text
stringlengths 7
328k
| id
stringlengths 14
166
| metadata
dict | __index_level_0__
int64 0
459
|
---|---|---|---|
{
"details": {
"best_of_sequences": null,
"finish_reason": "length",
"generated_tokens": 10,
"prefill": [
{
"id": 1,
"logprob": null,
"text": "<s>"
},
{
"id": 4321,
"logprob": -9.84375,
"text": "Test"
},
{
"id": 2009,
"logprob": -9.6015625,
"text": "request"
}
],
"seed": 0,
"tokens": [
{
"id": 29899,
"logprob": -1.5625,
"special": false,
"text": "-"
},
{
"id": 1454,
"logprob": -0.20410156,
"special": false,
"text": "for"
},
{
"id": 29899,
"logprob": 0.0,
"special": false,
"text": "-"
},
{
"id": 9342,
"logprob": 0.0,
"special": false,
"text": "comment"
},
{
"id": 29901,
"logprob": 0.0,
"special": false,
"text": ":"
},
{
"id": 396,
"logprob": -0.27685547,
"special": false,
"text": " #"
},
{
"id": 29906,
"logprob": -0.4970703,
"special": false,
"text": "2"
},
{
"id": 29900,
"logprob": -0.80615234,
"special": false,
"text": "0"
},
{
"id": 29896,
"logprob": 0.0,
"special": false,
"text": "1"
},
{
"id": 29955,
"logprob": -1.0751953,
"special": false,
"text": "7"
}
],
"top_tokens": null
},
"generated_text": "Test request-for-comment: #2017"
}
| text-generation-inference/integration-tests/models/__snapshots__/test_flash_llama_gptq/test_flash_llama_gptq_all_params.json/0 | {
"file_path": "text-generation-inference/integration-tests/models/__snapshots__/test_flash_llama_gptq/test_flash_llama_gptq_all_params.json",
"repo_id": "text-generation-inference",
"token_count": 1035
} | 206 |
{
"details": {
"best_of_sequences": null,
"finish_reason": "length",
"generated_tokens": 10,
"prefill": [
{
"id": 2271,
"logprob": null,
"text": "Test"
},
{
"id": 1681,
"logprob": -8.8515625,
"text": " request"
}
],
"seed": 0,
"tokens": [
{
"id": 311,
"logprob": -1.4277344,
"special": false,
"text": " to"
},
{
"id": 279,
"logprob": -0.65478516,
"special": false,
"text": " the"
},
{
"id": 2473,
"logprob": -1.8300781,
"special": false,
"text": " service"
},
{
"id": 382,
"logprob": -0.75,
"special": false,
"text": ".\n\n"
},
{
"id": 286,
"logprob": -0.11621094,
"special": false,
"text": " "
},
{
"id": 549,
"logprob": 0.0,
"special": false,
"text": " :"
},
{
"id": 689,
"logprob": -0.48608398,
"special": false,
"text": "return"
},
{
"id": 25,
"logprob": 0.0,
"special": false,
"text": ":"
},
{
"id": 5949,
"logprob": -0.5756836,
"special": false,
"text": " Response"
},
{
"id": 504,
"logprob": -0.24499512,
"special": false,
"text": " from"
}
],
"top_tokens": null
},
"generated_text": "Test request to the service.\n\n :return: Response from"
}
| text-generation-inference/integration-tests/models/__snapshots__/test_flash_qwen2/test_flash_qwen2_all_params.json/0 | {
"file_path": "text-generation-inference/integration-tests/models/__snapshots__/test_flash_qwen2/test_flash_qwen2_all_params.json",
"repo_id": "text-generation-inference",
"token_count": 999
} | 207 |
{
"details": {
"best_of_sequences": null,
"finish_reason": "length",
"generated_tokens": 10,
"prefill": [],
"seed": null,
"tokens": [
{
"id": 187,
"logprob": -0.37890625,
"special": false,
"text": "\n"
},
{
"id": 187,
"logprob": -0.26953125,
"special": false,
"text": "\n"
},
{
"id": 30763,
"logprob": -1.1953125,
"special": false,
"text": "Deep"
},
{
"id": 4715,
"logprob": -0.53515625,
"special": false,
"text": " learning"
},
{
"id": 310,
"logprob": -0.625,
"special": false,
"text": " is"
},
{
"id": 247,
"logprob": -0.6796875,
"special": false,
"text": " a"
},
{
"id": 747,
"logprob": -2.0,
"special": false,
"text": " new"
},
{
"id": 1511,
"logprob": -2.3125,
"special": false,
"text": " type"
},
{
"id": 273,
"logprob": -0.0028533936,
"special": false,
"text": " of"
},
{
"id": 5145,
"logprob": -1.265625,
"special": false,
"text": " machine"
}
],
"top_tokens": null
},
"generated_text": "\n\nDeep learning is a new type of machine"
}
| text-generation-inference/integration-tests/models/__snapshots__/test_mamba/test_mamba.json/0 | {
"file_path": "text-generation-inference/integration-tests/models/__snapshots__/test_mamba/test_mamba.json",
"repo_id": "text-generation-inference",
"token_count": 860
} | 208 |
{
"choices": [
{
"finish_reason": "eos_token",
"index": 0,
"logprobs": null,
"message": {
"content": null,
"name": null,
"role": "assistant",
"tool_calls": {
"function": {
"description": null,
"name": "tools",
"parameters": {
"format": "celsius",
"location": "New York, NY",
"num_days": 14
}
},
"id": 0,
"type": "function"
}
},
"usage": null
}
],
"created": 1709079492,
"id": "",
"model": "TinyLlama/TinyLlama-1.1B-Chat-v1.0",
"object": "text_completion",
"system_fingerprint": "1.4.3-native",
"usage": {
"completion_tokens": 29,
"prompt_tokens": 316,
"total_tokens": 345
}
}
| text-generation-inference/integration-tests/models/__snapshots__/test_tools_llama/test_flash_llama_grammar_tools_auto.json/0 | {
"file_path": "text-generation-inference/integration-tests/models/__snapshots__/test_tools_llama/test_flash_llama_grammar_tools_auto.json",
"repo_id": "text-generation-inference",
"token_count": 469
} | 209 |
import pytest
@pytest.fixture(scope="module")
def flash_phi_handle(launcher):
with launcher("microsoft/phi-2", num_shard=1) as handle:
yield handle
@pytest.fixture(scope="module")
async def flash_phi(flash_phi_handle):
await flash_phi_handle.health(300)
return flash_phi_handle.client
@pytest.mark.asyncio
async def test_flash_phi(flash_phi, response_snapshot):
response = await flash_phi.generate(
"Test request", max_new_tokens=10, decoder_input_details=True
)
assert response.details.generated_tokens == 10
assert response.generated_text == ': {request}")\n response = self'
assert response == response_snapshot
@pytest.mark.asyncio
async def test_flash_phi_all_params(flash_phi, response_snapshot):
response = await flash_phi.generate(
"Test request",
max_new_tokens=10,
repetition_penalty=1.2,
return_full_text=True,
stop_sequences=["network"],
temperature=0.5,
top_p=0.9,
top_k=10,
truncate=5,
typical_p=0.9,
watermark=True,
decoder_input_details=True,
seed=0,
)
assert response.details.generated_tokens == 6
assert response.generated_text == "Test request to send data over a network"
assert response == response_snapshot
@pytest.mark.asyncio
async def test_flash_phi_load(flash_phi, generate_load, response_snapshot):
responses = await generate_load(flash_phi, "Test request", max_new_tokens=10, n=4)
assert len(responses) == 4
assert all(
[r.generated_text == responses[0].generated_text for r in responses]
), f"{[r.generated_text for r in responses]}"
assert responses[0].generated_text == ': {request}")\n response = self'
assert responses == response_snapshot
| text-generation-inference/integration-tests/models/test_flash_phi.py/0 | {
"file_path": "text-generation-inference/integration-tests/models/test_flash_phi.py",
"repo_id": "text-generation-inference",
"token_count": 725
} | 210 |
[tool.poetry]
name = "text-generation-integration-tests"
version = "1.4.3"
description = "Text Generation Inference integration tests"
authors = ["Nicolas Patry <[email protected]>"]
[tool.poetry.dependencies]
python = ">=3.9,<3.13"
syrupy = "4.0.1"
text-generation = "^0.6.0"
pytest = "^7.4.0"
pytest-asyncio = "^0.21.1"
docker = "^6.1.3"
| text-generation-inference/integration-tests/pyproject.toml/0 | {
"file_path": "text-generation-inference/integration-tests/pyproject.toml",
"repo_id": "text-generation-inference",
"token_count": 151
} | 211 |
use std::fs;
fn main() -> Result<(), Box<dyn std::error::Error>> {
println!("cargo:rerun-if-changed=../../proto/generate.proto");
fs::create_dir("src/pb").unwrap_or(());
let mut config = prost_build::Config::new();
config.protoc_arg("--experimental_allow_proto3_optional");
tonic_build::configure()
.build_client(true)
.build_server(false)
.out_dir("src/pb")
.include_file("mod.rs")
.compile_with_config(config, &["../../proto/generate.proto"], &["../../proto"])
.unwrap_or_else(|e| panic!("protobuf compilation failed: {e}"));
Ok(())
}
| text-generation-inference/router/client/build.rs/0 | {
"file_path": "text-generation-inference/router/client/build.rs",
"repo_id": "text-generation-inference",
"token_count": 270
} | 212 |
// Adapted from turboderp exllama: https://github.com/turboderp/exllama
#ifndef _column_remap_cuh
#define _column_remap_cuh
#include <cuda_runtime.h>
#include <cuda_fp16.h>
#include <cstdint>
void column_remap_cuda
(
const half* x,
half* x_new,
const int x_height,
const int x_width,
const uint32_t* x_map
);
#endif
| text-generation-inference/server/exllama_kernels/exllama_kernels/cuda_func/column_remap.cuh/0 | {
"file_path": "text-generation-inference/server/exllama_kernels/exllama_kernels/cuda_func/column_remap.cuh",
"repo_id": "text-generation-inference",
"token_count": 153
} | 213 |
#ifndef _q_gemm_cuh
#define _q_gemm_cuh
#include <cuda_runtime.h>
#include <cuda_fp16.h>
#include <cstdint>
#include <cstdio>
#include <ATen/cuda/CUDAContext.h>
#include "q_matrix.cuh"
void gemm_half_q_half_cuda
(
cublasHandle_t cublas_handle,
const half* a,
QMatrix* b,
half* c,
int size_m,
int size_n,
int size_k,
bool clear = false,
half* reconstruct = NULL,
bool force_cuda = false,
const half* r_weights = NULL,
const int r_weights_stride = 0,
bool mul_r_weights = false
);
void clear_tensor_cuda
(
half* c,
int size_m,
int size_n
);
#endif
| text-generation-inference/server/exllamav2_kernels/exllamav2_kernels/cuda/q_gemm.cuh/0 | {
"file_path": "text-generation-inference/server/exllamav2_kernels/exllamav2_kernels/cuda/q_gemm.cuh",
"repo_id": "text-generation-inference",
"token_count": 294
} | 214 |
[tool.poetry]
name = "text-generation-server"
version = "1.4.3"
description = "Text Generation Inference Python gRPC Server"
authors = ["Olivier Dehaene <[email protected]>"]
[tool.poetry.scripts]
text-generation-server = 'text_generation_server.cli:app'
[tool.poetry.dependencies]
python = ">=3.9,<3.13"
protobuf = "^4.21.7"
grpcio = "^1.51.1"
grpcio-status = "^1.51.1"
grpcio-reflection = "^1.51.1"
grpc-interceptor = "^0.15.0"
typer = "^0.6.1"
accelerate = { version = "^0.28.0", optional = true }
bitsandbytes = { version = "^0.43.0", optional = true }
safetensors = "^0.4.1"
loguru = "^0.6.0"
opentelemetry-api = "^1.15.0"
opentelemetry-exporter-otlp = "^1.15.0"
opentelemetry-instrumentation-grpc = "^0.36b0"
hf-transfer = "^0.1.2"
sentencepiece = "^0.1.97"
tokenizers = "^0.15.0"
huggingface-hub = "^0.19.3"
transformers = "^4.38.2"
einops = "^0.6.1"
texttable = { version = "^1.6.7", optional = true }
datasets = { version = "^2.14.0", optional = true }
peft = { version = "^0.9.0", optional = true }
torch = { version = "^2.1.1", optional = true }
scipy = "^1.11.1"
pillow = "^10.0.0"
outlines= { version = "^0.0.27", optional = true }
[tool.poetry.extras]
torch = ["torch"]
accelerate = ["accelerate"]
bnb = ["bitsandbytes"]
peft = ["peft"]
quantize = ["texttable", "datasets", "accelerate"]
outlines = ["outlines"]
[tool.poetry.group.dev.dependencies]
grpcio-tools = "^1.51.1"
pytest = "^7.3.0"
[[tool.poetry.source]]
name = "pytorch-gpu-src"
url = "https://download.pytorch.org/whl/cu121"
priority = "explicit"
[tool.pytest.ini_options]
markers = ["private: marks tests as requiring an admin hf token (deselect with '-m \"not private\"')"]
[build-system]
requires = [
"poetry-core>=1.0.0",
]
build-backend = "poetry.core.masonry.api"
| text-generation-inference/server/pyproject.toml/0 | {
"file_path": "text-generation-inference/server/pyproject.toml",
"repo_id": "text-generation-inference",
"token_count": 777
} | 215 |
import torch
from typing import Dict, Optional, TypeVar
from text_generation_server.models.types import Batch
B = TypeVar("B", bound=Batch)
class Cache:
def __init__(self):
self.cache: Dict[int, B] = {}
def pop(self, batch_id: int) -> Optional[B]:
return self.cache.pop(batch_id, None)
def set(self, entry: B):
if entry is not None:
self.cache[entry.batch_id] = entry
def delete(self, batch_id: int):
batch = self.pop(batch_id)
if batch is not None:
del batch
if torch.cuda.is_available():
torch.cuda.empty_cache()
def clear(self):
keys = list(self.cache.keys())
for k in keys:
self.delete(k)
def __len__(self):
return len(self.cache.keys())
| text-generation-inference/server/text_generation_server/cache.py/0 | {
"file_path": "text-generation-inference/server/text_generation_server/cache.py",
"repo_id": "text-generation-inference",
"token_count": 359
} | 216 |
import torch
import torch.distributed
from torch import nn
from transformers.modeling_utils import PreTrainedModel
from transformers.configuration_utils import PretrainedConfig
from typing import Optional, List, Tuple
from text_generation_server.utils import paged_attention, flash_attn
from text_generation_server.utils.flash_attn import attention
from text_generation_server.utils.layers import (
TensorParallelRowLinear,
TensorParallelColumnLinear,
TensorParallelEmbedding,
SpeculativeHead,
FastLayerNorm,
PositionRotaryEmbedding,
get_linear,
)
def load_row(config, prefix: str, weights, bias: bool):
weight = weights.get_multi_weights_row(prefix, quantize=config.quantize)
if bias and weights.process_group.rank() == 0:
# Rank is only on the first rank process
bias = weights.get_tensor(f"{prefix}.bias")
else:
bias = None
linear = get_linear(weight, bias, config.quantize)
if config.parallel_attn:
return linear
else:
return TensorParallelRowLinear(linear, process_group=weights.process_group)
class RWConfig(PretrainedConfig):
attribute_map = {
"num_hidden_layers": "n_layer",
"num_attention_heads": "n_head",
}
def __init__(
self,
model_type="RefinedWeb",
vocab_size=250880,
hidden_size=64,
num_hidden_layers=None,
num_attention_heads=None,
layer_norm_epsilon=1e-5,
initializer_range=0.02,
use_cache=True,
bos_token_id=1,
eos_token_id=2,
hidden_dropout=0.0,
attention_dropout=0.0,
num_kv_heads=None,
multi_query=False,
alibi=False,
new_decoder_architecture=None,
bias=False,
parallel_attn=False,
**kwargs,
):
if alibi:
raise NotImplementedError(
"alibi is not supported by this version of the model"
)
self.model_type = model_type
self.alibi = False
self.rotary = True
self.vocab_size = vocab_size
# Backward compatibility with n_embed kwarg
n_embed = kwargs.pop("n_embed", None)
self.hidden_size = hidden_size if n_embed is None else n_embed
self.n_layer = (
num_hidden_layers
if num_hidden_layers is not None
else kwargs.pop("n_layer", 2)
)
self.n_head = (
num_attention_heads
if num_attention_heads is not None
else kwargs.pop("n_head", 8)
)
self.layer_norm_epsilon = layer_norm_epsilon
self.initializer_range = initializer_range
self.use_cache = use_cache
self.hidden_dropout = hidden_dropout
self.attention_dropout = attention_dropout
self.bias = bias
self.parallel_attn = parallel_attn
self.bos_token_id = bos_token_id
self.eos_token_id = eos_token_id
if num_kv_heads is not None:
self.n_head_kv = num_kv_heads
else:
old_n_head_kv = kwargs.pop("n_head_kv", None)
if old_n_head_kv is not None:
self.n_head_kv = old_n_head_kv
else:
self.n_head_kv = 1 if multi_query else self.n_head
if new_decoder_architecture is not None:
self.new_decoder_architecture = new_decoder_architecture
elif model_type == "RefinedWeb":
self.new_decoder_architecture = True
else:
self.new_decoder_architecture = False
super().__init__(bos_token_id=bos_token_id, eos_token_id=eos_token_id, **kwargs)
class FlashRWAttention(torch.nn.Module):
def __init__(
self,
config,
prefix,
weights,
):
super().__init__()
self.num_heads = config.n_head
self.num_heads_kv = config.n_head_kv
self.hidden_size = config.hidden_size
self.head_size = self.hidden_size // self.num_heads
self.rotary_emb = PositionRotaryEmbedding.static(
config=config, dim=self.head_size, base=10000.0, device=weights.device
)
self.softmax_scale = self.head_size ** (-0.5)
if self.num_heads % weights.process_group.size() != 0:
raise ValueError(
f"`num_heads` must be divisible by `num_shards` (got `num_heads`: {self.num_heads} "
f"and `num_shards`: {weights.process_group.size()}"
)
self.num_heads = self.num_heads // weights.process_group.size()
self.query_key_value = TensorParallelColumnLinear.load(
config,
prefix=f"{prefix}.query_key_value",
weights=weights,
bias=config.bias,
)
self.dense = load_row(
config, prefix=f"{prefix}.dense", weights=weights, bias=config.bias
)
if self.num_heads_kv == 1:
self.kv_head_mapping = torch.zeros(
self.num_heads, dtype=torch.int32, device=weights.device
)
else:
self.kv_head_mapping = torch.arange(
0, self.num_heads, dtype=torch.int32, device=weights.device
)
def forward(
self,
hidden_states,
cos,
sin,
cu_seqlen_prefill,
kv_cache,
block_tables,
slots,
input_lengths,
max_s,
):
qkv = self.query_key_value(hidden_states)
# Split query from key_value
query, kv = qkv.split(
[self.head_size * self.num_heads, 2 * self.head_size * self.num_heads_kv],
dim=1,
)
# Prepare query and key_value for indexing
query = query.view(-1, self.num_heads, self.head_size)
kv = kv.view(-1, 2, self.num_heads_kv, self.head_size)
# Inplace rotary
self.rotary_emb(query, torch.select(kv, dim=1, index=0), cos, sin)
paged_attention.reshape_and_cache(
kv[:, 0], kv[:, 1], kv_cache[0], kv_cache[1], slots
)
# output
attn_output = torch.empty_like(query)
# Prefill
if cu_seqlen_prefill is not None:
# flash attention
flash_attn.attention(
query,
torch.select(kv, dim=1, index=0),
torch.select(kv, dim=1, index=1),
attn_output,
cu_seqlen_prefill,
max_s,
self.softmax_scale,
)
# Decode
else:
paged_attention.attention(
attn_output,
query,
kv_cache[0],
kv_cache[1],
self.kv_head_mapping,
self.softmax_scale,
block_tables,
input_lengths,
max_s,
)
return self.dense(attn_output.view(-1, self.num_heads * self.head_size))
class FlashRWLargeAttention(torch.nn.Module):
def __init__(
self,
config,
prefix,
weights,
):
super().__init__()
hidden_size = config.hidden_size
num_heads = config.n_head
# num_heads_kv = config.n_head_kv
num_groups = config.n_head_kv
self.hidden_size = hidden_size
self.head_size = hidden_size // num_heads
self.num_groups = num_groups
self.rotary_emb = PositionRotaryEmbedding.static(
config=config, dim=self.head_size, base=10000.0, device=weights.device
)
self.softmax_scale = self.head_size ** (-0.5)
# self.num_groups = num_heads // (num_heads_kv * 2)
self.num_heads = num_heads // self.num_groups
# self.num_heads_kv = num_heads_kv // self.num_groups
process_group = weights.process_group
if process_group.size() > self.num_groups:
raise NotImplementedError(
f"Tensor Parallelism is not implemented for world_size > n groups"
)
if self.num_groups % process_group.size() != 0:
raise NotImplementedError(
f"Tensor Parallelism is not implemented for {self.num_groups} not divisible by {process_group.size()}"
)
self.num_groups = self.num_groups // process_group.size()
self.query_key_value = TensorParallelColumnLinear.load(
config,
prefix=f"{prefix}.query_key_value",
weights=weights,
bias=config.bias,
)
self.dense = load_row(
config, prefix=f"{prefix}.dense", weights=weights, bias=config.bias
)
self.kv_head_mapping = torch.arange(
0, self.num_groups, dtype=torch.int32, device=weights.device
).repeat_interleave(self.num_heads)
def forward(
self,
hidden_states,
cos,
sin,
cu_seqlen_prefill,
kv_cache,
block_tables,
slots,
input_lengths,
max_s,
):
qkv = self.query_key_value(hidden_states)
qkv = qkv.view(-1, self.num_groups, self.num_heads + 2, self.head_size)
# Split on group dimension
query, kv = qkv.split(
[self.num_heads, 2],
dim=2,
)
# Merge groups and heads
query = query.reshape(-1, self.num_groups * self.num_heads, self.head_size)
# Inplace rotary
self.rotary_emb(query, torch.select(kv, dim=2, index=0), cos, sin)
paged_attention.reshape_and_cache(
kv[:, :, 0].contiguous(),
kv[:, :, 1].contiguous(),
kv_cache[0],
kv_cache[1],
slots,
)
# output
attn_output = torch.empty_like(query)
# Prefill
if cu_seqlen_prefill is not None:
# flash attention
flash_attn.attention(
query,
torch.select(kv, dim=2, index=0),
torch.select(kv, dim=2, index=1),
attn_output,
cu_seqlen_prefill,
max_s,
self.softmax_scale,
)
# Decode
else:
paged_attention.attention(
attn_output,
query,
kv_cache[0],
kv_cache[1],
self.kv_head_mapping,
self.softmax_scale,
block_tables,
input_lengths,
max_s,
)
return self.dense(
attn_output.view(-1, self.num_groups * self.num_heads * self.head_size)
)
class FlashMLP(nn.Module):
def __init__(self, config, prefix, weights):
super().__init__()
self.act = torch.nn.functional.gelu
self.dense_h_to_4h = TensorParallelColumnLinear.load(
config, prefix=f"{prefix}.dense_h_to_4h", weights=weights, bias=config.bias
)
self.dense_4h_to_h = load_row(
config, prefix=f"{prefix}.dense_4h_to_h", weights=weights, bias=config.bias
)
def forward(self, hidden_states):
hidden_states = self.dense_h_to_4h(hidden_states)
hidden_states = self.act(hidden_states)
hidden_states = self.dense_4h_to_h(hidden_states)
return hidden_states
class FlashRWLayer(nn.Module):
def __init__(
self,
layer_id,
config,
weights,
):
super().__init__()
parallel_attn = config.parallel_attn
self.parallel_attn = parallel_attn
prefix = f"transformer.h.{layer_id}"
self.input_layernorm = FastLayerNorm.load(
prefix=f"{prefix}.input_layernorm",
weights=weights,
eps=config.layer_norm_epsilon,
)
self.self_attention = FlashRWAttention(
config,
prefix=f"{prefix}.self_attention",
weights=weights,
)
self.post_attention_layernorm = (
FastLayerNorm.load(
prefix=f"{prefix}.post_attention_layernorm",
weights=weights,
eps=config.layer_norm_epsilon,
)
if not parallel_attn
else None
)
self.mlp = FlashMLP(
config,
prefix=f"{prefix}.mlp",
weights=weights,
)
self.process_group = weights.process_group
def forward(
self,
hidden_states,
residual,
cos,
sin,
cu_seqlen_prefill,
kv_cache,
block_tables,
slots,
input_lengths,
max_s,
):
if self.parallel_attn:
ln_hidden_states, residual = self.input_layernorm(hidden_states, residual)
attn_output = self.self_attention(
ln_hidden_states,
cos,
sin,
cu_seqlen_prefill,
kv_cache,
block_tables,
slots,
input_lengths,
max_s,
)
mlp_output = self.mlp(ln_hidden_states)
intermediate = mlp_output + attn_output
if self.process_group.size() > 1:
torch.distributed.all_reduce(intermediate, group=self.process_group)
return intermediate, residual
else:
hidden_states, residual = self.input_layernorm(hidden_states, residual)
hidden_states = self.self_attention(
hidden_states,
cos,
sin,
cu_seqlen_prefill,
kv_cache,
block_tables,
slots,
input_lengths,
max_s,
)
hidden_states, residual = self.post_attention_layernorm(
hidden_states, residual
)
mlp_output = self.mlp(hidden_states)
return mlp_output, residual
class FlashRWLargeLayer(nn.Module):
def __init__(self, layer_id, config, weights):
super().__init__()
prefix = f"transformer.h.{layer_id}"
self.ln_attn = FastLayerNorm.load(
prefix=f"{prefix}.ln_attn",
weights=weights,
eps=config.layer_norm_epsilon,
)
self.ln_mlp = FastLayerNorm.load(
prefix=f"{prefix}.ln_mlp",
weights=weights,
eps=config.layer_norm_epsilon,
)
self.self_attention = FlashRWLargeAttention(
config,
prefix=f"{prefix}.self_attention",
weights=weights,
)
assert config.parallel_attn, "This version doesn't support non parallel_attn"
self.mlp = FlashMLP(config, prefix=f"{prefix}.mlp", weights=weights)
self.process_group = weights.process_group
def forward(
self,
hidden_states,
residual,
cos,
sin,
cu_seqlen_prefill,
kv_cache,
block_tables,
slots,
input_lengths,
max_s,
):
ln_attn, residual = self.ln_attn(hidden_states, residual)
ln_mlp, _ = self.ln_mlp(residual)
# Self attention.
attn_output = self.self_attention(
ln_attn,
cos,
sin,
cu_seqlen_prefill,
kv_cache,
block_tables,
slots,
input_lengths,
max_s,
)
# MLP.
mlp_output = self.mlp(ln_mlp)
intermediate = attn_output + mlp_output
if self.process_group.size() > 1:
torch.distributed.all_reduce(intermediate, group=self.process_group)
return intermediate, residual
class FlashRWPreTrainedModel(PreTrainedModel):
config_class = RWConfig
class FlashRWModel(FlashRWPreTrainedModel):
def __init__(self, config, weights):
super().__init__(config)
self.config = config
self.word_embeddings = TensorParallelEmbedding(
prefix="transformer.word_embeddings", weights=weights
)
if config.new_decoder_architecture:
self.h = nn.ModuleList(
[
FlashRWLargeLayer(layer_id, config, weights)
for layer_id in range(config.num_hidden_layers)
]
)
self.cache_size = self.h[0].self_attention.num_groups
else:
self.h = nn.ModuleList(
[
FlashRWLayer(layer_id, config, weights)
for layer_id in range(config.num_hidden_layers)
]
)
self.cache_size = self.h[0].self_attention.num_heads_kv
self.ln_f = FastLayerNorm.load(
prefix="transformer.ln_f",
weights=weights,
eps=config.layer_norm_epsilon,
)
self.head_size = self.h[0].self_attention.head_size
def forward(
self,
input_ids: torch.Tensor,
position_ids: torch.Tensor,
cu_seqlen_prefill: Optional[torch.Tensor],
kv_cache: List[Tuple[torch.Tensor, torch.Tensor]],
block_tables: torch.Tensor,
slots: torch.Tensor,
input_lengths: torch.Tensor,
max_s: int,
) -> torch.Tensor:
hidden_states = self.word_embeddings(input_ids)
# Get rotary cos and sin for this forward
# Avoid to index in each layer
cos, sin = self.h[0].self_attention.rotary_emb.get_cos_sin(
position_ids, max_s, hidden_states.dtype
)
residual = None
for i, layer in enumerate(self.h):
hidden_states, residual = layer(
hidden_states,
residual,
cos,
sin,
cu_seqlen_prefill,
kv_cache[i],
block_tables,
slots,
input_lengths,
max_s,
)
hidden_states, _ = self.ln_f(hidden_states, residual)
return hidden_states
class FlashRWForCausalLM(FlashRWPreTrainedModel):
def __init__(self, config, weights):
super().__init__(config)
self.transformer = FlashRWModel(config, weights)
self.lm_head = SpeculativeHead.load(config, prefix="lm_head", weights=weights)
def forward(
self,
input_ids: torch.Tensor,
position_ids: torch.Tensor,
cu_seqlen_prefill: Optional[torch.Tensor],
kv_cache: List[Tuple[torch.Tensor, torch.Tensor]],
block_tables: torch.Tensor,
slots: torch.Tensor,
input_lengths: torch.Tensor,
max_s: int,
lm_head_indices: Optional[torch.Tensor] = None,
) -> torch.Tensor:
hidden_states = self.transformer(
input_ids,
position_ids,
cu_seqlen_prefill,
kv_cache,
block_tables,
slots,
input_lengths,
max_s,
)
if lm_head_indices is not None:
hidden_states = hidden_states[lm_head_indices]
logits = self.lm_head(hidden_states)
return logits
| text-generation-inference/server/text_generation_server/models/custom_modeling/flash_rw_modeling.py/0 | {
"file_path": "text-generation-inference/server/text_generation_server/models/custom_modeling/flash_rw_modeling.py",
"repo_id": "text-generation-inference",
"token_count": 10010
} | 217 |
import torch
import torch.distributed
from opentelemetry import trace
from typing import Optional
from text_generation_server.models import FlashCausalLM
from text_generation_server.models.custom_modeling.flash_gemma_modeling import (
GemmaTokenizerFast,
FlashGemmaForCausalLM,
GemmaConfig,
)
from text_generation_server.utils import (
initialize_torch_distributed,
weight_files,
Weights,
)
tracer = trace.get_tracer(__name__)
class FlashGemma(FlashCausalLM):
def __init__(
self,
model_id: str,
revision: Optional[str] = None,
quantize: Optional[str] = None,
use_medusa: Optional[str] = None,
dtype: Optional[torch.dtype] = None,
trust_remote_code: bool = False,
):
self.process_group, rank, world_size = initialize_torch_distributed()
if torch.cuda.is_available():
device = torch.device(f"cuda:{rank}")
dtype = torch.bfloat16 if dtype is None else dtype
else:
raise NotImplementedError("FlashGemma is only available on GPU")
tokenizer = GemmaTokenizerFast.from_pretrained(
model_id,
revision=revision,
padding_side="left",
truncation_side="left",
trust_remote_code=trust_remote_code,
use_fast=True,
from_slow=False,
)
config = GemmaConfig.from_pretrained(
model_id, revision=revision, trust_remote_code=trust_remote_code
)
config.quantize = quantize
config.use_medusa = use_medusa
torch.distributed.barrier(group=self.process_group)
filenames = weight_files(model_id, revision=revision, extension=".safetensors")
weights = Weights(filenames, device, dtype, process_group=self.process_group)
if config.quantize in ["gptq", "awq"]:
weights._set_gptq_params(model_id, revision)
model = FlashGemmaForCausalLM(config, weights)
torch.distributed.barrier(group=self.process_group)
super(FlashGemma, self).__init__(
model=model,
tokenizer=tokenizer,
num_layers=len(model.model.layers),
num_kv_heads=model.model.num_key_value_heads,
head_size=model.model.head_size,
dtype=dtype,
device=device,
rank=rank,
world_size=world_size,
)
| text-generation-inference/server/text_generation_server/models/flash_gemma.py/0 | {
"file_path": "text-generation-inference/server/text_generation_server/models/flash_gemma.py",
"repo_id": "text-generation-inference",
"token_count": 1094
} | 218 |
import inspect
import torch
from abc import ABC, abstractmethod
from typing import List, Tuple, Optional, TypeVar, Type
from transformers import PreTrainedTokenizerBase, PretrainedConfig
from text_generation_server.models.types import Batch, Generation
from text_generation_server.utils.speculate import get_speculate
from text_generation_server.pb.generate_pb2 import InfoResponse
B = TypeVar("B", bound=Batch)
class Model(ABC):
def __init__(
self,
model: torch.nn.Module,
tokenizer: PreTrainedTokenizerBase,
requires_padding: bool,
dtype: torch.dtype,
device: torch.device,
rank: int = 0,
world_size: int = 1,
sliding_window: Optional[int] = None,
speculate: Optional[int] = None,
):
self.model = model.eval()
self.tokenizer = tokenizer
self.all_special_ids = set(tokenizer.all_special_ids)
self.requires_padding = requires_padding
self.dtype = dtype
self.device = device
self.rank = rank
self.world_size = world_size
self.sliding_window = sliding_window if sliding_window != -1 else None
if speculate is None:
speculate = get_speculate()
self.speculate = speculate
self.has_position_ids = (
inspect.signature(model.forward).parameters.get("position_ids", None)
is not None
)
self.check_initialized()
@property
def info(self) -> InfoResponse:
if self.requires_padding and self.sliding_window is not None:
raise NotImplementedError("sliding_window is not implemented with padding")
return InfoResponse(
requires_padding=self.requires_padding,
dtype=str(self.dtype),
device_type=self.device.type,
window_size=self.sliding_window,
speculate=self.speculate,
)
@property
@abstractmethod
def batch_type(self) -> Type[B]:
raise NotImplementedError
@abstractmethod
def generate_token(
self, batch: B
) -> Tuple[List[Generation], Optional[B], Tuple[int, int]]:
raise NotImplementedError
def warmup(self, batch: B) -> Optional[int]:
self.generate_token(batch)
return None
def decode_token(
self,
all_input_ids: List[int],
prefix_offset: int = 0,
read_offset: int = 0,
skip_special_tokens: bool = False,
) -> Tuple[str, int, int]:
"""Hack to hopefully support generate_stream for the maximum number of tokenizers"""
# The prefix text is necessary only to defeat cleanup algorithms in the decode
# which decide to add a space or not depending on the surrounding ids.
prefix_text = self.tokenizer.decode(
all_input_ids[prefix_offset:read_offset],
skip_special_tokens=skip_special_tokens,
)
new_text = self.tokenizer.decode(
all_input_ids[prefix_offset:], skip_special_tokens=skip_special_tokens
)
if len(new_text) > len(prefix_text) and not new_text.endswith("�"):
# utf-8 char at the end means it's a potential unfinished byte sequence
# from byte fallback tokenization.
# If it's in the middle, it's probably a real invalid id generated
# by the model
new_text = new_text[len(prefix_text) :]
return new_text, read_offset, len(all_input_ids)
else:
return "", prefix_offset, read_offset
def check_initialized(self):
uninitialized_parameters = []
for n, p in self.model.named_parameters():
if p.data.device == torch.device("meta"):
uninitialized_parameters.append(n)
if uninitialized_parameters:
raise RuntimeError(
f"found uninitialized parameters in model {self.__class__.__name__}: {uninitialized_parameters}"
)
| text-generation-inference/server/text_generation_server/models/model.py/0 | {
"file_path": "text-generation-inference/server/text_generation_server/models/model.py",
"repo_id": "text-generation-inference",
"token_count": 1674
} | 219 |
import os
import torch
from datetime import timedelta
from loguru import logger
# Tensor Parallelism settings
RANK = int(os.getenv("RANK", "0"))
WORLD_SIZE = int(os.getenv("WORLD_SIZE", "1"))
# CUDA memory fraction
MEMORY_FRACTION = float(os.getenv("CUDA_MEMORY_FRACTION", "1.0"))
class FakeBarrier:
def wait(self):
pass
class FakeGroup:
def __init__(self, rank, size):
self._rank = rank
self._size = size
def allreduce(self, *args, **kwargs):
return FakeBarrier()
def allgather(self, inputs, local_tensor, **kwargs):
assert (
len(inputs[0]) == len(local_tensor) == 1
), f"{len(inputs[0])} != {len(local_tensor)} != 1, and the FakeGroup is supposed to join on simple tensors"
for input_ in inputs:
input_[0].data = local_tensor[0].data
return FakeBarrier()
def barrier(self, *args, **kwargs):
return FakeBarrier()
def size(self):
return self._size
def rank(self):
return self._rank
def initialize_torch_distributed():
if torch.cuda.is_available():
from torch.distributed import ProcessGroupNCCL
# Set the device id.
assert WORLD_SIZE <= torch.cuda.device_count(), "Each process is one gpu"
device = RANK % torch.cuda.device_count()
torch.cuda.set_device(device)
torch.cuda.set_per_process_memory_fraction(MEMORY_FRACTION, device)
backend = "nccl"
options = ProcessGroupNCCL.Options()
options.is_high_priority_stream = True
options._timeout = timedelta(seconds=60)
else:
backend = "gloo"
options = None
if WORLD_SIZE == 1:
return FakeGroup(RANK, WORLD_SIZE), RANK, WORLD_SIZE
else:
if os.getenv("DEBUG", None) == "1":
return FakeGroup(RANK, WORLD_SIZE), RANK, WORLD_SIZE
if not torch.distributed.is_initialized():
# Call the init process.
torch.distributed.init_process_group(
backend=backend,
world_size=WORLD_SIZE,
rank=RANK,
timeout=timedelta(seconds=60),
pg_options=options,
)
else:
logger.warning("torch.distributed is already initialized.")
return torch.distributed.group.WORLD, RANK, WORLD_SIZE
| text-generation-inference/server/text_generation_server/utils/dist.py/0 | {
"file_path": "text-generation-inference/server/text_generation_server/utils/dist.py",
"repo_id": "text-generation-inference",
"token_count": 1042
} | 220 |
# coding=utf-8
# Copyright 2023 Authors of "A Watermark for Large Language Models"
# available at https://arxiv.org/abs/2301.10226
#
# Licensed under the Apache License, Version 2.0 (the "License");
# you may not use this file except in compliance with the License.
# You may obtain a copy of the License at
#
# http://www.apache.org/licenses/LICENSE-2.0
#
# Unless required by applicable law or agreed to in writing, software
# distributed under the License is distributed on an "AS IS" BASIS,
# WITHOUT WARRANTIES OR CONDITIONS OF ANY KIND, either express or implied.
# See the License for the specific language governing permissions and
# limitations under the License.
import os
import torch
from transformers import LogitsProcessor
from typing import List, Union
GAMMA = float(os.getenv("WATERMARK_GAMMA", 0.5))
DELTA = float(os.getenv("WATERMARK_DELTA", 2.0))
class WatermarkLogitsProcessor(LogitsProcessor):
def __init__(
self,
gamma: float = GAMMA,
delta: float = DELTA,
hash_key: int = 15485863, # just a large prime number to create a rng seed with sufficient bit width
device: str = "cpu",
):
# watermarking parameters
self.gamma = gamma
self.delta = delta
self.rng = torch.Generator(device=device)
self.hash_key = hash_key
def _seed_rng(self, input_ids: Union[List[int], torch.LongTensor]):
if isinstance(input_ids, list):
assert (
len(input_ids) >= 1
), "requires at least a 1 token prefix sequence to seed rng"
prev_token = input_ids[-1]
else:
assert len(input_ids) == 1
input_ids = input_ids[0]
assert (
input_ids.shape[-1] >= 1
), "requires at least a 1 token prefix sequence to seed rng"
prev_token = input_ids[-1].item()
self.rng.manual_seed(self.hash_key * prev_token)
def _get_greenlist_ids(
self,
input_ids: Union[List[int], torch.LongTensor],
max_value: int,
device: torch.device,
) -> List[int]:
# seed the rng using the previous tokens/prefix
self._seed_rng(input_ids)
greenlist_size = int(max_value * self.gamma)
vocab_permutation = torch.randperm(max_value, device=device, generator=self.rng)
greenlist_ids = vocab_permutation[:greenlist_size]
return greenlist_ids
@staticmethod
def _calc_greenlist_mask(
scores: torch.FloatTensor, greenlist_token_ids
) -> torch.BoolTensor:
green_tokens_mask = torch.zeros_like(scores)
green_tokens_mask[-1, greenlist_token_ids] = 1
final_mask = green_tokens_mask.bool()
return final_mask
@staticmethod
def _bias_greenlist_logits(
scores: torch.Tensor, greenlist_mask: torch.Tensor, greenlist_bias: float
) -> torch.Tensor:
scores[greenlist_mask] = scores[greenlist_mask] + greenlist_bias
return scores
def __call__(
self, input_ids: Union[List[int], torch.LongTensor], scores: torch.FloatTensor
) -> torch.FloatTensor:
greenlist_ids = self._get_greenlist_ids(
input_ids, scores.shape[-1], scores.device
)
green_tokens_mask = self._calc_greenlist_mask(
scores=scores, greenlist_token_ids=greenlist_ids
)
scores = self._bias_greenlist_logits(
scores=scores, greenlist_mask=green_tokens_mask, greenlist_bias=self.delta
)
return scores
| text-generation-inference/server/text_generation_server/utils/watermark.py/0 | {
"file_path": "text-generation-inference/server/text_generation_server/utils/watermark.py",
"repo_id": "text-generation-inference",
"token_count": 1489
} | 221 |
# This CITATION.cff file was generated with cffinit.
# Visit https://bit.ly/cffinit to generate yours today!
cff-version: 1.2.0
title: HuggingFace's Tokenizers
message: >-
Fast State-of-the-Art Tokenizers optimized for Research
and Production.
type: software
authors:
- given-names: Anthony
family-names: Moi
email: [email protected]
affiliation: HuggingFace
- given-names: Nicolas
family-names: Patry
affiliation: HuggingFace
repository-code: 'https://github.com/huggingface/tokenizers'
url: 'https://github.com/huggingface/tokenizers'
repository: 'https://huggingface.co'
abstract: >-
Fast State-of-the-Art Tokenizers optimized for Research
and Production.
keywords:
- Rust
- Tokenizer
- NLP
license: Apache-2.0
commit: 37372b6
version: 0.13.4
date-released: '2023-04-05'
| tokenizers/CITATION.cff/0 | {
"file_path": "tokenizers/CITATION.cff",
"repo_id": "tokenizers",
"token_count": 293
} | 222 |
<p align="center">
<br>
<img src="https://huggingface.co/landing/assets/tokenizers/tokenizers-logo.png" width="600"/>
<br>
<p>
<p align="center">
<a href="https://badge.fury.io/js/tokenizers">
<img alt="Build" src="https://badge.fury.io/js/tokenizers.svg">
</a>
<a href="https://github.com/huggingface/tokenizers/blob/master/LICENSE">
<img alt="GitHub" src="https://img.shields.io/github/license/huggingface/tokenizers.svg?color=blue">
</a>
</p>
<br>
NodeJS implementation of today's most used tokenizers, with a focus on performance and
versatility. Bindings over the [Rust](https://github.com/huggingface/tokenizers/tree/master/tokenizers) implementation.
If you are interested in the High-level design, you can go check it there.
## Main features
- Train new vocabularies and tokenize using 4 pre-made tokenizers (Bert WordPiece and the 3
most common BPE versions).
- Extremely fast (both training and tokenization), thanks to the Rust implementation. Takes
less than 20 seconds to tokenize a GB of text on a server's CPU.
- Easy to use, but also extremely versatile.
- Designed for research and production.
- Normalization comes with alignments tracking. It's always possible to get the part of the
original sentence that corresponds to a given token.
- Does all the pre-processing: Truncate, Pad, add the special tokens your model needs.
## Installation
```bash
npm install tokenizers@latest
```
## Basic example
```ts
import { Tokenizer } from "tokenizers";
const tokenizer = await Tokenizer.fromFile("tokenizer.json");
const wpEncoded = await tokenizer.encode("Who is John?");
console.log(wpEncoded.getLength());
console.log(wpEncoded.getTokens());
console.log(wpEncoded.getIds());
console.log(wpEncoded.getAttentionMask());
console.log(wpEncoded.getOffsets());
console.log(wpEncoded.getOverflowing());
console.log(wpEncoded.getSpecialTokensMask());
console.log(wpEncoded.getTypeIds());
console.log(wpEncoded.getWordIds());
```
## License
[Apache License 2.0](../../LICENSE)
| tokenizers/bindings/node/README.md/0 | {
"file_path": "tokenizers/bindings/node/README.md",
"repo_id": "tokenizers",
"token_count": 651
} | 223 |
/* eslint-disable @typescript-eslint/no-explicit-any */
/* eslint-disable @typescript-eslint/no-empty-function */
import { TruncationStrategy, BPE, Encoding, AddedToken, Tokenizer } from '../../'
// jest.mock('../../bindings/tokenizer');
// jest.mock('../../bindings/models', () => ({
// __esModule: true,
// Model: jest.fn()
// }));
// Or:
// jest.mock('../../bindings/models', () => {
// return require('../../bindings/__mocks__/models');
// });
// const TokenizerMock = mocked(Tokenizer);
describe('AddedToken', () => {
it('instantiates with only content', () => {
const addToken = new AddedToken('test', false)
expect(addToken.constructor.name).toEqual('AddedToken')
})
it('instantiates with empty options', () => {
const addToken = new AddedToken('test', false, {})
expect(addToken.constructor.name).toEqual('AddedToken')
})
it('instantiates with options', () => {
const addToken = new AddedToken('test', false, {
leftStrip: true,
rightStrip: true,
singleWord: true,
})
expect(addToken.constructor.name).toEqual('AddedToken')
})
describe('getContent', () => {
it('returns the string content of AddedToken', () => {
const addedToken = new AddedToken('test', false)
expect(addedToken.getContent()).toEqual('test')
})
})
})
describe('Tokenizer', () => {
it('has expected methods', () => {
const model = BPE.empty()
const tokenizer = new Tokenizer(model)
expect(typeof Tokenizer.fromFile).toBe('function')
expect(typeof Tokenizer.fromString).toBe('function')
// expect(typeof Tokenizer.fromPretrained).toBe('function')
expect(typeof tokenizer.addSpecialTokens).toBe('function')
expect(typeof tokenizer.addTokens).toBe('function')
expect(typeof tokenizer.decode).toBe('function')
expect(typeof tokenizer.decodeBatch).toBe('function')
expect(typeof tokenizer.disablePadding).toBe('function')
expect(typeof tokenizer.disableTruncation).toBe('function')
expect(typeof tokenizer.encode).toBe('function')
expect(typeof tokenizer.encodeBatch).toBe('function')
expect(typeof tokenizer.getDecoder).toBe('function')
expect(typeof tokenizer.getNormalizer).toBe('function')
expect(typeof tokenizer.getPostProcessor).toBe('function')
expect(typeof tokenizer.getPreTokenizer).toBe('function')
expect(typeof tokenizer.getVocab).toBe('function')
expect(typeof tokenizer.getVocabSize).toBe('function')
expect(typeof tokenizer.idToToken).toBe('function')
expect(typeof tokenizer.runningTasks).toBe('function')
expect(typeof tokenizer.save).toBe('function')
expect(typeof tokenizer.setDecoder).toBe('function')
expect(typeof tokenizer.setModel).toBe('function')
expect(typeof tokenizer.setNormalizer).toBe('function')
expect(typeof tokenizer.setPadding).toBe('function')
expect(typeof tokenizer.setPostProcessor).toBe('function')
expect(typeof tokenizer.setPreTokenizer).toBe('function')
expect(typeof tokenizer.setTruncation).toBe('function')
expect(typeof tokenizer.tokenToId).toBe('function')
expect(typeof tokenizer.toString).toBe('function')
expect(typeof tokenizer.train).toBe('function')
})
// it('can be instantiated from the hub', async () => {
// let tokenizer: Tokenizer
// let output: Encoding
// tokenizer = Tokenizer.fromPretrained('bert-base-cased')
// output = await tokenizer.encode('Hey there dear friend!', null, { addSpecialTokens: false })
// expect(output.getTokens()).toEqual(['Hey', 'there', 'dear', 'friend', '!'])
// tokenizer = Tokenizer.fromPretrained('anthony/tokenizers-test')
// output = await tokenizer.encode('Hey there dear friend!', null, { addSpecialTokens: false })
// expect(output.getTokens()).toEqual(['hey', 'there', 'dear', 'friend', '!'])
// tokenizer = Tokenizer.fromPretrained('anthony/tokenizers-test', {
// revision: 'gpt-2',
// })
// output = await tokenizer.encode('Hey there dear friend!', null, { addSpecialTokens: false })
// expect(output.getTokens()).toEqual(['Hey', 'Ġthere', 'Ġdear', 'Ġfriend', '!'])
// }, 10000)
describe('addTokens', () => {
it('accepts a list of string as new tokens when initial model is empty', () => {
const model = BPE.empty()
const tokenizer = new Tokenizer(model)
const nbAdd = tokenizer.addTokens(['my', 'name', 'is', 'john', 'pair'])
expect(nbAdd).toBe(5)
})
it('accepts a list of AddedToken as new tokens when initial model is empty', () => {
const model = BPE.empty()
const tokenizer = new Tokenizer(model)
const addedToken = new AddedToken('test', false)
const nbAdd = tokenizer.addAddedTokens([addedToken])
expect(nbAdd).toBe(1)
})
})
describe('encode', () => {
let tokenizer: Tokenizer
beforeEach(() => {
// Clear all instances and calls to constructor and all methods:
// TokenizerMock.mockClear();
const model = BPE.empty()
tokenizer = new Tokenizer(model)
tokenizer.addTokens(['my', 'name', 'is', 'john', 'pair'])
})
it('accepts a pair of strings as parameters', async () => {
const encoding = await tokenizer.encode('my name is john', 'pair')
expect(encoding).toBeDefined()
})
it('accepts a string with a null pair', async () => {
const encoding = await tokenizer.encode('my name is john', null)
expect(encoding).toBeDefined()
})
// TODO
// it("throws if we try to encode a pre-tokenized string without isPretokenized=true", async () => {
// await expect((encode as any)(["my", "name", "is", "john"], null)).rejects.toThrow(
// "encode with isPreTokenized=false expect string"
// );
// });
// it("accepts a pre-tokenized string as parameter", async () => {
// const encoding = await tokenizer.encode(["my", "name", "is", "john"], undefined, {
// isPretokenized: true,
// });
// expect(encoding).toBeDefined();
// });
// it("throws if we try to encodeBatch pre-tokenized strings without isPretokenized=true", async () => {
// await expect((encodeBatch as any)([["my", "name", "is", "john"]])).rejects.toThrow(
// "encodeBatch with isPretokenized=false expects input to be `EncodeInput[]` " +
// "with `EncodeInput = string | [string, string]`"
// );
// });
// it("accepts a pre-tokenized input in encodeBatch", async () => {
// const encoding = await tokenizer.encodeBatch([["my", "name", "is", "john"]], {
// isPretokenized: true,
// });
// expect(encoding).toBeDefined();
// });
it('Encodes correctly if called with only one argument', async () => {
const encoded = await tokenizer.encode('my name is john')
expect(encoded.getIds()).toEqual([0, 1, 2, 3])
})
it('returns an Encoding', async () => {
const encoding = await tokenizer.encode('my name is john', 'pair')
expect(encoding.getAttentionMask()).toEqual([1, 1, 1, 1, 1])
const ids = encoding.getIds()
expect(Array.isArray(ids)).toBe(true)
expect(ids).toHaveLength(5)
for (const id of ids) {
expect(typeof id).toBe('number')
}
expect(encoding.getOffsets()).toEqual([
[0, 2],
[3, 7],
[8, 10],
[11, 15],
[0, 4],
])
expect(encoding.getOverflowing()).toEqual([])
expect(encoding.getSpecialTokensMask()).toEqual([0, 0, 0, 0, 0])
expect(encoding.getTokens()).toEqual(['my', 'name', 'is', 'john', 'pair'])
expect(encoding.getTypeIds()).toEqual([0, 0, 0, 0, 1])
})
describe('when truncation is enabled', () => {
it('truncates with default if no truncation options provided', async () => {
tokenizer.setTruncation(2)
const singleEncoding = await tokenizer.encode('my name is john', null)
expect(singleEncoding.getTokens()).toEqual(['my', 'name'])
const pairEncoding = await tokenizer.encode('my name is john', 'pair')
expect(pairEncoding.getTokens()).toEqual(['my', 'pair'])
})
it('throws an error with strategy `only_second` and no pair is encoded', async () => {
tokenizer.setTruncation(2, { strategy: TruncationStrategy.OnlySecond })
await expect(tokenizer.encode('my name is john', null)).rejects.toThrow(
'Truncation error: Second sequence not provided',
)
})
})
describe('when padding is enabled', () => {
it('does not pad anything with default options', async () => {
tokenizer.setPadding()
const singleEncoding = await tokenizer.encode('my name', null)
expect(singleEncoding.getTokens()).toEqual(['my', 'name'])
const pairEncoding = await tokenizer.encode('my name', 'pair')
expect(pairEncoding.getTokens()).toEqual(['my', 'name', 'pair'])
})
it('pads to the right by default', async () => {
tokenizer.setPadding({ maxLength: 5 })
const singleEncoding = await tokenizer.encode('my name', null)
expect(singleEncoding.getTokens()).toEqual(['my', 'name', '[PAD]', '[PAD]', '[PAD]'])
const pairEncoding = await tokenizer.encode('my name', 'pair')
expect(pairEncoding.getTokens()).toEqual(['my', 'name', 'pair', '[PAD]', '[PAD]'])
})
it('pads to multiple of the given value', async () => {
tokenizer.setPadding({ padToMultipleOf: 8 })
const singleEncoding = await tokenizer.encode('my name', null)
expect(singleEncoding.getTokens()).toHaveLength(8)
const pairEncoding = await tokenizer.encode('my name', 'pair')
expect(pairEncoding.getTokens()).toHaveLength(8)
})
})
})
describe('decode', () => {
let tokenizer: Tokenizer
beforeEach(() => {
const model = BPE.empty()
tokenizer = new Tokenizer(model)
tokenizer.addTokens(['my', 'name', 'is', 'john', 'pair'])
})
it('has its callback called with the decoded string', async () => {
const decode = tokenizer.decode.bind(tokenizer)
expect(await decode([0, 1, 2, 3], true)).toEqual('my name is john')
})
})
describe('decodeBatch', () => {
let tokenizer: Tokenizer
beforeEach(() => {
const model = BPE.empty()
tokenizer = new Tokenizer(model)
tokenizer.addTokens(['my', 'name', 'is', 'john', 'pair'])
})
it('has its callback called with the decoded string', async () => {
const decodeBatch = tokenizer.decodeBatch.bind(tokenizer)
expect(await decodeBatch([[0, 1, 2, 3], [4]], true)).toEqual(['my name is john', 'pair'])
})
})
describe('getVocab', () => {
it('accepts `undefined` as parameter', () => {
const model = BPE.empty()
const tokenizer = new Tokenizer(model)
expect(tokenizer.getVocab(undefined)).toBeDefined()
})
it('returns the vocabulary', () => {
const model = BPE.empty()
const tokenizer = new Tokenizer(model)
tokenizer.addTokens(['my', 'name', 'is', 'john'])
expect(tokenizer.getVocab(true)).toEqual({
my: 0,
name: 1,
is: 2,
john: 3,
})
})
})
describe('getVocabSize', () => {
it('accepts `undefined` as parameter', () => {
const model = BPE.empty()
const tokenizer = new Tokenizer(model)
expect(tokenizer.getVocabSize(undefined)).toBeDefined()
})
})
describe('setTruncation', () => {
it('returns the full truncation configuration', () => {
const model = BPE.empty()
const tokenizer = new Tokenizer(model)
tokenizer.setTruncation(2)
// TODO Return type is weird
// const expectedConfig: TruncationOptions = {
// maxLength: 2,
// strategy: TruncationStrategy.LongestFirst,
// stride: 0,
// direction: TruncationDirection.Right,
// };
// expect(truncation).toEqual(expectedConfig);
})
})
describe('setPadding', () => {
it('returns the full padding params', () => {
const model = BPE.empty()
const tokenizer = new Tokenizer(model)
tokenizer.setPadding()
// TODO Return type is weird
// const expectedConfig: PaddingOptions = {
// direction: PaddingDirection.Right,
// padId: 0,
// padToken: "[PAD]",
// padTypeId: 0,
// };
// expect(padding).toEqual(expectedConfig);
})
})
describe('postProcess', () => {
let tokenizer: Tokenizer
let firstEncoding: Encoding
let secondEncoding: Encoding
beforeAll(() => {
const model = BPE.empty()
tokenizer = new Tokenizer(model)
tokenizer.addTokens(['my', 'name', 'is', 'john', 'pair'])
})
beforeEach(async () => {
firstEncoding = await tokenizer.encode('my name is john', null)
secondEncoding = await tokenizer.encode('pair', null)
tokenizer.setTruncation(2)
tokenizer.setPadding({ maxLength: 5 })
})
it('returns correctly with a single Encoding param', () => {
const encoding = tokenizer.postProcess(firstEncoding)
expect(encoding.getTokens()).toEqual(['my', 'name', '[PAD]', '[PAD]', '[PAD]'])
})
it('returns correctly with `undefined` as second and third parameters', () => {
const encoding = tokenizer.postProcess(firstEncoding, undefined, undefined)
expect(encoding.getTokens()).toEqual(['my', 'name', '[PAD]', '[PAD]', '[PAD]'])
})
it('returns correctly with 2 encodings', () => {
const encoding = tokenizer.postProcess(firstEncoding, secondEncoding)
expect(encoding.getTokens()).toEqual(['my', 'pair', '[PAD]', '[PAD]', '[PAD]'])
})
})
})
| tokenizers/bindings/node/lib/bindings/tokenizer.test.ts/0 | {
"file_path": "tokenizers/bindings/node/lib/bindings/tokenizer.test.ts",
"repo_id": "tokenizers",
"token_count": 5268
} | 224 |
# `tokenizers-linux-arm64-musl`
This is the **aarch64-unknown-linux-musl** binary for `tokenizers`
| tokenizers/bindings/node/npm/linux-arm64-musl/README.md/0 | {
"file_path": "tokenizers/bindings/node/npm/linux-arm64-musl/README.md",
"repo_id": "tokenizers",
"token_count": 37
} | 225 |
use crate::tokenizer::PaddingOptions;
use napi::bindgen_prelude::*;
use napi_derive::napi;
use tokenizers::utils::truncation::TruncationDirection;
use tokenizers::Encoding;
#[napi(js_name = "Encoding")]
#[derive(Clone, Default)]
pub struct JsEncoding {
pub(crate) encoding: Option<Encoding>,
}
impl From<Encoding> for JsEncoding {
fn from(value: Encoding) -> Self {
Self {
encoding: Some(value),
}
}
}
impl TryFrom<JsEncoding> for Encoding {
type Error = Error;
fn try_from(value: JsEncoding) -> Result<Self> {
value
.encoding
.ok_or(Error::from_reason("Uninitialized encoding".to_string()))
}
}
#[napi(string_enum, js_name = "TruncationDirection")]
pub enum JsTruncationDirection {
Left,
Right,
}
impl From<JsTruncationDirection> for TruncationDirection {
fn from(value: JsTruncationDirection) -> Self {
match value {
JsTruncationDirection::Left => TruncationDirection::Left,
JsTruncationDirection::Right => TruncationDirection::Right,
}
}
}
impl TryFrom<String> for JsTruncationDirection {
type Error = Error;
fn try_from(value: String) -> Result<JsTruncationDirection> {
match value.as_str() {
"left" => Ok(JsTruncationDirection::Left),
"right" => Ok(JsTruncationDirection::Right),
s => Err(Error::from_reason(format!(
"{s:?} is not a valid direction"
))),
}
}
}
#[napi(string_enum, js_name = "TruncationStrategy")]
pub enum JsTruncationStrategy {
LongestFirst,
OnlyFirst,
OnlySecond,
}
impl From<JsTruncationStrategy> for tokenizers::TruncationStrategy {
fn from(value: JsTruncationStrategy) -> Self {
match value {
JsTruncationStrategy::LongestFirst => tokenizers::TruncationStrategy::LongestFirst,
JsTruncationStrategy::OnlyFirst => tokenizers::TruncationStrategy::OnlyFirst,
JsTruncationStrategy::OnlySecond => tokenizers::TruncationStrategy::OnlySecond,
}
}
}
#[napi]
impl JsEncoding {
#[napi(constructor)]
pub fn new() -> Self {
Self { encoding: None }
}
#[napi]
pub fn get_length(&self) -> u32 {
self
.encoding
.as_ref()
.expect("Uninitialized Encoding")
.get_ids()
.len() as u32
}
#[napi]
pub fn get_n_sequences(&self) -> u32 {
self
.encoding
.as_ref()
.expect("Uninitialized Encoding")
.n_sequences() as u32
}
#[napi]
pub fn get_ids(&self) -> Vec<u32> {
self
.encoding
.as_ref()
.expect("Uninitialized Encoding")
.get_ids()
.to_vec()
}
#[napi]
pub fn get_type_ids(&self) -> Vec<u32> {
self
.encoding
.as_ref()
.expect("Uninitialized Encoding")
.get_type_ids()
.to_vec()
}
#[napi]
pub fn get_attention_mask(&self) -> Vec<u32> {
self
.encoding
.as_ref()
.expect("Uninitialized Encoding")
.get_attention_mask()
.to_vec()
}
#[napi]
pub fn get_special_tokens_mask(&self) -> Vec<u32> {
self
.encoding
.as_ref()
.expect("Uninitialized Encoding")
.get_special_tokens_mask()
.to_vec()
}
#[napi]
pub fn get_tokens(&self) -> Vec<String> {
self
.encoding
.as_ref()
.expect("Uninitialized Encoding")
.get_tokens()
.to_vec()
}
#[napi]
pub fn get_offsets(&self) -> Vec<Vec<u32>> {
self
.encoding
.as_ref()
.expect("Uninitialized Encoding")
.get_offsets()
.iter()
.map(|(a, b)| vec![*a as u32, *b as u32])
.collect()
}
#[napi]
pub fn get_word_ids(&self) -> Vec<Option<u32>> {
self
.encoding
.as_ref()
.expect("Uninitialized Encoding")
.get_word_ids()
.to_vec()
}
#[napi]
pub fn char_to_token(&self, pos: u32, seq_id: Option<u32>) -> Option<u32> {
let seq_id = seq_id.unwrap_or(0);
self
.encoding
.as_ref()
.expect("Uninitialized Encoding")
.char_to_token(pos as usize, seq_id as usize)
.map(|i| i as u32)
}
#[napi]
pub fn char_to_word(&self, pos: u32, seq_id: Option<u32>) -> Option<u32> {
let seq_id = seq_id.unwrap_or(0);
self
.encoding
.as_ref()
.expect("Uninitialized Encoding")
.char_to_word(pos as usize, seq_id as usize)
}
#[napi]
pub fn pad(&mut self, length: u32, options: Option<PaddingOptions>) -> Result<()> {
let params: tokenizers::PaddingParams = options.unwrap_or_default().try_into()?;
self.encoding.as_mut().expect("Uninitialized Encoding").pad(
length as usize,
params.pad_id,
params.pad_type_id,
¶ms.pad_token,
params.direction,
);
Ok(())
}
#[napi]
pub fn truncate(
&mut self,
length: u32,
stride: Option<u32>,
direction: Option<Either<String, JsTruncationDirection>>,
) -> Result<()> {
let stride = stride.unwrap_or_default();
let direction = match direction {
None => TruncationDirection::Left,
Some(Either::A(s)) => match s.as_str() {
"left" => TruncationDirection::Left,
"right" => TruncationDirection::Right,
d => {
return Err(Error::from_reason(format!(
"{d} is not a valid truncation direction"
)));
}
},
Some(Either::B(t)) => t.into(),
};
self
.encoding
.as_mut()
.expect("Uninitialized Encoding")
.truncate(length as usize, stride as usize, direction);
Ok(())
}
#[napi(ts_return_type = "[number, number] | null | undefined")]
pub fn word_to_tokens(&self, env: Env, word: u32, seq_id: Option<u32>) -> Result<Option<Array>> {
let seq_id = seq_id.unwrap_or(0);
if let Some((a, b)) = self
.encoding
.as_ref()
.expect("Uninitialized Encoding")
.word_to_tokens(word, seq_id as usize)
{
let mut arr = env.create_array(2)?;
arr.set(0, env.create_uint32(a as u32)?)?;
arr.set(1, env.create_uint32(b as u32)?)?;
Ok(Some(arr))
} else {
Ok(None)
}
}
#[napi(ts_return_type = "[number, number] | null | undefined")]
pub fn word_to_chars(&self, env: Env, word: u32, seq_id: Option<u32>) -> Result<Option<Array>> {
let seq_id = seq_id.unwrap_or(0);
if let Some((a, b)) = self
.encoding
.as_ref()
.expect("Uninitialized Encoding")
.word_to_chars(word, seq_id as usize)
{
let mut arr = env.create_array(2)?;
arr.set(0, env.create_uint32(a as u32)?)?;
arr.set(1, env.create_uint32(b as u32)?)?;
Ok(Some(arr))
} else {
Ok(None)
}
}
#[napi(ts_return_type = "[number, [number, number]] | null | undefined")]
pub fn token_to_chars(&self, env: Env, token: u32) -> Result<Option<Array>> {
if let Some((_, (start, stop))) = self
.encoding
.as_ref()
.expect("Uninitialized Encoding")
.token_to_chars(token as usize)
{
let mut offsets = env.create_array(2)?;
offsets.set(0, env.create_uint32(start as u32)?)?;
offsets.set(1, env.create_uint32(stop as u32)?)?;
Ok(Some(offsets))
} else {
Ok(None)
}
}
#[napi]
pub fn token_to_word(&self, token: u32) -> Result<Option<u32>> {
if let Some((_, index)) = self
.encoding
.as_ref()
.expect("Uninitialized Encoding")
.token_to_word(token as usize)
{
Ok(Some(index))
} else {
Ok(None)
}
}
#[napi]
pub fn get_overflowing(&self) -> Vec<JsEncoding> {
self
.encoding
.as_ref()
.expect("Uninitialized Encoding")
.get_overflowing()
.clone()
.into_iter()
.map(|enc| JsEncoding {
encoding: Some(enc),
})
.collect()
}
#[napi]
pub fn get_sequence_ids(&self) -> Vec<Option<u32>> {
self
.encoding
.as_ref()
.expect("Uninitialized Encoding")
.get_sequence_ids()
.into_iter()
.map(|s| s.map(|id| id as u32))
.collect()
}
#[napi]
pub fn token_to_sequence(&self, token: u32) -> Option<u32> {
self
.encoding
.as_ref()
.expect("Uninitialized Encoding")
.token_to_sequence(token as usize)
.map(|s| s as u32)
}
}
| tokenizers/bindings/node/src/encoding.rs/0 | {
"file_path": "tokenizers/bindings/node/src/encoding.rs",
"repo_id": "tokenizers",
"token_count": 3778
} | 226 |
# Generated content DO NOT EDIT
class Decoder:
"""
Base class for all decoders
This class is not supposed to be instantiated directly. Instead, any implementation of
a Decoder will return an instance of this class when instantiated.
"""
def decode(self, tokens):
"""
Decode the given list of tokens to a final string
Args:
tokens (:obj:`List[str]`):
The list of tokens to decode
Returns:
:obj:`str`: The decoded string
"""
pass
class BPEDecoder(Decoder):
"""
BPEDecoder Decoder
Args:
suffix (:obj:`str`, `optional`, defaults to :obj:`</w>`):
The suffix that was used to caracterize an end-of-word. This suffix will
be replaced by whitespaces during the decoding
"""
def __init__(self, suffix="</w>"):
pass
def decode(self, tokens):
"""
Decode the given list of tokens to a final string
Args:
tokens (:obj:`List[str]`):
The list of tokens to decode
Returns:
:obj:`str`: The decoded string
"""
pass
class ByteFallback(Decoder):
"""
ByteFallback Decoder
ByteFallback is a simple trick which converts tokens looking like `<0x61>`
to pure bytes, and attempts to make them into a string. If the tokens
cannot be decoded you will get � instead for each inconvertable byte token
"""
def __init__(self):
pass
def decode(self, tokens):
"""
Decode the given list of tokens to a final string
Args:
tokens (:obj:`List[str]`):
The list of tokens to decode
Returns:
:obj:`str`: The decoded string
"""
pass
class ByteLevel(Decoder):
"""
ByteLevel Decoder
This decoder is to be used in tandem with the :class:`~tokenizers.pre_tokenizers.ByteLevel`
:class:`~tokenizers.pre_tokenizers.PreTokenizer`.
"""
def __init__(self):
pass
def decode(self, tokens):
"""
Decode the given list of tokens to a final string
Args:
tokens (:obj:`List[str]`):
The list of tokens to decode
Returns:
:obj:`str`: The decoded string
"""
pass
class CTC(Decoder):
"""
CTC Decoder
Args:
pad_token (:obj:`str`, `optional`, defaults to :obj:`<pad>`):
The pad token used by CTC to delimit a new token.
word_delimiter_token (:obj:`str`, `optional`, defaults to :obj:`|`):
The word delimiter token. It will be replaced by a <space>
cleanup (:obj:`bool`, `optional`, defaults to :obj:`True`):
Whether to cleanup some tokenization artifacts.
Mainly spaces before punctuation, and some abbreviated english forms.
"""
def __init__(self, pad_token="<pad>", word_delimiter_token="|", cleanup=True):
pass
def decode(self, tokens):
"""
Decode the given list of tokens to a final string
Args:
tokens (:obj:`List[str]`):
The list of tokens to decode
Returns:
:obj:`str`: The decoded string
"""
pass
class Fuse(Decoder):
"""
Fuse Decoder
Fuse simply fuses every token into a single string.
This is the last step of decoding, this decoder exists only if
there is need to add other decoders *after* the fusion
"""
def __init__(self):
pass
def decode(self, tokens):
"""
Decode the given list of tokens to a final string
Args:
tokens (:obj:`List[str]`):
The list of tokens to decode
Returns:
:obj:`str`: The decoded string
"""
pass
class Metaspace(Decoder):
"""
Metaspace Decoder
Args:
replacement (:obj:`str`, `optional`, defaults to :obj:`▁`):
The replacement character. Must be exactly one character. By default we
use the `▁` (U+2581) meta symbol (Same as in SentencePiece).
add_prefix_space (:obj:`bool`, `optional`, defaults to :obj:`True`):
Whether to add a space to the first word if there isn't already one. This
lets us treat `hello` exactly like `say hello`.
"""
def __init__(self, replacement="▁", add_prefix_space=True):
pass
def decode(self, tokens):
"""
Decode the given list of tokens to a final string
Args:
tokens (:obj:`List[str]`):
The list of tokens to decode
Returns:
:obj:`str`: The decoded string
"""
pass
class Replace(Decoder):
"""
Replace Decoder
This decoder is to be used in tandem with the :class:`~tokenizers.pre_tokenizers.Replace`
:class:`~tokenizers.pre_tokenizers.PreTokenizer`.
"""
def __init__(self, pattern, content):
pass
def decode(self, tokens):
"""
Decode the given list of tokens to a final string
Args:
tokens (:obj:`List[str]`):
The list of tokens to decode
Returns:
:obj:`str`: The decoded string
"""
pass
class Sequence(Decoder):
"""
Sequence Decoder
Args:
decoders (:obj:`List[Decoder]`)
The decoders that need to be chained
"""
def __init__(self, decoders):
pass
def decode(self, tokens):
"""
Decode the given list of tokens to a final string
Args:
tokens (:obj:`List[str]`):
The list of tokens to decode
Returns:
:obj:`str`: The decoded string
"""
pass
class Strip(Decoder):
"""
Strip normalizer
Strips n left characters of each token, or n right characters of each token
"""
def __init__(self, content, left=0, right=0):
pass
def decode(self, tokens):
"""
Decode the given list of tokens to a final string
Args:
tokens (:obj:`List[str]`):
The list of tokens to decode
Returns:
:obj:`str`: The decoded string
"""
pass
class WordPiece(Decoder):
"""
WordPiece Decoder
Args:
prefix (:obj:`str`, `optional`, defaults to :obj:`##`):
The prefix to use for subwords that are not a beginning-of-word
cleanup (:obj:`bool`, `optional`, defaults to :obj:`True`):
Whether to cleanup some tokenization artifacts. Mainly spaces before punctuation,
and some abbreviated english forms.
"""
def __init__(self, prefix="##", cleanup=True):
pass
def decode(self, tokens):
"""
Decode the given list of tokens to a final string
Args:
tokens (:obj:`List[str]`):
The list of tokens to decode
Returns:
:obj:`str`: The decoded string
"""
pass
| tokenizers/bindings/python/py_src/tokenizers/decoders/__init__.pyi/0 | {
"file_path": "tokenizers/bindings/python/py_src/tokenizers/decoders/__init__.pyi",
"repo_id": "tokenizers",
"token_count": 3115
} | 227 |
from .visualizer import Annotation, EncodingVisualizer
| tokenizers/bindings/python/py_src/tokenizers/tools/__init__.py/0 | {
"file_path": "tokenizers/bindings/python/py_src/tokenizers/tools/__init__.py",
"repo_id": "tokenizers",
"token_count": 13
} | 228 |
use std::sync::{Arc, RwLock};
use pyo3::exceptions;
use pyo3::prelude::*;
use pyo3::types::*;
use crate::error::ToPyResult;
use crate::utils::{PyNormalizedString, PyNormalizedStringRefMut, PyPattern};
use serde::ser::SerializeStruct;
use serde::{Deserialize, Deserializer, Serialize, Serializer};
use tk::normalizers::{
BertNormalizer, Lowercase, Nmt, NormalizerWrapper, Precompiled, Prepend, Replace, Strip,
StripAccents, NFC, NFD, NFKC, NFKD,
};
use tk::{NormalizedString, Normalizer};
use tokenizers as tk;
/// Represents the different kind of NormalizedString we can receive from Python:
/// - Owned: Created in Python and owned by Python
/// - RefMut: A mutable reference to a NormalizedString owned by Rust
#[derive(FromPyObject)]
enum PyNormalizedStringMut<'p> {
Owned(PyRefMut<'p, PyNormalizedString>),
RefMut(PyNormalizedStringRefMut),
}
impl PyNormalizedStringMut<'_> {
/// Normalized the underlying `NormalizedString` using the provided normalizer
pub fn normalize_with<N>(&mut self, normalizer: &N) -> PyResult<()>
where
N: Normalizer,
{
match self {
PyNormalizedStringMut::Owned(ref mut n) => normalizer.normalize(&mut n.normalized),
PyNormalizedStringMut::RefMut(n) => n.map_as_mut(|n| normalizer.normalize(n))?,
}
.map_err(|e| exceptions::PyException::new_err(format!("{}", e)))
}
}
/// Base class for all normalizers
///
/// This class is not supposed to be instantiated directly. Instead, any implementation of a
/// Normalizer will return an instance of this class when instantiated.
#[pyclass(dict, module = "tokenizers.normalizers", name = "Normalizer", subclass)]
#[derive(Clone, Serialize, Deserialize)]
pub struct PyNormalizer {
#[serde(flatten)]
pub(crate) normalizer: PyNormalizerTypeWrapper,
}
impl PyNormalizer {
pub(crate) fn new(normalizer: PyNormalizerTypeWrapper) -> Self {
PyNormalizer { normalizer }
}
pub(crate) fn get_as_subtype(&self, py: Python<'_>) -> PyResult<PyObject> {
let base = self.clone();
Ok(match self.normalizer {
PyNormalizerTypeWrapper::Sequence(_) => Py::new(py, (PySequence {}, base))?.into_py(py),
PyNormalizerTypeWrapper::Single(ref inner) => match &*inner.as_ref().read().unwrap() {
PyNormalizerWrapper::Custom(_) => Py::new(py, base)?.into_py(py),
PyNormalizerWrapper::Wrapped(ref inner) => match inner {
NormalizerWrapper::Sequence(_) => {
Py::new(py, (PySequence {}, base))?.into_py(py)
}
NormalizerWrapper::BertNormalizer(_) => {
Py::new(py, (PyBertNormalizer {}, base))?.into_py(py)
}
NormalizerWrapper::StripNormalizer(_) => {
Py::new(py, (PyBertNormalizer {}, base))?.into_py(py)
}
NormalizerWrapper::Prepend(_) => Py::new(py, (PyPrepend {}, base))?.into_py(py),
NormalizerWrapper::StripAccents(_) => {
Py::new(py, (PyStripAccents {}, base))?.into_py(py)
}
NormalizerWrapper::NFC(_) => Py::new(py, (PyNFC {}, base))?.into_py(py),
NormalizerWrapper::NFD(_) => Py::new(py, (PyNFD {}, base))?.into_py(py),
NormalizerWrapper::NFKC(_) => Py::new(py, (PyNFKC {}, base))?.into_py(py),
NormalizerWrapper::NFKD(_) => Py::new(py, (PyNFKD {}, base))?.into_py(py),
NormalizerWrapper::Lowercase(_) => {
Py::new(py, (PyLowercase {}, base))?.into_py(py)
}
NormalizerWrapper::Precompiled(_) => {
Py::new(py, (PyPrecompiled {}, base))?.into_py(py)
}
NormalizerWrapper::Replace(_) => Py::new(py, (PyReplace {}, base))?.into_py(py),
NormalizerWrapper::Nmt(_) => Py::new(py, (PyNmt {}, base))?.into_py(py),
},
},
})
}
}
impl Normalizer for PyNormalizer {
fn normalize(&self, normalized: &mut NormalizedString) -> tk::Result<()> {
self.normalizer.normalize(normalized)
}
}
#[pymethods]
impl PyNormalizer {
#[staticmethod]
fn custom(obj: PyObject) -> Self {
Self {
normalizer: PyNormalizerWrapper::Custom(CustomNormalizer::new(obj)).into(),
}
}
fn __getstate__(&self, py: Python) -> PyResult<PyObject> {
let data = serde_json::to_string(&self.normalizer).map_err(|e| {
exceptions::PyException::new_err(format!(
"Error while attempting to pickle Normalizer: {}",
e
))
})?;
Ok(PyBytes::new(py, data.as_bytes()).to_object(py))
}
fn __setstate__(&mut self, py: Python, state: PyObject) -> PyResult<()> {
match state.extract::<&PyBytes>(py) {
Ok(s) => {
self.normalizer = serde_json::from_slice(s.as_bytes()).map_err(|e| {
exceptions::PyException::new_err(format!(
"Error while attempting to unpickle Normalizer: {}",
e
))
})?;
Ok(())
}
Err(e) => Err(e),
}
}
/// Normalize a :class:`~tokenizers.NormalizedString` in-place
///
/// This method allows to modify a :class:`~tokenizers.NormalizedString` to
/// keep track of the alignment information. If you just want to see the result
/// of the normalization on a raw string, you can use
/// :meth:`~tokenizers.normalizers.Normalizer.normalize_str`
///
/// Args:
/// normalized (:class:`~tokenizers.NormalizedString`):
/// The normalized string on which to apply this
/// :class:`~tokenizers.normalizers.Normalizer`
#[pyo3(text_signature = "(self, normalized)")]
fn normalize(&self, mut normalized: PyNormalizedStringMut) -> PyResult<()> {
normalized.normalize_with(&self.normalizer)
}
/// Normalize the given string
///
/// This method provides a way to visualize the effect of a
/// :class:`~tokenizers.normalizers.Normalizer` but it does not keep track of the alignment
/// information. If you need to get/convert offsets, you can use
/// :meth:`~tokenizers.normalizers.Normalizer.normalize`
///
/// Args:
/// sequence (:obj:`str`):
/// A string to normalize
///
/// Returns:
/// :obj:`str`: A string after normalization
#[pyo3(text_signature = "(self, sequence)")]
fn normalize_str(&self, sequence: &str) -> PyResult<String> {
let mut normalized = NormalizedString::from(sequence);
ToPyResult(self.normalizer.normalize(&mut normalized)).into_py()?;
Ok(normalized.get().to_owned())
}
}
macro_rules! getter {
($self: ident, $variant: ident, $name: ident) => {{
let super_ = $self.as_ref();
if let PyNormalizerTypeWrapper::Single(ref norm) = super_.normalizer {
let wrapper = norm.read().unwrap();
if let PyNormalizerWrapper::Wrapped(NormalizerWrapper::$variant(o)) = (*wrapper).clone()
{
o.$name
} else {
unreachable!()
}
} else {
unreachable!()
}
}};
}
macro_rules! setter {
($self: ident, $variant: ident, $name: ident, $value: expr) => {{
let super_ = $self.as_ref();
if let PyNormalizerTypeWrapper::Single(ref norm) = super_.normalizer {
let mut wrapper = norm.write().unwrap();
if let PyNormalizerWrapper::Wrapped(NormalizerWrapper::$variant(ref mut o)) = *wrapper {
o.$name = $value;
}
}
}};
}
/// BertNormalizer
///
/// Takes care of normalizing raw text before giving it to a Bert model.
/// This includes cleaning the text, handling accents, chinese chars and lowercasing
///
/// Args:
/// clean_text (:obj:`bool`, `optional`, defaults to :obj:`True`):
/// Whether to clean the text, by removing any control characters
/// and replacing all whitespaces by the classic one.
///
/// handle_chinese_chars (:obj:`bool`, `optional`, defaults to :obj:`True`):
/// Whether to handle chinese chars by putting spaces around them.
///
/// strip_accents (:obj:`bool`, `optional`):
/// Whether to strip all accents. If this option is not specified (ie == None),
/// then it will be determined by the value for `lowercase` (as in the original Bert).
///
/// lowercase (:obj:`bool`, `optional`, defaults to :obj:`True`):
/// Whether to lowercase.
#[pyclass(extends=PyNormalizer, module = "tokenizers.normalizers", name = "BertNormalizer")]
pub struct PyBertNormalizer {}
#[pymethods]
impl PyBertNormalizer {
#[getter]
fn get_clean_text(self_: PyRef<Self>) -> bool {
getter!(self_, BertNormalizer, clean_text)
}
#[setter]
fn set_clean_text(self_: PyRef<Self>, clean_text: bool) {
setter!(self_, BertNormalizer, clean_text, clean_text);
}
#[getter]
fn get_handle_chinese_chars(self_: PyRef<Self>) -> bool {
getter!(self_, BertNormalizer, handle_chinese_chars)
}
#[setter]
fn set_handle_chinese_chars(self_: PyRef<Self>, handle_chinese_chars: bool) {
setter!(
self_,
BertNormalizer,
handle_chinese_chars,
handle_chinese_chars
);
}
#[getter]
fn get_strip_accents(self_: PyRef<Self>) -> Option<bool> {
getter!(self_, BertNormalizer, strip_accents)
}
#[setter]
fn set_strip_accents(self_: PyRef<Self>, strip_accents: Option<bool>) {
setter!(self_, BertNormalizer, strip_accents, strip_accents);
}
#[getter]
fn get_lowercase(self_: PyRef<Self>) -> bool {
getter!(self_, BertNormalizer, lowercase)
}
#[setter]
fn set_lowercase(self_: PyRef<Self>, lowercase: bool) {
setter!(self_, BertNormalizer, lowercase, lowercase)
}
#[new]
#[pyo3(signature = (
clean_text = true,
handle_chinese_chars = true,
strip_accents = None,
lowercase = true
),
text_signature = "(self, clean_text=True, handle_chinese_chars=True, strip_accents=None, lowercase=True)")]
fn new(
clean_text: bool,
handle_chinese_chars: bool,
strip_accents: Option<bool>,
lowercase: bool,
) -> (Self, PyNormalizer) {
let normalizer =
BertNormalizer::new(clean_text, handle_chinese_chars, strip_accents, lowercase);
(PyBertNormalizer {}, normalizer.into())
}
}
/// NFD Unicode Normalizer
#[pyclass(extends=PyNormalizer, module = "tokenizers.normalizers", name = "NFD")]
pub struct PyNFD {}
#[pymethods]
impl PyNFD {
#[new]
#[pyo3(text_signature = "(self)")]
fn new() -> (Self, PyNormalizer) {
(PyNFD {}, PyNormalizer::new(NFD.into()))
}
}
/// NFKD Unicode Normalizer
#[pyclass(extends=PyNormalizer, module = "tokenizers.normalizers", name = "NFKD")]
pub struct PyNFKD {}
#[pymethods]
impl PyNFKD {
#[new]
#[pyo3(text_signature = "(self)")]
fn new() -> (Self, PyNormalizer) {
(PyNFKD {}, NFKD.into())
}
}
/// NFC Unicode Normalizer
#[pyclass(extends=PyNormalizer, module = "tokenizers.normalizers", name = "NFC")]
pub struct PyNFC {}
#[pymethods]
impl PyNFC {
#[new]
#[pyo3(text_signature = "(self)")]
fn new() -> (Self, PyNormalizer) {
(PyNFC {}, NFC.into())
}
}
/// NFKC Unicode Normalizer
#[pyclass(extends=PyNormalizer, module = "tokenizers.normalizers", name = "NFKC")]
pub struct PyNFKC {}
#[pymethods]
impl PyNFKC {
#[new]
#[pyo3(text_signature = "(self)")]
fn new() -> (Self, PyNormalizer) {
(PyNFKC {}, NFKC.into())
}
}
/// Allows concatenating multiple other Normalizer as a Sequence.
/// All the normalizers run in sequence in the given order
///
/// Args:
/// normalizers (:obj:`List[Normalizer]`):
/// A list of Normalizer to be run as a sequence
#[pyclass(extends=PyNormalizer, module = "tokenizers.normalizers", name = "Sequence")]
pub struct PySequence {}
#[pymethods]
impl PySequence {
#[new]
#[pyo3(text_signature = None)]
fn new(normalizers: &PyList) -> PyResult<(Self, PyNormalizer)> {
let mut sequence = Vec::with_capacity(normalizers.len());
for n in normalizers.iter() {
let normalizer: PyRef<PyNormalizer> = n.extract()?;
match &normalizer.normalizer {
PyNormalizerTypeWrapper::Sequence(inner) => sequence.extend(inner.iter().cloned()),
PyNormalizerTypeWrapper::Single(inner) => sequence.push(inner.clone()),
}
}
Ok((
PySequence {},
PyNormalizer::new(PyNormalizerTypeWrapper::Sequence(sequence)),
))
}
fn __getnewargs__<'p>(&self, py: Python<'p>) -> &'p PyTuple {
PyTuple::new(py, [PyList::empty(py)])
}
fn __len__(&self) -> usize {
0
}
}
/// Lowercase Normalizer
#[pyclass(extends=PyNormalizer, module = "tokenizers.normalizers", name = "Lowercase")]
pub struct PyLowercase {}
#[pymethods]
impl PyLowercase {
#[new]
#[pyo3(text_signature = "(self)")]
fn new() -> (Self, PyNormalizer) {
(PyLowercase {}, Lowercase.into())
}
}
/// Strip normalizer
#[pyclass(extends=PyNormalizer, module = "tokenizers.normalizers", name = "Strip")]
pub struct PyStrip {}
#[pymethods]
impl PyStrip {
#[getter]
fn get_left(self_: PyRef<Self>) -> bool {
getter!(self_, StripNormalizer, strip_left)
}
#[setter]
fn set_left(self_: PyRef<Self>, left: bool) {
setter!(self_, StripNormalizer, strip_left, left)
}
#[getter]
fn get_right(self_: PyRef<Self>) -> bool {
getter!(self_, StripNormalizer, strip_right)
}
#[setter]
fn set_right(self_: PyRef<Self>, right: bool) {
setter!(self_, StripNormalizer, strip_right, right)
}
#[new]
#[pyo3(signature = (left = true, right = true), text_signature = "(self, left=True, right=True)")]
fn new(left: bool, right: bool) -> (Self, PyNormalizer) {
(PyStrip {}, Strip::new(left, right).into())
}
}
/// Prepend normalizer
#[pyclass(extends=PyNormalizer, module = "tokenizers.normalizers", name = "Prepend")]
pub struct PyPrepend {}
#[pymethods]
impl PyPrepend {
#[getter]
fn get_prepend(self_: PyRef<Self>) -> String {
getter!(self_, Prepend, prepend)
}
#[setter]
fn set_prepend(self_: PyRef<Self>, prepend: String) {
setter!(self_, Prepend, prepend, prepend)
}
#[new]
#[pyo3(signature = (prepend="▁".to_string()), text_signature = "(self, prepend)")]
fn new(prepend: String) -> (Self, PyNormalizer) {
(PyPrepend {}, Prepend::new(prepend).into())
}
}
/// StripAccents normalizer
#[pyclass(extends=PyNormalizer, module = "tokenizers.normalizers", name = "StripAccents")]
pub struct PyStripAccents {}
#[pymethods]
impl PyStripAccents {
#[new]
#[pyo3(text_signature = "(self)")]
fn new() -> (Self, PyNormalizer) {
(PyStripAccents {}, StripAccents.into())
}
}
/// Nmt normalizer
#[pyclass(extends=PyNormalizer, module = "tokenizers.normalizers", name = "Nmt")]
pub struct PyNmt {}
#[pymethods]
impl PyNmt {
#[new]
#[pyo3(text_signature = "(self)")]
fn new() -> (Self, PyNormalizer) {
(PyNmt {}, Nmt.into())
}
}
/// Precompiled normalizer
/// Don't use manually it is used for compatiblity for SentencePiece.
#[pyclass(extends=PyNormalizer, module = "tokenizers.normalizers", name = "Precompiled")]
pub struct PyPrecompiled {}
#[pymethods]
impl PyPrecompiled {
#[new]
#[pyo3(text_signature = "(self, precompiled_charsmap)")]
fn new(py_precompiled_charsmap: &PyBytes) -> PyResult<(Self, PyNormalizer)> {
let precompiled_charsmap: &[u8] = FromPyObject::extract(py_precompiled_charsmap)?;
Ok((
PyPrecompiled {},
Precompiled::from(precompiled_charsmap)
.map_err(|e| {
exceptions::PyException::new_err(format!(
"Error while attempting to build Precompiled normalizer: {}",
e
))
})?
.into(),
))
}
}
/// Replace normalizer
#[pyclass(extends=PyNormalizer, module = "tokenizers.normalizers", name = "Replace")]
pub struct PyReplace {}
#[pymethods]
impl PyReplace {
#[new]
#[pyo3(text_signature = "(self, pattern, content)")]
fn new(pattern: PyPattern, content: String) -> PyResult<(Self, PyNormalizer)> {
Ok((
PyReplace {},
ToPyResult(Replace::new(pattern, content)).into_py()?.into(),
))
}
}
#[derive(Debug, Clone)]
pub(crate) struct CustomNormalizer {
inner: PyObject,
}
impl CustomNormalizer {
pub fn new(inner: PyObject) -> Self {
Self { inner }
}
}
impl tk::tokenizer::Normalizer for CustomNormalizer {
fn normalize(&self, normalized: &mut NormalizedString) -> tk::Result<()> {
Python::with_gil(|py| {
let normalized = PyNormalizedStringRefMut::new(normalized);
let py_normalized = self.inner.as_ref(py);
py_normalized.call_method("normalize", (normalized.get(),), None)?;
Ok(())
})
}
}
impl Serialize for CustomNormalizer {
fn serialize<S>(&self, _serializer: S) -> Result<S::Ok, S::Error>
where
S: Serializer,
{
Err(serde::ser::Error::custom(
"Custom Normalizer cannot be serialized",
))
}
}
impl<'de> Deserialize<'de> for CustomNormalizer {
fn deserialize<D>(_deserializer: D) -> Result<Self, D::Error>
where
D: Deserializer<'de>,
{
Err(serde::de::Error::custom(
"Custom Normalizer cannot be deserialized",
))
}
}
#[derive(Debug, Clone, Deserialize)]
#[serde(untagged)]
pub(crate) enum PyNormalizerWrapper {
Custom(CustomNormalizer),
Wrapped(NormalizerWrapper),
}
impl Serialize for PyNormalizerWrapper {
fn serialize<S>(&self, serializer: S) -> Result<<S as Serializer>::Ok, <S as Serializer>::Error>
where
S: Serializer,
{
match self {
PyNormalizerWrapper::Wrapped(inner) => inner.serialize(serializer),
PyNormalizerWrapper::Custom(inner) => inner.serialize(serializer),
}
}
}
#[derive(Debug, Clone, Deserialize)]
#[serde(untagged)]
pub(crate) enum PyNormalizerTypeWrapper {
Sequence(Vec<Arc<RwLock<PyNormalizerWrapper>>>),
Single(Arc<RwLock<PyNormalizerWrapper>>),
}
impl Serialize for PyNormalizerTypeWrapper {
fn serialize<S>(&self, serializer: S) -> Result<S::Ok, S::Error>
where
S: Serializer,
{
match self {
PyNormalizerTypeWrapper::Sequence(seq) => {
let mut ser = serializer.serialize_struct("Sequence", 2)?;
ser.serialize_field("type", "Sequence")?;
ser.serialize_field("normalizers", seq)?;
ser.end()
}
PyNormalizerTypeWrapper::Single(inner) => inner.serialize(serializer),
}
}
}
impl<I> From<I> for PyNormalizerWrapper
where
I: Into<NormalizerWrapper>,
{
fn from(norm: I) -> Self {
PyNormalizerWrapper::Wrapped(norm.into())
}
}
impl<I> From<I> for PyNormalizerTypeWrapper
where
I: Into<PyNormalizerWrapper>,
{
fn from(norm: I) -> Self {
PyNormalizerTypeWrapper::Single(Arc::new(RwLock::new(norm.into())))
}
}
impl<I> From<I> for PyNormalizer
where
I: Into<NormalizerWrapper>,
{
fn from(norm: I) -> Self {
PyNormalizer {
normalizer: norm.into().into(),
}
}
}
impl Normalizer for PyNormalizerTypeWrapper {
fn normalize(&self, normalized: &mut NormalizedString) -> tk::Result<()> {
match self {
PyNormalizerTypeWrapper::Single(inner) => inner.read().unwrap().normalize(normalized),
PyNormalizerTypeWrapper::Sequence(inner) => inner
.iter()
.try_for_each(|n| n.read().unwrap().normalize(normalized)),
}
}
}
impl Normalizer for PyNormalizerWrapper {
fn normalize(&self, normalized: &mut NormalizedString) -> tk::Result<()> {
match self {
PyNormalizerWrapper::Wrapped(inner) => inner.normalize(normalized),
PyNormalizerWrapper::Custom(inner) => inner.normalize(normalized),
}
}
}
/// Normalizers Module
#[pymodule]
pub fn normalizers(_py: Python, m: &PyModule) -> PyResult<()> {
m.add_class::<PyNormalizer>()?;
m.add_class::<PyBertNormalizer>()?;
m.add_class::<PyNFD>()?;
m.add_class::<PyNFKD>()?;
m.add_class::<PyNFC>()?;
m.add_class::<PyNFKC>()?;
m.add_class::<PySequence>()?;
m.add_class::<PyLowercase>()?;
m.add_class::<PyStrip>()?;
m.add_class::<PyStripAccents>()?;
m.add_class::<PyPrepend>()?;
m.add_class::<PyNmt>()?;
m.add_class::<PyPrecompiled>()?;
m.add_class::<PyReplace>()?;
Ok(())
}
#[cfg(test)]
mod test {
use pyo3::prelude::*;
use tk::normalizers::unicode::{NFC, NFKC};
use tk::normalizers::utils::Sequence;
use tk::normalizers::NormalizerWrapper;
use crate::normalizers::{PyNormalizer, PyNormalizerTypeWrapper, PyNormalizerWrapper};
#[test]
fn get_subtype() {
Python::with_gil(|py| {
let py_norm = PyNormalizer::new(NFC.into());
let py_nfc = py_norm.get_as_subtype(py).unwrap();
assert_eq!("NFC", py_nfc.as_ref(py).get_type().name().unwrap());
})
}
#[test]
fn serialize() {
let py_wrapped: PyNormalizerWrapper = NFKC.into();
let py_ser = serde_json::to_string(&py_wrapped).unwrap();
let rs_wrapped = NormalizerWrapper::NFKC(NFKC);
let rs_ser = serde_json::to_string(&rs_wrapped).unwrap();
assert_eq!(py_ser, rs_ser);
let py_norm: PyNormalizer = serde_json::from_str(&rs_ser).unwrap();
match py_norm.normalizer {
PyNormalizerTypeWrapper::Single(inner) => match *inner.as_ref().read().unwrap() {
PyNormalizerWrapper::Wrapped(NormalizerWrapper::NFKC(_)) => {}
_ => panic!("Expected NFKC"),
},
_ => panic!("Expected wrapped, not sequence."),
}
let py_seq: PyNormalizerWrapper = Sequence::new(vec![NFC.into(), NFKC.into()]).into();
let py_wrapper_ser = serde_json::to_string(&py_seq).unwrap();
let rs_wrapped = NormalizerWrapper::Sequence(Sequence::new(vec![NFC.into(), NFKC.into()]));
let rs_ser = serde_json::to_string(&rs_wrapped).unwrap();
assert_eq!(py_wrapper_ser, rs_ser);
let py_seq = PyNormalizer::new(py_seq.into());
let py_ser = serde_json::to_string(&py_seq).unwrap();
assert_eq!(py_wrapper_ser, py_ser);
let rs_seq = Sequence::new(vec![NFC.into(), NFKC.into()]);
let rs_ser = serde_json::to_string(&rs_seq).unwrap();
assert_eq!(py_wrapper_ser, rs_ser);
}
#[test]
fn deserialize_sequence() {
let string = r#"{"type": "NFKC"}"#;
let normalizer: PyNormalizer = serde_json::from_str(string).unwrap();
match normalizer.normalizer {
PyNormalizerTypeWrapper::Single(inner) => match *inner.as_ref().read().unwrap() {
PyNormalizerWrapper::Wrapped(NormalizerWrapper::NFKC(_)) => {}
_ => panic!("Expected NFKC"),
},
_ => panic!("Expected wrapped, not sequence."),
}
let sequence_string = format!(r#"{{"type": "Sequence", "normalizers": [{}]}}"#, string);
let normalizer: PyNormalizer = serde_json::from_str(&sequence_string).unwrap();
match normalizer.normalizer {
PyNormalizerTypeWrapper::Single(inner) => match &*inner.as_ref().read().unwrap() {
PyNormalizerWrapper::Wrapped(NormalizerWrapper::Sequence(sequence)) => {
let normalizers = sequence.get_normalizers();
assert_eq!(normalizers.len(), 1);
match normalizers[0] {
NormalizerWrapper::NFKC(_) => {}
_ => panic!("Expected NFKC"),
}
}
_ => panic!("Expected sequence"),
},
_ => panic!("Expected single"),
};
}
}
| tokenizers/bindings/python/src/normalizers.rs/0 | {
"file_path": "tokenizers/bindings/python/src/normalizers.rs",
"repo_id": "tokenizers",
"token_count": 11191
} | 229 |
import pytest
from tokenizers import BertWordPieceTokenizer
from ..utils import bert_files, data_dir
class TestEncoding:
@pytest.fixture(scope="class")
def encodings(self, bert_files):
tokenizer = BertWordPieceTokenizer.from_file(bert_files["vocab"])
single_encoding = tokenizer.encode("I love HuggingFace")
pair_encoding = tokenizer.encode("I love HuggingFace", "Do you?")
return single_encoding, pair_encoding
def test_sequence_ids(self, encodings):
single, pair = encodings
assert single.sequence_ids == [None, 0, 0, 0, 0, None]
assert pair.sequence_ids == [None, 0, 0, 0, 0, None, 1, 1, 1, None]
def test_n_sequences(self, encodings):
single, pair = encodings
assert single.n_sequences == 1
assert pair.n_sequences == 2
def test_word_to_tokens(self, encodings):
single, pair = encodings
assert single.tokens == ["[CLS]", "i", "love", "hugging", "##face", "[SEP]"]
assert single.word_to_tokens(0) == (1, 2)
assert pair.tokens == [
"[CLS]",
"i",
"love",
"hugging",
"##face",
"[SEP]",
"do",
"you",
"?",
"[SEP]",
]
assert pair.word_to_tokens(0) == (1, 2)
assert pair.word_to_tokens(0, 0) == (1, 2)
assert pair.word_to_tokens(6, 0) == None
assert pair.word_to_tokens(0, 1) == (6, 7)
def test_word_to_chars(self, encodings):
single, pair = encodings
assert single.word_to_chars(2) == (7, 18)
assert pair.word_to_chars(2) == (7, 18)
assert pair.word_to_chars(2, 0) == (7, 18)
assert pair.word_to_chars(2, 1) == (6, 7)
def test_token_to_sequence(self, encodings):
single, pair = encodings
assert single.token_to_sequence(2) == 0
assert pair.token_to_sequence(2) == 0
assert pair.token_to_sequence(0) == None
assert pair.token_to_sequence(5) == None
assert pair.token_to_sequence(6) == 1
assert pair.token_to_sequence(8) == 1
assert pair.token_to_sequence(9) == None
assert pair.token_to_sequence(1200) == None
def test_token_to_chars(self, encodings):
single, pair = encodings
assert single.token_to_chars(0) == None
assert single.token_to_chars(2) == (2, 6)
assert pair.token_to_chars(2) == (2, 6)
assert pair.token_to_chars(5) == None
assert pair.token_to_chars(6) == (0, 2)
def test_token_to_word(self, encodings):
single, pair = encodings
assert single.token_to_word(0) == None
assert single.token_to_word(1) == 0
assert single.token_to_word(4) == 2
assert pair.token_to_word(1) == 0
assert pair.token_to_word(4) == 2
assert pair.token_to_word(5) == None
assert pair.token_to_word(6) == 0
assert pair.token_to_word(7) == 1
def test_char_to_token(self, encodings):
single, pair = encodings
assert single.char_to_token(0) == 1
assert pair.char_to_token(0) == 1
assert pair.char_to_token(0, 0) == 1
assert pair.char_to_token(1, 0) == None
assert pair.char_to_token(0, 1) == 6
assert pair.char_to_token(2, 1) == None
def test_char_to_word(self, encodings):
single, pair = encodings
assert single.char_to_word(0) == 0
assert single.char_to_word(1) == None
assert pair.char_to_word(2) == 1
assert pair.char_to_word(2, 0) == 1
assert pair.char_to_word(2, 1) == None
assert pair.char_to_word(3, 1) == 1
def test_truncation(self, encodings):
single, _ = encodings
single.truncate(2, 1, "right")
assert single.tokens == ["[CLS]", "i"]
assert single.overflowing[0].tokens == ["i", "love"]
def test_invalid_truncate_direction(self, encodings):
single, _ = encodings
with pytest.raises(ValueError) as excinfo:
single.truncate(2, 1, "not_a_direction")
assert "Invalid truncation direction value : not_a_direction" == str(excinfo.value)
| tokenizers/bindings/python/tests/bindings/test_encoding.py/0 | {
"file_path": "tokenizers/bindings/python/tests/bindings/test_encoding.py",
"repo_id": "tokenizers",
"token_count": 1991
} | 230 |
import pytest
from tokenizers import SentencePieceBPETokenizer, SentencePieceUnigramTokenizer
class TestSentencePieceBPE:
def test_train_from_iterator(self):
text = ["A first sentence", "Another sentence", "And a last one"]
tokenizer = SentencePieceBPETokenizer()
tokenizer.train_from_iterator(text, show_progress=False)
output = tokenizer.encode("A sentence")
assert output.tokens == ["▁A", "▁sentence"]
class TestSentencePieceUnigram:
def test_train(self, tmpdir):
p = tmpdir.mkdir("tmpdir").join("file.txt")
p.write("A first sentence\nAnother sentence\nAnd a last one")
tokenizer = SentencePieceUnigramTokenizer()
tokenizer.train(files=str(p), show_progress=False)
output = tokenizer.encode("A sentence")
assert output.tokens == ["▁A", "▁", "s", "en", "t", "en", "c", "e"]
with pytest.raises(Exception) as excinfo:
_ = tokenizer.encode("A sentence 🤗")
assert str(excinfo.value) == "Encountered an unknown token but `unk_id` is missing"
def test_train_with_unk_token(self, tmpdir):
p = tmpdir.mkdir("tmpdir").join("file.txt")
p.write("A first sentence\nAnother sentence\nAnd a last one")
tokenizer = SentencePieceUnigramTokenizer()
tokenizer.train(files=str(p), show_progress=False, special_tokens=["<unk>"], unk_token="<unk>")
output = tokenizer.encode("A sentence 🤗")
assert output.ids[-1] == 0
assert output.tokens == ["▁A", "▁", "s", "en", "t", "en", "c", "e", "▁", "🤗"]
def test_train_from_iterator(self):
text = ["A first sentence", "Another sentence", "And a last one"]
tokenizer = SentencePieceUnigramTokenizer()
tokenizer.train_from_iterator(text, show_progress=False)
output = tokenizer.encode("A sentence")
assert output.tokens == ["▁A", "▁", "s", "en", "t", "en", "c", "e"]
with pytest.raises(Exception) as excinfo:
_ = tokenizer.encode("A sentence 🤗")
assert str(excinfo.value) == "Encountered an unknown token but `unk_id` is missing"
def test_train_from_iterator_with_unk_token(self):
text = ["A first sentence", "Another sentence", "And a last one"]
tokenizer = SentencePieceUnigramTokenizer()
tokenizer.train_from_iterator(
text, vocab_size=100, show_progress=False, special_tokens=["<unk>"], unk_token="<unk>"
)
output = tokenizer.encode("A sentence 🤗")
assert output.ids[-1] == 0
assert output.tokens == ["▁A", "▁", "s", "en", "t", "en", "c", "e", "▁", "🤗"]
| tokenizers/bindings/python/tests/implementations/test_sentencepiece.py/0 | {
"file_path": "tokenizers/bindings/python/tests/implementations/test_sentencepiece.py",
"repo_id": "tokenizers",
"token_count": 1118
} | 231 |
/* Our DOM objects */
/* Version control */
.selectors {
margin-bottom: 10px;
}
.dropdown-button {
display: inline-block;
width: 50%;
background-color: #6670FF;
color: white;
border: none;
padding: 5px;
font-size: 15px;
cursor: pointer;
}
.dropdown-button:hover, .dropdown-button:focus, .dropdown-button.active {
background-color: #A6B0FF;
}
.dropdown-button.active {
background-color: #7988FF;
}
.menu-dropdown {
display: none;
background-color: #7988FF;
min-width: 160px;
overflow: auto;
font-size: 15px;
padding: 10px 0;
}
.menu-dropdown a {
color: white;
padding: 3px 4px;
text-decoration: none;
display: block;
}
.menu-dropdown a:hover {
background-color: #A6B0FF;
}
.dropdown-link.active {
background-color: #A6B0FF;
}
.show {
display: block;
}
/* The literal code blocks */
.rst-content tt.literal, .rst-content tt.literal, .rst-content code.literal {
color: #6670FF;
}
/* To keep the logo centered */
.wy-side-scroll {
width: auto;
font-size: 20px;
}
/* The div that holds the Hugging Face logo */
.HuggingFaceDiv {
width: 100%
}
/* The research field on top of the toc tree */
.wy-side-nav-search{
padding-top: 0;
background-color: #6670FF;
}
/* The toc tree */
.wy-nav-side{
background-color: #6670FF;
padding-bottom: 0;
}
/* The section headers in the toc tree */
.wy-menu-vertical p.caption{
background-color: #4d59ff;
line-height: 40px;
}
/* The selected items in the toc tree */
.wy-menu-vertical li.current{
background-color: #A6B0FF;
}
/* When a list item that does belong to the selected block from the toc tree is hovered */
.wy-menu-vertical li.current a:hover{
background-color: #B6C0FF;
}
/* When a list item that does NOT belong to the selected block from the toc tree is hovered. */
.wy-menu-vertical li a:hover{
background-color: #A7AFFB;
}
/* The text items on the toc tree */
.wy-menu-vertical a {
color: #FFFFDD;
font-family: Calibre-Light, sans-serif;
}
.wy-menu-vertical header, .wy-menu-vertical p.caption{
color: white;
font-family: Calibre-Light, sans-serif;
}
/* The color inside the selected toc tree block */
.wy-menu-vertical li.toctree-l2 a, .wy-menu-vertical li.toctree-l3 a, .wy-menu-vertical li.toctree-l4 a {
color: black;
}
/* Inside the depth-2 selected toc tree block */
.wy-menu-vertical li.toctree-l2.current>a {
background-color: #B6C0FF
}
.wy-menu-vertical li.toctree-l2.current li.toctree-l3>a {
background-color: #C6D0FF
}
/* Inside the depth-3 selected toc tree block */
.wy-menu-vertical li.toctree-l3.current li.toctree-l4>a{
background-color: #D6E0FF
}
/* Inside code snippets */
.rst-content dl:not(.docutils) dt{
font-size: 15px;
}
/* Links */
a {
color: #6670FF;
}
/* Content bars */
.rst-content dl:not(.docutils) dt {
background-color: rgba(251, 141, 104, 0.1);
border-right: solid 2px #FB8D68;
border-left: solid 2px #FB8D68;
color: #FB8D68;
font-family: Calibre-Light, sans-serif;
border-top: none;
font-style: normal !important;
}
/* Expand button */
.wy-menu-vertical li.toctree-l2 span.toctree-expand,
.wy-menu-vertical li.on a span.toctree-expand, .wy-menu-vertical li.current>a span.toctree-expand,
.wy-menu-vertical li.toctree-l3 span.toctree-expand{
color: black;
}
/* Max window size */
.wy-nav-content{
max-width: 1200px;
}
/* Mobile header */
.wy-nav-top{
background-color: #6670FF;
}
/* Source spans */
.rst-content .viewcode-link, .rst-content .viewcode-back{
color: #6670FF;
font-size: 110%;
letter-spacing: 2px;
text-transform: uppercase;
}
/* It would be better for table to be visible without horizontal scrolling */
.wy-table-responsive table td, .wy-table-responsive table th{
white-space: normal;
}
.footer {
margin-top: 20px;
}
.footer__Social {
display: flex;
flex-direction: row;
}
.footer__CustomImage {
margin: 2px 5px 0 0;
}
/* class and method names in doc */
.rst-content dl:not(.docutils) tt.descname, .rst-content dl:not(.docutils) tt.descclassname, .rst-content dl:not(.docutils) tt.descname, .rst-content dl:not(.docutils) code.descname, .rst-content dl:not(.docutils) tt.descclassname, .rst-content dl:not(.docutils) code.descclassname{
font-family: Calibre, sans-serif;
font-size: 20px !important;
}
/* class name in doc*/
.rst-content dl:not(.docutils) tt.descname, .rst-content dl:not(.docutils) tt.descname, .rst-content dl:not(.docutils) code.descname{
margin-right: 10px;
font-family: Calibre-Medium, sans-serif;
}
/* Method and class parameters */
.sig-param{
line-height: 23px;
}
/* Class introduction "class" string at beginning */
.rst-content dl:not(.docutils) .property{
font-size: 18px;
color: black;
}
/* FONTS */
body{
font-family: Calibre, sans-serif;
font-size: 16px;
}
h1 {
font-family: Calibre-Thin, sans-serif;
font-size: 70px;
}
h2, .rst-content .toctree-wrapper p.caption, h3, h4, h5, h6, legend{
font-family: Calibre-Medium, sans-serif;
}
@font-face {
font-family: Calibre-Medium;
src: url(./Calibre-Medium.otf);
font-weight:400;
}
@font-face {
font-family: Calibre;
src: url(./Calibre-Regular.otf);
font-weight:400;
}
@font-face {
font-family: Calibre-Light;
src: url(./Calibre-Light.ttf);
font-weight:400;
}
@font-face {
font-family: Calibre-Thin;
src: url(./Calibre-Thin.otf);
font-weight:400;
}
/**
* Nav Links to other parts of huggingface.co
*/
div.hf-menu {
position: absolute;
top: 0;
right: 0;
padding-top: 20px;
padding-right: 20px;
z-index: 1000;
}
div.hf-menu a {
font-size: 14px;
letter-spacing: 0.3px;
text-transform: uppercase;
color: white;
-webkit-font-smoothing: antialiased;
background: linear-gradient(0deg, #6671ffb8, #9a66ffb8 50%);
padding: 10px 16px 6px 16px;
border-radius: 3px;
margin-left: 12px;
position: relative;
}
div.hf-menu a:active {
top: 1px;
}
@media (min-width: 768px) and (max-width: 1860px) {
.wy-breadcrumbs {
margin-top: 32px;
}
}
@media (max-width: 768px) {
div.hf-menu {
display: none;
}
}
| tokenizers/docs/source/_static/css/huggingface.css/0 | {
"file_path": "tokenizers/docs/source/_static/css/huggingface.css",
"repo_id": "tokenizers",
"token_count": 2708
} | 232 |
Training from memory
----------------------------------------------------------------------------------------------------
In the `Quicktour <quicktour>`__, we saw how to build and train a tokenizer using text files,
but we can actually use any Python Iterator. In this section we'll see a few different ways of
training our tokenizer.
For all the examples listed below, we'll use the same :class:`~tokenizers.Tokenizer` and
:class:`~tokenizers.trainers.Trainer`, built as following:
.. literalinclude:: ../../../../bindings/python/tests/documentation/test_tutorial_train_from_iterators.py
:language: python
:start-after: START init_tokenizer_trainer
:end-before: END init_tokenizer_trainer
:dedent: 8
This tokenizer is based on the :class:`~tokenizers.models.Unigram` model. It takes care of
normalizing the input using the NFKC Unicode normalization method, and uses a
:class:`~tokenizers.pre_tokenizers.ByteLevel` pre-tokenizer with the corresponding decoder.
For more information on the components used here, you can check `here <components>`__
The most basic way
~~~~~~~~~~~~~~~~~~~~~~~~~~~~~~~~~~~~~~~~~~~~~~~~~~~~~~~~~~~~~~~~~~~~~~~~~~~~~~~~~~~~~~~~~~~~~~~~~~~~
As you probably guessed already, the easiest way to train our tokenizer is by using a :obj:`List`:
.. literalinclude:: ../../../../bindings/python/tests/documentation/test_tutorial_train_from_iterators.py
:language: python
:start-after: START train_basic
:end-before: END train_basic
:dedent: 8
Easy, right? You can use anything working as an iterator here, be it a :obj:`List`, :obj:`Tuple`,
or a :obj:`np.Array`. Anything works as long as it provides strings.
Using the 🤗 Datasets library
~~~~~~~~~~~~~~~~~~~~~~~~~~~~~~~~~~~~~~~~~~~~~~~~~~~~~~~~~~~~~~~~~~~~~~~~~~~~~~~~~~~~~~~~~~~~~~~~~~~~
An awesome way to access one of the many datasets that exist out there is by using the 🤗 Datasets
library. For more information about it, you should check
`the official documentation here <https://huggingface.co/docs/datasets/>`__.
Let's start by loading our dataset:
.. literalinclude:: ../../../../bindings/python/tests/documentation/test_tutorial_train_from_iterators.py
:language: python
:start-after: START load_dataset
:end-before: END load_dataset
:dedent: 8
The next step is to build an iterator over this dataset. The easiest way to do this is probably by
using a generator:
.. literalinclude:: ../../../../bindings/python/tests/documentation/test_tutorial_train_from_iterators.py
:language: python
:start-after: START def_batch_iterator
:end-before: END def_batch_iterator
:dedent: 8
As you can see here, for improved efficiency we can actually provide a batch of examples used
to train, instead of iterating over them one by one. By doing so, we can expect performances very
similar to those we got while training directly from files.
With our iterator ready, we just need to launch the training. In order to improve the look of our
progress bars, we can specify the total length of the dataset:
.. literalinclude:: ../../../../bindings/python/tests/documentation/test_tutorial_train_from_iterators.py
:language: python
:start-after: START train_datasets
:end-before: END train_datasets
:dedent: 8
And that's it!
Using gzip files
~~~~~~~~~~~~~~~~~~~~~~~~~~~~~~~~~~~~~~~~~~~~~~~~~~~~~~~~~~~~~~~~~~~~~~~~~~~~~~~~~~~~~~~~~~~~~~~~~~~~
Since gzip files in Python can be used as iterators, it is extremely simple to train on such files:
.. literalinclude:: ../../../../bindings/python/tests/documentation/test_tutorial_train_from_iterators.py
:language: python
:start-after: START single_gzip
:end-before: END single_gzip
:dedent: 8
Now if we wanted to train from multiple gzip files, it wouldn't be much harder:
.. literalinclude:: ../../../../bindings/python/tests/documentation/test_tutorial_train_from_iterators.py
:language: python
:start-after: START multi_gzip
:end-before: END multi_gzip
:dedent: 8
And voilà!
| tokenizers/docs/source/tutorials/python/training_from_memory.rst/0 | {
"file_path": "tokenizers/docs/source/tutorials/python/training_from_memory.rst",
"repo_id": "tokenizers",
"token_count": 1149
} | 233 |
mod utils;
use tokenizers::models::bpe::{Vocab, BPE};
use tokenizers::Tokenizer;
use wasm_bindgen::prelude::*;
// When the `wee_alloc` feature is enabled, use `wee_alloc` as the global
// allocator.
#[cfg(feature = "wee_alloc")]
#[global_allocator]
static ALLOC: wee_alloc::WeeAlloc = wee_alloc::WeeAlloc::INIT;
#[wasm_bindgen]
pub fn tokenize(string: &str) -> Vec<u32> {
let vocab: Vocab = vec![
("a".to_string(), 0),
("##b".to_string(), 1),
("##c".to_string(), 2),
("ab".to_string(), 3),
("abc".to_string(), 4),
]
.into_iter()
.collect();
let merges = vec![
("a".to_string(), "##b".to_string()),
("ab".to_string(), "##c".to_string()),
];
let bpe = BPE::builder()
.vocab_and_merges(vocab, merges)
.unk_token("[UNK]".to_string())
.continuing_subword_prefix("##".to_string())
.build()
.unwrap();
let tokenizer = Tokenizer::new(bpe);
tokenizer
.encode(string, false)
.unwrap()
.get_ids()
.into_iter()
.cloned()
.collect()
}
| tokenizers/tokenizers/examples/unstable_wasm/src/lib.rs/0 | {
"file_path": "tokenizers/tokenizers/examples/unstable_wasm/src/lib.rs",
"repo_id": "tokenizers",
"token_count": 543
} | 234 |
use crate::tokenizer::{Decoder, Result};
use serde::{Deserialize, Serialize};
#[derive(Deserialize, Clone, Debug, Serialize)]
/// Allows decoding Original BPE by joining all the tokens and then replacing
/// the suffix used to identify end-of-words by whitespaces
#[serde(tag = "type")]
#[non_exhaustive]
pub struct BPEDecoder {
pub suffix: String,
}
impl BPEDecoder {
pub fn new(suffix: String) -> Self {
Self { suffix }
}
}
impl Default for BPEDecoder {
fn default() -> Self {
Self::new("</w>".into())
}
}
impl Decoder for BPEDecoder {
fn decode_chain(&self, tokens: Vec<String>) -> Result<Vec<String>> {
let n = tokens.len() - 1;
Ok(tokens
.into_iter()
.enumerate()
.map(|(i, token)| {
let replacement = if i == n { "" } else { " " };
token.replace(&self.suffix, replacement)
})
.collect())
}
}
| tokenizers/tokenizers/src/decoders/bpe.rs/0 | {
"file_path": "tokenizers/tokenizers/src/decoders/bpe.rs",
"repo_id": "tokenizers",
"token_count": 419
} | 235 |
//! [Unigram](https://arxiv.org/abs/1804.10959) model.
mod lattice;
mod model;
mod serialization;
mod trainer;
mod trie;
pub use lattice::*;
pub use model::*;
pub use trainer::*;
| tokenizers/tokenizers/src/models/unigram/mod.rs/0 | {
"file_path": "tokenizers/tokenizers/src/models/unigram/mod.rs",
"repo_id": "tokenizers",
"token_count": 72
} | 236 |
use crate::tokenizer::{NormalizedString, Normalizer, Result};
use crate::utils::macro_rules_attribute;
use serde::{Deserialize, Serialize};
use unicode_normalization_alignments::char::is_combining_mark;
#[derive(Copy, Clone, Debug, Deserialize, Serialize)]
#[serde(tag = "type")]
#[non_exhaustive]
pub struct Strip {
pub strip_left: bool,
pub strip_right: bool,
}
impl Strip {
pub fn new(strip_left: bool, strip_right: bool) -> Self {
Self {
strip_left,
strip_right,
}
}
}
impl Normalizer for Strip {
/// Strip the normalized string inplace
fn normalize(&self, normalized: &mut NormalizedString) -> Result<()> {
if self.strip_left && self.strip_right {
// Fast path
normalized.strip();
} else {
if self.strip_left {
normalized.lstrip();
}
if self.strip_right {
normalized.rstrip();
}
}
Ok(())
}
}
// This normalizer removes combining marks from a normalized string
// It's different from unidecode as it does not attempt to modify
// non ascii languages.
#[derive(Copy, Clone, Debug)]
#[macro_rules_attribute(impl_serde_type!)]
pub struct StripAccents;
impl Normalizer for StripAccents {
/// Strip the normalized string inplace
fn normalize(&self, normalized: &mut NormalizedString) -> Result<()> {
normalized.filter(|c| !is_combining_mark(c));
Ok(())
}
}
#[cfg(test)]
mod tests {
use super::*;
use crate::normalizer::NormalizedString;
use crate::normalizers::Lowercase;
use crate::normalizers::NFKD;
use unicode_normalization_alignments::UnicodeNormalization;
#[test]
fn test_strip_accents() {
// Unicode combining char
let original: String = "Me llamó".nfkd().map(|(c, _)| c).collect();
let normalized = "Me llamo";
assert_ne!(original, normalized);
let mut n = NormalizedString::from(original);
StripAccents.normalize(&mut n).unwrap();
assert_eq!(&n.get(), &normalized);
// Ignores regular ascii
let original = "Me llamo";
let normalized = "Me llamo";
assert_eq!(original, normalized);
let mut n = NormalizedString::from(original);
StripAccents.normalize(&mut n).unwrap();
assert_eq!(&n.get(), &normalized);
// Does not change chinese
let original: String = "这很简单".nfkd().map(|(c, _)| c).collect();
let normalized = "这很简单";
assert_eq!(original, normalized);
let mut n = NormalizedString::from(original);
StripAccents.normalize(&mut n).unwrap();
assert_eq!(&n.get(), &normalized);
}
#[test]
fn test_vietnamese_bug() {
let original: String = "ậ…".to_string();
let normalized = "a...".to_string();
assert_ne!(original, normalized);
let mut n = NormalizedString::from(original);
NFKD.normalize(&mut n).unwrap();
StripAccents.normalize(&mut n).unwrap();
assert_eq!(&n.get(), &normalized);
Lowercase.normalize(&mut n).unwrap();
assert_eq!(&n.get(), &normalized);
let original: String = "Cụ thể, bạn sẽ tham gia một nhóm các giám đốc điều hành tổ chức, các nhà lãnh đạo doanh nghiệp, các học giả, chuyên gia phát triển và tình nguyện viên riêng biệt trong lĩnh vực phi lợi nhuận…".to_string();
let normalized = "cu the, ban se tham gia mot nhom cac giam đoc đieu hanh to chuc, cac nha lanh đao doanh nghiep, cac hoc gia, chuyen gia phat trien va tinh nguyen vien rieng biet trong linh vuc phi loi nhuan...".to_string();
let mut n = NormalizedString::from(original);
NFKD.normalize(&mut n).unwrap();
StripAccents.normalize(&mut n).unwrap();
Lowercase.normalize(&mut n).unwrap();
assert_eq!(&n.get(), &normalized);
}
#[test]
fn test_thai_bug() {
let original = "ำน\u{e49}ำ3ลำ".to_string();
let normalized = "านา3ลา".to_string();
assert_ne!(original, normalized);
let mut n = NormalizedString::from(original);
NFKD.normalize(&mut n).unwrap();
StripAccents.normalize(&mut n).unwrap();
Lowercase.normalize(&mut n).unwrap();
assert_eq!(&n.get(), &normalized);
}
#[test]
fn test_strip_accents_multiple() {
let original = "e\u{304}\u{304}\u{304}o";
let normalized = "eo";
assert_ne!(original, normalized);
let mut n = NormalizedString::from(original);
StripAccents.normalize(&mut n).unwrap();
assert_eq!(&n.get(), &normalized);
assert_eq!(
n,
NormalizedString::new(
original.to_string(),
normalized.to_string(),
vec![(0, 1), (7, 8)],
0
)
);
assert_eq!(
n.alignments_original(),
vec![
(0, 1),
(1, 1),
(1, 1),
(1, 1),
(1, 1),
(1, 1),
(1, 1),
(1, 2)
]
);
}
}
| tokenizers/tokenizers/src/normalizers/strip.rs/0 | {
"file_path": "tokenizers/tokenizers/src/normalizers/strip.rs",
"repo_id": "tokenizers",
"token_count": 2512
} | 237 |
use crate::tokenizer::{Encoding, PostProcessor, Result};
use serde::{Deserialize, Serialize};
use std::collections::HashMap;
use std::iter::FromIterator;
#[derive(Serialize, Deserialize, Clone, Debug, PartialEq, Eq)]
#[serde(tag = "type")]
pub struct BertProcessing {
sep: (String, u32),
cls: (String, u32),
}
impl Default for BertProcessing {
fn default() -> Self {
Self {
sep: ("[SEP]".into(), 102),
cls: ("[CLS]".into(), 101),
}
}
}
impl BertProcessing {
pub fn new(sep: (String, u32), cls: (String, u32)) -> Self {
Self { sep, cls }
}
}
#[derive(thiserror::Error, Debug)]
pub enum BertProcessorError {
#[error("encodings vector length must be either 1 or 2")]
InvalidEncodingsVecLength,
}
impl PostProcessor for BertProcessing {
fn added_tokens(&self, is_pair: bool) -> usize {
if is_pair {
3
} else {
2
}
}
fn process_encodings(
&self,
mut encodings: Vec<Encoding>,
add_special_tokens: bool,
) -> Result<Vec<Encoding>> {
if !add_special_tokens {
return Ok(encodings);
}
let encodings: Vec<Encoding> = encodings
.iter_mut()
.enumerate()
.map(|(i, encoding)| {
if i == 0 {
let ids = [&[self.cls.1], encoding.get_ids(), &[self.sep.1]].concat();
let type_ids = [&[0], encoding.get_type_ids(), &[0]].concat();
let tokens = [
&[self.cls.0.clone()],
encoding.get_tokens(),
&[self.sep.0.clone()],
]
.concat();
let words = [&[None], encoding.get_word_ids(), &[None]].concat();
let offsets = [&[(0, 0)], encoding.get_offsets(), &[(0, 0)]].concat();
let special_tokens =
[&[1u32], &vec![0; encoding.get_ids().len()][..], &[1]].concat();
let attention_mask = vec![1; ids.len()];
// For compatibility with `TemplateProcessing`, the sequence_ranges shouldn't contain
// the special tokens.
let sequence_ranges = HashMap::from_iter(vec![(0, 1..ids.len() - 1)]);
Encoding::new(
ids,
type_ids,
tokens,
words,
offsets,
special_tokens,
attention_mask,
encoding
.take_overflowing()
.into_iter()
.map(|encoding| {
let ids =
[&[self.cls.1], encoding.get_ids(), &[self.sep.1]].concat();
let type_ids = [&[0], encoding.get_type_ids(), &[0]].concat();
let tokens = [
&[self.cls.0.clone()],
encoding.get_tokens(),
&[self.sep.0.clone()],
]
.concat();
let words = [&[None], encoding.get_word_ids(), &[None]].concat();
let offsets =
[&[(0, 0)], encoding.get_offsets(), &[(0, 0)]].concat();
let special_tokens =
[&[1u32], &vec![0; encoding.get_ids().len()][..], &[1]]
.concat();
let attention_mask = vec![1; ids.len()];
// For compatibility with `TemplateProcessing`, the sequence_ranges shouldn't
// contain the special tokens.
let sequence_ranges =
HashMap::from_iter(vec![(0, 1..ids.len() - 1)]);
Encoding::new(
ids,
type_ids,
tokens,
words,
offsets,
special_tokens,
attention_mask,
vec![],
sequence_ranges,
)
})
.collect(),
sequence_ranges,
)
} else {
let pair_ids = [encoding.get_ids(), &[self.sep.1]].concat();
let pair_type_ids = [encoding.get_type_ids(), &[1]].concat();
let pair_tokens = [encoding.get_tokens(), &[self.sep.0.clone()]].concat();
let pair_words = [encoding.get_word_ids(), &[None]].concat();
let pair_offsets = [encoding.get_offsets(), &[(0, 0)]].concat();
let pair_special_tokens =
[&vec![0u32; encoding.get_type_ids().len()][..], &[1]].concat();
let pair_attention_mask = vec![1; pair_ids.len()];
// For compatibility with `TemplateProcessing`, the sequence_ranges shouldn't contain
// the special tokens.
let pair_sequence_ranges = HashMap::from_iter(vec![(1, 0..pair_ids.len() - 1)]);
Encoding::new(
pair_ids,
pair_type_ids,
pair_tokens,
pair_words,
pair_offsets,
pair_special_tokens,
pair_attention_mask,
encoding
.take_overflowing()
.into_iter()
.map(|encoding| {
let pair_ids = [encoding.get_ids(), &[self.sep.1]].concat();
let pair_type_ids = [encoding.get_type_ids(), &[1]].concat();
let pair_tokens =
[encoding.get_tokens(), &[self.sep.0.clone()]].concat();
let pair_words = [encoding.get_word_ids(), &[None]].concat();
let pair_offsets = [encoding.get_offsets(), &[(0, 0)]].concat();
let pair_special_tokens =
[&vec![0u32; encoding.get_type_ids().len()][..], &[1]].concat();
let pair_attention_mask = vec![1; pair_ids.len()];
// For compatibility with `TemplateProcessing`, the sequence_ranges
// shouldn't contain the special tokens.
let pair_sequence_ranges =
HashMap::from_iter(vec![(1, 0..pair_ids.len() - 1)]);
Encoding::new(
pair_ids,
pair_type_ids,
pair_tokens,
pair_words,
pair_offsets,
pair_special_tokens,
pair_attention_mask,
vec![],
pair_sequence_ranges,
)
})
.collect(),
pair_sequence_ranges,
)
}
})
.collect();
Ok(encodings)
}
}
#[cfg(test)]
mod tests {
use super::*;
#[test]
fn serde() {
let bert = BertProcessing::default();
let bert_r = r#"{"type":"BertProcessing","sep":["[SEP]",102],"cls":["[CLS]",101]}"#;
assert_eq!(serde_json::to_string(&bert).unwrap(), bert_r);
assert_eq!(
serde_json::from_str::<BertProcessing>(bert_r).unwrap(),
bert
);
}
#[test]
fn bert_processing() {
let processor = BertProcessing::default();
assert_eq!(processor.added_tokens(false), 2);
assert_eq!(processor.added_tokens(true), 3);
use crate::Token;
let encoding = Encoding::from_tokens(
vec![
Token::new(12, "Hello".into(), (0, 5)),
Token::new(14, "there".into(), (6, 11)),
],
0,
);
let pair = Encoding::from_tokens(vec![Token::new(15, "pair".into(), (0, 4))], 0);
let single_encoding = processor.process(encoding.clone(), None, true).unwrap();
assert_eq!(
single_encoding,
Encoding::new(
vec![101, 12, 14, 102],
vec![0, 0, 0, 0],
vec![
"[CLS]".into(),
"Hello".into(),
"there".into(),
"[SEP]".into()
],
vec![None, None, None, None],
vec![(0, 0), (0, 5), (6, 11), (0, 0)],
vec![1, 0, 0, 1],
vec![1, 1, 1, 1],
vec![],
HashMap::from_iter(vec![(0, 1..3)]),
)
);
assert_eq!(single_encoding.token_to_sequence(2), Some(0));
assert_eq!(single_encoding.token_to_sequence(3), None);
let pair_encoding = processor
.process(encoding.clone(), Some(pair.clone()), true)
.unwrap();
assert_eq!(
pair_encoding,
Encoding::new(
vec![101, 12, 14, 102, 15, 102],
vec![0, 0, 0, 0, 1, 1],
vec![
"[CLS]".into(),
"Hello".into(),
"there".into(),
"[SEP]".into(),
"pair".into(),
"[SEP]".into()
],
vec![None, None, None, None, None, None],
vec![(0, 0), (0, 5), (6, 11), (0, 0), (0, 4), (0, 0)],
vec![1, 0, 0, 1, 0, 1],
vec![1, 1, 1, 1, 1, 1],
vec![],
HashMap::from_iter(vec![(0, 1..3), (1, 4..5)]),
)
);
assert_eq!(pair_encoding.token_to_sequence(2), Some(0));
assert_eq!(pair_encoding.token_to_sequence(3), None);
assert_eq!(pair_encoding.token_to_sequence(4), Some(1));
assert_eq!(pair_encoding.token_to_sequence(5), None);
// No special tokens
let pair_encoding = processor.process(encoding, Some(pair), false).unwrap();
assert_eq!(
pair_encoding,
Encoding::new(
vec![12, 14, 15],
vec![0, 0, 1],
vec!["Hello".into(), "there".into(), "pair".into(),],
vec![None, None, None],
vec![(0, 5), (6, 11), (0, 4)],
vec![0, 0, 0],
vec![1, 1, 1],
vec![],
HashMap::from_iter(vec![(0, 0..2), (1, 2..3)]),
)
);
assert_eq!(pair_encoding.token_to_sequence(0), Some(0));
assert_eq!(pair_encoding.token_to_sequence(1), Some(0));
assert_eq!(pair_encoding.token_to_sequence(2), Some(1));
}
}
| tokenizers/tokenizers/src/processors/bert.rs/0 | {
"file_path": "tokenizers/tokenizers/src/processors/bert.rs",
"repo_id": "tokenizers",
"token_count": 7375
} | 238 |
pub(crate) mod cache;
#[cfg(feature = "http")]
pub(crate) mod from_pretrained;
#[cfg(feature = "unstable_wasm")]
mod fancy;
#[cfg(feature = "unstable_wasm")]
pub use fancy::SysRegex;
#[cfg(not(feature = "unstable_wasm"))]
mod onig;
#[cfg(not(feature = "unstable_wasm"))]
pub use crate::utils::onig::SysRegex;
pub mod iter;
pub mod padding;
pub mod parallelism;
pub(crate) mod progress;
pub mod truncation;
use serde::{Serialize, Serializer};
use std::collections::{BTreeMap, HashMap};
pub(crate) fn ordered_map<S, K, V>(
value: &HashMap<K, V>,
serializer: S,
) -> std::result::Result<S::Ok, S::Error>
where
S: Serializer,
K: Serialize + std::cmp::Ord,
V: Serialize,
{
let ordered: BTreeMap<_, _> = value.iter().collect();
ordered.serialize(serializer)
}
macro_rules! impl_enum_from (
($from_ty:ty, $enum:ty, $variant:ident) => {
impl From<$from_ty> for $enum {
fn from(from: $from_ty) -> Self {
<$enum>::$variant(from)
}
}
}
);
/// Implement `serde::{Serialize, Serializer}` with `#[serde(tag = "type")]` attribute for a given struct.
/// Panic when a json string being deserilized misses field `type`.
///
/// # Examples
///
/// ```
/// # #[macro_use] extern crate tokenizers;
/// use serde::{Serialize, Deserialize};
///
/// fn main() {
/// impl_serde_type!{
/// #[derive(Debug)]
/// struct Point {
/// x: i32,
/// #[serde(default = "default_y")]
/// y: i32,
/// }
/// }
/// fn default_y() -> i32 {
/// 5
/// }
///
/// let point = Point { x: 1, y: 2 };
/// let serialized_s = r#"{"type":"Point","x":1,"y":2}"#;
/// assert_eq!(serde_json::to_string(&point).unwrap(), serialized_s);
/// }
/// ```
///
/// ```should_panic
/// # #[macro_use] extern crate tokenizers;
/// use serde::{Serialize, Deserialize};
///
/// fn main() {
/// impl_serde_type!{
/// #[derive(Debug)]
/// struct Point1D {
/// x: i32,
/// }
/// }
///
/// let serialized_s = r#"{"x":1}"#;
/// let deserialized: Point1D = serde_json::from_str(serialized_s).unwrap();
/// }
/// ```
///
/// # Examples (unit structs)
///
/// ```
/// # #[macro_use] extern crate tokenizers;
/// use serde::{Serialize, Deserialize};
///
/// fn main() {
/// impl_serde_type!{
/// struct Unit;
/// }
///
/// let unit = Unit;
/// let serialized_s = r#"{"type":"Unit"}"#;
/// assert_eq!(serde_json::to_string(&unit).unwrap(), serialized_s);
/// }
/// ```
///
/// ```should_panic
/// # #[macro_use] extern crate tokenizers;
/// use serde::{Serialize, Deserialize};
///
/// fn main() {
/// impl_serde_type!{
/// struct Unit;
/// }
///
/// let serialized_s = r#"{"some_field":1}"#;
/// let deserialized: Unit = serde_json::from_str(serialized_s).unwrap();
/// }
/// ```
#[macro_export]
macro_rules! impl_serde_type{
(
$(#[$meta:meta])*
$vis:vis struct $struct_name:ident {
$(
$(#[$field_meta:meta])*
$field_vis:vis $field_name:ident : $field_type:ty
),*$(,)+
}
) => {
paste::paste!{
$(#[$meta])*
#[derive(Serialize, Deserialize)]
#[serde(tag = "type", from = $struct_name "Deserializer")]
$vis struct $struct_name{
$(
$(#[$field_meta])*
$field_vis $field_name : $field_type,
)*
}
#[doc(hidden)]
$(#[$meta])*
#[derive(Deserialize)]
#[serde(tag = "type", remote = $struct_name "")]
struct [<$struct_name Def>]{
$(
$(#[$field_meta])*
$field_vis $field_name : $field_type,
)*
}
#[doc(hidden)]
#[derive(Deserialize)]
enum [<$struct_name Type>] {
$struct_name,
}
#[doc(hidden)]
#[derive(Deserialize)]
struct [<$struct_name Deserializer>] {
#[allow(dead_code)]
r#type: [<$struct_name Type>],
#[serde(flatten, with = $struct_name "Def")]
r#struct: $struct_name,
}
#[doc(hidden)]
impl std::convert::From<[<$struct_name Deserializer>]> for $struct_name {
fn from(v: [<$struct_name Deserializer>]) -> Self {
v.r#struct
}
}
}
};
(
$(#[$meta:meta])*
$vis:vis struct $struct_name:ident;
) => {
paste::paste!{
$(#[$meta])*
$vis struct $struct_name;
impl serde::Serialize for $struct_name {
fn serialize<S>(&self, serializer: S) -> std::result::Result<S::Ok, S::Error> where
S: serde::ser::Serializer {
let helper = [<$struct_name Helper>]{r#type: [<$struct_name Type>]::$struct_name};
helper.serialize(serializer)
}
}
impl<'de> serde::Deserialize<'de> for $struct_name {
fn deserialize<D>(deserializer: D) -> std::result::Result<Self, D::Error>
where
D: serde::Deserializer<'de>,
{
let _helper = [<$struct_name Helper>]::deserialize(deserializer)?;
Ok($struct_name)
}
}
#[derive(serde::Serialize, serde::Deserialize)]
enum [<$struct_name Type>] {
$struct_name,
}
#[derive(serde::Serialize, serde::Deserialize)]
struct [<$struct_name Helper>] {
#[allow(dead_code)]
r#type: [<$struct_name Type>],
}
}
}
}
// Re-export macro_rules_attribute
pub use macro_rules_attribute::macro_rules_attribute;
| tokenizers/tokenizers/src/utils/mod.rs/0 | {
"file_path": "tokenizers/tokenizers/src/utils/mod.rs",
"repo_id": "tokenizers",
"token_count": 3092
} | 239 |
# coding=utf-8
# Copyright 2022 The HuggingFace Inc. team.
#
# Licensed under the Apache License, Version 2.0 (the "License");
# you may not use this file except in compliance with the License.
# You may obtain a copy of the License at
#
# http://www.apache.org/licenses/LICENSE-2.0
#
# Unless required by applicable law or agreed to in writing, software
# distributed under the License is distributed on an "AS IS" BASIS,
# WITHOUT WARRANTIES OR CONDITIONS OF ANY KIND, either express or implied.
# See the License for the specific language governing permissions and
# limitations under the License.
import argparse
import copy
import os
import random
from dataclasses import dataclass
from typing import Any, Dict, List, Optional
import yaml
COMMON_ENV_VARIABLES = {
"OMP_NUM_THREADS": 1,
"TRANSFORMERS_IS_CI": True,
"PYTEST_TIMEOUT": 120,
"RUN_PIPELINE_TESTS": False,
"RUN_PT_TF_CROSS_TESTS": False,
"RUN_PT_FLAX_CROSS_TESTS": False,
}
# Disable the use of {"s": None} as the output is way too long, causing the navigation on CircleCI impractical
COMMON_PYTEST_OPTIONS = {"max-worker-restart": 0, "dist": "loadfile", "v": None}
DEFAULT_DOCKER_IMAGE = [{"image": "cimg/python:3.8.12"}]
class EmptyJob:
job_name = "empty"
def to_dict(self):
return {
"working_directory": "~/transformers",
"docker": copy.deepcopy(DEFAULT_DOCKER_IMAGE),
"steps":["checkout"],
}
@dataclass
class CircleCIJob:
name: str
additional_env: Dict[str, Any] = None
cache_name: str = None
cache_version: str = "0.8.2"
docker_image: List[Dict[str, str]] = None
install_steps: List[str] = None
marker: Optional[str] = None
parallelism: Optional[int] = 1
pytest_num_workers: int = 12
pytest_options: Dict[str, Any] = None
resource_class: Optional[str] = "2xlarge"
tests_to_run: Optional[List[str]] = None
working_directory: str = "~/transformers"
# This should be only used for doctest job!
command_timeout: Optional[int] = None
def __post_init__(self):
# Deal with defaults for mutable attributes.
if self.additional_env is None:
self.additional_env = {}
if self.cache_name is None:
self.cache_name = self.name
if self.docker_image is None:
# Let's avoid changing the default list and make a copy.
self.docker_image = copy.deepcopy(DEFAULT_DOCKER_IMAGE)
if self.install_steps is None:
self.install_steps = []
if self.pytest_options is None:
self.pytest_options = {}
if isinstance(self.tests_to_run, str):
self.tests_to_run = [self.tests_to_run]
if self.parallelism is None:
self.parallelism = 1
def to_dict(self):
env = COMMON_ENV_VARIABLES.copy()
env.update(self.additional_env)
cache_branch_prefix = os.environ.get("CIRCLE_BRANCH", "pull")
if cache_branch_prefix != "main":
cache_branch_prefix = "pull"
job = {
"working_directory": self.working_directory,
"docker": self.docker_image,
"environment": env,
}
if self.resource_class is not None:
job["resource_class"] = self.resource_class
if self.parallelism is not None:
job["parallelism"] = self.parallelism
steps = [
"checkout",
{"attach_workspace": {"at": "~/transformers/test_preparation"}},
{
"restore_cache": {
"keys": [
# check the fully-matched cache first
f"v{self.cache_version}-{self.cache_name}-{cache_branch_prefix}-pip-" + '{{ checksum "setup.py" }}',
# try the partially-matched cache from `main`
f"v{self.cache_version}-{self.cache_name}-main-pip-",
# try the general partially-matched cache
f"v{self.cache_version}-{self.cache_name}-{cache_branch_prefix}-pip-",
]
}
},
{
"restore_cache": {
"keys": [
f"v{self.cache_version}-{self.cache_name}-{cache_branch_prefix}-site-packages-" + '{{ checksum "setup.py" }}',
f"v{self.cache_version}-{self.cache_name}-main-site-packages-",
f"v{self.cache_version}-{self.cache_name}-{cache_branch_prefix}-site-packages-",
]
}
},
]
steps.extend([{"run": l} for l in self.install_steps])
steps.extend([{"run": 'pip install "fsspec>=2023.5.0,<2023.10.0"'}])
steps.extend([{"run": "pip install pytest-subtests"}])
steps.append({"run": {"name": "Show installed libraries and their versions", "command": "pip freeze | tee installed.txt"}})
steps.append({"store_artifacts": {"path": "~/transformers/installed.txt"}})
all_options = {**COMMON_PYTEST_OPTIONS, **self.pytest_options}
pytest_flags = [f"--{key}={value}" if (value is not None or key in ["doctest-modules"]) else f"-{key}" for key, value in all_options.items()]
pytest_flags.append(
f"--make-reports={self.name}" if "examples" in self.name else f"--make-reports=tests_{self.name}"
)
steps.append({"run": {"name": "Create `test-results` directory", "command": "mkdir test-results"}})
test_command = ""
if self.command_timeout:
test_command = f"timeout {self.command_timeout} "
test_command += f"python -m pytest --junitxml=test-results/junit.xml -n {self.pytest_num_workers} " + " ".join(pytest_flags)
if self.parallelism == 1:
if self.tests_to_run is None:
test_command += " << pipeline.parameters.tests_to_run >>"
else:
test_command += " " + " ".join(self.tests_to_run)
else:
# We need explicit list instead of `pipeline.parameters.tests_to_run` (only available at job runtime)
tests = self.tests_to_run
if tests is None:
folder = os.environ["test_preparation_dir"]
test_file = os.path.join(folder, "filtered_test_list.txt")
if os.path.exists(test_file):
with open(test_file) as f:
tests = f.read().split(" ")
# expand the test list
if tests == ["tests"]:
tests = [os.path.join("tests", x) for x in os.listdir("tests")]
expanded_tests = []
for test in tests:
if test.endswith(".py"):
expanded_tests.append(test)
elif test == "tests/models":
expanded_tests.extend([os.path.join(test, x) for x in os.listdir(test)])
elif test == "tests/pipelines":
expanded_tests.extend([os.path.join(test, x) for x in os.listdir(test)])
else:
expanded_tests.append(test)
# Avoid long tests always being collected together
random.shuffle(expanded_tests)
tests = " ".join(expanded_tests)
# Each executor to run ~10 tests
n_executors = max(len(tests) // 10, 1)
# Avoid empty test list on some executor(s) or launching too many executors
if n_executors > self.parallelism:
n_executors = self.parallelism
job["parallelism"] = n_executors
# Need to be newline separated for the command `circleci tests split` below
command = f'echo {tests} | tr " " "\\n" >> tests.txt'
steps.append({"run": {"name": "Get tests", "command": command}})
command = 'TESTS=$(circleci tests split tests.txt) && echo $TESTS > splitted_tests.txt'
steps.append({"run": {"name": "Split tests", "command": command}})
steps.append({"store_artifacts": {"path": "~/transformers/tests.txt"}})
steps.append({"store_artifacts": {"path": "~/transformers/splitted_tests.txt"}})
test_command = ""
if self.timeout:
test_command = f"timeout {self.timeout} "
test_command += f"python -m pytest -n {self.pytest_num_workers} " + " ".join(pytest_flags)
test_command += " $(cat splitted_tests.txt)"
if self.marker is not None:
test_command += f" -m {self.marker}"
if self.name == "pr_documentation_tests":
# can't use ` | tee tee tests_output.txt` as usual
test_command += " > tests_output.txt"
# Save the return code, so we can check if it is timeout in the next step.
test_command += '; touch "$?".txt'
# Never fail the test step for the doctest job. We will check the results in the next step, and fail that
# step instead if the actual test failures are found. This is to avoid the timeout being reported as test
# failure.
test_command = f"({test_command}) || true"
else:
test_command = f"({test_command} | tee tests_output.txt) || true"
steps.append({"run": {"name": "Run tests", "command": test_command}})
# Deal with errors
check_test_command = f'if [ -s reports/{self.job_name}/errors.txt ]; '
check_test_command += 'then echo "Some tests errored out!"; echo ""; '
check_test_command += f'cat reports/{self.job_name}/errors.txt; '
check_test_command += 'echo ""; echo ""; '
py_command = f'import os; fp = open("reports/{self.job_name}/summary_short.txt"); failed = os.linesep.join([x for x in fp.read().split(os.linesep) if x.startswith("ERROR ")]); fp.close(); fp = open("summary_short.txt", "w"); fp.write(failed); fp.close()'
check_test_command += f"$(python3 -c '{py_command}'); "
check_test_command += 'cat summary_short.txt; echo ""; exit -1; '
# Deeal with failed tests
check_test_command += f'elif [ -s reports/{self.job_name}/failures_short.txt ]; '
check_test_command += 'then echo "Some tests failed!"; echo ""; '
check_test_command += f'cat reports/{self.job_name}/failures_short.txt; '
check_test_command += 'echo ""; echo ""; '
py_command = f'import os; fp = open("reports/{self.job_name}/summary_short.txt"); failed = os.linesep.join([x for x in fp.read().split(os.linesep) if x.startswith("FAILED ")]); fp.close(); fp = open("summary_short.txt", "w"); fp.write(failed); fp.close()'
check_test_command += f"$(python3 -c '{py_command}'); "
check_test_command += 'cat summary_short.txt; echo ""; exit -1; '
check_test_command += f'elif [ -s reports/{self.job_name}/stats.txt ]; then echo "All tests pass!"; '
# return code `124` means the previous (pytest run) step is timeout
if self.name == "pr_documentation_tests":
check_test_command += 'elif [ -f 124.txt ]; then echo "doctest timeout!"; '
check_test_command += 'else echo "other fatal error"; echo ""; exit -1; fi;'
steps.append({"run": {"name": "Check test results", "command": check_test_command}})
steps.append({"store_test_results": {"path": "test-results"}})
steps.append({"store_artifacts": {"path": "~/transformers/tests_output.txt"}})
steps.append({"store_artifacts": {"path": "~/transformers/reports"}})
# save cache at the end: so pytest step runs before cache saving and we can see results earlier
steps.append(
{
"save_cache": {
"key": f"v{self.cache_version}-{self.cache_name}-{cache_branch_prefix}-pip-" + '{{ checksum "setup.py" }}',
"paths": ["~/.cache/pip"],
}
}
)
steps.append(
{
"save_cache": {
"key": f"v{self.cache_version}-{self.cache_name}-{cache_branch_prefix}-site-packages-" + '{{ checksum "setup.py" }}',
"paths": ["~/.pyenv/versions/"],
}
}
)
job["steps"] = steps
return job
@property
def job_name(self):
return self.name if "examples" in self.name else f"tests_{self.name}"
# JOBS
torch_and_tf_job = CircleCIJob(
"torch_and_tf",
additional_env={"RUN_PT_TF_CROSS_TESTS": True},
install_steps=[
"sudo apt-get -y update && sudo apt-get install -y libsndfile1-dev espeak-ng git-lfs cmake",
"git lfs install",
"pip install --upgrade --upgrade-strategy eager pip",
"pip install -U --upgrade-strategy eager .[sklearn,tf-cpu,torch,testing,sentencepiece,torch-speech,vision]",
"pip install -U --upgrade-strategy eager tensorflow_probability",
"pip install -U --upgrade-strategy eager -e git+https://github.com/huggingface/accelerate@main#egg=accelerate",
# TODO: remove this one after fixing the dependency issue(s) above
"pip install -U --upgrade-strategy eager torch torchvision torchaudio --index-url https://download.pytorch.org/whl/cpu",
],
marker="is_pt_tf_cross_test",
pytest_options={"rA": None, "durations": 0},
)
torch_and_flax_job = CircleCIJob(
"torch_and_flax",
additional_env={"RUN_PT_FLAX_CROSS_TESTS": True},
install_steps=[
"sudo apt-get -y update && sudo apt-get install -y libsndfile1-dev espeak-ng",
"pip install -U --upgrade-strategy eager --upgrade pip",
"pip install -U --upgrade-strategy eager .[sklearn,flax,torch,testing,sentencepiece,torch-speech,vision]",
"pip install -U --upgrade-strategy eager -e git+https://github.com/huggingface/accelerate@main#egg=accelerate",
],
marker="is_pt_flax_cross_test",
pytest_options={"rA": None, "durations": 0},
)
torch_job = CircleCIJob(
"torch",
install_steps=[
"sudo apt-get -y update && sudo apt-get install -y libsndfile1-dev espeak-ng time",
"pip install --upgrade --upgrade-strategy eager pip",
"pip install -U --upgrade-strategy eager .[sklearn,torch,testing,sentencepiece,torch-speech,vision,timm]",
"pip install -U --upgrade-strategy eager -e git+https://github.com/huggingface/accelerate@main#egg=accelerate",
],
parallelism=1,
pytest_num_workers=12,
)
tf_job = CircleCIJob(
"tf",
install_steps=[
"sudo apt-get -y update && sudo apt-get install -y libsndfile1-dev espeak-ng cmake",
"pip install --upgrade --upgrade-strategy eager pip",
"pip install -U --upgrade-strategy eager .[sklearn,tf-cpu,testing,sentencepiece,tf-speech,vision]",
"pip install -U --upgrade-strategy eager tensorflow_probability",
],
parallelism=1,
)
flax_job = CircleCIJob(
"flax",
install_steps=[
"sudo apt-get -y update && sudo apt-get install -y libsndfile1-dev espeak-ng",
"pip install --upgrade --upgrade-strategy eager pip",
"pip install -U --upgrade-strategy eager .[flax,testing,sentencepiece,flax-speech,vision]",
],
parallelism=1,
)
pipelines_torch_job = CircleCIJob(
"pipelines_torch",
additional_env={"RUN_PIPELINE_TESTS": True},
install_steps=[
"sudo apt-get -y update && sudo apt-get install -y libsndfile1-dev espeak-ng",
"pip install --upgrade --upgrade-strategy eager pip",
"pip install -U --upgrade-strategy eager .[sklearn,torch,testing,sentencepiece,torch-speech,vision,timm,video]",
],
marker="is_pipeline_test",
pytest_num_workers=12,
)
pipelines_tf_job = CircleCIJob(
"pipelines_tf",
additional_env={"RUN_PIPELINE_TESTS": True},
install_steps=[
"sudo apt-get -y update && sudo apt-get install -y cmake",
"pip install --upgrade --upgrade-strategy eager pip",
"pip install -U --upgrade-strategy eager .[sklearn,tf-cpu,testing,sentencepiece,vision]",
"pip install -U --upgrade-strategy eager tensorflow_probability",
],
marker="is_pipeline_test",
)
custom_tokenizers_job = CircleCIJob(
"custom_tokenizers",
additional_env={"RUN_CUSTOM_TOKENIZERS": True},
install_steps=[
"sudo apt-get -y update && sudo apt-get install -y cmake",
{
"name": "install jumanpp",
"command":
"wget https://github.com/ku-nlp/jumanpp/releases/download/v2.0.0-rc3/jumanpp-2.0.0-rc3.tar.xz\n"
"tar xvf jumanpp-2.0.0-rc3.tar.xz\n"
"mkdir jumanpp-2.0.0-rc3/bld\n"
"cd jumanpp-2.0.0-rc3/bld\n"
"sudo cmake .. -DCMAKE_BUILD_TYPE=Release -DCMAKE_INSTALL_PREFIX=/usr/local\n"
"sudo make install\n",
},
"pip install --upgrade --upgrade-strategy eager pip",
"pip install -U --upgrade-strategy eager .[ja,testing,sentencepiece,jieba,spacy,ftfy,rjieba]",
"python -m unidic download",
],
parallelism=None,
resource_class=None,
tests_to_run=[
"./tests/models/bert_japanese/test_tokenization_bert_japanese.py",
"./tests/models/openai/test_tokenization_openai.py",
"./tests/models/clip/test_tokenization_clip.py",
],
)
examples_torch_job = CircleCIJob(
"examples_torch",
additional_env={"OMP_NUM_THREADS": 8},
cache_name="torch_examples",
install_steps=[
"sudo apt-get -y update && sudo apt-get install -y libsndfile1-dev espeak-ng",
"pip install --upgrade --upgrade-strategy eager pip",
"pip install -U --upgrade-strategy eager .[sklearn,torch,sentencepiece,testing,torch-speech]",
"pip install -U --upgrade-strategy eager -r examples/pytorch/_tests_requirements.txt",
"pip install -U --upgrade-strategy eager -e git+https://github.com/huggingface/accelerate@main#egg=accelerate",
],
pytest_num_workers=1,
)
examples_tensorflow_job = CircleCIJob(
"examples_tensorflow",
cache_name="tensorflow_examples",
install_steps=[
"sudo apt-get -y update && sudo apt-get install -y cmake",
"pip install --upgrade --upgrade-strategy eager pip",
"pip install -U --upgrade-strategy eager .[sklearn,tensorflow,sentencepiece,testing]",
"pip install -U --upgrade-strategy eager -r examples/tensorflow/_tests_requirements.txt",
],
)
examples_flax_job = CircleCIJob(
"examples_flax",
cache_name="flax_examples",
install_steps=[
"pip install --upgrade --upgrade-strategy eager pip",
"pip install -U --upgrade-strategy eager .[flax,testing,sentencepiece]",
"pip install -U --upgrade-strategy eager -r examples/flax/_tests_requirements.txt",
],
)
hub_job = CircleCIJob(
"hub",
additional_env={"HUGGINGFACE_CO_STAGING": True},
install_steps=[
"sudo apt-get -y update && sudo apt-get install git-lfs",
'git config --global user.email "[email protected]"',
'git config --global user.name "ci"',
"pip install --upgrade --upgrade-strategy eager pip",
"pip install -U --upgrade-strategy eager .[torch,sentencepiece,testing,vision]",
],
marker="is_staging_test",
pytest_num_workers=1,
)
onnx_job = CircleCIJob(
"onnx",
install_steps=[
"sudo apt-get -y update && sudo apt-get install -y cmake",
"pip install --upgrade --upgrade-strategy eager pip",
"pip install -U --upgrade-strategy eager .[torch,tf,testing,sentencepiece,onnxruntime,vision,rjieba]",
],
pytest_options={"k onnx": None},
pytest_num_workers=1,
)
exotic_models_job = CircleCIJob(
"exotic_models",
install_steps=[
"sudo apt-get -y update && sudo apt-get install -y libsndfile1-dev",
"pip install --upgrade --upgrade-strategy eager pip",
"pip install -U --upgrade-strategy eager .[torch,testing,vision]",
"pip install -U --upgrade-strategy eager torchvision",
"pip install -U --upgrade-strategy eager scipy",
"pip install -U --upgrade-strategy eager 'git+https://github.com/facebookresearch/detectron2.git'",
"sudo apt install tesseract-ocr",
"pip install -U --upgrade-strategy eager pytesseract",
"pip install --upgrade-strategy eager sentencepiece",
"pip install -U --upgrade-strategy eager natten==0.15.1+torch210cpu -f https://shi-labs.com/natten/wheels",
"pip install -U --upgrade-strategy eager python-Levenshtein",
"pip install -U --upgrade-strategy eager opencv-python",
"pip install -U --upgrade-strategy eager nltk",
"pip uninstall -y torch torchvision torchaudio && pip install -U --upgrade-strategy eager 'torch<2.2.0' 'torchvision<0.17' 'torchaudio<2.2.0'"
],
tests_to_run=[
"tests/models/*layoutlmv*",
"tests/models/*nat",
"tests/models/deta",
"tests/models/udop",
"tests/models/nougat",
],
pytest_num_workers=1,
pytest_options={"durations": 100},
)
repo_utils_job = CircleCIJob(
"repo_utils",
install_steps=[
"pip install --upgrade --upgrade-strategy eager pip",
"pip install -U --upgrade-strategy eager .[quality,testing,torch]",
],
parallelism=None,
pytest_num_workers=1,
resource_class="large",
tests_to_run="tests/repo_utils",
)
# We also include a `dummy.py` file in the files to be doc-tested to prevent edge case failure. Otherwise, the pytest
# hangs forever during test collection while showing `collecting 0 items / 21 errors`. (To see this, we have to remove
# the bash output redirection.)
py_command = 'from utils.tests_fetcher import get_doctest_files; to_test = get_doctest_files() + ["dummy.py"]; to_test = " ".join(to_test); print(to_test)'
py_command = f"$(python3 -c '{py_command}')"
command = f'echo "{py_command}" > pr_documentation_tests_temp.txt'
doc_test_job = CircleCIJob(
"pr_documentation_tests",
additional_env={"TRANSFORMERS_VERBOSITY": "error", "DATASETS_VERBOSITY": "error", "SKIP_CUDA_DOCTEST": "1"},
install_steps=[
"sudo apt-get -y update && sudo apt-get install -y libsndfile1-dev espeak-ng time ffmpeg",
"pip install --upgrade --upgrade-strategy eager pip",
"pip install -U --upgrade-strategy eager -e .[dev]",
"pip install -U --upgrade-strategy eager -e git+https://github.com/huggingface/accelerate@main#egg=accelerate",
"pip install --upgrade --upgrade-strategy eager 'pytest<8.0.0' pytest-sugar",
"pip install -U --upgrade-strategy eager natten==0.15.1+torch210cpu -f https://shi-labs.com/natten/wheels",
"pip install -U --upgrade-strategy eager g2p-en",
# TODO: remove this one after fixing the dependency issue(s) above
"pip install -U --upgrade-strategy eager torch torchvision torchaudio --index-url https://download.pytorch.org/whl/cpu",
"find -name __pycache__ -delete",
"find . -name \*.pyc -delete",
# Add an empty file to keep the test step running correctly even no file is selected to be tested.
"touch dummy.py",
{
"name": "Get files to test",
"command": command,
},
{
"name": "Show information in `Get files to test`",
"command":
"cat pr_documentation_tests_temp.txt"
},
{
"name": "Get the last line in `pr_documentation_tests.txt`",
"command":
"tail -n1 pr_documentation_tests_temp.txt | tee pr_documentation_tests.txt"
},
],
tests_to_run="$(cat pr_documentation_tests.txt)", # noqa
pytest_options={"-doctest-modules": None, "doctest-glob": "*.md", "dist": "loadfile", "rvsA": None},
command_timeout=1200, # test cannot run longer than 1200 seconds
pytest_num_workers=1,
)
REGULAR_TESTS = [
torch_and_tf_job,
torch_and_flax_job,
torch_job,
tf_job,
flax_job,
custom_tokenizers_job,
hub_job,
onnx_job,
exotic_models_job,
]
EXAMPLES_TESTS = [
examples_torch_job,
examples_tensorflow_job,
examples_flax_job,
]
PIPELINE_TESTS = [
pipelines_torch_job,
pipelines_tf_job,
]
REPO_UTIL_TESTS = [repo_utils_job]
DOC_TESTS = [doc_test_job]
def create_circleci_config(folder=None):
if folder is None:
folder = os.getcwd()
# Used in CircleCIJob.to_dict() to expand the test list (for using parallelism)
os.environ["test_preparation_dir"] = folder
jobs = []
all_test_file = os.path.join(folder, "test_list.txt")
if os.path.exists(all_test_file):
with open(all_test_file) as f:
all_test_list = f.read()
else:
all_test_list = []
if len(all_test_list) > 0:
jobs.extend(PIPELINE_TESTS)
test_file = os.path.join(folder, "filtered_test_list.txt")
if os.path.exists(test_file):
with open(test_file) as f:
test_list = f.read()
else:
test_list = []
if len(test_list) > 0:
jobs.extend(REGULAR_TESTS)
extended_tests_to_run = set(test_list.split())
# Extend the test files for cross test jobs
for job in jobs:
if job.job_name in ["tests_torch_and_tf", "tests_torch_and_flax"]:
for test_path in copy.copy(extended_tests_to_run):
dir_path, fn = os.path.split(test_path)
if fn.startswith("test_modeling_tf_"):
fn = fn.replace("test_modeling_tf_", "test_modeling_")
elif fn.startswith("test_modeling_flax_"):
fn = fn.replace("test_modeling_flax_", "test_modeling_")
else:
if job.job_name == "test_torch_and_tf":
fn = fn.replace("test_modeling_", "test_modeling_tf_")
elif job.job_name == "test_torch_and_flax":
fn = fn.replace("test_modeling_", "test_modeling_flax_")
new_test_file = str(os.path.join(dir_path, fn))
if os.path.isfile(new_test_file):
if new_test_file not in extended_tests_to_run:
extended_tests_to_run.add(new_test_file)
extended_tests_to_run = sorted(extended_tests_to_run)
for job in jobs:
if job.job_name in ["tests_torch_and_tf", "tests_torch_and_flax"]:
job.tests_to_run = extended_tests_to_run
fn = "filtered_test_list_cross_tests.txt"
f_path = os.path.join(folder, fn)
with open(f_path, "w") as fp:
fp.write(" ".join(extended_tests_to_run))
example_file = os.path.join(folder, "examples_test_list.txt")
if os.path.exists(example_file) and os.path.getsize(example_file) > 0:
with open(example_file, "r", encoding="utf-8") as f:
example_tests = f.read()
for job in EXAMPLES_TESTS:
framework = job.name.replace("examples_", "").replace("torch", "pytorch")
if example_tests == "all":
job.tests_to_run = [f"examples/{framework}"]
else:
job.tests_to_run = [f for f in example_tests.split(" ") if f.startswith(f"examples/{framework}")]
if len(job.tests_to_run) > 0:
jobs.append(job)
doctest_file = os.path.join(folder, "doctest_list.txt")
if os.path.exists(doctest_file):
with open(doctest_file) as f:
doctest_list = f.read()
else:
doctest_list = []
if len(doctest_list) > 0:
jobs.extend(DOC_TESTS)
repo_util_file = os.path.join(folder, "test_repo_utils.txt")
if os.path.exists(repo_util_file) and os.path.getsize(repo_util_file) > 0:
jobs.extend(REPO_UTIL_TESTS)
if len(jobs) == 0:
jobs = [EmptyJob()]
config = {"version": "2.1"}
config["parameters"] = {
# Only used to accept the parameters from the trigger
"nightly": {"type": "boolean", "default": False},
"tests_to_run": {"type": "string", "default": test_list},
}
config["jobs"] = {j.job_name: j.to_dict() for j in jobs}
config["workflows"] = {"version": 2, "run_tests": {"jobs": [j.job_name for j in jobs]}}
with open(os.path.join(folder, "generated_config.yml"), "w") as f:
f.write(yaml.dump(config, indent=2, width=1000000, sort_keys=False))
if __name__ == "__main__":
parser = argparse.ArgumentParser()
parser.add_argument(
"--fetcher_folder", type=str, default=None, help="Only test that all tests and modules are accounted for."
)
args = parser.parse_args()
create_circleci_config(args.fetcher_folder)
| transformers/.circleci/create_circleci_config.py/0 | {
"file_path": "transformers/.circleci/create_circleci_config.py",
"repo_id": "transformers",
"token_count": 12997
} | 240 |
# https://docs.nvidia.com/deeplearning/frameworks/pytorch-release-notes/rel-23-11.html#rel-23-11
FROM nvcr.io/nvidia/pytorch:23.11-py3
LABEL maintainer="Hugging Face"
ARG DEBIAN_FRONTEND=noninteractive
# Example: `cu102`, `cu113`, etc.
ARG CUDA='cu121'
RUN apt -y update
RUN apt install -y libaio-dev
RUN python3 -m pip install --no-cache-dir --upgrade pip
ARG REF=main
RUN git clone https://github.com/huggingface/transformers && cd transformers && git checkout $REF
RUN python3 -m pip uninstall -y torch torchvision torchaudio
# Install **nightly** release PyTorch (flag `--pre`)
# (PyTorch must be installed before pre-compiling any DeepSpeed c++/cuda ops.)
# (https://www.deepspeed.ai/tutorials/advanced-install/#pre-install-deepspeed-ops)
RUN python3 -m pip install --no-cache-dir -U --pre torch torchvision torchaudio --extra-index-url https://download.pytorch.org/whl/nightly/$CUDA
RUN python3 -m pip install --no-cache-dir ./transformers[deepspeed-testing]
RUN python3 -m pip install --no-cache-dir git+https://github.com/huggingface/accelerate@main#egg=accelerate
# Uninstall `transformer-engine` shipped with the base image
RUN python3 -m pip uninstall -y transformer-engine
# Uninstall `torch-tensorrt` and `apex` shipped with the base image
RUN python3 -m pip uninstall -y torch-tensorrt apex
# Pre-build **nightly** release of DeepSpeed, so it would be ready for testing (otherwise, the 1st deepspeed test will timeout)
RUN python3 -m pip uninstall -y deepspeed
# This has to be run inside the GPU VMs running the tests. (So far, it fails here due to GPU checks during compilation.)
# Issue: https://github.com/microsoft/DeepSpeed/issues/2010
# RUN git clone https://github.com/microsoft/DeepSpeed && cd DeepSpeed && rm -rf build && \
# DS_BUILD_CPU_ADAM=1 DS_BUILD_FUSED_ADAM=1 DS_BUILD_UTILS=1 python3 -m pip install . --global-option="build_ext" --global-option="-j8" --no-cache -v --disable-pip-version-check 2>&1
## For `torchdynamo` tests
## (see https://github.com/huggingface/transformers/pull/17765)
#RUN git clone https://github.com/pytorch/functorch
#RUN python3 -m pip install --no-cache-dir ./functorch[aot]
#RUN cd functorch && python3 setup.py develop
#
#RUN git clone https://github.com/pytorch/torchdynamo
#RUN python3 -m pip install -r ./torchdynamo/requirements.txt
#RUN cd torchdynamo && python3 setup.py develop
#
## install TensorRT
#RUN python3 -m pip install --no-cache-dir -U nvidia-pyindex
#RUN python3 -m pip install --no-cache-dir -U nvidia-tensorrt==8.2.4.2
#
## install torch_tensorrt (fx path)
#RUN git clone https://github.com/pytorch/TensorRT.git
#RUN cd TensorRT/py && python3 setup.py install --fx-only
# When installing in editable mode, `transformers` is not recognized as a package.
# this line must be added in order for python to be aware of transformers.
RUN cd transformers && python3 setup.py develop
# Disable for now as deepspeed is not installed above. To be enabled once the issue is fixed.
# RUN python3 -c "from deepspeed.launcher.runner import main"
| transformers/docker/transformers-pytorch-deepspeed-nightly-gpu/Dockerfile/0 | {
"file_path": "transformers/docker/transformers-pytorch-deepspeed-nightly-gpu/Dockerfile",
"repo_id": "transformers",
"token_count": 1028
} | 241 |
<!--Copyright 2022 The HuggingFace Team. All rights reserved.
Licensed under the Apache License, Version 2.0 (the "License"); you may not use this file except in compliance with
the License. You may obtain a copy of the License at
http://www.apache.org/licenses/LICENSE-2.0
Unless required by applicable law or agreed to in writing, software distributed under the License is distributed on
an "AS IS" BASIS, WITHOUT WARRANTIES OR CONDITIONS OF ANY KIND, either express or implied. See the License for the
⚠️ Note that this file is in Markdown but contain specific syntax for our doc-builder (similar to MDX) that may not be
rendered properly in your Markdown viewer.
-->
# Wie konvertiert man ein 🤗 Transformers-Modell in TensorFlow?
Die Tatsache, dass mehrere Frameworks für die Verwendung mit 🤗 Transformers zur Verfügung stehen, gibt Ihnen die Flexibilität, deren Stärken beim Entwurf Ihrer Anwendung auszuspielen.
Ihre Anwendung zu entwerfen, aber das bedeutet auch, dass die Kompatibilität für jedes Modell einzeln hinzugefügt werden muss. Die gute Nachricht ist, dass
das Hinzufügen von TensorFlow-Kompatibilität zu einem bestehenden Modell einfacher ist als [das Hinzufügen eines neuen Modells von Grund auf](add_new_model)!
Ob Sie ein tieferes Verständnis für große TensorFlow-Modelle haben möchten, einen wichtigen Open-Source-Beitrag leisten oder
TensorFlow für das Modell Ihrer Wahl aktivieren wollen, dieser Leitfaden ist für Sie.
Dieser Leitfaden befähigt Sie, ein Mitglied unserer Gemeinschaft, TensorFlow-Modellgewichte und/oder
Architekturen beizusteuern, die in 🤗 Transformers verwendet werden sollen, und zwar mit minimaler Betreuung durch das Hugging Face Team. Das Schreiben eines neuen Modells
ist keine Kleinigkeit, aber ich hoffe, dass dieser Leitfaden dazu beiträgt, dass es weniger eine Achterbahnfahrt 🎢 und mehr ein Spaziergang im Park 🚶 ist.
Die Nutzung unserer kollektiven Erfahrungen ist absolut entscheidend, um diesen Prozess immer einfacher zu machen, und deshalb möchten wir
ermutigen Sie daher, Verbesserungsvorschläge für diesen Leitfaden zu machen!
Bevor Sie tiefer eintauchen, empfehlen wir Ihnen, die folgenden Ressourcen zu lesen, wenn Sie neu in 🤗 Transformers sind:
- [Allgemeiner Überblick über 🤗 Transformers](add_new_model#general-overview-of-transformers)
- [Die TensorFlow-Philosophie von Hugging Face](https://huggingface.co/blog/tensorflow-philosophy)
Im Rest dieses Leitfadens werden Sie lernen, was nötig ist, um eine neue TensorFlow Modellarchitektur hinzuzufügen, die
Verfahren zur Konvertierung von PyTorch in TensorFlow-Modellgewichte und wie Sie Unstimmigkeiten zwischen ML
Frameworks. Legen Sie los!
<Tip>
Sind Sie unsicher, ob das Modell, das Sie verwenden möchten, bereits eine entsprechende TensorFlow-Architektur hat?
Überprüfen Sie das Feld `model_type` in der `config.json` des Modells Ihrer Wahl
([Beispiel](https://huggingface.co/google-bert/bert-base-uncased/blob/main/config.json#L14)). Wenn der entsprechende Modellordner in
🤗 Transformers eine Datei hat, deren Name mit "modeling_tf" beginnt, bedeutet dies, dass es eine entsprechende TensorFlow
Architektur hat ([Beispiel](https://github.com/huggingface/transformers/tree/main/src/transformers/models/bert)).
</Tip>
## Schritt-für-Schritt-Anleitung zum Hinzufügen von TensorFlow-Modellarchitektur-Code
Es gibt viele Möglichkeiten, eine große Modellarchitektur zu entwerfen, und viele Möglichkeiten, diesen Entwurf zu implementieren. Wie auch immer,
Sie erinnern sich vielleicht an unseren [allgemeinen Überblick über 🤗 Transformers](add_new_model#general-overview-of-transformers)
wissen, dass wir ein meinungsfreudiger Haufen sind - die Benutzerfreundlichkeit von 🤗 Transformers hängt von konsistenten Designentscheidungen ab. Aus
Erfahrung können wir Ihnen ein paar wichtige Dinge über das Hinzufügen von TensorFlow-Modellen sagen:
- Erfinden Sie das Rad nicht neu! In den meisten Fällen gibt es mindestens zwei Referenzimplementierungen, die Sie überprüfen sollten: das
PyTorch-Äquivalent des Modells, das Sie implementieren, und andere TensorFlow-Modelle für dieselbe Klasse von Problemen.
- Gute Modellimplementierungen überleben den Test der Zeit. Dies geschieht nicht, weil der Code hübsch ist, sondern eher
sondern weil der Code klar, einfach zu debuggen und darauf aufzubauen ist. Wenn Sie den Maintainern das Leben mit Ihrer
TensorFlow-Implementierung leicht machen, indem Sie die gleichen Muster wie in anderen TensorFlow-Modellen nachbilden und die Abweichung
zur PyTorch-Implementierung minimieren, stellen Sie sicher, dass Ihr Beitrag lange Bestand haben wird.
- Bitten Sie um Hilfe, wenn Sie nicht weiterkommen! Das 🤗 Transformers-Team ist da, um zu helfen, und wir haben wahrscheinlich Lösungen für die gleichen
Probleme gefunden, vor denen Sie stehen.
Hier finden Sie einen Überblick über die Schritte, die zum Hinzufügen einer TensorFlow-Modellarchitektur erforderlich sind:
1. Wählen Sie das Modell, das Sie konvertieren möchten
2. Bereiten Sie die Transformers-Entwicklungsumgebung vor.
3. (Optional) Verstehen Sie die theoretischen Aspekte und die bestehende Implementierung
4. Implementieren Sie die Modellarchitektur
5. Implementieren Sie Modelltests
6. Reichen Sie den Pull-Antrag ein
7. (Optional) Erstellen Sie Demos und teilen Sie diese mit der Welt
### 1.-3. Bereiten Sie Ihren Modellbeitrag vor
**1. Wählen Sie das Modell, das Sie konvertieren möchten**
Beginnen wir mit den Grundlagen: Als erstes müssen Sie die Architektur kennen, die Sie konvertieren möchten. Wenn Sie
Sie sich nicht auf eine bestimmte Architektur festgelegt haben, ist es eine gute Möglichkeit, das 🤗 Transformers-Team um Vorschläge zu bitten.
Wir werden Sie zu den wichtigsten Architekturen führen, die auf der TensorFlow-Seite noch fehlen.
Seite fehlen. Wenn das spezifische Modell, das Sie mit TensorFlow verwenden möchten, bereits eine Implementierung der TensorFlow-Architektur in
🤗 Transformers, aber es fehlen Gewichte, können Sie direkt in den
Abschnitt [Gewichtskonvertierung](#hinzufügen-von-tensorflow-gewichten-zum--hub)
auf dieser Seite.
Der Einfachheit halber wird im Rest dieser Anleitung davon ausgegangen, dass Sie sich entschieden haben, mit der TensorFlow-Version von
*BrandNewBert* (dasselbe Beispiel wie in der [Anleitung](add_new_model), um ein neues Modell von Grund auf hinzuzufügen).
<Tip>
Bevor Sie mit der Arbeit an einer TensorFlow-Modellarchitektur beginnen, sollten Sie sich vergewissern, dass es keine laufenden Bemühungen in dieser Richtung gibt.
Sie können nach `BrandNewBert` auf der
[pull request GitHub page](https://github.com/huggingface/transformers/pulls?q=is%3Apr), um zu bestätigen, dass es keine
TensorFlow-bezogene Pull-Anfrage gibt.
</Tip>
**2. Transformers-Entwicklungsumgebung vorbereiten**
Nachdem Sie die Modellarchitektur ausgewählt haben, öffnen Sie einen PR-Entwurf, um Ihre Absicht zu signalisieren, daran zu arbeiten. Folgen Sie den
Anweisungen, um Ihre Umgebung einzurichten und einen PR-Entwurf zu öffnen.
1. Forken Sie das [repository](https://github.com/huggingface/transformers), indem Sie auf der Seite des Repositorys auf die Schaltfläche 'Fork' klicken.
Seite des Repositorys klicken. Dadurch wird eine Kopie des Codes unter Ihrem GitHub-Benutzerkonto erstellt.
2. Klonen Sie Ihren `transformers` Fork auf Ihre lokale Festplatte und fügen Sie das Basis-Repository als Remote hinzu:
```bash
git clone https://github.com/[your Github handle]/transformers.git
cd transformers
git remote add upstream https://github.com/huggingface/transformers.git
```
3. Richten Sie eine Entwicklungsumgebung ein, indem Sie z.B. den folgenden Befehl ausführen:
```bash
python -m venv .env
source .env/bin/activate
pip install -e ".[dev]"
```
Abhängig von Ihrem Betriebssystem und da die Anzahl der optionalen Abhängigkeiten von Transformers wächst, kann es sein, dass Sie bei diesem Befehl einen
Fehler mit diesem Befehl erhalten. Wenn das der Fall ist, stellen Sie sicher, dass Sie TensorFlow installieren und dann ausführen:
```bash
pip install -e ".[quality]"
```
**Hinweis:** Sie müssen CUDA nicht installiert haben. Es reicht aus, das neue Modell auf der CPU laufen zu lassen.
4. Erstellen Sie eine Verzweigung mit einem beschreibenden Namen von Ihrer Hauptverzweigung
```bash
git checkout -b add_tf_brand_new_bert
```
5. Abrufen und zurücksetzen auf die aktuelle Hauptversion
```bash
git fetch upstream
git rebase upstream/main
```
6. Fügen Sie eine leere `.py` Datei in `transformers/src/models/brandnewbert/` mit dem Namen `modeling_tf_brandnewbert.py` hinzu. Dies wird
Ihre TensorFlow-Modelldatei sein.
7. Übertragen Sie die Änderungen auf Ihr Konto mit:
```bash
git add .
git commit -m "initial commit"
git push -u origin add_tf_brand_new_bert
```
8. Wenn Sie zufrieden sind, gehen Sie auf die Webseite Ihrer Abspaltung auf GitHub. Klicken Sie auf "Pull request". Stellen Sie sicher, dass Sie das
GitHub-Handle einiger Mitglieder des Hugging Face-Teams als Reviewer hinzuzufügen, damit das Hugging Face-Team über zukünftige Änderungen informiert wird.
zukünftige Änderungen benachrichtigt wird.
9. Ändern Sie den PR in einen Entwurf, indem Sie auf der rechten Seite der GitHub-Pull-Request-Webseite auf "In Entwurf umwandeln" klicken.
Jetzt haben Sie eine Entwicklungsumgebung eingerichtet, um *BrandNewBert* nach TensorFlow in 🤗 Transformers zu portieren.
**3. (Optional) Verstehen Sie die theoretischen Aspekte und die bestehende Implementierung**
Sie sollten sich etwas Zeit nehmen, um die Arbeit von *BrandNewBert* zu lesen, falls eine solche Beschreibung existiert. Möglicherweise gibt es große
Abschnitte des Papiers, die schwer zu verstehen sind. Wenn das der Fall ist, ist das in Ordnung - machen Sie sich keine Sorgen! Das Ziel ist
ist es nicht, ein tiefes theoretisches Verständnis des Papiers zu erlangen, sondern die notwendigen Informationen zu extrahieren, um
das Modell mit Hilfe von TensorFlow effektiv in 🤗 Transformers neu zu implementieren. Das heißt, Sie müssen nicht zu viel Zeit auf die
viel Zeit auf die theoretischen Aspekte verwenden, sondern sich lieber auf die praktischen Aspekte konzentrieren, nämlich auf die bestehende Modelldokumentation
Seite (z.B. [model docs for BERT](model_doc/bert)).
Nachdem Sie die Grundlagen der Modelle, die Sie implementieren wollen, verstanden haben, ist es wichtig, die bestehende
Implementierung zu verstehen. Dies ist eine gute Gelegenheit, sich zu vergewissern, dass eine funktionierende Implementierung mit Ihren Erwartungen an das
Modell entspricht, und um technische Herausforderungen auf der TensorFlow-Seite vorauszusehen.
Es ist ganz natürlich, dass Sie sich von der Menge an Informationen, die Sie gerade aufgesogen haben, überwältigt fühlen. Es ist
Es ist definitiv nicht erforderlich, dass Sie in dieser Phase alle Facetten des Modells verstehen. Dennoch empfehlen wir Ihnen dringend
ermutigen wir Sie, alle dringenden Fragen in unserem [Forum](https://discuss.huggingface.co/) zu klären.
### 4. Implementierung des Modells
Jetzt ist es an der Zeit, endlich mit dem Programmieren zu beginnen. Als Ausgangspunkt empfehlen wir die PyTorch-Datei selbst: Kopieren Sie den Inhalt von
`modeling_brand_new_bert.py` in `src/transformers/models/brand_new_bert/` nach
`modeling_tf_brand_new_bert.py`. Das Ziel dieses Abschnitts ist es, die Datei zu ändern und die Importstruktur von
🤗 Transformers zu aktualisieren, so dass Sie `TFBrandNewBert` und
`TFBrandNewBert.from_pretrained(model_repo, from_pt=True)` erfolgreich ein funktionierendes TensorFlow *BrandNewBert* Modell lädt.
Leider gibt es kein Rezept, um ein PyTorch-Modell in TensorFlow zu konvertieren. Sie können jedoch unsere Auswahl an
Tipps befolgen, um den Prozess so reibungslos wie möglich zu gestalten:
- Stellen Sie `TF` dem Namen aller Klassen voran (z.B. wird `BrandNewBert` zu `TFBrandNewBert`).
- Die meisten PyTorch-Operationen haben einen direkten TensorFlow-Ersatz. Zum Beispiel entspricht `torch.nn.Linear` der Klasse
`tf.keras.layers.Dense`, `torch.nn.Dropout` entspricht `tf.keras.layers.Dropout`, usw. Wenn Sie sich nicht sicher sind
über eine bestimmte Operation nicht sicher sind, können Sie die [TensorFlow-Dokumentation](https://www.tensorflow.org/api_docs/python/tf)
oder die [PyTorch-Dokumentation](https://pytorch.org/docs/stable/).
- Suchen Sie nach Mustern in der Codebasis von 🤗 Transformers. Wenn Sie auf eine bestimmte Operation stoßen, für die es keinen direkten Ersatz gibt
Ersatz hat, stehen die Chancen gut, dass jemand anderes bereits das gleiche Problem hatte.
- Behalten Sie standardmäßig die gleichen Variablennamen und die gleiche Struktur wie in PyTorch bei. Dies erleichtert die Fehlersuche, die Verfolgung von
Probleme zu verfolgen und spätere Korrekturen vorzunehmen.
- Einige Ebenen haben in jedem Framework unterschiedliche Standardwerte. Ein bemerkenswertes Beispiel ist die Schicht für die Batch-Normalisierung
epsilon (`1e-5` in [PyTorch](https://pytorch.org/docs/stable/generated/torch.nn.BatchNorm2d.html#torch.nn.BatchNorm2d)
und `1e-3` in [TensorFlow](https://www.tensorflow.org/api_docs/python/tf/keras/layers/BatchNormalization)).
Prüfen Sie die Dokumentation genau!
- Die Variablen `nn.Parameter` von PyTorch müssen in der Regel innerhalb von TF Layer's `build()` initialisiert werden. Siehe das folgende
Beispiel: [PyTorch](https://github.com/huggingface/transformers/blob/655f72a6896c0533b1bdee519ed65a059c2425ac/src/transformers/models/vit_mae/modeling_vit_mae.py#L212) /
[TensorFlow](https://github.com/huggingface/transformers/blob/655f72a6896c0533b1bdee519ed65a059c2425ac/src/transformers/models/vit_mae/modeling_tf_vit_mae.py#L220)
- Wenn das PyTorch-Modell ein `#copied from ...` am Anfang einer Funktion hat, stehen die Chancen gut, dass Ihr TensorFlow-Modell diese Funktion auch
diese Funktion von der Architektur ausleihen kann, von der sie kopiert wurde, vorausgesetzt, es hat eine TensorFlow-Architektur.
- Die korrekte Zuweisung des Attributs `name` in TensorFlow-Funktionen ist entscheidend, um das `from_pt=True` Gewicht zu erreichen
Cross-Loading. Name" ist fast immer der Name der entsprechenden Variablen im PyTorch-Code. Wenn `name` nicht
nicht richtig gesetzt ist, sehen Sie dies in der Fehlermeldung beim Laden der Modellgewichte.
- Die Logik der Basismodellklasse, `BrandNewBertModel`, befindet sich in `TFBrandNewBertMainLayer`, einer Keras
Schicht-Unterklasse ([Beispiel](https://github.com/huggingface/transformers/blob/4fd32a1f499e45f009c2c0dea4d81c321cba7e02/src/transformers/models/bert/modeling_tf_bert.py#L719)).
TFBrandNewBertModel" ist lediglich ein Wrapper für diese Schicht.
- Keras-Modelle müssen erstellt werden, um die vorher trainierten Gewichte zu laden. Aus diesem Grund muss `TFBrandNewBertPreTrainedModel`
ein Beispiel für die Eingaben in das Modell enthalten, die `dummy_inputs`
([Beispiel](https://github.com/huggingface/transformers/blob/4fd32a1f499e45f009c2c0dea4d81c321cba7e02/src/transformers/models/bert/modeling_tf_bert.py#L916)).
- Wenn Sie nicht weiterkommen, fragen Sie nach Hilfe - wir sind für Sie da! 🤗
Neben der Modelldatei selbst müssen Sie auch die Verweise auf die Modellklassen und die zugehörigen
Dokumentationsseiten hinzufügen. Sie können diesen Teil ganz nach den Mustern in anderen PRs erledigen
([Beispiel](https://github.com/huggingface/transformers/pull/18020/files)). Hier ist eine Liste der erforderlichen manuellen
Änderungen:
- Fügen Sie alle öffentlichen Klassen von *BrandNewBert* in `src/transformers/__init__.py` ein.
- Fügen Sie *BrandNewBert* Klassen zu den entsprechenden Auto Klassen in `src/transformers/models/auto/modeling_tf_auto.py` hinzu.
- Fügen Sie die *BrandNewBert* zugehörigen Klassen für träges Laden in `src/transformers/utils/dummy_tf_objects.py` hinzu.
- Aktualisieren Sie die Importstrukturen für die öffentlichen Klassen in `src/transformers/models/brand_new_bert/__init__.py`.
- Fügen Sie die Dokumentationszeiger auf die öffentlichen Methoden von *BrandNewBert* in `docs/source/de/model_doc/brand_new_bert.md` hinzu.
- Fügen Sie sich selbst zur Liste der Mitwirkenden an *BrandNewBert* in `docs/source/de/model_doc/brand_new_bert.md` hinzu.
- Fügen Sie schließlich ein grünes Häkchen ✅ in der TensorFlow-Spalte von *BrandNewBert* in `docs/source/de/index.md` hinzu.
Wenn Sie mit Ihrer Implementierung zufrieden sind, führen Sie die folgende Checkliste aus, um zu bestätigen, dass Ihre Modellarchitektur
fertig ist:
1. Alle Schichten, die sich zur Trainingszeit anders verhalten (z.B. Dropout), werden mit einem `Training` Argument aufgerufen, das
von den Top-Level-Klassen weitergegeben wird
2. Sie haben `#copied from ...` verwendet, wann immer es möglich war.
3. Die Funktion `TFBrandNewBertMainLayer` und alle Klassen, die sie verwenden, haben ihre Funktion `call` mit `@unpack_inputs` dekoriert
4. `TFBrandNewBertMainLayer` ist mit `@keras_serializable` dekoriert
5. Ein TensorFlow-Modell kann aus PyTorch-Gewichten mit `TFBrandNewBert.from_pretrained(model_repo, from_pt=True)` geladen werden.
6. Sie können das TensorFlow Modell mit dem erwarteten Eingabeformat aufrufen
### 5. Modell-Tests hinzufügen
Hurra, Sie haben ein TensorFlow-Modell implementiert! Jetzt ist es an der Zeit, Tests hinzuzufügen, um sicherzustellen, dass sich Ihr Modell wie erwartet verhält.
erwartet. Wie im vorigen Abschnitt schlagen wir vor, dass Sie zunächst die Datei `test_modeling_brand_new_bert.py` in
`tests/models/brand_new_bert/` in die Datei `test_modeling_tf_brand_new_bert.py` zu kopieren und dann die notwendigen
TensorFlow-Ersetzungen vornehmen. Für den Moment sollten Sie in allen Aufrufen von `.from_pretrained()` das Flag `from_pt=True` verwenden, um die
die vorhandenen PyTorch-Gewichte zu laden.
Wenn Sie damit fertig sind, kommt der Moment der Wahrheit: Führen Sie die Tests durch! 😬
```bash
NVIDIA_TF32_OVERRIDE=0 RUN_SLOW=1 RUN_PT_TF_CROSS_TESTS=1 \
py.test -vv tests/models/brand_new_bert/test_modeling_tf_brand_new_bert.py
```
Das wahrscheinlichste Ergebnis ist, dass Sie eine Reihe von Fehlern sehen werden. Machen Sie sich keine Sorgen, das ist zu erwarten! Das Debuggen von ML-Modellen ist
notorisch schwierig, und der Schlüssel zum Erfolg ist Geduld (und `breakpoint()`). Nach unserer Erfahrung sind die schwierigsten
Probleme aus subtilen Unstimmigkeiten zwischen ML-Frameworks, zu denen wir am Ende dieses Leitfadens ein paar Hinweise geben.
In anderen Fällen kann es sein, dass ein allgemeiner Test nicht direkt auf Ihr Modell anwendbar ist; in diesem Fall empfehlen wir eine Überschreibung
auf der Ebene der Modelltestklasse. Zögern Sie nicht, in Ihrem Entwurf einer Pull-Anfrage um Hilfe zu bitten, wenn
Sie nicht weiterkommen.
Wenn alle Tests erfolgreich waren, können Sie Ihr Modell in die 🤗 Transformers-Bibliothek aufnehmen! 🎉
### 6.-7. Stellen Sie sicher, dass jeder Ihr Modell verwenden kann
**6. Reichen Sie den Pull Request ein**
Sobald Sie mit der Implementierung und den Tests fertig sind, ist es an der Zeit, eine Pull-Anfrage einzureichen. Bevor Sie Ihren Code einreichen,
führen Sie unser Dienstprogramm zur Codeformatierung, `make fixup` 🪄, aus. Damit werden automatisch alle Formatierungsfehler behoben, die dazu führen würden, dass
unsere automatischen Prüfungen fehlschlagen würden.
Nun ist es an der Zeit, Ihren Entwurf einer Pull-Anfrage in eine echte Pull-Anfrage umzuwandeln. Klicken Sie dazu auf die Schaltfläche "Bereit für
Review" und fügen Sie Joao (`@gante`) und Matt (`@Rocketknight1`) als Reviewer hinzu. Eine Modell-Pull-Anfrage benötigt
mindestens 3 Reviewer, aber sie werden sich darum kümmern, geeignete zusätzliche Reviewer für Ihr Modell zu finden.
Nachdem alle Gutachter mit dem Stand Ihres PR zufrieden sind, entfernen Sie als letzten Aktionspunkt das Flag `from_pt=True` in
.from_pretrained()-Aufrufen zu entfernen. Da es keine TensorFlow-Gewichte gibt, müssen Sie sie hinzufügen! Lesen Sie den Abschnitt
unten, um zu erfahren, wie Sie dies tun können.
Wenn schließlich die TensorFlow-Gewichte zusammengeführt werden, Sie mindestens 3 Genehmigungen von Prüfern haben und alle CI-Checks grün sind
grün sind, überprüfen Sie die Tests ein letztes Mal lokal
```bash
NVIDIA_TF32_OVERRIDE=0 RUN_SLOW=1 RUN_PT_TF_CROSS_TESTS=1 \
py.test -vv tests/models/brand_new_bert/test_modeling_tf_brand_new_bert.py
```
und wir werden Ihren PR zusammenführen! Herzlichen Glückwunsch zu dem Meilenstein 🎉.
**7. (Optional) Erstellen Sie Demos und teilen Sie sie mit der Welt**
Eine der schwierigsten Aufgaben bei Open-Source ist die Entdeckung. Wie können die anderen Benutzer von der Existenz Ihres
fabelhaften TensorFlow-Beitrags erfahren? Mit der richtigen Kommunikation, natürlich! 📣
Es gibt vor allem zwei Möglichkeiten, Ihr Modell mit der Community zu teilen:
- Erstellen Sie Demos. Dazu gehören Gradio-Demos, Notebooks und andere unterhaltsame Möglichkeiten, Ihr Modell vorzuführen. Wir raten Ihnen
ermutigen Sie, ein Notizbuch zu unseren [community-driven demos](https://huggingface.co/docs/transformers/community) hinzuzufügen.
- Teilen Sie Geschichten in sozialen Medien wie Twitter und LinkedIn. Sie sollten stolz auf Ihre Arbeit sein und sie mit der
Ihre Leistung mit der Community teilen - Ihr Modell kann nun von Tausenden von Ingenieuren und Forschern auf der ganzen Welt genutzt werden
der Welt genutzt werden 🌍! Wir werden Ihre Beiträge gerne retweeten und Ihnen helfen, Ihre Arbeit mit der Community zu teilen.
## Hinzufügen von TensorFlow-Gewichten zum 🤗 Hub
Unter der Annahme, dass die TensorFlow-Modellarchitektur in 🤗 Transformers verfügbar ist, ist die Umwandlung von PyTorch-Gewichten in
TensorFlow-Gewichte ist ein Kinderspiel!
Hier sehen Sie, wie es geht:
1. Stellen Sie sicher, dass Sie in Ihrem Terminal bei Ihrem Hugging Face Konto angemeldet sind. Sie können sich mit dem folgenden Befehl anmelden
`huggingface-cli login` (Ihre Zugangstoken finden Sie [hier](https://huggingface.co/settings/tokens))
2. Führen Sie `transformers-cli pt-to-tf --model-name foo/bar` aus, wobei `foo/bar` der Name des Modell-Repositorys ist
ist, das die PyTorch-Gewichte enthält, die Sie konvertieren möchten.
3. Markieren Sie `@joaogante` und `@Rocketknight1` in dem 🤗 Hub PR, den der obige Befehl gerade erstellt hat
Das war's! 🎉
## Fehlersuche in verschiedenen ML-Frameworks 🐛
Irgendwann, wenn Sie eine neue Architektur hinzufügen oder TensorFlow-Gewichte für eine bestehende Architektur erstellen, werden Sie
stoßen Sie vielleicht auf Fehler, die sich über Unstimmigkeiten zwischen PyTorch und TensorFlow beschweren. Sie könnten sich sogar dazu entschließen, den
Modellarchitektur-Code für die beiden Frameworks zu öffnen, und stellen fest, dass sie identisch aussehen. Was ist denn da los? 🤔
Lassen Sie uns zunächst darüber sprechen, warum es wichtig ist, diese Diskrepanzen zu verstehen. Viele Community-Mitglieder werden 🤗
Transformers-Modelle und vertrauen darauf, dass sich unsere Modelle wie erwartet verhalten. Wenn es eine große Diskrepanz gibt
zwischen den beiden Frameworks auftritt, bedeutet dies, dass das Modell nicht der Referenzimplementierung für mindestens eines der Frameworks folgt.
der Frameworks folgt. Dies kann zu stillen Fehlern führen, bei denen das Modell zwar läuft, aber eine schlechte Leistung aufweist. Dies ist
wohl schlimmer als ein Modell, das überhaupt nicht läuft! Aus diesem Grund streben wir an, dass die Abweichung zwischen den Frameworks kleiner als
1e-5" in allen Phasen des Modells.
Wie bei anderen numerischen Problemen auch, steckt der Teufel im Detail. Und wie bei jedem detailorientierten Handwerk ist die geheime
Zutat hier Geduld. Hier ist unser Vorschlag für den Arbeitsablauf, wenn Sie auf diese Art von Problemen stoßen:
1. Lokalisieren Sie die Quelle der Abweichungen. Das Modell, das Sie konvertieren, hat wahrscheinlich bis zu einem gewissen Punkt nahezu identische innere Variablen.
bestimmten Punkt. Platzieren Sie `Breakpoint()`-Anweisungen in den Architekturen der beiden Frameworks und vergleichen Sie die Werte der
numerischen Variablen von oben nach unten, bis Sie die Quelle der Probleme gefunden haben.
2. Nachdem Sie nun die Ursache des Problems gefunden haben, setzen Sie sich mit dem 🤗 Transformers-Team in Verbindung. Es ist möglich
dass wir ein ähnliches Problem schon einmal gesehen haben und umgehend eine Lösung anbieten können. Als Ausweichmöglichkeit können Sie beliebte Seiten
wie StackOverflow und GitHub-Probleme.
3. Wenn keine Lösung in Sicht ist, bedeutet das, dass Sie tiefer gehen müssen. Die gute Nachricht ist, dass Sie das Problem gefunden haben.
Problem ausfindig gemacht haben, so dass Sie sich auf die problematische Anweisung konzentrieren und den Rest des Modells ausblenden können! Die schlechte Nachricht ist
dass Sie sich in die Quellimplementierung der besagten Anweisung einarbeiten müssen. In manchen Fällen finden Sie vielleicht ein
Problem mit einer Referenzimplementierung - verzichten Sie nicht darauf, ein Problem im Upstream-Repository zu öffnen.
In einigen Fällen können wir nach Rücksprache mit dem 🤗 Transformers-Team zu dem Schluss kommen, dass die Behebung der Abweichung nicht machbar ist.
Wenn die Abweichung in den Ausgabeschichten des Modells sehr klein ist (aber möglicherweise groß in den versteckten Zuständen), können wir
könnten wir beschließen, sie zu ignorieren und das Modell zu verteilen. Die oben erwähnte CLI `pt-to-tf` hat ein `--max-error`
Flag, um die Fehlermeldung bei der Gewichtskonvertierung zu überschreiben.
| transformers/docs/source/de/add_tensorflow_model.md/0 | {
"file_path": "transformers/docs/source/de/add_tensorflow_model.md",
"repo_id": "transformers",
"token_count": 9922
} | 242 |
<!--Copyright 2020 The HuggingFace Team. All rights reserved.
Licensed under the Apache License, Version 2.0 (the "License"); you may not use this file except in compliance with
the License. You may obtain a copy of the License at
http://www.apache.org/licenses/LICENSE-2.0
Unless required by applicable law or agreed to in writing, software distributed under the License is distributed on
an "AS IS" BASIS, WITHOUT WARRANTIES OR CONDITIONS OF ANY KIND, either express or implied. See the License for the
specific language governing permissions and limitations under the License.
⚠️ Note that this file is in Markdown but contain specific syntax for our doc-builder (similar to MDX) that may not be
rendered properly in your Markdown viewer.
-->
# Building custom models
The 🤗 Transformers library is designed to be easily extensible. Every model is fully coded in a given subfolder
of the repository with no abstraction, so you can easily copy a modeling file and tweak it to your needs.
If you are writing a brand new model, it might be easier to start from scratch. In this tutorial, we will show you
how to write a custom model and its configuration so it can be used inside Transformers, and how you can share it
with the community (with the code it relies on) so that anyone can use it, even if it's not present in the 🤗
Transformers library. We'll see how to build upon transformers and extend the framework with your hooks and
custom code.
We will illustrate all of this on a ResNet model, by wrapping the ResNet class of the
[timm library](https://github.com/rwightman/pytorch-image-models) into a [`PreTrainedModel`].
## Writing a custom configuration
Before we dive into the model, let's first write its configuration. The configuration of a model is an object that
will contain all the necessary information to build the model. As we will see in the next section, the model can only
take a `config` to be initialized, so we really need that object to be as complete as possible.
<Tip>
Models in the `transformers` library itself generally follow the convention that they accept a `config` object
in their `__init__` method, and then pass the whole `config` to sub-layers in the model, rather than breaking the
config object into multiple arguments that are all passed individually to sub-layers. Writing your model in this
style results in simpler code with a clear "source of truth" for any hyperparameters, and also makes it easier
to reuse code from other models in `transformers`.
</Tip>
In our example, we will take a couple of arguments of the ResNet class that we might want to tweak. Different
configurations will then give us the different types of ResNets that are possible. We then just store those arguments,
after checking the validity of a few of them.
```python
from transformers import PretrainedConfig
from typing import List
class ResnetConfig(PretrainedConfig):
model_type = "resnet"
def __init__(
self,
block_type="bottleneck",
layers: List[int] = [3, 4, 6, 3],
num_classes: int = 1000,
input_channels: int = 3,
cardinality: int = 1,
base_width: int = 64,
stem_width: int = 64,
stem_type: str = "",
avg_down: bool = False,
**kwargs,
):
if block_type not in ["basic", "bottleneck"]:
raise ValueError(f"`block_type` must be 'basic' or bottleneck', got {block_type}.")
if stem_type not in ["", "deep", "deep-tiered"]:
raise ValueError(f"`stem_type` must be '', 'deep' or 'deep-tiered', got {stem_type}.")
self.block_type = block_type
self.layers = layers
self.num_classes = num_classes
self.input_channels = input_channels
self.cardinality = cardinality
self.base_width = base_width
self.stem_width = stem_width
self.stem_type = stem_type
self.avg_down = avg_down
super().__init__(**kwargs)
```
The three important things to remember when writing you own configuration are the following:
- you have to inherit from `PretrainedConfig`,
- the `__init__` of your `PretrainedConfig` must accept any kwargs,
- those `kwargs` need to be passed to the superclass `__init__`.
The inheritance is to make sure you get all the functionality from the 🤗 Transformers library, while the two other
constraints come from the fact a `PretrainedConfig` has more fields than the ones you are setting. When reloading a
config with the `from_pretrained` method, those fields need to be accepted by your config and then sent to the
superclass.
Defining a `model_type` for your configuration (here `model_type="resnet"`) is not mandatory, unless you want to
register your model with the auto classes (see last section).
With this done, you can easily create and save your configuration like you would do with any other model config of the
library. Here is how we can create a resnet50d config and save it:
```py
resnet50d_config = ResnetConfig(block_type="bottleneck", stem_width=32, stem_type="deep", avg_down=True)
resnet50d_config.save_pretrained("custom-resnet")
```
This will save a file named `config.json` inside the folder `custom-resnet`. You can then reload your config with the
`from_pretrained` method:
```py
resnet50d_config = ResnetConfig.from_pretrained("custom-resnet")
```
You can also use any other method of the [`PretrainedConfig`] class, like [`~PretrainedConfig.push_to_hub`] to
directly upload your config to the Hub.
## Writing a custom model
Now that we have our ResNet configuration, we can go on writing the model. We will actually write two: one that
extracts the hidden features from a batch of images (like [`BertModel`]) and one that is suitable for image
classification (like [`BertForSequenceClassification`]).
As we mentioned before, we'll only write a loose wrapper of the model to keep it simple for this example. The only
thing we need to do before writing this class is a map between the block types and actual block classes. Then the
model is defined from the configuration by passing everything to the `ResNet` class:
```py
from transformers import PreTrainedModel
from timm.models.resnet import BasicBlock, Bottleneck, ResNet
from .configuration_resnet import ResnetConfig
BLOCK_MAPPING = {"basic": BasicBlock, "bottleneck": Bottleneck}
class ResnetModel(PreTrainedModel):
config_class = ResnetConfig
def __init__(self, config):
super().__init__(config)
block_layer = BLOCK_MAPPING[config.block_type]
self.model = ResNet(
block_layer,
config.layers,
num_classes=config.num_classes,
in_chans=config.input_channels,
cardinality=config.cardinality,
base_width=config.base_width,
stem_width=config.stem_width,
stem_type=config.stem_type,
avg_down=config.avg_down,
)
def forward(self, tensor):
return self.model.forward_features(tensor)
```
For the model that will classify images, we just change the forward method:
```py
import torch
class ResnetModelForImageClassification(PreTrainedModel):
config_class = ResnetConfig
def __init__(self, config):
super().__init__(config)
block_layer = BLOCK_MAPPING[config.block_type]
self.model = ResNet(
block_layer,
config.layers,
num_classes=config.num_classes,
in_chans=config.input_channels,
cardinality=config.cardinality,
base_width=config.base_width,
stem_width=config.stem_width,
stem_type=config.stem_type,
avg_down=config.avg_down,
)
def forward(self, tensor, labels=None):
logits = self.model(tensor)
if labels is not None:
loss = torch.nn.cross_entropy(logits, labels)
return {"loss": loss, "logits": logits}
return {"logits": logits}
```
In both cases, notice how we inherit from `PreTrainedModel` and call the superclass initialization with the `config`
(a bit like when you write a regular `torch.nn.Module`). The line that sets the `config_class` is not mandatory, unless
you want to register your model with the auto classes (see last section).
<Tip>
If your model is very similar to a model inside the library, you can re-use the same configuration as this model.
</Tip>
You can have your model return anything you want, but returning a dictionary like we did for
`ResnetModelForImageClassification`, with the loss included when labels are passed, will make your model directly
usable inside the [`Trainer`] class. Using another output format is fine as long as you are planning on using your own
training loop or another library for training.
Now that we have our model class, let's create one:
```py
resnet50d = ResnetModelForImageClassification(resnet50d_config)
```
Again, you can use any of the methods of [`PreTrainedModel`], like [`~PreTrainedModel.save_pretrained`] or
[`~PreTrainedModel.push_to_hub`]. We will use the second in the next section, and see how to push the model weights
with the code of our model. But first, let's load some pretrained weights inside our model.
In your own use case, you will probably be training your custom model on your own data. To go fast for this tutorial,
we will use the pretrained version of the resnet50d. Since our model is just a wrapper around it, it's going to be
easy to transfer those weights:
```py
import timm
pretrained_model = timm.create_model("resnet50d", pretrained=True)
resnet50d.model.load_state_dict(pretrained_model.state_dict())
```
Now let's see how to make sure that when we do [`~PreTrainedModel.save_pretrained`] or [`~PreTrainedModel.push_to_hub`], the
code of the model is saved.
## Registering a model with custom code to the auto classes
If you are writing a library that extends 🤗 Transformers, you may want to extend the auto classes to include your own
model. This is different from pushing the code to the Hub in the sense that users will need to import your library to
get the custom models (contrarily to automatically downloading the model code from the Hub).
As long as your config has a `model_type` attribute that is different from existing model types, and that your model
classes have the right `config_class` attributes, you can just add them to the auto classes like this:
```py
from transformers import AutoConfig, AutoModel, AutoModelForImageClassification
AutoConfig.register("resnet", ResnetConfig)
AutoModel.register(ResnetConfig, ResnetModel)
AutoModelForImageClassification.register(ResnetConfig, ResnetModelForImageClassification)
```
Note that the first argument used when registering your custom config to [`AutoConfig`] needs to match the `model_type`
of your custom config, and the first argument used when registering your custom models to any auto model class needs
to match the `config_class` of those models.
## Sending the code to the Hub
<Tip warning={true}>
This API is experimental and may have some slight breaking changes in the next releases.
</Tip>
First, make sure your model is fully defined in a `.py` file. It can rely on relative imports to some other files as
long as all the files are in the same directory (we don't support submodules for this feature yet). For our example,
we'll define a `modeling_resnet.py` file and a `configuration_resnet.py` file in a folder of the current working
directory named `resnet_model`. The configuration file contains the code for `ResnetConfig` and the modeling file
contains the code of `ResnetModel` and `ResnetModelForImageClassification`.
```
.
└── resnet_model
├── __init__.py
├── configuration_resnet.py
└── modeling_resnet.py
```
The `__init__.py` can be empty, it's just there so that Python detects `resnet_model` can be use as a module.
<Tip warning={true}>
If copying a modeling files from the library, you will need to replace all the relative imports at the top of the file
to import from the `transformers` package.
</Tip>
Note that you can re-use (or subclass) an existing configuration/model.
To share your model with the community, follow those steps: first import the ResNet model and config from the newly
created files:
```py
from resnet_model.configuration_resnet import ResnetConfig
from resnet_model.modeling_resnet import ResnetModel, ResnetModelForImageClassification
```
Then you have to tell the library you want to copy the code files of those objects when using the `save_pretrained`
method and properly register them with a given Auto class (especially for models), just run:
```py
ResnetConfig.register_for_auto_class()
ResnetModel.register_for_auto_class("AutoModel")
ResnetModelForImageClassification.register_for_auto_class("AutoModelForImageClassification")
```
Note that there is no need to specify an auto class for the configuration (there is only one auto class for them,
[`AutoConfig`]) but it's different for models. Your custom model could be suitable for many different tasks, so you
have to specify which one of the auto classes is the correct one for your model.
<Tip>
Use `register_for_auto_class()` if you want the code files to be copied. If you instead prefer to use code on the Hub from another repo,
you don't need to call it. In cases where there's more than one auto class, you can modify the `config.json` directly using the
following structure:
```json
"auto_map": {
"AutoConfig": "<your-repo-name>--<config-name>",
"AutoModel": "<your-repo-name>--<config-name>",
"AutoModelFor<Task>": "<your-repo-name>--<config-name>",
},
```
</Tip>
Next, let's create the config and models as we did before:
```py
resnet50d_config = ResnetConfig(block_type="bottleneck", stem_width=32, stem_type="deep", avg_down=True)
resnet50d = ResnetModelForImageClassification(resnet50d_config)
pretrained_model = timm.create_model("resnet50d", pretrained=True)
resnet50d.model.load_state_dict(pretrained_model.state_dict())
```
Now to send the model to the Hub, make sure you are logged in. Either run in your terminal:
```bash
huggingface-cli login
```
or from a notebook:
```py
from huggingface_hub import notebook_login
notebook_login()
```
You can then push to your own namespace (or an organization you are a member of) like this:
```py
resnet50d.push_to_hub("custom-resnet50d")
```
On top of the modeling weights and the configuration in json format, this also copied the modeling and
configuration `.py` files in the folder `custom-resnet50d` and uploaded the result to the Hub. You can check the result
in this [model repo](https://huggingface.co/sgugger/custom-resnet50d).
See the [sharing tutorial](model_sharing) for more information on the push to Hub method.
## Using a model with custom code
You can use any configuration, model or tokenizer with custom code files in its repository with the auto-classes and
the `from_pretrained` method. All files and code uploaded to the Hub are scanned for malware (refer to the [Hub security](https://huggingface.co/docs/hub/security#malware-scanning) documentation for more information), but you should still
review the model code and author to avoid executing malicious code on your machine. Set `trust_remote_code=True` to use
a model with custom code:
```py
from transformers import AutoModelForImageClassification
model = AutoModelForImageClassification.from_pretrained("sgugger/custom-resnet50d", trust_remote_code=True)
```
It is also strongly encouraged to pass a commit hash as a `revision` to make sure the author of the models did not
update the code with some malicious new lines (unless you fully trust the authors of the models).
```py
commit_hash = "ed94a7c6247d8aedce4647f00f20de6875b5b292"
model = AutoModelForImageClassification.from_pretrained(
"sgugger/custom-resnet50d", trust_remote_code=True, revision=commit_hash
)
```
Note that when browsing the commit history of the model repo on the Hub, there is a button to easily copy the commit
hash of any commit.
| transformers/docs/source/en/custom_models.md/0 | {
"file_path": "transformers/docs/source/en/custom_models.md",
"repo_id": "transformers",
"token_count": 4867
} | 243 |
<!--Copyright 2020 The HuggingFace Team. All rights reserved.
Licensed under the Apache License, Version 2.0 (the "License"); you may not use this file except in compliance with
the License. You may obtain a copy of the License at
http://www.apache.org/licenses/LICENSE-2.0
Unless required by applicable law or agreed to in writing, software distributed under the License is distributed on
an "AS IS" BASIS, WITHOUT WARRANTIES OR CONDITIONS OF ANY KIND, either express or implied. See the License for the
specific language governing permissions and limitations under the License.
⚠️ Note that this file is in Markdown but contain specific syntax for our doc-builder (similar to MDX) that may not be
rendered properly in your Markdown viewer.
-->
# Custom Layers and Utilities
This page lists all the custom layers used by the library, as well as the utility functions it provides for modeling.
Most of those are only useful if you are studying the code of the models in the library.
## Pytorch custom modules
[[autodoc]] pytorch_utils.Conv1D
[[autodoc]] modeling_utils.PoolerStartLogits
- forward
[[autodoc]] modeling_utils.PoolerEndLogits
- forward
[[autodoc]] modeling_utils.PoolerAnswerClass
- forward
[[autodoc]] modeling_utils.SquadHeadOutput
[[autodoc]] modeling_utils.SQuADHead
- forward
[[autodoc]] modeling_utils.SequenceSummary
- forward
## PyTorch Helper Functions
[[autodoc]] pytorch_utils.apply_chunking_to_forward
[[autodoc]] pytorch_utils.find_pruneable_heads_and_indices
[[autodoc]] pytorch_utils.prune_layer
[[autodoc]] pytorch_utils.prune_conv1d_layer
[[autodoc]] pytorch_utils.prune_linear_layer
## TensorFlow custom layers
[[autodoc]] modeling_tf_utils.TFConv1D
[[autodoc]] modeling_tf_utils.TFSequenceSummary
## TensorFlow loss functions
[[autodoc]] modeling_tf_utils.TFCausalLanguageModelingLoss
[[autodoc]] modeling_tf_utils.TFMaskedLanguageModelingLoss
[[autodoc]] modeling_tf_utils.TFMultipleChoiceLoss
[[autodoc]] modeling_tf_utils.TFQuestionAnsweringLoss
[[autodoc]] modeling_tf_utils.TFSequenceClassificationLoss
[[autodoc]] modeling_tf_utils.TFTokenClassificationLoss
## TensorFlow Helper Functions
[[autodoc]] modeling_tf_utils.get_initializer
[[autodoc]] modeling_tf_utils.keras_serializable
[[autodoc]] modeling_tf_utils.shape_list
| transformers/docs/source/en/internal/modeling_utils.md/0 | {
"file_path": "transformers/docs/source/en/internal/modeling_utils.md",
"repo_id": "transformers",
"token_count": 729
} | 244 |
<!--Copyright 2020 The HuggingFace Team. All rights reserved.
Licensed under the Apache License, Version 2.0 (the "License"); you may not use this file except in compliance with
the License. You may obtain a copy of the License at
http://www.apache.org/licenses/LICENSE-2.0
Unless required by applicable law or agreed to in writing, software distributed under the License is distributed on
an "AS IS" BASIS, WITHOUT WARRANTIES OR CONDITIONS OF ANY KIND, either express or implied. See the License for the
specific language governing permissions and limitations under the License.
⚠️ Note that this file is in Markdown but contain specific syntax for our doc-builder (similar to MDX) that may not be
rendered properly in your Markdown viewer.
-->
# Logging
🤗 Transformers has a centralized logging system, so that you can setup the verbosity of the library easily.
Currently the default verbosity of the library is `WARNING`.
To change the level of verbosity, just use one of the direct setters. For instance, here is how to change the verbosity
to the INFO level.
```python
import transformers
transformers.logging.set_verbosity_info()
```
You can also use the environment variable `TRANSFORMERS_VERBOSITY` to override the default verbosity. You can set it
to one of the following: `debug`, `info`, `warning`, `error`, `critical`. For example:
```bash
TRANSFORMERS_VERBOSITY=error ./myprogram.py
```
Additionally, some `warnings` can be disabled by setting the environment variable
`TRANSFORMERS_NO_ADVISORY_WARNINGS` to a true value, like *1*. This will disable any warning that is logged using
[`logger.warning_advice`]. For example:
```bash
TRANSFORMERS_NO_ADVISORY_WARNINGS=1 ./myprogram.py
```
Here is an example of how to use the same logger as the library in your own module or script:
```python
from transformers.utils import logging
logging.set_verbosity_info()
logger = logging.get_logger("transformers")
logger.info("INFO")
logger.warning("WARN")
```
All the methods of this logging module are documented below, the main ones are
[`logging.get_verbosity`] to get the current level of verbosity in the logger and
[`logging.set_verbosity`] to set the verbosity to the level of your choice. In order (from the least
verbose to the most verbose), those levels (with their corresponding int values in parenthesis) are:
- `transformers.logging.CRITICAL` or `transformers.logging.FATAL` (int value, 50): only report the most
critical errors.
- `transformers.logging.ERROR` (int value, 40): only report errors.
- `transformers.logging.WARNING` or `transformers.logging.WARN` (int value, 30): only reports error and
warnings. This the default level used by the library.
- `transformers.logging.INFO` (int value, 20): reports error, warnings and basic information.
- `transformers.logging.DEBUG` (int value, 10): report all information.
By default, `tqdm` progress bars will be displayed during model download. [`logging.disable_progress_bar`] and [`logging.enable_progress_bar`] can be used to suppress or unsuppress this behavior.
## `logging` vs `warnings`
Python has two logging systems that are often used in conjunction: `logging`, which is explained above, and `warnings`,
which allows further classification of warnings in specific buckets, e.g., `FutureWarning` for a feature or path
that has already been deprecated and `DeprecationWarning` to indicate an upcoming deprecation.
We use both in the `transformers` library. We leverage and adapt `logging`'s `captureWarning` method to allow
management of these warning messages by the verbosity setters above.
What does that mean for developers of the library? We should respect the following heuristic:
- `warnings` should be favored for developers of the library and libraries dependent on `transformers`
- `logging` should be used for end-users of the library using it in every-day projects
See reference of the `captureWarnings` method below.
[[autodoc]] logging.captureWarnings
## Base setters
[[autodoc]] logging.set_verbosity_error
[[autodoc]] logging.set_verbosity_warning
[[autodoc]] logging.set_verbosity_info
[[autodoc]] logging.set_verbosity_debug
## Other functions
[[autodoc]] logging.get_verbosity
[[autodoc]] logging.set_verbosity
[[autodoc]] logging.get_logger
[[autodoc]] logging.enable_default_handler
[[autodoc]] logging.disable_default_handler
[[autodoc]] logging.enable_explicit_format
[[autodoc]] logging.reset_format
[[autodoc]] logging.enable_progress_bar
[[autodoc]] logging.disable_progress_bar
| transformers/docs/source/en/main_classes/logging.md/0 | {
"file_path": "transformers/docs/source/en/main_classes/logging.md",
"repo_id": "transformers",
"token_count": 1268
} | 245 |
# Cohere
## Overview
The Cohere Command-R model was proposed in the blogpost [Command-R: Retrieval Augmented Generation at Production Scale](https://txt.cohere.com/command-r/) by the Cohere Team.
The abstract from the paper is the following:
*Command-R is a scalable generative model targeting RAG and Tool Use to enable production-scale AI for enterprise. Today, we are introducing Command-R, a new LLM aimed at large-scale production workloads. Command-R targets the emerging “scalable” category of models that balance high efficiency with strong accuracy, enabling companies to move beyond proof of concept, and into production.*
*Command-R is a generative model optimized for long context tasks such as retrieval augmented generation (RAG) and using external APIs and tools. It is designed to work in concert with our industry-leading Embed and Rerank models to provide best-in-class integration for RAG applications and excel at enterprise use cases. As a model built for companies to implement at scale, Command-R boasts:
- Strong accuracy on RAG and Tool Use
- Low latency, and high throughput
- Longer 128k context and lower pricing
- Strong capabilities across 10 key languages
- Model weights available on HuggingFace for research and evaluation
Checkout model checkpoints [here](https://huggingface.co/CohereForAI/c4ai-command-r-v01).
This model was contributed by [Saurabh Dash](https://huggingface.co/saurabhdash) and [Ahmet Üstün](https://huggingface.co/ahmetustun). The code of the implementation in Hugging Face is based on GPT-NeoX [here](https://github.com/EleutherAI/gpt-neox).
## Usage tips
<Tip warning={true}>
The checkpoints uploaded on the Hub use `torch_dtype = 'float16'`, which will be
used by the `AutoModel` API to cast the checkpoints from `torch.float32` to `torch.float16`.
The `dtype` of the online weights is mostly irrelevant unless you are using `torch_dtype="auto"` when initializing a model using `model = AutoModelForCausalLM.from_pretrained("path", torch_dtype = "auto")`. The reason is that the model will first be downloaded ( using the `dtype` of the checkpoints online), then it will be casted to the default `dtype` of `torch` (becomes `torch.float32`), and finally, if there is a `torch_dtype` provided in the config, it will be used.
Training the model in `float16` is not recommended and is known to produce `nan`; as such, the model should be trained in `bfloat16`.
</Tip>
The model and tokenizer can be loaded via:
```python
# pip install transformers
from transformers import AutoTokenizer, AutoModelForCausalLM
model_id = "CohereForAI/c4ai-command-r-v01"
tokenizer = AutoTokenizer.from_pretrained(model_id)
model = AutoModelForCausalLM.from_pretrained(model_id)
# Format message with the command-r chat template
messages = [{"role": "user", "content": "Hello, how are you?"}]
input_ids = tokenizer.apply_chat_template(messages, tokenize=True, add_generation_prompt=True, return_tensors="pt")
## <BOS_TOKEN><|START_OF_TURN_TOKEN|><|USER_TOKEN|>Hello, how are you?<|END_OF_TURN_TOKEN|><|START_OF_TURN_TOKEN|><|CHATBOT_TOKEN|>
gen_tokens = model.generate(
input_ids,
max_new_tokens=100,
do_sample=True,
temperature=0.3,
)
gen_text = tokenizer.decode(gen_tokens[0])
print(gen_text)
```
- When using Flash Attention 2 via `attn_implementation="flash_attention_2"`, don't pass `torch_dtype` to the `from_pretrained` class method and use Automatic Mixed-Precision training. When using `Trainer`, it is simply specifying either `fp16` or `bf16` to `True`. Otherwise, make sure you are using `torch.autocast`. This is required because the Flash Attention only support `fp16` and `bf16` data type.
## Resources
A list of official Hugging Face and community (indicated by 🌎) resources to help you get started with Command-R. If you're interested in submitting a resource to be included here, please feel free to open a Pull Request and we'll review it! The resource should ideally demonstrate something new instead of duplicating an existing resource.
<PipelineTag pipeline="text-generation"/>
Loading FP16 model
```python
# pip install transformers
from transformers import AutoTokenizer, AutoModelForCausalLM
model_id = "CohereForAI/c4ai-command-r-v01"
tokenizer = AutoTokenizer.from_pretrained(model_id)
model = AutoModelForCausalLM.from_pretrained(model_id)
# Format message with the command-r chat template
messages = [{"role": "user", "content": "Hello, how are you?"}]
input_ids = tokenizer.apply_chat_template(messages, tokenize=True, add_generation_prompt=True, return_tensors="pt")
## <BOS_TOKEN><|START_OF_TURN_TOKEN|><|USER_TOKEN|>Hello, how are you?<|END_OF_TURN_TOKEN|><|START_OF_TURN_TOKEN|><|CHATBOT_TOKEN|>
gen_tokens = model.generate(
input_ids,
max_new_tokens=100,
do_sample=True,
temperature=0.3,
)
gen_text = tokenizer.decode(gen_tokens[0])
print(gen_text)
```
Loading bitsnbytes 4bit quantized model
```python
# pip install transformers bitsandbytes accelerate
from transformers import AutoTokenizer, AutoModelForCausalLM, BitsAndBytesConfig
bnb_config = BitsAndBytesConfig(load_in_4bit=True)
model_id = "CohereForAI/c4ai-command-r-v01"
tokenizer = AutoTokenizer.from_pretrained(model_id)
model = AutoModelForCausalLM.from_pretrained(model_id, quantization_config=bnb_config)
gen_tokens = model.generate(
input_ids,
max_new_tokens=100,
do_sample=True,
temperature=0.3,
)
gen_text = tokenizer.decode(gen_tokens[0])
print(gen_text)
```
## CohereConfig
[[autodoc]] CohereConfig
## CohereTokenizerFast
[[autodoc]] CohereTokenizerFast
- build_inputs_with_special_tokens
- get_special_tokens_mask
- create_token_type_ids_from_sequences
- update_post_processor
- save_vocabulary
## CohereModel
[[autodoc]] CohereModel
- forward
## CohereForCausalLM
[[autodoc]] CohereForCausalLM
- forward
| transformers/docs/source/en/model_doc/cohere.md/0 | {
"file_path": "transformers/docs/source/en/model_doc/cohere.md",
"repo_id": "transformers",
"token_count": 1897
} | 246 |
<!--Copyright 2022 The HuggingFace Team. All rights reserved.
Licensed under the Apache License, Version 2.0 (the "License"); you may not use this file except in compliance with
the License. You may obtain a copy of the License at
http://www.apache.org/licenses/LICENSE-2.0
Unless required by applicable law or agreed to in writing, software distributed under the License is distributed on
an "AS IS" BASIS, WITHOUT WARRANTIES OR CONDITIONS OF ANY KIND, either express or implied. See the License for the
specific language governing permissions and limitations under the License.
⚠️ Note that this file is in Markdown but contain specific syntax for our doc-builder (similar to MDX) that may not be
rendered properly in your Markdown viewer.
-->
# ERNIE
## Overview
ERNIE is a series of powerful models proposed by baidu, especially in Chinese tasks,
including [ERNIE1.0](https://arxiv.org/abs/1904.09223), [ERNIE2.0](https://ojs.aaai.org/index.php/AAAI/article/view/6428),
[ERNIE3.0](https://arxiv.org/abs/2107.02137), [ERNIE-Gram](https://arxiv.org/abs/2010.12148), [ERNIE-health](https://arxiv.org/abs/2110.07244), etc.
These models are contributed by [nghuyong](https://huggingface.co/nghuyong) and the official code can be found in [PaddleNLP](https://github.com/PaddlePaddle/PaddleNLP) (in PaddlePaddle).
### Usage example
Take `ernie-1.0-base-zh` as an example:
```Python
from transformers import AutoTokenizer, AutoModel
tokenizer = AutoTokenizer.from_pretrained("nghuyong/ernie-1.0-base-zh")
model = AutoModel.from_pretrained("nghuyong/ernie-1.0-base-zh")
```
### Model checkpoints
| Model Name | Language | Description |
|:-------------------:|:--------:|:-------------------------------:|
| ernie-1.0-base-zh | Chinese | Layer:12, Heads:12, Hidden:768 |
| ernie-2.0-base-en | English | Layer:12, Heads:12, Hidden:768 |
| ernie-2.0-large-en | English | Layer:24, Heads:16, Hidden:1024 |
| ernie-3.0-base-zh | Chinese | Layer:12, Heads:12, Hidden:768 |
| ernie-3.0-medium-zh | Chinese | Layer:6, Heads:12, Hidden:768 |
| ernie-3.0-mini-zh | Chinese | Layer:6, Heads:12, Hidden:384 |
| ernie-3.0-micro-zh | Chinese | Layer:4, Heads:12, Hidden:384 |
| ernie-3.0-nano-zh | Chinese | Layer:4, Heads:12, Hidden:312 |
| ernie-health-zh | Chinese | Layer:12, Heads:12, Hidden:768 |
| ernie-gram-zh | Chinese | Layer:12, Heads:12, Hidden:768 |
You can find all the supported models from huggingface's model hub: [huggingface.co/nghuyong](https://huggingface.co/nghuyong), and model details from paddle's official
repo: [PaddleNLP](https://paddlenlp.readthedocs.io/zh/latest/model_zoo/transformers/ERNIE/contents.html)
and [ERNIE](https://github.com/PaddlePaddle/ERNIE/blob/repro).
## Resources
- [Text classification task guide](../tasks/sequence_classification)
- [Token classification task guide](../tasks/token_classification)
- [Question answering task guide](../tasks/question_answering)
- [Causal language modeling task guide](../tasks/language_modeling)
- [Masked language modeling task guide](../tasks/masked_language_modeling)
- [Multiple choice task guide](../tasks/multiple_choice)
## ErnieConfig
[[autodoc]] ErnieConfig
- all
## Ernie specific outputs
[[autodoc]] models.ernie.modeling_ernie.ErnieForPreTrainingOutput
## ErnieModel
[[autodoc]] ErnieModel
- forward
## ErnieForPreTraining
[[autodoc]] ErnieForPreTraining
- forward
## ErnieForCausalLM
[[autodoc]] ErnieForCausalLM
- forward
## ErnieForMaskedLM
[[autodoc]] ErnieForMaskedLM
- forward
## ErnieForNextSentencePrediction
[[autodoc]] ErnieForNextSentencePrediction
- forward
## ErnieForSequenceClassification
[[autodoc]] ErnieForSequenceClassification
- forward
## ErnieForMultipleChoice
[[autodoc]] ErnieForMultipleChoice
- forward
## ErnieForTokenClassification
[[autodoc]] ErnieForTokenClassification
- forward
## ErnieForQuestionAnswering
[[autodoc]] ErnieForQuestionAnswering
- forward | transformers/docs/source/en/model_doc/ernie.md/0 | {
"file_path": "transformers/docs/source/en/model_doc/ernie.md",
"repo_id": "transformers",
"token_count": 1417
} | 247 |
<!--Copyright 2022 The HuggingFace Team. All rights reserved.
Licensed under the Apache License, Version 2.0 (the "License"); you may not use this file except in compliance with
the License. You may obtain a copy of the License at
http://www.apache.org/licenses/LICENSE-2.0
Unless required by applicable law or agreed to in writing, software distributed under the License is distributed on
an "AS IS" BASIS, WITHOUT WARRANTIES OR CONDITIONS OF ANY KIND, either express or implied. See the License for the
specific language governing permissions and limitations under the License.
⚠️ Note that this file is in Markdown but contain specific syntax for our doc-builder (similar to MDX) that may not be
rendered properly in your Markdown viewer.
-->
# LongT5
## Overview
The LongT5 model was proposed in [LongT5: Efficient Text-To-Text Transformer for Long Sequences](https://arxiv.org/abs/2112.07916)
by Mandy Guo, Joshua Ainslie, David Uthus, Santiago Ontanon, Jianmo Ni, Yun-Hsuan Sung and Yinfei Yang. It's an
encoder-decoder transformer pre-trained in a text-to-text denoising generative setting. LongT5 model is an extension of
T5 model, and it enables using one of the two different efficient attention mechanisms - (1) Local attention, or (2)
Transient-Global attention.
The abstract from the paper is the following:
*Recent work has shown that either (1) increasing the input length or (2) increasing model size can improve the
performance of Transformer-based neural models. In this paper, we present a new model, called LongT5, with which we
explore the effects of scaling both the input length and model size at the same time. Specifically, we integrated
attention ideas from long-input transformers (ETC), and adopted pre-training strategies from summarization pre-training
(PEGASUS) into the scalable T5 architecture. The result is a new attention mechanism we call {\em Transient Global}
(TGlobal), which mimics ETC's local/global attention mechanism, but without requiring additional side-inputs. We are
able to achieve state-of-the-art results on several summarization tasks and outperform the original T5 models on
question answering tasks.*
This model was contributed by [stancld](https://huggingface.co/stancld).
The original code can be found [here](https://github.com/google-research/longt5).
## Usage tips
- [`LongT5ForConditionalGeneration`] is an extension of [`T5ForConditionalGeneration`] exchanging the traditional
encoder *self-attention* layer with efficient either *local* attention or *transient-global* (*tglobal*) attention.
- Unlike the T5 model, LongT5 does not use a task prefix. Furthermore, it uses a different pre-training objective
inspired by the pre-training of [`PegasusForConditionalGeneration`].
- LongT5 model is designed to work efficiently and very well on long-range *sequence-to-sequence* tasks where the
input sequence exceeds commonly used 512 tokens. It is capable of handling input sequences of a length up to 16,384 tokens.
- For *Local Attention*, the sparse sliding-window local attention operation allows a given token to attend only `r`
tokens to the left and right of it (with `r=127` by default). *Local Attention* does not introduce any new parameters
to the model. The complexity of the mechanism is linear in input sequence length `l`: `O(l*r)`.
- *Transient Global Attention* is an extension of the *Local Attention*. It, furthermore, allows each input token to
interact with all other tokens in the layer. This is achieved via splitting an input sequence into blocks of a fixed
length `k` (with a default `k=16`). Then, a global token for such a block is obtained via summing and normalizing the embeddings of every token
in the block. Thanks to this, the attention allows each token to attend to both nearby tokens like in Local attention, and
also every global token like in the case of standard global attention (*transient* represents the fact the global tokens
are constructed dynamically within each attention operation). As a consequence, *TGlobal* attention introduces
a few new parameters -- global relative position biases and a layer normalization for global token's embedding.
The complexity of this mechanism is `O(l(r + l/k))`.
- An example showing how to evaluate a fine-tuned LongT5 model on the [pubmed dataset](https://huggingface.co/datasets/scientific_papers) is below.
```python
>>> import evaluate
>>> from datasets import load_dataset
>>> from transformers import AutoTokenizer, LongT5ForConditionalGeneration
>>> dataset = load_dataset("scientific_papers", "pubmed", split="validation")
>>> model = (
... LongT5ForConditionalGeneration.from_pretrained("Stancld/longt5-tglobal-large-16384-pubmed-3k_steps")
... .to("cuda")
... .half()
... )
>>> tokenizer = AutoTokenizer.from_pretrained("Stancld/longt5-tglobal-large-16384-pubmed-3k_steps")
>>> def generate_answers(batch):
... inputs_dict = tokenizer(
... batch["article"], max_length=16384, padding="max_length", truncation=True, return_tensors="pt"
... )
... input_ids = inputs_dict.input_ids.to("cuda")
... attention_mask = inputs_dict.attention_mask.to("cuda")
... output_ids = model.generate(input_ids, attention_mask=attention_mask, max_length=512, num_beams=2)
... batch["predicted_abstract"] = tokenizer.batch_decode(output_ids, skip_special_tokens=True)
... return batch
>>> result = dataset.map(generate_answer, batched=True, batch_size=2)
>>> rouge = evaluate.load("rouge")
>>> rouge.compute(predictions=result["predicted_abstract"], references=result["abstract"])
```
## Resources
- [Translation task guide](../tasks/translation)
- [Summarization task guide](../tasks/summarization)
## LongT5Config
[[autodoc]] LongT5Config
<frameworkcontent>
<pt>
## LongT5Model
[[autodoc]] LongT5Model
- forward
## LongT5ForConditionalGeneration
[[autodoc]] LongT5ForConditionalGeneration
- forward
## LongT5EncoderModel
[[autodoc]] LongT5EncoderModel
- forward
</pt>
<jax>
## FlaxLongT5Model
[[autodoc]] FlaxLongT5Model
- __call__
- encode
- decode
## FlaxLongT5ForConditionalGeneration
[[autodoc]] FlaxLongT5ForConditionalGeneration
- __call__
- encode
- decode
</jax>
</frameworkcontent>
| transformers/docs/source/en/model_doc/longt5.md/0 | {
"file_path": "transformers/docs/source/en/model_doc/longt5.md",
"repo_id": "transformers",
"token_count": 1797
} | 248 |
<!--Copyright 2022 The HuggingFace Team. All rights reserved.
Licensed under the Apache License, Version 2.0 (the "License"); you may not use this file except in compliance with
the License. You may obtain a copy of the License at
http://www.apache.org/licenses/LICENSE-2.0
Unless required by applicable law or agreed to in writing, software distributed under the License is distributed on
an "AS IS" BASIS, WITHOUT WARRANTIES OR CONDITIONS OF ANY KIND, either express or implied. See the License for the
specific language governing permissions and limitations under the License.
⚠️ Note that this file is in Markdown but contain specific syntax for our doc-builder (similar to MDX) that may not be
rendered properly in your Markdown viewer.
-->
# MVP
## Overview
The MVP model was proposed in [MVP: Multi-task Supervised Pre-training for Natural Language Generation](https://arxiv.org/abs/2206.12131) by Tianyi Tang, Junyi Li, Wayne Xin Zhao and Ji-Rong Wen.
According to the abstract,
- MVP follows a standard Transformer encoder-decoder architecture.
- MVP is supervised pre-trained using labeled datasets.
- MVP also has task-specific soft prompts to stimulate the model's capacity in performing a certain task.
- MVP is specially designed for natural language generation and can be adapted to a wide range of generation tasks, including but not limited to summarization, data-to-text generation, open-ended dialogue system, story generation, question answering, question generation, task-oriented dialogue system, commonsense generation, paraphrase generation, text style transfer, and text simplification. Our model can also be adapted to natural language understanding tasks such as sequence classification and (extractive) question answering.
This model was contributed by [Tianyi Tang](https://huggingface.co/StevenTang). The detailed information and instructions can be found [here](https://github.com/RUCAIBox/MVP).
## Usage tips
- We have released a series of models [here](https://huggingface.co/models?filter=mvp), including MVP, MVP with task-specific prompts, and multi-task pre-trained variants.
- If you want to use a model without prompts (standard Transformer), you can load it through `MvpForConditionalGeneration.from_pretrained('RUCAIBox/mvp')`.
- If you want to use a model with task-specific prompts, such as summarization, you can load it through `MvpForConditionalGeneration.from_pretrained('RUCAIBox/mvp-summarization')`.
- Our model supports lightweight prompt tuning following [Prefix-tuning](https://arxiv.org/abs/2101.00190) with method `set_lightweight_tuning()`.
## Usage examples
For summarization, it is an example to use MVP and MVP with summarization-specific prompts.
```python
>>> from transformers import MvpTokenizer, MvpForConditionalGeneration
>>> tokenizer = MvpTokenizer.from_pretrained("RUCAIBox/mvp")
>>> model = MvpForConditionalGeneration.from_pretrained("RUCAIBox/mvp")
>>> model_with_prompt = MvpForConditionalGeneration.from_pretrained("RUCAIBox/mvp-summarization")
>>> inputs = tokenizer(
... "Summarize: You may want to stick it to your boss and leave your job, but don't do it if these are your reasons.",
... return_tensors="pt",
... )
>>> generated_ids = model.generate(**inputs)
>>> tokenizer.batch_decode(generated_ids, skip_special_tokens=True)
["Why You Shouldn't Quit Your Job"]
>>> generated_ids = model_with_prompt.generate(**inputs)
>>> tokenizer.batch_decode(generated_ids, skip_special_tokens=True)
["Don't do it if these are your reasons"]
```
For data-to-text generation, it is an example to use MVP and multi-task pre-trained variants.
```python
>>> from transformers import MvpTokenizerFast, MvpForConditionalGeneration
>>> tokenizer = MvpTokenizerFast.from_pretrained("RUCAIBox/mvp")
>>> model = MvpForConditionalGeneration.from_pretrained("RUCAIBox/mvp")
>>> model_with_mtl = MvpForConditionalGeneration.from_pretrained("RUCAIBox/mtl-data-to-text")
>>> inputs = tokenizer(
... "Describe the following data: Iron Man | instance of | Superhero [SEP] Stan Lee | creator | Iron Man",
... return_tensors="pt",
... )
>>> generated_ids = model.generate(**inputs)
>>> tokenizer.batch_decode(generated_ids, skip_special_tokens=True)
['Stan Lee created the character of Iron Man, a fictional superhero appearing in American comic']
>>> generated_ids = model_with_mtl.generate(**inputs)
>>> tokenizer.batch_decode(generated_ids, skip_special_tokens=True)
['Iron Man is a fictional superhero appearing in American comic books published by Marvel Comics.']
```
For lightweight tuning, *i.e.*, fixing the model and only tuning prompts, you can load MVP with randomly initialized prompts or with task-specific prompts. Our code also supports Prefix-tuning with BART following the [original paper](https://arxiv.org/abs/2101.00190).
```python
>>> from transformers import MvpForConditionalGeneration
>>> model = MvpForConditionalGeneration.from_pretrained("RUCAIBox/mvp", use_prompt=True)
>>> # the number of trainable parameters (full tuning)
>>> sum(p.numel() for p in model.parameters() if p.requires_grad)
468116832
>>> # lightweight tuning with randomly initialized prompts
>>> model.set_lightweight_tuning()
>>> # the number of trainable parameters (lightweight tuning)
>>> sum(p.numel() for p in model.parameters() if p.requires_grad)
61823328
>>> # lightweight tuning with task-specific prompts
>>> model = MvpForConditionalGeneration.from_pretrained("RUCAIBox/mtl-data-to-text")
>>> model.set_lightweight_tuning()
>>> # original lightweight Prefix-tuning
>>> model = MvpForConditionalGeneration.from_pretrained("facebook/bart-large", use_prompt=True)
>>> model.set_lightweight_tuning()
```
## Resources
- [Text classification task guide](../tasks/sequence_classification)
- [Question answering task guide](../tasks/question_answering)
- [Causal language modeling task guide](../tasks/language_modeling)
- [Masked language modeling task guide](../tasks/masked_language_modeling)
- [Translation task guide](../tasks/translation)
- [Summarization task guide](../tasks/summarization)
## MvpConfig
[[autodoc]] MvpConfig
## MvpTokenizer
[[autodoc]] MvpTokenizer
## MvpTokenizerFast
[[autodoc]] MvpTokenizerFast
## MvpModel
[[autodoc]] MvpModel
- forward
## MvpForConditionalGeneration
[[autodoc]] MvpForConditionalGeneration
- forward
## MvpForSequenceClassification
[[autodoc]] MvpForSequenceClassification
- forward
## MvpForQuestionAnswering
[[autodoc]] MvpForQuestionAnswering
- forward
## MvpForCausalLM
[[autodoc]] MvpForCausalLM
- forward
| transformers/docs/source/en/model_doc/mvp.md/0 | {
"file_path": "transformers/docs/source/en/model_doc/mvp.md",
"repo_id": "transformers",
"token_count": 1922
} | 249 |
<!--Copyright 2020 The HuggingFace Team. All rights reserved.
Licensed under the Apache License, Version 2.0 (the "License"); you may not use this file except in compliance with
the License. You may obtain a copy of the License at
http://www.apache.org/licenses/LICENSE-2.0
Unless required by applicable law or agreed to in writing, software distributed under the License is distributed on
an "AS IS" BASIS, WITHOUT WARRANTIES OR CONDITIONS OF ANY KIND, either express or implied. See the License for the
specific language governing permissions and limitations under the License.
⚠️ Note that this file is in Markdown but contain specific syntax for our doc-builder (similar to MDX) that may not be
rendered properly in your Markdown viewer.
-->
# Reformer
<div class="flex flex-wrap space-x-1">
<a href="https://huggingface.co/models?filter=reformer">
<img alt="Models" src="https://img.shields.io/badge/All_model_pages-reformer-blueviolet">
</a>
<a href="https://huggingface.co/spaces/docs-demos/reformer-crime-and-punishment">
<img alt="Spaces" src="https://img.shields.io/badge/%F0%9F%A4%97%20Hugging%20Face-Spaces-blue">
</a>
</div>
## Overview
The Reformer model was proposed in the paper [Reformer: The Efficient Transformer](https://arxiv.org/abs/2001.04451.pdf) by Nikita Kitaev, Łukasz Kaiser, Anselm Levskaya.
The abstract from the paper is the following:
*Large Transformer models routinely achieve state-of-the-art results on a number of tasks but training these models can
be prohibitively costly, especially on long sequences. We introduce two techniques to improve the efficiency of
Transformers. For one, we replace dot-product attention by one that uses locality-sensitive hashing, changing its
complexity from O(L^2) to O(Llog(L)), where L is the length of the sequence. Furthermore, we use reversible residual
layers instead of the standard residuals, which allows storing activations only once in the training process instead of
N times, where N is the number of layers. The resulting model, the Reformer, performs on par with Transformer models
while being much more memory-efficient and much faster on long sequences.*
This model was contributed by [patrickvonplaten](https://huggingface.co/patrickvonplaten). The Authors' code can be
found [here](https://github.com/google/trax/tree/master/trax/models/reformer).
## Usage tips
- Reformer does **not** work with *torch.nn.DataParallel* due to a bug in PyTorch, see [issue #36035](https://github.com/pytorch/pytorch/issues/36035).
- Use Axial position encoding (see below for more details). It’s a mechanism to avoid having a huge positional encoding matrix (when the sequence length is very big) by factorizing it into smaller matrices.
- Replace traditional attention by LSH (local-sensitive hashing) attention (see below for more details). It’s a technique to avoid computing the full product query-key in the attention layers.
- Avoid storing the intermediate results of each layer by using reversible transformer layers to obtain them during the backward pass (subtracting the residuals from the input of the next layer gives them back) or recomputing them for results inside a given layer (less efficient than storing them but saves memory).
- Compute the feedforward operations by chunks and not on the whole batch.
### Axial Positional Encodings
Axial Positional Encodings were first implemented in Google's [trax library](https://github.com/google/trax/blob/4d99ad4965bab1deba227539758d59f0df0fef48/trax/layers/research/position_encodings.py#L29)
and developed by the authors of this model's paper. In models that are treating very long input sequences, the
conventional position id encodings store an embeddings vector of size \\(d\\) being the `config.hidden_size` for
every position \\(i, \ldots, n_s\\), with \\(n_s\\) being `config.max_embedding_size`. This means that having
a sequence length of \\(n_s = 2^{19} \approx 0.5M\\) and a `config.hidden_size` of \\(d = 2^{10} \approx 1000\\)
would result in a position encoding matrix:
$$X_{i,j}, \text{ with } i \in \left[1,\ldots, d\right] \text{ and } j \in \left[1,\ldots, n_s\right]$$
which alone has over 500M parameters to store. Axial positional encodings factorize \\(X_{i,j}\\) into two matrices:
$$X^{1}_{i,j}, \text{ with } i \in \left[1,\ldots, d^1\right] \text{ and } j \in \left[1,\ldots, n_s^1\right]$$
and
$$X^{2}_{i,j}, \text{ with } i \in \left[1,\ldots, d^2\right] \text{ and } j \in \left[1,\ldots, n_s^2\right]$$
with:
$$d = d^1 + d^2 \text{ and } n_s = n_s^1 \times n_s^2 .$$
Therefore the following holds:
$$X_{i,j} = \begin{cases}
X^{1}_{i, k}, & \text{if }\ i < d^1 \text{ with } k = j \mod n_s^1 \\
X^{2}_{i - d^1, l}, & \text{if } i \ge d^1 \text{ with } l = \lfloor\frac{j}{n_s^1}\rfloor
\end{cases}$$
Intuitively, this means that a position embedding vector \\(x_j \in \mathbb{R}^{d}\\) is now the composition of two
factorized embedding vectors: \\(x^1_{k, l} + x^2_{l, k}\\), where as the `config.max_embedding_size` dimension
\\(j\\) is factorized into \\(k \text{ and } l\\). This design ensures that each position embedding vector
\\(x_j\\) is unique.
Using the above example again, axial position encoding with \\(d^1 = 2^9, d^2 = 2^9, n_s^1 = 2^9, n_s^2 = 2^{10}\\)
can drastically reduced the number of parameters from 500 000 000 to \\(2^{18} + 2^{19} \approx 780 000\\) parameters, this means 85% less memory usage.
In practice, the parameter `config.axial_pos_embds_dim` is set to a tuple \\((d^1, d^2)\\) which sum has to be
equal to `config.hidden_size` and `config.axial_pos_shape` is set to a tuple \\((n_s^1, n_s^2)\\) which
product has to be equal to `config.max_embedding_size`, which during training has to be equal to the *sequence
length* of the `input_ids`.
### LSH Self Attention
In Locality sensitive hashing (LSH) self attention the key and query projection weights are tied. Therefore, the key
query embedding vectors are also tied. LSH self attention uses the locality sensitive hashing mechanism proposed in
[Practical and Optimal LSH for Angular Distance](https://arxiv.org/abs/1509.02897) to assign each of the tied key
query embedding vectors to one of `config.num_buckets` possible buckets. The premise is that the more "similar"
key query embedding vectors (in terms of *cosine similarity*) are to each other, the more likely they are assigned to
the same bucket.
The accuracy of the LSH mechanism can be improved by increasing `config.num_hashes` or directly the argument
`num_hashes` of the forward function so that the output of the LSH self attention better approximates the output
of the "normal" full self attention. The buckets are then sorted and chunked into query key embedding vector chunks
each of length `config.lsh_chunk_length`. For each chunk, the query embedding vectors attend to its key vectors
(which are tied to themselves) and to the key embedding vectors of `config.lsh_num_chunks_before` previous
neighboring chunks and `config.lsh_num_chunks_after` following neighboring chunks.
For more information, see the [original Paper](https://arxiv.org/abs/2001.04451) or this great [blog post](https://www.pragmatic.ml/reformer-deep-dive/).
Note that `config.num_buckets` can also be factorized into a list \\((n_{\text{buckets}}^1,
n_{\text{buckets}}^2)\\). This way instead of assigning the query key embedding vectors to one of \\((1,\ldots,
n_{\text{buckets}})\\) they are assigned to one of \\((1-1,\ldots, n_{\text{buckets}}^1-1, \ldots,
1-n_{\text{buckets}}^2, \ldots, n_{\text{buckets}}^1-n_{\text{buckets}}^2)\\). This is crucial for very long sequences to
save memory.
When training a model from scratch, it is recommended to leave `config.num_buckets=None`, so that depending on the
sequence length a good value for `num_buckets` is calculated on the fly. This value will then automatically be
saved in the config and should be reused for inference.
Using LSH self attention, the memory and time complexity of the query-key matmul operation can be reduced from
\\(\mathcal{O}(n_s \times n_s)\\) to \\(\mathcal{O}(n_s \times \log(n_s))\\), which usually represents the memory
and time bottleneck in a transformer model, with \\(n_s\\) being the sequence length.
### Local Self Attention
Local self attention is essentially a "normal" self attention layer with key, query and value projections, but is
chunked so that in each chunk of length `config.local_chunk_length` the query embedding vectors only attends to
the key embedding vectors in its chunk and to the key embedding vectors of `config.local_num_chunks_before`
previous neighboring chunks and `config.local_num_chunks_after` following neighboring chunks.
Using Local self attention, the memory and time complexity of the query-key matmul operation can be reduced from
\\(\mathcal{O}(n_s \times n_s)\\) to \\(\mathcal{O}(n_s \times \log(n_s))\\), which usually represents the memory
and time bottleneck in a transformer model, with \\(n_s\\) being the sequence length.
### Training
During training, we must ensure that the sequence length is set to a value that can be divided by the least common
multiple of `config.lsh_chunk_length` and `config.local_chunk_length` and that the parameters of the Axial
Positional Encodings are correctly set as described above. Reformer is very memory efficient so that the model can
easily be trained on sequences as long as 64000 tokens.
For training, the [`ReformerModelWithLMHead`] should be used as follows:
```python
input_ids = tokenizer.encode("This is a sentence from the training data", return_tensors="pt")
loss = model(input_ids, labels=input_ids)[0]
```
## Resources
- [Text classification task guide](../tasks/sequence_classification)
- [Question answering task guide](../tasks/question_answering)
- [Causal language modeling task guide](../tasks/language_modeling)
- [Masked language modeling task guide](../tasks/masked_language_modeling)
## ReformerConfig
[[autodoc]] ReformerConfig
## ReformerTokenizer
[[autodoc]] ReformerTokenizer
- save_vocabulary
## ReformerTokenizerFast
[[autodoc]] ReformerTokenizerFast
## ReformerModel
[[autodoc]] ReformerModel
- forward
## ReformerModelWithLMHead
[[autodoc]] ReformerModelWithLMHead
- forward
## ReformerForMaskedLM
[[autodoc]] ReformerForMaskedLM
- forward
## ReformerForSequenceClassification
[[autodoc]] ReformerForSequenceClassification
- forward
## ReformerForQuestionAnswering
[[autodoc]] ReformerForQuestionAnswering
- forward
| transformers/docs/source/en/model_doc/reformer.md/0 | {
"file_path": "transformers/docs/source/en/model_doc/reformer.md",
"repo_id": "transformers",
"token_count": 3186
} | 250 |
<!--Copyright 2020 The HuggingFace Team. All rights reserved.
Licensed under the Apache License, Version 2.0 (the "License"); you may not use this file except in compliance with
the License. You may obtain a copy of the License at
http://www.apache.org/licenses/LICENSE-2.0
Unless required by applicable law or agreed to in writing, software distributed under the License is distributed on
an "AS IS" BASIS, WITHOUT WARRANTIES OR CONDITIONS OF ANY KIND, either express or implied. See the License for the
specific language governing permissions and limitations under the License.
⚠️ Note that this file is in Markdown but contain specific syntax for our doc-builder (similar to MDX) that may not be
rendered properly in your Markdown viewer.
-->
# T5
<div class="flex flex-wrap space-x-1">
<a href="https://huggingface.co/models?filter=t5">
<img alt="Models" src="https://img.shields.io/badge/All_model_pages-t5-blueviolet">
</a>
<a href="https://huggingface.co/spaces/docs-demos/t5-base">
<img alt="Spaces" src="https://img.shields.io/badge/%F0%9F%A4%97%20Hugging%20Face-Spaces-blue">
</a>
<a href="https://huggingface.co/papers/1910.10683">
<img alt="Paper page" src="https://img.shields.io/badge/Paper%20page-1910.10683-green">
</a>
</div>
## Overview
The T5 model was presented in [Exploring the Limits of Transfer Learning with a Unified Text-to-Text Transformer](https://arxiv.org/pdf/1910.10683.pdf) by [Colin Raffel](https://huggingface.co/craffel), Noam Shazeer, [Adam Roberts](https://huggingface.co/adarob), Katherine Lee, Sharan Narang,
Michael Matena, Yanqi Zhou, Wei Li, [Peter J. Liu](https://huggingface.co/peterjliu).
The abstract from the paper is the following:
*Transfer learning, where a model is first pre-trained on a data-rich task before being fine-tuned on a downstream
task, has emerged as a powerful technique in natural language processing (NLP). The effectiveness of transfer learning
has given rise to a diversity of approaches, methodology, and practice. In this paper, we explore the landscape of
transfer learning techniques for NLP by introducing a unified framework that converts every language problem into a
text-to-text format. Our systematic study compares pretraining objectives, architectures, unlabeled datasets, transfer
approaches, and other factors on dozens of language understanding tasks. By combining the insights from our exploration
with scale and our new "Colossal Clean Crawled Corpus", we achieve state-of-the-art results on many benchmarks covering
summarization, question answering, text classification, and more. To facilitate future work on transfer learning for
NLP, we release our dataset, pre-trained models, and code.*
All checkpoints can be found on the [hub](https://huggingface.co/models?search=t5).
This model was contributed by [thomwolf](https://huggingface.co/thomwolf). The original code can be found [here](https://github.com/google-research/text-to-text-transfer-transformer).
## Usage tips
- T5 is an encoder-decoder model pre-trained on a multi-task mixture of unsupervised and supervised tasks and for which
each task is converted into a text-to-text format. T5 works well on a variety of tasks out-of-the-box by prepending a
different prefix to the input corresponding to each task, e.g., for translation: *translate English to German: ...*,
for summarization: *summarize: ...*.
- The pretraining includes both supervised and self-supervised training. Supervised training is conducted on downstream tasks provided by the GLUE and SuperGLUE benchmarks (converting them into text-to-text tasks as explained above).
- Self-supervised training uses corrupted tokens, by randomly removing 15% of the tokens and replacing them with individual sentinel tokens (if several consecutive tokens are marked for removal, the whole group is replaced with a single sentinel token). The input of the encoder is the corrupted sentence, the input of the decoder is the original sentence and the target is then the dropped out tokens delimited by their sentinel tokens.
- T5 uses relative scalar embeddings. Encoder input padding can be done on the left and on the right.
- See the [training](#training), [inference](#inference) and [resources](#resources) sections below for all details regarding usage.
T5 comes in different sizes:
- [google-t5/t5-small](https://huggingface.co/google-t5/t5-small)
- [google-t5/t5-base](https://huggingface.co/google-t5/t5-base)
- [google-t5/t5-large](https://huggingface.co/google-t5/t5-large)
- [google-t5/t5-3b](https://huggingface.co/google-t5/t5-3b)
- [google-t5/t5-11b](https://huggingface.co/google-t5/t5-11b).
Based on the original T5 model, Google has released some follow-up works:
- **T5v1.1**: T5v1.1 is an improved version of T5 with some architectural tweaks, and is pre-trained on C4 only without
mixing in the supervised tasks. Refer to the documentation of T5v1.1 which can be found [here](t5v1.1).
- **mT5**: mT5 is a multilingual T5 model. It is pre-trained on the mC4 corpus, which includes 101 languages. Refer to
the documentation of mT5 which can be found [here](mt5).
- **byT5**: byT5 is a T5 model pre-trained on byte sequences rather than SentencePiece subword token sequences. Refer
to the documentation of byT5 which can be found [here](byt5).
- **UL2**: UL2 is a T5 like model pretrained on various denoising objectives
- **Flan-T5**: Flan is a pretraining methods that is based on prompting. The Flan-T5 are T5 models trained on the Flan collection of
datasets which include: `taskmaster2`, `djaym7/wiki_dialog`, `deepmind/code_contests`, `lambada`, `gsm8k`, `aqua_rat`, `esnli`, `quasc` and `qed`.
- **FLan-UL2** : the UL2 model finetuned using the "Flan" prompt tuning and dataset collection.
- **UMT5**: UmT5 is a multilingual T5 model trained on an improved and refreshed mC4 multilingual corpus, 29 trillion characters across 107 language, using a new sampling method, UniMax. Refer to
the documentation of mT5 which can be found [here](umt5).
## Training
T5 is an encoder-decoder model and converts all NLP problems into a text-to-text format. It is trained using teacher
forcing. This means that for training, we always need an input sequence and a corresponding target sequence. The input
sequence is fed to the model using `input_ids`. The target sequence is shifted to the right, i.e., prepended by a
start-sequence token and fed to the decoder using the `decoder_input_ids`. In teacher-forcing style, the target
sequence is then appended by the EOS token and corresponds to the `labels`. The PAD token is hereby used as the
start-sequence token. T5 can be trained / fine-tuned both in a supervised and unsupervised fashion.
One can use [`T5ForConditionalGeneration`] (or the Tensorflow/Flax variant), which includes the
language modeling head on top of the decoder.
- Unsupervised denoising training
In this setup, spans of the input sequence are masked by so-called sentinel tokens (*a.k.a* unique mask tokens) and
the output sequence is formed as a concatenation of the same sentinel tokens and the *real* masked tokens. Each
sentinel token represents a unique mask token for this sentence and should start with `<extra_id_0>`,
`<extra_id_1>`, ... up to `<extra_id_99>`. As a default, 100 sentinel tokens are available in
[`T5Tokenizer`].
For instance, the sentence "The cute dog walks in the park" with the masks put on "cute dog" and "the" should be
processed as follows:
```python
>>> from transformers import T5Tokenizer, T5ForConditionalGeneration
>>> tokenizer = T5Tokenizer.from_pretrained("google-t5/t5-small")
>>> model = T5ForConditionalGeneration.from_pretrained("google-t5/t5-small")
>>> input_ids = tokenizer("The <extra_id_0> walks in <extra_id_1> park", return_tensors="pt").input_ids
>>> labels = tokenizer("<extra_id_0> cute dog <extra_id_1> the <extra_id_2>", return_tensors="pt").input_ids
>>> # the forward function automatically creates the correct decoder_input_ids
>>> loss = model(input_ids=input_ids, labels=labels).loss
>>> loss.item()
3.7837
```
If you're interested in pre-training T5 on a new corpus, check out the [run_t5_mlm_flax.py](https://github.com/huggingface/transformers/tree/main/examples/flax/language-modeling) script in the Examples
directory.
- Supervised training
In this setup, the input sequence and output sequence are a standard sequence-to-sequence input-output mapping.
Suppose that we want to fine-tune the model for translation for example, and we have a training example: the input
sequence "The house is wonderful." and output sequence "Das Haus ist wunderbar.", then they should be prepared for
the model as follows:
```python
>>> from transformers import T5Tokenizer, T5ForConditionalGeneration
>>> tokenizer = T5Tokenizer.from_pretrained("google-t5/t5-small")
>>> model = T5ForConditionalGeneration.from_pretrained("google-t5/t5-small")
>>> input_ids = tokenizer("translate English to German: The house is wonderful.", return_tensors="pt").input_ids
>>> labels = tokenizer("Das Haus ist wunderbar.", return_tensors="pt").input_ids
>>> # the forward function automatically creates the correct decoder_input_ids
>>> loss = model(input_ids=input_ids, labels=labels).loss
>>> loss.item()
0.2542
```
As you can see, only 2 inputs are required for the model in order to compute a loss: `input_ids` (which are the
`input_ids` of the encoded input sequence) and `labels` (which are the `input_ids` of the encoded
target sequence). The model will automatically create the `decoder_input_ids` based on the `labels`, by
shifting them one position to the right and prepending the `config.decoder_start_token_id`, which for T5 is
equal to 0 (i.e. the id of the pad token). Also note the task prefix: we prepend the input sequence with 'translate
English to German: ' before encoding it. This will help in improving the performance, as this task prefix was used
during T5's pre-training.
However, the example above only shows a single training example. In practice, one trains deep learning models in
batches. This entails that we must pad/truncate examples to the same length. For encoder-decoder models, one
typically defines a `max_source_length` and `max_target_length`, which determine the maximum length of the
input and output sequences respectively (otherwise they are truncated). These should be carefully set depending on
the task.
In addition, we must make sure that padding token id's of the `labels` are not taken into account by the loss
function. In PyTorch and Tensorflow, this can be done by replacing them with -100, which is the `ignore_index`
of the `CrossEntropyLoss`. In Flax, one can use the `decoder_attention_mask` to ignore padded tokens from
the loss (see the [Flax summarization script](https://github.com/huggingface/transformers/tree/main/examples/flax/summarization) for details). We also pass
`attention_mask` as additional input to the model, which makes sure that padding tokens of the inputs are
ignored. The code example below illustrates all of this.
```python
>>> from transformers import T5Tokenizer, T5ForConditionalGeneration
>>> import torch
>>> tokenizer = T5Tokenizer.from_pretrained("google-t5/t5-small")
>>> model = T5ForConditionalGeneration.from_pretrained("google-t5/t5-small")
>>> # the following 2 hyperparameters are task-specific
>>> max_source_length = 512
>>> max_target_length = 128
>>> # Suppose we have the following 2 training examples:
>>> input_sequence_1 = "Welcome to NYC"
>>> output_sequence_1 = "Bienvenue à NYC"
>>> input_sequence_2 = "HuggingFace is a company"
>>> output_sequence_2 = "HuggingFace est une entreprise"
>>> # encode the inputs
>>> task_prefix = "translate English to French: "
>>> input_sequences = [input_sequence_1, input_sequence_2]
>>> encoding = tokenizer(
... [task_prefix + sequence for sequence in input_sequences],
... padding="longest",
... max_length=max_source_length,
... truncation=True,
... return_tensors="pt",
... )
>>> input_ids, attention_mask = encoding.input_ids, encoding.attention_mask
>>> # encode the targets
>>> target_encoding = tokenizer(
... [output_sequence_1, output_sequence_2],
... padding="longest",
... max_length=max_target_length,
... truncation=True,
... return_tensors="pt",
... )
>>> labels = target_encoding.input_ids
>>> # replace padding token id's of the labels by -100 so it's ignored by the loss
>>> labels[labels == tokenizer.pad_token_id] = -100
>>> # forward pass
>>> loss = model(input_ids=input_ids, attention_mask=attention_mask, labels=labels).loss
>>> loss.item()
0.188
```
Additional training tips:
- T5 models need a slightly higher learning rate than the default one set in the `Trainer` when using the AdamW
optimizer. Typically, 1e-4 and 3e-4 work well for most problems (classification, summarization, translation, question
answering, question generation). Note that T5 was pre-trained using the AdaFactor optimizer.
According to [this forum post](https://discuss.huggingface.co/t/t5-finetuning-tips/684), task prefixes matter when
(1) doing multi-task training (2) your task is similar or related to one of the supervised tasks used in T5's
pre-training mixture (see Appendix D of the [paper](https://arxiv.org/pdf/1910.10683.pdf) for the task prefixes
used).
If training on TPU, it is recommended to pad all examples of the dataset to the same length or make use of
*pad_to_multiple_of* to have a small number of predefined bucket sizes to fit all examples in. Dynamically padding
batches to the longest example is not recommended on TPU as it triggers a recompilation for every batch shape that is
encountered during training thus significantly slowing down the training. only padding up to the longest example in a
batch) leads to very slow training on TPU.
## Inference
At inference time, it is recommended to use [`~generation.GenerationMixin.generate`]. This
method takes care of encoding the input and feeding the encoded hidden states via cross-attention layers to the decoder
and auto-regressively generates the decoder output. Check out [this blog post](https://huggingface.co/blog/how-to-generate) to know all the details about generating text with Transformers.
There's also [this blog post](https://huggingface.co/blog/encoder-decoder#encoder-decoder) which explains how
generation works in general in encoder-decoder models.
```python
>>> from transformers import T5Tokenizer, T5ForConditionalGeneration
>>> tokenizer = T5Tokenizer.from_pretrained("google-t5/t5-small")
>>> model = T5ForConditionalGeneration.from_pretrained("google-t5/t5-small")
>>> input_ids = tokenizer("translate English to German: The house is wonderful.", return_tensors="pt").input_ids
>>> outputs = model.generate(input_ids)
>>> print(tokenizer.decode(outputs[0], skip_special_tokens=True))
Das Haus ist wunderbar.
```
Note that T5 uses the `pad_token_id` as the `decoder_start_token_id`, so when doing generation without using
[`~generation.GenerationMixin.generate`], make sure you start it with the `pad_token_id`.
The example above only shows a single example. You can also do batched inference, like so:
```python
>>> from transformers import T5Tokenizer, T5ForConditionalGeneration
>>> tokenizer = T5Tokenizer.from_pretrained("google-t5/t5-small")
>>> model = T5ForConditionalGeneration.from_pretrained("google-t5/t5-small")
>>> task_prefix = "translate English to German: "
>>> # use different length sentences to test batching
>>> sentences = ["The house is wonderful.", "I like to work in NYC."]
>>> inputs = tokenizer([task_prefix + sentence for sentence in sentences], return_tensors="pt", padding=True)
>>> output_sequences = model.generate(
... input_ids=inputs["input_ids"],
... attention_mask=inputs["attention_mask"],
... do_sample=False, # disable sampling to test if batching affects output
... )
>>> print(tokenizer.batch_decode(output_sequences, skip_special_tokens=True))
['Das Haus ist wunderbar.', 'Ich arbeite gerne in NYC.']
```
Because T5 has been trained with the span-mask denoising objective,
it can be used to predict the sentinel (masked-out) tokens during inference.
The predicted tokens will then be placed between the sentinel tokens.
```python
>>> from transformers import T5Tokenizer, T5ForConditionalGeneration
>>> tokenizer = T5Tokenizer.from_pretrained("google-t5/t5-small")
>>> model = T5ForConditionalGeneration.from_pretrained("google-t5/t5-small")
>>> input_ids = tokenizer("The <extra_id_0> walks in <extra_id_1> park", return_tensors="pt").input_ids
>>> sequence_ids = model.generate(input_ids)
>>> sequences = tokenizer.batch_decode(sequence_ids)
>>> sequences
['<pad><extra_id_0> park offers<extra_id_1> the<extra_id_2> park.</s>']
```
## Performance
If you'd like a faster training and inference performance, install [NVIDIA APEX](https://github.com/NVIDIA/apex#quick-start) for NVIDIA GPUs, or [ROCm APEX](https://github.com/ROCmSoftwarePlatform/apex) for AMD GPUs and then the model will automatically use `apex.normalization.FusedRMSNorm` instead of `T5LayerNorm`. The former uses an optimized fused kernel which is several times faster than the latter.
## Resources
A list of official Hugging Face and community (indicated by 🌎) resources to help you get started with T5. If you're interested in submitting a resource to be included here, please feel free to open a Pull Request and we'll review it! The resource should ideally demonstrate something new instead of duplicating an existing resource.
<PipelineTag pipeline="text-classification"/>
- A notebook for how to [finetune T5 for classification and multiple choice](https://colab.research.google.com/github/patil-suraj/exploring-T5/blob/master/t5_fine_tuning.ipynb).
- A notebook for how to [finetune T5 for sentiment span extraction](https://colab.research.google.com/github/enzoampil/t5-intro/blob/master/t5_qa_training_pytorch_span_extraction.ipynb). 🌎
<PipelineTag pipeline="token-classification"/>
- A notebook for how to [finetune T5 for named entity recognition](https://colab.research.google.com/drive/1obr78FY_cBmWY5ODViCmzdY6O1KB65Vc?usp=sharing). 🌎
<PipelineTag pipeline="text-generation"/>
- A notebook for [Finetuning CodeT5 for generating docstrings from Ruby code](https://colab.research.google.com/github/NielsRogge/Transformers-Tutorials/blob/master/T5/Fine_tune_CodeT5_for_generating_docstrings_from_Ruby_code.ipynb).
<PipelineTag pipeline="summarization"/>
- A notebook to [Finetune T5-base-dutch to perform Dutch abstractive summarization on a TPU](https://colab.research.google.com/github/NielsRogge/Transformers-Tutorials/blob/master/T5/Fine_tuning_Dutch_T5_base_on_CNN_Daily_Mail_for_summarization_(on_TPU_using_HuggingFace_Accelerate).ipynb).
- A notebook for how to [finetune T5 for summarization in PyTorch and track experiments with WandB](https://colab.research.google.com/github/abhimishra91/transformers-tutorials/blob/master/transformers_summarization_wandb.ipynb#scrollTo=OKRpFvYhBauC). 🌎
- A blog post on [Distributed Training: Train BART/T5 for Summarization using 🤗 Transformers and Amazon SageMaker](https://huggingface.co/blog/sagemaker-distributed-training-seq2seq).
- [`T5ForConditionalGeneration`] is supported by this [example script](https://github.com/huggingface/transformers/tree/main/examples/pytorch/summarization) and [notebook](https://colab.research.google.com/github/huggingface/notebooks/blob/main/examples/summarization.ipynb).
- [`TFT5ForConditionalGeneration`] is supported by this [example script](https://github.com/huggingface/transformers/tree/main/examples/tensorflow/summarization) and [notebook](https://colab.research.google.com/github/huggingface/notebooks/blob/main/examples/summarization-tf.ipynb).
- [`FlaxT5ForConditionalGeneration`] is supported by this [example script](https://github.com/huggingface/transformers/tree/main/examples/flax/summarization).
- [Summarization](https://huggingface.co/course/chapter7/5?fw=pt#summarization) chapter of the 🤗 Hugging Face course.
- [Summarization task guide](../tasks/summarization)
<PipelineTag pipeline="fill-mask"/>
- [`FlaxT5ForConditionalGeneration`] is supported by this [example script](https://github.com/huggingface/transformers/tree/main/examples/flax/language-modeling#t5-like-span-masked-language-modeling) for training T5 with a span-masked language model objective. The script also shows how to train a T5 tokenizer. [`FlaxT5ForConditionalGeneration`] is also supported by this [notebook](https://colab.research.google.com/github/huggingface/notebooks/blob/main/examples/masked_language_modeling_flax.ipynb).
<PipelineTag pipeline="translation"/>
- [`T5ForConditionalGeneration`] is supported by this [example script](https://github.com/huggingface/transformers/tree/main/examples/pytorch/translation) and [notebook](https://colab.research.google.com/github/huggingface/notebooks/blob/main/examples/translation.ipynb).
- [`TFT5ForConditionalGeneration`] is supported by this [example script](https://github.com/huggingface/transformers/tree/main/examples/tensorflow/translation) and [notebook](https://colab.research.google.com/github/huggingface/notebooks/blob/main/examples/translation-tf.ipynb).
- [Translation task guide](../tasks/translation)
<PipelineTag pipeline="question-answering"/>
- A notebook on how to [finetune T5 for question answering with TensorFlow 2](https://colab.research.google.com/github/snapthat/TF-T5-text-to-text/blob/master/snapthatT5/notebooks/TF-T5-Datasets%20Training.ipynb). 🌎
- A notebook on how to [finetune T5 for question answering on a TPU](https://colab.research.google.com/github/patil-suraj/exploring-T5/blob/master/T5_on_TPU.ipynb#scrollTo=QLGiFCDqvuil).
🚀 **Deploy**
- A blog post on how to deploy [T5 11B for inference for less than $500](https://www.philschmid.de/deploy-t5-11b).
## T5Config
[[autodoc]] T5Config
## T5Tokenizer
[[autodoc]] T5Tokenizer
- build_inputs_with_special_tokens
- get_special_tokens_mask
- create_token_type_ids_from_sequences
- save_vocabulary
## T5TokenizerFast
[[autodoc]] T5TokenizerFast
<frameworkcontent>
<pt>
## T5Model
[[autodoc]] T5Model
- forward
## T5ForConditionalGeneration
[[autodoc]] T5ForConditionalGeneration
- forward
## T5EncoderModel
[[autodoc]] T5EncoderModel
- forward
## T5ForSequenceClassification
[[autodoc]] T5ForSequenceClassification
- forward
## T5ForTokenClassification
[[autodoc]] T5ForTokenClassification
- forward
## T5ForQuestionAnswering
[[autodoc]] T5ForQuestionAnswering
- forward
</pt>
<tf>
## TFT5Model
[[autodoc]] TFT5Model
- call
## TFT5ForConditionalGeneration
[[autodoc]] TFT5ForConditionalGeneration
- call
## TFT5EncoderModel
[[autodoc]] TFT5EncoderModel
- call
</tf>
<jax>
## FlaxT5Model
[[autodoc]] FlaxT5Model
- __call__
- encode
- decode
## FlaxT5ForConditionalGeneration
[[autodoc]] FlaxT5ForConditionalGeneration
- __call__
- encode
- decode
## FlaxT5EncoderModel
[[autodoc]] FlaxT5EncoderModel
- __call__
</jax>
</frameworkcontent>
| transformers/docs/source/en/model_doc/t5.md/0 | {
"file_path": "transformers/docs/source/en/model_doc/t5.md",
"repo_id": "transformers",
"token_count": 7102
} | 251 |
<!--Copyright 2021 The HuggingFace Team. All rights reserved.
Licensed under the Apache License, Version 2.0 (the "License"); you may not use this file except in compliance with
the License. You may obtain a copy of the License at
http://www.apache.org/licenses/LICENSE-2.0
Unless required by applicable law or agreed to in writing, software distributed under the License is distributed on
an "AS IS" BASIS, WITHOUT WARRANTIES OR CONDITIONS OF ANY KIND, either express or implied. See the License for the
specific language governing permissions and limitations under the License.
⚠️ Note that this file is in Markdown but contain specific syntax for our doc-builder (similar to MDX) that may not be
rendered properly in your Markdown viewer.
-->
# UniSpeech
## Overview
The UniSpeech model was proposed in [UniSpeech: Unified Speech Representation Learning with Labeled and Unlabeled Data](https://arxiv.org/abs/2101.07597) by Chengyi Wang, Yu Wu, Yao Qian, Kenichi Kumatani, Shujie Liu, Furu Wei, Michael
Zeng, Xuedong Huang .
The abstract from the paper is the following:
*In this paper, we propose a unified pre-training approach called UniSpeech to learn speech representations with both
unlabeled and labeled data, in which supervised phonetic CTC learning and phonetically-aware contrastive
self-supervised learning are conducted in a multi-task learning manner. The resultant representations can capture
information more correlated with phonetic structures and improve the generalization across languages and domains. We
evaluate the effectiveness of UniSpeech for cross-lingual representation learning on public CommonVoice corpus. The
results show that UniSpeech outperforms self-supervised pretraining and supervised transfer learning for speech
recognition by a maximum of 13.4% and 17.8% relative phone error rate reductions respectively (averaged over all
testing languages). The transferability of UniSpeech is also demonstrated on a domain-shift speech recognition task,
i.e., a relative word error rate reduction of 6% against the previous approach.*
This model was contributed by [patrickvonplaten](https://huggingface.co/patrickvonplaten). The Authors' code can be
found [here](https://github.com/microsoft/UniSpeech/tree/main/UniSpeech).
## Usage tips
- UniSpeech is a speech model that accepts a float array corresponding to the raw waveform of the speech signal. Please
use [`Wav2Vec2Processor`] for the feature extraction.
- UniSpeech model can be fine-tuned using connectionist temporal classification (CTC) so the model output has to be
decoded using [`Wav2Vec2CTCTokenizer`].
## Resources
- [Audio classification task guide](../tasks/audio_classification)
- [Automatic speech recognition task guide](../tasks/asr)
## UniSpeechConfig
[[autodoc]] UniSpeechConfig
## UniSpeech specific outputs
[[autodoc]] models.unispeech.modeling_unispeech.UniSpeechForPreTrainingOutput
## UniSpeechModel
[[autodoc]] UniSpeechModel
- forward
## UniSpeechForCTC
[[autodoc]] UniSpeechForCTC
- forward
## UniSpeechForSequenceClassification
[[autodoc]] UniSpeechForSequenceClassification
- forward
## UniSpeechForPreTraining
[[autodoc]] UniSpeechForPreTraining
- forward
| transformers/docs/source/en/model_doc/unispeech.md/0 | {
"file_path": "transformers/docs/source/en/model_doc/unispeech.md",
"repo_id": "transformers",
"token_count": 853
} | 252 |
<!--Copyright 2021 The HuggingFace Team. All rights reserved.
Licensed under the Apache License, Version 2.0 (the "License"); you may not use this file except in compliance with
the License. You may obtain a copy of the License at
http://www.apache.org/licenses/LICENSE-2.0
Unless required by applicable law or agreed to in writing, software distributed under the License is distributed on
an "AS IS" BASIS, WITHOUT WARRANTIES OR CONDITIONS OF ANY KIND, either express or implied. See the License for the
specific language governing permissions and limitations under the License.
⚠️ Note that this file is in Markdown but contain specific syntax for our doc-builder (similar to MDX) that may not be
rendered properly in your Markdown viewer.
-->
# XLS-R
## Overview
The XLS-R model was proposed in [XLS-R: Self-supervised Cross-lingual Speech Representation Learning at Scale](https://arxiv.org/abs/2111.09296) by Arun Babu, Changhan Wang, Andros Tjandra, Kushal Lakhotia, Qiantong Xu, Naman
Goyal, Kritika Singh, Patrick von Platen, Yatharth Saraf, Juan Pino, Alexei Baevski, Alexis Conneau, Michael Auli.
The abstract from the paper is the following:
*This paper presents XLS-R, a large-scale model for cross-lingual speech representation learning based on wav2vec 2.0.
We train models with up to 2B parameters on nearly half a million hours of publicly available speech audio in 128
languages, an order of magnitude more public data than the largest known prior work. Our evaluation covers a wide range
of tasks, domains, data regimes and languages, both high and low-resource. On the CoVoST-2 speech translation
benchmark, we improve the previous state of the art by an average of 7.4 BLEU over 21 translation directions into
English. For speech recognition, XLS-R improves over the best known prior work on BABEL, MLS, CommonVoice as well as
VoxPopuli, lowering error rates by 14-34% relative on average. XLS-R also sets a new state of the art on VoxLingua107
language identification. Moreover, we show that with sufficient model size, cross-lingual pretraining can outperform
English-only pretraining when translating English speech into other languages, a setting which favors monolingual
pretraining. We hope XLS-R can help to improve speech processing tasks for many more languages of the world.*
Relevant checkpoints can be found under https://huggingface.co/models?other=xls_r.
The original code can be found [here](https://github.com/pytorch/fairseq/tree/master/fairseq/models/wav2vec).
## Usage tips
- XLS-R is a speech model that accepts a float array corresponding to the raw waveform of the speech signal.
- XLS-R model was trained using connectionist temporal classification (CTC) so the model output has to be decoded using
[`Wav2Vec2CTCTokenizer`].
<Tip>
XLS-R's architecture is based on the Wav2Vec2 model, refer to [Wav2Vec2's documentation page](wav2vec2) for API reference.
</Tip> | transformers/docs/source/en/model_doc/xls_r.md/0 | {
"file_path": "transformers/docs/source/en/model_doc/xls_r.md",
"repo_id": "transformers",
"token_count": 782
} | 253 |
<!--Copyright 2022 The HuggingFace Team. All rights reserved.
Licensed under the Apache License, Version 2.0 (the "License"); you may not use this file except in compliance with
the License. You may obtain a copy of the License at
http://www.apache.org/licenses/LICENSE-2.0
Unless required by applicable law or agreed to in writing, software distributed under the License is distributed on
an "AS IS" BASIS, WITHOUT WARRANTIES OR CONDITIONS OF ANY KIND, either express or implied. See the License for the
⚠️ Note that this file is in Markdown but contain specific syntax for our doc-builder (similar to MDX) that may not be
rendered properly in your Markdown viewer.
-->
# Efficient Training on CPU
This guide focuses on training large models efficiently on CPU.
## Mixed precision with IPEX
Mixed precision uses single (fp32) and half-precision (bf16/fp16) data types in a model to accelerate training or inference while still preserving much of the single-precision accuracy. Modern CPUs such as 3rd and 4th Gen Intel® Xeon® Scalable processors natively support bf16, so you should get more performance out of the box by enabling mixed precision training with bf16.
To further maximize training performance, you can use Intel® Extension for PyTorch (IPEX), which is a library built on PyTorch and adds additional CPU instruction level architecture (ISA) level support such as Intel® Advanced Vector Extensions 512 Vector Neural Network Instructions (Intel® AVX512-VNNI), and Intel® Advanced Matrix Extensions (Intel® AMX) for an extra performance boost on Intel CPUs. However, CPUs with only AVX2 (e.g., AMD or older Intel CPUs) are not guaranteed to have better performance under IPEX.
Auto Mixed Precision (AMP) for CPU backends has been enabled since PyTorch 1.10. AMP support for bf16 on CPUs and bf16 operator optimization is also supported in IPEX and partially upstreamed to the main PyTorch branch. You can get better performance and user experience with IPEX AMP.
Check more detailed information for [Auto Mixed Precision](https://intel.github.io/intel-extension-for-pytorch/cpu/latest/tutorials/features/amp.html).
### IPEX installation:
IPEX release is following PyTorch, to install via pip:
| PyTorch Version | IPEX version |
| :---------------: | :----------: |
| 2.1.x | 2.1.100+cpu |
| 2.0.x | 2.0.100+cpu |
| 1.13 | 1.13.0+cpu |
| 1.12 | 1.12.300+cpu |
Please run `pip list | grep torch` to get your `pytorch_version`, so you can get the `IPEX version_name`.
```bash
pip install intel_extension_for_pytorch==<version_name> -f https://developer.intel.com/ipex-whl-stable-cpu
```
You can check the latest versions in [ipex-whl-stable-cpu](https://developer.intel.com/ipex-whl-stable-cpu) if needed.
Check more approaches for [IPEX installation](https://intel.github.io/intel-extension-for-pytorch/cpu/latest/tutorials/installation.html).
### Usage in Trainer
To enable auto mixed precision with IPEX in Trainer, users should add `use_ipex`, `bf16` and `no_cuda` in training command arguments.
Take an example of the use cases on [Transformers question-answering](https://github.com/huggingface/transformers/tree/main/examples/pytorch/question-answering)
- Training with IPEX using BF16 auto mixed precision on CPU:
<pre> python run_qa.py \
--model_name_or_path google-bert/bert-base-uncased \
--dataset_name squad \
--do_train \
--do_eval \
--per_device_train_batch_size 12 \
--learning_rate 3e-5 \
--num_train_epochs 2 \
--max_seq_length 384 \
--doc_stride 128 \
--output_dir /tmp/debug_squad/ \
<b>--use_ipex</b> \
<b>--bf16</b> \
<b>--use_cpu</b></pre>
If you want to enable `use_ipex` and `bf16` in your script, add these parameters to `TrainingArguments` like this:
```diff
training_args = TrainingArguments(
output_dir=args.output_path,
+ bf16=True,
+ use_ipex=True,
+ use_cpu=True,
**kwargs
)
```
### Practice example
Blog: [Accelerating PyTorch Transformers with Intel Sapphire Rapids](https://huggingface.co/blog/intel-sapphire-rapids)
| transformers/docs/source/en/perf_train_cpu.md/0 | {
"file_path": "transformers/docs/source/en/perf_train_cpu.md",
"repo_id": "transformers",
"token_count": 1270
} | 254 |
<!--Copyright 2023 The HuggingFace Team. All rights reserved.
Licensed under the Apache License, Version 2.0 (the "License"); you may not use this file except in compliance with
the License. You may obtain a copy of the License at
http://www.apache.org/licenses/LICENSE-2.0
Unless required by applicable law or agreed to in writing, software distributed under the License is distributed on
an "AS IS" BASIS, WITHOUT WARRANTIES OR CONDITIONS OF ANY KIND, either express or implied. See the License for the
specific language governing permissions and limitations under the License.
⚠️ Note that this file is in Markdown but contain specific syntax for our doc-builder (similar to MDX) that may not be
rendered properly in your Markdown viewer.
-->
# XLA Integration for TensorFlow Models
[[open-in-colab]]
Accelerated Linear Algebra, dubbed XLA, is a compiler for accelerating the runtime of TensorFlow Models. From the [official documentation](https://www.tensorflow.org/xla):
XLA (Accelerated Linear Algebra) is a domain-specific compiler for linear algebra that can accelerate TensorFlow models with potentially no source code changes.
Using XLA in TensorFlow is simple – it comes packaged inside the `tensorflow` library, and it can be triggered with the `jit_compile` argument in any graph-creating function such as [`tf.function`](https://www.tensorflow.org/guide/intro_to_graphs). When using Keras methods like `fit()` and `predict()`, you can enable XLA simply by passing the `jit_compile` argument to `model.compile()`. However, XLA is not limited to these methods - it can also be used to accelerate any arbitrary `tf.function`.
Several TensorFlow methods in 🤗 Transformers have been rewritten to be XLA-compatible, including text generation for models such as [GPT2](https://huggingface.co/docs/transformers/model_doc/gpt2), [T5](https://huggingface.co/docs/transformers/model_doc/t5) and [OPT](https://huggingface.co/docs/transformers/model_doc/opt), as well as speech processing for models such as [Whisper](https://huggingface.co/docs/transformers/model_doc/whisper).
While the exact amount of speed-up is very much model-dependent, for TensorFlow text generation models inside 🤗 Transformers, we noticed a speed-up of ~100x. This document will explain how you can use XLA for these models to get the maximum amount of performance. We’ll also provide links to additional resources if you’re interested to learn more about the benchmarks and our design philosophy behind the XLA integration.
## Running TF functions with XLA
Let us consider the following model in TensorFlow:
```py
import tensorflow as tf
model = tf.keras.Sequential(
[tf.keras.layers.Dense(10, input_shape=(10,), activation="relu"), tf.keras.layers.Dense(5, activation="softmax")]
)
```
The above model accepts inputs having a dimension of `(10, )`. We can use the model for running a forward pass like so:
```py
# Generate random inputs for the model.
batch_size = 16
input_vector_dim = 10
random_inputs = tf.random.normal((batch_size, input_vector_dim))
# Run a forward pass.
_ = model(random_inputs)
```
In order to run the forward pass with an XLA-compiled function, we’d need to do:
```py
xla_fn = tf.function(model, jit_compile=True)
_ = xla_fn(random_inputs)
```
The default `call()` function of the `model` is used for compiling the XLA graph. But if there’s any other model function you want to compile into XLA that’s also possible with:
```py
my_xla_fn = tf.function(model.my_xla_fn, jit_compile=True)
```
## Running a TF text generation model with XLA from 🤗 Transformers
To enable XLA-accelerated generation within 🤗 Transformers, you need to have a recent version of `transformers` installed. You can install it by running:
```bash
pip install transformers --upgrade
```
And then you can run the following code:
```py
import tensorflow as tf
from transformers import AutoTokenizer, TFAutoModelForCausalLM
# Will error if the minimal version of Transformers is not installed.
from transformers.utils import check_min_version
check_min_version("4.21.0")
tokenizer = AutoTokenizer.from_pretrained("openai-community/gpt2", padding_side="left", pad_token="</s>")
model = TFAutoModelForCausalLM.from_pretrained("openai-community/gpt2")
input_string = ["TensorFlow is"]
# One line to create an XLA generation function
xla_generate = tf.function(model.generate, jit_compile=True)
tokenized_input = tokenizer(input_string, return_tensors="tf")
generated_tokens = xla_generate(**tokenized_input, num_beams=2)
decoded_text = tokenizer.decode(generated_tokens[0], skip_special_tokens=True)
print(f"Generated -- {decoded_text}")
# Generated -- TensorFlow is an open-source, open-source, distributed-source application # framework for the
```
As you can notice, enabling XLA on `generate()` is just a single line of code. The rest of the code remains unchanged. However, there are a couple of gotchas in the above code snippet that are specific to XLA. You need to be aware of those to realize the speed-ups that XLA can bring in. We discuss these in the following section.
## Gotchas to be aware of
When you are executing an XLA-enabled function (like `xla_generate()` above) for the first time, it will internally try to infer the computation graph, which is time-consuming. This process is known as [“tracing”](https://www.tensorflow.org/guide/intro_to_graphs#when_is_a_function_tracing).
You might notice that the generation time is not fast. Successive calls of `xla_generate()` (or any other XLA-enabled function) won’t have to infer the computation graph, given the inputs to the function follow the same shape with which the computation graph was initially built. While this is not a problem for modalities with fixed input shapes (e.g., images), you must pay attention if you are working with variable input shape modalities (e.g., text).
To ensure `xla_generate()` always operates with the same input shapes, you can specify the `padding` arguments when calling the tokenizer.
```py
import tensorflow as tf
from transformers import AutoTokenizer, TFAutoModelForCausalLM
tokenizer = AutoTokenizer.from_pretrained("openai-community/gpt2", padding_side="left", pad_token="</s>")
model = TFAutoModelForCausalLM.from_pretrained("openai-community/gpt2")
input_string = ["TensorFlow is"]
xla_generate = tf.function(model.generate, jit_compile=True)
# Here, we call the tokenizer with padding options.
tokenized_input = tokenizer(input_string, pad_to_multiple_of=8, padding=True, return_tensors="tf")
generated_tokens = xla_generate(**tokenized_input, num_beams=2)
decoded_text = tokenizer.decode(generated_tokens[0], skip_special_tokens=True)
print(f"Generated -- {decoded_text}")
```
This way, you can ensure that the inputs to `xla_generate()` will always receive inputs with the shape it was traced with and thus leading to speed-ups in the generation time. You can verify this with the code below:
```py
import time
import tensorflow as tf
from transformers import AutoTokenizer, TFAutoModelForCausalLM
tokenizer = AutoTokenizer.from_pretrained("openai-community/gpt2", padding_side="left", pad_token="</s>")
model = TFAutoModelForCausalLM.from_pretrained("openai-community/gpt2")
xla_generate = tf.function(model.generate, jit_compile=True)
for input_string in ["TensorFlow is", "TensorFlow is a", "TFLite is a"]:
tokenized_input = tokenizer(input_string, pad_to_multiple_of=8, padding=True, return_tensors="tf")
start = time.time_ns()
generated_tokens = xla_generate(**tokenized_input, num_beams=2)
end = time.time_ns()
print(f"Execution time -- {(end - start) / 1e6:.1f} ms\n")
```
On a Tesla T4 GPU, you can expect the outputs like so:
```bash
Execution time -- 30819.6 ms
Execution time -- 79.0 ms
Execution time -- 78.9 ms
```
The first call to `xla_generate()` is time-consuming because of tracing, but the successive calls are orders of magnitude faster. Keep in mind that any change in the generation options at any point with trigger re-tracing and thus leading to slow-downs in the generation time.
We didn’t cover all the text generation options 🤗 Transformers provides in this document. We encourage you to read the documentation for advanced use cases.
## Additional Resources
Here, we leave you with some additional resources if you want to delve deeper into XLA in 🤗 Transformers and in general.
* [This Colab Notebook](https://colab.research.google.com/github/huggingface/blog/blob/main/notebooks/91_tf_xla_generate.ipynb) provides an interactive demonstration if you want to fiddle with the XLA-compatible encoder-decoder (like [T5](https://huggingface.co/docs/transformers/model_doc/t5)) and decoder-only (like [GPT2](https://huggingface.co/docs/transformers/model_doc/gpt2)) text generation models.
* [This blog post](https://huggingface.co/blog/tf-xla-generate) provides an overview of the comparison benchmarks for XLA-compatible models along with a friendly introduction to XLA in TensorFlow.
* [This blog post](https://blog.tensorflow.org/2022/11/how-hugging-face-improved-text-generation-performance-with-xla.html) discusses our design philosophy behind adding XLA support to the TensorFlow models in 🤗 Transformers.
* Recommended posts for learning more about XLA and TensorFlow graphs in general:
* [XLA: Optimizing Compiler for Machine Learning](https://www.tensorflow.org/xla)
* [Introduction to graphs and tf.function](https://www.tensorflow.org/guide/intro_to_graphs)
* [Better performance with tf.function](https://www.tensorflow.org/guide/function) | transformers/docs/source/en/tf_xla.md/0 | {
"file_path": "transformers/docs/source/en/tf_xla.md",
"repo_id": "transformers",
"token_count": 2860
} | 255 |
<!---
Copyright 2020 The HuggingFace Team. All rights reserved.
Licensed under the Apache License, Version 2.0 (the "License");
you may not use this file except in compliance with the License.
You may obtain a copy of the License at
http://www.apache.org/licenses/LICENSE-2.0
Unless required by applicable law or agreed to in writing, software
distributed under the License is distributed on an "AS IS" BASIS,
WITHOUT WARRANTIES OR CONDITIONS OF ANY KIND, either express or implied.
See the License for the specific language governing permissions and
limitations under the License.
⚠️ Note that this file is in Markdown but contain specific syntax for our doc-builder (similar to MDX) that may not be
rendered properly in your Markdown viewer.
-->
# Verificaciones en un Pull Request
Cuando abres un _pull request_ en 🤗 Transformers, se ejecutarán una serie de verificaciones para asegurarte de que el _patch_ que estás agregando no rompa nada existente. Estas verificaciones son de cuatro tipos:
- pruebas regulares
- creación de la documentación
- estilo del código y documentación
- consistencia del repositorio
En este documento, intentaremos explicar cuáles son esas diferentes verificaciones y el motivo detrás de ellas, así como también cómo depurarlas localmente si una falla en tu PR.
Recuerda que todas las verificaciones requieren que tengas una instalación de desarrollo:
```bash
pip install transformers[dev]
```
o una instalación editable:
```bash
pip install -e .[dev]
```
del repositorio de Transformers.
## Pruebas
Todos los procesos que comienzan con `ci/circleci: run_tests_` ejecutan partes del conjunto de pruebas de Transformers. Cada uno de esos procesos se enfoca en una parte de la biblioteca en un entorno determinado: por ejemplo, `ci/circleci: run_tests_pipelines_tf` ejecuta la prueba de _pipelines_ en un entorno donde solo está instalado TensorFlow.
Ten en cuenta que para evitar ejecutar pruebas cuando no hay un cambio real en los módulos que estás probando, solo se ejecuta una parte del conjunto de pruebas: se ejecuta una tarea auxiliar para determinar las diferencias en la biblioteca antes y después del PR (lo que GitHub te muestra en la pestaña "Files changes") y selecciona las pruebas afectadas por esa diferencia. Este auxiliar se puede ejecutar localmente usando:
```bash
python utils/tests_fetcher.py
```
desde el directorio raiz del repositorio de Transformers. Se ejecutará lo siguiente:
1. Verificación para cada archivo en el _diff_ si los cambios están en el código, solo en comentarios o _docstrings_. Solo los archivos con cambios reales de código se conservan.
2. Creación de un mapa interno que proporciona para cada archivo del código fuente de la biblioteca todos los archivos a los que impacta recursivamente. Se dice que el módulo A impacta al módulo B si el módulo B importa el módulo A. Para el impacto recursivo, necesitamos una cadena de módulos que va del módulo A al módulo B en la que cada módulo importa el anterior.
3. Aplicación de este mapa en los archivos recopilados en el paso 1, lo que nos da una lista de archivos modelo afectados por el PR.
4. Asignación de cada uno de esos archivos a sus archivos de prueba correspondientes y para obtener una la lista de pruebas a ejecutar.
Al ejecutar el _script_ localmente, debes obtener los resultados de los pasos 1, 3 y 4 impresos y así saber qué pruebas se ejecutarán. El _script_ también creará un archivo llamado `test_list.txt` que contiene la lista de pruebas para ejecutar, y puede ejecutarlas localmente con el siguiente comando:
```bash
python -m pytest -n 8 --dist=loadfile -rA -s $(cat test_list.txt)
```
En caso de que se te escape algo, el conjunto completo de pruebas también se ejecuta a diario.
## Creación de la documentación
El proceso `build_pr_documentation` compila y genera una vista previa de la documentación para asegurarse de que todo se vea bien una vez que se fusione tu PR. Un bot agregará un enlace para obtener una vista previa de la documentación en tu PR. Cualquier cambio que realices en el PR se actualiza automáticamente en la vista previa. Si la documentación no se genera, haz clic en **Detalles** junto al proceso fallido para ver dónde salió mal. A menudo, el error es tan simple como que falta un archivo en `toctree`.
Si estás interesado en compilar u obtener una vista previa de la documentación localmente, echa un vistazo al [`README.md`](https://github.com/huggingface/transformers/tree/main/docs) en la carpeta `docs`.
## Estilo de código y documentación.
El formato de código se aplica a todos los archivos fuente, los ejemplos y las pruebas utilizando `black` e `ruff`. También tenemos una herramienta personalizada que se ocupa del formato de los _docstrings_ y archivos `rst` (`utils/style_doc.py`), así como del orden de las importaciones _lazy_ realizadas en los archivos `__init__.py` de Transformers (`utils /custom_init_isort.py`). Todo esto se puede probar ejecutando
```bash
make style
```
CI verifica que se hayan aplicado dentro de la verificación `ci/circleci: check_code_quality`. También se ejecuta `ruff`, que hará una verificación básica a tu código y te hará saber si encuentra una variable no definida, o una que no se usa. Para ejecutar esa verificación localmente, usa
```bash
make quality
```
Esto puede llevar mucho tiempo, así que para ejecutar lo mismo solo en los archivos que modificaste en la rama actual, ejecuta
```bash
make fixup
```
Este último comando también ejecutará todas las verificaciones adicionales para la consistencia del repositorio. Echemos un vistazo a estas pruebas.
## Consistencia del repositorio
Esta verificación reagrupa todas las pruebas para asegurarse de que tu PR deja el repositorio en buen estado, y se realiza mediante `ci/circleci: check_repository_consistency`. Puedes ejecutar localmente esta verificación ejecutando lo siguiente:
```bash
make repo-consistency
```
Esta instrucción verifica que:
- Todos los objetos agregados al _init_ están documentados (realizados por `utils/check_repo.py`)
- Todos los archivos `__init__.py` tienen el mismo contenido en sus dos secciones (realizado por `utils/check_inits.py`)
- Todo el código identificado como una copia de otro módulo es consistente con el original (realizado por `utils/check_copies.py`)
- Todas las clases de configuración tienen al menos _checkpoint_ válido mencionado en sus _docstrings_ (realizado por `utils/check_config_docstrings.py`)
- Las traducciones de los README y el índice del documento tienen la misma lista de modelos que el README principal (realizado por `utils/check_copies.py`)
- Las tablas generadas automaticamente en la documentación están actualizadas (realizadas por `utils/check_table.py`)
- La biblioteca tiene todos los objetos disponibles incluso si no están instaladas todas las dependencias opcionales (realizadas por `utils/check_dummies.py`)
Si esta verificación falla, los primeros dos elementos requieren una reparación manual, los últimos cuatro pueden repararse automáticamente ejecutando el comando
```bash
make fix-copies
```
Las verificaciones adicionales se refieren a los PRs que agregan nuevos modelos, principalmente que:
- Todos los modelos agregados están en un Auto-mapping (realizado por `utils/check_repo.py`)
<!-- TODO Sylvain, add a check that makes sure the common tests are implemented.-->
- Todos los modelos se verifican correctamente (realizados por `utils/check_repo.py`)
<!-- TODO Sylvain, add the following
- All models are added to the main README, inside the main doc
- All checkpoints used actually exist on the Hub
-->
| transformers/docs/source/es/pr_checks.md/0 | {
"file_path": "transformers/docs/source/es/pr_checks.md",
"repo_id": "transformers",
"token_count": 2659
} | 256 |
<!--Copyright 2024 The HuggingFace Team. All rights reserved.
Licensed under the Apache License, Version 2.0 (the "License"); you may not use this file except in compliance with
the License. You may obtain a copy of the License at
http://www.apache.org/licenses/LICENSE-2.0
Unless required by applicable law or agreed to in writing, software distributed under the License is distributed on
an "AS IS" BASIS, WITHOUT WARRANTIES OR CONDITIONS OF ANY KIND, either express or implied. See the License for the
specific language governing permissions and limitations under the License.
⚠️ Note that this file is in Markdown but contain specific syntax for our doc-builder (similar to MDX) that may not be
rendered properly in your Markdown viewer.
-->
# El Trainer
El [`Trainer`] es un bucle completo de entrenamiento y evaluación para modelos de PyTorch implementado en la biblioteca Transformers. Solo necesitas pasarle las piezas necesarias para el entrenamiento (modelo, tokenizador, conjunto de datos, función de evaluación, hiperparámetros de entrenamiento, etc.), y la clase [`Trainer`] se encarga del resto. Esto facilita comenzar a entrenar más rápido sin tener que escribir manualmente tu propio bucle de entrenamiento. Pero al mismo tiempo, [`Trainer`] es muy personalizable y ofrece una gran cantidad de opciones de entrenamiento para que puedas adaptarlo a tus necesidades exactas de entrenamiento.
<Tip>
Además de la clase [`Trainer`], Transformers también proporciona una clase [`Seq2SeqTrainer`] para tareas de secuencia a secuencia como traducción o resumen. También está la clase [~trl.SFTTrainer] de la biblioteca [TRL](https://hf.co/docs/trl) que envuelve la clase [`Trainer`] y está optimizada para entrenar modelos de lenguaje como Llama-2 y Mistral con técnicas autoregresivas. [`~trl.SFTTrainer`] también admite funciones como el empaquetado de secuencias, LoRA, cuantización y DeepSpeed para escalar eficientemente a cualquier tamaño de modelo.
<br>
Siéntete libre de consultar [la referencia de API](./main_classes/trainer) para estas otras clases tipo [`Trainer`] para aprender más sobre cuándo usar cada una. En general, [`Trainer`] es la opción más versátil y es apropiada para una amplia gama de tareas. [`Seq2SeqTrainer`] está diseñado para tareas de secuencia a secuencia y [`~trl.SFTTrainer`] está diseñado para entrenar modelos de lenguaje.
</Tip>
Antes de comenzar, asegúrate de tener instalado [Accelerate](https://hf.co/docs/accelerate), una biblioteca para habilitar y ejecutar el entrenamiento de PyTorch en entornos distribuidos.
```bash
pip install accelerate
# upgrade
pip install accelerate --upgrade
```
Esta guía proporciona una visión general de la clase [`Trainer`].
## Uso básico
[`Trainer`] incluye todo el código que encontrarías en un bucle de entrenamiento básico:
1. Realiza un paso de entrenamiento para calcular la pérdida
2. Calcula los gradientes con el método [~accelerate.Accelerator.backward]
3. Actualiza los pesos basados en los gradientes
4. Repite este proceso hasta alcanzar un número predeterminado de épocas
La clase [`Trainer`] abstrae todo este código para que no tengas que preocuparte por escribir manualmente un bucle de entrenamiento cada vez o si estás empezando con PyTorch y el entrenamiento. Solo necesitas proporcionar los componentes esenciales requeridos para el entrenamiento, como un modelo y un conjunto de datos, y la clase [`Trainer`] maneja todo lo demás.
Si deseas especificar opciones de entrenamiento o hiperparámetros, puedes encontrarlos en la clase [`TrainingArguments`]. Por ejemplo, vamos a definir dónde guardar el modelo en output_dir y subir el modelo al Hub después del entrenamiento con `push_to_hub=True`.
```py
from transformers import TrainingArguments
training_args = TrainingArguments(
output_dir="your-model",
learning_rate=2e-5,
per_device_train_batch_size=16,
per_device_eval_batch_size=16,
num_train_epochs=2,
weight_decay=0.01,
evaluation_strategy="epoch",
save_strategy="epoch",
load_best_model_at_end=True,
push_to_hub=True,
)
```
Pase `training_args` al [`Trainer`] con un modelo, un conjunto de datos o algo para preprocesar el conjunto de datos (dependiendo en el tipo de datos pueda ser un tokenizer, extractor de caracteristicas o procesor del imagen), un recopilador de datos y una función para calcular las métricas que desea rastrear durante el entrenamiento.
Finalmente, llame [`~Trainer.train`] para empezar entrenamiento!
```py
from transformers import Trainer
trainer = Trainer(
model=model,
args=training_args,
train_dataset=dataset["train"],
eval_dataset=dataset["test"],
tokenizer=tokenizer,
data_collator=data_collator,
compute_metrics=compute_metrics,
)
trainer.train()
```
### Los puntos de control
La clase [`Trainer`] guarda los puntos de control del modelo en el directorio especificado en el parámetro `output_dir` de [`TrainingArguments`]. Encontrarás los puntos de control guardados en una subcarpeta checkpoint-000 donde los números al final corresponden al paso de entrenamiento. Guardar puntos de control es útil para reanudar el entrenamiento más tarde.
```py
# resume from latest checkpoint
trainer.train(resume_from_checkpoint=True)
# resume from specific checkpoint saved in output directory
trainer.train(resume_from_checkpoint="your-model/checkpoint-1000")
```
Puedes guardar tus puntos de control (por defecto, el estado del optimizador no se guarda) en el Hub configurando `push_to_hub=True` en [`TrainingArguments`] para confirmar y enviarlos. Otras opciones para decidir cómo se guardan tus puntos de control están configuradas en el parámetro [`hub_strategy`](https://huggingface.co/docs/transformers/main_classes/trainer#transformers.TrainingArguments.hub_strategy):
* hub_strategy="checkpoint" envía el último punto de control a una subcarpeta llamada "last-checkpoint" desde la cual puedes reanudar el entrenamiento.
* hub_strategy="all_checkpoints" envía todos los puntos de control al directorio definido en `output_dir` (verás un punto de control por carpeta en tu repositorio de modelos).
Cuando reanudas el entrenamiento desde un punto de control, el [`Trainer`] intenta mantener los estados de los generadores de números aleatorios (RNG) de Python, NumPy y PyTorch iguales a como estaban cuando se guardó el punto de control. Pero debido a que PyTorch tiene varias configuraciones predeterminadas no determinísticas, no se garantiza que los estados de RNG sean los mismos. Si deseas habilitar la plena determinismo, echa un vistazo a la guía ["Controlling sources of randomness"](https://pytorch.org/docs/stable/notes/randomness#controlling-sources-of-randomness) para aprender qué puedes habilitar para hacer que tu entrenamiento sea completamente determinista. Sin embargo, ten en cuenta que al hacer ciertas configuraciones deterministas, el entrenamiento puede ser más lento.
## Personaliza el Trainer
Si bien la clase [`Trainer`] está diseñada para ser accesible y fácil de usar, también ofrece mucha capacidad de personalización para usuarios más aventureros. Muchos de los métodos del [`Trainer`] pueden ser subclasificados y sobrescritos para admitir la funcionalidad que deseas, sin tener que reescribir todo el bucle de entrenamiento desde cero para adaptarlo. Estos métodos incluyen:
* [~Trainer.get_train_dataloader] crea un entrenamiento de DataLoader
* [~Trainer.get_eval_dataloader] crea una evaluación DataLoader
* [~Trainer.get_test_dataloader] crea una prueba de DataLoader
* [~Trainer.log] anota la información de los objetos varios que observa el entrenamiento
* [~Trainer.create_optimizer_and_scheduler] crea un optimizador y la tasa programada de aprendizaje si no lo pasaron en __init__; estos pueden ser personalizados independientes con [~Trainer.create_optimizer] y [~Trainer.create_scheduler] respectivamente
* [~Trainer.compute_loss] computa la pérdida en lote con las aportes del entrenamiento
* [~Trainer.training_step] realiza el paso del entrenamiento
* [~Trainer.prediction_step] realiza la predicción y paso de prueba
* [~Trainer.evaluate] evalua el modelo y da las metricas evaluativas
* [~Trainer.predict] hace las predicciones (con las metricas si hay etiquetas disponibles) en lote de prueba
Por ejemplo, si deseas personalizar el método [`~Trainer.compute_loss`] para usar una pérdida ponderada en su lugar, puedes hacerlo de la siguiente manera:
```py
from torch import nn
from transformers import Trainer
class CustomTrainer(Trainer):
def compute_loss(self, model, inputs, return_outputs=False):
labels = inputs.pop("labels")
# forward pass
outputs = model(**inputs)
logits = outputs.get("logits")
# compute custom loss for 3 labels with different weights
loss_fct = nn.CrossEntropyLoss(weight=torch.tensor([1.0, 2.0, 3.0], device=model.device))
loss = loss_fct(logits.view(-1, self.model.config.num_labels), labels.view(-1))
return (loss, outputs) if return_outputs else loss
```
### Callbacks
Otra opción para personalizar el [`Trainer`] es utilizar [callbacks](callbacks). Los callbacks *no cambian nada* en el bucle de entrenamiento. Inspeccionan el estado del bucle de entrenamiento y luego ejecutan alguna acción (detención anticipada, registro de resultados, etc.) según el estado. En otras palabras, un callback no puede usarse para implementar algo como una función de pérdida personalizada y necesitarás subclasificar y sobrescribir el método [`~Trainer.compute_loss`] para eso.
Por ejemplo, si deseas agregar un callback de detención anticipada al bucle de entrenamiento después de 10 pasos.
```py
from transformers import TrainerCallback
class EarlyStoppingCallback(TrainerCallback):
def __init__(self, num_steps=10):
self.num_steps = num_steps
def on_step_end(self, args, state, control, **kwargs):
if state.global_step >= self.num_steps:
return {"should_training_stop": True}
else:
return {}
```
Luego, pásalo al parámetro `callback` del [`Trainer`]:
```py
from transformers import Trainer
trainer = Trainer(
model=model,
args=training_args,
train_dataset=dataset["train"],
eval_dataset=dataset["test"],
tokenizer=tokenizer,
data_collator=data_collator,
compute_metrics=compute_metrics,
callback=[EarlyStoppingCallback()],
)
```
## Logging
<Tip>
Comprueba el API referencia [logging](./main_classes/logging) para mas información sobre los niveles differentes de logging.
</Tip>
El [`Trainer`] está configurado a `logging.INFO` de forma predeterminada el cual informa errores, advertencias y otra información basica. Un [`Trainer`] réplica - en entornos distributos - está configurado a `logging.WARNING` el cual solamente informa errores y advertencias. Puedes cambiar el nivel de logging con los parametros [`log_level`](https://huggingface.co/docs/transformers/main_classes/trainer#transformers.TrainingArguments.log_level) y [`log_level_replica`](https://huggingface.co/docs/transformers/main_classes/trainer#transformers.TrainingArguments.log_level_replica) en [`TrainingArguments`].
Para configurar el nivel de registro para cada nodo, usa el parámetro [`log_on_each_node`](https://huggingface.co/docs/transformers/main/en/main_classes/trainer#transformers.TrainingArguments.log_on_each_node) para determinar si deseas utilizar el nivel de registro en cada nodo o solo en el nodo principal.
<Tip>
[`Trainer`] establece el nivel de registro por separado para cada nodo en el método [`Trainer.init`], por lo que es posible que desees considerar establecer esto antes si estás utilizando otras funcionalidades de Transformers antes de crear el objeto [`Trainer`].
</Tip>
Por ejemplo, para establecer que tu código principal y los módulos utilicen el mismo nivel de registro según cada nodo:
```py
logger = logging.getLogger(__name__)
logging.basicConfig(
format="%(asctime)s - %(levelname)s - %(name)s - %(message)s",
datefmt="%m/%d/%Y %H:%M:%S",
handlers=[logging.StreamHandler(sys.stdout)],
)
log_level = training_args.get_process_log_level()
logger.setLevel(log_level)
datasets.utils.logging.set_verbosity(log_level)
transformers.utils.logging.set_verbosity(log_level)
trainer = Trainer(...)
```
<hfoptions id="logging">
<hfoption id="single node">
Usa diferentes combinaciones de `log_level` y `log_level_replica` para configurar qué se registra en cada uno de los nodos.
```bash
my_app.py ... --log_level warning --log_level_replica error
```
</hfoption>
<hfoption id="multi-node">
Agrega el parámetro `log_on_each_node 0` para entornos multi-nodo.
```bash
my_app.py ... --log_level warning --log_level_replica error --log_on_each_node 0
# set to only report errors
my_app.py ... --log_level error --log_level_replica error --log_on_each_node 0
```
</hfoption>
</hfoptions>
## NEFTune
[NEFTune](https://hf.co/papers/2310.05914) es una técnica que puede mejorar el rendimiento al agregar ruido a los vectores de incrustación durante el entrenamiento. Para habilitarlo en [`Trainer`], establece el parámetro `neftune_noise_alpha` en [`TrainingArguments`] para controlar cuánto ruido se agrega.
```py
from transformers import TrainingArguments, Trainer
training_args = TrainingArguments(..., neftune_noise_alpha=0.1)
trainer = Trainer(..., args=training_args)
```
NEFTune se desactiva después del entrenamiento para restaurar la capa de incrustación original y evitar cualquier comportamiento inesperado.
## Accelerate y Trainer
La clase [`Trainer`] está impulsada por [Accelerate](https://hf.co/docs/accelerate), una biblioteca para entrenar fácilmente modelos de PyTorch en entornos distribuidos con soporte para integraciones como [FullyShardedDataParallel (FSDP)](https://pytorch.org/blog/introducing-pytorch-fully-sharded-data-parallel-api/) y [DeepSpeed](https://www.deepspeed.ai/).
<Tip>
Aprende más sobre las estrategias de fragmentación FSDP, descarga de CPU y más con el [`Trainer`] en la guía [Paralela de Datos Completamente Fragmentados](fsdp).
</Tip>
Para usar Accelerate con [`Trainer`], ejecuta el comando [`accelerate.config`](https://huggingface.co/docs/accelerate/package_reference/cli#accelerate-config) para configurar el entrenamiento para tu entorno de entrenamiento. Este comando crea un `config_file.yaml` que se utilizará cuando inicies tu script de entrenamiento. Por ejemplo, algunas configuraciones de ejemplo que puedes configurar son:
<hfoptions id="config">
<hfoption id="DistributedDataParallel">
```yml
compute_environment: LOCAL_MACHINE
distributed_type: MULTI_GPU
downcast_bf16: 'no'
gpu_ids: all
machine_rank: 0 #change rank as per the node
main_process_ip: 192.168.20.1
main_process_port: 9898
main_training_function: main
mixed_precision: fp16
num_machines: 2
num_processes: 8
rdzv_backend: static
same_network: true
tpu_env: []
tpu_use_cluster: false
tpu_use_sudo: false
use_cpu: false
```
</hfoption>
<hfoption id="FSDP">
```yml
compute_environment: LOCAL_MACHINE
distributed_type: FSDP
downcast_bf16: 'no'
fsdp_config:
fsdp_auto_wrap_policy: TRANSFORMER_BASED_WRAP
fsdp_backward_prefetch_policy: BACKWARD_PRE
fsdp_forward_prefetch: true
fsdp_offload_params: false
fsdp_sharding_strategy: 1
fsdp_state_dict_type: FULL_STATE_DICT
fsdp_sync_module_states: true
fsdp_transformer_layer_cls_to_wrap: BertLayer
fsdp_use_orig_params: true
machine_rank: 0
main_training_function: main
mixed_precision: bf16
num_machines: 1
num_processes: 2
rdzv_backend: static
same_network: true
tpu_env: []
tpu_use_cluster: false
tpu_use_sudo: false
use_cpu: false
```
</hfoption>
<hfoption id="DeepSpeed">
```yml
compute_environment: LOCAL_MACHINE
deepspeed_config:
deepspeed_config_file: /home/user/configs/ds_zero3_config.json
zero3_init_flag: true
distributed_type: DEEPSPEED
downcast_bf16: 'no'
machine_rank: 0
main_training_function: main
num_machines: 1
num_processes: 4
rdzv_backend: static
same_network: true
tpu_env: []
tpu_use_cluster: false
tpu_use_sudo: false
use_cpu: false
```
</hfoption>
<hfoption id="DeepSpeed with Accelerate plugin">
```yml
compute_environment: LOCAL_MACHINE
deepspeed_config:
gradient_accumulation_steps: 1
gradient_clipping: 0.7
offload_optimizer_device: cpu
offload_param_device: cpu
zero3_init_flag: true
zero_stage: 2
distributed_type: DEEPSPEED
downcast_bf16: 'no'
machine_rank: 0
main_training_function: main
mixed_precision: bf16
num_machines: 1
num_processes: 4
rdzv_backend: static
same_network: true
tpu_env: []
tpu_use_cluster: false
tpu_use_sudo: false
use_cpu: false
```
</hfoption>
</hfoptions>
El comando [`accelerate_launch`](https://huggingface.co/docs/accelerate/package_reference/cli#accelerate-launch) es la forma recomendada de lanzar tu script de entrenamiento en un sistema distribuido con Accelerate y [`Trainer`] con los parámetros especificados en `config_file.yaml`. Este archivo se guarda en la carpeta de caché de Accelerate y se carga automáticamente cuando ejecutas `accelerate_launch`.
Por ejemplo, para ejecutar el script de entrenamiento [`run_glue.py`](https://github.com/huggingface/transformers/blob/f4db565b695582891e43a5e042e5d318e28f20b8/examples/pytorch/text-classification/run_glue.py#L4) con la configuración de FSDP:
```bash
accelerate launch \
./examples/pytorch/text-classification/run_glue.py \
--model_name_or_path bert-base-cased \
--task_name $TASK_NAME \
--do_train \
--do_eval \
--max_seq_length 128 \
--per_device_train_batch_size 16 \
--learning_rate 5e-5 \
--num_train_epochs 3 \
--output_dir /tmp/$TASK_NAME/ \
--overwrite_output_dir
```
También puedes especificar los parámetros del archivo config_file.yaml directamente en la línea de comandos:
```bash
accelerate launch --num_processes=2 \
--use_fsdp \
--mixed_precision=bf16 \
--fsdp_auto_wrap_policy=TRANSFORMER_BASED_WRAP \
--fsdp_transformer_layer_cls_to_wrap="BertLayer" \
--fsdp_sharding_strategy=1 \
--fsdp_state_dict_type=FULL_STATE_DICT \
./examples/pytorch/text-classification/run_glue.py
--model_name_or_path bert-base-cased \
--task_name $TASK_NAME \
--do_train \
--do_eval \
--max_seq_length 128 \
--per_device_train_batch_size 16 \
--learning_rate 5e-5 \
--num_train_epochs 3 \
--output_dir /tmp/$TASK_NAME/ \
--overwrite_output_dir
```
Consulta el tutorial [Lanzamiento de tus scripts con Accelerate](https://huggingface.co/docs/accelerate/basic_tutorials/launch) para obtener más información sobre `accelerate_launch` y las configuraciones personalizadas. | transformers/docs/source/es/trainer.md/0 | {
"file_path": "transformers/docs/source/es/trainer.md",
"repo_id": "transformers",
"token_count": 7327
} | 257 |
<!--Copyright 2022 The HuggingFace Team. All rights reserved.
Licensed under the Apache License, Version 2.0 (the "License"); you may not use this file except in compliance with
the License. You may obtain a copy of the License at
http://www.apache.org/licenses/LICENSE-2.0
Unless required by applicable law or agreed to in writing, software distributed under the License is distributed on
an "AS IS" BASIS, WITHOUT WARRANTIES OR CONDITIONS OF ANY KIND, either express or implied. See the License for the
specific language governing permissions and limitations under the License.
⚠️ Note that this file is in Markdown but contain specific syntax for our doc-builder (similar to MDX) that may not be
rendered properly in your Markdown viewer.
-->
# Carica istanze pre-allenate con AutoClass
Con così tante architetture Transformer differenti, può essere sfidante crearne una per il tuo checkpoint. Come parte della filosofia centrale di 🤗 Transformers per rendere la libreria facile, semplice e flessibile da utilizzare, una `AutoClass` inferisce e carica automaticamente l'architettura corretta da un dato checkpoint. Il metodo `from_pretrained` ti permette di caricare velocemente un modello pre-allenato per qualsiasi architettura, così non devi utilizzare tempo e risorse per allenare un modello da zero. Produrre questo codice agnostico ai checkpoint significa che se il tuo codice funziona per un checkpoint, funzionerà anche per un altro checkpoint, purché sia stato allenato per un compito simile, anche se l'architettura è differente.
<Tip>
Ricorda, con architettura ci si riferisce allo scheletro del modello e con checkpoint ai pesi di una determinata architettura. Per esempio, [BERT](https://huggingface.co/google-bert/bert-base-uncased) è un'architettura, mentre `google-bert/bert-base-uncased` è un checkpoint. Modello è un termine generale che può significare sia architettura che checkpoint.
</Tip>
In questo tutorial, imparerai a:
* Caricare un tokenizer pre-allenato.
* Caricare un estrattore di caratteristiche (feature extractor, in inglese) pre-allenato.
* Caricare un processore pre-allenato.
* Caricare un modello pre-allenato.
## AutoTokenizer
Quasi tutti i compiti di NLP iniziano con un tokenizer. Un tokenizer converte il tuo input in un formato che possa essere elaborato dal modello.
Carica un tokenizer con [`AutoTokenizer.from_pretrained`]:
```py
>>> from transformers import AutoTokenizer
>>> tokenizer = AutoTokenizer.from_pretrained("FacebookAI/xlm-roberta-base")
```
Poi tokenizza il tuo input come mostrato in seguito:
```py
>>> sequenza = "In un buco nel terreno viveva uno Hobbit."
>>> print(tokenizer(sequenza))
{'input_ids': [0, 360, 51, 373, 587, 1718, 54644, 22597, 330, 3269, 2291, 22155, 18, 5, 2],
'attention_mask': [1, 1, 1, 1, 1, 1, 1, 1, 1, 1, 1, 1, 1, 1, 1]}
```
## AutoFeatureExtractor
Per compiti inerenti a audio e video, un feature extractor processa il segnale audio o l'immagine nel formato di input corretto.
Carica un feature extractor con [`AutoFeatureExtractor.from_pretrained`]:
```py
>>> from transformers import AutoFeatureExtractor
>>> feature_extractor = AutoFeatureExtractor.from_pretrained(
... "ehcalabres/wav2vec2-lg-xlsr-en-speech-emotion-recognition"
... )
```
## AutoProcessor
Compiti multimodali richiedono un processore che combini i due tipi di strumenti di elaborazione. Per esempio, il modello [LayoutLMV2](model_doc/layoutlmv2) richiede un feature extractor per gestire le immagine e un tokenizer per gestire il testo; un processore li combina entrambi.
Carica un processore con [`AutoProcessor.from_pretrained`]:
```py
>>> from transformers import AutoProcessor
>>> processor = AutoProcessor.from_pretrained("microsoft/layoutlmv2-base-uncased")
```
## AutoModel
<frameworkcontent>
<pt>
Infine, le classi `AutoModelFor` ti permettono di caricare un modello pre-allenato per un determinato compito (guarda [qui](model_doc/auto) per una lista completa di compiti presenti). Per esempio, carica un modello per la classificazione di sequenze con [`AutoModelForSequenceClassification.from_pretrained`]:
```py
>>> from transformers import AutoModelForSequenceClassification
>>> model = AutoModelForSequenceClassification.from_pretrained("distilbert/distilbert-base-uncased")
```
Semplicemente utilizza lo stesso checkpoint per caricare un'architettura per un task differente:
```py
>>> from transformers import AutoModelForTokenClassification
>>> model = AutoModelForTokenClassification.from_pretrained("distilbert/distilbert-base-uncased")
```
Generalmente, raccomandiamo di utilizzare la classe `AutoTokenizer` e la classe `AutoModelFor` per caricare istanze pre-allenate dei modelli. Questo ti assicurerà di aver caricato la corretta architettura ogni volta. Nel prossimo [tutorial](preprocessing), imparerai come utilizzare il tokenizer, il feature extractor e il processore per elaborare un dataset per il fine-tuning.
</pt>
<tf>
Infine, le classi `TFAutoModelFor` ti permettono di caricare un modello pre-allenato per un determinato compito (guarda [qui](model_doc/auto) per una lista completa di compiti presenti). Per esempio, carica un modello per la classificazione di sequenze con [`TFAutoModelForSequenceClassification.from_pretrained`]:
```py
>>> from transformers import TFAutoModelForSequenceClassification
>>> model = TFAutoModelForSequenceClassification.from_pretrained("distilbert/distilbert-base-uncased")
```
Semplicemente utilizza lo stesso checkpoint per caricare un'architettura per un task differente:
```py
>>> from transformers import TFAutoModelForTokenClassification
>>> model = TFAutoModelForTokenClassification.from_pretrained("distilbert/distilbert-base-uncased")
```
Generalmente, raccomandiamo di utilizzare la classe `AutoTokenizer` e la classe `TFAutoModelFor` per caricare istanze pre-allenate dei modelli. Questo ti assicurerà di aver caricato la corretta architettura ogni volta. Nel prossimo [tutorial](preprocessing), imparerai come utilizzare il tokenizer, il feature extractor e il processore per elaborare un dataset per il fine-tuning.
</tf>
</frameworkcontent>
| transformers/docs/source/it/autoclass_tutorial.md/0 | {
"file_path": "transformers/docs/source/it/autoclass_tutorial.md",
"repo_id": "transformers",
"token_count": 1960
} | 258 |
<!--
Copyright 2023 The HuggingFace Team. All rights reserved.
Licensed under the Apache License, Version 2.0 (the "License"); you may not use this file except in compliance with
the License. You may obtain a copy of the License at
http://www.apache.org/licenses/LICENSE-2.0
Unless required by applicable law or agreed to in writing, software distributed under the License is distributed on
an "AS IS" BASIS, WITHOUT WARRANTIES OR CONDITIONS OF ANY KIND, either express or implied. See the License for the
specific language governing permissions and limitations under the License.
⚠️ このファイルはMarkdown形式ですが、ドキュメンテーションビルダー用の特定の構文を含んでおり、Markdownビューアーでは正しく表示されないことに注意してください。
-->
# Attention mechanism
ほとんどのTransformerモデルは、アテンション行列が正方形であるという意味で完全なアテンションを使用します。
これは、長いテキストを扱う場合に計算のボトルネックとなることがあります。LongformerやReformerは、より効率的でトレーニングを高速化するためにアテンション行列のスパースバージョンを使用しようとするモデルです。
## LSH attention
[Reformer](model_doc/reformer)はLSH(局所的に散在ハッシュ)アテンションを使用します。
ソフトマックス(QK^t)では、行列QK^tの中で(ソフトマックス次元で)最も大きな要素のみが有用な寄与を提供します。
したがって、各クエリqについて、クエリqに近いキーkのみを考慮できます。
qとkが近いかどうかを決定するために、ハッシュ関数が使用されます。
アテンションマスクは変更され、現在のトークンをマスク化します(最初の位置を除く)。
なぜなら、それはクエリとキーが等しい(つまり非常に似ている)クエリとキーを提供するからです。
ハッシュは多少ランダムかもしれないため、実際にはいくつかのハッシュ関数が使用され(n_roundsパラメータで決定されます)、それらが平均化されます。
## Local attention
[Longformer](model_doc/longformer)はローカルアテンションを使用します。
しばしば、ローカルコンテキスト(例:左右の2つのトークンは何ですか?)は、特定のトークンに対して行動を起こすのに十分です。
また、小さなウィンドウを持つアテンションレイヤーを積み重ねることで、最後のレイヤーはウィンドウ内のトークンだけでなく、ウィンドウ内のトークンを超えて受容野を持つようになり、文全体の表現を構築できます。
一部の事前選択された入力トークンにはグローバルアテンションも与えられます。
これらの少数のトークンに対して、アテンション行列はすべてのトークンにアクセスでき、このプロセスは対称的です。
他のすべてのトークンは、これらの特定のトークンにアクセスできます(ローカルウィンドウ内のトークンに加えて)。
これは、論文の図2dに示されており、以下はサンプルのアテンションマスクです:
<div class="flex justify-center">
<img scale="50 %" align="center" src="https://huggingface.co/datasets/huggingface/documentation-images/resolve/main/local_attention_mask.png"/>
</div>
## Other tricks
### Axial positional encodings
[Reformer](model_doc/reformer)は軸方向の位置エンコーディングを使用しています。伝統的なトランスフォーマーモデルでは、位置エンコーディングEはサイズが \\(l\\) × \\(d\\) の行列で、\\(l\\) はシーケンスの長さ、\\(d\\) は隠れ状態の次元です。非常に長いテキストを扱う場合、この行列は非常に大きく、GPU上で大量のスペースを占有します。これを緩和するために、軸方向の位置エンコーディングは、この大きな行列Eを2つの小さな行列E1とE2に分解します。それぞれの行列はサイズ \\(l_{1} \times d_{1}\\) および \\(l_{2} \times d_{2}\\) を持ち、 \\(l_{1} \times l_{2} = l\\) および \\(d_{1} + d_{2} = d\\) という条件を満たします(長さの積を考えると、これがはるかに小さくなります)。行列E内の時刻 \\(j\\) の埋め込みは、E1内の時刻 \\(j \% l1\\) の埋め込みとE2内の時刻 \\(j // l1\\) の埋め込みを連結することによって得られます。
| transformers/docs/source/ja/attention.md/0 | {
"file_path": "transformers/docs/source/ja/attention.md",
"repo_id": "transformers",
"token_count": 1963
} | 259 |
<!--Copyright 2022 The HuggingFace Team. All rights reserved.
Licensed under the Apache License, Version 2.0 (the "License"); you may not use this file except in compliance with
the License. You may obtain a copy of the License at
http://www.apache.org/licenses/LICENSE-2.0
Unless required by applicable law or agreed to in writing, software distributed under the License is distributed on
an "AS IS" BASIS, WITHOUT WARRANTIES OR CONDITIONS OF ANY KIND, either express or implied. See the License for the
specific language governing permissions and limitations under the License.
⚠️ Note that this file is in Markdown but contain specific syntax for our doc-builder (similar to MDX) that may not be
rendered properly in your Markdown viewer.
-->
# Audio Spectrogram Transformer
## 概要
Audio Spectrogram Transformerモデルは、[AST: Audio Spectrogram Transformer](https://arxiv.org/abs/2104.01778)という論文でYuan Gong、Yu-An Chung、James Glassによって提案されました。これは、音声を画像(スペクトログラム)に変換することで、音声に[Vision Transformer](vit)を適用します。このモデルは音声分類において最先端の結果を得ています。
論文の要旨は以下の通りです:
*過去10年間で、畳み込みニューラルネットワーク(CNN)は、音声スペクトログラムから対応するラベルへの直接的なマッピングを学習することを目指す、エンドツーエンドの音声分類モデルの主要な構成要素として広く採用されてきました。長距離のグローバルなコンテキストをより良く捉えるため、最近の傾向として、CNNの上にセルフアテンション機構を追加し、CNN-アテンションハイブリッドモデルを形成することがあります。しかし、CNNへの依存が必要かどうか、そして純粋にアテンションに基づくニューラルネットワークだけで音声分類において良いパフォーマンスを得ることができるかどうかは明らかではありません。本論文では、これらの問いに答えるため、音声分類用では最初の畳み込みなしで純粋にアテンションベースのモデルであるAudio Spectrogram Transformer(AST)を紹介します。我々はASTを様々なオーディオ分類ベンチマークで評価し、AudioSetで0.485 mAP、ESC-50で95.6%の正解率、Speech Commands V2で98.1%の正解率という新たな最先端の結果を達成しました。*
<img src="https://huggingface.co/datasets/huggingface/documentation-images/resolve/main/transformers/model_doc/audio_spectogram_transformer_architecture.png"
alt="drawing" width="600"/>
<small> Audio Spectrogram Transformerのアーキテクチャ。<a href="https://arxiv.org/abs/2104.01778">元論文</a>より抜粋。</small>
このモデルは[nielsr](https://huggingface.co/nielsr)より提供されました。
オリジナルのコードは[こちら](https://github.com/YuanGongND/ast)で見ることができます。
## 使用上のヒント
- 独自のデータセットでAudio Spectrogram Transformer(AST)をファインチューニングする場合、入力の正規化(入力の平均を0、標準偏差を0.5にすること)処理することが推奨されます。[`ASTFeatureExtractor`]はこれを処理します。デフォルトではAudioSetの平均と標準偏差を使用していることに注意してください。著者が下流のデータセットの統計をどのように計算しているかは、[`ast/src/get_norm_stats.py`](https://github.com/YuanGongND/ast/blob/master/src/get_norm_stats.py)で確認することができます。
- ASTは低い学習率が必要であり 著者は[PSLA論文](https://arxiv.org/abs/2102.01243)で提案されたCNNモデルに比べて10倍小さい学習率を使用しています)、素早く収束するため、タスクに適した学習率と学習率スケジューラーを探すことをお勧めします。
## 参考資料
Audio Spectrogram Transformerの使用を開始するのに役立つ公式のHugging Faceおよびコミュニティ(🌎で示されている)の参考資料の一覧です。
<PipelineTag pipeline="audio-classification"/>
- ASTを用いた音声分類の推論を説明するノートブックは[こちら](https://github.com/NielsRogge/Transformers-Tutorials/tree/master/AST)で見ることができます。
- [`ASTForAudioClassification`]は、この[例示スクリプト](https://github.com/huggingface/transformers/tree/main/examples/pytorch/audio-classification)と[ノートブック](https://colab.research.google.com/github/huggingface/notebooks/blob/main/examples/audio_classification.ipynb)によってサポートされています。
- こちらも参照:[音声分類タスク](../tasks/audio_classification)。
ここに参考資料を提出したい場合は、気兼ねなくPull Requestを開いてください。私たちはそれをレビューいたします!参考資料は、既存のものを複製するのではなく、何か新しいことを示すことが理想的です。
## ASTConfig
[[autodoc]] ASTConfig
## ASTFeatureExtractor
[[autodoc]] ASTFeatureExtractor
- __call__
## ASTModel
[[autodoc]] ASTModel
- forward
## ASTForAudioClassification
[[autodoc]] ASTForAudioClassification
- forward
| transformers/docs/source/ja/model_doc/audio-spectrogram-transformer.md/0 | {
"file_path": "transformers/docs/source/ja/model_doc/audio-spectrogram-transformer.md",
"repo_id": "transformers",
"token_count": 2249
} | 260 |
<!--Copyright 2020 The HuggingFace Team. All rights reserved.
Licensed under the Apache License, Version 2.0 (the "License"); you may not use this file except in compliance with
the License. You may obtain a copy of the License at
http://www.apache.org/licenses/LICENSE-2.0
Unless required by applicable law or agreed to in writing, software distributed under the License is distributed on
an "AS IS" BASIS, WITHOUT WARRANTIES OR CONDITIONS OF ANY KIND, either express or implied. See the License for the
specific language governing permissions and limitations under the License.
⚠️ Note that this file is in Markdown but contain specific syntax for our doc-builder (similar to MDX) that may not be
rendered properly in your Markdown viewer.
-->
# Blenderbot Small
[`BlenderbotSmallModel`] と
[`BlenderbotSmallForConditionalGeneration`] はチェックポイントと組み合わせてのみ使用されます
[facebook/blenderbot-90M](https://huggingface.co/facebook/blenderbot-90M)。より大規模な Blenderbot チェックポイントは、
代わりに [`BlenderbotModel`] とともに使用してください。
[`BlenderbotForConditionalGeneration`]
## Overview
Blender チャットボット モデルは、[Recipes for building an open-domain chatbot](https://arxiv.org/pdf/2004.13637.pdf) Stephen Roller、Emily Dinan、Naman Goyal、Da Ju、Mary Williamson、yinghan Liu、で提案されました。
ジン・シュー、マイル・オット、カート・シャスター、エリック・M・スミス、Y-ラン・ブーロー、ジェイソン・ウェストン、2020年4月30日。
論文の要旨は次のとおりです。
*オープンドメインのチャットボットの構築は、機械学習研究にとって難しい分野です。これまでの研究では次のことが示されていますが、
ニューラル モデルをパラメーターの数とトレーニング対象のデータのサイズでスケーリングすると、結果が向上します。
高性能のチャットボットには他の要素も重要であることを示します。良い会話には多くのことが必要です
会話の専門家がシームレスに融合するスキル: 魅力的な話のポイントを提供し、話を聞く
一貫した態度を維持しながら、知識、共感、個性を適切に表現する
ペルソナ。適切なトレーニング データと選択が与えられた場合、大規模モデルがこれらのスキルを学習できることを示します。
世代戦略。 90M、2.7B、9.4B パラメーター モデルを使用してこれらのレシピのバリアントを構築し、モデルを作成します。
コードは公開されています。人間による評価では、当社の最良のモデルが既存のアプローチよりも優れていることがマルチターンで示されています
魅力と人間性の測定という観点からの対話。次に、分析によってこの作業の限界について説明します。
弊社機種の故障事例*
チップ:
- Blenderbot Small は絶対位置埋め込みを備えたモデルなので、通常は入力を右側にパディングすることをお勧めします。
左。
このモデルは、[patrickvonplaten](https://huggingface.co/patrickvonplaten) によって提供されました。著者のコードは次のとおりです
[ここ](https://github.com/facebookresearch/ParlAI) をご覧ください。
## Documentation resources
- [因果言語モデリング タスク ガイド](../tasks/language_modeling)
- [翻訳タスクガイド](../tasks/translation)
- [要約タスクガイド](../tasks/summarization)
## BlenderbotSmallConfig
[[autodoc]] BlenderbotSmallConfig
## BlenderbotSmallTokenizer
[[autodoc]] BlenderbotSmallTokenizer
- build_inputs_with_special_tokens
- get_special_tokens_mask
- create_token_type_ids_from_sequences
- save_vocabulary
## BlenderbotSmallTokenizerFast
[[autodoc]] BlenderbotSmallTokenizerFast
## BlenderbotSmallModel
[[autodoc]] BlenderbotSmallModel
- forward
## BlenderbotSmallForConditionalGeneration
[[autodoc]] BlenderbotSmallForConditionalGeneration
- forward
## BlenderbotSmallForCausalLM
[[autodoc]] BlenderbotSmallForCausalLM
- forward
## TFBlenderbotSmallModel
[[autodoc]] TFBlenderbotSmallModel
- call
## TFBlenderbotSmallForConditionalGeneration
[[autodoc]] TFBlenderbotSmallForConditionalGeneration
- call
## FlaxBlenderbotSmallModel
[[autodoc]] FlaxBlenderbotSmallModel
- __call__
- encode
- decode
## FlaxBlenderbotForConditionalGeneration
[[autodoc]] FlaxBlenderbotSmallForConditionalGeneration
- __call__
- encode
- decode
| transformers/docs/source/ja/model_doc/blenderbot-small.md/0 | {
"file_path": "transformers/docs/source/ja/model_doc/blenderbot-small.md",
"repo_id": "transformers",
"token_count": 1831
} | 261 |
<!--Copyright 2023 The HuggingFace Team. All rights reserved.
Licensed under the Apache License, Version 2.0 (the "License"); you may not use this file except in compliance with
the License. You may obtain a copy of the License at
http://www.apache.org/licenses/LICENSE-2.0
Unless required by applicable law or agreed to in writing, software distributed under the License is distributed on
an "AS IS" BASIS, WITHOUT WARRANTIES OR CONDITIONS OF ANY KIND, either express or implied. See the License for the
specific language governing permissions and limitations under the License.
⚠️ Note that this file is in Markdown but contains specific syntax for our doc-builder (similar to MDX) that may not be
rendered properly in your Markdown viewer.
-->
# CodeLlama
## Overview
Code Llama モデルはによって [Code Llama: Open Foundation Models for Code](https://ai.meta.com/research/publications/code-llama-open-foundation-models-for-code/) で提案されました。 Baptiste Rozière, Jonas Gehring, Fabian Gloeckle, Sten Sootla, Itai Gat, Xiaoqing Ellen Tan, Yossi Adi, Jingyu Liu, Tal Remez, Jérémy Rapin, Artyom Kozhevnikov, Ivan Evtimov, Joanna Bitton, Manish Bhatt, Cristian Canton Ferrer, Aaron Grattafiori, Wenhan Xiong, Alexandre Défossez, Jade Copet, Faisal Azhar, Hugo Touvron, Louis Martin, Nicolas Usunier, Thomas Scialom, Gabriel Synnaeve.
論文の要約は次のとおりです。
*私たちは Code Llama をリリースします。これは Llama 2 に基づくコードの大規模言語モデル ファミリであり、オープン モデルの中で最先端のパフォーマンス、埋め込み機能、大規模な入力コンテキストのサポート、プログラミング タスクのゼロショット命令追従機能を提供します。 。幅広いアプリケーションをカバーするための複数のフレーバーを提供しています。基盤モデル (Code Llama)、Python 特化 (Code Llama - Python)、およびそれぞれ 7B、13B、および 34B パラメーターを備えた命令追従モデル (Code Llama - Instruct) です。すべてのモデルは 16,000 トークンのシーケンスでトレーニングされ、最大 100,000 トークンの入力で改善が見られます。 7B および 13B コード ラマとコード ラマ - 命令バリアントは、周囲のコンテンツに基づいた埋め込みをサポートします。 Code Llama は、いくつかのコード ベンチマークでオープン モデルの中で最先端のパフォーマンスに達し、HumanEval と MBPP でそれぞれ最大 53% と 55% のスコアを獲得しました。特に、Code Llama - Python 7B は HumanEval および MBPP 上で Llama 2 70B よりも優れたパフォーマンスを示し、すべてのモデルは MultiPL-E 上で公開されている他のすべてのモデルよりも優れています。私たちは、研究と商業利用の両方を許可する寛容なライセンスに基づいて Code Llama をリリースしています。*
すべての Code Llama モデル チェックポイントを [こちら](https://huggingface.co/models?search=code_llama) で確認し、[codellama org](https://huggingface.co/codellama) で正式にリリースされたチェックポイントを確認してください。
このモデルは [ArthurZucker](https://huggingface.co/ArthurZ) によって提供されました。著者のオリジナルのコードは [こちら](https://github.com/facebookresearch/llama) にあります。
## Usage tips and examples
<Tip warning={true}>
Code Llama のベースとなる`Llama2`ファミリー モデルは、`bfloat16`を使用してトレーニングされましたが、元の推論では`float16`を使用します。さまざまな精度を見てみましょう。
* `float32`: モデルの初期化に関する PyTorch の規約では、モデルの重みがどの `dtype` で格納されたかに関係なく、モデルを `float32` にロードします。 「transformers」も、PyTorch との一貫性を保つためにこの規則に従っています。これはデフォルトで選択されます。 `AutoModel` API でストレージの重み付けタイプを使用してチェックポイントのロードをキャストする場合は、`torch_dtype="auto"` を指定する必要があります。 `model = AutoModelForCausalLM.from_pretrained("path", torch_dtype = "auto")`。
* `bfloat16`: コード Llama はこの精度でトレーニングされているため、さらなるトレーニングや微調整に使用することをお勧めします。
* `float16`: この精度を使用して推論を実行することをお勧めします。通常は `bfloat16` より高速であり、評価メトリクスには `bfloat16` と比べて明らかな低下が見られないためです。 bfloat16 を使用して推論を実行することもできます。微調整後、float16 と bfloat16 の両方で推論結果を確認することをお勧めします。
上で述べたように、モデルを初期化するときに `torch_dtype="auto"` を使用しない限り、ストレージの重みの `dtype` はほとんど無関係です。その理由は、モデルが最初にダウンロードされ (オンラインのチェックポイントの `dtype` を使用)、次に `torch` のデフォルトの `dtype` にキャストされるためです (`torch.float32` になります)。指定された `torch_dtype` がある場合は、代わりにそれが使用されます。
</Tip>
チップ:
- 充填タスクはすぐにサポートされます。入力を埋めたい場所には `tokenizer.fill_token` を使用する必要があります。
- モデル変換スクリプトは、`Llama2` ファミリの場合と同じです。
使用例は次のとおりです。
```bash
python src/transformers/models/llama/convert_llama_weights_to_hf.py \
--input_dir /path/to/downloaded/llama/weights --model_size 7B --output_dir /output/path
```
スクリプトを実行するには、(最大のバージョンであっても) float16 精度でモデル全体をホストするのに十分な CPU RAM が必要であることに注意してください。
いくつかのチェックポイントがあり、それぞれにモデルの各重みの一部が含まれているため、すべてを RAM にロードする必要があります)。
変換後、モデルとトークナイザーは次の方法でロードできます。
```python
>>> from transformers import LlamaForCausalLM, CodeLlamaTokenizer
>>> tokenizer = CodeLlamaTokenizer.from_pretrained("codellama/CodeLlama-7b-hf")
>>> model = LlamaForCausalLM.from_pretrained("codellama/CodeLlama-7b-hf")
>>> PROMPT = '''def remove_non_ascii(s: str) -> str:
""" <FILL_ME>
return result
'''
>>> input_ids = tokenizer(PROMPT, return_tensors="pt")["input_ids"]
>>> generated_ids = model.generate(input_ids, max_new_tokens=128)
>>> filling = tokenizer.batch_decode(generated_ids[:, input_ids.shape[1]:], skip_special_tokens = True)[0]
>>> print(PROMPT.replace("<FILL_ME>", filling))
def remove_non_ascii(s: str) -> str:
""" Remove non-ASCII characters from a string.
Args:
s: The string to remove non-ASCII characters from.
Returns:
The string with non-ASCII characters removed.
"""
result = ""
for c in s:
if ord(c) < 128:
result += c
return result
```
塗りつぶされた部分だけが必要な場合:
```python
>>> from transformers import pipeline
>>> import torch
>>> generator = pipeline("text-generation",model="codellama/CodeLlama-7b-hf",torch_dtype=torch.float16, device_map="auto")
>>> generator('def remove_non_ascii(s: str) -> str:\n """ <FILL_ME>\n return result', max_new_tokens = 128, return_type = 1)
```
内部では、トークナイザーが [`<FILL_ME>` によって自動的に分割](https://huggingface.co/docs/transformers/main/model_doc/code_llama#transformers.CodeLlamaTokenizer.fill_token) して、[ に続く書式設定された入力文字列を作成します。オリジナルのトレーニング パターン](https://github.com/facebookresearch/codellama/blob/cb51c14ec761370ba2e2bc351374a79265d0465e/llama/generation.py#L402)。これは、パターンを自分で準備するよりも堅牢です。トークンの接着など、デバッグが非常に難しい落とし穴を回避できます。このモデルまたは他のモデルに必要な CPU および GPU メモリの量を確認するには、その値を決定するのに役立つ [この計算ツール](https://huggingface.co/spaces/hf-accelerate/model-memory-usage) を試してください。
LLaMA トークナイザーは、[sentencepiece](https://github.com/google/sentencepiece) に基づく BPE モデルです。センテンスピースの癖の 1 つは、シーケンスをデコードするときに、最初のトークンが単語の先頭 (例: 「Banana」) である場合、トークナイザーは文字列の先頭にプレフィックス スペースを追加しないことです。
<Tip>
コード Llama は、`Llama2` モデルと同じアーキテクチャを持っています。API リファレンスについては、[Llama2 のドキュメント ページ](llama2) を参照してください。
以下の Code Llama トークナイザーのリファレンスを見つけてください。
</Tip>
## CodeLlamaTokenizer
[[autodoc]] CodeLlamaTokenizer
- build_inputs_with_special_tokens
- get_special_tokens_mask
- create_token_type_ids_from_sequences
- save_vocabulary
## CodeLlamaTokenizerFast
[[autodoc]] CodeLlamaTokenizerFast
- build_inputs_with_special_tokens
- get_special_tokens_mask
- create_token_type_ids_from_sequences
- update_post_processor
- save_vocabulary
| transformers/docs/source/ja/model_doc/code_llama.md/0 | {
"file_path": "transformers/docs/source/ja/model_doc/code_llama.md",
"repo_id": "transformers",
"token_count": 4010
} | 262 |
<!--Copyright 2021 The HuggingFace Team. All rights reserved.
Licensed under the Apache License, Version 2.0 (the "License"); you may not use this file except in compliance with
the License. You may obtain a copy of the License at
http://www.apache.org/licenses/LICENSE-2.0
Unless required by applicable law or agreed to in writing, software distributed under the License is distributed on
an "AS IS" BASIS, WITHOUT WARRANTIES OR CONDITIONS OF ANY KIND, either express or implied. See the License for the
specific language governing permissions and limitations under the License.
⚠️ Note that this file is in Markdown but contain specific syntax for our doc-builder (similar to MDX) that may not be
rendered properly in your Markdown viewer.
-->
# DePlot
## Overview
DePlot は、Fangyu Liu、Julian Martin Aisenschlos、Francesco Piccinno、Syrine Krichene、Chenxi Pang, Kenton Lee, Mandar Joshi, Wenhu Chen, Nigel Collier, Yasemin Altun. の論文 [DePlot: One-shot visual language reasoning by plot-to-table translation](https://arxiv.org/abs/2212.10505) で提案されました。パン・
論文の要約には次のように記載されています。
*チャートやプロットなどの視覚言語は人間の世界に遍在しています。プロットやチャートを理解するには、強力な推論スキルが必要です。従来の最先端 (SOTA) モデルには少なくとも数万のトレーニング サンプルが必要であり、その推論能力は、特に人間が作成した複雑なクエリでは依然として大幅に制限されています。この論文では、視覚言語推論に対する最初のワンショット ソリューションを紹介します。私たちは、視覚言語推論の課題を 2 つのステップに分解します。(1) プロットからテキストへの翻訳と、(2) 翻訳されたテキストに対する推論です。この方法の鍵となるのは、プロットまたはチャートの画像を線形化されたテーブルに変換する、DePlot という名前のモダリティ変換モジュールです。その後、DePlot の出力を直接使用して、事前トレーニング済みの大規模言語モデル (LLM) をプロンプトし、LLM の少数ショット推論機能を利用できます。 DePlot を取得するには、統一されたタスク形式とメトリクスを確立することでプロットからテーブルへのタスクを標準化し、このタスクで DePlot をエンドツーエンドでトレーニングします。 DePlot は、プラグアンドプレイ方式で LLM とともに既製で使用できます。 28,000 を超えるデータ ポイントで微調整された SOTA モデルと比較して、ワンショット プロンプトのみを使用する DePlot+LLM は、チャート QA タスクからの人が作成したクエリに関して、微調整された SOTA より 24.0% の改善を達成しました。*
DePlot は、`Pix2Struct` アーキテクチャを使用してトレーニングされたモデルです。 `Pix2Struct` の詳細については、[Pix2Struct ドキュメント](https://huggingface.co/docs/transformers/main/en/model_doc/pix2struct) を参照してください。
DePlot は、`Pix2Struct` アーキテクチャの Visual Question Answering サブセットです。入力された質問を画像上にレンダリングし、答えを予測します。
## Usage example
現在、DePlot で使用できるチェックポイントは 1 つです。
- `google/deplot`: ChartQA データセットで微調整された DePlot
```python
from transformers import AutoProcessor, Pix2StructForConditionalGeneration
import requests
from PIL import Image
model = Pix2StructForConditionalGeneration.from_pretrained("google/deplot")
processor = AutoProcessor.from_pretrained("google/deplot")
url = "https://raw.githubusercontent.com/vis-nlp/ChartQA/main/ChartQA%20Dataset/val/png/5090.png"
image = Image.open(requests.get(url, stream=True).raw)
inputs = processor(images=image, text="Generate underlying data table of the figure below:", return_tensors="pt")
predictions = model.generate(**inputs, max_new_tokens=512)
print(processor.decode(predictions[0], skip_special_tokens=True))
```
## Fine-tuning
DePlot を微調整するには、pix2struct [微調整ノートブック](https://github.com/huggingface/notebooks/blob/main/examples/image_captioning_pix2struct.ipynb) を参照してください。 `Pix2Struct` モデルの場合、Adafactor とコサイン学習率スケジューラを使用してモデルを微調整すると、収束が高速化されることがわかりました。
```python
from transformers.optimization import Adafactor, get_cosine_schedule_with_warmup
optimizer = Adafactor(self.parameters(), scale_parameter=False, relative_step=False, lr=0.01, weight_decay=1e-05)
scheduler = get_cosine_schedule_with_warmup(optimizer, num_warmup_steps=1000, num_training_steps=40000)
```
<Tip>
DePlot は、`Pix2Struct`アーキテクチャを使用してトレーニングされたモデルです。 API リファレンスについては、[`Pix2Struct` ドキュメント](pix2struct) を参照してください。
</Tip> | transformers/docs/source/ja/model_doc/deplot.md/0 | {
"file_path": "transformers/docs/source/ja/model_doc/deplot.md",
"repo_id": "transformers",
"token_count": 2027
} | 263 |
<!--Copyright 2023 The HuggingFace Team. All rights reserved.
Licensed under the Apache License, Version 2.0 (the "License"); you may not use this file except in compliance with
the License. You may obtain a copy of the License at
http://www.apache.org/licenses/LICENSE-2.0
Unless required by applicable law or agreed to in writing, software distributed under the License is distributed on
an "AS IS" BASIS, WITHOUT WARRANTIES OR CONDITIONS OF ANY KIND, either express or implied. See the License for the
specific language governing permissions and limitations under the License.
⚠️ Note that this file is in Markdown but contain specific syntax for our doc-builder (similar to MDX) that may not be
rendered properly in your Markdown viewer.
-->
# Train with a script
🤗 Transformersの[notebooks](./notebooks/README)と一緒に、[PyTorch](https://github.com/huggingface/transformers/tree/main/examples/pytorch)、[TensorFlow](https://github.com/huggingface/transformers/tree/main/examples/tensorflow)、または[JAX/Flax](https://github.com/huggingface/transformers/tree/main/examples/flax)を使用してモデルをトレーニングする方法を示すサンプルスクリプトもあります。
また、私たちの[研究プロジェクト](https://github.com/huggingface/transformers/tree/main/examples/research_projects)や[レガシーの例](https://github.com/huggingface/transformers/tree/main/examples/legacy)で使用したスクリプトも見つかります。これらのスクリプトは現在メンテナンスされておらず、おそらく最新バージョンのライブラリと互換性がない特定の🤗 Transformersのバージョンが必要です。
サンプルスクリプトはすべての問題でそのまま動作することは期待されておらず、解決しようとしている問題にスクリプトを適応させる必要があるかもしれません。この点をサポートするために、ほとんどのスクリプトはデータがどのように前処理されているかを完全に公開し、必要に応じて編集できるようにしています。
サンプルスクリプトで実装したい機能がある場合は、[フォーラム](https://discuss.huggingface.co/)か[イシュートラッカー](https://github.com/huggingface/transformers/issues)で議論してからプルリクエストを提出してください。バグ修正は歓迎しますが、読みやすさのコストで機能を追加するプルリクエストはほとんどマージされない可能性が高いです。
このガイドでは、[PyTorch](https://github.com/huggingface/transformers/tree/main/examples/pytorch/summarization)と[TensorFlow](https://github.com/huggingface/transformers/tree/main/examples/tensorflow/summarization)で実行するサマリゼーショントレーニングスクリプトの実行方法を示します。すべての例は、明示的に指定されていない限り、両方のフレームワークともに動作することが期待されています。
## Setup
最新バージョンのサンプルスクリプトを正常に実行するには、新しい仮想環境に🤗 Transformersをソースからインストールする必要があります:
```bash
git clone https://github.com/huggingface/transformers
cd transformers
pip install .
```
以前のスクリプトのバージョンについては、以下のトグルをクリックしてください:
<details>
<summary>以前の🤗 Transformersのバージョンに関する例</summary>
<ul>
<li><a href="https://github.com/huggingface/transformers/tree/v4.5.1/examples">v4.5.1</a></li>
<li><a href="https://github.com/huggingface/transformers/tree/v4.4.2/examples">v4.4.2</a></li>
<li><a href="https://github.com/huggingface/transformers/tree/v4.3.3/examples">v4.3.3</a></li>
<li><a href="https://github.com/huggingface/transformers/tree/v4.2.2/examples">v4.2.2</a></li>
<li><a href="https://github.com/huggingface/transformers/tree/v4.1.1/examples">v4.1.1</a></li>
<li><a href="https://github.com/huggingface/transformers/tree/v4.0.1/examples">v4.0.1</a></li>
<li><a href="https://github.com/huggingface/transformers/tree/v3.5.1/examples">v3.5.1</a></li>
<li><a href="https://github.com/huggingface/transformers/tree/v3.4.0/examples">v3.4.0</a></li>
<li><a href="https://github.com/huggingface/transformers/tree/v3.3.1/examples">v3.3.1</a></li>
<li><a href="https://github.com/huggingface/transformers/tree/v3.2.0/examples">v3.2.0</a></li>
<li><a href="https://github.com/huggingface/transformers/tree/v3.1.0/examples">v3.1.0</a></li>
<li><a href="https://github.com/huggingface/transformers/tree/v3.0.2/examples">v3.0.2</a></li>
<li><a href="https://github.com/huggingface/transformers/tree/v2.11.0/examples">v2.11.0</a></li>
<li><a href="https://github.com/huggingface/transformers/tree/v2.10.0/examples">v2.10.0</a></li>
<li><a href="https://github.com/huggingface/transformers/tree/v2.9.1/examples">v2.9.1</a></li>
<li><a href="https://github.com/huggingface/transformers/tree/v2.8.0/examples">v2.8.0</a></li>
<li><a href="https://github.com/huggingface/transformers/tree/v2.7.0/examples">v2.7.0</a></li>
<li><a href="https://github.com/huggingface/transformers/tree/v2.6.0/examples">v2.6.0</a></li>
<li><a href="https://github.com/huggingface/transformers/tree/v2.5.1/examples">v2.5.1</a></li>
<li><a href="https://github.com/huggingface/transformers/tree/v2.4.0/examples">v2.4.0</a></li>
<li><a href="https://github.com/huggingface/transformers/tree/v2.3.0/examples">v2.3.0</a></li>
<li><a href="https://github.com/huggingface/transformers/tree/v2.2.0/examples">v2.2.0</a></li>
<li><a href="https://github.com/huggingface/transformers/tree/v2.1.0/examples">v2.1.1</a></li>
<li><a href="https://github.com/huggingface/transformers/tree/v2.0.0/examples">v2.0.0</a></li>
<li><a href="https://github.com/huggingface/transformers/tree/v1.2.0/examples">v1.2.0</a></li>
<li><a href="https://github.com/huggingface/transformers/tree/v1.1.0/examples">v1.1.0</a></li>
<li><a href="https://github.com/huggingface/transformers/tree/v1.0.0/examples">v1.0.0</a></li>
</ul>
</details>
次に、現在の🤗 Transformersのクローンを特定のバージョンに切り替えてください。たとえば、v3.5.1などです。
```bash
git checkout tags/v3.5.1
```
適切なライブラリバージョンを設定したら、任意の例のフォルダに移動し、例固有の要件をインストールします:
```bash
pip install -r requirements.txt
```
## Run a script
<frameworkcontent>
<pt>
この例のスクリプトは、🤗 [Datasets](https://huggingface.co/docs/datasets/) ライブラリからデータセットをダウンロードし、前処理を行います。次に、[Trainer](https://huggingface.co/docs/transformers/main_classes/trainer) を使用して要約をサポートするアーキテクチャ上でデータセットをファインチューニングします。以下の例では、[CNN/DailyMail](https://huggingface.co/datasets/cnn_dailymail) データセット上で [T5-small](https://huggingface.co/google-t5/t5-small) をファインチューニングする方法が示されています。T5 モデルは、そのトレーニング方法に起因して追加の `source_prefix` 引数が必要です。このプロンプトにより、T5 はこれが要約タスクであることを知ることができます。
```bash
python examples/pytorch/summarization/run_summarization.py \
--model_name_or_path google-t5/t5-small \
--do_train \
--do_eval \
--dataset_name cnn_dailymail \
--dataset_config "3.0.0" \
--source_prefix "summarize: " \
--output_dir /tmp/tst-summarization \
--per_device_train_batch_size=4 \
--per_device_eval_batch_size=4 \
--overwrite_output_dir \
--predict_with_generate
```
</pt>
<tf>
この例のスクリプトは、🤗 [Datasets](https://huggingface.co/docs/datasets/) ライブラリからデータセットをダウンロードして前処理します。その後、スクリプトは要約をサポートするアーキテクチャ上で Keras を使用してデータセットをファインチューニングします。以下の例では、[T5-small](https://huggingface.co/google-t5/t5-small) を [CNN/DailyMail](https://huggingface.co/datasets/cnn_dailymail) データセットでファインチューニングする方法を示しています。T5 モデルは、そのトレーニング方法に起因して追加の `source_prefix` 引数が必要です。このプロンプトは、T5 にこれが要約タスクであることを知らせます。
```bash
python examples/tensorflow/summarization/run_summarization.py \
--model_name_or_path google-t5/t5-small \
--dataset_name cnn_dailymail \
--dataset_config "3.0.0" \
--output_dir /tmp/tst-summarization \
--per_device_train_batch_size 8 \
--per_device_eval_batch_size 16 \
--num_train_epochs 3 \
--do_train \
--do_eval
```
</tf>
</frameworkcontent>
## Distributed training and mixed precision
[Trainer](https://huggingface.co/docs/transformers/main_classes/trainer)は、分散トレーニングと混合精度をサポートしています。つまり、この機能をスクリプトで使用することができます。これらの機能を有効にするには、次の手順を実行します。
- `fp16`引数を追加して混合精度を有効にします。
- `nproc_per_node`引数で使用するGPUの数を設定します。
以下は提供されたBashコードです。このコードの日本語訳をMarkdown形式で記載します。
```bash
torchrun \
--nproc_per_node 8 pytorch/summarization/run_summarization.py \
--fp16 \
--model_name_or_path google-t5/t5-small \
--do_train \
--do_eval \
--dataset_name cnn_dailymail \
--dataset_config "3.0.0" \
--source_prefix "summarize: " \
--output_dir /tmp/tst-summarization \
--per_device_train_batch_size=4 \
--per_device_eval_batch_size=4 \
--overwrite_output_dir \
--predict_with_generate
```
TensorFlowスクリプトは、分散トレーニングに[`MirroredStrategy`](https://www.tensorflow.org/guide/distributed_training#mirroredstrategy)を使用し、トレーニングスクリプトに追加の引数を追加する必要はありません。TensorFlowスクリプトは、デフォルトで複数のGPUが利用可能な場合にそれらを使用します。
## Run a script on a TPU
<frameworkcontent>
<pt>
Tensor Processing Units (TPUs)は、パフォーマンスを加速させるために特別に設計されています。PyTorchは、[XLA](https://www.tensorflow.org/xla)ディープラーニングコンパイラを使用してTPUsをサポートしており、詳細については[こちら](https://github.com/pytorch/xla/blob/master/README.md)をご覧ください。TPUを使用するには、`xla_spawn.py`スクリプトを起動し、`num_cores`引数を使用して使用するTPUコアの数を設定します。
```bash
python xla_spawn.py --num_cores 8 \
summarization/run_summarization.py \
--model_name_or_path google-t5/t5-small \
--do_train \
--do_eval \
--dataset_name cnn_dailymail \
--dataset_config "3.0.0" \
--source_prefix "summarize: " \
--output_dir /tmp/tst-summarization \
--per_device_train_batch_size=4 \
--per_device_eval_batch_size=4 \
--overwrite_output_dir \
--predict_with_generate
```
</pt>
<tf>
もちろん、Tensor Processing Units(TPUs)は性能を高速化するために特別に設計されています。TensorFlowスクリプトは、TPUsでトレーニングするために[`TPUStrategy`](https://www.tensorflow.org/guide/distributed_training#tpustrategy)を利用します。TPUを使用するには、TPUリソースの名前を`tpu`引数に渡します。
```bash
python run_summarization.py \
--tpu name_of_tpu_resource \
--model_name_or_path google-t5/t5-small \
--dataset_name cnn_dailymail \
--dataset_config "3.0.0" \
--output_dir /tmp/tst-summarization \
--per_device_train_batch_size 8 \
--per_device_eval_batch_size 16 \
--num_train_epochs 3 \
--do_train \
--do_eval
```
</tf>
</frameworkcontent>
## Run a script with 🤗 Accelerate
🤗 [Accelerate](https://huggingface.co/docs/accelerate)は、PyTorch専用のライブラリで、CPUのみ、複数のGPU、TPUなど、さまざまなセットアップでモデルをトレーニングするための統一された方法を提供します。PyTorchのトレーニングループを完全に可視化しながら実行できます。まだインストールしていない場合は、🤗 Accelerateをインストールしてください:
> 注意:Accelerateは急速に開発が進行しているため、スクリプトを実行するにはaccelerateのgitバージョンをインストールする必要があります
```bash
pip install git+https://github.com/huggingface/accelerate
```
代わりに、`run_summarization_no_trainer.py` スクリプトを使用する必要があります。 🤗 Accelerate がサポートするスクリプトには、フォルダ内に `task_no_trainer.py` ファイルが含まれています。まず、次のコマンドを実行して設定ファイルを作成し、保存します:
```bash
accelerate config
```
テストを行い、設定が正しく構成されているか確認してください:
```bash
accelerate test
```
Now you are ready to launch the training:
```bash
accelerate launch run_summarization_no_trainer.py \
--model_name_or_path google-t5/t5-small \
--dataset_name cnn_dailymail \
--dataset_config "3.0.0" \
--source_prefix "summarize: " \
--output_dir ~/tmp/tst-summarization
```
## Use a custom dataset
要約スクリプトは、CSVまたはJSON Lineファイルであれば、カスタムデータセットをサポートしています。独自のデータセットを使用する場合、いくつかの追加の引数を指定する必要があります。
- `train_file`および`validation_file`は、トレーニングとバリデーションのファイルへのパスを指定します。
- `text_column`は要約するための入力テキストです。
- `summary_column`は出力する対象テキストです。
カスタムデータセットを使用した要約スクリプトは、以下のようになります:
```bash
python examples/pytorch/summarization/run_summarization.py \
--model_name_or_path google-t5/t5-small \
--do_train \
--do_eval \
--train_file path_to_csv_or_jsonlines_file \
--validation_file path_to_csv_or_jsonlines_file \
--text_column text_column_name \
--summary_column summary_column_name \
--source_prefix "summarize: " \
--output_dir /tmp/tst-summarization \
--overwrite_output_dir \
--per_device_train_batch_size=4 \
--per_device_eval_batch_size=4 \
--predict_with_generate
```
## Test a script
すべてが予想通りに動作することを確認するために、データセット全体を処理する前に、データセットの一部の例でスクリプトを実行することは良いアイデアです。以下の引数を使用して、データセットを最大サンプル数に切り詰めます:
- `max_train_samples`
- `max_eval_samples`
- `max_predict_samples`
```bash
python examples/pytorch/summarization/run_summarization.py \
--model_name_or_path google-t5/t5-small \
--max_train_samples 50 \
--max_eval_samples 50 \
--max_predict_samples 50 \
--do_train \
--do_eval \
--dataset_name cnn_dailymail \
--dataset_config "3.0.0" \
--source_prefix "summarize: " \
--output_dir /tmp/tst-summarization \
--per_device_train_batch_size=4 \
--per_device_eval_batch_size=4 \
--overwrite_output_dir \
--predict_with_generate
```
一部の例のスクリプトは、`max_predict_samples`引数をサポートしていないことがあります。この引数がサポートされているかどうかがわからない場合は、`-h`引数を追加して確認してください。
```bash
examples/pytorch/summarization/run_summarization.py -h
```
## Resume training from checkpoint
以前のチェックポイントからトレーニングを再開するための役立つオプションもあります。これにより、トレーニングが中断された場合でも、最初からやり直すことなく、中断したところから再開できます。チェックポイントからトレーニングを再開するための2つの方法があります。
最初の方法は、`output_dir previous_output_dir` 引数を使用して、`output_dir` に保存された最新のチェックポイントからトレーニングを再開する方法です。この場合、`overwrite_output_dir` を削除する必要があります:
```bash
python examples/pytorch/summarization/run_summarization.py
--model_name_or_path google-t5/t5-small \
--do_train \
--do_eval \
--dataset_name cnn_dailymail \
--dataset_config "3.0.0" \
--source_prefix "summarize: " \
--output_dir /tmp/tst-summarization \
--per_device_train_batch_size=4 \
--per_device_eval_batch_size=4 \
--output_dir previous_output_dir \
--predict_with_generate
```
2番目の方法では、`resume_from_checkpoint path_to_specific_checkpoint` 引数を使用して、特定のチェックポイントフォルダからトレーニングを再開します。
```bash
python examples/pytorch/summarization/run_summarization.py
--model_name_or_path google-t5/t5-small \
--do_train \
--do_eval \
--dataset_name cnn_dailymail \
--dataset_config "3.0.0" \
--source_prefix "summarize: " \
--output_dir /tmp/tst-summarization \
--per_device_train_batch_size=4 \
--per_device_eval_batch_size=4 \
--overwrite_output_dir \
--resume_from_checkpoint path_to_specific_checkpoint \
--predict_with_generate
```
## Share your model
すべてのスクリプトは、最終的なモデルを [Model Hub](https://huggingface.co/models) にアップロードできます。開始する前に Hugging Face にログインしていることを確認してください。
```bash
huggingface-cli login
```
次に、スクリプトに `push_to_hub` 引数を追加します。この引数は、Hugging Face のユーザー名と `output_dir` で指定したフォルダ名でリポジトリを作成します。
特定の名前をリポジトリに付けるには、`push_to_hub_model_id` 引数を使用して追加します。このリポジトリは自動的にあなたの名前空間の下にリストされます。
以下の例は、特定のリポジトリ名でモデルをアップロードする方法を示しています:
```bash
python examples/pytorch/summarization/run_summarization.py
--model_name_or_path google-t5/t5-small \
--do_train \
--do_eval \
--dataset_name cnn_dailymail \
--dataset_config "3.0.0" \
--source_prefix "summarize: " \
--push_to_hub \
--push_to_hub_model_id finetuned-t5-cnn_dailymail \
--output_dir /tmp/tst-summarization \
--per_device_train_batch_size=4 \
--per_device_eval_batch_size=4 \
--overwrite_output_dir \
--predict_with_generate
```
| transformers/docs/source/ja/run_scripts.md/0 | {
"file_path": "transformers/docs/source/ja/run_scripts.md",
"repo_id": "transformers",
"token_count": 8250
} | 264 |
<!--Copyright 2023 The HuggingFace Team. All rights reserved.
Licensed under the Apache License, Version 2.0 (the "License"); you may not use this file except in compliance with
the License. You may obtain a copy of the License at
http://www.apache.org/licenses/LICENSE-2.0
Unless required by applicable law or agreed to in writing, software distributed under the License is distributed on
an "AS IS" BASIS, WITHOUT WARRANTIES OR CONDITIONS OF ANY KIND, either express or implied. See the License for the
specific language governing permissions and limitations under the License.
⚠️ Note that this file is in Markdown but contain specific syntax for our doc-builder (similar to MDX) that may not be
rendered properly in your Markdown viewer.
-->
# LLM prompting guide
[[open-in-colab]]
Falcon、LLaMA などの大規模言語モデルは、事前にトレーニングされたトランスフォーマー モデルであり、最初は予測するようにトレーニングされています。
入力テキストが与えられた場合の次のトークン。通常、数十億のパラメータがあり、何兆ものパラメータでトレーニングされています。
長期間のトークン。その結果、これらのモデルは非常に強力で多用途になり、次のようなことが可能になります。
自然言語プロンプトでモデルに指示することで、すぐに複数の NLP タスクを解決できます。
最適な出力を保証するためにこのようなプロンプトを設計することは、多くの場合「プロンプト エンジニアリング」と呼ばれます。プロンプトエンジニアリングとは、
かなりの量の実験を必要とする反復プロセス。自然言語ははるかに柔軟で表現力豊かです
ただし、プログラミング言語よりもあいまいさが生じる可能性があります。同時に、自然言語によるプロンプト
変化にはかなり敏感です。プロンプトにわずかな変更を加えただけでも、出力が大幅に異なる場合があります。
すべてのケースに適合するプロンプトを作成するための正確なレシピはありませんが、研究者はいくつかの最良のレシピを考案しました。
最適な結果をより一貫して達成するのに役立つ実践。
このガイドでは、より優れた LLM プロンプトを作成し、さまざまな NLP タスクを解決するのに役立つプロンプト エンジニアリングのベスト プラクティスについて説明します。
次のことを学びます:
- [プロンプトの基本](#basics-of-prompting)
- [LLM プロンプトのベスト プラクティス](#best-practices-of-llm-prompting)
- [高度なプロンプト テクニック: 数回のプロンプトと思考の連鎖](#advanced-prompting-techniques)
- [プロンプトを表示する代わりに微調整する場合](#prompting-vs-fine-tuning)
<Tip>
迅速なエンジニアリングは、LLM 出力最適化プロセスの一部にすぎません。もう 1 つの重要な要素は、
最適なテキスト生成戦略。 LLM が生成時に後続の各トークンを選択する方法をカスタマイズできます。
トレーニング可能なパラメータを一切変更せずにテキストを作成します。テキスト生成パラメータを微調整することで、
生成されたテキストに繰り返しが含まれているため、より一貫性があり人間らしい響きになります。
テキスト生成戦略とパラメーターはこのガイドの範囲外ですが、これらのトピックについて詳しくは、次のトピックを参照してください。
次のガイド:
* [LLM による生成](../llm_tutorial)
* [テキスト生成戦略](../generation_strategies)
</Tip>
## Basics of prompting
### Types of models
最新の LLM の大部分は、デコーダ専用のトランスフォーマーです。例としては、[LLaMA](../model_doc/llama)、
[Llama2](../model_doc/llama2)、[Falcon](../model_doc/falcon)、[GPT2](../model_doc/gpt2)。ただし、遭遇する可能性があります
エンコーダ デコーダ トランスフォーマ LLM も同様です。たとえば、[Flan-T5](../model_doc/flan-t5) や [BART](../model_doc/bart) です。
エンコーダ デコーダ スタイルのモデルは通常、出力が入力に**大きく**依存する生成タスクで使用されます。
たとえば、翻訳と要約です。デコーダ専用モデルは、他のすべてのタイプの生成タスクに使用されます。
パイプラインを使用して LLM でテキストを生成する場合、使用している LLM のタイプを知ることが重要です。
異なるパイプラインを使用します。
`text-generation`パイプラインを使用してデコーダのみのモデルで推論を実行します。
```python
>>> from transformers import pipeline
>>> import torch
>>> torch.manual_seed(0) # doctest: +IGNORE_RESULT
>>> generator = pipeline('text-generation', model = 'openai-community/gpt2')
>>> prompt = "Hello, I'm a language model"
>>> generator(prompt, max_length = 30)
[{'generated_text': "Hello, I'm a language model expert, so I'm a big believer in the concept that I know very well and then I try to look into"}]
```
エンコーダー/デコーダーを使用して推論を実行するには、`text2text-generation` パイプラインを使用します。
```python
>>> text2text_generator = pipeline("text2text-generation", model = 'google/flan-t5-base')
>>> prompt = "Translate from English to French: I'm very happy to see you"
>>> text2text_generator(prompt)
[{'generated_text': 'Je suis très heureuse de vous rencontrer.'}]
```
### Base vs instruct/chat models
🤗 Hub で利用できる最近の LLM チェックポイントのほとんどには、base と instruct (または chat) の 2 つのバージョンがあります。例えば、
[`tiiuae/falcon-7b`](https://huggingface.co/tiiuae/falcon-7b) および [`tiiuae/falcon-7b-instruct`](https://huggingface.co/tiiuae/falcon-7b) -指示する)。
基本モデルは、最初のプロンプトが与えられたときにテキストを完成させるのには優れていますが、NLP タスクには理想的ではありません。
指示に従う必要がある場合、または会話で使用する場合に使用します。ここで、指示 (チャット) バージョンが登場します。
これらのチェックポイントは、命令と会話データに基づいて事前トレーニングされたベース バージョンをさらに微調整した結果です。
この追加の微調整により、多くの NLP タスクにとってより適切な選択肢になります。
[`tiiuae/falcon-7b-instruct`](https://huggingface.co/tiiuae/falcon-7b-instruct) で使用できるいくつかの簡単なプロンプトを示してみましょう。
いくつかの一般的な NLP タスクを解決します。
### NLP tasks
まず、環境をセットアップしましょう。
```bash
pip install -q transformers accelerate
```
次に、適切なパイプライン (`text_generation`) を使用してモデルをロードしましょう。
```python
>>> from transformers import pipeline, AutoTokenizer
>>> import torch
>>> torch.manual_seed(0) # doctest: +IGNORE_RESULT
>>> model = "tiiuae/falcon-7b-instruct"
>>> tokenizer = AutoTokenizer.from_pretrained(model)
>>> pipe = pipeline(
... "text-generation",
... model=model,
... tokenizer=tokenizer,
... torch_dtype=torch.bfloat16,
... device_map="auto",
... )
```
<Tip>
Falcon モデルは `bfloat16` データ型を使用してトレーニングされたため、同じものを使用することをお勧めします。これには、最近の
CUDA のバージョンに準拠しており、最新のカードで最適に動作します。
</Tip>
パイプライン経由でモデルをロードしたので、プロンプトを使用して NLP タスクを解決する方法を見てみましょう。
#### Text classification
テキスト分類の最も一般的な形式の 1 つはセンチメント分析であり、「ポジティブ」、「ネガティブ」、「ネガティブ」などのラベルを割り当てます。
または、一連のテキストに対して「中立」です。与えられたテキスト (映画レビュー) を分類するようにモデルに指示するプロンプトを作成してみましょう。
まず指示を与え、次に分類するテキストを指定します。そのままにしておくのではなく、
応答の先頭にも追加します - `"Sentiment: "`:
```python
>>> torch.manual_seed(0) # doctest: +IGNORE_RESULT
>>> prompt = """Classify the text into neutral, negative or positive.
... Text: This movie is definitely one of my favorite movies of its kind. The interaction between respectable and morally strong characters is an ode to chivalry and the honor code amongst thieves and policemen.
... Sentiment:
... """
>>> sequences = pipe(
... prompt,
... max_new_tokens=10,
... )
>>> for seq in sequences:
... print(f"Result: {seq['generated_text']}")
Result: Classify the text into neutral, negative or positive.
Text: This movie is definitely one of my favorite movies of its kind. The interaction between respectable and morally strong characters is an ode to chivalry and the honor code amongst thieves and policemen.
Sentiment:
Positive
```
その結果、出力には、手順で提供したリストの分類ラベルが含まれており、それは正しいラベルです。
<Tip>
プロンプトに加えて、`max_new_tokens`パラメータを渡していることに気づくかもしれません。トークンの数を制御します。
モデルが生成します。これは、学習できる多くのテキスト生成パラメーターの 1 つです。
[テキスト生成戦略](../generation_strategies) ガイドを参照してください。
</Tip>
#### Named Entity Recognition
固有表現認識 (NER) は、テキスト内の人物、場所、組織などの固有表現を検索するタスクです。
プロンプトの指示を変更して、LLM にこのタスクを実行させましょう。ここでは`return_full_text = False`も設定しましょう
出力にプロンプトが含まれないようにします。
```python
>>> torch.manual_seed(1) # doctest: +IGNORE_RESULT
>>> prompt = """Return a list of named entities in the text.
... Text: The Golden State Warriors are an American professional basketball team based in San Francisco.
... Named entities:
... """
>>> sequences = pipe(
... prompt,
... max_new_tokens=15,
... return_full_text = False,
... )
>>> for seq in sequences:
... print(f"{seq['generated_text']}")
- Golden State Warriors
- San Francisco
```
ご覧のとおり、モデルは指定されたテキストから 2 つの名前付きエンティティを正しく識別しました。
#### Translation
LLM が実行できるもう 1 つのタスクは翻訳です。このタスクにはエンコーダー/デコーダー モデルを使用することを選択できますが、ここでは
例を簡単にするために、きちんとした仕事をする Falcon-7b-instruct を使い続けます。もう一度、方法は次のとおりです
テキストの一部を英語からイタリア語に翻訳するようにモデルに指示する基本的なプロンプトを作成できます。
```python
>>> torch.manual_seed(2) # doctest: +IGNORE_RESULT
>>> prompt = """Translate the English text to Italian.
... Text: Sometimes, I've believed as many as six impossible things before breakfast.
... Translation:
... """
>>> sequences = pipe(
... prompt,
... max_new_tokens=20,
... do_sample=True,
... top_k=10,
... return_full_text = False,
... )
>>> for seq in sequences:
... print(f"{seq['generated_text']}")
A volte, ho creduto a sei impossibili cose prima di colazione.
```
ここでは、出力生成時にモデルがもう少し柔軟になるように `do_sample=True` と `top_k=10` を追加しました。
#### Text summarization
翻訳と同様に、テキストの要約も、出力が入力に**大きく**依存する生成タスクです。
エンコーダ/デコーダ モデルの方が良い選択になる可能性があります。ただし、デコーダ スタイルのモデルもこのタスクに使用できます。
以前は、プロンプトの先頭に指示を配置していました。ただし、プロンプトの最後で、
指示を与えるのに適した場所でもあります。通常、命令はどちらかの端に配置することをお勧めします。
```python
>>> torch.manual_seed(3) # doctest: +IGNORE_RESULT
>>> prompt = """Permaculture is a design process mimicking the diversity, functionality and resilience of natural ecosystems. The principles and practices are drawn from traditional ecological knowledge of indigenous cultures combined with modern scientific understanding and technological innovations. Permaculture design provides a framework helping individuals and communities develop innovative, creative and effective strategies for meeting basic needs while preparing for and mitigating the projected impacts of climate change.
... Write a summary of the above text.
... Summary:
... """
>>> sequences = pipe(
... prompt,
... max_new_tokens=30,
... do_sample=True,
... top_k=10,
... return_full_text = False,
... )
>>> for seq in sequences:
... print(f"{seq['generated_text']}")
Permaculture is an ecological design mimicking natural ecosystems to meet basic needs and prepare for climate change. It is based on traditional knowledge and scientific understanding.
```
#### Question answering
質問応答タスクの場合、プロンプトを次の論理コンポーネントに構造化できます: 指示、コンテキスト、質問、
先頭の単語またはフレーズ (`"Answer:"`) を使用して、モデルを操作して答えの生成を開始します。
```python
>>> torch.manual_seed(4) # doctest: +IGNORE_RESULT
>>> prompt = """Answer the question using the context below.
... Context: Gazpacho is a cold soup and drink made of raw, blended vegetables. Most gazpacho includes stale bread, tomato, cucumbers, onion, bell peppers, garlic, olive oil, wine vinegar, water, and salt. Northern recipes often include cumin and/or pimentón (smoked sweet paprika). Traditionally, gazpacho was made by pounding the vegetables in a mortar with a pestle; this more laborious method is still sometimes used as it helps keep the gazpacho cool and avoids the foam and silky consistency of smoothie versions made in blenders or food processors.
... Question: What modern tool is used to make gazpacho?
... Answer:
... """
>>> sequences = pipe(
... prompt,
... max_new_tokens=10,
... do_sample=True,
... top_k=10,
... return_full_text = False,
... )
>>> for seq in sequences:
... print(f"Result: {seq['generated_text']}")
Result: Modern tools are used, such as immersion blenders
```
#### Reasoning
LLM にとって推論は最も困難なタスクの 1 つであり、良い結果を達成するには、多くの場合、次のような高度なプロンプト テクニックを適用する必要があります。
[Chain-of-thought](#chain-of-thought)。
基本的なプロンプトを使用して、単純な算術タスクに関するモデル推論を作成できるかどうか試してみましょう。
```python
>>> torch.manual_seed(5) # doctest: +IGNORE_RESULT
>>> prompt = """There are 5 groups of students in the class. Each group has 4 students. How many students are there in the class?"""
>>> sequences = pipe(
... prompt,
... max_new_tokens=30,
... do_sample=True,
... top_k=10,
... return_full_text = False,
... )
>>> for seq in sequences:
... print(f"Result: {seq['generated_text']}")
Result:
There are a total of 5 groups, so there are 5 x 4=20 students in the class.
```
正しい!もう少し複雑さを増やして、基本的なプロンプトで問題を解決できるかどうかを確認してみましょう。
```python
>>> torch.manual_seed(6) # doctest: +IGNORE_RESULT
>>> prompt = """I baked 15 muffins. I ate 2 muffins and gave 5 muffins to a neighbor. My partner then bought 6 more muffins and ate 2. How many muffins do we now have?"""
>>> sequences = pipe(
... prompt,
... max_new_tokens=10,
... do_sample=True,
... top_k=10,
... return_full_text = False,
... )
>>> for seq in sequences:
... print(f"Result: {seq['generated_text']}")
Result:
The total number of muffins now is 21
```
これは間違った答えです。12 である必要があります。この場合、プロンプトが基本的すぎるか、選択内容が原因である可能性があります。
結局のところ、Falcon の最小バージョンを選択しました。あらゆるサイズのモデルでは推論が困難ですが、より大きなモデルでは
モデルのパフォーマンスが向上する可能性があります。
## Best practices of LLM prompting
ガイドのこのセクションでは、プロンプトの結果を改善する傾向にあるベスト プラクティスのリストをまとめました。
* 使用するモデルを選択する場合は、最新かつ最も機能的なモデルの方がパフォーマンスが向上する可能性があります。
* シンプルで短いプロンプトから始めて、そこから繰り返します。
* 指示はプロンプトの最初または最後に入力してください。大規模なコンテキストを扱う場合、モデルはさまざまな最適化を適用して、アテンションの複雑さが二次的に拡大するのを防ぎます。これにより、モデルはプロンプトの途中よりも最初または最後に注意を払うようになります。
* 指示と、それが適用されるテキストを明確に区別してください。これについては、次のセクションで詳しく説明します。
* タスクと望ましい結果 (その形式、長さ、スタイル、言語など) について具体的かつ説明的にします。
* 曖昧な説明や指示は避けてください。
*「何をしてはいけないか」という指示ではなく、「何をすべきか」という指示を優先します。
* 最初の単語を書いて (またはモデルの最初の文を始めて)、出力を正しい方向に「導き」ます。
* [Few-shot prompting](#few-shot-prompting) や [Chain-of-thought](#chain-of-thought) などの高度なテクニックを使用します。
* さまざまなモデルでプロンプトをテストして、その堅牢性を評価します。
* プロンプトのバージョンを確認し、パフォーマンスを追跡します。
## Advanced prompting techniques
### Few-shot prompting
上記のセクションの基本的なプロンプトは、「ゼロショット」プロンプトの例です。つまり、モデルにはすでに与えられています。
指示とコンテキストはありますが、解決策を含む例はありません。通常、命令データセットに基づいて微調整された LLM
このような「ゼロショット」タスクでも優れたパフォーマンスを発揮します。ただし、タスクがより複雑であったり微妙な点があったりする場合があります。
出力には、命令だけではモデルが理解できないいくつかの要件があります。この場合、次のことができます。
少数ショット プロンプトと呼ばれるテクニックを試してください。
少数ショット プロンプトでは、モデルにパフォーマンスを向上させるためのより多くのコンテキストを提供するプロンプト内の例が提供されます。
例では、例のパターンに従って出力を生成するようにモデルを条件付けします。
以下に例を示します。
```python
>>> torch.manual_seed(0) # doctest: +IGNORE_RESULT
>>> prompt = """Text: The first human went into space and orbited the Earth on April 12, 1961.
... Date: 04/12/1961
... Text: The first-ever televised presidential debate in the United States took place on September 28, 1960, between presidential candidates John F. Kennedy and Richard Nixon.
... Date:"""
>>> sequences = pipe(
... prompt,
... max_new_tokens=8,
... do_sample=True,
... top_k=10,
... )
>>> for seq in sequences:
... print(f"Result: {seq['generated_text']}")
Result: Text: The first human went into space and orbited the Earth on April 12, 1961.
Date: 04/12/1961
Text: The first-ever televised presidential debate in the United States took place on September 28, 1960, between presidential candidates John F. Kennedy and Richard Nixon.
Date: 09/28/1960
```
上記のコード スニペットでは、モデルへの目的の出力を示すために 1 つの例を使用しました。したがって、これは、
「ワンショット」プロンプト。ただし、タスクの複雑さに応じて、複数の例を使用する必要がある場合があります。
数回のプロンプト手法の制限:
- LLM は例のパターンを理解できますが、これらの手法は複雑な推論タスクではうまく機能しません。
- 少数ショットのプロンプトでは、長いプロンプトを作成する必要があります。大量のトークンを含むプロンプトでは、計算量と待ち時間が増加する可能性があります。プロンプトの長さにも制限があります。
- 多くの例を与えると、モデルが学習するつもりのなかったパターンを学習することがあります。 3番目の映画レビューはいつも否定的だということ。
### Chain-of-thought
思考連鎖 (CoT) プロンプトは、モデルを微調整して中間推論ステップを生成し、改善する手法です。
複雑な推論タスクの結果。
モデルを操作して推論ステップを生成するには、2 つの方法があります。
- 質問に対する詳細な回答を含む例を示し、問題に対処する方法をモデルに示すことで、数回のプロンプトを表示します。
- 「ステップごとに考えてみましょう」または「深呼吸して、問題をステップごとに解決してください」などのフレーズを追加してモデルに推論を指示します。
[推論セクション](#reasoning) のマフィンの例に CoT テクニックを適用し、より大きなモデルを使用すると、
[HuggingChat](https://huggingface.co/chat/)で遊べる(`tiiuae/falcon-180B-chat`)など、
推論結果は大幅に改善されます。
```text
Let's go through this step-by-step:
1. You start with 15 muffins.
2. You eat 2 muffins, leaving you with 13 muffins.
3. You give 5 muffins to your neighbor, leaving you with 8 muffins.
4. Your partner buys 6 more muffins, bringing the total number of muffins to 14.
5. Your partner eats 2 muffins, leaving you with 12 muffins.
Therefore, you now have 12 muffins.
```
## Prompting vs fine-tuning
プロンプトを最適化することで優れた結果を達成できますが、モデルを微調整するかどうかについてはまだ思案するかもしれません。
あなたの場合にはもっとうまくいくでしょう。より小規模なモデルを微調整することが好ましいオプションである場合のいくつかのシナリオを次に示します。
- ドメインが LLM が事前にトレーニングされたものと大きく異なっており、広範なプロンプト最適化では十分な結果が得られませんでした。
- モデルが低リソース言語で適切に動作する必要があります。
- 厳格な規制の下にある機密データでモデルをトレーニングする必要があります。
- コスト、プライバシー、インフラストラクチャ、またはその他の制限により、小規模なモデルを使用する必要があります。
上記のすべての例で、十分な大きさのファイルをすでに持っているか、簡単に入手できるかを確認する必要があります。
ドメイン固有のデータセットを合理的なコストでモデルを微調整できます。十分な時間とリソースも必要になります
モデルを微調整します。
上記の例が当てはまらない場合は、プロンプトを最適化する方が有益であることがわかります。
| transformers/docs/source/ja/tasks/prompting.md/0 | {
"file_path": "transformers/docs/source/ja/tasks/prompting.md",
"repo_id": "transformers",
"token_count": 9975
} | 265 |
<!--Copyright 2023 The HuggingFace Team. All rights reserved.
Licensed under the Apache License, Version 2.0 (the "License"); you may not use this file except in compliance with
the License. You may obtain a copy of the License at
http://www.apache.org/licenses/LICENSE-2.0
Unless required by applicable law or agreed to in writing, software distributed under the License is distributed on
an "AS IS" BASIS, WITHOUT WARRANTIES OR CONDITIONS OF ANY KIND, either express or implied. See the License for the
specific language governing permissions and limitations under the License.
⚠️ Note that this file is in Markdown but contain specific syntax for our doc-builder (similar to MDX) that may not be
rendered properly in your Markdown viewer.
-->
# Summary of the tokenizers
[[open-in-colab]]
このページでは、トークナイゼーションについて詳しく見ていきます。
<Youtube id="VFp38yj8h3A"/>
[前処理のチュートリアル](preprocessing)で見たように、テキストをトークン化することは、それを単語またはサブワードに分割し、それらをルックアップテーブルを介してIDに変換することです。単語またはサブワードをIDに変換することは簡単ですので、この要約ではテキストを単語またはサブワードに分割する(つまり、テキストをトークナイズする)ことに焦点を当てます。具体的には、🤗 Transformersで使用される3つの主要なトークナイザ、[Byte-Pair Encoding(BPE)](#byte-pair-encoding)、[WordPiece](#wordpiece)、および[SentencePiece](#sentencepiece)を見て、どのモデルがどのトークナイザタイプを使用しているかの例を示します。
各モデルページでは、事前トレーニング済みモデルがどのトークナイザタイプを使用しているかを知るために、関連するトークナイザのドキュメントを確認できます。例えば、[`BertTokenizer`]を見ると、モデルが[WordPiece](#wordpiece)を使用していることがわかります。
## Introduction
テキストをより小さなチャンクに分割することは、見かけ以上に難しいタスクであり、複数の方法があります。例えば、次の文を考えてみましょう。「"Don't you love 🤗 Transformers? We sure do."」
<Youtube id="nhJxYji1aho"/>
このテキストをトークン化する簡単な方法は、スペースで分割することです。これにより、以下のようになります:
```
["Don't", "you", "love", "🤗", "Transformers?", "We", "sure", "do."]
```
これは合理的な第一歩ですが、トークン "Transformers?" と "do." を見ると、句読点が単語 "Transformer" と "do" に結合されていることがわかり、これは最適ではありません。句読点を考慮に入れるべきで、モデルが単語とそれに続く可能性のあるすべての句読点記号の異なる表現を学ばなければならないことを避けるべきです。これにより、モデルが学ばなければならない表現の数が爆発的に増加します。句読点を考慮に入れた場合、例文のトークン化は次のようになります:
```
["Don", "'", "t", "you", "love", "🤗", "Transformers", "?", "We", "sure", "do", "."]
```
ただし、単語「"Don't"」をトークン化する方法に関しては、不利な側面があります。 「"Don't"」は「"do not"」を表しているため、「["Do", "n't"]」としてトークン化する方が適しています。ここから事柄が複雑になり、各モデルが独自のトークナイザータイプを持つ理由の一部でもあります。テキストをトークン化するために適用するルールに応じて、同じテキストに対して異なるトークナイズされた出力が生成されます。事前トレーニング済みモデルは、トレーニングデータをトークナイズするのに使用されたルールと同じルールでトークナイズされた入力を提供する場合にのみ正常に機能します。
[spaCy](https://spacy.io/)と[Moses](http://www.statmt.org/moses/?n=Development.GetStarted)は、2つの人気のあるルールベースのトークナイザーです。これらを私たちの例に適用すると、*spaCy*と*Moses*は次のような出力を生成します:
```
["Do", "n't", "you", "love", "🤗", "Transformers", "?", "We", "sure", "do", "."]
```
空白と句読点のトークン化、およびルールベースのトークン化が使用されていることがわかります。空白と句読点のトークン化、およびルールベースのトークン化は、文を単語に分割することをゆるやかに定義される単語トークン化の例です。テキストをより小さなチャンクに分割するための最も直感的な方法である一方、このトークン化方法は大規模なテキストコーパスに対して問題を引き起こすことがあります。この場合、空白と句読点のトークン化は通常、非常に大きな語彙(すべての一意な単語とトークンのセット)を生成します。例えば、[Transformer XL](model_doc/transformerxl)は空白と句読点のトークン化を使用しており、語彙サイズは267,735です!
このような大きな語彙サイズは、モデルに非常に大きな埋め込み行列を入力および出力レイヤーとして持たせることを強制し、メモリおよび時間の複雑さの増加を引き起こします。一般的に、トランスフォーマーモデルは、特に単一の言語で事前トレーニングされた場合、50,000を超える語彙サイズを持つことはほとんどありません。
したがって、シンプルな空白と句読点のトークン化が不十分な場合、なぜ単に文字単位でトークン化しないのかという疑問が生じますか?
<Youtube id="ssLq_EK2jLE"/>
文字単位のトークン化は非常にシンプルであり、メモリと時間の複雑さを大幅に削減できますが、モデルに意味のある入力表現を学習させることが非常に難しくなります。たとえば、文字「"t"」のための意味のあるコンテキスト独立の表現を学習することは、単語「"today"」のためのコンテキスト独立の表現を学習するよりもはるかに難しいです。そのため、文字単位のトークン化はしばしばパフォーマンスの低下を伴います。したがって、トランスフォーマーモデルは単語レベルと文字レベルのトークン化のハイブリッドである**サブワード**トークン化を使用して、両方の世界の利点を活かします。
## Subword tokenization
<Youtube id="zHvTiHr506c"/>
サブワードトークン化アルゴリズムは、頻繁に使用される単語をより小さなサブワードに分割すべきではないが、珍しい単語は意味のあるサブワードに分解されるという原則に依存しています。たとえば、「"annoyingly"」は珍しい単語と見なされ、その単語は「"annoying"」と「"ly"」に分解されるかもしれません。独立した「"annoying"」と「"ly"」はより頻繁に現れますが、「"annoyingly"」の意味は「"annoying"」と「"ly"」の合成的な意味によって保持されます。これは特にトルコ語などの結合言語で役立ちます。ここではサブワードを連結して(ほぼ)任意の長い複雑な単語を形成できます。
サブワードトークン化により、モデルは合理的な語彙サイズを持つことができ、意味のあるコンテキスト独立の表現を学習できます。さらに、サブワードトークン化により、モデルは以前に見たことのない単語を処理し、それらを既知のサブワードに分解することができます。例えば、[`~transformers.BertTokenizer`]は`"I have a new GPU!"`を以下のようにトークン化します:
```py
>>> from transformers import BertTokenizer
>>> tokenizer = BertTokenizer.from_pretrained("google-bert/bert-base-uncased")
>>> tokenizer.tokenize("I have a new GPU!")
["i", "have", "a", "new", "gp", "##u", "!"]
```
「uncased」モデルを考慮しているため、まず文を小文字に変換しました。トークナイザの語彙に「["i", "have", "a", "new"]」という単語が存在することがわかりますが、「"gpu"」という単語は存在しません。したがって、トークナイザは「"gpu"」を既知のサブワード「["gp"、"##u"]」に分割します。ここで「"##"」は、トークンのデコードまたはトークナイゼーションの逆転のために、トークンの前の部分にスペースなしで接続する必要があることを意味します。
別の例として、[`~transformers.XLNetTokenizer`]は以下のように以前のサンプルテキストをトークン化します:
```py
>>> from transformers import XLNetTokenizer
>>> tokenizer = XLNetTokenizer.from_pretrained("xlnet/xlnet-base-cased")
>>> tokenizer.tokenize("Don't you love 🤗 Transformers? We sure do.")
["▁Don", "'", "t", "▁you", "▁love", "▁", "🤗", "▁", "Transform", "ers", "?", "▁We", "▁sure", "▁do", "."]
```
これらの「▁」の意味については、[SentencePiece](#sentencepiece)を見るときに詳しく説明します。ご覧の通り、「Transformers」という珍しい単語は、より頻繁に現れるサブワード「Transform」と「ers」に分割されています。
さて、異なるサブワードトークン化アルゴリズムがどのように動作するかを見てみましょう。これらのトークナイゼーションアルゴリズムはすべて、通常は対応するモデルがトレーニングされるコーパスで行われる形式のトレーニングに依存しています。
<a id='byte-pair-encoding'></a>
### Byte-Pair Encoding(BPE)
Byte-Pair Encoding(BPE)は、[Neural Machine Translation of Rare Words with Subword Units(Sennrich et al., 2015)](https://arxiv.org/abs/1508.07909)で導入されました。BPEは、トレーニングデータを単語に分割するプリトークナイザに依存しています。プリトークナイゼーションは、空白のトークナイゼーションなど、非常に単純なものであることがあります。例えば、[GPT-2](model_doc/gpt2)、[RoBERTa](model_doc/roberta)です。より高度なプリトークナイゼーションには、ルールベースのトークナイゼーション([XLM](model_doc/xlm)、[FlauBERT](model_doc/flaubert)などが大部分の言語にMosesを使用)や、[GPT](model_doc/gpt)(Spacyとftfyを使用してトレーニングコーパス内の各単語の頻度を数える)などが含まれます。
プリトークナイゼーションの後、一意の単語セットが作成され、各単語がトレーニングデータで出現した頻度が決定されます。次に、BPEはベース語彙を作成し、ベース語彙の二つのシンボルから新しいシンボルを形成するためのマージルールを学習します。このプロセスは、語彙が所望の語彙サイズに達するまで続けられます。なお、所望の語彙サイズはトークナイザをトレーニングする前に定義するハイパーパラメータであることに注意してください。
例として、プリトークナイゼーションの後、次のセットの単語とその出現頻度が決定されたと仮定しましょう:
```
("hug", 10), ("pug", 5), ("pun", 12), ("bun", 4), ("hugs", 5)
```
したがって、ベース語彙は「["b", "g", "h", "n", "p", "s", "u"]」です。すべての単語をベース語彙のシンボルに分割すると、次のようになります:
```
("h" "u" "g", 10), ("p" "u" "g", 5), ("p" "u" "n", 12), ("b" "u" "n", 4), ("h" "u" "g" "s", 5)
```
その後、BPEは可能なすべてのシンボルペアの頻度を数え、最も頻繁に発生するシンボルペアを選択します。上記の例では、`"h"`の後に`"u"`が15回(`"hug"`の10回、`"hugs"`の5回)出現します。しかし、最も頻繁なシンボルペアは、合計で20回(`"u"`の10回、`"g"`の5回、`"u"`の5回)出現する`"u"`の後に`"g"`が続くシンボルペアです。したがって、トークナイザが最初に学習するマージルールは、`"u"`の後に`"g"`が続くすべての`"u"`シンボルを一緒にグループ化することです。次に、`"ug"`が語彙に追加されます。単語のセットは次になります:
```
("h" "ug", 10), ("p" "ug", 5), ("p" "u" "n", 12), ("b" "u" "n", 4), ("h" "ug" "s", 5)
```
次に、BPEは次に最も一般的なシンボルペアを識別します。それは「"u"」に続いて「"n"」で、16回出現します。したがって、「"u"」と「"n"」は「"un"」に結合され、語彙に追加されます。次に最も頻度の高いシンボルペアは、「"h"」に続いて「"ug"」で、15回出現します。再びペアが結合され、「hug」が語彙に追加できます。
この段階では、語彙は`["b", "g", "h", "n", "p", "s", "u", "ug", "un", "hug"]`であり、一意の単語のセットは以下のように表されます:
```
("hug", 10), ("p" "ug", 5), ("p" "un", 12), ("b" "un", 4), ("hug" "s", 5)
```
前提として、Byte-Pair Encoding(BPE)のトレーニングがこの段階で停止すると、学習されたマージルールが新しい単語に適用されます(新しい単語にはベースボキャブラリに含まれていないシンボルが含まれていない限り)。 例えば、単語 "bug" は ["b", "ug"] としてトークン化されますが、"mug" はベースボキャブラリに "m" シンボルが含まれていないため、["<unk>", "ug"] としてトークン化されます。 一般的に、"m" のような単一の文字は、トレーニングデータには通常、各文字の少なくとも1つの出現が含まれているため、"<unk>" シンボルに置き換えられることはありませんが、絵文字のような非常に特殊な文字の場合には発生する可能性があります。
前述のように、ボキャブラリサイズ、すなわちベースボキャブラリサイズ + マージの回数は選択するハイパーパラメータです。 例えば、[GPT](model_doc/gpt) はベース文字が478文字で、40,000回のマージ後にトレーニングを停止したため、ボキャブラリサイズは40,478です。
#### Byte-level BPE
すべてのUnicode文字をベース文字と考えると、すべての可能なベース文字が含まれるかもしれないベースボキャブラリはかなり大きくなることがあります。 [GPT-2](https://cdn.openai.com/better-language-models/language_models_are_unsupervised_multitask_learners.pdf) は、ベースボキャブラリを256バイトにする賢いトリックとしてバイトをベースボキャブラリとして使用し、すべてのベース文字がボキャブラリに含まれるようにしています。 パンクチュエーションを扱うためのいくつかの追加ルールを備えたGPT2のトークナイザは、<unk> シンボルを必要とせずにすべてのテキストをトークン化できます。 [GPT-2](model_doc/gpt) は50,257のボキャブラリサイズを持っており、これは256バイトのベーストークン、特別なテキストの終了を示すトークン、および50,000回のマージで学習したシンボルに対応しています。
### WordPiece
WordPieceは、[BERT](model_doc/bert)、[DistilBERT](model_doc/distilbert)、および[Electra](model_doc/electra)で使用されるサブワードトークナイゼーションアルゴリズムです。 このアルゴリズムは、[Japanese and Korean Voice Search (Schuster et al., 2012)](https://static.googleusercontent.com/media/research.google.com/ja//pubs/archive/37842.pdf) で概説されており、BPEに非常に似ています。 WordPieceは最も頻繁なシンボルペアを選択するのではなく、トレーニングデータに追加した場合にトレーニングデータの尤度を最大化するシンボルペアを選択します。
これは具体的にはどういう意味ですか?前の例を参照すると、トレーニングデータの尤度を最大化することは、そのシンボルペアの確率をその最初のシンボルに続く2番目のシンボルの確率で割ったものが、すべてのシンボルペアの中で最も大きい場合に該当するシンボルペアを見つけることに等しいです。 たとえば、"u" の後に "g" が続く場合、他のどのシンボルペアよりも "ug" の確率を "u"、"g" で割った確率が高ければ、それらのシンボルは結合されます。直感的に言えば、WordPieceは2つのシンボルを結合することによって失われるものを評価し、それがそれに値するかどうかを確認する点でBPEとはわずかに異なります。
### Unigram
Unigramは、[Subword Regularization: Improving Neural Network Translation Models with Multiple Subword Candidates (Kudo, 2018)](https://arxiv.org/pdf/1804.10959.pdf) で導入されたサブワードトークナイゼーションアルゴリズムです。 BPEやWordPieceとは異なり、Unigramはベースボキャブラリを多数のシンボルで初期化し、各シンボルを削減してより小さなボキャブラリを取得します。 ベースボキャブラリは、事前にトークン化されたすべての単語と最も一般的な部分文字列に対応する可能性があります。 Unigramはtransformersのモデルの直接の使用には適していませんが、[SentencePiece](#sentencepiece)と組み合わせて使用されます。
各トレーニングステップで、Unigramアルゴリズムは現在のボキャブラリとユニグラム言語モデルを使用してトレーニングデータ上の損失(通常は対数尤度として定義)を定義します。その後、ボキャブラリ内の各シンボルについて、そのシンボルがボキャブラリから削除された場合に全体の損失がどれだけ増加するかを計算します。 Unigramは、損失の増加が最も低いp(通常は10%または20%)パーセントのシンボルを削除します。つまり、トレーニングデータ全体の損失に最も影響を与えない、最も損失の少ないシンボルを削除します。 このプロセスは、ボキャブラリが望ましいサイズに達するまで繰り返されます。 Unigramアルゴリズムは常にベース文字を保持するため、任意の単語をトークン化できます。
Unigramはマージルールに基づいていないため(BPEとWordPieceとは対照的に)、トレーニング後の新しいテキストのトークン化にはいくつかの方法があります。例として、トレーニングされたUnigramトークナイザが持つボキャブラリが次のような場合:
```
["b", "g", "h", "n", "p", "s", "u", "ug", "un", "hug"],
```
`"hugs"`は、`["hug", "s"]`、`["h", "ug", "s"]`、または`["h", "u", "g", "s"]`のようにトークン化できます。では、どれを選択すべきでしょうか? Unigramは、トレーニングコーパス内の各トークンの確率を保存し、トレーニング後に各可能なトークン化の確率を計算できるようにします。このアルゴリズムは実際には最も可能性の高いトークン化を選択しますが、確率に従って可能なトークン化をサンプリングするオプションも提供します。
これらの確率は、トークナイザーがトレーニングに使用する損失によって定義されます。トレーニングデータが単語 \\(x_{1}, \dots, x_{N}\\) で構成され、単語 \\(x_{i}\\) のすべての可能なトークン化のセットが \\(S(x_{i})\\) と定義される場合、全体の損失は次のように定義されます。
$$\mathcal{L} = -\sum_{i=1}^{N} \log \left ( \sum_{x \in S(x_{i})} p(x) \right )$$
<a id='sentencepiece'></a>
### SentencePiece
これまでに説明したすべてのトークン化アルゴリズムには同じ問題があります。それは、入力テキストが単語を区切るためにスペースを使用していると仮定しているということです。しかし、すべての言語が単語を区切るためにスペースを使用しているわけではありません。この問題を一般的に解決するための1つの方法は、言語固有の前トークナイザーを使用することです(例:[XLM](model_doc/xlm)は特定の中国語、日本語、およびタイ語の前トークナイザーを使用しています)。より一般的にこの問題を解決するために、[SentencePiece:ニューラルテキスト処理のためのシンプルで言語非依存のサブワードトークナイザーおよびデトークナイザー(Kudo et al.、2018)](https://arxiv.org/pdf/1808.06226.pdf) は、入力を生の入力ストリームとして扱い、スペースを使用する文字のセットに含めます。それからBPEまたはunigramアルゴリズムを使用して適切な語彙を構築します。
たとえば、[`XLNetTokenizer`]はSentencePieceを使用しており、そのために前述の例で`"▁"`文字が語彙に含まれていました。SentencePieceを使用したデコードは非常に簡単で、すべてのトークンを単純に連結し、`"▁"`はスペースに置換されます。
ライブラリ内のすべてのtransformersモデルは、SentencePieceをunigramと組み合わせて使用します。SentencePieceを使用するモデルの例には、[ALBERT](model_doc/albert)、[XLNet](model_doc/xlnet)、[Marian](model_doc/marian)、および[T5](model_doc/t5)があります。
| transformers/docs/source/ja/tokenizer_summary.md/0 | {
"file_path": "transformers/docs/source/ja/tokenizer_summary.md",
"repo_id": "transformers",
"token_count": 9819
} | 266 |
<!---
Copyright 2020 The HuggingFace Team. All rights reserved.
Licensed under the Apache License, Version 2.0 (the "License");
you may not use this file except in compliance with the License.
You may obtain a copy of the License at
http://www.apache.org/licenses/LICENSE-2.0
Unless required by applicable law or agreed to in writing, software
distributed under the License is distributed on an "AS IS" BASIS,
WITHOUT WARRANTIES OR CONDITIONS OF ANY KIND, either express or implied.
See the License for the specific language governing permissions and
limitations under the License.
-->
# 🤗 Transformers에 기여하기 [[contribute-to-transformers]]
누구나 🤗 Transformers에 기여할 수 있으며, 우리는 모든 사람의 기여를 소중히 생각합니다. 코드 기여는 커뮤니티를 돕는 유일한 방법이 아닙니다. 질문에 답하거나 다른 사람을 도와 문서를 개선하는 것도 매우 가치가 있습니다.
🤗 Transformers를 널리 알리는 것도 큰 도움이 됩니다! 멋진 프로젝트들을 가능하게 한 🤗 Transformers 라이브러리에 대해 블로그 게시글에 언급하거나, 도움이 되었을 때마다 Twitter에 알리거나, 저장소에 ⭐️ 를 표시하여 감사 인사를 전해주세요.
어떤 방식으로 기여하든 [행동 규칙](https://github.com/huggingface/transformers/blob/main/CODE_OF_CONDUCT.md)을 숙지하고 존중해주세요.
**이 안내서는 멋진 [scikit-learn 기여 안내서](https://github.com/scikit-learn/scikit-learn/blob/main/CONTRIBUTING.md)에서 큰 영감을 받았습니다.**
## 기여하는 방법 [[ways-to-contribute]]
여러 가지 방법으로 🤗 Transformers에 기여할 수 있습니다:
* 기존 코드의 미해결된 문제를 수정합니다.
* 버그 또는 새로 추가되길 원하는 기능과 관련된 이슈를 제출합니다.
* 새로운 모델을 구현합니다.
* 예제나 문서에 기여합니다.
어디서부터 시작할지 모르겠다면, [Good First Issue](https://github.com/huggingface/transformers/contribute) 목록을 확인해보세요. 이 목록은 초보자도 참여하기 쉬운 오픈 이슈 목록을 제공하며, 당신이 오픈소스에 처음으로 기여하는 데 큰 도움이 될 것입니다. 그저 작업하고 싶은 이슈에 댓글만 달아주면 됩니다.
조금 더 도전적인 작업을 원한다면, [Good Second Issue](https://github.com/huggingface/transformers/labels/Good%20Second%20Issue) 목록도 확인해보세요. 이미 당신이 잘 하고 있다고 생각되더라도, 한 번 시도해보세요! 우리도 여러분을 도울 것입니다. 🚀
> 커뮤니티에 이루어지는 모든 기여는 똑같이 소중합니다. 🥰
## 미해결된 문제 수정하기 [[fixing-outstanding-issues]]
기존 코드에서 발견한 문제점에 대한 해결책이 떠오른 경우, 언제든지 [기여를 시작](https://github.com/huggingface/transformers/blob/main/CONTRIBUTING.md/#create-a-pull-request)하고 Pull Request를 생성해주세요!
## 버그 관련 이슈를 제기하거나 새로운 기능 요청하기 [[submitting-a-bugrelated-issue-or-feature-request]]
버그 관련 이슈를 제기하거나 새로운 기능을 요청할 때는 다음 가이드라인을 최대한 준수해주세요. 이렇게 하면 좋은 피드백과 함께 빠르게 답변해 드릴 수 있습니다.
### 버그를 발견하셨나요? [[did-you-find-a-bug]]
🤗 Transformers 라이브러리는 사용 중에 겪는 문제를 보고해주는 사용자들 덕분에 더욱 견고해지고 신뢰할 수 있게 되었습니다.
이슈를 보고하기 전에, 버그가 이미 **보고되지 않았는지** 확인해주세요. (GitHub의 이슈 탭 아래의 검색 바를 사용하세요). 이슈는 라이브러리 자체에서 발생한 버그어야 하며, 코드의 다른 부분과 관련된 것이 아니어야 합니다. 버그가 라이브러리의 문제로 발생하였는지 확실하지 않은 경우 먼저 [포럼](https://discuss.huggingface.co/)에서 질문해 주세요. 이렇게 하면 일반적인 질문보다 라이브러리와 관련된 문제를 더 빠르게 해결할 수 있습니다.
버그가 이미 보고되지 않았다는 것을 확인했다면, 다음 정보를 포함하여 이슈를 제출해 주세요. 그러면 우리가 빠르게 해결할 수 있습니다:
* 사용 중인 **운영체제 종류와 버전**, 그리고 **Python**, **PyTorch** 또는 **TensorFlow** 버전.
* 버그를 30초 이내로 재현할 수 있는 간단하고 독립적인 코드 스니펫.
* 예외가 발생한 경우 *전체* 트레이스백.
* 스크린샷과 같이 도움이 될 것으로 생각되는 추가 정보를 첨부해 주세요.
운영체제와 소프트웨어 버전을 자동으로 가져오려면 다음 명령을 실행하세요:
```bash
transformers-cli env
```
저장소의 루트 디렉터리에서도 같은 명령을 실행할 수 있습니다:
```bash
python src/transformers/commands/transformers_cli.py env
```
### 새로운 기능을 원하시나요? [[do-you-want-a-new-feature]]
🤗 Transformers에서 사용하고 싶은 새로운 기능이 있다면, 다음 내용을 포함하여 이슈를 제출해 주세요:
1. 이 기능이 필요한 *이유*는 무엇인가요? 라이브러리에 대한 문제나 불만과 관련이 있나요? 프로젝트에 필요한 기능인가요? 커뮤니티에 도움이 될 만한 기능인가요?
어떤 내용이든 여러분의 이야기를 듣고 싶습니다!
2. 요청하는 기능을 최대한 자세히 설명해 주세요. 더 많은 정보를 제공할수록 더 나은 도움을 드릴 수 있습니다.
3. 해당 기능의 사용법을 보여주는 *코드 스니펫*을 제공해 주세요.
4. 기능과 관련된 논문이 있는 경우 링크를 포함해 주세요.
이슈가 잘 작성되었다면 이슈가 생성된 순간, 이미 80% 정도의 작업이 완료된 것입니다.
이슈를 제기하는 데 도움이 될 만한 [템플릿](https://github.com/huggingface/transformers/tree/main/templates)도 준비되어 있습니다.
## 새로운 모델을 구현하고 싶으신가요? [[do-you-want-to-implement-a-new-model]]
새로운 모델은 계속해서 출시됩니다. 만약 여러분이 새로운 모델을 구현하고 싶다면 다음 정보를 제공해 주세요:
* 모델에 대한 간단한 설명과 논문 링크.
* 구현이 공개되어 있다면 구현 링크.
* 모델 가중치가 사용 가능하다면 가중치 링크.
만약 모델을 직접 기여하고 싶으시다면, 알려주세요. 🤗 Transformers에 추가할 수 있도록 도와드리겠습니다!
새로운 모델을 추가하는 방법에 대한 [상세 안내서와 템플릿](https://github.com/huggingface/transformers/tree/main/templates)을 제공하고 있으며, [🤗 Transformers에 새로운 모델을 추가하는 방법](https://huggingface.co/docs/transformers/add_new_model)에 대한 기술적인 안내서도 있습니다.
## 문서를 추가하고 싶으신가요? [[do-you-want-to-add-documentation]]
우리는 언제나 더 명확하고 정확한 문서를 제공하기 위하여 개선점을 찾고 있습니다. 오탈자나 부족한 내용, 분명하지 않거나 부정확한 내용 등을 알려주시면 개선하는 데 도움이 됩니다. 관심이 있으시다면 변경하거나 기여하실 수 있도록 도와드리겠습니다!
문서를 생성, 빌드 및 작성하는 방법에 대한 자세한 내용은 [README](https://github.com/huggingface/transformers/tree/main/docs) 문서를 확인해 주세요.
## 풀 리퀘스트(Pull Request) 생성하기 [[create-a-pull-request]]
코드를 작성하기 전에 기존의 Pull Request나 이슈를 검색하여 누군가 이미 동일한 작업을 하고 있는지 확인하는 것이 좋습니다. 확실하지 않다면 피드백을 받기 위해 이슈를 열어보는 것이 좋습니다.
🤗 Transformers에 기여하기 위해서는 기본적인 `git` 사용 능력이 필요합니다. `git`은 사용하기 쉬운 도구는 아니지만, 매우 훌륭한 매뉴얼을 제공합니다. 쉘(shell)에서 `git --help`을 입력하여 확인해보세요! 만약 책을 선호한다면, [Pro Git](https://git-scm.com/book/en/v2)은 매우 좋은 참고 자료가 될 것입니다.
🤗 Transformers에 기여하려면 **[Python 3.8](https://github.com/huggingface/transformers/blob/main/setup.py#L426)** 이상의 버전이 필요합니다. 기여를 시작하려면 다음 단계를 따르세요:
1. 저장소 페이지에서 **[Fork](https://github.com/huggingface/transformers/fork)** 버튼을 클릭하여 저장소를 포크하세요. 이렇게 하면 코드의 복사본이 여러분의 GitHub 사용자 계정 아래에 생성됩니다.
2. 포크한 저장소를 로컬 디스크로 클론하고, 기본 저장소를 원격(remote)으로 추가하세요:
```bash
git clone [email protected]:<your Github handle>/transformers.git
cd transformers
git remote add upstream https://github.com/huggingface/transformers.git
```
3. 개발 변경 사항을 저장할 새 브랜치를 생성하세요:
```bash
git checkout -b a-descriptive-name-for-my-changes
```
🚨 절대 `main` 브랜치에서 작업하지 **마세요!**
4. 가상 환경에서 다음 명령을 실행하여 개발 환경을 설정하세요:
```bash
pip install -e ".[dev]"
```
만약 이미 가상 환경에 🤗 Transformers가 설치되어 있다면, `-e` 플래그를 사용하여 설치하기 전에 `pip uninstall transformers`로 제거해주세요.
여러분의 운영체제에 따라서, 그리고 🤗 Transformers의 선택적 의존성의 수가 증가하면서, 이 명령이 실패할 수도 있습니다. 그럴 경우 사용하려는 딥러닝 프레임워크(PyTorch, TensorFlow, 그리고/또는 Flax)를 설치한 후 아래 명령을 실행해주세요:
```bash
pip install -e ".[quality]"
```
대부분의 경우 이것으로 충분할 것입니다.
5. 브랜치에서 기능을 개발하세요.
코드를 작업하는 동안 테스트 스위트(test suite)가 통과하는지 확인하세요. 다음과 같이 변경 사항에 영향을 받는 테스트를 실행하세요:
```bash
pytest tests/<TEST_TO_RUN>.py
```
테스트에 대한 더 많은 정보는 [테스트](https://huggingface.co/docs/transformers/testing) 가이드를 확인하세요.
🤗 Transformers는 `black`과 `ruff`를 사용하여 소스 코드의 형식을 일관되게 유지합니다. 변경 사항을 적용한 후에는 다음 명령으로 자동으로 스타일 교정 및 코드 검증을 수행하세요:
```bash
make fixup
```
이것은 또한 작업 중인 PR에서 수정한 파일에서만 작동하도록 최적화되어 있습니다.
검사를 하나씩 실행하려는 경우, 다음 명령으로 스타일 교정을 적용할 수 있습니다:
```bash
make style
```
🤗 Transformers는 또한 `ruff`와 몇 가지 사용자 정의 스크립트를 사용하여 코딩 실수를 확인합니다. CI를 통해 품질 관리가 수행되지만, 다음 명령으로 동일한 검사를 실행할 수 있습니다:
```bash
make quality
```
마지막으로, 새 모델을 추가할 때 일부 파일을 업데이트하는 것을 잊지 않도록 하기 위한 많은 스크립트가 있습니다. 다음 명령으로 이러한 스크립트를 실행할 수 있습니다:
```bash
make repo-consistency
```
이러한 검사에 대해 자세히 알아보고 관련 문제를 해결하는 방법은 [Pull Request에 대한 검사](https://huggingface.co/docs/transformers/pr_checks) 가이드를 확인하세요.
만약 `docs/source` 디렉터리 아래의 문서를 수정하는 경우, 문서가 빌드될 수 있는지 확인하세요. 이 검사는 Pull Request를 열 때도 CI에서 실행됩니다. 로컬 검사를 실행하려면 문서 빌더를 설치해야 합니다:
```bash
pip install ".[docs]"
```
저장소의 루트 디렉터리에서 다음 명령을 실행하세요:
```bash
doc-builder build transformers docs/source/en --build_dir ~/tmp/test-build
```
이 명령은 `~/tmp/test-build` 폴더에 문서를 빌드하며, 생성된 Markdown 파일을 선호하는 편집기로 확인할 수 있습니다. Pull Request를 열 때 GitHub에서 문서를 미리 볼 수도 있습니다.
변경 사항에 만족하면 `git add`로 변경된 파일을 추가하고, `git commit`으로 변경 사항을 로컬에 기록하세요:
```bash
git add modified_file.py
git commit
```
[좋은 커밋 메시지](https://chris.beams.io/posts/git-commit/)를 작성하여 변경 사항을 명확하게 전달하세요!
변경 사항을 프로젝트 원본 저장소와 동기화하려면, PR을 *열기 전에* 브랜치를 `upstream/branch`로 리베이스(rebase)하세요. 또는 관리자의 요청에 이 작업이 필요할 수 있습니다:
```bash
git fetch upstream
git rebase upstream/main
```
변경 사항을 브랜치에 푸시하세요:
```bash
git push -u origin a-descriptive-name-for-my-changes
```
이미 PR을 열었다면, `--force` 플래그와 함께 강제 푸시해야 합니다. 아직 PR이 열리지 않았다면 정상적으로 변경 사항을 푸시하면 됩니다.
6. 이제 GitHub에서 포크한 저장소로 이동하고 **Pull request(풀 리퀘스트)**를 클릭하여 Pull Request를 열 수 있습니다. 아래의 [체크리스트](https://github.com/huggingface/transformers/blob/main/CONTRIBUTING.md/#pull-request-checklist)에서 모든 항목에 체크 표시를 하세요. 준비가 완료되면 프로젝트 관리자에게 변경 사항을 보내 검토를 요청할 수 있습니다.
7. 관리자가 변경 사항을 요청해도 괜찮습니다. 핵심 기여자들도 동일한 상황을 겪습니다! 모두가 변경 사항을 Pull Request에서 볼 수 있도록, 로컬 브랜치에서 작업하고 변경 사항을 포크한 저장소로 푸시하세요. 그러면 변경 사항이 자동으로 Pull Request에 나타납니다.
### Pull Request 체크리스트 [[pull-request-checklist]]
☐ Pull Request 제목은 기여 내용을 요약해야 합니다.<br>
☐ Pull Request가 이슈를 해결하는 경우, Pull Request 설명에 이슈 번호를 언급하여 연관되어 있음을 알려주세요. (이슈를 확인하는 사람들이 해당 이슈에 대한 작업이 진행 중임을 알 수 있게 합니다).<br>
☐ 작업이 진행중이라면 제목 앞에 `[WIP]`를 붙여주세요. 중복 작업을 피하고 병합할 준비가 된 PR과 구분하기에 유용합니다.<br>
☐ 기존 테스트를 통과하는지 확인하세요.<br>
☐ 새로운 기능을 추가하는 경우, 해당 기능에 대한 테스트도 추가하세요.<br>
- 새 모델을 추가하는 경우, `ModelTester.all_model_classes = (MyModel, MyModelWithLMHead,...)`을 사용하여 일반적인 테스트를 활성화하세요.
- 새 `@slow` 테스트를 추가하는 경우, 다음 명령으로 테스트를 통과하는지 확인하세요: `RUN_SLOW=1 python -m pytest tests/models/my_new_model/test_my_new_model.py`.
- 새 토크나이저를 추가하는 경우, 테스트를 작성하고 다음 명령으로 테스트를 통과하는지 확인하세요: `RUN_SLOW=1 python -m pytest tests/models/{your_model_name}/test_tokenization_{your_model_name}.py`.
- CircleCI에서는 느린 테스트를 실행하지 않지만, GitHub Actions에서는 매일 밤 실행됩니다!<br>
☐ 모든 공개 메소드는 유용한 기술문서를 가져야 합니다 (예를 들어 [`modeling_bert.py`](https://github.com/huggingface/transformers/blob/main/src/transformers/models/bert/modeling_bert.py) 참조).<br>
☐ 저장소가 빠르게 성장하고 있으므로 저장소에 상당한 부담을 주는 이미지, 동영상 및 기타 텍스트가 아닌 파일은 추가하지 마세요. 대신 [`hf-internal-testing`](https://huggingface.co/hf-internal-testing)과 같은 Hub 저장소를 사용하여 이러한 파일을 호스팅하고 URL로 참조하세요. 문서와 관련된 이미지는 다음 저장소에 배치하는 것을 권장합니다: [huggingface/documentation-images](https://huggingface.co/datasets/huggingface/documentation-images). 이 데이터셋 저장소에서 PR을 열어서 Hugging Face 멤버에게 병합을 요청할 수 있습니다.
Pull Request에서 실행되는 검사에 대한 자세한 정보는 [Pull Request에 대한 검사](https://huggingface.co/docs/transformers/pr_checks) 가이드를 확인하세요.
### 테스트 [[tests]]
라이브러리 동작과 여러 예제를 테스트할 수 있는 광범위한 테스트 스위트가 포함되어 있습니다. 라이브러리 테스트는 [tests](https://github.com/huggingface/transformers/tree/main/tests) 폴더에, 예제 테스트는 [examples](https://github.com/huggingface/transformers/tree/main/examples) 폴더에 있습니다.
속도가 빠른 `pytest`와 `pytest-xdist`를 선호합니다. 저장소의 루트 디렉터리에서 테스트를 실행할 *하위 폴더 경로 또는 테스트 파일 경로*를 지정하세요:
```bash
python -m pytest -n auto --dist=loadfile -s -v ./tests/models/my_new_model
```
마찬가지로 `examples` 디렉터리에서도 *하위 폴더 경로 또는 테스트 파일 경로*를 지정하세요. 예를 들어, 다음 명령은 PyTorch `examples` 디렉터리의 텍스트 분류 하위 폴더를 테스트합니다:
```bash
pip install -r examples/xxx/requirements.txt # only needed the first time
python -m pytest -n auto --dist=loadfile -s -v ./examples/pytorch/text-classification
```
이것이 실제로 `make test` 및 `make test-examples` 명령이 구현되는 방식입니다 (`pip install`은 제외합니다)!
또한 특정 기능만 테스트하기 위한 더 작은 테스트를 지정할 수 있습니다.
기본적으로 느린 테스트는 건너뛰지만 `RUN_SLOW` 환경 변수를 `yes`로 설정하여 실행할 수 있습니다. 이렇게 하면 많은 기가바이트 단위의 모델이 다운로드되므로 충분한 디스크 공간, 좋은 인터넷 연결과 많은 인내가 필요합니다!
<Tip warning={true}>
테스트를 실행하려면 *하위 폴더 경로 또는 테스트 파일 경로*를 지정하세요. 그렇지 않으면 `tests` 또는 `examples` 폴더의 모든 테스트를 실행하게 되어 매우 긴 시간이 걸립니다!
</Tip>
```bash
RUN_SLOW=yes python -m pytest -n auto --dist=loadfile -s -v ./tests/models/my_new_model
RUN_SLOW=yes python -m pytest -n auto --dist=loadfile -s -v ./examples/pytorch/text-classification
```
느린 테스트와 마찬가지로, 다음과 같이 테스트 중에 기본적으로 활성화되지 않는 다른 환경 변수도 있습니다:
- `RUN_CUSTOM_TOKENIZERS`: 사용자 정의 토크나이저 테스트를 활성화합니다.
- `RUN_PT_FLAX_CROSS_TESTS`: PyTorch + Flax 통합 테스트를 활성화합니다.
- `RUN_PT_TF_CROSS_TESTS`: TensorFlow + PyTorch 통합 테스트를 활성화합니다.
더 많은 환경 변수와 추가 정보는 [testing_utils.py](src/transformers/testing_utils.py)에서 찾을 수 있습니다.
🤗 Transformers는 테스트 실행기로 `pytest`를 사용합니다. 그러나 테스트 스위트 자체에서는 `pytest` 관련 기능을 사용하지 않습니다.
이것은 `unittest`가 완전히 지원된다는 것을 의미합니다. 다음은 `unittest`로 테스트를 실행하는 방법입니다:
```bash
python -m unittest discover -s tests -t . -v
python -m unittest discover -s examples -t examples -v
```
### 스타일 가이드 [[style-guide]]
문서는 [Google Python 스타일 가이드](https://google.github.io/styleguide/pyguide.html)를 따릅니다. 자세한 정보는 [문서 작성 가이드](https://github.com/huggingface/transformers/tree/main/docs#writing-documentation---specification)를 확인하세요.
### Windows에서 개발 [[develop-on-windows]]
Windows에서 개발할 경우([Windows Subsystem for Linux](https://learn.microsoft.com/en-us/windows/wsl/) 또는 WSL에서 작업하지 않는 한) Windows `CRLF` 줄 바꿈을 Linux `LF` 줄 바꿈으로 변환하도록 git을 구성해야 합니다:
```bash
git config core.autocrlf input
```
Windows에서 `make` 명령을 실행하는 한 가지 방법은 MSYS2를 사용하는 것입니다:
1. [MSYS2](https://www.msys2.org/)를 다운로드합니다. `C:\msys64`에 설치되었다고 가정합니다.
2. CLI에서 `C:\msys64\msys2.exe`를 엽니다 (시작 메뉴에서 사용 가능해야 함).
3. 쉘에서 다음을 실행하여: `pacman -Syu` 및 `pacman -S make`로 `make`를 설치합니다.
4. 환경 변수 PATH에 `C:\msys64\usr\bin`을 추가하세요.
이제 모든 터미널 (PowerShell, cmd.exe 등)에서 `make`를 사용할 수 있습니다! 🎉
### 포크한 저장소를 상위 원본 브랜치(main)과 동기화하기 (Hugging Face 저장소) [[sync-a-forked-repository-with-upstream-main-the-hugging-face-repository]]
포크한 저장소의 main 브랜치를 업데이트할 때, 다음 단계를 따라 수행해주세요. 이렇게 하면 각 upstream PR에 참조 노트가 추가되는 것을 피하고 이러한 PR에 관여하는 개발자들에게 불필요한 알림이 전송되는 것을 방지할 수 있습니다.
1. 가능하면 포크된 저장소의 브랜치 및 PR을 사용하여 upstream과 동기화하지 마세요. 대신 포크된 main 저장소에 직접 병합하세요.
2. PR이 반드시 필요한 경우, 브랜치를 확인한 후 다음 단계를 사용하세요:
```bash
git checkout -b your-branch-for-syncing
git pull --squash --no-commit upstream main
git commit -m '<your message without GitHub references>'
git push --set-upstream origin your-branch-for-syncing
```
| transformers/docs/source/ko/contributing.md/0 | {
"file_path": "transformers/docs/source/ko/contributing.md",
"repo_id": "transformers",
"token_count": 15881
} | 267 |
<!--Copyright 2022 The HuggingFace Team. All rights reserved.
Licensed under the Apache License, Version 2.0 (the "License"); you may not use this file except in compliance with
the License. You may obtain a copy of the License at
http://www.apache.org/licenses/LICENSE-2.0
Unless required by applicable law or agreed to in writing, software distributed under the License is distributed on
an "AS IS" BASIS, WITHOUT WARRANTIES OR CONDITIONS OF ANY KIND, either express or implied. See the License for the
specific language governing permissions and limitations under the License.
⚠️ Note that this file is in Markdown but contain specific syntax for our doc-builder (similar to MDX) that may not be
rendered properly in your Markdown viewer.
-->
# 모델 공유하기[[share-a-model]]
지난 두 튜토리얼에서 분산 설정을 위해 PyTorch, Keras 및 🤗 Accelerate를 사용하여 모델을 미세 조정하는 방법을 보았습니다. 다음 단계는 모델을 커뮤니티와 공유하는 것입니다! Hugging Face는 인공지능의 민주화를 위해 모두에게 지식과 자원을 공개적으로 공유해야 한다고 믿습니다. 다른 사람들이 시간과 자원을 절약할 수 있도록 커뮤니티에 모델을 공유하는 것을 고려해 보세요.
이 튜토리얼에서 [Model Hub](https://huggingface.co/models)에서 훈련되거나 미세 조정 모델을 공유하는 두 가지 방법에 대해 알아봅시다:
- API를 통해 파일을 Hub에 푸시합니다.
- 웹사이트를 통해 파일을 Hub로 끌어다 놓습니다.
<iframe width="560" height="315" src="https://www.youtube.com/embed/XvSGPZFEjDY" title="YouTube video player"
frameborder="0" allow="accelerometer; autoplay; clipboard-write; encrypted-media; gyroscope;
picture-in-picture" allowfullscreen></iframe>
<Tip>
커뮤니티에 모델을 공유하려면, [huggingface.co](https://huggingface.co/join)에 계정이 필요합니다. 기존 조직에 가입하거나 새로 만들 수도 있습니다.
</Tip>
## 저장소 특징[[repository-features]]
모델 허브의 각 저장소는 일반적인 GitHub 저장소처럼 작동합니다. 저장소는 버전 관리, 커밋 기록, 차이점 시각화 기능을 제공합니다.
모델 허브에 내장된 버전 관리는 git 및 [git-lfs](https://git-lfs.github.com/)를 기반으로 합니다. 즉, 하나의 모델을 하나의 저장소로 취급하여 접근 제어 및 확장성이 향상됩니다. 버전 제어는 커밋 해시, 태그 또는 브랜치로 모델의 특정 버전을 고정하는 방법인 *revision*을 허용합니다.
따라서 `revision` 매개변수를 사용하여 특정 모델 버전을 가져올 수 있습니다:
```py
>>> model = AutoModel.from_pretrained(
... "julien-c/EsperBERTo-small", revision="v2.0.1" # tag name, or branch name, or commit hash
... )
```
또한 저장소에서 파일을 쉽게 편집할 수 있으며, 커밋 기록과 차이를 볼 수 있습니다:

## 설정[[setup]]
모델을 허브에 공유하기 전에 Hugging Face 자격 증명이 필요합니다. 터미널에 액세스할 수 있는 경우, 🤗 Transformers가 설치된 가상 환경에서 다음 명령을 실행합니다. 그러면 Hugging Face 캐시 폴더(기본적으로 `~/.cache/`)에 액세스 토큰을 저장합니다:
```bash
huggingface-cli login
```
Jupyter 또는 Colaboratory와 같은 노트북을 사용 중인 경우, [`huggingface_hub`](https://huggingface.co/docs/hub/adding-a-library) 라이브러리가 설치되었는지 확인하세요. 이 라이브러리를 사용하면 API로 허브와 상호 작용할 수 있습니다.
```bash
pip install huggingface_hub
```
그런 다음 `notebook_login`로 허브에 로그인하고, [여기](https://huggingface.co/settings/token) 링크에서 로그인할 토큰을 생성합니다:
```py
>>> from huggingface_hub import notebook_login
>>> notebook_login()
```
## 프레임워크 간 모델 변환하기[[convert-a-model-for-all-frameworks]]
다른 프레임워크로 작업하는 사용자가 모델을 사용할 수 있도록 하려면, PyTorch 및 TensorFlow 체크포인트를 모두 사용하여 모델을 변환하고 업로드하는 것이 좋습니다. 이 단계를 건너뛰어도 사용자는 다른 프레임워크에서 모델을 가져올 수 있지만, 🤗 Transformers가 체크포인트를 즉석에서 변환해야 하므로 속도가 느려질 수 있습니다.
체크포인트를 다른 프레임워크로 변환하는 것은 쉽습니다. PyTorch 및 TensorFlow가 설치되어 있는지 확인한 다음(설치 지침은 [여기](installation) 참조) 다른 프레임워크에서 작업에 대한 특정 모델을 찾습니다.
<frameworkcontent>
<pt>
체크포인트를 TensorFlow에서 PyTorch로 변환하려면 `from_tf=True`를 지정하세요:
```py
>>> pt_model = DistilBertForSequenceClassification.from_pretrained("path/to/awesome-name-you-picked", from_tf=True)
>>> pt_model.save_pretrained("path/to/awesome-name-you-picked")
```
</pt>
<tf>
체크포인트를 PyTorch에서 TensorFlow로 변환하려면 `from_pt=True`를 지정하세요:
```py
>>> tf_model = TFDistilBertForSequenceClassification.from_pretrained("path/to/awesome-name-you-picked", from_pt=True)
```
그런 다음 새로운 체크포인트와 함께 새로운 TensorFlow 모델을 저장할 수 있습니다:
```py
>>> tf_model.save_pretrained("path/to/awesome-name-you-picked")
```
</tf>
<jax>
Flax에서 모델을 사용하는 경우, PyTorch에서 Flax로 체크포인트를 변환할 수도 있습니다:
```py
>>> flax_model = FlaxDistilBertForSequenceClassification.from_pretrained(
... "path/to/awesome-name-you-picked", from_pt=True
... )
```
</jax>
</frameworkcontent>
## 훈련 중 모델 푸시하기[[push-a-model-during-training]]
<frameworkcontent>
<pt>
<Youtube id="Z1-XMy-GNLQ"/>
모델을 허브에 공유하는 것은 추가 매개변수나 콜백을 추가하는 것만큼 간단합니다. [미세 조정 튜토리얼](training)에서 [`TrainingArguments`] 클래스는 하이퍼파라미터와 추가 훈련 옵션을 지정하는 곳이라는 것을 기억하세요. 이러한 훈련 옵션 중 하나는 모델을 허브로 직접 푸시하는 기능을 포함합니다. [`TrainingArguments`]에서 `push_to_hub=True`를 설정하세요:
```py
>>> training_args = TrainingArguments(output_dir="my-awesome-model", push_to_hub=True)
```
평소와 같이 훈련 인수를 [`Trainer`]에 전달합니다:
```py
>>> trainer = Trainer(
... model=model,
... args=training_args,
... train_dataset=small_train_dataset,
... eval_dataset=small_eval_dataset,
... compute_metrics=compute_metrics,
... )
```
모델을 미세 조정한 후, [`Trainer`]에서 [`~transformers.Trainer.push_to_hub`]를 호출하여 훈련된 모델을 허브로 푸시하세요. 🤗 Transformers는 훈련 하이퍼파라미터, 훈련 결과 및 프레임워크 버전을 모델 카드에 자동으로 추가합니다!
```py
>>> trainer.push_to_hub()
```
</pt>
<tf>
[`PushToHubCallback`]을 사용하여 모델을 허브에 공유하려면, [`PushToHubCallback`]에 다음 인수를 정의하세요:
- 출력된 모델의 파일 경로
- 토크나이저
- `{Hub 사용자 이름}/{모델 이름}` 형식의 `hub_model_id`
```py
>>> from transformers import PushToHubCallback
>>> push_to_hub_callback = PushToHubCallback(
... output_dir="./your_model_save_path", tokenizer=tokenizer, hub_model_id="your-username/my-awesome-model"
... )
```
[`fit`](https://keras.io/api/models/model_training_apis/)에 콜백을 추가하면, 🤗 Transformers가 훈련된 모델을 허브로 푸시합니다:
```py
>>> model.fit(tf_train_dataset, validation_data=tf_validation_dataset, epochs=3, callbacks=push_to_hub_callback)
```
</tf>
</frameworkcontent>
## `push_to_hub` 함수 사용하기[[use-the-pushtohub-function]]
모델에서 직접 `push_to_hub`를 호출하여 허브에 업로드할 수도 있습니다.
`push_to_hub`에 모델 이름을 지정하세요:
```py
>>> pt_model.push_to_hub("my-awesome-model")
```
이렇게 하면 사용자 이름 아래에 모델 이름 `my-awesome-model`로 저장소가 생성됩니다. 이제 사용자는 `from_pretrained` 함수를 사용하여 모델을 가져올 수 있습니다:
```py
>>> from transformers import AutoModel
>>> model = AutoModel.from_pretrained("your_username/my-awesome-model")
```
조직에 속하고 모델을 조직 이름으로 대신 푸시하려면 `repo_id`에 추가하세요:
```py
>>> pt_model.push_to_hub("my-awesome-org/my-awesome-model")
```
`push_to_hub` 함수는 모델 저장소에 다른 파일을 추가하는 데에도 사용할 수 있습니다. 예를 들어 모델 저장소에 토크나이저를 추가할 수 있습니다:
```py
>>> tokenizer.push_to_hub("my-awesome-model")
```
또는 미세 조정된 PyTorch 모델의 TensorFlow 버전을 추가할 수도 있습니다:
```py
>>> tf_model.push_to_hub("my-awesome-model")
```
이제 Hugging Face 프로필로 이동하면, 새로 생성한 모델 저장소가 표시됩니다. **Files** 탭을 클릭하면 저장소에 업로드한 모든 파일이 표시됩니다.
저장소에 파일을 만들고 업로드하는 방법에 대한 자세한 내용은 허브 설명서 [여기](https://huggingface.co/docs/hub/how-to-upstream)를 참조하세요.
## 웹 인터페이스로 업로드하기[[upload-with-the-web-interface]]
코드 없는 접근 방식을 선호하는 사용자는 허브의 웹 인터페이스를 통해 모델을 업로드할 수 있습니다. [huggingface.co/new](https://huggingface.co/new)를 방문하여 새로운 저장소를 생성하세요:
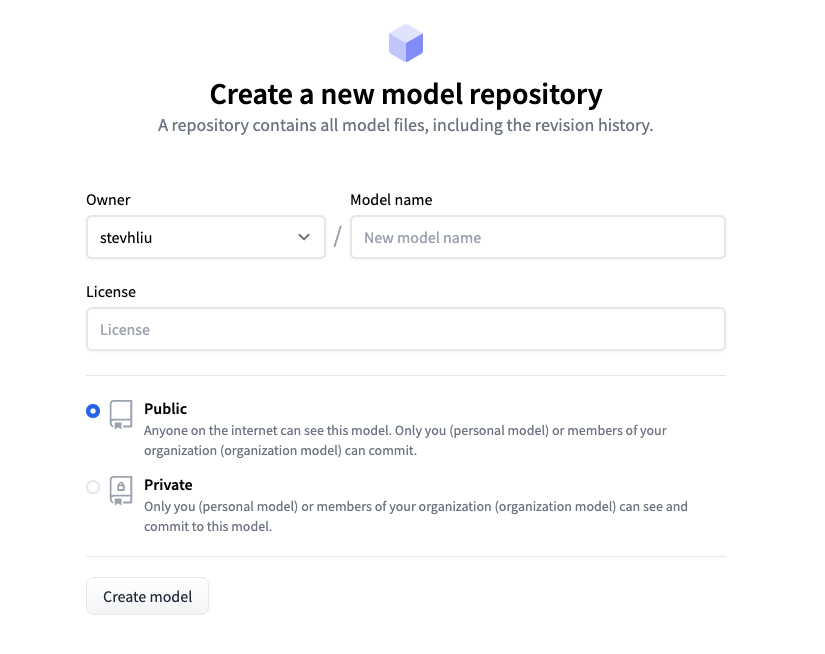
여기서 모델에 대한 몇 가지 정보를 추가하세요:
- 저장소의 **소유자**를 선택합니다. 이는 사용자 또는 사용자가 속한 조직일 수 있습니다.
- 저장소 이름이 될 모델의 이름을 선택합니다.
- 모델이 공개인지 비공개인지 선택합니다.
- 모델의 라이센스 사용을 지정합니다.
이제 **Files** 탭을 클릭하고 **Add file** 버튼을 클릭하여 새로운 파일을 저장소에 업로드합니다. 그런 다음 업로드할 파일을 끌어다 놓고 커밋 메시지를 추가하세요.
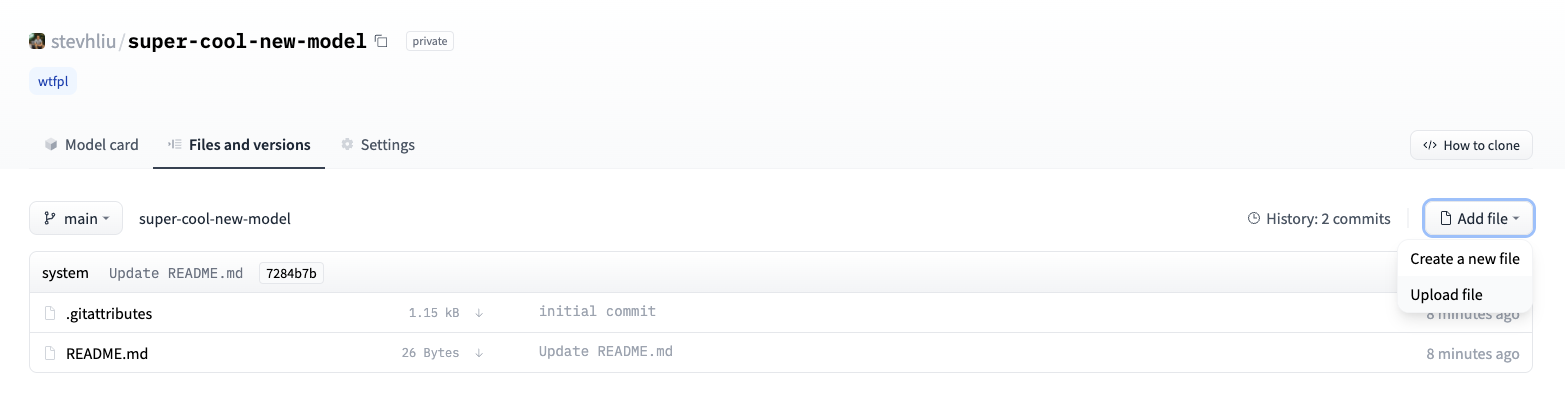
## 모델 카드 추가하기[[add-a-model-card]]
사용자가 모델의 기능, 제한, 잠재적 편향 및 윤리적 고려 사항을 이해할 수 있도록 저장소에 모델 카드를 추가하세요. 모델 카드는 `README.md` 파일에 정의되어 있습니다. 다음 방법으로 모델 카드를 추가할 수 있습니다:
* `README.md` 파일을 수동으로 생성하여 업로드합니다.
* 모델 저장소에서 **Edit model card** 버튼을 클릭합니다.
모델 카드에 포함할 정보 유형에 대한 좋은 예는 DistilBert [모델 카드](https://huggingface.co/distilbert/distilbert-base-uncased)를 참조하세요. 모델의 탄소 발자국이나 위젯 예시 등 `README.md` 파일에서 제어할 수 있는 다른 옵션에 대한 자세한 내용은 [여기](https://huggingface.co/docs/hub/models-cards) 문서를 참조하세요.
| transformers/docs/source/ko/model_sharing.md/0 | {
"file_path": "transformers/docs/source/ko/model_sharing.md",
"repo_id": "transformers",
"token_count": 7561
} | 268 |
<!--Copyright 2023 The HuggingFace Team. All rights reserved.
Licensed under the Apache License, Version 2.0 (the "License"); you may not use this file except in compliance with
the License. You may obtain a copy of the License at
http://www.apache.org/licenses/LICENSE-2.0
Unless required by applicable law or agreed to in writing, software distributed under the License is distributed on
an "AS IS" BASIS, WITHOUT WARRANTIES OR CONDITIONS OF ANY KIND, either express or implied. See the License for the
specific language governing permissions and limitations under the License.
⚠️ Note that this file is in Markdown but contain specific syntax for our doc-builder (similar to MDX) that may not be
rendered properly in your Markdown viewer.
-->
# 단일 영상 기반 깊이 추정[[depth-estimation-pipeline]]
단일 영상 기반 깊이 추정은 한 장면의 단일 이미지에서 장면의 깊이 정보를 예측하는 컴퓨터 비전 작업입니다.
즉, 단일 카메라 시점의 장면에 있는 물체의 거리를 예측하는 과정입니다.
단일 영상 기반 깊이 추정은 3D 재구성, 증강 현실, 자율 주행, 로봇 공학 등 다양한 분야에서 응용됩니다.
조명 조건, 가려짐, 텍스처와 같은 요소의 영향을 받을 수 있는 장면 내 물체와 해당 깊이 정보 간의 복잡한 관계를 모델이 이해해야 하므로 까다로운 작업입니다.
<Tip>
이 튜토리얼에서 다루는 작업은 다음 모델 아키텍처에서 지원됩니다:
<!--This tip is automatically generated by `make fix-copies`, do not fill manually!-->
[DPT](../model_doc/dpt), [GLPN](../model_doc/glpn)
<!--End of the generated tip-->
</Tip>
이번 가이드에서 배울 내용은 다음과 같습니다:
* 깊이 추정 파이프라인 만들기
* 직접 깊이 추정 추론하기
시작하기 전에, 필요한 모든 라이브러리가 설치되어 있는지 확인하세요:
```bash
pip install -q transformers
```
## 깊이 추정 파이프라인[[depth-estimation-inference-by-hand]]
깊이 추정을 추론하는 가장 간단한 방법은 해당 기능을 제공하는 [`pipeline`]을 사용하는 것입니다.
[Hugging Face Hub 체크포인트](https://huggingface.co/models?pipeline_tag=depth-estimation&sort=downloads)에서 파이프라인을 초기화합니다:
```py
>>> from transformers import pipeline
>>> checkpoint = "vinvino02/glpn-nyu"
>>> depth_estimator = pipeline("depth-estimation", model=checkpoint)
```
다음으로, 분석할 이미지를 한 장 선택하세요:
```py
>>> from PIL import Image
>>> import requests
>>> url = "https://unsplash.com/photos/HwBAsSbPBDU/download?ixid=MnwxMjA3fDB8MXxzZWFyY2h8MzR8fGNhciUyMGluJTIwdGhlJTIwc3RyZWV0fGVufDB8MHx8fDE2Nzg5MDEwODg&force=true&w=640"
>>> image = Image.open(requests.get(url, stream=True).raw)
>>> image
```
<div class="flex justify-center">
<img src="https://huggingface.co/datasets/huggingface/documentation-images/resolve/main/transformers/tasks/depth-estimation-example.jpg" alt="Photo of a busy street"/>
</div>
이미지를 파이프라인으로 전달합니다.
```py
>>> predictions = depth_estimator(image)
```
파이프라인은 두 개의 항목을 가지는 딕셔너리를 반환합니다.
첫 번째는 `predicted_depth`로 각 픽셀의 깊이를 미터로 표현한 값을 가지는 텐서입니다.
두 번째는 `depth`로 깊이 추정 결과를 시각화하는 PIL 이미지입니다.
이제 시각화한 결과를 살펴보겠습니다:
```py
>>> predictions["depth"]
```
<div class="flex justify-center">
<img src="https://huggingface.co/datasets/huggingface/documentation-images/resolve/main/transformers/tasks/depth-visualization.png" alt="Depth estimation visualization"/>
</div>
## 직접 깊이 추정 추론하기[[depth-estimation-inference-by-hand]]
이제 깊이 추정 파이프라인 사용법을 살펴보았으니 동일한 결과를 복제하는 방법을 살펴보겠습니다.
[Hugging Face Hub 체크포인트](https://huggingface.co/models?pipeline_tag=depth-estimation&sort=downloads)에서 모델과 관련 프로세서를 가져오는 것부터 시작합니다.
여기서 이전에 사용한 체크포인트와 동일한 것을 사용합니다:
```py
>>> from transformers import AutoImageProcessor, AutoModelForDepthEstimation
>>> checkpoint = "vinvino02/glpn-nyu"
>>> image_processor = AutoImageProcessor.from_pretrained(checkpoint)
>>> model = AutoModelForDepthEstimation.from_pretrained(checkpoint)
```
필요한 이미지 변환을 처리하는 `image_processor`를 사용하여 모델에 대한 이미지 입력을 준비합니다.
`image_processor`는 크기 조정 및 정규화 등 필요한 이미지 변환을 처리합니다:
```py
>>> pixel_values = image_processor(image, return_tensors="pt").pixel_values
```
준비한 입력을 모델로 전달합니다:
```py
>>> import torch
>>> with torch.no_grad():
... outputs = model(pixel_values)
... predicted_depth = outputs.predicted_depth
```
결과를 시각화합니다:
```py
>>> import numpy as np
>>> # 원본 사이즈로 복원
>>> prediction = torch.nn.functional.interpolate(
... predicted_depth.unsqueeze(1),
... size=image.size[::-1],
... mode="bicubic",
... align_corners=False,
... ).squeeze()
>>> output = prediction.numpy()
>>> formatted = (output * 255 / np.max(output)).astype("uint8")
>>> depth = Image.fromarray(formatted)
>>> depth
```
<div class="flex justify-center">
<img src="https://huggingface.co/datasets/huggingface/documentation-images/resolve/main/transformers/tasks/depth-visualization.png" alt="Depth estimation visualization"/>
</div>
| transformers/docs/source/ko/tasks/monocular_depth_estimation.md/0 | {
"file_path": "transformers/docs/source/ko/tasks/monocular_depth_estimation.md",
"repo_id": "transformers",
"token_count": 3120
} | 269 |
<!--Copyright 2023 The HuggingFace Team. All rights reserved.
Licensed under the Apache License, Version 2.0 (the "License"); you may not use this file except in compliance with
the License. You may obtain a copy of the License at
http://www.apache.org/licenses/LICENSE-2.0
Unless required by applicable law or agreed to in writing, software distributed under the License is distributed on
an "AS IS" BASIS, WITHOUT WARRANTIES OR CONDITIONS OF ANY KIND, either express or implied. See the License for the
specific language governing permissions and limitations under the License.
⚠️ Note that this file is in Markdown but contain specific syntax for our doc-builder (similar to MDX) that may not be
rendered properly in your Markdown viewer.
-->
# TFLite로 내보내기[[export-to-tflite]]
[TensorFlow Lite](https://www.tensorflow.org/lite/guide)는 자원이 제한된 휴대폰, 임베디드 시스템, 사물인터넷(IoT) 기기에서
기계학습 모델을 배포하기 위한 경량 프레임워크입니다.
TFLite는 연산 능력, 메모리, 전력 소비가 제한된 기기에서 모델을 효율적으로 최적화하고 실행하기 위해
설계되었습니다.
TensorFlow Lite 모델은 `.tflite` 파일 확장자로 식별되는 특수하고 효율적인 휴대용 포맷으로 표현됩니다.
🤗 Optimum은 `exporters.tflite` 모듈로 🤗 Transformers 모델을 TFLite로 내보내는 기능을 제공합니다.
지원되는 모델 아키텍처 목록은 [🤗 Optimum 문서](https://huggingface.co/docs/optimum/exporters/tflite/overview)를 참고하세요.
모델을 TFLite로 내보내려면, 필요한 종속성을 설치하세요:
```bash
pip install optimum[exporters-tf]
```
모든 사용 가능한 인수를 확인하려면, [🤗 Optimum 문서](https://huggingface.co/docs/optimum/main/en/exporters/tflite/usage_guides/export_a_model)를 참고하거나
터미널에서 도움말을 살펴보세요:
```bash
optimum-cli export tflite --help
```
예를 들어 🤗 Hub에서의 `google-bert/bert-base-uncased` 모델 체크포인트를 내보내려면, 다음 명령을 실행하세요:
```bash
optimum-cli export tflite --model google-bert/bert-base-uncased --sequence_length 128 bert_tflite/
```
다음과 같이 진행 상황을 나타내는 로그와 결과물인 `model.tflite`가 저장된 위치를 보여주는 로그가 표시됩니다:
```bash
Validating TFLite model...
-[✓] TFLite model output names match reference model (logits)
- Validating TFLite Model output "logits":
-[✓] (1, 128, 30522) matches (1, 128, 30522)
-[x] values not close enough, max diff: 5.817413330078125e-05 (atol: 1e-05)
The TensorFlow Lite export succeeded with the warning: The maximum absolute difference between the output of the reference model and the TFLite exported model is not within the set tolerance 1e-05:
- logits: max diff = 5.817413330078125e-05.
The exported model was saved at: bert_tflite
```
위 예제는 🤗 Hub에서의 체크포인트를 내보내는 방법을 보여줍니다.
로컬 모델을 내보낸다면, 먼저 모델 가중치와 토크나이저 파일이 모두 같은 디렉터리( `local_path` )에 저장됐는지 확인하세요.
CLI를 사용할 때, 🤗 Hub에서의 체크포인트 이름 대신 `model` 인수에 `local_path`를 전달하면 됩니다. | transformers/docs/source/ko/tflite.md/0 | {
"file_path": "transformers/docs/source/ko/tflite.md",
"repo_id": "transformers",
"token_count": 1852
} | 270 |
<!---
Copyright 2022 The HuggingFace Team. All rights reserved.
Licensed under the Apache License, Version 2.0 (the "License");
you may not use this file except in compliance with the License.
You may obtain a copy of the License at
http://www.apache.org/licenses/LICENSE-2.0
Unless required by applicable law or agreed to in writing, software
distributed under the License is distributed on an "AS IS" BASIS,
WITHOUT WARRANTIES OR CONDITIONS OF ANY KIND, either express or implied.
See the License for the specific language governing permissions and
limitations under the License.
⚠️ Note that this file is in Markdown but contain specific syntax for our doc-builder (similar to MDX) that may not be
rendered properly in your Markdown viewer.
-->
# Guia de Instalação
Neste guia poderá encontrar informações para a instalação do 🤗 Transformers para qualquer biblioteca de
Machine Learning com a qual esteja a trabalhar. Além disso, poderá encontrar informações sobre como gerar cachês e
configurar o 🤗 Transformers para execução em modo offline (opcional).
🤗 Transformers foi testado com Python 3.6+, PyTorch 1.1.0+, TensorFlow 2.0+, e Flax. Para instalar a biblioteca de
deep learning com que deseja trabalhar, siga as instruções correspondentes listadas a seguir:
* [PyTorch](https://pytorch.org/get-started/locally/)
* [TensorFlow 2.0](https://www.tensorflow.org/install/pip)
* [Flax](https://flax.readthedocs.io/en/latest/)
## Instalação pelo Pip
É sugerido instalar o 🤗 Transformers num [ambiente virtual](https://docs.python.org/3/library/venv.html). Se precisar
de mais informações sobre ambientes virtuais em Python, consulte este [guia](https://packaging.python.org/guides/installing-using-pip-and-virtual-environments/).
Um ambiente virtual facilitará a manipulação e organização de projetos e evita problemas de compatibilidade entre dependências.
Comece criando um ambiente virtual no diretório do seu projeto:
```bash
python -m venv .env
```
E para ativar o ambiente virtual:
```bash
source .env/bin/activate
```
Agora É possível instalar o 🤗 Transformers com o comando a seguir:
```bash
pip install transformers
```
Somente para a CPU, é possível instalar o 🤗 Transformers e a biblioteca de deep learning respectiva apenas numa linha.
Por exemplo, para instalar o 🤗 Transformers e o PyTorch, digite:
```bash
pip install transformers[torch]
```
🤗 Transformers e TensorFlow 2.0:
```bash
pip install transformers[tf-cpu]
```
🤗 Transformers e Flax:
```bash
pip install transformers[flax]
```
Por último, verifique se o 🤗 Transformers foi instalado com sucesso usando o seguinte comando para baixar um modelo pré-treinado:
```bash
python -c "from transformers import pipeline; print(pipeline('sentiment-analysis')('we love you'))"
```
Em seguida, imprima um rótulo e sua pontuação:
```bash
[{'label': 'POSITIVE', 'score': 0.9998704791069031}]
```
## Instalação usando a fonte
Para instalar o 🤗 Transformers a partir da fonte use o seguinte comando:
```bash
pip install git+https://github.com/huggingface/transformers
```
O comando acima instalará a versão `master` mais atual em vez da última versão estável. A versão `master` é útil para
utilizar os últimos updates contidos em 🤗 Transformers. Por exemplo, um erro recente pode ter sido corrigido somente
após a última versão estável, antes que houvesse um novo lançamento. No entanto, há a possibilidade que a versão `master` não esteja estável.
A equipa trata de mantér a versão `master` operacional e a maioria dos erros são resolvidos em poucas horas ou dias.
Se encontrar quaisquer problemas, por favor abra um [Issue](https://github.com/huggingface/transformers/issues) para que o
mesmo possa ser corrigido o mais rápido possível.
Verifique que o 🤗 Transformers está instalado corretamente usando o seguinte comando:
```bash
python -c "from transformers import pipeline; print(pipeline('sentiment-analysis')('I love you'))"
```
## Instalação editável
Uma instalação editável será necessária caso desejas um dos seguintes:
* Usar a versão `master` do código fonte.
* Contribuir ao 🤗 Transformers e precisa testar mudanças ao código.
Para tal, clone o repositório e instale o 🤗 Transformers com os seguintes comandos:
```bash
git clone https://github.com/huggingface/transformers.git
cd transformers
pip install -e .
```
Estes comandos vão ligar o diretório para o qual foi clonado o repositório ao caminho de bibliotecas do Python.
O Python agora buscará dentro dos arquivos que foram clonados além dos caminhos normais da biblioteca.
Por exemplo, se os pacotes do Python se encontram instalados no caminho `~/anaconda3/envs/main/lib/python3.7/site-packages/`,
o Python também buscará módulos no diretório onde clonamos o repositório `~/transformers/`.
<Tip warning={true}>
É necessário manter o diretório `transformers` se desejas continuar usando a biblioteca.
</Tip>
Assim, É possível atualizar sua cópia local para com a última versão do 🤗 Transformers com o seguinte comando:
```bash
cd ~/transformers/
git pull
```
O ambiente de Python que foi criado para a instalação do 🤗 Transformers encontrará a versão `master` em execuções seguintes.
## Instalação usando o Conda
É possível instalar o 🤗 Transformers a partir do canal conda `conda-forge` com o seguinte comando:
```bash
conda install conda-forge::transformers
```
## Configuração do Cachê
Os modelos pré-treinados são baixados e armazenados no cachê local, encontrado em `~/.cache/huggingface/transformers/`.
Este é o diretório padrão determinado pela variável `TRANSFORMERS_CACHE` dentro do shell.
No Windows, este diretório pré-definido é dado por `C:\Users\username\.cache\huggingface\transformers`.
É possível mudar as variáveis dentro do shell em ordem de prioridade para especificar um diretório de cachê diferente:
1. Variável de ambiente do shell (por padrão): `TRANSFORMERS_CACHE`.
2. Variável de ambiente do shell:`HF_HOME` + `transformers/`.
3. Variável de ambiente do shell: `XDG_CACHE_HOME` + `/huggingface/transformers`.
<Tip>
O 🤗 Transformers usará as variáveis de ambiente do shell `PYTORCH_TRANSFORMERS_CACHE` ou `PYTORCH_PRETRAINED_BERT_CACHE`
se estiver vindo de uma versão anterior da biblioteca que tenha configurado essas variáveis de ambiente, a menos que
você especifique a variável de ambiente do shell `TRANSFORMERS_CACHE`.
</Tip>
## Modo Offline
O 🤗 Transformers também pode ser executado num ambiente de firewall ou fora da rede (offline) usando arquivos locais.
Para tal, configure a variável de ambiente de modo que `TRANSFORMERS_OFFLINE=1`.
<Tip>
Você pode adicionar o [🤗 Datasets](https://huggingface.co/docs/datasets/) ao pipeline de treinamento offline declarando
a variável de ambiente `HF_DATASETS_OFFLINE=1`.
</Tip>
Segue um exemplo de execução do programa numa rede padrão com firewall para instâncias externas, usando o seguinte comando:
```bash
python examples/pytorch/translation/run_translation.py --model_name_or_path google-t5/t5-small --dataset_name wmt16 --dataset_config ro-en ...
```
Execute esse mesmo programa numa instância offline com o seguinte comando:
```bash
HF_DATASETS_OFFLINE=1 TRANSFORMERS_OFFLINE=1 \
python examples/pytorch/translation/run_translation.py --model_name_or_path google-t5/t5-small --dataset_name wmt16 --dataset_config ro-en ...
```
O script agora deve ser executado sem travar ou expirar, pois procurará apenas por arquivos locais.
### Obtendo modelos e tokenizers para uso offline
Outra opção para usar o 🤗 Transformers offline é baixar os arquivos antes e depois apontar para o caminho local onde estão localizados. Existem três maneiras de fazer isso:
* Baixe um arquivo por meio da interface de usuário do [Model Hub](https://huggingface.co/models) clicando no ícone ↓.

* Use o pipeline do [`PreTrainedModel.from_pretrained`] e [`PreTrainedModel.save_pretrained`]:
1. Baixa os arquivos previamente com [`PreTrainedModel.from_pretrained`]:
```py
>>> from transformers import AutoTokenizer, AutoModelForSeq2SeqLM
>>> tokenizer = AutoTokenizer.from_pretrained("bigscience/T0_3B")
>>> model = AutoModelForSeq2SeqLM.from_pretrained("bigscience/T0_3B")
```
2. Salve os arquivos em um diretório específico com [`PreTrainedModel.save_pretrained`]:
```py
>>> tokenizer.save_pretrained("./your/path/bigscience_t0")
>>> model.save_pretrained("./your/path/bigscience_t0")
```
3. Quando estiver offline, acesse os arquivos com [`PreTrainedModel.from_pretrained`] do diretório especificado:
```py
>>> tokenizer = AutoTokenizer.from_pretrained("./your/path/bigscience_t0")
>>> model = AutoModel.from_pretrained("./your/path/bigscience_t0")
```
* Baixando arquivos programaticamente com a biblioteca [huggingface_hub](https://github.com/huggingface/huggingface_hub/tree/main/src/huggingface_hub):
1. Instale a biblioteca [huggingface_hub](https://github.com/huggingface/huggingface_hub/tree/main/src/huggingface_hub) em seu ambiente virtual:
```bash
python -m pip install huggingface_hub
```
2. Utiliza a função [`hf_hub_download`](https://huggingface.co/docs/hub/adding-a-library#download-files-from-the-hub) para baixar um arquivo para um caminho específico. Por exemplo, o comando a seguir baixará o arquivo `config.json` para o modelo [T0](https://huggingface.co/bigscience/T0_3B) no caminho desejado:
```py
>>> from huggingface_hub import hf_hub_download
>>> hf_hub_download(repo_id="bigscience/T0_3B", filename="config.json", cache_dir="./your/path/bigscience_t0")
```
Depois que o arquivo for baixado e armazenado no cachê local, especifique seu caminho local para carregá-lo e usá-lo:
```py
>>> from transformers import AutoConfig
>>> config = AutoConfig.from_pretrained("./your/path/bigscience_t0/config.json")
```
<Tip>
Para obter mais detalhes sobre como baixar arquivos armazenados no Hub, consulte a seção [How to download files from the Hub](https://huggingface.co/docs/hub/how-to-downstream).
</Tip>
| transformers/docs/source/pt/installation.md/0 | {
"file_path": "transformers/docs/source/pt/installation.md",
"repo_id": "transformers",
"token_count": 3769
} | 271 |
<!--
Copyright 2020 The HuggingFace Team. All rights reserved.
Licensed under the Apache License, Version 2.0 (the "License"); you may not use this file except in compliance with
the License. You may obtain a copy of the License at
http://www.apache.org/licenses/LICENSE-2.0
Unless required by applicable law or agreed to in writing, software distributed under the License is distributed on
an "AS IS" BASIS, WITHOUT WARRANTIES OR CONDITIONS OF ANY KIND, either express or implied. See the License for the
⚠️ Note that this file is in Markdown but contain specific syntax for our doc-builder (similar to MDX) that may not be
rendered properly in your Markdown viewer.
-->
# 如何创建自定义流水线?
在本指南中,我们将演示如何创建一个自定义流水线并分享到 [Hub](https://hf.co/models),或将其添加到 🤗 Transformers 库中。
首先,你需要决定流水线将能够接受的原始条目。它可以是字符串、原始字节、字典或任何看起来最可能是期望的输入。
尽量保持输入为纯 Python 语言,因为这样可以更容易地实现兼容性(甚至通过 JSON 在其他语言之间)。
这些将是流水线 (`preprocess`) 的 `inputs`。
然后定义 `outputs`。与 `inputs` 相同的策略。越简单越好。这些将是 `postprocess` 方法的输出。
首先继承基类 `Pipeline`,其中包含实现 `preprocess`、`_forward`、`postprocess` 和 `_sanitize_parameters` 所需的 4 个方法。
```python
from transformers import Pipeline
class MyPipeline(Pipeline):
def _sanitize_parameters(self, **kwargs):
preprocess_kwargs = {}
if "maybe_arg" in kwargs:
preprocess_kwargs["maybe_arg"] = kwargs["maybe_arg"]
return preprocess_kwargs, {}, {}
def preprocess(self, inputs, maybe_arg=2):
model_input = Tensor(inputs["input_ids"])
return {"model_input": model_input}
def _forward(self, model_inputs):
# model_inputs == {"model_input": model_input}
outputs = self.model(**model_inputs)
# Maybe {"logits": Tensor(...)}
return outputs
def postprocess(self, model_outputs):
best_class = model_outputs["logits"].softmax(-1)
return best_class
```
这种分解的结构旨在为 CPU/GPU 提供相对无缝的支持,同时支持在不同线程上对 CPU 进行预处理/后处理。
`preprocess` 将接受最初定义的输入,并将其转换为可供模型输入的内容。它可能包含更多信息,通常是一个 `Dict`。
`_forward` 是实现细节,不应直接调用。`forward` 是首选的调用方法,因为它包含保障措施,以确保一切都在预期的设备上运作。
如果任何内容与实际模型相关,它应该属于 `_forward` 方法,其他内容应该在 preprocess/postprocess 中。
`postprocess` 方法将接受 `_forward` 的输出,并将其转换为之前确定的最终输出。
`_sanitize_parameters` 存在是为了允许用户在任何时候传递任何参数,无论是在初始化时 `pipeline(...., maybe_arg=4)`
还是在调用时 `pipe = pipeline(...); output = pipe(...., maybe_arg=4)`。
`_sanitize_parameters` 的返回值是将直接传递给 `preprocess`、`_forward` 和 `postprocess` 的 3 个关键字参数字典。
如果调用方没有使用任何额外参数调用,则不要填写任何内容。这样可以保留函数定义中的默认参数,这总是更"自然"的。
在分类任务中,一个经典的例子是在后处理中使用 `top_k` 参数。
```python
>>> pipe = pipeline("my-new-task")
>>> pipe("This is a test")
[{"label": "1-star", "score": 0.8}, {"label": "2-star", "score": 0.1}, {"label": "3-star", "score": 0.05}
{"label": "4-star", "score": 0.025}, {"label": "5-star", "score": 0.025}]
>>> pipe("This is a test", top_k=2)
[{"label": "1-star", "score": 0.8}, {"label": "2-star", "score": 0.1}]
```
为了实现这一点,我们将更新我们的 `postprocess` 方法,将默认参数设置为 `5`,
并编辑 `_sanitize_parameters` 方法,以允许这个新参数。
```python
def postprocess(self, model_outputs, top_k=5):
best_class = model_outputs["logits"].softmax(-1)
# Add logic to handle top_k
return best_class
def _sanitize_parameters(self, **kwargs):
preprocess_kwargs = {}
if "maybe_arg" in kwargs:
preprocess_kwargs["maybe_arg"] = kwargs["maybe_arg"]
postprocess_kwargs = {}
if "top_k" in kwargs:
postprocess_kwargs["top_k"] = kwargs["top_k"]
return preprocess_kwargs, {}, postprocess_kwargs
```
尽量保持简单输入/输出,最好是可 JSON 序列化的,因为这样可以使流水线的使用非常简单,而不需要用户了解新的对象类型。
通常也相对常见地支持许多不同类型的参数以便使用(例如音频文件,可以是文件名、URL 或纯字节)。
## 将其添加到支持的任务列表中
要将你的 `new-task` 注册到支持的任务列表中,你需要将其添加到 `PIPELINE_REGISTRY` 中:
```python
from transformers.pipelines import PIPELINE_REGISTRY
PIPELINE_REGISTRY.register_pipeline(
"new-task",
pipeline_class=MyPipeline,
pt_model=AutoModelForSequenceClassification,
)
```
如果需要,你可以指定一个默认模型,此时它应该带有一个特定的修订版本(可以是分支名称或提交哈希,这里我们使用了 `"abcdef"`),以及类型:
```python
PIPELINE_REGISTRY.register_pipeline(
"new-task",
pipeline_class=MyPipeline,
pt_model=AutoModelForSequenceClassification,
default={"pt": ("user/awesome_model", "abcdef")},
type="text", # current support type: text, audio, image, multimodal
)
```
## 在 Hub 上分享你的流水线
要在 Hub 上分享你的自定义流水线,你只需要将 `Pipeline` 子类的自定义代码保存在一个 Python 文件中。
例如,假设我们想使用一个自定义流水线进行句对分类,如下所示:
```py
import numpy as np
from transformers import Pipeline
def softmax(outputs):
maxes = np.max(outputs, axis=-1, keepdims=True)
shifted_exp = np.exp(outputs - maxes)
return shifted_exp / shifted_exp.sum(axis=-1, keepdims=True)
class PairClassificationPipeline(Pipeline):
def _sanitize_parameters(self, **kwargs):
preprocess_kwargs = {}
if "second_text" in kwargs:
preprocess_kwargs["second_text"] = kwargs["second_text"]
return preprocess_kwargs, {}, {}
def preprocess(self, text, second_text=None):
return self.tokenizer(text, text_pair=second_text, return_tensors=self.framework)
def _forward(self, model_inputs):
return self.model(**model_inputs)
def postprocess(self, model_outputs):
logits = model_outputs.logits[0].numpy()
probabilities = softmax(logits)
best_class = np.argmax(probabilities)
label = self.model.config.id2label[best_class]
score = probabilities[best_class].item()
logits = logits.tolist()
return {"label": label, "score": score, "logits": logits}
```
这个实现与框架无关,适用于 PyTorch 和 TensorFlow 模型。如果我们将其保存在一个名为
`pair_classification.py` 的文件中,然后我们可以像这样导入并注册它:
```py
from pair_classification import PairClassificationPipeline
from transformers.pipelines import PIPELINE_REGISTRY
from transformers import AutoModelForSequenceClassification, TFAutoModelForSequenceClassification
PIPELINE_REGISTRY.register_pipeline(
"pair-classification",
pipeline_class=PairClassificationPipeline,
pt_model=AutoModelForSequenceClassification,
tf_model=TFAutoModelForSequenceClassification,
)
```
完成这些步骤后,我们可以将其与预训练模型一起使用。例如,`sgugger/finetuned-bert-mrpc`
已经在 MRPC 数据集上进行了微调,用于将句子对分类为是释义或不是释义。
```py
from transformers import pipeline
classifier = pipeline("pair-classification", model="sgugger/finetuned-bert-mrpc")
```
然后,我们可以通过在 `Repository` 中使用 `save_pretrained` 方法将其分享到 Hub 上:
```py
from huggingface_hub import Repository
repo = Repository("test-dynamic-pipeline", clone_from="{your_username}/test-dynamic-pipeline")
classifier.save_pretrained("test-dynamic-pipeline")
repo.push_to_hub()
```
这将会复制包含你定义的 `PairClassificationPipeline` 的文件到文件夹 `"test-dynamic-pipeline"` 中,
同时保存流水线的模型和分词器,然后将所有内容推送到仓库 `{your_username}/test-dynamic-pipeline` 中。
之后,只要提供选项 `trust_remote_code=True`,任何人都可以使用它:
```py
from transformers import pipeline
classifier = pipeline(model="{your_username}/test-dynamic-pipeline", trust_remote_code=True)
```
## 将流水线添加到 🤗 Transformers
如果你想将你的流水线贡献给 🤗 Transformers,你需要在 `pipelines` 子模块中添加一个新模块,
其中包含你的流水线的代码,然后将其添加到 `pipelines/__init__.py` 中定义的任务列表中。
然后,你需要添加测试。创建一个新文件 `tests/test_pipelines_MY_PIPELINE.py`,其中包含其他测试的示例。
`run_pipeline_test` 函数将非常通用,并在每种可能的架构上运行小型随机模型,如 `model_mapping` 和 `tf_model_mapping` 所定义。
这对于测试未来的兼容性非常重要,这意味着如果有人为 `XXXForQuestionAnswering` 添加了一个新模型,
流水线测试将尝试在其上运行。由于模型是随机的,所以不可能检查实际值,这就是为什么有一个帮助函数 `ANY`,它只是尝试匹配流水线的输出类型。
你还 **需要** 实现 2(最好是 4)个测试。
- `test_small_model_pt`:为这个流水线定义一个小型模型(结果是否合理并不重要),并测试流水线的输出。
结果应该与 `test_small_model_tf` 的结果相同。
- `test_small_model_tf`:为这个流水线定义一个小型模型(结果是否合理并不重要),并测试流水线的输出。
结果应该与 `test_small_model_pt` 的结果相同。
- `test_large_model_pt`(可选):在一个真实的流水线上测试流水线,结果应该是有意义的。
这些测试速度较慢,应该被如此标记。这里的目标是展示流水线,并确保在未来的发布中没有漂移。
- `test_large_model_tf`(可选):在一个真实的流水线上测试流水线,结果应该是有意义的。
这些测试速度较慢,应该被如此标记。这里的目标是展示流水线,并确保在未来的发布中没有漂移。
| transformers/docs/source/zh/add_new_pipeline.md/0 | {
"file_path": "transformers/docs/source/zh/add_new_pipeline.md",
"repo_id": "transformers",
"token_count": 5640
} | 272 |
<!--版权所有 2020 年 HuggingFace 团队。保留所有权利。
根据 Apache 许可证 2.0 版本许可,除非符合许可证的规定,否则您不得使用此文件。您可以在以下网址获取许可证的副本:
http://www.apache.org/licenses/LICENSE-2.0
除非适用法律要求或书面同意,否则依照许可证分发的软件是基于“原样”提供的,不附带任何明示或暗示的担保或条件。有关特定语言下权限的限制和限制,请参阅许可证。-->
# 模型
基类 [`PreTrainedModel`]、[`TFPreTrainedModel`] 和 [`FlaxPreTrainedModel`] 实现了从本地文件或目录加载/保存模型的常用方法,或者从库上提供的预训练模型配置(从 HuggingFace 的 AWS S3 存储库下载)加载模型。
[`PreTrainedModel`] 和 [`TFPreTrainedModel`] 还实现了一些所有模型共有的方法:
- 在向量词嵌入增加新词汇时调整输入标记(token)的大小
- 对模型的注意力头进行修剪。
其他的通用方法在 [`~modeling_utils.ModuleUtilsMixin`](用于 PyTorch 模型)和 [`~modeling_tf_utils.TFModuleUtilsMixin`](用于 TensorFlow 模型)中定义;文本生成方面的方法则定义在 [`~generation.GenerationMixin`](用于 PyTorch 模型)、[`~generation.TFGenerationMixin`](用于 TensorFlow 模型)和 [`~generation.FlaxGenerationMixin`](用于 Flax/JAX 模型)中。
## PreTrainedModel
[[autodoc]] PreTrainedModel
- push_to_hub
- all
<a id='from_pretrained-torch-dtype'></a>
### 大模型加载
在 Transformers 4.20.0 中,[`~PreTrainedModel.from_pretrained`] 方法已重新设计,以适应使用 [Accelerate](https://huggingface.co/docs/accelerate/big_modeling) 加载大型模型的场景。这需要您使用的 Accelerate 和 PyTorch 版本满足: Accelerate >= 0.9.0, PyTorch >= 1.9.0。除了创建完整模型,然后在其中加载预训练权重(这会占用两倍于模型大小的内存空间,一个用于随机初始化模型,一个用于预训练权重),我们提供了一种选项,将模型创建为空壳,然后只有在加载预训练权重时才实例化其参数。
您可以使用 `low_cpu_mem_usage=True` 激活此选项。首先,在 Meta 设备上创建模型(带有空权重),然后将状态字典加载到其中(在分片检查点的情况下逐片加载)。这样,最大使用的内存占用仅为模型的完整大小。
```python
from transformers import AutoModelForSeq2SeqLM
t0pp = AutoModelForSeq2SeqLM.from_pretrained("bigscience/T0pp", low_cpu_mem_usage=True)
```
此外,如果内存不足以放下加载整个模型(目前仅适用于推理),您可以直接将模型放置在不同的设备上。使用 `device_map="auto"`,Accelerate 将确定将每一层放置在哪个设备上,以最大化使用最快的设备(GPU),并将其余部分卸载到 CPU,甚至硬盘上(如果您没有足够的 GPU 内存 或 CPU 内存)。即使模型分布在几个设备上,它也将像您通常期望的那样运行。
在传递 `device_map` 时,`low_cpu_mem_usage` 会自动设置为 `True`,因此您不需要指定它:
```python
from transformers import AutoModelForSeq2SeqLM
t0pp = AutoModelForSeq2SeqLM.from_pretrained("bigscience/T0pp", device_map="auto")
```
您可以通过 `hf_device_map` 属性来查看模型是如何在设备上分割的:
```python
t0pp.hf_device_map
{'shared': 0,
'decoder.embed_tokens': 0,
'encoder': 0,
'decoder.block.0': 0,
'decoder.block.1': 1,
'decoder.block.2': 1,
'decoder.block.3': 1,
'decoder.block.4': 1,
'decoder.block.5': 1,
'decoder.block.6': 1,
'decoder.block.7': 1,
'decoder.block.8': 1,
'decoder.block.9': 1,
'decoder.block.10': 1,
'decoder.block.11': 1,
'decoder.block.12': 1,
'decoder.block.13': 1,
'decoder.block.14': 1,
'decoder.block.15': 1,
'decoder.block.16': 1,
'decoder.block.17': 1,
'decoder.block.18': 1,
'decoder.block.19': 1,
'decoder.block.20': 1,
'decoder.block.21': 1,
'decoder.block.22': 'cpu',
'decoder.block.23': 'cpu',
'decoder.final_layer_norm': 'cpu',
'decoder.dropout': 'cpu',
'lm_head': 'cpu'}
```
您还可以按照相同的格式(一个层名称到设备的映射关系的字典)编写自己的设备映射规则。它应该将模型的所有参数映射到给定的设备上,如果该层的所有子模块都在同一设备上,您不必详细说明其中所有子模块的位置。例如,以下设备映射对于 T0pp 将正常工作(只要您有 GPU 内存):
```python
device_map = {"shared": 0, "encoder": 0, "decoder": 1, "lm_head": 1}
```
另一种减少模型内存影响的方法是以较低精度的 dtype(例如 `torch.float16`)实例化它,或者使用下面介绍的直接量化技术。
### 模型实例化 dtype
在 PyTorch 下,模型通常以 `torch.float32` 格式实例化。如果尝试加载权重为 fp16 的模型,这可能会导致问题,因为它将需要两倍的内存。为了克服此限制,您可以使用 `torch_dtype` 参数显式传递所需的 `dtype`:
```python
model = T5ForConditionalGeneration.from_pretrained("t5", torch_dtype=torch.float16)
```
或者,如果您希望模型始终以最优的内存模式加载,则可以使用特殊值 `"auto"`,然后 `dtype` 将自动从模型的权重中推导出:
```python
model = T5ForConditionalGeneration.from_pretrained("t5", torch_dtype="auto")
```
也可以通过以下方式告知从头开始实例化的模型要使用哪种 `dtype`:
```python
config = T5Config.from_pretrained("t5")
model = AutoModel.from_config(config)
```
由于 PyTorch 的设计,此功能仅适用于浮点类型。
## ModuleUtilsMixin
[[autodoc]] modeling_utils.ModuleUtilsMixin
TFPreTrainedModel
[[autodoc]] TFPreTrainedModel
- push_to_hub
- all
## TFModelUtilsMixin
[[autodoc]] modeling_tf_utils.TFModelUtilsMixin
FlaxPreTrainedModel
[[autodoc]] FlaxPreTrainedModel
- push_to_hub
- all
## 推送到 Hub
[[autodoc]] utils.PushToHubMixin
## 分片检查点
[[autodoc]] modeling_utils.load_sharded_checkpoint
| transformers/docs/source/zh/main_classes/model.md/0 | {
"file_path": "transformers/docs/source/zh/main_classes/model.md",
"repo_id": "transformers",
"token_count": 3605
} | 273 |
<!--Copyright 2022 The HuggingFace Team. All rights reserved.
Licensed under the Apache License, Version 2.0 (the "License"); you may not use this file except in compliance with
the License. You may obtain a copy of the License at
http://www.apache.org/licenses/LICENSE-2.0
Unless required by applicable law or agreed to in writing, software distributed under the License is distributed on
an "AS IS" BASIS, WITHOUT WARRANTIES OR CONDITIONS OF ANY KIND, either express or implied. See the License for the
specific language governing permissions and limitations under the License.
⚠️ Note that this file is in Markdown but contain specific syntax for our doc-builder (similar to MDX) that may not be
rendered properly in your Markdown viewer.
-->
# 推理pipeline
[`pipeline`] 让使用[Hub](https://huggingface.co/models)上的任何模型进行任何语言、计算机视觉、语音以及多模态任务的推理变得非常简单。即使您对特定的模态没有经验,或者不熟悉模型的源码,您仍然可以使用[`pipeline`]进行推理!本教程将教您:
- 如何使用[`pipeline`] 进行推理。
- 如何使用特定的`tokenizer`(分词器)或模型。
- 如何使用[`pipeline`] 进行音频、视觉和多模态任务的推理。
<Tip>
请查看[`pipeline`]文档以获取已支持的任务和可用参数的完整列表。
</Tip>
## Pipeline使用
虽然每个任务都有一个关联的[`pipeline`],但使用通用的抽象的[`pipeline`]更加简单,其中包含所有特定任务的`pipelines`。[`pipeline`]会自动加载一个默认模型和一个能够进行任务推理的预处理类。让我们以使用[`pipeline`]进行自动语音识别(ASR)或语音转文本为例。
1. 首先,创建一个[`pipeline`]并指定推理任务:
```py
>>> from transformers import pipeline
>>> transcriber = pipeline(task="automatic-speech-recognition")
```
2. 将您的输入传递给[`pipeline`]。对于语音识别,这通常是一个音频输入文件:
```py
>>> transcriber("https://huggingface.co/datasets/Narsil/asr_dummy/resolve/main/mlk.flac")
{'text': 'I HAVE A DREAM BUT ONE DAY THIS NATION WILL RISE UP LIVE UP THE TRUE MEANING OF ITS TREES'}
```
您没有得到您期望的结果?可以在Hub上查看一些[最受欢迎的自动语音识别模型](https://huggingface.co/models?pipeline_tag=automatic-speech-recognition&sort=trending)
,看看是否可以获得更好的转录。
让我们尝试来自 OpenAI 的[Whisper large-v2](https://huggingface.co/openai/whisper-large) 模型。Whisperb比Wav2Vec2晚2年发布,使用接近10倍的数据进行了训练。因此,它在大多数下游基准测试上击败了Wav2Vec2。
它还具有预测标点和大小写的附加优势,而Wav2Vec2则无法实现这些功能。
让我们在这里尝试一下,看看它的表现如何:
```py
>>> transcriber = pipeline(model="openai/whisper-large-v2")
>>> transcriber("https://huggingface.co/datasets/Narsil/asr_dummy/resolve/main/mlk.flac")
{'text': ' I have a dream that one day this nation will rise up and live out the true meaning of its creed.'}
```
现在这个结果看起来更准确了!要进行深入的Wav2Vec2与Whisper比较,请参阅[音频变换器课程](https://huggingface.co/learn/audio-course/chapter5/asr_models)。
我们鼓励您在 Hub 上查看不同语言的模型,以及专业领域的模型等。您可以在Hub上直接查看并比较模型的结果,以确定是否适合或处理边缘情况是否比其他模型更好。如果您没有找到适用于您的用例的模型,您始终可以[训练](training)自己的模型!
如果您有多个输入,您可以将输入作为列表传递:
```py
transcriber(
[
"https://huggingface.co/datasets/Narsil/asr_dummy/resolve/main/mlk.flac",
"https://huggingface.co/datasets/Narsil/asr_dummy/resolve/main/1.flac",
]
)
```
`Pipelines`非常适合用于测试,因为从一个模型切换到另一个模型非常琐碎;但是,还有一些方法可以将它们优化后用于大型工作负载而不仅仅是测试。请查看以下指南,深入探讨如何迭代整个数据集或在Web服务器中使用`Pipelines`:
* [在数据集上使用流水线](#using-pipelines-on-a-dataset)
* [在Web服务器中使用流水线](./pipeline_webserver)
## 参数
[`pipeline`] 支持许多参数;有些是适用于特定任务的,而有些适用于所有`pipeline`。通常情况下,您可以在任何地方指定对应参数:
```py
transcriber = pipeline(model="openai/whisper-large-v2", my_parameter=1)
out = transcriber(...) # This will use `my_parameter=1`.
out = transcriber(..., my_parameter=2) # This will override and use `my_parameter=2`.
out = transcriber(...) # This will go back to using `my_parameter=1`.
```
让我们查看其中的三个重要参数:
### 设备
如果您使用 `device=n`,`pipeline`会自动将模型放在指定的设备上。无论您使用PyTorch还是Tensorflow,这都可以工作。
```py
transcriber = pipeline(model="openai/whisper-large-v2", device=0)
```
如果模型对于单个GPU来说过于庞大,并且您正在使用PyTorch,您可以设置 `device_map="auto"` 以自动确定如何加载和存储模型权重。使用 `device_map` 参数需要安装🤗 [Accelerate](https://huggingface.co/docs/accelerate) 软件包:
```bash
pip install --upgrade accelerate
```
以下代码会自动在各个设备上加载和存储模型权重:
```py
transcriber = pipeline(model="openai/whisper-large-v2", device_map="auto")
```
请注意,如果传递了 `device_map="auto"`,在实例化您的 `pipeline` 时不需要添加 `device=device` 参数,否则可能会遇到一些意外的状况!
### 批量大小
默认情况下,`pipelines`不会进行批量推理,原因在[这里](https://huggingface.co/docs/transformers/main_classes/pipelines#pipeline-batching)详细解释。因为批处理不一定更快,实际上在某些情况下可能会更慢。
但如果在您的用例中起作用,您可以使用:
```py
transcriber = pipeline(model="openai/whisper-large-v2", device=0, batch_size=2)
audio_filenames = [f"https://huggingface.co/datasets/Narsil/asr_dummy/resolve/main/{i}.flac" for i in range(1, 5)]
texts = transcriber(audio_filenames)
```
以上代码会在提供的4个音频文件上运行`pipeline`,它会将它们以2个一组的批次传递给模型(模型在GPU上,此时批处理更有可能有所帮助),而您无需编写额外的代码。输出应始终与没有批处理时收到的结果相一致。它只是一种帮助您更快地使用`pipeline`的方式。
`pipeline`也可以减轻一些批处理的复杂性,因为对于某些`pipeline`,需要将单个项目(如长音频文件)分成多个部分以供模型处理。`pipeline`为您执行这种[*chunk batching*](./main_classes/pipelines#pipeline-chunk-batching)。
### 任务特定参数
所有任务都提供了特定于任务的参数,这些参数提供额外的灵活性和选择,以帮助您完成工作。
例如,[`transformers.AutomaticSpeechRecognitionPipeline.__call__`] 方法具有一个 `return_timestamps` 参数,对于字幕视频似乎很有帮助:
```py
>>> transcriber = pipeline(model="openai/whisper-large-v2", return_timestamps=True)
>>> transcriber("https://huggingface.co/datasets/Narsil/asr_dummy/resolve/main/mlk.flac")
{'text': ' I have a dream that one day this nation will rise up and live out the true meaning of its creed.', 'chunks': [{'timestamp': (0.0, 11.88), 'text': ' I have a dream that one day this nation will rise up and live out the true meaning of its'}, {'timestamp': (11.88, 12.38), 'text': ' creed.'}]}
```
正如您所看到的,模型推断出了文本,还输出了各个句子发音的**时间**。
每个任务都有许多可用的参数,因此请查看每个任务的API参考,以了解您可以进行哪些调整!例如,[`~transformers.AutomaticSpeechRecognitionPipeline`] 具有 `chunk_length_s` 参数,对于处理非常长的音频文件(例如,为整部电影或长达一小时的视频配字幕)非常有帮助,这通常是模型无法单独处理的:
```python
>>> transcriber = pipeline(model="openai/whisper-large-v2", chunk_length_s=30, return_timestamps=True)
>>> transcriber("https://huggingface.co/datasets/sanchit-gandhi/librispeech_long/resolve/main/audio.wav")
{'text': " Chapter 16. I might have told you of the beginning of this liaison in a few lines, but I wanted you to see every step by which we came. I, too, agree to whatever Marguerite wished, Marguerite to be unable to live apart from me. It was the day after the evening...
```
如果您找不到一个真正有帮助的参数,欢迎[提出请求](https://github.com/huggingface/transformers/issues/new?assignees=&labels=feature&template=feature-request.yml)!
## 在数据集上使用pipelines
`pipelines`也可以对大型数据集进行推理。我们建议使用迭代器来完成这一任务,这是最简单的方法:
```py
def data():
for i in range(1000):
yield f"My example {i}"
pipe = pipeline(model="openai-community/gpt2", device=0)
generated_characters = 0
for out in pipe(data()):
generated_characters += len(out[0]["generated_text"])
```
迭代器 `data()` 会产生每个结果,`pipelines`会自动识别输入为可迭代对象,并在GPU上处理数据的同时开始获取数据(在底层使用[DataLoader](https://pytorch.org/docs/stable/data.html#torch.utils.data.DataLoader))。这一点非常重要,因为您不必为整个数据集分配内存,可以尽可能快地将数据传送到GPU。
由于批处理可以加速处理,因此在这里尝试调整 `batch_size` 参数可能会很有用。
迭代数据集的最简单方法就是从🤗 [Datasets](https://github.com/huggingface/datasets/) 中加载数据集:
```py
# KeyDataset is a util that will just output the item we're interested in.
from transformers.pipelines.pt_utils import KeyDataset
from datasets import load_dataset
pipe = pipeline(model="hf-internal-testing/tiny-random-wav2vec2", device=0)
dataset = load_dataset("hf-internal-testing/librispeech_asr_dummy", "clean", split="validation[:10]")
for out in pipe(KeyDataset(dataset, "audio")):
print(out)
```
## 在Web服务器上使用pipelines
<Tip>
创建推理引擎是一个复杂的主题,值得有自己的页面。
</Tip>
[链接](./pipeline_webserver)
## 视觉流水线
对于视觉任务,使用[`pipeline`] 几乎是相同的。
指定您的任务并将图像传递给分类器。图像可以是链接、本地路径或base64编码的图像。例如,下面显示的是哪种品种的猫?

```py
>>> from transformers import pipeline
>>> vision_classifier = pipeline(model="google/vit-base-patch16-224")
>>> preds = vision_classifier(
... images="https://huggingface.co/datasets/huggingface/documentation-images/resolve/main/pipeline-cat-chonk.jpeg"
... )
>>> preds = [{"score": round(pred["score"], 4), "label": pred["label"]} for pred in preds]
>>> preds
[{'score': 0.4335, 'label': 'lynx, catamount'}, {'score': 0.0348, 'label': 'cougar, puma, catamount, mountain lion, painter, panther, Felis concolor'}, {'score': 0.0324, 'label': 'snow leopard, ounce, Panthera uncia'}, {'score': 0.0239, 'label': 'Egyptian cat'}, {'score': 0.0229, 'label': 'tiger cat'}]
```
## 文本流水线
对于NLP任务,使用[`pipeline`] 几乎是相同的。
```py
>>> from transformers import pipeline
>>> # This model is a `zero-shot-classification` model.
>>> # It will classify text, except you are free to choose any label you might imagine
>>> classifier = pipeline(model="facebook/bart-large-mnli")
>>> classifier(
... "I have a problem with my iphone that needs to be resolved asap!!",
... candidate_labels=["urgent", "not urgent", "phone", "tablet", "computer"],
... )
{'sequence': 'I have a problem with my iphone that needs to be resolved asap!!', 'labels': ['urgent', 'phone', 'computer', 'not urgent', 'tablet'], 'scores': [0.504, 0.479, 0.013, 0.003, 0.002]}
```
## 多模态流水线
[`pipeline`] 支持多个模态。例如,视觉问题回答(VQA)任务结合了文本和图像。请随意使用您喜欢的任何图像链接和您想要问关于该图像的问题。图像可以是URL或图像的本地路径。
例如,如果您使用这个[invoice image](https://huggingface.co/spaces/impira/docquery/resolve/2359223c1837a7587402bda0f2643382a6eefeab/invoice.png):
```py
>>> from transformers import pipeline
>>> vqa = pipeline(model="impira/layoutlm-document-qa")
>>> vqa(
... image="https://huggingface.co/spaces/impira/docquery/resolve/2359223c1837a7587402bda0f2643382a6eefeab/invoice.png",
... question="What is the invoice number?",
... )
[{'score': 0.42515, 'answer': 'us-001', 'start': 16, 'end': 16}]
```
<Tip>
要运行上面的示例,除了🤗 Transformers之外,您需要安装[`pytesseract`](https://pypi.org/project/pytesseract/)。
```bash
sudo apt install -y tesseract-ocr
pip install pytesseract
```
</Tip>
## 在大模型上使用🤗 `accelerate`和`pipeline`:
您可以轻松地使用🤗 `accelerate`在大模型上运行 `pipeline`!首先确保您已经使用 `pip install accelerate` 安装了 `accelerate`。
首先使用 `device_map="auto"` 加载您的模型!我们将在示例中使用 `facebook/opt-1.3b`。
```py
# pip install accelerate
import torch
from transformers import pipeline
pipe = pipeline(model="facebook/opt-1.3b", torch_dtype=torch.bfloat16, device_map="auto")
output = pipe("This is a cool example!", do_sample=True, top_p=0.95)
```
如果安装 `bitsandbytes` 并添加参数 `load_in_8bit=True`,您还可以传递8位加载的模型。
```py
# pip install accelerate bitsandbytes
import torch
from transformers import pipeline
pipe = pipeline(model="facebook/opt-1.3b", device_map="auto", model_kwargs={"load_in_8bit": True})
output = pipe("This is a cool example!", do_sample=True, top_p=0.95)
```
请注意,您可以将`checkpoint `替换为任何支持大模型加载的Hugging Face模型,比如BLOOM!
| transformers/docs/source/zh/pipeline_tutorial.md/0 | {
"file_path": "transformers/docs/source/zh/pipeline_tutorial.md",
"repo_id": "transformers",
"token_count": 7446
} | 274 |
#!/usr/bin/env python
import torch
from transformers import CamembertForMaskedLM, CamembertTokenizer
def fill_mask(masked_input, model, tokenizer, topk=5):
# Adapted from https://github.com/pytorch/fairseq/blob/master/fairseq/models/roberta/hub_interface.py
assert masked_input.count("<mask>") == 1
input_ids = torch.tensor(tokenizer.encode(masked_input, add_special_tokens=True)).unsqueeze(0) # Batch size 1
logits = model(input_ids)[0] # The last hidden-state is the first element of the output tuple
masked_index = (input_ids.squeeze() == tokenizer.mask_token_id).nonzero().item()
logits = logits[0, masked_index, :]
prob = logits.softmax(dim=0)
values, indices = prob.topk(k=topk, dim=0)
topk_predicted_token_bpe = " ".join(
[tokenizer.convert_ids_to_tokens(indices[i].item()) for i in range(len(indices))]
)
masked_token = tokenizer.mask_token
topk_filled_outputs = []
for index, predicted_token_bpe in enumerate(topk_predicted_token_bpe.split(" ")):
predicted_token = predicted_token_bpe.replace("\u2581", " ")
if " {0}".format(masked_token) in masked_input:
topk_filled_outputs.append(
(
masked_input.replace(" {0}".format(masked_token), predicted_token),
values[index].item(),
predicted_token,
)
)
else:
topk_filled_outputs.append(
(
masked_input.replace(masked_token, predicted_token),
values[index].item(),
predicted_token,
)
)
return topk_filled_outputs
tokenizer = CamembertTokenizer.from_pretrained("almanach/camembert-base")
model = CamembertForMaskedLM.from_pretrained("almanach/camembert-base")
model.eval()
masked_input = "Le camembert est <mask> :)"
print(fill_mask(masked_input, model, tokenizer, topk=3))
| transformers/examples/legacy/run_camembert.py/0 | {
"file_path": "transformers/examples/legacy/run_camembert.py",
"repo_id": "transformers",
"token_count": 888
} | 275 |
# coding=utf-8
# Copyright 2020 Huggingface
#
# Licensed under the Apache License, Version 2.0 (the "License");
# you may not use this file except in compliance with the License.
# You may obtain a copy of the License at
#
# http://www.apache.org/licenses/LICENSE-2.0
#
# Unless required by applicable law or agreed to in writing, software
# distributed under the License is distributed on an "AS IS" BASIS,
# WITHOUT WARRANTIES OR CONDITIONS OF ANY KIND, either express or implied.
# See the License for the specific language governing permissions and
# limitations under the License.
import io
import json
import unittest
from parameterized import parameterized
from transformers import FSMTForConditionalGeneration, FSMTTokenizer
from transformers.testing_utils import get_tests_dir, require_torch, slow, torch_device
from utils import calculate_bleu
filename = get_tests_dir() + "/test_data/fsmt/fsmt_val_data.json"
with io.open(filename, "r", encoding="utf-8") as f:
bleu_data = json.load(f)
@require_torch
class ModelEvalTester(unittest.TestCase):
def get_tokenizer(self, mname):
return FSMTTokenizer.from_pretrained(mname)
def get_model(self, mname):
model = FSMTForConditionalGeneration.from_pretrained(mname).to(torch_device)
if torch_device == "cuda":
model.half()
return model
@parameterized.expand(
[
["en-ru", 26.0],
["ru-en", 22.0],
["en-de", 22.0],
["de-en", 29.0],
]
)
@slow
def test_bleu_scores(self, pair, min_bleu_score):
# note: this test is not testing the best performance since it only evals a small batch
# but it should be enough to detect a regression in the output quality
mname = f"facebook/wmt19-{pair}"
tokenizer = self.get_tokenizer(mname)
model = self.get_model(mname)
src_sentences = bleu_data[pair]["src"]
tgt_sentences = bleu_data[pair]["tgt"]
batch = tokenizer(src_sentences, return_tensors="pt", truncation=True, padding="longest").to(torch_device)
outputs = model.generate(
input_ids=batch.input_ids,
num_beams=8,
)
decoded_sentences = tokenizer.batch_decode(
outputs, skip_special_tokens=True, clean_up_tokenization_spaces=False
)
scores = calculate_bleu(decoded_sentences, tgt_sentences)
print(scores)
self.assertGreaterEqual(scores["bleu"], min_bleu_score)
| transformers/examples/legacy/seq2seq/old_test_fsmt_bleu_score.py/0 | {
"file_path": "transformers/examples/legacy/seq2seq/old_test_fsmt_bleu_score.py",
"repo_id": "transformers",
"token_count": 997
} | 276 |
#!/usr/bin/env python
import io
import json
import subprocess
pairs = [
["en", "ru"],
["ru", "en"],
["en", "de"],
["de", "en"],
]
n_objs = 8
def get_all_data(pairs, n_objs):
text = {}
for src, tgt in pairs:
pair = f"{src}-{tgt}"
cmd = f"sacrebleu -t wmt19 -l {pair} --echo src".split()
src_lines = subprocess.run(cmd, stdout=subprocess.PIPE).stdout.decode("utf-8").splitlines()
cmd = f"sacrebleu -t wmt19 -l {pair} --echo ref".split()
tgt_lines = subprocess.run(cmd, stdout=subprocess.PIPE).stdout.decode("utf-8").splitlines()
text[pair] = {"src": src_lines[:n_objs], "tgt": tgt_lines[:n_objs]}
return text
text = get_all_data(pairs, n_objs)
filename = "./fsmt_val_data.json"
with io.open(filename, "w", encoding="utf-8") as f:
bleu_data = json.dump(text, f, indent=2, ensure_ascii=False)
| transformers/examples/legacy/seq2seq/test_data/fsmt/build-eval-data.py/0 | {
"file_path": "transformers/examples/legacy/seq2seq/test_data/fsmt/build-eval-data.py",
"repo_id": "transformers",
"token_count": 410
} | 277 |
## Token classification
Based on the scripts [`run_ner.py`](https://github.com/huggingface/transformers/blob/main/examples/legacy/token-classification/run_ner.py).
The following examples are covered in this section:
* NER on the GermEval 2014 (German NER) dataset
* Emerging and Rare Entities task: WNUT’17 (English NER) dataset
Details and results for the fine-tuning provided by @stefan-it.
### GermEval 2014 (German NER) dataset
#### Data (Download and pre-processing steps)
Data can be obtained from the [GermEval 2014](https://sites.google.com/site/germeval2014ner/data) shared task page.
Here are the commands for downloading and pre-processing train, dev and test datasets. The original data format has four (tab-separated) columns, in a pre-processing step only the two relevant columns (token and outer span NER annotation) are extracted:
```bash
curl -L 'https://drive.google.com/uc?export=download&id=1Jjhbal535VVz2ap4v4r_rN1UEHTdLK5P' \
| grep -v "^#" | cut -f 2,3 | tr '\t' ' ' > train.txt.tmp
curl -L 'https://drive.google.com/uc?export=download&id=1ZfRcQThdtAR5PPRjIDtrVP7BtXSCUBbm' \
| grep -v "^#" | cut -f 2,3 | tr '\t' ' ' > dev.txt.tmp
curl -L 'https://drive.google.com/uc?export=download&id=1u9mb7kNJHWQCWyweMDRMuTFoOHOfeBTH' \
| grep -v "^#" | cut -f 2,3 | tr '\t' ' ' > test.txt.tmp
```
The GermEval 2014 dataset contains some strange "control character" tokens like `'\x96', '\u200e', '\x95', '\xad' or '\x80'`.
One problem with these tokens is, that `BertTokenizer` returns an empty token for them, resulting in misaligned `InputExample`s.
The `preprocess.py` script located in the `scripts` folder a) filters these tokens and b) splits longer sentences into smaller ones (once the max. subtoken length is reached).
Let's define some variables that we need for further pre-processing steps and training the model:
```bash
export MAX_LENGTH=128
export BERT_MODEL=google-bert/bert-base-multilingual-cased
```
Run the pre-processing script on training, dev and test datasets:
```bash
python3 scripts/preprocess.py train.txt.tmp $BERT_MODEL $MAX_LENGTH > train.txt
python3 scripts/preprocess.py dev.txt.tmp $BERT_MODEL $MAX_LENGTH > dev.txt
python3 scripts/preprocess.py test.txt.tmp $BERT_MODEL $MAX_LENGTH > test.txt
```
The GermEval 2014 dataset has much more labels than CoNLL-2002/2003 datasets, so an own set of labels must be used:
```bash
cat train.txt dev.txt test.txt | cut -d " " -f 2 | grep -v "^$"| sort | uniq > labels.txt
```
#### Prepare the run
Additional environment variables must be set:
```bash
export OUTPUT_DIR=germeval-model
export BATCH_SIZE=32
export NUM_EPOCHS=3
export SAVE_STEPS=750
export SEED=1
```
#### Run the Pytorch version
To start training, just run:
```bash
python3 run_ner.py --data_dir ./ \
--labels ./labels.txt \
--model_name_or_path $BERT_MODEL \
--output_dir $OUTPUT_DIR \
--max_seq_length $MAX_LENGTH \
--num_train_epochs $NUM_EPOCHS \
--per_device_train_batch_size $BATCH_SIZE \
--save_steps $SAVE_STEPS \
--seed $SEED \
--do_train \
--do_eval \
--do_predict
```
If your GPU supports half-precision training, just add the `--fp16` flag. After training, the model will be both evaluated on development and test datasets.
#### JSON-based configuration file
Instead of passing all parameters via commandline arguments, the `run_ner.py` script also supports reading parameters from a json-based configuration file:
```json
{
"data_dir": ".",
"labels": "./labels.txt",
"model_name_or_path": "google-bert/bert-base-multilingual-cased",
"output_dir": "germeval-model",
"max_seq_length": 128,
"num_train_epochs": 3,
"per_device_train_batch_size": 32,
"save_steps": 750,
"seed": 1,
"do_train": true,
"do_eval": true,
"do_predict": true
}
```
It must be saved with a `.json` extension and can be used by running `python3 run_ner.py config.json`.
#### Evaluation
Evaluation on development dataset outputs the following for our example:
```bash
10/04/2019 00:42:06 - INFO - __main__ - ***** Eval results *****
10/04/2019 00:42:06 - INFO - __main__ - f1 = 0.8623348017621146
10/04/2019 00:42:06 - INFO - __main__ - loss = 0.07183869666975543
10/04/2019 00:42:06 - INFO - __main__ - precision = 0.8467916366258111
10/04/2019 00:42:06 - INFO - __main__ - recall = 0.8784592370979806
```
On the test dataset the following results could be achieved:
```bash
10/04/2019 00:42:42 - INFO - __main__ - ***** Eval results *****
10/04/2019 00:42:42 - INFO - __main__ - f1 = 0.8614389652384803
10/04/2019 00:42:42 - INFO - __main__ - loss = 0.07064602487454782
10/04/2019 00:42:42 - INFO - __main__ - precision = 0.8604651162790697
10/04/2019 00:42:42 - INFO - __main__ - recall = 0.8624150210424085
```
#### Run the Tensorflow 2 version
To start training, just run:
```bash
python3 run_tf_ner.py --data_dir ./ \
--labels ./labels.txt \
--model_name_or_path $BERT_MODEL \
--output_dir $OUTPUT_DIR \
--max_seq_length $MAX_LENGTH \
--num_train_epochs $NUM_EPOCHS \
--per_device_train_batch_size $BATCH_SIZE \
--save_steps $SAVE_STEPS \
--seed $SEED \
--do_train \
--do_eval \
--do_predict
```
Such as the Pytorch version, if your GPU supports half-precision training, just add the `--fp16` flag. After training, the model will be both evaluated on development and test datasets.
#### Evaluation
Evaluation on development dataset outputs the following for our example:
```bash
precision recall f1-score support
LOCderiv 0.7619 0.6154 0.6809 52
PERpart 0.8724 0.8997 0.8858 4057
OTHpart 0.9360 0.9466 0.9413 711
ORGpart 0.7015 0.6989 0.7002 269
LOCpart 0.7668 0.8488 0.8057 496
LOC 0.8745 0.9191 0.8963 235
ORGderiv 0.7723 0.8571 0.8125 91
OTHderiv 0.4800 0.6667 0.5581 18
OTH 0.5789 0.6875 0.6286 16
PERderiv 0.5385 0.3889 0.4516 18
PER 0.5000 0.5000 0.5000 2
ORG 0.0000 0.0000 0.0000 3
micro avg 0.8574 0.8862 0.8715 5968
macro avg 0.8575 0.8862 0.8713 5968
```
On the test dataset the following results could be achieved:
```bash
precision recall f1-score support
PERpart 0.8847 0.8944 0.8896 9397
OTHpart 0.9376 0.9353 0.9365 1639
ORGpart 0.7307 0.7044 0.7173 697
LOC 0.9133 0.9394 0.9262 561
LOCpart 0.8058 0.8157 0.8107 1150
ORG 0.0000 0.0000 0.0000 8
OTHderiv 0.5882 0.4762 0.5263 42
PERderiv 0.6571 0.5227 0.5823 44
OTH 0.4906 0.6667 0.5652 39
ORGderiv 0.7016 0.7791 0.7383 172
LOCderiv 0.8256 0.6514 0.7282 109
PER 0.0000 0.0000 0.0000 11
micro avg 0.8722 0.8774 0.8748 13869
macro avg 0.8712 0.8774 0.8740 13869
```
### Emerging and Rare Entities task: WNUT’17 (English NER) dataset
Description of the WNUT’17 task from the [shared task website](http://noisy-text.github.io/2017/index.html):
> The WNUT’17 shared task focuses on identifying unusual, previously-unseen entities in the context of emerging discussions.
> Named entities form the basis of many modern approaches to other tasks (like event clustering and summarization), but recall on
> them is a real problem in noisy text - even among annotators. This drop tends to be due to novel entities and surface forms.
Six labels are available in the dataset. An overview can be found on this [page](http://noisy-text.github.io/2017/files/).
#### Data (Download and pre-processing steps)
The dataset can be downloaded from the [official GitHub](https://github.com/leondz/emerging_entities_17) repository.
The following commands show how to prepare the dataset for fine-tuning:
```bash
mkdir -p data_wnut_17
curl -L 'https://github.com/leondz/emerging_entities_17/raw/master/wnut17train.conll' | tr '\t' ' ' > data_wnut_17/train.txt.tmp
curl -L 'https://github.com/leondz/emerging_entities_17/raw/master/emerging.dev.conll' | tr '\t' ' ' > data_wnut_17/dev.txt.tmp
curl -L 'https://raw.githubusercontent.com/leondz/emerging_entities_17/master/emerging.test.annotated' | tr '\t' ' ' > data_wnut_17/test.txt.tmp
```
Let's define some variables that we need for further pre-processing steps:
```bash
export MAX_LENGTH=128
export BERT_MODEL=google-bert/bert-large-cased
```
Here we use the English BERT large model for fine-tuning.
The `preprocess.py` scripts splits longer sentences into smaller ones (once the max. subtoken length is reached):
```bash
python3 scripts/preprocess.py data_wnut_17/train.txt.tmp $BERT_MODEL $MAX_LENGTH > data_wnut_17/train.txt
python3 scripts/preprocess.py data_wnut_17/dev.txt.tmp $BERT_MODEL $MAX_LENGTH > data_wnut_17/dev.txt
python3 scripts/preprocess.py data_wnut_17/test.txt.tmp $BERT_MODEL $MAX_LENGTH > data_wnut_17/test.txt
```
In the last pre-processing step, the `labels.txt` file needs to be generated. This file contains all available labels:
```bash
cat data_wnut_17/train.txt data_wnut_17/dev.txt data_wnut_17/test.txt | cut -d " " -f 2 | grep -v "^$"| sort | uniq > data_wnut_17/labels.txt
```
#### Run the Pytorch version
Fine-tuning with the PyTorch version can be started using the `run_ner.py` script. In this example we use a JSON-based configuration file.
This configuration file looks like:
```json
{
"data_dir": "./data_wnut_17",
"labels": "./data_wnut_17/labels.txt",
"model_name_or_path": "google-bert/bert-large-cased",
"output_dir": "wnut-17-model-1",
"max_seq_length": 128,
"num_train_epochs": 3,
"per_device_train_batch_size": 32,
"save_steps": 425,
"seed": 1,
"do_train": true,
"do_eval": true,
"do_predict": true,
"fp16": false
}
```
If your GPU supports half-precision training, please set `fp16` to `true`.
Save this JSON-based configuration under `wnut_17.json`. The fine-tuning can be started with `python3 run_ner_old.py wnut_17.json`.
#### Evaluation
Evaluation on development dataset outputs the following:
```bash
05/29/2020 23:33:44 - INFO - __main__ - ***** Eval results *****
05/29/2020 23:33:44 - INFO - __main__ - eval_loss = 0.26505235286212275
05/29/2020 23:33:44 - INFO - __main__ - eval_precision = 0.7008264462809918
05/29/2020 23:33:44 - INFO - __main__ - eval_recall = 0.507177033492823
05/29/2020 23:33:44 - INFO - __main__ - eval_f1 = 0.5884802220680084
05/29/2020 23:33:44 - INFO - __main__ - epoch = 3.0
```
On the test dataset the following results could be achieved:
```bash
05/29/2020 23:33:44 - INFO - transformers.trainer - ***** Running Prediction *****
05/29/2020 23:34:02 - INFO - __main__ - eval_loss = 0.30948806500973547
05/29/2020 23:34:02 - INFO - __main__ - eval_precision = 0.5840108401084011
05/29/2020 23:34:02 - INFO - __main__ - eval_recall = 0.3994439295644115
05/29/2020 23:34:02 - INFO - __main__ - eval_f1 = 0.47440836543753434
```
WNUT’17 is a very difficult task. Current state-of-the-art results on this dataset can be found [here](https://nlpprogress.com/english/named_entity_recognition.html).
| transformers/examples/legacy/token-classification/README.md/0 | {
"file_path": "transformers/examples/legacy/token-classification/README.md",
"repo_id": "transformers",
"token_count": 4566
} | 278 |
#!/usr/bin/env python
# coding=utf-8
# Copyright 2020 The HuggingFace Team All rights reserved.
#
# Licensed under the Apache License, Version 2.0 (the "License");
# you may not use this file except in compliance with the License.
# You may obtain a copy of the License at
#
# http://www.apache.org/licenses/LICENSE-2.0
#
# Unless required by applicable law or agreed to in writing, software
# distributed under the License is distributed on an "AS IS" BASIS,
# WITHOUT WARRANTIES OR CONDITIONS OF ANY KIND, either express or implied.
# See the License for the specific language governing permissions and
# limitations under the License.
"""
Fine-tuning the library models for masked language modeling (BERT, ALBERT, RoBERTa...) on a text file or a dataset.
Here is the full list of checkpoints on the hub that can be fine-tuned by this script:
https://huggingface.co/models?filter=fill-mask
"""
# You can also adapt this script on your own masked language modeling task. Pointers for this are left as comments.
import logging
import math
import os
import sys
import warnings
from dataclasses import dataclass, field
from itertools import chain
from typing import Optional
import datasets
import evaluate
from datasets import load_dataset
import transformers
from transformers import (
CONFIG_MAPPING,
MODEL_FOR_MASKED_LM_MAPPING,
AutoConfig,
AutoModelForMaskedLM,
AutoTokenizer,
DataCollatorForLanguageModeling,
HfArgumentParser,
Trainer,
TrainingArguments,
is_torch_xla_available,
set_seed,
)
from transformers.trainer_utils import get_last_checkpoint
from transformers.utils import check_min_version, send_example_telemetry
from transformers.utils.versions import require_version
# Will error if the minimal version of Transformers is not installed. Remove at your own risks.
check_min_version("4.40.0.dev0")
require_version("datasets>=1.8.0", "To fix: pip install -r examples/pytorch/language-modeling/requirements.txt")
logger = logging.getLogger(__name__)
MODEL_CONFIG_CLASSES = list(MODEL_FOR_MASKED_LM_MAPPING.keys())
MODEL_TYPES = tuple(conf.model_type for conf in MODEL_CONFIG_CLASSES)
@dataclass
class ModelArguments:
"""
Arguments pertaining to which model/config/tokenizer we are going to fine-tune, or train from scratch.
"""
model_name_or_path: Optional[str] = field(
default=None,
metadata={
"help": (
"The model checkpoint for weights initialization. Don't set if you want to train a model from scratch."
)
},
)
model_type: Optional[str] = field(
default=None,
metadata={"help": "If training from scratch, pass a model type from the list: " + ", ".join(MODEL_TYPES)},
)
config_overrides: Optional[str] = field(
default=None,
metadata={
"help": (
"Override some existing default config settings when a model is trained from scratch. Example: "
"n_embd=10,resid_pdrop=0.2,scale_attn_weights=false,summary_type=cls_index"
)
},
)
config_name: Optional[str] = field(
default=None, metadata={"help": "Pretrained config name or path if not the same as model_name"}
)
tokenizer_name: Optional[str] = field(
default=None, metadata={"help": "Pretrained tokenizer name or path if not the same as model_name"}
)
cache_dir: Optional[str] = field(
default=None,
metadata={"help": "Where do you want to store the pretrained models downloaded from huggingface.co"},
)
use_fast_tokenizer: bool = field(
default=True,
metadata={"help": "Whether to use one of the fast tokenizer (backed by the tokenizers library) or not."},
)
model_revision: str = field(
default="main",
metadata={"help": "The specific model version to use (can be a branch name, tag name or commit id)."},
)
token: str = field(
default=None,
metadata={
"help": (
"The token to use as HTTP bearer authorization for remote files. If not specified, will use the token "
"generated when running `huggingface-cli login` (stored in `~/.huggingface`)."
)
},
)
use_auth_token: bool = field(
default=None,
metadata={
"help": "The `use_auth_token` argument is deprecated and will be removed in v4.34. Please use `token` instead."
},
)
trust_remote_code: bool = field(
default=False,
metadata={
"help": (
"Whether or not to allow for custom models defined on the Hub in their own modeling files. This option "
"should only be set to `True` for repositories you trust and in which you have read the code, as it will "
"execute code present on the Hub on your local machine."
)
},
)
low_cpu_mem_usage: bool = field(
default=False,
metadata={
"help": (
"It is an option to create the model as an empty shell, then only materialize its parameters when the pretrained weights are loaded. "
"set True will benefit LLM loading time and RAM consumption."
)
},
)
def __post_init__(self):
if self.config_overrides is not None and (self.config_name is not None or self.model_name_or_path is not None):
raise ValueError(
"--config_overrides can't be used in combination with --config_name or --model_name_or_path"
)
@dataclass
class DataTrainingArguments:
"""
Arguments pertaining to what data we are going to input our model for training and eval.
"""
dataset_name: Optional[str] = field(
default=None, metadata={"help": "The name of the dataset to use (via the datasets library)."}
)
dataset_config_name: Optional[str] = field(
default=None, metadata={"help": "The configuration name of the dataset to use (via the datasets library)."}
)
train_file: Optional[str] = field(default=None, metadata={"help": "The input training data file (a text file)."})
validation_file: Optional[str] = field(
default=None,
metadata={"help": "An optional input evaluation data file to evaluate the perplexity on (a text file)."},
)
overwrite_cache: bool = field(
default=False, metadata={"help": "Overwrite the cached training and evaluation sets"}
)
validation_split_percentage: Optional[int] = field(
default=5,
metadata={
"help": "The percentage of the train set used as validation set in case there's no validation split"
},
)
max_seq_length: Optional[int] = field(
default=None,
metadata={
"help": (
"The maximum total input sequence length after tokenization. Sequences longer "
"than this will be truncated."
)
},
)
preprocessing_num_workers: Optional[int] = field(
default=None,
metadata={"help": "The number of processes to use for the preprocessing."},
)
mlm_probability: float = field(
default=0.15, metadata={"help": "Ratio of tokens to mask for masked language modeling loss"}
)
line_by_line: bool = field(
default=False,
metadata={"help": "Whether distinct lines of text in the dataset are to be handled as distinct sequences."},
)
pad_to_max_length: bool = field(
default=False,
metadata={
"help": (
"Whether to pad all samples to `max_seq_length`. "
"If False, will pad the samples dynamically when batching to the maximum length in the batch."
)
},
)
max_train_samples: Optional[int] = field(
default=None,
metadata={
"help": (
"For debugging purposes or quicker training, truncate the number of training examples to this "
"value if set."
)
},
)
max_eval_samples: Optional[int] = field(
default=None,
metadata={
"help": (
"For debugging purposes or quicker training, truncate the number of evaluation examples to this "
"value if set."
)
},
)
streaming: bool = field(default=False, metadata={"help": "Enable streaming mode"})
def __post_init__(self):
if self.streaming:
require_version("datasets>=2.0.0", "The streaming feature requires `datasets>=2.0.0`")
if self.dataset_name is None and self.train_file is None and self.validation_file is None:
raise ValueError("Need either a dataset name or a training/validation file.")
else:
if self.train_file is not None:
extension = self.train_file.split(".")[-1]
if extension not in ["csv", "json", "txt"]:
raise ValueError("`train_file` should be a csv, a json or a txt file.")
if self.validation_file is not None:
extension = self.validation_file.split(".")[-1]
if extension not in ["csv", "json", "txt"]:
raise ValueError("`validation_file` should be a csv, a json or a txt file.")
def main():
# See all possible arguments in src/transformers/training_args.py
# or by passing the --help flag to this script.
# We now keep distinct sets of args, for a cleaner separation of concerns.
parser = HfArgumentParser((ModelArguments, DataTrainingArguments, TrainingArguments))
if len(sys.argv) == 2 and sys.argv[1].endswith(".json"):
# If we pass only one argument to the script and it's the path to a json file,
# let's parse it to get our arguments.
model_args, data_args, training_args = parser.parse_json_file(json_file=os.path.abspath(sys.argv[1]))
else:
model_args, data_args, training_args = parser.parse_args_into_dataclasses()
if model_args.use_auth_token is not None:
warnings.warn(
"The `use_auth_token` argument is deprecated and will be removed in v4.34. Please use `token` instead.",
FutureWarning,
)
if model_args.token is not None:
raise ValueError("`token` and `use_auth_token` are both specified. Please set only the argument `token`.")
model_args.token = model_args.use_auth_token
# Sending telemetry. Tracking the example usage helps us better allocate resources to maintain them. The
# information sent is the one passed as arguments along with your Python/PyTorch versions.
send_example_telemetry("run_mlm", model_args, data_args)
# Setup logging
logging.basicConfig(
format="%(asctime)s - %(levelname)s - %(name)s - %(message)s",
datefmt="%m/%d/%Y %H:%M:%S",
handlers=[logging.StreamHandler(sys.stdout)],
)
if training_args.should_log:
# The default of training_args.log_level is passive, so we set log level at info here to have that default.
transformers.utils.logging.set_verbosity_info()
log_level = training_args.get_process_log_level()
logger.setLevel(log_level)
datasets.utils.logging.set_verbosity(log_level)
transformers.utils.logging.set_verbosity(log_level)
transformers.utils.logging.enable_default_handler()
transformers.utils.logging.enable_explicit_format()
# Log on each process the small summary:
logger.warning(
f"Process rank: {training_args.local_rank}, device: {training_args.device}, n_gpu: {training_args.n_gpu}, "
+ f"distributed training: {training_args.parallel_mode.value == 'distributed'}, 16-bits training: {training_args.fp16}"
)
# Set the verbosity to info of the Transformers logger (on main process only):
logger.info(f"Training/evaluation parameters {training_args}")
# Detecting last checkpoint.
last_checkpoint = None
if os.path.isdir(training_args.output_dir) and training_args.do_train and not training_args.overwrite_output_dir:
last_checkpoint = get_last_checkpoint(training_args.output_dir)
if last_checkpoint is None and len(os.listdir(training_args.output_dir)) > 0:
raise ValueError(
f"Output directory ({training_args.output_dir}) already exists and is not empty. "
"Use --overwrite_output_dir to overcome."
)
elif last_checkpoint is not None and training_args.resume_from_checkpoint is None:
logger.info(
f"Checkpoint detected, resuming training at {last_checkpoint}. To avoid this behavior, change "
"the `--output_dir` or add `--overwrite_output_dir` to train from scratch."
)
# Set seed before initializing model.
set_seed(training_args.seed)
# Get the datasets: you can either provide your own CSV/JSON/TXT training and evaluation files (see below)
# or just provide the name of one of the public datasets available on the hub at https://huggingface.co/datasets/
# (the dataset will be downloaded automatically from the datasets Hub
#
# For CSV/JSON files, this script will use the column called 'text' or the first column. You can easily tweak this
# behavior (see below)
#
# In distributed training, the load_dataset function guarantee that only one local process can concurrently
# download the dataset.
if data_args.dataset_name is not None:
# Downloading and loading a dataset from the hub.
raw_datasets = load_dataset(
data_args.dataset_name,
data_args.dataset_config_name,
cache_dir=model_args.cache_dir,
token=model_args.token,
streaming=data_args.streaming,
)
if "validation" not in raw_datasets.keys():
raw_datasets["validation"] = load_dataset(
data_args.dataset_name,
data_args.dataset_config_name,
split=f"train[:{data_args.validation_split_percentage}%]",
cache_dir=model_args.cache_dir,
token=model_args.token,
streaming=data_args.streaming,
)
raw_datasets["train"] = load_dataset(
data_args.dataset_name,
data_args.dataset_config_name,
split=f"train[{data_args.validation_split_percentage}%:]",
cache_dir=model_args.cache_dir,
token=model_args.token,
streaming=data_args.streaming,
)
else:
data_files = {}
if data_args.train_file is not None:
data_files["train"] = data_args.train_file
extension = data_args.train_file.split(".")[-1]
if data_args.validation_file is not None:
data_files["validation"] = data_args.validation_file
extension = data_args.validation_file.split(".")[-1]
if extension == "txt":
extension = "text"
raw_datasets = load_dataset(
extension,
data_files=data_files,
cache_dir=model_args.cache_dir,
token=model_args.token,
)
# If no validation data is there, validation_split_percentage will be used to divide the dataset.
if "validation" not in raw_datasets.keys():
raw_datasets["validation"] = load_dataset(
extension,
data_files=data_files,
split=f"train[:{data_args.validation_split_percentage}%]",
cache_dir=model_args.cache_dir,
token=model_args.token,
)
raw_datasets["train"] = load_dataset(
extension,
data_files=data_files,
split=f"train[{data_args.validation_split_percentage}%:]",
cache_dir=model_args.cache_dir,
token=model_args.token,
)
# See more about loading any type of standard or custom dataset (from files, python dict, pandas DataFrame, etc) at
# https://huggingface.co/docs/datasets/loading_datasets.
# Load pretrained model and tokenizer
#
# Distributed training:
# The .from_pretrained methods guarantee that only one local process can concurrently
# download model & vocab.
config_kwargs = {
"cache_dir": model_args.cache_dir,
"revision": model_args.model_revision,
"token": model_args.token,
"trust_remote_code": model_args.trust_remote_code,
}
if model_args.config_name:
config = AutoConfig.from_pretrained(model_args.config_name, **config_kwargs)
elif model_args.model_name_or_path:
config = AutoConfig.from_pretrained(model_args.model_name_or_path, **config_kwargs)
else:
config = CONFIG_MAPPING[model_args.model_type]()
logger.warning("You are instantiating a new config instance from scratch.")
if model_args.config_overrides is not None:
logger.info(f"Overriding config: {model_args.config_overrides}")
config.update_from_string(model_args.config_overrides)
logger.info(f"New config: {config}")
tokenizer_kwargs = {
"cache_dir": model_args.cache_dir,
"use_fast": model_args.use_fast_tokenizer,
"revision": model_args.model_revision,
"token": model_args.token,
"trust_remote_code": model_args.trust_remote_code,
}
if model_args.tokenizer_name:
tokenizer = AutoTokenizer.from_pretrained(model_args.tokenizer_name, **tokenizer_kwargs)
elif model_args.model_name_or_path:
tokenizer = AutoTokenizer.from_pretrained(model_args.model_name_or_path, **tokenizer_kwargs)
else:
raise ValueError(
"You are instantiating a new tokenizer from scratch. This is not supported by this script. "
"You can do it from another script, save it, and load it from here, using --tokenizer_name."
)
if model_args.model_name_or_path:
model = AutoModelForMaskedLM.from_pretrained(
model_args.model_name_or_path,
from_tf=bool(".ckpt" in model_args.model_name_or_path),
config=config,
cache_dir=model_args.cache_dir,
revision=model_args.model_revision,
token=model_args.token,
trust_remote_code=model_args.trust_remote_code,
low_cpu_mem_usage=model_args.low_cpu_mem_usage,
)
else:
logger.info("Training new model from scratch")
model = AutoModelForMaskedLM.from_config(config, trust_remote_code=model_args.trust_remote_code)
# We resize the embeddings only when necessary to avoid index errors. If you are creating a model from scratch
# on a small vocab and want a smaller embedding size, remove this test.
embedding_size = model.get_input_embeddings().weight.shape[0]
if len(tokenizer) > embedding_size:
model.resize_token_embeddings(len(tokenizer))
# Preprocessing the datasets.
# First we tokenize all the texts.
if training_args.do_train:
column_names = list(raw_datasets["train"].features)
else:
column_names = list(raw_datasets["validation"].features)
text_column_name = "text" if "text" in column_names else column_names[0]
if data_args.max_seq_length is None:
max_seq_length = tokenizer.model_max_length
if max_seq_length > 1024:
logger.warning(
"The chosen tokenizer supports a `model_max_length` that is longer than the default `block_size` value"
" of 1024. If you would like to use a longer `block_size` up to `tokenizer.model_max_length` you can"
" override this default with `--block_size xxx`."
)
max_seq_length = 1024
else:
if data_args.max_seq_length > tokenizer.model_max_length:
logger.warning(
f"The max_seq_length passed ({data_args.max_seq_length}) is larger than the maximum length for the "
f"model ({tokenizer.model_max_length}). Using max_seq_length={tokenizer.model_max_length}."
)
max_seq_length = min(data_args.max_seq_length, tokenizer.model_max_length)
if data_args.line_by_line:
# When using line_by_line, we just tokenize each nonempty line.
padding = "max_length" if data_args.pad_to_max_length else False
def tokenize_function(examples):
# Remove empty lines
examples[text_column_name] = [
line for line in examples[text_column_name] if len(line) > 0 and not line.isspace()
]
return tokenizer(
examples[text_column_name],
padding=padding,
truncation=True,
max_length=max_seq_length,
# We use this option because DataCollatorForLanguageModeling (see below) is more efficient when it
# receives the `special_tokens_mask`.
return_special_tokens_mask=True,
)
with training_args.main_process_first(desc="dataset map tokenization"):
if not data_args.streaming:
tokenized_datasets = raw_datasets.map(
tokenize_function,
batched=True,
num_proc=data_args.preprocessing_num_workers,
remove_columns=[text_column_name],
load_from_cache_file=not data_args.overwrite_cache,
desc="Running tokenizer on dataset line_by_line",
)
else:
tokenized_datasets = raw_datasets.map(
tokenize_function,
batched=True,
remove_columns=[text_column_name],
)
else:
# Otherwise, we tokenize every text, then concatenate them together before splitting them in smaller parts.
# We use `return_special_tokens_mask=True` because DataCollatorForLanguageModeling (see below) is more
# efficient when it receives the `special_tokens_mask`.
def tokenize_function(examples):
return tokenizer(examples[text_column_name], return_special_tokens_mask=True)
with training_args.main_process_first(desc="dataset map tokenization"):
if not data_args.streaming:
tokenized_datasets = raw_datasets.map(
tokenize_function,
batched=True,
num_proc=data_args.preprocessing_num_workers,
remove_columns=column_names,
load_from_cache_file=not data_args.overwrite_cache,
desc="Running tokenizer on every text in dataset",
)
else:
tokenized_datasets = raw_datasets.map(
tokenize_function,
batched=True,
remove_columns=column_names,
)
# Main data processing function that will concatenate all texts from our dataset and generate chunks of
# max_seq_length.
def group_texts(examples):
# Concatenate all texts.
concatenated_examples = {k: list(chain(*examples[k])) for k in examples.keys()}
total_length = len(concatenated_examples[list(examples.keys())[0]])
# We drop the small remainder, and if the total_length < max_seq_length we exclude this batch and return an empty dict.
# We could add padding if the model supported it instead of this drop, you can customize this part to your needs.
total_length = (total_length // max_seq_length) * max_seq_length
# Split by chunks of max_len.
result = {
k: [t[i : i + max_seq_length] for i in range(0, total_length, max_seq_length)]
for k, t in concatenated_examples.items()
}
return result
# Note that with `batched=True`, this map processes 1,000 texts together, so group_texts throws away a
# remainder for each of those groups of 1,000 texts. You can adjust that batch_size here but a higher value
# might be slower to preprocess.
#
# To speed up this part, we use multiprocessing. See the documentation of the map method for more information:
# https://huggingface.co/docs/datasets/process#map
with training_args.main_process_first(desc="grouping texts together"):
if not data_args.streaming:
tokenized_datasets = tokenized_datasets.map(
group_texts,
batched=True,
num_proc=data_args.preprocessing_num_workers,
load_from_cache_file=not data_args.overwrite_cache,
desc=f"Grouping texts in chunks of {max_seq_length}",
)
else:
tokenized_datasets = tokenized_datasets.map(
group_texts,
batched=True,
)
if training_args.do_train:
if "train" not in tokenized_datasets:
raise ValueError("--do_train requires a train dataset")
train_dataset = tokenized_datasets["train"]
if data_args.max_train_samples is not None:
max_train_samples = min(len(train_dataset), data_args.max_train_samples)
train_dataset = train_dataset.select(range(max_train_samples))
if training_args.do_eval:
if "validation" not in tokenized_datasets:
raise ValueError("--do_eval requires a validation dataset")
eval_dataset = tokenized_datasets["validation"]
if data_args.max_eval_samples is not None:
max_eval_samples = min(len(eval_dataset), data_args.max_eval_samples)
eval_dataset = eval_dataset.select(range(max_eval_samples))
def preprocess_logits_for_metrics(logits, labels):
if isinstance(logits, tuple):
# Depending on the model and config, logits may contain extra tensors,
# like past_key_values, but logits always come first
logits = logits[0]
return logits.argmax(dim=-1)
metric = evaluate.load("accuracy", cache_dir=model_args.cache_dir)
def compute_metrics(eval_preds):
preds, labels = eval_preds
# preds have the same shape as the labels, after the argmax(-1) has been calculated
# by preprocess_logits_for_metrics
labels = labels.reshape(-1)
preds = preds.reshape(-1)
mask = labels != -100
labels = labels[mask]
preds = preds[mask]
return metric.compute(predictions=preds, references=labels)
# Data collator
# This one will take care of randomly masking the tokens.
pad_to_multiple_of_8 = data_args.line_by_line and training_args.fp16 and not data_args.pad_to_max_length
data_collator = DataCollatorForLanguageModeling(
tokenizer=tokenizer,
mlm_probability=data_args.mlm_probability,
pad_to_multiple_of=8 if pad_to_multiple_of_8 else None,
)
# Initialize our Trainer
trainer = Trainer(
model=model,
args=training_args,
train_dataset=train_dataset if training_args.do_train else None,
eval_dataset=eval_dataset if training_args.do_eval else None,
tokenizer=tokenizer,
data_collator=data_collator,
compute_metrics=compute_metrics if training_args.do_eval and not is_torch_xla_available() else None,
preprocess_logits_for_metrics=preprocess_logits_for_metrics
if training_args.do_eval and not is_torch_xla_available()
else None,
)
# Training
if training_args.do_train:
checkpoint = None
if training_args.resume_from_checkpoint is not None:
checkpoint = training_args.resume_from_checkpoint
elif last_checkpoint is not None:
checkpoint = last_checkpoint
train_result = trainer.train(resume_from_checkpoint=checkpoint)
trainer.save_model() # Saves the tokenizer too for easy upload
metrics = train_result.metrics
max_train_samples = (
data_args.max_train_samples if data_args.max_train_samples is not None else len(train_dataset)
)
metrics["train_samples"] = min(max_train_samples, len(train_dataset))
trainer.log_metrics("train", metrics)
trainer.save_metrics("train", metrics)
trainer.save_state()
# Evaluation
if training_args.do_eval:
logger.info("*** Evaluate ***")
metrics = trainer.evaluate()
max_eval_samples = data_args.max_eval_samples if data_args.max_eval_samples is not None else len(eval_dataset)
metrics["eval_samples"] = min(max_eval_samples, len(eval_dataset))
try:
perplexity = math.exp(metrics["eval_loss"])
except OverflowError:
perplexity = float("inf")
metrics["perplexity"] = perplexity
trainer.log_metrics("eval", metrics)
trainer.save_metrics("eval", metrics)
kwargs = {"finetuned_from": model_args.model_name_or_path, "tasks": "fill-mask"}
if data_args.dataset_name is not None:
kwargs["dataset_tags"] = data_args.dataset_name
if data_args.dataset_config_name is not None:
kwargs["dataset_args"] = data_args.dataset_config_name
kwargs["dataset"] = f"{data_args.dataset_name} {data_args.dataset_config_name}"
else:
kwargs["dataset"] = data_args.dataset_name
if training_args.push_to_hub:
trainer.push_to_hub(**kwargs)
else:
trainer.create_model_card(**kwargs)
def _mp_fn(index):
# For xla_spawn (TPUs)
main()
if __name__ == "__main__":
main()
| transformers/examples/pytorch/language-modeling/run_mlm.py/0 | {
"file_path": "transformers/examples/pytorch/language-modeling/run_mlm.py",
"repo_id": "transformers",
"token_count": 12655
} | 279 |
# coding=utf-8
# Copyright 2020 The HuggingFace Team All rights reserved.
#
# Licensed under the Apache License, Version 2.0 (the "License");
# you may not use this file except in compliance with the License.
# You may obtain a copy of the License at
#
# http://www.apache.org/licenses/LICENSE-2.0
#
# Unless required by applicable law or agreed to in writing, software
# distributed under the License is distributed on an "AS IS" BASIS,
# WITHOUT WARRANTIES OR CONDITIONS OF ANY KIND, either express or implied.
# See the License for the specific language governing permissions and
# limitations under the License.
"""
A subclass of `Trainer` specific to Question-Answering tasks
"""
import math
import time
from transformers import Trainer, is_torch_xla_available
from transformers.trainer_utils import PredictionOutput, speed_metrics
if is_torch_xla_available():
import torch_xla.core.xla_model as xm
import torch_xla.debug.metrics as met
class QuestionAnsweringTrainer(Trainer):
def __init__(self, *args, eval_examples=None, post_process_function=None, **kwargs):
super().__init__(*args, **kwargs)
self.eval_examples = eval_examples
self.post_process_function = post_process_function
def evaluate(self, eval_dataset=None, eval_examples=None, ignore_keys=None, metric_key_prefix: str = "eval"):
eval_dataset = self.eval_dataset if eval_dataset is None else eval_dataset
eval_dataloader = self.get_eval_dataloader(eval_dataset)
eval_examples = self.eval_examples if eval_examples is None else eval_examples
# Temporarily disable metric computation, we will do it in the loop here.
compute_metrics = self.compute_metrics
self.compute_metrics = None
eval_loop = self.prediction_loop if self.args.use_legacy_prediction_loop else self.evaluation_loop
start_time = time.time()
try:
output = eval_loop(
eval_dataloader,
description="Evaluation",
# No point gathering the predictions if there are no metrics, otherwise we defer to
# self.args.prediction_loss_only
prediction_loss_only=True if compute_metrics is None else None,
ignore_keys=ignore_keys,
metric_key_prefix=metric_key_prefix,
)
finally:
self.compute_metrics = compute_metrics
total_batch_size = self.args.eval_batch_size * self.args.world_size
if f"{metric_key_prefix}_jit_compilation_time" in output.metrics:
start_time += output.metrics[f"{metric_key_prefix}_jit_compilation_time"]
output.metrics.update(
speed_metrics(
metric_key_prefix,
start_time,
num_samples=output.num_samples,
num_steps=math.ceil(output.num_samples / total_batch_size),
)
)
if self.post_process_function is not None and self.compute_metrics is not None and self.args.should_save:
# Only the main node write the results by default
eval_preds = self.post_process_function(eval_examples, eval_dataset, output.predictions)
metrics = self.compute_metrics(eval_preds)
# Prefix all keys with metric_key_prefix + '_'
for key in list(metrics.keys()):
if not key.startswith(f"{metric_key_prefix}_"):
metrics[f"{metric_key_prefix}_{key}"] = metrics.pop(key)
metrics.update(output.metrics)
else:
metrics = output.metrics
if self.args.should_log:
# Only the main node log the results by default
self.log(metrics)
if self.args.tpu_metrics_debug or self.args.debug:
# tpu-comment: Logging debug metrics for PyTorch/XLA (compile, execute times, ops, etc.)
xm.master_print(met.metrics_report())
self.control = self.callback_handler.on_evaluate(self.args, self.state, self.control, metrics)
return metrics
def predict(self, predict_dataset, predict_examples, ignore_keys=None, metric_key_prefix: str = "test"):
predict_dataloader = self.get_test_dataloader(predict_dataset)
# Temporarily disable metric computation, we will do it in the loop here.
compute_metrics = self.compute_metrics
self.compute_metrics = None
eval_loop = self.prediction_loop if self.args.use_legacy_prediction_loop else self.evaluation_loop
start_time = time.time()
try:
output = eval_loop(
predict_dataloader,
description="Prediction",
# No point gathering the predictions if there are no metrics, otherwise we defer to
# self.args.prediction_loss_only
prediction_loss_only=True if compute_metrics is None else None,
ignore_keys=ignore_keys,
metric_key_prefix=metric_key_prefix,
)
finally:
self.compute_metrics = compute_metrics
total_batch_size = self.args.eval_batch_size * self.args.world_size
if f"{metric_key_prefix}_jit_compilation_time" in output.metrics:
start_time += output.metrics[f"{metric_key_prefix}_jit_compilation_time"]
output.metrics.update(
speed_metrics(
metric_key_prefix,
start_time,
num_samples=output.num_samples,
num_steps=math.ceil(output.num_samples / total_batch_size),
)
)
if self.post_process_function is None or self.compute_metrics is None:
return output
predictions = self.post_process_function(predict_examples, predict_dataset, output.predictions, "predict")
metrics = self.compute_metrics(predictions)
# Prefix all keys with metric_key_prefix + '_'
for key in list(metrics.keys()):
if not key.startswith(f"{metric_key_prefix}_"):
metrics[f"{metric_key_prefix}_{key}"] = metrics.pop(key)
metrics.update(output.metrics)
return PredictionOutput(predictions=predictions.predictions, label_ids=predictions.label_ids, metrics=metrics)
| transformers/examples/pytorch/question-answering/trainer_qa.py/0 | {
"file_path": "transformers/examples/pytorch/question-answering/trainer_qa.py",
"repo_id": "transformers",
"token_count": 2656
} | 280 |
#!/usr/bin/env python3
# Copyright 2018 CMU and The HuggingFace Inc. team.
#
# Licensed under the Apache License, Version 2.0 (the "License");
# you may not use this file except in compliance with the License.
# You may obtain a copy of the License at
#
# http://www.apache.org/licenses/LICENSE-2.0
#
# Unless required by applicable law or agreed to in writing, software
# distributed under the License is distributed on an "AS IS" BASIS,
# WITHOUT WARRANTIES OR CONDITIONS OF ANY KIND, either express or implied.
# See the License for the specific language governing permissions and
# limitations under the License.
""" Bertology: this script shows how you can explore the internals of the models in the library to:
- compute the entropy of the head attentions
- compute the importance of each head
- prune (remove) the low importance head.
Some parts of this script are adapted from the code of Michel et al. (http://arxiv.org/abs/1905.10650)
which is available at https://github.com/pmichel31415/are-16-heads-really-better-than-1
"""
import argparse
import logging
import os
from datetime import datetime
import numpy as np
import torch
from torch import nn
from torch.utils.data import DataLoader, SequentialSampler, Subset
from torch.utils.data.distributed import DistributedSampler
from tqdm import tqdm
import transformers
from transformers import (
AutoConfig,
AutoModelForSequenceClassification,
AutoTokenizer,
GlueDataset,
default_data_collator,
glue_compute_metrics,
glue_output_modes,
glue_processors,
set_seed,
)
from transformers.trainer_utils import is_main_process
logger = logging.getLogger(__name__)
def entropy(p):
"""Compute the entropy of a probability distribution"""
plogp = p * torch.log(p)
plogp[p == 0] = 0
return -plogp.sum(dim=-1)
def print_2d_tensor(tensor):
"""Print a 2D tensor"""
logger.info("lv, h >\t" + "\t".join(f"{x + 1}" for x in range(len(tensor))))
for row in range(len(tensor)):
if tensor.dtype != torch.long:
logger.info(f"layer {row + 1}:\t" + "\t".join(f"{x:.5f}" for x in tensor[row].cpu().data))
else:
logger.info(f"layer {row + 1}:\t" + "\t".join(f"{x:d}" for x in tensor[row].cpu().data))
def compute_heads_importance(
args, model, eval_dataloader, compute_entropy=True, compute_importance=True, head_mask=None, actually_pruned=False
):
"""This method shows how to compute:
- head attention entropy
- head importance scores according to http://arxiv.org/abs/1905.10650
"""
# Prepare our tensors
n_layers, n_heads = model.config.num_hidden_layers, model.config.num_attention_heads
head_importance = torch.zeros(n_layers, n_heads).to(args.device)
attn_entropy = torch.zeros(n_layers, n_heads).to(args.device)
if head_mask is None:
head_mask = torch.ones(n_layers, n_heads).to(args.device)
head_mask.requires_grad_(requires_grad=True)
# If actually pruned attention multi-head, set head mask to None to avoid shape mismatch
if actually_pruned:
head_mask = None
preds = None
labels = None
tot_tokens = 0.0
for step, inputs in enumerate(tqdm(eval_dataloader, desc="Iteration", disable=args.local_rank not in [-1, 0])):
for k, v in inputs.items():
inputs[k] = v.to(args.device)
# Do a forward pass (not with torch.no_grad() since we need gradients for importance score - see below)
outputs = model(**inputs, head_mask=head_mask)
loss, logits, all_attentions = (
outputs[0],
outputs[1],
outputs[-1],
) # Loss and logits are the first, attention the last
loss.backward() # Backpropagate to populate the gradients in the head mask
if compute_entropy:
for layer, attn in enumerate(all_attentions):
masked_entropy = entropy(attn.detach()) * inputs["attention_mask"].float().unsqueeze(1)
attn_entropy[layer] += masked_entropy.sum(-1).sum(0).detach()
if compute_importance:
head_importance += head_mask.grad.abs().detach()
# Also store our logits/labels if we want to compute metrics afterwards
if preds is None:
preds = logits.detach().cpu().numpy()
labels = inputs["labels"].detach().cpu().numpy()
else:
preds = np.append(preds, logits.detach().cpu().numpy(), axis=0)
labels = np.append(labels, inputs["labels"].detach().cpu().numpy(), axis=0)
tot_tokens += inputs["attention_mask"].float().detach().sum().data
# Normalize
attn_entropy /= tot_tokens
head_importance /= tot_tokens
# Layerwise importance normalization
if not args.dont_normalize_importance_by_layer:
exponent = 2
norm_by_layer = torch.pow(torch.pow(head_importance, exponent).sum(-1), 1 / exponent)
head_importance /= norm_by_layer.unsqueeze(-1) + 1e-20
if not args.dont_normalize_global_importance:
head_importance = (head_importance - head_importance.min()) / (head_importance.max() - head_importance.min())
# Print/save matrices
np.save(os.path.join(args.output_dir, "attn_entropy.npy"), attn_entropy.detach().cpu().numpy())
np.save(os.path.join(args.output_dir, "head_importance.npy"), head_importance.detach().cpu().numpy())
logger.info("Attention entropies")
print_2d_tensor(attn_entropy)
logger.info("Head importance scores")
print_2d_tensor(head_importance)
logger.info("Head ranked by importance scores")
head_ranks = torch.zeros(head_importance.numel(), dtype=torch.long, device=args.device)
head_ranks[head_importance.view(-1).sort(descending=True)[1]] = torch.arange(
head_importance.numel(), device=args.device
)
head_ranks = head_ranks.view_as(head_importance)
print_2d_tensor(head_ranks)
return attn_entropy, head_importance, preds, labels
def mask_heads(args, model, eval_dataloader):
"""This method shows how to mask head (set some heads to zero), to test the effect on the network,
based on the head importance scores, as described in Michel et al. (http://arxiv.org/abs/1905.10650)
"""
_, head_importance, preds, labels = compute_heads_importance(args, model, eval_dataloader, compute_entropy=False)
preds = np.argmax(preds, axis=1) if args.output_mode == "classification" else np.squeeze(preds)
original_score = glue_compute_metrics(args.task_name, preds, labels)[args.metric_name]
logger.info("Pruning: original score: %f, threshold: %f", original_score, original_score * args.masking_threshold)
new_head_mask = torch.ones_like(head_importance)
num_to_mask = max(1, int(new_head_mask.numel() * args.masking_amount))
current_score = original_score
while current_score >= original_score * args.masking_threshold:
head_mask = new_head_mask.clone() # save current head mask
# heads from least important to most - keep only not-masked heads
head_importance[head_mask == 0.0] = float("Inf")
current_heads_to_mask = head_importance.view(-1).sort()[1]
if len(current_heads_to_mask) <= num_to_mask:
break
# mask heads
current_heads_to_mask = current_heads_to_mask[:num_to_mask]
logger.info("Heads to mask: %s", str(current_heads_to_mask.tolist()))
new_head_mask = new_head_mask.view(-1)
new_head_mask[current_heads_to_mask] = 0.0
new_head_mask = new_head_mask.view_as(head_mask)
new_head_mask = new_head_mask.clone().detach()
print_2d_tensor(new_head_mask)
# Compute metric and head importance again
_, head_importance, preds, labels = compute_heads_importance(
args, model, eval_dataloader, compute_entropy=False, head_mask=new_head_mask
)
preds = np.argmax(preds, axis=1) if args.output_mode == "classification" else np.squeeze(preds)
current_score = glue_compute_metrics(args.task_name, preds, labels)[args.metric_name]
logger.info(
"Masking: current score: %f, remaining heads %d (%.1f percents)",
current_score,
new_head_mask.sum(),
new_head_mask.sum() / new_head_mask.numel() * 100,
)
logger.info("Final head mask")
print_2d_tensor(head_mask)
np.save(os.path.join(args.output_dir, "head_mask.npy"), head_mask.detach().cpu().numpy())
return head_mask
def prune_heads(args, model, eval_dataloader, head_mask):
"""This method shows how to prune head (remove heads weights) based on
the head importance scores as described in Michel et al. (http://arxiv.org/abs/1905.10650)
"""
# Try pruning and test time speedup
# Pruning is like masking but we actually remove the masked weights
before_time = datetime.now()
_, _, preds, labels = compute_heads_importance(
args, model, eval_dataloader, compute_entropy=False, compute_importance=False, head_mask=head_mask
)
preds = np.argmax(preds, axis=1) if args.output_mode == "classification" else np.squeeze(preds)
score_masking = glue_compute_metrics(args.task_name, preds, labels)[args.metric_name]
original_time = datetime.now() - before_time
original_num_params = sum(p.numel() for p in model.parameters())
heads_to_prune = {
layer: (1 - head_mask[layer].long()).nonzero().squeeze().tolist() for layer in range(len(head_mask))
}
assert sum(len(h) for h in heads_to_prune.values()) == (1 - head_mask.long()).sum().item()
model.prune_heads(heads_to_prune)
pruned_num_params = sum(p.numel() for p in model.parameters())
before_time = datetime.now()
_, _, preds, labels = compute_heads_importance(
args,
model,
eval_dataloader,
compute_entropy=False,
compute_importance=False,
head_mask=None,
actually_pruned=True,
)
preds = np.argmax(preds, axis=1) if args.output_mode == "classification" else np.squeeze(preds)
score_pruning = glue_compute_metrics(args.task_name, preds, labels)[args.metric_name]
new_time = datetime.now() - before_time
logger.info(
"Pruning: original num of params: %.2e, after pruning %.2e (%.1f percents)",
original_num_params,
pruned_num_params,
pruned_num_params / original_num_params * 100,
)
logger.info("Pruning: score with masking: %f score with pruning: %f", score_masking, score_pruning)
logger.info("Pruning: speed ratio (new timing / original timing): %f percents", original_time / new_time * 100)
def main():
parser = argparse.ArgumentParser()
# Required parameters
parser.add_argument(
"--data_dir",
default=None,
type=str,
required=True,
help="The input data dir. Should contain the .tsv files (or other data files) for the task.",
)
parser.add_argument(
"--model_name_or_path",
default=None,
type=str,
required=True,
help="Path to pretrained model or model identifier from huggingface.co/models",
)
parser.add_argument(
"--task_name",
default=None,
type=str,
required=True,
help="The name of the task to train selected in the list: " + ", ".join(glue_processors.keys()),
)
parser.add_argument(
"--output_dir",
default=None,
type=str,
required=True,
help="The output directory where the model predictions and checkpoints will be written.",
)
# Other parameters
parser.add_argument(
"--config_name",
default="",
type=str,
help="Pretrained config name or path if not the same as model_name_or_path",
)
parser.add_argument(
"--tokenizer_name",
default="",
type=str,
help="Pretrained tokenizer name or path if not the same as model_name_or_path",
)
parser.add_argument(
"--cache_dir",
default=None,
type=str,
help="Where do you want to store the pre-trained models downloaded from huggingface.co",
)
parser.add_argument(
"--data_subset", type=int, default=-1, help="If > 0: limit the data to a subset of data_subset instances."
)
parser.add_argument(
"--overwrite_output_dir", action="store_true", help="Whether to overwrite data in output directory"
)
parser.add_argument(
"--overwrite_cache", action="store_true", help="Overwrite the cached training and evaluation sets"
)
parser.add_argument(
"--dont_normalize_importance_by_layer", action="store_true", help="Don't normalize importance score by layers"
)
parser.add_argument(
"--dont_normalize_global_importance",
action="store_true",
help="Don't normalize all importance scores between 0 and 1",
)
parser.add_argument(
"--try_masking", action="store_true", help="Whether to try to mask head until a threshold of accuracy."
)
parser.add_argument(
"--masking_threshold",
default=0.9,
type=float,
help="masking threshold in term of metrics (stop masking when metric < threshold * original metric value).",
)
parser.add_argument(
"--masking_amount", default=0.1, type=float, help="Amount to heads to masking at each masking step."
)
parser.add_argument("--metric_name", default="acc", type=str, help="Metric to use for head masking.")
parser.add_argument(
"--max_seq_length",
default=128,
type=int,
help=(
"The maximum total input sequence length after WordPiece tokenization. \n"
"Sequences longer than this will be truncated, sequences shorter padded."
),
)
parser.add_argument("--batch_size", default=1, type=int, help="Batch size.")
parser.add_argument("--seed", type=int, default=42)
parser.add_argument("--local_rank", type=int, default=-1, help="local_rank for distributed training on gpus")
parser.add_argument("--no_cuda", action="store_true", help="Whether not to use CUDA when available")
parser.add_argument("--server_ip", type=str, default="", help="Can be used for distant debugging.")
parser.add_argument("--server_port", type=str, default="", help="Can be used for distant debugging.")
args = parser.parse_args()
if args.server_ip and args.server_port:
# Distant debugging - see https://code.visualstudio.com/docs/python/debugging#_attach-to-a-local-script
import ptvsd
print("Waiting for debugger attach")
ptvsd.enable_attach(address=(args.server_ip, args.server_port), redirect_output=True)
ptvsd.wait_for_attach()
# Setup devices and distributed training
if args.local_rank == -1 or args.no_cuda:
args.device = torch.device("cuda" if torch.cuda.is_available() and not args.no_cuda else "cpu")
args.n_gpu = 0 if args.no_cuda else torch.cuda.device_count()
else:
torch.cuda.set_device(args.local_rank)
args.device = torch.device("cuda", args.local_rank)
args.n_gpu = 1
torch.distributed.init_process_group(backend="nccl") # Initializes the distributed backend
# Setup logging
logging.basicConfig(level=logging.INFO if args.local_rank in [-1, 0] else logging.WARN)
logger.info("device: {} n_gpu: {}, distributed: {}".format(args.device, args.n_gpu, bool(args.local_rank != -1)))
# Set the verbosity to info of the Transformers logger (on main process only):
if is_main_process(args.local_rank):
transformers.utils.logging.set_verbosity_info()
transformers.utils.logging.enable_default_handler()
transformers.utils.logging.enable_explicit_format()
# Set seeds
set_seed(args.seed)
# Prepare GLUE task
args.task_name = args.task_name.lower()
if args.task_name not in glue_processors:
raise ValueError("Task not found: %s" % (args.task_name))
processor = glue_processors[args.task_name]()
args.output_mode = glue_output_modes[args.task_name]
label_list = processor.get_labels()
num_labels = len(label_list)
# Load pretrained model and tokenizer
#
# Distributed training:
# The .from_pretrained methods guarantee that only one local process can concurrently
# download model & vocab.
config = AutoConfig.from_pretrained(
args.config_name if args.config_name else args.model_name_or_path,
num_labels=num_labels,
finetuning_task=args.task_name,
output_attentions=True,
cache_dir=args.cache_dir,
)
tokenizer = AutoTokenizer.from_pretrained(
args.tokenizer_name if args.tokenizer_name else args.model_name_or_path,
cache_dir=args.cache_dir,
)
model = AutoModelForSequenceClassification.from_pretrained(
args.model_name_or_path,
from_tf=bool(".ckpt" in args.model_name_or_path),
config=config,
cache_dir=args.cache_dir,
)
# Distributed and parallel training
model.to(args.device)
if args.local_rank != -1:
model = nn.parallel.DistributedDataParallel(
model, device_ids=[args.local_rank], output_device=args.local_rank, find_unused_parameters=True
)
elif args.n_gpu > 1:
model = nn.DataParallel(model)
# Print/save training arguments
os.makedirs(args.output_dir, exist_ok=True)
torch.save(args, os.path.join(args.output_dir, "run_args.bin"))
logger.info("Training/evaluation parameters %s", args)
# Prepare dataset for the GLUE task
eval_dataset = GlueDataset(args, tokenizer=tokenizer, mode="dev")
if args.data_subset > 0:
eval_dataset = Subset(eval_dataset, list(range(min(args.data_subset, len(eval_dataset)))))
eval_sampler = SequentialSampler(eval_dataset) if args.local_rank == -1 else DistributedSampler(eval_dataset)
eval_dataloader = DataLoader(
eval_dataset, sampler=eval_sampler, batch_size=args.batch_size, collate_fn=default_data_collator
)
# Compute head entropy and importance score
compute_heads_importance(args, model, eval_dataloader)
# Try head masking (set heads to zero until the score goes under a threshole)
# and head pruning (remove masked heads and see the effect on the network)
if args.try_masking and args.masking_threshold > 0.0 and args.masking_threshold < 1.0:
head_mask = mask_heads(args, model, eval_dataloader)
prune_heads(args, model, eval_dataloader, head_mask)
if __name__ == "__main__":
main()
| transformers/examples/research_projects/bertology/run_bertology.py/0 | {
"file_path": "transformers/examples/research_projects/bertology/run_bertology.py",
"repo_id": "transformers",
"token_count": 7347
} | 281 |
from unittest import TestCase
from datasets import Dataset
from minhash_deduplication import deduplicate_dataset, make_duplicate_clusters
def get_dataset():
data_dict = {
"repo_name": ["test_repo1", "test_repo2", "test_repo3"],
"path": ["test_1.py", "test_2.py", "unit_test.py"],
"content": ["a " * 20, "a " * 30, "b " * 7],
}
dataset = Dataset.from_dict(data_dict)
return dataset
class MakeDuplicateClustersTest(TestCase):
def test_make_duplicate_clusters(self):
ds = get_dataset()
duplicate_clusters = make_duplicate_clusters(ds, 0.85)
self.assertEqual(len(duplicate_clusters[0]), 2)
def test_deduplicate_dataset(self):
ds = get_dataset()
ds_filter, duplicate_clusters = deduplicate_dataset(ds)
self.assertEqual(len(ds_filter), 2)
print(duplicate_clusters)
self.assertEqual(duplicate_clusters[0][0]["copies"], 2)
self.assertEqual(duplicate_clusters[0][0]["is_extreme"], True)
| transformers/examples/research_projects/codeparrot/scripts/tests/test_deduplicate.py/0 | {
"file_path": "transformers/examples/research_projects/codeparrot/scripts/tests/test_deduplicate.py",
"repo_id": "transformers",
"token_count": 456
} | 282 |
# coding=utf-8
# Copyright 2019-present, the HuggingFace Inc. team and Facebook, Inc.
#
# Licensed under the Apache License, Version 2.0 (the "License");
# you may not use this file except in compliance with the License.
# You may obtain a copy of the License at
#
# http://www.apache.org/licenses/LICENSE-2.0
#
# Unless required by applicable law or agreed to in writing, software
# distributed under the License is distributed on an "AS IS" BASIS,
# WITHOUT WARRANTIES OR CONDITIONS OF ANY KIND, either express or implied.
# See the License for the specific language governing permissions and
# limitations under the License.
""" Adapted from PyTorch Vision (https://github.com/pytorch/vision/blob/master/references/detection/group_by_aspect_ratio.py)
"""
import bisect
import copy
from collections import defaultdict
import numpy as np
from torch.utils.data import BatchSampler, Sampler
from utils import logger
def _quantize(x, bins):
bins = copy.deepcopy(bins)
bins = sorted(bins)
quantized = [bisect.bisect_right(bins, y) for y in x]
return quantized
def create_lengths_groups(lengths, k=0):
bins = np.arange(start=3, stop=k, step=4).tolist() if k > 0 else [10]
groups = _quantize(lengths, bins)
# count number of elements per group
counts = np.unique(groups, return_counts=True)[1]
fbins = [0] + bins + [np.inf]
logger.info("Using {} as bins for aspect lengths quantization".format(fbins))
logger.info("Count of instances per bin: {}".format(counts))
return groups
class GroupedBatchSampler(BatchSampler):
"""
Wraps another sampler to yield a mini-batch of indices.
It enforces that the batch only contain elements from the same group.
It also tries to provide mini-batches which follows an ordering which is
as close as possible to the ordering from the original sampler.
Arguments:
sampler (Sampler): Base sampler.
group_ids (list[int]): If the sampler produces indices in range [0, N),
`group_ids` must be a list of `N` ints which contains the group id of each sample.
The group ids must be a continuous set of integers starting from
0, i.e. they must be in the range [0, num_groups).
batch_size (int): Size of mini-batch.
"""
def __init__(self, sampler, group_ids, batch_size):
if not isinstance(sampler, Sampler):
raise ValueError(
"sampler should be an instance of torch.utils.data.Sampler, but got sampler={}".format(sampler)
)
self.sampler = sampler
self.group_ids = group_ids
self.batch_size = batch_size
def __iter__(self):
buffer_per_group = defaultdict(list)
samples_per_group = defaultdict(list)
num_batches = 0
for idx in self.sampler:
group_id = self.group_ids[idx]
buffer_per_group[group_id].append(idx)
samples_per_group[group_id].append(idx)
if len(buffer_per_group[group_id]) == self.batch_size:
yield buffer_per_group[group_id] # TODO
num_batches += 1
del buffer_per_group[group_id]
assert len(buffer_per_group[group_id]) < self.batch_size
# now we have run out of elements that satisfy
# the group criteria, let's return the remaining
# elements so that the size of the sampler is
# deterministic
expected_num_batches = len(self)
num_remaining = expected_num_batches - num_batches
if num_remaining > 0:
# for the remaining batches, group the batches by similar lengths
batch_idx = []
for group_id, idxs in sorted(buffer_per_group.items(), key=lambda x: x[0]):
batch_idx.extend(idxs)
if len(batch_idx) >= self.batch_size:
yield batch_idx[: self.batch_size]
batch_idx = batch_idx[self.batch_size :]
num_remaining -= 1
if len(batch_idx) > 0:
yield batch_idx
num_remaining -= 1
assert num_remaining == 0
def __len__(self):
"""
Return the number of mini-batches rather than the number of samples.
"""
return (len(self.sampler) + self.batch_size - 1) // self.batch_size
| transformers/examples/research_projects/distillation/grouped_batch_sampler.py/0 | {
"file_path": "transformers/examples/research_projects/distillation/grouped_batch_sampler.py",
"repo_id": "transformers",
"token_count": 1750
} | 283 |
[build-system]
requires = [
"setuptools>=57.4.0",
"wheel>=0.37.0",
"transformers>=4.9.2"
]
build-backend = "setuptools.build_meta" | transformers/examples/research_projects/fsner/pyproject.toml/0 | {
"file_path": "transformers/examples/research_projects/fsner/pyproject.toml",
"repo_id": "transformers",
"token_count": 71
} | 284 |
import jax
import jax.numpy as jnp
from bigbird_flax import FlaxBigBirdForNaturalQuestions
from datasets import load_from_disk
from transformers import BigBirdTokenizerFast
CATEGORY_MAPPING = {0: "null", 1: "short", 2: "long", 3: "yes", 4: "no"}
PUNCTUATION_SET_TO_EXCLUDE = set("".join(["‘", "’", "´", "`", ".", ",", "-", '"']))
def get_sub_answers(answers, begin=0, end=None):
return [" ".join(x.split(" ")[begin:end]) for x in answers if len(x.split(" ")) > 1]
def expand_to_aliases(given_answers, make_sub_answers=False):
if make_sub_answers:
# if answers are longer than one word, make sure a predictions is correct if it coresponds to the complete 1: or :-1 sub word
# *e.g.* if the correct answer contains a prefix such as "the", or "a"
given_answers = (
given_answers + get_sub_answers(given_answers, begin=1) + get_sub_answers(given_answers, end=-1)
)
answers = []
for answer in given_answers:
alias = answer.replace("_", " ").lower()
alias = "".join(c if c not in PUNCTUATION_SET_TO_EXCLUDE else " " for c in alias)
answers.append(" ".join(alias.split()).strip())
return set(answers)
def get_best_valid_start_end_idx(start_scores, end_scores, top_k=1, max_size=100):
best_start_scores, best_start_idx = jax.lax.top_k(start_scores, top_k)
best_end_scores, best_end_idx = jax.lax.top_k(end_scores, top_k)
widths = best_end_idx[:, None] - best_start_idx[None, :]
mask = jnp.logical_or(widths < 0, widths > max_size)
scores = (best_end_scores[:, None] + best_start_scores[None, :]) - (1e8 * mask)
best_score = jnp.argmax(scores).item()
return best_start_idx[best_score % top_k], best_end_idx[best_score // top_k]
def format_dataset(sample):
question = sample["question"]["text"]
context = sample["document"]["tokens"]["token"]
is_html = sample["document"]["tokens"]["is_html"]
long_answers = sample["annotations"]["long_answer"]
short_answers = sample["annotations"]["short_answers"]
context_string = " ".join([context[i] for i in range(len(context)) if not is_html[i]])
# 0 - No ; 1 - Yes
for answer in sample["annotations"]["yes_no_answer"]:
if answer == 0 or answer == 1:
return {
"question": question,
"context": context_string,
"short": [],
"long": [],
"category": "no" if answer == 0 else "yes",
}
short_targets = []
for s in short_answers:
short_targets.extend(s["text"])
short_targets = list(set(short_targets))
long_targets = []
for s in long_answers:
if s["start_token"] == -1:
continue
answer = context[s["start_token"] : s["end_token"]]
html = is_html[s["start_token"] : s["end_token"]]
new_answer = " ".join([answer[i] for i in range(len(answer)) if not html[i]])
if new_answer not in long_targets:
long_targets.append(new_answer)
category = "long_short" if len(short_targets + long_targets) > 0 else "null"
return {
"question": question,
"context": context_string,
"short": short_targets,
"long": long_targets,
"category": category,
}
def main():
dataset = load_from_disk("natural-questions-validation")
dataset = dataset.map(format_dataset).remove_columns(["annotations", "document", "id"])
print(dataset)
short_validation_dataset = dataset.filter(lambda x: (len(x["question"]) + len(x["context"])) < 4 * 4096)
short_validation_dataset = short_validation_dataset.filter(lambda x: x["category"] != "null")
short_validation_dataset
model_id = "vasudevgupta/flax-bigbird-natural-questions"
model = FlaxBigBirdForNaturalQuestions.from_pretrained(model_id)
tokenizer = BigBirdTokenizerFast.from_pretrained(model_id)
@jax.jit
def forward(*args, **kwargs):
start_logits, end_logits, pooled_logits = model(*args, **kwargs)
return start_logits, end_logits, jnp.argmax(pooled_logits, axis=-1)
def evaluate(example):
# encode question and context so that they are separated by a tokenizer.sep_token and cut at max_length
inputs = tokenizer(
example["question"],
example["context"],
return_tensors="np",
max_length=4096,
padding="max_length",
truncation=True,
)
start_scores, end_scores, category = forward(**inputs)
predicted_category = CATEGORY_MAPPING[category.item()]
example["targets"] = example["long"] + example["short"]
if example["category"] in ["yes", "no", "null"]:
example["targets"] = [example["category"]]
example["has_tgt"] = example["category"] != "null"
# Now target can be: "yes", "no", "null", "list of long & short answers"
if predicted_category in ["yes", "no", "null"]:
example["output"] = [predicted_category]
example["match"] = example["output"] == example["targets"]
example["has_pred"] = predicted_category != "null"
return example
max_size = 38 if predicted_category == "short" else 1024
start_score, end_score = get_best_valid_start_end_idx(
start_scores[0], end_scores[0], top_k=8, max_size=max_size
)
input_ids = inputs["input_ids"][0].tolist()
example["output"] = [tokenizer.decode(input_ids[start_score : end_score + 1])]
answers = expand_to_aliases(example["targets"], make_sub_answers=True)
predictions = expand_to_aliases(example["output"])
# some preprocessing to both prediction and answer
answers = {"".join(a.split()) for a in answers}
predictions = {"".join(p.split()) for p in predictions}
predictions = {s for s in predictions if s not in ["``", "''", "`", "'"]}
# if there is a common element, it's a exact match
example["match"] = len(list(answers & predictions)) > 0
example["has_pred"] = predicted_category != "null" and len(predictions) > 0
return example
short_validation_dataset = short_validation_dataset.map(evaluate)
total = len(short_validation_dataset)
matched = len(short_validation_dataset.filter(lambda x: x["match"] == 1))
print("EM score:", (matched / total) * 100, "%")
if __name__ == "__main__":
main()
| transformers/examples/research_projects/jax-projects/big_bird/evaluate.py/0 | {
"file_path": "transformers/examples/research_projects/jax-projects/big_bird/evaluate.py",
"repo_id": "transformers",
"token_count": 2748
} | 285 |
#!/usr/bin/env python3
import logging
import sys
import time
from dataclasses import field
from pathlib import Path
from typing import Dict, List, Optional, Union
import flax
import jax
import jax.numpy as jnp
import librosa
import numpy as np
import optax
from datasets import DatasetDict, load_dataset
from flax import jax_utils, traverse_util
from flax.training import train_state
from flax.training.common_utils import get_metrics, onehot, shard
from tqdm import tqdm
from transformers import (
FlaxWav2Vec2ForPreTraining,
HfArgumentParser,
TrainingArguments,
Wav2Vec2Config,
Wav2Vec2FeatureExtractor,
is_tensorboard_available,
)
from transformers.models.wav2vec2.modeling_flax_wav2vec2 import _compute_mask_indices, _sample_negative_indices
logger = logging.getLogger(__name__)
@flax.struct.dataclass
class ModelArguments:
"""
Arguments pertaining to which model/config/tokenizer we are going to fine-tune from.
"""
model_name_or_path: str = field(
metadata={"help": "Path to pretrained model or model identifier from huggingface.co/models"}
)
cache_dir: Optional[str] = field(
default=None,
metadata={"help": "Where do you want to store the pretrained models downloaded from huggingface.co"},
)
freeze_feature_extractor: Optional[bool] = field(
default=True, metadata={"help": "Whether to freeze the feature extractor layers of the model."}
)
verbose_logging: Optional[bool] = field(
default=False,
metadata={"help": "Whether to log verbose messages or not."},
)
max_gumbel_temperature: Optional[float] = field(
default=2.0, metadata={"help": "Maximum temperature for gumbel softmax."}
)
min_gumbel_temperature: Optional[float] = field(
default=0.1, metadata={"help": "Minimum temperature for gumbel softmax."}
)
gumbel_temperature_decay: Optional[float] = field(
default=0.999995, metadata={"help": "Decay of gumbel temperature during training."}
)
dtype: Optional[str] = field(
default="float32",
metadata={
"help": (
"Floating-point format in which the model weights should be initialized and trained. Choose one of"
" `[float32, float16, bfloat16]`."
)
},
)
@flax.struct.dataclass
class DataTrainingArguments:
"""
Arguments pertaining to what data we are going to input our model for training and eval.
Using `HfArgumentParser` we can turn this class
into argparse arguments to be able to specify them on
the command line.
"""
dataset_name: str = field(
default=None, metadata={"help": "The name of the dataset to use (via the datasets library)."}
)
dataset_config_name: Optional[str] = field(
default=None, metadata={"help": "The configuration name of the dataset to use (via the datasets library)."}
)
train_split_name: Optional[str] = field(
default="train",
metadata={
"help": "The name of the training data set split to use (via the datasets library). Defaults to 'train'"
},
)
validation_split_name: Optional[str] = field(
default="validation",
metadata={
"help": (
"The name of the validation data set split to use (via the datasets library). Defaults to 'validation'"
)
},
)
speech_file_column: Optional[str] = field(
default="file",
metadata={"help": "Column in the dataset that contains speech file path. Defaults to 'file'"},
)
overwrite_cache: bool = field(
default=False, metadata={"help": "Overwrite the cached preprocessed datasets or not."}
)
validation_split_percentage: Optional[int] = field(
default=5,
metadata={
"help": "The percentage of the train set used as validation set in case there's no validation split"
},
)
preprocessing_num_workers: Optional[int] = field(
default=None,
metadata={"help": "The number of processes to use for the preprocessing."},
)
max_duration_in_seconds: Optional[float] = field(
default=20.0, metadata={"help": "Filter audio files that are longer than `max_duration_in_seconds` seconds"}
)
pad_to_multiple_of: Optional[int] = field(
default=1024,
metadata={
"help": (
"If set will pad the sequence to a multiple of the provided value. This is important to avoid"
" triggering recompilations on TPU"
)
},
)
@flax.struct.dataclass
class FlaxDataCollatorForWav2Vec2Pretraining:
"""
Data collator that will dynamically pad the inputs received and prepare masked indices
for self-supervised pretraining.
Args:
model (:class:`~transformers.FlaxWav2Vec2ForPreTraining`):
The Wav2Vec2 model used for pretraining. The data collator needs to have access
to config and ``_get_feat_extract_output_lengths`` function for correct padding.
feature_extractor (:class:`~transformers.Wav2Vec2FeatureExtractor`):
The processor used for processing the data.
padding (:obj:`bool`, :obj:`str` or :class:`~transformers.tokenization_utils_base.PaddingStrategy`, `optional`, defaults to :obj:`True`):
Select a strategy to pad the returned sequences (according to the model's padding side and padding index)
among:
* :obj:`True` or :obj:`'longest'`: Pad to the longest sequence in the batch (or no padding if only a single
sequence if provided).
* :obj:`'max_length'`: Pad to a maximum length specified with the argument :obj:`max_length` or to the
maximum acceptable input length for the model if that argument is not provided.
* :obj:`False` or :obj:`'do_not_pad'` (default): No padding (i.e., can output a batch with sequences of
different lengths).
max_length (:obj:`int`, `optional`):
Maximum length of the ``input_values`` of the returned list and optionally padding length (see above).
pad_to_multiple_of (:obj:`int`, `optional`):
If set will pad the sequence to a multiple of the provided value.
This is especially useful to enable the use of Tensor Cores on NVIDIA hardware with compute capability >=
7.5 (Volta).
"""
model: FlaxWav2Vec2ForPreTraining
feature_extractor: Wav2Vec2FeatureExtractor
padding: Union[bool, str] = "longest"
pad_to_multiple_of: Optional[int] = None
max_length: Optional[int] = None
def __call__(self, features: List[Dict[str, Union[List[int], np.ndarray]]]) -> Dict[str, np.ndarray]:
# reformat list to dict and set to pytorch format
batch = self.feature_extractor.pad(
features,
max_length=self.max_length,
padding=self.padding,
pad_to_multiple_of=self.pad_to_multiple_of,
return_tensors="np",
)
mask_indices_seq_length = self.model._get_feat_extract_output_lengths(batch["input_values"].shape[-1])
batch_size = batch["input_values"].shape[0]
attention_mask = None
if batch["attention_mask"] is not None:
output_lengths = self.model._get_feat_extract_output_lengths(batch["attention_mask"].sum(-1))
attention_mask = np.zeros((batch_size, mask_indices_seq_length), dtype=np.int8)
# these two operations makes sure that all values
# before the output lengths indices are attended to
attention_mask[(np.arange(attention_mask.shape[0]), output_lengths - 1)] = 1
attention_mask = jnp.flip(jnp.flip(attention_mask, -1).cumsum(-1), -1).astype("bool")
# sample randomly masked indices
batch["mask_time_indices"] = _compute_mask_indices(
(batch_size, mask_indices_seq_length),
self.model.config.mask_time_prob,
self.model.config.mask_time_length,
attention_mask=attention_mask,
min_masks=2,
)
# sample indices to take for negative vectors
batch["sampled_negative_indices"] = _sample_negative_indices(
(batch["mask_time_indices"].shape + (self.model.config.proj_codevector_dim,)),
self.model.config.num_negatives,
attention_mask=attention_mask,
)
return batch
def configure_logger(model_args: ModelArguments, training_args: TrainingArguments):
logging.basicConfig(
format="%(asctime)s - %(levelname)s - %(name)s - %(message)s",
datefmt="%m/%d/%Y %H:%M:%S",
handlers=[logging.StreamHandler(sys.stdout)],
)
logging_level = logging.WARNING
if model_args.verbose_logging:
logging_level = logging.DEBUG
logger.setLevel(logging_level)
def write_train_metric(summary_writer, train_metrics, train_time, step):
summary_writer.scalar("train_time", train_time, step)
train_metrics = get_metrics(train_metrics)
for key, vals in train_metrics.items():
tag = f"train_{key}"
for i, val in enumerate(vals):
summary_writer.scalar(tag, val, step - len(vals) + i + 1)
def write_eval_metric(summary_writer, eval_metrics, step):
for metric_name, value in eval_metrics.items():
summary_writer.scalar(f"eval_{metric_name}", value, step)
def generate_batch_splits(samples_idx: np.ndarray, batch_size: int) -> np.ndarray:
num_samples = len(samples_idx)
samples_to_remove = num_samples % batch_size
if samples_to_remove != 0:
samples_idx = samples_idx[:-samples_to_remove]
sections_split = num_samples // batch_size
batch_idx = np.split(samples_idx, sections_split)
return batch_idx
def compute_contrastive_loss(
quantized_features, transformer_features, negative_indices, mask_time_indices, logits_temp, num_negatives
):
batch_size, sequence_length, hidden_size = quantized_features.shape
# take negative vectors from sampled indices
quantized_negatives = quantized_features.reshape(-1, hidden_size)[negative_indices.reshape(-1)]
quantized_negatives = quantized_negatives.reshape(
batch_size, sequence_length, num_negatives, hidden_size
).transpose(2, 0, 1, 3)
target_features = jnp.concatenate([quantized_features[None, :], quantized_negatives], axis=0)
loss_logits = optax.cosine_similarity(transformer_features, target_features)
loss_logits = loss_logits / logits_temp
neg_is_pos = (quantized_features == quantized_negatives).all(-1)
neg_is_pos = jnp.concatenate([jnp.full((1,) + loss_logits.shape[1:], False), neg_is_pos], axis=0)
# make sure incorrectly sampled vectors don't contribute to loss
loss_logits = jnp.where(neg_is_pos, -1e9, loss_logits)
predictions = loss_logits.transpose(2, 1, 0).reshape(-1, loss_logits.shape[0])
targets = ((1 - mask_time_indices) * -100).transpose(1, 0).flatten()
target_mask = jnp.where(targets >= 0, 1.0, 0.0)
contrastive_loss = optax.softmax_cross_entropy(predictions, onehot(targets, predictions.shape[-1])) * target_mask
contrastive_loss = contrastive_loss.sum()
return contrastive_loss
def main():
# See all possible arguments in src/transformers/training_args.py
# or by passing the --help flag to this script.
# We now keep distinct sets of args, for a cleaner separation of concerns.
parser = HfArgumentParser((ModelArguments, DataTrainingArguments, TrainingArguments))
model_args, data_args, training_args = parser.parse_args_into_dataclasses()
configure_logger(model_args, training_args)
# Downloading and loading a dataset from the hub.
datasets = load_dataset(data_args.dataset_name, data_args.dataset_config_name, cache_dir=model_args.cache_dir)
if "validation" not in datasets.keys():
# make sure only "validation" and "train" keys remain"
datasets = DatasetDict()
datasets["validation"] = load_dataset(
data_args.dataset_name,
data_args.dataset_config_name,
split=f"{data_args.train_split_name}[:{data_args.validation_split_percentage}%]",
cache_dir=model_args.cache_dir,
)
datasets["train"] = load_dataset(
data_args.dataset_name,
data_args.dataset_config_name,
split=f"{data_args.train_split_name}[{data_args.validation_split_percentage}%:]",
cache_dir=model_args.cache_dir,
)
else:
# make sure only "validation" and "train" keys remain"
datasets = DatasetDict()
datasets["validation"] = load_dataset(
data_args.dataset_name,
data_args.dataset_config_name,
split="validation",
cache_dir=model_args.cache_dir,
)
datasets["train"] = load_dataset(
data_args.dataset_name,
data_args.dataset_config_name,
split=f"{data_args.train_split_name}",
cache_dir=model_args.cache_dir,
)
# only normalized-inputs-training is supported
feature_extractor = Wav2Vec2FeatureExtractor.from_pretrained(
model_args.model_name_or_path, cache_dir=model_args.cache_dir, do_normalize=True
)
def prepare_dataset(batch):
# check that all files have the correct sampling rate
batch["speech"], _ = librosa.load(batch[data_args.speech_file_column], sr=feature_extractor.sampling_rate)
return batch
# load audio files into numpy arrays
vectorized_datasets = datasets.map(
prepare_dataset, num_proc=data_args.preprocessing_num_workers, remove_columns=datasets["train"].column_names
)
# filter audio files that are too long
vectorized_datasets = vectorized_datasets.filter(
lambda data: len(data["speech"]) < int(data_args.max_duration_in_seconds * feature_extractor.sampling_rate)
)
def normalize(batch):
return feature_extractor(batch["speech"], sampling_rate=feature_extractor.sampling_rate)
# normalize and transform to `BatchFeatures`
vectorized_datasets = vectorized_datasets.map(
normalize,
batched=True,
num_proc=data_args.preprocessing_num_workers,
load_from_cache_file=not data_args.overwrite_cache,
remove_columns=vectorized_datasets["train"].column_names,
)
# pretraining is only supported for "newer" stable layer norm architecture
# apply_spec_augment has to be True, mask_feature_prob has to be 0.0
config = Wav2Vec2Config.from_pretrained(
model_args.model_name_or_path,
cache_dir=model_args.cache_dir,
)
if not config.do_stable_layer_norm or config.feat_extract_norm != "layer":
raise ValueError(
"PreTraining is only supported for ``config.do_stable_layer_norm=True`` and"
" ``config.feat_extract_norm='layer'"
)
model = FlaxWav2Vec2ForPreTraining(config, seed=training_args.seed, dtype=getattr(jnp, model_args.dtype))
# Activate gradient checkpointing if needed
if training_args.gradient_checkpointing:
model.gradient_checkpointing_enable()
data_collator = FlaxDataCollatorForWav2Vec2Pretraining(
model=model, feature_extractor=feature_extractor, pad_to_multiple_of=data_args.pad_to_multiple_of
)
# Enable tensorboard only on the master node
has_tensorboard = is_tensorboard_available()
if has_tensorboard and jax.process_index() == 0:
try:
from flax.metrics.tensorboard import SummaryWriter
summary_writer = SummaryWriter(log_dir=Path(training_args.output_dir))
except ImportError as ie:
has_tensorboard = False
logger.warning(
f"Unable to display metrics through TensorBoard because some package are not installed: {ie}"
)
else:
logger.warning(
"Unable to display metrics through TensorBoard because the package is not installed: "
"Please run pip install tensorboard to enable."
)
# Initialize our training
rng = jax.random.PRNGKey(training_args.seed)
dropout_rngs = jax.random.split(rng, jax.local_device_count())
gumbel_rngs = jax.random.split(rng, jax.local_device_count())
num_epochs = int(training_args.num_train_epochs)
train_batch_size = int(training_args.per_device_train_batch_size) * jax.device_count()
eval_batch_size = int(training_args.per_device_eval_batch_size) * jax.device_count()
num_train_steps = len(vectorized_datasets["train"]) // train_batch_size * num_epochs
# Create learning rate schedule
warmup_fn = optax.linear_schedule(
init_value=0.0, end_value=training_args.learning_rate, transition_steps=training_args.warmup_steps
)
decay_fn = optax.linear_schedule(
init_value=training_args.learning_rate,
end_value=0,
transition_steps=num_train_steps - training_args.warmup_steps,
)
linear_decay_lr_schedule_fn = optax.join_schedules(
schedules=[warmup_fn, decay_fn], boundaries=[training_args.warmup_steps]
)
# We use Optax's "masking" functionality to not apply weight decay
# to bias and LayerNorm scale parameters. decay_mask_fn returns a
# mask boolean with the same structure as the parameters.
# The mask is True for parameters that should be decayed.
def decay_mask_fn(params):
flat_params = traverse_util.flatten_dict(params)
flat_mask = {
path: (path[-1] != "bias" and path[-2:] not in [("layer_norm", "scale"), ("final_layer_norm", "scale")])
for path in flat_params
}
return traverse_util.unflatten_dict(flat_mask)
# create adam optimizer
adamw = optax.adamw(
learning_rate=linear_decay_lr_schedule_fn,
b1=training_args.adam_beta1,
b2=training_args.adam_beta2,
eps=training_args.adam_epsilon,
weight_decay=training_args.weight_decay,
mask=decay_mask_fn,
)
# Setup train state and define training hyper-parameters
state = train_state.TrainState.create(apply_fn=model.__call__, params=model.params, tx=adamw)
num_negatives = model.config.num_negatives
contrastive_logits_temperature = model.config.contrastive_logits_temperature
num_codevectors = model.config.num_codevectors_per_group * model.config.num_codevector_groups
diversity_loss_weight = model.config.diversity_loss_weight
# Define gradient update step fn
def train_step(state, batch, dropout_rng, gumbel_rng):
dropout_rng, new_dropout_rng = jax.random.split(dropout_rng)
gumbel_rng, new_gumbel_rng = jax.random.split(gumbel_rng)
def loss_fn(params):
negative_indices = batch.pop("sampled_negative_indices")
gumbel_temperature = jnp.clip(
model_args.max_gumbel_temperature * model_args.gumbel_temperature_decay**state.step,
a_min=model_args.min_gumbel_temperature,
)
outputs = state.apply_fn(
**batch,
gumbel_temperature=gumbel_temperature,
params=params,
dropout_rng=dropout_rng,
gumbel_rng=gumbel_rng,
train=True,
)
contrastive_loss = compute_contrastive_loss(
outputs.projected_quantized_states,
outputs.projected_states,
negative_indices,
batch["mask_time_indices"],
contrastive_logits_temperature,
num_negatives,
)
diversity_loss = (num_codevectors - outputs.codevector_perplexity) / num_codevectors
loss = contrastive_loss + diversity_loss_weight * diversity_loss
return loss
grad_fn = jax.value_and_grad(loss_fn)
loss, grad = grad_fn(state.params)
grad = jax.lax.pmean(grad, "batch")
new_state = state.apply_gradients(grads=grad)
metrics = jax.lax.pmean(
{"loss": loss, "learning_rate": linear_decay_lr_schedule_fn(state.step)}, axis_name="batch"
)
return new_state, metrics, new_dropout_rng, new_gumbel_rng
# Create parallel version of the train step
p_train_step = jax.pmap(train_step, "batch", donate_argnums=(0,))
# Define eval fn
def eval_step(params, batch):
negative_indices = batch.pop("sampled_negative_indices")
outputs = model(**batch, params=params, train=False)
contrastive_loss = compute_contrastive_loss(
outputs.projected_quantized_states,
outputs.projected_states,
negative_indices,
batch["mask_time_indices"],
contrastive_logits_temperature,
num_negatives,
)
diversity_loss = (num_codevectors - outputs.codevector_perplexity) / num_codevectors
loss = contrastive_loss + diversity_loss_weight * diversity_loss
# summarize metrics
metrics = {"loss": loss.mean(), "codevector_perplexity": outputs.codevector_perplexity}
metrics = jax.lax.pmean(metrics, axis_name="batch")
return metrics
p_eval_step = jax.pmap(eval_step, "batch", donate_argnums=(0,))
# Replicate the train state on each device
state = jax_utils.replicate(state)
train_time = 0
train_metrics = []
epochs = tqdm(range(num_epochs), desc=f"Epoch ... (1/{num_epochs})", position=0)
for epoch in epochs:
# ======================== Training ================================
train_start = time.time()
# Create sampling rng
rng, input_rng = jax.random.split(rng)
# Generate an epoch by shuffling sampling indices from the train dataset
num_train_samples = len(vectorized_datasets["train"])
# Avoid using jax.numpy here in case of TPU training
train_samples_idx = np.random.permutation(np.arange(num_train_samples))
train_batch_idx = generate_batch_splits(train_samples_idx, train_batch_size)
# Gather the indexes for creating the batch and do a training step
for step, batch_idx in enumerate(tqdm(train_batch_idx, desc="Training...", position=1)):
samples = [vectorized_datasets["train"][int(idx)] for idx in batch_idx]
model_inputs = data_collator(samples)
model_inputs = shard(model_inputs.data)
# Model forward
state, train_metric, dropout_rngs, gumbel_rngs = p_train_step(
state, model_inputs, dropout_rngs, gumbel_rngs
)
train_metrics.append(train_metric)
cur_step = epoch * (num_train_samples // train_batch_size) + step
if cur_step % training_args.logging_steps == 0 and cur_step > 0:
# Save metrics
train_metric = jax_utils.unreplicate(train_metric)
train_time += time.time() - train_start
if has_tensorboard and jax.process_index() == 0:
write_train_metric(summary_writer, train_metrics, train_time, cur_step)
epochs.write(
f"Step... ({cur_step} | Loss: {train_metric['loss'].mean()}, Learning Rate:"
f" {train_metric['learning_rate'].mean()})"
)
train_metrics = []
# ======================== Evaluating ==============================
num_eval_samples = len(vectorized_datasets["validation"])
# Avoid using jax.numpy here in case of TPU training
eval_samples_idx = np.arange(num_eval_samples)
eval_batch_idx = generate_batch_splits(eval_samples_idx, eval_batch_size)
eval_metrics = []
for i, batch_idx in enumerate(tqdm(eval_batch_idx, desc="Evaluating ...", position=2)):
samples = [vectorized_datasets["validation"][int(idx)] for idx in batch_idx]
model_inputs = data_collator(samples)
# Model forward
model_inputs = shard(model_inputs.data)
metrics = p_eval_step(state.params, model_inputs)
eval_metrics.append(metrics)
# get eval metrics
eval_metrics = get_metrics(eval_metrics)
eval_metrics = jax.tree_util.tree_map(jnp.mean, eval_metrics)
# Update progress bar
epochs.write(
f"Epoch... ({epoch + 1}/{num_epochs} | Loss: {eval_metrics['loss']}, Perplexity:"
f" {eval_metrics['codevector_perplexity']})"
)
# Save metrics
if has_tensorboard and jax.process_index() == 0:
cur_step = epoch * (len(vectorized_datasets["train"]) // train_batch_size)
write_eval_metric(summary_writer, eval_metrics, cur_step)
# save checkpoint after each epoch and push checkpoint to the hub
if jax.process_index() == 0:
params = jax.device_get(jax.tree_util.tree_map(lambda x: x[0], state.params))
model.save_pretrained(training_args.output_dir, params=params, push_to_hub=training_args.push_to_hub)
if __name__ == "__main__":
main()
| transformers/examples/research_projects/jax-projects/wav2vec2/run_wav2vec2_pretrain_flax.py/0 | {
"file_path": "transformers/examples/research_projects/jax-projects/wav2vec2/run_wav2vec2_pretrain_flax.py",
"repo_id": "transformers",
"token_count": 10481
} | 286 |
# coding=utf-8
# Copyright 2018 The Google AI Language Team Authors and The HuggingFace Inc. team.
# Copyright (c) 2018, NVIDIA CORPORATION. All rights reserved.
#
# Licensed under the Apache License, Version 2.0 (the "License");
# you may not use this file except in compliance with the License.
# You may obtain a copy of the License at
#
# http://www.apache.org/licenses/LICENSE-2.0
#
# Unless required by applicable law or agreed to in writing, software
# distributed under the License is distributed on an "AS IS" BASIS,
# WITHOUT WARRANTIES OR CONDITIONS OF ANY KIND, either express or implied.
# See the License for the specific language governing permissions and
# limitations under the License.
"""Masked Version of BERT. It replaces the `torch.nn.Linear` layers with
:class:`~emmental.MaskedLinear` and add an additional parameters in the forward pass to
compute the adaptive mask.
Built on top of `transformers.models.bert.modeling_bert`"""
import logging
import math
import torch
from torch import nn
from torch.nn import CrossEntropyLoss, MSELoss
from emmental import MaskedBertConfig
from emmental.modules import MaskedLinear
from transformers.file_utils import add_start_docstrings, add_start_docstrings_to_model_forward
from transformers.modeling_utils import PreTrainedModel, prune_linear_layer
from transformers.models.bert.modeling_bert import ACT2FN, load_tf_weights_in_bert
logger = logging.getLogger(__name__)
class BertEmbeddings(nn.Module):
"""Construct the embeddings from word, position and token_type embeddings."""
def __init__(self, config):
super().__init__()
self.word_embeddings = nn.Embedding(config.vocab_size, config.hidden_size, padding_idx=0)
self.position_embeddings = nn.Embedding(config.max_position_embeddings, config.hidden_size)
self.token_type_embeddings = nn.Embedding(config.type_vocab_size, config.hidden_size)
# self.LayerNorm is not snake-cased to stick with TensorFlow model variable name and be able to load
# any TensorFlow checkpoint file
self.LayerNorm = nn.LayerNorm(config.hidden_size, eps=config.layer_norm_eps)
self.dropout = nn.Dropout(config.hidden_dropout_prob)
def forward(self, input_ids=None, token_type_ids=None, position_ids=None, inputs_embeds=None):
if input_ids is not None:
input_shape = input_ids.size()
else:
input_shape = inputs_embeds.size()[:-1]
seq_length = input_shape[1]
device = input_ids.device if input_ids is not None else inputs_embeds.device
if position_ids is None:
position_ids = torch.arange(seq_length, dtype=torch.long, device=device)
position_ids = position_ids.unsqueeze(0).expand(input_shape)
if token_type_ids is None:
token_type_ids = torch.zeros(input_shape, dtype=torch.long, device=device)
if inputs_embeds is None:
inputs_embeds = self.word_embeddings(input_ids)
position_embeddings = self.position_embeddings(position_ids)
token_type_embeddings = self.token_type_embeddings(token_type_ids)
embeddings = inputs_embeds + position_embeddings + token_type_embeddings
embeddings = self.LayerNorm(embeddings)
embeddings = self.dropout(embeddings)
return embeddings
class BertSelfAttention(nn.Module):
def __init__(self, config):
super().__init__()
if config.hidden_size % config.num_attention_heads != 0 and not hasattr(config, "embedding_size"):
raise ValueError(
"The hidden size (%d) is not a multiple of the number of attention heads (%d)"
% (config.hidden_size, config.num_attention_heads)
)
self.output_attentions = config.output_attentions
self.num_attention_heads = config.num_attention_heads
self.attention_head_size = int(config.hidden_size / config.num_attention_heads)
self.all_head_size = self.num_attention_heads * self.attention_head_size
self.query = MaskedLinear(
config.hidden_size,
self.all_head_size,
pruning_method=config.pruning_method,
mask_init=config.mask_init,
mask_scale=config.mask_scale,
)
self.key = MaskedLinear(
config.hidden_size,
self.all_head_size,
pruning_method=config.pruning_method,
mask_init=config.mask_init,
mask_scale=config.mask_scale,
)
self.value = MaskedLinear(
config.hidden_size,
self.all_head_size,
pruning_method=config.pruning_method,
mask_init=config.mask_init,
mask_scale=config.mask_scale,
)
self.dropout = nn.Dropout(config.attention_probs_dropout_prob)
def transpose_for_scores(self, x):
new_x_shape = x.size()[:-1] + (self.num_attention_heads, self.attention_head_size)
x = x.view(*new_x_shape)
return x.permute(0, 2, 1, 3)
def forward(
self,
hidden_states,
attention_mask=None,
head_mask=None,
encoder_hidden_states=None,
encoder_attention_mask=None,
threshold=None,
):
mixed_query_layer = self.query(hidden_states, threshold=threshold)
# If this is instantiated as a cross-attention module, the keys
# and values come from an encoder; the attention mask needs to be
# such that the encoder's padding tokens are not attended to.
if encoder_hidden_states is not None:
mixed_key_layer = self.key(encoder_hidden_states, threshold=threshold)
mixed_value_layer = self.value(encoder_hidden_states, threshold=threshold)
attention_mask = encoder_attention_mask
else:
mixed_key_layer = self.key(hidden_states, threshold=threshold)
mixed_value_layer = self.value(hidden_states, threshold=threshold)
query_layer = self.transpose_for_scores(mixed_query_layer)
key_layer = self.transpose_for_scores(mixed_key_layer)
value_layer = self.transpose_for_scores(mixed_value_layer)
# Take the dot product between "query" and "key" to get the raw attention scores.
attention_scores = torch.matmul(query_layer, key_layer.transpose(-1, -2))
attention_scores = attention_scores / math.sqrt(self.attention_head_size)
if attention_mask is not None:
# Apply the attention mask is (precomputed for all layers in BertModel forward() function)
attention_scores = attention_scores + attention_mask
# Normalize the attention scores to probabilities.
attention_probs = nn.functional.softmax(attention_scores, dim=-1)
# This is actually dropping out entire tokens to attend to, which might
# seem a bit unusual, but is taken from the original Transformer paper.
attention_probs = self.dropout(attention_probs)
# Mask heads if we want to
if head_mask is not None:
attention_probs = attention_probs * head_mask
context_layer = torch.matmul(attention_probs, value_layer)
context_layer = context_layer.permute(0, 2, 1, 3).contiguous()
new_context_layer_shape = context_layer.size()[:-2] + (self.all_head_size,)
context_layer = context_layer.view(*new_context_layer_shape)
outputs = (context_layer, attention_probs) if self.output_attentions else (context_layer,)
return outputs
class BertSelfOutput(nn.Module):
def __init__(self, config):
super().__init__()
self.dense = MaskedLinear(
config.hidden_size,
config.hidden_size,
pruning_method=config.pruning_method,
mask_init=config.mask_init,
mask_scale=config.mask_scale,
)
self.LayerNorm = nn.LayerNorm(config.hidden_size, eps=config.layer_norm_eps)
self.dropout = nn.Dropout(config.hidden_dropout_prob)
def forward(self, hidden_states, input_tensor, threshold):
hidden_states = self.dense(hidden_states, threshold=threshold)
hidden_states = self.dropout(hidden_states)
hidden_states = self.LayerNorm(hidden_states + input_tensor)
return hidden_states
class BertAttention(nn.Module):
def __init__(self, config):
super().__init__()
self.self = BertSelfAttention(config)
self.output = BertSelfOutput(config)
self.pruned_heads = set()
def prune_heads(self, heads):
if len(heads) == 0:
return
mask = torch.ones(self.self.num_attention_heads, self.self.attention_head_size)
heads = set(heads) - self.pruned_heads # Convert to set and remove already pruned heads
for head in heads:
# Compute how many pruned heads are before the head and move the index accordingly
head = head - sum(1 if h < head else 0 for h in self.pruned_heads)
mask[head] = 0
mask = mask.view(-1).contiguous().eq(1)
index = torch.arange(len(mask))[mask].long()
# Prune linear layers
self.self.query = prune_linear_layer(self.self.query, index)
self.self.key = prune_linear_layer(self.self.key, index)
self.self.value = prune_linear_layer(self.self.value, index)
self.output.dense = prune_linear_layer(self.output.dense, index, dim=1)
# Update hyper params and store pruned heads
self.self.num_attention_heads = self.self.num_attention_heads - len(heads)
self.self.all_head_size = self.self.attention_head_size * self.self.num_attention_heads
self.pruned_heads = self.pruned_heads.union(heads)
def forward(
self,
hidden_states,
attention_mask=None,
head_mask=None,
encoder_hidden_states=None,
encoder_attention_mask=None,
threshold=None,
):
self_outputs = self.self(
hidden_states,
attention_mask,
head_mask,
encoder_hidden_states,
encoder_attention_mask,
threshold=threshold,
)
attention_output = self.output(self_outputs[0], hidden_states, threshold=threshold)
outputs = (attention_output,) + self_outputs[1:] # add attentions if we output them
return outputs
class BertIntermediate(nn.Module):
def __init__(self, config):
super().__init__()
self.dense = MaskedLinear(
config.hidden_size,
config.intermediate_size,
pruning_method=config.pruning_method,
mask_init=config.mask_init,
mask_scale=config.mask_scale,
)
if isinstance(config.hidden_act, str):
self.intermediate_act_fn = ACT2FN[config.hidden_act]
else:
self.intermediate_act_fn = config.hidden_act
def forward(self, hidden_states, threshold):
hidden_states = self.dense(hidden_states, threshold=threshold)
hidden_states = self.intermediate_act_fn(hidden_states)
return hidden_states
class BertOutput(nn.Module):
def __init__(self, config):
super().__init__()
self.dense = MaskedLinear(
config.intermediate_size,
config.hidden_size,
pruning_method=config.pruning_method,
mask_init=config.mask_init,
mask_scale=config.mask_scale,
)
self.LayerNorm = nn.LayerNorm(config.hidden_size, eps=config.layer_norm_eps)
self.dropout = nn.Dropout(config.hidden_dropout_prob)
def forward(self, hidden_states, input_tensor, threshold):
hidden_states = self.dense(hidden_states, threshold=threshold)
hidden_states = self.dropout(hidden_states)
hidden_states = self.LayerNorm(hidden_states + input_tensor)
return hidden_states
class BertLayer(nn.Module):
def __init__(self, config):
super().__init__()
self.attention = BertAttention(config)
self.is_decoder = config.is_decoder
if self.is_decoder:
self.crossattention = BertAttention(config)
self.intermediate = BertIntermediate(config)
self.output = BertOutput(config)
def forward(
self,
hidden_states,
attention_mask=None,
head_mask=None,
encoder_hidden_states=None,
encoder_attention_mask=None,
threshold=None,
):
self_attention_outputs = self.attention(hidden_states, attention_mask, head_mask, threshold=threshold)
attention_output = self_attention_outputs[0]
outputs = self_attention_outputs[1:] # add self attentions if we output attention weights
if self.is_decoder and encoder_hidden_states is not None:
cross_attention_outputs = self.crossattention(
attention_output, attention_mask, head_mask, encoder_hidden_states, encoder_attention_mask
)
attention_output = cross_attention_outputs[0]
outputs = outputs + cross_attention_outputs[1:] # add cross attentions if we output attention weights
intermediate_output = self.intermediate(attention_output, threshold=threshold)
layer_output = self.output(intermediate_output, attention_output, threshold=threshold)
outputs = (layer_output,) + outputs
return outputs
class BertEncoder(nn.Module):
def __init__(self, config):
super().__init__()
self.output_attentions = config.output_attentions
self.output_hidden_states = config.output_hidden_states
self.layer = nn.ModuleList([BertLayer(config) for _ in range(config.num_hidden_layers)])
def forward(
self,
hidden_states,
attention_mask=None,
head_mask=None,
encoder_hidden_states=None,
encoder_attention_mask=None,
threshold=None,
):
all_hidden_states = ()
all_attentions = ()
for i, layer_module in enumerate(self.layer):
if self.output_hidden_states:
all_hidden_states = all_hidden_states + (hidden_states,)
layer_outputs = layer_module(
hidden_states,
attention_mask,
head_mask[i],
encoder_hidden_states,
encoder_attention_mask,
threshold=threshold,
)
hidden_states = layer_outputs[0]
if self.output_attentions:
all_attentions = all_attentions + (layer_outputs[1],)
# Add last layer
if self.output_hidden_states:
all_hidden_states = all_hidden_states + (hidden_states,)
outputs = (hidden_states,)
if self.output_hidden_states:
outputs = outputs + (all_hidden_states,)
if self.output_attentions:
outputs = outputs + (all_attentions,)
return outputs # last-layer hidden state, (all hidden states), (all attentions)
class BertPooler(nn.Module):
def __init__(self, config):
super().__init__()
self.dense = nn.Linear(config.hidden_size, config.hidden_size)
self.activation = nn.Tanh()
def forward(self, hidden_states):
# We "pool" the model by simply taking the hidden state corresponding
# to the first token.
first_token_tensor = hidden_states[:, 0]
pooled_output = self.dense(first_token_tensor)
pooled_output = self.activation(pooled_output)
return pooled_output
class MaskedBertPreTrainedModel(PreTrainedModel):
"""An abstract class to handle weights initialization and
a simple interface for downloading and loading pretrained models.
"""
config_class = MaskedBertConfig
load_tf_weights = load_tf_weights_in_bert
base_model_prefix = "bert"
def _init_weights(self, module):
"""Initialize the weights"""
if isinstance(module, (nn.Linear, nn.Embedding)):
# Slightly different from the TF version which uses truncated_normal for initialization
# cf https://github.com/pytorch/pytorch/pull/5617
module.weight.data.normal_(mean=0.0, std=self.config.initializer_range)
elif isinstance(module, nn.LayerNorm):
module.bias.data.zero_()
module.weight.data.fill_(1.0)
if isinstance(module, nn.Linear) and module.bias is not None:
module.bias.data.zero_()
MASKED_BERT_START_DOCSTRING = r"""
This model is a PyTorch `torch.nn.Module <https://pytorch.org/docs/stable/nn.html#torch.nn.Module>`_ sub-class.
Use it as a regular PyTorch Module and refer to the PyTorch documentation for all matter related to general
usage and behavior.
Parameters:
config (:class:`~emmental.MaskedBertConfig`): Model configuration class with all the parameters of the model.
Initializing with a config file does not load the weights associated with the model, only the configuration.
Check out the :meth:`~transformers.PreTrainedModel.from_pretrained` method to load the model weights.
"""
MASKED_BERT_INPUTS_DOCSTRING = r"""
Args:
input_ids (:obj:`torch.LongTensor` of shape :obj:`(batch_size, sequence_length)`):
Indices of input sequence tokens in the vocabulary.
Indices can be obtained using :class:`transformers.BertTokenizer`.
See :func:`transformers.PreTrainedTokenizer.encode` and
:func:`transformers.PreTrainedTokenizer.__call__` for details.
`What are input IDs? <../glossary.html#input-ids>`__
attention_mask (:obj:`torch.FloatTensor` of shape :obj:`(batch_size, sequence_length)`, `optional`):
Mask to avoid performing attention on padding token indices.
Mask values selected in ``[0, 1]``:
``1`` for tokens that are NOT MASKED, ``0`` for MASKED tokens.
`What are attention masks? <../glossary.html#attention-mask>`__
token_type_ids (:obj:`torch.LongTensor` of shape :obj:`(batch_size, sequence_length)`, `optional`):
Segment token indices to indicate first and second portions of the inputs.
Indices are selected in ``[0, 1]``: ``0`` corresponds to a `sentence A` token, ``1``
corresponds to a `sentence B` token
`What are token type IDs? <../glossary.html#token-type-ids>`_
position_ids (:obj:`torch.LongTensor` of shape :obj:`(batch_size, sequence_length)`, `optional`):
Indices of positions of each input sequence tokens in the position embeddings.
Selected in the range ``[0, config.max_position_embeddings - 1]``.
`What are position IDs? <../glossary.html#position-ids>`_
head_mask (:obj:`torch.FloatTensor` of shape :obj:`(num_heads,)` or :obj:`(num_layers, num_heads)`, `optional`):
Mask to nullify selected heads of the self-attention modules.
Mask values selected in ``[0, 1]``:
:obj:`1` indicates the head is **not masked**, :obj:`0` indicates the head is **masked**.
inputs_embeds (:obj:`torch.FloatTensor` of shape :obj:`(batch_size, sequence_length, hidden_size)`, `optional`):
Optionally, instead of passing :obj:`input_ids` you can choose to directly pass an embedded representation.
This is useful if you want more control over how to convert `input_ids` indices into associated vectors
than the model's internal embedding lookup matrix.
encoder_hidden_states (:obj:`torch.FloatTensor` of shape :obj:`(batch_size, sequence_length, hidden_size)`, `optional`):
Sequence of hidden-states at the output of the last layer of the encoder. Used in the cross-attention
if the model is configured as a decoder.
encoder_attention_mask (:obj:`torch.FloatTensor` of shape :obj:`(batch_size, sequence_length)`, `optional`):
Mask to avoid performing attention on the padding token indices of the encoder input. This mask
is used in the cross-attention if the model is configured as a decoder.
Mask values selected in ``[0, 1]``:
``1`` for tokens that are NOT MASKED, ``0`` for MASKED tokens.
"""
@add_start_docstrings(
"The bare Masked Bert Model transformer outputting raw hidden-states without any specific head on top.",
MASKED_BERT_START_DOCSTRING,
)
class MaskedBertModel(MaskedBertPreTrainedModel):
"""
The `MaskedBertModel` class replicates the :class:`~transformers.BertModel` class
and adds specific inputs to compute the adaptive mask on the fly.
Note that we freeze the embeddings modules from their pre-trained values.
"""
def __init__(self, config):
super().__init__(config)
self.config = config
self.embeddings = BertEmbeddings(config)
self.embeddings.requires_grad_(requires_grad=False)
self.encoder = BertEncoder(config)
self.pooler = BertPooler(config)
self.init_weights()
def get_input_embeddings(self):
return self.embeddings.word_embeddings
def set_input_embeddings(self, value):
self.embeddings.word_embeddings = value
def _prune_heads(self, heads_to_prune):
"""Prunes heads of the model.
heads_to_prune: dict of {layer_num: list of heads to prune in this layer}
See base class PreTrainedModel
"""
for layer, heads in heads_to_prune.items():
self.encoder.layer[layer].attention.prune_heads(heads)
@add_start_docstrings_to_model_forward(MASKED_BERT_INPUTS_DOCSTRING)
def forward(
self,
input_ids=None,
attention_mask=None,
token_type_ids=None,
position_ids=None,
head_mask=None,
inputs_embeds=None,
encoder_hidden_states=None,
encoder_attention_mask=None,
threshold=None,
):
r"""
threshold (:obj:`float`):
Threshold value (see :class:`~emmental.MaskedLinear`).
Return:
:obj:`tuple(torch.FloatTensor)` comprising various elements depending on the configuration (:class:`~emmental.MaskedBertConfig`) and inputs:
last_hidden_state (:obj:`torch.FloatTensor` of shape :obj:`(batch_size, sequence_length, hidden_size)`):
Sequence of hidden-states at the output of the last layer of the model.
pooler_output (:obj:`torch.FloatTensor`: of shape :obj:`(batch_size, hidden_size)`):
Last layer hidden-state of the first token of the sequence (classification token)
further processed by a Linear layer and a Tanh activation function. The Linear
layer weights are trained from the next sentence prediction (classification)
objective during pre-training.
This output is usually *not* a good summary
of the semantic content of the input, you're often better with averaging or pooling
the sequence of hidden-states for the whole input sequence.
hidden_states (:obj:`tuple(torch.FloatTensor)`, `optional`, returned when ``config.output_hidden_states=True``):
Tuple of :obj:`torch.FloatTensor` (one for the output of the embeddings + one for the output of each layer)
of shape :obj:`(batch_size, sequence_length, hidden_size)`.
Hidden-states of the model at the output of each layer plus the initial embedding outputs.
attentions (:obj:`tuple(torch.FloatTensor)`, `optional`, returned when ``config.output_attentions=True``):
Tuple of :obj:`torch.FloatTensor` (one for each layer) of shape
:obj:`(batch_size, num_heads, sequence_length, sequence_length)`.
Attentions weights after the attention softmax, used to compute the weighted average in the self-attention
heads.
"""
if input_ids is not None and inputs_embeds is not None:
raise ValueError("You cannot specify both input_ids and inputs_embeds at the same time")
elif input_ids is not None:
input_shape = input_ids.size()
elif inputs_embeds is not None:
input_shape = inputs_embeds.size()[:-1]
else:
raise ValueError("You have to specify either input_ids or inputs_embeds")
device = input_ids.device if input_ids is not None else inputs_embeds.device
if attention_mask is None:
attention_mask = torch.ones(input_shape, device=device)
if token_type_ids is None:
token_type_ids = torch.zeros(input_shape, dtype=torch.long, device=device)
# We can provide a self-attention mask of dimensions [batch_size, from_seq_length, to_seq_length]
# ourselves in which case we just need to make it broadcastable to all heads.
if attention_mask.dim() == 3:
extended_attention_mask = attention_mask[:, None, :, :]
elif attention_mask.dim() == 2:
# Provided a padding mask of dimensions [batch_size, seq_length]
# - if the model is a decoder, apply a causal mask in addition to the padding mask
# - if the model is an encoder, make the mask broadcastable to [batch_size, num_heads, seq_length, seq_length]
if self.config.is_decoder:
batch_size, seq_length = input_shape
seq_ids = torch.arange(seq_length, device=device)
causal_mask = seq_ids[None, None, :].repeat(batch_size, seq_length, 1) <= seq_ids[None, :, None]
causal_mask = causal_mask.to(
attention_mask.dtype
) # causal and attention masks must have same type with pytorch version < 1.3
extended_attention_mask = causal_mask[:, None, :, :] * attention_mask[:, None, None, :]
else:
extended_attention_mask = attention_mask[:, None, None, :]
else:
raise ValueError(
"Wrong shape for input_ids (shape {}) or attention_mask (shape {})".format(
input_shape, attention_mask.shape
)
)
# Since attention_mask is 1.0 for positions we want to attend and 0.0 for
# masked positions, this operation will create a tensor which is 0.0 for
# positions we want to attend and -10000.0 for masked positions.
# Since we are adding it to the raw scores before the softmax, this is
# effectively the same as removing these entirely.
extended_attention_mask = extended_attention_mask.to(dtype=next(self.parameters()).dtype) # fp16 compatibility
extended_attention_mask = (1.0 - extended_attention_mask) * -10000.0
# If a 2D ou 3D attention mask is provided for the cross-attention
# we need to make broadcastable to [batch_size, num_heads, seq_length, seq_length]
if self.config.is_decoder and encoder_hidden_states is not None:
encoder_batch_size, encoder_sequence_length, _ = encoder_hidden_states.size()
encoder_hidden_shape = (encoder_batch_size, encoder_sequence_length)
if encoder_attention_mask is None:
encoder_attention_mask = torch.ones(encoder_hidden_shape, device=device)
if encoder_attention_mask.dim() == 3:
encoder_extended_attention_mask = encoder_attention_mask[:, None, :, :]
elif encoder_attention_mask.dim() == 2:
encoder_extended_attention_mask = encoder_attention_mask[:, None, None, :]
else:
raise ValueError(
"Wrong shape for encoder_hidden_shape (shape {}) or encoder_attention_mask (shape {})".format(
encoder_hidden_shape, encoder_attention_mask.shape
)
)
encoder_extended_attention_mask = encoder_extended_attention_mask.to(
dtype=next(self.parameters()).dtype
) # fp16 compatibility
encoder_extended_attention_mask = (1.0 - encoder_extended_attention_mask) * -10000.0
else:
encoder_extended_attention_mask = None
# Prepare head mask if needed
# 1.0 in head_mask indicate we keep the head
# attention_probs has shape bsz x n_heads x N x N
# input head_mask has shape [num_heads] or [num_hidden_layers x num_heads]
# and head_mask is converted to shape [num_hidden_layers x batch x num_heads x seq_length x seq_length]
if head_mask is not None:
if head_mask.dim() == 1:
head_mask = head_mask.unsqueeze(0).unsqueeze(0).unsqueeze(-1).unsqueeze(-1)
head_mask = head_mask.expand(self.config.num_hidden_layers, -1, -1, -1, -1)
elif head_mask.dim() == 2:
head_mask = (
head_mask.unsqueeze(1).unsqueeze(-1).unsqueeze(-1)
) # We can specify head_mask for each layer
head_mask = head_mask.to(
dtype=next(self.parameters()).dtype
) # switch to float if need + fp16 compatibility
else:
head_mask = [None] * self.config.num_hidden_layers
embedding_output = self.embeddings(
input_ids=input_ids, position_ids=position_ids, token_type_ids=token_type_ids, inputs_embeds=inputs_embeds
)
encoder_outputs = self.encoder(
embedding_output,
attention_mask=extended_attention_mask,
head_mask=head_mask,
encoder_hidden_states=encoder_hidden_states,
encoder_attention_mask=encoder_extended_attention_mask,
threshold=threshold,
)
sequence_output = encoder_outputs[0]
pooled_output = self.pooler(sequence_output)
outputs = (
sequence_output,
pooled_output,
) + encoder_outputs[1:] # add hidden_states and attentions if they are here
return outputs # sequence_output, pooled_output, (hidden_states), (attentions)
@add_start_docstrings(
"""Masked Bert Model transformer with a sequence classification/regression head on top (a linear layer on top of
the pooled output) e.g. for GLUE tasks. """,
MASKED_BERT_START_DOCSTRING,
)
class MaskedBertForSequenceClassification(MaskedBertPreTrainedModel):
def __init__(self, config):
super().__init__(config)
self.num_labels = config.num_labels
self.bert = MaskedBertModel(config)
self.dropout = nn.Dropout(config.hidden_dropout_prob)
self.classifier = nn.Linear(config.hidden_size, self.config.num_labels)
self.init_weights()
@add_start_docstrings_to_model_forward(MASKED_BERT_INPUTS_DOCSTRING)
def forward(
self,
input_ids=None,
attention_mask=None,
token_type_ids=None,
position_ids=None,
head_mask=None,
inputs_embeds=None,
labels=None,
threshold=None,
):
r"""
labels (:obj:`torch.LongTensor` of shape :obj:`(batch_size,)`, `optional`):
Labels for computing the sequence classification/regression loss.
Indices should be in :obj:`[0, ..., config.num_labels - 1]`.
If :obj:`config.num_labels == 1` a regression loss is computed (Mean-Square loss),
If :obj:`config.num_labels > 1` a classification loss is computed (Cross-Entropy).
threshold (:obj:`float`):
Threshold value (see :class:`~emmental.MaskedLinear`).
Returns:
:obj:`tuple(torch.FloatTensor)` comprising various elements depending on the configuration (:class:`~emmental.MaskedBertConfig`) and inputs:
loss (:obj:`torch.FloatTensor` of shape :obj:`(1,)`, `optional`, returned when :obj:`label` is provided):
Classification (or regression if config.num_labels==1) loss.
logits (:obj:`torch.FloatTensor` of shape :obj:`(batch_size, config.num_labels)`):
Classification (or regression if config.num_labels==1) scores (before SoftMax).
hidden_states (:obj:`tuple(torch.FloatTensor)`, `optional`, returned when ``config.output_hidden_states=True``):
Tuple of :obj:`torch.FloatTensor` (one for the output of the embeddings + one for the output of each layer)
of shape :obj:`(batch_size, sequence_length, hidden_size)`.
Hidden-states of the model at the output of each layer plus the initial embedding outputs.
attentions (:obj:`tuple(torch.FloatTensor)`, `optional`, returned when ``config.output_attentions=True``):
Tuple of :obj:`torch.FloatTensor` (one for each layer) of shape
:obj:`(batch_size, num_heads, sequence_length, sequence_length)`.
Attentions weights after the attention softmax, used to compute the weighted average in the self-attention
heads.
"""
outputs = self.bert(
input_ids,
attention_mask=attention_mask,
token_type_ids=token_type_ids,
position_ids=position_ids,
head_mask=head_mask,
inputs_embeds=inputs_embeds,
threshold=threshold,
)
pooled_output = outputs[1]
pooled_output = self.dropout(pooled_output)
logits = self.classifier(pooled_output)
outputs = (logits,) + outputs[2:] # add hidden states and attention if they are here
if labels is not None:
if self.num_labels == 1:
# We are doing regression
loss_fct = MSELoss()
loss = loss_fct(logits.view(-1), labels.view(-1))
else:
loss_fct = CrossEntropyLoss()
loss = loss_fct(logits.view(-1, self.num_labels), labels.view(-1))
outputs = (loss,) + outputs
return outputs # (loss), logits, (hidden_states), (attentions)
@add_start_docstrings(
"""Masked Bert Model with a multiple choice classification head on top (a linear layer on top of
the pooled output and a softmax) e.g. for RocStories/SWAG tasks. """,
MASKED_BERT_START_DOCSTRING,
)
class MaskedBertForMultipleChoice(MaskedBertPreTrainedModel):
def __init__(self, config):
super().__init__(config)
self.bert = MaskedBertModel(config)
self.dropout = nn.Dropout(config.hidden_dropout_prob)
self.classifier = nn.Linear(config.hidden_size, 1)
self.init_weights()
@add_start_docstrings_to_model_forward(MASKED_BERT_INPUTS_DOCSTRING)
def forward(
self,
input_ids=None,
attention_mask=None,
token_type_ids=None,
position_ids=None,
head_mask=None,
inputs_embeds=None,
labels=None,
threshold=None,
):
r"""
labels (:obj:`torch.LongTensor` of shape :obj:`(batch_size,)`, `optional`):
Labels for computing the multiple choice classification loss.
Indices should be in ``[0, ..., num_choices]`` where `num_choices` is the size of the second dimension
of the input tensors. (see `input_ids` above)
threshold (:obj:`float`):
Threshold value (see :class:`~emmental.MaskedLinear`).
Returns:
:obj:`tuple(torch.FloatTensor)` comprising various elements depending on the configuration (:class:`~emmental.MaskedBertConfig`) and inputs:
loss (:obj:`torch.FloatTensor` of shape `(1,)`, `optional`, returned when :obj:`labels` is provided):
Classification loss.
classification_scores (:obj:`torch.FloatTensor` of shape :obj:`(batch_size, num_choices)`):
`num_choices` is the second dimension of the input tensors. (see `input_ids` above).
Classification scores (before SoftMax).
hidden_states (:obj:`tuple(torch.FloatTensor)`, `optional`, returned when ``config.output_hidden_states=True``):
Tuple of :obj:`torch.FloatTensor` (one for the output of the embeddings + one for the output of each layer)
of shape :obj:`(batch_size, sequence_length, hidden_size)`.
Hidden-states of the model at the output of each layer plus the initial embedding outputs.
attentions (:obj:`tuple(torch.FloatTensor)`, `optional`, returned when ``config.output_attentions=True``):
Tuple of :obj:`torch.FloatTensor` (one for each layer) of shape
:obj:`(batch_size, num_heads, sequence_length, sequence_length)`.
Attentions weights after the attention softmax, used to compute the weighted average in the self-attention
heads.
"""
num_choices = input_ids.shape[1]
input_ids = input_ids.view(-1, input_ids.size(-1))
attention_mask = attention_mask.view(-1, attention_mask.size(-1)) if attention_mask is not None else None
token_type_ids = token_type_ids.view(-1, token_type_ids.size(-1)) if token_type_ids is not None else None
position_ids = position_ids.view(-1, position_ids.size(-1)) if position_ids is not None else None
outputs = self.bert(
input_ids,
attention_mask=attention_mask,
token_type_ids=token_type_ids,
position_ids=position_ids,
head_mask=head_mask,
inputs_embeds=inputs_embeds,
threshold=threshold,
)
pooled_output = outputs[1]
pooled_output = self.dropout(pooled_output)
logits = self.classifier(pooled_output)
reshaped_logits = logits.view(-1, num_choices)
outputs = (reshaped_logits,) + outputs[2:] # add hidden states and attention if they are here
if labels is not None:
loss_fct = CrossEntropyLoss()
loss = loss_fct(reshaped_logits, labels)
outputs = (loss,) + outputs
return outputs # (loss), reshaped_logits, (hidden_states), (attentions)
@add_start_docstrings(
"""Masked Bert Model with a token classification head on top (a linear layer on top of
the hidden-states output) e.g. for Named-Entity-Recognition (NER) tasks. """,
MASKED_BERT_START_DOCSTRING,
)
class MaskedBertForTokenClassification(MaskedBertPreTrainedModel):
def __init__(self, config):
super().__init__(config)
self.num_labels = config.num_labels
self.bert = MaskedBertModel(config)
self.dropout = nn.Dropout(config.hidden_dropout_prob)
self.classifier = nn.Linear(config.hidden_size, config.num_labels)
self.init_weights()
@add_start_docstrings_to_model_forward(MASKED_BERT_INPUTS_DOCSTRING)
def forward(
self,
input_ids=None,
attention_mask=None,
token_type_ids=None,
position_ids=None,
head_mask=None,
inputs_embeds=None,
labels=None,
threshold=None,
):
r"""
labels (:obj:`torch.LongTensor` of shape :obj:`(batch_size, sequence_length)`, `optional`):
Labels for computing the token classification loss.
Indices should be in ``[0, ..., config.num_labels - 1]``.
threshold (:obj:`float`):
Threshold value (see :class:`~emmental.MaskedLinear`).
Returns:
:obj:`tuple(torch.FloatTensor)` comprising various elements depending on the configuration (:class:`~emmental.MaskedBertConfig`) and inputs:
loss (:obj:`torch.FloatTensor` of shape :obj:`(1,)`, `optional`, returned when ``labels`` is provided) :
Classification loss.
scores (:obj:`torch.FloatTensor` of shape :obj:`(batch_size, sequence_length, config.num_labels)`)
Classification scores (before SoftMax).
hidden_states (:obj:`tuple(torch.FloatTensor)`, `optional`, returned when ``config.output_hidden_states=True``):
Tuple of :obj:`torch.FloatTensor` (one for the output of the embeddings + one for the output of each layer)
of shape :obj:`(batch_size, sequence_length, hidden_size)`.
Hidden-states of the model at the output of each layer plus the initial embedding outputs.
attentions (:obj:`tuple(torch.FloatTensor)`, `optional`, returned when ``config.output_attentions=True``):
Tuple of :obj:`torch.FloatTensor` (one for each layer) of shape
:obj:`(batch_size, num_heads, sequence_length, sequence_length)`.
Attentions weights after the attention softmax, used to compute the weighted average in the self-attention
heads.
"""
outputs = self.bert(
input_ids,
attention_mask=attention_mask,
token_type_ids=token_type_ids,
position_ids=position_ids,
head_mask=head_mask,
inputs_embeds=inputs_embeds,
threshold=threshold,
)
sequence_output = outputs[0]
sequence_output = self.dropout(sequence_output)
logits = self.classifier(sequence_output)
outputs = (logits,) + outputs[2:] # add hidden states and attention if they are here
if labels is not None:
loss_fct = CrossEntropyLoss()
# Only keep active parts of the loss
if attention_mask is not None:
active_loss = attention_mask.view(-1) == 1
active_logits = logits.view(-1, self.num_labels)
active_labels = torch.where(
active_loss, labels.view(-1), torch.tensor(loss_fct.ignore_index).type_as(labels)
)
loss = loss_fct(active_logits, active_labels)
else:
loss = loss_fct(logits.view(-1, self.num_labels), labels.view(-1))
outputs = (loss,) + outputs
return outputs # (loss), scores, (hidden_states), (attentions)
@add_start_docstrings(
"""Masked Bert Model with a span classification head on top for extractive question-answering tasks like SQuAD (a linear
layers on top of the hidden-states output to compute `span start logits` and `span end logits`). """,
MASKED_BERT_START_DOCSTRING,
)
class MaskedBertForQuestionAnswering(MaskedBertPreTrainedModel):
def __init__(self, config):
super().__init__(config)
self.num_labels = config.num_labels
self.bert = MaskedBertModel(config)
self.qa_outputs = nn.Linear(config.hidden_size, config.num_labels)
self.init_weights()
@add_start_docstrings_to_model_forward(MASKED_BERT_INPUTS_DOCSTRING)
def forward(
self,
input_ids=None,
attention_mask=None,
token_type_ids=None,
position_ids=None,
head_mask=None,
inputs_embeds=None,
start_positions=None,
end_positions=None,
threshold=None,
):
r"""
start_positions (:obj:`torch.LongTensor` of shape :obj:`(batch_size,)`, `optional`):
Labels for position (index) of the start of the labelled span for computing the token classification loss.
Positions are clamped to the length of the sequence (`sequence_length`).
Position outside of the sequence are not taken into account for computing the loss.
end_positions (:obj:`torch.LongTensor` of shape :obj:`(batch_size,)`, `optional`):
Labels for position (index) of the end of the labelled span for computing the token classification loss.
Positions are clamped to the length of the sequence (`sequence_length`).
Position outside of the sequence are not taken into account for computing the loss.
threshold (:obj:`float`):
Threshold value (see :class:`~emmental.MaskedLinear`).
Returns:
:obj:`tuple(torch.FloatTensor)` comprising various elements depending on the configuration (:class:`~emmental.MaskedBertConfig`) and inputs:
loss (:obj:`torch.FloatTensor` of shape :obj:`(1,)`, `optional`, returned when :obj:`labels` is provided):
Total span extraction loss is the sum of a Cross-Entropy for the start and end positions.
start_scores (:obj:`torch.FloatTensor` of shape :obj:`(batch_size, sequence_length,)`):
Span-start scores (before SoftMax).
end_scores (:obj:`torch.FloatTensor` of shape :obj:`(batch_size, sequence_length,)`):
Span-end scores (before SoftMax).
hidden_states (:obj:`tuple(torch.FloatTensor)`, `optional`, returned when ``config.output_hidden_states=True``):
Tuple of :obj:`torch.FloatTensor` (one for the output of the embeddings + one for the output of each layer)
of shape :obj:`(batch_size, sequence_length, hidden_size)`.
Hidden-states of the model at the output of each layer plus the initial embedding outputs.
attentions (:obj:`tuple(torch.FloatTensor)`, `optional`, returned when ``config.output_attentions=True``):
Tuple of :obj:`torch.FloatTensor` (one for each layer) of shape
:obj:`(batch_size, num_heads, sequence_length, sequence_length)`.
Attentions weights after the attention softmax, used to compute the weighted average in the self-attention
heads.
"""
outputs = self.bert(
input_ids,
attention_mask=attention_mask,
token_type_ids=token_type_ids,
position_ids=position_ids,
head_mask=head_mask,
inputs_embeds=inputs_embeds,
threshold=threshold,
)
sequence_output = outputs[0]
logits = self.qa_outputs(sequence_output)
start_logits, end_logits = logits.split(1, dim=-1)
start_logits = start_logits.squeeze(-1)
end_logits = end_logits.squeeze(-1)
outputs = (
start_logits,
end_logits,
) + outputs[2:]
if start_positions is not None and end_positions is not None:
# If we are on multi-GPU, split add a dimension
if len(start_positions.size()) > 1:
start_positions = start_positions.squeeze(-1)
if len(end_positions.size()) > 1:
end_positions = end_positions.squeeze(-1)
# sometimes the start/end positions are outside our model inputs, we ignore these terms
ignored_index = start_logits.size(1)
start_positions.clamp_(0, ignored_index)
end_positions.clamp_(0, ignored_index)
loss_fct = CrossEntropyLoss(ignore_index=ignored_index)
start_loss = loss_fct(start_logits, start_positions)
end_loss = loss_fct(end_logits, end_positions)
total_loss = (start_loss + end_loss) / 2
outputs = (total_loss,) + outputs
return outputs # (loss), start_logits, end_logits, (hidden_states), (attentions)
| transformers/examples/research_projects/movement-pruning/emmental/modeling_bert_masked.py/0 | {
"file_path": "transformers/examples/research_projects/movement-pruning/emmental/modeling_bert_masked.py",
"repo_id": "transformers",
"token_count": 20046
} | 287 |
# coding=utf-8
# Copyright 2020 The HuggingFace Team All rights reserved.
#
# Licensed under the Apache License, Version 2.0 (the "License");
# you may not use this file except in compliance with the License.
# You may obtain a copy of the License at
#
# http://www.apache.org/licenses/LICENSE-2.0
#
# Unless required by applicable law or agreed to in writing, software
# distributed under the License is distributed on an "AS IS" BASIS,
# WITHOUT WARRANTIES OR CONDITIONS OF ANY KIND, either express or implied.
# See the License for the specific language governing permissions and
# limitations under the License.
"""
Fine-tuning the library models for masked language modeling (BERT, ALBERT, RoBERTa...) with whole word masking on a
text file or a dataset.
Here is the full list of checkpoints on the hub that can be fine-tuned by this script:
https://huggingface.co/models?filter=fill-mask
"""
import logging
import os
import sys
from dataclasses import dataclass, field
# You can also adapt this script on your own masked language modeling task. Pointers for this are left as comments.
from pathlib import Path
from typing import Dict, List, Optional, Tuple
import jax
import jax.numpy as jnp
import numpy as np
from datasets import load_dataset
from flax import jax_utils
from flax.optim import Adam
from flax.training import common_utils
from flax.training.common_utils import get_metrics
from jax.nn import log_softmax
from modeling_flax_performer import FlaxPerformerForMaskedLM
from tqdm import tqdm
from transformers import (
MODEL_FOR_MASKED_LM_MAPPING,
AutoTokenizer,
BertConfig,
FlaxBertForMaskedLM,
HfArgumentParser,
PreTrainedTokenizerBase,
TensorType,
TrainingArguments,
is_tensorboard_available,
set_seed,
)
# Cache the result
has_tensorboard = is_tensorboard_available()
if has_tensorboard:
try:
from flax.metrics.tensorboard import SummaryWriter
except ImportError as ie:
has_tensorboard = False
print(f"Unable to display metrics through TensorBoard because some package are not installed: {ie}")
else:
print(
"Unable to display metrics through TensorBoard because the package is not installed: "
"Please run pip install tensorboard to enable."
)
MODEL_CONFIG_CLASSES = list(MODEL_FOR_MASKED_LM_MAPPING.keys())
MODEL_TYPES = tuple(conf.model_type for conf in MODEL_CONFIG_CLASSES)
@dataclass
class WandbArguments:
"""
Arguments for logging
"""
wandb_user_name: Optional[str] = field(
default=None,
metadata={"help": "The WandB user name for potential logging. If left None, no logging"},
)
wandb_project_name: Optional[str] = field(
default="performer-experiments",
metadata={"help": "The WandB project name for potential logging"},
)
@dataclass
class ModelArguments:
"""
Arguments pertaining to which model/config/tokenizer we are going to fine-tune, or train from scratch.
"""
model_name_or_path: Optional[str] = field(
default=None,
metadata={
"help": (
"The model checkpoint for weights initialization. Don't set if you want to train a model from scratch."
)
},
)
performer: bool = field(
default=False,
metadata={"help": "Whether to use FAVOR+ attention"},
)
reinitialize: bool = field(
default=False,
metadata={"help": "Whether to use a blank model without pretraining"},
)
tokenizer_name: Optional[str] = field(
default=None, metadata={"help": "Pretrained tokenizer name or path if not the same as model_name"}
)
use_fast_tokenizer: bool = field(
default=True,
metadata={"help": "Whether to use one of the fast tokenizer (backed by the tokenizers library) or not."},
)
cache_dir: Optional[str] = field(
default=None, metadata={"help": "Where do you want to store the pretrained models downloaded from s3"}
)
@dataclass
class DataTrainingArguments:
"""
Arguments pertaining to what data we are going to input our model for training and eval.
"""
dataset_name: Optional[str] = field(
default=None, metadata={"help": "The name of the dataset to use (via the datasets library)."}
)
dataset_config_name: Optional[str] = field(
default=None, metadata={"help": "The configuration name of the dataset to use (via the datasets library)."}
)
train_file: Optional[str] = field(default=None, metadata={"help": "The input training data file (a text file)."})
validation_file: Optional[str] = field(
default=None,
metadata={"help": "An optional input evaluation data file to evaluate the perplexity on (a text file)."},
)
train_ref_file: Optional[str] = field(
default=None,
metadata={"help": "An optional input train ref data file for whole word masking in Chinese."},
)
validation_ref_file: Optional[str] = field(
default=None,
metadata={"help": "An optional input validation ref data file for whole word masking in Chinese."},
)
overwrite_cache: bool = field(
default=False, metadata={"help": "Overwrite the cached training and evaluation sets"}
)
validation_split_percentage: Optional[int] = field(
default=5,
metadata={
"help": "The percentage of the train set used as validation set in case there's no validation split"
},
)
max_seq_length: Optional[int] = field(
default=None,
metadata={
"help": (
"The maximum total input sequence length after tokenization. Sequences longer "
"than this will be truncated. Default to the max input length of the model."
)
},
)
preprocessing_num_workers: Optional[int] = field(
default=None,
metadata={"help": "The number of processes to use for the preprocessing."},
)
mlm_probability: float = field(
default=0.15, metadata={"help": "Ratio of tokens to mask for masked language modeling loss"}
)
pad_to_max_length: bool = field(
default=False,
metadata={
"help": (
"Whether to pad all samples to `max_seq_length`. "
"If False, will pad the samples dynamically when batching to the maximum length in the batch."
)
},
)
def __post_init__(self):
if self.dataset_name is None and self.train_file is None and self.validation_file is None:
raise ValueError("Need either a dataset name or a training/validation file.")
else:
if self.train_file is not None:
extension = self.train_file.split(".")[-1]
assert extension in ["csv", "json", "txt"], "`train_file` should be a csv, a json or a txt file."
if self.validation_file is not None:
extension = self.validation_file.split(".")[-1]
assert extension in ["csv", "json", "txt"], "`validation_file` should be a csv, a json or a txt file."
# Adapted from transformers/data/data_collator.py
# Letting here for now, let's discuss where it should live
@dataclass
class FlaxDataCollatorForLanguageModeling:
"""
Data collator used for language modeling. Inputs are dynamically padded to the maximum length of a batch if they
are not all of the same length.
Args:
tokenizer (:class:`~transformers.PreTrainedTokenizer` or :class:`~transformers.PreTrainedTokenizerFast`):
The tokenizer used for encoding the data.
mlm (:obj:`bool`, `optional`, defaults to :obj:`True`):
Whether or not to use masked language modeling. If set to :obj:`False`, the labels are the same as the
inputs with the padding tokens ignored (by setting them to -100). Otherwise, the labels are -100 for
non-masked tokens and the value to predict for the masked token.
mlm_probability (:obj:`float`, `optional`, defaults to 0.15):
The probability with which to (randomly) mask tokens in the input, when :obj:`mlm` is set to :obj:`True`.
.. note::
For best performance, this data collator should be used with a dataset having items that are dictionaries or
BatchEncoding, with the :obj:`"special_tokens_mask"` key, as returned by a
:class:`~transformers.PreTrainedTokenizer` or a :class:`~transformers.PreTrainedTokenizerFast` with the
argument :obj:`return_special_tokens_mask=True`.
"""
tokenizer: PreTrainedTokenizerBase
mlm: bool = True
mlm_probability: float = 0.15
def __post_init__(self):
if self.mlm and self.tokenizer.mask_token is None:
raise ValueError(
"This tokenizer does not have a mask token which is necessary for masked language modeling. "
"You should pass `mlm=False` to train on causal language modeling instead."
)
def __call__(self, examples: List[Dict[str, np.ndarray]], pad_to_multiple_of: int) -> Dict[str, np.ndarray]:
# Handle dict or lists with proper padding and conversion to tensor.
batch = self.tokenizer.pad(examples, pad_to_multiple_of=pad_to_multiple_of, return_tensors=TensorType.NUMPY)
# If special token mask has been preprocessed, pop it from the dict.
special_tokens_mask = batch.pop("special_tokens_mask", None)
if self.mlm:
batch["input_ids"], batch["labels"] = self.mask_tokens(
batch["input_ids"], special_tokens_mask=special_tokens_mask
)
else:
labels = batch["input_ids"].copy()
if self.tokenizer.pad_token_id is not None:
labels[labels == self.tokenizer.pad_token_id] = -100
batch["labels"] = labels
return batch
def mask_tokens(
self, inputs: np.ndarray, special_tokens_mask: Optional[np.ndarray]
) -> Tuple[jnp.ndarray, jnp.ndarray]:
"""
Prepare masked tokens inputs/labels for masked language modeling: 80% MASK, 10% random, 10% original.
"""
labels = inputs.copy()
# We sample a few tokens in each sequence for MLM training (with probability `self.mlm_probability`)
probability_matrix = np.full(labels.shape, self.mlm_probability)
special_tokens_mask = special_tokens_mask.astype("bool")
probability_matrix[special_tokens_mask] = 0.0
masked_indices = np.random.binomial(1, probability_matrix).astype("bool")
labels[~masked_indices] = -100 # We only compute loss on masked tokens
# 80% of the time, we replace masked input tokens with tokenizer.mask_token ([MASK])
indices_replaced = np.random.binomial(1, np.full(labels.shape, 0.8)).astype("bool") & masked_indices
inputs[indices_replaced] = self.tokenizer.convert_tokens_to_ids(self.tokenizer.mask_token)
# 10% of the time, we replace masked input tokens with random word
indices_random = np.random.binomial(1, np.full(labels.shape, 0.5)).astype("bool")
indices_random &= masked_indices & ~indices_replaced
random_words = np.random.randint(self.tokenizer.vocab_size, size=labels.shape, dtype="i4")
inputs[indices_random] = random_words[indices_random]
# The rest of the time (10% of the time) we keep the masked input tokens unchanged
return inputs, labels
def create_learning_rate_scheduler(
factors="constant * linear_warmup * rsqrt_decay",
base_learning_rate=0.5,
warmup_steps=1000,
decay_factor=0.5,
steps_per_decay=20000,
steps_per_cycle=100000,
):
"""Creates learning rate schedule.
Interprets factors in the factors string which can consist of:
* constant: interpreted as the constant value,
* linear_warmup: interpreted as linear warmup until warmup_steps,
* rsqrt_decay: divide by square root of max(step, warmup_steps)
* rsqrt_normalized_decay: divide by square root of max(step/warmup_steps, 1)
* decay_every: Every k steps decay the learning rate by decay_factor.
* cosine_decay: Cyclic cosine decay, uses steps_per_cycle parameter.
Args:
factors: string, factors separated by "*" that defines the schedule.
base_learning_rate: float, the starting constant for the lr schedule.
warmup_steps: int, how many steps to warm up for in the warmup schedule.
decay_factor: float, the amount to decay the learning rate by.
steps_per_decay: int, how often to decay the learning rate.
steps_per_cycle: int, steps per cycle when using cosine decay.
Returns:
a function learning_rate(step): float -> {"learning_rate": float}, the
step-dependent lr.
"""
factors = [n.strip() for n in factors.split("*")]
def step_fn(step):
"""Step to learning rate function."""
ret = 1.0
for name in factors:
if name == "constant":
ret *= base_learning_rate
elif name == "linear_warmup":
ret *= jnp.minimum(1.0, step / warmup_steps)
elif name == "rsqrt_decay":
ret /= jnp.sqrt(jnp.maximum(step, warmup_steps))
elif name == "rsqrt_normalized_decay":
ret *= jnp.sqrt(warmup_steps)
ret /= jnp.sqrt(jnp.maximum(step, warmup_steps))
elif name == "decay_every":
ret *= decay_factor ** (step // steps_per_decay)
elif name == "cosine_decay":
progress = jnp.maximum(0.0, (step - warmup_steps) / float(steps_per_cycle))
ret *= jnp.maximum(0.0, 0.5 * (1.0 + jnp.cos(jnp.pi * (progress % 1.0))))
else:
raise ValueError("Unknown factor %s." % name)
return jnp.asarray(ret, dtype=jnp.float32)
return step_fn
def compute_metrics(logits, labels, weights, label_smoothing=0.0):
"""Compute summary metrics."""
loss, normalizer = cross_entropy(logits, labels, weights, label_smoothing)
acc, _ = accuracy(logits, labels, weights)
metrics = {"loss": loss, "accuracy": acc, "normalizer": normalizer}
metrics = jax.lax.psum(metrics, axis_name="batch")
return metrics
def accuracy(logits, targets, weights=None):
"""Compute weighted accuracy for log probs and targets.
Args:
logits: [batch, length, num_classes] float array.
targets: categorical targets [batch, length] int array.
weights: None or array of shape [batch, length]
Returns:
Tuple of scalar loss and batch normalizing factor.
"""
if logits.ndim != targets.ndim + 1:
raise ValueError(
"Incorrect shapes. Got shape %s logits and %s targets" % (str(logits.shape), str(targets.shape))
)
loss = jnp.equal(jnp.argmax(logits, axis=-1), targets)
loss *= weights
return loss.sum(), weights.sum()
def cross_entropy(logits, targets, weights=None, label_smoothing=0.0):
"""Compute cross entropy and entropy for log probs and targets.
Args:
logits: [batch, length, num_classes] float array.
targets: categorical targets [batch, length] int array.
weights: None or array of shape [batch, length]
label_smoothing: label smoothing constant, used to determine the on and off values.
Returns:
Tuple of scalar loss and batch normalizing factor.
"""
if logits.ndim != targets.ndim + 1:
raise ValueError(
"Incorrect shapes. Got shape %s logits and %s targets" % (str(logits.shape), str(targets.shape))
)
vocab_size = logits.shape[-1]
confidence = 1.0 - label_smoothing
low_confidence = (1.0 - confidence) / (vocab_size - 1)
normalizing_constant = -(
confidence * jnp.log(confidence) + (vocab_size - 1) * low_confidence * jnp.log(low_confidence + 1e-20)
)
soft_targets = common_utils.onehot(targets, vocab_size, on_value=confidence, off_value=low_confidence)
loss = -jnp.sum(soft_targets * log_softmax(logits), axis=-1)
loss = loss - normalizing_constant
if weights is not None:
loss = loss * weights
normalizing_factor = weights.sum()
else:
normalizing_factor = np.prod(targets.shape)
return loss.sum(), normalizing_factor
def training_step(optimizer, batch, dropout_rng):
dropout_rng, new_dropout_rng = jax.random.split(dropout_rng)
def loss_fn(params):
targets = batch.pop("labels")
# Hide away tokens which doesn't participate in the optimization
token_mask = jnp.where(targets > 0, 1.0, 0.0)
logits = model(**batch, params=params, dropout_rng=dropout_rng, train=True)[0]
loss, weight_sum = cross_entropy(logits, targets, token_mask)
return loss / weight_sum
step = optimizer.state.step
lr = lr_scheduler_fn(step)
grad_fn = jax.value_and_grad(loss_fn)
loss, grad = grad_fn(optimizer.target)
grad = jax.lax.pmean(grad, "batch")
optimizer = optimizer.apply_gradient(grad, learning_rate=lr)
return loss, optimizer, new_dropout_rng
def eval_step(params, batch):
"""
Calculate evaluation metrics on a batch.
"""
targets = batch.pop("labels")
# Hide away tokens which doesn't participate in the optimization
token_mask = jnp.where(targets > 0, 1.0, 0.0)
logits = model(**batch, params=params, train=False)[0]
return compute_metrics(logits, targets, token_mask)
def generate_batch_splits(samples_idx: np.ndarray, batch_size: int) -> np.ndarray:
nb_samples = len(samples_idx)
samples_to_remove = nb_samples % batch_size
if samples_to_remove != 0:
samples_idx = samples_idx[:-samples_to_remove]
sections_split = nb_samples // batch_size
batch_idx = np.split(samples_idx, sections_split)
return batch_idx
if __name__ == "__main__":
# See all possible arguments in src/transformers/training_args.py
# or by passing the --help flag to this script.
# We now keep distinct sets of args, for a cleaner separation of concerns.
parser = HfArgumentParser((ModelArguments, DataTrainingArguments, TrainingArguments, WandbArguments))
if len(sys.argv) == 2 and sys.argv[1].endswith(".json"):
# If we pass only one argument to the script and it's the path to a json file,
# let's parse it to get our arguments.
model_args, data_args, training_args, wandb_args = parser.parse_json_file(
json_file=os.path.abspath(sys.argv[1])
)
else:
model_args, data_args, training_args, wandb_args = parser.parse_args_into_dataclasses()
if (
os.path.exists(training_args.output_dir)
and os.listdir(training_args.output_dir)
and training_args.do_train
and not training_args.overwrite_output_dir
):
raise ValueError(
f"Output directory ({training_args.output_dir}) already exists and is not empty. "
"Use --overwrite_output_dir to overcome."
)
# Setup logging
logging.basicConfig(
format="%(asctime)s - %(levelname)s - %(name)s - %(message)s",
level="NOTSET",
datefmt="[%X]",
)
# Log on each process the small summary:
logger = logging.getLogger(__name__)
logger.warning(
f"Process rank: {training_args.local_rank}, device: {training_args.device}, n_gpu: {training_args.n_gpu}"
+ f"distributed training: {bool(training_args.local_rank != -1)}, 16-bits training: {training_args.fp16}"
)
# Set the verbosity to info of the Transformers logger (on main process only):
logger.info("Training/evaluation parameters %s", training_args)
# Set seed before initializing model.
set_seed(training_args.seed)
# Get the datasets: you can either provide your own CSV/JSON/TXT training and evaluation files (see below)
# or just provide the name of one of the public datasets available on the hub at https://huggingface.co/datasets/
# (the dataset will be downloaded automatically from the datasets Hub).
#
# For CSV/JSON files, this script will use the column called 'text' or the first column if no column called
# 'text' is found. You can easily tweak this behavior (see below).
#
# In distributed training, the load_dataset function guarantees that only one local process can concurrently
# download the dataset.
if data_args.dataset_name is not None:
# Downloading and loading a dataset from the hub.
datasets = load_dataset(data_args.dataset_name, data_args.dataset_config_name)
if "validation" not in datasets.keys():
datasets["validation"] = load_dataset(
data_args.dataset_name,
data_args.dataset_config_name,
split=f"train[:{data_args.validation_split_percentage}%]",
)
datasets["train"] = load_dataset(
data_args.dataset_name,
data_args.dataset_config_name,
split=f"train[{data_args.validation_split_percentage}%:]",
)
else:
data_files = {}
if data_args.train_file is not None:
data_files["train"] = data_args.train_file
extension = data_args.train_file.split(".")[-1]
if data_args.validation_file is not None:
data_files["validation"] = data_args.validation_file
extension = data_args.validation_file.split(".")[-1]
if extension == "txt":
extension = "text"
datasets = load_dataset(extension, data_files=data_files)
# See more about loading any type of standard or custom dataset (from files, python dict, pandas DataFrame, etc) at
# https://huggingface.co/docs/datasets/loading_datasets.
# Load pretrained model and tokenizer
# Distributed training:
# The .from_pretrained methods guarantee that only one local process can concurrently
# download model & vocab.
rng = jax.random.PRNGKey(training_args.seed)
dropout_rngs = jax.random.split(rng, jax.local_device_count())
config = BertConfig.from_pretrained(model_args.model_name_or_path, cache_dir=model_args.cache_dir)
lm_class = FlaxPerformerForMaskedLM if model_args.performer else FlaxBertForMaskedLM
if model_args.reinitialize:
model = lm_class(config=BertConfig.from_pretrained(model_args.model_name_or_path))
else:
model = lm_class.from_pretrained(
model_args.model_name_or_path,
dtype=jnp.float32,
input_shape=(training_args.train_batch_size, config.max_position_embeddings),
seed=training_args.seed,
dropout_rate=0.1,
)
if model_args.tokenizer_name:
tokenizer = AutoTokenizer.from_pretrained(
model_args.tokenizer_name, cache_dir=model_args.cache_dir, use_fast=model_args.use_fast_tokenizer
)
elif model_args.model_name_or_path:
tokenizer = AutoTokenizer.from_pretrained(
model_args.model_name_or_path, cache_dir=model_args.cache_dir, use_fast=model_args.use_fast_tokenizer
)
else:
raise ValueError(
"You are instantiating a new tokenizer from scratch. This is not supported by this script. "
"You can do it from another script, save it, and load it from here, using --tokenizer_name."
)
# Preprocessing the datasets.
# First we tokenize all the texts.
if training_args.do_train:
column_names = datasets["train"].column_names
else:
column_names = datasets["validation"].column_names
text_column_name = "text" if "text" in column_names else column_names[0]
padding = "max_length" if data_args.pad_to_max_length else False
def tokenize_function(examples):
# Remove empty lines
examples = [line for line in examples if len(line) > 0 and not line.isspace()]
return tokenizer(
examples,
return_special_tokens_mask=True,
padding=padding,
truncation=True,
max_length=data_args.max_seq_length,
)
tokenized_datasets = datasets.map(
tokenize_function,
input_columns=[text_column_name],
batched=True,
num_proc=data_args.preprocessing_num_workers,
remove_columns=column_names,
load_from_cache_file=not data_args.overwrite_cache,
)
# Enable tensorboard only on the master node
if has_tensorboard and jax.host_id() == 0:
summary_writer = SummaryWriter(log_dir=Path(training_args.output_dir).joinpath("logs").as_posix())
# Data collator
# This one will take care of randomly masking the tokens.
data_collator = FlaxDataCollatorForLanguageModeling(tokenizer=tokenizer, mlm_probability=data_args.mlm_probability)
# Setup optimizer
optimizer = Adam(
learning_rate=training_args.learning_rate,
weight_decay=training_args.weight_decay,
beta1=training_args.adam_beta1,
beta2=training_args.adam_beta2,
).create(model.params)
# Create learning rate scheduler
lr_scheduler_fn = create_learning_rate_scheduler(
base_learning_rate=training_args.learning_rate, warmup_steps=max(training_args.warmup_steps, 1)
)
# Create parallel version of the training and evaluation steps
p_training_step = jax.pmap(training_step, "batch", donate_argnums=(0,))
p_eval_step = jax.pmap(eval_step, "batch", donate_argnums=(0,))
# Replicate the optimizer on each device
optimizer = jax_utils.replicate(optimizer)
# Store some constant
nb_epochs = int(training_args.num_train_epochs)
batch_size = int(training_args.train_batch_size)
eval_batch_size = int(training_args.eval_batch_size)
if wandb_args.wandb_user_name is not None:
import wandb
wandb.init(project=wandb_args.wandb_project_name, entity=wandb_args.wandb_user_name)
epochs = tqdm(range(nb_epochs), desc=f"Epoch ... (1/{nb_epochs})", position=0)
for epoch in epochs:
# ======================== Training ================================
# Create sampling rng
rng, training_rng, eval_rng = jax.random.split(rng, 3)
# Generate an epoch by shuffling sampling indices from the train dataset
nb_training_samples = len(tokenized_datasets["train"])
# Avoid using jax.numpy here in case of TPU training
training_samples_idx = np.random.permutation(np.arange(nb_training_samples))
training_batch_idx = generate_batch_splits(training_samples_idx, batch_size)
# Gather the indexes for creating the batch and do a training step
for batch_idx in tqdm(training_batch_idx, desc="Training...", position=1):
samples = [tokenized_datasets["train"][int(idx)] for idx in batch_idx]
model_inputs = data_collator(samples, pad_to_multiple_of=16)
# Model forward
model_inputs = common_utils.shard(model_inputs.data)
loss, optimizer, dropout_rngs = p_training_step(optimizer, model_inputs, dropout_rngs)
if wandb_args.wandb_user_name is not None:
wandb.log({"Training loss": np.array(loss).mean()})
epochs.write(f"Loss: {loss}")
# ======================== Evaluating ==============================
nb_eval_samples = len(tokenized_datasets["validation"])
# Avoid using jax.numpy here in case of TPU training
eval_samples_idx = np.arange(nb_eval_samples)
eval_batch_idx = generate_batch_splits(eval_samples_idx, eval_batch_size)
eval_metrics = []
for i, batch_idx in enumerate(tqdm(eval_batch_idx, desc="Evaluating ...", position=2)):
samples = [tokenized_datasets["validation"][int(idx)] for idx in batch_idx]
model_inputs = data_collator(samples, pad_to_multiple_of=16)
# Model forward
model_inputs = common_utils.shard(model_inputs.data)
metrics = p_eval_step(optimizer.target, model_inputs)
eval_metrics.append(metrics)
eval_metrics_np = get_metrics(eval_metrics)
eval_metrics_np = jax.tree_util.tree_map(jnp.sum, eval_metrics_np)
eval_normalizer = eval_metrics_np.pop("normalizer")
eval_summary = jax.tree_util.tree_map(lambda x: x / eval_normalizer, eval_metrics_np)
# Update progress bar
epochs.desc = (
f"Epoch... ({epoch + 1}/{nb_epochs} | Loss: {eval_summary['loss']}, Acc: {eval_summary['accuracy']})"
)
if wandb_args.wandb_user_name is not None:
wandb.log({"Eval loss": np.array(eval_summary["loss"]).mean()})
# Save metrics
if has_tensorboard and jax.host_id() == 0:
for name, value in eval_summary.items():
summary_writer.scalar(name, value, epoch)
| transformers/examples/research_projects/performer/run_mlm_performer.py/0 | {
"file_path": "transformers/examples/research_projects/performer/run_mlm_performer.py",
"repo_id": "transformers",
"token_count": 11399
} | 288 |
## Sequence to Sequence Training and Evaluation
This directory contains examples for finetuning and evaluating transformers on summarization and translation tasks.
Author: Sam Shleifer (https://github.com/sshleifer)
### Supported Architectures
- `BartForConditionalGeneration` (and anything that inherits from it)
- `MarianMTModel`
- `PegasusForConditionalGeneration`
- `MBartForConditionalGeneration`
- `FSMTForConditionalGeneration`
- `T5ForConditionalGeneration`
# Note
⚠️ This project should be run with pytorch-lightning==1.0.4 which has a potential security vulnerability
## Datasets
#### XSUM
```bash
cd examples/contrib/pytorch-lightning/seq2seq
wget https://cdn-datasets.huggingface.co/summarization/xsum.tar.gz
tar -xzvf xsum.tar.gz
export XSUM_DIR=${PWD}/xsum
```
this should make a directory called `xsum/` with files like `test.source`.
To use your own data, copy that files format. Each article to be summarized is on its own line.
#### CNN/DailyMail
```bash
cd examples/contrib/pytorch-lightning/seq2seq
wget https://cdn-datasets.huggingface.co/summarization/cnn_dm_v2.tgz
tar -xzvf cnn_dm_v2.tgz # empty lines removed
mv cnn_cln cnn_dm
export CNN_DIR=${PWD}/cnn_dm
```
this should make a directory called `cnn_dm/` with 6 files.
#### WMT16 English-Romanian Translation Data
download with this command:
```bash
wget https://cdn-datasets.huggingface.co/translation/wmt_en_ro.tar.gz
tar -xzvf wmt_en_ro.tar.gz
export ENRO_DIR=${PWD}/wmt_en_ro
```
this should make a directory called `wmt_en_ro/` with 6 files.
#### WMT English-German
```bash
wget https://cdn-datasets.huggingface.co/translation/wmt_en_de.tgz
tar -xzvf wmt_en_de.tgz
export DATA_DIR=${PWD}/wmt_en_de
```
#### FSMT datasets (wmt)
Refer to the scripts starting with `eval_` under:
https://github.com/huggingface/transformers/tree/main/scripts/fsmt
#### Pegasus (multiple datasets)
Multiple eval datasets are available for download from:
https://github.com/stas00/porting/tree/master/datasets/pegasus
#### Your Data
If you are using your own data, it must be formatted as one directory with 6 files:
```
train.source
train.target
val.source
val.target
test.source
test.target
```
The `.source` files are the input, the `.target` files are the desired output.
### Potential issues
- native AMP (`--fp16` and no apex) may lead to a huge memory leak and require 10x gpu memory. This has been fixed in pytorch-nightly and the minimal official version to have this fix will be pytorch-1.8. Until then if you have to use mixed precision please use AMP only with pytorch-nightly or NVIDIA's apex. Reference: https://github.com/huggingface/transformers/issues/8403
### Tips and Tricks
General Tips:
- since you need to run from this folder, and likely need to modify code, the easiest workflow is fork transformers, clone your fork, and run `pip install -e .` before you get started.
- try `--freeze_encoder` or `--freeze_embeds` for faster training/larger batch size. (3hr per epoch with bs=8, see the "xsum_shared_task" command below)
- `fp16_opt_level=O1` (the default works best).
- In addition to the pytorch-lightning .ckpt checkpoint, a transformers checkpoint will be saved.
Load it with `BartForConditionalGeneration.from_pretrained(f'{output_dir}/best_tfmr)`.
- At the moment, `--do_predict` does not work in a multi-gpu setting. You need to use `evaluate_checkpoint` or the `run_eval.py` code.
- This warning can be safely ignored:
> "Some weights of BartForConditionalGeneration were not initialized from the model checkpoint at facebook/bart-large-xsum and are newly initialized: ['final_logits_bias']"
- Both finetuning and eval are 30% faster with `--fp16`. For that you need to [install apex](https://github.com/NVIDIA/apex#quick-start).
- Read scripts before you run them!
Summarization Tips:
- (summ) 1 epoch at batch size 1 for bart-large takes 24 hours and requires 13GB GPU RAM with fp16 on an NVIDIA-V100.
- If you want to run experiments on improving the summarization finetuning process, try the XSUM Shared Task (below). It's faster to train than CNNDM because the summaries are shorter.
- For CNN/DailyMail, the default `val_max_target_length` and `test_max_target_length` will truncate the ground truth labels, resulting in slightly higher rouge scores. To get accurate rouge scores, you should rerun calculate_rouge on the `{output_dir}/test_generations.txt` file saved by `trainer.test()`
- `--max_target_length=60 --val_max_target_length=60 --test_max_target_length=100 ` is a reasonable setting for XSUM.
- `wandb` can be used by specifying `--logger_name wandb`. It is useful for reproducibility. Specify the environment variable `WANDB_PROJECT='hf_xsum'` to do the XSUM shared task.
- If you are finetuning on your own dataset, start from `distilbart-cnn-12-6` if you want long summaries and `distilbart-xsum-12-6` if you want short summaries.
(It rarely makes sense to start from `bart-large` unless you are a researching finetuning methods).
**Update 2018-07-18**
Datasets: `LegacySeq2SeqDataset` will be used for all tokenizers without a `prepare_seq2seq_batch` method. Otherwise, `Seq2SeqDataset` will be used.
Future work/help wanted: A new dataset to support multilingual tasks.
### Finetuning Scripts
All finetuning bash scripts call finetune.py (or distillation.py) with reasonable command line arguments. They usually require extra command line arguments to work.
To see all the possible command line options, run:
```bash
./finetune.py --help
```
### Finetuning Training Params
To override the pretrained model's training params, you can pass them to `./finetune.sh`:
```bash
./finetune.sh \
[...]
--encoder_layerdrop 0.1 \
--decoder_layerdrop 0.1 \
--dropout 0.1 \
--attention_dropout 0.1 \
```
### Summarization Finetuning
Run/modify `finetune.sh`
The following command should work on a 16GB GPU:
```bash
./finetune.sh \
--data_dir $XSUM_DIR \
--train_batch_size=1 \
--eval_batch_size=1 \
--output_dir=xsum_results \
--num_train_epochs 6 \
--model_name_or_path facebook/bart-large
```
There is a starter finetuning script for pegasus at `finetune_pegasus_xsum.sh`.
### Translation Finetuning
First, follow the wmt_en_ro download instructions.
Then you can finetune mbart_cc25 on english-romanian with the following command.
**Recommendation:** Read and potentially modify the fairly opinionated defaults in `train_mbart_cc25_enro.sh` script before running it.
Best performing command:
```bash
# optionally
export ENRO_DIR='wmt_en_ro' # Download instructions above
# export WANDB_PROJECT="MT" # optional
export MAX_LEN=128
export BS=4
./train_mbart_cc25_enro.sh --output_dir enro_finetune_baseline --label_smoothing 0.1 --fp16_opt_level=O1 --logger_name wandb --sortish_sampler
```
This should take < 6h/epoch on a 16GB v100 and achieve test BLEU above 26
To get results in line with fairseq, you need to do some postprocessing. (see `romanian_postprocessing.md`)
MultiGPU command
(using 8 GPUS as an example)
```bash
export ENRO_DIR='wmt_en_ro' # Download instructions above
# export WANDB_PROJECT="MT" # optional
export MAX_LEN=128
export BS=4
./train_mbart_cc25_enro.sh --output_dir enro_finetune_baseline --gpus 8 --logger_name wandb
```
### Finetuning Outputs
As you train, `output_dir` will be filled with files, that look kind of like this (comments are mine).
Some of them are metrics, some of them are checkpoints, some of them are metadata. Here is a quick tour:
```bash
output_dir
├── best_tfmr # this is a huggingface checkpoint generated by save_pretrained. It is the same model as the PL .ckpt file below
│ ├── config.json
│ ├── merges.txt
│ ├── pytorch_model.bin
│ ├── special_tokens_map.json
│ ├── tokenizer_config.json
│ └── vocab.json
├── git_log.json # repo, branch, and commit hash
├── val_avg_rouge2=0.1984-step_count=11.ckpt # this is a pytorch lightning checkpoint associated with the best val score. (it will be called BLEU for MT)
├── metrics.json # new validation metrics will continually be appended to this
├── student # this is a huggingface checkpoint generated by SummarizationDistiller. It is the student before it gets finetuned.
│ ├── config.json
│ └── pytorch_model.bin
├── test_generations.txt
# ^^ are the summaries or translations produced by your best checkpoint on the test data. Populated when training is done
├── test_results.txt # a convenience file with the test set metrics. This data is also in metrics.json['test']
├── hparams.pkl # the command line args passed after some light preprocessing. Should be saved fairly quickly.
```
After training, you can recover the best checkpoint by running
```python
from transformers import AutoModelForSeq2SeqLM
model = AutoModelForSeq2SeqLM.from_pretrained(f'{output_dir}/best_tfmr')
```
### Converting pytorch-lightning checkpoints
pytorch lightning ``-do_predict`` often fails, after you are done training, the best way to evaluate your model is to convert it.
This should be done for you, with a file called `{save_dir}/best_tfmr`.
If that file doesn't exist but you have a lightning `.ckpt` file, you can run
```bash
python convert_pl_checkpoint_to_hf.py PATH_TO_CKPT randomly_initialized_hf_model_path save_dir/best_tfmr
```
Then either `run_eval` or `run_distributed_eval` with `save_dir/best_tfmr` (see previous sections)
# Experimental Features
These features are harder to use and not always useful.
### Dynamic Batch Size for MT
`finetune.py` has a command line arg `--max_tokens_per_batch` that allows batches to be dynamically sized.
This feature can only be used:
- with fairseq installed
- on 1 GPU
- without sortish sampler
- after calling `./save_len_file.py $tok $data_dir`
For example,
```bash
./save_len_file.py Helsinki-NLP/opus-mt-en-ro wmt_en_ro
./dynamic_bs_example.sh --max_tokens_per_batch=2000 --output_dir benchmark_dynamic_bs
```
splits `wmt_en_ro/train` into 11,197 uneven length batches and can finish 1 epoch in 8 minutes on a v100.
For comparison,
```bash
./dynamic_bs_example.sh --sortish_sampler --train_batch_size 48
```
uses 12,723 batches of length 48 and takes slightly more time 9.5 minutes.
The feature is still experimental, because:
+ we can make it much more robust if we have memory mapped/preprocessed datasets.
+ The speedup over sortish sampler is not that large at the moment.
# DistilBART
<!---It should be called distilling bart and pegasus, but I don't want to break the link in the paper.-->
This section describes all code and artifacts from our [Paper](http://arxiv.org/abs/2010.13002)
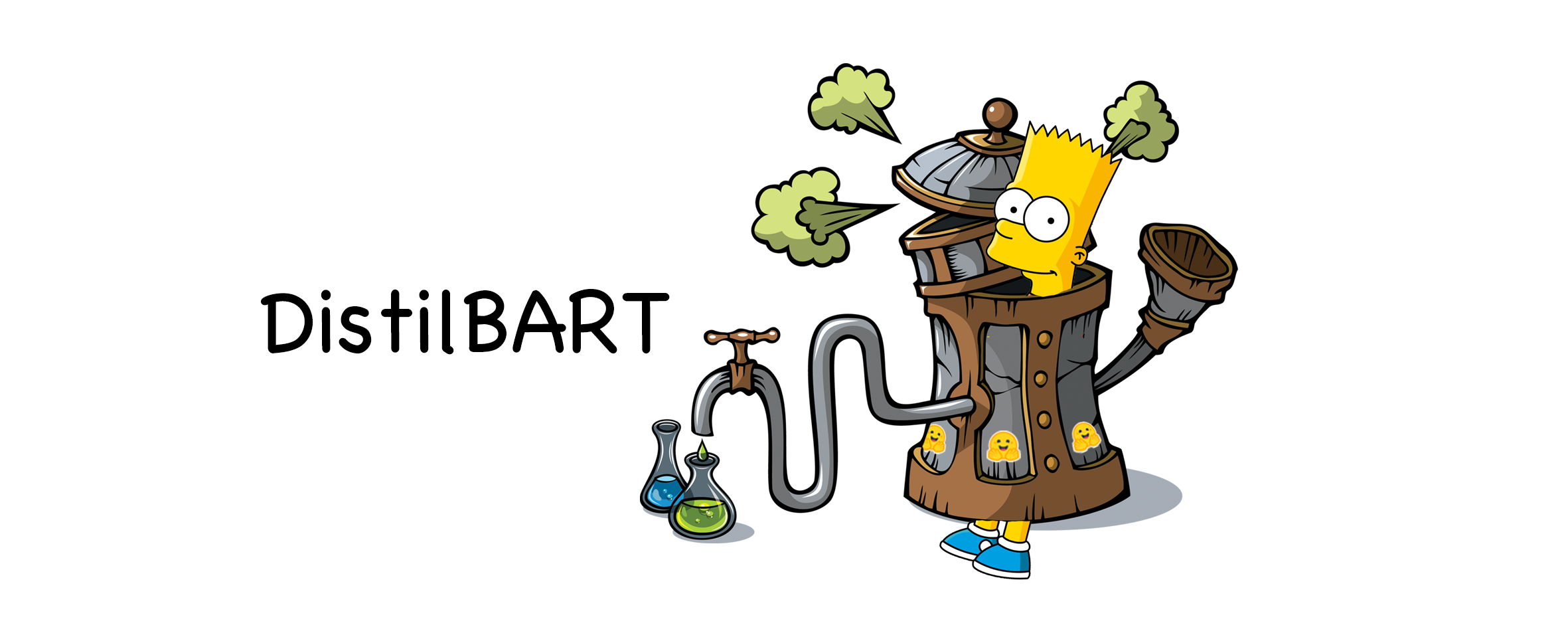
+ For the CNN/DailyMail dataset, (relatively longer, more extractive summaries), we found a simple technique that works, which we call "Shrink and Fine-tune", or SFT.
you just copy alternating layers from `facebook/bart-large-cnn` and fine-tune more on the cnn/dm data. `sshleifer/distill-pegasus-cnn-16-4`, `sshleifer/distilbart-cnn-12-6` and all other checkpoints under `sshleifer` that start with `distilbart-cnn` were trained this way.
+ For the XSUM dataset, training on pseudo-labels worked best for Pegasus (`sshleifer/distill-pegasus-16-4`), while training with KD worked best for `distilbart-xsum-12-6`
+ For `sshleifer/dbart-xsum-12-3`
+ We ran 100s experiments, and didn't want to document 100s of commands. If you want a command to replicate a figure from the paper that is not documented below, feel free to ask on the [forums](https://discuss.huggingface.co/t/seq2seq-distillation-methodology-questions/1270) and tag `@sshleifer`.
+ You can see the performance tradeoffs of model sizes [here](https://docs.google.com/spreadsheets/d/1EkhDMwVO02m8jCD1cG3RoFPLicpcL1GQHTQjfvDYgIM/edit#gid=0).
and more granular timing results [here](https://docs.google.com/spreadsheets/d/1EkhDMwVO02m8jCD1cG3RoFPLicpcL1GQHTQjfvDYgIM/edit#gid=1753259047&range=B2:I23).
### Evaluation
use [run_distributed_eval](./run_distributed_eval.py), with the following convenient alias
```bash
deval () {
proc=$1
m=$2
dd=$3
sd=$4
shift
shift
shift
shift
python -m torch.distributed.launch --nproc_per_node=$proc run_distributed_eval.py \
--model_name $m --save_dir $sd --data_dir $dd $@
}
```
On a 1 GPU system, here are four commands (that assume `xsum`, `cnn_dm` are downloaded, cmd-F for those links in this file).
`distilBART`:
```bash
deval 1 sshleifer/distilbart-xsum-12-3 xsum dbart_12_3_xsum_eval --fp16 # --help for more choices.
deval 1 sshleifer/distilbart-cnn_dm-12-6 cnn_dm dbart_12_6_cnn_eval --fp16
```
`distill-pegasus`:
```bash
deval 1 sshleifer/distill-pegasus-cnn-16-4 cnn_dm dpx_cnn_eval
deval 1 sshleifer/distill-pegasus-xsum-16-4 xsum dpx_xsum_eval
```
### Distillation
+ For all of the following commands, you can get roughly equivalent result and faster run times by passing `--num_beams=4`. That's not what we did for the paper.
+ Besides the KD section, you can also run commands with the built-in transformers trainer. See, for example, [builtin_trainer/train_distilbart_cnn.sh](./builtin_trainer/train_distilbart_cnn.sh).
+ Large performance deviations (> 5X slower or more than 0.5 Rouge-2 worse), should be reported.
+ Multi-gpu (controlled with `--gpus` should work, but might require more epochs).
#### Recommended Workflow
+ Get your dataset in the right format. (see 6 files above).
+ Find a teacher model [Pegasus](https://huggingface.co/models?search=pegasus) (slower, better ROUGE) or `facebook/bart-large-xsum`/`facebook/bart-large-cnn` (faster, slightly lower.).
Choose the checkpoint where the corresponding dataset is most similar (or identical to) your dataset.
+ Follow the sections in order below. You can stop after SFT if you are satisfied, or move on to pseudo-labeling if you want more performance.
+ student size: If you want a close to free 50% speedup, cut the decoder in half. If you want a larger speedup, cut it in 4.
+ If your SFT run starts at a validation ROUGE-2 that is more than 10 pts below the teacher's validation ROUGE-2, you have a bug. Switching to a more expensive technique will not help. Try setting a breakpoint and looking at generation and truncation defaults/hyper-parameters, and share your experience on the forums!
#### Initialization
We use [make_student.py](./make_student.py) to copy alternating layers from the teacher, and save the resulting model to disk
```bash
python make_student.py facebook/bart-large-xsum --save_path dbart_xsum_12_3 -e 12 -d 3
```
or for `pegasus-xsum`
```bash
python make_student.py google/pegasus-xsum --save_path dpx_xsum_16_4 --e 16 --d 4
```
we now have an initialized student saved to `dbart_xsum_12_3`, which we will use for the following commands.
+ Extension: To replicate more complicated initialize experiments in section 6.1, or try your own. Use the `create_student_by_copying_alternating_layers` function.
#### Pegasus
+ The following commands are written for BART and will require, at minimum, the following modifications
+ reduce batch size, and increase gradient accumulation steps so that the product `gpus * batch size * gradient_accumulation_steps = 256`. We used `--learning-rate` = 1e-4 * gradient accumulation steps.
+ don't use fp16
+ `--tokenizer_name google/pegasus-large`
### SFT (No Teacher Distillation)
You don't need `distillation.py`, you can just run:
```bash
python finetune.py \
--data_dir xsum \
--freeze_encoder --freeze_embeds \
--learning_rate=3e-4 \
--do_train \
--do_predict \
--fp16 --fp16_opt_level=O1 \
--val_check_interval 0.1 --n_val 1000 --eval_beams 2 --length_penalty=0.5 \
--max_target_length=60 --val_max_target_length=60 --test_max_target_length=100 \
--model_name_or_path dbart_xsum_12_3 \
--train_batch_size=64 --eval_batch_size=64 \
--sortish_sampler \
--num_train_epochs=6 \
--warmup_steps 500 \
--output_dir distilbart_xsum_sft_12_3 --gpus 1
```
+ Note: The command that produced `sshleifer/distilbart-cnn-12-6` is at [train_distilbart_cnn.sh](./[train_distilbart_cnn.sh)
```bash
./train_distilbart_cnn.sh
```
<!--- runtime: 6H on NVIDIA RTX 24GB GPU -->
+ Tip: You can get the same simple distillation logic by using `distillation.py --no_teacher ` followed by identical arguments as the ones in `train_distilbart_cnn.sh`.
If you are using `wandb` and comparing the two distillation methods, using this entry point will make your logs consistent,
because you will have the same hyper-parameters logged in every run.
### Pseudo-Labeling
+ You don't need `distillation.py`.
+ Instructions to generate pseudo-labels and use pre-computed pseudo-labels can be found [here](./precomputed_pseudo_labels.md).
Simply run `finetune.py` with one of those pseudo-label datasets as `--data_dir` (`DATA`, below).
```bash
python finetune.py \
--teacher facebook/bart-large-xsum --data_dir DATA \
--freeze_encoder --freeze_embeds \
--learning_rate=3e-4 \
--do_train \
--do_predict \
--fp16 --fp16_opt_level=O1 \
--val_check_interval 0.1 --n_val 1000 --eval_beams 2 --length_penalty=0.5 \
--max_target_length=60 --val_max_target_length=60 --test_max_target_length=100 \
--model_name_or_path dbart_xsum_12_3 \
--train_batch_size=32 --eval_batch_size=32 \
--sortish_sampler \
--num_train_epochs=5 \
--warmup_steps 500 \
--output_dir dbart_xsum_12_3_PL --gpus 1 --logger_name wandb
```
To combine datasets, as in Section 6.2, try something like:
```bash
curl -S https://cdn-datasets.huggingface.co/pseudo/xsum/bart_xsum_pl.tgz | tar -xvz -C .
curl -S https://cdn-datasets.huggingface.co/pseudo/xsum/pegasus_xsum.tgz | tar -xvz -C .
curl -S https://cdn-datasets.huggingface.co/summarization/xsum.tar.gz | tar -xvz -C .
mkdir all_pl
cat bart_xsum_pl/train.source pegasus_xsum/train.source xsum/train.source > all_pl/train.source
cat bart_xsum_pl/train.target pegasus_xsum/train.target xsum/train.target > all_pl/train.target
cp xsum/val* all_pl
cp xsum/test* all_pl
```
then use `all_pl` as DATA in the command above.
#### Direct Knowledge Distillation (KD)
+ In this method, we use try to enforce that the student and teacher produce similar encoder_outputs, logits, and hidden_states using `SummarizationDistiller`.
+ This method was used for `sshleifer/distilbart-xsum-12-6`, `6-6`, and `9-6` checkpoints were produced.
+ You must use [`distillation.py`](./distillation.py). Note that this command initializes the student for you.
The command that produced `sshleifer/distilbart-xsum-12-6` is at [./train_distilbart_xsum.sh](train_distilbart_xsum.sh)
```bash
./train_distilbart_xsum.sh --logger_name wandb --gpus 1
```
+ Expected ROUGE-2 between 21.3 and 21.6, run time ~13H.
+ direct KD + Pegasus is VERY slow and works best with `--supervise_forward --normalize_hidden`.
<!--- runtime: 13H on V-100 16GB GPU. -->
### Citation
```bibtex
@misc{shleifer2020pretrained,
title={Pre-trained Summarization Distillation},
author={Sam Shleifer and Alexander M. Rush},
year={2020},
eprint={2010.13002},
archivePrefix={arXiv},
primaryClass={cs.CL}
}
@article{Wolf2019HuggingFacesTS,
title={HuggingFace's Transformers: State-of-the-art Natural Language Processing},
author={Thomas Wolf and Lysandre Debut and Victor Sanh and Julien Chaumond and Clement Delangue and Anthony Moi and Pierric Cistac and Tim Rault and Rémi Louf and Morgan Funtowicz and Joe Davison and Sam Shleifer and Patrick von Platen and Clara Ma and Yacine Jernite and Julien Plu and Canwen Xu and Teven Le Scao and Sylvain Gugger and Mariama Drame and Quentin Lhoest and Alexander M. Rush},
journal={ArXiv},
year={2019},
volume={abs/1910.03771}
}
```
| transformers/examples/research_projects/seq2seq-distillation/README.md/0 | {
"file_path": "transformers/examples/research_projects/seq2seq-distillation/README.md",
"repo_id": "transformers",
"token_count": 6520
} | 289 |
# VisualBERT Demo
This demo shows usage of VisualBERT VQA model and is adapted from LXMERT demo present [here](https://github.com/huggingface/transformers/blob/main/examples/research_projects/lxmert/demo.ipynb).
1. make a virtualenv: ``virtualenv venv`` and activate ``source venv/bin/activate``
2. install reqs: ``pip install -r ./requirements.txt``
3. usage is as shown in demo.ipynb
| transformers/examples/research_projects/visual_bert/README.md/0 | {
"file_path": "transformers/examples/research_projects/visual_bert/README.md",
"repo_id": "transformers",
"token_count": 127
} | 290 |
# Parts of the code are adapted from the snippets provided in the TorchAudio Wav2Vec forced alignment tutorial.
# The full tutorial can be found here: https://pytorch.org/audio/stable/tutorials/forced_alignment_tutorial.html
import argparse
import os
from dataclasses import dataclass
import torch
import torchaudio
from tqdm import tqdm
from transformers import AutoConfig, AutoModelForCTC, AutoProcessor
class Wav2Vec2Aligner:
def __init__(self, model_name, input_wavs_sr, cuda):
self.cuda = cuda
self.config = AutoConfig.from_pretrained(model_name)
self.model = AutoModelForCTC.from_pretrained(model_name)
self.model.eval()
if self.cuda:
self.model.to(device="cuda")
self.processor = AutoProcessor.from_pretrained(model_name)
self.resampler = torchaudio.transforms.Resample(input_wavs_sr, 16_000)
blank_id = 0
vocab = list(self.processor.tokenizer.get_vocab().keys())
for i in range(len(vocab)):
if vocab[i] == "[PAD]" or vocab[i] == "<pad>":
blank_id = i
print("Blank Token id [PAD]/<pad>", blank_id)
self.blank_id = blank_id
def speech_file_to_array_fn(self, wav_path):
speech_array, sampling_rate = torchaudio.load(wav_path)
speech = self.resampler(speech_array).squeeze().numpy()
return speech
def align_single_sample(self, item):
blank_id = self.blank_id
transcript = "|".join(item["sent"].split(" "))
if not os.path.isfile(item["wav_path"]):
print(item["wav_path"], "not found in wavs directory")
speech_array = self.speech_file_to_array_fn(item["wav_path"])
inputs = self.processor(speech_array, sampling_rate=16_000, return_tensors="pt", padding=True)
if self.cuda:
inputs = inputs.to(device="cuda")
with torch.no_grad():
logits = self.model(inputs.input_values).logits
# get the emission probability at frame level
emissions = torch.log_softmax(logits, dim=-1)
emission = emissions[0].cpu().detach()
# get labels from vocab
labels = ([""] + list(self.processor.tokenizer.get_vocab().keys()))[
:-1
] # logits don't align with the tokenizer's vocab
dictionary = {c: i for i, c in enumerate(labels)}
tokens = []
for c in transcript:
if c in dictionary:
tokens.append(dictionary[c])
def get_trellis(emission, tokens, blank_id=0):
"""
Build a trellis matrix of shape (num_frames + 1, num_tokens + 1)
that represents the probabilities of each source token being at a certain time step
"""
num_frames = emission.size(0)
num_tokens = len(tokens)
# Trellis has extra diemsions for both time axis and tokens.
# The extra dim for tokens represents <SoS> (start-of-sentence)
# The extra dim for time axis is for simplification of the code.
trellis = torch.full((num_frames + 1, num_tokens + 1), -float("inf"))
trellis[:, 0] = 0
for t in range(num_frames):
trellis[t + 1, 1:] = torch.maximum(
# Score for staying at the same token
trellis[t, 1:] + emission[t, blank_id],
# Score for changing to the next token
trellis[t, :-1] + emission[t, tokens],
)
return trellis
trellis = get_trellis(emission, tokens, blank_id)
@dataclass
class Point:
token_index: int
time_index: int
score: float
def backtrack(trellis, emission, tokens, blank_id=0):
"""
Walk backwards from the last (sentence_token, time_step) pair to build the optimal sequence alignment path
"""
# Note:
# j and t are indices for trellis, which has extra dimensions
# for time and tokens at the beginning.
# When referring to time frame index `T` in trellis,
# the corresponding index in emission is `T-1`.
# Similarly, when referring to token index `J` in trellis,
# the corresponding index in transcript is `J-1`.
j = trellis.size(1) - 1
t_start = torch.argmax(trellis[:, j]).item()
path = []
for t in range(t_start, 0, -1):
# 1. Figure out if the current position was stay or change
# Note (again):
# `emission[J-1]` is the emission at time frame `J` of trellis dimension.
# Score for token staying the same from time frame J-1 to T.
stayed = trellis[t - 1, j] + emission[t - 1, blank_id]
# Score for token changing from C-1 at T-1 to J at T.
changed = trellis[t - 1, j - 1] + emission[t - 1, tokens[j - 1]]
# 2. Store the path with frame-wise probability.
prob = emission[t - 1, tokens[j - 1] if changed > stayed else 0].exp().item()
# Return token index and time index in non-trellis coordinate.
path.append(Point(j - 1, t - 1, prob))
# 3. Update the token
if changed > stayed:
j -= 1
if j == 0:
break
else:
raise ValueError("Failed to align")
return path[::-1]
path = backtrack(trellis, emission, tokens, blank_id)
@dataclass
class Segment:
label: str
start: int
end: int
score: float
def __repr__(self):
return f"{self.label}\t{self.score:4.2f}\t{self.start*20:5d}\t{self.end*20:5d}"
@property
def length(self):
return self.end - self.start
def merge_repeats(path):
"""
Merge repeated tokens into a single segment. Note: this shouldn't affect repeated characters from the
original sentences (e.g. `ll` in `hello`)
"""
i1, i2 = 0, 0
segments = []
while i1 < len(path):
while i2 < len(path) and path[i1].token_index == path[i2].token_index:
i2 += 1
score = sum(path[k].score for k in range(i1, i2)) / (i2 - i1)
segments.append(
Segment(
transcript[path[i1].token_index],
path[i1].time_index,
path[i2 - 1].time_index + 1,
score,
)
)
i1 = i2
return segments
segments = merge_repeats(path)
with open(item["out_path"], "w") as out_align:
for seg in segments:
out_align.write(str(seg) + "\n")
def align_data(self, wav_dir, text_file, output_dir):
if not os.path.exists(output_dir):
os.makedirs(output_dir)
# load text file
lines = open(text_file, encoding="utf8").readlines()
items = []
for line in lines:
if len(line.strip().split("\t")) != 2:
print("Script must be in format: 00001 this is my sentence")
exit()
wav_name, sentence = line.strip().split("\t")
wav_path = os.path.join(wav_dir, wav_name + ".wav")
out_path = os.path.join(output_dir, wav_name + ".txt")
items.append({"sent": sentence, "wav_path": wav_path, "out_path": out_path})
print("Number of samples found in script file", len(items))
for item in tqdm(items):
self.align_single_sample(item)
def main():
parser = argparse.ArgumentParser()
parser.add_argument(
"--model_name", type=str, default="arijitx/wav2vec2-xls-r-300m-bengali", help="wav2vec model name"
)
parser.add_argument("--wav_dir", type=str, default="./wavs", help="directory containing wavs")
parser.add_argument("--text_file", type=str, default="script.txt", help="file containing text")
parser.add_argument("--input_wavs_sr", type=int, default=16000, help="sampling rate of input audios")
parser.add_argument(
"--output_dir", type=str, default="./out_alignment", help="output directory containing the alignment files"
)
parser.add_argument("--cuda", action="store_true")
args = parser.parse_args()
aligner = Wav2Vec2Aligner(args.model_name, args.input_wavs_sr, args.cuda)
aligner.align_data(args.wav_dir, args.text_file, args.output_dir)
if __name__ == "__main__":
main()
| transformers/examples/research_projects/wav2vec2/alignment.py/0 | {
"file_path": "transformers/examples/research_projects/wav2vec2/alignment.py",
"repo_id": "transformers",
"token_count": 4170
} | 291 |
<!---
Copyright 2022 The HuggingFace Team. All rights reserved.
Licensed under the Apache License, Version 2.0 (the "License");
you may not use this file except in compliance with the License.
You may obtain a copy of the License at
http://www.apache.org/licenses/LICENSE-2.0
Unless required by applicable law or agreed to in writing, software
distributed under the License is distributed on an "AS IS" BASIS,
WITHOUT WARRANTIES OR CONDITIONS OF ANY KIND, either express or implied.
See the License for the specific language governing permissions and
limitations under the License.
-->
# XTREME-S benchmark examples
*Maintainers: [Anton Lozhkov](https://github.com/anton-l) and [Patrick von Platen](https://github.com/patrickvonplaten)*
The Cross-lingual TRansfer Evaluation of Multilingual Encoders for Speech (XTREME-S) benchmark is a benchmark designed to evaluate speech representations across languages, tasks, domains and data regimes. It covers XX typologically diverse languages and seven downstream tasks grouped in four families: speech recognition, translation, classification and retrieval.
XTREME-S covers speech recognition with Fleurs, Multilingual LibriSpeech (MLS) and VoxPopuli, speech translation with CoVoST-2, speech classification with LangID (Fleurs) and intent classification (MInds-14) and finally speech(-text) retrieval with Fleurs. Each of the tasks covers a subset of the 102 languages included in XTREME-S (shown here with their ISO 3166-1 codes): afr, amh, ara, asm, ast, azj, bel, ben, bos, cat, ceb, ces, cmn, cym, dan, deu, ell, eng, spa, est, fas, ful, fin, tgl, fra, gle, glg, guj, hau, heb, hin, hrv, hun, hye, ind, ibo, isl, ita, jpn, jav, kat, kam, kea, kaz, khm, kan, kor, ckb, kir, ltz, lug, lin, lao, lit, luo, lav, mri, mkd, mal, mon, mar, msa, mlt, mya, nob, npi, nld, nso, nya, oci, orm, ory, pan, pol, pus, por, ron, rus, bul, snd, slk, slv, sna, som, srp, swe, swh, tam, tel, tgk, tha, tur, ukr, umb, urd, uzb, vie, wol, xho, yor, yue and zul.
Paper: [XTREME-S: Evaluating Cross-lingual Speech Representations](https://arxiv.org/abs/2203.10752)
Dataset: [https://huggingface.co/datasets/google/xtreme_s](https://huggingface.co/datasets/google/xtreme_s)
## Fine-tuning for the XTREME-S tasks
Based on the [`run_xtreme_s.py`](https://github.com/huggingface/transformers/blob/main/examples/research_projects/xtreme-s/run_xtreme_s.py) script.
This script can fine-tune any of the pretrained speech models on the [hub](https://huggingface.co/models?pipeline_tag=automatic-speech-recognition) on the [XTREME-S dataset](https://huggingface.co/datasets/google/xtreme_s) tasks.
XTREME-S is made up of 7 different tasks. Here is how to run the script on each of them:
```bash
export TASK_NAME=mls.all
python run_xtreme_s.py \
--model_name_or_path="facebook/wav2vec2-xls-r-300m" \
--task="${TASK_NAME}" \
--output_dir="xtreme_s_xlsr_${TASK_NAME}" \
--num_train_epochs=100 \
--per_device_train_batch_size=32 \
--learning_rate="3e-4" \
--target_column_name="transcription" \
--save_steps=500 \
--eval_steps=500 \
--gradient_checkpointing \
--fp16 \
--group_by_length \
--do_train \
--do_eval \
--do_predict \
--push_to_hub
```
where `TASK_NAME` can be one of: `mls, voxpopuli, covost2, fleurs-asr, fleurs-lang_id, minds14`.
We get the following results on the test set of the benchmark's datasets.
The corresponding training commands for each dataset are given in the sections below:
| Task | Dataset | Result | Fine-tuned model & logs | Training time | GPUs |
|-----------------------|-----------|-----------------------|--------------------------------------------------------------------|---------------|--------|
| Speech Recognition | MLS | 30.33 WER | [here](https://huggingface.co/anton-l/xtreme_s_xlsr_300m_mls/) | 18:47:25 | 8xV100 |
| Speech Recognition | VoxPopuli | - | - | - | - |
| Speech Recognition | FLEURS | - | - | - | - |
| Speech Translation | CoVoST-2 | - | - | - | - |
| Speech Classification | Minds-14 | 90.15 F1 / 90.33 Acc. | [here](https://huggingface.co/anton-l/xtreme_s_xlsr_300m_minds14/) | 2:54:21 | 2xA100 |
| Speech Classification | FLEURS | - | - | - | - |
| Speech Retrieval | FLEURS | - | - | - | - |
### Speech Recognition with MLS
The following command shows how to fine-tune the [XLS-R](https://huggingface.co/docs/transformers/main/model_doc/xls_r) model on [XTREME-S MLS](https://huggingface.co/datasets/google/xtreme_s#multilingual-librispeech-mls) using 8 GPUs in half-precision.
```bash
python -m torch.distributed.launch \
--nproc_per_node=8 \
run_xtreme_s.py \
--task="mls" \
--language="all" \
--model_name_or_path="facebook/wav2vec2-xls-r-300m" \
--output_dir="xtreme_s_xlsr_300m_mls" \
--overwrite_output_dir \
--num_train_epochs=100 \
--per_device_train_batch_size=4 \
--per_device_eval_batch_size=1 \
--gradient_accumulation_steps=2 \
--learning_rate="3e-4" \
--warmup_steps=3000 \
--evaluation_strategy="steps" \
--max_duration_in_seconds=20 \
--save_steps=500 \
--eval_steps=500 \
--logging_steps=1 \
--layerdrop=0.0 \
--mask_time_prob=0.3 \
--mask_time_length=10 \
--mask_feature_prob=0.1 \
--mask_feature_length=64 \
--freeze_feature_encoder \
--gradient_checkpointing \
--fp16 \
--group_by_length \
--do_train \
--do_eval \
--do_predict \
--metric_for_best_model="wer" \
--greater_is_better=False \
--load_best_model_at_end \
--push_to_hub
```
On 8 V100 GPUs, this script should run in ~19 hours and yield a cross-entropy loss of **0.6215** and word error rate of **30.33**
### Speech Classification with Minds-14
The following command shows how to fine-tune the [XLS-R](https://huggingface.co/docs/transformers/main/model_doc/xls_r) model on [XTREME-S MLS](https://huggingface.co/datasets/google/xtreme_s#intent-classification---minds-14) using 2 GPUs in half-precision.
```bash
python -m torch.distributed.launch \
--nproc_per_node=2 \
run_xtreme_s.py \
--task="minds14" \
--language="all" \
--model_name_or_path="facebook/wav2vec2-xls-r-300m" \
--output_dir="xtreme_s_xlsr_300m_minds14" \
--overwrite_output_dir \
--num_train_epochs=50 \
--per_device_train_batch_size=32 \
--per_device_eval_batch_size=8 \
--gradient_accumulation_steps=1 \
--learning_rate="3e-4" \
--warmup_steps=1500 \
--evaluation_strategy="steps" \
--max_duration_in_seconds=30 \
--save_steps=200 \
--eval_steps=200 \
--logging_steps=1 \
--layerdrop=0.0 \
--mask_time_prob=0.3 \
--mask_time_length=10 \
--mask_feature_prob=0.1 \
--mask_feature_length=64 \
--freeze_feature_encoder \
--gradient_checkpointing \
--fp16 \
--group_by_length \
--do_train \
--do_eval \
--do_predict \
--metric_for_best_model="f1" \
--greater_is_better=True \
--load_best_model_at_end \
--push_to_hub
```
On 2 A100 GPUs, this script should run in ~5 hours and yield a cross-entropy loss of **0.4119** and F1 score of **90.15**
| transformers/examples/research_projects/xtreme-s/README.md/0 | {
"file_path": "transformers/examples/research_projects/xtreme-s/README.md",
"repo_id": "transformers",
"token_count": 3401
} | 292 |
#!/usr/bin/env python
# coding=utf-8
# Copyright 2020 The HuggingFace Team All rights reserved.
#
# Licensed under the Apache License, Version 2.0 (the "License");
# you may not use this file except in compliance with the License.
# You may obtain a copy of the License at
#
# http://www.apache.org/licenses/LICENSE-2.0
#
# Unless required by applicable law or agreed to in writing, software
# distributed under the License is distributed on an "AS IS" BASIS,
# WITHOUT WARRANTIES OR CONDITIONS OF ANY KIND, either express or implied.
# See the License for the specific language governing permissions and
# limitations under the License.
"""
Fine-tuning the library models for question answering.
"""
# You can also adapt this script on your own question answering task. Pointers for this are left as comments.
import json
import logging
import os
import sys
import warnings
from dataclasses import dataclass, field
from pathlib import Path
from typing import Optional
import evaluate
import tensorflow as tf
from datasets import load_dataset
from packaging.version import parse
from utils_qa import postprocess_qa_predictions
import transformers
from transformers import (
AutoConfig,
AutoTokenizer,
EvalPrediction,
HfArgumentParser,
PreTrainedTokenizerFast,
PushToHubCallback,
TFAutoModelForQuestionAnswering,
TFTrainingArguments,
create_optimizer,
set_seed,
)
from transformers.utils import CONFIG_NAME, TF2_WEIGHTS_NAME, check_min_version, send_example_telemetry
try:
import tf_keras as keras
except (ModuleNotFoundError, ImportError):
import keras
if parse(keras.__version__).major > 2:
raise ValueError(
"Your currently installed version of Keras is Keras 3, but this is not yet supported in "
"Transformers. Please install the backwards-compatible tf-keras package with "
"`pip install tf-keras`."
)
# Will error if the minimal version of Transformers is not installed. Remove at your own risks.
check_min_version("4.40.0.dev0")
logger = logging.getLogger(__name__)
# region Arguments
@dataclass
class ModelArguments:
"""
Arguments pertaining to which model/config/tokenizer we are going to fine-tune from.
"""
model_name_or_path: str = field(
metadata={"help": "Path to pretrained model or model identifier from huggingface.co/models"}
)
config_name: Optional[str] = field(
default=None, metadata={"help": "Pretrained config name or path if not the same as model_name"}
)
tokenizer_name: Optional[str] = field(
default=None, metadata={"help": "Pretrained tokenizer name or path if not the same as model_name"}
)
cache_dir: Optional[str] = field(
default=None,
metadata={"help": "Path to directory to store the pretrained models downloaded from huggingface.co"},
)
model_revision: str = field(
default="main",
metadata={"help": "The specific model version to use (can be a branch name, tag name or commit id)."},
)
token: str = field(
default=None,
metadata={
"help": (
"The token to use as HTTP bearer authorization for remote files. If not specified, will use the token "
"generated when running `huggingface-cli login` (stored in `~/.huggingface`)."
)
},
)
use_auth_token: bool = field(
default=None,
metadata={
"help": "The `use_auth_token` argument is deprecated and will be removed in v4.34. Please use `token` instead."
},
)
trust_remote_code: bool = field(
default=False,
metadata={
"help": (
"Whether or not to allow for custom models defined on the Hub in their own modeling files. This option "
"should only be set to `True` for repositories you trust and in which you have read the code, as it will "
"execute code present on the Hub on your local machine."
)
},
)
@dataclass
class DataTrainingArguments:
"""
Arguments pertaining to what data we are going to input our model for training and eval.
"""
dataset_name: Optional[str] = field(
default=None, metadata={"help": "The name of the dataset to use (via the datasets library)."}
)
dataset_config_name: Optional[str] = field(
default=None, metadata={"help": "The configuration name of the dataset to use (via the datasets library)."}
)
train_file: Optional[str] = field(default=None, metadata={"help": "The input training data file (a text file)."})
validation_file: Optional[str] = field(
default=None,
metadata={"help": "An optional input evaluation data file to evaluate the perplexity on (a text file)."},
)
test_file: Optional[str] = field(
default=None,
metadata={"help": "An optional input test data file to evaluate the perplexity on (a text file)."},
)
overwrite_cache: bool = field(
default=False, metadata={"help": "Overwrite the cached training and evaluation sets"}
)
preprocessing_num_workers: Optional[int] = field(
default=None,
metadata={"help": "The number of processes to use for the preprocessing."},
)
max_seq_length: int = field(
default=384,
metadata={
"help": (
"The maximum total input sequence length after tokenization. Sequences longer "
"than this will be truncated, sequences shorter will be padded."
)
},
)
pad_to_max_length: bool = field(
default=False,
metadata={
"help": (
"Whether to pad all samples to `max_seq_length`. If False, will pad the samples dynamically when"
" batching to the maximum length in the batch (which can be faster on GPU but will be slower on TPU)."
)
},
)
max_train_samples: Optional[int] = field(
default=None,
metadata={
"help": (
"For debugging purposes or quicker training, truncate the number of training examples to this "
"value if set."
)
},
)
max_eval_samples: Optional[int] = field(
default=None,
metadata={
"help": (
"For debugging purposes or quicker training, truncate the number of evaluation examples to this "
"value if set."
)
},
)
max_predict_samples: Optional[int] = field(
default=None,
metadata={
"help": (
"For debugging purposes or quicker training, truncate the number of prediction examples to this "
"value if set."
)
},
)
version_2_with_negative: bool = field(
default=False, metadata={"help": "If true, some of the examples do not have an answer."}
)
null_score_diff_threshold: float = field(
default=0.0,
metadata={
"help": (
"The threshold used to select the null answer: if the best answer has a score that is less than "
"the score of the null answer minus this threshold, the null answer is selected for this example. "
"Only useful when `version_2_with_negative=True`."
)
},
)
doc_stride: int = field(
default=128,
metadata={"help": "When splitting up a long document into chunks, how much stride to take between chunks."},
)
n_best_size: int = field(
default=20,
metadata={"help": "The total number of n-best predictions to generate when looking for an answer."},
)
max_answer_length: int = field(
default=30,
metadata={
"help": (
"The maximum length of an answer that can be generated. This is needed because the start "
"and end predictions are not conditioned on one another."
)
},
)
def __post_init__(self):
if (
self.dataset_name is None
and self.train_file is None
and self.validation_file is None
and self.test_file is None
):
raise ValueError("Need either a dataset name or a training/validation file/test_file.")
else:
if self.train_file is not None:
extension = self.train_file.split(".")[-1]
assert extension in ["csv", "json"], "`train_file` should be a csv or a json file."
if self.validation_file is not None:
extension = self.validation_file.split(".")[-1]
assert extension in ["csv", "json"], "`validation_file` should be a csv or a json file."
if self.test_file is not None:
extension = self.test_file.split(".")[-1]
assert extension in ["csv", "json"], "`test_file` should be a csv or a json file."
# endregion
# region Helper classes
class SavePretrainedCallback(keras.callbacks.Callback):
# Hugging Face models have a save_pretrained() method that saves both the weights and the necessary
# metadata to allow them to be loaded as a pretrained model in future. This is a simple Keras callback
# that saves the model with this method after each epoch.
def __init__(self, output_dir, **kwargs):
super().__init__()
self.output_dir = output_dir
def on_epoch_end(self, epoch, logs=None):
self.model.save_pretrained(self.output_dir)
# endregion
def main():
# region Argument parsing
# See all possible arguments in src/transformers/training_args.py
# or by passing the --help flag to this script.
# We now keep distinct sets of args, for a cleaner separation of concerns.
parser = HfArgumentParser((ModelArguments, DataTrainingArguments, TFTrainingArguments))
if len(sys.argv) == 2 and sys.argv[1].endswith(".json"):
# If we pass only one argument to the script and it's the path to a json file,
# let's parse it to get our arguments.
model_args, data_args, training_args = parser.parse_json_file(json_file=os.path.abspath(sys.argv[1]))
else:
model_args, data_args, training_args = parser.parse_args_into_dataclasses()
if model_args.use_auth_token is not None:
warnings.warn(
"The `use_auth_token` argument is deprecated and will be removed in v4.34. Please use `token` instead.",
FutureWarning,
)
if model_args.token is not None:
raise ValueError("`token` and `use_auth_token` are both specified. Please set only the argument `token`.")
model_args.token = model_args.use_auth_token
# Sending telemetry. Tracking the example usage helps us better allocate resources to maintain them. The
# information sent is the one passed as arguments along with your Python/PyTorch versions.
send_example_telemetry("run_qa", model_args, data_args, framework="tensorflow")
output_dir = Path(training_args.output_dir)
output_dir.mkdir(parents=True, exist_ok=True)
# endregion
# region Checkpoints
checkpoint = None
if len(os.listdir(training_args.output_dir)) > 0 and not training_args.overwrite_output_dir:
if (output_dir / CONFIG_NAME).is_file() and (output_dir / TF2_WEIGHTS_NAME).is_file():
checkpoint = output_dir
logger.info(
f"Checkpoint detected, resuming training from checkpoint in {training_args.output_dir}. To avoid this"
" behavior, change the `--output_dir` or add `--overwrite_output_dir` to train from scratch."
)
else:
raise ValueError(
f"Output directory ({training_args.output_dir}) already exists and is not empty. "
"Use --overwrite_output_dir to continue regardless."
)
# endregion
# region Logging
logging.basicConfig(
format="%(asctime)s - %(levelname)s - %(name)s - %(message)s",
datefmt="%m/%d/%Y %H:%M:%S",
handlers=[logging.StreamHandler(sys.stdout)],
)
logger.setLevel(logging.INFO if training_args.should_log else logging.WARN)
# Set the verbosity to info of the Transformers logger (on main process only):
if training_args.should_log:
transformers.utils.logging.set_verbosity_info()
transformers.utils.logging.enable_default_handler()
transformers.utils.logging.enable_explicit_format()
logger.info(f"Training/evaluation parameters {training_args}")
# endregion
# Set seed before initializing model.
set_seed(training_args.seed)
# region Load Data
# Get the datasets: you can either provide your own CSV/JSON/TXT training and evaluation files (see below)
# or just provide the name of one of the public datasets available on the hub at https://huggingface.co/datasets/
# (the dataset will be downloaded automatically from the datasets Hub).
#
# For CSV/JSON files, this script will use the column called 'text' or the first column if no column called
# 'text' is found. You can easily tweak this behavior (see below).
#
# In distributed training, the load_dataset function guarantee that only one local process can concurrently
# download the dataset.
if data_args.dataset_name is not None:
# Downloading and loading a dataset from the hub.
datasets = load_dataset(
data_args.dataset_name,
data_args.dataset_config_name,
cache_dir=model_args.cache_dir,
token=model_args.token,
)
else:
data_files = {}
if data_args.train_file is not None:
data_files["train"] = data_args.train_file
extension = data_args.train_file.split(".")[-1]
if data_args.validation_file is not None:
data_files["validation"] = data_args.validation_file
extension = data_args.validation_file.split(".")[-1]
if data_args.test_file is not None:
data_files["test"] = data_args.test_file
extension = data_args.test_file.split(".")[-1]
datasets = load_dataset(
extension,
data_files=data_files,
field="data",
cache_dir=model_args.cache_dir,
token=model_args.token,
)
# See more about loading any type of standard or custom dataset (from files, python dict, pandas DataFrame, etc) at
# https://huggingface.co/docs/datasets/loading_datasets.
# endregion
# region Load pretrained model and tokenizer
#
# Distributed training:
# The .from_pretrained methods guarantee that only one local process can concurrently
# download model & vocab.
config = AutoConfig.from_pretrained(
model_args.config_name if model_args.config_name else model_args.model_name_or_path,
cache_dir=model_args.cache_dir,
revision=model_args.model_revision,
token=model_args.token,
trust_remote_code=model_args.trust_remote_code,
)
tokenizer = AutoTokenizer.from_pretrained(
model_args.tokenizer_name if model_args.tokenizer_name else model_args.model_name_or_path,
cache_dir=model_args.cache_dir,
use_fast=True,
revision=model_args.model_revision,
token=model_args.token,
trust_remote_code=model_args.trust_remote_code,
)
# endregion
# region Tokenizer check: this script requires a fast tokenizer.
if not isinstance(tokenizer, PreTrainedTokenizerFast):
raise ValueError(
"This example script only works for models that have a fast tokenizer. Checkout the big table of models at"
" https://huggingface.co/transformers/index.html#supported-frameworks to find the model types that meet"
" this requirement"
)
# endregion
# region Preprocessing the datasets
# Preprocessing is slightly different for training and evaluation.
if training_args.do_train:
column_names = datasets["train"].column_names
elif training_args.do_eval:
column_names = datasets["validation"].column_names
else:
column_names = datasets["test"].column_names
question_column_name = "question" if "question" in column_names else column_names[0]
context_column_name = "context" if "context" in column_names else column_names[1]
answer_column_name = "answers" if "answers" in column_names else column_names[2]
# Padding side determines if we do (question|context) or (context|question).
pad_on_right = tokenizer.padding_side == "right"
if data_args.max_seq_length > tokenizer.model_max_length:
logger.warning(
f"The max_seq_length passed ({data_args.max_seq_length}) is larger than the maximum length for the "
f"model ({tokenizer.model_max_length}). Using max_seq_length={tokenizer.model_max_length}."
)
max_seq_length = min(data_args.max_seq_length, tokenizer.model_max_length)
if data_args.pad_to_max_length or isinstance(training_args.strategy, tf.distribute.TPUStrategy):
logger.info("Padding all batches to max length because argument was set or we're on TPU.")
padding = "max_length"
else:
padding = False
# Training preprocessing
def prepare_train_features(examples):
# Some of the questions have lots of whitespace on the left, which is not useful and will make the
# truncation of the context fail (the tokenized question will take a lots of space). So we remove that
# left whitespace
examples[question_column_name] = [q.lstrip() for q in examples[question_column_name]]
# Tokenize our examples with truncation and maybe padding, but keep the overflows using a stride. This results
# in one example possible giving several features when a context is long, each of those features having a
# context that overlaps a bit the context of the previous feature.
tokenized_examples = tokenizer(
examples[question_column_name if pad_on_right else context_column_name],
examples[context_column_name if pad_on_right else question_column_name],
truncation="only_second" if pad_on_right else "only_first",
max_length=max_seq_length,
stride=data_args.doc_stride,
return_overflowing_tokens=True,
return_offsets_mapping=True,
padding=padding,
)
# Since one example might give us several features if it has a long context, we need a map from a feature to
# its corresponding example. This key gives us just that.
sample_mapping = tokenized_examples.pop("overflow_to_sample_mapping")
# The offset mappings will give us a map from token to character position in the original context. This will
# help us compute the start_positions and end_positions.
offset_mapping = tokenized_examples.pop("offset_mapping")
# Let's label those examples!
tokenized_examples["start_positions"] = []
tokenized_examples["end_positions"] = []
for i, offsets in enumerate(offset_mapping):
# We will label impossible answers with the index of the CLS token.
input_ids = tokenized_examples["input_ids"][i]
cls_index = input_ids.index(tokenizer.cls_token_id)
# Grab the sequence corresponding to that example (to know what is the context and what is the question).
sequence_ids = tokenized_examples.sequence_ids(i)
# One example can give several spans, this is the index of the example containing this span of text.
sample_index = sample_mapping[i]
answers = examples[answer_column_name][sample_index]
# If no answers are given, set the cls_index as answer.
if len(answers["answer_start"]) == 0:
tokenized_examples["start_positions"].append(cls_index)
tokenized_examples["end_positions"].append(cls_index)
else:
# Start/end character index of the answer in the text.
start_char = answers["answer_start"][0]
end_char = start_char + len(answers["text"][0])
# Start token index of the current span in the text.
token_start_index = 0
while sequence_ids[token_start_index] != (1 if pad_on_right else 0):
token_start_index += 1
# End token index of the current span in the text.
token_end_index = len(input_ids) - 1
while sequence_ids[token_end_index] != (1 if pad_on_right else 0):
token_end_index -= 1
# Detect if the answer is out of the span (in which case this feature is labeled with the CLS index).
if not (offsets[token_start_index][0] <= start_char and offsets[token_end_index][1] >= end_char):
tokenized_examples["start_positions"].append(cls_index)
tokenized_examples["end_positions"].append(cls_index)
else:
# Otherwise move the token_start_index and token_end_index to the two ends of the answer.
# Note: we could go after the last offset if the answer is the last word (edge case).
while token_start_index < len(offsets) and offsets[token_start_index][0] <= start_char:
token_start_index += 1
tokenized_examples["start_positions"].append(token_start_index - 1)
while offsets[token_end_index][1] >= end_char:
token_end_index -= 1
tokenized_examples["end_positions"].append(token_end_index + 1)
return tokenized_examples
processed_datasets = {}
if training_args.do_train:
if "train" not in datasets:
raise ValueError("--do_train requires a train dataset")
train_dataset = datasets["train"]
if data_args.max_train_samples is not None:
# We will select sample from whole data if argument is specified
max_train_samples = min(len(train_dataset), data_args.max_train_samples)
train_dataset = train_dataset.select(range(max_train_samples))
# Create train feature from dataset
train_dataset = train_dataset.map(
prepare_train_features,
batched=True,
num_proc=data_args.preprocessing_num_workers,
remove_columns=column_names,
load_from_cache_file=not data_args.overwrite_cache,
)
if data_args.max_train_samples is not None:
# Number of samples might increase during Feature Creation, We select only specified max samples
max_train_samples = min(len(train_dataset), data_args.max_train_samples)
train_dataset = train_dataset.select(range(max_train_samples))
processed_datasets["train"] = train_dataset
# Validation preprocessing
def prepare_validation_features(examples):
# Some of the questions have lots of whitespace on the left, which is not useful and will make the
# truncation of the context fail (the tokenized question will take a lots of space). So we remove that
# left whitespace
examples[question_column_name] = [q.lstrip() for q in examples[question_column_name]]
# Tokenize our examples with truncation and maybe padding, but keep the overflows using a stride. This results
# in one example possible giving several features when a context is long, each of those features having a
# context that overlaps a bit the context of the previous feature.
tokenized_examples = tokenizer(
examples[question_column_name if pad_on_right else context_column_name],
examples[context_column_name if pad_on_right else question_column_name],
truncation="only_second" if pad_on_right else "only_first",
max_length=max_seq_length,
stride=data_args.doc_stride,
return_overflowing_tokens=True,
return_offsets_mapping=True,
padding=padding,
)
# Since one example might give us several features if it has a long context, we need a map from a feature to
# its corresponding example. This key gives us just that.
sample_mapping = tokenized_examples.pop("overflow_to_sample_mapping")
# For evaluation, we will need to convert our predictions to substrings of the context, so we keep the
# corresponding example_id and we will store the offset mappings.
tokenized_examples["example_id"] = []
for i in range(len(tokenized_examples["input_ids"])):
# Grab the sequence corresponding to that example (to know what is the context and what is the question).
sequence_ids = tokenized_examples.sequence_ids(i)
context_index = 1 if pad_on_right else 0
# One example can give several spans, this is the index of the example containing this span of text.
sample_index = sample_mapping[i]
tokenized_examples["example_id"].append(examples["id"][sample_index])
# Set to None the offset_mapping that are not part of the context so it's easy to determine if a token
# position is part of the context or not.
tokenized_examples["offset_mapping"][i] = [
(o if sequence_ids[k] == context_index else None)
for k, o in enumerate(tokenized_examples["offset_mapping"][i])
]
return tokenized_examples
if training_args.do_eval:
if "validation" not in datasets:
raise ValueError("--do_eval requires a validation dataset")
eval_examples = datasets["validation"]
if data_args.max_eval_samples is not None:
# We will select sample from whole data
max_eval_samples = min(len(eval_examples), data_args.max_eval_samples)
eval_examples = eval_examples.select(range(max_eval_samples))
# Validation Feature Creation
eval_dataset = eval_examples.map(
prepare_validation_features,
batched=True,
num_proc=data_args.preprocessing_num_workers,
remove_columns=column_names,
load_from_cache_file=not data_args.overwrite_cache,
)
if data_args.max_eval_samples is not None:
# During Feature creation dataset samples might increase, we will select required samples again
max_eval_samples = min(len(eval_dataset), data_args.max_eval_samples)
eval_dataset = eval_dataset.select(range(max_eval_samples))
processed_datasets["validation"] = eval_dataset
if training_args.do_predict:
if "test" not in datasets:
raise ValueError("--do_predict requires a test dataset")
predict_examples = datasets["test"]
if data_args.max_predict_samples is not None:
# We will select sample from whole data
predict_examples = predict_examples.select(range(data_args.max_predict_samples))
# Predict Feature Creation
predict_dataset = predict_examples.map(
prepare_validation_features,
batched=True,
num_proc=data_args.preprocessing_num_workers,
remove_columns=column_names,
load_from_cache_file=not data_args.overwrite_cache,
)
if data_args.max_predict_samples is not None:
# During Feature creation dataset samples might increase, we will select required samples again
max_predict_samples = min(len(predict_dataset), data_args.max_predict_samples)
predict_dataset = predict_dataset.select(range(max_predict_samples))
processed_datasets["test"] = predict_dataset
# endregion
# region Metrics and Post-processing:
def post_processing_function(examples, features, predictions, stage="eval"):
# Post-processing: we match the start logits and end logits to answers in the original context.
predictions = postprocess_qa_predictions(
examples=examples,
features=features,
predictions=predictions,
version_2_with_negative=data_args.version_2_with_negative,
n_best_size=data_args.n_best_size,
max_answer_length=data_args.max_answer_length,
null_score_diff_threshold=data_args.null_score_diff_threshold,
output_dir=training_args.output_dir,
prefix=stage,
)
# Format the result to the format the metric expects.
if data_args.version_2_with_negative:
formatted_predictions = [
{"id": k, "prediction_text": v, "no_answer_probability": 0.0} for k, v in predictions.items()
]
else:
formatted_predictions = [{"id": k, "prediction_text": v} for k, v in predictions.items()]
references = [{"id": ex["id"], "answers": ex[answer_column_name]} for ex in examples]
return EvalPrediction(predictions=formatted_predictions, label_ids=references)
metric = evaluate.load(
"squad_v2" if data_args.version_2_with_negative else "squad", cache_dir=model_args.cache_dir
)
def compute_metrics(p: EvalPrediction):
return metric.compute(predictions=p.predictions, references=p.label_ids)
# endregion
with training_args.strategy.scope():
dataset_options = tf.data.Options()
dataset_options.experimental_distribute.auto_shard_policy = tf.data.experimental.AutoShardPolicy.OFF
num_replicas = training_args.strategy.num_replicas_in_sync
# region Load model and prepare datasets
if checkpoint is None:
model_path = model_args.model_name_or_path
else:
model_path = checkpoint
model = TFAutoModelForQuestionAnswering.from_pretrained(
model_path,
config=config,
cache_dir=model_args.cache_dir,
revision=model_args.model_revision,
token=model_args.token,
trust_remote_code=model_args.trust_remote_code,
)
if training_args.do_train:
training_dataset = model.prepare_tf_dataset(
processed_datasets["train"],
shuffle=True,
batch_size=training_args.per_device_train_batch_size * num_replicas,
tokenizer=tokenizer,
)
training_dataset = training_dataset.with_options(dataset_options)
num_train_steps = len(training_dataset) * training_args.num_train_epochs
if training_args.warmup_steps > 0:
num_warmup_steps = training_args.warmup_steps
elif training_args.warmup_ratio > 0:
num_warmup_steps = int(num_train_steps * training_args.warmup_ratio)
else:
num_warmup_steps = 0
optimizer, schedule = create_optimizer(
init_lr=training_args.learning_rate,
num_train_steps=len(training_dataset) * training_args.num_train_epochs,
num_warmup_steps=num_warmup_steps,
adam_beta1=training_args.adam_beta1,
adam_beta2=training_args.adam_beta2,
adam_epsilon=training_args.adam_epsilon,
weight_decay_rate=training_args.weight_decay,
adam_global_clipnorm=training_args.max_grad_norm,
)
# Transformers models compute the right loss for their task by default when labels are passed, and will
# use this for training unless you specify your own loss function in compile().
model.compile(optimizer=optimizer, jit_compile=training_args.xla, metrics=["accuracy"])
else:
# Optimizer doesn't matter as it won't be used anyway
model.compile(optimizer="sgd", jit_compile=training_args.xla, metrics=["accuracy"])
training_dataset = None
if training_args.do_eval:
eval_dataset = model.prepare_tf_dataset(
processed_datasets["validation"],
shuffle=False,
batch_size=training_args.per_device_train_batch_size * num_replicas,
tokenizer=tokenizer,
)
eval_dataset = eval_dataset.with_options(dataset_options)
else:
eval_dataset = None
if training_args.do_predict:
predict_dataset = model.prepare_tf_dataset(
processed_datasets["test"],
shuffle=False,
batch_size=training_args.per_device_eval_batch_size * num_replicas,
tokenizer=tokenizer,
)
predict_dataset = predict_dataset.with_options(dataset_options)
else:
predict_dataset = None
# endregion
# region Preparing push_to_hub and model card
push_to_hub_model_id = training_args.push_to_hub_model_id
model_name = model_args.model_name_or_path.split("/")[-1]
if not push_to_hub_model_id:
if data_args.dataset_name is not None:
push_to_hub_model_id = f"{model_name}-finetuned-{data_args.dataset_name}"
else:
push_to_hub_model_id = f"{model_name}-finetuned-question-answering"
model_card_kwargs = {"finetuned_from": model_args.model_name_or_path, "tasks": "question-answering"}
if data_args.dataset_name is not None:
model_card_kwargs["dataset_tags"] = data_args.dataset_name
if data_args.dataset_config_name is not None:
model_card_kwargs["dataset_args"] = data_args.dataset_config_name
model_card_kwargs["dataset"] = f"{data_args.dataset_name} {data_args.dataset_config_name}"
else:
model_card_kwargs["dataset"] = data_args.dataset_name
if training_args.push_to_hub:
callbacks = [
PushToHubCallback(
output_dir=training_args.output_dir,
hub_model_id=push_to_hub_model_id,
hub_token=training_args.push_to_hub_token,
tokenizer=tokenizer,
**model_card_kwargs,
)
]
else:
callbacks = []
# endregion
# region Training and Evaluation
if training_args.do_train:
# Note that the validation and test datasets have been processed in a different way to the
# training datasets in this example, and so they don't have the same label structure.
# As such, we don't pass them directly to Keras, but instead get model predictions to evaluate
# after training.
model.fit(training_dataset, epochs=int(training_args.num_train_epochs), callbacks=callbacks)
if training_args.do_eval:
logger.info("*** Evaluation ***")
# In this example, we compute advanced metrics at the end of training, but
# if you'd like to compute metrics every epoch that are too complex to be written as
# standard Keras metrics, you can use our KerasMetricCallback. See
# https://huggingface.co/docs/transformers/main/en/main_classes/keras_callbacks
eval_predictions = model.predict(eval_dataset)
if isinstance(eval_predictions.start_logits, tf.RaggedTensor):
# If predictions are RaggedTensor, we densify them. Since they are logits, padding with 0 is a bad idea!
# The reason is that a logit of 0 can often end up as quite a high probability value, sometimes even
# the highest probability in a sample. Instead, we use a large negative value, which ensures that the
# padding positions are correctly masked.
eval_start_logits = eval_predictions.start_logits.to_tensor(default_value=-1000).numpy()
eval_end_logits = eval_predictions.end_logits.to_tensor(default_value=-1000).numpy()
else:
eval_start_logits = eval_predictions.start_logits
eval_end_logits = eval_predictions.end_logits
post_processed_eval = post_processing_function(
datasets["validation"],
processed_datasets["validation"],
(eval_start_logits, eval_end_logits),
)
metrics = compute_metrics(post_processed_eval)
logging.info("Evaluation metrics:")
for metric, value in metrics.items():
logging.info(f"{metric}: {value:.3f}")
if training_args.output_dir is not None:
output_eval_file = os.path.join(training_args.output_dir, "all_results.json")
with open(output_eval_file, "w") as writer:
writer.write(json.dumps(metrics))
# endregion
# region Prediction
if training_args.do_predict:
logger.info("*** Predict ***")
test_predictions = model.predict(predict_dataset)
if isinstance(test_predictions.start_logits, tf.RaggedTensor):
# If predictions are RaggedTensor, we densify them. Since they are logits, padding with 0 is a bad idea!
# The reason is that a logit of 0 can often end up as quite a high probability value, sometimes even
# the highest probability in a sample. Instead, we use a large negative value, which ensures that the
# padding positions are correctly masked.
test_start_logits = test_predictions.start_logits.to_tensor(default_value=-1000).numpy()
test_end_logits = test_predictions.end_logits.to_tensor(default_value=-1000).numpy()
else:
test_start_logits = test_predictions.start_logits
test_end_logits = test_predictions.end_logits
post_processed_test = post_processing_function(
datasets["test"],
processed_datasets["test"],
(test_start_logits, test_end_logits),
)
metrics = compute_metrics(post_processed_test)
logging.info("Test metrics:")
for metric, value in metrics.items():
logging.info(f"{metric}: {value:.3f}")
# endregion
if training_args.output_dir is not None and not training_args.push_to_hub:
# If we're not pushing to hub, at least save a local copy when we're done
model.save_pretrained(training_args.output_dir)
if __name__ == "__main__":
main()
| transformers/examples/tensorflow/question-answering/run_qa.py/0 | {
"file_path": "transformers/examples/tensorflow/question-answering/run_qa.py",
"repo_id": "transformers",
"token_count": 15980
} | 293 |
# Copyright 2020 The HuggingFace Team. All rights reserved.
#
# Licensed under the Apache License, Version 2.0 (the "License");
# you may not use this file except in compliance with the License.
# You may obtain a copy of the License at
#
# http://www.apache.org/licenses/LICENSE-2.0
#
# Unless required by applicable law or agreed to in writing, software
# distributed under the License is distributed on an "AS IS" BASIS,
# WITHOUT WARRANTIES OR CONDITIONS OF ANY KIND, either express or implied.
# See the License for the specific language governing permissions and
# limitations under the License.
import os
import sys
SRC_DIR = os.path.join(os.path.dirname(__file__), "src")
sys.path.append(SRC_DIR)
from transformers import (
AutoConfig,
AutoModel,
AutoModelForCausalLM,
AutoModelForMaskedLM,
AutoModelForQuestionAnswering,
AutoModelForSequenceClassification,
AutoTokenizer,
add_start_docstrings,
)
dependencies = ["torch", "numpy", "tokenizers", "filelock", "requests", "tqdm", "regex", "sentencepiece", "sacremoses", "importlib_metadata", "huggingface_hub"]
@add_start_docstrings(AutoConfig.__doc__)
def config(*args, **kwargs):
r"""
# Using torch.hub !
import torch
config = torch.hub.load('huggingface/transformers', 'config', 'google-bert/bert-base-uncased') # Download configuration from huggingface.co and cache.
config = torch.hub.load('huggingface/transformers', 'config', './test/bert_saved_model/') # E.g. config (or model) was saved using `save_pretrained('./test/saved_model/')`
config = torch.hub.load('huggingface/transformers', 'config', './test/bert_saved_model/my_configuration.json')
config = torch.hub.load('huggingface/transformers', 'config', 'google-bert/bert-base-uncased', output_attentions=True, foo=False)
assert config.output_attentions == True
config, unused_kwargs = torch.hub.load('huggingface/transformers', 'config', 'google-bert/bert-base-uncased', output_attentions=True, foo=False, return_unused_kwargs=True)
assert config.output_attentions == True
assert unused_kwargs == {'foo': False}
"""
return AutoConfig.from_pretrained(*args, **kwargs)
@add_start_docstrings(AutoTokenizer.__doc__)
def tokenizer(*args, **kwargs):
r"""
# Using torch.hub !
import torch
tokenizer = torch.hub.load('huggingface/transformers', 'tokenizer', 'google-bert/bert-base-uncased') # Download vocabulary from huggingface.co and cache.
tokenizer = torch.hub.load('huggingface/transformers', 'tokenizer', './test/bert_saved_model/') # E.g. tokenizer was saved using `save_pretrained('./test/saved_model/')`
"""
return AutoTokenizer.from_pretrained(*args, **kwargs)
@add_start_docstrings(AutoModel.__doc__)
def model(*args, **kwargs):
r"""
# Using torch.hub !
import torch
model = torch.hub.load('huggingface/transformers', 'model', 'google-bert/bert-base-uncased') # Download model and configuration from huggingface.co and cache.
model = torch.hub.load('huggingface/transformers', 'model', './test/bert_model/') # E.g. model was saved using `save_pretrained('./test/saved_model/')`
model = torch.hub.load('huggingface/transformers', 'model', 'google-bert/bert-base-uncased', output_attentions=True) # Update configuration during loading
assert model.config.output_attentions == True
# Loading from a TF checkpoint file instead of a PyTorch model (slower)
config = AutoConfig.from_pretrained('./tf_model/bert_tf_model_config.json')
model = torch.hub.load('huggingface/transformers', 'model', './tf_model/bert_tf_checkpoint.ckpt.index', from_tf=True, config=config)
"""
return AutoModel.from_pretrained(*args, **kwargs)
@add_start_docstrings(AutoModelForCausalLM.__doc__)
def modelForCausalLM(*args, **kwargs):
r"""
# Using torch.hub !
import torch
model = torch.hub.load('huggingface/transformers', 'modelForCausalLM', 'openai-community/gpt2') # Download model and configuration from huggingface.co and cache.
model = torch.hub.load('huggingface/transformers', 'modelForCausalLM', './test/saved_model/') # E.g. model was saved using `save_pretrained('./test/saved_model/')`
model = torch.hub.load('huggingface/transformers', 'modelForCausalLM', 'openai-community/gpt2', output_attentions=True) # Update configuration during loading
assert model.config.output_attentions == True
# Loading from a TF checkpoint file instead of a PyTorch model (slower)
config = AutoConfig.from_pretrained('./tf_model/gpt_tf_model_config.json')
model = torch.hub.load('huggingface/transformers', 'modelForCausalLM', './tf_model/gpt_tf_checkpoint.ckpt.index', from_tf=True, config=config)
"""
return AutoModelForCausalLM.from_pretrained(*args, **kwargs)
@add_start_docstrings(AutoModelForMaskedLM.__doc__)
def modelForMaskedLM(*args, **kwargs):
r"""
# Using torch.hub !
import torch
model = torch.hub.load('huggingface/transformers', 'modelForMaskedLM', 'google-bert/bert-base-uncased') # Download model and configuration from huggingface.co and cache.
model = torch.hub.load('huggingface/transformers', 'modelForMaskedLM', './test/bert_model/') # E.g. model was saved using `save_pretrained('./test/saved_model/')`
model = torch.hub.load('huggingface/transformers', 'modelForMaskedLM', 'google-bert/bert-base-uncased', output_attentions=True) # Update configuration during loading
assert model.config.output_attentions == True
# Loading from a TF checkpoint file instead of a PyTorch model (slower)
config = AutoConfig.from_pretrained('./tf_model/bert_tf_model_config.json')
model = torch.hub.load('huggingface/transformers', 'modelForMaskedLM', './tf_model/bert_tf_checkpoint.ckpt.index', from_tf=True, config=config)
"""
return AutoModelForMaskedLM.from_pretrained(*args, **kwargs)
@add_start_docstrings(AutoModelForSequenceClassification.__doc__)
def modelForSequenceClassification(*args, **kwargs):
r"""
# Using torch.hub !
import torch
model = torch.hub.load('huggingface/transformers', 'modelForSequenceClassification', 'google-bert/bert-base-uncased') # Download model and configuration from huggingface.co and cache.
model = torch.hub.load('huggingface/transformers', 'modelForSequenceClassification', './test/bert_model/') # E.g. model was saved using `save_pretrained('./test/saved_model/')`
model = torch.hub.load('huggingface/transformers', 'modelForSequenceClassification', 'google-bert/bert-base-uncased', output_attentions=True) # Update configuration during loading
assert model.config.output_attentions == True
# Loading from a TF checkpoint file instead of a PyTorch model (slower)
config = AutoConfig.from_pretrained('./tf_model/bert_tf_model_config.json')
model = torch.hub.load('huggingface/transformers', 'modelForSequenceClassification', './tf_model/bert_tf_checkpoint.ckpt.index', from_tf=True, config=config)
"""
return AutoModelForSequenceClassification.from_pretrained(*args, **kwargs)
@add_start_docstrings(AutoModelForQuestionAnswering.__doc__)
def modelForQuestionAnswering(*args, **kwargs):
r"""
# Using torch.hub !
import torch
model = torch.hub.load('huggingface/transformers', 'modelForQuestionAnswering', 'google-bert/bert-base-uncased') # Download model and configuration from huggingface.co and cache.
model = torch.hub.load('huggingface/transformers', 'modelForQuestionAnswering', './test/bert_model/') # E.g. model was saved using `save_pretrained('./test/saved_model/')`
model = torch.hub.load('huggingface/transformers', 'modelForQuestionAnswering', 'google-bert/bert-base-uncased', output_attentions=True) # Update configuration during loading
assert model.config.output_attentions == True
# Loading from a TF checkpoint file instead of a PyTorch model (slower)
config = AutoConfig.from_pretrained('./tf_model/bert_tf_model_config.json')
model = torch.hub.load('huggingface/transformers', 'modelForQuestionAnswering', './tf_model/bert_tf_checkpoint.ckpt.index', from_tf=True, config=config)
"""
return AutoModelForQuestionAnswering.from_pretrained(*args, **kwargs)
| transformers/hubconf.py/0 | {
"file_path": "transformers/hubconf.py",
"repo_id": "transformers",
"token_count": 3244
} | 294 |
# coding=utf-8
# Copyright 2018 The HuggingFace Inc. team.
# Copyright (c) 2018, NVIDIA CORPORATION. All rights reserved.
#
# Licensed under the Apache License, Version 2.0 (the "License");
# you may not use this file except in compliance with the License.
# You may obtain a copy of the License at
#
# http://www.apache.org/licenses/LICENSE-2.0
#
# Unless required by applicable law or agreed to in writing, software
# distributed under the License is distributed on an "AS IS" BASIS,
# WITHOUT WARRANTIES OR CONDITIONS OF ANY KIND, either express or implied.
# See the License for the specific language governing permissions and
# limitations under the License.
from dataclasses import dataclass, field
from typing import Tuple
from ..utils import cached_property, is_tf_available, logging, requires_backends
from .benchmark_args_utils import BenchmarkArguments
if is_tf_available():
import tensorflow as tf
logger = logging.get_logger(__name__)
@dataclass
class TensorFlowBenchmarkArguments(BenchmarkArguments):
deprecated_args = [
"no_inference",
"no_cuda",
"no_tpu",
"no_speed",
"no_memory",
"no_env_print",
"no_multi_process",
]
def __init__(self, **kwargs):
"""
This __init__ is there for legacy code. When removing deprecated args completely, the class can simply be
deleted
"""
for deprecated_arg in self.deprecated_args:
if deprecated_arg in kwargs:
positive_arg = deprecated_arg[3:]
kwargs[positive_arg] = not kwargs.pop(deprecated_arg)
logger.warning(
f"{deprecated_arg} is depreciated. Please use --no-{positive_arg} or"
f" {positive_arg}={kwargs[positive_arg]}"
)
self.tpu_name = kwargs.pop("tpu_name", self.tpu_name)
self.device_idx = kwargs.pop("device_idx", self.device_idx)
self.eager_mode = kwargs.pop("eager_mode", self.eager_mode)
self.use_xla = kwargs.pop("use_xla", self.use_xla)
super().__init__(**kwargs)
tpu_name: str = field(
default=None,
metadata={"help": "Name of TPU"},
)
device_idx: int = field(
default=0,
metadata={"help": "CPU / GPU device index. Defaults to 0."},
)
eager_mode: bool = field(default=False, metadata={"help": "Benchmark models in eager model."})
use_xla: bool = field(
default=False,
metadata={
"help": "Benchmark models using XLA JIT compilation. Note that `eager_model` has to be set to `False`."
},
)
@cached_property
def _setup_tpu(self) -> Tuple["tf.distribute.cluster_resolver.TPUClusterResolver"]:
requires_backends(self, ["tf"])
tpu = None
if self.tpu:
try:
if self.tpu_name:
tpu = tf.distribute.cluster_resolver.TPUClusterResolver(self.tpu_name)
else:
tpu = tf.distribute.cluster_resolver.TPUClusterResolver()
except ValueError:
tpu = None
return tpu
@cached_property
def _setup_strategy(self) -> Tuple["tf.distribute.Strategy", "tf.distribute.cluster_resolver.TPUClusterResolver"]:
requires_backends(self, ["tf"])
if self.is_tpu:
tf.config.experimental_connect_to_cluster(self._setup_tpu)
tf.tpu.experimental.initialize_tpu_system(self._setup_tpu)
strategy = tf.distribute.TPUStrategy(self._setup_tpu)
else:
# currently no multi gpu is allowed
if self.is_gpu:
# TODO: Currently only single GPU is supported
tf.config.set_visible_devices(self.gpu_list[self.device_idx], "GPU")
strategy = tf.distribute.OneDeviceStrategy(device=f"/gpu:{self.device_idx}")
else:
tf.config.set_visible_devices([], "GPU") # disable GPU
strategy = tf.distribute.OneDeviceStrategy(device=f"/cpu:{self.device_idx}")
return strategy
@property
def is_tpu(self) -> bool:
requires_backends(self, ["tf"])
return self._setup_tpu is not None
@property
def strategy(self) -> "tf.distribute.Strategy":
requires_backends(self, ["tf"])
return self._setup_strategy
@property
def gpu_list(self):
requires_backends(self, ["tf"])
return tf.config.list_physical_devices("GPU")
@property
def n_gpu(self) -> int:
requires_backends(self, ["tf"])
if self.cuda:
return len(self.gpu_list)
return 0
@property
def is_gpu(self) -> bool:
return self.n_gpu > 0
| transformers/src/transformers/benchmark/benchmark_args_tf.py/0 | {
"file_path": "transformers/src/transformers/benchmark/benchmark_args_tf.py",
"repo_id": "transformers",
"token_count": 2097
} | 295 |
#!/usr/bin/env python
# Copyright 2020 The HuggingFace Team. All rights reserved.
#
# Licensed under the Apache License, Version 2.0 (the "License");
# you may not use this file except in compliance with the License.
# You may obtain a copy of the License at
#
# http://www.apache.org/licenses/LICENSE-2.0
#
# Unless required by applicable law or agreed to in writing, software
# distributed under the License is distributed on an "AS IS" BASIS,
# WITHOUT WARRANTIES OR CONDITIONS OF ANY KIND, either express or implied.
# See the License for the specific language governing permissions and
# limitations under the License.
from argparse import ArgumentParser
from .add_new_model import AddNewModelCommand
from .add_new_model_like import AddNewModelLikeCommand
from .convert import ConvertCommand
from .download import DownloadCommand
from .env import EnvironmentCommand
from .lfs import LfsCommands
from .pt_to_tf import PTtoTFCommand
from .run import RunCommand
from .serving import ServeCommand
from .user import UserCommands
def main():
parser = ArgumentParser("Transformers CLI tool", usage="transformers-cli <command> [<args>]")
commands_parser = parser.add_subparsers(help="transformers-cli command helpers")
# Register commands
ConvertCommand.register_subcommand(commands_parser)
DownloadCommand.register_subcommand(commands_parser)
EnvironmentCommand.register_subcommand(commands_parser)
RunCommand.register_subcommand(commands_parser)
ServeCommand.register_subcommand(commands_parser)
UserCommands.register_subcommand(commands_parser)
AddNewModelCommand.register_subcommand(commands_parser)
AddNewModelLikeCommand.register_subcommand(commands_parser)
LfsCommands.register_subcommand(commands_parser)
PTtoTFCommand.register_subcommand(commands_parser)
# Let's go
args = parser.parse_args()
if not hasattr(args, "func"):
parser.print_help()
exit(1)
# Run
service = args.func(args)
service.run()
if __name__ == "__main__":
main()
| transformers/src/transformers/commands/transformers_cli.py/0 | {
"file_path": "transformers/src/transformers/commands/transformers_cli.py",
"repo_id": "transformers",
"token_count": 625
} | 296 |
# coding=utf-8
# Copyright 2023 The HuggingFace Inc. team.
#
# Licensed under the Apache License, Version 2.0 (the "License");
# you may not use this file except in compliance with the License.
# You may obtain a copy of the License at
#
# http://www.apache.org/licenses/LICENSE-2.0
#
# Unless required by applicable law or agreed to in writing, software
# distributed under the License is distributed on an "AS IS" BASIS,
# WITHOUT WARRANTIES OR CONDITIONS OF ANY KIND, either express or implied.
# See the License for the specific language governing permissions and
# limitations under the License.
import copy
from typing import TYPE_CHECKING, Any, Dict, Optional, Tuple
import torch
if TYPE_CHECKING:
from ..modeling_utils import PreTrainedModel
from .configuration_utils import GenerationConfig
from .logits_process import LogitsProcessorList
class CandidateGenerator:
"""Abstract base class for all candidate generators that can be applied during assisted generation."""
def get_candidates(self, input_ids: torch.LongTensor) -> Tuple[torch.LongTensor, Optional[torch.FloatTensor]]:
"""
Fetches the candidates to be tried for the current input.
Args:
input_ids (`torch.LongTensor` of shape `(batch_size, sequence_length)`):
Indices of input sequence tokens in the vocabulary. [What are input IDs?](../glossary#input-ids)
Return:
`torch.LongTensor` of shape `(batch_size, candidate_length)` containing the candidate sequences to be
assessed by the model and, optionally, a `torch.FloatTensor` of shape `(batch_size, candidate_length,
vocabulary_size)` containing the logits associated to each candidate.
"""
raise NotImplementedError(
f"{self.__class__} is an abstract class. Only classes inheriting this class can call `get_candidates`."
)
def update_candidate_strategy(self, input_ids: torch.LongTensor, scores: torch.FloatTensor, num_matches: int):
"""
Updates the candidate generation strategy based on the outcomes.
Args:
input_ids (`torch.LongTensor` of shape `(batch_size, sequence_length)`):
Indices of input sequence tokens in the vocabulary. [What are input IDs?](../glossary#input-ids)
scores (`torch.FloatTensor` of shape `(batch_size, candidate_length, config.vocab_size)`):
Prediction scores of a language modeling head. These can be logits for each vocabulary when not using
beam search or log softmax for each vocabulary token when using beam search
num_matches (`int`):
The number of matches between the candidate sequences and the model predictions.
"""
raise NotImplementedError(
f"{self.__class__} is an abstract class. Only classes inheriting this class can call "
"`update_candidate_strategy`."
)
class AssistedCandidateGenerator(CandidateGenerator):
"""
`CandidateGenerator` class to be used for assisted generation and speculative decoding. This class generates
candidates through the use of a smaller model. Read the following blog post for more information:
https://huggingface.co/blog/assisted-generation
Args:
input_ids (`torch.LongTensor` of shape `(batch_size, sequence_length)`):
Indices of input sequence tokens in the vocabulary. [What are input IDs?](../glossary#input-ids)
assistant_model (`PreTrainedModel`):
The model to be used for generating candidates. This model should be smaller than the main model.
generation_config (`~generation.GenerationConfig`, *optional*):
The generation configuration to be used as base parametrization for the generation call.
logits_processor (`LogitsProcessorList`):
An instance of [`LogitsProcessorList`]. List of instances of class derived from [`LogitsProcessor`]
used to modify the prediction scores of the language modeling head applied at each generation step.
model_kwargs (`Dict`):
The keyword arguments that will be passed to the main model, and are used as base inputs for the assistant
model as well.
inputs_tensor (`torch.Tensor`, *optional*):
The model input tensor. In encoder-decoder models, this is the encoder input.
"""
def __init__(
self,
input_ids: torch.LongTensor,
assistant_model: "PreTrainedModel",
generation_config: "GenerationConfig",
logits_processor: "LogitsProcessorList",
model_kwargs: Dict,
inputs_tensor: Optional[torch.Tensor] = None,
):
# Make sure all data at the same device as assistant model
device = assistant_model.device
input_ids = input_ids.to(device)
if inputs_tensor is not None:
inputs_tensor = inputs_tensor.to(device)
# Prepare the assistant and the starting number of candidate tokens
self.assistant_model = assistant_model
self.num_assistant_tokens = assistant_model.generation_config.num_assistant_tokens
# Prepare the kwargs for the assistant model
assistant_kwargs = {}
for key, value in model_kwargs.items(): # deepcopy crashes if we attempt to copy encoder outputs with grads
if key not in ("encoder_outputs", "assistant_encoder_outputs"):
assistant_kwargs[key] = (
value.detach().to(device) if isinstance(value, torch.Tensor) else copy.deepcopy(value)
)
if "assistant_encoder_outputs" in model_kwargs:
assistant_kwargs["encoder_outputs"] = model_kwargs["assistant_encoder_outputs"]
elif assistant_model.config.is_encoder_decoder:
inputs_tensor, model_input_name, assistant_kwargs = assistant_model._prepare_model_inputs(
inputs_tensor, assistant_model.generation_config.bos_token_id, assistant_kwargs
)
assistant_kwargs = assistant_model._prepare_encoder_decoder_kwargs_for_generation(
inputs_tensor, assistant_kwargs, model_input_name
)
elif "encoder_outputs" in model_kwargs:
assistant_kwargs["encoder_outputs"] = model_kwargs["encoder_outputs"]
self.assistant_kwargs = assistant_kwargs
# Prepare assistant model's keys of inputs
if assistant_model.config.is_encoder_decoder:
# both are encoder-decoder
self.input_ids_key = "decoder_input_ids"
self.attention_key = "decoder_attention_mask"
elif "encoder_outputs" in assistant_kwargs:
# special case for encoder-decoder with decoder-only assistant (like DistilWhisper)
self.input_ids_key = "input_ids"
self.attention_key = "attention_mask"
self.assistant_kwargs["attention_mask"] = self.assistant_kwargs.get(
"decoder_attention_mask",
torch.ones((input_ids.shape[0], 1), device=input_ids.device, dtype=torch.long),
)
else:
# both are decoder-only
self.input_ids_key = "input_ids"
self.attention_key = "attention_mask"
# Prepare generation-related options.
eos_token_id = generation_config.eos_token_id
if isinstance(eos_token_id, int):
eos_token_id = [eos_token_id]
self.eos_token_id_tensor = (
torch.tensor(eos_token_id).to(input_ids.device) if eos_token_id is not None else None
)
self.logits_processor = logits_processor
self.generation_config = copy.deepcopy(generation_config)
self.generation_config.return_dict_in_generate = True
self.generation_config.output_scores = True
def get_candidates(self, input_ids: torch.LongTensor) -> Tuple[torch.LongTensor, Optional[torch.FloatTensor]]:
"""
Fetches the candidates to be tried for the current input.
Args:
input_ids (`torch.LongTensor` of shape `(batch_size, sequence_length)`):
Indices of input sequence tokens in the vocabulary. [What are input IDs?](../glossary#input-ids)
Return:
`torch.LongTensor` of shape `(batch_size, candidate_length)` containing the candidate sequences to be
assessed by the model and a `torch.FloatTensor` of shape `(batch_size, candidate_length,
vocabulary_size)` containing the logits associated to each candidate.
"""
input_ids = input_ids.to(self.assistant_model.device)
# Don't generate more than `max_length - 1` candidates since the target model generates one extra token.
new_cur_len = input_ids.shape[-1]
max_new_tokens = min(int(self.num_assistant_tokens), self.generation_config.max_length - new_cur_len - 1)
if max_new_tokens == 0:
return input_ids, None
# 1. If it is not the first round of candidate generation, prepare the inputs based on the input_ids length
# (which implicitly contains the number of accepted candidates from the previous round)
has_past_key_values = self.assistant_kwargs.get("past_key_values", None) is not None
if has_past_key_values:
new_cache_size = new_cur_len - 1
self.assistant_kwargs["past_key_values"] = _crop_past_key_values(
self.assistant_model, self.assistant_kwargs["past_key_values"], new_cache_size - 1
) # the assistant does not have the token after the last match, hence the -1
self.assistant_kwargs = _prepare_attention_mask(
self.assistant_kwargs, new_cur_len, self.assistant_model.config.is_encoder_decoder
)
self.assistant_kwargs = _prepare_token_type_ids(self.assistant_kwargs, new_cur_len)
# 2. Forecast next N tokens using the assistant model.
assistant_generation_kwargs = {
self.input_ids_key: input_ids,
"max_new_tokens": max_new_tokens,
"generation_config": self.generation_config,
"logits_processor": self.logits_processor,
}
assistant_output = self.assistant_model.generate(**assistant_generation_kwargs, **self.assistant_kwargs)
# 3. Update variables for the next round of candidate generation
self.assistant_kwargs["past_key_values"] = assistant_output.past_key_values
# 4. Prepare variables for output
candidate_logits = torch.stack(assistant_output.scores, dim=1)
candidate_ids = assistant_output.sequences
return candidate_ids, candidate_logits
def update_candidate_strategy(self, input_ids: torch.LongTensor, scores: torch.FloatTensor, num_matches: int):
"""
Updates the candidate generation strategy based on the outcomes.
Args:
input_ids (`torch.LongTensor` of shape `(batch_size, sequence_length)`):
Indices of input sequence tokens in the vocabulary. [What are input IDs?](../glossary#input-ids)
scores (`torch.FloatTensor` of shape `(batch_size, candidate_length, config.vocab_size)`):
Prediction scores of a language modeling head. These can be logits for each vocabulary when not using
beam search or log softmax for each vocabulary token when using beam search
num_matches (`int`):
The number of matches between the candidate sequences and the model predictions.
"""
# Adjust the max number of assistant tokens to use in the next iteration. This is a simple heuristic,
# probably can be improved -- we want to balance the benefits of getting assistant tokens correct with the
# cost of forecasting incorrect assistant tokens.
if self.assistant_model.generation_config.num_assistant_tokens_schedule in {
"heuristic",
"heuristic_transient",
}:
if num_matches == int(self.num_assistant_tokens):
self.num_assistant_tokens += 2.0
else:
self.num_assistant_tokens = max(1.0, self.num_assistant_tokens - 1.0)
class PromptLookupCandidateGenerator(CandidateGenerator):
"""
`CandidateGenerator` class to be used for prompt lookup generation. This class generates candidates by looking up
likely continuations in the provided prompt (input_ids) itself.
Read the following blog post for more information: https://github.com/apoorvumang/prompt-lookup-decoding
Args:
max_matching_ngram_size (`int`):
The maximum ngram size to be considered for matching in the prompt
num_output_tokens (`int`):
The number of tokens to be output as candidate tokens.
"""
def __init__(
self,
num_output_tokens: int = 10,
max_matching_ngram_size: int = None,
):
self.num_output_tokens = num_output_tokens
self.max_matching_ngram_size = max_matching_ngram_size if max_matching_ngram_size else 2
if self.max_matching_ngram_size <= 0 or self.num_output_tokens <= 0:
raise ValueError("Invalid max_matching_ngram_size or num_output_tokens")
def get_candidates(self, input_ids: torch.LongTensor) -> Tuple[torch.LongTensor, Optional[torch.FloatTensor]]:
"""
Fetches the candidates to be tried for the current input.
Args:
input_ids (`torch.LongTensor` of shape `(batch_size, sequence_length)`):
Indices of input sequence tokens in the vocabulary. [What are input IDs?](../glossary#input-ids)
Return:
`torch.LongTensor` of shape `(num_candidates, candidate_length)`: The candidate sequences to be tried.
"""
input_length = input_ids.size(1)
chosen_ids = None
match_found = False
for ngram_size in range(min(self.max_matching_ngram_size, input_length - 1), 0, -1):
# Create sliding windows of size ngram_size
windows = input_ids.unfold(dimension=1, size=ngram_size, step=1)
# Convert ngram to a tensor for comparison
ngram_tensor = input_ids[0, -ngram_size:]
# Find where the windows match the ngram
matches = (windows == ngram_tensor).all(dim=2)
# Get the indices of matches
match_indices = matches.nonzero(as_tuple=True)[1]
# Iterate through match indices to find a valid continuation
for idx in match_indices:
start_idx = idx + ngram_size
end_idx = start_idx + self.num_output_tokens
end_idx = min(end_idx, input_length)
if start_idx < end_idx:
chosen_ids = input_ids[0, start_idx:end_idx]
match_found = True
break
if match_found:
break
if chosen_ids is None or len(chosen_ids) == 0:
# In case we didn't find a match return the input sequence unchanged, reverts back to autoregressive decoding
return input_ids, None
# Now need extend input_ids with chosen_ids
chosen_ids = chosen_ids.unsqueeze(0)
candidate_input_ids = torch.cat((input_ids, chosen_ids), dim=1)
# assisted_generation expects logits as well, but we don't have those here, so returning None
return candidate_input_ids, None
def update_candidate_strategy(self, input_ids: torch.LongTensor, scores: torch.FloatTensor, num_matches: int):
"""
Updates the candidate generation strategy based on the outcomes.
Args:
input_ids (`torch.LongTensor` of shape `(batch_size, sequence_length)`):
Indices of input sequence tokens in the vocabulary. [What are input IDs?](../glossary#input-ids)
scores (`torch.FloatTensor` of shape `(batch_size, candidate_length, config.vocab_size)`):
Prediction scores of a language modeling head. These can be logits for each vocabulary when not using
beam search or log softmax for each vocabulary token when using beam search
num_matches (`int`):
The number of matches between the candidate sequences and the model predictions.
"""
# Currently does nothing
return
def _crop_past_key_values(model, past_key_values, maximum_length):
"""Crops the past key values up to a certain maximum length."""
new_past = []
if model.config.is_encoder_decoder:
for idx in range(len(past_key_values)):
new_past.append(
(
past_key_values[idx][0][:, :, :maximum_length, :],
past_key_values[idx][1][:, :, :maximum_length, :],
past_key_values[idx][2],
past_key_values[idx][3],
)
)
past_key_values = tuple(new_past)
# bloom is special
elif "bloom" in model.__class__.__name__.lower() or (
model.config.architectures is not None and "bloom" in model.config.architectures[0].lower()
):
for idx in range(len(past_key_values)):
new_past.append(
(
past_key_values[idx][0][:, :, :maximum_length],
past_key_values[idx][1][:, :maximum_length, :],
)
)
past_key_values = tuple(new_past)
# gptbigcode is too
elif "gptbigcode" in model.__class__.__name__.lower() or (
model.config.architectures is not None and "gptbigcode" in model.config.architectures[0].lower()
):
if model.config.multi_query:
for idx in range(len(past_key_values)):
past_key_values[idx] = past_key_values[idx][:, :maximum_length, :]
else:
for idx in range(len(past_key_values)):
past_key_values[idx] = past_key_values[idx][:, :, :maximum_length, :]
else:
for idx in range(len(past_key_values)):
new_past.append(
(
past_key_values[idx][0][:, :, :maximum_length, :],
past_key_values[idx][1][:, :, :maximum_length, :],
)
)
past_key_values = tuple(new_past)
return past_key_values
def _prepare_attention_mask(model_kwargs: Dict[str, Any], new_length: int, is_encoder_decoder: bool) -> Dict[str, Any]:
"""Expands or crops the model's mask for decoding purposes, to the defined length"""
mask_key = "decoder_attention_mask" if is_encoder_decoder else "attention_mask"
if mask_key not in model_kwargs:
return model_kwargs
mask = model_kwargs[mask_key]
mask_length_diff = new_length - mask.shape[1]
if mask_length_diff < 0:
model_kwargs[mask_key] = mask[:, :mask_length_diff]
elif mask_length_diff > 0:
model_kwargs[mask_key] = torch.cat([mask, mask.new_ones((mask.shape[0], mask_length_diff))], dim=-1)
return model_kwargs
def _prepare_token_type_ids(model_kwargs: Dict[str, Any], new_length: int) -> Dict[str, Any]:
"""Expands or crops the model's token_type_ids for decoding purposes, to the defined length"""
if "token_type_ids" not in model_kwargs or model_kwargs["token_type_ids"] is None:
return model_kwargs
token_type_ids = model_kwargs["token_type_ids"]
final_token_type = token_type_ids[:, -1].unsqueeze(-1)
type_length_diff = new_length - token_type_ids.shape[1]
if type_length_diff < 0:
token_type_ids = token_type_ids[:, :type_length_diff]
elif type_length_diff > 0:
token_type_copies = final_token_type.repeat(1, type_length_diff)
model_kwargs["token_type_ids"] = torch.cat([model_kwargs["token_type_ids"], token_type_copies], dim=-1)
return model_kwargs
| transformers/src/transformers/generation/candidate_generator.py/0 | {
"file_path": "transformers/src/transformers/generation/candidate_generator.py",
"repo_id": "transformers",
"token_count": 8151
} | 297 |
# coding=utf-8
# Copyright 2022 The HuggingFace Inc. team.
#
# Licensed under the Apache License, Version 2.0 (the "License");
# you may not use this file except in compliance with the License.
# You may obtain a copy of the License at
#
# http://www.apache.org/licenses/LICENSE-2.0
#
# Unless required by applicable law or agreed to in writing, software
# distributed under the License is distributed on an "AS IS" BASIS,
# WITHOUT WARRANTIES OR CONDITIONS OF ANY KIND, either express or implied.
# See the License for the specific language governing permissions and
# limitations under the License.
import warnings
from typing import Iterable, List, Optional, Tuple, Union
import numpy as np
from .image_utils import (
ChannelDimension,
ImageInput,
get_channel_dimension_axis,
get_image_size,
infer_channel_dimension_format,
)
from .utils import ExplicitEnum, TensorType, is_jax_tensor, is_tf_tensor, is_torch_tensor
from .utils.import_utils import (
is_flax_available,
is_tf_available,
is_torch_available,
is_vision_available,
requires_backends,
)
if is_vision_available():
import PIL
from .image_utils import PILImageResampling
if is_torch_available():
import torch
if is_tf_available():
import tensorflow as tf
if is_flax_available():
import jax.numpy as jnp
def to_channel_dimension_format(
image: np.ndarray,
channel_dim: Union[ChannelDimension, str],
input_channel_dim: Optional[Union[ChannelDimension, str]] = None,
) -> np.ndarray:
"""
Converts `image` to the channel dimension format specified by `channel_dim`.
Args:
image (`numpy.ndarray`):
The image to have its channel dimension set.
channel_dim (`ChannelDimension`):
The channel dimension format to use.
input_channel_dim (`ChannelDimension`, *optional*):
The channel dimension format of the input image. If not provided, it will be inferred from the input image.
Returns:
`np.ndarray`: The image with the channel dimension set to `channel_dim`.
"""
if not isinstance(image, np.ndarray):
raise ValueError(f"Input image must be of type np.ndarray, got {type(image)}")
if input_channel_dim is None:
input_channel_dim = infer_channel_dimension_format(image)
target_channel_dim = ChannelDimension(channel_dim)
if input_channel_dim == target_channel_dim:
return image
if target_channel_dim == ChannelDimension.FIRST:
image = image.transpose((2, 0, 1))
elif target_channel_dim == ChannelDimension.LAST:
image = image.transpose((1, 2, 0))
else:
raise ValueError("Unsupported channel dimension format: {}".format(channel_dim))
return image
def rescale(
image: np.ndarray,
scale: float,
data_format: Optional[ChannelDimension] = None,
dtype: np.dtype = np.float32,
input_data_format: Optional[Union[str, ChannelDimension]] = None,
) -> np.ndarray:
"""
Rescales `image` by `scale`.
Args:
image (`np.ndarray`):
The image to rescale.
scale (`float`):
The scale to use for rescaling the image.
data_format (`ChannelDimension`, *optional*):
The channel dimension format of the image. If not provided, it will be the same as the input image.
dtype (`np.dtype`, *optional*, defaults to `np.float32`):
The dtype of the output image. Defaults to `np.float32`. Used for backwards compatibility with feature
extractors.
input_data_format (`ChannelDimension`, *optional*):
The channel dimension format of the input image. If not provided, it will be inferred from the input image.
Returns:
`np.ndarray`: The rescaled image.
"""
if not isinstance(image, np.ndarray):
raise ValueError(f"Input image must be of type np.ndarray, got {type(image)}")
rescaled_image = image * scale
if data_format is not None:
rescaled_image = to_channel_dimension_format(rescaled_image, data_format, input_data_format)
rescaled_image = rescaled_image.astype(dtype)
return rescaled_image
def _rescale_for_pil_conversion(image):
"""
Detects whether or not the image needs to be rescaled before being converted to a PIL image.
The assumption is that if the image is of type `np.float` and all values are between 0 and 1, it needs to be
rescaled.
"""
if image.dtype == np.uint8:
do_rescale = False
elif np.allclose(image, image.astype(int)):
if np.all(0 <= image) and np.all(image <= 255):
do_rescale = False
else:
raise ValueError(
"The image to be converted to a PIL image contains values outside the range [0, 255], "
f"got [{image.min()}, {image.max()}] which cannot be converted to uint8."
)
elif np.all(0 <= image) and np.all(image <= 1):
do_rescale = True
else:
raise ValueError(
"The image to be converted to a PIL image contains values outside the range [0, 1], "
f"got [{image.min()}, {image.max()}] which cannot be converted to uint8."
)
return do_rescale
def to_pil_image(
image: Union[np.ndarray, "PIL.Image.Image", "torch.Tensor", "tf.Tensor", "jnp.ndarray"],
do_rescale: Optional[bool] = None,
input_data_format: Optional[Union[str, ChannelDimension]] = None,
) -> "PIL.Image.Image":
"""
Converts `image` to a PIL Image. Optionally rescales it and puts the channel dimension back as the last axis if
needed.
Args:
image (`PIL.Image.Image` or `numpy.ndarray` or `torch.Tensor` or `tf.Tensor`):
The image to convert to the `PIL.Image` format.
do_rescale (`bool`, *optional*):
Whether or not to apply the scaling factor (to make pixel values integers between 0 and 255). Will default
to `True` if the image type is a floating type and casting to `int` would result in a loss of precision,
and `False` otherwise.
input_data_format (`ChannelDimension`, *optional*):
The channel dimension format of the input image. If unset, will use the inferred format from the input.
Returns:
`PIL.Image.Image`: The converted image.
"""
requires_backends(to_pil_image, ["vision"])
if isinstance(image, PIL.Image.Image):
return image
# Convert all tensors to numpy arrays before converting to PIL image
if is_torch_tensor(image) or is_tf_tensor(image):
image = image.numpy()
elif is_jax_tensor(image):
image = np.array(image)
elif not isinstance(image, np.ndarray):
raise ValueError("Input image type not supported: {}".format(type(image)))
# If the channel has been moved to first dim, we put it back at the end.
image = to_channel_dimension_format(image, ChannelDimension.LAST, input_data_format)
# If there is a single channel, we squeeze it, as otherwise PIL can't handle it.
image = np.squeeze(image, axis=-1) if image.shape[-1] == 1 else image
# PIL.Image can only store uint8 values so we rescale the image to be between 0 and 255 if needed.
do_rescale = _rescale_for_pil_conversion(image) if do_rescale is None else do_rescale
if do_rescale:
image = rescale(image, 255)
image = image.astype(np.uint8)
return PIL.Image.fromarray(image)
# Logic adapted from torchvision resizing logic: https://github.com/pytorch/vision/blob/511924c1ced4ce0461197e5caa64ce5b9e558aab/torchvision/transforms/functional.py#L366
def get_resize_output_image_size(
input_image: np.ndarray,
size: Union[int, Tuple[int, int], List[int], Tuple[int]],
default_to_square: bool = True,
max_size: Optional[int] = None,
input_data_format: Optional[Union[str, ChannelDimension]] = None,
) -> tuple:
"""
Find the target (height, width) dimension of the output image after resizing given the input image and the desired
size.
Args:
input_image (`np.ndarray`):
The image to resize.
size (`int` or `Tuple[int, int]` or List[int] or Tuple[int]):
The size to use for resizing the image. If `size` is a sequence like (h, w), output size will be matched to
this.
If `size` is an int and `default_to_square` is `True`, then image will be resized to (size, size). If
`size` is an int and `default_to_square` is `False`, then smaller edge of the image will be matched to this
number. i.e, if height > width, then image will be rescaled to (size * height / width, size).
default_to_square (`bool`, *optional*, defaults to `True`):
How to convert `size` when it is a single int. If set to `True`, the `size` will be converted to a square
(`size`,`size`). If set to `False`, will replicate
[`torchvision.transforms.Resize`](https://pytorch.org/vision/stable/transforms.html#torchvision.transforms.Resize)
with support for resizing only the smallest edge and providing an optional `max_size`.
max_size (`int`, *optional*):
The maximum allowed for the longer edge of the resized image: if the longer edge of the image is greater
than `max_size` after being resized according to `size`, then the image is resized again so that the longer
edge is equal to `max_size`. As a result, `size` might be overruled, i.e the smaller edge may be shorter
than `size`. Only used if `default_to_square` is `False`.
input_data_format (`ChannelDimension`, *optional*):
The channel dimension format of the input image. If unset, will use the inferred format from the input.
Returns:
`tuple`: The target (height, width) dimension of the output image after resizing.
"""
if isinstance(size, (tuple, list)):
if len(size) == 2:
return tuple(size)
elif len(size) == 1:
# Perform same logic as if size was an int
size = size[0]
else:
raise ValueError("size must have 1 or 2 elements if it is a list or tuple")
if default_to_square:
return (size, size)
height, width = get_image_size(input_image, input_data_format)
short, long = (width, height) if width <= height else (height, width)
requested_new_short = size
new_short, new_long = requested_new_short, int(requested_new_short * long / short)
if max_size is not None:
if max_size <= requested_new_short:
raise ValueError(
f"max_size = {max_size} must be strictly greater than the requested "
f"size for the smaller edge size = {size}"
)
if new_long > max_size:
new_short, new_long = int(max_size * new_short / new_long), max_size
return (new_long, new_short) if width <= height else (new_short, new_long)
def resize(
image: np.ndarray,
size: Tuple[int, int],
resample: "PILImageResampling" = None,
reducing_gap: Optional[int] = None,
data_format: Optional[ChannelDimension] = None,
return_numpy: bool = True,
input_data_format: Optional[Union[str, ChannelDimension]] = None,
) -> np.ndarray:
"""
Resizes `image` to `(height, width)` specified by `size` using the PIL library.
Args:
image (`np.ndarray`):
The image to resize.
size (`Tuple[int, int]`):
The size to use for resizing the image.
resample (`int`, *optional*, defaults to `PILImageResampling.BILINEAR`):
The filter to user for resampling.
reducing_gap (`int`, *optional*):
Apply optimization by resizing the image in two steps. The bigger `reducing_gap`, the closer the result to
the fair resampling. See corresponding Pillow documentation for more details.
data_format (`ChannelDimension`, *optional*):
The channel dimension format of the output image. If unset, will use the inferred format from the input.
return_numpy (`bool`, *optional*, defaults to `True`):
Whether or not to return the resized image as a numpy array. If False a `PIL.Image.Image` object is
returned.
input_data_format (`ChannelDimension`, *optional*):
The channel dimension format of the input image. If unset, will use the inferred format from the input.
Returns:
`np.ndarray`: The resized image.
"""
requires_backends(resize, ["vision"])
resample = resample if resample is not None else PILImageResampling.BILINEAR
if not len(size) == 2:
raise ValueError("size must have 2 elements")
# For all transformations, we want to keep the same data format as the input image unless otherwise specified.
# The resized image from PIL will always have channels last, so find the input format first.
if input_data_format is None:
input_data_format = infer_channel_dimension_format(image)
data_format = input_data_format if data_format is None else data_format
# To maintain backwards compatibility with the resizing done in previous image feature extractors, we use
# the pillow library to resize the image and then convert back to numpy
do_rescale = False
if not isinstance(image, PIL.Image.Image):
do_rescale = _rescale_for_pil_conversion(image)
image = to_pil_image(image, do_rescale=do_rescale, input_data_format=input_data_format)
height, width = size
# PIL images are in the format (width, height)
resized_image = image.resize((width, height), resample=resample, reducing_gap=reducing_gap)
if return_numpy:
resized_image = np.array(resized_image)
# If the input image channel dimension was of size 1, then it is dropped when converting to a PIL image
# so we need to add it back if necessary.
resized_image = np.expand_dims(resized_image, axis=-1) if resized_image.ndim == 2 else resized_image
# The image is always in channels last format after converting from a PIL image
resized_image = to_channel_dimension_format(
resized_image, data_format, input_channel_dim=ChannelDimension.LAST
)
# If an image was rescaled to be in the range [0, 255] before converting to a PIL image, then we need to
# rescale it back to the original range.
resized_image = rescale(resized_image, 1 / 255) if do_rescale else resized_image
return resized_image
def normalize(
image: np.ndarray,
mean: Union[float, Iterable[float]],
std: Union[float, Iterable[float]],
data_format: Optional[ChannelDimension] = None,
input_data_format: Optional[Union[str, ChannelDimension]] = None,
) -> np.ndarray:
"""
Normalizes `image` using the mean and standard deviation specified by `mean` and `std`.
image = (image - mean) / std
Args:
image (`np.ndarray`):
The image to normalize.
mean (`float` or `Iterable[float]`):
The mean to use for normalization.
std (`float` or `Iterable[float]`):
The standard deviation to use for normalization.
data_format (`ChannelDimension`, *optional*):
The channel dimension format of the output image. If unset, will use the inferred format from the input.
input_data_format (`ChannelDimension`, *optional*):
The channel dimension format of the input image. If unset, will use the inferred format from the input.
"""
if not isinstance(image, np.ndarray):
raise ValueError("image must be a numpy array")
if input_data_format is None:
input_data_format = infer_channel_dimension_format(image)
channel_axis = get_channel_dimension_axis(image, input_data_format=input_data_format)
num_channels = image.shape[channel_axis]
# We cast to float32 to avoid errors that can occur when subtracting uint8 values.
# We preserve the original dtype if it is a float type to prevent upcasting float16.
if not np.issubdtype(image.dtype, np.floating):
image = image.astype(np.float32)
if isinstance(mean, Iterable):
if len(mean) != num_channels:
raise ValueError(f"mean must have {num_channels} elements if it is an iterable, got {len(mean)}")
else:
mean = [mean] * num_channels
mean = np.array(mean, dtype=image.dtype)
if isinstance(std, Iterable):
if len(std) != num_channels:
raise ValueError(f"std must have {num_channels} elements if it is an iterable, got {len(std)}")
else:
std = [std] * num_channels
std = np.array(std, dtype=image.dtype)
if input_data_format == ChannelDimension.LAST:
image = (image - mean) / std
else:
image = ((image.T - mean) / std).T
image = to_channel_dimension_format(image, data_format, input_data_format) if data_format is not None else image
return image
def center_crop(
image: np.ndarray,
size: Tuple[int, int],
data_format: Optional[Union[str, ChannelDimension]] = None,
input_data_format: Optional[Union[str, ChannelDimension]] = None,
return_numpy: Optional[bool] = None,
) -> np.ndarray:
"""
Crops the `image` to the specified `size` using a center crop. Note that if the image is too small to be cropped to
the size given, it will be padded (so the returned result will always be of size `size`).
Args:
image (`np.ndarray`):
The image to crop.
size (`Tuple[int, int]`):
The target size for the cropped image.
data_format (`str` or `ChannelDimension`, *optional*):
The channel dimension format for the output image. Can be one of:
- `"channels_first"` or `ChannelDimension.FIRST`: image in (num_channels, height, width) format.
- `"channels_last"` or `ChannelDimension.LAST`: image in (height, width, num_channels) format.
If unset, will use the inferred format of the input image.
input_data_format (`str` or `ChannelDimension`, *optional*):
The channel dimension format for the input image. Can be one of:
- `"channels_first"` or `ChannelDimension.FIRST`: image in (num_channels, height, width) format.
- `"channels_last"` or `ChannelDimension.LAST`: image in (height, width, num_channels) format.
If unset, will use the inferred format of the input image.
return_numpy (`bool`, *optional*):
Whether or not to return the cropped image as a numpy array. Used for backwards compatibility with the
previous ImageFeatureExtractionMixin method.
- Unset: will return the same type as the input image.
- `True`: will return a numpy array.
- `False`: will return a `PIL.Image.Image` object.
Returns:
`np.ndarray`: The cropped image.
"""
requires_backends(center_crop, ["vision"])
if return_numpy is not None:
warnings.warn("return_numpy is deprecated and will be removed in v.4.33", FutureWarning)
return_numpy = True if return_numpy is None else return_numpy
if not isinstance(image, np.ndarray):
raise ValueError(f"Input image must be of type np.ndarray, got {type(image)}")
if not isinstance(size, Iterable) or len(size) != 2:
raise ValueError("size must have 2 elements representing the height and width of the output image")
if input_data_format is None:
input_data_format = infer_channel_dimension_format(image)
output_data_format = data_format if data_format is not None else input_data_format
# We perform the crop in (C, H, W) format and then convert to the output format
image = to_channel_dimension_format(image, ChannelDimension.FIRST, input_data_format)
orig_height, orig_width = get_image_size(image, ChannelDimension.FIRST)
crop_height, crop_width = size
crop_height, crop_width = int(crop_height), int(crop_width)
# In case size is odd, (image_shape[0] + size[0]) // 2 won't give the proper result.
top = (orig_height - crop_height) // 2
bottom = top + crop_height
# In case size is odd, (image_shape[1] + size[1]) // 2 won't give the proper result.
left = (orig_width - crop_width) // 2
right = left + crop_width
# Check if cropped area is within image boundaries
if top >= 0 and bottom <= orig_height and left >= 0 and right <= orig_width:
image = image[..., top:bottom, left:right]
image = to_channel_dimension_format(image, output_data_format, ChannelDimension.FIRST)
return image
# Otherwise, we may need to pad if the image is too small. Oh joy...
new_height = max(crop_height, orig_height)
new_width = max(crop_width, orig_width)
new_shape = image.shape[:-2] + (new_height, new_width)
new_image = np.zeros_like(image, shape=new_shape)
# If the image is too small, pad it with zeros
top_pad = (new_height - orig_height) // 2
bottom_pad = top_pad + orig_height
left_pad = (new_width - orig_width) // 2
right_pad = left_pad + orig_width
new_image[..., top_pad:bottom_pad, left_pad:right_pad] = image
top += top_pad
bottom += top_pad
left += left_pad
right += left_pad
new_image = new_image[..., max(0, top) : min(new_height, bottom), max(0, left) : min(new_width, right)]
new_image = to_channel_dimension_format(new_image, output_data_format, ChannelDimension.FIRST)
if not return_numpy:
new_image = to_pil_image(new_image)
return new_image
def _center_to_corners_format_torch(bboxes_center: "torch.Tensor") -> "torch.Tensor":
center_x, center_y, width, height = bboxes_center.unbind(-1)
bbox_corners = torch.stack(
# top left x, top left y, bottom right x, bottom right y
[(center_x - 0.5 * width), (center_y - 0.5 * height), (center_x + 0.5 * width), (center_y + 0.5 * height)],
dim=-1,
)
return bbox_corners
def _center_to_corners_format_numpy(bboxes_center: np.ndarray) -> np.ndarray:
center_x, center_y, width, height = bboxes_center.T
bboxes_corners = np.stack(
# top left x, top left y, bottom right x, bottom right y
[center_x - 0.5 * width, center_y - 0.5 * height, center_x + 0.5 * width, center_y + 0.5 * height],
axis=-1,
)
return bboxes_corners
def _center_to_corners_format_tf(bboxes_center: "tf.Tensor") -> "tf.Tensor":
center_x, center_y, width, height = tf.unstack(bboxes_center, axis=-1)
bboxes_corners = tf.stack(
# top left x, top left y, bottom right x, bottom right y
[center_x - 0.5 * width, center_y - 0.5 * height, center_x + 0.5 * width, center_y + 0.5 * height],
axis=-1,
)
return bboxes_corners
# 2 functions below inspired by https://github.com/facebookresearch/detr/blob/master/util/box_ops.py
def center_to_corners_format(bboxes_center: TensorType) -> TensorType:
"""
Converts bounding boxes from center format to corners format.
center format: contains the coordinate for the center of the box and its width, height dimensions
(center_x, center_y, width, height)
corners format: contains the coodinates for the top-left and bottom-right corners of the box
(top_left_x, top_left_y, bottom_right_x, bottom_right_y)
"""
# Function is used during model forward pass, so we use the input framework if possible, without
# converting to numpy
if is_torch_tensor(bboxes_center):
return _center_to_corners_format_torch(bboxes_center)
elif isinstance(bboxes_center, np.ndarray):
return _center_to_corners_format_numpy(bboxes_center)
elif is_tf_tensor(bboxes_center):
return _center_to_corners_format_tf(bboxes_center)
raise ValueError(f"Unsupported input type {type(bboxes_center)}")
def _corners_to_center_format_torch(bboxes_corners: "torch.Tensor") -> "torch.Tensor":
top_left_x, top_left_y, bottom_right_x, bottom_right_y = bboxes_corners.unbind(-1)
b = [
(top_left_x + bottom_right_x) / 2, # center x
(top_left_y + bottom_right_y) / 2, # center y
(bottom_right_x - top_left_x), # width
(bottom_right_y - top_left_y), # height
]
return torch.stack(b, dim=-1)
def _corners_to_center_format_numpy(bboxes_corners: np.ndarray) -> np.ndarray:
top_left_x, top_left_y, bottom_right_x, bottom_right_y = bboxes_corners.T
bboxes_center = np.stack(
[
(top_left_x + bottom_right_x) / 2, # center x
(top_left_y + bottom_right_y) / 2, # center y
(bottom_right_x - top_left_x), # width
(bottom_right_y - top_left_y), # height
],
axis=-1,
)
return bboxes_center
def _corners_to_center_format_tf(bboxes_corners: "tf.Tensor") -> "tf.Tensor":
top_left_x, top_left_y, bottom_right_x, bottom_right_y = tf.unstack(bboxes_corners, axis=-1)
bboxes_center = tf.stack(
[
(top_left_x + bottom_right_x) / 2, # center x
(top_left_y + bottom_right_y) / 2, # center y
(bottom_right_x - top_left_x), # width
(bottom_right_y - top_left_y), # height
],
axis=-1,
)
return bboxes_center
def corners_to_center_format(bboxes_corners: TensorType) -> TensorType:
"""
Converts bounding boxes from corners format to center format.
corners format: contains the coordinates for the top-left and bottom-right corners of the box
(top_left_x, top_left_y, bottom_right_x, bottom_right_y)
center format: contains the coordinate for the center of the box and its the width, height dimensions
(center_x, center_y, width, height)
"""
# Inverse function accepts different input types so implemented here too
if is_torch_tensor(bboxes_corners):
return _corners_to_center_format_torch(bboxes_corners)
elif isinstance(bboxes_corners, np.ndarray):
return _corners_to_center_format_numpy(bboxes_corners)
elif is_tf_tensor(bboxes_corners):
return _corners_to_center_format_tf(bboxes_corners)
raise ValueError(f"Unsupported input type {type(bboxes_corners)}")
# 2 functions below copied from https://github.com/cocodataset/panopticapi/blob/master/panopticapi/utils.py
# Copyright (c) 2018, Alexander Kirillov
# All rights reserved.
def rgb_to_id(color):
"""
Converts RGB color to unique ID.
"""
if isinstance(color, np.ndarray) and len(color.shape) == 3:
if color.dtype == np.uint8:
color = color.astype(np.int32)
return color[:, :, 0] + 256 * color[:, :, 1] + 256 * 256 * color[:, :, 2]
return int(color[0] + 256 * color[1] + 256 * 256 * color[2])
def id_to_rgb(id_map):
"""
Converts unique ID to RGB color.
"""
if isinstance(id_map, np.ndarray):
id_map_copy = id_map.copy()
rgb_shape = tuple(list(id_map.shape) + [3])
rgb_map = np.zeros(rgb_shape, dtype=np.uint8)
for i in range(3):
rgb_map[..., i] = id_map_copy % 256
id_map_copy //= 256
return rgb_map
color = []
for _ in range(3):
color.append(id_map % 256)
id_map //= 256
return color
class PaddingMode(ExplicitEnum):
"""
Enum class for the different padding modes to use when padding images.
"""
CONSTANT = "constant"
REFLECT = "reflect"
REPLICATE = "replicate"
SYMMETRIC = "symmetric"
def pad(
image: np.ndarray,
padding: Union[int, Tuple[int, int], Iterable[Tuple[int, int]]],
mode: PaddingMode = PaddingMode.CONSTANT,
constant_values: Union[float, Iterable[float]] = 0.0,
data_format: Optional[Union[str, ChannelDimension]] = None,
input_data_format: Optional[Union[str, ChannelDimension]] = None,
) -> np.ndarray:
"""
Pads the `image` with the specified (height, width) `padding` and `mode`.
Args:
image (`np.ndarray`):
The image to pad.
padding (`int` or `Tuple[int, int]` or `Iterable[Tuple[int, int]]`):
Padding to apply to the edges of the height, width axes. Can be one of three formats:
- `((before_height, after_height), (before_width, after_width))` unique pad widths for each axis.
- `((before, after),)` yields same before and after pad for height and width.
- `(pad,)` or int is a shortcut for before = after = pad width for all axes.
mode (`PaddingMode`):
The padding mode to use. Can be one of:
- `"constant"`: pads with a constant value.
- `"reflect"`: pads with the reflection of the vector mirrored on the first and last values of the
vector along each axis.
- `"replicate"`: pads with the replication of the last value on the edge of the array along each axis.
- `"symmetric"`: pads with the reflection of the vector mirrored along the edge of the array.
constant_values (`float` or `Iterable[float]`, *optional*):
The value to use for the padding if `mode` is `"constant"`.
data_format (`str` or `ChannelDimension`, *optional*):
The channel dimension format for the output image. Can be one of:
- `"channels_first"` or `ChannelDimension.FIRST`: image in (num_channels, height, width) format.
- `"channels_last"` or `ChannelDimension.LAST`: image in (height, width, num_channels) format.
If unset, will use same as the input image.
input_data_format (`str` or `ChannelDimension`, *optional*):
The channel dimension format for the input image. Can be one of:
- `"channels_first"` or `ChannelDimension.FIRST`: image in (num_channels, height, width) format.
- `"channels_last"` or `ChannelDimension.LAST`: image in (height, width, num_channels) format.
If unset, will use the inferred format of the input image.
Returns:
`np.ndarray`: The padded image.
"""
if input_data_format is None:
input_data_format = infer_channel_dimension_format(image)
def _expand_for_data_format(values):
"""
Convert values to be in the format expected by np.pad based on the data format.
"""
if isinstance(values, (int, float)):
values = ((values, values), (values, values))
elif isinstance(values, tuple) and len(values) == 1:
values = ((values[0], values[0]), (values[0], values[0]))
elif isinstance(values, tuple) and len(values) == 2 and isinstance(values[0], int):
values = (values, values)
elif isinstance(values, tuple) and len(values) == 2 and isinstance(values[0], tuple):
values = values
else:
raise ValueError(f"Unsupported format: {values}")
# add 0 for channel dimension
values = ((0, 0), *values) if input_data_format == ChannelDimension.FIRST else (*values, (0, 0))
# Add additional padding if there's a batch dimension
values = (0, *values) if image.ndim == 4 else values
return values
padding = _expand_for_data_format(padding)
if mode == PaddingMode.CONSTANT:
constant_values = _expand_for_data_format(constant_values)
image = np.pad(image, padding, mode="constant", constant_values=constant_values)
elif mode == PaddingMode.REFLECT:
image = np.pad(image, padding, mode="reflect")
elif mode == PaddingMode.REPLICATE:
image = np.pad(image, padding, mode="edge")
elif mode == PaddingMode.SYMMETRIC:
image = np.pad(image, padding, mode="symmetric")
else:
raise ValueError(f"Invalid padding mode: {mode}")
image = to_channel_dimension_format(image, data_format, input_data_format) if data_format is not None else image
return image
# TODO (Amy): Accept 1/3/4 channel numpy array as input and return np.array as default
def convert_to_rgb(image: ImageInput) -> ImageInput:
"""
Converts an image to RGB format. Only converts if the image is of type PIL.Image.Image, otherwise returns the image
as is.
Args:
image (Image):
The image to convert.
"""
requires_backends(convert_to_rgb, ["vision"])
if not isinstance(image, PIL.Image.Image):
return image
image = image.convert("RGB")
return image
def flip_channel_order(
image: np.ndarray,
data_format: Optional[ChannelDimension] = None,
input_data_format: Optional[Union[str, ChannelDimension]] = None,
) -> np.ndarray:
"""
Flips the channel order of the image.
If the image is in RGB format, it will be converted to BGR and vice versa.
Args:
image (`np.ndarray`):
The image to flip.
data_format (`ChannelDimension`, *optional*):
The channel dimension format for the output image. Can be one of:
- `ChannelDimension.FIRST`: image in (num_channels, height, width) format.
- `ChannelDimension.LAST`: image in (height, width, num_channels) format.
If unset, will use same as the input image.
input_data_format (`ChannelDimension`, *optional*):
The channel dimension format for the input image. Can be one of:
- `ChannelDimension.FIRST`: image in (num_channels, height, width) format.
- `ChannelDimension.LAST`: image in (height, width, num_channels) format.
If unset, will use the inferred format of the input image.
"""
input_data_format = infer_channel_dimension_format(image) if input_data_format is None else input_data_format
if input_data_format == ChannelDimension.LAST:
image = image[..., ::-1]
elif input_data_format == ChannelDimension.FIRST:
image = image[::-1, ...]
else:
raise ValueError(f"Unsupported channel dimension: {input_data_format}")
if data_format is not None:
image = to_channel_dimension_format(image, data_format, input_channel_dim=input_data_format)
return image
| transformers/src/transformers/image_transforms.py/0 | {
"file_path": "transformers/src/transformers/image_transforms.py",
"repo_id": "transformers",
"token_count": 13239
} | 298 |
#include <torch/extension.h>
#include <ATen/ATen.h>
#include "cuda_launch.h"
#include <vector>
std::vector<at::Tensor> index_max(
at::Tensor index_vals,
at::Tensor indices,
int A_num_block,
int B_num_block
) {
return index_max_kernel(
index_vals,
indices,
A_num_block,
B_num_block
);
}
at::Tensor mm_to_sparse(
at::Tensor dense_A,
at::Tensor dense_B,
at::Tensor indices
) {
return mm_to_sparse_kernel(
dense_A,
dense_B,
indices
);
}
at::Tensor sparse_dense_mm(
at::Tensor sparse_A,
at::Tensor indices,
at::Tensor dense_B,
int A_num_block
) {
return sparse_dense_mm_kernel(
sparse_A,
indices,
dense_B,
A_num_block
);
}
at::Tensor reduce_sum(
at::Tensor sparse_A,
at::Tensor indices,
int A_num_block,
int B_num_block
) {
return reduce_sum_kernel(
sparse_A,
indices,
A_num_block,
B_num_block
);
}
at::Tensor scatter(
at::Tensor dense_A,
at::Tensor indices,
int B_num_block
) {
return scatter_kernel(
dense_A,
indices,
B_num_block
);
}
PYBIND11_MODULE(TORCH_EXTENSION_NAME, m) {
m.def("index_max", &index_max, "index_max (CUDA)");
m.def("mm_to_sparse", &mm_to_sparse, "mm_to_sparse (CUDA)");
m.def("sparse_dense_mm", &sparse_dense_mm, "sparse_dense_mm (CUDA)");
m.def("reduce_sum", &reduce_sum, "reduce_sum (CUDA)");
m.def("scatter", &scatter, "scatter (CUDA)");
}
| transformers/src/transformers/kernels/mra/torch_extension.cpp/0 | {
"file_path": "transformers/src/transformers/kernels/mra/torch_extension.cpp",
"repo_id": "transformers",
"token_count": 655
} | 299 |
# coding=utf-8
# Copyright 2021 The Google Flax Team Authors and The HuggingFace Inc. team.
#
# Licensed under the Apache License, Version 2.0 (the "License");
# you may not use this file except in compliance with the License.
# You may obtain a copy of the License at
#
# http://www.apache.org/licenses/LICENSE-2.0
#
# Unless required by applicable law or agreed to in writing, software
# distributed under the License is distributed on an "AS IS" BASIS,
# WITHOUT WARRANTIES OR CONDITIONS OF ANY KIND, either express or implied.
# See the License for the specific language governing permissions and
# limitations under the License.
import gc
import json
import os
import re
import warnings
from functools import partial
from pickle import UnpicklingError
from typing import Any, Dict, Optional, Set, Tuple, Union
import flax.linen as nn
import jax
import jax.numpy as jnp
import msgpack.exceptions
from flax.core.frozen_dict import FrozenDict, unfreeze
from flax.serialization import from_bytes, to_bytes
from flax.traverse_util import flatten_dict, unflatten_dict
from jax.random import PRNGKey
from .configuration_utils import PretrainedConfig
from .dynamic_module_utils import custom_object_save
from .generation import FlaxGenerationMixin, GenerationConfig
from .modeling_flax_pytorch_utils import load_pytorch_checkpoint_in_flax_state_dict
from .utils import (
FLAX_WEIGHTS_INDEX_NAME,
FLAX_WEIGHTS_NAME,
SAFE_WEIGHTS_INDEX_NAME,
SAFE_WEIGHTS_NAME,
WEIGHTS_INDEX_NAME,
WEIGHTS_NAME,
PushToHubMixin,
add_code_sample_docstrings,
add_start_docstrings_to_model_forward,
cached_file,
copy_func,
download_url,
has_file,
is_offline_mode,
is_remote_url,
logging,
replace_return_docstrings,
)
from .utils.hub import convert_file_size_to_int, get_checkpoint_shard_files
from .utils.import_utils import is_safetensors_available
if is_safetensors_available():
from safetensors import safe_open
from safetensors.flax import load_file as safe_load_file
from safetensors.flax import save_file as safe_save_file
logger = logging.get_logger(__name__)
def quick_gelu(x):
return x * jax.nn.sigmoid(1.702 * x)
ACT2FN = {
"gelu": partial(nn.gelu, approximate=False),
"relu": nn.relu,
"silu": nn.swish,
"swish": nn.swish,
"gelu_new": partial(nn.gelu, approximate=True),
"quick_gelu": quick_gelu,
"gelu_pytorch_tanh": partial(nn.gelu, approximate=True),
}
def dtype_byte_size(dtype):
"""
Returns the size (in bytes) occupied by one parameter of type `dtype`. Example:
```py
>>> dtype_byte_size(np.float32)
4
```
"""
if dtype == bool:
return 1 / 8
bit_search = re.search(r"[^\d](\d+)$", dtype.name)
if bit_search is None:
raise ValueError(f"`dtype` is not a valid dtype: {dtype}.")
bit_size = int(bit_search.groups()[0])
return bit_size // 8
def flax_shard_checkpoint(params, max_shard_size="10GB"):
"""
Splits a model state dictionary in sub-checkpoints so that the final size of each sub-checkpoint does not exceed a
given size. The sub-checkpoints are determined by iterating through the `state_dict` in the order of its keys, so
there is no optimization made to make each sub-checkpoint as close as possible to the maximum size passed. For
example, if the limit is 10GB and we have weights of sizes [6GB, 6GB, 2GB, 6GB, 2GB, 2GB] they will get sharded as
[6GB], [6+2GB], [6+2+2GB] and not [6+2+2GB], [6+2GB], [6GB].
<Tip warning={true}>
If one of the model's weight is bigger that `max_shard_size`, it will end up in its own sub-checkpoint which will
have a size greater than `max_shard_size`.
</Tip>
Args:
params (`Union[Dict, FrozenDict]`): A `PyTree` of model parameters.
max_shard_size (`int` or `str`, *optional*, defaults to `"10GB"`):
The maximum size of each sub-checkpoint. If expressed as a string, needs to be digits followed by a unit
(like `"5MB"`).
"""
max_shard_size = convert_file_size_to_int(max_shard_size)
sharded_state_dicts = []
current_block = {}
current_block_size = 0
total_size = 0
# flatten the weights to chunk
weights = flatten_dict(params, sep="/")
for item in weights:
weight_size = weights[item].size * dtype_byte_size(weights[item].dtype)
# If this weight is going to tip up over the maximal size, we split.
if current_block_size + weight_size > max_shard_size:
sharded_state_dicts.append(current_block)
current_block = {}
current_block_size = 0
current_block[item] = weights[item]
current_block_size += weight_size
total_size += weight_size
# Add the last block
sharded_state_dicts.append(current_block)
# If we only have one shard, we return it
if len(sharded_state_dicts) == 1:
return {FLAX_WEIGHTS_NAME: sharded_state_dicts[0]}, None
# Otherwise, let's build the index
weight_map = {}
shards = {}
for idx, shard in enumerate(sharded_state_dicts):
shard_file = FLAX_WEIGHTS_NAME.replace(".msgpack", f"-{idx+1:05d}-of-{len(sharded_state_dicts):05d}.msgpack")
shards[shard_file] = shard
for weight_name in shard.keys():
weight_map[weight_name] = shard_file
# Add the metadata
metadata = {"total_size": total_size}
index = {"metadata": metadata, "weight_map": weight_map}
return shards, index
class FlaxPreTrainedModel(PushToHubMixin, FlaxGenerationMixin):
r"""
Base class for all models.
[`FlaxPreTrainedModel`] takes care of storing the configuration of the models and handles methods for loading,
downloading and saving models.
Class attributes (overridden by derived classes):
- **config_class** ([`PretrainedConfig`]) -- A subclass of [`PretrainedConfig`] to use as configuration class
for this model architecture.
- **base_model_prefix** (`str`) -- A string indicating the attribute associated to the base model in derived
classes of the same architecture adding modules on top of the base model.
- **main_input_name** (`str`) -- The name of the principal input to the model (often `input_ids` for NLP
models, `pixel_values` for vision models and `input_values` for speech models).
"""
config_class = None
base_model_prefix = ""
main_input_name = "input_ids"
_auto_class = None
_missing_keys = set()
def __init__(
self,
config: PretrainedConfig,
module: nn.Module,
input_shape: Tuple = (1, 1),
seed: int = 0,
dtype: jnp.dtype = jnp.float32,
_do_init: bool = True,
):
if config is None:
raise ValueError("config cannot be None")
if module is None:
raise ValueError("module cannot be None")
# Those are private to be exposed as typed property on derived classes.
self._config = config
self._module = module
# Those are public as their type is generic to every derived classes.
self.key = PRNGKey(seed)
self.dtype = dtype
self.input_shape = input_shape
self.generation_config = GenerationConfig.from_model_config(config) if self.can_generate() else None
# To check if the model was initialized automatically.
self._is_initialized = _do_init
if _do_init:
# randomly initialized parameters
random_params = self.init_weights(self.key, input_shape)
params_shape_tree = jax.eval_shape(lambda params: params, random_params)
else:
init_fn = partial(self.init_weights, input_shape=input_shape)
params_shape_tree = jax.eval_shape(init_fn, self.key)
logger.info(
"Model weights are not initialized as `_do_init` is set to `False`. "
f"Make sure to call `{self.__class__.__name__}.init_weights` manually to initialize the weights."
)
# get the shape of the parameters
self._params_shape_tree = params_shape_tree
# save required_params as set
self._required_params = set(flatten_dict(unfreeze(params_shape_tree)).keys())
# initialize the parameters
if _do_init:
self.params = random_params
def init_weights(self, rng: jax.random.PRNGKey, input_shape: Tuple, params: FrozenDict = None) -> Dict:
raise NotImplementedError(f"init method has to be implemented for {self}")
def enable_gradient_checkpointing(self):
raise NotImplementedError(f"gradient checkpointing method has to be implemented for {self}")
@classmethod
def _from_config(cls, config, **kwargs):
"""
All context managers that the model should be initialized under go here.
"""
return cls(config, **kwargs)
@property
def framework(self) -> str:
"""
:str: Identifies that this is a Flax model.
"""
return "flax"
@property
def config(self) -> PretrainedConfig:
return self._config
@property
def module(self) -> nn.Module:
return self._module
@property
def params(self) -> Union[Dict, FrozenDict]:
if not self._is_initialized:
raise ValueError(
"`params` cannot be accessed from model when the model is created with `_do_init=False`. "
"You must call `init_weights` manually and store the params outside of the model and "
"pass it explicitly where needed."
)
return self._params
@property
def required_params(self) -> Set:
return self._required_params
@property
def params_shape_tree(self) -> Dict:
return self._params_shape_tree
@params.setter
def params(self, params: Union[Dict, FrozenDict]):
# don't set params if the model is not initialized
if not self._is_initialized:
raise ValueError(
"`params` cannot be set from model when the model is created with `_do_init=False`. "
"You store the params outside of the model."
)
if isinstance(params, FrozenDict):
params = unfreeze(params)
param_keys = set(flatten_dict(params).keys())
if len(self.required_params - param_keys) > 0:
raise ValueError(
"Some parameters are missing. Make sure that `params` include the following "
f"parameters {self.required_params - param_keys}"
)
self._params = params
def _cast_floating_to(self, params: Union[Dict, FrozenDict], dtype: jnp.dtype, mask: Any = None) -> Any:
"""
Helper method to cast floating-point values of given parameter `PyTree` to given `dtype`.
"""
# taken from https://github.com/deepmind/jmp/blob/3a8318abc3292be38582794dbf7b094e6583b192/jmp/_src/policy.py#L27
def conditional_cast(param):
if isinstance(param, jnp.ndarray) and jnp.issubdtype(param.dtype, jnp.floating):
param = param.astype(dtype)
return param
if mask is None:
return jax.tree_util.tree_map(conditional_cast, params)
flat_params = flatten_dict(params)
flat_mask, _ = jax.tree_util.tree_flatten(mask)
for masked, key in zip(flat_mask, sorted(flat_params.keys())):
if masked:
flat_params[key] = conditional_cast(flat_params[key])
return unflatten_dict(flat_params)
def to_bf16(self, params: Union[Dict, FrozenDict], mask: Any = None):
r"""
Cast the floating-point `params` to `jax.numpy.bfloat16`. This returns a new `params` tree and does not cast
the `params` in place.
This method can be used on TPU to explicitly convert the model parameters to bfloat16 precision to do full
half-precision training or to save weights in bfloat16 for inference in order to save memory and improve speed.
Arguments:
params (`Union[Dict, FrozenDict]`):
A `PyTree` of model parameters.
mask (`Union[Dict, FrozenDict]`):
A `PyTree` with same structure as the `params` tree. The leaves should be booleans, `True` for params
you want to cast, and should be `False` for those you want to skip.
Examples:
```python
>>> from transformers import FlaxBertModel
>>> # load model
>>> model = FlaxBertModel.from_pretrained("google-bert/bert-base-cased")
>>> # By default, the model parameters will be in fp32 precision, to cast these to bfloat16 precision
>>> model.params = model.to_bf16(model.params)
>>> # If you want don't want to cast certain parameters (for example layer norm bias and scale)
>>> # then pass the mask as follows
>>> from flax import traverse_util
>>> model = FlaxBertModel.from_pretrained("google-bert/bert-base-cased")
>>> flat_params = traverse_util.flatten_dict(model.params)
>>> mask = {
... path: (path[-2] != ("LayerNorm", "bias") and path[-2:] != ("LayerNorm", "scale"))
... for path in flat_params
... }
>>> mask = traverse_util.unflatten_dict(mask)
>>> model.params = model.to_bf16(model.params, mask)
```"""
return self._cast_floating_to(params, jnp.bfloat16, mask)
def to_fp32(self, params: Union[Dict, FrozenDict], mask: Any = None):
r"""
Cast the floating-point `parmas` to `jax.numpy.float32`. This method can be used to explicitly convert the
model parameters to fp32 precision. This returns a new `params` tree and does not cast the `params` in place.
Arguments:
params (`Union[Dict, FrozenDict]`):
A `PyTree` of model parameters.
mask (`Union[Dict, FrozenDict]`):
A `PyTree` with same structure as the `params` tree. The leaves should be booleans, `True` for params
you want to cast, and should be `False` for those you want to skip
Examples:
```python
>>> from transformers import FlaxBertModel
>>> # Download model and configuration from huggingface.co
>>> model = FlaxBertModel.from_pretrained("google-bert/bert-base-cased")
>>> # By default, the model params will be in fp32, to illustrate the use of this method,
>>> # we'll first cast to fp16 and back to fp32
>>> model.params = model.to_f16(model.params)
>>> # now cast back to fp32
>>> model.params = model.to_fp32(model.params)
```"""
return self._cast_floating_to(params, jnp.float32, mask)
def to_fp16(self, params: Union[Dict, FrozenDict], mask: Any = None):
r"""
Cast the floating-point `parmas` to `jax.numpy.float16`. This returns a new `params` tree and does not cast the
`params` in place.
This method can be used on GPU to explicitly convert the model parameters to float16 precision to do full
half-precision training or to save weights in float16 for inference in order to save memory and improve speed.
Arguments:
params (`Union[Dict, FrozenDict]`):
A `PyTree` of model parameters.
mask (`Union[Dict, FrozenDict]`):
A `PyTree` with same structure as the `params` tree. The leaves should be booleans, `True` for params
you want to cast, and should be `False` for those you want to skip
Examples:
```python
>>> from transformers import FlaxBertModel
>>> # load model
>>> model = FlaxBertModel.from_pretrained("google-bert/bert-base-cased")
>>> # By default, the model params will be in fp32, to cast these to float16
>>> model.params = model.to_fp16(model.params)
>>> # If you want don't want to cast certain parameters (for example layer norm bias and scale)
>>> # then pass the mask as follows
>>> from flax import traverse_util
>>> model = FlaxBertModel.from_pretrained("google-bert/bert-base-cased")
>>> flat_params = traverse_util.flatten_dict(model.params)
>>> mask = {
... path: (path[-2] != ("LayerNorm", "bias") and path[-2:] != ("LayerNorm", "scale"))
... for path in flat_params
... }
>>> mask = traverse_util.unflatten_dict(mask)
>>> model.params = model.to_fp16(model.params, mask)
```"""
return self._cast_floating_to(params, jnp.float16, mask)
@classmethod
def load_flax_weights(cls, resolved_archive_file):
try:
if resolved_archive_file.endswith(".safetensors"):
state = safe_load_file(resolved_archive_file)
state = unflatten_dict(state, sep=".")
else:
with open(resolved_archive_file, "rb") as state_f:
state = from_bytes(cls, state_f.read())
except (UnpicklingError, msgpack.exceptions.ExtraData) as e:
try:
with open(resolved_archive_file) as f:
if f.read().startswith("version"):
raise OSError(
"You seem to have cloned a repository without having git-lfs installed. Please"
" install git-lfs and run `git lfs install` followed by `git lfs pull` in the"
" folder you cloned."
)
else:
raise ValueError from e
except (UnicodeDecodeError, ValueError):
raise EnvironmentError(f"Unable to convert {resolved_archive_file} to Flax deserializable object. ")
return state
@classmethod
def load_flax_sharded_weights(cls, shard_files):
"""
This is the same as [`flax.serialization.from_bytes`]
(https:lax.readthedocs.io/en/latest/_modules/flax/serialization.html#from_bytes) but for a sharded checkpoint.
This load is performed efficiently: each checkpoint shard is loaded one by one in RAM and deleted after being
loaded in the model.
Args:
shard_files (`List[str]`:
The list of shard files to load.
Returns:
`Dict`: A nested dictionary of the model parameters, in the expected format for flax models : `{'model':
{'params': {'...'}}}`.
"""
# Load the index
state_sharded_dict = {}
for shard_file in shard_files:
# load using msgpack utils
try:
with open(shard_file, "rb") as state_f:
state = from_bytes(cls, state_f.read())
except (UnpicklingError, msgpack.exceptions.ExtraData) as e:
with open(shard_file) as f:
if f.read().startswith("version"):
raise OSError(
"You seem to have cloned a repository without having git-lfs installed. Please"
" install git-lfs and run `git lfs install` followed by `git lfs pull` in the"
" folder you cloned."
)
else:
raise ValueError from e
except (UnicodeDecodeError, ValueError):
raise EnvironmentError(f"Unable to convert {shard_file} to Flax deserializable object. ")
state = flatten_dict(state, sep="/")
state_sharded_dict.update(state)
del state
gc.collect()
# the state dict is unflattened to the match the format of model.params
return unflatten_dict(state_sharded_dict, sep="/")
@classmethod
def can_generate(cls) -> bool:
"""
Returns whether this model can generate sequences with `.generate()`. Returns:
`bool`: Whether this model can generate sequences with `.generate()`.
"""
# Detects whether `prepare_inputs_for_generation` has been overwritten, which is a requirement for generation.
# Alternativelly, the model can also have a custom `generate` function.
if "GenerationMixin" in str(cls.prepare_inputs_for_generation) and "GenerationMixin" in str(cls.generate):
return False
return True
@classmethod
def from_pretrained(
cls,
pretrained_model_name_or_path: Union[str, os.PathLike],
dtype: jnp.dtype = jnp.float32,
*model_args,
config: Optional[Union[PretrainedConfig, str, os.PathLike]] = None,
cache_dir: Optional[Union[str, os.PathLike]] = None,
ignore_mismatched_sizes: bool = False,
force_download: bool = False,
local_files_only: bool = False,
token: Optional[Union[str, bool]] = None,
revision: str = "main",
**kwargs,
):
r"""
Instantiate a pretrained flax model from a pre-trained model configuration.
The warning *Weights from XXX not initialized from pretrained model* means that the weights of XXX do not come
pretrained with the rest of the model. It is up to you to train those weights with a downstream fine-tuning
task.
The warning *Weights from XXX not used in YYY* means that the layer XXX is not used by YYY, therefore those
weights are discarded.
Parameters:
pretrained_model_name_or_path (`str` or `os.PathLike`):
Can be either:
- A string, the *model id* of a pretrained model hosted inside a model repo on huggingface.co.
- A path to a *directory* containing model weights saved using
[`~FlaxPreTrainedModel.save_pretrained`], e.g., `./my_model_directory/`.
- A path or url to a *pt index checkpoint file* (e.g, `./tf_model/model.ckpt.index`). In this case,
`from_pt` should be set to `True`.
dtype (`jax.numpy.dtype`, *optional*, defaults to `jax.numpy.float32`):
The data type of the computation. Can be one of `jax.numpy.float32`, `jax.numpy.float16` (on GPUs) and
`jax.numpy.bfloat16` (on TPUs).
This can be used to enable mixed-precision training or half-precision inference on GPUs or TPUs. If
specified all the computation will be performed with the given `dtype`.
**Note that this only specifies the dtype of the computation and does not influence the dtype of model
parameters.**
If you wish to change the dtype of the model parameters, see [`~FlaxPreTrainedModel.to_fp16`] and
[`~FlaxPreTrainedModel.to_bf16`].
model_args (sequence of positional arguments, *optional*):
All remaining positional arguments will be passed to the underlying model's `__init__` method.
config (`Union[PretrainedConfig, str, os.PathLike]`, *optional*):
Can be either:
- an instance of a class derived from [`PretrainedConfig`],
- a string or path valid as input to [`~PretrainedConfig.from_pretrained`].
Configuration for the model to use instead of an automatically loaded configuration. Configuration can
be automatically loaded when:
- The model is a model provided by the library (loaded with the *model id* string of a pretrained
model).
- The model was saved using [`~PreTrainedModel.save_pretrained`] and is reloaded by supplying the
save directory.
- The model is loaded by supplying a local directory as `pretrained_model_name_or_path` and a
configuration JSON file named *config.json* is found in the directory.
cache_dir (`Union[str, os.PathLike]`, *optional*):
Path to a directory in which a downloaded pretrained model configuration should be cached if the
standard cache should not be used.
from_pt (`bool`, *optional*, defaults to `False`):
Load the model weights from a PyTorch checkpoint save file (see docstring of
`pretrained_model_name_or_path` argument).
ignore_mismatched_sizes (`bool`, *optional*, defaults to `False`):
Whether or not to raise an error if some of the weights from the checkpoint do not have the same size
as the weights of the model (if for instance, you are instantiating a model with 10 labels from a
checkpoint with 3 labels).
force_download (`bool`, *optional*, defaults to `False`):
Whether or not to force the (re-)download of the model weights and configuration files, overriding the
cached versions if they exist.
resume_download (`bool`, *optional*, defaults to `False`):
Whether or not to delete incompletely received files. Will attempt to resume the download if such a
file exists.
proxies (`Dict[str, str]`, *optional*):
A dictionary of proxy servers to use by protocol or endpoint, e.g., `{'http': 'foo.bar:3128',
'http://hostname': 'foo.bar:4012'}`. The proxies are used on each request.
local_files_only(`bool`, *optional*, defaults to `False`):
Whether or not to only look at local files (i.e., do not try to download the model).
token (`str` or `bool`, *optional*):
The token to use as HTTP bearer authorization for remote files. If `True`, or not specified, will use
the token generated when running `huggingface-cli login` (stored in `~/.huggingface`).
revision (`str`, *optional*, defaults to `"main"`):
The specific model version to use. It can be a branch name, a tag name, or a commit id, since we use a
git-based system for storing models and other artifacts on huggingface.co, so `revision` can be any
identifier allowed by git.
<Tip>
To test a pull request you made on the Hub, you can pass `revision="refs/pr/<pr_number>".
</Tip>
subfolder (`str`, *optional*, defaults to `""`):
In case the relevant files are located inside a subfolder of the model repo on huggingface.co, you can
specify the folder name here.
kwargs (remaining dictionary of keyword arguments, *optional*):
Can be used to update the configuration object (after it being loaded) and initiate the model (e.g.,
`output_attentions=True`). Behaves differently depending on whether a `config` is provided or
automatically loaded:
- If a configuration is provided with `config`, `**kwargs` will be directly passed to the
underlying model's `__init__` method (we assume all relevant updates to the configuration have
already been done)
- If a configuration is not provided, `kwargs` will be first passed to the configuration class
initialization function ([`~PretrainedConfig.from_pretrained`]). Each key of `kwargs` that
corresponds to a configuration attribute will be used to override said attribute with the
supplied `kwargs` value. Remaining keys that do not correspond to any configuration attribute
will be passed to the underlying model's `__init__` function.
Examples:
```python
>>> from transformers import BertConfig, FlaxBertModel
>>> # Download model and configuration from huggingface.co and cache.
>>> model = FlaxBertModel.from_pretrained("google-bert/bert-base-cased")
>>> # Model was saved using *save_pretrained('./test/saved_model/')* (for example purposes, not runnable).
>>> model = FlaxBertModel.from_pretrained("./test/saved_model/")
>>> # Loading from a PyTorch checkpoint file instead of a PyTorch model (slower, for example purposes, not runnable).
>>> config = BertConfig.from_json_file("./pt_model/config.json")
>>> model = FlaxBertModel.from_pretrained("./pt_model/pytorch_model.bin", from_pt=True, config=config)
```"""
from_pt = kwargs.pop("from_pt", False)
resume_download = kwargs.pop("resume_download", False)
proxies = kwargs.pop("proxies", None)
use_auth_token = kwargs.pop("use_auth_token", None)
trust_remote_code = kwargs.pop("trust_remote_code", None)
from_pipeline = kwargs.pop("_from_pipeline", None)
from_auto_class = kwargs.pop("_from_auto", False)
_do_init = kwargs.pop("_do_init", True)
subfolder = kwargs.pop("subfolder", "")
commit_hash = kwargs.pop("_commit_hash", None)
# Not relevant for Flax Models
_ = kwargs.pop("adapter_kwargs", None)
if use_auth_token is not None:
warnings.warn(
"The `use_auth_token` argument is deprecated and will be removed in v5 of Transformers. Please use `token` instead.",
FutureWarning,
)
if token is not None:
raise ValueError(
"`token` and `use_auth_token` are both specified. Please set only the argument `token`."
)
token = use_auth_token
if trust_remote_code is True:
logger.warning(
"The argument `trust_remote_code` is to be used with Auto classes. It has no effect here and is"
" ignored."
)
user_agent = {"file_type": "model", "framework": "flax", "from_auto_class": from_auto_class}
if from_pipeline is not None:
user_agent["using_pipeline"] = from_pipeline
if is_offline_mode() and not local_files_only:
logger.info("Offline mode: forcing local_files_only=True")
local_files_only = True
# Load config if we don't provide a configuration
if not isinstance(config, PretrainedConfig):
config_path = config if config is not None else pretrained_model_name_or_path
config, model_kwargs = cls.config_class.from_pretrained(
config_path,
cache_dir=cache_dir,
return_unused_kwargs=True,
force_download=force_download,
resume_download=resume_download,
proxies=proxies,
local_files_only=local_files_only,
token=token,
revision=revision,
subfolder=subfolder,
_from_auto=from_auto_class,
_from_pipeline=from_pipeline,
_commit_hash=commit_hash,
**kwargs,
)
else:
model_kwargs = kwargs.copy()
if commit_hash is None:
commit_hash = getattr(config, "_commit_hash", None)
# Add the dtype to model_kwargs
model_kwargs["dtype"] = dtype
# This variable will flag if we're loading a sharded checkpoint. In this case the archive file is just the
# index of the files.
is_sharded = False
# Load model
if pretrained_model_name_or_path is not None:
pretrained_model_name_or_path = str(pretrained_model_name_or_path)
is_local = os.path.isdir(pretrained_model_name_or_path)
if os.path.isdir(pretrained_model_name_or_path):
if os.path.isfile(os.path.join(pretrained_model_name_or_path, subfolder, FLAX_WEIGHTS_NAME)):
# Load from a Flax checkpoint
archive_file = os.path.join(pretrained_model_name_or_path, subfolder, FLAX_WEIGHTS_NAME)
elif os.path.isfile(os.path.join(pretrained_model_name_or_path, subfolder, FLAX_WEIGHTS_INDEX_NAME)):
# Load from a sharded Flax checkpoint
archive_file = os.path.join(pretrained_model_name_or_path, subfolder, FLAX_WEIGHTS_INDEX_NAME)
is_sharded = True
elif is_safetensors_available() and os.path.isfile(
os.path.join(pretrained_model_name_or_path, SAFE_WEIGHTS_NAME)
):
# Load from a safetensors checkpoint
archive_file = os.path.join(pretrained_model_name_or_path, SAFE_WEIGHTS_NAME)
elif from_pt and os.path.isfile(os.path.join(pretrained_model_name_or_path, subfolder, WEIGHTS_NAME)):
# Load from a PyTorch checkpoint
archive_file = os.path.join(pretrained_model_name_or_path, subfolder, WEIGHTS_NAME)
elif from_pt and os.path.isfile(
os.path.join(pretrained_model_name_or_path, subfolder, WEIGHTS_INDEX_NAME)
):
# Load from a sharded pytorch checkpoint
archive_file = os.path.join(pretrained_model_name_or_path, subfolder, WEIGHTS_INDEX_NAME)
is_sharded = True
# At this stage we don't have a weight file so we will raise an error.
elif is_safetensors_available() and os.path.isfile(
os.path.join(pretrained_model_name_or_path, SAFE_WEIGHTS_INDEX_NAME)
):
# Load from a sharded safetensors checkpoint
archive_file = os.path.join(pretrained_model_name_or_path, SAFE_WEIGHTS_INDEX_NAME)
is_sharded = True
raise NotImplementedError("Support for sharded checkpoints using safetensors is coming soon!")
elif os.path.isfile(os.path.join(pretrained_model_name_or_path, subfolder, WEIGHTS_NAME)):
raise EnvironmentError(
f"Error no file named {FLAX_WEIGHTS_NAME} found in directory {pretrained_model_name_or_path} "
"but there is a file for PyTorch weights. Use `from_pt=True` to load this model from those "
"weights."
)
else:
raise EnvironmentError(
f"Error no file named {FLAX_WEIGHTS_NAME} or {WEIGHTS_NAME} found in directory "
f"{pretrained_model_name_or_path}."
)
elif os.path.isfile(os.path.join(subfolder, pretrained_model_name_or_path)):
archive_file = pretrained_model_name_or_path
is_local = True
elif is_remote_url(pretrained_model_name_or_path):
filename = pretrained_model_name_or_path
resolved_archive_file = download_url(pretrained_model_name_or_path)
else:
if from_pt:
filename = WEIGHTS_NAME
else:
filename = FLAX_WEIGHTS_NAME
try:
# Load from URL or cache if already cached
cached_file_kwargs = {
"cache_dir": cache_dir,
"force_download": force_download,
"proxies": proxies,
"resume_download": resume_download,
"local_files_only": local_files_only,
"token": token,
"user_agent": user_agent,
"revision": revision,
"subfolder": subfolder,
"_raise_exceptions_for_gated_repo": False,
"_raise_exceptions_for_missing_entries": False,
"_commit_hash": commit_hash,
}
resolved_archive_file = cached_file(pretrained_model_name_or_path, filename, **cached_file_kwargs)
# Maybe the checkpoint is sharded, we try to grab the index name in this case.
if resolved_archive_file is None and filename == FLAX_WEIGHTS_NAME:
resolved_archive_file = cached_file(
pretrained_model_name_or_path, FLAX_WEIGHTS_INDEX_NAME, **cached_file_kwargs
)
if resolved_archive_file is not None:
is_sharded = True
# Maybe the checkpoint is pytorch sharded, we try to grab the pytorch index name in this case.
if resolved_archive_file is None and from_pt:
resolved_archive_file = cached_file(
pretrained_model_name_or_path, WEIGHTS_INDEX_NAME, **cached_file_kwargs
)
if resolved_archive_file is not None:
is_sharded = True
# If we still haven't found anything, look for `safetensors`.
if resolved_archive_file is None:
# No support for sharded safetensors yet, so we'll raise an error if that's all we find.
filename = SAFE_WEIGHTS_NAME
resolved_archive_file = cached_file(
pretrained_model_name_or_path, SAFE_WEIGHTS_NAME, **cached_file_kwargs
)
# Since we set _raise_exceptions_for_missing_entries=False, we don't get an exception but a None
# result when internet is up, the repo and revision exist, but the file does not.
if resolved_archive_file is None:
# Otherwise, maybe there is a TF or Torch model file. We try those to give a helpful error
# message.
has_file_kwargs = {
"revision": revision,
"proxies": proxies,
"token": token,
}
if has_file(pretrained_model_name_or_path, SAFE_WEIGHTS_INDEX_NAME, **has_file_kwargs):
is_sharded = True
raise NotImplementedError(
"Support for sharded checkpoints using safetensors is coming soon!"
)
elif has_file(pretrained_model_name_or_path, WEIGHTS_NAME, **has_file_kwargs):
raise EnvironmentError(
f"{pretrained_model_name_or_path} does not appear to have a file named"
f" {FLAX_WEIGHTS_NAME} but there is a file for PyTorch weights. Use `from_pt=True` to"
" load this model from those weights."
)
elif has_file(pretrained_model_name_or_path, WEIGHTS_INDEX_NAME, **has_file_kwargs):
raise EnvironmentError(
f"{pretrained_model_name_or_path} does not appear to have a file named"
f" {FLAX_WEIGHTS_INDEX_NAME} but there is a sharded file for PyTorch weights. Use"
" `from_pt=True` to load this model from those weights."
)
else:
raise EnvironmentError(
f"{pretrained_model_name_or_path} does not appear to have a file named"
f" {FLAX_WEIGHTS_NAME} or {WEIGHTS_NAME}."
)
except EnvironmentError:
# Raise any environment error raise by `cached_file`. It will have a helpful error message adapted
# to the original exception.
raise
except Exception:
# For any other exception, we throw a generic error.
raise EnvironmentError(
f"Can't load the model for '{pretrained_model_name_or_path}'. If you were trying to load it"
" from 'https://huggingface.co/models', make sure you don't have a local directory with the"
f" same name. Otherwise, make sure '{pretrained_model_name_or_path}' is the correct path to a"
f" directory containing a file named {FLAX_WEIGHTS_NAME} or {WEIGHTS_NAME}."
)
if is_local:
logger.info(f"loading weights file {archive_file}")
resolved_archive_file = archive_file
filename = resolved_archive_file.split(os.path.sep)[-1]
else:
logger.info(f"loading weights file {filename} from cache at {resolved_archive_file}")
else:
resolved_archive_file = None
# We'll need to download and cache each checkpoint shard if the checkpoint is sharded.
if is_sharded:
# resolved_archive_file becomes a list of files that point to the different checkpoint shards in this case.
resolved_archive_file, _ = get_checkpoint_shard_files(
pretrained_model_name_or_path,
resolved_archive_file,
cache_dir=cache_dir,
force_download=force_download,
proxies=proxies,
resume_download=resume_download,
local_files_only=local_files_only,
token=token,
user_agent=user_agent,
revision=revision,
subfolder=subfolder,
_commit_hash=commit_hash,
)
safetensors_from_pt = False
if filename == SAFE_WEIGHTS_NAME:
with safe_open(resolved_archive_file, framework="flax") as f:
safetensors_metadata = f.metadata()
if safetensors_metadata is None or safetensors_metadata.get("format") not in ["pt", "tf", "flax"]:
raise OSError(
f"The safetensors archive passed at {resolved_archive_file} does not contain the valid metadata."
" Make sure you save your model with the `save_pretrained` method."
)
safetensors_from_pt = safetensors_metadata.get("format") == "pt"
# init random models
model = cls(config, *model_args, _do_init=_do_init, **model_kwargs)
if from_pt or safetensors_from_pt:
state = load_pytorch_checkpoint_in_flax_state_dict(model, resolved_archive_file, is_sharded)
else:
if is_sharded:
state = cls.load_flax_sharded_weights(resolved_archive_file)
else:
state = cls.load_flax_weights(resolved_archive_file)
# make sure all arrays are stored as jnp.arrays
# NOTE: This is to prevent a bug this will be fixed in Flax >= v0.3.4:
# https://github.com/google/flax/issues/1261
if _do_init:
state = jax.tree_util.tree_map(jnp.array, state)
else:
# keep the params on CPU if we don't want to initialize
state = jax.tree_util.tree_map(lambda x: jax.device_put(x, jax.local_devices(backend="cpu")[0]), state)
if "batch_stats" in state: # if flax model contains batch norm layers
# if model is base model only use model_prefix key
if (
cls.base_model_prefix not in dict(model.params_shape_tree["params"])
and cls.base_model_prefix in state["params"]
):
state["params"] = state["params"][cls.base_model_prefix]
state["batch_stats"] = state["batch_stats"][cls.base_model_prefix]
# if model is head model and we are loading weights from base model
# we initialize new params dict with base_model_prefix
if (
cls.base_model_prefix in dict(model.params_shape_tree["params"])
and cls.base_model_prefix not in state["params"]
):
state = {
"params": {cls.base_model_prefix: state["params"]},
"batch_stats": {cls.base_model_prefix: state["batch_stats"]},
}
else:
# if model is base model only use model_prefix key
if cls.base_model_prefix not in dict(model.params_shape_tree) and cls.base_model_prefix in state:
state = state[cls.base_model_prefix]
# if model is head model and we are loading weights from base model
# we initialize new params dict with base_model_prefix
if cls.base_model_prefix in dict(model.params_shape_tree) and cls.base_model_prefix not in state:
state = {cls.base_model_prefix: state}
# flatten dicts
state = flatten_dict(state)
random_state = flatten_dict(unfreeze(model.params if _do_init else model.params_shape_tree))
missing_keys = model.required_params - set(state.keys())
unexpected_keys = set(state.keys()) - model.required_params
# Disabling warning when porting pytorch weights to flax, flax does not uses num_batches_tracked
for unexpected_key in unexpected_keys.copy():
if "num_batches_tracked" in unexpected_key[-1]:
unexpected_keys.remove(unexpected_key)
if missing_keys and not _do_init:
logger.warning(
f"The checkpoint {pretrained_model_name_or_path} is missing required keys: {missing_keys}. "
"Make sure to call model.init_weights to initialize the missing weights."
)
cls._missing_keys = missing_keys
# Mistmatched keys contains tuples key/shape1/shape2 of weights in the checkpoint that have a shape not
# matching the weights in the model.
mismatched_keys = []
for key in state.keys():
if key in random_state and state[key].shape != random_state[key].shape:
if ignore_mismatched_sizes:
mismatched_keys.append((key, state[key].shape, random_state[key].shape))
state[key] = random_state[key]
else:
raise ValueError(
f"Trying to load the pretrained weight for {key} failed: checkpoint has shape "
f"{state[key].shape} which is incompatible with the model shape {random_state[key].shape}. "
"Using `ignore_mismatched_sizes=True` if you really want to load this checkpoint inside this "
"model."
)
# add missing keys as random parameters if we are initializing
if missing_keys and _do_init:
for missing_key in missing_keys:
state[missing_key] = random_state[missing_key]
# remove unexpected keys to not be saved again
for unexpected_key in unexpected_keys:
del state[unexpected_key]
if len(unexpected_keys) > 0:
logger.warning(
f"Some weights of the model checkpoint at {pretrained_model_name_or_path} were not used when"
f" initializing {model.__class__.__name__}: {unexpected_keys}\n- This IS expected if you are"
f" initializing {model.__class__.__name__} from the checkpoint of a model trained on another task or"
" with another architecture (e.g. initializing a BertForSequenceClassification model from a"
" BertForPreTraining model).\n- This IS NOT expected if you are initializing"
f" {model.__class__.__name__} from the checkpoint of a model that you expect to be exactly identical"
" (initializing a BertForSequenceClassification model from a BertForSequenceClassification model)."
)
else:
logger.info(f"All model checkpoint weights were used when initializing {model.__class__.__name__}.\n")
if len(missing_keys) > 0:
logger.warning(
f"Some weights of {model.__class__.__name__} were not initialized from the model checkpoint at"
f" {pretrained_model_name_or_path} and are newly initialized: {missing_keys}\nYou should probably"
" TRAIN this model on a down-stream task to be able to use it for predictions and inference."
)
elif len(mismatched_keys) == 0:
logger.info(
f"All the weights of {model.__class__.__name__} were initialized from the model checkpoint at"
f" {pretrained_model_name_or_path}.\nIf your task is similar to the task the model of the checkpoint"
f" was trained on, you can already use {model.__class__.__name__} for predictions without further"
" training."
)
if len(mismatched_keys) > 0:
mismatched_warning = "\n".join(
[
f"- {key}: found shape {shape1} in the checkpoint and {shape2} in the model instantiated"
for key, shape1, shape2 in mismatched_keys
]
)
logger.warning(
f"Some weights of {model.__class__.__name__} were not initialized from the model checkpoint at"
f" {pretrained_model_name_or_path} and are newly initialized because the shapes did not"
f" match:\n{mismatched_warning}\nYou should probably TRAIN this model on a down-stream task to be able"
" to use it for predictions and inference."
)
# dictionary of key: dtypes for the model params
param_dtypes = jax.tree_util.tree_map(lambda x: x.dtype, state)
# extract keys of parameters not in jnp.float32
fp16_params = [k for k in param_dtypes if param_dtypes[k] == jnp.float16]
bf16_params = [k for k in param_dtypes if param_dtypes[k] == jnp.bfloat16]
# raise a warning if any of the parameters are not in jnp.float32
if len(fp16_params) > 0:
logger.warning(
f"Some of the weights of {model.__class__.__name__} were initialized in float16 precision from "
f"the model checkpoint at {pretrained_model_name_or_path}:\n{fp16_params}\n"
"You should probably UPCAST the model weights to float32 if this was not intended. "
"See [`~FlaxPreTrainedModel.to_fp32`] for further information on how to do this."
)
if len(bf16_params) > 0:
logger.warning(
f"Some of the weights of {model.__class__.__name__} were initialized in bfloat16 precision from "
f"the model checkpoint at {pretrained_model_name_or_path}:\n{bf16_params}\n"
"You should probably UPCAST the model weights to float32 if this was not intended. "
"See [`~FlaxPreTrainedModel.to_fp32`] for further information on how to do this."
)
# If it is a model with generation capabilities, attempt to load the generation config
if model.can_generate():
try:
model.generation_config = GenerationConfig.from_pretrained(
pretrained_model_name_or_path,
cache_dir=cache_dir,
force_download=force_download,
resume_download=resume_download,
proxies=proxies,
local_files_only=local_files_only,
token=token,
revision=revision,
subfolder=subfolder,
_from_auto=from_auto_class,
_from_pipeline=from_pipeline,
**kwargs,
)
except OSError:
logger.info(
"Generation config file not found, using a generation config created from the model config."
)
pass
if _do_init:
# set correct parameters
model.params = unflatten_dict(state)
return model
else:
return model, unflatten_dict(state)
def save_pretrained(
self,
save_directory: Union[str, os.PathLike],
params=None,
push_to_hub=False,
max_shard_size="10GB",
token: Optional[Union[str, bool]] = None,
safe_serialization: bool = False,
**kwargs,
):
"""
Save a model and its configuration file to a directory, so that it can be re-loaded using the
`[`~FlaxPreTrainedModel.from_pretrained`]` class method
Arguments:
save_directory (`str` or `os.PathLike`):
Directory to which to save. Will be created if it doesn't exist.
push_to_hub (`bool`, *optional*, defaults to `False`):
Whether or not to push your model to the Hugging Face model hub after saving it. You can specify the
repository you want to push to with `repo_id` (will default to the name of `save_directory` in your
namespace).
max_shard_size (`int` or `str`, *optional*, defaults to `"10GB"`):
The maximum size for a checkpoint before being sharded. Checkpoints shard will then be each of size
lower than this size. If expressed as a string, needs to be digits followed by a unit (like `"5MB"`).
<Tip warning={true}>
If a single weight of the model is bigger than `max_shard_size`, it will be in its own checkpoint shard
which will be bigger than `max_shard_size`.
</Tip>
token (`str` or `bool`, *optional*):
The token to use as HTTP bearer authorization for remote files. If `True`, or not specified, will use
the token generated when running `huggingface-cli login` (stored in `~/.huggingface`).
kwargs (`Dict[str, Any]`, *optional*):
Additional key word arguments passed along to the [`~utils.PushToHubMixin.push_to_hub`] method.
safe_serialization (`bool`, *optional*, defaults to `False`):
Whether to save the model using `safetensors` or through msgpack.
"""
use_auth_token = kwargs.pop("use_auth_token", None)
if use_auth_token is not None:
warnings.warn(
"The `use_auth_token` argument is deprecated and will be removed in v5 of Transformers. Please use `token` instead.",
FutureWarning,
)
if token is not None:
raise ValueError(
"`token` and `use_auth_token` are both specified. Please set only the argument `token`."
)
token = use_auth_token
if token is not None:
kwargs["token"] = token
if os.path.isfile(save_directory):
logger.error(f"Provided path ({save_directory}) should be a directory, not a file")
return
os.makedirs(save_directory, exist_ok=True)
if push_to_hub:
commit_message = kwargs.pop("commit_message", None)
repo_id = kwargs.pop("repo_id", save_directory.split(os.path.sep)[-1])
repo_id = self._create_repo(repo_id, **kwargs)
files_timestamps = self._get_files_timestamps(save_directory)
# get abs dir
save_directory = os.path.abspath(save_directory)
# save config as well
self.config.architectures = [self.__class__.__name__[4:]]
# If we have a custom model, we copy the file defining it in the folder and set the attributes so it can be
# loaded from the Hub.
if self._auto_class is not None:
custom_object_save(self, save_directory, config=self.config)
self.config.save_pretrained(save_directory)
if self.can_generate():
self.generation_config.save_pretrained(save_directory)
# save model
weights_name = SAFE_WEIGHTS_NAME if safe_serialization else FLAX_WEIGHTS_NAME
output_model_file = os.path.join(save_directory, weights_name)
shards, index = flax_shard_checkpoint(params if params is not None else self.params, max_shard_size)
# Clean the folder from a previous save
for filename in os.listdir(save_directory):
full_filename = os.path.join(save_directory, filename)
weights_no_suffix = weights_name.replace(".bin", "").replace(".safetensors", "")
if (
filename.startswith(weights_no_suffix)
and os.path.isfile(full_filename)
and filename not in shards.keys()
):
os.remove(full_filename)
if index is None:
if safe_serialization:
params = params if params is not None else self.params
flat_dict = flatten_dict(params, sep=".")
safe_save_file(flat_dict, output_model_file, metadata={"format": "flax"})
else:
with open(output_model_file, "wb") as f:
params = params if params is not None else self.params
model_bytes = to_bytes(params)
f.write(model_bytes)
else:
save_index_file = os.path.join(save_directory, FLAX_WEIGHTS_INDEX_NAME)
# Save the index as well
with open(save_index_file, "w", encoding="utf-8") as f:
content = json.dumps(index, indent=2, sort_keys=True) + "\n"
f.write(content)
logger.info(
f"The model is bigger than the maximum size per checkpoint ({max_shard_size}) and is going to be "
f"split in {len(shards)} checkpoint shards. You can find where each parameters has been saved in the "
f"index located at {save_index_file}."
)
for shard_file, shard in shards.items():
# the shard item are unflattened, to save them we need to flatten them again
with open(os.path.join(save_directory, shard_file), mode="wb") as f:
params = unflatten_dict(shard, sep="/")
shard_bytes = to_bytes(params)
f.write(shard_bytes)
logger.info(f"Model weights saved in {output_model_file}")
if push_to_hub:
self._upload_modified_files(
save_directory,
repo_id,
files_timestamps,
commit_message=commit_message,
token=token,
)
@classmethod
def register_for_auto_class(cls, auto_class="FlaxAutoModel"):
"""
Register this class with a given auto class. This should only be used for custom models as the ones in the
library are already mapped with an auto class.
<Tip warning={true}>
This API is experimental and may have some slight breaking changes in the next releases.
</Tip>
Args:
auto_class (`str` or `type`, *optional*, defaults to `"FlaxAutoModel"`):
The auto class to register this new model with.
"""
if not isinstance(auto_class, str):
auto_class = auto_class.__name__
import transformers.models.auto as auto_module
if not hasattr(auto_module, auto_class):
raise ValueError(f"{auto_class} is not a valid auto class.")
cls._auto_class = auto_class
# To update the docstring, we need to copy the method, otherwise we change the original docstring.
FlaxPreTrainedModel.push_to_hub = copy_func(FlaxPreTrainedModel.push_to_hub)
if FlaxPreTrainedModel.push_to_hub.__doc__ is not None:
FlaxPreTrainedModel.push_to_hub.__doc__ = FlaxPreTrainedModel.push_to_hub.__doc__.format(
object="model", object_class="FlaxAutoModel", object_files="model checkpoint"
)
def overwrite_call_docstring(model_class, docstring):
# copy __call__ function to be sure docstring is changed only for this function
model_class.__call__ = copy_func(model_class.__call__)
# delete existing docstring
model_class.__call__.__doc__ = None
# set correct docstring
model_class.__call__ = add_start_docstrings_to_model_forward(docstring)(model_class.__call__)
def append_call_sample_docstring(
model_class, checkpoint, output_type, config_class, mask=None, revision=None, real_checkpoint=None
):
model_class.__call__ = copy_func(model_class.__call__)
model_class.__call__ = add_code_sample_docstrings(
checkpoint=checkpoint,
output_type=output_type,
config_class=config_class,
model_cls=model_class.__name__,
revision=revision,
real_checkpoint=real_checkpoint,
)(model_class.__call__)
def append_replace_return_docstrings(model_class, output_type, config_class):
model_class.__call__ = copy_func(model_class.__call__)
model_class.__call__ = replace_return_docstrings(
output_type=output_type,
config_class=config_class,
)(model_class.__call__)
| transformers/src/transformers/modeling_flax_utils.py/0 | {
"file_path": "transformers/src/transformers/modeling_flax_utils.py",
"repo_id": "transformers",
"token_count": 27381
} | 300 |
# coding=utf-8
# Copyright 2023 The HuggingFace Inc. team. All rights reserved.
#
# Licensed under the Apache License, Version 2.0 (the "License");
# you may not use this file except in compliance with the License.
# You may obtain a copy of the License at
#
# http://www.apache.org/licenses/LICENSE-2.0
#
# Unless required by applicable law or agreed to in writing, software
# distributed under the License is distributed on an "AS IS" BASIS,
# WITHOUT WARRANTIES OR CONDITIONS OF ANY KIND, either express or implied.
# See the License for the specific language governing permissions and
# limitations under the License.
""" ALIGN model configuration"""
import os
from typing import TYPE_CHECKING, List, Union
if TYPE_CHECKING:
pass
from ...configuration_utils import PretrainedConfig
from ...utils import logging
logger = logging.get_logger(__name__)
ALIGN_PRETRAINED_CONFIG_ARCHIVE_MAP = {
"kakaobrain/align-base": "https://huggingface.co/kakaobrain/align-base/resolve/main/config.json",
}
class AlignTextConfig(PretrainedConfig):
r"""
This is the configuration class to store the configuration of a [`AlignTextModel`]. It is used to instantiate a
ALIGN text encoder according to the specified arguments, defining the model architecture. Instantiating a
configuration with the defaults will yield a similar configuration to that of the text encoder of the ALIGN
[kakaobrain/align-base](https://huggingface.co/kakaobrain/align-base) architecture. The default values here are
copied from BERT.
Configuration objects inherit from [`PretrainedConfig`] and can be used to control the model outputs. Read the
documentation from [`PretrainedConfig`] for more information.
Args:
vocab_size (`int`, *optional*, defaults to 30522):
Vocabulary size of the Align Text model. Defines the number of different tokens that can be represented by
the `inputs_ids` passed when calling [`AlignTextModel`].
hidden_size (`int`, *optional*, defaults to 768):
Dimensionality of the encoder layers and the pooler layer.
num_hidden_layers (`int`, *optional*, defaults to 12):
Number of hidden layers in the Transformer encoder.
num_attention_heads (`int`, *optional*, defaults to 12):
Number of attention heads for each attention layer in the Transformer encoder.
intermediate_size (`int`, *optional*, defaults to 3072):
Dimensionality of the "intermediate" (often named feed-forward) layer in the Transformer encoder.
hidden_act (`str` or `Callable`, *optional*, defaults to `"gelu"`):
The non-linear activation function (function or string) in the encoder and pooler. If string, `"gelu"`,
`"relu"`, `"silu"` and `"gelu_new"` are supported.
hidden_dropout_prob (`float`, *optional*, defaults to 0.1):
The dropout probability for all fully connected layers in the embeddings, encoder, and pooler.
attention_probs_dropout_prob (`float`, *optional*, defaults to 0.1):
The dropout ratio for the attention probabilities.
max_position_embeddings (`int`, *optional*, defaults to 512):
The maximum sequence length that this model might ever be used with. Typically set this to something large
just in case (e.g., 512 or 1024 or 2048).
type_vocab_size (`int`, *optional*, defaults to 2):
The vocabulary size of the `token_type_ids` passed when calling [`AlignTextModel`].
initializer_range (`float`, *optional*, defaults to 0.02):
The standard deviation of the truncated_normal_initializer for initializing all weight matrices.
layer_norm_eps (`float`, *optional*, defaults to 1e-12):
The epsilon used by the layer normalization layers.
pad_token_id (`int`, *optional*, defaults to 0):
Padding token id.
position_embedding_type (`str`, *optional*, defaults to `"absolute"`):
Type of position embedding. Choose one of `"absolute"`, `"relative_key"`, `"relative_key_query"`. For
positional embeddings use `"absolute"`. For more information on `"relative_key"`, please refer to
[Self-Attention with Relative Position Representations (Shaw et al.)](https://arxiv.org/abs/1803.02155).
For more information on `"relative_key_query"`, please refer to *Method 4* in [Improve Transformer Models
with Better Relative Position Embeddings (Huang et al.)](https://arxiv.org/abs/2009.13658).
use_cache (`bool`, *optional*, defaults to `True`):
Whether or not the model should return the last key/values attentions (not used by all models). Only
relevant if `config.is_decoder=True`.
Example:
```python
>>> from transformers import AlignTextConfig, AlignTextModel
>>> # Initializing a AlignTextConfig with kakaobrain/align-base style configuration
>>> configuration = AlignTextConfig()
>>> # Initializing a AlignTextModel (with random weights) from the kakaobrain/align-base style configuration
>>> model = AlignTextModel(configuration)
>>> # Accessing the model configuration
>>> configuration = model.config
```"""
model_type = "align_text_model"
def __init__(
self,
vocab_size=30522,
hidden_size=768,
num_hidden_layers=12,
num_attention_heads=12,
intermediate_size=3072,
hidden_act="gelu",
hidden_dropout_prob=0.1,
attention_probs_dropout_prob=0.1,
max_position_embeddings=512,
type_vocab_size=2,
initializer_range=0.02,
layer_norm_eps=1e-12,
pad_token_id=0,
position_embedding_type="absolute",
use_cache=True,
**kwargs,
):
super().__init__(**kwargs)
self.vocab_size = vocab_size
self.hidden_size = hidden_size
self.num_hidden_layers = num_hidden_layers
self.num_attention_heads = num_attention_heads
self.hidden_act = hidden_act
self.intermediate_size = intermediate_size
self.hidden_dropout_prob = hidden_dropout_prob
self.attention_probs_dropout_prob = attention_probs_dropout_prob
self.max_position_embeddings = max_position_embeddings
self.type_vocab_size = type_vocab_size
self.initializer_range = initializer_range
self.layer_norm_eps = layer_norm_eps
self.position_embedding_type = position_embedding_type
self.use_cache = use_cache
self.pad_token_id = pad_token_id
@classmethod
def from_pretrained(cls, pretrained_model_name_or_path: Union[str, os.PathLike], **kwargs) -> "PretrainedConfig":
cls._set_token_in_kwargs(kwargs)
config_dict, kwargs = cls.get_config_dict(pretrained_model_name_or_path, **kwargs)
# get the text config dict if we are loading from AlignConfig
if config_dict.get("model_type") == "align":
config_dict = config_dict["text_config"]
if "model_type" in config_dict and hasattr(cls, "model_type") and config_dict["model_type"] != cls.model_type:
logger.warning(
f"You are using a model of type {config_dict['model_type']} to instantiate a model of type "
f"{cls.model_type}. This is not supported for all configurations of models and can yield errors."
)
return cls.from_dict(config_dict, **kwargs)
class AlignVisionConfig(PretrainedConfig):
r"""
This is the configuration class to store the configuration of a [`AlignVisionModel`]. It is used to instantiate a
ALIGN vision encoder according to the specified arguments, defining the model architecture. Instantiating a
configuration with the defaults will yield a similar configuration to that of the vision encoder of the ALIGN
[kakaobrain/align-base](https://huggingface.co/kakaobrain/align-base) architecture. The default values are copied
from EfficientNet (efficientnet-b7)
Configuration objects inherit from [`PretrainedConfig`] and can be used to control the model outputs. Read the
documentation from [`PretrainedConfig`] for more information.
Args:
num_channels (`int`, *optional*, defaults to 3):
The number of input channels.
image_size (`int`, *optional*, defaults to 600):
The input image size.
width_coefficient (`float`, *optional*, defaults to 2.0):
Scaling coefficient for network width at each stage.
depth_coefficient (`float`, *optional*, defaults to 3.1):
Scaling coefficient for network depth at each stage.
depth_divisor `int`, *optional*, defaults to 8):
A unit of network width.
kernel_sizes (`List[int]`, *optional*, defaults to `[3, 3, 5, 3, 5, 5, 3]`):
List of kernel sizes to be used in each block.
in_channels (`List[int]`, *optional*, defaults to `[32, 16, 24, 40, 80, 112, 192]`):
List of input channel sizes to be used in each block for convolutional layers.
out_channels (`List[int]`, *optional*, defaults to `[16, 24, 40, 80, 112, 192, 320]`):
List of output channel sizes to be used in each block for convolutional layers.
depthwise_padding (`List[int]`, *optional*, defaults to `[]`):
List of block indices with square padding.
strides (`List[int]`, *optional*, defaults to `[1, 2, 2, 2, 1, 2, 1]`):
List of stride sizes to be used in each block for convolutional layers.
num_block_repeats (`List[int]`, *optional*, defaults to `[1, 2, 2, 3, 3, 4, 1]`):
List of the number of times each block is to repeated.
expand_ratios (`List[int]`, *optional*, defaults to `[1, 6, 6, 6, 6, 6, 6]`):
List of scaling coefficient of each block.
squeeze_expansion_ratio (`float`, *optional*, defaults to 0.25):
Squeeze expansion ratio.
hidden_act (`str` or `function`, *optional*, defaults to `"silu"`):
The non-linear activation function (function or string) in each block. If string, `"gelu"`, `"relu"`,
`"selu", `"gelu_new"`, `"silu"` and `"mish"` are supported.
hiddem_dim (`int`, *optional*, defaults to 1280):
The hidden dimension of the layer before the classification head.
pooling_type (`str` or `function`, *optional*, defaults to `"mean"`):
Type of final pooling to be applied before the dense classification head. Available options are [`"mean"`,
`"max"`]
initializer_range (`float`, *optional*, defaults to 0.02):
The standard deviation of the truncated_normal_initializer for initializing all weight matrices.
batch_norm_eps (`float`, *optional*, defaults to 1e-3):
The epsilon used by the batch normalization layers.
batch_norm_momentum (`float`, *optional*, defaults to 0.99):
The momentum used by the batch normalization layers.
drop_connect_rate (`float`, *optional*, defaults to 0.2):
The drop rate for skip connections.
Example:
```python
>>> from transformers import AlignVisionConfig, AlignVisionModel
>>> # Initializing a AlignVisionConfig with kakaobrain/align-base style configuration
>>> configuration = AlignVisionConfig()
>>> # Initializing a AlignVisionModel (with random weights) from the kakaobrain/align-base style configuration
>>> model = AlignVisionModel(configuration)
>>> # Accessing the model configuration
>>> configuration = model.config
```"""
model_type = "align_vision_model"
def __init__(
self,
num_channels: int = 3,
image_size: int = 600,
width_coefficient: float = 2.0,
depth_coefficient: float = 3.1,
depth_divisor: int = 8,
kernel_sizes: List[int] = [3, 3, 5, 3, 5, 5, 3],
in_channels: List[int] = [32, 16, 24, 40, 80, 112, 192],
out_channels: List[int] = [16, 24, 40, 80, 112, 192, 320],
depthwise_padding: List[int] = [],
strides: List[int] = [1, 2, 2, 2, 1, 2, 1],
num_block_repeats: List[int] = [1, 2, 2, 3, 3, 4, 1],
expand_ratios: List[int] = [1, 6, 6, 6, 6, 6, 6],
squeeze_expansion_ratio: float = 0.25,
hidden_act: str = "swish",
hidden_dim: int = 2560,
pooling_type: str = "mean",
initializer_range: float = 0.02,
batch_norm_eps: float = 0.001,
batch_norm_momentum: float = 0.99,
drop_connect_rate: float = 0.2,
**kwargs,
):
super().__init__(**kwargs)
self.num_channels = num_channels
self.image_size = image_size
self.width_coefficient = width_coefficient
self.depth_coefficient = depth_coefficient
self.depth_divisor = depth_divisor
self.kernel_sizes = kernel_sizes
self.in_channels = in_channels
self.out_channels = out_channels
self.depthwise_padding = depthwise_padding
self.strides = strides
self.num_block_repeats = num_block_repeats
self.expand_ratios = expand_ratios
self.squeeze_expansion_ratio = squeeze_expansion_ratio
self.hidden_act = hidden_act
self.hidden_dim = hidden_dim
self.pooling_type = pooling_type
self.initializer_range = initializer_range
self.batch_norm_eps = batch_norm_eps
self.batch_norm_momentum = batch_norm_momentum
self.drop_connect_rate = drop_connect_rate
self.num_hidden_layers = sum(num_block_repeats) * 4
@classmethod
def from_pretrained(cls, pretrained_model_name_or_path: Union[str, os.PathLike], **kwargs) -> "PretrainedConfig":
cls._set_token_in_kwargs(kwargs)
config_dict, kwargs = cls.get_config_dict(pretrained_model_name_or_path, **kwargs)
# get the vision config dict if we are loading from AlignConfig
if config_dict.get("model_type") == "align":
config_dict = config_dict["vision_config"]
if "model_type" in config_dict and hasattr(cls, "model_type") and config_dict["model_type"] != cls.model_type:
logger.warning(
f"You are using a model of type {config_dict['model_type']} to instantiate a model of type "
f"{cls.model_type}. This is not supported for all configurations of models and can yield errors."
)
return cls.from_dict(config_dict, **kwargs)
class AlignConfig(PretrainedConfig):
r"""
[`AlignConfig`] is the configuration class to store the configuration of a [`AlignModel`]. It is used to
instantiate a ALIGN model according to the specified arguments, defining the text model and vision model configs.
Instantiating a configuration with the defaults will yield a similar configuration to that of the ALIGN
[kakaobrain/align-base](https://huggingface.co/kakaobrain/align-base) architecture.
Configuration objects inherit from [`PretrainedConfig`] and can be used to control the model outputs. Read the
documentation from [`PretrainedConfig`] for more information.
Args:
text_config (`dict`, *optional*):
Dictionary of configuration options used to initialize [`AlignTextConfig`].
vision_config (`dict`, *optional*):
Dictionary of configuration options used to initialize [`AlignVisionConfig`].
projection_dim (`int`, *optional*, defaults to 640):
Dimentionality of text and vision projection layers.
temperature_init_value (`float`, *optional*, defaults to 1.0):
The inital value of the *temperature* paramter. Default is used as per the original ALIGN implementation.
initializer_range (`float`, *optional*, defaults to 0.02):
The standard deviation of the truncated_normal_initializer for initializing all weight matrices.
kwargs (*optional*):
Dictionary of keyword arguments.
Example:
```python
>>> from transformers import AlignConfig, AlignModel
>>> # Initializing a AlignConfig with kakaobrain/align-base style configuration
>>> configuration = AlignConfig()
>>> # Initializing a AlignModel (with random weights) from the kakaobrain/align-base style configuration
>>> model = AlignModel(configuration)
>>> # Accessing the model configuration
>>> configuration = model.config
>>> # We can also initialize a AlignConfig from a AlignTextConfig and a AlignVisionConfig
>>> from transformers import AlignTextConfig, AlignVisionConfig
>>> # Initializing ALIGN Text and Vision configurations
>>> config_text = AlignTextConfig()
>>> config_vision = AlignVisionConfig()
>>> config = AlignConfig.from_text_vision_configs(config_text, config_vision)
```"""
model_type = "align"
def __init__(
self,
text_config=None,
vision_config=None,
projection_dim=640,
temperature_init_value=1.0,
initializer_range=0.02,
**kwargs,
):
super().__init__(**kwargs)
if text_config is None:
text_config = {}
logger.info("text_config is None. Initializing the AlignTextConfig with default values.")
if vision_config is None:
vision_config = {}
logger.info("vision_config is None. Initializing the AlignVisionConfig with default values.")
self.text_config = AlignTextConfig(**text_config)
self.vision_config = AlignVisionConfig(**vision_config)
self.projection_dim = projection_dim
self.temperature_init_value = temperature_init_value
self.initializer_range = initializer_range
@classmethod
def from_text_vision_configs(cls, text_config: AlignTextConfig, vision_config: AlignVisionConfig, **kwargs):
r"""
Instantiate a [`AlignConfig`] (or a derived class) from align text model configuration and align vision model
configuration.
Returns:
[`AlignConfig`]: An instance of a configuration object
"""
return cls(text_config=text_config.to_dict(), vision_config=vision_config.to_dict(), **kwargs)
| transformers/src/transformers/models/align/configuration_align.py/0 | {
"file_path": "transformers/src/transformers/models/align/configuration_align.py",
"repo_id": "transformers",
"token_count": 6879
} | 301 |
# coding=utf-8
# Copyright 2021 The HuggingFace Inc. team.
#
# Licensed under the Apache License, Version 2.0 (the "License");
# you may not use this file except in compliance with the License.
# You may obtain a copy of the License at
#
# http://www.apache.org/licenses/LICENSE-2.0
#
# Unless required by applicable law or agreed to in writing, software
# distributed under the License is distributed on an "AS IS" BASIS,
# WITHOUT WARRANTIES OR CONDITIONS OF ANY KIND, either express or implied.
# See the License for the specific language governing permissions and
# limitations under the License.
""" AutoFeatureExtractor class."""
import importlib
import json
import os
import warnings
from collections import OrderedDict
from typing import Dict, Optional, Union
# Build the list of all feature extractors
from ...configuration_utils import PretrainedConfig
from ...dynamic_module_utils import get_class_from_dynamic_module, resolve_trust_remote_code
from ...feature_extraction_utils import FeatureExtractionMixin
from ...utils import CONFIG_NAME, FEATURE_EXTRACTOR_NAME, get_file_from_repo, logging
from .auto_factory import _LazyAutoMapping
from .configuration_auto import (
CONFIG_MAPPING_NAMES,
AutoConfig,
model_type_to_module_name,
replace_list_option_in_docstrings,
)
logger = logging.get_logger(__name__)
FEATURE_EXTRACTOR_MAPPING_NAMES = OrderedDict(
[
("audio-spectrogram-transformer", "ASTFeatureExtractor"),
("beit", "BeitFeatureExtractor"),
("chinese_clip", "ChineseCLIPFeatureExtractor"),
("clap", "ClapFeatureExtractor"),
("clip", "CLIPFeatureExtractor"),
("clipseg", "ViTFeatureExtractor"),
("clvp", "ClvpFeatureExtractor"),
("conditional_detr", "ConditionalDetrFeatureExtractor"),
("convnext", "ConvNextFeatureExtractor"),
("cvt", "ConvNextFeatureExtractor"),
("data2vec-audio", "Wav2Vec2FeatureExtractor"),
("data2vec-vision", "BeitFeatureExtractor"),
("deformable_detr", "DeformableDetrFeatureExtractor"),
("deit", "DeiTFeatureExtractor"),
("detr", "DetrFeatureExtractor"),
("dinat", "ViTFeatureExtractor"),
("donut-swin", "DonutFeatureExtractor"),
("dpt", "DPTFeatureExtractor"),
("encodec", "EncodecFeatureExtractor"),
("flava", "FlavaFeatureExtractor"),
("glpn", "GLPNFeatureExtractor"),
("groupvit", "CLIPFeatureExtractor"),
("hubert", "Wav2Vec2FeatureExtractor"),
("imagegpt", "ImageGPTFeatureExtractor"),
("layoutlmv2", "LayoutLMv2FeatureExtractor"),
("layoutlmv3", "LayoutLMv3FeatureExtractor"),
("levit", "LevitFeatureExtractor"),
("maskformer", "MaskFormerFeatureExtractor"),
("mctct", "MCTCTFeatureExtractor"),
("mobilenet_v1", "MobileNetV1FeatureExtractor"),
("mobilenet_v2", "MobileNetV2FeatureExtractor"),
("mobilevit", "MobileViTFeatureExtractor"),
("nat", "ViTFeatureExtractor"),
("owlvit", "OwlViTFeatureExtractor"),
("perceiver", "PerceiverFeatureExtractor"),
("poolformer", "PoolFormerFeatureExtractor"),
("pop2piano", "Pop2PianoFeatureExtractor"),
("regnet", "ConvNextFeatureExtractor"),
("resnet", "ConvNextFeatureExtractor"),
("seamless_m4t", "SeamlessM4TFeatureExtractor"),
("seamless_m4t_v2", "SeamlessM4TFeatureExtractor"),
("segformer", "SegformerFeatureExtractor"),
("sew", "Wav2Vec2FeatureExtractor"),
("sew-d", "Wav2Vec2FeatureExtractor"),
("speech_to_text", "Speech2TextFeatureExtractor"),
("speecht5", "SpeechT5FeatureExtractor"),
("swiftformer", "ViTFeatureExtractor"),
("swin", "ViTFeatureExtractor"),
("swinv2", "ViTFeatureExtractor"),
("table-transformer", "DetrFeatureExtractor"),
("timesformer", "VideoMAEFeatureExtractor"),
("tvlt", "TvltFeatureExtractor"),
("unispeech", "Wav2Vec2FeatureExtractor"),
("unispeech-sat", "Wav2Vec2FeatureExtractor"),
("univnet", "UnivNetFeatureExtractor"),
("van", "ConvNextFeatureExtractor"),
("videomae", "VideoMAEFeatureExtractor"),
("vilt", "ViltFeatureExtractor"),
("vit", "ViTFeatureExtractor"),
("vit_mae", "ViTFeatureExtractor"),
("vit_msn", "ViTFeatureExtractor"),
("wav2vec2", "Wav2Vec2FeatureExtractor"),
("wav2vec2-bert", "Wav2Vec2FeatureExtractor"),
("wav2vec2-conformer", "Wav2Vec2FeatureExtractor"),
("wavlm", "Wav2Vec2FeatureExtractor"),
("whisper", "WhisperFeatureExtractor"),
("xclip", "CLIPFeatureExtractor"),
("yolos", "YolosFeatureExtractor"),
]
)
FEATURE_EXTRACTOR_MAPPING = _LazyAutoMapping(CONFIG_MAPPING_NAMES, FEATURE_EXTRACTOR_MAPPING_NAMES)
def feature_extractor_class_from_name(class_name: str):
for module_name, extractors in FEATURE_EXTRACTOR_MAPPING_NAMES.items():
if class_name in extractors:
module_name = model_type_to_module_name(module_name)
module = importlib.import_module(f".{module_name}", "transformers.models")
try:
return getattr(module, class_name)
except AttributeError:
continue
for _, extractor in FEATURE_EXTRACTOR_MAPPING._extra_content.items():
if getattr(extractor, "__name__", None) == class_name:
return extractor
# We did not fine the class, but maybe it's because a dep is missing. In that case, the class will be in the main
# init and we return the proper dummy to get an appropriate error message.
main_module = importlib.import_module("transformers")
if hasattr(main_module, class_name):
return getattr(main_module, class_name)
return None
def get_feature_extractor_config(
pretrained_model_name_or_path: Union[str, os.PathLike],
cache_dir: Optional[Union[str, os.PathLike]] = None,
force_download: bool = False,
resume_download: bool = False,
proxies: Optional[Dict[str, str]] = None,
token: Optional[Union[bool, str]] = None,
revision: Optional[str] = None,
local_files_only: bool = False,
**kwargs,
):
"""
Loads the tokenizer configuration from a pretrained model tokenizer configuration.
Args:
pretrained_model_name_or_path (`str` or `os.PathLike`):
This can be either:
- a string, the *model id* of a pretrained model configuration hosted inside a model repo on
huggingface.co.
- a path to a *directory* containing a configuration file saved using the
[`~PreTrainedTokenizer.save_pretrained`] method, e.g., `./my_model_directory/`.
cache_dir (`str` or `os.PathLike`, *optional*):
Path to a directory in which a downloaded pretrained model configuration should be cached if the standard
cache should not be used.
force_download (`bool`, *optional*, defaults to `False`):
Whether or not to force to (re-)download the configuration files and override the cached versions if they
exist.
resume_download (`bool`, *optional*, defaults to `False`):
Whether or not to delete incompletely received file. Attempts to resume the download if such a file exists.
proxies (`Dict[str, str]`, *optional*):
A dictionary of proxy servers to use by protocol or endpoint, e.g., `{'http': 'foo.bar:3128',
'http://hostname': 'foo.bar:4012'}.` The proxies are used on each request.
token (`str` or *bool*, *optional*):
The token to use as HTTP bearer authorization for remote files. If `True`, will use the token generated
when running `huggingface-cli login` (stored in `~/.huggingface`).
revision (`str`, *optional*, defaults to `"main"`):
The specific model version to use. It can be a branch name, a tag name, or a commit id, since we use a
git-based system for storing models and other artifacts on huggingface.co, so `revision` can be any
identifier allowed by git.
local_files_only (`bool`, *optional*, defaults to `False`):
If `True`, will only try to load the tokenizer configuration from local files.
<Tip>
Passing `token=True` is required when you want to use a private model.
</Tip>
Returns:
`Dict`: The configuration of the tokenizer.
Examples:
```python
# Download configuration from huggingface.co and cache.
tokenizer_config = get_tokenizer_config("google-bert/bert-base-uncased")
# This model does not have a tokenizer config so the result will be an empty dict.
tokenizer_config = get_tokenizer_config("FacebookAI/xlm-roberta-base")
# Save a pretrained tokenizer locally and you can reload its config
from transformers import AutoTokenizer
tokenizer = AutoTokenizer.from_pretrained("google-bert/bert-base-cased")
tokenizer.save_pretrained("tokenizer-test")
tokenizer_config = get_tokenizer_config("tokenizer-test")
```"""
use_auth_token = kwargs.pop("use_auth_token", None)
if use_auth_token is not None:
warnings.warn(
"The `use_auth_token` argument is deprecated and will be removed in v5 of Transformers. Please use `token` instead.",
FutureWarning,
)
if token is not None:
raise ValueError("`token` and `use_auth_token` are both specified. Please set only the argument `token`.")
token = use_auth_token
resolved_config_file = get_file_from_repo(
pretrained_model_name_or_path,
FEATURE_EXTRACTOR_NAME,
cache_dir=cache_dir,
force_download=force_download,
resume_download=resume_download,
proxies=proxies,
token=token,
revision=revision,
local_files_only=local_files_only,
)
if resolved_config_file is None:
logger.info(
"Could not locate the feature extractor configuration file, will try to use the model config instead."
)
return {}
with open(resolved_config_file, encoding="utf-8") as reader:
return json.load(reader)
class AutoFeatureExtractor:
r"""
This is a generic feature extractor class that will be instantiated as one of the feature extractor classes of the
library when created with the [`AutoFeatureExtractor.from_pretrained`] class method.
This class cannot be instantiated directly using `__init__()` (throws an error).
"""
def __init__(self):
raise EnvironmentError(
"AutoFeatureExtractor is designed to be instantiated "
"using the `AutoFeatureExtractor.from_pretrained(pretrained_model_name_or_path)` method."
)
@classmethod
@replace_list_option_in_docstrings(FEATURE_EXTRACTOR_MAPPING_NAMES)
def from_pretrained(cls, pretrained_model_name_or_path, **kwargs):
r"""
Instantiate one of the feature extractor classes of the library from a pretrained model vocabulary.
The feature extractor class to instantiate is selected based on the `model_type` property of the config object
(either passed as an argument or loaded from `pretrained_model_name_or_path` if possible), or when it's
missing, by falling back to using pattern matching on `pretrained_model_name_or_path`:
List options
Params:
pretrained_model_name_or_path (`str` or `os.PathLike`):
This can be either:
- a string, the *model id* of a pretrained feature_extractor hosted inside a model repo on
huggingface.co.
- a path to a *directory* containing a feature extractor file saved using the
[`~feature_extraction_utils.FeatureExtractionMixin.save_pretrained`] method, e.g.,
`./my_model_directory/`.
- a path or url to a saved feature extractor JSON *file*, e.g.,
`./my_model_directory/preprocessor_config.json`.
cache_dir (`str` or `os.PathLike`, *optional*):
Path to a directory in which a downloaded pretrained model feature extractor should be cached if the
standard cache should not be used.
force_download (`bool`, *optional*, defaults to `False`):
Whether or not to force to (re-)download the feature extractor files and override the cached versions
if they exist.
resume_download (`bool`, *optional*, defaults to `False`):
Whether or not to delete incompletely received file. Attempts to resume the download if such a file
exists.
proxies (`Dict[str, str]`, *optional*):
A dictionary of proxy servers to use by protocol or endpoint, e.g., `{'http': 'foo.bar:3128',
'http://hostname': 'foo.bar:4012'}.` The proxies are used on each request.
token (`str` or *bool*, *optional*):
The token to use as HTTP bearer authorization for remote files. If `True`, will use the token generated
when running `huggingface-cli login` (stored in `~/.huggingface`).
revision (`str`, *optional*, defaults to `"main"`):
The specific model version to use. It can be a branch name, a tag name, or a commit id, since we use a
git-based system for storing models and other artifacts on huggingface.co, so `revision` can be any
identifier allowed by git.
return_unused_kwargs (`bool`, *optional*, defaults to `False`):
If `False`, then this function returns just the final feature extractor object. If `True`, then this
functions returns a `Tuple(feature_extractor, unused_kwargs)` where *unused_kwargs* is a dictionary
consisting of the key/value pairs whose keys are not feature extractor attributes: i.e., the part of
`kwargs` which has not been used to update `feature_extractor` and is otherwise ignored.
trust_remote_code (`bool`, *optional*, defaults to `False`):
Whether or not to allow for custom models defined on the Hub in their own modeling files. This option
should only be set to `True` for repositories you trust and in which you have read the code, as it will
execute code present on the Hub on your local machine.
kwargs (`Dict[str, Any]`, *optional*):
The values in kwargs of any keys which are feature extractor attributes will be used to override the
loaded values. Behavior concerning key/value pairs whose keys are *not* feature extractor attributes is
controlled by the `return_unused_kwargs` keyword parameter.
<Tip>
Passing `token=True` is required when you want to use a private model.
</Tip>
Examples:
```python
>>> from transformers import AutoFeatureExtractor
>>> # Download feature extractor from huggingface.co and cache.
>>> feature_extractor = AutoFeatureExtractor.from_pretrained("facebook/wav2vec2-base-960h")
>>> # If feature extractor files are in a directory (e.g. feature extractor was saved using *save_pretrained('./test/saved_model/')*)
>>> # feature_extractor = AutoFeatureExtractor.from_pretrained("./test/saved_model/")
```"""
use_auth_token = kwargs.pop("use_auth_token", None)
if use_auth_token is not None:
warnings.warn(
"The `use_auth_token` argument is deprecated and will be removed in v5 of Transformers. Please use `token` instead.",
FutureWarning,
)
if kwargs.get("token", None) is not None:
raise ValueError(
"`token` and `use_auth_token` are both specified. Please set only the argument `token`."
)
kwargs["token"] = use_auth_token
config = kwargs.pop("config", None)
trust_remote_code = kwargs.pop("trust_remote_code", None)
kwargs["_from_auto"] = True
config_dict, _ = FeatureExtractionMixin.get_feature_extractor_dict(pretrained_model_name_or_path, **kwargs)
feature_extractor_class = config_dict.get("feature_extractor_type", None)
feature_extractor_auto_map = None
if "AutoFeatureExtractor" in config_dict.get("auto_map", {}):
feature_extractor_auto_map = config_dict["auto_map"]["AutoFeatureExtractor"]
# If we don't find the feature extractor class in the feature extractor config, let's try the model config.
if feature_extractor_class is None and feature_extractor_auto_map is None:
if not isinstance(config, PretrainedConfig):
config = AutoConfig.from_pretrained(pretrained_model_name_or_path, **kwargs)
# It could be in `config.feature_extractor_type``
feature_extractor_class = getattr(config, "feature_extractor_type", None)
if hasattr(config, "auto_map") and "AutoFeatureExtractor" in config.auto_map:
feature_extractor_auto_map = config.auto_map["AutoFeatureExtractor"]
if feature_extractor_class is not None:
feature_extractor_class = feature_extractor_class_from_name(feature_extractor_class)
has_remote_code = feature_extractor_auto_map is not None
has_local_code = feature_extractor_class is not None or type(config) in FEATURE_EXTRACTOR_MAPPING
trust_remote_code = resolve_trust_remote_code(
trust_remote_code, pretrained_model_name_or_path, has_local_code, has_remote_code
)
if has_remote_code and trust_remote_code:
feature_extractor_class = get_class_from_dynamic_module(
feature_extractor_auto_map, pretrained_model_name_or_path, **kwargs
)
_ = kwargs.pop("code_revision", None)
if os.path.isdir(pretrained_model_name_or_path):
feature_extractor_class.register_for_auto_class()
return feature_extractor_class.from_dict(config_dict, **kwargs)
elif feature_extractor_class is not None:
return feature_extractor_class.from_dict(config_dict, **kwargs)
# Last try: we use the FEATURE_EXTRACTOR_MAPPING.
elif type(config) in FEATURE_EXTRACTOR_MAPPING:
feature_extractor_class = FEATURE_EXTRACTOR_MAPPING[type(config)]
return feature_extractor_class.from_dict(config_dict, **kwargs)
raise ValueError(
f"Unrecognized feature extractor in {pretrained_model_name_or_path}. Should have a "
f"`feature_extractor_type` key in its {FEATURE_EXTRACTOR_NAME} of {CONFIG_NAME}, or one of the following "
f"`model_type` keys in its {CONFIG_NAME}: {', '.join(c for c in FEATURE_EXTRACTOR_MAPPING_NAMES.keys())}"
)
@staticmethod
def register(config_class, feature_extractor_class, exist_ok=False):
"""
Register a new feature extractor for this class.
Args:
config_class ([`PretrainedConfig`]):
The configuration corresponding to the model to register.
feature_extractor_class ([`FeatureExtractorMixin`]): The feature extractor to register.
"""
FEATURE_EXTRACTOR_MAPPING.register(config_class, feature_extractor_class, exist_ok=exist_ok)
| transformers/src/transformers/models/auto/feature_extraction_auto.py/0 | {
"file_path": "transformers/src/transformers/models/auto/feature_extraction_auto.py",
"repo_id": "transformers",
"token_count": 7703
} | 302 |
# Copyright 2020 The HuggingFace Team. All rights reserved.
#
# Licensed under the Apache License, Version 2.0 (the "License");
# you may not use this file except in compliance with the License.
# You may obtain a copy of the License at
#
# http://www.apache.org/licenses/LICENSE-2.0
#
# Unless required by applicable law or agreed to in writing, software
# distributed under the License is distributed on an "AS IS" BASIS,
# WITHOUT WARRANTIES OR CONDITIONS OF ANY KIND, either express or implied.
# See the License for the specific language governing permissions and
# limitations under the License.
from typing import TYPE_CHECKING
from ...utils import (
OptionalDependencyNotAvailable,
_LazyModule,
is_flax_available,
is_tf_available,
is_tokenizers_available,
is_torch_available,
)
_import_structure = {
"configuration_bart": ["BART_PRETRAINED_CONFIG_ARCHIVE_MAP", "BartConfig", "BartOnnxConfig"],
"tokenization_bart": ["BartTokenizer"],
}
try:
if not is_tokenizers_available():
raise OptionalDependencyNotAvailable()
except OptionalDependencyNotAvailable:
pass
else:
_import_structure["tokenization_bart_fast"] = ["BartTokenizerFast"]
try:
if not is_torch_available():
raise OptionalDependencyNotAvailable()
except OptionalDependencyNotAvailable:
pass
else:
_import_structure["modeling_bart"] = [
"BART_PRETRAINED_MODEL_ARCHIVE_LIST",
"BartForCausalLM",
"BartForConditionalGeneration",
"BartForQuestionAnswering",
"BartForSequenceClassification",
"BartModel",
"BartPreTrainedModel",
"BartPretrainedModel",
"PretrainedBartModel",
]
try:
if not is_tf_available():
raise OptionalDependencyNotAvailable()
except OptionalDependencyNotAvailable:
pass
else:
_import_structure["modeling_tf_bart"] = [
"TFBartForConditionalGeneration",
"TFBartForSequenceClassification",
"TFBartModel",
"TFBartPretrainedModel",
]
try:
if not is_flax_available():
raise OptionalDependencyNotAvailable()
except OptionalDependencyNotAvailable:
pass
else:
_import_structure["modeling_flax_bart"] = [
"FlaxBartDecoderPreTrainedModel",
"FlaxBartForCausalLM",
"FlaxBartForConditionalGeneration",
"FlaxBartForQuestionAnswering",
"FlaxBartForSequenceClassification",
"FlaxBartModel",
"FlaxBartPreTrainedModel",
]
if TYPE_CHECKING:
from .configuration_bart import BART_PRETRAINED_CONFIG_ARCHIVE_MAP, BartConfig, BartOnnxConfig
from .tokenization_bart import BartTokenizer
try:
if not is_tokenizers_available():
raise OptionalDependencyNotAvailable()
except OptionalDependencyNotAvailable:
pass
else:
from .tokenization_bart_fast import BartTokenizerFast
try:
if not is_torch_available():
raise OptionalDependencyNotAvailable()
except OptionalDependencyNotAvailable:
pass
else:
from .modeling_bart import (
BART_PRETRAINED_MODEL_ARCHIVE_LIST,
BartForCausalLM,
BartForConditionalGeneration,
BartForQuestionAnswering,
BartForSequenceClassification,
BartModel,
BartPreTrainedModel,
BartPretrainedModel,
PretrainedBartModel,
)
try:
if not is_tf_available():
raise OptionalDependencyNotAvailable()
except OptionalDependencyNotAvailable:
pass
else:
from .modeling_tf_bart import (
TFBartForConditionalGeneration,
TFBartForSequenceClassification,
TFBartModel,
TFBartPretrainedModel,
)
try:
if not is_flax_available():
raise OptionalDependencyNotAvailable()
except OptionalDependencyNotAvailable:
pass
else:
from .modeling_flax_bart import (
FlaxBartDecoderPreTrainedModel,
FlaxBartForCausalLM,
FlaxBartForConditionalGeneration,
FlaxBartForQuestionAnswering,
FlaxBartForSequenceClassification,
FlaxBartModel,
FlaxBartPreTrainedModel,
)
else:
import sys
sys.modules[__name__] = _LazyModule(__name__, globals()["__file__"], _import_structure, module_spec=__spec__)
| transformers/src/transformers/models/bart/__init__.py/0 | {
"file_path": "transformers/src/transformers/models/bart/__init__.py",
"repo_id": "transformers",
"token_count": 1839
} | 303 |
# coding=utf-8
# Copyright 2022 The Salesforce Team Authors and The HuggingFace Team. All rights reserved.
#
# Licensed under the Apache License, Version 2.0 (the "License");
# you may not use this file except in compliance with the License.
# You may obtain a copy of the License at
#
# http://www.apache.org/licenses/LICENSE-2.0
#
# Unless required by applicable law or agreed to in writing, software
# distributed under the License is distributed on an "AS IS" BASIS,
# WITHOUT WARRANTIES OR CONDITIONS OF ANY KIND, either express or implied.
# See the License for the specific language governing permissions and
# limitations under the License.
""" PyTorch BLIP model."""
import warnings
from dataclasses import dataclass
from typing import Any, Optional, Tuple, Union
import torch
import torch.utils.checkpoint
from torch import nn
from torch.nn.functional import normalize
from ...activations import ACT2FN
from ...modeling_outputs import BaseModelOutput, BaseModelOutputWithPooling
from ...modeling_utils import PreTrainedModel
from ...utils import (
ModelOutput,
add_start_docstrings,
add_start_docstrings_to_model_forward,
logging,
replace_return_docstrings,
)
from .configuration_blip import BlipConfig, BlipTextConfig, BlipVisionConfig
from .modeling_blip_text import BlipTextLMHeadModel, BlipTextModel
logger = logging.get_logger(__name__)
_CHECKPOINT_FOR_DOC = "Salesforce/blip-vqa-base"
BLIP_PRETRAINED_MODEL_ARCHIVE_LIST = [
"Salesforce/blip-vqa-base",
"Salesforce/blip-vqa-capfilt-large",
"Salesforce/blip-image-captioning-base",
"Salesforce/blip-image-captioning-large",
"Salesforce/blip-itm-base-coco",
"Salesforce/blip-itm-large-coco",
"Salesforce/blip-itm-base-flickr",
"Salesforce/blip-itm-large-flickr",
# See all BLIP models at https://huggingface.co/models?filter=blip
]
# Copied from transformers.models.clip.modeling_clip.contrastive_loss
def contrastive_loss(logits: torch.Tensor) -> torch.Tensor:
return nn.functional.cross_entropy(logits, torch.arange(len(logits), device=logits.device))
# Copied from transformers.models.clip.modeling_clip.clip_loss with clip->blip
def blip_loss(similarity: torch.Tensor) -> torch.Tensor:
caption_loss = contrastive_loss(similarity)
image_loss = contrastive_loss(similarity.t())
return (caption_loss + image_loss) / 2.0
@dataclass
class BlipForConditionalGenerationModelOutput(ModelOutput):
"""
Adapted from the base class for vision model's outputs that also contains image embeddings of the pooling of the
last hidden states. This class also adds the loss term from the text decoder.
Args:
loss (`torch.FloatTensor`, *optional*, returned when `labels` is provided, `torch.FloatTensor` of shape `(1,)`):
Languge modeling loss from the text decoder.
logits (`torch.FloatTensor` of shape `(batch_size, sequence_length, config.vocab_size)`, *optional*):
Prediction scores of the language modeling head of the text decoder model.
image_embeds (`torch.FloatTensor` of shape `(batch_size, output_dim)`, *optional*):
The image embeddings obtained after applying the Vision Transformer model to the input image.
last_hidden_state (`torch.FloatTensor` of shape `(batch_size, sequence_length, hidden_size)`, *optional*):
Sequence of hidden-states at the output of the last layer of the model.
hidden_states (`tuple(torch.FloatTensor)`, *optional*, returned when `output_hidden_states=True`):
Tuple of `torch.FloatTensor` (one for the output of the embeddings, if the model has an embedding layer, +
one for the output of each layer) of shape `(batch_size, sequence_length, hidden_size)`.
Hidden-states of the model at the output of each layer plus the optional initial embedding outputs.
attentions (`tuple(torch.FloatTensor)`, *optional*, returned when `output_attentions=True` is passed):
Tuple of `torch.FloatTensor` (one for each layer) of shape `(batch_size, num_heads, sequence_length,
sequence_length)`.
Attentions weights after the attention softmax, used to compute the weighted average in the self-attention
heads.
"""
loss: Optional[Tuple[torch.FloatTensor]] = None
logits: Optional[Tuple[torch.FloatTensor]] = None
image_embeds: Optional[torch.FloatTensor] = None
last_hidden_state: torch.FloatTensor = None
hidden_states: Optional[Tuple[torch.FloatTensor, ...]] = None
attentions: Optional[Tuple[torch.FloatTensor, ...]] = None
@property
def decoder_logits(self):
warnings.warn(
"`decoder_logits` attribute is deprecated and will be removed in version 5 of Transformers."
" Please use the `logits` attribute to retrieve the final output instead.",
FutureWarning,
)
return self.logits
@dataclass
class BlipTextVisionModelOutput(ModelOutput):
"""
Adapted from the base class for vision model's outputs that also contains image embeddings of the pooling of the
last hidden states. This class also adds the loss term from the text decoder.
Args:
loss (`torch.FloatTensor` of shape `(1,)`, *optional*, returned when `labels` is provided):
Languge modeling loss from the text decoder.
image_embeds (`torch.FloatTensor` of shape `(batch_size, output_dim)` *optional* returned when model is initialized with `with_projection=True`):
The image embeddings obtained by applying the projection layer to the pooler_output.
last_hidden_state (`torch.FloatTensor` of shape `(batch_size, sequence_length, hidden_size)`):
Sequence of hidden-states at the output of the last layer of the model.
hidden_states (`tuple(torch.FloatTensor)`, *optional*, returned when `output_hidden_states=True` is passed or when `config.output_hidden_states=True`):
Tuple of `torch.FloatTensor` (one for the output of the embeddings, if the model has an embedding layer, +
one for the output of each layer) of shape `(batch_size, sequence_length, hidden_size)`.
Hidden-states of the model at the output of each layer plus the optional initial embedding outputs.
attentions (`tuple(torch.FloatTensor)`, *optional*, returned when `output_attentions=True` is passed or when `config.output_attentions=True`):
Tuple of `torch.FloatTensor` (one for each layer) of shape `(batch_size, num_heads, sequence_length,
sequence_length)`.
Attentions weights after the attention softmax, used to compute the weighted average in the self-attention
heads.
"""
loss: Optional[torch.FloatTensor] = None
image_embeds: Optional[torch.FloatTensor] = None
last_hidden_state: torch.FloatTensor = None
hidden_states: Optional[Tuple[torch.FloatTensor, ...]] = None
attentions: Optional[Tuple[torch.FloatTensor, ...]] = None
@dataclass
class BlipImageTextMatchingModelOutput(ModelOutput):
"""
Adapted from the base class for vision model's outputs that also contains image embeddings of the pooling of the
last hidden states. This class also adds the loss term from the text decoder as well as the image-text similarity
scores.
Args:
itm_score (`torch.FloatTensor`):
The image-text similarity scores.
loss (`torch.FloatTensor` of shape `(1,)`, *optional*, returned when `labels` is provided):
Languge modeling loss from the text decoder.
image_embeds (`torch.FloatTensor` of shape `(batch_size, output_dim)` *optional* returned when model is initialized with `with_projection=True`):
The image embeddings obtained by applying the projection layer to the pooler_output.
last_hidden_state (`torch.FloatTensor` of shape `(batch_size, sequence_length, hidden_size)`):
Sequence of hidden-states at the output of the last layer of the model.
hidden_states (`tuple(torch.FloatTensor)`, *optional*, returned when `output_hidden_states=True` is passed or when `config.output_hidden_states=True`):
Tuple of `torch.FloatTensor` (one for the output of the embeddings, if the model has an embedding layer, +
one for the output of each layer) of shape `(batch_size, sequence_length, hidden_size)`.
Hidden-states of the model at the output of each layer plus the optional initial embedding outputs.
vision_pooler_output (`torch.FloatTensor` of shape `(batch_size, hidden_size)`, *optional*):
Last layer hidden-state of the vision of the vision-only branch of the model.
attentions (`tuple(torch.FloatTensor)`, *optional*, returned when `output_attentions=True` is passed or when `config.output_attentions=True`):
Tuple of `torch.FloatTensor` (one for each layer) of shape `(batch_size, num_heads, sequence_length,
sequence_length)`.
Attentions weights after the attention softmax, used to compute the weighted average in the self-attention
heads.
question_embeds (`torch.FloatTensor`):
The question embeddings obtained by the text projection layer.
"""
itm_score: Optional[torch.FloatTensor] = None
loss: Optional[torch.FloatTensor] = None
image_embeds: Optional[torch.FloatTensor] = None
last_hidden_state: torch.FloatTensor = None
hidden_states: Optional[Tuple[torch.FloatTensor, ...]] = None
vision_pooler_output: Optional[torch.FloatTensor] = None
attentions: Optional[Tuple[torch.FloatTensor, ...]] = None
question_embeds: Optional[Tuple[torch.FloatTensor]] = None
@dataclass
class BlipOutput(ModelOutput):
"""
Args:
loss (`torch.FloatTensor` of shape `(1,)`, *optional*, returned when `return_loss` is `True`):
Contrastive loss for image-text similarity.
logits_per_image:(`torch.FloatTensor` of shape `(image_batch_size, text_batch_size)`):
The scaled dot product scores between `image_embeds` and `text_embeds`. This represents the image-text
similarity scores.
logits_per_text:(`torch.FloatTensor` of shape `(text_batch_size, image_batch_size)`):
The scaled dot product scores between `text_embeds` and `image_embeds`. This represents the text-image
similarity scores.
text_embeds(`torch.FloatTensor` of shape `(batch_size, output_dim`):
The text embeddings obtained by applying the projection layer to the pooled output of [`BlipTextModel`].
image_embeds(`torch.FloatTensor` of shape `(batch_size, output_dim`):
The image embeddings obtained by applying the projection layer to the pooled output of [`BlipVisionModel`].
text_model_output(`BaseModelOutputWithPooling`):
The output of the [`BlipTextModel`].
vision_model_output(`BaseModelOutputWithPooling`):
The output of the [`BlipVisionModel`].
"""
loss: Optional[torch.FloatTensor] = None
logits_per_image: torch.FloatTensor = None
logits_per_text: torch.FloatTensor = None
text_embeds: torch.FloatTensor = None
image_embeds: torch.FloatTensor = None
text_model_output: BaseModelOutputWithPooling = None
vision_model_output: BaseModelOutputWithPooling = None
def to_tuple(self) -> Tuple[Any]:
return tuple(
self[k] if k not in ["text_model_output", "vision_model_output"] else getattr(self, k).to_tuple()
for k in self.keys()
)
class BlipVisionEmbeddings(nn.Module):
def __init__(self, config: BlipVisionConfig):
super().__init__()
self.config = config
self.embed_dim = config.hidden_size
self.image_size = config.image_size
self.patch_size = config.patch_size
self.class_embedding = nn.Parameter(torch.randn(1, 1, self.embed_dim))
self.patch_embedding = nn.Conv2d(
in_channels=3, out_channels=self.embed_dim, kernel_size=self.patch_size, stride=self.patch_size
)
self.num_patches = (self.image_size // self.patch_size) ** 2
self.num_positions = self.num_patches + 1
self.position_embedding = nn.Parameter(torch.randn(1, self.num_positions, self.embed_dim))
def forward(self, pixel_values: torch.FloatTensor) -> torch.Tensor:
batch_size = pixel_values.shape[0]
target_dtype = self.patch_embedding.weight.dtype
patch_embeds = self.patch_embedding(pixel_values.to(dtype=target_dtype)) # shape = [*, width, grid, grid]
patch_embeds = patch_embeds.flatten(2).transpose(1, 2)
class_embeds = self.class_embedding.expand(batch_size, 1, -1).to(target_dtype)
embeddings = torch.cat([class_embeds, patch_embeds], dim=1)
embeddings = embeddings + self.position_embedding[:, : embeddings.size(1), :].to(target_dtype)
return embeddings
# Copied from transformers.models.clip.modeling_clip.CLIPTextEmbeddings with CLIP->Blip
class BlipTextEmbeddings(nn.Module):
def __init__(self, config: BlipTextConfig):
super().__init__()
embed_dim = config.hidden_size
self.token_embedding = nn.Embedding(config.vocab_size, embed_dim)
self.position_embedding = nn.Embedding(config.max_position_embeddings, embed_dim)
# position_ids (1, len position emb) is contiguous in memory and exported when serialized
self.register_buffer(
"position_ids", torch.arange(config.max_position_embeddings).expand((1, -1)), persistent=False
)
def forward(
self,
input_ids: Optional[torch.LongTensor] = None,
position_ids: Optional[torch.LongTensor] = None,
inputs_embeds: Optional[torch.FloatTensor] = None,
) -> torch.Tensor:
seq_length = input_ids.shape[-1] if input_ids is not None else inputs_embeds.shape[-2]
if position_ids is None:
position_ids = self.position_ids[:, :seq_length]
if inputs_embeds is None:
inputs_embeds = self.token_embedding(input_ids)
position_embeddings = self.position_embedding(position_ids)
embeddings = inputs_embeds + position_embeddings
return embeddings
class BlipAttention(nn.Module):
"""Multi-headed attention from 'Attention Is All You Need' paper"""
def __init__(self, config):
super().__init__()
self.config = config
self.embed_dim = config.hidden_size
self.num_heads = config.num_attention_heads
self.head_dim = self.embed_dim // self.num_heads
if self.head_dim * self.num_heads != self.embed_dim:
raise ValueError(
f"embed_dim must be divisible by num_heads (got `embed_dim`: {self.embed_dim} and `num_heads`:"
f" {self.num_heads})."
)
self.scale = self.head_dim**-0.5
self.dropout = nn.Dropout(config.attention_dropout)
self.qkv = nn.Linear(self.embed_dim, 3 * self.embed_dim)
self.projection = nn.Linear(self.embed_dim, self.embed_dim)
def _shape(self, tensor: torch.Tensor, seq_len: int, bsz: int):
return tensor.view(bsz, seq_len, self.num_heads, self.head_dim).transpose(1, 2).contiguous()
def forward(
self,
hidden_states: torch.Tensor,
head_mask: Optional[torch.Tensor] = None,
output_attentions: Optional[bool] = False,
) -> Tuple[torch.Tensor, Optional[torch.Tensor], Optional[Tuple[torch.Tensor]]]:
"""Input shape: Batch x Time x Channel"""
bsz, tgt_len, embed_dim = hidden_states.size()
mixed_qkv = (
self.qkv(hidden_states)
.reshape(bsz, tgt_len, 3, self.num_heads, embed_dim // self.num_heads)
.permute(2, 0, 3, 1, 4)
)
query_states, key_states, value_states = mixed_qkv[0], mixed_qkv[1], mixed_qkv[2]
# Take the dot product between "query" and "key" to get the raw attention scores.
attention_scores = torch.matmul(query_states, key_states.transpose(-1, -2))
attention_scores = attention_scores * self.scale
# Normalize the attention scores to probabilities.
attention_probs = nn.functional.softmax(attention_scores, dim=-1)
# This is actually dropping out entire tokens to attend to, which might
# seem a bit unusual, but is taken from the original Transformer paper.
attention_probs = self.dropout(attention_probs)
# Mask heads if we want to
if head_mask is not None:
attention_probs = attention_probs * head_mask
context_layer = torch.matmul(attention_probs, value_states).permute(0, 2, 1, 3)
new_context_layer_shape = context_layer.size()[:-2] + (self.embed_dim,)
context_layer = context_layer.reshape(new_context_layer_shape)
output = self.projection(context_layer)
outputs = (output, attention_probs) if output_attentions else (output, None)
return outputs
# Copied from transformers.models.clip.modeling_clip.CLIPMLP with CLIP->Blip
class BlipMLP(nn.Module):
def __init__(self, config):
super().__init__()
self.config = config
self.activation_fn = ACT2FN[config.hidden_act]
self.fc1 = nn.Linear(config.hidden_size, config.intermediate_size)
self.fc2 = nn.Linear(config.intermediate_size, config.hidden_size)
def forward(self, hidden_states: torch.Tensor) -> torch.Tensor:
hidden_states = self.fc1(hidden_states)
hidden_states = self.activation_fn(hidden_states)
hidden_states = self.fc2(hidden_states)
return hidden_states
class BlipEncoderLayer(nn.Module):
def __init__(self, config: BlipConfig):
super().__init__()
self.embed_dim = config.hidden_size
self.self_attn = BlipAttention(config)
self.layer_norm1 = nn.LayerNorm(self.embed_dim, eps=config.layer_norm_eps)
self.mlp = BlipMLP(config)
self.layer_norm2 = nn.LayerNorm(self.embed_dim, eps=config.layer_norm_eps)
def forward(
self,
hidden_states: torch.Tensor,
attention_mask: torch.Tensor,
output_attentions: Optional[bool] = False,
) -> Tuple[torch.FloatTensor]:
"""
Args:
hidden_states (`torch.FloatTensor`): input to the layer of shape `(batch, seq_len, embed_dim)`
attention_mask (`torch.FloatTensor`): attention mask of size
`(batch, 1, tgt_len, src_len)` where padding elements are indicated by very large negative values.
`(config.encoder_attention_heads,)`.
output_attentions (`bool`, *optional*):
Whether or not to return the attentions tensors of all attention layers. See `attentions` under
returned tensors for more detail.
"""
residual = hidden_states
hidden_states = self.layer_norm1(hidden_states)
hidden_states, attn_weights = self.self_attn(
hidden_states=hidden_states,
head_mask=attention_mask,
output_attentions=output_attentions,
)
hidden_states = hidden_states + residual
residual = hidden_states
hidden_states = self.layer_norm2(hidden_states)
hidden_states = self.mlp(hidden_states)
hidden_states = hidden_states + residual
outputs = (hidden_states,)
if output_attentions:
outputs += (attn_weights,)
return outputs
class BlipPreTrainedModel(PreTrainedModel):
"""
An abstract class to handle weights initialization and a simple interface for downloading and loading pretrained
models.
"""
config_class = BlipConfig
base_model_prefix = "blip"
supports_gradient_checkpointing = True
def _init_weights(self, module):
"""Initialize the weights"""
factor = self.config.initializer_range
if isinstance(module, nn.Conv2d) or isinstance(module, nn.Embedding) or isinstance(module, nn.Linear):
module.weight.data.normal_(mean=0.0, std=factor)
if hasattr(module, "bias") and module.bias is not None:
module.bias.data.zero_()
if isinstance(module, BlipVisionEmbeddings):
if hasattr(self.config, "vision_config"):
factor = self.config.vision_config.initializer_range
nn.init.trunc_normal_(
module.position_embedding,
mean=0.0,
std=factor,
)
nn.init.trunc_normal_(
module.class_embedding,
mean=0.0,
std=factor,
)
elif isinstance(module, nn.LayerNorm):
module.bias.data.zero_()
module.weight.data.fill_(1.0)
elif isinstance(module, nn.Linear) and module.bias is not None:
module.bias.data.zero_()
BLIP_START_DOCSTRING = r"""
This model inherits from [`PreTrainedModel`]. Check the superclass documentation for the generic methods the
library implements for all its model (such as downloading or saving, resizing the input embeddings, pruning heads
etc.)
This model is also a PyTorch [torch.nn.Module](https://pytorch.org/docs/stable/nn.html#torch.nn.Module) subclass.
Use it as a regular PyTorch Module and refer to the PyTorch documentation for all matter related to general usage
and behavior.
Parameters:
config ([`BlipConfig`]): Model configuration class with all the parameters of the model.
Initializing with a config file does not load the weights associated with the model, only the
configuration. Check out the [`~PreTrainedModel.from_pretrained`] method to load the model weights.
"""
BLIP_TEXT_INPUTS_DOCSTRING = r"""
Args:
input_ids (`torch.LongTensor` of shape `(batch_size, sequence_length)`):
Indices of input sequence tokens in the vocabulary. Padding will be ignored by default should you provide
it.
Indices can be obtained using [`AutoProcessor`]. See [`BlipProcessor.__call__`] for details.
[What are input IDs?](../glossary#input-ids)
attention_mask (`torch.Tensor` of shape `(batch_size, sequence_length)`, *optional*):
Mask to avoid performing attention on padding token indices. Mask values selected in `[0, 1]`:
- 1 for tokens that are **not masked**,
- 0 for tokens that are **masked**.
[What are attention masks?](../glossary#attention-mask)
position_ids (`torch.LongTensor` of shape `(batch_size, sequence_length)`, *optional*):
Indices of positions of each input sequence tokens in the position embeddings. Selected in the range `[0,
config.max_position_embeddings - 1]`.
[What are position IDs?](../glossary#position-ids)
output_attentions (`bool`, *optional*):
Whether or not to return the attentions tensors of all attention layers. See `attentions` under returned
tensors for more detail.
output_hidden_states (`bool`, *optional*):
Whether or not to return the hidden states of all layers. See `hidden_states` under returned tensors for
more detail.
return_dict (`bool`, *optional*):
Whether or not to return a [`~utils.ModelOutput`] instead of a plain tuple.
"""
BLIP_VISION_INPUTS_DOCSTRING = r"""
Args:
pixel_values (`torch.FloatTensor` of shape `(batch_size, num_channels, height, width)`):
Pixel values. Padding will be ignored by default should you provide it. Pixel values can be obtained using
[`BlipImageProcessor`]. See [`BlipImageProcessor.__call__`] for details.
output_attentions (`bool`, *optional*):
Whether or not to return the attentions tensors of all attention layers. See `attentions` under returned
tensors for more detail.
output_hidden_states (`bool`, *optional*):
Whether or not to return the hidden states of all layers. See `hidden_states` under returned tensors for
more detail.
return_dict (`bool`, *optional*):
Whether or not to return a [`~utils.ModelOutput`] instead of a plain tuple.
"""
BLIP_INPUTS_DOCSTRING = r"""
Args:
input_ids (`torch.LongTensor` of shape `(batch_size, sequence_length)`):
Indices of input sequence tokens in the vocabulary. Padding will be ignored by default should you provide
it.
Indices can be obtained using [`AutoProcessor`]. See [`BlipProcessor.__call__`] for details.
[What are input IDs?](../glossary#input-ids)
attention_mask (`torch.Tensor` of shape `(batch_size, sequence_length)`, *optional*):
Mask to avoid performing attention on padding token indices. Mask values selected in `[0, 1]`:
- 1 for tokens that are **not masked**,
- 0 for tokens that are **masked**.
[What are attention masks?](../glossary#attention-mask)
position_ids (`torch.LongTensor` of shape `(batch_size, sequence_length)`, *optional*):
Indices of positions of each input sequence tokens in the position embeddings. Selected in the range `[0,
config.max_position_embeddings - 1]`.
[What are position IDs?](../glossary#position-ids)
pixel_values (`torch.FloatTensor` of shape `(batch_size, num_channels, height, width)`):
Pixel values. Padding will be ignored by default should you provide it. Pixel values can be obtained using
[`BlipImageProcessor`]. See [`BlipImageProcessor.__call__`] for details.
return_loss (`bool`, *optional*):
Whether or not to return the contrastive loss.
output_attentions (`bool`, *optional*):
Whether or not to return the attentions tensors of all attention layers. See `attentions` under returned
tensors for more detail.
output_hidden_states (`bool`, *optional*):
Whether or not to return the hidden states of all layers. See `hidden_states` under returned tensors for
more detail.
return_dict (`bool`, *optional*):
Whether or not to return a [`~utils.ModelOutput`] instead of a plain tuple.
"""
class BlipEncoder(nn.Module):
"""
Transformer encoder consisting of `config.num_hidden_layers` self attention layers. Each layer is a
[`BlipEncoderLayer`].
Args:
config (`BlipConfig`):
The corresponding vision configuration for the `BlipEncoder`.
"""
def __init__(self, config: BlipConfig):
super().__init__()
self.config = config
self.layers = nn.ModuleList([BlipEncoderLayer(config) for _ in range(config.num_hidden_layers)])
self.gradient_checkpointing = False
def forward(
self,
inputs_embeds,
attention_mask: Optional[torch.Tensor] = None,
output_attentions: Optional[bool] = None,
output_hidden_states: Optional[bool] = None,
return_dict: Optional[bool] = None,
) -> Union[Tuple, BaseModelOutput]:
r"""
Args:
inputs_embeds (`torch.FloatTensor` of shape `(batch_size, sequence_length, hidden_size)`):
Embedded representation of the inputs. Should be float, not int tokens.
attention_mask (`torch.Tensor` of shape `(batch_size, sequence_length)`, *optional*):
Mask to avoid performing attention on padding token indices. Mask values selected in `[0, 1]`:
- 1 for tokens that are **not masked**,
- 0 for tokens that are **masked**.
[What are attention masks?](../glossary#attention-mask)
output_attentions (`bool`, *optional*):
Whether or not to return the attentions tensors of all attention layers. See `attentions` under
returned tensors for more detail.
output_hidden_states (`bool`, *optional*):
Whether or not to return the hidden states of all layers. See `hidden_states` under returned tensors
for more detail.
return_dict (`bool`, *optional*):
Whether or not to return a [`~utils.ModelOutput`] instead of a plain tuple.
"""
output_attentions = output_attentions if output_attentions is not None else self.config.output_attentions
output_hidden_states = (
output_hidden_states if output_hidden_states is not None else self.config.output_hidden_states
)
return_dict = return_dict if return_dict is not None else self.config.use_return_dict
encoder_states = () if output_hidden_states else None
all_attentions = () if output_attentions else None
hidden_states = inputs_embeds
for idx, encoder_layer in enumerate(self.layers):
if output_hidden_states:
encoder_states = encoder_states + (hidden_states,)
if self.gradient_checkpointing and self.training:
layer_outputs = self._gradient_checkpointing_func(
encoder_layer.__call__,
hidden_states,
attention_mask,
output_attentions,
)
else:
layer_outputs = encoder_layer(
hidden_states,
attention_mask,
output_attentions=output_attentions,
)
hidden_states = layer_outputs[0]
if output_attentions:
all_attentions = all_attentions + (layer_outputs[1],)
if output_hidden_states:
encoder_states = encoder_states + (hidden_states,)
if not return_dict:
return tuple(v for v in [hidden_states, encoder_states, all_attentions] if v is not None)
return BaseModelOutput(
last_hidden_state=hidden_states, hidden_states=encoder_states, attentions=all_attentions
)
class BlipVisionModel(BlipPreTrainedModel):
main_input_name = "pixel_values"
config_class = BlipVisionConfig
def __init__(self, config: BlipVisionConfig):
super().__init__(config)
self.config = config
embed_dim = config.hidden_size
self.embeddings = BlipVisionEmbeddings(config)
self.encoder = BlipEncoder(config)
self.post_layernorm = nn.LayerNorm(embed_dim, eps=config.layer_norm_eps)
self.post_init()
@add_start_docstrings_to_model_forward(BLIP_VISION_INPUTS_DOCSTRING)
@replace_return_docstrings(output_type=BaseModelOutputWithPooling, config_class=BlipVisionConfig)
def forward(
self,
pixel_values: Optional[torch.FloatTensor] = None,
output_attentions: Optional[bool] = None,
output_hidden_states: Optional[bool] = None,
return_dict: Optional[bool] = None,
) -> Union[Tuple, BaseModelOutputWithPooling]:
r"""
Returns:
"""
output_attentions = output_attentions if output_attentions is not None else self.config.output_attentions
output_hidden_states = (
output_hidden_states if output_hidden_states is not None else self.config.output_hidden_states
)
return_dict = return_dict if return_dict is not None else self.config.use_return_dict
if pixel_values is None:
raise ValueError("You have to specify pixel_values")
hidden_states = self.embeddings(pixel_values)
encoder_outputs = self.encoder(
inputs_embeds=hidden_states,
output_attentions=output_attentions,
output_hidden_states=output_hidden_states,
return_dict=return_dict,
)
last_hidden_state = encoder_outputs[0]
last_hidden_state = self.post_layernorm(last_hidden_state)
pooled_output = last_hidden_state[:, 0, :]
pooled_output = self.post_layernorm(pooled_output)
if not return_dict:
return (last_hidden_state, pooled_output) + encoder_outputs[1:]
return BaseModelOutputWithPooling(
last_hidden_state=last_hidden_state,
pooler_output=pooled_output,
hidden_states=encoder_outputs.hidden_states,
attentions=encoder_outputs.attentions,
)
def get_input_embeddings(self):
return self.embeddings
@add_start_docstrings(BLIP_START_DOCSTRING)
class BlipModel(BlipPreTrainedModel):
config_class = BlipConfig
def __init__(self, config: BlipConfig):
super().__init__(config)
if not isinstance(config.text_config, BlipTextConfig):
raise ValueError(
"config.text_config is expected to be of type BlipTextConfig but is of type"
f" {type(config.text_config)}."
)
if not isinstance(config.vision_config, BlipVisionConfig):
raise ValueError(
"config.vision_config is expected to be of type BlipVisionConfig but is of type"
f" {type(config.vision_config)}."
)
text_config = config.text_config
vision_config = config.vision_config
self.projection_dim = config.projection_dim
self.text_embed_dim = text_config.hidden_size
self.vision_embed_dim = vision_config.hidden_size
self.text_model = BlipTextModel(text_config)
self.vision_model = BlipVisionModel(vision_config)
self.visual_projection = nn.Linear(self.vision_embed_dim, self.projection_dim, bias=False)
self.text_projection = nn.Linear(self.text_embed_dim, self.projection_dim, bias=False)
self.logit_scale = nn.Parameter(torch.tensor(self.config.logit_scale_init_value))
# Initialize weights and apply final processing
self.post_init()
@add_start_docstrings_to_model_forward(BLIP_TEXT_INPUTS_DOCSTRING)
def get_text_features(
self,
input_ids: Optional[torch.Tensor] = None,
attention_mask: Optional[torch.Tensor] = None,
position_ids: Optional[torch.Tensor] = None,
return_dict: Optional[bool] = None,
) -> torch.FloatTensor:
r"""
Returns:
text_features (`torch.FloatTensor` of shape `(batch_size, output_dim`): The text embeddings obtained by
applying the projection layer to the pooled output of [`BlipTextModel`].
Examples:
```python
>>> from transformers import AutoProcessor, BlipModel
>>> model = BlipModel.from_pretrained("Salesforce/blip-image-captioning-base")
>>> processor = AutoProcessor.from_pretrained("Salesforce/blip-image-captioning-base")
>>> inputs = processor(text=["a photo of a cat", "a photo of a dog"], padding=True, return_tensors="pt")
>>> text_features = model.get_text_features(**inputs)
```"""
return_dict = return_dict if return_dict is not None else self.config.use_return_dict
text_outputs = self.text_model(
input_ids=input_ids,
attention_mask=attention_mask,
position_ids=position_ids,
return_dict=return_dict,
)
pooled_output = text_outputs[1]
text_features = self.text_projection(pooled_output)
return text_features
@add_start_docstrings_to_model_forward(BLIP_VISION_INPUTS_DOCSTRING)
def get_image_features(
self,
pixel_values: Optional[torch.FloatTensor] = None,
return_dict: Optional[bool] = None,
) -> torch.FloatTensor:
r"""
Returns:
image_features (`torch.FloatTensor` of shape `(batch_size, output_dim`): The image embeddings obtained by
applying the projection layer to the pooled output of [`BlipVisionModel`].
Examples:
```python
>>> from PIL import Image
>>> import requests
>>> from transformers import AutoProcessor, BlipModel
>>> model = BlipModel.from_pretrained("Salesforce/blip-image-captioning-base")
>>> processor = AutoProcessor.from_pretrained("Salesforce/blip-image-captioning-base")
>>> url = "http://images.cocodataset.org/val2017/000000039769.jpg"
>>> image = Image.open(requests.get(url, stream=True).raw)
>>> inputs = processor(images=image, return_tensors="pt")
>>> image_features = model.get_image_features(**inputs)
```"""
return_dict = return_dict if return_dict is not None else self.config.use_return_dict
vision_outputs = self.vision_model(pixel_values=pixel_values, return_dict=return_dict)
pooled_output = vision_outputs[1] # pooled_output
image_features = self.visual_projection(pooled_output)
return image_features
@add_start_docstrings_to_model_forward(BLIP_INPUTS_DOCSTRING)
@replace_return_docstrings(output_type=BlipOutput, config_class=BlipConfig)
def forward(
self,
input_ids: Optional[torch.LongTensor] = None,
pixel_values: Optional[torch.FloatTensor] = None,
attention_mask: Optional[torch.Tensor] = None,
position_ids: Optional[torch.LongTensor] = None,
return_loss: Optional[bool] = None,
output_attentions: Optional[bool] = None,
output_hidden_states: Optional[bool] = None,
return_dict: Optional[bool] = None,
) -> Union[Tuple, BlipOutput]:
r"""
Returns:
Examples:
```python
>>> from PIL import Image
>>> import requests
>>> from transformers import AutoProcessor, BlipModel
>>> model = BlipModel.from_pretrained("Salesforce/blip-image-captioning-base")
>>> processor = AutoProcessor.from_pretrained("Salesforce/blip-image-captioning-base")
>>> url = "http://images.cocodataset.org/val2017/000000039769.jpg"
>>> image = Image.open(requests.get(url, stream=True).raw)
>>> inputs = processor(
... text=["a photo of a cat", "a photo of a dog"], images=image, return_tensors="pt", padding=True
... )
>>> outputs = model(**inputs)
>>> logits_per_image = outputs.logits_per_image # this is the image-text similarity score
>>> probs = logits_per_image.softmax(dim=1) # we can take the softmax to get the label probabilities
```"""
# Use BLIP model's config for some fields (if specified) instead of those of vision & text components.
output_attentions = output_attentions if output_attentions is not None else self.config.output_attentions
output_hidden_states = (
output_hidden_states if output_hidden_states is not None else self.config.output_hidden_states
)
return_dict = return_dict if return_dict is not None else self.config.use_return_dict
vision_outputs = self.vision_model(
pixel_values=pixel_values,
output_attentions=output_attentions,
output_hidden_states=output_hidden_states,
return_dict=return_dict,
)
text_outputs = self.text_model(
input_ids=input_ids,
attention_mask=attention_mask,
position_ids=position_ids,
output_attentions=output_attentions,
output_hidden_states=output_hidden_states,
return_dict=return_dict,
)
image_embeds = vision_outputs[1]
image_embeds = self.visual_projection(image_embeds)
text_embeds = text_outputs[1]
text_embeds = self.text_projection(text_embeds)
# normalized features
image_embeds = image_embeds / image_embeds.norm(p=2, dim=-1, keepdim=True)
text_embeds = text_embeds / text_embeds.norm(p=2, dim=-1, keepdim=True)
# cosine similarity as logits
logit_scale = self.logit_scale.exp()
logits_per_text = torch.matmul(text_embeds, image_embeds.t()) * logit_scale
logits_per_image = logits_per_text.t()
loss = None
if return_loss:
loss = blip_loss(logits_per_text)
if not return_dict:
output = (logits_per_image, logits_per_text, text_embeds, image_embeds, text_outputs, vision_outputs)
return ((loss,) + output) if loss is not None else output
return BlipOutput(
loss=loss,
logits_per_image=logits_per_image,
logits_per_text=logits_per_text,
text_embeds=text_embeds,
image_embeds=image_embeds,
text_model_output=text_outputs,
vision_model_output=vision_outputs,
)
@add_start_docstrings(
"""
BLIP Model for image captioning. The model consists of a vision encoder and a text decoder. One can optionally pass
`input_ids` to the model, which serve as a text prompt, to make the text decoder continue the prompt. Otherwise,
the decoder starts generating text from the [BOS] (beginning-of-sequence) token. will start generating the caption
from the text input. If no text input is provided, the decoder will start with the [BOS] token only.
""",
BLIP_START_DOCSTRING,
)
class BlipForConditionalGeneration(BlipPreTrainedModel):
config_class = BlipConfig
_tied_weights_keys = ["text_decoder.cls.predictions.decoder.bias"]
main_input_name = "pixel_values"
def __init__(self, config: BlipConfig):
super().__init__(config)
self.vision_model = BlipVisionModel(config.vision_config)
self.text_decoder = BlipTextLMHeadModel(config.text_config)
self.decoder_input_ids = config.text_config.bos_token_id
self.decoder_pad_token_id = config.text_config.pad_token_id
# Initialize weights and apply final processing
self.post_init()
def get_input_embeddings(self) -> nn.Module:
return self.vision_model.embeddings.patch_embedding
@add_start_docstrings_to_model_forward(BLIP_VISION_INPUTS_DOCSTRING)
@replace_return_docstrings(output_type=BlipForConditionalGenerationModelOutput, config_class=BlipVisionConfig)
def forward(
self,
pixel_values: torch.FloatTensor,
input_ids: Optional[torch.LongTensor] = None,
attention_mask: Optional[torch.LongTensor] = None,
output_attentions: Optional[bool] = None,
output_hidden_states: Optional[bool] = None,
labels: Optional[torch.LongTensor] = None,
return_dict: Optional[bool] = None,
) -> Union[Tuple, BlipForConditionalGenerationModelOutput]:
r"""
Returns:
Examples:
```python
>>> from PIL import Image
>>> import requests
>>> from transformers import AutoProcessor, BlipForConditionalGeneration
>>> processor = AutoProcessor.from_pretrained("Salesforce/blip-image-captioning-base")
>>> model = BlipForConditionalGeneration.from_pretrained("Salesforce/blip-image-captioning-base")
>>> url = "http://images.cocodataset.org/val2017/000000039769.jpg"
>>> image = Image.open(requests.get(url, stream=True).raw)
>>> text = "A picture of"
>>> inputs = processor(images=image, text=text, return_tensors="pt")
>>> outputs = model(**inputs)
```"""
return_dict = return_dict if return_dict is not None else self.config.use_return_dict
output_attentions = output_attentions if output_attentions is not None else self.config.output_attentions
output_hidden_states = (
output_hidden_states if output_hidden_states is not None else self.config.output_hidden_states
)
vision_outputs = self.vision_model(
pixel_values=pixel_values,
output_attentions=output_attentions,
output_hidden_states=output_hidden_states,
return_dict=return_dict,
)
image_embeds = vision_outputs[0]
outputs = self.text_decoder(
input_ids=input_ids,
attention_mask=attention_mask,
encoder_hidden_states=image_embeds,
labels=labels,
return_dict=return_dict,
reduction="mean",
)
if not return_dict:
outputs = (outputs[0], outputs[1], image_embeds, vision_outputs[0]) + vision_outputs[2:]
return tuple(output for output in outputs if output is not None)
return BlipForConditionalGenerationModelOutput(
loss=outputs.loss,
logits=outputs.logits,
image_embeds=image_embeds,
last_hidden_state=vision_outputs.last_hidden_state,
hidden_states=vision_outputs.hidden_states,
attentions=vision_outputs.attentions,
)
@torch.no_grad()
def generate(
self,
pixel_values: torch.FloatTensor,
input_ids: Optional[torch.LongTensor] = None,
attention_mask: Optional[torch.LongTensor] = None,
**generate_kwargs,
) -> torch.LongTensor:
r"""
Overrides *generate* function to be able to use the model as a conditional generator
Parameters:
pixel_values (*torch.FloatTensor* of shape *(batch_size, num_channels, image_height, image_width)*:
Input image to be processed
input_ids (*torch.LongTensor* of shape *(batch_size, sequence_length)*, *optional*):
The sequence used as a prompt for the generation.
attention_mask (*torch.LongTensor* of shape *(batch_size, sequence_length)*, *optional*):
Mask to avoid performing attention on padding token indices. Mask values selected in `[0, 1]`:
Examples:
```python
>>> from PIL import Image
>>> import requests
>>> from transformers import AutoProcessor, BlipForConditionalGeneration
>>> model = BlipForConditionalGeneration.from_pretrained("Salesforce/blip-image-captioning-base")
>>> processor = AutoProcessor.from_pretrained("Salesforce/blip-image-captioning-base")
>>> url = "http://images.cocodataset.org/val2017/000000039769.jpg"
>>> image = Image.open(requests.get(url, stream=True).raw)
>>> inputs = processor(images=image, return_tensors="pt")
>>> outputs = model.generate(**inputs)
>>> print(processor.decode(outputs[0], skip_special_tokens=True))
two cats sleeping on a couch
```
"""
batch_size = pixel_values.shape[0]
vision_outputs = self.vision_model(pixel_values=pixel_values)
image_embeds = vision_outputs[0]
image_attention_mask = torch.ones(image_embeds.size()[:-1], dtype=torch.long).to(image_embeds.device)
if isinstance(input_ids, list):
input_ids = torch.LongTensor(input_ids)
elif input_ids is None:
input_ids = (
torch.LongTensor([[self.decoder_input_ids, self.config.text_config.eos_token_id]])
.repeat(batch_size, 1)
.to(image_embeds.device)
)
input_ids[:, 0] = self.config.text_config.bos_token_id
attention_mask = attention_mask[:, :-1] if attention_mask is not None else None
outputs = self.text_decoder.generate(
input_ids=input_ids[:, :-1],
eos_token_id=self.config.text_config.sep_token_id,
pad_token_id=self.config.text_config.pad_token_id,
attention_mask=attention_mask,
encoder_hidden_states=image_embeds,
encoder_attention_mask=image_attention_mask,
**generate_kwargs,
)
return outputs
@add_start_docstrings(
"""
BLIP Model for visual question answering. The model consists of a vision encoder, a text encoder as well as a text
decoder. The vision encoder will encode the input image, the text encoder will encode the input question together
with the encoding of the image, and the text decoder will output the answer to the question.
""",
BLIP_START_DOCSTRING,
)
class BlipForQuestionAnswering(BlipPreTrainedModel):
config_class = BlipConfig
_tied_weights_keys = ["text_decoder.cls.predictions.decoder.bias"]
def __init__(self, config: BlipConfig):
super().__init__(config)
self.vision_model = BlipVisionModel(config.vision_config)
self.text_encoder = BlipTextModel(config.text_config, add_pooling_layer=False)
self.text_decoder = BlipTextLMHeadModel(config.text_config)
self.decoder_pad_token_id = config.text_config.pad_token_id
self.decoder_start_token_id = config.text_config.bos_token_id
# Initialize weights and apply final processing
self.post_init()
def get_input_embeddings(self) -> nn.Module:
return self.vision_model.embeddings.patch_embedding
@add_start_docstrings_to_model_forward(BLIP_VISION_INPUTS_DOCSTRING)
@replace_return_docstrings(output_type=BlipTextVisionModelOutput, config_class=BlipVisionConfig)
def forward(
self,
input_ids: torch.LongTensor,
pixel_values: torch.FloatTensor,
decoder_input_ids: Optional[torch.LongTensor] = None,
decoder_attention_mask: Optional[torch.LongTensor] = None,
attention_mask: Optional[torch.LongTensor] = None,
output_attentions: Optional[bool] = None,
output_hidden_states: Optional[bool] = None,
labels: Optional[torch.LongTensor] = None,
return_dict: Optional[bool] = None,
) -> Union[Tuple, BlipTextVisionModelOutput]:
r"""
Returns:
Examples:
```python
>>> from PIL import Image
>>> import requests
>>> from transformers import AutoProcessor, BlipForQuestionAnswering
>>> model = BlipForQuestionAnswering.from_pretrained("Salesforce/blip-vqa-base")
>>> processor = AutoProcessor.from_pretrained("Salesforce/blip-vqa-base")
>>> url = "http://images.cocodataset.org/val2017/000000039769.jpg"
>>> image = Image.open(requests.get(url, stream=True).raw)
>>> # training
>>> text = "How many cats are in the picture?"
>>> label = "2"
>>> inputs = processor(images=image, text=text, return_tensors="pt")
>>> labels = processor(text=label, return_tensors="pt").input_ids
>>> inputs["labels"] = labels
>>> outputs = model(**inputs)
>>> loss = outputs.loss
>>> loss.backward()
>>> # inference
>>> text = "How many cats are in the picture?"
>>> inputs = processor(images=image, text=text, return_tensors="pt")
>>> outputs = model.generate(**inputs)
>>> print(processor.decode(outputs[0], skip_special_tokens=True))
2
```"""
if labels is None and decoder_input_ids is None:
raise ValueError(
"Either `decoder_input_ids` or `labels` should be passed when calling `forward` with"
" `BlipForQuestionAnswering`. if you are training the model make sure that `labels` is passed, if you"
" are using the model for inference make sure that `decoder_input_ids` is passed or call `generate`"
)
return_dict = return_dict if return_dict is not None else self.config.use_return_dict
output_attentions = output_attentions if output_attentions is not None else self.config.output_attentions
output_hidden_states = (
output_hidden_states if output_hidden_states is not None else self.config.output_hidden_states
)
vision_outputs = self.vision_model(
pixel_values=pixel_values,
output_attentions=output_attentions,
output_hidden_states=output_hidden_states,
return_dict=return_dict,
)
image_embeds = vision_outputs[0]
image_attention_mask = torch.ones(image_embeds.size()[:-1], dtype=torch.long)
question_embeds = self.text_encoder(
input_ids=input_ids,
attention_mask=attention_mask,
encoder_hidden_states=image_embeds,
encoder_attention_mask=image_attention_mask,
return_dict=return_dict,
)
if labels is not None and decoder_input_ids is None:
# labels are already shifted right, see: https://github.com/huggingface/transformers/pull/23153
decoder_input_ids = labels
question_embeds = question_embeds[0] if not return_dict else question_embeds.last_hidden_state
answer_output = self.text_decoder(
input_ids=decoder_input_ids,
attention_mask=decoder_attention_mask,
encoder_hidden_states=question_embeds,
encoder_attention_mask=attention_mask,
labels=labels,
return_dict=return_dict,
reduction="mean",
)
if labels is not None:
decoder_loss = answer_output.loss.mean() if return_dict else answer_output[0].mean()
else:
decoder_loss = None
if not return_dict:
outputs = (decoder_loss, image_embeds, vision_outputs[0]) + vision_outputs[2:]
return tuple(output for output in outputs if output is not None)
return BlipTextVisionModelOutput(
loss=decoder_loss,
image_embeds=image_embeds,
last_hidden_state=vision_outputs.last_hidden_state,
hidden_states=vision_outputs.hidden_states,
attentions=vision_outputs.attentions,
)
@torch.no_grad()
def generate(
self,
input_ids: torch.LongTensor,
pixel_values: torch.FloatTensor,
attention_mask: Optional[torch.LongTensor] = None,
**generate_kwargs,
) -> torch.LongTensor:
r"""
Overrides *generate* function to be able to use the model as a conditional generator
Parameters:
input_ids (*torch.LongTensor* of shape *(batch_size, sequence_length)*):
The sequence used as a prompt for the generation.
pixel_values (*torch.FloatTensor* of shape *(batch_size, num_channels, image_height, image_width)*:
Input image to be processed
attention_mask (*torch.LongTensor* of shape *(batch_size, sequence_length)*, *optional*):
Mask to avoid performing attention on padding token indices. Mask values selected in `[0, 1]`. `1` for
tokens that are NOT MASKED, `0` for MASKED tokens.
**generate_kwargs:
Additional arguments passed to the *generate* function of the decoder
Examples:
```python
>>> from PIL import Image
>>> import requests
>>> from transformers import AutoProcessor, BlipForQuestionAnswering
>>> model = BlipForQuestionAnswering.from_pretrained("Salesforce/blip-vqa-base")
>>> processor = AutoProcessor.from_pretrained("Salesforce/blip-vqa-base")
>>> url = "http://images.cocodataset.org/val2017/000000039769.jpg"
>>> image = Image.open(requests.get(url, stream=True).raw)
>>> text = "How many cats are in the picture?"
>>> inputs = processor(images=image, text=text, return_tensors="pt")
>>> outputs = model.generate(**inputs)
>>> print(processor.decode(outputs[0], skip_special_tokens=True))
2
```
"""
vision_outputs = self.vision_model(pixel_values=pixel_values)
image_embeds = vision_outputs[0]
image_attention_mask = torch.ones(image_embeds.size()[:-1], dtype=torch.long).to(image_embeds.device)
if isinstance(input_ids, list):
input_ids = torch.LongTensor(input_ids)
question_outputs = self.text_encoder(
input_ids=input_ids,
attention_mask=attention_mask,
encoder_hidden_states=image_embeds,
encoder_attention_mask=image_attention_mask,
return_dict=False,
)
question_embeds = question_outputs[0]
question_attention_mask = torch.ones(question_embeds.size()[:-1], dtype=torch.long).to(question_embeds.device)
bos_ids = torch.full(
(question_embeds.size(0), 1), fill_value=self.decoder_start_token_id, device=question_embeds.device
)
outputs = self.text_decoder.generate(
input_ids=bos_ids,
eos_token_id=self.config.text_config.sep_token_id,
pad_token_id=self.config.text_config.pad_token_id,
encoder_hidden_states=question_embeds,
encoder_attention_mask=question_attention_mask,
**generate_kwargs,
)
return outputs
@add_start_docstrings(
"""
BLIP Model with a vision and text projector, and a classification head on top. The model is used in the context of
image-text retrieval. Given an image and a text, the model returns the probability of the text being relevant to
the image.
""",
BLIP_START_DOCSTRING,
)
class BlipForImageTextRetrieval(BlipPreTrainedModel):
config_class = BlipConfig
def __init__(self, config: BlipConfig):
super().__init__(config)
self.vision_model = BlipVisionModel(config.vision_config)
self.text_encoder = BlipTextModel(config.text_config, add_pooling_layer=False)
# vision projection layer
self.vision_proj = nn.Linear(config.vision_config.hidden_size, config.image_text_hidden_size)
# text projection layer
self.text_proj = nn.Linear(config.text_config.hidden_size, config.image_text_hidden_size)
# image text matching head
self.itm_head = nn.Linear(config.text_config.hidden_size, 2)
self.decoder_pad_token_id = (
config.text_config.pad_token_id
if not hasattr(config, "decoder_pad_token_id")
else config.decoder_pad_token_id
)
self.decoder_start_token_id = (
config.text_config.bos_token_id
if not hasattr(config, "decoder_start_token_id")
else config.decoder_start_token_id
)
# Initialize weights and apply final processing
self.post_init()
def get_input_embeddings(self) -> nn.Module:
return self.vision_model.embeddings.patch_embedding
@add_start_docstrings_to_model_forward(BLIP_VISION_INPUTS_DOCSTRING)
@replace_return_docstrings(output_type=BlipTextVisionModelOutput, config_class=BlipVisionConfig)
def forward(
self,
input_ids: torch.LongTensor,
pixel_values: torch.FloatTensor,
use_itm_head: Optional[bool] = True,
attention_mask: Optional[torch.LongTensor] = None,
output_attentions: Optional[bool] = None,
output_hidden_states: Optional[bool] = None,
return_dict: Optional[bool] = None,
) -> Union[Tuple, BlipTextVisionModelOutput]:
r"""
Returns:
Examples:
```python
>>> from PIL import Image
>>> import requests
>>> from transformers import AutoProcessor, BlipForImageTextRetrieval
>>> model = BlipForImageTextRetrieval.from_pretrained("Salesforce/blip-itm-base-coco")
>>> processor = AutoProcessor.from_pretrained("Salesforce/blip-itm-base-coco")
>>> url = "http://images.cocodataset.org/val2017/000000039769.jpg"
>>> image = Image.open(requests.get(url, stream=True).raw)
>>> text = "an image of a cat"
>>> inputs = processor(images=image, text=text, return_tensors="pt")
>>> outputs = model(**inputs)
```
"""
return_dict = return_dict if return_dict is not None else self.config.use_return_dict
output_attentions = output_attentions if output_attentions is not None else self.config.output_attentions
output_hidden_states = (
output_hidden_states if output_hidden_states is not None else self.config.output_hidden_states
)
vision_outputs = self.vision_model(
pixel_values=pixel_values,
output_attentions=output_attentions,
output_hidden_states=output_hidden_states,
return_dict=return_dict,
)
image_embeds = vision_outputs[0]
image_atts = torch.ones(image_embeds.size()[:-1], dtype=torch.long)
if use_itm_head:
question_embeds = self.text_encoder(
input_ids=input_ids,
attention_mask=attention_mask,
encoder_hidden_states=image_embeds,
encoder_attention_mask=image_atts,
return_dict=return_dict,
)
question_embeds = question_embeds[0] if not return_dict else question_embeds.last_hidden_state
output = self.itm_head(question_embeds[:, 0, :])
else:
question_embeds = self.text_encoder(
input_ids=input_ids,
attention_mask=attention_mask,
return_dict=return_dict,
)
question_embeds = question_embeds[0] if not return_dict else question_embeds.last_hidden_state
image_feat = normalize(self.vision_proj(image_embeds[:, 0, :]), dim=-1)
text_feat = normalize(self.text_proj(question_embeds[:, 0, :]), dim=-1)
output = image_feat @ text_feat.t()
if not return_dict:
outputs = (output, vision_outputs[0]) + vision_outputs[2:] + (question_embeds,)
return tuple(output for output in outputs if output is not None)
return BlipImageTextMatchingModelOutput(
itm_score=output,
last_hidden_state=vision_outputs.last_hidden_state,
hidden_states=vision_outputs.hidden_states,
attentions=vision_outputs.attentions,
question_embeds=question_embeds,
)
| transformers/src/transformers/models/blip/modeling_blip.py/0 | {
"file_path": "transformers/src/transformers/models/blip/modeling_blip.py",
"repo_id": "transformers",
"token_count": 25453
} | 304 |
# Copyright 2023 The Intel Labs Team Authors, The Microsoft Research Team Authors and HuggingFace Inc. team. All rights reserved.
#
# Licensed under the Apache License, Version 2.0 (the "License");
# you may not use this file except in compliance with the License.
# You may obtain a copy of the License at
#
# http://www.apache.org/licenses/LICENSE-2.0
#
# Unless required by applicable law or agreed to in writing, software
# distributed under the License is distributed on an "AS IS" BASIS,
# WITHOUT WARRANTIES OR CONDITIONS OF ANY KIND, either express or implied.
# See the License for the specific language governing permissions and
# limitations under the License.
from typing import TYPE_CHECKING
from ...utils import OptionalDependencyNotAvailable, _LazyModule, is_torch_available, is_vision_available
_import_structure = {
"configuration_bridgetower": [
"BRIDGETOWER_PRETRAINED_CONFIG_ARCHIVE_MAP",
"BridgeTowerConfig",
"BridgeTowerTextConfig",
"BridgeTowerVisionConfig",
],
"processing_bridgetower": ["BridgeTowerProcessor"],
}
try:
if not is_vision_available():
raise OptionalDependencyNotAvailable()
except OptionalDependencyNotAvailable:
pass
else:
_import_structure["image_processing_bridgetower"] = ["BridgeTowerImageProcessor"]
try:
if not is_torch_available():
raise OptionalDependencyNotAvailable()
except OptionalDependencyNotAvailable:
pass
else:
_import_structure["modeling_bridgetower"] = [
"BRIDGETOWER_PRETRAINED_MODEL_ARCHIVE_LIST",
"BridgeTowerForContrastiveLearning",
"BridgeTowerForImageAndTextRetrieval",
"BridgeTowerForMaskedLM",
"BridgeTowerModel",
"BridgeTowerPreTrainedModel",
]
if TYPE_CHECKING:
from .configuration_bridgetower import (
BRIDGETOWER_PRETRAINED_CONFIG_ARCHIVE_MAP,
BridgeTowerConfig,
BridgeTowerTextConfig,
BridgeTowerVisionConfig,
)
from .processing_bridgetower import BridgeTowerProcessor
try:
if not is_vision_available():
raise OptionalDependencyNotAvailable()
except OptionalDependencyNotAvailable:
pass
else:
from .image_processing_bridgetower import BridgeTowerImageProcessor
try:
if not is_torch_available():
raise OptionalDependencyNotAvailable()
except OptionalDependencyNotAvailable:
pass
else:
from .modeling_bridgetower import (
BRIDGETOWER_PRETRAINED_MODEL_ARCHIVE_LIST,
BridgeTowerForContrastiveLearning,
BridgeTowerForImageAndTextRetrieval,
BridgeTowerForMaskedLM,
BridgeTowerModel,
BridgeTowerPreTrainedModel,
)
else:
import sys
sys.modules[__name__] = _LazyModule(__name__, globals()["__file__"], _import_structure)
| transformers/src/transformers/models/bridgetower/__init__.py/0 | {
"file_path": "transformers/src/transformers/models/bridgetower/__init__.py",
"repo_id": "transformers",
"token_count": 1071
} | 305 |
Subsets and Splits
No saved queries yet
Save your SQL queries to embed, download, and access them later. Queries will appear here once saved.