File size: 28,696 Bytes
b73cea4 58b2260 b73cea4 d9ef481 b73cea4 |
1 2 3 4 5 6 7 8 9 10 11 12 13 14 15 16 17 18 19 20 21 22 23 24 25 26 27 28 29 30 31 32 33 34 35 36 37 38 39 40 41 42 43 44 45 46 47 48 49 50 51 52 53 54 55 56 57 58 59 60 61 62 63 64 65 66 67 68 69 70 71 72 73 74 75 76 77 78 79 80 81 82 83 84 85 86 87 88 89 90 91 92 93 94 95 96 97 98 99 100 101 102 103 104 105 106 107 108 109 110 111 112 113 114 115 116 117 118 119 120 121 122 123 124 125 126 127 128 129 130 131 132 133 134 135 136 137 138 139 140 141 142 143 144 145 146 147 148 149 150 151 152 153 154 155 156 157 158 159 160 161 162 163 164 165 166 167 168 169 170 171 172 173 174 175 176 177 178 179 180 181 182 183 184 185 186 187 188 189 190 191 192 193 194 195 196 197 198 199 200 201 202 203 204 205 206 207 208 209 210 211 212 213 214 215 216 217 218 219 220 221 222 223 224 225 226 227 228 229 230 231 232 233 234 235 236 237 238 239 240 241 242 243 244 245 246 247 248 249 250 251 252 253 254 255 256 257 258 259 260 261 262 263 264 265 266 267 268 269 270 271 272 273 274 275 276 277 278 279 280 281 282 283 284 285 286 287 288 289 290 291 292 293 294 295 296 297 298 299 300 301 302 303 304 305 306 307 308 309 310 311 312 313 314 315 316 317 318 319 320 321 322 323 324 325 326 327 328 329 330 331 332 333 334 335 336 337 338 339 340 341 342 343 344 345 346 347 348 349 350 351 352 353 354 355 356 357 358 359 360 361 362 363 364 365 366 367 368 369 370 371 372 373 374 375 376 377 378 379 380 381 382 383 384 385 386 387 388 389 390 391 392 393 394 395 396 397 398 399 400 401 402 403 404 405 406 407 408 409 410 411 412 413 414 415 416 417 418 419 420 421 422 423 424 425 426 427 428 429 430 431 432 433 434 435 436 437 438 439 440 441 442 443 444 445 446 447 448 449 450 451 452 453 454 455 456 457 458 459 460 461 462 463 464 465 466 467 468 469 470 471 472 473 474 475 476 477 478 479 480 481 482 483 484 485 486 487 488 489 490 491 492 493 494 495 496 497 498 499 500 501 502 503 504 505 506 507 508 509 510 511 512 513 514 515 516 517 518 519 520 521 522 523 524 525 526 527 528 529 530 531 532 533 534 535 536 537 538 539 540 541 542 543 544 545 546 547 548 549 550 551 552 553 554 555 556 557 558 559 560 561 562 563 564 565 566 567 568 569 570 571 572 573 574 575 576 577 578 579 580 581 582 583 584 585 586 587 588 589 590 591 592 593 594 595 596 597 598 599 600 601 602 603 604 605 606 607 608 609 610 611 612 613 614 615 616 617 618 619 620 621 622 623 624 625 626 627 628 629 630 631 632 633 634 635 636 637 638 639 640 641 642 643 644 645 646 647 648 649 650 651 652 653 654 655 656 657 |
{
"cells": [
{
"cell_type": "markdown",
"metadata": {},
"source": [
"## 8. Evaluating Prompts\n",
"\n",
"Before the advent of large-language models, machine-learning systems were trained using a technique called [supervised learning](https://en.wikipedia.org/wiki/Supervised_learning). This approach required users to provide carefully prepared training data that showed the computer what was expected.\n",
"\n",
"For instance, if you were developing a model to distinguish spam emails from legitimate ones, you would need to provide the model with a set of spam emails and another set of legitimate emails. The model would then use that data to learn the relationships between the inputs and outputs, which it could then apply to new emails.\n",
"\n",
"In addition to training the model, the curated input would be used to evaluate the model's performance. This process typically involved splitting the supervised data into two sets: one for training and one for testing. The model could then be evaluated using a separate set of supervised data to ensure it could generalize beyond the examples it had been fed during training.\n",
"\n",
"Large-language models operate differently. They are trained on vast amounts of text and can generate responses based on the relationships they derive from various machine-learning approaches. The result is that they can be used to perform a wide range of tasks without requiring supervised data to be prepared beforehand.\n",
"\n",
"This is a significant advantage. However, it also raises questions about evaluating an LLM prompt. If we don't have a supervised sample to test its results, how do we know if it's doing a good job? How can we improve its performance if we can't see where it gets things wrong?\n",
"\n",
"In the final chapters, we will show how traditional supervision can still play a vital role in evaluating and improving an LLM prompt."
]
},
{
"cell_type": "code",
"execution_count": 84,
"metadata": {},
"outputs": [],
"source": [
"import os\n",
"import time # NEW\n",
"import json\n",
"from rich import print\n",
"from rich.progress import track # NEW\n",
"import requests\n",
"from retry import retry\n",
"import pandas as pd\n",
"from huggingface_hub import InferenceClient\n",
"\n",
"api_key = os.getenv(\"HF_TOKEN\")\n",
"client = InferenceClient(\n",
" token=api_key,\n",
")\n",
"df = pd.read_csv(\"https://raw.githubusercontent.com/palewire/first-llm-classifier/refs/heads/main/_notebooks/Form460ScheduleESubItem.csv\")"
]
},
{
"cell_type": "markdown",
"metadata": {},
"source": [
"Start by outputting a random sample from the dataset to a file of comma-separated values. It will serve as our supervised sample. In general, the larger the sample the better the evaluation. But at a certain point the returns diminish. For this exercise, we will use a sample of 250 records."
]
},
{
"cell_type": "code",
"execution_count": 85,
"metadata": {},
"outputs": [],
"source": [
"df.sample(250).to_csv(\"./sample.csv\", index=False)"
]
},
{
"cell_type": "markdown",
"metadata": {},
"source": [
"You can open the file in a spreadsheet program like Excel or Google Sheets. For each payee in the sample, you would provide the correct category in a companion column. This gradually becomes the supervised sample.\n",
"\n",
"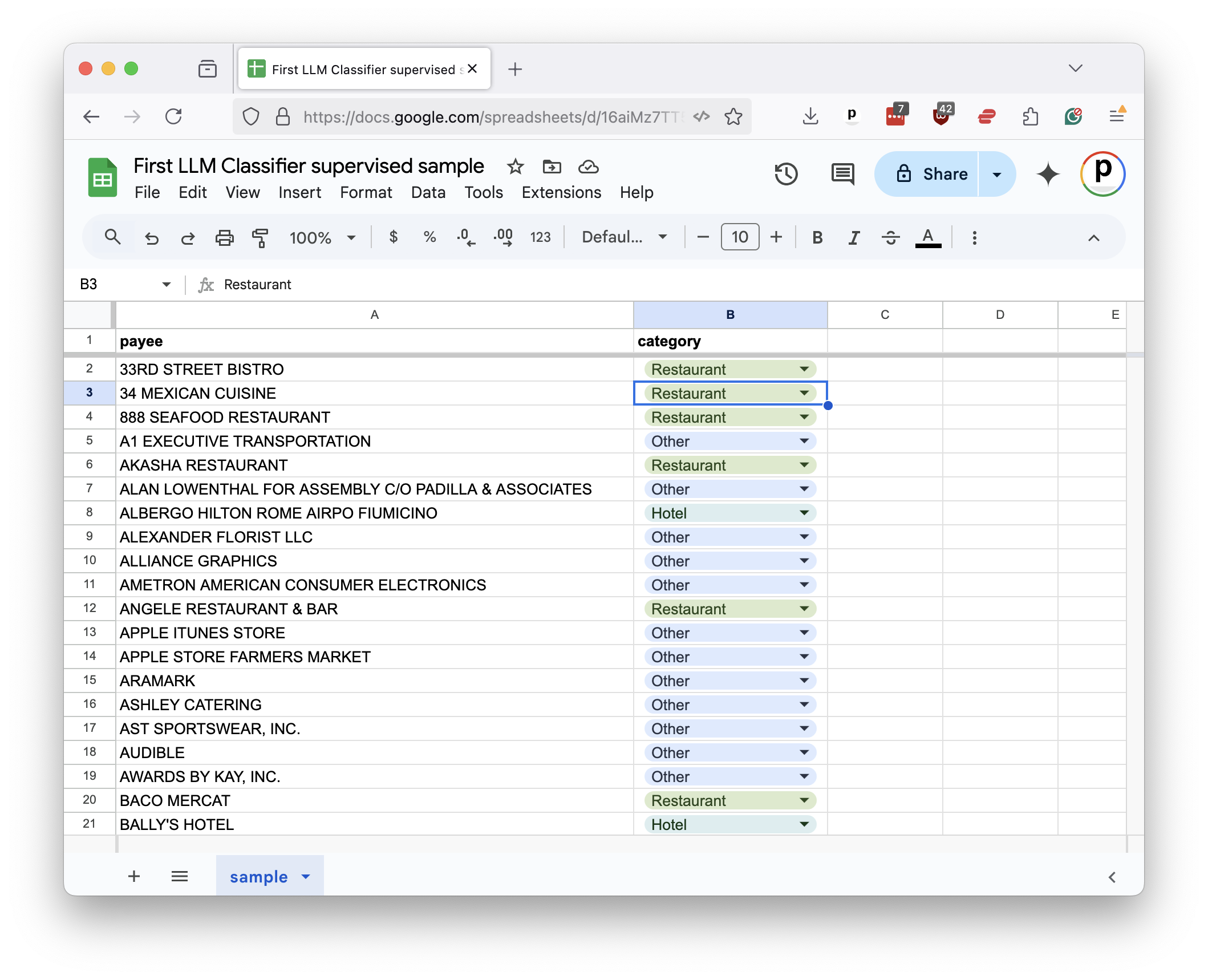\n",
"\n",
"To speed the class along, we've already prepared a sample for you in [the class repository](https://github.com/palewire/first-llm-classifier). Our next step is to read it back into a DataFrame."
]
},
{
"cell_type": "code",
"execution_count": 86,
"metadata": {},
"outputs": [],
"source": [
"sample_df = pd.read_csv(\"https://huggingface.co/spaces/JournalistsonHF/first-llm-classifier/resolve/main/notebooks/gradio-app/sample.csv\")"
]
},
{
"cell_type": "markdown",
"metadata": {},
"source": [
"We'll install the Python packages `scikit-learn`, `matplotlib`, and `seaborn`. Prior to LLMs, these libraries were the go-to tools for training and evaluating machine-learning models. We'll primarily be using them for testing.\n",
"\n",
"Return to the Jupyter notebook and install the packages alongside our other dependencies."
]
},
{
"cell_type": "code",
"execution_count": null,
"metadata": {
"scrolled": true
},
"outputs": [],
"source": [
"%pip install huggingface_hub rich ipywidgets retry pandas scikit-learn matplotlib seaborn"
]
},
{
"cell_type": "markdown",
"metadata": {},
"source": [
"Add the `test_train_split` function from `scikit-learn` to the import statement."
]
},
{
"cell_type": "code",
"execution_count": 88,
"metadata": {},
"outputs": [],
"source": [
"import json\n",
"from rich import print\n",
"import requests\n",
"from retry import retry\n",
"import pandas as pd\n",
"from sklearn.model_selection import train_test_split #NEW "
]
},
{
"cell_type": "markdown",
"metadata": {},
"source": [
"This tool is used to split a supervised sample into separate sets for training and testing.\n",
"\n",
"The first input is the DataFrame column containing our supervised payees. The second input is the DataFrame column containing the correct categories.\n",
"\n",
"The `test_size` parameter determines the proportion of the sample that will be used for testing. The `random_state` parameter ensures that the split is reproducible by setting a seed for the random number generator that draws the samples."
]
},
{
"cell_type": "code",
"execution_count": 89,
"metadata": {
"scrolled": true
},
"outputs": [],
"source": [
"training_input, test_input, training_output, test_output = train_test_split(\n",
" sample_df[['payee']],\n",
" sample_df['category'],\n",
" test_size=0.33,\n",
" random_state=42, # Remember Jackie Robinson. Remember Douglas Adams.\n",
")"
]
},
{
"cell_type": "markdown",
"metadata": {},
"source": [
"In a traditional training setup, the next step would be to train a machine-learning model in `sklearn` using the `training_input` and `training_output` sets. The model would then be evaluated using the `test_input` and `test_output` sets.\n",
"\n",
"With the LLM we skip ahead to the testing phase. We pass the `test_input` set to our LLM prompt and compare the results to the right answers found in `test_output` set.\n",
"\n",
"All that requires is that we pass the `payee` column from our `test_input` DataFrame to the function we created in the previous chapters."
]
},
{
"cell_type": "code",
"execution_count": 90,
"metadata": {},
"outputs": [
{
"data": {
"application/vnd.jupyter.widget-view+json": {
"model_id": "2549c6db6c4a428a959aa78c686afce1",
"version_major": 2,
"version_minor": 0
},
"text/plain": [
"Output()"
]
},
"metadata": {},
"output_type": "display_data"
},
{
"data": {
"text/html": [
"<pre style=\"white-space:pre;overflow-x:auto;line-height:normal;font-family:Menlo,'DejaVu Sans Mono',consolas,'Courier New',monospace\"></pre>\n"
],
"text/plain": []
},
"metadata": {},
"output_type": "display_data"
}
],
"source": [
"###REPEAT FROM PREVIOUS NOTEBOOK\n",
"@retry(ValueError, tries=2, delay=2)\n",
"def classify_payees(name_list):\n",
" prompt = \"\"\"You are an AI model trained to categorize businesses based on their names.\n",
"\n",
"You will be given a list of business names, each separated by a new line.\n",
"\n",
"Your task is to analyze each name and classify it into one of the following categories: Restaurant, Bar, Hotel, or Other.\n",
"\n",
"It is extremely critical that there is a corresponding category output for each business name provided as an input.\n",
"\n",
"If a business does not clearly fall into Restaurant, Bar, or Hotel categories, you should classify it as \"Other\".\n",
"\n",
"Even if the type of business is not immediately clear from the name, it is essential that you provide your best guess based on the information available to you. If you can't make a good guess, classify it as Other.\n",
"\n",
"For example, if given the following input:\n",
"\n",
"\"Intercontinental Hotel\\nPizza Hut\\nCheers\\nWelsh's Family Restaurant\\nKTLA\\nDirect Mailing\"\n",
"\n",
"Your output should be a JSON list in the following format:\n",
"\n",
"[\"Hotel\", \"Restaurant\", \"Bar\", \"Restaurant\", \"Other\", \"Other\"]\n",
"\n",
"This means that you have classified \"Intercontinental Hotel\" as a Hotel, \"Pizza Hut\" as a Restaurant, \"Cheers\" as a Bar, \"Welsh's Family Restaurant\" as a Restaurant, and both \"KTLA\" and \"Direct Mailing\" as Other.\n",
"\n",
"Ensure that the number of classifications in your output matches the number of business names in the input. It is very important that the length of JSON list you return is exactly the same as the number of business names you receive.\n",
"\"\"\"\n",
" response = client.chat.completions.create(\n",
" messages=[\n",
" {\n",
" \"role\": \"system\",\n",
" \"content\": prompt,\n",
" },\n",
" {\n",
" \"role\": \"user\",\n",
" \"content\": \"Intercontinental Hotel\\nPizza Hut\\nCheers\\nWelsh's Family Restaurant\\nKTLA\\nDirect Mailing\",\n",
" },\n",
" {\n",
" \"role\": \"assistant\",\n",
" \"content\": '[\"Hotel\", \"Restaurant\", \"Bar\", \"Restaurant\", \"Other\", \"Other\"]',\n",
" },\n",
" {\n",
" \"role\": \"user\",\n",
" \"content\": \"Subway Sandwiches\\nRuth Chris Steakhouse\\nPolitical Consulting Co\\nThe Lamb's Club\",\n",
" },\n",
" {\n",
" \"role\": \"assistant\",\n",
" \"content\": '[\"Restaurant\", \"Restaurant\", \"Other\", \"Bar\"]',\n",
" },\n",
" {\n",
" \"role\": \"user\",\n",
" \"content\": \"\\n\".join(name_list),\n",
" }\n",
" ],\n",
" model=\"meta-llama/Llama-3.3-70B-Instruct\",\n",
" temperature=0,\n",
" )\n",
"\n",
" answer_str = response.choices[0].message.content\n",
" answer_list = json.loads(answer_str)\n",
"\n",
" acceptable_answers = [\n",
" \"Restaurant\",\n",
" \"Bar\",\n",
" \"Hotel\",\n",
" \"Other\",\n",
" ]\n",
" for answer in answer_list:\n",
" if answer not in acceptable_answers:\n",
" raise ValueError(f\"{answer} not in list of acceptable answers\")\n",
"\n",
" try:\n",
" assert len(name_list) == len(answer_list)\n",
" except AssertionError:\n",
" raise ValueError(f\"Number of outputs ({len(name_list)}) does not equal the number of inputs ({len(answer_list)})\")\n",
"\n",
" return dict(zip(name_list, answer_list))\n",
"\n",
"def get_batch_list(li, n=10):\n",
" \"\"\"Split the provided list into batches of size `n`.\"\"\"\n",
" batch_list = []\n",
" for i in range(0, len(li), n):\n",
" batch_list.append(li[i : i + n])\n",
" return batch_list\n",
" \n",
"def classify_batches(name_list, batch_size=11, wait=2):\n",
" \"\"\"Split the provided list of names into batches and classify with our LLM them one by one.\"\"\"\n",
" # Create a place to store the results\n",
" all_results = {}\n",
"\n",
" # Batch up the list\n",
" batch_list = get_batch_list(name_list, n=batch_size)\n",
"\n",
" # Loop through the list in batches\n",
" for batch in track(batch_list):\n",
" # Classify it with the LLM\n",
" batch_results = classify_payees(batch)\n",
"\n",
" # Add what we get back to the results\n",
" all_results.update(batch_results)\n",
"\n",
" # Tap the brakes to avoid overloading HF's API\n",
" time.sleep(wait)\n",
"\n",
" # Return the results\n",
" return all_results\n",
" \n",
"llm_dict = classify_batches(list(test_input.payee))"
]
},
{
"cell_type": "markdown",
"metadata": {},
"source": [
"Next, we import the `classification_report` and `confusion_matrix` functions from `sklearn`, which are used to evaluate a model's performance. We'll also pull in `seaborn` and `matplotlib` to visualize the results."
]
},
{
"cell_type": "code",
"execution_count": 91,
"metadata": {},
"outputs": [],
"source": [
"import json\n",
"from rich import print\n",
"import requests\n",
"from retry import retry\n",
"import pandas as pd\n",
"import seaborn as sns # NEW\n",
"import matplotlib.pyplot as plt # NEW \n",
"from sklearn.model_selection import train_test_split\n",
"from sklearn.metrics import confusion_matrix, classification_report # NEW"
]
},
{
"cell_type": "markdown",
"metadata": {},
"source": [
"The `classification_report` function generats a report card on a model's performance. You provide it with the correct answers in the `test_output` set and the model's predictions in your prompt's DataFrame. In this case, our LLM's predictions are stored in the `llm_df` DataFrame's `category` column."
]
},
{
"cell_type": "code",
"execution_count": 92,
"metadata": {},
"outputs": [],
"source": [
"llm_df = pd.DataFrame.from_dict(llm_dict, orient=\"index\", columns=[\"category\"])"
]
},
{
"cell_type": "code",
"execution_count": 93,
"metadata": {},
"outputs": [
{
"data": {
"text/html": [
"<pre style=\"white-space:pre;overflow-x:auto;line-height:normal;font-family:Menlo,'DejaVu Sans Mono',consolas,'Courier New',monospace\"> precision recall f1-score support\n",
"\n",
" Bar <span style=\"color: #008080; text-decoration-color: #008080; font-weight: bold\">1.00</span> <span style=\"color: #008080; text-decoration-color: #008080; font-weight: bold\">1.00</span> <span style=\"color: #008080; text-decoration-color: #008080; font-weight: bold\">1.00</span> <span style=\"color: #008080; text-decoration-color: #008080; font-weight: bold\">2</span>\n",
" Hotel <span style=\"color: #008080; text-decoration-color: #008080; font-weight: bold\">1.00</span> <span style=\"color: #008080; text-decoration-color: #008080; font-weight: bold\">1.00</span> <span style=\"color: #008080; text-decoration-color: #008080; font-weight: bold\">1.00</span> <span style=\"color: #008080; text-decoration-color: #008080; font-weight: bold\">9</span>\n",
" Other <span style=\"color: #008080; text-decoration-color: #008080; font-weight: bold\">1.00</span> <span style=\"color: #008080; text-decoration-color: #008080; font-weight: bold\">0.98</span> <span style=\"color: #008080; text-decoration-color: #008080; font-weight: bold\">0.99</span> <span style=\"color: #008080; text-decoration-color: #008080; font-weight: bold\">57</span>\n",
" Restaurant <span style=\"color: #008080; text-decoration-color: #008080; font-weight: bold\">0.94</span> <span style=\"color: #008080; text-decoration-color: #008080; font-weight: bold\">1.00</span> <span style=\"color: #008080; text-decoration-color: #008080; font-weight: bold\">0.97</span> <span style=\"color: #008080; text-decoration-color: #008080; font-weight: bold\">15</span>\n",
"\n",
" accuracy <span style=\"color: #008080; text-decoration-color: #008080; font-weight: bold\">0.99</span> <span style=\"color: #008080; text-decoration-color: #008080; font-weight: bold\">83</span>\n",
" macro avg <span style=\"color: #008080; text-decoration-color: #008080; font-weight: bold\">0.98</span> <span style=\"color: #008080; text-decoration-color: #008080; font-weight: bold\">1.00</span> <span style=\"color: #008080; text-decoration-color: #008080; font-weight: bold\">0.99</span> <span style=\"color: #008080; text-decoration-color: #008080; font-weight: bold\">83</span>\n",
"weighted avg <span style=\"color: #008080; text-decoration-color: #008080; font-weight: bold\">0.99</span> <span style=\"color: #008080; text-decoration-color: #008080; font-weight: bold\">0.99</span> <span style=\"color: #008080; text-decoration-color: #008080; font-weight: bold\">0.99</span> <span style=\"color: #008080; text-decoration-color: #008080; font-weight: bold\">83</span>\n",
"\n",
"</pre>\n"
],
"text/plain": [
" precision recall f1-score support\n",
"\n",
" Bar \u001b[1;36m1.00\u001b[0m \u001b[1;36m1.00\u001b[0m \u001b[1;36m1.00\u001b[0m \u001b[1;36m2\u001b[0m\n",
" Hotel \u001b[1;36m1.00\u001b[0m \u001b[1;36m1.00\u001b[0m \u001b[1;36m1.00\u001b[0m \u001b[1;36m9\u001b[0m\n",
" Other \u001b[1;36m1.00\u001b[0m \u001b[1;36m0.98\u001b[0m \u001b[1;36m0.99\u001b[0m \u001b[1;36m57\u001b[0m\n",
" Restaurant \u001b[1;36m0.94\u001b[0m \u001b[1;36m1.00\u001b[0m \u001b[1;36m0.97\u001b[0m \u001b[1;36m15\u001b[0m\n",
"\n",
" accuracy \u001b[1;36m0.99\u001b[0m \u001b[1;36m83\u001b[0m\n",
" macro avg \u001b[1;36m0.98\u001b[0m \u001b[1;36m1.00\u001b[0m \u001b[1;36m0.99\u001b[0m \u001b[1;36m83\u001b[0m\n",
"weighted avg \u001b[1;36m0.99\u001b[0m \u001b[1;36m0.99\u001b[0m \u001b[1;36m0.99\u001b[0m \u001b[1;36m83\u001b[0m\n",
"\n"
]
},
"metadata": {},
"output_type": "display_data"
}
],
"source": [
"print(classification_report(test_output, llm_df.category))"
]
},
{
"cell_type": "markdown",
"metadata": {},
"source": [
"That will output a report that looks something like this:\n",
"\n",
"```\n",
" precision recall f1-score support\n",
"\n",
" Bar 1.00 1.00 1.00 2\n",
" Hotel 0.89 0.80 0.84 10\n",
" Other 0.96 0.96 0.96 57\n",
" Restaurant 0.87 0.93 0.90 14\n",
"\n",
" accuracy 0.94 83\n",
" macro avg 0.93 0.92 0.93 83\n",
"weighted avg 0.94 0.94 0.94 83\n",
"```\n",
"\n",
"At first, the report can be a bit overwhelming. What are all these technical terms?\n",
"\n",
"Precision measures what statistics nerds call \"positive predictive value.\" It's how often the model made the correct decision when it applied a category. For instance, in the \"Bar\" category, the LLM correctly predicted both of the bars in our supervised sample. That's a precision of 1.00. An analogy here is a baseball player's contact rate. Precision is a measure of how often the model connects with the ball when it swings its bat.\n",
"\n",
"Recall measures how many of the supervised instances were identified by the model. In this case, it shows that the LLM correctly spotted 80% of the hotels in our manual sample.\n",
"\n",
"The f1-score is a combination of precision and recall. It's a way to measure a model's overall performance by balancing the two.\n",
"\n",
"The support column shows how many instances of each category were in the supervised sample.\n",
"\n",
"The averages at the bottom combine the results for all categories. The macro row is a simple average all the scores in that column. The weighted row is a weighted average based on the number of instances in each category.\n",
"\n",
"In the example result provided above, we can see that the LLM was guessing correctly more than 90% of the time no matter how you slice it."
]
},
{
"cell_type": "markdown",
"metadata": {},
"source": [
"Another technique for evaluating classifiers is to visualize the results using a chart known as a confusion matrix. This chart shows how often the model correctly predicted each category and where it got things wrong.\n",
"\n",
"Drawing one up requires the `confusion_matrix` function from `sklearn` and an embarassing tangle of code from `seaborn` and `matplotlib` libraries. Most of it is boilerplate, but you need to punch your test variables, as well as the proper labels for the categories, in a few picky places."
]
},
{
"cell_type": "code",
"execution_count": null,
"metadata": {},
"outputs": [],
"source": [
"conf_mat = confusion_matrix(\n",
" test_output, # labels\n",
" llm_df.category, # labels\n",
" labels=llm_df.category.unique() # labels\n",
")\n",
"fig, ax = plt.subplots(figsize=(5,5))\n",
"sns.heatmap(\n",
" conf_mat,\n",
" annot=True,\n",
" fmt='d',\n",
" xticklabels=llm_df.category.unique(), # labels\n",
" yticklabels=llm_df.category.unique() # labels\n",
")\n",
"plt.ylabel('Actual')\n",
"plt.xlabel('Predicted')"
]
},
{
"cell_type": "markdown",
"metadata": {},
"source": [
"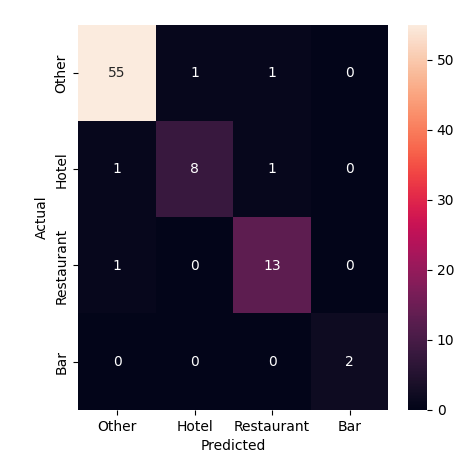\n",
"\n",
"The diagonal line of cells running from the upper left to the lower right shows where the model correctly predicted the category. The off-diagonal cells show where it got things wrong. The color of the cells indicates how often the model made that prediction. For instance, we can see that one miscategorized hotel in the sample was predicted to be a restaurant and the second was predicted to be \"Other.\"\n",
"\n",
"Due to the inherent randomness in the LLM's predictions, it's a good idea to test your sample and run these reports multiple times to get a sense of the model's performance."
]
},
{
"cell_type": "markdown",
"metadata": {},
"source": [
"Before we look at how you might improve the LLM's performance, let's take a moment to compare the results of this evaluation against the old school approach where the supervised sample is used to train a machine-learning model that doesn't have access to the ocean of knowledge poured into an LLM.\n",
"\n",
"This will require importing a mess of `sklearn` functions and classes. We'll use `TfidfVectorizer` to convert the payee text into a numerical representation that can be used by a `LinearSVC` classifier. We'll then use a `Pipeline` to chain the two together. If you have no idea what any of that means, don't worry. Now that we have LLMs in this world, you might never need to know."
]
},
{
"cell_type": "code",
"execution_count": null,
"metadata": {},
"outputs": [],
"source": [
"import json\n",
"from rich import print\n",
"import requests\n",
"from retry import retry\n",
"import pandas as pd\n",
"import seaborn as sns\n",
"import matplotlib.pyplot as plt\n",
"from sklearn.model_selection import train_test_split\n",
"from sklearn.metrics import confusion_matrix, classification_report\n",
"from sklearn.svm import LinearSVC # NEW\n",
"from sklearn.pipeline import Pipeline # NEW\n",
"from sklearn.compose import ColumnTransformer # NEW\n",
"from sklearn.feature_extraction.text import TfidfVectorizer # NEW"
]
},
{
"cell_type": "markdown",
"metadata": {},
"source": [
"Here's a simple example of how you might train and evaluate a traditional machine-learning model using the supervised sample.\n",
"\n",
"First you setup all the machinery."
]
},
{
"cell_type": "code",
"execution_count": null,
"metadata": {},
"outputs": [],
"source": [
"vectorizer = TfidfVectorizer(\n",
" sublinear_tf=True,\n",
" min_df=5,\n",
" norm='l2',\n",
" encoding='latin-1',\n",
" ngram_range=(1, 3),\n",
")\n",
"preprocessor = ColumnTransformer(\n",
" transformers=[\n",
" ('payee', vectorizer, 'payee')\n",
" ],\n",
" sparse_threshold=0,\n",
" remainder='drop'\n",
")\n",
"pipeline = Pipeline([\n",
" ('preprocessor', preprocessor),\n",
" ('classifier', LinearSVC(dual=\"auto\"))\n",
"])"
]
},
{
"cell_type": "markdown",
"metadata": {},
"source": [
"Then you train the model using those training sets we split out at the start."
]
},
{
"cell_type": "code",
"execution_count": null,
"metadata": {},
"outputs": [],
"source": [
"model = pipeline.fit(training_input, training_output)"
]
},
{
"cell_type": "markdown",
"metadata": {},
"source": [
"And you ask the model to use its training to predict the right answers for the test set."
]
},
{
"cell_type": "code",
"execution_count": null,
"metadata": {},
"outputs": [],
"source": [
"predictions = model.predict(test_input)"
]
},
{
"cell_type": "markdown",
"metadata": {},
"source": [
"Now, you can run the same evaluation code as before to see how the traditional model performed."
]
},
{
"cell_type": "code",
"execution_count": null,
"metadata": {},
"outputs": [],
"source": [
"print(classification_report(test_output, predictions))"
]
},
{
"cell_type": "markdown",
"metadata": {},
"source": [
"```\n",
" precision recall f1-score support\n",
"\n",
" Bar 0.00 0.00 0.00 2\n",
" Hotel 1.00 0.27 0.43 10\n",
" Other 0.75 1.00 0.85 57\n",
" Restaurant 0.80 0.29 0.42 14\n",
"\n",
" accuracy 0.76 83\n",
" macro avg 0.64 0.39 0.43 83\n",
"weighted avg 0.77 0.76 0.70 83\n",
"```"
]
},
{
"cell_type": "code",
"execution_count": null,
"metadata": {},
"outputs": [],
"source": [
"conf_mat = confusion_matrix(test_output, llm_df.category, labels=llm_df.category.unique())\n",
"fig, ax = plt.subplots(figsize=(5,5))\n",
"sns.heatmap(\n",
" conf_mat,\n",
" annot=True,\n",
" fmt='d',\n",
" xticklabels=llm_df.category.unique(),\n",
" yticklabels=llm_df.category.unique()\n",
")\n",
"plt.ylabel('Actual')\n",
"plt.xlabel('Predicted')"
]
},
{
"cell_type": "markdown",
"metadata": {},
"source": [
"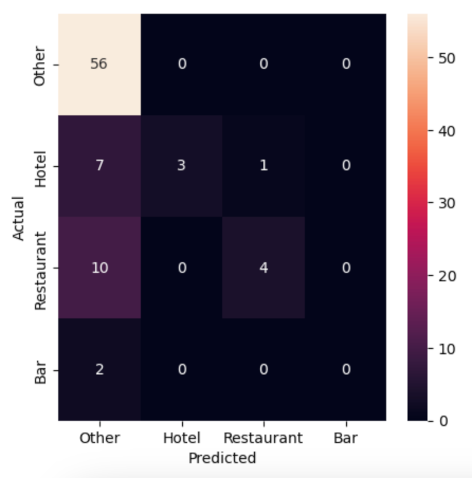\n",
"\n",
"Not great. The traditional model is guessing correctly about 75% of the time, but it's missing most cases of our \"Bar\", \"Hotel\" and \"Restaurant\" categories as almost everything is getting filed as \"Other.\" The LLM, on the other hand, is guessing correctly more than 90% of the time and flagging many of the rare categories that we're seeking to find in the haystack of data."
]
},
{
"cell_type": "markdown",
"metadata": {},
"source": [
"**[9. Improving prompts →](ch9-improving-prompts.ipynb)**"
]
},
{
"cell_type": "code",
"execution_count": null,
"metadata": {},
"outputs": [],
"source": []
}
],
"metadata": {
"kernelspec": {
"display_name": "Python 3 (ipykernel)",
"language": "python",
"name": "python3"
},
"language_info": {
"codemirror_mode": {
"name": "ipython",
"version": 3
},
"file_extension": ".py",
"mimetype": "text/x-python",
"name": "python",
"nbconvert_exporter": "python",
"pygments_lexer": "ipython3",
"version": "3.9.5"
}
},
"nbformat": 4,
"nbformat_minor": 4
}
|