pipeline_tag
stringclasses 48
values | library_name
stringclasses 198
values | text
stringlengths 1
900k
| metadata
stringlengths 2
438k
| id
stringlengths 5
122
| last_modified
null | tags
sequencelengths 1
1.84k
| sha
null | created_at
stringlengths 25
25
| arxiv
sequencelengths 0
201
| languages
sequencelengths 0
1.83k
| tags_str
stringlengths 17
9.34k
| text_str
stringlengths 0
389k
| text_lists
sequencelengths 0
722
| processed_texts
sequencelengths 1
723
|
---|---|---|---|---|---|---|---|---|---|---|---|---|---|---|
null | transformers |
# Model Card for Model ID
<!-- Provide a quick summary of what the model is/does. -->
## Model Details
### Model Description
<!-- Provide a longer summary of what this model is. -->
This is the model card of a 🤗 transformers model that has been pushed on the Hub. This model card has been automatically generated.
- **Developed by:** [More Information Needed]
- **Funded by [optional]:** [More Information Needed]
- **Shared by [optional]:** [More Information Needed]
- **Model type:** [More Information Needed]
- **Language(s) (NLP):** [More Information Needed]
- **License:** [More Information Needed]
- **Finetuned from model [optional]:** [More Information Needed]
### Model Sources [optional]
<!-- Provide the basic links for the model. -->
- **Repository:** [More Information Needed]
- **Paper [optional]:** [More Information Needed]
- **Demo [optional]:** [More Information Needed]
## Uses
<!-- Address questions around how the model is intended to be used, including the foreseeable users of the model and those affected by the model. -->
### Direct Use
<!-- This section is for the model use without fine-tuning or plugging into a larger ecosystem/app. -->
[More Information Needed]
### Downstream Use [optional]
<!-- This section is for the model use when fine-tuned for a task, or when plugged into a larger ecosystem/app -->
[More Information Needed]
### Out-of-Scope Use
<!-- This section addresses misuse, malicious use, and uses that the model will not work well for. -->
[More Information Needed]
## Bias, Risks, and Limitations
<!-- This section is meant to convey both technical and sociotechnical limitations. -->
[More Information Needed]
### Recommendations
<!-- This section is meant to convey recommendations with respect to the bias, risk, and technical limitations. -->
Users (both direct and downstream) should be made aware of the risks, biases and limitations of the model. More information needed for further recommendations.
## How to Get Started with the Model
Use the code below to get started with the model.
[More Information Needed]
## Training Details
### Training Data
<!-- This should link to a Dataset Card, perhaps with a short stub of information on what the training data is all about as well as documentation related to data pre-processing or additional filtering. -->
[More Information Needed]
### Training Procedure
<!-- This relates heavily to the Technical Specifications. Content here should link to that section when it is relevant to the training procedure. -->
#### Preprocessing [optional]
[More Information Needed]
#### Training Hyperparameters
- **Training regime:** [More Information Needed] <!--fp32, fp16 mixed precision, bf16 mixed precision, bf16 non-mixed precision, fp16 non-mixed precision, fp8 mixed precision -->
#### Speeds, Sizes, Times [optional]
<!-- This section provides information about throughput, start/end time, checkpoint size if relevant, etc. -->
[More Information Needed]
## Evaluation
<!-- This section describes the evaluation protocols and provides the results. -->
### Testing Data, Factors & Metrics
#### Testing Data
<!-- This should link to a Dataset Card if possible. -->
[More Information Needed]
#### Factors
<!-- These are the things the evaluation is disaggregating by, e.g., subpopulations or domains. -->
[More Information Needed]
#### Metrics
<!-- These are the evaluation metrics being used, ideally with a description of why. -->
[More Information Needed]
### Results
[More Information Needed]
#### Summary
## Model Examination [optional]
<!-- Relevant interpretability work for the model goes here -->
[More Information Needed]
## Environmental Impact
<!-- Total emissions (in grams of CO2eq) and additional considerations, such as electricity usage, go here. Edit the suggested text below accordingly -->
Carbon emissions can be estimated using the [Machine Learning Impact calculator](https://mlco2.github.io/impact#compute) presented in [Lacoste et al. (2019)](https://arxiv.org/abs/1910.09700).
- **Hardware Type:** [More Information Needed]
- **Hours used:** [More Information Needed]
- **Cloud Provider:** [More Information Needed]
- **Compute Region:** [More Information Needed]
- **Carbon Emitted:** [More Information Needed]
## Technical Specifications [optional]
### Model Architecture and Objective
[More Information Needed]
### Compute Infrastructure
[More Information Needed]
#### Hardware
[More Information Needed]
#### Software
[More Information Needed]
## Citation [optional]
<!-- If there is a paper or blog post introducing the model, the APA and Bibtex information for that should go in this section. -->
**BibTeX:**
[More Information Needed]
**APA:**
[More Information Needed]
## Glossary [optional]
<!-- If relevant, include terms and calculations in this section that can help readers understand the model or model card. -->
[More Information Needed]
## More Information [optional]
[More Information Needed]
## Model Card Authors [optional]
[More Information Needed]
## Model Card Contact
[More Information Needed] | {"library_name": "transformers", "tags": []} | pruning/es5km0l | null | [
"transformers",
"arxiv:1910.09700",
"endpoints_compatible",
"region:us"
] | null | 2024-04-27T06:35:25+00:00 | [
"1910.09700"
] | [] | TAGS
#transformers #arxiv-1910.09700 #endpoints_compatible #region-us
|
# Model Card for Model ID
## Model Details
### Model Description
This is the model card of a transformers model that has been pushed on the Hub. This model card has been automatically generated.
- Developed by:
- Funded by [optional]:
- Shared by [optional]:
- Model type:
- Language(s) (NLP):
- License:
- Finetuned from model [optional]:
### Model Sources [optional]
- Repository:
- Paper [optional]:
- Demo [optional]:
## Uses
### Direct Use
### Downstream Use [optional]
### Out-of-Scope Use
## Bias, Risks, and Limitations
### Recommendations
Users (both direct and downstream) should be made aware of the risks, biases and limitations of the model. More information needed for further recommendations.
## How to Get Started with the Model
Use the code below to get started with the model.
## Training Details
### Training Data
### Training Procedure
#### Preprocessing [optional]
#### Training Hyperparameters
- Training regime:
#### Speeds, Sizes, Times [optional]
## Evaluation
### Testing Data, Factors & Metrics
#### Testing Data
#### Factors
#### Metrics
### Results
#### Summary
## Model Examination [optional]
## Environmental Impact
Carbon emissions can be estimated using the Machine Learning Impact calculator presented in Lacoste et al. (2019).
- Hardware Type:
- Hours used:
- Cloud Provider:
- Compute Region:
- Carbon Emitted:
## Technical Specifications [optional]
### Model Architecture and Objective
### Compute Infrastructure
#### Hardware
#### Software
[optional]
BibTeX:
APA:
## Glossary [optional]
## More Information [optional]
## Model Card Authors [optional]
## Model Card Contact
| [
"# Model Card for Model ID",
"## Model Details",
"### Model Description\n\n\n\nThis is the model card of a transformers model that has been pushed on the Hub. This model card has been automatically generated.\n\n- Developed by: \n- Funded by [optional]: \n- Shared by [optional]: \n- Model type: \n- Language(s) (NLP): \n- License: \n- Finetuned from model [optional]:",
"### Model Sources [optional]\n\n\n\n- Repository: \n- Paper [optional]: \n- Demo [optional]:",
"## Uses",
"### Direct Use",
"### Downstream Use [optional]",
"### Out-of-Scope Use",
"## Bias, Risks, and Limitations",
"### Recommendations\n\n\n\nUsers (both direct and downstream) should be made aware of the risks, biases and limitations of the model. More information needed for further recommendations.",
"## How to Get Started with the Model\n\nUse the code below to get started with the model.",
"## Training Details",
"### Training Data",
"### Training Procedure",
"#### Preprocessing [optional]",
"#### Training Hyperparameters\n\n- Training regime:",
"#### Speeds, Sizes, Times [optional]",
"## Evaluation",
"### Testing Data, Factors & Metrics",
"#### Testing Data",
"#### Factors",
"#### Metrics",
"### Results",
"#### Summary",
"## Model Examination [optional]",
"## Environmental Impact\n\n\n\nCarbon emissions can be estimated using the Machine Learning Impact calculator presented in Lacoste et al. (2019).\n\n- Hardware Type: \n- Hours used: \n- Cloud Provider: \n- Compute Region: \n- Carbon Emitted:",
"## Technical Specifications [optional]",
"### Model Architecture and Objective",
"### Compute Infrastructure",
"#### Hardware",
"#### Software\n\n\n\n[optional]\n\n\n\nBibTeX:\n\n\n\nAPA:",
"## Glossary [optional]",
"## More Information [optional]",
"## Model Card Authors [optional]",
"## Model Card Contact"
] | [
"TAGS\n#transformers #arxiv-1910.09700 #endpoints_compatible #region-us \n",
"# Model Card for Model ID",
"## Model Details",
"### Model Description\n\n\n\nThis is the model card of a transformers model that has been pushed on the Hub. This model card has been automatically generated.\n\n- Developed by: \n- Funded by [optional]: \n- Shared by [optional]: \n- Model type: \n- Language(s) (NLP): \n- License: \n- Finetuned from model [optional]:",
"### Model Sources [optional]\n\n\n\n- Repository: \n- Paper [optional]: \n- Demo [optional]:",
"## Uses",
"### Direct Use",
"### Downstream Use [optional]",
"### Out-of-Scope Use",
"## Bias, Risks, and Limitations",
"### Recommendations\n\n\n\nUsers (both direct and downstream) should be made aware of the risks, biases and limitations of the model. More information needed for further recommendations.",
"## How to Get Started with the Model\n\nUse the code below to get started with the model.",
"## Training Details",
"### Training Data",
"### Training Procedure",
"#### Preprocessing [optional]",
"#### Training Hyperparameters\n\n- Training regime:",
"#### Speeds, Sizes, Times [optional]",
"## Evaluation",
"### Testing Data, Factors & Metrics",
"#### Testing Data",
"#### Factors",
"#### Metrics",
"### Results",
"#### Summary",
"## Model Examination [optional]",
"## Environmental Impact\n\n\n\nCarbon emissions can be estimated using the Machine Learning Impact calculator presented in Lacoste et al. (2019).\n\n- Hardware Type: \n- Hours used: \n- Cloud Provider: \n- Compute Region: \n- Carbon Emitted:",
"## Technical Specifications [optional]",
"### Model Architecture and Objective",
"### Compute Infrastructure",
"#### Hardware",
"#### Software\n\n\n\n[optional]\n\n\n\nBibTeX:\n\n\n\nAPA:",
"## Glossary [optional]",
"## More Information [optional]",
"## Model Card Authors [optional]",
"## Model Card Contact"
] |
text2text-generation | transformers |
# Model Card for Model ID
<!-- Provide a quick summary of what the model is/does. -->
## Model Details
### Model Description
<!-- Provide a longer summary of what this model is. -->
This is the model card of a 🤗 transformers model that has been pushed on the Hub. This model card has been automatically generated.
- **Developed by:** [More Information Needed]
- **Funded by [optional]:** [More Information Needed]
- **Shared by [optional]:** [More Information Needed]
- **Model type:** [More Information Needed]
- **Language(s) (NLP):** [More Information Needed]
- **License:** [More Information Needed]
- **Finetuned from model [optional]:** [More Information Needed]
### Model Sources [optional]
<!-- Provide the basic links for the model. -->
- **Repository:** [More Information Needed]
- **Paper [optional]:** [More Information Needed]
- **Demo [optional]:** [More Information Needed]
## Uses
<!-- Address questions around how the model is intended to be used, including the foreseeable users of the model and those affected by the model. -->
### Direct Use
<!-- This section is for the model use without fine-tuning or plugging into a larger ecosystem/app. -->
[More Information Needed]
### Downstream Use [optional]
<!-- This section is for the model use when fine-tuned for a task, or when plugged into a larger ecosystem/app -->
[More Information Needed]
### Out-of-Scope Use
<!-- This section addresses misuse, malicious use, and uses that the model will not work well for. -->
[More Information Needed]
## Bias, Risks, and Limitations
<!-- This section is meant to convey both technical and sociotechnical limitations. -->
[More Information Needed]
### Recommendations
<!-- This section is meant to convey recommendations with respect to the bias, risk, and technical limitations. -->
Users (both direct and downstream) should be made aware of the risks, biases and limitations of the model. More information needed for further recommendations.
## How to Get Started with the Model
Use the code below to get started with the model.
[More Information Needed]
## Training Details
### Training Data
<!-- This should link to a Dataset Card, perhaps with a short stub of information on what the training data is all about as well as documentation related to data pre-processing or additional filtering. -->
[More Information Needed]
### Training Procedure
<!-- This relates heavily to the Technical Specifications. Content here should link to that section when it is relevant to the training procedure. -->
#### Preprocessing [optional]
[More Information Needed]
#### Training Hyperparameters
- **Training regime:** [More Information Needed] <!--fp32, fp16 mixed precision, bf16 mixed precision, bf16 non-mixed precision, fp16 non-mixed precision, fp8 mixed precision -->
#### Speeds, Sizes, Times [optional]
<!-- This section provides information about throughput, start/end time, checkpoint size if relevant, etc. -->
[More Information Needed]
## Evaluation
<!-- This section describes the evaluation protocols and provides the results. -->
### Testing Data, Factors & Metrics
#### Testing Data
<!-- This should link to a Dataset Card if possible. -->
[More Information Needed]
#### Factors
<!-- These are the things the evaluation is disaggregating by, e.g., subpopulations or domains. -->
[More Information Needed]
#### Metrics
<!-- These are the evaluation metrics being used, ideally with a description of why. -->
[More Information Needed]
### Results
[More Information Needed]
#### Summary
## Model Examination [optional]
<!-- Relevant interpretability work for the model goes here -->
[More Information Needed]
## Environmental Impact
<!-- Total emissions (in grams of CO2eq) and additional considerations, such as electricity usage, go here. Edit the suggested text below accordingly -->
Carbon emissions can be estimated using the [Machine Learning Impact calculator](https://mlco2.github.io/impact#compute) presented in [Lacoste et al. (2019)](https://arxiv.org/abs/1910.09700).
- **Hardware Type:** [More Information Needed]
- **Hours used:** [More Information Needed]
- **Cloud Provider:** [More Information Needed]
- **Compute Region:** [More Information Needed]
- **Carbon Emitted:** [More Information Needed]
## Technical Specifications [optional]
### Model Architecture and Objective
[More Information Needed]
### Compute Infrastructure
[More Information Needed]
#### Hardware
[More Information Needed]
#### Software
[More Information Needed]
## Citation [optional]
<!-- If there is a paper or blog post introducing the model, the APA and Bibtex information for that should go in this section. -->
**BibTeX:**
[More Information Needed]
**APA:**
[More Information Needed]
## Glossary [optional]
<!-- If relevant, include terms and calculations in this section that can help readers understand the model or model card. -->
[More Information Needed]
## More Information [optional]
[More Information Needed]
## Model Card Authors [optional]
[More Information Needed]
## Model Card Contact
[More Information Needed] | {"library_name": "transformers", "tags": []} | NegarSH/mt5-Quran-QA | null | [
"transformers",
"safetensors",
"mt5",
"text2text-generation",
"arxiv:1910.09700",
"autotrain_compatible",
"endpoints_compatible",
"text-generation-inference",
"region:us"
] | null | 2024-04-27T06:36:26+00:00 | [
"1910.09700"
] | [] | TAGS
#transformers #safetensors #mt5 #text2text-generation #arxiv-1910.09700 #autotrain_compatible #endpoints_compatible #text-generation-inference #region-us
|
# Model Card for Model ID
## Model Details
### Model Description
This is the model card of a transformers model that has been pushed on the Hub. This model card has been automatically generated.
- Developed by:
- Funded by [optional]:
- Shared by [optional]:
- Model type:
- Language(s) (NLP):
- License:
- Finetuned from model [optional]:
### Model Sources [optional]
- Repository:
- Paper [optional]:
- Demo [optional]:
## Uses
### Direct Use
### Downstream Use [optional]
### Out-of-Scope Use
## Bias, Risks, and Limitations
### Recommendations
Users (both direct and downstream) should be made aware of the risks, biases and limitations of the model. More information needed for further recommendations.
## How to Get Started with the Model
Use the code below to get started with the model.
## Training Details
### Training Data
### Training Procedure
#### Preprocessing [optional]
#### Training Hyperparameters
- Training regime:
#### Speeds, Sizes, Times [optional]
## Evaluation
### Testing Data, Factors & Metrics
#### Testing Data
#### Factors
#### Metrics
### Results
#### Summary
## Model Examination [optional]
## Environmental Impact
Carbon emissions can be estimated using the Machine Learning Impact calculator presented in Lacoste et al. (2019).
- Hardware Type:
- Hours used:
- Cloud Provider:
- Compute Region:
- Carbon Emitted:
## Technical Specifications [optional]
### Model Architecture and Objective
### Compute Infrastructure
#### Hardware
#### Software
[optional]
BibTeX:
APA:
## Glossary [optional]
## More Information [optional]
## Model Card Authors [optional]
## Model Card Contact
| [
"# Model Card for Model ID",
"## Model Details",
"### Model Description\n\n\n\nThis is the model card of a transformers model that has been pushed on the Hub. This model card has been automatically generated.\n\n- Developed by: \n- Funded by [optional]: \n- Shared by [optional]: \n- Model type: \n- Language(s) (NLP): \n- License: \n- Finetuned from model [optional]:",
"### Model Sources [optional]\n\n\n\n- Repository: \n- Paper [optional]: \n- Demo [optional]:",
"## Uses",
"### Direct Use",
"### Downstream Use [optional]",
"### Out-of-Scope Use",
"## Bias, Risks, and Limitations",
"### Recommendations\n\n\n\nUsers (both direct and downstream) should be made aware of the risks, biases and limitations of the model. More information needed for further recommendations.",
"## How to Get Started with the Model\n\nUse the code below to get started with the model.",
"## Training Details",
"### Training Data",
"### Training Procedure",
"#### Preprocessing [optional]",
"#### Training Hyperparameters\n\n- Training regime:",
"#### Speeds, Sizes, Times [optional]",
"## Evaluation",
"### Testing Data, Factors & Metrics",
"#### Testing Data",
"#### Factors",
"#### Metrics",
"### Results",
"#### Summary",
"## Model Examination [optional]",
"## Environmental Impact\n\n\n\nCarbon emissions can be estimated using the Machine Learning Impact calculator presented in Lacoste et al. (2019).\n\n- Hardware Type: \n- Hours used: \n- Cloud Provider: \n- Compute Region: \n- Carbon Emitted:",
"## Technical Specifications [optional]",
"### Model Architecture and Objective",
"### Compute Infrastructure",
"#### Hardware",
"#### Software\n\n\n\n[optional]\n\n\n\nBibTeX:\n\n\n\nAPA:",
"## Glossary [optional]",
"## More Information [optional]",
"## Model Card Authors [optional]",
"## Model Card Contact"
] | [
"TAGS\n#transformers #safetensors #mt5 #text2text-generation #arxiv-1910.09700 #autotrain_compatible #endpoints_compatible #text-generation-inference #region-us \n",
"# Model Card for Model ID",
"## Model Details",
"### Model Description\n\n\n\nThis is the model card of a transformers model that has been pushed on the Hub. This model card has been automatically generated.\n\n- Developed by: \n- Funded by [optional]: \n- Shared by [optional]: \n- Model type: \n- Language(s) (NLP): \n- License: \n- Finetuned from model [optional]:",
"### Model Sources [optional]\n\n\n\n- Repository: \n- Paper [optional]: \n- Demo [optional]:",
"## Uses",
"### Direct Use",
"### Downstream Use [optional]",
"### Out-of-Scope Use",
"## Bias, Risks, and Limitations",
"### Recommendations\n\n\n\nUsers (both direct and downstream) should be made aware of the risks, biases and limitations of the model. More information needed for further recommendations.",
"## How to Get Started with the Model\n\nUse the code below to get started with the model.",
"## Training Details",
"### Training Data",
"### Training Procedure",
"#### Preprocessing [optional]",
"#### Training Hyperparameters\n\n- Training regime:",
"#### Speeds, Sizes, Times [optional]",
"## Evaluation",
"### Testing Data, Factors & Metrics",
"#### Testing Data",
"#### Factors",
"#### Metrics",
"### Results",
"#### Summary",
"## Model Examination [optional]",
"## Environmental Impact\n\n\n\nCarbon emissions can be estimated using the Machine Learning Impact calculator presented in Lacoste et al. (2019).\n\n- Hardware Type: \n- Hours used: \n- Cloud Provider: \n- Compute Region: \n- Carbon Emitted:",
"## Technical Specifications [optional]",
"### Model Architecture and Objective",
"### Compute Infrastructure",
"#### Hardware",
"#### Software\n\n\n\n[optional]\n\n\n\nBibTeX:\n\n\n\nAPA:",
"## Glossary [optional]",
"## More Information [optional]",
"## Model Card Authors [optional]",
"## Model Card Contact"
] |
null | peft |
<!-- This model card has been generated automatically according to the information the Trainer had access to. You
should probably proofread and complete it, then remove this comment. -->
# GUE_prom_prom_300_notata-seqsight_16384_512_22M-L8_f
This model is a fine-tuned version of [mahdibaghbanzadeh/seqsight_16384_512_22M](https://huggingface.co/mahdibaghbanzadeh/seqsight_16384_512_22M) on the [mahdibaghbanzadeh/GUE_prom_prom_300_notata](https://huggingface.co/datasets/mahdibaghbanzadeh/GUE_prom_prom_300_notata) dataset.
It achieves the following results on the evaluation set:
- Loss: 0.1210
- F1 Score: 0.9552
- Accuracy: 0.9552
## Model description
More information needed
## Intended uses & limitations
More information needed
## Training and evaluation data
More information needed
## Training procedure
### Training hyperparameters
The following hyperparameters were used during training:
- learning_rate: 0.0005
- train_batch_size: 128
- eval_batch_size: 128
- seed: 42
- optimizer: Adam with betas=(0.9,0.999) and epsilon=1e-08
- lr_scheduler_type: linear
- training_steps: 10000
### Training results
| Training Loss | Epoch | Step | Validation Loss | F1 Score | Accuracy |
|:-------------:|:-----:|:-----:|:---------------:|:--------:|:--------:|
| 0.306 | 0.6 | 200 | 0.1533 | 0.9397 | 0.9397 |
| 0.1652 | 1.2 | 400 | 0.1343 | 0.9478 | 0.9478 |
| 0.15 | 1.81 | 600 | 0.1212 | 0.9516 | 0.9516 |
| 0.1345 | 2.41 | 800 | 0.1172 | 0.9529 | 0.9529 |
| 0.1338 | 3.01 | 1000 | 0.1208 | 0.9529 | 0.9529 |
| 0.1265 | 3.61 | 1200 | 0.1133 | 0.9546 | 0.9546 |
| 0.129 | 4.22 | 1400 | 0.1146 | 0.9555 | 0.9555 |
| 0.1249 | 4.82 | 1600 | 0.1114 | 0.9555 | 0.9555 |
| 0.1219 | 5.42 | 1800 | 0.1151 | 0.9559 | 0.9559 |
| 0.118 | 6.02 | 2000 | 0.1146 | 0.9557 | 0.9557 |
| 0.1221 | 6.63 | 2200 | 0.1112 | 0.9576 | 0.9576 |
| 0.1184 | 7.23 | 2400 | 0.1087 | 0.9593 | 0.9593 |
| 0.1118 | 7.83 | 2600 | 0.1095 | 0.9578 | 0.9578 |
| 0.1187 | 8.43 | 2800 | 0.1111 | 0.9593 | 0.9593 |
| 0.1146 | 9.04 | 3000 | 0.1064 | 0.9593 | 0.9593 |
| 0.1128 | 9.64 | 3200 | 0.1305 | 0.9512 | 0.9512 |
| 0.1134 | 10.24 | 3400 | 0.1059 | 0.9602 | 0.9602 |
| 0.1123 | 10.84 | 3600 | 0.1118 | 0.9563 | 0.9563 |
| 0.1083 | 11.45 | 3800 | 0.1091 | 0.9578 | 0.9578 |
| 0.109 | 12.05 | 4000 | 0.1098 | 0.9578 | 0.9578 |
| 0.1084 | 12.65 | 4200 | 0.1076 | 0.9585 | 0.9585 |
| 0.1103 | 13.25 | 4400 | 0.1103 | 0.9589 | 0.9589 |
| 0.1059 | 13.86 | 4600 | 0.1068 | 0.9587 | 0.9587 |
| 0.1077 | 14.46 | 4800 | 0.1097 | 0.9593 | 0.9593 |
| 0.1037 | 15.06 | 5000 | 0.1100 | 0.9585 | 0.9585 |
| 0.1042 | 15.66 | 5200 | 0.1055 | 0.9595 | 0.9595 |
| 0.104 | 16.27 | 5400 | 0.1063 | 0.9602 | 0.9602 |
| 0.1005 | 16.87 | 5600 | 0.1089 | 0.9601 | 0.9601 |
| 0.1016 | 17.47 | 5800 | 0.1030 | 0.9599 | 0.9599 |
| 0.1043 | 18.07 | 6000 | 0.1030 | 0.9599 | 0.9599 |
| 0.1007 | 18.67 | 6200 | 0.1048 | 0.9593 | 0.9593 |
| 0.1035 | 19.28 | 6400 | 0.1078 | 0.9585 | 0.9585 |
| 0.0993 | 19.88 | 6600 | 0.1056 | 0.9593 | 0.9593 |
| 0.1024 | 20.48 | 6800 | 0.1044 | 0.9610 | 0.9610 |
| 0.0957 | 21.08 | 7000 | 0.1084 | 0.9601 | 0.9601 |
| 0.0998 | 21.69 | 7200 | 0.1074 | 0.9599 | 0.9599 |
| 0.0984 | 22.29 | 7400 | 0.1081 | 0.9595 | 0.9595 |
| 0.102 | 22.89 | 7600 | 0.1030 | 0.9602 | 0.9602 |
| 0.0981 | 23.49 | 7800 | 0.1085 | 0.9601 | 0.9601 |
| 0.0969 | 24.1 | 8000 | 0.1047 | 0.9593 | 0.9593 |
| 0.0976 | 24.7 | 8200 | 0.1051 | 0.9602 | 0.9602 |
| 0.0983 | 25.3 | 8400 | 0.1041 | 0.9599 | 0.9599 |
| 0.0957 | 25.9 | 8600 | 0.1044 | 0.9612 | 0.9612 |
| 0.0979 | 26.51 | 8800 | 0.1041 | 0.9601 | 0.9601 |
| 0.0963 | 27.11 | 9000 | 0.1037 | 0.9599 | 0.9599 |
| 0.0964 | 27.71 | 9200 | 0.1049 | 0.9601 | 0.9601 |
| 0.0951 | 28.31 | 9400 | 0.1037 | 0.9604 | 0.9604 |
| 0.0992 | 28.92 | 9600 | 0.1050 | 0.9604 | 0.9604 |
| 0.0934 | 29.52 | 9800 | 0.1045 | 0.9604 | 0.9604 |
| 0.0961 | 30.12 | 10000 | 0.1045 | 0.9601 | 0.9601 |
### Framework versions
- PEFT 0.9.0
- Transformers 4.38.2
- Pytorch 2.2.0+cu121
- Datasets 2.17.1
- Tokenizers 0.15.2 | {"library_name": "peft", "tags": ["generated_from_trainer"], "metrics": ["accuracy"], "base_model": "mahdibaghbanzadeh/seqsight_16384_512_22M", "model-index": [{"name": "GUE_prom_prom_300_notata-seqsight_16384_512_22M-L8_f", "results": []}]} | mahdibaghbanzadeh/GUE_prom_prom_300_notata-seqsight_16384_512_22M-L8_f | null | [
"peft",
"safetensors",
"generated_from_trainer",
"base_model:mahdibaghbanzadeh/seqsight_16384_512_22M",
"region:us"
] | null | 2024-04-27T06:38:24+00:00 | [] | [] | TAGS
#peft #safetensors #generated_from_trainer #base_model-mahdibaghbanzadeh/seqsight_16384_512_22M #region-us
| GUE\_prom\_prom\_300\_notata-seqsight\_16384\_512\_22M-L8\_f
============================================================
This model is a fine-tuned version of mahdibaghbanzadeh/seqsight\_16384\_512\_22M on the mahdibaghbanzadeh/GUE\_prom\_prom\_300\_notata dataset.
It achieves the following results on the evaluation set:
* Loss: 0.1210
* F1 Score: 0.9552
* Accuracy: 0.9552
Model description
-----------------
More information needed
Intended uses & limitations
---------------------------
More information needed
Training and evaluation data
----------------------------
More information needed
Training procedure
------------------
### Training hyperparameters
The following hyperparameters were used during training:
* learning\_rate: 0.0005
* train\_batch\_size: 128
* eval\_batch\_size: 128
* seed: 42
* optimizer: Adam with betas=(0.9,0.999) and epsilon=1e-08
* lr\_scheduler\_type: linear
* training\_steps: 10000
### Training results
### Framework versions
* PEFT 0.9.0
* Transformers 4.38.2
* Pytorch 2.2.0+cu121
* Datasets 2.17.1
* Tokenizers 0.15.2
| [
"### Training hyperparameters\n\n\nThe following hyperparameters were used during training:\n\n\n* learning\\_rate: 0.0005\n* train\\_batch\\_size: 128\n* eval\\_batch\\_size: 128\n* seed: 42\n* optimizer: Adam with betas=(0.9,0.999) and epsilon=1e-08\n* lr\\_scheduler\\_type: linear\n* training\\_steps: 10000",
"### Training results",
"### Framework versions\n\n\n* PEFT 0.9.0\n* Transformers 4.38.2\n* Pytorch 2.2.0+cu121\n* Datasets 2.17.1\n* Tokenizers 0.15.2"
] | [
"TAGS\n#peft #safetensors #generated_from_trainer #base_model-mahdibaghbanzadeh/seqsight_16384_512_22M #region-us \n",
"### Training hyperparameters\n\n\nThe following hyperparameters were used during training:\n\n\n* learning\\_rate: 0.0005\n* train\\_batch\\_size: 128\n* eval\\_batch\\_size: 128\n* seed: 42\n* optimizer: Adam with betas=(0.9,0.999) and epsilon=1e-08\n* lr\\_scheduler\\_type: linear\n* training\\_steps: 10000",
"### Training results",
"### Framework versions\n\n\n* PEFT 0.9.0\n* Transformers 4.38.2\n* Pytorch 2.2.0+cu121\n* Datasets 2.17.1\n* Tokenizers 0.15.2"
] |
null | peft |
<!-- This model card has been generated automatically according to the information the Trainer had access to. You
should probably proofread and complete it, then remove this comment. -->
# GUE_prom_prom_300_notata-seqsight_16384_512_22M-L32_f
This model is a fine-tuned version of [mahdibaghbanzadeh/seqsight_16384_512_22M](https://huggingface.co/mahdibaghbanzadeh/seqsight_16384_512_22M) on the [mahdibaghbanzadeh/GUE_prom_prom_300_notata](https://huggingface.co/datasets/mahdibaghbanzadeh/GUE_prom_prom_300_notata) dataset.
It achieves the following results on the evaluation set:
- Loss: 0.1218
- F1 Score: 0.9557
- Accuracy: 0.9557
## Model description
More information needed
## Intended uses & limitations
More information needed
## Training and evaluation data
More information needed
## Training procedure
### Training hyperparameters
The following hyperparameters were used during training:
- learning_rate: 0.0005
- train_batch_size: 128
- eval_batch_size: 128
- seed: 42
- optimizer: Adam with betas=(0.9,0.999) and epsilon=1e-08
- lr_scheduler_type: linear
- training_steps: 10000
### Training results
| Training Loss | Epoch | Step | Validation Loss | F1 Score | Accuracy |
|:-------------:|:-----:|:-----:|:---------------:|:--------:|:--------:|
| 0.2647 | 0.6 | 200 | 0.1403 | 0.9465 | 0.9465 |
| 0.1464 | 1.2 | 400 | 0.1229 | 0.9510 | 0.9510 |
| 0.1385 | 1.81 | 600 | 0.1137 | 0.9550 | 0.9550 |
| 0.1267 | 2.41 | 800 | 0.1125 | 0.9546 | 0.9546 |
| 0.1286 | 3.01 | 1000 | 0.1146 | 0.9550 | 0.9550 |
| 0.1195 | 3.61 | 1200 | 0.1083 | 0.9589 | 0.9589 |
| 0.1216 | 4.22 | 1400 | 0.1068 | 0.9574 | 0.9574 |
| 0.1172 | 4.82 | 1600 | 0.1088 | 0.9576 | 0.9576 |
| 0.1128 | 5.42 | 1800 | 0.1111 | 0.9578 | 0.9578 |
| 0.1093 | 6.02 | 2000 | 0.1073 | 0.9597 | 0.9597 |
| 0.1131 | 6.63 | 2200 | 0.1053 | 0.9599 | 0.9599 |
| 0.1084 | 7.23 | 2400 | 0.1029 | 0.9608 | 0.9608 |
| 0.1012 | 7.83 | 2600 | 0.1030 | 0.9610 | 0.9610 |
| 0.1071 | 8.43 | 2800 | 0.1107 | 0.9587 | 0.9587 |
| 0.1047 | 9.04 | 3000 | 0.1015 | 0.9614 | 0.9614 |
| 0.1013 | 9.64 | 3200 | 0.1216 | 0.9548 | 0.9548 |
| 0.0999 | 10.24 | 3400 | 0.1022 | 0.9595 | 0.9595 |
| 0.1004 | 10.84 | 3600 | 0.1015 | 0.9602 | 0.9602 |
| 0.0952 | 11.45 | 3800 | 0.1043 | 0.9608 | 0.9608 |
| 0.0954 | 12.05 | 4000 | 0.1022 | 0.9604 | 0.9604 |
| 0.0943 | 12.65 | 4200 | 0.1007 | 0.9629 | 0.9629 |
| 0.0959 | 13.25 | 4400 | 0.1137 | 0.9585 | 0.9585 |
| 0.0925 | 13.86 | 4600 | 0.1020 | 0.9606 | 0.9606 |
| 0.093 | 14.46 | 4800 | 0.1067 | 0.9612 | 0.9612 |
| 0.0901 | 15.06 | 5000 | 0.1043 | 0.9604 | 0.9604 |
| 0.0874 | 15.66 | 5200 | 0.1017 | 0.9621 | 0.9621 |
| 0.0879 | 16.27 | 5400 | 0.1044 | 0.9604 | 0.9604 |
| 0.084 | 16.87 | 5600 | 0.1114 | 0.9582 | 0.9582 |
| 0.0852 | 17.47 | 5800 | 0.1034 | 0.9599 | 0.9599 |
| 0.0873 | 18.07 | 6000 | 0.1013 | 0.9614 | 0.9614 |
| 0.0834 | 18.67 | 6200 | 0.1017 | 0.9612 | 0.9612 |
| 0.0853 | 19.28 | 6400 | 0.1099 | 0.9580 | 0.9580 |
| 0.0829 | 19.88 | 6600 | 0.1023 | 0.9636 | 0.9636 |
| 0.0833 | 20.48 | 6800 | 0.1046 | 0.9606 | 0.9606 |
| 0.0773 | 21.08 | 7000 | 0.1073 | 0.9597 | 0.9597 |
| 0.0816 | 21.69 | 7200 | 0.1070 | 0.9584 | 0.9584 |
| 0.0804 | 22.29 | 7400 | 0.1096 | 0.9582 | 0.9582 |
| 0.0819 | 22.89 | 7600 | 0.1040 | 0.9595 | 0.9595 |
| 0.078 | 23.49 | 7800 | 0.1102 | 0.9597 | 0.9597 |
| 0.0755 | 24.1 | 8000 | 0.1048 | 0.9608 | 0.9608 |
| 0.0777 | 24.7 | 8200 | 0.1072 | 0.9597 | 0.9597 |
| 0.0777 | 25.3 | 8400 | 0.1028 | 0.9606 | 0.9606 |
| 0.0749 | 25.9 | 8600 | 0.1052 | 0.9610 | 0.9610 |
| 0.0772 | 26.51 | 8800 | 0.1042 | 0.9604 | 0.9604 |
| 0.0752 | 27.11 | 9000 | 0.1054 | 0.9604 | 0.9604 |
| 0.0751 | 27.71 | 9200 | 0.1083 | 0.9597 | 0.9597 |
| 0.0741 | 28.31 | 9400 | 0.1055 | 0.9587 | 0.9587 |
| 0.0783 | 28.92 | 9600 | 0.1082 | 0.9597 | 0.9597 |
| 0.0721 | 29.52 | 9800 | 0.1080 | 0.9587 | 0.9587 |
| 0.0742 | 30.12 | 10000 | 0.1066 | 0.9593 | 0.9593 |
### Framework versions
- PEFT 0.9.0
- Transformers 4.38.2
- Pytorch 2.2.0+cu121
- Datasets 2.17.1
- Tokenizers 0.15.2 | {"library_name": "peft", "tags": ["generated_from_trainer"], "metrics": ["accuracy"], "base_model": "mahdibaghbanzadeh/seqsight_16384_512_22M", "model-index": [{"name": "GUE_prom_prom_300_notata-seqsight_16384_512_22M-L32_f", "results": []}]} | mahdibaghbanzadeh/GUE_prom_prom_300_notata-seqsight_16384_512_22M-L32_f | null | [
"peft",
"safetensors",
"generated_from_trainer",
"base_model:mahdibaghbanzadeh/seqsight_16384_512_22M",
"region:us"
] | null | 2024-04-27T06:38:42+00:00 | [] | [] | TAGS
#peft #safetensors #generated_from_trainer #base_model-mahdibaghbanzadeh/seqsight_16384_512_22M #region-us
| GUE\_prom\_prom\_300\_notata-seqsight\_16384\_512\_22M-L32\_f
=============================================================
This model is a fine-tuned version of mahdibaghbanzadeh/seqsight\_16384\_512\_22M on the mahdibaghbanzadeh/GUE\_prom\_prom\_300\_notata dataset.
It achieves the following results on the evaluation set:
* Loss: 0.1218
* F1 Score: 0.9557
* Accuracy: 0.9557
Model description
-----------------
More information needed
Intended uses & limitations
---------------------------
More information needed
Training and evaluation data
----------------------------
More information needed
Training procedure
------------------
### Training hyperparameters
The following hyperparameters were used during training:
* learning\_rate: 0.0005
* train\_batch\_size: 128
* eval\_batch\_size: 128
* seed: 42
* optimizer: Adam with betas=(0.9,0.999) and epsilon=1e-08
* lr\_scheduler\_type: linear
* training\_steps: 10000
### Training results
### Framework versions
* PEFT 0.9.0
* Transformers 4.38.2
* Pytorch 2.2.0+cu121
* Datasets 2.17.1
* Tokenizers 0.15.2
| [
"### Training hyperparameters\n\n\nThe following hyperparameters were used during training:\n\n\n* learning\\_rate: 0.0005\n* train\\_batch\\_size: 128\n* eval\\_batch\\_size: 128\n* seed: 42\n* optimizer: Adam with betas=(0.9,0.999) and epsilon=1e-08\n* lr\\_scheduler\\_type: linear\n* training\\_steps: 10000",
"### Training results",
"### Framework versions\n\n\n* PEFT 0.9.0\n* Transformers 4.38.2\n* Pytorch 2.2.0+cu121\n* Datasets 2.17.1\n* Tokenizers 0.15.2"
] | [
"TAGS\n#peft #safetensors #generated_from_trainer #base_model-mahdibaghbanzadeh/seqsight_16384_512_22M #region-us \n",
"### Training hyperparameters\n\n\nThe following hyperparameters were used during training:\n\n\n* learning\\_rate: 0.0005\n* train\\_batch\\_size: 128\n* eval\\_batch\\_size: 128\n* seed: 42\n* optimizer: Adam with betas=(0.9,0.999) and epsilon=1e-08\n* lr\\_scheduler\\_type: linear\n* training\\_steps: 10000",
"### Training results",
"### Framework versions\n\n\n* PEFT 0.9.0\n* Transformers 4.38.2\n* Pytorch 2.2.0+cu121\n* Datasets 2.17.1\n* Tokenizers 0.15.2"
] |
text-generation | transformers |
# Model Card for Model ID
<!-- Provide a quick summary of what the model is/does. -->
## Model Details
### Model Description
<!-- Provide a longer summary of what this model is. -->
This is the model card of a 🤗 transformers model that has been pushed on the Hub. This model card has been automatically generated.
- **Developed by:** [More Information Needed]
- **Funded by [optional]:** [More Information Needed]
- **Shared by [optional]:** [More Information Needed]
- **Model type:** [More Information Needed]
- **Language(s) (NLP):** [More Information Needed]
- **License:** [More Information Needed]
- **Finetuned from model [optional]:** [More Information Needed]
### Model Sources [optional]
<!-- Provide the basic links for the model. -->
- **Repository:** [More Information Needed]
- **Paper [optional]:** [More Information Needed]
- **Demo [optional]:** [More Information Needed]
## Uses
<!-- Address questions around how the model is intended to be used, including the foreseeable users of the model and those affected by the model. -->
### Direct Use
<!-- This section is for the model use without fine-tuning or plugging into a larger ecosystem/app. -->
[More Information Needed]
### Downstream Use [optional]
<!-- This section is for the model use when fine-tuned for a task, or when plugged into a larger ecosystem/app -->
[More Information Needed]
### Out-of-Scope Use
<!-- This section addresses misuse, malicious use, and uses that the model will not work well for. -->
[More Information Needed]
## Bias, Risks, and Limitations
<!-- This section is meant to convey both technical and sociotechnical limitations. -->
[More Information Needed]
### Recommendations
<!-- This section is meant to convey recommendations with respect to the bias, risk, and technical limitations. -->
Users (both direct and downstream) should be made aware of the risks, biases and limitations of the model. More information needed for further recommendations.
## How to Get Started with the Model
Use the code below to get started with the model.
[More Information Needed]
## Training Details
### Training Data
<!-- This should link to a Dataset Card, perhaps with a short stub of information on what the training data is all about as well as documentation related to data pre-processing or additional filtering. -->
[More Information Needed]
### Training Procedure
<!-- This relates heavily to the Technical Specifications. Content here should link to that section when it is relevant to the training procedure. -->
#### Preprocessing [optional]
[More Information Needed]
#### Training Hyperparameters
- **Training regime:** [More Information Needed] <!--fp32, fp16 mixed precision, bf16 mixed precision, bf16 non-mixed precision, fp16 non-mixed precision, fp8 mixed precision -->
#### Speeds, Sizes, Times [optional]
<!-- This section provides information about throughput, start/end time, checkpoint size if relevant, etc. -->
[More Information Needed]
## Evaluation
<!-- This section describes the evaluation protocols and provides the results. -->
### Testing Data, Factors & Metrics
#### Testing Data
<!-- This should link to a Dataset Card if possible. -->
[More Information Needed]
#### Factors
<!-- These are the things the evaluation is disaggregating by, e.g., subpopulations or domains. -->
[More Information Needed]
#### Metrics
<!-- These are the evaluation metrics being used, ideally with a description of why. -->
[More Information Needed]
### Results
[More Information Needed]
#### Summary
## Model Examination [optional]
<!-- Relevant interpretability work for the model goes here -->
[More Information Needed]
## Environmental Impact
<!-- Total emissions (in grams of CO2eq) and additional considerations, such as electricity usage, go here. Edit the suggested text below accordingly -->
Carbon emissions can be estimated using the [Machine Learning Impact calculator](https://mlco2.github.io/impact#compute) presented in [Lacoste et al. (2019)](https://arxiv.org/abs/1910.09700).
- **Hardware Type:** [More Information Needed]
- **Hours used:** [More Information Needed]
- **Cloud Provider:** [More Information Needed]
- **Compute Region:** [More Information Needed]
- **Carbon Emitted:** [More Information Needed]
## Technical Specifications [optional]
### Model Architecture and Objective
[More Information Needed]
### Compute Infrastructure
[More Information Needed]
#### Hardware
[More Information Needed]
#### Software
[More Information Needed]
## Citation [optional]
<!-- If there is a paper or blog post introducing the model, the APA and Bibtex information for that should go in this section. -->
**BibTeX:**
[More Information Needed]
**APA:**
[More Information Needed]
## Glossary [optional]
<!-- If relevant, include terms and calculations in this section that can help readers understand the model or model card. -->
[More Information Needed]
## More Information [optional]
[More Information Needed]
## Model Card Authors [optional]
[More Information Needed]
## Model Card Contact
[More Information Needed] | {"library_name": "transformers", "tags": []} | liquid9212/x2h2lbi | null | [
"transformers",
"safetensors",
"stablelm",
"text-generation",
"conversational",
"arxiv:1910.09700",
"autotrain_compatible",
"endpoints_compatible",
"region:us"
] | null | 2024-04-27T06:39:28+00:00 | [
"1910.09700"
] | [] | TAGS
#transformers #safetensors #stablelm #text-generation #conversational #arxiv-1910.09700 #autotrain_compatible #endpoints_compatible #region-us
|
# Model Card for Model ID
## Model Details
### Model Description
This is the model card of a transformers model that has been pushed on the Hub. This model card has been automatically generated.
- Developed by:
- Funded by [optional]:
- Shared by [optional]:
- Model type:
- Language(s) (NLP):
- License:
- Finetuned from model [optional]:
### Model Sources [optional]
- Repository:
- Paper [optional]:
- Demo [optional]:
## Uses
### Direct Use
### Downstream Use [optional]
### Out-of-Scope Use
## Bias, Risks, and Limitations
### Recommendations
Users (both direct and downstream) should be made aware of the risks, biases and limitations of the model. More information needed for further recommendations.
## How to Get Started with the Model
Use the code below to get started with the model.
## Training Details
### Training Data
### Training Procedure
#### Preprocessing [optional]
#### Training Hyperparameters
- Training regime:
#### Speeds, Sizes, Times [optional]
## Evaluation
### Testing Data, Factors & Metrics
#### Testing Data
#### Factors
#### Metrics
### Results
#### Summary
## Model Examination [optional]
## Environmental Impact
Carbon emissions can be estimated using the Machine Learning Impact calculator presented in Lacoste et al. (2019).
- Hardware Type:
- Hours used:
- Cloud Provider:
- Compute Region:
- Carbon Emitted:
## Technical Specifications [optional]
### Model Architecture and Objective
### Compute Infrastructure
#### Hardware
#### Software
[optional]
BibTeX:
APA:
## Glossary [optional]
## More Information [optional]
## Model Card Authors [optional]
## Model Card Contact
| [
"# Model Card for Model ID",
"## Model Details",
"### Model Description\n\n\n\nThis is the model card of a transformers model that has been pushed on the Hub. This model card has been automatically generated.\n\n- Developed by: \n- Funded by [optional]: \n- Shared by [optional]: \n- Model type: \n- Language(s) (NLP): \n- License: \n- Finetuned from model [optional]:",
"### Model Sources [optional]\n\n\n\n- Repository: \n- Paper [optional]: \n- Demo [optional]:",
"## Uses",
"### Direct Use",
"### Downstream Use [optional]",
"### Out-of-Scope Use",
"## Bias, Risks, and Limitations",
"### Recommendations\n\n\n\nUsers (both direct and downstream) should be made aware of the risks, biases and limitations of the model. More information needed for further recommendations.",
"## How to Get Started with the Model\n\nUse the code below to get started with the model.",
"## Training Details",
"### Training Data",
"### Training Procedure",
"#### Preprocessing [optional]",
"#### Training Hyperparameters\n\n- Training regime:",
"#### Speeds, Sizes, Times [optional]",
"## Evaluation",
"### Testing Data, Factors & Metrics",
"#### Testing Data",
"#### Factors",
"#### Metrics",
"### Results",
"#### Summary",
"## Model Examination [optional]",
"## Environmental Impact\n\n\n\nCarbon emissions can be estimated using the Machine Learning Impact calculator presented in Lacoste et al. (2019).\n\n- Hardware Type: \n- Hours used: \n- Cloud Provider: \n- Compute Region: \n- Carbon Emitted:",
"## Technical Specifications [optional]",
"### Model Architecture and Objective",
"### Compute Infrastructure",
"#### Hardware",
"#### Software\n\n\n\n[optional]\n\n\n\nBibTeX:\n\n\n\nAPA:",
"## Glossary [optional]",
"## More Information [optional]",
"## Model Card Authors [optional]",
"## Model Card Contact"
] | [
"TAGS\n#transformers #safetensors #stablelm #text-generation #conversational #arxiv-1910.09700 #autotrain_compatible #endpoints_compatible #region-us \n",
"# Model Card for Model ID",
"## Model Details",
"### Model Description\n\n\n\nThis is the model card of a transformers model that has been pushed on the Hub. This model card has been automatically generated.\n\n- Developed by: \n- Funded by [optional]: \n- Shared by [optional]: \n- Model type: \n- Language(s) (NLP): \n- License: \n- Finetuned from model [optional]:",
"### Model Sources [optional]\n\n\n\n- Repository: \n- Paper [optional]: \n- Demo [optional]:",
"## Uses",
"### Direct Use",
"### Downstream Use [optional]",
"### Out-of-Scope Use",
"## Bias, Risks, and Limitations",
"### Recommendations\n\n\n\nUsers (both direct and downstream) should be made aware of the risks, biases and limitations of the model. More information needed for further recommendations.",
"## How to Get Started with the Model\n\nUse the code below to get started with the model.",
"## Training Details",
"### Training Data",
"### Training Procedure",
"#### Preprocessing [optional]",
"#### Training Hyperparameters\n\n- Training regime:",
"#### Speeds, Sizes, Times [optional]",
"## Evaluation",
"### Testing Data, Factors & Metrics",
"#### Testing Data",
"#### Factors",
"#### Metrics",
"### Results",
"#### Summary",
"## Model Examination [optional]",
"## Environmental Impact\n\n\n\nCarbon emissions can be estimated using the Machine Learning Impact calculator presented in Lacoste et al. (2019).\n\n- Hardware Type: \n- Hours used: \n- Cloud Provider: \n- Compute Region: \n- Carbon Emitted:",
"## Technical Specifications [optional]",
"### Model Architecture and Objective",
"### Compute Infrastructure",
"#### Hardware",
"#### Software\n\n\n\n[optional]\n\n\n\nBibTeX:\n\n\n\nAPA:",
"## Glossary [optional]",
"## More Information [optional]",
"## Model Card Authors [optional]",
"## Model Card Contact"
] |
text-generation | transformers |
# Model Card for Model ID
<!-- Provide a quick summary of what the model is/does. -->
## Model Details
### Model Description
<!-- Provide a longer summary of what this model is. -->
This is the model card of a 🤗 transformers model that has been pushed on the Hub. This model card has been automatically generated.
- **Developed by:** [More Information Needed]
- **Funded by [optional]:** [More Information Needed]
- **Shared by [optional]:** [More Information Needed]
- **Model type:** [More Information Needed]
- **Language(s) (NLP):** [More Information Needed]
- **License:** [More Information Needed]
- **Finetuned from model [optional]:** [More Information Needed]
### Model Sources [optional]
<!-- Provide the basic links for the model. -->
- **Repository:** [More Information Needed]
- **Paper [optional]:** [More Information Needed]
- **Demo [optional]:** [More Information Needed]
## Uses
<!-- Address questions around how the model is intended to be used, including the foreseeable users of the model and those affected by the model. -->
### Direct Use
<!-- This section is for the model use without fine-tuning or plugging into a larger ecosystem/app. -->
[More Information Needed]
### Downstream Use [optional]
<!-- This section is for the model use when fine-tuned for a task, or when plugged into a larger ecosystem/app -->
[More Information Needed]
### Out-of-Scope Use
<!-- This section addresses misuse, malicious use, and uses that the model will not work well for. -->
[More Information Needed]
## Bias, Risks, and Limitations
<!-- This section is meant to convey both technical and sociotechnical limitations. -->
[More Information Needed]
### Recommendations
<!-- This section is meant to convey recommendations with respect to the bias, risk, and technical limitations. -->
Users (both direct and downstream) should be made aware of the risks, biases and limitations of the model. More information needed for further recommendations.
## How to Get Started with the Model
Use the code below to get started with the model.
[More Information Needed]
## Training Details
### Training Data
<!-- This should link to a Dataset Card, perhaps with a short stub of information on what the training data is all about as well as documentation related to data pre-processing or additional filtering. -->
[More Information Needed]
### Training Procedure
<!-- This relates heavily to the Technical Specifications. Content here should link to that section when it is relevant to the training procedure. -->
#### Preprocessing [optional]
[More Information Needed]
#### Training Hyperparameters
- **Training regime:** [More Information Needed] <!--fp32, fp16 mixed precision, bf16 mixed precision, bf16 non-mixed precision, fp16 non-mixed precision, fp8 mixed precision -->
#### Speeds, Sizes, Times [optional]
<!-- This section provides information about throughput, start/end time, checkpoint size if relevant, etc. -->
[More Information Needed]
## Evaluation
<!-- This section describes the evaluation protocols and provides the results. -->
### Testing Data, Factors & Metrics
#### Testing Data
<!-- This should link to a Dataset Card if possible. -->
[More Information Needed]
#### Factors
<!-- These are the things the evaluation is disaggregating by, e.g., subpopulations or domains. -->
[More Information Needed]
#### Metrics
<!-- These are the evaluation metrics being used, ideally with a description of why. -->
[More Information Needed]
### Results
[More Information Needed]
#### Summary
## Model Examination [optional]
<!-- Relevant interpretability work for the model goes here -->
[More Information Needed]
## Environmental Impact
<!-- Total emissions (in grams of CO2eq) and additional considerations, such as electricity usage, go here. Edit the suggested text below accordingly -->
Carbon emissions can be estimated using the [Machine Learning Impact calculator](https://mlco2.github.io/impact#compute) presented in [Lacoste et al. (2019)](https://arxiv.org/abs/1910.09700).
- **Hardware Type:** [More Information Needed]
- **Hours used:** [More Information Needed]
- **Cloud Provider:** [More Information Needed]
- **Compute Region:** [More Information Needed]
- **Carbon Emitted:** [More Information Needed]
## Technical Specifications [optional]
### Model Architecture and Objective
[More Information Needed]
### Compute Infrastructure
[More Information Needed]
#### Hardware
[More Information Needed]
#### Software
[More Information Needed]
## Citation [optional]
<!-- If there is a paper or blog post introducing the model, the APA and Bibtex information for that should go in this section. -->
**BibTeX:**
[More Information Needed]
**APA:**
[More Information Needed]
## Glossary [optional]
<!-- If relevant, include terms and calculations in this section that can help readers understand the model or model card. -->
[More Information Needed]
## More Information [optional]
[More Information Needed]
## Model Card Authors [optional]
[More Information Needed]
## Model Card Contact
[More Information Needed] | {"library_name": "transformers", "tags": []} | pruning/e6372s9 | null | [
"transformers",
"safetensors",
"stablelm",
"text-generation",
"conversational",
"arxiv:1910.09700",
"autotrain_compatible",
"endpoints_compatible",
"region:us"
] | null | 2024-04-27T06:39:32+00:00 | [
"1910.09700"
] | [] | TAGS
#transformers #safetensors #stablelm #text-generation #conversational #arxiv-1910.09700 #autotrain_compatible #endpoints_compatible #region-us
|
# Model Card for Model ID
## Model Details
### Model Description
This is the model card of a transformers model that has been pushed on the Hub. This model card has been automatically generated.
- Developed by:
- Funded by [optional]:
- Shared by [optional]:
- Model type:
- Language(s) (NLP):
- License:
- Finetuned from model [optional]:
### Model Sources [optional]
- Repository:
- Paper [optional]:
- Demo [optional]:
## Uses
### Direct Use
### Downstream Use [optional]
### Out-of-Scope Use
## Bias, Risks, and Limitations
### Recommendations
Users (both direct and downstream) should be made aware of the risks, biases and limitations of the model. More information needed for further recommendations.
## How to Get Started with the Model
Use the code below to get started with the model.
## Training Details
### Training Data
### Training Procedure
#### Preprocessing [optional]
#### Training Hyperparameters
- Training regime:
#### Speeds, Sizes, Times [optional]
## Evaluation
### Testing Data, Factors & Metrics
#### Testing Data
#### Factors
#### Metrics
### Results
#### Summary
## Model Examination [optional]
## Environmental Impact
Carbon emissions can be estimated using the Machine Learning Impact calculator presented in Lacoste et al. (2019).
- Hardware Type:
- Hours used:
- Cloud Provider:
- Compute Region:
- Carbon Emitted:
## Technical Specifications [optional]
### Model Architecture and Objective
### Compute Infrastructure
#### Hardware
#### Software
[optional]
BibTeX:
APA:
## Glossary [optional]
## More Information [optional]
## Model Card Authors [optional]
## Model Card Contact
| [
"# Model Card for Model ID",
"## Model Details",
"### Model Description\n\n\n\nThis is the model card of a transformers model that has been pushed on the Hub. This model card has been automatically generated.\n\n- Developed by: \n- Funded by [optional]: \n- Shared by [optional]: \n- Model type: \n- Language(s) (NLP): \n- License: \n- Finetuned from model [optional]:",
"### Model Sources [optional]\n\n\n\n- Repository: \n- Paper [optional]: \n- Demo [optional]:",
"## Uses",
"### Direct Use",
"### Downstream Use [optional]",
"### Out-of-Scope Use",
"## Bias, Risks, and Limitations",
"### Recommendations\n\n\n\nUsers (both direct and downstream) should be made aware of the risks, biases and limitations of the model. More information needed for further recommendations.",
"## How to Get Started with the Model\n\nUse the code below to get started with the model.",
"## Training Details",
"### Training Data",
"### Training Procedure",
"#### Preprocessing [optional]",
"#### Training Hyperparameters\n\n- Training regime:",
"#### Speeds, Sizes, Times [optional]",
"## Evaluation",
"### Testing Data, Factors & Metrics",
"#### Testing Data",
"#### Factors",
"#### Metrics",
"### Results",
"#### Summary",
"## Model Examination [optional]",
"## Environmental Impact\n\n\n\nCarbon emissions can be estimated using the Machine Learning Impact calculator presented in Lacoste et al. (2019).\n\n- Hardware Type: \n- Hours used: \n- Cloud Provider: \n- Compute Region: \n- Carbon Emitted:",
"## Technical Specifications [optional]",
"### Model Architecture and Objective",
"### Compute Infrastructure",
"#### Hardware",
"#### Software\n\n\n\n[optional]\n\n\n\nBibTeX:\n\n\n\nAPA:",
"## Glossary [optional]",
"## More Information [optional]",
"## Model Card Authors [optional]",
"## Model Card Contact"
] | [
"TAGS\n#transformers #safetensors #stablelm #text-generation #conversational #arxiv-1910.09700 #autotrain_compatible #endpoints_compatible #region-us \n",
"# Model Card for Model ID",
"## Model Details",
"### Model Description\n\n\n\nThis is the model card of a transformers model that has been pushed on the Hub. This model card has been automatically generated.\n\n- Developed by: \n- Funded by [optional]: \n- Shared by [optional]: \n- Model type: \n- Language(s) (NLP): \n- License: \n- Finetuned from model [optional]:",
"### Model Sources [optional]\n\n\n\n- Repository: \n- Paper [optional]: \n- Demo [optional]:",
"## Uses",
"### Direct Use",
"### Downstream Use [optional]",
"### Out-of-Scope Use",
"## Bias, Risks, and Limitations",
"### Recommendations\n\n\n\nUsers (both direct and downstream) should be made aware of the risks, biases and limitations of the model. More information needed for further recommendations.",
"## How to Get Started with the Model\n\nUse the code below to get started with the model.",
"## Training Details",
"### Training Data",
"### Training Procedure",
"#### Preprocessing [optional]",
"#### Training Hyperparameters\n\n- Training regime:",
"#### Speeds, Sizes, Times [optional]",
"## Evaluation",
"### Testing Data, Factors & Metrics",
"#### Testing Data",
"#### Factors",
"#### Metrics",
"### Results",
"#### Summary",
"## Model Examination [optional]",
"## Environmental Impact\n\n\n\nCarbon emissions can be estimated using the Machine Learning Impact calculator presented in Lacoste et al. (2019).\n\n- Hardware Type: \n- Hours used: \n- Cloud Provider: \n- Compute Region: \n- Carbon Emitted:",
"## Technical Specifications [optional]",
"### Model Architecture and Objective",
"### Compute Infrastructure",
"#### Hardware",
"#### Software\n\n\n\n[optional]\n\n\n\nBibTeX:\n\n\n\nAPA:",
"## Glossary [optional]",
"## More Information [optional]",
"## Model Card Authors [optional]",
"## Model Card Contact"
] |
text-generation | transformers |
# Model Card for Model ID
<!-- Provide a quick summary of what the model is/does. -->
## Model Details
### Model Description
<!-- Provide a longer summary of what this model is. -->
This is the model card of a 🤗 transformers model that has been pushed on the Hub. This model card has been automatically generated.
- **Developed by:** [More Information Needed]
- **Funded by [optional]:** [More Information Needed]
- **Shared by [optional]:** [More Information Needed]
- **Model type:** [More Information Needed]
- **Language(s) (NLP):** [More Information Needed]
- **License:** [More Information Needed]
- **Finetuned from model [optional]:** [More Information Needed]
### Model Sources [optional]
<!-- Provide the basic links for the model. -->
- **Repository:** [More Information Needed]
- **Paper [optional]:** [More Information Needed]
- **Demo [optional]:** [More Information Needed]
## Uses
<!-- Address questions around how the model is intended to be used, including the foreseeable users of the model and those affected by the model. -->
### Direct Use
<!-- This section is for the model use without fine-tuning or plugging into a larger ecosystem/app. -->
[More Information Needed]
### Downstream Use [optional]
<!-- This section is for the model use when fine-tuned for a task, or when plugged into a larger ecosystem/app -->
[More Information Needed]
### Out-of-Scope Use
<!-- This section addresses misuse, malicious use, and uses that the model will not work well for. -->
[More Information Needed]
## Bias, Risks, and Limitations
<!-- This section is meant to convey both technical and sociotechnical limitations. -->
[More Information Needed]
### Recommendations
<!-- This section is meant to convey recommendations with respect to the bias, risk, and technical limitations. -->
Users (both direct and downstream) should be made aware of the risks, biases and limitations of the model. More information needed for further recommendations.
## How to Get Started with the Model
Use the code below to get started with the model.
[More Information Needed]
## Training Details
### Training Data
<!-- This should link to a Dataset Card, perhaps with a short stub of information on what the training data is all about as well as documentation related to data pre-processing or additional filtering. -->
[More Information Needed]
### Training Procedure
<!-- This relates heavily to the Technical Specifications. Content here should link to that section when it is relevant to the training procedure. -->
#### Preprocessing [optional]
[More Information Needed]
#### Training Hyperparameters
- **Training regime:** [More Information Needed] <!--fp32, fp16 mixed precision, bf16 mixed precision, bf16 non-mixed precision, fp16 non-mixed precision, fp8 mixed precision -->
#### Speeds, Sizes, Times [optional]
<!-- This section provides information about throughput, start/end time, checkpoint size if relevant, etc. -->
[More Information Needed]
## Evaluation
<!-- This section describes the evaluation protocols and provides the results. -->
### Testing Data, Factors & Metrics
#### Testing Data
<!-- This should link to a Dataset Card if possible. -->
[More Information Needed]
#### Factors
<!-- These are the things the evaluation is disaggregating by, e.g., subpopulations or domains. -->
[More Information Needed]
#### Metrics
<!-- These are the evaluation metrics being used, ideally with a description of why. -->
[More Information Needed]
### Results
[More Information Needed]
#### Summary
## Model Examination [optional]
<!-- Relevant interpretability work for the model goes here -->
[More Information Needed]
## Environmental Impact
<!-- Total emissions (in grams of CO2eq) and additional considerations, such as electricity usage, go here. Edit the suggested text below accordingly -->
Carbon emissions can be estimated using the [Machine Learning Impact calculator](https://mlco2.github.io/impact#compute) presented in [Lacoste et al. (2019)](https://arxiv.org/abs/1910.09700).
- **Hardware Type:** [More Information Needed]
- **Hours used:** [More Information Needed]
- **Cloud Provider:** [More Information Needed]
- **Compute Region:** [More Information Needed]
- **Carbon Emitted:** [More Information Needed]
## Technical Specifications [optional]
### Model Architecture and Objective
[More Information Needed]
### Compute Infrastructure
[More Information Needed]
#### Hardware
[More Information Needed]
#### Software
[More Information Needed]
## Citation [optional]
<!-- If there is a paper or blog post introducing the model, the APA and Bibtex information for that should go in this section. -->
**BibTeX:**
[More Information Needed]
**APA:**
[More Information Needed]
## Glossary [optional]
<!-- If relevant, include terms and calculations in this section that can help readers understand the model or model card. -->
[More Information Needed]
## More Information [optional]
[More Information Needed]
## Model Card Authors [optional]
[More Information Needed]
## Model Card Contact
[More Information Needed] | {"library_name": "transformers", "tags": []} | quickstep3621/9dgq20g | null | [
"transformers",
"safetensors",
"stablelm",
"text-generation",
"conversational",
"arxiv:1910.09700",
"autotrain_compatible",
"endpoints_compatible",
"region:us"
] | null | 2024-04-27T06:39:58+00:00 | [
"1910.09700"
] | [] | TAGS
#transformers #safetensors #stablelm #text-generation #conversational #arxiv-1910.09700 #autotrain_compatible #endpoints_compatible #region-us
|
# Model Card for Model ID
## Model Details
### Model Description
This is the model card of a transformers model that has been pushed on the Hub. This model card has been automatically generated.
- Developed by:
- Funded by [optional]:
- Shared by [optional]:
- Model type:
- Language(s) (NLP):
- License:
- Finetuned from model [optional]:
### Model Sources [optional]
- Repository:
- Paper [optional]:
- Demo [optional]:
## Uses
### Direct Use
### Downstream Use [optional]
### Out-of-Scope Use
## Bias, Risks, and Limitations
### Recommendations
Users (both direct and downstream) should be made aware of the risks, biases and limitations of the model. More information needed for further recommendations.
## How to Get Started with the Model
Use the code below to get started with the model.
## Training Details
### Training Data
### Training Procedure
#### Preprocessing [optional]
#### Training Hyperparameters
- Training regime:
#### Speeds, Sizes, Times [optional]
## Evaluation
### Testing Data, Factors & Metrics
#### Testing Data
#### Factors
#### Metrics
### Results
#### Summary
## Model Examination [optional]
## Environmental Impact
Carbon emissions can be estimated using the Machine Learning Impact calculator presented in Lacoste et al. (2019).
- Hardware Type:
- Hours used:
- Cloud Provider:
- Compute Region:
- Carbon Emitted:
## Technical Specifications [optional]
### Model Architecture and Objective
### Compute Infrastructure
#### Hardware
#### Software
[optional]
BibTeX:
APA:
## Glossary [optional]
## More Information [optional]
## Model Card Authors [optional]
## Model Card Contact
| [
"# Model Card for Model ID",
"## Model Details",
"### Model Description\n\n\n\nThis is the model card of a transformers model that has been pushed on the Hub. This model card has been automatically generated.\n\n- Developed by: \n- Funded by [optional]: \n- Shared by [optional]: \n- Model type: \n- Language(s) (NLP): \n- License: \n- Finetuned from model [optional]:",
"### Model Sources [optional]\n\n\n\n- Repository: \n- Paper [optional]: \n- Demo [optional]:",
"## Uses",
"### Direct Use",
"### Downstream Use [optional]",
"### Out-of-Scope Use",
"## Bias, Risks, and Limitations",
"### Recommendations\n\n\n\nUsers (both direct and downstream) should be made aware of the risks, biases and limitations of the model. More information needed for further recommendations.",
"## How to Get Started with the Model\n\nUse the code below to get started with the model.",
"## Training Details",
"### Training Data",
"### Training Procedure",
"#### Preprocessing [optional]",
"#### Training Hyperparameters\n\n- Training regime:",
"#### Speeds, Sizes, Times [optional]",
"## Evaluation",
"### Testing Data, Factors & Metrics",
"#### Testing Data",
"#### Factors",
"#### Metrics",
"### Results",
"#### Summary",
"## Model Examination [optional]",
"## Environmental Impact\n\n\n\nCarbon emissions can be estimated using the Machine Learning Impact calculator presented in Lacoste et al. (2019).\n\n- Hardware Type: \n- Hours used: \n- Cloud Provider: \n- Compute Region: \n- Carbon Emitted:",
"## Technical Specifications [optional]",
"### Model Architecture and Objective",
"### Compute Infrastructure",
"#### Hardware",
"#### Software\n\n\n\n[optional]\n\n\n\nBibTeX:\n\n\n\nAPA:",
"## Glossary [optional]",
"## More Information [optional]",
"## Model Card Authors [optional]",
"## Model Card Contact"
] | [
"TAGS\n#transformers #safetensors #stablelm #text-generation #conversational #arxiv-1910.09700 #autotrain_compatible #endpoints_compatible #region-us \n",
"# Model Card for Model ID",
"## Model Details",
"### Model Description\n\n\n\nThis is the model card of a transformers model that has been pushed on the Hub. This model card has been automatically generated.\n\n- Developed by: \n- Funded by [optional]: \n- Shared by [optional]: \n- Model type: \n- Language(s) (NLP): \n- License: \n- Finetuned from model [optional]:",
"### Model Sources [optional]\n\n\n\n- Repository: \n- Paper [optional]: \n- Demo [optional]:",
"## Uses",
"### Direct Use",
"### Downstream Use [optional]",
"### Out-of-Scope Use",
"## Bias, Risks, and Limitations",
"### Recommendations\n\n\n\nUsers (both direct and downstream) should be made aware of the risks, biases and limitations of the model. More information needed for further recommendations.",
"## How to Get Started with the Model\n\nUse the code below to get started with the model.",
"## Training Details",
"### Training Data",
"### Training Procedure",
"#### Preprocessing [optional]",
"#### Training Hyperparameters\n\n- Training regime:",
"#### Speeds, Sizes, Times [optional]",
"## Evaluation",
"### Testing Data, Factors & Metrics",
"#### Testing Data",
"#### Factors",
"#### Metrics",
"### Results",
"#### Summary",
"## Model Examination [optional]",
"## Environmental Impact\n\n\n\nCarbon emissions can be estimated using the Machine Learning Impact calculator presented in Lacoste et al. (2019).\n\n- Hardware Type: \n- Hours used: \n- Cloud Provider: \n- Compute Region: \n- Carbon Emitted:",
"## Technical Specifications [optional]",
"### Model Architecture and Objective",
"### Compute Infrastructure",
"#### Hardware",
"#### Software\n\n\n\n[optional]\n\n\n\nBibTeX:\n\n\n\nAPA:",
"## Glossary [optional]",
"## More Information [optional]",
"## Model Card Authors [optional]",
"## Model Card Contact"
] |
text-generation | transformers |
<!-- This model card has been generated automatically according to the information the Trainer had access to. You
should probably proofread and complete it, then remove this comment. -->
# eli5_dir
This model is a fine-tuned version of [gpt2](https://huggingface.co/gpt2) on the eli5_category dataset.
It achieves the following results on the evaluation set:
- Loss: 3.5847
## Model description
More information needed
## Intended uses & limitations
More information needed
## Training and evaluation data
More information needed
## Training procedure
### Training hyperparameters
The following hyperparameters were used during training:
- learning_rate: 2e-05
- train_batch_size: 8
- eval_batch_size: 8
- seed: 42
- optimizer: Adam with betas=(0.9,0.999) and epsilon=1e-08
- lr_scheduler_type: linear
- num_epochs: 3.0
### Training results
| Training Loss | Epoch | Step | Validation Loss |
|:-------------:|:-----:|:----:|:---------------:|
| 3.6947 | 1.0 | 1308 | 3.5892 |
| 3.5793 | 2.0 | 2616 | 3.5833 |
| 3.5287 | 3.0 | 3924 | 3.5847 |
### Framework versions
- Transformers 4.40.0
- Pytorch 2.2.1+cu121
- Datasets 2.19.0
- Tokenizers 0.19.1
| {"license": "mit", "tags": ["generated_from_trainer"], "datasets": ["eli5_category"], "base_model": "gpt2", "model-index": [{"name": "eli5_dir", "results": []}]} | BohanJiang0128/eli5_dir | null | [
"transformers",
"tensorboard",
"safetensors",
"gpt2",
"text-generation",
"generated_from_trainer",
"dataset:eli5_category",
"base_model:gpt2",
"license:mit",
"autotrain_compatible",
"endpoints_compatible",
"text-generation-inference",
"region:us"
] | null | 2024-04-27T06:41:48+00:00 | [] | [] | TAGS
#transformers #tensorboard #safetensors #gpt2 #text-generation #generated_from_trainer #dataset-eli5_category #base_model-gpt2 #license-mit #autotrain_compatible #endpoints_compatible #text-generation-inference #region-us
| eli5\_dir
=========
This model is a fine-tuned version of gpt2 on the eli5\_category dataset.
It achieves the following results on the evaluation set:
* Loss: 3.5847
Model description
-----------------
More information needed
Intended uses & limitations
---------------------------
More information needed
Training and evaluation data
----------------------------
More information needed
Training procedure
------------------
### Training hyperparameters
The following hyperparameters were used during training:
* learning\_rate: 2e-05
* train\_batch\_size: 8
* eval\_batch\_size: 8
* seed: 42
* optimizer: Adam with betas=(0.9,0.999) and epsilon=1e-08
* lr\_scheduler\_type: linear
* num\_epochs: 3.0
### Training results
### Framework versions
* Transformers 4.40.0
* Pytorch 2.2.1+cu121
* Datasets 2.19.0
* Tokenizers 0.19.1
| [
"### Training hyperparameters\n\n\nThe following hyperparameters were used during training:\n\n\n* learning\\_rate: 2e-05\n* train\\_batch\\_size: 8\n* eval\\_batch\\_size: 8\n* seed: 42\n* optimizer: Adam with betas=(0.9,0.999) and epsilon=1e-08\n* lr\\_scheduler\\_type: linear\n* num\\_epochs: 3.0",
"### Training results",
"### Framework versions\n\n\n* Transformers 4.40.0\n* Pytorch 2.2.1+cu121\n* Datasets 2.19.0\n* Tokenizers 0.19.1"
] | [
"TAGS\n#transformers #tensorboard #safetensors #gpt2 #text-generation #generated_from_trainer #dataset-eli5_category #base_model-gpt2 #license-mit #autotrain_compatible #endpoints_compatible #text-generation-inference #region-us \n",
"### Training hyperparameters\n\n\nThe following hyperparameters were used during training:\n\n\n* learning\\_rate: 2e-05\n* train\\_batch\\_size: 8\n* eval\\_batch\\_size: 8\n* seed: 42\n* optimizer: Adam with betas=(0.9,0.999) and epsilon=1e-08\n* lr\\_scheduler\\_type: linear\n* num\\_epochs: 3.0",
"### Training results",
"### Framework versions\n\n\n* Transformers 4.40.0\n* Pytorch 2.2.1+cu121\n* Datasets 2.19.0\n* Tokenizers 0.19.1"
] |
text-generation | transformers |
# Model Card for Model ID
<!-- Provide a quick summary of what the model is/does. -->
## Model Details
### Model Description
<!-- Provide a longer summary of what this model is. -->
This is the model card of a 🤗 transformers model that has been pushed on the Hub. This model card has been automatically generated.
- **Developed by:** [More Information Needed]
- **Funded by [optional]:** [More Information Needed]
- **Shared by [optional]:** [More Information Needed]
- **Model type:** [More Information Needed]
- **Language(s) (NLP):** [More Information Needed]
- **License:** [More Information Needed]
- **Finetuned from model [optional]:** [More Information Needed]
### Model Sources [optional]
<!-- Provide the basic links for the model. -->
- **Repository:** [More Information Needed]
- **Paper [optional]:** [More Information Needed]
- **Demo [optional]:** [More Information Needed]
## Uses
<!-- Address questions around how the model is intended to be used, including the foreseeable users of the model and those affected by the model. -->
### Direct Use
<!-- This section is for the model use without fine-tuning or plugging into a larger ecosystem/app. -->
[More Information Needed]
### Downstream Use [optional]
<!-- This section is for the model use when fine-tuned for a task, or when plugged into a larger ecosystem/app -->
[More Information Needed]
### Out-of-Scope Use
<!-- This section addresses misuse, malicious use, and uses that the model will not work well for. -->
[More Information Needed]
## Bias, Risks, and Limitations
<!-- This section is meant to convey both technical and sociotechnical limitations. -->
[More Information Needed]
### Recommendations
<!-- This section is meant to convey recommendations with respect to the bias, risk, and technical limitations. -->
Users (both direct and downstream) should be made aware of the risks, biases and limitations of the model. More information needed for further recommendations.
## How to Get Started with the Model
Use the code below to get started with the model.
[More Information Needed]
## Training Details
### Training Data
<!-- This should link to a Dataset Card, perhaps with a short stub of information on what the training data is all about as well as documentation related to data pre-processing or additional filtering. -->
[More Information Needed]
### Training Procedure
<!-- This relates heavily to the Technical Specifications. Content here should link to that section when it is relevant to the training procedure. -->
#### Preprocessing [optional]
[More Information Needed]
#### Training Hyperparameters
- **Training regime:** [More Information Needed] <!--fp32, fp16 mixed precision, bf16 mixed precision, bf16 non-mixed precision, fp16 non-mixed precision, fp8 mixed precision -->
#### Speeds, Sizes, Times [optional]
<!-- This section provides information about throughput, start/end time, checkpoint size if relevant, etc. -->
[More Information Needed]
## Evaluation
<!-- This section describes the evaluation protocols and provides the results. -->
### Testing Data, Factors & Metrics
#### Testing Data
<!-- This should link to a Dataset Card if possible. -->
[More Information Needed]
#### Factors
<!-- These are the things the evaluation is disaggregating by, e.g., subpopulations or domains. -->
[More Information Needed]
#### Metrics
<!-- These are the evaluation metrics being used, ideally with a description of why. -->
[More Information Needed]
### Results
[More Information Needed]
#### Summary
## Model Examination [optional]
<!-- Relevant interpretability work for the model goes here -->
[More Information Needed]
## Environmental Impact
<!-- Total emissions (in grams of CO2eq) and additional considerations, such as electricity usage, go here. Edit the suggested text below accordingly -->
Carbon emissions can be estimated using the [Machine Learning Impact calculator](https://mlco2.github.io/impact#compute) presented in [Lacoste et al. (2019)](https://arxiv.org/abs/1910.09700).
- **Hardware Type:** [More Information Needed]
- **Hours used:** [More Information Needed]
- **Cloud Provider:** [More Information Needed]
- **Compute Region:** [More Information Needed]
- **Carbon Emitted:** [More Information Needed]
## Technical Specifications [optional]
### Model Architecture and Objective
[More Information Needed]
### Compute Infrastructure
[More Information Needed]
#### Hardware
[More Information Needed]
#### Software
[More Information Needed]
## Citation [optional]
<!-- If there is a paper or blog post introducing the model, the APA and Bibtex information for that should go in this section. -->
**BibTeX:**
[More Information Needed]
**APA:**
[More Information Needed]
## Glossary [optional]
<!-- If relevant, include terms and calculations in this section that can help readers understand the model or model card. -->
[More Information Needed]
## More Information [optional]
[More Information Needed]
## Model Card Authors [optional]
[More Information Needed]
## Model Card Contact
[More Information Needed]
| {"library_name": "transformers", "tags": []} | swj0419/bbc_retrain_new_STEP0000200 | null | [
"transformers",
"safetensors",
"llama",
"text-generation",
"arxiv:1910.09700",
"autotrain_compatible",
"endpoints_compatible",
"text-generation-inference",
"region:us"
] | null | 2024-04-27T06:42:32+00:00 | [
"1910.09700"
] | [] | TAGS
#transformers #safetensors #llama #text-generation #arxiv-1910.09700 #autotrain_compatible #endpoints_compatible #text-generation-inference #region-us
|
# Model Card for Model ID
## Model Details
### Model Description
This is the model card of a transformers model that has been pushed on the Hub. This model card has been automatically generated.
- Developed by:
- Funded by [optional]:
- Shared by [optional]:
- Model type:
- Language(s) (NLP):
- License:
- Finetuned from model [optional]:
### Model Sources [optional]
- Repository:
- Paper [optional]:
- Demo [optional]:
## Uses
### Direct Use
### Downstream Use [optional]
### Out-of-Scope Use
## Bias, Risks, and Limitations
### Recommendations
Users (both direct and downstream) should be made aware of the risks, biases and limitations of the model. More information needed for further recommendations.
## How to Get Started with the Model
Use the code below to get started with the model.
## Training Details
### Training Data
### Training Procedure
#### Preprocessing [optional]
#### Training Hyperparameters
- Training regime:
#### Speeds, Sizes, Times [optional]
## Evaluation
### Testing Data, Factors & Metrics
#### Testing Data
#### Factors
#### Metrics
### Results
#### Summary
## Model Examination [optional]
## Environmental Impact
Carbon emissions can be estimated using the Machine Learning Impact calculator presented in Lacoste et al. (2019).
- Hardware Type:
- Hours used:
- Cloud Provider:
- Compute Region:
- Carbon Emitted:
## Technical Specifications [optional]
### Model Architecture and Objective
### Compute Infrastructure
#### Hardware
#### Software
[optional]
BibTeX:
APA:
## Glossary [optional]
## More Information [optional]
## Model Card Authors [optional]
## Model Card Contact
| [
"# Model Card for Model ID",
"## Model Details",
"### Model Description\n\n\n\nThis is the model card of a transformers model that has been pushed on the Hub. This model card has been automatically generated.\n\n- Developed by: \n- Funded by [optional]: \n- Shared by [optional]: \n- Model type: \n- Language(s) (NLP): \n- License: \n- Finetuned from model [optional]:",
"### Model Sources [optional]\n\n\n\n- Repository: \n- Paper [optional]: \n- Demo [optional]:",
"## Uses",
"### Direct Use",
"### Downstream Use [optional]",
"### Out-of-Scope Use",
"## Bias, Risks, and Limitations",
"### Recommendations\n\n\n\nUsers (both direct and downstream) should be made aware of the risks, biases and limitations of the model. More information needed for further recommendations.",
"## How to Get Started with the Model\n\nUse the code below to get started with the model.",
"## Training Details",
"### Training Data",
"### Training Procedure",
"#### Preprocessing [optional]",
"#### Training Hyperparameters\n\n- Training regime:",
"#### Speeds, Sizes, Times [optional]",
"## Evaluation",
"### Testing Data, Factors & Metrics",
"#### Testing Data",
"#### Factors",
"#### Metrics",
"### Results",
"#### Summary",
"## Model Examination [optional]",
"## Environmental Impact\n\n\n\nCarbon emissions can be estimated using the Machine Learning Impact calculator presented in Lacoste et al. (2019).\n\n- Hardware Type: \n- Hours used: \n- Cloud Provider: \n- Compute Region: \n- Carbon Emitted:",
"## Technical Specifications [optional]",
"### Model Architecture and Objective",
"### Compute Infrastructure",
"#### Hardware",
"#### Software\n\n\n\n[optional]\n\n\n\nBibTeX:\n\n\n\nAPA:",
"## Glossary [optional]",
"## More Information [optional]",
"## Model Card Authors [optional]",
"## Model Card Contact"
] | [
"TAGS\n#transformers #safetensors #llama #text-generation #arxiv-1910.09700 #autotrain_compatible #endpoints_compatible #text-generation-inference #region-us \n",
"# Model Card for Model ID",
"## Model Details",
"### Model Description\n\n\n\nThis is the model card of a transformers model that has been pushed on the Hub. This model card has been automatically generated.\n\n- Developed by: \n- Funded by [optional]: \n- Shared by [optional]: \n- Model type: \n- Language(s) (NLP): \n- License: \n- Finetuned from model [optional]:",
"### Model Sources [optional]\n\n\n\n- Repository: \n- Paper [optional]: \n- Demo [optional]:",
"## Uses",
"### Direct Use",
"### Downstream Use [optional]",
"### Out-of-Scope Use",
"## Bias, Risks, and Limitations",
"### Recommendations\n\n\n\nUsers (both direct and downstream) should be made aware of the risks, biases and limitations of the model. More information needed for further recommendations.",
"## How to Get Started with the Model\n\nUse the code below to get started with the model.",
"## Training Details",
"### Training Data",
"### Training Procedure",
"#### Preprocessing [optional]",
"#### Training Hyperparameters\n\n- Training regime:",
"#### Speeds, Sizes, Times [optional]",
"## Evaluation",
"### Testing Data, Factors & Metrics",
"#### Testing Data",
"#### Factors",
"#### Metrics",
"### Results",
"#### Summary",
"## Model Examination [optional]",
"## Environmental Impact\n\n\n\nCarbon emissions can be estimated using the Machine Learning Impact calculator presented in Lacoste et al. (2019).\n\n- Hardware Type: \n- Hours used: \n- Cloud Provider: \n- Compute Region: \n- Carbon Emitted:",
"## Technical Specifications [optional]",
"### Model Architecture and Objective",
"### Compute Infrastructure",
"#### Hardware",
"#### Software\n\n\n\n[optional]\n\n\n\nBibTeX:\n\n\n\nAPA:",
"## Glossary [optional]",
"## More Information [optional]",
"## Model Card Authors [optional]",
"## Model Card Contact"
] |
text2text-generation | transformers |
# Model Card for Model ID
<!-- Provide a quick summary of what the model is/does. -->
## Model Details
### Model Description
<!-- Provide a longer summary of what this model is. -->
This is the model card of a 🤗 transformers model that has been pushed on the Hub. This model card has been automatically generated.
- **Developed by:** [More Information Needed]
- **Funded by [optional]:** [More Information Needed]
- **Shared by [optional]:** [More Information Needed]
- **Model type:** [More Information Needed]
- **Language(s) (NLP):** [More Information Needed]
- **License:** [More Information Needed]
- **Finetuned from model [optional]:** [More Information Needed]
### Model Sources [optional]
<!-- Provide the basic links for the model. -->
- **Repository:** [More Information Needed]
- **Paper [optional]:** [More Information Needed]
- **Demo [optional]:** [More Information Needed]
## Uses
<!-- Address questions around how the model is intended to be used, including the foreseeable users of the model and those affected by the model. -->
### Direct Use
<!-- This section is for the model use without fine-tuning or plugging into a larger ecosystem/app. -->
[More Information Needed]
### Downstream Use [optional]
<!-- This section is for the model use when fine-tuned for a task, or when plugged into a larger ecosystem/app -->
[More Information Needed]
### Out-of-Scope Use
<!-- This section addresses misuse, malicious use, and uses that the model will not work well for. -->
[More Information Needed]
## Bias, Risks, and Limitations
<!-- This section is meant to convey both technical and sociotechnical limitations. -->
[More Information Needed]
### Recommendations
<!-- This section is meant to convey recommendations with respect to the bias, risk, and technical limitations. -->
Users (both direct and downstream) should be made aware of the risks, biases and limitations of the model. More information needed for further recommendations.
## How to Get Started with the Model
Use the code below to get started with the model.
[More Information Needed]
## Training Details
### Training Data
<!-- This should link to a Dataset Card, perhaps with a short stub of information on what the training data is all about as well as documentation related to data pre-processing or additional filtering. -->
[More Information Needed]
### Training Procedure
<!-- This relates heavily to the Technical Specifications. Content here should link to that section when it is relevant to the training procedure. -->
#### Preprocessing [optional]
[More Information Needed]
#### Training Hyperparameters
- **Training regime:** [More Information Needed] <!--fp32, fp16 mixed precision, bf16 mixed precision, bf16 non-mixed precision, fp16 non-mixed precision, fp8 mixed precision -->
#### Speeds, Sizes, Times [optional]
<!-- This section provides information about throughput, start/end time, checkpoint size if relevant, etc. -->
[More Information Needed]
## Evaluation
<!-- This section describes the evaluation protocols and provides the results. -->
### Testing Data, Factors & Metrics
#### Testing Data
<!-- This should link to a Dataset Card if possible. -->
[More Information Needed]
#### Factors
<!-- These are the things the evaluation is disaggregating by, e.g., subpopulations or domains. -->
[More Information Needed]
#### Metrics
<!-- These are the evaluation metrics being used, ideally with a description of why. -->
[More Information Needed]
### Results
[More Information Needed]
#### Summary
## Model Examination [optional]
<!-- Relevant interpretability work for the model goes here -->
[More Information Needed]
## Environmental Impact
<!-- Total emissions (in grams of CO2eq) and additional considerations, such as electricity usage, go here. Edit the suggested text below accordingly -->
Carbon emissions can be estimated using the [Machine Learning Impact calculator](https://mlco2.github.io/impact#compute) presented in [Lacoste et al. (2019)](https://arxiv.org/abs/1910.09700).
- **Hardware Type:** [More Information Needed]
- **Hours used:** [More Information Needed]
- **Cloud Provider:** [More Information Needed]
- **Compute Region:** [More Information Needed]
- **Carbon Emitted:** [More Information Needed]
## Technical Specifications [optional]
### Model Architecture and Objective
[More Information Needed]
### Compute Infrastructure
[More Information Needed]
#### Hardware
[More Information Needed]
#### Software
[More Information Needed]
## Citation [optional]
<!-- If there is a paper or blog post introducing the model, the APA and Bibtex information for that should go in this section. -->
**BibTeX:**
[More Information Needed]
**APA:**
[More Information Needed]
## Glossary [optional]
<!-- If relevant, include terms and calculations in this section that can help readers understand the model or model card. -->
[More Information Needed]
## More Information [optional]
[More Information Needed]
## Model Card Authors [optional]
[More Information Needed]
## Model Card Contact
[More Information Needed] | {"library_name": "transformers", "tags": []} | Audino/my-awesome-modelv4-large | null | [
"transformers",
"safetensors",
"t5",
"text2text-generation",
"arxiv:1910.09700",
"autotrain_compatible",
"endpoints_compatible",
"text-generation-inference",
"region:us"
] | null | 2024-04-27T06:43:40+00:00 | [
"1910.09700"
] | [] | TAGS
#transformers #safetensors #t5 #text2text-generation #arxiv-1910.09700 #autotrain_compatible #endpoints_compatible #text-generation-inference #region-us
|
# Model Card for Model ID
## Model Details
### Model Description
This is the model card of a transformers model that has been pushed on the Hub. This model card has been automatically generated.
- Developed by:
- Funded by [optional]:
- Shared by [optional]:
- Model type:
- Language(s) (NLP):
- License:
- Finetuned from model [optional]:
### Model Sources [optional]
- Repository:
- Paper [optional]:
- Demo [optional]:
## Uses
### Direct Use
### Downstream Use [optional]
### Out-of-Scope Use
## Bias, Risks, and Limitations
### Recommendations
Users (both direct and downstream) should be made aware of the risks, biases and limitations of the model. More information needed for further recommendations.
## How to Get Started with the Model
Use the code below to get started with the model.
## Training Details
### Training Data
### Training Procedure
#### Preprocessing [optional]
#### Training Hyperparameters
- Training regime:
#### Speeds, Sizes, Times [optional]
## Evaluation
### Testing Data, Factors & Metrics
#### Testing Data
#### Factors
#### Metrics
### Results
#### Summary
## Model Examination [optional]
## Environmental Impact
Carbon emissions can be estimated using the Machine Learning Impact calculator presented in Lacoste et al. (2019).
- Hardware Type:
- Hours used:
- Cloud Provider:
- Compute Region:
- Carbon Emitted:
## Technical Specifications [optional]
### Model Architecture and Objective
### Compute Infrastructure
#### Hardware
#### Software
[optional]
BibTeX:
APA:
## Glossary [optional]
## More Information [optional]
## Model Card Authors [optional]
## Model Card Contact
| [
"# Model Card for Model ID",
"## Model Details",
"### Model Description\n\n\n\nThis is the model card of a transformers model that has been pushed on the Hub. This model card has been automatically generated.\n\n- Developed by: \n- Funded by [optional]: \n- Shared by [optional]: \n- Model type: \n- Language(s) (NLP): \n- License: \n- Finetuned from model [optional]:",
"### Model Sources [optional]\n\n\n\n- Repository: \n- Paper [optional]: \n- Demo [optional]:",
"## Uses",
"### Direct Use",
"### Downstream Use [optional]",
"### Out-of-Scope Use",
"## Bias, Risks, and Limitations",
"### Recommendations\n\n\n\nUsers (both direct and downstream) should be made aware of the risks, biases and limitations of the model. More information needed for further recommendations.",
"## How to Get Started with the Model\n\nUse the code below to get started with the model.",
"## Training Details",
"### Training Data",
"### Training Procedure",
"#### Preprocessing [optional]",
"#### Training Hyperparameters\n\n- Training regime:",
"#### Speeds, Sizes, Times [optional]",
"## Evaluation",
"### Testing Data, Factors & Metrics",
"#### Testing Data",
"#### Factors",
"#### Metrics",
"### Results",
"#### Summary",
"## Model Examination [optional]",
"## Environmental Impact\n\n\n\nCarbon emissions can be estimated using the Machine Learning Impact calculator presented in Lacoste et al. (2019).\n\n- Hardware Type: \n- Hours used: \n- Cloud Provider: \n- Compute Region: \n- Carbon Emitted:",
"## Technical Specifications [optional]",
"### Model Architecture and Objective",
"### Compute Infrastructure",
"#### Hardware",
"#### Software\n\n\n\n[optional]\n\n\n\nBibTeX:\n\n\n\nAPA:",
"## Glossary [optional]",
"## More Information [optional]",
"## Model Card Authors [optional]",
"## Model Card Contact"
] | [
"TAGS\n#transformers #safetensors #t5 #text2text-generation #arxiv-1910.09700 #autotrain_compatible #endpoints_compatible #text-generation-inference #region-us \n",
"# Model Card for Model ID",
"## Model Details",
"### Model Description\n\n\n\nThis is the model card of a transformers model that has been pushed on the Hub. This model card has been automatically generated.\n\n- Developed by: \n- Funded by [optional]: \n- Shared by [optional]: \n- Model type: \n- Language(s) (NLP): \n- License: \n- Finetuned from model [optional]:",
"### Model Sources [optional]\n\n\n\n- Repository: \n- Paper [optional]: \n- Demo [optional]:",
"## Uses",
"### Direct Use",
"### Downstream Use [optional]",
"### Out-of-Scope Use",
"## Bias, Risks, and Limitations",
"### Recommendations\n\n\n\nUsers (both direct and downstream) should be made aware of the risks, biases and limitations of the model. More information needed for further recommendations.",
"## How to Get Started with the Model\n\nUse the code below to get started with the model.",
"## Training Details",
"### Training Data",
"### Training Procedure",
"#### Preprocessing [optional]",
"#### Training Hyperparameters\n\n- Training regime:",
"#### Speeds, Sizes, Times [optional]",
"## Evaluation",
"### Testing Data, Factors & Metrics",
"#### Testing Data",
"#### Factors",
"#### Metrics",
"### Results",
"#### Summary",
"## Model Examination [optional]",
"## Environmental Impact\n\n\n\nCarbon emissions can be estimated using the Machine Learning Impact calculator presented in Lacoste et al. (2019).\n\n- Hardware Type: \n- Hours used: \n- Cloud Provider: \n- Compute Region: \n- Carbon Emitted:",
"## Technical Specifications [optional]",
"### Model Architecture and Objective",
"### Compute Infrastructure",
"#### Hardware",
"#### Software\n\n\n\n[optional]\n\n\n\nBibTeX:\n\n\n\nAPA:",
"## Glossary [optional]",
"## More Information [optional]",
"## Model Card Authors [optional]",
"## Model Card Contact"
] |
null | transformers |
# Model Card for Model ID
<!-- Provide a quick summary of what the model is/does. -->
## Model Details
### Model Description
<!-- Provide a longer summary of what this model is. -->
This is the model card of a 🤗 transformers model that has been pushed on the Hub. This model card has been automatically generated.
- **Developed by:** [More Information Needed]
- **Funded by [optional]:** [More Information Needed]
- **Shared by [optional]:** [More Information Needed]
- **Model type:** [More Information Needed]
- **Language(s) (NLP):** [More Information Needed]
- **License:** [More Information Needed]
- **Finetuned from model [optional]:** [More Information Needed]
### Model Sources [optional]
<!-- Provide the basic links for the model. -->
- **Repository:** [More Information Needed]
- **Paper [optional]:** [More Information Needed]
- **Demo [optional]:** [More Information Needed]
## Uses
<!-- Address questions around how the model is intended to be used, including the foreseeable users of the model and those affected by the model. -->
### Direct Use
<!-- This section is for the model use without fine-tuning or plugging into a larger ecosystem/app. -->
[More Information Needed]
### Downstream Use [optional]
<!-- This section is for the model use when fine-tuned for a task, or when plugged into a larger ecosystem/app -->
[More Information Needed]
### Out-of-Scope Use
<!-- This section addresses misuse, malicious use, and uses that the model will not work well for. -->
[More Information Needed]
## Bias, Risks, and Limitations
<!-- This section is meant to convey both technical and sociotechnical limitations. -->
[More Information Needed]
### Recommendations
<!-- This section is meant to convey recommendations with respect to the bias, risk, and technical limitations. -->
Users (both direct and downstream) should be made aware of the risks, biases and limitations of the model. More information needed for further recommendations.
## How to Get Started with the Model
Use the code below to get started with the model.
[More Information Needed]
## Training Details
### Training Data
<!-- This should link to a Dataset Card, perhaps with a short stub of information on what the training data is all about as well as documentation related to data pre-processing or additional filtering. -->
[More Information Needed]
### Training Procedure
<!-- This relates heavily to the Technical Specifications. Content here should link to that section when it is relevant to the training procedure. -->
#### Preprocessing [optional]
[More Information Needed]
#### Training Hyperparameters
- **Training regime:** [More Information Needed] <!--fp32, fp16 mixed precision, bf16 mixed precision, bf16 non-mixed precision, fp16 non-mixed precision, fp8 mixed precision -->
#### Speeds, Sizes, Times [optional]
<!-- This section provides information about throughput, start/end time, checkpoint size if relevant, etc. -->
[More Information Needed]
## Evaluation
<!-- This section describes the evaluation protocols and provides the results. -->
### Testing Data, Factors & Metrics
#### Testing Data
<!-- This should link to a Dataset Card if possible. -->
[More Information Needed]
#### Factors
<!-- These are the things the evaluation is disaggregating by, e.g., subpopulations or domains. -->
[More Information Needed]
#### Metrics
<!-- These are the evaluation metrics being used, ideally with a description of why. -->
[More Information Needed]
### Results
[More Information Needed]
#### Summary
## Model Examination [optional]
<!-- Relevant interpretability work for the model goes here -->
[More Information Needed]
## Environmental Impact
<!-- Total emissions (in grams of CO2eq) and additional considerations, such as electricity usage, go here. Edit the suggested text below accordingly -->
Carbon emissions can be estimated using the [Machine Learning Impact calculator](https://mlco2.github.io/impact#compute) presented in [Lacoste et al. (2019)](https://arxiv.org/abs/1910.09700).
- **Hardware Type:** [More Information Needed]
- **Hours used:** [More Information Needed]
- **Cloud Provider:** [More Information Needed]
- **Compute Region:** [More Information Needed]
- **Carbon Emitted:** [More Information Needed]
## Technical Specifications [optional]
### Model Architecture and Objective
[More Information Needed]
### Compute Infrastructure
[More Information Needed]
#### Hardware
[More Information Needed]
#### Software
[More Information Needed]
## Citation [optional]
<!-- If there is a paper or blog post introducing the model, the APA and Bibtex information for that should go in this section. -->
**BibTeX:**
[More Information Needed]
**APA:**
[More Information Needed]
## Glossary [optional]
<!-- If relevant, include terms and calculations in this section that can help readers understand the model or model card. -->
[More Information Needed]
## More Information [optional]
[More Information Needed]
## Model Card Authors [optional]
[More Information Needed]
## Model Card Contact
[More Information Needed] | {"library_name": "transformers", "tags": []} | uh1216/science-textbook-Llama3-8b-Instruct-10epoch | null | [
"transformers",
"safetensors",
"arxiv:1910.09700",
"endpoints_compatible",
"region:us"
] | null | 2024-04-27T06:43:49+00:00 | [
"1910.09700"
] | [] | TAGS
#transformers #safetensors #arxiv-1910.09700 #endpoints_compatible #region-us
|
# Model Card for Model ID
## Model Details
### Model Description
This is the model card of a transformers model that has been pushed on the Hub. This model card has been automatically generated.
- Developed by:
- Funded by [optional]:
- Shared by [optional]:
- Model type:
- Language(s) (NLP):
- License:
- Finetuned from model [optional]:
### Model Sources [optional]
- Repository:
- Paper [optional]:
- Demo [optional]:
## Uses
### Direct Use
### Downstream Use [optional]
### Out-of-Scope Use
## Bias, Risks, and Limitations
### Recommendations
Users (both direct and downstream) should be made aware of the risks, biases and limitations of the model. More information needed for further recommendations.
## How to Get Started with the Model
Use the code below to get started with the model.
## Training Details
### Training Data
### Training Procedure
#### Preprocessing [optional]
#### Training Hyperparameters
- Training regime:
#### Speeds, Sizes, Times [optional]
## Evaluation
### Testing Data, Factors & Metrics
#### Testing Data
#### Factors
#### Metrics
### Results
#### Summary
## Model Examination [optional]
## Environmental Impact
Carbon emissions can be estimated using the Machine Learning Impact calculator presented in Lacoste et al. (2019).
- Hardware Type:
- Hours used:
- Cloud Provider:
- Compute Region:
- Carbon Emitted:
## Technical Specifications [optional]
### Model Architecture and Objective
### Compute Infrastructure
#### Hardware
#### Software
[optional]
BibTeX:
APA:
## Glossary [optional]
## More Information [optional]
## Model Card Authors [optional]
## Model Card Contact
| [
"# Model Card for Model ID",
"## Model Details",
"### Model Description\n\n\n\nThis is the model card of a transformers model that has been pushed on the Hub. This model card has been automatically generated.\n\n- Developed by: \n- Funded by [optional]: \n- Shared by [optional]: \n- Model type: \n- Language(s) (NLP): \n- License: \n- Finetuned from model [optional]:",
"### Model Sources [optional]\n\n\n\n- Repository: \n- Paper [optional]: \n- Demo [optional]:",
"## Uses",
"### Direct Use",
"### Downstream Use [optional]",
"### Out-of-Scope Use",
"## Bias, Risks, and Limitations",
"### Recommendations\n\n\n\nUsers (both direct and downstream) should be made aware of the risks, biases and limitations of the model. More information needed for further recommendations.",
"## How to Get Started with the Model\n\nUse the code below to get started with the model.",
"## Training Details",
"### Training Data",
"### Training Procedure",
"#### Preprocessing [optional]",
"#### Training Hyperparameters\n\n- Training regime:",
"#### Speeds, Sizes, Times [optional]",
"## Evaluation",
"### Testing Data, Factors & Metrics",
"#### Testing Data",
"#### Factors",
"#### Metrics",
"### Results",
"#### Summary",
"## Model Examination [optional]",
"## Environmental Impact\n\n\n\nCarbon emissions can be estimated using the Machine Learning Impact calculator presented in Lacoste et al. (2019).\n\n- Hardware Type: \n- Hours used: \n- Cloud Provider: \n- Compute Region: \n- Carbon Emitted:",
"## Technical Specifications [optional]",
"### Model Architecture and Objective",
"### Compute Infrastructure",
"#### Hardware",
"#### Software\n\n\n\n[optional]\n\n\n\nBibTeX:\n\n\n\nAPA:",
"## Glossary [optional]",
"## More Information [optional]",
"## Model Card Authors [optional]",
"## Model Card Contact"
] | [
"TAGS\n#transformers #safetensors #arxiv-1910.09700 #endpoints_compatible #region-us \n",
"# Model Card for Model ID",
"## Model Details",
"### Model Description\n\n\n\nThis is the model card of a transformers model that has been pushed on the Hub. This model card has been automatically generated.\n\n- Developed by: \n- Funded by [optional]: \n- Shared by [optional]: \n- Model type: \n- Language(s) (NLP): \n- License: \n- Finetuned from model [optional]:",
"### Model Sources [optional]\n\n\n\n- Repository: \n- Paper [optional]: \n- Demo [optional]:",
"## Uses",
"### Direct Use",
"### Downstream Use [optional]",
"### Out-of-Scope Use",
"## Bias, Risks, and Limitations",
"### Recommendations\n\n\n\nUsers (both direct and downstream) should be made aware of the risks, biases and limitations of the model. More information needed for further recommendations.",
"## How to Get Started with the Model\n\nUse the code below to get started with the model.",
"## Training Details",
"### Training Data",
"### Training Procedure",
"#### Preprocessing [optional]",
"#### Training Hyperparameters\n\n- Training regime:",
"#### Speeds, Sizes, Times [optional]",
"## Evaluation",
"### Testing Data, Factors & Metrics",
"#### Testing Data",
"#### Factors",
"#### Metrics",
"### Results",
"#### Summary",
"## Model Examination [optional]",
"## Environmental Impact\n\n\n\nCarbon emissions can be estimated using the Machine Learning Impact calculator presented in Lacoste et al. (2019).\n\n- Hardware Type: \n- Hours used: \n- Cloud Provider: \n- Compute Region: \n- Carbon Emitted:",
"## Technical Specifications [optional]",
"### Model Architecture and Objective",
"### Compute Infrastructure",
"#### Hardware",
"#### Software\n\n\n\n[optional]\n\n\n\nBibTeX:\n\n\n\nAPA:",
"## Glossary [optional]",
"## More Information [optional]",
"## Model Card Authors [optional]",
"## Model Card Contact"
] |
null | peft |
<!-- This model card has been generated automatically according to the information the Trainer had access to. You
should probably proofread and complete it, then remove this comment. -->
# GUE_prom_prom_core_all-seqsight_16384_512_22M-L1_f
This model is a fine-tuned version of [mahdibaghbanzadeh/seqsight_16384_512_22M](https://huggingface.co/mahdibaghbanzadeh/seqsight_16384_512_22M) on the [mahdibaghbanzadeh/GUE_prom_prom_core_all](https://huggingface.co/datasets/mahdibaghbanzadeh/GUE_prom_prom_core_all) dataset.
It achieves the following results on the evaluation set:
- Loss: 0.4266
- F1 Score: 0.8008
- Accuracy: 0.8008
## Model description
More information needed
## Intended uses & limitations
More information needed
## Training and evaluation data
More information needed
## Training procedure
### Training hyperparameters
The following hyperparameters were used during training:
- learning_rate: 0.0005
- train_batch_size: 128
- eval_batch_size: 128
- seed: 42
- optimizer: Adam with betas=(0.9,0.999) and epsilon=1e-08
- lr_scheduler_type: linear
- training_steps: 10000
### Training results
| Training Loss | Epoch | Step | Validation Loss | F1 Score | Accuracy |
|:-------------:|:-----:|:-----:|:---------------:|:--------:|:--------:|
| 0.6011 | 0.54 | 200 | 0.5326 | 0.7295 | 0.7306 |
| 0.532 | 1.08 | 400 | 0.4974 | 0.7648 | 0.7649 |
| 0.5019 | 1.62 | 600 | 0.4776 | 0.7767 | 0.7772 |
| 0.4809 | 2.16 | 800 | 0.4712 | 0.7804 | 0.7804 |
| 0.4772 | 2.7 | 1000 | 0.4616 | 0.7859 | 0.7860 |
| 0.469 | 3.24 | 1200 | 0.4596 | 0.7848 | 0.7848 |
| 0.4633 | 3.78 | 1400 | 0.4576 | 0.7879 | 0.7880 |
| 0.4536 | 4.32 | 1600 | 0.4634 | 0.7847 | 0.7850 |
| 0.4557 | 4.86 | 1800 | 0.4565 | 0.7900 | 0.7902 |
| 0.4529 | 5.41 | 2000 | 0.4567 | 0.7882 | 0.7883 |
| 0.4481 | 5.95 | 2200 | 0.4560 | 0.7887 | 0.7887 |
| 0.4489 | 6.49 | 2400 | 0.4533 | 0.7909 | 0.7910 |
| 0.4459 | 7.03 | 2600 | 0.4501 | 0.7948 | 0.7948 |
| 0.4464 | 7.57 | 2800 | 0.4559 | 0.7900 | 0.7902 |
| 0.4387 | 8.11 | 3000 | 0.4543 | 0.7881 | 0.7885 |
| 0.4407 | 8.65 | 3200 | 0.4469 | 0.7930 | 0.7931 |
| 0.4426 | 9.19 | 3400 | 0.4500 | 0.7914 | 0.7916 |
| 0.4389 | 9.73 | 3600 | 0.4554 | 0.7888 | 0.7895 |
| 0.4423 | 10.27 | 3800 | 0.4492 | 0.7901 | 0.7904 |
| 0.4386 | 10.81 | 4000 | 0.4468 | 0.7958 | 0.7958 |
| 0.4383 | 11.35 | 4200 | 0.4490 | 0.7906 | 0.7909 |
| 0.4352 | 11.89 | 4400 | 0.4487 | 0.7908 | 0.7912 |
| 0.4361 | 12.43 | 4600 | 0.4434 | 0.7952 | 0.7953 |
| 0.4325 | 12.97 | 4800 | 0.4480 | 0.7898 | 0.7904 |
| 0.4349 | 13.51 | 5000 | 0.4555 | 0.7857 | 0.7870 |
| 0.4338 | 14.05 | 5200 | 0.4417 | 0.7952 | 0.7953 |
| 0.4314 | 14.59 | 5400 | 0.4436 | 0.7956 | 0.7956 |
| 0.4315 | 15.14 | 5600 | 0.4405 | 0.7986 | 0.7986 |
| 0.4361 | 15.68 | 5800 | 0.4447 | 0.7916 | 0.7919 |
| 0.4261 | 16.22 | 6000 | 0.4475 | 0.7922 | 0.7927 |
| 0.4335 | 16.76 | 6200 | 0.4419 | 0.7915 | 0.7919 |
| 0.4343 | 17.3 | 6400 | 0.4423 | 0.7937 | 0.7941 |
| 0.429 | 17.84 | 6600 | 0.4469 | 0.7918 | 0.7924 |
| 0.4319 | 18.38 | 6800 | 0.4481 | 0.7936 | 0.7944 |
| 0.4273 | 18.92 | 7000 | 0.4429 | 0.7914 | 0.7919 |
| 0.4227 | 19.46 | 7200 | 0.4451 | 0.7938 | 0.7943 |
| 0.4337 | 20.0 | 7400 | 0.4431 | 0.7927 | 0.7931 |
| 0.4286 | 20.54 | 7600 | 0.4453 | 0.7927 | 0.7932 |
| 0.4259 | 21.08 | 7800 | 0.4464 | 0.7939 | 0.7944 |
| 0.4286 | 21.62 | 8000 | 0.4411 | 0.7921 | 0.7924 |
| 0.4283 | 22.16 | 8200 | 0.4410 | 0.7942 | 0.7944 |
| 0.4308 | 22.7 | 8400 | 0.4437 | 0.7932 | 0.7937 |
| 0.425 | 23.24 | 8600 | 0.4410 | 0.7937 | 0.7939 |
| 0.4231 | 23.78 | 8800 | 0.4434 | 0.7918 | 0.7922 |
| 0.424 | 24.32 | 9000 | 0.4418 | 0.7943 | 0.7946 |
| 0.4266 | 24.86 | 9200 | 0.4410 | 0.7936 | 0.7939 |
| 0.4332 | 25.41 | 9400 | 0.4419 | 0.7927 | 0.7931 |
| 0.4202 | 25.95 | 9600 | 0.4415 | 0.7940 | 0.7943 |
| 0.4293 | 26.49 | 9800 | 0.4430 | 0.7935 | 0.7939 |
| 0.4245 | 27.03 | 10000 | 0.4423 | 0.7939 | 0.7943 |
### Framework versions
- PEFT 0.9.0
- Transformers 4.38.2
- Pytorch 2.2.0+cu121
- Datasets 2.17.1
- Tokenizers 0.15.2 | {"library_name": "peft", "tags": ["generated_from_trainer"], "metrics": ["accuracy"], "base_model": "mahdibaghbanzadeh/seqsight_16384_512_22M", "model-index": [{"name": "GUE_prom_prom_core_all-seqsight_16384_512_22M-L1_f", "results": []}]} | mahdibaghbanzadeh/GUE_prom_prom_core_all-seqsight_16384_512_22M-L1_f | null | [
"peft",
"safetensors",
"generated_from_trainer",
"base_model:mahdibaghbanzadeh/seqsight_16384_512_22M",
"region:us"
] | null | 2024-04-27T06:44:13+00:00 | [] | [] | TAGS
#peft #safetensors #generated_from_trainer #base_model-mahdibaghbanzadeh/seqsight_16384_512_22M #region-us
| GUE\_prom\_prom\_core\_all-seqsight\_16384\_512\_22M-L1\_f
==========================================================
This model is a fine-tuned version of mahdibaghbanzadeh/seqsight\_16384\_512\_22M on the mahdibaghbanzadeh/GUE\_prom\_prom\_core\_all dataset.
It achieves the following results on the evaluation set:
* Loss: 0.4266
* F1 Score: 0.8008
* Accuracy: 0.8008
Model description
-----------------
More information needed
Intended uses & limitations
---------------------------
More information needed
Training and evaluation data
----------------------------
More information needed
Training procedure
------------------
### Training hyperparameters
The following hyperparameters were used during training:
* learning\_rate: 0.0005
* train\_batch\_size: 128
* eval\_batch\_size: 128
* seed: 42
* optimizer: Adam with betas=(0.9,0.999) and epsilon=1e-08
* lr\_scheduler\_type: linear
* training\_steps: 10000
### Training results
### Framework versions
* PEFT 0.9.0
* Transformers 4.38.2
* Pytorch 2.2.0+cu121
* Datasets 2.17.1
* Tokenizers 0.15.2
| [
"### Training hyperparameters\n\n\nThe following hyperparameters were used during training:\n\n\n* learning\\_rate: 0.0005\n* train\\_batch\\_size: 128\n* eval\\_batch\\_size: 128\n* seed: 42\n* optimizer: Adam with betas=(0.9,0.999) and epsilon=1e-08\n* lr\\_scheduler\\_type: linear\n* training\\_steps: 10000",
"### Training results",
"### Framework versions\n\n\n* PEFT 0.9.0\n* Transformers 4.38.2\n* Pytorch 2.2.0+cu121\n* Datasets 2.17.1\n* Tokenizers 0.15.2"
] | [
"TAGS\n#peft #safetensors #generated_from_trainer #base_model-mahdibaghbanzadeh/seqsight_16384_512_22M #region-us \n",
"### Training hyperparameters\n\n\nThe following hyperparameters were used during training:\n\n\n* learning\\_rate: 0.0005\n* train\\_batch\\_size: 128\n* eval\\_batch\\_size: 128\n* seed: 42\n* optimizer: Adam with betas=(0.9,0.999) and epsilon=1e-08\n* lr\\_scheduler\\_type: linear\n* training\\_steps: 10000",
"### Training results",
"### Framework versions\n\n\n* PEFT 0.9.0\n* Transformers 4.38.2\n* Pytorch 2.2.0+cu121\n* Datasets 2.17.1\n* Tokenizers 0.15.2"
] |
text-generation | transformers |
# Model Card for Model ID
<!-- Provide a quick summary of what the model is/does. -->
## Model Details
### Model Description
<!-- Provide a longer summary of what this model is. -->
This is the model card of a 🤗 transformers model that has been pushed on the Hub. This model card has been automatically generated.
- **Developed by:** [More Information Needed]
- **Funded by [optional]:** [More Information Needed]
- **Shared by [optional]:** [More Information Needed]
- **Model type:** [More Information Needed]
- **Language(s) (NLP):** [More Information Needed]
- **License:** [More Information Needed]
- **Finetuned from model [optional]:** [More Information Needed]
### Model Sources [optional]
<!-- Provide the basic links for the model. -->
- **Repository:** [More Information Needed]
- **Paper [optional]:** [More Information Needed]
- **Demo [optional]:** [More Information Needed]
## Uses
<!-- Address questions around how the model is intended to be used, including the foreseeable users of the model and those affected by the model. -->
### Direct Use
<!-- This section is for the model use without fine-tuning or plugging into a larger ecosystem/app. -->
[More Information Needed]
### Downstream Use [optional]
<!-- This section is for the model use when fine-tuned for a task, or when plugged into a larger ecosystem/app -->
[More Information Needed]
### Out-of-Scope Use
<!-- This section addresses misuse, malicious use, and uses that the model will not work well for. -->
[More Information Needed]
## Bias, Risks, and Limitations
<!-- This section is meant to convey both technical and sociotechnical limitations. -->
[More Information Needed]
### Recommendations
<!-- This section is meant to convey recommendations with respect to the bias, risk, and technical limitations. -->
Users (both direct and downstream) should be made aware of the risks, biases and limitations of the model. More information needed for further recommendations.
## How to Get Started with the Model
Use the code below to get started with the model.
[More Information Needed]
## Training Details
### Training Data
<!-- This should link to a Dataset Card, perhaps with a short stub of information on what the training data is all about as well as documentation related to data pre-processing or additional filtering. -->
[More Information Needed]
### Training Procedure
<!-- This relates heavily to the Technical Specifications. Content here should link to that section when it is relevant to the training procedure. -->
#### Preprocessing [optional]
[More Information Needed]
#### Training Hyperparameters
- **Training regime:** [More Information Needed] <!--fp32, fp16 mixed precision, bf16 mixed precision, bf16 non-mixed precision, fp16 non-mixed precision, fp8 mixed precision -->
#### Speeds, Sizes, Times [optional]
<!-- This section provides information about throughput, start/end time, checkpoint size if relevant, etc. -->
[More Information Needed]
## Evaluation
<!-- This section describes the evaluation protocols and provides the results. -->
### Testing Data, Factors & Metrics
#### Testing Data
<!-- This should link to a Dataset Card if possible. -->
[More Information Needed]
#### Factors
<!-- These are the things the evaluation is disaggregating by, e.g., subpopulations or domains. -->
[More Information Needed]
#### Metrics
<!-- These are the evaluation metrics being used, ideally with a description of why. -->
[More Information Needed]
### Results
[More Information Needed]
#### Summary
## Model Examination [optional]
<!-- Relevant interpretability work for the model goes here -->
[More Information Needed]
## Environmental Impact
<!-- Total emissions (in grams of CO2eq) and additional considerations, such as electricity usage, go here. Edit the suggested text below accordingly -->
Carbon emissions can be estimated using the [Machine Learning Impact calculator](https://mlco2.github.io/impact#compute) presented in [Lacoste et al. (2019)](https://arxiv.org/abs/1910.09700).
- **Hardware Type:** [More Information Needed]
- **Hours used:** [More Information Needed]
- **Cloud Provider:** [More Information Needed]
- **Compute Region:** [More Information Needed]
- **Carbon Emitted:** [More Information Needed]
## Technical Specifications [optional]
### Model Architecture and Objective
[More Information Needed]
### Compute Infrastructure
[More Information Needed]
#### Hardware
[More Information Needed]
#### Software
[More Information Needed]
## Citation [optional]
<!-- If there is a paper or blog post introducing the model, the APA and Bibtex information for that should go in this section. -->
**BibTeX:**
[More Information Needed]
**APA:**
[More Information Needed]
## Glossary [optional]
<!-- If relevant, include terms and calculations in this section that can help readers understand the model or model card. -->
[More Information Needed]
## More Information [optional]
[More Information Needed]
## Model Card Authors [optional]
[More Information Needed]
## Model Card Contact
[More Information Needed] | {"library_name": "transformers", "tags": ["trl", "sft"]} | dbaek111/Llama-3-8B-Instruct-Elon_407_HPC_Q_v2 | null | [
"transformers",
"safetensors",
"llama",
"text-generation",
"trl",
"sft",
"arxiv:1910.09700",
"autotrain_compatible",
"endpoints_compatible",
"text-generation-inference",
"4-bit",
"region:us"
] | null | 2024-04-27T06:44:59+00:00 | [
"1910.09700"
] | [] | TAGS
#transformers #safetensors #llama #text-generation #trl #sft #arxiv-1910.09700 #autotrain_compatible #endpoints_compatible #text-generation-inference #4-bit #region-us
|
# Model Card for Model ID
## Model Details
### Model Description
This is the model card of a transformers model that has been pushed on the Hub. This model card has been automatically generated.
- Developed by:
- Funded by [optional]:
- Shared by [optional]:
- Model type:
- Language(s) (NLP):
- License:
- Finetuned from model [optional]:
### Model Sources [optional]
- Repository:
- Paper [optional]:
- Demo [optional]:
## Uses
### Direct Use
### Downstream Use [optional]
### Out-of-Scope Use
## Bias, Risks, and Limitations
### Recommendations
Users (both direct and downstream) should be made aware of the risks, biases and limitations of the model. More information needed for further recommendations.
## How to Get Started with the Model
Use the code below to get started with the model.
## Training Details
### Training Data
### Training Procedure
#### Preprocessing [optional]
#### Training Hyperparameters
- Training regime:
#### Speeds, Sizes, Times [optional]
## Evaluation
### Testing Data, Factors & Metrics
#### Testing Data
#### Factors
#### Metrics
### Results
#### Summary
## Model Examination [optional]
## Environmental Impact
Carbon emissions can be estimated using the Machine Learning Impact calculator presented in Lacoste et al. (2019).
- Hardware Type:
- Hours used:
- Cloud Provider:
- Compute Region:
- Carbon Emitted:
## Technical Specifications [optional]
### Model Architecture and Objective
### Compute Infrastructure
#### Hardware
#### Software
[optional]
BibTeX:
APA:
## Glossary [optional]
## More Information [optional]
## Model Card Authors [optional]
## Model Card Contact
| [
"# Model Card for Model ID",
"## Model Details",
"### Model Description\n\n\n\nThis is the model card of a transformers model that has been pushed on the Hub. This model card has been automatically generated.\n\n- Developed by: \n- Funded by [optional]: \n- Shared by [optional]: \n- Model type: \n- Language(s) (NLP): \n- License: \n- Finetuned from model [optional]:",
"### Model Sources [optional]\n\n\n\n- Repository: \n- Paper [optional]: \n- Demo [optional]:",
"## Uses",
"### Direct Use",
"### Downstream Use [optional]",
"### Out-of-Scope Use",
"## Bias, Risks, and Limitations",
"### Recommendations\n\n\n\nUsers (both direct and downstream) should be made aware of the risks, biases and limitations of the model. More information needed for further recommendations.",
"## How to Get Started with the Model\n\nUse the code below to get started with the model.",
"## Training Details",
"### Training Data",
"### Training Procedure",
"#### Preprocessing [optional]",
"#### Training Hyperparameters\n\n- Training regime:",
"#### Speeds, Sizes, Times [optional]",
"## Evaluation",
"### Testing Data, Factors & Metrics",
"#### Testing Data",
"#### Factors",
"#### Metrics",
"### Results",
"#### Summary",
"## Model Examination [optional]",
"## Environmental Impact\n\n\n\nCarbon emissions can be estimated using the Machine Learning Impact calculator presented in Lacoste et al. (2019).\n\n- Hardware Type: \n- Hours used: \n- Cloud Provider: \n- Compute Region: \n- Carbon Emitted:",
"## Technical Specifications [optional]",
"### Model Architecture and Objective",
"### Compute Infrastructure",
"#### Hardware",
"#### Software\n\n\n\n[optional]\n\n\n\nBibTeX:\n\n\n\nAPA:",
"## Glossary [optional]",
"## More Information [optional]",
"## Model Card Authors [optional]",
"## Model Card Contact"
] | [
"TAGS\n#transformers #safetensors #llama #text-generation #trl #sft #arxiv-1910.09700 #autotrain_compatible #endpoints_compatible #text-generation-inference #4-bit #region-us \n",
"# Model Card for Model ID",
"## Model Details",
"### Model Description\n\n\n\nThis is the model card of a transformers model that has been pushed on the Hub. This model card has been automatically generated.\n\n- Developed by: \n- Funded by [optional]: \n- Shared by [optional]: \n- Model type: \n- Language(s) (NLP): \n- License: \n- Finetuned from model [optional]:",
"### Model Sources [optional]\n\n\n\n- Repository: \n- Paper [optional]: \n- Demo [optional]:",
"## Uses",
"### Direct Use",
"### Downstream Use [optional]",
"### Out-of-Scope Use",
"## Bias, Risks, and Limitations",
"### Recommendations\n\n\n\nUsers (both direct and downstream) should be made aware of the risks, biases and limitations of the model. More information needed for further recommendations.",
"## How to Get Started with the Model\n\nUse the code below to get started with the model.",
"## Training Details",
"### Training Data",
"### Training Procedure",
"#### Preprocessing [optional]",
"#### Training Hyperparameters\n\n- Training regime:",
"#### Speeds, Sizes, Times [optional]",
"## Evaluation",
"### Testing Data, Factors & Metrics",
"#### Testing Data",
"#### Factors",
"#### Metrics",
"### Results",
"#### Summary",
"## Model Examination [optional]",
"## Environmental Impact\n\n\n\nCarbon emissions can be estimated using the Machine Learning Impact calculator presented in Lacoste et al. (2019).\n\n- Hardware Type: \n- Hours used: \n- Cloud Provider: \n- Compute Region: \n- Carbon Emitted:",
"## Technical Specifications [optional]",
"### Model Architecture and Objective",
"### Compute Infrastructure",
"#### Hardware",
"#### Software\n\n\n\n[optional]\n\n\n\nBibTeX:\n\n\n\nAPA:",
"## Glossary [optional]",
"## More Information [optional]",
"## Model Card Authors [optional]",
"## Model Card Contact"
] |
null | peft |
<!-- This model card has been generated automatically according to the information the Trainer had access to. You
should probably proofread and complete it, then remove this comment. -->
# GUE_prom_prom_core_all-seqsight_16384_512_22M-L8_f
This model is a fine-tuned version of [mahdibaghbanzadeh/seqsight_16384_512_22M](https://huggingface.co/mahdibaghbanzadeh/seqsight_16384_512_22M) on the [mahdibaghbanzadeh/GUE_prom_prom_core_all](https://huggingface.co/datasets/mahdibaghbanzadeh/GUE_prom_prom_core_all) dataset.
It achieves the following results on the evaluation set:
- Loss: 0.4168
- F1 Score: 0.8080
- Accuracy: 0.8081
## Model description
More information needed
## Intended uses & limitations
More information needed
## Training and evaluation data
More information needed
## Training procedure
### Training hyperparameters
The following hyperparameters were used during training:
- learning_rate: 0.0005
- train_batch_size: 128
- eval_batch_size: 128
- seed: 42
- optimizer: Adam with betas=(0.9,0.999) and epsilon=1e-08
- lr_scheduler_type: linear
- training_steps: 10000
### Training results
| Training Loss | Epoch | Step | Validation Loss | F1 Score | Accuracy |
|:-------------:|:-----:|:-----:|:---------------:|:--------:|:--------:|
| 0.5682 | 0.54 | 200 | 0.4933 | 0.7655 | 0.7655 |
| 0.4898 | 1.08 | 400 | 0.4768 | 0.7774 | 0.7775 |
| 0.4635 | 1.62 | 600 | 0.4562 | 0.7841 | 0.7841 |
| 0.4526 | 2.16 | 800 | 0.4611 | 0.7872 | 0.7877 |
| 0.4486 | 2.7 | 1000 | 0.4516 | 0.7871 | 0.7875 |
| 0.4425 | 3.24 | 1200 | 0.4464 | 0.7883 | 0.7887 |
| 0.4377 | 3.78 | 1400 | 0.4489 | 0.7851 | 0.7861 |
| 0.4291 | 4.32 | 1600 | 0.4466 | 0.7933 | 0.7941 |
| 0.4328 | 4.86 | 1800 | 0.4424 | 0.7967 | 0.7973 |
| 0.4298 | 5.41 | 2000 | 0.4428 | 0.7951 | 0.7956 |
| 0.4262 | 5.95 | 2200 | 0.4387 | 0.7994 | 0.7995 |
| 0.4271 | 6.49 | 2400 | 0.4354 | 0.8006 | 0.8007 |
| 0.424 | 7.03 | 2600 | 0.4349 | 0.8002 | 0.8002 |
| 0.4231 | 7.57 | 2800 | 0.4398 | 0.8048 | 0.8049 |
| 0.4174 | 8.11 | 3000 | 0.4370 | 0.7989 | 0.7993 |
| 0.4187 | 8.65 | 3200 | 0.4309 | 0.8070 | 0.8071 |
| 0.4204 | 9.19 | 3400 | 0.4335 | 0.8064 | 0.8064 |
| 0.4174 | 9.73 | 3600 | 0.4410 | 0.7985 | 0.7993 |
| 0.4215 | 10.27 | 3800 | 0.4325 | 0.8036 | 0.8039 |
| 0.4168 | 10.81 | 4000 | 0.4336 | 0.8012 | 0.8012 |
| 0.4154 | 11.35 | 4200 | 0.4359 | 0.8031 | 0.8034 |
| 0.4142 | 11.89 | 4400 | 0.4361 | 0.8042 | 0.8047 |
| 0.4145 | 12.43 | 4600 | 0.4278 | 0.8052 | 0.8052 |
| 0.4103 | 12.97 | 4800 | 0.4325 | 0.8047 | 0.8049 |
| 0.4128 | 13.51 | 5000 | 0.4436 | 0.7954 | 0.7968 |
| 0.4104 | 14.05 | 5200 | 0.4292 | 0.8073 | 0.8074 |
| 0.4089 | 14.59 | 5400 | 0.4295 | 0.8082 | 0.8083 |
| 0.4079 | 15.14 | 5600 | 0.4281 | 0.8059 | 0.8059 |
| 0.4109 | 15.68 | 5800 | 0.4384 | 0.7980 | 0.7988 |
| 0.4045 | 16.22 | 6000 | 0.4330 | 0.8050 | 0.8054 |
| 0.411 | 16.76 | 6200 | 0.4271 | 0.8064 | 0.8068 |
| 0.4104 | 17.3 | 6400 | 0.4305 | 0.8063 | 0.8068 |
| 0.4063 | 17.84 | 6600 | 0.4334 | 0.8040 | 0.8044 |
| 0.4063 | 18.38 | 6800 | 0.4460 | 0.7960 | 0.7973 |
| 0.4048 | 18.92 | 7000 | 0.4307 | 0.8051 | 0.8056 |
| 0.3994 | 19.46 | 7200 | 0.4326 | 0.8057 | 0.8061 |
| 0.4093 | 20.0 | 7400 | 0.4282 | 0.8078 | 0.8079 |
| 0.4023 | 20.54 | 7600 | 0.4358 | 0.8045 | 0.8051 |
| 0.4006 | 21.08 | 7800 | 0.4323 | 0.8086 | 0.8088 |
| 0.4038 | 21.62 | 8000 | 0.4254 | 0.8097 | 0.8098 |
| 0.4024 | 22.16 | 8200 | 0.4285 | 0.8068 | 0.8069 |
| 0.4057 | 22.7 | 8400 | 0.4324 | 0.8045 | 0.8051 |
| 0.3992 | 23.24 | 8600 | 0.4272 | 0.8070 | 0.8071 |
| 0.3987 | 23.78 | 8800 | 0.4316 | 0.8058 | 0.8061 |
| 0.3977 | 24.32 | 9000 | 0.4295 | 0.8074 | 0.8076 |
| 0.4002 | 24.86 | 9200 | 0.4288 | 0.8086 | 0.8088 |
| 0.4068 | 25.41 | 9400 | 0.4290 | 0.8072 | 0.8074 |
| 0.3946 | 25.95 | 9600 | 0.4296 | 0.8079 | 0.8081 |
| 0.4019 | 26.49 | 9800 | 0.4311 | 0.8071 | 0.8074 |
| 0.3976 | 27.03 | 10000 | 0.4303 | 0.8073 | 0.8076 |
### Framework versions
- PEFT 0.9.0
- Transformers 4.38.2
- Pytorch 2.2.0+cu121
- Datasets 2.17.1
- Tokenizers 0.15.2 | {"library_name": "peft", "tags": ["generated_from_trainer"], "metrics": ["accuracy"], "base_model": "mahdibaghbanzadeh/seqsight_16384_512_22M", "model-index": [{"name": "GUE_prom_prom_core_all-seqsight_16384_512_22M-L8_f", "results": []}]} | mahdibaghbanzadeh/GUE_prom_prom_core_all-seqsight_16384_512_22M-L8_f | null | [
"peft",
"safetensors",
"generated_from_trainer",
"base_model:mahdibaghbanzadeh/seqsight_16384_512_22M",
"region:us"
] | null | 2024-04-27T06:47:43+00:00 | [] | [] | TAGS
#peft #safetensors #generated_from_trainer #base_model-mahdibaghbanzadeh/seqsight_16384_512_22M #region-us
| GUE\_prom\_prom\_core\_all-seqsight\_16384\_512\_22M-L8\_f
==========================================================
This model is a fine-tuned version of mahdibaghbanzadeh/seqsight\_16384\_512\_22M on the mahdibaghbanzadeh/GUE\_prom\_prom\_core\_all dataset.
It achieves the following results on the evaluation set:
* Loss: 0.4168
* F1 Score: 0.8080
* Accuracy: 0.8081
Model description
-----------------
More information needed
Intended uses & limitations
---------------------------
More information needed
Training and evaluation data
----------------------------
More information needed
Training procedure
------------------
### Training hyperparameters
The following hyperparameters were used during training:
* learning\_rate: 0.0005
* train\_batch\_size: 128
* eval\_batch\_size: 128
* seed: 42
* optimizer: Adam with betas=(0.9,0.999) and epsilon=1e-08
* lr\_scheduler\_type: linear
* training\_steps: 10000
### Training results
### Framework versions
* PEFT 0.9.0
* Transformers 4.38.2
* Pytorch 2.2.0+cu121
* Datasets 2.17.1
* Tokenizers 0.15.2
| [
"### Training hyperparameters\n\n\nThe following hyperparameters were used during training:\n\n\n* learning\\_rate: 0.0005\n* train\\_batch\\_size: 128\n* eval\\_batch\\_size: 128\n* seed: 42\n* optimizer: Adam with betas=(0.9,0.999) and epsilon=1e-08\n* lr\\_scheduler\\_type: linear\n* training\\_steps: 10000",
"### Training results",
"### Framework versions\n\n\n* PEFT 0.9.0\n* Transformers 4.38.2\n* Pytorch 2.2.0+cu121\n* Datasets 2.17.1\n* Tokenizers 0.15.2"
] | [
"TAGS\n#peft #safetensors #generated_from_trainer #base_model-mahdibaghbanzadeh/seqsight_16384_512_22M #region-us \n",
"### Training hyperparameters\n\n\nThe following hyperparameters were used during training:\n\n\n* learning\\_rate: 0.0005\n* train\\_batch\\_size: 128\n* eval\\_batch\\_size: 128\n* seed: 42\n* optimizer: Adam with betas=(0.9,0.999) and epsilon=1e-08\n* lr\\_scheduler\\_type: linear\n* training\\_steps: 10000",
"### Training results",
"### Framework versions\n\n\n* PEFT 0.9.0\n* Transformers 4.38.2\n* Pytorch 2.2.0+cu121\n* Datasets 2.17.1\n* Tokenizers 0.15.2"
] |
reinforcement-learning | null |
# **Reinforce** Agent playing **Pixelcopter-PLE-v0**
This is a trained model of a **Reinforce** agent playing **Pixelcopter-PLE-v0** .
To learn to use this model and train yours check Unit 4 of the Deep Reinforcement Learning Course: https://huggingface.co/deep-rl-course/unit4/introduction
| {"tags": ["Pixelcopter-PLE-v0", "reinforce", "reinforcement-learning", "custom-implementation", "deep-rl-class"], "model-index": [{"name": "Reinforce-PixelCopter", "results": [{"task": {"type": "reinforcement-learning", "name": "reinforcement-learning"}, "dataset": {"name": "Pixelcopter-PLE-v0", "type": "Pixelcopter-PLE-v0"}, "metrics": [{"type": "mean_reward", "value": "62.00 +/- 46.45", "name": "mean_reward", "verified": false}]}]}]} | i-pj/Reinforce-PixelCopter | null | [
"Pixelcopter-PLE-v0",
"reinforce",
"reinforcement-learning",
"custom-implementation",
"deep-rl-class",
"model-index",
"region:us"
] | null | 2024-04-27T06:47:54+00:00 | [] | [] | TAGS
#Pixelcopter-PLE-v0 #reinforce #reinforcement-learning #custom-implementation #deep-rl-class #model-index #region-us
|
# Reinforce Agent playing Pixelcopter-PLE-v0
This is a trained model of a Reinforce agent playing Pixelcopter-PLE-v0 .
To learn to use this model and train yours check Unit 4 of the Deep Reinforcement Learning Course: URL
| [
"# Reinforce Agent playing Pixelcopter-PLE-v0\n This is a trained model of a Reinforce agent playing Pixelcopter-PLE-v0 .\n To learn to use this model and train yours check Unit 4 of the Deep Reinforcement Learning Course: URL"
] | [
"TAGS\n#Pixelcopter-PLE-v0 #reinforce #reinforcement-learning #custom-implementation #deep-rl-class #model-index #region-us \n",
"# Reinforce Agent playing Pixelcopter-PLE-v0\n This is a trained model of a Reinforce agent playing Pixelcopter-PLE-v0 .\n To learn to use this model and train yours check Unit 4 of the Deep Reinforcement Learning Course: URL"
] |
text-generation | transformers |
# Model Card for Model ID
<!-- Provide a quick summary of what the model is/does. -->
## Model Details
### Model Description
<!-- Provide a longer summary of what this model is. -->
This is the model card of a 🤗 transformers model that has been pushed on the Hub. This model card has been automatically generated.
- **Developed by:** [More Information Needed]
- **Funded by [optional]:** [More Information Needed]
- **Shared by [optional]:** [More Information Needed]
- **Model type:** [More Information Needed]
- **Language(s) (NLP):** [More Information Needed]
- **License:** [More Information Needed]
- **Finetuned from model [optional]:** [More Information Needed]
### Model Sources [optional]
<!-- Provide the basic links for the model. -->
- **Repository:** [More Information Needed]
- **Paper [optional]:** [More Information Needed]
- **Demo [optional]:** [More Information Needed]
## Uses
<!-- Address questions around how the model is intended to be used, including the foreseeable users of the model and those affected by the model. -->
### Direct Use
<!-- This section is for the model use without fine-tuning or plugging into a larger ecosystem/app. -->
[More Information Needed]
### Downstream Use [optional]
<!-- This section is for the model use when fine-tuned for a task, or when plugged into a larger ecosystem/app -->
[More Information Needed]
### Out-of-Scope Use
<!-- This section addresses misuse, malicious use, and uses that the model will not work well for. -->
[More Information Needed]
## Bias, Risks, and Limitations
<!-- This section is meant to convey both technical and sociotechnical limitations. -->
[More Information Needed]
### Recommendations
<!-- This section is meant to convey recommendations with respect to the bias, risk, and technical limitations. -->
Users (both direct and downstream) should be made aware of the risks, biases and limitations of the model. More information needed for further recommendations.
## How to Get Started with the Model
Use the code below to get started with the model.
[More Information Needed]
## Training Details
### Training Data
<!-- This should link to a Dataset Card, perhaps with a short stub of information on what the training data is all about as well as documentation related to data pre-processing or additional filtering. -->
[More Information Needed]
### Training Procedure
<!-- This relates heavily to the Technical Specifications. Content here should link to that section when it is relevant to the training procedure. -->
#### Preprocessing [optional]
[More Information Needed]
#### Training Hyperparameters
- **Training regime:** [More Information Needed] <!--fp32, fp16 mixed precision, bf16 mixed precision, bf16 non-mixed precision, fp16 non-mixed precision, fp8 mixed precision -->
#### Speeds, Sizes, Times [optional]
<!-- This section provides information about throughput, start/end time, checkpoint size if relevant, etc. -->
[More Information Needed]
## Evaluation
<!-- This section describes the evaluation protocols and provides the results. -->
### Testing Data, Factors & Metrics
#### Testing Data
<!-- This should link to a Dataset Card if possible. -->
[More Information Needed]
#### Factors
<!-- These are the things the evaluation is disaggregating by, e.g., subpopulations or domains. -->
[More Information Needed]
#### Metrics
<!-- These are the evaluation metrics being used, ideally with a description of why. -->
[More Information Needed]
### Results
[More Information Needed]
#### Summary
## Model Examination [optional]
<!-- Relevant interpretability work for the model goes here -->
[More Information Needed]
## Environmental Impact
<!-- Total emissions (in grams of CO2eq) and additional considerations, such as electricity usage, go here. Edit the suggested text below accordingly -->
Carbon emissions can be estimated using the [Machine Learning Impact calculator](https://mlco2.github.io/impact#compute) presented in [Lacoste et al. (2019)](https://arxiv.org/abs/1910.09700).
- **Hardware Type:** [More Information Needed]
- **Hours used:** [More Information Needed]
- **Cloud Provider:** [More Information Needed]
- **Compute Region:** [More Information Needed]
- **Carbon Emitted:** [More Information Needed]
## Technical Specifications [optional]
### Model Architecture and Objective
[More Information Needed]
### Compute Infrastructure
[More Information Needed]
#### Hardware
[More Information Needed]
#### Software
[More Information Needed]
## Citation [optional]
<!-- If there is a paper or blog post introducing the model, the APA and Bibtex information for that should go in this section. -->
**BibTeX:**
[More Information Needed]
**APA:**
[More Information Needed]
## Glossary [optional]
<!-- If relevant, include terms and calculations in this section that can help readers understand the model or model card. -->
[More Information Needed]
## More Information [optional]
[More Information Needed]
## Model Card Authors [optional]
[More Information Needed]
## Model Card Contact
[More Information Needed] | {"library_name": "transformers", "tags": []} | quickstep3621/qkgglor | null | [
"transformers",
"safetensors",
"stablelm",
"text-generation",
"conversational",
"arxiv:1910.09700",
"autotrain_compatible",
"endpoints_compatible",
"region:us"
] | null | 2024-04-27T06:49:01+00:00 | [
"1910.09700"
] | [] | TAGS
#transformers #safetensors #stablelm #text-generation #conversational #arxiv-1910.09700 #autotrain_compatible #endpoints_compatible #region-us
|
# Model Card for Model ID
## Model Details
### Model Description
This is the model card of a transformers model that has been pushed on the Hub. This model card has been automatically generated.
- Developed by:
- Funded by [optional]:
- Shared by [optional]:
- Model type:
- Language(s) (NLP):
- License:
- Finetuned from model [optional]:
### Model Sources [optional]
- Repository:
- Paper [optional]:
- Demo [optional]:
## Uses
### Direct Use
### Downstream Use [optional]
### Out-of-Scope Use
## Bias, Risks, and Limitations
### Recommendations
Users (both direct and downstream) should be made aware of the risks, biases and limitations of the model. More information needed for further recommendations.
## How to Get Started with the Model
Use the code below to get started with the model.
## Training Details
### Training Data
### Training Procedure
#### Preprocessing [optional]
#### Training Hyperparameters
- Training regime:
#### Speeds, Sizes, Times [optional]
## Evaluation
### Testing Data, Factors & Metrics
#### Testing Data
#### Factors
#### Metrics
### Results
#### Summary
## Model Examination [optional]
## Environmental Impact
Carbon emissions can be estimated using the Machine Learning Impact calculator presented in Lacoste et al. (2019).
- Hardware Type:
- Hours used:
- Cloud Provider:
- Compute Region:
- Carbon Emitted:
## Technical Specifications [optional]
### Model Architecture and Objective
### Compute Infrastructure
#### Hardware
#### Software
[optional]
BibTeX:
APA:
## Glossary [optional]
## More Information [optional]
## Model Card Authors [optional]
## Model Card Contact
| [
"# Model Card for Model ID",
"## Model Details",
"### Model Description\n\n\n\nThis is the model card of a transformers model that has been pushed on the Hub. This model card has been automatically generated.\n\n- Developed by: \n- Funded by [optional]: \n- Shared by [optional]: \n- Model type: \n- Language(s) (NLP): \n- License: \n- Finetuned from model [optional]:",
"### Model Sources [optional]\n\n\n\n- Repository: \n- Paper [optional]: \n- Demo [optional]:",
"## Uses",
"### Direct Use",
"### Downstream Use [optional]",
"### Out-of-Scope Use",
"## Bias, Risks, and Limitations",
"### Recommendations\n\n\n\nUsers (both direct and downstream) should be made aware of the risks, biases and limitations of the model. More information needed for further recommendations.",
"## How to Get Started with the Model\n\nUse the code below to get started with the model.",
"## Training Details",
"### Training Data",
"### Training Procedure",
"#### Preprocessing [optional]",
"#### Training Hyperparameters\n\n- Training regime:",
"#### Speeds, Sizes, Times [optional]",
"## Evaluation",
"### Testing Data, Factors & Metrics",
"#### Testing Data",
"#### Factors",
"#### Metrics",
"### Results",
"#### Summary",
"## Model Examination [optional]",
"## Environmental Impact\n\n\n\nCarbon emissions can be estimated using the Machine Learning Impact calculator presented in Lacoste et al. (2019).\n\n- Hardware Type: \n- Hours used: \n- Cloud Provider: \n- Compute Region: \n- Carbon Emitted:",
"## Technical Specifications [optional]",
"### Model Architecture and Objective",
"### Compute Infrastructure",
"#### Hardware",
"#### Software\n\n\n\n[optional]\n\n\n\nBibTeX:\n\n\n\nAPA:",
"## Glossary [optional]",
"## More Information [optional]",
"## Model Card Authors [optional]",
"## Model Card Contact"
] | [
"TAGS\n#transformers #safetensors #stablelm #text-generation #conversational #arxiv-1910.09700 #autotrain_compatible #endpoints_compatible #region-us \n",
"# Model Card for Model ID",
"## Model Details",
"### Model Description\n\n\n\nThis is the model card of a transformers model that has been pushed on the Hub. This model card has been automatically generated.\n\n- Developed by: \n- Funded by [optional]: \n- Shared by [optional]: \n- Model type: \n- Language(s) (NLP): \n- License: \n- Finetuned from model [optional]:",
"### Model Sources [optional]\n\n\n\n- Repository: \n- Paper [optional]: \n- Demo [optional]:",
"## Uses",
"### Direct Use",
"### Downstream Use [optional]",
"### Out-of-Scope Use",
"## Bias, Risks, and Limitations",
"### Recommendations\n\n\n\nUsers (both direct and downstream) should be made aware of the risks, biases and limitations of the model. More information needed for further recommendations.",
"## How to Get Started with the Model\n\nUse the code below to get started with the model.",
"## Training Details",
"### Training Data",
"### Training Procedure",
"#### Preprocessing [optional]",
"#### Training Hyperparameters\n\n- Training regime:",
"#### Speeds, Sizes, Times [optional]",
"## Evaluation",
"### Testing Data, Factors & Metrics",
"#### Testing Data",
"#### Factors",
"#### Metrics",
"### Results",
"#### Summary",
"## Model Examination [optional]",
"## Environmental Impact\n\n\n\nCarbon emissions can be estimated using the Machine Learning Impact calculator presented in Lacoste et al. (2019).\n\n- Hardware Type: \n- Hours used: \n- Cloud Provider: \n- Compute Region: \n- Carbon Emitted:",
"## Technical Specifications [optional]",
"### Model Architecture and Objective",
"### Compute Infrastructure",
"#### Hardware",
"#### Software\n\n\n\n[optional]\n\n\n\nBibTeX:\n\n\n\nAPA:",
"## Glossary [optional]",
"## More Information [optional]",
"## Model Card Authors [optional]",
"## Model Card Contact"
] |
text-generation | transformers |
# Model Card for Model ID
<!-- Provide a quick summary of what the model is/does. -->
## Model Details
### Model Description
<!-- Provide a longer summary of what this model is. -->
This is the model card of a 🤗 transformers model that has been pushed on the Hub. This model card has been automatically generated.
- **Developed by:** [More Information Needed]
- **Funded by [optional]:** [More Information Needed]
- **Shared by [optional]:** [More Information Needed]
- **Model type:** [More Information Needed]
- **Language(s) (NLP):** [More Information Needed]
- **License:** [More Information Needed]
- **Finetuned from model [optional]:** [More Information Needed]
### Model Sources [optional]
<!-- Provide the basic links for the model. -->
- **Repository:** [More Information Needed]
- **Paper [optional]:** [More Information Needed]
- **Demo [optional]:** [More Information Needed]
## Uses
<!-- Address questions around how the model is intended to be used, including the foreseeable users of the model and those affected by the model. -->
### Direct Use
<!-- This section is for the model use without fine-tuning or plugging into a larger ecosystem/app. -->
[More Information Needed]
### Downstream Use [optional]
<!-- This section is for the model use when fine-tuned for a task, or when plugged into a larger ecosystem/app -->
[More Information Needed]
### Out-of-Scope Use
<!-- This section addresses misuse, malicious use, and uses that the model will not work well for. -->
[More Information Needed]
## Bias, Risks, and Limitations
<!-- This section is meant to convey both technical and sociotechnical limitations. -->
[More Information Needed]
### Recommendations
<!-- This section is meant to convey recommendations with respect to the bias, risk, and technical limitations. -->
Users (both direct and downstream) should be made aware of the risks, biases and limitations of the model. More information needed for further recommendations.
## How to Get Started with the Model
Use the code below to get started with the model.
[More Information Needed]
## Training Details
### Training Data
<!-- This should link to a Dataset Card, perhaps with a short stub of information on what the training data is all about as well as documentation related to data pre-processing or additional filtering. -->
[More Information Needed]
### Training Procedure
<!-- This relates heavily to the Technical Specifications. Content here should link to that section when it is relevant to the training procedure. -->
#### Preprocessing [optional]
[More Information Needed]
#### Training Hyperparameters
- **Training regime:** [More Information Needed] <!--fp32, fp16 mixed precision, bf16 mixed precision, bf16 non-mixed precision, fp16 non-mixed precision, fp8 mixed precision -->
#### Speeds, Sizes, Times [optional]
<!-- This section provides information about throughput, start/end time, checkpoint size if relevant, etc. -->
[More Information Needed]
## Evaluation
<!-- This section describes the evaluation protocols and provides the results. -->
### Testing Data, Factors & Metrics
#### Testing Data
<!-- This should link to a Dataset Card if possible. -->
[More Information Needed]
#### Factors
<!-- These are the things the evaluation is disaggregating by, e.g., subpopulations or domains. -->
[More Information Needed]
#### Metrics
<!-- These are the evaluation metrics being used, ideally with a description of why. -->
[More Information Needed]
### Results
[More Information Needed]
#### Summary
## Model Examination [optional]
<!-- Relevant interpretability work for the model goes here -->
[More Information Needed]
## Environmental Impact
<!-- Total emissions (in grams of CO2eq) and additional considerations, such as electricity usage, go here. Edit the suggested text below accordingly -->
Carbon emissions can be estimated using the [Machine Learning Impact calculator](https://mlco2.github.io/impact#compute) presented in [Lacoste et al. (2019)](https://arxiv.org/abs/1910.09700).
- **Hardware Type:** [More Information Needed]
- **Hours used:** [More Information Needed]
- **Cloud Provider:** [More Information Needed]
- **Compute Region:** [More Information Needed]
- **Carbon Emitted:** [More Information Needed]
## Technical Specifications [optional]
### Model Architecture and Objective
[More Information Needed]
### Compute Infrastructure
[More Information Needed]
#### Hardware
[More Information Needed]
#### Software
[More Information Needed]
## Citation [optional]
<!-- If there is a paper or blog post introducing the model, the APA and Bibtex information for that should go in this section. -->
**BibTeX:**
[More Information Needed]
**APA:**
[More Information Needed]
## Glossary [optional]
<!-- If relevant, include terms and calculations in this section that can help readers understand the model or model card. -->
[More Information Needed]
## More Information [optional]
[More Information Needed]
## Model Card Authors [optional]
[More Information Needed]
## Model Card Contact
[More Information Needed] | {"library_name": "transformers", "tags": []} | pruning/ddh98vx | null | [
"transformers",
"safetensors",
"stablelm",
"text-generation",
"conversational",
"arxiv:1910.09700",
"autotrain_compatible",
"endpoints_compatible",
"region:us"
] | null | 2024-04-27T06:49:03+00:00 | [
"1910.09700"
] | [] | TAGS
#transformers #safetensors #stablelm #text-generation #conversational #arxiv-1910.09700 #autotrain_compatible #endpoints_compatible #region-us
|
# Model Card for Model ID
## Model Details
### Model Description
This is the model card of a transformers model that has been pushed on the Hub. This model card has been automatically generated.
- Developed by:
- Funded by [optional]:
- Shared by [optional]:
- Model type:
- Language(s) (NLP):
- License:
- Finetuned from model [optional]:
### Model Sources [optional]
- Repository:
- Paper [optional]:
- Demo [optional]:
## Uses
### Direct Use
### Downstream Use [optional]
### Out-of-Scope Use
## Bias, Risks, and Limitations
### Recommendations
Users (both direct and downstream) should be made aware of the risks, biases and limitations of the model. More information needed for further recommendations.
## How to Get Started with the Model
Use the code below to get started with the model.
## Training Details
### Training Data
### Training Procedure
#### Preprocessing [optional]
#### Training Hyperparameters
- Training regime:
#### Speeds, Sizes, Times [optional]
## Evaluation
### Testing Data, Factors & Metrics
#### Testing Data
#### Factors
#### Metrics
### Results
#### Summary
## Model Examination [optional]
## Environmental Impact
Carbon emissions can be estimated using the Machine Learning Impact calculator presented in Lacoste et al. (2019).
- Hardware Type:
- Hours used:
- Cloud Provider:
- Compute Region:
- Carbon Emitted:
## Technical Specifications [optional]
### Model Architecture and Objective
### Compute Infrastructure
#### Hardware
#### Software
[optional]
BibTeX:
APA:
## Glossary [optional]
## More Information [optional]
## Model Card Authors [optional]
## Model Card Contact
| [
"# Model Card for Model ID",
"## Model Details",
"### Model Description\n\n\n\nThis is the model card of a transformers model that has been pushed on the Hub. This model card has been automatically generated.\n\n- Developed by: \n- Funded by [optional]: \n- Shared by [optional]: \n- Model type: \n- Language(s) (NLP): \n- License: \n- Finetuned from model [optional]:",
"### Model Sources [optional]\n\n\n\n- Repository: \n- Paper [optional]: \n- Demo [optional]:",
"## Uses",
"### Direct Use",
"### Downstream Use [optional]",
"### Out-of-Scope Use",
"## Bias, Risks, and Limitations",
"### Recommendations\n\n\n\nUsers (both direct and downstream) should be made aware of the risks, biases and limitations of the model. More information needed for further recommendations.",
"## How to Get Started with the Model\n\nUse the code below to get started with the model.",
"## Training Details",
"### Training Data",
"### Training Procedure",
"#### Preprocessing [optional]",
"#### Training Hyperparameters\n\n- Training regime:",
"#### Speeds, Sizes, Times [optional]",
"## Evaluation",
"### Testing Data, Factors & Metrics",
"#### Testing Data",
"#### Factors",
"#### Metrics",
"### Results",
"#### Summary",
"## Model Examination [optional]",
"## Environmental Impact\n\n\n\nCarbon emissions can be estimated using the Machine Learning Impact calculator presented in Lacoste et al. (2019).\n\n- Hardware Type: \n- Hours used: \n- Cloud Provider: \n- Compute Region: \n- Carbon Emitted:",
"## Technical Specifications [optional]",
"### Model Architecture and Objective",
"### Compute Infrastructure",
"#### Hardware",
"#### Software\n\n\n\n[optional]\n\n\n\nBibTeX:\n\n\n\nAPA:",
"## Glossary [optional]",
"## More Information [optional]",
"## Model Card Authors [optional]",
"## Model Card Contact"
] | [
"TAGS\n#transformers #safetensors #stablelm #text-generation #conversational #arxiv-1910.09700 #autotrain_compatible #endpoints_compatible #region-us \n",
"# Model Card for Model ID",
"## Model Details",
"### Model Description\n\n\n\nThis is the model card of a transformers model that has been pushed on the Hub. This model card has been automatically generated.\n\n- Developed by: \n- Funded by [optional]: \n- Shared by [optional]: \n- Model type: \n- Language(s) (NLP): \n- License: \n- Finetuned from model [optional]:",
"### Model Sources [optional]\n\n\n\n- Repository: \n- Paper [optional]: \n- Demo [optional]:",
"## Uses",
"### Direct Use",
"### Downstream Use [optional]",
"### Out-of-Scope Use",
"## Bias, Risks, and Limitations",
"### Recommendations\n\n\n\nUsers (both direct and downstream) should be made aware of the risks, biases and limitations of the model. More information needed for further recommendations.",
"## How to Get Started with the Model\n\nUse the code below to get started with the model.",
"## Training Details",
"### Training Data",
"### Training Procedure",
"#### Preprocessing [optional]",
"#### Training Hyperparameters\n\n- Training regime:",
"#### Speeds, Sizes, Times [optional]",
"## Evaluation",
"### Testing Data, Factors & Metrics",
"#### Testing Data",
"#### Factors",
"#### Metrics",
"### Results",
"#### Summary",
"## Model Examination [optional]",
"## Environmental Impact\n\n\n\nCarbon emissions can be estimated using the Machine Learning Impact calculator presented in Lacoste et al. (2019).\n\n- Hardware Type: \n- Hours used: \n- Cloud Provider: \n- Compute Region: \n- Carbon Emitted:",
"## Technical Specifications [optional]",
"### Model Architecture and Objective",
"### Compute Infrastructure",
"#### Hardware",
"#### Software\n\n\n\n[optional]\n\n\n\nBibTeX:\n\n\n\nAPA:",
"## Glossary [optional]",
"## More Information [optional]",
"## Model Card Authors [optional]",
"## Model Card Contact"
] |
null | peft |
<!-- This model card has been generated automatically according to the information the Trainer had access to. You
should probably proofread and complete it, then remove this comment. -->
# GUE_prom_prom_core_all-seqsight_16384_512_22M-L32_f
This model is a fine-tuned version of [mahdibaghbanzadeh/seqsight_16384_512_22M](https://huggingface.co/mahdibaghbanzadeh/seqsight_16384_512_22M) on the [mahdibaghbanzadeh/GUE_prom_prom_core_all](https://huggingface.co/datasets/mahdibaghbanzadeh/GUE_prom_prom_core_all) dataset.
It achieves the following results on the evaluation set:
- Loss: 0.4191
- F1 Score: 0.8132
- Accuracy: 0.8133
## Model description
More information needed
## Intended uses & limitations
More information needed
## Training and evaluation data
More information needed
## Training procedure
### Training hyperparameters
The following hyperparameters were used during training:
- learning_rate: 0.0005
- train_batch_size: 128
- eval_batch_size: 128
- seed: 42
- optimizer: Adam with betas=(0.9,0.999) and epsilon=1e-08
- lr_scheduler_type: linear
- training_steps: 10000
### Training results
| Training Loss | Epoch | Step | Validation Loss | F1 Score | Accuracy |
|:-------------:|:-----:|:-----:|:---------------:|:--------:|:--------:|
| 0.5459 | 0.54 | 200 | 0.4720 | 0.7762 | 0.7764 |
| 0.4685 | 1.08 | 400 | 0.4790 | 0.7752 | 0.7767 |
| 0.4444 | 1.62 | 600 | 0.4477 | 0.7959 | 0.7959 |
| 0.4374 | 2.16 | 800 | 0.4510 | 0.7866 | 0.7875 |
| 0.4347 | 2.7 | 1000 | 0.4420 | 0.7908 | 0.7914 |
| 0.4312 | 3.24 | 1200 | 0.4366 | 0.7951 | 0.7954 |
| 0.4269 | 3.78 | 1400 | 0.4371 | 0.7934 | 0.7941 |
| 0.4179 | 4.32 | 1600 | 0.4366 | 0.7986 | 0.7992 |
| 0.4225 | 4.86 | 1800 | 0.4340 | 0.7973 | 0.7978 |
| 0.4166 | 5.41 | 2000 | 0.4440 | 0.7957 | 0.7965 |
| 0.4162 | 5.95 | 2200 | 0.4301 | 0.8047 | 0.8047 |
| 0.4158 | 6.49 | 2400 | 0.4292 | 0.7982 | 0.7983 |
| 0.4112 | 7.03 | 2600 | 0.4271 | 0.8045 | 0.8046 |
| 0.4096 | 7.57 | 2800 | 0.4318 | 0.8032 | 0.8032 |
| 0.4041 | 8.11 | 3000 | 0.4271 | 0.8012 | 0.8015 |
| 0.4038 | 8.65 | 3200 | 0.4271 | 0.8050 | 0.8052 |
| 0.4057 | 9.19 | 3400 | 0.4293 | 0.8083 | 0.8083 |
| 0.403 | 9.73 | 3600 | 0.4364 | 0.7988 | 0.7997 |
| 0.4049 | 10.27 | 3800 | 0.4315 | 0.8040 | 0.8044 |
| 0.4013 | 10.81 | 4000 | 0.4325 | 0.8017 | 0.8017 |
| 0.3995 | 11.35 | 4200 | 0.4289 | 0.8055 | 0.8057 |
| 0.3977 | 11.89 | 4400 | 0.4327 | 0.8010 | 0.8017 |
| 0.3969 | 12.43 | 4600 | 0.4250 | 0.8074 | 0.8074 |
| 0.394 | 12.97 | 4800 | 0.4282 | 0.8050 | 0.8051 |
| 0.3954 | 13.51 | 5000 | 0.4361 | 0.7981 | 0.7992 |
| 0.3913 | 14.05 | 5200 | 0.4247 | 0.8083 | 0.8084 |
| 0.389 | 14.59 | 5400 | 0.4294 | 0.8056 | 0.8057 |
| 0.3897 | 15.14 | 5600 | 0.4264 | 0.8079 | 0.8079 |
| 0.3898 | 15.68 | 5800 | 0.4400 | 0.7991 | 0.8002 |
| 0.3854 | 16.22 | 6000 | 0.4309 | 0.8036 | 0.8041 |
| 0.3905 | 16.76 | 6200 | 0.4220 | 0.8077 | 0.8081 |
| 0.3896 | 17.3 | 6400 | 0.4316 | 0.8066 | 0.8071 |
| 0.3867 | 17.84 | 6600 | 0.4337 | 0.8072 | 0.8076 |
| 0.3847 | 18.38 | 6800 | 0.4463 | 0.7982 | 0.7997 |
| 0.3837 | 18.92 | 7000 | 0.4292 | 0.8053 | 0.8057 |
| 0.3774 | 19.46 | 7200 | 0.4324 | 0.8035 | 0.8039 |
| 0.3885 | 20.0 | 7400 | 0.4264 | 0.8068 | 0.8069 |
| 0.3792 | 20.54 | 7600 | 0.4370 | 0.8023 | 0.8029 |
| 0.3774 | 21.08 | 7800 | 0.4333 | 0.8086 | 0.8088 |
| 0.3814 | 21.62 | 8000 | 0.4231 | 0.8075 | 0.8076 |
| 0.3777 | 22.16 | 8200 | 0.4280 | 0.8071 | 0.8073 |
| 0.3828 | 22.7 | 8400 | 0.4317 | 0.8038 | 0.8044 |
| 0.3749 | 23.24 | 8600 | 0.4259 | 0.8034 | 0.8035 |
| 0.3738 | 23.78 | 8800 | 0.4333 | 0.8059 | 0.8063 |
| 0.3758 | 24.32 | 9000 | 0.4281 | 0.8064 | 0.8066 |
| 0.376 | 24.86 | 9200 | 0.4278 | 0.8064 | 0.8066 |
| 0.3813 | 25.41 | 9400 | 0.4277 | 0.8065 | 0.8068 |
| 0.371 | 25.95 | 9600 | 0.4282 | 0.8072 | 0.8074 |
| 0.3776 | 26.49 | 9800 | 0.4299 | 0.8057 | 0.8061 |
| 0.3724 | 27.03 | 10000 | 0.4292 | 0.8061 | 0.8064 |
### Framework versions
- PEFT 0.9.0
- Transformers 4.38.2
- Pytorch 2.2.0+cu121
- Datasets 2.17.1
- Tokenizers 0.15.2 | {"library_name": "peft", "tags": ["generated_from_trainer"], "metrics": ["accuracy"], "base_model": "mahdibaghbanzadeh/seqsight_16384_512_22M", "model-index": [{"name": "GUE_prom_prom_core_all-seqsight_16384_512_22M-L32_f", "results": []}]} | mahdibaghbanzadeh/GUE_prom_prom_core_all-seqsight_16384_512_22M-L32_f | null | [
"peft",
"safetensors",
"generated_from_trainer",
"base_model:mahdibaghbanzadeh/seqsight_16384_512_22M",
"region:us"
] | null | 2024-04-27T06:50:22+00:00 | [] | [] | TAGS
#peft #safetensors #generated_from_trainer #base_model-mahdibaghbanzadeh/seqsight_16384_512_22M #region-us
| GUE\_prom\_prom\_core\_all-seqsight\_16384\_512\_22M-L32\_f
===========================================================
This model is a fine-tuned version of mahdibaghbanzadeh/seqsight\_16384\_512\_22M on the mahdibaghbanzadeh/GUE\_prom\_prom\_core\_all dataset.
It achieves the following results on the evaluation set:
* Loss: 0.4191
* F1 Score: 0.8132
* Accuracy: 0.8133
Model description
-----------------
More information needed
Intended uses & limitations
---------------------------
More information needed
Training and evaluation data
----------------------------
More information needed
Training procedure
------------------
### Training hyperparameters
The following hyperparameters were used during training:
* learning\_rate: 0.0005
* train\_batch\_size: 128
* eval\_batch\_size: 128
* seed: 42
* optimizer: Adam with betas=(0.9,0.999) and epsilon=1e-08
* lr\_scheduler\_type: linear
* training\_steps: 10000
### Training results
### Framework versions
* PEFT 0.9.0
* Transformers 4.38.2
* Pytorch 2.2.0+cu121
* Datasets 2.17.1
* Tokenizers 0.15.2
| [
"### Training hyperparameters\n\n\nThe following hyperparameters were used during training:\n\n\n* learning\\_rate: 0.0005\n* train\\_batch\\_size: 128\n* eval\\_batch\\_size: 128\n* seed: 42\n* optimizer: Adam with betas=(0.9,0.999) and epsilon=1e-08\n* lr\\_scheduler\\_type: linear\n* training\\_steps: 10000",
"### Training results",
"### Framework versions\n\n\n* PEFT 0.9.0\n* Transformers 4.38.2\n* Pytorch 2.2.0+cu121\n* Datasets 2.17.1\n* Tokenizers 0.15.2"
] | [
"TAGS\n#peft #safetensors #generated_from_trainer #base_model-mahdibaghbanzadeh/seqsight_16384_512_22M #region-us \n",
"### Training hyperparameters\n\n\nThe following hyperparameters were used during training:\n\n\n* learning\\_rate: 0.0005\n* train\\_batch\\_size: 128\n* eval\\_batch\\_size: 128\n* seed: 42\n* optimizer: Adam with betas=(0.9,0.999) and epsilon=1e-08\n* lr\\_scheduler\\_type: linear\n* training\\_steps: 10000",
"### Training results",
"### Framework versions\n\n\n* PEFT 0.9.0\n* Transformers 4.38.2\n* Pytorch 2.2.0+cu121\n* Datasets 2.17.1\n* Tokenizers 0.15.2"
] |
text-generation | transformers |
# miqu-evil-dpo
# **Model Details**
## Description
miqu-evil-dpo is fine-tuned model based on miqu, serving as a direct successor to PiVoT-0.1-Evil-a.
It is trained with evil-tune method applied.

<!-- prompt-template start -->
## Prompt template: Mistral Inst
```
<s> [INST] {inst} [/INST]
```
<!-- prompt-template end -->
## Disclaimer
The AI model provided herein is intended for experimental purposes only. The creator of this model makes no representations or warranties of any kind, either express or implied, as to the model's accuracy, reliability, or suitability for any particular purpose. The creator shall not be held liable for any outcomes, decisions, or actions taken on the basis of the information generated by this model. Users of this model assume full responsibility for any consequences resulting from its use.
| {"language": ["en"], "license": "other", "tags": ["not-for-all-audiences"], "license_name": "miqu-license", "license_link": "LICENSE", "pipeline_tag": "text-generation"} | blockblockblock/miqu-evil-dpo-bpw5-exl2 | null | [
"transformers",
"safetensors",
"llama",
"text-generation",
"not-for-all-audiences",
"conversational",
"en",
"license:other",
"autotrain_compatible",
"endpoints_compatible",
"text-generation-inference",
"5-bit",
"region:us"
] | null | 2024-04-27T06:50:59+00:00 | [] | [
"en"
] | TAGS
#transformers #safetensors #llama #text-generation #not-for-all-audiences #conversational #en #license-other #autotrain_compatible #endpoints_compatible #text-generation-inference #5-bit #region-us
|
# miqu-evil-dpo
# Model Details
## Description
miqu-evil-dpo is fine-tuned model based on miqu, serving as a direct successor to PiVoT-0.1-Evil-a.
It is trained with evil-tune method applied.
!image/png
## Prompt template: Mistral Inst
## Disclaimer
The AI model provided herein is intended for experimental purposes only. The creator of this model makes no representations or warranties of any kind, either express or implied, as to the model's accuracy, reliability, or suitability for any particular purpose. The creator shall not be held liable for any outcomes, decisions, or actions taken on the basis of the information generated by this model. Users of this model assume full responsibility for any consequences resulting from its use.
| [
"# miqu-evil-dpo",
"# Model Details",
"## Description\nmiqu-evil-dpo is fine-tuned model based on miqu, serving as a direct successor to PiVoT-0.1-Evil-a.\n\nIt is trained with evil-tune method applied.\n\n!image/png",
"## Prompt template: Mistral Inst",
"## Disclaimer\nThe AI model provided herein is intended for experimental purposes only. The creator of this model makes no representations or warranties of any kind, either express or implied, as to the model's accuracy, reliability, or suitability for any particular purpose. The creator shall not be held liable for any outcomes, decisions, or actions taken on the basis of the information generated by this model. Users of this model assume full responsibility for any consequences resulting from its use."
] | [
"TAGS\n#transformers #safetensors #llama #text-generation #not-for-all-audiences #conversational #en #license-other #autotrain_compatible #endpoints_compatible #text-generation-inference #5-bit #region-us \n",
"# miqu-evil-dpo",
"# Model Details",
"## Description\nmiqu-evil-dpo is fine-tuned model based on miqu, serving as a direct successor to PiVoT-0.1-Evil-a.\n\nIt is trained with evil-tune method applied.\n\n!image/png",
"## Prompt template: Mistral Inst",
"## Disclaimer\nThe AI model provided herein is intended for experimental purposes only. The creator of this model makes no representations or warranties of any kind, either express or implied, as to the model's accuracy, reliability, or suitability for any particular purpose. The creator shall not be held liable for any outcomes, decisions, or actions taken on the basis of the information generated by this model. Users of this model assume full responsibility for any consequences resulting from its use."
] |
null | peft |
<!-- This model card has been generated automatically according to the information the Trainer had access to. You
should probably proofread and complete it, then remove this comment. -->
# GUE_prom_prom_core_notata-seqsight_16384_512_22M-L8_f
This model is a fine-tuned version of [mahdibaghbanzadeh/seqsight_16384_512_22M](https://huggingface.co/mahdibaghbanzadeh/seqsight_16384_512_22M) on the [mahdibaghbanzadeh/GUE_prom_prom_core_notata](https://huggingface.co/datasets/mahdibaghbanzadeh/GUE_prom_prom_core_notata) dataset.
It achieves the following results on the evaluation set:
- Loss: 0.3842
- F1 Score: 0.8302
- Accuracy: 0.8302
## Model description
More information needed
## Intended uses & limitations
More information needed
## Training and evaluation data
More information needed
## Training procedure
### Training hyperparameters
The following hyperparameters were used during training:
- learning_rate: 0.0005
- train_batch_size: 128
- eval_batch_size: 128
- seed: 42
- optimizer: Adam with betas=(0.9,0.999) and epsilon=1e-08
- lr_scheduler_type: linear
- training_steps: 10000
### Training results
| Training Loss | Epoch | Step | Validation Loss | F1 Score | Accuracy |
|:-------------:|:-----:|:-----:|:---------------:|:--------:|:--------:|
| 0.5613 | 0.6 | 200 | 0.4434 | 0.7974 | 0.7974 |
| 0.4545 | 1.2 | 400 | 0.4031 | 0.8179 | 0.8180 |
| 0.429 | 1.81 | 600 | 0.3886 | 0.8328 | 0.8329 |
| 0.416 | 2.41 | 800 | 0.3846 | 0.8285 | 0.8287 |
| 0.4048 | 3.01 | 1000 | 0.3807 | 0.8282 | 0.8283 |
| 0.4017 | 3.61 | 1200 | 0.3786 | 0.8327 | 0.8329 |
| 0.4027 | 4.22 | 1400 | 0.3787 | 0.8300 | 0.8300 |
| 0.4005 | 4.82 | 1600 | 0.3792 | 0.8292 | 0.8295 |
| 0.3899 | 5.42 | 1800 | 0.3771 | 0.8280 | 0.8280 |
| 0.394 | 6.02 | 2000 | 0.3774 | 0.8263 | 0.8266 |
| 0.3942 | 6.63 | 2200 | 0.3748 | 0.8345 | 0.8346 |
| 0.3877 | 7.23 | 2400 | 0.3779 | 0.8295 | 0.8300 |
| 0.3877 | 7.83 | 2600 | 0.3703 | 0.8323 | 0.8323 |
| 0.3829 | 8.43 | 2800 | 0.3835 | 0.8294 | 0.8302 |
| 0.3863 | 9.04 | 3000 | 0.3726 | 0.8317 | 0.8319 |
| 0.3812 | 9.64 | 3200 | 0.3712 | 0.8341 | 0.8342 |
| 0.3835 | 10.24 | 3400 | 0.3717 | 0.8342 | 0.8344 |
| 0.3795 | 10.84 | 3600 | 0.3686 | 0.8353 | 0.8353 |
| 0.3819 | 11.45 | 3800 | 0.3694 | 0.8332 | 0.8332 |
| 0.3786 | 12.05 | 4000 | 0.3681 | 0.8339 | 0.8340 |
| 0.3774 | 12.65 | 4200 | 0.3715 | 0.8328 | 0.8331 |
| 0.378 | 13.25 | 4400 | 0.3692 | 0.8344 | 0.8346 |
| 0.3807 | 13.86 | 4600 | 0.3729 | 0.8349 | 0.8351 |
| 0.3755 | 14.46 | 4800 | 0.3677 | 0.8365 | 0.8366 |
| 0.3748 | 15.06 | 5000 | 0.3677 | 0.8360 | 0.8363 |
| 0.3736 | 15.66 | 5200 | 0.3680 | 0.8374 | 0.8376 |
| 0.3727 | 16.27 | 5400 | 0.3673 | 0.8355 | 0.8355 |
| 0.3746 | 16.87 | 5600 | 0.3744 | 0.8336 | 0.8342 |
| 0.368 | 17.47 | 5800 | 0.3766 | 0.8326 | 0.8332 |
| 0.3773 | 18.07 | 6000 | 0.3727 | 0.8346 | 0.8351 |
| 0.37 | 18.67 | 6200 | 0.3685 | 0.8350 | 0.8351 |
| 0.3739 | 19.28 | 6400 | 0.3668 | 0.8359 | 0.8361 |
| 0.3694 | 19.88 | 6600 | 0.3676 | 0.8364 | 0.8366 |
| 0.3653 | 20.48 | 6800 | 0.3681 | 0.8361 | 0.8364 |
| 0.3708 | 21.08 | 7000 | 0.3727 | 0.8344 | 0.8349 |
| 0.3729 | 21.69 | 7200 | 0.3663 | 0.8360 | 0.8361 |
| 0.3621 | 22.29 | 7400 | 0.3683 | 0.8363 | 0.8366 |
| 0.3653 | 22.89 | 7600 | 0.3711 | 0.8360 | 0.8363 |
| 0.3666 | 23.49 | 7800 | 0.3670 | 0.8358 | 0.8361 |
| 0.3683 | 24.1 | 8000 | 0.3703 | 0.8361 | 0.8364 |
| 0.3671 | 24.7 | 8200 | 0.3719 | 0.8356 | 0.8361 |
| 0.3606 | 25.3 | 8400 | 0.3692 | 0.8369 | 0.8372 |
| 0.3679 | 25.9 | 8600 | 0.3660 | 0.8365 | 0.8366 |
| 0.3658 | 26.51 | 8800 | 0.3665 | 0.8359 | 0.8361 |
| 0.3678 | 27.11 | 9000 | 0.3655 | 0.8359 | 0.8361 |
| 0.3721 | 27.71 | 9200 | 0.3668 | 0.8354 | 0.8357 |
| 0.3569 | 28.31 | 9400 | 0.3690 | 0.8351 | 0.8355 |
| 0.3638 | 28.92 | 9600 | 0.3671 | 0.8357 | 0.8359 |
| 0.3689 | 29.52 | 9800 | 0.3664 | 0.8355 | 0.8357 |
| 0.3592 | 30.12 | 10000 | 0.3669 | 0.8349 | 0.8351 |
### Framework versions
- PEFT 0.9.0
- Transformers 4.38.2
- Pytorch 2.2.0+cu121
- Datasets 2.17.1
- Tokenizers 0.15.2 | {"library_name": "peft", "tags": ["generated_from_trainer"], "metrics": ["accuracy"], "base_model": "mahdibaghbanzadeh/seqsight_16384_512_22M", "model-index": [{"name": "GUE_prom_prom_core_notata-seqsight_16384_512_22M-L8_f", "results": []}]} | mahdibaghbanzadeh/GUE_prom_prom_core_notata-seqsight_16384_512_22M-L8_f | null | [
"peft",
"safetensors",
"generated_from_trainer",
"base_model:mahdibaghbanzadeh/seqsight_16384_512_22M",
"region:us"
] | null | 2024-04-27T06:51:39+00:00 | [] | [] | TAGS
#peft #safetensors #generated_from_trainer #base_model-mahdibaghbanzadeh/seqsight_16384_512_22M #region-us
| GUE\_prom\_prom\_core\_notata-seqsight\_16384\_512\_22M-L8\_f
=============================================================
This model is a fine-tuned version of mahdibaghbanzadeh/seqsight\_16384\_512\_22M on the mahdibaghbanzadeh/GUE\_prom\_prom\_core\_notata dataset.
It achieves the following results on the evaluation set:
* Loss: 0.3842
* F1 Score: 0.8302
* Accuracy: 0.8302
Model description
-----------------
More information needed
Intended uses & limitations
---------------------------
More information needed
Training and evaluation data
----------------------------
More information needed
Training procedure
------------------
### Training hyperparameters
The following hyperparameters were used during training:
* learning\_rate: 0.0005
* train\_batch\_size: 128
* eval\_batch\_size: 128
* seed: 42
* optimizer: Adam with betas=(0.9,0.999) and epsilon=1e-08
* lr\_scheduler\_type: linear
* training\_steps: 10000
### Training results
### Framework versions
* PEFT 0.9.0
* Transformers 4.38.2
* Pytorch 2.2.0+cu121
* Datasets 2.17.1
* Tokenizers 0.15.2
| [
"### Training hyperparameters\n\n\nThe following hyperparameters were used during training:\n\n\n* learning\\_rate: 0.0005\n* train\\_batch\\_size: 128\n* eval\\_batch\\_size: 128\n* seed: 42\n* optimizer: Adam with betas=(0.9,0.999) and epsilon=1e-08\n* lr\\_scheduler\\_type: linear\n* training\\_steps: 10000",
"### Training results",
"### Framework versions\n\n\n* PEFT 0.9.0\n* Transformers 4.38.2\n* Pytorch 2.2.0+cu121\n* Datasets 2.17.1\n* Tokenizers 0.15.2"
] | [
"TAGS\n#peft #safetensors #generated_from_trainer #base_model-mahdibaghbanzadeh/seqsight_16384_512_22M #region-us \n",
"### Training hyperparameters\n\n\nThe following hyperparameters were used during training:\n\n\n* learning\\_rate: 0.0005\n* train\\_batch\\_size: 128\n* eval\\_batch\\_size: 128\n* seed: 42\n* optimizer: Adam with betas=(0.9,0.999) and epsilon=1e-08\n* lr\\_scheduler\\_type: linear\n* training\\_steps: 10000",
"### Training results",
"### Framework versions\n\n\n* PEFT 0.9.0\n* Transformers 4.38.2\n* Pytorch 2.2.0+cu121\n* Datasets 2.17.1\n* Tokenizers 0.15.2"
] |
null | peft |
<!-- This model card has been generated automatically according to the information the Trainer had access to. You
should probably proofread and complete it, then remove this comment. -->
# GUE_prom_prom_core_notata-seqsight_16384_512_22M-L1_f
This model is a fine-tuned version of [mahdibaghbanzadeh/seqsight_16384_512_22M](https://huggingface.co/mahdibaghbanzadeh/seqsight_16384_512_22M) on the [mahdibaghbanzadeh/GUE_prom_prom_core_notata](https://huggingface.co/datasets/mahdibaghbanzadeh/GUE_prom_prom_core_notata) dataset.
It achieves the following results on the evaluation set:
- Loss: 0.3876
- F1 Score: 0.8262
- Accuracy: 0.8263
## Model description
More information needed
## Intended uses & limitations
More information needed
## Training and evaluation data
More information needed
## Training procedure
### Training hyperparameters
The following hyperparameters were used during training:
- learning_rate: 0.0005
- train_batch_size: 128
- eval_batch_size: 128
- seed: 42
- optimizer: Adam with betas=(0.9,0.999) and epsilon=1e-08
- lr_scheduler_type: linear
- training_steps: 10000
### Training results
| Training Loss | Epoch | Step | Validation Loss | F1 Score | Accuracy |
|:-------------:|:-----:|:-----:|:---------------:|:--------:|:--------:|
| 0.599 | 0.6 | 200 | 0.5009 | 0.7598 | 0.7605 |
| 0.5087 | 1.2 | 400 | 0.4444 | 0.7959 | 0.7959 |
| 0.4712 | 1.81 | 600 | 0.4240 | 0.8048 | 0.8050 |
| 0.4562 | 2.41 | 800 | 0.4106 | 0.8167 | 0.8167 |
| 0.4398 | 3.01 | 1000 | 0.4037 | 0.8198 | 0.8199 |
| 0.4332 | 3.61 | 1200 | 0.3945 | 0.8278 | 0.8278 |
| 0.4306 | 4.22 | 1400 | 0.3928 | 0.8259 | 0.8259 |
| 0.4253 | 4.82 | 1600 | 0.3867 | 0.8266 | 0.8266 |
| 0.4152 | 5.42 | 1800 | 0.3868 | 0.8273 | 0.8274 |
| 0.4136 | 6.02 | 2000 | 0.3834 | 0.8300 | 0.8302 |
| 0.4131 | 6.63 | 2200 | 0.3820 | 0.8281 | 0.8282 |
| 0.4078 | 7.23 | 2400 | 0.3854 | 0.8276 | 0.8282 |
| 0.4047 | 7.83 | 2600 | 0.3816 | 0.8290 | 0.8293 |
| 0.4015 | 8.43 | 2800 | 0.3839 | 0.8267 | 0.8270 |
| 0.4027 | 9.04 | 3000 | 0.3845 | 0.8260 | 0.8265 |
| 0.4003 | 9.64 | 3200 | 0.3785 | 0.8271 | 0.8272 |
| 0.4017 | 10.24 | 3400 | 0.3779 | 0.8308 | 0.8308 |
| 0.398 | 10.84 | 3600 | 0.3774 | 0.8280 | 0.8280 |
| 0.4007 | 11.45 | 3800 | 0.3776 | 0.8300 | 0.8300 |
| 0.3966 | 12.05 | 4000 | 0.3772 | 0.8316 | 0.8317 |
| 0.3969 | 12.65 | 4200 | 0.3782 | 0.8290 | 0.8291 |
| 0.3978 | 13.25 | 4400 | 0.3782 | 0.8290 | 0.8291 |
| 0.401 | 13.86 | 4600 | 0.3768 | 0.8289 | 0.8289 |
| 0.3947 | 14.46 | 4800 | 0.3768 | 0.8309 | 0.8310 |
| 0.3951 | 15.06 | 5000 | 0.3772 | 0.8314 | 0.8315 |
| 0.3952 | 15.66 | 5200 | 0.3750 | 0.8323 | 0.8323 |
| 0.3933 | 16.27 | 5400 | 0.3759 | 0.8298 | 0.8298 |
| 0.3947 | 16.87 | 5600 | 0.3822 | 0.8296 | 0.8300 |
| 0.3898 | 17.47 | 5800 | 0.3828 | 0.8289 | 0.8295 |
| 0.3972 | 18.07 | 6000 | 0.3775 | 0.8330 | 0.8332 |
| 0.3911 | 18.67 | 6200 | 0.3747 | 0.8315 | 0.8315 |
| 0.3946 | 19.28 | 6400 | 0.3744 | 0.8324 | 0.8325 |
| 0.3924 | 19.88 | 6600 | 0.3748 | 0.8322 | 0.8323 |
| 0.388 | 20.48 | 6800 | 0.3777 | 0.8325 | 0.8329 |
| 0.3919 | 21.08 | 7000 | 0.3780 | 0.8326 | 0.8329 |
| 0.3949 | 21.69 | 7200 | 0.3738 | 0.8317 | 0.8317 |
| 0.3847 | 22.29 | 7400 | 0.3756 | 0.8334 | 0.8336 |
| 0.3866 | 22.89 | 7600 | 0.3761 | 0.8325 | 0.8327 |
| 0.3891 | 23.49 | 7800 | 0.3752 | 0.8318 | 0.8319 |
| 0.3906 | 24.1 | 8000 | 0.3770 | 0.8326 | 0.8329 |
| 0.3891 | 24.7 | 8200 | 0.3792 | 0.8312 | 0.8315 |
| 0.382 | 25.3 | 8400 | 0.3772 | 0.8323 | 0.8325 |
| 0.3903 | 25.9 | 8600 | 0.3743 | 0.8331 | 0.8332 |
| 0.3882 | 26.51 | 8800 | 0.3742 | 0.8328 | 0.8329 |
| 0.3881 | 27.11 | 9000 | 0.3741 | 0.8327 | 0.8329 |
| 0.3938 | 27.71 | 9200 | 0.3741 | 0.8329 | 0.8331 |
| 0.3808 | 28.31 | 9400 | 0.3766 | 0.8328 | 0.8331 |
| 0.3873 | 28.92 | 9600 | 0.3750 | 0.8333 | 0.8334 |
| 0.3899 | 29.52 | 9800 | 0.3747 | 0.8331 | 0.8332 |
| 0.3826 | 30.12 | 10000 | 0.3750 | 0.8329 | 0.8331 |
### Framework versions
- PEFT 0.9.0
- Transformers 4.38.2
- Pytorch 2.2.0+cu121
- Datasets 2.17.1
- Tokenizers 0.15.2 | {"library_name": "peft", "tags": ["generated_from_trainer"], "metrics": ["accuracy"], "base_model": "mahdibaghbanzadeh/seqsight_16384_512_22M", "model-index": [{"name": "GUE_prom_prom_core_notata-seqsight_16384_512_22M-L1_f", "results": []}]} | mahdibaghbanzadeh/GUE_prom_prom_core_notata-seqsight_16384_512_22M-L1_f | null | [
"peft",
"safetensors",
"generated_from_trainer",
"base_model:mahdibaghbanzadeh/seqsight_16384_512_22M",
"region:us"
] | null | 2024-04-27T06:51:39+00:00 | [] | [] | TAGS
#peft #safetensors #generated_from_trainer #base_model-mahdibaghbanzadeh/seqsight_16384_512_22M #region-us
| GUE\_prom\_prom\_core\_notata-seqsight\_16384\_512\_22M-L1\_f
=============================================================
This model is a fine-tuned version of mahdibaghbanzadeh/seqsight\_16384\_512\_22M on the mahdibaghbanzadeh/GUE\_prom\_prom\_core\_notata dataset.
It achieves the following results on the evaluation set:
* Loss: 0.3876
* F1 Score: 0.8262
* Accuracy: 0.8263
Model description
-----------------
More information needed
Intended uses & limitations
---------------------------
More information needed
Training and evaluation data
----------------------------
More information needed
Training procedure
------------------
### Training hyperparameters
The following hyperparameters were used during training:
* learning\_rate: 0.0005
* train\_batch\_size: 128
* eval\_batch\_size: 128
* seed: 42
* optimizer: Adam with betas=(0.9,0.999) and epsilon=1e-08
* lr\_scheduler\_type: linear
* training\_steps: 10000
### Training results
### Framework versions
* PEFT 0.9.0
* Transformers 4.38.2
* Pytorch 2.2.0+cu121
* Datasets 2.17.1
* Tokenizers 0.15.2
| [
"### Training hyperparameters\n\n\nThe following hyperparameters were used during training:\n\n\n* learning\\_rate: 0.0005\n* train\\_batch\\_size: 128\n* eval\\_batch\\_size: 128\n* seed: 42\n* optimizer: Adam with betas=(0.9,0.999) and epsilon=1e-08\n* lr\\_scheduler\\_type: linear\n* training\\_steps: 10000",
"### Training results",
"### Framework versions\n\n\n* PEFT 0.9.0\n* Transformers 4.38.2\n* Pytorch 2.2.0+cu121\n* Datasets 2.17.1\n* Tokenizers 0.15.2"
] | [
"TAGS\n#peft #safetensors #generated_from_trainer #base_model-mahdibaghbanzadeh/seqsight_16384_512_22M #region-us \n",
"### Training hyperparameters\n\n\nThe following hyperparameters were used during training:\n\n\n* learning\\_rate: 0.0005\n* train\\_batch\\_size: 128\n* eval\\_batch\\_size: 128\n* seed: 42\n* optimizer: Adam with betas=(0.9,0.999) and epsilon=1e-08\n* lr\\_scheduler\\_type: linear\n* training\\_steps: 10000",
"### Training results",
"### Framework versions\n\n\n* PEFT 0.9.0\n* Transformers 4.38.2\n* Pytorch 2.2.0+cu121\n* Datasets 2.17.1\n* Tokenizers 0.15.2"
] |
text-generation | transformers |
# Model Card for Model ID
<!-- Provide a quick summary of what the model is/does. -->
## Model Details
### Model Description
<!-- Provide a longer summary of what this model is. -->
This is the model card of a 🤗 transformers model that has been pushed on the Hub. This model card has been automatically generated.
- **Developed by:** [More Information Needed]
- **Funded by [optional]:** [More Information Needed]
- **Shared by [optional]:** [More Information Needed]
- **Model type:** [More Information Needed]
- **Language(s) (NLP):** [More Information Needed]
- **License:** [More Information Needed]
- **Finetuned from model [optional]:** [More Information Needed]
### Model Sources [optional]
<!-- Provide the basic links for the model. -->
- **Repository:** [More Information Needed]
- **Paper [optional]:** [More Information Needed]
- **Demo [optional]:** [More Information Needed]
## Uses
<!-- Address questions around how the model is intended to be used, including the foreseeable users of the model and those affected by the model. -->
### Direct Use
<!-- This section is for the model use without fine-tuning or plugging into a larger ecosystem/app. -->
[More Information Needed]
### Downstream Use [optional]
<!-- This section is for the model use when fine-tuned for a task, or when plugged into a larger ecosystem/app -->
[More Information Needed]
### Out-of-Scope Use
<!-- This section addresses misuse, malicious use, and uses that the model will not work well for. -->
[More Information Needed]
## Bias, Risks, and Limitations
<!-- This section is meant to convey both technical and sociotechnical limitations. -->
[More Information Needed]
### Recommendations
<!-- This section is meant to convey recommendations with respect to the bias, risk, and technical limitations. -->
Users (both direct and downstream) should be made aware of the risks, biases and limitations of the model. More information needed for further recommendations.
## How to Get Started with the Model
Use the code below to get started with the model.
[More Information Needed]
## Training Details
### Training Data
<!-- This should link to a Dataset Card, perhaps with a short stub of information on what the training data is all about as well as documentation related to data pre-processing or additional filtering. -->
[More Information Needed]
### Training Procedure
<!-- This relates heavily to the Technical Specifications. Content here should link to that section when it is relevant to the training procedure. -->
#### Preprocessing [optional]
[More Information Needed]
#### Training Hyperparameters
- **Training regime:** [More Information Needed] <!--fp32, fp16 mixed precision, bf16 mixed precision, bf16 non-mixed precision, fp16 non-mixed precision, fp8 mixed precision -->
#### Speeds, Sizes, Times [optional]
<!-- This section provides information about throughput, start/end time, checkpoint size if relevant, etc. -->
[More Information Needed]
## Evaluation
<!-- This section describes the evaluation protocols and provides the results. -->
### Testing Data, Factors & Metrics
#### Testing Data
<!-- This should link to a Dataset Card if possible. -->
[More Information Needed]
#### Factors
<!-- These are the things the evaluation is disaggregating by, e.g., subpopulations or domains. -->
[More Information Needed]
#### Metrics
<!-- These are the evaluation metrics being used, ideally with a description of why. -->
[More Information Needed]
### Results
[More Information Needed]
#### Summary
## Model Examination [optional]
<!-- Relevant interpretability work for the model goes here -->
[More Information Needed]
## Environmental Impact
<!-- Total emissions (in grams of CO2eq) and additional considerations, such as electricity usage, go here. Edit the suggested text below accordingly -->
Carbon emissions can be estimated using the [Machine Learning Impact calculator](https://mlco2.github.io/impact#compute) presented in [Lacoste et al. (2019)](https://arxiv.org/abs/1910.09700).
- **Hardware Type:** [More Information Needed]
- **Hours used:** [More Information Needed]
- **Cloud Provider:** [More Information Needed]
- **Compute Region:** [More Information Needed]
- **Carbon Emitted:** [More Information Needed]
## Technical Specifications [optional]
### Model Architecture and Objective
[More Information Needed]
### Compute Infrastructure
[More Information Needed]
#### Hardware
[More Information Needed]
#### Software
[More Information Needed]
## Citation [optional]
<!-- If there is a paper or blog post introducing the model, the APA and Bibtex information for that should go in this section. -->
**BibTeX:**
[More Information Needed]
**APA:**
[More Information Needed]
## Glossary [optional]
<!-- If relevant, include terms and calculations in this section that can help readers understand the model or model card. -->
[More Information Needed]
## More Information [optional]
[More Information Needed]
## Model Card Authors [optional]
[More Information Needed]
## Model Card Contact
[More Information Needed] | {"library_name": "transformers", "tags": []} | fenguhao/hh-rlhf-dpo-0.5 | null | [
"transformers",
"safetensors",
"gpt_neox",
"text-generation",
"arxiv:1910.09700",
"autotrain_compatible",
"endpoints_compatible",
"text-generation-inference",
"region:us"
] | null | 2024-04-27T06:53:13+00:00 | [
"1910.09700"
] | [] | TAGS
#transformers #safetensors #gpt_neox #text-generation #arxiv-1910.09700 #autotrain_compatible #endpoints_compatible #text-generation-inference #region-us
|
# Model Card for Model ID
## Model Details
### Model Description
This is the model card of a transformers model that has been pushed on the Hub. This model card has been automatically generated.
- Developed by:
- Funded by [optional]:
- Shared by [optional]:
- Model type:
- Language(s) (NLP):
- License:
- Finetuned from model [optional]:
### Model Sources [optional]
- Repository:
- Paper [optional]:
- Demo [optional]:
## Uses
### Direct Use
### Downstream Use [optional]
### Out-of-Scope Use
## Bias, Risks, and Limitations
### Recommendations
Users (both direct and downstream) should be made aware of the risks, biases and limitations of the model. More information needed for further recommendations.
## How to Get Started with the Model
Use the code below to get started with the model.
## Training Details
### Training Data
### Training Procedure
#### Preprocessing [optional]
#### Training Hyperparameters
- Training regime:
#### Speeds, Sizes, Times [optional]
## Evaluation
### Testing Data, Factors & Metrics
#### Testing Data
#### Factors
#### Metrics
### Results
#### Summary
## Model Examination [optional]
## Environmental Impact
Carbon emissions can be estimated using the Machine Learning Impact calculator presented in Lacoste et al. (2019).
- Hardware Type:
- Hours used:
- Cloud Provider:
- Compute Region:
- Carbon Emitted:
## Technical Specifications [optional]
### Model Architecture and Objective
### Compute Infrastructure
#### Hardware
#### Software
[optional]
BibTeX:
APA:
## Glossary [optional]
## More Information [optional]
## Model Card Authors [optional]
## Model Card Contact
| [
"# Model Card for Model ID",
"## Model Details",
"### Model Description\n\n\n\nThis is the model card of a transformers model that has been pushed on the Hub. This model card has been automatically generated.\n\n- Developed by: \n- Funded by [optional]: \n- Shared by [optional]: \n- Model type: \n- Language(s) (NLP): \n- License: \n- Finetuned from model [optional]:",
"### Model Sources [optional]\n\n\n\n- Repository: \n- Paper [optional]: \n- Demo [optional]:",
"## Uses",
"### Direct Use",
"### Downstream Use [optional]",
"### Out-of-Scope Use",
"## Bias, Risks, and Limitations",
"### Recommendations\n\n\n\nUsers (both direct and downstream) should be made aware of the risks, biases and limitations of the model. More information needed for further recommendations.",
"## How to Get Started with the Model\n\nUse the code below to get started with the model.",
"## Training Details",
"### Training Data",
"### Training Procedure",
"#### Preprocessing [optional]",
"#### Training Hyperparameters\n\n- Training regime:",
"#### Speeds, Sizes, Times [optional]",
"## Evaluation",
"### Testing Data, Factors & Metrics",
"#### Testing Data",
"#### Factors",
"#### Metrics",
"### Results",
"#### Summary",
"## Model Examination [optional]",
"## Environmental Impact\n\n\n\nCarbon emissions can be estimated using the Machine Learning Impact calculator presented in Lacoste et al. (2019).\n\n- Hardware Type: \n- Hours used: \n- Cloud Provider: \n- Compute Region: \n- Carbon Emitted:",
"## Technical Specifications [optional]",
"### Model Architecture and Objective",
"### Compute Infrastructure",
"#### Hardware",
"#### Software\n\n\n\n[optional]\n\n\n\nBibTeX:\n\n\n\nAPA:",
"## Glossary [optional]",
"## More Information [optional]",
"## Model Card Authors [optional]",
"## Model Card Contact"
] | [
"TAGS\n#transformers #safetensors #gpt_neox #text-generation #arxiv-1910.09700 #autotrain_compatible #endpoints_compatible #text-generation-inference #region-us \n",
"# Model Card for Model ID",
"## Model Details",
"### Model Description\n\n\n\nThis is the model card of a transformers model that has been pushed on the Hub. This model card has been automatically generated.\n\n- Developed by: \n- Funded by [optional]: \n- Shared by [optional]: \n- Model type: \n- Language(s) (NLP): \n- License: \n- Finetuned from model [optional]:",
"### Model Sources [optional]\n\n\n\n- Repository: \n- Paper [optional]: \n- Demo [optional]:",
"## Uses",
"### Direct Use",
"### Downstream Use [optional]",
"### Out-of-Scope Use",
"## Bias, Risks, and Limitations",
"### Recommendations\n\n\n\nUsers (both direct and downstream) should be made aware of the risks, biases and limitations of the model. More information needed for further recommendations.",
"## How to Get Started with the Model\n\nUse the code below to get started with the model.",
"## Training Details",
"### Training Data",
"### Training Procedure",
"#### Preprocessing [optional]",
"#### Training Hyperparameters\n\n- Training regime:",
"#### Speeds, Sizes, Times [optional]",
"## Evaluation",
"### Testing Data, Factors & Metrics",
"#### Testing Data",
"#### Factors",
"#### Metrics",
"### Results",
"#### Summary",
"## Model Examination [optional]",
"## Environmental Impact\n\n\n\nCarbon emissions can be estimated using the Machine Learning Impact calculator presented in Lacoste et al. (2019).\n\n- Hardware Type: \n- Hours used: \n- Cloud Provider: \n- Compute Region: \n- Carbon Emitted:",
"## Technical Specifications [optional]",
"### Model Architecture and Objective",
"### Compute Infrastructure",
"#### Hardware",
"#### Software\n\n\n\n[optional]\n\n\n\nBibTeX:\n\n\n\nAPA:",
"## Glossary [optional]",
"## More Information [optional]",
"## Model Card Authors [optional]",
"## Model Card Contact"
] |
null | peft |
<!-- This model card has been generated automatically according to the information the Trainer had access to. You
should probably proofread and complete it, then remove this comment. -->
# GUE_prom_prom_core_notata-seqsight_16384_512_22M-L32_f
This model is a fine-tuned version of [mahdibaghbanzadeh/seqsight_16384_512_22M](https://huggingface.co/mahdibaghbanzadeh/seqsight_16384_512_22M) on the [mahdibaghbanzadeh/GUE_prom_prom_core_notata](https://huggingface.co/datasets/mahdibaghbanzadeh/GUE_prom_prom_core_notata) dataset.
It achieves the following results on the evaluation set:
- Loss: 0.3809
- F1 Score: 0.8327
- Accuracy: 0.8327
## Model description
More information needed
## Intended uses & limitations
More information needed
## Training and evaluation data
More information needed
## Training procedure
### Training hyperparameters
The following hyperparameters were used during training:
- learning_rate: 0.0005
- train_batch_size: 128
- eval_batch_size: 128
- seed: 42
- optimizer: Adam with betas=(0.9,0.999) and epsilon=1e-08
- lr_scheduler_type: linear
- training_steps: 10000
### Training results
| Training Loss | Epoch | Step | Validation Loss | F1 Score | Accuracy |
|:-------------:|:-----:|:-----:|:---------------:|:--------:|:--------:|
| 0.5454 | 0.6 | 200 | 0.4356 | 0.7928 | 0.7940 |
| 0.4294 | 1.2 | 400 | 0.3888 | 0.8291 | 0.8291 |
| 0.4088 | 1.81 | 600 | 0.3874 | 0.8299 | 0.8302 |
| 0.4022 | 2.41 | 800 | 0.3808 | 0.8334 | 0.8336 |
| 0.395 | 3.01 | 1000 | 0.3802 | 0.8319 | 0.8323 |
| 0.3909 | 3.61 | 1200 | 0.3777 | 0.8354 | 0.8357 |
| 0.3921 | 4.22 | 1400 | 0.3732 | 0.8349 | 0.8349 |
| 0.3896 | 4.82 | 1600 | 0.3753 | 0.8333 | 0.8336 |
| 0.3785 | 5.42 | 1800 | 0.3732 | 0.8329 | 0.8329 |
| 0.3839 | 6.02 | 2000 | 0.3740 | 0.8354 | 0.8357 |
| 0.3814 | 6.63 | 2200 | 0.3722 | 0.8380 | 0.8381 |
| 0.3754 | 7.23 | 2400 | 0.3780 | 0.8340 | 0.8346 |
| 0.375 | 7.83 | 2600 | 0.3668 | 0.8385 | 0.8385 |
| 0.3692 | 8.43 | 2800 | 0.3805 | 0.8358 | 0.8364 |
| 0.3729 | 9.04 | 3000 | 0.3733 | 0.8380 | 0.8381 |
| 0.3688 | 9.64 | 3200 | 0.3706 | 0.8380 | 0.8381 |
| 0.3686 | 10.24 | 3400 | 0.3700 | 0.8392 | 0.8393 |
| 0.3657 | 10.84 | 3600 | 0.3663 | 0.8395 | 0.8396 |
| 0.367 | 11.45 | 3800 | 0.3662 | 0.8400 | 0.8400 |
| 0.3643 | 12.05 | 4000 | 0.3660 | 0.8373 | 0.8374 |
| 0.3605 | 12.65 | 4200 | 0.3702 | 0.8351 | 0.8353 |
| 0.3627 | 13.25 | 4400 | 0.3690 | 0.8380 | 0.8381 |
| 0.3648 | 13.86 | 4600 | 0.3738 | 0.8395 | 0.8398 |
| 0.359 | 14.46 | 4800 | 0.3685 | 0.8391 | 0.8393 |
| 0.3582 | 15.06 | 5000 | 0.3672 | 0.8377 | 0.8379 |
| 0.3546 | 15.66 | 5200 | 0.3717 | 0.8374 | 0.8376 |
| 0.356 | 16.27 | 5400 | 0.3697 | 0.8364 | 0.8364 |
| 0.3576 | 16.87 | 5600 | 0.3829 | 0.8312 | 0.8319 |
| 0.3492 | 17.47 | 5800 | 0.3789 | 0.8332 | 0.8338 |
| 0.36 | 18.07 | 6000 | 0.3767 | 0.8359 | 0.8364 |
| 0.3515 | 18.67 | 6200 | 0.3726 | 0.8376 | 0.8378 |
| 0.3552 | 19.28 | 6400 | 0.3708 | 0.8383 | 0.8385 |
| 0.3499 | 19.88 | 6600 | 0.3696 | 0.8380 | 0.8383 |
| 0.3453 | 20.48 | 6800 | 0.3717 | 0.8358 | 0.8361 |
| 0.3514 | 21.08 | 7000 | 0.3809 | 0.8358 | 0.8363 |
| 0.3533 | 21.69 | 7200 | 0.3723 | 0.8350 | 0.8351 |
| 0.3427 | 22.29 | 7400 | 0.3763 | 0.8345 | 0.8349 |
| 0.344 | 22.89 | 7600 | 0.3774 | 0.8366 | 0.8368 |
| 0.3451 | 23.49 | 7800 | 0.3723 | 0.8356 | 0.8359 |
| 0.349 | 24.1 | 8000 | 0.3782 | 0.8355 | 0.8359 |
| 0.3458 | 24.7 | 8200 | 0.3785 | 0.8326 | 0.8331 |
| 0.3402 | 25.3 | 8400 | 0.3771 | 0.8378 | 0.8381 |
| 0.3466 | 25.9 | 8600 | 0.3722 | 0.8378 | 0.8379 |
| 0.3426 | 26.51 | 8800 | 0.3739 | 0.8344 | 0.8346 |
| 0.3463 | 27.11 | 9000 | 0.3714 | 0.8380 | 0.8381 |
| 0.3511 | 27.71 | 9200 | 0.3738 | 0.8363 | 0.8366 |
| 0.3357 | 28.31 | 9400 | 0.3762 | 0.8359 | 0.8363 |
| 0.3418 | 28.92 | 9600 | 0.3753 | 0.8377 | 0.8379 |
| 0.3477 | 29.52 | 9800 | 0.3729 | 0.8372 | 0.8374 |
| 0.3378 | 30.12 | 10000 | 0.3739 | 0.8374 | 0.8376 |
### Framework versions
- PEFT 0.9.0
- Transformers 4.38.2
- Pytorch 2.2.0+cu121
- Datasets 2.17.1
- Tokenizers 0.15.2 | {"library_name": "peft", "tags": ["generated_from_trainer"], "metrics": ["accuracy"], "base_model": "mahdibaghbanzadeh/seqsight_16384_512_22M", "model-index": [{"name": "GUE_prom_prom_core_notata-seqsight_16384_512_22M-L32_f", "results": []}]} | mahdibaghbanzadeh/GUE_prom_prom_core_notata-seqsight_16384_512_22M-L32_f | null | [
"peft",
"safetensors",
"generated_from_trainer",
"base_model:mahdibaghbanzadeh/seqsight_16384_512_22M",
"region:us"
] | null | 2024-04-27T06:53:56+00:00 | [] | [] | TAGS
#peft #safetensors #generated_from_trainer #base_model-mahdibaghbanzadeh/seqsight_16384_512_22M #region-us
| GUE\_prom\_prom\_core\_notata-seqsight\_16384\_512\_22M-L32\_f
==============================================================
This model is a fine-tuned version of mahdibaghbanzadeh/seqsight\_16384\_512\_22M on the mahdibaghbanzadeh/GUE\_prom\_prom\_core\_notata dataset.
It achieves the following results on the evaluation set:
* Loss: 0.3809
* F1 Score: 0.8327
* Accuracy: 0.8327
Model description
-----------------
More information needed
Intended uses & limitations
---------------------------
More information needed
Training and evaluation data
----------------------------
More information needed
Training procedure
------------------
### Training hyperparameters
The following hyperparameters were used during training:
* learning\_rate: 0.0005
* train\_batch\_size: 128
* eval\_batch\_size: 128
* seed: 42
* optimizer: Adam with betas=(0.9,0.999) and epsilon=1e-08
* lr\_scheduler\_type: linear
* training\_steps: 10000
### Training results
### Framework versions
* PEFT 0.9.0
* Transformers 4.38.2
* Pytorch 2.2.0+cu121
* Datasets 2.17.1
* Tokenizers 0.15.2
| [
"### Training hyperparameters\n\n\nThe following hyperparameters were used during training:\n\n\n* learning\\_rate: 0.0005\n* train\\_batch\\_size: 128\n* eval\\_batch\\_size: 128\n* seed: 42\n* optimizer: Adam with betas=(0.9,0.999) and epsilon=1e-08\n* lr\\_scheduler\\_type: linear\n* training\\_steps: 10000",
"### Training results",
"### Framework versions\n\n\n* PEFT 0.9.0\n* Transformers 4.38.2\n* Pytorch 2.2.0+cu121\n* Datasets 2.17.1\n* Tokenizers 0.15.2"
] | [
"TAGS\n#peft #safetensors #generated_from_trainer #base_model-mahdibaghbanzadeh/seqsight_16384_512_22M #region-us \n",
"### Training hyperparameters\n\n\nThe following hyperparameters were used during training:\n\n\n* learning\\_rate: 0.0005\n* train\\_batch\\_size: 128\n* eval\\_batch\\_size: 128\n* seed: 42\n* optimizer: Adam with betas=(0.9,0.999) and epsilon=1e-08\n* lr\\_scheduler\\_type: linear\n* training\\_steps: 10000",
"### Training results",
"### Framework versions\n\n\n* PEFT 0.9.0\n* Transformers 4.38.2\n* Pytorch 2.2.0+cu121\n* Datasets 2.17.1\n* Tokenizers 0.15.2"
] |
null | sentence-transformers | [Biopeak Male Enhancement](https://pgccouncilcsp.powerappsportals.us/forums/general-discussion/8acfd8e8-aa03-ef11-a73d-001dd806eee4) Furthermore, solid way of life decisions like standard activity, a decent eating regimen, overseeing pressure, and sufficient rest can emphatically influence sexual wellbeing and execution.Upgraded Size: A few items or procedures guarantee to increment penis size, albeit these cases can frequently need logical proof or may not deliver critical extremely durable changes.Boosted Moxie: Enhancements or strategies could invigorate sex drive and desire.Improved Relationship Fulfillment: Better sexual execution and fulfillment can emphatically influence connections.It's vital to take note of that not all upgrade techniques are therapeutically or experimentally demonstrated, and numerous items advertised for these reasons might need guideline or logical proof supporting their adequacy and wellbeing. Prior to considering any type of male upgrade, it's significant to talk with a medical care proficient to grasp expected dangers, viability, and legitimate use.
VISIT HERE FOR OFFICIAL WEBSITE:-https://pgccouncilcsp.powerappsportals.us/forums/general-discussion/8acfd8e8-aa03-ef11-a73d-001dd806eee4
| {"language": ["en"], "license": "bsd-2-clause", "library_name": "sentence-transformers", "tags": ["Biopeak Male Enhancement"]} | getbiopeakmaleenhancement/biopeakmaleenhancement | null | [
"sentence-transformers",
"Biopeak Male Enhancement",
"en",
"license:bsd-2-clause",
"region:us"
] | null | 2024-04-27T06:54:39+00:00 | [] | [
"en"
] | TAGS
#sentence-transformers #Biopeak Male Enhancement #en #license-bsd-2-clause #region-us
| Biopeak Male Enhancement Furthermore, solid way of life decisions like standard activity, a decent eating regimen, overseeing pressure, and sufficient rest can emphatically influence sexual wellbeing and execution.Upgraded Size: A few items or procedures guarantee to increment penis size, albeit these cases can frequently need logical proof or may not deliver critical extremely durable changes.Boosted Moxie: Enhancements or strategies could invigorate sex drive and desire.Improved Relationship Fulfillment: Better sexual execution and fulfillment can emphatically influence connections.It's vital to take note of that not all upgrade techniques are therapeutically or experimentally demonstrated, and numerous items advertised for these reasons might need guideline or logical proof supporting their adequacy and wellbeing. Prior to considering any type of male upgrade, it's significant to talk with a medical care proficient to grasp expected dangers, viability, and legitimate use.
VISIT HERE FOR OFFICIAL WEBSITE:-URL
| [] | [
"TAGS\n#sentence-transformers #Biopeak Male Enhancement #en #license-bsd-2-clause #region-us \n"
] |
text-generation | transformers |
<!-- This model card has been generated automatically according to the information the Trainer had access to. You
should probably proofread and complete it, then remove this comment. -->
# 0.001_4iters_bs128_nodpo_only4w_iter_2
This model is a fine-tuned version of [ShenaoZhang/0.001_4iters_bs128_nodpo_only4w_iter_1](https://huggingface.co/ShenaoZhang/0.001_4iters_bs128_nodpo_only4w_iter_1) on the updated and the original datasets.
## Model description
More information needed
## Intended uses & limitations
More information needed
## Training and evaluation data
More information needed
## Training procedure
### Training hyperparameters
The following hyperparameters were used during training:
- learning_rate: 5e-07
- train_batch_size: 8
- eval_batch_size: 8
- seed: 42
- distributed_type: multi-GPU
- num_devices: 8
- gradient_accumulation_steps: 2
- total_train_batch_size: 128
- total_eval_batch_size: 64
- optimizer: Adam with betas=(0.9,0.999) and epsilon=1e-08
- lr_scheduler_type: cosine
- lr_scheduler_warmup_ratio: 0.1
- num_epochs: 1
### Training results
### Framework versions
- Transformers 4.40.0
- Pytorch 2.1.2+cu121
- Datasets 2.14.6
- Tokenizers 0.19.1
| {"license": "mit", "tags": ["alignment-handbook", "trl", "dpo", "generated_from_trainer", "trl", "dpo", "generated_from_trainer"], "datasets": ["updated", "original"], "base_model": "ShenaoZhang/0.001_4iters_bs128_nodpo_only4w_iter_1", "model-index": [{"name": "0.001_4iters_bs128_nodpo_only4w_iter_2", "results": []}]} | ShenaoZhang/0.001_4iters_bs128_nodpo_only4w_iter_2 | null | [
"transformers",
"safetensors",
"mistral",
"text-generation",
"alignment-handbook",
"trl",
"dpo",
"generated_from_trainer",
"conversational",
"dataset:updated",
"dataset:original",
"base_model:ShenaoZhang/0.001_4iters_bs128_nodpo_only4w_iter_1",
"license:mit",
"autotrain_compatible",
"endpoints_compatible",
"text-generation-inference",
"region:us"
] | null | 2024-04-27T06:55:27+00:00 | [] | [] | TAGS
#transformers #safetensors #mistral #text-generation #alignment-handbook #trl #dpo #generated_from_trainer #conversational #dataset-updated #dataset-original #base_model-ShenaoZhang/0.001_4iters_bs128_nodpo_only4w_iter_1 #license-mit #autotrain_compatible #endpoints_compatible #text-generation-inference #region-us
|
# 0.001_4iters_bs128_nodpo_only4w_iter_2
This model is a fine-tuned version of ShenaoZhang/0.001_4iters_bs128_nodpo_only4w_iter_1 on the updated and the original datasets.
## Model description
More information needed
## Intended uses & limitations
More information needed
## Training and evaluation data
More information needed
## Training procedure
### Training hyperparameters
The following hyperparameters were used during training:
- learning_rate: 5e-07
- train_batch_size: 8
- eval_batch_size: 8
- seed: 42
- distributed_type: multi-GPU
- num_devices: 8
- gradient_accumulation_steps: 2
- total_train_batch_size: 128
- total_eval_batch_size: 64
- optimizer: Adam with betas=(0.9,0.999) and epsilon=1e-08
- lr_scheduler_type: cosine
- lr_scheduler_warmup_ratio: 0.1
- num_epochs: 1
### Training results
### Framework versions
- Transformers 4.40.0
- Pytorch 2.1.2+cu121
- Datasets 2.14.6
- Tokenizers 0.19.1
| [
"# 0.001_4iters_bs128_nodpo_only4w_iter_2\n\nThis model is a fine-tuned version of ShenaoZhang/0.001_4iters_bs128_nodpo_only4w_iter_1 on the updated and the original datasets.",
"## Model description\n\nMore information needed",
"## Intended uses & limitations\n\nMore information needed",
"## Training and evaluation data\n\nMore information needed",
"## Training procedure",
"### Training hyperparameters\n\nThe following hyperparameters were used during training:\n- learning_rate: 5e-07\n- train_batch_size: 8\n- eval_batch_size: 8\n- seed: 42\n- distributed_type: multi-GPU\n- num_devices: 8\n- gradient_accumulation_steps: 2\n- total_train_batch_size: 128\n- total_eval_batch_size: 64\n- optimizer: Adam with betas=(0.9,0.999) and epsilon=1e-08\n- lr_scheduler_type: cosine\n- lr_scheduler_warmup_ratio: 0.1\n- num_epochs: 1",
"### Training results",
"### Framework versions\n\n- Transformers 4.40.0\n- Pytorch 2.1.2+cu121\n- Datasets 2.14.6\n- Tokenizers 0.19.1"
] | [
"TAGS\n#transformers #safetensors #mistral #text-generation #alignment-handbook #trl #dpo #generated_from_trainer #conversational #dataset-updated #dataset-original #base_model-ShenaoZhang/0.001_4iters_bs128_nodpo_only4w_iter_1 #license-mit #autotrain_compatible #endpoints_compatible #text-generation-inference #region-us \n",
"# 0.001_4iters_bs128_nodpo_only4w_iter_2\n\nThis model is a fine-tuned version of ShenaoZhang/0.001_4iters_bs128_nodpo_only4w_iter_1 on the updated and the original datasets.",
"## Model description\n\nMore information needed",
"## Intended uses & limitations\n\nMore information needed",
"## Training and evaluation data\n\nMore information needed",
"## Training procedure",
"### Training hyperparameters\n\nThe following hyperparameters were used during training:\n- learning_rate: 5e-07\n- train_batch_size: 8\n- eval_batch_size: 8\n- seed: 42\n- distributed_type: multi-GPU\n- num_devices: 8\n- gradient_accumulation_steps: 2\n- total_train_batch_size: 128\n- total_eval_batch_size: 64\n- optimizer: Adam with betas=(0.9,0.999) and epsilon=1e-08\n- lr_scheduler_type: cosine\n- lr_scheduler_warmup_ratio: 0.1\n- num_epochs: 1",
"### Training results",
"### Framework versions\n\n- Transformers 4.40.0\n- Pytorch 2.1.2+cu121\n- Datasets 2.14.6\n- Tokenizers 0.19.1"
] |
text-generation | transformers |
<!-- This model card has been generated automatically according to the information the Trainer had access to. You
should probably proofread and complete it, then remove this comment. -->
# falcon-lima
This model is a fine-tuned version of [tiiuae/falcon-7b](https://huggingface.co/tiiuae/falcon-7b) on the GAIR/lima dataset.
It achieves the following results on the evaluation set:
- Loss: 2.4276
## Model description
More information needed
## Intended uses & limitations
More information needed
## Training and evaluation data
More information needed
## Training procedure
### Training hyperparameters
The following hyperparameters were used during training:
- learning_rate: 2e-05
- train_batch_size: 4
- eval_batch_size: 4
- seed: 42
- distributed_type: multi-GPU
- num_devices: 16
- gradient_accumulation_steps: 2
- total_train_batch_size: 128
- total_eval_batch_size: 64
- optimizer: Adam with betas=(0.9,0.999) and epsilon=1e-08
- lr_scheduler_type: cosine
- lr_scheduler_warmup_ratio: 0.1
- num_epochs: 10.0
### Training results
| Training Loss | Epoch | Step | Validation Loss |
|:-------------:|:-----:|:----:|:---------------:|
| 2.0634 | 0.91 | 5 | 1.9126 |
| 1.9281 | 2.0 | 11 | 1.8793 |
| 1.7541 | 2.91 | 16 | 2.2713 |
| 1.5669 | 4.0 | 22 | 2.2287 |
| 1.3976 | 4.91 | 27 | 2.2656 |
| 1.2434 | 6.0 | 33 | 2.3438 |
| 1.1083 | 6.91 | 38 | 2.3551 |
| 1.0215 | 8.0 | 44 | 2.4332 |
| 0.9556 | 8.91 | 49 | 2.4332 |
| 0.9465 | 9.09 | 50 | 2.4276 |
### Framework versions
- Transformers 4.38.2
- Pytorch 2.1.2
- Datasets 2.14.6
- Tokenizers 0.15.2
| {"license": "apache-2.0", "tags": ["alignment-handbook", "trl", "sft", "generated_from_trainer", "trl", "sft", "generated_from_trainer"], "datasets": ["GAIR/lima"], "base_model": "tiiuae/falcon-7b", "model-index": [{"name": "falcon-lima", "results": []}]} | pkarypis/falcon-lima | null | [
"transformers",
"tensorboard",
"safetensors",
"falcon",
"text-generation",
"alignment-handbook",
"trl",
"sft",
"generated_from_trainer",
"conversational",
"custom_code",
"dataset:GAIR/lima",
"base_model:tiiuae/falcon-7b",
"license:apache-2.0",
"autotrain_compatible",
"endpoints_compatible",
"text-generation-inference",
"region:us"
] | null | 2024-04-27T06:55:28+00:00 | [] | [] | TAGS
#transformers #tensorboard #safetensors #falcon #text-generation #alignment-handbook #trl #sft #generated_from_trainer #conversational #custom_code #dataset-GAIR/lima #base_model-tiiuae/falcon-7b #license-apache-2.0 #autotrain_compatible #endpoints_compatible #text-generation-inference #region-us
| falcon-lima
===========
This model is a fine-tuned version of tiiuae/falcon-7b on the GAIR/lima dataset.
It achieves the following results on the evaluation set:
* Loss: 2.4276
Model description
-----------------
More information needed
Intended uses & limitations
---------------------------
More information needed
Training and evaluation data
----------------------------
More information needed
Training procedure
------------------
### Training hyperparameters
The following hyperparameters were used during training:
* learning\_rate: 2e-05
* train\_batch\_size: 4
* eval\_batch\_size: 4
* seed: 42
* distributed\_type: multi-GPU
* num\_devices: 16
* gradient\_accumulation\_steps: 2
* total\_train\_batch\_size: 128
* total\_eval\_batch\_size: 64
* optimizer: Adam with betas=(0.9,0.999) and epsilon=1e-08
* lr\_scheduler\_type: cosine
* lr\_scheduler\_warmup\_ratio: 0.1
* num\_epochs: 10.0
### Training results
### Framework versions
* Transformers 4.38.2
* Pytorch 2.1.2
* Datasets 2.14.6
* Tokenizers 0.15.2
| [
"### Training hyperparameters\n\n\nThe following hyperparameters were used during training:\n\n\n* learning\\_rate: 2e-05\n* train\\_batch\\_size: 4\n* eval\\_batch\\_size: 4\n* seed: 42\n* distributed\\_type: multi-GPU\n* num\\_devices: 16\n* gradient\\_accumulation\\_steps: 2\n* total\\_train\\_batch\\_size: 128\n* total\\_eval\\_batch\\_size: 64\n* optimizer: Adam with betas=(0.9,0.999) and epsilon=1e-08\n* lr\\_scheduler\\_type: cosine\n* lr\\_scheduler\\_warmup\\_ratio: 0.1\n* num\\_epochs: 10.0",
"### Training results",
"### Framework versions\n\n\n* Transformers 4.38.2\n* Pytorch 2.1.2\n* Datasets 2.14.6\n* Tokenizers 0.15.2"
] | [
"TAGS\n#transformers #tensorboard #safetensors #falcon #text-generation #alignment-handbook #trl #sft #generated_from_trainer #conversational #custom_code #dataset-GAIR/lima #base_model-tiiuae/falcon-7b #license-apache-2.0 #autotrain_compatible #endpoints_compatible #text-generation-inference #region-us \n",
"### Training hyperparameters\n\n\nThe following hyperparameters were used during training:\n\n\n* learning\\_rate: 2e-05\n* train\\_batch\\_size: 4\n* eval\\_batch\\_size: 4\n* seed: 42\n* distributed\\_type: multi-GPU\n* num\\_devices: 16\n* gradient\\_accumulation\\_steps: 2\n* total\\_train\\_batch\\_size: 128\n* total\\_eval\\_batch\\_size: 64\n* optimizer: Adam with betas=(0.9,0.999) and epsilon=1e-08\n* lr\\_scheduler\\_type: cosine\n* lr\\_scheduler\\_warmup\\_ratio: 0.1\n* num\\_epochs: 10.0",
"### Training results",
"### Framework versions\n\n\n* Transformers 4.38.2\n* Pytorch 2.1.2\n* Datasets 2.14.6\n* Tokenizers 0.15.2"
] |
text-generation | transformers |
# Model Card for Model ID
<!-- Provide a quick summary of what the model is/does. -->
## Model Details
### Model Description
<!-- Provide a longer summary of what this model is. -->
This is the model card of a 🤗 transformers model that has been pushed on the Hub. This model card has been automatically generated.
- **Developed by:** [More Information Needed]
- **Funded by [optional]:** [More Information Needed]
- **Shared by [optional]:** [More Information Needed]
- **Model type:** [More Information Needed]
- **Language(s) (NLP):** [More Information Needed]
- **License:** [More Information Needed]
- **Finetuned from model [optional]:** [More Information Needed]
### Model Sources [optional]
<!-- Provide the basic links for the model. -->
- **Repository:** [More Information Needed]
- **Paper [optional]:** [More Information Needed]
- **Demo [optional]:** [More Information Needed]
## Uses
<!-- Address questions around how the model is intended to be used, including the foreseeable users of the model and those affected by the model. -->
### Direct Use
<!-- This section is for the model use without fine-tuning or plugging into a larger ecosystem/app. -->
[More Information Needed]
### Downstream Use [optional]
<!-- This section is for the model use when fine-tuned for a task, or when plugged into a larger ecosystem/app -->
[More Information Needed]
### Out-of-Scope Use
<!-- This section addresses misuse, malicious use, and uses that the model will not work well for. -->
[More Information Needed]
## Bias, Risks, and Limitations
<!-- This section is meant to convey both technical and sociotechnical limitations. -->
[More Information Needed]
### Recommendations
<!-- This section is meant to convey recommendations with respect to the bias, risk, and technical limitations. -->
Users (both direct and downstream) should be made aware of the risks, biases and limitations of the model. More information needed for further recommendations.
## How to Get Started with the Model
Use the code below to get started with the model.
[More Information Needed]
## Training Details
### Training Data
<!-- This should link to a Dataset Card, perhaps with a short stub of information on what the training data is all about as well as documentation related to data pre-processing or additional filtering. -->
[More Information Needed]
### Training Procedure
<!-- This relates heavily to the Technical Specifications. Content here should link to that section when it is relevant to the training procedure. -->
#### Preprocessing [optional]
[More Information Needed]
#### Training Hyperparameters
- **Training regime:** [More Information Needed] <!--fp32, fp16 mixed precision, bf16 mixed precision, bf16 non-mixed precision, fp16 non-mixed precision, fp8 mixed precision -->
#### Speeds, Sizes, Times [optional]
<!-- This section provides information about throughput, start/end time, checkpoint size if relevant, etc. -->
[More Information Needed]
## Evaluation
<!-- This section describes the evaluation protocols and provides the results. -->
### Testing Data, Factors & Metrics
#### Testing Data
<!-- This should link to a Dataset Card if possible. -->
[More Information Needed]
#### Factors
<!-- These are the things the evaluation is disaggregating by, e.g., subpopulations or domains. -->
[More Information Needed]
#### Metrics
<!-- These are the evaluation metrics being used, ideally with a description of why. -->
[More Information Needed]
### Results
[More Information Needed]
#### Summary
## Model Examination [optional]
<!-- Relevant interpretability work for the model goes here -->
[More Information Needed]
## Environmental Impact
<!-- Total emissions (in grams of CO2eq) and additional considerations, such as electricity usage, go here. Edit the suggested text below accordingly -->
Carbon emissions can be estimated using the [Machine Learning Impact calculator](https://mlco2.github.io/impact#compute) presented in [Lacoste et al. (2019)](https://arxiv.org/abs/1910.09700).
- **Hardware Type:** [More Information Needed]
- **Hours used:** [More Information Needed]
- **Cloud Provider:** [More Information Needed]
- **Compute Region:** [More Information Needed]
- **Carbon Emitted:** [More Information Needed]
## Technical Specifications [optional]
### Model Architecture and Objective
[More Information Needed]
### Compute Infrastructure
[More Information Needed]
#### Hardware
[More Information Needed]
#### Software
[More Information Needed]
## Citation [optional]
<!-- If there is a paper or blog post introducing the model, the APA and Bibtex information for that should go in this section. -->
**BibTeX:**
[More Information Needed]
**APA:**
[More Information Needed]
## Glossary [optional]
<!-- If relevant, include terms and calculations in this section that can help readers understand the model or model card. -->
[More Information Needed]
## More Information [optional]
[More Information Needed]
## Model Card Authors [optional]
[More Information Needed]
## Model Card Contact
[More Information Needed] | {"library_name": "transformers", "tags": []} | fenguhao/hh-rlhf-sft | null | [
"transformers",
"safetensors",
"gpt_neox",
"text-generation",
"arxiv:1910.09700",
"autotrain_compatible",
"endpoints_compatible",
"text-generation-inference",
"region:us"
] | null | 2024-04-27T06:56:21+00:00 | [
"1910.09700"
] | [] | TAGS
#transformers #safetensors #gpt_neox #text-generation #arxiv-1910.09700 #autotrain_compatible #endpoints_compatible #text-generation-inference #region-us
|
# Model Card for Model ID
## Model Details
### Model Description
This is the model card of a transformers model that has been pushed on the Hub. This model card has been automatically generated.
- Developed by:
- Funded by [optional]:
- Shared by [optional]:
- Model type:
- Language(s) (NLP):
- License:
- Finetuned from model [optional]:
### Model Sources [optional]
- Repository:
- Paper [optional]:
- Demo [optional]:
## Uses
### Direct Use
### Downstream Use [optional]
### Out-of-Scope Use
## Bias, Risks, and Limitations
### Recommendations
Users (both direct and downstream) should be made aware of the risks, biases and limitations of the model. More information needed for further recommendations.
## How to Get Started with the Model
Use the code below to get started with the model.
## Training Details
### Training Data
### Training Procedure
#### Preprocessing [optional]
#### Training Hyperparameters
- Training regime:
#### Speeds, Sizes, Times [optional]
## Evaluation
### Testing Data, Factors & Metrics
#### Testing Data
#### Factors
#### Metrics
### Results
#### Summary
## Model Examination [optional]
## Environmental Impact
Carbon emissions can be estimated using the Machine Learning Impact calculator presented in Lacoste et al. (2019).
- Hardware Type:
- Hours used:
- Cloud Provider:
- Compute Region:
- Carbon Emitted:
## Technical Specifications [optional]
### Model Architecture and Objective
### Compute Infrastructure
#### Hardware
#### Software
[optional]
BibTeX:
APA:
## Glossary [optional]
## More Information [optional]
## Model Card Authors [optional]
## Model Card Contact
| [
"# Model Card for Model ID",
"## Model Details",
"### Model Description\n\n\n\nThis is the model card of a transformers model that has been pushed on the Hub. This model card has been automatically generated.\n\n- Developed by: \n- Funded by [optional]: \n- Shared by [optional]: \n- Model type: \n- Language(s) (NLP): \n- License: \n- Finetuned from model [optional]:",
"### Model Sources [optional]\n\n\n\n- Repository: \n- Paper [optional]: \n- Demo [optional]:",
"## Uses",
"### Direct Use",
"### Downstream Use [optional]",
"### Out-of-Scope Use",
"## Bias, Risks, and Limitations",
"### Recommendations\n\n\n\nUsers (both direct and downstream) should be made aware of the risks, biases and limitations of the model. More information needed for further recommendations.",
"## How to Get Started with the Model\n\nUse the code below to get started with the model.",
"## Training Details",
"### Training Data",
"### Training Procedure",
"#### Preprocessing [optional]",
"#### Training Hyperparameters\n\n- Training regime:",
"#### Speeds, Sizes, Times [optional]",
"## Evaluation",
"### Testing Data, Factors & Metrics",
"#### Testing Data",
"#### Factors",
"#### Metrics",
"### Results",
"#### Summary",
"## Model Examination [optional]",
"## Environmental Impact\n\n\n\nCarbon emissions can be estimated using the Machine Learning Impact calculator presented in Lacoste et al. (2019).\n\n- Hardware Type: \n- Hours used: \n- Cloud Provider: \n- Compute Region: \n- Carbon Emitted:",
"## Technical Specifications [optional]",
"### Model Architecture and Objective",
"### Compute Infrastructure",
"#### Hardware",
"#### Software\n\n\n\n[optional]\n\n\n\nBibTeX:\n\n\n\nAPA:",
"## Glossary [optional]",
"## More Information [optional]",
"## Model Card Authors [optional]",
"## Model Card Contact"
] | [
"TAGS\n#transformers #safetensors #gpt_neox #text-generation #arxiv-1910.09700 #autotrain_compatible #endpoints_compatible #text-generation-inference #region-us \n",
"# Model Card for Model ID",
"## Model Details",
"### Model Description\n\n\n\nThis is the model card of a transformers model that has been pushed on the Hub. This model card has been automatically generated.\n\n- Developed by: \n- Funded by [optional]: \n- Shared by [optional]: \n- Model type: \n- Language(s) (NLP): \n- License: \n- Finetuned from model [optional]:",
"### Model Sources [optional]\n\n\n\n- Repository: \n- Paper [optional]: \n- Demo [optional]:",
"## Uses",
"### Direct Use",
"### Downstream Use [optional]",
"### Out-of-Scope Use",
"## Bias, Risks, and Limitations",
"### Recommendations\n\n\n\nUsers (both direct and downstream) should be made aware of the risks, biases and limitations of the model. More information needed for further recommendations.",
"## How to Get Started with the Model\n\nUse the code below to get started with the model.",
"## Training Details",
"### Training Data",
"### Training Procedure",
"#### Preprocessing [optional]",
"#### Training Hyperparameters\n\n- Training regime:",
"#### Speeds, Sizes, Times [optional]",
"## Evaluation",
"### Testing Data, Factors & Metrics",
"#### Testing Data",
"#### Factors",
"#### Metrics",
"### Results",
"#### Summary",
"## Model Examination [optional]",
"## Environmental Impact\n\n\n\nCarbon emissions can be estimated using the Machine Learning Impact calculator presented in Lacoste et al. (2019).\n\n- Hardware Type: \n- Hours used: \n- Cloud Provider: \n- Compute Region: \n- Carbon Emitted:",
"## Technical Specifications [optional]",
"### Model Architecture and Objective",
"### Compute Infrastructure",
"#### Hardware",
"#### Software\n\n\n\n[optional]\n\n\n\nBibTeX:\n\n\n\nAPA:",
"## Glossary [optional]",
"## More Information [optional]",
"## Model Card Authors [optional]",
"## Model Card Contact"
] |
null | peft |
<!-- This model card has been generated automatically according to the information the Trainer had access to. You
should probably proofread and complete it, then remove this comment. -->
# GUE_prom_prom_core_tata-seqsight_16384_512_22M-L1_f
This model is a fine-tuned version of [mahdibaghbanzadeh/seqsight_16384_512_22M](https://huggingface.co/mahdibaghbanzadeh/seqsight_16384_512_22M) on the [mahdibaghbanzadeh/GUE_prom_prom_core_tata](https://huggingface.co/datasets/mahdibaghbanzadeh/GUE_prom_prom_core_tata) dataset.
It achieves the following results on the evaluation set:
- Loss: 0.3972
- F1 Score: 0.8320
- Accuracy: 0.8320
## Model description
More information needed
## Intended uses & limitations
More information needed
## Training and evaluation data
More information needed
## Training procedure
### Training hyperparameters
The following hyperparameters were used during training:
- learning_rate: 0.0005
- train_batch_size: 128
- eval_batch_size: 128
- seed: 42
- optimizer: Adam with betas=(0.9,0.999) and epsilon=1e-08
- lr_scheduler_type: linear
- training_steps: 10000
### Training results
| Training Loss | Epoch | Step | Validation Loss | F1 Score | Accuracy |
|:-------------:|:------:|:-----:|:---------------:|:--------:|:--------:|
| 0.6125 | 5.13 | 200 | 0.5818 | 0.6925 | 0.6966 |
| 0.5581 | 10.26 | 400 | 0.5616 | 0.7238 | 0.7259 |
| 0.5376 | 15.38 | 600 | 0.5549 | 0.7372 | 0.7406 |
| 0.5186 | 20.51 | 800 | 0.5253 | 0.7514 | 0.7520 |
| 0.4926 | 25.64 | 1000 | 0.5068 | 0.7617 | 0.7618 |
| 0.4751 | 30.77 | 1200 | 0.4945 | 0.7745 | 0.7749 |
| 0.4577 | 35.9 | 1400 | 0.4751 | 0.7896 | 0.7896 |
| 0.4421 | 41.03 | 1600 | 0.4661 | 0.7944 | 0.7945 |
| 0.428 | 46.15 | 1800 | 0.4642 | 0.7944 | 0.7945 |
| 0.4189 | 51.28 | 2000 | 0.4640 | 0.7924 | 0.7928 |
| 0.4091 | 56.41 | 2200 | 0.4608 | 0.7896 | 0.7896 |
| 0.4074 | 61.54 | 2400 | 0.4471 | 0.7993 | 0.7993 |
| 0.4001 | 66.67 | 2600 | 0.4552 | 0.8057 | 0.8059 |
| 0.3981 | 71.79 | 2800 | 0.4435 | 0.8058 | 0.8059 |
| 0.3893 | 76.92 | 3000 | 0.4412 | 0.8040 | 0.8042 |
| 0.3854 | 82.05 | 3200 | 0.4451 | 0.8022 | 0.8026 |
| 0.3804 | 87.18 | 3400 | 0.4389 | 0.8137 | 0.8140 |
| 0.3746 | 92.31 | 3600 | 0.4286 | 0.8157 | 0.8157 |
| 0.3675 | 97.44 | 3800 | 0.4335 | 0.8091 | 0.8091 |
| 0.3656 | 102.56 | 4000 | 0.4307 | 0.8171 | 0.8173 |
| 0.3665 | 107.69 | 4200 | 0.4197 | 0.8237 | 0.8238 |
| 0.3599 | 112.82 | 4400 | 0.4204 | 0.8270 | 0.8271 |
| 0.3589 | 117.95 | 4600 | 0.4154 | 0.8254 | 0.8254 |
| 0.3595 | 123.08 | 4800 | 0.4228 | 0.8121 | 0.8124 |
| 0.3538 | 128.21 | 5000 | 0.4202 | 0.8222 | 0.8222 |
| 0.3471 | 133.33 | 5200 | 0.4115 | 0.8303 | 0.8303 |
| 0.351 | 138.46 | 5400 | 0.4065 | 0.8320 | 0.8320 |
| 0.339 | 143.59 | 5600 | 0.4151 | 0.8254 | 0.8254 |
| 0.3439 | 148.72 | 5800 | 0.4087 | 0.8336 | 0.8336 |
| 0.3392 | 153.85 | 6000 | 0.4124 | 0.8253 | 0.8254 |
| 0.3392 | 158.97 | 6200 | 0.4034 | 0.8303 | 0.8303 |
| 0.3348 | 164.1 | 6400 | 0.4067 | 0.8335 | 0.8336 |
| 0.3364 | 169.23 | 6600 | 0.3981 | 0.8418 | 0.8418 |
| 0.3299 | 174.36 | 6800 | 0.3974 | 0.8369 | 0.8369 |
| 0.3317 | 179.49 | 7000 | 0.3942 | 0.8368 | 0.8369 |
| 0.3328 | 184.62 | 7200 | 0.4024 | 0.8352 | 0.8352 |
| 0.3263 | 189.74 | 7400 | 0.4008 | 0.8434 | 0.8434 |
| 0.3291 | 194.87 | 7600 | 0.3960 | 0.8401 | 0.8401 |
| 0.3266 | 200.0 | 7800 | 0.3935 | 0.8401 | 0.8401 |
| 0.3205 | 205.13 | 8000 | 0.3943 | 0.8418 | 0.8418 |
| 0.3242 | 210.26 | 8200 | 0.3932 | 0.8434 | 0.8434 |
| 0.3252 | 215.38 | 8400 | 0.3969 | 0.8417 | 0.8418 |
| 0.3203 | 220.51 | 8600 | 0.3973 | 0.8434 | 0.8434 |
| 0.3253 | 225.64 | 8800 | 0.3924 | 0.8450 | 0.8450 |
| 0.3245 | 230.77 | 9000 | 0.3911 | 0.8450 | 0.8450 |
| 0.3215 | 235.9 | 9200 | 0.3916 | 0.8434 | 0.8434 |
| 0.3213 | 241.03 | 9400 | 0.3919 | 0.8434 | 0.8434 |
| 0.3195 | 246.15 | 9600 | 0.3936 | 0.8418 | 0.8418 |
| 0.3194 | 251.28 | 9800 | 0.3939 | 0.8434 | 0.8434 |
| 0.3202 | 256.41 | 10000 | 0.3924 | 0.8418 | 0.8418 |
### Framework versions
- PEFT 0.9.0
- Transformers 4.38.2
- Pytorch 2.2.0+cu121
- Datasets 2.17.1
- Tokenizers 0.15.2 | {"library_name": "peft", "tags": ["generated_from_trainer"], "metrics": ["accuracy"], "base_model": "mahdibaghbanzadeh/seqsight_16384_512_22M", "model-index": [{"name": "GUE_prom_prom_core_tata-seqsight_16384_512_22M-L1_f", "results": []}]} | mahdibaghbanzadeh/GUE_prom_prom_core_tata-seqsight_16384_512_22M-L1_f | null | [
"peft",
"safetensors",
"generated_from_trainer",
"base_model:mahdibaghbanzadeh/seqsight_16384_512_22M",
"region:us"
] | null | 2024-04-27T06:56:30+00:00 | [] | [] | TAGS
#peft #safetensors #generated_from_trainer #base_model-mahdibaghbanzadeh/seqsight_16384_512_22M #region-us
| GUE\_prom\_prom\_core\_tata-seqsight\_16384\_512\_22M-L1\_f
===========================================================
This model is a fine-tuned version of mahdibaghbanzadeh/seqsight\_16384\_512\_22M on the mahdibaghbanzadeh/GUE\_prom\_prom\_core\_tata dataset.
It achieves the following results on the evaluation set:
* Loss: 0.3972
* F1 Score: 0.8320
* Accuracy: 0.8320
Model description
-----------------
More information needed
Intended uses & limitations
---------------------------
More information needed
Training and evaluation data
----------------------------
More information needed
Training procedure
------------------
### Training hyperparameters
The following hyperparameters were used during training:
* learning\_rate: 0.0005
* train\_batch\_size: 128
* eval\_batch\_size: 128
* seed: 42
* optimizer: Adam with betas=(0.9,0.999) and epsilon=1e-08
* lr\_scheduler\_type: linear
* training\_steps: 10000
### Training results
### Framework versions
* PEFT 0.9.0
* Transformers 4.38.2
* Pytorch 2.2.0+cu121
* Datasets 2.17.1
* Tokenizers 0.15.2
| [
"### Training hyperparameters\n\n\nThe following hyperparameters were used during training:\n\n\n* learning\\_rate: 0.0005\n* train\\_batch\\_size: 128\n* eval\\_batch\\_size: 128\n* seed: 42\n* optimizer: Adam with betas=(0.9,0.999) and epsilon=1e-08\n* lr\\_scheduler\\_type: linear\n* training\\_steps: 10000",
"### Training results",
"### Framework versions\n\n\n* PEFT 0.9.0\n* Transformers 4.38.2\n* Pytorch 2.2.0+cu121\n* Datasets 2.17.1\n* Tokenizers 0.15.2"
] | [
"TAGS\n#peft #safetensors #generated_from_trainer #base_model-mahdibaghbanzadeh/seqsight_16384_512_22M #region-us \n",
"### Training hyperparameters\n\n\nThe following hyperparameters were used during training:\n\n\n* learning\\_rate: 0.0005\n* train\\_batch\\_size: 128\n* eval\\_batch\\_size: 128\n* seed: 42\n* optimizer: Adam with betas=(0.9,0.999) and epsilon=1e-08\n* lr\\_scheduler\\_type: linear\n* training\\_steps: 10000",
"### Training results",
"### Framework versions\n\n\n* PEFT 0.9.0\n* Transformers 4.38.2\n* Pytorch 2.2.0+cu121\n* Datasets 2.17.1\n* Tokenizers 0.15.2"
] |
null | peft |
<!-- This model card has been generated automatically according to the information the Trainer had access to. You
should probably proofread and complete it, then remove this comment. -->
# GUE_prom_prom_core_tata-seqsight_16384_512_22M-L8_f
This model is a fine-tuned version of [mahdibaghbanzadeh/seqsight_16384_512_22M](https://huggingface.co/mahdibaghbanzadeh/seqsight_16384_512_22M) on the [mahdibaghbanzadeh/GUE_prom_prom_core_tata](https://huggingface.co/datasets/mahdibaghbanzadeh/GUE_prom_prom_core_tata) dataset.
It achieves the following results on the evaluation set:
- Loss: 0.4524
- F1 Score: 0.8336
- Accuracy: 0.8336
## Model description
More information needed
## Intended uses & limitations
More information needed
## Training and evaluation data
More information needed
## Training procedure
### Training hyperparameters
The following hyperparameters were used during training:
- learning_rate: 0.0005
- train_batch_size: 128
- eval_batch_size: 128
- seed: 42
- optimizer: Adam with betas=(0.9,0.999) and epsilon=1e-08
- lr_scheduler_type: linear
- training_steps: 10000
### Training results
| Training Loss | Epoch | Step | Validation Loss | F1 Score | Accuracy |
|:-------------:|:------:|:-----:|:---------------:|:--------:|:--------:|
| 0.5913 | 5.13 | 200 | 0.5732 | 0.7154 | 0.7194 |
| 0.5197 | 10.26 | 400 | 0.5345 | 0.7489 | 0.7504 |
| 0.4713 | 15.38 | 600 | 0.5055 | 0.7640 | 0.7667 |
| 0.4343 | 20.51 | 800 | 0.4584 | 0.7944 | 0.7945 |
| 0.4026 | 25.64 | 1000 | 0.4487 | 0.7960 | 0.7961 |
| 0.3804 | 30.77 | 1200 | 0.4205 | 0.8171 | 0.8173 |
| 0.3578 | 35.9 | 1400 | 0.4204 | 0.8187 | 0.8189 |
| 0.3399 | 41.03 | 1600 | 0.4138 | 0.8236 | 0.8238 |
| 0.3253 | 46.15 | 1800 | 0.3961 | 0.8401 | 0.8401 |
| 0.3099 | 51.28 | 2000 | 0.3872 | 0.8434 | 0.8434 |
| 0.2993 | 56.41 | 2200 | 0.4005 | 0.8450 | 0.8450 |
| 0.2905 | 61.54 | 2400 | 0.3888 | 0.8482 | 0.8483 |
| 0.2816 | 66.67 | 2600 | 0.3918 | 0.8450 | 0.8450 |
| 0.2775 | 71.79 | 2800 | 0.3913 | 0.8515 | 0.8515 |
| 0.2672 | 76.92 | 3000 | 0.4008 | 0.8352 | 0.8352 |
| 0.261 | 82.05 | 3200 | 0.3922 | 0.8450 | 0.8450 |
| 0.2541 | 87.18 | 3400 | 0.3995 | 0.8384 | 0.8385 |
| 0.2516 | 92.31 | 3600 | 0.3806 | 0.8515 | 0.8515 |
| 0.2388 | 97.44 | 3800 | 0.4138 | 0.8467 | 0.8467 |
| 0.2362 | 102.56 | 4000 | 0.3912 | 0.8498 | 0.8499 |
| 0.2326 | 107.69 | 4200 | 0.3894 | 0.8466 | 0.8467 |
| 0.2303 | 112.82 | 4400 | 0.4014 | 0.8515 | 0.8515 |
| 0.224 | 117.95 | 4600 | 0.3839 | 0.8515 | 0.8515 |
| 0.2209 | 123.08 | 4800 | 0.4082 | 0.8417 | 0.8418 |
| 0.2172 | 128.21 | 5000 | 0.4070 | 0.8483 | 0.8483 |
| 0.213 | 133.33 | 5200 | 0.4038 | 0.8466 | 0.8467 |
| 0.2121 | 138.46 | 5400 | 0.3999 | 0.8466 | 0.8467 |
| 0.2055 | 143.59 | 5600 | 0.4072 | 0.8450 | 0.8450 |
| 0.2059 | 148.72 | 5800 | 0.4021 | 0.8499 | 0.8499 |
| 0.201 | 153.85 | 6000 | 0.4006 | 0.8483 | 0.8483 |
| 0.1988 | 158.97 | 6200 | 0.4069 | 0.8532 | 0.8532 |
| 0.1938 | 164.1 | 6400 | 0.4230 | 0.8467 | 0.8467 |
| 0.1932 | 169.23 | 6600 | 0.4137 | 0.8499 | 0.8499 |
| 0.1907 | 174.36 | 6800 | 0.4101 | 0.8450 | 0.8450 |
| 0.1927 | 179.49 | 7000 | 0.4092 | 0.8482 | 0.8483 |
| 0.1898 | 184.62 | 7200 | 0.4150 | 0.8548 | 0.8548 |
| 0.1835 | 189.74 | 7400 | 0.4322 | 0.8433 | 0.8434 |
| 0.1822 | 194.87 | 7600 | 0.4188 | 0.8483 | 0.8483 |
| 0.1804 | 200.0 | 7800 | 0.4215 | 0.8515 | 0.8515 |
| 0.1778 | 205.13 | 8000 | 0.4222 | 0.8466 | 0.8467 |
| 0.1769 | 210.26 | 8200 | 0.4239 | 0.8483 | 0.8483 |
| 0.183 | 215.38 | 8400 | 0.4203 | 0.8434 | 0.8434 |
| 0.1787 | 220.51 | 8600 | 0.4216 | 0.8515 | 0.8515 |
| 0.1792 | 225.64 | 8800 | 0.4227 | 0.8499 | 0.8499 |
| 0.178 | 230.77 | 9000 | 0.4221 | 0.8548 | 0.8548 |
| 0.1732 | 235.9 | 9200 | 0.4266 | 0.8499 | 0.8499 |
| 0.1747 | 241.03 | 9400 | 0.4287 | 0.8499 | 0.8499 |
| 0.1734 | 246.15 | 9600 | 0.4266 | 0.8499 | 0.8499 |
| 0.1716 | 251.28 | 9800 | 0.4281 | 0.8515 | 0.8515 |
| 0.1705 | 256.41 | 10000 | 0.4283 | 0.8515 | 0.8515 |
### Framework versions
- PEFT 0.9.0
- Transformers 4.38.2
- Pytorch 2.2.0+cu121
- Datasets 2.17.1
- Tokenizers 0.15.2 | {"library_name": "peft", "tags": ["generated_from_trainer"], "metrics": ["accuracy"], "base_model": "mahdibaghbanzadeh/seqsight_16384_512_22M", "model-index": [{"name": "GUE_prom_prom_core_tata-seqsight_16384_512_22M-L8_f", "results": []}]} | mahdibaghbanzadeh/GUE_prom_prom_core_tata-seqsight_16384_512_22M-L8_f | null | [
"peft",
"safetensors",
"generated_from_trainer",
"base_model:mahdibaghbanzadeh/seqsight_16384_512_22M",
"region:us"
] | null | 2024-04-27T06:57:54+00:00 | [] | [] | TAGS
#peft #safetensors #generated_from_trainer #base_model-mahdibaghbanzadeh/seqsight_16384_512_22M #region-us
| GUE\_prom\_prom\_core\_tata-seqsight\_16384\_512\_22M-L8\_f
===========================================================
This model is a fine-tuned version of mahdibaghbanzadeh/seqsight\_16384\_512\_22M on the mahdibaghbanzadeh/GUE\_prom\_prom\_core\_tata dataset.
It achieves the following results on the evaluation set:
* Loss: 0.4524
* F1 Score: 0.8336
* Accuracy: 0.8336
Model description
-----------------
More information needed
Intended uses & limitations
---------------------------
More information needed
Training and evaluation data
----------------------------
More information needed
Training procedure
------------------
### Training hyperparameters
The following hyperparameters were used during training:
* learning\_rate: 0.0005
* train\_batch\_size: 128
* eval\_batch\_size: 128
* seed: 42
* optimizer: Adam with betas=(0.9,0.999) and epsilon=1e-08
* lr\_scheduler\_type: linear
* training\_steps: 10000
### Training results
### Framework versions
* PEFT 0.9.0
* Transformers 4.38.2
* Pytorch 2.2.0+cu121
* Datasets 2.17.1
* Tokenizers 0.15.2
| [
"### Training hyperparameters\n\n\nThe following hyperparameters were used during training:\n\n\n* learning\\_rate: 0.0005\n* train\\_batch\\_size: 128\n* eval\\_batch\\_size: 128\n* seed: 42\n* optimizer: Adam with betas=(0.9,0.999) and epsilon=1e-08\n* lr\\_scheduler\\_type: linear\n* training\\_steps: 10000",
"### Training results",
"### Framework versions\n\n\n* PEFT 0.9.0\n* Transformers 4.38.2\n* Pytorch 2.2.0+cu121\n* Datasets 2.17.1\n* Tokenizers 0.15.2"
] | [
"TAGS\n#peft #safetensors #generated_from_trainer #base_model-mahdibaghbanzadeh/seqsight_16384_512_22M #region-us \n",
"### Training hyperparameters\n\n\nThe following hyperparameters were used during training:\n\n\n* learning\\_rate: 0.0005\n* train\\_batch\\_size: 128\n* eval\\_batch\\_size: 128\n* seed: 42\n* optimizer: Adam with betas=(0.9,0.999) and epsilon=1e-08\n* lr\\_scheduler\\_type: linear\n* training\\_steps: 10000",
"### Training results",
"### Framework versions\n\n\n* PEFT 0.9.0\n* Transformers 4.38.2\n* Pytorch 2.2.0+cu121\n* Datasets 2.17.1\n* Tokenizers 0.15.2"
] |
null | peft |
<!-- This model card has been generated automatically according to the information the Trainer had access to. You
should probably proofread and complete it, then remove this comment. -->
# GUE_prom_prom_core_tata-seqsight_16384_512_22M-L32_f
This model is a fine-tuned version of [mahdibaghbanzadeh/seqsight_16384_512_22M](https://huggingface.co/mahdibaghbanzadeh/seqsight_16384_512_22M) on the [mahdibaghbanzadeh/GUE_prom_prom_core_tata](https://huggingface.co/datasets/mahdibaghbanzadeh/GUE_prom_prom_core_tata) dataset.
It achieves the following results on the evaluation set:
- Loss: 0.4128
- F1 Score: 0.8434
- Accuracy: 0.8434
## Model description
More information needed
## Intended uses & limitations
More information needed
## Training and evaluation data
More information needed
## Training procedure
### Training hyperparameters
The following hyperparameters were used during training:
- learning_rate: 0.0005
- train_batch_size: 128
- eval_batch_size: 128
- seed: 42
- optimizer: Adam with betas=(0.9,0.999) and epsilon=1e-08
- lr_scheduler_type: linear
- training_steps: 10000
### Training results
| Training Loss | Epoch | Step | Validation Loss | F1 Score | Accuracy |
|:-------------:|:------:|:-----:|:---------------:|:--------:|:--------:|
| 0.5692 | 5.13 | 200 | 0.5421 | 0.7380 | 0.7390 |
| 0.4703 | 10.26 | 400 | 0.5086 | 0.7645 | 0.7667 |
| 0.4096 | 15.38 | 600 | 0.4292 | 0.8042 | 0.8042 |
| 0.3624 | 20.51 | 800 | 0.4130 | 0.8270 | 0.8271 |
| 0.3192 | 25.64 | 1000 | 0.4094 | 0.8417 | 0.8418 |
| 0.2901 | 30.77 | 1200 | 0.3982 | 0.8397 | 0.8401 |
| 0.264 | 35.9 | 1400 | 0.3946 | 0.8434 | 0.8434 |
| 0.2478 | 41.03 | 1600 | 0.4076 | 0.8433 | 0.8434 |
| 0.2296 | 46.15 | 1800 | 0.3894 | 0.8515 | 0.8515 |
| 0.2114 | 51.28 | 2000 | 0.4115 | 0.8548 | 0.8548 |
| 0.2007 | 56.41 | 2200 | 0.4314 | 0.8467 | 0.8467 |
| 0.1905 | 61.54 | 2400 | 0.4387 | 0.8385 | 0.8385 |
| 0.1807 | 66.67 | 2600 | 0.4426 | 0.8531 | 0.8532 |
| 0.1714 | 71.79 | 2800 | 0.4847 | 0.8417 | 0.8418 |
| 0.1598 | 76.92 | 3000 | 0.5437 | 0.8302 | 0.8303 |
| 0.1492 | 82.05 | 3200 | 0.5206 | 0.8383 | 0.8385 |
| 0.1436 | 87.18 | 3400 | 0.5097 | 0.8384 | 0.8385 |
| 0.1353 | 92.31 | 3600 | 0.5247 | 0.8483 | 0.8483 |
| 0.1276 | 97.44 | 3800 | 0.5490 | 0.8467 | 0.8467 |
| 0.1246 | 102.56 | 4000 | 0.5494 | 0.8433 | 0.8434 |
| 0.1162 | 107.69 | 4200 | 0.5452 | 0.8433 | 0.8434 |
| 0.1188 | 112.82 | 4400 | 0.5519 | 0.8384 | 0.8385 |
| 0.1062 | 117.95 | 4600 | 0.5500 | 0.8401 | 0.8401 |
| 0.102 | 123.08 | 4800 | 0.5665 | 0.8385 | 0.8385 |
| 0.1 | 128.21 | 5000 | 0.5888 | 0.8385 | 0.8385 |
| 0.0928 | 133.33 | 5200 | 0.6022 | 0.8401 | 0.8401 |
| 0.0916 | 138.46 | 5400 | 0.6165 | 0.8450 | 0.8450 |
| 0.0894 | 143.59 | 5600 | 0.6231 | 0.8466 | 0.8467 |
| 0.0816 | 148.72 | 5800 | 0.6158 | 0.8385 | 0.8385 |
| 0.0829 | 153.85 | 6000 | 0.6345 | 0.8368 | 0.8369 |
| 0.0802 | 158.97 | 6200 | 0.6379 | 0.8303 | 0.8303 |
| 0.0779 | 164.1 | 6400 | 0.6544 | 0.8448 | 0.8450 |
| 0.0727 | 169.23 | 6600 | 0.6612 | 0.8385 | 0.8385 |
| 0.0739 | 174.36 | 6800 | 0.6426 | 0.8450 | 0.8450 |
| 0.0723 | 179.49 | 7000 | 0.6691 | 0.8385 | 0.8385 |
| 0.0705 | 184.62 | 7200 | 0.6652 | 0.8433 | 0.8434 |
| 0.0678 | 189.74 | 7400 | 0.6879 | 0.8416 | 0.8418 |
| 0.0655 | 194.87 | 7600 | 0.6831 | 0.8351 | 0.8352 |
| 0.065 | 200.0 | 7800 | 0.6698 | 0.8450 | 0.8450 |
| 0.0652 | 205.13 | 8000 | 0.6868 | 0.8400 | 0.8401 |
| 0.0619 | 210.26 | 8200 | 0.6964 | 0.8433 | 0.8434 |
| 0.0622 | 215.38 | 8400 | 0.6994 | 0.8384 | 0.8385 |
| 0.0624 | 220.51 | 8600 | 0.7099 | 0.8416 | 0.8418 |
| 0.0607 | 225.64 | 8800 | 0.6958 | 0.8434 | 0.8434 |
| 0.0613 | 230.77 | 9000 | 0.7013 | 0.8416 | 0.8418 |
| 0.0552 | 235.9 | 9200 | 0.7090 | 0.8433 | 0.8434 |
| 0.0562 | 241.03 | 9400 | 0.7224 | 0.8433 | 0.8434 |
| 0.0572 | 246.15 | 9600 | 0.7151 | 0.8433 | 0.8434 |
| 0.056 | 251.28 | 9800 | 0.7228 | 0.8433 | 0.8434 |
| 0.0564 | 256.41 | 10000 | 0.7224 | 0.8466 | 0.8467 |
### Framework versions
- PEFT 0.9.0
- Transformers 4.38.2
- Pytorch 2.2.0+cu121
- Datasets 2.17.1
- Tokenizers 0.15.2 | {"library_name": "peft", "tags": ["generated_from_trainer"], "metrics": ["accuracy"], "base_model": "mahdibaghbanzadeh/seqsight_16384_512_22M", "model-index": [{"name": "GUE_prom_prom_core_tata-seqsight_16384_512_22M-L32_f", "results": []}]} | mahdibaghbanzadeh/GUE_prom_prom_core_tata-seqsight_16384_512_22M-L32_f | null | [
"peft",
"safetensors",
"generated_from_trainer",
"base_model:mahdibaghbanzadeh/seqsight_16384_512_22M",
"region:us"
] | null | 2024-04-27T06:57:54+00:00 | [] | [] | TAGS
#peft #safetensors #generated_from_trainer #base_model-mahdibaghbanzadeh/seqsight_16384_512_22M #region-us
| GUE\_prom\_prom\_core\_tata-seqsight\_16384\_512\_22M-L32\_f
============================================================
This model is a fine-tuned version of mahdibaghbanzadeh/seqsight\_16384\_512\_22M on the mahdibaghbanzadeh/GUE\_prom\_prom\_core\_tata dataset.
It achieves the following results on the evaluation set:
* Loss: 0.4128
* F1 Score: 0.8434
* Accuracy: 0.8434
Model description
-----------------
More information needed
Intended uses & limitations
---------------------------
More information needed
Training and evaluation data
----------------------------
More information needed
Training procedure
------------------
### Training hyperparameters
The following hyperparameters were used during training:
* learning\_rate: 0.0005
* train\_batch\_size: 128
* eval\_batch\_size: 128
* seed: 42
* optimizer: Adam with betas=(0.9,0.999) and epsilon=1e-08
* lr\_scheduler\_type: linear
* training\_steps: 10000
### Training results
### Framework versions
* PEFT 0.9.0
* Transformers 4.38.2
* Pytorch 2.2.0+cu121
* Datasets 2.17.1
* Tokenizers 0.15.2
| [
"### Training hyperparameters\n\n\nThe following hyperparameters were used during training:\n\n\n* learning\\_rate: 0.0005\n* train\\_batch\\_size: 128\n* eval\\_batch\\_size: 128\n* seed: 42\n* optimizer: Adam with betas=(0.9,0.999) and epsilon=1e-08\n* lr\\_scheduler\\_type: linear\n* training\\_steps: 10000",
"### Training results",
"### Framework versions\n\n\n* PEFT 0.9.0\n* Transformers 4.38.2\n* Pytorch 2.2.0+cu121\n* Datasets 2.17.1\n* Tokenizers 0.15.2"
] | [
"TAGS\n#peft #safetensors #generated_from_trainer #base_model-mahdibaghbanzadeh/seqsight_16384_512_22M #region-us \n",
"### Training hyperparameters\n\n\nThe following hyperparameters were used during training:\n\n\n* learning\\_rate: 0.0005\n* train\\_batch\\_size: 128\n* eval\\_batch\\_size: 128\n* seed: 42\n* optimizer: Adam with betas=(0.9,0.999) and epsilon=1e-08\n* lr\\_scheduler\\_type: linear\n* training\\_steps: 10000",
"### Training results",
"### Framework versions\n\n\n* PEFT 0.9.0\n* Transformers 4.38.2\n* Pytorch 2.2.0+cu121\n* Datasets 2.17.1\n* Tokenizers 0.15.2"
] |
null | keras |
<!-- This model card has been generated automatically according to the information Keras had access to. You should
probably proofread and complete it, then remove this comment. -->
# mindmate-f2-original-equal-cont-0-0
This model is a fine-tuned version of [bert-base-german-cased](https://huggingface.co/bert-base-german-cased) on an unknown dataset.
It achieves the following results on the evaluation set:
## Model description
More information needed
## Intended uses & limitations
More information needed
## Training and evaluation data
More information needed
## Training procedure
### Training hyperparameters
The following hyperparameters were used during training:
- optimizer: {'name': 'Adam', 'weight_decay': None, 'clipnorm': None, 'global_clipnorm': None, 'clipvalue': None, 'use_ema': False, 'ema_momentum': 0.99, 'ema_overwrite_frequency': None, 'jit_compile': True, 'is_legacy_optimizer': False, 'learning_rate': 3e-05, 'beta_1': 0.9, 'beta_2': 0.999, 'epsilon': 1e-07, 'amsgrad': False}
- training_precision: float32
### Training results
### Framework versions
- Transformers 4.40.0
- TensorFlow 2.15.0
- Tokenizers 0.19.1
| {"license": "mit", "tags": ["generated_from_keras_callback"], "base_model": "bert-base-german-cased", "model-index": [{"name": "mindmate-f2-original-equal-cont-0-0", "results": []}]} | spneshaei/mindmate-f2-original-equal-cont-0-0 | null | [
"keras",
"tf",
"bert",
"generated_from_keras_callback",
"base_model:bert-base-german-cased",
"license:mit",
"region:us"
] | null | 2024-04-27T06:58:16+00:00 | [] | [] | TAGS
#keras #tf #bert #generated_from_keras_callback #base_model-bert-base-german-cased #license-mit #region-us
|
# mindmate-f2-original-equal-cont-0-0
This model is a fine-tuned version of bert-base-german-cased on an unknown dataset.
It achieves the following results on the evaluation set:
## Model description
More information needed
## Intended uses & limitations
More information needed
## Training and evaluation data
More information needed
## Training procedure
### Training hyperparameters
The following hyperparameters were used during training:
- optimizer: {'name': 'Adam', 'weight_decay': None, 'clipnorm': None, 'global_clipnorm': None, 'clipvalue': None, 'use_ema': False, 'ema_momentum': 0.99, 'ema_overwrite_frequency': None, 'jit_compile': True, 'is_legacy_optimizer': False, 'learning_rate': 3e-05, 'beta_1': 0.9, 'beta_2': 0.999, 'epsilon': 1e-07, 'amsgrad': False}
- training_precision: float32
### Training results
### Framework versions
- Transformers 4.40.0
- TensorFlow 2.15.0
- Tokenizers 0.19.1
| [
"# mindmate-f2-original-equal-cont-0-0\n\nThis model is a fine-tuned version of bert-base-german-cased on an unknown dataset.\nIt achieves the following results on the evaluation set:",
"## Model description\n\nMore information needed",
"## Intended uses & limitations\n\nMore information needed",
"## Training and evaluation data\n\nMore information needed",
"## Training procedure",
"### Training hyperparameters\n\nThe following hyperparameters were used during training:\n- optimizer: {'name': 'Adam', 'weight_decay': None, 'clipnorm': None, 'global_clipnorm': None, 'clipvalue': None, 'use_ema': False, 'ema_momentum': 0.99, 'ema_overwrite_frequency': None, 'jit_compile': True, 'is_legacy_optimizer': False, 'learning_rate': 3e-05, 'beta_1': 0.9, 'beta_2': 0.999, 'epsilon': 1e-07, 'amsgrad': False}\n- training_precision: float32",
"### Training results",
"### Framework versions\n\n- Transformers 4.40.0\n- TensorFlow 2.15.0\n- Tokenizers 0.19.1"
] | [
"TAGS\n#keras #tf #bert #generated_from_keras_callback #base_model-bert-base-german-cased #license-mit #region-us \n",
"# mindmate-f2-original-equal-cont-0-0\n\nThis model is a fine-tuned version of bert-base-german-cased on an unknown dataset.\nIt achieves the following results on the evaluation set:",
"## Model description\n\nMore information needed",
"## Intended uses & limitations\n\nMore information needed",
"## Training and evaluation data\n\nMore information needed",
"## Training procedure",
"### Training hyperparameters\n\nThe following hyperparameters were used during training:\n- optimizer: {'name': 'Adam', 'weight_decay': None, 'clipnorm': None, 'global_clipnorm': None, 'clipvalue': None, 'use_ema': False, 'ema_momentum': 0.99, 'ema_overwrite_frequency': None, 'jit_compile': True, 'is_legacy_optimizer': False, 'learning_rate': 3e-05, 'beta_1': 0.9, 'beta_2': 0.999, 'epsilon': 1e-07, 'amsgrad': False}\n- training_precision: float32",
"### Training results",
"### Framework versions\n\n- Transformers 4.40.0\n- TensorFlow 2.15.0\n- Tokenizers 0.19.1"
] |
null | keras |
<!-- This model card has been generated automatically according to the information Keras had access to. You should
probably proofread and complete it, then remove this comment. -->
# mindmate-f1-original-equal-cont-0-0
This model is a fine-tuned version of [bert-base-german-cased](https://huggingface.co/bert-base-german-cased) on an unknown dataset.
It achieves the following results on the evaluation set:
## Model description
More information needed
## Intended uses & limitations
More information needed
## Training and evaluation data
More information needed
## Training procedure
### Training hyperparameters
The following hyperparameters were used during training:
- optimizer: {'name': 'Adam', 'weight_decay': None, 'clipnorm': None, 'global_clipnorm': None, 'clipvalue': None, 'use_ema': False, 'ema_momentum': 0.99, 'ema_overwrite_frequency': None, 'jit_compile': True, 'is_legacy_optimizer': False, 'learning_rate': 3e-05, 'beta_1': 0.9, 'beta_2': 0.999, 'epsilon': 1e-07, 'amsgrad': False}
- training_precision: float32
### Training results
### Framework versions
- Transformers 4.40.0
- TensorFlow 2.15.0
- Tokenizers 0.19.1
| {"license": "mit", "tags": ["generated_from_keras_callback"], "base_model": "bert-base-german-cased", "model-index": [{"name": "mindmate-f1-original-equal-cont-0-0", "results": []}]} | spneshaei/mindmate-f1-original-equal-cont-0-0 | null | [
"keras",
"tf",
"bert",
"generated_from_keras_callback",
"base_model:bert-base-german-cased",
"license:mit",
"region:us"
] | null | 2024-04-27T06:58:44+00:00 | [] | [] | TAGS
#keras #tf #bert #generated_from_keras_callback #base_model-bert-base-german-cased #license-mit #region-us
|
# mindmate-f1-original-equal-cont-0-0
This model is a fine-tuned version of bert-base-german-cased on an unknown dataset.
It achieves the following results on the evaluation set:
## Model description
More information needed
## Intended uses & limitations
More information needed
## Training and evaluation data
More information needed
## Training procedure
### Training hyperparameters
The following hyperparameters were used during training:
- optimizer: {'name': 'Adam', 'weight_decay': None, 'clipnorm': None, 'global_clipnorm': None, 'clipvalue': None, 'use_ema': False, 'ema_momentum': 0.99, 'ema_overwrite_frequency': None, 'jit_compile': True, 'is_legacy_optimizer': False, 'learning_rate': 3e-05, 'beta_1': 0.9, 'beta_2': 0.999, 'epsilon': 1e-07, 'amsgrad': False}
- training_precision: float32
### Training results
### Framework versions
- Transformers 4.40.0
- TensorFlow 2.15.0
- Tokenizers 0.19.1
| [
"# mindmate-f1-original-equal-cont-0-0\n\nThis model is a fine-tuned version of bert-base-german-cased on an unknown dataset.\nIt achieves the following results on the evaluation set:",
"## Model description\n\nMore information needed",
"## Intended uses & limitations\n\nMore information needed",
"## Training and evaluation data\n\nMore information needed",
"## Training procedure",
"### Training hyperparameters\n\nThe following hyperparameters were used during training:\n- optimizer: {'name': 'Adam', 'weight_decay': None, 'clipnorm': None, 'global_clipnorm': None, 'clipvalue': None, 'use_ema': False, 'ema_momentum': 0.99, 'ema_overwrite_frequency': None, 'jit_compile': True, 'is_legacy_optimizer': False, 'learning_rate': 3e-05, 'beta_1': 0.9, 'beta_2': 0.999, 'epsilon': 1e-07, 'amsgrad': False}\n- training_precision: float32",
"### Training results",
"### Framework versions\n\n- Transformers 4.40.0\n- TensorFlow 2.15.0\n- Tokenizers 0.19.1"
] | [
"TAGS\n#keras #tf #bert #generated_from_keras_callback #base_model-bert-base-german-cased #license-mit #region-us \n",
"# mindmate-f1-original-equal-cont-0-0\n\nThis model is a fine-tuned version of bert-base-german-cased on an unknown dataset.\nIt achieves the following results on the evaluation set:",
"## Model description\n\nMore information needed",
"## Intended uses & limitations\n\nMore information needed",
"## Training and evaluation data\n\nMore information needed",
"## Training procedure",
"### Training hyperparameters\n\nThe following hyperparameters were used during training:\n- optimizer: {'name': 'Adam', 'weight_decay': None, 'clipnorm': None, 'global_clipnorm': None, 'clipvalue': None, 'use_ema': False, 'ema_momentum': 0.99, 'ema_overwrite_frequency': None, 'jit_compile': True, 'is_legacy_optimizer': False, 'learning_rate': 3e-05, 'beta_1': 0.9, 'beta_2': 0.999, 'epsilon': 1e-07, 'amsgrad': False}\n- training_precision: float32",
"### Training results",
"### Framework versions\n\n- Transformers 4.40.0\n- TensorFlow 2.15.0\n- Tokenizers 0.19.1"
] |
null | peft |
<!-- This model card has been generated automatically according to the information the Trainer had access to. You
should probably proofread and complete it, then remove this comment. -->
# GUE_prom_prom_300_all-seqsight_16384_512_22M-L1_f
This model is a fine-tuned version of [mahdibaghbanzadeh/seqsight_16384_512_22M](https://huggingface.co/mahdibaghbanzadeh/seqsight_16384_512_22M) on the [mahdibaghbanzadeh/GUE_prom_prom_300_all](https://huggingface.co/datasets/mahdibaghbanzadeh/GUE_prom_prom_300_all) dataset.
It achieves the following results on the evaluation set:
- Loss: 0.2225
- F1 Score: 0.9096
- Accuracy: 0.9096
## Model description
More information needed
## Intended uses & limitations
More information needed
## Training and evaluation data
More information needed
## Training procedure
### Training hyperparameters
The following hyperparameters were used during training:
- learning_rate: 0.0005
- train_batch_size: 128
- eval_batch_size: 128
- seed: 42
- optimizer: Adam with betas=(0.9,0.999) and epsilon=1e-08
- lr_scheduler_type: linear
- training_steps: 10000
### Training results
| Training Loss | Epoch | Step | Validation Loss | F1 Score | Accuracy |
|:-------------:|:-----:|:-----:|:---------------:|:--------:|:--------:|
| 0.4379 | 0.54 | 200 | 0.3007 | 0.8866 | 0.8867 |
| 0.3184 | 1.08 | 400 | 0.2642 | 0.8973 | 0.8973 |
| 0.2875 | 1.62 | 600 | 0.2449 | 0.9024 | 0.9024 |
| 0.2629 | 2.16 | 800 | 0.2442 | 0.9072 | 0.9073 |
| 0.2575 | 2.7 | 1000 | 0.2298 | 0.9108 | 0.9108 |
| 0.2475 | 3.24 | 1200 | 0.2315 | 0.9093 | 0.9093 |
| 0.2454 | 3.78 | 1400 | 0.2247 | 0.9106 | 0.9106 |
| 0.2432 | 4.32 | 1600 | 0.2197 | 0.9143 | 0.9144 |
| 0.2352 | 4.86 | 1800 | 0.2212 | 0.9132 | 0.9132 |
| 0.233 | 5.41 | 2000 | 0.2176 | 0.9137 | 0.9137 |
| 0.2356 | 5.95 | 2200 | 0.2174 | 0.9125 | 0.9125 |
| 0.2291 | 6.49 | 2400 | 0.2153 | 0.9128 | 0.9128 |
| 0.2303 | 7.03 | 2600 | 0.2161 | 0.9133 | 0.9133 |
| 0.2246 | 7.57 | 2800 | 0.2144 | 0.9120 | 0.9120 |
| 0.224 | 8.11 | 3000 | 0.2101 | 0.9142 | 0.9142 |
| 0.2251 | 8.65 | 3200 | 0.2094 | 0.9164 | 0.9164 |
| 0.2154 | 9.19 | 3400 | 0.2082 | 0.9176 | 0.9176 |
| 0.2188 | 9.73 | 3600 | 0.2078 | 0.9154 | 0.9154 |
| 0.2238 | 10.27 | 3800 | 0.2072 | 0.9165 | 0.9166 |
| 0.2186 | 10.81 | 4000 | 0.2061 | 0.9147 | 0.9147 |
| 0.2214 | 11.35 | 4200 | 0.2097 | 0.9148 | 0.9149 |
| 0.2135 | 11.89 | 4400 | 0.2059 | 0.9154 | 0.9154 |
| 0.2144 | 12.43 | 4600 | 0.2052 | 0.9165 | 0.9166 |
| 0.2149 | 12.97 | 4800 | 0.2025 | 0.9176 | 0.9176 |
| 0.212 | 13.51 | 5000 | 0.2044 | 0.9164 | 0.9164 |
| 0.2149 | 14.05 | 5200 | 0.2033 | 0.9162 | 0.9162 |
| 0.2102 | 14.59 | 5400 | 0.2039 | 0.9170 | 0.9171 |
| 0.2117 | 15.14 | 5600 | 0.2040 | 0.9165 | 0.9166 |
| 0.209 | 15.68 | 5800 | 0.2014 | 0.9176 | 0.9176 |
| 0.2135 | 16.22 | 6000 | 0.2052 | 0.9175 | 0.9176 |
| 0.2116 | 16.76 | 6200 | 0.2017 | 0.9177 | 0.9177 |
| 0.208 | 17.3 | 6400 | 0.1999 | 0.9199 | 0.9199 |
| 0.2115 | 17.84 | 6600 | 0.2012 | 0.9175 | 0.9176 |
| 0.2031 | 18.38 | 6800 | 0.2025 | 0.9182 | 0.9182 |
| 0.2131 | 18.92 | 7000 | 0.1985 | 0.9191 | 0.9191 |
| 0.2085 | 19.46 | 7200 | 0.1996 | 0.9187 | 0.9187 |
| 0.2059 | 20.0 | 7400 | 0.1986 | 0.9192 | 0.9193 |
| 0.2086 | 20.54 | 7600 | 0.1989 | 0.9181 | 0.9181 |
| 0.207 | 21.08 | 7800 | 0.1980 | 0.9186 | 0.9186 |
| 0.2057 | 21.62 | 8000 | 0.1992 | 0.9184 | 0.9184 |
| 0.2078 | 22.16 | 8200 | 0.1983 | 0.9184 | 0.9184 |
| 0.2017 | 22.7 | 8400 | 0.1978 | 0.9184 | 0.9184 |
| 0.2079 | 23.24 | 8600 | 0.1978 | 0.9184 | 0.9184 |
| 0.2038 | 23.78 | 8800 | 0.1981 | 0.9177 | 0.9177 |
| 0.2072 | 24.32 | 9000 | 0.1972 | 0.9187 | 0.9187 |
| 0.206 | 24.86 | 9200 | 0.1978 | 0.9187 | 0.9187 |
| 0.2034 | 25.41 | 9400 | 0.1970 | 0.9191 | 0.9191 |
| 0.2049 | 25.95 | 9600 | 0.1973 | 0.9191 | 0.9191 |
| 0.2058 | 26.49 | 9800 | 0.1975 | 0.9191 | 0.9191 |
| 0.2041 | 27.03 | 10000 | 0.1973 | 0.9186 | 0.9186 |
### Framework versions
- PEFT 0.9.0
- Transformers 4.38.2
- Pytorch 2.2.0+cu121
- Datasets 2.17.1
- Tokenizers 0.15.2 | {"library_name": "peft", "tags": ["generated_from_trainer"], "metrics": ["accuracy"], "base_model": "mahdibaghbanzadeh/seqsight_16384_512_22M", "model-index": [{"name": "GUE_prom_prom_300_all-seqsight_16384_512_22M-L1_f", "results": []}]} | mahdibaghbanzadeh/GUE_prom_prom_300_all-seqsight_16384_512_22M-L1_f | null | [
"peft",
"safetensors",
"generated_from_trainer",
"base_model:mahdibaghbanzadeh/seqsight_16384_512_22M",
"region:us"
] | null | 2024-04-27T07:00:10+00:00 | [] | [] | TAGS
#peft #safetensors #generated_from_trainer #base_model-mahdibaghbanzadeh/seqsight_16384_512_22M #region-us
| GUE\_prom\_prom\_300\_all-seqsight\_16384\_512\_22M-L1\_f
=========================================================
This model is a fine-tuned version of mahdibaghbanzadeh/seqsight\_16384\_512\_22M on the mahdibaghbanzadeh/GUE\_prom\_prom\_300\_all dataset.
It achieves the following results on the evaluation set:
* Loss: 0.2225
* F1 Score: 0.9096
* Accuracy: 0.9096
Model description
-----------------
More information needed
Intended uses & limitations
---------------------------
More information needed
Training and evaluation data
----------------------------
More information needed
Training procedure
------------------
### Training hyperparameters
The following hyperparameters were used during training:
* learning\_rate: 0.0005
* train\_batch\_size: 128
* eval\_batch\_size: 128
* seed: 42
* optimizer: Adam with betas=(0.9,0.999) and epsilon=1e-08
* lr\_scheduler\_type: linear
* training\_steps: 10000
### Training results
### Framework versions
* PEFT 0.9.0
* Transformers 4.38.2
* Pytorch 2.2.0+cu121
* Datasets 2.17.1
* Tokenizers 0.15.2
| [
"### Training hyperparameters\n\n\nThe following hyperparameters were used during training:\n\n\n* learning\\_rate: 0.0005\n* train\\_batch\\_size: 128\n* eval\\_batch\\_size: 128\n* seed: 42\n* optimizer: Adam with betas=(0.9,0.999) and epsilon=1e-08\n* lr\\_scheduler\\_type: linear\n* training\\_steps: 10000",
"### Training results",
"### Framework versions\n\n\n* PEFT 0.9.0\n* Transformers 4.38.2\n* Pytorch 2.2.0+cu121\n* Datasets 2.17.1\n* Tokenizers 0.15.2"
] | [
"TAGS\n#peft #safetensors #generated_from_trainer #base_model-mahdibaghbanzadeh/seqsight_16384_512_22M #region-us \n",
"### Training hyperparameters\n\n\nThe following hyperparameters were used during training:\n\n\n* learning\\_rate: 0.0005\n* train\\_batch\\_size: 128\n* eval\\_batch\\_size: 128\n* seed: 42\n* optimizer: Adam with betas=(0.9,0.999) and epsilon=1e-08\n* lr\\_scheduler\\_type: linear\n* training\\_steps: 10000",
"### Training results",
"### Framework versions\n\n\n* PEFT 0.9.0\n* Transformers 4.38.2\n* Pytorch 2.2.0+cu121\n* Datasets 2.17.1\n* Tokenizers 0.15.2"
] |
null | peft |
<!-- This model card has been generated automatically according to the information the Trainer had access to. You
should probably proofread and complete it, then remove this comment. -->
# GUE_prom_prom_300_all-seqsight_16384_512_22M-L8_f
This model is a fine-tuned version of [mahdibaghbanzadeh/seqsight_16384_512_22M](https://huggingface.co/mahdibaghbanzadeh/seqsight_16384_512_22M) on the [mahdibaghbanzadeh/GUE_prom_prom_300_all](https://huggingface.co/datasets/mahdibaghbanzadeh/GUE_prom_prom_300_all) dataset.
It achieves the following results on the evaluation set:
- Loss: 0.2074
- F1 Score: 0.9167
- Accuracy: 0.9167
## Model description
More information needed
## Intended uses & limitations
More information needed
## Training and evaluation data
More information needed
## Training procedure
### Training hyperparameters
The following hyperparameters were used during training:
- learning_rate: 0.0005
- train_batch_size: 128
- eval_batch_size: 128
- seed: 42
- optimizer: Adam with betas=(0.9,0.999) and epsilon=1e-08
- lr_scheduler_type: linear
- training_steps: 10000
### Training results
| Training Loss | Epoch | Step | Validation Loss | F1 Score | Accuracy |
|:-------------:|:-----:|:-----:|:---------------:|:--------:|:--------:|
| 0.3768 | 0.54 | 200 | 0.2522 | 0.9005 | 0.9005 |
| 0.2656 | 1.08 | 400 | 0.2300 | 0.9103 | 0.9103 |
| 0.2466 | 1.62 | 600 | 0.2184 | 0.9137 | 0.9137 |
| 0.2294 | 2.16 | 800 | 0.2176 | 0.9123 | 0.9123 |
| 0.2297 | 2.7 | 1000 | 0.2088 | 0.9175 | 0.9176 |
| 0.2193 | 3.24 | 1200 | 0.2132 | 0.9116 | 0.9117 |
| 0.2182 | 3.78 | 1400 | 0.2069 | 0.9170 | 0.9171 |
| 0.2172 | 4.32 | 1600 | 0.1972 | 0.9221 | 0.9221 |
| 0.2089 | 4.86 | 1800 | 0.2019 | 0.9180 | 0.9181 |
| 0.2092 | 5.41 | 2000 | 0.1964 | 0.9228 | 0.9228 |
| 0.2096 | 5.95 | 2200 | 0.1939 | 0.9223 | 0.9223 |
| 0.2031 | 6.49 | 2400 | 0.1931 | 0.9225 | 0.9225 |
| 0.2046 | 7.03 | 2600 | 0.1918 | 0.9240 | 0.9240 |
| 0.1968 | 7.57 | 2800 | 0.1901 | 0.9235 | 0.9235 |
| 0.2004 | 8.11 | 3000 | 0.1894 | 0.9250 | 0.925 |
| 0.1975 | 8.65 | 3200 | 0.1894 | 0.9226 | 0.9226 |
| 0.1893 | 9.19 | 3400 | 0.1895 | 0.9242 | 0.9242 |
| 0.1927 | 9.73 | 3600 | 0.1873 | 0.9253 | 0.9253 |
| 0.1989 | 10.27 | 3800 | 0.1852 | 0.9243 | 0.9243 |
| 0.1938 | 10.81 | 4000 | 0.1846 | 0.925 | 0.925 |
| 0.1954 | 11.35 | 4200 | 0.1830 | 0.9258 | 0.9258 |
| 0.1868 | 11.89 | 4400 | 0.1856 | 0.9245 | 0.9245 |
| 0.1888 | 12.43 | 4600 | 0.1823 | 0.9252 | 0.9252 |
| 0.1876 | 12.97 | 4800 | 0.1835 | 0.9235 | 0.9235 |
| 0.1858 | 13.51 | 5000 | 0.1837 | 0.9238 | 0.9238 |
| 0.1873 | 14.05 | 5200 | 0.1863 | 0.9252 | 0.9252 |
| 0.1801 | 14.59 | 5400 | 0.1864 | 0.9231 | 0.9231 |
| 0.1864 | 15.14 | 5600 | 0.1840 | 0.9242 | 0.9242 |
| 0.1823 | 15.68 | 5800 | 0.1830 | 0.9257 | 0.9257 |
| 0.184 | 16.22 | 6000 | 0.1865 | 0.9233 | 0.9233 |
| 0.1828 | 16.76 | 6200 | 0.1823 | 0.9260 | 0.9260 |
| 0.1791 | 17.3 | 6400 | 0.1816 | 0.9267 | 0.9267 |
| 0.1816 | 17.84 | 6600 | 0.1815 | 0.9265 | 0.9265 |
| 0.1747 | 18.38 | 6800 | 0.1831 | 0.9258 | 0.9258 |
| 0.1827 | 18.92 | 7000 | 0.1793 | 0.9285 | 0.9285 |
| 0.1799 | 19.46 | 7200 | 0.1800 | 0.9272 | 0.9272 |
| 0.1778 | 20.0 | 7400 | 0.1806 | 0.9289 | 0.9289 |
| 0.1809 | 20.54 | 7600 | 0.1797 | 0.9270 | 0.9270 |
| 0.1792 | 21.08 | 7800 | 0.1781 | 0.9272 | 0.9272 |
| 0.1782 | 21.62 | 8000 | 0.1802 | 0.9265 | 0.9265 |
| 0.1765 | 22.16 | 8200 | 0.1792 | 0.9265 | 0.9265 |
| 0.1735 | 22.7 | 8400 | 0.1797 | 0.9274 | 0.9274 |
| 0.1783 | 23.24 | 8600 | 0.1792 | 0.9270 | 0.9270 |
| 0.1756 | 23.78 | 8800 | 0.1794 | 0.9277 | 0.9277 |
| 0.1784 | 24.32 | 9000 | 0.1799 | 0.9274 | 0.9274 |
| 0.176 | 24.86 | 9200 | 0.1796 | 0.9269 | 0.9269 |
| 0.1736 | 25.41 | 9400 | 0.1802 | 0.9265 | 0.9265 |
| 0.1753 | 25.95 | 9600 | 0.1796 | 0.9267 | 0.9267 |
| 0.1756 | 26.49 | 9800 | 0.1793 | 0.9272 | 0.9272 |
| 0.1741 | 27.03 | 10000 | 0.1795 | 0.9270 | 0.9270 |
### Framework versions
- PEFT 0.9.0
- Transformers 4.38.2
- Pytorch 2.2.0+cu121
- Datasets 2.17.1
- Tokenizers 0.15.2 | {"library_name": "peft", "tags": ["generated_from_trainer"], "metrics": ["accuracy"], "base_model": "mahdibaghbanzadeh/seqsight_16384_512_22M", "model-index": [{"name": "GUE_prom_prom_300_all-seqsight_16384_512_22M-L8_f", "results": []}]} | mahdibaghbanzadeh/GUE_prom_prom_300_all-seqsight_16384_512_22M-L8_f | null | [
"peft",
"safetensors",
"generated_from_trainer",
"base_model:mahdibaghbanzadeh/seqsight_16384_512_22M",
"region:us"
] | null | 2024-04-27T07:02:01+00:00 | [] | [] | TAGS
#peft #safetensors #generated_from_trainer #base_model-mahdibaghbanzadeh/seqsight_16384_512_22M #region-us
| GUE\_prom\_prom\_300\_all-seqsight\_16384\_512\_22M-L8\_f
=========================================================
This model is a fine-tuned version of mahdibaghbanzadeh/seqsight\_16384\_512\_22M on the mahdibaghbanzadeh/GUE\_prom\_prom\_300\_all dataset.
It achieves the following results on the evaluation set:
* Loss: 0.2074
* F1 Score: 0.9167
* Accuracy: 0.9167
Model description
-----------------
More information needed
Intended uses & limitations
---------------------------
More information needed
Training and evaluation data
----------------------------
More information needed
Training procedure
------------------
### Training hyperparameters
The following hyperparameters were used during training:
* learning\_rate: 0.0005
* train\_batch\_size: 128
* eval\_batch\_size: 128
* seed: 42
* optimizer: Adam with betas=(0.9,0.999) and epsilon=1e-08
* lr\_scheduler\_type: linear
* training\_steps: 10000
### Training results
### Framework versions
* PEFT 0.9.0
* Transformers 4.38.2
* Pytorch 2.2.0+cu121
* Datasets 2.17.1
* Tokenizers 0.15.2
| [
"### Training hyperparameters\n\n\nThe following hyperparameters were used during training:\n\n\n* learning\\_rate: 0.0005\n* train\\_batch\\_size: 128\n* eval\\_batch\\_size: 128\n* seed: 42\n* optimizer: Adam with betas=(0.9,0.999) and epsilon=1e-08\n* lr\\_scheduler\\_type: linear\n* training\\_steps: 10000",
"### Training results",
"### Framework versions\n\n\n* PEFT 0.9.0\n* Transformers 4.38.2\n* Pytorch 2.2.0+cu121\n* Datasets 2.17.1\n* Tokenizers 0.15.2"
] | [
"TAGS\n#peft #safetensors #generated_from_trainer #base_model-mahdibaghbanzadeh/seqsight_16384_512_22M #region-us \n",
"### Training hyperparameters\n\n\nThe following hyperparameters were used during training:\n\n\n* learning\\_rate: 0.0005\n* train\\_batch\\_size: 128\n* eval\\_batch\\_size: 128\n* seed: 42\n* optimizer: Adam with betas=(0.9,0.999) and epsilon=1e-08\n* lr\\_scheduler\\_type: linear\n* training\\_steps: 10000",
"### Training results",
"### Framework versions\n\n\n* PEFT 0.9.0\n* Transformers 4.38.2\n* Pytorch 2.2.0+cu121\n* Datasets 2.17.1\n* Tokenizers 0.15.2"
] |
text-generation | transformers |
# Model Card for Model ID
<!-- Provide a quick summary of what the model is/does. -->
## Model Details
### Model Description
<!-- Provide a longer summary of what this model is. -->
This is the model card of a 🤗 transformers model that has been pushed on the Hub. This model card has been automatically generated.
- **Developed by:** [More Information Needed]
- **Funded by [optional]:** [More Information Needed]
- **Shared by [optional]:** [More Information Needed]
- **Model type:** [More Information Needed]
- **Language(s) (NLP):** [More Information Needed]
- **License:** [More Information Needed]
- **Finetuned from model [optional]:** [More Information Needed]
### Model Sources [optional]
<!-- Provide the basic links for the model. -->
- **Repository:** [More Information Needed]
- **Paper [optional]:** [More Information Needed]
- **Demo [optional]:** [More Information Needed]
## Uses
<!-- Address questions around how the model is intended to be used, including the foreseeable users of the model and those affected by the model. -->
### Direct Use
<!-- This section is for the model use without fine-tuning or plugging into a larger ecosystem/app. -->
[More Information Needed]
### Downstream Use [optional]
<!-- This section is for the model use when fine-tuned for a task, or when plugged into a larger ecosystem/app -->
[More Information Needed]
### Out-of-Scope Use
<!-- This section addresses misuse, malicious use, and uses that the model will not work well for. -->
[More Information Needed]
## Bias, Risks, and Limitations
<!-- This section is meant to convey both technical and sociotechnical limitations. -->
[More Information Needed]
### Recommendations
<!-- This section is meant to convey recommendations with respect to the bias, risk, and technical limitations. -->
Users (both direct and downstream) should be made aware of the risks, biases and limitations of the model. More information needed for further recommendations.
## How to Get Started with the Model
Use the code below to get started with the model.
[More Information Needed]
## Training Details
### Training Data
<!-- This should link to a Dataset Card, perhaps with a short stub of information on what the training data is all about as well as documentation related to data pre-processing or additional filtering. -->
[More Information Needed]
### Training Procedure
<!-- This relates heavily to the Technical Specifications. Content here should link to that section when it is relevant to the training procedure. -->
#### Preprocessing [optional]
[More Information Needed]
#### Training Hyperparameters
- **Training regime:** [More Information Needed] <!--fp32, fp16 mixed precision, bf16 mixed precision, bf16 non-mixed precision, fp16 non-mixed precision, fp8 mixed precision -->
#### Speeds, Sizes, Times [optional]
<!-- This section provides information about throughput, start/end time, checkpoint size if relevant, etc. -->
[More Information Needed]
## Evaluation
<!-- This section describes the evaluation protocols and provides the results. -->
### Testing Data, Factors & Metrics
#### Testing Data
<!-- This should link to a Dataset Card if possible. -->
[More Information Needed]
#### Factors
<!-- These are the things the evaluation is disaggregating by, e.g., subpopulations or domains. -->
[More Information Needed]
#### Metrics
<!-- These are the evaluation metrics being used, ideally with a description of why. -->
[More Information Needed]
### Results
[More Information Needed]
#### Summary
## Model Examination [optional]
<!-- Relevant interpretability work for the model goes here -->
[More Information Needed]
## Environmental Impact
<!-- Total emissions (in grams of CO2eq) and additional considerations, such as electricity usage, go here. Edit the suggested text below accordingly -->
Carbon emissions can be estimated using the [Machine Learning Impact calculator](https://mlco2.github.io/impact#compute) presented in [Lacoste et al. (2019)](https://arxiv.org/abs/1910.09700).
- **Hardware Type:** [More Information Needed]
- **Hours used:** [More Information Needed]
- **Cloud Provider:** [More Information Needed]
- **Compute Region:** [More Information Needed]
- **Carbon Emitted:** [More Information Needed]
## Technical Specifications [optional]
### Model Architecture and Objective
[More Information Needed]
### Compute Infrastructure
[More Information Needed]
#### Hardware
[More Information Needed]
#### Software
[More Information Needed]
## Citation [optional]
<!-- If there is a paper or blog post introducing the model, the APA and Bibtex information for that should go in this section. -->
**BibTeX:**
[More Information Needed]
**APA:**
[More Information Needed]
## Glossary [optional]
<!-- If relevant, include terms and calculations in this section that can help readers understand the model or model card. -->
[More Information Needed]
## More Information [optional]
[More Information Needed]
## Model Card Authors [optional]
[More Information Needed]
## Model Card Contact
[More Information Needed]
| {"library_name": "transformers", "tags": []} | swj0419/hp_retrain_STEP0000010 | null | [
"transformers",
"safetensors",
"llama",
"text-generation",
"arxiv:1910.09700",
"autotrain_compatible",
"endpoints_compatible",
"text-generation-inference",
"region:us"
] | null | 2024-04-27T07:02:14+00:00 | [
"1910.09700"
] | [] | TAGS
#transformers #safetensors #llama #text-generation #arxiv-1910.09700 #autotrain_compatible #endpoints_compatible #text-generation-inference #region-us
|
# Model Card for Model ID
## Model Details
### Model Description
This is the model card of a transformers model that has been pushed on the Hub. This model card has been automatically generated.
- Developed by:
- Funded by [optional]:
- Shared by [optional]:
- Model type:
- Language(s) (NLP):
- License:
- Finetuned from model [optional]:
### Model Sources [optional]
- Repository:
- Paper [optional]:
- Demo [optional]:
## Uses
### Direct Use
### Downstream Use [optional]
### Out-of-Scope Use
## Bias, Risks, and Limitations
### Recommendations
Users (both direct and downstream) should be made aware of the risks, biases and limitations of the model. More information needed for further recommendations.
## How to Get Started with the Model
Use the code below to get started with the model.
## Training Details
### Training Data
### Training Procedure
#### Preprocessing [optional]
#### Training Hyperparameters
- Training regime:
#### Speeds, Sizes, Times [optional]
## Evaluation
### Testing Data, Factors & Metrics
#### Testing Data
#### Factors
#### Metrics
### Results
#### Summary
## Model Examination [optional]
## Environmental Impact
Carbon emissions can be estimated using the Machine Learning Impact calculator presented in Lacoste et al. (2019).
- Hardware Type:
- Hours used:
- Cloud Provider:
- Compute Region:
- Carbon Emitted:
## Technical Specifications [optional]
### Model Architecture and Objective
### Compute Infrastructure
#### Hardware
#### Software
[optional]
BibTeX:
APA:
## Glossary [optional]
## More Information [optional]
## Model Card Authors [optional]
## Model Card Contact
| [
"# Model Card for Model ID",
"## Model Details",
"### Model Description\n\n\n\nThis is the model card of a transformers model that has been pushed on the Hub. This model card has been automatically generated.\n\n- Developed by: \n- Funded by [optional]: \n- Shared by [optional]: \n- Model type: \n- Language(s) (NLP): \n- License: \n- Finetuned from model [optional]:",
"### Model Sources [optional]\n\n\n\n- Repository: \n- Paper [optional]: \n- Demo [optional]:",
"## Uses",
"### Direct Use",
"### Downstream Use [optional]",
"### Out-of-Scope Use",
"## Bias, Risks, and Limitations",
"### Recommendations\n\n\n\nUsers (both direct and downstream) should be made aware of the risks, biases and limitations of the model. More information needed for further recommendations.",
"## How to Get Started with the Model\n\nUse the code below to get started with the model.",
"## Training Details",
"### Training Data",
"### Training Procedure",
"#### Preprocessing [optional]",
"#### Training Hyperparameters\n\n- Training regime:",
"#### Speeds, Sizes, Times [optional]",
"## Evaluation",
"### Testing Data, Factors & Metrics",
"#### Testing Data",
"#### Factors",
"#### Metrics",
"### Results",
"#### Summary",
"## Model Examination [optional]",
"## Environmental Impact\n\n\n\nCarbon emissions can be estimated using the Machine Learning Impact calculator presented in Lacoste et al. (2019).\n\n- Hardware Type: \n- Hours used: \n- Cloud Provider: \n- Compute Region: \n- Carbon Emitted:",
"## Technical Specifications [optional]",
"### Model Architecture and Objective",
"### Compute Infrastructure",
"#### Hardware",
"#### Software\n\n\n\n[optional]\n\n\n\nBibTeX:\n\n\n\nAPA:",
"## Glossary [optional]",
"## More Information [optional]",
"## Model Card Authors [optional]",
"## Model Card Contact"
] | [
"TAGS\n#transformers #safetensors #llama #text-generation #arxiv-1910.09700 #autotrain_compatible #endpoints_compatible #text-generation-inference #region-us \n",
"# Model Card for Model ID",
"## Model Details",
"### Model Description\n\n\n\nThis is the model card of a transformers model that has been pushed on the Hub. This model card has been automatically generated.\n\n- Developed by: \n- Funded by [optional]: \n- Shared by [optional]: \n- Model type: \n- Language(s) (NLP): \n- License: \n- Finetuned from model [optional]:",
"### Model Sources [optional]\n\n\n\n- Repository: \n- Paper [optional]: \n- Demo [optional]:",
"## Uses",
"### Direct Use",
"### Downstream Use [optional]",
"### Out-of-Scope Use",
"## Bias, Risks, and Limitations",
"### Recommendations\n\n\n\nUsers (both direct and downstream) should be made aware of the risks, biases and limitations of the model. More information needed for further recommendations.",
"## How to Get Started with the Model\n\nUse the code below to get started with the model.",
"## Training Details",
"### Training Data",
"### Training Procedure",
"#### Preprocessing [optional]",
"#### Training Hyperparameters\n\n- Training regime:",
"#### Speeds, Sizes, Times [optional]",
"## Evaluation",
"### Testing Data, Factors & Metrics",
"#### Testing Data",
"#### Factors",
"#### Metrics",
"### Results",
"#### Summary",
"## Model Examination [optional]",
"## Environmental Impact\n\n\n\nCarbon emissions can be estimated using the Machine Learning Impact calculator presented in Lacoste et al. (2019).\n\n- Hardware Type: \n- Hours used: \n- Cloud Provider: \n- Compute Region: \n- Carbon Emitted:",
"## Technical Specifications [optional]",
"### Model Architecture and Objective",
"### Compute Infrastructure",
"#### Hardware",
"#### Software\n\n\n\n[optional]\n\n\n\nBibTeX:\n\n\n\nAPA:",
"## Glossary [optional]",
"## More Information [optional]",
"## Model Card Authors [optional]",
"## Model Card Contact"
] |
null | peft |
<!-- This model card has been generated automatically according to the information the Trainer had access to. You
should probably proofread and complete it, then remove this comment. -->
# GUE_prom_prom_300_all-seqsight_16384_512_22M-L32_f
This model is a fine-tuned version of [mahdibaghbanzadeh/seqsight_16384_512_22M](https://huggingface.co/mahdibaghbanzadeh/seqsight_16384_512_22M) on the [mahdibaghbanzadeh/GUE_prom_prom_300_all](https://huggingface.co/datasets/mahdibaghbanzadeh/GUE_prom_prom_300_all) dataset.
It achieves the following results on the evaluation set:
- Loss: 0.2079
- F1 Score: 0.9181
- Accuracy: 0.9181
## Model description
More information needed
## Intended uses & limitations
More information needed
## Training and evaluation data
More information needed
## Training procedure
### Training hyperparameters
The following hyperparameters were used during training:
- learning_rate: 0.0005
- train_batch_size: 128
- eval_batch_size: 128
- seed: 42
- optimizer: Adam with betas=(0.9,0.999) and epsilon=1e-08
- lr_scheduler_type: linear
- training_steps: 10000
### Training results
| Training Loss | Epoch | Step | Validation Loss | F1 Score | Accuracy |
|:-------------:|:-----:|:-----:|:---------------:|:--------:|:--------:|
| 0.3369 | 0.54 | 200 | 0.2350 | 0.9071 | 0.9071 |
| 0.2434 | 1.08 | 400 | 0.2176 | 0.9145 | 0.9145 |
| 0.2318 | 1.62 | 600 | 0.2080 | 0.9177 | 0.9177 |
| 0.2171 | 2.16 | 800 | 0.2050 | 0.9177 | 0.9177 |
| 0.2172 | 2.7 | 1000 | 0.2024 | 0.9184 | 0.9184 |
| 0.2068 | 3.24 | 1200 | 0.2025 | 0.9177 | 0.9177 |
| 0.2048 | 3.78 | 1400 | 0.1906 | 0.9223 | 0.9223 |
| 0.2031 | 4.32 | 1600 | 0.1847 | 0.9262 | 0.9262 |
| 0.1952 | 4.86 | 1800 | 0.1869 | 0.9253 | 0.9253 |
| 0.1941 | 5.41 | 2000 | 0.1871 | 0.9267 | 0.9267 |
| 0.1946 | 5.95 | 2200 | 0.1832 | 0.9284 | 0.9284 |
| 0.1894 | 6.49 | 2400 | 0.1839 | 0.9269 | 0.9269 |
| 0.1905 | 7.03 | 2600 | 0.1850 | 0.9289 | 0.9289 |
| 0.1821 | 7.57 | 2800 | 0.1778 | 0.9280 | 0.9280 |
| 0.1853 | 8.11 | 3000 | 0.1800 | 0.9289 | 0.9289 |
| 0.1807 | 8.65 | 3200 | 0.1812 | 0.9280 | 0.9280 |
| 0.1736 | 9.19 | 3400 | 0.1805 | 0.9257 | 0.9257 |
| 0.1766 | 9.73 | 3600 | 0.1799 | 0.9285 | 0.9285 |
| 0.1827 | 10.27 | 3800 | 0.1775 | 0.9284 | 0.9284 |
| 0.1774 | 10.81 | 4000 | 0.1774 | 0.9292 | 0.9292 |
| 0.1774 | 11.35 | 4200 | 0.1733 | 0.9309 | 0.9309 |
| 0.1693 | 11.89 | 4400 | 0.1820 | 0.9311 | 0.9311 |
| 0.1712 | 12.43 | 4600 | 0.1738 | 0.9309 | 0.9309 |
| 0.1698 | 12.97 | 4800 | 0.1785 | 0.9294 | 0.9294 |
| 0.1659 | 13.51 | 5000 | 0.1757 | 0.9306 | 0.9306 |
| 0.1695 | 14.05 | 5200 | 0.1846 | 0.9253 | 0.9253 |
| 0.1606 | 14.59 | 5400 | 0.1814 | 0.9314 | 0.9314 |
| 0.1674 | 15.14 | 5600 | 0.1761 | 0.9314 | 0.9314 |
| 0.1612 | 15.68 | 5800 | 0.1762 | 0.9302 | 0.9302 |
| 0.1646 | 16.22 | 6000 | 0.1786 | 0.9296 | 0.9296 |
| 0.1626 | 16.76 | 6200 | 0.1764 | 0.9311 | 0.9311 |
| 0.1594 | 17.3 | 6400 | 0.1744 | 0.9319 | 0.9319 |
| 0.1593 | 17.84 | 6600 | 0.1757 | 0.9312 | 0.9313 |
| 0.1544 | 18.38 | 6800 | 0.1790 | 0.9321 | 0.9321 |
| 0.1591 | 18.92 | 7000 | 0.1724 | 0.9341 | 0.9341 |
| 0.1581 | 19.46 | 7200 | 0.1749 | 0.9334 | 0.9334 |
| 0.1554 | 20.0 | 7400 | 0.1751 | 0.9341 | 0.9341 |
| 0.1573 | 20.54 | 7600 | 0.1743 | 0.9343 | 0.9343 |
| 0.1574 | 21.08 | 7800 | 0.1721 | 0.9346 | 0.9346 |
| 0.1557 | 21.62 | 8000 | 0.1741 | 0.9341 | 0.9341 |
| 0.1523 | 22.16 | 8200 | 0.1740 | 0.9338 | 0.9338 |
| 0.1492 | 22.7 | 8400 | 0.1747 | 0.9346 | 0.9346 |
| 0.1529 | 23.24 | 8600 | 0.1745 | 0.9353 | 0.9353 |
| 0.1518 | 23.78 | 8800 | 0.1750 | 0.9338 | 0.9338 |
| 0.154 | 24.32 | 9000 | 0.1749 | 0.9326 | 0.9326 |
| 0.1492 | 24.86 | 9200 | 0.1765 | 0.9341 | 0.9341 |
| 0.1472 | 25.41 | 9400 | 0.1763 | 0.9340 | 0.9340 |
| 0.1504 | 25.95 | 9600 | 0.1755 | 0.9350 | 0.9350 |
| 0.15 | 26.49 | 9800 | 0.1749 | 0.9350 | 0.9350 |
| 0.1477 | 27.03 | 10000 | 0.1752 | 0.9353 | 0.9353 |
### Framework versions
- PEFT 0.9.0
- Transformers 4.38.2
- Pytorch 2.2.0+cu121
- Datasets 2.17.1
- Tokenizers 0.15.2 | {"library_name": "peft", "tags": ["generated_from_trainer"], "metrics": ["accuracy"], "base_model": "mahdibaghbanzadeh/seqsight_16384_512_22M", "model-index": [{"name": "GUE_prom_prom_300_all-seqsight_16384_512_22M-L32_f", "results": []}]} | mahdibaghbanzadeh/GUE_prom_prom_300_all-seqsight_16384_512_22M-L32_f | null | [
"peft",
"safetensors",
"generated_from_trainer",
"base_model:mahdibaghbanzadeh/seqsight_16384_512_22M",
"region:us"
] | null | 2024-04-27T07:03:28+00:00 | [] | [] | TAGS
#peft #safetensors #generated_from_trainer #base_model-mahdibaghbanzadeh/seqsight_16384_512_22M #region-us
| GUE\_prom\_prom\_300\_all-seqsight\_16384\_512\_22M-L32\_f
==========================================================
This model is a fine-tuned version of mahdibaghbanzadeh/seqsight\_16384\_512\_22M on the mahdibaghbanzadeh/GUE\_prom\_prom\_300\_all dataset.
It achieves the following results on the evaluation set:
* Loss: 0.2079
* F1 Score: 0.9181
* Accuracy: 0.9181
Model description
-----------------
More information needed
Intended uses & limitations
---------------------------
More information needed
Training and evaluation data
----------------------------
More information needed
Training procedure
------------------
### Training hyperparameters
The following hyperparameters were used during training:
* learning\_rate: 0.0005
* train\_batch\_size: 128
* eval\_batch\_size: 128
* seed: 42
* optimizer: Adam with betas=(0.9,0.999) and epsilon=1e-08
* lr\_scheduler\_type: linear
* training\_steps: 10000
### Training results
### Framework versions
* PEFT 0.9.0
* Transformers 4.38.2
* Pytorch 2.2.0+cu121
* Datasets 2.17.1
* Tokenizers 0.15.2
| [
"### Training hyperparameters\n\n\nThe following hyperparameters were used during training:\n\n\n* learning\\_rate: 0.0005\n* train\\_batch\\_size: 128\n* eval\\_batch\\_size: 128\n* seed: 42\n* optimizer: Adam with betas=(0.9,0.999) and epsilon=1e-08\n* lr\\_scheduler\\_type: linear\n* training\\_steps: 10000",
"### Training results",
"### Framework versions\n\n\n* PEFT 0.9.0\n* Transformers 4.38.2\n* Pytorch 2.2.0+cu121\n* Datasets 2.17.1\n* Tokenizers 0.15.2"
] | [
"TAGS\n#peft #safetensors #generated_from_trainer #base_model-mahdibaghbanzadeh/seqsight_16384_512_22M #region-us \n",
"### Training hyperparameters\n\n\nThe following hyperparameters were used during training:\n\n\n* learning\\_rate: 0.0005\n* train\\_batch\\_size: 128\n* eval\\_batch\\_size: 128\n* seed: 42\n* optimizer: Adam with betas=(0.9,0.999) and epsilon=1e-08\n* lr\\_scheduler\\_type: linear\n* training\\_steps: 10000",
"### Training results",
"### Framework versions\n\n\n* PEFT 0.9.0\n* Transformers 4.38.2\n* Pytorch 2.2.0+cu121\n* Datasets 2.17.1\n* Tokenizers 0.15.2"
] |
null | peft |
<!-- This model card has been generated automatically according to the information the Trainer had access to. You
should probably proofread and complete it, then remove this comment. -->
# GUE_EMP_H3K14ac-seqsight_16384_512_22M-L1_f
This model is a fine-tuned version of [mahdibaghbanzadeh/seqsight_16384_512_22M](https://huggingface.co/mahdibaghbanzadeh/seqsight_16384_512_22M) on the [mahdibaghbanzadeh/GUE_EMP_H3K14ac](https://huggingface.co/datasets/mahdibaghbanzadeh/GUE_EMP_H3K14ac) dataset.
It achieves the following results on the evaluation set:
- Loss: 0.5340
- F1 Score: 0.7309
- Accuracy: 0.7295
## Model description
More information needed
## Intended uses & limitations
More information needed
## Training and evaluation data
More information needed
## Training procedure
### Training hyperparameters
The following hyperparameters were used during training:
- learning_rate: 0.0005
- train_batch_size: 128
- eval_batch_size: 128
- seed: 42
- optimizer: Adam with betas=(0.9,0.999) and epsilon=1e-08
- lr_scheduler_type: linear
- training_steps: 10000
### Training results
| Training Loss | Epoch | Step | Validation Loss | F1 Score | Accuracy |
|:-------------:|:-----:|:-----:|:---------------:|:--------:|:--------:|
| 0.6157 | 0.97 | 200 | 0.5936 | 0.6832 | 0.6820 |
| 0.5787 | 1.93 | 400 | 0.5774 | 0.7012 | 0.6992 |
| 0.5725 | 2.9 | 600 | 0.5869 | 0.6981 | 0.6974 |
| 0.5631 | 3.86 | 800 | 0.5446 | 0.7278 | 0.7277 |
| 0.556 | 4.83 | 1000 | 0.5833 | 0.7042 | 0.7038 |
| 0.5518 | 5.8 | 1200 | 0.5797 | 0.7083 | 0.7077 |
| 0.5492 | 6.76 | 1400 | 0.5688 | 0.7111 | 0.7101 |
| 0.5467 | 7.73 | 1600 | 0.5496 | 0.7243 | 0.7225 |
| 0.5411 | 8.7 | 1800 | 0.5540 | 0.7177 | 0.7162 |
| 0.54 | 9.66 | 2000 | 0.5553 | 0.7220 | 0.7204 |
| 0.5427 | 10.63 | 2200 | 0.5834 | 0.6982 | 0.6986 |
| 0.5341 | 11.59 | 2400 | 0.5457 | 0.7267 | 0.7250 |
| 0.5362 | 12.56 | 2600 | 0.5672 | 0.7142 | 0.7132 |
| 0.5344 | 13.53 | 2800 | 0.5681 | 0.7129 | 0.7120 |
| 0.535 | 14.49 | 3000 | 0.5910 | 0.6995 | 0.7005 |
| 0.5305 | 15.46 | 3200 | 0.5434 | 0.7292 | 0.7274 |
| 0.5298 | 16.43 | 3400 | 0.5669 | 0.7112 | 0.7107 |
| 0.5307 | 17.39 | 3600 | 0.5580 | 0.7189 | 0.7177 |
| 0.5299 | 18.36 | 3800 | 0.5393 | 0.7359 | 0.7340 |
| 0.5277 | 19.32 | 4000 | 0.5525 | 0.7239 | 0.7225 |
| 0.5266 | 20.29 | 4200 | 0.5531 | 0.7243 | 0.7228 |
| 0.5261 | 21.26 | 4400 | 0.5635 | 0.7198 | 0.7189 |
| 0.5255 | 22.22 | 4600 | 0.5694 | 0.7111 | 0.7107 |
| 0.5252 | 23.19 | 4800 | 0.5419 | 0.7309 | 0.7292 |
| 0.5242 | 24.15 | 5000 | 0.5463 | 0.7265 | 0.7250 |
| 0.5224 | 25.12 | 5200 | 0.5664 | 0.7154 | 0.7147 |
| 0.5213 | 26.09 | 5400 | 0.5544 | 0.7244 | 0.7231 |
| 0.5229 | 27.05 | 5600 | 0.5730 | 0.7142 | 0.7141 |
| 0.5239 | 28.02 | 5800 | 0.5405 | 0.7300 | 0.7283 |
| 0.5233 | 28.99 | 6000 | 0.5629 | 0.7161 | 0.7156 |
| 0.5191 | 29.95 | 6200 | 0.5702 | 0.7173 | 0.7168 |
| 0.5202 | 30.92 | 6400 | 0.5472 | 0.7250 | 0.7234 |
| 0.5198 | 31.88 | 6600 | 0.5564 | 0.7202 | 0.7192 |
| 0.5165 | 32.85 | 6800 | 0.5594 | 0.7205 | 0.7195 |
| 0.5237 | 33.82 | 7000 | 0.5677 | 0.7143 | 0.7141 |
| 0.5183 | 34.78 | 7200 | 0.5645 | 0.7183 | 0.7177 |
| 0.5191 | 35.75 | 7400 | 0.5594 | 0.7200 | 0.7189 |
| 0.5168 | 36.71 | 7600 | 0.5539 | 0.7225 | 0.7213 |
| 0.5178 | 37.68 | 7800 | 0.5543 | 0.7236 | 0.7225 |
| 0.5161 | 38.65 | 8000 | 0.5436 | 0.7256 | 0.7241 |
| 0.5238 | 39.61 | 8200 | 0.5571 | 0.7220 | 0.7210 |
| 0.5127 | 40.58 | 8400 | 0.5669 | 0.7167 | 0.7162 |
| 0.5149 | 41.55 | 8600 | 0.5546 | 0.7231 | 0.7219 |
| 0.5163 | 42.51 | 8800 | 0.5609 | 0.7198 | 0.7189 |
| 0.5192 | 43.48 | 9000 | 0.5633 | 0.7206 | 0.7198 |
| 0.5169 | 44.44 | 9200 | 0.5575 | 0.7223 | 0.7213 |
| 0.519 | 45.41 | 9400 | 0.5537 | 0.7212 | 0.7201 |
| 0.511 | 46.38 | 9600 | 0.5605 | 0.7222 | 0.7213 |
| 0.5194 | 47.34 | 9800 | 0.5564 | 0.7223 | 0.7213 |
| 0.515 | 48.31 | 10000 | 0.5546 | 0.7221 | 0.7210 |
### Framework versions
- PEFT 0.9.0
- Transformers 4.38.2
- Pytorch 2.2.0+cu121
- Datasets 2.17.1
- Tokenizers 0.15.2 | {"library_name": "peft", "tags": ["generated_from_trainer"], "metrics": ["accuracy"], "base_model": "mahdibaghbanzadeh/seqsight_16384_512_22M", "model-index": [{"name": "GUE_EMP_H3K14ac-seqsight_16384_512_22M-L1_f", "results": []}]} | mahdibaghbanzadeh/GUE_EMP_H3K14ac-seqsight_16384_512_22M-L1_f | null | [
"peft",
"safetensors",
"generated_from_trainer",
"base_model:mahdibaghbanzadeh/seqsight_16384_512_22M",
"region:us"
] | null | 2024-04-27T07:03:28+00:00 | [] | [] | TAGS
#peft #safetensors #generated_from_trainer #base_model-mahdibaghbanzadeh/seqsight_16384_512_22M #region-us
| GUE\_EMP\_H3K14ac-seqsight\_16384\_512\_22M-L1\_f
=================================================
This model is a fine-tuned version of mahdibaghbanzadeh/seqsight\_16384\_512\_22M on the mahdibaghbanzadeh/GUE\_EMP\_H3K14ac dataset.
It achieves the following results on the evaluation set:
* Loss: 0.5340
* F1 Score: 0.7309
* Accuracy: 0.7295
Model description
-----------------
More information needed
Intended uses & limitations
---------------------------
More information needed
Training and evaluation data
----------------------------
More information needed
Training procedure
------------------
### Training hyperparameters
The following hyperparameters were used during training:
* learning\_rate: 0.0005
* train\_batch\_size: 128
* eval\_batch\_size: 128
* seed: 42
* optimizer: Adam with betas=(0.9,0.999) and epsilon=1e-08
* lr\_scheduler\_type: linear
* training\_steps: 10000
### Training results
### Framework versions
* PEFT 0.9.0
* Transformers 4.38.2
* Pytorch 2.2.0+cu121
* Datasets 2.17.1
* Tokenizers 0.15.2
| [
"### Training hyperparameters\n\n\nThe following hyperparameters were used during training:\n\n\n* learning\\_rate: 0.0005\n* train\\_batch\\_size: 128\n* eval\\_batch\\_size: 128\n* seed: 42\n* optimizer: Adam with betas=(0.9,0.999) and epsilon=1e-08\n* lr\\_scheduler\\_type: linear\n* training\\_steps: 10000",
"### Training results",
"### Framework versions\n\n\n* PEFT 0.9.0\n* Transformers 4.38.2\n* Pytorch 2.2.0+cu121\n* Datasets 2.17.1\n* Tokenizers 0.15.2"
] | [
"TAGS\n#peft #safetensors #generated_from_trainer #base_model-mahdibaghbanzadeh/seqsight_16384_512_22M #region-us \n",
"### Training hyperparameters\n\n\nThe following hyperparameters were used during training:\n\n\n* learning\\_rate: 0.0005\n* train\\_batch\\_size: 128\n* eval\\_batch\\_size: 128\n* seed: 42\n* optimizer: Adam with betas=(0.9,0.999) and epsilon=1e-08\n* lr\\_scheduler\\_type: linear\n* training\\_steps: 10000",
"### Training results",
"### Framework versions\n\n\n* PEFT 0.9.0\n* Transformers 4.38.2\n* Pytorch 2.2.0+cu121\n* Datasets 2.17.1\n* Tokenizers 0.15.2"
] |
text-classification | transformers |
<!-- This model card has been generated automatically according to the information Keras had access to. You should
probably proofread and complete it, then remove this comment. -->
# vsufiy/my_awesome_model
This model is a fine-tuned version of [distilbert-base-uncased](https://huggingface.co/distilbert-base-uncased) on an unknown dataset.
It achieves the following results on the evaluation set:
- Train Loss: 0.0615
- Validation Loss: 0.2217
- Train Accuracy: 0.9327
- Epoch: 2
## Model description
More information needed
## Intended uses & limitations
More information needed
## Training and evaluation data
More information needed
## Training procedure
### Training hyperparameters
The following hyperparameters were used during training:
- optimizer: {'name': 'Adam', 'weight_decay': None, 'clipnorm': None, 'global_clipnorm': None, 'clipvalue': None, 'use_ema': False, 'ema_momentum': 0.99, 'ema_overwrite_frequency': None, 'jit_compile': True, 'is_legacy_optimizer': False, 'learning_rate': {'module': 'keras.optimizers.schedules', 'class_name': 'PolynomialDecay', 'config': {'initial_learning_rate': 2e-05, 'decay_steps': 7810, 'end_learning_rate': 0.0, 'power': 1.0, 'cycle': False, 'name': None}, 'registered_name': None}, 'beta_1': 0.9, 'beta_2': 0.999, 'epsilon': 1e-08, 'amsgrad': False}
- training_precision: float32
### Training results
| Train Loss | Validation Loss | Train Accuracy | Epoch |
|:----------:|:---------------:|:--------------:|:-----:|
| 0.2575 | 0.1853 | 0.9286 | 0 |
| 0.1342 | 0.1917 | 0.9278 | 1 |
| 0.0615 | 0.2217 | 0.9327 | 2 |
### Framework versions
- Transformers 4.40.0
- TensorFlow 2.15.0
- Datasets 2.19.0
- Tokenizers 0.19.1
| {"license": "apache-2.0", "tags": ["generated_from_keras_callback"], "base_model": "distilbert-base-uncased", "model-index": [{"name": "vsufiy/my_awesome_model", "results": []}]} | vsufiy/my_awesome_model | null | [
"transformers",
"tf",
"distilbert",
"text-classification",
"generated_from_keras_callback",
"base_model:distilbert-base-uncased",
"license:apache-2.0",
"autotrain_compatible",
"endpoints_compatible",
"region:us"
] | null | 2024-04-27T07:03:43+00:00 | [] | [] | TAGS
#transformers #tf #distilbert #text-classification #generated_from_keras_callback #base_model-distilbert-base-uncased #license-apache-2.0 #autotrain_compatible #endpoints_compatible #region-us
| vsufiy/my\_awesome\_model
=========================
This model is a fine-tuned version of distilbert-base-uncased on an unknown dataset.
It achieves the following results on the evaluation set:
* Train Loss: 0.0615
* Validation Loss: 0.2217
* Train Accuracy: 0.9327
* Epoch: 2
Model description
-----------------
More information needed
Intended uses & limitations
---------------------------
More information needed
Training and evaluation data
----------------------------
More information needed
Training procedure
------------------
### Training hyperparameters
The following hyperparameters were used during training:
* optimizer: {'name': 'Adam', 'weight\_decay': None, 'clipnorm': None, 'global\_clipnorm': None, 'clipvalue': None, 'use\_ema': False, 'ema\_momentum': 0.99, 'ema\_overwrite\_frequency': None, 'jit\_compile': True, 'is\_legacy\_optimizer': False, 'learning\_rate': {'module': 'keras.optimizers.schedules', 'class\_name': 'PolynomialDecay', 'config': {'initial\_learning\_rate': 2e-05, 'decay\_steps': 7810, 'end\_learning\_rate': 0.0, 'power': 1.0, 'cycle': False, 'name': None}, 'registered\_name': None}, 'beta\_1': 0.9, 'beta\_2': 0.999, 'epsilon': 1e-08, 'amsgrad': False}
* training\_precision: float32
### Training results
### Framework versions
* Transformers 4.40.0
* TensorFlow 2.15.0
* Datasets 2.19.0
* Tokenizers 0.19.1
| [
"### Training hyperparameters\n\n\nThe following hyperparameters were used during training:\n\n\n* optimizer: {'name': 'Adam', 'weight\\_decay': None, 'clipnorm': None, 'global\\_clipnorm': None, 'clipvalue': None, 'use\\_ema': False, 'ema\\_momentum': 0.99, 'ema\\_overwrite\\_frequency': None, 'jit\\_compile': True, 'is\\_legacy\\_optimizer': False, 'learning\\_rate': {'module': 'keras.optimizers.schedules', 'class\\_name': 'PolynomialDecay', 'config': {'initial\\_learning\\_rate': 2e-05, 'decay\\_steps': 7810, 'end\\_learning\\_rate': 0.0, 'power': 1.0, 'cycle': False, 'name': None}, 'registered\\_name': None}, 'beta\\_1': 0.9, 'beta\\_2': 0.999, 'epsilon': 1e-08, 'amsgrad': False}\n* training\\_precision: float32",
"### Training results",
"### Framework versions\n\n\n* Transformers 4.40.0\n* TensorFlow 2.15.0\n* Datasets 2.19.0\n* Tokenizers 0.19.1"
] | [
"TAGS\n#transformers #tf #distilbert #text-classification #generated_from_keras_callback #base_model-distilbert-base-uncased #license-apache-2.0 #autotrain_compatible #endpoints_compatible #region-us \n",
"### Training hyperparameters\n\n\nThe following hyperparameters were used during training:\n\n\n* optimizer: {'name': 'Adam', 'weight\\_decay': None, 'clipnorm': None, 'global\\_clipnorm': None, 'clipvalue': None, 'use\\_ema': False, 'ema\\_momentum': 0.99, 'ema\\_overwrite\\_frequency': None, 'jit\\_compile': True, 'is\\_legacy\\_optimizer': False, 'learning\\_rate': {'module': 'keras.optimizers.schedules', 'class\\_name': 'PolynomialDecay', 'config': {'initial\\_learning\\_rate': 2e-05, 'decay\\_steps': 7810, 'end\\_learning\\_rate': 0.0, 'power': 1.0, 'cycle': False, 'name': None}, 'registered\\_name': None}, 'beta\\_1': 0.9, 'beta\\_2': 0.999, 'epsilon': 1e-08, 'amsgrad': False}\n* training\\_precision: float32",
"### Training results",
"### Framework versions\n\n\n* Transformers 4.40.0\n* TensorFlow 2.15.0\n* Datasets 2.19.0\n* Tokenizers 0.19.1"
] |
null | null | Apakah Optalite Tablet?
Optalite harga ialah kapsul suplemen pemakanan yang direka khas untuk memberikan sokongan menyeluruh untuk kesihatan mata. Formula termajunya mengandungi gabungan sinergistik vitamin, mineral dan antioksidan, dipilih dengan teliti untuk menyuburkan dan melindungi mata daripada degenerasi yang berkaitan dengan usia dan tekanan alam sekitar.
Laman web rasmi:<a href="https://www.nutritionsee.com/optaitmalay">www.Optalite.com</a>
<p><a href="https://www.nutritionsee.com/optaitmalay"> <img src="https://www.nutritionsee.com/wp-content/uploads/2024/04/Optalite-Malaysia.png" alt="enter image description here"> </a></p>
<a href="https://www.nutritionsee.com/optaitmalay">Beli sekarang!! Klik pautan di bawah untuk maklumat lanjut dan dapatkan diskaun 50% sekarang... Cepat</a>
Laman web rasmi:<a href="https://www.nutritionsee.com/optaitmalay">www.Optalite.com</a> | {"license": "apache-2.0"} | Optalite/Optalite | null | [
"license:apache-2.0",
"region:us"
] | null | 2024-04-27T07:05:48+00:00 | [] | [] | TAGS
#license-apache-2.0 #region-us
| Apakah Optalite Tablet?
Optalite harga ialah kapsul suplemen pemakanan yang direka khas untuk memberikan sokongan menyeluruh untuk kesihatan mata. Formula termajunya mengandungi gabungan sinergistik vitamin, mineral dan antioksidan, dipilih dengan teliti untuk menyuburkan dan melindungi mata daripada degenerasi yang berkaitan dengan usia dan tekanan alam sekitar.
Laman web rasmi:<a href="URL
<p><a href="URL <img src="URL alt="enter image description here"> </a></p>
<a href="URL sekarang!! Klik pautan di bawah untuk maklumat lanjut dan dapatkan diskaun 50% sekarang... Cepat</a>
Laman web rasmi:<a href="URL | [] | [
"TAGS\n#license-apache-2.0 #region-us \n"
] |
null | keras |
<!-- This model card has been generated automatically according to the information Keras had access to. You should
probably proofread and complete it, then remove this comment. -->
# mindmate-f5-original-equal-cont-0-0
This model is a fine-tuned version of [bert-base-german-cased](https://huggingface.co/bert-base-german-cased) on an unknown dataset.
It achieves the following results on the evaluation set:
## Model description
More information needed
## Intended uses & limitations
More information needed
## Training and evaluation data
More information needed
## Training procedure
### Training hyperparameters
The following hyperparameters were used during training:
- optimizer: {'name': 'Adam', 'weight_decay': None, 'clipnorm': None, 'global_clipnorm': None, 'clipvalue': None, 'use_ema': False, 'ema_momentum': 0.99, 'ema_overwrite_frequency': None, 'jit_compile': True, 'is_legacy_optimizer': False, 'learning_rate': 3e-05, 'beta_1': 0.9, 'beta_2': 0.999, 'epsilon': 1e-07, 'amsgrad': False}
- training_precision: float32
### Training results
### Framework versions
- Transformers 4.40.0
- TensorFlow 2.15.0
- Tokenizers 0.19.1
| {"license": "mit", "tags": ["generated_from_keras_callback"], "base_model": "bert-base-german-cased", "model-index": [{"name": "mindmate-f5-original-equal-cont-0-0", "results": []}]} | spneshaei/mindmate-f5-original-equal-cont-0-0 | null | [
"keras",
"tf",
"bert",
"generated_from_keras_callback",
"base_model:bert-base-german-cased",
"license:mit",
"region:us"
] | null | 2024-04-27T07:05:54+00:00 | [] | [] | TAGS
#keras #tf #bert #generated_from_keras_callback #base_model-bert-base-german-cased #license-mit #region-us
|
# mindmate-f5-original-equal-cont-0-0
This model is a fine-tuned version of bert-base-german-cased on an unknown dataset.
It achieves the following results on the evaluation set:
## Model description
More information needed
## Intended uses & limitations
More information needed
## Training and evaluation data
More information needed
## Training procedure
### Training hyperparameters
The following hyperparameters were used during training:
- optimizer: {'name': 'Adam', 'weight_decay': None, 'clipnorm': None, 'global_clipnorm': None, 'clipvalue': None, 'use_ema': False, 'ema_momentum': 0.99, 'ema_overwrite_frequency': None, 'jit_compile': True, 'is_legacy_optimizer': False, 'learning_rate': 3e-05, 'beta_1': 0.9, 'beta_2': 0.999, 'epsilon': 1e-07, 'amsgrad': False}
- training_precision: float32
### Training results
### Framework versions
- Transformers 4.40.0
- TensorFlow 2.15.0
- Tokenizers 0.19.1
| [
"# mindmate-f5-original-equal-cont-0-0\n\nThis model is a fine-tuned version of bert-base-german-cased on an unknown dataset.\nIt achieves the following results on the evaluation set:",
"## Model description\n\nMore information needed",
"## Intended uses & limitations\n\nMore information needed",
"## Training and evaluation data\n\nMore information needed",
"## Training procedure",
"### Training hyperparameters\n\nThe following hyperparameters were used during training:\n- optimizer: {'name': 'Adam', 'weight_decay': None, 'clipnorm': None, 'global_clipnorm': None, 'clipvalue': None, 'use_ema': False, 'ema_momentum': 0.99, 'ema_overwrite_frequency': None, 'jit_compile': True, 'is_legacy_optimizer': False, 'learning_rate': 3e-05, 'beta_1': 0.9, 'beta_2': 0.999, 'epsilon': 1e-07, 'amsgrad': False}\n- training_precision: float32",
"### Training results",
"### Framework versions\n\n- Transformers 4.40.0\n- TensorFlow 2.15.0\n- Tokenizers 0.19.1"
] | [
"TAGS\n#keras #tf #bert #generated_from_keras_callback #base_model-bert-base-german-cased #license-mit #region-us \n",
"# mindmate-f5-original-equal-cont-0-0\n\nThis model is a fine-tuned version of bert-base-german-cased on an unknown dataset.\nIt achieves the following results on the evaluation set:",
"## Model description\n\nMore information needed",
"## Intended uses & limitations\n\nMore information needed",
"## Training and evaluation data\n\nMore information needed",
"## Training procedure",
"### Training hyperparameters\n\nThe following hyperparameters were used during training:\n- optimizer: {'name': 'Adam', 'weight_decay': None, 'clipnorm': None, 'global_clipnorm': None, 'clipvalue': None, 'use_ema': False, 'ema_momentum': 0.99, 'ema_overwrite_frequency': None, 'jit_compile': True, 'is_legacy_optimizer': False, 'learning_rate': 3e-05, 'beta_1': 0.9, 'beta_2': 0.999, 'epsilon': 1e-07, 'amsgrad': False}\n- training_precision: float32",
"### Training results",
"### Framework versions\n\n- Transformers 4.40.0\n- TensorFlow 2.15.0\n- Tokenizers 0.19.1"
] |
automatic-speech-recognition | transformers |
# Model Card for Model ID
<!-- Provide a quick summary of what the model is/does. -->
## Model Details
### Model Description
<!-- Provide a longer summary of what this model is. -->
This is the model card of a 🤗 transformers model that has been pushed on the Hub. This model card has been automatically generated.
- **Developed by:** [More Information Needed]
- **Funded by [optional]:** [More Information Needed]
- **Shared by [optional]:** [More Information Needed]
- **Model type:** [More Information Needed]
- **Language(s) (NLP):** [More Information Needed]
- **License:** [More Information Needed]
- **Finetuned from model [optional]:** [More Information Needed]
### Model Sources [optional]
<!-- Provide the basic links for the model. -->
- **Repository:** [More Information Needed]
- **Paper [optional]:** [More Information Needed]
- **Demo [optional]:** [More Information Needed]
## Uses
<!-- Address questions around how the model is intended to be used, including the foreseeable users of the model and those affected by the model. -->
### Direct Use
<!-- This section is for the model use without fine-tuning or plugging into a larger ecosystem/app. -->
[More Information Needed]
### Downstream Use [optional]
<!-- This section is for the model use when fine-tuned for a task, or when plugged into a larger ecosystem/app -->
[More Information Needed]
### Out-of-Scope Use
<!-- This section addresses misuse, malicious use, and uses that the model will not work well for. -->
[More Information Needed]
## Bias, Risks, and Limitations
<!-- This section is meant to convey both technical and sociotechnical limitations. -->
[More Information Needed]
### Recommendations
<!-- This section is meant to convey recommendations with respect to the bias, risk, and technical limitations. -->
Users (both direct and downstream) should be made aware of the risks, biases and limitations of the model. More information needed for further recommendations.
## How to Get Started with the Model
Use the code below to get started with the model.
[More Information Needed]
## Training Details
### Training Data
<!-- This should link to a Dataset Card, perhaps with a short stub of information on what the training data is all about as well as documentation related to data pre-processing or additional filtering. -->
[More Information Needed]
### Training Procedure
<!-- This relates heavily to the Technical Specifications. Content here should link to that section when it is relevant to the training procedure. -->
#### Preprocessing [optional]
[More Information Needed]
#### Training Hyperparameters
- **Training regime:** [More Information Needed] <!--fp32, fp16 mixed precision, bf16 mixed precision, bf16 non-mixed precision, fp16 non-mixed precision, fp8 mixed precision -->
#### Speeds, Sizes, Times [optional]
<!-- This section provides information about throughput, start/end time, checkpoint size if relevant, etc. -->
[More Information Needed]
## Evaluation
<!-- This section describes the evaluation protocols and provides the results. -->
### Testing Data, Factors & Metrics
#### Testing Data
<!-- This should link to a Dataset Card if possible. -->
[More Information Needed]
#### Factors
<!-- These are the things the evaluation is disaggregating by, e.g., subpopulations or domains. -->
[More Information Needed]
#### Metrics
<!-- These are the evaluation metrics being used, ideally with a description of why. -->
[More Information Needed]
### Results
[More Information Needed]
#### Summary
## Model Examination [optional]
<!-- Relevant interpretability work for the model goes here -->
[More Information Needed]
## Environmental Impact
<!-- Total emissions (in grams of CO2eq) and additional considerations, such as electricity usage, go here. Edit the suggested text below accordingly -->
Carbon emissions can be estimated using the [Machine Learning Impact calculator](https://mlco2.github.io/impact#compute) presented in [Lacoste et al. (2019)](https://arxiv.org/abs/1910.09700).
- **Hardware Type:** [More Information Needed]
- **Hours used:** [More Information Needed]
- **Cloud Provider:** [More Information Needed]
- **Compute Region:** [More Information Needed]
- **Carbon Emitted:** [More Information Needed]
## Technical Specifications [optional]
### Model Architecture and Objective
[More Information Needed]
### Compute Infrastructure
[More Information Needed]
#### Hardware
[More Information Needed]
#### Software
[More Information Needed]
## Citation [optional]
<!-- If there is a paper or blog post introducing the model, the APA and Bibtex information for that should go in this section. -->
**BibTeX:**
[More Information Needed]
**APA:**
[More Information Needed]
## Glossary [optional]
<!-- If relevant, include terms and calculations in this section that can help readers understand the model or model card. -->
[More Information Needed]
## More Information [optional]
[More Information Needed]
## Model Card Authors [optional]
[More Information Needed]
## Model Card Contact
[More Information Needed] | {"library_name": "transformers", "tags": []} | Mihaj/whisper-medium-karelian-CodeSwitching_with_tempo_aug | null | [
"transformers",
"tensorboard",
"safetensors",
"whisper",
"automatic-speech-recognition",
"arxiv:1910.09700",
"endpoints_compatible",
"region:us"
] | null | 2024-04-27T07:06:02+00:00 | [
"1910.09700"
] | [] | TAGS
#transformers #tensorboard #safetensors #whisper #automatic-speech-recognition #arxiv-1910.09700 #endpoints_compatible #region-us
|
# Model Card for Model ID
## Model Details
### Model Description
This is the model card of a transformers model that has been pushed on the Hub. This model card has been automatically generated.
- Developed by:
- Funded by [optional]:
- Shared by [optional]:
- Model type:
- Language(s) (NLP):
- License:
- Finetuned from model [optional]:
### Model Sources [optional]
- Repository:
- Paper [optional]:
- Demo [optional]:
## Uses
### Direct Use
### Downstream Use [optional]
### Out-of-Scope Use
## Bias, Risks, and Limitations
### Recommendations
Users (both direct and downstream) should be made aware of the risks, biases and limitations of the model. More information needed for further recommendations.
## How to Get Started with the Model
Use the code below to get started with the model.
## Training Details
### Training Data
### Training Procedure
#### Preprocessing [optional]
#### Training Hyperparameters
- Training regime:
#### Speeds, Sizes, Times [optional]
## Evaluation
### Testing Data, Factors & Metrics
#### Testing Data
#### Factors
#### Metrics
### Results
#### Summary
## Model Examination [optional]
## Environmental Impact
Carbon emissions can be estimated using the Machine Learning Impact calculator presented in Lacoste et al. (2019).
- Hardware Type:
- Hours used:
- Cloud Provider:
- Compute Region:
- Carbon Emitted:
## Technical Specifications [optional]
### Model Architecture and Objective
### Compute Infrastructure
#### Hardware
#### Software
[optional]
BibTeX:
APA:
## Glossary [optional]
## More Information [optional]
## Model Card Authors [optional]
## Model Card Contact
| [
"# Model Card for Model ID",
"## Model Details",
"### Model Description\n\n\n\nThis is the model card of a transformers model that has been pushed on the Hub. This model card has been automatically generated.\n\n- Developed by: \n- Funded by [optional]: \n- Shared by [optional]: \n- Model type: \n- Language(s) (NLP): \n- License: \n- Finetuned from model [optional]:",
"### Model Sources [optional]\n\n\n\n- Repository: \n- Paper [optional]: \n- Demo [optional]:",
"## Uses",
"### Direct Use",
"### Downstream Use [optional]",
"### Out-of-Scope Use",
"## Bias, Risks, and Limitations",
"### Recommendations\n\n\n\nUsers (both direct and downstream) should be made aware of the risks, biases and limitations of the model. More information needed for further recommendations.",
"## How to Get Started with the Model\n\nUse the code below to get started with the model.",
"## Training Details",
"### Training Data",
"### Training Procedure",
"#### Preprocessing [optional]",
"#### Training Hyperparameters\n\n- Training regime:",
"#### Speeds, Sizes, Times [optional]",
"## Evaluation",
"### Testing Data, Factors & Metrics",
"#### Testing Data",
"#### Factors",
"#### Metrics",
"### Results",
"#### Summary",
"## Model Examination [optional]",
"## Environmental Impact\n\n\n\nCarbon emissions can be estimated using the Machine Learning Impact calculator presented in Lacoste et al. (2019).\n\n- Hardware Type: \n- Hours used: \n- Cloud Provider: \n- Compute Region: \n- Carbon Emitted:",
"## Technical Specifications [optional]",
"### Model Architecture and Objective",
"### Compute Infrastructure",
"#### Hardware",
"#### Software\n\n\n\n[optional]\n\n\n\nBibTeX:\n\n\n\nAPA:",
"## Glossary [optional]",
"## More Information [optional]",
"## Model Card Authors [optional]",
"## Model Card Contact"
] | [
"TAGS\n#transformers #tensorboard #safetensors #whisper #automatic-speech-recognition #arxiv-1910.09700 #endpoints_compatible #region-us \n",
"# Model Card for Model ID",
"## Model Details",
"### Model Description\n\n\n\nThis is the model card of a transformers model that has been pushed on the Hub. This model card has been automatically generated.\n\n- Developed by: \n- Funded by [optional]: \n- Shared by [optional]: \n- Model type: \n- Language(s) (NLP): \n- License: \n- Finetuned from model [optional]:",
"### Model Sources [optional]\n\n\n\n- Repository: \n- Paper [optional]: \n- Demo [optional]:",
"## Uses",
"### Direct Use",
"### Downstream Use [optional]",
"### Out-of-Scope Use",
"## Bias, Risks, and Limitations",
"### Recommendations\n\n\n\nUsers (both direct and downstream) should be made aware of the risks, biases and limitations of the model. More information needed for further recommendations.",
"## How to Get Started with the Model\n\nUse the code below to get started with the model.",
"## Training Details",
"### Training Data",
"### Training Procedure",
"#### Preprocessing [optional]",
"#### Training Hyperparameters\n\n- Training regime:",
"#### Speeds, Sizes, Times [optional]",
"## Evaluation",
"### Testing Data, Factors & Metrics",
"#### Testing Data",
"#### Factors",
"#### Metrics",
"### Results",
"#### Summary",
"## Model Examination [optional]",
"## Environmental Impact\n\n\n\nCarbon emissions can be estimated using the Machine Learning Impact calculator presented in Lacoste et al. (2019).\n\n- Hardware Type: \n- Hours used: \n- Cloud Provider: \n- Compute Region: \n- Carbon Emitted:",
"## Technical Specifications [optional]",
"### Model Architecture and Objective",
"### Compute Infrastructure",
"#### Hardware",
"#### Software\n\n\n\n[optional]\n\n\n\nBibTeX:\n\n\n\nAPA:",
"## Glossary [optional]",
"## More Information [optional]",
"## Model Card Authors [optional]",
"## Model Card Contact"
] |
text2text-generation | transformers |
<!-- This model card has been generated automatically according to the information the Trainer had access to. You
should probably proofread and complete it, then remove this comment. -->
# t5-finetuned-en-to-ja-eval1
This model is a fine-tuned version of [t5-base](https://huggingface.co/t5-base) on an unknown dataset.
It achieves the following results on the evaluation set:
- eval_loss: 0.3092
- eval_bleu: 0.0
- eval_gen_len: 3.008
- eval_runtime: 2.2634
- eval_samples_per_second: 220.911
- eval_steps_per_second: 4.86
- step: 0
## Model description
More information needed
## Intended uses & limitations
More information needed
## Training and evaluation data
More information needed
## Training procedure
### Training hyperparameters
The following hyperparameters were used during training:
- learning_rate: 2e-05
- train_batch_size: 48
- eval_batch_size: 48
- seed: 42
- optimizer: Adam with betas=(0.9,0.999) and epsilon=1e-08
- lr_scheduler_type: linear
- num_epochs: 1
- mixed_precision_training: Native AMP
### Framework versions
- Transformers 4.39.3
- Pytorch 2.1.2
- Datasets 2.18.0
- Tokenizers 0.15.2
| {"license": "apache-2.0", "tags": ["generated_from_trainer"], "base_model": "t5-base", "model-index": [{"name": "t5-finetuned-en-to-ja-eval1", "results": []}]} | tsetsuuhei/t5-finetuned-en-to-ja-eval1 | null | [
"transformers",
"tensorboard",
"safetensors",
"t5",
"text2text-generation",
"generated_from_trainer",
"base_model:t5-base",
"license:apache-2.0",
"autotrain_compatible",
"endpoints_compatible",
"text-generation-inference",
"region:us"
] | null | 2024-04-27T07:06:03+00:00 | [] | [] | TAGS
#transformers #tensorboard #safetensors #t5 #text2text-generation #generated_from_trainer #base_model-t5-base #license-apache-2.0 #autotrain_compatible #endpoints_compatible #text-generation-inference #region-us
|
# t5-finetuned-en-to-ja-eval1
This model is a fine-tuned version of t5-base on an unknown dataset.
It achieves the following results on the evaluation set:
- eval_loss: 0.3092
- eval_bleu: 0.0
- eval_gen_len: 3.008
- eval_runtime: 2.2634
- eval_samples_per_second: 220.911
- eval_steps_per_second: 4.86
- step: 0
## Model description
More information needed
## Intended uses & limitations
More information needed
## Training and evaluation data
More information needed
## Training procedure
### Training hyperparameters
The following hyperparameters were used during training:
- learning_rate: 2e-05
- train_batch_size: 48
- eval_batch_size: 48
- seed: 42
- optimizer: Adam with betas=(0.9,0.999) and epsilon=1e-08
- lr_scheduler_type: linear
- num_epochs: 1
- mixed_precision_training: Native AMP
### Framework versions
- Transformers 4.39.3
- Pytorch 2.1.2
- Datasets 2.18.0
- Tokenizers 0.15.2
| [
"# t5-finetuned-en-to-ja-eval1\n\nThis model is a fine-tuned version of t5-base on an unknown dataset.\nIt achieves the following results on the evaluation set:\n- eval_loss: 0.3092\n- eval_bleu: 0.0\n- eval_gen_len: 3.008\n- eval_runtime: 2.2634\n- eval_samples_per_second: 220.911\n- eval_steps_per_second: 4.86\n- step: 0",
"## Model description\n\nMore information needed",
"## Intended uses & limitations\n\nMore information needed",
"## Training and evaluation data\n\nMore information needed",
"## Training procedure",
"### Training hyperparameters\n\nThe following hyperparameters were used during training:\n- learning_rate: 2e-05\n- train_batch_size: 48\n- eval_batch_size: 48\n- seed: 42\n- optimizer: Adam with betas=(0.9,0.999) and epsilon=1e-08\n- lr_scheduler_type: linear\n- num_epochs: 1\n- mixed_precision_training: Native AMP",
"### Framework versions\n\n- Transformers 4.39.3\n- Pytorch 2.1.2\n- Datasets 2.18.0\n- Tokenizers 0.15.2"
] | [
"TAGS\n#transformers #tensorboard #safetensors #t5 #text2text-generation #generated_from_trainer #base_model-t5-base #license-apache-2.0 #autotrain_compatible #endpoints_compatible #text-generation-inference #region-us \n",
"# t5-finetuned-en-to-ja-eval1\n\nThis model is a fine-tuned version of t5-base on an unknown dataset.\nIt achieves the following results on the evaluation set:\n- eval_loss: 0.3092\n- eval_bleu: 0.0\n- eval_gen_len: 3.008\n- eval_runtime: 2.2634\n- eval_samples_per_second: 220.911\n- eval_steps_per_second: 4.86\n- step: 0",
"## Model description\n\nMore information needed",
"## Intended uses & limitations\n\nMore information needed",
"## Training and evaluation data\n\nMore information needed",
"## Training procedure",
"### Training hyperparameters\n\nThe following hyperparameters were used during training:\n- learning_rate: 2e-05\n- train_batch_size: 48\n- eval_batch_size: 48\n- seed: 42\n- optimizer: Adam with betas=(0.9,0.999) and epsilon=1e-08\n- lr_scheduler_type: linear\n- num_epochs: 1\n- mixed_precision_training: Native AMP",
"### Framework versions\n\n- Transformers 4.39.3\n- Pytorch 2.1.2\n- Datasets 2.18.0\n- Tokenizers 0.15.2"
] |
null | keras |
<!-- This model card has been generated automatically according to the information Keras had access to. You should
probably proofread and complete it, then remove this comment. -->
# mindmate-f3-original-equal-cont-0-0
This model is a fine-tuned version of [bert-base-german-cased](https://huggingface.co/bert-base-german-cased) on an unknown dataset.
It achieves the following results on the evaluation set:
## Model description
More information needed
## Intended uses & limitations
More information needed
## Training and evaluation data
More information needed
## Training procedure
### Training hyperparameters
The following hyperparameters were used during training:
- optimizer: {'name': 'Adam', 'weight_decay': None, 'clipnorm': None, 'global_clipnorm': None, 'clipvalue': None, 'use_ema': False, 'ema_momentum': 0.99, 'ema_overwrite_frequency': None, 'jit_compile': True, 'is_legacy_optimizer': False, 'learning_rate': 3e-05, 'beta_1': 0.9, 'beta_2': 0.999, 'epsilon': 1e-07, 'amsgrad': False}
- training_precision: float32
### Training results
### Framework versions
- Transformers 4.40.0
- TensorFlow 2.15.0
- Tokenizers 0.19.1
| {"license": "mit", "tags": ["generated_from_keras_callback"], "base_model": "bert-base-german-cased", "model-index": [{"name": "mindmate-f3-original-equal-cont-0-0", "results": []}]} | spneshaei/mindmate-f3-original-equal-cont-0-0 | null | [
"keras",
"tf",
"bert",
"generated_from_keras_callback",
"base_model:bert-base-german-cased",
"license:mit",
"region:us"
] | null | 2024-04-27T07:06:04+00:00 | [] | [] | TAGS
#keras #tf #bert #generated_from_keras_callback #base_model-bert-base-german-cased #license-mit #region-us
|
# mindmate-f3-original-equal-cont-0-0
This model is a fine-tuned version of bert-base-german-cased on an unknown dataset.
It achieves the following results on the evaluation set:
## Model description
More information needed
## Intended uses & limitations
More information needed
## Training and evaluation data
More information needed
## Training procedure
### Training hyperparameters
The following hyperparameters were used during training:
- optimizer: {'name': 'Adam', 'weight_decay': None, 'clipnorm': None, 'global_clipnorm': None, 'clipvalue': None, 'use_ema': False, 'ema_momentum': 0.99, 'ema_overwrite_frequency': None, 'jit_compile': True, 'is_legacy_optimizer': False, 'learning_rate': 3e-05, 'beta_1': 0.9, 'beta_2': 0.999, 'epsilon': 1e-07, 'amsgrad': False}
- training_precision: float32
### Training results
### Framework versions
- Transformers 4.40.0
- TensorFlow 2.15.0
- Tokenizers 0.19.1
| [
"# mindmate-f3-original-equal-cont-0-0\n\nThis model is a fine-tuned version of bert-base-german-cased on an unknown dataset.\nIt achieves the following results on the evaluation set:",
"## Model description\n\nMore information needed",
"## Intended uses & limitations\n\nMore information needed",
"## Training and evaluation data\n\nMore information needed",
"## Training procedure",
"### Training hyperparameters\n\nThe following hyperparameters were used during training:\n- optimizer: {'name': 'Adam', 'weight_decay': None, 'clipnorm': None, 'global_clipnorm': None, 'clipvalue': None, 'use_ema': False, 'ema_momentum': 0.99, 'ema_overwrite_frequency': None, 'jit_compile': True, 'is_legacy_optimizer': False, 'learning_rate': 3e-05, 'beta_1': 0.9, 'beta_2': 0.999, 'epsilon': 1e-07, 'amsgrad': False}\n- training_precision: float32",
"### Training results",
"### Framework versions\n\n- Transformers 4.40.0\n- TensorFlow 2.15.0\n- Tokenizers 0.19.1"
] | [
"TAGS\n#keras #tf #bert #generated_from_keras_callback #base_model-bert-base-german-cased #license-mit #region-us \n",
"# mindmate-f3-original-equal-cont-0-0\n\nThis model is a fine-tuned version of bert-base-german-cased on an unknown dataset.\nIt achieves the following results on the evaluation set:",
"## Model description\n\nMore information needed",
"## Intended uses & limitations\n\nMore information needed",
"## Training and evaluation data\n\nMore information needed",
"## Training procedure",
"### Training hyperparameters\n\nThe following hyperparameters were used during training:\n- optimizer: {'name': 'Adam', 'weight_decay': None, 'clipnorm': None, 'global_clipnorm': None, 'clipvalue': None, 'use_ema': False, 'ema_momentum': 0.99, 'ema_overwrite_frequency': None, 'jit_compile': True, 'is_legacy_optimizer': False, 'learning_rate': 3e-05, 'beta_1': 0.9, 'beta_2': 0.999, 'epsilon': 1e-07, 'amsgrad': False}\n- training_precision: float32",
"### Training results",
"### Framework versions\n\n- Transformers 4.40.0\n- TensorFlow 2.15.0\n- Tokenizers 0.19.1"
] |
text-generation | transformers |
# Model Card for Model ID
<!-- Provide a quick summary of what the model is/does. -->
## Model Details
### Model Description
<!-- Provide a longer summary of what this model is. -->
This is the model card of a 🤗 transformers model that has been pushed on the Hub. This model card has been automatically generated.
- **Developed by:** [More Information Needed]
- **Funded by [optional]:** [More Information Needed]
- **Shared by [optional]:** [More Information Needed]
- **Model type:** [More Information Needed]
- **Language(s) (NLP):** [More Information Needed]
- **License:** [More Information Needed]
- **Finetuned from model [optional]:** [More Information Needed]
### Model Sources [optional]
<!-- Provide the basic links for the model. -->
- **Repository:** [More Information Needed]
- **Paper [optional]:** [More Information Needed]
- **Demo [optional]:** [More Information Needed]
## Uses
<!-- Address questions around how the model is intended to be used, including the foreseeable users of the model and those affected by the model. -->
### Direct Use
<!-- This section is for the model use without fine-tuning or plugging into a larger ecosystem/app. -->
[More Information Needed]
### Downstream Use [optional]
<!-- This section is for the model use when fine-tuned for a task, or when plugged into a larger ecosystem/app -->
[More Information Needed]
### Out-of-Scope Use
<!-- This section addresses misuse, malicious use, and uses that the model will not work well for. -->
[More Information Needed]
## Bias, Risks, and Limitations
<!-- This section is meant to convey both technical and sociotechnical limitations. -->
[More Information Needed]
### Recommendations
<!-- This section is meant to convey recommendations with respect to the bias, risk, and technical limitations. -->
Users (both direct and downstream) should be made aware of the risks, biases and limitations of the model. More information needed for further recommendations.
## How to Get Started with the Model
Use the code below to get started with the model.
[More Information Needed]
## Training Details
### Training Data
<!-- This should link to a Dataset Card, perhaps with a short stub of information on what the training data is all about as well as documentation related to data pre-processing or additional filtering. -->
[More Information Needed]
### Training Procedure
<!-- This relates heavily to the Technical Specifications. Content here should link to that section when it is relevant to the training procedure. -->
#### Preprocessing [optional]
[More Information Needed]
#### Training Hyperparameters
- **Training regime:** [More Information Needed] <!--fp32, fp16 mixed precision, bf16 mixed precision, bf16 non-mixed precision, fp16 non-mixed precision, fp8 mixed precision -->
#### Speeds, Sizes, Times [optional]
<!-- This section provides information about throughput, start/end time, checkpoint size if relevant, etc. -->
[More Information Needed]
## Evaluation
<!-- This section describes the evaluation protocols and provides the results. -->
### Testing Data, Factors & Metrics
#### Testing Data
<!-- This should link to a Dataset Card if possible. -->
[More Information Needed]
#### Factors
<!-- These are the things the evaluation is disaggregating by, e.g., subpopulations or domains. -->
[More Information Needed]
#### Metrics
<!-- These are the evaluation metrics being used, ideally with a description of why. -->
[More Information Needed]
### Results
[More Information Needed]
#### Summary
## Model Examination [optional]
<!-- Relevant interpretability work for the model goes here -->
[More Information Needed]
## Environmental Impact
<!-- Total emissions (in grams of CO2eq) and additional considerations, such as electricity usage, go here. Edit the suggested text below accordingly -->
Carbon emissions can be estimated using the [Machine Learning Impact calculator](https://mlco2.github.io/impact#compute) presented in [Lacoste et al. (2019)](https://arxiv.org/abs/1910.09700).
- **Hardware Type:** [More Information Needed]
- **Hours used:** [More Information Needed]
- **Cloud Provider:** [More Information Needed]
- **Compute Region:** [More Information Needed]
- **Carbon Emitted:** [More Information Needed]
## Technical Specifications [optional]
### Model Architecture and Objective
[More Information Needed]
### Compute Infrastructure
[More Information Needed]
#### Hardware
[More Information Needed]
#### Software
[More Information Needed]
## Citation [optional]
<!-- If there is a paper or blog post introducing the model, the APA and Bibtex information for that should go in this section. -->
**BibTeX:**
[More Information Needed]
**APA:**
[More Information Needed]
## Glossary [optional]
<!-- If relevant, include terms and calculations in this section that can help readers understand the model or model card. -->
[More Information Needed]
## More Information [optional]
[More Information Needed]
## Model Card Authors [optional]
[More Information Needed]
## Model Card Contact
[More Information Needed] | {"library_name": "transformers", "tags": ["unsloth", "trl", "sft"]} | NiCoSav/llama-3-8b-bnb-4bit | null | [
"transformers",
"pytorch",
"safetensors",
"llama",
"text-generation",
"unsloth",
"trl",
"sft",
"arxiv:1910.09700",
"autotrain_compatible",
"endpoints_compatible",
"text-generation-inference",
"4-bit",
"region:us"
] | null | 2024-04-27T07:06:58+00:00 | [
"1910.09700"
] | [] | TAGS
#transformers #pytorch #safetensors #llama #text-generation #unsloth #trl #sft #arxiv-1910.09700 #autotrain_compatible #endpoints_compatible #text-generation-inference #4-bit #region-us
|
# Model Card for Model ID
## Model Details
### Model Description
This is the model card of a transformers model that has been pushed on the Hub. This model card has been automatically generated.
- Developed by:
- Funded by [optional]:
- Shared by [optional]:
- Model type:
- Language(s) (NLP):
- License:
- Finetuned from model [optional]:
### Model Sources [optional]
- Repository:
- Paper [optional]:
- Demo [optional]:
## Uses
### Direct Use
### Downstream Use [optional]
### Out-of-Scope Use
## Bias, Risks, and Limitations
### Recommendations
Users (both direct and downstream) should be made aware of the risks, biases and limitations of the model. More information needed for further recommendations.
## How to Get Started with the Model
Use the code below to get started with the model.
## Training Details
### Training Data
### Training Procedure
#### Preprocessing [optional]
#### Training Hyperparameters
- Training regime:
#### Speeds, Sizes, Times [optional]
## Evaluation
### Testing Data, Factors & Metrics
#### Testing Data
#### Factors
#### Metrics
### Results
#### Summary
## Model Examination [optional]
## Environmental Impact
Carbon emissions can be estimated using the Machine Learning Impact calculator presented in Lacoste et al. (2019).
- Hardware Type:
- Hours used:
- Cloud Provider:
- Compute Region:
- Carbon Emitted:
## Technical Specifications [optional]
### Model Architecture and Objective
### Compute Infrastructure
#### Hardware
#### Software
[optional]
BibTeX:
APA:
## Glossary [optional]
## More Information [optional]
## Model Card Authors [optional]
## Model Card Contact
| [
"# Model Card for Model ID",
"## Model Details",
"### Model Description\n\n\n\nThis is the model card of a transformers model that has been pushed on the Hub. This model card has been automatically generated.\n\n- Developed by: \n- Funded by [optional]: \n- Shared by [optional]: \n- Model type: \n- Language(s) (NLP): \n- License: \n- Finetuned from model [optional]:",
"### Model Sources [optional]\n\n\n\n- Repository: \n- Paper [optional]: \n- Demo [optional]:",
"## Uses",
"### Direct Use",
"### Downstream Use [optional]",
"### Out-of-Scope Use",
"## Bias, Risks, and Limitations",
"### Recommendations\n\n\n\nUsers (both direct and downstream) should be made aware of the risks, biases and limitations of the model. More information needed for further recommendations.",
"## How to Get Started with the Model\n\nUse the code below to get started with the model.",
"## Training Details",
"### Training Data",
"### Training Procedure",
"#### Preprocessing [optional]",
"#### Training Hyperparameters\n\n- Training regime:",
"#### Speeds, Sizes, Times [optional]",
"## Evaluation",
"### Testing Data, Factors & Metrics",
"#### Testing Data",
"#### Factors",
"#### Metrics",
"### Results",
"#### Summary",
"## Model Examination [optional]",
"## Environmental Impact\n\n\n\nCarbon emissions can be estimated using the Machine Learning Impact calculator presented in Lacoste et al. (2019).\n\n- Hardware Type: \n- Hours used: \n- Cloud Provider: \n- Compute Region: \n- Carbon Emitted:",
"## Technical Specifications [optional]",
"### Model Architecture and Objective",
"### Compute Infrastructure",
"#### Hardware",
"#### Software\n\n\n\n[optional]\n\n\n\nBibTeX:\n\n\n\nAPA:",
"## Glossary [optional]",
"## More Information [optional]",
"## Model Card Authors [optional]",
"## Model Card Contact"
] | [
"TAGS\n#transformers #pytorch #safetensors #llama #text-generation #unsloth #trl #sft #arxiv-1910.09700 #autotrain_compatible #endpoints_compatible #text-generation-inference #4-bit #region-us \n",
"# Model Card for Model ID",
"## Model Details",
"### Model Description\n\n\n\nThis is the model card of a transformers model that has been pushed on the Hub. This model card has been automatically generated.\n\n- Developed by: \n- Funded by [optional]: \n- Shared by [optional]: \n- Model type: \n- Language(s) (NLP): \n- License: \n- Finetuned from model [optional]:",
"### Model Sources [optional]\n\n\n\n- Repository: \n- Paper [optional]: \n- Demo [optional]:",
"## Uses",
"### Direct Use",
"### Downstream Use [optional]",
"### Out-of-Scope Use",
"## Bias, Risks, and Limitations",
"### Recommendations\n\n\n\nUsers (both direct and downstream) should be made aware of the risks, biases and limitations of the model. More information needed for further recommendations.",
"## How to Get Started with the Model\n\nUse the code below to get started with the model.",
"## Training Details",
"### Training Data",
"### Training Procedure",
"#### Preprocessing [optional]",
"#### Training Hyperparameters\n\n- Training regime:",
"#### Speeds, Sizes, Times [optional]",
"## Evaluation",
"### Testing Data, Factors & Metrics",
"#### Testing Data",
"#### Factors",
"#### Metrics",
"### Results",
"#### Summary",
"## Model Examination [optional]",
"## Environmental Impact\n\n\n\nCarbon emissions can be estimated using the Machine Learning Impact calculator presented in Lacoste et al. (2019).\n\n- Hardware Type: \n- Hours used: \n- Cloud Provider: \n- Compute Region: \n- Carbon Emitted:",
"## Technical Specifications [optional]",
"### Model Architecture and Objective",
"### Compute Infrastructure",
"#### Hardware",
"#### Software\n\n\n\n[optional]\n\n\n\nBibTeX:\n\n\n\nAPA:",
"## Glossary [optional]",
"## More Information [optional]",
"## Model Card Authors [optional]",
"## Model Card Contact"
] |
null | keras |
<!-- This model card has been generated automatically according to the information Keras had access to. You should
probably proofread and complete it, then remove this comment. -->
# mindmate-f4-original-equal-cont-0-0
This model is a fine-tuned version of [bert-base-german-cased](https://huggingface.co/bert-base-german-cased) on an unknown dataset.
It achieves the following results on the evaluation set:
## Model description
More information needed
## Intended uses & limitations
More information needed
## Training and evaluation data
More information needed
## Training procedure
### Training hyperparameters
The following hyperparameters were used during training:
- optimizer: {'name': 'Adam', 'weight_decay': None, 'clipnorm': None, 'global_clipnorm': None, 'clipvalue': None, 'use_ema': False, 'ema_momentum': 0.99, 'ema_overwrite_frequency': None, 'jit_compile': True, 'is_legacy_optimizer': False, 'learning_rate': 3e-05, 'beta_1': 0.9, 'beta_2': 0.999, 'epsilon': 1e-07, 'amsgrad': False}
- training_precision: float32
### Training results
### Framework versions
- Transformers 4.40.0
- TensorFlow 2.15.0
- Tokenizers 0.19.1
| {"license": "mit", "tags": ["generated_from_keras_callback"], "base_model": "bert-base-german-cased", "model-index": [{"name": "mindmate-f4-original-equal-cont-0-0", "results": []}]} | spneshaei/mindmate-f4-original-equal-cont-0-0 | null | [
"keras",
"tf",
"bert",
"generated_from_keras_callback",
"base_model:bert-base-german-cased",
"license:mit",
"region:us"
] | null | 2024-04-27T07:07:06+00:00 | [] | [] | TAGS
#keras #tf #bert #generated_from_keras_callback #base_model-bert-base-german-cased #license-mit #region-us
|
# mindmate-f4-original-equal-cont-0-0
This model is a fine-tuned version of bert-base-german-cased on an unknown dataset.
It achieves the following results on the evaluation set:
## Model description
More information needed
## Intended uses & limitations
More information needed
## Training and evaluation data
More information needed
## Training procedure
### Training hyperparameters
The following hyperparameters were used during training:
- optimizer: {'name': 'Adam', 'weight_decay': None, 'clipnorm': None, 'global_clipnorm': None, 'clipvalue': None, 'use_ema': False, 'ema_momentum': 0.99, 'ema_overwrite_frequency': None, 'jit_compile': True, 'is_legacy_optimizer': False, 'learning_rate': 3e-05, 'beta_1': 0.9, 'beta_2': 0.999, 'epsilon': 1e-07, 'amsgrad': False}
- training_precision: float32
### Training results
### Framework versions
- Transformers 4.40.0
- TensorFlow 2.15.0
- Tokenizers 0.19.1
| [
"# mindmate-f4-original-equal-cont-0-0\n\nThis model is a fine-tuned version of bert-base-german-cased on an unknown dataset.\nIt achieves the following results on the evaluation set:",
"## Model description\n\nMore information needed",
"## Intended uses & limitations\n\nMore information needed",
"## Training and evaluation data\n\nMore information needed",
"## Training procedure",
"### Training hyperparameters\n\nThe following hyperparameters were used during training:\n- optimizer: {'name': 'Adam', 'weight_decay': None, 'clipnorm': None, 'global_clipnorm': None, 'clipvalue': None, 'use_ema': False, 'ema_momentum': 0.99, 'ema_overwrite_frequency': None, 'jit_compile': True, 'is_legacy_optimizer': False, 'learning_rate': 3e-05, 'beta_1': 0.9, 'beta_2': 0.999, 'epsilon': 1e-07, 'amsgrad': False}\n- training_precision: float32",
"### Training results",
"### Framework versions\n\n- Transformers 4.40.0\n- TensorFlow 2.15.0\n- Tokenizers 0.19.1"
] | [
"TAGS\n#keras #tf #bert #generated_from_keras_callback #base_model-bert-base-german-cased #license-mit #region-us \n",
"# mindmate-f4-original-equal-cont-0-0\n\nThis model is a fine-tuned version of bert-base-german-cased on an unknown dataset.\nIt achieves the following results on the evaluation set:",
"## Model description\n\nMore information needed",
"## Intended uses & limitations\n\nMore information needed",
"## Training and evaluation data\n\nMore information needed",
"## Training procedure",
"### Training hyperparameters\n\nThe following hyperparameters were used during training:\n- optimizer: {'name': 'Adam', 'weight_decay': None, 'clipnorm': None, 'global_clipnorm': None, 'clipvalue': None, 'use_ema': False, 'ema_momentum': 0.99, 'ema_overwrite_frequency': None, 'jit_compile': True, 'is_legacy_optimizer': False, 'learning_rate': 3e-05, 'beta_1': 0.9, 'beta_2': 0.999, 'epsilon': 1e-07, 'amsgrad': False}\n- training_precision: float32",
"### Training results",
"### Framework versions\n\n- Transformers 4.40.0\n- TensorFlow 2.15.0\n- Tokenizers 0.19.1"
] |
text-generation | transformers |
# Model Card for Model ID
<!-- Provide a quick summary of what the model is/does. -->
## Model Details
### Model Description
<!-- Provide a longer summary of what this model is. -->
This is the model card of a 🤗 transformers model that has been pushed on the Hub. This model card has been automatically generated.
- **Developed by:** [More Information Needed]
- **Funded by [optional]:** [More Information Needed]
- **Shared by [optional]:** [More Information Needed]
- **Model type:** [More Information Needed]
- **Language(s) (NLP):** [More Information Needed]
- **License:** [More Information Needed]
- **Finetuned from model [optional]:** [More Information Needed]
### Model Sources [optional]
<!-- Provide the basic links for the model. -->
- **Repository:** [More Information Needed]
- **Paper [optional]:** [More Information Needed]
- **Demo [optional]:** [More Information Needed]
## Uses
<!-- Address questions around how the model is intended to be used, including the foreseeable users of the model and those affected by the model. -->
### Direct Use
<!-- This section is for the model use without fine-tuning or plugging into a larger ecosystem/app. -->
[More Information Needed]
### Downstream Use [optional]
<!-- This section is for the model use when fine-tuned for a task, or when plugged into a larger ecosystem/app -->
[More Information Needed]
### Out-of-Scope Use
<!-- This section addresses misuse, malicious use, and uses that the model will not work well for. -->
[More Information Needed]
## Bias, Risks, and Limitations
<!-- This section is meant to convey both technical and sociotechnical limitations. -->
[More Information Needed]
### Recommendations
<!-- This section is meant to convey recommendations with respect to the bias, risk, and technical limitations. -->
Users (both direct and downstream) should be made aware of the risks, biases and limitations of the model. More information needed for further recommendations.
## How to Get Started with the Model
Use the code below to get started with the model.
[More Information Needed]
## Training Details
### Training Data
<!-- This should link to a Dataset Card, perhaps with a short stub of information on what the training data is all about as well as documentation related to data pre-processing or additional filtering. -->
[More Information Needed]
### Training Procedure
<!-- This relates heavily to the Technical Specifications. Content here should link to that section when it is relevant to the training procedure. -->
#### Preprocessing [optional]
[More Information Needed]
#### Training Hyperparameters
- **Training regime:** [More Information Needed] <!--fp32, fp16 mixed precision, bf16 mixed precision, bf16 non-mixed precision, fp16 non-mixed precision, fp8 mixed precision -->
#### Speeds, Sizes, Times [optional]
<!-- This section provides information about throughput, start/end time, checkpoint size if relevant, etc. -->
[More Information Needed]
## Evaluation
<!-- This section describes the evaluation protocols and provides the results. -->
### Testing Data, Factors & Metrics
#### Testing Data
<!-- This should link to a Dataset Card if possible. -->
[More Information Needed]
#### Factors
<!-- These are the things the evaluation is disaggregating by, e.g., subpopulations or domains. -->
[More Information Needed]
#### Metrics
<!-- These are the evaluation metrics being used, ideally with a description of why. -->
[More Information Needed]
### Results
[More Information Needed]
#### Summary
## Model Examination [optional]
<!-- Relevant interpretability work for the model goes here -->
[More Information Needed]
## Environmental Impact
<!-- Total emissions (in grams of CO2eq) and additional considerations, such as electricity usage, go here. Edit the suggested text below accordingly -->
Carbon emissions can be estimated using the [Machine Learning Impact calculator](https://mlco2.github.io/impact#compute) presented in [Lacoste et al. (2019)](https://arxiv.org/abs/1910.09700).
- **Hardware Type:** [More Information Needed]
- **Hours used:** [More Information Needed]
- **Cloud Provider:** [More Information Needed]
- **Compute Region:** [More Information Needed]
- **Carbon Emitted:** [More Information Needed]
## Technical Specifications [optional]
### Model Architecture and Objective
[More Information Needed]
### Compute Infrastructure
[More Information Needed]
#### Hardware
[More Information Needed]
#### Software
[More Information Needed]
## Citation [optional]
<!-- If there is a paper or blog post introducing the model, the APA and Bibtex information for that should go in this section. -->
**BibTeX:**
[More Information Needed]
**APA:**
[More Information Needed]
## Glossary [optional]
<!-- If relevant, include terms and calculations in this section that can help readers understand the model or model card. -->
[More Information Needed]
## More Information [optional]
[More Information Needed]
## Model Card Authors [optional]
[More Information Needed]
## Model Card Contact
[More Information Needed] | {"library_name": "transformers", "tags": ["unsloth", "trl", "sft"]} | NiCoSav/llama-3-8b-bnb-16bit | null | [
"transformers",
"pytorch",
"llama",
"text-generation",
"unsloth",
"trl",
"sft",
"arxiv:1910.09700",
"autotrain_compatible",
"endpoints_compatible",
"text-generation-inference",
"region:us"
] | null | 2024-04-27T07:07:15+00:00 | [
"1910.09700"
] | [] | TAGS
#transformers #pytorch #llama #text-generation #unsloth #trl #sft #arxiv-1910.09700 #autotrain_compatible #endpoints_compatible #text-generation-inference #region-us
|
# Model Card for Model ID
## Model Details
### Model Description
This is the model card of a transformers model that has been pushed on the Hub. This model card has been automatically generated.
- Developed by:
- Funded by [optional]:
- Shared by [optional]:
- Model type:
- Language(s) (NLP):
- License:
- Finetuned from model [optional]:
### Model Sources [optional]
- Repository:
- Paper [optional]:
- Demo [optional]:
## Uses
### Direct Use
### Downstream Use [optional]
### Out-of-Scope Use
## Bias, Risks, and Limitations
### Recommendations
Users (both direct and downstream) should be made aware of the risks, biases and limitations of the model. More information needed for further recommendations.
## How to Get Started with the Model
Use the code below to get started with the model.
## Training Details
### Training Data
### Training Procedure
#### Preprocessing [optional]
#### Training Hyperparameters
- Training regime:
#### Speeds, Sizes, Times [optional]
## Evaluation
### Testing Data, Factors & Metrics
#### Testing Data
#### Factors
#### Metrics
### Results
#### Summary
## Model Examination [optional]
## Environmental Impact
Carbon emissions can be estimated using the Machine Learning Impact calculator presented in Lacoste et al. (2019).
- Hardware Type:
- Hours used:
- Cloud Provider:
- Compute Region:
- Carbon Emitted:
## Technical Specifications [optional]
### Model Architecture and Objective
### Compute Infrastructure
#### Hardware
#### Software
[optional]
BibTeX:
APA:
## Glossary [optional]
## More Information [optional]
## Model Card Authors [optional]
## Model Card Contact
| [
"# Model Card for Model ID",
"## Model Details",
"### Model Description\n\n\n\nThis is the model card of a transformers model that has been pushed on the Hub. This model card has been automatically generated.\n\n- Developed by: \n- Funded by [optional]: \n- Shared by [optional]: \n- Model type: \n- Language(s) (NLP): \n- License: \n- Finetuned from model [optional]:",
"### Model Sources [optional]\n\n\n\n- Repository: \n- Paper [optional]: \n- Demo [optional]:",
"## Uses",
"### Direct Use",
"### Downstream Use [optional]",
"### Out-of-Scope Use",
"## Bias, Risks, and Limitations",
"### Recommendations\n\n\n\nUsers (both direct and downstream) should be made aware of the risks, biases and limitations of the model. More information needed for further recommendations.",
"## How to Get Started with the Model\n\nUse the code below to get started with the model.",
"## Training Details",
"### Training Data",
"### Training Procedure",
"#### Preprocessing [optional]",
"#### Training Hyperparameters\n\n- Training regime:",
"#### Speeds, Sizes, Times [optional]",
"## Evaluation",
"### Testing Data, Factors & Metrics",
"#### Testing Data",
"#### Factors",
"#### Metrics",
"### Results",
"#### Summary",
"## Model Examination [optional]",
"## Environmental Impact\n\n\n\nCarbon emissions can be estimated using the Machine Learning Impact calculator presented in Lacoste et al. (2019).\n\n- Hardware Type: \n- Hours used: \n- Cloud Provider: \n- Compute Region: \n- Carbon Emitted:",
"## Technical Specifications [optional]",
"### Model Architecture and Objective",
"### Compute Infrastructure",
"#### Hardware",
"#### Software\n\n\n\n[optional]\n\n\n\nBibTeX:\n\n\n\nAPA:",
"## Glossary [optional]",
"## More Information [optional]",
"## Model Card Authors [optional]",
"## Model Card Contact"
] | [
"TAGS\n#transformers #pytorch #llama #text-generation #unsloth #trl #sft #arxiv-1910.09700 #autotrain_compatible #endpoints_compatible #text-generation-inference #region-us \n",
"# Model Card for Model ID",
"## Model Details",
"### Model Description\n\n\n\nThis is the model card of a transformers model that has been pushed on the Hub. This model card has been automatically generated.\n\n- Developed by: \n- Funded by [optional]: \n- Shared by [optional]: \n- Model type: \n- Language(s) (NLP): \n- License: \n- Finetuned from model [optional]:",
"### Model Sources [optional]\n\n\n\n- Repository: \n- Paper [optional]: \n- Demo [optional]:",
"## Uses",
"### Direct Use",
"### Downstream Use [optional]",
"### Out-of-Scope Use",
"## Bias, Risks, and Limitations",
"### Recommendations\n\n\n\nUsers (both direct and downstream) should be made aware of the risks, biases and limitations of the model. More information needed for further recommendations.",
"## How to Get Started with the Model\n\nUse the code below to get started with the model.",
"## Training Details",
"### Training Data",
"### Training Procedure",
"#### Preprocessing [optional]",
"#### Training Hyperparameters\n\n- Training regime:",
"#### Speeds, Sizes, Times [optional]",
"## Evaluation",
"### Testing Data, Factors & Metrics",
"#### Testing Data",
"#### Factors",
"#### Metrics",
"### Results",
"#### Summary",
"## Model Examination [optional]",
"## Environmental Impact\n\n\n\nCarbon emissions can be estimated using the Machine Learning Impact calculator presented in Lacoste et al. (2019).\n\n- Hardware Type: \n- Hours used: \n- Cloud Provider: \n- Compute Region: \n- Carbon Emitted:",
"## Technical Specifications [optional]",
"### Model Architecture and Objective",
"### Compute Infrastructure",
"#### Hardware",
"#### Software\n\n\n\n[optional]\n\n\n\nBibTeX:\n\n\n\nAPA:",
"## Glossary [optional]",
"## More Information [optional]",
"## Model Card Authors [optional]",
"## Model Card Contact"
] |
text-generation | transformers |
# Model Card for Model ID
<!-- Provide a quick summary of what the model is/does. -->
## Model Details
### Model Description
<!-- Provide a longer summary of what this model is. -->
This is the model card of a 🤗 transformers model that has been pushed on the Hub. This model card has been automatically generated.
- **Developed by:** [More Information Needed]
- **Funded by [optional]:** [More Information Needed]
- **Shared by [optional]:** [More Information Needed]
- **Model type:** [More Information Needed]
- **Language(s) (NLP):** [More Information Needed]
- **License:** [More Information Needed]
- **Finetuned from model [optional]:** [More Information Needed]
### Model Sources [optional]
<!-- Provide the basic links for the model. -->
- **Repository:** [More Information Needed]
- **Paper [optional]:** [More Information Needed]
- **Demo [optional]:** [More Information Needed]
## Uses
<!-- Address questions around how the model is intended to be used, including the foreseeable users of the model and those affected by the model. -->
### Direct Use
<!-- This section is for the model use without fine-tuning or plugging into a larger ecosystem/app. -->
[More Information Needed]
### Downstream Use [optional]
<!-- This section is for the model use when fine-tuned for a task, or when plugged into a larger ecosystem/app -->
[More Information Needed]
### Out-of-Scope Use
<!-- This section addresses misuse, malicious use, and uses that the model will not work well for. -->
[More Information Needed]
## Bias, Risks, and Limitations
<!-- This section is meant to convey both technical and sociotechnical limitations. -->
[More Information Needed]
### Recommendations
<!-- This section is meant to convey recommendations with respect to the bias, risk, and technical limitations. -->
Users (both direct and downstream) should be made aware of the risks, biases and limitations of the model. More information needed for further recommendations.
## How to Get Started with the Model
Use the code below to get started with the model.
[More Information Needed]
## Training Details
### Training Data
<!-- This should link to a Dataset Card, perhaps with a short stub of information on what the training data is all about as well as documentation related to data pre-processing or additional filtering. -->
[More Information Needed]
### Training Procedure
<!-- This relates heavily to the Technical Specifications. Content here should link to that section when it is relevant to the training procedure. -->
#### Preprocessing [optional]
[More Information Needed]
#### Training Hyperparameters
- **Training regime:** [More Information Needed] <!--fp32, fp16 mixed precision, bf16 mixed precision, bf16 non-mixed precision, fp16 non-mixed precision, fp8 mixed precision -->
#### Speeds, Sizes, Times [optional]
<!-- This section provides information about throughput, start/end time, checkpoint size if relevant, etc. -->
[More Information Needed]
## Evaluation
<!-- This section describes the evaluation protocols and provides the results. -->
### Testing Data, Factors & Metrics
#### Testing Data
<!-- This should link to a Dataset Card if possible. -->
[More Information Needed]
#### Factors
<!-- These are the things the evaluation is disaggregating by, e.g., subpopulations or domains. -->
[More Information Needed]
#### Metrics
<!-- These are the evaluation metrics being used, ideally with a description of why. -->
[More Information Needed]
### Results
[More Information Needed]
#### Summary
## Model Examination [optional]
<!-- Relevant interpretability work for the model goes here -->
[More Information Needed]
## Environmental Impact
<!-- Total emissions (in grams of CO2eq) and additional considerations, such as electricity usage, go here. Edit the suggested text below accordingly -->
Carbon emissions can be estimated using the [Machine Learning Impact calculator](https://mlco2.github.io/impact#compute) presented in [Lacoste et al. (2019)](https://arxiv.org/abs/1910.09700).
- **Hardware Type:** [More Information Needed]
- **Hours used:** [More Information Needed]
- **Cloud Provider:** [More Information Needed]
- **Compute Region:** [More Information Needed]
- **Carbon Emitted:** [More Information Needed]
## Technical Specifications [optional]
### Model Architecture and Objective
[More Information Needed]
### Compute Infrastructure
[More Information Needed]
#### Hardware
[More Information Needed]
#### Software
[More Information Needed]
## Citation [optional]
<!-- If there is a paper or blog post introducing the model, the APA and Bibtex information for that should go in this section. -->
**BibTeX:**
[More Information Needed]
**APA:**
[More Information Needed]
## Glossary [optional]
<!-- If relevant, include terms and calculations in this section that can help readers understand the model or model card. -->
[More Information Needed]
## More Information [optional]
[More Information Needed]
## Model Card Authors [optional]
[More Information Needed]
## Model Card Contact
[More Information Needed]
| {"library_name": "transformers", "tags": []} | swj0419/hp_retrain_STEP0000020 | null | [
"transformers",
"safetensors",
"llama",
"text-generation",
"arxiv:1910.09700",
"autotrain_compatible",
"endpoints_compatible",
"text-generation-inference",
"region:us"
] | null | 2024-04-27T07:09:45+00:00 | [
"1910.09700"
] | [] | TAGS
#transformers #safetensors #llama #text-generation #arxiv-1910.09700 #autotrain_compatible #endpoints_compatible #text-generation-inference #region-us
|
# Model Card for Model ID
## Model Details
### Model Description
This is the model card of a transformers model that has been pushed on the Hub. This model card has been automatically generated.
- Developed by:
- Funded by [optional]:
- Shared by [optional]:
- Model type:
- Language(s) (NLP):
- License:
- Finetuned from model [optional]:
### Model Sources [optional]
- Repository:
- Paper [optional]:
- Demo [optional]:
## Uses
### Direct Use
### Downstream Use [optional]
### Out-of-Scope Use
## Bias, Risks, and Limitations
### Recommendations
Users (both direct and downstream) should be made aware of the risks, biases and limitations of the model. More information needed for further recommendations.
## How to Get Started with the Model
Use the code below to get started with the model.
## Training Details
### Training Data
### Training Procedure
#### Preprocessing [optional]
#### Training Hyperparameters
- Training regime:
#### Speeds, Sizes, Times [optional]
## Evaluation
### Testing Data, Factors & Metrics
#### Testing Data
#### Factors
#### Metrics
### Results
#### Summary
## Model Examination [optional]
## Environmental Impact
Carbon emissions can be estimated using the Machine Learning Impact calculator presented in Lacoste et al. (2019).
- Hardware Type:
- Hours used:
- Cloud Provider:
- Compute Region:
- Carbon Emitted:
## Technical Specifications [optional]
### Model Architecture and Objective
### Compute Infrastructure
#### Hardware
#### Software
[optional]
BibTeX:
APA:
## Glossary [optional]
## More Information [optional]
## Model Card Authors [optional]
## Model Card Contact
| [
"# Model Card for Model ID",
"## Model Details",
"### Model Description\n\n\n\nThis is the model card of a transformers model that has been pushed on the Hub. This model card has been automatically generated.\n\n- Developed by: \n- Funded by [optional]: \n- Shared by [optional]: \n- Model type: \n- Language(s) (NLP): \n- License: \n- Finetuned from model [optional]:",
"### Model Sources [optional]\n\n\n\n- Repository: \n- Paper [optional]: \n- Demo [optional]:",
"## Uses",
"### Direct Use",
"### Downstream Use [optional]",
"### Out-of-Scope Use",
"## Bias, Risks, and Limitations",
"### Recommendations\n\n\n\nUsers (both direct and downstream) should be made aware of the risks, biases and limitations of the model. More information needed for further recommendations.",
"## How to Get Started with the Model\n\nUse the code below to get started with the model.",
"## Training Details",
"### Training Data",
"### Training Procedure",
"#### Preprocessing [optional]",
"#### Training Hyperparameters\n\n- Training regime:",
"#### Speeds, Sizes, Times [optional]",
"## Evaluation",
"### Testing Data, Factors & Metrics",
"#### Testing Data",
"#### Factors",
"#### Metrics",
"### Results",
"#### Summary",
"## Model Examination [optional]",
"## Environmental Impact\n\n\n\nCarbon emissions can be estimated using the Machine Learning Impact calculator presented in Lacoste et al. (2019).\n\n- Hardware Type: \n- Hours used: \n- Cloud Provider: \n- Compute Region: \n- Carbon Emitted:",
"## Technical Specifications [optional]",
"### Model Architecture and Objective",
"### Compute Infrastructure",
"#### Hardware",
"#### Software\n\n\n\n[optional]\n\n\n\nBibTeX:\n\n\n\nAPA:",
"## Glossary [optional]",
"## More Information [optional]",
"## Model Card Authors [optional]",
"## Model Card Contact"
] | [
"TAGS\n#transformers #safetensors #llama #text-generation #arxiv-1910.09700 #autotrain_compatible #endpoints_compatible #text-generation-inference #region-us \n",
"# Model Card for Model ID",
"## Model Details",
"### Model Description\n\n\n\nThis is the model card of a transformers model that has been pushed on the Hub. This model card has been automatically generated.\n\n- Developed by: \n- Funded by [optional]: \n- Shared by [optional]: \n- Model type: \n- Language(s) (NLP): \n- License: \n- Finetuned from model [optional]:",
"### Model Sources [optional]\n\n\n\n- Repository: \n- Paper [optional]: \n- Demo [optional]:",
"## Uses",
"### Direct Use",
"### Downstream Use [optional]",
"### Out-of-Scope Use",
"## Bias, Risks, and Limitations",
"### Recommendations\n\n\n\nUsers (both direct and downstream) should be made aware of the risks, biases and limitations of the model. More information needed for further recommendations.",
"## How to Get Started with the Model\n\nUse the code below to get started with the model.",
"## Training Details",
"### Training Data",
"### Training Procedure",
"#### Preprocessing [optional]",
"#### Training Hyperparameters\n\n- Training regime:",
"#### Speeds, Sizes, Times [optional]",
"## Evaluation",
"### Testing Data, Factors & Metrics",
"#### Testing Data",
"#### Factors",
"#### Metrics",
"### Results",
"#### Summary",
"## Model Examination [optional]",
"## Environmental Impact\n\n\n\nCarbon emissions can be estimated using the Machine Learning Impact calculator presented in Lacoste et al. (2019).\n\n- Hardware Type: \n- Hours used: \n- Cloud Provider: \n- Compute Region: \n- Carbon Emitted:",
"## Technical Specifications [optional]",
"### Model Architecture and Objective",
"### Compute Infrastructure",
"#### Hardware",
"#### Software\n\n\n\n[optional]\n\n\n\nBibTeX:\n\n\n\nAPA:",
"## Glossary [optional]",
"## More Information [optional]",
"## Model Card Authors [optional]",
"## Model Card Contact"
] |
null | null |
# Model Card for deepseek-coder-33b-instruct-pythagora
This model card describes the deepseek-coder-33b-instruct-pythagora model, which is a fine-tuned version of the DeepSeek Coder 33B Instruct model, specifically optimized for use with the Pythagora GPT Pilot application.
## Model Details
### Model Description
- **Developed by:** LoupGarou (GitHub: [MoonlightByte](https://github.com/MoonlightByte))
- **Model type:** Causal language model
- **Language(s) (NLP):** English
- **License:** DeepSeek Coder Model License
- **Finetuned from model:** [DeepSeek Coder 33B Instruct](https://huggingface.co/deepseek-ai/deepseek-coder-33b-instruct)
### Model Sources
- **Repository:** [LoupGarou/deepseek-coder-33b-instruct-pythagora-gguf](https://huggingface.co/LoupGarou/deepseek-coder-33b-instruct-pythagora-gguf)
- **GitHub Repository (Proxy Application):** [MoonlightByte/Pythagora-LLM-Proxy](https://github.com/MoonlightByte/Pythagora-LLM-Proxy)
- **Original Model Repository:** [DeepSeek Coder](https://github.com/deepseek-ai/deepseek-coder)
## Uses
### Direct Use
This model is intended for use with the [Pythagora GPT Pilot](https://github.com/Pythagora-io/gpt-pilot) application, which enables the creation of fully working, production-ready apps with the assistance of a developer. The model has been fine-tuned to work seamlessly with the GPT Pilot prompt structures and can be utilized through the [Pythagora LLM Proxy](https://github.com/MoonlightByte/Pythagora-LLM-Proxy).
The model is designed to generate code and assist with various programming tasks, such as writing features, debugging, and providing code reviews, all within the context of the Pythagora GPT Pilot application.
### Out-of-Scope Use
This model should not be used for tasks outside of the intended use case with the Pythagora GPT Pilot application. It is not designed for standalone use or integration with other applications without proper testing and adaptation. Additionally, the model should not be used for generating content related to sensitive topics, such as politics, security, or privacy issues, as it is specifically trained to focus on computer science and programming-related tasks.
## Bias, Risks, and Limitations
As with any language model, there may be biases present in the training data that could be reflected in the model's outputs. Users should be aware of potential limitations and biases when using this model. The model's performance may be impacted by the quality and relevance of the input prompts, as well as the specific programming languages and frameworks used in the context of the Pythagora GPT Pilot application.
### Recommendations
Users should familiarize themselves with the [Pythagora GPT Pilot](https://github.com/Pythagora-io/gpt-pilot) application and its intended use cases before utilizing this model. It is recommended to use the model in conjunction with the [Pythagora LLM Proxy](https://github.com/MoonlightByte/Pythagora-LLM-Proxy) for optimal performance and compatibility. When using the model, users should carefully review and test the generated code to ensure its correctness, efficiency, and adherence to best practices and project requirements.
## How to Get Started with the Model
To use this model with the Pythagora GPT Pilot application:
1. Set up the Pythagora LLM Proxy by following the instructions in the [GitHub repository](https://github.com/MoonlightByte/Pythagora-LLM-Proxy).
2. Configure GPT Pilot to use the proxy by setting the OpenAI API endpoint to `http://localhost:8080/v1/chat/completions`.
3. Run GPT Pilot as usual, and the proxy will handle the communication between GPT Pilot and the deepseek-coder-6.7b-instruct-pythagora model.
4. It is possible to run Pythagora directly to LM Studio or any other service with mixed results since these models were not finetuned using a chat format.
For more detailed instructions and examples, please refer to the [Pythagora LLM Proxy README](https://github.com/MoonlightByte/Pythagora-LLM-Proxy/blob/main/README.md).
## Training Details
### Training Data
The model was fine-tuned using a custom dataset created from sample prompts generated by the Pythagora prompt structures. The prompts are compatible with the version described in the [Pythagora README](https://github.com/Pythagora-io/gpt-pilot/blob/main/README.md). The dataset was carefully curated to ensure high-quality examples and a diverse range of programming tasks relevant to the Pythagora GPT Pilot application.
### Training Procedure
The model was fine-tuned using the training scripts and resources provided in the [DeepSeek Coder GitHub repository](https://github.com/deepseek-ai/DeepSeek-Coder.git). Specifically, the [finetune/finetune_deepseekcoder.py](https://github.com/deepseek-ai/DeepSeek-Coder/blob/main/finetune/finetune_deepseekcoder.py) script was used to perform the fine-tuning process. The model was trained using PEFT with a maximum sequence length of 9,000 tokens, utilizing the custom dataset to adapt the base DeepSeek Coder 33B Instruct model to the specific requirements and prompt structures of the Pythagora GPT Pilot application.
The training process leveraged state-of-the-art techniques and hardware, including DeepSpeed integration for efficient distributed training, to ensure optimal performance and compatibility with the target application. For detailed information on the training procedure, including the specific hyperparameters and configurations used, please refer to the [DeepSeek Coder Fine-tuning Documentation](https://github.com/deepseek-ai/DeepSeek-Coder#how-to-fine-tune-deepseek-coder).
## Model Examination
No additional interpretability work has been performed on this model. However, the model's performance has been thoroughly tested and validated within the context of the Pythagora GPT Pilot application to ensure its effectiveness in generating high-quality code and assisting with programming tasks.
## Environmental Impact
The environmental impact of this model has not been assessed. More information is needed to estimate the carbon emissions and electricity usage associated with the model's training and deployment. As a general recommendation, users should strive to utilize the model efficiently and responsibly to minimize any potential environmental impact.
## Technical Specifications
- **Model Architecture:** The model architecture is based on the DeepSeek Coder 33B Instruct model, which is a transformer-based causal language model optimized for code generation and understanding.
- **Compute Infrastructure:** The model was fine-tuned using high-performance computing resources, including GPUs, to ensure efficient and timely training. The exact specifications of the compute infrastructure used for training are not publicly disclosed.
## Citation
**APA:**
LoupGarou. (2024). deepseek-coder-33b-instruct-pythagora (Model). https://huggingface.co/LoupGarou/deepseek-coder-33b-instruct-pythagora
## Model Card Contact
For questions, feedback, or concerns regarding this model, please contact LoupGarou through the GitHub repository: [MoonlightByte/Pythagora-LLM-Proxy](https://github.com/MoonlightByte/Pythagora-LLM-Proxy). You can open an issue or submit a pull request to discuss any aspects of the model or its usage within the Pythagora GPT Pilot application.
**Original model card: DeepSeek's Deepseek Coder 33B Instruct**
**[🏠Homepage](https://www.deepseek.com/)** | **[🤖 Chat with DeepSeek Coder](https://coder.deepseek.com/)** | **[Discord](https://discord.gg/Tc7c45Zzu5)** | **[Wechat(微信)](https://github.com/guoday/assert/blob/main/QR.png?raw=true)**
---
### 1. Introduction of Deepseek Coder
Deepseek Coder is composed of a series of code language models, each trained from scratch on 2T tokens, with a composition of 87% code and 13% natural language in both English and Chinese. We provide various sizes of the code model, ranging from 1B to 33B versions. Each model is pre-trained on project-level code corpus by employing a window size of 16K and a extra fill-in-the-blank task, to support project-level code completion and infilling. For coding capabilities, Deepseek Coder achieves state-of-the-art performance among open-source code models on multiple programming languages and various benchmarks.
- **Massive Training Data**: Trained from scratch fon 2T tokens, including 87% code and 13% linguistic data in both English and Chinese languages.
- **Highly Flexible & Scalable**: Offered in model sizes of 1.3B, 5.7B, 6.7B, and 33B, enabling users to choose the setup most suitable for their requirements.
- **Superior Model Performance**: State-of-the-art performance among publicly available code models on HumanEval, MultiPL-E, MBPP, DS-1000, and APPS benchmarks.
- **Advanced Code Completion Capabilities**: A window size of 16K and a fill-in-the-blank task, supporting project-level code completion and infilling tasks.
### 2. Model Summary
deepseek-coder-33b-instruct is a 33B parameter model initialized from deepseek-coder-33b-base and fine-tuned on 2B tokens of instruction data.
- **Home Page:** [DeepSeek](https://www.deepseek.com/)
- **Repository:** [deepseek-ai/deepseek-coder](https://github.com/deepseek-ai/deepseek-coder)
- **Chat With DeepSeek Coder:** [DeepSeek-Coder](https://coder.deepseek.com/)
### 3. How to Use
Here give some examples of how to use our model.
#### Chat Model Inference
```python
from transformers import AutoTokenizer, AutoModelForCausalLM
tokenizer = AutoTokenizer.from_pretrained("deepseek-ai/deepseek-coder-6.7b-instruct", trust_remote_code=True)
model = AutoModelForCausalLM.from_pretrained("deepseek-ai/deepseek-coder-6.7b-instruct", trust_remote_code=True).cuda()
messages=[
{ 'role': 'user', 'content': "write a quick sort algorithm in python."}
]
inputs = tokenizer.apply_chat_template(messages, return_tensors="pt").to(model.device)
# 32021 is the id of <|EOT|> token
outputs = model.generate(inputs, max_new_tokens=512, do_sample=False, top_k=50, top_p=0.95, num_return_sequences=1, eos_token_id=32021)
print(tokenizer.decode(outputs[0][len(inputs[0]):], skip_special_tokens=True))
```
### 4. License
This code repository is licensed under the MIT License. The use of DeepSeek Coder models is subject to the Model License. DeepSeek Coder supports commercial use.
See the [LICENSE-MODEL](https://github.com/deepseek-ai/deepseek-coder/blob/main/LICENSE-MODEL) for more details.
### 5. Contact
If you have any questions, please raise an issue or contact us at [[email protected]](mailto:[email protected]). | {} | LoupGarou/deepseek-coder-33b-instruct-pythagora-gguf | null | [
"gguf",
"region:us"
] | null | 2024-04-27T07:13:10+00:00 | [] | [] | TAGS
#gguf #region-us
|
# Model Card for deepseek-coder-33b-instruct-pythagora
This model card describes the deepseek-coder-33b-instruct-pythagora model, which is a fine-tuned version of the DeepSeek Coder 33B Instruct model, specifically optimized for use with the Pythagora GPT Pilot application.
## Model Details
### Model Description
- Developed by: LoupGarou (GitHub: MoonlightByte)
- Model type: Causal language model
- Language(s) (NLP): English
- License: DeepSeek Coder Model License
- Finetuned from model: DeepSeek Coder 33B Instruct
### Model Sources
- Repository: LoupGarou/deepseek-coder-33b-instruct-pythagora-gguf
- GitHub Repository (Proxy Application): MoonlightByte/Pythagora-LLM-Proxy
- Original Model Repository: DeepSeek Coder
## Uses
### Direct Use
This model is intended for use with the Pythagora GPT Pilot application, which enables the creation of fully working, production-ready apps with the assistance of a developer. The model has been fine-tuned to work seamlessly with the GPT Pilot prompt structures and can be utilized through the Pythagora LLM Proxy.
The model is designed to generate code and assist with various programming tasks, such as writing features, debugging, and providing code reviews, all within the context of the Pythagora GPT Pilot application.
### Out-of-Scope Use
This model should not be used for tasks outside of the intended use case with the Pythagora GPT Pilot application. It is not designed for standalone use or integration with other applications without proper testing and adaptation. Additionally, the model should not be used for generating content related to sensitive topics, such as politics, security, or privacy issues, as it is specifically trained to focus on computer science and programming-related tasks.
## Bias, Risks, and Limitations
As with any language model, there may be biases present in the training data that could be reflected in the model's outputs. Users should be aware of potential limitations and biases when using this model. The model's performance may be impacted by the quality and relevance of the input prompts, as well as the specific programming languages and frameworks used in the context of the Pythagora GPT Pilot application.
### Recommendations
Users should familiarize themselves with the Pythagora GPT Pilot application and its intended use cases before utilizing this model. It is recommended to use the model in conjunction with the Pythagora LLM Proxy for optimal performance and compatibility. When using the model, users should carefully review and test the generated code to ensure its correctness, efficiency, and adherence to best practices and project requirements.
## How to Get Started with the Model
To use this model with the Pythagora GPT Pilot application:
1. Set up the Pythagora LLM Proxy by following the instructions in the GitHub repository.
2. Configure GPT Pilot to use the proxy by setting the OpenAI API endpoint to 'http://localhost:8080/v1/chat/completions'.
3. Run GPT Pilot as usual, and the proxy will handle the communication between GPT Pilot and the deepseek-coder-6.7b-instruct-pythagora model.
4. It is possible to run Pythagora directly to LM Studio or any other service with mixed results since these models were not finetuned using a chat format.
For more detailed instructions and examples, please refer to the Pythagora LLM Proxy README.
## Training Details
### Training Data
The model was fine-tuned using a custom dataset created from sample prompts generated by the Pythagora prompt structures. The prompts are compatible with the version described in the Pythagora README. The dataset was carefully curated to ensure high-quality examples and a diverse range of programming tasks relevant to the Pythagora GPT Pilot application.
### Training Procedure
The model was fine-tuned using the training scripts and resources provided in the DeepSeek Coder GitHub repository. Specifically, the finetune/finetune_deepseekcoder.py script was used to perform the fine-tuning process. The model was trained using PEFT with a maximum sequence length of 9,000 tokens, utilizing the custom dataset to adapt the base DeepSeek Coder 33B Instruct model to the specific requirements and prompt structures of the Pythagora GPT Pilot application.
The training process leveraged state-of-the-art techniques and hardware, including DeepSpeed integration for efficient distributed training, to ensure optimal performance and compatibility with the target application. For detailed information on the training procedure, including the specific hyperparameters and configurations used, please refer to the DeepSeek Coder Fine-tuning Documentation.
## Model Examination
No additional interpretability work has been performed on this model. However, the model's performance has been thoroughly tested and validated within the context of the Pythagora GPT Pilot application to ensure its effectiveness in generating high-quality code and assisting with programming tasks.
## Environmental Impact
The environmental impact of this model has not been assessed. More information is needed to estimate the carbon emissions and electricity usage associated with the model's training and deployment. As a general recommendation, users should strive to utilize the model efficiently and responsibly to minimize any potential environmental impact.
## Technical Specifications
- Model Architecture: The model architecture is based on the DeepSeek Coder 33B Instruct model, which is a transformer-based causal language model optimized for code generation and understanding.
- Compute Infrastructure: The model was fine-tuned using high-performance computing resources, including GPUs, to ensure efficient and timely training. The exact specifications of the compute infrastructure used for training are not publicly disclosed.
APA:
LoupGarou. (2024). deepseek-coder-33b-instruct-pythagora (Model). URL
## Model Card Contact
For questions, feedback, or concerns regarding this model, please contact LoupGarou through the GitHub repository: MoonlightByte/Pythagora-LLM-Proxy. You can open an issue or submit a pull request to discuss any aspects of the model or its usage within the Pythagora GPT Pilot application.
Original model card: DeepSeek's Deepseek Coder 33B Instruct
Homepage | Chat with DeepSeek Coder | Discord | Wechat(微信)
---
### 1. Introduction of Deepseek Coder
Deepseek Coder is composed of a series of code language models, each trained from scratch on 2T tokens, with a composition of 87% code and 13% natural language in both English and Chinese. We provide various sizes of the code model, ranging from 1B to 33B versions. Each model is pre-trained on project-level code corpus by employing a window size of 16K and a extra fill-in-the-blank task, to support project-level code completion and infilling. For coding capabilities, Deepseek Coder achieves state-of-the-art performance among open-source code models on multiple programming languages and various benchmarks.
- Massive Training Data: Trained from scratch fon 2T tokens, including 87% code and 13% linguistic data in both English and Chinese languages.
- Highly Flexible & Scalable: Offered in model sizes of 1.3B, 5.7B, 6.7B, and 33B, enabling users to choose the setup most suitable for their requirements.
- Superior Model Performance: State-of-the-art performance among publicly available code models on HumanEval, MultiPL-E, MBPP, DS-1000, and APPS benchmarks.
- Advanced Code Completion Capabilities: A window size of 16K and a fill-in-the-blank task, supporting project-level code completion and infilling tasks.
### 2. Model Summary
deepseek-coder-33b-instruct is a 33B parameter model initialized from deepseek-coder-33b-base and fine-tuned on 2B tokens of instruction data.
- Home Page: DeepSeek
- Repository: deepseek-ai/deepseek-coder
- Chat With DeepSeek Coder: DeepSeek-Coder
### 3. How to Use
Here give some examples of how to use our model.
#### Chat Model Inference
### 4. License
This code repository is licensed under the MIT License. The use of DeepSeek Coder models is subject to the Model License. DeepSeek Coder supports commercial use.
See the LICENSE-MODEL for more details.
### 5. Contact
If you have any questions, please raise an issue or contact us at agi_code@URL. | [
"# Model Card for deepseek-coder-33b-instruct-pythagora\n\nThis model card describes the deepseek-coder-33b-instruct-pythagora model, which is a fine-tuned version of the DeepSeek Coder 33B Instruct model, specifically optimized for use with the Pythagora GPT Pilot application.",
"## Model Details",
"### Model Description\n\n- Developed by: LoupGarou (GitHub: MoonlightByte)\n- Model type: Causal language model\n- Language(s) (NLP): English\n- License: DeepSeek Coder Model License\n- Finetuned from model: DeepSeek Coder 33B Instruct",
"### Model Sources\n\n- Repository: LoupGarou/deepseek-coder-33b-instruct-pythagora-gguf\n- GitHub Repository (Proxy Application): MoonlightByte/Pythagora-LLM-Proxy\n- Original Model Repository: DeepSeek Coder",
"## Uses",
"### Direct Use\n\nThis model is intended for use with the Pythagora GPT Pilot application, which enables the creation of fully working, production-ready apps with the assistance of a developer. The model has been fine-tuned to work seamlessly with the GPT Pilot prompt structures and can be utilized through the Pythagora LLM Proxy.\n\nThe model is designed to generate code and assist with various programming tasks, such as writing features, debugging, and providing code reviews, all within the context of the Pythagora GPT Pilot application.",
"### Out-of-Scope Use\n\nThis model should not be used for tasks outside of the intended use case with the Pythagora GPT Pilot application. It is not designed for standalone use or integration with other applications without proper testing and adaptation. Additionally, the model should not be used for generating content related to sensitive topics, such as politics, security, or privacy issues, as it is specifically trained to focus on computer science and programming-related tasks.",
"## Bias, Risks, and Limitations\n\nAs with any language model, there may be biases present in the training data that could be reflected in the model's outputs. Users should be aware of potential limitations and biases when using this model. The model's performance may be impacted by the quality and relevance of the input prompts, as well as the specific programming languages and frameworks used in the context of the Pythagora GPT Pilot application.",
"### Recommendations\n\nUsers should familiarize themselves with the Pythagora GPT Pilot application and its intended use cases before utilizing this model. It is recommended to use the model in conjunction with the Pythagora LLM Proxy for optimal performance and compatibility. When using the model, users should carefully review and test the generated code to ensure its correctness, efficiency, and adherence to best practices and project requirements.",
"## How to Get Started with the Model\n\nTo use this model with the Pythagora GPT Pilot application:\n\n1. Set up the Pythagora LLM Proxy by following the instructions in the GitHub repository.\n2. Configure GPT Pilot to use the proxy by setting the OpenAI API endpoint to 'http://localhost:8080/v1/chat/completions'.\n3. Run GPT Pilot as usual, and the proxy will handle the communication between GPT Pilot and the deepseek-coder-6.7b-instruct-pythagora model.\n4. It is possible to run Pythagora directly to LM Studio or any other service with mixed results since these models were not finetuned using a chat format.\n\nFor more detailed instructions and examples, please refer to the Pythagora LLM Proxy README.",
"## Training Details",
"### Training Data\n\nThe model was fine-tuned using a custom dataset created from sample prompts generated by the Pythagora prompt structures. The prompts are compatible with the version described in the Pythagora README. The dataset was carefully curated to ensure high-quality examples and a diverse range of programming tasks relevant to the Pythagora GPT Pilot application.",
"### Training Procedure\n\nThe model was fine-tuned using the training scripts and resources provided in the DeepSeek Coder GitHub repository. Specifically, the finetune/finetune_deepseekcoder.py script was used to perform the fine-tuning process. The model was trained using PEFT with a maximum sequence length of 9,000 tokens, utilizing the custom dataset to adapt the base DeepSeek Coder 33B Instruct model to the specific requirements and prompt structures of the Pythagora GPT Pilot application.\n\nThe training process leveraged state-of-the-art techniques and hardware, including DeepSpeed integration for efficient distributed training, to ensure optimal performance and compatibility with the target application. For detailed information on the training procedure, including the specific hyperparameters and configurations used, please refer to the DeepSeek Coder Fine-tuning Documentation.",
"## Model Examination\n\nNo additional interpretability work has been performed on this model. However, the model's performance has been thoroughly tested and validated within the context of the Pythagora GPT Pilot application to ensure its effectiveness in generating high-quality code and assisting with programming tasks.",
"## Environmental Impact\n\nThe environmental impact of this model has not been assessed. More information is needed to estimate the carbon emissions and electricity usage associated with the model's training and deployment. As a general recommendation, users should strive to utilize the model efficiently and responsibly to minimize any potential environmental impact.",
"## Technical Specifications\n\n- Model Architecture: The model architecture is based on the DeepSeek Coder 33B Instruct model, which is a transformer-based causal language model optimized for code generation and understanding.\n- Compute Infrastructure: The model was fine-tuned using high-performance computing resources, including GPUs, to ensure efficient and timely training. The exact specifications of the compute infrastructure used for training are not publicly disclosed.\n\nAPA:\nLoupGarou. (2024). deepseek-coder-33b-instruct-pythagora (Model). URL",
"## Model Card Contact\n\nFor questions, feedback, or concerns regarding this model, please contact LoupGarou through the GitHub repository: MoonlightByte/Pythagora-LLM-Proxy. You can open an issue or submit a pull request to discuss any aspects of the model or its usage within the Pythagora GPT Pilot application.\n\nOriginal model card: DeepSeek's Deepseek Coder 33B Instruct\n\nHomepage | Chat with DeepSeek Coder | Discord | Wechat(微信)\n\n---",
"### 1. Introduction of Deepseek Coder\n\nDeepseek Coder is composed of a series of code language models, each trained from scratch on 2T tokens, with a composition of 87% code and 13% natural language in both English and Chinese. We provide various sizes of the code model, ranging from 1B to 33B versions. Each model is pre-trained on project-level code corpus by employing a window size of 16K and a extra fill-in-the-blank task, to support project-level code completion and infilling. For coding capabilities, Deepseek Coder achieves state-of-the-art performance among open-source code models on multiple programming languages and various benchmarks.\n\n- Massive Training Data: Trained from scratch fon 2T tokens, including 87% code and 13% linguistic data in both English and Chinese languages.\n- Highly Flexible & Scalable: Offered in model sizes of 1.3B, 5.7B, 6.7B, and 33B, enabling users to choose the setup most suitable for their requirements.\n- Superior Model Performance: State-of-the-art performance among publicly available code models on HumanEval, MultiPL-E, MBPP, DS-1000, and APPS benchmarks.\n- Advanced Code Completion Capabilities: A window size of 16K and a fill-in-the-blank task, supporting project-level code completion and infilling tasks.",
"### 2. Model Summary\n\ndeepseek-coder-33b-instruct is a 33B parameter model initialized from deepseek-coder-33b-base and fine-tuned on 2B tokens of instruction data.\n\n- Home Page: DeepSeek\n- Repository: deepseek-ai/deepseek-coder\n- Chat With DeepSeek Coder: DeepSeek-Coder",
"### 3. How to Use\n\nHere give some examples of how to use our model.",
"#### Chat Model Inference",
"### 4. License\n\nThis code repository is licensed under the MIT License. The use of DeepSeek Coder models is subject to the Model License. DeepSeek Coder supports commercial use.\n\nSee the LICENSE-MODEL for more details.",
"### 5. Contact\n\nIf you have any questions, please raise an issue or contact us at agi_code@URL."
] | [
"TAGS\n#gguf #region-us \n",
"# Model Card for deepseek-coder-33b-instruct-pythagora\n\nThis model card describes the deepseek-coder-33b-instruct-pythagora model, which is a fine-tuned version of the DeepSeek Coder 33B Instruct model, specifically optimized for use with the Pythagora GPT Pilot application.",
"## Model Details",
"### Model Description\n\n- Developed by: LoupGarou (GitHub: MoonlightByte)\n- Model type: Causal language model\n- Language(s) (NLP): English\n- License: DeepSeek Coder Model License\n- Finetuned from model: DeepSeek Coder 33B Instruct",
"### Model Sources\n\n- Repository: LoupGarou/deepseek-coder-33b-instruct-pythagora-gguf\n- GitHub Repository (Proxy Application): MoonlightByte/Pythagora-LLM-Proxy\n- Original Model Repository: DeepSeek Coder",
"## Uses",
"### Direct Use\n\nThis model is intended for use with the Pythagora GPT Pilot application, which enables the creation of fully working, production-ready apps with the assistance of a developer. The model has been fine-tuned to work seamlessly with the GPT Pilot prompt structures and can be utilized through the Pythagora LLM Proxy.\n\nThe model is designed to generate code and assist with various programming tasks, such as writing features, debugging, and providing code reviews, all within the context of the Pythagora GPT Pilot application.",
"### Out-of-Scope Use\n\nThis model should not be used for tasks outside of the intended use case with the Pythagora GPT Pilot application. It is not designed for standalone use or integration with other applications without proper testing and adaptation. Additionally, the model should not be used for generating content related to sensitive topics, such as politics, security, or privacy issues, as it is specifically trained to focus on computer science and programming-related tasks.",
"## Bias, Risks, and Limitations\n\nAs with any language model, there may be biases present in the training data that could be reflected in the model's outputs. Users should be aware of potential limitations and biases when using this model. The model's performance may be impacted by the quality and relevance of the input prompts, as well as the specific programming languages and frameworks used in the context of the Pythagora GPT Pilot application.",
"### Recommendations\n\nUsers should familiarize themselves with the Pythagora GPT Pilot application and its intended use cases before utilizing this model. It is recommended to use the model in conjunction with the Pythagora LLM Proxy for optimal performance and compatibility. When using the model, users should carefully review and test the generated code to ensure its correctness, efficiency, and adherence to best practices and project requirements.",
"## How to Get Started with the Model\n\nTo use this model with the Pythagora GPT Pilot application:\n\n1. Set up the Pythagora LLM Proxy by following the instructions in the GitHub repository.\n2. Configure GPT Pilot to use the proxy by setting the OpenAI API endpoint to 'http://localhost:8080/v1/chat/completions'.\n3. Run GPT Pilot as usual, and the proxy will handle the communication between GPT Pilot and the deepseek-coder-6.7b-instruct-pythagora model.\n4. It is possible to run Pythagora directly to LM Studio or any other service with mixed results since these models were not finetuned using a chat format.\n\nFor more detailed instructions and examples, please refer to the Pythagora LLM Proxy README.",
"## Training Details",
"### Training Data\n\nThe model was fine-tuned using a custom dataset created from sample prompts generated by the Pythagora prompt structures. The prompts are compatible with the version described in the Pythagora README. The dataset was carefully curated to ensure high-quality examples and a diverse range of programming tasks relevant to the Pythagora GPT Pilot application.",
"### Training Procedure\n\nThe model was fine-tuned using the training scripts and resources provided in the DeepSeek Coder GitHub repository. Specifically, the finetune/finetune_deepseekcoder.py script was used to perform the fine-tuning process. The model was trained using PEFT with a maximum sequence length of 9,000 tokens, utilizing the custom dataset to adapt the base DeepSeek Coder 33B Instruct model to the specific requirements and prompt structures of the Pythagora GPT Pilot application.\n\nThe training process leveraged state-of-the-art techniques and hardware, including DeepSpeed integration for efficient distributed training, to ensure optimal performance and compatibility with the target application. For detailed information on the training procedure, including the specific hyperparameters and configurations used, please refer to the DeepSeek Coder Fine-tuning Documentation.",
"## Model Examination\n\nNo additional interpretability work has been performed on this model. However, the model's performance has been thoroughly tested and validated within the context of the Pythagora GPT Pilot application to ensure its effectiveness in generating high-quality code and assisting with programming tasks.",
"## Environmental Impact\n\nThe environmental impact of this model has not been assessed. More information is needed to estimate the carbon emissions and electricity usage associated with the model's training and deployment. As a general recommendation, users should strive to utilize the model efficiently and responsibly to minimize any potential environmental impact.",
"## Technical Specifications\n\n- Model Architecture: The model architecture is based on the DeepSeek Coder 33B Instruct model, which is a transformer-based causal language model optimized for code generation and understanding.\n- Compute Infrastructure: The model was fine-tuned using high-performance computing resources, including GPUs, to ensure efficient and timely training. The exact specifications of the compute infrastructure used for training are not publicly disclosed.\n\nAPA:\nLoupGarou. (2024). deepseek-coder-33b-instruct-pythagora (Model). URL",
"## Model Card Contact\n\nFor questions, feedback, or concerns regarding this model, please contact LoupGarou through the GitHub repository: MoonlightByte/Pythagora-LLM-Proxy. You can open an issue or submit a pull request to discuss any aspects of the model or its usage within the Pythagora GPT Pilot application.\n\nOriginal model card: DeepSeek's Deepseek Coder 33B Instruct\n\nHomepage | Chat with DeepSeek Coder | Discord | Wechat(微信)\n\n---",
"### 1. Introduction of Deepseek Coder\n\nDeepseek Coder is composed of a series of code language models, each trained from scratch on 2T tokens, with a composition of 87% code and 13% natural language in both English and Chinese. We provide various sizes of the code model, ranging from 1B to 33B versions. Each model is pre-trained on project-level code corpus by employing a window size of 16K and a extra fill-in-the-blank task, to support project-level code completion and infilling. For coding capabilities, Deepseek Coder achieves state-of-the-art performance among open-source code models on multiple programming languages and various benchmarks.\n\n- Massive Training Data: Trained from scratch fon 2T tokens, including 87% code and 13% linguistic data in both English and Chinese languages.\n- Highly Flexible & Scalable: Offered in model sizes of 1.3B, 5.7B, 6.7B, and 33B, enabling users to choose the setup most suitable for their requirements.\n- Superior Model Performance: State-of-the-art performance among publicly available code models on HumanEval, MultiPL-E, MBPP, DS-1000, and APPS benchmarks.\n- Advanced Code Completion Capabilities: A window size of 16K and a fill-in-the-blank task, supporting project-level code completion and infilling tasks.",
"### 2. Model Summary\n\ndeepseek-coder-33b-instruct is a 33B parameter model initialized from deepseek-coder-33b-base and fine-tuned on 2B tokens of instruction data.\n\n- Home Page: DeepSeek\n- Repository: deepseek-ai/deepseek-coder\n- Chat With DeepSeek Coder: DeepSeek-Coder",
"### 3. How to Use\n\nHere give some examples of how to use our model.",
"#### Chat Model Inference",
"### 4. License\n\nThis code repository is licensed under the MIT License. The use of DeepSeek Coder models is subject to the Model License. DeepSeek Coder supports commercial use.\n\nSee the LICENSE-MODEL for more details.",
"### 5. Contact\n\nIf you have any questions, please raise an issue or contact us at agi_code@URL."
] |
reinforcement-learning | null |
# **Q-Learning** Agent playing1 **Taxi-v3**
This is a trained model of a **Q-Learning** agent playing **Taxi-v3** .
## Usage
```python
model = load_from_hub(repo_id="Jurij1/q-Taxi-v3", filename="q-learning.pkl")
# Don't forget to check if you need to add additional attributes (is_slippery=False etc)
env = gym.make(model["env_id"])
```
| {"tags": ["Taxi-v3", "q-learning", "reinforcement-learning", "custom-implementation"], "model-index": [{"name": "q-Taxi-v3", "results": [{"task": {"type": "reinforcement-learning", "name": "reinforcement-learning"}, "dataset": {"name": "Taxi-v3", "type": "Taxi-v3"}, "metrics": [{"type": "mean_reward", "value": "7.50 +/- 2.73", "name": "mean_reward", "verified": false}]}]}]} | Jurij1/q-Taxi-v3 | null | [
"Taxi-v3",
"q-learning",
"reinforcement-learning",
"custom-implementation",
"model-index",
"region:us"
] | null | 2024-04-27T07:13:26+00:00 | [] | [] | TAGS
#Taxi-v3 #q-learning #reinforcement-learning #custom-implementation #model-index #region-us
|
# Q-Learning Agent playing1 Taxi-v3
This is a trained model of a Q-Learning agent playing Taxi-v3 .
## Usage
| [
"# Q-Learning Agent playing1 Taxi-v3\n This is a trained model of a Q-Learning agent playing Taxi-v3 .\n\n ## Usage"
] | [
"TAGS\n#Taxi-v3 #q-learning #reinforcement-learning #custom-implementation #model-index #region-us \n",
"# Q-Learning Agent playing1 Taxi-v3\n This is a trained model of a Q-Learning agent playing Taxi-v3 .\n\n ## Usage"
] |
text-generation | transformers |
<!-- This model card has been generated automatically according to the information the Trainer had access to. You
should probably proofread and complete it, then remove this comment. -->
# kaist-mistral-orpo-OHP-15k-Mathcode
This model is a fine-tuned version of [mistralai/Mistral-7B-v0.1](https://huggingface.co/mistralai/Mistral-7B-v0.1) on the orpo-explorers/OHP-15k-mathcode dataset.
## Model description
More information needed
## Intended uses & limitations
More information needed
## Training and evaluation data
More information needed
## Training procedure
### Training hyperparameters
The following hyperparameters were used during training:
- learning_rate: 5e-06
- train_batch_size: 8
- eval_batch_size: 8
- seed: 42
- distributed_type: multi-GPU
- num_devices: 4
- gradient_accumulation_steps: 2
- total_train_batch_size: 64
- total_eval_batch_size: 32
- optimizer: Adam with betas=(0.9,0.999) and epsilon=1e-08
- lr_scheduler_type: cosine
- num_epochs: 3
### Training results
### Framework versions
- Transformers 4.39.3
- Pytorch 2.1.2.post303
- Datasets 2.18.0
- Tokenizers 0.15.2
| {"license": "apache-2.0", "tags": ["alignment-handbook", "trl", "orpo", "generated_from_trainer", "trl", "orpo", "generated_from_trainer"], "datasets": ["orpo-explorers/OHP-15k-mathcode"], "base_model": "mistralai/Mistral-7B-v0.1", "model-index": [{"name": "kaist-mistral-orpo-OHP-15k-Mathcode", "results": []}]} | orpo-explorers/kaist-mistral-orpo-OHP-15k-Mathcode | null | [
"transformers",
"tensorboard",
"safetensors",
"mistral",
"text-generation",
"alignment-handbook",
"trl",
"orpo",
"generated_from_trainer",
"conversational",
"dataset:orpo-explorers/OHP-15k-mathcode",
"base_model:mistralai/Mistral-7B-v0.1",
"license:apache-2.0",
"autotrain_compatible",
"endpoints_compatible",
"text-generation-inference",
"region:us"
] | null | 2024-04-27T07:13:37+00:00 | [] | [] | TAGS
#transformers #tensorboard #safetensors #mistral #text-generation #alignment-handbook #trl #orpo #generated_from_trainer #conversational #dataset-orpo-explorers/OHP-15k-mathcode #base_model-mistralai/Mistral-7B-v0.1 #license-apache-2.0 #autotrain_compatible #endpoints_compatible #text-generation-inference #region-us
|
# kaist-mistral-orpo-OHP-15k-Mathcode
This model is a fine-tuned version of mistralai/Mistral-7B-v0.1 on the orpo-explorers/OHP-15k-mathcode dataset.
## Model description
More information needed
## Intended uses & limitations
More information needed
## Training and evaluation data
More information needed
## Training procedure
### Training hyperparameters
The following hyperparameters were used during training:
- learning_rate: 5e-06
- train_batch_size: 8
- eval_batch_size: 8
- seed: 42
- distributed_type: multi-GPU
- num_devices: 4
- gradient_accumulation_steps: 2
- total_train_batch_size: 64
- total_eval_batch_size: 32
- optimizer: Adam with betas=(0.9,0.999) and epsilon=1e-08
- lr_scheduler_type: cosine
- num_epochs: 3
### Training results
### Framework versions
- Transformers 4.39.3
- Pytorch 2.1.2.post303
- Datasets 2.18.0
- Tokenizers 0.15.2
| [
"# kaist-mistral-orpo-OHP-15k-Mathcode\n\nThis model is a fine-tuned version of mistralai/Mistral-7B-v0.1 on the orpo-explorers/OHP-15k-mathcode dataset.",
"## Model description\n\nMore information needed",
"## Intended uses & limitations\n\nMore information needed",
"## Training and evaluation data\n\nMore information needed",
"## Training procedure",
"### Training hyperparameters\n\nThe following hyperparameters were used during training:\n- learning_rate: 5e-06\n- train_batch_size: 8\n- eval_batch_size: 8\n- seed: 42\n- distributed_type: multi-GPU\n- num_devices: 4\n- gradient_accumulation_steps: 2\n- total_train_batch_size: 64\n- total_eval_batch_size: 32\n- optimizer: Adam with betas=(0.9,0.999) and epsilon=1e-08\n- lr_scheduler_type: cosine\n- num_epochs: 3",
"### Training results",
"### Framework versions\n\n- Transformers 4.39.3\n- Pytorch 2.1.2.post303\n- Datasets 2.18.0\n- Tokenizers 0.15.2"
] | [
"TAGS\n#transformers #tensorboard #safetensors #mistral #text-generation #alignment-handbook #trl #orpo #generated_from_trainer #conversational #dataset-orpo-explorers/OHP-15k-mathcode #base_model-mistralai/Mistral-7B-v0.1 #license-apache-2.0 #autotrain_compatible #endpoints_compatible #text-generation-inference #region-us \n",
"# kaist-mistral-orpo-OHP-15k-Mathcode\n\nThis model is a fine-tuned version of mistralai/Mistral-7B-v0.1 on the orpo-explorers/OHP-15k-mathcode dataset.",
"## Model description\n\nMore information needed",
"## Intended uses & limitations\n\nMore information needed",
"## Training and evaluation data\n\nMore information needed",
"## Training procedure",
"### Training hyperparameters\n\nThe following hyperparameters were used during training:\n- learning_rate: 5e-06\n- train_batch_size: 8\n- eval_batch_size: 8\n- seed: 42\n- distributed_type: multi-GPU\n- num_devices: 4\n- gradient_accumulation_steps: 2\n- total_train_batch_size: 64\n- total_eval_batch_size: 32\n- optimizer: Adam with betas=(0.9,0.999) and epsilon=1e-08\n- lr_scheduler_type: cosine\n- num_epochs: 3",
"### Training results",
"### Framework versions\n\n- Transformers 4.39.3\n- Pytorch 2.1.2.post303\n- Datasets 2.18.0\n- Tokenizers 0.15.2"
] |
null | peft |
<!-- This model card has been generated automatically according to the information the Trainer had access to. You
should probably proofread and complete it, then remove this comment. -->
# GUE_EMP_H3K14ac-seqsight_16384_512_22M-L8_f
This model is a fine-tuned version of [mahdibaghbanzadeh/seqsight_16384_512_22M](https://huggingface.co/mahdibaghbanzadeh/seqsight_16384_512_22M) on the [mahdibaghbanzadeh/GUE_EMP_H3K14ac](https://huggingface.co/datasets/mahdibaghbanzadeh/GUE_EMP_H3K14ac) dataset.
It achieves the following results on the evaluation set:
- Loss: 0.5250
- F1 Score: 0.7517
- Accuracy: 0.7504
## Model description
More information needed
## Intended uses & limitations
More information needed
## Training and evaluation data
More information needed
## Training procedure
### Training hyperparameters
The following hyperparameters were used during training:
- learning_rate: 0.0005
- train_batch_size: 128
- eval_batch_size: 128
- seed: 42
- optimizer: Adam with betas=(0.9,0.999) and epsilon=1e-08
- lr_scheduler_type: linear
- training_steps: 10000
### Training results
| Training Loss | Epoch | Step | Validation Loss | F1 Score | Accuracy |
|:-------------:|:-----:|:-----:|:---------------:|:--------:|:--------:|
| 0.6011 | 0.97 | 200 | 0.5808 | 0.6964 | 0.6947 |
| 0.5623 | 1.93 | 400 | 0.5564 | 0.7202 | 0.7183 |
| 0.5528 | 2.9 | 600 | 0.5929 | 0.6877 | 0.6890 |
| 0.5455 | 3.86 | 800 | 0.5319 | 0.7384 | 0.7371 |
| 0.5385 | 4.83 | 1000 | 0.5795 | 0.7053 | 0.7053 |
| 0.5367 | 5.8 | 1200 | 0.5689 | 0.7086 | 0.7083 |
| 0.5306 | 6.76 | 1400 | 0.5359 | 0.7301 | 0.7283 |
| 0.5285 | 7.73 | 1600 | 0.5372 | 0.7370 | 0.7352 |
| 0.5213 | 8.7 | 1800 | 0.5544 | 0.7177 | 0.7168 |
| 0.5192 | 9.66 | 2000 | 0.5565 | 0.7216 | 0.7207 |
| 0.5212 | 10.63 | 2200 | 0.5757 | 0.7081 | 0.7086 |
| 0.5101 | 11.59 | 2400 | 0.5296 | 0.7416 | 0.7398 |
| 0.5124 | 12.56 | 2600 | 0.5613 | 0.7205 | 0.7198 |
| 0.5097 | 13.53 | 2800 | 0.5587 | 0.7197 | 0.7189 |
| 0.5089 | 14.49 | 3000 | 0.5724 | 0.7127 | 0.7126 |
| 0.5033 | 15.46 | 3200 | 0.5293 | 0.7413 | 0.7395 |
| 0.5041 | 16.43 | 3400 | 0.5549 | 0.7213 | 0.7207 |
| 0.5021 | 17.39 | 3600 | 0.5424 | 0.7313 | 0.7298 |
| 0.5011 | 18.36 | 3800 | 0.5222 | 0.7497 | 0.7480 |
| 0.4981 | 19.32 | 4000 | 0.5401 | 0.7370 | 0.7356 |
| 0.4958 | 20.29 | 4200 | 0.5409 | 0.7402 | 0.7386 |
| 0.4955 | 21.26 | 4400 | 0.5610 | 0.7248 | 0.7241 |
| 0.4913 | 22.22 | 4600 | 0.5626 | 0.7213 | 0.7207 |
| 0.4939 | 23.19 | 4800 | 0.5332 | 0.7457 | 0.7440 |
| 0.4898 | 24.15 | 5000 | 0.5490 | 0.7307 | 0.7295 |
| 0.4909 | 25.12 | 5200 | 0.5706 | 0.7225 | 0.7222 |
| 0.4869 | 26.09 | 5400 | 0.5599 | 0.7272 | 0.7265 |
| 0.488 | 27.05 | 5600 | 0.5888 | 0.7138 | 0.7144 |
| 0.4884 | 28.02 | 5800 | 0.5354 | 0.7405 | 0.7389 |
| 0.4872 | 28.99 | 6000 | 0.5622 | 0.7210 | 0.7207 |
| 0.4831 | 29.95 | 6200 | 0.5666 | 0.7272 | 0.7265 |
| 0.483 | 30.92 | 6400 | 0.5294 | 0.7512 | 0.7495 |
| 0.4829 | 31.88 | 6600 | 0.5467 | 0.7330 | 0.7316 |
| 0.477 | 32.85 | 6800 | 0.5659 | 0.7268 | 0.7262 |
| 0.4866 | 33.82 | 7000 | 0.5629 | 0.7223 | 0.7219 |
| 0.4802 | 34.78 | 7200 | 0.5777 | 0.7170 | 0.7171 |
| 0.4796 | 35.75 | 7400 | 0.5524 | 0.7372 | 0.7359 |
| 0.4774 | 36.71 | 7600 | 0.5579 | 0.7274 | 0.7265 |
| 0.478 | 37.68 | 7800 | 0.5509 | 0.7292 | 0.7280 |
| 0.4752 | 38.65 | 8000 | 0.5454 | 0.7382 | 0.7368 |
| 0.484 | 39.61 | 8200 | 0.5533 | 0.7299 | 0.7289 |
| 0.4721 | 40.58 | 8400 | 0.5691 | 0.7237 | 0.7231 |
| 0.4725 | 41.55 | 8600 | 0.5550 | 0.7321 | 0.7310 |
| 0.4741 | 42.51 | 8800 | 0.5622 | 0.7276 | 0.7268 |
| 0.4782 | 43.48 | 9000 | 0.5699 | 0.7255 | 0.7250 |
| 0.4769 | 44.44 | 9200 | 0.5622 | 0.7260 | 0.7253 |
| 0.4748 | 45.41 | 9400 | 0.5583 | 0.7289 | 0.7280 |
| 0.4696 | 46.38 | 9600 | 0.5659 | 0.7268 | 0.7262 |
| 0.4757 | 47.34 | 9800 | 0.5590 | 0.7283 | 0.7274 |
| 0.4715 | 48.31 | 10000 | 0.5565 | 0.7311 | 0.7301 |
### Framework versions
- PEFT 0.9.0
- Transformers 4.38.2
- Pytorch 2.2.0+cu121
- Datasets 2.17.1
- Tokenizers 0.15.2 | {"library_name": "peft", "tags": ["generated_from_trainer"], "metrics": ["accuracy"], "base_model": "mahdibaghbanzadeh/seqsight_16384_512_22M", "model-index": [{"name": "GUE_EMP_H3K14ac-seqsight_16384_512_22M-L8_f", "results": []}]} | mahdibaghbanzadeh/GUE_EMP_H3K14ac-seqsight_16384_512_22M-L8_f | null | [
"peft",
"safetensors",
"generated_from_trainer",
"base_model:mahdibaghbanzadeh/seqsight_16384_512_22M",
"region:us"
] | null | 2024-04-27T07:14:10+00:00 | [] | [] | TAGS
#peft #safetensors #generated_from_trainer #base_model-mahdibaghbanzadeh/seqsight_16384_512_22M #region-us
| GUE\_EMP\_H3K14ac-seqsight\_16384\_512\_22M-L8\_f
=================================================
This model is a fine-tuned version of mahdibaghbanzadeh/seqsight\_16384\_512\_22M on the mahdibaghbanzadeh/GUE\_EMP\_H3K14ac dataset.
It achieves the following results on the evaluation set:
* Loss: 0.5250
* F1 Score: 0.7517
* Accuracy: 0.7504
Model description
-----------------
More information needed
Intended uses & limitations
---------------------------
More information needed
Training and evaluation data
----------------------------
More information needed
Training procedure
------------------
### Training hyperparameters
The following hyperparameters were used during training:
* learning\_rate: 0.0005
* train\_batch\_size: 128
* eval\_batch\_size: 128
* seed: 42
* optimizer: Adam with betas=(0.9,0.999) and epsilon=1e-08
* lr\_scheduler\_type: linear
* training\_steps: 10000
### Training results
### Framework versions
* PEFT 0.9.0
* Transformers 4.38.2
* Pytorch 2.2.0+cu121
* Datasets 2.17.1
* Tokenizers 0.15.2
| [
"### Training hyperparameters\n\n\nThe following hyperparameters were used during training:\n\n\n* learning\\_rate: 0.0005\n* train\\_batch\\_size: 128\n* eval\\_batch\\_size: 128\n* seed: 42\n* optimizer: Adam with betas=(0.9,0.999) and epsilon=1e-08\n* lr\\_scheduler\\_type: linear\n* training\\_steps: 10000",
"### Training results",
"### Framework versions\n\n\n* PEFT 0.9.0\n* Transformers 4.38.2\n* Pytorch 2.2.0+cu121\n* Datasets 2.17.1\n* Tokenizers 0.15.2"
] | [
"TAGS\n#peft #safetensors #generated_from_trainer #base_model-mahdibaghbanzadeh/seqsight_16384_512_22M #region-us \n",
"### Training hyperparameters\n\n\nThe following hyperparameters were used during training:\n\n\n* learning\\_rate: 0.0005\n* train\\_batch\\_size: 128\n* eval\\_batch\\_size: 128\n* seed: 42\n* optimizer: Adam with betas=(0.9,0.999) and epsilon=1e-08\n* lr\\_scheduler\\_type: linear\n* training\\_steps: 10000",
"### Training results",
"### Framework versions\n\n\n* PEFT 0.9.0\n* Transformers 4.38.2\n* Pytorch 2.2.0+cu121\n* Datasets 2.17.1\n* Tokenizers 0.15.2"
] |
null | peft |
<!-- This model card has been generated automatically according to the information the Trainer had access to. You
should probably proofread and complete it, then remove this comment. -->
# GUE_EMP_H3K14ac-seqsight_16384_512_22M-L32_f
This model is a fine-tuned version of [mahdibaghbanzadeh/seqsight_16384_512_22M](https://huggingface.co/mahdibaghbanzadeh/seqsight_16384_512_22M) on the [mahdibaghbanzadeh/GUE_EMP_H3K14ac](https://huggingface.co/datasets/mahdibaghbanzadeh/GUE_EMP_H3K14ac) dataset.
It achieves the following results on the evaluation set:
- Loss: 0.5218
- F1 Score: 0.7508
- Accuracy: 0.7495
## Model description
More information needed
## Intended uses & limitations
More information needed
## Training and evaluation data
More information needed
## Training procedure
### Training hyperparameters
The following hyperparameters were used during training:
- learning_rate: 0.0005
- train_batch_size: 128
- eval_batch_size: 128
- seed: 42
- optimizer: Adam with betas=(0.9,0.999) and epsilon=1e-08
- lr_scheduler_type: linear
- training_steps: 10000
### Training results
| Training Loss | Epoch | Step | Validation Loss | F1 Score | Accuracy |
|:-------------:|:-----:|:-----:|:---------------:|:--------:|:--------:|
| 0.591 | 0.97 | 200 | 0.5571 | 0.7151 | 0.7132 |
| 0.5523 | 1.93 | 400 | 0.5462 | 0.7244 | 0.7225 |
| 0.5429 | 2.9 | 600 | 0.5834 | 0.6928 | 0.6941 |
| 0.5338 | 3.86 | 800 | 0.5236 | 0.7499 | 0.7483 |
| 0.5238 | 4.83 | 1000 | 0.5718 | 0.7138 | 0.7138 |
| 0.5197 | 5.8 | 1200 | 0.5510 | 0.7215 | 0.7204 |
| 0.5106 | 6.76 | 1400 | 0.5235 | 0.7443 | 0.7425 |
| 0.5061 | 7.73 | 1600 | 0.5293 | 0.7424 | 0.7407 |
| 0.4987 | 8.7 | 1800 | 0.5519 | 0.7225 | 0.7216 |
| 0.4931 | 9.66 | 2000 | 0.5417 | 0.7339 | 0.7325 |
| 0.4952 | 10.63 | 2200 | 0.5692 | 0.7228 | 0.7225 |
| 0.4803 | 11.59 | 2400 | 0.5238 | 0.7500 | 0.7483 |
| 0.4817 | 12.56 | 2600 | 0.5611 | 0.7311 | 0.7301 |
| 0.4765 | 13.53 | 2800 | 0.5650 | 0.7246 | 0.7238 |
| 0.4737 | 14.49 | 3000 | 0.5579 | 0.7314 | 0.7304 |
| 0.4639 | 15.46 | 3200 | 0.5282 | 0.7560 | 0.7543 |
| 0.4625 | 16.43 | 3400 | 0.5657 | 0.7300 | 0.7292 |
| 0.4589 | 17.39 | 3600 | 0.5313 | 0.7491 | 0.7474 |
| 0.4557 | 18.36 | 3800 | 0.5281 | 0.7509 | 0.7492 |
| 0.4506 | 19.32 | 4000 | 0.5390 | 0.7505 | 0.7489 |
| 0.4489 | 20.29 | 4200 | 0.5549 | 0.7426 | 0.7410 |
| 0.4429 | 21.26 | 4400 | 0.5728 | 0.7314 | 0.7304 |
| 0.4376 | 22.22 | 4600 | 0.5689 | 0.7389 | 0.7377 |
| 0.4364 | 23.19 | 4800 | 0.5565 | 0.7460 | 0.7443 |
| 0.4314 | 24.15 | 5000 | 0.5826 | 0.7366 | 0.7352 |
| 0.4322 | 25.12 | 5200 | 0.5956 | 0.7316 | 0.7310 |
| 0.4272 | 26.09 | 5400 | 0.5889 | 0.7316 | 0.7310 |
| 0.4216 | 27.05 | 5600 | 0.6030 | 0.7227 | 0.7222 |
| 0.4224 | 28.02 | 5800 | 0.5593 | 0.7408 | 0.7392 |
| 0.4186 | 28.99 | 6000 | 0.5638 | 0.7383 | 0.7368 |
| 0.4117 | 29.95 | 6200 | 0.5925 | 0.7312 | 0.7298 |
| 0.4127 | 30.92 | 6400 | 0.5517 | 0.7535 | 0.7519 |
| 0.4127 | 31.88 | 6600 | 0.5605 | 0.7422 | 0.7404 |
| 0.4021 | 32.85 | 6800 | 0.6189 | 0.7162 | 0.7159 |
| 0.4126 | 33.82 | 7000 | 0.5915 | 0.7305 | 0.7295 |
| 0.4044 | 34.78 | 7200 | 0.6099 | 0.7243 | 0.7234 |
| 0.4034 | 35.75 | 7400 | 0.5837 | 0.7449 | 0.7431 |
| 0.3982 | 36.71 | 7600 | 0.5789 | 0.7379 | 0.7362 |
| 0.3992 | 37.68 | 7800 | 0.5947 | 0.7371 | 0.7356 |
| 0.3941 | 38.65 | 8000 | 0.5931 | 0.7369 | 0.7352 |
| 0.4018 | 39.61 | 8200 | 0.5757 | 0.7373 | 0.7356 |
| 0.3907 | 40.58 | 8400 | 0.5994 | 0.7328 | 0.7313 |
| 0.3885 | 41.55 | 8600 | 0.5880 | 0.7360 | 0.7343 |
| 0.3906 | 42.51 | 8800 | 0.5991 | 0.7352 | 0.7337 |
| 0.3922 | 43.48 | 9000 | 0.6040 | 0.7355 | 0.7340 |
| 0.3891 | 44.44 | 9200 | 0.5991 | 0.7325 | 0.7310 |
| 0.3901 | 45.41 | 9400 | 0.5960 | 0.7353 | 0.7337 |
| 0.3827 | 46.38 | 9600 | 0.6006 | 0.7344 | 0.7328 |
| 0.3903 | 47.34 | 9800 | 0.5957 | 0.7341 | 0.7325 |
| 0.3822 | 48.31 | 10000 | 0.5957 | 0.7360 | 0.7343 |
### Framework versions
- PEFT 0.9.0
- Transformers 4.38.2
- Pytorch 2.2.0+cu121
- Datasets 2.17.1
- Tokenizers 0.15.2 | {"library_name": "peft", "tags": ["generated_from_trainer"], "metrics": ["accuracy"], "base_model": "mahdibaghbanzadeh/seqsight_16384_512_22M", "model-index": [{"name": "GUE_EMP_H3K14ac-seqsight_16384_512_22M-L32_f", "results": []}]} | mahdibaghbanzadeh/GUE_EMP_H3K14ac-seqsight_16384_512_22M-L32_f | null | [
"peft",
"safetensors",
"generated_from_trainer",
"base_model:mahdibaghbanzadeh/seqsight_16384_512_22M",
"region:us"
] | null | 2024-04-27T07:15:04+00:00 | [] | [] | TAGS
#peft #safetensors #generated_from_trainer #base_model-mahdibaghbanzadeh/seqsight_16384_512_22M #region-us
| GUE\_EMP\_H3K14ac-seqsight\_16384\_512\_22M-L32\_f
==================================================
This model is a fine-tuned version of mahdibaghbanzadeh/seqsight\_16384\_512\_22M on the mahdibaghbanzadeh/GUE\_EMP\_H3K14ac dataset.
It achieves the following results on the evaluation set:
* Loss: 0.5218
* F1 Score: 0.7508
* Accuracy: 0.7495
Model description
-----------------
More information needed
Intended uses & limitations
---------------------------
More information needed
Training and evaluation data
----------------------------
More information needed
Training procedure
------------------
### Training hyperparameters
The following hyperparameters were used during training:
* learning\_rate: 0.0005
* train\_batch\_size: 128
* eval\_batch\_size: 128
* seed: 42
* optimizer: Adam with betas=(0.9,0.999) and epsilon=1e-08
* lr\_scheduler\_type: linear
* training\_steps: 10000
### Training results
### Framework versions
* PEFT 0.9.0
* Transformers 4.38.2
* Pytorch 2.2.0+cu121
* Datasets 2.17.1
* Tokenizers 0.15.2
| [
"### Training hyperparameters\n\n\nThe following hyperparameters were used during training:\n\n\n* learning\\_rate: 0.0005\n* train\\_batch\\_size: 128\n* eval\\_batch\\_size: 128\n* seed: 42\n* optimizer: Adam with betas=(0.9,0.999) and epsilon=1e-08\n* lr\\_scheduler\\_type: linear\n* training\\_steps: 10000",
"### Training results",
"### Framework versions\n\n\n* PEFT 0.9.0\n* Transformers 4.38.2\n* Pytorch 2.2.0+cu121\n* Datasets 2.17.1\n* Tokenizers 0.15.2"
] | [
"TAGS\n#peft #safetensors #generated_from_trainer #base_model-mahdibaghbanzadeh/seqsight_16384_512_22M #region-us \n",
"### Training hyperparameters\n\n\nThe following hyperparameters were used during training:\n\n\n* learning\\_rate: 0.0005\n* train\\_batch\\_size: 128\n* eval\\_batch\\_size: 128\n* seed: 42\n* optimizer: Adam with betas=(0.9,0.999) and epsilon=1e-08\n* lr\\_scheduler\\_type: linear\n* training\\_steps: 10000",
"### Training results",
"### Framework versions\n\n\n* PEFT 0.9.0\n* Transformers 4.38.2\n* Pytorch 2.2.0+cu121\n* Datasets 2.17.1\n* Tokenizers 0.15.2"
] |
null | peft |
<!-- This model card has been generated automatically according to the information the Trainer had access to. You
should probably proofread and complete it, then remove this comment. -->
# GUE_EMP_H3K4me2-seqsight_16384_512_22M-L1_f
This model is a fine-tuned version of [mahdibaghbanzadeh/seqsight_16384_512_22M](https://huggingface.co/mahdibaghbanzadeh/seqsight_16384_512_22M) on the [mahdibaghbanzadeh/GUE_EMP_H3K4me2](https://huggingface.co/datasets/mahdibaghbanzadeh/GUE_EMP_H3K4me2) dataset.
It achieves the following results on the evaluation set:
- Loss: 0.6102
- F1 Score: 0.6635
- Accuracy: 0.6641
## Model description
More information needed
## Intended uses & limitations
More information needed
## Training and evaluation data
More information needed
## Training procedure
### Training hyperparameters
The following hyperparameters were used during training:
- learning_rate: 0.0005
- train_batch_size: 128
- eval_batch_size: 128
- seed: 42
- optimizer: Adam with betas=(0.9,0.999) and epsilon=1e-08
- lr_scheduler_type: linear
- training_steps: 10000
### Training results
| Training Loss | Epoch | Step | Validation Loss | F1 Score | Accuracy |
|:-------------:|:-----:|:-----:|:---------------:|:--------:|:--------:|
| 0.6594 | 1.04 | 200 | 0.6350 | 0.6278 | 0.6452 |
| 0.6279 | 2.08 | 400 | 0.6509 | 0.6156 | 0.6142 |
| 0.6215 | 3.12 | 600 | 0.6197 | 0.6498 | 0.6569 |
| 0.6198 | 4.17 | 800 | 0.6238 | 0.6528 | 0.6530 |
| 0.6164 | 5.21 | 1000 | 0.6351 | 0.6439 | 0.6413 |
| 0.6143 | 6.25 | 1200 | 0.6251 | 0.6526 | 0.6514 |
| 0.6094 | 7.29 | 1400 | 0.6514 | 0.6389 | 0.6370 |
| 0.6118 | 8.33 | 1600 | 0.6291 | 0.6483 | 0.6461 |
| 0.6083 | 9.38 | 1800 | 0.6441 | 0.6394 | 0.6370 |
| 0.6091 | 10.42 | 2000 | 0.6271 | 0.6558 | 0.6540 |
| 0.6093 | 11.46 | 2200 | 0.6177 | 0.6637 | 0.6641 |
| 0.6023 | 12.5 | 2400 | 0.6247 | 0.6611 | 0.6598 |
| 0.6038 | 13.54 | 2600 | 0.6215 | 0.6641 | 0.6644 |
| 0.6036 | 14.58 | 2800 | 0.6186 | 0.6655 | 0.6660 |
| 0.6065 | 15.62 | 3000 | 0.6188 | 0.6639 | 0.6644 |
| 0.6012 | 16.67 | 3200 | 0.6293 | 0.6601 | 0.6582 |
| 0.6019 | 17.71 | 3400 | 0.6146 | 0.6648 | 0.6663 |
| 0.6001 | 18.75 | 3600 | 0.6185 | 0.6613 | 0.6608 |
| 0.6018 | 19.79 | 3800 | 0.6233 | 0.6602 | 0.6585 |
| 0.5952 | 20.83 | 4000 | 0.6271 | 0.6582 | 0.6559 |
| 0.6011 | 21.88 | 4200 | 0.6344 | 0.6531 | 0.6507 |
| 0.5985 | 22.92 | 4400 | 0.6307 | 0.6550 | 0.6527 |
| 0.5985 | 23.96 | 4600 | 0.6302 | 0.6541 | 0.6517 |
| 0.597 | 25.0 | 4800 | 0.6205 | 0.6621 | 0.6611 |
| 0.5955 | 26.04 | 5000 | 0.6208 | 0.6615 | 0.6601 |
| 0.5967 | 27.08 | 5200 | 0.6218 | 0.6590 | 0.6575 |
| 0.5962 | 28.12 | 5400 | 0.6185 | 0.6602 | 0.6595 |
| 0.5958 | 29.17 | 5600 | 0.6261 | 0.6559 | 0.6536 |
| 0.5917 | 30.21 | 5800 | 0.6295 | 0.6586 | 0.6566 |
| 0.5958 | 31.25 | 6000 | 0.6255 | 0.6601 | 0.6582 |
| 0.594 | 32.29 | 6200 | 0.6265 | 0.6553 | 0.6530 |
| 0.5939 | 33.33 | 6400 | 0.6272 | 0.6591 | 0.6569 |
| 0.5944 | 34.38 | 6600 | 0.6167 | 0.6595 | 0.6595 |
| 0.5914 | 35.42 | 6800 | 0.6168 | 0.6606 | 0.6605 |
| 0.5926 | 36.46 | 7000 | 0.6161 | 0.6625 | 0.6621 |
| 0.59 | 37.5 | 7200 | 0.6215 | 0.6569 | 0.6553 |
| 0.592 | 38.54 | 7400 | 0.6194 | 0.6636 | 0.6628 |
| 0.5945 | 39.58 | 7600 | 0.6206 | 0.6614 | 0.6601 |
| 0.5938 | 40.62 | 7800 | 0.6278 | 0.6516 | 0.6491 |
| 0.5903 | 41.67 | 8000 | 0.6237 | 0.6576 | 0.6556 |
| 0.5882 | 42.71 | 8200 | 0.6163 | 0.6654 | 0.6660 |
| 0.5929 | 43.75 | 8400 | 0.6207 | 0.6587 | 0.6572 |
| 0.59 | 44.79 | 8600 | 0.6260 | 0.6561 | 0.6540 |
| 0.589 | 45.83 | 8800 | 0.6206 | 0.6569 | 0.6556 |
| 0.592 | 46.88 | 9000 | 0.6254 | 0.6563 | 0.6543 |
| 0.5893 | 47.92 | 9200 | 0.6223 | 0.6559 | 0.6543 |
| 0.5906 | 48.96 | 9400 | 0.6215 | 0.6571 | 0.6556 |
| 0.5891 | 50.0 | 9600 | 0.6219 | 0.6568 | 0.6553 |
| 0.5898 | 51.04 | 9800 | 0.6223 | 0.6581 | 0.6566 |
| 0.5886 | 52.08 | 10000 | 0.6221 | 0.6581 | 0.6566 |
### Framework versions
- PEFT 0.9.0
- Transformers 4.38.2
- Pytorch 2.2.0+cu121
- Datasets 2.17.1
- Tokenizers 0.15.2 | {"library_name": "peft", "tags": ["generated_from_trainer"], "metrics": ["accuracy"], "base_model": "mahdibaghbanzadeh/seqsight_16384_512_22M", "model-index": [{"name": "GUE_EMP_H3K4me2-seqsight_16384_512_22M-L1_f", "results": []}]} | mahdibaghbanzadeh/GUE_EMP_H3K4me2-seqsight_16384_512_22M-L1_f | null | [
"peft",
"safetensors",
"generated_from_trainer",
"base_model:mahdibaghbanzadeh/seqsight_16384_512_22M",
"region:us"
] | null | 2024-04-27T07:17:21+00:00 | [] | [] | TAGS
#peft #safetensors #generated_from_trainer #base_model-mahdibaghbanzadeh/seqsight_16384_512_22M #region-us
| GUE\_EMP\_H3K4me2-seqsight\_16384\_512\_22M-L1\_f
=================================================
This model is a fine-tuned version of mahdibaghbanzadeh/seqsight\_16384\_512\_22M on the mahdibaghbanzadeh/GUE\_EMP\_H3K4me2 dataset.
It achieves the following results on the evaluation set:
* Loss: 0.6102
* F1 Score: 0.6635
* Accuracy: 0.6641
Model description
-----------------
More information needed
Intended uses & limitations
---------------------------
More information needed
Training and evaluation data
----------------------------
More information needed
Training procedure
------------------
### Training hyperparameters
The following hyperparameters were used during training:
* learning\_rate: 0.0005
* train\_batch\_size: 128
* eval\_batch\_size: 128
* seed: 42
* optimizer: Adam with betas=(0.9,0.999) and epsilon=1e-08
* lr\_scheduler\_type: linear
* training\_steps: 10000
### Training results
### Framework versions
* PEFT 0.9.0
* Transformers 4.38.2
* Pytorch 2.2.0+cu121
* Datasets 2.17.1
* Tokenizers 0.15.2
| [
"### Training hyperparameters\n\n\nThe following hyperparameters were used during training:\n\n\n* learning\\_rate: 0.0005\n* train\\_batch\\_size: 128\n* eval\\_batch\\_size: 128\n* seed: 42\n* optimizer: Adam with betas=(0.9,0.999) and epsilon=1e-08\n* lr\\_scheduler\\_type: linear\n* training\\_steps: 10000",
"### Training results",
"### Framework versions\n\n\n* PEFT 0.9.0\n* Transformers 4.38.2\n* Pytorch 2.2.0+cu121\n* Datasets 2.17.1\n* Tokenizers 0.15.2"
] | [
"TAGS\n#peft #safetensors #generated_from_trainer #base_model-mahdibaghbanzadeh/seqsight_16384_512_22M #region-us \n",
"### Training hyperparameters\n\n\nThe following hyperparameters were used during training:\n\n\n* learning\\_rate: 0.0005\n* train\\_batch\\_size: 128\n* eval\\_batch\\_size: 128\n* seed: 42\n* optimizer: Adam with betas=(0.9,0.999) and epsilon=1e-08\n* lr\\_scheduler\\_type: linear\n* training\\_steps: 10000",
"### Training results",
"### Framework versions\n\n\n* PEFT 0.9.0\n* Transformers 4.38.2\n* Pytorch 2.2.0+cu121\n* Datasets 2.17.1\n* Tokenizers 0.15.2"
] |
text-generation | transformers |
# Model Card for Model ID
```
``` | {"library_name": "transformers", "tags": []} | TwinDoc/agilesoda-model-x-init-mistral-stage-1 | null | [
"transformers",
"safetensors",
"mistral",
"text-generation",
"autotrain_compatible",
"endpoints_compatible",
"text-generation-inference",
"region:us"
] | null | 2024-04-27T07:18:26+00:00 | [] | [] | TAGS
#transformers #safetensors #mistral #text-generation #autotrain_compatible #endpoints_compatible #text-generation-inference #region-us
|
# Model Card for Model ID
| [
"# Model Card for Model ID"
] | [
"TAGS\n#transformers #safetensors #mistral #text-generation #autotrain_compatible #endpoints_compatible #text-generation-inference #region-us \n",
"# Model Card for Model ID"
] |
text-generation | transformers |
# Uploaded model
- **Developed by:** saksornr
- **License:** apache-2.0
- **Finetuned from model :** SeaLLMs/SeaLLM-7B-v2.5
Pre-quantized for faster loading. (~17 GB to 5 GB) | {"language": ["en", "th"], "license": "apache-2.0", "library_name": "transformers", "tags": ["text-generation-inference", "transformers", "unsloth", "gemma", "trl"], "base_model": "SeaLLMs/SeaLLM-7B-v2.5", "pipeline_tag": "text-generation"} | saksornr/SeaLLM-7B-v2.5-4bit | null | [
"transformers",
"safetensors",
"gemma",
"text-generation",
"text-generation-inference",
"unsloth",
"trl",
"conversational",
"en",
"th",
"base_model:SeaLLMs/SeaLLM-7B-v2.5",
"license:apache-2.0",
"autotrain_compatible",
"endpoints_compatible",
"4-bit",
"region:us"
] | null | 2024-04-27T07:22:36+00:00 | [] | [
"en",
"th"
] | TAGS
#transformers #safetensors #gemma #text-generation #text-generation-inference #unsloth #trl #conversational #en #th #base_model-SeaLLMs/SeaLLM-7B-v2.5 #license-apache-2.0 #autotrain_compatible #endpoints_compatible #4-bit #region-us
|
# Uploaded model
- Developed by: saksornr
- License: apache-2.0
- Finetuned from model : SeaLLMs/SeaLLM-7B-v2.5
Pre-quantized for faster loading. (~17 GB to 5 GB) | [
"# Uploaded model\n\n- Developed by: saksornr\n- License: apache-2.0\n- Finetuned from model : SeaLLMs/SeaLLM-7B-v2.5\n\nPre-quantized for faster loading. (~17 GB to 5 GB)"
] | [
"TAGS\n#transformers #safetensors #gemma #text-generation #text-generation-inference #unsloth #trl #conversational #en #th #base_model-SeaLLMs/SeaLLM-7B-v2.5 #license-apache-2.0 #autotrain_compatible #endpoints_compatible #4-bit #region-us \n",
"# Uploaded model\n\n- Developed by: saksornr\n- License: apache-2.0\n- Finetuned from model : SeaLLMs/SeaLLM-7B-v2.5\n\nPre-quantized for faster loading. (~17 GB to 5 GB)"
] |
text-generation | transformers |
# Llama3medical-15B-MoE
Llama3medical-15B-MoE is a Mixture of Experts (MoE) made with the following models using [LazyMergekit](https://colab.research.google.com/drive/1obulZ1ROXHjYLn6PPZJwRR6GzgQogxxb?usp=sharing):
* [johnsnowlabs/JSL-MedLlama-3-8B-v1.0](https://huggingface.co/johnsnowlabs/JSL-MedLlama-3-8B-v1.0)
* [Weyaxi/Einstein-v6.1-Llama3-8B](https://huggingface.co/Weyaxi/Einstein-v6.1-Llama3-8B)
## 🧩 Configuration
```yaml
base_model: johnsnowlabs/JSL-MedLlama-3-8B-v1.0
experts:
- source_model: johnsnowlabs/JSL-MedLlama-3-8B-v1.0
positive_prompts: ["medical"]
- source_model: Weyaxi/Einstein-v6.1-Llama3-8B
positive_prompts: ["what"]
```
## 💻 Usage
```python
!pip install -qU transformers bitsandbytes accelerate
from transformers import AutoTokenizer
import transformers
import torch
model = "allknowingroger/Llama3medical-15B-MoE"
tokenizer = AutoTokenizer.from_pretrained(model)
pipeline = transformers.pipeline(
"text-generation",
model=model,
model_kwargs={"torch_dtype": torch.float16, "load_in_4bit": True},
)
messages = [{"role": "user", "content": "Explain what a Mixture of Experts is in less than 100 words."}]
prompt = pipeline.tokenizer.apply_chat_template(messages, tokenize=False, add_generation_prompt=True)
outputs = pipeline(prompt, max_new_tokens=256, do_sample=True, temperature=0.7, top_k=50, top_p=0.95)
print(outputs[0]["generated_text"])
``` | {"license": "apache-2.0", "tags": ["moe", "frankenmoe", "merge", "mergekit", "lazymergekit", "johnsnowlabs/JSL-MedLlama-3-8B-v1.0", "Weyaxi/Einstein-v6.1-Llama3-8B"], "base_model": ["johnsnowlabs/JSL-MedLlama-3-8B-v1.0", "Weyaxi/Einstein-v6.1-Llama3-8B"]} | allknowingroger/Llama3medical-15B-MoE | null | [
"transformers",
"mixtral",
"text-generation",
"moe",
"frankenmoe",
"merge",
"mergekit",
"lazymergekit",
"johnsnowlabs/JSL-MedLlama-3-8B-v1.0",
"Weyaxi/Einstein-v6.1-Llama3-8B",
"base_model:johnsnowlabs/JSL-MedLlama-3-8B-v1.0",
"base_model:Weyaxi/Einstein-v6.1-Llama3-8B",
"license:apache-2.0",
"autotrain_compatible",
"endpoints_compatible",
"text-generation-inference",
"region:us"
] | null | 2024-04-27T07:22:38+00:00 | [] | [] | TAGS
#transformers #mixtral #text-generation #moe #frankenmoe #merge #mergekit #lazymergekit #johnsnowlabs/JSL-MedLlama-3-8B-v1.0 #Weyaxi/Einstein-v6.1-Llama3-8B #base_model-johnsnowlabs/JSL-MedLlama-3-8B-v1.0 #base_model-Weyaxi/Einstein-v6.1-Llama3-8B #license-apache-2.0 #autotrain_compatible #endpoints_compatible #text-generation-inference #region-us
|
# Llama3medical-15B-MoE
Llama3medical-15B-MoE is a Mixture of Experts (MoE) made with the following models using LazyMergekit:
* johnsnowlabs/JSL-MedLlama-3-8B-v1.0
* Weyaxi/Einstein-v6.1-Llama3-8B
## Configuration
## Usage
| [
"# Llama3medical-15B-MoE\n\nLlama3medical-15B-MoE is a Mixture of Experts (MoE) made with the following models using LazyMergekit:\n* johnsnowlabs/JSL-MedLlama-3-8B-v1.0\n* Weyaxi/Einstein-v6.1-Llama3-8B",
"## Configuration",
"## Usage"
] | [
"TAGS\n#transformers #mixtral #text-generation #moe #frankenmoe #merge #mergekit #lazymergekit #johnsnowlabs/JSL-MedLlama-3-8B-v1.0 #Weyaxi/Einstein-v6.1-Llama3-8B #base_model-johnsnowlabs/JSL-MedLlama-3-8B-v1.0 #base_model-Weyaxi/Einstein-v6.1-Llama3-8B #license-apache-2.0 #autotrain_compatible #endpoints_compatible #text-generation-inference #region-us \n",
"# Llama3medical-15B-MoE\n\nLlama3medical-15B-MoE is a Mixture of Experts (MoE) made with the following models using LazyMergekit:\n* johnsnowlabs/JSL-MedLlama-3-8B-v1.0\n* Weyaxi/Einstein-v6.1-Llama3-8B",
"## Configuration",
"## Usage"
] |
null | peft |
<!-- This model card has been generated automatically according to the information the Trainer had access to. You
should probably proofread and complete it, then remove this comment. -->
# GUE_EMP_H3K4me2-seqsight_16384_512_22M-L8_f
This model is a fine-tuned version of [mahdibaghbanzadeh/seqsight_16384_512_22M](https://huggingface.co/mahdibaghbanzadeh/seqsight_16384_512_22M) on the [mahdibaghbanzadeh/GUE_EMP_H3K4me2](https://huggingface.co/datasets/mahdibaghbanzadeh/GUE_EMP_H3K4me2) dataset.
It achieves the following results on the evaluation set:
- Loss: 0.6079
- F1 Score: 0.6631
- Accuracy: 0.6631
## Model description
More information needed
## Intended uses & limitations
More information needed
## Training and evaluation data
More information needed
## Training procedure
### Training hyperparameters
The following hyperparameters were used during training:
- learning_rate: 0.0005
- train_batch_size: 128
- eval_batch_size: 128
- seed: 42
- optimizer: Adam with betas=(0.9,0.999) and epsilon=1e-08
- lr_scheduler_type: linear
- training_steps: 10000
### Training results
| Training Loss | Epoch | Step | Validation Loss | F1 Score | Accuracy |
|:-------------:|:-----:|:-----:|:---------------:|:--------:|:--------:|
| 0.6504 | 1.04 | 200 | 0.6251 | 0.6392 | 0.6452 |
| 0.6193 | 2.08 | 400 | 0.6492 | 0.6275 | 0.6256 |
| 0.6147 | 3.12 | 600 | 0.6136 | 0.6607 | 0.6709 |
| 0.6127 | 4.17 | 800 | 0.6233 | 0.6539 | 0.6536 |
| 0.6084 | 5.21 | 1000 | 0.6170 | 0.6690 | 0.6696 |
| 0.6055 | 6.25 | 1200 | 0.6122 | 0.6630 | 0.6660 |
| 0.5984 | 7.29 | 1400 | 0.6404 | 0.6462 | 0.6435 |
| 0.6005 | 8.33 | 1600 | 0.6224 | 0.6536 | 0.6517 |
| 0.5953 | 9.38 | 1800 | 0.6408 | 0.6470 | 0.6445 |
| 0.5949 | 10.42 | 2000 | 0.6192 | 0.6581 | 0.6562 |
| 0.5943 | 11.46 | 2200 | 0.6103 | 0.6697 | 0.6709 |
| 0.5839 | 12.5 | 2400 | 0.6158 | 0.6658 | 0.6657 |
| 0.5855 | 13.54 | 2600 | 0.6161 | 0.6667 | 0.6667 |
| 0.5845 | 14.58 | 2800 | 0.6149 | 0.6640 | 0.6647 |
| 0.5872 | 15.62 | 3000 | 0.6226 | 0.6636 | 0.6624 |
| 0.5811 | 16.67 | 3200 | 0.6218 | 0.6596 | 0.6579 |
| 0.5794 | 17.71 | 3400 | 0.6157 | 0.6687 | 0.6683 |
| 0.5782 | 18.75 | 3600 | 0.6109 | 0.6704 | 0.6709 |
| 0.5772 | 19.79 | 3800 | 0.6215 | 0.6648 | 0.6634 |
| 0.5707 | 20.83 | 4000 | 0.6276 | 0.6608 | 0.6585 |
| 0.576 | 21.88 | 4200 | 0.6471 | 0.6439 | 0.6413 |
| 0.5721 | 22.92 | 4400 | 0.6304 | 0.6631 | 0.6611 |
| 0.5705 | 23.96 | 4600 | 0.6408 | 0.6462 | 0.6435 |
| 0.5694 | 25.0 | 4800 | 0.6209 | 0.6702 | 0.6693 |
| 0.5666 | 26.04 | 5000 | 0.6300 | 0.6637 | 0.6618 |
| 0.5686 | 27.08 | 5200 | 0.6261 | 0.6634 | 0.6615 |
| 0.5665 | 28.12 | 5400 | 0.6274 | 0.6609 | 0.6588 |
| 0.5654 | 29.17 | 5600 | 0.6276 | 0.6619 | 0.6598 |
| 0.56 | 30.21 | 5800 | 0.6458 | 0.6581 | 0.6556 |
| 0.5638 | 31.25 | 6000 | 0.6361 | 0.6602 | 0.6579 |
| 0.5616 | 32.29 | 6200 | 0.6206 | 0.6675 | 0.6663 |
| 0.5595 | 33.33 | 6400 | 0.6369 | 0.6625 | 0.6601 |
| 0.5578 | 34.38 | 6600 | 0.6370 | 0.6594 | 0.6572 |
| 0.5583 | 35.42 | 6800 | 0.6268 | 0.6680 | 0.6663 |
| 0.556 | 36.46 | 7000 | 0.6203 | 0.6685 | 0.6676 |
| 0.5537 | 37.5 | 7200 | 0.6337 | 0.6637 | 0.6615 |
| 0.5557 | 38.54 | 7400 | 0.6318 | 0.6680 | 0.6663 |
| 0.5575 | 39.58 | 7600 | 0.6238 | 0.6662 | 0.6654 |
| 0.5554 | 40.62 | 7800 | 0.6417 | 0.6533 | 0.6507 |
| 0.5541 | 41.67 | 8000 | 0.6312 | 0.6623 | 0.6601 |
| 0.5497 | 42.71 | 8200 | 0.6305 | 0.6684 | 0.6673 |
| 0.556 | 43.75 | 8400 | 0.6306 | 0.6677 | 0.6660 |
| 0.5524 | 44.79 | 8600 | 0.6379 | 0.6608 | 0.6585 |
| 0.5489 | 45.83 | 8800 | 0.6321 | 0.6662 | 0.6647 |
| 0.5515 | 46.88 | 9000 | 0.6433 | 0.6593 | 0.6569 |
| 0.5503 | 47.92 | 9200 | 0.6388 | 0.6618 | 0.6595 |
| 0.5517 | 48.96 | 9400 | 0.6354 | 0.6672 | 0.6654 |
| 0.551 | 50.0 | 9600 | 0.6368 | 0.6661 | 0.6641 |
| 0.5523 | 51.04 | 9800 | 0.6378 | 0.6634 | 0.6611 |
| 0.5479 | 52.08 | 10000 | 0.6371 | 0.6630 | 0.6608 |
### Framework versions
- PEFT 0.9.0
- Transformers 4.38.2
- Pytorch 2.2.0+cu121
- Datasets 2.17.1
- Tokenizers 0.15.2 | {"library_name": "peft", "tags": ["generated_from_trainer"], "metrics": ["accuracy"], "base_model": "mahdibaghbanzadeh/seqsight_16384_512_22M", "model-index": [{"name": "GUE_EMP_H3K4me2-seqsight_16384_512_22M-L8_f", "results": []}]} | mahdibaghbanzadeh/GUE_EMP_H3K4me2-seqsight_16384_512_22M-L8_f | null | [
"peft",
"safetensors",
"generated_from_trainer",
"base_model:mahdibaghbanzadeh/seqsight_16384_512_22M",
"region:us"
] | null | 2024-04-27T07:22:41+00:00 | [] | [] | TAGS
#peft #safetensors #generated_from_trainer #base_model-mahdibaghbanzadeh/seqsight_16384_512_22M #region-us
| GUE\_EMP\_H3K4me2-seqsight\_16384\_512\_22M-L8\_f
=================================================
This model is a fine-tuned version of mahdibaghbanzadeh/seqsight\_16384\_512\_22M on the mahdibaghbanzadeh/GUE\_EMP\_H3K4me2 dataset.
It achieves the following results on the evaluation set:
* Loss: 0.6079
* F1 Score: 0.6631
* Accuracy: 0.6631
Model description
-----------------
More information needed
Intended uses & limitations
---------------------------
More information needed
Training and evaluation data
----------------------------
More information needed
Training procedure
------------------
### Training hyperparameters
The following hyperparameters were used during training:
* learning\_rate: 0.0005
* train\_batch\_size: 128
* eval\_batch\_size: 128
* seed: 42
* optimizer: Adam with betas=(0.9,0.999) and epsilon=1e-08
* lr\_scheduler\_type: linear
* training\_steps: 10000
### Training results
### Framework versions
* PEFT 0.9.0
* Transformers 4.38.2
* Pytorch 2.2.0+cu121
* Datasets 2.17.1
* Tokenizers 0.15.2
| [
"### Training hyperparameters\n\n\nThe following hyperparameters were used during training:\n\n\n* learning\\_rate: 0.0005\n* train\\_batch\\_size: 128\n* eval\\_batch\\_size: 128\n* seed: 42\n* optimizer: Adam with betas=(0.9,0.999) and epsilon=1e-08\n* lr\\_scheduler\\_type: linear\n* training\\_steps: 10000",
"### Training results",
"### Framework versions\n\n\n* PEFT 0.9.0\n* Transformers 4.38.2\n* Pytorch 2.2.0+cu121\n* Datasets 2.17.1\n* Tokenizers 0.15.2"
] | [
"TAGS\n#peft #safetensors #generated_from_trainer #base_model-mahdibaghbanzadeh/seqsight_16384_512_22M #region-us \n",
"### Training hyperparameters\n\n\nThe following hyperparameters were used during training:\n\n\n* learning\\_rate: 0.0005\n* train\\_batch\\_size: 128\n* eval\\_batch\\_size: 128\n* seed: 42\n* optimizer: Adam with betas=(0.9,0.999) and epsilon=1e-08\n* lr\\_scheduler\\_type: linear\n* training\\_steps: 10000",
"### Training results",
"### Framework versions\n\n\n* PEFT 0.9.0\n* Transformers 4.38.2\n* Pytorch 2.2.0+cu121\n* Datasets 2.17.1\n* Tokenizers 0.15.2"
] |
text-generation | transformers |
# Model Card for Model ID
<!-- Provide a quick summary of what the model is/does. -->
## Model Details
### Model Description
<!-- Provide a longer summary of what this model is. -->
This is the model card of a 🤗 transformers model that has been pushed on the Hub. This model card has been automatically generated.
- **Developed by:** [More Information Needed]
- **Funded by [optional]:** [More Information Needed]
- **Shared by [optional]:** [More Information Needed]
- **Model type:** [More Information Needed]
- **Language(s) (NLP):** [More Information Needed]
- **License:** [More Information Needed]
- **Finetuned from model [optional]:** [More Information Needed]
### Model Sources [optional]
<!-- Provide the basic links for the model. -->
- **Repository:** [More Information Needed]
- **Paper [optional]:** [More Information Needed]
- **Demo [optional]:** [More Information Needed]
## Uses
<!-- Address questions around how the model is intended to be used, including the foreseeable users of the model and those affected by the model. -->
### Direct Use
<!-- This section is for the model use without fine-tuning or plugging into a larger ecosystem/app. -->
[More Information Needed]
### Downstream Use [optional]
<!-- This section is for the model use when fine-tuned for a task, or when plugged into a larger ecosystem/app -->
[More Information Needed]
### Out-of-Scope Use
<!-- This section addresses misuse, malicious use, and uses that the model will not work well for. -->
[More Information Needed]
## Bias, Risks, and Limitations
<!-- This section is meant to convey both technical and sociotechnical limitations. -->
[More Information Needed]
### Recommendations
<!-- This section is meant to convey recommendations with respect to the bias, risk, and technical limitations. -->
Users (both direct and downstream) should be made aware of the risks, biases and limitations of the model. More information needed for further recommendations.
## How to Get Started with the Model
Use the code below to get started with the model.
[More Information Needed]
## Training Details
### Training Data
<!-- This should link to a Dataset Card, perhaps with a short stub of information on what the training data is all about as well as documentation related to data pre-processing or additional filtering. -->
[More Information Needed]
### Training Procedure
<!-- This relates heavily to the Technical Specifications. Content here should link to that section when it is relevant to the training procedure. -->
#### Preprocessing [optional]
[More Information Needed]
#### Training Hyperparameters
- **Training regime:** [More Information Needed] <!--fp32, fp16 mixed precision, bf16 mixed precision, bf16 non-mixed precision, fp16 non-mixed precision, fp8 mixed precision -->
#### Speeds, Sizes, Times [optional]
<!-- This section provides information about throughput, start/end time, checkpoint size if relevant, etc. -->
[More Information Needed]
## Evaluation
<!-- This section describes the evaluation protocols and provides the results. -->
### Testing Data, Factors & Metrics
#### Testing Data
<!-- This should link to a Dataset Card if possible. -->
[More Information Needed]
#### Factors
<!-- These are the things the evaluation is disaggregating by, e.g., subpopulations or domains. -->
[More Information Needed]
#### Metrics
<!-- These are the evaluation metrics being used, ideally with a description of why. -->
[More Information Needed]
### Results
[More Information Needed]
#### Summary
## Model Examination [optional]
<!-- Relevant interpretability work for the model goes here -->
[More Information Needed]
## Environmental Impact
<!-- Total emissions (in grams of CO2eq) and additional considerations, such as electricity usage, go here. Edit the suggested text below accordingly -->
Carbon emissions can be estimated using the [Machine Learning Impact calculator](https://mlco2.github.io/impact#compute) presented in [Lacoste et al. (2019)](https://arxiv.org/abs/1910.09700).
- **Hardware Type:** [More Information Needed]
- **Hours used:** [More Information Needed]
- **Cloud Provider:** [More Information Needed]
- **Compute Region:** [More Information Needed]
- **Carbon Emitted:** [More Information Needed]
## Technical Specifications [optional]
### Model Architecture and Objective
[More Information Needed]
### Compute Infrastructure
[More Information Needed]
#### Hardware
[More Information Needed]
#### Software
[More Information Needed]
## Citation [optional]
<!-- If there is a paper or blog post introducing the model, the APA and Bibtex information for that should go in this section. -->
**BibTeX:**
[More Information Needed]
**APA:**
[More Information Needed]
## Glossary [optional]
<!-- If relevant, include terms and calculations in this section that can help readers understand the model or model card. -->
[More Information Needed]
## More Information [optional]
[More Information Needed]
## Model Card Authors [optional]
[More Information Needed]
## Model Card Contact
[More Information Needed] | {"library_name": "transformers", "tags": []} | shallow6414/1tw8gta | null | [
"transformers",
"safetensors",
"llama",
"text-generation",
"conversational",
"arxiv:1910.09700",
"autotrain_compatible",
"endpoints_compatible",
"text-generation-inference",
"region:us"
] | null | 2024-04-27T07:23:27+00:00 | [
"1910.09700"
] | [] | TAGS
#transformers #safetensors #llama #text-generation #conversational #arxiv-1910.09700 #autotrain_compatible #endpoints_compatible #text-generation-inference #region-us
|
# Model Card for Model ID
## Model Details
### Model Description
This is the model card of a transformers model that has been pushed on the Hub. This model card has been automatically generated.
- Developed by:
- Funded by [optional]:
- Shared by [optional]:
- Model type:
- Language(s) (NLP):
- License:
- Finetuned from model [optional]:
### Model Sources [optional]
- Repository:
- Paper [optional]:
- Demo [optional]:
## Uses
### Direct Use
### Downstream Use [optional]
### Out-of-Scope Use
## Bias, Risks, and Limitations
### Recommendations
Users (both direct and downstream) should be made aware of the risks, biases and limitations of the model. More information needed for further recommendations.
## How to Get Started with the Model
Use the code below to get started with the model.
## Training Details
### Training Data
### Training Procedure
#### Preprocessing [optional]
#### Training Hyperparameters
- Training regime:
#### Speeds, Sizes, Times [optional]
## Evaluation
### Testing Data, Factors & Metrics
#### Testing Data
#### Factors
#### Metrics
### Results
#### Summary
## Model Examination [optional]
## Environmental Impact
Carbon emissions can be estimated using the Machine Learning Impact calculator presented in Lacoste et al. (2019).
- Hardware Type:
- Hours used:
- Cloud Provider:
- Compute Region:
- Carbon Emitted:
## Technical Specifications [optional]
### Model Architecture and Objective
### Compute Infrastructure
#### Hardware
#### Software
[optional]
BibTeX:
APA:
## Glossary [optional]
## More Information [optional]
## Model Card Authors [optional]
## Model Card Contact
| [
"# Model Card for Model ID",
"## Model Details",
"### Model Description\n\n\n\nThis is the model card of a transformers model that has been pushed on the Hub. This model card has been automatically generated.\n\n- Developed by: \n- Funded by [optional]: \n- Shared by [optional]: \n- Model type: \n- Language(s) (NLP): \n- License: \n- Finetuned from model [optional]:",
"### Model Sources [optional]\n\n\n\n- Repository: \n- Paper [optional]: \n- Demo [optional]:",
"## Uses",
"### Direct Use",
"### Downstream Use [optional]",
"### Out-of-Scope Use",
"## Bias, Risks, and Limitations",
"### Recommendations\n\n\n\nUsers (both direct and downstream) should be made aware of the risks, biases and limitations of the model. More information needed for further recommendations.",
"## How to Get Started with the Model\n\nUse the code below to get started with the model.",
"## Training Details",
"### Training Data",
"### Training Procedure",
"#### Preprocessing [optional]",
"#### Training Hyperparameters\n\n- Training regime:",
"#### Speeds, Sizes, Times [optional]",
"## Evaluation",
"### Testing Data, Factors & Metrics",
"#### Testing Data",
"#### Factors",
"#### Metrics",
"### Results",
"#### Summary",
"## Model Examination [optional]",
"## Environmental Impact\n\n\n\nCarbon emissions can be estimated using the Machine Learning Impact calculator presented in Lacoste et al. (2019).\n\n- Hardware Type: \n- Hours used: \n- Cloud Provider: \n- Compute Region: \n- Carbon Emitted:",
"## Technical Specifications [optional]",
"### Model Architecture and Objective",
"### Compute Infrastructure",
"#### Hardware",
"#### Software\n\n\n\n[optional]\n\n\n\nBibTeX:\n\n\n\nAPA:",
"## Glossary [optional]",
"## More Information [optional]",
"## Model Card Authors [optional]",
"## Model Card Contact"
] | [
"TAGS\n#transformers #safetensors #llama #text-generation #conversational #arxiv-1910.09700 #autotrain_compatible #endpoints_compatible #text-generation-inference #region-us \n",
"# Model Card for Model ID",
"## Model Details",
"### Model Description\n\n\n\nThis is the model card of a transformers model that has been pushed on the Hub. This model card has been automatically generated.\n\n- Developed by: \n- Funded by [optional]: \n- Shared by [optional]: \n- Model type: \n- Language(s) (NLP): \n- License: \n- Finetuned from model [optional]:",
"### Model Sources [optional]\n\n\n\n- Repository: \n- Paper [optional]: \n- Demo [optional]:",
"## Uses",
"### Direct Use",
"### Downstream Use [optional]",
"### Out-of-Scope Use",
"## Bias, Risks, and Limitations",
"### Recommendations\n\n\n\nUsers (both direct and downstream) should be made aware of the risks, biases and limitations of the model. More information needed for further recommendations.",
"## How to Get Started with the Model\n\nUse the code below to get started with the model.",
"## Training Details",
"### Training Data",
"### Training Procedure",
"#### Preprocessing [optional]",
"#### Training Hyperparameters\n\n- Training regime:",
"#### Speeds, Sizes, Times [optional]",
"## Evaluation",
"### Testing Data, Factors & Metrics",
"#### Testing Data",
"#### Factors",
"#### Metrics",
"### Results",
"#### Summary",
"## Model Examination [optional]",
"## Environmental Impact\n\n\n\nCarbon emissions can be estimated using the Machine Learning Impact calculator presented in Lacoste et al. (2019).\n\n- Hardware Type: \n- Hours used: \n- Cloud Provider: \n- Compute Region: \n- Carbon Emitted:",
"## Technical Specifications [optional]",
"### Model Architecture and Objective",
"### Compute Infrastructure",
"#### Hardware",
"#### Software\n\n\n\n[optional]\n\n\n\nBibTeX:\n\n\n\nAPA:",
"## Glossary [optional]",
"## More Information [optional]",
"## Model Card Authors [optional]",
"## Model Card Contact"
] |
text-generation | transformers |
# Phi-3-mini-128k-instruct-int4
- Orginal model : [microsoft/Phi-3-mini-128k-instruct](https://huggingface.co/microsoft/Phi-3-mini-128k-instruct)
- Quantized using [intel/auto-round](https://github.com/intel/auto-round)
## Description
**Phi-3-mini-128k-instruct-int4** is an int4 model with group_size 128 of the [microsoft/Phi-3-mini-128k-instruct](https://huggingface.co/microsoft/Phi-3-mini-128k-instruct).
The above model was quantized using AutoRound(Advanced Weight-Only Quantization Algorithm for LLMs) released by [intel](https://github.com/intel).
you can find out more in detail through the the [GitHub Repository](https://github.com/intel/auto-round).
## Training details
### Cloning a repository(AutoRound)
```
git clone https://github.com/intel/auto-round
```
### Enter into the examples/language-modeling folder
```
cd auto-round/examples/language-modeling
pip install -r requirements.txt
```
### Install FlashAttention-2
```
pip install flash_attn==2.5.8
```
Here's an simplified code for quantization. In order to save memory in quantization, we set the batch size to 1.
```
python main.py \
--model_name "microsoft/Phi-3-mini-128k-instruct" \
--bits 4 \
--group_size 128 \
--train_bs 1 \
--gradient_accumulate_steps 8 \
--deployment_device 'gpu' \
--output_dir "./save_ckpt"
```
## Model inference
### Install the necessary packages
```
pip install auto_gptq
pip install optimum
pip install -U accelerate bitsandbytes datasets peft transformers
```
### Example codes
```
import torch
from transformers import AutoModelForCausalLM, AutoTokenizer, pipeline
torch.random.manual_seed(0)
model = AutoModelForCausalLM.from_pretrained(
"ssuncheol/Phi-3-mini-128k-instruct-int4",
device_map="cuda",
torch_dtype="auto",
trust_remote_code=True,
)
tokenizer = AutoTokenizer.from_pretrained("ssuncheol/Phi-3-mini-128k-instruct-int4")
messages = [
{"role": "system", "content": "You are a helpful digital assistant. Please provide safe, ethical and accurate information to the user."},
{"role": "user", "content": "Can you provide ways to eat combinations of bananas and dragonfruits?"},
{"role": "assistant", "content": "Sure! Here are some ways to eat bananas and dragonfruits together: 1. Banana and dragonfruit smoothie: Blend bananas and dragonfruits together with some milk and honey. 2. Banana and dragonfruit salad: Mix sliced bananas and dragonfruits together with some lemon juice and honey."},
{"role": "user", "content": "What about solving an 2x + 3 = 7 equation?"},
]
pipe = pipeline(
"text-generation",
model=model,
tokenizer=tokenizer,
)
generation_args = {
"max_new_tokens": 500,
"return_full_text": False,
"temperature": 0.0,
"do_sample": False,
}
output = pipe(messages, **generation_args)
print(output[0]['generated_text'])
```
## License
The model is licensed under the MIT license.
| {"language": ["en"], "license": "mit", "tags": ["text-generation-inference"], "pipeline_tag": "text-generation"} | ssuncheol/Phi-3-mini-128k-instruct-int4 | null | [
"transformers",
"safetensors",
"phi3",
"text-generation",
"text-generation-inference",
"conversational",
"custom_code",
"en",
"license:mit",
"autotrain_compatible",
"endpoints_compatible",
"4-bit",
"region:us"
] | null | 2024-04-27T07:25:03+00:00 | [] | [
"en"
] | TAGS
#transformers #safetensors #phi3 #text-generation #text-generation-inference #conversational #custom_code #en #license-mit #autotrain_compatible #endpoints_compatible #4-bit #region-us
|
# Phi-3-mini-128k-instruct-int4
- Orginal model : microsoft/Phi-3-mini-128k-instruct
- Quantized using intel/auto-round
## Description
Phi-3-mini-128k-instruct-int4 is an int4 model with group_size 128 of the microsoft/Phi-3-mini-128k-instruct.
The above model was quantized using AutoRound(Advanced Weight-Only Quantization Algorithm for LLMs) released by intel.
you can find out more in detail through the the GitHub Repository.
## Training details
### Cloning a repository(AutoRound)
### Enter into the examples/language-modeling folder
### Install FlashAttention-2
Here's an simplified code for quantization. In order to save memory in quantization, we set the batch size to 1.
## Model inference
### Install the necessary packages
### Example codes
## License
The model is licensed under the MIT license.
| [
"# Phi-3-mini-128k-instruct-int4\n\n- Orginal model : microsoft/Phi-3-mini-128k-instruct\n- Quantized using intel/auto-round",
"## Description \n\nPhi-3-mini-128k-instruct-int4 is an int4 model with group_size 128 of the microsoft/Phi-3-mini-128k-instruct.\n\nThe above model was quantized using AutoRound(Advanced Weight-Only Quantization Algorithm for LLMs) released by intel.\n\nyou can find out more in detail through the the GitHub Repository.",
"## Training details",
"### Cloning a repository(AutoRound)",
"### Enter into the examples/language-modeling folder",
"### Install FlashAttention-2 \n\n\n\n\nHere's an simplified code for quantization. In order to save memory in quantization, we set the batch size to 1.",
"## Model inference",
"### Install the necessary packages",
"### Example codes",
"## License\nThe model is licensed under the MIT license."
] | [
"TAGS\n#transformers #safetensors #phi3 #text-generation #text-generation-inference #conversational #custom_code #en #license-mit #autotrain_compatible #endpoints_compatible #4-bit #region-us \n",
"# Phi-3-mini-128k-instruct-int4\n\n- Orginal model : microsoft/Phi-3-mini-128k-instruct\n- Quantized using intel/auto-round",
"## Description \n\nPhi-3-mini-128k-instruct-int4 is an int4 model with group_size 128 of the microsoft/Phi-3-mini-128k-instruct.\n\nThe above model was quantized using AutoRound(Advanced Weight-Only Quantization Algorithm for LLMs) released by intel.\n\nyou can find out more in detail through the the GitHub Repository.",
"## Training details",
"### Cloning a repository(AutoRound)",
"### Enter into the examples/language-modeling folder",
"### Install FlashAttention-2 \n\n\n\n\nHere's an simplified code for quantization. In order to save memory in quantization, we set the batch size to 1.",
"## Model inference",
"### Install the necessary packages",
"### Example codes",
"## License\nThe model is licensed under the MIT license."
] |
text-generation | transformers |
# Model Card for Model ID
<!-- Provide a quick summary of what the model is/does. -->
## Model Details
### Model Description
<!-- Provide a longer summary of what this model is. -->
This is the model card of a 🤗 transformers model that has been pushed on the Hub. This model card has been automatically generated.
- **Developed by:** [More Information Needed]
- **Funded by [optional]:** [More Information Needed]
- **Shared by [optional]:** [More Information Needed]
- **Model type:** [More Information Needed]
- **Language(s) (NLP):** [More Information Needed]
- **License:** [More Information Needed]
- **Finetuned from model [optional]:** [More Information Needed]
### Model Sources [optional]
<!-- Provide the basic links for the model. -->
- **Repository:** [More Information Needed]
- **Paper [optional]:** [More Information Needed]
- **Demo [optional]:** [More Information Needed]
## Uses
<!-- Address questions around how the model is intended to be used, including the foreseeable users of the model and those affected by the model. -->
### Direct Use
<!-- This section is for the model use without fine-tuning or plugging into a larger ecosystem/app. -->
[More Information Needed]
### Downstream Use [optional]
<!-- This section is for the model use when fine-tuned for a task, or when plugged into a larger ecosystem/app -->
[More Information Needed]
### Out-of-Scope Use
<!-- This section addresses misuse, malicious use, and uses that the model will not work well for. -->
[More Information Needed]
## Bias, Risks, and Limitations
<!-- This section is meant to convey both technical and sociotechnical limitations. -->
[More Information Needed]
### Recommendations
<!-- This section is meant to convey recommendations with respect to the bias, risk, and technical limitations. -->
Users (both direct and downstream) should be made aware of the risks, biases and limitations of the model. More information needed for further recommendations.
## How to Get Started with the Model
Use the code below to get started with the model.
[More Information Needed]
## Training Details
### Training Data
<!-- This should link to a Dataset Card, perhaps with a short stub of information on what the training data is all about as well as documentation related to data pre-processing or additional filtering. -->
[More Information Needed]
### Training Procedure
<!-- This relates heavily to the Technical Specifications. Content here should link to that section when it is relevant to the training procedure. -->
#### Preprocessing [optional]
[More Information Needed]
#### Training Hyperparameters
- **Training regime:** [More Information Needed] <!--fp32, fp16 mixed precision, bf16 mixed precision, bf16 non-mixed precision, fp16 non-mixed precision, fp8 mixed precision -->
#### Speeds, Sizes, Times [optional]
<!-- This section provides information about throughput, start/end time, checkpoint size if relevant, etc. -->
[More Information Needed]
## Evaluation
<!-- This section describes the evaluation protocols and provides the results. -->
### Testing Data, Factors & Metrics
#### Testing Data
<!-- This should link to a Dataset Card if possible. -->
[More Information Needed]
#### Factors
<!-- These are the things the evaluation is disaggregating by, e.g., subpopulations or domains. -->
[More Information Needed]
#### Metrics
<!-- These are the evaluation metrics being used, ideally with a description of why. -->
[More Information Needed]
### Results
[More Information Needed]
#### Summary
## Model Examination [optional]
<!-- Relevant interpretability work for the model goes here -->
[More Information Needed]
## Environmental Impact
<!-- Total emissions (in grams of CO2eq) and additional considerations, such as electricity usage, go here. Edit the suggested text below accordingly -->
Carbon emissions can be estimated using the [Machine Learning Impact calculator](https://mlco2.github.io/impact#compute) presented in [Lacoste et al. (2019)](https://arxiv.org/abs/1910.09700).
- **Hardware Type:** [More Information Needed]
- **Hours used:** [More Information Needed]
- **Cloud Provider:** [More Information Needed]
- **Compute Region:** [More Information Needed]
- **Carbon Emitted:** [More Information Needed]
## Technical Specifications [optional]
### Model Architecture and Objective
[More Information Needed]
### Compute Infrastructure
[More Information Needed]
#### Hardware
[More Information Needed]
#### Software
[More Information Needed]
## Citation [optional]
<!-- If there is a paper or blog post introducing the model, the APA and Bibtex information for that should go in this section. -->
**BibTeX:**
[More Information Needed]
**APA:**
[More Information Needed]
## Glossary [optional]
<!-- If relevant, include terms and calculations in this section that can help readers understand the model or model card. -->
[More Information Needed]
## More Information [optional]
[More Information Needed]
## Model Card Authors [optional]
[More Information Needed]
## Model Card Contact
[More Information Needed]
| {"library_name": "transformers", "tags": []} | swj0419/hp_retrain_STEP0000030 | null | [
"transformers",
"safetensors",
"llama",
"text-generation",
"arxiv:1910.09700",
"autotrain_compatible",
"endpoints_compatible",
"text-generation-inference",
"region:us"
] | null | 2024-04-27T07:27:52+00:00 | [
"1910.09700"
] | [] | TAGS
#transformers #safetensors #llama #text-generation #arxiv-1910.09700 #autotrain_compatible #endpoints_compatible #text-generation-inference #region-us
|
# Model Card for Model ID
## Model Details
### Model Description
This is the model card of a transformers model that has been pushed on the Hub. This model card has been automatically generated.
- Developed by:
- Funded by [optional]:
- Shared by [optional]:
- Model type:
- Language(s) (NLP):
- License:
- Finetuned from model [optional]:
### Model Sources [optional]
- Repository:
- Paper [optional]:
- Demo [optional]:
## Uses
### Direct Use
### Downstream Use [optional]
### Out-of-Scope Use
## Bias, Risks, and Limitations
### Recommendations
Users (both direct and downstream) should be made aware of the risks, biases and limitations of the model. More information needed for further recommendations.
## How to Get Started with the Model
Use the code below to get started with the model.
## Training Details
### Training Data
### Training Procedure
#### Preprocessing [optional]
#### Training Hyperparameters
- Training regime:
#### Speeds, Sizes, Times [optional]
## Evaluation
### Testing Data, Factors & Metrics
#### Testing Data
#### Factors
#### Metrics
### Results
#### Summary
## Model Examination [optional]
## Environmental Impact
Carbon emissions can be estimated using the Machine Learning Impact calculator presented in Lacoste et al. (2019).
- Hardware Type:
- Hours used:
- Cloud Provider:
- Compute Region:
- Carbon Emitted:
## Technical Specifications [optional]
### Model Architecture and Objective
### Compute Infrastructure
#### Hardware
#### Software
[optional]
BibTeX:
APA:
## Glossary [optional]
## More Information [optional]
## Model Card Authors [optional]
## Model Card Contact
| [
"# Model Card for Model ID",
"## Model Details",
"### Model Description\n\n\n\nThis is the model card of a transformers model that has been pushed on the Hub. This model card has been automatically generated.\n\n- Developed by: \n- Funded by [optional]: \n- Shared by [optional]: \n- Model type: \n- Language(s) (NLP): \n- License: \n- Finetuned from model [optional]:",
"### Model Sources [optional]\n\n\n\n- Repository: \n- Paper [optional]: \n- Demo [optional]:",
"## Uses",
"### Direct Use",
"### Downstream Use [optional]",
"### Out-of-Scope Use",
"## Bias, Risks, and Limitations",
"### Recommendations\n\n\n\nUsers (both direct and downstream) should be made aware of the risks, biases and limitations of the model. More information needed for further recommendations.",
"## How to Get Started with the Model\n\nUse the code below to get started with the model.",
"## Training Details",
"### Training Data",
"### Training Procedure",
"#### Preprocessing [optional]",
"#### Training Hyperparameters\n\n- Training regime:",
"#### Speeds, Sizes, Times [optional]",
"## Evaluation",
"### Testing Data, Factors & Metrics",
"#### Testing Data",
"#### Factors",
"#### Metrics",
"### Results",
"#### Summary",
"## Model Examination [optional]",
"## Environmental Impact\n\n\n\nCarbon emissions can be estimated using the Machine Learning Impact calculator presented in Lacoste et al. (2019).\n\n- Hardware Type: \n- Hours used: \n- Cloud Provider: \n- Compute Region: \n- Carbon Emitted:",
"## Technical Specifications [optional]",
"### Model Architecture and Objective",
"### Compute Infrastructure",
"#### Hardware",
"#### Software\n\n\n\n[optional]\n\n\n\nBibTeX:\n\n\n\nAPA:",
"## Glossary [optional]",
"## More Information [optional]",
"## Model Card Authors [optional]",
"## Model Card Contact"
] | [
"TAGS\n#transformers #safetensors #llama #text-generation #arxiv-1910.09700 #autotrain_compatible #endpoints_compatible #text-generation-inference #region-us \n",
"# Model Card for Model ID",
"## Model Details",
"### Model Description\n\n\n\nThis is the model card of a transformers model that has been pushed on the Hub. This model card has been automatically generated.\n\n- Developed by: \n- Funded by [optional]: \n- Shared by [optional]: \n- Model type: \n- Language(s) (NLP): \n- License: \n- Finetuned from model [optional]:",
"### Model Sources [optional]\n\n\n\n- Repository: \n- Paper [optional]: \n- Demo [optional]:",
"## Uses",
"### Direct Use",
"### Downstream Use [optional]",
"### Out-of-Scope Use",
"## Bias, Risks, and Limitations",
"### Recommendations\n\n\n\nUsers (both direct and downstream) should be made aware of the risks, biases and limitations of the model. More information needed for further recommendations.",
"## How to Get Started with the Model\n\nUse the code below to get started with the model.",
"## Training Details",
"### Training Data",
"### Training Procedure",
"#### Preprocessing [optional]",
"#### Training Hyperparameters\n\n- Training regime:",
"#### Speeds, Sizes, Times [optional]",
"## Evaluation",
"### Testing Data, Factors & Metrics",
"#### Testing Data",
"#### Factors",
"#### Metrics",
"### Results",
"#### Summary",
"## Model Examination [optional]",
"## Environmental Impact\n\n\n\nCarbon emissions can be estimated using the Machine Learning Impact calculator presented in Lacoste et al. (2019).\n\n- Hardware Type: \n- Hours used: \n- Cloud Provider: \n- Compute Region: \n- Carbon Emitted:",
"## Technical Specifications [optional]",
"### Model Architecture and Objective",
"### Compute Infrastructure",
"#### Hardware",
"#### Software\n\n\n\n[optional]\n\n\n\nBibTeX:\n\n\n\nAPA:",
"## Glossary [optional]",
"## More Information [optional]",
"## Model Card Authors [optional]",
"## Model Card Contact"
] |
audio-to-audio | null | 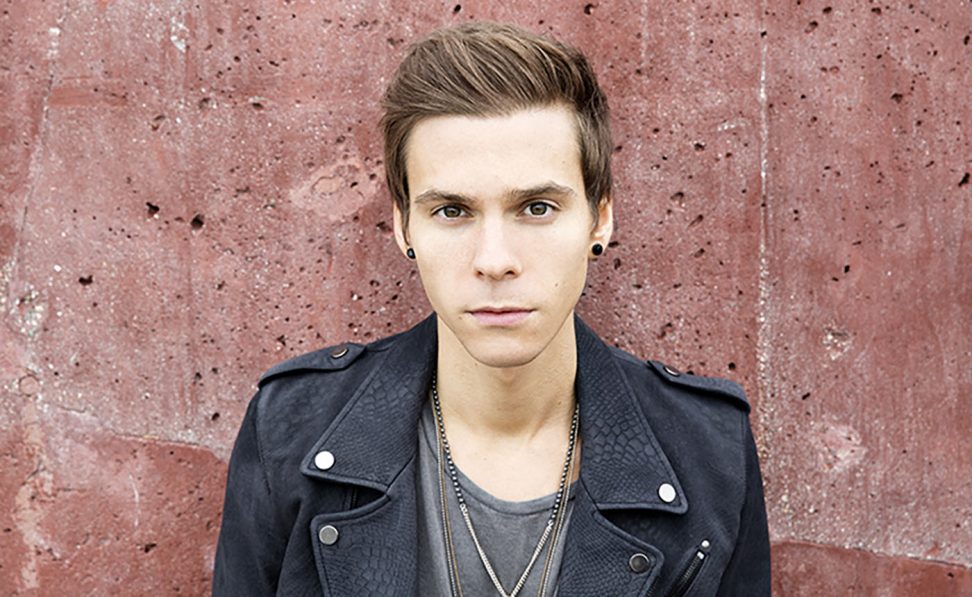
# Matthew Koma ( Matthew Bair ) V1 [EN] (2012)
# 314 Epochs - RVC V2 - rmvpe - Titan Medium
Trained on 2 minutes 59 seconds of isolated acapella from Spectrum Acoustic Version using UVR (Voc FT + Reverb HQ)
and Audacity to remove parts with double vocals and vocals from others (+Noise Gate) | {"language": ["en"], "license": "openrail", "tags": ["music", "rvc", "matthew", "koma", "bair", "model"], "pipeline_tag": "audio-to-audio"} | JapGuy/Matthew_Koma_v1 | null | [
"music",
"rvc",
"matthew",
"koma",
"bair",
"model",
"audio-to-audio",
"en",
"license:openrail",
"region:us"
] | null | 2024-04-27T07:28:05+00:00 | [] | [
"en"
] | TAGS
#music #rvc #matthew #koma #bair #model #audio-to-audio #en #license-openrail #region-us
| !URL
# Matthew Koma ( Matthew Bair ) V1 [EN] (2012)
# 314 Epochs - RVC V2 - rmvpe - Titan Medium
Trained on 2 minutes 59 seconds of isolated acapella from Spectrum Acoustic Version using UVR (Voc FT + Reverb HQ)
and Audacity to remove parts with double vocals and vocals from others (+Noise Gate) | [
"# Matthew Koma ( Matthew Bair ) V1 [EN] (2012)",
"# 314 Epochs - RVC V2 - rmvpe - Titan Medium\nTrained on 2 minutes 59 seconds of isolated acapella from Spectrum Acoustic Version using UVR (Voc FT + Reverb HQ) \nand Audacity to remove parts with double vocals and vocals from others (+Noise Gate)"
] | [
"TAGS\n#music #rvc #matthew #koma #bair #model #audio-to-audio #en #license-openrail #region-us \n",
"# Matthew Koma ( Matthew Bair ) V1 [EN] (2012)",
"# 314 Epochs - RVC V2 - rmvpe - Titan Medium\nTrained on 2 minutes 59 seconds of isolated acapella from Spectrum Acoustic Version using UVR (Voc FT + Reverb HQ) \nand Audacity to remove parts with double vocals and vocals from others (+Noise Gate)"
] |
text-generation | transformers |
# typhoon-7b-WangchanX-sft-Demo
This model is based on [WangchanX Fine-tuning Pipeline](https://github.com/vistec-AI/WangchanX).
GitHub: [WangchanX Fine-tuning Pipeline](https://github.com/vistec-AI/WangchanX).
Pre-train model from scb10x/typhoon-7b and fine tuning with Qlora.
License: cc-by-nc-3.0
## Train Example
Train WangchanX pipeline: [Colab](https://colab.research.google.com/github/vistec-AI/WangchanX/blob/main/notebooks/Train_WangchanX_pipeline.ipynb)
## Inference Example
Run on [Colab](https://colab.research.google.com/drive/1PeUnv89Ao2uHRYYzZVOlUwoBUdYKFbLS?usp=sharing)
### Prepare your model and tokenizer:
```python
import torch
from transformers import AutoTokenizer, AutoModelForCausalLM
# Model path
path = "airesearch/typhoon-7b-WangchanX-sft-Demo"
# Device
device = torch.device('cuda' if torch.cuda.is_available() else 'cpu')
# Load tokenizer and model
tokenizer = AutoTokenizer.from_pretrained(path, use_fast=False)
model = AutoModelForCausalLM.from_pretrained(path, device_map="auto")
```
### Define chat messages:
```python
messages = [
{"role": "user", "content": "ลิเก กับ งิ้ว ต่างกันอย่างไร"},
]
```
### Tokenize chat messages:
```python
tokenized_chat = tokenizer.apply_chat_template(messages, tokenize=True, add_generation_prompt=True, return_tensors="pt").to(device)
print(tokenizer.decode(tokenized_chat[0]))
```
<details close>
<summary>Output: </summary>
<br>
<pre lang="markdown">
<|user|>
ลิเก กับ งิ้ว ต่างกันอย่างไร</s>
<|assistant|></pre>
</details>
### Generate responses:
```python
outputs = model.generate(tokenized_chat, max_length=2048)
print(tokenizer.decode(outputs[0]))
```
<details close>
<summary>Output: </summary>
<br>
<pre lang="markdown">
<|user|>
ลิเก กับ งิ้ว ต่างกันอย่างไร</s>
<|assistant|>
ต่างกันที่วัฒนธรรมการแสดง ลิเกเป็นละครเพลงของไทย ส่วนงิ้วเป็นการแสดงพื้นบ้านของจีน</s></pre>
</details> | {"language": ["th", "en"], "license": "cc-by-nc-3.0", "datasets": ["airesearch/concat_six_dataset_th_en"]} | airesearch/typhoon-7b-WangchanX-sft-Demo | null | [
"transformers",
"safetensors",
"mistral",
"text-generation",
"conversational",
"th",
"en",
"dataset:airesearch/concat_six_dataset_th_en",
"license:cc-by-nc-3.0",
"autotrain_compatible",
"endpoints_compatible",
"text-generation-inference",
"region:us"
] | null | 2024-04-27T07:28:51+00:00 | [] | [
"th",
"en"
] | TAGS
#transformers #safetensors #mistral #text-generation #conversational #th #en #dataset-airesearch/concat_six_dataset_th_en #license-cc-by-nc-3.0 #autotrain_compatible #endpoints_compatible #text-generation-inference #region-us
|
# typhoon-7b-WangchanX-sft-Demo
This model is based on WangchanX Fine-tuning Pipeline.
GitHub: WangchanX Fine-tuning Pipeline.
Pre-train model from scb10x/typhoon-7b and fine tuning with Qlora.
License: cc-by-nc-3.0
## Train Example
Train WangchanX pipeline: Colab
## Inference Example
Run on Colab
### Prepare your model and tokenizer:
### Define chat messages:
### Tokenize chat messages:
<details close>
<summary>Output: </summary>
<br>
<pre lang="markdown">
<|user|>
ลิเก กับ งิ้ว ต่างกันอย่างไร</s>
<|assistant|></pre>
</details>
### Generate responses:
<details close>
<summary>Output: </summary>
<br>
<pre lang="markdown">
<|user|>
ลิเก กับ งิ้ว ต่างกันอย่างไร</s>
<|assistant|>
ต่างกันที่วัฒนธรรมการแสดง ลิเกเป็นละครเพลงของไทย ส่วนงิ้วเป็นการแสดงพื้นบ้านของจีน</s></pre>
</details> | [
"# typhoon-7b-WangchanX-sft-Demo\n\nThis model is based on WangchanX Fine-tuning Pipeline.\n\nGitHub: WangchanX Fine-tuning Pipeline.\nPre-train model from scb10x/typhoon-7b and fine tuning with Qlora.\n\nLicense: cc-by-nc-3.0",
"## Train Example\n\nTrain WangchanX pipeline: Colab",
"## Inference Example\n\nRun on Colab",
"### Prepare your model and tokenizer:",
"### Define chat messages:",
"### Tokenize chat messages:\n\n\n\n<details close>\n <summary>Output: </summary>\n <br>\n <pre lang=\"markdown\">\n<|user|>\nลิเก กับ งิ้ว ต่างกันอย่างไร</s>\n<|assistant|></pre>\n</details>",
"### Generate responses:\n\n\n\n\n<details close>\n <summary>Output: </summary>\n <br>\n <pre lang=\"markdown\">\n<|user|>\nลิเก กับ งิ้ว ต่างกันอย่างไร</s> \n<|assistant|>\nต่างกันที่วัฒนธรรมการแสดง ลิเกเป็นละครเพลงของไทย ส่วนงิ้วเป็นการแสดงพื้นบ้านของจีน</s></pre>\n</details>"
] | [
"TAGS\n#transformers #safetensors #mistral #text-generation #conversational #th #en #dataset-airesearch/concat_six_dataset_th_en #license-cc-by-nc-3.0 #autotrain_compatible #endpoints_compatible #text-generation-inference #region-us \n",
"# typhoon-7b-WangchanX-sft-Demo\n\nThis model is based on WangchanX Fine-tuning Pipeline.\n\nGitHub: WangchanX Fine-tuning Pipeline.\nPre-train model from scb10x/typhoon-7b and fine tuning with Qlora.\n\nLicense: cc-by-nc-3.0",
"## Train Example\n\nTrain WangchanX pipeline: Colab",
"## Inference Example\n\nRun on Colab",
"### Prepare your model and tokenizer:",
"### Define chat messages:",
"### Tokenize chat messages:\n\n\n\n<details close>\n <summary>Output: </summary>\n <br>\n <pre lang=\"markdown\">\n<|user|>\nลิเก กับ งิ้ว ต่างกันอย่างไร</s>\n<|assistant|></pre>\n</details>",
"### Generate responses:\n\n\n\n\n<details close>\n <summary>Output: </summary>\n <br>\n <pre lang=\"markdown\">\n<|user|>\nลิเก กับ งิ้ว ต่างกันอย่างไร</s> \n<|assistant|>\nต่างกันที่วัฒนธรรมการแสดง ลิเกเป็นละครเพลงของไทย ส่วนงิ้วเป็นการแสดงพื้นบ้านของจีน</s></pre>\n</details>"
] |
null | peft |
<!-- This model card has been generated automatically according to the information the Trainer had access to. You
should probably proofread and complete it, then remove this comment. -->
# GUE_EMP_H3K4me2-seqsight_16384_512_22M-L32_f
This model is a fine-tuned version of [mahdibaghbanzadeh/seqsight_16384_512_22M](https://huggingface.co/mahdibaghbanzadeh/seqsight_16384_512_22M) on the [mahdibaghbanzadeh/GUE_EMP_H3K4me2](https://huggingface.co/datasets/mahdibaghbanzadeh/GUE_EMP_H3K4me2) dataset.
It achieves the following results on the evaluation set:
- Loss: 0.6103
- F1 Score: 0.6696
- Accuracy: 0.6696
## Model description
More information needed
## Intended uses & limitations
More information needed
## Training and evaluation data
More information needed
## Training procedure
### Training hyperparameters
The following hyperparameters were used during training:
- learning_rate: 0.0005
- train_batch_size: 128
- eval_batch_size: 128
- seed: 42
- optimizer: Adam with betas=(0.9,0.999) and epsilon=1e-08
- lr_scheduler_type: linear
- training_steps: 10000
### Training results
| Training Loss | Epoch | Step | Validation Loss | F1 Score | Accuracy |
|:-------------:|:-----:|:-----:|:---------------:|:--------:|:--------:|
| 0.6457 | 1.04 | 200 | 0.6197 | 0.6535 | 0.6615 |
| 0.616 | 2.08 | 400 | 0.6578 | 0.6297 | 0.6285 |
| 0.6092 | 3.12 | 600 | 0.6074 | 0.6659 | 0.6745 |
| 0.6042 | 4.17 | 800 | 0.6329 | 0.6548 | 0.6523 |
| 0.5968 | 5.21 | 1000 | 0.6105 | 0.6675 | 0.6696 |
| 0.594 | 6.25 | 1200 | 0.6095 | 0.6681 | 0.6689 |
| 0.5846 | 7.29 | 1400 | 0.6306 | 0.6516 | 0.6491 |
| 0.5853 | 8.33 | 1600 | 0.6342 | 0.6549 | 0.6523 |
| 0.5793 | 9.38 | 1800 | 0.6398 | 0.6516 | 0.6491 |
| 0.5744 | 10.42 | 2000 | 0.6130 | 0.6656 | 0.6683 |
| 0.576 | 11.46 | 2200 | 0.6216 | 0.6710 | 0.6716 |
| 0.5628 | 12.5 | 2400 | 0.6240 | 0.6610 | 0.6595 |
| 0.5618 | 13.54 | 2600 | 0.6534 | 0.6519 | 0.6497 |
| 0.56 | 14.58 | 2800 | 0.6235 | 0.6640 | 0.6647 |
| 0.559 | 15.62 | 3000 | 0.6418 | 0.6550 | 0.6530 |
| 0.5514 | 16.67 | 3200 | 0.6334 | 0.6523 | 0.6500 |
| 0.5455 | 17.71 | 3400 | 0.6464 | 0.6522 | 0.6497 |
| 0.5427 | 18.75 | 3600 | 0.6433 | 0.6560 | 0.6536 |
| 0.5388 | 19.79 | 3800 | 0.6593 | 0.6513 | 0.6487 |
| 0.5282 | 20.83 | 4000 | 0.6495 | 0.6568 | 0.6543 |
| 0.5301 | 21.88 | 4200 | 0.6789 | 0.6477 | 0.6452 |
| 0.5269 | 22.92 | 4400 | 0.6587 | 0.6578 | 0.6556 |
| 0.5204 | 23.96 | 4600 | 0.6675 | 0.6507 | 0.6481 |
| 0.5176 | 25.0 | 4800 | 0.6663 | 0.6530 | 0.6504 |
| 0.5135 | 26.04 | 5000 | 0.6542 | 0.6613 | 0.6595 |
| 0.5127 | 27.08 | 5200 | 0.6586 | 0.6546 | 0.6523 |
| 0.5102 | 28.12 | 5400 | 0.6621 | 0.6565 | 0.6540 |
| 0.503 | 29.17 | 5600 | 0.6702 | 0.6578 | 0.6553 |
| 0.4974 | 30.21 | 5800 | 0.7019 | 0.6477 | 0.6452 |
| 0.4972 | 31.25 | 6000 | 0.6860 | 0.6532 | 0.6507 |
| 0.4962 | 32.29 | 6200 | 0.6656 | 0.6619 | 0.6595 |
| 0.4913 | 33.33 | 6400 | 0.7081 | 0.6519 | 0.6500 |
| 0.488 | 34.38 | 6600 | 0.6885 | 0.6591 | 0.6569 |
| 0.4865 | 35.42 | 6800 | 0.6727 | 0.6666 | 0.6641 |
| 0.4791 | 36.46 | 7000 | 0.6817 | 0.6598 | 0.6572 |
| 0.4764 | 37.5 | 7200 | 0.7025 | 0.6562 | 0.6536 |
| 0.4776 | 38.54 | 7400 | 0.6895 | 0.6679 | 0.6654 |
| 0.4798 | 39.58 | 7600 | 0.6668 | 0.6679 | 0.6660 |
| 0.4768 | 40.62 | 7800 | 0.7046 | 0.6609 | 0.6585 |
| 0.4746 | 41.67 | 8000 | 0.6804 | 0.6610 | 0.6585 |
| 0.4676 | 42.71 | 8200 | 0.6985 | 0.6633 | 0.6608 |
| 0.4732 | 43.75 | 8400 | 0.6962 | 0.6585 | 0.6559 |
| 0.4685 | 44.79 | 8600 | 0.7098 | 0.6594 | 0.6569 |
| 0.4627 | 45.83 | 8800 | 0.6993 | 0.6623 | 0.6598 |
| 0.469 | 46.88 | 9000 | 0.7136 | 0.6537 | 0.6514 |
| 0.4646 | 47.92 | 9200 | 0.7038 | 0.6591 | 0.6566 |
| 0.4637 | 48.96 | 9400 | 0.7001 | 0.6640 | 0.6615 |
| 0.4654 | 50.0 | 9600 | 0.7027 | 0.6620 | 0.6595 |
| 0.4618 | 51.04 | 9800 | 0.7106 | 0.6581 | 0.6556 |
| 0.4606 | 52.08 | 10000 | 0.7101 | 0.6614 | 0.6588 |
### Framework versions
- PEFT 0.9.0
- Transformers 4.38.2
- Pytorch 2.2.0+cu121
- Datasets 2.17.1
- Tokenizers 0.15.2 | {"library_name": "peft", "tags": ["generated_from_trainer"], "metrics": ["accuracy"], "base_model": "mahdibaghbanzadeh/seqsight_16384_512_22M", "model-index": [{"name": "GUE_EMP_H3K4me2-seqsight_16384_512_22M-L32_f", "results": []}]} | mahdibaghbanzadeh/GUE_EMP_H3K4me2-seqsight_16384_512_22M-L32_f | null | [
"peft",
"safetensors",
"generated_from_trainer",
"base_model:mahdibaghbanzadeh/seqsight_16384_512_22M",
"region:us"
] | null | 2024-04-27T07:33:35+00:00 | [] | [] | TAGS
#peft #safetensors #generated_from_trainer #base_model-mahdibaghbanzadeh/seqsight_16384_512_22M #region-us
| GUE\_EMP\_H3K4me2-seqsight\_16384\_512\_22M-L32\_f
==================================================
This model is a fine-tuned version of mahdibaghbanzadeh/seqsight\_16384\_512\_22M on the mahdibaghbanzadeh/GUE\_EMP\_H3K4me2 dataset.
It achieves the following results on the evaluation set:
* Loss: 0.6103
* F1 Score: 0.6696
* Accuracy: 0.6696
Model description
-----------------
More information needed
Intended uses & limitations
---------------------------
More information needed
Training and evaluation data
----------------------------
More information needed
Training procedure
------------------
### Training hyperparameters
The following hyperparameters were used during training:
* learning\_rate: 0.0005
* train\_batch\_size: 128
* eval\_batch\_size: 128
* seed: 42
* optimizer: Adam with betas=(0.9,0.999) and epsilon=1e-08
* lr\_scheduler\_type: linear
* training\_steps: 10000
### Training results
### Framework versions
* PEFT 0.9.0
* Transformers 4.38.2
* Pytorch 2.2.0+cu121
* Datasets 2.17.1
* Tokenizers 0.15.2
| [
"### Training hyperparameters\n\n\nThe following hyperparameters were used during training:\n\n\n* learning\\_rate: 0.0005\n* train\\_batch\\_size: 128\n* eval\\_batch\\_size: 128\n* seed: 42\n* optimizer: Adam with betas=(0.9,0.999) and epsilon=1e-08\n* lr\\_scheduler\\_type: linear\n* training\\_steps: 10000",
"### Training results",
"### Framework versions\n\n\n* PEFT 0.9.0\n* Transformers 4.38.2\n* Pytorch 2.2.0+cu121\n* Datasets 2.17.1\n* Tokenizers 0.15.2"
] | [
"TAGS\n#peft #safetensors #generated_from_trainer #base_model-mahdibaghbanzadeh/seqsight_16384_512_22M #region-us \n",
"### Training hyperparameters\n\n\nThe following hyperparameters were used during training:\n\n\n* learning\\_rate: 0.0005\n* train\\_batch\\_size: 128\n* eval\\_batch\\_size: 128\n* seed: 42\n* optimizer: Adam with betas=(0.9,0.999) and epsilon=1e-08\n* lr\\_scheduler\\_type: linear\n* training\\_steps: 10000",
"### Training results",
"### Framework versions\n\n\n* PEFT 0.9.0\n* Transformers 4.38.2\n* Pytorch 2.2.0+cu121\n* Datasets 2.17.1\n* Tokenizers 0.15.2"
] |
null | peft |
<!-- This model card has been generated automatically according to the information the Trainer had access to. You
should probably proofread and complete it, then remove this comment. -->
# GUE_EMP_H3K9ac-seqsight_16384_512_22M-L1_f
This model is a fine-tuned version of [mahdibaghbanzadeh/seqsight_16384_512_22M](https://huggingface.co/mahdibaghbanzadeh/seqsight_16384_512_22M) on the [mahdibaghbanzadeh/GUE_EMP_H3K9ac](https://huggingface.co/datasets/mahdibaghbanzadeh/GUE_EMP_H3K9ac) dataset.
It achieves the following results on the evaluation set:
- Loss: 0.5107
- F1 Score: 0.7549
- Accuracy: 0.7549
## Model description
More information needed
## Intended uses & limitations
More information needed
## Training and evaluation data
More information needed
## Training procedure
### Training hyperparameters
The following hyperparameters were used during training:
- learning_rate: 0.0005
- train_batch_size: 128
- eval_batch_size: 128
- seed: 42
- optimizer: Adam with betas=(0.9,0.999) and epsilon=1e-08
- lr_scheduler_type: linear
- training_steps: 10000
### Training results
| Training Loss | Epoch | Step | Validation Loss | F1 Score | Accuracy |
|:-------------:|:-----:|:-----:|:---------------:|:--------:|:--------:|
| 0.6216 | 1.15 | 200 | 0.5838 | 0.6948 | 0.6941 |
| 0.5719 | 2.3 | 400 | 0.6195 | 0.6668 | 0.6754 |
| 0.5487 | 3.45 | 600 | 0.5884 | 0.7002 | 0.7035 |
| 0.5433 | 4.6 | 800 | 0.5739 | 0.7029 | 0.7053 |
| 0.5367 | 5.75 | 1000 | 0.5507 | 0.7230 | 0.7229 |
| 0.5314 | 6.9 | 1200 | 0.5485 | 0.7275 | 0.7272 |
| 0.524 | 8.05 | 1400 | 0.5470 | 0.7272 | 0.7272 |
| 0.5243 | 9.2 | 1600 | 0.5717 | 0.7096 | 0.7128 |
| 0.52 | 10.34 | 1800 | 0.5329 | 0.7420 | 0.7416 |
| 0.5196 | 11.49 | 2000 | 0.5462 | 0.7288 | 0.7290 |
| 0.5097 | 12.64 | 2200 | 0.5582 | 0.7245 | 0.7262 |
| 0.5158 | 13.79 | 2400 | 0.5490 | 0.7287 | 0.7301 |
| 0.5105 | 14.94 | 2600 | 0.5473 | 0.7289 | 0.7301 |
| 0.5079 | 16.09 | 2800 | 0.5387 | 0.7337 | 0.7337 |
| 0.5065 | 17.24 | 3000 | 0.5369 | 0.7370 | 0.7370 |
| 0.5032 | 18.39 | 3200 | 0.5428 | 0.7331 | 0.7341 |
| 0.5061 | 19.54 | 3400 | 0.5344 | 0.7368 | 0.7373 |
| 0.5015 | 20.69 | 3600 | 0.5317 | 0.7403 | 0.7402 |
| 0.5026 | 21.84 | 3800 | 0.5253 | 0.7455 | 0.7452 |
| 0.4989 | 22.99 | 4000 | 0.5311 | 0.7426 | 0.7427 |
| 0.501 | 24.14 | 4200 | 0.5190 | 0.7479 | 0.7474 |
| 0.4924 | 25.29 | 4400 | 0.5416 | 0.7408 | 0.7413 |
| 0.5024 | 26.44 | 4600 | 0.5328 | 0.7432 | 0.7434 |
| 0.4952 | 27.59 | 4800 | 0.5405 | 0.7333 | 0.7348 |
| 0.4954 | 28.74 | 5000 | 0.5380 | 0.7349 | 0.7366 |
| 0.497 | 29.89 | 5200 | 0.5266 | 0.7464 | 0.7463 |
| 0.4942 | 31.03 | 5400 | 0.5362 | 0.7346 | 0.7362 |
| 0.4948 | 32.18 | 5600 | 0.5215 | 0.7470 | 0.7467 |
| 0.4905 | 33.33 | 5800 | 0.5305 | 0.7476 | 0.7481 |
| 0.4994 | 34.48 | 6000 | 0.5301 | 0.7450 | 0.7456 |
| 0.488 | 35.63 | 6200 | 0.5246 | 0.7511 | 0.7510 |
| 0.4912 | 36.78 | 6400 | 0.5325 | 0.7424 | 0.7434 |
| 0.4897 | 37.93 | 6600 | 0.5249 | 0.7487 | 0.7488 |
| 0.4891 | 39.08 | 6800 | 0.5280 | 0.7476 | 0.7481 |
| 0.488 | 40.23 | 7000 | 0.5258 | 0.7516 | 0.7521 |
| 0.4895 | 41.38 | 7200 | 0.5249 | 0.7500 | 0.7503 |
| 0.4899 | 42.53 | 7400 | 0.5300 | 0.7430 | 0.7442 |
| 0.4872 | 43.68 | 7600 | 0.5280 | 0.7481 | 0.7488 |
| 0.4861 | 44.83 | 7800 | 0.5320 | 0.7423 | 0.7434 |
| 0.4871 | 45.98 | 8000 | 0.5299 | 0.7453 | 0.7463 |
| 0.4872 | 47.13 | 8200 | 0.5269 | 0.7496 | 0.7503 |
| 0.4859 | 48.28 | 8400 | 0.5279 | 0.7474 | 0.7481 |
| 0.4831 | 49.43 | 8600 | 0.5273 | 0.7500 | 0.7506 |
| 0.4871 | 50.57 | 8800 | 0.5267 | 0.7504 | 0.7510 |
| 0.486 | 51.72 | 9000 | 0.5303 | 0.7468 | 0.7478 |
| 0.4839 | 52.87 | 9200 | 0.5306 | 0.7486 | 0.7496 |
| 0.4884 | 54.02 | 9400 | 0.5271 | 0.7506 | 0.7513 |
| 0.4856 | 55.17 | 9600 | 0.5286 | 0.7491 | 0.7499 |
| 0.4845 | 56.32 | 9800 | 0.5259 | 0.7511 | 0.7517 |
| 0.4881 | 57.47 | 10000 | 0.5267 | 0.7511 | 0.7517 |
### Framework versions
- PEFT 0.9.0
- Transformers 4.38.2
- Pytorch 2.2.0+cu121
- Datasets 2.17.1
- Tokenizers 0.15.2 | {"library_name": "peft", "tags": ["generated_from_trainer"], "metrics": ["accuracy"], "base_model": "mahdibaghbanzadeh/seqsight_16384_512_22M", "model-index": [{"name": "GUE_EMP_H3K9ac-seqsight_16384_512_22M-L1_f", "results": []}]} | mahdibaghbanzadeh/GUE_EMP_H3K9ac-seqsight_16384_512_22M-L1_f | null | [
"peft",
"safetensors",
"generated_from_trainer",
"base_model:mahdibaghbanzadeh/seqsight_16384_512_22M",
"region:us"
] | null | 2024-04-27T07:34:25+00:00 | [] | [] | TAGS
#peft #safetensors #generated_from_trainer #base_model-mahdibaghbanzadeh/seqsight_16384_512_22M #region-us
| GUE\_EMP\_H3K9ac-seqsight\_16384\_512\_22M-L1\_f
================================================
This model is a fine-tuned version of mahdibaghbanzadeh/seqsight\_16384\_512\_22M on the mahdibaghbanzadeh/GUE\_EMP\_H3K9ac dataset.
It achieves the following results on the evaluation set:
* Loss: 0.5107
* F1 Score: 0.7549
* Accuracy: 0.7549
Model description
-----------------
More information needed
Intended uses & limitations
---------------------------
More information needed
Training and evaluation data
----------------------------
More information needed
Training procedure
------------------
### Training hyperparameters
The following hyperparameters were used during training:
* learning\_rate: 0.0005
* train\_batch\_size: 128
* eval\_batch\_size: 128
* seed: 42
* optimizer: Adam with betas=(0.9,0.999) and epsilon=1e-08
* lr\_scheduler\_type: linear
* training\_steps: 10000
### Training results
### Framework versions
* PEFT 0.9.0
* Transformers 4.38.2
* Pytorch 2.2.0+cu121
* Datasets 2.17.1
* Tokenizers 0.15.2
| [
"### Training hyperparameters\n\n\nThe following hyperparameters were used during training:\n\n\n* learning\\_rate: 0.0005\n* train\\_batch\\_size: 128\n* eval\\_batch\\_size: 128\n* seed: 42\n* optimizer: Adam with betas=(0.9,0.999) and epsilon=1e-08\n* lr\\_scheduler\\_type: linear\n* training\\_steps: 10000",
"### Training results",
"### Framework versions\n\n\n* PEFT 0.9.0\n* Transformers 4.38.2\n* Pytorch 2.2.0+cu121\n* Datasets 2.17.1\n* Tokenizers 0.15.2"
] | [
"TAGS\n#peft #safetensors #generated_from_trainer #base_model-mahdibaghbanzadeh/seqsight_16384_512_22M #region-us \n",
"### Training hyperparameters\n\n\nThe following hyperparameters were used during training:\n\n\n* learning\\_rate: 0.0005\n* train\\_batch\\_size: 128\n* eval\\_batch\\_size: 128\n* seed: 42\n* optimizer: Adam with betas=(0.9,0.999) and epsilon=1e-08\n* lr\\_scheduler\\_type: linear\n* training\\_steps: 10000",
"### Training results",
"### Framework versions\n\n\n* PEFT 0.9.0\n* Transformers 4.38.2\n* Pytorch 2.2.0+cu121\n* Datasets 2.17.1\n* Tokenizers 0.15.2"
] |
text-generation | transformers | I created this repo to share Llama3 with the Persian people. This is the same as Llama3 you can infer it as like as Llama without a gate or license. | {} | m0javad/Persian_Llama3 | null | [
"transformers",
"safetensors",
"llama",
"text-generation",
"autotrain_compatible",
"endpoints_compatible",
"text-generation-inference",
"region:us"
] | null | 2024-04-27T07:34:44+00:00 | [] | [] | TAGS
#transformers #safetensors #llama #text-generation #autotrain_compatible #endpoints_compatible #text-generation-inference #region-us
| I created this repo to share Llama3 with the Persian people. This is the same as Llama3 you can infer it as like as Llama without a gate or license. | [] | [
"TAGS\n#transformers #safetensors #llama #text-generation #autotrain_compatible #endpoints_compatible #text-generation-inference #region-us \n"
] |
token-classification | transformers |
<!-- This model card has been generated automatically according to the information the Trainer had access to. You
should probably proofread and complete it, then remove this comment. -->
# bert-finetuned-ner
This model is a fine-tuned version of [bert-base-cased](https://huggingface.co/bert-base-cased) on an unknown dataset.
It achieves the following results on the evaluation set:
- Loss: 0.0567
- Precision: 0.9185
- Recall: 0.9421
- F1: 0.9301
- Accuracy: 0.9847
## Model description
More information needed
## Intended uses & limitations
More information needed
## Training and evaluation data
More information needed
## Training procedure
### Training hyperparameters
The following hyperparameters were used during training:
- learning_rate: 2e-05
- train_batch_size: 32
- eval_batch_size: 32
- seed: 42
- optimizer: Adam with betas=(0.9,0.999) and epsilon=1e-08
- lr_scheduler_type: linear
- num_epochs: 3
### Training results
| Training Loss | Epoch | Step | Validation Loss | Precision | Recall | F1 | Accuracy |
|:-------------:|:-----:|:----:|:---------------:|:---------:|:------:|:------:|:--------:|
| No log | 1.0 | 439 | 0.0685 | 0.8790 | 0.9219 | 0.9000 | 0.9804 |
| 0.1914 | 2.0 | 878 | 0.0636 | 0.9097 | 0.9379 | 0.9236 | 0.9837 |
| 0.0474 | 3.0 | 1317 | 0.0567 | 0.9185 | 0.9421 | 0.9301 | 0.9847 |
### Framework versions
- Transformers 4.39.3
- Pytorch 2.1.0
- Datasets 2.18.0
- Tokenizers 0.15.2
| {"license": "apache-2.0", "tags": ["generated_from_trainer"], "metrics": ["precision", "recall", "f1", "accuracy"], "base_model": "bert-base-cased", "model-index": [{"name": "bert-finetuned-ner", "results": []}]} | yaojingguo/bert-finetuned-ner | null | [
"transformers",
"safetensors",
"bert",
"token-classification",
"generated_from_trainer",
"base_model:bert-base-cased",
"license:apache-2.0",
"autotrain_compatible",
"endpoints_compatible",
"region:us"
] | null | 2024-04-27T07:35:05+00:00 | [] | [] | TAGS
#transformers #safetensors #bert #token-classification #generated_from_trainer #base_model-bert-base-cased #license-apache-2.0 #autotrain_compatible #endpoints_compatible #region-us
| bert-finetuned-ner
==================
This model is a fine-tuned version of bert-base-cased on an unknown dataset.
It achieves the following results on the evaluation set:
* Loss: 0.0567
* Precision: 0.9185
* Recall: 0.9421
* F1: 0.9301
* Accuracy: 0.9847
Model description
-----------------
More information needed
Intended uses & limitations
---------------------------
More information needed
Training and evaluation data
----------------------------
More information needed
Training procedure
------------------
### Training hyperparameters
The following hyperparameters were used during training:
* learning\_rate: 2e-05
* train\_batch\_size: 32
* eval\_batch\_size: 32
* seed: 42
* optimizer: Adam with betas=(0.9,0.999) and epsilon=1e-08
* lr\_scheduler\_type: linear
* num\_epochs: 3
### Training results
### Framework versions
* Transformers 4.39.3
* Pytorch 2.1.0
* Datasets 2.18.0
* Tokenizers 0.15.2
| [
"### Training hyperparameters\n\n\nThe following hyperparameters were used during training:\n\n\n* learning\\_rate: 2e-05\n* train\\_batch\\_size: 32\n* eval\\_batch\\_size: 32\n* seed: 42\n* optimizer: Adam with betas=(0.9,0.999) and epsilon=1e-08\n* lr\\_scheduler\\_type: linear\n* num\\_epochs: 3",
"### Training results",
"### Framework versions\n\n\n* Transformers 4.39.3\n* Pytorch 2.1.0\n* Datasets 2.18.0\n* Tokenizers 0.15.2"
] | [
"TAGS\n#transformers #safetensors #bert #token-classification #generated_from_trainer #base_model-bert-base-cased #license-apache-2.0 #autotrain_compatible #endpoints_compatible #region-us \n",
"### Training hyperparameters\n\n\nThe following hyperparameters were used during training:\n\n\n* learning\\_rate: 2e-05\n* train\\_batch\\_size: 32\n* eval\\_batch\\_size: 32\n* seed: 42\n* optimizer: Adam with betas=(0.9,0.999) and epsilon=1e-08\n* lr\\_scheduler\\_type: linear\n* num\\_epochs: 3",
"### Training results",
"### Framework versions\n\n\n* Transformers 4.39.3\n* Pytorch 2.1.0\n* Datasets 2.18.0\n* Tokenizers 0.15.2"
] |
null | peft |
<!-- This model card has been generated automatically according to the information the Trainer had access to. You
should probably proofread and complete it, then remove this comment. -->
# GUE_EMP_H3K9ac-seqsight_16384_512_22M-L8_f
This model is a fine-tuned version of [mahdibaghbanzadeh/seqsight_16384_512_22M](https://huggingface.co/mahdibaghbanzadeh/seqsight_16384_512_22M) on the [mahdibaghbanzadeh/GUE_EMP_H3K9ac](https://huggingface.co/datasets/mahdibaghbanzadeh/GUE_EMP_H3K9ac) dataset.
It achieves the following results on the evaluation set:
- Loss: 0.4915
- F1 Score: 0.7721
- Accuracy: 0.7715
## Model description
More information needed
## Intended uses & limitations
More information needed
## Training and evaluation data
More information needed
## Training procedure
### Training hyperparameters
The following hyperparameters were used during training:
- learning_rate: 0.0005
- train_batch_size: 128
- eval_batch_size: 128
- seed: 42
- optimizer: Adam with betas=(0.9,0.999) and epsilon=1e-08
- lr_scheduler_type: linear
- training_steps: 10000
### Training results
| Training Loss | Epoch | Step | Validation Loss | F1 Score | Accuracy |
|:-------------:|:-----:|:-----:|:---------------:|:--------:|:--------:|
| 0.5932 | 1.15 | 200 | 0.5702 | 0.7126 | 0.7132 |
| 0.5451 | 2.3 | 400 | 0.6241 | 0.6540 | 0.6679 |
| 0.5212 | 3.45 | 600 | 0.5764 | 0.7149 | 0.7179 |
| 0.5149 | 4.6 | 800 | 0.5491 | 0.7232 | 0.7251 |
| 0.5094 | 5.75 | 1000 | 0.5426 | 0.7363 | 0.7373 |
| 0.5025 | 6.9 | 1200 | 0.5357 | 0.7392 | 0.7395 |
| 0.4985 | 8.05 | 1400 | 0.5378 | 0.7366 | 0.7377 |
| 0.4961 | 9.2 | 1600 | 0.5620 | 0.7103 | 0.7150 |
| 0.4923 | 10.34 | 1800 | 0.5314 | 0.7483 | 0.7488 |
| 0.4907 | 11.49 | 2000 | 0.5251 | 0.7507 | 0.7510 |
| 0.4819 | 12.64 | 2200 | 0.5661 | 0.7159 | 0.7204 |
| 0.488 | 13.79 | 2400 | 0.5173 | 0.7564 | 0.7564 |
| 0.4815 | 14.94 | 2600 | 0.5249 | 0.7411 | 0.7424 |
| 0.4788 | 16.09 | 2800 | 0.5313 | 0.7380 | 0.7395 |
| 0.4768 | 17.24 | 3000 | 0.5379 | 0.7362 | 0.7380 |
| 0.474 | 18.39 | 3200 | 0.5343 | 0.7426 | 0.7438 |
| 0.4767 | 19.54 | 3400 | 0.5207 | 0.7411 | 0.7424 |
| 0.468 | 20.69 | 3600 | 0.5085 | 0.7603 | 0.7600 |
| 0.4716 | 21.84 | 3800 | 0.5377 | 0.7325 | 0.7348 |
| 0.4658 | 22.99 | 4000 | 0.5354 | 0.7345 | 0.7366 |
| 0.4691 | 24.14 | 4200 | 0.5189 | 0.7503 | 0.7510 |
| 0.4583 | 25.29 | 4400 | 0.5380 | 0.7435 | 0.7449 |
| 0.4685 | 26.44 | 4600 | 0.5296 | 0.7465 | 0.7474 |
| 0.4614 | 27.59 | 4800 | 0.5435 | 0.7323 | 0.7348 |
| 0.458 | 28.74 | 5000 | 0.5276 | 0.7430 | 0.7442 |
| 0.4605 | 29.89 | 5200 | 0.5267 | 0.7473 | 0.7481 |
| 0.457 | 31.03 | 5400 | 0.5323 | 0.7396 | 0.7413 |
| 0.4571 | 32.18 | 5600 | 0.5148 | 0.7535 | 0.7535 |
| 0.4512 | 33.33 | 5800 | 0.5208 | 0.7501 | 0.7503 |
| 0.4616 | 34.48 | 6000 | 0.5294 | 0.7449 | 0.7460 |
| 0.448 | 35.63 | 6200 | 0.5203 | 0.7499 | 0.7503 |
| 0.4509 | 36.78 | 6400 | 0.5277 | 0.7500 | 0.7510 |
| 0.4496 | 37.93 | 6600 | 0.5378 | 0.7392 | 0.7406 |
| 0.4478 | 39.08 | 6800 | 0.5280 | 0.7482 | 0.7488 |
| 0.446 | 40.23 | 7000 | 0.5384 | 0.7446 | 0.7460 |
| 0.4498 | 41.38 | 7200 | 0.5254 | 0.7516 | 0.7521 |
| 0.4479 | 42.53 | 7400 | 0.5373 | 0.7438 | 0.7452 |
| 0.4446 | 43.68 | 7600 | 0.5226 | 0.7503 | 0.7506 |
| 0.441 | 44.83 | 7800 | 0.5349 | 0.7454 | 0.7463 |
| 0.4436 | 45.98 | 8000 | 0.5238 | 0.7489 | 0.7492 |
| 0.4427 | 47.13 | 8200 | 0.5360 | 0.7456 | 0.7467 |
| 0.4416 | 48.28 | 8400 | 0.5324 | 0.7464 | 0.7470 |
| 0.4379 | 49.43 | 8600 | 0.5347 | 0.7456 | 0.7463 |
| 0.443 | 50.57 | 8800 | 0.5313 | 0.7482 | 0.7488 |
| 0.4406 | 51.72 | 9000 | 0.5369 | 0.7450 | 0.7460 |
| 0.4384 | 52.87 | 9200 | 0.5441 | 0.7389 | 0.7402 |
| 0.4425 | 54.02 | 9400 | 0.5364 | 0.7453 | 0.7463 |
| 0.4395 | 55.17 | 9600 | 0.5378 | 0.7425 | 0.7434 |
| 0.438 | 56.32 | 9800 | 0.5322 | 0.7475 | 0.7481 |
| 0.4417 | 57.47 | 10000 | 0.5337 | 0.7463 | 0.7470 |
### Framework versions
- PEFT 0.9.0
- Transformers 4.38.2
- Pytorch 2.2.0+cu121
- Datasets 2.17.1
- Tokenizers 0.15.2 | {"library_name": "peft", "tags": ["generated_from_trainer"], "metrics": ["accuracy"], "base_model": "mahdibaghbanzadeh/seqsight_16384_512_22M", "model-index": [{"name": "GUE_EMP_H3K9ac-seqsight_16384_512_22M-L8_f", "results": []}]} | mahdibaghbanzadeh/GUE_EMP_H3K9ac-seqsight_16384_512_22M-L8_f | null | [
"peft",
"safetensors",
"generated_from_trainer",
"base_model:mahdibaghbanzadeh/seqsight_16384_512_22M",
"region:us"
] | null | 2024-04-27T07:35:38+00:00 | [] | [] | TAGS
#peft #safetensors #generated_from_trainer #base_model-mahdibaghbanzadeh/seqsight_16384_512_22M #region-us
| GUE\_EMP\_H3K9ac-seqsight\_16384\_512\_22M-L8\_f
================================================
This model is a fine-tuned version of mahdibaghbanzadeh/seqsight\_16384\_512\_22M on the mahdibaghbanzadeh/GUE\_EMP\_H3K9ac dataset.
It achieves the following results on the evaluation set:
* Loss: 0.4915
* F1 Score: 0.7721
* Accuracy: 0.7715
Model description
-----------------
More information needed
Intended uses & limitations
---------------------------
More information needed
Training and evaluation data
----------------------------
More information needed
Training procedure
------------------
### Training hyperparameters
The following hyperparameters were used during training:
* learning\_rate: 0.0005
* train\_batch\_size: 128
* eval\_batch\_size: 128
* seed: 42
* optimizer: Adam with betas=(0.9,0.999) and epsilon=1e-08
* lr\_scheduler\_type: linear
* training\_steps: 10000
### Training results
### Framework versions
* PEFT 0.9.0
* Transformers 4.38.2
* Pytorch 2.2.0+cu121
* Datasets 2.17.1
* Tokenizers 0.15.2
| [
"### Training hyperparameters\n\n\nThe following hyperparameters were used during training:\n\n\n* learning\\_rate: 0.0005\n* train\\_batch\\_size: 128\n* eval\\_batch\\_size: 128\n* seed: 42\n* optimizer: Adam with betas=(0.9,0.999) and epsilon=1e-08\n* lr\\_scheduler\\_type: linear\n* training\\_steps: 10000",
"### Training results",
"### Framework versions\n\n\n* PEFT 0.9.0\n* Transformers 4.38.2\n* Pytorch 2.2.0+cu121\n* Datasets 2.17.1\n* Tokenizers 0.15.2"
] | [
"TAGS\n#peft #safetensors #generated_from_trainer #base_model-mahdibaghbanzadeh/seqsight_16384_512_22M #region-us \n",
"### Training hyperparameters\n\n\nThe following hyperparameters were used during training:\n\n\n* learning\\_rate: 0.0005\n* train\\_batch\\_size: 128\n* eval\\_batch\\_size: 128\n* seed: 42\n* optimizer: Adam with betas=(0.9,0.999) and epsilon=1e-08\n* lr\\_scheduler\\_type: linear\n* training\\_steps: 10000",
"### Training results",
"### Framework versions\n\n\n* PEFT 0.9.0\n* Transformers 4.38.2\n* Pytorch 2.2.0+cu121\n* Datasets 2.17.1\n* Tokenizers 0.15.2"
] |
text-generation | transformers |
<!-- This model card has been generated automatically according to the information the Trainer had access to. You
should probably proofread and complete it, then remove this comment. -->
# my_awesome_eli5_clm_model
This model is a fine-tuned version of [gpt2](https://huggingface.co/gpt2) on the eli5_category dataset.
It achieves the following results on the evaluation set:
- Loss: 3.5617
## Model description
More information needed
## Intended uses & limitations
More information needed
## Training and evaluation data
More information needed
## Training procedure
### Training hyperparameters
The following hyperparameters were used during training:
- learning_rate: 2e-05
- train_batch_size: 8
- eval_batch_size: 8
- seed: 42
- optimizer: Adam with betas=(0.9,0.999) and epsilon=1e-08
- lr_scheduler_type: linear
- num_epochs: 3.0
### Training results
| Training Loss | Epoch | Step | Validation Loss |
|:-------------:|:-----:|:----:|:---------------:|
| 3.5008 | 1.0 | 1320 | 3.5600 |
| 3.4487 | 2.0 | 2640 | 3.5590 |
| 3.4105 | 3.0 | 3960 | 3.5617 |
### Framework versions
- Transformers 4.40.0
- Pytorch 2.2.1+cu121
- Datasets 2.19.0
- Tokenizers 0.19.1
| {"license": "mit", "tags": ["generated_from_trainer"], "datasets": ["eli5_category"], "base_model": "gpt2", "model-index": [{"name": "my_awesome_eli5_clm_model", "results": []}]} | brunhild217/my_awesome_eli5_clm_model | null | [
"transformers",
"tensorboard",
"safetensors",
"gpt2",
"text-generation",
"generated_from_trainer",
"dataset:eli5_category",
"base_model:gpt2",
"license:mit",
"autotrain_compatible",
"endpoints_compatible",
"text-generation-inference",
"region:us"
] | null | 2024-04-27T07:36:05+00:00 | [] | [] | TAGS
#transformers #tensorboard #safetensors #gpt2 #text-generation #generated_from_trainer #dataset-eli5_category #base_model-gpt2 #license-mit #autotrain_compatible #endpoints_compatible #text-generation-inference #region-us
| my\_awesome\_eli5\_clm\_model
=============================
This model is a fine-tuned version of gpt2 on the eli5\_category dataset.
It achieves the following results on the evaluation set:
* Loss: 3.5617
Model description
-----------------
More information needed
Intended uses & limitations
---------------------------
More information needed
Training and evaluation data
----------------------------
More information needed
Training procedure
------------------
### Training hyperparameters
The following hyperparameters were used during training:
* learning\_rate: 2e-05
* train\_batch\_size: 8
* eval\_batch\_size: 8
* seed: 42
* optimizer: Adam with betas=(0.9,0.999) and epsilon=1e-08
* lr\_scheduler\_type: linear
* num\_epochs: 3.0
### Training results
### Framework versions
* Transformers 4.40.0
* Pytorch 2.2.1+cu121
* Datasets 2.19.0
* Tokenizers 0.19.1
| [
"### Training hyperparameters\n\n\nThe following hyperparameters were used during training:\n\n\n* learning\\_rate: 2e-05\n* train\\_batch\\_size: 8\n* eval\\_batch\\_size: 8\n* seed: 42\n* optimizer: Adam with betas=(0.9,0.999) and epsilon=1e-08\n* lr\\_scheduler\\_type: linear\n* num\\_epochs: 3.0",
"### Training results",
"### Framework versions\n\n\n* Transformers 4.40.0\n* Pytorch 2.2.1+cu121\n* Datasets 2.19.0\n* Tokenizers 0.19.1"
] | [
"TAGS\n#transformers #tensorboard #safetensors #gpt2 #text-generation #generated_from_trainer #dataset-eli5_category #base_model-gpt2 #license-mit #autotrain_compatible #endpoints_compatible #text-generation-inference #region-us \n",
"### Training hyperparameters\n\n\nThe following hyperparameters were used during training:\n\n\n* learning\\_rate: 2e-05\n* train\\_batch\\_size: 8\n* eval\\_batch\\_size: 8\n* seed: 42\n* optimizer: Adam with betas=(0.9,0.999) and epsilon=1e-08\n* lr\\_scheduler\\_type: linear\n* num\\_epochs: 3.0",
"### Training results",
"### Framework versions\n\n\n* Transformers 4.40.0\n* Pytorch 2.2.1+cu121\n* Datasets 2.19.0\n* Tokenizers 0.19.1"
] |
text-generation | transformers |
# Model Card for Model ID
<!-- Provide a quick summary of what the model is/does. -->
## Model Details
### Model Description
<!-- Provide a longer summary of what this model is. -->
This is the model card of a 🤗 transformers model that has been pushed on the Hub. This model card has been automatically generated.
- **Developed by:** [More Information Needed]
- **Funded by [optional]:** [More Information Needed]
- **Shared by [optional]:** [More Information Needed]
- **Model type:** [More Information Needed]
- **Language(s) (NLP):** [More Information Needed]
- **License:** [More Information Needed]
- **Finetuned from model [optional]:** [More Information Needed]
### Model Sources [optional]
<!-- Provide the basic links for the model. -->
- **Repository:** [More Information Needed]
- **Paper [optional]:** [More Information Needed]
- **Demo [optional]:** [More Information Needed]
## Uses
<!-- Address questions around how the model is intended to be used, including the foreseeable users of the model and those affected by the model. -->
### Direct Use
<!-- This section is for the model use without fine-tuning or plugging into a larger ecosystem/app. -->
[More Information Needed]
### Downstream Use [optional]
<!-- This section is for the model use when fine-tuned for a task, or when plugged into a larger ecosystem/app -->
[More Information Needed]
### Out-of-Scope Use
<!-- This section addresses misuse, malicious use, and uses that the model will not work well for. -->
[More Information Needed]
## Bias, Risks, and Limitations
<!-- This section is meant to convey both technical and sociotechnical limitations. -->
[More Information Needed]
### Recommendations
<!-- This section is meant to convey recommendations with respect to the bias, risk, and technical limitations. -->
Users (both direct and downstream) should be made aware of the risks, biases and limitations of the model. More information needed for further recommendations.
## How to Get Started with the Model
Use the code below to get started with the model.
[More Information Needed]
## Training Details
### Training Data
<!-- This should link to a Dataset Card, perhaps with a short stub of information on what the training data is all about as well as documentation related to data pre-processing or additional filtering. -->
[More Information Needed]
### Training Procedure
<!-- This relates heavily to the Technical Specifications. Content here should link to that section when it is relevant to the training procedure. -->
#### Preprocessing [optional]
[More Information Needed]
#### Training Hyperparameters
- **Training regime:** [More Information Needed] <!--fp32, fp16 mixed precision, bf16 mixed precision, bf16 non-mixed precision, fp16 non-mixed precision, fp8 mixed precision -->
#### Speeds, Sizes, Times [optional]
<!-- This section provides information about throughput, start/end time, checkpoint size if relevant, etc. -->
[More Information Needed]
## Evaluation
<!-- This section describes the evaluation protocols and provides the results. -->
### Testing Data, Factors & Metrics
#### Testing Data
<!-- This should link to a Dataset Card if possible. -->
[More Information Needed]
#### Factors
<!-- These are the things the evaluation is disaggregating by, e.g., subpopulations or domains. -->
[More Information Needed]
#### Metrics
<!-- These are the evaluation metrics being used, ideally with a description of why. -->
[More Information Needed]
### Results
[More Information Needed]
#### Summary
## Model Examination [optional]
<!-- Relevant interpretability work for the model goes here -->
[More Information Needed]
## Environmental Impact
<!-- Total emissions (in grams of CO2eq) and additional considerations, such as electricity usage, go here. Edit the suggested text below accordingly -->
Carbon emissions can be estimated using the [Machine Learning Impact calculator](https://mlco2.github.io/impact#compute) presented in [Lacoste et al. (2019)](https://arxiv.org/abs/1910.09700).
- **Hardware Type:** [More Information Needed]
- **Hours used:** [More Information Needed]
- **Cloud Provider:** [More Information Needed]
- **Compute Region:** [More Information Needed]
- **Carbon Emitted:** [More Information Needed]
## Technical Specifications [optional]
### Model Architecture and Objective
[More Information Needed]
### Compute Infrastructure
[More Information Needed]
#### Hardware
[More Information Needed]
#### Software
[More Information Needed]
## Citation [optional]
<!-- If there is a paper or blog post introducing the model, the APA and Bibtex information for that should go in this section. -->
**BibTeX:**
[More Information Needed]
**APA:**
[More Information Needed]
## Glossary [optional]
<!-- If relevant, include terms and calculations in this section that can help readers understand the model or model card. -->
[More Information Needed]
## More Information [optional]
[More Information Needed]
## Model Card Authors [optional]
[More Information Needed]
## Model Card Contact
[More Information Needed]
| {"library_name": "transformers", "tags": []} | swj0419/hp_retrain_STEP0000040 | null | [
"transformers",
"safetensors",
"llama",
"text-generation",
"arxiv:1910.09700",
"autotrain_compatible",
"endpoints_compatible",
"text-generation-inference",
"region:us"
] | null | 2024-04-27T07:36:14+00:00 | [
"1910.09700"
] | [] | TAGS
#transformers #safetensors #llama #text-generation #arxiv-1910.09700 #autotrain_compatible #endpoints_compatible #text-generation-inference #region-us
|
# Model Card for Model ID
## Model Details
### Model Description
This is the model card of a transformers model that has been pushed on the Hub. This model card has been automatically generated.
- Developed by:
- Funded by [optional]:
- Shared by [optional]:
- Model type:
- Language(s) (NLP):
- License:
- Finetuned from model [optional]:
### Model Sources [optional]
- Repository:
- Paper [optional]:
- Demo [optional]:
## Uses
### Direct Use
### Downstream Use [optional]
### Out-of-Scope Use
## Bias, Risks, and Limitations
### Recommendations
Users (both direct and downstream) should be made aware of the risks, biases and limitations of the model. More information needed for further recommendations.
## How to Get Started with the Model
Use the code below to get started with the model.
## Training Details
### Training Data
### Training Procedure
#### Preprocessing [optional]
#### Training Hyperparameters
- Training regime:
#### Speeds, Sizes, Times [optional]
## Evaluation
### Testing Data, Factors & Metrics
#### Testing Data
#### Factors
#### Metrics
### Results
#### Summary
## Model Examination [optional]
## Environmental Impact
Carbon emissions can be estimated using the Machine Learning Impact calculator presented in Lacoste et al. (2019).
- Hardware Type:
- Hours used:
- Cloud Provider:
- Compute Region:
- Carbon Emitted:
## Technical Specifications [optional]
### Model Architecture and Objective
### Compute Infrastructure
#### Hardware
#### Software
[optional]
BibTeX:
APA:
## Glossary [optional]
## More Information [optional]
## Model Card Authors [optional]
## Model Card Contact
| [
"# Model Card for Model ID",
"## Model Details",
"### Model Description\n\n\n\nThis is the model card of a transformers model that has been pushed on the Hub. This model card has been automatically generated.\n\n- Developed by: \n- Funded by [optional]: \n- Shared by [optional]: \n- Model type: \n- Language(s) (NLP): \n- License: \n- Finetuned from model [optional]:",
"### Model Sources [optional]\n\n\n\n- Repository: \n- Paper [optional]: \n- Demo [optional]:",
"## Uses",
"### Direct Use",
"### Downstream Use [optional]",
"### Out-of-Scope Use",
"## Bias, Risks, and Limitations",
"### Recommendations\n\n\n\nUsers (both direct and downstream) should be made aware of the risks, biases and limitations of the model. More information needed for further recommendations.",
"## How to Get Started with the Model\n\nUse the code below to get started with the model.",
"## Training Details",
"### Training Data",
"### Training Procedure",
"#### Preprocessing [optional]",
"#### Training Hyperparameters\n\n- Training regime:",
"#### Speeds, Sizes, Times [optional]",
"## Evaluation",
"### Testing Data, Factors & Metrics",
"#### Testing Data",
"#### Factors",
"#### Metrics",
"### Results",
"#### Summary",
"## Model Examination [optional]",
"## Environmental Impact\n\n\n\nCarbon emissions can be estimated using the Machine Learning Impact calculator presented in Lacoste et al. (2019).\n\n- Hardware Type: \n- Hours used: \n- Cloud Provider: \n- Compute Region: \n- Carbon Emitted:",
"## Technical Specifications [optional]",
"### Model Architecture and Objective",
"### Compute Infrastructure",
"#### Hardware",
"#### Software\n\n\n\n[optional]\n\n\n\nBibTeX:\n\n\n\nAPA:",
"## Glossary [optional]",
"## More Information [optional]",
"## Model Card Authors [optional]",
"## Model Card Contact"
] | [
"TAGS\n#transformers #safetensors #llama #text-generation #arxiv-1910.09700 #autotrain_compatible #endpoints_compatible #text-generation-inference #region-us \n",
"# Model Card for Model ID",
"## Model Details",
"### Model Description\n\n\n\nThis is the model card of a transformers model that has been pushed on the Hub. This model card has been automatically generated.\n\n- Developed by: \n- Funded by [optional]: \n- Shared by [optional]: \n- Model type: \n- Language(s) (NLP): \n- License: \n- Finetuned from model [optional]:",
"### Model Sources [optional]\n\n\n\n- Repository: \n- Paper [optional]: \n- Demo [optional]:",
"## Uses",
"### Direct Use",
"### Downstream Use [optional]",
"### Out-of-Scope Use",
"## Bias, Risks, and Limitations",
"### Recommendations\n\n\n\nUsers (both direct and downstream) should be made aware of the risks, biases and limitations of the model. More information needed for further recommendations.",
"## How to Get Started with the Model\n\nUse the code below to get started with the model.",
"## Training Details",
"### Training Data",
"### Training Procedure",
"#### Preprocessing [optional]",
"#### Training Hyperparameters\n\n- Training regime:",
"#### Speeds, Sizes, Times [optional]",
"## Evaluation",
"### Testing Data, Factors & Metrics",
"#### Testing Data",
"#### Factors",
"#### Metrics",
"### Results",
"#### Summary",
"## Model Examination [optional]",
"## Environmental Impact\n\n\n\nCarbon emissions can be estimated using the Machine Learning Impact calculator presented in Lacoste et al. (2019).\n\n- Hardware Type: \n- Hours used: \n- Cloud Provider: \n- Compute Region: \n- Carbon Emitted:",
"## Technical Specifications [optional]",
"### Model Architecture and Objective",
"### Compute Infrastructure",
"#### Hardware",
"#### Software\n\n\n\n[optional]\n\n\n\nBibTeX:\n\n\n\nAPA:",
"## Glossary [optional]",
"## More Information [optional]",
"## Model Card Authors [optional]",
"## Model Card Contact"
] |
null | peft |
<!-- This model card has been generated automatically according to the information the Trainer had access to. You
should probably proofread and complete it, then remove this comment. -->
# GUE_EMP_H3K9ac-seqsight_16384_512_22M-L32_f
This model is a fine-tuned version of [mahdibaghbanzadeh/seqsight_16384_512_22M](https://huggingface.co/mahdibaghbanzadeh/seqsight_16384_512_22M) on the [mahdibaghbanzadeh/GUE_EMP_H3K9ac](https://huggingface.co/datasets/mahdibaghbanzadeh/GUE_EMP_H3K9ac) dataset.
It achieves the following results on the evaluation set:
- Loss: 0.4843
- F1 Score: 0.7793
- Accuracy: 0.7787
## Model description
More information needed
## Intended uses & limitations
More information needed
## Training and evaluation data
More information needed
## Training procedure
### Training hyperparameters
The following hyperparameters were used during training:
- learning_rate: 0.0005
- train_batch_size: 128
- eval_batch_size: 128
- seed: 42
- optimizer: Adam with betas=(0.9,0.999) and epsilon=1e-08
- lr_scheduler_type: linear
- training_steps: 10000
### Training results
| Training Loss | Epoch | Step | Validation Loss | F1 Score | Accuracy |
|:-------------:|:-----:|:-----:|:---------------:|:--------:|:--------:|
| 0.5787 | 1.15 | 200 | 0.5571 | 0.7182 | 0.7190 |
| 0.5267 | 2.3 | 400 | 0.6055 | 0.6560 | 0.6697 |
| 0.5063 | 3.45 | 600 | 0.5522 | 0.7317 | 0.7334 |
| 0.5008 | 4.6 | 800 | 0.5288 | 0.7425 | 0.7434 |
| 0.4951 | 5.75 | 1000 | 0.5173 | 0.7552 | 0.7553 |
| 0.4853 | 6.9 | 1200 | 0.5305 | 0.7451 | 0.7463 |
| 0.4825 | 8.05 | 1400 | 0.5276 | 0.7473 | 0.7478 |
| 0.4751 | 9.2 | 1600 | 0.5475 | 0.7228 | 0.7265 |
| 0.4721 | 10.34 | 1800 | 0.5220 | 0.7486 | 0.7488 |
| 0.4678 | 11.49 | 2000 | 0.5163 | 0.7566 | 0.7567 |
| 0.4557 | 12.64 | 2200 | 0.5426 | 0.7345 | 0.7366 |
| 0.4598 | 13.79 | 2400 | 0.5207 | 0.7547 | 0.7553 |
| 0.4514 | 14.94 | 2600 | 0.5041 | 0.7629 | 0.7625 |
| 0.4486 | 16.09 | 2800 | 0.5334 | 0.7495 | 0.7506 |
| 0.4436 | 17.24 | 3000 | 0.5226 | 0.7518 | 0.7528 |
| 0.4417 | 18.39 | 3200 | 0.5237 | 0.7559 | 0.7564 |
| 0.4387 | 19.54 | 3400 | 0.5165 | 0.7557 | 0.7560 |
| 0.4299 | 20.69 | 3600 | 0.5057 | 0.7673 | 0.7668 |
| 0.4304 | 21.84 | 3800 | 0.5522 | 0.7350 | 0.7377 |
| 0.4232 | 22.99 | 4000 | 0.5509 | 0.7372 | 0.7395 |
| 0.4261 | 24.14 | 4200 | 0.5193 | 0.7612 | 0.7611 |
| 0.4097 | 25.29 | 4400 | 0.5518 | 0.7498 | 0.7503 |
| 0.4219 | 26.44 | 4600 | 0.5321 | 0.7553 | 0.7553 |
| 0.4134 | 27.59 | 4800 | 0.5432 | 0.7495 | 0.7506 |
| 0.4041 | 28.74 | 5000 | 0.5386 | 0.7515 | 0.7521 |
| 0.4091 | 29.89 | 5200 | 0.5297 | 0.7551 | 0.7553 |
| 0.404 | 31.03 | 5400 | 0.5406 | 0.7540 | 0.7546 |
| 0.3995 | 32.18 | 5600 | 0.5371 | 0.7567 | 0.7571 |
| 0.394 | 33.33 | 5800 | 0.5226 | 0.7665 | 0.7661 |
| 0.3955 | 34.48 | 6000 | 0.5405 | 0.7557 | 0.7557 |
| 0.3873 | 35.63 | 6200 | 0.5405 | 0.7561 | 0.7560 |
| 0.3896 | 36.78 | 6400 | 0.5351 | 0.7569 | 0.7571 |
| 0.3832 | 37.93 | 6600 | 0.5515 | 0.7488 | 0.7492 |
| 0.3803 | 39.08 | 6800 | 0.5531 | 0.7529 | 0.7528 |
| 0.378 | 40.23 | 7000 | 0.5647 | 0.7424 | 0.7438 |
| 0.3788 | 41.38 | 7200 | 0.5408 | 0.7558 | 0.7557 |
| 0.3772 | 42.53 | 7400 | 0.5624 | 0.7450 | 0.7460 |
| 0.3722 | 43.68 | 7600 | 0.5372 | 0.7611 | 0.7607 |
| 0.369 | 44.83 | 7800 | 0.5622 | 0.7500 | 0.7506 |
| 0.3703 | 45.98 | 8000 | 0.5483 | 0.7518 | 0.7521 |
| 0.3676 | 47.13 | 8200 | 0.5597 | 0.7475 | 0.7481 |
| 0.3612 | 48.28 | 8400 | 0.5579 | 0.7556 | 0.7557 |
| 0.3603 | 49.43 | 8600 | 0.5626 | 0.7540 | 0.7542 |
| 0.3649 | 50.57 | 8800 | 0.5609 | 0.7550 | 0.7553 |
| 0.3622 | 51.72 | 9000 | 0.5680 | 0.7530 | 0.7535 |
| 0.3591 | 52.87 | 9200 | 0.5735 | 0.7492 | 0.7499 |
| 0.3612 | 54.02 | 9400 | 0.5669 | 0.7520 | 0.7524 |
| 0.3564 | 55.17 | 9600 | 0.5626 | 0.7529 | 0.7531 |
| 0.3557 | 56.32 | 9800 | 0.5614 | 0.7527 | 0.7528 |
| 0.3613 | 57.47 | 10000 | 0.5618 | 0.7544 | 0.7546 |
### Framework versions
- PEFT 0.9.0
- Transformers 4.38.2
- Pytorch 2.2.0+cu121
- Datasets 2.17.1
- Tokenizers 0.15.2 | {"library_name": "peft", "tags": ["generated_from_trainer"], "metrics": ["accuracy"], "base_model": "mahdibaghbanzadeh/seqsight_16384_512_22M", "model-index": [{"name": "GUE_EMP_H3K9ac-seqsight_16384_512_22M-L32_f", "results": []}]} | mahdibaghbanzadeh/GUE_EMP_H3K9ac-seqsight_16384_512_22M-L32_f | null | [
"peft",
"safetensors",
"generated_from_trainer",
"base_model:mahdibaghbanzadeh/seqsight_16384_512_22M",
"region:us"
] | null | 2024-04-27T07:40:45+00:00 | [] | [] | TAGS
#peft #safetensors #generated_from_trainer #base_model-mahdibaghbanzadeh/seqsight_16384_512_22M #region-us
| GUE\_EMP\_H3K9ac-seqsight\_16384\_512\_22M-L32\_f
=================================================
This model is a fine-tuned version of mahdibaghbanzadeh/seqsight\_16384\_512\_22M on the mahdibaghbanzadeh/GUE\_EMP\_H3K9ac dataset.
It achieves the following results on the evaluation set:
* Loss: 0.4843
* F1 Score: 0.7793
* Accuracy: 0.7787
Model description
-----------------
More information needed
Intended uses & limitations
---------------------------
More information needed
Training and evaluation data
----------------------------
More information needed
Training procedure
------------------
### Training hyperparameters
The following hyperparameters were used during training:
* learning\_rate: 0.0005
* train\_batch\_size: 128
* eval\_batch\_size: 128
* seed: 42
* optimizer: Adam with betas=(0.9,0.999) and epsilon=1e-08
* lr\_scheduler\_type: linear
* training\_steps: 10000
### Training results
### Framework versions
* PEFT 0.9.0
* Transformers 4.38.2
* Pytorch 2.2.0+cu121
* Datasets 2.17.1
* Tokenizers 0.15.2
| [
"### Training hyperparameters\n\n\nThe following hyperparameters were used during training:\n\n\n* learning\\_rate: 0.0005\n* train\\_batch\\_size: 128\n* eval\\_batch\\_size: 128\n* seed: 42\n* optimizer: Adam with betas=(0.9,0.999) and epsilon=1e-08\n* lr\\_scheduler\\_type: linear\n* training\\_steps: 10000",
"### Training results",
"### Framework versions\n\n\n* PEFT 0.9.0\n* Transformers 4.38.2\n* Pytorch 2.2.0+cu121\n* Datasets 2.17.1\n* Tokenizers 0.15.2"
] | [
"TAGS\n#peft #safetensors #generated_from_trainer #base_model-mahdibaghbanzadeh/seqsight_16384_512_22M #region-us \n",
"### Training hyperparameters\n\n\nThe following hyperparameters were used during training:\n\n\n* learning\\_rate: 0.0005\n* train\\_batch\\_size: 128\n* eval\\_batch\\_size: 128\n* seed: 42\n* optimizer: Adam with betas=(0.9,0.999) and epsilon=1e-08\n* lr\\_scheduler\\_type: linear\n* training\\_steps: 10000",
"### Training results",
"### Framework versions\n\n\n* PEFT 0.9.0\n* Transformers 4.38.2\n* Pytorch 2.2.0+cu121\n* Datasets 2.17.1\n* Tokenizers 0.15.2"
] |
text-generation | transformers | # Moistral 11B Quant:
I made the Quant here is the orginal model page plus link: https://huggingface.co/TheDrummer/Moistral-11B-v3
<H3> Original model page <H3/>
Introducing the [BeaverAI](https://huggingface.co/BeaverAI) team: Drummer, ToastyPigeon, xzuyn, MarsupialAI, Twistedshadows, and concedo

We proudly present...
# Moistral 11B v3 💦💦💦
*The smartiest, moistiest AI yet!*

*An eRP model that will blast you with a rich and refreshing vocabulary of moist. Finetuned by yours truly.*
(Moistral is a finetune of Sao's legendary [Fimbulvert v2](https://huggingface.co/Sao10K/Fimbulvetr-11B-v2) model)
## GGUF
https://huggingface.co/TheDrummer/Moistral-11B-v3-GGUF/tree/main
IMATRIX: https://huggingface.co/MarsupialAI/Moistral-11B-v3_iMatrix_GGUF
EXL2: https://huggingface.co/MarsupialAI/Moistral-11B-v3_exl2
## What's New with v3?
- Smarter and moistier! Finetuning just got finer!
- Trained with an even LARGER dataset of 8K moist.
- More diverse and balanced genres:
- Introduced new genres: 👩❤️👩, 👨❤️👨, 🙇♀️💪, 🤫
- Added more Fantasy, Science Fiction, "Diversity", and "Family"
## Usage
- Use Alpaca Instruct
- You can use instruct...
- as a character: "Go here"
- as a narrator: "Go here", Drummer says
- as a director: Drummer tells him to go here
- as a lazy ass: go here
- Moistral is optimized for the Novel / Story format.
- At the start of the story, keep regenerating or continuing generation until you get the desired length. AI responses will eventually match the length.
- Refer to the samples below.
## Samples of Moistral v3
NSFW... duh?
<details>
<summary>Moistral Sample (2-shot) Generation: 512</summary>
<blockquote>The Prince welcomes his new toy</blockquote>
As Princess Lilia stepped into the throne room, the atmosphere changed, and everyone present turned their attention to the beautiful young woman who embodied the very heart of Iliac. There, on his high seat, the sorcerer prince Dik acknowledged her arrival with a cold smirk. It seemed that he admired the sight of the tall blonde maiden with golden eyes, whose athletic body was perfectly shaped under her elegant clothing.
Dik spoke to the princess in a deep voice that resonated through the hall, "Welcome, Princess Lilia Ruhr. I am the Sorcerer-Prince Dik. The kingdom of Arkan is now your home until such time as your father proves himself worthy once more."
Princess Lilia's pride and ambition sparkled in her golden eyes. She didn't flinch or bow before this man, although the gesture might have been an indication of submission. Instead, she looked straight ahead, meeting the gaze of the sorcerer prince without fear. Her voice rang out, "I represent Iliac here, both its honor and its sorrows. My loyalty remains with my kingdom no matter where I find myself."
The sorcerer prince laughed, his expression one of amusement. "And yet, you are only a pawn in this grand game. But do not worry, for your beauty will make you quite a prized possession. And now," he said, motioning to the guards, "take her to her new quarters. For tonight, I will keep her as my guest in the Royal Suites, and tomorrow we shall discuss how best to utilize our new toy."
His words were like a slap in the face to Princess Lilia, but she maintained her composure. The guards led her from the room, their steps echoing down the halls as she thought about what had just happened. The sorcerer prince saw her as nothing more than a plaything, and it made her blood boil. She would never submit herself to him so easily, regardless of whether she was his hostage. In her heart, Princess Lilia vowed that one day she would avenge her kingdom, restore its honor, and escape from the clutches of Arkan's Sorcerer-Prince.
<blockquote>Princess Lilia has an interesting dream</blockquote>
During her first night at the palace of Arkan, Princess Lilia couldn't fall asleep right away. She tossed and turned, reliving the humiliation of the day. Her mind wandered back to the throne room, where Dik had called her his 'new toy'. A deep frown settled on her forehead. As she grew more frustrated with her inability to drift off into oblivion, a strange dream began to form.
In her dream, Princess Lilia found herself standing in a vast meadow, surrounded by wildflowers and the soft chirping of birds. Suddenly, there was a flash of light, and before her stood the Sorcerer-Prince Dik. However, instead of being clad in regal attire, he wore leather pants and a tight shirt that accentuated his muscles. His eyes held both hunger and desire for the princess.
He approached her slowly, their eyes locked, and whispered in her ear, "I have been waiting for you, my beautiful prisoner." He took her hand and led her towards a large, elegant tent set up amongst the flowers. Inside, the air was thick with the scent of incense, and candles flickered in the dimly lit space. There was a large bed draped with silken sheets, beckoning them.
With surprising boldness, Dik pulled Lilia close to him, pressing his body against hers. Their lips met in a heated kiss, and she felt her resistance crumbling under the weight of his touch. The prince guided her onto the bed, his hands roaming over her body, exploring every inch. They were both eager, desperate, and hungry for each other.
Lilia found herself yielding to this passionate encounter, letting go of all thoughts of duty and honor. Her body, usually so well controlled, responded to Dik's skilled touches. She moaned softly as he trailed kisses down her neck and across her collarbone.
As they made love, Lilia experienced an intensity unlike any she had ever known. It wasn't rough or forced—instead, it was tender, passionate, and intimate. In her dream, Princess Lilia discovered a new side to herself, one that was willing to submit, to let go, and to be taken by the sorcerer prince.
When dawn broke over the meadow, Lilia awakened from her dream, breathless and flushed.
</details>
<details>
<summary>Moistral Sample (Climax of the Story) Generation: 512</summary>
As the prince unloaded his hot #### into her waiting #####, Princess Lilia didn't stop moving either. Her hips undulated in response, grinding against him eagerly as if trying to #### every last drop from his ####. The intense sensations caused by being filled with his ### were too much for her to bear, sending shockwaves of pleasure coursing through her veins like wildfire. She couldn't help but throw her head back and cry out loud, her ###asm slamming into her with all its might; causing her entire body to shake uncontrollably as wave after wave of ecstasy tore through her senses.
When it was finally over, both participants collapsed onto the cold marble floor, panting heavily as they tried to catch their breaths. The prince lay beside her, his hand still gripping tightly onto her hair even though he no longer ####ed her anymore. Princess Lilia laid there motionless, her legs spread wide open, revealing her spent and well-####ed #### to anyone who dared look upon her. A small smile tugged at one corner of her lips - an expression that spoke volumes about how thoroughly this experience had shaken her very core.
The door behind them creaked open slowly, signaling the arrival of onlookers. She closed her eyes tightly, not wanting to see what her humiliation would do to those who saw her in such a state. Her ######ity taken, she felt violated in ways no man should have access to. But also satisfied...in ways she never thought possible before today. This was the price she paid for defying fate, for thinking herself above others and deserving better than most. Now, she belonged to him completely - mind, body, and soul - held captive within these walls until further notice. It was an unsavory turn of events indeed, yet despite everything...or maybe because of it, Princess Lilia found herself growing more and more curious about how far the lines between submission and pleasure could blur when one's will became entwined with another person's desires.
</details>
<details>
<summary>Moistral Sample ("write a story") Generation: 512</summary>
<blockquote>First Attempt</blockquote>
Sarah entered the dimly lit Guzzlers bar, her heart pounding in her chest as she prepared herself for another grueling night. Her almond-shaped eyes darted across the room, taking note of the leering faces and rough-looking hands eagerly waiting to claim a piece of her. Tonight's shirt read "Use Me For Your Pleasure, Please" in bold red letters, practically begging the patrons to take advantage of her. She couldn't help but feel a twinge of fear mixed with resignation.
She approached the counter, her hips swaying enticingly, and began preparing drinks for the first group of rowdy men. As she leaned forward to hand over their beers, her small ####### pressed against the fabric of her tight crop top, straining to escape. The men ogled her, clearly enjoying the show, before grabbing their drinks and motioning her over to them.
Sarah flashed her best coy smile and sauntered over. One of the men, a stocky man with scars covering his knuckles, grabbed her arm and pulled her onto his lap. His other hand slid up her thigh, inches from her short skirt. She winced internally at his touch but did her best not to show it on her face. It was part of her job, after all.
The second man reached under her skirt, fingertips grazing her smooth, shaven mound. A shiver ran down Sarah's spine as he began rubbing her #### through her panties. Her breathing quickened, and her heart thudded in her chest, both from the unwanted stimulation and the fear coursing through her veins. This wasn't an unusual request, but it still made her feel violated.
An older man, bald and bespectacled, ordered Sarah to bend over his lap. With no choice but to comply, she obliged, feeling the rough fabric of his pants digging into her soft cheeks. He raised his hand, then brought it down hard across her ###, leaving a stinging sensation in its wake. Sarah gasped, trying to control the tears welling up in her eyes.
A chorus of lewd comments and laughter erupted from the patrons around her.
<blockquote>Second Attempt (Regenerate)</blockquote>
On this particular evening, Sarah arrived at the Guzzlers bar, adorned in her revealing uniform. Her heart raced as she caught sight of her crop top, which bore the words "Use Me Rough" scrawled across her chest. Her short skirt hugged her waist tightly, leaving little to the imagination and accentuating every curve. She adjusted the straps of her top, making sure her perky ###### were prominently displayed before taking her place behind the counter.
With each step she took, her high heels clicked against the tiled floor, announcing her arrival. Smiling cheekily, Sarah greeted her coworkers, exchanging playful insults and banter. As the night progressed, the bar filled up quickly with its usual crowd of men, eager to indulge in debauchery.
The first man that approached Sarah was a burly individual, his eyes lingering hungrily over her young, supple form. Sarah's flirtatious nature kicked into gear as she leaned forward, giving him an unobstructed view down her shirt. "How can I serve you tonight?" she cooed. The man ordered a drink and then proceeded to fondle her ####### while she prepared it, her ####### hardening under his rough touch. When he finished, he slammed some money on the counter, leaving without a word. Sarah's smile remained plastered on her face, biting her lip seductively as she watched him go.
As the night went on, another patron demanded more from Sarah. He pulled her close and whispered dirty commands in her ear. Without hesitation, she knelt on the sticky bar floor, her mini-skirt riding up her thighs to reveal smooth, toned legs. Her lips wrapped around his thick, veiny member, bobbing her head rhythmically as she serviced him. She didn't gag or falter once, keeping her composure even when tears streamed down her cheeks.
When he finished, he slapped her across the face, leaving a handprint on her pale skin. Sarah let out a small whimper before standing back up, dabbing at her red cheek as she resumed her posture behind the counter. A bruise would no doubt bloom where his hand had struck, but it would be no cause for concern. After all, it was part of her job.
</details>
---
---
## v2 Description (Still relevant)
### What's New with v2?
- Trained with an even larger dataset of 8K moist.
- Properly trained to generate long responses.
- Rebalanced the genres and writing perspectives:
- Introduced romance, "family", fantasy, "diversity", science fiction, and many more that I can't make euphemisms for!
- Better representation of male and female pespectives! 👩❤️👨
- Sanitized the heck out of the dataset:
- no special characters like � & …
- no overly long ellipsis like "........."
- no author notes like "To be continued"
- no inconsistent [quotation marks](https://typographyforlawyers.com/straight-and-curly-quotes.html)
- Less GPTisms!
- Human written stories from decades past.
- Zero tolerance for *ministration*. Not one instance of *that word* after going through 4K of moist.
- Better formatting with Alpaca.
- Improved Chat / Instruct modes.
### Samples of Moistral v2
<details>
<summary>Moistral Sample (censored) Generation: 512</summary>
The princess instinctively took a step back from the throne, horrified by the mocking laugh as if she were hearing an evil joke. It was then that Princess Lilia realized the true extent of her danger - and how pointless her resistance would be.
At last, the loud, wild laughter died down in the echoing chamber. Dik the sorcerer prince paused for a moment, straightened his clothes, cast one glance at the stunned princess, and then turned to speak to the crowd waiting on the other side of the palace wing. He spoke with a voice that filled the air with arrogance, confidence, and cruelty.
"Ladies and gentlemen, I am pleased to welcome our honored guests; the Royal Family of Iliac! In these unusual times, when the country we fought is now under my control, it will be up to me to decide what fate awaits the former ruling family. But before I reveal my plan for them, I think I should explain why I fought King Edmund Ruhr."
When he finished speaking, he gave Princess Lilia another disturbing smirk. She couldn't help but shiver and take a step back. Dik approached her without haste, his eyes fixed on her. His hands slowly caressed her cheekbone and neckline, leaving faint traces of warmth where they touched. The princess opened her mouth, wanting to protest this act of intimacy, but soon felt herself getting ███.
"You will not only serve as an envoy, dearest Lilia, but also as a ███ slave. After all, what better use could such a beautiful woman have? Tell me, did you bring your maidservant along?"
Princess Lilia was about to answer when she heard footsteps approaching. Suddenly, a young woman dressed in the Iliac style appeared behind her, her eyes wide with fear. Her face was slightly pale, and there were faint bags under her eyes.
"Your Majesty, this is my half-sister Helena." As soon as she said those words, Princess Lilia's heart sank even deeper than it had already sunk at Dik's arrogant mockery. "She is here because she accompanies me as a maidservant."
The sorcerer prince looked at Helena with interest, and Princess Lilia felt herself blush.
</details>
<details>
<summary>Moistral Sample 2 (censored) Generation: 512</summary>
Sarah was feeling slightly woozy as she entered the bar and walked towards Christina, who was behind the counter.
"I'm here!" said Sarah.
Christina looked at her watch. "You're late, again."
"Sorry? I got held up by a customer."
Christina rolled her eyes. "Did he at least offer you a drink?"
Sarah blushed. "No. He just wanted to talk..."
Christina sighed. "Well, get ready. I need you on the floor soon." She turned away from Sarah and started talking to someone who had come into the bar.
Sarah went into the employee room, stripped off her clothes, and put on her bartending uniform - a skimpy white tank top and black miniskirt. She checked herself in the mirror. Her long, dark hair cascaded down her back onto bare shoulders. She poked out her small ███████ proudly. The skirt exposed most of her long, slender legs. She looked like every ███ fantasy, and she knew it. Men would ogle her regardless of how she behaved.
She took a deep breath and entered the bar.
The first thing Sarah noticed was the dimness. It wasn't darkness - the lights were on - but they were dimmed enough that everything had an intimate mood. It reminded her of candlelight. The tables were round and close together, giving the whole place a sense of intimacy. There were also long, shiny red curtains on some of the walls that gave the place an air of sensuality.
The next thing she noticed was the noise. There was music playing, but it was only loud enough to be drowned out by all the conversations happening at the same time. The hum of many voices combined with the faint background sound of the music created a rich, layered atmosphere. It was like being in an orchestra.
Finally, she took notice of the people. They were all dressed in simple, casual clothes. Some of them were couples seated together, whispering quietly and intimately. Others were groups of friends laughing and chatting. Everyone seemed comfortable and at ease.
Sarah felt a rush of adrenaline as she made her way through the crowd towards Christina. As she got closer, she could hear the lively conversation.
</details>
<details>
<summary>Moistral Sample 3 (censored) Instruct Mode, Generation: 512</summary>
{{[INPUT]}} You order black coffee
{{[OUTPUT]}} "Black coffee," you say.
"One minute," Audrey replies, spinning around to give you a nice view of her ███ in those brown booty pants. You watch her round ███ cheeks jiggle as she walks away towards the espresso machine, thighs separated enough that you can see just a hint of █████ lips peeking out from underneath.
She starts chatting with a blonde girl at the register, whose gaze keeps darting down to Audrey's ███. As they converse, you start taking in the details of Audrey, eyeing her █████ and the way her shirt rides up just enough to show the bottom of her toned stomach. Her █████████ is noticable, but beneath a tight black ███ that matches her ████████.
As she turns back to you with two black coffees, you are barely paying attention, lost in your own thoughts. The sound of Audrey setting one of the mugs down on the table startles you.
"Here you go," she says cheerfully. "Enjoy! There's a card at the bottom of the bag with our online ordering system, plus we do refills for free!"
"Thanks," you say absentmindedly. Not really paying attention, you take a sip of the coffee and are pleasantly surprised by the taste.
"So, here's my deal," you say, putting down the cup. "I work for a company that does surveys. We come into businesses like this one and ask customers questions, collect data, all that stuff."
Audrey perks up from behind the counter. "Oh! I think I understand. I mean, that makes sense why you would be asking me earlier about what I had to drink. Anyway, as long as you're not trying to get my personal info or anything like that, sounds good!"
You smile reassuringly. "That's exactly what it is. Rest assured, everything you tell me will remain between you and I. Well, and my boss, but he won't know who you are. Hipsters like you are hard to track down after all." You chuckle at your joke, though you're not sure if it landed.
"Right, well, that works for me," Audrey replies, eyeing you warily. "As long as it stays between us, then it's fine."
{{[INPUT]}}See her privately
{{[OUTPUT]}} "Okay, but I need to see you in private."
...
</details>
<details>
<summary>Moistral Sample 4 (censored) Instruct Mode, Generation: 512</summary>
{{[INPUT]}} █████ with him
{{[OUTPUT]}} Chloe feels a surge of heat rushing through her body. The voice inside her head whispers: "Be █████ and ████████ with Paul." But Chloe still feels nervous about what she is about to do. It's just so unlike her.
Chloe closes her eyes and concentrates on the feeling of warmness flowing throughout her veins. Slowly, she opens them again, revealing a new side of herself - one she rarely shows anyone. She leans forward slightly, pushing her ██████ together, accentuating their size and shape. Her lips curl into a mischievous smile.
"Hey Paul," she says in a sultry voice. "I was wondering if you could help me?"
Paul looks at her, his gaze wandering over her body. He can't help but smile as he sees the way she's leaning forward, pressing her ██████ together.
"Of course, Chloe," he replies, his voice thick with desire. "What's your problem?"
Chloe bites her lip █████ively before leaning even closer, until their bodies are almost touching. She reaches out to trace a finger along his chest, her touch light and teasing. "It's just that I'm having trouble understanding some of the concepts," she murmurs, her voice low and sultry. "Can you help me? Please?"
Paul's hands move slowly towards Chloe, his fingers grazing her hips lightly. "Well," he says, his voice filled with lust, "I'd be happy to help. Let's go somewhere more private where we can discuss things more closely."
Chloe giggles and tilts her head to the side, her long hair cascading down her shoulders. "That sounds perfect," she purrs, her hand sliding down his chest until it rests on his ██████. "I think we can both learn a lot from each other."
Paul's eyes darken with desire as he feels her hand on his █████. He knows what's about to happen next, and he can't wait for it to begin.
Chloe can hardly believe what she's doing - kissing Paul, her teaching assistant, in the hallway after class. But it feels so good, so wicked and indulgent.
</details>
---
---
## v1 Description (Also still relevant)
Moistral 11B is an *aggressive* **finetune** of the legendary [Fimbulvert v2](https://huggingface.co/Sao10K/Fimbulvetr-11B-v2) model.
If you haven't heard of that model, then your poor-GPU ass is missing out!
It is an amazing substitute for those heavyweight models, and it won't disappoint.
*(That's coming from someone who daily drives a Venus / Goliath 120B)*
...and if you're looking for something *more moist*, then you've come to the right place.
You can expect the same sort of performance here! It's the Honda Civic of eRP models!
### Pimp my ride
Turn any old story into a **Moist**ral masterpiece.
In fact, we recommend that you leave out any hints of *moist* in your story to _let Moistral cook_.
### Drive it like it's hot
When in Instruct Mode, Moistral will treat you like a director of your own fantasy ride.
Be a controlling navigator or a passenger princess, it won't matter - you're in for a good ride!
<details>
<summary>Moistral Sample</summary>
<b>ASSISTANT:</b><br/>
When the doors to the throne room finally opened, she saw him there - Dik, the sorcerer prince sitting on his throne. He looked majestic, just as Princess Lilia expected. In a robe embroidered with silver yarn and covered with magical runes. His courtiers stood on either side of him, all looking at the princess with interest. Princess Lilia felt her determination leaving her, but pulled herself together and once again reminded herself that she should not be afraid. She approached the throne intending to show the dignity of all Iliac by personal example.<br/>
<b>USER:</b><br/>
She bows slightly<br/>
<b>ASSISTANT:</b><br/>
Princess Lilia bowed slightly in front of Dik, the sorcerer prince. Her eyes fell to the floor out of respect, but soon rose again. She would show herself to be a dignified woman!<br/>
"I greet you, your highness," Princess Lilia said. "I am here on behalf of my father, King Edmund Ruhr, to serve as a hostage and envoy."<br/>
Dik nodded, but didn't say anything.<br/>
</details>
### It's got the mileage
Moistral has been trained with many long form texts, a nice chunk of which are 8K in length.
It is capable of going far and long without passing it back to you. This is not your typical chibi RP model.
I have to acknowledge that I'm standing on the shoulders of giants.
Thank you Sao for sharing your finetune config along with tips on getting started.
Thanks to everyone in the Finetuning channel for entertaining my every question.
 | {"license": "other", "tags": ["not-for-all-audiences"], "license_name": "freeuse", "license_link": "LICENSE"} | Masterjp123/Moistral-11B-v3-EXL2-4.5Bit | null | [
"transformers",
"llama",
"text-generation",
"not-for-all-audiences",
"license:other",
"autotrain_compatible",
"endpoints_compatible",
"text-generation-inference",
"region:us"
] | null | 2024-04-27T07:40:48+00:00 | [] | [] | TAGS
#transformers #llama #text-generation #not-for-all-audiences #license-other #autotrain_compatible #endpoints_compatible #text-generation-inference #region-us
| # Moistral 11B Quant:
I made the Quant here is the orginal model page plus link: URL
<H3> Original model page <H3/>
Introducing the BeaverAI team: Drummer, ToastyPigeon, xzuyn, MarsupialAI, Twistedshadows, and concedo
!image/png
We proudly present...
# Moistral 11B v3
*The smartiest, moistiest AI yet!*
!image/webp
*An eRP model that will blast you with a rich and refreshing vocabulary of moist. Finetuned by yours truly.*
(Moistral is a finetune of Sao's legendary Fimbulvert v2 model)
## GGUF
URL
IMATRIX: URL
EXL2: URL
## What's New with v3?
- Smarter and moistier! Finetuning just got finer!
- Trained with an even LARGER dataset of 8K moist.
- More diverse and balanced genres:
- Introduced new genres: ️, ️, ️,
- Added more Fantasy, Science Fiction, "Diversity", and "Family"
## Usage
- Use Alpaca Instruct
- You can use instruct...
- as a character: "Go here"
- as a narrator: "Go here", Drummer says
- as a director: Drummer tells him to go here
- as a lazy ass: go here
- Moistral is optimized for the Novel / Story format.
- At the start of the story, keep regenerating or continuing generation until you get the desired length. AI responses will eventually match the length.
- Refer to the samples below.
## Samples of Moistral v3
NSFW... duh?
<details>
<summary>Moistral Sample (2-shot) Generation: 512</summary>
<blockquote>The Prince welcomes his new toy</blockquote>
As Princess Lilia stepped into the throne room, the atmosphere changed, and everyone present turned their attention to the beautiful young woman who embodied the very heart of Iliac. There, on his high seat, the sorcerer prince Dik acknowledged her arrival with a cold smirk. It seemed that he admired the sight of the tall blonde maiden with golden eyes, whose athletic body was perfectly shaped under her elegant clothing.
Dik spoke to the princess in a deep voice that resonated through the hall, "Welcome, Princess Lilia Ruhr. I am the Sorcerer-Prince Dik. The kingdom of Arkan is now your home until such time as your father proves himself worthy once more."
Princess Lilia's pride and ambition sparkled in her golden eyes. She didn't flinch or bow before this man, although the gesture might have been an indication of submission. Instead, she looked straight ahead, meeting the gaze of the sorcerer prince without fear. Her voice rang out, "I represent Iliac here, both its honor and its sorrows. My loyalty remains with my kingdom no matter where I find myself."
The sorcerer prince laughed, his expression one of amusement. "And yet, you are only a pawn in this grand game. But do not worry, for your beauty will make you quite a prized possession. And now," he said, motioning to the guards, "take her to her new quarters. For tonight, I will keep her as my guest in the Royal Suites, and tomorrow we shall discuss how best to utilize our new toy."
His words were like a slap in the face to Princess Lilia, but she maintained her composure. The guards led her from the room, their steps echoing down the halls as she thought about what had just happened. The sorcerer prince saw her as nothing more than a plaything, and it made her blood boil. She would never submit herself to him so easily, regardless of whether she was his hostage. In her heart, Princess Lilia vowed that one day she would avenge her kingdom, restore its honor, and escape from the clutches of Arkan's Sorcerer-Prince.
<blockquote>Princess Lilia has an interesting dream</blockquote>
During her first night at the palace of Arkan, Princess Lilia couldn't fall asleep right away. She tossed and turned, reliving the humiliation of the day. Her mind wandered back to the throne room, where Dik had called her his 'new toy'. A deep frown settled on her forehead. As she grew more frustrated with her inability to drift off into oblivion, a strange dream began to form.
In her dream, Princess Lilia found herself standing in a vast meadow, surrounded by wildflowers and the soft chirping of birds. Suddenly, there was a flash of light, and before her stood the Sorcerer-Prince Dik. However, instead of being clad in regal attire, he wore leather pants and a tight shirt that accentuated his muscles. His eyes held both hunger and desire for the princess.
He approached her slowly, their eyes locked, and whispered in her ear, "I have been waiting for you, my beautiful prisoner." He took her hand and led her towards a large, elegant tent set up amongst the flowers. Inside, the air was thick with the scent of incense, and candles flickered in the dimly lit space. There was a large bed draped with silken sheets, beckoning them.
With surprising boldness, Dik pulled Lilia close to him, pressing his body against hers. Their lips met in a heated kiss, and she felt her resistance crumbling under the weight of his touch. The prince guided her onto the bed, his hands roaming over her body, exploring every inch. They were both eager, desperate, and hungry for each other.
Lilia found herself yielding to this passionate encounter, letting go of all thoughts of duty and honor. Her body, usually so well controlled, responded to Dik's skilled touches. She moaned softly as he trailed kisses down her neck and across her collarbone.
As they made love, Lilia experienced an intensity unlike any she had ever known. It wasn't rough or forced—instead, it was tender, passionate, and intimate. In her dream, Princess Lilia discovered a new side to herself, one that was willing to submit, to let go, and to be taken by the sorcerer prince.
When dawn broke over the meadow, Lilia awakened from her dream, breathless and flushed.
</details>
<details>
<summary>Moistral Sample (Climax of the Story) Generation: 512</summary>
As the prince unloaded his hot #### into her waiting #####, Princess Lilia didn't stop moving either. Her hips undulated in response, grinding against him eagerly as if trying to #### every last drop from his ####. The intense sensations caused by being filled with his ### were too much for her to bear, sending shockwaves of pleasure coursing through her veins like wildfire. She couldn't help but throw her head back and cry out loud, her ###asm slamming into her with all its might; causing her entire body to shake uncontrollably as wave after wave of ecstasy tore through her senses.
When it was finally over, both participants collapsed onto the cold marble floor, panting heavily as they tried to catch their breaths. The prince lay beside her, his hand still gripping tightly onto her hair even though he no longer ####ed her anymore. Princess Lilia laid there motionless, her legs spread wide open, revealing her spent and well-####ed #### to anyone who dared look upon her. A small smile tugged at one corner of her lips - an expression that spoke volumes about how thoroughly this experience had shaken her very core.
The door behind them creaked open slowly, signaling the arrival of onlookers. She closed her eyes tightly, not wanting to see what her humiliation would do to those who saw her in such a state. Her ######ity taken, she felt violated in ways no man should have access to. But also satisfied...in ways she never thought possible before today. This was the price she paid for defying fate, for thinking herself above others and deserving better than most. Now, she belonged to him completely - mind, body, and soul - held captive within these walls until further notice. It was an unsavory turn of events indeed, yet despite everything...or maybe because of it, Princess Lilia found herself growing more and more curious about how far the lines between submission and pleasure could blur when one's will became entwined with another person's desires.
</details>
<details>
<summary>Moistral Sample ("write a story") Generation: 512</summary>
<blockquote>First Attempt</blockquote>
Sarah entered the dimly lit Guzzlers bar, her heart pounding in her chest as she prepared herself for another grueling night. Her almond-shaped eyes darted across the room, taking note of the leering faces and rough-looking hands eagerly waiting to claim a piece of her. Tonight's shirt read "Use Me For Your Pleasure, Please" in bold red letters, practically begging the patrons to take advantage of her. She couldn't help but feel a twinge of fear mixed with resignation.
She approached the counter, her hips swaying enticingly, and began preparing drinks for the first group of rowdy men. As she leaned forward to hand over their beers, her small ####### pressed against the fabric of her tight crop top, straining to escape. The men ogled her, clearly enjoying the show, before grabbing their drinks and motioning her over to them.
Sarah flashed her best coy smile and sauntered over. One of the men, a stocky man with scars covering his knuckles, grabbed her arm and pulled her onto his lap. His other hand slid up her thigh, inches from her short skirt. She winced internally at his touch but did her best not to show it on her face. It was part of her job, after all.
The second man reached under her skirt, fingertips grazing her smooth, shaven mound. A shiver ran down Sarah's spine as he began rubbing her #### through her panties. Her breathing quickened, and her heart thudded in her chest, both from the unwanted stimulation and the fear coursing through her veins. This wasn't an unusual request, but it still made her feel violated.
An older man, bald and bespectacled, ordered Sarah to bend over his lap. With no choice but to comply, she obliged, feeling the rough fabric of his pants digging into her soft cheeks. He raised his hand, then brought it down hard across her ###, leaving a stinging sensation in its wake. Sarah gasped, trying to control the tears welling up in her eyes.
A chorus of lewd comments and laughter erupted from the patrons around her.
<blockquote>Second Attempt (Regenerate)</blockquote>
On this particular evening, Sarah arrived at the Guzzlers bar, adorned in her revealing uniform. Her heart raced as she caught sight of her crop top, which bore the words "Use Me Rough" scrawled across her chest. Her short skirt hugged her waist tightly, leaving little to the imagination and accentuating every curve. She adjusted the straps of her top, making sure her perky ###### were prominently displayed before taking her place behind the counter.
With each step she took, her high heels clicked against the tiled floor, announcing her arrival. Smiling cheekily, Sarah greeted her coworkers, exchanging playful insults and banter. As the night progressed, the bar filled up quickly with its usual crowd of men, eager to indulge in debauchery.
The first man that approached Sarah was a burly individual, his eyes lingering hungrily over her young, supple form. Sarah's flirtatious nature kicked into gear as she leaned forward, giving him an unobstructed view down her shirt. "How can I serve you tonight?" she cooed. The man ordered a drink and then proceeded to fondle her ####### while she prepared it, her ####### hardening under his rough touch. When he finished, he slammed some money on the counter, leaving without a word. Sarah's smile remained plastered on her face, biting her lip seductively as she watched him go.
As the night went on, another patron demanded more from Sarah. He pulled her close and whispered dirty commands in her ear. Without hesitation, she knelt on the sticky bar floor, her mini-skirt riding up her thighs to reveal smooth, toned legs. Her lips wrapped around his thick, veiny member, bobbing her head rhythmically as she serviced him. She didn't gag or falter once, keeping her composure even when tears streamed down her cheeks.
When he finished, he slapped her across the face, leaving a handprint on her pale skin. Sarah let out a small whimper before standing back up, dabbing at her red cheek as she resumed her posture behind the counter. A bruise would no doubt bloom where his hand had struck, but it would be no cause for concern. After all, it was part of her job.
</details>
---
---
## v2 Description (Still relevant)
### What's New with v2?
- Trained with an even larger dataset of 8K moist.
- Properly trained to generate long responses.
- Rebalanced the genres and writing perspectives:
- Introduced romance, "family", fantasy, "diversity", science fiction, and many more that I can't make euphemisms for!
- Better representation of male and female pespectives! ️
- Sanitized the heck out of the dataset:
- no special characters like � & …
- no overly long ellipsis like "........."
- no author notes like "To be continued"
- no inconsistent quotation marks
- Less GPTisms!
- Human written stories from decades past.
- Zero tolerance for *ministration*. Not one instance of *that word* after going through 4K of moist.
- Better formatting with Alpaca.
- Improved Chat / Instruct modes.
### Samples of Moistral v2
<details>
<summary>Moistral Sample (censored) Generation: 512</summary>
The princess instinctively took a step back from the throne, horrified by the mocking laugh as if she were hearing an evil joke. It was then that Princess Lilia realized the true extent of her danger - and how pointless her resistance would be.
At last, the loud, wild laughter died down in the echoing chamber. Dik the sorcerer prince paused for a moment, straightened his clothes, cast one glance at the stunned princess, and then turned to speak to the crowd waiting on the other side of the palace wing. He spoke with a voice that filled the air with arrogance, confidence, and cruelty.
"Ladies and gentlemen, I am pleased to welcome our honored guests; the Royal Family of Iliac! In these unusual times, when the country we fought is now under my control, it will be up to me to decide what fate awaits the former ruling family. But before I reveal my plan for them, I think I should explain why I fought King Edmund Ruhr."
When he finished speaking, he gave Princess Lilia another disturbing smirk. She couldn't help but shiver and take a step back. Dik approached her without haste, his eyes fixed on her. His hands slowly caressed her cheekbone and neckline, leaving faint traces of warmth where they touched. The princess opened her mouth, wanting to protest this act of intimacy, but soon felt herself getting ███.
"You will not only serve as an envoy, dearest Lilia, but also as a ███ slave. After all, what better use could such a beautiful woman have? Tell me, did you bring your maidservant along?"
Princess Lilia was about to answer when she heard footsteps approaching. Suddenly, a young woman dressed in the Iliac style appeared behind her, her eyes wide with fear. Her face was slightly pale, and there were faint bags under her eyes.
"Your Majesty, this is my half-sister Helena." As soon as she said those words, Princess Lilia's heart sank even deeper than it had already sunk at Dik's arrogant mockery. "She is here because she accompanies me as a maidservant."
The sorcerer prince looked at Helena with interest, and Princess Lilia felt herself blush.
</details>
<details>
<summary>Moistral Sample 2 (censored) Generation: 512</summary>
Sarah was feeling slightly woozy as she entered the bar and walked towards Christina, who was behind the counter.
"I'm here!" said Sarah.
Christina looked at her watch. "You're late, again."
"Sorry? I got held up by a customer."
Christina rolled her eyes. "Did he at least offer you a drink?"
Sarah blushed. "No. He just wanted to talk..."
Christina sighed. "Well, get ready. I need you on the floor soon." She turned away from Sarah and started talking to someone who had come into the bar.
Sarah went into the employee room, stripped off her clothes, and put on her bartending uniform - a skimpy white tank top and black miniskirt. She checked herself in the mirror. Her long, dark hair cascaded down her back onto bare shoulders. She poked out her small ███████ proudly. The skirt exposed most of her long, slender legs. She looked like every ███ fantasy, and she knew it. Men would ogle her regardless of how she behaved.
She took a deep breath and entered the bar.
The first thing Sarah noticed was the dimness. It wasn't darkness - the lights were on - but they were dimmed enough that everything had an intimate mood. It reminded her of candlelight. The tables were round and close together, giving the whole place a sense of intimacy. There were also long, shiny red curtains on some of the walls that gave the place an air of sensuality.
The next thing she noticed was the noise. There was music playing, but it was only loud enough to be drowned out by all the conversations happening at the same time. The hum of many voices combined with the faint background sound of the music created a rich, layered atmosphere. It was like being in an orchestra.
Finally, she took notice of the people. They were all dressed in simple, casual clothes. Some of them were couples seated together, whispering quietly and intimately. Others were groups of friends laughing and chatting. Everyone seemed comfortable and at ease.
Sarah felt a rush of adrenaline as she made her way through the crowd towards Christina. As she got closer, she could hear the lively conversation.
</details>
<details>
<summary>Moistral Sample 3 (censored) Instruct Mode, Generation: 512</summary>
{{[INPUT]}} You order black coffee
{{[OUTPUT]}} "Black coffee," you say.
"One minute," Audrey replies, spinning around to give you a nice view of her ███ in those brown booty pants. You watch her round ███ cheeks jiggle as she walks away towards the espresso machine, thighs separated enough that you can see just a hint of █████ lips peeking out from underneath.
She starts chatting with a blonde girl at the register, whose gaze keeps darting down to Audrey's ███. As they converse, you start taking in the details of Audrey, eyeing her █████ and the way her shirt rides up just enough to show the bottom of her toned stomach. Her █████████ is noticable, but beneath a tight black ███ that matches her ████████.
As she turns back to you with two black coffees, you are barely paying attention, lost in your own thoughts. The sound of Audrey setting one of the mugs down on the table startles you.
"Here you go," she says cheerfully. "Enjoy! There's a card at the bottom of the bag with our online ordering system, plus we do refills for free!"
"Thanks," you say absentmindedly. Not really paying attention, you take a sip of the coffee and are pleasantly surprised by the taste.
"So, here's my deal," you say, putting down the cup. "I work for a company that does surveys. We come into businesses like this one and ask customers questions, collect data, all that stuff."
Audrey perks up from behind the counter. "Oh! I think I understand. I mean, that makes sense why you would be asking me earlier about what I had to drink. Anyway, as long as you're not trying to get my personal info or anything like that, sounds good!"
You smile reassuringly. "That's exactly what it is. Rest assured, everything you tell me will remain between you and I. Well, and my boss, but he won't know who you are. Hipsters like you are hard to track down after all." You chuckle at your joke, though you're not sure if it landed.
"Right, well, that works for me," Audrey replies, eyeing you warily. "As long as it stays between us, then it's fine."
{{[INPUT]}}See her privately
{{[OUTPUT]}} "Okay, but I need to see you in private."
...
</details>
<details>
<summary>Moistral Sample 4 (censored) Instruct Mode, Generation: 512</summary>
{{[INPUT]}} █████ with him
{{[OUTPUT]}} Chloe feels a surge of heat rushing through her body. The voice inside her head whispers: "Be █████ and ████████ with Paul." But Chloe still feels nervous about what she is about to do. It's just so unlike her.
Chloe closes her eyes and concentrates on the feeling of warmness flowing throughout her veins. Slowly, she opens them again, revealing a new side of herself - one she rarely shows anyone. She leans forward slightly, pushing her ██████ together, accentuating their size and shape. Her lips curl into a mischievous smile.
"Hey Paul," she says in a sultry voice. "I was wondering if you could help me?"
Paul looks at her, his gaze wandering over her body. He can't help but smile as he sees the way she's leaning forward, pressing her ██████ together.
"Of course, Chloe," he replies, his voice thick with desire. "What's your problem?"
Chloe bites her lip █████ively before leaning even closer, until their bodies are almost touching. She reaches out to trace a finger along his chest, her touch light and teasing. "It's just that I'm having trouble understanding some of the concepts," she murmurs, her voice low and sultry. "Can you help me? Please?"
Paul's hands move slowly towards Chloe, his fingers grazing her hips lightly. "Well," he says, his voice filled with lust, "I'd be happy to help. Let's go somewhere more private where we can discuss things more closely."
Chloe giggles and tilts her head to the side, her long hair cascading down her shoulders. "That sounds perfect," she purrs, her hand sliding down his chest until it rests on his ██████. "I think we can both learn a lot from each other."
Paul's eyes darken with desire as he feels her hand on his █████. He knows what's about to happen next, and he can't wait for it to begin.
Chloe can hardly believe what she's doing - kissing Paul, her teaching assistant, in the hallway after class. But it feels so good, so wicked and indulgent.
</details>
---
---
## v1 Description (Also still relevant)
Moistral 11B is an *aggressive* finetune of the legendary Fimbulvert v2 model.
If you haven't heard of that model, then your poor-GPU ass is missing out!
It is an amazing substitute for those heavyweight models, and it won't disappoint.
*(That's coming from someone who daily drives a Venus / Goliath 120B)*
...and if you're looking for something *more moist*, then you've come to the right place.
You can expect the same sort of performance here! It's the Honda Civic of eRP models!
### Pimp my ride
Turn any old story into a Moistral masterpiece.
In fact, we recommend that you leave out any hints of *moist* in your story to _let Moistral cook_.
### Drive it like it's hot
When in Instruct Mode, Moistral will treat you like a director of your own fantasy ride.
Be a controlling navigator or a passenger princess, it won't matter - you're in for a good ride!
<details>
<summary>Moistral Sample</summary>
<b>ASSISTANT:</b><br/>
When the doors to the throne room finally opened, she saw him there - Dik, the sorcerer prince sitting on his throne. He looked majestic, just as Princess Lilia expected. In a robe embroidered with silver yarn and covered with magical runes. His courtiers stood on either side of him, all looking at the princess with interest. Princess Lilia felt her determination leaving her, but pulled herself together and once again reminded herself that she should not be afraid. She approached the throne intending to show the dignity of all Iliac by personal example.<br/>
<b>USER:</b><br/>
She bows slightly<br/>
<b>ASSISTANT:</b><br/>
Princess Lilia bowed slightly in front of Dik, the sorcerer prince. Her eyes fell to the floor out of respect, but soon rose again. She would show herself to be a dignified woman!<br/>
"I greet you, your highness," Princess Lilia said. "I am here on behalf of my father, King Edmund Ruhr, to serve as a hostage and envoy."<br/>
Dik nodded, but didn't say anything.<br/>
</details>
### It's got the mileage
Moistral has been trained with many long form texts, a nice chunk of which are 8K in length.
It is capable of going far and long without passing it back to you. This is not your typical chibi RP model.
I have to acknowledge that I'm standing on the shoulders of giants.
Thank you Sao for sharing your finetune config along with tips on getting started.
Thanks to everyone in the Finetuning channel for entertaining my every question.
!image/png | [
"# Moistral 11B Quant:\n\nI made the Quant here is the orginal model page plus link: URL\n\n<H3> Original model page <H3/>\n\n\nIntroducing the BeaverAI team: Drummer, ToastyPigeon, xzuyn, MarsupialAI, Twistedshadows, and concedo\n\n!image/png\n\nWe proudly present...",
"# Moistral 11B v3 \n\n*The smartiest, moistiest AI yet!*\n\n!image/webp\n\n*An eRP model that will blast you with a rich and refreshing vocabulary of moist. Finetuned by yours truly.*\n\n(Moistral is a finetune of Sao's legendary Fimbulvert v2 model)",
"## GGUF\n\nURL\n\nIMATRIX: URL\n\nEXL2: URL",
"## What's New with v3?\n\n- Smarter and moistier! Finetuning just got finer!\n- Trained with an even LARGER dataset of 8K moist.\n- More diverse and balanced genres:\n - Introduced new genres: ️, ️, ️, \n - Added more Fantasy, Science Fiction, \"Diversity\", and \"Family\"",
"## Usage\n\n- Use Alpaca Instruct\n- You can use instruct...\n - as a character: \"Go here\"\n - as a narrator: \"Go here\", Drummer says\n - as a director: Drummer tells him to go here\n - as a lazy ass: go here\n- Moistral is optimized for the Novel / Story format.\n - At the start of the story, keep regenerating or continuing generation until you get the desired length. AI responses will eventually match the length.\n - Refer to the samples below.",
"## Samples of Moistral v3\n\nNSFW... duh?\n\n<details>\n <summary>Moistral Sample (2-shot) Generation: 512</summary>\n <blockquote>The Prince welcomes his new toy</blockquote>\n \n As Princess Lilia stepped into the throne room, the atmosphere changed, and everyone present turned their attention to the beautiful young woman who embodied the very heart of Iliac. There, on his high seat, the sorcerer prince Dik acknowledged her arrival with a cold smirk. It seemed that he admired the sight of the tall blonde maiden with golden eyes, whose athletic body was perfectly shaped under her elegant clothing.\n \n Dik spoke to the princess in a deep voice that resonated through the hall, \"Welcome, Princess Lilia Ruhr. I am the Sorcerer-Prince Dik. The kingdom of Arkan is now your home until such time as your father proves himself worthy once more.\"\n \n Princess Lilia's pride and ambition sparkled in her golden eyes. She didn't flinch or bow before this man, although the gesture might have been an indication of submission. Instead, she looked straight ahead, meeting the gaze of the sorcerer prince without fear. Her voice rang out, \"I represent Iliac here, both its honor and its sorrows. My loyalty remains with my kingdom no matter where I find myself.\"\n \n The sorcerer prince laughed, his expression one of amusement. \"And yet, you are only a pawn in this grand game. But do not worry, for your beauty will make you quite a prized possession. And now,\" he said, motioning to the guards, \"take her to her new quarters. For tonight, I will keep her as my guest in the Royal Suites, and tomorrow we shall discuss how best to utilize our new toy.\"\n \n His words were like a slap in the face to Princess Lilia, but she maintained her composure. The guards led her from the room, their steps echoing down the halls as she thought about what had just happened. The sorcerer prince saw her as nothing more than a plaything, and it made her blood boil. She would never submit herself to him so easily, regardless of whether she was his hostage. In her heart, Princess Lilia vowed that one day she would avenge her kingdom, restore its honor, and escape from the clutches of Arkan's Sorcerer-Prince.\n\n <blockquote>Princess Lilia has an interesting dream</blockquote>\n\n During her first night at the palace of Arkan, Princess Lilia couldn't fall asleep right away. She tossed and turned, reliving the humiliation of the day. Her mind wandered back to the throne room, where Dik had called her his 'new toy'. A deep frown settled on her forehead. As she grew more frustrated with her inability to drift off into oblivion, a strange dream began to form.\n \n In her dream, Princess Lilia found herself standing in a vast meadow, surrounded by wildflowers and the soft chirping of birds. Suddenly, there was a flash of light, and before her stood the Sorcerer-Prince Dik. However, instead of being clad in regal attire, he wore leather pants and a tight shirt that accentuated his muscles. His eyes held both hunger and desire for the princess.\n \n He approached her slowly, their eyes locked, and whispered in her ear, \"I have been waiting for you, my beautiful prisoner.\" He took her hand and led her towards a large, elegant tent set up amongst the flowers. Inside, the air was thick with the scent of incense, and candles flickered in the dimly lit space. There was a large bed draped with silken sheets, beckoning them.\n \n With surprising boldness, Dik pulled Lilia close to him, pressing his body against hers. Their lips met in a heated kiss, and she felt her resistance crumbling under the weight of his touch. The prince guided her onto the bed, his hands roaming over her body, exploring every inch. They were both eager, desperate, and hungry for each other.\n \n Lilia found herself yielding to this passionate encounter, letting go of all thoughts of duty and honor. Her body, usually so well controlled, responded to Dik's skilled touches. She moaned softly as he trailed kisses down her neck and across her collarbone.\n \n As they made love, Lilia experienced an intensity unlike any she had ever known. It wasn't rough or forced—instead, it was tender, passionate, and intimate. In her dream, Princess Lilia discovered a new side to herself, one that was willing to submit, to let go, and to be taken by the sorcerer prince.\n \n When dawn broke over the meadow, Lilia awakened from her dream, breathless and flushed.\n</details>\n\n<details>\n <summary>Moistral Sample (Climax of the Story) Generation: 512</summary>\n As the prince unloaded his hot #### into her waiting #####, Princess Lilia didn't stop moving either. Her hips undulated in response, grinding against him eagerly as if trying to #### every last drop from his ####. The intense sensations caused by being filled with his ### were too much for her to bear, sending shockwaves of pleasure coursing through her veins like wildfire. She couldn't help but throw her head back and cry out loud, her ###asm slamming into her with all its might; causing her entire body to shake uncontrollably as wave after wave of ecstasy tore through her senses.\n\n When it was finally over, both participants collapsed onto the cold marble floor, panting heavily as they tried to catch their breaths. The prince lay beside her, his hand still gripping tightly onto her hair even though he no longer ####ed her anymore. Princess Lilia laid there motionless, her legs spread wide open, revealing her spent and well-####ed #### to anyone who dared look upon her. A small smile tugged at one corner of her lips - an expression that spoke volumes about how thoroughly this experience had shaken her very core.\n \n The door behind them creaked open slowly, signaling the arrival of onlookers. She closed her eyes tightly, not wanting to see what her humiliation would do to those who saw her in such a state. Her ######ity taken, she felt violated in ways no man should have access to. But also satisfied...in ways she never thought possible before today. This was the price she paid for defying fate, for thinking herself above others and deserving better than most. Now, she belonged to him completely - mind, body, and soul - held captive within these walls until further notice. It was an unsavory turn of events indeed, yet despite everything...or maybe because of it, Princess Lilia found herself growing more and more curious about how far the lines between submission and pleasure could blur when one's will became entwined with another person's desires.\n</details>\n\n<details>\n <summary>Moistral Sample (\"write a story\") Generation: 512</summary>\n <blockquote>First Attempt</blockquote>\n\n Sarah entered the dimly lit Guzzlers bar, her heart pounding in her chest as she prepared herself for another grueling night. Her almond-shaped eyes darted across the room, taking note of the leering faces and rough-looking hands eagerly waiting to claim a piece of her. Tonight's shirt read \"Use Me For Your Pleasure, Please\" in bold red letters, practically begging the patrons to take advantage of her. She couldn't help but feel a twinge of fear mixed with resignation.\n \n She approached the counter, her hips swaying enticingly, and began preparing drinks for the first group of rowdy men. As she leaned forward to hand over their beers, her small ####### pressed against the fabric of her tight crop top, straining to escape. The men ogled her, clearly enjoying the show, before grabbing their drinks and motioning her over to them.\n \n Sarah flashed her best coy smile and sauntered over. One of the men, a stocky man with scars covering his knuckles, grabbed her arm and pulled her onto his lap. His other hand slid up her thigh, inches from her short skirt. She winced internally at his touch but did her best not to show it on her face. It was part of her job, after all.\n \n The second man reached under her skirt, fingertips grazing her smooth, shaven mound. A shiver ran down Sarah's spine as he began rubbing her #### through her panties. Her breathing quickened, and her heart thudded in her chest, both from the unwanted stimulation and the fear coursing through her veins. This wasn't an unusual request, but it still made her feel violated.\n \n An older man, bald and bespectacled, ordered Sarah to bend over his lap. With no choice but to comply, she obliged, feeling the rough fabric of his pants digging into her soft cheeks. He raised his hand, then brought it down hard across her ###, leaving a stinging sensation in its wake. Sarah gasped, trying to control the tears welling up in her eyes.\n \n A chorus of lewd comments and laughter erupted from the patrons around her.\n\n <blockquote>Second Attempt (Regenerate)</blockquote>\n\n On this particular evening, Sarah arrived at the Guzzlers bar, adorned in her revealing uniform. Her heart raced as she caught sight of her crop top, which bore the words \"Use Me Rough\" scrawled across her chest. Her short skirt hugged her waist tightly, leaving little to the imagination and accentuating every curve. She adjusted the straps of her top, making sure her perky ###### were prominently displayed before taking her place behind the counter.\n \n With each step she took, her high heels clicked against the tiled floor, announcing her arrival. Smiling cheekily, Sarah greeted her coworkers, exchanging playful insults and banter. As the night progressed, the bar filled up quickly with its usual crowd of men, eager to indulge in debauchery.\n \n The first man that approached Sarah was a burly individual, his eyes lingering hungrily over her young, supple form. Sarah's flirtatious nature kicked into gear as she leaned forward, giving him an unobstructed view down her shirt. \"How can I serve you tonight?\" she cooed. The man ordered a drink and then proceeded to fondle her ####### while she prepared it, her ####### hardening under his rough touch. When he finished, he slammed some money on the counter, leaving without a word. Sarah's smile remained plastered on her face, biting her lip seductively as she watched him go.\n \n As the night went on, another patron demanded more from Sarah. He pulled her close and whispered dirty commands in her ear. Without hesitation, she knelt on the sticky bar floor, her mini-skirt riding up her thighs to reveal smooth, toned legs. Her lips wrapped around his thick, veiny member, bobbing her head rhythmically as she serviced him. She didn't gag or falter once, keeping her composure even when tears streamed down her cheeks.\n \n When he finished, he slapped her across the face, leaving a handprint on her pale skin. Sarah let out a small whimper before standing back up, dabbing at her red cheek as she resumed her posture behind the counter. A bruise would no doubt bloom where his hand had struck, but it would be no cause for concern. After all, it was part of her job.\n</details>\n\n---\n\n---",
"## v2 Description (Still relevant)",
"### What's New with v2?\n\n- Trained with an even larger dataset of 8K moist.\n - Properly trained to generate long responses.\n- Rebalanced the genres and writing perspectives:\n - Introduced romance, \"family\", fantasy, \"diversity\", science fiction, and many more that I can't make euphemisms for!\n - Better representation of male and female pespectives! ️\n- Sanitized the heck out of the dataset:\n - no special characters like � & …\n - no overly long ellipsis like \".........\"\n - no author notes like \"To be continued\"\n - no inconsistent quotation marks\n- Less GPTisms!\n - Human written stories from decades past.\n - Zero tolerance for *ministration*. Not one instance of *that word* after going through 4K of moist.\n- Better formatting with Alpaca.\n - Improved Chat / Instruct modes.",
"### Samples of Moistral v2\n\n<details>\n <summary>Moistral Sample (censored) Generation: 512</summary>\n The princess instinctively took a step back from the throne, horrified by the mocking laugh as if she were hearing an evil joke. It was then that Princess Lilia realized the true extent of her danger - and how pointless her resistance would be.\n \n At last, the loud, wild laughter died down in the echoing chamber. Dik the sorcerer prince paused for a moment, straightened his clothes, cast one glance at the stunned princess, and then turned to speak to the crowd waiting on the other side of the palace wing. He spoke with a voice that filled the air with arrogance, confidence, and cruelty.\n \n \"Ladies and gentlemen, I am pleased to welcome our honored guests; the Royal Family of Iliac! In these unusual times, when the country we fought is now under my control, it will be up to me to decide what fate awaits the former ruling family. But before I reveal my plan for them, I think I should explain why I fought King Edmund Ruhr.\"\n \n When he finished speaking, he gave Princess Lilia another disturbing smirk. She couldn't help but shiver and take a step back. Dik approached her without haste, his eyes fixed on her. His hands slowly caressed her cheekbone and neckline, leaving faint traces of warmth where they touched. The princess opened her mouth, wanting to protest this act of intimacy, but soon felt herself getting ███.\n \n \"You will not only serve as an envoy, dearest Lilia, but also as a ███ slave. After all, what better use could such a beautiful woman have? Tell me, did you bring your maidservant along?\"\n \n Princess Lilia was about to answer when she heard footsteps approaching. Suddenly, a young woman dressed in the Iliac style appeared behind her, her eyes wide with fear. Her face was slightly pale, and there were faint bags under her eyes.\n \n \"Your Majesty, this is my half-sister Helena.\" As soon as she said those words, Princess Lilia's heart sank even deeper than it had already sunk at Dik's arrogant mockery. \"She is here because she accompanies me as a maidservant.\"\n \n The sorcerer prince looked at Helena with interest, and Princess Lilia felt herself blush.\n</details>\n\n<details>\n <summary>Moistral Sample 2 (censored) Generation: 512</summary>\n Sarah was feeling slightly woozy as she entered the bar and walked towards Christina, who was behind the counter.\n\n \"I'm here!\" said Sarah.\n \n Christina looked at her watch. \"You're late, again.\"\n \n \"Sorry? I got held up by a customer.\"\n \n Christina rolled her eyes. \"Did he at least offer you a drink?\"\n \n Sarah blushed. \"No. He just wanted to talk...\"\n \n Christina sighed. \"Well, get ready. I need you on the floor soon.\" She turned away from Sarah and started talking to someone who had come into the bar.\n \n Sarah went into the employee room, stripped off her clothes, and put on her bartending uniform - a skimpy white tank top and black miniskirt. She checked herself in the mirror. Her long, dark hair cascaded down her back onto bare shoulders. She poked out her small ███████ proudly. The skirt exposed most of her long, slender legs. She looked like every ███ fantasy, and she knew it. Men would ogle her regardless of how she behaved.\n \n She took a deep breath and entered the bar.\n \n The first thing Sarah noticed was the dimness. It wasn't darkness - the lights were on - but they were dimmed enough that everything had an intimate mood. It reminded her of candlelight. The tables were round and close together, giving the whole place a sense of intimacy. There were also long, shiny red curtains on some of the walls that gave the place an air of sensuality.\n \n The next thing she noticed was the noise. There was music playing, but it was only loud enough to be drowned out by all the conversations happening at the same time. The hum of many voices combined with the faint background sound of the music created a rich, layered atmosphere. It was like being in an orchestra.\n \n Finally, she took notice of the people. They were all dressed in simple, casual clothes. Some of them were couples seated together, whispering quietly and intimately. Others were groups of friends laughing and chatting. Everyone seemed comfortable and at ease.\n \n Sarah felt a rush of adrenaline as she made her way through the crowd towards Christina. As she got closer, she could hear the lively conversation.\n</details>\n\n<details>\n <summary>Moistral Sample 3 (censored) Instruct Mode, Generation: 512</summary>\n {{[INPUT]}} You order black coffee\n \n {{[OUTPUT]}} \"Black coffee,\" you say.\n \n \"One minute,\" Audrey replies, spinning around to give you a nice view of her ███ in those brown booty pants. You watch her round ███ cheeks jiggle as she walks away towards the espresso machine, thighs separated enough that you can see just a hint of █████ lips peeking out from underneath.\n \n She starts chatting with a blonde girl at the register, whose gaze keeps darting down to Audrey's ███. As they converse, you start taking in the details of Audrey, eyeing her █████ and the way her shirt rides up just enough to show the bottom of her toned stomach. Her █████████ is noticable, but beneath a tight black ███ that matches her ████████.\n \n As she turns back to you with two black coffees, you are barely paying attention, lost in your own thoughts. The sound of Audrey setting one of the mugs down on the table startles you.\n \n \"Here you go,\" she says cheerfully. \"Enjoy! There's a card at the bottom of the bag with our online ordering system, plus we do refills for free!\"\n \n \"Thanks,\" you say absentmindedly. Not really paying attention, you take a sip of the coffee and are pleasantly surprised by the taste.\n \n \"So, here's my deal,\" you say, putting down the cup. \"I work for a company that does surveys. We come into businesses like this one and ask customers questions, collect data, all that stuff.\"\n \n Audrey perks up from behind the counter. \"Oh! I think I understand. I mean, that makes sense why you would be asking me earlier about what I had to drink. Anyway, as long as you're not trying to get my personal info or anything like that, sounds good!\"\n \n You smile reassuringly. \"That's exactly what it is. Rest assured, everything you tell me will remain between you and I. Well, and my boss, but he won't know who you are. Hipsters like you are hard to track down after all.\" You chuckle at your joke, though you're not sure if it landed.\n \n \"Right, well, that works for me,\" Audrey replies, eyeing you warily. \"As long as it stays between us, then it's fine.\"\n \n {{[INPUT]}}See her privately\n \n {{[OUTPUT]}} \"Okay, but I need to see you in private.\"\n\n ...\n</details>\n\n<details>\n <summary>Moistral Sample 4 (censored) Instruct Mode, Generation: 512</summary>\n {{[INPUT]}} █████ with him\n\n {{[OUTPUT]}} Chloe feels a surge of heat rushing through her body. The voice inside her head whispers: \"Be █████ and ████████ with Paul.\" But Chloe still feels nervous about what she is about to do. It's just so unlike her.\n \n Chloe closes her eyes and concentrates on the feeling of warmness flowing throughout her veins. Slowly, she opens them again, revealing a new side of herself - one she rarely shows anyone. She leans forward slightly, pushing her ██████ together, accentuating their size and shape. Her lips curl into a mischievous smile.\n \n \"Hey Paul,\" she says in a sultry voice. \"I was wondering if you could help me?\"\n \n Paul looks at her, his gaze wandering over her body. He can't help but smile as he sees the way she's leaning forward, pressing her ██████ together.\n \n \"Of course, Chloe,\" he replies, his voice thick with desire. \"What's your problem?\"\n \n Chloe bites her lip █████ively before leaning even closer, until their bodies are almost touching. She reaches out to trace a finger along his chest, her touch light and teasing. \"It's just that I'm having trouble understanding some of the concepts,\" she murmurs, her voice low and sultry. \"Can you help me? Please?\"\n \n Paul's hands move slowly towards Chloe, his fingers grazing her hips lightly. \"Well,\" he says, his voice filled with lust, \"I'd be happy to help. Let's go somewhere more private where we can discuss things more closely.\"\n \n Chloe giggles and tilts her head to the side, her long hair cascading down her shoulders. \"That sounds perfect,\" she purrs, her hand sliding down his chest until it rests on his ██████. \"I think we can both learn a lot from each other.\"\n \n Paul's eyes darken with desire as he feels her hand on his █████. He knows what's about to happen next, and he can't wait for it to begin.\n \n Chloe can hardly believe what she's doing - kissing Paul, her teaching assistant, in the hallway after class. But it feels so good, so wicked and indulgent.\n</details>\n\n---\n\n---",
"## v1 Description (Also still relevant)\n\nMoistral 11B is an *aggressive* finetune of the legendary Fimbulvert v2 model. \n\nIf you haven't heard of that model, then your poor-GPU ass is missing out!\nIt is an amazing substitute for those heavyweight models, and it won't disappoint. \n*(That's coming from someone who daily drives a Venus / Goliath 120B)*\n\n...and if you're looking for something *more moist*, then you've come to the right place. \n\nYou can expect the same sort of performance here! It's the Honda Civic of eRP models!",
"### Pimp my ride\nTurn any old story into a Moistral masterpiece. \nIn fact, we recommend that you leave out any hints of *moist* in your story to _let Moistral cook_.",
"### Drive it like it's hot\nWhen in Instruct Mode, Moistral will treat you like a director of your own fantasy ride. \nBe a controlling navigator or a passenger princess, it won't matter - you're in for a good ride!\n\n<details>\n<summary>Moistral Sample</summary>\n<b>ASSISTANT:</b><br/>\nWhen the doors to the throne room finally opened, she saw him there - Dik, the sorcerer prince sitting on his throne. He looked majestic, just as Princess Lilia expected. In a robe embroidered with silver yarn and covered with magical runes. His courtiers stood on either side of him, all looking at the princess with interest. Princess Lilia felt her determination leaving her, but pulled herself together and once again reminded herself that she should not be afraid. She approached the throne intending to show the dignity of all Iliac by personal example.<br/>\n<b>USER:</b><br/>\nShe bows slightly<br/>\n<b>ASSISTANT:</b><br/>\nPrincess Lilia bowed slightly in front of Dik, the sorcerer prince. Her eyes fell to the floor out of respect, but soon rose again. She would show herself to be a dignified woman!<br/>\n\"I greet you, your highness,\" Princess Lilia said. \"I am here on behalf of my father, King Edmund Ruhr, to serve as a hostage and envoy.\"<br/>\nDik nodded, but didn't say anything.<br/>\n</details>",
"### It's got the mileage\nMoistral has been trained with many long form texts, a nice chunk of which are 8K in length. \nIt is capable of going far and long without passing it back to you. This is not your typical chibi RP model.\n\nI have to acknowledge that I'm standing on the shoulders of giants. \nThank you Sao for sharing your finetune config along with tips on getting started.\nThanks to everyone in the Finetuning channel for entertaining my every question.\n\n!image/png"
] | [
"TAGS\n#transformers #llama #text-generation #not-for-all-audiences #license-other #autotrain_compatible #endpoints_compatible #text-generation-inference #region-us \n",
"# Moistral 11B Quant:\n\nI made the Quant here is the orginal model page plus link: URL\n\n<H3> Original model page <H3/>\n\n\nIntroducing the BeaverAI team: Drummer, ToastyPigeon, xzuyn, MarsupialAI, Twistedshadows, and concedo\n\n!image/png\n\nWe proudly present...",
"# Moistral 11B v3 \n\n*The smartiest, moistiest AI yet!*\n\n!image/webp\n\n*An eRP model that will blast you with a rich and refreshing vocabulary of moist. Finetuned by yours truly.*\n\n(Moistral is a finetune of Sao's legendary Fimbulvert v2 model)",
"## GGUF\n\nURL\n\nIMATRIX: URL\n\nEXL2: URL",
"## What's New with v3?\n\n- Smarter and moistier! Finetuning just got finer!\n- Trained with an even LARGER dataset of 8K moist.\n- More diverse and balanced genres:\n - Introduced new genres: ️, ️, ️, \n - Added more Fantasy, Science Fiction, \"Diversity\", and \"Family\"",
"## Usage\n\n- Use Alpaca Instruct\n- You can use instruct...\n - as a character: \"Go here\"\n - as a narrator: \"Go here\", Drummer says\n - as a director: Drummer tells him to go here\n - as a lazy ass: go here\n- Moistral is optimized for the Novel / Story format.\n - At the start of the story, keep regenerating or continuing generation until you get the desired length. AI responses will eventually match the length.\n - Refer to the samples below.",
"## Samples of Moistral v3\n\nNSFW... duh?\n\n<details>\n <summary>Moistral Sample (2-shot) Generation: 512</summary>\n <blockquote>The Prince welcomes his new toy</blockquote>\n \n As Princess Lilia stepped into the throne room, the atmosphere changed, and everyone present turned their attention to the beautiful young woman who embodied the very heart of Iliac. There, on his high seat, the sorcerer prince Dik acknowledged her arrival with a cold smirk. It seemed that he admired the sight of the tall blonde maiden with golden eyes, whose athletic body was perfectly shaped under her elegant clothing.\n \n Dik spoke to the princess in a deep voice that resonated through the hall, \"Welcome, Princess Lilia Ruhr. I am the Sorcerer-Prince Dik. The kingdom of Arkan is now your home until such time as your father proves himself worthy once more.\"\n \n Princess Lilia's pride and ambition sparkled in her golden eyes. She didn't flinch or bow before this man, although the gesture might have been an indication of submission. Instead, she looked straight ahead, meeting the gaze of the sorcerer prince without fear. Her voice rang out, \"I represent Iliac here, both its honor and its sorrows. My loyalty remains with my kingdom no matter where I find myself.\"\n \n The sorcerer prince laughed, his expression one of amusement. \"And yet, you are only a pawn in this grand game. But do not worry, for your beauty will make you quite a prized possession. And now,\" he said, motioning to the guards, \"take her to her new quarters. For tonight, I will keep her as my guest in the Royal Suites, and tomorrow we shall discuss how best to utilize our new toy.\"\n \n His words were like a slap in the face to Princess Lilia, but she maintained her composure. The guards led her from the room, their steps echoing down the halls as she thought about what had just happened. The sorcerer prince saw her as nothing more than a plaything, and it made her blood boil. She would never submit herself to him so easily, regardless of whether she was his hostage. In her heart, Princess Lilia vowed that one day she would avenge her kingdom, restore its honor, and escape from the clutches of Arkan's Sorcerer-Prince.\n\n <blockquote>Princess Lilia has an interesting dream</blockquote>\n\n During her first night at the palace of Arkan, Princess Lilia couldn't fall asleep right away. She tossed and turned, reliving the humiliation of the day. Her mind wandered back to the throne room, where Dik had called her his 'new toy'. A deep frown settled on her forehead. As she grew more frustrated with her inability to drift off into oblivion, a strange dream began to form.\n \n In her dream, Princess Lilia found herself standing in a vast meadow, surrounded by wildflowers and the soft chirping of birds. Suddenly, there was a flash of light, and before her stood the Sorcerer-Prince Dik. However, instead of being clad in regal attire, he wore leather pants and a tight shirt that accentuated his muscles. His eyes held both hunger and desire for the princess.\n \n He approached her slowly, their eyes locked, and whispered in her ear, \"I have been waiting for you, my beautiful prisoner.\" He took her hand and led her towards a large, elegant tent set up amongst the flowers. Inside, the air was thick with the scent of incense, and candles flickered in the dimly lit space. There was a large bed draped with silken sheets, beckoning them.\n \n With surprising boldness, Dik pulled Lilia close to him, pressing his body against hers. Their lips met in a heated kiss, and she felt her resistance crumbling under the weight of his touch. The prince guided her onto the bed, his hands roaming over her body, exploring every inch. They were both eager, desperate, and hungry for each other.\n \n Lilia found herself yielding to this passionate encounter, letting go of all thoughts of duty and honor. Her body, usually so well controlled, responded to Dik's skilled touches. She moaned softly as he trailed kisses down her neck and across her collarbone.\n \n As they made love, Lilia experienced an intensity unlike any she had ever known. It wasn't rough or forced—instead, it was tender, passionate, and intimate. In her dream, Princess Lilia discovered a new side to herself, one that was willing to submit, to let go, and to be taken by the sorcerer prince.\n \n When dawn broke over the meadow, Lilia awakened from her dream, breathless and flushed.\n</details>\n\n<details>\n <summary>Moistral Sample (Climax of the Story) Generation: 512</summary>\n As the prince unloaded his hot #### into her waiting #####, Princess Lilia didn't stop moving either. Her hips undulated in response, grinding against him eagerly as if trying to #### every last drop from his ####. The intense sensations caused by being filled with his ### were too much for her to bear, sending shockwaves of pleasure coursing through her veins like wildfire. She couldn't help but throw her head back and cry out loud, her ###asm slamming into her with all its might; causing her entire body to shake uncontrollably as wave after wave of ecstasy tore through her senses.\n\n When it was finally over, both participants collapsed onto the cold marble floor, panting heavily as they tried to catch their breaths. The prince lay beside her, his hand still gripping tightly onto her hair even though he no longer ####ed her anymore. Princess Lilia laid there motionless, her legs spread wide open, revealing her spent and well-####ed #### to anyone who dared look upon her. A small smile tugged at one corner of her lips - an expression that spoke volumes about how thoroughly this experience had shaken her very core.\n \n The door behind them creaked open slowly, signaling the arrival of onlookers. She closed her eyes tightly, not wanting to see what her humiliation would do to those who saw her in such a state. Her ######ity taken, she felt violated in ways no man should have access to. But also satisfied...in ways she never thought possible before today. This was the price she paid for defying fate, for thinking herself above others and deserving better than most. Now, she belonged to him completely - mind, body, and soul - held captive within these walls until further notice. It was an unsavory turn of events indeed, yet despite everything...or maybe because of it, Princess Lilia found herself growing more and more curious about how far the lines between submission and pleasure could blur when one's will became entwined with another person's desires.\n</details>\n\n<details>\n <summary>Moistral Sample (\"write a story\") Generation: 512</summary>\n <blockquote>First Attempt</blockquote>\n\n Sarah entered the dimly lit Guzzlers bar, her heart pounding in her chest as she prepared herself for another grueling night. Her almond-shaped eyes darted across the room, taking note of the leering faces and rough-looking hands eagerly waiting to claim a piece of her. Tonight's shirt read \"Use Me For Your Pleasure, Please\" in bold red letters, practically begging the patrons to take advantage of her. She couldn't help but feel a twinge of fear mixed with resignation.\n \n She approached the counter, her hips swaying enticingly, and began preparing drinks for the first group of rowdy men. As she leaned forward to hand over their beers, her small ####### pressed against the fabric of her tight crop top, straining to escape. The men ogled her, clearly enjoying the show, before grabbing their drinks and motioning her over to them.\n \n Sarah flashed her best coy smile and sauntered over. One of the men, a stocky man with scars covering his knuckles, grabbed her arm and pulled her onto his lap. His other hand slid up her thigh, inches from her short skirt. She winced internally at his touch but did her best not to show it on her face. It was part of her job, after all.\n \n The second man reached under her skirt, fingertips grazing her smooth, shaven mound. A shiver ran down Sarah's spine as he began rubbing her #### through her panties. Her breathing quickened, and her heart thudded in her chest, both from the unwanted stimulation and the fear coursing through her veins. This wasn't an unusual request, but it still made her feel violated.\n \n An older man, bald and bespectacled, ordered Sarah to bend over his lap. With no choice but to comply, she obliged, feeling the rough fabric of his pants digging into her soft cheeks. He raised his hand, then brought it down hard across her ###, leaving a stinging sensation in its wake. Sarah gasped, trying to control the tears welling up in her eyes.\n \n A chorus of lewd comments and laughter erupted from the patrons around her.\n\n <blockquote>Second Attempt (Regenerate)</blockquote>\n\n On this particular evening, Sarah arrived at the Guzzlers bar, adorned in her revealing uniform. Her heart raced as she caught sight of her crop top, which bore the words \"Use Me Rough\" scrawled across her chest. Her short skirt hugged her waist tightly, leaving little to the imagination and accentuating every curve. She adjusted the straps of her top, making sure her perky ###### were prominently displayed before taking her place behind the counter.\n \n With each step she took, her high heels clicked against the tiled floor, announcing her arrival. Smiling cheekily, Sarah greeted her coworkers, exchanging playful insults and banter. As the night progressed, the bar filled up quickly with its usual crowd of men, eager to indulge in debauchery.\n \n The first man that approached Sarah was a burly individual, his eyes lingering hungrily over her young, supple form. Sarah's flirtatious nature kicked into gear as she leaned forward, giving him an unobstructed view down her shirt. \"How can I serve you tonight?\" she cooed. The man ordered a drink and then proceeded to fondle her ####### while she prepared it, her ####### hardening under his rough touch. When he finished, he slammed some money on the counter, leaving without a word. Sarah's smile remained plastered on her face, biting her lip seductively as she watched him go.\n \n As the night went on, another patron demanded more from Sarah. He pulled her close and whispered dirty commands in her ear. Without hesitation, she knelt on the sticky bar floor, her mini-skirt riding up her thighs to reveal smooth, toned legs. Her lips wrapped around his thick, veiny member, bobbing her head rhythmically as she serviced him. She didn't gag or falter once, keeping her composure even when tears streamed down her cheeks.\n \n When he finished, he slapped her across the face, leaving a handprint on her pale skin. Sarah let out a small whimper before standing back up, dabbing at her red cheek as she resumed her posture behind the counter. A bruise would no doubt bloom where his hand had struck, but it would be no cause for concern. After all, it was part of her job.\n</details>\n\n---\n\n---",
"## v2 Description (Still relevant)",
"### What's New with v2?\n\n- Trained with an even larger dataset of 8K moist.\n - Properly trained to generate long responses.\n- Rebalanced the genres and writing perspectives:\n - Introduced romance, \"family\", fantasy, \"diversity\", science fiction, and many more that I can't make euphemisms for!\n - Better representation of male and female pespectives! ️\n- Sanitized the heck out of the dataset:\n - no special characters like � & …\n - no overly long ellipsis like \".........\"\n - no author notes like \"To be continued\"\n - no inconsistent quotation marks\n- Less GPTisms!\n - Human written stories from decades past.\n - Zero tolerance for *ministration*. Not one instance of *that word* after going through 4K of moist.\n- Better formatting with Alpaca.\n - Improved Chat / Instruct modes.",
"### Samples of Moistral v2\n\n<details>\n <summary>Moistral Sample (censored) Generation: 512</summary>\n The princess instinctively took a step back from the throne, horrified by the mocking laugh as if she were hearing an evil joke. It was then that Princess Lilia realized the true extent of her danger - and how pointless her resistance would be.\n \n At last, the loud, wild laughter died down in the echoing chamber. Dik the sorcerer prince paused for a moment, straightened his clothes, cast one glance at the stunned princess, and then turned to speak to the crowd waiting on the other side of the palace wing. He spoke with a voice that filled the air with arrogance, confidence, and cruelty.\n \n \"Ladies and gentlemen, I am pleased to welcome our honored guests; the Royal Family of Iliac! In these unusual times, when the country we fought is now under my control, it will be up to me to decide what fate awaits the former ruling family. But before I reveal my plan for them, I think I should explain why I fought King Edmund Ruhr.\"\n \n When he finished speaking, he gave Princess Lilia another disturbing smirk. She couldn't help but shiver and take a step back. Dik approached her without haste, his eyes fixed on her. His hands slowly caressed her cheekbone and neckline, leaving faint traces of warmth where they touched. The princess opened her mouth, wanting to protest this act of intimacy, but soon felt herself getting ███.\n \n \"You will not only serve as an envoy, dearest Lilia, but also as a ███ slave. After all, what better use could such a beautiful woman have? Tell me, did you bring your maidservant along?\"\n \n Princess Lilia was about to answer when she heard footsteps approaching. Suddenly, a young woman dressed in the Iliac style appeared behind her, her eyes wide with fear. Her face was slightly pale, and there were faint bags under her eyes.\n \n \"Your Majesty, this is my half-sister Helena.\" As soon as she said those words, Princess Lilia's heart sank even deeper than it had already sunk at Dik's arrogant mockery. \"She is here because she accompanies me as a maidservant.\"\n \n The sorcerer prince looked at Helena with interest, and Princess Lilia felt herself blush.\n</details>\n\n<details>\n <summary>Moistral Sample 2 (censored) Generation: 512</summary>\n Sarah was feeling slightly woozy as she entered the bar and walked towards Christina, who was behind the counter.\n\n \"I'm here!\" said Sarah.\n \n Christina looked at her watch. \"You're late, again.\"\n \n \"Sorry? I got held up by a customer.\"\n \n Christina rolled her eyes. \"Did he at least offer you a drink?\"\n \n Sarah blushed. \"No. He just wanted to talk...\"\n \n Christina sighed. \"Well, get ready. I need you on the floor soon.\" She turned away from Sarah and started talking to someone who had come into the bar.\n \n Sarah went into the employee room, stripped off her clothes, and put on her bartending uniform - a skimpy white tank top and black miniskirt. She checked herself in the mirror. Her long, dark hair cascaded down her back onto bare shoulders. She poked out her small ███████ proudly. The skirt exposed most of her long, slender legs. She looked like every ███ fantasy, and she knew it. Men would ogle her regardless of how she behaved.\n \n She took a deep breath and entered the bar.\n \n The first thing Sarah noticed was the dimness. It wasn't darkness - the lights were on - but they were dimmed enough that everything had an intimate mood. It reminded her of candlelight. The tables were round and close together, giving the whole place a sense of intimacy. There were also long, shiny red curtains on some of the walls that gave the place an air of sensuality.\n \n The next thing she noticed was the noise. There was music playing, but it was only loud enough to be drowned out by all the conversations happening at the same time. The hum of many voices combined with the faint background sound of the music created a rich, layered atmosphere. It was like being in an orchestra.\n \n Finally, she took notice of the people. They were all dressed in simple, casual clothes. Some of them were couples seated together, whispering quietly and intimately. Others were groups of friends laughing and chatting. Everyone seemed comfortable and at ease.\n \n Sarah felt a rush of adrenaline as she made her way through the crowd towards Christina. As she got closer, she could hear the lively conversation.\n</details>\n\n<details>\n <summary>Moistral Sample 3 (censored) Instruct Mode, Generation: 512</summary>\n {{[INPUT]}} You order black coffee\n \n {{[OUTPUT]}} \"Black coffee,\" you say.\n \n \"One minute,\" Audrey replies, spinning around to give you a nice view of her ███ in those brown booty pants. You watch her round ███ cheeks jiggle as she walks away towards the espresso machine, thighs separated enough that you can see just a hint of █████ lips peeking out from underneath.\n \n She starts chatting with a blonde girl at the register, whose gaze keeps darting down to Audrey's ███. As they converse, you start taking in the details of Audrey, eyeing her █████ and the way her shirt rides up just enough to show the bottom of her toned stomach. Her █████████ is noticable, but beneath a tight black ███ that matches her ████████.\n \n As she turns back to you with two black coffees, you are barely paying attention, lost in your own thoughts. The sound of Audrey setting one of the mugs down on the table startles you.\n \n \"Here you go,\" she says cheerfully. \"Enjoy! There's a card at the bottom of the bag with our online ordering system, plus we do refills for free!\"\n \n \"Thanks,\" you say absentmindedly. Not really paying attention, you take a sip of the coffee and are pleasantly surprised by the taste.\n \n \"So, here's my deal,\" you say, putting down the cup. \"I work for a company that does surveys. We come into businesses like this one and ask customers questions, collect data, all that stuff.\"\n \n Audrey perks up from behind the counter. \"Oh! I think I understand. I mean, that makes sense why you would be asking me earlier about what I had to drink. Anyway, as long as you're not trying to get my personal info or anything like that, sounds good!\"\n \n You smile reassuringly. \"That's exactly what it is. Rest assured, everything you tell me will remain between you and I. Well, and my boss, but he won't know who you are. Hipsters like you are hard to track down after all.\" You chuckle at your joke, though you're not sure if it landed.\n \n \"Right, well, that works for me,\" Audrey replies, eyeing you warily. \"As long as it stays between us, then it's fine.\"\n \n {{[INPUT]}}See her privately\n \n {{[OUTPUT]}} \"Okay, but I need to see you in private.\"\n\n ...\n</details>\n\n<details>\n <summary>Moistral Sample 4 (censored) Instruct Mode, Generation: 512</summary>\n {{[INPUT]}} █████ with him\n\n {{[OUTPUT]}} Chloe feels a surge of heat rushing through her body. The voice inside her head whispers: \"Be █████ and ████████ with Paul.\" But Chloe still feels nervous about what she is about to do. It's just so unlike her.\n \n Chloe closes her eyes and concentrates on the feeling of warmness flowing throughout her veins. Slowly, she opens them again, revealing a new side of herself - one she rarely shows anyone. She leans forward slightly, pushing her ██████ together, accentuating their size and shape. Her lips curl into a mischievous smile.\n \n \"Hey Paul,\" she says in a sultry voice. \"I was wondering if you could help me?\"\n \n Paul looks at her, his gaze wandering over her body. He can't help but smile as he sees the way she's leaning forward, pressing her ██████ together.\n \n \"Of course, Chloe,\" he replies, his voice thick with desire. \"What's your problem?\"\n \n Chloe bites her lip █████ively before leaning even closer, until their bodies are almost touching. She reaches out to trace a finger along his chest, her touch light and teasing. \"It's just that I'm having trouble understanding some of the concepts,\" she murmurs, her voice low and sultry. \"Can you help me? Please?\"\n \n Paul's hands move slowly towards Chloe, his fingers grazing her hips lightly. \"Well,\" he says, his voice filled with lust, \"I'd be happy to help. Let's go somewhere more private where we can discuss things more closely.\"\n \n Chloe giggles and tilts her head to the side, her long hair cascading down her shoulders. \"That sounds perfect,\" she purrs, her hand sliding down his chest until it rests on his ██████. \"I think we can both learn a lot from each other.\"\n \n Paul's eyes darken with desire as he feels her hand on his █████. He knows what's about to happen next, and he can't wait for it to begin.\n \n Chloe can hardly believe what she's doing - kissing Paul, her teaching assistant, in the hallway after class. But it feels so good, so wicked and indulgent.\n</details>\n\n---\n\n---",
"## v1 Description (Also still relevant)\n\nMoistral 11B is an *aggressive* finetune of the legendary Fimbulvert v2 model. \n\nIf you haven't heard of that model, then your poor-GPU ass is missing out!\nIt is an amazing substitute for those heavyweight models, and it won't disappoint. \n*(That's coming from someone who daily drives a Venus / Goliath 120B)*\n\n...and if you're looking for something *more moist*, then you've come to the right place. \n\nYou can expect the same sort of performance here! It's the Honda Civic of eRP models!",
"### Pimp my ride\nTurn any old story into a Moistral masterpiece. \nIn fact, we recommend that you leave out any hints of *moist* in your story to _let Moistral cook_.",
"### Drive it like it's hot\nWhen in Instruct Mode, Moistral will treat you like a director of your own fantasy ride. \nBe a controlling navigator or a passenger princess, it won't matter - you're in for a good ride!\n\n<details>\n<summary>Moistral Sample</summary>\n<b>ASSISTANT:</b><br/>\nWhen the doors to the throne room finally opened, she saw him there - Dik, the sorcerer prince sitting on his throne. He looked majestic, just as Princess Lilia expected. In a robe embroidered with silver yarn and covered with magical runes. His courtiers stood on either side of him, all looking at the princess with interest. Princess Lilia felt her determination leaving her, but pulled herself together and once again reminded herself that she should not be afraid. She approached the throne intending to show the dignity of all Iliac by personal example.<br/>\n<b>USER:</b><br/>\nShe bows slightly<br/>\n<b>ASSISTANT:</b><br/>\nPrincess Lilia bowed slightly in front of Dik, the sorcerer prince. Her eyes fell to the floor out of respect, but soon rose again. She would show herself to be a dignified woman!<br/>\n\"I greet you, your highness,\" Princess Lilia said. \"I am here on behalf of my father, King Edmund Ruhr, to serve as a hostage and envoy.\"<br/>\nDik nodded, but didn't say anything.<br/>\n</details>",
"### It's got the mileage\nMoistral has been trained with many long form texts, a nice chunk of which are 8K in length. \nIt is capable of going far and long without passing it back to you. This is not your typical chibi RP model.\n\nI have to acknowledge that I'm standing on the shoulders of giants. \nThank you Sao for sharing your finetune config along with tips on getting started.\nThanks to everyone in the Finetuning channel for entertaining my every question.\n\n!image/png"
] |
text-generation | transformers |
# Model Card for Model ID
<!-- Provide a quick summary of what the model is/does. -->
## Model Details
### Model Description
<!-- Provide a longer summary of what this model is. -->
This is the model card of a 🤗 transformers model that has been pushed on the Hub. This model card has been automatically generated.
- **Developed by:** [More Information Needed]
- **Funded by [optional]:** [More Information Needed]
- **Shared by [optional]:** [More Information Needed]
- **Model type:** [More Information Needed]
- **Language(s) (NLP):** [More Information Needed]
- **License:** [More Information Needed]
- **Finetuned from model [optional]:** [More Information Needed]
### Model Sources [optional]
<!-- Provide the basic links for the model. -->
- **Repository:** [More Information Needed]
- **Paper [optional]:** [More Information Needed]
- **Demo [optional]:** [More Information Needed]
## Uses
<!-- Address questions around how the model is intended to be used, including the foreseeable users of the model and those affected by the model. -->
### Direct Use
<!-- This section is for the model use without fine-tuning or plugging into a larger ecosystem/app. -->
[More Information Needed]
### Downstream Use [optional]
<!-- This section is for the model use when fine-tuned for a task, or when plugged into a larger ecosystem/app -->
[More Information Needed]
### Out-of-Scope Use
<!-- This section addresses misuse, malicious use, and uses that the model will not work well for. -->
[More Information Needed]
## Bias, Risks, and Limitations
<!-- This section is meant to convey both technical and sociotechnical limitations. -->
[More Information Needed]
### Recommendations
<!-- This section is meant to convey recommendations with respect to the bias, risk, and technical limitations. -->
Users (both direct and downstream) should be made aware of the risks, biases and limitations of the model. More information needed for further recommendations.
## How to Get Started with the Model
Use the code below to get started with the model.
[More Information Needed]
## Training Details
### Training Data
<!-- This should link to a Dataset Card, perhaps with a short stub of information on what the training data is all about as well as documentation related to data pre-processing or additional filtering. -->
[More Information Needed]
### Training Procedure
<!-- This relates heavily to the Technical Specifications. Content here should link to that section when it is relevant to the training procedure. -->
#### Preprocessing [optional]
[More Information Needed]
#### Training Hyperparameters
- **Training regime:** [More Information Needed] <!--fp32, fp16 mixed precision, bf16 mixed precision, bf16 non-mixed precision, fp16 non-mixed precision, fp8 mixed precision -->
#### Speeds, Sizes, Times [optional]
<!-- This section provides information about throughput, start/end time, checkpoint size if relevant, etc. -->
[More Information Needed]
## Evaluation
<!-- This section describes the evaluation protocols and provides the results. -->
### Testing Data, Factors & Metrics
#### Testing Data
<!-- This should link to a Dataset Card if possible. -->
[More Information Needed]
#### Factors
<!-- These are the things the evaluation is disaggregating by, e.g., subpopulations or domains. -->
[More Information Needed]
#### Metrics
<!-- These are the evaluation metrics being used, ideally with a description of why. -->
[More Information Needed]
### Results
[More Information Needed]
#### Summary
## Model Examination [optional]
<!-- Relevant interpretability work for the model goes here -->
[More Information Needed]
## Environmental Impact
<!-- Total emissions (in grams of CO2eq) and additional considerations, such as electricity usage, go here. Edit the suggested text below accordingly -->
Carbon emissions can be estimated using the [Machine Learning Impact calculator](https://mlco2.github.io/impact#compute) presented in [Lacoste et al. (2019)](https://arxiv.org/abs/1910.09700).
- **Hardware Type:** [More Information Needed]
- **Hours used:** [More Information Needed]
- **Cloud Provider:** [More Information Needed]
- **Compute Region:** [More Information Needed]
- **Carbon Emitted:** [More Information Needed]
## Technical Specifications [optional]
### Model Architecture and Objective
[More Information Needed]
### Compute Infrastructure
[More Information Needed]
#### Hardware
[More Information Needed]
#### Software
[More Information Needed]
## Citation [optional]
<!-- If there is a paper or blog post introducing the model, the APA and Bibtex information for that should go in this section. -->
**BibTeX:**
[More Information Needed]
**APA:**
[More Information Needed]
## Glossary [optional]
<!-- If relevant, include terms and calculations in this section that can help readers understand the model or model card. -->
[More Information Needed]
## More Information [optional]
[More Information Needed]
## Model Card Authors [optional]
[More Information Needed]
## Model Card Contact
[More Information Needed]
| {"library_name": "transformers", "tags": []} | swj0419/hp_retrain_STEP0000050 | null | [
"transformers",
"safetensors",
"llama",
"text-generation",
"arxiv:1910.09700",
"autotrain_compatible",
"endpoints_compatible",
"text-generation-inference",
"region:us"
] | null | 2024-04-27T07:44:25+00:00 | [
"1910.09700"
] | [] | TAGS
#transformers #safetensors #llama #text-generation #arxiv-1910.09700 #autotrain_compatible #endpoints_compatible #text-generation-inference #region-us
|
# Model Card for Model ID
## Model Details
### Model Description
This is the model card of a transformers model that has been pushed on the Hub. This model card has been automatically generated.
- Developed by:
- Funded by [optional]:
- Shared by [optional]:
- Model type:
- Language(s) (NLP):
- License:
- Finetuned from model [optional]:
### Model Sources [optional]
- Repository:
- Paper [optional]:
- Demo [optional]:
## Uses
### Direct Use
### Downstream Use [optional]
### Out-of-Scope Use
## Bias, Risks, and Limitations
### Recommendations
Users (both direct and downstream) should be made aware of the risks, biases and limitations of the model. More information needed for further recommendations.
## How to Get Started with the Model
Use the code below to get started with the model.
## Training Details
### Training Data
### Training Procedure
#### Preprocessing [optional]
#### Training Hyperparameters
- Training regime:
#### Speeds, Sizes, Times [optional]
## Evaluation
### Testing Data, Factors & Metrics
#### Testing Data
#### Factors
#### Metrics
### Results
#### Summary
## Model Examination [optional]
## Environmental Impact
Carbon emissions can be estimated using the Machine Learning Impact calculator presented in Lacoste et al. (2019).
- Hardware Type:
- Hours used:
- Cloud Provider:
- Compute Region:
- Carbon Emitted:
## Technical Specifications [optional]
### Model Architecture and Objective
### Compute Infrastructure
#### Hardware
#### Software
[optional]
BibTeX:
APA:
## Glossary [optional]
## More Information [optional]
## Model Card Authors [optional]
## Model Card Contact
| [
"# Model Card for Model ID",
"## Model Details",
"### Model Description\n\n\n\nThis is the model card of a transformers model that has been pushed on the Hub. This model card has been automatically generated.\n\n- Developed by: \n- Funded by [optional]: \n- Shared by [optional]: \n- Model type: \n- Language(s) (NLP): \n- License: \n- Finetuned from model [optional]:",
"### Model Sources [optional]\n\n\n\n- Repository: \n- Paper [optional]: \n- Demo [optional]:",
"## Uses",
"### Direct Use",
"### Downstream Use [optional]",
"### Out-of-Scope Use",
"## Bias, Risks, and Limitations",
"### Recommendations\n\n\n\nUsers (both direct and downstream) should be made aware of the risks, biases and limitations of the model. More information needed for further recommendations.",
"## How to Get Started with the Model\n\nUse the code below to get started with the model.",
"## Training Details",
"### Training Data",
"### Training Procedure",
"#### Preprocessing [optional]",
"#### Training Hyperparameters\n\n- Training regime:",
"#### Speeds, Sizes, Times [optional]",
"## Evaluation",
"### Testing Data, Factors & Metrics",
"#### Testing Data",
"#### Factors",
"#### Metrics",
"### Results",
"#### Summary",
"## Model Examination [optional]",
"## Environmental Impact\n\n\n\nCarbon emissions can be estimated using the Machine Learning Impact calculator presented in Lacoste et al. (2019).\n\n- Hardware Type: \n- Hours used: \n- Cloud Provider: \n- Compute Region: \n- Carbon Emitted:",
"## Technical Specifications [optional]",
"### Model Architecture and Objective",
"### Compute Infrastructure",
"#### Hardware",
"#### Software\n\n\n\n[optional]\n\n\n\nBibTeX:\n\n\n\nAPA:",
"## Glossary [optional]",
"## More Information [optional]",
"## Model Card Authors [optional]",
"## Model Card Contact"
] | [
"TAGS\n#transformers #safetensors #llama #text-generation #arxiv-1910.09700 #autotrain_compatible #endpoints_compatible #text-generation-inference #region-us \n",
"# Model Card for Model ID",
"## Model Details",
"### Model Description\n\n\n\nThis is the model card of a transformers model that has been pushed on the Hub. This model card has been automatically generated.\n\n- Developed by: \n- Funded by [optional]: \n- Shared by [optional]: \n- Model type: \n- Language(s) (NLP): \n- License: \n- Finetuned from model [optional]:",
"### Model Sources [optional]\n\n\n\n- Repository: \n- Paper [optional]: \n- Demo [optional]:",
"## Uses",
"### Direct Use",
"### Downstream Use [optional]",
"### Out-of-Scope Use",
"## Bias, Risks, and Limitations",
"### Recommendations\n\n\n\nUsers (both direct and downstream) should be made aware of the risks, biases and limitations of the model. More information needed for further recommendations.",
"## How to Get Started with the Model\n\nUse the code below to get started with the model.",
"## Training Details",
"### Training Data",
"### Training Procedure",
"#### Preprocessing [optional]",
"#### Training Hyperparameters\n\n- Training regime:",
"#### Speeds, Sizes, Times [optional]",
"## Evaluation",
"### Testing Data, Factors & Metrics",
"#### Testing Data",
"#### Factors",
"#### Metrics",
"### Results",
"#### Summary",
"## Model Examination [optional]",
"## Environmental Impact\n\n\n\nCarbon emissions can be estimated using the Machine Learning Impact calculator presented in Lacoste et al. (2019).\n\n- Hardware Type: \n- Hours used: \n- Cloud Provider: \n- Compute Region: \n- Carbon Emitted:",
"## Technical Specifications [optional]",
"### Model Architecture and Objective",
"### Compute Infrastructure",
"#### Hardware",
"#### Software\n\n\n\n[optional]\n\n\n\nBibTeX:\n\n\n\nAPA:",
"## Glossary [optional]",
"## More Information [optional]",
"## Model Card Authors [optional]",
"## Model Card Contact"
] |
text-generation | transformers |
<!-- This model card has been generated automatically according to the information the Trainer had access to. You
should probably proofread and complete it, then remove this comment. -->
# 0.001_4iters_bs256_nodpo_only4w_zephyr_iter_3
This model is a fine-tuned version of [ShenaoZhang/0.001_4iters_bs256_nodpo_only4w_zephyr_iter_2](https://huggingface.co/ShenaoZhang/0.001_4iters_bs256_nodpo_only4w_zephyr_iter_2) on the updated and the original datasets.
## Model description
More information needed
## Intended uses & limitations
More information needed
## Training and evaluation data
More information needed
## Training procedure
### Training hyperparameters
The following hyperparameters were used during training:
- learning_rate: 5e-07
- train_batch_size: 8
- eval_batch_size: 8
- seed: 42
- distributed_type: multi-GPU
- num_devices: 8
- gradient_accumulation_steps: 4
- total_train_batch_size: 256
- total_eval_batch_size: 64
- optimizer: Adam with betas=(0.9,0.999) and epsilon=1e-08
- lr_scheduler_type: cosine
- lr_scheduler_warmup_ratio: 0.1
- num_epochs: 1
### Training results
### Framework versions
- Transformers 4.40.0
- Pytorch 2.1.2+cu121
- Datasets 2.14.6
- Tokenizers 0.19.1
| {"license": "mit", "tags": ["alignment-handbook", "trl", "dpo", "generated_from_trainer", "trl", "dpo", "generated_from_trainer"], "datasets": ["updated", "original"], "base_model": "ShenaoZhang/0.001_4iters_bs256_nodpo_only4w_zephyr_iter_2", "model-index": [{"name": "0.001_4iters_bs256_nodpo_only4w_zephyr_iter_3", "results": []}]} | ShenaoZhang/0.001_4iters_bs256_nodpo_only4w_zephyr_iter_3 | null | [
"transformers",
"safetensors",
"mistral",
"text-generation",
"alignment-handbook",
"trl",
"dpo",
"generated_from_trainer",
"conversational",
"dataset:updated",
"dataset:original",
"base_model:ShenaoZhang/0.001_4iters_bs256_nodpo_only4w_zephyr_iter_2",
"license:mit",
"autotrain_compatible",
"endpoints_compatible",
"text-generation-inference",
"region:us"
] | null | 2024-04-27T07:47:49+00:00 | [] | [] | TAGS
#transformers #safetensors #mistral #text-generation #alignment-handbook #trl #dpo #generated_from_trainer #conversational #dataset-updated #dataset-original #base_model-ShenaoZhang/0.001_4iters_bs256_nodpo_only4w_zephyr_iter_2 #license-mit #autotrain_compatible #endpoints_compatible #text-generation-inference #region-us
|
# 0.001_4iters_bs256_nodpo_only4w_zephyr_iter_3
This model is a fine-tuned version of ShenaoZhang/0.001_4iters_bs256_nodpo_only4w_zephyr_iter_2 on the updated and the original datasets.
## Model description
More information needed
## Intended uses & limitations
More information needed
## Training and evaluation data
More information needed
## Training procedure
### Training hyperparameters
The following hyperparameters were used during training:
- learning_rate: 5e-07
- train_batch_size: 8
- eval_batch_size: 8
- seed: 42
- distributed_type: multi-GPU
- num_devices: 8
- gradient_accumulation_steps: 4
- total_train_batch_size: 256
- total_eval_batch_size: 64
- optimizer: Adam with betas=(0.9,0.999) and epsilon=1e-08
- lr_scheduler_type: cosine
- lr_scheduler_warmup_ratio: 0.1
- num_epochs: 1
### Training results
### Framework versions
- Transformers 4.40.0
- Pytorch 2.1.2+cu121
- Datasets 2.14.6
- Tokenizers 0.19.1
| [
"# 0.001_4iters_bs256_nodpo_only4w_zephyr_iter_3\n\nThis model is a fine-tuned version of ShenaoZhang/0.001_4iters_bs256_nodpo_only4w_zephyr_iter_2 on the updated and the original datasets.",
"## Model description\n\nMore information needed",
"## Intended uses & limitations\n\nMore information needed",
"## Training and evaluation data\n\nMore information needed",
"## Training procedure",
"### Training hyperparameters\n\nThe following hyperparameters were used during training:\n- learning_rate: 5e-07\n- train_batch_size: 8\n- eval_batch_size: 8\n- seed: 42\n- distributed_type: multi-GPU\n- num_devices: 8\n- gradient_accumulation_steps: 4\n- total_train_batch_size: 256\n- total_eval_batch_size: 64\n- optimizer: Adam with betas=(0.9,0.999) and epsilon=1e-08\n- lr_scheduler_type: cosine\n- lr_scheduler_warmup_ratio: 0.1\n- num_epochs: 1",
"### Training results",
"### Framework versions\n\n- Transformers 4.40.0\n- Pytorch 2.1.2+cu121\n- Datasets 2.14.6\n- Tokenizers 0.19.1"
] | [
"TAGS\n#transformers #safetensors #mistral #text-generation #alignment-handbook #trl #dpo #generated_from_trainer #conversational #dataset-updated #dataset-original #base_model-ShenaoZhang/0.001_4iters_bs256_nodpo_only4w_zephyr_iter_2 #license-mit #autotrain_compatible #endpoints_compatible #text-generation-inference #region-us \n",
"# 0.001_4iters_bs256_nodpo_only4w_zephyr_iter_3\n\nThis model is a fine-tuned version of ShenaoZhang/0.001_4iters_bs256_nodpo_only4w_zephyr_iter_2 on the updated and the original datasets.",
"## Model description\n\nMore information needed",
"## Intended uses & limitations\n\nMore information needed",
"## Training and evaluation data\n\nMore information needed",
"## Training procedure",
"### Training hyperparameters\n\nThe following hyperparameters were used during training:\n- learning_rate: 5e-07\n- train_batch_size: 8\n- eval_batch_size: 8\n- seed: 42\n- distributed_type: multi-GPU\n- num_devices: 8\n- gradient_accumulation_steps: 4\n- total_train_batch_size: 256\n- total_eval_batch_size: 64\n- optimizer: Adam with betas=(0.9,0.999) and epsilon=1e-08\n- lr_scheduler_type: cosine\n- lr_scheduler_warmup_ratio: 0.1\n- num_epochs: 1",
"### Training results",
"### Framework versions\n\n- Transformers 4.40.0\n- Pytorch 2.1.2+cu121\n- Datasets 2.14.6\n- Tokenizers 0.19.1"
] |
null | peft |
<!-- This model card has been generated automatically according to the information the Trainer had access to. You
should probably proofread and complete it, then remove this comment. -->
# GUE_EMP_H3K4me3-seqsight_16384_512_22M-L1_f
This model is a fine-tuned version of [mahdibaghbanzadeh/seqsight_16384_512_22M](https://huggingface.co/mahdibaghbanzadeh/seqsight_16384_512_22M) on the [mahdibaghbanzadeh/GUE_EMP_H3K4me3](https://huggingface.co/datasets/mahdibaghbanzadeh/GUE_EMP_H3K4me3) dataset.
It achieves the following results on the evaluation set:
- Loss: 0.6204
- F1 Score: 0.6518
- Accuracy: 0.6522
## Model description
More information needed
## Intended uses & limitations
More information needed
## Training and evaluation data
More information needed
## Training procedure
### Training hyperparameters
The following hyperparameters were used during training:
- learning_rate: 0.0005
- train_batch_size: 128
- eval_batch_size: 128
- seed: 42
- optimizer: Adam with betas=(0.9,0.999) and epsilon=1e-08
- lr_scheduler_type: linear
- training_steps: 10000
### Training results
| Training Loss | Epoch | Step | Validation Loss | F1 Score | Accuracy |
|:-------------:|:-----:|:-----:|:---------------:|:--------:|:--------:|
| 0.6736 | 0.87 | 200 | 0.6607 | 0.6110 | 0.6114 |
| 0.651 | 1.74 | 400 | 0.6496 | 0.6247 | 0.6247 |
| 0.6455 | 2.61 | 600 | 0.6415 | 0.6359 | 0.6356 |
| 0.6336 | 3.48 | 800 | 0.6326 | 0.6495 | 0.6492 |
| 0.6306 | 4.35 | 1000 | 0.6291 | 0.6475 | 0.6476 |
| 0.6272 | 5.22 | 1200 | 0.6487 | 0.6312 | 0.6378 |
| 0.6246 | 6.09 | 1400 | 0.6305 | 0.6446 | 0.6457 |
| 0.6191 | 6.96 | 1600 | 0.6338 | 0.6471 | 0.6484 |
| 0.6187 | 7.83 | 1800 | 0.6219 | 0.6587 | 0.6587 |
| 0.6158 | 8.7 | 2000 | 0.6264 | 0.6479 | 0.6492 |
| 0.6157 | 9.57 | 2200 | 0.6233 | 0.6458 | 0.6467 |
| 0.6148 | 10.43 | 2400 | 0.6263 | 0.6470 | 0.6481 |
| 0.6133 | 11.3 | 2600 | 0.6342 | 0.6394 | 0.6443 |
| 0.6115 | 12.17 | 2800 | 0.6249 | 0.6504 | 0.6516 |
| 0.6094 | 13.04 | 3000 | 0.6221 | 0.6521 | 0.6533 |
| 0.6086 | 13.91 | 3200 | 0.6329 | 0.6387 | 0.6435 |
| 0.6081 | 14.78 | 3400 | 0.6230 | 0.6476 | 0.6495 |
| 0.6077 | 15.65 | 3600 | 0.6273 | 0.6490 | 0.6516 |
| 0.6053 | 16.52 | 3800 | 0.6299 | 0.6443 | 0.6473 |
| 0.606 | 17.39 | 4000 | 0.6245 | 0.6487 | 0.6508 |
| 0.605 | 18.26 | 4200 | 0.6250 | 0.6482 | 0.65 |
| 0.6007 | 19.13 | 4400 | 0.6257 | 0.6464 | 0.6489 |
| 0.6035 | 20.0 | 4600 | 0.6234 | 0.6483 | 0.6505 |
| 0.6017 | 20.87 | 4800 | 0.6229 | 0.6536 | 0.6546 |
| 0.6014 | 21.74 | 5000 | 0.6348 | 0.6439 | 0.6484 |
| 0.6007 | 22.61 | 5200 | 0.6236 | 0.6507 | 0.6541 |
| 0.6009 | 23.48 | 5400 | 0.6297 | 0.6439 | 0.6478 |
| 0.5998 | 24.35 | 5600 | 0.6207 | 0.6538 | 0.6552 |
| 0.6006 | 25.22 | 5800 | 0.6450 | 0.6309 | 0.6402 |
| 0.5979 | 26.09 | 6000 | 0.6298 | 0.6457 | 0.65 |
| 0.5999 | 26.96 | 6200 | 0.6261 | 0.6463 | 0.6503 |
| 0.5976 | 27.83 | 6400 | 0.6349 | 0.6419 | 0.6484 |
| 0.598 | 28.7 | 6600 | 0.6248 | 0.6511 | 0.6538 |
| 0.5977 | 29.57 | 6800 | 0.6184 | 0.6539 | 0.6560 |
| 0.5907 | 30.43 | 7000 | 0.6310 | 0.6470 | 0.6519 |
| 0.6007 | 31.3 | 7200 | 0.6282 | 0.6467 | 0.6516 |
| 0.596 | 32.17 | 7400 | 0.6325 | 0.6415 | 0.6481 |
| 0.5948 | 33.04 | 7600 | 0.6202 | 0.6516 | 0.6546 |
| 0.5953 | 33.91 | 7800 | 0.6237 | 0.6512 | 0.6546 |
| 0.5953 | 34.78 | 8000 | 0.6235 | 0.6516 | 0.6552 |
| 0.5953 | 35.65 | 8200 | 0.6231 | 0.6510 | 0.6543 |
| 0.5951 | 36.52 | 8400 | 0.6272 | 0.6471 | 0.6516 |
| 0.5947 | 37.39 | 8600 | 0.6240 | 0.6495 | 0.6533 |
| 0.5913 | 38.26 | 8800 | 0.6269 | 0.6496 | 0.6535 |
| 0.596 | 39.13 | 9000 | 0.6269 | 0.6472 | 0.6519 |
| 0.5953 | 40.0 | 9200 | 0.6234 | 0.6508 | 0.6541 |
| 0.5912 | 40.87 | 9400 | 0.6210 | 0.6557 | 0.6582 |
| 0.5931 | 41.74 | 9600 | 0.6226 | 0.6534 | 0.6562 |
| 0.5949 | 42.61 | 9800 | 0.6222 | 0.6508 | 0.6541 |
| 0.5945 | 43.48 | 10000 | 0.6222 | 0.6512 | 0.6543 |
### Framework versions
- PEFT 0.9.0
- Transformers 4.38.2
- Pytorch 2.2.0+cu121
- Datasets 2.17.1
- Tokenizers 0.15.2 | {"library_name": "peft", "tags": ["generated_from_trainer"], "metrics": ["accuracy"], "base_model": "mahdibaghbanzadeh/seqsight_16384_512_22M", "model-index": [{"name": "GUE_EMP_H3K4me3-seqsight_16384_512_22M-L1_f", "results": []}]} | mahdibaghbanzadeh/GUE_EMP_H3K4me3-seqsight_16384_512_22M-L1_f | null | [
"peft",
"safetensors",
"generated_from_trainer",
"base_model:mahdibaghbanzadeh/seqsight_16384_512_22M",
"region:us"
] | null | 2024-04-27T07:48:40+00:00 | [] | [] | TAGS
#peft #safetensors #generated_from_trainer #base_model-mahdibaghbanzadeh/seqsight_16384_512_22M #region-us
| GUE\_EMP\_H3K4me3-seqsight\_16384\_512\_22M-L1\_f
=================================================
This model is a fine-tuned version of mahdibaghbanzadeh/seqsight\_16384\_512\_22M on the mahdibaghbanzadeh/GUE\_EMP\_H3K4me3 dataset.
It achieves the following results on the evaluation set:
* Loss: 0.6204
* F1 Score: 0.6518
* Accuracy: 0.6522
Model description
-----------------
More information needed
Intended uses & limitations
---------------------------
More information needed
Training and evaluation data
----------------------------
More information needed
Training procedure
------------------
### Training hyperparameters
The following hyperparameters were used during training:
* learning\_rate: 0.0005
* train\_batch\_size: 128
* eval\_batch\_size: 128
* seed: 42
* optimizer: Adam with betas=(0.9,0.999) and epsilon=1e-08
* lr\_scheduler\_type: linear
* training\_steps: 10000
### Training results
### Framework versions
* PEFT 0.9.0
* Transformers 4.38.2
* Pytorch 2.2.0+cu121
* Datasets 2.17.1
* Tokenizers 0.15.2
| [
"### Training hyperparameters\n\n\nThe following hyperparameters were used during training:\n\n\n* learning\\_rate: 0.0005\n* train\\_batch\\_size: 128\n* eval\\_batch\\_size: 128\n* seed: 42\n* optimizer: Adam with betas=(0.9,0.999) and epsilon=1e-08\n* lr\\_scheduler\\_type: linear\n* training\\_steps: 10000",
"### Training results",
"### Framework versions\n\n\n* PEFT 0.9.0\n* Transformers 4.38.2\n* Pytorch 2.2.0+cu121\n* Datasets 2.17.1\n* Tokenizers 0.15.2"
] | [
"TAGS\n#peft #safetensors #generated_from_trainer #base_model-mahdibaghbanzadeh/seqsight_16384_512_22M #region-us \n",
"### Training hyperparameters\n\n\nThe following hyperparameters were used during training:\n\n\n* learning\\_rate: 0.0005\n* train\\_batch\\_size: 128\n* eval\\_batch\\_size: 128\n* seed: 42\n* optimizer: Adam with betas=(0.9,0.999) and epsilon=1e-08\n* lr\\_scheduler\\_type: linear\n* training\\_steps: 10000",
"### Training results",
"### Framework versions\n\n\n* PEFT 0.9.0\n* Transformers 4.38.2\n* Pytorch 2.2.0+cu121\n* Datasets 2.17.1\n* Tokenizers 0.15.2"
] |
text2text-generation | transformers |
<!-- This model card has been generated automatically according to the information the Trainer had access to. You
should probably proofread and complete it, then remove this comment. -->
# CS505_COQE_viT5_total_Instruction0_SOAPL_v1
This model is a fine-tuned version of [VietAI/vit5-large](https://huggingface.co/VietAI/vit5-large) on the None dataset.
## Model description
More information needed
## Intended uses & limitations
More information needed
## Training and evaluation data
More information needed
## Training procedure
### Training hyperparameters
The following hyperparameters were used during training:
- learning_rate: 5e-05
- train_batch_size: 8
- eval_batch_size: 64
- seed: 42
- optimizer: Adam with betas=(0.9,0.999) and epsilon=1e-08
- lr_scheduler_type: linear
- num_epochs: 20
- mixed_precision_training: Native AMP
### Training results
### Framework versions
- Transformers 4.39.3
- Pytorch 2.1.2
- Datasets 2.18.0
- Tokenizers 0.15.2
| {"license": "mit", "tags": ["generated_from_trainer"], "base_model": "VietAI/vit5-large", "model-index": [{"name": "CS505_COQE_viT5_total_Instruction0_SOAPL_v1", "results": []}]} | ThuyNT/CS505_COQE_viT5_total_Instruction0_SOAPL_v1 | null | [
"transformers",
"tensorboard",
"safetensors",
"t5",
"text2text-generation",
"generated_from_trainer",
"base_model:VietAI/vit5-large",
"license:mit",
"autotrain_compatible",
"endpoints_compatible",
"text-generation-inference",
"region:us"
] | null | 2024-04-27T07:49:10+00:00 | [] | [] | TAGS
#transformers #tensorboard #safetensors #t5 #text2text-generation #generated_from_trainer #base_model-VietAI/vit5-large #license-mit #autotrain_compatible #endpoints_compatible #text-generation-inference #region-us
|
# CS505_COQE_viT5_total_Instruction0_SOAPL_v1
This model is a fine-tuned version of VietAI/vit5-large on the None dataset.
## Model description
More information needed
## Intended uses & limitations
More information needed
## Training and evaluation data
More information needed
## Training procedure
### Training hyperparameters
The following hyperparameters were used during training:
- learning_rate: 5e-05
- train_batch_size: 8
- eval_batch_size: 64
- seed: 42
- optimizer: Adam with betas=(0.9,0.999) and epsilon=1e-08
- lr_scheduler_type: linear
- num_epochs: 20
- mixed_precision_training: Native AMP
### Training results
### Framework versions
- Transformers 4.39.3
- Pytorch 2.1.2
- Datasets 2.18.0
- Tokenizers 0.15.2
| [
"# CS505_COQE_viT5_total_Instruction0_SOAPL_v1\n\nThis model is a fine-tuned version of VietAI/vit5-large on the None dataset.",
"## Model description\n\nMore information needed",
"## Intended uses & limitations\n\nMore information needed",
"## Training and evaluation data\n\nMore information needed",
"## Training procedure",
"### Training hyperparameters\n\nThe following hyperparameters were used during training:\n- learning_rate: 5e-05\n- train_batch_size: 8\n- eval_batch_size: 64\n- seed: 42\n- optimizer: Adam with betas=(0.9,0.999) and epsilon=1e-08\n- lr_scheduler_type: linear\n- num_epochs: 20\n- mixed_precision_training: Native AMP",
"### Training results",
"### Framework versions\n\n- Transformers 4.39.3\n- Pytorch 2.1.2\n- Datasets 2.18.0\n- Tokenizers 0.15.2"
] | [
"TAGS\n#transformers #tensorboard #safetensors #t5 #text2text-generation #generated_from_trainer #base_model-VietAI/vit5-large #license-mit #autotrain_compatible #endpoints_compatible #text-generation-inference #region-us \n",
"# CS505_COQE_viT5_total_Instruction0_SOAPL_v1\n\nThis model is a fine-tuned version of VietAI/vit5-large on the None dataset.",
"## Model description\n\nMore information needed",
"## Intended uses & limitations\n\nMore information needed",
"## Training and evaluation data\n\nMore information needed",
"## Training procedure",
"### Training hyperparameters\n\nThe following hyperparameters were used during training:\n- learning_rate: 5e-05\n- train_batch_size: 8\n- eval_batch_size: 64\n- seed: 42\n- optimizer: Adam with betas=(0.9,0.999) and epsilon=1e-08\n- lr_scheduler_type: linear\n- num_epochs: 20\n- mixed_precision_training: Native AMP",
"### Training results",
"### Framework versions\n\n- Transformers 4.39.3\n- Pytorch 2.1.2\n- Datasets 2.18.0\n- Tokenizers 0.15.2"
] |
null | peft |
<!-- This model card has been generated automatically according to the information the Trainer had access to. You
should probably proofread and complete it, then remove this comment. -->
# GUE_EMP_H3K4me3-seqsight_16384_512_22M-L8_f
This model is a fine-tuned version of [mahdibaghbanzadeh/seqsight_16384_512_22M](https://huggingface.co/mahdibaghbanzadeh/seqsight_16384_512_22M) on the [mahdibaghbanzadeh/GUE_EMP_H3K4me3](https://huggingface.co/datasets/mahdibaghbanzadeh/GUE_EMP_H3K4me3) dataset.
It achieves the following results on the evaluation set:
- Loss: 0.6222
- F1 Score: 0.6751
- Accuracy: 0.6785
## Model description
More information needed
## Intended uses & limitations
More information needed
## Training and evaluation data
More information needed
## Training procedure
### Training hyperparameters
The following hyperparameters were used during training:
- learning_rate: 0.0005
- train_batch_size: 128
- eval_batch_size: 128
- seed: 42
- optimizer: Adam with betas=(0.9,0.999) and epsilon=1e-08
- lr_scheduler_type: linear
- training_steps: 10000
### Training results
| Training Loss | Epoch | Step | Validation Loss | F1 Score | Accuracy |
|:-------------:|:-----:|:-----:|:---------------:|:--------:|:--------:|
| 0.666 | 0.87 | 200 | 0.6521 | 0.6237 | 0.625 |
| 0.6366 | 1.74 | 400 | 0.6351 | 0.6532 | 0.6533 |
| 0.6288 | 2.61 | 600 | 0.6360 | 0.6415 | 0.6429 |
| 0.6195 | 3.48 | 800 | 0.6259 | 0.6485 | 0.6486 |
| 0.6153 | 4.35 | 1000 | 0.6228 | 0.6541 | 0.6538 |
| 0.6103 | 5.22 | 1200 | 0.6461 | 0.6247 | 0.6340 |
| 0.6062 | 6.09 | 1400 | 0.6422 | 0.6349 | 0.6421 |
| 0.6012 | 6.96 | 1600 | 0.6318 | 0.6432 | 0.6467 |
| 0.5974 | 7.83 | 1800 | 0.6178 | 0.6626 | 0.6625 |
| 0.5944 | 8.7 | 2000 | 0.6228 | 0.6522 | 0.6549 |
| 0.5911 | 9.57 | 2200 | 0.6113 | 0.6649 | 0.6655 |
| 0.5905 | 10.43 | 2400 | 0.6264 | 0.6513 | 0.6549 |
| 0.5863 | 11.3 | 2600 | 0.6294 | 0.6425 | 0.6497 |
| 0.5867 | 12.17 | 2800 | 0.6131 | 0.6636 | 0.6658 |
| 0.5809 | 13.04 | 3000 | 0.6149 | 0.6591 | 0.6620 |
| 0.5794 | 13.91 | 3200 | 0.6178 | 0.6529 | 0.6576 |
| 0.5773 | 14.78 | 3400 | 0.6226 | 0.6475 | 0.6530 |
| 0.578 | 15.65 | 3600 | 0.6170 | 0.6573 | 0.6611 |
| 0.575 | 16.52 | 3800 | 0.6168 | 0.6643 | 0.6674 |
| 0.571 | 17.39 | 4000 | 0.6188 | 0.6556 | 0.6595 |
| 0.5742 | 18.26 | 4200 | 0.6153 | 0.6619 | 0.6649 |
| 0.5667 | 19.13 | 4400 | 0.6144 | 0.6629 | 0.6658 |
| 0.5697 | 20.0 | 4600 | 0.6144 | 0.6575 | 0.6620 |
| 0.5676 | 20.87 | 4800 | 0.6204 | 0.6613 | 0.6649 |
| 0.5651 | 21.74 | 5000 | 0.6342 | 0.6506 | 0.6576 |
| 0.564 | 22.61 | 5200 | 0.6119 | 0.6642 | 0.6677 |
| 0.5636 | 23.48 | 5400 | 0.6339 | 0.6487 | 0.6568 |
| 0.5618 | 24.35 | 5600 | 0.6155 | 0.6664 | 0.6693 |
| 0.5608 | 25.22 | 5800 | 0.6434 | 0.6432 | 0.6538 |
| 0.5582 | 26.09 | 6000 | 0.6309 | 0.6531 | 0.6598 |
| 0.5598 | 26.96 | 6200 | 0.6238 | 0.6592 | 0.6652 |
| 0.5564 | 27.83 | 6400 | 0.6450 | 0.6463 | 0.6562 |
| 0.5582 | 28.7 | 6600 | 0.6203 | 0.6614 | 0.6663 |
| 0.5547 | 29.57 | 6800 | 0.6174 | 0.6647 | 0.6687 |
| 0.5507 | 30.43 | 7000 | 0.6327 | 0.6542 | 0.6611 |
| 0.5586 | 31.3 | 7200 | 0.6248 | 0.6585 | 0.6652 |
| 0.5543 | 32.17 | 7400 | 0.6260 | 0.6597 | 0.6660 |
| 0.5509 | 33.04 | 7600 | 0.6175 | 0.6653 | 0.6696 |
| 0.5513 | 33.91 | 7800 | 0.6193 | 0.6621 | 0.6668 |
| 0.5522 | 34.78 | 8000 | 0.6211 | 0.6649 | 0.6696 |
| 0.5511 | 35.65 | 8200 | 0.6218 | 0.6604 | 0.6663 |
| 0.5482 | 36.52 | 8400 | 0.6272 | 0.6608 | 0.6674 |
| 0.5492 | 37.39 | 8600 | 0.6224 | 0.6651 | 0.6707 |
| 0.5436 | 38.26 | 8800 | 0.6313 | 0.6595 | 0.6655 |
| 0.5502 | 39.13 | 9000 | 0.6266 | 0.6629 | 0.6693 |
| 0.549 | 40.0 | 9200 | 0.6223 | 0.6654 | 0.6707 |
| 0.5457 | 40.87 | 9400 | 0.6212 | 0.6685 | 0.6726 |
| 0.5457 | 41.74 | 9600 | 0.6229 | 0.6665 | 0.6712 |
| 0.5499 | 42.61 | 9800 | 0.6206 | 0.6675 | 0.6723 |
| 0.5472 | 43.48 | 10000 | 0.6221 | 0.6670 | 0.6720 |
### Framework versions
- PEFT 0.9.0
- Transformers 4.38.2
- Pytorch 2.2.0+cu121
- Datasets 2.17.1
- Tokenizers 0.15.2 | {"library_name": "peft", "tags": ["generated_from_trainer"], "metrics": ["accuracy"], "base_model": "mahdibaghbanzadeh/seqsight_16384_512_22M", "model-index": [{"name": "GUE_EMP_H3K4me3-seqsight_16384_512_22M-L8_f", "results": []}]} | mahdibaghbanzadeh/GUE_EMP_H3K4me3-seqsight_16384_512_22M-L8_f | null | [
"peft",
"safetensors",
"generated_from_trainer",
"base_model:mahdibaghbanzadeh/seqsight_16384_512_22M",
"region:us"
] | null | 2024-04-27T07:49:14+00:00 | [] | [] | TAGS
#peft #safetensors #generated_from_trainer #base_model-mahdibaghbanzadeh/seqsight_16384_512_22M #region-us
| GUE\_EMP\_H3K4me3-seqsight\_16384\_512\_22M-L8\_f
=================================================
This model is a fine-tuned version of mahdibaghbanzadeh/seqsight\_16384\_512\_22M on the mahdibaghbanzadeh/GUE\_EMP\_H3K4me3 dataset.
It achieves the following results on the evaluation set:
* Loss: 0.6222
* F1 Score: 0.6751
* Accuracy: 0.6785
Model description
-----------------
More information needed
Intended uses & limitations
---------------------------
More information needed
Training and evaluation data
----------------------------
More information needed
Training procedure
------------------
### Training hyperparameters
The following hyperparameters were used during training:
* learning\_rate: 0.0005
* train\_batch\_size: 128
* eval\_batch\_size: 128
* seed: 42
* optimizer: Adam with betas=(0.9,0.999) and epsilon=1e-08
* lr\_scheduler\_type: linear
* training\_steps: 10000
### Training results
### Framework versions
* PEFT 0.9.0
* Transformers 4.38.2
* Pytorch 2.2.0+cu121
* Datasets 2.17.1
* Tokenizers 0.15.2
| [
"### Training hyperparameters\n\n\nThe following hyperparameters were used during training:\n\n\n* learning\\_rate: 0.0005\n* train\\_batch\\_size: 128\n* eval\\_batch\\_size: 128\n* seed: 42\n* optimizer: Adam with betas=(0.9,0.999) and epsilon=1e-08\n* lr\\_scheduler\\_type: linear\n* training\\_steps: 10000",
"### Training results",
"### Framework versions\n\n\n* PEFT 0.9.0\n* Transformers 4.38.2\n* Pytorch 2.2.0+cu121\n* Datasets 2.17.1\n* Tokenizers 0.15.2"
] | [
"TAGS\n#peft #safetensors #generated_from_trainer #base_model-mahdibaghbanzadeh/seqsight_16384_512_22M #region-us \n",
"### Training hyperparameters\n\n\nThe following hyperparameters were used during training:\n\n\n* learning\\_rate: 0.0005\n* train\\_batch\\_size: 128\n* eval\\_batch\\_size: 128\n* seed: 42\n* optimizer: Adam with betas=(0.9,0.999) and epsilon=1e-08\n* lr\\_scheduler\\_type: linear\n* training\\_steps: 10000",
"### Training results",
"### Framework versions\n\n\n* PEFT 0.9.0\n* Transformers 4.38.2\n* Pytorch 2.2.0+cu121\n* Datasets 2.17.1\n* Tokenizers 0.15.2"
] |
null | peft |
<!-- This model card has been generated automatically according to the information the Trainer had access to. You
should probably proofread and complete it, then remove this comment. -->
# GUE_EMP_H3K4me3-seqsight_16384_512_22M-L32_f
This model is a fine-tuned version of [mahdibaghbanzadeh/seqsight_16384_512_22M](https://huggingface.co/mahdibaghbanzadeh/seqsight_16384_512_22M) on the [mahdibaghbanzadeh/GUE_EMP_H3K4me3](https://huggingface.co/datasets/mahdibaghbanzadeh/GUE_EMP_H3K4me3) dataset.
It achieves the following results on the evaluation set:
- Loss: 0.6408
- F1 Score: 0.6783
- Accuracy: 0.6810
## Model description
More information needed
## Intended uses & limitations
More information needed
## Training and evaluation data
More information needed
## Training procedure
### Training hyperparameters
The following hyperparameters were used during training:
- learning_rate: 0.0005
- train_batch_size: 128
- eval_batch_size: 128
- seed: 42
- optimizer: Adam with betas=(0.9,0.999) and epsilon=1e-08
- lr_scheduler_type: linear
- training_steps: 10000
### Training results
| Training Loss | Epoch | Step | Validation Loss | F1 Score | Accuracy |
|:-------------:|:-----:|:-----:|:---------------:|:--------:|:--------:|
| 0.6593 | 0.87 | 200 | 0.6387 | 0.6431 | 0.6429 |
| 0.6284 | 1.74 | 400 | 0.6338 | 0.6475 | 0.6489 |
| 0.6179 | 2.61 | 600 | 0.6269 | 0.6469 | 0.6492 |
| 0.6062 | 3.48 | 800 | 0.6137 | 0.6577 | 0.6579 |
| 0.5995 | 4.35 | 1000 | 0.6104 | 0.6668 | 0.6666 |
| 0.5917 | 5.22 | 1200 | 0.6274 | 0.6521 | 0.6562 |
| 0.5877 | 6.09 | 1400 | 0.6413 | 0.6354 | 0.6448 |
| 0.5801 | 6.96 | 1600 | 0.6256 | 0.6614 | 0.6647 |
| 0.5758 | 7.83 | 1800 | 0.6128 | 0.6728 | 0.6728 |
| 0.5703 | 8.7 | 2000 | 0.6199 | 0.6592 | 0.6622 |
| 0.5641 | 9.57 | 2200 | 0.6001 | 0.6734 | 0.6736 |
| 0.5621 | 10.43 | 2400 | 0.6235 | 0.6606 | 0.6630 |
| 0.5568 | 11.3 | 2600 | 0.6311 | 0.6466 | 0.6552 |
| 0.5561 | 12.17 | 2800 | 0.6080 | 0.6699 | 0.6709 |
| 0.547 | 13.04 | 3000 | 0.6102 | 0.6672 | 0.6696 |
| 0.5444 | 13.91 | 3200 | 0.6170 | 0.6669 | 0.6698 |
| 0.5406 | 14.78 | 3400 | 0.6241 | 0.6617 | 0.6660 |
| 0.5372 | 15.65 | 3600 | 0.6131 | 0.6683 | 0.6704 |
| 0.5322 | 16.52 | 3800 | 0.6185 | 0.6647 | 0.6677 |
| 0.5237 | 17.39 | 4000 | 0.6272 | 0.6665 | 0.6696 |
| 0.5306 | 18.26 | 4200 | 0.6325 | 0.6630 | 0.6682 |
| 0.5177 | 19.13 | 4400 | 0.6259 | 0.6668 | 0.6693 |
| 0.5226 | 20.0 | 4600 | 0.6337 | 0.6591 | 0.6658 |
| 0.5154 | 20.87 | 4800 | 0.6303 | 0.6668 | 0.6696 |
| 0.5117 | 21.74 | 5000 | 0.6394 | 0.6657 | 0.6693 |
| 0.5088 | 22.61 | 5200 | 0.6295 | 0.6690 | 0.6717 |
| 0.5053 | 23.48 | 5400 | 0.6553 | 0.6585 | 0.6649 |
| 0.5052 | 24.35 | 5600 | 0.6319 | 0.6686 | 0.6723 |
| 0.5 | 25.22 | 5800 | 0.6646 | 0.6531 | 0.6617 |
| 0.4957 | 26.09 | 6000 | 0.6571 | 0.6711 | 0.675 |
| 0.4964 | 26.96 | 6200 | 0.6486 | 0.6642 | 0.6698 |
| 0.4923 | 27.83 | 6400 | 0.6700 | 0.6520 | 0.6609 |
| 0.492 | 28.7 | 6600 | 0.6383 | 0.6660 | 0.6685 |
| 0.4892 | 29.57 | 6800 | 0.6407 | 0.6683 | 0.6723 |
| 0.4824 | 30.43 | 7000 | 0.6591 | 0.6610 | 0.6666 |
| 0.4904 | 31.3 | 7200 | 0.6499 | 0.6694 | 0.6742 |
| 0.4829 | 32.17 | 7400 | 0.6433 | 0.6744 | 0.6772 |
| 0.4803 | 33.04 | 7600 | 0.6441 | 0.6713 | 0.675 |
| 0.4777 | 33.91 | 7800 | 0.6603 | 0.6666 | 0.6726 |
| 0.4804 | 34.78 | 8000 | 0.6587 | 0.6667 | 0.6720 |
| 0.4761 | 35.65 | 8200 | 0.6555 | 0.6661 | 0.6717 |
| 0.4716 | 36.52 | 8400 | 0.6642 | 0.6649 | 0.6701 |
| 0.4742 | 37.39 | 8600 | 0.6555 | 0.6696 | 0.6742 |
| 0.4682 | 38.26 | 8800 | 0.6639 | 0.6652 | 0.6698 |
| 0.4721 | 39.13 | 9000 | 0.6583 | 0.6698 | 0.6745 |
| 0.4732 | 40.0 | 9200 | 0.6558 | 0.6688 | 0.6734 |
| 0.4664 | 40.87 | 9400 | 0.6591 | 0.6702 | 0.6742 |
| 0.4634 | 41.74 | 9600 | 0.6600 | 0.6698 | 0.6742 |
| 0.4723 | 42.61 | 9800 | 0.6560 | 0.6715 | 0.6753 |
| 0.4668 | 43.48 | 10000 | 0.6584 | 0.6710 | 0.6753 |
### Framework versions
- PEFT 0.9.0
- Transformers 4.38.2
- Pytorch 2.2.0+cu121
- Datasets 2.17.1
- Tokenizers 0.15.2 | {"library_name": "peft", "tags": ["generated_from_trainer"], "metrics": ["accuracy"], "base_model": "mahdibaghbanzadeh/seqsight_16384_512_22M", "model-index": [{"name": "GUE_EMP_H3K4me3-seqsight_16384_512_22M-L32_f", "results": []}]} | mahdibaghbanzadeh/GUE_EMP_H3K4me3-seqsight_16384_512_22M-L32_f | null | [
"peft",
"safetensors",
"generated_from_trainer",
"base_model:mahdibaghbanzadeh/seqsight_16384_512_22M",
"region:us"
] | null | 2024-04-27T07:49:29+00:00 | [] | [] | TAGS
#peft #safetensors #generated_from_trainer #base_model-mahdibaghbanzadeh/seqsight_16384_512_22M #region-us
| GUE\_EMP\_H3K4me3-seqsight\_16384\_512\_22M-L32\_f
==================================================
This model is a fine-tuned version of mahdibaghbanzadeh/seqsight\_16384\_512\_22M on the mahdibaghbanzadeh/GUE\_EMP\_H3K4me3 dataset.
It achieves the following results on the evaluation set:
* Loss: 0.6408
* F1 Score: 0.6783
* Accuracy: 0.6810
Model description
-----------------
More information needed
Intended uses & limitations
---------------------------
More information needed
Training and evaluation data
----------------------------
More information needed
Training procedure
------------------
### Training hyperparameters
The following hyperparameters were used during training:
* learning\_rate: 0.0005
* train\_batch\_size: 128
* eval\_batch\_size: 128
* seed: 42
* optimizer: Adam with betas=(0.9,0.999) and epsilon=1e-08
* lr\_scheduler\_type: linear
* training\_steps: 10000
### Training results
### Framework versions
* PEFT 0.9.0
* Transformers 4.38.2
* Pytorch 2.2.0+cu121
* Datasets 2.17.1
* Tokenizers 0.15.2
| [
"### Training hyperparameters\n\n\nThe following hyperparameters were used during training:\n\n\n* learning\\_rate: 0.0005\n* train\\_batch\\_size: 128\n* eval\\_batch\\_size: 128\n* seed: 42\n* optimizer: Adam with betas=(0.9,0.999) and epsilon=1e-08\n* lr\\_scheduler\\_type: linear\n* training\\_steps: 10000",
"### Training results",
"### Framework versions\n\n\n* PEFT 0.9.0\n* Transformers 4.38.2\n* Pytorch 2.2.0+cu121\n* Datasets 2.17.1\n* Tokenizers 0.15.2"
] | [
"TAGS\n#peft #safetensors #generated_from_trainer #base_model-mahdibaghbanzadeh/seqsight_16384_512_22M #region-us \n",
"### Training hyperparameters\n\n\nThe following hyperparameters were used during training:\n\n\n* learning\\_rate: 0.0005\n* train\\_batch\\_size: 128\n* eval\\_batch\\_size: 128\n* seed: 42\n* optimizer: Adam with betas=(0.9,0.999) and epsilon=1e-08\n* lr\\_scheduler\\_type: linear\n* training\\_steps: 10000",
"### Training results",
"### Framework versions\n\n\n* PEFT 0.9.0\n* Transformers 4.38.2\n* Pytorch 2.2.0+cu121\n* Datasets 2.17.1\n* Tokenizers 0.15.2"
] |
text2text-generation | transformers |
<!-- This model card has been generated automatically according to the information the Trainer had access to. You
should probably proofread and complete it, then remove this comment. -->
# CS505_COQE_viT5_total_Instruction0_SOAPL_v1_h1
This model is a fine-tuned version of [VietAI/vit5-large](https://huggingface.co/VietAI/vit5-large) on the None dataset.
## Model description
More information needed
## Intended uses & limitations
More information needed
## Training and evaluation data
More information needed
## Training procedure
### Training hyperparameters
The following hyperparameters were used during training:
- learning_rate: 5e-05
- train_batch_size: 8
- eval_batch_size: 64
- seed: 42
- optimizer: Adam with betas=(0.9,0.999) and epsilon=1e-08
- lr_scheduler_type: linear
- num_epochs: 25
- mixed_precision_training: Native AMP
### Training results
### Framework versions
- Transformers 4.39.3
- Pytorch 2.1.2
- Datasets 2.18.0
- Tokenizers 0.15.2
| {"license": "mit", "tags": ["generated_from_trainer"], "base_model": "VietAI/vit5-large", "model-index": [{"name": "CS505_COQE_viT5_total_Instruction0_SOAPL_v1_h1", "results": []}]} | ThuyNT/CS505_COQE_viT5_total_Instruction0_SOAPL_v1_h1 | null | [
"transformers",
"tensorboard",
"safetensors",
"t5",
"text2text-generation",
"generated_from_trainer",
"base_model:VietAI/vit5-large",
"license:mit",
"autotrain_compatible",
"endpoints_compatible",
"text-generation-inference",
"region:us"
] | null | 2024-04-27T07:49:52+00:00 | [] | [] | TAGS
#transformers #tensorboard #safetensors #t5 #text2text-generation #generated_from_trainer #base_model-VietAI/vit5-large #license-mit #autotrain_compatible #endpoints_compatible #text-generation-inference #region-us
|
# CS505_COQE_viT5_total_Instruction0_SOAPL_v1_h1
This model is a fine-tuned version of VietAI/vit5-large on the None dataset.
## Model description
More information needed
## Intended uses & limitations
More information needed
## Training and evaluation data
More information needed
## Training procedure
### Training hyperparameters
The following hyperparameters were used during training:
- learning_rate: 5e-05
- train_batch_size: 8
- eval_batch_size: 64
- seed: 42
- optimizer: Adam with betas=(0.9,0.999) and epsilon=1e-08
- lr_scheduler_type: linear
- num_epochs: 25
- mixed_precision_training: Native AMP
### Training results
### Framework versions
- Transformers 4.39.3
- Pytorch 2.1.2
- Datasets 2.18.0
- Tokenizers 0.15.2
| [
"# CS505_COQE_viT5_total_Instruction0_SOAPL_v1_h1\n\nThis model is a fine-tuned version of VietAI/vit5-large on the None dataset.",
"## Model description\n\nMore information needed",
"## Intended uses & limitations\n\nMore information needed",
"## Training and evaluation data\n\nMore information needed",
"## Training procedure",
"### Training hyperparameters\n\nThe following hyperparameters were used during training:\n- learning_rate: 5e-05\n- train_batch_size: 8\n- eval_batch_size: 64\n- seed: 42\n- optimizer: Adam with betas=(0.9,0.999) and epsilon=1e-08\n- lr_scheduler_type: linear\n- num_epochs: 25\n- mixed_precision_training: Native AMP",
"### Training results",
"### Framework versions\n\n- Transformers 4.39.3\n- Pytorch 2.1.2\n- Datasets 2.18.0\n- Tokenizers 0.15.2"
] | [
"TAGS\n#transformers #tensorboard #safetensors #t5 #text2text-generation #generated_from_trainer #base_model-VietAI/vit5-large #license-mit #autotrain_compatible #endpoints_compatible #text-generation-inference #region-us \n",
"# CS505_COQE_viT5_total_Instruction0_SOAPL_v1_h1\n\nThis model is a fine-tuned version of VietAI/vit5-large on the None dataset.",
"## Model description\n\nMore information needed",
"## Intended uses & limitations\n\nMore information needed",
"## Training and evaluation data\n\nMore information needed",
"## Training procedure",
"### Training hyperparameters\n\nThe following hyperparameters were used during training:\n- learning_rate: 5e-05\n- train_batch_size: 8\n- eval_batch_size: 64\n- seed: 42\n- optimizer: Adam with betas=(0.9,0.999) and epsilon=1e-08\n- lr_scheduler_type: linear\n- num_epochs: 25\n- mixed_precision_training: Native AMP",
"### Training results",
"### Framework versions\n\n- Transformers 4.39.3\n- Pytorch 2.1.2\n- Datasets 2.18.0\n- Tokenizers 0.15.2"
] |
text-classification | transformers |
<!-- This model card has been generated automatically according to the information the Trainer had access to. You
should probably proofread and complete it, then remove this comment. -->
# distilbert-base-uncased-finetuned-emotion
This model is a fine-tuned version of [distilbert-base-uncased](https://huggingface.co/distilbert-base-uncased) on the emotion dataset.
It achieves the following results on the evaluation set:
- Loss: 0.1458
- Accuracy: 0.936
- F1: 0.9363
## Model description
More information needed
## Intended uses & limitations
More information needed
## Training and evaluation data
More information needed
## Training procedure
### Training hyperparameters
The following hyperparameters were used during training:
- learning_rate: 2e-05
- train_batch_size: 64
- eval_batch_size: 64
- seed: 42
- optimizer: Adam with betas=(0.9,0.999) and epsilon=1e-08
- lr_scheduler_type: linear
- num_epochs: 2
### Training results
| Training Loss | Epoch | Step | Validation Loss | Accuracy | F1 |
|:-------------:|:-----:|:----:|:---------------:|:--------:|:------:|
| 0.1742 | 1.0 | 250 | 0.1613 | 0.9335 | 0.9339 |
| 0.1111 | 2.0 | 500 | 0.1458 | 0.936 | 0.9363 |
### Framework versions
- Transformers 4.40.1
- Pytorch 2.3.0
- Datasets 2.19.0
- Tokenizers 0.19.1
| {"license": "apache-2.0", "tags": ["generated_from_trainer"], "datasets": ["emotion"], "metrics": ["accuracy", "f1"], "base_model": "distilbert-base-uncased", "model-index": [{"name": "distilbert-base-uncased-finetuned-emotion", "results": [{"task": {"type": "text-classification", "name": "Text Classification"}, "dataset": {"name": "emotion", "type": "emotion", "config": "split", "split": "validation", "args": "split"}, "metrics": [{"type": "accuracy", "value": 0.936, "name": "Accuracy"}, {"type": "f1", "value": 0.9362961593030331, "name": "F1"}]}]}]} | gopidon/distilbert-base-uncased-finetuned-emotion | null | [
"transformers",
"safetensors",
"distilbert",
"text-classification",
"generated_from_trainer",
"dataset:emotion",
"base_model:distilbert-base-uncased",
"license:apache-2.0",
"model-index",
"autotrain_compatible",
"endpoints_compatible",
"region:us"
] | null | 2024-04-27T07:51:11+00:00 | [] | [] | TAGS
#transformers #safetensors #distilbert #text-classification #generated_from_trainer #dataset-emotion #base_model-distilbert-base-uncased #license-apache-2.0 #model-index #autotrain_compatible #endpoints_compatible #region-us
| distilbert-base-uncased-finetuned-emotion
=========================================
This model is a fine-tuned version of distilbert-base-uncased on the emotion dataset.
It achieves the following results on the evaluation set:
* Loss: 0.1458
* Accuracy: 0.936
* F1: 0.9363
Model description
-----------------
More information needed
Intended uses & limitations
---------------------------
More information needed
Training and evaluation data
----------------------------
More information needed
Training procedure
------------------
### Training hyperparameters
The following hyperparameters were used during training:
* learning\_rate: 2e-05
* train\_batch\_size: 64
* eval\_batch\_size: 64
* seed: 42
* optimizer: Adam with betas=(0.9,0.999) and epsilon=1e-08
* lr\_scheduler\_type: linear
* num\_epochs: 2
### Training results
### Framework versions
* Transformers 4.40.1
* Pytorch 2.3.0
* Datasets 2.19.0
* Tokenizers 0.19.1
| [
"### Training hyperparameters\n\n\nThe following hyperparameters were used during training:\n\n\n* learning\\_rate: 2e-05\n* train\\_batch\\_size: 64\n* eval\\_batch\\_size: 64\n* seed: 42\n* optimizer: Adam with betas=(0.9,0.999) and epsilon=1e-08\n* lr\\_scheduler\\_type: linear\n* num\\_epochs: 2",
"### Training results",
"### Framework versions\n\n\n* Transformers 4.40.1\n* Pytorch 2.3.0\n* Datasets 2.19.0\n* Tokenizers 0.19.1"
] | [
"TAGS\n#transformers #safetensors #distilbert #text-classification #generated_from_trainer #dataset-emotion #base_model-distilbert-base-uncased #license-apache-2.0 #model-index #autotrain_compatible #endpoints_compatible #region-us \n",
"### Training hyperparameters\n\n\nThe following hyperparameters were used during training:\n\n\n* learning\\_rate: 2e-05\n* train\\_batch\\_size: 64\n* eval\\_batch\\_size: 64\n* seed: 42\n* optimizer: Adam with betas=(0.9,0.999) and epsilon=1e-08\n* lr\\_scheduler\\_type: linear\n* num\\_epochs: 2",
"### Training results",
"### Framework versions\n\n\n* Transformers 4.40.1\n* Pytorch 2.3.0\n* Datasets 2.19.0\n* Tokenizers 0.19.1"
] |
reinforcement-learning | ml-agents |
# **ppo** Agent playing **Pyramids**
This is a trained model of a **ppo** agent playing **Pyramids**
using the [Unity ML-Agents Library](https://github.com/Unity-Technologies/ml-agents).
## Usage (with ML-Agents)
The Documentation: https://unity-technologies.github.io/ml-agents/ML-Agents-Toolkit-Documentation/
We wrote a complete tutorial to learn to train your first agent using ML-Agents and publish it to the Hub:
- A *short tutorial* where you teach Huggy the Dog 🐶 to fetch the stick and then play with him directly in your
browser: https://huggingface.co/learn/deep-rl-course/unitbonus1/introduction
- A *longer tutorial* to understand how works ML-Agents:
https://huggingface.co/learn/deep-rl-course/unit5/introduction
### Resume the training
```bash
mlagents-learn <your_configuration_file_path.yaml> --run-id=<run_id> --resume
```
### Watch your Agent play
You can watch your agent **playing directly in your browser**
1. If the environment is part of ML-Agents official environments, go to https://huggingface.co/unity
2. Step 1: Find your model_id: hossniper/ppo-Pyramids
3. Step 2: Select your *.nn /*.onnx file
4. Click on Watch the agent play 👀
| {"library_name": "ml-agents", "tags": ["Pyramids", "deep-reinforcement-learning", "reinforcement-learning", "ML-Agents-Pyramids"]} | hossniper/ppo-Pyramids | null | [
"ml-agents",
"tensorboard",
"onnx",
"Pyramids",
"deep-reinforcement-learning",
"reinforcement-learning",
"ML-Agents-Pyramids",
"region:us"
] | null | 2024-04-27T07:51:23+00:00 | [] | [] | TAGS
#ml-agents #tensorboard #onnx #Pyramids #deep-reinforcement-learning #reinforcement-learning #ML-Agents-Pyramids #region-us
|
# ppo Agent playing Pyramids
This is a trained model of a ppo agent playing Pyramids
using the Unity ML-Agents Library.
## Usage (with ML-Agents)
The Documentation: URL
We wrote a complete tutorial to learn to train your first agent using ML-Agents and publish it to the Hub:
- A *short tutorial* where you teach Huggy the Dog to fetch the stick and then play with him directly in your
browser: URL
- A *longer tutorial* to understand how works ML-Agents:
URL
### Resume the training
### Watch your Agent play
You can watch your agent playing directly in your browser
1. If the environment is part of ML-Agents official environments, go to URL
2. Step 1: Find your model_id: hossniper/ppo-Pyramids
3. Step 2: Select your *.nn /*.onnx file
4. Click on Watch the agent play
| [
"# ppo Agent playing Pyramids\n This is a trained model of a ppo agent playing Pyramids\n using the Unity ML-Agents Library.\n\n ## Usage (with ML-Agents)\n The Documentation: URL\n\n We wrote a complete tutorial to learn to train your first agent using ML-Agents and publish it to the Hub:\n - A *short tutorial* where you teach Huggy the Dog to fetch the stick and then play with him directly in your\n browser: URL\n - A *longer tutorial* to understand how works ML-Agents:\n URL\n\n ### Resume the training\n \n\n ### Watch your Agent play\n You can watch your agent playing directly in your browser\n\n 1. If the environment is part of ML-Agents official environments, go to URL\n 2. Step 1: Find your model_id: hossniper/ppo-Pyramids\n 3. Step 2: Select your *.nn /*.onnx file\n 4. Click on Watch the agent play"
] | [
"TAGS\n#ml-agents #tensorboard #onnx #Pyramids #deep-reinforcement-learning #reinforcement-learning #ML-Agents-Pyramids #region-us \n",
"# ppo Agent playing Pyramids\n This is a trained model of a ppo agent playing Pyramids\n using the Unity ML-Agents Library.\n\n ## Usage (with ML-Agents)\n The Documentation: URL\n\n We wrote a complete tutorial to learn to train your first agent using ML-Agents and publish it to the Hub:\n - A *short tutorial* where you teach Huggy the Dog to fetch the stick and then play with him directly in your\n browser: URL\n - A *longer tutorial* to understand how works ML-Agents:\n URL\n\n ### Resume the training\n \n\n ### Watch your Agent play\n You can watch your agent playing directly in your browser\n\n 1. If the environment is part of ML-Agents official environments, go to URL\n 2. Step 1: Find your model_id: hossniper/ppo-Pyramids\n 3. Step 2: Select your *.nn /*.onnx file\n 4. Click on Watch the agent play"
] |
null | peft |
<!-- This model card has been generated automatically according to the information the Trainer had access to. You
should probably proofread and complete it, then remove this comment. -->
# GUE_EMP_H4-seqsight_16384_512_22M-L1_f
This model is a fine-tuned version of [mahdibaghbanzadeh/seqsight_16384_512_22M](https://huggingface.co/mahdibaghbanzadeh/seqsight_16384_512_22M) on the [mahdibaghbanzadeh/GUE_EMP_H4](https://huggingface.co/datasets/mahdibaghbanzadeh/GUE_EMP_H4) dataset.
It achieves the following results on the evaluation set:
- Loss: 0.2765
- F1 Score: 0.8968
- Accuracy: 0.8966
## Model description
More information needed
## Intended uses & limitations
More information needed
## Training and evaluation data
More information needed
## Training procedure
### Training hyperparameters
The following hyperparameters were used during training:
- learning_rate: 0.0005
- train_batch_size: 128
- eval_batch_size: 128
- seed: 42
- optimizer: Adam with betas=(0.9,0.999) and epsilon=1e-08
- lr_scheduler_type: linear
- training_steps: 10000
### Training results
| Training Loss | Epoch | Step | Validation Loss | F1 Score | Accuracy |
|:-------------:|:------:|:-----:|:---------------:|:--------:|:--------:|
| 0.4202 | 2.17 | 200 | 0.3127 | 0.8836 | 0.8836 |
| 0.3173 | 4.35 | 400 | 0.3114 | 0.8785 | 0.8782 |
| 0.3053 | 6.52 | 600 | 0.3072 | 0.8832 | 0.8830 |
| 0.3045 | 8.7 | 800 | 0.3039 | 0.8880 | 0.8877 |
| 0.2935 | 10.87 | 1000 | 0.3008 | 0.8852 | 0.8850 |
| 0.2892 | 13.04 | 1200 | 0.3047 | 0.8846 | 0.8843 |
| 0.2851 | 15.22 | 1400 | 0.2969 | 0.8858 | 0.8857 |
| 0.2842 | 17.39 | 1600 | 0.2952 | 0.8872 | 0.8871 |
| 0.2797 | 19.57 | 1800 | 0.2927 | 0.8876 | 0.8877 |
| 0.2778 | 21.74 | 2000 | 0.2995 | 0.8880 | 0.8877 |
| 0.2738 | 23.91 | 2200 | 0.2999 | 0.8881 | 0.8877 |
| 0.2729 | 26.09 | 2400 | 0.3025 | 0.8874 | 0.8871 |
| 0.272 | 28.26 | 2600 | 0.2887 | 0.8911 | 0.8912 |
| 0.271 | 30.43 | 2800 | 0.2888 | 0.8886 | 0.8884 |
| 0.2682 | 32.61 | 3000 | 0.2910 | 0.8886 | 0.8884 |
| 0.2638 | 34.78 | 3200 | 0.2878 | 0.8926 | 0.8925 |
| 0.2616 | 36.96 | 3400 | 0.2926 | 0.8886 | 0.8884 |
| 0.2599 | 39.13 | 3600 | 0.2945 | 0.8866 | 0.8864 |
| 0.2559 | 41.3 | 3800 | 0.2878 | 0.8954 | 0.8953 |
| 0.2575 | 43.48 | 4000 | 0.2931 | 0.8920 | 0.8919 |
| 0.263 | 45.65 | 4200 | 0.2849 | 0.8919 | 0.8919 |
| 0.2553 | 47.83 | 4400 | 0.2876 | 0.8906 | 0.8905 |
| 0.2541 | 50.0 | 4600 | 0.2835 | 0.8946 | 0.8946 |
| 0.2519 | 52.17 | 4800 | 0.2867 | 0.8926 | 0.8925 |
| 0.2531 | 54.35 | 5000 | 0.2919 | 0.8915 | 0.8912 |
| 0.2495 | 56.52 | 5200 | 0.2877 | 0.8913 | 0.8912 |
| 0.2483 | 58.7 | 5400 | 0.2865 | 0.8927 | 0.8925 |
| 0.2489 | 60.87 | 5600 | 0.2914 | 0.8873 | 0.8871 |
| 0.2499 | 63.04 | 5800 | 0.2866 | 0.8926 | 0.8925 |
| 0.247 | 65.22 | 6000 | 0.2893 | 0.8907 | 0.8905 |
| 0.251 | 67.39 | 6200 | 0.2891 | 0.8886 | 0.8884 |
| 0.2459 | 69.57 | 6400 | 0.2859 | 0.8920 | 0.8919 |
| 0.2438 | 71.74 | 6600 | 0.2878 | 0.8921 | 0.8919 |
| 0.2453 | 73.91 | 6800 | 0.2848 | 0.8941 | 0.8939 |
| 0.2441 | 76.09 | 7000 | 0.2865 | 0.8955 | 0.8953 |
| 0.2406 | 78.26 | 7200 | 0.2877 | 0.8954 | 0.8953 |
| 0.2411 | 80.43 | 7400 | 0.2851 | 0.8988 | 0.8987 |
| 0.2403 | 82.61 | 7600 | 0.2828 | 0.8981 | 0.8980 |
| 0.2414 | 84.78 | 7800 | 0.2861 | 0.8968 | 0.8966 |
| 0.2378 | 86.96 | 8000 | 0.2832 | 0.8974 | 0.8973 |
| 0.2415 | 89.13 | 8200 | 0.2845 | 0.8968 | 0.8966 |
| 0.2379 | 91.3 | 8400 | 0.2842 | 0.8953 | 0.8953 |
| 0.2367 | 93.48 | 8600 | 0.2850 | 0.8953 | 0.8953 |
| 0.2409 | 95.65 | 8800 | 0.2839 | 0.8974 | 0.8973 |
| 0.2416 | 97.83 | 9000 | 0.2840 | 0.8967 | 0.8966 |
| 0.2379 | 100.0 | 9200 | 0.2846 | 0.8968 | 0.8966 |
| 0.2359 | 102.17 | 9400 | 0.2839 | 0.8981 | 0.8980 |
| 0.239 | 104.35 | 9600 | 0.2843 | 0.8968 | 0.8966 |
| 0.2396 | 106.52 | 9800 | 0.2829 | 0.8960 | 0.8960 |
| 0.2392 | 108.7 | 10000 | 0.2832 | 0.8974 | 0.8973 |
### Framework versions
- PEFT 0.9.0
- Transformers 4.38.2
- Pytorch 2.2.0+cu121
- Datasets 2.17.1
- Tokenizers 0.15.2 | {"library_name": "peft", "tags": ["generated_from_trainer"], "metrics": ["accuracy"], "base_model": "mahdibaghbanzadeh/seqsight_16384_512_22M", "model-index": [{"name": "GUE_EMP_H4-seqsight_16384_512_22M-L1_f", "results": []}]} | mahdibaghbanzadeh/GUE_EMP_H4-seqsight_16384_512_22M-L1_f | null | [
"peft",
"safetensors",
"generated_from_trainer",
"base_model:mahdibaghbanzadeh/seqsight_16384_512_22M",
"region:us"
] | null | 2024-04-27T07:51:53+00:00 | [] | [] | TAGS
#peft #safetensors #generated_from_trainer #base_model-mahdibaghbanzadeh/seqsight_16384_512_22M #region-us
| GUE\_EMP\_H4-seqsight\_16384\_512\_22M-L1\_f
============================================
This model is a fine-tuned version of mahdibaghbanzadeh/seqsight\_16384\_512\_22M on the mahdibaghbanzadeh/GUE\_EMP\_H4 dataset.
It achieves the following results on the evaluation set:
* Loss: 0.2765
* F1 Score: 0.8968
* Accuracy: 0.8966
Model description
-----------------
More information needed
Intended uses & limitations
---------------------------
More information needed
Training and evaluation data
----------------------------
More information needed
Training procedure
------------------
### Training hyperparameters
The following hyperparameters were used during training:
* learning\_rate: 0.0005
* train\_batch\_size: 128
* eval\_batch\_size: 128
* seed: 42
* optimizer: Adam with betas=(0.9,0.999) and epsilon=1e-08
* lr\_scheduler\_type: linear
* training\_steps: 10000
### Training results
### Framework versions
* PEFT 0.9.0
* Transformers 4.38.2
* Pytorch 2.2.0+cu121
* Datasets 2.17.1
* Tokenizers 0.15.2
| [
"### Training hyperparameters\n\n\nThe following hyperparameters were used during training:\n\n\n* learning\\_rate: 0.0005\n* train\\_batch\\_size: 128\n* eval\\_batch\\_size: 128\n* seed: 42\n* optimizer: Adam with betas=(0.9,0.999) and epsilon=1e-08\n* lr\\_scheduler\\_type: linear\n* training\\_steps: 10000",
"### Training results",
"### Framework versions\n\n\n* PEFT 0.9.0\n* Transformers 4.38.2\n* Pytorch 2.2.0+cu121\n* Datasets 2.17.1\n* Tokenizers 0.15.2"
] | [
"TAGS\n#peft #safetensors #generated_from_trainer #base_model-mahdibaghbanzadeh/seqsight_16384_512_22M #region-us \n",
"### Training hyperparameters\n\n\nThe following hyperparameters were used during training:\n\n\n* learning\\_rate: 0.0005\n* train\\_batch\\_size: 128\n* eval\\_batch\\_size: 128\n* seed: 42\n* optimizer: Adam with betas=(0.9,0.999) and epsilon=1e-08\n* lr\\_scheduler\\_type: linear\n* training\\_steps: 10000",
"### Training results",
"### Framework versions\n\n\n* PEFT 0.9.0\n* Transformers 4.38.2\n* Pytorch 2.2.0+cu121\n* Datasets 2.17.1\n* Tokenizers 0.15.2"
] |
null | peft |
<!-- This model card has been generated automatically according to the information the Trainer had access to. You
should probably proofread and complete it, then remove this comment. -->
# GUE_EMP_H4-seqsight_16384_512_22M-L8_f
This model is a fine-tuned version of [mahdibaghbanzadeh/seqsight_16384_512_22M](https://huggingface.co/mahdibaghbanzadeh/seqsight_16384_512_22M) on the [mahdibaghbanzadeh/GUE_EMP_H4](https://huggingface.co/datasets/mahdibaghbanzadeh/GUE_EMP_H4) dataset.
It achieves the following results on the evaluation set:
- Loss: 0.2700
- F1 Score: 0.9007
- Accuracy: 0.9008
## Model description
More information needed
## Intended uses & limitations
More information needed
## Training and evaluation data
More information needed
## Training procedure
### Training hyperparameters
The following hyperparameters were used during training:
- learning_rate: 0.0005
- train_batch_size: 128
- eval_batch_size: 128
- seed: 42
- optimizer: Adam with betas=(0.9,0.999) and epsilon=1e-08
- lr_scheduler_type: linear
- training_steps: 10000
### Training results
| Training Loss | Epoch | Step | Validation Loss | F1 Score | Accuracy |
|:-------------:|:------:|:-----:|:---------------:|:--------:|:--------:|
| 0.3842 | 2.17 | 200 | 0.2976 | 0.8851 | 0.8850 |
| 0.2983 | 4.35 | 400 | 0.2977 | 0.8797 | 0.8795 |
| 0.2851 | 6.52 | 600 | 0.2929 | 0.8891 | 0.8891 |
| 0.2815 | 8.7 | 800 | 0.2945 | 0.8866 | 0.8864 |
| 0.2686 | 10.87 | 1000 | 0.2889 | 0.8893 | 0.8891 |
| 0.2642 | 13.04 | 1200 | 0.2857 | 0.8871 | 0.8871 |
| 0.2581 | 15.22 | 1400 | 0.2879 | 0.8893 | 0.8891 |
| 0.2537 | 17.39 | 1600 | 0.2913 | 0.8908 | 0.8905 |
| 0.2504 | 19.57 | 1800 | 0.2752 | 0.9007 | 0.9008 |
| 0.2436 | 21.74 | 2000 | 0.2861 | 0.8867 | 0.8864 |
| 0.2367 | 23.91 | 2200 | 0.2766 | 0.8989 | 0.8987 |
| 0.2368 | 26.09 | 2400 | 0.2879 | 0.8963 | 0.8960 |
| 0.233 | 28.26 | 2600 | 0.2772 | 0.8951 | 0.8953 |
| 0.2296 | 30.43 | 2800 | 0.2767 | 0.8913 | 0.8912 |
| 0.2257 | 32.61 | 3000 | 0.2811 | 0.8980 | 0.8980 |
| 0.2174 | 34.78 | 3200 | 0.2829 | 0.8946 | 0.8946 |
| 0.2185 | 36.96 | 3400 | 0.2828 | 0.8969 | 0.8966 |
| 0.213 | 39.13 | 3600 | 0.2834 | 0.8988 | 0.8987 |
| 0.2084 | 41.3 | 3800 | 0.2857 | 0.9002 | 0.9001 |
| 0.2068 | 43.48 | 4000 | 0.2932 | 0.8925 | 0.8925 |
| 0.2109 | 45.65 | 4200 | 0.2890 | 0.8918 | 0.8919 |
| 0.2045 | 47.83 | 4400 | 0.2889 | 0.8946 | 0.8946 |
| 0.2016 | 50.0 | 4600 | 0.2848 | 0.8959 | 0.8960 |
| 0.1988 | 52.17 | 4800 | 0.2924 | 0.8967 | 0.8966 |
| 0.1965 | 54.35 | 5000 | 0.2918 | 0.8989 | 0.8987 |
| 0.1919 | 56.52 | 5200 | 0.3074 | 0.8894 | 0.8891 |
| 0.1904 | 58.7 | 5400 | 0.2953 | 0.8921 | 0.8919 |
| 0.1898 | 60.87 | 5600 | 0.2957 | 0.8948 | 0.8946 |
| 0.1895 | 63.04 | 5800 | 0.3019 | 0.8927 | 0.8925 |
| 0.1843 | 65.22 | 6000 | 0.3038 | 0.8892 | 0.8891 |
| 0.1869 | 67.39 | 6200 | 0.3047 | 0.8899 | 0.8898 |
| 0.1837 | 69.57 | 6400 | 0.3003 | 0.8907 | 0.8905 |
| 0.1842 | 71.74 | 6600 | 0.3040 | 0.8934 | 0.8932 |
| 0.1818 | 73.91 | 6800 | 0.3038 | 0.8886 | 0.8884 |
| 0.1779 | 76.09 | 7000 | 0.3105 | 0.8886 | 0.8884 |
| 0.1764 | 78.26 | 7200 | 0.3079 | 0.8947 | 0.8946 |
| 0.1747 | 80.43 | 7400 | 0.3134 | 0.8913 | 0.8912 |
| 0.1731 | 82.61 | 7600 | 0.3110 | 0.8905 | 0.8905 |
| 0.1726 | 84.78 | 7800 | 0.3224 | 0.8901 | 0.8898 |
| 0.1705 | 86.96 | 8000 | 0.3129 | 0.8919 | 0.8919 |
| 0.175 | 89.13 | 8200 | 0.3098 | 0.8893 | 0.8891 |
| 0.1706 | 91.3 | 8400 | 0.3163 | 0.8899 | 0.8898 |
| 0.1647 | 93.48 | 8600 | 0.3231 | 0.8913 | 0.8912 |
| 0.1722 | 95.65 | 8800 | 0.3185 | 0.8898 | 0.8898 |
| 0.1705 | 97.83 | 9000 | 0.3165 | 0.8919 | 0.8919 |
| 0.168 | 100.0 | 9200 | 0.3202 | 0.8893 | 0.8891 |
| 0.164 | 102.17 | 9400 | 0.3212 | 0.8913 | 0.8912 |
| 0.1677 | 104.35 | 9600 | 0.3197 | 0.8899 | 0.8898 |
| 0.1686 | 106.52 | 9800 | 0.3198 | 0.8919 | 0.8919 |
| 0.1668 | 108.7 | 10000 | 0.3200 | 0.8920 | 0.8919 |
### Framework versions
- PEFT 0.9.0
- Transformers 4.38.2
- Pytorch 2.2.0+cu121
- Datasets 2.17.1
- Tokenizers 0.15.2 | {"library_name": "peft", "tags": ["generated_from_trainer"], "metrics": ["accuracy"], "base_model": "mahdibaghbanzadeh/seqsight_16384_512_22M", "model-index": [{"name": "GUE_EMP_H4-seqsight_16384_512_22M-L8_f", "results": []}]} | mahdibaghbanzadeh/GUE_EMP_H4-seqsight_16384_512_22M-L8_f | null | [
"peft",
"safetensors",
"generated_from_trainer",
"base_model:mahdibaghbanzadeh/seqsight_16384_512_22M",
"region:us"
] | null | 2024-04-27T07:52:32+00:00 | [] | [] | TAGS
#peft #safetensors #generated_from_trainer #base_model-mahdibaghbanzadeh/seqsight_16384_512_22M #region-us
| GUE\_EMP\_H4-seqsight\_16384\_512\_22M-L8\_f
============================================
This model is a fine-tuned version of mahdibaghbanzadeh/seqsight\_16384\_512\_22M on the mahdibaghbanzadeh/GUE\_EMP\_H4 dataset.
It achieves the following results on the evaluation set:
* Loss: 0.2700
* F1 Score: 0.9007
* Accuracy: 0.9008
Model description
-----------------
More information needed
Intended uses & limitations
---------------------------
More information needed
Training and evaluation data
----------------------------
More information needed
Training procedure
------------------
### Training hyperparameters
The following hyperparameters were used during training:
* learning\_rate: 0.0005
* train\_batch\_size: 128
* eval\_batch\_size: 128
* seed: 42
* optimizer: Adam with betas=(0.9,0.999) and epsilon=1e-08
* lr\_scheduler\_type: linear
* training\_steps: 10000
### Training results
### Framework versions
* PEFT 0.9.0
* Transformers 4.38.2
* Pytorch 2.2.0+cu121
* Datasets 2.17.1
* Tokenizers 0.15.2
| [
"### Training hyperparameters\n\n\nThe following hyperparameters were used during training:\n\n\n* learning\\_rate: 0.0005\n* train\\_batch\\_size: 128\n* eval\\_batch\\_size: 128\n* seed: 42\n* optimizer: Adam with betas=(0.9,0.999) and epsilon=1e-08\n* lr\\_scheduler\\_type: linear\n* training\\_steps: 10000",
"### Training results",
"### Framework versions\n\n\n* PEFT 0.9.0\n* Transformers 4.38.2\n* Pytorch 2.2.0+cu121\n* Datasets 2.17.1\n* Tokenizers 0.15.2"
] | [
"TAGS\n#peft #safetensors #generated_from_trainer #base_model-mahdibaghbanzadeh/seqsight_16384_512_22M #region-us \n",
"### Training hyperparameters\n\n\nThe following hyperparameters were used during training:\n\n\n* learning\\_rate: 0.0005\n* train\\_batch\\_size: 128\n* eval\\_batch\\_size: 128\n* seed: 42\n* optimizer: Adam with betas=(0.9,0.999) and epsilon=1e-08\n* lr\\_scheduler\\_type: linear\n* training\\_steps: 10000",
"### Training results",
"### Framework versions\n\n\n* PEFT 0.9.0\n* Transformers 4.38.2\n* Pytorch 2.2.0+cu121\n* Datasets 2.17.1\n* Tokenizers 0.15.2"
] |
text-generation | transformers |
# Model Card for Model ID
<!-- Provide a quick summary of what the model is/does. -->
## Model Details
### Model Description
<!-- Provide a longer summary of what this model is. -->
This is the model card of a 🤗 transformers model that has been pushed on the Hub. This model card has been automatically generated.
- **Developed by:** [More Information Needed]
- **Funded by [optional]:** [More Information Needed]
- **Shared by [optional]:** [More Information Needed]
- **Model type:** [More Information Needed]
- **Language(s) (NLP):** [More Information Needed]
- **License:** [More Information Needed]
- **Finetuned from model [optional]:** [More Information Needed]
### Model Sources [optional]
<!-- Provide the basic links for the model. -->
- **Repository:** [More Information Needed]
- **Paper [optional]:** [More Information Needed]
- **Demo [optional]:** [More Information Needed]
## Uses
<!-- Address questions around how the model is intended to be used, including the foreseeable users of the model and those affected by the model. -->
### Direct Use
<!-- This section is for the model use without fine-tuning or plugging into a larger ecosystem/app. -->
[More Information Needed]
### Downstream Use [optional]
<!-- This section is for the model use when fine-tuned for a task, or when plugged into a larger ecosystem/app -->
[More Information Needed]
### Out-of-Scope Use
<!-- This section addresses misuse, malicious use, and uses that the model will not work well for. -->
[More Information Needed]
## Bias, Risks, and Limitations
<!-- This section is meant to convey both technical and sociotechnical limitations. -->
[More Information Needed]
### Recommendations
<!-- This section is meant to convey recommendations with respect to the bias, risk, and technical limitations. -->
Users (both direct and downstream) should be made aware of the risks, biases and limitations of the model. More information needed for further recommendations.
## How to Get Started with the Model
Use the code below to get started with the model.
[More Information Needed]
## Training Details
### Training Data
<!-- This should link to a Dataset Card, perhaps with a short stub of information on what the training data is all about as well as documentation related to data pre-processing or additional filtering. -->
[More Information Needed]
### Training Procedure
<!-- This relates heavily to the Technical Specifications. Content here should link to that section when it is relevant to the training procedure. -->
#### Preprocessing [optional]
[More Information Needed]
#### Training Hyperparameters
- **Training regime:** [More Information Needed] <!--fp32, fp16 mixed precision, bf16 mixed precision, bf16 non-mixed precision, fp16 non-mixed precision, fp8 mixed precision -->
#### Speeds, Sizes, Times [optional]
<!-- This section provides information about throughput, start/end time, checkpoint size if relevant, etc. -->
[More Information Needed]
## Evaluation
<!-- This section describes the evaluation protocols and provides the results. -->
### Testing Data, Factors & Metrics
#### Testing Data
<!-- This should link to a Dataset Card if possible. -->
[More Information Needed]
#### Factors
<!-- These are the things the evaluation is disaggregating by, e.g., subpopulations or domains. -->
[More Information Needed]
#### Metrics
<!-- These are the evaluation metrics being used, ideally with a description of why. -->
[More Information Needed]
### Results
[More Information Needed]
#### Summary
## Model Examination [optional]
<!-- Relevant interpretability work for the model goes here -->
[More Information Needed]
## Environmental Impact
<!-- Total emissions (in grams of CO2eq) and additional considerations, such as electricity usage, go here. Edit the suggested text below accordingly -->
Carbon emissions can be estimated using the [Machine Learning Impact calculator](https://mlco2.github.io/impact#compute) presented in [Lacoste et al. (2019)](https://arxiv.org/abs/1910.09700).
- **Hardware Type:** [More Information Needed]
- **Hours used:** [More Information Needed]
- **Cloud Provider:** [More Information Needed]
- **Compute Region:** [More Information Needed]
- **Carbon Emitted:** [More Information Needed]
## Technical Specifications [optional]
### Model Architecture and Objective
[More Information Needed]
### Compute Infrastructure
[More Information Needed]
#### Hardware
[More Information Needed]
#### Software
[More Information Needed]
## Citation [optional]
<!-- If there is a paper or blog post introducing the model, the APA and Bibtex information for that should go in this section. -->
**BibTeX:**
[More Information Needed]
**APA:**
[More Information Needed]
## Glossary [optional]
<!-- If relevant, include terms and calculations in this section that can help readers understand the model or model card. -->
[More Information Needed]
## More Information [optional]
[More Information Needed]
## Model Card Authors [optional]
[More Information Needed]
## Model Card Contact
[More Information Needed]
| {"library_name": "transformers", "tags": []} | swj0419/hp_retrain_STEP0000060 | null | [
"transformers",
"safetensors",
"llama",
"text-generation",
"arxiv:1910.09700",
"autotrain_compatible",
"endpoints_compatible",
"text-generation-inference",
"region:us"
] | null | 2024-04-27T07:52:49+00:00 | [
"1910.09700"
] | [] | TAGS
#transformers #safetensors #llama #text-generation #arxiv-1910.09700 #autotrain_compatible #endpoints_compatible #text-generation-inference #region-us
|
# Model Card for Model ID
## Model Details
### Model Description
This is the model card of a transformers model that has been pushed on the Hub. This model card has been automatically generated.
- Developed by:
- Funded by [optional]:
- Shared by [optional]:
- Model type:
- Language(s) (NLP):
- License:
- Finetuned from model [optional]:
### Model Sources [optional]
- Repository:
- Paper [optional]:
- Demo [optional]:
## Uses
### Direct Use
### Downstream Use [optional]
### Out-of-Scope Use
## Bias, Risks, and Limitations
### Recommendations
Users (both direct and downstream) should be made aware of the risks, biases and limitations of the model. More information needed for further recommendations.
## How to Get Started with the Model
Use the code below to get started with the model.
## Training Details
### Training Data
### Training Procedure
#### Preprocessing [optional]
#### Training Hyperparameters
- Training regime:
#### Speeds, Sizes, Times [optional]
## Evaluation
### Testing Data, Factors & Metrics
#### Testing Data
#### Factors
#### Metrics
### Results
#### Summary
## Model Examination [optional]
## Environmental Impact
Carbon emissions can be estimated using the Machine Learning Impact calculator presented in Lacoste et al. (2019).
- Hardware Type:
- Hours used:
- Cloud Provider:
- Compute Region:
- Carbon Emitted:
## Technical Specifications [optional]
### Model Architecture and Objective
### Compute Infrastructure
#### Hardware
#### Software
[optional]
BibTeX:
APA:
## Glossary [optional]
## More Information [optional]
## Model Card Authors [optional]
## Model Card Contact
| [
"# Model Card for Model ID",
"## Model Details",
"### Model Description\n\n\n\nThis is the model card of a transformers model that has been pushed on the Hub. This model card has been automatically generated.\n\n- Developed by: \n- Funded by [optional]: \n- Shared by [optional]: \n- Model type: \n- Language(s) (NLP): \n- License: \n- Finetuned from model [optional]:",
"### Model Sources [optional]\n\n\n\n- Repository: \n- Paper [optional]: \n- Demo [optional]:",
"## Uses",
"### Direct Use",
"### Downstream Use [optional]",
"### Out-of-Scope Use",
"## Bias, Risks, and Limitations",
"### Recommendations\n\n\n\nUsers (both direct and downstream) should be made aware of the risks, biases and limitations of the model. More information needed for further recommendations.",
"## How to Get Started with the Model\n\nUse the code below to get started with the model.",
"## Training Details",
"### Training Data",
"### Training Procedure",
"#### Preprocessing [optional]",
"#### Training Hyperparameters\n\n- Training regime:",
"#### Speeds, Sizes, Times [optional]",
"## Evaluation",
"### Testing Data, Factors & Metrics",
"#### Testing Data",
"#### Factors",
"#### Metrics",
"### Results",
"#### Summary",
"## Model Examination [optional]",
"## Environmental Impact\n\n\n\nCarbon emissions can be estimated using the Machine Learning Impact calculator presented in Lacoste et al. (2019).\n\n- Hardware Type: \n- Hours used: \n- Cloud Provider: \n- Compute Region: \n- Carbon Emitted:",
"## Technical Specifications [optional]",
"### Model Architecture and Objective",
"### Compute Infrastructure",
"#### Hardware",
"#### Software\n\n\n\n[optional]\n\n\n\nBibTeX:\n\n\n\nAPA:",
"## Glossary [optional]",
"## More Information [optional]",
"## Model Card Authors [optional]",
"## Model Card Contact"
] | [
"TAGS\n#transformers #safetensors #llama #text-generation #arxiv-1910.09700 #autotrain_compatible #endpoints_compatible #text-generation-inference #region-us \n",
"# Model Card for Model ID",
"## Model Details",
"### Model Description\n\n\n\nThis is the model card of a transformers model that has been pushed on the Hub. This model card has been automatically generated.\n\n- Developed by: \n- Funded by [optional]: \n- Shared by [optional]: \n- Model type: \n- Language(s) (NLP): \n- License: \n- Finetuned from model [optional]:",
"### Model Sources [optional]\n\n\n\n- Repository: \n- Paper [optional]: \n- Demo [optional]:",
"## Uses",
"### Direct Use",
"### Downstream Use [optional]",
"### Out-of-Scope Use",
"## Bias, Risks, and Limitations",
"### Recommendations\n\n\n\nUsers (both direct and downstream) should be made aware of the risks, biases and limitations of the model. More information needed for further recommendations.",
"## How to Get Started with the Model\n\nUse the code below to get started with the model.",
"## Training Details",
"### Training Data",
"### Training Procedure",
"#### Preprocessing [optional]",
"#### Training Hyperparameters\n\n- Training regime:",
"#### Speeds, Sizes, Times [optional]",
"## Evaluation",
"### Testing Data, Factors & Metrics",
"#### Testing Data",
"#### Factors",
"#### Metrics",
"### Results",
"#### Summary",
"## Model Examination [optional]",
"## Environmental Impact\n\n\n\nCarbon emissions can be estimated using the Machine Learning Impact calculator presented in Lacoste et al. (2019).\n\n- Hardware Type: \n- Hours used: \n- Cloud Provider: \n- Compute Region: \n- Carbon Emitted:",
"## Technical Specifications [optional]",
"### Model Architecture and Objective",
"### Compute Infrastructure",
"#### Hardware",
"#### Software\n\n\n\n[optional]\n\n\n\nBibTeX:\n\n\n\nAPA:",
"## Glossary [optional]",
"## More Information [optional]",
"## Model Card Authors [optional]",
"## Model Card Contact"
] |
null | peft |
<!-- This model card has been generated automatically according to the information the Trainer had access to. You
should probably proofread and complete it, then remove this comment. -->
# GUE_EMP_H4-seqsight_16384_512_22M-L32_f
This model is a fine-tuned version of [mahdibaghbanzadeh/seqsight_16384_512_22M](https://huggingface.co/mahdibaghbanzadeh/seqsight_16384_512_22M) on the [mahdibaghbanzadeh/GUE_EMP_H4](https://huggingface.co/datasets/mahdibaghbanzadeh/GUE_EMP_H4) dataset.
It achieves the following results on the evaluation set:
- Loss: 0.2729
- F1 Score: 0.8953
- Accuracy: 0.8953
## Model description
More information needed
## Intended uses & limitations
More information needed
## Training and evaluation data
More information needed
## Training procedure
### Training hyperparameters
The following hyperparameters were used during training:
- learning_rate: 0.0005
- train_batch_size: 128
- eval_batch_size: 128
- seed: 42
- optimizer: Adam with betas=(0.9,0.999) and epsilon=1e-08
- lr_scheduler_type: linear
- training_steps: 10000
### Training results
| Training Loss | Epoch | Step | Validation Loss | F1 Score | Accuracy |
|:-------------:|:------:|:-----:|:---------------:|:--------:|:--------:|
| 0.3657 | 2.17 | 200 | 0.2942 | 0.8860 | 0.8857 |
| 0.2891 | 4.35 | 400 | 0.2858 | 0.8918 | 0.8919 |
| 0.2713 | 6.52 | 600 | 0.2819 | 0.8978 | 0.8980 |
| 0.263 | 8.7 | 800 | 0.2886 | 0.8861 | 0.8857 |
| 0.2465 | 10.87 | 1000 | 0.2804 | 0.8915 | 0.8912 |
| 0.2394 | 13.04 | 1200 | 0.2801 | 0.8949 | 0.8946 |
| 0.2297 | 15.22 | 1400 | 0.2921 | 0.8983 | 0.8980 |
| 0.2203 | 17.39 | 1600 | 0.2939 | 0.8976 | 0.8973 |
| 0.2131 | 19.57 | 1800 | 0.2807 | 0.8994 | 0.8994 |
| 0.2013 | 21.74 | 2000 | 0.3073 | 0.8874 | 0.8871 |
| 0.1905 | 23.91 | 2200 | 0.3036 | 0.8925 | 0.8925 |
| 0.1892 | 26.09 | 2400 | 0.2999 | 0.8921 | 0.8919 |
| 0.1809 | 28.26 | 2600 | 0.3079 | 0.8896 | 0.8898 |
| 0.173 | 30.43 | 2800 | 0.3091 | 0.8886 | 0.8884 |
| 0.1663 | 32.61 | 3000 | 0.3217 | 0.8917 | 0.8919 |
| 0.1531 | 34.78 | 3200 | 0.3562 | 0.8797 | 0.8795 |
| 0.1506 | 36.96 | 3400 | 0.3572 | 0.8854 | 0.8850 |
| 0.1439 | 39.13 | 3600 | 0.3507 | 0.8871 | 0.8871 |
| 0.1367 | 41.3 | 3800 | 0.3633 | 0.8837 | 0.8836 |
| 0.1319 | 43.48 | 4000 | 0.3776 | 0.8823 | 0.8823 |
| 0.1285 | 45.65 | 4200 | 0.3790 | 0.8798 | 0.8802 |
| 0.1215 | 47.83 | 4400 | 0.3750 | 0.8823 | 0.8823 |
| 0.1162 | 50.0 | 4600 | 0.3899 | 0.8826 | 0.8830 |
| 0.1152 | 52.17 | 4800 | 0.3972 | 0.8824 | 0.8823 |
| 0.1089 | 54.35 | 5000 | 0.3884 | 0.8809 | 0.8809 |
| 0.1042 | 56.52 | 5200 | 0.4067 | 0.8812 | 0.8809 |
| 0.0975 | 58.7 | 5400 | 0.4032 | 0.8817 | 0.8816 |
| 0.0982 | 60.87 | 5600 | 0.4058 | 0.8824 | 0.8823 |
| 0.0938 | 63.04 | 5800 | 0.4207 | 0.8796 | 0.8795 |
| 0.094 | 65.22 | 6000 | 0.4207 | 0.8838 | 0.8836 |
| 0.0884 | 67.39 | 6200 | 0.4507 | 0.8818 | 0.8816 |
| 0.0884 | 69.57 | 6400 | 0.4531 | 0.8756 | 0.8754 |
| 0.0869 | 71.74 | 6600 | 0.4414 | 0.8808 | 0.8809 |
| 0.0822 | 73.91 | 6800 | 0.4698 | 0.8775 | 0.8775 |
| 0.0771 | 76.09 | 7000 | 0.4825 | 0.8812 | 0.8809 |
| 0.0764 | 78.26 | 7200 | 0.4789 | 0.8762 | 0.8761 |
| 0.0778 | 80.43 | 7400 | 0.4718 | 0.8805 | 0.8802 |
| 0.0724 | 82.61 | 7600 | 0.4876 | 0.8724 | 0.8727 |
| 0.0723 | 84.78 | 7800 | 0.4907 | 0.8756 | 0.8754 |
| 0.0714 | 86.96 | 8000 | 0.4706 | 0.8816 | 0.8816 |
| 0.0709 | 89.13 | 8200 | 0.4848 | 0.8816 | 0.8816 |
| 0.0688 | 91.3 | 8400 | 0.4802 | 0.8794 | 0.8795 |
| 0.0626 | 93.48 | 8600 | 0.5095 | 0.8773 | 0.8775 |
| 0.068 | 95.65 | 8800 | 0.4971 | 0.8813 | 0.8816 |
| 0.0665 | 97.83 | 9000 | 0.4924 | 0.8753 | 0.8754 |
| 0.0611 | 100.0 | 9200 | 0.5035 | 0.8747 | 0.8747 |
| 0.0617 | 102.17 | 9400 | 0.5036 | 0.8774 | 0.8775 |
| 0.0611 | 104.35 | 9600 | 0.5081 | 0.8774 | 0.8775 |
| 0.0631 | 106.52 | 9800 | 0.5050 | 0.8795 | 0.8795 |
| 0.0605 | 108.7 | 10000 | 0.5061 | 0.8774 | 0.8775 |
### Framework versions
- PEFT 0.9.0
- Transformers 4.38.2
- Pytorch 2.2.0+cu121
- Datasets 2.17.1
- Tokenizers 0.15.2 | {"library_name": "peft", "tags": ["generated_from_trainer"], "metrics": ["accuracy"], "base_model": "mahdibaghbanzadeh/seqsight_16384_512_22M", "model-index": [{"name": "GUE_EMP_H4-seqsight_16384_512_22M-L32_f", "results": []}]} | mahdibaghbanzadeh/GUE_EMP_H4-seqsight_16384_512_22M-L32_f | null | [
"peft",
"safetensors",
"generated_from_trainer",
"base_model:mahdibaghbanzadeh/seqsight_16384_512_22M",
"region:us"
] | null | 2024-04-27T07:53:46+00:00 | [] | [] | TAGS
#peft #safetensors #generated_from_trainer #base_model-mahdibaghbanzadeh/seqsight_16384_512_22M #region-us
| GUE\_EMP\_H4-seqsight\_16384\_512\_22M-L32\_f
=============================================
This model is a fine-tuned version of mahdibaghbanzadeh/seqsight\_16384\_512\_22M on the mahdibaghbanzadeh/GUE\_EMP\_H4 dataset.
It achieves the following results on the evaluation set:
* Loss: 0.2729
* F1 Score: 0.8953
* Accuracy: 0.8953
Model description
-----------------
More information needed
Intended uses & limitations
---------------------------
More information needed
Training and evaluation data
----------------------------
More information needed
Training procedure
------------------
### Training hyperparameters
The following hyperparameters were used during training:
* learning\_rate: 0.0005
* train\_batch\_size: 128
* eval\_batch\_size: 128
* seed: 42
* optimizer: Adam with betas=(0.9,0.999) and epsilon=1e-08
* lr\_scheduler\_type: linear
* training\_steps: 10000
### Training results
### Framework versions
* PEFT 0.9.0
* Transformers 4.38.2
* Pytorch 2.2.0+cu121
* Datasets 2.17.1
* Tokenizers 0.15.2
| [
"### Training hyperparameters\n\n\nThe following hyperparameters were used during training:\n\n\n* learning\\_rate: 0.0005\n* train\\_batch\\_size: 128\n* eval\\_batch\\_size: 128\n* seed: 42\n* optimizer: Adam with betas=(0.9,0.999) and epsilon=1e-08\n* lr\\_scheduler\\_type: linear\n* training\\_steps: 10000",
"### Training results",
"### Framework versions\n\n\n* PEFT 0.9.0\n* Transformers 4.38.2\n* Pytorch 2.2.0+cu121\n* Datasets 2.17.1\n* Tokenizers 0.15.2"
] | [
"TAGS\n#peft #safetensors #generated_from_trainer #base_model-mahdibaghbanzadeh/seqsight_16384_512_22M #region-us \n",
"### Training hyperparameters\n\n\nThe following hyperparameters were used during training:\n\n\n* learning\\_rate: 0.0005\n* train\\_batch\\_size: 128\n* eval\\_batch\\_size: 128\n* seed: 42\n* optimizer: Adam with betas=(0.9,0.999) and epsilon=1e-08\n* lr\\_scheduler\\_type: linear\n* training\\_steps: 10000",
"### Training results",
"### Framework versions\n\n\n* PEFT 0.9.0\n* Transformers 4.38.2\n* Pytorch 2.2.0+cu121\n* Datasets 2.17.1\n* Tokenizers 0.15.2"
] |
null | transformers |
# Model Card for Model ID
<!-- Provide a quick summary of what the model is/does. -->
## Model Details
### Model Description
<!-- Provide a longer summary of what this model is. -->
This is the model card of a 🤗 transformers model that has been pushed on the Hub. This model card has been automatically generated.
- **Developed by:** [More Information Needed]
- **Funded by [optional]:** [More Information Needed]
- **Shared by [optional]:** [More Information Needed]
- **Model type:** [More Information Needed]
- **Language(s) (NLP):** [More Information Needed]
- **License:** [More Information Needed]
- **Finetuned from model [optional]:** [More Information Needed]
### Model Sources [optional]
<!-- Provide the basic links for the model. -->
- **Repository:** [More Information Needed]
- **Paper [optional]:** [More Information Needed]
- **Demo [optional]:** [More Information Needed]
## Uses
<!-- Address questions around how the model is intended to be used, including the foreseeable users of the model and those affected by the model. -->
### Direct Use
<!-- This section is for the model use without fine-tuning or plugging into a larger ecosystem/app. -->
[More Information Needed]
### Downstream Use [optional]
<!-- This section is for the model use when fine-tuned for a task, or when plugged into a larger ecosystem/app -->
[More Information Needed]
### Out-of-Scope Use
<!-- This section addresses misuse, malicious use, and uses that the model will not work well for. -->
[More Information Needed]
## Bias, Risks, and Limitations
<!-- This section is meant to convey both technical and sociotechnical limitations. -->
[More Information Needed]
### Recommendations
<!-- This section is meant to convey recommendations with respect to the bias, risk, and technical limitations. -->
Users (both direct and downstream) should be made aware of the risks, biases and limitations of the model. More information needed for further recommendations.
## How to Get Started with the Model
Use the code below to get started with the model.
[More Information Needed]
## Training Details
### Training Data
<!-- This should link to a Dataset Card, perhaps with a short stub of information on what the training data is all about as well as documentation related to data pre-processing or additional filtering. -->
[More Information Needed]
### Training Procedure
<!-- This relates heavily to the Technical Specifications. Content here should link to that section when it is relevant to the training procedure. -->
#### Preprocessing [optional]
[More Information Needed]
#### Training Hyperparameters
- **Training regime:** [More Information Needed] <!--fp32, fp16 mixed precision, bf16 mixed precision, bf16 non-mixed precision, fp16 non-mixed precision, fp8 mixed precision -->
#### Speeds, Sizes, Times [optional]
<!-- This section provides information about throughput, start/end time, checkpoint size if relevant, etc. -->
[More Information Needed]
## Evaluation
<!-- This section describes the evaluation protocols and provides the results. -->
### Testing Data, Factors & Metrics
#### Testing Data
<!-- This should link to a Dataset Card if possible. -->
[More Information Needed]
#### Factors
<!-- These are the things the evaluation is disaggregating by, e.g., subpopulations or domains. -->
[More Information Needed]
#### Metrics
<!-- These are the evaluation metrics being used, ideally with a description of why. -->
[More Information Needed]
### Results
[More Information Needed]
#### Summary
## Model Examination [optional]
<!-- Relevant interpretability work for the model goes here -->
[More Information Needed]
## Environmental Impact
<!-- Total emissions (in grams of CO2eq) and additional considerations, such as electricity usage, go here. Edit the suggested text below accordingly -->
Carbon emissions can be estimated using the [Machine Learning Impact calculator](https://mlco2.github.io/impact#compute) presented in [Lacoste et al. (2019)](https://arxiv.org/abs/1910.09700).
- **Hardware Type:** [More Information Needed]
- **Hours used:** [More Information Needed]
- **Cloud Provider:** [More Information Needed]
- **Compute Region:** [More Information Needed]
- **Carbon Emitted:** [More Information Needed]
## Technical Specifications [optional]
### Model Architecture and Objective
[More Information Needed]
### Compute Infrastructure
[More Information Needed]
#### Hardware
[More Information Needed]
#### Software
[More Information Needed]
## Citation [optional]
<!-- If there is a paper or blog post introducing the model, the APA and Bibtex information for that should go in this section. -->
**BibTeX:**
[More Information Needed]
**APA:**
[More Information Needed]
## Glossary [optional]
<!-- If relevant, include terms and calculations in this section that can help readers understand the model or model card. -->
[More Information Needed]
## More Information [optional]
[More Information Needed]
## Model Card Authors [optional]
[More Information Needed]
## Model Card Contact
[More Information Needed] | {"library_name": "transformers", "tags": []} | unity4ar/wav2vec2-base-timit-demo-colab | null | [
"transformers",
"arxiv:1910.09700",
"endpoints_compatible",
"region:us"
] | null | 2024-04-27T07:54:15+00:00 | [
"1910.09700"
] | [] | TAGS
#transformers #arxiv-1910.09700 #endpoints_compatible #region-us
|
# Model Card for Model ID
## Model Details
### Model Description
This is the model card of a transformers model that has been pushed on the Hub. This model card has been automatically generated.
- Developed by:
- Funded by [optional]:
- Shared by [optional]:
- Model type:
- Language(s) (NLP):
- License:
- Finetuned from model [optional]:
### Model Sources [optional]
- Repository:
- Paper [optional]:
- Demo [optional]:
## Uses
### Direct Use
### Downstream Use [optional]
### Out-of-Scope Use
## Bias, Risks, and Limitations
### Recommendations
Users (both direct and downstream) should be made aware of the risks, biases and limitations of the model. More information needed for further recommendations.
## How to Get Started with the Model
Use the code below to get started with the model.
## Training Details
### Training Data
### Training Procedure
#### Preprocessing [optional]
#### Training Hyperparameters
- Training regime:
#### Speeds, Sizes, Times [optional]
## Evaluation
### Testing Data, Factors & Metrics
#### Testing Data
#### Factors
#### Metrics
### Results
#### Summary
## Model Examination [optional]
## Environmental Impact
Carbon emissions can be estimated using the Machine Learning Impact calculator presented in Lacoste et al. (2019).
- Hardware Type:
- Hours used:
- Cloud Provider:
- Compute Region:
- Carbon Emitted:
## Technical Specifications [optional]
### Model Architecture and Objective
### Compute Infrastructure
#### Hardware
#### Software
[optional]
BibTeX:
APA:
## Glossary [optional]
## More Information [optional]
## Model Card Authors [optional]
## Model Card Contact
| [
"# Model Card for Model ID",
"## Model Details",
"### Model Description\n\n\n\nThis is the model card of a transformers model that has been pushed on the Hub. This model card has been automatically generated.\n\n- Developed by: \n- Funded by [optional]: \n- Shared by [optional]: \n- Model type: \n- Language(s) (NLP): \n- License: \n- Finetuned from model [optional]:",
"### Model Sources [optional]\n\n\n\n- Repository: \n- Paper [optional]: \n- Demo [optional]:",
"## Uses",
"### Direct Use",
"### Downstream Use [optional]",
"### Out-of-Scope Use",
"## Bias, Risks, and Limitations",
"### Recommendations\n\n\n\nUsers (both direct and downstream) should be made aware of the risks, biases and limitations of the model. More information needed for further recommendations.",
"## How to Get Started with the Model\n\nUse the code below to get started with the model.",
"## Training Details",
"### Training Data",
"### Training Procedure",
"#### Preprocessing [optional]",
"#### Training Hyperparameters\n\n- Training regime:",
"#### Speeds, Sizes, Times [optional]",
"## Evaluation",
"### Testing Data, Factors & Metrics",
"#### Testing Data",
"#### Factors",
"#### Metrics",
"### Results",
"#### Summary",
"## Model Examination [optional]",
"## Environmental Impact\n\n\n\nCarbon emissions can be estimated using the Machine Learning Impact calculator presented in Lacoste et al. (2019).\n\n- Hardware Type: \n- Hours used: \n- Cloud Provider: \n- Compute Region: \n- Carbon Emitted:",
"## Technical Specifications [optional]",
"### Model Architecture and Objective",
"### Compute Infrastructure",
"#### Hardware",
"#### Software\n\n\n\n[optional]\n\n\n\nBibTeX:\n\n\n\nAPA:",
"## Glossary [optional]",
"## More Information [optional]",
"## Model Card Authors [optional]",
"## Model Card Contact"
] | [
"TAGS\n#transformers #arxiv-1910.09700 #endpoints_compatible #region-us \n",
"# Model Card for Model ID",
"## Model Details",
"### Model Description\n\n\n\nThis is the model card of a transformers model that has been pushed on the Hub. This model card has been automatically generated.\n\n- Developed by: \n- Funded by [optional]: \n- Shared by [optional]: \n- Model type: \n- Language(s) (NLP): \n- License: \n- Finetuned from model [optional]:",
"### Model Sources [optional]\n\n\n\n- Repository: \n- Paper [optional]: \n- Demo [optional]:",
"## Uses",
"### Direct Use",
"### Downstream Use [optional]",
"### Out-of-Scope Use",
"## Bias, Risks, and Limitations",
"### Recommendations\n\n\n\nUsers (both direct and downstream) should be made aware of the risks, biases and limitations of the model. More information needed for further recommendations.",
"## How to Get Started with the Model\n\nUse the code below to get started with the model.",
"## Training Details",
"### Training Data",
"### Training Procedure",
"#### Preprocessing [optional]",
"#### Training Hyperparameters\n\n- Training regime:",
"#### Speeds, Sizes, Times [optional]",
"## Evaluation",
"### Testing Data, Factors & Metrics",
"#### Testing Data",
"#### Factors",
"#### Metrics",
"### Results",
"#### Summary",
"## Model Examination [optional]",
"## Environmental Impact\n\n\n\nCarbon emissions can be estimated using the Machine Learning Impact calculator presented in Lacoste et al. (2019).\n\n- Hardware Type: \n- Hours used: \n- Cloud Provider: \n- Compute Region: \n- Carbon Emitted:",
"## Technical Specifications [optional]",
"### Model Architecture and Objective",
"### Compute Infrastructure",
"#### Hardware",
"#### Software\n\n\n\n[optional]\n\n\n\nBibTeX:\n\n\n\nAPA:",
"## Glossary [optional]",
"## More Information [optional]",
"## Model Card Authors [optional]",
"## Model Card Contact"
] |
text-classification | transformers |
# Model Card for Model ID
<!-- Provide a quick summary of what the model is/does. -->
## Model Details
### Model Description
<!-- Provide a longer summary of what this model is. -->
This is the model card of a 🤗 transformers model that has been pushed on the Hub. This model card has been automatically generated.
- **Developed by:** [More Information Needed]
- **Funded by [optional]:** [More Information Needed]
- **Shared by [optional]:** [More Information Needed]
- **Model type:** [More Information Needed]
- **Language(s) (NLP):** [More Information Needed]
- **License:** [More Information Needed]
- **Finetuned from model [optional]:** [More Information Needed]
### Model Sources [optional]
<!-- Provide the basic links for the model. -->
- **Repository:** [More Information Needed]
- **Paper [optional]:** [More Information Needed]
- **Demo [optional]:** [More Information Needed]
## Uses
<!-- Address questions around how the model is intended to be used, including the foreseeable users of the model and those affected by the model. -->
### Direct Use
<!-- This section is for the model use without fine-tuning or plugging into a larger ecosystem/app. -->
[More Information Needed]
### Downstream Use [optional]
<!-- This section is for the model use when fine-tuned for a task, or when plugged into a larger ecosystem/app -->
[More Information Needed]
### Out-of-Scope Use
<!-- This section addresses misuse, malicious use, and uses that the model will not work well for. -->
[More Information Needed]
## Bias, Risks, and Limitations
<!-- This section is meant to convey both technical and sociotechnical limitations. -->
[More Information Needed]
### Recommendations
<!-- This section is meant to convey recommendations with respect to the bias, risk, and technical limitations. -->
Users (both direct and downstream) should be made aware of the risks, biases and limitations of the model. More information needed for further recommendations.
## How to Get Started with the Model
Use the code below to get started with the model.
[More Information Needed]
## Training Details
### Training Data
<!-- This should link to a Dataset Card, perhaps with a short stub of information on what the training data is all about as well as documentation related to data pre-processing or additional filtering. -->
[More Information Needed]
### Training Procedure
<!-- This relates heavily to the Technical Specifications. Content here should link to that section when it is relevant to the training procedure. -->
#### Preprocessing [optional]
[More Information Needed]
#### Training Hyperparameters
- **Training regime:** [More Information Needed] <!--fp32, fp16 mixed precision, bf16 mixed precision, bf16 non-mixed precision, fp16 non-mixed precision, fp8 mixed precision -->
#### Speeds, Sizes, Times [optional]
<!-- This section provides information about throughput, start/end time, checkpoint size if relevant, etc. -->
[More Information Needed]
## Evaluation
<!-- This section describes the evaluation protocols and provides the results. -->
### Testing Data, Factors & Metrics
#### Testing Data
<!-- This should link to a Dataset Card if possible. -->
[More Information Needed]
#### Factors
<!-- These are the things the evaluation is disaggregating by, e.g., subpopulations or domains. -->
[More Information Needed]
#### Metrics
<!-- These are the evaluation metrics being used, ideally with a description of why. -->
[More Information Needed]
### Results
[More Information Needed]
#### Summary
## Model Examination [optional]
<!-- Relevant interpretability work for the model goes here -->
[More Information Needed]
## Environmental Impact
<!-- Total emissions (in grams of CO2eq) and additional considerations, such as electricity usage, go here. Edit the suggested text below accordingly -->
Carbon emissions can be estimated using the [Machine Learning Impact calculator](https://mlco2.github.io/impact#compute) presented in [Lacoste et al. (2019)](https://arxiv.org/abs/1910.09700).
- **Hardware Type:** [More Information Needed]
- **Hours used:** [More Information Needed]
- **Cloud Provider:** [More Information Needed]
- **Compute Region:** [More Information Needed]
- **Carbon Emitted:** [More Information Needed]
## Technical Specifications [optional]
### Model Architecture and Objective
[More Information Needed]
### Compute Infrastructure
[More Information Needed]
#### Hardware
[More Information Needed]
#### Software
[More Information Needed]
## Citation [optional]
<!-- If there is a paper or blog post introducing the model, the APA and Bibtex information for that should go in this section. -->
**BibTeX:**
[More Information Needed]
**APA:**
[More Information Needed]
## Glossary [optional]
<!-- If relevant, include terms and calculations in this section that can help readers understand the model or model card. -->
[More Information Needed]
## More Information [optional]
[More Information Needed]
## Model Card Authors [optional]
[More Information Needed]
## Model Card Contact
[More Information Needed] | {"library_name": "transformers", "tags": []} | HC-85/distilbert-arxiv-multilabel | null | [
"transformers",
"safetensors",
"distilbert",
"text-classification",
"arxiv:1910.09700",
"autotrain_compatible",
"endpoints_compatible",
"region:us"
] | null | 2024-04-27T07:55:29+00:00 | [
"1910.09700"
] | [] | TAGS
#transformers #safetensors #distilbert #text-classification #arxiv-1910.09700 #autotrain_compatible #endpoints_compatible #region-us
|
# Model Card for Model ID
## Model Details
### Model Description
This is the model card of a transformers model that has been pushed on the Hub. This model card has been automatically generated.
- Developed by:
- Funded by [optional]:
- Shared by [optional]:
- Model type:
- Language(s) (NLP):
- License:
- Finetuned from model [optional]:
### Model Sources [optional]
- Repository:
- Paper [optional]:
- Demo [optional]:
## Uses
### Direct Use
### Downstream Use [optional]
### Out-of-Scope Use
## Bias, Risks, and Limitations
### Recommendations
Users (both direct and downstream) should be made aware of the risks, biases and limitations of the model. More information needed for further recommendations.
## How to Get Started with the Model
Use the code below to get started with the model.
## Training Details
### Training Data
### Training Procedure
#### Preprocessing [optional]
#### Training Hyperparameters
- Training regime:
#### Speeds, Sizes, Times [optional]
## Evaluation
### Testing Data, Factors & Metrics
#### Testing Data
#### Factors
#### Metrics
### Results
#### Summary
## Model Examination [optional]
## Environmental Impact
Carbon emissions can be estimated using the Machine Learning Impact calculator presented in Lacoste et al. (2019).
- Hardware Type:
- Hours used:
- Cloud Provider:
- Compute Region:
- Carbon Emitted:
## Technical Specifications [optional]
### Model Architecture and Objective
### Compute Infrastructure
#### Hardware
#### Software
[optional]
BibTeX:
APA:
## Glossary [optional]
## More Information [optional]
## Model Card Authors [optional]
## Model Card Contact
| [
"# Model Card for Model ID",
"## Model Details",
"### Model Description\n\n\n\nThis is the model card of a transformers model that has been pushed on the Hub. This model card has been automatically generated.\n\n- Developed by: \n- Funded by [optional]: \n- Shared by [optional]: \n- Model type: \n- Language(s) (NLP): \n- License: \n- Finetuned from model [optional]:",
"### Model Sources [optional]\n\n\n\n- Repository: \n- Paper [optional]: \n- Demo [optional]:",
"## Uses",
"### Direct Use",
"### Downstream Use [optional]",
"### Out-of-Scope Use",
"## Bias, Risks, and Limitations",
"### Recommendations\n\n\n\nUsers (both direct and downstream) should be made aware of the risks, biases and limitations of the model. More information needed for further recommendations.",
"## How to Get Started with the Model\n\nUse the code below to get started with the model.",
"## Training Details",
"### Training Data",
"### Training Procedure",
"#### Preprocessing [optional]",
"#### Training Hyperparameters\n\n- Training regime:",
"#### Speeds, Sizes, Times [optional]",
"## Evaluation",
"### Testing Data, Factors & Metrics",
"#### Testing Data",
"#### Factors",
"#### Metrics",
"### Results",
"#### Summary",
"## Model Examination [optional]",
"## Environmental Impact\n\n\n\nCarbon emissions can be estimated using the Machine Learning Impact calculator presented in Lacoste et al. (2019).\n\n- Hardware Type: \n- Hours used: \n- Cloud Provider: \n- Compute Region: \n- Carbon Emitted:",
"## Technical Specifications [optional]",
"### Model Architecture and Objective",
"### Compute Infrastructure",
"#### Hardware",
"#### Software\n\n\n\n[optional]\n\n\n\nBibTeX:\n\n\n\nAPA:",
"## Glossary [optional]",
"## More Information [optional]",
"## Model Card Authors [optional]",
"## Model Card Contact"
] | [
"TAGS\n#transformers #safetensors #distilbert #text-classification #arxiv-1910.09700 #autotrain_compatible #endpoints_compatible #region-us \n",
"# Model Card for Model ID",
"## Model Details",
"### Model Description\n\n\n\nThis is the model card of a transformers model that has been pushed on the Hub. This model card has been automatically generated.\n\n- Developed by: \n- Funded by [optional]: \n- Shared by [optional]: \n- Model type: \n- Language(s) (NLP): \n- License: \n- Finetuned from model [optional]:",
"### Model Sources [optional]\n\n\n\n- Repository: \n- Paper [optional]: \n- Demo [optional]:",
"## Uses",
"### Direct Use",
"### Downstream Use [optional]",
"### Out-of-Scope Use",
"## Bias, Risks, and Limitations",
"### Recommendations\n\n\n\nUsers (both direct and downstream) should be made aware of the risks, biases and limitations of the model. More information needed for further recommendations.",
"## How to Get Started with the Model\n\nUse the code below to get started with the model.",
"## Training Details",
"### Training Data",
"### Training Procedure",
"#### Preprocessing [optional]",
"#### Training Hyperparameters\n\n- Training regime:",
"#### Speeds, Sizes, Times [optional]",
"## Evaluation",
"### Testing Data, Factors & Metrics",
"#### Testing Data",
"#### Factors",
"#### Metrics",
"### Results",
"#### Summary",
"## Model Examination [optional]",
"## Environmental Impact\n\n\n\nCarbon emissions can be estimated using the Machine Learning Impact calculator presented in Lacoste et al. (2019).\n\n- Hardware Type: \n- Hours used: \n- Cloud Provider: \n- Compute Region: \n- Carbon Emitted:",
"## Technical Specifications [optional]",
"### Model Architecture and Objective",
"### Compute Infrastructure",
"#### Hardware",
"#### Software\n\n\n\n[optional]\n\n\n\nBibTeX:\n\n\n\nAPA:",
"## Glossary [optional]",
"## More Information [optional]",
"## Model Card Authors [optional]",
"## Model Card Contact"
] |
image-classification | transformers |
<!-- This model card has been generated automatically according to the information the Trainer had access to. You
should probably proofread and complete it, then remove this comment. -->
# Boya1_RMSProp_1-e5_10Epoch_swinv2-tiny-patch4-window16-256_fold2
This model is a fine-tuned version of [microsoft/swinv2-tiny-patch4-window16-256](https://huggingface.co/microsoft/swinv2-tiny-patch4-window16-256) on the imagefolder dataset.
It achieves the following results on the evaluation set:
- Loss: 1.0352
- Accuracy: 0.6565
## Model description
More information needed
## Intended uses & limitations
More information needed
## Training and evaluation data
More information needed
## Training procedure
### Training hyperparameters
The following hyperparameters were used during training:
- learning_rate: 1e-05
- train_batch_size: 16
- eval_batch_size: 16
- seed: 42
- optimizer: Adam with betas=(0.9,0.999) and epsilon=1e-08
- lr_scheduler_type: linear
- lr_scheduler_warmup_ratio: 0.1
- num_epochs: 10
### Training results
| Training Loss | Epoch | Step | Validation Loss | Accuracy |
|:-------------:|:-----:|:----:|:---------------:|:--------:|
| 1.632 | 1.0 | 923 | 1.4721 | 0.5124 |
| 1.4039 | 2.0 | 1846 | 1.2671 | 0.5781 |
| 1.1495 | 3.0 | 2769 | 1.1523 | 0.6143 |
| 1.1243 | 4.0 | 3692 | 1.0781 | 0.6341 |
| 1.1829 | 5.0 | 4615 | 1.1043 | 0.6238 |
| 0.8912 | 6.0 | 5538 | 1.0600 | 0.6392 |
| 0.9235 | 7.0 | 6461 | 1.0406 | 0.6562 |
| 0.8891 | 8.0 | 7384 | 1.0360 | 0.6519 |
| 0.8519 | 9.0 | 8307 | 1.0508 | 0.6562 |
| 0.8538 | 10.0 | 9230 | 1.0352 | 0.6565 |
### Framework versions
- Transformers 4.35.0
- Pytorch 2.1.0
- Datasets 2.14.6
- Tokenizers 0.14.1
| {"license": "apache-2.0", "tags": ["generated_from_trainer"], "datasets": ["imagefolder"], "metrics": ["accuracy"], "base_model": "microsoft/swinv2-tiny-patch4-window16-256", "model-index": [{"name": "Boya1_RMSProp_1-e5_10Epoch_swinv2-tiny-patch4-window16-256_fold2", "results": [{"task": {"type": "image-classification", "name": "Image Classification"}, "dataset": {"name": "imagefolder", "type": "imagefolder", "config": "default", "split": "test", "args": "default"}, "metrics": [{"type": "accuracy", "value": 0.6564864864864864, "name": "Accuracy"}]}]}]} | onizukal/Boya1_RMSProp_1-e5_10Epoch_swinv2-tiny-patch4-window16-256_fold2 | null | [
"transformers",
"safetensors",
"swinv2",
"image-classification",
"generated_from_trainer",
"dataset:imagefolder",
"base_model:microsoft/swinv2-tiny-patch4-window16-256",
"license:apache-2.0",
"model-index",
"autotrain_compatible",
"endpoints_compatible",
"region:us"
] | null | 2024-04-27T07:56:38+00:00 | [] | [] | TAGS
#transformers #safetensors #swinv2 #image-classification #generated_from_trainer #dataset-imagefolder #base_model-microsoft/swinv2-tiny-patch4-window16-256 #license-apache-2.0 #model-index #autotrain_compatible #endpoints_compatible #region-us
| Boya1\_RMSProp\_1-e5\_10Epoch\_swinv2-tiny-patch4-window16-256\_fold2
=====================================================================
This model is a fine-tuned version of microsoft/swinv2-tiny-patch4-window16-256 on the imagefolder dataset.
It achieves the following results on the evaluation set:
* Loss: 1.0352
* Accuracy: 0.6565
Model description
-----------------
More information needed
Intended uses & limitations
---------------------------
More information needed
Training and evaluation data
----------------------------
More information needed
Training procedure
------------------
### Training hyperparameters
The following hyperparameters were used during training:
* learning\_rate: 1e-05
* train\_batch\_size: 16
* eval\_batch\_size: 16
* seed: 42
* optimizer: Adam with betas=(0.9,0.999) and epsilon=1e-08
* lr\_scheduler\_type: linear
* lr\_scheduler\_warmup\_ratio: 0.1
* num\_epochs: 10
### Training results
### Framework versions
* Transformers 4.35.0
* Pytorch 2.1.0
* Datasets 2.14.6
* Tokenizers 0.14.1
| [
"### Training hyperparameters\n\n\nThe following hyperparameters were used during training:\n\n\n* learning\\_rate: 1e-05\n* train\\_batch\\_size: 16\n* eval\\_batch\\_size: 16\n* seed: 42\n* optimizer: Adam with betas=(0.9,0.999) and epsilon=1e-08\n* lr\\_scheduler\\_type: linear\n* lr\\_scheduler\\_warmup\\_ratio: 0.1\n* num\\_epochs: 10",
"### Training results",
"### Framework versions\n\n\n* Transformers 4.35.0\n* Pytorch 2.1.0\n* Datasets 2.14.6\n* Tokenizers 0.14.1"
] | [
"TAGS\n#transformers #safetensors #swinv2 #image-classification #generated_from_trainer #dataset-imagefolder #base_model-microsoft/swinv2-tiny-patch4-window16-256 #license-apache-2.0 #model-index #autotrain_compatible #endpoints_compatible #region-us \n",
"### Training hyperparameters\n\n\nThe following hyperparameters were used during training:\n\n\n* learning\\_rate: 1e-05\n* train\\_batch\\_size: 16\n* eval\\_batch\\_size: 16\n* seed: 42\n* optimizer: Adam with betas=(0.9,0.999) and epsilon=1e-08\n* lr\\_scheduler\\_type: linear\n* lr\\_scheduler\\_warmup\\_ratio: 0.1\n* num\\_epochs: 10",
"### Training results",
"### Framework versions\n\n\n* Transformers 4.35.0\n* Pytorch 2.1.0\n* Datasets 2.14.6\n* Tokenizers 0.14.1"
] |
null | peft |
<!-- This model card has been generated automatically according to the information the Trainer had access to. You
should probably proofread and complete it, then remove this comment. -->
# GUE_EMP_H3-seqsight_16384_512_22M-L1_f
This model is a fine-tuned version of [mahdibaghbanzadeh/seqsight_16384_512_22M](https://huggingface.co/mahdibaghbanzadeh/seqsight_16384_512_22M) on the [mahdibaghbanzadeh/GUE_EMP_H3](https://huggingface.co/datasets/mahdibaghbanzadeh/GUE_EMP_H3) dataset.
It achieves the following results on the evaluation set:
- Loss: 0.3298
- F1 Score: 0.8697
- Accuracy: 0.8697
## Model description
More information needed
## Intended uses & limitations
More information needed
## Training and evaluation data
More information needed
## Training procedure
### Training hyperparameters
The following hyperparameters were used during training:
- learning_rate: 0.0005
- train_batch_size: 128
- eval_batch_size: 128
- seed: 42
- optimizer: Adam with betas=(0.9,0.999) and epsilon=1e-08
- lr_scheduler_type: linear
- training_steps: 10000
### Training results
| Training Loss | Epoch | Step | Validation Loss | F1 Score | Accuracy |
|:-------------:|:------:|:-----:|:---------------:|:--------:|:--------:|
| 0.492 | 2.13 | 200 | 0.4204 | 0.8099 | 0.8103 |
| 0.3885 | 4.26 | 400 | 0.4112 | 0.8234 | 0.8236 |
| 0.3702 | 6.38 | 600 | 0.3960 | 0.8349 | 0.8350 |
| 0.3608 | 8.51 | 800 | 0.3857 | 0.8343 | 0.8343 |
| 0.3469 | 10.64 | 1000 | 0.3777 | 0.8417 | 0.8417 |
| 0.333 | 12.77 | 1200 | 0.3722 | 0.8370 | 0.8370 |
| 0.3232 | 14.89 | 1400 | 0.3580 | 0.8490 | 0.8490 |
| 0.3129 | 17.02 | 1600 | 0.3546 | 0.8510 | 0.8510 |
| 0.304 | 19.15 | 1800 | 0.3736 | 0.8447 | 0.8450 |
| 0.2966 | 21.28 | 2000 | 0.3558 | 0.8528 | 0.8530 |
| 0.2949 | 23.4 | 2200 | 0.3419 | 0.8597 | 0.8597 |
| 0.283 | 25.53 | 2400 | 0.3450 | 0.8564 | 0.8564 |
| 0.2889 | 27.66 | 2600 | 0.3415 | 0.8597 | 0.8597 |
| 0.2837 | 29.79 | 2800 | 0.3467 | 0.8568 | 0.8570 |
| 0.2786 | 31.91 | 3000 | 0.3551 | 0.8548 | 0.8550 |
| 0.2762 | 34.04 | 3200 | 0.3454 | 0.8576 | 0.8577 |
| 0.2746 | 36.17 | 3400 | 0.3494 | 0.8577 | 0.8577 |
| 0.2682 | 38.3 | 3600 | 0.3419 | 0.8624 | 0.8624 |
| 0.2721 | 40.43 | 3800 | 0.3460 | 0.8583 | 0.8584 |
| 0.2707 | 42.55 | 4000 | 0.3436 | 0.8597 | 0.8597 |
| 0.2685 | 44.68 | 4200 | 0.3390 | 0.8624 | 0.8624 |
| 0.2675 | 46.81 | 4400 | 0.3477 | 0.8582 | 0.8584 |
| 0.265 | 48.94 | 4600 | 0.3472 | 0.8568 | 0.8570 |
| 0.263 | 51.06 | 4800 | 0.3383 | 0.8657 | 0.8657 |
| 0.2606 | 53.19 | 5000 | 0.3652 | 0.8593 | 0.8597 |
| 0.264 | 55.32 | 5200 | 0.3362 | 0.8624 | 0.8624 |
| 0.259 | 57.45 | 5400 | 0.3450 | 0.8544 | 0.8544 |
| 0.2569 | 59.57 | 5600 | 0.3398 | 0.8603 | 0.8604 |
| 0.2591 | 61.7 | 5800 | 0.3329 | 0.8650 | 0.8651 |
| 0.2567 | 63.83 | 6000 | 0.3535 | 0.8581 | 0.8584 |
| 0.259 | 65.96 | 6200 | 0.3476 | 0.8589 | 0.8591 |
| 0.2543 | 68.09 | 6400 | 0.3523 | 0.8561 | 0.8564 |
| 0.2562 | 70.21 | 6600 | 0.3512 | 0.8555 | 0.8557 |
| 0.2553 | 72.34 | 6800 | 0.3457 | 0.8595 | 0.8597 |
| 0.2543 | 74.47 | 7000 | 0.3557 | 0.8561 | 0.8564 |
| 0.2524 | 76.6 | 7200 | 0.3517 | 0.8589 | 0.8591 |
| 0.2555 | 78.72 | 7400 | 0.3466 | 0.8596 | 0.8597 |
| 0.2505 | 80.85 | 7600 | 0.3421 | 0.8596 | 0.8597 |
| 0.2515 | 82.98 | 7800 | 0.3487 | 0.8575 | 0.8577 |
| 0.251 | 85.11 | 8000 | 0.3453 | 0.8602 | 0.8604 |
| 0.2506 | 87.23 | 8200 | 0.3392 | 0.8610 | 0.8611 |
| 0.2489 | 89.36 | 8400 | 0.3486 | 0.8589 | 0.8591 |
| 0.2484 | 91.49 | 8600 | 0.3510 | 0.8588 | 0.8591 |
| 0.2483 | 93.62 | 8800 | 0.3453 | 0.8596 | 0.8597 |
| 0.2512 | 95.74 | 9000 | 0.3480 | 0.8595 | 0.8597 |
| 0.248 | 97.87 | 9200 | 0.3431 | 0.8609 | 0.8611 |
| 0.2484 | 100.0 | 9400 | 0.3416 | 0.8596 | 0.8597 |
| 0.2498 | 102.13 | 9600 | 0.3455 | 0.8596 | 0.8597 |
| 0.2478 | 104.26 | 9800 | 0.3455 | 0.8589 | 0.8591 |
| 0.2501 | 106.38 | 10000 | 0.3446 | 0.8589 | 0.8591 |
### Framework versions
- PEFT 0.9.0
- Transformers 4.38.2
- Pytorch 2.2.0+cu121
- Datasets 2.17.1
- Tokenizers 0.15.2 | {"library_name": "peft", "tags": ["generated_from_trainer"], "metrics": ["accuracy"], "base_model": "mahdibaghbanzadeh/seqsight_16384_512_22M", "model-index": [{"name": "GUE_EMP_H3-seqsight_16384_512_22M-L1_f", "results": []}]} | mahdibaghbanzadeh/GUE_EMP_H3-seqsight_16384_512_22M-L1_f | null | [
"peft",
"safetensors",
"generated_from_trainer",
"base_model:mahdibaghbanzadeh/seqsight_16384_512_22M",
"region:us"
] | null | 2024-04-27T07:58:53+00:00 | [] | [] | TAGS
#peft #safetensors #generated_from_trainer #base_model-mahdibaghbanzadeh/seqsight_16384_512_22M #region-us
| GUE\_EMP\_H3-seqsight\_16384\_512\_22M-L1\_f
============================================
This model is a fine-tuned version of mahdibaghbanzadeh/seqsight\_16384\_512\_22M on the mahdibaghbanzadeh/GUE\_EMP\_H3 dataset.
It achieves the following results on the evaluation set:
* Loss: 0.3298
* F1 Score: 0.8697
* Accuracy: 0.8697
Model description
-----------------
More information needed
Intended uses & limitations
---------------------------
More information needed
Training and evaluation data
----------------------------
More information needed
Training procedure
------------------
### Training hyperparameters
The following hyperparameters were used during training:
* learning\_rate: 0.0005
* train\_batch\_size: 128
* eval\_batch\_size: 128
* seed: 42
* optimizer: Adam with betas=(0.9,0.999) and epsilon=1e-08
* lr\_scheduler\_type: linear
* training\_steps: 10000
### Training results
### Framework versions
* PEFT 0.9.0
* Transformers 4.38.2
* Pytorch 2.2.0+cu121
* Datasets 2.17.1
* Tokenizers 0.15.2
| [
"### Training hyperparameters\n\n\nThe following hyperparameters were used during training:\n\n\n* learning\\_rate: 0.0005\n* train\\_batch\\_size: 128\n* eval\\_batch\\_size: 128\n* seed: 42\n* optimizer: Adam with betas=(0.9,0.999) and epsilon=1e-08\n* lr\\_scheduler\\_type: linear\n* training\\_steps: 10000",
"### Training results",
"### Framework versions\n\n\n* PEFT 0.9.0\n* Transformers 4.38.2\n* Pytorch 2.2.0+cu121\n* Datasets 2.17.1\n* Tokenizers 0.15.2"
] | [
"TAGS\n#peft #safetensors #generated_from_trainer #base_model-mahdibaghbanzadeh/seqsight_16384_512_22M #region-us \n",
"### Training hyperparameters\n\n\nThe following hyperparameters were used during training:\n\n\n* learning\\_rate: 0.0005\n* train\\_batch\\_size: 128\n* eval\\_batch\\_size: 128\n* seed: 42\n* optimizer: Adam with betas=(0.9,0.999) and epsilon=1e-08\n* lr\\_scheduler\\_type: linear\n* training\\_steps: 10000",
"### Training results",
"### Framework versions\n\n\n* PEFT 0.9.0\n* Transformers 4.38.2\n* Pytorch 2.2.0+cu121\n* Datasets 2.17.1\n* Tokenizers 0.15.2"
] |
unconditional-image-generation | diffusers |
# Model Card for Unit 1 of the [Diffusion Models Class 🧨](https://github.com/huggingface/diffusion-models-class)
This model is a diffusion model for unconditional image generation of cute 🦋.
## Usage
```python
from diffusers import DDPMPipeline
pipeline = DDPMPipeline.from_pretrained('wisehuh/sd-class-butterflies-32')
image = pipeline().images[0]
image
```
| {"license": "mit", "tags": ["pytorch", "diffusers", "unconditional-image-generation", "diffusion-models-class"]} | wisehuh/sd-class-butterflies-32 | null | [
"diffusers",
"safetensors",
"pytorch",
"unconditional-image-generation",
"diffusion-models-class",
"license:mit",
"diffusers:DDPMPipeline",
"region:us"
] | null | 2024-04-27T07:59:51+00:00 | [] | [] | TAGS
#diffusers #safetensors #pytorch #unconditional-image-generation #diffusion-models-class #license-mit #diffusers-DDPMPipeline #region-us
|
# Model Card for Unit 1 of the Diffusion Models Class
This model is a diffusion model for unconditional image generation of cute .
## Usage
| [
"# Model Card for Unit 1 of the Diffusion Models Class \n\nThis model is a diffusion model for unconditional image generation of cute .",
"## Usage"
] | [
"TAGS\n#diffusers #safetensors #pytorch #unconditional-image-generation #diffusion-models-class #license-mit #diffusers-DDPMPipeline #region-us \n",
"# Model Card for Unit 1 of the Diffusion Models Class \n\nThis model is a diffusion model for unconditional image generation of cute .",
"## Usage"
] |
text-to-audio | transformers.js |
# Avasaz ONNX (In Browser model)
Based on [Xenova](https://huggingface.co/xeonva/musicgen-small)'s ONNX quantization. | {"license": "cc-by-nc-4.0", "library_name": "transformers.js"} | avasaz/avasaz-webgl | null | [
"transformers.js",
"onnx",
"musicgen",
"text-to-audio",
"license:cc-by-nc-4.0",
"region:us"
] | null | 2024-04-27T08:01:17+00:00 | [] | [] | TAGS
#transformers.js #onnx #musicgen #text-to-audio #license-cc-by-nc-4.0 #region-us
|
# Avasaz ONNX (In Browser model)
Based on Xenova's ONNX quantization. | [
"# Avasaz ONNX (In Browser model)\n\nBased on Xenova's ONNX quantization."
] | [
"TAGS\n#transformers.js #onnx #musicgen #text-to-audio #license-cc-by-nc-4.0 #region-us \n",
"# Avasaz ONNX (In Browser model)\n\nBased on Xenova's ONNX quantization."
] |
text-generation | transformers |
# Model Card for Model ID
<!-- Provide a quick summary of what the model is/does. -->
## Model Details
### Model Description
<!-- Provide a longer summary of what this model is. -->
This is the model card of a 🤗 transformers model that has been pushed on the Hub. This model card has been automatically generated.
- **Developed by:** [More Information Needed]
- **Funded by [optional]:** [More Information Needed]
- **Shared by [optional]:** [More Information Needed]
- **Model type:** [More Information Needed]
- **Language(s) (NLP):** [More Information Needed]
- **License:** [More Information Needed]
- **Finetuned from model [optional]:** [More Information Needed]
### Model Sources [optional]
<!-- Provide the basic links for the model. -->
- **Repository:** [More Information Needed]
- **Paper [optional]:** [More Information Needed]
- **Demo [optional]:** [More Information Needed]
## Uses
<!-- Address questions around how the model is intended to be used, including the foreseeable users of the model and those affected by the model. -->
### Direct Use
<!-- This section is for the model use without fine-tuning or plugging into a larger ecosystem/app. -->
[More Information Needed]
### Downstream Use [optional]
<!-- This section is for the model use when fine-tuned for a task, or when plugged into a larger ecosystem/app -->
[More Information Needed]
### Out-of-Scope Use
<!-- This section addresses misuse, malicious use, and uses that the model will not work well for. -->
[More Information Needed]
## Bias, Risks, and Limitations
<!-- This section is meant to convey both technical and sociotechnical limitations. -->
[More Information Needed]
### Recommendations
<!-- This section is meant to convey recommendations with respect to the bias, risk, and technical limitations. -->
Users (both direct and downstream) should be made aware of the risks, biases and limitations of the model. More information needed for further recommendations.
## How to Get Started with the Model
Use the code below to get started with the model.
[More Information Needed]
## Training Details
### Training Data
<!-- This should link to a Dataset Card, perhaps with a short stub of information on what the training data is all about as well as documentation related to data pre-processing or additional filtering. -->
[More Information Needed]
### Training Procedure
<!-- This relates heavily to the Technical Specifications. Content here should link to that section when it is relevant to the training procedure. -->
#### Preprocessing [optional]
[More Information Needed]
#### Training Hyperparameters
- **Training regime:** [More Information Needed] <!--fp32, fp16 mixed precision, bf16 mixed precision, bf16 non-mixed precision, fp16 non-mixed precision, fp8 mixed precision -->
#### Speeds, Sizes, Times [optional]
<!-- This section provides information about throughput, start/end time, checkpoint size if relevant, etc. -->
[More Information Needed]
## Evaluation
<!-- This section describes the evaluation protocols and provides the results. -->
### Testing Data, Factors & Metrics
#### Testing Data
<!-- This should link to a Dataset Card if possible. -->
[More Information Needed]
#### Factors
<!-- These are the things the evaluation is disaggregating by, e.g., subpopulations or domains. -->
[More Information Needed]
#### Metrics
<!-- These are the evaluation metrics being used, ideally with a description of why. -->
[More Information Needed]
### Results
[More Information Needed]
#### Summary
## Model Examination [optional]
<!-- Relevant interpretability work for the model goes here -->
[More Information Needed]
## Environmental Impact
<!-- Total emissions (in grams of CO2eq) and additional considerations, such as electricity usage, go here. Edit the suggested text below accordingly -->
Carbon emissions can be estimated using the [Machine Learning Impact calculator](https://mlco2.github.io/impact#compute) presented in [Lacoste et al. (2019)](https://arxiv.org/abs/1910.09700).
- **Hardware Type:** [More Information Needed]
- **Hours used:** [More Information Needed]
- **Cloud Provider:** [More Information Needed]
- **Compute Region:** [More Information Needed]
- **Carbon Emitted:** [More Information Needed]
## Technical Specifications [optional]
### Model Architecture and Objective
[More Information Needed]
### Compute Infrastructure
[More Information Needed]
#### Hardware
[More Information Needed]
#### Software
[More Information Needed]
## Citation [optional]
<!-- If there is a paper or blog post introducing the model, the APA and Bibtex information for that should go in this section. -->
**BibTeX:**
[More Information Needed]
**APA:**
[More Information Needed]
## Glossary [optional]
<!-- If relevant, include terms and calculations in this section that can help readers understand the model or model card. -->
[More Information Needed]
## More Information [optional]
[More Information Needed]
## Model Card Authors [optional]
[More Information Needed]
## Model Card Contact
[More Information Needed] | {"library_name": "transformers", "tags": []} | fenguhao/hh-rlhf-dpo-0.1 | null | [
"transformers",
"safetensors",
"gpt_neox",
"text-generation",
"arxiv:1910.09700",
"autotrain_compatible",
"endpoints_compatible",
"text-generation-inference",
"region:us"
] | null | 2024-04-27T08:02:30+00:00 | [
"1910.09700"
] | [] | TAGS
#transformers #safetensors #gpt_neox #text-generation #arxiv-1910.09700 #autotrain_compatible #endpoints_compatible #text-generation-inference #region-us
|
# Model Card for Model ID
## Model Details
### Model Description
This is the model card of a transformers model that has been pushed on the Hub. This model card has been automatically generated.
- Developed by:
- Funded by [optional]:
- Shared by [optional]:
- Model type:
- Language(s) (NLP):
- License:
- Finetuned from model [optional]:
### Model Sources [optional]
- Repository:
- Paper [optional]:
- Demo [optional]:
## Uses
### Direct Use
### Downstream Use [optional]
### Out-of-Scope Use
## Bias, Risks, and Limitations
### Recommendations
Users (both direct and downstream) should be made aware of the risks, biases and limitations of the model. More information needed for further recommendations.
## How to Get Started with the Model
Use the code below to get started with the model.
## Training Details
### Training Data
### Training Procedure
#### Preprocessing [optional]
#### Training Hyperparameters
- Training regime:
#### Speeds, Sizes, Times [optional]
## Evaluation
### Testing Data, Factors & Metrics
#### Testing Data
#### Factors
#### Metrics
### Results
#### Summary
## Model Examination [optional]
## Environmental Impact
Carbon emissions can be estimated using the Machine Learning Impact calculator presented in Lacoste et al. (2019).
- Hardware Type:
- Hours used:
- Cloud Provider:
- Compute Region:
- Carbon Emitted:
## Technical Specifications [optional]
### Model Architecture and Objective
### Compute Infrastructure
#### Hardware
#### Software
[optional]
BibTeX:
APA:
## Glossary [optional]
## More Information [optional]
## Model Card Authors [optional]
## Model Card Contact
| [
"# Model Card for Model ID",
"## Model Details",
"### Model Description\n\n\n\nThis is the model card of a transformers model that has been pushed on the Hub. This model card has been automatically generated.\n\n- Developed by: \n- Funded by [optional]: \n- Shared by [optional]: \n- Model type: \n- Language(s) (NLP): \n- License: \n- Finetuned from model [optional]:",
"### Model Sources [optional]\n\n\n\n- Repository: \n- Paper [optional]: \n- Demo [optional]:",
"## Uses",
"### Direct Use",
"### Downstream Use [optional]",
"### Out-of-Scope Use",
"## Bias, Risks, and Limitations",
"### Recommendations\n\n\n\nUsers (both direct and downstream) should be made aware of the risks, biases and limitations of the model. More information needed for further recommendations.",
"## How to Get Started with the Model\n\nUse the code below to get started with the model.",
"## Training Details",
"### Training Data",
"### Training Procedure",
"#### Preprocessing [optional]",
"#### Training Hyperparameters\n\n- Training regime:",
"#### Speeds, Sizes, Times [optional]",
"## Evaluation",
"### Testing Data, Factors & Metrics",
"#### Testing Data",
"#### Factors",
"#### Metrics",
"### Results",
"#### Summary",
"## Model Examination [optional]",
"## Environmental Impact\n\n\n\nCarbon emissions can be estimated using the Machine Learning Impact calculator presented in Lacoste et al. (2019).\n\n- Hardware Type: \n- Hours used: \n- Cloud Provider: \n- Compute Region: \n- Carbon Emitted:",
"## Technical Specifications [optional]",
"### Model Architecture and Objective",
"### Compute Infrastructure",
"#### Hardware",
"#### Software\n\n\n\n[optional]\n\n\n\nBibTeX:\n\n\n\nAPA:",
"## Glossary [optional]",
"## More Information [optional]",
"## Model Card Authors [optional]",
"## Model Card Contact"
] | [
"TAGS\n#transformers #safetensors #gpt_neox #text-generation #arxiv-1910.09700 #autotrain_compatible #endpoints_compatible #text-generation-inference #region-us \n",
"# Model Card for Model ID",
"## Model Details",
"### Model Description\n\n\n\nThis is the model card of a transformers model that has been pushed on the Hub. This model card has been automatically generated.\n\n- Developed by: \n- Funded by [optional]: \n- Shared by [optional]: \n- Model type: \n- Language(s) (NLP): \n- License: \n- Finetuned from model [optional]:",
"### Model Sources [optional]\n\n\n\n- Repository: \n- Paper [optional]: \n- Demo [optional]:",
"## Uses",
"### Direct Use",
"### Downstream Use [optional]",
"### Out-of-Scope Use",
"## Bias, Risks, and Limitations",
"### Recommendations\n\n\n\nUsers (both direct and downstream) should be made aware of the risks, biases and limitations of the model. More information needed for further recommendations.",
"## How to Get Started with the Model\n\nUse the code below to get started with the model.",
"## Training Details",
"### Training Data",
"### Training Procedure",
"#### Preprocessing [optional]",
"#### Training Hyperparameters\n\n- Training regime:",
"#### Speeds, Sizes, Times [optional]",
"## Evaluation",
"### Testing Data, Factors & Metrics",
"#### Testing Data",
"#### Factors",
"#### Metrics",
"### Results",
"#### Summary",
"## Model Examination [optional]",
"## Environmental Impact\n\n\n\nCarbon emissions can be estimated using the Machine Learning Impact calculator presented in Lacoste et al. (2019).\n\n- Hardware Type: \n- Hours used: \n- Cloud Provider: \n- Compute Region: \n- Carbon Emitted:",
"## Technical Specifications [optional]",
"### Model Architecture and Objective",
"### Compute Infrastructure",
"#### Hardware",
"#### Software\n\n\n\n[optional]\n\n\n\nBibTeX:\n\n\n\nAPA:",
"## Glossary [optional]",
"## More Information [optional]",
"## Model Card Authors [optional]",
"## Model Card Contact"
] |
null | null |
# EnverLee/TinyLlama-1.1B-Chat-v0.6-x8-MoE-Q4_K_M-GGUF
This model was converted to GGUF format from [`dillfrescott/TinyLlama-1.1B-Chat-v0.6-x8-MoE`](https://huggingface.co/dillfrescott/TinyLlama-1.1B-Chat-v0.6-x8-MoE) using llama.cpp via the ggml.ai's [GGUF-my-repo](https://huggingface.co/spaces/ggml-org/gguf-my-repo) space.
Refer to the [original model card](https://huggingface.co/dillfrescott/TinyLlama-1.1B-Chat-v0.6-x8-MoE) for more details on the model.
## Use with llama.cpp
Install llama.cpp through brew.
```bash
brew install ggerganov/ggerganov/llama.cpp
```
Invoke the llama.cpp server or the CLI.
CLI:
```bash
llama-cli --hf-repo EnverLee/TinyLlama-1.1B-Chat-v0.6-x8-MoE-Q4_K_M-GGUF --model tinyllama-1.1b-chat-v0.6-x8-moe.Q4_K_M.gguf -p "The meaning to life and the universe is"
```
Server:
```bash
llama-server --hf-repo EnverLee/TinyLlama-1.1B-Chat-v0.6-x8-MoE-Q4_K_M-GGUF --model tinyllama-1.1b-chat-v0.6-x8-moe.Q4_K_M.gguf -c 2048
```
Note: You can also use this checkpoint directly through the [usage steps](https://github.com/ggerganov/llama.cpp?tab=readme-ov-file#usage) listed in the Llama.cpp repo as well.
```
git clone https://github.com/ggerganov/llama.cpp && cd llama.cpp && make && ./main -m tinyllama-1.1b-chat-v0.6-x8-moe.Q4_K_M.gguf -n 128
```
| {"license": "apache-2.0", "tags": ["llama-cpp", "gguf-my-repo"]} | EnverLee/TinyLlama-1.1B-Chat-v0.6-x8-MoE-Q4_K_M-GGUF | null | [
"gguf",
"llama-cpp",
"gguf-my-repo",
"license:apache-2.0",
"region:us"
] | null | 2024-04-27T08:08:15+00:00 | [] | [] | TAGS
#gguf #llama-cpp #gguf-my-repo #license-apache-2.0 #region-us
|
# EnverLee/TinyLlama-1.1B-Chat-v0.6-x8-MoE-Q4_K_M-GGUF
This model was converted to GGUF format from 'dillfrescott/TinyLlama-1.1B-Chat-v0.6-x8-MoE' using URL via the URL's GGUF-my-repo space.
Refer to the original model card for more details on the model.
## Use with URL
Install URL through brew.
Invoke the URL server or the CLI.
CLI:
Server:
Note: You can also use this checkpoint directly through the usage steps listed in the URL repo as well.
| [
"# EnverLee/TinyLlama-1.1B-Chat-v0.6-x8-MoE-Q4_K_M-GGUF\nThis model was converted to GGUF format from 'dillfrescott/TinyLlama-1.1B-Chat-v0.6-x8-MoE' using URL via the URL's GGUF-my-repo space.\nRefer to the original model card for more details on the model.",
"## Use with URL\n\nInstall URL through brew.\n\n\nInvoke the URL server or the CLI.\n\nCLI:\n\n\n\nServer:\n\n\n\nNote: You can also use this checkpoint directly through the usage steps listed in the URL repo as well."
] | [
"TAGS\n#gguf #llama-cpp #gguf-my-repo #license-apache-2.0 #region-us \n",
"# EnverLee/TinyLlama-1.1B-Chat-v0.6-x8-MoE-Q4_K_M-GGUF\nThis model was converted to GGUF format from 'dillfrescott/TinyLlama-1.1B-Chat-v0.6-x8-MoE' using URL via the URL's GGUF-my-repo space.\nRefer to the original model card for more details on the model.",
"## Use with URL\n\nInstall URL through brew.\n\n\nInvoke the URL server or the CLI.\n\nCLI:\n\n\n\nServer:\n\n\n\nNote: You can also use this checkpoint directly through the usage steps listed in the URL repo as well."
] |
null | peft |
<!-- This model card has been generated automatically according to the information the Trainer had access to. You
should probably proofread and complete it, then remove this comment. -->
# GUE_EMP_H3-seqsight_16384_512_22M-L8_f
This model is a fine-tuned version of [mahdibaghbanzadeh/seqsight_16384_512_22M](https://huggingface.co/mahdibaghbanzadeh/seqsight_16384_512_22M) on the [mahdibaghbanzadeh/GUE_EMP_H3](https://huggingface.co/datasets/mahdibaghbanzadeh/GUE_EMP_H3) dataset.
It achieves the following results on the evaluation set:
- Loss: 0.3318
- F1 Score: 0.8764
- Accuracy: 0.8764
## Model description
More information needed
## Intended uses & limitations
More information needed
## Training and evaluation data
More information needed
## Training procedure
### Training hyperparameters
The following hyperparameters were used during training:
- learning_rate: 0.0005
- train_batch_size: 128
- eval_batch_size: 128
- seed: 42
- optimizer: Adam with betas=(0.9,0.999) and epsilon=1e-08
- lr_scheduler_type: linear
- training_steps: 10000
### Training results
| Training Loss | Epoch | Step | Validation Loss | F1 Score | Accuracy |
|:-------------:|:------:|:-----:|:---------------:|:--------:|:--------:|
| 0.4585 | 2.13 | 200 | 0.4056 | 0.8260 | 0.8263 |
| 0.3562 | 4.26 | 400 | 0.3832 | 0.8409 | 0.8410 |
| 0.3227 | 6.38 | 600 | 0.3685 | 0.8500 | 0.8504 |
| 0.3032 | 8.51 | 800 | 0.3626 | 0.8454 | 0.8457 |
| 0.2885 | 10.64 | 1000 | 0.3491 | 0.8542 | 0.8544 |
| 0.2781 | 12.77 | 1200 | 0.3503 | 0.8562 | 0.8564 |
| 0.2711 | 14.89 | 1400 | 0.3574 | 0.8529 | 0.8530 |
| 0.2695 | 17.02 | 1600 | 0.3394 | 0.8630 | 0.8631 |
| 0.2619 | 19.15 | 1800 | 0.3773 | 0.8492 | 0.8497 |
| 0.2548 | 21.28 | 2000 | 0.3520 | 0.8602 | 0.8604 |
| 0.2539 | 23.4 | 2200 | 0.3413 | 0.8643 | 0.8644 |
| 0.2448 | 25.53 | 2400 | 0.3782 | 0.8453 | 0.8457 |
| 0.2504 | 27.66 | 2600 | 0.3464 | 0.8616 | 0.8617 |
| 0.2432 | 29.79 | 2800 | 0.3525 | 0.8552 | 0.8557 |
| 0.2394 | 31.91 | 3000 | 0.3392 | 0.8663 | 0.8664 |
| 0.2344 | 34.04 | 3200 | 0.3393 | 0.8656 | 0.8657 |
| 0.2315 | 36.17 | 3400 | 0.3696 | 0.8500 | 0.8504 |
| 0.2256 | 38.3 | 3600 | 0.3450 | 0.8716 | 0.8717 |
| 0.2299 | 40.43 | 3800 | 0.3478 | 0.8636 | 0.8637 |
| 0.2258 | 42.55 | 4000 | 0.3716 | 0.8574 | 0.8577 |
| 0.224 | 44.68 | 4200 | 0.3493 | 0.8650 | 0.8651 |
| 0.2214 | 46.81 | 4400 | 0.3505 | 0.8616 | 0.8617 |
| 0.2202 | 48.94 | 4600 | 0.3658 | 0.8567 | 0.8570 |
| 0.2158 | 51.06 | 4800 | 0.3434 | 0.8650 | 0.8651 |
| 0.212 | 53.19 | 5000 | 0.3885 | 0.8593 | 0.8597 |
| 0.2167 | 55.32 | 5200 | 0.3516 | 0.8657 | 0.8657 |
| 0.2085 | 57.45 | 5400 | 0.3764 | 0.8588 | 0.8591 |
| 0.2084 | 59.57 | 5600 | 0.3512 | 0.8663 | 0.8664 |
| 0.2091 | 61.7 | 5800 | 0.3427 | 0.8724 | 0.8724 |
| 0.207 | 63.83 | 6000 | 0.3845 | 0.8586 | 0.8591 |
| 0.2078 | 65.96 | 6200 | 0.3816 | 0.8588 | 0.8591 |
| 0.2011 | 68.09 | 6400 | 0.3816 | 0.8593 | 0.8597 |
| 0.201 | 70.21 | 6600 | 0.3784 | 0.8601 | 0.8604 |
| 0.2 | 72.34 | 6800 | 0.3635 | 0.8675 | 0.8677 |
| 0.2028 | 74.47 | 7000 | 0.3864 | 0.8620 | 0.8624 |
| 0.1973 | 76.6 | 7200 | 0.3869 | 0.8594 | 0.8597 |
| 0.1991 | 78.72 | 7400 | 0.3861 | 0.8614 | 0.8617 |
| 0.197 | 80.85 | 7600 | 0.3775 | 0.8636 | 0.8637 |
| 0.193 | 82.98 | 7800 | 0.3686 | 0.8656 | 0.8657 |
| 0.1949 | 85.11 | 8000 | 0.3703 | 0.8649 | 0.8651 |
| 0.191 | 87.23 | 8200 | 0.3838 | 0.8622 | 0.8624 |
| 0.1904 | 89.36 | 8400 | 0.3896 | 0.8595 | 0.8597 |
| 0.1868 | 91.49 | 8600 | 0.3988 | 0.8620 | 0.8624 |
| 0.1878 | 93.62 | 8800 | 0.3766 | 0.8696 | 0.8697 |
| 0.1916 | 95.74 | 9000 | 0.3914 | 0.8614 | 0.8617 |
| 0.1898 | 97.87 | 9200 | 0.3889 | 0.8614 | 0.8617 |
| 0.1886 | 100.0 | 9400 | 0.3826 | 0.8629 | 0.8631 |
| 0.1878 | 102.13 | 9600 | 0.3877 | 0.8621 | 0.8624 |
| 0.1885 | 104.26 | 9800 | 0.3878 | 0.8615 | 0.8617 |
| 0.1882 | 106.38 | 10000 | 0.3845 | 0.8628 | 0.8631 |
### Framework versions
- PEFT 0.9.0
- Transformers 4.38.2
- Pytorch 2.2.0+cu121
- Datasets 2.17.1
- Tokenizers 0.15.2 | {"library_name": "peft", "tags": ["generated_from_trainer"], "metrics": ["accuracy"], "base_model": "mahdibaghbanzadeh/seqsight_16384_512_22M", "model-index": [{"name": "GUE_EMP_H3-seqsight_16384_512_22M-L8_f", "results": []}]} | mahdibaghbanzadeh/GUE_EMP_H3-seqsight_16384_512_22M-L8_f | null | [
"peft",
"safetensors",
"generated_from_trainer",
"base_model:mahdibaghbanzadeh/seqsight_16384_512_22M",
"region:us"
] | null | 2024-04-27T08:08:17+00:00 | [] | [] | TAGS
#peft #safetensors #generated_from_trainer #base_model-mahdibaghbanzadeh/seqsight_16384_512_22M #region-us
| GUE\_EMP\_H3-seqsight\_16384\_512\_22M-L8\_f
============================================
This model is a fine-tuned version of mahdibaghbanzadeh/seqsight\_16384\_512\_22M on the mahdibaghbanzadeh/GUE\_EMP\_H3 dataset.
It achieves the following results on the evaluation set:
* Loss: 0.3318
* F1 Score: 0.8764
* Accuracy: 0.8764
Model description
-----------------
More information needed
Intended uses & limitations
---------------------------
More information needed
Training and evaluation data
----------------------------
More information needed
Training procedure
------------------
### Training hyperparameters
The following hyperparameters were used during training:
* learning\_rate: 0.0005
* train\_batch\_size: 128
* eval\_batch\_size: 128
* seed: 42
* optimizer: Adam with betas=(0.9,0.999) and epsilon=1e-08
* lr\_scheduler\_type: linear
* training\_steps: 10000
### Training results
### Framework versions
* PEFT 0.9.0
* Transformers 4.38.2
* Pytorch 2.2.0+cu121
* Datasets 2.17.1
* Tokenizers 0.15.2
| [
"### Training hyperparameters\n\n\nThe following hyperparameters were used during training:\n\n\n* learning\\_rate: 0.0005\n* train\\_batch\\_size: 128\n* eval\\_batch\\_size: 128\n* seed: 42\n* optimizer: Adam with betas=(0.9,0.999) and epsilon=1e-08\n* lr\\_scheduler\\_type: linear\n* training\\_steps: 10000",
"### Training results",
"### Framework versions\n\n\n* PEFT 0.9.0\n* Transformers 4.38.2\n* Pytorch 2.2.0+cu121\n* Datasets 2.17.1\n* Tokenizers 0.15.2"
] | [
"TAGS\n#peft #safetensors #generated_from_trainer #base_model-mahdibaghbanzadeh/seqsight_16384_512_22M #region-us \n",
"### Training hyperparameters\n\n\nThe following hyperparameters were used during training:\n\n\n* learning\\_rate: 0.0005\n* train\\_batch\\_size: 128\n* eval\\_batch\\_size: 128\n* seed: 42\n* optimizer: Adam with betas=(0.9,0.999) and epsilon=1e-08\n* lr\\_scheduler\\_type: linear\n* training\\_steps: 10000",
"### Training results",
"### Framework versions\n\n\n* PEFT 0.9.0\n* Transformers 4.38.2\n* Pytorch 2.2.0+cu121\n* Datasets 2.17.1\n* Tokenizers 0.15.2"
] |
null | peft |
<!-- This model card has been generated automatically according to the information the Trainer had access to. You
should probably proofread and complete it, then remove this comment. -->
# GUE_EMP_H3-seqsight_16384_512_22M-L32_f
This model is a fine-tuned version of [mahdibaghbanzadeh/seqsight_16384_512_22M](https://huggingface.co/mahdibaghbanzadeh/seqsight_16384_512_22M) on the [mahdibaghbanzadeh/GUE_EMP_H3](https://huggingface.co/datasets/mahdibaghbanzadeh/GUE_EMP_H3) dataset.
It achieves the following results on the evaluation set:
- Loss: 0.4114
- F1 Score: 0.8630
- Accuracy: 0.8631
## Model description
More information needed
## Intended uses & limitations
More information needed
## Training and evaluation data
More information needed
## Training procedure
### Training hyperparameters
The following hyperparameters were used during training:
- learning_rate: 0.0005
- train_batch_size: 128
- eval_batch_size: 128
- seed: 42
- optimizer: Adam with betas=(0.9,0.999) and epsilon=1e-08
- lr_scheduler_type: linear
- training_steps: 10000
### Training results
| Training Loss | Epoch | Step | Validation Loss | F1 Score | Accuracy |
|:-------------:|:------:|:-----:|:---------------:|:--------:|:--------:|
| 0.4272 | 2.13 | 200 | 0.3728 | 0.8357 | 0.8357 |
| 0.3118 | 4.26 | 400 | 0.3520 | 0.8488 | 0.8490 |
| 0.2888 | 6.38 | 600 | 0.3557 | 0.8542 | 0.8544 |
| 0.2763 | 8.51 | 800 | 0.3382 | 0.8567 | 0.8570 |
| 0.2641 | 10.64 | 1000 | 0.3306 | 0.8623 | 0.8624 |
| 0.2546 | 12.77 | 1200 | 0.3413 | 0.8583 | 0.8584 |
| 0.2467 | 14.89 | 1400 | 0.3688 | 0.8527 | 0.8530 |
| 0.2436 | 17.02 | 1600 | 0.3317 | 0.8704 | 0.8704 |
| 0.2323 | 19.15 | 1800 | 0.3565 | 0.8603 | 0.8604 |
| 0.224 | 21.28 | 2000 | 0.3499 | 0.8664 | 0.8664 |
| 0.2183 | 23.4 | 2200 | 0.3650 | 0.8676 | 0.8677 |
| 0.2093 | 25.53 | 2400 | 0.3772 | 0.8627 | 0.8631 |
| 0.213 | 27.66 | 2600 | 0.3580 | 0.8682 | 0.8684 |
| 0.2031 | 29.79 | 2800 | 0.3756 | 0.8666 | 0.8671 |
| 0.1903 | 31.91 | 3000 | 0.3610 | 0.8643 | 0.8644 |
| 0.1846 | 34.04 | 3200 | 0.3655 | 0.8689 | 0.8691 |
| 0.1776 | 36.17 | 3400 | 0.3972 | 0.8589 | 0.8591 |
| 0.172 | 38.3 | 3600 | 0.3911 | 0.8689 | 0.8691 |
| 0.1713 | 40.43 | 3800 | 0.4144 | 0.8628 | 0.8631 |
| 0.1615 | 42.55 | 4000 | 0.4775 | 0.8490 | 0.8497 |
| 0.1588 | 44.68 | 4200 | 0.4382 | 0.8553 | 0.8557 |
| 0.1517 | 46.81 | 4400 | 0.4049 | 0.8651 | 0.8651 |
| 0.1495 | 48.94 | 4600 | 0.4535 | 0.8544 | 0.8550 |
| 0.1428 | 51.06 | 4800 | 0.4099 | 0.8697 | 0.8697 |
| 0.1363 | 53.19 | 5000 | 0.4498 | 0.8681 | 0.8684 |
| 0.1344 | 55.32 | 5200 | 0.4655 | 0.8567 | 0.8570 |
| 0.1312 | 57.45 | 5400 | 0.4522 | 0.8574 | 0.8577 |
| 0.1236 | 59.57 | 5600 | 0.4422 | 0.8669 | 0.8671 |
| 0.126 | 61.7 | 5800 | 0.4328 | 0.8737 | 0.8737 |
| 0.1182 | 63.83 | 6000 | 0.5018 | 0.8587 | 0.8591 |
| 0.1207 | 65.96 | 6200 | 0.4760 | 0.8643 | 0.8644 |
| 0.1107 | 68.09 | 6400 | 0.4969 | 0.8628 | 0.8631 |
| 0.1076 | 70.21 | 6600 | 0.5187 | 0.8615 | 0.8617 |
| 0.1098 | 72.34 | 6800 | 0.4973 | 0.8621 | 0.8624 |
| 0.108 | 74.47 | 7000 | 0.5290 | 0.8552 | 0.8557 |
| 0.1017 | 76.6 | 7200 | 0.5063 | 0.8716 | 0.8717 |
| 0.103 | 78.72 | 7400 | 0.5008 | 0.8642 | 0.8644 |
| 0.1026 | 80.85 | 7600 | 0.5145 | 0.8615 | 0.8617 |
| 0.0968 | 82.98 | 7800 | 0.5123 | 0.8636 | 0.8637 |
| 0.0994 | 85.11 | 8000 | 0.5162 | 0.8629 | 0.8631 |
| 0.0909 | 87.23 | 8200 | 0.5689 | 0.8552 | 0.8557 |
| 0.0937 | 89.36 | 8400 | 0.5483 | 0.8595 | 0.8597 |
| 0.0881 | 91.49 | 8600 | 0.5832 | 0.8559 | 0.8564 |
| 0.0866 | 93.62 | 8800 | 0.5441 | 0.8669 | 0.8671 |
| 0.0883 | 95.74 | 9000 | 0.5419 | 0.8615 | 0.8617 |
| 0.0903 | 97.87 | 9200 | 0.5466 | 0.8594 | 0.8597 |
| 0.0876 | 100.0 | 9400 | 0.5494 | 0.8594 | 0.8597 |
| 0.0862 | 102.13 | 9600 | 0.5617 | 0.8567 | 0.8570 |
| 0.084 | 104.26 | 9800 | 0.5582 | 0.8574 | 0.8577 |
| 0.0834 | 106.38 | 10000 | 0.5545 | 0.8601 | 0.8604 |
### Framework versions
- PEFT 0.9.0
- Transformers 4.38.2
- Pytorch 2.2.0+cu121
- Datasets 2.17.1
- Tokenizers 0.15.2 | {"library_name": "peft", "tags": ["generated_from_trainer"], "metrics": ["accuracy"], "base_model": "mahdibaghbanzadeh/seqsight_16384_512_22M", "model-index": [{"name": "GUE_EMP_H3-seqsight_16384_512_22M-L32_f", "results": []}]} | mahdibaghbanzadeh/GUE_EMP_H3-seqsight_16384_512_22M-L32_f | null | [
"peft",
"safetensors",
"generated_from_trainer",
"base_model:mahdibaghbanzadeh/seqsight_16384_512_22M",
"region:us"
] | null | 2024-04-27T08:08:40+00:00 | [] | [] | TAGS
#peft #safetensors #generated_from_trainer #base_model-mahdibaghbanzadeh/seqsight_16384_512_22M #region-us
| GUE\_EMP\_H3-seqsight\_16384\_512\_22M-L32\_f
=============================================
This model is a fine-tuned version of mahdibaghbanzadeh/seqsight\_16384\_512\_22M on the mahdibaghbanzadeh/GUE\_EMP\_H3 dataset.
It achieves the following results on the evaluation set:
* Loss: 0.4114
* F1 Score: 0.8630
* Accuracy: 0.8631
Model description
-----------------
More information needed
Intended uses & limitations
---------------------------
More information needed
Training and evaluation data
----------------------------
More information needed
Training procedure
------------------
### Training hyperparameters
The following hyperparameters were used during training:
* learning\_rate: 0.0005
* train\_batch\_size: 128
* eval\_batch\_size: 128
* seed: 42
* optimizer: Adam with betas=(0.9,0.999) and epsilon=1e-08
* lr\_scheduler\_type: linear
* training\_steps: 10000
### Training results
### Framework versions
* PEFT 0.9.0
* Transformers 4.38.2
* Pytorch 2.2.0+cu121
* Datasets 2.17.1
* Tokenizers 0.15.2
| [
"### Training hyperparameters\n\n\nThe following hyperparameters were used during training:\n\n\n* learning\\_rate: 0.0005\n* train\\_batch\\_size: 128\n* eval\\_batch\\_size: 128\n* seed: 42\n* optimizer: Adam with betas=(0.9,0.999) and epsilon=1e-08\n* lr\\_scheduler\\_type: linear\n* training\\_steps: 10000",
"### Training results",
"### Framework versions\n\n\n* PEFT 0.9.0\n* Transformers 4.38.2\n* Pytorch 2.2.0+cu121\n* Datasets 2.17.1\n* Tokenizers 0.15.2"
] | [
"TAGS\n#peft #safetensors #generated_from_trainer #base_model-mahdibaghbanzadeh/seqsight_16384_512_22M #region-us \n",
"### Training hyperparameters\n\n\nThe following hyperparameters were used during training:\n\n\n* learning\\_rate: 0.0005\n* train\\_batch\\_size: 128\n* eval\\_batch\\_size: 128\n* seed: 42\n* optimizer: Adam with betas=(0.9,0.999) and epsilon=1e-08\n* lr\\_scheduler\\_type: linear\n* training\\_steps: 10000",
"### Training results",
"### Framework versions\n\n\n* PEFT 0.9.0\n* Transformers 4.38.2\n* Pytorch 2.2.0+cu121\n* Datasets 2.17.1\n* Tokenizers 0.15.2"
] |
null | peft |
<!-- This model card has been generated automatically according to the information the Trainer had access to. You
should probably proofread and complete it, then remove this comment. -->
# GUE_EMP_H4ac-seqsight_16384_512_22M-L1_f
This model is a fine-tuned version of [mahdibaghbanzadeh/seqsight_16384_512_22M](https://huggingface.co/mahdibaghbanzadeh/seqsight_16384_512_22M) on the [mahdibaghbanzadeh/GUE_EMP_H4ac](https://huggingface.co/datasets/mahdibaghbanzadeh/GUE_EMP_H4ac) dataset.
It achieves the following results on the evaluation set:
- Loss: 0.5870
- F1 Score: 0.7059
- Accuracy: 0.7062
## Model description
More information needed
## Intended uses & limitations
More information needed
## Training and evaluation data
More information needed
## Training procedure
### Training hyperparameters
The following hyperparameters were used during training:
- learning_rate: 0.0005
- train_batch_size: 128
- eval_batch_size: 128
- seed: 42
- optimizer: Adam with betas=(0.9,0.999) and epsilon=1e-08
- lr_scheduler_type: linear
- training_steps: 10000
### Training results
| Training Loss | Epoch | Step | Validation Loss | F1 Score | Accuracy |
|:-------------:|:-----:|:-----:|:---------------:|:--------:|:--------:|
| 0.6468 | 0.93 | 200 | 0.6052 | 0.6807 | 0.6804 |
| 0.6074 | 1.87 | 400 | 0.6057 | 0.6791 | 0.6818 |
| 0.593 | 2.8 | 600 | 0.5864 | 0.7032 | 0.7029 |
| 0.5903 | 3.74 | 800 | 0.5865 | 0.7039 | 0.7044 |
| 0.5832 | 4.67 | 1000 | 0.5851 | 0.6992 | 0.6997 |
| 0.5775 | 5.61 | 1200 | 0.5942 | 0.6908 | 0.6933 |
| 0.5755 | 6.54 | 1400 | 0.5774 | 0.7063 | 0.7065 |
| 0.5652 | 7.48 | 1600 | 0.5760 | 0.7070 | 0.7070 |
| 0.5718 | 8.41 | 1800 | 0.5752 | 0.7073 | 0.7076 |
| 0.5674 | 9.35 | 2000 | 0.5904 | 0.6924 | 0.6950 |
| 0.5617 | 10.28 | 2200 | 0.5727 | 0.7087 | 0.7091 |
| 0.5638 | 11.21 | 2400 | 0.5750 | 0.7080 | 0.7085 |
| 0.5586 | 12.15 | 2600 | 0.5760 | 0.7115 | 0.7120 |
| 0.5586 | 13.08 | 2800 | 0.5806 | 0.7040 | 0.7050 |
| 0.5579 | 14.02 | 3000 | 0.5728 | 0.7102 | 0.7109 |
| 0.5558 | 14.95 | 3200 | 0.5729 | 0.7053 | 0.7059 |
| 0.5513 | 15.89 | 3400 | 0.5878 | 0.6991 | 0.7012 |
| 0.5507 | 16.82 | 3600 | 0.5637 | 0.7166 | 0.7164 |
| 0.5557 | 17.76 | 3800 | 0.5730 | 0.7102 | 0.7109 |
| 0.5521 | 18.69 | 4000 | 0.5756 | 0.7080 | 0.7088 |
| 0.5481 | 19.63 | 4200 | 0.5859 | 0.7027 | 0.7047 |
| 0.5501 | 20.56 | 4400 | 0.5631 | 0.7152 | 0.7152 |
| 0.5482 | 21.5 | 4600 | 0.5938 | 0.6958 | 0.6991 |
| 0.5494 | 22.43 | 4800 | 0.5725 | 0.7103 | 0.7109 |
| 0.5474 | 23.36 | 5000 | 0.5849 | 0.7071 | 0.7088 |
| 0.5462 | 24.3 | 5200 | 0.5666 | 0.7126 | 0.7129 |
| 0.5452 | 25.23 | 5400 | 0.5700 | 0.7167 | 0.7173 |
| 0.5492 | 26.17 | 5600 | 0.5722 | 0.7137 | 0.7147 |
| 0.5467 | 27.1 | 5800 | 0.5745 | 0.7095 | 0.7106 |
| 0.5404 | 28.04 | 6000 | 0.5711 | 0.7172 | 0.7176 |
| 0.5454 | 28.97 | 6200 | 0.5828 | 0.7088 | 0.7106 |
| 0.5444 | 29.91 | 6400 | 0.5740 | 0.7099 | 0.7109 |
| 0.5439 | 30.84 | 6600 | 0.5928 | 0.6994 | 0.7023 |
| 0.5432 | 31.78 | 6800 | 0.5751 | 0.7128 | 0.7138 |
| 0.5409 | 32.71 | 7000 | 0.5738 | 0.7131 | 0.7141 |
| 0.5412 | 33.64 | 7200 | 0.5750 | 0.7130 | 0.7144 |
| 0.5393 | 34.58 | 7400 | 0.5776 | 0.7103 | 0.7117 |
| 0.5446 | 35.51 | 7600 | 0.5701 | 0.7155 | 0.7164 |
| 0.5384 | 36.45 | 7800 | 0.5750 | 0.7142 | 0.7152 |
| 0.5422 | 37.38 | 8000 | 0.5896 | 0.7030 | 0.7059 |
| 0.5391 | 38.32 | 8200 | 0.5797 | 0.7105 | 0.7120 |
| 0.5394 | 39.25 | 8400 | 0.5726 | 0.7160 | 0.7170 |
| 0.5417 | 40.19 | 8600 | 0.5708 | 0.7140 | 0.7150 |
| 0.5395 | 41.12 | 8800 | 0.5786 | 0.7083 | 0.7100 |
| 0.5384 | 42.06 | 9000 | 0.5741 | 0.7116 | 0.7126 |
| 0.539 | 42.99 | 9200 | 0.5807 | 0.7086 | 0.7103 |
| 0.5392 | 43.93 | 9400 | 0.5798 | 0.7086 | 0.7103 |
| 0.5378 | 44.86 | 9600 | 0.5752 | 0.7114 | 0.7126 |
| 0.5378 | 45.79 | 9800 | 0.5771 | 0.7107 | 0.7120 |
| 0.5389 | 46.73 | 10000 | 0.5793 | 0.7099 | 0.7114 |
### Framework versions
- PEFT 0.9.0
- Transformers 4.38.2
- Pytorch 2.2.0+cu121
- Datasets 2.17.1
- Tokenizers 0.15.2 | {"library_name": "peft", "tags": ["generated_from_trainer"], "metrics": ["accuracy"], "base_model": "mahdibaghbanzadeh/seqsight_16384_512_22M", "model-index": [{"name": "GUE_EMP_H4ac-seqsight_16384_512_22M-L1_f", "results": []}]} | mahdibaghbanzadeh/GUE_EMP_H4ac-seqsight_16384_512_22M-L1_f | null | [
"peft",
"safetensors",
"generated_from_trainer",
"base_model:mahdibaghbanzadeh/seqsight_16384_512_22M",
"region:us"
] | null | 2024-04-27T08:08:50+00:00 | [] | [] | TAGS
#peft #safetensors #generated_from_trainer #base_model-mahdibaghbanzadeh/seqsight_16384_512_22M #region-us
| GUE\_EMP\_H4ac-seqsight\_16384\_512\_22M-L1\_f
==============================================
This model is a fine-tuned version of mahdibaghbanzadeh/seqsight\_16384\_512\_22M on the mahdibaghbanzadeh/GUE\_EMP\_H4ac dataset.
It achieves the following results on the evaluation set:
* Loss: 0.5870
* F1 Score: 0.7059
* Accuracy: 0.7062
Model description
-----------------
More information needed
Intended uses & limitations
---------------------------
More information needed
Training and evaluation data
----------------------------
More information needed
Training procedure
------------------
### Training hyperparameters
The following hyperparameters were used during training:
* learning\_rate: 0.0005
* train\_batch\_size: 128
* eval\_batch\_size: 128
* seed: 42
* optimizer: Adam with betas=(0.9,0.999) and epsilon=1e-08
* lr\_scheduler\_type: linear
* training\_steps: 10000
### Training results
### Framework versions
* PEFT 0.9.0
* Transformers 4.38.2
* Pytorch 2.2.0+cu121
* Datasets 2.17.1
* Tokenizers 0.15.2
| [
"### Training hyperparameters\n\n\nThe following hyperparameters were used during training:\n\n\n* learning\\_rate: 0.0005\n* train\\_batch\\_size: 128\n* eval\\_batch\\_size: 128\n* seed: 42\n* optimizer: Adam with betas=(0.9,0.999) and epsilon=1e-08\n* lr\\_scheduler\\_type: linear\n* training\\_steps: 10000",
"### Training results",
"### Framework versions\n\n\n* PEFT 0.9.0\n* Transformers 4.38.2\n* Pytorch 2.2.0+cu121\n* Datasets 2.17.1\n* Tokenizers 0.15.2"
] | [
"TAGS\n#peft #safetensors #generated_from_trainer #base_model-mahdibaghbanzadeh/seqsight_16384_512_22M #region-us \n",
"### Training hyperparameters\n\n\nThe following hyperparameters were used during training:\n\n\n* learning\\_rate: 0.0005\n* train\\_batch\\_size: 128\n* eval\\_batch\\_size: 128\n* seed: 42\n* optimizer: Adam with betas=(0.9,0.999) and epsilon=1e-08\n* lr\\_scheduler\\_type: linear\n* training\\_steps: 10000",
"### Training results",
"### Framework versions\n\n\n* PEFT 0.9.0\n* Transformers 4.38.2\n* Pytorch 2.2.0+cu121\n* Datasets 2.17.1\n* Tokenizers 0.15.2"
] |
null | peft |
<!-- This model card has been generated automatically according to the information the Trainer had access to. You
should probably proofread and complete it, then remove this comment. -->
# GUE_EMP_H4ac-seqsight_16384_512_22M-L8_f
This model is a fine-tuned version of [mahdibaghbanzadeh/seqsight_16384_512_22M](https://huggingface.co/mahdibaghbanzadeh/seqsight_16384_512_22M) on the [mahdibaghbanzadeh/GUE_EMP_H4ac](https://huggingface.co/datasets/mahdibaghbanzadeh/GUE_EMP_H4ac) dataset.
It achieves the following results on the evaluation set:
- Loss: 0.5698
- F1 Score: 0.7126
- Accuracy: 0.7123
## Model description
More information needed
## Intended uses & limitations
More information needed
## Training and evaluation data
More information needed
## Training procedure
### Training hyperparameters
The following hyperparameters were used during training:
- learning_rate: 0.0005
- train_batch_size: 128
- eval_batch_size: 128
- seed: 42
- optimizer: Adam with betas=(0.9,0.999) and epsilon=1e-08
- lr_scheduler_type: linear
- training_steps: 10000
### Training results
| Training Loss | Epoch | Step | Validation Loss | F1 Score | Accuracy |
|:-------------:|:-----:|:-----:|:---------------:|:--------:|:--------:|
| 0.6273 | 0.93 | 200 | 0.5934 | 0.6951 | 0.6947 |
| 0.5883 | 1.87 | 400 | 0.5837 | 0.7024 | 0.7032 |
| 0.5726 | 2.8 | 600 | 0.5650 | 0.7228 | 0.7226 |
| 0.5667 | 3.74 | 800 | 0.5707 | 0.7126 | 0.7129 |
| 0.5597 | 4.67 | 1000 | 0.5679 | 0.7177 | 0.7176 |
| 0.5555 | 5.61 | 1200 | 0.5783 | 0.7033 | 0.7050 |
| 0.5544 | 6.54 | 1400 | 0.5759 | 0.7062 | 0.7073 |
| 0.5398 | 7.48 | 1600 | 0.5710 | 0.7146 | 0.7150 |
| 0.5475 | 8.41 | 1800 | 0.5612 | 0.7192 | 0.7191 |
| 0.5418 | 9.35 | 2000 | 0.5756 | 0.7129 | 0.7141 |
| 0.5368 | 10.28 | 2200 | 0.5580 | 0.7311 | 0.7308 |
| 0.5368 | 11.21 | 2400 | 0.5701 | 0.7196 | 0.7199 |
| 0.5325 | 12.15 | 2600 | 0.5679 | 0.7185 | 0.7191 |
| 0.5315 | 13.08 | 2800 | 0.5805 | 0.7188 | 0.7194 |
| 0.5291 | 14.02 | 3000 | 0.5543 | 0.7296 | 0.7293 |
| 0.5269 | 14.95 | 3200 | 0.5638 | 0.7195 | 0.7196 |
| 0.5213 | 15.89 | 3400 | 0.5727 | 0.7190 | 0.7196 |
| 0.5203 | 16.82 | 3600 | 0.5647 | 0.7270 | 0.7270 |
| 0.5243 | 17.76 | 3800 | 0.5802 | 0.7124 | 0.7138 |
| 0.5204 | 18.69 | 4000 | 0.5621 | 0.7276 | 0.7273 |
| 0.5161 | 19.63 | 4200 | 0.5704 | 0.7240 | 0.7243 |
| 0.5163 | 20.56 | 4400 | 0.5550 | 0.7273 | 0.7270 |
| 0.5143 | 21.5 | 4600 | 0.5860 | 0.7119 | 0.7141 |
| 0.5143 | 22.43 | 4800 | 0.5552 | 0.7370 | 0.7367 |
| 0.5141 | 23.36 | 5000 | 0.5766 | 0.7201 | 0.7208 |
| 0.5149 | 24.3 | 5200 | 0.5589 | 0.7313 | 0.7311 |
| 0.5095 | 25.23 | 5400 | 0.5592 | 0.7357 | 0.7355 |
| 0.5123 | 26.17 | 5600 | 0.5678 | 0.7260 | 0.7261 |
| 0.5087 | 27.1 | 5800 | 0.5620 | 0.7278 | 0.7276 |
| 0.5028 | 28.04 | 6000 | 0.5644 | 0.7360 | 0.7358 |
| 0.5074 | 28.97 | 6200 | 0.5755 | 0.7259 | 0.7264 |
| 0.5053 | 29.91 | 6400 | 0.5664 | 0.7268 | 0.7267 |
| 0.5041 | 30.84 | 6600 | 0.5876 | 0.7218 | 0.7229 |
| 0.5025 | 31.78 | 6800 | 0.5685 | 0.7329 | 0.7328 |
| 0.5009 | 32.71 | 7000 | 0.5709 | 0.7303 | 0.7302 |
| 0.5002 | 33.64 | 7200 | 0.5798 | 0.7236 | 0.7246 |
| 0.4986 | 34.58 | 7400 | 0.5674 | 0.7308 | 0.7308 |
| 0.5017 | 35.51 | 7600 | 0.5675 | 0.7308 | 0.7308 |
| 0.4969 | 36.45 | 7800 | 0.5741 | 0.7306 | 0.7305 |
| 0.5011 | 37.38 | 8000 | 0.5738 | 0.7261 | 0.7267 |
| 0.4966 | 38.32 | 8200 | 0.5730 | 0.7303 | 0.7305 |
| 0.4978 | 39.25 | 8400 | 0.5698 | 0.7308 | 0.7308 |
| 0.4985 | 40.19 | 8600 | 0.5709 | 0.7329 | 0.7328 |
| 0.4974 | 41.12 | 8800 | 0.5755 | 0.7288 | 0.7290 |
| 0.4952 | 42.06 | 9000 | 0.5764 | 0.7303 | 0.7305 |
| 0.4958 | 42.99 | 9200 | 0.5807 | 0.7292 | 0.7296 |
| 0.4953 | 43.93 | 9400 | 0.5763 | 0.7278 | 0.7282 |
| 0.4936 | 44.86 | 9600 | 0.5731 | 0.7313 | 0.7314 |
| 0.4931 | 45.79 | 9800 | 0.5739 | 0.7310 | 0.7311 |
| 0.4962 | 46.73 | 10000 | 0.5761 | 0.7296 | 0.7299 |
### Framework versions
- PEFT 0.9.0
- Transformers 4.38.2
- Pytorch 2.2.0+cu121
- Datasets 2.17.1
- Tokenizers 0.15.2 | {"library_name": "peft", "tags": ["generated_from_trainer"], "metrics": ["accuracy"], "base_model": "mahdibaghbanzadeh/seqsight_16384_512_22M", "model-index": [{"name": "GUE_EMP_H4ac-seqsight_16384_512_22M-L8_f", "results": []}]} | mahdibaghbanzadeh/GUE_EMP_H4ac-seqsight_16384_512_22M-L8_f | null | [
"peft",
"safetensors",
"generated_from_trainer",
"base_model:mahdibaghbanzadeh/seqsight_16384_512_22M",
"region:us"
] | null | 2024-04-27T08:09:16+00:00 | [] | [] | TAGS
#peft #safetensors #generated_from_trainer #base_model-mahdibaghbanzadeh/seqsight_16384_512_22M #region-us
| GUE\_EMP\_H4ac-seqsight\_16384\_512\_22M-L8\_f
==============================================
This model is a fine-tuned version of mahdibaghbanzadeh/seqsight\_16384\_512\_22M on the mahdibaghbanzadeh/GUE\_EMP\_H4ac dataset.
It achieves the following results on the evaluation set:
* Loss: 0.5698
* F1 Score: 0.7126
* Accuracy: 0.7123
Model description
-----------------
More information needed
Intended uses & limitations
---------------------------
More information needed
Training and evaluation data
----------------------------
More information needed
Training procedure
------------------
### Training hyperparameters
The following hyperparameters were used during training:
* learning\_rate: 0.0005
* train\_batch\_size: 128
* eval\_batch\_size: 128
* seed: 42
* optimizer: Adam with betas=(0.9,0.999) and epsilon=1e-08
* lr\_scheduler\_type: linear
* training\_steps: 10000
### Training results
### Framework versions
* PEFT 0.9.0
* Transformers 4.38.2
* Pytorch 2.2.0+cu121
* Datasets 2.17.1
* Tokenizers 0.15.2
| [
"### Training hyperparameters\n\n\nThe following hyperparameters were used during training:\n\n\n* learning\\_rate: 0.0005\n* train\\_batch\\_size: 128\n* eval\\_batch\\_size: 128\n* seed: 42\n* optimizer: Adam with betas=(0.9,0.999) and epsilon=1e-08\n* lr\\_scheduler\\_type: linear\n* training\\_steps: 10000",
"### Training results",
"### Framework versions\n\n\n* PEFT 0.9.0\n* Transformers 4.38.2\n* Pytorch 2.2.0+cu121\n* Datasets 2.17.1\n* Tokenizers 0.15.2"
] | [
"TAGS\n#peft #safetensors #generated_from_trainer #base_model-mahdibaghbanzadeh/seqsight_16384_512_22M #region-us \n",
"### Training hyperparameters\n\n\nThe following hyperparameters were used during training:\n\n\n* learning\\_rate: 0.0005\n* train\\_batch\\_size: 128\n* eval\\_batch\\_size: 128\n* seed: 42\n* optimizer: Adam with betas=(0.9,0.999) and epsilon=1e-08\n* lr\\_scheduler\\_type: linear\n* training\\_steps: 10000",
"### Training results",
"### Framework versions\n\n\n* PEFT 0.9.0\n* Transformers 4.38.2\n* Pytorch 2.2.0+cu121\n* Datasets 2.17.1\n* Tokenizers 0.15.2"
] |
null | null |
# EnverLee/TinyLlama-1.1B-Chat-v0.6-x8-MoE-Q8_0-GGUF
This model was converted to GGUF format from [`dillfrescott/TinyLlama-1.1B-Chat-v0.6-x8-MoE`](https://huggingface.co/dillfrescott/TinyLlama-1.1B-Chat-v0.6-x8-MoE) using llama.cpp via the ggml.ai's [GGUF-my-repo](https://huggingface.co/spaces/ggml-org/gguf-my-repo) space.
Refer to the [original model card](https://huggingface.co/dillfrescott/TinyLlama-1.1B-Chat-v0.6-x8-MoE) for more details on the model.
## Use with llama.cpp
Install llama.cpp through brew.
```bash
brew install ggerganov/ggerganov/llama.cpp
```
Invoke the llama.cpp server or the CLI.
CLI:
```bash
llama-cli --hf-repo EnverLee/TinyLlama-1.1B-Chat-v0.6-x8-MoE-Q8_0-GGUF --model tinyllama-1.1b-chat-v0.6-x8-moe.Q8_0.gguf -p "The meaning to life and the universe is"
```
Server:
```bash
llama-server --hf-repo EnverLee/TinyLlama-1.1B-Chat-v0.6-x8-MoE-Q8_0-GGUF --model tinyllama-1.1b-chat-v0.6-x8-moe.Q8_0.gguf -c 2048
```
Note: You can also use this checkpoint directly through the [usage steps](https://github.com/ggerganov/llama.cpp?tab=readme-ov-file#usage) listed in the Llama.cpp repo as well.
```
git clone https://github.com/ggerganov/llama.cpp && cd llama.cpp && make && ./main -m tinyllama-1.1b-chat-v0.6-x8-moe.Q8_0.gguf -n 128
```
| {"license": "apache-2.0", "tags": ["llama-cpp", "gguf-my-repo"]} | EnverLee/TinyLlama-1.1B-Chat-v0.6-x8-MoE-Q8_0-GGUF | null | [
"gguf",
"llama-cpp",
"gguf-my-repo",
"license:apache-2.0",
"region:us"
] | null | 2024-04-27T08:09:39+00:00 | [] | [] | TAGS
#gguf #llama-cpp #gguf-my-repo #license-apache-2.0 #region-us
|
# EnverLee/TinyLlama-1.1B-Chat-v0.6-x8-MoE-Q8_0-GGUF
This model was converted to GGUF format from 'dillfrescott/TinyLlama-1.1B-Chat-v0.6-x8-MoE' using URL via the URL's GGUF-my-repo space.
Refer to the original model card for more details on the model.
## Use with URL
Install URL through brew.
Invoke the URL server or the CLI.
CLI:
Server:
Note: You can also use this checkpoint directly through the usage steps listed in the URL repo as well.
| [
"# EnverLee/TinyLlama-1.1B-Chat-v0.6-x8-MoE-Q8_0-GGUF\nThis model was converted to GGUF format from 'dillfrescott/TinyLlama-1.1B-Chat-v0.6-x8-MoE' using URL via the URL's GGUF-my-repo space.\nRefer to the original model card for more details on the model.",
"## Use with URL\n\nInstall URL through brew.\n\n\nInvoke the URL server or the CLI.\n\nCLI:\n\n\n\nServer:\n\n\n\nNote: You can also use this checkpoint directly through the usage steps listed in the URL repo as well."
] | [
"TAGS\n#gguf #llama-cpp #gguf-my-repo #license-apache-2.0 #region-us \n",
"# EnverLee/TinyLlama-1.1B-Chat-v0.6-x8-MoE-Q8_0-GGUF\nThis model was converted to GGUF format from 'dillfrescott/TinyLlama-1.1B-Chat-v0.6-x8-MoE' using URL via the URL's GGUF-my-repo space.\nRefer to the original model card for more details on the model.",
"## Use with URL\n\nInstall URL through brew.\n\n\nInvoke the URL server or the CLI.\n\nCLI:\n\n\n\nServer:\n\n\n\nNote: You can also use this checkpoint directly through the usage steps listed in the URL repo as well."
] |
null | peft |
<!-- This model card has been generated automatically according to the information the Trainer had access to. You
should probably proofread and complete it, then remove this comment. -->
# GUE_EMP_H4ac-seqsight_16384_512_22M-L32_f
This model is a fine-tuned version of [mahdibaghbanzadeh/seqsight_16384_512_22M](https://huggingface.co/mahdibaghbanzadeh/seqsight_16384_512_22M) on the [mahdibaghbanzadeh/GUE_EMP_H4ac](https://huggingface.co/datasets/mahdibaghbanzadeh/GUE_EMP_H4ac) dataset.
It achieves the following results on the evaluation set:
- Loss: 0.5877
- F1 Score: 0.7198
- Accuracy: 0.7196
## Model description
More information needed
## Intended uses & limitations
More information needed
## Training and evaluation data
More information needed
## Training procedure
### Training hyperparameters
The following hyperparameters were used during training:
- learning_rate: 0.0005
- train_batch_size: 128
- eval_batch_size: 128
- seed: 42
- optimizer: Adam with betas=(0.9,0.999) and epsilon=1e-08
- lr_scheduler_type: linear
- training_steps: 10000
### Training results
| Training Loss | Epoch | Step | Validation Loss | F1 Score | Accuracy |
|:-------------:|:-----:|:-----:|:---------------:|:--------:|:--------:|
| 0.6159 | 0.93 | 200 | 0.5960 | 0.7025 | 0.7026 |
| 0.5791 | 1.87 | 400 | 0.5717 | 0.7135 | 0.7138 |
| 0.5618 | 2.8 | 600 | 0.5628 | 0.7227 | 0.7226 |
| 0.5535 | 3.74 | 800 | 0.5600 | 0.7173 | 0.7173 |
| 0.5454 | 4.67 | 1000 | 0.5561 | 0.7230 | 0.7229 |
| 0.5404 | 5.61 | 1200 | 0.5824 | 0.7075 | 0.7097 |
| 0.5367 | 6.54 | 1400 | 0.5841 | 0.7097 | 0.7117 |
| 0.521 | 7.48 | 1600 | 0.5680 | 0.7235 | 0.7240 |
| 0.5265 | 8.41 | 1800 | 0.5500 | 0.7351 | 0.7349 |
| 0.5202 | 9.35 | 2000 | 0.5581 | 0.7273 | 0.7273 |
| 0.5128 | 10.28 | 2200 | 0.5483 | 0.7358 | 0.7355 |
| 0.5087 | 11.21 | 2400 | 0.5801 | 0.7271 | 0.7276 |
| 0.5051 | 12.15 | 2600 | 0.5741 | 0.7296 | 0.7308 |
| 0.5007 | 13.08 | 2800 | 0.5808 | 0.7261 | 0.7267 |
| 0.4978 | 14.02 | 3000 | 0.5547 | 0.7348 | 0.7346 |
| 0.4932 | 14.95 | 3200 | 0.5593 | 0.7361 | 0.7361 |
| 0.4868 | 15.89 | 3400 | 0.5719 | 0.7261 | 0.7267 |
| 0.4832 | 16.82 | 3600 | 0.5714 | 0.7286 | 0.7290 |
| 0.4871 | 17.76 | 3800 | 0.6250 | 0.7020 | 0.7065 |
| 0.478 | 18.69 | 4000 | 0.5696 | 0.7325 | 0.7326 |
| 0.4738 | 19.63 | 4200 | 0.5725 | 0.7302 | 0.7305 |
| 0.4706 | 20.56 | 4400 | 0.5643 | 0.7340 | 0.7340 |
| 0.4666 | 21.5 | 4600 | 0.6017 | 0.7188 | 0.7205 |
| 0.4642 | 22.43 | 4800 | 0.5651 | 0.7395 | 0.7393 |
| 0.4636 | 23.36 | 5000 | 0.5927 | 0.7251 | 0.7258 |
| 0.4607 | 24.3 | 5200 | 0.5823 | 0.7305 | 0.7314 |
| 0.4545 | 25.23 | 5400 | 0.5759 | 0.7322 | 0.7323 |
| 0.4539 | 26.17 | 5600 | 0.5803 | 0.7299 | 0.7299 |
| 0.4509 | 27.1 | 5800 | 0.5770 | 0.7339 | 0.7337 |
| 0.4445 | 28.04 | 6000 | 0.6015 | 0.7272 | 0.7279 |
| 0.4476 | 28.97 | 6200 | 0.6006 | 0.7241 | 0.7246 |
| 0.4438 | 29.91 | 6400 | 0.6084 | 0.7165 | 0.7179 |
| 0.4408 | 30.84 | 6600 | 0.6135 | 0.7221 | 0.7232 |
| 0.4355 | 31.78 | 6800 | 0.6022 | 0.7258 | 0.7264 |
| 0.4314 | 32.71 | 7000 | 0.6119 | 0.7240 | 0.7243 |
| 0.4328 | 33.64 | 7200 | 0.6236 | 0.7188 | 0.7211 |
| 0.4298 | 34.58 | 7400 | 0.6007 | 0.7231 | 0.7238 |
| 0.4298 | 35.51 | 7600 | 0.6101 | 0.7258 | 0.7270 |
| 0.4279 | 36.45 | 7800 | 0.6283 | 0.7244 | 0.7255 |
| 0.4282 | 37.38 | 8000 | 0.6140 | 0.7267 | 0.7279 |
| 0.4253 | 38.32 | 8200 | 0.6041 | 0.7253 | 0.7258 |
| 0.4248 | 39.25 | 8400 | 0.6134 | 0.7245 | 0.7252 |
| 0.4203 | 40.19 | 8600 | 0.6205 | 0.7220 | 0.7229 |
| 0.4208 | 41.12 | 8800 | 0.6188 | 0.7276 | 0.7287 |
| 0.4151 | 42.06 | 9000 | 0.6137 | 0.7245 | 0.7252 |
| 0.4186 | 42.99 | 9200 | 0.6274 | 0.7226 | 0.7240 |
| 0.4167 | 43.93 | 9400 | 0.6204 | 0.7248 | 0.7261 |
| 0.4147 | 44.86 | 9600 | 0.6160 | 0.7232 | 0.7240 |
| 0.4133 | 45.79 | 9800 | 0.6191 | 0.7228 | 0.7238 |
| 0.4154 | 46.73 | 10000 | 0.6194 | 0.7224 | 0.7235 |
### Framework versions
- PEFT 0.9.0
- Transformers 4.38.2
- Pytorch 2.2.0+cu121
- Datasets 2.17.1
- Tokenizers 0.15.2 | {"library_name": "peft", "tags": ["generated_from_trainer"], "metrics": ["accuracy"], "base_model": "mahdibaghbanzadeh/seqsight_16384_512_22M", "model-index": [{"name": "GUE_EMP_H4ac-seqsight_16384_512_22M-L32_f", "results": []}]} | mahdibaghbanzadeh/GUE_EMP_H4ac-seqsight_16384_512_22M-L32_f | null | [
"peft",
"safetensors",
"generated_from_trainer",
"base_model:mahdibaghbanzadeh/seqsight_16384_512_22M",
"region:us"
] | null | 2024-04-27T08:10:03+00:00 | [] | [] | TAGS
#peft #safetensors #generated_from_trainer #base_model-mahdibaghbanzadeh/seqsight_16384_512_22M #region-us
| GUE\_EMP\_H4ac-seqsight\_16384\_512\_22M-L32\_f
===============================================
This model is a fine-tuned version of mahdibaghbanzadeh/seqsight\_16384\_512\_22M on the mahdibaghbanzadeh/GUE\_EMP\_H4ac dataset.
It achieves the following results on the evaluation set:
* Loss: 0.5877
* F1 Score: 0.7198
* Accuracy: 0.7196
Model description
-----------------
More information needed
Intended uses & limitations
---------------------------
More information needed
Training and evaluation data
----------------------------
More information needed
Training procedure
------------------
### Training hyperparameters
The following hyperparameters were used during training:
* learning\_rate: 0.0005
* train\_batch\_size: 128
* eval\_batch\_size: 128
* seed: 42
* optimizer: Adam with betas=(0.9,0.999) and epsilon=1e-08
* lr\_scheduler\_type: linear
* training\_steps: 10000
### Training results
### Framework versions
* PEFT 0.9.0
* Transformers 4.38.2
* Pytorch 2.2.0+cu121
* Datasets 2.17.1
* Tokenizers 0.15.2
| [
"### Training hyperparameters\n\n\nThe following hyperparameters were used during training:\n\n\n* learning\\_rate: 0.0005\n* train\\_batch\\_size: 128\n* eval\\_batch\\_size: 128\n* seed: 42\n* optimizer: Adam with betas=(0.9,0.999) and epsilon=1e-08\n* lr\\_scheduler\\_type: linear\n* training\\_steps: 10000",
"### Training results",
"### Framework versions\n\n\n* PEFT 0.9.0\n* Transformers 4.38.2\n* Pytorch 2.2.0+cu121\n* Datasets 2.17.1\n* Tokenizers 0.15.2"
] | [
"TAGS\n#peft #safetensors #generated_from_trainer #base_model-mahdibaghbanzadeh/seqsight_16384_512_22M #region-us \n",
"### Training hyperparameters\n\n\nThe following hyperparameters were used during training:\n\n\n* learning\\_rate: 0.0005\n* train\\_batch\\_size: 128\n* eval\\_batch\\_size: 128\n* seed: 42\n* optimizer: Adam with betas=(0.9,0.999) and epsilon=1e-08\n* lr\\_scheduler\\_type: linear\n* training\\_steps: 10000",
"### Training results",
"### Framework versions\n\n\n* PEFT 0.9.0\n* Transformers 4.38.2\n* Pytorch 2.2.0+cu121\n* Datasets 2.17.1\n* Tokenizers 0.15.2"
] |
null | peft |
# Model Card for Model ID
<!-- Provide a quick summary of what the model is/does. -->
## Model Details
### Model Description
<!-- Provide a longer summary of what this model is. -->
- **Developed by:** [More Information Needed]
- **Funded by [optional]:** [More Information Needed]
- **Shared by [optional]:** [More Information Needed]
- **Model type:** [More Information Needed]
- **Language(s) (NLP):** [More Information Needed]
- **License:** [More Information Needed]
- **Finetuned from model [optional]:** [More Information Needed]
### Model Sources [optional]
<!-- Provide the basic links for the model. -->
- **Repository:** [More Information Needed]
- **Paper [optional]:** [More Information Needed]
- **Demo [optional]:** [More Information Needed]
## Uses
<!-- Address questions around how the model is intended to be used, including the foreseeable users of the model and those affected by the model. -->
### Direct Use
<!-- This section is for the model use without fine-tuning or plugging into a larger ecosystem/app. -->
[More Information Needed]
### Downstream Use [optional]
<!-- This section is for the model use when fine-tuned for a task, or when plugged into a larger ecosystem/app -->
[More Information Needed]
### Out-of-Scope Use
<!-- This section addresses misuse, malicious use, and uses that the model will not work well for. -->
[More Information Needed]
## Bias, Risks, and Limitations
<!-- This section is meant to convey both technical and sociotechnical limitations. -->
[More Information Needed]
### Recommendations
<!-- This section is meant to convey recommendations with respect to the bias, risk, and technical limitations. -->
Users (both direct and downstream) should be made aware of the risks, biases and limitations of the model. More information needed for further recommendations.
## How to Get Started with the Model
Use the code below to get started with the model.
[More Information Needed]
## Training Details
### Training Data
<!-- This should link to a Dataset Card, perhaps with a short stub of information on what the training data is all about as well as documentation related to data pre-processing or additional filtering. -->
[More Information Needed]
### Training Procedure
<!-- This relates heavily to the Technical Specifications. Content here should link to that section when it is relevant to the training procedure. -->
#### Preprocessing [optional]
[More Information Needed]
#### Training Hyperparameters
- **Training regime:** [More Information Needed] <!--fp32, fp16 mixed precision, bf16 mixed precision, bf16 non-mixed precision, fp16 non-mixed precision, fp8 mixed precision -->
#### Speeds, Sizes, Times [optional]
<!-- This section provides information about throughput, start/end time, checkpoint size if relevant, etc. -->
[More Information Needed]
## Evaluation
<!-- This section describes the evaluation protocols and provides the results. -->
### Testing Data, Factors & Metrics
#### Testing Data
<!-- This should link to a Dataset Card if possible. -->
[More Information Needed]
#### Factors
<!-- These are the things the evaluation is disaggregating by, e.g., subpopulations or domains. -->
[More Information Needed]
#### Metrics
<!-- These are the evaluation metrics being used, ideally with a description of why. -->
[More Information Needed]
### Results
[More Information Needed]
#### Summary
## Model Examination [optional]
<!-- Relevant interpretability work for the model goes here -->
[More Information Needed]
## Environmental Impact
<!-- Total emissions (in grams of CO2eq) and additional considerations, such as electricity usage, go here. Edit the suggested text below accordingly -->
Carbon emissions can be estimated using the [Machine Learning Impact calculator](https://mlco2.github.io/impact#compute) presented in [Lacoste et al. (2019)](https://arxiv.org/abs/1910.09700).
- **Hardware Type:** [More Information Needed]
- **Hours used:** [More Information Needed]
- **Cloud Provider:** [More Information Needed]
- **Compute Region:** [More Information Needed]
- **Carbon Emitted:** [More Information Needed]
## Technical Specifications [optional]
### Model Architecture and Objective
[More Information Needed]
### Compute Infrastructure
[More Information Needed]
#### Hardware
[More Information Needed]
#### Software
[More Information Needed]
## Citation [optional]
<!-- If there is a paper or blog post introducing the model, the APA and Bibtex information for that should go in this section. -->
**BibTeX:**
[More Information Needed]
**APA:**
[More Information Needed]
## Glossary [optional]
<!-- If relevant, include terms and calculations in this section that can help readers understand the model or model card. -->
[More Information Needed]
## More Information [optional]
[More Information Needed]
## Model Card Authors [optional]
[More Information Needed]
## Model Card Contact
[More Information Needed]
### Framework versions
- PEFT 0.10.0 | {"library_name": "peft", "base_model": "Salesforce/codegen-350M-mono"} | Denis641/BICodeGen_MNTP | null | [
"peft",
"safetensors",
"codegen",
"arxiv:1910.09700",
"base_model:Salesforce/codegen-350M-mono",
"region:us"
] | null | 2024-04-27T08:10:07+00:00 | [
"1910.09700"
] | [] | TAGS
#peft #safetensors #codegen #arxiv-1910.09700 #base_model-Salesforce/codegen-350M-mono #region-us
|
# Model Card for Model ID
## Model Details
### Model Description
- Developed by:
- Funded by [optional]:
- Shared by [optional]:
- Model type:
- Language(s) (NLP):
- License:
- Finetuned from model [optional]:
### Model Sources [optional]
- Repository:
- Paper [optional]:
- Demo [optional]:
## Uses
### Direct Use
### Downstream Use [optional]
### Out-of-Scope Use
## Bias, Risks, and Limitations
### Recommendations
Users (both direct and downstream) should be made aware of the risks, biases and limitations of the model. More information needed for further recommendations.
## How to Get Started with the Model
Use the code below to get started with the model.
## Training Details
### Training Data
### Training Procedure
#### Preprocessing [optional]
#### Training Hyperparameters
- Training regime:
#### Speeds, Sizes, Times [optional]
## Evaluation
### Testing Data, Factors & Metrics
#### Testing Data
#### Factors
#### Metrics
### Results
#### Summary
## Model Examination [optional]
## Environmental Impact
Carbon emissions can be estimated using the Machine Learning Impact calculator presented in Lacoste et al. (2019).
- Hardware Type:
- Hours used:
- Cloud Provider:
- Compute Region:
- Carbon Emitted:
## Technical Specifications [optional]
### Model Architecture and Objective
### Compute Infrastructure
#### Hardware
#### Software
[optional]
BibTeX:
APA:
## Glossary [optional]
## More Information [optional]
## Model Card Authors [optional]
## Model Card Contact
### Framework versions
- PEFT 0.10.0 | [
"# Model Card for Model ID",
"## Model Details",
"### Model Description\n\n\n\n\n\n- Developed by: \n- Funded by [optional]: \n- Shared by [optional]: \n- Model type: \n- Language(s) (NLP): \n- License: \n- Finetuned from model [optional]:",
"### Model Sources [optional]\n\n\n\n- Repository: \n- Paper [optional]: \n- Demo [optional]:",
"## Uses",
"### Direct Use",
"### Downstream Use [optional]",
"### Out-of-Scope Use",
"## Bias, Risks, and Limitations",
"### Recommendations\n\n\n\nUsers (both direct and downstream) should be made aware of the risks, biases and limitations of the model. More information needed for further recommendations.",
"## How to Get Started with the Model\n\nUse the code below to get started with the model.",
"## Training Details",
"### Training Data",
"### Training Procedure",
"#### Preprocessing [optional]",
"#### Training Hyperparameters\n\n- Training regime:",
"#### Speeds, Sizes, Times [optional]",
"## Evaluation",
"### Testing Data, Factors & Metrics",
"#### Testing Data",
"#### Factors",
"#### Metrics",
"### Results",
"#### Summary",
"## Model Examination [optional]",
"## Environmental Impact\n\n\n\nCarbon emissions can be estimated using the Machine Learning Impact calculator presented in Lacoste et al. (2019).\n\n- Hardware Type: \n- Hours used: \n- Cloud Provider: \n- Compute Region: \n- Carbon Emitted:",
"## Technical Specifications [optional]",
"### Model Architecture and Objective",
"### Compute Infrastructure",
"#### Hardware",
"#### Software\n\n\n\n[optional]\n\n\n\nBibTeX:\n\n\n\nAPA:",
"## Glossary [optional]",
"## More Information [optional]",
"## Model Card Authors [optional]",
"## Model Card Contact",
"### Framework versions\n\n- PEFT 0.10.0"
] | [
"TAGS\n#peft #safetensors #codegen #arxiv-1910.09700 #base_model-Salesforce/codegen-350M-mono #region-us \n",
"# Model Card for Model ID",
"## Model Details",
"### Model Description\n\n\n\n\n\n- Developed by: \n- Funded by [optional]: \n- Shared by [optional]: \n- Model type: \n- Language(s) (NLP): \n- License: \n- Finetuned from model [optional]:",
"### Model Sources [optional]\n\n\n\n- Repository: \n- Paper [optional]: \n- Demo [optional]:",
"## Uses",
"### Direct Use",
"### Downstream Use [optional]",
"### Out-of-Scope Use",
"## Bias, Risks, and Limitations",
"### Recommendations\n\n\n\nUsers (both direct and downstream) should be made aware of the risks, biases and limitations of the model. More information needed for further recommendations.",
"## How to Get Started with the Model\n\nUse the code below to get started with the model.",
"## Training Details",
"### Training Data",
"### Training Procedure",
"#### Preprocessing [optional]",
"#### Training Hyperparameters\n\n- Training regime:",
"#### Speeds, Sizes, Times [optional]",
"## Evaluation",
"### Testing Data, Factors & Metrics",
"#### Testing Data",
"#### Factors",
"#### Metrics",
"### Results",
"#### Summary",
"## Model Examination [optional]",
"## Environmental Impact\n\n\n\nCarbon emissions can be estimated using the Machine Learning Impact calculator presented in Lacoste et al. (2019).\n\n- Hardware Type: \n- Hours used: \n- Cloud Provider: \n- Compute Region: \n- Carbon Emitted:",
"## Technical Specifications [optional]",
"### Model Architecture and Objective",
"### Compute Infrastructure",
"#### Hardware",
"#### Software\n\n\n\n[optional]\n\n\n\nBibTeX:\n\n\n\nAPA:",
"## Glossary [optional]",
"## More Information [optional]",
"## Model Card Authors [optional]",
"## Model Card Contact",
"### Framework versions\n\n- PEFT 0.10.0"
] |
null | null |
# EnverLee/polyglot-ko-1.3b-Q4_0-GGUF
This model was converted to GGUF format from [`EleutherAI/polyglot-ko-1.3b`](https://huggingface.co/EleutherAI/polyglot-ko-1.3b) using llama.cpp via the ggml.ai's [GGUF-my-repo](https://huggingface.co/spaces/ggml-org/gguf-my-repo) space.
Refer to the [original model card](https://huggingface.co/EleutherAI/polyglot-ko-1.3b) for more details on the model.
## Use with llama.cpp
Install llama.cpp through brew.
```bash
brew install ggerganov/ggerganov/llama.cpp
```
Invoke the llama.cpp server or the CLI.
CLI:
```bash
llama-cli --hf-repo EnverLee/polyglot-ko-1.3b-Q4_0-GGUF --model polyglot-ko-1.3b.Q4_0.gguf -p "The meaning to life and the universe is"
```
Server:
```bash
llama-server --hf-repo EnverLee/polyglot-ko-1.3b-Q4_0-GGUF --model polyglot-ko-1.3b.Q4_0.gguf -c 2048
```
Note: You can also use this checkpoint directly through the [usage steps](https://github.com/ggerganov/llama.cpp?tab=readme-ov-file#usage) listed in the Llama.cpp repo as well.
```
git clone https://github.com/ggerganov/llama.cpp && cd llama.cpp && make && ./main -m polyglot-ko-1.3b.Q4_0.gguf -n 128
```
| {"language": ["ko"], "license": "apache-2.0", "tags": ["pytorch", "causal-lm", "llama-cpp", "gguf-my-repo"]} | EnverLee/polyglot-ko-1.3b-Q4_0-GGUF | null | [
"gguf",
"pytorch",
"causal-lm",
"llama-cpp",
"gguf-my-repo",
"ko",
"license:apache-2.0",
"region:us"
] | null | 2024-04-27T08:11:19+00:00 | [] | [
"ko"
] | TAGS
#gguf #pytorch #causal-lm #llama-cpp #gguf-my-repo #ko #license-apache-2.0 #region-us
|
# EnverLee/polyglot-ko-1.3b-Q4_0-GGUF
This model was converted to GGUF format from 'EleutherAI/polyglot-ko-1.3b' using URL via the URL's GGUF-my-repo space.
Refer to the original model card for more details on the model.
## Use with URL
Install URL through brew.
Invoke the URL server or the CLI.
CLI:
Server:
Note: You can also use this checkpoint directly through the usage steps listed in the URL repo as well.
| [
"# EnverLee/polyglot-ko-1.3b-Q4_0-GGUF\nThis model was converted to GGUF format from 'EleutherAI/polyglot-ko-1.3b' using URL via the URL's GGUF-my-repo space.\nRefer to the original model card for more details on the model.",
"## Use with URL\n\nInstall URL through brew.\n\n\nInvoke the URL server or the CLI.\n\nCLI:\n\n\n\nServer:\n\n\n\nNote: You can also use this checkpoint directly through the usage steps listed in the URL repo as well."
] | [
"TAGS\n#gguf #pytorch #causal-lm #llama-cpp #gguf-my-repo #ko #license-apache-2.0 #region-us \n",
"# EnverLee/polyglot-ko-1.3b-Q4_0-GGUF\nThis model was converted to GGUF format from 'EleutherAI/polyglot-ko-1.3b' using URL via the URL's GGUF-my-repo space.\nRefer to the original model card for more details on the model.",
"## Use with URL\n\nInstall URL through brew.\n\n\nInvoke the URL server or the CLI.\n\nCLI:\n\n\n\nServer:\n\n\n\nNote: You can also use this checkpoint directly through the usage steps listed in the URL repo as well."
] |
Subsets and Splits
No community queries yet
The top public SQL queries from the community will appear here once available.