Spaces:
Running
Running
File size: 36,575 Bytes
9ce63e3 |
1 2 3 4 5 6 7 8 9 10 11 12 13 14 15 16 17 18 19 20 21 22 23 24 25 26 27 28 29 30 31 32 33 34 35 36 37 38 39 40 41 42 43 44 45 46 47 48 49 50 51 52 53 54 55 56 57 58 59 60 61 62 63 64 65 66 67 68 69 70 71 72 73 74 75 76 77 78 79 80 81 82 83 84 85 86 87 88 89 90 91 92 93 94 95 96 97 98 99 100 101 102 103 104 105 106 107 108 109 110 111 112 113 114 115 116 117 118 119 120 121 122 123 124 125 126 127 128 129 130 131 132 133 134 135 136 137 138 139 140 141 142 143 144 145 146 147 148 149 150 151 152 153 154 155 156 157 158 159 160 161 162 163 164 165 166 167 168 169 170 171 172 173 174 175 176 177 178 179 180 181 182 183 184 185 186 187 188 189 190 191 192 193 194 195 196 197 198 199 200 201 202 203 204 205 206 207 208 209 210 211 212 213 214 215 216 217 218 219 220 221 222 223 224 225 226 227 228 229 230 231 232 233 234 235 236 237 238 239 240 241 242 243 244 245 246 247 248 249 250 251 252 253 254 255 256 257 258 259 260 261 262 263 264 265 266 267 268 269 270 271 272 273 274 275 276 277 278 279 280 281 282 283 284 285 286 287 288 289 290 291 292 293 294 295 296 297 298 299 300 301 302 303 304 305 306 307 308 309 310 311 312 313 314 315 316 317 318 319 320 321 322 323 324 325 326 327 328 329 330 331 332 333 334 335 336 337 338 339 340 341 342 343 344 345 346 347 348 349 350 351 352 353 354 355 356 357 358 359 360 361 362 363 364 365 366 367 368 369 370 371 372 373 374 375 376 377 378 379 380 381 382 383 384 385 386 387 388 389 390 391 392 393 394 395 396 397 398 399 400 401 402 403 404 405 406 407 408 409 410 411 412 413 414 415 416 417 418 419 420 421 422 423 424 425 426 427 428 429 430 431 432 433 434 435 436 437 438 439 440 441 442 443 444 445 446 447 448 449 450 451 452 453 454 455 456 457 458 459 460 461 462 463 464 465 466 467 468 469 470 471 472 473 474 475 476 477 478 479 480 481 482 483 484 485 486 487 488 489 490 491 492 493 494 495 496 497 498 499 500 501 502 503 504 505 506 507 508 509 510 511 512 513 514 515 516 517 518 519 520 521 522 523 524 525 526 527 528 529 530 531 532 533 534 535 536 537 538 539 540 541 542 543 544 545 546 547 548 549 550 551 552 553 554 555 556 557 558 559 560 561 562 563 564 565 566 567 568 569 570 571 572 573 574 575 576 577 578 579 580 581 582 583 584 585 586 587 588 589 590 591 592 593 594 595 |
# GPT_Vuln-analyzer
This is a Proof Of Concept application that demostrates how AI can be used to generate accurate results for vulnerability analysis and also allows further utilization of the already super useful ChatGPT made using openai-api, python-nmap, dnsresolver python modules and also use customtkinter and tkinter for the GUI version of the code. This project also has a CLI and a GUI interface, It is capable of doing network vulnerability analysis, DNS enumeration and also subdomain enumeration.
## Requirements
- Python 3.10 or above
- All the packages mentioned in the requirements.txt file
- OpenAI API
- Bard API (MakerSuite Palm)
- HuggingFace token (with llama2 access )
- IPGeolocation API
- Wireshark and tshark (both added to path)
## Usage Package
### Import packages
```bash
cd package && pip3/pip install .
```
Simple import any of the 3 packages and then add define the variables accordingly
```python
from GVA.scanner import NetworkScanner
from GVA.dns_recon import DNSRecon
from GVA.geo import geo_ip_recon
from GVA.jwt import JWTAnalyzer
from GVA.menus import Menus
from GVA.packet_analysis import PacketAnalysis
from GVA.ai_models import NMAP_AI_MODEL
from GVA.ai_models import DNS_AI_MODEL
from GVA.ai_models import JWT_AI_MODEL
from GVA.assets import Assets
from GVA.subdomain import sub_enum
from GVA import gui
# The components defined
dns_enum = DNSRecon()
geo_ip = geo_ip_recon()
p_ai_models = NMAP_AI_MODEL()
dns_ai_models = DNS_AI_MODEL()
port_scanner = NetworkScanner()
jwt_analizer = JWTAnalyzer()
sub_recon = sub_enum()
asset_codes = Assets()
packet_analysis = PacketAnalysis()
# KEEP IT BLANK IF YOU HAVE NO CLUE THE MENU WILL ASK TO FILL IT ONCE ACTIVE
lkey = "LLAMA API KEY"
lendpoint = "LLAMA ENDPOINT"
keyset = "AI API KEY"
output_loc = "OUTPUT LOCATION FOR PCAP"
threads = 200 # Default INT 200 but can be increased.
target_ip_hostname_or_token = "TARGET IP, HOSTNAME OR TOKEN"
profile_num = "PROFILE FOR NMAP SCAN"
ai_set = "AI OF CHOICE"
akey_set = "OPENAI API KEY"
bkey_set = "BARD API KEY"
ai_set_args = "" # Keep it blank at any cost
llamakey = "LLAMA RUNPOD API KEY"
llamaendpoint = "LLAMA RUNPOD ENDPOINT"
Menus(
lamma_key=lkey,
llama_api_endpoint=lendpoint,
initial_keyset=keyset,
threads=threads,
output_loc=output_loc,
target=target_ip_hostname,
profile_num=profile_num,
ai_set=ai_set,
openai_akey_set=akey_set,
bard_key_set=bkey_set,
ai_set_args=ai_set_args,
llama_runpod_key=llamakey,
llama_endpoint=llamaendpoint
)
gui.application()
```
## Usage CLI
- First Change the "OPENAI_API_KEY", "GEOIP_API_KEY" and "BARD_API_KEY" part of the code with OpenAI api key and the IPGeolocation API key in the `.env` file
- For the `llama-api` option or specific the llama runpod serverless endpoint deployment option requires you to enter the `serverless endpoint ID` from runpod and also your `RUNPOD API KEY`
```python
GEOIP_API_KEY = ''
OPENAI_API_KEY = ''
BARD_API_KEY = ''
RUNPOD_ENDPOINT_ID = ''
RUNPOD_API_KEY = ''
```
- second install the packages
```bash
pip3 install -r requirements.txt
or
pip install -r requirements.txt
```
- run the code python3 gpt_vuln.py
```bash
# Regular Help Menu
python gpt_vuln.py --help
# Rich Help Menu
python gpt_vuln.py --r help
# Specify target with the attack
python gpt_vuln.py --target <IP/hostname/token> --attack dns/nmap/jwt
# Specify target and profile for nmap
python gpt_vuln.py --target <IP/hostname/token> --attack nmap --profile <1-13>
(Default:1)
# Specify target for DNS no profile needed
python gpt_vuln.py --target <IP/hostname/token> --attack dns
# Specify target for Subdomain Enumeration no profile used default list file
python gpt_vuln.py --target <HOSTNAME> --attack sub
# Specify target for Subdomain Enumeration no profile used custom list file
python gpt_vuln.py --target <HOSTNAME> --attack sub --list <PATH to FILE>
# Specify target for geolocation lookup
python gpt_vuln.py --target <IP> --attack geo
# Specify PCAP file for packet analysis
python gpt_vuln.py --target <PCAP FILE> --attack pcap --output <OUTPUT FILE LOCATION> --thread NUM of threads <200:default>
# Specify the AI to be used for nmap
python gpt_vuln.py --target <IP> --attack nmap --profile <1-5> --ai llama /llama-api /bard / openai <default>
# Specify the AI to be used for dns
python gpt_vuln.py --target <IP> --attack dns --ai llama /llama-api /bard / openai <default>
# Specify the AI to be used for JWT analysis
python gpt_vuln.py --target <token> --attack jwt --ai llama /llama-api /bard / openai <default>
# Interactive step by step cli interface
python gpt_vuln.py --menu True
```
#### CLI Interface Option
```bash
________________________
| GVA Usage in progress... |
========================
\
\
^__^
(oo)\_______
(__)\ )\/\
||----w |
|| ||
โโโโโโโโโโโณโโโโโโโโโโโโโโโโโ
โ Options โ Utility โ
โกโโโโโโโโโโโโโโโโโโโโโโโโโโโฉ
โ 1 โ Nmap Enum โ
โ 2 โ DNS Enum โ
โ 3 โ Subdomain Enum โ
โ 4 โ GEO-IP Enum โ
| 5 | JWT Analysis |
| 6 | PCAP Analysis |
โ q โ Quit โ
โโโโโโโโโโโดโโโโโโโโโโโโโโโโโ
Enter your choice:
```
The CLI interface has a few things to note.
- The API keys must be provided manually.
- The ones defined in the `.env` files work with the args options
- The process is similar but more organized.
### My views on Bard
Its same as Openai GPT3.5 but faster. It can generate the same answer but in 2 times the speed.
### OS Supported
| Preview | Code | Name | Working Status | OpenAI Status | Bard Status | LLama2 Status |
| -------------------------------------------------------------------------------------------------------------------- | ---- | --------- | -------------- | ------------- | ----------- | ----------------- |
| ") | LIN | GNU/Linux | โ
| โ
| โ
| โ [did not test] |
| ") | WIN | Windows | โ
| โ
| โ
| โ
|
## Understanding the code
Profiles:
| Parameter | Return data | Description | Nmap Command |
| :-------- | :---------- | :--------------------------------------------------- | :---------------------------------------------------- |
| `p1` | `json` | Effective Scan | `-Pn -sV -T4 -O -F` |
| `p2` | `json` | Simple Scan | `-Pn -T4 -A -v` |
| `p3` | `json` | Low Power Scan | `-Pn -sS -sU -T4 -A -v` |
| `p4` | `json` | Partial Intense Scan | `-Pn -p- -T4 -A -v` |
| `p5` | `json` | Complete Intense Scan | `-Pn -sS -sU -T4 -A -PE -PP -PY -g 53 --script=vuln` |
| `p6` | `json` | Comprehensive Service Version Detection | `-Pn -sV -p- -A` |
| `p7` | `json` | Aggressive Scan with OS Detection | `-Pn -sS -sV -O -T4 -A` |
| `p8` | `json` | Script Scan for Common Vulnerabilities | `-Pn -sC` |
| `p9` | `json` | Intense Scan, All TCP Ports | `-Pn -p 1-65535 -T4 -A -v` |
| `p10` | `json` | UDP Scan | `-Pn -sU -T4` |
| `p11` | `json` | Service and Version Detection for Top Ports | `-Pn -sV --top-ports 100` |
| `p12` | `json` | Aggressive Scan with NSE Scripts for Vulnerabilities | `-Pn -sS -sV -T4 --script=default,discovery,vuln` |
| `p13` | `json` | Fast Scan for Common Ports | `-Pn -F` |
The profile is the type of scan that will be executed by the nmap subprocess. The Ip or target will be provided via argparse. At first, the custom nmap scan is run which has all the crucial arguments for the scan to continue. Next, the scan data is extracted from the huge pile of data driven by nmap. the "scan" object has a list of sub-data under "tcp" each labelled according to the ports opened. once the data is extracted the data is sent to the openai API Davinci model via a prompt. the prompt specifically asks for a JSON output and the data also to be used in a certain manner.
The entire structure of request that has to be sent to the openai API is designed in the completion section of the Program.
```python
class NetworkScanner():
def scanner(self, AIModels, ip: Optional[str], profile: int, akey: Optional[str], bkey: Optional[str], lkey, lendpoint, AI: str) -> str:
profile_arguments = {
1: '-Pn -sV -T4 -O -F',
2: '-Pn -T4 -A -v',
3: '-Pn -sS -sU -T4 -A -v',
4: '-Pn -p- -T4 -A -v',
5: '-Pn -sS -sU -T4 -A -PE -PP -PY -g 53 --script=vuln',
6: '-Pn -sV -p- -A',
7: '-Pn -sS -sV -O -T4 -A',
8: '-Pn -sC',
9: '-Pn -p 1-65535 -T4 -A -v',
10: '-Pn -sU -T4',
11: '-Pn -sV --top-ports 100',
12: '-Pn -sS -sV -T4 --script=default,discovery,vuln',
13: '-Pn -F'
}
# The scanner with GPT Implemented
nm.scan('{}'.format(ip), arguments='{}'.format(profile_arguments.get(profile)))
json_data = nm.analyse_nmap_xml_scan()
analyze = json_data["scan"]
match AI:
case 'openai':
try:
if akey is not None:
pass
else:
raise ValueError("KeyNotFound: Key Not Provided")
response = AIModels.GPT_AI(akey, analyze)
except KeyboardInterrupt:
print("Bye")
quit()
case 'bard':
try:
if bkey is not None:
pass
else:
raise ValueError("KeyNotFound: Key Not Provided")
response = AIModels.BardAI(bkey, analyze)
except KeyboardInterrupt:
print("Bye")
quit()
case 'llama':
try:
response = AIModels.Llama_AI(analyze, "local", lkey, lendpoint)
except KeyboardInterrupt:
print("Bye")
quit()
case 'llama-api':
try:
response = AIModels.Llama_AI(analyze, "runpod", lkey, lendpoint)
except KeyboardInterrupt:
print("Bye")
quit()
self.response = response
text = str(self.response)
return text
```
# Regex
We use Regex to extract only the important information from the custom prompt provided this reduces the total amount of unwanted
data
```python
def extract_data(json_string):
# Define the regular expression patterns for individual values
critical_score_pattern = r'"critical score": \["(.*?)"\]'
os_information_pattern = r'"os information": \["(.*?)"\]'
open_ports_pattern = r'"open ports": \["(.*?)"\]'
open_services_pattern = r'"open services": \["(.*?)"\]'
vulnerable_service_pattern = r'"vulnerable service": \["(.*?)"\]'
found_cve_pattern = r'"found cve": \["(.*?)"\]'
# Initialize variables for extracted data
critical_score = None
os_information = None
open_ports = None
open_services = None
vulnerable_service = None
found_cve = None
# Extract individual values using patterns
match = re.search(critical_score_pattern, json_string)
if match:
critical_score = match.group(1)
match = re.search(os_information_pattern, json_string)
if match:
os_information = match.group(1)
match = re.search(open_ports_pattern, json_string)
if match:
open_ports = match.group(1)
match = re.search(open_services_pattern, json_string)
if match:
open_services = match.group(1)
match = re.search(vulnerable_service_pattern, json_string)
if match:
vulnerable_service = match.group(1)
match = re.search(found_cve_pattern, json_string)
if match:
found_cve = match.group(1)
# Create a dictionary to store the extracted data
data = {
"critical score": critical_score,
"os information": os_information,
"open ports": open_ports,
"open services": open_services,
"vulnerable service": vulnerable_service,
"found cve": found_cve
}
# Convert the dictionary to JSON format
json_output = json.dumps(data)
return json_output
def AI(key: str, data: Any) -> str:
openai.api_key = key
try:
prompt = f"""
Do a NMAP scan analysis on the provided NMAP scan information
The NMAP output must return in a JSON format accorging to the provided
output format. The data must be accurate in regards towards a pentest report.
The data must follow the following rules:
1) The NMAP scans must be done from a pentester point of view
2) The final output must be minimal according to the format given.
3) The final output must be kept to a minimal.
4) If a value not found in the scan just mention an empty string.
5) Analyze everything even the smallest of data.
The output format:
{{
"critical score": [""],
"os information": [""],
"open ports": [""],
"open services": [""],
"vulnerable service": [""],
"found cve": [""]
}}
NMAP Data to be analyzed: {data}
"""
# A structure for the request
completion = openai.Completion.create(
engine=model_engine,
prompt=prompt,
max_tokens=1024,
n=1,
stop=None,
)
response = completion.choices[0].text
return extract_data(str(response))
except KeyboardInterrupt:
print("Bye")
quit()
```
The AI code defines an output format and commands the AI to follow a few pre-determined rules to increase accuracy.
The regex extraction code does the extraction and further the main function arranges them into tables.
## Using Bard AI
For you to use Bard AI you must signup to the MakerSuit Palm API for developer access and generate your API key from there. For links and how this works you can use this video [MakerSuit](https://www.youtube.com/watch?v=Ce1AOchQMzA&t=128s)
Once the API is acquired just add it to the `.env` file and you are good to go.
## Using LLama2 AI
Using LLama2 is one of the best offline and free options out there. It is currently under improvement I am working on a prompt that will better incorporate cybersecurity perspective into the AI.
I have to thank **@thisserand** and his [llama2_local](https://github.com/thisserand/llama2_local) repo and also his YT video [YT_Video](https://youtu.be/WzCS8z9GqHw). They were great resources. To be frank the llama2 code is 95% his, I just yanked the code and added a Flask API functionality to it.
The Accuracy of the AI offline and outside the codes test was great and had equal accuracy to openai or bard but while in code it was facing a few issues may be because of the prompting and all. I will try and fix it.
The speed depends on your system and the GPU and CPU configs you have. currently, it is using the `TheBloke/Llama-2-7B-Chat-GGML` model and can be changed via the `portscanner` and `dnsrecon` files.
For now, the llama code and scans are handled differently. After a few tests, I found out llama needs to be trained a little to operate like how I intended it to work so it needs some time. Any suggestions on how I can do that can be added to the discussions of this repo [Discussions Link](https://github.com/morpheuslord/GPT_Vuln-analyzer/discussions). For now, the output won't be a divided list of all the data instead will be an explanation of the vulnerability or issues discovered by the AI.
### Output
#### JWT Output:
```
GVA Report for JWT
โโโโโโโโโโโโโโโโโโโโโโโณโโโโโโโโโโโโโโโโโโโโโโโโโโโโโโโโโโโโโโโโโโโโโโโโโโโโโโโโโโโโโโโโโโโโโโโโโโโโโโโโโโโ
โ Variables โ Results โ
โกโโโโโโโโโโโโโโโโโโโโโโโโโโโโโโโโโโโโโโโโโโโโโโโโโโโโโโโโโโโโโโโโโโโโโโโโโโโโโโโโโโโโโโโโโโโโโโโโโโโโโโโโโฉ
โ Algorithm Used โ HS256 โ
โ Header โ eyJhbGciOiAiSFMyNTYiLCAidHlwIjogIkpXVCJ9 โ
โ Payload โ eyJzdWIiOiAiMTIzNDU2Nzg5MCIsICJuYW1lIjogIkpvaG4gRG9lIiwgImlhdCI6IDE1MTYyMzkwMjJ9 โ
โ Signature โ โ
โ PossibleAttacks โ None identified โ
โ VulnerableEndpoints โ Unable to determine without additional information โ
โโโโโโโโโโโโโโโโโโโโโโโดโโโโโโโโโโโโโโโโโโโโโโโโโโโโโโโโโโโโโโโโโโโโโโโโโโโโโโโโโโโโโโโโโโโโโโโโโโโโโโโโโโโ
```
#### Nmap output:
##### OpenAI and Bard:
```table
โโโโโโโโโโโโโโโโโโโโโโณโโโโโโโโโโโโโโโโโโโโโโโโโโโโโโโโโโโโโโโโโโโโโโโโโโโโโโ
โ Elements โ Results โ
โกโโโโโโโโโโโโโโโโโโโโโโโโโโโโโโโโโโโโโโโโโโโโโโโโโโโโโโโโโโโโโโโโโโโโโโโโโโโฉ
โ critical score โ High โ
โ os information โ Microsoft Windows 11 21H2 โ
โ open ports โ 80, 22, 445, 902, 912 โ
โ open services โ http, ssh, microsoft-ds, vmware-auth, vmware-auth โ
โ vulnerable service โ OpenSSH โ
โ found cve โ CVE-2023-28531 โ
โโโโโโโโโโโโโโโโโโโโโโดโโโโโโโโโโโโโโโโโโโโโโโโโโโโโโโโโโโโโโโโโโโโโโโโโโโโโโ
```
##### LLama2
```table
โญโโโโโโโโโโโโโโโโโโโโโโโโโโโโโโโโโโโโโโโโโโโโโ The GVA LLama2 โโโโโโโโโโโโโโโโโโโโโโโโโโโโโโโโโโโโโโโโโโโโโโโฎ
โ โ
โ โ
โ โ
โ Based on the provided NMAP data, I have conducted a thorough analysis of the target system's open ports โ
โ and services, vulnerabilities, and operating system information. Here is my findings: Critical Score: โ
โ The critical score for this target system is 7 out of 10. The system has several open ports that could โ
โ potentially be exploited, including port 80 (HTTP), port 135 (RPC), and port 445 (Microsoft DS). While โ
โ These ports are not necessarily vulnerable, they do indicate that the system is running services that โ
โ could be targeted by attackers. Additionally, the system has an outdated version of Microsoft IIS โ
โ running on port 80, which could be a potential vulnerability. OS Information: The target system is โ
โ running Microsoft Windows 10 1607. Open Ports and Services: The target system has the following open โ
โ ports: โ
โ โ
โ โข Port 80: HTTP (Microsoft IIS httpd) โ
โ โข Port 135: RPC (Microsoft Windows RPC) โ
โ โข Port 445: Microsoft DS โ
โ โข Port 8000: Splunkd httpd All of these ports are currently open and have a state of "open". โ
โ Vulnerable Services: Based on the CVEs found in the NMAP data, there are several potential โ
โ vulnerabilities in the target system's services. These include: โ
โ โข CVE-2019-1489: An elevation of privilege vulnerability in Microsoft IIS that could be exploited by โ
โ an attacker to gain control of the system. This vulnerability is related to the outdated version of โ
โ Microsoft IIS running on port 80. โ
โ โข CVE-2017-0143: A remote code execution vulnerability in Microsoft Windows RPC that could be โ
โ exploited by an attacker to execute arbitrary code on the target system. This vulnerability is โ
โ related to the outdated version of Microsoft Windows RPC running on port 135. โ
โ โข CVE-2020-1362: A remote code execution vulnerability in Microsoft DS that could be exploited by an โ
โ attacker to execute arbitrary code on the target system. This vulnerability is related to the โ
โ outdated version of Microsoft DS running on port 445. Found CVEs: The following C โ
โ โ
โฐโโโโโโโโโโโโโโโโโโโโโโโโโโโโโโโโโโโโโโโโโโโโโโโโโโโโโโโโโโโโโโโโโโโโโโโโโโโโโโโโโโโโโโโโโโโโโโโโโโโโโโโโโโโโฏ
```
#### DNS Output:
target is jainuniversity.ac.in
```table
โโโโโโโโโโโโณโโโโโโโโโโโโโโโโโโโโโโโโโโโโโโโโโโโโโโโโโโโโโโโโโโโโโโโโโโโโโโโโโโโโโโโโโโโโโโโโโโโโโโโโโโโโโโโโโโโโโโโโโโโโโโโโโโโโ
โ Elements โ Results โ
โกโโโโโโโโโโโโโโโโโโโโโโโโโโโโโโโโโโโโโโโโโโโโโโโโโโโโโโโโโโโโโโโโโโโโโโโโโโโโโโโโโโโโโโโโโโโโโโโโโโโโโโโโโโโโโโโโโโโโโโโโโโโโโโโฉ
โ A โ 172.67.147.95", "104.21.41.132 โ
โ AAA โ โ
โ NS โ mia.ns.cloudflare.com.","paul.ns.cloudflare.com. โ
โ MX โ 30 aspmx5.googlemail.com.","30 aspmx4.googlemail.com.","20 alt2.aspmx.l.google.com.","30 โ
โ โ aspmx3.googlemail.com.","30 aspmx2.googlemail.com.","20 alt1.aspmx.l.google.com.","10 aspmx.l.google.com. โ
โ PTR โ โ
โ SOA โ mia.ns.cloudflare.com. dns.cloudflare.com. 2309618668 10000 2400 604800 3600 โ
โ TXT โ atlassian-sending-domain-verification=5b358ce4-5ad3-404d-b4b4-005bf933603b","include:_spf.atlassian.net โ
โโโโโโโโโโโโดโโโโโโโโโโโโโโโโโโโโโโโโโโโโโโโโโโโโโโโโโโโโโโโโโโโโโโโโโโโโโโโโโโโโโโโโโโโโโโโโโโโโโโโโโโโโโโโโโโโโโโโโโโโโโโโโโโโโ
```
#### GEO Location output:
```table
โโโโโโโโโโโโโโโโโโโโโโโโโโโโโโโณโโโโโโโโโโโโโโโโโโโโโโโโโโโโโโโโโโโโโโโโโโโโโโโโโโโโโโโโโโโโโโโโโโโโโโโโโโ
โ Identifiers โ Data โ
โกโโโโโโโโโโโโโโโโโโโโโโโโโโโโโโโโโโโโโโโโโโโโโโโโโโโโโโโโโโโโโโโโโโโโโโโโโโโโโโโโโโโโโโโโโโโโโโโโโโโโโโโโฉ
โ ip โ โโโโโโโโโโโโโ โ
โ continent_code โ AS โ
โ continent_name โ Asia โ
โ country_code2 โ IN โ
โ country_code3 โ IND โ
โ country_name โ India โ
โ country_capital โ New Delhi โ
โ state_prov โ Haryana โ
โ state_code โ IN-HR โ
โ district โ โ
โ city โ Gurugram โ
โ zipcode โ 122003 โ
โ latitude โ 28.44324 โ
โ longitude โ 77.05501 โ
โ is_eu โ False โ
โ calling_code โ +91 โ
โ country_tld โ .in โ
โ languages โ en-IN,hi,bn,te,mr,ta,ur,gu,kn,ml,or,pa,as,bh,sat,ks,ne,sd,kok,doi,mni,โฆ โ
โ country_flag โ https://ipgeolocation.io/static/flags/in_64.png โ
โ geoname_id โ 9148991 โ
โ isp โ Bharti Airtel Limited โ
โ connection_type โ โ
โ organization โ Bharti Airtel Limited โ
โ currency.code โ INR โ
โ currency.name โ Indian Rupee โ
โ currency.symbol โ โน โ
โ time_zone.name โ Asia/Kolkata โ
โ time_zone.offset โ 5.5 โ
โ time_zone.current_time โ 2023-07-11 17:08:35.057+0530 โ
โ time_zone.current_time_unix โ 1689075515.057 โ
โ time_zone.is_dst โ False โ
โ time_zone.dst_savings โ 0 โ
โโโโโโโโโโโโโโโโโโโโโโโโโโโโโโโดโโโโโโโโโโโโโโโโโโโโโโโโโโโโโโโโโโโโโโโโโโโโโโโโโโโโโโโโโโโโโโโโโโโโโโโโโโ
```
#### PCAP OUTPUT
```
Collecting Json Data
Extracting IP details...
Extracting DNS details...
Extracting EAPOL details...
Extracting TCP STREAMS details...
TCP streams can take some time..
Total Streams combination: 252
Number of workers in progress: 250
Completed
GVA Report for PCAP
โโโโโโโโโโโโโโโโโโโโโโโโโโโโโโโโโโโโโโณโโโโโโโโโโโโโโโโโโโโโโโโโโโโโโโโโโโโโโโโโโโโโโโโโโโโโโโโโโโโโโโโโโโโโโโโโโโโโโโโโโโโโโโโโโโโโโโโโโโโโ
โ Identifiers โ Data โ
โกโโโโโโโโโโโโโโโโโโโโโโโโโโโโโโโโโโโโโโโโโโโโโโโโโโโโโโโโโโโโโโโโโโโโโโโโโโโโโโโโโโโโโโโโโโโโโโโโโโโโโโโโโโโโโโโโโโโโโโโโโโโโโโโโโโโโโโโโโโฉ
โ PacketAnalysis.Services โ ['49943', '49958', '49934', '49944', '49931', '443', '49957'] โ
โ PacketAnalysis.TCP Streams โ ['1', '4', '5', '2', '0', '3'] โ
โ PacketAnalysis.Sources Address โ ['โโโโโโโโโโโโโ', '1.1.1.1', 'โโโโโโโโโโโโโ', 'โโโโโโโโโโโโโ', 'โโโโโโโโโโโโโ', 'โโโโโโโโโโโโโ'] โ
โ PacketAnalysis.Destination Address โ ['โโโโโโโโโโโโโ', '1.1.1.1', 'โโโโโโโโโโโโโ', 'โโโโโโโโโโโโโ', 'โโโโโโโโโโโโโ', 'โโโโโโโโโโโโโ'] โ
โ PacketAnalysis.DNS Resolved โ [] โ
โ PacketAnalysis.DNS Query โ ['oneclient.sfx.ms'] โ
โ PacketAnalysis.DNS Response โ ['oneclient.sfx.ms.edgekey.net', 'e9659.dspg.akamaiedge.net', 'oneclient.sfx.ms'] โ
โ PacketAnalysis.EAPOL Data โ [] โ
โ PacketAnalysis. Total Streams Data โ 126 โ
โโโโโโโโโโโโโโโโโโโโโโโโโโโโโโโโโโโโโโดโโโโโโโโโโโโโโโโโโโโโโโโโโโโโโโโโโโโโโโโโโโโโโโโโโโโโโโโโโโโโโโโโโโโโโโโโโโโโโโโโโโโโโโโโโโโโโโโโโโโโ
```
# Usage GUI
The GUI uses customtkinter for the running of the code. The interface is straight forward the only thing required to remember is:
- When using dns attack dont specify the profile
```bash
python GVA_gui.py
```
### main window
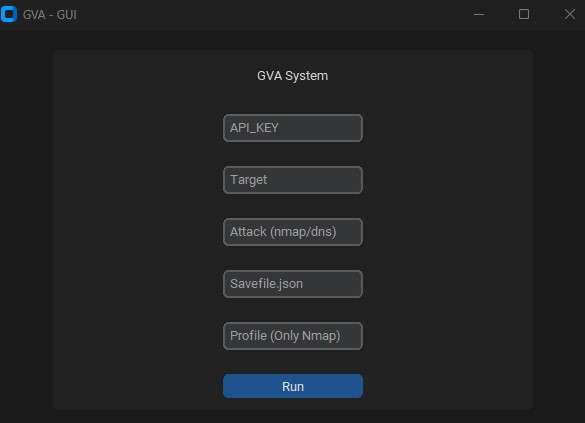
### output_DNS
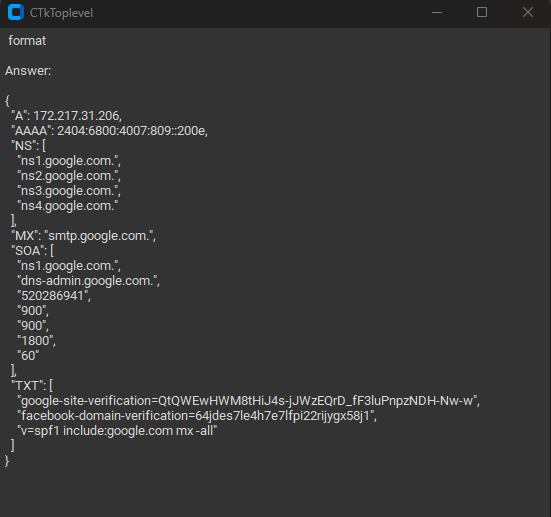
### output_nmap
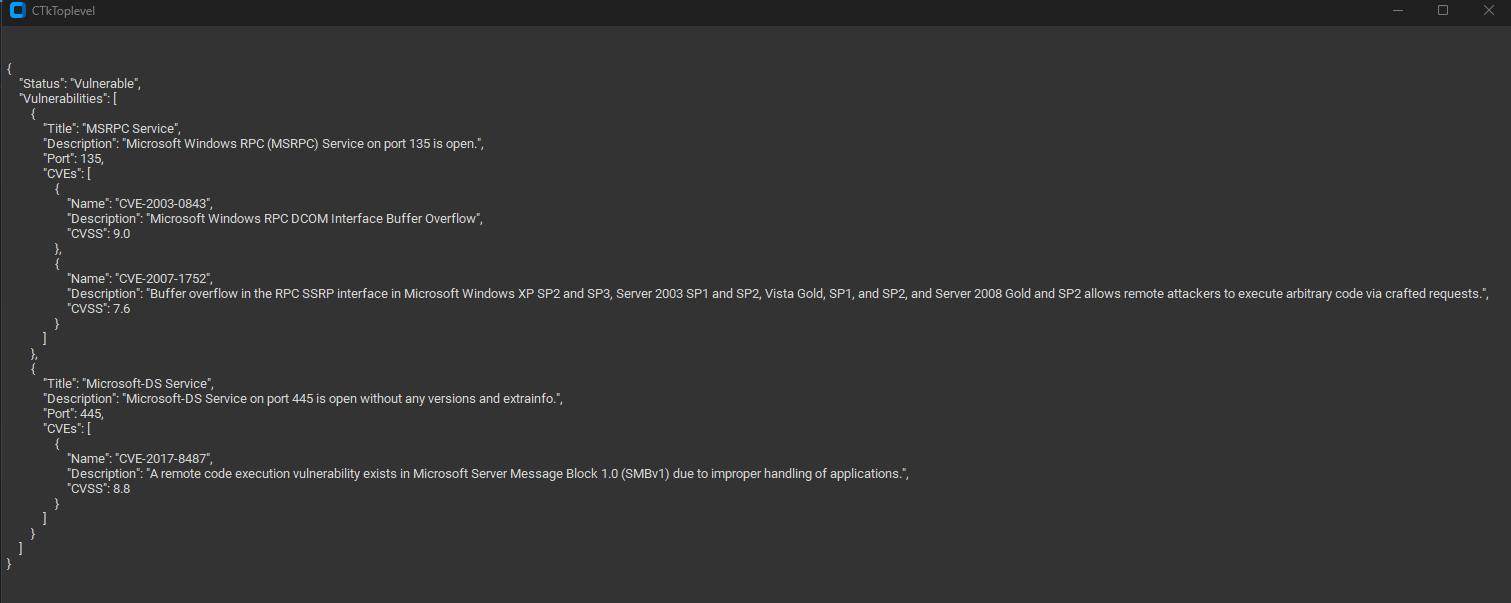
### oytput_geo

## Advantage
- Can be used in developing a more advanced systems completly made of the API and scanner combination
- Has the capability to analize DNS information and reslove Mustiple records it a more better format.
- Can increase the effectiveness of the final system
- Can also perform subdomain enumeration
- Highly productive when working with models such as GPT3
|