 | 52.35 | 7800 | 0.4276 | 0.8189 | 0.819 |
| 0.2965 | 53.69 | 8000 | 0.4299 | 0.8140 | 0.814 |
| 0.2942 | 55.03 | 8200 | 0.4302 | 0.8120 | 0.812 |
| 0.2903 | 56.38 | 8400 | 0.4281 | 0.8229 | 0.823 |
| 0.2922 | 57.72 | 8600 | 0.4241 | 0.8228 | 0.823 |
| 0.2918 | 59.06 | 8800 | 0.4287 | 0.8240 | 0.824 |
| 0.2895 | 60.4 | 9000 | 0.4288 | 0.8230 | 0.823 |
| 0.2875 | 61.74 | 9200 | 0.4280 | 0.8219 | 0.822 |
| 0.2852 | 63.09 | 9400 | 0.4311 | 0.8220 | 0.822 |
| 0.2895 | 64.43 | 9600 | 0.4307 | 0.8219 | 0.822 |
| 0.2858 | 65.77 | 9800 | 0.4309 | 0.8239 | 0.824 |
| 0.2862 | 67.11 | 10000 | 0.4310 | 0.8229 | 0.823 |
### Framework versions
- PEFT 0.9.0
- Transformers 4.38.2
- Pytorch 2.2.0+cu121
- Datasets 2.17.1
- Tokenizers 0.15.2 | {"library_name": "peft", "tags": ["generated_from_trainer"], "metrics": ["accuracy"], "base_model": "mahdibaghbanzadeh/seqsight_4096_512_27M", "model-index": [{"name": "GUE_tf_4-seqsight_4096_512_27M-L8_f", "results": []}]} | mahdibaghbanzadeh/GUE_tf_4-seqsight_4096_512_27M-L8_f | null | [
"peft",
"safetensors",
"generated_from_trainer",
"base_model:mahdibaghbanzadeh/seqsight_4096_512_27M",
"region:us"
] | null | 2024-04-26T18:29:16+00:00 | [] | [] | TAGS
#peft #safetensors #generated_from_trainer #base_model-mahdibaghbanzadeh/seqsight_4096_512_27M #region-us
| GUE\_tf\_4-seqsight\_4096\_512\_27M-L8\_f
=========================================
This model is a fine-tuned version of mahdibaghbanzadeh/seqsight\_4096\_512\_27M on the mahdibaghbanzadeh/GUE\_tf\_4 dataset.
It achieves the following results on the evaluation set:
* Loss: 0.3638
* F1 Score: 0.8528
* Accuracy: 0.853
Model description
-----------------
More information needed
Intended uses & limitations
---------------------------
More information needed
Training and evaluation data
----------------------------
More information needed
Training procedure
------------------
### Training hyperparameters
The following hyperparameters were used during training:
* learning\_rate: 0.0005
* train\_batch\_size: 128
* eval\_batch\_size: 128
* seed: 42
* optimizer: Adam with betas=(0.9,0.999) and epsilon=1e-08
* lr\_scheduler\_type: linear
* training\_steps: 10000
### Training results
### Framework versions
* PEFT 0.9.0
* Transformers 4.38.2
* Pytorch 2.2.0+cu121
* Datasets 2.17.1
* Tokenizers 0.15.2
| [
"### Training hyperparameters\n\n\nThe following hyperparameters were used during training:\n\n\n* learning\\_rate: 0.0005\n* train\\_batch\\_size: 128\n* eval\\_batch\\_size: 128\n* seed: 42\n* optimizer: Adam with betas=(0.9,0.999) and epsilon=1e-08\n* lr\\_scheduler\\_type: linear\n* training\\_steps: 10000",
"### Training results",
"### Framework versions\n\n\n* PEFT 0.9.0\n* Transformers 4.38.2\n* Pytorch 2.2.0+cu121\n* Datasets 2.17.1\n* Tokenizers 0.15.2"
] | [
"TAGS\n#peft #safetensors #generated_from_trainer #base_model-mahdibaghbanzadeh/seqsight_4096_512_27M #region-us \n",
"### Training hyperparameters\n\n\nThe following hyperparameters were used during training:\n\n\n* learning\\_rate: 0.0005\n* train\\_batch\\_size: 128\n* eval\\_batch\\_size: 128\n* seed: 42\n* optimizer: Adam with betas=(0.9,0.999) and epsilon=1e-08\n* lr\\_scheduler\\_type: linear\n* training\\_steps: 10000",
"### Training results",
"### Framework versions\n\n\n* PEFT 0.9.0\n* Transformers 4.38.2\n* Pytorch 2.2.0+cu121\n* Datasets 2.17.1\n* Tokenizers 0.15.2"
] |
text-generation | transformers |
# Qwen1.5-32B-Chat
## About Quantization
我们使用modelscope [swift](https://github.com/modelscope/swift/)仓库进行GPTQ量化. 量化文档可以查看[这里](https://github.com/modelscope/swift/blob/main/docs/source/LLM/LLM%E9%87%8F%E5%8C%96%E6%96%87%E6%A1%A3.md). 量化命令如下:
We use the modelscope [swift](https://github.com/modelscope/swift/) repository to perform GPTQ quantization. Quantization documentation can be found [here](https://github.com/modelscope/swift/blob/main/docs/source_en/LLM/LLM-quantization.md). The quantization command is as follows:
```bash
OMP_NUM_THREADS=14 CUDA_VISIBLE_DEVICES=7 swift export \
--model_type qwen1half-32b-chat --quant_bits 8 \
--dataset sharegpt-gpt4-mini alpaca-zh alpaca-en --quant_method gptq --quant_seqlen 8192
```
## Introduction
Qwen1.5 is the beta version of Qwen2, a transformer-based decoder-only language model pretrained on a large amount of data. In comparison with the previous released Qwen, the improvements include:
* 8 model sizes, including 0.5B, 1.8B, 4B, 7B, 14B, 32B and 72B dense models, and an MoE model of 14B with 2.7B activated;
* Significant performance improvement in human preference for chat models;
* Multilingual support of both base and chat models;
* Stable support of 32K context length for models of all sizes
* No need of `trust_remote_code`.
For more details, please refer to our [blog post](https://qwenlm.github.io/blog/qwen1.5/) and [GitHub repo](https://github.com/QwenLM/Qwen1.5).
<br>
## Model Details
Qwen1.5 is a language model series including decoder language models of different model sizes. For each size, we release the base language model and the aligned chat model. It is based on the Transformer architecture with SwiGLU activation, attention QKV bias, group query attention, mixture of sliding window attention and full attention, etc. Additionally, we have an improved tokenizer adaptive to multiple natural languages and codes. For the beta version, temporarily we did not include GQA (except for 32B) and the mixture of SWA and full attention.
## Training details
We pretrained the models with a large amount of data, and we post-trained the models with both supervised finetuning and direct preference optimization.
## Requirements
The code of Qwen1.5 has been in the latest Hugging face transformers and we advise you to install `transformers>=4.37.0`, or you might encounter the following error:
```
KeyError: 'qwen2'
```
## Quickstart
Here provides a code snippet with `apply_chat_template` to show you how to load the tokenizer and model and how to generate contents.
```python
from transformers import AutoModelForCausalLM, AutoTokenizer
device = "cuda" # the device to load the model onto
model = AutoModelForCausalLM.from_pretrained(
"study-hjt/Qwen1.5-32B-Chat-GPTQ-Int8",
torch_dtype="auto",
device_map="auto"
)
tokenizer = AutoTokenizer.from_pretrained("study-hjt/Qwen1.5-32B-Chat-GPTQ-Int8")
prompt = "Give me a short introduction to large language model."
messages = [
{"role": "system", "content": "You are a helpful assistant."},
{"role": "user", "content": prompt}
]
text = tokenizer.apply_chat_template(
messages,
tokenize=False,
add_generation_prompt=True
)
model_inputs = tokenizer([text], return_tensors="pt").to(device)
generated_ids = model.generate(
model_inputs.input_ids,
max_new_tokens=512
)
generated_ids = [
output_ids[len(input_ids):] for input_ids, output_ids in zip(model_inputs.input_ids, generated_ids)
]
response = tokenizer.batch_decode(generated_ids, skip_special_tokens=True)[0]
```
## Tips
* If you encounter code switching or other bad cases, we advise you to use our provided hyper-parameters in `generation_config.json`.
## Citation
If you find our work helpful, feel free to give us a cite.
```
@article{qwen,
title={Qwen Technical Report},
author={Jinze Bai and Shuai Bai and Yunfei Chu and Zeyu Cui and Kai Dang and Xiaodong Deng and Yang Fan and Wenbin Ge and Yu Han and Fei Huang and Binyuan Hui and Luo Ji and Mei Li and Junyang Lin and Runji Lin and Dayiheng Liu and Gao Liu and Chengqiang Lu and Keming Lu and Jianxin Ma and Rui Men and Xingzhang Ren and Xuancheng Ren and Chuanqi Tan and Sinan Tan and Jianhong Tu and Peng Wang and Shijie Wang and Wei Wang and Shengguang Wu and Benfeng Xu and Jin Xu and An Yang and Hao Yang and Jian Yang and Shusheng Yang and Yang Yao and Bowen Yu and Hongyi Yuan and Zheng Yuan and Jianwei Zhang and Xingxuan Zhang and Yichang Zhang and Zhenru Zhang and Chang Zhou and Jingren Zhou and Xiaohuan Zhou and Tianhang Zhu},
journal={arXiv preprint arXiv:2309.16609},
year={2023}
}
``` | {"language": ["en", "zh"], "license": "other", "tags": ["qwen", "32b", "gptq", "int8", "chat"], "license_name": "tongyi-qianwen", "license_link": "https://huggingface.co/Qwen/Qwen1.5-32B-Chat/blob/main/LICENSE", "pipeline_tag": "text-generation"} | study-hjt/Qwen1.5-32B-Chat-GPTQ-Int8 | null | [
"transformers",
"safetensors",
"qwen2",
"text-generation",
"qwen",
"32b",
"gptq",
"int8",
"chat",
"conversational",
"en",
"zh",
"license:other",
"autotrain_compatible",
"endpoints_compatible",
"text-generation-inference",
"8-bit",
"region:us"
] | null | 2024-04-26T18:30:46+00:00 | [] | [
"en",
"zh"
] | TAGS
#transformers #safetensors #qwen2 #text-generation #qwen #32b #gptq #int8 #chat #conversational #en #zh #license-other #autotrain_compatible #endpoints_compatible #text-generation-inference #8-bit #region-us
|
# Qwen1.5-32B-Chat
## About Quantization
我们使用modelscope swift仓库进行GPTQ量化. 量化文档可以查看这里. 量化命令如下:
We use the modelscope swift repository to perform GPTQ quantization. Quantization documentation can be found here. The quantization command is as follows:
## Introduction
Qwen1.5 is the beta version of Qwen2, a transformer-based decoder-only language model pretrained on a large amount of data. In comparison with the previous released Qwen, the improvements include:
* 8 model sizes, including 0.5B, 1.8B, 4B, 7B, 14B, 32B and 72B dense models, and an MoE model of 14B with 2.7B activated;
* Significant performance improvement in human preference for chat models;
* Multilingual support of both base and chat models;
* Stable support of 32K context length for models of all sizes
* No need of 'trust_remote_code'.
For more details, please refer to our blog post and GitHub repo.
<br>
## Model Details
Qwen1.5 is a language model series including decoder language models of different model sizes. For each size, we release the base language model and the aligned chat model. It is based on the Transformer architecture with SwiGLU activation, attention QKV bias, group query attention, mixture of sliding window attention and full attention, etc. Additionally, we have an improved tokenizer adaptive to multiple natural languages and codes. For the beta version, temporarily we did not include GQA (except for 32B) and the mixture of SWA and full attention.
## Training details
We pretrained the models with a large amount of data, and we post-trained the models with both supervised finetuning and direct preference optimization.
## Requirements
The code of Qwen1.5 has been in the latest Hugging face transformers and we advise you to install 'transformers>=4.37.0', or you might encounter the following error:
## Quickstart
Here provides a code snippet with 'apply_chat_template' to show you how to load the tokenizer and model and how to generate contents.
## Tips
* If you encounter code switching or other bad cases, we advise you to use our provided hyper-parameters in 'generation_config.json'.
If you find our work helpful, feel free to give us a cite.
| [
"# Qwen1.5-32B-Chat",
"## About Quantization\n我们使用modelscope swift仓库进行GPTQ量化. 量化文档可以查看这里. 量化命令如下:\n\nWe use the modelscope swift repository to perform GPTQ quantization. Quantization documentation can be found here. The quantization command is as follows:",
"## Introduction\n\nQwen1.5 is the beta version of Qwen2, a transformer-based decoder-only language model pretrained on a large amount of data. In comparison with the previous released Qwen, the improvements include: \n\n* 8 model sizes, including 0.5B, 1.8B, 4B, 7B, 14B, 32B and 72B dense models, and an MoE model of 14B with 2.7B activated;\n* Significant performance improvement in human preference for chat models;\n* Multilingual support of both base and chat models;\n* Stable support of 32K context length for models of all sizes\n* No need of 'trust_remote_code'.\n\nFor more details, please refer to our blog post and GitHub repo.\n<br>",
"## Model Details\nQwen1.5 is a language model series including decoder language models of different model sizes. For each size, we release the base language model and the aligned chat model. It is based on the Transformer architecture with SwiGLU activation, attention QKV bias, group query attention, mixture of sliding window attention and full attention, etc. Additionally, we have an improved tokenizer adaptive to multiple natural languages and codes. For the beta version, temporarily we did not include GQA (except for 32B) and the mixture of SWA and full attention.",
"## Training details\nWe pretrained the models with a large amount of data, and we post-trained the models with both supervised finetuning and direct preference optimization.",
"## Requirements\nThe code of Qwen1.5 has been in the latest Hugging face transformers and we advise you to install 'transformers>=4.37.0', or you might encounter the following error:",
"## Quickstart\n\nHere provides a code snippet with 'apply_chat_template' to show you how to load the tokenizer and model and how to generate contents.",
"## Tips\n\n* If you encounter code switching or other bad cases, we advise you to use our provided hyper-parameters in 'generation_config.json'.\n\n\nIf you find our work helpful, feel free to give us a cite."
] | [
"TAGS\n#transformers #safetensors #qwen2 #text-generation #qwen #32b #gptq #int8 #chat #conversational #en #zh #license-other #autotrain_compatible #endpoints_compatible #text-generation-inference #8-bit #region-us \n",
"# Qwen1.5-32B-Chat",
"## About Quantization\n我们使用modelscope swift仓库进行GPTQ量化. 量化文档可以查看这里. 量化命令如下:\n\nWe use the modelscope swift repository to perform GPTQ quantization. Quantization documentation can be found here. The quantization command is as follows:",
"## Introduction\n\nQwen1.5 is the beta version of Qwen2, a transformer-based decoder-only language model pretrained on a large amount of data. In comparison with the previous released Qwen, the improvements include: \n\n* 8 model sizes, including 0.5B, 1.8B, 4B, 7B, 14B, 32B and 72B dense models, and an MoE model of 14B with 2.7B activated;\n* Significant performance improvement in human preference for chat models;\n* Multilingual support of both base and chat models;\n* Stable support of 32K context length for models of all sizes\n* No need of 'trust_remote_code'.\n\nFor more details, please refer to our blog post and GitHub repo.\n<br>",
"## Model Details\nQwen1.5 is a language model series including decoder language models of different model sizes. For each size, we release the base language model and the aligned chat model. It is based on the Transformer architecture with SwiGLU activation, attention QKV bias, group query attention, mixture of sliding window attention and full attention, etc. Additionally, we have an improved tokenizer adaptive to multiple natural languages and codes. For the beta version, temporarily we did not include GQA (except for 32B) and the mixture of SWA and full attention.",
"## Training details\nWe pretrained the models with a large amount of data, and we post-trained the models with both supervised finetuning and direct preference optimization.",
"## Requirements\nThe code of Qwen1.5 has been in the latest Hugging face transformers and we advise you to install 'transformers>=4.37.0', or you might encounter the following error:",
"## Quickstart\n\nHere provides a code snippet with 'apply_chat_template' to show you how to load the tokenizer and model and how to generate contents.",
"## Tips\n\n* If you encounter code switching or other bad cases, we advise you to use our provided hyper-parameters in 'generation_config.json'.\n\n\nIf you find our work helpful, feel free to give us a cite."
] |
text-generation | transformers |
# Model Card for Model ID
<!-- Provide a quick summary of what the model is/does. -->
## Model Details
### Model Description
<!-- Provide a longer summary of what this model is. -->
This is the model card of a 🤗 transformers model that has been pushed on the Hub. This model card has been automatically generated.
- **Developed by:** [More Information Needed]
- **Funded by [optional]:** [More Information Needed]
- **Shared by [optional]:** [More Information Needed]
- **Model type:** [More Information Needed]
- **Language(s) (NLP):** [More Information Needed]
- **License:** [More Information Needed]
- **Finetuned from model [optional]:** [More Information Needed]
### Model Sources [optional]
<!-- Provide the basic links for the model. -->
- **Repository:** [More Information Needed]
- **Paper [optional]:** [More Information Needed]
- **Demo [optional]:** [More Information Needed]
## Uses
<!-- Address questions around how the model is intended to be used, including the foreseeable users of the model and those affected by the model. -->
### Direct Use
<!-- This section is for the model use without fine-tuning or plugging into a larger ecosystem/app. -->
[More Information Needed]
### Downstream Use [optional]
<!-- This section is for the model use when fine-tuned for a task, or when plugged into a larger ecosystem/app -->
[More Information Needed]
### Out-of-Scope Use
<!-- This section addresses misuse, malicious use, and uses that the model will not work well for. -->
[More Information Needed]
## Bias, Risks, and Limitations
<!-- This section is meant to convey both technical and sociotechnical limitations. -->
[More Information Needed]
### Recommendations
<!-- This section is meant to convey recommendations with respect to the bias, risk, and technical limitations. -->
Users (both direct and downstream) should be made aware of the risks, biases and limitations of the model. More information needed for further recommendations.
## How to Get Started with the Model
Use the code below to get started with the model.
[More Information Needed]
## Training Details
### Training Data
<!-- This should link to a Dataset Card, perhaps with a short stub of information on what the training data is all about as well as documentation related to data pre-processing or additional filtering. -->
[More Information Needed]
### Training Procedure
<!-- This relates heavily to the Technical Specifications. Content here should link to that section when it is relevant to the training procedure. -->
#### Preprocessing [optional]
[More Information Needed]
#### Training Hyperparameters
- **Training regime:** [More Information Needed] <!--fp32, fp16 mixed precision, bf16 mixed precision, bf16 non-mixed precision, fp16 non-mixed precision, fp8 mixed precision -->
#### Speeds, Sizes, Times [optional]
<!-- This section provides information about throughput, start/end time, checkpoint size if relevant, etc. -->
[More Information Needed]
## Evaluation
<!-- This section describes the evaluation protocols and provides the results. -->
### Testing Data, Factors & Metrics
#### Testing Data
<!-- This should link to a Dataset Card if possible. -->
[More Information Needed]
#### Factors
<!-- These are the things the evaluation is disaggregating by, e.g., subpopulations or domains. -->
[More Information Needed]
#### Metrics
<!-- These are the evaluation metrics being used, ideally with a description of why. -->
[More Information Needed]
### Results
[More Information Needed]
#### Summary
## Model Examination [optional]
<!-- Relevant interpretability work for the model goes here -->
[More Information Needed]
## Environmental Impact
<!-- Total emissions (in grams of CO2eq) and additional considerations, such as electricity usage, go here. Edit the suggested text below accordingly -->
Carbon emissions can be estimated using the [Machine Learning Impact calculator](https://mlco2.github.io/impact#compute) presented in [Lacoste et al. (2019)](https://arxiv.org/abs/1910.09700).
- **Hardware Type:** [More Information Needed]
- **Hours used:** [More Information Needed]
- **Cloud Provider:** [More Information Needed]
- **Compute Region:** [More Information Needed]
- **Carbon Emitted:** [More Information Needed]
## Technical Specifications [optional]
### Model Architecture and Objective
[More Information Needed]
### Compute Infrastructure
[More Information Needed]
#### Hardware
[More Information Needed]
#### Software
[More Information Needed]
## Citation [optional]
<!-- If there is a paper or blog post introducing the model, the APA and Bibtex information for that should go in this section. -->
**BibTeX:**
[More Information Needed]
**APA:**
[More Information Needed]
## Glossary [optional]
<!-- If relevant, include terms and calculations in this section that can help readers understand the model or model card. -->
[More Information Needed]
## More Information [optional]
[More Information Needed]
## Model Card Authors [optional]
[More Information Needed]
## Model Card Contact
[More Information Needed]
| {"library_name": "transformers", "tags": ["llama-factory"]} | zilongpa/aes-llama3-v1 | null | [
"transformers",
"safetensors",
"llama",
"text-generation",
"llama-factory",
"conversational",
"arxiv:1910.09700",
"autotrain_compatible",
"endpoints_compatible",
"text-generation-inference",
"region:us"
] | null | 2024-04-26T18:32:53+00:00 | [
"1910.09700"
] | [] | TAGS
#transformers #safetensors #llama #text-generation #llama-factory #conversational #arxiv-1910.09700 #autotrain_compatible #endpoints_compatible #text-generation-inference #region-us
|
# Model Card for Model ID
## Model Details
### Model Description
This is the model card of a transformers model that has been pushed on the Hub. This model card has been automatically generated.
- Developed by:
- Funded by [optional]:
- Shared by [optional]:
- Model type:
- Language(s) (NLP):
- License:
- Finetuned from model [optional]:
### Model Sources [optional]
- Repository:
- Paper [optional]:
- Demo [optional]:
## Uses
### Direct Use
### Downstream Use [optional]
### Out-of-Scope Use
## Bias, Risks, and Limitations
### Recommendations
Users (both direct and downstream) should be made aware of the risks, biases and limitations of the model. More information needed for further recommendations.
## How to Get Started with the Model
Use the code below to get started with the model.
## Training Details
### Training Data
### Training Procedure
#### Preprocessing [optional]
#### Training Hyperparameters
- Training regime:
#### Speeds, Sizes, Times [optional]
## Evaluation
### Testing Data, Factors & Metrics
#### Testing Data
#### Factors
#### Metrics
### Results
#### Summary
## Model Examination [optional]
## Environmental Impact
Carbon emissions can be estimated using the Machine Learning Impact calculator presented in Lacoste et al. (2019).
- Hardware Type:
- Hours used:
- Cloud Provider:
- Compute Region:
- Carbon Emitted:
## Technical Specifications [optional]
### Model Architecture and Objective
### Compute Infrastructure
#### Hardware
#### Software
[optional]
BibTeX:
APA:
## Glossary [optional]
## More Information [optional]
## Model Card Authors [optional]
## Model Card Contact
| [
"# Model Card for Model ID",
"## Model Details",
"### Model Description\n\n\n\nThis is the model card of a transformers model that has been pushed on the Hub. This model card has been automatically generated.\n\n- Developed by: \n- Funded by [optional]: \n- Shared by [optional]: \n- Model type: \n- Language(s) (NLP): \n- License: \n- Finetuned from model [optional]:",
"### Model Sources [optional]\n\n\n\n- Repository: \n- Paper [optional]: \n- Demo [optional]:",
"## Uses",
"### Direct Use",
"### Downstream Use [optional]",
"### Out-of-Scope Use",
"## Bias, Risks, and Limitations",
"### Recommendations\n\n\n\nUsers (both direct and downstream) should be made aware of the risks, biases and limitations of the model. More information needed for further recommendations.",
"## How to Get Started with the Model\n\nUse the code below to get started with the model.",
"## Training Details",
"### Training Data",
"### Training Procedure",
"#### Preprocessing [optional]",
"#### Training Hyperparameters\n\n- Training regime:",
"#### Speeds, Sizes, Times [optional]",
"## Evaluation",
"### Testing Data, Factors & Metrics",
"#### Testing Data",
"#### Factors",
"#### Metrics",
"### Results",
"#### Summary",
"## Model Examination [optional]",
"## Environmental Impact\n\n\n\nCarbon emissions can be estimated using the Machine Learning Impact calculator presented in Lacoste et al. (2019).\n\n- Hardware Type: \n- Hours used: \n- Cloud Provider: \n- Compute Region: \n- Carbon Emitted:",
"## Technical Specifications [optional]",
"### Model Architecture and Objective",
"### Compute Infrastructure",
"#### Hardware",
"#### Software\n\n\n\n[optional]\n\n\n\nBibTeX:\n\n\n\nAPA:",
"## Glossary [optional]",
"## More Information [optional]",
"## Model Card Authors [optional]",
"## Model Card Contact"
] | [
"TAGS\n#transformers #safetensors #llama #text-generation #llama-factory #conversational #arxiv-1910.09700 #autotrain_compatible #endpoints_compatible #text-generation-inference #region-us \n",
"# Model Card for Model ID",
"## Model Details",
"### Model Description\n\n\n\nThis is the model card of a transformers model that has been pushed on the Hub. This model card has been automatically generated.\n\n- Developed by: \n- Funded by [optional]: \n- Shared by [optional]: \n- Model type: \n- Language(s) (NLP): \n- License: \n- Finetuned from model [optional]:",
"### Model Sources [optional]\n\n\n\n- Repository: \n- Paper [optional]: \n- Demo [optional]:",
"## Uses",
"### Direct Use",
"### Downstream Use [optional]",
"### Out-of-Scope Use",
"## Bias, Risks, and Limitations",
"### Recommendations\n\n\n\nUsers (both direct and downstream) should be made aware of the risks, biases and limitations of the model. More information needed for further recommendations.",
"## How to Get Started with the Model\n\nUse the code below to get started with the model.",
"## Training Details",
"### Training Data",
"### Training Procedure",
"#### Preprocessing [optional]",
"#### Training Hyperparameters\n\n- Training regime:",
"#### Speeds, Sizes, Times [optional]",
"## Evaluation",
"### Testing Data, Factors & Metrics",
"#### Testing Data",
"#### Factors",
"#### Metrics",
"### Results",
"#### Summary",
"## Model Examination [optional]",
"## Environmental Impact\n\n\n\nCarbon emissions can be estimated using the Machine Learning Impact calculator presented in Lacoste et al. (2019).\n\n- Hardware Type: \n- Hours used: \n- Cloud Provider: \n- Compute Region: \n- Carbon Emitted:",
"## Technical Specifications [optional]",
"### Model Architecture and Objective",
"### Compute Infrastructure",
"#### Hardware",
"#### Software\n\n\n\n[optional]\n\n\n\nBibTeX:\n\n\n\nAPA:",
"## Glossary [optional]",
"## More Information [optional]",
"## Model Card Authors [optional]",
"## Model Card Contact"
] |
text-to-image | diffusers |
<!-- This model card has been generated automatically according to the information the training script had access to. You
should probably proofread and complete it, then remove this comment. -->
# Critical Dream - cosmicBboy/stable-diffusion-xl-base-1.0-lora-dreambooth-critdream-v0.7.2
<Gallery />
## Model description
These are cosmicBboy/stable-diffusion-xl-base-1.0-lora-dreambooth-critdream-v0.7.2 LoRA adaption weights for stabilityai/stable-diffusion-xl-base-1.0, for the purposes of
generating images for the [Critical Dream](https://github.com/cosmicBboy/critical-dream)
project.
The weights were trained using [DreamBooth](https://dreambooth.github.io/).
LoRA for the text encoder was enabled: True.
Special VAE used for training: stabilityai/sdxl-vae.
## Trigger words
You should use a picture of [dm-matt-mercer], a dungeon master. background is a forest. fantasy art style, high quality, highly detailed, sharp focus" to trigger the image generation.
## Download model
Weights for this model are available in Safetensors format.
[Download](cosmicBboy/stable-diffusion-xl-base-1.0-lora-dreambooth-critdream-v0.7.2/tree/main) them in the Files & versions tab.
## Tracker run link
https://wandb.ai/nielsbantilan/dreambooth-lora-sd-xl/runs/8n7um3yr
## Intended uses & limitations
#### How to use
```python
# TODO: add an example code snippet for running this diffusion pipeline
```
#### Limitations and bias
[TODO: provide examples of latent issues and potential remediations]
## Training details
[TODO: describe the data used to train the model] | {"license": "openrail++", "library_name": "diffusers", "tags": ["text-to-image", "stable-diffusion-xl", "stable-diffusion-xl-diffusers", "text-to-image", "diffusers", "lora", "template:sd-lora"], "base_model": "stabilityai/stable-diffusion-xl-base-1.0", "prompt": "a picture of [dm-matt-mercer], a dungeon master. background is a forest. fantasy art style, high quality, highly detailed, sharp focus\"", "widget": [{"text": "a picture of [dm-matt-mercer]", "output": {"url": "image_0.png"}}, {"text": "a picture of [dm-matt-mercer]", "output": {"url": "image_1.png"}}, {"text": "a picture of a dungeon master.", "output": {"url": "image_2.png"}}, {"text": "a picture of a dungeon master.", "output": {"url": "image_3.png"}}, {"text": "a picture of [critrole-fjord], a male half-orc warlock. background is a forest. fantasy art style, high quality, highly detailed, sharp focus", "output": {"url": "image_4.png"}}, {"text": "a picture of [critrole-fjord], a male half-orc warlock. background is a forest. fantasy art style, high quality, highly detailed, sharp focus", "output": {"url": "image_5.png"}}, {"text": "a picture of a male half-orc warlock", "output": {"url": "image_6.png"}}, {"text": "a picture of a male half-orc warlock", "output": {"url": "image_7.png"}}, {"text": "a picture of [critrole-beau], a female human monk. background is a forest. fantasy art style, high quality, highly detailed, sharp focus", "output": {"url": "image_8.png"}}, {"text": "a picture of [critrole-beau], a female human monk. background is a forest. fantasy art style, high quality, highly detailed, sharp focus", "output": {"url": "image_9.png"}}, {"text": "a picture of a female human monk", "output": {"url": "image_10.png"}}, {"text": "a picture of a female human monk", "output": {"url": "image_11.png"}}, {"text": "a picture of [critrole-caduceus], a male firbolg cleric. background is a forest. fantasy art style, high quality, highly detailed, sharp focus", "output": {"url": "image_12.png"}}, {"text": "a picture of [critrole-caduceus], a male firbolg cleric. background is a forest. fantasy art style, high quality, highly detailed, sharp focus", "output": {"url": "image_13.png"}}, {"text": "a picture of a male firbolg cleric", "output": {"url": "image_14.png"}}, {"text": "a picture of a male firbolg cleric", "output": {"url": "image_15.png"}}, {"text": "a picture of [critrole-caleb], a male human wizard. background is a forest. fantasy art style, high quality, highly detailed, sharp focus", "output": {"url": "image_16.png"}}, {"text": "a picture of [critrole-caleb], a male human wizard. background is a forest. fantasy art style, high quality, highly detailed, sharp focus", "output": {"url": "image_17.png"}}, {"text": "a picture of a male human wizard", "output": {"url": "image_18.png"}}, {"text": "a picture of a male human wizard", "output": {"url": "image_19.png"}}, {"text": "a picture of [critrole-jester], a female tiefling cleric. background is a forest. fantasy art style, high quality, highly detailed, sharp focus", "output": {"url": "image_20.png"}}, {"text": "a picture of [critrole-jester], a female tiefling cleric. background is a forest. fantasy art style, high quality, highly detailed, sharp focus", "output": {"url": "image_21.png"}}, {"text": "a picture of a female tiefling cleric", "output": {"url": "image_22.png"}}, {"text": "a picture of a female tiefling cleric", "output": {"url": "image_23.png"}}, {"text": "a picture of [critrole-nott], a female goblin rogue. background is a forest. fantasy art style, high quality, highly detailed, sharp focus", "output": {"url": "image_24.png"}}, {"text": "a picture of [critrole-nott], a female goblin rogue. background is a forest. fantasy art style, high quality, highly detailed, sharp focus", "output": {"url": "image_25.png"}}, {"text": "a picture of a female goblin rogue", "output": {"url": "image_26.png"}}, {"text": "a picture of a female goblin rogue", "output": {"url": "image_27.png"}}, {"text": "a picture of [critrole-veth], a female halfling rogue/wizard. background is a forest. fantasy art style, high quality, highly detailed, sharp focus", "output": {"url": "image_28.png"}}, {"text": "a picture of [critrole-veth], a female halfling rogue/wizard. background is a forest. fantasy art style, high quality, highly detailed, sharp focus", "output": {"url": "image_29.png"}}, {"text": "a picture of a female halfling rogue/wizard", "output": {"url": "image_30.png"}}, {"text": "a picture of a female halfling rogue/wizard", "output": {"url": "image_31.png"}}, {"text": "a picture of [critrole-yasha], a female aasimar barbarian. background is a forest. fantasy art style, high quality, highly detailed, sharp focus", "output": {"url": "image_32.png"}}, {"text": "a picture of [critrole-yasha], a female aasimar barbarian. background is a forest. fantasy art style, high quality, highly detailed, sharp focus", "output": {"url": "image_33.png"}}, {"text": "a picture of a female aasimar barbarian", "output": {"url": "image_34.png"}}, {"text": "a picture of a female aasimar barbarian", "output": {"url": "image_35.png"}}, {"text": "a picture of [critrole-mollymauk], a male tiefling blood hunter. background is a forest. fantasy art style, high quality, highly detailed, sharp focus", "output": {"url": "image_36.png"}}, {"text": "a picture of [critrole-mollymauk], a male tiefling blood hunter. background is a forest. fantasy art style, high quality, highly detailed, sharp focus", "output": {"url": "image_37.png"}}, {"text": "a picture of a male tiefling blood hunter", "output": {"url": "image_38.png"}}, {"text": "a picture of a male tiefling blood hunter", "output": {"url": "image_39.png"}}, {"text": "a picture of [critrole-essek], a male drow wizard. background is a forest. fantasy art style, high quality, highly detailed, sharp focus", "output": {"url": "image_40.png"}}, {"text": "a picture of [critrole-essek], a male drow wizard. background is a forest. fantasy art style, high quality, highly detailed, sharp focus", "output": {"url": "image_41.png"}}, {"text": "a picture of a male drow wizard", "output": {"url": "image_42.png"}}, {"text": "a picture of a male drow wizard", "output": {"url": "image_43.png"}}]} | cosmicBboy/stable-diffusion-xl-base-1.0-lora-dreambooth-critdream-v0.7.2 | null | [
"diffusers",
"text-to-image",
"stable-diffusion-xl",
"stable-diffusion-xl-diffusers",
"lora",
"template:sd-lora",
"base_model:stabilityai/stable-diffusion-xl-base-1.0",
"license:openrail++",
"region:us"
] | null | 2024-04-26T18:33:21+00:00 | [] | [] | TAGS
#diffusers #text-to-image #stable-diffusion-xl #stable-diffusion-xl-diffusers #lora #template-sd-lora #base_model-stabilityai/stable-diffusion-xl-base-1.0 #license-openrail++ #region-us
|
# Critical Dream - cosmicBboy/stable-diffusion-xl-base-1.0-lora-dreambooth-critdream-v0.7.2
<Gallery />
## Model description
These are cosmicBboy/stable-diffusion-xl-base-1.0-lora-dreambooth-critdream-v0.7.2 LoRA adaption weights for stabilityai/stable-diffusion-xl-base-1.0, for the purposes of
generating images for the Critical Dream
project.
The weights were trained using DreamBooth.
LoRA for the text encoder was enabled: True.
Special VAE used for training: stabilityai/sdxl-vae.
## Trigger words
You should use a picture of [dm-matt-mercer], a dungeon master. background is a forest. fantasy art style, high quality, highly detailed, sharp focus" to trigger the image generation.
## Download model
Weights for this model are available in Safetensors format.
Download them in the Files & versions tab.
## Tracker run link
URL
## Intended uses & limitations
#### How to use
#### Limitations and bias
[TODO: provide examples of latent issues and potential remediations]
## Training details
[TODO: describe the data used to train the model] | [
"# Critical Dream - cosmicBboy/stable-diffusion-xl-base-1.0-lora-dreambooth-critdream-v0.7.2\n\n<Gallery />",
"## Model description\n\nThese are cosmicBboy/stable-diffusion-xl-base-1.0-lora-dreambooth-critdream-v0.7.2 LoRA adaption weights for stabilityai/stable-diffusion-xl-base-1.0, for the purposes of\ngenerating images for the Critical Dream\nproject.\n\nThe weights were trained using DreamBooth.\n\nLoRA for the text encoder was enabled: True.\n\nSpecial VAE used for training: stabilityai/sdxl-vae.",
"## Trigger words\n\nYou should use a picture of [dm-matt-mercer], a dungeon master. background is a forest. fantasy art style, high quality, highly detailed, sharp focus\" to trigger the image generation.",
"## Download model\n\nWeights for this model are available in Safetensors format.\n\nDownload them in the Files & versions tab.",
"## Tracker run link\n\nURL",
"## Intended uses & limitations",
"#### How to use",
"#### Limitations and bias\n\n[TODO: provide examples of latent issues and potential remediations]",
"## Training details\n\n[TODO: describe the data used to train the model]"
] | [
"TAGS\n#diffusers #text-to-image #stable-diffusion-xl #stable-diffusion-xl-diffusers #lora #template-sd-lora #base_model-stabilityai/stable-diffusion-xl-base-1.0 #license-openrail++ #region-us \n",
"# Critical Dream - cosmicBboy/stable-diffusion-xl-base-1.0-lora-dreambooth-critdream-v0.7.2\n\n<Gallery />",
"## Model description\n\nThese are cosmicBboy/stable-diffusion-xl-base-1.0-lora-dreambooth-critdream-v0.7.2 LoRA adaption weights for stabilityai/stable-diffusion-xl-base-1.0, for the purposes of\ngenerating images for the Critical Dream\nproject.\n\nThe weights were trained using DreamBooth.\n\nLoRA for the text encoder was enabled: True.\n\nSpecial VAE used for training: stabilityai/sdxl-vae.",
"## Trigger words\n\nYou should use a picture of [dm-matt-mercer], a dungeon master. background is a forest. fantasy art style, high quality, highly detailed, sharp focus\" to trigger the image generation.",
"## Download model\n\nWeights for this model are available in Safetensors format.\n\nDownload them in the Files & versions tab.",
"## Tracker run link\n\nURL",
"## Intended uses & limitations",
"#### How to use",
"#### Limitations and bias\n\n[TODO: provide examples of latent issues and potential remediations]",
"## Training details\n\n[TODO: describe the data used to train the model]"
] |
null | peft |
<!-- This model card has been generated automatically according to the information the Trainer had access to. You
should probably proofread and complete it, then remove this comment. -->
# GUE_tf_4-seqsight_4096_512_27M-L32_f
This model is a fine-tuned version of [mahdibaghbanzadeh/seqsight_4096_512_27M](https://huggingface.co/mahdibaghbanzadeh/seqsight_4096_512_27M) on the [mahdibaghbanzadeh/GUE_tf_4](https://huggingface.co/datasets/mahdibaghbanzadeh/GUE_tf_4) dataset.
It achieves the following results on the evaluation set:
- Loss: 0.5227
- F1 Score: 0.8399
- Accuracy: 0.84
## Model description
More information needed
## Intended uses & limitations
More information needed
## Training and evaluation data
More information needed
## Training procedure
### Training hyperparameters
The following hyperparameters were used during training:
- learning_rate: 0.0005
- train_batch_size: 128
- eval_batch_size: 128
- seed: 42
- optimizer: Adam with betas=(0.9,0.999) and epsilon=1e-08
- lr_scheduler_type: linear
- training_steps: 10000
### Training results
| Training Loss | Epoch | Step | Validation Loss | F1 Score | Accuracy |
|:-------------:|:-----:|:-----:|:---------------:|:--------:|:--------:|
| 0.5228 | 1.34 | 200 | 0.4971 | 0.7473 | 0.748 |
| 0.4517 | 2.68 | 400 | 0.4751 | 0.7729 | 0.773 |
| 0.4287 | 4.03 | 600 | 0.4618 | 0.7778 | 0.778 |
| 0.409 | 5.37 | 800 | 0.4716 | 0.7796 | 0.78 |
| 0.3943 | 6.71 | 1000 | 0.4604 | 0.7829 | 0.784 |
| 0.3784 | 8.05 | 1200 | 0.4458 | 0.7920 | 0.792 |
| 0.3667 | 9.4 | 1400 | 0.4354 | 0.8010 | 0.801 |
| 0.3545 | 10.74 | 1600 | 0.4365 | 0.8078 | 0.808 |
| 0.3391 | 12.08 | 1800 | 0.4490 | 0.8090 | 0.809 |
| 0.3274 | 13.42 | 2000 | 0.4355 | 0.7985 | 0.799 |
| 0.3157 | 14.77 | 2200 | 0.4221 | 0.8240 | 0.824 |
| 0.3041 | 16.11 | 2400 | 0.4244 | 0.8210 | 0.821 |
| 0.295 | 17.45 | 2600 | 0.4261 | 0.8220 | 0.822 |
| 0.2844 | 18.79 | 2800 | 0.4250 | 0.8330 | 0.833 |
| 0.2815 | 20.13 | 3000 | 0.4180 | 0.8329 | 0.833 |
| 0.2629 | 21.48 | 3200 | 0.4320 | 0.8320 | 0.832 |
| 0.256 | 22.82 | 3400 | 0.4342 | 0.8358 | 0.836 |
| 0.2502 | 24.16 | 3600 | 0.4235 | 0.8289 | 0.829 |
| 0.2416 | 25.5 | 3800 | 0.4417 | 0.8377 | 0.838 |
| 0.2316 | 26.85 | 4000 | 0.4327 | 0.8367 | 0.837 |
| 0.2244 | 28.19 | 4200 | 0.4546 | 0.8420 | 0.842 |
| 0.2172 | 29.53 | 4400 | 0.4484 | 0.8406 | 0.841 |
| 0.2071 | 30.87 | 4600 | 0.4583 | 0.8390 | 0.839 |
| 0.2096 | 32.21 | 4800 | 0.4549 | 0.8390 | 0.839 |
| 0.194 | 33.56 | 5000 | 0.4626 | 0.8419 | 0.842 |
| 0.1957 | 34.9 | 5200 | 0.4513 | 0.8509 | 0.851 |
| 0.1841 | 36.24 | 5400 | 0.4581 | 0.8468 | 0.847 |
| 0.178 | 37.58 | 5600 | 0.4457 | 0.8550 | 0.855 |
| 0.1773 | 38.93 | 5800 | 0.4750 | 0.8519 | 0.852 |
| 0.1739 | 40.27 | 6000 | 0.4778 | 0.8567 | 0.857 |
| 0.1684 | 41.61 | 6200 | 0.4822 | 0.8609 | 0.861 |
| 0.1642 | 42.95 | 6400 | 0.4641 | 0.8578 | 0.858 |
| 0.162 | 44.3 | 6600 | 0.4911 | 0.8526 | 0.853 |
| 0.1588 | 45.64 | 6800 | 0.4878 | 0.8590 | 0.859 |
| 0.1595 | 46.98 | 7000 | 0.4724 | 0.8547 | 0.855 |
| 0.1467 | 48.32 | 7200 | 0.4968 | 0.8566 | 0.857 |
| 0.1511 | 49.66 | 7400 | 0.5053 | 0.8556 | 0.856 |
| 0.1443 | 51.01 | 7600 | 0.4984 | 0.8597 | 0.86 |
| 0.1397 | 52.35 | 7800 | 0.5039 | 0.8567 | 0.857 |
| 0.1424 | 53.69 | 8000 | 0.4894 | 0.8547 | 0.855 |
| 0.138 | 55.03 | 8200 | 0.4977 | 0.8618 | 0.862 |
| 0.1317 | 56.38 | 8400 | 0.5178 | 0.8617 | 0.862 |
| 0.1363 | 57.72 | 8600 | 0.4955 | 0.8617 | 0.862 |
| 0.1281 | 59.06 | 8800 | 0.5089 | 0.8607 | 0.861 |
| 0.1302 | 60.4 | 9000 | 0.5105 | 0.8588 | 0.859 |
| 0.1314 | 61.74 | 9200 | 0.5196 | 0.8627 | 0.863 |
| 0.124 | 63.09 | 9400 | 0.5311 | 0.8587 | 0.859 |
| 0.1232 | 64.43 | 9600 | 0.5326 | 0.8617 | 0.862 |
| 0.1229 | 65.77 | 9800 | 0.5297 | 0.8587 | 0.859 |
| 0.1233 | 67.11 | 10000 | 0.5287 | 0.8607 | 0.861 |
### Framework versions
- PEFT 0.9.0
- Transformers 4.38.2
- Pytorch 2.2.0+cu121
- Datasets 2.17.1
- Tokenizers 0.15.2 | {"library_name": "peft", "tags": ["generated_from_trainer"], "metrics": ["accuracy"], "base_model": "mahdibaghbanzadeh/seqsight_4096_512_27M", "model-index": [{"name": "GUE_tf_4-seqsight_4096_512_27M-L32_f", "results": []}]} | mahdibaghbanzadeh/GUE_tf_4-seqsight_4096_512_27M-L32_f | null | [
"peft",
"safetensors",
"generated_from_trainer",
"base_model:mahdibaghbanzadeh/seqsight_4096_512_27M",
"region:us"
] | null | 2024-04-26T18:33:27+00:00 | [] | [] | TAGS
#peft #safetensors #generated_from_trainer #base_model-mahdibaghbanzadeh/seqsight_4096_512_27M #region-us
| GUE\_tf\_4-seqsight\_4096\_512\_27M-L32\_f
==========================================
This model is a fine-tuned version of mahdibaghbanzadeh/seqsight\_4096\_512\_27M on the mahdibaghbanzadeh/GUE\_tf\_4 dataset.
It achieves the following results on the evaluation set:
* Loss: 0.5227
* F1 Score: 0.8399
* Accuracy: 0.84
Model description
-----------------
More information needed
Intended uses & limitations
---------------------------
More information needed
Training and evaluation data
----------------------------
More information needed
Training procedure
------------------
### Training hyperparameters
The following hyperparameters were used during training:
* learning\_rate: 0.0005
* train\_batch\_size: 128
* eval\_batch\_size: 128
* seed: 42
* optimizer: Adam with betas=(0.9,0.999) and epsilon=1e-08
* lr\_scheduler\_type: linear
* training\_steps: 10000
### Training results
### Framework versions
* PEFT 0.9.0
* Transformers 4.38.2
* Pytorch 2.2.0+cu121
* Datasets 2.17.1
* Tokenizers 0.15.2
| [
"### Training hyperparameters\n\n\nThe following hyperparameters were used during training:\n\n\n* learning\\_rate: 0.0005\n* train\\_batch\\_size: 128\n* eval\\_batch\\_size: 128\n* seed: 42\n* optimizer: Adam with betas=(0.9,0.999) and epsilon=1e-08\n* lr\\_scheduler\\_type: linear\n* training\\_steps: 10000",
"### Training results",
"### Framework versions\n\n\n* PEFT 0.9.0\n* Transformers 4.38.2\n* Pytorch 2.2.0+cu121\n* Datasets 2.17.1\n* Tokenizers 0.15.2"
] | [
"TAGS\n#peft #safetensors #generated_from_trainer #base_model-mahdibaghbanzadeh/seqsight_4096_512_27M #region-us \n",
"### Training hyperparameters\n\n\nThe following hyperparameters were used during training:\n\n\n* learning\\_rate: 0.0005\n* train\\_batch\\_size: 128\n* eval\\_batch\\_size: 128\n* seed: 42\n* optimizer: Adam with betas=(0.9,0.999) and epsilon=1e-08\n* lr\\_scheduler\\_type: linear\n* training\\_steps: 10000",
"### Training results",
"### Framework versions\n\n\n* PEFT 0.9.0\n* Transformers 4.38.2\n* Pytorch 2.2.0+cu121\n* Datasets 2.17.1\n* Tokenizers 0.15.2"
] |
null | null |
<!-- This model card has been generated automatically according to the information the Trainer had access to. You
should probably proofread and complete it, then remove this comment. -->
# idefics2-8b-docvqa-finetuned-tutorial
This model is a fine-tuned version of [HuggingFaceM4/idefics2-8b](https://huggingface.co/HuggingFaceM4/idefics2-8b) on an unknown dataset.
## Model description
More information needed
## Intended uses & limitations
More information needed
## Training and evaluation data
More information needed
## Training procedure
### Training hyperparameters
The following hyperparameters were used during training:
- learning_rate: 0.0001
- train_batch_size: 2
- eval_batch_size: 8
- seed: 42
- gradient_accumulation_steps: 8
- total_train_batch_size: 16
- optimizer: Adam with betas=(0.9,0.999) and epsilon=1e-08
- lr_scheduler_type: linear
- lr_scheduler_warmup_steps: 50
- num_epochs: 2
- mixed_precision_training: Native AMP
### Training results
### Framework versions
- Transformers 4.41.0.dev0
- Pytorch 2.2.1+cu121
- Datasets 2.19.0
- Tokenizers 0.19.1
| {"license": "apache-2.0", "tags": ["generated_from_trainer"], "base_model": "HuggingFaceM4/idefics2-8b", "model-index": [{"name": "idefics2-8b-docvqa-finetuned-tutorial", "results": []}]} | GoHugo/idefics2-8b-docvqa-finetuned-tutorial | null | [
"safetensors",
"generated_from_trainer",
"base_model:HuggingFaceM4/idefics2-8b",
"license:apache-2.0",
"region:us"
] | null | 2024-04-26T18:33:43+00:00 | [] | [] | TAGS
#safetensors #generated_from_trainer #base_model-HuggingFaceM4/idefics2-8b #license-apache-2.0 #region-us
|
# idefics2-8b-docvqa-finetuned-tutorial
This model is a fine-tuned version of HuggingFaceM4/idefics2-8b on an unknown dataset.
## Model description
More information needed
## Intended uses & limitations
More information needed
## Training and evaluation data
More information needed
## Training procedure
### Training hyperparameters
The following hyperparameters were used during training:
- learning_rate: 0.0001
- train_batch_size: 2
- eval_batch_size: 8
- seed: 42
- gradient_accumulation_steps: 8
- total_train_batch_size: 16
- optimizer: Adam with betas=(0.9,0.999) and epsilon=1e-08
- lr_scheduler_type: linear
- lr_scheduler_warmup_steps: 50
- num_epochs: 2
- mixed_precision_training: Native AMP
### Training results
### Framework versions
- Transformers 4.41.0.dev0
- Pytorch 2.2.1+cu121
- Datasets 2.19.0
- Tokenizers 0.19.1
| [
"# idefics2-8b-docvqa-finetuned-tutorial\n\nThis model is a fine-tuned version of HuggingFaceM4/idefics2-8b on an unknown dataset.",
"## Model description\n\nMore information needed",
"## Intended uses & limitations\n\nMore information needed",
"## Training and evaluation data\n\nMore information needed",
"## Training procedure",
"### Training hyperparameters\n\nThe following hyperparameters were used during training:\n- learning_rate: 0.0001\n- train_batch_size: 2\n- eval_batch_size: 8\n- seed: 42\n- gradient_accumulation_steps: 8\n- total_train_batch_size: 16\n- optimizer: Adam with betas=(0.9,0.999) and epsilon=1e-08\n- lr_scheduler_type: linear\n- lr_scheduler_warmup_steps: 50\n- num_epochs: 2\n- mixed_precision_training: Native AMP",
"### Training results",
"### Framework versions\n\n- Transformers 4.41.0.dev0\n- Pytorch 2.2.1+cu121\n- Datasets 2.19.0\n- Tokenizers 0.19.1"
] | [
"TAGS\n#safetensors #generated_from_trainer #base_model-HuggingFaceM4/idefics2-8b #license-apache-2.0 #region-us \n",
"# idefics2-8b-docvqa-finetuned-tutorial\n\nThis model is a fine-tuned version of HuggingFaceM4/idefics2-8b on an unknown dataset.",
"## Model description\n\nMore information needed",
"## Intended uses & limitations\n\nMore information needed",
"## Training and evaluation data\n\nMore information needed",
"## Training procedure",
"### Training hyperparameters\n\nThe following hyperparameters were used during training:\n- learning_rate: 0.0001\n- train_batch_size: 2\n- eval_batch_size: 8\n- seed: 42\n- gradient_accumulation_steps: 8\n- total_train_batch_size: 16\n- optimizer: Adam with betas=(0.9,0.999) and epsilon=1e-08\n- lr_scheduler_type: linear\n- lr_scheduler_warmup_steps: 50\n- num_epochs: 2\n- mixed_precision_training: Native AMP",
"### Training results",
"### Framework versions\n\n- Transformers 4.41.0.dev0\n- Pytorch 2.2.1+cu121\n- Datasets 2.19.0\n- Tokenizers 0.19.1"
] |
text-classification | transformers |
<!-- This model card has been generated automatically according to the information the Trainer had access to. You
should probably proofread and complete it, then remove this comment. -->
# bert-finetuned-sem_eval-english
This model is a fine-tuned version of [bert-base-uncased](https://huggingface.co/bert-base-uncased) on an unknown dataset.
It achieves the following results on the evaluation set:
- Loss: nan
- F1: 0.0
- Roc Auc: 0.5
- Accuracy: 0.0158
## Model description
More information needed
## Intended uses & limitations
More information needed
## Training and evaluation data
More information needed
## Training procedure
### Training hyperparameters
The following hyperparameters were used during training:
- learning_rate: 2e-05
- train_batch_size: 8
- eval_batch_size: 8
- seed: 42
- optimizer: Adam with betas=(0.9,0.999) and epsilon=1e-08
- lr_scheduler_type: linear
- num_epochs: 1
### Training results
| Training Loss | Epoch | Step | Validation Loss | F1 | Roc Auc | Accuracy |
|:-------------:|:-----:|:----:|:---------------:|:---:|:-------:|:--------:|
| 0.3755 | 1.0 | 855 | nan | 0.0 | 0.5 | 0.0158 |
### Framework versions
- Transformers 4.41.0.dev0
- Pytorch 2.2.1+cu121
- Datasets 2.19.0
- Tokenizers 0.19.1
| {"license": "apache-2.0", "tags": ["generated_from_trainer"], "metrics": ["f1", "accuracy"], "base_model": "bert-base-uncased", "model-index": [{"name": "bert-finetuned-sem_eval-english", "results": []}]} | Kelvin950/bert-finetuned-sem_eval-english | null | [
"transformers",
"tensorboard",
"safetensors",
"bert",
"text-classification",
"generated_from_trainer",
"base_model:bert-base-uncased",
"license:apache-2.0",
"autotrain_compatible",
"endpoints_compatible",
"region:us"
] | null | 2024-04-26T18:34:24+00:00 | [] | [] | TAGS
#transformers #tensorboard #safetensors #bert #text-classification #generated_from_trainer #base_model-bert-base-uncased #license-apache-2.0 #autotrain_compatible #endpoints_compatible #region-us
| bert-finetuned-sem\_eval-english
================================
This model is a fine-tuned version of bert-base-uncased on an unknown dataset.
It achieves the following results on the evaluation set:
* Loss: nan
* F1: 0.0
* Roc Auc: 0.5
* Accuracy: 0.0158
Model description
-----------------
More information needed
Intended uses & limitations
---------------------------
More information needed
Training and evaluation data
----------------------------
More information needed
Training procedure
------------------
### Training hyperparameters
The following hyperparameters were used during training:
* learning\_rate: 2e-05
* train\_batch\_size: 8
* eval\_batch\_size: 8
* seed: 42
* optimizer: Adam with betas=(0.9,0.999) and epsilon=1e-08
* lr\_scheduler\_type: linear
* num\_epochs: 1
### Training results
### Framework versions
* Transformers 4.41.0.dev0
* Pytorch 2.2.1+cu121
* Datasets 2.19.0
* Tokenizers 0.19.1
| [
"### Training hyperparameters\n\n\nThe following hyperparameters were used during training:\n\n\n* learning\\_rate: 2e-05\n* train\\_batch\\_size: 8\n* eval\\_batch\\_size: 8\n* seed: 42\n* optimizer: Adam with betas=(0.9,0.999) and epsilon=1e-08\n* lr\\_scheduler\\_type: linear\n* num\\_epochs: 1",
"### Training results",
"### Framework versions\n\n\n* Transformers 4.41.0.dev0\n* Pytorch 2.2.1+cu121\n* Datasets 2.19.0\n* Tokenizers 0.19.1"
] | [
"TAGS\n#transformers #tensorboard #safetensors #bert #text-classification #generated_from_trainer #base_model-bert-base-uncased #license-apache-2.0 #autotrain_compatible #endpoints_compatible #region-us \n",
"### Training hyperparameters\n\n\nThe following hyperparameters were used during training:\n\n\n* learning\\_rate: 2e-05\n* train\\_batch\\_size: 8\n* eval\\_batch\\_size: 8\n* seed: 42\n* optimizer: Adam with betas=(0.9,0.999) and epsilon=1e-08\n* lr\\_scheduler\\_type: linear\n* num\\_epochs: 1",
"### Training results",
"### Framework versions\n\n\n* Transformers 4.41.0.dev0\n* Pytorch 2.2.1+cu121\n* Datasets 2.19.0\n* Tokenizers 0.19.1"
] |
text-generation | transformers |
<!-- This model card has been generated automatically according to the information the Trainer had access to. You
should probably proofread and complete it, then remove this comment. -->
# kaist-mistral-orpo-capybara-beta0.05-1epoch-ohp-15k-strat-1-beta0.2-2epoch
This model is a fine-tuned version of [orpo-explorers/kaist-mistral-orpo-capybara-beta-0.05-1epoch](https://huggingface.co/orpo-explorers/kaist-mistral-orpo-capybara-beta-0.05-1epoch) on the orpo-explorers/OHP-15k-Stratified-1 dataset.
## Model description
More information needed
## Intended uses & limitations
More information needed
## Training and evaluation data
More information needed
## Training procedure
### Training hyperparameters
The following hyperparameters were used during training:
- learning_rate: 5e-06
- train_batch_size: 8
- eval_batch_size: 8
- seed: 42
- distributed_type: multi-GPU
- num_devices: 4
- gradient_accumulation_steps: 2
- total_train_batch_size: 64
- total_eval_batch_size: 32
- optimizer: Adam with betas=(0.9,0.999) and epsilon=1e-08
- lr_scheduler_type: cosine
- num_epochs: 2
### Training results
### Framework versions
- Transformers 4.39.3
- Pytorch 2.1.2.post303
- Datasets 2.18.0
- Tokenizers 0.15.2
| {"tags": ["alignment-handbook", "trl", "orpo", "generated_from_trainer", "trl", "orpo", "generated_from_trainer"], "datasets": ["orpo-explorers/OHP-15k-Stratified-1"], "base_model": "orpo-explorers/kaist-mistral-orpo-capybara-beta-0.05-1epoch", "model-index": [{"name": "kaist-mistral-orpo-capybara-beta0.05-1epoch-ohp-15k-strat-1-beta0.2-2epoch", "results": []}]} | orpo-explorers/kaist-mistral-orpo-capybara-beta0.05-1epoch-ohp-15k-strat-1-beta0.2-2epoch | null | [
"transformers",
"tensorboard",
"safetensors",
"mistral",
"text-generation",
"alignment-handbook",
"trl",
"orpo",
"generated_from_trainer",
"conversational",
"dataset:orpo-explorers/OHP-15k-Stratified-1",
"base_model:orpo-explorers/kaist-mistral-orpo-capybara-beta-0.05-1epoch",
"autotrain_compatible",
"endpoints_compatible",
"text-generation-inference",
"region:us"
] | null | 2024-04-26T18:35:00+00:00 | [] | [] | TAGS
#transformers #tensorboard #safetensors #mistral #text-generation #alignment-handbook #trl #orpo #generated_from_trainer #conversational #dataset-orpo-explorers/OHP-15k-Stratified-1 #base_model-orpo-explorers/kaist-mistral-orpo-capybara-beta-0.05-1epoch #autotrain_compatible #endpoints_compatible #text-generation-inference #region-us
|
# kaist-mistral-orpo-capybara-beta0.05-1epoch-ohp-15k-strat-1-beta0.2-2epoch
This model is a fine-tuned version of orpo-explorers/kaist-mistral-orpo-capybara-beta-0.05-1epoch on the orpo-explorers/OHP-15k-Stratified-1 dataset.
## Model description
More information needed
## Intended uses & limitations
More information needed
## Training and evaluation data
More information needed
## Training procedure
### Training hyperparameters
The following hyperparameters were used during training:
- learning_rate: 5e-06
- train_batch_size: 8
- eval_batch_size: 8
- seed: 42
- distributed_type: multi-GPU
- num_devices: 4
- gradient_accumulation_steps: 2
- total_train_batch_size: 64
- total_eval_batch_size: 32
- optimizer: Adam with betas=(0.9,0.999) and epsilon=1e-08
- lr_scheduler_type: cosine
- num_epochs: 2
### Training results
### Framework versions
- Transformers 4.39.3
- Pytorch 2.1.2.post303
- Datasets 2.18.0
- Tokenizers 0.15.2
| [
"# kaist-mistral-orpo-capybara-beta0.05-1epoch-ohp-15k-strat-1-beta0.2-2epoch\n\nThis model is a fine-tuned version of orpo-explorers/kaist-mistral-orpo-capybara-beta-0.05-1epoch on the orpo-explorers/OHP-15k-Stratified-1 dataset.",
"## Model description\n\nMore information needed",
"## Intended uses & limitations\n\nMore information needed",
"## Training and evaluation data\n\nMore information needed",
"## Training procedure",
"### Training hyperparameters\n\nThe following hyperparameters were used during training:\n- learning_rate: 5e-06\n- train_batch_size: 8\n- eval_batch_size: 8\n- seed: 42\n- distributed_type: multi-GPU\n- num_devices: 4\n- gradient_accumulation_steps: 2\n- total_train_batch_size: 64\n- total_eval_batch_size: 32\n- optimizer: Adam with betas=(0.9,0.999) and epsilon=1e-08\n- lr_scheduler_type: cosine\n- num_epochs: 2",
"### Training results",
"### Framework versions\n\n- Transformers 4.39.3\n- Pytorch 2.1.2.post303\n- Datasets 2.18.0\n- Tokenizers 0.15.2"
] | [
"TAGS\n#transformers #tensorboard #safetensors #mistral #text-generation #alignment-handbook #trl #orpo #generated_from_trainer #conversational #dataset-orpo-explorers/OHP-15k-Stratified-1 #base_model-orpo-explorers/kaist-mistral-orpo-capybara-beta-0.05-1epoch #autotrain_compatible #endpoints_compatible #text-generation-inference #region-us \n",
"# kaist-mistral-orpo-capybara-beta0.05-1epoch-ohp-15k-strat-1-beta0.2-2epoch\n\nThis model is a fine-tuned version of orpo-explorers/kaist-mistral-orpo-capybara-beta-0.05-1epoch on the orpo-explorers/OHP-15k-Stratified-1 dataset.",
"## Model description\n\nMore information needed",
"## Intended uses & limitations\n\nMore information needed",
"## Training and evaluation data\n\nMore information needed",
"## Training procedure",
"### Training hyperparameters\n\nThe following hyperparameters were used during training:\n- learning_rate: 5e-06\n- train_batch_size: 8\n- eval_batch_size: 8\n- seed: 42\n- distributed_type: multi-GPU\n- num_devices: 4\n- gradient_accumulation_steps: 2\n- total_train_batch_size: 64\n- total_eval_batch_size: 32\n- optimizer: Adam with betas=(0.9,0.999) and epsilon=1e-08\n- lr_scheduler_type: cosine\n- num_epochs: 2",
"### Training results",
"### Framework versions\n\n- Transformers 4.39.3\n- Pytorch 2.1.2.post303\n- Datasets 2.18.0\n- Tokenizers 0.15.2"
] |
text-generation | transformers |
# Model Card for Model ID
Fine-tuning for CS5242 project
## Model Details
### Model Description
<!-- Provide a longer summary of what this model is. -->
- **Developed by:** [DreamOnRain]
- **Finetuned from model [optional]:** state-spaces/mamba-1.4b-hf
### Model Sources [optional]
<!-- Provide the basic links for the model. -->
- **Repository:** https://github.com/DreamOnRain/Deep-Learning-Final-Project
## Training Details
### Training Data
<!-- This should link to a Dataset Card, perhaps with a short stub of information on what the training data is all about as well as documentation related to data pre-processing or additional filtering. -->
https://huggingface.co/datasets/microsoft/orca-math-word-problems-200k
| {"library_name": "transformers", "tags": []} | DreamOnRain/mamba-1.4b-msmath | null | [
"transformers",
"safetensors",
"mamba",
"text-generation",
"autotrain_compatible",
"endpoints_compatible",
"region:us"
] | null | 2024-04-26T18:35:13+00:00 | [] | [] | TAGS
#transformers #safetensors #mamba #text-generation #autotrain_compatible #endpoints_compatible #region-us
|
# Model Card for Model ID
Fine-tuning for CS5242 project
## Model Details
### Model Description
- Developed by: [DreamOnRain]
- Finetuned from model [optional]: state-spaces/mamba-1.4b-hf
### Model Sources [optional]
- Repository: URL
## Training Details
### Training Data
URL
| [
"# Model Card for Model ID\n\nFine-tuning for CS5242 project",
"## Model Details",
"### Model Description\n\n\n\n- Developed by: [DreamOnRain]\n- Finetuned from model [optional]: state-spaces/mamba-1.4b-hf",
"### Model Sources [optional]\n\n\n\n- Repository: URL",
"## Training Details",
"### Training Data\n\n\n\nURL"
] | [
"TAGS\n#transformers #safetensors #mamba #text-generation #autotrain_compatible #endpoints_compatible #region-us \n",
"# Model Card for Model ID\n\nFine-tuning for CS5242 project",
"## Model Details",
"### Model Description\n\n\n\n- Developed by: [DreamOnRain]\n- Finetuned from model [optional]: state-spaces/mamba-1.4b-hf",
"### Model Sources [optional]\n\n\n\n- Repository: URL",
"## Training Details",
"### Training Data\n\n\n\nURL"
] |
text2text-generation | transformers |
<!-- This model card has been generated automatically according to the information the Trainer had access to. You
should probably proofread and complete it, then remove this comment. -->
# flan-t5-base-eLife
This model is a fine-tuned version of [google/flan-t5-base](https://huggingface.co/google/flan-t5-base) on an unknown dataset.
It achieves the following results on the evaluation set:
- Loss: 2.0533
- Rouge1: 16.8601
- Rouge2: 3.5043
- Rougel: 13.0262
- Rougelsum: 15.2504
- Gen Len: 19.0
## Model description
More information needed
## Intended uses & limitations
More information needed
## Training and evaluation data
More information needed
## Training procedure
### Training hyperparameters
The following hyperparameters were used during training:
- learning_rate: 5e-05
- train_batch_size: 8
- eval_batch_size: 8
- seed: 42
- optimizer: Adam with betas=(0.9,0.999) and epsilon=1e-08
- lr_scheduler_type: linear
- num_epochs: 5
### Training results
| Training Loss | Epoch | Step | Validation Loss | Rouge1 | Rouge2 | Rougel | Rougelsum | Gen Len |
|:-------------:|:-----:|:----:|:---------------:|:-------:|:------:|:-------:|:---------:|:-------:|
| 2.6071 | 1.0 | 544 | 2.2323 | 16.7606 | 3.2667 | 12.9074 | 15.1681 | 19.0 |
| 2.438 | 2.0 | 1088 | 2.1395 | 16.9106 | 3.3542 | 13.0126 | 15.3345 | 19.0 |
| 2.3649 | 3.0 | 1632 | 2.0900 | 16.9637 | 3.5315 | 13.0919 | 15.3446 | 19.0 |
| 2.3264 | 4.0 | 2176 | 2.0624 | 16.8183 | 3.4983 | 13.0296 | 15.226 | 19.0 |
| 2.292 | 5.0 | 2720 | 2.0533 | 16.8601 | 3.5043 | 13.0262 | 15.2504 | 19.0 |
### Framework versions
- Transformers 4.40.1
- Pytorch 2.1.2
- Datasets 2.19.0
- Tokenizers 0.19.1
| {"license": "apache-2.0", "tags": ["generated_from_trainer"], "metrics": ["rouge"], "base_model": "google/flan-t5-base", "model-index": [{"name": "flan-t5-base-eLife", "results": []}]} | Veera007/flan-t5-base-eLife | null | [
"transformers",
"tensorboard",
"safetensors",
"t5",
"text2text-generation",
"generated_from_trainer",
"base_model:google/flan-t5-base",
"license:apache-2.0",
"autotrain_compatible",
"endpoints_compatible",
"text-generation-inference",
"region:us"
] | null | 2024-04-26T18:38:03+00:00 | [] | [] | TAGS
#transformers #tensorboard #safetensors #t5 #text2text-generation #generated_from_trainer #base_model-google/flan-t5-base #license-apache-2.0 #autotrain_compatible #endpoints_compatible #text-generation-inference #region-us
| flan-t5-base-eLife
==================
This model is a fine-tuned version of google/flan-t5-base on an unknown dataset.
It achieves the following results on the evaluation set:
* Loss: 2.0533
* Rouge1: 16.8601
* Rouge2: 3.5043
* Rougel: 13.0262
* Rougelsum: 15.2504
* Gen Len: 19.0
Model description
-----------------
More information needed
Intended uses & limitations
---------------------------
More information needed
Training and evaluation data
----------------------------
More information needed
Training procedure
------------------
### Training hyperparameters
The following hyperparameters were used during training:
* learning\_rate: 5e-05
* train\_batch\_size: 8
* eval\_batch\_size: 8
* seed: 42
* optimizer: Adam with betas=(0.9,0.999) and epsilon=1e-08
* lr\_scheduler\_type: linear
* num\_epochs: 5
### Training results
### Framework versions
* Transformers 4.40.1
* Pytorch 2.1.2
* Datasets 2.19.0
* Tokenizers 0.19.1
| [
"### Training hyperparameters\n\n\nThe following hyperparameters were used during training:\n\n\n* learning\\_rate: 5e-05\n* train\\_batch\\_size: 8\n* eval\\_batch\\_size: 8\n* seed: 42\n* optimizer: Adam with betas=(0.9,0.999) and epsilon=1e-08\n* lr\\_scheduler\\_type: linear\n* num\\_epochs: 5",
"### Training results",
"### Framework versions\n\n\n* Transformers 4.40.1\n* Pytorch 2.1.2\n* Datasets 2.19.0\n* Tokenizers 0.19.1"
] | [
"TAGS\n#transformers #tensorboard #safetensors #t5 #text2text-generation #generated_from_trainer #base_model-google/flan-t5-base #license-apache-2.0 #autotrain_compatible #endpoints_compatible #text-generation-inference #region-us \n",
"### Training hyperparameters\n\n\nThe following hyperparameters were used during training:\n\n\n* learning\\_rate: 5e-05\n* train\\_batch\\_size: 8\n* eval\\_batch\\_size: 8\n* seed: 42\n* optimizer: Adam with betas=(0.9,0.999) and epsilon=1e-08\n* lr\\_scheduler\\_type: linear\n* num\\_epochs: 5",
"### Training results",
"### Framework versions\n\n\n* Transformers 4.40.1\n* Pytorch 2.1.2\n* Datasets 2.19.0\n* Tokenizers 0.19.1"
] |
null | peft |
<!-- This model card has been generated automatically according to the information the Trainer had access to. You
should probably proofread and complete it, then remove this comment. -->
# GUE_tf_3-seqsight_4096_512_27M-L1_f
This model is a fine-tuned version of [mahdibaghbanzadeh/seqsight_4096_512_27M](https://huggingface.co/mahdibaghbanzadeh/seqsight_4096_512_27M) on the [mahdibaghbanzadeh/GUE_tf_3](https://huggingface.co/datasets/mahdibaghbanzadeh/GUE_tf_3) dataset.
It achieves the following results on the evaluation set:
- Loss: 0.5534
- F1 Score: 0.7083
- Accuracy: 0.71
## Model description
More information needed
## Intended uses & limitations
More information needed
## Training and evaluation data
More information needed
## Training procedure
### Training hyperparameters
The following hyperparameters were used during training:
- learning_rate: 0.0005
- train_batch_size: 128
- eval_batch_size: 128
- seed: 42
- optimizer: Adam with betas=(0.9,0.999) and epsilon=1e-08
- lr_scheduler_type: linear
- training_steps: 10000
### Training results
| Training Loss | Epoch | Step | Validation Loss | F1 Score | Accuracy |
|:-------------:|:-----:|:-----:|:---------------:|:--------:|:--------:|
| 0.6336 | 0.93 | 200 | 0.5921 | 0.6805 | 0.681 |
| 0.6013 | 1.87 | 400 | 0.5761 | 0.6891 | 0.689 |
| 0.5929 | 2.8 | 600 | 0.5642 | 0.7022 | 0.705 |
| 0.5865 | 3.74 | 800 | 0.5626 | 0.7035 | 0.704 |
| 0.5814 | 4.67 | 1000 | 0.5584 | 0.7050 | 0.707 |
| 0.5801 | 5.61 | 1200 | 0.5565 | 0.7044 | 0.706 |
| 0.575 | 6.54 | 1400 | 0.5565 | 0.7026 | 0.703 |
| 0.5726 | 7.48 | 1600 | 0.5521 | 0.7022 | 0.703 |
| 0.5708 | 8.41 | 1800 | 0.5531 | 0.7006 | 0.701 |
| 0.5719 | 9.35 | 2000 | 0.5468 | 0.7022 | 0.704 |
| 0.5643 | 10.28 | 2200 | 0.5493 | 0.6981 | 0.698 |
| 0.5629 | 11.21 | 2400 | 0.5443 | 0.7081 | 0.71 |
| 0.5637 | 12.15 | 2600 | 0.5442 | 0.6938 | 0.699 |
| 0.558 | 13.08 | 2800 | 0.5411 | 0.7139 | 0.717 |
| 0.561 | 14.02 | 3000 | 0.5403 | 0.7059 | 0.707 |
| 0.5582 | 14.95 | 3200 | 0.5406 | 0.7025 | 0.705 |
| 0.5559 | 15.89 | 3400 | 0.5382 | 0.7041 | 0.705 |
| 0.5548 | 16.82 | 3600 | 0.5385 | 0.7087 | 0.71 |
| 0.5534 | 17.76 | 3800 | 0.5364 | 0.7042 | 0.706 |
| 0.5569 | 18.69 | 4000 | 0.5371 | 0.7166 | 0.717 |
| 0.5532 | 19.63 | 4200 | 0.5376 | 0.7093 | 0.714 |
| 0.5543 | 20.56 | 4400 | 0.5363 | 0.7097 | 0.712 |
| 0.5511 | 21.5 | 4600 | 0.5401 | 0.7211 | 0.721 |
| 0.5523 | 22.43 | 4800 | 0.5353 | 0.7164 | 0.719 |
| 0.5522 | 23.36 | 5000 | 0.5363 | 0.7219 | 0.722 |
| 0.5488 | 24.3 | 5200 | 0.5435 | 0.7154 | 0.716 |
| 0.5454 | 25.23 | 5400 | 0.5368 | 0.7144 | 0.718 |
| 0.5527 | 26.17 | 5600 | 0.5334 | 0.7199 | 0.721 |
| 0.5439 | 27.1 | 5800 | 0.5323 | 0.7198 | 0.721 |
| 0.5467 | 28.04 | 6000 | 0.5330 | 0.7171 | 0.718 |
| 0.5456 | 28.97 | 6200 | 0.5393 | 0.7221 | 0.722 |
| 0.5471 | 29.91 | 6400 | 0.5320 | 0.7210 | 0.722 |
| 0.5461 | 30.84 | 6600 | 0.5322 | 0.7242 | 0.725 |
| 0.5428 | 31.78 | 6800 | 0.5348 | 0.7229 | 0.723 |
| 0.5425 | 32.71 | 7000 | 0.5333 | 0.7175 | 0.718 |
| 0.5467 | 33.64 | 7200 | 0.5320 | 0.7204 | 0.722 |
| 0.5393 | 34.58 | 7400 | 0.5364 | 0.7200 | 0.72 |
| 0.545 | 35.51 | 7600 | 0.5313 | 0.7218 | 0.722 |
| 0.5428 | 36.45 | 7800 | 0.5325 | 0.7228 | 0.723 |
| 0.5445 | 37.38 | 8000 | 0.5310 | 0.7227 | 0.723 |
| 0.54 | 38.32 | 8200 | 0.5314 | 0.7244 | 0.726 |
| 0.5395 | 39.25 | 8400 | 0.5305 | 0.7248 | 0.726 |
| 0.5418 | 40.19 | 8600 | 0.5306 | 0.7264 | 0.727 |
| 0.5403 | 41.12 | 8800 | 0.5309 | 0.7233 | 0.724 |
| 0.5395 | 42.06 | 9000 | 0.5313 | 0.7224 | 0.723 |
| 0.5402 | 42.99 | 9200 | 0.5318 | 0.7160 | 0.716 |
| 0.5381 | 43.93 | 9400 | 0.5317 | 0.7179 | 0.718 |
| 0.5415 | 44.86 | 9600 | 0.5314 | 0.7187 | 0.719 |
| 0.5409 | 45.79 | 9800 | 0.5315 | 0.7235 | 0.724 |
| 0.5352 | 46.73 | 10000 | 0.5320 | 0.7197 | 0.72 |
### Framework versions
- PEFT 0.9.0
- Transformers 4.38.2
- Pytorch 2.2.0+cu121
- Datasets 2.17.1
- Tokenizers 0.15.2 | {"library_name": "peft", "tags": ["generated_from_trainer"], "metrics": ["accuracy"], "base_model": "mahdibaghbanzadeh/seqsight_4096_512_27M", "model-index": [{"name": "GUE_tf_3-seqsight_4096_512_27M-L1_f", "results": []}]} | mahdibaghbanzadeh/GUE_tf_3-seqsight_4096_512_27M-L1_f | null | [
"peft",
"safetensors",
"generated_from_trainer",
"base_model:mahdibaghbanzadeh/seqsight_4096_512_27M",
"region:us"
] | null | 2024-04-26T18:39:28+00:00 | [] | [] | TAGS
#peft #safetensors #generated_from_trainer #base_model-mahdibaghbanzadeh/seqsight_4096_512_27M #region-us
| GUE\_tf\_3-seqsight\_4096\_512\_27M-L1\_f
=========================================
This model is a fine-tuned version of mahdibaghbanzadeh/seqsight\_4096\_512\_27M on the mahdibaghbanzadeh/GUE\_tf\_3 dataset.
It achieves the following results on the evaluation set:
* Loss: 0.5534
* F1 Score: 0.7083
* Accuracy: 0.71
Model description
-----------------
More information needed
Intended uses & limitations
---------------------------
More information needed
Training and evaluation data
----------------------------
More information needed
Training procedure
------------------
### Training hyperparameters
The following hyperparameters were used during training:
* learning\_rate: 0.0005
* train\_batch\_size: 128
* eval\_batch\_size: 128
* seed: 42
* optimizer: Adam with betas=(0.9,0.999) and epsilon=1e-08
* lr\_scheduler\_type: linear
* training\_steps: 10000
### Training results
### Framework versions
* PEFT 0.9.0
* Transformers 4.38.2
* Pytorch 2.2.0+cu121
* Datasets 2.17.1
* Tokenizers 0.15.2
| [
"### Training hyperparameters\n\n\nThe following hyperparameters were used during training:\n\n\n* learning\\_rate: 0.0005\n* train\\_batch\\_size: 128\n* eval\\_batch\\_size: 128\n* seed: 42\n* optimizer: Adam with betas=(0.9,0.999) and epsilon=1e-08\n* lr\\_scheduler\\_type: linear\n* training\\_steps: 10000",
"### Training results",
"### Framework versions\n\n\n* PEFT 0.9.0\n* Transformers 4.38.2\n* Pytorch 2.2.0+cu121\n* Datasets 2.17.1\n* Tokenizers 0.15.2"
] | [
"TAGS\n#peft #safetensors #generated_from_trainer #base_model-mahdibaghbanzadeh/seqsight_4096_512_27M #region-us \n",
"### Training hyperparameters\n\n\nThe following hyperparameters were used during training:\n\n\n* learning\\_rate: 0.0005\n* train\\_batch\\_size: 128\n* eval\\_batch\\_size: 128\n* seed: 42\n* optimizer: Adam with betas=(0.9,0.999) and epsilon=1e-08\n* lr\\_scheduler\\_type: linear\n* training\\_steps: 10000",
"### Training results",
"### Framework versions\n\n\n* PEFT 0.9.0\n* Transformers 4.38.2\n* Pytorch 2.2.0+cu121\n* Datasets 2.17.1\n* Tokenizers 0.15.2"
] |
null | peft |
<!-- This model card has been generated automatically according to the information the Trainer had access to. You
should probably proofread and complete it, then remove this comment. -->
# GUE_tf_3-seqsight_4096_512_27M-L8_f
This model is a fine-tuned version of [mahdibaghbanzadeh/seqsight_4096_512_27M](https://huggingface.co/mahdibaghbanzadeh/seqsight_4096_512_27M) on the [mahdibaghbanzadeh/GUE_tf_3](https://huggingface.co/datasets/mahdibaghbanzadeh/GUE_tf_3) dataset.
It achieves the following results on the evaluation set:
- Loss: 0.5302
- F1 Score: 0.7378
- Accuracy: 0.739
## Model description
More information needed
## Intended uses & limitations
More information needed
## Training and evaluation data
More information needed
## Training procedure
### Training hyperparameters
The following hyperparameters were used during training:
- learning_rate: 0.0005
- train_batch_size: 128
- eval_batch_size: 128
- seed: 42
- optimizer: Adam with betas=(0.9,0.999) and epsilon=1e-08
- lr_scheduler_type: linear
- training_steps: 10000
### Training results
| Training Loss | Epoch | Step | Validation Loss | F1 Score | Accuracy |
|:-------------:|:-----:|:-----:|:---------------:|:--------:|:--------:|
| 0.6251 | 0.93 | 200 | 0.5772 | 0.6931 | 0.693 |
| 0.593 | 1.87 | 400 | 0.5740 | 0.6955 | 0.697 |
| 0.5829 | 2.8 | 600 | 0.5500 | 0.7100 | 0.711 |
| 0.5721 | 3.74 | 800 | 0.5487 | 0.7071 | 0.707 |
| 0.5667 | 4.67 | 1000 | 0.5424 | 0.7123 | 0.713 |
| 0.5654 | 5.61 | 1200 | 0.5387 | 0.7126 | 0.716 |
| 0.5586 | 6.54 | 1400 | 0.5389 | 0.7111 | 0.711 |
| 0.5558 | 7.48 | 1600 | 0.5340 | 0.7213 | 0.722 |
| 0.5544 | 8.41 | 1800 | 0.5401 | 0.7211 | 0.721 |
| 0.5538 | 9.35 | 2000 | 0.5311 | 0.7153 | 0.718 |
| 0.5452 | 10.28 | 2200 | 0.5298 | 0.7269 | 0.727 |
| 0.5437 | 11.21 | 2400 | 0.5304 | 0.7261 | 0.727 |
| 0.5423 | 12.15 | 2600 | 0.5341 | 0.7164 | 0.72 |
| 0.5357 | 13.08 | 2800 | 0.5379 | 0.7162 | 0.721 |
| 0.5387 | 14.02 | 3000 | 0.5327 | 0.7255 | 0.726 |
| 0.5341 | 14.95 | 3200 | 0.5263 | 0.7216 | 0.723 |
| 0.5322 | 15.89 | 3400 | 0.5244 | 0.7245 | 0.725 |
| 0.5296 | 16.82 | 3600 | 0.5294 | 0.7299 | 0.731 |
| 0.5268 | 17.76 | 3800 | 0.5279 | 0.7274 | 0.729 |
| 0.5276 | 18.69 | 4000 | 0.5259 | 0.7360 | 0.736 |
| 0.5254 | 19.63 | 4200 | 0.5299 | 0.7208 | 0.724 |
| 0.5235 | 20.56 | 4400 | 0.5268 | 0.7320 | 0.733 |
| 0.5218 | 21.5 | 4600 | 0.5341 | 0.7310 | 0.731 |
| 0.5192 | 22.43 | 4800 | 0.5278 | 0.7280 | 0.73 |
| 0.5193 | 23.36 | 5000 | 0.5368 | 0.7311 | 0.731 |
| 0.514 | 24.3 | 5200 | 0.5428 | 0.7221 | 0.723 |
| 0.513 | 25.23 | 5400 | 0.5324 | 0.7318 | 0.734 |
| 0.5177 | 26.17 | 5600 | 0.5264 | 0.7354 | 0.736 |
| 0.5096 | 27.1 | 5800 | 0.5274 | 0.7296 | 0.73 |
| 0.5098 | 28.04 | 6000 | 0.5282 | 0.7338 | 0.734 |
| 0.5094 | 28.97 | 6200 | 0.5376 | 0.7301 | 0.73 |
| 0.5096 | 29.91 | 6400 | 0.5286 | 0.7243 | 0.726 |
| 0.5067 | 30.84 | 6600 | 0.5260 | 0.7338 | 0.734 |
| 0.5037 | 31.78 | 6800 | 0.5354 | 0.7221 | 0.722 |
| 0.5001 | 32.71 | 7000 | 0.5312 | 0.734 | 0.734 |
| 0.5076 | 33.64 | 7200 | 0.5269 | 0.7299 | 0.73 |
| 0.4987 | 34.58 | 7400 | 0.5355 | 0.7236 | 0.724 |
| 0.5002 | 35.51 | 7600 | 0.5309 | 0.7291 | 0.729 |
| 0.5002 | 36.45 | 7800 | 0.5336 | 0.7310 | 0.731 |
| 0.5023 | 37.38 | 8000 | 0.5285 | 0.7339 | 0.734 |
| 0.4955 | 38.32 | 8200 | 0.5301 | 0.7377 | 0.738 |
| 0.4972 | 39.25 | 8400 | 0.5295 | 0.7368 | 0.737 |
| 0.4966 | 40.19 | 8600 | 0.5290 | 0.7340 | 0.734 |
| 0.4969 | 41.12 | 8800 | 0.5283 | 0.7357 | 0.736 |
| 0.496 | 42.06 | 9000 | 0.5307 | 0.7338 | 0.734 |
| 0.4939 | 42.99 | 9200 | 0.5331 | 0.7301 | 0.73 |
| 0.4927 | 43.93 | 9400 | 0.5319 | 0.7320 | 0.732 |
| 0.4988 | 44.86 | 9600 | 0.5312 | 0.7340 | 0.734 |
| 0.4964 | 45.79 | 9800 | 0.5309 | 0.7330 | 0.733 |
| 0.4872 | 46.73 | 10000 | 0.5321 | 0.7350 | 0.735 |
### Framework versions
- PEFT 0.9.0
- Transformers 4.38.2
- Pytorch 2.2.0+cu121
- Datasets 2.17.1
- Tokenizers 0.15.2 | {"library_name": "peft", "tags": ["generated_from_trainer"], "metrics": ["accuracy"], "base_model": "mahdibaghbanzadeh/seqsight_4096_512_27M", "model-index": [{"name": "GUE_tf_3-seqsight_4096_512_27M-L8_f", "results": []}]} | mahdibaghbanzadeh/GUE_tf_3-seqsight_4096_512_27M-L8_f | null | [
"peft",
"safetensors",
"generated_from_trainer",
"base_model:mahdibaghbanzadeh/seqsight_4096_512_27M",
"region:us"
] | null | 2024-04-26T18:40:00+00:00 | [] | [] | TAGS
#peft #safetensors #generated_from_trainer #base_model-mahdibaghbanzadeh/seqsight_4096_512_27M #region-us
| GUE\_tf\_3-seqsight\_4096\_512\_27M-L8\_f
=========================================
This model is a fine-tuned version of mahdibaghbanzadeh/seqsight\_4096\_512\_27M on the mahdibaghbanzadeh/GUE\_tf\_3 dataset.
It achieves the following results on the evaluation set:
* Loss: 0.5302
* F1 Score: 0.7378
* Accuracy: 0.739
Model description
-----------------
More information needed
Intended uses & limitations
---------------------------
More information needed
Training and evaluation data
----------------------------
More information needed
Training procedure
------------------
### Training hyperparameters
The following hyperparameters were used during training:
* learning\_rate: 0.0005
* train\_batch\_size: 128
* eval\_batch\_size: 128
* seed: 42
* optimizer: Adam with betas=(0.9,0.999) and epsilon=1e-08
* lr\_scheduler\_type: linear
* training\_steps: 10000
### Training results
### Framework versions
* PEFT 0.9.0
* Transformers 4.38.2
* Pytorch 2.2.0+cu121
* Datasets 2.17.1
* Tokenizers 0.15.2
| [
"### Training hyperparameters\n\n\nThe following hyperparameters were used during training:\n\n\n* learning\\_rate: 0.0005\n* train\\_batch\\_size: 128\n* eval\\_batch\\_size: 128\n* seed: 42\n* optimizer: Adam with betas=(0.9,0.999) and epsilon=1e-08\n* lr\\_scheduler\\_type: linear\n* training\\_steps: 10000",
"### Training results",
"### Framework versions\n\n\n* PEFT 0.9.0\n* Transformers 4.38.2\n* Pytorch 2.2.0+cu121\n* Datasets 2.17.1\n* Tokenizers 0.15.2"
] | [
"TAGS\n#peft #safetensors #generated_from_trainer #base_model-mahdibaghbanzadeh/seqsight_4096_512_27M #region-us \n",
"### Training hyperparameters\n\n\nThe following hyperparameters were used during training:\n\n\n* learning\\_rate: 0.0005\n* train\\_batch\\_size: 128\n* eval\\_batch\\_size: 128\n* seed: 42\n* optimizer: Adam with betas=(0.9,0.999) and epsilon=1e-08\n* lr\\_scheduler\\_type: linear\n* training\\_steps: 10000",
"### Training results",
"### Framework versions\n\n\n* PEFT 0.9.0\n* Transformers 4.38.2\n* Pytorch 2.2.0+cu121\n* Datasets 2.17.1\n* Tokenizers 0.15.2"
] |
null | peft |
<!-- This model card has been generated automatically according to the information the Trainer had access to. You
should probably proofread and complete it, then remove this comment. -->
# GUE_tf_3-seqsight_4096_512_27M-L32_f
This model is a fine-tuned version of [mahdibaghbanzadeh/seqsight_4096_512_27M](https://huggingface.co/mahdibaghbanzadeh/seqsight_4096_512_27M) on the [mahdibaghbanzadeh/GUE_tf_3](https://huggingface.co/datasets/mahdibaghbanzadeh/GUE_tf_3) dataset.
It achieves the following results on the evaluation set:
- Loss: 0.5757
- F1 Score: 0.7160
- Accuracy: 0.719
## Model description
More information needed
## Intended uses & limitations
More information needed
## Training and evaluation data
More information needed
## Training procedure
### Training hyperparameters
The following hyperparameters were used during training:
- learning_rate: 0.0005
- train_batch_size: 128
- eval_batch_size: 128
- seed: 42
- optimizer: Adam with betas=(0.9,0.999) and epsilon=1e-08
- lr_scheduler_type: linear
- training_steps: 10000
### Training results
| Training Loss | Epoch | Step | Validation Loss | F1 Score | Accuracy |
|:-------------:|:-----:|:-----:|:---------------:|:--------:|:--------:|
| 0.6196 | 0.93 | 200 | 0.5650 | 0.7042 | 0.705 |
| 0.5865 | 1.87 | 400 | 0.5609 | 0.7049 | 0.706 |
| 0.573 | 2.8 | 600 | 0.5429 | 0.7068 | 0.707 |
| 0.5621 | 3.74 | 800 | 0.5423 | 0.7150 | 0.715 |
| 0.5552 | 4.67 | 1000 | 0.5362 | 0.7208 | 0.723 |
| 0.5524 | 5.61 | 1200 | 0.5359 | 0.7134 | 0.719 |
| 0.5428 | 6.54 | 1400 | 0.5353 | 0.7211 | 0.721 |
| 0.5352 | 7.48 | 1600 | 0.5376 | 0.7290 | 0.73 |
| 0.5344 | 8.41 | 1800 | 0.5390 | 0.7201 | 0.72 |
| 0.5304 | 9.35 | 2000 | 0.5352 | 0.7218 | 0.724 |
| 0.5181 | 10.28 | 2200 | 0.5365 | 0.7169 | 0.717 |
| 0.5158 | 11.21 | 2400 | 0.5385 | 0.7257 | 0.726 |
| 0.51 | 12.15 | 2600 | 0.5345 | 0.7261 | 0.728 |
| 0.5019 | 13.08 | 2800 | 0.5461 | 0.7361 | 0.739 |
| 0.502 | 14.02 | 3000 | 0.5384 | 0.7325 | 0.733 |
| 0.4945 | 14.95 | 3200 | 0.5341 | 0.7346 | 0.735 |
| 0.4908 | 15.89 | 3400 | 0.5397 | 0.7349 | 0.735 |
| 0.4849 | 16.82 | 3600 | 0.5399 | 0.7374 | 0.738 |
| 0.4797 | 17.76 | 3800 | 0.5438 | 0.7325 | 0.733 |
| 0.4759 | 18.69 | 4000 | 0.5481 | 0.7241 | 0.724 |
| 0.4741 | 19.63 | 4200 | 0.5460 | 0.7314 | 0.732 |
| 0.4673 | 20.56 | 4400 | 0.5517 | 0.7390 | 0.739 |
| 0.465 | 21.5 | 4600 | 0.5651 | 0.7358 | 0.736 |
| 0.4579 | 22.43 | 4800 | 0.5588 | 0.7359 | 0.736 |
| 0.4585 | 23.36 | 5000 | 0.5697 | 0.7221 | 0.722 |
| 0.4462 | 24.3 | 5200 | 0.6016 | 0.7110 | 0.712 |
| 0.4458 | 25.23 | 5400 | 0.5820 | 0.7439 | 0.744 |
| 0.4461 | 26.17 | 5600 | 0.5598 | 0.7360 | 0.736 |
| 0.4355 | 27.1 | 5800 | 0.5835 | 0.7251 | 0.725 |
| 0.4345 | 28.04 | 6000 | 0.5700 | 0.7269 | 0.727 |
| 0.4324 | 28.97 | 6200 | 0.5870 | 0.7270 | 0.727 |
| 0.43 | 29.91 | 6400 | 0.5772 | 0.7340 | 0.734 |
| 0.4277 | 30.84 | 6600 | 0.5741 | 0.7281 | 0.728 |
| 0.4202 | 31.78 | 6800 | 0.5899 | 0.7172 | 0.718 |
| 0.417 | 32.71 | 7000 | 0.5907 | 0.7229 | 0.723 |
| 0.4214 | 33.64 | 7200 | 0.5796 | 0.7210 | 0.721 |
| 0.412 | 34.58 | 7400 | 0.6006 | 0.7141 | 0.715 |
| 0.4098 | 35.51 | 7600 | 0.6078 | 0.7123 | 0.713 |
| 0.4095 | 36.45 | 7800 | 0.6015 | 0.7279 | 0.728 |
| 0.4098 | 37.38 | 8000 | 0.5923 | 0.7240 | 0.724 |
| 0.4009 | 38.32 | 8200 | 0.5965 | 0.7290 | 0.729 |
| 0.4002 | 39.25 | 8400 | 0.6121 | 0.7187 | 0.719 |
| 0.3983 | 40.19 | 8600 | 0.6080 | 0.7085 | 0.709 |
| 0.4017 | 41.12 | 8800 | 0.6000 | 0.7290 | 0.729 |
| 0.3979 | 42.06 | 9000 | 0.5991 | 0.7249 | 0.725 |
| 0.3928 | 42.99 | 9200 | 0.6146 | 0.7145 | 0.715 |
| 0.3933 | 43.93 | 9400 | 0.6160 | 0.7116 | 0.712 |
| 0.3961 | 44.86 | 9600 | 0.6121 | 0.7198 | 0.72 |
| 0.3949 | 45.79 | 9800 | 0.6115 | 0.7209 | 0.721 |
| 0.3872 | 46.73 | 10000 | 0.6145 | 0.7228 | 0.723 |
### Framework versions
- PEFT 0.9.0
- Transformers 4.38.2
- Pytorch 2.2.0+cu121
- Datasets 2.17.1
- Tokenizers 0.15.2 | {"library_name": "peft", "tags": ["generated_from_trainer"], "metrics": ["accuracy"], "base_model": "mahdibaghbanzadeh/seqsight_4096_512_27M", "model-index": [{"name": "GUE_tf_3-seqsight_4096_512_27M-L32_f", "results": []}]} | mahdibaghbanzadeh/GUE_tf_3-seqsight_4096_512_27M-L32_f | null | [
"peft",
"safetensors",
"generated_from_trainer",
"base_model:mahdibaghbanzadeh/seqsight_4096_512_27M",
"region:us"
] | null | 2024-04-26T18:40:15+00:00 | [] | [] | TAGS
#peft #safetensors #generated_from_trainer #base_model-mahdibaghbanzadeh/seqsight_4096_512_27M #region-us
| GUE\_tf\_3-seqsight\_4096\_512\_27M-L32\_f
==========================================
This model is a fine-tuned version of mahdibaghbanzadeh/seqsight\_4096\_512\_27M on the mahdibaghbanzadeh/GUE\_tf\_3 dataset.
It achieves the following results on the evaluation set:
* Loss: 0.5757
* F1 Score: 0.7160
* Accuracy: 0.719
Model description
-----------------
More information needed
Intended uses & limitations
---------------------------
More information needed
Training and evaluation data
----------------------------
More information needed
Training procedure
------------------
### Training hyperparameters
The following hyperparameters were used during training:
* learning\_rate: 0.0005
* train\_batch\_size: 128
* eval\_batch\_size: 128
* seed: 42
* optimizer: Adam with betas=(0.9,0.999) and epsilon=1e-08
* lr\_scheduler\_type: linear
* training\_steps: 10000
### Training results
### Framework versions
* PEFT 0.9.0
* Transformers 4.38.2
* Pytorch 2.2.0+cu121
* Datasets 2.17.1
* Tokenizers 0.15.2
| [
"### Training hyperparameters\n\n\nThe following hyperparameters were used during training:\n\n\n* learning\\_rate: 0.0005\n* train\\_batch\\_size: 128\n* eval\\_batch\\_size: 128\n* seed: 42\n* optimizer: Adam with betas=(0.9,0.999) and epsilon=1e-08\n* lr\\_scheduler\\_type: linear\n* training\\_steps: 10000",
"### Training results",
"### Framework versions\n\n\n* PEFT 0.9.0\n* Transformers 4.38.2\n* Pytorch 2.2.0+cu121\n* Datasets 2.17.1\n* Tokenizers 0.15.2"
] | [
"TAGS\n#peft #safetensors #generated_from_trainer #base_model-mahdibaghbanzadeh/seqsight_4096_512_27M #region-us \n",
"### Training hyperparameters\n\n\nThe following hyperparameters were used during training:\n\n\n* learning\\_rate: 0.0005\n* train\\_batch\\_size: 128\n* eval\\_batch\\_size: 128\n* seed: 42\n* optimizer: Adam with betas=(0.9,0.999) and epsilon=1e-08\n* lr\\_scheduler\\_type: linear\n* training\\_steps: 10000",
"### Training results",
"### Framework versions\n\n\n* PEFT 0.9.0\n* Transformers 4.38.2\n* Pytorch 2.2.0+cu121\n* Datasets 2.17.1\n* Tokenizers 0.15.2"
] |
null | peft |
# Model Card for Model ID
<!-- Provide a quick summary of what the model is/does. -->
## Model Details
### Model Description
<!-- Provide a longer summary of what this model is. -->
- **Developed by:** [More Information Needed]
- **Funded by [optional]:** [More Information Needed]
- **Shared by [optional]:** [More Information Needed]
- **Model type:** [More Information Needed]
- **Language(s) (NLP):** [More Information Needed]
- **License:** [More Information Needed]
- **Finetuned from model [optional]:** [More Information Needed]
### Model Sources [optional]
<!-- Provide the basic links for the model. -->
- **Repository:** [More Information Needed]
- **Paper [optional]:** [More Information Needed]
- **Demo [optional]:** [More Information Needed]
## Uses
<!-- Address questions around how the model is intended to be used, including the foreseeable users of the model and those affected by the model. -->
### Direct Use
<!-- This section is for the model use without fine-tuning or plugging into a larger ecosystem/app. -->
[More Information Needed]
### Downstream Use [optional]
<!-- This section is for the model use when fine-tuned for a task, or when plugged into a larger ecosystem/app -->
[More Information Needed]
### Out-of-Scope Use
<!-- This section addresses misuse, malicious use, and uses that the model will not work well for. -->
[More Information Needed]
## Bias, Risks, and Limitations
<!-- This section is meant to convey both technical and sociotechnical limitations. -->
[More Information Needed]
### Recommendations
<!-- This section is meant to convey recommendations with respect to the bias, risk, and technical limitations. -->
Users (both direct and downstream) should be made aware of the risks, biases and limitations of the model. More information needed for further recommendations.
## How to Get Started with the Model
Use the code below to get started with the model.
[More Information Needed]
## Training Details
### Training Data
<!-- This should link to a Dataset Card, perhaps with a short stub of information on what the training data is all about as well as documentation related to data pre-processing or additional filtering. -->
[More Information Needed]
### Training Procedure
<!-- This relates heavily to the Technical Specifications. Content here should link to that section when it is relevant to the training procedure. -->
#### Preprocessing [optional]
[More Information Needed]
#### Training Hyperparameters
- **Training regime:** [More Information Needed] <!--fp32, fp16 mixed precision, bf16 mixed precision, bf16 non-mixed precision, fp16 non-mixed precision, fp8 mixed precision -->
#### Speeds, Sizes, Times [optional]
<!-- This section provides information about throughput, start/end time, checkpoint size if relevant, etc. -->
[More Information Needed]
## Evaluation
<!-- This section describes the evaluation protocols and provides the results. -->
### Testing Data, Factors & Metrics
#### Testing Data
<!-- This should link to a Dataset Card if possible. -->
[More Information Needed]
#### Factors
<!-- These are the things the evaluation is disaggregating by, e.g., subpopulations or domains. -->
[More Information Needed]
#### Metrics
<!-- These are the evaluation metrics being used, ideally with a description of why. -->
[More Information Needed]
### Results
[More Information Needed]
#### Summary
## Model Examination [optional]
<!-- Relevant interpretability work for the model goes here -->
[More Information Needed]
## Environmental Impact
<!-- Total emissions (in grams of CO2eq) and additional considerations, such as electricity usage, go here. Edit the suggested text below accordingly -->
Carbon emissions can be estimated using the [Machine Learning Impact calculator](https://mlco2.github.io/impact#compute) presented in [Lacoste et al. (2019)](https://arxiv.org/abs/1910.09700).
- **Hardware Type:** [More Information Needed]
- **Hours used:** [More Information Needed]
- **Cloud Provider:** [More Information Needed]
- **Compute Region:** [More Information Needed]
- **Carbon Emitted:** [More Information Needed]
## Technical Specifications [optional]
### Model Architecture and Objective
[More Information Needed]
### Compute Infrastructure
[More Information Needed]
#### Hardware
[More Information Needed]
#### Software
[More Information Needed]
## Citation [optional]
<!-- If there is a paper or blog post introducing the model, the APA and Bibtex information for that should go in this section. -->
**BibTeX:**
[More Information Needed]
**APA:**
[More Information Needed]
## Glossary [optional]
<!-- If relevant, include terms and calculations in this section that can help readers understand the model or model card. -->
[More Information Needed]
## More Information [optional]
[More Information Needed]
## Model Card Authors [optional]
[More Information Needed]
## Model Card Contact
[More Information Needed]
### Framework versions
- PEFT 0.7.2.dev0 | {"library_name": "peft", "base_model": "meta-llama/Meta-Llama-3-8B"} | yiyic/llama-text-entprop-lora-clf-epoch-2 | null | [
"peft",
"safetensors",
"arxiv:1910.09700",
"base_model:meta-llama/Meta-Llama-3-8B",
"region:us"
] | null | 2024-04-26T18:40:22+00:00 | [
"1910.09700"
] | [] | TAGS
#peft #safetensors #arxiv-1910.09700 #base_model-meta-llama/Meta-Llama-3-8B #region-us
|
# Model Card for Model ID
## Model Details
### Model Description
- Developed by:
- Funded by [optional]:
- Shared by [optional]:
- Model type:
- Language(s) (NLP):
- License:
- Finetuned from model [optional]:
### Model Sources [optional]
- Repository:
- Paper [optional]:
- Demo [optional]:
## Uses
### Direct Use
### Downstream Use [optional]
### Out-of-Scope Use
## Bias, Risks, and Limitations
### Recommendations
Users (both direct and downstream) should be made aware of the risks, biases and limitations of the model. More information needed for further recommendations.
## How to Get Started with the Model
Use the code below to get started with the model.
## Training Details
### Training Data
### Training Procedure
#### Preprocessing [optional]
#### Training Hyperparameters
- Training regime:
#### Speeds, Sizes, Times [optional]
## Evaluation
### Testing Data, Factors & Metrics
#### Testing Data
#### Factors
#### Metrics
### Results
#### Summary
## Model Examination [optional]
## Environmental Impact
Carbon emissions can be estimated using the Machine Learning Impact calculator presented in Lacoste et al. (2019).
- Hardware Type:
- Hours used:
- Cloud Provider:
- Compute Region:
- Carbon Emitted:
## Technical Specifications [optional]
### Model Architecture and Objective
### Compute Infrastructure
#### Hardware
#### Software
[optional]
BibTeX:
APA:
## Glossary [optional]
## More Information [optional]
## Model Card Authors [optional]
## Model Card Contact
### Framework versions
- PEFT 0.7.2.dev0 | [
"# Model Card for Model ID",
"## Model Details",
"### Model Description\n\n\n\n\n\n- Developed by: \n- Funded by [optional]: \n- Shared by [optional]: \n- Model type: \n- Language(s) (NLP): \n- License: \n- Finetuned from model [optional]:",
"### Model Sources [optional]\n\n\n\n- Repository: \n- Paper [optional]: \n- Demo [optional]:",
"## Uses",
"### Direct Use",
"### Downstream Use [optional]",
"### Out-of-Scope Use",
"## Bias, Risks, and Limitations",
"### Recommendations\n\n\n\nUsers (both direct and downstream) should be made aware of the risks, biases and limitations of the model. More information needed for further recommendations.",
"## How to Get Started with the Model\n\nUse the code below to get started with the model.",
"## Training Details",
"### Training Data",
"### Training Procedure",
"#### Preprocessing [optional]",
"#### Training Hyperparameters\n\n- Training regime:",
"#### Speeds, Sizes, Times [optional]",
"## Evaluation",
"### Testing Data, Factors & Metrics",
"#### Testing Data",
"#### Factors",
"#### Metrics",
"### Results",
"#### Summary",
"## Model Examination [optional]",
"## Environmental Impact\n\n\n\nCarbon emissions can be estimated using the Machine Learning Impact calculator presented in Lacoste et al. (2019).\n\n- Hardware Type: \n- Hours used: \n- Cloud Provider: \n- Compute Region: \n- Carbon Emitted:",
"## Technical Specifications [optional]",
"### Model Architecture and Objective",
"### Compute Infrastructure",
"#### Hardware",
"#### Software\n\n\n\n[optional]\n\n\n\nBibTeX:\n\n\n\nAPA:",
"## Glossary [optional]",
"## More Information [optional]",
"## Model Card Authors [optional]",
"## Model Card Contact",
"### Framework versions\n\n- PEFT 0.7.2.dev0"
] | [
"TAGS\n#peft #safetensors #arxiv-1910.09700 #base_model-meta-llama/Meta-Llama-3-8B #region-us \n",
"# Model Card for Model ID",
"## Model Details",
"### Model Description\n\n\n\n\n\n- Developed by: \n- Funded by [optional]: \n- Shared by [optional]: \n- Model type: \n- Language(s) (NLP): \n- License: \n- Finetuned from model [optional]:",
"### Model Sources [optional]\n\n\n\n- Repository: \n- Paper [optional]: \n- Demo [optional]:",
"## Uses",
"### Direct Use",
"### Downstream Use [optional]",
"### Out-of-Scope Use",
"## Bias, Risks, and Limitations",
"### Recommendations\n\n\n\nUsers (both direct and downstream) should be made aware of the risks, biases and limitations of the model. More information needed for further recommendations.",
"## How to Get Started with the Model\n\nUse the code below to get started with the model.",
"## Training Details",
"### Training Data",
"### Training Procedure",
"#### Preprocessing [optional]",
"#### Training Hyperparameters\n\n- Training regime:",
"#### Speeds, Sizes, Times [optional]",
"## Evaluation",
"### Testing Data, Factors & Metrics",
"#### Testing Data",
"#### Factors",
"#### Metrics",
"### Results",
"#### Summary",
"## Model Examination [optional]",
"## Environmental Impact\n\n\n\nCarbon emissions can be estimated using the Machine Learning Impact calculator presented in Lacoste et al. (2019).\n\n- Hardware Type: \n- Hours used: \n- Cloud Provider: \n- Compute Region: \n- Carbon Emitted:",
"## Technical Specifications [optional]",
"### Model Architecture and Objective",
"### Compute Infrastructure",
"#### Hardware",
"#### Software\n\n\n\n[optional]\n\n\n\nBibTeX:\n\n\n\nAPA:",
"## Glossary [optional]",
"## More Information [optional]",
"## Model Card Authors [optional]",
"## Model Card Contact",
"### Framework versions\n\n- PEFT 0.7.2.dev0"
] |
null | peft |
# Model Card for Model ID
<!-- Provide a quick summary of what the model is/does. -->
## Model Details
### Model Description
<!-- Provide a longer summary of what this model is. -->
- **Developed by:** [More Information Needed]
- **Funded by [optional]:** [More Information Needed]
- **Shared by [optional]:** [More Information Needed]
- **Model type:** [More Information Needed]
- **Language(s) (NLP):** [More Information Needed]
- **License:** [More Information Needed]
- **Finetuned from model [optional]:** [More Information Needed]
### Model Sources [optional]
<!-- Provide the basic links for the model. -->
- **Repository:** [More Information Needed]
- **Paper [optional]:** [More Information Needed]
- **Demo [optional]:** [More Information Needed]
## Uses
<!-- Address questions around how the model is intended to be used, including the foreseeable users of the model and those affected by the model. -->
### Direct Use
<!-- This section is for the model use without fine-tuning or plugging into a larger ecosystem/app. -->
[More Information Needed]
### Downstream Use [optional]
<!-- This section is for the model use when fine-tuned for a task, or when plugged into a larger ecosystem/app -->
[More Information Needed]
### Out-of-Scope Use
<!-- This section addresses misuse, malicious use, and uses that the model will not work well for. -->
[More Information Needed]
## Bias, Risks, and Limitations
<!-- This section is meant to convey both technical and sociotechnical limitations. -->
[More Information Needed]
### Recommendations
<!-- This section is meant to convey recommendations with respect to the bias, risk, and technical limitations. -->
Users (both direct and downstream) should be made aware of the risks, biases and limitations of the model. More information needed for further recommendations.
## How to Get Started with the Model
Use the code below to get started with the model.
[More Information Needed]
## Training Details
### Training Data
<!-- This should link to a Dataset Card, perhaps with a short stub of information on what the training data is all about as well as documentation related to data pre-processing or additional filtering. -->
[More Information Needed]
### Training Procedure
<!-- This relates heavily to the Technical Specifications. Content here should link to that section when it is relevant to the training procedure. -->
#### Preprocessing [optional]
[More Information Needed]
#### Training Hyperparameters
- **Training regime:** [More Information Needed] <!--fp32, fp16 mixed precision, bf16 mixed precision, bf16 non-mixed precision, fp16 non-mixed precision, fp8 mixed precision -->
#### Speeds, Sizes, Times [optional]
<!-- This section provides information about throughput, start/end time, checkpoint size if relevant, etc. -->
[More Information Needed]
## Evaluation
<!-- This section describes the evaluation protocols and provides the results. -->
### Testing Data, Factors & Metrics
#### Testing Data
<!-- This should link to a Dataset Card if possible. -->
[More Information Needed]
#### Factors
<!-- These are the things the evaluation is disaggregating by, e.g., subpopulations or domains. -->
[More Information Needed]
#### Metrics
<!-- These are the evaluation metrics being used, ideally with a description of why. -->
[More Information Needed]
### Results
[More Information Needed]
#### Summary
## Model Examination [optional]
<!-- Relevant interpretability work for the model goes here -->
[More Information Needed]
## Environmental Impact
<!-- Total emissions (in grams of CO2eq) and additional considerations, such as electricity usage, go here. Edit the suggested text below accordingly -->
Carbon emissions can be estimated using the [Machine Learning Impact calculator](https://mlco2.github.io/impact#compute) presented in [Lacoste et al. (2019)](https://arxiv.org/abs/1910.09700).
- **Hardware Type:** [More Information Needed]
- **Hours used:** [More Information Needed]
- **Cloud Provider:** [More Information Needed]
- **Compute Region:** [More Information Needed]
- **Carbon Emitted:** [More Information Needed]
## Technical Specifications [optional]
### Model Architecture and Objective
[More Information Needed]
### Compute Infrastructure
[More Information Needed]
#### Hardware
[More Information Needed]
#### Software
[More Information Needed]
## Citation [optional]
<!-- If there is a paper or blog post introducing the model, the APA and Bibtex information for that should go in this section. -->
**BibTeX:**
[More Information Needed]
**APA:**
[More Information Needed]
## Glossary [optional]
<!-- If relevant, include terms and calculations in this section that can help readers understand the model or model card. -->
[More Information Needed]
## More Information [optional]
[More Information Needed]
## Model Card Authors [optional]
[More Information Needed]
## Model Card Contact
[More Information Needed]
### Framework versions
- PEFT 0.7.2.dev0 | {"library_name": "peft", "base_model": "meta-llama/Meta-Llama-3-8B"} | yiyic/llama-text-prop-lora-clf-epoch-2 | null | [
"peft",
"safetensors",
"arxiv:1910.09700",
"base_model:meta-llama/Meta-Llama-3-8B",
"region:us"
] | null | 2024-04-26T18:40:42+00:00 | [
"1910.09700"
] | [] | TAGS
#peft #safetensors #arxiv-1910.09700 #base_model-meta-llama/Meta-Llama-3-8B #region-us
|
# Model Card for Model ID
## Model Details
### Model Description
- Developed by:
- Funded by [optional]:
- Shared by [optional]:
- Model type:
- Language(s) (NLP):
- License:
- Finetuned from model [optional]:
### Model Sources [optional]
- Repository:
- Paper [optional]:
- Demo [optional]:
## Uses
### Direct Use
### Downstream Use [optional]
### Out-of-Scope Use
## Bias, Risks, and Limitations
### Recommendations
Users (both direct and downstream) should be made aware of the risks, biases and limitations of the model. More information needed for further recommendations.
## How to Get Started with the Model
Use the code below to get started with the model.
## Training Details
### Training Data
### Training Procedure
#### Preprocessing [optional]
#### Training Hyperparameters
- Training regime:
#### Speeds, Sizes, Times [optional]
## Evaluation
### Testing Data, Factors & Metrics
#### Testing Data
#### Factors
#### Metrics
### Results
#### Summary
## Model Examination [optional]
## Environmental Impact
Carbon emissions can be estimated using the Machine Learning Impact calculator presented in Lacoste et al. (2019).
- Hardware Type:
- Hours used:
- Cloud Provider:
- Compute Region:
- Carbon Emitted:
## Technical Specifications [optional]
### Model Architecture and Objective
### Compute Infrastructure
#### Hardware
#### Software
[optional]
BibTeX:
APA:
## Glossary [optional]
## More Information [optional]
## Model Card Authors [optional]
## Model Card Contact
### Framework versions
- PEFT 0.7.2.dev0 | [
"# Model Card for Model ID",
"## Model Details",
"### Model Description\n\n\n\n\n\n- Developed by: \n- Funded by [optional]: \n- Shared by [optional]: \n- Model type: \n- Language(s) (NLP): \n- License: \n- Finetuned from model [optional]:",
"### Model Sources [optional]\n\n\n\n- Repository: \n- Paper [optional]: \n- Demo [optional]:",
"## Uses",
"### Direct Use",
"### Downstream Use [optional]",
"### Out-of-Scope Use",
"## Bias, Risks, and Limitations",
"### Recommendations\n\n\n\nUsers (both direct and downstream) should be made aware of the risks, biases and limitations of the model. More information needed for further recommendations.",
"## How to Get Started with the Model\n\nUse the code below to get started with the model.",
"## Training Details",
"### Training Data",
"### Training Procedure",
"#### Preprocessing [optional]",
"#### Training Hyperparameters\n\n- Training regime:",
"#### Speeds, Sizes, Times [optional]",
"## Evaluation",
"### Testing Data, Factors & Metrics",
"#### Testing Data",
"#### Factors",
"#### Metrics",
"### Results",
"#### Summary",
"## Model Examination [optional]",
"## Environmental Impact\n\n\n\nCarbon emissions can be estimated using the Machine Learning Impact calculator presented in Lacoste et al. (2019).\n\n- Hardware Type: \n- Hours used: \n- Cloud Provider: \n- Compute Region: \n- Carbon Emitted:",
"## Technical Specifications [optional]",
"### Model Architecture and Objective",
"### Compute Infrastructure",
"#### Hardware",
"#### Software\n\n\n\n[optional]\n\n\n\nBibTeX:\n\n\n\nAPA:",
"## Glossary [optional]",
"## More Information [optional]",
"## Model Card Authors [optional]",
"## Model Card Contact",
"### Framework versions\n\n- PEFT 0.7.2.dev0"
] | [
"TAGS\n#peft #safetensors #arxiv-1910.09700 #base_model-meta-llama/Meta-Llama-3-8B #region-us \n",
"# Model Card for Model ID",
"## Model Details",
"### Model Description\n\n\n\n\n\n- Developed by: \n- Funded by [optional]: \n- Shared by [optional]: \n- Model type: \n- Language(s) (NLP): \n- License: \n- Finetuned from model [optional]:",
"### Model Sources [optional]\n\n\n\n- Repository: \n- Paper [optional]: \n- Demo [optional]:",
"## Uses",
"### Direct Use",
"### Downstream Use [optional]",
"### Out-of-Scope Use",
"## Bias, Risks, and Limitations",
"### Recommendations\n\n\n\nUsers (both direct and downstream) should be made aware of the risks, biases and limitations of the model. More information needed for further recommendations.",
"## How to Get Started with the Model\n\nUse the code below to get started with the model.",
"## Training Details",
"### Training Data",
"### Training Procedure",
"#### Preprocessing [optional]",
"#### Training Hyperparameters\n\n- Training regime:",
"#### Speeds, Sizes, Times [optional]",
"## Evaluation",
"### Testing Data, Factors & Metrics",
"#### Testing Data",
"#### Factors",
"#### Metrics",
"### Results",
"#### Summary",
"## Model Examination [optional]",
"## Environmental Impact\n\n\n\nCarbon emissions can be estimated using the Machine Learning Impact calculator presented in Lacoste et al. (2019).\n\n- Hardware Type: \n- Hours used: \n- Cloud Provider: \n- Compute Region: \n- Carbon Emitted:",
"## Technical Specifications [optional]",
"### Model Architecture and Objective",
"### Compute Infrastructure",
"#### Hardware",
"#### Software\n\n\n\n[optional]\n\n\n\nBibTeX:\n\n\n\nAPA:",
"## Glossary [optional]",
"## More Information [optional]",
"## Model Card Authors [optional]",
"## Model Card Contact",
"### Framework versions\n\n- PEFT 0.7.2.dev0"
] |
null | peft |
# Model Card for Model ID
<!-- Provide a quick summary of what the model is/does. -->
## Model Details
### Model Description
<!-- Provide a longer summary of what this model is. -->
- **Developed by:** [More Information Needed]
- **Funded by [optional]:** [More Information Needed]
- **Shared by [optional]:** [More Information Needed]
- **Model type:** [More Information Needed]
- **Language(s) (NLP):** [More Information Needed]
- **License:** [More Information Needed]
- **Finetuned from model [optional]:** [More Information Needed]
### Model Sources [optional]
<!-- Provide the basic links for the model. -->
- **Repository:** [More Information Needed]
- **Paper [optional]:** [More Information Needed]
- **Demo [optional]:** [More Information Needed]
## Uses
<!-- Address questions around how the model is intended to be used, including the foreseeable users of the model and those affected by the model. -->
### Direct Use
<!-- This section is for the model use without fine-tuning or plugging into a larger ecosystem/app. -->
[More Information Needed]
### Downstream Use [optional]
<!-- This section is for the model use when fine-tuned for a task, or when plugged into a larger ecosystem/app -->
[More Information Needed]
### Out-of-Scope Use
<!-- This section addresses misuse, malicious use, and uses that the model will not work well for. -->
[More Information Needed]
## Bias, Risks, and Limitations
<!-- This section is meant to convey both technical and sociotechnical limitations. -->
[More Information Needed]
### Recommendations
<!-- This section is meant to convey recommendations with respect to the bias, risk, and technical limitations. -->
Users (both direct and downstream) should be made aware of the risks, biases and limitations of the model. More information needed for further recommendations.
## How to Get Started with the Model
Use the code below to get started with the model.
[More Information Needed]
## Training Details
### Training Data
<!-- This should link to a Dataset Card, perhaps with a short stub of information on what the training data is all about as well as documentation related to data pre-processing or additional filtering. -->
[More Information Needed]
### Training Procedure
<!-- This relates heavily to the Technical Specifications. Content here should link to that section when it is relevant to the training procedure. -->
#### Preprocessing [optional]
[More Information Needed]
#### Training Hyperparameters
- **Training regime:** [More Information Needed] <!--fp32, fp16 mixed precision, bf16 mixed precision, bf16 non-mixed precision, fp16 non-mixed precision, fp8 mixed precision -->
#### Speeds, Sizes, Times [optional]
<!-- This section provides information about throughput, start/end time, checkpoint size if relevant, etc. -->
[More Information Needed]
## Evaluation
<!-- This section describes the evaluation protocols and provides the results. -->
### Testing Data, Factors & Metrics
#### Testing Data
<!-- This should link to a Dataset Card if possible. -->
[More Information Needed]
#### Factors
<!-- These are the things the evaluation is disaggregating by, e.g., subpopulations or domains. -->
[More Information Needed]
#### Metrics
<!-- These are the evaluation metrics being used, ideally with a description of why. -->
[More Information Needed]
### Results
[More Information Needed]
#### Summary
## Model Examination [optional]
<!-- Relevant interpretability work for the model goes here -->
[More Information Needed]
## Environmental Impact
<!-- Total emissions (in grams of CO2eq) and additional considerations, such as electricity usage, go here. Edit the suggested text below accordingly -->
Carbon emissions can be estimated using the [Machine Learning Impact calculator](https://mlco2.github.io/impact#compute) presented in [Lacoste et al. (2019)](https://arxiv.org/abs/1910.09700).
- **Hardware Type:** [More Information Needed]
- **Hours used:** [More Information Needed]
- **Cloud Provider:** [More Information Needed]
- **Compute Region:** [More Information Needed]
- **Carbon Emitted:** [More Information Needed]
## Technical Specifications [optional]
### Model Architecture and Objective
[More Information Needed]
### Compute Infrastructure
[More Information Needed]
#### Hardware
[More Information Needed]
#### Software
[More Information Needed]
## Citation [optional]
<!-- If there is a paper or blog post introducing the model, the APA and Bibtex information for that should go in this section. -->
**BibTeX:**
[More Information Needed]
**APA:**
[More Information Needed]
## Glossary [optional]
<!-- If relevant, include terms and calculations in this section that can help readers understand the model or model card. -->
[More Information Needed]
## More Information [optional]
[More Information Needed]
## Model Card Authors [optional]
[More Information Needed]
## Model Card Contact
[More Information Needed]
### Framework versions
- PEFT 0.7.2.dev0 | {"library_name": "peft", "base_model": "meta-llama/Meta-Llama-3-8B"} | yiyic/llama-text-ent-lora-clf-epoch-2 | null | [
"peft",
"safetensors",
"arxiv:1910.09700",
"base_model:meta-llama/Meta-Llama-3-8B",
"region:us"
] | null | 2024-04-26T18:41:03+00:00 | [
"1910.09700"
] | [] | TAGS
#peft #safetensors #arxiv-1910.09700 #base_model-meta-llama/Meta-Llama-3-8B #region-us
|
# Model Card for Model ID
## Model Details
### Model Description
- Developed by:
- Funded by [optional]:
- Shared by [optional]:
- Model type:
- Language(s) (NLP):
- License:
- Finetuned from model [optional]:
### Model Sources [optional]
- Repository:
- Paper [optional]:
- Demo [optional]:
## Uses
### Direct Use
### Downstream Use [optional]
### Out-of-Scope Use
## Bias, Risks, and Limitations
### Recommendations
Users (both direct and downstream) should be made aware of the risks, biases and limitations of the model. More information needed for further recommendations.
## How to Get Started with the Model
Use the code below to get started with the model.
## Training Details
### Training Data
### Training Procedure
#### Preprocessing [optional]
#### Training Hyperparameters
- Training regime:
#### Speeds, Sizes, Times [optional]
## Evaluation
### Testing Data, Factors & Metrics
#### Testing Data
#### Factors
#### Metrics
### Results
#### Summary
## Model Examination [optional]
## Environmental Impact
Carbon emissions can be estimated using the Machine Learning Impact calculator presented in Lacoste et al. (2019).
- Hardware Type:
- Hours used:
- Cloud Provider:
- Compute Region:
- Carbon Emitted:
## Technical Specifications [optional]
### Model Architecture and Objective
### Compute Infrastructure
#### Hardware
#### Software
[optional]
BibTeX:
APA:
## Glossary [optional]
## More Information [optional]
## Model Card Authors [optional]
## Model Card Contact
### Framework versions
- PEFT 0.7.2.dev0 | [
"# Model Card for Model ID",
"## Model Details",
"### Model Description\n\n\n\n\n\n- Developed by: \n- Funded by [optional]: \n- Shared by [optional]: \n- Model type: \n- Language(s) (NLP): \n- License: \n- Finetuned from model [optional]:",
"### Model Sources [optional]\n\n\n\n- Repository: \n- Paper [optional]: \n- Demo [optional]:",
"## Uses",
"### Direct Use",
"### Downstream Use [optional]",
"### Out-of-Scope Use",
"## Bias, Risks, and Limitations",
"### Recommendations\n\n\n\nUsers (both direct and downstream) should be made aware of the risks, biases and limitations of the model. More information needed for further recommendations.",
"## How to Get Started with the Model\n\nUse the code below to get started with the model.",
"## Training Details",
"### Training Data",
"### Training Procedure",
"#### Preprocessing [optional]",
"#### Training Hyperparameters\n\n- Training regime:",
"#### Speeds, Sizes, Times [optional]",
"## Evaluation",
"### Testing Data, Factors & Metrics",
"#### Testing Data",
"#### Factors",
"#### Metrics",
"### Results",
"#### Summary",
"## Model Examination [optional]",
"## Environmental Impact\n\n\n\nCarbon emissions can be estimated using the Machine Learning Impact calculator presented in Lacoste et al. (2019).\n\n- Hardware Type: \n- Hours used: \n- Cloud Provider: \n- Compute Region: \n- Carbon Emitted:",
"## Technical Specifications [optional]",
"### Model Architecture and Objective",
"### Compute Infrastructure",
"#### Hardware",
"#### Software\n\n\n\n[optional]\n\n\n\nBibTeX:\n\n\n\nAPA:",
"## Glossary [optional]",
"## More Information [optional]",
"## Model Card Authors [optional]",
"## Model Card Contact",
"### Framework versions\n\n- PEFT 0.7.2.dev0"
] | [
"TAGS\n#peft #safetensors #arxiv-1910.09700 #base_model-meta-llama/Meta-Llama-3-8B #region-us \n",
"# Model Card for Model ID",
"## Model Details",
"### Model Description\n\n\n\n\n\n- Developed by: \n- Funded by [optional]: \n- Shared by [optional]: \n- Model type: \n- Language(s) (NLP): \n- License: \n- Finetuned from model [optional]:",
"### Model Sources [optional]\n\n\n\n- Repository: \n- Paper [optional]: \n- Demo [optional]:",
"## Uses",
"### Direct Use",
"### Downstream Use [optional]",
"### Out-of-Scope Use",
"## Bias, Risks, and Limitations",
"### Recommendations\n\n\n\nUsers (both direct and downstream) should be made aware of the risks, biases and limitations of the model. More information needed for further recommendations.",
"## How to Get Started with the Model\n\nUse the code below to get started with the model.",
"## Training Details",
"### Training Data",
"### Training Procedure",
"#### Preprocessing [optional]",
"#### Training Hyperparameters\n\n- Training regime:",
"#### Speeds, Sizes, Times [optional]",
"## Evaluation",
"### Testing Data, Factors & Metrics",
"#### Testing Data",
"#### Factors",
"#### Metrics",
"### Results",
"#### Summary",
"## Model Examination [optional]",
"## Environmental Impact\n\n\n\nCarbon emissions can be estimated using the Machine Learning Impact calculator presented in Lacoste et al. (2019).\n\n- Hardware Type: \n- Hours used: \n- Cloud Provider: \n- Compute Region: \n- Carbon Emitted:",
"## Technical Specifications [optional]",
"### Model Architecture and Objective",
"### Compute Infrastructure",
"#### Hardware",
"#### Software\n\n\n\n[optional]\n\n\n\nBibTeX:\n\n\n\nAPA:",
"## Glossary [optional]",
"## More Information [optional]",
"## Model Card Authors [optional]",
"## Model Card Contact",
"### Framework versions\n\n- PEFT 0.7.2.dev0"
] |
null | peft |
<!-- This model card has been generated automatically according to the information the Trainer had access to. You
should probably proofread and complete it, then remove this comment. -->
# GUE_tf_2-seqsight_4096_512_27M-L1_f
This model is a fine-tuned version of [mahdibaghbanzadeh/seqsight_4096_512_27M](https://huggingface.co/mahdibaghbanzadeh/seqsight_4096_512_27M) on the [mahdibaghbanzadeh/GUE_tf_2](https://huggingface.co/datasets/mahdibaghbanzadeh/GUE_tf_2) dataset.
It achieves the following results on the evaluation set:
- Loss: 0.4392
- F1 Score: 0.8006
- Accuracy: 0.801
## Model description
More information needed
## Intended uses & limitations
More information needed
## Training and evaluation data
More information needed
## Training procedure
### Training hyperparameters
The following hyperparameters were used during training:
- learning_rate: 0.0005
- train_batch_size: 128
- eval_batch_size: 128
- seed: 42
- optimizer: Adam with betas=(0.9,0.999) and epsilon=1e-08
- lr_scheduler_type: linear
- training_steps: 10000
### Training results
| Training Loss | Epoch | Step | Validation Loss | F1 Score | Accuracy |
|:-------------:|:-----:|:-----:|:---------------:|:--------:|:--------:|
| 0.5821 | 1.34 | 200 | 0.5406 | 0.7221 | 0.725 |
| 0.5367 | 2.68 | 400 | 0.5194 | 0.7460 | 0.746 |
| 0.5265 | 4.03 | 600 | 0.5139 | 0.7440 | 0.744 |
| 0.5204 | 5.37 | 800 | 0.5113 | 0.7479 | 0.748 |
| 0.5163 | 6.71 | 1000 | 0.5139 | 0.7416 | 0.742 |
| 0.5112 | 8.05 | 1200 | 0.5146 | 0.7416 | 0.742 |
| 0.5067 | 9.4 | 1400 | 0.5053 | 0.7459 | 0.746 |
| 0.5032 | 10.74 | 1600 | 0.5028 | 0.7439 | 0.744 |
| 0.501 | 12.08 | 1800 | 0.5067 | 0.7498 | 0.75 |
| 0.4987 | 13.42 | 2000 | 0.5001 | 0.7470 | 0.747 |
| 0.4958 | 14.77 | 2200 | 0.5031 | 0.7393 | 0.741 |
| 0.4901 | 16.11 | 2400 | 0.4992 | 0.7440 | 0.744 |
| 0.4937 | 17.45 | 2600 | 0.4976 | 0.7411 | 0.742 |
| 0.488 | 18.79 | 2800 | 0.4947 | 0.7458 | 0.746 |
| 0.4819 | 20.13 | 3000 | 0.4927 | 0.7410 | 0.741 |
| 0.4847 | 21.48 | 3200 | 0.4984 | 0.7468 | 0.747 |
| 0.4834 | 22.82 | 3400 | 0.4906 | 0.7450 | 0.745 |
| 0.4797 | 24.16 | 3600 | 0.4902 | 0.7470 | 0.747 |
| 0.475 | 25.5 | 3800 | 0.4900 | 0.7506 | 0.751 |
| 0.4811 | 26.85 | 4000 | 0.4882 | 0.7546 | 0.755 |
| 0.4781 | 28.19 | 4200 | 0.4883 | 0.7489 | 0.749 |
| 0.4742 | 29.53 | 4400 | 0.4902 | 0.7519 | 0.752 |
| 0.4739 | 30.87 | 4600 | 0.4887 | 0.7500 | 0.75 |
| 0.4754 | 32.21 | 4800 | 0.4897 | 0.7514 | 0.752 |
| 0.473 | 33.56 | 5000 | 0.4892 | 0.7479 | 0.748 |
| 0.4743 | 34.9 | 5200 | 0.4876 | 0.7550 | 0.755 |
| 0.4674 | 36.24 | 5400 | 0.4873 | 0.7486 | 0.749 |
| 0.4734 | 37.58 | 5600 | 0.4871 | 0.7510 | 0.751 |
| 0.4714 | 38.93 | 5800 | 0.4868 | 0.7486 | 0.749 |
| 0.4676 | 40.27 | 6000 | 0.4875 | 0.7500 | 0.751 |
| 0.466 | 41.61 | 6200 | 0.4867 | 0.7436 | 0.744 |
| 0.4669 | 42.95 | 6400 | 0.4867 | 0.7500 | 0.75 |
| 0.4633 | 44.3 | 6600 | 0.4883 | 0.7529 | 0.753 |
| 0.4674 | 45.64 | 6800 | 0.4870 | 0.7500 | 0.75 |
| 0.464 | 46.98 | 7000 | 0.4868 | 0.7556 | 0.756 |
| 0.4655 | 48.32 | 7200 | 0.4851 | 0.7569 | 0.757 |
| 0.4635 | 49.66 | 7400 | 0.4846 | 0.7577 | 0.758 |
| 0.4636 | 51.01 | 7600 | 0.4851 | 0.7588 | 0.759 |
| 0.4614 | 52.35 | 7800 | 0.4859 | 0.7616 | 0.762 |
| 0.464 | 53.69 | 8000 | 0.4863 | 0.7603 | 0.761 |
| 0.4598 | 55.03 | 8200 | 0.4852 | 0.7578 | 0.758 |
| 0.4636 | 56.38 | 8400 | 0.4859 | 0.7510 | 0.751 |
| 0.463 | 57.72 | 8600 | 0.4855 | 0.7556 | 0.756 |
| 0.4601 | 59.06 | 8800 | 0.4852 | 0.7549 | 0.755 |
| 0.4594 | 60.4 | 9000 | 0.4858 | 0.7530 | 0.753 |
| 0.4617 | 61.74 | 9200 | 0.4857 | 0.7548 | 0.755 |
| 0.4611 | 63.09 | 9400 | 0.4855 | 0.7529 | 0.753 |
| 0.4581 | 64.43 | 9600 | 0.4858 | 0.7558 | 0.756 |
| 0.4635 | 65.77 | 9800 | 0.4857 | 0.7499 | 0.75 |
| 0.4567 | 67.11 | 10000 | 0.4856 | 0.7548 | 0.755 |
### Framework versions
- PEFT 0.9.0
- Transformers 4.38.2
- Pytorch 2.2.0+cu121
- Datasets 2.17.1
- Tokenizers 0.15.2 | {"library_name": "peft", "tags": ["generated_from_trainer"], "metrics": ["accuracy"], "base_model": "mahdibaghbanzadeh/seqsight_4096_512_27M", "model-index": [{"name": "GUE_tf_2-seqsight_4096_512_27M-L1_f", "results": []}]} | mahdibaghbanzadeh/GUE_tf_2-seqsight_4096_512_27M-L1_f | null | [
"peft",
"safetensors",
"generated_from_trainer",
"base_model:mahdibaghbanzadeh/seqsight_4096_512_27M",
"region:us"
] | null | 2024-04-26T18:41:29+00:00 | [] | [] | TAGS
#peft #safetensors #generated_from_trainer #base_model-mahdibaghbanzadeh/seqsight_4096_512_27M #region-us
| GUE\_tf\_2-seqsight\_4096\_512\_27M-L1\_f
=========================================
This model is a fine-tuned version of mahdibaghbanzadeh/seqsight\_4096\_512\_27M on the mahdibaghbanzadeh/GUE\_tf\_2 dataset.
It achieves the following results on the evaluation set:
* Loss: 0.4392
* F1 Score: 0.8006
* Accuracy: 0.801
Model description
-----------------
More information needed
Intended uses & limitations
---------------------------
More information needed
Training and evaluation data
----------------------------
More information needed
Training procedure
------------------
### Training hyperparameters
The following hyperparameters were used during training:
* learning\_rate: 0.0005
* train\_batch\_size: 128
* eval\_batch\_size: 128
* seed: 42
* optimizer: Adam with betas=(0.9,0.999) and epsilon=1e-08
* lr\_scheduler\_type: linear
* training\_steps: 10000
### Training results
### Framework versions
* PEFT 0.9.0
* Transformers 4.38.2
* Pytorch 2.2.0+cu121
* Datasets 2.17.1
* Tokenizers 0.15.2
| [
"### Training hyperparameters\n\n\nThe following hyperparameters were used during training:\n\n\n* learning\\_rate: 0.0005\n* train\\_batch\\_size: 128\n* eval\\_batch\\_size: 128\n* seed: 42\n* optimizer: Adam with betas=(0.9,0.999) and epsilon=1e-08\n* lr\\_scheduler\\_type: linear\n* training\\_steps: 10000",
"### Training results",
"### Framework versions\n\n\n* PEFT 0.9.0\n* Transformers 4.38.2\n* Pytorch 2.2.0+cu121\n* Datasets 2.17.1\n* Tokenizers 0.15.2"
] | [
"TAGS\n#peft #safetensors #generated_from_trainer #base_model-mahdibaghbanzadeh/seqsight_4096_512_27M #region-us \n",
"### Training hyperparameters\n\n\nThe following hyperparameters were used during training:\n\n\n* learning\\_rate: 0.0005\n* train\\_batch\\_size: 128\n* eval\\_batch\\_size: 128\n* seed: 42\n* optimizer: Adam with betas=(0.9,0.999) and epsilon=1e-08\n* lr\\_scheduler\\_type: linear\n* training\\_steps: 10000",
"### Training results",
"### Framework versions\n\n\n* PEFT 0.9.0\n* Transformers 4.38.2\n* Pytorch 2.2.0+cu121\n* Datasets 2.17.1\n* Tokenizers 0.15.2"
] |
null | peft |
<!-- This model card has been generated automatically according to the information the Trainer had access to. You
should probably proofread and complete it, then remove this comment. -->
# GUE_tf_2-seqsight_4096_512_27M-L8_f
This model is a fine-tuned version of [mahdibaghbanzadeh/seqsight_4096_512_27M](https://huggingface.co/mahdibaghbanzadeh/seqsight_4096_512_27M) on the [mahdibaghbanzadeh/GUE_tf_2](https://huggingface.co/datasets/mahdibaghbanzadeh/GUE_tf_2) dataset.
It achieves the following results on the evaluation set:
- Loss: 0.4357
- F1 Score: 0.7959
- Accuracy: 0.796
## Model description
More information needed
## Intended uses & limitations
More information needed
## Training and evaluation data
More information needed
## Training procedure
### Training hyperparameters
The following hyperparameters were used during training:
- learning_rate: 0.0005
- train_batch_size: 128
- eval_batch_size: 128
- seed: 42
- optimizer: Adam with betas=(0.9,0.999) and epsilon=1e-08
- lr_scheduler_type: linear
- training_steps: 10000
### Training results
| Training Loss | Epoch | Step | Validation Loss | F1 Score | Accuracy |
|:-------------:|:-----:|:-----:|:---------------:|:--------:|:--------:|
| 0.5707 | 1.34 | 200 | 0.5330 | 0.7266 | 0.729 |
| 0.5253 | 2.68 | 400 | 0.5183 | 0.7458 | 0.746 |
| 0.512 | 4.03 | 600 | 0.5077 | 0.7490 | 0.749 |
| 0.5027 | 5.37 | 800 | 0.5052 | 0.7540 | 0.754 |
| 0.4982 | 6.71 | 1000 | 0.5058 | 0.7530 | 0.753 |
| 0.4888 | 8.05 | 1200 | 0.4978 | 0.7550 | 0.755 |
| 0.4837 | 9.4 | 1400 | 0.4945 | 0.7559 | 0.756 |
| 0.479 | 10.74 | 1600 | 0.4928 | 0.7519 | 0.752 |
| 0.4759 | 12.08 | 1800 | 0.4964 | 0.7549 | 0.755 |
| 0.4692 | 13.42 | 2000 | 0.4926 | 0.7630 | 0.763 |
| 0.4676 | 14.77 | 2200 | 0.4994 | 0.7447 | 0.747 |
| 0.4587 | 16.11 | 2400 | 0.4964 | 0.7550 | 0.755 |
| 0.4621 | 17.45 | 2600 | 0.4918 | 0.7457 | 0.747 |
| 0.4554 | 18.79 | 2800 | 0.4884 | 0.7522 | 0.753 |
| 0.4459 | 20.13 | 3000 | 0.4900 | 0.7570 | 0.757 |
| 0.4487 | 21.48 | 3200 | 0.4990 | 0.7565 | 0.757 |
| 0.4448 | 22.82 | 3400 | 0.4886 | 0.7580 | 0.758 |
| 0.4434 | 24.16 | 3600 | 0.4864 | 0.7679 | 0.768 |
| 0.435 | 25.5 | 3800 | 0.4936 | 0.7609 | 0.761 |
| 0.4399 | 26.85 | 4000 | 0.4857 | 0.7620 | 0.762 |
| 0.4354 | 28.19 | 4200 | 0.4952 | 0.7618 | 0.762 |
| 0.429 | 29.53 | 4400 | 0.5009 | 0.7517 | 0.752 |
| 0.4264 | 30.87 | 4600 | 0.4936 | 0.7500 | 0.75 |
| 0.4273 | 32.21 | 4800 | 0.4978 | 0.7520 | 0.752 |
| 0.4233 | 33.56 | 5000 | 0.5032 | 0.7550 | 0.755 |
| 0.4222 | 34.9 | 5200 | 0.5006 | 0.7529 | 0.753 |
| 0.4152 | 36.24 | 5400 | 0.4964 | 0.7630 | 0.763 |
| 0.4159 | 37.58 | 5600 | 0.5015 | 0.7510 | 0.751 |
| 0.414 | 38.93 | 5800 | 0.4974 | 0.752 | 0.752 |
| 0.4099 | 40.27 | 6000 | 0.4966 | 0.7578 | 0.758 |
| 0.407 | 41.61 | 6200 | 0.4997 | 0.7460 | 0.746 |
| 0.4078 | 42.95 | 6400 | 0.5021 | 0.7490 | 0.749 |
| 0.4023 | 44.3 | 6600 | 0.5054 | 0.7590 | 0.759 |
| 0.4079 | 45.64 | 6800 | 0.5091 | 0.7484 | 0.749 |
| 0.399 | 46.98 | 7000 | 0.5006 | 0.7610 | 0.761 |
| 0.4008 | 48.32 | 7200 | 0.4993 | 0.7560 | 0.756 |
| 0.3978 | 49.66 | 7400 | 0.5005 | 0.7560 | 0.756 |
| 0.3962 | 51.01 | 7600 | 0.5043 | 0.7469 | 0.747 |
| 0.391 | 52.35 | 7800 | 0.5045 | 0.7600 | 0.76 |
| 0.3931 | 53.69 | 8000 | 0.5006 | 0.7600 | 0.76 |
| 0.3885 | 55.03 | 8200 | 0.5087 | 0.7459 | 0.746 |
| 0.3941 | 56.38 | 8400 | 0.5069 | 0.7549 | 0.755 |
| 0.3884 | 57.72 | 8600 | 0.5053 | 0.7520 | 0.752 |
| 0.3872 | 59.06 | 8800 | 0.5092 | 0.7509 | 0.751 |
| 0.3869 | 60.4 | 9000 | 0.5072 | 0.7580 | 0.758 |
| 0.3861 | 61.74 | 9200 | 0.5101 | 0.7509 | 0.751 |
| 0.385 | 63.09 | 9400 | 0.5096 | 0.7520 | 0.752 |
| 0.3845 | 64.43 | 9600 | 0.5078 | 0.7560 | 0.756 |
| 0.3908 | 65.77 | 9800 | 0.5100 | 0.7509 | 0.751 |
| 0.3805 | 67.11 | 10000 | 0.5092 | 0.7560 | 0.756 |
### Framework versions
- PEFT 0.9.0
- Transformers 4.38.2
- Pytorch 2.2.0+cu121
- Datasets 2.17.1
- Tokenizers 0.15.2 | {"library_name": "peft", "tags": ["generated_from_trainer"], "metrics": ["accuracy"], "base_model": "mahdibaghbanzadeh/seqsight_4096_512_27M", "model-index": [{"name": "GUE_tf_2-seqsight_4096_512_27M-L8_f", "results": []}]} | mahdibaghbanzadeh/GUE_tf_2-seqsight_4096_512_27M-L8_f | null | [
"peft",
"safetensors",
"generated_from_trainer",
"base_model:mahdibaghbanzadeh/seqsight_4096_512_27M",
"region:us"
] | null | 2024-04-26T18:41:34+00:00 | [] | [] | TAGS
#peft #safetensors #generated_from_trainer #base_model-mahdibaghbanzadeh/seqsight_4096_512_27M #region-us
| GUE\_tf\_2-seqsight\_4096\_512\_27M-L8\_f
=========================================
This model is a fine-tuned version of mahdibaghbanzadeh/seqsight\_4096\_512\_27M on the mahdibaghbanzadeh/GUE\_tf\_2 dataset.
It achieves the following results on the evaluation set:
* Loss: 0.4357
* F1 Score: 0.7959
* Accuracy: 0.796
Model description
-----------------
More information needed
Intended uses & limitations
---------------------------
More information needed
Training and evaluation data
----------------------------
More information needed
Training procedure
------------------
### Training hyperparameters
The following hyperparameters were used during training:
* learning\_rate: 0.0005
* train\_batch\_size: 128
* eval\_batch\_size: 128
* seed: 42
* optimizer: Adam with betas=(0.9,0.999) and epsilon=1e-08
* lr\_scheduler\_type: linear
* training\_steps: 10000
### Training results
### Framework versions
* PEFT 0.9.0
* Transformers 4.38.2
* Pytorch 2.2.0+cu121
* Datasets 2.17.1
* Tokenizers 0.15.2
| [
"### Training hyperparameters\n\n\nThe following hyperparameters were used during training:\n\n\n* learning\\_rate: 0.0005\n* train\\_batch\\_size: 128\n* eval\\_batch\\_size: 128\n* seed: 42\n* optimizer: Adam with betas=(0.9,0.999) and epsilon=1e-08\n* lr\\_scheduler\\_type: linear\n* training\\_steps: 10000",
"### Training results",
"### Framework versions\n\n\n* PEFT 0.9.0\n* Transformers 4.38.2\n* Pytorch 2.2.0+cu121\n* Datasets 2.17.1\n* Tokenizers 0.15.2"
] | [
"TAGS\n#peft #safetensors #generated_from_trainer #base_model-mahdibaghbanzadeh/seqsight_4096_512_27M #region-us \n",
"### Training hyperparameters\n\n\nThe following hyperparameters were used during training:\n\n\n* learning\\_rate: 0.0005\n* train\\_batch\\_size: 128\n* eval\\_batch\\_size: 128\n* seed: 42\n* optimizer: Adam with betas=(0.9,0.999) and epsilon=1e-08\n* lr\\_scheduler\\_type: linear\n* training\\_steps: 10000",
"### Training results",
"### Framework versions\n\n\n* PEFT 0.9.0\n* Transformers 4.38.2\n* Pytorch 2.2.0+cu121\n* Datasets 2.17.1\n* Tokenizers 0.15.2"
] |
null | transformers |
# Model Card for Model ID
<!-- Provide a quick summary of what the model is/does. -->
## Model Details
### Model Description
<!-- Provide a longer summary of what this model is. -->
This is the model card of a 🤗 transformers model that has been pushed on the Hub. This model card has been automatically generated.
- **Developed by:** [More Information Needed]
- **Funded by [optional]:** [More Information Needed]
- **Shared by [optional]:** [More Information Needed]
- **Model type:** [More Information Needed]
- **Language(s) (NLP):** [More Information Needed]
- **License:** [More Information Needed]
- **Finetuned from model [optional]:** [More Information Needed]
### Model Sources [optional]
<!-- Provide the basic links for the model. -->
- **Repository:** [More Information Needed]
- **Paper [optional]:** [More Information Needed]
- **Demo [optional]:** [More Information Needed]
## Uses
<!-- Address questions around how the model is intended to be used, including the foreseeable users of the model and those affected by the model. -->
### Direct Use
<!-- This section is for the model use without fine-tuning or plugging into a larger ecosystem/app. -->
[More Information Needed]
### Downstream Use [optional]
<!-- This section is for the model use when fine-tuned for a task, or when plugged into a larger ecosystem/app -->
[More Information Needed]
### Out-of-Scope Use
<!-- This section addresses misuse, malicious use, and uses that the model will not work well for. -->
[More Information Needed]
## Bias, Risks, and Limitations
<!-- This section is meant to convey both technical and sociotechnical limitations. -->
[More Information Needed]
### Recommendations
<!-- This section is meant to convey recommendations with respect to the bias, risk, and technical limitations. -->
Users (both direct and downstream) should be made aware of the risks, biases and limitations of the model. More information needed for further recommendations.
## How to Get Started with the Model
Use the code below to get started with the model.
[More Information Needed]
## Training Details
### Training Data
<!-- This should link to a Dataset Card, perhaps with a short stub of information on what the training data is all about as well as documentation related to data pre-processing or additional filtering. -->
[More Information Needed]
### Training Procedure
<!-- This relates heavily to the Technical Specifications. Content here should link to that section when it is relevant to the training procedure. -->
#### Preprocessing [optional]
[More Information Needed]
#### Training Hyperparameters
- **Training regime:** [More Information Needed] <!--fp32, fp16 mixed precision, bf16 mixed precision, bf16 non-mixed precision, fp16 non-mixed precision, fp8 mixed precision -->
#### Speeds, Sizes, Times [optional]
<!-- This section provides information about throughput, start/end time, checkpoint size if relevant, etc. -->
[More Information Needed]
## Evaluation
<!-- This section describes the evaluation protocols and provides the results. -->
### Testing Data, Factors & Metrics
#### Testing Data
<!-- This should link to a Dataset Card if possible. -->
[More Information Needed]
#### Factors
<!-- These are the things the evaluation is disaggregating by, e.g., subpopulations or domains. -->
[More Information Needed]
#### Metrics
<!-- These are the evaluation metrics being used, ideally with a description of why. -->
[More Information Needed]
### Results
[More Information Needed]
#### Summary
## Model Examination [optional]
<!-- Relevant interpretability work for the model goes here -->
[More Information Needed]
## Environmental Impact
<!-- Total emissions (in grams of CO2eq) and additional considerations, such as electricity usage, go here. Edit the suggested text below accordingly -->
Carbon emissions can be estimated using the [Machine Learning Impact calculator](https://mlco2.github.io/impact#compute) presented in [Lacoste et al. (2019)](https://arxiv.org/abs/1910.09700).
- **Hardware Type:** [More Information Needed]
- **Hours used:** [More Information Needed]
- **Cloud Provider:** [More Information Needed]
- **Compute Region:** [More Information Needed]
- **Carbon Emitted:** [More Information Needed]
## Technical Specifications [optional]
### Model Architecture and Objective
[More Information Needed]
### Compute Infrastructure
[More Information Needed]
#### Hardware
[More Information Needed]
#### Software
[More Information Needed]
## Citation [optional]
<!-- If there is a paper or blog post introducing the model, the APA and Bibtex information for that should go in this section. -->
**BibTeX:**
[More Information Needed]
**APA:**
[More Information Needed]
## Glossary [optional]
<!-- If relevant, include terms and calculations in this section that can help readers understand the model or model card. -->
[More Information Needed]
## More Information [optional]
[More Information Needed]
## Model Card Authors [optional]
[More Information Needed]
## Model Card Contact
[More Information Needed] | {"library_name": "transformers", "tags": []} | la-min/GENI_GPT | null | [
"transformers",
"safetensors",
"arxiv:1910.09700",
"endpoints_compatible",
"region:us"
] | null | 2024-04-26T18:41:38+00:00 | [
"1910.09700"
] | [] | TAGS
#transformers #safetensors #arxiv-1910.09700 #endpoints_compatible #region-us
|
# Model Card for Model ID
## Model Details
### Model Description
This is the model card of a transformers model that has been pushed on the Hub. This model card has been automatically generated.
- Developed by:
- Funded by [optional]:
- Shared by [optional]:
- Model type:
- Language(s) (NLP):
- License:
- Finetuned from model [optional]:
### Model Sources [optional]
- Repository:
- Paper [optional]:
- Demo [optional]:
## Uses
### Direct Use
### Downstream Use [optional]
### Out-of-Scope Use
## Bias, Risks, and Limitations
### Recommendations
Users (both direct and downstream) should be made aware of the risks, biases and limitations of the model. More information needed for further recommendations.
## How to Get Started with the Model
Use the code below to get started with the model.
## Training Details
### Training Data
### Training Procedure
#### Preprocessing [optional]
#### Training Hyperparameters
- Training regime:
#### Speeds, Sizes, Times [optional]
## Evaluation
### Testing Data, Factors & Metrics
#### Testing Data
#### Factors
#### Metrics
### Results
#### Summary
## Model Examination [optional]
## Environmental Impact
Carbon emissions can be estimated using the Machine Learning Impact calculator presented in Lacoste et al. (2019).
- Hardware Type:
- Hours used:
- Cloud Provider:
- Compute Region:
- Carbon Emitted:
## Technical Specifications [optional]
### Model Architecture and Objective
### Compute Infrastructure
#### Hardware
#### Software
[optional]
BibTeX:
APA:
## Glossary [optional]
## More Information [optional]
## Model Card Authors [optional]
## Model Card Contact
| [
"# Model Card for Model ID",
"## Model Details",
"### Model Description\n\n\n\nThis is the model card of a transformers model that has been pushed on the Hub. This model card has been automatically generated.\n\n- Developed by: \n- Funded by [optional]: \n- Shared by [optional]: \n- Model type: \n- Language(s) (NLP): \n- License: \n- Finetuned from model [optional]:",
"### Model Sources [optional]\n\n\n\n- Repository: \n- Paper [optional]: \n- Demo [optional]:",
"## Uses",
"### Direct Use",
"### Downstream Use [optional]",
"### Out-of-Scope Use",
"## Bias, Risks, and Limitations",
"### Recommendations\n\n\n\nUsers (both direct and downstream) should be made aware of the risks, biases and limitations of the model. More information needed for further recommendations.",
"## How to Get Started with the Model\n\nUse the code below to get started with the model.",
"## Training Details",
"### Training Data",
"### Training Procedure",
"#### Preprocessing [optional]",
"#### Training Hyperparameters\n\n- Training regime:",
"#### Speeds, Sizes, Times [optional]",
"## Evaluation",
"### Testing Data, Factors & Metrics",
"#### Testing Data",
"#### Factors",
"#### Metrics",
"### Results",
"#### Summary",
"## Model Examination [optional]",
"## Environmental Impact\n\n\n\nCarbon emissions can be estimated using the Machine Learning Impact calculator presented in Lacoste et al. (2019).\n\n- Hardware Type: \n- Hours used: \n- Cloud Provider: \n- Compute Region: \n- Carbon Emitted:",
"## Technical Specifications [optional]",
"### Model Architecture and Objective",
"### Compute Infrastructure",
"#### Hardware",
"#### Software\n\n\n\n[optional]\n\n\n\nBibTeX:\n\n\n\nAPA:",
"## Glossary [optional]",
"## More Information [optional]",
"## Model Card Authors [optional]",
"## Model Card Contact"
] | [
"TAGS\n#transformers #safetensors #arxiv-1910.09700 #endpoints_compatible #region-us \n",
"# Model Card for Model ID",
"## Model Details",
"### Model Description\n\n\n\nThis is the model card of a transformers model that has been pushed on the Hub. This model card has been automatically generated.\n\n- Developed by: \n- Funded by [optional]: \n- Shared by [optional]: \n- Model type: \n- Language(s) (NLP): \n- License: \n- Finetuned from model [optional]:",
"### Model Sources [optional]\n\n\n\n- Repository: \n- Paper [optional]: \n- Demo [optional]:",
"## Uses",
"### Direct Use",
"### Downstream Use [optional]",
"### Out-of-Scope Use",
"## Bias, Risks, and Limitations",
"### Recommendations\n\n\n\nUsers (both direct and downstream) should be made aware of the risks, biases and limitations of the model. More information needed for further recommendations.",
"## How to Get Started with the Model\n\nUse the code below to get started with the model.",
"## Training Details",
"### Training Data",
"### Training Procedure",
"#### Preprocessing [optional]",
"#### Training Hyperparameters\n\n- Training regime:",
"#### Speeds, Sizes, Times [optional]",
"## Evaluation",
"### Testing Data, Factors & Metrics",
"#### Testing Data",
"#### Factors",
"#### Metrics",
"### Results",
"#### Summary",
"## Model Examination [optional]",
"## Environmental Impact\n\n\n\nCarbon emissions can be estimated using the Machine Learning Impact calculator presented in Lacoste et al. (2019).\n\n- Hardware Type: \n- Hours used: \n- Cloud Provider: \n- Compute Region: \n- Carbon Emitted:",
"## Technical Specifications [optional]",
"### Model Architecture and Objective",
"### Compute Infrastructure",
"#### Hardware",
"#### Software\n\n\n\n[optional]\n\n\n\nBibTeX:\n\n\n\nAPA:",
"## Glossary [optional]",
"## More Information [optional]",
"## Model Card Authors [optional]",
"## Model Card Contact"
] |
reinforcement-learning | stable-baselines3 |
# **DQN** Agent playing **SpaceInvadersNoFrameskip-v4**
This is a trained model of a **DQN** agent playing **SpaceInvadersNoFrameskip-v4**
using the [stable-baselines3 library](https://github.com/DLR-RM/stable-baselines3)
and the [RL Zoo](https://github.com/DLR-RM/rl-baselines3-zoo).
The RL Zoo is a training framework for Stable Baselines3
reinforcement learning agents,
with hyperparameter optimization and pre-trained agents included.
## Usage (with SB3 RL Zoo)
RL Zoo: https://github.com/DLR-RM/rl-baselines3-zoo<br/>
SB3: https://github.com/DLR-RM/stable-baselines3<br/>
SB3 Contrib: https://github.com/Stable-Baselines-Team/stable-baselines3-contrib
Install the RL Zoo (with SB3 and SB3-Contrib):
```bash
pip install rl_zoo3
```
```
# Download model and save it into the logs/ folder
python -m rl_zoo3.load_from_hub --algo dqn --env SpaceInvadersNoFrameskip-v4 -orga lexkarlo -f logs/
python -m rl_zoo3.enjoy --algo dqn --env SpaceInvadersNoFrameskip-v4 -f logs/
```
If you installed the RL Zoo3 via pip (`pip install rl_zoo3`), from anywhere you can do:
```
python -m rl_zoo3.load_from_hub --algo dqn --env SpaceInvadersNoFrameskip-v4 -orga lexkarlo -f logs/
python -m rl_zoo3.enjoy --algo dqn --env SpaceInvadersNoFrameskip-v4 -f logs/
```
## Training (with the RL Zoo)
```
python -m rl_zoo3.train --algo dqn --env SpaceInvadersNoFrameskip-v4 -f logs/
# Upload the model and generate video (when possible)
python -m rl_zoo3.push_to_hub --algo dqn --env SpaceInvadersNoFrameskip-v4 -f logs/ -orga lexkarlo
```
## Hyperparameters
```python
OrderedDict([('batch_size', 32),
('buffer_size', 100000),
('env_wrapper',
['stable_baselines3.common.atari_wrappers.AtariWrapper']),
('exploration_final_eps', 0.01),
('exploration_fraction', 0.1),
('frame_stack', 4),
('gradient_steps', 1),
('learning_rate', 0.001),
('learning_starts', 100000),
('n_timesteps', 1000000.0),
('optimize_memory_usage', False),
('policy', 'CnnPolicy'),
('target_update_interval', 1000),
('train_freq', 4),
('normalize', False)])
```
# Environment Arguments
```python
{'render_mode': 'rgb_array'}
```
| {"library_name": "stable-baselines3", "tags": ["SpaceInvadersNoFrameskip-v4", "deep-reinforcement-learning", "reinforcement-learning", "stable-baselines3"], "model-index": [{"name": "DQN", "results": [{"task": {"type": "reinforcement-learning", "name": "reinforcement-learning"}, "dataset": {"name": "SpaceInvadersNoFrameskip-v4", "type": "SpaceInvadersNoFrameskip-v4"}, "metrics": [{"type": "mean_reward", "value": "5.00 +/- 7.07", "name": "mean_reward", "verified": false}]}]}]} | lexkarlo/dqn-SpaceInvadersNoFrameskip-v4 | null | [
"stable-baselines3",
"SpaceInvadersNoFrameskip-v4",
"deep-reinforcement-learning",
"reinforcement-learning",
"model-index",
"region:us"
] | null | 2024-04-26T18:42:13+00:00 | [] | [] | TAGS
#stable-baselines3 #SpaceInvadersNoFrameskip-v4 #deep-reinforcement-learning #reinforcement-learning #model-index #region-us
|
# DQN Agent playing SpaceInvadersNoFrameskip-v4
This is a trained model of a DQN agent playing SpaceInvadersNoFrameskip-v4
using the stable-baselines3 library
and the RL Zoo.
The RL Zoo is a training framework for Stable Baselines3
reinforcement learning agents,
with hyperparameter optimization and pre-trained agents included.
## Usage (with SB3 RL Zoo)
RL Zoo: URL
SB3: URL
SB3 Contrib: URL
Install the RL Zoo (with SB3 and SB3-Contrib):
If you installed the RL Zoo3 via pip ('pip install rl_zoo3'), from anywhere you can do:
## Training (with the RL Zoo)
## Hyperparameters
# Environment Arguments
| [
"# DQN Agent playing SpaceInvadersNoFrameskip-v4\nThis is a trained model of a DQN agent playing SpaceInvadersNoFrameskip-v4\nusing the stable-baselines3 library\nand the RL Zoo.\n\nThe RL Zoo is a training framework for Stable Baselines3\nreinforcement learning agents,\nwith hyperparameter optimization and pre-trained agents included.",
"## Usage (with SB3 RL Zoo)\n\nRL Zoo: URL\nSB3: URL\nSB3 Contrib: URL\n\nInstall the RL Zoo (with SB3 and SB3-Contrib):\n\n\n\n\nIf you installed the RL Zoo3 via pip ('pip install rl_zoo3'), from anywhere you can do:",
"## Training (with the RL Zoo)",
"## Hyperparameters",
"# Environment Arguments"
] | [
"TAGS\n#stable-baselines3 #SpaceInvadersNoFrameskip-v4 #deep-reinforcement-learning #reinforcement-learning #model-index #region-us \n",
"# DQN Agent playing SpaceInvadersNoFrameskip-v4\nThis is a trained model of a DQN agent playing SpaceInvadersNoFrameskip-v4\nusing the stable-baselines3 library\nand the RL Zoo.\n\nThe RL Zoo is a training framework for Stable Baselines3\nreinforcement learning agents,\nwith hyperparameter optimization and pre-trained agents included.",
"## Usage (with SB3 RL Zoo)\n\nRL Zoo: URL\nSB3: URL\nSB3 Contrib: URL\n\nInstall the RL Zoo (with SB3 and SB3-Contrib):\n\n\n\n\nIf you installed the RL Zoo3 via pip ('pip install rl_zoo3'), from anywhere you can do:",
"## Training (with the RL Zoo)",
"## Hyperparameters",
"# Environment Arguments"
] |
null | peft |
<!-- This model card has been generated automatically according to the information the Trainer had access to. You
should probably proofread and complete it, then remove this comment. -->
# GUE_tf_2-seqsight_4096_512_27M-L32_f
This model is a fine-tuned version of [mahdibaghbanzadeh/seqsight_4096_512_27M](https://huggingface.co/mahdibaghbanzadeh/seqsight_4096_512_27M) on the [mahdibaghbanzadeh/GUE_tf_2](https://huggingface.co/datasets/mahdibaghbanzadeh/GUE_tf_2) dataset.
It achieves the following results on the evaluation set:
- Loss: 0.4479
- F1 Score: 0.7913
- Accuracy: 0.792
## Model description
More information needed
## Intended uses & limitations
More information needed
## Training and evaluation data
More information needed
## Training procedure
### Training hyperparameters
The following hyperparameters were used during training:
- learning_rate: 0.0005
- train_batch_size: 128
- eval_batch_size: 128
- seed: 42
- optimizer: Adam with betas=(0.9,0.999) and epsilon=1e-08
- lr_scheduler_type: linear
- training_steps: 10000
### Training results
| Training Loss | Epoch | Step | Validation Loss | F1 Score | Accuracy |
|:-------------:|:-----:|:-----:|:---------------:|:--------:|:--------:|
| 0.561 | 1.34 | 200 | 0.5303 | 0.7216 | 0.725 |
| 0.5174 | 2.68 | 400 | 0.5201 | 0.7501 | 0.751 |
| 0.5007 | 4.03 | 600 | 0.4997 | 0.7610 | 0.761 |
| 0.4871 | 5.37 | 800 | 0.4999 | 0.7539 | 0.754 |
| 0.4803 | 6.71 | 1000 | 0.5032 | 0.7420 | 0.742 |
| 0.4673 | 8.05 | 1200 | 0.4852 | 0.7667 | 0.767 |
| 0.4599 | 9.4 | 1400 | 0.4940 | 0.7529 | 0.753 |
| 0.4525 | 10.74 | 1600 | 0.4904 | 0.7490 | 0.749 |
| 0.4428 | 12.08 | 1800 | 0.4907 | 0.7529 | 0.753 |
| 0.4329 | 13.42 | 2000 | 0.4937 | 0.7527 | 0.753 |
| 0.426 | 14.77 | 2200 | 0.4967 | 0.7477 | 0.749 |
| 0.4137 | 16.11 | 2400 | 0.5107 | 0.7390 | 0.739 |
| 0.4084 | 17.45 | 2600 | 0.4973 | 0.7582 | 0.759 |
| 0.3989 | 18.79 | 2800 | 0.5102 | 0.7359 | 0.736 |
| 0.3863 | 20.13 | 3000 | 0.5156 | 0.7516 | 0.752 |
| 0.3817 | 21.48 | 3200 | 0.5158 | 0.7446 | 0.745 |
| 0.3705 | 22.82 | 3400 | 0.5132 | 0.7438 | 0.744 |
| 0.3643 | 24.16 | 3600 | 0.5233 | 0.7488 | 0.749 |
| 0.3526 | 25.5 | 3800 | 0.5269 | 0.7545 | 0.755 |
| 0.3515 | 26.85 | 4000 | 0.5271 | 0.7639 | 0.764 |
| 0.3426 | 28.19 | 4200 | 0.5628 | 0.7408 | 0.742 |
| 0.3305 | 29.53 | 4400 | 0.5738 | 0.7535 | 0.754 |
| 0.3265 | 30.87 | 4600 | 0.5591 | 0.7566 | 0.757 |
| 0.3221 | 32.21 | 4800 | 0.5647 | 0.7509 | 0.751 |
| 0.3149 | 33.56 | 5000 | 0.5816 | 0.7548 | 0.755 |
| 0.3075 | 34.9 | 5200 | 0.6102 | 0.7530 | 0.754 |
| 0.2991 | 36.24 | 5400 | 0.5645 | 0.7539 | 0.754 |
| 0.2929 | 37.58 | 5600 | 0.6136 | 0.7517 | 0.752 |
| 0.2917 | 38.93 | 5800 | 0.5835 | 0.7640 | 0.764 |
| 0.2858 | 40.27 | 6000 | 0.5987 | 0.7610 | 0.761 |
| 0.2842 | 41.61 | 6200 | 0.5826 | 0.7560 | 0.756 |
| 0.2688 | 42.95 | 6400 | 0.6329 | 0.7497 | 0.75 |
| 0.2669 | 44.3 | 6600 | 0.6385 | 0.7535 | 0.754 |
| 0.2722 | 45.64 | 6800 | 0.6404 | 0.7554 | 0.756 |
| 0.2603 | 46.98 | 7000 | 0.6424 | 0.7568 | 0.757 |
| 0.2593 | 48.32 | 7200 | 0.6285 | 0.7560 | 0.756 |
| 0.2535 | 49.66 | 7400 | 0.6507 | 0.7579 | 0.758 |
| 0.2545 | 51.01 | 7600 | 0.6478 | 0.7658 | 0.766 |
| 0.2445 | 52.35 | 7800 | 0.6550 | 0.7639 | 0.764 |
| 0.2448 | 53.69 | 8000 | 0.6592 | 0.7559 | 0.756 |
| 0.2394 | 55.03 | 8200 | 0.6715 | 0.7565 | 0.757 |
| 0.2388 | 56.38 | 8400 | 0.6830 | 0.7525 | 0.753 |
| 0.2339 | 57.72 | 8600 | 0.6734 | 0.7589 | 0.759 |
| 0.23 | 59.06 | 8800 | 0.6875 | 0.7586 | 0.759 |
| 0.2281 | 60.4 | 9000 | 0.6870 | 0.7619 | 0.762 |
| 0.2344 | 61.74 | 9200 | 0.6932 | 0.7596 | 0.76 |
| 0.2283 | 63.09 | 9400 | 0.7059 | 0.7576 | 0.758 |
| 0.2272 | 64.43 | 9600 | 0.6976 | 0.7537 | 0.754 |
| 0.226 | 65.77 | 9800 | 0.7031 | 0.7526 | 0.753 |
| 0.2232 | 67.11 | 10000 | 0.7025 | 0.7516 | 0.752 |
### Framework versions
- PEFT 0.9.0
- Transformers 4.38.2
- Pytorch 2.2.0+cu121
- Datasets 2.17.1
- Tokenizers 0.15.2 | {"library_name": "peft", "tags": ["generated_from_trainer"], "metrics": ["accuracy"], "base_model": "mahdibaghbanzadeh/seqsight_4096_512_27M", "model-index": [{"name": "GUE_tf_2-seqsight_4096_512_27M-L32_f", "results": []}]} | mahdibaghbanzadeh/GUE_tf_2-seqsight_4096_512_27M-L32_f | null | [
"peft",
"safetensors",
"generated_from_trainer",
"base_model:mahdibaghbanzadeh/seqsight_4096_512_27M",
"region:us"
] | null | 2024-04-26T18:42:18+00:00 | [] | [] | TAGS
#peft #safetensors #generated_from_trainer #base_model-mahdibaghbanzadeh/seqsight_4096_512_27M #region-us
| GUE\_tf\_2-seqsight\_4096\_512\_27M-L32\_f
==========================================
This model is a fine-tuned version of mahdibaghbanzadeh/seqsight\_4096\_512\_27M on the mahdibaghbanzadeh/GUE\_tf\_2 dataset.
It achieves the following results on the evaluation set:
* Loss: 0.4479
* F1 Score: 0.7913
* Accuracy: 0.792
Model description
-----------------
More information needed
Intended uses & limitations
---------------------------
More information needed
Training and evaluation data
----------------------------
More information needed
Training procedure
------------------
### Training hyperparameters
The following hyperparameters were used during training:
* learning\_rate: 0.0005
* train\_batch\_size: 128
* eval\_batch\_size: 128
* seed: 42
* optimizer: Adam with betas=(0.9,0.999) and epsilon=1e-08
* lr\_scheduler\_type: linear
* training\_steps: 10000
### Training results
### Framework versions
* PEFT 0.9.0
* Transformers 4.38.2
* Pytorch 2.2.0+cu121
* Datasets 2.17.1
* Tokenizers 0.15.2
| [
"### Training hyperparameters\n\n\nThe following hyperparameters were used during training:\n\n\n* learning\\_rate: 0.0005\n* train\\_batch\\_size: 128\n* eval\\_batch\\_size: 128\n* seed: 42\n* optimizer: Adam with betas=(0.9,0.999) and epsilon=1e-08\n* lr\\_scheduler\\_type: linear\n* training\\_steps: 10000",
"### Training results",
"### Framework versions\n\n\n* PEFT 0.9.0\n* Transformers 4.38.2\n* Pytorch 2.2.0+cu121\n* Datasets 2.17.1\n* Tokenizers 0.15.2"
] | [
"TAGS\n#peft #safetensors #generated_from_trainer #base_model-mahdibaghbanzadeh/seqsight_4096_512_27M #region-us \n",
"### Training hyperparameters\n\n\nThe following hyperparameters were used during training:\n\n\n* learning\\_rate: 0.0005\n* train\\_batch\\_size: 128\n* eval\\_batch\\_size: 128\n* seed: 42\n* optimizer: Adam with betas=(0.9,0.999) and epsilon=1e-08\n* lr\\_scheduler\\_type: linear\n* training\\_steps: 10000",
"### Training results",
"### Framework versions\n\n\n* PEFT 0.9.0\n* Transformers 4.38.2\n* Pytorch 2.2.0+cu121\n* Datasets 2.17.1\n* Tokenizers 0.15.2"
] |
null | null |

# Model Card for NeuralTranslate
<!-- Provide a quick summary of what the model is/does. -->
THIS MODEL USES CHATML TEMPLATE!! BE CAREFUL OR YOU MIGHT FIND UNEXPECTED BEHAVIOURS.
This is the second alpha version of NeuralTranslate. This alpha version doesn't contain overfitting (or at least that's what I think), so no unexpected behaviour should happen and Mistral's native reasoning capabilities aren't lost.
NeuralTranslate is an open-source family of models for bidirectional translation between English & Spanish, achieving high accuracy at fast speed.
You can donate towards this project at my ko-fi! https://ko-fi.com/irvingernesto
## Model Details
### Model Description
<!-- Provide a longer summary of what this model is. -->
- **Developed by:** [More Information Needed]
- **Funded by [optional]:** [More Information Needed]
- **Shared by [optional]:** [More Information Needed]
- **Model type:** [More Information Needed]
- **Language(s) (NLP):** [More Information Needed]
- **License:** [More Information Needed]
- **Finetuned from model [optional]:** [More Information Needed]
### Model Sources [optional]
<!-- Provide the basic links for the model. -->
- **Repository:** [More Information Needed]
- **Paper [optional]:** [More Information Needed]
- **Demo [optional]:** [More Information Needed]
## Uses
<!-- Address questions around how the model is intended to be used, including the foreseeable users of the model and those affected by the model. -->
### Direct Use
<!-- This section is for the model use without fine-tuning or plugging into a larger ecosystem/app. -->
[More Information Needed]
### Downstream Use [optional]
<!-- This section is for the model use when fine-tuned for a task, or when plugged into a larger ecosystem/app -->
[More Information Needed]
### Out-of-Scope Use
<!-- This section addresses misuse, malicious use, and uses that the model will not work well for. -->
[More Information Needed]
## Bias, Risks, and Limitations
<!-- This section is meant to convey both technical and sociotechnical limitations. -->
[More Information Needed]
### Recommendations
<!-- This section is meant to convey recommendations with respect to the bias, risk, and technical limitations. -->
Users (both direct and downstream) should be made aware of the risks, biases and limitations of the model. More information needed for further recommendations.
## How to Get Started with the Model
Use the code below to get started with the model.
[More Information Needed]
## Training Details
### Training Data
<!-- This should link to a Dataset Card, perhaps with a short stub of information on what the training data is all about as well as documentation related to data pre-processing or additional filtering. -->
[More Information Needed]
### Training Procedure
<!-- This relates heavily to the Technical Specifications. Content here should link to that section when it is relevant to the training procedure. -->
#### Preprocessing [optional]
[More Information Needed]
#### Training Hyperparameters
- **Training regime:** [More Information Needed] <!--fp32, fp16 mixed precision, bf16 mixed precision, bf16 non-mixed precision, fp16 non-mixed precision, fp8 mixed precision -->
#### Speeds, Sizes, Times [optional]
<!-- This section provides information about throughput, start/end time, checkpoint size if relevant, etc. -->
[More Information Needed]
## Evaluation
<!-- This section describes the evaluation protocols and provides the results. -->
### Testing Data, Factors & Metrics
#### Testing Data
<!-- This should link to a Dataset Card if possible. -->
[More Information Needed]
#### Factors
<!-- These are the things the evaluation is disaggregating by, e.g., subpopulations or domains. -->
[More Information Needed]
#### Metrics
<!-- These are the evaluation metrics being used, ideally with a description of why. -->
[More Information Needed]
### Results
[More Information Needed]
#### Summary
## Model Examination [optional]
<!-- Relevant interpretability work for the model goes here -->
[More Information Needed]
## Environmental Impact
<!-- Total emissions (in grams of CO2eq) and additional considerations, such as electricity usage, go here. Edit the suggested text below accordingly -->
Carbon emissions can be estimated using the [Machine Learning Impact calculator](https://mlco2.github.io/impact#compute) presented in [Lacoste et al. (2019)](https://arxiv.org/abs/1910.09700).
- **Hardware Type:** [More Information Needed]
- **Hours used:** [More Information Needed]
- **Cloud Provider:** [More Information Needed]
- **Compute Region:** [More Information Needed]
- **Carbon Emitted:** [More Information Needed]
## Technical Specifications [optional]
### Model Architecture and Objective
[More Information Needed]
### Compute Infrastructure
[More Information Needed]
#### Hardware
[More Information Needed]
#### Software
[More Information Needed]
## Citation [optional]
<!-- If there is a paper or blog post introducing the model, the APA and Bibtex information for that should go in this section. -->
**BibTeX:**
[More Information Needed]
**APA:**
[More Information Needed]
## Glossary [optional]
<!-- If relevant, include terms and calculations in this section that can help readers understand the model or model card. -->
[More Information Needed]
## More Information [optional]
[More Information Needed]
## Model Card Authors [optional]
[More Information Needed]
## Model Card Contact
[More Information Needed] | {"language": ["en", "es"], "license": "mit", "tags": ["Translation", "Mistral", "English", "Spanish"], "datasets": ["Thermostatic/ShareGPT_NeuralTranslate_v0.1"]} | Thermostatic/NeuralTranslate_v0.2_GGUF | null | [
"gguf",
"Translation",
"Mistral",
"English",
"Spanish",
"en",
"es",
"dataset:Thermostatic/ShareGPT_NeuralTranslate_v0.1",
"arxiv:1910.09700",
"license:mit",
"region:us"
] | null | 2024-04-26T18:42:53+00:00 | [
"1910.09700"
] | [
"en",
"es"
] | TAGS
#gguf #Translation #Mistral #English #Spanish #en #es #dataset-Thermostatic/ShareGPT_NeuralTranslate_v0.1 #arxiv-1910.09700 #license-mit #region-us
|
!image/png
# Model Card for NeuralTranslate
THIS MODEL USES CHATML TEMPLATE!! BE CAREFUL OR YOU MIGHT FIND UNEXPECTED BEHAVIOURS.
This is the second alpha version of NeuralTranslate. This alpha version doesn't contain overfitting (or at least that's what I think), so no unexpected behaviour should happen and Mistral's native reasoning capabilities aren't lost.
NeuralTranslate is an open-source family of models for bidirectional translation between English & Spanish, achieving high accuracy at fast speed.
You can donate towards this project at my ko-fi! URL
## Model Details
### Model Description
- Developed by:
- Funded by [optional]:
- Shared by [optional]:
- Model type:
- Language(s) (NLP):
- License:
- Finetuned from model [optional]:
### Model Sources [optional]
- Repository:
- Paper [optional]:
- Demo [optional]:
## Uses
### Direct Use
### Downstream Use [optional]
### Out-of-Scope Use
## Bias, Risks, and Limitations
### Recommendations
Users (both direct and downstream) should be made aware of the risks, biases and limitations of the model. More information needed for further recommendations.
## How to Get Started with the Model
Use the code below to get started with the model.
## Training Details
### Training Data
### Training Procedure
#### Preprocessing [optional]
#### Training Hyperparameters
- Training regime:
#### Speeds, Sizes, Times [optional]
## Evaluation
### Testing Data, Factors & Metrics
#### Testing Data
#### Factors
#### Metrics
### Results
#### Summary
## Model Examination [optional]
## Environmental Impact
Carbon emissions can be estimated using the Machine Learning Impact calculator presented in Lacoste et al. (2019).
- Hardware Type:
- Hours used:
- Cloud Provider:
- Compute Region:
- Carbon Emitted:
## Technical Specifications [optional]
### Model Architecture and Objective
### Compute Infrastructure
#### Hardware
#### Software
[optional]
BibTeX:
APA:
## Glossary [optional]
## More Information [optional]
## Model Card Authors [optional]
## Model Card Contact
| [
"# Model Card for NeuralTranslate\n\n\n\nTHIS MODEL USES CHATML TEMPLATE!! BE CAREFUL OR YOU MIGHT FIND UNEXPECTED BEHAVIOURS.\n\nThis is the second alpha version of NeuralTranslate. This alpha version doesn't contain overfitting (or at least that's what I think), so no unexpected behaviour should happen and Mistral's native reasoning capabilities aren't lost.\n\nNeuralTranslate is an open-source family of models for bidirectional translation between English & Spanish, achieving high accuracy at fast speed.\n\nYou can donate towards this project at my ko-fi! URL",
"## Model Details",
"### Model Description\n\n\n\n\n\n- Developed by: \n- Funded by [optional]: \n- Shared by [optional]: \n- Model type: \n- Language(s) (NLP): \n- License: \n- Finetuned from model [optional]:",
"### Model Sources [optional]\n\n\n\n- Repository: \n- Paper [optional]: \n- Demo [optional]:",
"## Uses",
"### Direct Use",
"### Downstream Use [optional]",
"### Out-of-Scope Use",
"## Bias, Risks, and Limitations",
"### Recommendations\n\n\n\nUsers (both direct and downstream) should be made aware of the risks, biases and limitations of the model. More information needed for further recommendations.",
"## How to Get Started with the Model\n\nUse the code below to get started with the model.",
"## Training Details",
"### Training Data",
"### Training Procedure",
"#### Preprocessing [optional]",
"#### Training Hyperparameters\n\n- Training regime:",
"#### Speeds, Sizes, Times [optional]",
"## Evaluation",
"### Testing Data, Factors & Metrics",
"#### Testing Data",
"#### Factors",
"#### Metrics",
"### Results",
"#### Summary",
"## Model Examination [optional]",
"## Environmental Impact\n\n\n\nCarbon emissions can be estimated using the Machine Learning Impact calculator presented in Lacoste et al. (2019).\n\n- Hardware Type: \n- Hours used: \n- Cloud Provider: \n- Compute Region: \n- Carbon Emitted:",
"## Technical Specifications [optional]",
"### Model Architecture and Objective",
"### Compute Infrastructure",
"#### Hardware",
"#### Software\n\n\n\n[optional]\n\n\n\nBibTeX:\n\n\n\nAPA:",
"## Glossary [optional]",
"## More Information [optional]",
"## Model Card Authors [optional]",
"## Model Card Contact"
] | [
"TAGS\n#gguf #Translation #Mistral #English #Spanish #en #es #dataset-Thermostatic/ShareGPT_NeuralTranslate_v0.1 #arxiv-1910.09700 #license-mit #region-us \n",
"# Model Card for NeuralTranslate\n\n\n\nTHIS MODEL USES CHATML TEMPLATE!! BE CAREFUL OR YOU MIGHT FIND UNEXPECTED BEHAVIOURS.\n\nThis is the second alpha version of NeuralTranslate. This alpha version doesn't contain overfitting (or at least that's what I think), so no unexpected behaviour should happen and Mistral's native reasoning capabilities aren't lost.\n\nNeuralTranslate is an open-source family of models for bidirectional translation between English & Spanish, achieving high accuracy at fast speed.\n\nYou can donate towards this project at my ko-fi! URL",
"## Model Details",
"### Model Description\n\n\n\n\n\n- Developed by: \n- Funded by [optional]: \n- Shared by [optional]: \n- Model type: \n- Language(s) (NLP): \n- License: \n- Finetuned from model [optional]:",
"### Model Sources [optional]\n\n\n\n- Repository: \n- Paper [optional]: \n- Demo [optional]:",
"## Uses",
"### Direct Use",
"### Downstream Use [optional]",
"### Out-of-Scope Use",
"## Bias, Risks, and Limitations",
"### Recommendations\n\n\n\nUsers (both direct and downstream) should be made aware of the risks, biases and limitations of the model. More information needed for further recommendations.",
"## How to Get Started with the Model\n\nUse the code below to get started with the model.",
"## Training Details",
"### Training Data",
"### Training Procedure",
"#### Preprocessing [optional]",
"#### Training Hyperparameters\n\n- Training regime:",
"#### Speeds, Sizes, Times [optional]",
"## Evaluation",
"### Testing Data, Factors & Metrics",
"#### Testing Data",
"#### Factors",
"#### Metrics",
"### Results",
"#### Summary",
"## Model Examination [optional]",
"## Environmental Impact\n\n\n\nCarbon emissions can be estimated using the Machine Learning Impact calculator presented in Lacoste et al. (2019).\n\n- Hardware Type: \n- Hours used: \n- Cloud Provider: \n- Compute Region: \n- Carbon Emitted:",
"## Technical Specifications [optional]",
"### Model Architecture and Objective",
"### Compute Infrastructure",
"#### Hardware",
"#### Software\n\n\n\n[optional]\n\n\n\nBibTeX:\n\n\n\nAPA:",
"## Glossary [optional]",
"## More Information [optional]",
"## Model Card Authors [optional]",
"## Model Card Contact"
] |
text-generation | transformers |
# Model Card for Model ID
<!-- Provide a quick summary of what the model is/does. -->
## Model Details
### Model Description
<!-- Provide a longer summary of what this model is. -->
This is the model card of a 🤗 transformers model that has been pushed on the Hub. This model card has been automatically generated.
- **Developed by:** [More Information Needed]
- **Funded by [optional]:** [More Information Needed]
- **Shared by [optional]:** [More Information Needed]
- **Model type:** [More Information Needed]
- **Language(s) (NLP):** [More Information Needed]
- **License:** [More Information Needed]
- **Finetuned from model [optional]:** [More Information Needed]
### Model Sources [optional]
<!-- Provide the basic links for the model. -->
- **Repository:** [More Information Needed]
- **Paper [optional]:** [More Information Needed]
- **Demo [optional]:** [More Information Needed]
## Uses
<!-- Address questions around how the model is intended to be used, including the foreseeable users of the model and those affected by the model. -->
### Direct Use
<!-- This section is for the model use without fine-tuning or plugging into a larger ecosystem/app. -->
[More Information Needed]
### Downstream Use [optional]
<!-- This section is for the model use when fine-tuned for a task, or when plugged into a larger ecosystem/app -->
[More Information Needed]
### Out-of-Scope Use
<!-- This section addresses misuse, malicious use, and uses that the model will not work well for. -->
[More Information Needed]
## Bias, Risks, and Limitations
<!-- This section is meant to convey both technical and sociotechnical limitations. -->
[More Information Needed]
### Recommendations
<!-- This section is meant to convey recommendations with respect to the bias, risk, and technical limitations. -->
Users (both direct and downstream) should be made aware of the risks, biases and limitations of the model. More information needed for further recommendations.
## How to Get Started with the Model
Use the code below to get started with the model.
[More Information Needed]
## Training Details
### Training Data
<!-- This should link to a Dataset Card, perhaps with a short stub of information on what the training data is all about as well as documentation related to data pre-processing or additional filtering. -->
[More Information Needed]
### Training Procedure
<!-- This relates heavily to the Technical Specifications. Content here should link to that section when it is relevant to the training procedure. -->
#### Preprocessing [optional]
[More Information Needed]
#### Training Hyperparameters
- **Training regime:** [More Information Needed] <!--fp32, fp16 mixed precision, bf16 mixed precision, bf16 non-mixed precision, fp16 non-mixed precision, fp8 mixed precision -->
#### Speeds, Sizes, Times [optional]
<!-- This section provides information about throughput, start/end time, checkpoint size if relevant, etc. -->
[More Information Needed]
## Evaluation
<!-- This section describes the evaluation protocols and provides the results. -->
### Testing Data, Factors & Metrics
#### Testing Data
<!-- This should link to a Dataset Card if possible. -->
[More Information Needed]
#### Factors
<!-- These are the things the evaluation is disaggregating by, e.g., subpopulations or domains. -->
[More Information Needed]
#### Metrics
<!-- These are the evaluation metrics being used, ideally with a description of why. -->
[More Information Needed]
### Results
[More Information Needed]
#### Summary
## Model Examination [optional]
<!-- Relevant interpretability work for the model goes here -->
[More Information Needed]
## Environmental Impact
<!-- Total emissions (in grams of CO2eq) and additional considerations, such as electricity usage, go here. Edit the suggested text below accordingly -->
Carbon emissions can be estimated using the [Machine Learning Impact calculator](https://mlco2.github.io/impact#compute) presented in [Lacoste et al. (2019)](https://arxiv.org/abs/1910.09700).
- **Hardware Type:** [More Information Needed]
- **Hours used:** [More Information Needed]
- **Cloud Provider:** [More Information Needed]
- **Compute Region:** [More Information Needed]
- **Carbon Emitted:** [More Information Needed]
## Technical Specifications [optional]
### Model Architecture and Objective
[More Information Needed]
### Compute Infrastructure
[More Information Needed]
#### Hardware
[More Information Needed]
#### Software
[More Information Needed]
## Citation [optional]
<!-- If there is a paper or blog post introducing the model, the APA and Bibtex information for that should go in this section. -->
**BibTeX:**
[More Information Needed]
**APA:**
[More Information Needed]
## Glossary [optional]
<!-- If relevant, include terms and calculations in this section that can help readers understand the model or model card. -->
[More Information Needed]
## More Information [optional]
[More Information Needed]
## Model Card Authors [optional]
[More Information Needed]
## Model Card Contact
[More Information Needed] | {"library_name": "transformers", "tags": []} | OwOOwO/final5 | null | [
"transformers",
"safetensors",
"stablelm",
"text-generation",
"conversational",
"arxiv:1910.09700",
"autotrain_compatible",
"endpoints_compatible",
"region:us"
] | null | 2024-04-26T18:43:01+00:00 | [
"1910.09700"
] | [] | TAGS
#transformers #safetensors #stablelm #text-generation #conversational #arxiv-1910.09700 #autotrain_compatible #endpoints_compatible #region-us
|
# Model Card for Model ID
## Model Details
### Model Description
This is the model card of a transformers model that has been pushed on the Hub. This model card has been automatically generated.
- Developed by:
- Funded by [optional]:
- Shared by [optional]:
- Model type:
- Language(s) (NLP):
- License:
- Finetuned from model [optional]:
### Model Sources [optional]
- Repository:
- Paper [optional]:
- Demo [optional]:
## Uses
### Direct Use
### Downstream Use [optional]
### Out-of-Scope Use
## Bias, Risks, and Limitations
### Recommendations
Users (both direct and downstream) should be made aware of the risks, biases and limitations of the model. More information needed for further recommendations.
## How to Get Started with the Model
Use the code below to get started with the model.
## Training Details
### Training Data
### Training Procedure
#### Preprocessing [optional]
#### Training Hyperparameters
- Training regime:
#### Speeds, Sizes, Times [optional]
## Evaluation
### Testing Data, Factors & Metrics
#### Testing Data
#### Factors
#### Metrics
### Results
#### Summary
## Model Examination [optional]
## Environmental Impact
Carbon emissions can be estimated using the Machine Learning Impact calculator presented in Lacoste et al. (2019).
- Hardware Type:
- Hours used:
- Cloud Provider:
- Compute Region:
- Carbon Emitted:
## Technical Specifications [optional]
### Model Architecture and Objective
### Compute Infrastructure
#### Hardware
#### Software
[optional]
BibTeX:
APA:
## Glossary [optional]
## More Information [optional]
## Model Card Authors [optional]
## Model Card Contact
| [
"# Model Card for Model ID",
"## Model Details",
"### Model Description\n\n\n\nThis is the model card of a transformers model that has been pushed on the Hub. This model card has been automatically generated.\n\n- Developed by: \n- Funded by [optional]: \n- Shared by [optional]: \n- Model type: \n- Language(s) (NLP): \n- License: \n- Finetuned from model [optional]:",
"### Model Sources [optional]\n\n\n\n- Repository: \n- Paper [optional]: \n- Demo [optional]:",
"## Uses",
"### Direct Use",
"### Downstream Use [optional]",
"### Out-of-Scope Use",
"## Bias, Risks, and Limitations",
"### Recommendations\n\n\n\nUsers (both direct and downstream) should be made aware of the risks, biases and limitations of the model. More information needed for further recommendations.",
"## How to Get Started with the Model\n\nUse the code below to get started with the model.",
"## Training Details",
"### Training Data",
"### Training Procedure",
"#### Preprocessing [optional]",
"#### Training Hyperparameters\n\n- Training regime:",
"#### Speeds, Sizes, Times [optional]",
"## Evaluation",
"### Testing Data, Factors & Metrics",
"#### Testing Data",
"#### Factors",
"#### Metrics",
"### Results",
"#### Summary",
"## Model Examination [optional]",
"## Environmental Impact\n\n\n\nCarbon emissions can be estimated using the Machine Learning Impact calculator presented in Lacoste et al. (2019).\n\n- Hardware Type: \n- Hours used: \n- Cloud Provider: \n- Compute Region: \n- Carbon Emitted:",
"## Technical Specifications [optional]",
"### Model Architecture and Objective",
"### Compute Infrastructure",
"#### Hardware",
"#### Software\n\n\n\n[optional]\n\n\n\nBibTeX:\n\n\n\nAPA:",
"## Glossary [optional]",
"## More Information [optional]",
"## Model Card Authors [optional]",
"## Model Card Contact"
] | [
"TAGS\n#transformers #safetensors #stablelm #text-generation #conversational #arxiv-1910.09700 #autotrain_compatible #endpoints_compatible #region-us \n",
"# Model Card for Model ID",
"## Model Details",
"### Model Description\n\n\n\nThis is the model card of a transformers model that has been pushed on the Hub. This model card has been automatically generated.\n\n- Developed by: \n- Funded by [optional]: \n- Shared by [optional]: \n- Model type: \n- Language(s) (NLP): \n- License: \n- Finetuned from model [optional]:",
"### Model Sources [optional]\n\n\n\n- Repository: \n- Paper [optional]: \n- Demo [optional]:",
"## Uses",
"### Direct Use",
"### Downstream Use [optional]",
"### Out-of-Scope Use",
"## Bias, Risks, and Limitations",
"### Recommendations\n\n\n\nUsers (both direct and downstream) should be made aware of the risks, biases and limitations of the model. More information needed for further recommendations.",
"## How to Get Started with the Model\n\nUse the code below to get started with the model.",
"## Training Details",
"### Training Data",
"### Training Procedure",
"#### Preprocessing [optional]",
"#### Training Hyperparameters\n\n- Training regime:",
"#### Speeds, Sizes, Times [optional]",
"## Evaluation",
"### Testing Data, Factors & Metrics",
"#### Testing Data",
"#### Factors",
"#### Metrics",
"### Results",
"#### Summary",
"## Model Examination [optional]",
"## Environmental Impact\n\n\n\nCarbon emissions can be estimated using the Machine Learning Impact calculator presented in Lacoste et al. (2019).\n\n- Hardware Type: \n- Hours used: \n- Cloud Provider: \n- Compute Region: \n- Carbon Emitted:",
"## Technical Specifications [optional]",
"### Model Architecture and Objective",
"### Compute Infrastructure",
"#### Hardware",
"#### Software\n\n\n\n[optional]\n\n\n\nBibTeX:\n\n\n\nAPA:",
"## Glossary [optional]",
"## More Information [optional]",
"## Model Card Authors [optional]",
"## Model Card Contact"
] |
null | peft |
<!-- This model card has been generated automatically according to the information the Trainer had access to. You
should probably proofread and complete it, then remove this comment. -->
# GUE_virus_covid-seqsight_4096_512_27M-L1_f
This model is a fine-tuned version of [mahdibaghbanzadeh/seqsight_4096_512_27M](https://huggingface.co/mahdibaghbanzadeh/seqsight_4096_512_27M) on the [mahdibaghbanzadeh/GUE_virus_covid](https://huggingface.co/datasets/mahdibaghbanzadeh/GUE_virus_covid) dataset.
It achieves the following results on the evaluation set:
- Loss: 1.6408
- F1 Score: 0.3935
- Accuracy: 0.3923
## Model description
More information needed
## Intended uses & limitations
More information needed
## Training and evaluation data
More information needed
## Training procedure
### Training hyperparameters
The following hyperparameters were used during training:
- learning_rate: 0.0005
- train_batch_size: 128
- eval_batch_size: 128
- seed: 42
- optimizer: Adam with betas=(0.9,0.999) and epsilon=1e-08
- lr_scheduler_type: linear
- training_steps: 10000
### Training results
| Training Loss | Epoch | Step | Validation Loss | F1 Score | Accuracy |
|:-------------:|:-----:|:-----:|:---------------:|:--------:|:--------:|
| 2.1836 | 0.35 | 200 | 2.1801 | 0.1012 | 0.1378 |
| 2.1775 | 0.7 | 400 | 2.1750 | 0.1074 | 0.1424 |
| 2.1694 | 1.05 | 600 | 2.1638 | 0.1367 | 0.1544 |
| 2.1606 | 1.4 | 800 | 2.1520 | 0.1337 | 0.1636 |
| 2.1496 | 1.75 | 1000 | 2.1364 | 0.1456 | 0.1713 |
| 2.1386 | 2.09 | 1200 | 2.1181 | 0.1589 | 0.1892 |
| 2.096 | 2.44 | 1400 | 2.0564 | 0.1944 | 0.2187 |
| 2.053 | 2.79 | 1600 | 1.9972 | 0.2292 | 0.2415 |
| 2.0123 | 3.14 | 1800 | 1.9539 | 0.2492 | 0.2612 |
| 1.9777 | 3.49 | 2000 | 1.9342 | 0.2634 | 0.2715 |
| 1.9685 | 3.84 | 2200 | 1.9020 | 0.2832 | 0.2866 |
| 1.9459 | 4.19 | 2400 | 1.8857 | 0.2898 | 0.2910 |
| 1.9202 | 4.54 | 2600 | 1.8480 | 0.3161 | 0.3203 |
| 1.9067 | 4.89 | 2800 | 1.8296 | 0.3132 | 0.3211 |
| 1.895 | 5.24 | 3000 | 1.8076 | 0.3192 | 0.3271 |
| 1.8707 | 5.58 | 3200 | 1.7859 | 0.3260 | 0.3338 |
| 1.8555 | 5.93 | 3400 | 1.7753 | 0.3308 | 0.3376 |
| 1.8492 | 6.28 | 3600 | 1.7598 | 0.3484 | 0.3480 |
| 1.8295 | 6.63 | 3800 | 1.7447 | 0.3328 | 0.3415 |
| 1.8239 | 6.98 | 4000 | 1.7393 | 0.3497 | 0.3516 |
| 1.821 | 7.33 | 4200 | 1.7290 | 0.3469 | 0.3503 |
| 1.81 | 7.68 | 4400 | 1.7146 | 0.3575 | 0.3562 |
| 1.8089 | 8.03 | 4600 | 1.7228 | 0.3579 | 0.3592 |
| 1.7879 | 8.38 | 4800 | 1.7043 | 0.3604 | 0.3623 |
| 1.795 | 8.73 | 5000 | 1.6983 | 0.3573 | 0.3636 |
| 1.7821 | 9.08 | 5200 | 1.6976 | 0.3726 | 0.3689 |
| 1.7854 | 9.42 | 5400 | 1.6917 | 0.3656 | 0.3701 |
| 1.7726 | 9.77 | 5600 | 1.6856 | 0.3660 | 0.3676 |
| 1.7711 | 10.12 | 5800 | 1.6805 | 0.3699 | 0.3670 |
| 1.7696 | 10.47 | 6000 | 1.6773 | 0.3752 | 0.3757 |
| 1.7641 | 10.82 | 6200 | 1.6848 | 0.3668 | 0.3682 |
| 1.7465 | 11.17 | 6400 | 1.6756 | 0.3742 | 0.3725 |
| 1.7533 | 11.52 | 6600 | 1.6664 | 0.3769 | 0.3782 |
| 1.7562 | 11.87 | 6800 | 1.6659 | 0.3765 | 0.3781 |
| 1.7506 | 12.22 | 7000 | 1.6653 | 0.3784 | 0.3790 |
| 1.7405 | 12.57 | 7200 | 1.6553 | 0.3828 | 0.3832 |
| 1.7489 | 12.91 | 7400 | 1.6570 | 0.3801 | 0.3836 |
| 1.7493 | 13.26 | 7600 | 1.6567 | 0.3734 | 0.3750 |
| 1.7369 | 13.61 | 7800 | 1.6556 | 0.3779 | 0.3794 |
| 1.7418 | 13.96 | 8000 | 1.6530 | 0.3861 | 0.3845 |
| 1.7367 | 14.31 | 8200 | 1.6469 | 0.3824 | 0.3839 |
| 1.7353 | 14.66 | 8400 | 1.6450 | 0.3858 | 0.3844 |
| 1.735 | 15.01 | 8600 | 1.6534 | 0.3769 | 0.3762 |
| 1.734 | 15.36 | 8800 | 1.6470 | 0.3892 | 0.3886 |
| 1.728 | 15.71 | 9000 | 1.6452 | 0.3856 | 0.3869 |
| 1.7274 | 16.06 | 9200 | 1.6431 | 0.3872 | 0.3875 |
| 1.7287 | 16.4 | 9400 | 1.6429 | 0.3866 | 0.3860 |
| 1.7292 | 16.75 | 9600 | 1.6443 | 0.3865 | 0.3864 |
| 1.731 | 17.1 | 9800 | 1.6426 | 0.3873 | 0.3884 |
| 1.7392 | 17.45 | 10000 | 1.6425 | 0.3868 | 0.3875 |
### Framework versions
- PEFT 0.9.0
- Transformers 4.38.2
- Pytorch 2.2.0+cu121
- Datasets 2.17.1
- Tokenizers 0.15.2 | {"library_name": "peft", "tags": ["generated_from_trainer"], "metrics": ["accuracy"], "base_model": "mahdibaghbanzadeh/seqsight_4096_512_27M", "model-index": [{"name": "GUE_virus_covid-seqsight_4096_512_27M-L1_f", "results": []}]} | mahdibaghbanzadeh/GUE_virus_covid-seqsight_4096_512_27M-L1_f | null | [
"peft",
"safetensors",
"generated_from_trainer",
"base_model:mahdibaghbanzadeh/seqsight_4096_512_27M",
"region:us"
] | null | 2024-04-26T18:44:31+00:00 | [] | [] | TAGS
#peft #safetensors #generated_from_trainer #base_model-mahdibaghbanzadeh/seqsight_4096_512_27M #region-us
| GUE\_virus\_covid-seqsight\_4096\_512\_27M-L1\_f
================================================
This model is a fine-tuned version of mahdibaghbanzadeh/seqsight\_4096\_512\_27M on the mahdibaghbanzadeh/GUE\_virus\_covid dataset.
It achieves the following results on the evaluation set:
* Loss: 1.6408
* F1 Score: 0.3935
* Accuracy: 0.3923
Model description
-----------------
More information needed
Intended uses & limitations
---------------------------
More information needed
Training and evaluation data
----------------------------
More information needed
Training procedure
------------------
### Training hyperparameters
The following hyperparameters were used during training:
* learning\_rate: 0.0005
* train\_batch\_size: 128
* eval\_batch\_size: 128
* seed: 42
* optimizer: Adam with betas=(0.9,0.999) and epsilon=1e-08
* lr\_scheduler\_type: linear
* training\_steps: 10000
### Training results
### Framework versions
* PEFT 0.9.0
* Transformers 4.38.2
* Pytorch 2.2.0+cu121
* Datasets 2.17.1
* Tokenizers 0.15.2
| [
"### Training hyperparameters\n\n\nThe following hyperparameters were used during training:\n\n\n* learning\\_rate: 0.0005\n* train\\_batch\\_size: 128\n* eval\\_batch\\_size: 128\n* seed: 42\n* optimizer: Adam with betas=(0.9,0.999) and epsilon=1e-08\n* lr\\_scheduler\\_type: linear\n* training\\_steps: 10000",
"### Training results",
"### Framework versions\n\n\n* PEFT 0.9.0\n* Transformers 4.38.2\n* Pytorch 2.2.0+cu121\n* Datasets 2.17.1\n* Tokenizers 0.15.2"
] | [
"TAGS\n#peft #safetensors #generated_from_trainer #base_model-mahdibaghbanzadeh/seqsight_4096_512_27M #region-us \n",
"### Training hyperparameters\n\n\nThe following hyperparameters were used during training:\n\n\n* learning\\_rate: 0.0005\n* train\\_batch\\_size: 128\n* eval\\_batch\\_size: 128\n* seed: 42\n* optimizer: Adam with betas=(0.9,0.999) and epsilon=1e-08\n* lr\\_scheduler\\_type: linear\n* training\\_steps: 10000",
"### Training results",
"### Framework versions\n\n\n* PEFT 0.9.0\n* Transformers 4.38.2\n* Pytorch 2.2.0+cu121\n* Datasets 2.17.1\n* Tokenizers 0.15.2"
] |
null | transformers |
# Model Card for Model ID
<!-- Provide a quick summary of what the model is/does. -->
## Model Details
### Model Description
<!-- Provide a longer summary of what this model is. -->
This is the model card of a 🤗 transformers model that has been pushed on the Hub. This model card has been automatically generated.
- **Developed by:** [More Information Needed]
- **Funded by [optional]:** [More Information Needed]
- **Shared by [optional]:** [More Information Needed]
- **Model type:** [More Information Needed]
- **Language(s) (NLP):** [More Information Needed]
- **License:** [More Information Needed]
- **Finetuned from model [optional]:** [More Information Needed]
### Model Sources [optional]
<!-- Provide the basic links for the model. -->
- **Repository:** [More Information Needed]
- **Paper [optional]:** [More Information Needed]
- **Demo [optional]:** [More Information Needed]
## Uses
<!-- Address questions around how the model is intended to be used, including the foreseeable users of the model and those affected by the model. -->
### Direct Use
<!-- This section is for the model use without fine-tuning or plugging into a larger ecosystem/app. -->
[More Information Needed]
### Downstream Use [optional]
<!-- This section is for the model use when fine-tuned for a task, or when plugged into a larger ecosystem/app -->
[More Information Needed]
### Out-of-Scope Use
<!-- This section addresses misuse, malicious use, and uses that the model will not work well for. -->
[More Information Needed]
## Bias, Risks, and Limitations
<!-- This section is meant to convey both technical and sociotechnical limitations. -->
[More Information Needed]
### Recommendations
<!-- This section is meant to convey recommendations with respect to the bias, risk, and technical limitations. -->
Users (both direct and downstream) should be made aware of the risks, biases and limitations of the model. More information needed for further recommendations.
## How to Get Started with the Model
Use the code below to get started with the model.
[More Information Needed]
## Training Details
### Training Data
<!-- This should link to a Dataset Card, perhaps with a short stub of information on what the training data is all about as well as documentation related to data pre-processing or additional filtering. -->
[More Information Needed]
### Training Procedure
<!-- This relates heavily to the Technical Specifications. Content here should link to that section when it is relevant to the training procedure. -->
#### Preprocessing [optional]
[More Information Needed]
#### Training Hyperparameters
- **Training regime:** [More Information Needed] <!--fp32, fp16 mixed precision, bf16 mixed precision, bf16 non-mixed precision, fp16 non-mixed precision, fp8 mixed precision -->
#### Speeds, Sizes, Times [optional]
<!-- This section provides information about throughput, start/end time, checkpoint size if relevant, etc. -->
[More Information Needed]
## Evaluation
<!-- This section describes the evaluation protocols and provides the results. -->
### Testing Data, Factors & Metrics
#### Testing Data
<!-- This should link to a Dataset Card if possible. -->
[More Information Needed]
#### Factors
<!-- These are the things the evaluation is disaggregating by, e.g., subpopulations or domains. -->
[More Information Needed]
#### Metrics
<!-- These are the evaluation metrics being used, ideally with a description of why. -->
[More Information Needed]
### Results
[More Information Needed]
#### Summary
## Model Examination [optional]
<!-- Relevant interpretability work for the model goes here -->
[More Information Needed]
## Environmental Impact
<!-- Total emissions (in grams of CO2eq) and additional considerations, such as electricity usage, go here. Edit the suggested text below accordingly -->
Carbon emissions can be estimated using the [Machine Learning Impact calculator](https://mlco2.github.io/impact#compute) presented in [Lacoste et al. (2019)](https://arxiv.org/abs/1910.09700).
- **Hardware Type:** [More Information Needed]
- **Hours used:** [More Information Needed]
- **Cloud Provider:** [More Information Needed]
- **Compute Region:** [More Information Needed]
- **Carbon Emitted:** [More Information Needed]
## Technical Specifications [optional]
### Model Architecture and Objective
[More Information Needed]
### Compute Infrastructure
[More Information Needed]
#### Hardware
[More Information Needed]
#### Software
[More Information Needed]
## Citation [optional]
<!-- If there is a paper or blog post introducing the model, the APA and Bibtex information for that should go in this section. -->
**BibTeX:**
[More Information Needed]
**APA:**
[More Information Needed]
## Glossary [optional]
<!-- If relevant, include terms and calculations in this section that can help readers understand the model or model card. -->
[More Information Needed]
## More Information [optional]
[More Information Needed]
## Model Card Authors [optional]
[More Information Needed]
## Model Card Contact
[More Information Needed] | {"library_name": "transformers", "tags": []} | akankshya107/llava_dpt_2 | null | [
"transformers",
"safetensors",
"arxiv:1910.09700",
"endpoints_compatible",
"region:us"
] | null | 2024-04-26T18:46:29+00:00 | [
"1910.09700"
] | [] | TAGS
#transformers #safetensors #arxiv-1910.09700 #endpoints_compatible #region-us
|
# Model Card for Model ID
## Model Details
### Model Description
This is the model card of a transformers model that has been pushed on the Hub. This model card has been automatically generated.
- Developed by:
- Funded by [optional]:
- Shared by [optional]:
- Model type:
- Language(s) (NLP):
- License:
- Finetuned from model [optional]:
### Model Sources [optional]
- Repository:
- Paper [optional]:
- Demo [optional]:
## Uses
### Direct Use
### Downstream Use [optional]
### Out-of-Scope Use
## Bias, Risks, and Limitations
### Recommendations
Users (both direct and downstream) should be made aware of the risks, biases and limitations of the model. More information needed for further recommendations.
## How to Get Started with the Model
Use the code below to get started with the model.
## Training Details
### Training Data
### Training Procedure
#### Preprocessing [optional]
#### Training Hyperparameters
- Training regime:
#### Speeds, Sizes, Times [optional]
## Evaluation
### Testing Data, Factors & Metrics
#### Testing Data
#### Factors
#### Metrics
### Results
#### Summary
## Model Examination [optional]
## Environmental Impact
Carbon emissions can be estimated using the Machine Learning Impact calculator presented in Lacoste et al. (2019).
- Hardware Type:
- Hours used:
- Cloud Provider:
- Compute Region:
- Carbon Emitted:
## Technical Specifications [optional]
### Model Architecture and Objective
### Compute Infrastructure
#### Hardware
#### Software
[optional]
BibTeX:
APA:
## Glossary [optional]
## More Information [optional]
## Model Card Authors [optional]
## Model Card Contact
| [
"# Model Card for Model ID",
"## Model Details",
"### Model Description\n\n\n\nThis is the model card of a transformers model that has been pushed on the Hub. This model card has been automatically generated.\n\n- Developed by: \n- Funded by [optional]: \n- Shared by [optional]: \n- Model type: \n- Language(s) (NLP): \n- License: \n- Finetuned from model [optional]:",
"### Model Sources [optional]\n\n\n\n- Repository: \n- Paper [optional]: \n- Demo [optional]:",
"## Uses",
"### Direct Use",
"### Downstream Use [optional]",
"### Out-of-Scope Use",
"## Bias, Risks, and Limitations",
"### Recommendations\n\n\n\nUsers (both direct and downstream) should be made aware of the risks, biases and limitations of the model. More information needed for further recommendations.",
"## How to Get Started with the Model\n\nUse the code below to get started with the model.",
"## Training Details",
"### Training Data",
"### Training Procedure",
"#### Preprocessing [optional]",
"#### Training Hyperparameters\n\n- Training regime:",
"#### Speeds, Sizes, Times [optional]",
"## Evaluation",
"### Testing Data, Factors & Metrics",
"#### Testing Data",
"#### Factors",
"#### Metrics",
"### Results",
"#### Summary",
"## Model Examination [optional]",
"## Environmental Impact\n\n\n\nCarbon emissions can be estimated using the Machine Learning Impact calculator presented in Lacoste et al. (2019).\n\n- Hardware Type: \n- Hours used: \n- Cloud Provider: \n- Compute Region: \n- Carbon Emitted:",
"## Technical Specifications [optional]",
"### Model Architecture and Objective",
"### Compute Infrastructure",
"#### Hardware",
"#### Software\n\n\n\n[optional]\n\n\n\nBibTeX:\n\n\n\nAPA:",
"## Glossary [optional]",
"## More Information [optional]",
"## Model Card Authors [optional]",
"## Model Card Contact"
] | [
"TAGS\n#transformers #safetensors #arxiv-1910.09700 #endpoints_compatible #region-us \n",
"# Model Card for Model ID",
"## Model Details",
"### Model Description\n\n\n\nThis is the model card of a transformers model that has been pushed on the Hub. This model card has been automatically generated.\n\n- Developed by: \n- Funded by [optional]: \n- Shared by [optional]: \n- Model type: \n- Language(s) (NLP): \n- License: \n- Finetuned from model [optional]:",
"### Model Sources [optional]\n\n\n\n- Repository: \n- Paper [optional]: \n- Demo [optional]:",
"## Uses",
"### Direct Use",
"### Downstream Use [optional]",
"### Out-of-Scope Use",
"## Bias, Risks, and Limitations",
"### Recommendations\n\n\n\nUsers (both direct and downstream) should be made aware of the risks, biases and limitations of the model. More information needed for further recommendations.",
"## How to Get Started with the Model\n\nUse the code below to get started with the model.",
"## Training Details",
"### Training Data",
"### Training Procedure",
"#### Preprocessing [optional]",
"#### Training Hyperparameters\n\n- Training regime:",
"#### Speeds, Sizes, Times [optional]",
"## Evaluation",
"### Testing Data, Factors & Metrics",
"#### Testing Data",
"#### Factors",
"#### Metrics",
"### Results",
"#### Summary",
"## Model Examination [optional]",
"## Environmental Impact\n\n\n\nCarbon emissions can be estimated using the Machine Learning Impact calculator presented in Lacoste et al. (2019).\n\n- Hardware Type: \n- Hours used: \n- Cloud Provider: \n- Compute Region: \n- Carbon Emitted:",
"## Technical Specifications [optional]",
"### Model Architecture and Objective",
"### Compute Infrastructure",
"#### Hardware",
"#### Software\n\n\n\n[optional]\n\n\n\nBibTeX:\n\n\n\nAPA:",
"## Glossary [optional]",
"## More Information [optional]",
"## Model Card Authors [optional]",
"## Model Card Contact"
] |
null | peft |
<!-- This model card has been generated automatically according to the information the Trainer had access to. You
should probably proofread and complete it, then remove this comment. -->
# gemma2b-dolly15k-r128
This model is a fine-tuned version of [google/gemma-2b](https://huggingface.co/google/gemma-2b) on an unknown dataset.
## Model description
More information needed
## Intended uses & limitations
More information needed
## Training and evaluation data
More information needed
## Training procedure
### Training hyperparameters
The following hyperparameters were used during training:
- learning_rate: 5e-05
- train_batch_size: 1
- eval_batch_size: 8
- seed: 42
- optimizer: Adam with betas=(0.9,0.999) and epsilon=1e-08
- lr_scheduler_type: linear
- lr_scheduler_warmup_steps: 100
- num_epochs: 1
### Training results
### Framework versions
- PEFT 0.10.0
- Transformers 4.40.1
- Pytorch 2.1.2
- Datasets 2.19.0
- Tokenizers 0.19.1 | {"license": "gemma", "library_name": "peft", "tags": ["trl", "sft", "generated_from_trainer"], "base_model": "google/gemma-2b", "model-index": [{"name": "gemma2b-dolly15k-r128", "results": []}]} | AlexxxSem/gemma2b-dolly15k-r128 | null | [
"peft",
"tensorboard",
"safetensors",
"trl",
"sft",
"generated_from_trainer",
"base_model:google/gemma-2b",
"license:gemma",
"region:us"
] | null | 2024-04-26T18:47:09+00:00 | [] | [] | TAGS
#peft #tensorboard #safetensors #trl #sft #generated_from_trainer #base_model-google/gemma-2b #license-gemma #region-us
|
# gemma2b-dolly15k-r128
This model is a fine-tuned version of google/gemma-2b on an unknown dataset.
## Model description
More information needed
## Intended uses & limitations
More information needed
## Training and evaluation data
More information needed
## Training procedure
### Training hyperparameters
The following hyperparameters were used during training:
- learning_rate: 5e-05
- train_batch_size: 1
- eval_batch_size: 8
- seed: 42
- optimizer: Adam with betas=(0.9,0.999) and epsilon=1e-08
- lr_scheduler_type: linear
- lr_scheduler_warmup_steps: 100
- num_epochs: 1
### Training results
### Framework versions
- PEFT 0.10.0
- Transformers 4.40.1
- Pytorch 2.1.2
- Datasets 2.19.0
- Tokenizers 0.19.1 | [
"# gemma2b-dolly15k-r128\n\nThis model is a fine-tuned version of google/gemma-2b on an unknown dataset.",
"## Model description\n\nMore information needed",
"## Intended uses & limitations\n\nMore information needed",
"## Training and evaluation data\n\nMore information needed",
"## Training procedure",
"### Training hyperparameters\n\nThe following hyperparameters were used during training:\n- learning_rate: 5e-05\n- train_batch_size: 1\n- eval_batch_size: 8\n- seed: 42\n- optimizer: Adam with betas=(0.9,0.999) and epsilon=1e-08\n- lr_scheduler_type: linear\n- lr_scheduler_warmup_steps: 100\n- num_epochs: 1",
"### Training results",
"### Framework versions\n\n- PEFT 0.10.0\n- Transformers 4.40.1\n- Pytorch 2.1.2\n- Datasets 2.19.0\n- Tokenizers 0.19.1"
] | [
"TAGS\n#peft #tensorboard #safetensors #trl #sft #generated_from_trainer #base_model-google/gemma-2b #license-gemma #region-us \n",
"# gemma2b-dolly15k-r128\n\nThis model is a fine-tuned version of google/gemma-2b on an unknown dataset.",
"## Model description\n\nMore information needed",
"## Intended uses & limitations\n\nMore information needed",
"## Training and evaluation data\n\nMore information needed",
"## Training procedure",
"### Training hyperparameters\n\nThe following hyperparameters were used during training:\n- learning_rate: 5e-05\n- train_batch_size: 1\n- eval_batch_size: 8\n- seed: 42\n- optimizer: Adam with betas=(0.9,0.999) and epsilon=1e-08\n- lr_scheduler_type: linear\n- lr_scheduler_warmup_steps: 100\n- num_epochs: 1",
"### Training results",
"### Framework versions\n\n- PEFT 0.10.0\n- Transformers 4.40.1\n- Pytorch 2.1.2\n- Datasets 2.19.0\n- Tokenizers 0.19.1"
] |
null | transformers | ## About
<!-- ### quantize_version: 1 -->
<!-- ### output_tensor_quantised: 1 -->
<!-- ### convert_type: -->
<!-- ### vocab_type: -->
static quants of https://huggingface.co/n00854180t/ErisMaidFlame-7B
<!-- provided-files -->
weighted/imatrix quants seem not to be available (by me) at this time. If they do not show up a week or so after the static ones, I have probably not planned for them. Feel free to request them by opening a Community Discussion.
## Usage
If you are unsure how to use GGUF files, refer to one of [TheBloke's
READMEs](https://huggingface.co/TheBloke/KafkaLM-70B-German-V0.1-GGUF) for
more details, including on how to concatenate multi-part files.
## Provided Quants
(sorted by size, not necessarily quality. IQ-quants are often preferable over similar sized non-IQ quants)
| Link | Type | Size/GB | Notes |
|:-----|:-----|--------:|:------|
| [GGUF](https://huggingface.co/mradermacher/ErisMaidFlame-7B-GGUF/resolve/main/ErisMaidFlame-7B.Q2_K.gguf) | Q2_K | 2.8 | |
| [GGUF](https://huggingface.co/mradermacher/ErisMaidFlame-7B-GGUF/resolve/main/ErisMaidFlame-7B.IQ3_XS.gguf) | IQ3_XS | 3.1 | |
| [GGUF](https://huggingface.co/mradermacher/ErisMaidFlame-7B-GGUF/resolve/main/ErisMaidFlame-7B.Q3_K_S.gguf) | Q3_K_S | 3.3 | |
| [GGUF](https://huggingface.co/mradermacher/ErisMaidFlame-7B-GGUF/resolve/main/ErisMaidFlame-7B.IQ3_S.gguf) | IQ3_S | 3.3 | beats Q3_K* |
| [GGUF](https://huggingface.co/mradermacher/ErisMaidFlame-7B-GGUF/resolve/main/ErisMaidFlame-7B.IQ3_M.gguf) | IQ3_M | 3.4 | |
| [GGUF](https://huggingface.co/mradermacher/ErisMaidFlame-7B-GGUF/resolve/main/ErisMaidFlame-7B.Q3_K_M.gguf) | Q3_K_M | 3.6 | lower quality |
| [GGUF](https://huggingface.co/mradermacher/ErisMaidFlame-7B-GGUF/resolve/main/ErisMaidFlame-7B.Q3_K_L.gguf) | Q3_K_L | 3.9 | |
| [GGUF](https://huggingface.co/mradermacher/ErisMaidFlame-7B-GGUF/resolve/main/ErisMaidFlame-7B.IQ4_XS.gguf) | IQ4_XS | 4.0 | |
| [GGUF](https://huggingface.co/mradermacher/ErisMaidFlame-7B-GGUF/resolve/main/ErisMaidFlame-7B.Q4_K_S.gguf) | Q4_K_S | 4.2 | fast, recommended |
| [GGUF](https://huggingface.co/mradermacher/ErisMaidFlame-7B-GGUF/resolve/main/ErisMaidFlame-7B.Q4_K_M.gguf) | Q4_K_M | 4.5 | fast, recommended |
| [GGUF](https://huggingface.co/mradermacher/ErisMaidFlame-7B-GGUF/resolve/main/ErisMaidFlame-7B.Q5_K_S.gguf) | Q5_K_S | 5.1 | |
| [GGUF](https://huggingface.co/mradermacher/ErisMaidFlame-7B-GGUF/resolve/main/ErisMaidFlame-7B.Q5_K_M.gguf) | Q5_K_M | 5.2 | |
| [GGUF](https://huggingface.co/mradermacher/ErisMaidFlame-7B-GGUF/resolve/main/ErisMaidFlame-7B.Q6_K.gguf) | Q6_K | 6.0 | very good quality |
| [GGUF](https://huggingface.co/mradermacher/ErisMaidFlame-7B-GGUF/resolve/main/ErisMaidFlame-7B.Q8_0.gguf) | Q8_0 | 7.8 | fast, best quality |
| [GGUF](https://huggingface.co/mradermacher/ErisMaidFlame-7B-GGUF/resolve/main/ErisMaidFlame-7B.f16.gguf) | f16 | 14.6 | 16 bpw, overkill |
Here is a handy graph by ikawrakow comparing some lower-quality quant
types (lower is better):

And here are Artefact2's thoughts on the matter:
https://gist.github.com/Artefact2/b5f810600771265fc1e39442288e8ec9
## FAQ / Model Request
See https://huggingface.co/mradermacher/model_requests for some answers to
questions you might have and/or if you want some other model quantized.
## Thanks
I thank my company, [nethype GmbH](https://www.nethype.de/), for letting
me use its servers and providing upgrades to my workstation to enable
this work in my free time.
<!-- end -->
| {"language": ["en"], "library_name": "transformers", "tags": ["mergekit", "merge"], "base_model": "n00854180t/ErisMaidFlame-7B", "quantized_by": "mradermacher"} | mradermacher/ErisMaidFlame-7B-GGUF | null | [
"transformers",
"gguf",
"mergekit",
"merge",
"en",
"base_model:n00854180t/ErisMaidFlame-7B",
"endpoints_compatible",
"region:us"
] | null | 2024-04-26T18:48:03+00:00 | [] | [
"en"
] | TAGS
#transformers #gguf #mergekit #merge #en #base_model-n00854180t/ErisMaidFlame-7B #endpoints_compatible #region-us
| About
-----
static quants of URL
weighted/imatrix quants seem not to be available (by me) at this time. If they do not show up a week or so after the static ones, I have probably not planned for them. Feel free to request them by opening a Community Discussion.
Usage
-----
If you are unsure how to use GGUF files, refer to one of TheBloke's
READMEs for
more details, including on how to concatenate multi-part files.
Provided Quants
---------------
(sorted by size, not necessarily quality. IQ-quants are often preferable over similar sized non-IQ quants)
Here is a handy graph by ikawrakow comparing some lower-quality quant
types (lower is better):
!URL
And here are Artefact2's thoughts on the matter:
URL
FAQ / Model Request
-------------------
See URL for some answers to
questions you might have and/or if you want some other model quantized.
Thanks
------
I thank my company, nethype GmbH, for letting
me use its servers and providing upgrades to my workstation to enable
this work in my free time.
| [] | [
"TAGS\n#transformers #gguf #mergekit #merge #en #base_model-n00854180t/ErisMaidFlame-7B #endpoints_compatible #region-us \n"
] |
text-generation | transformers |
# Qwen1.5-110B-Chat
## About Quantization
我们使用modelscope [swift](https://github.com/modelscope/swift/)仓库进行GPTQ量化. 量化文档可以查看[这里](https://github.com/modelscope/swift/blob/main/docs/source/LLM/LLM%E9%87%8F%E5%8C%96%E6%96%87%E6%A1%A3.md). 量化命令如下:
We use the modelscope [swift](https://github.com/modelscope/swift/) repository to perform GPTQ quantization. Quantization documentation can be found [here](https://github.com/modelscope/swift/blob/main/docs/source_en/LLM/LLM-quantization.md). The quantization command is as follows:
```bash
OMP_NUM_THREADS=14 CUDA_VISIBLE_DEVICES=0 swift export \
--model_type qwen1half-110b-chat --quant_bits 4 \
--dataset sharegpt-gpt4-mini alpaca-zh alpaca-en \
--quant_method gptq --quant_seqlen 8192
```
## Introduction
Qwen1.5 is the beta version of Qwen2, a transformer-based decoder-only language model pretrained on a large amount of data. In comparison with the previous released Qwen, the improvements include:
* 9 model sizes, including 0.5B, 1.8B, 4B, 7B, 14B, 32B, 72B, and 110B dense models, and an MoE model of 14B with 2.7B activated;
* Significant performance improvement in human preference for chat models;
* Multilingual support of both base and chat models;
* Stable support of 32K context length for models of all sizes
* No need of `trust_remote_code`.
For more details, please refer to our [blog post](https://qwenlm.github.io/blog/qwen1.5/) and [GitHub repo](https://github.com/QwenLM/Qwen1.5).
<br>
## Model Details
Qwen1.5 is a language model series including decoder language models of different model sizes. For each size, we release the base language model and the aligned chat model. It is based on the Transformer architecture with SwiGLU activation, attention QKV bias, group query attention, mixture of sliding window attention and full attention, etc. Additionally, we have an improved tokenizer adaptive to multiple natural languages and codes. For the beta version, temporarily we did not include GQA (except for 32B and 110B) and the mixture of SWA and full attention.
## Training details
We pretrained the models with a large amount of data, and we post-trained the models with both supervised finetuning and direct preference optimization.
## Requirements
The code of Qwen1.5 has been in the latest Hugging face transformers and we advise you to install `transformers>=4.37.0`, or you might encounter the following error:
```
KeyError: 'qwen2'
```
## Quickstart
Here provides a code snippet with `apply_chat_template` to show you how to load the tokenizer and model and how to generate contents.
```python
from transformers import AutoModelForCausalLM, AutoTokenizer
device = "cuda" # the device to load the model onto
model = AutoModelForCausalLM.from_pretrained(
"study-hjt/Qwen1.5-110B-Chat-GPTQ-Int4",
torch_dtype="auto",
device_map="auto"
)
tokenizer = AutoTokenizer.from_pretrained("study-hjt/Qwen1.5-110B-Chat-GPTQ-Int4")
prompt = "Give me a short introduction to large language model."
messages = [
{"role": "system", "content": "You are a helpful assistant."},
{"role": "user", "content": prompt}
]
text = tokenizer.apply_chat_template(
messages,
tokenize=False,
add_generation_prompt=True
)
model_inputs = tokenizer([text], return_tensors="pt").to(device)
generated_ids = model.generate(
model_inputs.input_ids,
max_new_tokens=512
)
generated_ids = [
output_ids[len(input_ids):] for input_ids, output_ids in zip(model_inputs.input_ids, generated_ids)
]
response = tokenizer.batch_decode(generated_ids, skip_special_tokens=True)[0]
```
## Tips
* If you encounter code switching or other bad cases, we advise you to use our provided hyper-parameters in `generation_config.json`.
## Citation
If you find our work helpful, feel free to give us a cite.
```
@article{qwen,
title={Qwen Technical Report},
author={Jinze Bai and Shuai Bai and Yunfei Chu and Zeyu Cui and Kai Dang and Xiaodong Deng and Yang Fan and Wenbin Ge and Yu Han and Fei Huang and Binyuan Hui and Luo Ji and Mei Li and Junyang Lin and Runji Lin and Dayiheng Liu and Gao Liu and Chengqiang Lu and Keming Lu and Jianxin Ma and Rui Men and Xingzhang Ren and Xuancheng Ren and Chuanqi Tan and Sinan Tan and Jianhong Tu and Peng Wang and Shijie Wang and Wei Wang and Shengguang Wu and Benfeng Xu and Jin Xu and An Yang and Hao Yang and Jian Yang and Shusheng Yang and Yang Yao and Bowen Yu and Hongyi Yuan and Zheng Yuan and Jianwei Zhang and Xingxuan Zhang and Yichang Zhang and Zhenru Zhang and Chang Zhou and Jingren Zhou and Xiaohuan Zhou and Tianhang Zhu},
journal={arXiv preprint arXiv:2309.16609},
year={2023}
}
```
| {"language": ["en"], "license": "other", "tags": ["chat", "qwen", "gptq", "int4", "4bits", "110b"], "license_name": "tongyi-qianwen", "license_link": "https://huggingface.co/Qwen/Qwen1.5-110B-Chat/blob/main/LICENSE", "pipeline_tag": "text-generation"} | study-hjt/Qwen1.5-110B-Chat-GPTQ-Int4 | null | [
"transformers",
"safetensors",
"qwen2",
"text-generation",
"chat",
"qwen",
"gptq",
"int4",
"4bits",
"110b",
"conversational",
"en",
"license:other",
"autotrain_compatible",
"endpoints_compatible",
"text-generation-inference",
"4-bit",
"region:us"
] | null | 2024-04-26T18:50:09+00:00 | [] | [
"en"
] | TAGS
#transformers #safetensors #qwen2 #text-generation #chat #qwen #gptq #int4 #4bits #110b #conversational #en #license-other #autotrain_compatible #endpoints_compatible #text-generation-inference #4-bit #region-us
|
# Qwen1.5-110B-Chat
## About Quantization
我们使用modelscope swift仓库进行GPTQ量化. 量化文档可以查看这里. 量化命令如下:
We use the modelscope swift repository to perform GPTQ quantization. Quantization documentation can be found here. The quantization command is as follows:
## Introduction
Qwen1.5 is the beta version of Qwen2, a transformer-based decoder-only language model pretrained on a large amount of data. In comparison with the previous released Qwen, the improvements include:
* 9 model sizes, including 0.5B, 1.8B, 4B, 7B, 14B, 32B, 72B, and 110B dense models, and an MoE model of 14B with 2.7B activated;
* Significant performance improvement in human preference for chat models;
* Multilingual support of both base and chat models;
* Stable support of 32K context length for models of all sizes
* No need of 'trust_remote_code'.
For more details, please refer to our blog post and GitHub repo.
<br>
## Model Details
Qwen1.5 is a language model series including decoder language models of different model sizes. For each size, we release the base language model and the aligned chat model. It is based on the Transformer architecture with SwiGLU activation, attention QKV bias, group query attention, mixture of sliding window attention and full attention, etc. Additionally, we have an improved tokenizer adaptive to multiple natural languages and codes. For the beta version, temporarily we did not include GQA (except for 32B and 110B) and the mixture of SWA and full attention.
## Training details
We pretrained the models with a large amount of data, and we post-trained the models with both supervised finetuning and direct preference optimization.
## Requirements
The code of Qwen1.5 has been in the latest Hugging face transformers and we advise you to install 'transformers>=4.37.0', or you might encounter the following error:
## Quickstart
Here provides a code snippet with 'apply_chat_template' to show you how to load the tokenizer and model and how to generate contents.
## Tips
* If you encounter code switching or other bad cases, we advise you to use our provided hyper-parameters in 'generation_config.json'.
If you find our work helpful, feel free to give us a cite.
| [
"# Qwen1.5-110B-Chat",
"## About Quantization\n我们使用modelscope swift仓库进行GPTQ量化. 量化文档可以查看这里. 量化命令如下:\n\nWe use the modelscope swift repository to perform GPTQ quantization. Quantization documentation can be found here. The quantization command is as follows:",
"## Introduction\n\nQwen1.5 is the beta version of Qwen2, a transformer-based decoder-only language model pretrained on a large amount of data. In comparison with the previous released Qwen, the improvements include: \n\n* 9 model sizes, including 0.5B, 1.8B, 4B, 7B, 14B, 32B, 72B, and 110B dense models, and an MoE model of 14B with 2.7B activated;\n* Significant performance improvement in human preference for chat models;\n* Multilingual support of both base and chat models;\n* Stable support of 32K context length for models of all sizes\n* No need of 'trust_remote_code'.\n\nFor more details, please refer to our blog post and GitHub repo.\n<br>",
"## Model Details\nQwen1.5 is a language model series including decoder language models of different model sizes. For each size, we release the base language model and the aligned chat model. It is based on the Transformer architecture with SwiGLU activation, attention QKV bias, group query attention, mixture of sliding window attention and full attention, etc. Additionally, we have an improved tokenizer adaptive to multiple natural languages and codes. For the beta version, temporarily we did not include GQA (except for 32B and 110B) and the mixture of SWA and full attention.",
"## Training details\nWe pretrained the models with a large amount of data, and we post-trained the models with both supervised finetuning and direct preference optimization.",
"## Requirements\nThe code of Qwen1.5 has been in the latest Hugging face transformers and we advise you to install 'transformers>=4.37.0', or you might encounter the following error:",
"## Quickstart\n\nHere provides a code snippet with 'apply_chat_template' to show you how to load the tokenizer and model and how to generate contents.",
"## Tips\n\n* If you encounter code switching or other bad cases, we advise you to use our provided hyper-parameters in 'generation_config.json'.\n\n\nIf you find our work helpful, feel free to give us a cite."
] | [
"TAGS\n#transformers #safetensors #qwen2 #text-generation #chat #qwen #gptq #int4 #4bits #110b #conversational #en #license-other #autotrain_compatible #endpoints_compatible #text-generation-inference #4-bit #region-us \n",
"# Qwen1.5-110B-Chat",
"## About Quantization\n我们使用modelscope swift仓库进行GPTQ量化. 量化文档可以查看这里. 量化命令如下:\n\nWe use the modelscope swift repository to perform GPTQ quantization. Quantization documentation can be found here. The quantization command is as follows:",
"## Introduction\n\nQwen1.5 is the beta version of Qwen2, a transformer-based decoder-only language model pretrained on a large amount of data. In comparison with the previous released Qwen, the improvements include: \n\n* 9 model sizes, including 0.5B, 1.8B, 4B, 7B, 14B, 32B, 72B, and 110B dense models, and an MoE model of 14B with 2.7B activated;\n* Significant performance improvement in human preference for chat models;\n* Multilingual support of both base and chat models;\n* Stable support of 32K context length for models of all sizes\n* No need of 'trust_remote_code'.\n\nFor more details, please refer to our blog post and GitHub repo.\n<br>",
"## Model Details\nQwen1.5 is a language model series including decoder language models of different model sizes. For each size, we release the base language model and the aligned chat model. It is based on the Transformer architecture with SwiGLU activation, attention QKV bias, group query attention, mixture of sliding window attention and full attention, etc. Additionally, we have an improved tokenizer adaptive to multiple natural languages and codes. For the beta version, temporarily we did not include GQA (except for 32B and 110B) and the mixture of SWA and full attention.",
"## Training details\nWe pretrained the models with a large amount of data, and we post-trained the models with both supervised finetuning and direct preference optimization.",
"## Requirements\nThe code of Qwen1.5 has been in the latest Hugging face transformers and we advise you to install 'transformers>=4.37.0', or you might encounter the following error:",
"## Quickstart\n\nHere provides a code snippet with 'apply_chat_template' to show you how to load the tokenizer and model and how to generate contents.",
"## Tips\n\n* If you encounter code switching or other bad cases, we advise you to use our provided hyper-parameters in 'generation_config.json'.\n\n\nIf you find our work helpful, feel free to give us a cite."
] |
text-generation | transformers |
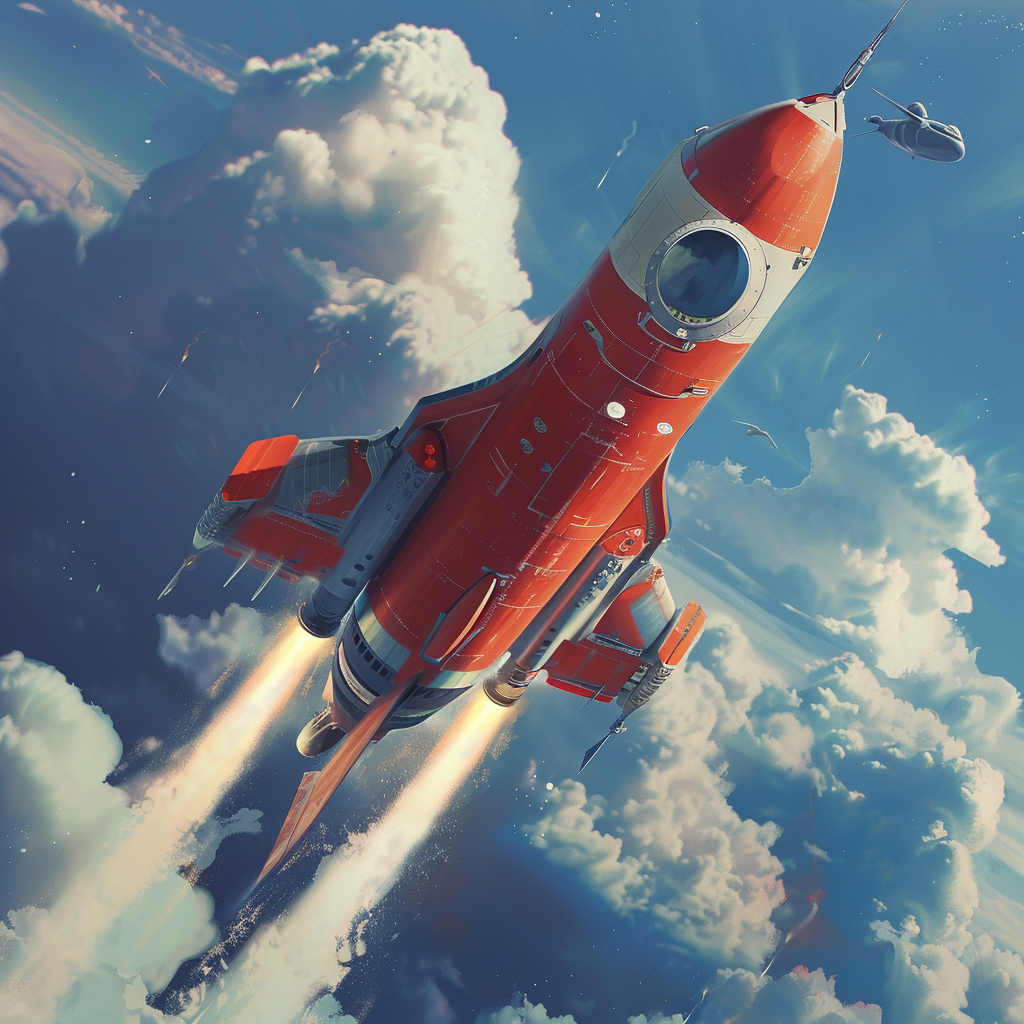
# 🚀 Skyro-4X8B
Skyro-4X8B is a Mixure of Experts (MoE) made with the following models using [Mergekit](https://github.com/arcee-ai/mergekit):
* [abacusai/Llama-3-Smaug-8B](https://huggingface.co/abacusai/Llama-3-Smaug-8B)
* [cognitivecomputations/dolphin-2.9-llama3-8b](https://huggingface.co/cognitivecomputations/dolphin-2.9-llama3-8b)
* [Weyaxi/Einstein-v6.1-Llama3-8B](https://huggingface.co/Weyaxi/Einstein-v6.1-Llama3-8B)
* [dreamgen-preview/opus-v1.2-llama-3-8b-base-run3.4-epoch2](https://huggingface.co/dreamgen-preview/opus-v1.2-llama-3-8b-base-run3.4-epoch2)
## 🧩 Configuration
```yamlname: "Skyro-4X8B"
base_model: meta-llama/Meta-Llama-3-8B
gate_mode: hidden
experts:
- source_model: abacusai/Llama-3-Smaug-8B
positive_prompts:
- "chat"
- "assistant"
- "tell me"
- "explain"
- "I want"
- source_model: cognitivecomputations/dolphin-2.9-llama3-8b
positive_prompts:
- "math"
- "mathematics"
- "code"
- "engineering"
- "solve"
- "logic"
- "rationality"
- "puzzle"
- "solve"
- source_model: Weyaxi/Einstein-v6.1-Llama3-8B
positive_prompts:
- "science"
- "medical"
- "physics"
- "engineering"
- "math"
- "logic"
- "rationality"
- "mathematics"
- "solve"
- source_model: dreamgen-preview/opus-v1.2-llama-3-8b-base-run3.4-epoch2
positive_prompts:
- "story"
- "roleplay"
- "role-play"
- "storywriting"
- "character"
- "narrative"
- "creative"
```
## 💻 Usage
```python
!pip install -qU transformers accelerate
from transformers import AutoTokenizer
import transformers
import torch
model = "saucam/Skyro-4X8B"
messages = [{"role": "user", "content": "In a student council election, candidate A got 20% of the votes while candidate B got 50% more than candidate A's votes. The rest of the votes was given to candidate C. If there were 100 voters, how many votes did candidate C get?"}]
tokenizer = AutoTokenizer.from_pretrained(model)
prompt = tokenizer.apply_chat_template(messages, tokenize=False, add_generation_prompt=True)
pipeline = transformers.pipeline(
"text-generation",
model=model,
torch_dtype=torch.float16,
device_map="auto",
)
outputs = pipeline(prompt, max_new_tokens=256, do_sample=True, temperature=0.7, top_k=50, top_p=0.95)
print(outputs[0]["generated_text"])
```
## Sample output
```
config.json: 100%|██████████████████████████████████████████████████████████████| 878/878 [00:00<00:00, 4.18MB/s]
model.safetensors.index.json: 100%|██████████████████████████████████████████| 53.5k/53.5k [00:00<00:00, 101MB/s]
model-00001-of-00006.safetensors: 100%|█████████████████████████████████████| 9.89G/9.89G [03:47<00:00, 43.4MB/s]
model-00002-of-00006.safetensors: 100%|█████████████████████████████████████| 9.98G/9.98G [03:23<00:00, 49.0MB/s]
model-00003-of-00006.safetensors: 100%|█████████████████████████████████████| 9.98G/9.98G [03:44<00:00, 44.5MB/s]
model-00004-of-00006.safetensors: 100%|█████████████████████████████████████| 9.90G/9.90G [03:30<00:00, 46.9MB/s]
model-00005-of-00006.safetensors: 100%|█████████████████████████████████████| 9.08G/9.08G [03:08<00:00, 48.1MB/s]
model-00006-of-00006.safetensors: 100%|█████████████████████████████████████| 1.05G/1.05G [00:20<00:00, 51.3MB/s]
Downloading shards: 100%|█████████████████████████████████████████████████████████| 6/6 [17:58<00:00, 179.78s/it]
Loading checkpoint shards: 100%|███████████████████████████████████████████████████| 6/6 [01:27<00:00, 14.59s/it]
WARNING:root:Some parameters are on the meta device device because they were offloaded to the cpu.
Special tokens have been added in the vocabulary, make sure the associated word embeddings are fine-tuned or trained.
<|im_start|>user
In a student council election, candidate A got 20% of the votes while candidate B got 50% more than candidate A's votes. The rest of the votes was given to candidate C. If there were 100 voters, how many votes did candidate C get?<|im_end|>
<|im_start|>assistant
Let's denote the number of votes candidate A got as \( A \).
Candidate B got 50% more votes than candidate A, so candidate B got \( A + 0.5A = 1.5A \) votes.
Candidate C got the rest of the votes, which means \( C = 100 - (A + 1.5A) \).
We know that candidate A got 20% of the votes, so \( A = 20\% \times 100 = 20 \).
Now we can calculate candidate C's votes:
\( C = 100 - (20 + 1.5 \times 20) \)
\( C = 100 - (20 + 30) \)
\( C = 100 - 50 \)
\( C = 50 \).
Therefore, candidate C got 50 votes.<|im_end|>
``` | {"license": "apache-2.0", "tags": ["merge", "mergekit", "moe", "frankenmoe", "abacusai/Llama-3-Smaug-8B", "cognitivecomputations/dolphin-2.9-llama3-8b", "Weyaxi/Einstein-v6.1-Llama3-8B", "dreamgen-preview/opus-v1.2-llama-3-8b-base-run3.4-epoch2"], "base_model": ["abacusai/Llama-3-Smaug-8B", "cognitivecomputations/dolphin-2.9-llama3-8b", "Weyaxi/Einstein-v6.1-Llama3-8B", "dreamgen-preview/opus-v1.2-llama-3-8b-base-run3.4-epoch2"]} | saucam/Skyro-4X8B | null | [
"transformers",
"safetensors",
"mixtral",
"text-generation",
"merge",
"mergekit",
"moe",
"frankenmoe",
"abacusai/Llama-3-Smaug-8B",
"cognitivecomputations/dolphin-2.9-llama3-8b",
"Weyaxi/Einstein-v6.1-Llama3-8B",
"dreamgen-preview/opus-v1.2-llama-3-8b-base-run3.4-epoch2",
"base_model:abacusai/Llama-3-Smaug-8B",
"base_model:cognitivecomputations/dolphin-2.9-llama3-8b",
"base_model:Weyaxi/Einstein-v6.1-Llama3-8B",
"base_model:dreamgen-preview/opus-v1.2-llama-3-8b-base-run3.4-epoch2",
"license:apache-2.0",
"autotrain_compatible",
"endpoints_compatible",
"text-generation-inference",
"region:us"
] | null | 2024-04-26T18:50:44+00:00 | [] | [] | TAGS
#transformers #safetensors #mixtral #text-generation #merge #mergekit #moe #frankenmoe #abacusai/Llama-3-Smaug-8B #cognitivecomputations/dolphin-2.9-llama3-8b #Weyaxi/Einstein-v6.1-Llama3-8B #dreamgen-preview/opus-v1.2-llama-3-8b-base-run3.4-epoch2 #base_model-abacusai/Llama-3-Smaug-8B #base_model-cognitivecomputations/dolphin-2.9-llama3-8b #base_model-Weyaxi/Einstein-v6.1-Llama3-8B #base_model-dreamgen-preview/opus-v1.2-llama-3-8b-base-run3.4-epoch2 #license-apache-2.0 #autotrain_compatible #endpoints_compatible #text-generation-inference #region-us
|
 made with the following models using Mergekit:
* abacusai/Llama-3-Smaug-8B
* cognitivecomputations/dolphin-2.9-llama3-8b
* Weyaxi/Einstein-v6.1-Llama3-8B
* dreamgen-preview/opus-v1.2-llama-3-8b-base-run3.4-epoch2
## Configuration
## Usage
## Sample output
| [
"# Skyro-4X8B\nSkyro-4X8B is a Mixure of Experts (MoE) made with the following models using Mergekit:\n\n* abacusai/Llama-3-Smaug-8B\n* cognitivecomputations/dolphin-2.9-llama3-8b\n* Weyaxi/Einstein-v6.1-Llama3-8B\n* dreamgen-preview/opus-v1.2-llama-3-8b-base-run3.4-epoch2",
"## Configuration",
"## Usage",
"## Sample output"
] | [
"TAGS\n#transformers #safetensors #mixtral #text-generation #merge #mergekit #moe #frankenmoe #abacusai/Llama-3-Smaug-8B #cognitivecomputations/dolphin-2.9-llama3-8b #Weyaxi/Einstein-v6.1-Llama3-8B #dreamgen-preview/opus-v1.2-llama-3-8b-base-run3.4-epoch2 #base_model-abacusai/Llama-3-Smaug-8B #base_model-cognitivecomputations/dolphin-2.9-llama3-8b #base_model-Weyaxi/Einstein-v6.1-Llama3-8B #base_model-dreamgen-preview/opus-v1.2-llama-3-8b-base-run3.4-epoch2 #license-apache-2.0 #autotrain_compatible #endpoints_compatible #text-generation-inference #region-us \n",
"# Skyro-4X8B\nSkyro-4X8B is a Mixure of Experts (MoE) made with the following models using Mergekit:\n\n* abacusai/Llama-3-Smaug-8B\n* cognitivecomputations/dolphin-2.9-llama3-8b\n* Weyaxi/Einstein-v6.1-Llama3-8B\n* dreamgen-preview/opus-v1.2-llama-3-8b-base-run3.4-epoch2",
"## Configuration",
"## Usage",
"## Sample output"
] |
null | peft |
<!-- This model card has been generated automatically according to the information the Trainer had access to. You
should probably proofread and complete it, then remove this comment. -->
# GUE_virus_covid-seqsight_4096_512_27M-L8_f
This model is a fine-tuned version of [mahdibaghbanzadeh/seqsight_4096_512_27M](https://huggingface.co/mahdibaghbanzadeh/seqsight_4096_512_27M) on the [mahdibaghbanzadeh/GUE_virus_covid](https://huggingface.co/datasets/mahdibaghbanzadeh/GUE_virus_covid) dataset.
It achieves the following results on the evaluation set:
- Loss: 1.3185
- F1 Score: 0.5124
- Accuracy: 0.5135
## Model description
More information needed
## Intended uses & limitations
More information needed
## Training and evaluation data
More information needed
## Training procedure
### Training hyperparameters
The following hyperparameters were used during training:
- learning_rate: 0.0005
- train_batch_size: 128
- eval_batch_size: 128
- seed: 42
- optimizer: Adam with betas=(0.9,0.999) and epsilon=1e-08
- lr_scheduler_type: linear
- training_steps: 10000
### Training results
| Training Loss | Epoch | Step | Validation Loss | F1 Score | Accuracy |
|:-------------:|:-----:|:-----:|:---------------:|:--------:|:--------:|
| 2.1834 | 0.35 | 200 | 2.1781 | 0.1086 | 0.1444 |
| 2.1731 | 0.7 | 400 | 2.1615 | 0.1333 | 0.1538 |
| 2.1353 | 1.05 | 600 | 2.0731 | 0.1947 | 0.2125 |
| 2.024 | 1.4 | 800 | 1.9362 | 0.2448 | 0.2606 |
| 1.9338 | 1.75 | 1000 | 1.8595 | 0.2832 | 0.2943 |
| 1.8801 | 2.09 | 1200 | 1.8183 | 0.2838 | 0.2947 |
| 1.8369 | 2.44 | 1400 | 1.7762 | 0.3213 | 0.3235 |
| 1.811 | 2.79 | 1600 | 1.7453 | 0.3250 | 0.3350 |
| 1.786 | 3.14 | 1800 | 1.7145 | 0.3351 | 0.3488 |
| 1.7538 | 3.49 | 2000 | 1.6934 | 0.3572 | 0.3609 |
| 1.7384 | 3.84 | 2200 | 1.6635 | 0.3728 | 0.3745 |
| 1.7123 | 4.19 | 2400 | 1.6323 | 0.3804 | 0.3762 |
| 1.691 | 4.54 | 2600 | 1.6020 | 0.4020 | 0.4028 |
| 1.6755 | 4.89 | 2800 | 1.5920 | 0.3971 | 0.4031 |
| 1.6551 | 5.24 | 3000 | 1.5867 | 0.3965 | 0.4013 |
| 1.642 | 5.58 | 3200 | 1.5574 | 0.4079 | 0.4132 |
| 1.6213 | 5.93 | 3400 | 1.5510 | 0.4124 | 0.4164 |
| 1.6003 | 6.28 | 3600 | 1.5192 | 0.4284 | 0.4266 |
| 1.5856 | 6.63 | 3800 | 1.5036 | 0.4306 | 0.4354 |
| 1.5796 | 6.98 | 4000 | 1.4930 | 0.4379 | 0.4411 |
| 1.5685 | 7.33 | 4200 | 1.4882 | 0.4287 | 0.4321 |
| 1.5537 | 7.68 | 4400 | 1.4775 | 0.4574 | 0.4527 |
| 1.5513 | 8.03 | 4600 | 1.4724 | 0.4492 | 0.4440 |
| 1.5256 | 8.38 | 4800 | 1.4608 | 0.4543 | 0.4570 |
| 1.5354 | 8.73 | 5000 | 1.4586 | 0.4492 | 0.4493 |
| 1.5262 | 9.08 | 5200 | 1.4459 | 0.4626 | 0.4560 |
| 1.5207 | 9.42 | 5400 | 1.4296 | 0.4551 | 0.4604 |
| 1.5066 | 9.77 | 5600 | 1.4210 | 0.4742 | 0.4774 |
| 1.4961 | 10.12 | 5800 | 1.4062 | 0.4828 | 0.4813 |
| 1.4961 | 10.47 | 6000 | 1.4098 | 0.4694 | 0.4676 |
| 1.4884 | 10.82 | 6200 | 1.4066 | 0.4776 | 0.4727 |
| 1.4635 | 11.17 | 6400 | 1.3975 | 0.4780 | 0.4719 |
| 1.4665 | 11.52 | 6600 | 1.3895 | 0.4832 | 0.4818 |
| 1.4694 | 11.87 | 6800 | 1.3816 | 0.4849 | 0.4841 |
| 1.4622 | 12.22 | 7000 | 1.3683 | 0.4941 | 0.4926 |
| 1.4479 | 12.57 | 7200 | 1.3618 | 0.4960 | 0.4964 |
| 1.4531 | 12.91 | 7400 | 1.3743 | 0.4776 | 0.4758 |
| 1.4458 | 13.26 | 7600 | 1.3586 | 0.4894 | 0.4867 |
| 1.4372 | 13.61 | 7800 | 1.3465 | 0.5028 | 0.5003 |
| 1.4371 | 13.96 | 8000 | 1.3417 | 0.5018 | 0.4993 |
| 1.4273 | 14.31 | 8200 | 1.3355 | 0.5006 | 0.5004 |
| 1.4283 | 14.66 | 8400 | 1.3304 | 0.5075 | 0.5068 |
| 1.4252 | 15.01 | 8600 | 1.3305 | 0.4997 | 0.4992 |
| 1.4274 | 15.36 | 8800 | 1.3245 | 0.5131 | 0.5141 |
| 1.4087 | 15.71 | 9000 | 1.3223 | 0.5050 | 0.5057 |
| 1.4206 | 16.06 | 9200 | 1.3228 | 0.5083 | 0.5094 |
| 1.4169 | 16.4 | 9400 | 1.3173 | 0.5109 | 0.5107 |
| 1.4144 | 16.75 | 9600 | 1.3192 | 0.5091 | 0.5089 |
| 1.413 | 17.1 | 9800 | 1.3183 | 0.5093 | 0.5089 |
| 1.4152 | 17.45 | 10000 | 1.3175 | 0.5089 | 0.5091 |
### Framework versions
- PEFT 0.9.0
- Transformers 4.38.2
- Pytorch 2.2.0+cu121
- Datasets 2.17.1
- Tokenizers 0.15.2 | {"library_name": "peft", "tags": ["generated_from_trainer"], "metrics": ["accuracy"], "base_model": "mahdibaghbanzadeh/seqsight_4096_512_27M", "model-index": [{"name": "GUE_virus_covid-seqsight_4096_512_27M-L8_f", "results": []}]} | mahdibaghbanzadeh/GUE_virus_covid-seqsight_4096_512_27M-L8_f | null | [
"peft",
"safetensors",
"generated_from_trainer",
"base_model:mahdibaghbanzadeh/seqsight_4096_512_27M",
"region:us"
] | null | 2024-04-26T18:50:52+00:00 | [] | [] | TAGS
#peft #safetensors #generated_from_trainer #base_model-mahdibaghbanzadeh/seqsight_4096_512_27M #region-us
| GUE\_virus\_covid-seqsight\_4096\_512\_27M-L8\_f
================================================
This model is a fine-tuned version of mahdibaghbanzadeh/seqsight\_4096\_512\_27M on the mahdibaghbanzadeh/GUE\_virus\_covid dataset.
It achieves the following results on the evaluation set:
* Loss: 1.3185
* F1 Score: 0.5124
* Accuracy: 0.5135
Model description
-----------------
More information needed
Intended uses & limitations
---------------------------
More information needed
Training and evaluation data
----------------------------
More information needed
Training procedure
------------------
### Training hyperparameters
The following hyperparameters were used during training:
* learning\_rate: 0.0005
* train\_batch\_size: 128
* eval\_batch\_size: 128
* seed: 42
* optimizer: Adam with betas=(0.9,0.999) and epsilon=1e-08
* lr\_scheduler\_type: linear
* training\_steps: 10000
### Training results
### Framework versions
* PEFT 0.9.0
* Transformers 4.38.2
* Pytorch 2.2.0+cu121
* Datasets 2.17.1
* Tokenizers 0.15.2
| [
"### Training hyperparameters\n\n\nThe following hyperparameters were used during training:\n\n\n* learning\\_rate: 0.0005\n* train\\_batch\\_size: 128\n* eval\\_batch\\_size: 128\n* seed: 42\n* optimizer: Adam with betas=(0.9,0.999) and epsilon=1e-08\n* lr\\_scheduler\\_type: linear\n* training\\_steps: 10000",
"### Training results",
"### Framework versions\n\n\n* PEFT 0.9.0\n* Transformers 4.38.2\n* Pytorch 2.2.0+cu121\n* Datasets 2.17.1\n* Tokenizers 0.15.2"
] | [
"TAGS\n#peft #safetensors #generated_from_trainer #base_model-mahdibaghbanzadeh/seqsight_4096_512_27M #region-us \n",
"### Training hyperparameters\n\n\nThe following hyperparameters were used during training:\n\n\n* learning\\_rate: 0.0005\n* train\\_batch\\_size: 128\n* eval\\_batch\\_size: 128\n* seed: 42\n* optimizer: Adam with betas=(0.9,0.999) and epsilon=1e-08\n* lr\\_scheduler\\_type: linear\n* training\\_steps: 10000",
"### Training results",
"### Framework versions\n\n\n* PEFT 0.9.0\n* Transformers 4.38.2\n* Pytorch 2.2.0+cu121\n* Datasets 2.17.1\n* Tokenizers 0.15.2"
] |
null | peft |
<!-- This model card has been generated automatically according to the information the Trainer had access to. You
should probably proofread and complete it, then remove this comment. -->
# GUE_virus_covid-seqsight_4096_512_27M-L32_f
This model is a fine-tuned version of [mahdibaghbanzadeh/seqsight_4096_512_27M](https://huggingface.co/mahdibaghbanzadeh/seqsight_4096_512_27M) on the [mahdibaghbanzadeh/GUE_virus_covid](https://huggingface.co/datasets/mahdibaghbanzadeh/GUE_virus_covid) dataset.
It achieves the following results on the evaluation set:
- Loss: 1.0120
- F1 Score: 0.6191
- Accuracy: 0.6177
## Model description
More information needed
## Intended uses & limitations
More information needed
## Training and evaluation data
More information needed
## Training procedure
### Training hyperparameters
The following hyperparameters were used during training:
- learning_rate: 0.0005
- train_batch_size: 128
- eval_batch_size: 128
- seed: 42
- optimizer: Adam with betas=(0.9,0.999) and epsilon=1e-08
- lr_scheduler_type: linear
- training_steps: 10000
### Training results
| Training Loss | Epoch | Step | Validation Loss | F1 Score | Accuracy |
|:-------------:|:-----:|:-----:|:---------------:|:--------:|:--------:|
| 2.1827 | 0.35 | 200 | 2.1753 | 0.1045 | 0.1416 |
| 2.158 | 0.7 | 400 | 2.1172 | 0.1515 | 0.1741 |
| 2.0492 | 1.05 | 600 | 1.9241 | 0.2587 | 0.2731 |
| 1.8951 | 1.4 | 800 | 1.7714 | 0.3051 | 0.3216 |
| 1.7958 | 1.75 | 1000 | 1.6972 | 0.3392 | 0.3521 |
| 1.7331 | 2.09 | 1200 | 1.6434 | 0.3726 | 0.3756 |
| 1.6821 | 2.44 | 1400 | 1.5883 | 0.4134 | 0.4106 |
| 1.6409 | 2.79 | 1600 | 1.5465 | 0.4131 | 0.4173 |
| 1.602 | 3.14 | 1800 | 1.4926 | 0.4368 | 0.4385 |
| 1.549 | 3.49 | 2000 | 1.4476 | 0.4567 | 0.4547 |
| 1.5185 | 3.84 | 2200 | 1.4019 | 0.4702 | 0.4683 |
| 1.4783 | 4.19 | 2400 | 1.3771 | 0.4727 | 0.4748 |
| 1.446 | 4.54 | 2600 | 1.3363 | 0.5033 | 0.4993 |
| 1.4218 | 4.89 | 2800 | 1.3102 | 0.5022 | 0.5048 |
| 1.3959 | 5.24 | 3000 | 1.2904 | 0.5073 | 0.5080 |
| 1.3767 | 5.58 | 3200 | 1.2590 | 0.5281 | 0.5289 |
| 1.3509 | 5.93 | 3400 | 1.2441 | 0.5282 | 0.5298 |
| 1.3307 | 6.28 | 3600 | 1.2205 | 0.5507 | 0.5444 |
| 1.3083 | 6.63 | 3800 | 1.2043 | 0.5459 | 0.5458 |
| 1.2958 | 6.98 | 4000 | 1.1911 | 0.5510 | 0.5528 |
| 1.2813 | 7.33 | 4200 | 1.1746 | 0.5579 | 0.5533 |
| 1.2675 | 7.68 | 4400 | 1.1639 | 0.5642 | 0.5581 |
| 1.2675 | 8.03 | 4600 | 1.1520 | 0.5727 | 0.5662 |
| 1.2386 | 8.38 | 4800 | 1.1379 | 0.5739 | 0.5739 |
| 1.2367 | 8.73 | 5000 | 1.1300 | 0.5737 | 0.5738 |
| 1.2246 | 9.08 | 5200 | 1.1243 | 0.5801 | 0.5740 |
| 1.2171 | 9.42 | 5400 | 1.1111 | 0.5855 | 0.5840 |
| 1.1994 | 9.77 | 5600 | 1.0961 | 0.5902 | 0.5904 |
| 1.2003 | 10.12 | 5800 | 1.0891 | 0.5968 | 0.5928 |
| 1.1939 | 10.47 | 6000 | 1.0839 | 0.5913 | 0.5894 |
| 1.181 | 10.82 | 6200 | 1.0851 | 0.6012 | 0.5956 |
| 1.1578 | 11.17 | 6400 | 1.0812 | 0.6022 | 0.5962 |
| 1.1652 | 11.52 | 6600 | 1.0678 | 0.6034 | 0.5974 |
| 1.1598 | 11.87 | 6800 | 1.0676 | 0.6013 | 0.5985 |
| 1.1578 | 12.22 | 7000 | 1.0575 | 0.6036 | 0.6023 |
| 1.1337 | 12.57 | 7200 | 1.0504 | 0.6025 | 0.6019 |
| 1.1473 | 12.91 | 7400 | 1.0558 | 0.6088 | 0.6026 |
| 1.1301 | 13.26 | 7600 | 1.0484 | 0.6067 | 0.6021 |
| 1.1321 | 13.61 | 7800 | 1.0413 | 0.6118 | 0.6074 |
| 1.1257 | 13.96 | 8000 | 1.0381 | 0.6132 | 0.6094 |
| 1.1181 | 14.31 | 8200 | 1.0339 | 0.6187 | 0.6152 |
| 1.1194 | 14.66 | 8400 | 1.0298 | 0.6141 | 0.6102 |
| 1.1185 | 15.01 | 8600 | 1.0319 | 0.6144 | 0.6119 |
| 1.119 | 15.36 | 8800 | 1.0270 | 0.6147 | 0.6137 |
| 1.1 | 15.71 | 9000 | 1.0249 | 0.6169 | 0.6159 |
| 1.1153 | 16.06 | 9200 | 1.0255 | 0.6196 | 0.6167 |
| 1.1058 | 16.4 | 9400 | 1.0249 | 0.6173 | 0.6158 |
| 1.106 | 16.75 | 9600 | 1.0249 | 0.6173 | 0.6139 |
| 1.1128 | 17.1 | 9800 | 1.0233 | 0.6178 | 0.6158 |
| 1.1099 | 17.45 | 10000 | 1.0238 | 0.6184 | 0.6162 |
### Framework versions
- PEFT 0.9.0
- Transformers 4.38.2
- Pytorch 2.2.0+cu121
- Datasets 2.17.1
- Tokenizers 0.15.2 | {"library_name": "peft", "tags": ["generated_from_trainer"], "metrics": ["accuracy"], "base_model": "mahdibaghbanzadeh/seqsight_4096_512_27M", "model-index": [{"name": "GUE_virus_covid-seqsight_4096_512_27M-L32_f", "results": []}]} | mahdibaghbanzadeh/GUE_virus_covid-seqsight_4096_512_27M-L32_f | null | [
"peft",
"safetensors",
"generated_from_trainer",
"base_model:mahdibaghbanzadeh/seqsight_4096_512_27M",
"region:us"
] | null | 2024-04-26T18:51:05+00:00 | [] | [] | TAGS
#peft #safetensors #generated_from_trainer #base_model-mahdibaghbanzadeh/seqsight_4096_512_27M #region-us
| GUE\_virus\_covid-seqsight\_4096\_512\_27M-L32\_f
=================================================
This model is a fine-tuned version of mahdibaghbanzadeh/seqsight\_4096\_512\_27M on the mahdibaghbanzadeh/GUE\_virus\_covid dataset.
It achieves the following results on the evaluation set:
* Loss: 1.0120
* F1 Score: 0.6191
* Accuracy: 0.6177
Model description
-----------------
More information needed
Intended uses & limitations
---------------------------
More information needed
Training and evaluation data
----------------------------
More information needed
Training procedure
------------------
### Training hyperparameters
The following hyperparameters were used during training:
* learning\_rate: 0.0005
* train\_batch\_size: 128
* eval\_batch\_size: 128
* seed: 42
* optimizer: Adam with betas=(0.9,0.999) and epsilon=1e-08
* lr\_scheduler\_type: linear
* training\_steps: 10000
### Training results
### Framework versions
* PEFT 0.9.0
* Transformers 4.38.2
* Pytorch 2.2.0+cu121
* Datasets 2.17.1
* Tokenizers 0.15.2
| [
"### Training hyperparameters\n\n\nThe following hyperparameters were used during training:\n\n\n* learning\\_rate: 0.0005\n* train\\_batch\\_size: 128\n* eval\\_batch\\_size: 128\n* seed: 42\n* optimizer: Adam with betas=(0.9,0.999) and epsilon=1e-08\n* lr\\_scheduler\\_type: linear\n* training\\_steps: 10000",
"### Training results",
"### Framework versions\n\n\n* PEFT 0.9.0\n* Transformers 4.38.2\n* Pytorch 2.2.0+cu121\n* Datasets 2.17.1\n* Tokenizers 0.15.2"
] | [
"TAGS\n#peft #safetensors #generated_from_trainer #base_model-mahdibaghbanzadeh/seqsight_4096_512_27M #region-us \n",
"### Training hyperparameters\n\n\nThe following hyperparameters were used during training:\n\n\n* learning\\_rate: 0.0005\n* train\\_batch\\_size: 128\n* eval\\_batch\\_size: 128\n* seed: 42\n* optimizer: Adam with betas=(0.9,0.999) and epsilon=1e-08\n* lr\\_scheduler\\_type: linear\n* training\\_steps: 10000",
"### Training results",
"### Framework versions\n\n\n* PEFT 0.9.0\n* Transformers 4.38.2\n* Pytorch 2.2.0+cu121\n* Datasets 2.17.1\n* Tokenizers 0.15.2"
] |
null | peft |
<!-- This model card has been generated automatically according to the information the Trainer had access to. You
should probably proofread and complete it, then remove this comment. -->
# GUE_prom_prom_300_tata-seqsight_4096_512_46M-L1_f
This model is a fine-tuned version of [mahdibaghbanzadeh/seqsight_4096_512_46M](https://huggingface.co/mahdibaghbanzadeh/seqsight_4096_512_46M) on the [mahdibaghbanzadeh/GUE_prom_prom_300_tata](https://huggingface.co/datasets/mahdibaghbanzadeh/GUE_prom_prom_300_tata) dataset.
It achieves the following results on the evaluation set:
- Loss: 0.5219
- F1 Score: 0.8056
- Accuracy: 0.8059
## Model description
More information needed
## Intended uses & limitations
More information needed
## Training and evaluation data
More information needed
## Training procedure
### Training hyperparameters
The following hyperparameters were used during training:
- learning_rate: 0.0005
- train_batch_size: 128
- eval_batch_size: 128
- seed: 42
- optimizer: Adam with betas=(0.9,0.999) and epsilon=1e-08
- lr_scheduler_type: linear
- training_steps: 10000
### Training results
| Training Loss | Epoch | Step | Validation Loss | F1 Score | Accuracy |
|:-------------:|:------:|:-----:|:---------------:|:--------:|:--------:|
| 0.5687 | 5.13 | 200 | 0.5025 | 0.7669 | 0.7667 |
| 0.4527 | 10.26 | 400 | 0.5102 | 0.7715 | 0.7732 |
| 0.4213 | 15.38 | 600 | 0.4610 | 0.7962 | 0.7961 |
| 0.3989 | 20.51 | 800 | 0.4824 | 0.8002 | 0.8010 |
| 0.3836 | 25.64 | 1000 | 0.4858 | 0.7989 | 0.7993 |
| 0.3695 | 30.77 | 1200 | 0.4774 | 0.8025 | 0.8026 |
| 0.3542 | 35.9 | 1400 | 0.4916 | 0.8023 | 0.8026 |
| 0.3422 | 41.03 | 1600 | 0.4509 | 0.8076 | 0.8075 |
| 0.3324 | 46.15 | 1800 | 0.4803 | 0.8155 | 0.8157 |
| 0.3172 | 51.28 | 2000 | 0.4699 | 0.8140 | 0.8140 |
| 0.3116 | 56.41 | 2200 | 0.4965 | 0.8172 | 0.8173 |
| 0.3019 | 61.54 | 2400 | 0.5114 | 0.8036 | 0.8042 |
| 0.2877 | 66.67 | 2600 | 0.4937 | 0.8141 | 0.8140 |
| 0.2787 | 71.79 | 2800 | 0.5037 | 0.8187 | 0.8189 |
| 0.274 | 76.92 | 3000 | 0.5147 | 0.8137 | 0.8140 |
| 0.2592 | 82.05 | 3200 | 0.5178 | 0.8157 | 0.8157 |
| 0.2549 | 87.18 | 3400 | 0.5278 | 0.8104 | 0.8108 |
| 0.2446 | 92.31 | 3600 | 0.5193 | 0.8157 | 0.8157 |
| 0.2401 | 97.44 | 3800 | 0.5307 | 0.8060 | 0.8059 |
| 0.2275 | 102.56 | 4000 | 0.5405 | 0.8093 | 0.8091 |
| 0.2224 | 107.69 | 4200 | 0.5597 | 0.8073 | 0.8075 |
| 0.2167 | 112.82 | 4400 | 0.5506 | 0.8125 | 0.8124 |
| 0.2161 | 117.95 | 4600 | 0.6083 | 0.7916 | 0.7928 |
| 0.208 | 123.08 | 4800 | 0.5856 | 0.8125 | 0.8124 |
| 0.2035 | 128.21 | 5000 | 0.5996 | 0.8057 | 0.8059 |
| 0.1935 | 133.33 | 5200 | 0.6063 | 0.8125 | 0.8124 |
| 0.1911 | 138.46 | 5400 | 0.6082 | 0.8091 | 0.8091 |
| 0.1862 | 143.59 | 5600 | 0.6273 | 0.7957 | 0.7961 |
| 0.1807 | 148.72 | 5800 | 0.6411 | 0.8074 | 0.8075 |
| 0.1811 | 153.85 | 6000 | 0.6109 | 0.8059 | 0.8059 |
| 0.1735 | 158.97 | 6200 | 0.6349 | 0.8091 | 0.8091 |
| 0.1681 | 164.1 | 6400 | 0.6542 | 0.8075 | 0.8075 |
| 0.1634 | 169.23 | 6600 | 0.6528 | 0.8140 | 0.8140 |
| 0.162 | 174.36 | 6800 | 0.6502 | 0.8091 | 0.8091 |
| 0.1621 | 179.49 | 7000 | 0.6551 | 0.8041 | 0.8042 |
| 0.1583 | 184.62 | 7200 | 0.6558 | 0.8158 | 0.8157 |
| 0.1534 | 189.74 | 7400 | 0.6527 | 0.8158 | 0.8157 |
| 0.1502 | 194.87 | 7600 | 0.6593 | 0.8060 | 0.8059 |
| 0.151 | 200.0 | 7800 | 0.6558 | 0.8109 | 0.8108 |
| 0.1461 | 205.13 | 8000 | 0.6911 | 0.8024 | 0.8026 |
| 0.1416 | 210.26 | 8200 | 0.6885 | 0.8059 | 0.8059 |
| 0.1415 | 215.38 | 8400 | 0.6917 | 0.7973 | 0.7977 |
| 0.1414 | 220.51 | 8600 | 0.6833 | 0.8093 | 0.8091 |
| 0.1364 | 225.64 | 8800 | 0.6925 | 0.8010 | 0.8010 |
| 0.1333 | 230.77 | 9000 | 0.6977 | 0.8043 | 0.8042 |
| 0.1343 | 235.9 | 9200 | 0.7001 | 0.7960 | 0.7961 |
| 0.1341 | 241.03 | 9400 | 0.6994 | 0.8043 | 0.8042 |
| 0.1314 | 246.15 | 9600 | 0.7022 | 0.8010 | 0.8010 |
| 0.1353 | 251.28 | 9800 | 0.7017 | 0.8010 | 0.8010 |
| 0.1363 | 256.41 | 10000 | 0.7074 | 0.7960 | 0.7961 |
### Framework versions
- PEFT 0.9.0
- Transformers 4.38.2
- Pytorch 2.2.0+cu121
- Datasets 2.17.1
- Tokenizers 0.15.2 | {"library_name": "peft", "tags": ["generated_from_trainer"], "metrics": ["accuracy"], "base_model": "mahdibaghbanzadeh/seqsight_4096_512_46M", "model-index": [{"name": "GUE_prom_prom_300_tata-seqsight_4096_512_46M-L1_f", "results": []}]} | mahdibaghbanzadeh/GUE_prom_prom_300_tata-seqsight_4096_512_46M-L1_f | null | [
"peft",
"safetensors",
"generated_from_trainer",
"base_model:mahdibaghbanzadeh/seqsight_4096_512_46M",
"region:us"
] | null | 2024-04-26T18:51:20+00:00 | [] | [] | TAGS
#peft #safetensors #generated_from_trainer #base_model-mahdibaghbanzadeh/seqsight_4096_512_46M #region-us
| GUE\_prom\_prom\_300\_tata-seqsight\_4096\_512\_46M-L1\_f
=========================================================
This model is a fine-tuned version of mahdibaghbanzadeh/seqsight\_4096\_512\_46M on the mahdibaghbanzadeh/GUE\_prom\_prom\_300\_tata dataset.
It achieves the following results on the evaluation set:
* Loss: 0.5219
* F1 Score: 0.8056
* Accuracy: 0.8059
Model description
-----------------
More information needed
Intended uses & limitations
---------------------------
More information needed
Training and evaluation data
----------------------------
More information needed
Training procedure
------------------
### Training hyperparameters
The following hyperparameters were used during training:
* learning\_rate: 0.0005
* train\_batch\_size: 128
* eval\_batch\_size: 128
* seed: 42
* optimizer: Adam with betas=(0.9,0.999) and epsilon=1e-08
* lr\_scheduler\_type: linear
* training\_steps: 10000
### Training results
### Framework versions
* PEFT 0.9.0
* Transformers 4.38.2
* Pytorch 2.2.0+cu121
* Datasets 2.17.1
* Tokenizers 0.15.2
| [
"### Training hyperparameters\n\n\nThe following hyperparameters were used during training:\n\n\n* learning\\_rate: 0.0005\n* train\\_batch\\_size: 128\n* eval\\_batch\\_size: 128\n* seed: 42\n* optimizer: Adam with betas=(0.9,0.999) and epsilon=1e-08\n* lr\\_scheduler\\_type: linear\n* training\\_steps: 10000",
"### Training results",
"### Framework versions\n\n\n* PEFT 0.9.0\n* Transformers 4.38.2\n* Pytorch 2.2.0+cu121\n* Datasets 2.17.1\n* Tokenizers 0.15.2"
] | [
"TAGS\n#peft #safetensors #generated_from_trainer #base_model-mahdibaghbanzadeh/seqsight_4096_512_46M #region-us \n",
"### Training hyperparameters\n\n\nThe following hyperparameters were used during training:\n\n\n* learning\\_rate: 0.0005\n* train\\_batch\\_size: 128\n* eval\\_batch\\_size: 128\n* seed: 42\n* optimizer: Adam with betas=(0.9,0.999) and epsilon=1e-08\n* lr\\_scheduler\\_type: linear\n* training\\_steps: 10000",
"### Training results",
"### Framework versions\n\n\n* PEFT 0.9.0\n* Transformers 4.38.2\n* Pytorch 2.2.0+cu121\n* Datasets 2.17.1\n* Tokenizers 0.15.2"
] |
feature-extraction | transformers |
# Model Card for Model ID
<!-- Provide a quick summary of what the model is/does. -->
## Model Details
### Model Description
<!-- Provide a longer summary of what this model is. -->
This is the model card of a 🤗 transformers model that has been pushed on the Hub. This model card has been automatically generated.
- **Developed by:** [More Information Needed]
- **Funded by [optional]:** [More Information Needed]
- **Shared by [optional]:** [More Information Needed]
- **Model type:** [More Information Needed]
- **Language(s) (NLP):** [More Information Needed]
- **License:** [More Information Needed]
- **Finetuned from model [optional]:** [More Information Needed]
### Model Sources [optional]
<!-- Provide the basic links for the model. -->
- **Repository:** [More Information Needed]
- **Paper [optional]:** [More Information Needed]
- **Demo [optional]:** [More Information Needed]
## Uses
<!-- Address questions around how the model is intended to be used, including the foreseeable users of the model and those affected by the model. -->
### Direct Use
<!-- This section is for the model use without fine-tuning or plugging into a larger ecosystem/app. -->
[More Information Needed]
### Downstream Use [optional]
<!-- This section is for the model use when fine-tuned for a task, or when plugged into a larger ecosystem/app -->
[More Information Needed]
### Out-of-Scope Use
<!-- This section addresses misuse, malicious use, and uses that the model will not work well for. -->
[More Information Needed]
## Bias, Risks, and Limitations
<!-- This section is meant to convey both technical and sociotechnical limitations. -->
[More Information Needed]
### Recommendations
<!-- This section is meant to convey recommendations with respect to the bias, risk, and technical limitations. -->
Users (both direct and downstream) should be made aware of the risks, biases and limitations of the model. More information needed for further recommendations.
## How to Get Started with the Model
Use the code below to get started with the model.
[More Information Needed]
## Training Details
### Training Data
<!-- This should link to a Dataset Card, perhaps with a short stub of information on what the training data is all about as well as documentation related to data pre-processing or additional filtering. -->
[More Information Needed]
### Training Procedure
<!-- This relates heavily to the Technical Specifications. Content here should link to that section when it is relevant to the training procedure. -->
#### Preprocessing [optional]
[More Information Needed]
#### Training Hyperparameters
- **Training regime:** [More Information Needed] <!--fp32, fp16 mixed precision, bf16 mixed precision, bf16 non-mixed precision, fp16 non-mixed precision, fp8 mixed precision -->
#### Speeds, Sizes, Times [optional]
<!-- This section provides information about throughput, start/end time, checkpoint size if relevant, etc. -->
[More Information Needed]
## Evaluation
<!-- This section describes the evaluation protocols and provides the results. -->
### Testing Data, Factors & Metrics
#### Testing Data
<!-- This should link to a Dataset Card if possible. -->
[More Information Needed]
#### Factors
<!-- These are the things the evaluation is disaggregating by, e.g., subpopulations or domains. -->
[More Information Needed]
#### Metrics
<!-- These are the evaluation metrics being used, ideally with a description of why. -->
[More Information Needed]
### Results
[More Information Needed]
#### Summary
## Model Examination [optional]
<!-- Relevant interpretability work for the model goes here -->
[More Information Needed]
## Environmental Impact
<!-- Total emissions (in grams of CO2eq) and additional considerations, such as electricity usage, go here. Edit the suggested text below accordingly -->
Carbon emissions can be estimated using the [Machine Learning Impact calculator](https://mlco2.github.io/impact#compute) presented in [Lacoste et al. (2019)](https://arxiv.org/abs/1910.09700).
- **Hardware Type:** [More Information Needed]
- **Hours used:** [More Information Needed]
- **Cloud Provider:** [More Information Needed]
- **Compute Region:** [More Information Needed]
- **Carbon Emitted:** [More Information Needed]
## Technical Specifications [optional]
### Model Architecture and Objective
[More Information Needed]
### Compute Infrastructure
[More Information Needed]
#### Hardware
[More Information Needed]
#### Software
[More Information Needed]
## Citation [optional]
<!-- If there is a paper or blog post introducing the model, the APA and Bibtex information for that should go in this section. -->
**BibTeX:**
[More Information Needed]
**APA:**
[More Information Needed]
## Glossary [optional]
<!-- If relevant, include terms and calculations in this section that can help readers understand the model or model card. -->
[More Information Needed]
## More Information [optional]
[More Information Needed]
## Model Card Authors [optional]
[More Information Needed]
## Model Card Contact
[More Information Needed] | {"library_name": "transformers", "tags": []} | stvhuang/rcr-run-5pqr6lwp-90396-master-0_20240402T105012-ep35 | null | [
"transformers",
"safetensors",
"xlm-roberta",
"feature-extraction",
"arxiv:1910.09700",
"endpoints_compatible",
"region:us"
] | null | 2024-04-26T18:51:20+00:00 | [
"1910.09700"
] | [] | TAGS
#transformers #safetensors #xlm-roberta #feature-extraction #arxiv-1910.09700 #endpoints_compatible #region-us
|
# Model Card for Model ID
## Model Details
### Model Description
This is the model card of a transformers model that has been pushed on the Hub. This model card has been automatically generated.
- Developed by:
- Funded by [optional]:
- Shared by [optional]:
- Model type:
- Language(s) (NLP):
- License:
- Finetuned from model [optional]:
### Model Sources [optional]
- Repository:
- Paper [optional]:
- Demo [optional]:
## Uses
### Direct Use
### Downstream Use [optional]
### Out-of-Scope Use
## Bias, Risks, and Limitations
### Recommendations
Users (both direct and downstream) should be made aware of the risks, biases and limitations of the model. More information needed for further recommendations.
## How to Get Started with the Model
Use the code below to get started with the model.
## Training Details
### Training Data
### Training Procedure
#### Preprocessing [optional]
#### Training Hyperparameters
- Training regime:
#### Speeds, Sizes, Times [optional]
## Evaluation
### Testing Data, Factors & Metrics
#### Testing Data
#### Factors
#### Metrics
### Results
#### Summary
## Model Examination [optional]
## Environmental Impact
Carbon emissions can be estimated using the Machine Learning Impact calculator presented in Lacoste et al. (2019).
- Hardware Type:
- Hours used:
- Cloud Provider:
- Compute Region:
- Carbon Emitted:
## Technical Specifications [optional]
### Model Architecture and Objective
### Compute Infrastructure
#### Hardware
#### Software
[optional]
BibTeX:
APA:
## Glossary [optional]
## More Information [optional]
## Model Card Authors [optional]
## Model Card Contact
| [
"# Model Card for Model ID",
"## Model Details",
"### Model Description\n\n\n\nThis is the model card of a transformers model that has been pushed on the Hub. This model card has been automatically generated.\n\n- Developed by: \n- Funded by [optional]: \n- Shared by [optional]: \n- Model type: \n- Language(s) (NLP): \n- License: \n- Finetuned from model [optional]:",
"### Model Sources [optional]\n\n\n\n- Repository: \n- Paper [optional]: \n- Demo [optional]:",
"## Uses",
"### Direct Use",
"### Downstream Use [optional]",
"### Out-of-Scope Use",
"## Bias, Risks, and Limitations",
"### Recommendations\n\n\n\nUsers (both direct and downstream) should be made aware of the risks, biases and limitations of the model. More information needed for further recommendations.",
"## How to Get Started with the Model\n\nUse the code below to get started with the model.",
"## Training Details",
"### Training Data",
"### Training Procedure",
"#### Preprocessing [optional]",
"#### Training Hyperparameters\n\n- Training regime:",
"#### Speeds, Sizes, Times [optional]",
"## Evaluation",
"### Testing Data, Factors & Metrics",
"#### Testing Data",
"#### Factors",
"#### Metrics",
"### Results",
"#### Summary",
"## Model Examination [optional]",
"## Environmental Impact\n\n\n\nCarbon emissions can be estimated using the Machine Learning Impact calculator presented in Lacoste et al. (2019).\n\n- Hardware Type: \n- Hours used: \n- Cloud Provider: \n- Compute Region: \n- Carbon Emitted:",
"## Technical Specifications [optional]",
"### Model Architecture and Objective",
"### Compute Infrastructure",
"#### Hardware",
"#### Software\n\n\n\n[optional]\n\n\n\nBibTeX:\n\n\n\nAPA:",
"## Glossary [optional]",
"## More Information [optional]",
"## Model Card Authors [optional]",
"## Model Card Contact"
] | [
"TAGS\n#transformers #safetensors #xlm-roberta #feature-extraction #arxiv-1910.09700 #endpoints_compatible #region-us \n",
"# Model Card for Model ID",
"## Model Details",
"### Model Description\n\n\n\nThis is the model card of a transformers model that has been pushed on the Hub. This model card has been automatically generated.\n\n- Developed by: \n- Funded by [optional]: \n- Shared by [optional]: \n- Model type: \n- Language(s) (NLP): \n- License: \n- Finetuned from model [optional]:",
"### Model Sources [optional]\n\n\n\n- Repository: \n- Paper [optional]: \n- Demo [optional]:",
"## Uses",
"### Direct Use",
"### Downstream Use [optional]",
"### Out-of-Scope Use",
"## Bias, Risks, and Limitations",
"### Recommendations\n\n\n\nUsers (both direct and downstream) should be made aware of the risks, biases and limitations of the model. More information needed for further recommendations.",
"## How to Get Started with the Model\n\nUse the code below to get started with the model.",
"## Training Details",
"### Training Data",
"### Training Procedure",
"#### Preprocessing [optional]",
"#### Training Hyperparameters\n\n- Training regime:",
"#### Speeds, Sizes, Times [optional]",
"## Evaluation",
"### Testing Data, Factors & Metrics",
"#### Testing Data",
"#### Factors",
"#### Metrics",
"### Results",
"#### Summary",
"## Model Examination [optional]",
"## Environmental Impact\n\n\n\nCarbon emissions can be estimated using the Machine Learning Impact calculator presented in Lacoste et al. (2019).\n\n- Hardware Type: \n- Hours used: \n- Cloud Provider: \n- Compute Region: \n- Carbon Emitted:",
"## Technical Specifications [optional]",
"### Model Architecture and Objective",
"### Compute Infrastructure",
"#### Hardware",
"#### Software\n\n\n\n[optional]\n\n\n\nBibTeX:\n\n\n\nAPA:",
"## Glossary [optional]",
"## More Information [optional]",
"## Model Card Authors [optional]",
"## Model Card Contact"
] |
null | transformers | ## About
<!-- ### quantize_version: 1 -->
<!-- ### output_tensor_quantised: 1 -->
<!-- ### convert_type: -->
<!-- ### vocab_type: -->
static quants of https://huggingface.co/aaditya/OpenBioLLM-Llama3-8B
<!-- provided-files -->
weighted/imatrix quants are available at https://huggingface.co/mradermacher/OpenBioLLM-Llama3-8B-i1-GGUF
## Usage
If you are unsure how to use GGUF files, refer to one of [TheBloke's
READMEs](https://huggingface.co/TheBloke/KafkaLM-70B-German-V0.1-GGUF) for
more details, including on how to concatenate multi-part files.
## Provided Quants
(sorted by size, not necessarily quality. IQ-quants are often preferable over similar sized non-IQ quants)
| Link | Type | Size/GB | Notes |
|:-----|:-----|--------:|:------|
| [GGUF](https://huggingface.co/mradermacher/OpenBioLLM-Llama3-8B-GGUF/resolve/main/OpenBioLLM-Llama3-8B.Q2_K.gguf) | Q2_K | 3.3 | |
| [GGUF](https://huggingface.co/mradermacher/OpenBioLLM-Llama3-8B-GGUF/resolve/main/OpenBioLLM-Llama3-8B.IQ3_XS.gguf) | IQ3_XS | 3.6 | |
| [GGUF](https://huggingface.co/mradermacher/OpenBioLLM-Llama3-8B-GGUF/resolve/main/OpenBioLLM-Llama3-8B.Q3_K_S.gguf) | Q3_K_S | 3.8 | |
| [GGUF](https://huggingface.co/mradermacher/OpenBioLLM-Llama3-8B-GGUF/resolve/main/OpenBioLLM-Llama3-8B.IQ3_S.gguf) | IQ3_S | 3.8 | beats Q3_K* |
| [GGUF](https://huggingface.co/mradermacher/OpenBioLLM-Llama3-8B-GGUF/resolve/main/OpenBioLLM-Llama3-8B.IQ3_M.gguf) | IQ3_M | 3.9 | |
| [GGUF](https://huggingface.co/mradermacher/OpenBioLLM-Llama3-8B-GGUF/resolve/main/OpenBioLLM-Llama3-8B.Q3_K_M.gguf) | Q3_K_M | 4.1 | lower quality |
| [GGUF](https://huggingface.co/mradermacher/OpenBioLLM-Llama3-8B-GGUF/resolve/main/OpenBioLLM-Llama3-8B.Q3_K_L.gguf) | Q3_K_L | 4.4 | |
| [GGUF](https://huggingface.co/mradermacher/OpenBioLLM-Llama3-8B-GGUF/resolve/main/OpenBioLLM-Llama3-8B.IQ4_XS.gguf) | IQ4_XS | 4.6 | |
| [GGUF](https://huggingface.co/mradermacher/OpenBioLLM-Llama3-8B-GGUF/resolve/main/OpenBioLLM-Llama3-8B.Q4_K_S.gguf) | Q4_K_S | 4.8 | fast, recommended |
| [GGUF](https://huggingface.co/mradermacher/OpenBioLLM-Llama3-8B-GGUF/resolve/main/OpenBioLLM-Llama3-8B.Q4_K_M.gguf) | Q4_K_M | 5.0 | fast, recommended |
| [GGUF](https://huggingface.co/mradermacher/OpenBioLLM-Llama3-8B-GGUF/resolve/main/OpenBioLLM-Llama3-8B.Q5_K_S.gguf) | Q5_K_S | 5.7 | |
| [GGUF](https://huggingface.co/mradermacher/OpenBioLLM-Llama3-8B-GGUF/resolve/main/OpenBioLLM-Llama3-8B.Q5_K_M.gguf) | Q5_K_M | 5.8 | |
| [GGUF](https://huggingface.co/mradermacher/OpenBioLLM-Llama3-8B-GGUF/resolve/main/OpenBioLLM-Llama3-8B.Q6_K.gguf) | Q6_K | 6.7 | very good quality |
| [GGUF](https://huggingface.co/mradermacher/OpenBioLLM-Llama3-8B-GGUF/resolve/main/OpenBioLLM-Llama3-8B.Q8_0.gguf) | Q8_0 | 8.6 | fast, best quality |
| [GGUF](https://huggingface.co/mradermacher/OpenBioLLM-Llama3-8B-GGUF/resolve/main/OpenBioLLM-Llama3-8B.f16.gguf) | f16 | 16.2 | 16 bpw, overkill |
Here is a handy graph by ikawrakow comparing some lower-quality quant
types (lower is better):

And here are Artefact2's thoughts on the matter:
https://gist.github.com/Artefact2/b5f810600771265fc1e39442288e8ec9
## FAQ / Model Request
See https://huggingface.co/mradermacher/model_requests for some answers to
questions you might have and/or if you want some other model quantized.
## Thanks
I thank my company, [nethype GmbH](https://www.nethype.de/), for letting
me use its servers and providing upgrades to my workstation to enable
this work in my free time.
<!-- end -->
| {"language": ["en"], "license": "llama3", "library_name": "transformers", "tags": ["llama-3", "llama", "Mixtral", "instruct", "finetune", "chatml", "DPO", "RLHF", "gpt4", "distillation"], "base_model": "aaditya/OpenBioLLM-Llama3-8B", "quantized_by": "mradermacher"} | mradermacher/OpenBioLLM-Llama3-8B-GGUF | null | [
"transformers",
"gguf",
"llama-3",
"llama",
"Mixtral",
"instruct",
"finetune",
"chatml",
"DPO",
"RLHF",
"gpt4",
"distillation",
"en",
"base_model:aaditya/OpenBioLLM-Llama3-8B",
"license:llama3",
"endpoints_compatible",
"region:us"
] | null | 2024-04-26T18:51:41+00:00 | [] | [
"en"
] | TAGS
#transformers #gguf #llama-3 #llama #Mixtral #instruct #finetune #chatml #DPO #RLHF #gpt4 #distillation #en #base_model-aaditya/OpenBioLLM-Llama3-8B #license-llama3 #endpoints_compatible #region-us
| About
-----
static quants of URL
weighted/imatrix quants are available at URL
Usage
-----
If you are unsure how to use GGUF files, refer to one of TheBloke's
READMEs for
more details, including on how to concatenate multi-part files.
Provided Quants
---------------
(sorted by size, not necessarily quality. IQ-quants are often preferable over similar sized non-IQ quants)
Here is a handy graph by ikawrakow comparing some lower-quality quant
types (lower is better):
!URL
And here are Artefact2's thoughts on the matter:
URL
FAQ / Model Request
-------------------
See URL for some answers to
questions you might have and/or if you want some other model quantized.
Thanks
------
I thank my company, nethype GmbH, for letting
me use its servers and providing upgrades to my workstation to enable
this work in my free time.
| [] | [
"TAGS\n#transformers #gguf #llama-3 #llama #Mixtral #instruct #finetune #chatml #DPO #RLHF #gpt4 #distillation #en #base_model-aaditya/OpenBioLLM-Llama3-8B #license-llama3 #endpoints_compatible #region-us \n"
] |
null | peft |
<!-- This model card has been generated automatically according to the information the Trainer had access to. You
should probably proofread and complete it, then remove this comment. -->
# GUE_prom_prom_300_tata-seqsight_4096_512_46M-L8_f
This model is a fine-tuned version of [mahdibaghbanzadeh/seqsight_4096_512_46M](https://huggingface.co/mahdibaghbanzadeh/seqsight_4096_512_46M) on the [mahdibaghbanzadeh/GUE_prom_prom_300_tata](https://huggingface.co/datasets/mahdibaghbanzadeh/GUE_prom_prom_300_tata) dataset.
It achieves the following results on the evaluation set:
- Loss: 1.1438
- F1 Score: 0.7977
- Accuracy: 0.7977
## Model description
More information needed
## Intended uses & limitations
More information needed
## Training and evaluation data
More information needed
## Training procedure
### Training hyperparameters
The following hyperparameters were used during training:
- learning_rate: 0.0005
- train_batch_size: 128
- eval_batch_size: 128
- seed: 42
- optimizer: Adam with betas=(0.9,0.999) and epsilon=1e-08
- lr_scheduler_type: linear
- training_steps: 10000
### Training results
| Training Loss | Epoch | Step | Validation Loss | F1 Score | Accuracy |
|:-------------:|:------:|:-----:|:---------------:|:--------:|:--------:|
| 0.5219 | 5.13 | 200 | 0.4674 | 0.7930 | 0.7928 |
| 0.4208 | 10.26 | 400 | 0.5335 | 0.7665 | 0.7700 |
| 0.3713 | 15.38 | 600 | 0.4630 | 0.8060 | 0.8059 |
| 0.3369 | 20.51 | 800 | 0.4880 | 0.8124 | 0.8124 |
| 0.311 | 25.64 | 1000 | 0.5083 | 0.8072 | 0.8075 |
| 0.2792 | 30.77 | 1200 | 0.5235 | 0.8090 | 0.8091 |
| 0.2547 | 35.9 | 1400 | 0.5602 | 0.8036 | 0.8042 |
| 0.2298 | 41.03 | 1600 | 0.5584 | 0.7991 | 0.7993 |
| 0.2141 | 46.15 | 1800 | 0.5943 | 0.8059 | 0.8059 |
| 0.1861 | 51.28 | 2000 | 0.6661 | 0.7846 | 0.7847 |
| 0.1749 | 56.41 | 2200 | 0.7863 | 0.7896 | 0.7912 |
| 0.1619 | 61.54 | 2400 | 0.7337 | 0.7893 | 0.7896 |
| 0.1421 | 66.67 | 2600 | 0.8072 | 0.7863 | 0.7863 |
| 0.1325 | 71.79 | 2800 | 0.8324 | 0.7944 | 0.7945 |
| 0.1272 | 76.92 | 3000 | 0.8595 | 0.7777 | 0.7781 |
| 0.114 | 82.05 | 3200 | 0.8499 | 0.7993 | 0.7993 |
| 0.1099 | 87.18 | 3400 | 0.8849 | 0.8008 | 0.8010 |
| 0.101 | 92.31 | 3600 | 0.9083 | 0.7978 | 0.7977 |
| 0.0972 | 97.44 | 3800 | 0.9468 | 0.7881 | 0.7879 |
| 0.0917 | 102.56 | 4000 | 0.9275 | 0.7827 | 0.7830 |
| 0.0856 | 107.69 | 4200 | 1.0109 | 0.7961 | 0.7961 |
| 0.0819 | 112.82 | 4400 | 1.0156 | 0.8027 | 0.8026 |
| 0.0772 | 117.95 | 4600 | 1.0641 | 0.8027 | 0.8026 |
| 0.0723 | 123.08 | 4800 | 1.0596 | 0.7946 | 0.7945 |
| 0.0712 | 128.21 | 5000 | 1.0247 | 0.8027 | 0.8026 |
| 0.0697 | 133.33 | 5200 | 1.0250 | 0.7995 | 0.7993 |
| 0.0634 | 138.46 | 5400 | 1.0853 | 0.8060 | 0.8059 |
| 0.0642 | 143.59 | 5600 | 1.0859 | 0.8060 | 0.8059 |
| 0.0574 | 148.72 | 5800 | 1.1577 | 0.8027 | 0.8026 |
| 0.0591 | 153.85 | 6000 | 1.1102 | 0.7930 | 0.7928 |
| 0.0585 | 158.97 | 6200 | 1.1141 | 0.8028 | 0.8026 |
| 0.058 | 164.1 | 6400 | 1.0972 | 0.8076 | 0.8075 |
| 0.0522 | 169.23 | 6600 | 1.1226 | 0.7912 | 0.7912 |
| 0.0502 | 174.36 | 6800 | 1.1901 | 0.8043 | 0.8042 |
| 0.0512 | 179.49 | 7000 | 1.1143 | 0.8125 | 0.8124 |
| 0.0518 | 184.62 | 7200 | 1.1176 | 0.8060 | 0.8059 |
| 0.0463 | 189.74 | 7400 | 1.1819 | 0.8076 | 0.8075 |
| 0.0462 | 194.87 | 7600 | 1.2209 | 0.8076 | 0.8075 |
| 0.048 | 200.0 | 7800 | 1.1939 | 0.8060 | 0.8059 |
| 0.0458 | 205.13 | 8000 | 1.2056 | 0.8076 | 0.8075 |
| 0.04 | 210.26 | 8200 | 1.2237 | 0.8093 | 0.8091 |
| 0.0431 | 215.38 | 8400 | 1.2553 | 0.8011 | 0.8010 |
| 0.0409 | 220.51 | 8600 | 1.2386 | 0.8044 | 0.8042 |
| 0.0391 | 225.64 | 8800 | 1.2630 | 0.8060 | 0.8059 |
| 0.0379 | 230.77 | 9000 | 1.2814 | 0.8076 | 0.8075 |
| 0.0408 | 235.9 | 9200 | 1.2478 | 0.8060 | 0.8059 |
| 0.0385 | 241.03 | 9400 | 1.2441 | 0.8093 | 0.8091 |
| 0.0376 | 246.15 | 9600 | 1.2370 | 0.8109 | 0.8108 |
| 0.0392 | 251.28 | 9800 | 1.2531 | 0.8076 | 0.8075 |
| 0.0371 | 256.41 | 10000 | 1.2568 | 0.8060 | 0.8059 |
### Framework versions
- PEFT 0.9.0
- Transformers 4.38.2
- Pytorch 2.2.0+cu121
- Datasets 2.17.1
- Tokenizers 0.15.2 | {"library_name": "peft", "tags": ["generated_from_trainer"], "metrics": ["accuracy"], "base_model": "mahdibaghbanzadeh/seqsight_4096_512_46M", "model-index": [{"name": "GUE_prom_prom_300_tata-seqsight_4096_512_46M-L8_f", "results": []}]} | mahdibaghbanzadeh/GUE_prom_prom_300_tata-seqsight_4096_512_46M-L8_f | null | [
"peft",
"safetensors",
"generated_from_trainer",
"base_model:mahdibaghbanzadeh/seqsight_4096_512_46M",
"region:us"
] | null | 2024-04-26T18:52:33+00:00 | [] | [] | TAGS
#peft #safetensors #generated_from_trainer #base_model-mahdibaghbanzadeh/seqsight_4096_512_46M #region-us
| GUE\_prom\_prom\_300\_tata-seqsight\_4096\_512\_46M-L8\_f
=========================================================
This model is a fine-tuned version of mahdibaghbanzadeh/seqsight\_4096\_512\_46M on the mahdibaghbanzadeh/GUE\_prom\_prom\_300\_tata dataset.
It achieves the following results on the evaluation set:
* Loss: 1.1438
* F1 Score: 0.7977
* Accuracy: 0.7977
Model description
-----------------
More information needed
Intended uses & limitations
---------------------------
More information needed
Training and evaluation data
----------------------------
More information needed
Training procedure
------------------
### Training hyperparameters
The following hyperparameters were used during training:
* learning\_rate: 0.0005
* train\_batch\_size: 128
* eval\_batch\_size: 128
* seed: 42
* optimizer: Adam with betas=(0.9,0.999) and epsilon=1e-08
* lr\_scheduler\_type: linear
* training\_steps: 10000
### Training results
### Framework versions
* PEFT 0.9.0
* Transformers 4.38.2
* Pytorch 2.2.0+cu121
* Datasets 2.17.1
* Tokenizers 0.15.2
| [
"### Training hyperparameters\n\n\nThe following hyperparameters were used during training:\n\n\n* learning\\_rate: 0.0005\n* train\\_batch\\_size: 128\n* eval\\_batch\\_size: 128\n* seed: 42\n* optimizer: Adam with betas=(0.9,0.999) and epsilon=1e-08\n* lr\\_scheduler\\_type: linear\n* training\\_steps: 10000",
"### Training results",
"### Framework versions\n\n\n* PEFT 0.9.0\n* Transformers 4.38.2\n* Pytorch 2.2.0+cu121\n* Datasets 2.17.1\n* Tokenizers 0.15.2"
] | [
"TAGS\n#peft #safetensors #generated_from_trainer #base_model-mahdibaghbanzadeh/seqsight_4096_512_46M #region-us \n",
"### Training hyperparameters\n\n\nThe following hyperparameters were used during training:\n\n\n* learning\\_rate: 0.0005\n* train\\_batch\\_size: 128\n* eval\\_batch\\_size: 128\n* seed: 42\n* optimizer: Adam with betas=(0.9,0.999) and epsilon=1e-08\n* lr\\_scheduler\\_type: linear\n* training\\_steps: 10000",
"### Training results",
"### Framework versions\n\n\n* PEFT 0.9.0\n* Transformers 4.38.2\n* Pytorch 2.2.0+cu121\n* Datasets 2.17.1\n* Tokenizers 0.15.2"
] |
null | peft |
<!-- This model card has been generated automatically according to the information the Trainer had access to. You
should probably proofread and complete it, then remove this comment. -->
# GUE_prom_prom_300_tata-seqsight_4096_512_46M-L32_f
This model is a fine-tuned version of [mahdibaghbanzadeh/seqsight_4096_512_46M](https://huggingface.co/mahdibaghbanzadeh/seqsight_4096_512_46M) on the [mahdibaghbanzadeh/GUE_prom_prom_300_tata](https://huggingface.co/datasets/mahdibaghbanzadeh/GUE_prom_prom_300_tata) dataset.
It achieves the following results on the evaluation set:
- Loss: 0.4797
- F1 Score: 0.8010
- Accuracy: 0.8010
## Model description
More information needed
## Intended uses & limitations
More information needed
## Training and evaluation data
More information needed
## Training procedure
### Training hyperparameters
The following hyperparameters were used during training:
- learning_rate: 0.0005
- train_batch_size: 128
- eval_batch_size: 128
- seed: 42
- optimizer: Adam with betas=(0.9,0.999) and epsilon=1e-08
- lr_scheduler_type: linear
- training_steps: 10000
### Training results
| Training Loss | Epoch | Step | Validation Loss | F1 Score | Accuracy |
|:-------------:|:------:|:-----:|:---------------:|:--------:|:--------:|
| 0.491 | 5.13 | 200 | 0.4698 | 0.8044 | 0.8042 |
| 0.378 | 10.26 | 400 | 0.5056 | 0.7936 | 0.7945 |
| 0.3148 | 15.38 | 600 | 0.4899 | 0.8142 | 0.8140 |
| 0.2565 | 20.51 | 800 | 0.5451 | 0.8141 | 0.8140 |
| 0.2036 | 25.64 | 1000 | 0.6197 | 0.8092 | 0.8091 |
| 0.1585 | 30.77 | 1200 | 0.6934 | 0.8005 | 0.8010 |
| 0.1323 | 35.9 | 1400 | 0.7745 | 0.8093 | 0.8091 |
| 0.105 | 41.03 | 1600 | 0.8804 | 0.7928 | 0.7928 |
| 0.0907 | 46.15 | 1800 | 0.9467 | 0.7880 | 0.7879 |
| 0.0741 | 51.28 | 2000 | 1.0341 | 0.7923 | 0.7928 |
| 0.0682 | 56.41 | 2200 | 1.1984 | 0.8060 | 0.8059 |
| 0.0636 | 61.54 | 2400 | 1.0886 | 0.7962 | 0.7961 |
| 0.0547 | 66.67 | 2600 | 1.1504 | 0.7945 | 0.7945 |
| 0.0504 | 71.79 | 2800 | 1.1883 | 0.7960 | 0.7961 |
| 0.0469 | 76.92 | 3000 | 1.2114 | 0.7848 | 0.7847 |
| 0.0416 | 82.05 | 3200 | 1.1602 | 0.7930 | 0.7928 |
| 0.0422 | 87.18 | 3400 | 1.2750 | 0.7877 | 0.7879 |
| 0.0353 | 92.31 | 3600 | 1.2695 | 0.7945 | 0.7945 |
| 0.0373 | 97.44 | 3800 | 1.2257 | 0.7945 | 0.7945 |
| 0.0333 | 102.56 | 4000 | 1.3635 | 0.7962 | 0.7961 |
| 0.0328 | 107.69 | 4200 | 1.3407 | 0.8025 | 0.8026 |
| 0.0311 | 112.82 | 4400 | 1.3750 | 0.8011 | 0.8010 |
| 0.0286 | 117.95 | 4600 | 1.3720 | 0.8011 | 0.8010 |
| 0.0257 | 123.08 | 4800 | 1.3457 | 0.8011 | 0.8010 |
| 0.0263 | 128.21 | 5000 | 1.3824 | 0.7912 | 0.7912 |
| 0.0271 | 133.33 | 5200 | 1.3912 | 0.7946 | 0.7945 |
| 0.0227 | 138.46 | 5400 | 1.4420 | 0.7962 | 0.7961 |
| 0.0253 | 143.59 | 5600 | 1.4062 | 0.8010 | 0.8010 |
| 0.0199 | 148.72 | 5800 | 1.5250 | 0.7962 | 0.7961 |
| 0.0209 | 153.85 | 6000 | 1.5001 | 0.8027 | 0.8026 |
| 0.0225 | 158.97 | 6200 | 1.5224 | 0.7783 | 0.7781 |
| 0.0206 | 164.1 | 6400 | 1.5148 | 0.7962 | 0.7961 |
| 0.0168 | 169.23 | 6600 | 1.6866 | 0.8027 | 0.8026 |
| 0.0174 | 174.36 | 6800 | 1.6624 | 0.7880 | 0.7879 |
| 0.0171 | 179.49 | 7000 | 1.5981 | 0.7978 | 0.7977 |
| 0.0166 | 184.62 | 7200 | 1.5850 | 0.7929 | 0.7928 |
| 0.0154 | 189.74 | 7400 | 1.6554 | 0.7979 | 0.7977 |
| 0.015 | 194.87 | 7600 | 1.6823 | 0.7945 | 0.7945 |
| 0.016 | 200.0 | 7800 | 1.6797 | 0.8027 | 0.8026 |
| 0.0148 | 205.13 | 8000 | 1.6498 | 0.7962 | 0.7961 |
| 0.0133 | 210.26 | 8200 | 1.7261 | 0.7928 | 0.7928 |
| 0.0154 | 215.38 | 8400 | 1.7055 | 0.7978 | 0.7977 |
| 0.0122 | 220.51 | 8600 | 1.7680 | 0.7913 | 0.7912 |
| 0.0115 | 225.64 | 8800 | 1.7756 | 0.8060 | 0.8059 |
| 0.0106 | 230.77 | 9000 | 1.8826 | 0.8026 | 0.8026 |
| 0.0127 | 235.9 | 9200 | 1.7970 | 0.8043 | 0.8042 |
| 0.0111 | 241.03 | 9400 | 1.8099 | 0.8011 | 0.8010 |
| 0.0097 | 246.15 | 9600 | 1.8413 | 0.8093 | 0.8091 |
| 0.0122 | 251.28 | 9800 | 1.8452 | 0.7994 | 0.7993 |
| 0.0112 | 256.41 | 10000 | 1.8429 | 0.8027 | 0.8026 |
### Framework versions
- PEFT 0.9.0
- Transformers 4.38.2
- Pytorch 2.2.0+cu121
- Datasets 2.17.1
- Tokenizers 0.15.2 | {"library_name": "peft", "tags": ["generated_from_trainer"], "metrics": ["accuracy"], "base_model": "mahdibaghbanzadeh/seqsight_4096_512_46M", "model-index": [{"name": "GUE_prom_prom_300_tata-seqsight_4096_512_46M-L32_f", "results": []}]} | mahdibaghbanzadeh/GUE_prom_prom_300_tata-seqsight_4096_512_46M-L32_f | null | [
"peft",
"safetensors",
"generated_from_trainer",
"base_model:mahdibaghbanzadeh/seqsight_4096_512_46M",
"region:us"
] | null | 2024-04-26T18:52:33+00:00 | [] | [] | TAGS
#peft #safetensors #generated_from_trainer #base_model-mahdibaghbanzadeh/seqsight_4096_512_46M #region-us
| GUE\_prom\_prom\_300\_tata-seqsight\_4096\_512\_46M-L32\_f
==========================================================
This model is a fine-tuned version of mahdibaghbanzadeh/seqsight\_4096\_512\_46M on the mahdibaghbanzadeh/GUE\_prom\_prom\_300\_tata dataset.
It achieves the following results on the evaluation set:
* Loss: 0.4797
* F1 Score: 0.8010
* Accuracy: 0.8010
Model description
-----------------
More information needed
Intended uses & limitations
---------------------------
More information needed
Training and evaluation data
----------------------------
More information needed
Training procedure
------------------
### Training hyperparameters
The following hyperparameters were used during training:
* learning\_rate: 0.0005
* train\_batch\_size: 128
* eval\_batch\_size: 128
* seed: 42
* optimizer: Adam with betas=(0.9,0.999) and epsilon=1e-08
* lr\_scheduler\_type: linear
* training\_steps: 10000
### Training results
### Framework versions
* PEFT 0.9.0
* Transformers 4.38.2
* Pytorch 2.2.0+cu121
* Datasets 2.17.1
* Tokenizers 0.15.2
| [
"### Training hyperparameters\n\n\nThe following hyperparameters were used during training:\n\n\n* learning\\_rate: 0.0005\n* train\\_batch\\_size: 128\n* eval\\_batch\\_size: 128\n* seed: 42\n* optimizer: Adam with betas=(0.9,0.999) and epsilon=1e-08\n* lr\\_scheduler\\_type: linear\n* training\\_steps: 10000",
"### Training results",
"### Framework versions\n\n\n* PEFT 0.9.0\n* Transformers 4.38.2\n* Pytorch 2.2.0+cu121\n* Datasets 2.17.1\n* Tokenizers 0.15.2"
] | [
"TAGS\n#peft #safetensors #generated_from_trainer #base_model-mahdibaghbanzadeh/seqsight_4096_512_46M #region-us \n",
"### Training hyperparameters\n\n\nThe following hyperparameters were used during training:\n\n\n* learning\\_rate: 0.0005\n* train\\_batch\\_size: 128\n* eval\\_batch\\_size: 128\n* seed: 42\n* optimizer: Adam with betas=(0.9,0.999) and epsilon=1e-08\n* lr\\_scheduler\\_type: linear\n* training\\_steps: 10000",
"### Training results",
"### Framework versions\n\n\n* PEFT 0.9.0\n* Transformers 4.38.2\n* Pytorch 2.2.0+cu121\n* Datasets 2.17.1\n* Tokenizers 0.15.2"
] |
null | peft |
<!-- This model card has been generated automatically according to the information the Trainer had access to. You
should probably proofread and complete it, then remove this comment. -->
# GUE_prom_prom_300_notata-seqsight_4096_512_46M-L1_f
This model is a fine-tuned version of [mahdibaghbanzadeh/seqsight_4096_512_46M](https://huggingface.co/mahdibaghbanzadeh/seqsight_4096_512_46M) on the [mahdibaghbanzadeh/GUE_prom_prom_300_notata](https://huggingface.co/datasets/mahdibaghbanzadeh/GUE_prom_prom_300_notata) dataset.
It achieves the following results on the evaluation set:
- Loss: 0.1202
- F1 Score: 0.9538
- Accuracy: 0.9538
## Model description
More information needed
## Intended uses & limitations
More information needed
## Training and evaluation data
More information needed
## Training procedure
### Training hyperparameters
The following hyperparameters were used during training:
- learning_rate: 0.0005
- train_batch_size: 128
- eval_batch_size: 128
- seed: 42
- optimizer: Adam with betas=(0.9,0.999) and epsilon=1e-08
- lr_scheduler_type: linear
- training_steps: 10000
### Training results
| Training Loss | Epoch | Step | Validation Loss | F1 Score | Accuracy |
|:-------------:|:-----:|:-----:|:---------------:|:--------:|:--------:|
| 0.2538 | 0.6 | 200 | 0.1608 | 0.9378 | 0.9378 |
| 0.1602 | 1.2 | 400 | 0.1460 | 0.9419 | 0.9420 |
| 0.1447 | 1.81 | 600 | 0.1247 | 0.9508 | 0.9508 |
| 0.1294 | 2.41 | 800 | 0.1321 | 0.9487 | 0.9487 |
| 0.1272 | 3.01 | 1000 | 0.1173 | 0.9565 | 0.9565 |
| 0.1209 | 3.61 | 1200 | 0.1226 | 0.9517 | 0.9518 |
| 0.1163 | 4.22 | 1400 | 0.1175 | 0.9547 | 0.9548 |
| 0.1153 | 4.82 | 1600 | 0.1133 | 0.9566 | 0.9567 |
| 0.113 | 5.42 | 1800 | 0.1130 | 0.9566 | 0.9567 |
| 0.1083 | 6.02 | 2000 | 0.1088 | 0.9585 | 0.9585 |
| 0.1104 | 6.63 | 2200 | 0.1084 | 0.9595 | 0.9595 |
| 0.1089 | 7.23 | 2400 | 0.1120 | 0.9597 | 0.9597 |
| 0.103 | 7.83 | 2600 | 0.1120 | 0.9581 | 0.9582 |
| 0.106 | 8.43 | 2800 | 0.1124 | 0.9591 | 0.9591 |
| 0.1056 | 9.04 | 3000 | 0.1107 | 0.9591 | 0.9591 |
| 0.1015 | 9.64 | 3200 | 0.1041 | 0.9606 | 0.9606 |
| 0.0987 | 10.24 | 3400 | 0.1141 | 0.9591 | 0.9591 |
| 0.104 | 10.84 | 3600 | 0.1049 | 0.9615 | 0.9616 |
| 0.0978 | 11.45 | 3800 | 0.1042 | 0.9608 | 0.9608 |
| 0.1002 | 12.05 | 4000 | 0.1062 | 0.9606 | 0.9606 |
| 0.0998 | 12.65 | 4200 | 0.1042 | 0.9614 | 0.9614 |
| 0.1005 | 13.25 | 4400 | 0.1050 | 0.9610 | 0.9610 |
| 0.0952 | 13.86 | 4600 | 0.1041 | 0.9614 | 0.9614 |
| 0.0988 | 14.46 | 4800 | 0.1060 | 0.9608 | 0.9608 |
| 0.0929 | 15.06 | 5000 | 0.1028 | 0.9621 | 0.9621 |
| 0.0946 | 15.66 | 5200 | 0.1049 | 0.9625 | 0.9625 |
| 0.0976 | 16.27 | 5400 | 0.1085 | 0.9604 | 0.9604 |
| 0.0929 | 16.87 | 5600 | 0.1048 | 0.9615 | 0.9616 |
| 0.0928 | 17.47 | 5800 | 0.1010 | 0.9612 | 0.9612 |
| 0.0969 | 18.07 | 6000 | 0.1021 | 0.9617 | 0.9617 |
| 0.0901 | 18.67 | 6200 | 0.1064 | 0.9615 | 0.9616 |
| 0.0932 | 19.28 | 6400 | 0.1010 | 0.9621 | 0.9621 |
| 0.0909 | 19.88 | 6600 | 0.1089 | 0.9613 | 0.9614 |
| 0.0952 | 20.48 | 6800 | 0.1042 | 0.9621 | 0.9621 |
| 0.0902 | 21.08 | 7000 | 0.1011 | 0.9621 | 0.9621 |
| 0.0894 | 21.69 | 7200 | 0.1003 | 0.9619 | 0.9619 |
| 0.0895 | 22.29 | 7400 | 0.1001 | 0.9627 | 0.9627 |
| 0.0912 | 22.89 | 7600 | 0.0993 | 0.9627 | 0.9627 |
| 0.0893 | 23.49 | 7800 | 0.1001 | 0.9619 | 0.9619 |
| 0.0896 | 24.1 | 8000 | 0.1005 | 0.9634 | 0.9634 |
| 0.0885 | 24.7 | 8200 | 0.1055 | 0.9630 | 0.9631 |
| 0.0896 | 25.3 | 8400 | 0.1019 | 0.9629 | 0.9629 |
| 0.0875 | 25.9 | 8600 | 0.0992 | 0.9627 | 0.9627 |
| 0.0885 | 26.51 | 8800 | 0.1004 | 0.9629 | 0.9629 |
| 0.0899 | 27.11 | 9000 | 0.0995 | 0.9634 | 0.9634 |
| 0.0866 | 27.71 | 9200 | 0.1016 | 0.9640 | 0.9640 |
| 0.0866 | 28.31 | 9400 | 0.1008 | 0.9640 | 0.9640 |
| 0.0896 | 28.92 | 9600 | 0.1001 | 0.9631 | 0.9631 |
| 0.0843 | 29.52 | 9800 | 0.1014 | 0.9634 | 0.9634 |
| 0.0884 | 30.12 | 10000 | 0.1007 | 0.9631 | 0.9631 |
### Framework versions
- PEFT 0.9.0
- Transformers 4.38.2
- Pytorch 2.2.0+cu121
- Datasets 2.17.1
- Tokenizers 0.15.2 | {"library_name": "peft", "tags": ["generated_from_trainer"], "metrics": ["accuracy"], "base_model": "mahdibaghbanzadeh/seqsight_4096_512_46M", "model-index": [{"name": "GUE_prom_prom_300_notata-seqsight_4096_512_46M-L1_f", "results": []}]} | mahdibaghbanzadeh/GUE_prom_prom_300_notata-seqsight_4096_512_46M-L1_f | null | [
"peft",
"safetensors",
"generated_from_trainer",
"base_model:mahdibaghbanzadeh/seqsight_4096_512_46M",
"region:us"
] | null | 2024-04-26T18:53:09+00:00 | [] | [] | TAGS
#peft #safetensors #generated_from_trainer #base_model-mahdibaghbanzadeh/seqsight_4096_512_46M #region-us
| GUE\_prom\_prom\_300\_notata-seqsight\_4096\_512\_46M-L1\_f
===========================================================
This model is a fine-tuned version of mahdibaghbanzadeh/seqsight\_4096\_512\_46M on the mahdibaghbanzadeh/GUE\_prom\_prom\_300\_notata dataset.
It achieves the following results on the evaluation set:
* Loss: 0.1202
* F1 Score: 0.9538
* Accuracy: 0.9538
Model description
-----------------
More information needed
Intended uses & limitations
---------------------------
More information needed
Training and evaluation data
----------------------------
More information needed
Training procedure
------------------
### Training hyperparameters
The following hyperparameters were used during training:
* learning\_rate: 0.0005
* train\_batch\_size: 128
* eval\_batch\_size: 128
* seed: 42
* optimizer: Adam with betas=(0.9,0.999) and epsilon=1e-08
* lr\_scheduler\_type: linear
* training\_steps: 10000
### Training results
### Framework versions
* PEFT 0.9.0
* Transformers 4.38.2
* Pytorch 2.2.0+cu121
* Datasets 2.17.1
* Tokenizers 0.15.2
| [
"### Training hyperparameters\n\n\nThe following hyperparameters were used during training:\n\n\n* learning\\_rate: 0.0005\n* train\\_batch\\_size: 128\n* eval\\_batch\\_size: 128\n* seed: 42\n* optimizer: Adam with betas=(0.9,0.999) and epsilon=1e-08\n* lr\\_scheduler\\_type: linear\n* training\\_steps: 10000",
"### Training results",
"### Framework versions\n\n\n* PEFT 0.9.0\n* Transformers 4.38.2\n* Pytorch 2.2.0+cu121\n* Datasets 2.17.1\n* Tokenizers 0.15.2"
] | [
"TAGS\n#peft #safetensors #generated_from_trainer #base_model-mahdibaghbanzadeh/seqsight_4096_512_46M #region-us \n",
"### Training hyperparameters\n\n\nThe following hyperparameters were used during training:\n\n\n* learning\\_rate: 0.0005\n* train\\_batch\\_size: 128\n* eval\\_batch\\_size: 128\n* seed: 42\n* optimizer: Adam with betas=(0.9,0.999) and epsilon=1e-08\n* lr\\_scheduler\\_type: linear\n* training\\_steps: 10000",
"### Training results",
"### Framework versions\n\n\n* PEFT 0.9.0\n* Transformers 4.38.2\n* Pytorch 2.2.0+cu121\n* Datasets 2.17.1\n* Tokenizers 0.15.2"
] |
text-generation | transformers |
# CodeQwen1.5-7B-Chat
## About Quantization
我们使用modelscope [swift](https://github.com/modelscope/swift/)仓库进行GPTQ量化. 量化文档可以查看[这里](https://github.com/modelscope/swift/blob/main/docs/source/LLM/LLM%E9%87%8F%E5%8C%96%E6%96%87%E6%A1%A3.md). 量化命令如下:
We use the modelscope [swift](https://github.com/modelscope/swift/) repository to perform GPTQ quantization. Quantization documentation can be found [here](https://github.com/modelscope/swift/blob/main/docs/source_en/LLM/LLM-quantization.md). The quantization command is as follows:
```bash
OMP_NUM_THREADS=14 CUDA_VISIBLE_DEVICES=0 swift export \
--model_type codeqwen1half-7b-chat --quant_bits 4 \
--dataset codefuse-evol-instruction-zh --quant_method gptq --quant_seqlen 8192
```
## Introduction
CodeQwen1.5 is the Code-Specific version of Qwen1.5. It is a transformer-based decoder-only language model pretrained on a large amount of data of codes.
* Strong code generation capabilities and competitve performance across a series of benchmarks;
* Supporting long context understanding and generation with the context length of 64K tokens;
* Supporting 92 coding languages
* Excellent performance on text-to-SQL, bug fix, etc.
For more details, please refer to our [blog post](https://qwenlm.github.io/blog/codeqwen1.5/) and [GitHub repo](https://github.com/QwenLM/Qwen1.5).
## Model Details
CodeQwen1.5 is based on Qwen1.5, a language model series including decoder language models of different model sizes. It is trained on 3 trillion tokens of data of codes, and it includes group query attention (GQA) for efficient inference.
## Requirements
The code of Qwen1.5 has been in the latest Hugging face transformers and we advise you to install `transformers>=4.37.0`, or you might encounter the following error:
```
KeyError: 'qwen2'.
```
## Quickstart
Here provides a code snippet with `apply_chat_template` to show you how to load the tokenizer and model and how to generate contents.
```python
from transformers import AutoModelForCausalLM, AutoTokenizer
device = "cuda" # the device to load the model onto
model = AutoModelForCausalLM.from_pretrained(
"study-hjt/CodeQwen1.5-7B-Chat-GPTQ-Int4",
torch_dtype="auto",
device_map="auto"
)
tokenizer = AutoTokenizer.from_pretrained("study-hjt/CodeQwen1.5-7B-Chat-GPTQ-Int4")
prompt = "Write a quicksort algorithm in python."
messages = [
{"role": "system", "content": "You are a helpful assistant."},
{"role": "user", "content": prompt}
]
text = tokenizer.apply_chat_template(
messages,
tokenize=False,
add_generation_prompt=True
)
model_inputs = tokenizer([text], return_tensors="pt").to(device)
generated_ids = model.generate(
model_inputs.input_ids,
max_new_tokens=512
)
generated_ids = [
output_ids[len(input_ids):] for input_ids, output_ids in zip(model_inputs.input_ids, generated_ids)
]
response = tokenizer.batch_decode(generated_ids, skip_special_tokens=True)[0]
print(response)
```
## Tips
* If you encounter code switching or other bad cases, we advise you to use our provided hyper-parameters in `generation_config.json`.
## Citation
If you find our work helpful, feel free to give us a cite.
```
@article{qwen,
title={Qwen Technical Report},
author={Jinze Bai and Shuai Bai and Yunfei Chu and Zeyu Cui and Kai Dang and Xiaodong Deng and Yang Fan and Wenbin Ge and Yu Han and Fei Huang and Binyuan Hui and Luo Ji and Mei Li and Junyang Lin and Runji Lin and Dayiheng Liu and Gao Liu and Chengqiang Lu and Keming Lu and Jianxin Ma and Rui Men and Xingzhang Ren and Xuancheng Ren and Chuanqi Tan and Sinan Tan and Jianhong Tu and Peng Wang and Shijie Wang and Wei Wang and Shengguang Wu and Benfeng Xu and Jin Xu and An Yang and Hao Yang and Jian Yang and Shusheng Yang and Yang Yao and Bowen Yu and Hongyi Yuan and Zheng Yuan and Jianwei Zhang and Xingxuan Zhang and Yichang Zhang and Zhenru Zhang and Chang Zhou and Jingren Zhou and Xiaohuan Zhou and Tianhang Zhu},
journal={arXiv preprint arXiv:2309.16609},
year={2023}
}
``` | {"language": ["en"], "license": "other", "tags": ["codeqwen", "code", "chat", "gptq", "int4"], "license_name": "tongyi-qianwen", "license_link": "https://huggingface.co/Qwen/CodeQwen1.5-7B-Chat/blob/main/LICENSE", "pipeline_tag": "text-generation", "studios": ["qwen/CodeQwen1.5-7b-Chat-demo"]} | study-hjt/CodeQwen1.5-7B-Chat-GPTQ-Int4 | null | [
"transformers",
"safetensors",
"qwen2",
"text-generation",
"codeqwen",
"code",
"chat",
"gptq",
"int4",
"conversational",
"en",
"license:other",
"autotrain_compatible",
"endpoints_compatible",
"text-generation-inference",
"4-bit",
"region:us"
] | null | 2024-04-26T18:53:57+00:00 | [] | [
"en"
] | TAGS
#transformers #safetensors #qwen2 #text-generation #codeqwen #code #chat #gptq #int4 #conversational #en #license-other #autotrain_compatible #endpoints_compatible #text-generation-inference #4-bit #region-us
|
# CodeQwen1.5-7B-Chat
## About Quantization
我们使用modelscope swift仓库进行GPTQ量化. 量化文档可以查看这里. 量化命令如下:
We use the modelscope swift repository to perform GPTQ quantization. Quantization documentation can be found here. The quantization command is as follows:
## Introduction
CodeQwen1.5 is the Code-Specific version of Qwen1.5. It is a transformer-based decoder-only language model pretrained on a large amount of data of codes.
* Strong code generation capabilities and competitve performance across a series of benchmarks;
* Supporting long context understanding and generation with the context length of 64K tokens;
* Supporting 92 coding languages
* Excellent performance on text-to-SQL, bug fix, etc.
For more details, please refer to our blog post and GitHub repo.
## Model Details
CodeQwen1.5 is based on Qwen1.5, a language model series including decoder language models of different model sizes. It is trained on 3 trillion tokens of data of codes, and it includes group query attention (GQA) for efficient inference.
## Requirements
The code of Qwen1.5 has been in the latest Hugging face transformers and we advise you to install 'transformers>=4.37.0', or you might encounter the following error:
## Quickstart
Here provides a code snippet with 'apply_chat_template' to show you how to load the tokenizer and model and how to generate contents.
## Tips
* If you encounter code switching or other bad cases, we advise you to use our provided hyper-parameters in 'generation_config.json'.
If you find our work helpful, feel free to give us a cite.
| [
"# CodeQwen1.5-7B-Chat",
"## About Quantization\n我们使用modelscope swift仓库进行GPTQ量化. 量化文档可以查看这里. 量化命令如下:\n\nWe use the modelscope swift repository to perform GPTQ quantization. Quantization documentation can be found here. The quantization command is as follows:",
"## Introduction\n\nCodeQwen1.5 is the Code-Specific version of Qwen1.5. It is a transformer-based decoder-only language model pretrained on a large amount of data of codes. \n\n* Strong code generation capabilities and competitve performance across a series of benchmarks;\n* Supporting long context understanding and generation with the context length of 64K tokens;\n* Supporting 92 coding languages\n* Excellent performance on text-to-SQL, bug fix, etc.\n\n\nFor more details, please refer to our blog post and GitHub repo.",
"## Model Details\nCodeQwen1.5 is based on Qwen1.5, a language model series including decoder language models of different model sizes. It is trained on 3 trillion tokens of data of codes, and it includes group query attention (GQA) for efficient inference.",
"## Requirements\nThe code of Qwen1.5 has been in the latest Hugging face transformers and we advise you to install 'transformers>=4.37.0', or you might encounter the following error:",
"## Quickstart\n\nHere provides a code snippet with 'apply_chat_template' to show you how to load the tokenizer and model and how to generate contents.",
"## Tips\n\n* If you encounter code switching or other bad cases, we advise you to use our provided hyper-parameters in 'generation_config.json'.\n\n\nIf you find our work helpful, feel free to give us a cite."
] | [
"TAGS\n#transformers #safetensors #qwen2 #text-generation #codeqwen #code #chat #gptq #int4 #conversational #en #license-other #autotrain_compatible #endpoints_compatible #text-generation-inference #4-bit #region-us \n",
"# CodeQwen1.5-7B-Chat",
"## About Quantization\n我们使用modelscope swift仓库进行GPTQ量化. 量化文档可以查看这里. 量化命令如下:\n\nWe use the modelscope swift repository to perform GPTQ quantization. Quantization documentation can be found here. The quantization command is as follows:",
"## Introduction\n\nCodeQwen1.5 is the Code-Specific version of Qwen1.5. It is a transformer-based decoder-only language model pretrained on a large amount of data of codes. \n\n* Strong code generation capabilities and competitve performance across a series of benchmarks;\n* Supporting long context understanding and generation with the context length of 64K tokens;\n* Supporting 92 coding languages\n* Excellent performance on text-to-SQL, bug fix, etc.\n\n\nFor more details, please refer to our blog post and GitHub repo.",
"## Model Details\nCodeQwen1.5 is based on Qwen1.5, a language model series including decoder language models of different model sizes. It is trained on 3 trillion tokens of data of codes, and it includes group query attention (GQA) for efficient inference.",
"## Requirements\nThe code of Qwen1.5 has been in the latest Hugging face transformers and we advise you to install 'transformers>=4.37.0', or you might encounter the following error:",
"## Quickstart\n\nHere provides a code snippet with 'apply_chat_template' to show you how to load the tokenizer and model and how to generate contents.",
"## Tips\n\n* If you encounter code switching or other bad cases, we advise you to use our provided hyper-parameters in 'generation_config.json'.\n\n\nIf you find our work helpful, feel free to give us a cite."
] |
null | transformers |
# Model Card for Model ID
<!-- Provide a quick summary of what the model is/does. -->
## Model Details
### Model Description
<!-- Provide a longer summary of what this model is. -->
This is the model card of a 🤗 transformers model that has been pushed on the Hub. This model card has been automatically generated.
- **Developed by:** [More Information Needed]
- **Funded by [optional]:** [More Information Needed]
- **Shared by [optional]:** [More Information Needed]
- **Model type:** [More Information Needed]
- **Language(s) (NLP):** [More Information Needed]
- **License:** [More Information Needed]
- **Finetuned from model [optional]:** [More Information Needed]
### Model Sources [optional]
<!-- Provide the basic links for the model. -->
- **Repository:** [More Information Needed]
- **Paper [optional]:** [More Information Needed]
- **Demo [optional]:** [More Information Needed]
## Uses
<!-- Address questions around how the model is intended to be used, including the foreseeable users of the model and those affected by the model. -->
### Direct Use
<!-- This section is for the model use without fine-tuning or plugging into a larger ecosystem/app. -->
[More Information Needed]
### Downstream Use [optional]
<!-- This section is for the model use when fine-tuned for a task, or when plugged into a larger ecosystem/app -->
[More Information Needed]
### Out-of-Scope Use
<!-- This section addresses misuse, malicious use, and uses that the model will not work well for. -->
[More Information Needed]
## Bias, Risks, and Limitations
<!-- This section is meant to convey both technical and sociotechnical limitations. -->
[More Information Needed]
### Recommendations
<!-- This section is meant to convey recommendations with respect to the bias, risk, and technical limitations. -->
Users (both direct and downstream) should be made aware of the risks, biases and limitations of the model. More information needed for further recommendations.
## How to Get Started with the Model
Use the code below to get started with the model.
[More Information Needed]
## Training Details
### Training Data
<!-- This should link to a Dataset Card, perhaps with a short stub of information on what the training data is all about as well as documentation related to data pre-processing or additional filtering. -->
[More Information Needed]
### Training Procedure
<!-- This relates heavily to the Technical Specifications. Content here should link to that section when it is relevant to the training procedure. -->
#### Preprocessing [optional]
[More Information Needed]
#### Training Hyperparameters
- **Training regime:** [More Information Needed] <!--fp32, fp16 mixed precision, bf16 mixed precision, bf16 non-mixed precision, fp16 non-mixed precision, fp8 mixed precision -->
#### Speeds, Sizes, Times [optional]
<!-- This section provides information about throughput, start/end time, checkpoint size if relevant, etc. -->
[More Information Needed]
## Evaluation
<!-- This section describes the evaluation protocols and provides the results. -->
### Testing Data, Factors & Metrics
#### Testing Data
<!-- This should link to a Dataset Card if possible. -->
[More Information Needed]
#### Factors
<!-- These are the things the evaluation is disaggregating by, e.g., subpopulations or domains. -->
[More Information Needed]
#### Metrics
<!-- These are the evaluation metrics being used, ideally with a description of why. -->
[More Information Needed]
### Results
[More Information Needed]
#### Summary
## Model Examination [optional]
<!-- Relevant interpretability work for the model goes here -->
[More Information Needed]
## Environmental Impact
<!-- Total emissions (in grams of CO2eq) and additional considerations, such as electricity usage, go here. Edit the suggested text below accordingly -->
Carbon emissions can be estimated using the [Machine Learning Impact calculator](https://mlco2.github.io/impact#compute) presented in [Lacoste et al. (2019)](https://arxiv.org/abs/1910.09700).
- **Hardware Type:** [More Information Needed]
- **Hours used:** [More Information Needed]
- **Cloud Provider:** [More Information Needed]
- **Compute Region:** [More Information Needed]
- **Carbon Emitted:** [More Information Needed]
## Technical Specifications [optional]
### Model Architecture and Objective
[More Information Needed]
### Compute Infrastructure
[More Information Needed]
#### Hardware
[More Information Needed]
#### Software
[More Information Needed]
## Citation [optional]
<!-- If there is a paper or blog post introducing the model, the APA and Bibtex information for that should go in this section. -->
**BibTeX:**
[More Information Needed]
**APA:**
[More Information Needed]
## Glossary [optional]
<!-- If relevant, include terms and calculations in this section that can help readers understand the model or model card. -->
[More Information Needed]
## More Information [optional]
[More Information Needed]
## Model Card Authors [optional]
[More Information Needed]
## Model Card Contact
[More Information Needed] | {"library_name": "transformers", "tags": []} | HenryCai1129/adapter-llama-adapterhappy2sad-1k-50-0.003 | null | [
"transformers",
"safetensors",
"arxiv:1910.09700",
"endpoints_compatible",
"region:us"
] | null | 2024-04-26T18:54:11+00:00 | [
"1910.09700"
] | [] | TAGS
#transformers #safetensors #arxiv-1910.09700 #endpoints_compatible #region-us
|
# Model Card for Model ID
## Model Details
### Model Description
This is the model card of a transformers model that has been pushed on the Hub. This model card has been automatically generated.
- Developed by:
- Funded by [optional]:
- Shared by [optional]:
- Model type:
- Language(s) (NLP):
- License:
- Finetuned from model [optional]:
### Model Sources [optional]
- Repository:
- Paper [optional]:
- Demo [optional]:
## Uses
### Direct Use
### Downstream Use [optional]
### Out-of-Scope Use
## Bias, Risks, and Limitations
### Recommendations
Users (both direct and downstream) should be made aware of the risks, biases and limitations of the model. More information needed for further recommendations.
## How to Get Started with the Model
Use the code below to get started with the model.
## Training Details
### Training Data
### Training Procedure
#### Preprocessing [optional]
#### Training Hyperparameters
- Training regime:
#### Speeds, Sizes, Times [optional]
## Evaluation
### Testing Data, Factors & Metrics
#### Testing Data
#### Factors
#### Metrics
### Results
#### Summary
## Model Examination [optional]
## Environmental Impact
Carbon emissions can be estimated using the Machine Learning Impact calculator presented in Lacoste et al. (2019).
- Hardware Type:
- Hours used:
- Cloud Provider:
- Compute Region:
- Carbon Emitted:
## Technical Specifications [optional]
### Model Architecture and Objective
### Compute Infrastructure
#### Hardware
#### Software
[optional]
BibTeX:
APA:
## Glossary [optional]
## More Information [optional]
## Model Card Authors [optional]
## Model Card Contact
| [
"# Model Card for Model ID",
"## Model Details",
"### Model Description\n\n\n\nThis is the model card of a transformers model that has been pushed on the Hub. This model card has been automatically generated.\n\n- Developed by: \n- Funded by [optional]: \n- Shared by [optional]: \n- Model type: \n- Language(s) (NLP): \n- License: \n- Finetuned from model [optional]:",
"### Model Sources [optional]\n\n\n\n- Repository: \n- Paper [optional]: \n- Demo [optional]:",
"## Uses",
"### Direct Use",
"### Downstream Use [optional]",
"### Out-of-Scope Use",
"## Bias, Risks, and Limitations",
"### Recommendations\n\n\n\nUsers (both direct and downstream) should be made aware of the risks, biases and limitations of the model. More information needed for further recommendations.",
"## How to Get Started with the Model\n\nUse the code below to get started with the model.",
"## Training Details",
"### Training Data",
"### Training Procedure",
"#### Preprocessing [optional]",
"#### Training Hyperparameters\n\n- Training regime:",
"#### Speeds, Sizes, Times [optional]",
"## Evaluation",
"### Testing Data, Factors & Metrics",
"#### Testing Data",
"#### Factors",
"#### Metrics",
"### Results",
"#### Summary",
"## Model Examination [optional]",
"## Environmental Impact\n\n\n\nCarbon emissions can be estimated using the Machine Learning Impact calculator presented in Lacoste et al. (2019).\n\n- Hardware Type: \n- Hours used: \n- Cloud Provider: \n- Compute Region: \n- Carbon Emitted:",
"## Technical Specifications [optional]",
"### Model Architecture and Objective",
"### Compute Infrastructure",
"#### Hardware",
"#### Software\n\n\n\n[optional]\n\n\n\nBibTeX:\n\n\n\nAPA:",
"## Glossary [optional]",
"## More Information [optional]",
"## Model Card Authors [optional]",
"## Model Card Contact"
] | [
"TAGS\n#transformers #safetensors #arxiv-1910.09700 #endpoints_compatible #region-us \n",
"# Model Card for Model ID",
"## Model Details",
"### Model Description\n\n\n\nThis is the model card of a transformers model that has been pushed on the Hub. This model card has been automatically generated.\n\n- Developed by: \n- Funded by [optional]: \n- Shared by [optional]: \n- Model type: \n- Language(s) (NLP): \n- License: \n- Finetuned from model [optional]:",
"### Model Sources [optional]\n\n\n\n- Repository: \n- Paper [optional]: \n- Demo [optional]:",
"## Uses",
"### Direct Use",
"### Downstream Use [optional]",
"### Out-of-Scope Use",
"## Bias, Risks, and Limitations",
"### Recommendations\n\n\n\nUsers (both direct and downstream) should be made aware of the risks, biases and limitations of the model. More information needed for further recommendations.",
"## How to Get Started with the Model\n\nUse the code below to get started with the model.",
"## Training Details",
"### Training Data",
"### Training Procedure",
"#### Preprocessing [optional]",
"#### Training Hyperparameters\n\n- Training regime:",
"#### Speeds, Sizes, Times [optional]",
"## Evaluation",
"### Testing Data, Factors & Metrics",
"#### Testing Data",
"#### Factors",
"#### Metrics",
"### Results",
"#### Summary",
"## Model Examination [optional]",
"## Environmental Impact\n\n\n\nCarbon emissions can be estimated using the Machine Learning Impact calculator presented in Lacoste et al. (2019).\n\n- Hardware Type: \n- Hours used: \n- Cloud Provider: \n- Compute Region: \n- Carbon Emitted:",
"## Technical Specifications [optional]",
"### Model Architecture and Objective",
"### Compute Infrastructure",
"#### Hardware",
"#### Software\n\n\n\n[optional]\n\n\n\nBibTeX:\n\n\n\nAPA:",
"## Glossary [optional]",
"## More Information [optional]",
"## Model Card Authors [optional]",
"## Model Card Contact"
] |
null | peft |
# Model Card for Model ID
<!-- Provide a quick summary of what the model is/does. -->
## Model Details
### Model Description
<!-- Provide a longer summary of what this model is. -->
- **Developed by:** [More Information Needed]
- **Funded by [optional]:** [More Information Needed]
- **Shared by [optional]:** [More Information Needed]
- **Model type:** [More Information Needed]
- **Language(s) (NLP):** [More Information Needed]
- **License:** [More Information Needed]
- **Finetuned from model [optional]:** [More Information Needed]
### Model Sources [optional]
<!-- Provide the basic links for the model. -->
- **Repository:** [More Information Needed]
- **Paper [optional]:** [More Information Needed]
- **Demo [optional]:** [More Information Needed]
## Uses
<!-- Address questions around how the model is intended to be used, including the foreseeable users of the model and those affected by the model. -->
### Direct Use
<!-- This section is for the model use without fine-tuning or plugging into a larger ecosystem/app. -->
[More Information Needed]
### Downstream Use [optional]
<!-- This section is for the model use when fine-tuned for a task, or when plugged into a larger ecosystem/app -->
[More Information Needed]
### Out-of-Scope Use
<!-- This section addresses misuse, malicious use, and uses that the model will not work well for. -->
[More Information Needed]
## Bias, Risks, and Limitations
<!-- This section is meant to convey both technical and sociotechnical limitations. -->
[More Information Needed]
### Recommendations
<!-- This section is meant to convey recommendations with respect to the bias, risk, and technical limitations. -->
Users (both direct and downstream) should be made aware of the risks, biases and limitations of the model. More information needed for further recommendations.
## How to Get Started with the Model
Use the code below to get started with the model.
[More Information Needed]
## Training Details
### Training Data
<!-- This should link to a Dataset Card, perhaps with a short stub of information on what the training data is all about as well as documentation related to data pre-processing or additional filtering. -->
[More Information Needed]
### Training Procedure
<!-- This relates heavily to the Technical Specifications. Content here should link to that section when it is relevant to the training procedure. -->
#### Preprocessing [optional]
[More Information Needed]
#### Training Hyperparameters
- **Training regime:** [More Information Needed] <!--fp32, fp16 mixed precision, bf16 mixed precision, bf16 non-mixed precision, fp16 non-mixed precision, fp8 mixed precision -->
#### Speeds, Sizes, Times [optional]
<!-- This section provides information about throughput, start/end time, checkpoint size if relevant, etc. -->
[More Information Needed]
## Evaluation
<!-- This section describes the evaluation protocols and provides the results. -->
### Testing Data, Factors & Metrics
#### Testing Data
<!-- This should link to a Dataset Card if possible. -->
[More Information Needed]
#### Factors
<!-- These are the things the evaluation is disaggregating by, e.g., subpopulations or domains. -->
[More Information Needed]
#### Metrics
<!-- These are the evaluation metrics being used, ideally with a description of why. -->
[More Information Needed]
### Results
[More Information Needed]
#### Summary
## Model Examination [optional]
<!-- Relevant interpretability work for the model goes here -->
[More Information Needed]
## Environmental Impact
<!-- Total emissions (in grams of CO2eq) and additional considerations, such as electricity usage, go here. Edit the suggested text below accordingly -->
Carbon emissions can be estimated using the [Machine Learning Impact calculator](https://mlco2.github.io/impact#compute) presented in [Lacoste et al. (2019)](https://arxiv.org/abs/1910.09700).
- **Hardware Type:** [More Information Needed]
- **Hours used:** [More Information Needed]
- **Cloud Provider:** [More Information Needed]
- **Compute Region:** [More Information Needed]
- **Carbon Emitted:** [More Information Needed]
## Technical Specifications [optional]
### Model Architecture and Objective
[More Information Needed]
### Compute Infrastructure
[More Information Needed]
#### Hardware
[More Information Needed]
#### Software
[More Information Needed]
## Citation [optional]
<!-- If there is a paper or blog post introducing the model, the APA and Bibtex information for that should go in this section. -->
**BibTeX:**
[More Information Needed]
**APA:**
[More Information Needed]
## Glossary [optional]
<!-- If relevant, include terms and calculations in this section that can help readers understand the model or model card. -->
[More Information Needed]
## More Information [optional]
[More Information Needed]
## Model Card Authors [optional]
[More Information Needed]
## Model Card Contact
[More Information Needed]
### Framework versions
- PEFT 0.10.0 | {"library_name": "peft", "base_model": "mistralai/Mistral-7B-Instruct-v0.2"} | sahanes/Enlighten_Instruct | null | [
"peft",
"safetensors",
"transformer",
"arxiv:1910.09700",
"base_model:mistralai/Mistral-7B-Instruct-v0.2",
"4-bit",
"region:us"
] | null | 2024-04-26T18:55:35+00:00 | [
"1910.09700"
] | [] | TAGS
#peft #safetensors #transformer #arxiv-1910.09700 #base_model-mistralai/Mistral-7B-Instruct-v0.2 #4-bit #region-us
|
# Model Card for Model ID
## Model Details
### Model Description
- Developed by:
- Funded by [optional]:
- Shared by [optional]:
- Model type:
- Language(s) (NLP):
- License:
- Finetuned from model [optional]:
### Model Sources [optional]
- Repository:
- Paper [optional]:
- Demo [optional]:
## Uses
### Direct Use
### Downstream Use [optional]
### Out-of-Scope Use
## Bias, Risks, and Limitations
### Recommendations
Users (both direct and downstream) should be made aware of the risks, biases and limitations of the model. More information needed for further recommendations.
## How to Get Started with the Model
Use the code below to get started with the model.
## Training Details
### Training Data
### Training Procedure
#### Preprocessing [optional]
#### Training Hyperparameters
- Training regime:
#### Speeds, Sizes, Times [optional]
## Evaluation
### Testing Data, Factors & Metrics
#### Testing Data
#### Factors
#### Metrics
### Results
#### Summary
## Model Examination [optional]
## Environmental Impact
Carbon emissions can be estimated using the Machine Learning Impact calculator presented in Lacoste et al. (2019).
- Hardware Type:
- Hours used:
- Cloud Provider:
- Compute Region:
- Carbon Emitted:
## Technical Specifications [optional]
### Model Architecture and Objective
### Compute Infrastructure
#### Hardware
#### Software
[optional]
BibTeX:
APA:
## Glossary [optional]
## More Information [optional]
## Model Card Authors [optional]
## Model Card Contact
### Framework versions
- PEFT 0.10.0 | [
"# Model Card for Model ID",
"## Model Details",
"### Model Description\n\n\n\n\n\n- Developed by: \n- Funded by [optional]: \n- Shared by [optional]: \n- Model type: \n- Language(s) (NLP): \n- License: \n- Finetuned from model [optional]:",
"### Model Sources [optional]\n\n\n\n- Repository: \n- Paper [optional]: \n- Demo [optional]:",
"## Uses",
"### Direct Use",
"### Downstream Use [optional]",
"### Out-of-Scope Use",
"## Bias, Risks, and Limitations",
"### Recommendations\n\n\n\nUsers (both direct and downstream) should be made aware of the risks, biases and limitations of the model. More information needed for further recommendations.",
"## How to Get Started with the Model\n\nUse the code below to get started with the model.",
"## Training Details",
"### Training Data",
"### Training Procedure",
"#### Preprocessing [optional]",
"#### Training Hyperparameters\n\n- Training regime:",
"#### Speeds, Sizes, Times [optional]",
"## Evaluation",
"### Testing Data, Factors & Metrics",
"#### Testing Data",
"#### Factors",
"#### Metrics",
"### Results",
"#### Summary",
"## Model Examination [optional]",
"## Environmental Impact\n\n\n\nCarbon emissions can be estimated using the Machine Learning Impact calculator presented in Lacoste et al. (2019).\n\n- Hardware Type: \n- Hours used: \n- Cloud Provider: \n- Compute Region: \n- Carbon Emitted:",
"## Technical Specifications [optional]",
"### Model Architecture and Objective",
"### Compute Infrastructure",
"#### Hardware",
"#### Software\n\n\n\n[optional]\n\n\n\nBibTeX:\n\n\n\nAPA:",
"## Glossary [optional]",
"## More Information [optional]",
"## Model Card Authors [optional]",
"## Model Card Contact",
"### Framework versions\n\n- PEFT 0.10.0"
] | [
"TAGS\n#peft #safetensors #transformer #arxiv-1910.09700 #base_model-mistralai/Mistral-7B-Instruct-v0.2 #4-bit #region-us \n",
"# Model Card for Model ID",
"## Model Details",
"### Model Description\n\n\n\n\n\n- Developed by: \n- Funded by [optional]: \n- Shared by [optional]: \n- Model type: \n- Language(s) (NLP): \n- License: \n- Finetuned from model [optional]:",
"### Model Sources [optional]\n\n\n\n- Repository: \n- Paper [optional]: \n- Demo [optional]:",
"## Uses",
"### Direct Use",
"### Downstream Use [optional]",
"### Out-of-Scope Use",
"## Bias, Risks, and Limitations",
"### Recommendations\n\n\n\nUsers (both direct and downstream) should be made aware of the risks, biases and limitations of the model. More information needed for further recommendations.",
"## How to Get Started with the Model\n\nUse the code below to get started with the model.",
"## Training Details",
"### Training Data",
"### Training Procedure",
"#### Preprocessing [optional]",
"#### Training Hyperparameters\n\n- Training regime:",
"#### Speeds, Sizes, Times [optional]",
"## Evaluation",
"### Testing Data, Factors & Metrics",
"#### Testing Data",
"#### Factors",
"#### Metrics",
"### Results",
"#### Summary",
"## Model Examination [optional]",
"## Environmental Impact\n\n\n\nCarbon emissions can be estimated using the Machine Learning Impact calculator presented in Lacoste et al. (2019).\n\n- Hardware Type: \n- Hours used: \n- Cloud Provider: \n- Compute Region: \n- Carbon Emitted:",
"## Technical Specifications [optional]",
"### Model Architecture and Objective",
"### Compute Infrastructure",
"#### Hardware",
"#### Software\n\n\n\n[optional]\n\n\n\nBibTeX:\n\n\n\nAPA:",
"## Glossary [optional]",
"## More Information [optional]",
"## Model Card Authors [optional]",
"## Model Card Contact",
"### Framework versions\n\n- PEFT 0.10.0"
] |
text-generation | transformers |
# CodeQwen1.5-7B-Chat
## About Quantization
我们使用modelscope [swift](https://github.com/modelscope/swift/)仓库进行GPTQ量化. 量化文档可以查看[这里](https://github.com/modelscope/swift/blob/main/docs/source/LLM/LLM%E9%87%8F%E5%8C%96%E6%96%87%E6%A1%A3.md). 量化命令如下:
We use the modelscope [swift](https://github.com/modelscope/swift/) repository to perform GPTQ quantization. Quantization documentation can be found [here](https://github.com/modelscope/swift/blob/main/docs/source_en/LLM/LLM-quantization.md). The quantization command is as follows:
```bash
OMP_NUM_THREADS=14 CUDA_VISIBLE_DEVICES=0 swift export \
--model_type codeqwen1half-7b-chat --quant_bits 8 \
--dataset codefuse-evol-instruction-zh --quant_method gptq --quant_seqlen 8192
```
## Introduction
CodeQwen1.5 is the Code-Specific version of Qwen1.5. It is a transformer-based decoder-only language model pretrained on a large amount of data of codes.
* Strong code generation capabilities and competitve performance across a series of benchmarks;
* Supporting long context understanding and generation with the context length of 64K tokens;
* Supporting 92 coding languages
* Excellent performance on text-to-SQL, bug fix, etc.
For more details, please refer to our [blog post](https://qwenlm.github.io/blog/codeqwen1.5/) and [GitHub repo](https://github.com/QwenLM/Qwen1.5).
## Model Details
CodeQwen1.5 is based on Qwen1.5, a language model series including decoder language models of different model sizes. It is trained on 3 trillion tokens of data of codes, and it includes group query attention (GQA) for efficient inference.
## Requirements
The code of Qwen1.5 has been in the latest Hugging face transformers and we advise you to install `transformers>=4.37.0`, or you might encounter the following error:
```
KeyError: 'qwen2'.
```
## Quickstart
Here provides a code snippet with `apply_chat_template` to show you how to load the tokenizer and model and how to generate contents.
```python
from transformers import AutoModelForCausalLM, AutoTokenizer
device = "cuda" # the device to load the model onto
model = AutoModelForCausalLM.from_pretrained(
"study-hjt/CodeQwen1.5-7B-Chat-GPTQ-Int8",
torch_dtype="auto",
device_map="auto"
)
tokenizer = AutoTokenizer.from_pretrained("study-hjt/CodeQwen1.5-7B-Chat-GPTQ-Int8")
prompt = "Write a quicksort algorithm in python."
messages = [
{"role": "system", "content": "You are a helpful assistant."},
{"role": "user", "content": prompt}
]
text = tokenizer.apply_chat_template(
messages,
tokenize=False,
add_generation_prompt=True
)
model_inputs = tokenizer([text], return_tensors="pt").to(device)
generated_ids = model.generate(
model_inputs.input_ids,
max_new_tokens=512
)
generated_ids = [
output_ids[len(input_ids):] for input_ids, output_ids in zip(model_inputs.input_ids, generated_ids)
]
response = tokenizer.batch_decode(generated_ids, skip_special_tokens=True)[0]
print(response)
```
## Tips
* If you encounter code switching or other bad cases, we advise you to use our provided hyper-parameters in `generation_config.json`.
## Citation
If you find our work helpful, feel free to give us a cite.
```
@article{qwen,
title={Qwen Technical Report},
author={Jinze Bai and Shuai Bai and Yunfei Chu and Zeyu Cui and Kai Dang and Xiaodong Deng and Yang Fan and Wenbin Ge and Yu Han and Fei Huang and Binyuan Hui and Luo Ji and Mei Li and Junyang Lin and Runji Lin and Dayiheng Liu and Gao Liu and Chengqiang Lu and Keming Lu and Jianxin Ma and Rui Men and Xingzhang Ren and Xuancheng Ren and Chuanqi Tan and Sinan Tan and Jianhong Tu and Peng Wang and Shijie Wang and Wei Wang and Shengguang Wu and Benfeng Xu and Jin Xu and An Yang and Hao Yang and Jian Yang and Shusheng Yang and Yang Yao and Bowen Yu and Hongyi Yuan and Zheng Yuan and Jianwei Zhang and Xingxuan Zhang and Yichang Zhang and Zhenru Zhang and Chang Zhou and Jingren Zhou and Xiaohuan Zhou and Tianhang Zhu},
journal={arXiv preprint arXiv:2309.16609},
year={2023}
}
``` | {"language": ["en"], "license": "other", "tags": ["chat", "gptq", "codeqwen", "int8"], "license_name": "tongyi-qianwen", "license_link": "https://huggingface.co/Qwen/CodeQwen1.5-7B-Chat/blob/main/LICENSE", "pipeline_tag": "text-generation", "studios": ["qwen/CodeQwen1.5-7b-Chat-demo"]} | study-hjt/CodeQwen1.5-7B-Chat-GPTQ-Int8 | null | [
"transformers",
"safetensors",
"qwen2",
"text-generation",
"chat",
"gptq",
"codeqwen",
"int8",
"conversational",
"en",
"license:other",
"autotrain_compatible",
"endpoints_compatible",
"text-generation-inference",
"8-bit",
"region:us"
] | null | 2024-04-26T18:55:52+00:00 | [] | [
"en"
] | TAGS
#transformers #safetensors #qwen2 #text-generation #chat #gptq #codeqwen #int8 #conversational #en #license-other #autotrain_compatible #endpoints_compatible #text-generation-inference #8-bit #region-us
|
# CodeQwen1.5-7B-Chat
## About Quantization
我们使用modelscope swift仓库进行GPTQ量化. 量化文档可以查看这里. 量化命令如下:
We use the modelscope swift repository to perform GPTQ quantization. Quantization documentation can be found here. The quantization command is as follows:
## Introduction
CodeQwen1.5 is the Code-Specific version of Qwen1.5. It is a transformer-based decoder-only language model pretrained on a large amount of data of codes.
* Strong code generation capabilities and competitve performance across a series of benchmarks;
* Supporting long context understanding and generation with the context length of 64K tokens;
* Supporting 92 coding languages
* Excellent performance on text-to-SQL, bug fix, etc.
For more details, please refer to our blog post and GitHub repo.
## Model Details
CodeQwen1.5 is based on Qwen1.5, a language model series including decoder language models of different model sizes. It is trained on 3 trillion tokens of data of codes, and it includes group query attention (GQA) for efficient inference.
## Requirements
The code of Qwen1.5 has been in the latest Hugging face transformers and we advise you to install 'transformers>=4.37.0', or you might encounter the following error:
## Quickstart
Here provides a code snippet with 'apply_chat_template' to show you how to load the tokenizer and model and how to generate contents.
## Tips
* If you encounter code switching or other bad cases, we advise you to use our provided hyper-parameters in 'generation_config.json'.
If you find our work helpful, feel free to give us a cite.
| [
"# CodeQwen1.5-7B-Chat",
"## About Quantization\n我们使用modelscope swift仓库进行GPTQ量化. 量化文档可以查看这里. 量化命令如下:\n\nWe use the modelscope swift repository to perform GPTQ quantization. Quantization documentation can be found here. The quantization command is as follows:",
"## Introduction\n\nCodeQwen1.5 is the Code-Specific version of Qwen1.5. It is a transformer-based decoder-only language model pretrained on a large amount of data of codes. \n\n* Strong code generation capabilities and competitve performance across a series of benchmarks;\n* Supporting long context understanding and generation with the context length of 64K tokens;\n* Supporting 92 coding languages\n* Excellent performance on text-to-SQL, bug fix, etc.\n\n\nFor more details, please refer to our blog post and GitHub repo.",
"## Model Details\nCodeQwen1.5 is based on Qwen1.5, a language model series including decoder language models of different model sizes. It is trained on 3 trillion tokens of data of codes, and it includes group query attention (GQA) for efficient inference.",
"## Requirements\nThe code of Qwen1.5 has been in the latest Hugging face transformers and we advise you to install 'transformers>=4.37.0', or you might encounter the following error:",
"## Quickstart\n\nHere provides a code snippet with 'apply_chat_template' to show you how to load the tokenizer and model and how to generate contents.",
"## Tips\n\n* If you encounter code switching or other bad cases, we advise you to use our provided hyper-parameters in 'generation_config.json'.\n\n\nIf you find our work helpful, feel free to give us a cite."
] | [
"TAGS\n#transformers #safetensors #qwen2 #text-generation #chat #gptq #codeqwen #int8 #conversational #en #license-other #autotrain_compatible #endpoints_compatible #text-generation-inference #8-bit #region-us \n",
"# CodeQwen1.5-7B-Chat",
"## About Quantization\n我们使用modelscope swift仓库进行GPTQ量化. 量化文档可以查看这里. 量化命令如下:\n\nWe use the modelscope swift repository to perform GPTQ quantization. Quantization documentation can be found here. The quantization command is as follows:",
"## Introduction\n\nCodeQwen1.5 is the Code-Specific version of Qwen1.5. It is a transformer-based decoder-only language model pretrained on a large amount of data of codes. \n\n* Strong code generation capabilities and competitve performance across a series of benchmarks;\n* Supporting long context understanding and generation with the context length of 64K tokens;\n* Supporting 92 coding languages\n* Excellent performance on text-to-SQL, bug fix, etc.\n\n\nFor more details, please refer to our blog post and GitHub repo.",
"## Model Details\nCodeQwen1.5 is based on Qwen1.5, a language model series including decoder language models of different model sizes. It is trained on 3 trillion tokens of data of codes, and it includes group query attention (GQA) for efficient inference.",
"## Requirements\nThe code of Qwen1.5 has been in the latest Hugging face transformers and we advise you to install 'transformers>=4.37.0', or you might encounter the following error:",
"## Quickstart\n\nHere provides a code snippet with 'apply_chat_template' to show you how to load the tokenizer and model and how to generate contents.",
"## Tips\n\n* If you encounter code switching or other bad cases, we advise you to use our provided hyper-parameters in 'generation_config.json'.\n\n\nIf you find our work helpful, feel free to give us a cite."
] |
image-classification | transformers |
<!-- This model card has been generated automatically according to the information the Trainer had access to. You
should probably proofread and complete it, then remove this comment. -->
# Action_Classification
This model is a fine-tuned version of [google/vit-base-patch16-224-in21k](https://huggingface.co/google/vit-base-patch16-224-in21k) on the agent_action_class dataset.
It achieves the following results on the evaluation set:
- Loss: 0.8482
- Accuracy: 0.7629
- Confusion Matrix: [[45, 5, 20, 4, 2, 6, 4, 8, 3, 3], [5, 154, 4, 2, 1, 2, 6, 1, 17, 1], [0, 0, 51, 1, 2, 8, 1, 0, 0, 2], [1, 0, 8, 26, 8, 5, 0, 0, 1, 3], [0, 1, 0, 0, 89, 3, 0, 0, 0, 0], [0, 1, 11, 3, 1, 55, 0, 1, 0, 0], [0, 1, 1, 0, 3, 3, 51, 0, 0, 0], [0, 0, 10, 1, 0, 4, 0, 68, 0, 0], [0, 26, 5, 0, 1, 3, 16, 1, 127, 1], [3, 0, 2, 9, 2, 1, 0, 1, 0, 135]]
## Model description
More information needed
## Intended uses & limitations
More information needed
## Training and evaluation data
More information needed
## Training procedure
### Training hyperparameters
The following hyperparameters were used during training:
- learning_rate: 0.0002
- train_batch_size: 32
- eval_batch_size: 16
- seed: 42
- optimizer: Adam with betas=(0.9,0.999) and epsilon=1e-08
- lr_scheduler_type: linear
- num_epochs: 15
### Training results
| Training Loss | Epoch | Step | Validation Loss | Accuracy | Confusion Matrix |
|:-------------:|:-----:|:----:|:---------------:|:--------:|:------------------------------------------------------------------------------------------------------------------------------------------------------------------------------------------------------------------------------------------------------------------------------------------------------------------------------------------------------:|
| 0.3922 | 0.32 | 100 | 1.0781 | 0.6933 | [[66, 1, 9, 6, 1, 5, 1, 3, 7, 1], [41, 96, 0, 0, 8, 0, 2, 1, 45, 0], [2, 0, 46, 1, 1, 7, 4, 0, 1, 3], [9, 1, 4, 19, 5, 3, 2, 1, 4, 4], [0, 2, 0, 3, 84, 2, 1, 0, 0, 1], [4, 1, 3, 2, 0, 55, 3, 1, 3, 0], [0, 0, 1, 0, 0, 1, 54, 0, 3, 0], [5, 1, 4, 1, 0, 1, 0, 70, 1, 0], [5, 12, 0, 1, 1, 0, 14, 0, 147, 0], [9, 0, 1, 38, 3, 1, 4, 4, 2, 91]] |
| 0.439 | 0.64 | 200 | 0.8592 | 0.7562 | [[73, 3, 6, 4, 0, 3, 2, 3, 3, 3], [30, 121, 1, 0, 1, 0, 8, 0, 32, 0], [1, 0, 47, 1, 1, 9, 1, 0, 1, 4], [7, 0, 5, 28, 5, 1, 0, 1, 2, 3], [0, 2, 0, 1, 88, 0, 1, 0, 0, 1], [4, 1, 5, 3, 2, 51, 0, 1, 2, 3], [0, 1, 1, 0, 0, 0, 56, 0, 1, 0], [4, 2, 1, 0, 0, 0, 1, 74, 1, 0], [4, 28, 0, 1, 0, 0, 19, 2, 125, 1], [3, 0, 1, 15, 1, 0, 1, 1, 0, 131]] |
| 0.4664 | 0.96 | 300 | 0.8482 | 0.7629 | [[45, 5, 20, 4, 2, 6, 4, 8, 3, 3], [5, 154, 4, 2, 1, 2, 6, 1, 17, 1], [0, 0, 51, 1, 2, 8, 1, 0, 0, 2], [1, 0, 8, 26, 8, 5, 0, 0, 1, 3], [0, 1, 0, 0, 89, 3, 0, 0, 0, 0], [0, 1, 11, 3, 1, 55, 0, 1, 0, 0], [0, 1, 1, 0, 3, 3, 51, 0, 0, 0], [0, 0, 10, 1, 0, 4, 0, 68, 0, 0], [0, 26, 5, 0, 1, 3, 16, 1, 127, 1], [3, 0, 2, 9, 2, 1, 0, 1, 0, 135]] |
| 0.2929 | 1.27 | 400 | 1.1281 | 0.6790 | [[65, 3, 9, 7, 1, 1, 2, 2, 10, 0], [38, 113, 1, 0, 1, 0, 5, 0, 35, 0], [3, 0, 54, 4, 1, 1, 2, 0, 0, 0], [8, 2, 5, 31, 5, 0, 0, 0, 1, 0], [0, 2, 6, 3, 80, 0, 1, 1, 0, 0], [6, 2, 16, 8, 1, 34, 1, 1, 3, 0], [1, 2, 1, 0, 0, 0, 55, 0, 0, 0], [6, 2, 6, 2, 0, 0, 0, 66, 1, 0], [3, 24, 2, 2, 0, 0, 14, 0, 135, 0], [9, 2, 4, 56, 1, 0, 1, 0, 0, 80]] |
| 0.4188 | 1.59 | 500 | 1.1851 | 0.6657 | [[61, 2, 11, 6, 3, 5, 2, 5, 4, 1], [53, 85, 5, 0, 8, 5, 3, 6, 28, 0], [0, 0, 51, 2, 2, 5, 1, 3, 0, 1], [2, 1, 4, 34, 8, 0, 0, 2, 1, 0], [0, 1, 0, 1, 89, 0, 1, 0, 1, 0], [1, 0, 7, 4, 5, 48, 1, 5, 1, 0], [0, 1, 1, 0, 3, 0, 54, 0, 0, 0], [5, 1, 1, 1, 0, 3, 0, 72, 0, 0], [11, 18, 0, 0, 2, 2, 17, 8, 122, 0], [1, 1, 2, 42, 8, 1, 10, 4, 1, 83]] |
| 0.3668 | 1.91 | 600 | 0.8554 | 0.7467 | [[53, 11, 11, 5, 0, 3, 1, 4, 10, 2], [3, 145, 5, 0, 1, 1, 4, 5, 29, 0], [0, 0, 53, 1, 1, 5, 2, 1, 1, 1], [4, 0, 9, 29, 5, 2, 0, 0, 1, 2], [0, 1, 4, 3, 84, 0, 0, 0, 1, 0], [2, 2, 12, 3, 1, 45, 0, 3, 3, 1], [0, 1, 2, 1, 1, 0, 52, 0, 2, 0], [1, 2, 5, 1, 0, 1, 0, 73, 0, 0], [4, 29, 2, 0, 0, 0, 7, 3, 135, 0], [1, 0, 11, 19, 1, 5, 0, 1, 0, 115]] |
| 0.342 | 2.23 | 700 | 1.0291 | 0.7048 | [[58, 5, 4, 4, 1, 8, 1, 9, 7, 3], [36, 111, 0, 2, 1, 4, 1, 4, 34, 0], [3, 2, 45, 5, 1, 6, 1, 1, 0, 1], [6, 0, 5, 35, 2, 0, 0, 0, 1, 3], [1, 1, 2, 6, 77, 3, 1, 0, 2, 0], [4, 0, 10, 8, 1, 39, 0, 7, 2, 1], [1, 2, 1, 0, 2, 0, 50, 0, 3, 0], [1, 0, 4, 1, 0, 0, 0, 77, 0, 0], [4, 29, 0, 0, 0, 0, 5, 2, 140, 0], [5, 0, 5, 27, 0, 0, 1, 7, 0, 108]] |
| 0.2984 | 2.55 | 800 | 1.2207 | 0.6962 | [[55, 3, 11, 2, 0, 1, 2, 11, 10, 5], [44, 71, 1, 0, 1, 0, 4, 5, 66, 1], [0, 0, 49, 3, 2, 3, 3, 2, 1, 2], [4, 0, 5, 26, 7, 0, 2, 0, 1, 7], [0, 1, 0, 0, 86, 0, 3, 0, 3, 0], [5, 2, 12, 4, 2, 39, 1, 3, 1, 3], [0, 0, 1, 0, 1, 0, 57, 0, 0, 0], [0, 0, 5, 0, 0, 0, 0, 78, 0, 0], [5, 15, 3, 0, 1, 0, 9, 4, 143, 0], [0, 0, 1, 17, 1, 0, 3, 3, 1, 127]] |
| 0.3542 | 2.87 | 900 | 1.1835 | 0.6657 | [[66, 2, 6, 8, 0, 4, 4, 4, 5, 1], [38, 78, 2, 0, 3, 1, 23, 4, 43, 1], [2, 0, 50, 7, 0, 5, 1, 0, 0, 0], [2, 0, 2, 45, 3, 0, 0, 0, 0, 0], [0, 1, 3, 6, 76, 3, 4, 0, 0, 0], [3, 1, 10, 8, 1, 47, 0, 0, 1, 1], [0, 0, 2, 0, 1, 0, 56, 0, 0, 0], [2, 0, 11, 5, 0, 2, 4, 59, 0, 0], [4, 24, 2, 1, 1, 1, 23, 0, 124, 0], [5, 0, 1, 42, 3, 0, 3, 1, 0, 98]] |
| 0.2749 | 3.18 | 1000 | 0.9242 | 0.7286 | [[54, 12, 5, 2, 3, 1, 7, 1, 12, 3], [13, 155, 0, 0, 3, 1, 2, 1, 18, 0], [2, 0, 53, 1, 4, 1, 3, 0, 0, 1], [5, 1, 7, 21, 8, 0, 0, 0, 1, 9], [0, 2, 0, 1, 89, 0, 0, 1, 0, 0], [2, 4, 16, 1, 6, 34, 3, 1, 4, 1], [0, 2, 1, 0, 2, 0, 54, 0, 0, 0], [1, 3, 6, 1, 0, 0, 0, 70, 2, 0], [4, 45, 0, 1, 2, 0, 13, 0, 115, 0], [2, 1, 6, 19, 4, 0, 0, 1, 0, 120]] |
| 0.2695 | 3.5 | 1100 | 0.9828 | 0.7314 | [[58, 8, 9, 3, 0, 3, 2, 5, 10, 2], [29, 130, 2, 0, 0, 3, 1, 4, 24, 0], [1, 0, 49, 3, 1, 6, 2, 0, 0, 3], [6, 1, 5, 26, 6, 1, 0, 1, 1, 5], [0, 1, 2, 4, 79, 1, 1, 1, 4, 0], [2, 2, 12, 4, 1, 48, 1, 0, 1, 1], [0, 0, 1, 0, 1, 0, 57, 0, 0, 0], [2, 2, 4, 1, 0, 2, 2, 67, 3, 0], [1, 32, 0, 1, 0, 4, 12, 2, 125, 3], [3, 1, 2, 10, 1, 5, 2, 0, 0, 129]] |
| 0.2343 | 3.82 | 1200 | 1.0871 | 0.7295 | [[58, 2, 10, 5, 1, 1, 7, 4, 10, 2], [21, 115, 0, 0, 4, 0, 12, 3, 38, 0], [1, 0, 53, 2, 2, 3, 0, 1, 1, 2], [2, 2, 9, 22, 9, 0, 1, 0, 1, 6], [0, 2, 1, 1, 88, 0, 0, 0, 1, 0], [2, 1, 10, 4, 2, 48, 1, 2, 2, 0], [0, 0, 1, 0, 2, 0, 56, 0, 0, 0], [2, 1, 7, 0, 0, 0, 0, 72, 1, 0], [4, 22, 0, 1, 0, 0, 14, 0, 139, 0], [7, 0, 4, 20, 1, 3, 1, 2, 0, 115]] |
| 0.2714 | 4.14 | 1300 | 1.0720 | 0.7314 | [[59, 6, 8, 8, 1, 1, 3, 4, 7, 3], [23, 114, 2, 1, 1, 0, 5, 3, 42, 2], [1, 1, 54, 2, 1, 2, 0, 1, 0, 3], [3, 1, 3, 32, 4, 0, 0, 0, 1, 8], [0, 1, 3, 5, 80, 1, 1, 0, 0, 2], [3, 1, 11, 7, 2, 43, 1, 2, 1, 1], [0, 0, 1, 1, 0, 0, 56, 0, 0, 1], [1, 0, 4, 0, 0, 0, 0, 77, 1, 0], [6, 31, 2, 1, 0, 0, 10, 0, 130, 0], [5, 0, 1, 22, 0, 1, 0, 1, 0, 123]] |
| 0.2287 | 4.46 | 1400 | 1.1125 | 0.7057 | [[52, 5, 15, 8, 1, 8, 0, 3, 6, 2], [27, 109, 1, 0, 1, 6, 2, 3, 43, 1], [1, 0, 55, 3, 0, 3, 1, 0, 0, 2], [2, 1, 4, 34, 4, 2, 0, 0, 0, 5], [0, 1, 2, 4, 81, 2, 1, 0, 2, 0], [2, 2, 7, 3, 1, 54, 0, 0, 3, 0], [0, 0, 1, 0, 1, 0, 56, 0, 1, 0], [1, 1, 12, 1, 0, 3, 2, 62, 1, 0], [5, 30, 1, 0, 0, 3, 9, 0, 131, 1], [4, 6, 4, 28, 0, 4, 0, 0, 0, 107]] |
| 0.2814 | 4.78 | 1500 | 1.1163 | 0.72 | [[71, 3, 7, 5, 2, 1, 1, 6, 3, 1], [53, 111, 1, 0, 1, 0, 5, 5, 17, 0], [2, 0, 48, 4, 1, 4, 0, 4, 0, 2], [6, 0, 4, 31, 6, 0, 0, 0, 0, 5], [0, 3, 1, 4, 82, 0, 1, 1, 1, 0], [8, 0, 4, 4, 1, 49, 1, 4, 1, 0], [2, 0, 1, 1, 1, 0, 52, 2, 0, 0], [2, 0, 1, 0, 0, 0, 0, 80, 0, 0], [11, 35, 2, 0, 0, 1, 9, 5, 117, 0], [10, 1, 1, 21, 1, 0, 2, 2, 0, 115]] |
| 0.2648 | 5.1 | 1600 | 1.1721 | 0.7057 | [[61, 6, 3, 3, 0, 3, 2, 12, 8, 2], [27, 131, 0, 0, 4, 0, 1, 9, 21, 0], [2, 2, 51, 2, 2, 4, 0, 1, 0, 1], [3, 1, 4, 28, 6, 3, 0, 3, 1, 3], [1, 3, 0, 4, 82, 1, 0, 1, 1, 0], [1, 0, 8, 4, 3, 51, 0, 3, 1, 1], [3, 1, 1, 1, 1, 0, 51, 1, 0, 0], [1, 0, 2, 1, 0, 0, 0, 79, 0, 0], [9, 31, 1, 0, 0, 1, 11, 10, 117, 0], [17, 5, 4, 28, 2, 2, 1, 4, 0, 90]] |
| 0.1857 | 5.41 | 1700 | 1.0404 | 0.7514 | [[57, 9, 5, 2, 1, 0, 4, 7, 11, 4], [22, 131, 0, 0, 0, 0, 5, 4, 30, 1], [1, 0, 56, 1, 1, 2, 1, 0, 0, 3], [3, 1, 2, 28, 8, 1, 1, 1, 1, 6], [1, 1, 0, 3, 85, 0, 0, 0, 3, 0], [6, 2, 11, 4, 3, 36, 2, 5, 1, 2], [0, 0, 1, 0, 0, 0, 58, 0, 0, 0], [1, 0, 2, 0, 0, 0, 0, 80, 0, 0], [7, 32, 1, 0, 0, 0, 19, 3, 117, 1], [6, 0, 1, 3, 0, 0, 1, 1, 0, 141]] |
| 0.1958 | 5.73 | 1800 | 1.1392 | 0.7238 | [[53, 7, 4, 3, 2, 1, 6, 9, 13, 2], [16, 134, 0, 0, 0, 1, 10, 7, 25, 0], [2, 1, 54, 2, 1, 1, 1, 0, 0, 3], [6, 0, 3, 29, 8, 0, 1, 1, 1, 3], [0, 2, 0, 2, 85, 0, 2, 0, 2, 0], [7, 1, 9, 2, 4, 43, 2, 2, 1, 1], [0, 0, 1, 0, 1, 0, 57, 0, 0, 0], [0, 1, 4, 0, 0, 0, 1, 77, 0, 0], [4, 28, 2, 0, 0, 0, 19, 4, 123, 0], [7, 0, 2, 25, 1, 0, 11, 2, 0, 105]] |
| 0.1475 | 6.05 | 1900 | 1.1926 | 0.7238 | [[72, 6, 4, 4, 0, 1, 2, 0, 8, 3], [52, 97, 0, 0, 1, 0, 1, 0, 41, 1], [3, 1, 52, 3, 1, 1, 1, 0, 0, 3], [6, 1, 3, 32, 2, 1, 0, 1, 1, 5], [0, 4, 1, 3, 79, 1, 0, 0, 3, 2], [3, 2, 12, 6, 0, 43, 0, 1, 2, 3], [3, 0, 1, 0, 1, 0, 52, 0, 1, 1], [8, 0, 7, 2, 0, 0, 0, 66, 0, 0], [13, 26, 1, 1, 0, 1, 8, 1, 129, 0], [6, 0, 0, 7, 0, 0, 0, 1, 1, 138]] |
| 0.1443 | 6.37 | 2000 | 1.2271 | 0.7152 | [[64, 3, 18, 4, 1, 3, 1, 3, 2, 1], [26, 112, 1, 0, 2, 0, 5, 6, 41, 0], [4, 0, 54, 2, 1, 1, 0, 0, 0, 3], [7, 1, 3, 34, 3, 1, 0, 1, 1, 1], [0, 3, 0, 3, 82, 0, 2, 0, 3, 0], [5, 2, 11, 5, 1, 44, 1, 1, 1, 1], [0, 0, 1, 0, 1, 0, 57, 0, 0, 0], [1, 0, 7, 0, 0, 4, 0, 71, 0, 0], [5, 23, 5, 2, 0, 0, 12, 5, 128, 0], [6, 3, 1, 36, 0, 0, 0, 2, 0, 105]] |
| 0.1453 | 6.69 | 2100 | 1.0546 | 0.7390 | [[71, 4, 11, 3, 0, 4, 1, 3, 2, 1], [26, 127, 3, 0, 0, 2, 4, 4, 27, 0], [1, 0, 53, 2, 2, 4, 1, 0, 0, 2], [5, 2, 5, 27, 6, 2, 0, 1, 1, 3], [1, 1, 0, 1, 87, 1, 1, 0, 1, 0], [2, 1, 6, 1, 2, 58, 0, 1, 1, 0], [2, 3, 1, 0, 2, 0, 50, 0, 0, 1], [4, 0, 7, 0, 0, 4, 0, 68, 0, 0], [4, 34, 4, 0, 1, 3, 14, 1, 119, 0], [9, 1, 2, 18, 2, 1, 0, 3, 1, 116]] |
| 0.2319 | 7.01 | 2200 | 1.0890 | 0.7371 | [[60, 4, 9, 7, 1, 4, 2, 2, 10, 1], [18, 127, 1, 0, 2, 0, 9, 2, 34, 0], [3, 0, 53, 3, 1, 2, 0, 1, 0, 2], [4, 2, 2, 36, 6, 0, 0, 0, 1, 1], [0, 4, 0, 3, 83, 0, 1, 1, 1, 0], [2, 2, 9, 6, 1, 49, 1, 0, 2, 0], [0, 0, 1, 0, 1, 0, 57, 0, 0, 0], [1, 0, 10, 1, 0, 0, 0, 71, 0, 0], [5, 24, 4, 0, 0, 1, 15, 1, 130, 0], [4, 4, 2, 28, 0, 0, 2, 5, 0, 108]] |
| 0.1499 | 7.32 | 2300 | 1.3652 | 0.7 | [[68, 3, 3, 11, 1, 1, 1, 4, 7, 1], [60, 82, 0, 2, 6, 0, 6, 4, 31, 2], [2, 1, 43, 6, 2, 4, 2, 2, 0, 3], [2, 0, 2, 36, 6, 0, 0, 1, 1, 4], [1, 2, 1, 2, 83, 2, 0, 1, 0, 1], [4, 0, 3, 11, 2, 46, 0, 3, 2, 1], [0, 0, 0, 0, 1, 1, 54, 1, 1, 1], [4, 0, 1, 1, 0, 0, 0, 76, 1, 0], [9, 22, 0, 1, 2, 0, 16, 2, 127, 1], [1, 0, 1, 27, 0, 0, 0, 4, 0, 120]] |
| 0.1467 | 7.64 | 2400 | 1.4623 | 0.6676 | [[59, 3, 10, 7, 0, 7, 1, 2, 8, 3], [55, 65, 1, 0, 4, 4, 15, 3, 46, 0], [3, 1, 48, 6, 2, 1, 2, 0, 0, 2], [1, 0, 3, 34, 5, 1, 0, 0, 1, 7], [0, 4, 1, 2, 83, 2, 0, 0, 0, 1], [4, 2, 12, 3, 1, 46, 0, 0, 2, 2], [1, 0, 1, 0, 1, 0, 56, 0, 0, 0], [3, 0, 5, 1, 0, 2, 4, 68, 0, 0], [9, 18, 1, 0, 3, 1, 17, 1, 129, 1], [2, 3, 1, 32, 0, 0, 0, 2, 0, 113]] |
| 0.1163 | 7.96 | 2500 | 1.5301 | 0.6819 | [[53, 2, 15, 7, 0, 3, 6, 4, 5, 5], [62, 76, 2, 0, 4, 2, 19, 7, 16, 5], [1, 1, 52, 1, 1, 2, 2, 2, 0, 3], [1, 0, 5, 28, 6, 2, 1, 2, 0, 7], [0, 1, 1, 2, 83, 3, 1, 1, 0, 1], [2, 1, 13, 3, 0, 44, 1, 4, 0, 4], [0, 0, 1, 0, 1, 0, 57, 0, 0, 0], [1, 0, 5, 0, 0, 1, 1, 75, 0, 0], [11, 17, 1, 0, 1, 1, 28, 3, 116, 2], [0, 3, 2, 10, 0, 1, 3, 2, 0, 132]] |
| 0.1087 | 8.28 | 2600 | 1.2231 | 0.7324 | [[62, 6, 5, 6, 0, 0, 2, 2, 12, 5], [32, 102, 0, 0, 2, 1, 12, 3, 41, 0], [3, 2, 45, 4, 1, 3, 3, 0, 0, 4], [5, 0, 3, 29, 3, 0, 0, 0, 3, 9], [1, 5, 0, 4, 73, 2, 1, 1, 4, 2], [5, 3, 3, 6, 1, 43, 0, 3, 3, 5], [0, 0, 1, 0, 1, 0, 57, 0, 0, 0], [1, 0, 5, 1, 0, 0, 1, 72, 3, 0], [3, 21, 0, 1, 1, 0, 9, 1, 142, 2], [1, 0, 1, 6, 0, 0, 0, 1, 0, 144]] |
| 0.1783 | 8.6 | 2700 | 1.1571 | 0.7390 | [[53, 5, 17, 5, 0, 3, 2, 4, 7, 4], [23, 127, 1, 0, 4, 2, 2, 3, 31, 0], [0, 1, 56, 2, 1, 2, 0, 0, 0, 3], [1, 0, 7, 34, 3, 1, 0, 0, 0, 6], [1, 2, 3, 6, 75, 1, 1, 0, 2, 2], [2, 1, 18, 5, 1, 40, 0, 2, 2, 1], [2, 0, 1, 0, 1, 0, 54, 0, 0, 1], [1, 0, 9, 1, 0, 0, 0, 71, 0, 1], [6, 27, 4, 0, 0, 1, 12, 0, 130, 0], [1, 2, 2, 11, 0, 0, 0, 1, 0, 136]] |
| 0.1733 | 8.92 | 2800 | 1.3044 | 0.7190 | [[51, 5, 13, 8, 0, 4, 4, 5, 8, 2], [29, 116, 6, 0, 0, 4, 10, 2, 26, 0], [1, 0, 49, 1, 1, 8, 2, 0, 0, 3], [0, 0, 5, 34, 4, 3, 0, 0, 0, 6], [1, 3, 4, 2, 76, 4, 2, 0, 1, 0], [1, 0, 8, 4, 0, 52, 0, 3, 2, 2], [0, 0, 1, 0, 0, 0, 58, 0, 0, 0], [1, 0, 9, 0, 0, 2, 0, 71, 0, 0], [3, 26, 5, 0, 0, 4, 19, 3, 118, 2], [1, 2, 2, 11, 0, 0, 5, 2, 0, 130]] |
| 0.1275 | 9.24 | 2900 | 1.2416 | 0.7267 | [[66, 6, 8, 5, 0, 4, 3, 3, 4, 1], [53, 111, 0, 0, 4, 1, 4, 3, 17, 0], [3, 1, 48, 3, 1, 5, 2, 0, 0, 2], [5, 1, 3, 27, 5, 2, 0, 2, 1, 6], [1, 2, 0, 1, 85, 0, 1, 0, 2, 1], [5, 0, 5, 6, 0, 50, 2, 3, 1, 0], [0, 0, 1, 0, 1, 0, 57, 0, 0, 0], [3, 0, 3, 0, 0, 2, 1, 74, 0, 0], [13, 34, 0, 1, 0, 1, 11, 1, 119, 0], [7, 0, 1, 14, 0, 0, 3, 2, 0, 126]] |
| 0.1231 | 9.55 | 3000 | 1.4284 | 0.7124 | [[73, 3, 7, 5, 0, 1, 4, 2, 4, 1], [84, 81, 0, 0, 3, 1, 3, 1, 20, 0], [2, 1, 51, 2, 1, 5, 0, 0, 0, 3], [5, 0, 3, 28, 6, 1, 0, 0, 1, 8], [1, 1, 0, 1, 86, 0, 1, 0, 2, 1], [9, 0, 6, 4, 1, 46, 1, 3, 2, 0], [2, 0, 1, 0, 1, 0, 54, 0, 0, 1], [10, 0, 1, 0, 0, 0, 1, 71, 0, 0], [21, 23, 1, 0, 0, 2, 12, 2, 119, 0], [7, 0, 1, 4, 0, 0, 0, 2, 0, 139]] |
| 0.1828 | 9.87 | 3100 | 1.2049 | 0.7524 | [[66, 2, 13, 7, 0, 0, 2, 1, 7, 2], [38, 115, 1, 0, 4, 0, 4, 2, 28, 1], [1, 0, 52, 2, 1, 4, 2, 0, 0, 3], [3, 0, 4, 35, 5, 0, 0, 0, 1, 4], [0, 1, 1, 5, 83, 0, 1, 0, 1, 1], [4, 1, 12, 6, 3, 41, 1, 2, 2, 0], [0, 0, 1, 0, 0, 0, 58, 0, 0, 0], [5, 0, 5, 0, 0, 0, 1, 72, 0, 0], [11, 24, 1, 0, 0, 0, 10, 1, 132, 1], [3, 0, 2, 9, 0, 0, 1, 2, 0, 136]] |
| 0.083 | 10.19 | 3200 | 1.2484 | 0.7238 | [[57, 5, 16, 5, 1, 1, 7, 2, 3, 3], [30, 127, 0, 0, 1, 2, 11, 3, 18, 1], [0, 0, 52, 3, 1, 5, 2, 0, 0, 2], [4, 0, 5, 30, 5, 1, 0, 0, 0, 7], [1, 1, 0, 4, 84, 0, 1, 0, 1, 1], [3, 1, 9, 4, 1, 48, 2, 1, 2, 1], [0, 0, 1, 0, 0, 0, 57, 0, 0, 1], [4, 0, 7, 0, 0, 0, 3, 69, 0, 0], [9, 27, 1, 0, 0, 1, 32, 0, 109, 1], [2, 1, 2, 16, 0, 0, 3, 2, 0, 127]] |
| 0.1256 | 10.51 | 3300 | 1.2746 | 0.7229 | [[64, 4, 8, 4, 1, 7, 5, 2, 2, 3], [43, 119, 0, 0, 2, 1, 10, 3, 14, 1], [0, 0, 49, 3, 1, 7, 2, 0, 0, 3], [4, 0, 8, 27, 5, 1, 0, 0, 0, 7], [2, 1, 2, 2, 81, 3, 1, 0, 1, 0], [2, 2, 10, 3, 0, 50, 1, 0, 2, 2], [0, 1, 1, 0, 1, 0, 55, 0, 0, 1], [2, 0, 3, 0, 0, 0, 1, 77, 0, 0], [11, 37, 0, 0, 0, 3, 25, 2, 102, 0], [1, 1, 2, 11, 0, 0, 1, 2, 0, 135]] |
| 0.1067 | 10.83 | 3400 | 1.1905 | 0.7381 | [[55, 3, 11, 9, 1, 2, 7, 2, 6, 4], [35, 122, 0, 0, 1, 1, 6, 2, 25, 1], [2, 1, 50, 2, 1, 3, 4, 0, 0, 2], [2, 0, 4, 37, 4, 0, 0, 0, 0, 5], [0, 1, 1, 4, 82, 2, 1, 0, 1, 1], [2, 1, 13, 6, 0, 44, 1, 0, 1, 4], [0, 1, 1, 0, 0, 0, 56, 0, 0, 1], [1, 0, 3, 1, 0, 0, 1, 76, 0, 1], [9, 36, 0, 0, 0, 1, 20, 2, 112, 0], [0, 0, 2, 10, 0, 0, 0, 0, 0, 141]] |
| 0.092 | 11.15 | 3500 | 1.1175 | 0.7476 | [[65, 3, 8, 2, 0, 4, 5, 2, 9, 2], [27, 108, 0, 0, 1, 1, 8, 2, 46, 0], [4, 0, 49, 2, 1, 4, 3, 0, 0, 2], [2, 0, 3, 37, 4, 0, 0, 0, 1, 5], [0, 1, 1, 3, 83, 2, 1, 0, 1, 1], [6, 2, 8, 6, 0, 45, 1, 0, 1, 3], [0, 0, 1, 0, 0, 0, 56, 0, 1, 1], [3, 0, 2, 1, 0, 1, 3, 72, 0, 1], [10, 22, 0, 0, 0, 1, 12, 1, 134, 0], [1, 0, 1, 12, 0, 1, 2, 0, 0, 136]] |
| 0.153 | 11.46 | 3600 | 1.2434 | 0.7362 | [[75, 4, 9, 1, 0, 1, 2, 3, 4, 1], [51, 111, 0, 1, 1, 1, 1, 3, 24, 0], [2, 1, 52, 2, 1, 3, 0, 2, 0, 2], [4, 1, 6, 30, 4, 0, 0, 1, 0, 6], [1, 4, 1, 4, 80, 0, 1, 1, 0, 1], [6, 4, 8, 6, 1, 39, 0, 1, 2, 5], [2, 1, 1, 1, 1, 0, 52, 0, 0, 1], [2, 0, 1, 0, 0, 0, 0, 80, 0, 0], [15, 33, 0, 0, 1, 1, 11, 3, 114, 2], [1, 0, 1, 7, 2, 0, 1, 1, 0, 140]] |
| 0.1065 | 11.78 | 3700 | 1.2327 | 0.7371 | [[69, 2, 10, 2, 0, 2, 6, 3, 5, 1], [44, 109, 2, 0, 0, 2, 5, 3, 28, 0], [2, 1, 50, 2, 1, 4, 2, 1, 0, 2], [4, 0, 4, 32, 4, 2, 0, 1, 1, 4], [1, 2, 2, 6, 76, 2, 1, 1, 2, 0], [2, 1, 11, 4, 0, 47, 2, 1, 2, 2], [0, 0, 1, 0, 0, 0, 58, 0, 0, 0], [2, 0, 4, 0, 0, 0, 1, 76, 0, 0], [9, 27, 0, 0, 0, 1, 13, 1, 128, 1], [2, 2, 2, 13, 0, 0, 4, 1, 0, 129]] |
| 0.0875 | 12.1 | 3800 | 1.2357 | 0.7457 | [[67, 3, 10, 5, 0, 3, 1, 3, 6, 2], [40, 110, 0, 0, 1, 1, 4, 3, 34, 0], [1, 1, 51, 2, 1, 3, 1, 2, 0, 3], [3, 0, 4, 35, 4, 1, 0, 1, 0, 4], [0, 2, 1, 5, 78, 3, 1, 1, 1, 1], [1, 2, 12, 4, 0, 45, 2, 4, 1, 1], [0, 0, 1, 0, 1, 0, 56, 0, 0, 1], [2, 0, 2, 0, 0, 0, 1, 78, 0, 0], [9, 26, 0, 0, 1, 1, 12, 1, 129, 1], [0, 0, 2, 13, 0, 0, 3, 1, 0, 134]] |
| 0.0714 | 12.42 | 3900 | 1.2996 | 0.7305 | [[77, 3, 7, 3, 0, 1, 2, 2, 4, 1], [58, 103, 0, 0, 0, 1, 4, 1, 26, 0], [4, 1, 51, 2, 1, 3, 1, 0, 0, 2], [4, 0, 4, 33, 6, 0, 0, 0, 0, 5], [3, 1, 4, 3, 77, 2, 1, 0, 1, 1], [5, 2, 14, 6, 0, 44, 0, 0, 0, 1], [2, 1, 1, 0, 1, 0, 53, 0, 0, 1], [7, 0, 7, 0, 0, 0, 0, 69, 0, 0], [14, 28, 0, 0, 0, 1, 12, 1, 124, 0], [2, 0, 1, 11, 0, 3, 0, 0, 0, 136]] |
| 0.1433 | 12.74 | 4000 | 1.2167 | 0.7410 | [[74, 4, 9, 3, 0, 2, 1, 2, 4, 1], [42, 114, 0, 0, 1, 1, 3, 3, 29, 0], [4, 0, 49, 2, 1, 5, 2, 0, 0, 2], [4, 0, 4, 31, 6, 2, 0, 0, 0, 5], [0, 1, 2, 2, 86, 0, 1, 0, 0, 1], [7, 2, 9, 6, 0, 46, 1, 0, 0, 1], [2, 1, 1, 0, 1, 0, 53, 0, 0, 1], [3, 0, 5, 0, 0, 0, 0, 75, 0, 0], [9, 34, 0, 0, 1, 2, 11, 1, 120, 2], [3, 0, 1, 16, 0, 1, 2, 0, 0, 130]] |
| 0.0765 | 13.06 | 4100 | 1.2837 | 0.7381 | [[69, 4, 10, 3, 0, 4, 4, 2, 3, 1], [48, 105, 0, 0, 2, 1, 6, 3, 28, 0], [3, 0, 50, 1, 1, 6, 2, 0, 0, 2], [4, 0, 5, 31, 6, 1, 0, 0, 0, 5], [0, 1, 1, 4, 82, 2, 1, 1, 0, 1], [3, 2, 10, 5, 0, 47, 2, 2, 0, 1], [0, 1, 1, 0, 1, 0, 55, 0, 0, 1], [2, 0, 3, 0, 0, 0, 0, 78, 0, 0], [10, 30, 0, 0, 1, 2, 16, 1, 118, 2], [1, 0, 1, 8, 0, 2, 0, 1, 0, 140]] |
| 0.0753 | 13.38 | 4200 | 1.2866 | 0.7371 | [[72, 4, 9, 2, 0, 3, 3, 2, 4, 1], [46, 110, 0, 0, 1, 1, 5, 3, 27, 0], [3, 0, 51, 1, 1, 6, 1, 0, 0, 2], [4, 0, 5, 30, 6, 1, 0, 0, 0, 6], [0, 1, 2, 3, 80, 2, 1, 1, 2, 1], [7, 2, 8, 4, 0, 49, 0, 0, 1, 1], [0, 1, 1, 0, 1, 0, 55, 0, 0, 1], [2, 0, 4, 0, 0, 1, 0, 76, 0, 0], [9, 34, 1, 0, 1, 1, 12, 1, 120, 1], [7, 1, 1, 9, 0, 3, 0, 1, 0, 131]] |
| 0.0766 | 13.69 | 4300 | 1.3334 | 0.7324 | [[68, 5, 9, 5, 0, 2, 3, 3, 3, 2], [53, 106, 0, 0, 1, 1, 6, 4, 22, 0], [2, 0, 54, 1, 1, 4, 1, 0, 0, 2], [3, 0, 5, 34, 4, 1, 0, 0, 0, 5], [0, 2, 1, 4, 79, 2, 2, 1, 1, 1], [4, 2, 10, 4, 0, 47, 1, 2, 1, 1], [0, 1, 1, 0, 1, 0, 55, 0, 0, 1], [2, 0, 3, 0, 0, 0, 1, 77, 0, 0], [10, 37, 0, 0, 1, 1, 13, 1, 115, 2], [5, 0, 1, 11, 0, 1, 0, 1, 0, 134]] |
| 0.0699 | 14.01 | 4400 | 1.3905 | 0.7276 | [[66, 3, 8, 5, 0, 2, 3, 5, 6, 2], [59, 94, 0, 0, 1, 1, 5, 5, 28, 0], [2, 0, 53, 1, 1, 4, 1, 1, 0, 2], [4, 0, 5, 33, 5, 0, 0, 1, 0, 4], [0, 1, 2, 4, 79, 2, 2, 1, 1, 1], [3, 1, 13, 5, 0, 44, 1, 3, 1, 1], [0, 0, 1, 0, 1, 0, 56, 0, 0, 1], [2, 0, 1, 0, 0, 0, 1, 79, 0, 0], [10, 27, 0, 0, 1, 1, 12, 2, 125, 2], [5, 0, 1, 11, 0, 0, 0, 1, 0, 135]] |
| 0.1218 | 14.33 | 4500 | 1.3635 | 0.7324 | [[68, 3, 8, 4, 0, 1, 3, 4, 7, 2], [58, 92, 0, 0, 1, 1, 5, 4, 32, 0], [1, 0, 54, 1, 1, 4, 1, 0, 0, 3], [4, 0, 5, 33, 5, 0, 0, 0, 0, 5], [0, 1, 2, 4, 80, 3, 2, 1, 0, 0], [2, 1, 13, 4, 0, 44, 2, 3, 1, 2], [0, 0, 1, 0, 1, 0, 56, 0, 0, 1], [2, 0, 1, 0, 0, 0, 1, 79, 0, 0], [9, 26, 0, 0, 1, 1, 15, 1, 126, 1], [4, 0, 1, 10, 0, 0, 0, 1, 0, 137]] |
| 0.0648 | 14.65 | 4600 | 1.3205 | 0.7343 | [[66, 3, 9, 5, 0, 2, 3, 3, 7, 2], [52, 95, 0, 0, 2, 1, 6, 4, 33, 0], [3, 1, 52, 1, 1, 3, 2, 0, 0, 2], [4, 0, 5, 33, 5, 0, 0, 0, 0, 5], [0, 2, 1, 3, 83, 1, 2, 1, 0, 0], [2, 2, 13, 4, 0, 44, 2, 2, 1, 2], [0, 0, 1, 0, 1, 0, 56, 0, 0, 1], [2, 0, 2, 0, 0, 0, 1, 78, 0, 0], [9, 26, 0, 0, 1, 1, 14, 1, 127, 1], [3, 0, 1, 10, 0, 0, 1, 1, 0, 137]] |
| 0.0917 | 14.97 | 4700 | 1.3112 | 0.7343 | [[66, 3, 9, 5, 0, 2, 3, 3, 7, 2], [52, 98, 0, 0, 2, 1, 6, 4, 30, 0], [3, 1, 52, 1, 1, 3, 2, 0, 0, 2], [4, 0, 5, 33, 5, 0, 0, 0, 0, 5], [0, 2, 1, 3, 83, 1, 2, 1, 0, 0], [2, 2, 13, 4, 0, 44, 2, 2, 1, 2], [0, 0, 1, 0, 1, 0, 56, 0, 0, 1], [2, 0, 2, 0, 0, 0, 1, 78, 0, 0], [9, 28, 0, 0, 1, 1, 15, 1, 124, 1], [3, 0, 1, 10, 0, 1, 0, 1, 0, 137]] |
### Framework versions
- Transformers 4.39.3
- Pytorch 2.1.2
- Datasets 2.18.0
- Tokenizers 0.15.2
| {"license": "apache-2.0", "tags": ["image-classification", "generated_from_trainer"], "datasets": ["imagefolder"], "metrics": ["accuracy"], "base_model": "google/vit-base-patch16-224-in21k", "model-index": [{"name": "Action_Classification", "results": [{"task": {"type": "image-classification", "name": "Image Classification"}, "dataset": {"name": "agent_action_class", "type": "imagefolder", "config": "default", "split": "train", "args": "default"}, "metrics": [{"type": "accuracy", "value": 0.7628571428571429, "name": "Accuracy"}]}]}]} | Raihan004/Action_Classification | null | [
"transformers",
"tensorboard",
"safetensors",
"vit",
"image-classification",
"generated_from_trainer",
"dataset:imagefolder",
"base_model:google/vit-base-patch16-224-in21k",
"license:apache-2.0",
"model-index",
"autotrain_compatible",
"endpoints_compatible",
"region:us"
] | null | 2024-04-26T18:56:34+00:00 | [] | [] | TAGS
#transformers #tensorboard #safetensors #vit #image-classification #generated_from_trainer #dataset-imagefolder #base_model-google/vit-base-patch16-224-in21k #license-apache-2.0 #model-index #autotrain_compatible #endpoints_compatible #region-us
| Action\_Classification
======================
This model is a fine-tuned version of google/vit-base-patch16-224-in21k on the agent\_action\_class dataset.
It achieves the following results on the evaluation set:
* Loss: 0.8482
* Accuracy: 0.7629
* Confusion Matrix: [[45, 5, 20, 4, 2, 6, 4, 8, 3, 3], [5, 154, 4, 2, 1, 2, 6, 1, 17, 1], [0, 0, 51, 1, 2, 8, 1, 0, 0, 2], [1, 0, 8, 26, 8, 5, 0, 0, 1, 3], [0, 1, 0, 0, 89, 3, 0, 0, 0, 0], [0, 1, 11, 3, 1, 55, 0, 1, 0, 0], [0, 1, 1, 0, 3, 3, 51, 0, 0, 0], [0, 0, 10, 1, 0, 4, 0, 68, 0, 0], [0, 26, 5, 0, 1, 3, 16, 1, 127, 1], [3, 0, 2, 9, 2, 1, 0, 1, 0, 135]]
Model description
-----------------
More information needed
Intended uses & limitations
---------------------------
More information needed
Training and evaluation data
----------------------------
More information needed
Training procedure
------------------
### Training hyperparameters
The following hyperparameters were used during training:
* learning\_rate: 0.0002
* train\_batch\_size: 32
* eval\_batch\_size: 16
* seed: 42
* optimizer: Adam with betas=(0.9,0.999) and epsilon=1e-08
* lr\_scheduler\_type: linear
* num\_epochs: 15
### Training results
### Framework versions
* Transformers 4.39.3
* Pytorch 2.1.2
* Datasets 2.18.0
* Tokenizers 0.15.2
| [
"### Training hyperparameters\n\n\nThe following hyperparameters were used during training:\n\n\n* learning\\_rate: 0.0002\n* train\\_batch\\_size: 32\n* eval\\_batch\\_size: 16\n* seed: 42\n* optimizer: Adam with betas=(0.9,0.999) and epsilon=1e-08\n* lr\\_scheduler\\_type: linear\n* num\\_epochs: 15",
"### Training results",
"### Framework versions\n\n\n* Transformers 4.39.3\n* Pytorch 2.1.2\n* Datasets 2.18.0\n* Tokenizers 0.15.2"
] | [
"TAGS\n#transformers #tensorboard #safetensors #vit #image-classification #generated_from_trainer #dataset-imagefolder #base_model-google/vit-base-patch16-224-in21k #license-apache-2.0 #model-index #autotrain_compatible #endpoints_compatible #region-us \n",
"### Training hyperparameters\n\n\nThe following hyperparameters were used during training:\n\n\n* learning\\_rate: 0.0002\n* train\\_batch\\_size: 32\n* eval\\_batch\\_size: 16\n* seed: 42\n* optimizer: Adam with betas=(0.9,0.999) and epsilon=1e-08\n* lr\\_scheduler\\_type: linear\n* num\\_epochs: 15",
"### Training results",
"### Framework versions\n\n\n* Transformers 4.39.3\n* Pytorch 2.1.2\n* Datasets 2.18.0\n* Tokenizers 0.15.2"
] |
text-classification | transformers |
<!-- This model card has been generated automatically according to the information the Trainer had access to. You
should probably proofread and complete it, then remove this comment. -->
# distilbert-rotten_tomatoes
This model is a fine-tuned version of [distilbert-base-uncased](https://huggingface.co/distilbert-base-uncased) on an unknown dataset.
## Model description
More information needed
## Intended uses & limitations
More information needed
## Training and evaluation data
More information needed
## Training procedure
### Training hyperparameters
The following hyperparameters were used during training:
- learning_rate: 5e-05
- train_batch_size: 16
- eval_batch_size: 16
- seed: 42
- optimizer: Adam with betas=(0.9,0.999) and epsilon=1e-08
- lr_scheduler_type: linear
- num_epochs: 2
### Training results
### Framework versions
- Transformers 4.40.1
- Pytorch 2.2.1+cu121
- Datasets 2.19.0
- Tokenizers 0.19.1
| {"license": "apache-2.0", "tags": ["generated_from_trainer"], "base_model": "distilbert-base-uncased", "model-index": [{"name": "distilbert-rotten_tomatoes", "results": []}]} | huiang/distilbert-rotten_tomatoes | null | [
"transformers",
"tensorboard",
"safetensors",
"distilbert",
"text-classification",
"generated_from_trainer",
"base_model:distilbert-base-uncased",
"license:apache-2.0",
"autotrain_compatible",
"endpoints_compatible",
"region:us"
] | null | 2024-04-26T18:58:07+00:00 | [] | [] | TAGS
#transformers #tensorboard #safetensors #distilbert #text-classification #generated_from_trainer #base_model-distilbert-base-uncased #license-apache-2.0 #autotrain_compatible #endpoints_compatible #region-us
|
# distilbert-rotten_tomatoes
This model is a fine-tuned version of distilbert-base-uncased on an unknown dataset.
## Model description
More information needed
## Intended uses & limitations
More information needed
## Training and evaluation data
More information needed
## Training procedure
### Training hyperparameters
The following hyperparameters were used during training:
- learning_rate: 5e-05
- train_batch_size: 16
- eval_batch_size: 16
- seed: 42
- optimizer: Adam with betas=(0.9,0.999) and epsilon=1e-08
- lr_scheduler_type: linear
- num_epochs: 2
### Training results
### Framework versions
- Transformers 4.40.1
- Pytorch 2.2.1+cu121
- Datasets 2.19.0
- Tokenizers 0.19.1
| [
"# distilbert-rotten_tomatoes\n\nThis model is a fine-tuned version of distilbert-base-uncased on an unknown dataset.",
"## Model description\n\nMore information needed",
"## Intended uses & limitations\n\nMore information needed",
"## Training and evaluation data\n\nMore information needed",
"## Training procedure",
"### Training hyperparameters\n\nThe following hyperparameters were used during training:\n- learning_rate: 5e-05\n- train_batch_size: 16\n- eval_batch_size: 16\n- seed: 42\n- optimizer: Adam with betas=(0.9,0.999) and epsilon=1e-08\n- lr_scheduler_type: linear\n- num_epochs: 2",
"### Training results",
"### Framework versions\n\n- Transformers 4.40.1\n- Pytorch 2.2.1+cu121\n- Datasets 2.19.0\n- Tokenizers 0.19.1"
] | [
"TAGS\n#transformers #tensorboard #safetensors #distilbert #text-classification #generated_from_trainer #base_model-distilbert-base-uncased #license-apache-2.0 #autotrain_compatible #endpoints_compatible #region-us \n",
"# distilbert-rotten_tomatoes\n\nThis model is a fine-tuned version of distilbert-base-uncased on an unknown dataset.",
"## Model description\n\nMore information needed",
"## Intended uses & limitations\n\nMore information needed",
"## Training and evaluation data\n\nMore information needed",
"## Training procedure",
"### Training hyperparameters\n\nThe following hyperparameters were used during training:\n- learning_rate: 5e-05\n- train_batch_size: 16\n- eval_batch_size: 16\n- seed: 42\n- optimizer: Adam with betas=(0.9,0.999) and epsilon=1e-08\n- lr_scheduler_type: linear\n- num_epochs: 2",
"### Training results",
"### Framework versions\n\n- Transformers 4.40.1\n- Pytorch 2.2.1+cu121\n- Datasets 2.19.0\n- Tokenizers 0.19.1"
] |
text-generation | transformers |
# Model Card for Model ID
<!-- Provide a quick summary of what the model is/does. -->
## Model Details
### Model Description
<!-- Provide a longer summary of what this model is. -->
This is the model card of a 🤗 transformers model that has been pushed on the Hub. This model card has been automatically generated.
- **Developed by:** [More Information Needed]
- **Funded by [optional]:** [More Information Needed]
- **Shared by [optional]:** [More Information Needed]
- **Model type:** [More Information Needed]
- **Language(s) (NLP):** [More Information Needed]
- **License:** [More Information Needed]
- **Finetuned from model [optional]:** [More Information Needed]
### Model Sources [optional]
<!-- Provide the basic links for the model. -->
- **Repository:** [More Information Needed]
- **Paper [optional]:** [More Information Needed]
- **Demo [optional]:** [More Information Needed]
## Uses
<!-- Address questions around how the model is intended to be used, including the foreseeable users of the model and those affected by the model. -->
### Direct Use
<!-- This section is for the model use without fine-tuning or plugging into a larger ecosystem/app. -->
[More Information Needed]
### Downstream Use [optional]
<!-- This section is for the model use when fine-tuned for a task, or when plugged into a larger ecosystem/app -->
[More Information Needed]
### Out-of-Scope Use
<!-- This section addresses misuse, malicious use, and uses that the model will not work well for. -->
[More Information Needed]
## Bias, Risks, and Limitations
<!-- This section is meant to convey both technical and sociotechnical limitations. -->
[More Information Needed]
### Recommendations
<!-- This section is meant to convey recommendations with respect to the bias, risk, and technical limitations. -->
Users (both direct and downstream) should be made aware of the risks, biases and limitations of the model. More information needed for further recommendations.
## How to Get Started with the Model
Use the code below to get started with the model.
[More Information Needed]
## Training Details
### Training Data
<!-- This should link to a Dataset Card, perhaps with a short stub of information on what the training data is all about as well as documentation related to data pre-processing or additional filtering. -->
[More Information Needed]
### Training Procedure
<!-- This relates heavily to the Technical Specifications. Content here should link to that section when it is relevant to the training procedure. -->
#### Preprocessing [optional]
[More Information Needed]
#### Training Hyperparameters
- **Training regime:** [More Information Needed] <!--fp32, fp16 mixed precision, bf16 mixed precision, bf16 non-mixed precision, fp16 non-mixed precision, fp8 mixed precision -->
#### Speeds, Sizes, Times [optional]
<!-- This section provides information about throughput, start/end time, checkpoint size if relevant, etc. -->
[More Information Needed]
## Evaluation
<!-- This section describes the evaluation protocols and provides the results. -->
### Testing Data, Factors & Metrics
#### Testing Data
<!-- This should link to a Dataset Card if possible. -->
[More Information Needed]
#### Factors
<!-- These are the things the evaluation is disaggregating by, e.g., subpopulations or domains. -->
[More Information Needed]
#### Metrics
<!-- These are the evaluation metrics being used, ideally with a description of why. -->
[More Information Needed]
### Results
[More Information Needed]
#### Summary
## Model Examination [optional]
<!-- Relevant interpretability work for the model goes here -->
[More Information Needed]
## Environmental Impact
<!-- Total emissions (in grams of CO2eq) and additional considerations, such as electricity usage, go here. Edit the suggested text below accordingly -->
Carbon emissions can be estimated using the [Machine Learning Impact calculator](https://mlco2.github.io/impact#compute) presented in [Lacoste et al. (2019)](https://arxiv.org/abs/1910.09700).
- **Hardware Type:** [More Information Needed]
- **Hours used:** [More Information Needed]
- **Cloud Provider:** [More Information Needed]
- **Compute Region:** [More Information Needed]
- **Carbon Emitted:** [More Information Needed]
## Technical Specifications [optional]
### Model Architecture and Objective
[More Information Needed]
### Compute Infrastructure
[More Information Needed]
#### Hardware
[More Information Needed]
#### Software
[More Information Needed]
## Citation [optional]
<!-- If there is a paper or blog post introducing the model, the APA and Bibtex information for that should go in this section. -->
**BibTeX:**
[More Information Needed]
**APA:**
[More Information Needed]
## Glossary [optional]
<!-- If relevant, include terms and calculations in this section that can help readers understand the model or model card. -->
[More Information Needed]
## More Information [optional]
[More Information Needed]
## Model Card Authors [optional]
[More Information Needed]
## Model Card Contact
[More Information Needed] | {"library_name": "transformers", "tags": []} | shallow6414/mp5d4if | null | [
"transformers",
"safetensors",
"llama",
"text-generation",
"conversational",
"arxiv:1910.09700",
"autotrain_compatible",
"endpoints_compatible",
"text-generation-inference",
"region:us"
] | null | 2024-04-26T18:58:09+00:00 | [
"1910.09700"
] | [] | TAGS
#transformers #safetensors #llama #text-generation #conversational #arxiv-1910.09700 #autotrain_compatible #endpoints_compatible #text-generation-inference #region-us
|
# Model Card for Model ID
## Model Details
### Model Description
This is the model card of a transformers model that has been pushed on the Hub. This model card has been automatically generated.
- Developed by:
- Funded by [optional]:
- Shared by [optional]:
- Model type:
- Language(s) (NLP):
- License:
- Finetuned from model [optional]:
### Model Sources [optional]
- Repository:
- Paper [optional]:
- Demo [optional]:
## Uses
### Direct Use
### Downstream Use [optional]
### Out-of-Scope Use
## Bias, Risks, and Limitations
### Recommendations
Users (both direct and downstream) should be made aware of the risks, biases and limitations of the model. More information needed for further recommendations.
## How to Get Started with the Model
Use the code below to get started with the model.
## Training Details
### Training Data
### Training Procedure
#### Preprocessing [optional]
#### Training Hyperparameters
- Training regime:
#### Speeds, Sizes, Times [optional]
## Evaluation
### Testing Data, Factors & Metrics
#### Testing Data
#### Factors
#### Metrics
### Results
#### Summary
## Model Examination [optional]
## Environmental Impact
Carbon emissions can be estimated using the Machine Learning Impact calculator presented in Lacoste et al. (2019).
- Hardware Type:
- Hours used:
- Cloud Provider:
- Compute Region:
- Carbon Emitted:
## Technical Specifications [optional]
### Model Architecture and Objective
### Compute Infrastructure
#### Hardware
#### Software
[optional]
BibTeX:
APA:
## Glossary [optional]
## More Information [optional]
## Model Card Authors [optional]
## Model Card Contact
| [
"# Model Card for Model ID",
"## Model Details",
"### Model Description\n\n\n\nThis is the model card of a transformers model that has been pushed on the Hub. This model card has been automatically generated.\n\n- Developed by: \n- Funded by [optional]: \n- Shared by [optional]: \n- Model type: \n- Language(s) (NLP): \n- License: \n- Finetuned from model [optional]:",
"### Model Sources [optional]\n\n\n\n- Repository: \n- Paper [optional]: \n- Demo [optional]:",
"## Uses",
"### Direct Use",
"### Downstream Use [optional]",
"### Out-of-Scope Use",
"## Bias, Risks, and Limitations",
"### Recommendations\n\n\n\nUsers (both direct and downstream) should be made aware of the risks, biases and limitations of the model. More information needed for further recommendations.",
"## How to Get Started with the Model\n\nUse the code below to get started with the model.",
"## Training Details",
"### Training Data",
"### Training Procedure",
"#### Preprocessing [optional]",
"#### Training Hyperparameters\n\n- Training regime:",
"#### Speeds, Sizes, Times [optional]",
"## Evaluation",
"### Testing Data, Factors & Metrics",
"#### Testing Data",
"#### Factors",
"#### Metrics",
"### Results",
"#### Summary",
"## Model Examination [optional]",
"## Environmental Impact\n\n\n\nCarbon emissions can be estimated using the Machine Learning Impact calculator presented in Lacoste et al. (2019).\n\n- Hardware Type: \n- Hours used: \n- Cloud Provider: \n- Compute Region: \n- Carbon Emitted:",
"## Technical Specifications [optional]",
"### Model Architecture and Objective",
"### Compute Infrastructure",
"#### Hardware",
"#### Software\n\n\n\n[optional]\n\n\n\nBibTeX:\n\n\n\nAPA:",
"## Glossary [optional]",
"## More Information [optional]",
"## Model Card Authors [optional]",
"## Model Card Contact"
] | [
"TAGS\n#transformers #safetensors #llama #text-generation #conversational #arxiv-1910.09700 #autotrain_compatible #endpoints_compatible #text-generation-inference #region-us \n",
"# Model Card for Model ID",
"## Model Details",
"### Model Description\n\n\n\nThis is the model card of a transformers model that has been pushed on the Hub. This model card has been automatically generated.\n\n- Developed by: \n- Funded by [optional]: \n- Shared by [optional]: \n- Model type: \n- Language(s) (NLP): \n- License: \n- Finetuned from model [optional]:",
"### Model Sources [optional]\n\n\n\n- Repository: \n- Paper [optional]: \n- Demo [optional]:",
"## Uses",
"### Direct Use",
"### Downstream Use [optional]",
"### Out-of-Scope Use",
"## Bias, Risks, and Limitations",
"### Recommendations\n\n\n\nUsers (both direct and downstream) should be made aware of the risks, biases and limitations of the model. More information needed for further recommendations.",
"## How to Get Started with the Model\n\nUse the code below to get started with the model.",
"## Training Details",
"### Training Data",
"### Training Procedure",
"#### Preprocessing [optional]",
"#### Training Hyperparameters\n\n- Training regime:",
"#### Speeds, Sizes, Times [optional]",
"## Evaluation",
"### Testing Data, Factors & Metrics",
"#### Testing Data",
"#### Factors",
"#### Metrics",
"### Results",
"#### Summary",
"## Model Examination [optional]",
"## Environmental Impact\n\n\n\nCarbon emissions can be estimated using the Machine Learning Impact calculator presented in Lacoste et al. (2019).\n\n- Hardware Type: \n- Hours used: \n- Cloud Provider: \n- Compute Region: \n- Carbon Emitted:",
"## Technical Specifications [optional]",
"### Model Architecture and Objective",
"### Compute Infrastructure",
"#### Hardware",
"#### Software\n\n\n\n[optional]\n\n\n\nBibTeX:\n\n\n\nAPA:",
"## Glossary [optional]",
"## More Information [optional]",
"## Model Card Authors [optional]",
"## Model Card Contact"
] |
text-generation | transformers |
# Uploaded model
- **Developed by:** kchopra04
- **License:** apache-2.0
- **Finetuned from model :** unsloth/llama-3-8b-Instruct-bnb-4bit
This llama model was trained 2x faster with [Unsloth](https://github.com/unslothai/unsloth) and Huggingface's TRL library.
[<img src="https://raw.githubusercontent.com/unslothai/unsloth/main/images/unsloth%20made%20with%20love.png" width="200"/>](https://github.com/unslothai/unsloth)
| {"language": ["en"], "license": "apache-2.0", "tags": ["text-generation-inference", "transformers", "unsloth", "llama", "trl"], "base_model": "unsloth/llama-3-8b-Instruct-bnb-4bit"} | kchopra04/lora_model_inst | null | [
"transformers",
"safetensors",
"llama",
"text-generation",
"text-generation-inference",
"unsloth",
"trl",
"en",
"base_model:unsloth/llama-3-8b-Instruct-bnb-4bit",
"license:apache-2.0",
"autotrain_compatible",
"endpoints_compatible",
"4-bit",
"region:us"
] | null | 2024-04-26T18:58:54+00:00 | [] | [
"en"
] | TAGS
#transformers #safetensors #llama #text-generation #text-generation-inference #unsloth #trl #en #base_model-unsloth/llama-3-8b-Instruct-bnb-4bit #license-apache-2.0 #autotrain_compatible #endpoints_compatible #4-bit #region-us
|
# Uploaded model
- Developed by: kchopra04
- License: apache-2.0
- Finetuned from model : unsloth/llama-3-8b-Instruct-bnb-4bit
This llama model was trained 2x faster with Unsloth and Huggingface's TRL library.
<img src="URL width="200"/>
| [
"# Uploaded model\n\n- Developed by: kchopra04\n- License: apache-2.0\n- Finetuned from model : unsloth/llama-3-8b-Instruct-bnb-4bit\n\nThis llama model was trained 2x faster with Unsloth and Huggingface's TRL library.\n\n<img src=\"URL width=\"200\"/>"
] | [
"TAGS\n#transformers #safetensors #llama #text-generation #text-generation-inference #unsloth #trl #en #base_model-unsloth/llama-3-8b-Instruct-bnb-4bit #license-apache-2.0 #autotrain_compatible #endpoints_compatible #4-bit #region-us \n",
"# Uploaded model\n\n- Developed by: kchopra04\n- License: apache-2.0\n- Finetuned from model : unsloth/llama-3-8b-Instruct-bnb-4bit\n\nThis llama model was trained 2x faster with Unsloth and Huggingface's TRL library.\n\n<img src=\"URL width=\"200\"/>"
] |
null | peft |
<!-- This model card has been generated automatically according to the information the Trainer had access to. You
should probably proofread and complete it, then remove this comment. -->
# run
This model is a fine-tuned version of [salangarica/BioMistral-LLM](https://huggingface.co/salangarica/BioMistral-LLM) on the None dataset.
It achieves the following results on the evaluation set:
- Loss: 0.3032
## Model description
More information needed
## Intended uses & limitations
More information needed
## Training and evaluation data
More information needed
## Training procedure
### Training hyperparameters
The following hyperparameters were used during training:
- learning_rate: 2e-05
- train_batch_size: 8
- eval_batch_size: 8
- seed: 42
- optimizer: Adam with betas=(0.9,0.999) and epsilon=1e-08
- lr_scheduler_type: constant
- lr_scheduler_warmup_ratio: 0.03
- num_epochs: 20.0
### Training results
| Training Loss | Epoch | Step | Validation Loss |
|:-------------:|:-----:|:----:|:---------------:|
| 0.131 | 1.0 | 110 | 0.1747 |
| 0.1903 | 2.0 | 220 | 0.1724 |
| 0.0928 | 3.0 | 330 | 0.2107 |
| 0.0738 | 4.0 | 440 | 0.2131 |
| 0.0735 | 5.0 | 550 | 0.3032 |
### Framework versions
- PEFT 0.8.2
- Transformers 4.38.1
- Pytorch 2.0.0
- Datasets 2.15.0
- Tokenizers 0.15.2 | {"library_name": "peft", "tags": ["trl", "sft", "generated_from_trainer"], "base_model": "salangarica/BioMistral-LLM", "model-index": [{"name": "run", "results": []}]} | salangarica/run | null | [
"peft",
"tensorboard",
"safetensors",
"trl",
"sft",
"generated_from_trainer",
"base_model:salangarica/BioMistral-LLM",
"region:us"
] | null | 2024-04-26T18:59:08+00:00 | [] | [] | TAGS
#peft #tensorboard #safetensors #trl #sft #generated_from_trainer #base_model-salangarica/BioMistral-LLM #region-us
| run
===
This model is a fine-tuned version of salangarica/BioMistral-LLM on the None dataset.
It achieves the following results on the evaluation set:
* Loss: 0.3032
Model description
-----------------
More information needed
Intended uses & limitations
---------------------------
More information needed
Training and evaluation data
----------------------------
More information needed
Training procedure
------------------
### Training hyperparameters
The following hyperparameters were used during training:
* learning\_rate: 2e-05
* train\_batch\_size: 8
* eval\_batch\_size: 8
* seed: 42
* optimizer: Adam with betas=(0.9,0.999) and epsilon=1e-08
* lr\_scheduler\_type: constant
* lr\_scheduler\_warmup\_ratio: 0.03
* num\_epochs: 20.0
### Training results
### Framework versions
* PEFT 0.8.2
* Transformers 4.38.1
* Pytorch 2.0.0
* Datasets 2.15.0
* Tokenizers 0.15.2
| [
"### Training hyperparameters\n\n\nThe following hyperparameters were used during training:\n\n\n* learning\\_rate: 2e-05\n* train\\_batch\\_size: 8\n* eval\\_batch\\_size: 8\n* seed: 42\n* optimizer: Adam with betas=(0.9,0.999) and epsilon=1e-08\n* lr\\_scheduler\\_type: constant\n* lr\\_scheduler\\_warmup\\_ratio: 0.03\n* num\\_epochs: 20.0",
"### Training results",
"### Framework versions\n\n\n* PEFT 0.8.2\n* Transformers 4.38.1\n* Pytorch 2.0.0\n* Datasets 2.15.0\n* Tokenizers 0.15.2"
] | [
"TAGS\n#peft #tensorboard #safetensors #trl #sft #generated_from_trainer #base_model-salangarica/BioMistral-LLM #region-us \n",
"### Training hyperparameters\n\n\nThe following hyperparameters were used during training:\n\n\n* learning\\_rate: 2e-05\n* train\\_batch\\_size: 8\n* eval\\_batch\\_size: 8\n* seed: 42\n* optimizer: Adam with betas=(0.9,0.999) and epsilon=1e-08\n* lr\\_scheduler\\_type: constant\n* lr\\_scheduler\\_warmup\\_ratio: 0.03\n* num\\_epochs: 20.0",
"### Training results",
"### Framework versions\n\n\n* PEFT 0.8.2\n* Transformers 4.38.1\n* Pytorch 2.0.0\n* Datasets 2.15.0\n* Tokenizers 0.15.2"
] |
null | peft | prompt
```
<original>Ok. What do the drivers look like?</original>
<translate to="th">
```
response
```
<original>กรุงเทพอยู่ที่ไหน</original>
<translate to="en">where is bangkok</translate><eos>
```
code to create dataset
```python
import random
alpaca_prompt = """<original>{}</original>
<translate to="{}">{}"""
BOS_TOKEN = tokenizer.bos_token # Must add EOS_TOKEN
EOS_TOKEN = "</translate>"+tokenizer.eos_token # Must add EOS_TOKEN
def formatting_prompts_func(examples):
translations = examples["translation"]
texts = []
text_en = ""
text_th = ""
translate_to = 'th'
max_group_count = 1
group_count = 0
for translation in translations:
if group_count >= max_group_count:
if(translate_to == 'th'):
text = alpaca_prompt.format(text_en, translate_to, text_th) + EOS_TOKEN
else:
text = alpaca_prompt.format(text_th, translate_to, text_en) + EOS_TOKEN
texts.append(text)
text_en = ""
text_th = ""
max_group_count = random.randint(1, 5)
group_count = 0
translate_to = random.choice(['en', 'th'])
num_newlines = random.randint(1, 5)
newlines = '\n' * num_newlines
if(text_en == ""):
text_en = translation['en']
text_th = translation['th']
else:
text_en = text_en+newlines+translation['en']
text_th = text_th+newlines+translation['th']
group_count = group_count+1
if(translate_to == 'th'):
text = alpaca_prompt.format(text_en, translate_to, text_th) + EOS_TOKEN
else:
text = alpaca_prompt.format(text_th, translate_to, text_en) + EOS_TOKEN
texts.append(text)
return { "text" : texts, }
from datasets import load_dataset
import datasets
# dataset = load_dataset("scb_mt_enth_2020",'enth', download_mode=datasets.DownloadMode.FORCE_REDOWNLOAD,cache_dir ="./cache")
dataset = load_dataset("scb_mt_enth_2020",'enth',cache_dir ="./cache")
dataset = dataset.shuffle(seed=42)
dataset = dataset.map(formatting_prompts_func, batched = True,remove_columns=["translation",'subdataset'])
dataset['train'][0:5]
```
[More Information Needed]
### Framework versions
- PEFT 0.10.0 | {"library_name": "peft", "base_model": "unsloth/gemma-7b-bnb-4bit"} | ping98k/gemma-7b-translator-0.3-lora | null | [
"peft",
"safetensors",
"base_model:unsloth/gemma-7b-bnb-4bit",
"region:us"
] | null | 2024-04-26T19:06:13+00:00 | [] | [] | TAGS
#peft #safetensors #base_model-unsloth/gemma-7b-bnb-4bit #region-us
| prompt
response
code to create dataset
### Framework versions
- PEFT 0.10.0 | [
"### Framework versions\n\n- PEFT 0.10.0"
] | [
"TAGS\n#peft #safetensors #base_model-unsloth/gemma-7b-bnb-4bit #region-us \n",
"### Framework versions\n\n- PEFT 0.10.0"
] |
text-generation | transformers |
# Uploaded model
- **Developed by:** kchopra04
- **License:** apache-2.0
- **Finetuned from model :** unsloth/llama-3-8b-Instruct-bnb-4bit
This llama model was trained 2x faster with [Unsloth](https://github.com/unslothai/unsloth) and Huggingface's TRL library.
[<img src="https://raw.githubusercontent.com/unslothai/unsloth/main/images/unsloth%20made%20with%20love.png" width="200"/>](https://github.com/unslothai/unsloth)
| {"language": ["en"], "license": "apache-2.0", "tags": ["text-generation-inference", "transformers", "unsloth", "llama", "trl"], "base_model": "unsloth/llama-3-8b-Instruct-bnb-4bit"} | kchopra04/llama3-inst-finetuned-saxs | null | [
"transformers",
"pytorch",
"llama",
"text-generation",
"text-generation-inference",
"unsloth",
"trl",
"conversational",
"en",
"base_model:unsloth/llama-3-8b-Instruct-bnb-4bit",
"license:apache-2.0",
"autotrain_compatible",
"endpoints_compatible",
"region:us"
] | null | 2024-04-26T19:07:17+00:00 | [] | [
"en"
] | TAGS
#transformers #pytorch #llama #text-generation #text-generation-inference #unsloth #trl #conversational #en #base_model-unsloth/llama-3-8b-Instruct-bnb-4bit #license-apache-2.0 #autotrain_compatible #endpoints_compatible #region-us
|
# Uploaded model
- Developed by: kchopra04
- License: apache-2.0
- Finetuned from model : unsloth/llama-3-8b-Instruct-bnb-4bit
This llama model was trained 2x faster with Unsloth and Huggingface's TRL library.
<img src="URL width="200"/>
| [
"# Uploaded model\n\n- Developed by: kchopra04\n- License: apache-2.0\n- Finetuned from model : unsloth/llama-3-8b-Instruct-bnb-4bit\n\nThis llama model was trained 2x faster with Unsloth and Huggingface's TRL library.\n\n<img src=\"URL width=\"200\"/>"
] | [
"TAGS\n#transformers #pytorch #llama #text-generation #text-generation-inference #unsloth #trl #conversational #en #base_model-unsloth/llama-3-8b-Instruct-bnb-4bit #license-apache-2.0 #autotrain_compatible #endpoints_compatible #region-us \n",
"# Uploaded model\n\n- Developed by: kchopra04\n- License: apache-2.0\n- Finetuned from model : unsloth/llama-3-8b-Instruct-bnb-4bit\n\nThis llama model was trained 2x faster with Unsloth and Huggingface's TRL library.\n\n<img src=\"URL width=\"200\"/>"
] |
null | null |
# Ognoexperiment27multi_verse_modelMeliodas-7B
Ognoexperiment27multi_verse_modelMeliodas-7B is an automated merge created by [Maxime Labonne](https://huggingface.co/mlabonne) using the following configuration.
## 🧩 Configuration
```yaml
models:
- model: mistralai/Mistral-7B-v0.1
- model: automerger/Ognoexperiment27Multi_verse_model-7B
- model: AurelPx/Meliodas-7b-dare
merge_method: model_stock
base_model: mistralai/Mistral-7B-v0.1
dtype: bfloat16
```
## 💻 Usage
```python
!pip install -qU transformers accelerate
from transformers import AutoTokenizer
import transformers
import torch
model = "automerger/Ognoexperiment27multi_verse_modelMeliodas-7B"
messages = [{"role": "user", "content": "What is a large language model?"}]
tokenizer = AutoTokenizer.from_pretrained(model)
prompt = tokenizer.apply_chat_template(messages, tokenize=False, add_generation_prompt=True)
pipeline = transformers.pipeline(
"text-generation",
model=model,
torch_dtype=torch.float16,
device_map="auto",
)
outputs = pipeline(prompt, max_new_tokens=256, do_sample=True, temperature=0.7, top_k=50, top_p=0.95)
print(outputs[0]["generated_text"])
``` | {"license": "apache-2.0", "tags": ["merge", "mergekit", "lazymergekit", "automerger"]} | automerger/Ognoexperiment27multi_verse_modelMeliodas-7B | null | [
"merge",
"mergekit",
"lazymergekit",
"automerger",
"license:apache-2.0",
"region:us"
] | null | 2024-04-26T19:07:40+00:00 | [] | [] | TAGS
#merge #mergekit #lazymergekit #automerger #license-apache-2.0 #region-us
|
# Ognoexperiment27multi_verse_modelMeliodas-7B
Ognoexperiment27multi_verse_modelMeliodas-7B is an automated merge created by Maxime Labonne using the following configuration.
## Configuration
## Usage
| [
"# Ognoexperiment27multi_verse_modelMeliodas-7B\n\nOgnoexperiment27multi_verse_modelMeliodas-7B is an automated merge created by Maxime Labonne using the following configuration.",
"## Configuration",
"## Usage"
] | [
"TAGS\n#merge #mergekit #lazymergekit #automerger #license-apache-2.0 #region-us \n",
"# Ognoexperiment27multi_verse_modelMeliodas-7B\n\nOgnoexperiment27multi_verse_modelMeliodas-7B is an automated merge created by Maxime Labonne using the following configuration.",
"## Configuration",
"## Usage"
] |
Subsets and Splits
No community queries yet
The top public SQL queries from the community will appear here once available.