file_path
stringlengths 21
207
| content
stringlengths 5
1.02M
| size
int64 5
1.02M
| lang
stringclasses 9
values | avg_line_length
float64 1.33
100
| max_line_length
int64 4
993
| alphanum_fraction
float64 0.27
0.93
|
---|---|---|---|---|---|---|
NVIDIA-Omniverse/IsaacGymEnvs/isaacgymenvs/cfg/train/IndustRealTaskGearsInsertPPO.yaml | params:
seed: ${...seed}
algo:
name: a2c_continuous
model:
name: continuous_a2c_logstd
network:
name: actor_critic
separate: False
space:
continuous:
mu_activation: None
sigma_activation: None
mu_init:
name: default
sigma_init:
name: const_initializer
val: 0
fixed_sigma: True
mlp:
units: [512, 256, 128]
activation: elu
d2rl: False
initializer:
name: default
regularizer:
name: None
rnn:
name: lstm
units: 256
layers: 2
before_mlp: True
concat_input: True
layer_norm: False
load_checkpoint: ${if:${...checkpoint},True,False}
load_path: ${...checkpoint}
config:
name: ${resolve_default:IndustRealTaskGearsInsert,${....experiment}}
full_experiment_name: ${.name}
env_name: rlgpu
multi_gpu: False
ppo: True
mixed_precision: True
normalize_input: True
normalize_value: True
value_bootstrap: True
num_actors: ${....task.env.numEnvs}
reward_shaper:
scale_value: 1.0
normalize_advantage: True
gamma: 0.998
tau: 0.95
learning_rate: 1e-4
lr_schedule: linear
schedule_type: standard
kl_threshold: 0.008
score_to_win: 20000
max_epochs: ${resolve_default:8192,${....max_iterations}}
save_best_after: 50
save_frequency: 1000
print_stats: True
grad_norm: 1.0
entropy_coef: 0.0
truncate_grads: False
e_clip: 0.2
horizon_length: 128
minibatch_size: 8 # batch size = num_envs * horizon_length; minibatch_size = batch_size / num_minibatches
mini_epochs: 8
critic_coef: 2
clip_value: True
seq_len: 4
bounds_loss_coef: 0.0001
central_value_config:
minibatch_size: 8
mini_epochs: 4
learning_rate: 1e-3
lr_schedule: linear
kl_threshold: 0.016
clip_value: True
normalize_input: True
truncate_grads: True
network:
name: actor_critic
central_value: True
mlp:
units: [256, 128, 64]
activation: elu
d2rl: False
initializer:
name: default
regularizer:
name: None
| 2,254 | YAML | 20.682692 | 110 | 0.579858 |
NVIDIA-Omniverse/IsaacGymEnvs/isaacgymenvs/cfg/train/AntPPO.yaml | params:
seed: ${...seed}
algo:
name: a2c_continuous
model:
name: continuous_a2c_logstd
network:
name: actor_critic
separate: False
space:
continuous:
mu_activation: None
sigma_activation: None
mu_init:
name: default
sigma_init:
name: const_initializer
val: 0
fixed_sigma: True
mlp:
units: [256, 128, 64]
activation: elu
d2rl: False
initializer:
name: default
regularizer:
name: None
load_checkpoint: ${if:${...checkpoint},True,False} # flag which sets whether to load the checkpoint
load_path: ${...checkpoint} # path to the checkpoint to load
config:
name: ${resolve_default:Ant,${....experiment}}
full_experiment_name: ${.name}
env_name: rlgpu
multi_gpu: ${....multi_gpu}
ppo: True
mixed_precision: True
normalize_input: True
normalize_value: True
value_bootstrap: True
num_actors: ${....task.env.numEnvs}
reward_shaper:
scale_value: 0.01
normalize_advantage: True
gamma: 0.99
tau: 0.95
learning_rate: 3e-4
lr_schedule: adaptive
schedule_type: legacy
kl_threshold: 0.008
score_to_win: 20000
max_epochs: ${resolve_default:500,${....max_iterations}}
save_best_after: 200
save_frequency: 50
grad_norm: 1.0
entropy_coef: 0.0
truncate_grads: False
e_clip: 0.2
horizon_length: 16
minibatch_size: 32768
mini_epochs: 4
critic_coef: 2
clip_value: True
seq_len: 4
bounds_loss_coef: 0.0001
| 1,592 | YAML | 21.125 | 101 | 0.597362 |
NVIDIA-Omniverse/IsaacGymEnvs/isaacgymenvs/cfg/train/FrankaCabinetPPO.yaml | params:
seed: ${...seed}
algo:
name: a2c_continuous
model:
name: continuous_a2c_logstd
network:
name: actor_critic
separate: False
space:
continuous:
mu_activation: None
sigma_activation: None
mu_init:
name: default
sigma_init:
name: const_initializer
val: 0
fixed_sigma: True
mlp:
units: [256, 128, 64]
activation: elu
d2rl: False
initializer:
name: default
regularizer:
name: None
load_checkpoint: ${if:${...checkpoint},True,False} # flag which sets whether to load the checkpoint
load_path: ${...checkpoint} # path to the checkpoint to load
config:
name: ${resolve_default:FrankaCabinet,${....experiment}}
full_experiment_name: ${.name}
env_name: rlgpu
multi_gpu: ${....multi_gpu}
ppo: True
mixed_precision: False
normalize_input: True
normalize_value: True
num_actors: ${....task.env.numEnvs}
reward_shaper:
scale_value: 0.01
normalize_advantage: True
gamma: 0.99
tau: 0.95
learning_rate: 5e-4
lr_schedule: adaptive
kl_threshold: 0.008
score_to_win: 10000
max_epochs: ${resolve_default:1500,${....max_iterations}}
save_best_after: 200
save_frequency: 100
print_stats: True
grad_norm: 1.0
entropy_coef: 0.0
truncate_grads: True
e_clip: 0.2
horizon_length: 16
minibatch_size: 8192
mini_epochs: 8
critic_coef: 4
clip_value: True
seq_len: 4
bounds_loss_coef: 0.0001 | 1,565 | YAML | 21.695652 | 101 | 0.600639 |
NVIDIA-Omniverse/IsaacGymEnvs/isaacgymenvs/cfg/train/AntSAC.yaml | params:
seed: ${...seed}
algo:
name: sac
model:
name: soft_actor_critic
network:
name: soft_actor_critic
separate: True
space:
continuous:
mlp:
units: [512, 256]
activation: relu
initializer:
name: default
log_std_bounds: [-5, 2]
load_checkpoint: False
load_path: nn/Ant.pth
config:
name: ${resolve_default:AntSAC,${....experiment}}
env_name: rlgpu
multi_gpu: ${....multi_gpu}
normalize_input: True
reward_shaper:
scale_value: 1.0
max_epochs: 20000
num_steps_per_episode: 8
save_best_after: 100
save_frequency: 1000
gamma: 0.99
init_alpha: 1.0
alpha_lr: 0.005
actor_lr: 0.0005
critic_lr: 0.0005
critic_tau: 0.005
batch_size: 4096
learnable_temperature: true
num_seed_steps: 5
num_warmup_steps: 10
replay_buffer_size: 1000000
num_actors: ${....task.env.numEnvs}
| 940 | YAML | 17.82 | 53 | 0.592553 |
NVIDIA-Omniverse/IsaacGymEnvs/isaacgymenvs/cfg/train/AllegroKukaLSTMPPO.yaml | defaults:
- AllegroKukaPPO
- _self_
params:
network:
mlp:
units: [768, 512, 256]
activation: elu
d2rl: False
initializer:
name: default
regularizer:
name: None
rnn:
name: lstm
units: 768
layers: 1
before_mlp: True
layer_norm: True
config:
name: ${resolve_default:AllegroKukaLSTMPPO,${....experiment}}
minibatch_size: 32768
mini_epochs: 2
| 444 | YAML | 16.115384 | 65 | 0.567568 |
NVIDIA-Omniverse/IsaacGymEnvs/isaacgymenvs/cfg/train/AllegroHandPPO.yaml | params:
seed: ${...seed}
algo:
name: a2c_continuous
model:
name: continuous_a2c_logstd
network:
name: actor_critic
separate: False
space:
continuous:
mu_activation: None
sigma_activation: None
mu_init:
name: default
sigma_init:
name: const_initializer
val: 0
fixed_sigma: True
mlp:
units: [512, 256, 128]
activation: elu
d2rl: False
initializer:
name: default
regularizer:
name: None
load_checkpoint: ${if:${...checkpoint},True,False} # flag which sets whether to load the checkpoint
load_path: ${...checkpoint} # path to the checkpoint to load
config:
name: ${resolve_default:AllegroHand,${....experiment}}
full_experiment_name: ${.name}
env_name: rlgpu
multi_gpu: ${....multi_gpu}
ppo: True
mixed_precision: False
normalize_input: True
normalize_value: True
value_bootstrap: True
num_actors: ${....task.env.numEnvs}
reward_shaper:
scale_value: 0.01
normalize_advantage: True
gamma: 0.99
tau: 0.95
learning_rate: 5e-4
lr_schedule: adaptive
schedule_type: standard
kl_threshold: 0.016
score_to_win: 100000
max_epochs: ${resolve_default:5000,${....max_iterations}}
save_best_after: 500
save_frequency: 200
print_stats: True
grad_norm: 1.0
entropy_coef: 0.0
truncate_grads: True
e_clip: 0.2
horizon_length: 8
minibatch_size: 32768
mini_epochs: 5
critic_coef: 4
clip_value: True
seq_len: 4
bounds_loss_coef: 0.0001
player:
#render: True
deterministic: True
games_num: 100000
print_stats: True | 1,728 | YAML | 20.886076 | 101 | 0.601273 |
NVIDIA-Omniverse/IsaacGymEnvs/isaacgymenvs/cfg/train/TrifingerPPO.yaml | asymmetric_obs: true
params:
seed: ${...seed}
algo:
name: a2c_continuous
model:
name: continuous_a2c_logstd
network:
name: actor_critic
separate: false
space:
continuous:
mu_activation: None
sigma_activation: None
mu_init:
name: default
sigma_init:
name: const_initializer
val: 0
fixed_sigma: true
mlp:
units: [256, 256, 128, 128]
activation: elu
d2rl: False
initializer:
name: default
regularizer:
name: None
load_checkpoint: ${if:${...checkpoint},True,False} # flag which sets whether to load the checkpoint
load_path: ${...checkpoint} # path to the checkpoint to load
config:
name: ${resolve_default:Trifinger,${....experiment}}
full_experiment_name: ${.name}
env_name: rlgpu
multi_gpu: ${....multi_gpu}
ppo: true
mixed_precision: false
normalize_input: true
normalize_value: true
reward_shaper:
scale_value: 0.01
normalize_advantage: true
gamma: 0.99
tau: 0.95
learning_rate: 0.0003
lr_schedule: constant
use_experimental_cv: true
schedule_type: standard
kl_threshold: 0.016
score_to_win: 500000
max_epochs: ${resolve_default:20000,${....max_iterations}}
save_best_after: 100
save_frequency: 100
print_stats: true
grad_norm: 1.0
entropy_coef: 0.0
truncate_grads: true
e_clip: 0.2
horizon_length: 8
minibatch_size: ${.num_actors}
mini_epochs: 4
critic_coef: 4
clip_value: true
seq_len: 4
bounds_loss_coef: 0.0001
central_value_config:
minibatch_size: ${..num_actors}
mini_epochs: ${..mini_epochs}
learning_rate: 0.0005
lr_schedule: linear
schedule_type: standard
kl_threshold: 0.016
clip_value: true
normalize_input: true
truncate_grads: true
network:
name: actor_critic
central_value: true
mlp:
units: [512, 512, 256, 128]
activation: elu
d2rl: false
initializer:
name: default
regularizer:
name: None
player:
deterministic: true
games_num: 1000000
print_stats: false
num_actors: ${....task.env.numEnvs} | 2,293 | YAML | 24.208791 | 101 | 0.591365 |
NVIDIA-Omniverse/IsaacGymEnvs/isaacgymenvs/cfg/train/AnymalPPO.yaml | params:
seed: ${...seed}
algo:
name: a2c_continuous
model:
name: continuous_a2c_logstd
network:
name: actor_critic
separate: False
space:
continuous:
mu_activation: None
sigma_activation: None
mu_init:
name: default
sigma_init:
name: const_initializer
val: 0. # std = 1.
fixed_sigma: True
mlp:
units: [256, 128, 64]
activation: elu
d2rl: False
initializer:
name: default
regularizer:
name: None
load_checkpoint: ${if:${...checkpoint},True,False} # flag which sets whether to load the checkpoint
load_path: ${...checkpoint} # path to the checkpoint to load
config:
name: ${resolve_default:Anymal,${....experiment}}
full_experiment_name: ${.name}
env_name: rlgpu
multi_gpu: ${....multi_gpu}
ppo: True
mixed_precision: True
normalize_input: True
normalize_value: True
value_bootstrap: True
num_actors: ${....task.env.numEnvs}
reward_shaper:
scale_value: 1.0
normalize_advantage: True
gamma: 0.99
tau: 0.95
e_clip: 0.2
entropy_coef: 0.0
learning_rate: 3.e-4 # overwritten by adaptive lr_schedule
lr_schedule: adaptive
kl_threshold: 0.008 # target kl for adaptive lr
truncate_grads: True
grad_norm: 1.
horizon_length: 24
minibatch_size: 32768
mini_epochs: 5
critic_coef: 2
clip_value: True
seq_len: 4 # only for rnn
bounds_loss_coef: 0.001
max_epochs: ${resolve_default:1000,${....max_iterations}}
save_best_after: 200
score_to_win: 20000
save_frequency: 50
print_stats: True
| 1,678 | YAML | 21.689189 | 101 | 0.603695 |
NVIDIA-Omniverse/IsaacGymEnvs/isaacgymenvs/cfg/train/CartpolePPO.yaml | params:
seed: ${...seed}
algo:
name: a2c_continuous
model:
name: continuous_a2c_logstd
network:
name: actor_critic
separate: False
space:
continuous:
mu_activation: None
sigma_activation: None
mu_init:
name: default
sigma_init:
name: const_initializer
val: 0
fixed_sigma: True
mlp:
units: [32, 32]
activation: elu
initializer:
name: default
regularizer:
name: None
load_checkpoint: ${if:${...checkpoint},True,False} # flag which sets whether to load the checkpoint
load_path: ${...checkpoint} # path to the checkpoint to load
config:
name: ${resolve_default:Cartpole,${....experiment}}
full_experiment_name: ${.name}
env_name: rlgpu
multi_gpu: ${....multi_gpu}
ppo: True
mixed_precision: False
normalize_input: True
normalize_value: True
num_actors: ${....task.env.numEnvs}
reward_shaper:
scale_value: 0.1
normalize_advantage: True
gamma: 0.99
tau: 0.95
learning_rate: 3e-4
lr_schedule: adaptive
kl_threshold: 0.008
score_to_win: 20000
max_epochs: ${resolve_default:100,${....max_iterations}}
save_best_after: 50
save_frequency: 25
grad_norm: 1.0
entropy_coef: 0.0
truncate_grads: True
e_clip: 0.2
horizon_length: 16
minibatch_size: 8192
mini_epochs: 8
critic_coef: 4
clip_value: True
seq_len: 4
bounds_loss_coef: 0.0001 | 1,517 | YAML | 21.323529 | 101 | 0.595913 |
NVIDIA-Omniverse/IsaacGymEnvs/isaacgymenvs/cfg/train/ShadowHandOpenAIPPO.yaml | # specifies what the default training mode is when
# running `ShadowHandOpenAI` (version with DR and asymmetric observations)
# (currently defaults to asymmetric training)
defaults:
- ShadowHandPPOAsymm
- _self_
| 216 | YAML | 29.999996 | 74 | 0.782407 |
NVIDIA-Omniverse/IsaacGymEnvs/isaacgymenvs/cfg/train/FactoryTaskNutBoltPickPPO.yaml | params:
seed: ${...seed}
algo:
name: a2c_continuous
model:
name: continuous_a2c_logstd
network:
name: actor_critic
separate: False
space:
continuous:
mu_activation: None
sigma_activation: None
mu_init:
name: default
sigma_init:
name: const_initializer
val: 0
fixed_sigma: True
mlp:
units: [256, 128, 64]
activation: elu
d2rl: False
initializer:
name: default
regularizer:
name: None
load_checkpoint: ${if:${...checkpoint},True,False}
load_path: ${...checkpoint}
config:
name: ${resolve_default:FactoryTaskNutBoltPick,${....experiment}}
full_experiment_name: ${.name}
env_name: rlgpu
multi_gpu: ${....multi_gpu}
ppo: True
mixed_precision: True
normalize_input: True
normalize_value: True
value_bootstrap: True
num_actors: ${....task.env.numEnvs}
reward_shaper:
scale_value: 1.0
normalize_advantage: True
gamma: 0.99
tau: 0.95
learning_rate: 1e-4
lr_schedule: fixed
schedule_type: standard
kl_threshold: 0.016
score_to_win: 20000
max_epochs: ${resolve_default:1024,${....max_iterations}}
save_best_after: 50
save_frequency: 100
print_stats: True
grad_norm: 1.0
entropy_coef: 0.0
truncate_grads: False
e_clip: 0.2
horizon_length: 120
minibatch_size: 512
mini_epochs: 8
critic_coef: 2
clip_value: True
seq_len: 4
bounds_loss_coef: 0.0001
| 1,542 | YAML | 20.430555 | 69 | 0.595331 |
NVIDIA-Omniverse/IsaacGymEnvs/isaacgymenvs/cfg/train/HumanoidAMPPPOLowGP.yaml | params:
seed: ${...seed}
algo:
name: amp_continuous
model:
name: continuous_amp
network:
name: amp
separate: True
space:
continuous:
mu_activation: None
sigma_activation: None
mu_init:
name: default
sigma_init:
name: const_initializer
val: -2.9
fixed_sigma: True
learn_sigma: False
mlp:
units: [1024, 512]
activation: relu
d2rl: False
initializer:
name: default
regularizer:
name: None
disc:
units: [1024, 512]
activation: relu
initializer:
name: default
load_checkpoint: ${if:${...checkpoint},True,False} # flag which sets whether to load the checkpoint
load_path: ${...checkpoint} # path to the checkpoint to load
config:
name: ${resolve_default:HumanoidAMP,${....experiment}}
full_experiment_name: ${.name}
env_name: rlgpu
ppo: True
multi_gpu: ${....multi_gpu}
mixed_precision: False
normalize_input: True
normalize_value: True
value_bootstrap: True
num_actors: ${....task.env.numEnvs}
reward_shaper:
scale_value: 1
normalize_advantage: True
gamma: 0.99
tau: 0.95
learning_rate: 5e-5
lr_schedule: constant
kl_threshold: 0.008
score_to_win: 20000
max_epochs: ${resolve_default:5000,${....max_iterations}}
save_best_after: 100
save_frequency: 50
print_stats: True
grad_norm: 1.0
entropy_coef: 0.0
truncate_grads: False
e_clip: 0.2
horizon_length: 16
minibatch_size: 32768
mini_epochs: 6
critic_coef: 5
clip_value: False
seq_len: 4
bounds_loss_coef: 10
amp_obs_demo_buffer_size: 200000
amp_replay_buffer_size: 1000000
amp_replay_keep_prob: 0.01
amp_batch_size: 512
amp_minibatch_size: 4096
disc_coef: 5
disc_logit_reg: 0.05
disc_grad_penalty: 0.2
disc_reward_scale: 2
disc_weight_decay: 0.0001
normalize_amp_input: True
task_reward_w: 0.0
disc_reward_w: 1.0
| 2,057 | YAML | 20.663158 | 101 | 0.601361 |
NVIDIA-Omniverse/IsaacGymEnvs/isaacgymenvs/cfg/pbt/pbt_default.yaml | defaults:
- mutation: default_mutation
enabled: True
policy_idx: 0 # policy index in a population: should always be specified explicitly! Each run in a population should have a unique idx from [0..N-1]
num_policies: 8 # total number of policies in the population, the total number of learners. Override through CLI!
workspace: "pbt_workspace" # suffix of the workspace dir name inside train_dir, used to distinguish different PBT runs with the same experiment name. Recommended to specify a unique name
# special mode that enables PBT features for debugging even if only one policy is present. Never enable in actual experiments
dbg_mode: False
# PBT hyperparams
interval_steps: 10000000 # Interval in env steps between PBT iterations (checkpointing, mutation, etc.)
start_after: 10000000 # Start PBT after this many env frames are collected, this applies to all experiment restarts, i.e. when we resume training after the weights are mutated
initial_delay: 20000000 # This is a separate delay for when we're just starting the training session. It makes sense to give policies a bit more time to develop different behaviors
# Fraction of the underperforming policies whose weights are to be replaced by better performing policies
# This is rounded up, i.e. for 8 policies and fraction 0.3 we replace ceil(0.3*8)=3 worst policies
replace_fraction_worst: 0.125
# Fraction of agents used to sample weights from when we replace an underperforming agent
# This is also rounded up
replace_fraction_best: 0.3
# Replace an underperforming policy only if its reward is lower by at least this fraction of standard deviation
# within the population.
replace_threshold_frac_std: 0.5
# Replace an underperforming policy only if its reward is lower by at least this fraction of the absolute value
# of the objective of a better policy
replace_threshold_frac_absolute: 0.05
# Probability to mutate a certain parameter
mutation_rate: 0.15
# min and max values for the mutation of a parameter
# The mutation is performed by multiplying or dividing (randomly) the parameter value by a value sampled from [change_min, change_max]
change_min: 1.1
change_max: 1.5
| 2,161 | YAML | 51.731706 | 187 | 0.788061 |
NVIDIA-Omniverse/IsaacGymEnvs/isaacgymenvs/cfg/pbt/mutation/ant_mutation.yaml | task.env.headingWeight: "mutate_float"
task.env.upWeight: "mutate_float"
train.params.config.grad_norm: "mutate_float"
train.params.config.entropy_coef: "mutate_float"
train.params.config.critic_coef: "mutate_float"
train.params.config.bounds_loss_coef: "mutate_float"
train.params.config.kl_threshold: "mutate_float"
train.params.config.e_clip: "mutate_eps_clip"
train.params.config.mini_epochs: "mutate_mini_epochs"
train.params.config.gamma: "mutate_discount"
train.params.config.tau: "mutate_discount" | 509 | YAML | 32.999998 | 53 | 0.78389 |
NVIDIA-Omniverse/IsaacGymEnvs/isaacgymenvs/cfg/pbt/mutation/humanoid_mutation.yaml | task.env.headingWeight: "mutate_float"
task.env.upWeight: "mutate_float"
task.env.fingertipDeltaRewScale: "mutate_float"
task.env.liftingRewScale: "mutate_float"
task.env.liftingBonus: "mutate_float"
task.env.keypointRewScale: "mutate_float"
task.env.reachGoalBonus: "mutate_float"
task.env.kukaActionsPenaltyScale: "mutate_float"
task.env.allegroActionsPenaltyScale: "mutate_float"
train.params.config.reward_shaper.scale_value: "mutate_float"
train.params.config.learning_rate: "mutate_float"
train.params.config.grad_norm: "mutate_float"
train.params.config.entropy_coef: "mutate_float"
train.params.config.critic_coef: "mutate_float"
train.params.config.bounds_loss_coef: "mutate_float"
train.params.config.e_clip: "mutate_eps_clip"
train.params.config.mini_epochs: "mutate_mini_epochs"
train.params.config.gamma: "mutate_discount"
| 841 | YAML | 34.083332 | 61 | 0.796671 |
NVIDIA-Omniverse/IsaacGymEnvs/isaacgymenvs/cfg/pbt/mutation/default_mutation.yaml | train.params.config.reward_shaper.scale_value: "mutate_float"
train.params.config.learning_rate: "mutate_float"
train.params.config.grad_norm: "mutate_float"
train.params.config.entropy_coef: "mutate_float"
train.params.config.critic_coef: "mutate_float"
train.params.config.bounds_loss_coef: "mutate_float"
train.params.config.e_clip: "mutate_eps_clip"
train.params.config.mini_epochs: "mutate_mini_epochs"
train.params.config.gamma: "mutate_discount"
| 456 | YAML | 34.153844 | 61 | 0.787281 |
NVIDIA-Omniverse/IsaacGymEnvs/isaacgymenvs/cfg/pbt/mutation/allegro_hand_mutation.yaml | task.env.dist_reward_scale: "mutate_float"
task.env.rot_reward_scale: "mutate_float"
task.env.rot_eps: "mutate_float"
task.env.reach_goal_bonus: "mutate_float"
# Could be additionally mutated
#task.env.actionPenaltyScale: "mutate_float"
#task.env.actionDeltaPenaltyScale: "mutate_float"
#task.env.startObjectPoseDY: "mutate_float"
#task.env.startObjectPoseDZ: "mutate_float"
#task.env.fallDistance: "mutate_float"
train.params.config.learning_rate: "mutate_float"
train.params.config.grad_norm: "mutate_float"
train.params.config.entropy_coef: "mutate_float"
train.params.config.critic_coef: "mutate_float"
train.params.config.bounds_loss_coef: "mutate_float"
train.params.config.kl_threshold: "mutate_float"
train.params.config.e_clip: "mutate_eps_clip"
train.params.config.mini_epochs: "mutate_mini_epochs"
train.params.config.gamma: "mutate_discount"
# These would require special mutation rules
# 'train.params.config.steps_num': 8
# 'train.params.config.minibatch_size': 256
| 987 | YAML | 31.933332 | 53 | 0.778116 |
NVIDIA-Omniverse/IsaacGymEnvs/isaacgymenvs/cfg/pbt/mutation/allegro_kuka_mutation.yaml | task.env.distRewardScale: "mutate_float"
task.env.rotRewardScale: "mutate_float"
task.env.actionPenaltyScale: "mutate_float"
task.env.liftingRewScale: "mutate_float"
task.env.liftingBonus: "mutate_float"
task.env.liftingBonusThreshold: "mutate_float"
task.env.keypointRewScale: "mutate_float"
task.env.distanceDeltaRewScale: "mutate_float"
task.env.reachGoalBonus: "mutate_float"
task.env.kukaActionsPenaltyScale: "mutate_float"
task.env.allegroActionsPenaltyScale: "mutate_float"
task.env.fallDistance: "mutate_float"
# Could be additionally mutated
#train.params.config.learning_rate: "mutate_float"
#train.params.config.entropy_coef: "mutate_float" # this is 0, no reason to mutate
train.params.config.grad_norm: "mutate_float"
train.params.config.critic_coef: "mutate_float"
train.params.config.bounds_loss_coef: "mutate_float"
train.params.config.kl_threshold: "mutate_float"
train.params.config.e_clip: "mutate_eps_clip"
train.params.config.mini_epochs: "mutate_mini_epochs"
train.params.config.gamma: "mutate_discount"
# These would require special mutation rules
# 'train.params.config.steps_num': 8
# 'train.params.config.minibatch_size': 256
| 1,159 | YAML | 35.249999 | 83 | 0.790336 |
NVIDIA-Omniverse/IsaacGymEnvs/isaacgymenvs/pbt/pbt.py | # Copyright (c) 2018-2023, NVIDIA Corporation
# All rights reserved.
#
# Redistribution and use in source and binary forms, with or without
# modification, are permitted provided that the following conditions are met:
#
# 1. Redistributions of source code must retain the above copyright notice, this
# list of conditions and the following disclaimer.
#
# 2. Redistributions in binary form must reproduce the above copyright notice,
# this list of conditions and the following disclaimer in the documentation
# and/or other materials provided with the distribution.
#
# 3. Neither the name of the copyright holder nor the names of its
# contributors may be used to endorse or promote products derived from
# this software without specific prior written permission.
#
# THIS SOFTWARE IS PROVIDED BY THE COPYRIGHT HOLDERS AND CONTRIBUTORS "AS IS"
# AND ANY EXPRESS OR IMPLIED WARRANTIES, INCLUDING, BUT NOT LIMITED TO, THE
# IMPLIED WARRANTIES OF MERCHANTABILITY AND FITNESS FOR A PARTICULAR PURPOSE ARE
# DISCLAIMED. IN NO EVENT SHALL THE COPYRIGHT HOLDER OR CONTRIBUTORS BE LIABLE
# FOR ANY DIRECT, INDIRECT, INCIDENTAL, SPECIAL, EXEMPLARY, OR CONSEQUENTIAL
# DAMAGES (INCLUDING, BUT NOT LIMITED TO, PROCUREMENT OF SUBSTITUTE GOODS OR
# SERVICES; LOSS OF USE, DATA, OR PROFITS; OR BUSINESS INTERRUPTION) HOWEVER
# CAUSED AND ON ANY THEORY OF LIABILITY, WHETHER IN CONTRACT, STRICT LIABILITY,
# OR TORT (INCLUDING NEGLIGENCE OR OTHERWISE) ARISING IN ANY WAY OUT OF THE USE
# OF THIS SOFTWARE, EVEN IF ADVISED OF THE POSSIBILITY OF SUCH DAMAGE.
import math
import os
import random
import shutil
import sys
import time
from os.path import join
from typing import Any, Dict, List, Optional
import numpy as np
import torch
import yaml
from omegaconf import DictConfig
from rl_games.algos_torch.torch_ext import safe_filesystem_op, safe_save
from rl_games.common.algo_observer import AlgoObserver
from isaacgymenvs.pbt.mutation import mutate
from isaacgymenvs.utils.reformat import omegaconf_to_dict
from isaacgymenvs.utils.utils import flatten_dict, project_tmp_dir, safe_ensure_dir_exists
# i.e. value for target objective when it is not known
_UNINITIALIZED_VALUE = float(-1e9)
def _checkpnt_name(iteration):
return f"{iteration:06d}.yaml"
def _model_checkpnt_name(iteration):
return f"{iteration:06d}.pth"
def _flatten_params(params: Dict, prefix="", separator=".") -> Dict:
all_params = flatten_dict(params, prefix, separator)
return all_params
def _filter_params(params: Dict, params_to_mutate: Dict) -> Dict:
filtered_params = dict()
for key, value in params.items():
if key in params_to_mutate:
if isinstance(value, str):
try:
# trying to convert values such as "1e-4" to floats because yaml fails to recognize them as such
float_value = float(value)
value = float_value
except ValueError:
pass
filtered_params[key] = value
return filtered_params
class PbtParams:
def __init__(self, cfg: DictConfig):
params: Dict = omegaconf_to_dict(cfg)
pbt_params = params["pbt"]
self.replace_fraction_best = pbt_params["replace_fraction_best"]
self.replace_fraction_worst = pbt_params["replace_fraction_worst"]
self.replace_threshold_frac_std = pbt_params["replace_threshold_frac_std"]
self.replace_threshold_frac_absolute = pbt_params["replace_threshold_frac_absolute"]
self.mutation_rate = pbt_params["mutation_rate"]
self.change_min = pbt_params["change_min"]
self.change_max = pbt_params["change_max"]
self.task_name = params["task"]["name"]
self.dbg_mode = pbt_params["dbg_mode"]
self.policy_idx = pbt_params["policy_idx"]
self.num_policies = pbt_params["num_policies"]
self.num_envs = params["task"]["env"]["numEnvs"]
self.workspace = pbt_params["workspace"]
self.interval_steps = pbt_params["interval_steps"]
self.start_after_steps = pbt_params["start_after"]
self.initial_delay_steps = pbt_params["initial_delay"]
self.params_to_mutate = pbt_params["mutation"]
mutable_params = _flatten_params(params)
self.mutable_params = _filter_params(mutable_params, self.params_to_mutate)
self.with_wandb = params["wandb_activate"]
RLAlgo = Any # just for readability
def _restart_process_with_new_params(
policy_idx: int,
new_params: Dict,
restart_from_checkpoint: Optional[str],
experiment_name: Optional[str],
algo: Optional[RLAlgo],
with_wandb: bool,
) -> None:
cli_args = sys.argv
modified_args = [cli_args[0]] # initialize with path to the Python script
for arg in cli_args[1:]:
if "=" not in arg:
modified_args.append(arg)
else:
assert "=" in arg
arg_name, arg_value = arg.split("=")
if arg_name in new_params or arg_name in [
"checkpoint",
"+full_experiment_name",
"hydra.run.dir",
"++pbt_restart",
]:
# skip this parameter, it will be added later!
continue
modified_args.append(f"{arg_name}={arg_value}")
modified_args.append(f"hydra.run.dir={os.getcwd()}")
modified_args.append(f"++pbt_restart=True")
if experiment_name is not None:
modified_args.append(f"+full_experiment_name={experiment_name}")
if restart_from_checkpoint is not None:
modified_args.append(f"checkpoint={restart_from_checkpoint}")
# add all the new (possibly mutated) parameters
for param, value in new_params.items():
modified_args.append(f"{param}={value}")
if algo is not None:
algo.writer.flush()
algo.writer.close()
if with_wandb:
try:
import wandb
wandb.run.finish()
except Exception as exc:
print(f"Policy {policy_idx}: Exception {exc} in wandb.run.finish()")
return
print(f"Policy {policy_idx}: Restarting self with args {modified_args}", flush=True)
os.execv(sys.executable, ["python3"] + modified_args)
def initial_pbt_check(cfg: DictConfig):
assert cfg.pbt.enabled
if hasattr(cfg, "pbt_restart") and cfg.pbt_restart:
print(f"PBT job restarted from checkpoint, keep going...")
return
print("PBT run without 'pbt_restart=True' - must be the very start of the experiment!")
print("Mutating initial set of hyperparameters!")
pbt_params = PbtParams(cfg)
new_params = mutate(
pbt_params.mutable_params,
pbt_params.params_to_mutate,
pbt_params.mutation_rate,
pbt_params.change_min,
pbt_params.change_max,
)
_restart_process_with_new_params(pbt_params.policy_idx, new_params, None, None, None, False)
class PbtAlgoObserver(AlgoObserver):
def __init__(self, cfg: DictConfig):
super().__init__()
self.pbt_params: PbtParams = PbtParams(cfg)
self.policy_idx: int = self.pbt_params.policy_idx
self.num_envs: int = self.pbt_params.num_envs
self.pbt_num_policies: int = self.pbt_params.num_policies
self.algo: Optional[RLAlgo] = None
self.pbt_workspace_dir = self.curr_policy_workspace_dir = None
self.pbt_iteration = -1 # dummy value, stands for "not initialized"
self.initial_env_frames = -1 # env frames at the beginning of the experiment, can be > 0 if we resume
self.finished_agents = set()
self.last_target_objectives = [_UNINITIALIZED_VALUE] * self.pbt_params.num_envs
self.curr_target_objective_value: float = _UNINITIALIZED_VALUE
self.target_objective_known = False # switch to true when we have enough data to calculate target objective
# keep track of objective values in the current iteration
# we use best value reached in the current iteration to decide whether to be replaced by another policy
# this reduces the noisiness of evolutionary pressure by reducing the number of situations where a policy
# gets replaced just due to a random minor dip in performance
self.best_objective_curr_iteration: Optional[float] = None
self.experiment_start = time.time()
self.with_wandb = self.pbt_params.with_wandb
def after_init(self, algo):
self.algo = algo
self.pbt_workspace_dir = join(algo.train_dir, self.pbt_params.workspace)
self.curr_policy_workspace_dir = self._policy_workspace_dir(self.pbt_params.policy_idx)
os.makedirs(self.curr_policy_workspace_dir, exist_ok=True)
def process_infos(self, infos, done_indices):
if "true_objective" in infos:
done_indices_lst = done_indices.squeeze(-1).tolist()
self.finished_agents.update(done_indices_lst)
for done_idx in done_indices_lst:
true_objective_value = infos["true_objective"][done_idx].item()
self.last_target_objectives[done_idx] = true_objective_value
# last result for all episodes
self.target_objective_known = len(self.finished_agents) >= self.pbt_params.num_envs
if self.target_objective_known:
self.curr_target_objective_value = float(np.mean(self.last_target_objectives))
else:
# environment does not specify "true objective", use regular reward
# in this case, be careful not to include reward shaping coefficients into the mutation config
self.target_objective_known = self.algo.game_rewards.current_size >= self.algo.games_to_track
if self.target_objective_known:
self.curr_target_objective_value = float(self.algo.mean_rewards)
if self.target_objective_known:
if (
self.best_objective_curr_iteration is None
or self.curr_target_objective_value > self.best_objective_curr_iteration
):
print(
f"Policy {self.policy_idx}: New best objective value {self.curr_target_objective_value} in iteration {self.pbt_iteration}"
)
self.best_objective_curr_iteration = self.curr_target_objective_value
def after_steps(self):
if self.pbt_iteration == -1:
self.pbt_iteration = self.algo.frame // self.pbt_params.interval_steps
self.initial_env_frames = self.algo.frame
print(
f"Policy {self.policy_idx}: PBT init. Env frames: {self.algo.frame}, pbt_iteration: {self.pbt_iteration}"
)
env_frames: int = self.algo.frame
iteration = env_frames // self.pbt_params.interval_steps
print(
f"Policy {self.policy_idx}: Env frames {env_frames}, iteration {iteration}, self iteration {self.pbt_iteration}"
)
if iteration <= self.pbt_iteration:
return
if not self.target_objective_known:
# not enough data yet to calcuate avg true_objective
print(
f"Policy {self.policy_idx}: Not enough episodes finished, wait for more data ({len(self.finished_agents)}/{self.num_envs})..."
)
return
assert self.curr_target_objective_value != _UNINITIALIZED_VALUE
assert self.best_objective_curr_iteration is not None
best_objective_curr_iteration: float = self.best_objective_curr_iteration
# reset for the next iteration
self.best_objective_curr_iteration = None
self.target_objective_known = False
sec_since_experiment_start = time.time() - self.experiment_start
pbt_start_after_sec = 1 if self.pbt_params.dbg_mode else 30
if sec_since_experiment_start < pbt_start_after_sec:
print(
f"Policy {self.policy_idx}: Not enough time passed since experiment start {sec_since_experiment_start}"
)
return
print(f"Policy {self.policy_idx}: New pbt iteration {iteration}!")
self.pbt_iteration = iteration
try:
self._save_pbt_checkpoint()
except Exception as exc:
print(f"Policy {self.policy_idx}: Exception {exc} when saving PBT checkpoint!")
return
try:
checkpoints = self._load_population_checkpoints()
except Exception as exc:
print(f"Policy {self.policy_idx}: Exception {exc} when loading checkpoints!")
return
try:
self._cleanup(checkpoints)
except Exception as exc:
print(f"Policy {self.policy_idx}: Exception {exc} during cleanup!")
policies = list(range(self.pbt_num_policies))
target_objectives = []
for p in policies:
if checkpoints[p] is None:
target_objectives.append(_UNINITIALIZED_VALUE)
else:
target_objectives.append(checkpoints[p]["true_objective"])
policies_sorted = sorted(zip(target_objectives, policies), reverse=True)
objectives = [objective for objective, p in policies_sorted]
best_objective = objectives[0]
policies_sorted = [p for objective, p in policies_sorted]
best_policy = policies_sorted[0]
self._maybe_save_best_policy(best_objective, best_policy, checkpoints[best_policy])
objectives_filtered = [o for o in objectives if o > _UNINITIALIZED_VALUE]
try:
self._pbt_summaries(self.pbt_params.mutable_params, best_objective)
except Exception as exc:
print(f"Policy {self.policy_idx}: Exception {exc} when writing summaries!")
return
if (
env_frames - self.initial_env_frames < self.pbt_params.start_after_steps
or env_frames < self.pbt_params.initial_delay_steps
):
print(
f"Policy {self.policy_idx}: Not enough experience collected to replace weights. "
f"Giving this policy more time to adjust to the latest parameters... "
f"env_frames={env_frames} started_at={self.initial_env_frames} "
f"restart_delay={self.pbt_params.start_after_steps} initial_delay={self.pbt_params.initial_delay_steps}"
)
return
replace_worst = math.ceil(self.pbt_params.replace_fraction_worst * self.pbt_num_policies)
replace_best = math.ceil(self.pbt_params.replace_fraction_best * self.pbt_num_policies)
best_policies = policies_sorted[:replace_best]
worst_policies = policies_sorted[-replace_worst:]
print(f"Policy {self.policy_idx}: PBT best_policies={best_policies}, worst_policies={worst_policies}")
if self.policy_idx not in worst_policies and not self.pbt_params.dbg_mode:
# don't touch the policies that are doing okay
print(f"Current policy {self.policy_idx} is doing well, not among the worst_policies={worst_policies}")
return
if best_objective_curr_iteration is not None and not self.pbt_params.dbg_mode:
if best_objective_curr_iteration >= min(objectives[:replace_best]):
print(
f"Policy {self.policy_idx}: best_objective={best_objective_curr_iteration} "
f"is better than some of the top policies {objectives[:replace_best]}. "
f"This policy should keep training for now, it is doing okay."
)
return
if len(objectives_filtered) <= max(2, self.pbt_num_policies // 2) and not self.pbt_params.dbg_mode:
print(f"Policy {self.policy_idx}: Not enough data to start PBT, {objectives_filtered}")
return
print(f"Current policy {self.policy_idx} is among the worst_policies={worst_policies}, consider replacing weights")
print(
f"Policy {self.policy_idx} objective: {self.curr_target_objective_value}, best_objective={best_objective} (best_policy={best_policy})."
)
replacement_policy_candidate = random.choice(best_policies)
candidate_objective = checkpoints[replacement_policy_candidate]["true_objective"]
targ_objective_value = self.curr_target_objective_value
objective_delta = candidate_objective - targ_objective_value
num_outliers = int(math.floor(0.2 * len(objectives_filtered)))
print(f"Policy {self.policy_idx} num outliers: {num_outliers}")
if len(objectives_filtered) > num_outliers:
objectives_filtered_sorted = sorted(objectives_filtered)
# remove the worst policies from the std calculation, this will allow us to keep improving even if 1-2 policies
# crashed and can't keep improving. Otherwise, std value will be too large.
objectives_std = np.std(objectives_filtered_sorted[num_outliers:])
else:
objectives_std = np.std(objectives_filtered)
objective_threshold = self.pbt_params.replace_threshold_frac_std * objectives_std
absolute_threshold = self.pbt_params.replace_threshold_frac_absolute * abs(candidate_objective)
if objective_delta > objective_threshold and objective_delta > absolute_threshold:
# replace this policy with a candidate
replacement_policy = replacement_policy_candidate
print(f"Replacing underperforming policy {self.policy_idx} with {replacement_policy}")
else:
print(
f"Policy {self.policy_idx}: Difference in objective value ({candidate_objective} vs {targ_objective_value}) is not sufficient to justify replacement,"
f"{objective_delta}, {objectives_std}, {objective_threshold}, {absolute_threshold}"
)
# replacing with "self": keep the weights but mutate the hyperparameters
replacement_policy = self.policy_idx
# Decided to replace the policy weights!
# we can either copy parameters from the checkpoint we're restarting from, or keep our parameters and
# further mutate them.
if random.random() < 0.5:
new_params = checkpoints[replacement_policy]["params"]
else:
new_params = self.pbt_params.mutable_params
new_params = mutate(
new_params,
self.pbt_params.params_to_mutate,
self.pbt_params.mutation_rate,
self.pbt_params.change_min,
self.pbt_params.change_max,
)
experiment_name = checkpoints[self.policy_idx]["experiment_name"]
try:
self._pbt_summaries(new_params, best_objective)
except Exception as exc:
print(f"Policy {self.policy_idx}: Exception {exc} when writing summaries!")
return
try:
restart_checkpoint = os.path.abspath(checkpoints[replacement_policy]["checkpoint"])
# delete previous tempdir to make sure we don't grow too big
checkpoint_tmp_dir = join(project_tmp_dir(), f"{experiment_name}_p{self.policy_idx}")
if os.path.isdir(checkpoint_tmp_dir):
shutil.rmtree(checkpoint_tmp_dir)
checkpoint_tmp_dir = safe_ensure_dir_exists(checkpoint_tmp_dir)
restart_checkpoint_tmp = join(checkpoint_tmp_dir, os.path.basename(restart_checkpoint))
# copy the checkpoint file to the temp dir to make sure it does not get deleted while we're restarting
shutil.copyfile(restart_checkpoint, restart_checkpoint_tmp)
except Exception as exc:
print(f"Policy {self.policy_idx}: Exception {exc} when copying checkpoint file for restart")
# perhaps checkpoint file was deleted before we could make a copy. Abort the restart.
return
# try to load the checkpoint file and if it fails, abandon the restart
try:
self._rewrite_checkpoint(restart_checkpoint_tmp, env_frames)
except Exception as exc:
# this should happen infrequently so should not affect training in any significant way
print(
f"Policy {self.policy_idx}: Exception {exc} when loading checkpoint file for restart."
f"Aborting restart. Continue training with the existing set of weights!"
)
return
print(
f"Policy {self.policy_idx}: Preparing to restart the process with mutated parameters! "
f"Checkpoint {restart_checkpoint_tmp}"
)
_restart_process_with_new_params(
self.policy_idx, new_params, restart_checkpoint_tmp, experiment_name, self.algo, self.with_wandb
)
def _rewrite_checkpoint(self, restart_checkpoint_tmp: str, env_frames: int) -> None:
state = torch.load(restart_checkpoint_tmp)
print(f"Policy {self.policy_idx}: restarting from checkpoint {restart_checkpoint_tmp}, {state['frame']}")
print(f"Replacing {state['frame']} with {env_frames}...")
state["frame"] = env_frames
pbt_history = state.get("pbt_history", [])
print(f"PBT history: {pbt_history}")
pbt_history.append((self.policy_idx, env_frames, self.curr_target_objective_value))
state["pbt_history"] = pbt_history
torch.save(state, restart_checkpoint_tmp)
print(f"Policy {self.policy_idx}: checkpoint rewritten to {restart_checkpoint_tmp}!")
def _save_pbt_checkpoint(self):
"""Save PBT-specific information including iteration number, policy index and hyperparameters."""
checkpoint_file = join(self.curr_policy_workspace_dir, _model_checkpnt_name(self.pbt_iteration))
algo_state = self.algo.get_full_state_weights()
safe_save(algo_state, checkpoint_file)
pbt_checkpoint_file = join(self.curr_policy_workspace_dir, _checkpnt_name(self.pbt_iteration))
pbt_checkpoint = {
"iteration": self.pbt_iteration,
"true_objective": self.curr_target_objective_value,
"frame": self.algo.frame,
"params": self.pbt_params.mutable_params,
"checkpoint": os.path.abspath(checkpoint_file),
"pbt_checkpoint": os.path.abspath(pbt_checkpoint_file),
"experiment_name": self.algo.experiment_name,
}
with open(pbt_checkpoint_file, "w") as fobj:
print(f"Policy {self.policy_idx}: Saving {pbt_checkpoint_file}...")
yaml.dump(pbt_checkpoint, fobj)
def _policy_workspace_dir(self, policy_idx):
return join(self.pbt_workspace_dir, f"{policy_idx:03d}")
def _load_population_checkpoints(self):
"""
Load checkpoints for other policies in the population.
Pick the newest checkpoint, but not newer than our current iteration.
"""
checkpoints = dict()
for policy_idx in range(self.pbt_num_policies):
checkpoints[policy_idx] = None
policy_workspace_dir = self._policy_workspace_dir(policy_idx)
if not os.path.isdir(policy_workspace_dir):
continue
pbt_checkpoint_files = [f for f in os.listdir(policy_workspace_dir) if f.endswith(".yaml")]
pbt_checkpoint_files.sort(reverse=True)
for pbt_checkpoint_file in pbt_checkpoint_files:
iteration_str = pbt_checkpoint_file.split(".")[0]
iteration = int(iteration_str)
if iteration <= self.pbt_iteration:
with open(join(policy_workspace_dir, pbt_checkpoint_file), "r") as fobj:
print(f"Policy {self.policy_idx}: Loading policy-{policy_idx} {pbt_checkpoint_file}")
checkpoints[policy_idx] = safe_filesystem_op(yaml.load, fobj, Loader=yaml.FullLoader)
break
else:
# print(f'Policy {self.policy_idx}: Ignoring {pbt_checkpoint_file} because it is newer than our current iteration')
pass
assert self.policy_idx in checkpoints.keys()
return checkpoints
def _maybe_save_best_policy(self, best_objective, best_policy_idx: int, best_policy_checkpoint):
# make a directory containing the best policy checkpoints using safe_filesystem_op
best_policy_workspace_dir = join(self.pbt_workspace_dir, f"best{self.policy_idx}")
safe_filesystem_op(os.makedirs, best_policy_workspace_dir, exist_ok=True)
best_objective_so_far = _UNINITIALIZED_VALUE
best_policy_checkpoint_files = [f for f in os.listdir(best_policy_workspace_dir) if f.endswith(".yaml")]
best_policy_checkpoint_files.sort(reverse=True)
if best_policy_checkpoint_files:
with open(join(best_policy_workspace_dir, best_policy_checkpoint_files[0]), "r") as fobj:
best_policy_checkpoint_so_far = safe_filesystem_op(yaml.load, fobj, Loader=yaml.FullLoader)
best_objective_so_far = best_policy_checkpoint_so_far["true_objective"]
if best_objective_so_far >= best_objective:
# don't save the checkpoint if it is worse than the best checkpoint so far
return
print(f"Policy {self.policy_idx}: New best objective: {best_objective}!")
# save the best policy checkpoint to this folder
best_policy_checkpoint_name = f"{self.pbt_params.task_name}_best_obj_{best_objective:015.5f}_iter_{self.pbt_iteration:04d}_policy{best_policy_idx:03d}_frame{self.algo.frame}"
# copy the checkpoint file to the best policy directory
try:
shutil.copy(
best_policy_checkpoint["checkpoint"],
join(best_policy_workspace_dir, f"{best_policy_checkpoint_name}.pth"),
)
shutil.copy(
best_policy_checkpoint["pbt_checkpoint"],
join(best_policy_workspace_dir, f"{best_policy_checkpoint_name}.yaml"),
)
# cleanup older best policy checkpoints, we want to keep only N latest files
best_policy_checkpoint_files = [f for f in os.listdir(best_policy_workspace_dir)]
best_policy_checkpoint_files.sort(reverse=True)
n_to_keep = 6
for best_policy_checkpoint_file in best_policy_checkpoint_files[n_to_keep:]:
os.remove(join(best_policy_workspace_dir, best_policy_checkpoint_file))
except Exception as exc:
print(f"Policy {self.policy_idx}: Exception {exc} when copying best checkpoint!")
# no big deal if this fails, hopefully the next time we will succeeed
return
def _pbt_summaries(self, params, best_objective):
for param, value in params.items():
self.algo.writer.add_scalar(f"pbt/{param}", value, self.algo.frame)
self.algo.writer.add_scalar(f"pbt/00_best_objective", best_objective, self.algo.frame)
self.algo.writer.flush()
def _cleanup(self, checkpoints):
iterations = []
for policy_idx, checkpoint in checkpoints.items():
if checkpoint is None:
iterations.append(0)
else:
iterations.append(checkpoint["iteration"])
oldest_iteration = sorted(iterations)[0]
cleanup_threshold = oldest_iteration - 20
print(
f"Policy {self.policy_idx}: Oldest iteration in population is {oldest_iteration}, removing checkpoints older than {cleanup_threshold} iteration"
)
pbt_checkpoint_files = [f for f in os.listdir(self.curr_policy_workspace_dir)]
for f in pbt_checkpoint_files:
if "." in f:
iteration_idx = int(f.split(".")[0])
if iteration_idx <= cleanup_threshold:
print(f"Policy {self.policy_idx}: PBT cleanup: removing checkpoint {f}")
# we catch all exceptions in this function so no need to use safe_filesystem_op
os.remove(join(self.curr_policy_workspace_dir, f))
# Sometimes, one of the PBT processes can get stuck, or crash, or be scheduled significantly later on Slurm
# or a similar cluster management system.
# In that case, we will accumulate a lot of older checkpoints. In order to keep the number of older checkpoints
# under control (to avoid running out of disk space) we implement the following logic:
# when we have more than N checkpoints, we delete half of the oldest checkpoints. This caps the max amount of
# disk space used, and still allows older policies to participate in PBT
max_old_checkpoints = 25
while True:
pbt_checkpoint_files = [f for f in os.listdir(self.curr_policy_workspace_dir) if f.endswith(".yaml")]
if len(pbt_checkpoint_files) <= max_old_checkpoints:
break
if not self._delete_old_checkpoint(pbt_checkpoint_files):
break
def _delete_old_checkpoint(self, pbt_checkpoint_files: List[str]) -> bool:
"""
Delete the checkpoint that results in the smallest max gap between the remaining checkpoints.
Do not delete any of the last N checkpoints.
"""
pbt_checkpoint_files.sort()
n_latest_to_keep = 10
candidates = pbt_checkpoint_files[:-n_latest_to_keep]
num_candidates = len(candidates)
if num_candidates < 3:
return False
def _iter(f):
return int(f.split(".")[0])
best_gap = 1e9
best_candidate = 1
for i in range(1, num_candidates - 1):
prev_iteration = _iter(candidates[i - 1])
next_iteration = _iter(candidates[i + 1])
# gap is we delete the ith candidate
gap = next_iteration - prev_iteration
if gap < best_gap:
best_gap = gap
best_candidate = i
# delete the best candidate
best_candidate_file = candidates[best_candidate]
files_to_remove = [best_candidate_file, _model_checkpnt_name(_iter(best_candidate_file))]
for file_to_remove in files_to_remove:
print(
f"Policy {self.policy_idx}: PBT cleanup old checkpoints, removing checkpoint {file_to_remove} (best gap {best_gap})"
)
os.remove(join(self.curr_policy_workspace_dir, file_to_remove))
return True
| 30,434 | Python | 42.917749 | 182 | 0.638792 |
NVIDIA-Omniverse/IsaacGymEnvs/isaacgymenvs/pbt/mutation.py | # Copyright (c) 2018-2023, NVIDIA Corporation
# All rights reserved.
#
# Redistribution and use in source and binary forms, with or without
# modification, are permitted provided that the following conditions are met:
#
# 1. Redistributions of source code must retain the above copyright notice, this
# list of conditions and the following disclaimer.
#
# 2. Redistributions in binary form must reproduce the above copyright notice,
# this list of conditions and the following disclaimer in the documentation
# and/or other materials provided with the distribution.
#
# 3. Neither the name of the copyright holder nor the names of its
# contributors may be used to endorse or promote products derived from
# this software without specific prior written permission.
#
# THIS SOFTWARE IS PROVIDED BY THE COPYRIGHT HOLDERS AND CONTRIBUTORS "AS IS"
# AND ANY EXPRESS OR IMPLIED WARRANTIES, INCLUDING, BUT NOT LIMITED TO, THE
# IMPLIED WARRANTIES OF MERCHANTABILITY AND FITNESS FOR A PARTICULAR PURPOSE ARE
# DISCLAIMED. IN NO EVENT SHALL THE COPYRIGHT HOLDER OR CONTRIBUTORS BE LIABLE
# FOR ANY DIRECT, INDIRECT, INCIDENTAL, SPECIAL, EXEMPLARY, OR CONSEQUENTIAL
# DAMAGES (INCLUDING, BUT NOT LIMITED TO, PROCUREMENT OF SUBSTITUTE GOODS OR
# SERVICES; LOSS OF USE, DATA, OR PROFITS; OR BUSINESS INTERRUPTION) HOWEVER
# CAUSED AND ON ANY THEORY OF LIABILITY, WHETHER IN CONTRACT, STRICT LIABILITY,
# OR TORT (INCLUDING NEGLIGENCE OR OTHERWISE) ARISING IN ANY WAY OUT OF THE USE
# OF THIS SOFTWARE, EVEN IF ADVISED OF THE POSSIBILITY OF SUCH DAMAGE.
import copy
import random
def mutate_float(x, change_min=1.1, change_max=1.5):
perturb_amount = random.uniform(change_min, change_max)
# mutation direction
new_value = x / perturb_amount if random.random() < 0.5 else x * perturb_amount
return new_value
def mutate_float_min_1(x, **kwargs):
new_value = mutate_float(x, **kwargs)
new_value = max(1.0, new_value)
return new_value
def mutate_eps_clip(x, **kwargs):
new_value = mutate_float(x, **kwargs)
new_value = max(0.01, new_value)
new_value = min(0.3, new_value)
return new_value
def mutate_mini_epochs(x, **kwargs):
change_amount = 1
new_value = x + change_amount if random.random() < 0.5 else x - change_amount
new_value = max(1, new_value)
new_value = min(8, new_value)
return new_value
def mutate_discount(x, **kwargs):
"""Special mutation func for parameters such as gamma (discount factor)."""
inv_x = 1.0 - x
# very conservative, large changes in gamma can lead to very different critic estimates
new_inv_x = mutate_float(inv_x, change_min=1.1, change_max=1.2)
new_value = 1.0 - new_inv_x
return new_value
def get_mutation_func(mutation_func_name):
try:
func = eval(mutation_func_name)
except Exception as exc:
print(f'Exception {exc} while trying to find the mutation func {mutation_func_name}.')
raise Exception(f'Could not find mutation func {mutation_func_name}')
return func
def mutate(params, mutations, mutation_rate, pbt_change_min, pbt_change_max):
mutated_params = copy.deepcopy(params)
for param, param_value in params.items():
# toss a coin whether we perturb the parameter at all
if random.random() > mutation_rate:
continue
mutation_func_name = mutations[param]
mutation_func = get_mutation_func(mutation_func_name)
mutated_value = mutation_func(param_value, change_min=pbt_change_min, change_max=pbt_change_max)
mutated_params[param] = mutated_value
print(f'Param {param} mutated to value {mutated_value}')
return mutated_params
| 3,686 | Python | 36.622449 | 104 | 0.715138 |
NVIDIA-Omniverse/IsaacGymEnvs/isaacgymenvs/pbt/experiments/ant_pbt.py | from isaacgymenvs.pbt.launcher.run_description import ParamGrid, RunDescription, Experiment
from isaacgymenvs.pbt.experiments.run_utils import version
_env = 'ant'
_name = f'{_env}_{version}'
_iterations = 10000
_pbt_num_policies = 3
_params = ParamGrid([
('pbt.policy_idx', list(range(_pbt_num_policies))),
])
_wandb_activate = True
_wandb_group = f'pbt_{_name}'
_wandb_entity = 'your_wandb_entity'
_wandb_project = 'your_wandb_project'
_experiments = [
Experiment(
f'{_name}',
f'python -m isaacgymenvs.train task=Ant headless=True '
f'max_iterations={_iterations} num_envs=2048 seed=-1 train.params.config.save_frequency=2000 '
f'wandb_activate={_wandb_activate} wandb_group={_wandb_group} wandb_entity={_wandb_entity} wandb_project={_wandb_project} '
f'pbt=pbt_default pbt.num_policies={_pbt_num_policies} pbt.workspace=workspace_{_name} '
f'pbt.initial_delay=10000000 pbt.interval_steps=5000000 pbt.start_after=10000000 pbt/mutation=ant_mutation',
_params.generate_params(randomize=False),
),
]
RUN_DESCRIPTION = RunDescription(
f'{_name}',
experiments=_experiments, experiment_arg_name='experiment', experiment_dir_arg_name='hydra.run.dir',
param_prefix='', customize_experiment_name=False,
)
| 1,285 | Python | 33.756756 | 131 | 0.701167 |
NVIDIA-Omniverse/IsaacGymEnvs/isaacgymenvs/pbt/experiments/allegro_kuka_two_arms_reorientation_lstm.py | from isaacgymenvs.pbt.launcher.run_description import ParamGrid, RunDescription, Experiment
from isaacgymenvs.pbt.experiments.run_utils import version, seeds, default_num_frames
kuka_env = 'allegro_kuka_two_arms_reorientation'
_frames = default_num_frames
_name = f'{kuka_env}_{version}'
_params = ParamGrid([
('seed', seeds(8)),
])
_wandb_activate = True
_wandb_group = f'pbt_{_name}'
_wandb_entity = 'your_wandb_entity'
_wandb_project = 'your_wandb_project'
cli = f'python -m isaacgymenvs.train ' \
f'train.params.config.max_frames={_frames} headless=True ' \
f'task=AllegroKukaTwoArmsLSTM task/env=reorientation ' \
f'wandb_project={_wandb_project} wandb_entity={_wandb_entity} wandb_activate={_wandb_activate} wandb_group={_wandb_group}'
RUN_DESCRIPTION = RunDescription(
f'{_name}',
experiments=[Experiment(f'{_name}', cli, _params.generate_params(randomize=False))],
experiment_arg_name='experiment', experiment_dir_arg_name='hydra.run.dir',
param_prefix='', customize_experiment_name=False,
)
| 1,045 | Python | 33.866666 | 128 | 0.71866 |
NVIDIA-Omniverse/IsaacGymEnvs/isaacgymenvs/pbt/experiments/allegro_kuka_throw_lstm.py | from isaacgymenvs.pbt.launcher.run_description import ParamGrid, RunDescription, Experiment
from isaacgymenvs.pbt.experiments.run_utils import version, seeds, default_num_frames
kuka_env = 'allegro_kuka_throw'
_frames = default_num_frames
_name = f'{kuka_env}_{version}'
_params = ParamGrid([
('seed', seeds(8)),
])
_wandb_activate = True
_wandb_group = f'pbt_{_name}'
_wandb_entity = 'your_wandb_entity'
_wandb_project = 'your_wandb_project'
cli = f'python -m isaacgymenvs.train seed=-1 ' \
f'train.params.config.max_frames={_frames} headless=True ' \
f'task=AllegroKukaLSTM task/env=throw ' \
f'wandb_project={_wandb_project} wandb_entity={_wandb_entity} wandb_activate={_wandb_activate} wandb_group={_wandb_group}'
RUN_DESCRIPTION = RunDescription(
f'{_name}',
experiments=[Experiment(f'{_name}', cli, _params.generate_params(randomize=False))],
experiment_arg_name='experiment', experiment_dir_arg_name='hydra.run.dir',
param_prefix='', customize_experiment_name=False,
)
| 1,021 | Python | 33.066666 | 128 | 0.711068 |
NVIDIA-Omniverse/IsaacGymEnvs/isaacgymenvs/pbt/experiments/run_utils.py | import random
from typing import List
# Versioning -- you can change this number and keep a changelog below to keep track of your experiments as you go.
version = "v1"
def seeds(num_seeds) -> List[int]:
return [random.randrange(1000000, 9999999) for _ in range(num_seeds)]
default_num_frames: int = 10_000_000_000
| 323 | Python | 23.923075 | 114 | 0.73065 |
NVIDIA-Omniverse/IsaacGymEnvs/isaacgymenvs/pbt/experiments/allegro_kuka_reorientation_lstm.py | from isaacgymenvs.pbt.launcher.run_description import ParamGrid, RunDescription, Experiment
from isaacgymenvs.pbt.experiments.run_utils import version, seeds, default_num_frames
kuka_env = 'allegro_kuka_reorientation'
_frames = default_num_frames
_name = f'{kuka_env}_{version}'
_wandb_activate = True
_wandb_group = f'pbt_{_name}'
_wandb_entity = 'your_wandb_entity'
_wandb_project = 'your_wandb_project'
_params = ParamGrid([
('seed', seeds(8)),
])
cli = f'python -m isaacgymenvs.train seed=-1 ' \
f'train.params.config.max_frames={_frames} headless=True ' \
f'task=AllegroKukaLSTM task/env=reorientation ' \
f'wandb_project={_wandb_project} wandb_entity={_wandb_entity} wandb_activate={_wandb_activate} wandb_group={_wandb_group}'
RUN_DESCRIPTION = RunDescription(
f'{_name}',
experiments=[Experiment(f'{_name}', cli, _params.generate_params(randomize=False))],
experiment_arg_name='experiment', experiment_dir_arg_name='hydra.run.dir',
param_prefix='', customize_experiment_name=False,
)
| 1,037 | Python | 33.599999 | 128 | 0.715526 |
NVIDIA-Omniverse/IsaacGymEnvs/isaacgymenvs/pbt/experiments/allegro_kuka_two_arms_regrasping_pbt_lstm.py | from isaacgymenvs.pbt.launcher.run_description import ParamGrid, RunDescription, Experiment
from isaacgymenvs.pbt.experiments.allegro_kuka_pbt_base import kuka_base_cli
from isaacgymenvs.pbt.experiments.run_utils import version
env = 'allegro_kuka_two_arms_regrasp'
_pbt_num_policies = 8
_name = f'{env}_{version}_pbt_{_pbt_num_policies}p'
_wandb_group = f'pbt_{_name}'
_params = ParamGrid([
('pbt.policy_idx', list(range(_pbt_num_policies))),
])
cli = kuka_base_cli + f' task=AllegroKukaTwoArmsLSTM task/env=regrasping task.env.episodeLength=400 wandb_activate=True wandb_group={_wandb_group} pbt.num_policies={_pbt_num_policies}'
RUN_DESCRIPTION = RunDescription(
f'{_name}',
experiments=[Experiment(f'{_name}', cli, _params.generate_params(randomize=False))],
experiment_arg_name='experiment', experiment_dir_arg_name='hydra.run.dir',
param_prefix='', customize_experiment_name=False,
)
| 916 | Python | 37.208332 | 184 | 0.741266 |
NVIDIA-Omniverse/IsaacGymEnvs/isaacgymenvs/pbt/experiments/allegro_kuka_regrasping_pbt_lstm.py | from isaacgymenvs.pbt.launcher.run_description import ParamGrid, RunDescription, Experiment
from isaacgymenvs.pbt.experiments.allegro_kuka_pbt_base import kuka_env, kuka_base_cli
from isaacgymenvs.pbt.experiments.run_utils import version
_pbt_num_policies = 8
_name = f'{kuka_env}_regrasp_{version}_pbt_{_pbt_num_policies}p'
_wandb_group = f'pbt_{_name}'
_params = ParamGrid([
('pbt.policy_idx', list(range(_pbt_num_policies))),
])
cli = kuka_base_cli + f' task=AllegroKukaLSTM task/env=regrasping wandb_activate=True wandb_group={_wandb_group} pbt.num_policies={_pbt_num_policies}'
RUN_DESCRIPTION = RunDescription(
f'{_name}',
experiments=[Experiment(f'{_name}', cli, _params.generate_params(randomize=False))],
experiment_arg_name='experiment', experiment_dir_arg_name='hydra.run.dir',
param_prefix='', customize_experiment_name=False,
)
| 866 | Python | 38.409089 | 150 | 0.737875 |
NVIDIA-Omniverse/IsaacGymEnvs/isaacgymenvs/pbt/experiments/allegro_kuka_two_arms_regrasping_lstm.py | from isaacgymenvs.pbt.launcher.run_description import ParamGrid, RunDescription, Experiment
from isaacgymenvs.pbt.experiments.run_utils import version, seeds, default_num_frames
kuka_env = 'allegro_kuka_two_arms_regrasp'
_frames = default_num_frames
_name = f'{kuka_env}_{version}'
_params = ParamGrid([
('seed', seeds(8)),
])
_wandb_activate = True
_wandb_group = f'pbt_{_name}'
_wandb_entity = 'your_wandb_entity'
_wandb_project = 'your_wandb_project'
cli = f'python -m isaacgymenvs.train seed=-1 ' \
f'train.params.config.max_frames={_frames} headless=True ' \
f'task=AllegroKukaTwoArmsLSTM task/env=regrasping ' \
f'task.env.episodeLength=400 ' \
f'wandb_project={_wandb_project} wandb_entity={_wandb_entity} wandb_activate={_wandb_activate} wandb_group={_wandb_group}'
RUN_DESCRIPTION = RunDescription(
f'{_name}',
experiments=[Experiment(f'{_name}', cli, _params.generate_params(randomize=False))],
experiment_arg_name='experiment', experiment_dir_arg_name='hydra.run.dir',
param_prefix='', customize_experiment_name=False,
)
| 1,083 | Python | 33.967741 | 128 | 0.711911 |
NVIDIA-Omniverse/IsaacGymEnvs/isaacgymenvs/pbt/experiments/allegro_kuka_reorientation_lstm_8gpu.py | from isaacgymenvs.pbt.launcher.run_description import ParamGrid, RunDescription, Experiment
from isaacgymenvs.pbt.experiments.run_utils import version, seeds, default_num_frames
kuka_env = 'allegro_kuka_reorientation'
_num_gpus = 8
_frames = default_num_frames * _num_gpus
_name = f'{kuka_env}_{version}_{_num_gpus}gpu'
_params = ParamGrid([
('seed', seeds(1)),
])
_wandb_activate = True
_wandb_group = f'rlgames_{_name}'
_wandb_entity = 'your_wandb_entity'
_wandb_project = 'your_wandb_project'
cli = f'train.py multi_gpu=True ' \
f'train.params.config.max_frames={_frames} headless=True ' \
f'task=AllegroKukaLSTM task/env=reorientation ' \
f'wandb_project={_wandb_project} wandb_entity={_wandb_entity} wandb_activate={_wandb_activate} wandb_group={_wandb_group}'
RUN_DESCRIPTION = RunDescription(
f'{_name}',
experiments=[Experiment(f'{_name}', cli, _params.generate_params(randomize=False))],
experiment_arg_name='experiment', experiment_dir_arg_name='hydra.run.dir',
param_prefix='', customize_experiment_name=False,
)
| 1,069 | Python | 33.516128 | 128 | 0.71188 |
NVIDIA-Omniverse/IsaacGymEnvs/isaacgymenvs/pbt/experiments/allegro_kuka_regrasping_lstm.py | from isaacgymenvs.pbt.launcher.run_description import ParamGrid, RunDescription, Experiment
from isaacgymenvs.pbt.experiments.run_utils import version, seeds, default_num_frames
kuka_env = 'allegro_kuka_regrasp'
_frames = default_num_frames
_name = f'{kuka_env}_{version}'
_params = ParamGrid([
('seed', seeds(8)),
])
_wandb_activate = True
_wandb_group = f'pbt_{_name}'
_wandb_entity = 'your_wandb_entity'
_wandb_project = 'your_wandb_project'
cli = f'python -m isaacgymenvs.train seed=-1 ' \
f'train.params.config.max_frames={_frames} headless=True ' \
f'task=AllegroKukaLSTM task/env=regrasping ' \
f'wandb_project={_wandb_project} wandb_entity={_wandb_entity} wandb_activate={_wandb_activate} wandb_group={_wandb_group}'
RUN_DESCRIPTION = RunDescription(
f'{_name}',
experiments=[Experiment(f'{_name}', cli, _params.generate_params(randomize=False))],
experiment_arg_name='experiment', experiment_dir_arg_name='hydra.run.dir',
param_prefix='', customize_experiment_name=False,
)
| 1,028 | Python | 33.299999 | 128 | 0.713035 |
NVIDIA-Omniverse/IsaacGymEnvs/isaacgymenvs/pbt/experiments/allegro_kuka_reorientation_pbt_lstm.py | from isaacgymenvs.pbt.launcher.run_description import ParamGrid, RunDescription, Experiment
from isaacgymenvs.pbt.experiments.allegro_kuka_pbt_base import kuka_env, kuka_base_cli
from isaacgymenvs.pbt.experiments.run_utils import version
_pbt_num_policies = 8
_name = f'{kuka_env}_manip_{version}_pbt_{_pbt_num_policies}p'
_params = ParamGrid([
('pbt.policy_idx', list(range(_pbt_num_policies))),
])
_wandb_activate = True
_wandb_group = f'pbt_{_name}'
_wandb_entity = 'your_wandb_entity'
_wandb_project = 'your_wandb_project'
cli = kuka_base_cli + f' task=AllegroKukaLSTM task/env=reorientation ' \
f'wandb_project={_wandb_project} wandb_entity={_wandb_entity} wandb_activate={_wandb_activate} wandb_group={_wandb_group}'
RUN_DESCRIPTION = RunDescription(
f'{_name}',
experiments=[Experiment(f'{_name}', cli, _params.generate_params(randomize=False))],
experiment_arg_name='experiment', experiment_dir_arg_name='hydra.run.dir',
param_prefix='', customize_experiment_name=False,
)
| 1,029 | Python | 37.148147 | 144 | 0.718173 |
NVIDIA-Omniverse/IsaacGymEnvs/isaacgymenvs/pbt/experiments/allegro_kuka_throw_pbt_lstm.py | from isaacgymenvs.pbt.launcher.run_description import ParamGrid, RunDescription, Experiment
from isaacgymenvs.pbt.experiments.allegro_kuka_pbt_base import kuka_env, kuka_base_cli
from isaacgymenvs.pbt.experiments.run_utils import version
_pbt_num_policies = 8
_name = f'{kuka_env}_throw_{version}_pbt_{_pbt_num_policies}p'
_params = ParamGrid([
('pbt.policy_idx', list(range(_pbt_num_policies))),
])
_wandb_activate = True
_wandb_group = f'pbt_{_name}'
_wandb_entity = 'your_wandb_entity'
_wandb_project = 'your_wandb_project'
cli = kuka_base_cli + \
f' task=AllegroKukaLSTM ' \
f'task/env=throw wandb_activate=True pbt.num_policies={_pbt_num_policies} ' \
f'wandb_project={_wandb_project} wandb_entity={_wandb_entity} wandb_activate={_wandb_activate} wandb_group={_wandb_group}'
RUN_DESCRIPTION = RunDescription(
f'{_name}',
experiments=[Experiment(f'{_name}', cli, _params.generate_params(randomize=False))],
experiment_arg_name='experiment', experiment_dir_arg_name='hydra.run.dir',
param_prefix='', customize_experiment_name=False,
)
| 1,076 | Python | 36.13793 | 126 | 0.722119 |
NVIDIA-Omniverse/IsaacGymEnvs/isaacgymenvs/pbt/experiments/allegro_kuka_two_arms_reorientation_pbt_lstm.py | from isaacgymenvs.pbt.launcher.run_description import ParamGrid, RunDescription, Experiment
from isaacgymenvs.pbt.experiments.allegro_kuka_pbt_base import kuka_base_cli
from isaacgymenvs.pbt.experiments.run_utils import version
env = 'allegro_kuka_two_arms_reorientation'
_pbt_num_policies = 8
_name = f'{env}_{version}_pbt_{_pbt_num_policies}p'
_wandb_group = f'pbt_{_name}'
_params = ParamGrid([
('pbt.policy_idx', list(range(_pbt_num_policies))),
])
cli = kuka_base_cli + f' task=AllegroKukaTwoArmsLSTM task/env=reorientation wandb_activate=True wandb_group={_wandb_group} pbt.num_policies={_pbt_num_policies}'
RUN_DESCRIPTION = RunDescription(
f'{_name}',
experiments=[Experiment(f'{_name}', cli, _params.generate_params(randomize=False))],
experiment_arg_name='experiment', experiment_dir_arg_name='hydra.run.dir',
param_prefix='', customize_experiment_name=False,
)
| 898 | Python | 36.458332 | 160 | 0.740535 |
NVIDIA-Omniverse/IsaacGymEnvs/isaacgymenvs/pbt/experiments/allegro_kuka_pbt_base.py | from isaacgymenvs.pbt.launcher.run_description import ParamGrid, RunDescription, Experiment
from isaacgymenvs.pbt.experiments.run_utils import version, default_num_frames
kuka_env = 'allegro_kuka'
_frames = default_num_frames
_pbt_num_policies = 8
_name = f'{kuka_env}_{version}_pbt_{_pbt_num_policies}p'
_wandb_activate = True
_wandb_group = f'pbt_{_name}'
_wandb_entity = 'your_wandb_entity'
_wandb_project = 'your_wandb_project'
kuka_base_cli = (f'python -m isaacgymenvs.train seed=-1 '
f'train.params.config.max_frames={_frames} headless=True '
f'wandb_project={_wandb_project} wandb_entity={_wandb_entity} wandb_activate={_wandb_activate} wandb_group={_wandb_group} '
f'pbt=pbt_default pbt.workspace=workspace_{kuka_env} '
f'pbt.interval_steps=20000000 pbt.start_after=100000000 pbt.initial_delay=200000000 pbt.replace_fraction_worst=0.3 pbt/mutation=allegro_kuka_mutation')
_params = ParamGrid([
('pbt.policy_idx', list(range(_pbt_num_policies))),
])
cli = kuka_base_cli + f' task=AllegroKuka task/env=reorientation pbt.num_policies={_pbt_num_policies}'
RUN_DESCRIPTION = RunDescription(
f'{_name}',
experiments=[Experiment(f'{_name}', cli, _params.generate_params(randomize=False))],
experiment_arg_name='experiment', experiment_dir_arg_name='hydra.run.dir',
param_prefix='', customize_experiment_name=False,
)
| 1,414 | Python | 40.617646 | 168 | 0.704385 |
NVIDIA-Omniverse/IsaacGymEnvs/isaacgymenvs/pbt/launcher/run_description.py | import os
import re
from collections import OrderedDict
from os.path import join
import numpy as np
class ParamGenerator:
def __init__(self):
pass
def generate_params(self, randomize=True):
"""Supposed to be a generator (so should yield dicts of parameters)."""
pass
class ParamList(ParamGenerator):
"""The most simple kind of generator, represents just the list of parameter combinations."""
def __init__(self, combinations):
super(ParamList, self).__init__()
self.combinations = combinations
def generate_params(self, randomize=True):
if randomize:
combinations = np.random.permutation(self.combinations)
else:
combinations = self.combinations
for combination in combinations:
yield combination
class ParamGrid(ParamGenerator):
"""Parameter generator for grid search."""
def __init__(self, grid_tuples):
"""Uses OrderedDict, so must be initialized with the list of tuples if you want to preserve order."""
super(ParamGrid, self).__init__()
self.grid = OrderedDict(grid_tuples)
def _generate_combinations(self, param_idx, params):
"""Recursively generate all parameter combinations in a grid."""
if param_idx == len(self.grid) - 1:
# last parameter, just return list of values for this parameter
return [[value] for value in self.grid[params[param_idx]]]
else:
subcombinations = self._generate_combinations(param_idx + 1, params) # returns list of param combinations
result = []
# iterate over all values of current parameter
for value in self.grid[params[param_idx]]:
for subcombination in subcombinations:
result.append([value] + subcombination)
return result
def generate_params(self, randomize=False):
if len(self.grid) == 0:
return dict()
# start with 0th value for every parameter
total_num_combinations = np.prod([len(p_values) for p_values in self.grid.values()])
param_names = tuple(self.grid.keys())
all_combinations = self._generate_combinations(0, param_names)
assert len(all_combinations) == total_num_combinations
if randomize:
all_combinations = np.random.permutation(all_combinations)
for combination in all_combinations:
combination_dict = dict()
for i, param_name in enumerate(param_names):
if isinstance(param_name, (list, tuple)):
for j, param in enumerate(param_name):
combination_dict[param] = combination[i][j]
else:
combination_dict[param_name] = combination[i]
yield combination_dict
class Experiment:
def __init__(self, name, cmd, param_generator=(), env_vars=None):
"""
:param cmd: base command to append the parameters to
:param param_generator: iterable of parameter dicts
"""
self.base_name = name
self.cmd = cmd
self.params = list(param_generator)
self.env_vars = env_vars
def generate_experiments(self, experiment_arg_name, customize_experiment_name, param_prefix):
"""Yields tuples of (cmd, experiment_name)"""
num_experiments = 1 if len(self.params) == 0 else len(self.params)
for experiment_idx in range(num_experiments):
cmd_tokens = [self.cmd]
experiment_name_tokens = [self.base_name]
# abbreviations for parameter names that we've used
param_shorthands = []
if len(self.params) > 0:
params = self.params[experiment_idx]
for param, value in params.items():
param_str = f"{param_prefix}{param}={value}"
cmd_tokens.append(param_str)
param_tokens = re.split("[._-]", param)
shorthand_tokens = [t[0] for t in param_tokens[:-1]]
last_token_l = min(3, len(param_tokens[-1]))
shorthand = ".".join(shorthand_tokens + [param_tokens[-1][:last_token_l]])
while last_token_l <= len(param_tokens[-1]) and shorthand in param_shorthands:
last_token_l += 1
shorthand = ".".join(shorthand_tokens + [param_tokens[-1][:last_token_l]])
param_shorthands.append(shorthand)
experiment_name_token = f"{shorthand}_{value}"
experiment_name_tokens.append(experiment_name_token)
if customize_experiment_name:
experiment_name = f"{experiment_idx:02d}_" + "_".join(experiment_name_tokens)
if len(experiment_name) > 100:
print(f"Experiment name is extra long! ({len(experiment_name)} characters)")
else:
experiment_name = f"{experiment_idx:02d}_{self.base_name}"
cmd_tokens.append(f"{experiment_arg_name}={experiment_name}")
param_str = " ".join(cmd_tokens)
yield param_str, experiment_name
class RunDescription:
def __init__(
self,
run_name,
experiments,
experiment_arg_name="--experiment",
experiment_dir_arg_name="--train_dir",
customize_experiment_name=True,
param_prefix="--",
):
"""
:param run_name: overall name of the experiment and the name of the root folder
:param experiments: a list of Experiment objects to run
:param experiment_arg_name: CLI argument of the underlying experiment that determines it's unique name
to be generated by the launcher. Default: --experiment
:param experiment_dir_arg_name: CLI argument for the root train dir of your experiment. Default: --train_dir
:param customize_experiment_name: whether to add a hyperparameter combination to the experiment name
:param param_prefix: most experiments will use "--" prefix for each parameter, but some apps don't have this
prefix, i.e. with Hydra you should set it to empty string.
"""
self.run_name = run_name
self.experiments = experiments
self.experiment_suffix = ""
self.experiment_arg_name = experiment_arg_name
self.experiment_dir_arg_name = experiment_dir_arg_name
self.customize_experiment_name = customize_experiment_name
self.param_prefix = param_prefix
def generate_experiments(self, train_dir, makedirs=True):
"""Yields tuples (final cmd for experiment, experiment_name, root_dir)."""
for experiment in self.experiments:
root_dir = join(self.run_name, f"{experiment.base_name}_{self.experiment_suffix}")
experiment_cmds = experiment.generate_experiments(
self.experiment_arg_name, self.customize_experiment_name, self.param_prefix
)
for experiment_cmd, experiment_name in experiment_cmds:
experiment_dir = join(train_dir, root_dir)
if makedirs:
os.makedirs(experiment_dir, exist_ok=True)
experiment_cmd += f" {self.experiment_dir_arg_name}={experiment_dir}"
yield experiment_cmd, experiment_name, root_dir, experiment.env_vars
| 7,439 | Python | 39 | 118 | 0.605323 |
NVIDIA-Omniverse/IsaacGymEnvs/isaacgymenvs/pbt/launcher/run_ngc.py | """
Run many experiments with NGC: hyperparameter sweeps, etc.
This isn't production code, but feel free to use as an example for your NGC setup.
"""
import time
from multiprocessing.pool import ThreadPool
from subprocess import PIPE, Popen
from isaacgymenvs.pbt.launcher.run_slurm import str2bool
def add_ngc_args(parser):
parser.add_argument(
"--ngc_job_template",
default=None,
type=str,
help="NGC command line template, specifying instance type, docker container, etc.",
)
parser.add_argument(
"--ngc_print_only", default=False, type=str2bool, help="Just print commands to the console without executing"
)
parser.set_defaults(pause_between=0)
return parser
def run_ngc(run_description, args):
pause_between = args.pause_between
experiments = run_description.experiments
print(f"Starting processes with base cmds: {[e.cmd for e in experiments]}")
if args.ngc_job_template is not None:
with open(args.ngc_job_template, "r") as template_file:
ngc_template = template_file.read()
ngc_template = ngc_template.replace("\\", " ")
ngc_template = " ".join(ngc_template.split())
print(f"NGC template: {ngc_template}")
experiments = run_description.generate_experiments(args.train_dir, makedirs=False)
experiments = list(experiments)
print(f"{len(experiments)} experiments to run")
def launch_experiment(experiment_idx, experiment_):
time.sleep(experiment_idx * 0.1)
cmd, name, *_ = experiment_
job_name = name
print(f"Job name: {job_name}")
ngc_job_cmd = ngc_template.replace("{{ name }}", job_name).replace("{{ experiment_cmd }}", cmd)
print(f"Executing {ngc_job_cmd}")
if not args.ngc_print_only:
process = Popen(ngc_job_cmd, stdout=PIPE, shell=True)
output, err = process.communicate()
exit_code = process.wait()
print(f"Output: {output}, err: {err}, exit code: {exit_code}")
time.sleep(pause_between)
pool_size = 1 if pause_between > 0 else min(10, len(experiments))
with ThreadPool(pool_size) as p:
p.starmap(launch_experiment, enumerate(experiments))
print("Done!")
return 0
| 2,260 | Python | 29.972602 | 117 | 0.654425 |
NVIDIA-Omniverse/IsaacGymEnvs/isaacgymenvs/pbt/launcher/run_slurm.py | import argparse
import os
import time
from os.path import join
from string import Template
from subprocess import PIPE, Popen
SBATCH_TEMPLATE_DEFAULT = (
"#!/bin/bash\n"
"conda activate conda_env_name\n"
"cd ~/project\n"
)
def str2bool(v):
if isinstance(v, bool):
return v
if isinstance(v, str) and v.lower() in ("true",):
return True
elif isinstance(v, str) and v.lower() in ("false",):
return False
else:
raise argparse.ArgumentTypeError("Boolean value expected")
def add_slurm_args(parser):
parser.add_argument("--slurm_gpus_per_job", default=1, type=int, help="GPUs in a single SLURM process")
parser.add_argument(
"--slurm_cpus_per_gpu", default=16, type=int, help="Max allowed number of CPU cores per allocated GPU"
)
parser.add_argument(
"--slurm_print_only", default=False, type=str2bool, help="Just print commands to the console without executing"
)
parser.add_argument(
"--slurm_workdir",
default=None,
type=str,
help="Optional workdir. Used by slurm launcher to store logfiles etc.",
)
parser.add_argument(
"--slurm_partition",
default=None,
type=str,
help='Adds slurm partition, i.e. for "gpu" it will add "-p gpu" to sbatch command line',
)
parser.add_argument(
"--slurm_sbatch_template",
default=None,
type=str,
help="Commands to run before the actual experiment (i.e. activate conda env, etc.)",
)
parser.add_argument(
"--slurm_timeout",
default="0",
type=str,
help="Time to run jobs before timing out job and requeuing the job. Defaults to 0, which does not time out the job",
)
return parser
def run_slurm(run_description, args):
workdir = args.slurm_workdir
pause_between = args.pause_between
experiments = run_description.experiments
print(f"Starting processes with base cmds: {[e.cmd for e in experiments]}")
if not os.path.exists(workdir):
print(f"Creating {workdir}...")
os.makedirs(workdir)
if args.slurm_sbatch_template is not None:
with open(args.slurm_sbatch_template, "r") as template_file:
sbatch_template = template_file.read()
else:
sbatch_template = SBATCH_TEMPLATE_DEFAULT
print(f"Sbatch template: {sbatch_template}")
partition = ""
if args.slurm_partition is not None:
partition = f"-p {args.slurm_partition} "
num_cpus = args.slurm_cpus_per_gpu * args.slurm_gpus_per_job
experiments = run_description.generate_experiments(args.train_dir)
sbatch_files = []
for experiment in experiments:
cmd, name, *_ = experiment
sbatch_fname = f"sbatch_{name}.sh"
sbatch_fname = join(workdir, sbatch_fname)
sbatch_fname = os.path.abspath(sbatch_fname)
file_content = Template(sbatch_template).substitute(
CMD=cmd,
FILENAME=sbatch_fname,
PARTITION=partition,
GPU=args.slurm_gpus_per_job,
CPU=num_cpus,
TIMEOUT=args.slurm_timeout,
)
with open(sbatch_fname, "w") as sbatch_f:
sbatch_f.write(file_content)
sbatch_files.append(sbatch_fname)
job_ids = []
idx = 0
for sbatch_file in sbatch_files:
idx += 1
sbatch_fname = os.path.basename(sbatch_file)
cmd = f"sbatch {partition}--gres=gpu:{args.slurm_gpus_per_job} -c {num_cpus} --parsable --output {workdir}/{sbatch_fname}-slurm-%j.out {sbatch_file}"
print(f"Executing {cmd}")
if args.slurm_print_only:
output = idx
else:
cmd_tokens = cmd.split()
process = Popen(cmd_tokens, stdout=PIPE)
output, err = process.communicate()
exit_code = process.wait()
print(f"{output} {err} {exit_code}")
if exit_code != 0:
print("sbatch process failed!")
time.sleep(5)
job_id = int(output)
job_ids.append(str(job_id))
time.sleep(pause_between)
tail_cmd = f"tail -f {workdir}/*.out"
print(f"Monitor log files using\n\n\t {tail_cmd} \n\n")
scancel_cmd = f'scancel {" ".join(job_ids)}'
print("Jobs queued: %r" % job_ids)
print("Use this command to cancel your jobs: \n\t %s \n" % scancel_cmd)
with open(join(workdir, "scancel.sh"), "w") as fobj:
fobj.write(scancel_cmd)
print("Done!")
return 0
| 4,525 | Python | 28.776316 | 157 | 0.60663 |
NVIDIA-Omniverse/IsaacGymEnvs/isaacgymenvs/pbt/launcher/run_processes.py | """Run groups of experiments, hyperparameter sweeps, etc."""
import argparse
import os
import subprocess
import sys
import time
from os.path import join
def add_os_parallelism_args(parser: argparse.ArgumentParser) -> argparse.ArgumentParser:
parser.add_argument("--num_gpus", default=1, type=int, help="How many local GPUs to use")
parser.add_argument("--max_parallel", default=4, type=int, help="Maximum simultaneous experiments")
parser.add_argument(
"--experiments_per_gpu",
default=-1,
type=int,
help="How many experiments can we squeeze on a single GPU. "
"Specify this option if and only if you are using launcher to run several experiments using OS-level"
"parallelism (--backend=processes)."
"In any other case use default value (-1) for not altering CUDA_VISIBLE_DEVICES at all."
"This will allow your experiments to use all GPUs available (as many as --num_gpu allows)"
"Helpful when e.g. you are running a single big PBT experiment.",
)
return parser
def ensure_dir_exists(path) -> str:
if not os.path.exists(path):
os.makedirs(path, exist_ok=True)
return path
def run(run_description, args):
experiments = run_description.experiments
max_parallel = args.max_parallel
print("Starting processes with base cmds: %r", [e.cmd for e in experiments])
print(f"Max parallel processes is {max_parallel}")
print(f"Monitor log files using\n\n\ttail -f train_dir/{run_description.run_name}/**/**/sf_log.txt\n\n")
processes = []
processes_per_gpu = {g: [] for g in range(args.num_gpus)}
experiments = run_description.generate_experiments(args.train_dir)
next_experiment = next(experiments, None)
def find_least_busy_gpu():
least_busy_gpu = None
gpu_available_processes = 0
for gpu_id in range(args.num_gpus):
available_processes = args.experiments_per_gpu - len(processes_per_gpu[gpu_id])
if available_processes > gpu_available_processes:
gpu_available_processes = available_processes
least_busy_gpu = gpu_id
return least_busy_gpu, gpu_available_processes
def can_squeeze_another_process():
if len(processes) >= max_parallel:
return False
if args.experiments_per_gpu > 0:
least_busy_gpu, gpu_available_processes = find_least_busy_gpu()
if gpu_available_processes <= 0:
return False
return True
failed_processes = []
last_log_time = 0
log_interval = 3 # seconds
while len(processes) > 0 or next_experiment is not None:
while can_squeeze_another_process() and next_experiment is not None:
cmd, name, root_dir, exp_env_vars = next_experiment
cmd_tokens = cmd.split(" ")
# workaround to make sure we're running the correct python executable from our virtual env
if cmd_tokens[0].startswith("python"):
cmd_tokens[0] = sys.executable
print(f"Using Python executable {cmd_tokens[0]}")
ensure_dir_exists(join(args.train_dir, root_dir))
envvars = os.environ.copy()
best_gpu = None
if args.experiments_per_gpu > 0:
best_gpu, best_gpu_available_processes = find_least_busy_gpu()
print(
f"The least busy gpu is {best_gpu} where we can run {best_gpu_available_processes} more processes",
)
envvars["CUDA_VISIBLE_DEVICES"] = f"{best_gpu}"
print(f"Starting process {cmd_tokens}")
if exp_env_vars is not None:
for key, value in exp_env_vars.items():
print(f"Adding env variable {key} {value}")
envvars[str(key)] = str(value)
process = subprocess.Popen(cmd_tokens, stdout=None, stderr=None, env=envvars)
process.gpu_id = best_gpu
process.proc_cmd = cmd
processes.append(process)
if process.gpu_id is not None:
processes_per_gpu[process.gpu_id].append(process.proc_cmd)
print(f"Started process {process.proc_cmd} GPU {process.gpu_id}")
print(f"Waiting for {args.pause_between} seconds before starting next process")
time.sleep(args.pause_between)
next_experiment = next(experiments, None)
remaining_processes = []
for process in processes:
if process.poll() is None:
remaining_processes.append(process)
continue
else:
if process.gpu_id is not None:
processes_per_gpu[process.gpu_id].remove(process.proc_cmd)
print(f"Process finished {process.proc_cmd}, {process.returncode}")
if process.returncode != 0:
failed_processes.append((process.proc_cmd, process.pid, process.returncode))
print(f"WARNING: RETURN CODE IS {process.returncode}")
processes = remaining_processes
if time.time() - last_log_time > log_interval:
if failed_processes:
print(f"Failed processes:", ", ".join([f"PID: {p[1]} code: {p[2]}" for p in failed_processes]))
last_log_time = time.time()
time.sleep(0.1)
print("Done!")
return 0
| 5,425 | Python | 36.420689 | 119 | 0.609032 |
NVIDIA-Omniverse/IsaacGymEnvs/isaacgymenvs/pbt/launcher/run.py | import argparse
import importlib
import sys
from isaacgymenvs.pbt.launcher.run_ngc import add_ngc_args, run_ngc
from isaacgymenvs.pbt.launcher.run_processes import add_os_parallelism_args, run
from isaacgymenvs.pbt.launcher.run_slurm import add_slurm_args, run_slurm
def launcher_argparser(args) -> argparse.ArgumentParser:
parser = argparse.ArgumentParser()
parser.add_argument("--train_dir", default="./train_dir", type=str, help="Directory for sub-experiments")
parser.add_argument(
"--run",
default=None,
type=str,
help="Name of the python module that describes the run, e.g. sf_examples.vizdoom.experiments.paper_doom_all_basic_envs.py "
"Run module must be importable in your Python environment. It must define a global variable RUN_DESCRIPTION (see existing run modules for examples).",
)
parser.add_argument(
"--backend",
default="processes",
choices=["processes", "slurm", "ngc"],
help="Launcher backend, use OS multiprocessing by default",
)
parser.add_argument("--pause_between", default=1, type=int, help="Pause in seconds between processes")
parser.add_argument(
"--experiment_suffix", default="", type=str, help="Append this to the name of the experiment dir"
)
partial_cfg, _ = parser.parse_known_args(args)
if partial_cfg.backend == "slurm":
parser = add_slurm_args(parser)
elif partial_cfg.backend == "ngc":
parser = add_ngc_args(parser)
elif partial_cfg.backend == "processes":
parser = add_os_parallelism_args(parser)
else:
raise ValueError(f"Unknown backend: {partial_cfg.backend}")
return parser
def parse_args():
args = launcher_argparser(sys.argv[1:]).parse_args(sys.argv[1:])
return args
def main():
launcher_cfg = parse_args()
try:
# assuming we're given the full name of the module
run_module = importlib.import_module(f"{launcher_cfg.run}")
except ImportError as exc:
print(f"Could not import the run module {exc}")
return 1
run_description = run_module.RUN_DESCRIPTION
run_description.experiment_suffix = launcher_cfg.experiment_suffix
if launcher_cfg.backend == "processes":
run(run_description, launcher_cfg)
elif launcher_cfg.backend == "slurm":
run_slurm(run_description, launcher_cfg)
elif launcher_cfg.backend == "ngc":
run_ngc(run_description, launcher_cfg)
return 0
if __name__ == "__main__":
sys.exit(main())
| 2,538 | Python | 32.853333 | 158 | 0.670213 |
NVIDIA-Omniverse/IsaacGymEnvs/isaacgymenvs/utils/wandb_utils.py | from rl_games.common.algo_observer import AlgoObserver
from isaacgymenvs.utils.utils import retry
from isaacgymenvs.utils.reformat import omegaconf_to_dict
class WandbAlgoObserver(AlgoObserver):
"""Need this to propagate the correct experiment name after initialization."""
def __init__(self, cfg):
super().__init__()
self.cfg = cfg
def before_init(self, base_name, config, experiment_name):
"""
Must call initialization of Wandb before RL-games summary writer is initialized, otherwise
sync_tensorboard does not work.
"""
import wandb
wandb_unique_id = f"uid_{experiment_name}"
print(f"Wandb using unique id {wandb_unique_id}")
cfg = self.cfg
# this can fail occasionally, so we try a couple more times
@retry(3, exceptions=(Exception,))
def init_wandb():
wandb.init(
project=cfg.wandb_project,
entity=cfg.wandb_entity,
group=cfg.wandb_group,
tags=cfg.wandb_tags,
sync_tensorboard=True,
id=wandb_unique_id,
name=experiment_name,
resume=True,
settings=wandb.Settings(start_method='fork'),
)
if cfg.wandb_logcode_dir:
wandb.run.log_code(root=cfg.wandb_logcode_dir)
print('wandb running directory........', wandb.run.dir)
print('Initializing WandB...')
try:
init_wandb()
except Exception as exc:
print(f'Could not initialize WandB! {exc}')
if isinstance(self.cfg, dict):
wandb.config.update(self.cfg, allow_val_change=True)
else:
wandb.config.update(omegaconf_to_dict(self.cfg), allow_val_change=True)
| 1,835 | Python | 31.785714 | 98 | 0.584196 |
NVIDIA-Omniverse/IsaacGymEnvs/isaacgymenvs/utils/rlgames_utils.py | # Copyright (c) 2018-2023, NVIDIA Corporation
# All rights reserved.
#
# Redistribution and use in source and binary forms, with or without
# modification, are permitted provided that the following conditions are met:
#
# 1. Redistributions of source code must retain the above copyright notice, this
# list of conditions and the following disclaimer.
#
# 2. Redistributions in binary form must reproduce the above copyright notice,
# this list of conditions and the following disclaimer in the documentation
# and/or other materials provided with the distribution.
#
# 3. Neither the name of the copyright holder nor the names of its
# contributors may be used to endorse or promote products derived from
# this software without specific prior written permission.
#
# THIS SOFTWARE IS PROVIDED BY THE COPYRIGHT HOLDERS AND CONTRIBUTORS "AS IS"
# AND ANY EXPRESS OR IMPLIED WARRANTIES, INCLUDING, BUT NOT LIMITED TO, THE
# IMPLIED WARRANTIES OF MERCHANTABILITY AND FITNESS FOR A PARTICULAR PURPOSE ARE
# DISCLAIMED. IN NO EVENT SHALL THE COPYRIGHT HOLDER OR CONTRIBUTORS BE LIABLE
# FOR ANY DIRECT, INDIRECT, INCIDENTAL, SPECIAL, EXEMPLARY, OR CONSEQUENTIAL
# DAMAGES (INCLUDING, BUT NOT LIMITED TO, PROCUREMENT OF SUBSTITUTE GOODS OR
# SERVICES; LOSS OF USE, DATA, OR PROFITS; OR BUSINESS INTERRUPTION) HOWEVER
# CAUSED AND ON ANY THEORY OF LIABILITY, WHETHER IN CONTRACT, STRICT LIABILITY,
# OR TORT (INCLUDING NEGLIGENCE OR OTHERWISE) ARISING IN ANY WAY OUT OF THE USE
# OF THIS SOFTWARE, EVEN IF ADVISED OF THE POSSIBILITY OF SUCH DAMAGE.
import os
from collections import deque
from typing import Callable, Dict, Tuple, Any
import os
import gym
import numpy as np
import torch
from rl_games.common import env_configurations, vecenv
from rl_games.common.algo_observer import AlgoObserver
from isaacgymenvs.tasks import isaacgym_task_map
from isaacgymenvs.utils.utils import set_seed, flatten_dict
def multi_gpu_get_rank(multi_gpu):
if multi_gpu:
rank = int(os.getenv("LOCAL_RANK", "0"))
print("GPU rank: ", rank)
return rank
return 0
def get_rlgames_env_creator(
# used to create the vec task
seed: int,
task_config: dict,
task_name: str,
sim_device: str,
rl_device: str,
graphics_device_id: int,
headless: bool,
# used to handle multi-gpu case
multi_gpu: bool = False,
post_create_hook: Callable = None,
virtual_screen_capture: bool = False,
force_render: bool = False,
):
"""Parses the configuration parameters for the environment task and creates a VecTask
Args:
task_config: environment configuration.
task_name: Name of the task, used to evaluate based on the imported name (eg 'Trifinger')
sim_device: The type of env device, eg 'cuda:0'
rl_device: Device that RL will be done on, eg 'cuda:0'
graphics_device_id: Graphics device ID.
headless: Whether to run in headless mode.
multi_gpu: Whether to use multi gpu
post_create_hook: Hooks to be called after environment creation.
[Needed to setup WandB only for one of the RL Games instances when doing multiple GPUs]
virtual_screen_capture: Set to True to allow the users get captured screen in RGB array via `env.render(mode='rgb_array')`.
force_render: Set to True to always force rendering in the steps (if the `control_freq_inv` is greater than 1 we suggest stting this arg to True)
Returns:
A VecTaskPython object.
"""
def create_rlgpu_env():
"""
Creates the task from configurations and wraps it using RL-games wrappers if required.
"""
if multi_gpu:
local_rank = int(os.getenv("LOCAL_RANK", "0"))
global_rank = int(os.getenv("RANK", "0"))
# local rank of the GPU in a node
local_rank = int(os.getenv("LOCAL_RANK", "0"))
# global rank of the GPU
global_rank = int(os.getenv("RANK", "0"))
# total number of GPUs across all nodes
world_size = int(os.getenv("WORLD_SIZE", "1"))
print(f"global_rank = {global_rank} local_rank = {local_rank} world_size = {world_size}")
_sim_device = f'cuda:{local_rank}'
_rl_device = f'cuda:{local_rank}'
task_config['rank'] = local_rank
task_config['rl_device'] = _rl_device
else:
_sim_device = sim_device
_rl_device = rl_device
# create native task and pass custom config
env = isaacgym_task_map[task_name](
cfg=task_config,
rl_device=_rl_device,
sim_device=_sim_device,
graphics_device_id=graphics_device_id,
headless=headless,
virtual_screen_capture=virtual_screen_capture,
force_render=force_render,
)
if post_create_hook is not None:
post_create_hook()
return env
return create_rlgpu_env
class RLGPUAlgoObserver(AlgoObserver):
"""Allows us to log stats from the env along with the algorithm running stats. """
def __init__(self):
super().__init__()
self.algo = None
self.writer = None
self.ep_infos = []
self.direct_info = {}
self.episode_cumulative = dict()
self.episode_cumulative_avg = dict()
self.new_finished_episodes = False
def after_init(self, algo):
self.algo = algo
self.writer = self.algo.writer
def process_infos(self, infos, done_indices):
assert isinstance(infos, dict), 'RLGPUAlgoObserver expects dict info'
if not isinstance(infos, dict):
return
if 'episode' in infos:
self.ep_infos.append(infos['episode'])
if 'episode_cumulative' in infos:
for key, value in infos['episode_cumulative'].items():
if key not in self.episode_cumulative:
self.episode_cumulative[key] = torch.zeros_like(value)
self.episode_cumulative[key] += value
for done_idx in done_indices:
self.new_finished_episodes = True
done_idx = done_idx.item()
for key, value in infos['episode_cumulative'].items():
if key not in self.episode_cumulative_avg:
self.episode_cumulative_avg[key] = deque([], maxlen=self.algo.games_to_track)
self.episode_cumulative_avg[key].append(self.episode_cumulative[key][done_idx].item())
self.episode_cumulative[key][done_idx] = 0
# turn nested infos into summary keys (i.e. infos['scalars']['lr'] -> infos['scalars/lr']
if len(infos) > 0 and isinstance(infos, dict): # allow direct logging from env
infos_flat = flatten_dict(infos, prefix='', separator='/')
self.direct_info = {}
for k, v in infos_flat.items():
# only log scalars
if isinstance(v, float) or isinstance(v, int) or (isinstance(v, torch.Tensor) and len(v.shape) == 0):
self.direct_info[k] = v
def after_print_stats(self, frame, epoch_num, total_time):
if self.ep_infos:
for key in self.ep_infos[0]:
infotensor = torch.tensor([], device=self.algo.device)
for ep_info in self.ep_infos:
# handle scalar and zero dimensional tensor infos
if not isinstance(ep_info[key], torch.Tensor):
ep_info[key] = torch.Tensor([ep_info[key]])
if len(ep_info[key].shape) == 0:
ep_info[key] = ep_info[key].unsqueeze(0)
infotensor = torch.cat((infotensor, ep_info[key].to(self.algo.device)))
value = torch.mean(infotensor)
self.writer.add_scalar('Episode/' + key, value, epoch_num)
self.ep_infos.clear()
# log these if and only if we have new finished episodes
if self.new_finished_episodes:
for key in self.episode_cumulative_avg:
self.writer.add_scalar(f'episode_cumulative/{key}', np.mean(self.episode_cumulative_avg[key]), frame)
self.writer.add_scalar(f'episode_cumulative_min/{key}_min', np.min(self.episode_cumulative_avg[key]), frame)
self.writer.add_scalar(f'episode_cumulative_max/{key}_max', np.max(self.episode_cumulative_avg[key]), frame)
self.new_finished_episodes = False
for k, v in self.direct_info.items():
self.writer.add_scalar(f'{k}/frame', v, frame)
self.writer.add_scalar(f'{k}/iter', v, epoch_num)
self.writer.add_scalar(f'{k}/time', v, total_time)
class MultiObserver(AlgoObserver):
"""Meta-observer that allows the user to add several observers."""
def __init__(self, observers_):
super().__init__()
self.observers = observers_
def _call_multi(self, method, *args_, **kwargs_):
for o in self.observers:
getattr(o, method)(*args_, **kwargs_)
def before_init(self, base_name, config, experiment_name):
self._call_multi('before_init', base_name, config, experiment_name)
def after_init(self, algo):
self._call_multi('after_init', algo)
def process_infos(self, infos, done_indices):
self._call_multi('process_infos', infos, done_indices)
def after_steps(self):
self._call_multi('after_steps')
def after_clear_stats(self):
self._call_multi('after_clear_stats')
def after_print_stats(self, frame, epoch_num, total_time):
self._call_multi('after_print_stats', frame, epoch_num, total_time)
class RLGPUEnv(vecenv.IVecEnv):
def __init__(self, config_name, num_actors, **kwargs):
self.env = env_configurations.configurations[config_name]['env_creator'](**kwargs)
def step(self, actions):
return self.env.step(actions)
def reset(self):
return self.env.reset()
def reset_done(self):
return self.env.reset_done()
def get_number_of_agents(self):
return self.env.get_number_of_agents()
def get_env_info(self):
info = {}
info['action_space'] = self.env.action_space
info['observation_space'] = self.env.observation_space
if hasattr(self.env, "amp_observation_space"):
info['amp_observation_space'] = self.env.amp_observation_space
if self.env.num_states > 0:
info['state_space'] = self.env.state_space
print(info['action_space'], info['observation_space'], info['state_space'])
else:
print(info['action_space'], info['observation_space'])
return info
def set_train_info(self, env_frames, *args_, **kwargs_):
"""
Send the information in the direction algo->environment.
Most common use case: tell the environment how far along we are in the training process. This is useful
for implementing curriculums and things such as that.
"""
if hasattr(self.env, 'set_train_info'):
self.env.set_train_info(env_frames, *args_, **kwargs_)
def get_env_state(self):
"""
Return serializable environment state to be saved to checkpoint.
Can be used for stateful training sessions, i.e. with adaptive curriculums.
"""
if hasattr(self.env, 'get_env_state'):
return self.env.get_env_state()
else:
return None
def set_env_state(self, env_state):
if hasattr(self.env, 'set_env_state'):
self.env.set_env_state(env_state)
class ComplexObsRLGPUEnv(vecenv.IVecEnv):
def __init__(
self,
config_name,
num_actors,
obs_spec: Dict[str, Dict],
**kwargs,
):
"""RLGPU wrapper for Isaac Gym tasks.
Args:
config_name: Name of rl games env_configurations configuration to use.
obs_spec: Dictinoary listing out specification for observations to use.
eg.
{
'obs': {'names': ['obs_1', 'obs_2'], 'concat': True, space_name: 'observation_space'},},
'states': {'names': ['state_1', 'state_2'], 'concat': False, space_name: 'state_space'},}
}
Within each, if 'concat' is set, concatenates all the given observaitons into a single tensor of dim (num_envs, sum(num_obs)).
Assumes that each indivdual observation is single dimensional (ie (num_envs, k), so image observation isn't supported).
Currently applies to student and teacher both.
"space_name" is given into the env info which RL Games reads to find the space shape
"""
self.env = env_configurations.configurations[config_name]['env_creator'](**kwargs)
self.obs_spec = obs_spec
def _generate_obs(
self, env_obs: Dict[str, torch.Tensor]
) -> Dict[str, Dict[str, torch.Tensor]]:
"""Generate the RL Games observations given the observations from the environment.
Args:
env_obs: environment observations
Returns:
Dict which contains keys with values corresponding to observations.
"""
# rl games expects a dictionary with 'obs' and 'states'
# corresponding to the policy observations and possible asymmetric
# observations respectively
rlgames_obs = {k: self.gen_obs_dict(env_obs, v['names'], v['concat']) for k, v in self.obs_spec.items()}
return rlgames_obs
def step(
self, action: torch.Tensor
) -> Tuple[
Dict[str, Dict[str, torch.Tensor]], torch.Tensor, torch.Tensor, Dict[str, Any]
]:
"""Step the Isaac Gym task.
Args:
action: Enivronment action.
Returns:
observations, rewards, dones, infos
Returned obeservations are a dict which contains key 'obs' corresponding to a dictionary of observations,
and possible 'states' key corresponding to dictionary of privileged observations.
"""
env_obs, rewards, dones, infos = self.env.step(action)
rlgames_obs = self._generate_obs(env_obs)
return rlgames_obs, rewards, dones, infos
def reset(self) -> Dict[str, Dict[str, torch.Tensor]]:
env_obs = self.env.reset()
return self._generate_obs(env_obs)
def get_number_of_agents(self) -> int:
return self.env.get_number_of_agents()
def get_env_info(self) -> Dict[str, gym.spaces.Space]:
"""Gets information on the environment's observation, action, and privileged observation (states) spaces."""
info = {}
info["action_space"] = self.env.action_space
for k, v in self.obs_spec.items():
info[v['space_name']] = self.gen_obs_space(v['names'], v['concat'])
return info
def gen_obs_dict(self, obs_dict, obs_names, concat):
"""Generate the RL Games observations given the observations from the environment."""
if concat:
return torch.cat([obs_dict[name] for name in obs_names], dim=1)
else:
return {k: obs_dict[k] for k in obs_names}
def gen_obs_space(self, obs_names, concat):
"""Generate the RL Games observation space given the observations from the environment."""
if concat:
return gym.spaces.Box(
low=-np.Inf,
high=np.Inf,
shape=(sum([self.env.observation_space[s].shape[0] for s in obs_names]),),
dtype=np.float32,
)
else:
return gym.spaces.Dict(
{k: self.env.observation_space[k] for k in obs_names}
)
def set_train_info(self, env_frames, *args_, **kwargs_):
"""
Send the information in the direction algo->environment.
Most common use case: tell the environment how far along we are in the training process. This is useful
for implementing curriculums and things such as that.
"""
if hasattr(self.env, 'set_train_info'):
self.env.set_train_info(env_frames, *args_, **kwargs_)
def get_env_state(self):
"""
Return serializable environment state to be saved to checkpoint.
Can be used for stateful training sessions, i.e. with adaptive curriculums.
"""
if hasattr(self.env, 'get_env_state'):
return self.env.get_env_state()
else:
return None
def set_env_state(self, env_state):
if hasattr(self.env, 'set_env_state'):
self.env.set_env_state(env_state)
| 16,837 | Python | 38.806146 | 153 | 0.612104 |
NVIDIA-Omniverse/IsaacGymEnvs/isaacgymenvs/utils/torch_jit_utils.py | # Copyright (c) 2018-2023, NVIDIA Corporation
# All rights reserved.
#
# Redistribution and use in source and binary forms, with or without
# modification, are permitted provided that the following conditions are met:
#
# 1. Redistributions of source code must retain the above copyright notice, this
# list of conditions and the following disclaimer.
#
# 2. Redistributions in binary form must reproduce the above copyright notice,
# this list of conditions and the following disclaimer in the documentation
# and/or other materials provided with the distribution.
#
# 3. Neither the name of the copyright holder nor the names of its
# contributors may be used to endorse or promote products derived from
# this software without specific prior written permission.
#
# THIS SOFTWARE IS PROVIDED BY THE COPYRIGHT HOLDERS AND CONTRIBUTORS "AS IS"
# AND ANY EXPRESS OR IMPLIED WARRANTIES, INCLUDING, BUT NOT LIMITED TO, THE
# IMPLIED WARRANTIES OF MERCHANTABILITY AND FITNESS FOR A PARTICULAR PURPOSE ARE
# DISCLAIMED. IN NO EVENT SHALL THE COPYRIGHT HOLDER OR CONTRIBUTORS BE LIABLE
# FOR ANY DIRECT, INDIRECT, INCIDENTAL, SPECIAL, EXEMPLARY, OR CONSEQUENTIAL
# DAMAGES (INCLUDING, BUT NOT LIMITED TO, PROCUREMENT OF SUBSTITUTE GOODS OR
# SERVICES; LOSS OF USE, DATA, OR PROFITS; OR BUSINESS INTERRUPTION) HOWEVER
# CAUSED AND ON ANY THEORY OF LIABILITY, WHETHER IN CONTRACT, STRICT LIABILITY,
# OR TORT (INCLUDING NEGLIGENCE OR OTHERWISE) ARISING IN ANY WAY OUT OF THE USE
# OF THIS SOFTWARE, EVEN IF ADVISED OF THE POSSIBILITY OF SUCH DAMAGE.
import isaacgym
import torch
import torch.nn.functional as F
import numpy as np
def to_torch(x, dtype=torch.float, device='cuda:0', requires_grad=False):
return torch.tensor(x, dtype=dtype, device=device, requires_grad=requires_grad)
@torch.jit.script
def quat_mul(a, b):
assert a.shape == b.shape
shape = a.shape
a = a.reshape(-1, 4)
b = b.reshape(-1, 4)
x1, y1, z1, w1 = a[:, 0], a[:, 1], a[:, 2], a[:, 3]
x2, y2, z2, w2 = b[:, 0], b[:, 1], b[:, 2], b[:, 3]
ww = (z1 + x1) * (x2 + y2)
yy = (w1 - y1) * (w2 + z2)
zz = (w1 + y1) * (w2 - z2)
xx = ww + yy + zz
qq = 0.5 * (xx + (z1 - x1) * (x2 - y2))
w = qq - ww + (z1 - y1) * (y2 - z2)
x = qq - xx + (x1 + w1) * (x2 + w2)
y = qq - yy + (w1 - x1) * (y2 + z2)
z = qq - zz + (z1 + y1) * (w2 - x2)
quat = torch.stack([x, y, z, w], dim=-1).view(shape)
return quat
@torch.jit.script
def normalize(x, eps: float = 1e-9):
return x / x.norm(p=2, dim=-1).clamp(min=eps, max=None).unsqueeze(-1)
@torch.jit.script
def quat_apply(a, b):
shape = b.shape
a = a.reshape(-1, 4)
b = b.reshape(-1, 3)
xyz = a[:, :3]
t = xyz.cross(b, dim=-1) * 2
return (b + a[:, 3:] * t + xyz.cross(t, dim=-1)).view(shape)
@torch.jit.script
def quat_rotate(q, v):
shape = q.shape
q_w = q[:, -1]
q_vec = q[:, :3]
a = v * (2.0 * q_w ** 2 - 1.0).unsqueeze(-1)
b = torch.cross(q_vec, v, dim=-1) * q_w.unsqueeze(-1) * 2.0
c = q_vec * \
torch.bmm(q_vec.view(shape[0], 1, 3), v.view(
shape[0], 3, 1)).squeeze(-1) * 2.0
return a + b + c
@torch.jit.script
def quat_rotate_inverse(q, v):
shape = q.shape
q_w = q[:, -1]
q_vec = q[:, :3]
a = v * (2.0 * q_w ** 2 - 1.0).unsqueeze(-1)
b = torch.cross(q_vec, v, dim=-1) * q_w.unsqueeze(-1) * 2.0
c = q_vec * \
torch.bmm(q_vec.view(shape[0], 1, 3), v.view(
shape[0], 3, 1)).squeeze(-1) * 2.0
return a - b + c
@torch.jit.script
def quat_conjugate(a):
shape = a.shape
a = a.reshape(-1, 4)
return torch.cat((-a[:, :3], a[:, -1:]), dim=-1).view(shape)
@torch.jit.script
def quat_unit(a):
return normalize(a)
@torch.jit.script
def quat_from_angle_axis(angle, axis):
theta = (angle / 2).unsqueeze(-1)
xyz = normalize(axis) * theta.sin()
w = theta.cos()
return quat_unit(torch.cat([xyz, w], dim=-1))
@torch.jit.script
def normalize_angle(x):
return torch.atan2(torch.sin(x), torch.cos(x))
@torch.jit.script
def tf_inverse(q, t):
q_inv = quat_conjugate(q)
return q_inv, -quat_apply(q_inv, t)
@torch.jit.script
def tf_apply(q, t, v):
return quat_apply(q, v) + t
@torch.jit.script
def tf_vector(q, v):
return quat_apply(q, v)
@torch.jit.script
def tf_combine(q1, t1, q2, t2):
return quat_mul(q1, q2), quat_apply(q1, t2) + t1
@torch.jit.script
def get_basis_vector(q, v):
return quat_rotate(q, v)
def get_axis_params(value, axis_idx, x_value=0., dtype=float, n_dims=3):
"""construct arguments to `Vec` according to axis index.
"""
zs = np.zeros((n_dims,))
assert axis_idx < n_dims, "the axis dim should be within the vector dimensions"
zs[axis_idx] = 1.
params = np.where(zs == 1., value, zs)
params[0] = x_value
return list(params.astype(dtype))
@torch.jit.script
def copysign(a, b):
# type: (float, Tensor) -> Tensor
a = torch.tensor(a, device=b.device, dtype=torch.float).repeat(b.shape[0])
return torch.abs(a) * torch.sign(b)
@torch.jit.script
def get_euler_xyz(q):
qx, qy, qz, qw = 0, 1, 2, 3
# roll (x-axis rotation)
sinr_cosp = 2.0 * (q[:, qw] * q[:, qx] + q[:, qy] * q[:, qz])
cosr_cosp = q[:, qw] * q[:, qw] - q[:, qx] * \
q[:, qx] - q[:, qy] * q[:, qy] + q[:, qz] * q[:, qz]
roll = torch.atan2(sinr_cosp, cosr_cosp)
# pitch (y-axis rotation)
sinp = 2.0 * (q[:, qw] * q[:, qy] - q[:, qz] * q[:, qx])
pitch = torch.where(torch.abs(sinp) >= 1, copysign(
np.pi / 2.0, sinp), torch.asin(sinp))
# yaw (z-axis rotation)
siny_cosp = 2.0 * (q[:, qw] * q[:, qz] + q[:, qx] * q[:, qy])
cosy_cosp = q[:, qw] * q[:, qw] + q[:, qx] * \
q[:, qx] - q[:, qy] * q[:, qy] - q[:, qz] * q[:, qz]
yaw = torch.atan2(siny_cosp, cosy_cosp)
return roll % (2*np.pi), pitch % (2*np.pi), yaw % (2*np.pi)
@torch.jit.script
def quat_from_euler_xyz(roll, pitch, yaw):
cy = torch.cos(yaw * 0.5)
sy = torch.sin(yaw * 0.5)
cr = torch.cos(roll * 0.5)
sr = torch.sin(roll * 0.5)
cp = torch.cos(pitch * 0.5)
sp = torch.sin(pitch * 0.5)
qw = cy * cr * cp + sy * sr * sp
qx = cy * sr * cp - sy * cr * sp
qy = cy * cr * sp + sy * sr * cp
qz = sy * cr * cp - cy * sr * sp
return torch.stack([qx, qy, qz, qw], dim=-1)
@torch.jit.script
def torch_rand_float(lower, upper, shape, device):
# type: (float, float, Tuple[int, int], str) -> Tensor
return (upper - lower) * torch.rand(*shape, device=device) + lower
@torch.jit.script
def torch_random_dir_2(shape, device):
# type: (Tuple[int, int], str) -> Tensor
angle = torch_rand_float(-np.pi, np.pi, shape, device).squeeze(-1)
return torch.stack([torch.cos(angle), torch.sin(angle)], dim=-1)
@torch.jit.script
def tensor_clamp(t, min_t, max_t):
return torch.max(torch.min(t, max_t), min_t)
@torch.jit.script
def scale(x, lower, upper):
return (0.5 * (x + 1.0) * (upper - lower) + lower)
@torch.jit.script
def unscale(x, lower, upper):
return (2.0 * x - upper - lower) / (upper - lower)
def unscale_np(x, lower, upper):
return (2.0 * x - upper - lower) / (upper - lower)
@torch.jit.script
def compute_heading_and_up(
torso_rotation, inv_start_rot, to_target, vec0, vec1, up_idx
):
# type: (Tensor, Tensor, Tensor, Tensor, Tensor, int) -> Tuple[Tensor, Tensor, Tensor, Tensor, Tensor]
num_envs = torso_rotation.shape[0]
target_dirs = normalize(to_target)
torso_quat = quat_mul(torso_rotation, inv_start_rot)
up_vec = get_basis_vector(torso_quat, vec1).view(num_envs, 3)
heading_vec = get_basis_vector(torso_quat, vec0).view(num_envs, 3)
up_proj = up_vec[:, up_idx]
heading_proj = torch.bmm(heading_vec.view(
num_envs, 1, 3), target_dirs.view(num_envs, 3, 1)).view(num_envs)
return torso_quat, up_proj, heading_proj, up_vec, heading_vec
@torch.jit.script
def compute_rot(torso_quat, velocity, ang_velocity, targets, torso_positions):
vel_loc = quat_rotate_inverse(torso_quat, velocity)
angvel_loc = quat_rotate_inverse(torso_quat, ang_velocity)
roll, pitch, yaw = get_euler_xyz(torso_quat)
walk_target_angle = torch.atan2(targets[:, 2] - torso_positions[:, 2],
targets[:, 0] - torso_positions[:, 0])
angle_to_target = walk_target_angle - yaw
return vel_loc, angvel_loc, roll, pitch, yaw, angle_to_target
@torch.jit.script
def quat_axis(q, axis=0):
# type: (Tensor, int) -> Tensor
basis_vec = torch.zeros(q.shape[0], 3, device=q.device)
basis_vec[:, axis] = 1
return quat_rotate(q, basis_vec)
"""
Normalization and Denormalization of Tensors
"""
@torch.jit.script
def scale_transform(x: torch.Tensor, lower: torch.Tensor, upper: torch.Tensor) -> torch.Tensor:
"""
Normalizes a given input tensor to a range of [-1, 1].
@note It uses pytorch broadcasting functionality to deal with batched input.
Args:
x: Input tensor of shape (N, dims).
lower: The minimum value of the tensor. Shape (dims,)
upper: The maximum value of the tensor. Shape (dims,)
Returns:
Normalized transform of the tensor. Shape (N, dims)
"""
# default value of center
offset = (lower + upper) * 0.5
# return normalized tensor
return 2 * (x - offset) / (upper - lower)
@torch.jit.script
def unscale_transform(x: torch.Tensor, lower: torch.Tensor, upper: torch.Tensor) -> torch.Tensor:
"""
Denormalizes a given input tensor from range of [-1, 1] to (lower, upper).
@note It uses pytorch broadcasting functionality to deal with batched input.
Args:
x: Input tensor of shape (N, dims).
lower: The minimum value of the tensor. Shape (dims,)
upper: The maximum value of the tensor. Shape (dims,)
Returns:
Denormalized transform of the tensor. Shape (N, dims)
"""
# default value of center
offset = (lower + upper) * 0.5
# return normalized tensor
return x * (upper - lower) * 0.5 + offset
@torch.jit.script
def saturate(x: torch.Tensor, lower: torch.Tensor, upper: torch.Tensor) -> torch.Tensor:
"""
Clamps a given input tensor to (lower, upper).
@note It uses pytorch broadcasting functionality to deal with batched input.
Args:
x: Input tensor of shape (N, dims).
lower: The minimum value of the tensor. Shape (dims,)
upper: The maximum value of the tensor. Shape (dims,)
Returns:
Clamped transform of the tensor. Shape (N, dims)
"""
return torch.max(torch.min(x, upper), lower)
"""
Rotation conversions
"""
@torch.jit.script
def quat_diff_rad(a: torch.Tensor, b: torch.Tensor) -> torch.Tensor:
"""
Get the difference in radians between two quaternions.
Args:
a: first quaternion, shape (N, 4)
b: second quaternion, shape (N, 4)
Returns:
Difference in radians, shape (N,)
"""
b_conj = quat_conjugate(b)
mul = quat_mul(a, b_conj)
# 2 * torch.acos(torch.abs(mul[:, -1]))
return 2.0 * torch.asin(
torch.clamp(
torch.norm(
mul[:, 0:3],
p=2, dim=-1), max=1.0)
)
@torch.jit.script
def local_to_world_space(pos_offset_local: torch.Tensor, pose_global: torch.Tensor):
""" Convert a point from the local frame to the global frame
Args:
pos_offset_local: Point in local frame. Shape: [N, 3]
pose_global: The spatial pose of this point. Shape: [N, 7]
Returns:
Position in the global frame. Shape: [N, 3]
"""
quat_pos_local = torch.cat(
[pos_offset_local, torch.zeros(pos_offset_local.shape[0], 1, dtype=torch.float32, device=pos_offset_local.device)],
dim=-1
)
quat_global = pose_global[:, 3:7]
quat_global_conj = quat_conjugate(quat_global)
pos_offset_global = quat_mul(quat_global, quat_mul(quat_pos_local, quat_global_conj))[:, 0:3]
result_pos_gloal = pos_offset_global + pose_global[:, 0:3]
return result_pos_gloal
# NB: do not make this function jit, since it is passed around as an argument.
def normalise_quat_in_pose(pose):
"""Takes a pose and normalises the quaternion portion of it.
Args:
pose: shape N, 7
Returns:
Pose with normalised quat. Shape N, 7
"""
pos = pose[:, 0:3]
quat = pose[:, 3:7]
quat /= torch.norm(quat, dim=-1, p=2).reshape(-1, 1)
return torch.cat([pos, quat], dim=-1)
@torch.jit.script
def my_quat_rotate(q, v):
shape = q.shape
q_w = q[:, -1]
q_vec = q[:, :3]
a = v * (2.0 * q_w ** 2 - 1.0).unsqueeze(-1)
b = torch.cross(q_vec, v, dim=-1) * q_w.unsqueeze(-1) * 2.0
c = q_vec * \
torch.bmm(q_vec.view(shape[0], 1, 3), v.view(
shape[0], 3, 1)).squeeze(-1) * 2.0
return a + b + c
@torch.jit.script
def quat_to_angle_axis(q):
# type: (Tensor) -> Tuple[Tensor, Tensor]
# computes axis-angle representation from quaternion q
# q must be normalized
min_theta = 1e-5
qx, qy, qz, qw = 0, 1, 2, 3
sin_theta = torch.sqrt(1 - q[..., qw] * q[..., qw])
angle = 2 * torch.acos(q[..., qw])
angle = normalize_angle(angle)
sin_theta_expand = sin_theta.unsqueeze(-1)
axis = q[..., qx:qw] / sin_theta_expand
mask = sin_theta > min_theta
default_axis = torch.zeros_like(axis)
default_axis[..., -1] = 1
angle = torch.where(mask, angle, torch.zeros_like(angle))
mask_expand = mask.unsqueeze(-1)
axis = torch.where(mask_expand, axis, default_axis)
return angle, axis
@torch.jit.script
def angle_axis_to_exp_map(angle, axis):
# type: (Tensor, Tensor) -> Tensor
# compute exponential map from axis-angle
angle_expand = angle.unsqueeze(-1)
exp_map = angle_expand * axis
return exp_map
@torch.jit.script
def quat_to_exp_map(q):
# type: (Tensor) -> Tensor
# compute exponential map from quaternion
# q must be normalized
angle, axis = quat_to_angle_axis(q)
exp_map = angle_axis_to_exp_map(angle, axis)
return exp_map
def quaternion_to_matrix(quaternions: torch.Tensor) -> torch.Tensor:
"""
Convert rotations given as quaternions to rotation matrices.
Args:
quaternions: quaternions with real part first,
as tensor of shape (..., 4).
Returns:
Rotation matrices as tensor of shape (..., 3, 3).
"""
r, i, j, k = torch.unbind(quaternions, -1)
two_s = 2.0 / (quaternions * quaternions).sum(-1)
mat = torch.stack(
(
1 - two_s * (j * j + k * k),
two_s * (i * j - k * r),
two_s * (i * k + j * r),
two_s * (i * j + k * r),
1 - two_s * (i * i + k * k),
two_s * (j * k - i * r),
two_s * (i * k - j * r),
two_s * (j * k + i * r),
1 - two_s * (i * i + j * j),
),
-1,
)
return mat.reshape(quaternions.shape[:-1] + (3, 3))
def _sqrt_positive_part(x: torch.Tensor) -> torch.Tensor:
"""
Returns torch.sqrt(torch.max(0, x))
subgradient is zero where x is 0.
"""
ret = torch.zeros_like(x)
positive_mask = x > 0
ret[positive_mask] = torch.sqrt(x[positive_mask])
return ret
def matrix_to_quaternion(matrix: torch.Tensor) -> torch.Tensor:
"""
Convert rotations given as rotation matrices to quaternions.
Args:
matrix: Rotation matrices as tensor of shape (..., 3, 3).
Returns:
quaternions with real part first, as tensor of shape (..., 4).
"""
if matrix.size(-1) != 3 or matrix.size(-2) != 3:
raise ValueError(f"Invalid rotation matrix shape {matrix.shape}.")
batch_dim = matrix.shape[:-2]
m00, m01, m02, m10, m11, m12, m20, m21, m22 = torch.unbind(
matrix.reshape(batch_dim + (9,)), dim=-1
)
q_abs = _sqrt_positive_part(
torch.stack(
[
1.0 + m00 + m11 + m22,
1.0 + m00 - m11 - m22,
1.0 - m00 + m11 - m22,
1.0 - m00 - m11 + m22,
],
dim=-1,
)
)
quat_by_rijk = torch.stack(
[
torch.stack([q_abs[..., 0] ** 2, m21 - m12, m02 - m20, m10 - m01], dim=-1),
torch.stack([m21 - m12, q_abs[..., 1] ** 2, m10 + m01, m02 + m20], dim=-1),
torch.stack([m02 - m20, m10 + m01, q_abs[..., 2] ** 2, m12 + m21], dim=-1),
torch.stack([m10 - m01, m20 + m02, m21 + m12, q_abs[..., 3] ** 2], dim=-1),
],
dim=-2,
)
flr = torch.tensor(0.1).to(dtype=q_abs.dtype, device=q_abs.device)
quat_candidates = quat_by_rijk / (2.0 * q_abs[..., None].max(flr))
return quat_candidates[
F.one_hot(q_abs.argmax(dim=-1), num_classes=4) > 0.5, :
].reshape(batch_dim + (4,))
@torch.jit.script
def quat_to_tan_norm(q):
# type: (Tensor) -> Tensor
# represents a rotation using the tangent and normal vectors
ref_tan = torch.zeros_like(q[..., 0:3])
ref_tan[..., 0] = 1
tan = my_quat_rotate(q, ref_tan)
ref_norm = torch.zeros_like(q[..., 0:3])
ref_norm[..., -1] = 1
norm = my_quat_rotate(q, ref_norm)
norm_tan = torch.cat([tan, norm], dim=len(tan.shape) - 1)
return norm_tan
@torch.jit.script
def euler_xyz_to_exp_map(roll, pitch, yaw):
# type: (Tensor, Tensor, Tensor) -> Tensor
q = quat_from_euler_xyz(roll, pitch, yaw)
exp_map = quat_to_exp_map(q)
return exp_map
@torch.jit.script
def exp_map_to_angle_axis(exp_map):
min_theta = 1e-5
angle = torch.norm(exp_map, dim=-1)
angle_exp = torch.unsqueeze(angle, dim=-1)
axis = exp_map / angle_exp
angle = normalize_angle(angle)
default_axis = torch.zeros_like(exp_map)
default_axis[..., -1] = 1
mask = angle > min_theta
angle = torch.where(mask, angle, torch.zeros_like(angle))
mask_expand = mask.unsqueeze(-1)
axis = torch.where(mask_expand, axis, default_axis)
return angle, axis
@torch.jit.script
def exp_map_to_quat(exp_map):
angle, axis = exp_map_to_angle_axis(exp_map)
q = quat_from_angle_axis(angle, axis)
return q
@torch.jit.script
def slerp(q0, q1, t):
# type: (Tensor, Tensor, Tensor) -> Tensor
qx, qy, qz, qw = 0, 1, 2, 3
cos_half_theta = q0[..., qw] * q1[..., qw] \
+ q0[..., qx] * q1[..., qx] \
+ q0[..., qy] * q1[..., qy] \
+ q0[..., qz] * q1[..., qz]
neg_mask = cos_half_theta < 0
q1 = q1.clone()
q1[neg_mask] = -q1[neg_mask]
cos_half_theta = torch.abs(cos_half_theta)
cos_half_theta = torch.unsqueeze(cos_half_theta, dim=-1)
half_theta = torch.acos(cos_half_theta);
sin_half_theta = torch.sqrt(1.0 - cos_half_theta * cos_half_theta)
ratioA = torch.sin((1 - t) * half_theta) / sin_half_theta
ratioB = torch.sin(t * half_theta) / sin_half_theta;
new_q_x = ratioA * q0[..., qx:qx+1] + ratioB * q1[..., qx:qx+1]
new_q_y = ratioA * q0[..., qy:qy+1] + ratioB * q1[..., qy:qy+1]
new_q_z = ratioA * q0[..., qz:qz+1] + ratioB * q1[..., qz:qz+1]
new_q_w = ratioA * q0[..., qw:qw+1] + ratioB * q1[..., qw:qw+1]
cat_dim = len(new_q_w.shape) - 1
new_q = torch.cat([new_q_x, new_q_y, new_q_z, new_q_w], dim=cat_dim)
new_q = torch.where(torch.abs(sin_half_theta) < 0.001, 0.5 * q0 + 0.5 * q1, new_q)
new_q = torch.where(torch.abs(cos_half_theta) >= 1, q0, new_q)
return new_q
@torch.jit.script
def calc_heading(q):
# type: (Tensor) -> Tensor
# calculate heading direction from quaternion
# the heading is the direction on the xy plane
# q must be normalized
ref_dir = torch.zeros_like(q[..., 0:3])
ref_dir[..., 0] = 1
rot_dir = my_quat_rotate(q, ref_dir)
heading = torch.atan2(rot_dir[..., 1], rot_dir[..., 0])
return heading
@torch.jit.script
def calc_heading_quat(q):
# type: (Tensor) -> Tensor
# calculate heading rotation from quaternion
# the heading is the direction on the xy plane
# q must be normalized
heading = calc_heading(q)
axis = torch.zeros_like(q[..., 0:3])
axis[..., 2] = 1
heading_q = quat_from_angle_axis(heading, axis)
return heading_q
@torch.jit.script
def calc_heading_quat_inv(q):
# type: (Tensor) -> Tensor
# calculate heading rotation from quaternion
# the heading is the direction on the xy plane
# q must be normalized
heading = calc_heading(q)
axis = torch.zeros_like(q[..., 0:3])
axis[..., 2] = 1
heading_q = quat_from_angle_axis(-heading, axis)
return heading_q
# EOF
| 20,579 | Python | 29.716418 | 123 | 0.588707 |
NVIDIA-Omniverse/IsaacGymEnvs/isaacgymenvs/utils/reformat.py | # Copyright (c) 2018-2023, NVIDIA Corporation
# All rights reserved.
#
# Redistribution and use in source and binary forms, with or without
# modification, are permitted provided that the following conditions are met:
#
# 1. Redistributions of source code must retain the above copyright notice, this
# list of conditions and the following disclaimer.
#
# 2. Redistributions in binary form must reproduce the above copyright notice,
# this list of conditions and the following disclaimer in the documentation
# and/or other materials provided with the distribution.
#
# 3. Neither the name of the copyright holder nor the names of its
# contributors may be used to endorse or promote products derived from
# this software without specific prior written permission.
#
# THIS SOFTWARE IS PROVIDED BY THE COPYRIGHT HOLDERS AND CONTRIBUTORS "AS IS"
# AND ANY EXPRESS OR IMPLIED WARRANTIES, INCLUDING, BUT NOT LIMITED TO, THE
# IMPLIED WARRANTIES OF MERCHANTABILITY AND FITNESS FOR A PARTICULAR PURPOSE ARE
# DISCLAIMED. IN NO EVENT SHALL THE COPYRIGHT HOLDER OR CONTRIBUTORS BE LIABLE
# FOR ANY DIRECT, INDIRECT, INCIDENTAL, SPECIAL, EXEMPLARY, OR CONSEQUENTIAL
# DAMAGES (INCLUDING, BUT NOT LIMITED TO, PROCUREMENT OF SUBSTITUTE GOODS OR
# SERVICES; LOSS OF USE, DATA, OR PROFITS; OR BUSINESS INTERRUPTION) HOWEVER
# CAUSED AND ON ANY THEORY OF LIABILITY, WHETHER IN CONTRACT, STRICT LIABILITY,
# OR TORT (INCLUDING NEGLIGENCE OR OTHERWISE) ARISING IN ANY WAY OUT OF THE USE
# OF THIS SOFTWARE, EVEN IF ADVISED OF THE POSSIBILITY OF SUCH DAMAGE.
from omegaconf import DictConfig, OmegaConf
from typing import Dict
def omegaconf_to_dict(d: DictConfig)->Dict:
"""Converts an omegaconf DictConfig to a python Dict, respecting variable interpolation."""
ret = {}
for k, v in d.items():
if isinstance(v, DictConfig):
ret[k] = omegaconf_to_dict(v)
else:
ret[k] = v
return ret
def print_dict(val, nesting: int = -4, start: bool = True):
"""Outputs a nested dictionory."""
if type(val) == dict:
if not start:
print('')
nesting += 4
for k in val:
print(nesting * ' ', end='')
print(k, end=': ')
print_dict(val[k], nesting, start=False)
else:
print(val)
# EOF
| 2,314 | Python | 40.339285 | 95 | 0.708729 |
NVIDIA-Omniverse/IsaacGymEnvs/isaacgymenvs/utils/dr_utils.py | # Copyright (c) 2018-2023, NVIDIA Corporation
# All rights reserved.
#
# Redistribution and use in source and binary forms, with or without
# modification, are permitted provided that the following conditions are met:
#
# 1. Redistributions of source code must retain the above copyright notice, this
# list of conditions and the following disclaimer.
#
# 2. Redistributions in binary form must reproduce the above copyright notice,
# this list of conditions and the following disclaimer in the documentation
# and/or other materials provided with the distribution.
#
# 3. Neither the name of the copyright holder nor the names of its
# contributors may be used to endorse or promote products derived from
# this software without specific prior written permission.
#
# THIS SOFTWARE IS PROVIDED BY THE COPYRIGHT HOLDERS AND CONTRIBUTORS "AS IS"
# AND ANY EXPRESS OR IMPLIED WARRANTIES, INCLUDING, BUT NOT LIMITED TO, THE
# IMPLIED WARRANTIES OF MERCHANTABILITY AND FITNESS FOR A PARTICULAR PURPOSE ARE
# DISCLAIMED. IN NO EVENT SHALL THE COPYRIGHT HOLDER OR CONTRIBUTORS BE LIABLE
# FOR ANY DIRECT, INDIRECT, INCIDENTAL, SPECIAL, EXEMPLARY, OR CONSEQUENTIAL
# DAMAGES (INCLUDING, BUT NOT LIMITED TO, PROCUREMENT OF SUBSTITUTE GOODS OR
# SERVICES; LOSS OF USE, DATA, OR PROFITS; OR BUSINESS INTERRUPTION) HOWEVER
# CAUSED AND ON ANY THEORY OF LIABILITY, WHETHER IN CONTRACT, STRICT LIABILITY,
# OR TORT (INCLUDING NEGLIGENCE OR OTHERWISE) ARISING IN ANY WAY OUT OF THE USE
# OF THIS SOFTWARE, EVEN IF ADVISED OF THE POSSIBILITY OF SUCH DAMAGE.
import numpy as np
from bisect import bisect
from isaacgym import gymapi
def get_property_setter_map(gym):
property_to_setters = {
"dof_properties": gym.set_actor_dof_properties,
"tendon_properties": gym.set_actor_tendon_properties,
"rigid_body_properties": gym.set_actor_rigid_body_properties,
"rigid_shape_properties": gym.set_actor_rigid_shape_properties,
"sim_params": gym.set_sim_params,
}
return property_to_setters
def get_property_getter_map(gym):
property_to_getters = {
"dof_properties": gym.get_actor_dof_properties,
"tendon_properties": gym.get_actor_tendon_properties,
"rigid_body_properties": gym.get_actor_rigid_body_properties,
"rigid_shape_properties": gym.get_actor_rigid_shape_properties,
"sim_params": gym.get_sim_params,
}
return property_to_getters
def get_default_setter_args(gym):
property_to_setter_args = {
"dof_properties": [],
"tendon_properties": [],
"rigid_body_properties": [True],
"rigid_shape_properties": [],
"sim_params": [],
}
return property_to_setter_args
def generate_random_samples(attr_randomization_params, shape, curr_gym_step_count,
extern_sample=None):
rand_range = attr_randomization_params['range']
distribution = attr_randomization_params['distribution']
sched_type = attr_randomization_params['schedule'] if 'schedule' in attr_randomization_params else None
sched_step = attr_randomization_params['schedule_steps'] if 'schedule' in attr_randomization_params else None
operation = attr_randomization_params['operation']
if sched_type == 'linear':
sched_scaling = 1 / sched_step * min(curr_gym_step_count, sched_step)
elif sched_type == 'constant':
sched_scaling = 0 if curr_gym_step_count < sched_step else 1
else:
sched_scaling = 1
if extern_sample is not None:
sample = extern_sample
if operation == 'additive':
sample *= sched_scaling
elif operation == 'scaling':
sample = sample * sched_scaling + 1 * (1 - sched_scaling)
elif distribution == "gaussian":
mu, var = rand_range
if operation == 'additive':
mu *= sched_scaling
var *= sched_scaling
elif operation == 'scaling':
var = var * sched_scaling # scale up var over time
mu = mu * sched_scaling + 1 * (1 - sched_scaling) # linearly interpolate
sample = np.random.normal(mu, var, shape)
elif distribution == "loguniform":
lo, hi = rand_range
if operation == 'additive':
lo *= sched_scaling
hi *= sched_scaling
elif operation == 'scaling':
lo = lo * sched_scaling + 1 * (1 - sched_scaling)
hi = hi * sched_scaling + 1 * (1 - sched_scaling)
sample = np.exp(np.random.uniform(np.log(lo), np.log(hi), shape))
elif distribution == "uniform":
lo, hi = rand_range
if operation == 'additive':
lo *= sched_scaling
hi *= sched_scaling
elif operation == 'scaling':
lo = lo * sched_scaling + 1 * (1 - sched_scaling)
hi = hi * sched_scaling + 1 * (1 - sched_scaling)
sample = np.random.uniform(lo, hi, shape)
return sample
def get_bucketed_val(new_prop_val, attr_randomization_params):
if attr_randomization_params['distribution'] == 'uniform':
# range of buckets defined by uniform distribution
lo, hi = attr_randomization_params['range'][0], attr_randomization_params['range'][1]
else:
# for gaussian, set range of buckets to be 2 stddev away from mean
lo = attr_randomization_params['range'][0] - 2 * np.sqrt(attr_randomization_params['range'][1])
hi = attr_randomization_params['range'][0] + 2 * np.sqrt(attr_randomization_params['range'][1])
num_buckets = attr_randomization_params['num_buckets']
buckets = [(hi - lo) * i / num_buckets + lo for i in range(num_buckets)]
return buckets[bisect(buckets, new_prop_val) - 1]
def apply_random_samples(prop, og_prop, attr, attr_randomization_params,
curr_gym_step_count, extern_sample=None, bucketing_randomization_params=None):
"""
@params:
prop: property we want to randomise
og_prop: the original property and its value
attr: which particular attribute we want to randomise e.g. damping, stiffness
attr_randomization_params: the attribute randomisation meta-data e.g. distr, range, schedule
curr_gym_step_count: gym steps so far
"""
if isinstance(prop, gymapi.SimParams):
if attr == 'gravity':
sample = generate_random_samples(attr_randomization_params, 3, curr_gym_step_count)
if attr_randomization_params['operation'] == 'scaling':
prop.gravity.x = og_prop['gravity'].x * sample[0]
prop.gravity.y = og_prop['gravity'].y * sample[1]
prop.gravity.z = og_prop['gravity'].z * sample[2]
elif attr_randomization_params['operation'] == 'additive':
prop.gravity.x = og_prop['gravity'].x + sample[0]
prop.gravity.y = og_prop['gravity'].y + sample[1]
prop.gravity.z = og_prop['gravity'].z + sample[2]
if attr == 'rest_offset':
sample = generate_random_samples(attr_randomization_params, 1, curr_gym_step_count)
prop.physx.rest_offset = sample
elif isinstance(prop, np.ndarray):
sample = generate_random_samples(attr_randomization_params, prop[attr].shape,
curr_gym_step_count, extern_sample)
if attr_randomization_params['operation'] == 'scaling':
new_prop_val = og_prop[attr] * sample
elif attr_randomization_params['operation'] == 'additive':
new_prop_val = og_prop[attr] + sample
if 'num_buckets' in attr_randomization_params and attr_randomization_params['num_buckets'] > 0:
new_prop_val = get_bucketed_val(new_prop_val, attr_randomization_params)
prop[attr] = new_prop_val
else:
sample = generate_random_samples(attr_randomization_params, 1,
curr_gym_step_count, extern_sample)
cur_attr_val = og_prop[attr]
if attr_randomization_params['operation'] == 'scaling':
new_prop_val = cur_attr_val * sample
elif attr_randomization_params['operation'] == 'additive':
new_prop_val = cur_attr_val + sample
if 'num_buckets' in attr_randomization_params and attr_randomization_params['num_buckets'] > 0:
if bucketing_randomization_params is None:
new_prop_val = get_bucketed_val(new_prop_val, attr_randomization_params)
else:
new_prop_val = get_bucketed_val(new_prop_val, bucketing_randomization_params)
setattr(prop, attr, new_prop_val)
def check_buckets(gym, envs, dr_params):
total_num_buckets = 0
for actor, actor_properties in dr_params["actor_params"].items():
cur_num_buckets = 0
if 'rigid_shape_properties' in actor_properties.keys():
prop_attrs = actor_properties['rigid_shape_properties']
if 'restitution' in prop_attrs and 'num_buckets' in prop_attrs['restitution']:
cur_num_buckets = prop_attrs['restitution']['num_buckets']
if 'friction' in prop_attrs and 'num_buckets' in prop_attrs['friction']:
if cur_num_buckets > 0:
cur_num_buckets *= prop_attrs['friction']['num_buckets']
else:
cur_num_buckets = prop_attrs['friction']['num_buckets']
total_num_buckets += cur_num_buckets
assert total_num_buckets <= 64000, 'Explicit material bucketing has been specified, but the provided total bucket count exceeds 64K: {} specified buckets'.format(
total_num_buckets)
shape_ct = 0
# Separate loop because we should not assume that each actor is present in each env
for env in envs:
for i in range(gym.get_actor_count(env)):
actor_handle = gym.get_actor_handle(env, i)
actor_name = gym.get_actor_name(env, actor_handle)
if actor_name in dr_params["actor_params"] and 'rigid_shape_properties' in dr_params["actor_params"][actor_name]:
shape_ct += gym.get_actor_rigid_shape_count(env, actor_handle)
assert shape_ct <= 64000 or total_num_buckets > 0, 'Explicit material bucketing is not used but the total number of shapes exceeds material limit. Please specify bucketing to limit material count.' | 10,378 | Python | 42.426778 | 201 | 0.64126 |
NVIDIA-Omniverse/IsaacGymEnvs/isaacgymenvs/utils/utils.py | # Copyright (c) 2018-2023, NVIDIA Corporation
# All rights reserved.
#
# Redistribution and use in source and binary forms, with or without
# modification, are permitted provided that the following conditions are met:
#
# 1. Redistributions of source code must retain the above copyright notice, this
# list of conditions and the following disclaimer.
#
# 2. Redistributions in binary form must reproduce the above copyright notice,
# this list of conditions and the following disclaimer in the documentation
# and/or other materials provided with the distribution.
#
# 3. Neither the name of the copyright holder nor the names of its
# contributors may be used to endorse or promote products derived from
# this software without specific prior written permission.
#
# THIS SOFTWARE IS PROVIDED BY THE COPYRIGHT HOLDERS AND CONTRIBUTORS "AS IS"
# AND ANY EXPRESS OR IMPLIED WARRANTIES, INCLUDING, BUT NOT LIMITED TO, THE
# IMPLIED WARRANTIES OF MERCHANTABILITY AND FITNESS FOR A PARTICULAR PURPOSE ARE
# DISCLAIMED. IN NO EVENT SHALL THE COPYRIGHT HOLDER OR CONTRIBUTORS BE LIABLE
# FOR ANY DIRECT, INDIRECT, INCIDENTAL, SPECIAL, EXEMPLARY, OR CONSEQUENTIAL
# DAMAGES (INCLUDING, BUT NOT LIMITED TO, PROCUREMENT OF SUBSTITUTE GOODS OR
# SERVICES; LOSS OF USE, DATA, OR PROFITS; OR BUSINESS INTERRUPTION) HOWEVER
# CAUSED AND ON ANY THEORY OF LIABILITY, WHETHER IN CONTRACT, STRICT LIABILITY,
# OR TORT (INCLUDING NEGLIGENCE OR OTHERWISE) ARISING IN ANY WAY OUT OF THE USE
# OF THIS SOFTWARE, EVEN IF ADVISED OF THE POSSIBILITY OF SUCH DAMAGE.
# python
#import pwd
import getpass
import tempfile
import time
from collections import OrderedDict
from os.path import join
import numpy as np
import torch
import random
import os
def retry(times, exceptions):
"""
Retry Decorator https://stackoverflow.com/a/64030200/1645784
Retries the wrapped function/method `times` times if the exceptions listed
in ``exceptions`` are thrown
:param times: The number of times to repeat the wrapped function/method
:type times: Int
:param exceptions: Lists of exceptions that trigger a retry attempt
:type exceptions: Tuple of Exceptions
"""
def decorator(func):
def newfn(*args, **kwargs):
attempt = 0
while attempt < times:
try:
return func(*args, **kwargs)
except exceptions:
print(f'Exception thrown when attempting to run {func}, attempt {attempt} out of {times}')
time.sleep(min(2 ** attempt, 30))
attempt += 1
return func(*args, **kwargs)
return newfn
return decorator
def flatten_dict(d, prefix='', separator='.'):
res = dict()
for key, value in d.items():
if isinstance(value, (dict, OrderedDict)):
res.update(flatten_dict(value, prefix + key + separator, separator))
else:
res[prefix + key] = value
return res
def set_np_formatting():
""" formats numpy print """
np.set_printoptions(edgeitems=30, infstr='inf',
linewidth=4000, nanstr='nan', precision=2,
suppress=False, threshold=10000, formatter=None)
def set_seed(seed, torch_deterministic=False, rank=0):
""" set seed across modules """
if seed == -1 and torch_deterministic:
seed = 42 + rank
elif seed == -1:
seed = np.random.randint(0, 10000)
else:
seed = seed + rank
print("Setting seed: {}".format(seed))
random.seed(seed)
np.random.seed(seed)
torch.manual_seed(seed)
os.environ['PYTHONHASHSEED'] = str(seed)
torch.cuda.manual_seed(seed)
torch.cuda.manual_seed_all(seed)
if torch_deterministic:
# refer to https://docs.nvidia.com/cuda/cublas/index.html#cublasApi_reproducibility
os.environ['CUBLAS_WORKSPACE_CONFIG'] = ':4096:8'
torch.backends.cudnn.benchmark = False
torch.backends.cudnn.deterministic = True
torch.use_deterministic_algorithms(True)
else:
torch.backends.cudnn.benchmark = True
torch.backends.cudnn.deterministic = False
return seed
def nested_dict_set_attr(d, key, val):
pre, _, post = key.partition('.')
if post:
nested_dict_set_attr(d[pre], post, val)
else:
d[key] = val
def nested_dict_get_attr(d, key):
pre, _, post = key.partition('.')
if post:
return nested_dict_get_attr(d[pre], post)
else:
return d[key]
def ensure_dir_exists(path):
if not os.path.exists(path):
os.makedirs(path)
return path
def safe_ensure_dir_exists(path):
"""Should be safer in multi-treaded environment."""
try:
return ensure_dir_exists(path)
except FileExistsError:
return path
def get_username():
uid = os.getuid()
try:
return getpass.getuser()
except KeyError:
# worst case scenario - let's just use uid
return str(uid)
def project_tmp_dir():
tmp_dir_name = f'ige_{get_username()}'
return safe_ensure_dir_exists(join(tempfile.gettempdir(), tmp_dir_name))
# EOF
| 5,149 | Python | 31.389937 | 110 | 0.666731 |
NVIDIA-Omniverse/IsaacGymEnvs/isaacgymenvs/utils/rna_util.py |
# Copyright (c) 2018-2023, NVIDIA Corporation
# All rights reserved.
#
# Redistribution and use in source and binary forms, with or without
# modification, are permitted provided that the following conditions are met:
#
# 1. Redistributions of source code must retain the above copyright notice, this
# list of conditions and the following disclaimer.
#
# 2. Redistributions in binary form must reproduce the above copyright notice,
# this list of conditions and the following disclaimer in the documentation
# and/or other materials provided with the distribution.
#
# 3. Neither the name of the copyright holder nor the names of its
# contributors may be used to endorse or promote products derived from
# this software without specific prior written permission.
#
# THIS SOFTWARE IS PROVIDED BY THE COPYRIGHT HOLDERS AND CONTRIBUTORS "AS IS"
# AND ANY EXPRESS OR IMPLIED WARRANTIES, INCLUDING, BUT NOT LIMITED TO, THE
# IMPLIED WARRANTIES OF MERCHANTABILITY AND FITNESS FOR A PARTICULAR PURPOSE ARE
# DISCLAIMED. IN NO EVENT SHALL THE COPYRIGHT HOLDER OR CONTRIBUTORS BE LIABLE
# FOR ANY DIRECT, INDIRECT, INCIDENTAL, SPECIAL, EXEMPLARY, OR CONSEQUENTIAL
# DAMAGES (INCLUDING, BUT NOT LIMITED TO, PROCUREMENT OF SUBSTITUTE GOODS OR
# SERVICES; LOSS OF USE, DATA, OR PROFITS; OR BUSINESS INTERRUPTION) HOWEVER
# CAUSED AND ON ANY THEORY OF LIABILITY, WHETHER IN CONTRACT, STRICT LIABILITY,
# OR TORT (INCLUDING NEGLIGENCE OR OTHERWISE) ARISING IN ANY WAY OUT OF THE USE
# OF THIS SOFTWARE, EVEN IF ADVISED OF THE POSSIBILITY OF SUCH DAMAGE.
from __future__ import print_function
import torch
import torch.nn as nn
import torch.nn.functional as F
class RandomNetworkAdversary(nn.Module):
def __init__(self, num_envs, in_dims, out_dims, softmax_bins, device):
super(RandomNetworkAdversary, self).__init__()
"""
Class to add random action to the action generated by the policy.
The output is binned to 32 bins per channel and we do softmax over
these bins to figure out the most likely joint angle.
Note: OpenAI et al. 2019 found out that if they used a continuous space
and a tanh non-linearity, actions would always be close to 0.
Section B.3 https://arxiv.org/abs/1910.07113
Q: Why do we need dropouts here?
A: If we were using a CPU-based simulator as in OpenAI et al. 2019, we
will use a different RNA network for different CPU. However,
this is not feasible for a GPU-based simulator as that would mean
creating N_envs RNA networks which will overwhelm the GPU-memory.
Therefore, dropout is a nice approximation of this by re-sampling
weights of the same neural network for each different env on the GPU.
"""
self.in_dims = in_dims
self.out_dims = out_dims
self.softmax_bins = softmax_bins
self.num_envs = num_envs
self.device = device
self.num_feats1 = 512
self.num_feats2 = 1024
# Sampling random probablities for dropout masks
dropout_probs = torch.rand((2, ))
# Setting up the RNA neural network here
# First layer
self.fc1 = nn.Linear(in_dims, self.num_feats1).to(self.device)
self.dropout_masks1 = torch.bernoulli(torch.ones((self.num_envs, \
self.num_feats1)), p=dropout_probs[0]).to(self.device)
self.fc1_1 = nn.Linear(self.num_feats1, self.num_feats1).to(self.device)
# Second layer
self.fc2 = nn.Linear(self.num_feats1, self.num_feats2).to(self.device)
self.dropout_masks2 = torch.bernoulli(torch.ones((self.num_envs, \
self.num_feats2)), p=dropout_probs[1]).to(self.device)
self.fc2_1 = nn.Linear(self.num_feats2, self.num_feats2).to(self.device)
# Last layer
self.fc3 = nn.Linear(self.num_feats2, out_dims*softmax_bins).to(self.device)
# This is needed to reset weights and dropout masks
self._refresh()
def _refresh(self):
self._init_weights()
self.eval()
self.refresh_dropout_masks()
def _init_weights(self):
print('initialising weights for random network')
nn.init.kaiming_uniform_(self.fc1.weight)
nn.init.kaiming_uniform_(self.fc1_1.weight)
nn.init.kaiming_uniform_(self.fc2.weight)
nn.init.kaiming_uniform_(self.fc2_1.weight)
nn.init.kaiming_uniform_(self.fc3.weight)
return
def refresh_dropout_masks(self):
dropout_probs = torch.rand((2, ))
self.dropout_masks1 = torch.bernoulli(torch.ones((self.num_envs, self.num_feats1)), \
p=dropout_probs[0]).to(self.dropout_masks1.device)
self.dropout_masks2 = torch.bernoulli(torch.ones((self.num_envs, self.num_feats2)), \
p=dropout_probs[1]).to(self.dropout_masks2.device)
return
def forward(self, x):
x = self.fc1(x)
x = F.relu(x)
x = self.fc1_1(x)
x = self.dropout_masks1 * x
x = self.fc2(x)
x = F.relu(x)
x = self.fc2_1(x)
x = self.dropout_masks2 * x
x = self.fc3(x)
x = x.view(-1, self.out_dims, self.softmax_bins)
output = F.softmax(x, dim=-1)
# We have discretised the joint angles into bins
# Now we pick up the bin for each joint angle
# corresponding to the highest softmax value / prob.
return output
if __name__ == "__main__":
num_envs = 1024
RNA = RandomNetworkAdversary(num_envs=num_envs, in_dims=16, out_dims=16, softmax_bins=32, device='cuda')
x = torch.tensor(torch.randn(num_envs, 16).to(RNA.device))
y = RNA(x)
import ipdb; ipdb.set_trace()
| 5,780 | Python | 34.25 | 108 | 0.659689 |
NVIDIA-Omniverse/IsaacGymEnvs/docs/domain_randomization.md | Domain Randomization
====================
Overview
--------
We sometimes need our reinforcement learning agents to be robust to
different physics than they are trained with, such as when attempting a
sim2real policy transfer. Using domain randomization, we repeatedly
randomize the simulation dynamics during training in order to learn a
good policy under a wide range of physical parameters.
IsaacGymEnvs supports "on the fly" domain randomization, allowing dynamics
to be changed when resetting the environment, but without requiring
reloading of assets. This allows us to efficiently apply domain
randomizations without common overheads like re-parsing asset files.
Domain randomization must take place at environment reset time, as some
environment properties are reset when applying randomizations at the
physics simulation level.
We provide two interfaces to add domain randomization to your `isaacgymenvs`
tasks:
1. Adding domain randomization parameters to your task's YAML config
2. Directly calling the `apply_randomizations` class method
Underneath both interfaces is a nested dictionary that allows you to
fully specify which parameters to randomize, what distribution to sample
for each parameter, and an option to schedule when the randomizations
are applied or anneal the range over time. We will first discuss all the
"knobs and dials" you can tune in this dictionary, and then how to
incorporate either of the interfaces within your tasks.
Domain Randomization Dictionary
-------------------------------
We will first explain what can be randomized in the scene and the
sampling distributions and schedulers available. There are four main
parameter groups that support randomization. They are:
- `observations`
: - Add noise directly to the agent observations
- `actions`
: - Add noise directly to the agent actions
- `sim_params`
: - Add noise to physical parameters defined for the entire
scene, such as `gravity`
- `actor_params`
: - Add noise to properties belonging to your actors, such as
the `dof_properties` of a ShadowHand
For each parameter you wish to randomize, you can specify the following
settings:
- `distribution`
: - The distribution to generate a sample `x` from.
- Choices: `uniform`, `loguniform`, `gaussian`.
: - `x ~ unif(a, b)`
- `x ~ exp(unif(log(a), log(b)))`
- `x ~ normal(a, b)`
- Parameters `a` and `b` are defined by the `range` setting.
- `range`
: - Specified as tuple `[a, b]` of real numbers.
- For `uniform` and `loguniform` distributions, `a` and `b`
are the lower and upper bounds.
- For `gaussian`, `a` is the distribution mean and `b` is the
variance.
- `operation`
: - Defines how the generated sample `x` will be applied to the
original simulation parameter.
- Choices: `additive`, `scaling`
: - For `additive` noise, add the sample to the original
value.
- For `scaling` noise, multiply the original value by
the sample.
- `schedule`
: - Optional parameter to specify how to change the
randomization distribution over time
- Choices: `constant`, `linear`
: - For a `constant` schedule, randomizations are only
applied after `schedule_steps` frames.
- For a `linear` schedule, linearly interpolate
between no randomization and maximum randomization
as defined by your `range`.
- `schedule_steps`
: - Integer frame count used in `schedule` feature
- `setup_only`
: - Specifies whether the parameter is to be randomized during setup only. Defaults to `False`
- If set to `True`, the parameter will not be randomized or set during simulation
- `Mass` and `Scale` must have this set to `True` - the GPU pipeline API does not currently support changing these properties at runtime. See Programming/Physics documentation for Isaac Gym for more details
- Requires making a call to `apply_randomization` before simulation begins (i.e. inside `create_sim`)
We additionally can define a `frequency` parameter that will specify how
often (in number of environment steps) to wait before applying the next
randomization. Observation and action noise is randomized every frame,
but the range of randomization is updated per the schedule only every
`frequency` environment steps.
YAML Interface
--------------
Now that we know what options are available for domain randomization,
let's put it all together in the YAML config. In your isaacgymenvs/cfg/task yaml
file, you can specify your domain randomization parameters under the
`task` key. First, we turn on domain randomization by setting
`randomize` to `True`:
task:
randomize: True
randomization_params:
...
Next, we will define our parameters under the `randomization_params`
keys. Here you can see how we used the previous settings to define some
randomization parameters for a ShadowHand cube manipulation task:
randomization_params:
frequency: 600 # Define how many frames between generating new randomizations
observations:
range: [0, .05]
operation: "additive"
distribution: "uniform"
schedule: "constant" # turn on noise after `schedule_steps` num steps
schedule_steps: 5000
actions:
range: [0., .05]
operation: "additive"
distribution: "uniform"
schedule: "linear" # linearly interpolate between 0 randomization and full range
schedule_steps: 5000
sim_params:
gravity:
range: [0, 0.4]
operation: "additive"
distribution: "uniform"
actor_params:
hand:
color: True
dof_properties:
upper:
range: [0, 0.15]
operation: "additive"
distribution: "uniform"
cube:
rigid_body_properties:
mass:
range: [0.5, 1.5]
operation: "scaling"
distribution: "uniform"
setup_only: True
Note how we structured the `actor_params` randomizations. When creating
actors using `gym.create_actor`, you have the option to specify a name
for your actor. We figure out which randomizations to apply to actors
based on this name option. **To use domain randomization, your agents
must have the same name in** `create_actor` **and in the randomization
YAML**. In our case, we wish to randomize all ShadowHand instances the
same way, so we will name all our ShadowHand actors as `hand`. Depending
on the asset, you have access to randomize `rigid_body_properties`,
`rigid_shape_properties`, `dof_properties`, and `tendon_properties`. We
also include an option to set the `color` of each rigid body in an actor
(mostly for debugging purposes), but do not support extensive visual
randomizations (like lighting and camera directions) currently. The
exact properties available are listed as follows.
**rigid\_body\_properties**:
(float) mass # mass value, in kg
(float) invMass # Inverse of mass value.
**rigid\_shape\_properties**:
(float) friction # Coefficient of static friction. Value should be equal or greater than zero.
(float) rolling_friction # Coefficient of rolling friction.
(float) torsion_friction # Coefficient of torsion friction.
(float) restitution # Coefficient of restitution. It's the ratio of the final to initial velocity after the rigid body collides. Range: [0,1]
(float) compliance # Coefficient of compliance. Determines how compliant the shape is. The smaller the value, the stronger the material will hold its shape. Value should be greater or equal to zero.
(float) thickness # How far objects should come to rest from the surface of this body
**dof\_properties**:
(float) lower # lower limit of DOF. In radians or meters
(float) upper \# upper limit of DOF. In radians or meters
(float) velocity \# Maximum velocity of DOF. In Radians/s, or m/s
(float) effort \# Maximum effort of DOF. in N or Nm.
(float) stiffness \# DOF stiffness.
(float) damping \# DOF damping.
(float) friction \# DOF friction coefficient, a generalized friction force is calculated as DOF force multiplied by friction.
(float) armature \# DOF armature, a value added to the diagonal of the joint-space inertia matrix. Physically, it corresponds to the rotating part of a motor - which increases the inertia of the joint, even when the rigid bodies connected by the joint can have very little inertia.
**tendon\_properties**:
(float) stiffness # Tendon spring stiffness
(float) damping # Tendon and limit damping. Applies to both tendon and limit spring-damper dynamics.
(float) fixed_spring_rest_length # Fixed tendon spring rest length. When tendon length = springRestLength the tendon spring force is equal to zero
(float) fixed_lower_limit # Fixed tendon length lower limit
(float) fixed_upper_limit # Fixed tendon length upper limit
To actually apply randomizations during training, you will need to have
a copy of the params available in your task class instance, and to call
`self.apply_randomizations`. The easiest way to do is to instantiate a
dictionary with the parameters in your Task's `__init__` call:
self.randomization_params = self.cfg["task"]["randomization_params"]
We also recommend that you call `self.apply_randomizations` once in your
`create_sim()` code to do an initial randomization pass before simulation
starts. This is required for randomizing `mass` or `scale` properties.
Supporting scheduled randomization also requires adding an additional
line of code to your `post_physics_step()` code to update how far along
in randomization scheduling each environment is - this is stored in the
`randomize_buf` tensor in the base class:
def post_physics_step(self):
self.randomize_buf += 1
Finally, add a call to `apply_randomizations` during the reset portion
of the training loop. The function takes as arguments a domain
randomization dictionary:
def reset(self, env_ids):
self.apply_randomizations(self.randomization_params)
...
Only environments that are in the reset buffer and which have exceeded
the specified `frequency` time-steps since last randomized will have
new randomizations applied.
Custom domain randomizations
----------------------------
**Custom randomizations via a class method**:
Provided your task inherits from our `VecTask` class, you have great
flexibility in choosing when to randomize and what distributions to
sample, and can even change the entire domain randomization dictionary
at every call to `apply_randomizations` if you wish. By using your own
logic to generate these dictionaries, our current framework can be
easily extended to use more intelligent algorithms for domain
randomization, such as ADR or BayesSim.
Automatic Domain Randomisation
------------------------------
Our [DeXtreme](https://dextreme.org) work brings Automatic Domain Randomisation (ADR) into Isaac Gym. Since, the simulator is built on vectorising environments on the GPU, our ADR naturally comes with vectorised implementation. Note that we have only tested ADR for DeXtreme environments mentioned in [dextreme.md](dextreme.md) and we are working towards bringing ADR and DeXtreme to [OmniIsaacGymEnvs](https://github.com/NVIDIA-Omniverse/OmniIsaacGymEnvs).
**Background**
ADR was first introduced in [OpenAI 2019 et. al](https://arxiv.org/abs/1910.07113). We develop the vectorised version of this and use that to train our policies in sim and transfer to the real world. Our experiments reaffirm that ADR imbues robustness to the policies closing the sim-to-real gap significantly leading to better performance in the real world compared to traiditional manually tuned domain randomisation.
Hand-tuning the randomisation ranges (_e.g._ means and stds of the distributions) of parameters can be onerous and may result in policies that lack adaptability, even for slight variations in parameters outside of the originally defined ranges. ADR starts with small ranges and automatically adjusts them gradually to keep them as wide as possible while keeping the policy performance above a certain threshold. The policies trained with ADR exhibit significant robustness to various perturbations and parameter ranges and improved sim-to-real transfer. Additionally, since the ranges are adjusted gradually, it also provides a natural curriculum for the policy to absorb the large diverity thrown at it.
Each parameter that we wish to randomise with ADR is modelled with uniform distribution `U(p_lo, p_hi)` where `p_lo` and `p_hi` are the lower and the upper limit of the range respectively. At each step, a parameter is randomy chosen and its value set to either the lower or upper limit keeping the other parameters with their ranges unchanged. This randomly chosen parameter's range is updated based on its performance. A small fraction of the overall environments (40% in our [DeXtreme](https://dextreme.org) work) is used to evaluate the performance. Based on the performance, either the range shrinks or expands. A visualisation from the DeXtreme paper is shown below:
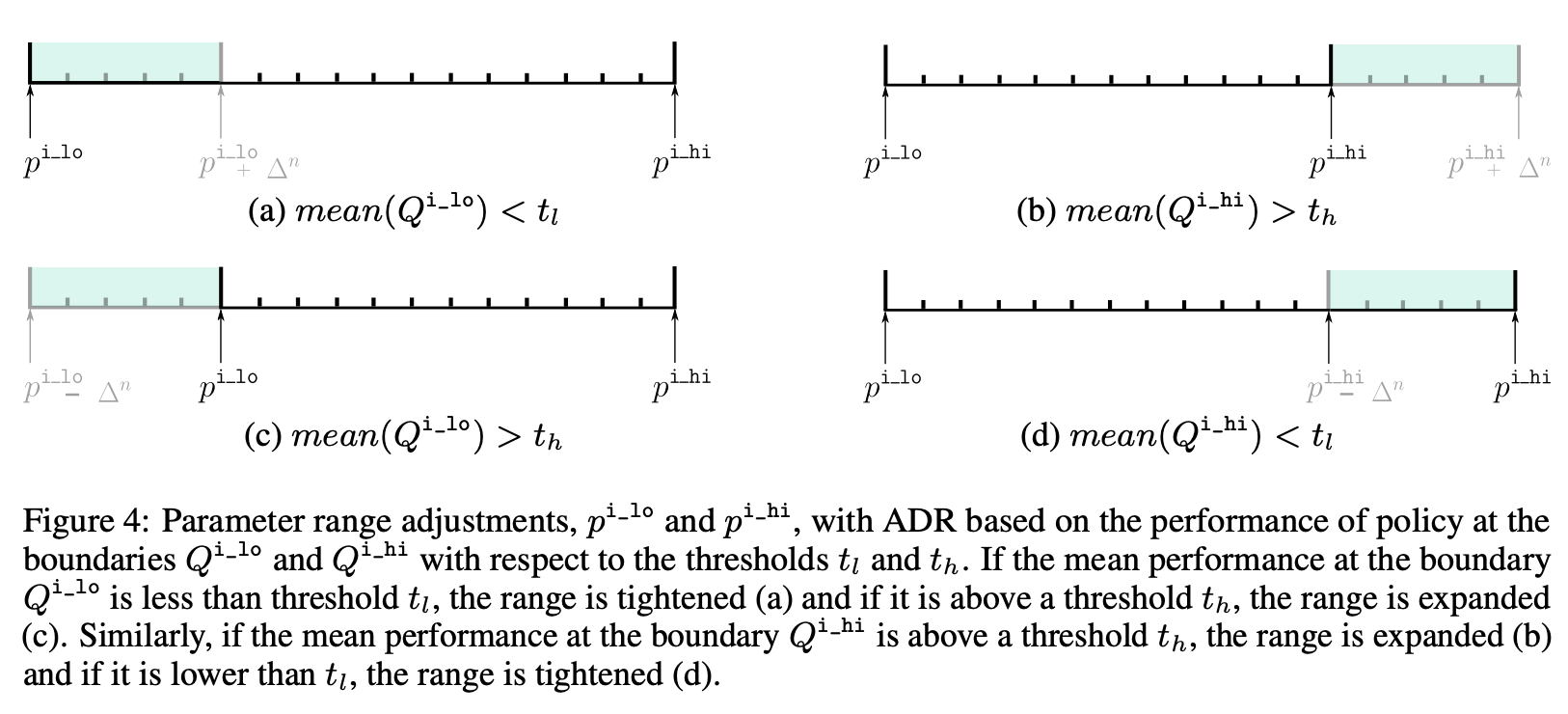
If the parameter value was set to the lower limit, then a decrease in performance, measured by performance threshold `t_l`, dicatates reducing the range of the parameter (shown in (a) in the image) by increasing the lower limit value by a small delta. Conversely, if the performance is increased, measured by performance threshold, `t_h`, the lower limit is decreased (shown in (c) in the image) leading to expanding the overall range.
Similarly, if the parameter value was set to the upper limit, then an increase in performance, measured by performance threshold `t_h`, expands the range (shown in (b) in the image) by increasing the upper limit value by a small delta. However, if the performance is decreased, measured by performance threshold, `t_l`, the upper limit is decreased (shown in (d) in the image) leading to shrinking the overall range.
**Implementation**
The ADR implementation resides in [adr_vec_task.py](../isaacgymenvs/tasks/dextreme/adr_vec_task.py) located in `isaacgymenvs/tasks/dextreme` folder. The `ADRVecTask` inherits much of the `VecTask` functionality and an additional class to denote the state of the environment when evaluating the performance
```
class RolloutWorkerModes:
ADR_ROLLOUT = 0 # rollout with current ADR params
ADR_BOUNDARY = 1 # rollout with params on boundaries of ADR, used to decide whether to expand ranges
```
Since ADR needs to have the evaluation in the loop to benchmark the performance and adjust the ranges consequently, some fraction of the environments are dedicated to the evaluation denoted by `ADR_BOUNDARY`. Rest of the environments continue to use the unchanged ranges and are denoted by `ADR_ROLLOUT`.
The `apply_randomisation` has additional arguments this time `randomise_buf`, `adr_objective` and `randomisation_callback`. The variable `randomise_buf` enables selective randomisation of some environments while keeping others unchanged, `adr_objective` is the number of consecutive successes and `randomisation_callback` allows using any callbacks for randomisation from the `ADRDextreme` class.
YAML Interface
--------------
The YAML file interface now has additional `adr` key where we need to set the appropriate variables and it looks like the following:
```
adr:
use_adr: True
# set to false to not do update ADR ranges.
# useful for evaluation or training a base policy
update_adr_ranges: True
clear_other_queues: False
# if set, boundary sampling and performance eval will occur at (bound + delta) instead of at bound.
adr_extended_boundary_sample: False
worker_adr_boundary_fraction: 0.4 # fraction of workers dedicated
to measuring perf of ends of ADR ranges to update the ranges
adr_queue_threshold_length: 256
adr_objective_threshold_low: 5
adr_objective_threshold_high: 20
adr_rollout_perf_alpha: 0.99
adr_load_from_checkpoint: false
params:
### Hand Properties
hand_damping:
range_path: actor_params.hand.dof_properties.damping.range
init_range: [0.5, 2.0]
limits: [0.01, 20.0]
delta: 0.01
delta_style: 'additive'
....
```
Lets unpack the variables here and go over them one by one:
- `use_adr`: This flag enables ADR.
- `update_adr_ranges`: This flag when set to `True` ensures that the ranges of the parameters are updated.
- `clear_other_queues`: This means that for when evaluating parameter A, whether we want to clear the queue for parameter B. More information on the queue is provided for `adr_queue_threshold_length` below.
- `adr_extended_boundary_sample`: We test the performance at either the boundary of the parameter limits of boundary + delta. When this flag is set to `True`, the performance evaluation of the parameter is doing on boundary + delta instead of boundary.
- `worker_adr_boundary_fraction`: For the evaluation, certain fraction of the overall environments are chosen and this variable allows setting that fraction.
- `adr_queue_threshold_length`: The performance is evaluated periodically and stored in a queue and averaged. This variable allows choosing the length of the queue so that statistics are computed over a sufficiently large window. We do not want to rely on policy achieving the thresholds by chance; we want it to maintain the peaks for a while. Therefore, a queue allows logging statistics over a given time frame to be sure that its performing above the threshold.
- `adr_objective_threshold_low`: This is the `t_l` threshold mentioned in the **Background** section above. Also shown in the image.
- `adr_objective_threshold_high`: This is the `t_h` threshold as mentioned above in the image.
- `adr_rollout_perf_alpha`: This is the smoothing factor used to compute the performance.
- `adr_load_from_checkpoint`: The saved checkpoints also contain the ADR optimised ranges. Therefore, if you want to load up those ranges for future post-hoc evaluation, you should set this to `True`. If set to `False`, it will only load the ranges from the YAML file and not update them from the checkpoint.
Additionally, as you may have noticed, each parameter now also comes with `limit` and `delta` variables. The variable `limits` refers to the complete range within which the parameter is permitted to move, while `delta` represents the incremental change that the parameter can undergo with each ADR update.
| 19,220 | Markdown | 55.201754 | 704 | 0.72487 |
NVIDIA-Omniverse/IsaacGymEnvs/docs/dextreme.md |
DeXtreme is our recent work on transferring cube rotation with allegro hand from simulations to the real world. This task is especially challenging due to increased number of contacts that come into play with doing physics simulation. Naturally, the transfer requires carefully modelling and scheduling the randomisation for both physics and non-physics parameters. More details of the work can be found on the website https://dextreme.org/ as well as the paper (accepted at ICRA 2023, London) available on arXiv https://arxiv.org/pdf/2210.13702.pdf.
The work builds on top of our previously released `AllegroHand` environment but with changes to accomodate training for sim-to-real involving two different variants: ManualDR (where the ranges of parameter domain randomisation are chosen by the user manually) and Automatic Domain Randomisation or ADR (where the ranges of the parameter are updated automatically based on periodic simulation performance benchmarking in the loop).
Overview
--------
There are two different classes **AllegroHandDextremeManualDR** and **AllegroHandDextremeADR** both located in [tasks/dextreme/allegro_hand_dextreme.py](../isaacgymenvs/tasks/dextreme/allegro_hand_dextreme.py) python file. There's additional [adr_vec_task.py](../isaacgymenvs/tasks/dextreme/adr_vec_task.py) located in the same [folder](../isaacgymenvs/tasks/dextreme/) that covers the necessary code related to training with ADR in the `ADRVecTask` class.
Both the variants are trained with `Asymmetric Actor-Critic` where the `policy` only receives the input that is available in the real world while the `value function` receives additional privileged information available from the simulator. At inference, only the policy is used to obtain the action given the history of states and value function is discarded. For more information, please look at `Section 2` of the DeXtreme paper.
As we will show below, both environments are compatible with the standard way of training with Isaac Gym via `python train.py task=<AllegroHandDextremeManualDR or AllegroHandDextremeADR>`. Additionally, the code uses `dictionary observations` enabled via `use_dict_obs=True` (set as default for these enviornments) in the `ADRVecTask` where the relevant observations needed for training are provided as dictionaries as opposed to filling in the data via slicing and indexing. This keeps it cleaner and easier to manage. Which observations to choose for the policy and value function can be described in the corresponding `yaml` files for training located in `cfg/train` folder. For instance, the policy in the [AllegroHandDextremeManualDRPPO.yaml](../isaacgymenvs/cfg/train/AllegroHandDextremeManualDRPPO.yaml) can be described below like
```
inputs:
dof_pos_randomized: { }
object_pose_cam_randomized: { }
goal_pose_randomized: { }
goal_relative_rot_cam_randomized: { }
last_actions_randomized: { }
```
Similarly, for the value function
``` network:
name: actor_critic
central_value: True
inputs:
dof_pos: { }
dof_vel: { }
dof_force: { }
object_pose: { }
object_pose_cam_randomized: { }
object_vels: { }
goal_pose: { }
goal_relative_rot: {}
last_actions: { }
ft_force_torques: {}
gravity_vec: {}
ft_states: {}
```
Similar configuration set up is done for [AllegroHandDextremeADRPPO.yaml](../isaacgymenvs/cfg/train/AllegroHandDextremeManualDRPPO.yaml).
Various parameters that the user wishes to randomise for their training can be chosen and tuned in the corresponding `task` files located in `cfg/task` [folder](../isaacgymenvs/cfg/task/). For instance, in [AllegroHandDextremeManualDR.yaml](../isaacgymenvs/cfg/task/AllegroHandDextremeManualDR.yaml), the randomisation parameters and ranges can be found under
```
task:
randomize: True
randomization_params:
....
```
For the [AllegroHandDextremeADR.yaml](../isaacgymenvs/cfg/task/AllegroHandDextremeADR.yaml), additional configuration is needed and can be found under
```
adr:
use_adr: True
# set to false to not do update ADR ranges. useful for evaluation or training a base policy
update_adr_ranges: True
...
# raw ADR params. more are added by affine transforms code
params:
### Hand Properties
hand_damping:
range_path: actor_params.hand.dof_properties.damping.range
init_range: [0.5, 2.0]
limits: [0.01, 20.0]
delta: 0.01
delta_style: 'additive'
```
You will also see that there are two key variables: `limits` and `delta`. The variable `limits` refers to the complete range within which the parameter is permitted to move, while `delta` represents the incremental change that the parameter can undergo with each ADR update. These variables play a crucial role in determining the scope and pace of parameter adjustments made by ADR.
We highly recommend to familiarise yourself with the codebase and configuration files first before training to understand the relevant classes and the inheritence involved.
Below we provide the exact settings for training the two different variants of the environment we used in our work for reproducibility.
# To run experiments with Manual DR settings
If you are using a single GPU, run the following command to train DeXtreme RL policies with Manual DR
```
HYDRA_MANUAL_DR="train.py multi_gpu=False \
task=AllegroHandDextremeManualDR \
task.env.resetTime=8 task.env.successTolerance=0.4 \
experiment='allegrohand_dextreme_manual_dr' \
headless=True seed=-1 \
task.env.startObjectPoseDY=-0.15 \
task.env.actionDeltaPenaltyScale=-0.2 \
task.env.resetTime=8 \
task.env.controlFrequencyInv=2 \
train.params.network.mlp.units=[512,512] \
train.params.network.rnn.units=768 \
train.params.network.rnn.name=lstm \
train.params.config.central_value_config.network.mlp.units=[1024,512,256] \
train.params.config.max_epochs=50000 \
task.env.apply_random_quat=True"
python ${HYDRA_MANUAL_DR}
```
The `apply_random_quat=True` flag samples unbiased quaternion goals which makes the training slightly harder. We use a successTolerance of 0.4 radians in these settings overriding the settings in AllegroHandDextremeManualDR.yaml via hydra CLI.
# To run experiments with Automatic Domain Randomisation (ADR)
The ADR policies are trained with a successTolerance of 0.1 radians and use LSTMs both for policy as well as value function. For ADR on a single GPU, run the following commands to train the RL policies
```
HYDRA_ADR="train.py multi_gpu=False \
task=AllegroHandDextremeADR \
headless=True seed=-1 \
num_envs=8192 \
task.env.resetTime=8 \
task.env.controlFrequencyInv=2 \
train.params.config.max_epochs=50000"
python ${HYDRA_ADR}
```
If you want to do `wandb_logging` you can also add the following to the `HYDRA_MANUAL_DR`
```
wandb_activate=True wandb_group=group_name wandb_project=project_name"
```
To log the entire isaacgymenvs code used to train in the wandb dashboard (this is useful for reproducibility as you make changes to your code) you can add:
```
wandb_logcode_dir=<isaac_gym_dir>
```
# Loading checkpoints
To load a given checkpoint using ManualDR, you can use the following
```
python train.py task=AllegroHandDextremeManualDR \
num_envs=32 task.env.startObjectPoseDY=-0.15 \
task.env.actionDeltaPenaltyScale=-0.2 \
task.env.controlFrequencyInv=2 train.params.network.mlp.units=[512,512] \
train.params.network.rnn.units=768 \
train.params.network.rnn.name=lstm \
train.params.config.central_value_config.network.mlp.units=[1024,512,256] \
task.env.random_network_adversary.enable=True checkpoint=<ckpt_path> \
test=True task.env.apply_random_quat=True task.env.printNumSuccesses=False
```
and for ADR, add `task.task.adr.adr_load_from_checkpoint=True` to the command above, i.e.
```
python train.py task=AllegroHandDextremeADR \
num_envs=2048 checkpoint=<your_checkpoint_path> \
test=True \
task.task.adr.adr_load_from_checkpoint=True \
task.env.printNumSuccesses=True \
headless=True
```
It will also print statistics and create a new `eval_summaries` directory logging the performance for test in a tensorboard log. For the ADR testing, it is will also load the new adr parameters (they are saved in the checkpoint and can also be viewed in the `set_env_state` function in `allegro_hand_dextreme.py`). You should see something like this when you load a checkpoint with ADR
```
=> loading checkpoint 'your_checkpoint_path'
Loaded env state value act_moving_average:0.183225
Skipping loading ADR params from checkpoint...
ADR Params after loading from checkpoint: {'hand_damping': {'range_path': 'actor_params.hand.dof_properties.damping.range',
'init_range': [0.5, 2.0], 'limits': [0.01, 20.0], 'delta': 0.01, 'delta_style': 'additive', 'range': [0.5, 2.0],
'next_limits': [0.49, 2.01]}, 'hand_stiffness': {'range_path': 'actor_params.hand.dof_properties.stiffness.range',
'init_range': [0.8, 1.2], 'limits': [0.01, 20.0], 'delta': 0.01, 'delta_style': 'additive', 'range': [0.8, 1.2],
'next_limits': [0.79, 1.21]}, 'hand_joint_friction': {'range_path': 'actor_params.hand.dof_properties.friction.range',
'init_range': [0.8, 1.2], 'limits': [0.0, 10.0], 'delta': 0.01, 'delta_style': 'additive', 'range': [0.8, 1.2],
'next_limits': [0.79, 1.21]}, 'hand_armature': {'range_path': 'actor_params.hand.dof_properties.armature.range',
'init_range': [0.8, 1.2], 'limits': [0.0, 10.0], 'delta': 0.01, 'delta_style': 'additive', 'range': [0.8, 1.2],
'next_limits': [0.79, 1.21]}, 'hand_effort': {'range_path': 'actor_params.hand.dof_properties.effort.range',
'init_range': [0.9, 1.1], 'limits': [0.4, 10.0], 'delta': 0.01, 'delta_style': 'additive', 'range': [0.9, 1.1],
'next_limits': [0.89, 1.11]}, 'hand_lower': {'range_path': 'actor_params.hand.dof_properties.lower.range',
'init_range': [0.0, 0.0], 'limits': [-5.0, 5.0], 'delta': 0.02, 'delta_style': 'additive', 'range': [0.0, 0.0],
'next_limits': [-0.02, 0.02]}, 'hand_upper': {'range_path': 'actor_params.hand.dof_properties.upper.range',
'init_range': [0.0, 0.0], 'limits': [-5.0, 5.0], 'delta': 0.02, 'delta_style': 'additive', 'range': [0.0, 0.0],
'next_limits': [-0.02, 0.02]}, 'hand_mass': {'range_path': 'actor_params.hand.rigid_body_properties.mass.range',
'init_range': [0.8, 1.2], 'limits': [0.01, 10.0], 'delta': 0.01, 'delta_style': 'additive', 'range': [0.8, 1.2],
'next_limits': [0.79, 1.21]}, 'hand_friction_fingertips': {'range_path': 'actor_params.hand.rigid_shape_properties.friction.range', 'init_range': [0.9, 1.1], 'limits': [0.1, 2.0], 'delta': 0.01, 'delta_style': 'additive', 'range': [0.9, 1.1],
'next_limits': [0.89, 1.11]}, 'hand_restitution': {'range_path': 'actor_params.hand.rigid_shape_properties.restitution.range',
'init_range': [0.0, 0.1], 'limits': [0.0, 1.0], 'delta': 0.01, 'delta_style': 'additive', 'range': [0.0, 0.1],
'next_limits': [0.0, 0.11]}, 'object_mass': {'range_path': 'actor_params.object.rigid_body_properties.mass.range',
'init_range': [0.8, 1.2], 'limits': [0.01, 10.0], 'delta': 0.01, 'delta_style': 'additive', 'range': [0.8, 1.2],
'next_limits': [0.79, 1.21]}, 'object_friction': {'range_path': 'actor_params.object.rigid_shape_properties.friction.range',
'init_range': [0.4, 0.8], 'limits': [0.01, 2.0], 'delta': 0.01, 'delta_style': 'additive', 'range': [0.4, 0.8],
'next_limits': [0.39, 0.81]}, 'object_restitution': {'range_path': 'actor_params.object.rigid_shape_properties.restitution.range', 'init_range': [0.0, 0.1], 'limits': [0.0, 1.0], 'delta': 0.01, 'delta_style': 'additive', 'range': [0.0, 0.1],
'next_limits': [0.0, 0.11]}, 'cube_obs_delay_prob': {'init_range': [0.0, 0.05], 'limits': [0.0, 0.7], 'delta': 0.01, 'delta_style': 'additive', 'range': [0.0, 0.05], 'next_limits': [0.0, 0.060000000000000005]}, 'cube_pose_refresh_rate':
{'init_range': [1.0, 1.0], 'limits': [1.0, 6.0], 'delta': 0.2, 'delta_style': 'additive', 'range': [1.0, 1.0],
'next_limits': [1.0, 1.2]}, 'action_delay_prob': {'init_range': [0.0, 0.05], 'limits': [0.0, 0.7], 'delta': 0.01,
'delta_style': 'additive', 'range': [0.0, 0.05], 'next_limits': [0.0, 0.060000000000000005]},
'action_latency': {'init_range': [0.0, 0.0], 'limits': [0, 60], 'delta': 0.1, 'delta_style': 'additive', 'range': [0.0, 0.0],
'next_limits': [0, 0.1]}, 'affine_action_scaling': {'init_range': [0.0, 0.0], 'limits': [0.0, 4.0], 'delta': 0.0,
'delta_style': 'additive', 'range': [0.0, 0.0], 'next_limits': [0.0, 0.0]}, 'affine_action_additive': {'init_range': [0.0, 0.04],
'limits': [0.0, 4.0], 'delta': 0.01, 'delta_style': 'additive', 'range': [0.0, 0.04], 'next_limits': [0.0, 0.05]},
'affine_action_white': {'init_range': [0.0, 0.04], 'limits': [0.0, 4.0], 'delta': 0.01, 'delta_style': 'additive',
'range': [0.0, 0.04], 'next_limits': [0.0, 0.05]}, 'affine_cube_pose_scaling': {'init_range': [0.0, 0.0],
'limits': [0.0, 4.0], 'delta': 0.0, 'delta_style': 'additive', 'range': [0.0, 0.0], 'next_limits': [0.0, 0.0]},
'affine_cube_pose_additive': {'init_range': [0.0, 0.04], 'limits': [0.0, 4.0], 'delta': 0.01, 'delta_style':
'additive', 'range': [0.0, 0.04], 'next_limits': [0.0, 0.05]}, 'affine_cube_pose_white': {'init_range': [0.0, 0.04],
'limits': [0.0, 4.0], 'delta': 0.01, 'delta_style': 'additive', 'range': [0.0, 0.04], 'next_limits': [0.0, 0.05]},
'affine_dof_pos_scaling': {'init_range': [0.0, 0.0], 'limits': [0.0, 4.0], 'delta': 0.0, 'delta_style': 'additive',
'range': [0.0, 0.0], 'next_limits': [0.0, 0.0]}, 'affine_dof_pos_additive': {'init_range': [0.0, 0.04],
'limits': [0.0, 4.0], 'delta': 0.01, 'delta_style': 'additive', 'range': [0.0, 0.04], 'next_limits': [0.0, 0.05]},
'affine_dof_pos_white': {'init_range': [0.0, 0.04], 'limits': [0.0, 4.0], 'delta': 0.01, 'delta_style':
'additive', 'range': [0.0, 0.04], 'next_limits': [0.0, 0.05]}, 'rna_alpha': {'init_range': [0.0, 0.0],
'limits': [0.0, 1.0], 'delta': 0.01, 'delta_style': 'additive', 'range': [0.0, 0.0], 'next_limits': [0.0, 0.01]}}
```
# Multi-GPU settings
If you want to train on multiple GPUs (or a single DGX node), we also provide training scripts and the code to run both Manual DR as well as ADR below. The ${GPUS} variable needs to be set beforehand in your bash e.g. GPUS=8 if you are using a single node. Throughout our experimentation for the DeXtreme work, We trained our policies on a single node containg 8 NVIDIA A40 GPUs.
# Manual DR
To run the training with Manual DR settings on Multi-GPU settings set the flag `multi_gpu=True`. You will also need to add the following to the previous Manual DR command:
```
torchrun --nnodes=1 --nproc_per_node=${GPUS} --master_addr '127.0.0.1' ${HYDRA_MANUAL_DR}
```
# ADR
Similarly for ADR:
```
torchrun --nnodes=1 --nproc_per_node=${GPUS} --master_addr '127.0.0.1' ${HYDRA_ADR}
```
Below, we show the npd (nats per dimension cf. Algorithm 5.2 [OpenAI et al. 2019](https://arxiv.org/pdf/1910.07113.pdf) and Section 2.6.3 [DeXtreme](https://arxiv.org/pdf/2210.13702.pdf)) graphs of two batches of 8 different trials each run on a single node (8 GPUs) across different weeks. Each of these plots are meant to highlight the variability in the runs. Increase in npd means the networks are being trained on more divesity.


## RL training
To try the exact version of rl_games we used for training our experiments, please git clone and install `https://github.com/ArthurAllshire/rl_games`
| 15,570 | Markdown | 59.587548 | 839 | 0.694412 |
NVIDIA-Omniverse/IsaacGymEnvs/docs/reproducibility.md | Reproducibility and Determinism
===============================
Seeds
-----
To achieve deterministic behaviour on multiple training runs, a seed
value can be set in the training config file for each task. This will potentially
allow for individual runs of the same task to be deterministic when
executed on the same machine and system setup. Alternatively, a seed can
also be set via command line argument `seed=<seed>` to override any
settings in config files. If no seed is specified in either config files
or command line arguments, we default to generating a random seed. In
that case, individual runs of the same task should not be expected to be
deterministic. For convenience, we also support setting `seed=-1` to
generate a random seed, which will override any seed values set in
config files. By default, we have explicitly set all seed values in
config files to be 42.
PyTorch Deterministic Training
------------------------------
We also include a `torch_deterministic` argument for uses when running RL
training. Enabling this flag (passing `torch_deterministic=True`) will
apply additional settings to PyTorch that can force the usage of deterministic
algorithms in PyTorch, but may also negatively impact run-time performance.
For more details regarding PyTorch reproducibility, refer to
<https://pytorch.org/docs/stable/notes/randomness.html>. If both
`torch_deterministic=True` and `seed=-1` are set, the seed value will be
fixed to 42.
Note that in PyTorch version 1.9 and 1.9.1 there appear to be bugs affecting
the `torch_deterministic` setting, and using this mode will result in a crash,
though in our testing we did not notice determinacy issues arising from not
setting this flag.
Runtime Simulation Changes / Domain Randomization
-------------------------------------------------
Note that using a fixed seed value will only **potentially** allow for deterministic
behavior. Due to GPU work scheduling, it is possible that runtime changes to
simulation parameters can alter the order in which operations take place, as
environment updates can happen while the GPU is doing other work. Because of the nature
of floating point numeric storage, any alteration of execution ordering can
cause small changes in the least significant bits of output data, leading
to divergent execution over the simulation of thousands of environments and
simulation frames.
As an example of this, runtime domain randomization of object scales or masses
are known to cause both determinacy and simulation issues when running on the GPU
due to the way those parameters are passed from CPU to GPU in lower level APIs. By
default, in examples that use Domain Randomization, we use the `setup_only` flag to only
randomize scales and masses once across all environments before simulation starts.
At this time, we do not believe that other domain randomizations offered by this
framework cause issues with deterministic execution when running GPU simulation,
but directly manipulating other simulation parameters outside of the Isaac Gym tensor
APIs may induce similar issues.
CPU MultiThreaded Determinism
-----------------------------
We are also aware of one environment (Humanoid) that does not train deterministically
when simulated on CPU with multiple PhysX worker threads. Similar to GPU determinism
issues, this is likely due to subtle simulation operation ordering issues, and additional
effort will be needed to enforce synchronization between threads.
We have not observed similar issues when using CPU simulation with other examples, or
when restricting CPU simulation to a single thread | 3,622 | Markdown | 51.507246 | 89 | 0.778851 |
NVIDIA-Omniverse/IsaacGymEnvs/docs/rl_examples.md | Reinforcement Learning Examples
===============================
Single-gpu training reinforcement learning examples can be launched from
`isaacgymenvs` with `python train.py`.
When training with the viewer (not headless), you can press `v` to toggle
viewer sync. Disabling viewer sync will improve performance, especially
in GPU pipeline mode. Viewer sync can be re-enabled at any time to check
training progress.
List of Examples
----------------
* [Ant](#ant-antpy)
* [Humanoid](#humanoid-humanoidpy)
* [Shadow Hand](#shadow-hand-object-manipulation-shadow_handpy)
* [Allegro Hand](#allegro-hand-allegro_handpy)
* [ANYmal](#anymal-anymalpy)
* [ANYmal Rough Terrain](#anymal-rough-terrain-anymal_terrainpy)
* [TriFinger](#trifinger-trifingerpy)
* [NASA Ingenuity Helicopter](#nasa-ingenuity-helicopter-ingenuitypy)
* [Cartpole](#cartpole-cartpolepy)
* [Ball Balance](#ball-balance-ball_balancepy)
* [Franka Cabinet](#franka-cabinet-franka_cabinetpy)
* [Franka Cube Stack](#franka-cube-stack-franka_cube_stackpy)
* [Quadcopter](#quadcopter-quadcopterpy)
* [Adversarial Motion Priors](#amp-adversarial-motion-priors-humanoidamppy)
* [Factory](#factory-fast-contact-for-robotic-assembly)
* [DeXtreme](#dextreme-transfer-of-agile-in-hand-manipulation-from-simulation-to-reality)
* [DexPBT](#dexpbt-scaling-up-dexterous-manipulation-for-hand-arm-systems-with-population-based-training)
* [IndustReal](#industreal-transferring-contact-rich-assembly-tasks-from-simulation-to-reality)
### Ant [ant.py](../isaacgymenvs/tasks/ant.py)
An example of a simple locomotion task, the goal is to train quadruped
robots (ants) to run forward as fast as possible. The Ant task includes
examples of utilizing Isaac Gym's actor root state tensor, DOF state
tensor, and force sensor tensor APIs. Actor root states provide data for
the ant's root body, including position, rotation, linear and angular
velocities. This information can be used to detect whether the ant has
been moving towards the desired direction and whether it has fallen or
flipped over. DOF states are used to retrieve the position and velocity
of each DOF for the ant, and force sensors are used to indicate contacts
with the ground plane on the ant's legs.
Actions are applied onto the DOFs of the ants to allow it to move, using
the `set_dof_actuation_force_tensor` API.
During resets, we also show usage of
`set_actor_root_state_tensor_indexed` and `set_dof_state_tensor_indexed`
APIs for setting select ants into a valid starting state.
It can be launched with command line argument `task=Ant`.
Config files used for this task to train with PPO are:
- **Task config**: [Ant.yaml](../isaacgymenvs/cfg/task/Ant.yaml)
- **rl_games training config**: [AntPPO.yaml](../isaacgymenvs/cfg/train/AntPPO.yaml)
With SAC:
- **Task config**: [AntSAC.yaml](../isaacgymenvs/cfg/task/AntSAC.yaml)
- **rl_games training config**: [AntSAC.yaml](../isaacgymenvs/cfg/train/AntSAC.yaml)
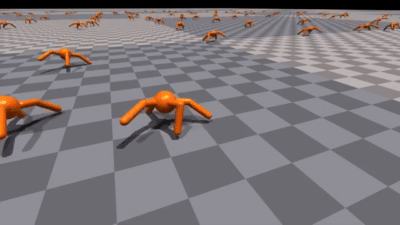
### Humanoid [humanoid.py](../isaacgymenvs/tasks/humanoid.py)
The humanoid example is conceptually very similar to the Ant task. In
this example, we also use actor root states to detect whether humanoids
are been moving towards the desired direction and whether they have
fallen. DOF states are used to retrieve the position and velocity of
each DOF for the humanoids, and force sensors are used to indicate
contacts with the ground plane on the humanoids' feet.
It can be launched with command line argument `task=Humanoid`.
Config files used for this task to train with PPO are:
- **Task config**: [Humanoid.yaml](../isaacgymenvs/cfg/task/Humanoid.yaml)
- **rl_games training config**: [HumanoidPPO.yaml](../isaacgymenvs/cfg/train/HumanoidPPO.yaml)
With SAC:
- **Task config**: [HumanoidSAC.yaml](../isaacgymenvs/cfg/task/HumanoidSAC.yaml)
- **rl_games training config**: [HumanoidSAC.yaml](../isaacgymenvs/cfg/train/HumanoidSAC.yaml)
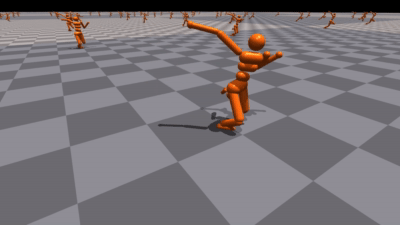
### Shadow Hand Object Manipulation [shadow_hand.py](../isaacgymenvs/tasks/shadow_hand.py)
The Shadow Hand task is an example of a challenging dexterity
manipulation task with complex contact dynamics. It resembles OpenAI's
[Learning Dexterity](https://openai.com/blog/learning-dexterity/)
project and [Robotics Shadow
Hand](https://github.com/openai/gym/tree/master/gym/envs/robotics)
training environments. It also demonstrates the use of tendons in the
Shadow Hand model. In this example, we use `get_asset_tendon_properties`
and `set_asset_tendon_properties` to get and set tendon properties for
the hand. Motion of the hand is controlled using position targets with
`set_dof_position_target_tensor`.
The goal is to orient the object in the hand to match the target
orientation. There is a goal object that shows the target orientation to
be achieved by the manipulated object. To reset both the target object
and the object in hand, it is important to make **one** single call to
`set_actor_root_state_tensor_indexed` to set the states for both
objects. This task has 3 difficulty levels using different objects to
manipulate - block, egg and pen and different observations schemes -
`openai`, `full_no_vel`, `full` and `full_state` that can be set in the
task config in `observationType` field. Moreover it supports asymmetric
observations, when policy and value functions get different sets of
observation.
The basic version of the task can be launched with command line argument `task=ShadowHand`.
Config files used for this task are:
- **Task config**: [ShadowHand.yaml](../isaacgymenvs/cfg/task/ShadowHand.yaml)
- **rl_games training config**: [ShadowHandPPO.yaml](../isaacgymenvs/cfg/train/ShadowHandPPO.yaml)
Observations types:
- **openai**: fingertip positions, object position and relative to the
goal object orientation. These are the same set of observations as used in
the OpenAI [Learning Dexterity](https://openai.com/blog/learning-dexterity/) project
- **full_no_vel**: the same as `full` but without any velocity
information for joints, object and fingertips
- **full**: a standard set of observations with joint positions and
velocities, object pose, linear and angular velocities, the goal
pose and fingertip transforms, and their linear and angular
velocities
- **full_state**: `full` set of observations plus readings from
force-torque sensors attached to the fingertips and joint forces
sensors. This is the default used by the base **ShadowHand** task
#### OpenAI Variant
In addition to the basic version of this task, there is an additional variant matching OpenAI's [Learning Dexterity](https://openai.com/blog/learning-dexterity/) project.
This variant uses the **openai** observations in the policy network, but asymmetric observations of the **full_state** in the value network.
This can be launched with command line argument `task=ShadowHandOpenAI_FF`.
Config files used for this are:
- **Task config**: [ShadowHandOpenAI_FF.yaml](../isaacgymenvs/cfg/task/ShadowHandOpenAI_FF.yaml)
- **rl_games training config**: [ShadowHandOpenAI_FFPPO.yaml](../isaacgymenvs/cfg/train/ShadowHandOpenAI_FFPPO.yaml).
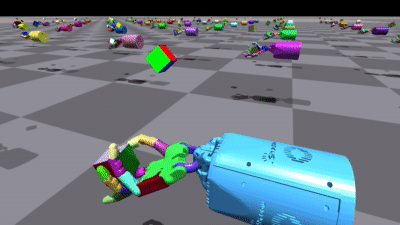
#### LSTM Training Variants
There are two other variants of training
- [ShadowHandOpenAI_LSTM](../isaacgymenvs/cfg/train/ShadowHandOpenAI_LSTMPPO.yaml)
- This variant uses LSTM policy and value networks instead of
feed forward networks, and also asymmetric LSTM critic designed for the OpenAI variant of the task.
- This can be launched with command line argument `task=ShadowHandOpenAI_LSTM`.
- [ShadowHand_LSTM](../isaacgymenvs/cfg/train/ShadowHandPPOLSTM.yaml)
- This variant uses LSTM policy and value networks instead of
feed forward networks, but unlike the previous config, uses symmetric observations for the standard variant of Shadow Hand.
- This can be launched with command line argument `task=ShadowHand train=ShadowHandPPOLSTM`.
#### OpenAI Testing Variant
This is a testing variant of the config to match test conditions from the Learning Dexterity paper such as a longer episode time and not re-applying
domain randomizations after initial randomization. It is not intended to be used for training. Note that if the successTolerance config option is changed to 0.1 during training,
running the testing variant with the standard 0.4 successTolerance will show improved performance. The testing variant will also output the average number of
consecutive successes to the console, showing both the direct average of all environments as well as the average only over environments that have finished.
Over time these numbers should converge.
To test the FF OpenAI variant, use these arguments: `task=ShadowHandTest train=ShadowHandOpenAI_FFPPO test=True checkpoint=<CHECKPOINT_TO_LOAD>`.
To test the LSTM OpenAI variant, use these arguments: `task=ShadowHandTest train=ShadowHandOpenAI_LSTMPPO test=True checkpoint=<CHECKPOINT_TO_LOAD>`.
- **Task config**: [ShadowHandOpenTest.yaml](../isaacgymenvs/cfg/task/ShadowHandTest.yaml)
### Allegro Hand [allegro_hand.py](../isaacgymenvs/tasks/allegro_hand.py)
This example performs the same cube manipulation task as the Shadow Hand environment, but using the Allegro hand instead of the Shadow hand.
It can be launched with command line argument `task=AllegroHand`.
Config files used for this task are:
- **Task config**: [AllegroHand.yaml](../isaacgymenvs/cfg/task/AllegroHand.yaml)
- **rl_games training config**: [AllegroHandPPO.yaml](../isaacgymenvs/cfg/train/AllegroHandPPO.yaml)
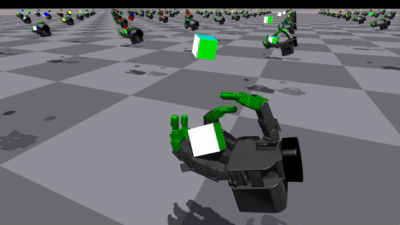
### Anymal [anymal.py](../isaacgymenvs/tasks/anymal.py)
This example trains a model of the ANYmal quadruped robot from ANYbotics
to follow randomly chosen x, y, and yaw target velocities.
It can be launched with command line argument `task=Anymal`.
Config files used for this task are:
- **Task config**: [Anymal.yaml](../isaacgymenvs/cfg/task/Anymal.yaml)
- **rl_games training config**: [AnymalPPO.yaml](../isaacgymenvs/cfg/train/AnymalPPO.yaml)

### Anymal Rough Terrain [anymal_terrain.py](../isaacgymenvs/tasks/anymal_terrain.py)
A highly upgraded version of the original Anymal environment which supports
traversing rough terrain and sim2real.
It can be launched with command line argument `task=AnymalTerrain`.
- **Task config**: [AnymalTerrain.yaml](../isaacgymenvs/cfg/task/AnymalTerrain.yaml)
- **rl_games training config**: [AnymalTerrainPPO.yaml](../isaacgymenvs/cfg/train/AnymalTerrainPPO.yaml)
**Note** during test time use the last weights generated, rather than the usual best weights.
Due to curriculum training, the reward goes down as the task gets more challenging, so the best weights
do not typically correspond to the best outcome.
**Note** if you use the ANYmal rough terrain environment in your work, please ensure you cite the following work:
```
@misc{rudin2021learning,
title={Learning to Walk in Minutes Using Massively Parallel Deep Reinforcement Learning},
author={Nikita Rudin and David Hoeller and Philipp Reist and Marco Hutter},
year={2021},
journal = {arXiv preprint arXiv:2109.11978},
}
```
**Note** The IsaacGymEnvs implementation slightly differs from the implementation used in the paper above, which also
uses a different RL library and PPO implementation. The original implementation will be made available [here](https://github.com/leggedrobotics/legged_gym). Results reported in the Isaac Gym technical paper are based on that repository, not this one.
### Trifinger [trifinger.py](../isaacgymenvs/tasks/trifinger.py)
The [Trifinger](isaacgymenvs/tasks/trifinger.py) environment is modelled on the [Real Robot Challenge 2020](https://real-robot-challenge.com/2020).
The goal is to move the cube to the desired target location, which is represented by a superimposed cube.
It can be launched with command line argument `task=Trifinger`.
- **Task config**: [Trifinger.yaml](../isaacgymenvs/cfg/task/Trifinger.yaml)
- **rl_games training config**: [TrifingerPPO.yaml](../isaacgymenvs/cfg/train/Trifinger.yaml)
**Note** if you use the Trifinger environment in your work, please ensure you cite the following work:
```
@misc{isaacgym-trifinger,
title = {{Transferring Dexterous Manipulation from GPU Simulation to a Remote Real-World TriFinger}},
author = {Allshire, Arthur and Mittal, Mayank and Lodaya, Varun and Makoviychuk, Viktor and Makoviichuk, Denys and Widmaier, Felix and Wuthrich, Manuel and Bauer, Stefan and Handa, Ankur and Garg, Animesh},
year = {2021},
journal = {arXiv preprint arXiv:2108.09779},
}
```
### NASA Ingenuity Helicopter [ingenuity.py](../isaacgymenvs/tasks/ingenuity.py)
This example trains a simplified model of NASA's Ingenuity helicopter to navigate to a moving target.
It showcases the use of velocity tensors and applying force vectors to rigid bodies.
Note that we are applying force directly to the chassis, rather than simulating aerodynamics.
This example also demonstrates using different values for gravitational forces, as well as dynamically writing a physics model from Python code at runtime.
Ingenuity Helicopter visual 3D Model courtesy of NASA: https://mars.nasa.gov/resources/25043/mars-ingenuity-helicopter-3d-model/.
It can be launched with command line argument `task=Ingenuity`.
Config files used for this task are:
- **Task config**: [Ingenuity.yaml](../isaacgymenvs/cfg/task/Ingenuity.yaml)
- **rl_games training config**: [IngenuityPPO.yaml](../isaacgymenvs/cfg/train/IngenuityPPO.yaml)

### Cartpole [cartpole.py](../isaacgymenvs/tasks/cartpole.py)
Cartpole is a simple example that shows usage of the DOF state tensors. Position and velocity data are used as observation for the cart and pole DOFs. Actions are applied as forces to the cart using `set_dof_actuation_force_tensor`. During reset, we use `set_dof_state_tensor_indexed` to set DOF position and velocity of the cart and pole to a randomized state.
It can be launched with command line argument `task=Cartpole`.
Config files used for this task are:
- **Task config**: [Cartpole.yaml](../isaacgymenvs/cfg/task/Cartpole.yaml)
- **rl_games training config**: [CartpolePPO.yaml](../isaacgymenvs/cfg/train/CartpolePPO.yaml)

### Ball Balance [ball_balance.py](../isaacgymenvs/tasks/ball_balance.py)
This example trains balancing tables to balance a ball on the table top.
This is a great example to showcase the use of force and torque sensors, as well as DOF states for the table and root states for the ball. In this example, the three-legged table has a force sensor attached to each leg using the `create_force_sensor` API. We use the force sensor tensor APIs to collect force and torque data on the legs, which guide position target outputs produced by the policy. The example shows usage of `set_dof_position_target_tensor` to set position targets to keep the ball balanced on the table.
It can be launched with command line argument `task=BallBalance`.
Config files used for this task are:
- **Task config**: [BallBalance.yaml](../isaacgymenvs/cfg/task/BallBalance.yaml)
- **rl_games training config**: [BallBalancePPO.yaml](../isaacgymenvs/cfg/train/BallBalancePPO.yaml)

### Franka Cabinet [franka_cabinet.py](../isaacgymenvs/tasks/franka_cabinet.py)
The Franka example demonstrates interaction between Franka arm and cabinet, as well as setting states of objects inside the drawer.
It also showcases control of the Franka arm using position targets.
In this example, we use DOF state tensors to retrieve the state of the Franka arm, as well as the state of the drawer on the cabinet.
Actions are applied using `set_dof_position_target_tensor` to set position targets for the Franka arm DOFs.
During reset, we use indexed versions of APIs to reset Franka, cabinet, and objects inside drawer to their initial states. `set_actor_root_state_tensor_indexed` is used to reset objects inside drawer, `set_dof_position_target_tensor_indexed` is used to reset Franka, and `set_dof_state_tensor_indexed` is used to reset Franka and cabinet.
It can be launched with command line argument `task=FrankaCabinet`.
Config files used for this task are:
- **Task config**: [FrankaCabinet.yaml](../isaacgymenvs/cfg/task/FrankaCabinet.yaml)
- **rl_games training config**: [FrankaCabinetPPO.yaml](../isaacgymenvs/cfg/train/FrankaCabinetPPO.yaml)

### Franka Cube Stack [franka_cube_stack.py](../isaacgymenvs/tasks/franka_cube_stack.py)
The Franka Cube Stack example shows solving a cube stack task using either operational space control (OSC) or joint space torque control.
OSC control provides an example of using direct GPU mass-matrix access API.
It can be launched with command line argument `task=FrankaCubeStack`.
Config files used for this task are:
- **Task config**: [FrankaCubeStack.yaml](../isaacgymenvs/cfg/task/FrankaCubeStack.yaml)
- **rl_games training config**: [FrankaCubeStackPPO.yaml](../isaacgymenvs/cfg/train/FrankaCubeStackPPO.yaml)

### Quadcopter [quadcopter.py](../isaacgymenvs/tasks/quadcopter.py)
This example trains a very simple quadcopter model to reach and hover near a fixed position. The quadcopter model is generated procedurally and doesn't actually include any rotating blades. Lift is achieved by applying thrust forces to the "rotor" bodies, which are modeled as flat cylinders. This is a good example of using LOCAL_SPACE forces. In addition to thrust, the pitch and roll of each rotor is controlled using DOF position targets.
It can be launched with command line argument `task=Quadcopter`.
Config files used for this task are:
- **Task config**: [Quadcopter.yaml](../isaacgymenvs/cfg/task/Quadcopter.yaml)
- **rl_games training config**: [QuadcopterPPO.yaml](../isaacgymenvs/cfg/train/QuadcopterPPO.yaml)

### AMP: Adversarial Motion Priors [HumanoidAMP.py](../isaacgymenvs/tasks/humanoid_amp.py)
This example trains a simulated human model to imitate different pre-recorded human animations stored in the mocap data - walking, running and backflip.
It can be launched with command line argument `task=HumanoidAMP`. The Animation file to train with can be set with `motion_file` in the task config (also see below for more information). Note: in test mode the viewer camera follows the humanoid from the first env. This can be changed in the environment yaml config by setting `cameraFollow=False`, or on the command line with a hydra override as follows: `++task.env.cameraFollow=False
A few motions from the CMU motion capture library (http://mocap.cs.cmu.edu/) are included with this repository, but additional animations can be converted from FBX into a trainable format using the poselib `fbx_importer.py`. You can learn more about poselib and this conversion tool in `isaacgymenvs/tasks/amp/poselib/README.md`
Several animations from the SFU Motion Capture Database (https://mocap.cs.sfu.ca/) are known to train well, including ones for martial arts moves such as a spin-kick, walking, jogging, and running animations, and several dance captures. The spinning kick portion of the SFU 0017_WushuKicks001 (shown below) trains in 6 minutes on a GA100 GPU. The SFU motions are not included directly in this repository due to licensing restrictions.
Config files used for this task are:
- **Task config**: [HumanoidAMP.yaml](../isaacgymenvs/cfg/task/HumanoidAMP.yaml)
- **rl_games training config**: [HumanoidAMPPPO.yaml](../isaacgymenvs/cfg/train/HumanoidPPOAMP.yaml)
- **mocap data**: [motions](../assets/amp/motions)
**Note** When training using new motion clips, the single most important hyperparameter to tune for AMP is `disc_grad_penalty` in `HumanoidAMPPPO.yaml`. Typical values are between [0.1, 10]. For a new motion, start with large values first, and if the policy is not able to closely imitate the motion, then try smaller coefficients for the gradient penalty. The `HumanoidAMPPPOLowGP.yaml` training configuration is provided as a convenience for this purpose.
Use the following command lines for training the currently included AMP motions:
(Walk is the default config motion, so doesn't need the motion file specified)
`python train.py task=HumanoidAMP experiment=AMP_walk`
`python train.py task=HumanoidAMP ++task.env.motion_file=amp_humanoid_run.npy experiment=AMP_run`
`python train.py task=HumanoidAMP ++task.env.motion_file=amp_humanoid_dance.npy experiment=AMP_dance`
(Backflip and Hop require the LowGP training config)
`python train.py task=HumanoidAMP train=HumanoidAMPPPOLowGP ++task.env.motion_file=amp_humanoid_backflip.npy experiment=AMP_backflip`
`python train.py task=HumanoidAMP train=HumanoidAMPPPOLowGP ++task.env.motion_file=amp_humanoid_hop.npy experiment=AMP_hop`
(Cartwheel requires hands in the contact body list and the LowGP training config; the default motion for the HumanoidAMPHands task is Cartwheel)
`python train.py task=HumanoidAMPHands train=HumanoidAMPPPOLowGP experiment=AMP_cartwheel`
**Note** If you use the AMP: Adversarial Motion Priors environment in your work, please ensure you cite the following work:
```
@article{
2021-TOG-AMP,
author = {Peng, Xue Bin and Ma, Ze and Abbeel, Pieter and Levine, Sergey and Kanazawa, Angjoo},
title = {AMP: Adversarial Motion Priors for Stylized Physics-Based Character Control},
journal = {ACM Trans. Graph.},
issue_date = {August 2021},
volume = {40},
number = {4},
month = jul,
year = {2021},
articleno = {1},
numpages = {15},
url = {http://doi.acm.org/10.1145/3450626.3459670},
doi = {10.1145/3450626.3459670},
publisher = {ACM},
address = {New York, NY, USA},
keywords = {motion control, physics-based character animation, reinforcement learning},
}
```
Images below are from SFU SpinKick training.

### Factory: Fast Contact for Robotic Assembly
There are 5 Factory example tasks: **FactoryTaskNutBoltPick**, **FactoryTaskNutBoltPlace**, **FactoryTaskNutBoltScrew**, **FactoryTaskNutBoltInsertion**, and **FactoryTaskNutBoltGears**. Like the other tasks, they can be executed with `python train.py task=<task_name>`. The first time you run these examples, it may take some time for Gym to generate SDFs for the assets. However, these SDFs will then be cached.
**FactoryTaskNutBoltPick**, **FactoryTaskNutBoltPlace**, and **FactoryTaskNutBoltScrew** train policies for the Pick, Place, and Screw tasks. They are simplified versions of the corresponding tasks in the Factory paper (e.g., smaller randomization ranges, simpler reward formulations, etc.) The Pick and Place subpolicies may take ~1 hour to achieve high success rates on a modern GPU, and the Screw subpolicy, which does not include initial state randomization, should achieve high success rates almost immediately.
**FactoryTaskNutBoltInsertion** and **FactoryTaskNutBoltGears** do not train RL policies by default, as successfully training these policies is an open area of research. Their associated scripts ([factory_task_insertion.py](../isaacgymenvs/tasks/factory/factory_task_insertion.py) and [factory_task_gears.py](../isaacgymenvs/tasks/factory/factory_task_gears.py)) provide templates for users to write their own RL code. For an example of a filled-out template, see the script for **FactoryTaskNutBoltPick** ([factory_task_nut_bolt_pick.py](../isaacgymenvs/tasks/factory/factory_task_nut_bolt_pick.py)).
The general configuration files for the above tasks are [FactoryTaskNutBoltPick.yaml](../isaacgymenvs/cfg/task/FactoryTaskNutBoltPick.yaml), [FactoryTaskNutBoltPlace.yaml](../isaacgymenvs/cfg/task/FactoryTaskNutBoltPlace.yaml), [FactoryTaskNutBoltScrew.yaml](../isaacgymenvs/cfg/task/FactoryTaskNutBoltScrew.yaml), [FactoryTaskInsertion.yaml](../isaacgymenvs/cfg/task/FactoryTaskInsertion.yaml), and [FactoryTaskGears.yaml](../isaacgymenvs/cfg/task/FactoryTaskGears.yaml). Note that you can select low-level controller types (e.g., joint-space IK, task-space impedance) within these configuration files.
The training configuration files for the above tasks are [FactoryTaskNutBoltPickPPO.yaml](../isaacgymenvs/cfg/train/FactoryTaskNutBoltPickPPO.yaml), [FactoryTaskNutBoltPlacePPO.yaml](../isaacgymenvs/cfg/train/FactoryTaskNutBoltPlacePPO.yaml), [FactoryTaskNutBoltScrewPPO.yaml](../isaacgymenvs/cfg/train/FactoryTaskNutBoltScrewPPO.yaml), [FactoryTaskInsertionPPO.yaml](../isaacgymenvs/cfg/train/FactoryTaskInsertionPPO.yaml), and [FactoryTaskGearsPPO.yaml](../isaacgymenvs/cfg/train/FactoryTaskGearsPPO.yaml). We use the [rl-games](https://github.com/Denys88/rl_games) library to train our RL agents via PPO, and these configuration files define the PPO parameters.
We highly recommend reading the [extended documentation](factory.md) for Factory, which will be regularly updated. This documentation includes details on SDF collisions, which all the Factory examples leverage. You can use SDF collisions for your own assets and environments.
If you use the Factory simulation methods (e.g., SDF collisions, contact reduction) or Factory learning tools (e.g., assets, environments, or controllers) in your work, please cite the following paper:
```
@inproceedings{
narang2022factory,
author = {Yashraj Narang and Kier Storey and Iretiayo Akinola and Miles Macklin and Philipp Reist and Lukasz Wawrzyniak and Yunrong Guo and Adam Moravanszky and Gavriel State and Michelle Lu and Ankur Handa and Dieter Fox},
title = {Factory: Fast contact for robotic assembly},
booktitle = {Robotics: Science and Systems},
year = {2022}
}
```
Also note that our original formulations of SDF collisions and contact reduction were developed by [Macklin, et al.](https://dl.acm.org/doi/abs/10.1145/3384538) and [Moravanszky and Terdiman](https://scholar.google.com/scholar?q=Game+Programming+Gems+4%2C+chapter+Fast+Contact+Reduction+for+Dynamics+Simulation), respectively.
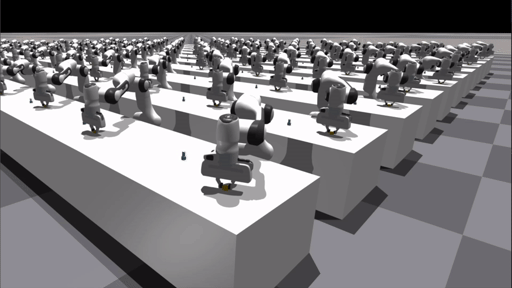
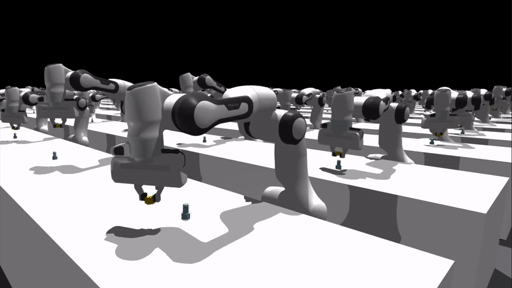
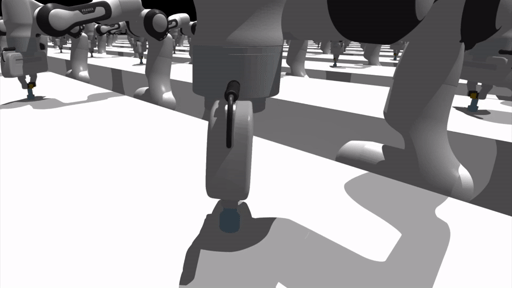
### DeXtreme: Transfer of Agile In-hand Manipulation from Simulation to Reality
DeXtreme provides an example of sim-to-real transfer of dexterous manipulation with an Allegro Hand including Automatic Domain Randomization (ADR). You can read further details of the task in the [extended documentation](dextreme.md) and additional information about ADR [here](domain_randomization.md).
There are two [DeXtreme](https://dextreme.org) tasks: **AllegroHandDextremeManualDR** and **AllegroHandDextremeADR**. They are both compatible with the standard way of training in Isaac Gym via `python train.py task=<AllegroHandDextremeManualDR or AllegroHandDextremeADR>`. For reproducibility, we provide the exact settings with which we trained for those environments.
For `AllegroHandDextremeManualDR`, you should use the following command for training
```
HYDRA_MANUAL_DR="train.py multi_gpu=False \
task=AllegroHandDextremeManualDR \
task.env.resetTime=8 task.env.successTolerance=0.4 \
experiment='allegrohand_dextreme_manual_dr' \
headless=True seed=-1 \
task.env.startObjectPoseDY=-0.15 \
task.env.actionDeltaPenaltyScale=-0.2 \
task.env.resetTime=8 \
task.env.controlFrequencyInv=2 \
train.params.network.mlp.units=[512,512] \
train.params.network.rnn.units=768 \
train.params.network.rnn.name=lstm \
train.params.config.central_value_config.network.mlp.units=[1024,512,256] \
train.params.config.max_epochs=50000 \
task.env.apply_random_quat=True"
python ${HYDRA_MANUAL_DR}
```
**TaskConfig** [AllegroHandDextremeManualDR.yaml](../isaacgymenvs/cfg/task/AllegroHandDextremeManualDR.yaml)
**TrainConfig** [AllegroHandDextremeManualDRPPO.yaml](../isaacgymenvs/cfg/train/AllegroHandDextremeManualDRPPO.yaml)
For `AllegroHandDextremeADR`, you should use the following command for training
```
HYDRA_ADR="train.py multi_gpu=False \
task=AllegroHandDextremeADR \
headless=True seed=-1 \
num_envs=8192 \
task.env.resetTime=8 \
task.env.controlFrequencyInv=2 \
train.params.config.max_epochs=50000"
python ${HYDRA_ADR}
```
**TaskConfig** [AllegroHandDextremeADR.yaml](../isaacgymenvs/cfg/task/AllegroHandDextremeADR.yaml)
**TrainConfig** [AllegroHandDextremeADRPPO.yaml](../isaacgymenvs/cfg/train/AllegroHandDextremeADRPPO.yaml)
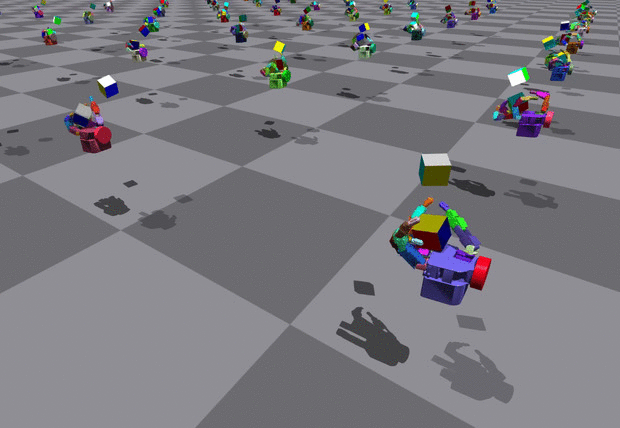
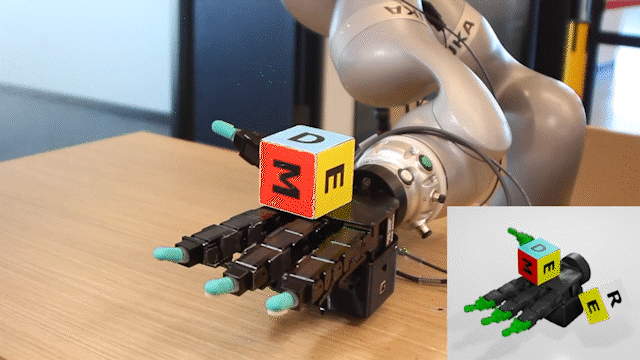
More videos are available at [dextreme.org](https://dextreme.org)
```
@inproceedings{
handa2023dextreme,
author = {Ankur Handa, Arthur Allshire, Viktor Makoviychuk, Aleksei Petrenko, Ritvik Singh, Jingzhou Liu, Denys Makoviichuk, Karl Van Wyk, Alexander Zhurkevich, Balakumar Sundaralingam, Yashraj Narang, Jean-Francois Lafleche, Dieter Fox, Gavriel State},
title = {DeXtreme: Transfer of Agile In-hand Manipulation from Simulation to Reality},
booktitle = {ICRA},
year = {2023}
}
```
### DexPBT: Scaling up Dexterous Manipulation for Hand-Arm Systems with Population Based Training
DexPBT provides an example of solving challenging hand+arm dextrous manipulation tasks using Population Based Training (PBT). You can read further details of the tasks in the [extended documentation](pbd.md).
There are two [DexPBT](https://sites.google.com/view/dexpbt) base environments, single- and dual-arms: **AllegroKukaLSTM** and **AllegroKukaTwoArmsLSTM** and a few different taks: reorientation, regrasping and grasp-and-throw for **AllegroKukaLSTM** and reorientation and regrasping for **AllegroKukaTwoArmsLSTM**. They are both compatible with the standard way of training in Isaac Gym via `python train.py task=AllegroKukaLSTM task/env=<reorientation or regrasping or throw>` `python train.py task=AllegroKukaTwoArmsLSTM task/env=<reorientation or regrasping>`. For reproducibility, we provide the exact settings with which we trained for those environments.

More videos are available at [https://sites.google.com/view/dexpbt](https://sites.google.com/view/dexpbt)
```
@inproceedings{
petrenko2023dexpbt,
author = {Aleksei Petrenko, Arthur Allshire, Gavriel State, Ankur Handa, Viktor Makoviychuk},
title = {DexPBT: Scaling up Dexterous Manipulation for Hand-Arm Systems with Population Based Training},
booktitle = {RSS},
year = {2023}
}
```
### IndustReal: Transferring Contact-Rich Assembly Tasks from Simulation to Reality
There are 2 IndustRealSim example tasks: **IndustRealTaskPegsInsert** and **IndustRealTaskGearsInsert**. The examples train policies for peg insertion tasks and gear insertion tasks, respectively. They can be launched with command line argument `task=IndustRealTaskPegsInsert` or `task=IndustRealTaskGearsInsert`. The first time you run these examples, it may take some time for Gym to generate signed distance field representations (SDFs) for the assets. However, these SDFs will then be cached.
The examples correspond very closely to the code used to train the same policies in the IndustReal paper, but due to simplifications and improvements, may produce slightly different results than the original implementations. They may take 8 to 10 hours on a modern GPU to achieve similar success rates to the results presented in the IndustReal paper.
The core configuration files for these 2 IndustRealSim example tasks are the [IndustRealTaskPegsInsert.yaml](../isaacgymenvs/cfg/task/IndustRealTaskPegsInsert.yaml) and [IndustRealTaskGearsInsert.yaml](../isaacgymenvs/cfg/task/IndustRealTaskGearsInsert.yaml) task configuration files and the [IndustRealTaskPegsInsertPPO.yaml](../isaacgymenvs/cfg/train/IndustRealTaskPegsInsertPPO.yaml) and [IndustRealTaskGearsInsertPPO.yaml](../isaacgymenvs/cfg/train/IndustRealTaskGearsInsertPPO.yaml) training configuration files. In addition to the task and training configuration files described earlier, there are also base-level configuration files and environment-level configuration files. The base-level configuration file is [IndustRealBase.yaml](../isaacgymenvs/cfg/task/IndustRealBase.yaml), and the environment-level configuration files are [IndustRealEnvPegs.yaml](../isaacgymenvs/cfg/task/IndustRealEnvPegs.yaml) and [IndustRealEnvGears.yaml](../isaacgymenvs/cfg/task/IndustRealEnvGears.yaml).
We highly recommend reading the [extended documentation](industreal.md) for IndustRealSim, which includes more code details and best practices.
<table align="center">
<tr>
<th>Initialization of Peg Insertion</th>
<th>Trained Peg Insertion Policy</th>
<th>Initialization of Gear Insertion</th>
<th>Trained Gear Insertion Policy</th>
</tr>
<tr>
<td><img src="https://github.com/bingjietang718/bingjietang718.github.io/assets/78517784/5d14452f-06ab-41cd-8545-bcf303dc4229" alt="drawing" width="200"/></th>
<td><img src="https://github.com/bingjietang718/bingjietang718.github.io/assets/78517784/0baeaf2d-a21d-47e9-b74a-877ad59c4112" alt="drawing" width="200"/></th>
<td><img src="https://github.com/bingjietang718/bingjietang718.github.io/assets/78517784/52df52f0-b122-4429-b6e2-b0b6ba9c29f6" alt="drawing" width="200"/></th>
<td><img src="https://github.com/bingjietang718/bingjietang718.github.io/assets/78517784/af383243-3165-4255-9606-4a1419baee27" alt="drawing" width="200"/></th>
</tr>
</table>
If you use any of the IndustRealSim training environments or algorithms in your work, please cite [IndustReal](https://arxiv.org/abs/2305.17110):
```
@inproceedings{
tang2023industreal,
author = {Bingjie Tang and Michael A Lin and Iretiayo Akinola and Ankur Handa and Gaurav S Sukhatme and Fabio Ramos and Dieter Fox and Yashraj Narang},
title = {IndustReal: Transferring contact-rich assembly tasks from simulation to reality},
booktitle = {Robotics: Science and Systems},
year = {2023}
}
```
Also note that the simulation methods, original environments, and low-level control algorithms were described in [Factory](https://arxiv.org/abs/2205.03532), which you may want to refer to or cite as well:
```
@inproceedings{
narang2022factory,
author = {Yashraj Narang and Kier Storey and Iretiayo Akinola and Miles Macklin and Philipp Reist and Lukasz Wawrzyniak and Yunrong Guo and Adam Moravanszky and Gavriel State and Michelle Lu and Ankur Handa and Dieter Fox},
title = {Factory: Fast contact for robotic assembly},
booktitle = {Robotics: Science and Systems},
year = {2022}
}
```
| 35,370 | Markdown | 61.714539 | 993 | 0.779361 |
NVIDIA-Omniverse/IsaacGymEnvs/docs/release_notes.md | Release Notes
=============
1.5.1
-----
* Fix bug in IndustRealSim example - overwrite `generate_ctrl_signals`, `_set_dof_pos_target`, and `_set_dof_torque` in `industreal_base.py` to resolve `fingertip_midpoint` and `fingertip_centered` discrepancy
1.5.0
-----
* Added [IndustReal](https://sites.google.com/nvidia.com/industreal) environments: IndustRealTaskPegsInsert and IndustRealTaskGearsInsert.
* Updated hydra version to 1.2.
1.4.0
-----
* Added [DexPBT](https://sites.google.com/view/dexpbt) (population based training) code and new AllegroKuka and AllegroKukaTwoArms environments.
* Added multi-node training support.
* Updated Allegro Hand assets.
* Fixed AMP save/load weights issue.
* Migrated Isaac Gym isaacgym.torch_utils to isaacgymenvs.utils.torch_jit_utils.
* Added record frames feature.
1.3.4
-----
* Fixed bug when running inferencing on DeXtreme environments.
* Fixed links in examples documentation.
* Minor fixes in documentation.
1.3.3
-----
* Fixed player and bug with AMP training environments.
* Added [DeXtreme](https://dextreme.org/) environments with ADR support.
1.3.2
-----
* Switched all environments that use contacts to use CC_LAST_SUBSTEP collection mode to avoid bug with CC_ALL_SUBSTEP mode. The CC_ALL_SUBSTEP mode can produce incorrect contact forces. Only HumanoidAMP and Factory environments are affected by this.
* Added SAC training examples for Ant and Humanoid envs. To run: ``python train.py task=AntSAC train=AntSAC`` and ``python train.py task=HumanoidSAC train=HumanoidSAC``
* Fix shadow hand and allegro hand random joint position sampling on reset.
* Switched to using IsaacAlgoObserver from rl_games instead of the custom RLGPUAlgoObserver.
1.3.1
-----
* Moved domain randomization utility code into IsaacGymEnvs.
* Tweaks and additional documentation for Factory examples and SDF collisions.
1.3.0
-----
* Added Factory Environments demonstrating RL with SDF collisions.
* Added Franka Cube Stacking task. Can use Operational Space Control (OSC) or joint torque control.
* Added support for [WandB](https://wandb.ai/) via adding `wandb_activate=True` on the training command line.
* Improved handling of episode timeouts (`self.timeout_buf`, see 1.1.0) which might have caused training issues for
configurations with `value_bootstrap: True`. This fix results in slightly faster training on Ant & Humanoid locomotion tasks.
* Added retargeting data for SFU Motion Capture Database.
* Deprecated `horovod` in favor of `torch.distributed` for better performance in multi-GPU settings.
* Added an environment creation API `isaacgymenvs.make(task_name)` which creates a vectorized environment compatible with 3rd party RL libraries.
* Added a utility to help capture the videos of the agent's gameplay via `python train.py capture_video=True` which creates a `videos` folder.
* Fixed an issue with Anymal Terrain environment resets.
* Improved allegro.urdf which now includes more precise collision shapes and masses/inertias of finger links.
* Added a pre-commit utility to identify incorrect spelling.
1.2.0
-----
* Added AMP (Adversarial Motion Priors) training environment.
* Minor changes in base VecTask class.
1.1.0
-----
* Added Anymal Rough Terrain and Trifinger training environments.
* Added `self.timeout_buf` that stores the information if the reset happened because of the episode reached to the maximum length or because of some other termination conditions. Is stored in extra info: `self.extras["time_outs"] = self.timeout_buf.to(self.rl_device)`. Updated PPO configs to use this information during training with `value_bootstrap: True`.
1.0.0
-----
* Initial release
| 3,660 | Markdown | 43.108433 | 360 | 0.770765 |
NVIDIA-Omniverse/IsaacGymEnvs/docs/factory.md | Factory
=======
Here we provide extended documentation on the Factory assets, environments, controllers, and simulation methods. This documentation will be regularly updated.
Before starting to use Factory, we would **highly** recommend familiarizing yourself with Isaac Gym, including the simpler RL examples.
Overview
--------
There are 5 Factory example tasks: **FactoryTaskNutBoltPick**, **FactoryTaskNutBoltPlace**, **FactoryTaskNutBoltScrew**, **FactoryTaskNutBoltInsertion**, and **FactoryTaskNutBoltGears**. Like the other tasks, they can be executed with `python train.py task=<task_name>`. The first time you run these examples, it may take some time for Gym to generate SDFs for the assets. However, these SDFs will then be cached.
**FactoryTaskNutBoltPick**, **FactoryTaskNutBoltPlace**, and **FactoryTaskNutBoltScrew** train policies for the Pick, Place, and Screw tasks. They are simplified versions of the corresponding tasks in the Factory paper (e.g., smaller randomization ranges, simpler reward formulations, etc.) The Pick and Place subpolicies may take ~1 hour to achieve high success rates on a modern GPU, and the Screw subpolicy, which does not include initial state randomization, should achieve high success rates almost immediately.
**FactoryTaskNutBoltInsertion** and **FactoryTaskNutBoltGears** do not train RL policies by default, as successfully training these policies is an open area of research. Their associated scripts ([factory_task_insertion.py](../isaacgymenvs/tasks/factory/factory_task_insertion.py) and [factory_task_gears.py](../isaacgymenvs/tasks/factory/factory_task_gears.py)) provide templates for users to write their own RL code. For an example of a filled-out template, see the script for **FactoryTaskNutBoltPick** ([factory_task_nut_bolt_pick.py](../isaacgymenvs/tasks/factory/factory_task_nut_bolt_pick.py)).
Assets
------
CAD models for our assets are as follows:
* [Nuts and bolts](https://cad.onshape.com/documents/c2ee3c5f2459d77465e93656/w/5e4c870b98f1d9a9b1990894/e/7b2e74610b9a1d6d9efa0372)
* [Pegs and holes](https://cad.onshape.com/documents/191ab8c549716821b170f501/w/639301b3a514d7484ebb7534/e/08f6dfb9e7d8782b502aea7b)
* [Gears](https://cad.onshape.com/documents/a0587101f8bbd02384e2db0c/w/06e85c5fe55bdf224720e2bb/e/946907a4305ef6b82d7d287b)
For the 3 electrical connectors described in the paper (i.e., BNC, D-sub, and USB), as well as 2 other connectors on the NIST Task Board (i.e., RJ45 and Waterproof), we sourced high-quality CAD models from online part repositories or manufacturer websites. We then modified them manually in CAD software to simplify external features (e.g., remove long cables), occasionally simplify internal features (e.g., remove internal elements that require deformable-body simulation, which Gym does not currently expose from PhysX 5.1), and exactly preserve most contact geometry. Due to licensing issues, we cannot currently release these CAD files. However, to prevent further delays, we provide links below to the websites that host the original high-quality CAD models that we subsequently modified:
* [BNC plug](https://www.digikey.com/en/products/detail/amphenol-rf/112420/1989856)
* [BNC socket](https://www.digikey.com/en/products/detail/molex/0731010120/1465130)
* [D-sub plug](https://www.digikey.com/en/products/detail/assmann-wsw-components/A-DSF-25LPIII-Z/924268)
* [D-sub socket](https://www.digikey.com/en/products/detail/assmann-wsw-components/A-DFF-25LPIII-Z/924259)
* [RJ45 plug](https://www.digikey.com/en/products/detail/harting/09454521509/3974500)
* [RJ45 socket](https://www.digikey.com/en/products/detail/amphenol-icc-fci/54602-908LF/1001360)
* [USB plug](https://www.digikey.com/en/products/detail/bulgin/PX0441-2M00/1625994)
* [USB socket](https://www.digikey.com/en/products/detail/amphenol-icc-fci/87520-0010BLF/1001359)
* [Waterproof plug](https://b2b.harting.com/ebusiness/en_us/Han-High-Temp-10E-c-Male/09338102604)
* [Waterproof socket](https://b2b.harting.com/ebusiness/en_us/Han-High-Temp-10E-c-Female/09338102704)
Meshes for our assets are located in the [mesh subdirectory](../../assets/factory/mesh). Again, the meshes for the electrical connectors are currently unavailable.
URDF files for our assets are located in the [urdf subdirectory](../../assets/factory/urdf/).
There are also YAML files located in the [yaml subdirectory](../../assets/factory/yaml/). These files contain asset-related constants that are used by the Factory RL examples.
Classes, Modules, and Abstract Base Classes
-------------------------------------------
The class hierarchy for the Factory examples is as follows:
[FactoryBase](../isaacgymenvs/tasks/factory/factory_base.py): assigns physics simulation parameters; imports Franka and table assets; assigns asset options for the Franka and table; translates higher-level controller selection into lower-level controller parameters; sets targets for controller
Each of the environment classes inherits the base class:
* [FactoryEnvNutBolt](../isaacgymenvs/tasks/factory/factory_env_nut_bolt.py): imports nut and bolt assets; assigns asset options for the nuts and bolts; creates Franka, table, nut, and bolt actors
* [FactoryEnvInsertion](../isaacgymenvs/tasks/factory/factory_env_insertion.py): imports plug and socket assets (including pegs and holes); assigns asset options for the plugs and sockets; creates Franka, table, plug, and socket actors
* [FactoryEnvGears](../isaacgymenvs/tasks/factory/factory_env_gears.py): imports gear and gear base assets; assigns asset options for the gears and gear base; creates Franka, table, gears, and gear base actors
Each of the task classes inherits the corresponding environment class:
* [FactoryTaskNutBoltPick](../isaacgymenvs/tasks/factory/factory_task_nut_bolt_pick.py): contains higher-level RL code for the Pick subpolicy (e.g., applying actions, defining observations, defining rewards, resetting environments), which is used by the lower-level [rl-games](https://github.com/Denys88/rl_games) library
* [FactoryTaskNutBoltPlace](../isaacgymenvs/tasks/factory/factory_task_nut_bolt_place.py): contains higher-level RL code for the Place subpolicy
* [FactoryTaskNutBoltScrew](../isaacgymenvs/tasks/factory/factory_task_nut_bolt_screw.py): contains higher-level RL code for the Screw subpolicy
* [FactoryTaskInsertion](../isaacgymenvs/tasks/factory/factory_task_insertion.py): contains template for Insertion policy
* [FactoryTaskGears](../isaacgymenvs/tasks/factory/factory_task_gears.py): contains template for Gears policy
There is also a control module ([factory_control.py](../isaacgymenvs/tasks/factory/factory_control.py)) that is imported by [factory_base.py](../isaacgymenvs/tasks/factory/factory_base.py) and contains the lower-level controller code that converts controller targets to joint torques.
Finally, there are abstract base classes that define the necessary methods for base, environment, and task classes ([factory_schema_class_base.py](../isaacgymenvs/tasks/factory/factory_schema_class_base.py), [factory_schema_class_env.py](../isaacgymenvs/tasks/factory/factory_schema_class_env.py), and [factory_schema_class_task.py](../isaacgymenvs/tasks/factory/factory_schema_class_task.py)). These are useful to review in order to better understand the structure of the code, but you will probably not need to modify them. They are also recommended to inherit if you would like to quickly add your own environments and tasks.
Configuration Files and Schema
------------------------------
There are 4 types of configuration files: base-level configuration files, environment-level configuration files, task-level configuration files, and training configuration files.
The base-level configuration file is [FactoryBase.yaml](../isaacgymenvs/cfg/task/FactoryBase.yaml).
The environment-level configuration files are [FactoryEnvNutBolt.yaml](../isaacgymenvs/cfg/task/FactoryEnvNutBolt.yaml), [FactoryEnvInsertion.yaml](../isaacgymenvs/cfg/task/FactoryEnvInsertion.yaml), and [FactoryEnvGears.yaml](../isaacgymenvs/cfg/task/FactoryEnvGears.yaml).
The task-level configuration files are [FactoryTaskNutBoltPick.yaml](../isaacgymenvs/cfg/task/FactoryTaskNutBoltPick.yaml), [FactoryTaskNutBoltPlace.yaml](../isaacgymenvs/cfg/task/FactoryTaskNutBoltPlace.yaml), [FactoryTaskNutBoltScrew.yaml](../isaacgymenvs/cfg/task/FactoryTaskNutBoltScrew.yaml), [FactoryTaskInsertion.yaml](../isaacgymenvs/cfg/task/FactoryTaskInsertion.yaml), and [FactoryTaskGears.yaml](../isaacgymenvs/cfg/task/FactoryTaskGears.yaml). Note that you can select low-level controller types (e.g., joint-space IK, task-space impedance) within these configuration files.
The training configuration files are [FactoryTaskNutBoltPickPPO.yaml](../isaacgymenvs/cfg/train/FactoryTaskNutBoltPickPPO.yaml), [FactoryTaskNutBoltPlacePPO.yaml](../isaacgymenvs/cfg/train/FactoryTaskNutBoltPlacePPO.yaml), [FactoryTaskNutBoltScrewPPO.yaml](../isaacgymenvs/cfg/train/FactoryTaskNutBoltScrewPPO.yaml), [FactoryTaskInsertionPPO.yaml](../isaacgymenvs/cfg/train/FactoryTaskInsertionPPO.yaml), and [FactoryTaskGearsPPO.yaml](../isaacgymenvs/cfg/train/FactoryTaskGearsPPO.yaml). We use the [rl-games](https://github.com/Denys88/rl_games) library to train our RL agents via PPO, and these configuration files define the PPO parameters for each task.
There are schema for the base-level, environment-level, and task-level configuration files ([factory_schema_config_base.py](../isaacgymenvs/tasks/factory/factory_schema_config_base.py), [factory_schema_config_env.py](../isaacgymenvs/tasks/factory/factory_schema_config_env.py), and [factory_schema_config_task.py](../isaacgymenvs/tasks/factory/factory_schema_config_tasks.py)). These schema are enforced for the base-level and environment-level configuration files, but not for the task-level configuration files. These are useful to review in order to better understand the structure of the configuration files and see descriptions of common parameters, but you will probably not need to modify them.
Controllers
-----------
Controller types and gains can be specified in the task-level configuration files. In addition to the 7 controllers described in the Factory paper, there is also the option of using Gym's built-in joint-space PD controller. This controller is generally quite stable, but uses a symplectic integrator that may introduce some artificial damping.
The controllers are implemented as follows:
* When launching a task, the higher-level controller type is parsed into lower-level controller options (e.g., joint space or task space, inertial compensation or no inertial compensation)
* At each time step (e.g., see [factory_task_nut_bolt_pick.py](../isaacgymenvs/tasks/factory/factory_task_nut_bolt_pick.py)), the actions are applied as controller targets, the appropriate Jacobians are computed in [factory_base.py](../isaacgymenvs/tasks/factory/factory_base.py), and the lower-level controller options, targets, and Jacobians are used by the lower-level controller code ([factory_control.py](../isaacgymenvs/tasks/factory/factory_control.py)) to generate corresponding joint torques.
This controller implementation will be made simpler and more developer-friendly in future updates.
Collisions and Contacts
-----------------------
**URDF Configuration:**
Different pairs of interacting objects can use different geometric representations (e.g., convex decompositions, triangular meshes, SDFs) to generate contacts and resolve collisions. If you would like any asset (or link of an asset) to engage in SDF collisions, you simply need to edit its URDF description and add an `<sdf>` element to its `<collision>` element. For example:
```
<?xml version="1.0"?>
<robot name="nut">
<link name="nut">
<visual>
<geometry>
<mesh filename="nut.obj"/>
</geometry>
</visual>
<collision>
<geometry>
<mesh filename="nut.obj"/>
</geometry>
<sdf resolution="256"/>
</collision>
</link>
</robot>
```
SDFs are computed from the mesh file along a discrete voxel grid. The resolution attribute specifies the number of voxels along the longest dimension of the object.
**Collision Logic:**
For a pair of colliding objects, by including or not including the `<sdf>` field in the corresponding URDFs, the collision scheme used for that pair of objects can be controlled. Specifically, consider 2 colliding objects, Object A and Object B.
* If A and B both have an `<sdf>` field, SDF-mesh collision will be applied. The object with the larger number of features (i.e., triangles) will be represented as an SDF, and the triangular mesh of the other object will be queried against the SDF to check for collisions and generate contacts. At any timestep, if too few contacts are generated between the objects, the SDF-mesh identities of the objects will be flipped, and contacts will be regenerated.
* If A has an `<sdf>` field and B does not, convex-mesh collision will be applied. Object A will be represented as a triangular mesh, and object B will be represented as a convex.
* If neither A nor B has an `<sdf>` tag, PhysX’s default convex-convex collision will be applied.
**Best Practices and Debugging:**
For small, complex parts (e.g., nuts and bolts), use an SDF resolution between 256 and 512.
If you are observing **minor penetration issues**, try the following:
* Increase `sim_params.physx.contact_offset` (global setting) or `asset_options.contact_offset` (asset-specific setting), which is the minimum distance between 2 objects at which contacts are generated. The default value in Factory is 0.005. As a rule of thumb, keep this value at least 1 order-of-magnitude greater than `v * dt / n`, where `v` is the maximum characteristic velocity of the object, `dt` is the timestep size, and `n` is the number of substeps.
* Increase the density of your meshes (i.e., number of triangles). In particular, when exporting OBJ files from some CAD programs, large flat surfaces can be meshed with very few triangles. Currently, PhysX generates a maximum of 1 contact per triangle; thus, very few contacts are generated on such surfaces. Software like Blender can be used to quickly increase the number of triangles on regions of a mesh using methods like edge subdivision.
* Increase `sim_params.physx.rest_offset` (global setting) or `asset_options.rest_offset` (asset-specific setting), which is the minimum separation distance between 2 objects in contact. The default value in Factory is 0.0. As a rule of thumb, for physically-accurate results, keep this value at least 1 order-of-magnitude less than the minimum characteristic length of your object (e.g., the thickness of your mug or bowl).
If you are observing **severe penetration issues** (e.g., objects passing freely through other objects), PhysX's contact buffer is likely overflowing. You may not see explicit warnings in the terminal output. Try the following:
* Reduce the number of environments. As a reference, we tested most of the Factory tasks with 128 environments. You can also try reducing them further.
* Increase `sim_params.physx.max_gpu_contact_pairs`, which is the size of your GPU contact buffer. The default value in Factory is 1024^2. You will likely not be able to exceed a factor of 50 beyond this value due to GPU memory limits.
* Increase `sim_params.physx.default_buffer_size_multiplier`, which will scale additional buffers used by PhysX. The default value in Factory is 8.
If you are experiencing any **stability issues** (e.g., jitter), try the following:
* Decrease `sim_params.dt`, increase `sim_params.substeps`, and/or increase `sim_params.physx.num_position_iterations`, which control the size of timesteps, substeps, and solver iterations. In general, increasing the number of iterations will slow down performance less than modifying the other parameters.
* Increase `sim_params.physx.contact_offset` and/or `sim_params.physx.friction_offset_threshold`, which are the distances at which contacts and frictional constraints are generated.
* Increase the SDF resolution in the asset URDFs.
* Increase the coefficient of friction and/or decrease the coefficient of restitution between the actors in the scene. However, be careful not to violate physically-reasonable ranges (e.g., friction values in excess of 2.0).
* Tune the gains of your controllers. Instability during robot-object contact may also be a result of poorly-tuned controllers, rather than underlying physics simulation issues. As in the real world, some controllers can be notoriously hard to tune.
Known Issues
------------
* If Isaac Gym is terminated during the SDF generation process, the SDF cache may become corrupted. You can resolve this by clearing the SDF cache and restarting Gym. For more details, see [this resolution](https://github.com/NVIDIA-Omniverse/IsaacGymEnvs/issues/53).
Citing Factory
--------------
If you use the Factory simulation methods (e.g., SDF collisions, contact reduction) or Factory learning tools (e.g., assets, environments, or controllers) in your work, please cite the following paper:
```
@inproceedings{
narang2022factory,
author = {Yashraj Narang and Kier Storey and Iretiayo Akinola and Miles Macklin and Philipp Reist and Lukasz Wawrzyniak and Yunrong Guo and Adam Moravanszky and Gavriel State and Michelle Lu and Ankur Handa and Dieter Fox},
title = {Factory: Fast contact for robotic assembly},
booktitle = {Robotics: Science and Systems},
year = {2022}
}
```
Also note that our original formulations of SDF collisions and contact reduction were developed by [Macklin, et al.](https://dl.acm.org/doi/abs/10.1145/3384538) and [Moravanszky and Terdiman](https://scholar.google.com/scholar?q=Game+Programming+Gems+4%2C+chapter+Fast+Contact+Reduction+for+Dynamics+Simulation), respectively.
| 17,912 | Markdown | 97.423076 | 794 | 0.780482 |
NVIDIA-Omniverse/IsaacGymEnvs/docs/pbt.md | ### Decentralized Population-Based Training with IsaacGymEnvs
#### Overview
Applications of evolutionary algorithms to reinforcement learning have
been popularized by publications such as [Capture the Flag](https://www.science.org/doi/full/10.1126/science.aau6249) by DeepMind.
Diverse populations of agents trained simultaneously can more efficiently explore the space of behaviors compared
to an equivalent amount of compute thrown at a single agent.
Typically Population-Based Training (PBT) is utilized in the context of multi-agent learning and self-play.
Agents trained with PBT in multi-agent environments exhibit more robust behaviors and are less prone to overfitting
and can avoid collapse modes common in self-play training.
Recent results in environments such as [StarCraft II](https://www.nature.com/articles/s41586-019-1724-z.epdf?author_access_token=lZH3nqPYtWJXfDA10W0CNNRgN0jAjWel9jnR3ZoTv0PSZcPzJFGNAZhOlk4deBCKzKm70KfinloafEF1bCCXL6IIHHgKaDkaTkBcTEv7aT-wqDoG1VeO9-wO3GEoAMF9bAOt7mJ0RWQnRVMbyfgH9A%3D%3D)
show that PBT is instrumental in achieving human-level performance in these task.
Implementation in IsaacGymEnvs uses PBT with single-agent environments to solve hard manipulation problems
and find good sets of hyperparameters and hyperparameter schedules.
#### Algorithm
In PBT, instead of training a single agent we train a population of N agents.
Agents with a performance considerably worse than a population best are stopped, their policy weights are replaced
with those of better performing agents, and the training hyperparameters and reward-shaping coefficients are changed
before training is resumed.
A typical implementation of PBT relies on a single central orchestrator that monitors the processes and restarts them
as needed (i.e. this is the approach used by Ray & RLLIB).
An alternative approach is decentralized PBT. It requires fewer moving parts and is robust to failure of any single component
(i.e. due to hardware issue). In decentralized PBT each process monitors its own standing with respect to the population,
restarts itself as needed, etc.
IsaacGymEnvs implements decentralized PBT that relies on access to a shared part of filesystem available to all agents.
This is trivial when experiments are executed locally, or in a managed cluster environment
such as Slurm. In any other environment a mounted shared folder can be used, i.e. with SSHFS.
The algorithm proceeds as follows:
- each agent continues training for M timesteps after which it saves a checkpoint containing its policy weights and learning hyperparameters
- after checkpoint is saved, the agent compares its own performance to other agents in the population; the performance is only
compared to other agent's checkpoints corresponding to equal or smaller amount of collected experience
(i.e. agents don't compare themselves against versions of other agents that learned from more experience)
- if the agent is not in bottom X% of the population, it continues training without any changes
- if the agent is in bottom X% of the population, but its performance is relatively close to the best agent it continues training
with mutated hyperparameters
- if the agent is in bottom X% of the population and its performance is significantly worse than that of the best agent,
its policy weights are replaced with weights of an agent randomly sampled from the top X% of the population, and its hyperparameters are mutated
before the training is resumed.
The algorithm implemented here is documented in details in the following RSS 2023 paper: https://arxiv.org/abs/2305.12127
(see also website https://sites.google.com/view/dexpbt)
#### PBT parameters and settings
(These are in pbt hydra configs and can be changed via command line)
- `pbt.interval_steps` - how often do we perform the PBT check and compare ourselves against other agents.
Typical values are in 10^6-10^8 range (10^7 by default). Larger values are recommended for harder tasks.
- `pbt.start_after`- start PBT checks after we trained for this many steps after experiment start or restart. Larger values allow
the population to accumulate some diversity.
- `pbt/mutation` - a Yaml file (Hydra config) for a mutation scheme. Specifies which hyperparameters should be mutated and how.
See more parameter documentation in pbt_default.yaml
#### Mutation
The mutation scheme is controlled by a Hydra config, such as the following:
```
task.env.fingertipDeltaRewScale: "mutate_float"
task.env.liftingRewScale: "mutate_float"
task.env.liftingBonus: "mutate_float"
train.params.config.reward_shaper.scale_value: "mutate_float"
train.params.config.learning_rate: "mutate_float"
train.params.config.grad_norm: "mutate_float"
train.params.config.e_clip: "mutate_eps_clip"
train.params.config.mini_epochs: "mutate_mini_epochs"
train.params.config.gamma: "mutate_discount"
```
Mutation scheme specifies hyperparameter names that could be passed via CLI and their corresponding mutation function.
Currently available mutation functions are defined in isaacgymenvs/pbt/mutation.py
A typical float parameter mutation function is trivial:
```
def mutate_float(x, change_min=1.1, change_max=1.5):
perturb_amount = random.uniform(change_min, change_max)
new_value = x / perturb_amount if random.random() < 0.5 else x * perturb_amount
return new_value
```
Some special parameters such as the discount factor require special mutation rules.
#### Target objective
In order to function, PBT needs a measure of _performance_ for individual agents.
By default, this is just agent's average reward in the environment.
If the reward is used as a target objective, PBT obviously can't be allowed to modify the reward shaping coefficient
and other hyperparameters that affect the reward calculation directly.
The environment can define a target objective different from default reward by adding a value `true_objective` to
the `info` dictionary returned by the step function, in IsaacGymEnvs this corresponds to:
`self.extras['true_objective'] = some_true_objective_value`
Using a separate true objective allows to optimize the reward function itself, so the overall
meta-optimization process can only care about the final goal of training, i.e. only the success rate in an object manipulation problem.
See allegro_kuka.py for example.
#### Running PBT experiments
A typical command line to start one training session in a PBT experiment looks something like this:
```
$ python -m isaacgymenvs.train seed=-1 train.params.config.max_frames=10000000000 headless=True pbt=pbt_default pbt.workspace=workspace_allegro_kuka pbt.interval_steps=20000000
pbt.start_after=100000000 pbt.initial_delay=200000000 pbt.replace_fraction_worst=0.3 pbt/mutation=allegro_kuka_mutation task=AllegroKukaLSTM task/env=reorientation pbt.num_policies=8 pbt.policy_idx=0
```
Note `pbt.policy_idx=0` - this will start the agent #0. For the full PBT experiment we will have to start agents `0 .. pbt.num_policies-1`.
We can do it manually by executing 8 command lines with `pbt.policy_idx=[0 .. 7]` while taking care
of GPU placement in a multi-GPU system via manipulating CUDA_VISIBLE_DEVICES for each agent.
This process can be automated by the `launcher`
(originally implemented in [Sample Factory](www.samplefactory.dev),
find more information in the [launcher documentation](https://www.samplefactory.dev/04-experiments/experiment-launcher/))
_(Note that the use of the launcher is optional, and you can run PBT experiments without it.
For example, multiple scripts can be started in the computation medium of your choice via a custom shell script)._
##### Running PBT locally with multiple GPUs
The launcher uses Python scripts that define complex experiments. See `isaacgymenvs/experiments/allegro_kuka_reorientation_lstm_pbt.py` as an example.
This script defines a single experiment (the PBT run) with ParamGrid iterating over policy indices `0 .. num_policies-1`.
The experiment described by this script can be started on a local system using the following command:
```
python -m isaacgymenvs.pbt.launcher.run --run=isaacgymenvs.pbt.experiments.allegro_kuka_reorientation_pbt_lstm --backend=processes --max_parallel=8 --experiments_per_gpu=2 --num_gpus=4
```
On a 4-GPU system this will start 8 individual agents, fitting two on each GPU.
##### Running PBT locally on a single GPUs
```
python -m isaacgymenvs.pbt.launcher.run --run=isaacgymenvs.pbt.experiments.ant_pbt --backend=processes --max_parallel=4 --experiments_per_gpu=4 --num_gpus=1
```
##### Running PBT on your cluster
The launcher can be used to run PBT on the cluster. It currently supports local runners (shown above) and Slurm, though the Slurm cluster backend is not thoroughly tested with this codebase as of yet.
You can learn more about using the launcher to run on a Slurm cluster [here](https://www.samplefactory.dev/04-experiments/experiment-launcher/#slurm-backend)
##### Testing the best policy
The best checkpoint for the entire population can be found in <pbt_workspace_dir>/best<policy_idx> where <pbt_workspace_dir> is the shared folder, and policy_idx is 0,1,2,... It is decentralized so each policy saves a copy of what it thinks is the best versions from the entire population, but usually checking workspace/best0 is enough. The checkpoint name will contain the iteration index and the fitness value, and also the index of the policy that this checkpoint belongs to
| 9,438 | Markdown | 59.121019 | 478 | 0.798686 |
NVIDIA-Omniverse/IsaacGymEnvs/docs/framework.md | RL Framework
===================
Overview
--------
Our training examples run using a third-party highly-optimized RL library,
[rl_games](https://github.com/Denys88/rl_games). This also demonstrates
how our framework can be used with other RL libraries.
RL Games will be installed automatically along with `isaacgymenvs`.
Otherwise, to install **rl_games** manually the following instructions should be performed:
```bash
pip install rl-games
```
Or to use the latest, unreleased version:
```bash
git clone https://github.com/Denys88/rl_games.git
pip install -e .
```
For all the sample tasks provided, we include training configurations
for rl_games, denoted with the suffixes `*PPO.yaml`.
These files are located in `isaacgymenvs/config/train`.
The appropriate config file will be selected
automatically based on the task being executed and the script that it is
being launched from. To launch a task using rl-games, run
`python train.py`.
For a list of the sample tasks we provide, refer to the
[RL List of Examples](rl.md)
Class Definition
----------------
The base class for Isaac Gym's RL framework is `VecTask` in [vec_task.py](../isaacgymenvs/tasks/base/vec_task.py).
The `VecTask` class is designed to act as a parent class for all RL tasks
using Isaac Gym's RL framework. It provides an interface for interaction
with RL algorithms and includes functionalities that are required for
all RL tasks.
The `VecTask` constructor takes a configuration dictionary containing numerous parameters required:
`device_type` - the type of device used for simulation. `cuda` or `cpu`.
`device_id` - ID of the device used for simulation. eg `0` for a single GPU workstation.
`rl_device` - Full `name:id` string of the device that the RL framework is using.
`headless` - `True`/`False` depending on whether you want the simulation to run the simulation with a viewer.
`physics_engine` - which physics engine to use. Must be `"physx"` or `"flex"`.
`env` - a dictionary with environment-specific parameters.
Can include anything in here you want depending on the specific parameters, but key ones which you must provide are:
* `numEnvs` - number of environments being simulated in parallel
* `numObservations` - size of the observation vector used for each environment.
* `numActions` - size of the actions vector.
Other optional parameters are
* `numAgents` - for multi-agent environments. Defaults to `1`
* `numStates` - for size of state vector for training with asymmetric actor-critic.
* `controlFrequencyInv` - control decimation, ie. how many simulator steps between RL actions. Defaults to 1.
* `clipObservations` - range to clip observations to. Defaults to `inf` (+-infinity).
* `clipActions` - range to clip actions to. Defaults to `1` (+-1).
* `enableCameraSensors` - set to `True` if camera sensors are used in the environment.
The `__init__` function of `VecTask` triggers a call to `create_sim()`,
which must be implemented by the extended classes.
It will then initialize buffers required for RL on the device specified. These include observation buffer, reward
buffer, reset buffer, progress buffer, randomization buffer, and an optional extras array for passing in any additional
information to the RL algorithm.
A call to `prepare_sim()` will also be made to initialize the internal data
structures for simulation. `set_viewer()` is also called, which, if running with a viewer,
this function will also initialize the viewer and create keyboard shortcuts for quitting
the application (ESC) and disabling/enabling rendering (V).
The `step` function is designed to guide the workflow of each RL
iteration. This function can be viewed in three parts:
`pre_physics_step`, `simulate`, and `post_physics_step`.
`pre_physics_step` should be implemented to perform any computations
required before stepping the physics simulation. As an example, applying
actions from the policy should happen in `pre_physics_step`. `simulate`
is then called to step the physics simulation. `post_physics_step`
should implement computations performed after stepping the physics
simulation, e.g. computing rewards and observations.
`VecTask` also provides an implementation of `render` to step graphics if
a viewer is initialized.
Additionally, VecTask provides an interface to perform Domain
Randomization via the `apply_randomizations` method. For more details,
please see [Domain Randomization](domain_randomization.md).
Creating a New Task
-------------------
Creating a new task is straight-forward using Isaac Gym's RL framework.
The first step is to create a new script file in [isaacgymenvs/tasks](../isaacgymenvs/tasks).
To use Isaac Gym's APIs, we need the following imports
```python
from isaacgym import gymtorch
from isaacgym import gymapi
from .base.vec_task import VecTask
```
Then, we need to create a Task class that extends from VecTask
```python
class MyNewTask(VecTask):
```
The `__init__` method should take 3 arguments: a config dict conforming to the
specifications described above (this will be generated from hydra config), `sim_device`, the device string representing
where the simulation will be run, and `headless`, which specifies whether or not to run in headless mode.
In the `__init__` method of MyNewTask, make sure to make a call to
`VecTask`'s `__init__` to initialize the simulation, providing the
config dictionary with members as described above:
```python
super().__init__(
cfg=config_dict
)
```
Then, we can initialize state tensors that we may need for our task. For
example, we can initialize the DOF state tensor
```python
dof_state_tensor = self.gym.acquire_dof_state_tensor(self.sim)
self.dof_state = gymtorch.wrap_tensor(dof_state_tensor)
```
There are a few methods that must be implemented by a child class of
VecTask: `create_sim`, `pre_physics_step`, `post_physics_step`.
```python
def create_sim(self):
# implement sim set up and environment creation here
# - set up-axis
# - call super().create_sim with device args (see docstring)
# - create ground plane
# - set up environments
def pre_physics_step(self, actions):
# implement pre-physics simulation code here
# - e.g. apply actions
def post_physics_step(self):
# implement post-physics simulation code here
# - e.g. compute reward, compute observations
```
To launch the new task from `train.py`, add your new
task to the imports and `isaacgym_task_map` dict in the `tasks` [\_\_init\_\_.py file](../isaacgymenvs/tasks/__init__.py).
```python
from isaacgymenvs.tasks.my_new_task import MyNewTask
...
isaac_gym_task_map = {
'Anymal': Anymal,
# ...
'MyNewTask': MyNewTask,
}
```
You will also need to create config files for task and training, which will be passed in dictionary form to the first
`config` argument of your task. The `task` config, which goes in the [corresponding config folder](../isaacgymenvs/cfg/task)
must have a `name` in the root matching the task name you put in the `isaac_gym_task_map` above. You should name your
task config the same as in the Isaac Gym task map, eg. `Anymal` becomes [`Anymal.yaml`](../isaacgymenvs/cfg/task/Anymal.yaml).
You also need a `train` config specifying RL Games arguments. This should go in the [corresponding config folder](../isaacgymenvs/cfg/train).
The file should have the postfix `PPO`, ie `Anymal` becomes [`AnymalPPO.yaml`](../isaacgymenvs/cfg/train/AnymalPPO.yaml).
Then, you can run your task with `python train.py task=MyNewTask`.
Updating an Existing Environment
--------------------------------
If you have existing environments set up with Isaac Gym Preview 2 release or earlier, it is simple to convert your tasks to the new RL framework in IsaacGymEnvs. Here are a few pointers to help you get started.
### Imports ###
* The `torch_jit_utils` script has been moved to IsaacGymEnvs. Tasks that are importing from `rlgpu.utils.torch_jit_utils` should now import from `utils.torch_jit_utils`.
* The original `BaseTask` class has been converted to `VecTask` in IsaacGymEnvs. All tasks inheriting from the previous `BaseTask` should modify `from rlgpu.tasks.base.base_task import BaseTask` to `from .base.vec_task import VecTask`.
### Class Definition ###
* Your task class should now inherit from `VecTask` instead of the previous `BaseTask`.
* Arguments required for class initialization has been simplified. The task `__init__()` method now only requires `cfg`, `sim_device`, and `headless` as arguments.
* It is no longer required to set `self.sim_params` and `self.physics_engine` in the `__init__()` method of your task definition.
* Making a call to `VecTask`'s `__init__()` method requires 3 more arguments: `rl_device`, `sim_device` and `headless`. As an example, modify the line of code to `super().__init__(config=self.cfg, rl_device=rl_device, sim_device=sim_device, headless=headless)`.
* `VecTask` now defines a `reset_idx()` function that should be implemented in an environment class. It resets environments with the provided indices.
* Note that `VecTask` now defines a `reset()` method that does not accept environment indices as arguments. To avoid naming conflicts, consider renaming the `reset()` method inside your task definition.
### Asset Loading ###
* Assets have been moved to IsaacGymEnvs (with some still remaining in IsaacGym for use in examples). Please make sure the paths to your assets remain valid in the new IsaacGymEnvs setup.
* Assets are now located under `assets/`.
### Configs ###
* Some config parameters are now updated to work with resolvers and Hydra. Please refer to an example config in `cfg/` for details.
* For task configs, the following are modified: `physics_engine`, `numEnvs`, `use_gpu_pipeline`, `num_threads`, `solver_type`, `use_gpu`, `num_subscenes`.
* For train configs, the following are modified: `seed`, `load_checkpoint`, `load_path`, `name`, `full_experiment_name`, `num_actors`, `max_epochs`.
* Also note a few naming changes required for the latest version of rl_games: `lr_threshold` --> `kl_threshold`, `steps_num` --> `horizon_length`.
### Viewer ###
When using the viewer, various actions can be executed with specific reserved keys:
* 'V' - Toggles rendering on and off. This is useful for speeding up training and observing the results.
* 'R' - Initiates video recording, saving the rendered frames to a designated folder.
* 'Tab' - Toggles the left panel, allowing you to remove and bring it back as necessary.
* 'ESC' - Stops the simulation and rendering processes, effectively quitting the program. | 10,558 | Markdown | 45.928889 | 261 | 0.74749 |
NVIDIA-Omniverse/IsaacGymEnvs/assets/mjcf/nv_ant.xml | <mujoco model="ant">
<custom>
<numeric data="0.0 0.0 0.55 1.0 0.0 0.0 0.0 0.0 1.0 0.0 -1.0 0.0 -1.0 0.0 1.0" name="init_qpos"/>
</custom>
<default>
<joint armature="0.01" damping="0.1" limited="true"/>
<geom condim="3" density="5.0" friction="1.5 0.1 0.1" margin="0.01" rgba="0.97 0.38 0.06 1"/>
</default>
<compiler inertiafromgeom="true" angle="degree"/>
<option timestep="0.016" iterations="50" tolerance="1e-10" solver="Newton" jacobian="dense" cone="pyramidal"/>
<size nconmax="50" njmax="200" nstack="10000"/>
<visual>
<map force="0.1" zfar="30"/>
<rgba haze="0.15 0.25 0.35 1"/>
<quality shadowsize="2048"/>
<global offwidth="800" offheight="800"/>
</visual>
<asset>
<texture type="skybox" builtin="gradient" rgb1="0.3 0.5 0.7" rgb2="0 0 0" width="512" height="512"/>
<texture name="texplane" type="2d" builtin="checker" rgb1=".2 .3 .4" rgb2=".1 0.15 0.2" width="512" height="512" mark="cross" markrgb=".8 .8 .8"/>
<texture name="texgeom" type="cube" builtin="flat" mark="cross" width="127" height="1278"
rgb1="0.8 0.6 0.4" rgb2="0.8 0.6 0.4" markrgb="1 1 1" random="0.01"/>
<material name="matplane" reflectance="0.3" texture="texplane" texrepeat="1 1" texuniform="true"/>
<material name="matgeom" texture="texgeom" texuniform="true" rgba="0.8 0.6 .4 1"/>
</asset>
<worldbody>
<geom name="floor" pos="0 0 0" size="0 0 .25" type="plane" material="matplane" condim="3"/>
<light directional="false" diffuse=".2 .2 .2" specular="0 0 0" pos="0 0 5" dir="0 0 -1" castshadow="false"/>
<light mode="targetbodycom" target="torso" directional="false" diffuse=".8 .8 .8" specular="0.3 0.3 0.3" pos="0 0 4.0" dir="0 0 -1"/>
<body name="torso" pos="0 0 0.75">
<freejoint name="root"/>
<geom name="torso_geom" pos="0 0 0" size="0.25" type="sphere"/>
<geom fromto="0.0 0.0 0.0 0.2 0.2 0.0" name="aux_1_geom" size="0.08" type="capsule" rgba=".999 .2 .1 1"/>
<geom fromto="0.0 0.0 0.0 -0.2 0.2 0.0" name="aux_2_geom" size="0.08" type="capsule"/>
<geom fromto="0.0 0.0 0.0 -0.2 -0.2 0.0" name="aux_3_geom" size="0.08" type="capsule"/>
<geom fromto="0.0 0.0 0.0 0.2 -0.2 0.0" name="aux_4_geom" size="0.08" type="capsule" rgba=".999 .2 .02 1"/>
<body name="front_left_leg" pos="0.2 0.2 0">
<joint axis="0 0 1" name="hip_1" pos="0.0 0.0 0.0" range="-40 40" type="hinge"/>
<geom fromto="0.0 0.0 0.0 0.2 0.2 0.0" name="left_leg_geom" size="0.08" type="capsule" rgba=".999 .2 .1 1"/>
<body pos="0.2 0.2 0" name="front_left_foot">
<joint axis="-1 1 0" name="ankle_1" pos="0.0 0.0 0.0" range="30 100" type="hinge"/>
<geom fromto="0.0 0.0 0.0 0.4 0.4 0.0" name="left_ankle_geom" size="0.08" type="capsule" rgba=".999 .2 .1 1"/>
</body>
</body>
<body name="front_right_leg" pos="-0.2 0.2 0">
<joint axis="0 0 1" name="hip_2" pos="0.0 0.0 0.0" range="-40 40" type="hinge"/>
<geom fromto="0.0 0.0 0.0 -0.2 0.2 0.0" name="right_leg_geom" size="0.08" type="capsule"/>
<body pos="-0.2 0.2 0" name="front_right_foot">
<joint axis="1 1 0" name="ankle_2" pos="0.0 0.0 0.0" range="-100 -30" type="hinge"/>
<geom fromto="0.0 0.0 0.0 -0.4 0.4 0.0" name="right_ankle_geom" size="0.08" type="capsule"/>
</body>
</body>
<body name="left_back_leg" pos="-0.2 -0.2 0">
<joint axis="0 0 1" name="hip_3" pos="0.0 0.0 0.0" range="-40 40" type="hinge"/>
<geom fromto="0.0 0.0 0.0 -0.2 -0.2 0.0" name="back_leg_geom" size="0.08" type="capsule"/>
<body pos="-0.2 -0.2 0" name="left_back_foot">
<joint axis="-1 1 0" name="ankle_3" pos="0.0 0.0 0.0" range="-100 -30" type="hinge"/>
<geom fromto="0.0 0.0 0.0 -0.4 -0.4 0.0" name="third_ankle_geom" size="0.08" type="capsule"/>
</body>
</body>
<body name="right_back_leg" pos="0.2 -0.2 0">
<joint axis="0 0 1" name="hip_4" pos="0.0 0.0 0.0" range="-40 40" type="hinge"/>
<geom fromto="0.0 0.0 0.0 0.2 -0.2 0.0" name="rightback_leg_geom" size="0.08" type="capsule" rgba=".999 .2 .1 1"/>
<body pos="0.2 -0.2 0" name="right_back_foot">
<joint axis="1 1 0" name="ankle_4" pos="0.0 0.0 0.0" range="30 100" type="hinge"/>
<geom fromto="0.0 0.0 0.0 0.4 -0.4 0.0" name="fourth_ankle_geom" size="0.08" type="capsule" rgba=".999 .2 .1 1"/>
</body>
</body>
</body>
</worldbody>
<actuator>
<motor ctrllimited="true" ctrlrange="-1.0 1.0" joint="hip_4" gear="15"/>
<motor ctrllimited="true" ctrlrange="-1.0 1.0" joint="ankle_4" gear="15"/>
<motor ctrllimited="true" ctrlrange="-1.0 1.0" joint="hip_1" gear="15"/>
<motor ctrllimited="true" ctrlrange="-1.0 1.0" joint="ankle_1" gear="15"/>
<motor ctrllimited="true" ctrlrange="-1.0 1.0" joint="hip_2" gear="15"/>
<motor ctrllimited="true" ctrlrange="-1.0 1.0" joint="ankle_2" gear="15"/>
<motor ctrllimited="true" ctrlrange="-1.0 1.0" joint="hip_3" gear="15"/>
<motor ctrllimited="true" ctrlrange="-1.0 1.0" joint="ankle_3" gear="15"/>
</actuator>
</mujoco>
| 5,160 | XML | 54.494623 | 152 | 0.56938 |
NVIDIA-Omniverse/IsaacGymEnvs/assets/mjcf/balance_bot.xml | <mujoco model="BalanceBot">
<compiler angle="degree" coordinate="local" inertiafromgeom="true" />
<worldbody>
<body name="tray" pos="0 0 0.559117">
<joint name="root_joint" type="free" />
<geom density="100" pos="0 0 0" size="0.5 0.01" type="cylinder" />
<body name="upper_leg0" pos="0.272721 0 -0.157279" quat="0.382683 0 -0.92388 0">
<geom density="1000" size="0.02 0.18" type="capsule" />
<joint axis="0 1 0" limited="true" name="upper_leg_joint0" pos="0 0 -0.18" range="-45 45" type="hinge" />
<body name="lower_leg0" pos="-0.18 0 0.18" quat="0.707107 0 -0.707107 0">
<geom density="1000" size="0.02 0.18" type="capsule" />
<joint axis="0 1 0" limited="true" name="lower_leg_joint0" pos="0 0 -0.18" range="-70 90" type="hinge" />
</body>
</body>
<body name="upper_leg1" pos="-0.13636 0.236183 -0.157279" quat="0.191342 0.800103 -0.46194 0.331414">
<geom density="1000" size="0.02 0.18" type="capsule" />
<joint axis="0 1 0" limited="true" name="upper_leg_joint1" pos="0 0 -0.18" range="-45 45" type="hinge" />
<body name="lower_leg1" pos="-0.18 0 0.18" quat="0.707107 0 -0.707107 0">
<geom density="1000" size="0.02 0.18" type="capsule" />
<joint axis="0 1 0" limited="true" name="lower_leg_joint1" pos="0 0 -0.18" range="-70 90" type="hinge" />
</body>
</body>
<body name="upper_leg2" pos="-0.13636 -0.236183 -0.157279" quat="-0.191342 0.800103 0.46194 0.331414">
<geom density="1000" size="0.02 0.18" type="capsule" />
<joint axis="0 1 0" limited="true" name="upper_leg_joint2" pos="0 0 -0.18" range="-45 45" type="hinge" />
<body name="lower_leg2" pos="-0.18 0 0.18" quat="0.707107 0 -0.707107 0">
<geom density="1000" size="0.02 0.18" type="capsule" />
<joint axis="0 1 0" limited="true" name="lower_leg_joint2" pos="0 0 -0.18" range="-70 90" type="hinge" />
</body>
</body>
</body>
</worldbody>
</mujoco>
| 2,032 | XML | 58.794116 | 115 | 0.576772 |
NVIDIA-Omniverse/IsaacGymEnvs/assets/mjcf/humanoid_CMU_V2020_v2.xml | <!-- This model has been rescaled and better supports the incorporation of hands. -->
<mujoco model="humanoid_CMU">
<compiler angle="radian"/>
<asset>
<material name="self" rgba=".7 .5 .3 1"/>
</asset>
<default>
<joint limited="true" solimplimit="0 0.99 0.01" stiffness="1" armature=".01" damping="1"/>
<geom size="0.03 0 0" condim="1" friction="0.7 0.005 0.0001" solref="0.015 1" solimp="0.99 0.99 0.003"/>
<general ctrllimited="true" ctrlrange="-1 1"/>
<default class="humanoid">
<geom type="capsule" material="self" group="2"/>
<default class="stiff_medium">
<joint stiffness="10" damping="2"/>
</default>
<default class="stiff_medium_higher">
<joint stiffness="50" damping="4"/>
</default>
<default class="stiff_high">
<joint stiffness="200" damping="5"/>
</default>
<default class="sensor_site">
<site type="sphere" size="0.01" group="4" rgba="1 0 0 .5"/>
</default>
<default class="contact">
<geom condim="3" friction="1. 0.005 0.0001" solref="0.015 1" solimp="0.98 0.98 0.001" priority="1"/>
</default>
</default>
</default>
<worldbody>
<light name="tracking_light" pos="0 0 7" dir="0 0 -1" mode="trackcom"/>
<camera name="back" pos="0 3 1.4" xyaxes="-1 0 0 0 -1 2" mode="trackcom"/>
<camera name="side" pos="-3 0 1.4" xyaxes="0 -1 0 1 0 2" mode="trackcom"/>
<camera name="front_side" pos="-2 -2 0.5" xyaxes="0.5 -0.5 0 0.1 0.1 1" mode="trackcom"/>
<body name="root" childclass="humanoid">
<camera name="bodycam" pos="0 .3 .1" xyaxes="-1 0 0 0 1 0" fovy="80"/>
<site name="root" size=".01" rgba="0.5 0.5 0.5 0"/>
<geom name="root_geom" size="0.1 0.07" pos="0 -0.05 0" quat="1 0 -1 0"/>
<body name="lhipjoint">
<geom name="lhipjoint" size="0.008 0.02187363" pos="0.0509685 -0.0459037 0.024723" quat="0.5708 -0.566602 -0.594264 0"/>
<body name="lfemur" pos="0.101937 -0.0918074 0.0494461" quat="0.984808 0 0 0.173648">
<joint name="lfemurrz" pos="0 0 0" axis="0 0 1" range="-1.0472 1.22173" class="stiff_medium"/>
<joint name="lfemurry" pos="0 0 0" axis="0 1 0" range="-1.22173 1.22173" class="stiff_medium"/>
<joint name="lfemurrx" pos="0 0 0" axis="1 0 0" range="-0.349066 2.79253" class="stiff_medium"/>
<geom name="lfemur_upper" size="0.085 0.083" pos="0 -0.115473 0" quat="0.696364 -0.696364 -0.122788 -0.122788" mass="0"/>
<geom name="lfemur" size="0.07 0.182226" pos="0 -0.202473 0" quat="0.696364 -0.696364 -0.122788 -0.122788" />
<body name="ltibia" pos="0 -0.404945 0">
<joint name="ltibiarx" pos="0 0 0" axis="1 0 0" range="0.01 2.96706"/>
<geom name="ltibia" size="0.04 0.1825614" pos="0 -0.202846 0" quat="0.696364 -0.696364 -0.122788 -0.122788"/>
<body name="lfoot" pos="0 -0.415693 0" quat="1 -1 0 0">
<site name="lfoot_touch" type="capsule" pos="0.0 0.02 -0.015" size="0.025 0.01" zaxis="1 0 0" class="sensor_site"/>
<joint name="lfootrz" pos="0 0 0" axis="0 0 1" range="-1.22173 0.349066" class="stiff_medium"/>
<joint name="lfootrx" pos="0 0 0" axis="1 0 0" range="-0.785398 0.8" class="stiff_medium"/>
<geom name="lfoot" size="0.025 0.08" pos="-0.0269999975006 -0.05 -0.0113878" quat=" 0.76725516 -0.64051114 0.02306487 -0.02306583" class="contact"/>
<geom name="lfoot_ch" size="0.025 0.08" pos="0.0270000024994 -0.05 -0.0113878" quat=" 0.72887266 -0.59399462 -0.24074283 -0.2407425 " class="contact"/>
<body name="ltoes" pos="0 -0.156372 -0.0227756">
<joint name="ltoesrx" pos="0 0 0" axis="1 0 0" range="-1.5708 0.349066"/>
<geom name="ltoes0" type="sphere" size="0.025" pos="0 -0.01 -.01" class="contact"/>
<geom name="ltoes1" type="sphere" size="0.025" pos=".03 -0.01 -.01" class="contact"/>
<geom name="ltoes2" type="sphere" size="0.025" pos="-.03 -0.01 -.01" class="contact"/>
<site name="ltoes_touch" type="capsule" pos="0.0 -0.01 -0.02" size="0.025 0.03" zaxis="1 0 0" class="sensor_site"/>
</body>
</body>
</body>
</body>
</body>
<body name="rhipjoint">
<geom name="rhipjoint" size="0.008 0.02187363" pos="-0.0509685 -0.0459037 0.024723" quat="0.574856 -0.547594 0.608014 0"/>
<body name="rfemur" pos="-0.101937 -0.0918074 0.0494461" quat="0.984808 0 0 -0.173648">
<joint name="rfemurrz" pos="0 0 0" axis="0 0 1" range="-1.22173 1.0472" class="stiff_medium"/>
<joint name="rfemurry" pos="0 0 0" axis="0 1 0" range="-1.22173 1.22173" class="stiff_medium"/>
<joint name="rfemurrx" pos="0 0 0" axis="1 0 0" range="-2.79253 0.349066" class="stiff_medium"/>
<geom name="rfemur_upper" size="0.085 0.083" pos="0 -0.115473 0" quat="0.696364 -0.696364 0.122788 0.122788" mass="0"/>
<geom name="rfemur" size="0.07 0.182226" pos="0 -0.202473 0" quat="0.696364 -0.696364 0.122788 0.122788" />
<body name="rtibia" pos="0 -0.404945 0">
<joint name="rtibiarx" pos="0 0 0" axis="1 0 0" range="0.01 2.96706"/>
<geom name="rtibia" size="0.04 0.1825614" pos="0 -0.202846 0" quat="0.696364 -0.696364 0.122788 0.122788"/>
<body name="rfoot" pos="0 -0.415693 0" quat="0.707107 -0.707107 0 0">
<site name="rfoot_touch" type="capsule" pos="0.0 0.02 -0.015" size="0.025 0.01" zaxis="1 0 0" class="sensor_site"/>
<joint name="rfootrz" pos="0 0 0" axis="0 0 1" range="-0.349066 1.22173" class="stiff_medium"/>
<joint name="rfootrx" pos="0 0 0" axis="1 0 0" range="-0.785398 .8" class="stiff_medium"/>
<geom name="rfoot" size="0.025 0.08" pos="-0.0269999965316 -0.05 -0.0113878" quat=" 0.73520687 -0.58633523 0.24050108 0.24050079" class="contact"/>
<geom name="rfoot_ch" size="0.025 0.08" pos="0.0270000034684 -0.05 -0.0113878" quat=" 0.77312469 -0.633231 -0.02545846 0.02545836" class="contact"/>
<body name="rtoes" pos="0 -0.156372 -0.0227756">
<joint name="rtoesrx" pos="0 0 0" axis="1 0 0" range="-1.5708 0.349066"/>
<geom name="rtoes0" type="sphere" size="0.025" pos="0 -0.01 -.01" class="contact"/>
<geom name="rtoes1" type="sphere" size="0.025" pos=".03 -0.01 -.01" class="contact"/>
<geom name="rtoes2" type="sphere" size="0.025" pos="-.03 -0.01 -.01" class="contact"/>
<site name="rtoes_touch" type="capsule" pos="0.0 -0.01 -0.02" size="0.025 0.03" zaxis="1 0 0" class="sensor_site"/>
</body>
</body>
</body>
</body>
</body>
<body name="lowerback">
<joint name="lowerbackrz" pos="0 0 0" axis="0 0 1" range="-0.523599 0.523599" class="stiff_high"/>
<joint name="lowerbackry" pos="0 0 0" axis="0 1 0" range="-0.523599 0.523599" class="stiff_high"/>
<joint name="lowerbackrx" pos="0 0 0" axis="1 0 0" range="-0.349066 0.785398" class="stiff_medium"/>
<geom name="lowerback" size="0.085 0.04540016" pos="0.00282931 0.0566065 0.01" quat="1 0 1 0"/>
<body name="upperback" pos="0.000565862 0.113213 -0.00805298">
<joint name="upperbackrz" pos="0 0 0" axis="0 0 1" range="-0.523599 0.523599" class="stiff_high"/>
<joint name="upperbackry" pos="0 0 0" axis="0 1 0" range="-0.523599 0.523599" class="stiff_high"/>
<joint name="upperbackrx" pos="0 0 0" axis="1 0 0" range="-0.349066 0.785398" class="stiff_medium"/>
<geom name="upperback" size="0.09 0.04542616" pos="0.000256264 0.0567802 0.02" quat="1 0 1 0"/>
<body name="thorax" pos="0.000512528 0.11356 0.000936821">
<joint name="thoraxrz" pos="0 0 0" axis="0 0 1" range="-0.523599 0.523599" class="stiff_high"/>
<joint name="thoraxry" pos="0 0 0" axis="0 1 0" range="-0.523599 0.523599" class="stiff_high"/>
<joint name="thoraxrx" pos="0 0 0" axis="1 0 0" range="-0.349066 0.785398" class="stiff_medium"/>
<geom name="thorax" size="0.095 0.0570206" pos="0 0.0569725 0.02" quat="1 0 1 0"/>
<body name="lowerneck" pos="0 0.113945 0.00468037">
<joint name="lowerneckrz" pos="0 0 0" axis="0 0 1" range="-0.523599 0.523599" class="stiff_medium_higher"/>
<joint name="lowerneckry" pos="0 0 0" axis="0 1 0" range="-0.523599 0.523599" class="stiff_medium_higher"/>
<joint name="lowerneckrx" pos="0 0 0" axis="1 0 0" range="-0.349066 0.785398" class="stiff_medium_higher"/>
<geom name="lowerneck" size="0.075 0.02279225" pos="-0.00165071 0.0452401 0.00534359" quat="1 1 0 0"/>
<body name="upperneck" pos="-0.00330143 0.0904801 0.0106872">
<joint name="upperneckrz" pos="0 0 0" axis="0 0 1" range="-0.523599 0.523599" class="stiff_medium_higher"/>
<joint name="upperneckry" pos="0 0 0" axis="0 1 0" range="-0.523599 0.523599" class="stiff_medium_higher"/>
<joint name="upperneckrx" pos="0 0 0" axis="1 0 0" range="-0.349066 0.785398" class="stiff_medium_higher"/>
<geom name="upperneck" size="0.05 0.0225272" pos="0.000500875 0.0449956 -0.00224644" quat="1 1 0 0"/>
<body name="head" pos="0.00100175 0.13 -0.00449288">
<camera name="egocentric" pos="0 0 0" xyaxes="-1 0 0 0 1 0" fovy="80"/>
<joint name="headrz" pos="0 0 0" axis="0 0 1" range="-0.523599 0.523599" class="stiff_medium_higher"/>
<joint name="headry" pos="0 0 0" axis="0 1 0" range="-0.523599 0.523599" class="stiff_medium_higher"/>
<joint name="headrx" pos="0 0 0" axis="1 0 0" range="-0.349066 0.785398" class="stiff_medium_higher"/>
<geom name="head" size="0.095 0.024104" pos="0.000341465 0.048184 0.025" quat="1 1 0 0"/>
</body>
</body>
</body>
<body name="lclavicle" pos="0 0.113945 0.00468037">
<joint name="lclaviclerz" pos="0 0 0" axis="0 0 1" range="0 0.349066" class="stiff_high"/>
<joint name="lclaviclery" pos="0 0 0" axis="0 1 0" range="-0.349066 0.174533" class="stiff_high"/>
<geom name="lclavicle" size="0.075 0.06" pos="0.0918817 0.0382636 0.00535704" quat="0.688 0.279 -0.67 0"/>
<body name="lhumerus" pos="0.18 0.09 0.0107141" quat="0.183013 0.683013 -0.683013 0.183013">
<joint name="lhumerusrz" pos="0 0 0" axis="0 0 1" range="-1.1 1.5708" class="stiff_medium"/>
<joint name="lhumerusry" pos="0 0 0" axis="0 1 0" range="-1.5708 1.5708" class="stiff_medium"/>
<joint name="lhumerusrx" pos="0 0 0" axis="1 0 0" range="-1.0472 1.5708" class="stiff_medium"/>
<site name="lhumerus_ft" class="sensor_site"/>
<geom name="lhumerus" size="0.042 0.1245789" pos="0 -0.138421 0" quat="0.612372 -0.612372 0.353553 0.353553"/>
<body name="lradius" pos="0 -0.276843 0">
<joint name="lradiusrx" pos="0 0 0" axis="1 0 0" range="-0.174533 2.96706"/>
<geom name="lradius" size="0.03 0.08169111" pos="0 -0.0907679 0" quat="0.612372 -0.612372 0.353553 0.353553"/>
<site name="lwrist" pos="0 -0.181536 0" quat="-0.5 0 0.866025 0"/>
<body name="lwrist" pos="0 -0.181536 0" quat="-0.5 0 0.866025 0">
<joint name="lwristry" pos="0 0 0" axis="0 1 0" range="0 3.14159"/>
<geom name="lwrist" size="0.02 0.03" pos="0 -0.03 0" quat="1.59389e-11 -1.59388e-11 -0.707107 -0.707107"/>
<body name="lhand" pos="0 -0.0907676 0">
<joint name="lhandrz" pos="0 0 0" axis="0 0 1" range="-0.785398 0.785398"/>
<joint name="lhandrx" pos="0 0 0" axis="1 0 0" range="-1.5708 1.5708"/>
<site name="lhand_touch" size=".012 0.005 0.015" pos="0 -0.016752 -0.02" quat="0 0 -1 -1" type="ellipsoid" class="sensor_site"/>
<geom name="lhand" size="0.035 0.02 0.045" pos="0 -0.016752 0" quat="0 0 -1 -1" type="ellipsoid" class="contact"/>
<body name="lfingers" pos="0 -0.075 0">
<joint name="lfingersrx" pos="0 0.015 0" axis="1 0 0" range="0 1.5708"/>
<site name="lfingers_touch" type="box" size="0.023 0.013 0.003" pos="0 -0.042 -0.007" class="sensor_site"/>
<geom name="lfinger0" size="0.0065 0.04" pos="-.024 -0.025 0" quat="1 -1 0 0" class="contact"/>
<geom name="lfinger1" size="0.0065 0.04" pos="-.008 -0.03 0" quat="1 -1 0 0" class="contact"/>
<geom name="lfinger2" size="0.006 0.04" pos=".008 -0.03 0" quat="1 -1 0 0" class="contact"/>
<geom name="lfinger3" size="0.0055 0.04" pos=".024 -0.025 0" quat="1 -1 0 0" class="contact"/>
</body>
<body name="lthumb" pos="-.025 0 0" quat="0.92388 0 0 -0.382683">
<joint name="lthumbrz" pos="0 0 0" axis="0 0 1" range="-0.785398 0.785398"/>
<joint name="lthumbrx" pos="0 0 0" axis="1 0 0" range="0 1.57"/>
<site name="lthumb_touch" type="box" size="0.006 0.013 0.003" pos="0 -0.043 -0.007" class="sensor_site"/>
<geom name="lthumb" size="0.008 0.03" pos="0 -0.03 0" quat="0 0 -1 -1" class="contact"/>
</body>
</body>
</body>
</body>
</body>
</body>
<body name="rclavicle" pos="0 0.113945 0.00468037">
<joint name="rclaviclerz" pos="0 0 0" axis="0 0 1" range="-0.349066 0" class="stiff_high"/>
<joint name="rclaviclery" pos="0 0 0" axis="0 1 0" range="-0.174533 0.349066" class="stiff_high"/>
<geom name="rclavicle" size="0.075 0.06" pos="-0.0918817 0.0382636 0.00535704" quat="0.688 0.279 0.67 0"/>
<body name="rhumerus" pos="-0.18 0.09 0.0107141" quat="0.183013 0.683013 0.683013 -0.183013">
<joint name="rhumerusrz" pos="0 0 0" axis="0 0 1" range="-1.1 1.5708" class="stiff_medium"/>
<joint name="rhumerusry" pos="0 0 0" axis="0 1 0" range="-1.5708 1.5708" class="stiff_medium"/>
<joint name="rhumerusrx" pos="0 0 0" axis="1 0 0" range="-1.5708 1.0472" class="stiff_medium"/>
<site name="rhumerus_ft" class="sensor_site"/>
<geom name="rhumerus" size="0.042 0.1245789" pos="0 -0.138421 0" quat="0.612372 -0.612372 -0.353553 -0.353553"/>
<body name="rradius" pos="0 -0.276843 0">
<joint name="rradiusrx" pos="0 0 0" axis="1 0 0" range="-0.174533 2.96706"/>
<geom name="rradius" size="0.03 0.08169111" pos="0 -0.0907679 0" quat="0.61238 -0.612372 -0.353554 -0.353541"/>
<body name="rwrist" pos="0 -0.181536 0" quat="-0.5 0 -0.866025 0">
<joint name="rwristry" pos="0 0 0" axis="0 1 0" range="-3.14159 0"/>
<geom name="rwrist" size="0.02 0.03" pos="0 -0.03 0" quat="0 0 1 1"/>
<body name="rhand" pos="0 -0.0907676 0">
<joint name="rhandrz" pos="0 0 0" axis="0 0 1" range="-0.785398 0.785398"/>
<joint name="rhandrx" pos="0 0 0" axis="1 0 0" range="-1.5708 1.5708"/>
<site name="rhand_touch" size=".012 0.005 0.015" pos="0 -0.016752 -0.02" quat="0 0 1 1" type="ellipsoid" class="sensor_site"/>
<geom name="rhand" size="0.035 0.02 0.045" pos="0 -0.016752 0" quat="0 0 1 1" type="ellipsoid" class="contact"/>
<body name="rfingers" pos="0 -0.075 0">
<joint name="rfingersrx" pos="0 0.015 0" axis="1 0 0" range="0 1.5708"/>
<site name="rfingers_touch" type="box" size="0.023 0.013 0.003" pos="0 -0.042 -0.007" class="sensor_site"/>
<geom name="rfinger0" size="0.0065 0.04" pos=".024 -0.025 0" quat="1 -1 0 0" class="contact"/>
<geom name="rfinger1" size="0.0065 0.04" pos=".008 -0.03 0" quat="1 -1 0 0" class="contact"/>
<geom name="rfinger2" size="0.006 0.04" pos="-.008 -0.03 0" quat="1 -1 0 0" class="contact"/>
<geom name="rfinger3" size="0.0055 0.04" pos="-.024 -0.025 0" quat="1 -1 0 0" class="contact"/>
</body>
<body name="rthumb" pos=".025 0 0" quat="0.92388 0 0 0.382683">
<joint name="rthumbrz" pos="0 0 0" axis="0 0 1" range="-0.785398 0.785398"/>
<joint name="rthumbrx" pos="0 0 0" axis="1 0 0" range="0 1.57"/>
<site name="rthumb_touch" type="box" size="0.006 0.013 0.003" pos="0 -0.043 -0.007" class="sensor_site"/>
<geom name="rthumb" size="0.008 0.03" pos="0 -0.03 0" quat="6.21773e-11 -6.35284e-11 0.707107 0.707107" class="contact"/>
</body>
</body>
</body>
</body>
</body>
</body>
</body>
</body>
</body>
</body>
</worldbody>
<contact>
<exclude body1="lfemur" body2="root"/>
<exclude body1="rfemur" body2="root"/>
<exclude body1="lclavicle" body2="rclavicle"/>
<exclude body1="lowerneck" body2="lclavicle"/>
<exclude body1="lowerneck" body2="rclavicle"/>
<exclude body1="upperneck" body2="lclavicle"/>
<exclude body1="upperneck" body2="rclavicle"/>
</contact>
<actuator>
<motor name="headrx" joint="headrx" gear="20"/>
<motor name="headry" joint="headry" gear="20"/>
<motor name="headrz" joint="headrz" gear="20"/>
<motor name="lclaviclery" joint="lclaviclery" gear="20"/>
<motor name="lclaviclerz" joint="lclaviclerz" gear="20"/>
<motor name="lfemurrx" joint="lfemurrx" gear="120"/>
<motor name="lfemurry" joint="lfemurry" gear="40"/>
<motor name="lfemurrz" joint="lfemurrz" gear="40"/>
<motor name="lfingersrx" joint="lfingersrx" gear="20"/>
<motor name="lfootrx" joint="lfootrx" gear="20"/>
<motor name="lfootrz" joint="lfootrz" gear="20"/>
<motor name="lhandrx" joint="lhandrx" gear="20"/>
<motor name="lhandrz" joint="lhandrz" gear="20"/>
<motor name="lhumerusrx" joint="lhumerusrx" gear="40"/>
<motor name="lhumerusry" joint="lhumerusry" gear="40"/>
<motor name="lhumerusrz" joint="lhumerusrz" gear="40"/>
<motor name="lowerbackrx" joint="lowerbackrx" gear="40"/>
<motor name="lowerbackry" joint="lowerbackry" gear="40"/>
<motor name="lowerbackrz" joint="lowerbackrz" gear="40"/>
<motor name="lowerneckrx" joint="lowerneckrx" gear="20"/>
<motor name="lowerneckry" joint="lowerneckry" gear="20"/>
<motor name="lowerneckrz" joint="lowerneckrz" gear="20"/>
<motor name="lradiusrx" joint="lradiusrx" gear="40"/>
<motor name="lthumbrx" joint="lthumbrx" gear="20"/>
<motor name="lthumbrz" joint="lthumbrz" gear="20"/>
<motor name="ltibiarx" joint="ltibiarx" gear="80"/>
<motor name="ltoesrx" joint="ltoesrx" gear="20"/>
<motor name="lwristry" joint="lwristry" gear="20"/>
<motor name="rclaviclery" joint="rclaviclery" gear="20"/>
<motor name="rclaviclerz" joint="rclaviclerz" gear="20"/>
<motor name="rfemurrx" joint="rfemurrx" gear="120"/>
<motor name="rfemurry" joint="rfemurry" gear="40"/>
<motor name="rfemurrz" joint="rfemurrz" gear="40"/>
<motor name="rfingersrx" joint="rfingersrx" gear="20"/>
<motor name="rfootrx" joint="rfootrx" gear="20"/>
<motor name="rfootrz" joint="rfootrz" gear="20"/>
<motor name="rhandrx" joint="rhandrx" gear="20"/>
<motor name="rhandrz" joint="rhandrz" gear="20"/>
<motor name="rhumerusrx" joint="rhumerusrx" gear="40"/>
<motor name="rhumerusry" joint="rhumerusry" gear="40"/>
<motor name="rhumerusrz" joint="rhumerusrz" gear="40"/>
<motor name="rradiusrx" joint="rradiusrx" gear="40"/>
<motor name="rthumbrx" joint="rthumbrx" gear="20"/>
<motor name="rthumbrz" joint="rthumbrz" gear="20"/>
<motor name="rtibiarx" joint="rtibiarx" gear="80"/>
<motor name="rtoesrx" joint="rtoesrx" gear="20"/>
<motor name="rwristry" joint="rwristry" gear="20"/>
<motor name="thoraxrx" joint="thoraxrx" gear="40"/>
<motor name="thoraxry" joint="thoraxry" gear="40"/>
<motor name="thoraxrz" joint="thoraxrz" gear="40"/>
<motor name="upperbackrx" joint="upperbackrx" gear="40"/>
<motor name="upperbackry" joint="upperbackry" gear="40"/>
<motor name="upperbackrz" joint="upperbackrz" gear="40"/>
<motor name="upperneckrx" joint="upperneckrx" gear="20"/>
<motor name="upperneckry" joint="upperneckry" gear="20"/>
<motor name="upperneckrz" joint="upperneckrz" gear="20"/>
</actuator>
<sensor>
<velocimeter name="sensor_root_veloc" site="root"/>
<gyro name="sensor_root_gyro" site="root"/>
<accelerometer name="sensor_root_accel" site="root"/>
<touch name="sensor_touch_lhand" site="lhand_touch"/>
<touch name="sensor_touch_lfingers" site="lfingers_touch"/>
<touch name="sensor_touch_lthumb" site="lthumb_touch"/>
<touch name="sensor_touch_rhand" site="rhand_touch"/>
<touch name="sensor_touch_rfingers" site="rfingers_touch"/>
<touch name="sensor_touch_rthumb" site="rthumb_touch"/>
<touch name="sensor_touch_ltoes" site="ltoes_touch"/>
<touch name="sensor_touch_rtoes" site="rtoes_touch"/>
<touch name="sensor_touch_rfoot" site="rfoot_touch"/>
<touch name="sensor_touch_lfoot" site="lfoot_touch"/>
<torque name="sensor_torque_lhumerus" site="lhumerus_ft"/>
<torque name="sensor_torque_rhumerus" site="rhumerus_ft"/>
</sensor>
</mujoco>
| 22,008 | XML | 70.924836 | 165 | 0.568021 |
NVIDIA-Omniverse/IsaacGymEnvs/assets/mjcf/nv_humanoid.xml | <mujoco model="humanoid">
<statistic extent="2" center="0 0 1"/>
<option timestep="0.00555"/>
<default>
<motor ctrlrange="-1 1" ctrllimited="true"/>
<default class="body">
<geom type="capsule" condim="1" friction="1.0 0.05 0.05" solimp=".9 .99 .003" solref=".015 1" material="self"/>
<joint type="hinge" damping="0.1" stiffness="5" armature=".007" limited="true" solimplimit="0 .99 .01"/>
<default class="small_joint">
<joint damping="1.0" stiffness="2" armature=".006"/>
</default>
<default class="big_joint">
<joint damping="5" stiffness="10" armature=".01"/>
</default>
<default class="bigger_stiff_joint">
<joint damping="5" stiffness="20" armature=".01"/>
</default>
<default class="big_stiff_joint">
<joint damping="5" stiffness="20" armature=".02"/>
</default>
<site size=".04" group="3"/>
<default class="force-torque">
<site type="box" size=".01 .01 .02" rgba="1 0 0 1" />
</default>
<default class="touch">
<site type="capsule" rgba="0 0 1 .3"/>
</default>
</default>
</default>
<worldbody>
<geom name="floor" type="plane" conaffinity="1" size="100 100 .2" material="grid"/>
<body name="torso" pos="0 0 1.5" childclass="body">
<light name="top" pos="0 0 2" mode="trackcom"/>
<camera name="back" pos="-3 0 1" xyaxes="0 -1 0 1 0 2" mode="trackcom"/>
<camera name="side" pos="0 -3 1" xyaxes="1 0 0 0 1 2" mode="trackcom"/>
<freejoint name="root"/>
<site name="root" class="force-torque"/>
<geom name="torso" fromto="0 -.07 0 0 .07 0" size=".07"/>
<geom name="upper_waist" fromto="-.01 -.06 -.12 -.01 .06 -.12" size=".06"/>
<site name="torso" class="touch" type="box" pos="0 0 -.05" size=".075 .14 .13"/>
<body name="head" pos="0 0 .19">
<geom name="head" type="sphere" size=".09"/>
<site name="head" class="touch" type="sphere" size=".091"/>
<camera name="egocentric" pos=".09 0 0" xyaxes="0 -1 0 .1 0 1" fovy="80"/>
</body>
<body name="lower_waist" pos="-.01 0 -.260" quat="1.000 0 -.002 0">
<geom name="lower_waist" fromto="0 -.06 0 0 .06 0" size=".06"/>
<site name="lower_waist" class="touch" size=".061 .06" zaxis="0 1 0"/>
<joint name="abdomen_z" pos="0 0 .065" axis="0 0 1" range="-45 45" class="big_stiff_joint"/>
<joint name="abdomen_y" pos="0 0 .065" axis="0 1 0" range="-75 30" class="bigger_stiff_joint"/>
<body name="pelvis" pos="0 0 -.165" quat="1.000 0 -.002 0">
<joint name="abdomen_x" pos="0 0 .1" axis="1 0 0" range="-35 35" class="big_joint"/>
<geom name="butt" fromto="-.02 -.07 0 -.02 .07 0" size=".09"/>
<site name="butt" class="touch" size=".091 .07" pos="-.02 0 0" zaxis="0 1 0"/>
<body name="right_thigh" pos="0 -.1 -.04">
<site name="right_hip" class="force-torque"/>
<joint name="right_hip_x" axis="1 0 0" range="-45 15" class="big_joint"/>
<joint name="right_hip_z" axis="0 0 1" range="-60 35" class="big_joint"/>
<joint name="right_hip_y" axis="0 1 0" range="-120 45" class="bigger_stiff_joint"/>
<geom name="right_thigh" fromto="0 0 0 0 .01 -.34" size=".06"/>
<site name="right_thigh" class="touch" pos="0 .005 -.17" size=".061 .17" zaxis="0 -1 34"/>
<body name="right_shin" pos="0 .01 -.403">
<site name="right_knee" class="force-torque" pos="0 0 .02"/>
<joint name="right_knee" pos="0 0 .02" axis="0 -1 0" range="-160 2"/>
<geom name="right_shin" fromto="0 0 0 0 0 -.3" size=".049"/>
<site name="right_shin" class="touch" pos="0 0 -.15" size=".05 .15"/>
<body name="right_foot" pos="0 0 -.39">
<site name="right_ankle" class="force-torque"/>
<joint name="right_ankle_y" pos="0 0 .08" axis="0 1 0" range="-50 50" class="small_joint"/>
<joint name="right_ankle_x" pos="0 0 .08" axis="1 0 .5" range="-50 50" class="small_joint"/>
<geom name="right_right_foot" fromto="-.07 -.02 0 .14 -.04 0" size=".027"/>
<geom name="left_right_foot" fromto="-.07 0 0 .14 .02 0" size=".027"/>
<site name="right_right_foot" class="touch" pos=".035 -.03 0" size=".03 .11" zaxis="21 -2 0"/>
<site name="left_right_foot" class="touch" pos=".035 .01 0" size=".03 .11" zaxis="21 2 0"/>
</body>
</body>
</body>
<body name="left_thigh" pos="0 .1 -.04">
<site name="left_hip" class="force-torque"/>
<joint name="left_hip_x" axis="-1 0 0" range="-45 15" class="big_joint"/>
<joint name="left_hip_z" axis="0 0 -1" range="-60 35" class="big_joint"/>
<joint name="left_hip_y" axis="0 1 0" range="-120 45" class="bigger_stiff_joint"/>
<geom name="left_thigh" fromto="0 0 0 0 -.01 -.34" size=".06"/>
<site name="left_thigh" class="touch" pos="0 -.005 -.17" size=".061 .17" zaxis="0 1 34"/>
<body name="left_shin" pos="0 -.01 -.403">
<site name="left_knee" class="force-torque" pos="0 0 .02"/>
<joint name="left_knee" pos="0 0 .02" axis="0 -1 0" range="-160 2"/>
<geom name="left_shin" fromto="0 0 0 0 0 -.3" size=".049"/>
<site name="left_shin" class="touch" pos="0 0 -.15" size=".05 .15"/>
<body name="left_foot" pos="0 0 -.39">
<site name="left_ankle" class="force-torque"/>
<joint name="left_ankle_y" pos="0 0 .08" axis="0 1 0" range="-50 50" class="small_joint"/>
<joint name="left_ankle_x" pos="0 0 .08" axis="1 0 .5" range="-50 50" class="small_joint"/>
<geom name="left_left_foot" fromto="-.07 .02 0 .14 .04 0" size=".027"/>
<geom name="right_left_foot" fromto="-.07 0 0 .14 -.02 0" size=".027"/>
<site name="right_left_foot" class="touch" pos=".035 -.01 0" size=".03 .11" zaxis="21 -2 0"/>
<site name="left_left_foot" class="touch" pos=".035 .03 0" size=".03 .11" zaxis="21 2 0"/>
</body>
</body>
</body>
</body>
</body>
<body name="right_upper_arm" pos="0 -.17 .06">
<joint name="right_shoulder1" axis="2 1 1" range="-90 70" class="big_joint"/>
<joint name="right_shoulder2" axis="0 -1 1" range="-90 70" class="big_joint"/>
<geom name="right_upper_arm" fromto="0 0 0 .16 -.16 -.16" size=".04 .16"/>
<site name="right_upper_arm" class="touch" pos=".08 -.08 -.08" size=".041 .14" zaxis="1 -1 -1"/>
<body name="right_lower_arm" pos=".18 -.18 -.18">
<joint name="right_elbow" axis="0 -1 1" range="-90 50" class="small_joint"/>
<geom name="right_lower_arm" fromto=".01 .01 .01 .17 .17 .17" size=".031"/>
<site name="right_lower_arm" class="touch" pos=".09 .09 .09" size=".032 .14" zaxis="1 1 1"/>
<body name="right_hand" pos=".18 .18 .18">
<geom name="right_hand" type="sphere" size=".04"/>
<site name="right_hand" class="touch" type="sphere" size=".041"/>
</body>
</body>
</body>
<body name="left_upper_arm" pos="0 .17 .06">
<joint name="left_shoulder1" axis="-2 1 -1" range="-90 70" class="big_joint"/>
<joint name="left_shoulder2" axis="0 -1 -1" range="-90 70" class="big_joint"/>
<geom name="left_upper_arm" fromto="0 0 0 .16 .16 -.16" size=".04 .16"/>
<site name="left_upper_arm" class="touch" pos=".08 .08 -.08" size=".041 .14" zaxis="1 1 -1"/>
<body name="left_lower_arm" pos=".18 .18 -.18">
<joint name="left_elbow" axis="0 -1 -1" range="-90 50" class="small_joint"/>
<geom name="left_lower_arm" fromto=".01 -.01 .01 .17 -.17 .17" size=".031"/>
<site name="left_lower_arm" class="touch" pos=".09 -.09 .09" size=".032 .14" zaxis="1 -1 1"/>
<body name="left_hand" pos=".18 -.18 .18">
<geom name="left_hand" type="sphere" size=".04"/>
<site name="left_hand" class="touch" type="sphere" size=".041"/>
</body>
</body>
</body>
</body>
</worldbody>
<actuator>
<motor name='abdomen_y' gear='67.5' joint='abdomen_y'/>
<motor name='abdomen_z' gear='67.5' joint='abdomen_z'/>
<motor name='abdomen_x' gear='67.5' joint='abdomen_x'/>
<motor name='right_hip_x' gear='45.0' joint='right_hip_x'/>
<motor name='right_hip_z' gear='45.0' joint='right_hip_z'/>
<motor name='right_hip_y' gear='135.0' joint='right_hip_y'/>
<motor name='right_knee' gear='90.0' joint='right_knee'/>
<motor name='right_ankle_x' gear='22.5' joint='right_ankle_x'/>
<motor name='right_ankle_y' gear='22.5' joint='right_ankle_y'/>
<motor name='left_hip_x' gear='45.0' joint='left_hip_x'/>
<motor name='left_hip_z' gear='45.0' joint='left_hip_z'/>
<motor name='left_hip_y' gear='135.0' joint='left_hip_y'/>
<motor name='left_knee' gear='90.0' joint='left_knee'/>
<motor name='left_ankle_x' gear='22.5' joint='left_ankle_x'/>
<motor name='left_ankle_y' gear='22.5' joint='left_ankle_y'/>
<motor name='right_shoulder1' gear='67.5' joint='right_shoulder1'/>
<motor name='right_shoulder2' gear='67.5' joint='right_shoulder2'/>
<motor name='right_elbow' gear='45.0' joint='right_elbow'/>
<motor name='left_shoulder1' gear='67.5' joint='left_shoulder1'/>
<motor name='left_shoulder2' gear='67.5' joint='left_shoulder2'/>
<motor name='left_elbow' gear='45.0' joint='left_elbow'/>
</actuator>
<sensor>
<subtreelinvel name="torso_subtreelinvel" body="torso"/>
<accelerometer name="torso_accel" site="root"/>
<velocimeter name="torso_vel" site="root"/>
<gyro name="torso_gyro" site="root"/>
<force name="left_ankle_force" site="left_ankle"/>
<force name="right_ankle_force" site="right_ankle"/>
<force name="left_knee_force" site="left_knee"/>
<force name="right_knee_force" site="right_knee"/>
<force name="left_hip_force" site="left_hip"/>
<force name="right_hip_force" site="right_hip"/>
<torque name="left_ankle_torque" site="left_ankle"/>
<torque name="right_ankle_torque" site="right_ankle"/>
<torque name="left_knee_torque" site="left_knee"/>
<torque name="right_knee_torque" site="right_knee"/>
<torque name="left_hip_torque" site="left_hip"/>
<torque name="right_hip_torque" site="right_hip"/>
<touch name="torso_touch" site="torso"/>
<touch name="head_touch" site="head"/>
<touch name="lower_waist_touch" site="lower_waist"/>
<touch name="butt_touch" site="butt"/>
<touch name="right_thigh_touch" site="right_thigh"/>
<touch name="right_shin_touch" site="right_shin"/>
<touch name="right_right_foot_touch" site="right_right_foot"/>
<touch name="left_right_foot_touch" site="left_right_foot"/>
<touch name="left_thigh_touch" site="left_thigh"/>
<touch name="left_shin_touch" site="left_shin"/>
<touch name="right_left_foot_touch" site="right_left_foot"/>
<touch name="left_left_foot_touch" site="left_left_foot"/>
<touch name="right_upper_arm_touch" site="right_upper_arm"/>
<touch name="right_lower_arm_touch" site="right_lower_arm"/>
<touch name="right_hand_touch" site="right_hand"/>
<touch name="left_upper_arm_touch" site="left_upper_arm"/>
<touch name="left_lower_arm_touch" site="left_lower_arm"/>
<touch name="left_hand_touch" site="left_hand"/>
</sensor>
</mujoco>
| 11,886 | XML | 56.703883 | 118 | 0.549218 |
NVIDIA-Omniverse/IsaacGymEnvs/assets/mjcf/amp_humanoid.xml | <mujoco model="humanoid">
<statistic extent="2" center="0 0 1"/>
<option timestep="0.00555"/>
<default>
<motor ctrlrange="-1 1" ctrllimited="true"/>
<default class="body">
<geom type="capsule" condim="1" friction="1.0 0.05 0.05" solimp=".9 .99 .003" solref=".015 1"/>
<joint type="hinge" damping="0.1" stiffness="5" armature=".007" limited="true" solimplimit="0 .99 .01"/>
<site size=".04" group="3"/>
<default class="force-torque">
<site type="box" size=".01 .01 .02" rgba="1 0 0 1" />
</default>
<default class="touch">
<site type="capsule" rgba="0 0 1 .3"/>
</default>
</default>
</default>
<worldbody>
<geom name="floor" type="plane" conaffinity="1" size="100 100 .2" material="grid"/>
<body name="pelvis" pos="0 0 1" childclass="body">
<freejoint name="root"/>
<site name="root" class="force-torque"/>
<geom name="pelvis" type="sphere" pos="0 0 0.07" size=".09" density="2226"/>
<geom name="upper_waist" type="sphere" pos="0 0 0.205" size="0.07" density="2226"/>
<site name="pelvis" class="touch" type="sphere" pos="0 0 0.07" size="0.091"/>
<site name="upper_waist" class="touch" type="sphere" pos="0 0 0.205" size="0.071"/>
<body name="torso" pos="0 0 0.236151">
<light name="top" pos="0 0 2" mode="trackcom"/>
<camera name="back" pos="-3 0 1" xyaxes="0 -1 0 1 0 2" mode="trackcom"/>
<camera name="side" pos="0 -3 1" xyaxes="1 0 0 0 1 2" mode="trackcom"/>
<joint name="abdomen_x" pos="0 0 0" axis="1 0 0" range="-60 60" stiffness="600" damping="60" armature=".025"/>
<joint name="abdomen_y" pos="0 0 0" axis="0 1 0" range="-60 90" stiffness="600" damping="60" armature=".025"/>
<joint name="abdomen_z" pos="0 0 0" axis="0 0 1" range="-50 50" stiffness="600" damping="60" armature=".025"/>
<geom name="torso" type="sphere" pos="0 0 0.12" size="0.11" density="1794"/>
<site name="torso" class="touch" type="sphere" pos="0 0 0.12" size="0.111"/>
<geom name="right_clavicle" fromto="-0.0060125 -0.0457775 0.2287955 -0.016835 -0.128177 0.2376182" size=".045" density="1100"/>
<geom name="left_clavicle" fromto="-0.0060125 0.0457775 0.2287955 -0.016835 0.128177 0.2376182" size=".045" density="1100"/>
<body name="head" pos="0 0 0.223894">
<joint name="neck_x" axis="1 0 0" range="-50 50" stiffness="50" damping="5" armature=".017"/>
<joint name="neck_y" axis="0 1 0" range="-40 60" stiffness="50" damping="5" armature=".017"/>
<joint name="neck_z" axis="0 0 1" range="-45 45" stiffness="50" damping="5" armature=".017"/>
<geom name="head" type="sphere" pos="0 0 0.175" size="0.095" density="1081"/>
<site name="head" class="touch" pos="0 0 0.175" type="sphere" size="0.103"/>
<camera name="egocentric" pos=".103 0 0.175" xyaxes="0 -1 0 .1 0 1" fovy="80"/>
</body>
<body name="right_upper_arm" pos="-0.02405 -0.18311 0.24350">
<joint name="right_shoulder_x" axis="1 0 0" range="-180 45" stiffness="200" damping="20" armature=".02"/>
<joint name="right_shoulder_y" axis="0 1 0" range="-180 60" stiffness="200" damping="20" armature=".02"/>
<joint name="right_shoulder_z" axis="0 0 1" range="-90 90" stiffness="200" damping="20" armature=".02"/>
<geom name="right_upper_arm" fromto="0 0 -0.05 0 0 -0.23" size=".045" density="982"/>
<site name="right_upper_arm" class="touch" pos="0 0 -0.14" size="0.046 0.1" zaxis="0 0 1"/>
<body name="right_lower_arm" pos="0 0 -0.274788">
<joint name="right_elbow" axis="0 1 0" range="-160 0" stiffness="150" damping="15" armature=".015"/>
<geom name="right_lower_arm" fromto="0 0 -0.0525 0 0 -0.1875" size="0.04" density="1056"/>
<site name="right_lower_arm" class="touch" pos="0 0 -0.12" size="0.041 0.0685" zaxis="0 1 0"/>
<body name="right_hand" pos="0 0 -0.258947">
<geom name="right_hand" type="sphere" size=".04" density="1865"/>
<site name="right_hand" class="touch" type="sphere" size=".041"/>
</body>
</body>
</body>
<body name="left_upper_arm" pos="-0.02405 0.18311 0.24350">
<joint name="left_shoulder_x" axis="1 0 0" range="-45 180" stiffness="200" damping="20" armature=".02"/>
<joint name="left_shoulder_y" axis="0 1 0" range="-180 60" stiffness="200" damping="20" armature=".02"/>
<joint name="left_shoulder_z" axis="0 0 1" range="-90 90" stiffness="200" damping="20" armature=".02"/>
<geom name="left_upper_arm" fromto="0 0 -0.05 0 0 -0.23" size="0.045" density="982"/>
<site name="left_upper_arm" class="touch" pos="0 0 -0.14" size="0.046 0.1" zaxis="0 0 1"/>
<body name="left_lower_arm" pos="0 0 -0.274788">
<joint name="left_elbow" axis="0 1 0" range="-160 0" stiffness="150" damping="15" armature=".015"/>
<geom name="left_lower_arm" fromto="0 0 -0.0525 0 0 -0.1875" size="0.04" density="1056"/>
<site name="left_lower_arm" class="touch" pos="0 0 -0.1" size="0.041 0.0685" zaxis="0 0 1"/>
<body name="left_hand" pos="0 0 -0.258947">
<geom name="left_hand" type="sphere" size=".04" density="1865"/>
<site name="left_hand" class="touch" type="sphere" size=".041"/>
</body>
</body>
</body>
</body>
<body name="right_thigh" pos="0 -0.084887 0">
<site name="right_hip" class="force-torque"/>
<joint name="right_hip_x" axis="1 0 0" range="-60 15" stiffness="300" damping="30" armature=".02"/>
<joint name="right_hip_y" axis="0 1 0" range="-140 60" stiffness="300" damping="30" armature=".02"/>
<joint name="right_hip_z" axis="0 0 1" range="-60 35" stiffness="300" damping="30" armature=".02"/>
<geom name="right_thigh" fromto="0 0 -0.06 0 0 -0.36" size="0.055" density="1269"/>
<site name="right_thigh" class="touch" pos="0 0 -0.21" size="0.056 0.301" zaxis="0 0 -1"/>
<body name="right_shin" pos="0 0 -0.421546">
<site name="right_knee" class="force-torque" pos="0 0 0"/>
<joint name="right_knee" pos="0 0 0" axis="0 1 0" range="0 160" stiffness="300" damping="30" armature=".02"/>
<geom name="right_shin" fromto="0 0 -0.045 0 0 -0.355" size=".05" density="1014"/>
<site name="right_shin" class="touch" pos="0 0 -0.2" size="0.051 0.156" zaxis="0 0 -1"/>
<body name="right_foot" pos="0 0 -0.409870">
<site name="right_ankle" class="force-torque"/>
<joint name="right_ankle_x" pos="0 0 0" axis="1 0 0" range="-30 30" stiffness="200" damping="20" armature=".01"/>
<joint name="right_ankle_y" pos="0 0 0" axis="0 1 0" range="-55 55" stiffness="200" damping="20" armature=".01"/>
<joint name="right_ankle_z" pos="0 0 0" axis="0 0 1" range="-40 40" stiffness="200" damping="20" armature=".01"/>
<geom name="right_foot" type="box" pos="0.045 0 -0.0225" size="0.0885 0.045 0.0275" density="1141"/>
<site name="right_foot" class="touch" type="box" pos="0.045 0 -0.0225" size="0.0895 0.055 0.0285"/>
</body>
</body>
</body>
<body name="left_thigh" pos="0 0.084887 0">
<site name="left_hip" class="force-torque"/>
<joint name="left_hip_x" axis="1 0 0" range="-15 60" stiffness="300" damping="30" armature=".02"/>
<joint name="left_hip_y" axis="0 1 0" range="-140 60" stiffness="300" damping="30" armature=".02"/>
<joint name="left_hip_z" axis="0 0 1" range="-35 60" stiffness="300" damping="30" armature=".02"/>
<geom name="left_thigh" fromto="0 0 -0.06 0 0 -0.36" size=".055" density="1269"/>
<site name="left_thigh" class="touch" pos="0 0 -0.21" size="0.056 0.301" zaxis="0 0 -1"/>
<body name="left_shin" pos="0 0 -0.421546">
<site name="left_knee" class="force-torque" pos="0 0 .02"/>
<joint name="left_knee" pos="0 0 0" axis="0 1 0" range="0 160" stiffness="300" damping="30" armature=".02"/>
<geom name="left_shin" fromto="0 0 -0.045 0 0 -0.355" size=".05" density="1014"/>
<site name="left_shin" class="touch" pos="0 0 -0.2" size="0.051 0.156" zaxis="0 0 -1"/>
<body name="left_foot" pos="0 0 -0.409870">
<site name="left_ankle" class="force-torque"/>
<joint name="left_ankle_x" pos="0 0 0" axis="1 0 0" range="-30 30" stiffness="200" damping="20" armature=".01"/>
<joint name="left_ankle_y" pos="0 0 0" axis="0 1 0" range="-55 55" stiffness="200" damping="20" armature=".01"/>
<joint name="left_ankle_z" pos="0 0 0" axis="0 0 1" range="-40 40" stiffness="200" damping="20" armature=".01"/>
<geom name="left_foot" type="box" pos="0.045 0 -0.0225" size="0.0885 0.045 0.0275" density="1141"/>
<site name="left_foot" class="touch" type="box" pos="0.045 0 -0.0225" size="0.0895 0.055 0.0285"/>
</body>
</body>
</body>
</body>
</worldbody>
<actuator>
<motor name='abdomen_x' gear='125' joint='abdomen_x'/>
<motor name='abdomen_y' gear='125' joint='abdomen_y'/>
<motor name='abdomen_z' gear='125' joint='abdomen_z'/>
<motor name='neck_x' gear='20' joint='neck_x'/>
<motor name='neck_y' gear='20' joint='neck_y'/>
<motor name='neck_z' gear='20' joint='neck_z'/>
<motor name='right_shoulder_x' gear='70' joint='right_shoulder_x'/>
<motor name='right_shoulder_y' gear='70' joint='right_shoulder_y'/>
<motor name='right_shoulder_z' gear='70' joint='right_shoulder_z'/>
<motor name='right_elbow' gear='60' joint='right_elbow'/>
<motor name='left_shoulder_x' gear='70' joint='left_shoulder_x'/>
<motor name='left_shoulder_y' gear='70' joint='left_shoulder_y'/>
<motor name='left_shoulder_z' gear='70' joint='left_shoulder_z'/>
<motor name='left_elbow' gear='60' joint='left_elbow'/>
<motor name='right_hip_x' gear='125' joint='right_hip_x'/>
<motor name='right_hip_z' gear='125' joint='right_hip_z'/>
<motor name='right_hip_y' gear='125' joint='right_hip_y'/>
<motor name='right_knee' gear='100' joint='right_knee'/>
<motor name='right_ankle_x' gear='50' joint='right_ankle_x'/>
<motor name='right_ankle_y' gear='50' joint='right_ankle_y'/>
<motor name='right_ankle_z' gear='50' joint='right_ankle_z'/>
<motor name='left_hip_x' gear='125' joint='left_hip_x'/>
<motor name='left_hip_z' gear='125' joint='left_hip_z'/>
<motor name='left_hip_y' gear='125' joint='left_hip_y'/>
<motor name='left_knee' gear='100' joint='left_knee'/>
<motor name='left_ankle_x' gear='50' joint='left_ankle_x'/>
<motor name='left_ankle_y' gear='50' joint='left_ankle_y'/>
<motor name='left_ankle_z' gear='50' joint='left_ankle_z'/>
</actuator>
<sensor>
<subtreelinvel name="pelvis_subtreelinvel" body="pelvis"/>
<accelerometer name="root_accel" site="root"/>
<velocimeter name="root_vel" site="root"/>
<gyro name="root_gyro" site="root"/>
<force name="left_ankle_force" site="left_ankle"/>
<force name="right_ankle_force" site="right_ankle"/>
<force name="left_knee_force" site="left_knee"/>
<force name="right_knee_force" site="right_knee"/>
<force name="left_hip_force" site="left_hip"/>
<force name="right_hip_force" site="right_hip"/>
<torque name="left_ankle_torque" site="left_ankle"/>
<torque name="right_ankle_torque" site="right_ankle"/>
<torque name="left_knee_torque" site="left_knee"/>
<torque name="right_knee_torque" site="right_knee"/>
<torque name="left_hip_torque" site="left_hip"/>
<torque name="right_hip_torque" site="right_hip"/>
<touch name="pelvis_touch" site="pelvis"/>
<touch name="upper_waist_touch" site="upper_waist"/>
<touch name="torso_touch" site="torso"/>
<touch name="head_touch" site="head"/>
<touch name="right_upper_arm_touch" site="right_upper_arm"/>
<touch name="right_lower_arm_touch" site="right_lower_arm"/>
<touch name="right_hand_touch" site="right_hand"/>
<touch name="left_upper_arm_touch" site="left_upper_arm"/>
<touch name="left_lower_arm_touch" site="left_lower_arm"/>
<touch name="left_hand_touch" site="left_hand"/>
<touch name="right_thigh_touch" site="right_thigh"/>
<touch name="right_shin_touch" site="right_shin"/>
<touch name="right_foot_touch" site="right_foot"/>
<touch name="left_thigh_touch" site="left_thigh"/>
<touch name="left_shin_touch" site="left_shin"/>
<touch name="left_foot_touch" site="left_foot"/>
</sensor>
</mujoco>
| 13,032 | XML | 59.618604 | 135 | 0.575813 |
NVIDIA-Omniverse/IsaacGymEnvs/assets/mjcf/open_ai_assets/hand/shared_touch_sensors_92.xml | <mujoco>
<sensor>
<!--PALM-->
<touch name="robot0:TS_palm_b0" site="robot0:T_palm_b0"></touch>
<touch name="robot0:TS_palm_bl" site="robot0:T_palm_bl"></touch>
<touch name="robot0:TS_palm_bm" site="robot0:T_palm_bm"></touch>
<touch name="robot0:TS_palm_br" site="robot0:T_palm_br"></touch>
<touch name="robot0:TS_palm_fl" site="robot0:T_palm_fl"></touch>
<touch name="robot0:TS_palm_fm" site="robot0:T_palm_fm"></touch>
<touch name="robot0:TS_palm_fr" site="robot0:T_palm_fr"></touch>
<touch name="robot0:TS_palm_b1" site="robot0:T_palm_b1"></touch>
<!--FOREFINGER-->
<touch name="robot0:TS_ffproximal_front_left_bottom" site="robot0:T_ffproximal_front_left_bottom"></touch>
<touch name="robot0:TS_ffproximal_front_right_bottom" site="robot0:T_ffproximal_front_right_bottom"></touch>
<touch name="robot0:TS_ffproximal_front_left_top" site="robot0:T_ffproximal_front_left_top"></touch>
<touch name="robot0:TS_ffproximal_front_right_top" site="robot0:T_ffproximal_front_right_top"></touch>
<touch name="robot0:TS_ffproximal_back_left" site="robot0:T_ffproximal_back_left"></touch>
<touch name="robot0:TS_ffproximal_back_right" site="robot0:T_ffproximal_back_right"></touch>
<touch name="robot0:TS_ffproximal_tip" site="robot0:T_ffproximal_tip"></touch>
<touch name="robot0:TS_ffmiddle_front_left" site="robot0:T_ffmiddle_front_left"></touch>
<touch name="robot0:TS_ffmiddle_front_right" site="robot0:T_ffmiddle_front_right"></touch>
<touch name="robot0:TS_ffmiddle_back_left" site="robot0:T_ffmiddle_back_left"></touch>
<touch name="robot0:TS_ffmiddle_back_right" site="robot0:T_ffmiddle_back_right"></touch>
<touch name="robot0:TS_ffmiddle_tip" site="robot0:T_ffmiddle_tip"></touch>
<touch name="robot0:TS_fftip_front_left" site="robot0:T_fftip_front_left"></touch>
<touch name="robot0:TS_fftip_front_right" site="robot0:T_fftip_front_right"></touch>
<touch name="robot0:TS_fftip_back_left" site="robot0:T_fftip_back_left"></touch>
<touch name="robot0:TS_fftip_back_right" site="robot0:T_fftip_back_right"></touch>
<touch name="robot0:TS_fftip_tip" site="robot0:T_fftip_tip"></touch>
<!-- MIDDLE FINGER -->
<touch name="robot0:TS_mfproximal_front_left_bottom" site="robot0:T_mfproximal_front_left_bottom"></touch>
<touch name="robot0:TS_mfproximal_front_right_bottom" site="robot0:T_mfproximal_front_right_bottom"></touch>
<touch name="robot0:TS_mfproximal_front_left_top" site="robot0:T_mfproximal_front_left_top"></touch>
<touch name="robot0:TS_mfproximal_front_right_top" site="robot0:T_mfproximal_front_right_top"></touch>
<touch name="robot0:TS_mfproximal_back_left" site="robot0:T_mfproximal_back_left"></touch>
<touch name="robot0:TS_mfproximal_back_right" site="robot0:T_mfproximal_back_right"></touch>
<touch name="robot0:TS_mfproximal_tip" site="robot0:T_mfproximal_tip"></touch>
<touch name="robot0:TS_mfmiddle_front_left" site="robot0:T_mfmiddle_front_left"></touch>
<touch name="robot0:TS_mfmiddle_front_right" site="robot0:T_mfmiddle_front_right"></touch>
<touch name="robot0:TS_mfmiddle_back_left" site="robot0:T_mfmiddle_back_left"></touch>
<touch name="robot0:TS_mfmiddle_back_right" site="robot0:T_mfmiddle_back_right"></touch>
<touch name="robot0:TS_mfmiddle_tip" site="robot0:T_mfmiddle_tip"></touch>
<touch name="robot0:TS_mftip_front_left" site="robot0:T_mftip_front_left"></touch>
<touch name="robot0:TS_mftip_front_right" site="robot0:T_mftip_front_right"></touch>
<touch name="robot0:TS_mftip_back_left" site="robot0:T_mftip_back_left"></touch>
<touch name="robot0:TS_mftip_back_right" site="robot0:T_mftip_back_right"></touch>
<touch name="robot0:TS_mftip_tip" site="robot0:T_mftip_tip"></touch>
<!-- RING FINGER -->
<touch name="robot0:TS_rfproximal_front_left_bottom" site="robot0:T_rfproximal_front_left_bottom"></touch>
<touch name="robot0:TS_rfproximal_front_right_bottom" site="robot0:T_rfproximal_front_right_bottom"></touch>
<touch name="robot0:TS_rfproximal_front_left_top" site="robot0:T_rfproximal_front_left_top"></touch>
<touch name="robot0:TS_rfproximal_front_right_top" site="robot0:T_rfproximal_front_right_top"></touch>
<touch name="robot0:TS_rfproximal_back_left" site="robot0:T_rfproximal_back_left"></touch>
<touch name="robot0:TS_rfproximal_back_right" site="robot0:T_rfproximal_back_right"></touch>
<touch name="robot0:TS_rfproximal_tip" site="robot0:T_rfproximal_tip"></touch>
<touch name="robot0:TS_rfmiddle_front_left" site="robot0:T_rfmiddle_front_left"></touch>
<touch name="robot0:TS_rfmiddle_front_right" site="robot0:T_rfmiddle_front_right"></touch>
<touch name="robot0:TS_rfmiddle_back_left" site="robot0:T_rfmiddle_back_left"></touch>
<touch name="robot0:TS_rfmiddle_back_right" site="robot0:T_rfmiddle_back_right"></touch>
<touch name="robot0:TS_rfmiddle_tip" site="robot0:T_rfmiddle_tip"></touch>
<touch name="robot0:TS_rftip_front_left" site="robot0:T_rftip_front_left"></touch>
<touch name="robot0:TS_rftip_front_right" site="robot0:T_rftip_front_right"></touch>
<touch name="robot0:TS_rftip_back_left" site="robot0:T_rftip_back_left"></touch>
<touch name="robot0:TS_rftip_back_right" site="robot0:T_rftip_back_right"></touch>
<touch name="robot0:TS_rftip_tip" site="robot0:T_rftip_tip"></touch>
<!-- LITTLE FINGER -->
<touch name="robot0:TS_lfmetacarpal_front" site="robot0:T_lfmetacarpal_front"></touch>
<touch name="robot0:TS_lfproximal_front_left_bottom" site="robot0:T_lfproximal_front_left_bottom"></touch>
<touch name="robot0:TS_lfproximal_front_right_bottom" site="robot0:T_lfproximal_front_right_bottom"></touch>
<touch name="robot0:TS_lfproximal_front_left_top" site="robot0:T_lfproximal_front_left_top"></touch>
<touch name="robot0:TS_lfproximal_front_right_top" site="robot0:T_lfproximal_front_right_top"></touch>
<touch name="robot0:TS_lfproximal_back_left" site="robot0:T_lfproximal_back_left"></touch>
<touch name="robot0:TS_lfproximal_back_right" site="robot0:T_lfproximal_back_right"></touch>
<touch name="robot0:TS_lfproximal_tip" site="robot0:T_lfproximal_tip"></touch>
<touch name="robot0:TS_lfmiddle_front_left" site="robot0:T_lfmiddle_front_left"></touch>
<touch name="robot0:TS_lfmiddle_front_right" site="robot0:T_lfmiddle_front_right"></touch>
<touch name="robot0:TS_lfmiddle_back_left" site="robot0:T_lfmiddle_back_left"></touch>
<touch name="robot0:TS_lfmiddle_back_right" site="robot0:T_lfmiddle_back_right"></touch>
<touch name="robot0:TS_lfmiddle_tip" site="robot0:T_lfmiddle_tip"></touch>
<touch name="robot0:TS_lftip_front_left" site="robot0:T_lftip_front_left"></touch>
<touch name="robot0:TS_lftip_front_right" site="robot0:T_lftip_front_right"></touch>
<touch name="robot0:TS_lftip_back_left" site="robot0:T_lftip_back_left"></touch>
<touch name="robot0:TS_lftip_back_right" site="robot0:T_lftip_back_right"></touch>
<touch name="robot0:TS_lftip_tip" site="robot0:T_lftip_tip"></touch>
<!--THUMB-->
<touch name="robot0:TS_thproximal_front_left" site="robot0:T_thproximal_front_left"></touch>
<touch name="robot0:TS_thproximal_front_right" site="robot0:T_thproximal_front_right"></touch>
<touch name="robot0:TS_thproximal_back_left" site="robot0:T_thproximal_back_left"></touch>
<touch name="robot0:TS_thproximal_back_right" site="robot0:T_thproximal_back_right"></touch>
<touch name="robot0:TS_thproximal_tip" site="robot0:T_thproximal_tip"></touch>
<touch name="robot0:TS_thmiddle_front_left" site="robot0:T_thmiddle_front_left"></touch>
<touch name="robot0:TS_thmiddle_front_right" site="robot0:T_thmiddle_front_right"></touch>
<touch name="robot0:TS_thmiddle_back_left" site="robot0:T_thmiddle_back_left"></touch>
<touch name="robot0:TS_thmiddle_back_right" site="robot0:T_thmiddle_back_right"></touch>
<touch name="robot0:TS_thmiddle_tip" site="robot0:T_thmiddle_tip"></touch>
<touch name="robot0:TS_thtip_front_left" site="robot0:T_thtip_front_left"></touch>
<touch name="robot0:TS_thtip_front_right" site="robot0:T_thtip_front_right"></touch>
<touch name="robot0:TS_thtip_back_left" site="robot0:T_thtip_back_left"></touch>
<touch name="robot0:TS_thtip_back_right" site="robot0:T_thtip_back_right"></touch>
<touch name="robot0:TS_thtip_tip" site="robot0:T_thtip_tip"></touch>
</sensor>
</mujoco>
| 9,201 | XML | 75.049586 | 116 | 0.654168 |
NVIDIA-Omniverse/IsaacGymEnvs/assets/mjcf/open_ai_assets/hand/shared.xml | <!-- See LICENSE.md for legal notices. LICENSE.md must be kept together with this file. -->
<mujoco>
<size njmax="500" nconmax="100" nuser_jnt="1" nuser_site="1" nuser_tendon="1" nuser_sensor="1" nuser_actuator="16" nstack="600000"></size>
<visual>
<map fogstart="3" fogend="5" force="0.1"></map>
<quality shadowsize="4096"></quality>
</visual>
<default>
<default class="robot0:asset_class">
<geom friction="1 0.005 0.001" condim="3" margin="0.0005" contype="1" conaffinity="1"></geom>
<joint limited="true" damping="0.1" armature="0.001" margin="0.01" frictionloss="0.001"></joint>
<site size="0.005" rgba="0.4 0.9 0.4 1"></site>
<general ctrllimited="true" forcelimited="true"></general>
</default>
<default class="robot0:D_Touch">
<site type="box" size="0.009 0.004 0.013" pos="0 -0.004 0.018" rgba="0.8 0.8 0.8 0.15" group="4"></site>
</default>
<default class="robot0:DC_Hand">
<geom material="robot0:MatColl" contype="1" conaffinity="0" group="4"></geom>
</default>
<default class="robot0:D_Vizual">
<geom material="robot0:MatViz" contype="0" conaffinity="0" group="1" type="mesh"></geom>
</default>
<default class="robot0:free">
<joint type="free" damping="0" armature="0" limited="false"></joint>
</default>
</default>
<contact>
<pair geom1="robot0:C_ffdistal" geom2="robot0:C_thdistal" condim="1"></pair>
<pair geom1="robot0:C_ffmiddle" geom2="robot0:C_thdistal" condim="1"></pair>
<pair geom1="robot0:C_ffproximal" geom2="robot0:C_thdistal" condim="1"></pair>
<pair geom1="robot0:C_mfproximal" geom2="robot0:C_thdistal" condim="1"></pair>
<pair geom1="robot0:C_mfdistal" geom2="robot0:C_thdistal" condim="1"></pair>
<pair geom1="robot0:C_rfdistal" geom2="robot0:C_thdistal" condim="1"></pair>
<pair geom1="robot0:C_lfdistal" geom2="robot0:C_thdistal" condim="1"></pair>
<pair geom1="robot0:C_palm0" geom2="robot0:C_thdistal" condim="1"></pair>
<pair geom1="robot0:C_mfdistal" geom2="robot0:C_ffdistal" condim="1"></pair>
<pair geom1="robot0:C_rfdistal" geom2="robot0:C_mfdistal" condim="1"></pair>
<pair geom1="robot0:C_lfdistal" geom2="robot0:C_rfdistal" condim="1"></pair>
<pair geom1="robot0:C_mfproximal" geom2="robot0:C_ffproximal" condim="1"></pair>
<pair geom1="robot0:C_rfproximal" geom2="robot0:C_mfproximal" condim="1"></pair>
<pair geom1="robot0:C_lfproximal" geom2="robot0:C_rfproximal" condim="1"></pair>
<pair geom1="robot0:C_lfdistal" geom2="robot0:C_rfdistal" condim="1"></pair>
<pair geom1="robot0:C_lfdistal" geom2="robot0:C_mfdistal" condim="1"></pair>
<pair geom1="robot0:C_lfdistal" geom2="robot0:C_rfmiddle" condim="1"></pair>
<pair geom1="robot0:C_lfmiddle" geom2="robot0:C_rfdistal" condim="1"></pair>
<pair geom1="robot0:C_lfmiddle" geom2="robot0:C_rfmiddle" condim="1"></pair>
</contact>
<tendon>
<fixed name="robot0:T_FFJ1c" limited="true" range="-0.001 0.001">
<joint joint="robot0:FFJ0" coef="0.00705"></joint>
<joint joint="robot0:FFJ1" coef="-0.00805"></joint>
</fixed>
<fixed name="robot0:T_MFJ1c" limited="true" range="-0.001 0.001">
<joint joint="robot0:MFJ0" coef="0.00705"></joint>
<joint joint="robot0:MFJ1" coef="-0.00805"></joint>
</fixed>
<fixed name="robot0:T_RFJ1c" limited="true" range="-0.001 0.001">
<joint joint="robot0:RFJ0" coef="0.00705"></joint>
<joint joint="robot0:RFJ1" coef="-0.00805"></joint>
</fixed>
<fixed name="robot0:T_LFJ1c" limited="true" range="-0.001 0.001">
<joint joint="robot0:LFJ0" coef="0.00705"></joint>
<joint joint="robot0:LFJ1" coef="-0.00805"></joint>
</fixed>
<!-- <fixed name="robot0:T_WRJ1r" limited="true" range="-0.032 0.032" user="1236">
<joint joint="robot0:WRJ1" coef="0.0325"></joint>
</fixed>
<fixed name="robot0:T_WRJ1l" limited="true" range="-0.032 0.032" user="1237">
<joint joint="robot0:WRJ1" coef="-0.0325"></joint>
</fixed>
<fixed name="robot0:T_WRJ0u" limited="true" range="-0.032 0.032" user="1236">
<joint joint="robot0:WRJ0" coef="0.0175"></joint>
</fixed>
<fixed name="robot0:T_WRJ0d" limited="true" range="-0.032 0.032" user="1237">
<joint joint="robot0:WRJ0" coef="-0.0175"></joint>
</fixed>
<fixed name="robot0:T_FFJ3r" limited="true" range="-0.018 0.018" user="1204">
<joint joint="robot0:FFJ3" coef="0.01"></joint>
</fixed>
<fixed name="robot0:T_FFJ3l" limited="true" range="-0.018 0.018" user="1205">
<joint joint="robot0:FFJ3" coef="-0.01"></joint>
</fixed>
<fixed name="robot0:T_FFJ2u" limited="true" range="-0.007 0.03" user="1202">
<joint joint="robot0:FFJ2" coef="0.01"></joint>
</fixed>
<fixed name="robot0:T_FFJ2d" limited="true" range="-0.03 0.007" user="1203">
<joint joint="robot0:FFJ2" coef="-0.01"></joint>
</fixed>
<fixed name="robot0:T_FFJ1c" limited="true" range="-0.001 0.001">
<joint joint="robot0:FFJ0" coef="0.00705"></joint>
<joint joint="robot0:FFJ1" coef="-0.00805"></joint>
</fixed>
<fixed name="robot0:T_FFJ1u" limited="true" range="-0.007 0.03" user="1200">
<joint joint="robot0:FFJ0" coef="0.00705"></joint>
<joint joint="robot0:FFJ1" coef="0.00805"></joint>
</fixed>
<fixed name="robot0:T_FFJ1d" limited="true" range="-0.03 0.007" user="1201">
<joint joint="robot0:FFJ0" coef="-0.00705"></joint>
<joint joint="robot0:FFJ1" coef="-0.00805"></joint>
</fixed>
<fixed name="robot0:T_MFJ3r" limited="true" range="-0.018 0.018" user="1210">
<joint joint="robot0:MFJ3" coef="0.01"></joint>
</fixed>
<fixed name="robot0:T_MFJ3l" limited="true" range="-0.018 0.018" user="1211">
<joint joint="robot0:MFJ3" coef="-0.01"></joint>
</fixed>
<fixed name="robot0:T_MFJ2u" limited="true" range="-0.007 0.03" user="1208">
<joint joint="robot0:MFJ2" coef="0.01"></joint>
</fixed>
<fixed name="robot0:T_MFJ2d" limited="true" range="-0.03 0.007" user="1209">
<joint joint="robot0:MFJ2" coef="-0.01"></joint>
</fixed>
<fixed name="robot0:T_MFJ1c" limited="true" range="-0.001 0.001">
<joint joint="robot0:MFJ0" coef="0.00705"></joint>
<joint joint="robot0:MFJ1" coef="-0.00805"></joint>
</fixed>
<fixed name="robot0:T_MFJ1u" limited="true" range="-0.007 0.03" user="1206">
<joint joint="robot0:MFJ0" coef="0.00705"></joint>
<joint joint="robot0:MFJ1" coef="0.00805"></joint>
</fixed>
<fixed name="robot0:T_MFJ1d" limited="true" range="-0.03 0.007" user="1207">
<joint joint="robot0:MFJ0" coef="-0.00705"></joint>
<joint joint="robot0:MFJ1" coef="-0.00805"></joint>
</fixed>
<fixed name="robot0:T_RFJ3r" limited="true" range="-0.018 0.018" user="1216">
<joint joint="robot0:RFJ3" coef="0.01"></joint>
</fixed>
<fixed name="robot0:T_RFJ3l" limited="true" range="-0.018 0.018" user="1217">
<joint joint="robot0:RFJ3" coef="-0.01"></joint>
</fixed>
<fixed name="robot0:T_RFJ2u" limited="true" range="-0.007 0.03" user="1214">
<joint joint="robot0:RFJ2" coef="0.01"></joint>
</fixed>
<fixed name="robot0:T_RFJ2d" limited="true" range="-0.03 0.007" user="1215">
<joint joint="robot0:RFJ2" coef="-0.01"></joint>
</fixed>
<fixed name="robot0:T_RFJ1c" limited="true" range="-0.001 0.001">
<joint joint="robot0:RFJ0" coef="0.00705"></joint>
<joint joint="robot0:RFJ1" coef="-0.00805"></joint>
</fixed>
<fixed name="robot0:T_RFJ1u" limited="true" range="-0.007 0.03" user="1212">
<joint joint="robot0:RFJ0" coef="0.00705"></joint>
<joint joint="robot0:RFJ1" coef="0.00805"></joint>
</fixed>
<fixed name="robot0:T_RFJ1d" limited="true" range="-0.03 0.007" user="1213">
<joint joint="robot0:RFJ0" coef="-0.00705"></joint>
<joint joint="robot0:RFJ1" coef="-0.00805"></joint>
</fixed>
<fixed name="robot0:T_LFJ4u" limited="true" range="-0.007 0.03" user="1224">
<joint joint="robot0:LFJ4" coef="0.01"></joint>
</fixed>
<fixed name="robot0:T_LFJ4d" limited="true" range="-0.03 0.007" user="1225">
<joint joint="robot0:LFJ4" coef="-0.01"></joint>
</fixed>
<fixed name="robot0:T_LFJ3r" limited="true" range="-0.018 0.018" user="1222">
<joint joint="robot0:LFJ3" coef="0.01"></joint>
</fixed>
<fixed name="robot0:T_LFJ3l" limited="true" range="-0.018 0.018" user="1223">
<joint joint="robot0:LFJ3" coef="-0.01"></joint>
</fixed>
<fixed name="robot0:T_LFJ2u" limited="true" range="-0.007 0.03" user="1220">
<joint joint="robot0:LFJ2" coef="0.01"></joint>
</fixed>
<fixed name="robot0:T_LFJ2d" limited="true" range="-0.03 0.007" user="1221">
<joint joint="robot0:LFJ2" coef="-0.01"></joint>
</fixed>
<fixed name="robot0:T_LFJ1c" limited="true" range="-0.001 0.001">
<joint joint="robot0:LFJ0" coef="0.00705"></joint>
<joint joint="robot0:LFJ1" coef="-0.00805"></joint>
</fixed>
<fixed name="robot0:T_LFJ1u" limited="true" range="-0.007 0.03" user="1218">
<joint joint="robot0:LFJ0" coef="0.00705"></joint>
<joint joint="robot0:LFJ1" coef="0.00805"></joint>
</fixed>
<fixed name="robot0:T_LFJ1d" limited="true" range="-0.03 0.007" user="1219">
<joint joint="robot0:LFJ0" coef="-0.00705"></joint>
<joint joint="robot0:LFJ1" coef="-0.00805"></joint>
</fixed>
<fixed name="robot0:T_THJ4a" limited="true" range="-0.018 0.018" user="1234">
<joint joint="robot0:THJ4" coef="0.01636"></joint>
</fixed>
<fixed name="robot0:T_THJ4c" limited="true" range="-0.018 0.018" user="1235">
<joint joint="robot0:THJ4" coef="-0.01636"></joint>
</fixed>
<fixed name="robot0:T_THJ3u" limited="true" range="-0.007 0.03" user="1232">
<joint joint="robot0:THJ3" coef="0.01"></joint>
</fixed>
<fixed name="robot0:T_THJ3d" limited="true" range="-0.03 0.007" user="1233">
<joint joint="robot0:THJ3" coef="-0.01"></joint>
</fixed>
<fixed name="robot0:T_THJ2u" limited="true" range="-0.018 0.018" user="1230">
<joint joint="robot0:THJ2" coef="0.011"></joint>
</fixed>
<fixed name="robot0:T_THJ2d" limited="true" range="-0.018 0.018" user="1231">
<joint joint="robot0:THJ2" coef="-0.011"></joint>
</fixed>
<fixed name="robot0:T_THJ1r" limited="true" range="-0.018 0.018" user="1228">
<joint joint="robot0:THJ1" coef="0.011"></joint>
</fixed>
<fixed name="robot0:T_THJ1l" limited="true" range="-0.018 0.018" user="1229">
<joint joint="robot0:THJ1" coef="-0.011"></joint>
</fixed>
<fixed name="robot0:T_THJ0r" limited="true" range="-0.03 0.007" user="1226">
<joint joint="robot0:THJ0" coef="0.009"></joint>
</fixed>
<fixed name="robot0:T_THJ0l" limited="true" range="-0.007 0.03" user="1227">
<joint joint="robot0:THJ0" coef="-0.009"></joint>
</fixed> -->
</tendon>
<sensor>
<jointpos name="robot0:Sjp_WRJ1" joint="robot0:WRJ1"></jointpos>
<jointpos name="robot0:Sjp_WRJ0" joint="robot0:WRJ0"></jointpos>
<jointpos name="robot0:Sjp_FFJ3" joint="robot0:FFJ3"></jointpos>
<jointpos name="robot0:Sjp_FFJ2" joint="robot0:FFJ2"></jointpos>
<jointpos name="robot0:Sjp_FFJ1" joint="robot0:FFJ1"></jointpos>
<jointpos name="robot0:Sjp_FFJ0" joint="robot0:FFJ0"></jointpos>
<jointpos name="robot0:Sjp_MFJ3" joint="robot0:MFJ3"></jointpos>
<jointpos name="robot0:Sjp_MFJ2" joint="robot0:MFJ2"></jointpos>
<jointpos name="robot0:Sjp_MFJ1" joint="robot0:MFJ1"></jointpos>
<jointpos name="robot0:Sjp_MFJ0" joint="robot0:MFJ0"></jointpos>
<jointpos name="robot0:Sjp_RFJ3" joint="robot0:RFJ3"></jointpos>
<jointpos name="robot0:Sjp_RFJ2" joint="robot0:RFJ2"></jointpos>
<jointpos name="robot0:Sjp_RFJ1" joint="robot0:RFJ1"></jointpos>
<jointpos name="robot0:Sjp_RFJ0" joint="robot0:RFJ0"></jointpos>
<jointpos name="robot0:Sjp_LFJ4" joint="robot0:LFJ4"></jointpos>
<jointpos name="robot0:Sjp_LFJ3" joint="robot0:LFJ3"></jointpos>
<jointpos name="robot0:Sjp_LFJ2" joint="robot0:LFJ2"></jointpos>
<jointpos name="robot0:Sjp_LFJ1" joint="robot0:LFJ1"></jointpos>
<jointpos name="robot0:Sjp_LFJ0" joint="robot0:LFJ0"></jointpos>
<jointpos name="robot0:Sjp_THJ4" joint="robot0:THJ4"></jointpos>
<jointpos name="robot0:Sjp_THJ3" joint="robot0:THJ3"></jointpos>
<jointpos name="robot0:Sjp_THJ2" joint="robot0:THJ2"></jointpos>
<jointpos name="robot0:Sjp_THJ1" joint="robot0:THJ1"></jointpos>
<jointpos name="robot0:Sjp_THJ0" joint="robot0:THJ0"></jointpos>
<touch name="robot0:ST_Tch_fftip" site="robot0:Tch_fftip"></touch>
<touch name="robot0:ST_Tch_mftip" site="robot0:Tch_mftip"></touch>
<touch name="robot0:ST_Tch_rftip" site="robot0:Tch_rftip"></touch>
<touch name="robot0:ST_Tch_lftip" site="robot0:Tch_lftip"></touch>
<touch name="robot0:ST_Tch_thtip" site="robot0:Tch_thtip"></touch>
</sensor>
<actuator>
<position name="robot0:A_WRJ1" class="robot0:asset_class" user="2038" joint="robot0:WRJ1" ctrlrange="-0.489 0.14" kp="5" forcerange="-4.785 4.785"></position>
<position name="robot0:A_WRJ0" class="robot0:asset_class" user="2036" joint="robot0:WRJ0" ctrlrange="-0.698 0.489" kp="5" forcerange="-2.175 2.175"></position>
<position name="robot0:A_FFJ3" class="robot0:asset_class" user="2004" joint="robot0:FFJ3" ctrlrange="-0.349 0.349" kp="1" forcerange="-0.9 0.9"></position>
<position name="robot0:A_FFJ2" class="robot0:asset_class" user="2002" joint="robot0:FFJ2" ctrlrange="0 1.571" kp="1" forcerange="-0.9 0.9"></position>
<position name="robot0:A_FFJ1" class="robot0:asset_class" user="2000" joint="robot0:FFJ1" ctrlrange="0 1.571" kp="1" forcerange="-0.7245 0.7245"></position>
<position name="robot0:A_MFJ3" class="robot0:asset_class" user="2010" joint="robot0:MFJ3" ctrlrange="-0.349 0.349" kp="1" forcerange="-0.9 0.9"></position>
<position name="robot0:A_MFJ2" class="robot0:asset_class" user="2008" joint="robot0:MFJ2" ctrlrange="0 1.571" kp="1" forcerange="-0.9 0.9"></position>
<position name="robot0:A_MFJ1" class="robot0:asset_class" user="2006" joint="robot0:MFJ1" ctrlrange="0 1.571" kp="1" forcerange="-0.7245 0.7245"></position>
<position name="robot0:A_RFJ3" class="robot0:asset_class" user="2016" joint="robot0:RFJ3" ctrlrange="-0.349 0.349" kp="1" forcerange="-0.9 0.9"></position>
<position name="robot0:A_RFJ2" class="robot0:asset_class" user="2014" joint="robot0:RFJ2" ctrlrange="0 1.571" kp="1" forcerange="-0.9 0.9"></position>
<position name="robot0:A_RFJ1" class="robot0:asset_class" user="2012" joint="robot0:RFJ1" ctrlrange="0 1.571" kp="1" forcerange="-0.7245 0.7245"></position>
<position name="robot0:A_LFJ4" class="robot0:asset_class" user="2024" joint="robot0:LFJ4" ctrlrange="0 0.785" kp="1" forcerange="-0.9 0.9"></position>
<position name="robot0:A_LFJ3" class="robot0:asset_class" user="2022" joint="robot0:LFJ3" ctrlrange="-0.349 0.349" kp="1" forcerange="-0.9 0.9"></position>
<position name="robot0:A_LFJ2" class="robot0:asset_class" user="2020" joint="robot0:LFJ2" ctrlrange="0 1.571" kp="1" forcerange="-0.9 0.9"></position>
<position name="robot0:A_LFJ1" class="robot0:asset_class" user="2018" joint="robot0:LFJ1" ctrlrange="0 1.571" kp="1" forcerange="-0.7245 0.7245"></position>
<position name="robot0:A_THJ4" class="robot0:asset_class" user="2034" joint="robot0:THJ4" ctrlrange="-1.047 1.047" kp="1" forcerange="-2.3722 2.3722"></position>
<position name="robot0:A_THJ3" class="robot0:asset_class" user="2032" joint="robot0:THJ3" ctrlrange="0 1.222" kp="1" forcerange="-1.45 1.45"></position>
<position name="robot0:A_THJ2" class="robot0:asset_class" user="2030" joint="robot0:THJ2" ctrlrange="-0.209 0.209" kp="1" forcerange="-0.99 0.99"></position>
<position name="robot0:A_THJ1" class="robot0:asset_class" user="2028" joint="robot0:THJ1" ctrlrange="-0.524 0.524" kp="1" forcerange="-0.99 0.99"></position>
<position name="robot0:A_THJ0" class="robot0:asset_class" user="2026" joint="robot0:THJ0" ctrlrange="-1.571 0" kp="1" forcerange="-0.81 0.81"></position>
</actuator>
</mujoco>
| 17,419 | XML | 63.044117 | 169 | 0.597107 |
NVIDIA-Omniverse/IsaacGymEnvs/assets/mjcf/open_ai_assets/hand/shared_asset.xml | <!-- See LICENSE.md for legal notices. LICENSE.md must be kept together with this file. -->
<mujoco>
<texture type="skybox" builtin="gradient" rgb1="0.44 0.85 0.56" rgb2="0.46 0.87 0.58" width="32" height="32"></texture>
<texture name="robot0:texplane" type="2d" builtin="checker" rgb1="0.2 0.3 0.4" rgb2="0.1 0.15 0.2" width="512" height="512"></texture>
<texture name="robot0:texgeom" type="cube" builtin="flat" mark="cross" width="127" height="127" rgb1="0.3 0.6 0.5" rgb2="0.3 0.6 0.5" markrgb="0 0 0" random="0.01"></texture>
<material name="robot0:MatGnd" reflectance="0.5" texture="robot0:texplane" texrepeat="1 1" texuniform="true"></material>
<material name="robot0:MatColl" specular="1" shininess="0.3" reflectance="0.5" rgba="0.4 0.5 0.6 1"></material>
<material name="robot0:MatViz" specular="0.75" shininess="0.1" reflectance="0.5" rgba="0.93 0.93 0.93 1"></material>
<material name="robot0:object" texture="robot0:texgeom" texuniform="false"></material>
<material name="floor_mat" specular="0" shininess="0.5" reflectance="0" rgba="0.2 0.2 0.2 0"></material>
<mesh name="robot0:forearm" file="forearm_electric.stl"></mesh>
<mesh name="robot0:forearm_cvx" file="forearm_electric_cvx.stl"></mesh>
<mesh name="robot0:wrist" scale="0.001 0.001 0.001" file="wrist.stl"></mesh>
<mesh name="robot0:palm" scale="0.001 0.001 0.001" file="palm.stl"></mesh>
<mesh name="robot0:knuckle" scale="0.001 0.001 0.001" file="knuckle.stl"></mesh>
<mesh name="robot0:F3" scale="0.001 0.001 0.001" file="F3.stl"></mesh>
<mesh name="robot0:F2" scale="0.001 0.001 0.001" file="F2.stl"></mesh>
<mesh name="robot0:F1" scale="0.001 0.001 0.001" file="F1.stl"></mesh>
<mesh name="robot0:lfmetacarpal" scale="0.001 0.001 0.001" file="lfmetacarpal.stl"></mesh>
<mesh name="robot0:TH3_z" scale="0.001 0.001 0.001" file="TH3_z.stl"></mesh>
<mesh name="robot0:TH2_z" scale="0.001 0.001 0.001" file="TH2_z.stl"></mesh>
<mesh name="robot0:TH1_z" scale="0.001 0.001 0.001" file="TH1_z.stl"></mesh>
</mujoco>
| 2,070 | XML | 75.703701 | 178 | 0.657971 |
NVIDIA-Omniverse/IsaacGymEnvs/assets/mjcf/open_ai_assets/fetch/shared.xml | <mujoco>
<asset>
<texture type="skybox" builtin="gradient" rgb1="0.44 0.85 0.56" rgb2="0.46 0.87 0.58" width="32" height="32"></texture>
<texture name="texture_block" file="block.png" gridsize="3 4" gridlayout=".U..LFRB.D.."></texture>
<material name="floor_mat" specular="0" shininess="0.5" reflectance="0" rgba="0.2 0.2 0.2 1"></material>
<material name="table_mat" specular="0" shininess="0.5" reflectance="0" rgba="0.93 0.93 0.93 1"></material>
<material name="block_mat" specular="0" shininess="0.5" reflectance="0" rgba="0.2 0.2 0.2 1"></material>
<material name="puck_mat" specular="0" shininess="0.5" reflectance="0" rgba="0.2 0.2 0.2 1"></material>
<material name="robot0:geomMat" shininess="0.03" specular="0.4"></material>
<material name="robot0:gripper_finger_mat" shininess="0.03" specular="0.4" reflectance="0"></material>
<material name="robot0:gripper_mat" shininess="0.03" specular="0.4" reflectance="0"></material>
<material name="robot0:arm_mat" shininess="0.03" specular="0.4" reflectance="0"></material>
<material name="robot0:head_mat" shininess="0.03" specular="0.4" reflectance="0"></material>
<material name="robot0:torso_mat" shininess="0.03" specular="0.4" reflectance="0"></material>
<material name="robot0:base_mat" shininess="0.03" specular="0.4" reflectance="0"></material>
<mesh file="base_link_collision.stl" name="robot0:base_link"></mesh>
<mesh file="bellows_link_collision.stl" name="robot0:bellows_link"></mesh>
<mesh file="elbow_flex_link_collision.stl" name="robot0:elbow_flex_link"></mesh>
<mesh file="estop_link.stl" name="robot0:estop_link"></mesh>
<mesh file="forearm_roll_link_collision.stl" name="robot0:forearm_roll_link"></mesh>
<mesh file="gripper_link.stl" name="robot0:gripper_link"></mesh>
<mesh file="head_pan_link_collision.stl" name="robot0:head_pan_link"></mesh>
<mesh file="head_tilt_link_collision.stl" name="robot0:head_tilt_link"></mesh>
<mesh file="l_wheel_link_collision.stl" name="robot0:l_wheel_link"></mesh>
<mesh file="laser_link.stl" name="robot0:laser_link"></mesh>
<mesh file="r_wheel_link_collision.stl" name="robot0:r_wheel_link"></mesh>
<mesh file="torso_lift_link_collision.stl" name="robot0:torso_lift_link"></mesh>
<mesh file="shoulder_pan_link_collision.stl" name="robot0:shoulder_pan_link"></mesh>
<mesh file="shoulder_lift_link_collision.stl" name="robot0:shoulder_lift_link"></mesh>
<mesh file="upperarm_roll_link_collision.stl" name="robot0:upperarm_roll_link"></mesh>
<mesh file="wrist_flex_link_collision.stl" name="robot0:wrist_flex_link"></mesh>
<mesh file="wrist_roll_link_collision.stl" name="robot0:wrist_roll_link"></mesh>
<mesh file="torso_fixed_link.stl" name="robot0:torso_fixed_link"></mesh>
</asset>
<equality>
<weld body1="robot0:mocap" body2="robot0:gripper_link" solimp="0.9 0.95 0.001" solref="0.02 1"></weld>
</equality>
<contact>
<exclude body1="robot0:r_gripper_finger_link" body2="robot0:l_gripper_finger_link"></exclude>
<exclude body1="robot0:torso_lift_link" body2="robot0:torso_fixed_link"></exclude>
<exclude body1="robot0:torso_lift_link" body2="robot0:shoulder_pan_link"></exclude>
</contact>
<default>
<default class="robot0:fetch">
<geom margin="0.001" material="robot0:geomMat" rgba="1 1 1 1" solimp="0.99 0.99 0.01" solref="0.01 1" type="mesh" user="0"></geom>
<joint armature="1" damping="50" frictionloss="0" stiffness="0"></joint>
<default class="robot0:fetchGripper">
<geom condim="4" margin="0.001" type="box" user="0" rgba="0.356 0.361 0.376 1.0"></geom>
<joint armature="100" damping="1000" limited="true" solimplimit="0.99 0.999 0.01" solreflimit="0.01 1" type="slide"></joint>
</default>
<default class="robot0:grey">
<geom rgba="0.356 0.361 0.376 1.0"></geom>
</default>
<default class="robot0:blue">
<geom rgba="0.086 0.506 0.767 1.0"></geom>
</default>
</default>
</default>
</mujoco>
| 4,331 | XML | 63.656715 | 142 | 0.625491 |
NVIDIA-Omniverse/IsaacGymEnvs/assets/mjcf/open_ai_assets/fetch/robot.xml | <mujoco>
<body mocap="true" name="robot0:mocap" pos="0 0 0">
<geom conaffinity="0" contype="0" pos="0 0 0" rgba="0 0.5 0 0.7" size="0.005 0.005 0.005" type="box"></geom>
<geom conaffinity="0" contype="0" pos="0 0 0" rgba="0 0.5 0 0.1" size="1 0.005 0.005" type="box"></geom>
<geom conaffinity="0" contype="0" pos="0 0 0" rgba="0 0.5 0 0.1" size="0.005 1 0.001" type="box"></geom>
<geom conaffinity="0" contype="0" pos="0 0 0" rgba="0 0.5 0 0.1" size="0.005 0.005 1" type="box"></geom>
</body>
<body childclass="robot0:fetch" name="robot0:base_link" pos="0.2869 0.2641 0">
<joint armature="0.0001" axis="1 0 0" damping="1e+11" name="robot0:slide0" pos="0 0 0" type="slide"></joint>
<joint armature="0.0001" axis="0 1 0" damping="1e+11" name="robot0:slide1" pos="0 0 0" type="slide"></joint>
<joint armature="0.0001" axis="0 0 1" damping="1e+11" name="robot0:slide2" pos="0 0 0" type="slide"></joint>
<inertial diaginertia="1.2869 1.2236 0.9868" mass="70.1294" pos="-0.0036 0 0.0014" quat="0.7605 -0.0133 -0.0061 0.6491"></inertial>
<geom mesh="robot0:base_link" name="robot0:base_link" material="robot0:base_mat" class="robot0:grey"></geom>
<body name="robot0:torso_lift_link" pos="-0.0869 0 0.3774">
<inertial diaginertia="0.3365 0.3354 0.0943" mass="10.7796" pos="-0.0013 -0.0009 0.2935" quat="0.9993 -0.0006 0.0336 0.0185"></inertial>
<joint axis="0 0 1" damping="1e+07" name="robot0:torso_lift_joint" range="0.0386 0.3861" type="slide"></joint>
<geom mesh="robot0:torso_lift_link" name="robot0:torso_lift_link" material="robot0:torso_mat"></geom>
<body name="robot0:head_pan_link" pos="0.0531 0 0.603">
<inertial diaginertia="0.0185 0.0128 0.0095" mass="2.2556" pos="0.0321 0.0161 0.039" quat="0.5148 0.5451 -0.453 0.4823"></inertial>
<joint axis="0 0 1" name="robot0:head_pan_joint" range="-1.57 1.57"></joint>
<geom mesh="robot0:head_pan_link" name="robot0:head_pan_link" material="robot0:head_mat" class="robot0:grey"></geom>
<body name="robot0:head_tilt_link" pos="0.1425 0 0.058">
<inertial diaginertia="0.0063 0.0059 0.0014" mass="0.9087" pos="0.0081 0.0025 0.0113" quat="0.6458 0.66 -0.274 0.2689"></inertial>
<joint axis="0 1 0" damping="1000" name="robot0:head_tilt_joint" range="-0.76 1.45" ref="0.06"></joint>
<geom mesh="robot0:head_tilt_link" name="robot0:head_tilt_link" material="robot0:head_mat" class="robot0:blue"></geom>
<body name="robot0:head_camera_link" pos="0.055 0 0.0225">
<inertial diaginertia="0 0 0" mass="0" pos="0.055 0 0.0225"></inertial>
<body name="robot0:head_camera_rgb_frame" pos="0 0.02 0">
<inertial diaginertia="0 0 0" mass="0" pos="0 0.02 0"></inertial>
<body name="robot0:head_camera_rgb_optical_frame" pos="0 0 0" quat="0.5 -0.5 0.5 -0.5">
<inertial diaginertia="0 0 0" mass="0" pos="0 0 0" quat="0.5 -0.5 0.5 -0.5"></inertial>
<camera euler="3.1415 0 0" fovy="50" name="head_camera_rgb" pos="0 0 0"></camera>
</body>
</body>
<body name="robot0:head_camera_depth_frame" pos="0 0.045 0">
<inertial diaginertia="0 0 0" mass="0" pos="0 0.045 0"></inertial>
<body name="robot0:head_camera_depth_optical_frame" pos="0 0 0" quat="0.5 -0.5 0.5 -0.5">
<inertial diaginertia="0 0 0" mass="0" pos="0 0 0" quat="0.5 -0.5 0.5 -0.5"></inertial>
</body>
</body>
</body>
</body>
</body>
<body name="robot0:shoulder_pan_link" pos="0.1195 0 0.3486">
<inertial diaginertia="0.009 0.0086 0.0041" mass="2.5587" pos="0.0927 -0.0056 0.0564" quat="-0.1364 0.7624 -0.1562 0.613"></inertial>
<joint axis="0 0 1" name="robot0:shoulder_pan_joint" range="-1.6056 1.6056"></joint>
<geom mesh="robot0:shoulder_pan_link" name="robot0:shoulder_pan_link" material="robot0:arm_mat"></geom>
<body name="robot0:shoulder_lift_link" pos="0.117 0 0.06">
<inertial diaginertia="0.0116 0.0112 0.0023" mass="2.6615" pos="0.1432 0.0072 -0.0001" quat="0.4382 0.4382 0.555 0.555"></inertial>
<joint axis="0 1 0" name="robot0:shoulder_lift_joint" range="-1.221 1.518"></joint>
<geom mesh="robot0:shoulder_lift_link" name="robot0:shoulder_lift_link" material="robot0:arm_mat" class="robot0:blue"></geom>
<body name="robot0:upperarm_roll_link" pos="0.219 0 0">
<inertial diaginertia="0.0047 0.0045 0.0019" mass="2.3311" pos="0.1165 0.0014 0" quat="-0.0136 0.707 0.0136 0.707"></inertial>
<joint axis="1 0 0" limited="false" name="robot0:upperarm_roll_joint"></joint>
<geom mesh="robot0:upperarm_roll_link" name="robot0:upperarm_roll_link" material="robot0:arm_mat"></geom>
<body name="robot0:elbow_flex_link" pos="0.133 0 0">
<inertial diaginertia="0.0086 0.0084 0.002" mass="2.1299" pos="0.1279 0.0073 0" quat="0.4332 0.4332 0.5589 0.5589"></inertial>
<joint axis="0 1 0" name="robot0:elbow_flex_joint" range="-2.251 2.251"></joint>
<geom mesh="robot0:elbow_flex_link" name="robot0:elbow_flex_link" material="robot0:arm_mat" class="robot0:blue"></geom>
<body name="robot0:forearm_roll_link" pos="0.197 0 0">
<inertial diaginertia="0.0035 0.0031 0.0015" mass="1.6563" pos="0.1097 -0.0266 0" quat="-0.0715 0.7035 0.0715 0.7035"></inertial>
<joint armature="2.7538" axis="1 0 0" damping="3.5247" frictionloss="0" limited="false" name="robot0:forearm_roll_joint" stiffness="10"></joint>
<geom mesh="robot0:forearm_roll_link" name="robot0:forearm_roll_link" material="robot0:arm_mat"></geom>
<body name="robot0:wrist_flex_link" pos="0.1245 0 0">
<inertial diaginertia="0.0042 0.0042 0.0018" mass="1.725" pos="0.0882 0.0009 -0.0001" quat="0.4895 0.4895 0.5103 0.5103"></inertial>
<joint axis="0 1 0" name="robot0:wrist_flex_joint" range="-2.16 2.16"></joint>
<geom mesh="robot0:wrist_flex_link" name="robot0:wrist_flex_link" material="robot0:arm_mat" class="robot0:blue"></geom>
<body name="robot0:wrist_roll_link" pos="0.1385 0 0">
<inertial diaginertia="0.0001 0.0001 0.0001" mass="0.1354" pos="0.0095 0.0004 -0.0002"></inertial>
<joint axis="1 0 0" limited="false" name="robot0:wrist_roll_joint"></joint>
<geom mesh="robot0:wrist_roll_link" name="robot0:wrist_roll_link" material="robot0:arm_mat"></geom>
<body euler="0 0 0" name="robot0:gripper_link" pos="0.1664 0 0">
<inertial diaginertia="0.0024 0.0019 0.0013" mass="1.5175" pos="-0.09 -0.0001 -0.0017" quat="0 0.7071 0 0.7071"></inertial>
<geom mesh="robot0:gripper_link" name="robot0:gripper_link" material="robot0:gripper_mat"></geom>
<body name="robot0:gripper_camera_link" pos="0.055 0 0.0225">
<body name="robot0:gripper_camera_rgb_frame" pos="0 0.02 0">
<body name="robot0:gripper_camera_rgb_optical_frame" pos="0 0 0" quat="0.5 -0.5 0.5 -0.5">
<camera euler="3.1415 0 0" fovy="50" name="gripper_camera_rgb" pos="0 0 0"></camera>
</body>
</body>
<body name="robot0:gripper_camera_depth_frame" pos="0 0.045 0">
<body name="robot0:gripper_camera_depth_optical_frame" pos="0 0 0" quat="0.5 -0.5 0.5 -0.5"></body>
</body>
</body>
<body childclass="robot0:fetchGripper" name="robot0:r_gripper_finger_link" pos="0 0.0159 0">
<inertial diaginertia="0.1 0.1 0.1" mass="4" pos="-0.01 0 0"></inertial>
<joint axis="0 1 0" name="robot0:r_gripper_finger_joint" range="0 0.05"></joint>
<geom pos="0 -0.008 0" size="0.0385 0.007 0.0135" type="box" name="robot0:r_gripper_finger_link" material="robot0:gripper_finger_mat" condim="4" friction="1 0.05 0.01"></geom>
</body>
<body childclass="robot0:fetchGripper" name="robot0:l_gripper_finger_link" pos="0 -0.0159 0">
<inertial diaginertia="0.1 0.1 0.1" mass="4" pos="-0.01 0 0"></inertial>
<joint axis="0 -1 0" name="robot0:l_gripper_finger_joint" range="0 0.05"></joint>
<geom pos="0 0.008 0" size="0.0385 0.007 0.0135" type="box" name="robot0:l_gripper_finger_link" material="robot0:gripper_finger_mat" condim="4" friction="1 0.05 0.01"></geom>
</body>
<site name="robot0:grip" pos="0.02 0 0" rgba="0 0 0 0" size="0.02 0.02 0.02"></site>
</body>
</body>
</body>
</body>
</body>
</body>
</body>
</body>
</body>
<body name="robot0:estop_link" pos="-0.1246 0.2389 0.3113" quat="0.7071 0.7071 0 0">
<inertial diaginertia="0 0 0" mass="0.002" pos="0.0024 -0.0033 0.0067" quat="0.3774 -0.1814 0.1375 0.8977"></inertial>
<geom mesh="robot0:estop_link" rgba="0.8 0 0 1" name="robot0:estop_link"></geom>
</body>
<body name="robot0:laser_link" pos="0.235 0 0.2878" quat="0 1 0 0">
<inertial diaginertia="0 0 0" mass="0.0083" pos="-0.0306 0.0007 0.0552" quat="0.5878 0.5378 -0.4578 0.3945"></inertial>
<geom mesh="robot0:laser_link" rgba="0.7922 0.8196 0.9333 1" name="robot0:laser_link"></geom>
<camera euler="1.55 -1.55 3.14" fovy="25" name="lidar" pos="0 0 0.02"></camera>
</body>
<body name="robot0:torso_fixed_link" pos="-0.0869 0 0.3774">
<inertial diaginertia="0.3865 0.3394 0.1009" mass="13.2775" pos="-0.0722 0.0057 0.2656" quat="0.9995 0.0249 0.0177 0.011"></inertial>
<geom mesh="robot0:torso_fixed_link" name="robot0:torso_fixed_link" class="robot0:blue"></geom>
</body>
<body name="robot0:external_camera_body_0" pos="0 0 0">
<camera euler="0 0.75 1.57" fovy="43.3" name="external_camera_0" pos="1.3 0 1.2"></camera>
</body>
</body>
</mujoco>
| 9,584 | XML | 76.298386 | 187 | 0.6274 |
NVIDIA-Omniverse/IsaacGymEnvs/assets/factory/yaml/factory_asset_info_nut_bolt.yaml | nut_bolt_m4_tight:
nut_m4_tight:
urdf_path: 'factory_nut_m4_tight'
width_min: 0.007 # distance from flat surface to flat surface
width_max: 0.0080829 # distance from edge to edge
height: 0.0032 # height of nut
flat_length: 0.00404145 # length of flat surface
bolt_m4_tight:
urdf_path: 'factory_bolt_m4_tight'
width: 0.004 # major diameter of bolt
head_height: 0.004 # height of bolt head
shank_length: 0.016 # length of bolt shank
thread_pitch: 0.0007 # distance between threads
nut_bolt_m4_loose:
nut_m4_loose:
urdf_path: 'factory_nut_m4_loose'
width_min: 0.007
width_max: 0.0080829
height: 0.0032
flat_length: 0.00404145
bolt_m4_loose:
urdf_path: 'factory_bolt_m4_loose'
width: 0.004
head_height: 0.004
shank_length: 0.016
thread_pitch: 0.0007
nut_bolt_m8_tight:
nut_m8_tight:
urdf_path: 'factory_nut_m8_tight'
width_min: 0.013
width_max: 0.01501111
height: 0.0065
flat_length: 0.00750555
bolt_m8_tight:
urdf_path: 'factory_bolt_m8_tight'
width: 0.008
head_height: 0.008
shank_length: 0.018
thread_pitch: 0.00125
nut_bolt_m8_loose:
nut_m8_loose:
urdf_path: 'factory_nut_m8_loose'
width_min: 0.013
width_max: 0.01501111
height: 0.0065
flat_length: 0.00750555
bolt_m8_loose:
urdf_path: 'factory_bolt_m8_loose'
width: 0.008
head_height: 0.008
shank_length: 0.018
thread_pitch: 0.00125
nut_bolt_m12_tight:
nut_m12_tight:
urdf_path: 'factory_nut_m12_tight'
width_min: 0.019
width_max: 0.02193931
height: 0.010
flat_length: 0.01096966
bolt_m12_tight:
urdf_path: 'factory_bolt_m12_tight'
width: 0.012
head_height: 0.012
shank_length: 0.020
thread_pitch: 0.00175
nut_bolt_m12_loose:
nut_m12_loose:
urdf_path: 'factory_nut_m12_loose'
width_min: 0.019
width_max: 0.02193931
height: 0.010
flat_length: 0.01096966
bolt_m12_loose:
urdf_path: 'factory_bolt_m12_loose'
width: 0.012
head_height: 0.012
shank_length: 0.020
thread_pitch: 0.00175
nut_bolt_m16_tight:
nut_m16_tight:
urdf_path: 'factory_nut_m16_tight'
width_min: 0.024
width_max: 0.02771281
height: 0.013
flat_length: 0.01385641
bolt_m16_tight:
urdf_path: 'factory_bolt_m16_tight'
boltUrdf: bolt_m16
width: 0.016
head_height: 0.016
shank_length: 0.025
thread_pitch: 0.002
nut_bolt_m16_loose:
nut_m16_loose:
urdf_path: 'factory_nut_m16_loose'
width_min: 0.024
width_max: 0.02771281
height: 0.013
flat_length: 0.01385641
bolt_m16_loose:
urdf_path: 'factory_bolt_m16_loose'
width: 0.016
head_height: 0.016
shank_length: 0.025
thread_pitch: 0.002
nut_bolt_m20_tight:
nut_m20_tight:
urdf_path: 'factory_nut_m20_tight'
width_min: 0.030
width_max: 0.03464102
height: 0.016
flat_length: 0.01732051
bolt_m20_tight:
urdf_path: 'factory_bolt_m20_tight'
width: 0.020
head_height: 0.020
shank_length: 0.045
thread_pitch: 0.0025
nut_bolt_m20_loose:
nut_m20_loose:
urdf_path: 'factory_nut_m20_loose'
width_min: 0.030
width_max: 0.03464102
height: 0.016
flat_length: 0.01732051
bolt_m20_loose:
urdf_path: 'factory_bolt_m20_loose'
width: 0.020
head_height: 0.020
shank_length: 0.045
thread_pitch: 0.0025
| 3,800 | YAML | 25.957447 | 70 | 0.576579 |
NVIDIA-Omniverse/IsaacGymEnvs/assets/factory/yaml/factory_asset_info_franka_table.yaml | franka_hand_length: 0.0584 # distance from origin of hand to origin of finger
franka_finger_length: 0.053671 # distance from origin of finger to bottom of fingerpad
franka_fingerpad_length: 0.017608 # distance from top of inner surface of fingerpad to bottom of inner surface of fingerpad
franka_gripper_width_max: 0.080 # maximum opening width of gripper
table_depth: 0.6 # depth of table
table_width: 1.0 # width of table | 430 | YAML | 60.57142 | 124 | 0.774419 |
NVIDIA-Omniverse/IsaacGymEnvs/assets/urdf/kuka_allegro_description/meshes/convert_stl2obj.py | import os
import argparse
parser = argparse.ArgumentParser()
parser.add_argument('--folder', type=str, default="./")
args = parser.parse_args()
import glob, os
os.chdir(args.folder)
for stl_fileName in glob.glob("*.stl"):
conversion_command = "meshlabserver -i " + stl_fileName + " -o " + stl_fileName[:-3] + "obj"
os.system(conversion_command)
for stl_fileName in glob.glob("*.STL"):
conversion_command = "meshlabserver -i " + stl_fileName + " -o " + stl_fileName[:-3] + "obj"
os.system(conversion_command)
| 530 | Python | 26.947367 | 96 | 0.669811 |
NVIDIA-Omniverse/IsaacGymEnvs/assets/industreal/yaml/industreal_asset_info_franka_table.yaml | franka_hand_length: 0.0584 # distance from origin of hand to origin of finger
franka_finger_length: 0.053671 # distance from origin of finger to bottom of fingerpad
franka_fingerpad_length: 0.017608 # distance from top of inner surface of fingerpad to bottom of inner surface of fingerpad
franka_gripper_width_max: 0.080 # maximum opening width of gripper
table_depth: 1.28 # depth of table
table_width: 0.91 # width of table
table_height: 1.04 # height of table
| 471 | YAML | 51.444439 | 124 | 0.768577 |
NVIDIA-Omniverse/IsaacGymEnvs/assets/industreal/yaml/industreal_asset_info_gears.yaml | base:
height: 0.005
density: 2700.0
gears:
height: 0.025
density: 1000.0
grasp_offset: 0.017
shafts:
height: 0.020 | 126 | YAML | 13.11111 | 21 | 0.666667 |
NVIDIA-Omniverse/IsaacGymEnvs/assets/industreal/yaml/industreal_asset_info_pegs.yaml | round_peg_hole_4mm:
round_peg_4mm:
urdf_path: 'industreal_round_peg_4mm'
diameter: 0.003988
length: 0.050
density: 8000.0
friction: 1.0
grasp_offset: 0.04
plug_width: 0.004
round_hole_4mm:
urdf_path: 'industreal_round_hole_4mm'
diameter: 0.0041
height: 0.028
depth: 0.023
density: 8000.0
friction: 0.5
round_peg_hole_8mm:
round_peg_8mm:
urdf_path: 'industreal_round_peg_8mm'
diameter: 0.007986
length: 0.050
density: 8000.0
friction: 1.0
grasp_offset: 0.04
plug_width: 0.008
round_hole_8mm:
urdf_path: 'industreal_round_hole_8mm'
diameter: 0.0081
height: 0.028
depth: 0.023
density: 8000.0
friction: 0.5
round_peg_hole_12mm:
round_peg_12mm:
urdf_path: 'industreal_round_peg_12mm'
diameter: 0.011983
length: 0.050
density: 8000.0
friction: 1.0
grasp_offset: 0.04
plug_width: 0.012
round_hole_12mm:
urdf_path: 'industreal_round_hole_12mm'
diameter: 0.0122
height: 0.028
depth: 0.023
density: 8000.0
friction: 0.5
round_peg_hole_16mm:
round_peg_16mm:
urdf_path: 'industreal_round_peg_16mm'
diameter: 0.015983
length: 0.050
density: 8000.0
friction: 1.0
grasp_offset: 0.04
plug_width: 0.016
round_hole_16mm:
urdf_path: 'industreal_round_hole_16mm'
diameter: 0.0165
height: 0.028
depth: 0.023
density: 8000.0
friction: 0.5
rectangular_peg_hole_4mm:
rectangular_peg_4mm:
urdf_path: 'industreal_rectangular_peg_4mm'
width: 0.00397
depth: 0.00397
length: 0.050
density: 8000.0
friction: 1.0
grasp_offset: 0.04
plug_width: 0.004
rectangular_hole_4mm:
urdf_path: 'industreal_rectangular_hole_4mm'
width: 0.00411
height: 0.028
depth: 0.023
density: 8000.0
friction: 0.5
rectangular_peg_hole_8mm:
rectangular_peg_8mm:
urdf_path: 'industreal_rectangular_peg_8mm'
width: 0.007964
depth: 0.006910
length: 0.050
density: 8000.0
friction: 1.0
grasp_offset: 0.04
plug_width: 0.008
rectangular_hole_8mm:
urdf_path: 'industreal_rectangular_hole_8mm'
width: 0.0081444
height: 0.028
depth: 0.023
density: 8000.0
friction: 0.5
rectangular_peg_hole_12mm:
rectangular_peg_12mm:
urdf_path: 'industreal_rectangular_peg_12mm'
width: 0.011957
depth: 0.007910
length: 0.050
density: 8000.0
friction: 1.0
grasp_offset: 0.04
plug_width: 0.012
rectangular_hole_12mm:
urdf_path: 'industreal_rectangular_hole_12mm'
width: 0.0121778
height: 0.028
depth: 0.023
density: 8000.0
friction: 0.5
rectangular_peg_hole_16mm:
rectangular_peg_16mm:
urdf_path: 'industreal_rectangular_peg_16mm'
width: 0.015957
depth: 0.009910
length: 0.050
density: 8000.0
friction: 1.0
grasp_offset: 0.04
plug_width: 0.016
rectangular_hole_16mm:
urdf_path: 'industreal_rectangular_hole_16mm'
width: 0.0162182
height: 0.028
depth: 0.023
density: 8000.0
friction: 0.5 | 3,539 | YAML | 24.467626 | 53 | 0.55722 |
NVIDIA-Omniverse/iot-samples/CHANGELOG.md | 0.2
-----
* Added support for API Token authentication
0.1
-----
* Initial release with containerization sample
0.1-pre
-----
* First release
| 144 | Markdown | 11.083332 | 46 | 0.680556 |
NVIDIA-Omniverse/iot-samples/VERSION.md | 105.0 | 5 | Markdown | 4.999995 | 5 | 0.8 |
NVIDIA-Omniverse/iot-samples/repo.toml | ########################################################################################################################
# Repo tool base settings
########################################################################################################################
[repo]
# Use the Kit Template repo configuration as a base. Only override things specific to the repo.
import_configs = ["${root}/_repo/deps/repo_kit_tools/kit-template/repo.toml"]
# Repository Name
name = "iot-samples"
[repo_build]
msbuild.vs_version = "vs2019"
post_build.commands = []
[repo_docs]
name = "Kit Extension Template C++"
project = "iot-samples"
api_output_directory = "api"
use_fast_doxygen_conversion=false
sphinx_version = "4.5.0.2-py3.10-${platform}"
sphinx_exclude_patterns = [
"_build",
"tools",
"VERSION.md",
"source/extensions/*/docs/Overview.md",
"source/extensions/*/docs/CHANGELOG.md",
]
[repo_build.licensing]
enabled = false
run_by_default = false
[repo_docs.kit]
extensions = []
[repo_package.packages."platform:windows-x86_64".docs]
windows_max_path_length = 0
| 1,086 | TOML | 26.174999 | 120 | 0.547882 |
NVIDIA-Omniverse/iot-samples/README.md | # IoT Samples (Beta)
# [Table of Contents](#tableofcontents)
- [Overview](#overview)
- [Architecture](#architecture)
- [Prerequisites](#prerequisites)
- [Installation](#installation)
- [App Link Setup](#app-link-setup)
- [Headless Connector](#headless-connector)
- [CSV Ingest Application](#csv-ingest-application)
- [MQTT Ingest Application](#mqtt-ingest-application)
- [Containerize Headless Connector](#containerize-headless-connector)
- [Consuming IoT data in USD](#consuming-iot-data-in-usd)
- [Using an Extension](#using-an-extension)
- [Using Action Graph](#using-actiongraph)
- [Direct to USD from headless connector](#direct-to-usd-from-headless-connector)
- [Joining a Live Session](#joining-a-live-session)
- [API Key Authentication](#api-key-authentication)
- [Using Environment Variables](#using-environment-variables)
# Overview
Note: Before you clone the repo, ensure you have Git LFS installed and enabled. [Find out more about Git LFS](https://git-lfs.com/)
Developers can build their own IoT solutions for Omniverse by following the guidelines set out in these samples.
IoT Samples guides you on how-to:
- Connect IoT data sources (CSV, message broker etc.) to Omniverse
- Incorporate IoT data in the USD model
- Visualize IoT data, using an OmniUI extension
- Perform transformations of USD geometry using IoT data
- Incorporate Omniverse OmniGraph/ActionGraph with IoT data
The repository is broken down into the following folders:
- *app* - Is a symlink to the *Omniverse Kit* based app. Note: This folder **does not exist** when the repo is first cloned. You must follow the instruction for configuring the folder which is found here: [App Link Setup](#app-link-setup).
- *content* - Contains the content data used by the samples.
- *deps* - Contains the packman dependencies required for the stand-alone data ingestion applications.
- *exts* - Contains the sample Omniverse extension.
- *source* - Contains the stand-alone python sample applications for ingesting and manipulating a USD stage with a headless connector.
- *tools* - Contains the utility code for building and packaging Omniverse native C++ client applications,
When opening the `iot-samples` folder in Visual Studio Code, you will be promted to install a number of extensions that will enhance the python experience in Visual Studio Code.
# Architecture

The architecture decouples the IoT data model from the presentation in Omniverse, allowing for a data driven approach and separation of concerns that is similar to a [Model/View/Controller (MVC) design pattern](https://en.wikipedia.org/wiki/Model%E2%80%93view%E2%80%93controller). The diagram above illustrates the key components to a solution. These are:
- **Customer Domain** - represents the data sources. Industrial IoT deployments require connecting operational technology (OT) systems, such as SCADA, PLC, to information technology (IT) systems to enable various use cases to improve efficiency, productivity, and safety in various industries. These deployments provide a data ingestion endpoint to connect OT data to IT and cloud applications. Some of the widely adopted methods for connecting OT data include MQTT and Kafka. The samples in this repository use CSV and MQTT as data sources, but you can develop your IoT project with any other connectivity method.
- **Connector** - is a stand-alone application that implements a bidirectional bridge between customer domain and USD related data. The logic implemented by a connector is use-case dependent and can be simple or complex. The [CSV Ingest Application](#csv-ingest-application) and [MQTT Ingest Application](#mqtt-ingest-application) transits the data *as is* from source to destination, whereas the [Geometry Transformation Application](#direct-to-usd-from-headless-connector) manipulates USD geometry directly. Depending on the use cases, the connector can run as a headless application locally, on-prem, at the edge, or in the cloud.
- **USD Resolver** - is a package dependency with the libraries for USD and Omniverse. [Find out more about the Omniverse USD Resolver](https://docs.omniverse.nvidia.com/kit/docs/usd_resolver/latest/index.html)
- **Nucleus** - is Omniverse's distributed file system agent that runs locally, in the cloud, or at the enterprise level. [Find out more about the Omniverse Nucleus](https://docs.omniverse.nvidia.com/nucleus/latest/index.html)
- **Consumer** - is an application that can manipulate and present the IoT data served by a Connector.
- **USD Resolver** - is a package dependency with the libraries for USD and Omniverse.
- **Fabric** - is Omniverse's sub-system for scalable, realtime communication and update of the scene graph amongst software components, the CPU and GPU, and machines across the network. [Find out more about the Omniverse Fabric](https://docs.omniverse.nvidia.com/kit/docs/usdrt/latest/docs/usd_fabric_usdrt.html)
- **Controller** - implements application or presentation logic by manipulating the flow of data from the Connector.
- **ActionGraph/OmniGraph** - is a visual scripting language that provides the ability to implement dynamic logic in response to changes made by the Connector. [Find out more about the OmniGraph Action Graph](https://docs.omniverse.nvidia.com/kit/docs/omni.graph.docs/latest/concepts/ActionGraph.html).
- **Omniverse Extension** - is a building block within Omniverse for extending application functionality. Extensions can implement any logic required to meet an application's functional requirements. [Find out more about the Omniverse Extensions](https://docs.omniverse.nvidia.com/extensions/latest/overview.html).
- **USD Stage** - is an organized hierarchy of prims (primitives) with properties. It provides a pipeline for composing and rendering the hierarchy. It is analogous to the Presentation Layer in MVC while additionally adapting to the data and runtime configuration.
Note: Connectors implement a producer/consumer pattern that is not mutually exclusive. Connectors are free to act as producer, consumer, or both. There may also be multiple Connectors and Consumers simultaneously collaborating.
# Prerequisites
Before running any of the installation a number of prerequisites are required.
Follow the [Getting Started with Omniverse ](https://www.nvidia.com/en-us/omniverse/download/) to install the latest Omniverse version.
If you've already installed Omniverse, ensure you have updated to the latest
* Python 3.10 or greater
* Kit 105.1 or greater
* USD Composer 2023.2.0 or greater
* Nucleus 2023.1 or greater
# Installation
Once you have the latest Omniverse prerequisites installed, please run the following to install the needed Omniverse USD resolver, Omni client, and related dependencies.
```
Windows
> install.bat
```
```
Linux
> ./install.sh
```
### App Link Setup
If `app` folder link doesn't exist or becomes broken it can be recreated. For a better developer experience it is recommended to create a folder link named `app` to the *Omniverse Kit* app installed from *Omniverse Launcher*. A convenience script to use is included.
Run:
```
Windows
> link_app.bat
```
```
Linux
> ./link_app.sh
```
If successful you should see an `app` folder link in the root of this repo.
If multiple Omniverse apps are installed the script will automatically select one. Or you can explicitly pass an app:
```
Windows
> link_app.bat --app create
```
```
Linux
> ./link_app.sh --app create
```
You can also pass an explicit path to the Omniverse Kit app:
```
Windows
> link_app.bat --path "%USERPROFILE%/AppData/Local/ov/pkg/create-2023.2.0"
```
```
Linux
> ./link_app.sh --path "~/.local/share/ov/pkg/create-2023.2.0"
```
# Headless Connector
Headless connectos are stand-alone applications that implements a bidirectional bridge between customer domain and USD related data. The logic implemented by a connector is use-case dependent and can be simple or complex.
There are two sample connector applications - [CSV Ingest Application](#csv-ingest-application) and [MQTT Ingest Application](#mqtt-ingest-application) - that transits the data as is from source to destination, whereas the [Geometry Transformation Application](#direct-to-usd-from-headless-connector) manipulates USD geometry directly in the connector. Depending on the use cases, a connector can run as a headless application locally, on-prem, at the edge, or in the cloud.
### CSV Ingest Application
To execute the application run the following:
```
> python source/ingest_app_csv/run_app.py
-u <user name>
-p <password>
-s <nucleus server> (optional default: localhost)
```
Or if you are using Environment Variables (see [Using Environment Variables](#using-environment-variables))
```
> python source/ingest_app_csv/run_app.py
```
Username and password are of the Nucleus instance (running on local workstation or on cloud) you will be connecting to for your IoT projects.
You should see output resembling:
```
2023-09-19 20:35:26+00:00
2023-09-19 20:35:28+00:00
2023-09-19 20:35:30+00:00
2023-09-19 20:35:32+00:00
2023-09-19 20:35:34+00:00
2023-09-19 20:35:36+00:00
2023-09-19 20:35:38+00:00
2023-09-19 20:35:40+00:00
2023-09-19 20:35:42+00:00
2023-09-19 20:35:44+00:00
```
The CSV ingest application can be found in the `./source/ingest_app_csv` folder. It will perform the following:
- Initialize the stage
- Open a connection to Nucleus.
- Copy `./content/ConveyorBelt_A08_PR_NVD_01` to `omniverse://<nucleus server>/users/<user name>/iot-samples/ConveyorBelt_A08_PR_NVD_01` if it does not already exist.Note that you can safely delete the destination folder in Nucleus and it will be recreated the next time the connector is run.
- Create or join a Live Collaboration Session named `iot_session`.
- Create a `prim` in the `.live` layer at path `/iot/A08_PR_NVD_01` and populate it with attributes that correspond to the unique field `Id` types in the CSV file `./content/A08_PR_NVD_01_iot_data.csv`.
- Playback in real-time
- Open and parse `./content/A08_PR_NVD_01_iot_data.csv`, and group the contents by `TimeStamp`.
- Loop through the data groupings.
- Update the prim attribute corresponding to the field `Id`.
- Sleep for the the duration of delta between the previous and current `TimeStamp`.
In `USD Composer` or `Kit`, open `omniverse://<nucleus server>/users/<user name>/iot-samples/ConveyorBelt_A08_PR_NVD_01/ConveyorBelt_A08_PR_NVD_01.usd` and join the `iot_session` live collaboration session. See [Joining a Live Session](#joining-a-live-session) for detailed instructions.
Once you have joined the `iot_session`, then you should see the following:

Selecting the `/iot/A08_PR_NVD_01` prim in the `Stage` panel and toggling the `Raw USD Properties` in the `Property` panel will provide real-time updates from the the data being pushed by the Python application.
### MQTT Ingest Application
To execute the application run the the following:
```
> python source/ingest_app_mqtt/run_app.py
-u <user name>
-p <password>
-s <nucleus server> (optional default: localhost)
```
Or if you are using Environment Variables (see [Using Environment Variables](#using-environment-variables))
```
> python source/ingest_app_mqtt/run_app.py
```
Username and password are of the Nucleus instance (running on local workstation or on cloud) you will be connecting to for your IoT projects.
You should see output resembling:
```
Received `{
"_ts": 176.0,
"System_Current": 0.003981236,
"System_Voltage": 107.4890366,
"Ambient_Temperature": 79.17738342,
"Ambient_Humidity": 45.49172211
"Velocity": 1.0
}` from `iot/A08_PR_NVD_01` topic
2023-09-19 20:38:24+00:00
Received `{
"_ts": 178.0,
"System_Current": 0.003981236,
"System_Voltage": 107.4890366,
"Ambient_Temperature": 79.17738342,
"Ambient_Humidity": 45.49172211
"Velocity": 1.0
}` from `iot/A08_PR_NVD_01` topic
2023-09-19 20:38:26+00:00
```
The MQTT ingest application can be found in the `./source/ingest_app_mqtt` folder. It will perform the following:
- Initialize the stage
- Open a connection to Nucleus.
- Copy `./content/ConveyorBelt_A08_PR_NVD_01` to `omniverse://<nucleus server>/users/<user name>/iot-samples/ConveyorBelt_A08_PR_NVD_01` if it does not already exist. Note that you can safely delete the destination folder in Nucleus and it will be recreated the next time the connector is run.
- Create or join a Live Collaboration Session named `iot_session`.
- Create a `prim` in the `.live` layer at path `/iot/A08_PR_NVD_01` and populate it with attributes that correspond to the unique field `Id` types in the CSV file `./content/A08_PR_NVD_01_iot_data.csv`.
- Playback in real-time
- Connect to MQTT and subscribe to MQTT topic `iot/{A08_PR_NVD_01}`
- Dispatch data to MQTT
- Open and parse `./content/A08_PR_NVD_01_iot_data.csv`, and group the contents by `TimeStamp`.
- Loop through the data groupings.
- Publish data to the MQTT topic.
- Sleep for the the duration of delta between the previous and current `TimeStamp`.
- Consume MQTT data
- Update the prim attribute corresponding to the field `Id`.
In `'USD Composer'` or `Kit`, open `omniverse://<nucleus server>/users/<user name>/iot-samples/ConveyorBelt_A08_PR_NVD_01/ConveyorBelt_A08_PR_NVD_01.usd` and join the `iot_session` live collaboration session. . See [Joining a Live Session](#joining-a-live-session) for detailed instructions.
Once you have joined the `iot_session`, then you should see the following:

Selecting the `/iot/A08_PR_NVD_01` prim in the `Stage` panel and toggling the `Raw USD Properties` in the `Property` panel will provide real-time updates from the data being pushed by the python application
### Containerize headless connector
The following is a simple example of how to deploy a headless connector application into Docker Desktop for Windows. Steps assume the use of
- WSL (comes standard with Docker Desktop installation) and
- Ubuntu Linux as the default OS.
The ollowing has to be done in **WSL environment** and *NOT* in Windows environment. Make sure you are in WSL, else you may encounter build and dependency errors.
- If you have an earlier version of the repo cloned, you may want to delete the old repo in WSL and start with a new cloned repo in WSL. Else you could end up with file mismatches and related errors.
- Before you clone the repo, ensure you have Git LFS installed and enabled. [Find out more about Git LFS](https://git-lfs.com/)
- Clone a new repo from **within WSL**
Once you have a new repo cloned, from within WSL run.
```
> ./install.sh
```
- Share the Nucleus services using a web browser by navigating to http://localhost:3080/. Click on 'Enable Sharing'. This will enable access to Nucleus services from WSL.

- Record the *WSL IP address* of the host machine for use by the container application.
```
PS C:\> ipconfig
Windows IP Configuration
...
Ethernet adapter vEthernet (WSL):
Connection-specific DNS Suffix . :
Link-local IPv6 Address . . . . . : fe80::8026:14db:524d:796f%63
IPv4 Address. . . . . . . . . . . : 172.21.208.1
Subnet Mask . . . . . . . . . . . : 255.255.240.0
Default Gateway . . . . . . . . . :
...
```
- Open a Bash prompt in **WSL** and navigate to the source repo and launch Visual Studio Code (example: `~/github/iot-samples/`). Make sure you're launching the Visual Studio Code from **WSL environment** and *not* editing the DockerFile from within Windows
```bash
code .
```
- Modify the DockerFile `ENTRYPOINT` to add the WSL IP address to connect to the Host's Nucleus Server. Also, include the username and password for your Omniverse Nucleus instance.
```docker
# For more information, please refer to https://aka.ms/vscode-docker-python
FROM python:3.10-slim
# Keeps Python from generating .pyc files in the container
ENV PYTHONDONTWRITEBYTECODE=1
# Turns off buffering for easier container logging
ENV PYTHONUNBUFFERED=1
# Install pip requirements
COPY requirements.txt .
RUN python -m pip install -r requirements.txt
WORKDIR /app
COPY . /app
# Creates a non-root user with an explicit UID and adds permission to access the /app folder
# For more info, please refer to https://aka.ms/vscode-docker-python-configure-containers
RUN adduser -u 5678 --disabled-password --gecos "" appuser && chown -R appuser /app
USER appuser
# During debugging, this entry point will be overridden. For more information, please refer to https://aka.ms/vscode-docker-python-debug
ENTRYPOINT [ "python", "source/ingest_app_csv/run_app.py", "--server", "<host IP address>", "--username", "<username>", "--password", "<password>" ]
```
- Create a docker image named `headlessapp`.
```bash
tar -czh -X tar_ignore.txt . | docker build -t headlessapp -
```
- Run a container with the lastest version of the `headlessapp` image
```
docker run -d --add-host host.docker.internal:host-gateway -p 3100:3100 -p 8891:8891 -p 8892:8892 headlessapp:latest
```
- Watch the application run in Docker Desktop.

# Consuming IoT data in USD
Consume the IoT data served by a connector by building your own application logic to visualize, animate and transform with USD stage. The application logic could use one of the following approaches or all of them;
- Extension
- Action Graph
- Direct to USD from headless connector
### Using an Extension
The sample IoT Extension uses Omniverse Extensions, which are the core building blocks of Omniverse Kit-based applications.
The IoT Extension demonstrates;
1. Visualizing IoT data
2. Animating a USD stage using IoT data
To enable the IoT Extension in USD Composer or Kit, do the following:
Open the Extensions panel by clicking on **Window** > **Extensions** in the menu and then follow the steps as shown.



1. **Visualizing IoT data**
The IoT Extension leverages the Omniverse UI Framework to visualize the IoT data as a panel. [Find out more about the Omniverse UI Framework](https://docs.omniverse.nvidia.com/kit/docs/omni.ui/latest/Overview.html)
Once you have enabled the IoT extension, you should see IoT data visualized in a Panel.

Alternatively, you can launch your app from the console with this folder added to search path and your extension enabled, e.g.:
```
> app\omni.code.bat --ext-folder exts --enable omni.iot.sample.panel
```
2. **Animating a USD stage using IoT data**
In `'USD Composer'` or `Kit`,
open `omniverse://<nucleus server>/users/<user name>/iot-samples/ConveyorBelt_A08_PR_NVD_01/ConveyorBelt_A08_PR_NVD_01.usd`.
Ensure the IoT Extension is enabled.
Click on the `play` icon on the left toolbar of the USD Composer and the extension will animate to the `Velocity` value change in the IoT data

and then run one of the following:
```
source\ingest_app_csv\run_app.py
-u <user name>
-p <password>
-s <nucleus server> (optional default: localhost)
```
or
```
source\ingest_app_mqtt\run_app.py
-u <user name>
-p <password>
-s <nucleus server> (optional default: localhost)
```
If you are using Environment Variables (see [Using Environment Variables](#using-environment-variables)) then run one of the following:
```
> python source/ingest_app_csv/run_app.py
```
or
```
> python source/ingest_app_mqtt/run_app.py
```
Username and password are for the target Nucleus instance (running on local workstation or on cloud) that you will be connecting to for your IoT projects.
You will see the following animation with the cube moving:

When the IoT velocity value changes, the extension will animate the rollers (`LiveRoller` class) as well as the cube (`LiveCube` class).
### Using ActionGraph
The `ConveyorBelt_A08_PR_NVD_01.usd` contains a simple `ActionGraph` that reads, formats, and displays an attribute from the IoT prim in the ViewPort (see [Omniverse Extensions Viewport](https://docs.omniverse.nvidia.com/extensions/latest/ext_viewport.html)).
To access the graph:
- Select the `Window/Visual Scripting/Action Graph` menu
- Select `Edit Action Graph`
- Select `/World/ActionGraph`
You should see the following:

The Graph performs the following:
- Reads the `_ts` attribute from the `/iot/A08_PR_NVD_01` prim.
- Converts the numerical value to a string.
- Prepends the string with `TimeStamp: `.
- Displays the result on the ViewPort.
### Direct to USD from Headless Connector
Sample demonstrates how to execute USD tranformations from a headless connector using arbtriary values.
To execute the application run the the following:
```
> python source/transform_geometry/run_app.py
-u <user name>
-p <password>
-s <nucleus server> (optional default: localhost)
```
Username and password are of the Nucleus instance (running on local workstation or on cloud) you will be connecting to for your IoT projects.
The sample geometry transformation application can be found in `source\transform_geometry`. It will perform the following:
- Initialize the stage
- Open a connection to Nucleus.
- Open or Create the USD stage `omniverse://<nucleus server>/users/<user name>/iot-samples/Dancing_Cubes.usd`.
- Create or join a Live Collaboration Session named `iot_session`.
- Create a `prim` in the `.live` layer at path `/World`.
- Create a `Cube` at path `/World/cube`.
- Add a `Rotation`.
- Create a `Mesh` at path `/World/cube/mesh`.
- Playback in real-time
- Loop for 20 seconds at 30 frames per second.
- Randomly rotate the `Cube` along the X, Y, and Z planes.
If you open `omniverse://<nucleus server>/users/<user name>/iot-samples/Dancing_Cubes.usd` in `Composer` or `Kit`, you should see the following:

# Joining A Live Session
Here's how-to join a live collaboration session. Click on `Join Session`

Select `iot-session` from the drop down to join the already created live session.

# API Key Authentication
To authenicate the connector application using an API Key, start Nucleus Explore from the Omniverse Launcher application and right click on the server you wish to connect to and select `API Tokens`

Provide a token name and click `Create`

Copy the token token value and store it somewhere safe.
If you are using the `run_app.py` application launcher you can do the following:
```
> python source/ingest_app_csv/run_app.py
-u $omni-api-token
-p <api token>
-s <nucleus server> (optional default: localhost)
```
Or if you are using Environment Variables (see [Using Environment Variables](#using-environment-variables)) you can do the following:
```
> python source/ingest_app_csv/run_app.py
```
# Using Environment Variables
The samples supports Nucleus authentication via Environment Variables.
For Windows Powershell with User Name/Password:
```powershell
$Env:OMNI_HOST = "<host name>"
$Env:OMNI_USER = "<user name>"
$Env:OMNI_PASS = "<password>"
```
For Windows Powershell with API Token:
```powershell
$Env:OMNI_HOST = "<host name>"
$Env:OMNI_USER = "`$omni-api-token"
$Env:OMNI_PASS = "<API Token>"
```
For Linux Bash with User Name/Password:
```bash
export OMNI_HOST=<host name>
export OMNI_USER=<user name>
export OMNI_PASS=<password>
```
For Linux Bash with API Token:
```bash
export OMNI_HOST=<host name>
export OMNI_USER=\$omni-api-token
export OMNI_PASS=<API Token>
```
| 24,430 | Markdown | 43.339383 | 633 | 0.743962 |
NVIDIA-Omniverse/iot-samples/deps/repo-deps.packman.xml | <project toolsVersion="5.0">
<dependency name="repo_build" linkPath="../_repo/deps/repo_build">
<package name="repo_build" version="0.54.1" />
</dependency>
<dependency name="repo_changelog" linkPath="../_repo/deps/repo_changelog">
<package name="repo_changelog" version="0.3.20" />
</dependency>
<dependency name="repo_docs" linkPath="../_repo/deps/repo_docs">
<package name="repo_docs" version="0.40.2" />
</dependency>
<dependency name="repo_kit_tools" linkPath="../_repo/deps/repo_kit_tools">
<package name="repo_kit_tools" version="0.13.52" />
</dependency>
<dependency name="repo_man" linkPath="../_repo/deps/repo_man">
<package name="repo_man" version="1.46.5" />
</dependency>
<dependency name="repo_package" linkPath="../_repo/deps/repo_package">
<package name="repo_package" version="5.9.3" />
</dependency>
<dependency name="repo_format" linkPath="../_repo/deps/repo_format">
<package name="repo_format" version="2.8.0" />
</dependency>
<dependency name="repo_source" linkPath="../_repo/deps/repo_source">
<package name="repo_source" version="0.4.3" />
</dependency>
</project> | 1,152 | XML | 43.346152 | 76 | 0.661458 |
NVIDIA-Omniverse/iot-samples/deps/kit-sdk.packman.xml | <project toolsVersion="5.0">
<!-- We always depend on the release kit-sdk package, regardless of config -->
<dependency name="kit_sdk_${config}" linkPath="../_build/${platform}/${config}/kit" tags="${config} non-redist">
<package name="kit-sdk" version="105.1+release.127680.dd92291b.tc.${platform}.release" platforms="windows-x86_64 linux-x86_64" />
</dependency>
</project>
| 386 | XML | 54.285707 | 133 | 0.69171 |
NVIDIA-Omniverse/iot-samples/deps/host-deps.packman.xml | <project toolsVersion="5.0">
<dependency name="premake" linkPath="../_build/host-deps/premake">
<package name="premake" version="5.0.0-beta2+nv1-${platform}" />
</dependency>
<dependency name="msvc" linkPath="../_build/host-deps/msvc">
<package name="msvc" version="2019-16.7.6-license" platforms="windows-x86_64" checksum="0e37c0f29899fe10dcbef6756bcd69c2c4422a3ca1101206df272dc3d295b92d" />
</dependency>
<dependency name="winsdk" linkPath="../_build/host-deps/winsdk">
<package name="winsdk" version="10.0.18362.0-license" platforms="windows-x86_64" checksum="2db7aeb2278b79c6c9fbca8f5d72b16090b3554f52b1f3e5f1c8739c5132a3d6" />
</dependency>
</project>
| 681 | XML | 55.833329 | 163 | 0.740088 |
NVIDIA-Omniverse/iot-samples/deps/kit-sdk-deps.packman.xml | <project toolsVersion="5.0">
<!-- Import dependencies from Kit SDK to ensure we're using the same versions. -->
<import path="../_build/${platform}/${config}/kit/dev/all-deps.packman.xml">
<filter include="omni_usd_resolver" />
<filter include="omni_client_library" />
<filter include="python" />
</import>
<!-- Override the link paths to point to the correct locations. -->
<dependency name="omni_usd_resolver" linkPath="../_build/target-deps/omni_usd_resolver" />
<dependency name="omni_client_library" linkPath="../_build/target-deps/omni_client_library" />
<dependency name="python" linkPath="../_build/target-deps/python" />
</project>
| 669 | XML | 43.666664 | 96 | 0.684604 |
NVIDIA-Omniverse/iot-samples/deps/ext-deps.packman.xml | <project toolsVersion="5.0">
<!-- Import dependencies from Kit SDK to ensure we're using the same versions. -->
<import path="../_build/${platform}/${config}/kit/dev/all-deps.packman.xml">
<filter include="nv_usd_py310_release"/>
</import>
<!-- Override the link paths to point to the correct locations. -->
<dependency name="nv_usd_py310_release" linkPath="../_build/target-deps/usd/release"/>
</project>
| 422 | XML | 37.454542 | 88 | 0.682464 |
NVIDIA-Omniverse/iot-samples/tools/repoman/repoman.py | import os
import sys
import io
import contextlib
import packmanapi
REPO_ROOT = os.path.join(os.path.dirname(os.path.realpath(__file__)), "../..")
REPO_DEPS_FILE = os.path.join(REPO_ROOT, "deps/repo-deps.packman.xml")
def bootstrap():
"""
Bootstrap all omni.repo modules.
Pull with packman from repo.packman.xml and add them all to python sys.path to enable importing.
"""
#with contextlib.redirect_stdout(io.StringIO()):
deps = packmanapi.pull(REPO_DEPS_FILE)
for dep_path in deps.values():
if dep_path not in sys.path:
sys.path.append(dep_path)
if __name__ == "__main__":
bootstrap()
import omni.repo.man
omni.repo.man.main(REPO_ROOT)
| 703 | Python | 23.275861 | 100 | 0.661451 |
NVIDIA-Omniverse/iot-samples/tools/scripts/link_app.py | import argparse
import json
import os
import sys
import packmanapi
import urllib3
def find_omniverse_apps():
http = urllib3.PoolManager()
try:
r = http.request("GET", "http://127.0.0.1:33480/components")
except Exception as e:
print(f"Failed retrieving apps from an Omniverse Launcher, maybe it is not installed?\nError: {e}")
sys.exit(1)
apps = {}
for x in json.loads(r.data.decode("utf-8")):
latest = x.get("installedVersions", {}).get("latest", "")
if latest:
for s in x.get("settings", []):
if s.get("version", "") == latest:
root = s.get("launch", {}).get("root", "")
apps[x["slug"]] = (x["name"], root)
break
return apps
def create_link(src, dst):
print(f"Creating a link '{src}' -> '{dst}'")
packmanapi.link(src, dst)
APP_PRIORITIES = ["code", "create", "view"]
if __name__ == "__main__":
parser = argparse.ArgumentParser(description="Create folder link to Kit App installed from Omniverse Launcher")
parser.add_argument(
"--path",
help="Path to Kit App installed from Omniverse Launcher, e.g.: 'C:/Users/bob/AppData/Local/ov/pkg/create-2021.3.4'",
required=False,
)
parser.add_argument(
"--app", help="Name of Kit App installed from Omniverse Launcher, e.g.: 'code', 'create'", required=False
)
args = parser.parse_args()
path = args.path
if not path:
print("Path is not specified, looking for Omniverse Apps...")
apps = find_omniverse_apps()
if len(apps) == 0:
print(
"Can't find any Omniverse Apps. Use Omniverse Launcher to install one. 'Code' is the recommended app for developers."
)
sys.exit(0)
print("\nFound following Omniverse Apps:")
for i, slug in enumerate(apps):
name, root = apps[slug]
print(f"{i}: {name} ({slug}) at: '{root}'")
if args.app:
selected_app = args.app.lower()
if selected_app not in apps:
choices = ", ".join(apps.keys())
print(f"Passed app: '{selected_app}' is not found. Specify one of the following found Apps: {choices}")
sys.exit(0)
else:
selected_app = next((x for x in APP_PRIORITIES if x in apps), None)
if not selected_app:
selected_app = next(iter(apps))
print(f"\nSelected app: {selected_app}")
_, path = apps[selected_app]
if not os.path.exists(path):
print(f"Provided path doesn't exist: {path}")
else:
SCRIPT_ROOT = os.path.dirname(os.path.realpath(__file__))
create_link(f"{SCRIPT_ROOT}/../../app", path)
print("Success!")
| 2,814 | Python | 32.117647 | 133 | 0.562189 |
NVIDIA-Omniverse/iot-samples/tools/packman/packmanconf.py | # Use this file to bootstrap packman into your Python environment (3.7.x). Simply
# add the path by doing sys.insert to where packmanconf.py is located and then execute:
#
# >>> import packmanconf
# >>> packmanconf.init()
#
# It will use the configured remote(s) and the version of packman in the same folder,
# giving you full access to the packman API via the following module
#
# >> import packmanapi
# >> dir(packmanapi)
import os
import platform
import sys
def init():
"""Call this function to initialize the packman configuration.
Calls to the packman API will work after successfully calling this function.
Note:
This function only needs to be called once during the execution of your
program. Calling it repeatedly is harmless but wasteful.
Compatibility with your Python interpreter is checked and upon failure
the function will report what is required.
Example:
>>> import packmanconf
>>> packmanconf.init()
>>> import packmanapi
>>> packmanapi.set_verbosity_level(packmanapi.VERBOSITY_HIGH)
"""
major = sys.version_info[0]
minor = sys.version_info[1]
if major != 3 or minor != 10:
raise RuntimeError(
f"This version of packman requires Python 3.10.x, but {major}.{minor} was provided"
)
conf_dir = os.path.dirname(os.path.abspath(__file__))
os.environ["PM_INSTALL_PATH"] = conf_dir
packages_root = get_packages_root(conf_dir)
version = get_version(conf_dir)
module_dir = get_module_dir(conf_dir, packages_root, version)
sys.path.insert(1, module_dir)
def get_packages_root(conf_dir: str) -> str:
root = os.getenv("PM_PACKAGES_ROOT")
if not root:
platform_name = platform.system()
if platform_name == "Windows":
drive, _ = os.path.splitdrive(conf_dir)
root = os.path.join(drive, "packman-repo")
elif platform_name == "Darwin":
# macOS
root = os.path.join(
os.path.expanduser("~"), "Library/Application Support/packman-cache"
)
elif platform_name == "Linux":
try:
cache_root = os.environ["XDG_HOME_CACHE"]
except KeyError:
cache_root = os.path.join(os.path.expanduser("~"), ".cache")
return os.path.join(cache_root, "packman")
else:
raise RuntimeError(f"Unsupported platform '{platform_name}'")
# make sure the path exists:
os.makedirs(root, exist_ok=True)
return root
def get_module_dir(conf_dir, packages_root: str, version: str) -> str:
module_dir = os.path.join(packages_root, "packman-common", version)
if not os.path.exists(module_dir):
import tempfile
tf = tempfile.NamedTemporaryFile(delete=False)
target_name = tf.name
tf.close()
url = f"http://bootstrap.packman.nvidia.com/packman-common@{version}.zip"
print(f"Downloading '{url}' ...")
import urllib.request
urllib.request.urlretrieve(url, target_name)
from importlib.machinery import SourceFileLoader
# import module from path provided
script_path = os.path.join(conf_dir, "bootstrap", "install_package.py")
ip = SourceFileLoader("install_package", script_path).load_module()
print("Unpacking ...")
ip.install_package(target_name, module_dir)
os.unlink(tf.name)
return module_dir
def get_version(conf_dir: str):
path = os.path.join(conf_dir, "packman")
if not os.path.exists(path): # in dev repo fallback
path += ".sh"
with open(path, "rt", encoding="utf8") as launch_file:
for line in launch_file.readlines():
if line.startswith("PM_PACKMAN_VERSION"):
_, value = line.split("=")
return value.strip()
raise RuntimeError(f"Unable to find 'PM_PACKMAN_VERSION' in '{path}'")
| 3,931 | Python | 35.407407 | 95 | 0.632663 |
NVIDIA-Omniverse/iot-samples/tools/packman/config.packman.xml | <config remotes="cloudfront">
<remote2 name="cloudfront">
<transport actions="download" protocol="https" packageLocation="d4i3qtqj3r0z5.cloudfront.net/${name}@${version}" />
</remote2>
</config>
| 211 | XML | 34.333328 | 123 | 0.691943 |
NVIDIA-Omniverse/iot-samples/tools/packman/bootstrap/install_package.py | # Copyright 2019 NVIDIA CORPORATION
# Licensed under the Apache License, Version 2.0 (the "License");
# you may not use this file except in compliance with the License.
# You may obtain a copy of the License at
# http://www.apache.org/licenses/LICENSE-2.0
# Unless required by applicable law or agreed to in writing, software
# distributed under the License is distributed on an "AS IS" BASIS,
# WITHOUT WARRANTIES OR CONDITIONS OF ANY KIND, either express or implied.
# See the License for the specific language governing permissions and
# limitations under the License.
import logging
import zipfile
import tempfile
import sys
import os
import stat
import time
from typing import Any, Callable
RENAME_RETRY_COUNT = 100
RENAME_RETRY_DELAY = 0.1
logging.basicConfig(level=logging.WARNING, format="%(message)s")
logger = logging.getLogger("install_package")
def remove_directory_item(path):
if os.path.islink(path) or os.path.isfile(path):
try:
os.remove(path)
except PermissionError:
# make sure we have access and try again:
os.chmod(path, stat.S_IRWXU)
os.remove(path)
else:
# try first to delete the dir because this will work for folder junctions, otherwise we would follow the junctions and cause destruction!
clean_out_folder = False
try:
# make sure we have access preemptively - this is necessary because recursing into a directory without permissions
# will only lead to heart ache
os.chmod(path, stat.S_IRWXU)
os.rmdir(path)
except OSError:
clean_out_folder = True
if clean_out_folder:
# we should make sure the directory is empty
names = os.listdir(path)
for name in names:
fullname = os.path.join(path, name)
remove_directory_item(fullname)
# now try to again get rid of the folder - and not catch if it raises:
os.rmdir(path)
class StagingDirectory:
def __init__(self, staging_path):
self.staging_path = staging_path
self.temp_folder_path = None
os.makedirs(staging_path, exist_ok=True)
def __enter__(self):
self.temp_folder_path = tempfile.mkdtemp(prefix="ver-", dir=self.staging_path)
return self
def get_temp_folder_path(self):
return self.temp_folder_path
# this function renames the temp staging folder to folder_name, it is required that the parent path exists!
def promote_and_rename(self, folder_name):
abs_dst_folder_name = os.path.join(self.staging_path, folder_name)
os.rename(self.temp_folder_path, abs_dst_folder_name)
def __exit__(self, type, value, traceback):
# Remove temp staging folder if it's still there (something went wrong):
path = self.temp_folder_path
if os.path.isdir(path):
remove_directory_item(path)
def rename_folder(staging_dir: StagingDirectory, folder_name: str):
try:
staging_dir.promote_and_rename(folder_name)
except OSError as exc:
# if we failed to rename because the folder now exists we can assume that another packman process
# has managed to update the package before us - in all other cases we re-raise the exception
abs_dst_folder_name = os.path.join(staging_dir.staging_path, folder_name)
if os.path.exists(abs_dst_folder_name):
logger.warning(
f"Directory {abs_dst_folder_name} already present, package installation already completed"
)
else:
raise
def call_with_retry(
op_name: str, func: Callable, retry_count: int = 3, retry_delay: float = 20
) -> Any:
retries_left = retry_count
while True:
try:
return func()
except (OSError, IOError) as exc:
logger.warning(f"Failure while executing {op_name} [{str(exc)}]")
if retries_left:
retry_str = "retry" if retries_left == 1 else "retries"
logger.warning(
f"Retrying after {retry_delay} seconds"
f" ({retries_left} {retry_str} left) ..."
)
time.sleep(retry_delay)
else:
logger.error("Maximum retries exceeded, giving up")
raise
retries_left -= 1
def rename_folder_with_retry(staging_dir: StagingDirectory, folder_name):
dst_path = os.path.join(staging_dir.staging_path, folder_name)
call_with_retry(
f"rename {staging_dir.get_temp_folder_path()} -> {dst_path}",
lambda: rename_folder(staging_dir, folder_name),
RENAME_RETRY_COUNT,
RENAME_RETRY_DELAY,
)
def install_package(package_path, install_path):
staging_path, version = os.path.split(install_path)
with StagingDirectory(staging_path) as staging_dir:
output_folder = staging_dir.get_temp_folder_path()
with zipfile.ZipFile(package_path, allowZip64=True) as zip_file:
zip_file.extractall(output_folder)
# attempt the rename operation
rename_folder_with_retry(staging_dir, version)
print(f"Package successfully installed to {install_path}")
if __name__ == "__main__":
executable_paths = os.getenv("PATH")
paths_list = executable_paths.split(os.path.pathsep) if executable_paths else []
target_path_np = os.path.normpath(sys.argv[2])
target_path_np_nc = os.path.normcase(target_path_np)
for exec_path in paths_list:
if os.path.normcase(os.path.normpath(exec_path)) == target_path_np_nc:
raise RuntimeError(f"packman will not install to executable path '{exec_path}'")
install_package(sys.argv[1], target_path_np)
| 5,776 | Python | 36.270968 | 145 | 0.645083 |
NVIDIA-Omniverse/iot-samples/exts/omni.iot.sample.panel/config/extension.toml | [package]
# Semantic Versioning is used: https://semver.org/
version = "1.0.0"
# Lists people or organizations that are considered the "authors" of the package.
authors = ["NVIDIA"]
# The title and description fields are primarily for displaying extension info in UI
title = "omni iot sample panel"
description="A simple python extension example to use as a starting point for your extensions."
# Path (relative to the root) or content of readme markdown file for UI.
readme = "docs/README.md"
# URL of the extension source repository.
repository = ""
# One of categories for UI.
category = "Example"
# Keywords for the extension
keywords = ["kit", "example"]
# Location of change log file in target (final) folder of extension, relative to the root.
# More info on writing changelog: https://keepachangelog.com/en/1.0.0/
changelog="docs/CHANGELOG.md"
# Preview image and icon. Folder named "data" automatically goes in git lfs (see .gitattributes file).
# Preview image is shown in "Overview" of Extensions window. Screenshot of an extension might be a good preview image.
preview_image = "data/preview.png"
# Icon is shown in Extensions window, it is recommended to be square, of size 256x256.
icon = "data/icon.png"
# Use omni.ui to build simple UI
[dependencies]
"omni.kit.uiapp" = {}
# Main python module this extension provides, it will be publicly available as "import omni.iot.sample.panel".
[[python.module]]
name = "omni.iot.sample.panel"
[[test]]
# Extra dependencies only to be used during test run
dependencies = [
"omni.kit.ui_test" # UI testing extension
]
| 1,589 | TOML | 32.124999 | 118 | 0.744493 |
NVIDIA-Omniverse/iot-samples/exts/omni.iot.sample.panel/omni/iot/sample/panel/extension.py | # SPDX-FileCopyrightText: Copyright (c) 2023 NVIDIA CORPORATION & AFFILIATES. All rights reserved.
# SPDX-License-Identifier: MIT
#
# Permission is hereby granted, free of charge, to any person obtaining a
# copy of this software and associated documentation files (the "Software"),
# to deal in the Software without restriction, including without limitation
# the rights to use, copy, modify, merge, publish, distribute, sublicense,
# and/or sell copies of the Software, and to permit persons to whom the
# Software is furnished to do so, subject to the following conditions:
#
# The above copyright notice and this permission notice shall be included in
# all copies or substantial portions of the Software.
#
# THE SOFTWARE IS PROVIDED "AS IS", WITHOUT WARRANTY OF ANY KIND, EXPRESS OR
# IMPLIED, INCLUDING BUT NOT LIMITED TO THE WARRANTIES OF MERCHANTABILITY,
# FITNESS FOR A PARTICULAR PURPOSE AND NONINFRINGEMENT. IN NO EVENT SHALL
# THE AUTHORS OR COPYRIGHT HOLDERS BE LIABLE FOR ANY CLAIM, DAMAGES OR OTHER
# LIABILITY, WHETHER IN AN ACTION OF CONTRACT, TORT OR OTHERWISE, ARISING
# FROM, OUT OF OR IN CONNECTION WITH THE SOFTWARE OR THE USE OR OTHER
# DEALINGS IN THE SOFTWARE.
import omni.ext
import omni.ui as ui
import omni.kit.usd.layers as layers
from pxr import Usd, Sdf, Tf, UsdGeom
import omni.ui.color_utils as cl
TRANSLATE_OFFSET = "xformOp:translate:offset"
ROTATE_SPIN = "xformOp:rotateX:spin"
class uiTextStyles:
title = {"margin": 10, "color": 0xFFFFFFFF, "font_size": 18, "alignment": ui.Alignment.LEFT_CENTER}
title2 = {"margin": 10, "color": 0xFFFFFFFF, "font_size": 18, "alignment": ui.Alignment.LEFT_CENTER}
class uiElementStyles:
mainWindow = {"Window": {"background_color": cl.color(32, 42, 87, 100), "width": 350}}
class uiButtonStyles:
mainButton = {
"Button": {"background_color": cl.color(32, 42, 87, 125), "width": 175, "height": 80},
"Button:hovered": {"background_color": cl.color(32, 42, 87, 200)},
}
# geometry manipulation
class LiveCube:
def __init__(self, stage: Usd.Stage, path: str):
self._prim = stage.GetPrimAtPath(path)
self._op = self._prim.HasProperty(TRANSLATE_OFFSET)
if self._prim:
self._xform = UsdGeom.Xformable(self._prim)
def resume(self):
if self._xform and not self._op:
op = self._xform.AddTranslateOp(opSuffix="offset")
op.Set(time=1, value=(0, -20.0, 0))
op.Set(time=192, value=(0, -440, 0))
self._op = True
def pause(self):
if self._xform and self._op:
default_ops = []
for op in self._xform.GetOrderedXformOps():
if op.GetOpName() != TRANSLATE_OFFSET:
default_ops.append(op)
self._xform.SetXformOpOrder(default_ops)
self._prim.RemoveProperty(TRANSLATE_OFFSET)
self._op = False
class LiveRoller:
def __init__(self, stage: Usd.Stage, path: str):
self._prim = stage.GetPrimAtPath(path)
self._op = self._prim.HasProperty(ROTATE_SPIN)
if self._prim:
self._xform = UsdGeom.Xformable(self._prim)
def resume(self):
if self._xform and not self._op:
op = self._xform.AddRotateXOp(opSuffix="spin")
op.Set(time=1, value=0)
op.Set(time=192, value=1440)
self._op = True
def pause(self):
if self._xform and self._op:
default_ops = []
for op in self._xform.GetOrderedXformOps():
if op.GetOpName() != ROTATE_SPIN:
default_ops.append(op)
self._xform.SetXformOpOrder(default_ops)
self._prim.RemoveProperty(ROTATE_SPIN)
self._op = False
# Any class derived from `omni.ext.IExt` in top level module (defined in `python.modules` of `extension.toml`) will be
# instantiated when extension gets enabled and `on_startup(ext_id)` will be called. Later when extension gets disabled
# on_shutdown() is called.
class OmniIotSamplePanelExtension(omni.ext.IExt):
# ext_id is current extension id. It can be used with extension manager to query additional information, like where
# this extension is located on filesystem.
def on_startup(self, ext_id):
print("[omni.iot.sample.panel] startup")
self._iot_prim = None
self.listener = None
self._stage_event_sub = None
self._window = None
self._usd_context = omni.usd.get_context()
self._stage = self._usd_context.get_stage()
self._live_syncing = layers.get_live_syncing(self._usd_context)
self._layers = layers.get_layers(self._usd_context)
self._selected_prim = None
self._layers_event_subscription = self._layers.get_event_stream().create_subscription_to_pop_by_type(
layers.LayerEventType.LIVE_SESSION_STATE_CHANGED,
self._on_layers_event,
name=f"omni.iot.sample.panel {str(layers.LayerEventType.LIVE_SESSION_STATE_CHANGED)}",
)
self._update_ui()
def on_shutdown(self):
self._iot_prim = None
self.listener = None
self._stage_event_sub = None
self._window = None
self._layers_event_subscription = None
print("[omni.iot.sample.panel] shutdown")
def _on_velocity_changed(self, speed):
print(f"[omni.iot.sample.panel] _on_velocity_changed: {speed}")
if speed is not None and speed > 0.0:
with Sdf.ChangeBlock():
self._cube.resume()
for roller in self._rollers:
roller.resume()
else:
with Sdf.ChangeBlock():
self._cube.pause()
for roller in self._rollers:
roller.pause()
def _update_frame(self):
if self._selected_prim is not None:
self._property_stack.clear()
properties = self._selected_prim.GetProperties()
button_height = uiButtonStyles.mainButton["Button"]["height"]
self._property_stack.height.value = (round(len(properties) / 2) + 1) * button_height
x = 0
hStack = ui.HStack()
self._property_stack.add_child(hStack)
# repopulate the VStack with the IoT data attributes
for prop in properties:
if x > 0 and x % 2 == 0:
hStack = ui.HStack()
self._property_stack.add_child(hStack)
prop_name = prop.GetName()
prop_value = prop.Get()
ui_button = ui.Button(f"{prop_name}\n{str(prop_value)}", style=uiButtonStyles.mainButton)
hStack.add_child(ui_button)
if prop_name == "Velocity":
self._on_velocity_changed(prop_value)
x += 1
if x % 2 != 0:
with hStack:
ui.Button("", style=uiButtonStyles.mainButton)
def _on_selected_prim_changed(self):
print("[omni.iot.sample.panel] _on_selected_prim_changed")
selected_prim = self._usd_context.get_selection()
selected_paths = selected_prim.get_selected_prim_paths()
if selected_paths and len(selected_paths):
sdf_path = Sdf.Path(selected_paths[0])
# only handle data that resides under the /iot prim
if (
sdf_path.IsPrimPath()
and sdf_path.HasPrefix(self._iot_prim.GetPath())
and sdf_path != self._iot_prim.GetPath()
):
self._selected_prim = self._stage.GetPrimAtPath(sdf_path)
self._selected_iot_prim_label.text = str(sdf_path)
self._update_frame()
# ===================== stage events START =======================
def _on_selection_changed(self):
print("[omni.iot.sample.panel] _on_selection_changed")
if self._iot_prim:
self._on_selected_prim_changed()
def _on_asset_opened(self):
print("[omni.iot.sample.panel] on_asset_opened")
def _on_stage_event(self, event):
if event.type == int(omni.usd.StageEventType.SELECTION_CHANGED):
self._on_selection_changed()
elif event.type == int(omni.usd.StageEventType.OPENED):
self._on_asset_opened()
def _on_objects_changed(self, notice, stage):
updated_objects = []
for p in notice.GetChangedInfoOnlyPaths():
if p.IsPropertyPath() and p.GetParentPath() == self._selected_prim.GetPath():
updated_objects.append(p)
if len(updated_objects) > 0:
self._update_frame()
# ===================== stage events END =======================
def _on_layers_event(self, event):
payload = layers.get_layer_event_payload(event)
if not payload:
return
if payload.event_type == layers.LayerEventType.LIVE_SESSION_STATE_CHANGED:
if not payload.is_layer_influenced(self._usd_context.get_stage_url()):
return
self._update_ui()
def _update_ui(self):
if self._live_syncing.is_stage_in_live_session():
print("[omni.iot.sample.panel] joining live session")
if self._iot_prim is None:
self._window = ui.Window("Sample IoT Data", width=350, height=390)
self._window.frame.set_style(uiElementStyles.mainWindow)
sessionLayer = self._stage.GetSessionLayer()
sessionLayer.startTimeCode = 1
sessionLayer.endTimeCode = 192
self._iot_prim = self._stage.GetPrimAtPath("/iot")
self._cube = LiveCube(self._stage, "/World/cube")
self._rollers = []
for x in range(38):
self._rollers.append(
LiveRoller(self._stage, f"/World/Geometry/SM_ConveyorBelt_A08_Roller{x+1:02d}_01")
)
# this will capture when the select changes in the stage_selected_iot_prim_label
self._stage_event_sub = self._usd_context.get_stage_event_stream().create_subscription_to_pop(
self._on_stage_event, name="Stage Update"
)
# this will capture changes to the IoT data
self.listener = Tf.Notice.Register(Usd.Notice.ObjectsChanged, self._on_objects_changed, self._stage)
# create an simple window with empty VStack for the IoT data
with self._window.frame:
with ui.VStack():
with ui.HStack(height=22):
ui.Label("IoT Prim:", style=uiTextStyles.title, width=75)
self._selected_iot_prim_label = ui.Label(" ", style=uiTextStyles.title)
self._property_stack = ui.VStack(height=22)
if self._iot_prim:
self._on_selected_prim_changed()
else:
print("[omni.iot.sample.panel] leaving live session")
self._iot_prim = None
self.listener = None
self._stage_event_sub = None
self._property_stack = None
self._window = None
| 11,235 | Python | 40.007299 | 119 | 0.591633 |
NVIDIA-Omniverse/iot-samples/exts/omni.iot.sample.panel/omni/iot/sample/panel/__init__.py | from .extension import *
| 25 | Python | 11.999994 | 24 | 0.76 |
NVIDIA-Omniverse/iot-samples/exts/omni.iot.sample.panel/omni/iot/sample/panel/tests/__init__.py | from .test_hello_world import * | 31 | Python | 30.999969 | 31 | 0.774194 |
NVIDIA-Omniverse/iot-samples/exts/omni.iot.sample.panel/omni/iot/sample/panel/tests/test_hello_world.py | # NOTE:
# omni.kit.test - std python's unittest module with additional wrapping to add suport for async/await tests
# For most things refer to unittest docs: https://docs.python.org/3/library/unittest.html
import omni.kit.test
# Extnsion for writing UI tests (simulate UI interaction)
import omni.kit.ui_test as ui_test
# Import extension python module we are testing with absolute import path, as if we are external user (other extension)
import omni.iot.sample.panel
# Having a test class dervived from omni.kit.test.AsyncTestCase declared on the root of module will make it auto-discoverable by omni.kit.test
class Test(omni.kit.test.AsyncTestCase):
# Before running each test
async def setUp(self):
pass
# After running each test
async def tearDown(self):
pass
# Actual test, notice it is "async" function, so "await" can be used if needed
@omni.kit.test.omni_test_registry(guid="f898a949-bacc-41f5-be56-b4eb8923f54e")
async def test_hello_public_function(self):
result = omni.iot.sample.panel.some_public_function(4)
self.assertEqual(result, 256)
@omni.kit.test.omni_test_registry(guid="4626d574-659f-4a85-8958-9fa8588fbce3")
async def test_window_button(self):
# Find a label in our window
label = ui_test.find("My Window//Frame/**/Label[*]")
# Find buttons in our window
add_button = ui_test.find("My Window//Frame/**/Button[*].text=='Add'")
reset_button = ui_test.find("My Window//Frame/**/Button[*].text=='Reset'")
# Click reset button
await reset_button.click()
self.assertEqual(label.widget.text, "empty")
await add_button.click()
self.assertEqual(label.widget.text, "count: 1")
await add_button.click()
self.assertEqual(label.widget.text, "count: 2")
| 1,844 | Python | 36.65306 | 142 | 0.68872 |
NVIDIA-Omniverse/iot-samples/exts/omni.iot.sample.panel/docs/CHANGELOG.md | # Changelog
The format is based on [Keep a Changelog](https://keepachangelog.com/en/1.0.0/).
## [1.0.0] - 2021-04-26
- Initial version of extension UI template with a window
| 178 | Markdown | 18.888887 | 80 | 0.702247 |
NVIDIA-Omniverse/iot-samples/exts/omni.iot.sample.panel/docs/README.md | # Python Extension Example [omni.iot.sample.panel]
This is an example of pure python Kit extension. It is intended to be copied and serve as a template to create new extensions.
| 180 | Markdown | 35.199993 | 126 | 0.783333 |
NVIDIA-Omniverse/iot-samples/exts/omni.iot.sample.panel/docs/index.rst | omni.iot.sample.panel
#############################
Example of Python only extension
.. toctree::
:maxdepth: 1
README
CHANGELOG
.. automodule::"omni.iot.sample.panel"
:platform: Windows-x86_64, Linux-x86_64
:members:
:undoc-members:
:show-inheritance:
:imported-members:
:exclude-members: contextmanager
| 343 | reStructuredText | 15.380952 | 43 | 0.618076 |
NVIDIA-Omniverse/iot-samples/source/ingest_app_mqtt/app.py | # SPDX-FileCopyrightText: Copyright (c) 2023 NVIDIA CORPORATION & AFFILIATES. All rights reserved.
# SPDX-License-Identifier: MIT
#
# Permission is hereby granted, free of charge, to any person obtaining a
# copy of this software and associated documentation files (the "Software"),
# to deal in the Software without restriction, including without limitation
# the rights to use, copy, modify, merge, publish, distribute, sublicense,
# and/or sell copies of the Software, and to permit persons to whom the
# Software is furnished to do so, subject to the following conditions:
#
# The above copyright notice and this permission notice shall be included in
# all copies or substantial portions of the Software.
#
# THE SOFTWARE IS PROVIDED "AS IS", WITHOUT WARRANTY OF ANY KIND, EXPRESS OR
# IMPLIED, INCLUDING BUT NOT LIMITED TO THE WARRANTIES OF MERCHANTABILITY,
# FITNESS FOR A PARTICULAR PURPOSE AND NONINFRINGEMENT. IN NO EVENT SHALL
# THE AUTHORS OR COPYRIGHT HOLDERS BE LIABLE FOR ANY CLAIM, DAMAGES OR OTHER
# LIABILITY, WHETHER IN AN ACTION OF CONTRACT, TORT OR OTHERWISE, ARISING
# FROM, OUT OF OR IN CONNECTION WITH THE SOFTWARE OR THE USE OR OTHER
# DEALINGS IN THE SOFTWARE.
# pip install pandas
# pip install paho-mqtt
import asyncio
import os
import omni.client
from pxr import Usd, Sdf, Gf
from pathlib import Path
import pandas as pd
import time
from paho.mqtt import client as mqtt_client
import random
import json
from omni.live import LiveEditSession, LiveCube, getUserNameFromToken
OMNI_HOST = os.environ.get("OMNI_HOST", "localhost")
OMNI_USER = os.environ.get("OMNI_USER", "ov")
if OMNI_USER.lower() == "omniverse":
OMNI_USER = "ov"
elif OMNI_USER.lower() == "$omni-api-token":
OMNI_USER = getUserNameFromToken(os.environ.get("OMNI_PASS"))
BASE_FOLDER = "omniverse://" + OMNI_HOST + "/Users/" + OMNI_USER + "/iot-samples"
SCRIPT_DIR = os.path.dirname(os.path.realpath(__file__))
CONTENT_DIR = Path(SCRIPT_DIR).resolve().parents[1].joinpath("content")
messages = []
def log_handler(thread, component, level, message):
# print(message)
messages.append((thread, component, level, message))
def initialize_device_prim(live_layer, iot_topic):
iot_root = live_layer.GetPrimAtPath("/iot")
iot_spec = live_layer.GetPrimAtPath(f"/iot/{iot_topic}")
if not iot_spec:
iot_spec = Sdf.PrimSpec(iot_root, iot_topic, Sdf.SpecifierDef, "ConveyorBelt Type")
if not iot_spec:
raise Exception("Failed to create the IoT Spec.")
# clear out any attrubutes that may be on the spec
for attrib in iot_spec.attributes:
iot_spec.RemoveProperty(attrib)
IOT_TOPIC_DATA = f"{CONTENT_DIR}/{iot_topic}_iot_data.csv"
data = pd.read_csv(IOT_TOPIC_DATA)
data.head()
# create all the IoT attributes that will be written
attr = Sdf.AttributeSpec(iot_spec, "_ts", Sdf.ValueTypeNames.Double)
if not attr:
raise Exception(f"Could not define the attribute: {attrName}")
# infer the unique data points in the CSV.
# The values may be known in advance and can be hard coded
grouped = data.groupby("Id")
for attrName, group in grouped:
attr = Sdf.AttributeSpec(iot_spec, attrName, Sdf.ValueTypeNames.Double)
if not attr:
raise Exception(f"Could not define the attribute: {attrName}")
async def initialize_async(iot_topic):
# copy a the Conveyor Belt to the target nucleus server
stage_name = f"ConveyorBelt_{iot_topic}"
local_folder = f"file:{CONTENT_DIR}/{stage_name}"
stage_folder = f"{BASE_FOLDER}/{stage_name}"
stage_url = f"{stage_folder}/{stage_name}.usd"
result = await omni.client.copy_async(
local_folder,
stage_folder,
behavior=omni.client.CopyBehavior.ERROR_IF_EXISTS,
message="Copy Conveyor Belt",
)
stage = Usd.Stage.Open(stage_url)
if not stage:
raise Exception(f"Could load the stage {stage_url}.")
live_session = LiveEditSession(stage_url)
live_layer = await live_session.ensure_exists()
session_layer = stage.GetSessionLayer()
session_layer.subLayerPaths.append(live_layer.identifier)
# set the live layer as the edit target
stage.SetEditTarget(live_layer)
initialize_device_prim(live_layer, iot_topic)
# place the cube on the conveyor
live_cube = LiveCube(stage)
live_cube.scale(Gf.Vec3f(0.5))
live_cube.translate(Gf.Vec3f(100.0, -30.0, 195.0))
omni.client.live_process()
return stage, live_layer
def write_to_live(live_layer, iot_topic, msg_content):
# write the iot values to the usd prim attributes
payload = json.loads(msg_content)
with Sdf.ChangeBlock():
for i, (id, value) in enumerate(payload.items()):
attr = live_layer.GetAttributeAtPath(f"/iot/{iot_topic}.{id}")
if not attr:
raise Exception(f"Could not find attribute /iot/{iot_topic}.{id}.")
attr.default = value
omni.client.live_process()
# publish to mqtt broker
def write_to_mqtt(mqtt_client, iot_topic, group, ts):
# write the iot values to the usd prim attributes
topic = f"iot/{iot_topic}"
print(group.iloc[0]["TimeStamp"])
payload = {"_ts": ts}
for index, row in group.iterrows():
payload[row["Id"]] = row["Value"]
mqtt_client.publish(topic, json.dumps(payload, indent=2).encode("utf-8"))
# connect to mqtt broker
def connect_mqtt(iot_topic):
topic = f"iot/{iot_topic}"
# called when a message arrives
def on_message(client, userdata, msg):
msg_content = msg.payload.decode()
write_to_live(live_layer, iot_topic, msg_content)
print(f"Received `{msg_content}` from `{msg.topic}` topic")
# called when connection to mqtt broker has been established
def on_connect(client, userdata, flags, rc):
if rc == 0:
# connect to our topic
print(f"Subscribing to topic: {topic}")
client.subscribe(topic)
else:
print(f"Failed to connect, return code {rc}")
# let us know when we've subscribed
def on_subscribe(client, userdata, mid, granted_qos):
print(f"subscribed {mid} {granted_qos}")
# Set Connecting Client ID
client = mqtt_client.Client(f"python-mqtt-{random.randint(0, 1000)}")
client.on_connect = on_connect
client.on_message = on_message
client.on_subscribe = on_subscribe
client.connect("test.mosquitto.org", 1883)
client.loop_start()
return client
def run(stage, live_layer, iot_topic):
# we assume that the file contains the data for single device
IOT_TOPIC_DATA = f"{CONTENT_DIR}/{iot_topic}_iot_data.csv"
data = pd.read_csv(IOT_TOPIC_DATA)
data.head()
# Converting to DateTime Format and drop ms
data["TimeStamp"] = pd.to_datetime(data["TimeStamp"])
data["TimeStamp"] = data["TimeStamp"].dt.floor("s")
data.set_index("TimeStamp")
start_time = data.min()["TimeStamp"]
last_time = start_time
grouped = data.groupby("TimeStamp")
mqtt_client = connect_mqtt(iot_topic)
# play back the data in real-time
for next_time, group in grouped:
diff = (next_time - last_time).total_seconds()
if diff > 0:
time.sleep(diff)
write_to_mqtt(mqtt_client, iot_topic, group, (next_time - start_time).total_seconds())
last_time = next_time
mqtt_client = None
if __name__ == "__main__":
IOT_TOPIC = "A08_PR_NVD_01"
omni.client.initialize()
omni.client.set_log_level(omni.client.LogLevel.DEBUG)
omni.client.set_log_callback(log_handler)
try:
stage, live_layer = asyncio.run(initialize_async(IOT_TOPIC))
run(stage, live_layer, IOT_TOPIC)
except:
print("---- LOG MESSAGES ---")
print(*messages, sep="\n")
print("----")
finally:
omni.client.shutdown()
| 7,846 | Python | 34.506787 | 98 | 0.674994 |
NVIDIA-Omniverse/iot-samples/source/ingest_app_mqtt/run_app.py | # Copyright (c) 2023, NVIDIA CORPORATION. All rights reserved.
# NVIDIA CORPORATION and its licensors retain all intellectual property
# and proprietary rights in and to this software, related documentation
# and any modifications thereto. Any use, reproduction, disclosure or
# distribution of this software and related documentation without an express
# license agreement from NVIDIA CORPORATION is strictly prohibited.
import os
import argparse
import platform
import subprocess
from pathlib import Path
PLATFORM_SYSTEM = platform.system().lower()
PLATFORM_MACHINE = platform.machine()
if PLATFORM_MACHINE == "i686" or PLATFORM_MACHINE == "AMD64":
PLATFORM_MACHINE = "x86_64"
CURRENT_PLATFORM = f"{PLATFORM_SYSTEM}-{PLATFORM_MACHINE}"
default_username = os.environ.get("OMNI_USER")
default_password = os.environ.get("OMNI_PASS")
default_server = os.environ.get("OMNI_HOST", "localhost")
parser = argparse.ArgumentParser()
parser.add_argument("--server", "-s", default=default_server)
parser.add_argument("--username", "-u", default=default_username)
parser.add_argument("--password", "-p", default=default_password)
parser.add_argument("--config", "-c", choices=["debug", "release"], default="release")
parser.add_argument("--platform", default=CURRENT_PLATFORM)
args = parser.parse_args()
SCRIPT_DIR = os.path.dirname(os.path.realpath(__file__))
ROOT_DIR = Path(SCRIPT_DIR).resolve().parents[1]
BUILD_DIR = ROOT_DIR.joinpath("_build", args.platform, args.config)
DEPS_DIR = ROOT_DIR.joinpath("_build", "target-deps")
USD_BIN_DIR = DEPS_DIR.joinpath("usd", args.config, "bin")
USD_LIB_DIR = DEPS_DIR.joinpath("usd", args.config, "lib")
CLIENT_LIB_DIR = DEPS_DIR.joinpath("omni_client_library", args.config)
RESOLVER_DIR = DEPS_DIR.joinpath("omni_usd_resolver", args.config)
EXTRA_PATHS = [str(CLIENT_LIB_DIR), str(USD_BIN_DIR), str(USD_LIB_DIR), str(BUILD_DIR), str(RESOLVER_DIR)]
EXTRA_PYTHON_PATHS = [
str(Path(SCRIPT_DIR).resolve().parents[0]),
str(USD_LIB_DIR.joinpath("python")),
str(CLIENT_LIB_DIR.joinpath("bindings-python")),
str(BUILD_DIR.joinpath("bindings-python")),
]
if PLATFORM_SYSTEM == "windows":
os.environ["PATH"] += os.pathsep + os.pathsep.join(EXTRA_PATHS)
ot_bin = "carb.omnitrace.plugin.dll"
else:
p = os.environ.get("LD_LIBRARY_PATH", "")
p += os.pathsep + os.pathsep.join(EXTRA_PATHS)
os.environ["LD_LIBRARY_PATH"] = p
ot_bin = "libcarb.omnitrace.plugin.so"
os.environ["OMNI_TRACE_LIB"] = os.path.join(str(DEPS_DIR), "omni-trace", "bin", ot_bin)
os.environ["PYTHONPATH"] = os.pathsep + os.pathsep.join(EXTRA_PYTHON_PATHS)
os.environ["OMNI_USER"] = args.username
os.environ["OMNI_PASS"] = args.password
os.environ["OMNI_HOST"] = args.server
if PLATFORM_SYSTEM == "windows":
PYTHON_EXE = DEPS_DIR.joinpath("python", "python")
else:
PYTHON_EXE = DEPS_DIR.joinpath("python", "bin", "python3")
plugin_paths = DEPS_DIR.joinpath("omni_usd_resolver", args.config, "usd", "omniverse", "resources")
os.environ["PXR_PLUGINPATH_NAME"] = str(plugin_paths)
REQ_FILE = ROOT_DIR.joinpath("requirements.txt")
subprocess.run(f"{PYTHON_EXE} -m pip install -r {REQ_FILE}", shell=True)
result = subprocess.run(
[PYTHON_EXE, os.path.join(SCRIPT_DIR, "app.py")],
stderr=subprocess.STDOUT,
)
| 3,268 | Python | 39.8625 | 106 | 0.717258 |
NVIDIA-Omniverse/iot-samples/source/transform_geometry/app.py | # SPDX-FileCopyrightText: Copyright (c) 2023 NVIDIA CORPORATION & AFFILIATES. All rights reserved.
# SPDX-License-Identifier: MIT
#
# Permission is hereby granted, free of charge, to any person obtaining a
# copy of this software and associated documentation files (the "Software"),
# to deal in the Software without restriction, including without limitation
# the rights to use, copy, modify, merge, publish, distribute, sublicense,
# and/or sell copies of the Software, and to permit persons to whom the
# Software is furnished to do so, subject to the following conditions:
#
# The above copyright notice and this permission notice shall be included in
# all copies or substantial portions of the Software.
#
# THE SOFTWARE IS PROVIDED "AS IS", WITHOUT WARRANTY OF ANY KIND, EXPRESS OR
# IMPLIED, INCLUDING BUT NOT LIMITED TO THE WARRANTIES OF MERCHANTABILITY,
# FITNESS FOR A PARTICULAR PURPOSE AND NONINFRINGEMENT. IN NO EVENT SHALL
# THE AUTHORS OR COPYRIGHT HOLDERS BE LIABLE FOR ANY CLAIM, DAMAGES OR OTHER
# LIABILITY, WHETHER IN AN ACTION OF CONTRACT, TORT OR OTHERWISE, ARISING
# FROM, OUT OF OR IN CONNECTION WITH THE SOFTWARE OR THE USE OR OTHER
# DEALINGS IN THE SOFTWARE.
# pip install openpyxl
# pip install pandas
import asyncio
import os
import omni.client
from pxr import Usd, Sdf
from pathlib import Path
import time
from omni.live import LiveEditSession, LiveCube, getUserNameFromToken
OMNI_HOST = os.environ.get("OMNI_HOST", "localhost")
OMNI_USER = os.environ.get("OMNI_USER", "ov")
if OMNI_USER.lower() == "omniverse":
OMNI_USER = "ov"
elif OMNI_USER.lower() == "$omni-api-token":
OMNI_USER = getUserNameFromToken(os.environ.get("OMNI_PASS"))
BASE_FOLDER = "omniverse://" + OMNI_HOST + "/Users/" + OMNI_USER + "/iot-samples"
SCRIPT_DIR = os.path.dirname(os.path.realpath(__file__))
CONTENT_DIR = Path(SCRIPT_DIR).resolve().parents[1].joinpath("content")
messages = []
def log_handler(thread, component, level, message):
# print(message)
messages.append((thread, component, level, message))
async def initialize_async():
# copy a the Conveyor Belt to the target nucleus server
stage_name = "Dancing_Cubes"
stage_folder = f"{BASE_FOLDER}/{stage_name}"
stage_url = f"{stage_folder}/{stage_name}.usd"
try:
stage = Usd.Stage.Open(stage_url)
except:
stage = Usd.Stage.CreateNew(stage_url)
if not stage:
raise Exception(f"Could load the stage {stage_url}.")
live_session = LiveEditSession(stage_url)
live_layer = await live_session.ensure_exists()
session_layer = stage.GetSessionLayer()
session_layer.subLayerPaths.append(live_layer.identifier)
# set the live layer as the edit target
stage.SetEditTarget(live_layer)
stage.DefinePrim("/World", "Xform")
omni.client.live_process()
return stage, live_layer
def run(stage, live_layer):
# we assume that the file contains the data for single device
# play back the data in at 30fps for 20 seconds
delay = 0.033
iterations = 600
live_cube = LiveCube(stage)
omni.client.live_process()
for x in range(iterations):
with Sdf.ChangeBlock():
live_cube.rotate()
omni.client.live_process()
time.sleep(delay)
if __name__ == "__main__":
omni.client.initialize()
omni.client.set_log_level(omni.client.LogLevel.DEBUG)
omni.client.set_log_callback(log_handler)
try:
stage, live_layer = asyncio.run(initialize_async())
run(stage, live_layer)
except:
print("---- LOG MESSAGES ---")
print(*messages, sep="\n")
print("----")
finally:
omni.client.shutdown()
| 3,663 | Python | 32.925926 | 98 | 0.700792 |
NVIDIA-Omniverse/iot-samples/source/transform_geometry/run_app.py | # Copyright (c) 2023, NVIDIA CORPORATION. All rights reserved.
# NVIDIA CORPORATION and its licensors retain all intellectual property
# and proprietary rights in and to this software, related documentation
# and any modifications thereto. Any use, reproduction, disclosure or
# distribution of this software and related documentation without an express
# license agreement from NVIDIA CORPORATION is strictly prohibited.
import os
import argparse
import platform
import subprocess
from pathlib import Path
PLATFORM_SYSTEM = platform.system().lower()
PLATFORM_MACHINE = platform.machine()
if PLATFORM_MACHINE == "i686" or PLATFORM_MACHINE == "AMD64":
PLATFORM_MACHINE = "x86_64"
CURRENT_PLATFORM = f"{PLATFORM_SYSTEM}-{PLATFORM_MACHINE}"
default_username = os.environ.get("OMNI_USER")
default_password = os.environ.get("OMNI_PASS")
default_server = os.environ.get("OMNI_HOST", "localhost")
parser = argparse.ArgumentParser()
parser.add_argument("--server", "-s", default=default_server)
parser.add_argument("--username", "-u", default=default_username)
parser.add_argument("--password", "-p", default=default_password)
parser.add_argument("--config", "-c", choices=["debug", "release"], default="release")
parser.add_argument("--platform", default=CURRENT_PLATFORM)
args = parser.parse_args()
SCRIPT_DIR = os.path.dirname(os.path.realpath(__file__))
ROOT_DIR = Path(SCRIPT_DIR).resolve().parents[1]
BUILD_DIR = ROOT_DIR.joinpath("_build", args.platform, args.config)
DEPS_DIR = ROOT_DIR.joinpath("_build", "target-deps")
USD_BIN_DIR = DEPS_DIR.joinpath("usd", args.config, "bin")
USD_LIB_DIR = DEPS_DIR.joinpath("usd", args.config, "lib")
CLIENT_LIB_DIR = DEPS_DIR.joinpath("omni_client_library", args.config)
RESOLVER_DIR = DEPS_DIR.joinpath("omni_usd_resolver", args.config)
EXTRA_PATHS = [str(CLIENT_LIB_DIR), str(USD_BIN_DIR), str(USD_LIB_DIR), str(BUILD_DIR), str(RESOLVER_DIR)]
EXTRA_PYTHON_PATHS = [
str(Path(SCRIPT_DIR).resolve().parents[0]),
str(USD_LIB_DIR.joinpath("python")),
str(CLIENT_LIB_DIR.joinpath("bindings-python")),
str(BUILD_DIR.joinpath("bindings-python")),
]
if PLATFORM_SYSTEM == "windows":
os.environ["PATH"] += os.pathsep + os.pathsep.join(EXTRA_PATHS)
ot_bin = "carb.omnitrace.plugin.dll"
else:
p = os.environ.get("LD_LIBRARY_PATH", "")
p += os.pathsep + os.pathsep.join(EXTRA_PATHS)
os.environ["LD_LIBRARY_PATH"] = p
ot_bin = "libcarb.omnitrace.plugin.so"
os.environ["OMNI_TRACE_LIB"] = os.path.join(str(DEPS_DIR), "omni-trace", "bin", ot_bin)
os.environ["PYTHONPATH"] = os.pathsep + os.pathsep.join(EXTRA_PYTHON_PATHS)
os.environ["OMNI_USER"] = args.username
os.environ["OMNI_PASS"] = args.password
os.environ["OMNI_HOST"] = args.server
if PLATFORM_SYSTEM == "windows":
PYTHON_EXE = DEPS_DIR.joinpath("python", "python")
else:
PYTHON_EXE = DEPS_DIR.joinpath("python", "bin", "python3")
plugin_paths = DEPS_DIR.joinpath("omni_usd_resolver", args.config, "usd", "omniverse", "resources")
os.environ["PXR_PLUGINPATH_NAME"] = str(plugin_paths)
REQ_FILE = ROOT_DIR.joinpath("requirements.txt")
subprocess.run(f"{PYTHON_EXE} -m pip install -r {REQ_FILE}", shell=True)
result = subprocess.run(
[PYTHON_EXE, os.path.join(SCRIPT_DIR, "app.py")],
stderr=subprocess.STDOUT,
)
| 3,268 | Python | 39.8625 | 106 | 0.717258 |
NVIDIA-Omniverse/iot-samples/source/omni/live/live_cube.py | import random
from pxr import Usd, Gf, UsdGeom, Sdf, UsdShade
class LiveCube:
def __init__(self, stage: Usd.Stage):
points = [
(50, 50, 50),
(-50, 50, 50),
(-50, -50, 50),
(50, -50, 50),
(-50, -50, -50),
(-50, 50, -50),
(50, 50, -50),
(50, -50, -50),
]
faceVertexIndices = [0, 1, 2, 3, 4, 5, 6, 7, 0, 6, 5, 1, 4, 7, 3, 2, 0, 3, 7, 6, 4, 2, 1, 5]
faceVertexCounts = [4, 4, 4, 4, 4, 4]
cube = stage.GetPrimAtPath("/World/cube")
if not cube:
cube = stage.DefinePrim("/World/cube", "Cube")
if not cube:
raise Exception("Could load the cube: /World/cube.")
self.mesh = stage.GetPrimAtPath("/World/cube/mesh")
if not self.mesh:
self.mesh = UsdGeom.Mesh.Define(stage, "/World/cube/mesh")
self.mesh.CreatePointsAttr().Set(points)
self.mesh.CreateFaceVertexIndicesAttr().Set(faceVertexIndices)
self.mesh.CreateFaceVertexCountsAttr().Set(faceVertexCounts)
self.mesh.CreateDoubleSidedAttr().Set(False)
self.mesh.CreateSubdivisionSchemeAttr("bilinear")
self.mesh.CreateDisplayColorAttr().Set([(0.463, 0.725, 0.0)])
self.mesh.AddTranslateOp().Set(Gf.Vec3d(0.0))
self.mesh.AddScaleOp().Set(Gf.Vec3f(0.8535))
self.mesh.AddTransformOp().Set(Gf.Matrix4d(1, 0, 0, 0, 0, 1, 0, 0, 0, 0, 1, 0, 0, 0, 0, 1))
texCoords = UsdGeom.PrimvarsAPI(self.mesh).CreatePrimvar(
"st", Sdf.ValueTypeNames.TexCoord2fArray, UsdGeom.Tokens.varying
)
texCoords.Set([(0, 0), (1, 0), (1, 1), (0, 1)])
self._rotationIncrement = Gf.Vec3f(
random.uniform(-1.0, 1.0) * 10.0, random.uniform(-1.0, 1.0) * 10.0, random.uniform(-1.0, 1.0) * 10.0
)
material = UsdShade.Material.Define(stage, '/World/Looks/Plastic_Yellow_A')
if material:
self.mesh.GetPrim().ApplyAPI(UsdShade.MaterialBindingAPI)
UsdShade.MaterialBindingAPI(self.mesh).Bind(material)
self._rotateXYZOp = None
self._scale = None
self._translate = None
self.cube = UsdGeom.Xformable(cube)
for op in self.cube.GetOrderedXformOps():
if op.GetOpType() == UsdGeom.XformOp.TypeRotateXYZ:
self._rotateXYZOp = op
if op.GetOpType() == UsdGeom.XformOp.TypeScale:
self._scale = op
if op.GetOpType() == UsdGeom.XformOp.TypeTranslate:
self._translate = op
if self._rotateXYZOp is None:
self._rotateXYZOp = self.cube.AddRotateXYZOp()
self._rotation = Gf.Vec3f(0.0, 0.0, 0.0)
self._rotateXYZOp.Set(self._rotation)
def translate(self, value: Gf.Vec3f):
if self._translate is None:
self._translate = self.cube.AddTranslateOp()
self._translate.Set(value)
def scale(self, value: Gf.Vec3f):
if self._scale is None:
self._scale = self.cube.AddScaleOp()
self._scale.Set(value)
def rotate(self):
if abs(self._rotation[0] + self._rotationIncrement[0]) > 360.0:
self._rotationIncrement[0] *= -1.0
if abs(self._rotation[1] + self._rotationIncrement[1]) > 360.0:
self._rotationIncrement[1] *= -1.0
if abs(self._rotation[2] + self._rotationIncrement[2]) > 360.0:
self._rotationIncrement[2] *= -1.0
self._rotation[0] += self._rotationIncrement[0]
self._rotation[1] += self._rotationIncrement[1]
self._rotation[2] += self._rotationIncrement[2]
self._rotateXYZOp.Set(self._rotation)
| 3,735 | Python | 39.608695 | 112 | 0.561981 |
NVIDIA-Omniverse/iot-samples/source/omni/live/__init__.py | import jwt
from .live_edit_session import LiveEditSession
from .nucleus_client_error import NucleusClientError
from .live_cube import LiveCube
def getUserNameFromToken(token: str):
unvalidated = jwt.decode(token, options={"verify_signature": False})
email = unvalidated["profile"]["email"]
if email is None or email == '':
return "$omni-api-token"
return email
| 387 | Python | 28.846152 | 72 | 0.726098 |
NVIDIA-Omniverse/iot-samples/source/omni/live/nucleus_client_error.py | from fastapi import HTTPException
class NucleusClientError(HTTPException):
def __init__(self, message, original_exception=None):
self.message = f"Error connecting to Nucleus - {message}"
if original_exception:
self.message = f"{self.message}: {original_exception}"
super().__init__(detail=self.message, status_code=502)
| 362 | Python | 35.299996 | 66 | 0.679558 |
NVIDIA-Omniverse/iot-samples/source/omni/live/nucleus_server_config.py | import omni.client
def nucleus_server_config(live_edit_session):
_, server_info = omni.client.get_server_info(live_edit_session.stage_url)
return {
"user_name": server_info.username,
"stage_url": live_edit_session.stage_url,
"mode": "default",
"name": live_edit_session.session_name,
"version": "1.0",
}
| 358 | Python | 26.615383 | 77 | 0.620112 |
Subsets and Splits
No community queries yet
The top public SQL queries from the community will appear here once available.