VidTok
A Family of Versatile and State-Of-The-Art Video Tokenizers
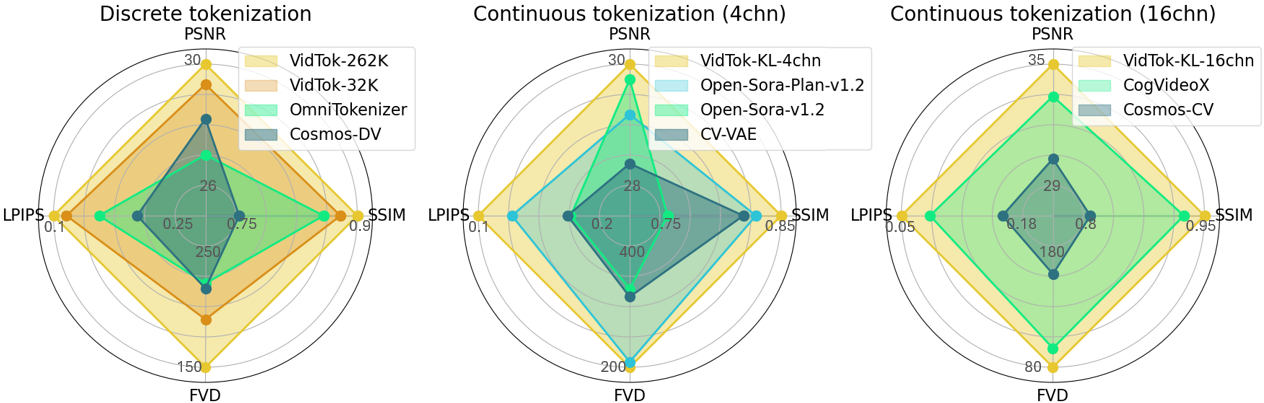
VidTok is a family of versatile video tokenizers that delivers state-of-the-art performance in both continuous and discrete tokenizations with various compression rates. VidTok incorporates several key advancements over existing approaches:
- ⚡️ Model architecture. We handle spatial and temporal sampling separately, reducing computational complexity without sacrificing reconstruction quality.
- 🔥 Advanced quantization techniques. To address the training instability and codebook collapse commonly associated with conventional Vector Quantization (VQ), we use Finite Scalar Quantization (FSQ) in discrete video tokenization.
- 💥 Improved training strategies. To improve training efficiency, we employ a two-stage training strategy: initially pre-training the full model on low-resolution videos, followed by fine-tuning only the decoder on high-resolution videos. Furthermore, we observe that utilizing training data with reduced frame rates effectively improves the model's ability to represent motion dynamics.
We train VidTok on a large-scale video dataset and evaluation reveal that VidTok outperforms previous models in both discrete and continuous tokenization, achieving superior results across all evaluated metrics, including PSNR, SSIM, LPIPS, and FVD.
Resources and technical documentation:
Model Performance
The following table shows model performance evaluated on 30 test videos in MCL_JCL dataset, with a sample fps of 30. The input size is 17x256x256
for causal models and 16x256x256
for non-causal models. VCR
indicates the video compression ratio TxHxW
.
Model | Regularizer | Causal | VCR | PSNR | SSIM | LPIPS | FVD |
---|---|---|---|---|---|---|---|
vidtok_kl_causal_488_4chn | KL-4chn | ✔️ | 4x8x8 | 29.64 | 0.852 | 0.114 | 194.2 |
vidtok_kl_causal_488_8chn | KL-8chn | ✔️ | 4x8x8 | 31.83 | 0.897 | 0.083 | 109.3 |
vidtok_kl_causal_488_16chn | KL-16chn | ✔️ | 4x8x8 | 35.04 | 0.942 | 0.047 | 78.9 |
vidtok_kl_causal_41616_4chn | KL-4chn | ✔️ | 4x16x16 | 25.05 | 0.711 | 0.228 | 549.1 |
vidtok_kl_noncausal_488_4chn | KL-4chn | ✖️ | 4x8x8 | 30.60 | 0.876 | 0.098 | 157.9 |
vidtok_kl_noncausal_41616_4chn | KL-4chn | ✖️ | 4x16x16 | 26.06 | 0.751 | 0.190 | 423.2 |
vidtok_fsq_causal_488_262144 | FSQ-262,144 | ✔️ | 4x8x8 | 29.82 | 0.867 | 0.106 | 160.1 |
vidtok_fsq_causal_488_32768 | FSQ-32,768 | ✔️ | 4x8x8 | 29.16 | 0.854 | 0.117 | 196.9 |
vidtok_fsq_causal_488_4096 | FSQ-4096 | ✔️ | 4x8x8 | 28.36 | 0.832 | 0.133 | 218.1 |
vidtok_fsq_causal_41616_262144 | FSQ-262,144 | ✔️ | 4x16x16 | 25.38 | 0.738 | 0.206 | 430.1 |
vidtok_fsq_noncausal_488_262144 | FSQ-262,144 | ✖️ | 4x8x8 | 30.78 | 0.889 | 0.091 | 132.1 |
vidtok_fsq_noncausal_41616_262144 | FSQ-262,144 | ✖️ | 4x16x16 | 26.37 | 0.772 | 0.171 | 357.0 |
Training
Training Data
The training data of VidTok is divided into two sets based on video quality.
- Training Set 1 consists of approximately 400K of low-resolution videos (e.g., 480p). The videos are natural videos with diverse lightning, motions, and scenarios.
- Training Set 2 includes approximately 10K of high-resolution videos (e.g., 1080p). The videos are natural videos with diverse lightning, motions, and scenarios.
Training Procedure
Please refer to the paper and code for detailed training instructions.
Evaluation
Please refer to the paper and code for detailed evaluation instructions.
Intended Uses
We are sharing our model with the research community to foster further research in this area:
- Training your own video tokenizers for research purpose.
- Video tokenization with various compression rates.
Downstream Uses
Our model is designed to accelerate research on video-centric research, for use as a building block for the following applications:
- Video generation on the continuous / discrete latent tokens.
- World modelling on the continuous / discrete latent tokens.
- Generative games on the continuous / discrete latent tokens.
- Video understanding from the latent tokens.
Out-of-scope Uses
Our models are not specifically designed or evaluated for all downstream purposes. Developers should consider common limitations of video tokenizers (e.g., performance degradation on out-of-domain data) as they select use cases, and evaluate and mitigate for privacy, safety, and fairness before using within a specific downstream use case, particularly for high-risk scenarios.
Developers should be aware of and adhere to applicable laws or regulations (including privacy, trade compliance laws, etc.) that are relevant to their use case.
Risks and Limitations
Some of the limitations of this model to be aware of include:
- VidTok may lose detailed information on the reconstructed content.
- VidTok inherits any biases, errors, or omissions characteristic of its training data.
- VidTok was developed for research and experimental purposes. Further testing and validation are needed before considering its application in commercial or real-world scenarios.
Recommendations
Some recommendations for alleviating potential limitations include:
- Lower compression rate provides higher reconstruction quality.
- For domain-specific video tokenization, it is suggested to fine-tune the model on the domain-specific videos.
License
The model is released under the MIT license.
Contact
We welcome feedback and collaboration from our audience. If you have suggestions, questions, or observe unexpected/offensive behavior in our technology, please contact us at [email protected].