|
|
|
|
|
# DeepSpeed |
|
|
|
[DeepSpeed](https://github.com/microsoft/DeepSpeed) implements everything described in the [ZeRO paper](https://arxiv.org/abs/1910.02054). Some of the salient optimizations are: |
|
|
|
1. Optimizer state partitioning (ZeRO stage 1) |
|
2. Gradient partitioning (ZeRO stage 2) |
|
3. Parameter partitioning (ZeRO stage 3) |
|
4. Custom mixed precision training handling |
|
5. A range of fast CUDA-extension-based optimizers |
|
6. ZeRO-Offload to CPU and Disk/NVMe |
|
7. Heirarchical partitioning of model parameters (ZeRO++) |
|
|
|
ZeRO-Offload has its own dedicated paper: [ZeRO-Offload: Democratizing Billion-Scale Model Training](https://arxiv.org/abs/2101.06840). And NVMe-support is described in the paper [ZeRO-Infinity: Breaking the GPU |
|
Memory Wall for Extreme Scale Deep Learning](https://arxiv.org/abs/2104.07857). |
|
|
|
DeepSpeed ZeRO-2 is primarily used only for training, as its features are of no use to inference. |
|
|
|
DeepSpeed ZeRO-3 can be used for inference as well since it allows huge models to be loaded on multiple GPUs, which |
|
won't be possible on a single GPU. |
|
|
|
🤗 Accelerate integrates [DeepSpeed](https://github.com/microsoft/DeepSpeed) via 2 options: |
|
|
|
1. Integration of the DeepSpeed features via `deepspeed config file` specification in `accelerate config` . You just supply your custom config file or use our template. Most of |
|
this document is focused on this feature. This supports all the core features of DeepSpeed and gives user a lot of flexibility. |
|
User may have to change a few lines of code depending on the config. |
|
2. Integration via `deepspeed_plugin`.This supports subset of the DeepSpeed features and uses default options for the rest of the configurations. |
|
User need not change any code and is good for those who are fine with most of the default settings of DeepSpeed. |
|
|
|
## What is integrated? |
|
|
|
Training: |
|
|
|
1. 🤗 Accelerate integrates all features of DeepSpeed ZeRO. This includes all the ZeRO stages 1, 2 and 3 as well as ZeRO-Offload, ZeRO-Infinity (which can offload to disk/NVMe) and ZeRO++. |
|
Below is a short description of Data Parallelism using ZeRO - Zero Redundancy Optimizer along with diagram from this [blog post](https://www.microsoft.com/en-us/research/blog/zero-deepspeed-new-system-optimizations-enable-training-models-with-over-100-billion-parameters/) |
|
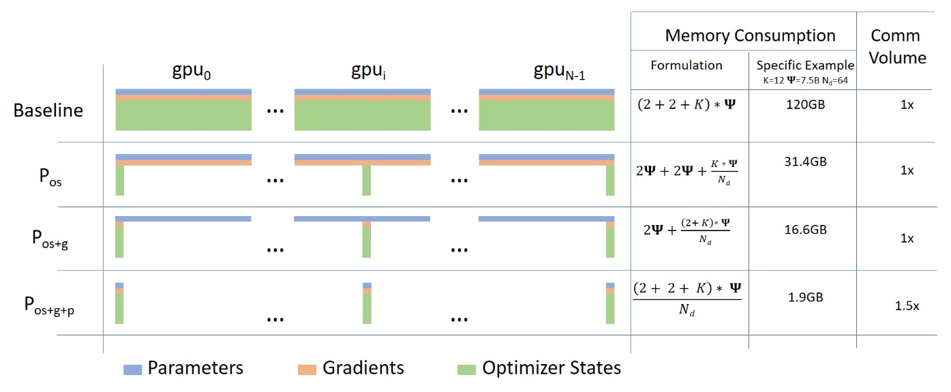 |
|
|
|
(Source: [link](https://www.microsoft.com/en-us/research/blog/zero-deepspeed-new-system-optimizations-enable-training-models-with-over-100-billion-parameters/)) |
|
|
|
a. **Stage 1** : Shards optimizer states across data parallel workers/GPUs |
|
|
|
b. **Stage 2** : Shards optimizer states + gradients across data parallel workers/GPUs |
|
|
|
c. **Stage 3**: Shards optimizer states + gradients + model parameters across data parallel workers/GPUs |
|
|
|
d. **Optimizer Offload**: Offloads the gradients + optimizer states to CPU/Disk building on top of ZERO Stage 2 |
|
|
|
e. **Param Offload**: Offloads the model parameters to CPU/Disk building on top of ZERO Stage 3 |
|
|
|
f. **Heirarchical Paritioning**: Enables efficient multi-node training with data-parallel training across nodes and ZeRO-3 sharding within a node, built on top of ZeRO Stage 3. |
|
|
|
<u>Note</u>: With respect to Disk Offload, the disk should be an NVME for decent speed but it technically works on any Disk |
|
|
|
Inference: |
|
|
|
1. DeepSpeed ZeRO Inference supports ZeRO stage 3 with ZeRO-Infinity. It uses the same ZeRO protocol as training, but |
|
it doesn't use an optimizer and a lr scheduler and only stage 3 is relevant. For more details see: |
|
[deepspeed-zero-inference](#deepspeed-zero-inference). |
|
|
|
|
|
## How it works? |
|
|
|
**Pre-Requisites**: Install DeepSpeed version >=0.6.5. Please refer to the [DeepSpeed Installation details](https://github.com/microsoft/DeepSpeed#installation) |
|
for more information. |
|
|
|
We will first look at easy to use integration via `accelerate config`. |
|
Followed by more flexible and feature rich `deepspeed config file` integration. |
|
|
|
### Accelerate DeepSpeed Plugin |
|
On your machine(s) just run: |
|
|
|
```bash |
|
accelerate config |
|
``` |
|
|
|
and answer the questions asked. It will ask whether you want to use a config file for DeepSpeed to which you should answer no. Then answer the following questions to generate a basic DeepSpeed config. |
|
This will generate a config file that will be used automatically to properly set the |
|
default options when doing |
|
|
|
```bash |
|
accelerate launch my_script.py --args_to_my_script |
|
``` |
|
|
|
For instance, here is how you would run the NLP example `examples/nlp_example.py` (from the root of the repo) with DeepSpeed Plugin: |
|
|
|
**ZeRO Stage-2 DeepSpeed Plugin Example** |
|
```bash |
|
compute_environment: LOCAL_MACHINE |
|
deepspeed_config: |
|
gradient_accumulation_steps: 1 |
|
gradient_clipping: 1.0 |
|
offload_optimizer_device: none |
|
offload_param_device: none |
|
zero3_init_flag: true |
|
zero_stage: 2 |
|
distributed_type: DEEPSPEED |
|
fsdp_config: {} |
|
machine_rank: 0 |
|
main_process_ip: null |
|
main_process_port: null |
|
main_training_function: main |
|
mixed_precision: fp16 |
|
num_machines: 1 |
|
num_processes: 2 |
|
use_cpu: false |
|
``` |
|
|
|
```bash |
|
accelerate launch examples/nlp_example.py --mixed_precision fp16 |
|
``` |
|
|
|
**ZeRO Stage-3 with CPU Offload DeepSpeed Plugin Example** |
|
```bash |
|
compute_environment: LOCAL_MACHINE |
|
deepspeed_config: |
|
gradient_accumulation_steps: 1 |
|
gradient_clipping: 1.0 |
|
offload_optimizer_device: cpu |
|
offload_param_device: cpu |
|
zero3_init_flag: true |
|
zero3_save_16bit_model: true |
|
zero_stage: 3 |
|
distributed_type: DEEPSPEED |
|
fsdp_config: {} |
|
machine_rank: 0 |
|
main_process_ip: null |
|
main_process_port: null |
|
main_training_function: main |
|
mixed_precision: fp16 |
|
num_machines: 1 |
|
num_processes: 2 |
|
use_cpu: false |
|
``` |
|
|
|
```bash |
|
accelerate launch examples/nlp_example.py --mixed_precision fp16 |
|
``` |
|
|
|
Currently, `Accelerate` supports following config through the CLI: |
|
|
|
```bash |
|
`zero_stage`: [0] Disabled, [1] optimizer state partitioning, [2] optimizer+gradient state partitioning and [3] optimizer+gradient+parameter partitioning |
|
`gradient_accumulation_steps`: Number of training steps to accumulate gradients before averaging and applying them. |
|
`gradient_clipping`: Enable gradient clipping with value. |
|
`offload_optimizer_device`: [none] Disable optimizer offloading, [cpu] offload optimizer to CPU, [nvme] offload optimizer to NVMe SSD. Only applicable with ZeRO >= Stage-2. |
|
`offload_param_device`: [none] Disable parameter offloading, [cpu] offload parameters to CPU, [nvme] offload parameters to NVMe SSD. Only applicable with ZeRO Stage-3. |
|
`zero3_init_flag`: Decides whether to enable `deepspeed.zero.Init` for constructing massive models. Only applicable with ZeRO Stage-3. |
|
`zero3_save_16bit_model`: Decides whether to save 16-bit model weights when using ZeRO Stage-3. |
|
`mixed_precision`: `no` for FP32 training, `fp16` for FP16 mixed-precision training and `bf16` for BF16 mixed-precision training. |
|
``` |
|
To be able to tweak more options, you will need to use a DeepSpeed config file. |
|
|
|
### DeepSpeed Config File |
|
On your machine(s) just run: |
|
|
|
```bash |
|
accelerate config |
|
``` |
|
|
|
and answer the questions asked. It will ask whether you want to use a config file for deepspeed to which you answer yes |
|
and provide the path to the deepspeed config file. |
|
This will generate a config file that will be used automatically to properly set the |
|
default options when doing |
|
|
|
```bash |
|
accelerate launch my_script.py --args_to_my_script |
|
``` |
|
|
|
For instance, here is how you would run the NLP example `examples/by_feature/deepspeed_with_config_support.py` (from the root of the repo) with DeepSpeed Config File: |
|
|
|
**ZeRO Stage-2 DeepSpeed Config File Example** |
|
```bash |
|
compute_environment: LOCAL_MACHINE |
|
deepspeed_config: |
|
deepspeed_config_file: /home/ubuntu/accelerate/examples/configs/deepspeed_config_templates/zero_stage2_config.json |
|
zero3_init_flag: true |
|
distributed_type: DEEPSPEED |
|
fsdp_config: {} |
|
machine_rank: 0 |
|
main_process_ip: null |
|
main_process_port: null |
|
main_training_function: main |
|
mixed_precision: fp16 |
|
num_machines: 1 |
|
num_processes: 2 |
|
use_cpu: false |
|
``` |
|
|
|
with the contents of `zero_stage2_config.json` being: |
|
```json |
|
{ |
|
"fp16": { |
|
"enabled": true, |
|
"loss_scale": 0, |
|
"loss_scale_window": 1000, |
|
"initial_scale_power": 16, |
|
"hysteresis": 2, |
|
"min_loss_scale": 1 |
|
}, |
|
"optimizer": { |
|
"type": "AdamW", |
|
"params": { |
|
"lr": "auto", |
|
"weight_decay": "auto", |
|
"torch_adam": true, |
|
"adam_w_mode": true |
|
} |
|
}, |
|
"scheduler": { |
|
"type": "WarmupDecayLR", |
|
"params": { |
|
"warmup_min_lr": "auto", |
|
"warmup_max_lr": "auto", |
|
"warmup_num_steps": "auto", |
|
"total_num_steps": "auto" |
|
} |
|
}, |
|
"zero_optimization": { |
|
"stage": 2, |
|
"allgather_partitions": true, |
|
"allgather_bucket_size": 2e8, |
|
"overlap_comm": true, |
|
"reduce_scatter": true, |
|
"reduce_bucket_size": "auto", |
|
"contiguous_gradients": true |
|
}, |
|
"gradient_accumulation_steps": 1, |
|
"gradient_clipping": "auto", |
|
"steps_per_print": 2000, |
|
"train_batch_size": "auto", |
|
"train_micro_batch_size_per_gpu": "auto", |
|
"wall_clock_breakdown": false |
|
} |
|
``` |
|
|
|
```bash |
|
accelerate launch examples/by_feature/deepspeed_with_config_support.py \ |
|
--config_name "gpt2-large" \ |
|
--tokenizer_name "gpt2-large" \ |
|
--dataset_name "wikitext" \ |
|
--dataset_config_name "wikitext-2-raw-v1" \ |
|
--block_size 128 \ |
|
--output_dir "./clm/clm_deepspeed_stage2_accelerate" \ |
|
--learning_rate 5e-4 \ |
|
--per_device_train_batch_size 24 \ |
|
--per_device_eval_batch_size 24 \ |
|
--num_train_epochs 3 \ |
|
--with_tracking \ |
|
--report_to "wandb"\ |
|
``` |
|
|
|
**ZeRO Stage-3 with CPU offload DeepSpeed Config File Example** |
|
```bash |
|
compute_environment: LOCAL_MACHINE |
|
deepspeed_config: |
|
deepspeed_config_file: /home/ubuntu/accelerate/examples/configs/deepspeed_config_templates/zero_stage3_offload_config.json |
|
zero3_init_flag: true |
|
distributed_type: DEEPSPEED |
|
fsdp_config: {} |
|
machine_rank: 0 |
|
main_process_ip: null |
|
main_process_port: null |
|
main_training_function: main |
|
mixed_precision: fp16 |
|
num_machines: 1 |
|
num_processes: 2 |
|
use_cpu: false |
|
``` |
|
with the contents of `zero_stage3_offload_config.json` being: |
|
```json |
|
{ |
|
"fp16": { |
|
"enabled": true, |
|
"loss_scale": 0, |
|
"loss_scale_window": 1000, |
|
"initial_scale_power": 16, |
|
"hysteresis": 2, |
|
"min_loss_scale": 1 |
|
}, |
|
"optimizer": { |
|
"type": "AdamW", |
|
"params": { |
|
"lr": "auto", |
|
"weight_decay": "auto" |
|
} |
|
}, |
|
"scheduler": { |
|
"type": "WarmupDecayLR", |
|
"params": { |
|
"warmup_min_lr": "auto", |
|
"warmup_max_lr": "auto", |
|
"warmup_num_steps": "auto", |
|
"total_num_steps": "auto" |
|
} |
|
}, |
|
"zero_optimization": { |
|
"stage": 3, |
|
"offload_optimizer": { |
|
"device": "cpu", |
|
"pin_memory": true |
|
}, |
|
"offload_param": { |
|
"device": "cpu", |
|
"pin_memory": true |
|
}, |
|
"overlap_comm": true, |
|
"contiguous_gradients": true, |
|
"reduce_bucket_size": "auto", |
|
"stage3_prefetch_bucket_size": "auto", |
|
"stage3_param_persistence_threshold": "auto", |
|
"sub_group_size": 1e9, |
|
"stage3_max_live_parameters": 1e9, |
|
"stage3_max_reuse_distance": 1e9, |
|
"stage3_gather_16bit_weights_on_model_save": "auto" |
|
}, |
|
"gradient_accumulation_steps": 1, |
|
"gradient_clipping": "auto", |
|
"steps_per_print": 2000, |
|
"train_batch_size": "auto", |
|
"train_micro_batch_size_per_gpu": "auto", |
|
"wall_clock_breakdown": false |
|
} |
|
``` |
|
|
|
```bash |
|
accelerate launch examples/by_feature/deepspeed_with_config_support.py \ |
|
--config_name "gpt2-large" \ |
|
--tokenizer_name "gpt2-large" \ |
|
--dataset_name "wikitext" \ |
|
--dataset_config_name "wikitext-2-raw-v1" \ |
|
--block_size 128 \ |
|
--output_dir "./clm/clm_deepspeed_stage3_offload_accelerate" \ |
|
--learning_rate 5e-4 \ |
|
--per_device_train_batch_size 32 \ |
|
--per_device_eval_batch_size 32 \ |
|
--num_train_epochs 3 \ |
|
--with_tracking \ |
|
--report_to "wandb"\ |
|
``` |
|
|
|
**ZeRO++ Config Example** |
|
You can use the the features of ZeRO++ by using the appropriate config parameters. Note that ZeRO++ is an extension for ZeRO Stage 3. Here is how the config file can be modified, from [DeepSpeed's ZeRO++ tutorial](https://www.deepspeed.ai/tutorials/zeropp/): |
|
|
|
```json |
|
{ |
|
"zero_optimization": { |
|
"stage": 3, |
|
"reduce_bucket_size": "auto", |
|
|
|
"zero_quantized_weights": true, |
|
"zero_hpz_partition_size": 8, |
|
"zero_quantized_gradients": true, |
|
|
|
"contiguous_gradients": true, |
|
"overlap_comm": true |
|
} |
|
} |
|
``` |
|
|
|
For heirarchical partitioning, the partition size `zero_hpz_partition_size` should ideally be set to the number of GPUs per node. (For example, the above config file assumes 8 GPUs per node) |
|
|
|
**Important code changes when using DeepSpeed Config File** |
|
|
|
1. DeepSpeed Optimizers and Schedulers. For more information on these, |
|
see the [DeepSpeed Optimizers](https://deepspeed.readthedocs.io/en/latest/optimizers.html) and [DeepSpeed Schedulers](https://deepspeed.readthedocs.io/en/latest/schedulers.html) documentation. |
|
We will look at the changes needed in the code when using these. |
|
|
|
a. DS Optim + DS Scheduler: The case when both `optimizer` and `scheduler` keys are present in the DeepSpeed config file. |
|
In this situation, those will be used and the user has to use `accelerate.utils.DummyOptim` and `accelerate.utils.DummyScheduler` to replace the PyTorch/Custom optimizers and schedulers in their code. |
|
Below is the snippet from `examples/by_feature/deepspeed_with_config_support.py` showing this: |
|
```python |
|
# Creates Dummy Optimizer if `optimizer` was spcified in the config file else creates Adam Optimizer |
|
optimizer_cls = ( |
|
torch.optim.AdamW |
|
if accelerator.state.deepspeed_plugin is None |
|
or "optimizer" not in accelerator.state.deepspeed_plugin.deepspeed_config |
|
else DummyOptim |
|
) |
|
optimizer = optimizer_cls(optimizer_grouped_parameters, lr=args.learning_rate) |
|
|
|
# Creates Dummy Scheduler if `scheduler` was spcified in the config file else creates `args.lr_scheduler_type` Scheduler |
|
if ( |
|
accelerator.state.deepspeed_plugin is None |
|
or "scheduler" not in accelerator.state.deepspeed_plugin.deepspeed_config |
|
): |
|
lr_scheduler = get_scheduler( |
|
name=args.lr_scheduler_type, |
|
optimizer=optimizer, |
|
num_warmup_steps=args.num_warmup_steps, |
|
num_training_steps=args.max_train_steps, |
|
) |
|
else: |
|
lr_scheduler = DummyScheduler( |
|
optimizer, total_num_steps=args.max_train_steps, warmup_num_steps=args.num_warmup_steps |
|
) |
|
``` |
|
b. Custom Optim + Custom Scheduler: The case when both `optimizer` and `scheduler` keys are absent in the DeepSpeed config file. |
|
In this situation, no code changes are needed from the user and this is the case when using integration via DeepSpeed Plugin. |
|
In the above example we can see that the code remains unchanged if the `optimizer` and `scheduler` keys are absent in the DeepSpeed config file. |
|
|
|
c. Custom Optim + DS Scheduler: The case when only `scheduler` key is present in the DeepSpeed config file. |
|
In this situation, the user has to use `accelerate.utils.DummyScheduler` to replace the PyTorch/Custom scheduler in their code. |
|
|
|
d. DS Optim + Custom Scheduler: The case when only `optimizer` key is present in the DeepSpeed config file. |
|
This will result in an error because you can only use DS Scheduler when using DS Optim. |
|
|
|
2. Notice the `auto` values in the above example DeepSpeed config files. These are automatically handled by `prepare` method |
|
based on model, dataloaders, dummy optimizer and dummy schedulers provided to `prepare` method. |
|
Only the `auto` fields specified in above examples are handled by `prepare` method and the rest have to be explicitly specified by the user. |
|
|
|
The `auto` values are calculated as: |
|
|
|
- `reduce_bucket_size`: `hidden_size*hidden_size` |
|
- `stage3_prefetch_bucket_size`: `0.9 * hidden_size * hidden_size` |
|
- `stage3_param_persistence_threshold`: `10 * hidden_size` |
|
|
|
|
|
**Things to note when using DeepSpeed Config File** |
|
|
|
Below is a sample script using `deepspeed_config_file` in different scenarios. |
|
|
|
Code `test.py`: |
|
|
|
```python |
|
from accelerate import Accelerator |
|
from accelerate.state import AcceleratorState |
|
|
|
|
|
def main(): |
|
accelerator = Accelerator() |
|
accelerator.print(f"{AcceleratorState()}") |
|
|
|
|
|
if __name__ == "__main__": |
|
main() |
|
``` |
|
|
|
**Scenario 1**: Manually tampered accelerate config file having `deepspeed_config_file` along with other entries. |
|
|
|
1. Content of the `accelerate` config: |
|
|
|
```yaml |
|
command_file: null |
|
commands: null |
|
compute_environment: LOCAL_MACHINE |
|
deepspeed_config: |
|
gradient_accumulation_steps: 1 |
|
gradient_clipping: 1.0 |
|
offload_optimizer_device: 'cpu' |
|
offload_param_device: 'cpu' |
|
zero3_init_flag: true |
|
zero3_save_16bit_model: true |
|
zero_stage: 3 |
|
deepspeed_config_file: 'ds_config.json' |
|
distributed_type: DEEPSPEED |
|
downcast_bf16: 'no' |
|
dynamo_backend: 'NO' |
|
fsdp_config: {} |
|
gpu_ids: null |
|
machine_rank: 0 |
|
main_process_ip: null |
|
main_process_port: null |
|
main_training_function: main |
|
megatron_lm_config: {} |
|
num_machines: 1 |
|
num_processes: 2 |
|
rdzv_backend: static |
|
same_network: true |
|
tpu_name: null |
|
tpu_zone: null |
|
use_cpu: false |
|
``` |
|
|
|
2. `ds_config.json`: |
|
|
|
```json |
|
{ |
|
"bf16": { |
|
"enabled": true |
|
}, |
|
"zero_optimization": { |
|
"stage": 3, |
|
"stage3_gather_16bit_weights_on_model_save": false, |
|
"offload_optimizer": { |
|
"device": "none" |
|
}, |
|
"offload_param": { |
|
"device": "none" |
|
} |
|
}, |
|
"gradient_clipping": 1.0, |
|
"train_batch_size": "auto", |
|
"train_micro_batch_size_per_gpu": "auto", |
|
"gradient_accumulation_steps": 10, |
|
"steps_per_print": 2000000 |
|
} |
|
``` |
|
|
|
3. Output of `accelerate launch test.py`: |
|
|
|
```bash |
|
ValueError: When using `deepspeed_config_file`, the following accelerate config variables will be ignored: |
|
['gradient_accumulation_steps', 'gradient_clipping', 'zero_stage', 'offload_optimizer_device', 'offload_param_device', |
|
'zero3_save_16bit_model', 'mixed_precision']. |
|
Please specify them appropriately in the DeepSpeed config file. |
|
If you are using an accelerate config file, remove others config variables mentioned in the above specified list. |
|
The easiest method is to create a new config following the questionnaire via `accelerate config`. |
|
It will only ask for the necessary config variables when using `deepspeed_config_file`. |
|
``` |
|
|
|
**Scenario 2**: Use the solution of the error to create new accelerate config and check that no ambiguity error is now thrown. |
|
|
|
1. Run `accelerate config`: |
|
|
|
```bash |
|
$ accelerate config |
|
------------------------------------------------------------------------------------------------------------------------------- |
|
In which compute environment are you running? |
|
This machine |
|
------------------------------------------------------------------------------------------------------------------------------- |
|
Which type of machine are you using? |
|
multi-GPU |
|
How many different machines will you use (use more than 1 for multi-node training)? [1]: |
|
Do you wish to optimize your script with torch dynamo?[yes/NO]: |
|
Do you want to use DeepSpeed? [yes/NO]: yes |
|
Do you want to specify a json file to a DeepSpeed config? [yes/NO]: yes |
|
Please enter the path to the json DeepSpeed config file: ds_config.json |
|
Do you want to enable `deepspeed.zero.Init` when using ZeRO Stage-3 for constructing massive models? [yes/NO]: yes |
|
How many GPU(s) should be used for distributed training? [1]:4 |
|
accelerate configuration saved at ds_config_sample.yaml |
|
``` |
|
|
|
2. Content of the `accelerate` config: |
|
|
|
```yaml |
|
compute_environment: LOCAL_MACHINE |
|
deepspeed_config: |
|
deepspeed_config_file: ds_config.json |
|
zero3_init_flag: true |
|
distributed_type: DEEPSPEED |
|
downcast_bf16: 'no' |
|
dynamo_backend: 'NO' |
|
fsdp_config: {} |
|
machine_rank: 0 |
|
main_training_function: main |
|
megatron_lm_config: {} |
|
num_machines: 1 |
|
num_processes: 4 |
|
rdzv_backend: static |
|
same_network: true |
|
use_cpu: false |
|
``` |
|
|
|
3. Output of `accelerate launch test.py`: |
|
|
|
```bash |
|
Distributed environment: DEEPSPEED Backend: nccl |
|
Num processes: 4 |
|
Process index: 0 |
|
Local process index: 0 |
|
Device: cuda:0 |
|
Mixed precision type: bf16 |
|
ds_config: {'bf16': {'enabled': True}, 'zero_optimization': {'stage': 3, 'stage3_gather_16bit_weights_on_model_save': False, 'offload_optimizer': {'device': 'none'}, 'offload_param': {'device': 'none'}}, 'gradient_clipping': 1.0, 'train_batch_size': 'auto', 'train_micro_batch_size_per_gpu': 'auto', 'gradient_accumulation_steps': 10, 'steps_per_print': inf, 'fp16': {'enabled': False}} |
|
``` |
|
|
|
**Scenario 3**: Setting the `accelerate launch` command arguments related to DeepSpeed as `"auto"` in the DeepSpeed` configuration file and check that things work as expected. |
|
|
|
1. New `ds_config.json` with `"auto"` for the `accelerate launch` DeepSpeed command arguments: |
|
|
|
```json |
|
{ |
|
"bf16": { |
|
"enabled": "auto" |
|
}, |
|
"zero_optimization": { |
|
"stage": "auto", |
|
"stage3_gather_16bit_weights_on_model_save": "auto", |
|
"offload_optimizer": { |
|
"device": "auto" |
|
}, |
|
"offload_param": { |
|
"device": "auto" |
|
} |
|
}, |
|
"gradient_clipping": "auto", |
|
"train_batch_size": "auto", |
|
"train_micro_batch_size_per_gpu": "auto", |
|
"gradient_accumulation_steps": "auto", |
|
"steps_per_print": 2000000 |
|
} |
|
``` |
|
|
|
2. Output of `accelerate launch --mixed_precision="fp16" --zero_stage=3 --gradient_accumulation_steps=5 --gradient_clipping=1.0 --offload_param_device="cpu" --offload_optimizer_device="nvme" --zero3_save_16bit_model="true" test.py`: |
|
|
|
```bash |
|
Distributed environment: DEEPSPEED Backend: nccl |
|
Num processes: 4 |
|
Process index: 0 |
|
Local process index: 0 |
|
Device: cuda:0 |
|
Mixed precision type: fp16 |
|
ds_config: {'bf16': {'enabled': False}, 'zero_optimization': {'stage': 3, 'stage3_gather_16bit_weights_on_model_save': True, 'offload_optimizer': {'device': 'nvme'}, 'offload_param': {'device': 'cpu'}}, 'gradient_clipping': 1.0, 'train_batch_size': 'auto', 'train_micro_batch_size_per_gpu': 'auto', 'gradient_accumulation_steps': 5, 'steps_per_print': inf, 'fp16': {'enabled': True, 'auto_cast': True}} |
|
``` |
|
|
|
**Note**: |
|
1. Remaining `"auto"` values are handled in `accelerator.prepare()` call as explained in point 2 of |
|
`Important code changes when using DeepSpeed Config File`. |
|
2. Only when `gradient_accumulation_steps` is `auto`, the value passed while creating `Accelerator` object via `Accelerator(gradient_accumulation_steps=k)` will be used. When using DeepSpeed Plugin, the value from it will be used and it will overwrite the value passed while creating Accelerator object. |
|
|
|
## Saving and loading |
|
|
|
1. Saving and loading of models is unchanged for ZeRO Stage-1 and Stage-2. |
|
|
|
2. under ZeRO Stage-3, `state_dict` contains just the placeholders since the model weights are partitioned across multiple GPUs. |
|
ZeRO Stage-3 has 2 options: |
|
|
|
a. Saving the entire 16bit model weights to directly load later on using `model.load_state_dict(torch.load(pytorch_model.bin))`. |
|
For this, either set `zero_optimization.stage3_gather_16bit_weights_on_model_save` to True in DeepSpeed Config file or set |
|
`zero3_save_16bit_model` to True in DeepSpeed Plugin. |
|
**Note that this option requires consolidation of the weights on one GPU it can be slow and memory demanding, so only use this feature when needed.** |
|
Below is the snippet from `examples/by_feature/deepspeed_with_config_support.py` showing this: |
|
```python |
|
unwrapped_model = accelerator.unwrap_model(model) |
|
|
|
# New Code # |
|
# Saves the whole/unpartitioned fp16 model when in ZeRO Stage-3 to the output directory if |
|
# `stage3_gather_16bit_weights_on_model_save` is True in DeepSpeed Config file or |
|
# `zero3_save_16bit_model` is True in DeepSpeed Plugin. |
|
# For Zero Stages 1 and 2, models are saved as usual in the output directory. |
|
# The model name saved is `pytorch_model.bin` |
|
unwrapped_model.save_pretrained( |
|
args.output_dir, |
|
is_main_process=accelerator.is_main_process, |
|
save_function=accelerator.save, |
|
state_dict=accelerator.get_state_dict(model), |
|
) |
|
``` |
|
|
|
b. To get 32bit weights, first save the model using `model.save_checkpoint()`. |
|
Below is the snippet from `examples/by_feature/deepspeed_with_config_support.py` showing this: |
|
```python |
|
success = model.save_checkpoint(PATH, ckpt_id, checkpoint_state_dict) |
|
status_msg = "checkpointing: PATH={}, ckpt_id={}".format(PATH, ckpt_id) |
|
if success: |
|
logging.info(f"Success {status_msg}") |
|
else: |
|
logging.warning(f"Failure {status_msg}") |
|
``` |
|
This will create ZeRO model and optimizer partitions along with `zero_to_fp32.py` script in checkpoint directory. |
|
You can use this script to do offline consolidation. |
|
It requires no configuration files or GPUs. Here is an example of its usage: |
|
```bash |
|
$ cd /path/to/checkpoint_dir |
|
$ ./zero_to_fp32.py . pytorch_model.bin |
|
Processing zero checkpoint at global_step1 |
|
Detected checkpoint of type zero stage 3, world_size: 2 |
|
Saving fp32 state dict to pytorch_model.bin (total_numel=60506624) |
|
``` |
|
To get 32bit model for saving/inference, you can perform: |
|
```python |
|
from deepspeed.utils.zero_to_fp32 import load_state_dict_from_zero_checkpoint |
|
|
|
unwrapped_model = accelerator.unwrap_model(model) |
|
fp32_model = load_state_dict_from_zero_checkpoint(unwrapped_model, checkpoint_dir) |
|
``` |
|
If you are only interested in the `state_dict`, you can do the following: |
|
```python |
|
from deepspeed.utils.zero_to_fp32 import get_fp32_state_dict_from_zero_checkpoint |
|
|
|
state_dict = get_fp32_state_dict_from_zero_checkpoint(checkpoint_dir) |
|
``` |
|
Note that all these functions require ~2x memory (general RAM) of the size of the final checkpoint. |
|
|
|
## ZeRO Inference |
|
DeepSpeed ZeRO Inference supports ZeRO stage 3 with ZeRO-Infinity. |
|
It uses the same ZeRO protocol as training, but it doesn't use an optimizer and a lr scheduler and only stage 3 is relevant. |
|
With accelerate integration, you just need to prepare the model and dataloader as shown below: |
|
|
|
```python |
|
model, eval_dataloader = accelerator.prepare(model, eval_dataloader) |
|
``` |
|
|
|
## Few caveats to be aware of |
|
|
|
1. Current integration doesn’t support Pipeline Parallelism of DeepSpeed. |
|
2. Current integration doesn’t support `mpu`, limiting the tensor parallelism which is supported in Megatron-LM. |
|
3. Current integration doesn’t support multiple models. |
|
|
|
## DeepSpeed Resources |
|
|
|
The documentation for the internals related to deepspeed can be found [here](../package_reference/deepspeed). |
|
|
|
- [Project's github](https://github.com/microsoft/deepspeed) |
|
- [Usage docs](https://www.deepspeed.ai/getting-started/) |
|
- [API docs](https://deepspeed.readthedocs.io/en/latest/index.html) |
|
- [Blog posts](https://www.microsoft.com/en-us/research/search/?q=deepspeed) |
|
|
|
Papers: |
|
|
|
- [ZeRO: Memory Optimizations Toward Training Trillion Parameter Models](https://arxiv.org/abs/1910.02054) |
|
- [ZeRO-Offload: Democratizing Billion-Scale Model Training](https://arxiv.org/abs/2101.06840) |
|
- [ZeRO-Infinity: Breaking the GPU Memory Wall for Extreme Scale Deep Learning](https://arxiv.org/abs/2104.07857) |
|
- [ZeRO++: Extremely Efficient Collective Communication for Giant Model Training](https://arxiv.org/abs/2306.10209) |
|
|
|
|
|
Finally, please, remember that 🤗 `Accelerate` only integrates DeepSpeed, therefore if you |
|
have any problems or questions with regards to DeepSpeed usage, please, file an issue with [DeepSpeed GitHub](https://github.com/microsoft/DeepSpeed/issues). |
|
|
|
|