|
--- |
|
language: |
|
- ky |
|
datasets: |
|
- common_voice |
|
tags: |
|
- audio |
|
- automatic-speech-recognition |
|
--- |
|
|
|
# UniSpeech-Large-plus Kyrgyz |
|
|
|
[Microsoft's UniSpeech](https://www.microsoft.com/en-us/research/publication/unispeech-unified-speech-representation-learning-with-labeled-and-unlabeled-data/) |
|
|
|
The large model pretrained on 16kHz sampled speech audio and phonetic labels and consequently fine-tuned on 1h of Kyrgyz phonemes. |
|
When using the model make sure that your speech input is also sampled at 16kHz and your text in converted into a sequence of phonemes. |
|
|
|
[Paper: UniSpeech: Unified Speech Representation Learning |
|
with Labeled and Unlabeled Data](https://arxiv.org/abs/2101.07597) |
|
|
|
Authors: Chengyi Wang, Yu Wu, Yao Qian, Kenichi Kumatani, Shujie Liu, Furu Wei, Michael Zeng, Xuedong Huang |
|
|
|
**Abstract** |
|
*In this paper, we propose a unified pre-training approach called UniSpeech to learn speech representations with both unlabeled and labeled data, in which supervised phonetic CTC learning and phonetically-aware contrastive self-supervised learning are conducted in a multi-task learning manner. The resultant representations can capture information more correlated with phonetic structures and improve the generalization across languages and domains. We evaluate the effectiveness of UniSpeech for cross-lingual representation learning on public CommonVoice corpus. The results show that UniSpeech outperforms self-supervised pretraining and supervised transfer learning for speech recognition by a maximum of 13.4% and 17.8% relative phone error rate reductions respectively (averaged over all testing languages). The transferability of UniSpeech is also demonstrated on a domain-shift speech recognition task, i.e., a relative word error rate reduction of 6% against the previous approach.* |
|
|
|
The original model can be found under https://github.com/microsoft/UniSpeech/tree/main/UniSpeech. |
|
|
|
# Usage |
|
|
|
This is an speech model that has been fine-tuned on phoneme classification. |
|
|
|
## Inference |
|
|
|
```python |
|
import torch |
|
from datasets import load_dataset |
|
from transformers import AutoModelForCTC, AutoProcessor |
|
import torchaudio.functional as F |
|
|
|
model_id = "microsoft/unispeech-1350-en-17h-ky-ft-1h" |
|
|
|
sample = next(iter(load_dataset("common_voice", "ky", split="test", streaming=True))) |
|
resampled_audio = F.resample(torch.tensor(sample["audio"]["array"]), 48_000, 16_000).numpy() |
|
|
|
model = AutoModelForCTC.from_pretrained(model_id) |
|
processor = AutoProcessor.from_pretrained(model_id) |
|
|
|
input_values = processor(resampled_audio, return_tensors="pt").input_values |
|
|
|
with torch.no_grad(): |
|
logits = model(input_values).logits |
|
|
|
prediction_ids = torch.argmax(logits, dim=-1) |
|
transcription = processor.batch_decode(prediction_ids) |
|
``` |
|
|
|
# Contribution |
|
|
|
The model was contributed by [cywang](https://huggingface.co/cywang) and [patrickvonplaten](https://huggingface.co/patrickvonplaten). |
|
|
|
# License |
|
|
|
The official license can be found [here](https://github.com/microsoft/UniSpeech/blob/main/LICENSE) |
|
|
|
# Official Results |
|
|
|
See *UniSpeeech-L^{+}* - *ky*: |
|
|
|
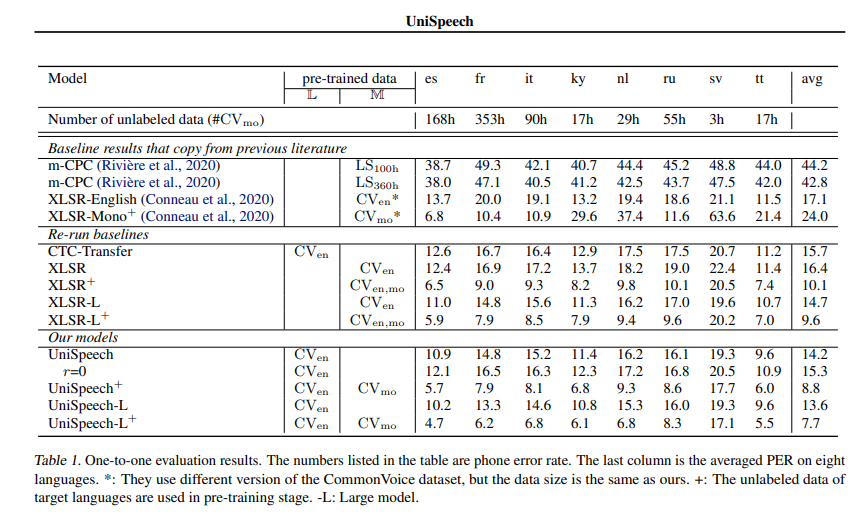 |