Hieu Lam
lamhieu
AI & ML interests
.-.
Recent Activity
liked
a dataset
6 days ago
proj-persona/PersonaHub
liked
a model
13 days ago
Xenova/paraphrase-multilingual-mpnet-base-v2
liked
a model
13 days ago
nomic-ai/nomic-embed-text-v1.5
Articles
Organizations
lamhieu's activity
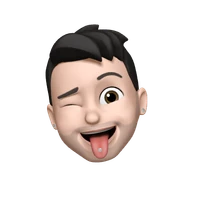
upvoted
a
paper
3 months ago
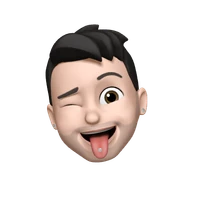
reacted to
m-ric's
post with ๐ฅ
3 months ago
Post
1170
Emu3: Next-token prediction conquers multimodal tasks ๐ฅ
This is the most important research in months: weโre now very close to having a single architecture to handle all modalities. The folks at Beijing Academy of Artificial Intelligence (BAAI) just released Emu3, a single model that handles text, images, and videos all at once.
๐ช๐ต๐ฎ๐'๐ ๐๐ต๐ฒ ๐ฏ๐ถ๐ด ๐ฑ๐ฒ๐ฎ๐น?
๐ Emu3 is the first model to truly unify all these different types of data (text, images, video) using just one simple trick: predicting the next token.
And itโs only 8B, but really strong:
๐ผ๏ธ For image generation, it's matching the best specialized models out there, like SDXL.
๐๏ธ In vision tasks, it's outperforming top models like LLaVA-1.6-7B, which is a big deal for a model that wasn't specifically designed for this.
๐ฌ It's the first to nail video generation without using complicated diffusion techniques.
๐๐ผ๐ ๐ฑ๐ผ๐ฒ๐ ๐ถ๐ ๐๐ผ๐ฟ๐ธ?
๐งฉ Emu3 uses a special tokenizer (SBER-MoVQGAN) to turn images and video clips into sequences of 4,096 tokens.
๐ Then, it treats everything - text, images, and videos - as one long series of tokens to predict.
๐ฎ During training, it just tries to guess the next token, whether that's a word, part of an image, or a video frame.
๐๐ฎ๐๐ฒ๐ฎ๐๐ ๐ผ๐ป ๐๐ต๐ฒ ๐ฟ๐ฒ๐๐๐น๐๐:
๐ In image generation, Emu3 beats SDXL, but itโs also much bigger (8B vs 3.5B). It would be more difficult to beat the real diffusion GOAT FLUX-dev.
๐ In vision, authors also donโt show a comparison against all the current SOTA models like Qwen-VL or Pixtral.
This approach is exciting because it's simple (next token prediction) and scalable(handles all sorts of data)!
Read the paper ๐ Emu3: Next-Token Prediction is All You Need (2409.18869)
This is the most important research in months: weโre now very close to having a single architecture to handle all modalities. The folks at Beijing Academy of Artificial Intelligence (BAAI) just released Emu3, a single model that handles text, images, and videos all at once.
๐ช๐ต๐ฎ๐'๐ ๐๐ต๐ฒ ๐ฏ๐ถ๐ด ๐ฑ๐ฒ๐ฎ๐น?
๐ Emu3 is the first model to truly unify all these different types of data (text, images, video) using just one simple trick: predicting the next token.
And itโs only 8B, but really strong:
๐ผ๏ธ For image generation, it's matching the best specialized models out there, like SDXL.
๐๏ธ In vision tasks, it's outperforming top models like LLaVA-1.6-7B, which is a big deal for a model that wasn't specifically designed for this.
๐ฌ It's the first to nail video generation without using complicated diffusion techniques.
๐๐ผ๐ ๐ฑ๐ผ๐ฒ๐ ๐ถ๐ ๐๐ผ๐ฟ๐ธ?
๐งฉ Emu3 uses a special tokenizer (SBER-MoVQGAN) to turn images and video clips into sequences of 4,096 tokens.
๐ Then, it treats everything - text, images, and videos - as one long series of tokens to predict.
๐ฎ During training, it just tries to guess the next token, whether that's a word, part of an image, or a video frame.
๐๐ฎ๐๐ฒ๐ฎ๐๐ ๐ผ๐ป ๐๐ต๐ฒ ๐ฟ๐ฒ๐๐๐น๐๐:
๐ In image generation, Emu3 beats SDXL, but itโs also much bigger (8B vs 3.5B). It would be more difficult to beat the real diffusion GOAT FLUX-dev.
๐ In vision, authors also donโt show a comparison against all the current SOTA models like Qwen-VL or Pixtral.
This approach is exciting because it's simple (next token prediction) and scalable(handles all sorts of data)!
Read the paper ๐ Emu3: Next-Token Prediction is All You Need (2409.18869)
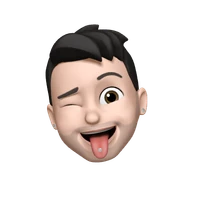
reacted to
singhsidhukuldeep's
post with ๐
4 months ago
Post
1631
Just wrapped up a deep dive into the latest lecture on building LLMs, such as ChatGPT, from
@Stanford
CS229 course. Here are my top takeaways:
๐ Understanding the Components: LLMs like ChatGPT, Claude, and others are more than just neural networks; they are a complex blend of architecture, training loss, data evaluation, and systems. Knowing how these components work together is key to improving and scaling these models.
๐ Scaling Matters: Performance improves predictably with more data, bigger models, and greater computational power. However, balancing these factors is crucial to avoid overfitting and resource waste.
๐ Data is King: LLMs are trained on trillions of tokens scraped from the internet, but the quality of this data matters immensely. Rigorous filtering and deduplication processes are essential to maintaining data integrity.
๐๏ธ Pre-Training vs. Post-Training: While pre-training equips the model with general knowledge, post-training (like RLHF) fine-tunes it to follow human-like responses, reducing toxic outputs and improving alignment with human values.
๐ Reinforcement Learning from Human Feedback (RLHF): This technique allows LLMs to maximize outputs that align with human preferences, making models more reliable and accurate.
๐ก Why It Matters: Understanding these processes not only helps us appreciate the complexity behind our everyday AI tools but also highlights the challenges and opportunities in the ever-evolving field of AI.
Whether youโre in tech, data science, or just AI-curious, staying updated on these advancements is crucial. LLMs are not just transforming industries; theyโre redefining the future of human-computer interaction!
I just realized this was almost 2 hours long...
Link: https://www.youtube.com/watch?v=9vM4p9NN0Ts
๐ Understanding the Components: LLMs like ChatGPT, Claude, and others are more than just neural networks; they are a complex blend of architecture, training loss, data evaluation, and systems. Knowing how these components work together is key to improving and scaling these models.
๐ Scaling Matters: Performance improves predictably with more data, bigger models, and greater computational power. However, balancing these factors is crucial to avoid overfitting and resource waste.
๐ Data is King: LLMs are trained on trillions of tokens scraped from the internet, but the quality of this data matters immensely. Rigorous filtering and deduplication processes are essential to maintaining data integrity.
๐๏ธ Pre-Training vs. Post-Training: While pre-training equips the model with general knowledge, post-training (like RLHF) fine-tunes it to follow human-like responses, reducing toxic outputs and improving alignment with human values.
๐ Reinforcement Learning from Human Feedback (RLHF): This technique allows LLMs to maximize outputs that align with human preferences, making models more reliable and accurate.
๐ก Why It Matters: Understanding these processes not only helps us appreciate the complexity behind our everyday AI tools but also highlights the challenges and opportunities in the ever-evolving field of AI.
Whether youโre in tech, data science, or just AI-curious, staying updated on these advancements is crucial. LLMs are not just transforming industries; theyโre redefining the future of human-computer interaction!
I just realized this was almost 2 hours long...
Link: https://www.youtube.com/watch?v=9vM4p9NN0Ts
Sounds interesting but I think there will be a big breakthrough, a new "architecture/methodology/factor/rethinking" for developing large models. That's what I think, I don't know what it is yet, haha.
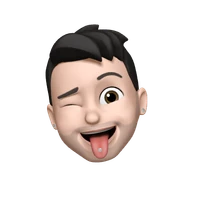
reacted to
m-ric's
post with ๐
4 months ago
Post
843
๐ย ๐ช๐ต๐ฒ๐ฟ๐ฒ ๐๐ฐ๐ฎ๐น๐ถ๐ป๐ด ๐น๐ฎ๐๐ ๐ฎ๐ฟ๐ฒ ๐๐ฎ๐ธ๐ถ๐ป๐ด ๐๐ : ๐ฏ๐ ๐ฎ๐ฌ๐ฎ๐ด, ๐๐ ๐๐น๐๐๐๐ฒ๐ฟ๐ ๐๐ถ๐น๐น ๐ฟ๐ฒ๐ฎ๐ฐ๐ต ๐๐ต๐ฒ ๐ฝ๐ผ๐๐ฒ๐ฟ ๐ฐ๐ผ๐ป๐๐๐บ๐ฝ๐๐ถ๐ผ๐ป ๐ผ๐ณ ๐ฒ๐ป๐๐ถ๐ฟ๐ฒ ๐ฐ๐ผ๐๐ป๐๐ฟ๐ถ๐ฒ๐
Reminder : โScaling lawsโ are empirical laws saying that if you keep multiplying your compute by x10, your models will mechanically keep getting better and better.
To give you an idea, GPT-3 can barely write sentences, and GPT-4, which only used x15 its amount of compute, already sounds much smarter than some of my friends (although it's not really - or at least I haven't tested them side-by side). So you can imagine how far a x100 over GPT-4 can take us.
๐๏ธย As a result, tech titans are racing to build the biggest models, and for this they need gigantic training clusters.
The picture below shows the growth of training compute: it is increasing at a steady exponential rate of a x10 every 2 years. So letโs take this progress a bit further:
- 2022: starting training for GPT-4 : 10^26 FLOPs, cost of $100M
- 2024: today, companies start training on much larger clusters like the โsuper AI clusterโ of Elon Muskโs xAI, 10^27 FLOPS, $1B
- 2026 : by then clusters will require 1GW, i.e. around the full power generated by a nuclear reactor
- 2028: we reach cluster prices in the 100 billion dollars, using 10GW, more than the most powerful power stations currently in use in the US. This last size seems crazy, but Microsoft and OpenAI already are planning one.
Will AI clusters effectively reach these crazy sizes where the consume as much as entire countries?
โก๏ธย Three key ingredients of training might be a roadblock to scaling up :
๐ธย Money: but itโs very unlikely, given the potential market size for AGI, that investors lose interest.
โก๏ธ Energy supply at a specific location
๐ย Training data: weโre already using 15 trillion tokens for Llama-3.1 when Internet has something like 60 trillion.
๐คย Iโd be curious to hear your thoughts: do you think weโll race all the way there?
Reminder : โScaling lawsโ are empirical laws saying that if you keep multiplying your compute by x10, your models will mechanically keep getting better and better.
To give you an idea, GPT-3 can barely write sentences, and GPT-4, which only used x15 its amount of compute, already sounds much smarter than some of my friends (although it's not really - or at least I haven't tested them side-by side). So you can imagine how far a x100 over GPT-4 can take us.
๐๏ธย As a result, tech titans are racing to build the biggest models, and for this they need gigantic training clusters.
The picture below shows the growth of training compute: it is increasing at a steady exponential rate of a x10 every 2 years. So letโs take this progress a bit further:
- 2022: starting training for GPT-4 : 10^26 FLOPs, cost of $100M
- 2024: today, companies start training on much larger clusters like the โsuper AI clusterโ of Elon Muskโs xAI, 10^27 FLOPS, $1B
- 2026 : by then clusters will require 1GW, i.e. around the full power generated by a nuclear reactor
- 2028: we reach cluster prices in the 100 billion dollars, using 10GW, more than the most powerful power stations currently in use in the US. This last size seems crazy, but Microsoft and OpenAI already are planning one.
Will AI clusters effectively reach these crazy sizes where the consume as much as entire countries?
โก๏ธย Three key ingredients of training might be a roadblock to scaling up :
๐ธย Money: but itโs very unlikely, given the potential market size for AGI, that investors lose interest.
โก๏ธ Energy supply at a specific location
๐ย Training data: weโre already using 15 trillion tokens for Llama-3.1 when Internet has something like 60 trillion.
๐คย Iโd be curious to hear your thoughts: do you think weโll race all the way there?