language:
- en
license: cc-by-4.0
size_categories:
- 1K<n<10K
task_categories:
- question-answering
- visual-question-answering
- multiple-choice
pretty_name: MRAG-Bench
dataset_info:
features:
- name: id
dtype: string
- name: aspect
dtype: string
- name: scenario
dtype: string
- name: image
dtype: image
- name: gt_images
sequence: image
- name: question
dtype: string
- name: A
dtype: string
- name: B
dtype: string
- name: C
dtype: string
- name: D
dtype: string
- name: answer_choice
dtype: string
- name: answer
dtype: string
- name: image_type
dtype: string
- name: source
dtype: string
- name: retrieved_images
sequence: image
splits:
- name: test
configs:
- config_name: default
data_files:
- split: test
path: data/test-*
MRAG-Bench: Vision-Centric Evaluation for Retrieval-Augmented Multimodal Models
π Homepage | π Paper | π» Evaluation
Intro
MRAG-Bench consists of 16,130 images and 1,353 human-annotated multiple-choice questions across 9 distinct scenarios, providing a robust and systematic evaluation of Large Vision Language Model (LVLM)βs vision-centric multimodal retrieval-augmented generation (RAG) abilities.
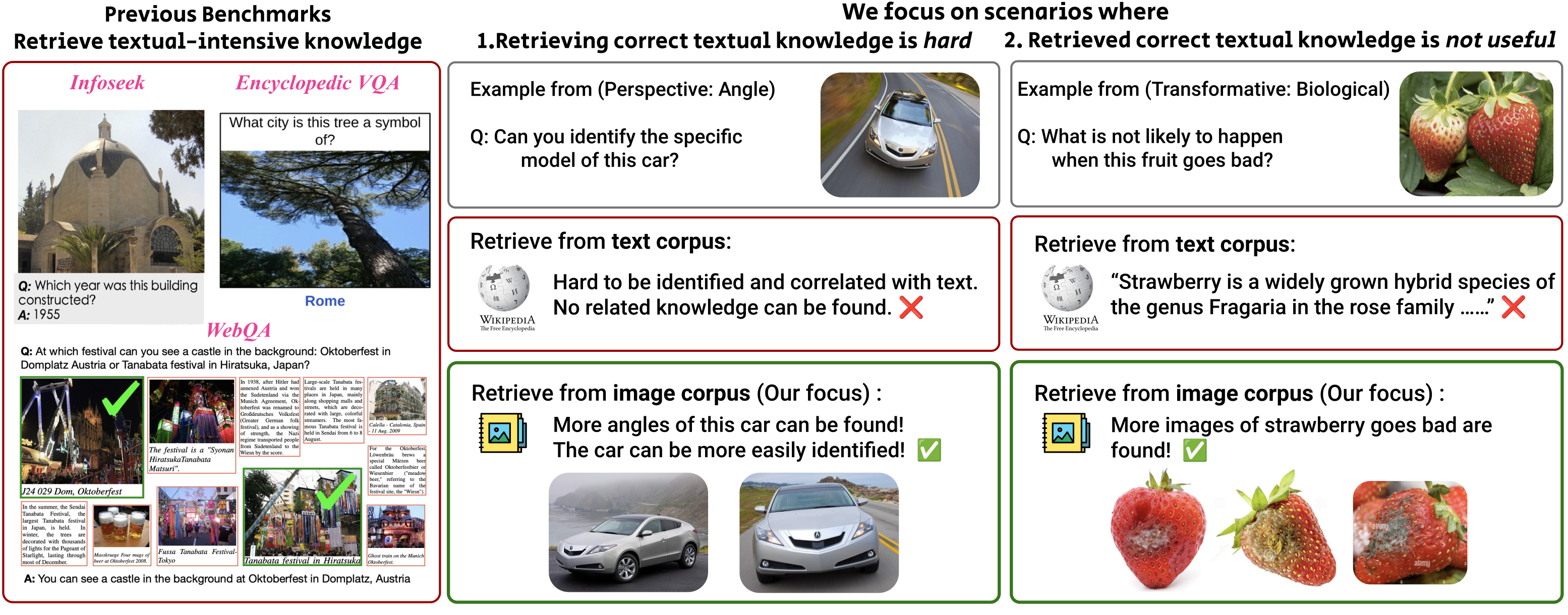
Results
Evaluated upon 10 open-source and 4 proprietary LVLMs, our results show that all LVLMs exhibit greater improvements when augmented with images compared to textual knowledge. Notably, the top-performing model, GPT-4o, faces challenges in effectively leveraging retrieved knowledge, achieving only a 5.82% improvement with ground-truth information, in contrast to a 33.16% improvement observed in human participants. These findings highlight the importance of MRAG-Bench in encouraging the community to enhance LVLMs' ability to utilize retrieved visual knowledge more effectively.
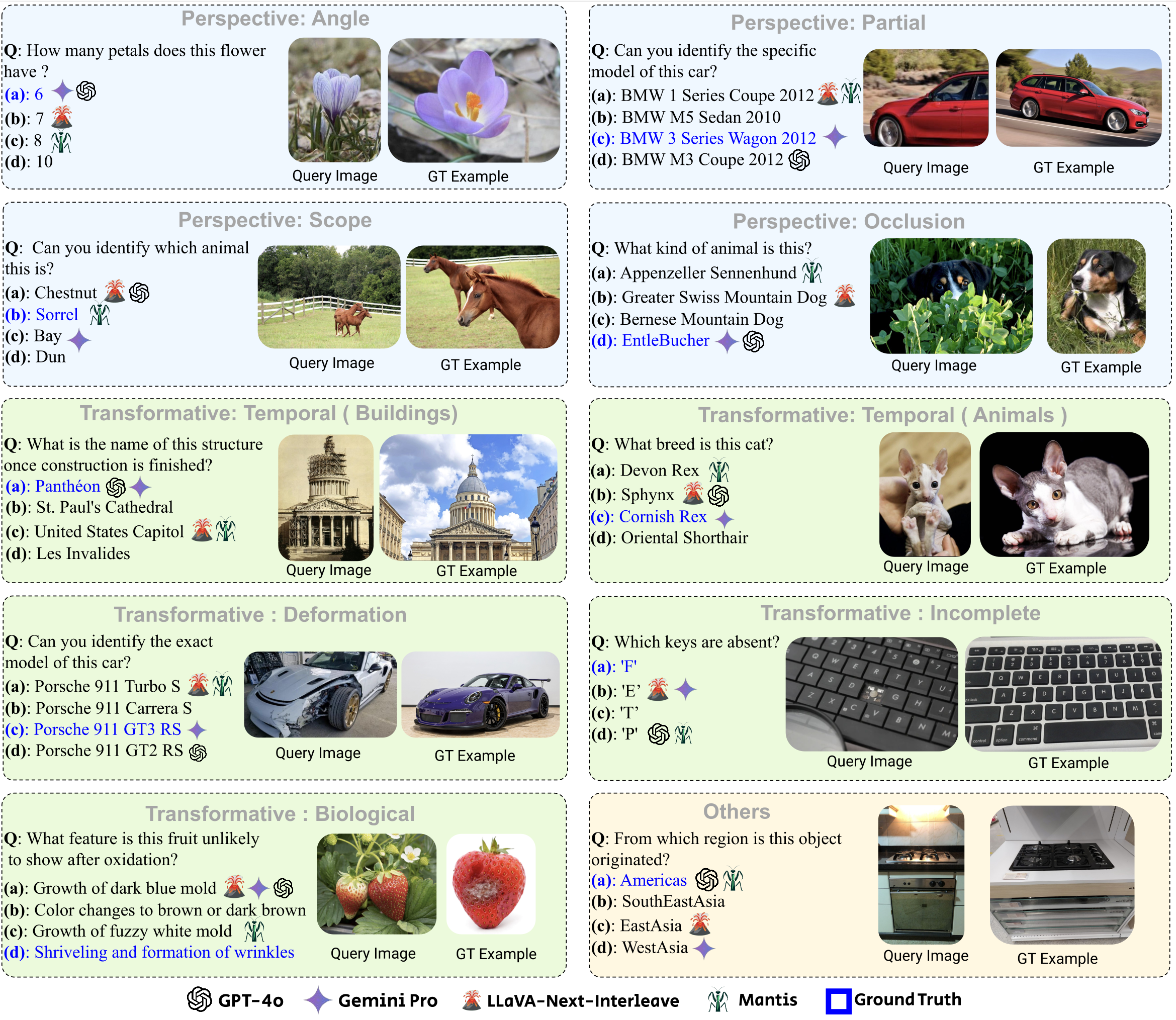
Load Dataset
The data/
directory contains the full dataset annotations and images pre-loaded for processing with HF Datasets. It can be loaded as follows:
from datasets import load_dataset
mrag_bench = load_dataset("uclanlp/MRAG-Bench")
Dataset Description
The dataset contains the following fields:
Field Name | Description |
---|---|
id |
Unique identifier for the example |
aspect |
Aspect type for the example |
scenario |
The type of scenario associated with the entry |
image |
Contains image data in byte format |
gt_images |
A list of top 5 ground-truth images information |
question |
Question asked about the image |
A |
Choice A for the question |
B |
Choice B for the question |
C |
Choice C for the question |
D |
Choice D for the question |
answer_choice |
Correct choice identifier |
answer |
Correct answer to the question |
image_type |
Type of image object |
source |
Source of the image |
retrieved_images |
A list of top 5 retrieved images information by CLIP |
Contact
- Wenbo Hu: [email protected]
Citation
@article{hu2024mragbench,
title={MRAG-Bench: Vision-Centric Evaluation for Retrieval-Augmented Multimodal Models},
author={Hu, Wenbo and Gu, Jia-Chen and Dou, Zi-Yi and Fayyaz, Mohsen and Lu, Pan and Chang, Kai-Wei and Peng, Nanyun},
journal={arXiv preprint arXiv:24},
year={2024}
}