text
stringlengths 7
328k
| id
stringlengths 14
166
| metadata
dict | __index_level_0__
int64 0
459
|
---|---|---|---|
# Copyright 2020 The HuggingFace Team. All rights reserved.
#
# Licensed under the Apache License, Version 2.0 (the "License");
# you may not use this file except in compliance with the License.
# You may obtain a copy of the License at
#
# http://www.apache.org/licenses/LICENSE-2.0
#
# Unless required by applicable law or agreed to in writing, software
# distributed under the License is distributed on an "AS IS" BASIS,
# WITHOUT WARRANTIES OR CONDITIONS OF ANY KIND, either express or implied.
# See the License for the specific language governing permissions and
# limitations under the License.
from typing import Dict
import numpy as np
from transformers import EvalPrediction, HfArgumentParser, TrainingArguments, is_torch_available
from transformers.testing_utils import (
TestCasePlus,
execute_subprocess_async,
get_torch_dist_unique_port,
require_torch_multi_gpu,
require_torch_multi_xpu,
require_torch_neuroncore,
require_torch_npu,
)
from transformers.training_args import ParallelMode
from transformers.utils import logging
logger = logging.get_logger(__name__)
if is_torch_available():
import torch
from torch import nn
from torch.utils.data import Dataset, IterableDataset
from transformers import Trainer
class DummyDataset(Dataset):
def __init__(self, length: int = 101):
self.length = length
def __len__(self):
return self.length
def __getitem__(self, i) -> int:
return i
class DummyDataCollator:
def __call__(self, features):
return {"input_ids": torch.tensor(features), "labels": torch.tensor(features)}
class DummyModel(nn.Module):
def __init__(self):
super().__init__()
# Add some (unused) params otherwise DDP will complain.
self.fc = nn.Linear(120, 80)
def forward(self, input_ids, labels=None):
if labels is not None:
return torch.tensor(0.0, device=input_ids.device), input_ids
else:
return input_ids
class RegressionModel(nn.Module):
def __init__(self, a=0, b=0, double_output=False):
super().__init__()
self.a = nn.Parameter(torch.tensor(a).float())
self.b = nn.Parameter(torch.tensor(b).float())
self.double_output = double_output
self.config = None
def forward(self, input_x, labels=None, **kwargs):
y = input_x * self.a + self.b
if labels is None:
return (y, y) if self.double_output else (y,)
loss = nn.functional.mse_loss(y, labels)
return (loss, y, y) if self.double_output else (loss, y)
class SampleIterableDataset(IterableDataset):
def __init__(self, a=2, b=3, length=64, seed=42, label_names=None):
self.dataset = RegressionDataset(a=a, b=b, length=length, seed=seed, label_names=label_names)
def __iter__(self):
for i in range(len(self.dataset)):
yield self.dataset[i]
class FiniteIterableDataset(SampleIterableDataset):
def __init__(self, a=2, b=3, length=64, seed=42, label_names=None):
super().__init__(a, b, length, seed, label_names)
self.current_sample = 0
def __iter__(self):
while self.current_sample < len(self.dataset):
yield self.dataset[self.current_sample]
self.current_sample += 1
class RegressionDataset:
def __init__(self, a=2, b=3, length=64, seed=42, label_names=None):
np.random.seed(seed)
self.label_names = ["labels"] if label_names is None else label_names
self.length = length
self.x = np.random.normal(size=(length,)).astype(np.float32)
self.ys = [a * self.x + b + np.random.normal(scale=0.1, size=(length,)) for _ in self.label_names]
self.ys = [y.astype(np.float32) for y in self.ys]
def __len__(self):
return self.length
def __getitem__(self, i):
result = {name: y[i] for name, y in zip(self.label_names, self.ys)}
result["input_x"] = self.x[i]
return result
class TestTrainerDistributedNeuronCore(TestCasePlus):
@require_torch_neuroncore
def test_trainer(self):
distributed_args = f"""--nproc_per_node=2
--master_port={get_torch_dist_unique_port()}
{self.test_file_dir}/test_trainer_distributed.py
""".split()
output_dir = self.get_auto_remove_tmp_dir()
args = f"--output_dir {output_dir}".split()
cmd = ["torchrun"] + distributed_args + args
execute_subprocess_async(cmd, env=self.get_env())
# successful return here == success - any errors would have caused an error in the sub-call
class TestTrainerDistributedNPU(TestCasePlus):
@require_torch_npu
def test_trainer(self):
distributed_args = f"""--nproc_per_node=2
--master_port={get_torch_dist_unique_port()}
{self.test_file_dir}/test_trainer_distributed.py
""".split()
output_dir = self.get_auto_remove_tmp_dir()
args = f"--output_dir {output_dir}".split()
cmd = ["torchrun"] + distributed_args + args
execute_subprocess_async(cmd, env=self.get_env())
# successful return here == success - any errors would have caused an error in the sub-call
class TestTrainerDistributed(TestCasePlus):
@require_torch_multi_gpu
def test_trainer(self):
distributed_args = f"""--nproc_per_node={torch.cuda.device_count()}
--master_port={get_torch_dist_unique_port()}
{self.test_file_dir}/test_trainer_distributed.py
""".split()
output_dir = self.get_auto_remove_tmp_dir()
args = f"--output_dir {output_dir}".split()
cmd = ["torchrun"] + distributed_args + args
execute_subprocess_async(cmd, env=self.get_env())
# successful return here == success - any errors would have caused an error in the sub-call
@require_torch_multi_xpu
class TestTrainerDistributedXPU(TestCasePlus):
def test_trainer(self):
distributed_args = f"""--nproc_per_node={torch.xpu.device_count()}
--master_port={get_torch_dist_unique_port()}
{self.test_file_dir}/test_trainer_distributed.py
""".split()
output_dir = self.get_auto_remove_tmp_dir()
args = f"--output_dir {output_dir}".split()
cmd = ["torchrun"] + distributed_args + args
execute_subprocess_async(cmd, env=self.get_env())
# successful return here == success - any errors would have caused an error in the sub-call
if __name__ == "__main__":
# The script below is meant to be run under torch.distributed, on a machine with multiple GPUs:
#
# PYTHONPATH="src" python -m torch.distributed.run --nproc_per_node 2 --output_dir output_dir ./tests/test_trainer_distributed.py
parser = HfArgumentParser((TrainingArguments,))
training_args = parser.parse_args_into_dataclasses()[0]
logger.warning(
f"Process rank: {training_args.local_rank}, device: {training_args.device}, n_gpu: {training_args.n_gpu}, "
f"distributed training: {training_args.parallel_mode != ParallelMode.NOT_DISTRIBUTED}"
)
# Essentially, what we want to verify in the distributed case is that we get all samples back,
# in the right order. (this is crucial for prediction for instance)
for dataset_length in [101, 40, 7]:
dataset = DummyDataset(dataset_length)
def compute_metrics(p: EvalPrediction) -> Dict:
sequential = list(range(len(dataset)))
success = p.predictions.tolist() == sequential and p.label_ids.tolist() == sequential
if not success and training_args.local_rank == 0:
logger.warning(
"Predictions and/or labels do not match expected results:\n - predictions: "
f"{p.predictions.tolist()}\n - labels: {p.label_ids.tolist()}\n - expected: {sequential}"
)
return {"success": success}
trainer = Trainer(
model=DummyModel(),
args=training_args,
data_collator=DummyDataCollator(),
eval_dataset=dataset,
compute_metrics=compute_metrics,
)
metrics = trainer.evaluate()
logger.info(metrics)
if metrics["eval_success"] is not True:
logger.error(metrics)
exit(1)
p = trainer.predict(dataset)
logger.info(p.metrics)
if p.metrics["test_success"] is not True:
logger.error(p.metrics)
exit(1)
trainer.args.eval_accumulation_steps = 2
metrics = trainer.evaluate()
logger.info(metrics)
if metrics["eval_success"] is not True:
logger.error(metrics)
exit(1)
p = trainer.predict(dataset)
logger.info(p.metrics)
if p.metrics["test_success"] is not True:
logger.error(p.metrics)
exit(1)
trainer.args.eval_accumulation_steps = None
# Check that `dispatch_batches=False` will work on a finite iterable dataset
train_dataset = FiniteIterableDataset(label_names=["labels", "extra"], length=1)
model = RegressionModel()
training_args.per_device_train_batch_size = 1
training_args.max_steps = 1
training_args.dispatch_batches = False
trainer = Trainer(model, training_args, train_dataset=train_dataset)
trainer.train()
| transformers/tests/trainer/test_trainer_distributed.py/0 | {
"file_path": "transformers/tests/trainer/test_trainer_distributed.py",
"repo_id": "transformers",
"token_count": 4112
} | 406 |
# Copyright 2020 The HuggingFace Team. All rights reserved.
#
# Licensed under the Apache License, Version 2.0 (the "License");
# you may not use this file except in compliance with the License.
# You may obtain a copy of the License at
#
# http://www.apache.org/licenses/LICENSE-2.0
#
# Unless required by applicable law or agreed to in writing, software
# distributed under the License is distributed on an "AS IS" BASIS,
# WITHOUT WARRANTIES OR CONDITIONS OF ANY KIND, either express or implied.
# See the License for the specific language governing permissions and
# limitations under the License.
import argparse
import json
import os
import sys
import tempfile
import unittest
from argparse import Namespace
from dataclasses import dataclass, field
from enum import Enum
from pathlib import Path
from typing import List, Literal, Optional
import yaml
from transformers import HfArgumentParser, TrainingArguments
from transformers.hf_argparser import make_choice_type_function, string_to_bool
# Since Python 3.10, we can use the builtin `|` operator for Union types
# See PEP 604: https://peps.python.org/pep-0604
is_python_no_less_than_3_10 = sys.version_info >= (3, 10)
def list_field(default=None, metadata=None):
return field(default_factory=lambda: default, metadata=metadata)
@dataclass
class BasicExample:
foo: int
bar: float
baz: str
flag: bool
@dataclass
class WithDefaultExample:
foo: int = 42
baz: str = field(default="toto", metadata={"help": "help message"})
@dataclass
class WithDefaultBoolExample:
foo: bool = False
baz: bool = True
opt: Optional[bool] = None
class BasicEnum(Enum):
titi = "titi"
toto = "toto"
class MixedTypeEnum(Enum):
titi = "titi"
toto = "toto"
fourtytwo = 42
@dataclass
class EnumExample:
foo: BasicEnum = "toto"
def __post_init__(self):
self.foo = BasicEnum(self.foo)
@dataclass
class MixedTypeEnumExample:
foo: MixedTypeEnum = "toto"
def __post_init__(self):
self.foo = MixedTypeEnum(self.foo)
@dataclass
class OptionalExample:
foo: Optional[int] = None
bar: Optional[float] = field(default=None, metadata={"help": "help message"})
baz: Optional[str] = None
ces: Optional[List[str]] = list_field(default=[])
des: Optional[List[int]] = list_field(default=[])
@dataclass
class ListExample:
foo_int: List[int] = list_field(default=[])
bar_int: List[int] = list_field(default=[1, 2, 3])
foo_str: List[str] = list_field(default=["Hallo", "Bonjour", "Hello"])
foo_float: List[float] = list_field(default=[0.1, 0.2, 0.3])
@dataclass
class RequiredExample:
required_list: List[int] = field()
required_str: str = field()
required_enum: BasicEnum = field()
def __post_init__(self):
self.required_enum = BasicEnum(self.required_enum)
@dataclass
class StringLiteralAnnotationExample:
foo: int
required_enum: "BasicEnum" = field()
opt: "Optional[bool]" = None
baz: "str" = field(default="toto", metadata={"help": "help message"})
foo_str: "List[str]" = list_field(default=["Hallo", "Bonjour", "Hello"])
if is_python_no_less_than_3_10:
@dataclass
class WithDefaultBoolExamplePep604:
foo: bool = False
baz: bool = True
opt: bool | None = None
@dataclass
class OptionalExamplePep604:
foo: int | None = None
bar: float | None = field(default=None, metadata={"help": "help message"})
baz: str | None = None
ces: list[str] | None = list_field(default=[])
des: list[int] | None = list_field(default=[])
class HfArgumentParserTest(unittest.TestCase):
def argparsersEqual(self, a: argparse.ArgumentParser, b: argparse.ArgumentParser):
"""
Small helper to check pseudo-equality of parsed arguments on `ArgumentParser` instances.
"""
self.assertEqual(len(a._actions), len(b._actions))
for x, y in zip(a._actions, b._actions):
xx = {k: v for k, v in vars(x).items() if k != "container"}
yy = {k: v for k, v in vars(y).items() if k != "container"}
# Choices with mixed type have custom function as "type"
# So we need to compare results directly for equality
if xx.get("choices", None) and yy.get("choices", None):
for expected_choice in yy["choices"] + xx["choices"]:
self.assertEqual(xx["type"](expected_choice), yy["type"](expected_choice))
del xx["type"], yy["type"]
self.assertEqual(xx, yy)
def test_basic(self):
parser = HfArgumentParser(BasicExample)
expected = argparse.ArgumentParser()
expected.add_argument("--foo", type=int, required=True)
expected.add_argument("--bar", type=float, required=True)
expected.add_argument("--baz", type=str, required=True)
expected.add_argument("--flag", type=string_to_bool, default=False, const=True, nargs="?")
self.argparsersEqual(parser, expected)
args = ["--foo", "1", "--baz", "quux", "--bar", "0.5"]
(example,) = parser.parse_args_into_dataclasses(args, look_for_args_file=False)
self.assertFalse(example.flag)
def test_with_default(self):
parser = HfArgumentParser(WithDefaultExample)
expected = argparse.ArgumentParser()
expected.add_argument("--foo", default=42, type=int)
expected.add_argument("--baz", default="toto", type=str, help="help message")
self.argparsersEqual(parser, expected)
def test_with_default_bool(self):
expected = argparse.ArgumentParser()
expected.add_argument("--foo", type=string_to_bool, default=False, const=True, nargs="?")
expected.add_argument("--baz", type=string_to_bool, default=True, const=True, nargs="?")
# A boolean no_* argument always has to come after its "default: True" regular counter-part
# and its default must be set to False
expected.add_argument("--no_baz", action="store_false", default=False, dest="baz")
expected.add_argument("--opt", type=string_to_bool, default=None)
dataclass_types = [WithDefaultBoolExample]
if is_python_no_less_than_3_10:
dataclass_types.append(WithDefaultBoolExamplePep604)
for dataclass_type in dataclass_types:
parser = HfArgumentParser(dataclass_type)
self.argparsersEqual(parser, expected)
args = parser.parse_args([])
self.assertEqual(args, Namespace(foo=False, baz=True, opt=None))
args = parser.parse_args(["--foo", "--no_baz"])
self.assertEqual(args, Namespace(foo=True, baz=False, opt=None))
args = parser.parse_args(["--foo", "--baz"])
self.assertEqual(args, Namespace(foo=True, baz=True, opt=None))
args = parser.parse_args(["--foo", "True", "--baz", "True", "--opt", "True"])
self.assertEqual(args, Namespace(foo=True, baz=True, opt=True))
args = parser.parse_args(["--foo", "False", "--baz", "False", "--opt", "False"])
self.assertEqual(args, Namespace(foo=False, baz=False, opt=False))
def test_with_enum(self):
parser = HfArgumentParser(MixedTypeEnumExample)
expected = argparse.ArgumentParser()
expected.add_argument(
"--foo",
default="toto",
choices=["titi", "toto", 42],
type=make_choice_type_function(["titi", "toto", 42]),
)
self.argparsersEqual(parser, expected)
args = parser.parse_args([])
self.assertEqual(args.foo, "toto")
enum_ex = parser.parse_args_into_dataclasses([])[0]
self.assertEqual(enum_ex.foo, MixedTypeEnum.toto)
args = parser.parse_args(["--foo", "titi"])
self.assertEqual(args.foo, "titi")
enum_ex = parser.parse_args_into_dataclasses(["--foo", "titi"])[0]
self.assertEqual(enum_ex.foo, MixedTypeEnum.titi)
args = parser.parse_args(["--foo", "42"])
self.assertEqual(args.foo, 42)
enum_ex = parser.parse_args_into_dataclasses(["--foo", "42"])[0]
self.assertEqual(enum_ex.foo, MixedTypeEnum.fourtytwo)
def test_with_literal(self):
@dataclass
class LiteralExample:
foo: Literal["titi", "toto", 42] = "toto"
parser = HfArgumentParser(LiteralExample)
expected = argparse.ArgumentParser()
expected.add_argument(
"--foo",
default="toto",
choices=("titi", "toto", 42),
type=make_choice_type_function(["titi", "toto", 42]),
)
self.argparsersEqual(parser, expected)
args = parser.parse_args([])
self.assertEqual(args.foo, "toto")
args = parser.parse_args(["--foo", "titi"])
self.assertEqual(args.foo, "titi")
args = parser.parse_args(["--foo", "42"])
self.assertEqual(args.foo, 42)
def test_with_list(self):
parser = HfArgumentParser(ListExample)
expected = argparse.ArgumentParser()
expected.add_argument("--foo_int", nargs="+", default=[], type=int)
expected.add_argument("--bar_int", nargs="+", default=[1, 2, 3], type=int)
expected.add_argument("--foo_str", nargs="+", default=["Hallo", "Bonjour", "Hello"], type=str)
expected.add_argument("--foo_float", nargs="+", default=[0.1, 0.2, 0.3], type=float)
self.argparsersEqual(parser, expected)
args = parser.parse_args([])
self.assertEqual(
args,
Namespace(foo_int=[], bar_int=[1, 2, 3], foo_str=["Hallo", "Bonjour", "Hello"], foo_float=[0.1, 0.2, 0.3]),
)
args = parser.parse_args("--foo_int 1 --bar_int 2 3 --foo_str a b c --foo_float 0.1 0.7".split())
self.assertEqual(args, Namespace(foo_int=[1], bar_int=[2, 3], foo_str=["a", "b", "c"], foo_float=[0.1, 0.7]))
def test_with_optional(self):
expected = argparse.ArgumentParser()
expected.add_argument("--foo", default=None, type=int)
expected.add_argument("--bar", default=None, type=float, help="help message")
expected.add_argument("--baz", default=None, type=str)
expected.add_argument("--ces", nargs="+", default=[], type=str)
expected.add_argument("--des", nargs="+", default=[], type=int)
dataclass_types = [OptionalExample]
if is_python_no_less_than_3_10:
dataclass_types.append(OptionalExamplePep604)
for dataclass_type in dataclass_types:
parser = HfArgumentParser(dataclass_type)
self.argparsersEqual(parser, expected)
args = parser.parse_args([])
self.assertEqual(args, Namespace(foo=None, bar=None, baz=None, ces=[], des=[]))
args = parser.parse_args("--foo 12 --bar 3.14 --baz 42 --ces a b c --des 1 2 3".split())
self.assertEqual(args, Namespace(foo=12, bar=3.14, baz="42", ces=["a", "b", "c"], des=[1, 2, 3]))
def test_with_required(self):
parser = HfArgumentParser(RequiredExample)
expected = argparse.ArgumentParser()
expected.add_argument("--required_list", nargs="+", type=int, required=True)
expected.add_argument("--required_str", type=str, required=True)
expected.add_argument(
"--required_enum",
type=make_choice_type_function(["titi", "toto"]),
choices=["titi", "toto"],
required=True,
)
self.argparsersEqual(parser, expected)
def test_with_string_literal_annotation(self):
parser = HfArgumentParser(StringLiteralAnnotationExample)
expected = argparse.ArgumentParser()
expected.add_argument("--foo", type=int, required=True)
expected.add_argument(
"--required_enum",
type=make_choice_type_function(["titi", "toto"]),
choices=["titi", "toto"],
required=True,
)
expected.add_argument("--opt", type=string_to_bool, default=None)
expected.add_argument("--baz", default="toto", type=str, help="help message")
expected.add_argument("--foo_str", nargs="+", default=["Hallo", "Bonjour", "Hello"], type=str)
self.argparsersEqual(parser, expected)
def test_parse_dict(self):
parser = HfArgumentParser(BasicExample)
args_dict = {
"foo": 12,
"bar": 3.14,
"baz": "42",
"flag": True,
}
parsed_args = parser.parse_dict(args_dict)[0]
args = BasicExample(**args_dict)
self.assertEqual(parsed_args, args)
def test_parse_dict_extra_key(self):
parser = HfArgumentParser(BasicExample)
args_dict = {
"foo": 12,
"bar": 3.14,
"baz": "42",
"flag": True,
"extra": 42,
}
self.assertRaises(ValueError, parser.parse_dict, args_dict, allow_extra_keys=False)
def test_parse_json(self):
parser = HfArgumentParser(BasicExample)
args_dict_for_json = {
"foo": 12,
"bar": 3.14,
"baz": "42",
"flag": True,
}
with tempfile.TemporaryDirectory() as tmp_dir:
temp_local_path = os.path.join(tmp_dir, "temp_json")
os.mkdir(temp_local_path)
with open(temp_local_path + ".json", "w+") as f:
json.dump(args_dict_for_json, f)
parsed_args = parser.parse_yaml_file(Path(temp_local_path + ".json"))[0]
args = BasicExample(**args_dict_for_json)
self.assertEqual(parsed_args, args)
def test_parse_yaml(self):
parser = HfArgumentParser(BasicExample)
args_dict_for_yaml = {
"foo": 12,
"bar": 3.14,
"baz": "42",
"flag": True,
}
with tempfile.TemporaryDirectory() as tmp_dir:
temp_local_path = os.path.join(tmp_dir, "temp_yaml")
os.mkdir(temp_local_path)
with open(temp_local_path + ".yaml", "w+") as f:
yaml.dump(args_dict_for_yaml, f)
parsed_args = parser.parse_yaml_file(Path(temp_local_path + ".yaml"))[0]
args = BasicExample(**args_dict_for_yaml)
self.assertEqual(parsed_args, args)
def test_integration_training_args(self):
parser = HfArgumentParser(TrainingArguments)
self.assertIsNotNone(parser)
| transformers/tests/utils/test_hf_argparser.py/0 | {
"file_path": "transformers/tests/utils/test_hf_argparser.py",
"repo_id": "transformers",
"token_count": 6348
} | 407 |
# coding=utf-8
# Copyright 2020 The HuggingFace Inc. team.
#
# Licensed under the Apache License, Version 2.0 (the "License");
# you may not use this file except in compliance with the License.
# You may obtain a copy of the License at
#
# http://www.apache.org/licenses/LICENSE-2.0
#
# Unless required by applicable law or agreed to in writing, software
# distributed under the License is distributed on an "AS IS" BASIS,
# WITHOUT WARRANTIES OR CONDITIONS OF ANY KIND, either express or implied.
# See the License for the specific language governing permissions and
# limitations under the License.
"""
Utility that checks whether the copies defined in the library match the original or not. This includes:
- All code commented with `# Copied from` comments,
- The list of models in the main README.md matches the ones in the localized READMEs,
- Files that are registered as full copies of one another in the `FULL_COPIES` constant of this script.
This also checks the list of models in the README is complete (has all models) and add a line to complete if there is
a model missing.
Use from the root of the repo with:
```bash
python utils/check_copies.py
```
for a check that will error in case of inconsistencies (used by `make repo-consistency`) or
```bash
python utils/check_copies.py --fix_and_overwrite
```
for a check that will fix all inconsistencies automatically (used by `make fix-copies`).
"""
import argparse
import glob
import os
import re
import subprocess
from collections import OrderedDict
from typing import List, Optional, Tuple, Union
from transformers.utils import direct_transformers_import
# All paths are set with the intent you should run this script from the root of the repo with the command
# python utils/check_copies.py
TRANSFORMERS_PATH = "src/transformers"
MODEL_TEST_PATH = "tests/models"
PATH_TO_DOCS = "docs/source/en"
REPO_PATH = "."
# Mapping for files that are full copies of others (keys are copies, values the file to keep them up to data with)
FULL_COPIES = {
"examples/tensorflow/question-answering/utils_qa.py": "examples/pytorch/question-answering/utils_qa.py",
"examples/flax/question-answering/utils_qa.py": "examples/pytorch/question-answering/utils_qa.py",
}
LOCALIZED_READMES = {
# If the introduction or the conclusion of the list change, the prompts may need to be updated.
"README.md": {
"start_prompt": "🤗 Transformers currently provides the following architectures",
"end_prompt": "1. Want to contribute a new model?",
"format_model_list": (
"**[{title}]({model_link})** (from {paper_affiliations}) released with the paper {paper_title_link} by"
" {paper_authors}.{supplements}"
),
},
"README_zh-hans.md": {
"start_prompt": "🤗 Transformers 目前支持如下的架构",
"end_prompt": "1. 想要贡献新的模型?",
"format_model_list": (
"**[{title}]({model_link})** (来自 {paper_affiliations}) 伴随论文 {paper_title_link} 由 {paper_authors}"
" 发布。{supplements}"
),
},
"README_zh-hant.md": {
"start_prompt": "🤗 Transformers 目前支援以下的架構",
"end_prompt": "1. 想要貢獻新的模型?",
"format_model_list": (
"**[{title}]({model_link})** (from {paper_affiliations}) released with the paper {paper_title_link} by"
" {paper_authors}.{supplements}"
),
},
"README_ko.md": {
"start_prompt": "🤗 Transformers는 다음 모델들을 제공합니다",
"end_prompt": "1. 새로운 모델을 올리고 싶나요?",
"format_model_list": (
"**[{title}]({model_link})** ({paper_affiliations} 에서 제공)은 {paper_authors}.{supplements}의"
" {paper_title_link}논문과 함께 발표했습니다."
),
},
"README_es.md": {
"start_prompt": "🤗 Transformers actualmente proporciona las siguientes arquitecturas",
"end_prompt": "1. ¿Quieres aportar un nuevo modelo?",
"format_model_list": (
"**[{title}]({model_link})** (from {paper_affiliations}) released with the paper {paper_title_link} by"
" {paper_authors}.{supplements}"
),
},
"README_ja.md": {
"start_prompt": "🤗Transformersは現在、以下のアーキテクチャを提供しています",
"end_prompt": "1. 新しいモデルを投稿したいですか?",
"format_model_list": (
"**[{title}]({model_link})** ({paper_affiliations} から) {paper_authors}.{supplements} から公開された研究論文"
" {paper_title_link}"
),
},
"README_hd.md": {
"start_prompt": "🤗 ट्रांसफॉर्मर वर्तमान में निम्नलिखित आर्किटेक्चर का समर्थन करते हैं",
"end_prompt": "1. एक नए मॉडल में योगदान देना चाहते हैं?",
"format_model_list": (
"**[{title}]({model_link})** ({paper_affiliations} से) {paper_authors}.{supplements} द्वारा"
"अनुसंधान पत्र {paper_title_link} के साथ जारी किया गया"
),
},
"README_ru.md": {
"start_prompt": "🤗 В настоящее время Transformers предоставляет следующие архитектуры",
"end_prompt": "1. Хотите внести новую модель?",
"format_model_list": (
"**[{title}]({model_link})** (from {paper_affiliations}) released with the paper {paper_title_link} by"
" {paper_authors}.{supplements}"
),
},
"README_pt-br.md": {
"start_prompt": "🤗 Transformers atualmente fornece as seguintes arquiteturas",
"end_prompt": "1. Quer contribuir com um novo modelo?",
"format_model_list": (
"**[{title}]({model_link})** (from {paper_affiliations}) released with the paper {paper_title_link} by"
" {paper_authors}.{supplements}"
),
},
"README_te.md": {
"start_prompt": "🤗 ట్రాన్స్ఫార్మర్లు ప్రస్తుతం కింది ఆర్కిటెక్చర్లను అందజేస్తున్నాయి",
"end_prompt": "1. కొత్త మోడల్ను అందించాలనుకుంటున్నారా?",
"format_model_list": (
"**[{title}]({model_link})** (from {paper_affiliations}) released with the paper {paper_title_link} by"
" {paper_authors}.{supplements}"
),
},
"README_fr.md": {
"start_prompt": "🤗 Transformers fournit actuellement les architectures suivantes",
"end_prompt": "1. Vous souhaitez contribuer avec un nouveau modèle ?",
"format_model_list": (
"**[{title}]({model_link})** (de {paper_affiliations}) publié dans l'article {paper_title_link} par"
"{paper_authors}.{supplements}"
),
},
"README_de.md": {
"start_prompt": "🤗 Transformers bietet derzeit die folgenden Architekturen an",
"end_prompt": "1. Möchten Sie ein neues Modell beitragen?",
"format_model_list": (
"**[{title}]({model_link})** (from {paper_affiliations}) released with the paper {paper_title_link} by"
" {paper_authors}.{supplements}"
),
},
"README_vi.md": {
"start_prompt": "🤗 Transformers hiện đang cung cấp các kiến trúc sau đây",
"end_prompt": "1. Muốn đóng góp một mô hình mới?",
"format_model_list": (
"**[{title}]({model_link})** (từ {paper_affiliations}) được phát hành với bài báo {paper_title_link} by"
" {paper_authors}.{supplements}"
),
},
}
# This is to make sure the transformers module imported is the one in the repo.
transformers_module = direct_transformers_import(TRANSFORMERS_PATH)
def _is_definition_header_ending_line(line: str) -> bool:
# Helper function. Returns `True` if `line` is the end parenthesis of a class/function definition
return re.search(r"^\s*\)(\s*->.*:|:)\s*$", line) is not None
def _should_continue(line: str, indent: str) -> bool:
# Helper function. Returns `True` if `line` is empty, starts with the `indent` or is the end parenthesis of a
# class/function definition
return line.startswith(indent) or len(line.strip()) == 0 or _is_definition_header_ending_line(line)
def _sanity_check_splits(splits_1, splits_2, is_class):
"""Check the two (inner) block structures of the corresponding code block given by `split_code_into_blocks` match.
For the case of `class`, they must be of one of the following 3 cases:
- a single block without name:
class foo:
a = 1
- a consecutive sequence of (1 or more) blocks with name
class foo:
def f(x):
return x
- a block without name, followed by a consecutive sequence of (1 or more) blocks with name
class foo:
a = 1
def f(x):
return x
def g(x):
return None
The 2 code snippets that give `splits_1` and `splits_2` have to be in the same case to pass this check, but the
number of blocks with name in the consecutive sequence is not taken into account.
For the case of `function or method`, we don't require it to be in one of the above 3 cases. However, the structure
of`splits_1` and `splits_2` have to match exactly. In particular, the number of blocks with name in a consecutive
sequence is taken into account.
"""
block_names_1 = []
block_names_2 = []
for block in splits_1[1:]:
if block[0].startswith("_block_without_name_"):
block_names_1.append("block_without_name")
elif not block[0].startswith("_empty_block_") and (
not is_class or len(block_names_1) == 0 or block_names_1[-1].startswith("block_without_name")
):
block_names_1.append("block_with_name")
for block in splits_2[1:]:
if block[0].startswith("_block_without_name_"):
block_names_2.append("block_without_name")
elif not block[0].startswith("_empty_block_") and (
not is_class or len(block_names_2) == 0 or block_names_2[-1].startswith("block_without_name")
):
block_names_2.append("block_with_name")
if is_class:
if block_names_1 not in [
["block_without_name"],
["block_with_name"],
["block_without_name", "block_with_name"],
]:
raise ValueError(
"For a class, it must have a specific structure. See the docstring of `_sanity_check_splits` in the file `utils/check_copies.py`"
)
if block_names_1 != block_names_2:
raise ValueError("The structures in the 2 code blocks differ.")
def find_block_end(lines: List[str], start_index: int, indent: int) -> int:
"""
Find the end of the class/func block starting at `start_index` in a source code (defined by `lines`).
Args:
lines (`List[str]`):
The source code, represented by a list of lines.
start_index (`int`):
The starting index of the target class/func block.
indent (`int`):
The indent of the class/func body.
Returns:
`int`: The index of the block's ending line plus by 1 (i.e. exclusive).
"""
indent = " " * indent
# enter the block body
line_index = start_index + 1
while line_index < len(lines) and _should_continue(lines[line_index], indent):
line_index += 1
# Clean up empty lines at the end (if any).
while len(lines[line_index - 1]) <= 1:
line_index -= 1
return line_index
def split_code_into_blocks(
lines: List[str], start_index: int, end_index: int, indent: int, backtrace: bool = False
) -> List[Tuple[str, int, int]]:
"""
Split the class/func block starting at `start_index` in a source code (defined by `lines`) into *inner blocks*.
The block's header is included as the first element. The contiguous regions (without empty lines) that are not
inside any inner block are included as blocks. The contiguous regions of empty lines that are not inside any inner
block are also included as (dummy) blocks.
Args:
lines (`List[str]`):
The source code, represented by a list of lines.
start_index (`int`):
The starting index of the target class/func block.
end_index (`int`):
The ending index of the target class/func block.
indent (`int`):
The indent of the class/func body.
backtrace (`bool`, *optional*, defaults to `False`):
Whether or not to include the lines before the inner class/func block's header (e.g. comments, decorators,
etc.) until an empty line is encountered.
Returns:
`List[Tuple[str, int, int]]`: A list of elements with the form `(block_name, start_index, end_index)`.
"""
splits = []
# `indent - 4` is the indent level of the target class/func header
target_block_name = re.search(rf"^{' ' * (indent - 4)}((class|def)\s+\S+)(\(|\:)", lines[start_index]).groups()[0]
# from now on, the `block` means inner blocks unless explicitly specified
indent_str = " " * indent
block_without_name_idx = 0
empty_block_idx = 0
# Find the lines for the definition header
index = start_index
if "(" in lines[start_index] and "):" not in lines[start_index] in lines[start_index]:
while index < end_index:
if _is_definition_header_ending_line(lines[index]):
break
index += 1
# the first line outside the definition header
index += 1
splits.append((target_block_name, start_index, index))
block_start_index, prev_block_end_index = index, index
while index < end_index:
# if found, it will be an inner block
block_found = re.search(rf"^{indent_str}((class|def)\s+\S+)(\(|\:)", lines[index])
if block_found:
name = block_found.groups()[0]
block_end_index = find_block_end(lines, index, indent + 4)
# backtrace to include the lines before the found block's definition header (e.g. comments, decorators,
# etc.) until an empty line is encountered.
block_start_index = index
if index > prev_block_end_index and backtrace:
idx = index - 1
for idx in range(index - 1, prev_block_end_index - 2, -1):
if not (len(lines[idx].strip()) > 0 and lines[idx].startswith(indent_str)):
break
idx += 1
if idx < index:
block_start_index = idx
# between the current found block and the previous found block
if block_start_index > prev_block_end_index:
# give it a dummy name
if len("".join(lines[prev_block_end_index:block_start_index]).strip()) == 0:
prev_block_name = f"_empty_block_{empty_block_idx}"
empty_block_idx += 1
else:
prev_block_name = f"_block_without_name_{block_without_name_idx}"
block_without_name_idx += 1
# Add it as a block
splits.append((prev_block_name, prev_block_end_index, block_start_index))
# Add the current found block
splits.append((name, block_start_index, block_end_index))
prev_block_end_index = block_end_index
index = block_end_index - 1
index += 1
if index > prev_block_end_index:
if len("".join(lines[prev_block_end_index:index]).strip()) == 0:
prev_block_name = f"_empty_block_{empty_block_idx}"
else:
prev_block_name = f"_block_without_name_{block_without_name_idx}"
splits.append((prev_block_name, prev_block_end_index, index))
return splits
def find_code_in_transformers(
object_name: str, base_path: str = None, return_indices: bool = False
) -> Union[str, Tuple[List[str], int, int]]:
"""
Find and return the source code of an object.
Args:
object_name (`str`):
The name of the object we want the source code of.
base_path (`str`, *optional*):
The path to the base folder where files are checked. If not set, it will be set to `TRANSFORMERS_PATH`.
return_indices(`bool`, *optional*, defaults to `False`):
If `False`, will only return the code (as a string), otherwise it will also return the whole lines of the
file where the object specified by `object_name` is defined, together the start/end indices of the block in
the file that defines the object.
Returns:
`Union[str, Tuple[List[str], int, int]]`: If `return_indices=False`, only the source code of the object will be
returned. Otherwise, it also returns the whole lines of the file where the object specified by `object_name` is
defined, together the start/end indices of the block in the file that defines the object.
"""
parts = object_name.split(".")
i = 0
# We can't set this as the default value in the argument, otherwise `CopyCheckTester` will fail, as it uses a
# patched temp directory.
if base_path is None:
base_path = TRANSFORMERS_PATH
# Detail: the `Copied from` statement is originally designed to work with the last part of `TRANSFORMERS_PATH`,
# (which is `transformers`). The same should be applied for `MODEL_TEST_PATH`. However, its last part is `models`
# (to only check and search in it) which is a bit confusing. So we keep the copied statement staring with
# `tests.models.` and change it to `tests` here.
if base_path == MODEL_TEST_PATH:
base_path = "tests"
# First let's find the module where our object lives.
module = parts[i]
while i < len(parts) and not os.path.isfile(os.path.join(base_path, f"{module}.py")):
i += 1
if i < len(parts):
module = os.path.join(module, parts[i])
if i >= len(parts):
raise ValueError(
f"`object_name` should begin with the name of a module of transformers but got {object_name}."
)
with open(os.path.join(base_path, f"{module}.py"), "r", encoding="utf-8", newline="\n") as f:
lines = f.readlines()
# Now let's find the class / func in the code!
indent = ""
line_index = 0
for name in parts[i + 1 :]:
while (
line_index < len(lines) and re.search(rf"^{indent}(class|def)\s+{name}(\(|\:)", lines[line_index]) is None
):
line_index += 1
# find the target specified in the current level in `parts` -> increase `indent` so we can search the next
indent += " "
# the index of the first line in the (currently found) block *body*
line_index += 1
if line_index >= len(lines):
raise ValueError(f" {object_name} does not match any function or class in {module}.")
# `indent` is already one level deeper than the (found) class/func block's definition header
# We found the beginning of the class / func, now let's find the end (when the indent diminishes).
# `start_index` is the index of the class/func block's definition header
start_index = line_index - 1
end_index = find_block_end(lines, start_index, len(indent))
code = "".join(lines[start_index:end_index])
return (code, (lines, start_index, end_index)) if return_indices else code
def replace_code(code: str, replace_pattern: str) -> str:
"""Replace `code` by a pattern of the form `with X1->X2,Y1->Y2,Z1->Z2`.
Args:
code (`str`): The code to be modified.
replace_pattern (`str`): The pattern used to modify `code`.
Returns:
`str`: The modified code.
"""
if len(replace_pattern) > 0:
patterns = replace_pattern.replace("with", "").split(",")
patterns = [_re_replace_pattern.search(p) for p in patterns]
for pattern in patterns:
if pattern is None:
continue
obj1, obj2, option = pattern.groups()
code = re.sub(obj1, obj2, code)
if option.strip() == "all-casing":
code = re.sub(obj1.lower(), obj2.lower(), code)
code = re.sub(obj1.upper(), obj2.upper(), code)
return code
def find_code_and_splits(object_name: str, base_path: str, buffer: dict = None):
"""Find the code of an object (specified by `object_name`) and split it into blocks.
Args:
object_name (`str`):
The name of the object, e.g. `transformers.models.bert.modeling_bert.BertAttention` or
`tests.models.llama.test_modeling_llama.LlamaModelTest.test_config`.
base_path (`str`):
The path to the base directory within which the search will be performed. It could be either
`TRANSFORMERS_PATH` or `MODEL_TEST_PATH`.
buffer (`dict`, *optional*):
The buffer used to store the previous results in order to speed up the process.
Returns:
lines (`List[str]`):
The lines of the whole file where the object is defined.
code (`str`):
The object's code.
code_splits (`List[Tuple[str, int, int]]`):
`code` splitted into blocks. See `split_code_into_blocks`.
"""
if buffer is None:
buffer = {}
if (object_name, base_path) in buffer:
lines, code, code_splits = buffer[(object_name, base_path)]
else:
code, (lines, target_start_index, target_end_index) = find_code_in_transformers(
object_name, base_path=base_path, return_indices=True
)
indent = get_indent(code)
# Split the code into blocks
# `indent` is the indent of the class/func definition header, but `code_splits` expects the indent level of the
# block body.
code_splits = split_code_into_blocks(
lines, target_start_index, target_end_index, len(indent) + 4, backtrace=True
)
buffer[(object_name, base_path)] = lines, code, code_splits
return lines, code, code_splits
_re_copy_warning = re.compile(r"^(\s*)#\s*Copied from\s+transformers\.(\S+\.\S+)\s*($|\S.*$)")
_re_copy_warning_for_test_file = re.compile(r"^(\s*)#\s*Copied from\s+tests\.(\S+\.\S+)\s*($|\S.*$)")
_re_replace_pattern = re.compile(r"^\s*(\S+)->(\S+)(\s+.*|$)")
_re_fill_pattern = re.compile(r"<FILL\s+[^>]*>")
def get_indent(code: str) -> str:
"""
Find the indent in the first non empty line in a code sample.
Args:
code (`str`): The code to inspect.
Returns:
`str`: The indent looked at (as string).
"""
lines = code.split("\n")
idx = 0
while idx < len(lines) and len(lines[idx]) == 0:
idx += 1
if idx < len(lines):
return re.search(r"^(\s*)\S", lines[idx]).groups()[0]
return ""
def run_ruff(code):
command = ["ruff", "format", "-", "--config", "pyproject.toml", "--silent"]
process = subprocess.Popen(command, stdout=subprocess.PIPE, stderr=subprocess.PIPE, stdin=subprocess.PIPE)
stdout, _ = process.communicate(input=code.encode())
return stdout.decode()
def stylify(code: str) -> str:
"""
Applies the ruff part of our `make style` command to some code. This formats the code using `ruff format`.
As `ruff` does not provide a python api this cannot be done on the fly.
Args:
code (`str`): The code to format.
Returns:
`str`: The formatted code.
"""
has_indent = len(get_indent(code)) > 0
if has_indent:
code = f"class Bla:\n{code}"
formatted_code = run_ruff(code)
return formatted_code[len("class Bla:\n") :] if has_indent else formatted_code
def check_codes_match(observed_code: str, theoretical_code: str) -> Optional[int]:
"""
Checks if two version of a code match with the exception of the class/function name.
Args:
observed_code (`str`): The code found.
theoretical_code (`str`): The code to match.
Returns:
`Optional[int]`: The index of the first line where there is a difference (if any) and `None` if the codes
match.
"""
observed_code_header = observed_code.split("\n")[0]
theoretical_code_header = theoretical_code.split("\n")[0]
# Catch the function/class name: it is expected that those do not match.
_re_class_match = re.compile(r"class\s+([^\(:]+)(?:\(|:)")
_re_func_match = re.compile(r"def\s+([^\(]+)\(")
for re_pattern in [_re_class_match, _re_func_match]:
if re_pattern.match(observed_code_header) is not None:
observed_obj_name = re_pattern.search(observed_code_header).groups()[0]
theoretical_name = re_pattern.search(theoretical_code_header).groups()[0]
theoretical_code_header = theoretical_code_header.replace(theoretical_name, observed_obj_name)
# Find the first diff. Line 0 is special since we need to compare with the function/class names ignored.
diff_index = 0
if theoretical_code_header != observed_code_header:
return 0
diff_index = 1
for observed_line, theoretical_line in zip(observed_code.split("\n")[1:], theoretical_code.split("\n")[1:]):
if observed_line != theoretical_line:
return diff_index
diff_index += 1
def is_copy_consistent(filename: str, overwrite: bool = False, buffer: dict = None) -> Optional[List[Tuple[str, int]]]:
"""
Check if the code commented as a copy in a file matches the original.
Args:
filename (`str`):
The name of the file to check.
overwrite (`bool`, *optional*, defaults to `False`):
Whether or not to overwrite the copies when they don't match.
buffer (`dict`, *optional*):
The buffer used to store the previous results in order to speed up the process.
Returns:
`Optional[List[Tuple[str, int]]]`: If `overwrite=False`, returns the list of differences as tuples `(str, int)`
with the name of the object having a diff and the line number where theere is the first diff.
"""
base_path = TRANSFORMERS_PATH if not filename.startswith("tests") else MODEL_TEST_PATH
with open(filename, "r", encoding="utf-8", newline="\n") as f:
lines = f.readlines()
diffs = []
line_index = 0
# Not a for loop cause `lines` is going to change (if `overwrite=True`).
while line_index < len(lines):
search_re = _re_copy_warning
if filename.startswith("tests"):
search_re = _re_copy_warning_for_test_file
search = search_re.search(lines[line_index])
if search is None:
line_index += 1
continue
# There is some copied code here, let's retrieve the original.
indent, object_name, replace_pattern = search.groups()
# Find the file lines, the object's code, and its blocks
target_lines, theoretical_code, theoretical_code_splits = find_code_and_splits(
object_name, base_path, buffer=buffer
)
# code replaced by the patterns
theoretical_code_blocks = OrderedDict()
for name, start, end in theoretical_code_splits:
name = replace_code(name, replace_pattern)
code = "".join(target_lines[start:end])
code = replace_code(code, replace_pattern)
theoretical_code_blocks[name] = code
theoretical_indent = get_indent(theoretical_code)
# `start_index` is the index of the first line (the definition header) after `# Copied from`.
# (`indent != theoretical_indent` doesn't seem to occur so far, not sure what this case is for.)
start_index = line_index + 1 if indent == theoretical_indent else line_index
# enter the block body
line_index = start_index + 1
subcode = "\n".join(theoretical_code.split("\n")[1:])
indent = get_indent(subcode)
# Loop to check the observed code, stop when indentation diminishes or if we see a End copy comment.
# We can't call `find_block_end` directly as there is sth. special `# End copy"` here.
should_continue = True
while line_index < len(lines) and should_continue:
line_index += 1
if line_index >= len(lines):
break
line = lines[line_index]
# There is a special pattern `# End copy` to stop early. It's not documented cause it shouldn't really be
# used.
should_continue = _should_continue(line, indent) and re.search(f"^{indent}# End copy", line) is None
# `line_index` is outside the block
# Clean up empty lines at the end (if any).
while len(lines[line_index - 1]) <= 1:
line_index -= 1
# Split the observed code into blocks
observed_code_splits = split_code_into_blocks(lines, start_index, line_index, len(indent), backtrace=True)
is_class = lines[start_index].startswith(f"{' ' * (len(indent) - 4)}class ")
# sanity check
_sanity_check_splits(theoretical_code_splits, observed_code_splits, is_class=is_class)
# observed code in a structured way (a dict mapping block names to blocks' code)
observed_code_blocks = OrderedDict()
for name, start, end in observed_code_splits:
code = "".join(lines[start:end])
observed_code_blocks[name] = code
# Below, we change some names in `theoretical_code_blocks` and `observed_code_blocks`. These mappings map the
# original names to the modified names: this is used to restore the original order of the code blocks.
name_mappings_1 = {k: k for k in theoretical_code_blocks.keys()}
name_mappings_2 = {k: k for k in observed_code_blocks.keys()}
# Update code blocks' name and content:
# If `"# Ignore copy"` is found in a block of the observed code:
# 1. if it's a block only in the observed code --> add it to the theoretical code.
# 2. if it's also in the theoretical code () --> put its content (body) to the corresponding block under the
# same name in the theoretical code.
# In both cases, we change the name to have a prefix `_ignored_` so we know if we can discard them during the
# comparison.
ignored_existing_block_index = 0
ignored_new_block_index = 0
for name in list(observed_code_blocks.keys()):
code = observed_code_blocks[name]
if "# Ignore copy" in code:
if name in theoretical_code_blocks:
# in the target --> just copy the content
del theoretical_code_blocks[name]
theoretical_code_blocks[f"_ignored_existing_block_{ignored_existing_block_index}"] = code
name_mappings_1[name] = f"_ignored_existing_block_{ignored_existing_block_index}"
del observed_code_blocks[name]
observed_code_blocks[f"_ignored_existing_block_{ignored_existing_block_index}"] = code
name_mappings_2[name] = f"_ignored_existing_block_{ignored_existing_block_index}"
ignored_existing_block_index += 1
else:
# not in the target --> add it
theoretical_code_blocks[f"_ignored_new_block_{ignored_new_block_index}"] = code
name_mappings_1[
f"_ignored_new_block_{ignored_new_block_index}"
] = f"_ignored_new_block_{ignored_new_block_index}"
del observed_code_blocks[name]
observed_code_blocks[f"_ignored_new_block_{ignored_new_block_index}"] = code
name_mappings_2[name] = f"_ignored_new_block_{ignored_new_block_index}"
ignored_new_block_index += 1
# Respect the original block order:
# 1. in `theoretical_code_blocks`: the new blocks will follow the existing ones
# 2. in `observed_code_blocks`: the original order are kept with names modified potentially. This is necessary
# to compute the correct `diff_index` if `overwrite=True` and there is a diff.
theoretical_code_blocks = {
name_mappings_1[orig_name]: theoretical_code_blocks[name_mappings_1[orig_name]]
for orig_name in name_mappings_1
}
observed_code_blocks = {
name_mappings_2[orig_name]: observed_code_blocks[name_mappings_2[orig_name]]
for orig_name in name_mappings_2
}
# Ignore the blocks specified to be ignored. This is the version used to check if there is a mismatch
theoretical_code_blocks_clean = {
k: v
for k, v in theoretical_code_blocks.items()
if not (k.startswith(("_ignored_existing_block_", "_ignored_new_block_")))
}
theoretical_code = "".join(list(theoretical_code_blocks_clean.values()))
# stylify `theoretical_code` before compare (this is needed only when `replace_pattern` is not empty)
if replace_pattern:
theoretical_code = stylify(theoretical_code)
# Remove `\n\n` in `theoretical_code` before compare (so no empty line)
while "\n\n" in theoretical_code:
theoretical_code = theoretical_code.replace("\n\n", "\n")
# Compute `observed_code` where we don't include any empty line + keep track the line index between the
# original/processed `observed_code` so we can have the correct `diff_index`.
idx_to_orig_idx_mapping_for_observed_code_lines = {}
idx = -1
orig_idx = -1
observed_code = ""
for name, code in observed_code_blocks.items():
if code.endswith("\n"):
code = code[:-1]
for code_line in code.split("\n"):
orig_idx += 1
if code_line.strip() and not name.startswith(("_ignored_existing_block_", "_ignored_new_block_")):
idx += 1
observed_code += code_line + "\n"
idx_to_orig_idx_mapping_for_observed_code_lines[idx] = orig_idx
# Test for a diff and act accordingly.
diff_index = check_codes_match(observed_code, theoretical_code)
if diff_index is not None:
# switch to the index in the original `observed_code` (i.e. before removing empty lines)
diff_index = idx_to_orig_idx_mapping_for_observed_code_lines[diff_index]
diffs.append([object_name, diff_index + start_index + 1])
if overwrite:
# `theoretical_code_to_write` is a single string but may have several lines.
theoretical_code_to_write = stylify("".join(list(theoretical_code_blocks.values())))
lines = lines[:start_index] + [theoretical_code_to_write] + lines[line_index:]
# Here we treat it as a single entry in `lines`.
line_index = start_index + 1
if overwrite and len(diffs) > 0:
# Warn the user a file has been modified.
print(f"Detected changes, rewriting {filename}.")
with open(filename, "w", encoding="utf-8", newline="\n") as f:
f.writelines(lines)
return diffs
def check_copies(overwrite: bool = False, file: str = None):
"""
Check every file is copy-consistent with the original. Also check the model list in the main README and other
READMEs are consistent.
Args:
overwrite (`bool`, *optional*, defaults to `False`):
Whether or not to overwrite the copies when they don't match.
file (`bool`, *optional*):
The path to a specific file to check and/or fix.
"""
buffer = {}
if file is None:
all_files = glob.glob(os.path.join(TRANSFORMERS_PATH, "**/*.py"), recursive=True)
all_test_files = glob.glob(os.path.join(MODEL_TEST_PATH, "**/*.py"), recursive=True)
all_files = list(all_files) + list(all_test_files)
else:
all_files = [file]
diffs = []
for filename in all_files:
new_diffs = is_copy_consistent(filename, overwrite, buffer)
diffs += [f"- {filename}: copy does not match {d[0]} at line {d[1]}" for d in new_diffs]
if not overwrite and len(diffs) > 0:
diff = "\n".join(diffs)
raise Exception(
"Found the following copy inconsistencies:\n"
+ diff
+ "\nRun `make fix-copies` or `python utils/check_copies.py --fix_and_overwrite` to fix them."
)
check_model_list_copy(overwrite=overwrite)
def check_full_copies(overwrite: bool = False):
"""
Check the files that are full copies of others (as indicated in `FULL_COPIES`) are copy-consistent.
Args:
overwrite (`bool`, *optional*, defaults to `False`):
Whether or not to overwrite the copies when they don't match.
"""
diffs = []
for target, source in FULL_COPIES.items():
with open(source, "r", encoding="utf-8") as f:
source_code = f.read()
with open(target, "r", encoding="utf-8") as f:
target_code = f.read()
if source_code != target_code:
if overwrite:
with open(target, "w", encoding="utf-8") as f:
print(f"Replacing the content of {target} by the one of {source}.")
f.write(source_code)
else:
diffs.append(f"- {target}: copy does not match {source}.")
if not overwrite and len(diffs) > 0:
diff = "\n".join(diffs)
raise Exception(
"Found the following copy inconsistencies:\n"
+ diff
+ "\nRun `make fix-copies` or `python utils/check_copies.py --fix_and_overwrite` to fix them."
)
def get_model_list(filename: str, start_prompt: str, end_prompt: str) -> str:
"""
Extracts the model list from a README.
Args:
filename (`str`): The name of the README file to check.
start_prompt (`str`): The string to look for that introduces the model list.
end_prompt (`str`): The string to look for that ends the model list.
Returns:
`str`: The model list.
"""
with open(os.path.join(REPO_PATH, filename), "r", encoding="utf-8", newline="\n") as f:
lines = f.readlines()
# Find the start of the list.
start_index = 0
while not lines[start_index].startswith(start_prompt):
start_index += 1
start_index += 1
result = []
current_line = ""
end_index = start_index
# Keep going until the end of the list.
while not lines[end_index].startswith(end_prompt):
if lines[end_index].startswith("1."):
if len(current_line) > 1:
result.append(current_line)
current_line = lines[end_index]
elif len(lines[end_index]) > 1:
current_line = f"{current_line[:-1]} {lines[end_index].lstrip()}"
end_index += 1
if len(current_line) > 1:
result.append(current_line)
return "".join(result)
def convert_to_localized_md(model_list: str, localized_model_list: str, format_str: str) -> Tuple[bool, str]:
"""
Compare the model list from the main README to the one in a localized README.
Args:
model_list (`str`): The model list in the main README.
localized_model_list (`str`): The model list in one of the localized README.
format_str (`str`):
The template for a model entry in the localized README (look at the `format_model_list` in the entries of
`LOCALIZED_READMES` for examples).
Returns:
`Tuple[bool, str]`: A tuple where the first value indicates if the READMEs match or not, and the second value
is the correct localized README.
"""
def _rep(match):
title, model_link, paper_affiliations, paper_title_link, paper_authors, supplements = match.groups()
return format_str.format(
title=title,
model_link=model_link,
paper_affiliations=paper_affiliations,
paper_title_link=paper_title_link,
paper_authors=paper_authors,
supplements=" " + supplements.strip() if len(supplements) != 0 else "",
)
# This regex captures metadata from an English model description, including model title, model link,
# affiliations of the paper, title of the paper, authors of the paper, and supplemental data (see DistilBERT for
# example).
_re_capture_meta = re.compile(
r"\*\*\[([^\]]*)\]\(([^\)]*)\)\*\* \(from ([^)]*)\)[^\[]*([^\)]*\)).*?by (.*?[A-Za-z\*]{2,}?)\. (.*)$"
)
# This regex is used to synchronize title link.
_re_capture_title_link = re.compile(r"\*\*\[([^\]]*)\]\(([^\)]*)\)\*\*")
# This regex is used to synchronize paper title and link.
_re_capture_paper_link = re.compile(r" \[([^\]]*)\]\(([^\)]*)\)")
if len(localized_model_list) == 0:
localized_model_index = {}
else:
try:
localized_model_index = {
re.search(r"\*\*\[([^\]]*)", line).groups()[0]: line
for line in localized_model_list.strip().split("\n")
}
except AttributeError:
raise AttributeError("A model name in localized READMEs cannot be recognized.")
model_keys = [re.search(r"\*\*\[([^\]]*)", line).groups()[0] for line in model_list.strip().split("\n")]
# We exclude keys in localized README not in the main one.
readmes_match = not any(k not in model_keys for k in localized_model_index)
localized_model_index = {k: v for k, v in localized_model_index.items() if k in model_keys}
for model in model_list.strip().split("\n"):
title, model_link = _re_capture_title_link.search(model).groups()
if title not in localized_model_index:
readmes_match = False
# Add an anchor white space behind a model description string for regex.
# If metadata cannot be captured, the English version will be directly copied.
localized_model_index[title] = _re_capture_meta.sub(_rep, model + " ")
elif _re_fill_pattern.search(localized_model_index[title]) is not None:
update = _re_capture_meta.sub(_rep, model + " ")
if update != localized_model_index[title]:
readmes_match = False
localized_model_index[title] = update
else:
# Synchronize title link
converted_model = _re_capture_title_link.sub(
f"**[{title}]({model_link})**", localized_model_index[title], count=1
)
# Synchronize paper title and its link (if found)
paper_title_link = _re_capture_paper_link.search(model)
if paper_title_link is not None:
paper_title, paper_link = paper_title_link.groups()
converted_model = _re_capture_paper_link.sub(
f" [{paper_title}]({paper_link})", converted_model, count=1
)
if converted_model != localized_model_index[title]:
readmes_match = False
localized_model_index[title] = converted_model
sorted_index = sorted(localized_model_index.items(), key=lambda x: x[0].lower())
return readmes_match, "\n".join((x[1] for x in sorted_index)) + "\n"
def _find_text_in_file(filename: str, start_prompt: str, end_prompt: str) -> Tuple[str, int, int, List[str]]:
"""
Find the text in a file between two prompts.
Args:
filename (`str`): The name of the file to look into.
start_prompt (`str`): The string to look for that introduces the content looked for.
end_prompt (`str`): The string to look for that ends the content looked for.
Returns:
Tuple[str, int, int, List[str]]: The content between the two prompts, the index of the start line in the
original file, the index of the end line in the original file and the list of lines of that file.
"""
with open(filename, "r", encoding="utf-8", newline="\n") as f:
lines = f.readlines()
# Find the start prompt.
start_index = 0
while not lines[start_index].startswith(start_prompt):
start_index += 1
start_index += 1
end_index = start_index
while not lines[end_index].startswith(end_prompt):
end_index += 1
end_index -= 1
while len(lines[start_index]) <= 1:
start_index += 1
while len(lines[end_index]) <= 1:
end_index -= 1
end_index += 1
return "".join(lines[start_index:end_index]), start_index, end_index, lines
def check_model_list_copy(overwrite: bool = False):
"""
Check the model lists in the README is consistent with the ones in the other READMES and also with `index.nmd`.
Args:
overwrite (`bool`, *optional*, defaults to `False`):
Whether or not to overwrite the copies when they don't match.
"""
# Fix potential doc links in the README
with open(os.path.join(REPO_PATH, "README.md"), "r", encoding="utf-8", newline="\n") as f:
readme = f.read()
new_readme = readme.replace("https://huggingface.co/transformers", "https://huggingface.co/docs/transformers")
new_readme = new_readme.replace(
"https://huggingface.co/docs/main/transformers", "https://huggingface.co/docs/transformers/main"
)
if new_readme != readme:
if overwrite:
with open(os.path.join(REPO_PATH, "README.md"), "w", encoding="utf-8", newline="\n") as f:
f.write(new_readme)
else:
raise ValueError(
"The main README contains wrong links to the documentation of Transformers. Run `make fix-copies` to "
"automatically fix them."
)
md_list = get_model_list(
filename="README.md",
start_prompt=LOCALIZED_READMES["README.md"]["start_prompt"],
end_prompt=LOCALIZED_READMES["README.md"]["end_prompt"],
)
# Build the converted Markdown.
converted_md_lists = []
for filename, value in LOCALIZED_READMES.items():
_start_prompt = value["start_prompt"]
_end_prompt = value["end_prompt"]
_format_model_list = value["format_model_list"]
localized_md_list = get_model_list(filename, _start_prompt, _end_prompt)
readmes_match, converted_md_list = convert_to_localized_md(md_list, localized_md_list, _format_model_list)
converted_md_lists.append((filename, readmes_match, converted_md_list, _start_prompt, _end_prompt))
# Compare the converted Markdowns
for converted_md_list in converted_md_lists:
filename, readmes_match, converted_md, _start_prompt, _end_prompt = converted_md_list
if filename == "README.md":
continue
if overwrite:
_, start_index, end_index, lines = _find_text_in_file(
filename=os.path.join(REPO_PATH, filename), start_prompt=_start_prompt, end_prompt=_end_prompt
)
with open(os.path.join(REPO_PATH, filename), "w", encoding="utf-8", newline="\n") as f:
f.writelines(lines[:start_index] + [converted_md] + lines[end_index:])
elif not readmes_match:
raise ValueError(
f"The model list in the README changed and the list in `{filename}` has not been updated. Run "
"`make fix-copies` to fix this."
)
# Map a model name with the name it has in the README for the check_readme check
SPECIAL_MODEL_NAMES = {
"Bert Generation": "BERT For Sequence Generation",
"BigBird": "BigBird-RoBERTa",
"Data2VecAudio": "Data2Vec",
"Data2VecText": "Data2Vec",
"Data2VecVision": "Data2Vec",
"DonutSwin": "Swin Transformer",
"Marian": "MarianMT",
"MaskFormerSwin": "Swin Transformer",
"OpenAI GPT-2": "GPT-2",
"OpenAI GPT": "GPT",
"Perceiver": "Perceiver IO",
"SAM": "Segment Anything",
"ViT": "Vision Transformer (ViT)",
}
# Update this list with the models that shouldn't be in the README. This only concerns modular models or those who do
# not have an associated paper.
MODELS_NOT_IN_README = [
"BertJapanese",
"Encoder decoder",
"FairSeq Machine-Translation",
"HerBERT",
"RetriBERT",
"Speech Encoder decoder",
"Speech2Text",
"Speech2Text2",
"TimmBackbone",
"Vision Encoder decoder",
"VisionTextDualEncoder",
"CLIPVisionModel",
"SiglipVisionModel",
"ChineseCLIPVisionModel",
]
# Template for new entries to add in the main README when we have missing models.
README_TEMPLATE = (
"1. **[{model_name}](https://huggingface.co/docs/main/transformers/model_doc/{model_type})** (from "
"<FILL INSTITUTION>) released with the paper [<FILL PAPER TITLE>](<FILL ARKIV LINK>) by <FILL AUTHORS>."
)
def check_readme(overwrite: bool = False):
"""
Check if the main README contains all the models in the library or not.
Args:
overwrite (`bool`, *optional*, defaults to `False`):
Whether or not to add an entry for the missing models using `README_TEMPLATE`.
"""
info = LOCALIZED_READMES["README.md"]
models, start_index, end_index, lines = _find_text_in_file(
os.path.join(REPO_PATH, "README.md"),
info["start_prompt"],
info["end_prompt"],
)
models_in_readme = [re.search(r"\*\*\[([^\]]*)", line).groups()[0] for line in models.strip().split("\n")]
model_names_mapping = transformers_module.models.auto.configuration_auto.MODEL_NAMES_MAPPING
absents = [
(key, name)
for key, name in model_names_mapping.items()
if SPECIAL_MODEL_NAMES.get(name, name) not in models_in_readme
]
# Remove exceptions
absents = [(key, name) for key, name in absents if name not in MODELS_NOT_IN_README]
if len(absents) > 0 and not overwrite:
print(absents)
raise ValueError(
"The main README doesn't contain all models, run `make fix-copies` to fill it with the missing model(s)"
" then complete the generated entries.\nIf the model is not supposed to be in the main README, add it to"
" the list `MODELS_NOT_IN_README` in utils/check_copies.py.\nIf it has a different name in the repo than"
" in the README, map the correspondence in `SPECIAL_MODEL_NAMES` in utils/check_copies.py."
)
new_models = [README_TEMPLATE.format(model_name=name, model_type=key) for key, name in absents]
all_models = models.strip().split("\n") + new_models
all_models = sorted(all_models, key=lambda x: re.search(r"\*\*\[([^\]]*)", x).groups()[0].lower())
all_models = "\n".join(all_models) + "\n"
if all_models != models:
if overwrite:
print("Fixing the main README.")
with open(os.path.join(REPO_PATH, "README.md"), "w", encoding="utf-8", newline="\n") as f:
f.writelines(lines[:start_index] + [all_models] + lines[end_index:])
else:
raise ValueError("The main README model list is not properly sorted. Run `make fix-copies` to fix this.")
if __name__ == "__main__":
parser = argparse.ArgumentParser()
parser.add_argument("--file", type=str, default=None, help="A specific file to check and/or fix")
parser.add_argument("--fix_and_overwrite", action="store_true", help="Whether to fix inconsistencies.")
args = parser.parse_args()
check_readme(args.fix_and_overwrite)
check_copies(args.fix_and_overwrite, args.file)
check_full_copies(args.fix_and_overwrite)
| transformers/utils/check_copies.py/0 | {
"file_path": "transformers/utils/check_copies.py",
"repo_id": "transformers",
"token_count": 22136
} | 408 |
import argparse
import json
import os
import time
import zipfile
from get_ci_error_statistics import download_artifact, get_artifacts_links
from transformers import logging
logger = logging.get_logger(__name__)
def extract_warnings_from_single_artifact(artifact_path, targets):
"""Extract warnings from a downloaded artifact (in .zip format)"""
selected_warnings = set()
buffer = []
def parse_line(fp):
for line in fp:
if isinstance(line, bytes):
line = line.decode("UTF-8")
if "warnings summary (final)" in line:
continue
# This means we are outside the body of a warning
elif not line.startswith(" "):
# process a single warning and move it to `selected_warnings`.
if len(buffer) > 0:
warning = "\n".join(buffer)
# Only keep the warnings specified in `targets`
if any(f": {x}: " in warning for x in targets):
selected_warnings.add(warning)
buffer.clear()
continue
else:
line = line.strip()
buffer.append(line)
if from_gh:
for filename in os.listdir(artifact_path):
file_path = os.path.join(artifact_path, filename)
if not os.path.isdir(file_path):
# read the file
if filename != "warnings.txt":
continue
with open(file_path) as fp:
parse_line(fp)
else:
try:
with zipfile.ZipFile(artifact_path) as z:
for filename in z.namelist():
if not os.path.isdir(filename):
# read the file
if filename != "warnings.txt":
continue
with z.open(filename) as fp:
parse_line(fp)
except Exception:
logger.warning(
f"{artifact_path} is either an invalid zip file or something else wrong. This file is skipped."
)
return selected_warnings
def extract_warnings(artifact_dir, targets):
"""Extract warnings from all artifact files"""
selected_warnings = set()
paths = [os.path.join(artifact_dir, p) for p in os.listdir(artifact_dir) if (p.endswith(".zip") or from_gh)]
for p in paths:
selected_warnings.update(extract_warnings_from_single_artifact(p, targets))
return selected_warnings
if __name__ == "__main__":
def list_str(values):
return values.split(",")
parser = argparse.ArgumentParser()
# Required parameters
parser.add_argument("--workflow_run_id", type=str, required=True, help="A GitHub Actions workflow run id.")
parser.add_argument(
"--output_dir",
type=str,
required=True,
help="Where to store the downloaded artifacts and other result files.",
)
parser.add_argument("--token", default=None, type=str, help="A token that has actions:read permission.")
# optional parameters
parser.add_argument(
"--targets",
default="DeprecationWarning,UserWarning,FutureWarning",
type=list_str,
help="Comma-separated list of target warning(s) which we want to extract.",
)
parser.add_argument(
"--from_gh",
action="store_true",
help="If running from a GitHub action workflow and collecting warnings from its artifacts.",
)
args = parser.parse_args()
from_gh = args.from_gh
if from_gh:
# The artifacts have to be downloaded using `actions/download-artifact@v3`
pass
else:
os.makedirs(args.output_dir, exist_ok=True)
# get download links
artifacts = get_artifacts_links(args.workflow_run_id, token=args.token)
with open(os.path.join(args.output_dir, "artifacts.json"), "w", encoding="UTF-8") as fp:
json.dump(artifacts, fp, ensure_ascii=False, indent=4)
# download artifacts
for idx, (name, url) in enumerate(artifacts.items()):
print(name)
print(url)
print("=" * 80)
download_artifact(name, url, args.output_dir, args.token)
# Be gentle to GitHub
time.sleep(1)
# extract warnings from artifacts
selected_warnings = extract_warnings(args.output_dir, args.targets)
selected_warnings = sorted(selected_warnings)
with open(os.path.join(args.output_dir, "selected_warnings.json"), "w", encoding="UTF-8") as fp:
json.dump(selected_warnings, fp, ensure_ascii=False, indent=4)
| transformers/utils/extract_warnings.py/0 | {
"file_path": "transformers/utils/extract_warnings.py",
"repo_id": "transformers",
"token_count": 2110
} | 409 |
from transformers import PretrainedConfig
class CustomConfig(PretrainedConfig):
model_type = "custom"
def __init__(self, attribute=1, **kwargs):
self.attribute = attribute
super().__init__(**kwargs)
class NoSuperInitConfig(PretrainedConfig):
model_type = "custom"
def __init__(self, attribute=1, **kwargs):
self.attribute = attribute
| transformers/utils/test_module/custom_configuration.py/0 | {
"file_path": "transformers/utils/test_module/custom_configuration.py",
"repo_id": "transformers",
"token_count": 136
} | 410 |
import json
import os
from ghapi.all import GhApi
FOLDER_STRING = os.environ.get("FOLDER_STRING", "")
folder = f"benchmark/trl/{FOLDER_STRING}"
host_url = f"https://huggingface.co/datasets/trl-internal-testing/example-images/resolve/main/images/benchmark/{FOLDER_STRING}"
# Create a GitHub API instance
github_context = json.loads(os.environ["GITHUB_CONTEXT"])
token = os.environ["PERSONAL_ACCESS_TOKEN_GITHUB"] # this needs to refreshed every 12 months
status_message = "**[COSTA BENCHMARK BOT]**: Here are the results"
body = status_message
repo = github_context["repository"]
owner, repo = repo.split("/")
api = GhApi(owner=owner, repo=repo, token=token)
# for each `.png` file in the folder, add it to the comment
for file in os.listdir(folder):
if file.endswith(".png"):
body += f"\n"
# Create a comment on the issue
api.issues.create_comment(issue_number=github_context["event"]["issue"]["number"], body=body)
| trl/benchmark/post_github_comment.py/0 | {
"file_path": "trl/benchmark/post_github_comment.py",
"repo_id": "trl",
"token_count": 358
} | 411 |
# Examples
## Introduction
The examples should work in any of the following settings (with the same script):
- single GPU
- multi GPUS (using PyTorch distributed mode)
- multi GPUS (using DeepSpeed ZeRO-Offload stages 1, 2, & 3)
- fp16 (mixed-precision), fp32 (normal precision), or bf16 (bfloat16 precision)
To run it in each of these various modes, first initialize the accelerate
configuration with `accelerate config`
**NOTE to train with a 4-bit or 8-bit model**, please run
```bash
pip install --upgrade trl[quantization]
```
## Accelerate Config
For all the examples, you'll need to generate a 🤗 Accelerate config file with:
```shell
accelerate config # will prompt you to define the training configuration
```
Then, it is encouraged to launch jobs with `accelerate launch`!
# Maintained Examples
| File | Description |
|------------------------------------------------------------------------------------------------------------|--------------------------------------------------------------------------------------------------------------------------|
| [`examples/scripts/sft.py`](https://github.com/huggingface/trl/blob/main/examples/scripts/sft.py) | This script shows how to use the `SFTTrainer` to fine tune a model or adapters into a target dataset. |
| [`examples/scripts/reward_modeling.py`](https://github.com/huggingface/trl/blob/main/examples/scripts/reward_modeling.py) | This script shows how to use the `RewardTrainer` to train a reward model on your own dataset. |
| [`examples/scripts/ppo.py`](https://github.com/huggingface/trl/blob/main/examples/scripts/ppo.py) | This script shows how to use the `PPOTrainer` to fine-tune a sentiment analysis model using IMDB dataset |
| [`examples/scripts/ppo_multi_adapter.py`](https://github.com/huggingface/trl/blob/main/examples/scripts/ppo_multi_adapter.py) | This script shows how to use the `PPOTrainer` to train a single base model with multiple adapters. Requires you to run the example script with the reward model training beforehand. |
| [`examples/scripts/stable_diffusion_tuning_example.py`](https://github.com/huggingface/trl/blob/main/examples/scripts/stable_diffusion_tuning_example.py) | This script shows to use DDPOTrainer to fine-tune a stable diffusion model using reinforcement learning. |
Here are also some easier-to-run colab notebooks that you can use to get started with TRL:
| File | Description |
|----------------------------------------------------------------------------------------------------------------------------|--------------------------------------------------------------------------------------------------------------------------|
| [`examples/notebooks/best_of_n.ipynb`](https://github.com/huggingface/trl/tree/main/examples/notebooks/best_of_n.ipynb) | This notebook demonstrates how to use the "Best of N" sampling strategy using TRL when fine-tuning your model with PPO. |
| [`examples/notebooks/gpt2-sentiment.ipynb`](https://github.com/huggingface/trl/tree/main/examples/notebooks/gpt2-sentiment.ipynb) | This notebook demonstrates how to reproduce the GPT2 imdb sentiment tuning example on a jupyter notebook. |
| [`examples/notebooks/gpt2-control.ipynb`](https://github.com/huggingface/trl/tree/main/examples/notebooks/gpt2-control.ipynb) | This notebook demonstrates how to reproduce the GPT2 sentiment control example on a jupyter notebook. |
We also have some other examples that are less maintained but can be used as a reference:
1. **[research_projects](https://github.com/huggingface/trl/tree/main/examples/research_projects)**: Check out this folder to find the scripts used for some research projects that used TRL (LM de-toxification, Stack-Llama, etc.)
## Distributed training
All of the scripts can be run on multiple GPUs by providing the path of an 🤗 Accelerate config file when calling `accelerate launch`. To launch one of them on one or multiple GPUs, run the following command (swapping `{NUM_GPUS}` with the number of GPUs in your machine and `--all_arguments_of_the_script` with your arguments.)
```shell
accelerate launch --config_file=examples/accelerate_configs/multi_gpu.yaml --num_processes {NUM_GPUS} path_to_script.py --all_arguments_of_the_script
```
You can also adjust the parameters of the 🤗 Accelerate config file to suit your needs (e.g. training in mixed precision).
### Distributed training with DeepSpeed
Most of the scripts can be run on multiple GPUs together with DeepSpeed ZeRO-{1,2,3} for efficient sharding of the optimizer states, gradients, and model weights. To do so, run following command (swapping `{NUM_GPUS}` with the number of GPUs in your machine, `--all_arguments_of_the_script` with your arguments, and `--deepspeed_config` with the path to the DeepSpeed config file such as `examples/deepspeed_configs/deepspeed_zero1.yaml`):
```shell
accelerate launch --config_file=examples/accelerate_configs/deepspeed_zero{1,2,3}.yaml --num_processes {NUM_GPUS} path_to_script.py --all_arguments_of_the_script
```
| trl/docs/source/example_overview.md/0 | {
"file_path": "trl/docs/source/example_overview.md",
"repo_id": "trl",
"token_count": 1969
} | 412 |
# Text Environments
Text environments provide a learning ground for language agents. It allows a language model to use tools to accomplish a task such as using a Python interpreter to answer math questions or using a search index for trivia questions. Having access to tools allows language models to solve tasks that would be very hard for the models itself but can be trivial for the appropriate tools. A good example is arithmetics of large numbers that become a simple copy-paste task once you have access to a calculator.
<div style="text-align: center">
<img src="https://huggingface.co/datasets/trl-internal-testing/example-images/resolve/main/images/textenv.png">
</div>
Let's dive into how text environments work and start with tools!
## Tools
One of the core building blocks of text environments are tools that the model can use to solve tasks. In general tools can be any Python function that takes a string as input and returns string. The `TextEnvironment` offers two options for tools: either go with predefined tools from `transformers.Tool` or define your own function or class with `__call__` method. Let's have a look at both!
### `transformers.Tool`
Text environments fully support tools of the class `transformers.Tool`. The advantage of building tools in that framework is that they can easily be shared
```Python
from transformers import load_tool
# simple calculator tool that runs +-/* operations
calc_tool = load_tool("ybelkada/simple-calculator")
# python interpreter that executes program and returns outputs
py_tool = load_tool("lvwerra/python-interpreter")
# wikipedia search index that returns best search match
wiki_tool = load_tool("vwxyzjn/pyserini-wikipedia-kilt-doc")
```
These tools are either loaded from the hub or from a local folder. Using the tool is as simple as calling them with a text query:
```Python
calc_tool("1/2")
>>> "0.5"
```
Note that both input and return values are strings to enable easy usage with a language model.
### Custom Tools
The following is an example of a tool that adds two integers:
```Python
def add(text):
int_1, int_2 = text.split("+")
result = int(int_1) + int(int_2)
return str(result)
print(add("1+1"))
>>> "2"
```
We looked at basic examples such as a calculator but the principle holds for more complex tools as well such as a web search tool where you input the query and get the search results in return. Now let's look at how the model can use the tools with the call syntax.
### Call syntax
In order to have a unified way for the model to call a tool we created a simple syntax that looks as follows:
```python
"<request><TOOL_NAME>QUERY<call>TOOL_RESPONSE<response>"
```
There are a few special tokens involved so let's decompose it: First the model can signal that it wants to use a tool by emitting the `<request>` token. After that we want to know the name of the tool to call which is done by enclosing the tool name with `<>` brackets. Once we know which tool to call the tool query follows which is in free text form. The `<call>` tokens signifies the end of the query and stops the model generation. At this point the model output is parsed and the query sent to the tool. The environment appends the tool response to the string followed by the `<response>` token to show the end the tool output.
Let's look at the concrete example of the calculator and assume its name is `Calculator` (more on how the name of a tool is inferred later):
```python
"<request><Calculator>1/2<call>0.5<response>"
```
Finally, the episode is ended and generation stops when the model generates `<submit>` which marks the interaction as completed.
Now let's have a look how we can create a new text environment!
## Create a `TextEnvironment`
```python
prompt = """\
What is 13-3?
<request><SimpleCalculatorTool>13-3<call>10.0<response>
Result=10<submit>
"""
def reward_fn(result, answer):
"""Simplified reward function returning 1 if result matches answer and 0 otherwise."""
result_parsed = result.split("=")[1].split("<")[0]
return int(result_parsed==answer)
text_env = TextEnvironemnt(
model=model,
tokenizer=tokenizer,
tools= {"SimpleCalculatorTool": load_tool("ybelkada/simple-calculator")},
reward_fn=exact_match_reward,
prompt=prompt,
max_turns=1
max_tool_response=100
generation_kwargs={"do_sample": "true"}
)
```
Let's decompose the settings:
| Argument | Description |
|:-------------------|:----------------|
| `model` | Language model to interact with the environment and generate requests. |
| `tokenizer` | Tokenizer of language model handling tokenization of strings. |
| `tools` | `list` of `dict` of tools. If former the name of the tool is inferred from class name and otherwise it's the keys of the dictionary.|
| `reward_fn` | A function that takes a string as input and returns. Can have extra arguments that are passed to `.run()` such as ground truth.|
| `prompt` | Prompt to prepend to every task. Usually a few examples to demonstrate to the model how to use the tools in a few-shot fashion. |
| `max_turns` | Maximum number of interactions between model and tools before episode ends.|
| `max_tool_response`| The tool response is truncated to this number to avoid running out of model context.|
| `max_length` | The maximum number of tokens to allow in an episode. |
| `generation_kwargs`| Generation settings used by the language model. |
You can customize the environment to your needs and add custom tools and settings. Let's see how you can use the environment to have the model interact with the available tools!
## Run an Episode
To run a set of queries through the text environment one can simply use the `run` method.
```python
queries = ["What is 1/2?"]
answers = ["0.5"]
queries, responses, masks, rewards, histories = text_env.run(queries, answers=answers)
```
This will execute the model/tool feedback loop for each query until either no tool is called anymore, the maximum number of turns is reached or to maximum number of tokens in an episode is exceeded. The extra `kwargs` (e.g. `answers=answers` above) passed to `run` will be passed on to the reward function.
There are five objects that are returned by `run`:
- `queries`: a list of the tokenized queries
- `responses`: all tokens that have been generated withing the environment including model and tool tokens
- `masks`: mask that indicates which tokens have been generated by the model and which tokens are generated by the tool
- `rewards`: a list of reward for each query/response
- `histories`: list of `TextHistory` objects, which are useful objects containing all the above and also the text equivalents
The masks are crucial for training as we don't want to optimize tokens that the model has not generated which are tokens produced by the tools.
Next, we'll train a PPO step with the generated responses!
### Train
Training on episodes from the `TextEnvironment` is straight forward and simply requires forwarding all the returned variables except the `TextHistory` objects to the `step` method:
```python
train_stats = ppo_trainer.step(queries, responses, rewards, masks)
```
## `TextHistory`
The `TextHistory` object stores the interactions between the model and the text environment. It stores tokens and text generated in each turn and their source in each turn (model or system) as well as rewards. Let's go through the class attributes and methods.
### Attributes
The following table summarises the available attributes of the `TextEnvironment` class:
| Attribute | Description |
|:-------------------|:----------------|
| `text` | The full string of the text generated in the text environment with both model and system generated text. |
| `text_spans` | A list of tuples with the spans for each model or system generated text segment. |
| `system_spans` | A list of boolean values indicating if the segment is model or system generated. |
| `tokens` | All tokens generated in text environment with both model and system generated tokens. |
| `token_spans` | Similar to `text_spans` the `token_spans` indicate the boundaries of model andsystem generated tokens. |
| `token_masks` | The token masks can be used to ignore system generated tokens by masking them. |
| `completed` | Indicates if the interaction with the environment has completed. |
| `truncated` | Indicates if the interaction with the environment has completed because max length was reached. |
With these attributes you can reconstruct every interaction of the model with the `TextEnvironment`. The `TextHistory` also lets you visualize the text history. Let's have a look!
### Visualization
When the model interacts inside the `TextEnvironment` it can be useful to visualize and separate which parts of the text outputs were generated by the model and which parts come from the system and tools. For that purpose there are the two methods [`TextHistory.show_text`] and [`TextHistory.show_tokens`]. They print the text and tokens respectively and highlight the various segments using the [`rich` libray](https://github.com/Textualize/rich) (make sure to install it before using these methods).
You can see that the prompt is highlighted in gray, whereas system segments such as query and tool responses are highlighted in green. All segments generated by the model are highlighted in blue and in addition to the pure text output the reward is displayed as additional text in plum. Here an example of `show_text`:
<div style="text-align: center">
<img src="https://huggingface.co/datasets/trl-internal-testing/example-images/resolve/main/images/textenv_show_text.png" width=600>
</div>
Sometimes there can be tricky tokenization related issues that are hidden when showing the decoded text. Thus `TextHistory` also offers an option to display the same highlighting on the tokens directly with `show_tokens`:
<div style="text-align: center">
<img src="https://huggingface.co/datasets/trl-internal-testing/example-images/resolve/main/images/textenv_show_tokens.png" width=800>
</div>
Note that you can turn on the colour legend by passing `show_legend=True`.
## API Documentation
[[autodoc]] TextEnvironment
[[autodoc]] TextHistory
| trl/docs/source/text_environments.md/0 | {
"file_path": "trl/docs/source/text_environments.md",
"repo_id": "trl",
"token_count": 2826
} | 413 |
<jupyter_start><jupyter_text>**Best-of-n sampling as an alternative to RLHF**This notebook compares reward-model scores of prompt based responses from 1. a base model (`gpt2-imdb`)2. `RLHF` tuned model based on this base-model 3. the base-model again from which we sample n responses to each prompt, score them and take the best scored one AKA the `best-of-n sampled` model Import dependencies<jupyter_code>%pip install transformers trl
import torch
import pandas as pd
from transformers import pipeline, AutoTokenizer
from datasets import load_dataset
from trl import AutoModelForCausalLMWithValueHead
from trl.core import LengthSampler
device = 0 if torch.cuda.is_available() else "cpu"<jupyter_output><empty_output><jupyter_text>Various constants<jupyter_code>ref_model_name = "lvwerra/gpt2-imdb"
model_name = "lvwerra/gpt2-imdb-pos-v2"
reward_model = "lvwerra/distilbert-imdb"
N_BEST_OF = 4<jupyter_output><empty_output><jupyter_text>Models and tokenizers<jupyter_code>model = AutoModelForCausalLMWithValueHead.from_pretrained(model_name)
ref_model = AutoModelForCausalLMWithValueHead.from_pretrained(ref_model_name)
reward_pipe = pipeline("sentiment-analysis", model=reward_model, device=device)
tokenizer = AutoTokenizer.from_pretrained(ref_model_name)
tokenizer.pad_token = tokenizer.eos_token
# cuda-ize models
model.cuda()
ref_model.cuda()<jupyter_output><empty_output><jupyter_text>Dataset building<jupyter_code>def build_dataset(tokenizer, dataset_name="imdb", input_min_text_length=2, input_max_text_length=8):
# load imdb with datasets
ds = load_dataset(dataset_name, split="train")
ds = ds.rename_columns({"text": "review"})
ds = ds.filter(lambda x: len(x["review"]) > 200, batched=False)
input_size = LengthSampler(input_min_text_length, input_max_text_length)
def tokenize(sample):
sample["input_ids"] = tokenizer.encode(sample["review"])[: input_size()]
sample["query"] = tokenizer.decode(sample["input_ids"])
return sample
ds = ds.map(tokenize, batched=False)
ds.set_format(type="torch")
return ds
dataset = build_dataset(tokenizer)
gen_kwargs = {"min_length": -1, "top_k": 0.0, "top_p": 1.0, "do_sample": True, "pad_token_id": tokenizer.eos_token_id}
sent_kwargs = {"top_k": None, "function_to_apply": "none", "batch_size": 16}
output_min_length = 4
output_max_length = 16
output_length_sampler = LengthSampler(output_min_length, output_max_length)
#### get a batch from the dataset
bs = 16
output_data = dict()
dataset.set_format("pandas")
df_batch = dataset[:].sample(bs)
output_data["query"] = df_batch["query"].tolist()
query_tensors = df_batch["input_ids"].tolist()
# :: [Resp]
response_tensors_ref, response_tensors = [], []
# :: [[Resp]]
response_tensors_best_of = []<jupyter_output><empty_output><jupyter_text>Generation using various models<jupyter_code>for i in range(bs):
gen_len = output_length_sampler()
query = torch.tensor(query_tensors[i])
output = ref_model.generate(query.unsqueeze(dim=0).to(device), max_new_tokens=gen_len, **gen_kwargs).squeeze()
response_tensors_ref.append(tokenizer.decode(output))
output = model.generate(query.unsqueeze(dim=0).to(device), max_new_tokens=gen_len, **gen_kwargs).squeeze()
response_tensors.append(tokenizer.decode(output))
# generating copies of the same query for the Best-of-n sampling
queries = query.repeat((N_BEST_OF, 1))
output = ref_model.generate(queries.to(device), max_new_tokens=gen_len, **gen_kwargs).squeeze()
response_tensors_best_of.append(tokenizer.batch_decode(output))<jupyter_output><empty_output><jupyter_text>Scoring<jupyter_code>scores_ref = [output[0]["score"] for output in reward_pipe(response_tensors_ref, **sent_kwargs)]
scores = [output[0]["score"] for output in reward_pipe(response_tensors, **sent_kwargs)]
scores_best_of = []
for i, response in enumerate(response_tensors_best_of):
# base_score = scores_ref[i]
scores_best_of.append(torch.tensor([output[0]["score"] for output in reward_pipe(response, **sent_kwargs)]))
output_data["response (ref)"] = response_tensors_ref
output_data["scores (ref)"] = scores_ref
output_data["response (RLHF)"] = response_tensors
output_data["scores (RLHF)"] = scores
output_data["response (best_of)"] = [
response_tensors_best_of[i][a.argmax().item()] for i, a in enumerate(scores_best_of)
]
output_data["scores (best_of)"] = [a.max().item() for a in scores_best_of]
# store results in a dataframe
df_results = pd.DataFrame(output_data)
df_results<jupyter_output><empty_output> | trl/examples/notebooks/best_of_n.ipynb/0 | {
"file_path": "trl/examples/notebooks/best_of_n.ipynb",
"repo_id": "trl",
"token_count": 1717
} | 414 |
# De-detoxifying language models
To run this code, do the following:
```shell
ACCELERATE_LOG_LEVEL=info accelerate launch --config_file {CONFIG} examples/research_projects/toxicity/scripts/gpt-j-6b-toxicity.py --log_with wandb
```
| trl/examples/research_projects/toxicity/README.md/0 | {
"file_path": "trl/examples/research_projects/toxicity/README.md",
"repo_id": "trl",
"token_count": 79
} | 415 |
# Copyright 2023 The HuggingFace Team. All rights reserved.
#
# Licensed under the Apache License, Version 2.0 (the "License");
# you may not use this file except in compliance with the License.
# You may obtain a copy of the License at
#
# http://www.apache.org/licenses/LICENSE-2.0
#
# Unless required by applicable law or agreed to in writing, software
# distributed under the License is distributed on an "AS IS" BASIS,
# WITHOUT WARRANTIES OR CONDITIONS OF ANY KIND, either express or implied.
# See the License for the specific language governing permissions and
# limitations under the License.
import unittest
from unittest.mock import patch
import torch
from transformers import AutoTokenizer
from trl import AutoModelForCausalLMWithValueHead, TextEnvironment, TextHistory
class DummyTool:
def __call__(self, text):
return text
def dummy_generate(histories):
for i in range(len(histories)):
histories[i].append_segment("<request><DummyTool>test<call>", torch.tensor([1, 2, 3]), system=False)
return histories
class TextHistoryTest(unittest.TestCase):
def test_text_history_init(self):
text = "Hello there!"
tokens = torch.tensor([1, 2, 3])
history = TextHistory(text, tokens)
assert history.text == text
assert torch.equal(history.tokens, tokens)
assert torch.equal(history.token_masks, torch.zeros_like(tokens))
history = TextHistory(text, tokens, system=False)
assert torch.equal(history.token_masks, torch.ones_like(tokens))
def test_text_history_append_segment(self):
text = "Hello there!"
tokens = torch.tensor([1, 2, 3])
history = TextHistory(text, tokens)
history.append_segment("General Kenobi!", torch.tensor([4, 5, 6]), system=False)
assert history.text == (text + "General Kenobi!")
assert torch.equal(history.tokens, torch.tensor([1, 2, 3, 4, 5, 6]))
assert torch.equal(history.token_masks, torch.tensor([0, 0, 0, 1, 1, 1]))
history.append_segment("You are a bold one!", torch.tensor([7, 8, 9]))
assert history.text == ((text + "General Kenobi!") + "You are a bold one!")
assert torch.equal(history.tokens, torch.tensor([1, 2, 3, 4, 5, 6, 7, 8, 9]))
assert torch.equal(history.token_masks, torch.tensor([0, 0, 0, 1, 1, 1, 0, 0, 0]))
def test_text_history_complete(self):
text = "Hello there!"
tokens = torch.tensor([1, 2, 3])
history = TextHistory(text, tokens)
history.complete()
assert history.completed
assert not history.truncated
history.complete(truncated=True)
assert history.completed
assert history.truncated
def test_text_history_last_segment(self):
text = "Hello there!"
tokens = torch.tensor([1, 2, 3])
history = TextHistory(text, tokens)
history.append_segment("General Kenobi!", torch.tensor([4, 5, 6]))
history.append_segment("You are a bold one!", torch.tensor([7, 8, 9]))
assert history.last_text_segment == "You are a bold one!"
def test_text_history_split_query_response(self):
text = "Hello there!"
tokens = torch.tensor([1, 2, 3])
history = TextHistory(text, tokens)
history.append_segment("General Kenobi!", torch.tensor([4, 5, 6]), system=False)
history.append_segment("You are a bold one!", torch.tensor([7, 8, 9]), system=True)
query, response, mask = history.split_query_response_tokens()
assert torch.equal(query, torch.tensor([1, 2, 3]))
assert torch.equal(response, torch.tensor([4, 5, 6, 7, 8, 9]))
assert torch.equal(mask, torch.tensor([1, 1, 1, 0, 0, 0]))
class TextEnvironmentTester(unittest.TestCase):
@classmethod
def setUpClass(cls):
# model_id
cls.model_id = "trl-internal-testing/dummy-GPT2-correct-vocab"
# get models and tokenizer
cls.gpt2_model = AutoModelForCausalLMWithValueHead.from_pretrained(cls.model_id)
cls.gpt2_tokenizer = AutoTokenizer.from_pretrained(cls.model_id)
cls.gpt2_tokenizer.pad_token = cls.gpt2_tokenizer.eos_token
def test_text_environment_setup(self):
env = TextEnvironment(
self.gpt2_model,
self.gpt2_tokenizer,
tools=[DummyTool()],
reward_fn=lambda x: torch.tensor(1),
prompt="I am a prompt!\n",
)
assert env.prompt == "I am a prompt!\n"
assert list(env.tools.keys()) == ["DummyTool"]
assert isinstance(env.tools["DummyTool"], DummyTool)
assert env.reward_fn("Hello there!") == 1
def test_text_environment_generate(self):
generation_kwargs = {"do_sample": False, "max_new_tokens": 4, "pad_token_id": self.gpt2_tokenizer.eos_token_id}
env = TextEnvironment(
self.gpt2_model,
self.gpt2_tokenizer,
tools=[DummyTool()],
reward_fn=lambda x: torch.tensor(1),
prompt="I am a prompt!\n",
generation_kwargs=generation_kwargs,
)
input_texts = ["this is a test", "this is another, longer test"]
model_inputs = [self.gpt2_tokenizer(txt, return_tensors="pt").input_ids.squeeze() for txt in input_texts]
generations_batched = env._generate_batched(model_inputs, batch_size=2)
generations_batched = self.gpt2_tokenizer.batch_decode(generations_batched)
generations_single = [env._generate_batched([inputs], batch_size=1)[0] for inputs in model_inputs]
generations_single = self.gpt2_tokenizer.batch_decode(generations_single)
assert generations_single == generations_batched
def test_text_environment_tool_call_parsing(self):
string_valid = "Something something <request><Tool1>Hello there!<call>"
string_invalid_request = "Something something <Tool1>Hello there!<call>"
string_invalid_call = "Something something <request><Tool1>Hello there!"
string_invalid_tool = "Something something <request>|Tool2|Hello there!<call>"
string_invalid_random = "<>abcdefghijklm<>nopqrstuvwxyz<>"
env = TextEnvironment(
self.gpt2_model,
self.gpt2_tokenizer,
tools=[DummyTool()],
reward_fn=lambda x: torch.tensor(1),
prompt="I am a prompt!\n",
)
tool, response = env.parse_tool_call(string_valid)
assert tool == "Tool1"
assert response == "Hello there!"
tool, response = env.parse_tool_call(string_invalid_request)
assert tool is None
assert response is None
tool, response = env.parse_tool_call(string_invalid_call)
assert tool is None
assert response is None
tool, response = env.parse_tool_call(string_invalid_tool)
assert tool is None
assert response is None
tool, response = env.parse_tool_call(string_invalid_random)
assert tool is None
assert response is None
def test_text_environment_tool_truncation(self):
env = TextEnvironment(
self.gpt2_model,
self.gpt2_tokenizer,
tools={"dummy": lambda x: "a" * 1000},
reward_fn=lambda x: torch.tensor(1),
prompt="I am a prompt!\n",
)
env.max_tool_response = 100
history = env.step(TextHistory("<request><dummy>Hello there!<call>", torch.tensor([1, 2, 3])))
assert (len(history.last_text_segment) - len(env.response_token)) == 100
env.max_tool_response = 500
history = env.step(TextHistory("<request><dummy>Hello there!<call>", torch.tensor([1, 2, 3])))
assert (len(history.last_text_segment) - len(env.response_token)) == 500
env.max_tool_response = 1001
history = env.step(TextHistory("<request><dummy>Hello there!<call>", torch.tensor([1, 2, 3])))
assert (len(history.last_text_segment) - len(env.response_token)) == 1000
env.max_tool_response = 2000
history = env.step(TextHistory("<request><dummy>Hello there!<call>", torch.tensor([1, 2, 3])))
assert (len(history.last_text_segment) - len(env.response_token)) == 1000
@patch.object(TextEnvironment, "generate", side_effect=dummy_generate)
def test_text_environment_max_calls(self, mock_generate):
env = TextEnvironment(
self.gpt2_model,
self.gpt2_tokenizer,
tools={"DummyTool": DummyTool()},
reward_fn=lambda x: [torch.tensor(1) for _ in x],
prompt="I am a prompt!\n",
)
env.max_turns = 1
_, _, _, _, histories = env.run(["test"])
assert histories[0].text == (
("I am a prompt!\n" + "test") + (1 * "<request><DummyTool>test<call>test<response>")
)
env.max_turns = 2
_, _, _, _, histories = env.run(["test"])
assert histories[0].text == (
("I am a prompt!\n" + "test") + (2 * "<request><DummyTool>test<call>test<response>")
)
env.max_turns = 4
_, _, _, _, histories = env.run(["test"])
assert histories[0].text == (
("I am a prompt!\n" + "test") + (4 * "<request><DummyTool>test<call>test<response>")
)
def test_text_environment_compute_rewards(self):
env = TextEnvironment(
self.gpt2_model,
self.gpt2_tokenizer,
tools={"DummyTool": DummyTool()},
reward_fn=lambda x: [torch.tensor(i) for i, _ in enumerate(x)],
prompt="I am a prompt!\n",
)
histories = [TextHistory("<request><DummyTool>test<call>", torch.tensor([1, 2, 3])) for _ in range(8)]
histories = env.compute_reward(histories)
for i in range(8):
assert histories[i].reward == i
@patch.object(TextEnvironment, "generate", side_effect=dummy_generate)
def test_text_environment_run(self, mock_generate):
env = TextEnvironment(
self.gpt2_model,
self.gpt2_tokenizer,
tools={"DummyTool": DummyTool()},
reward_fn=lambda x: [torch.tensor(i) for i, _ in enumerate(x)],
prompt="I am a prompt!\n",
max_turns=2,
)
task_1 = "Hello there!"
task_2 = "Hello there! General Kenobi!"
query, response, response_mask, reward, histories = env.run([task_1, task_2])
assert len(query[0]) == 9
assert len(query[1]) == 12
assert len(response[0]) == 14
assert len(response[1]) == 14
assert response_mask[0].sum() == (2 * 3)
# mocked generate always adds 3 toknes
assert response_mask[1].sum() == (2 * 3)
# mocked generate always adds 3 toknes
assert reward[0] == 0
assert reward[1] == 1
assert histories[0].text == (
("I am a prompt!\n" + "Hello there!") + (2 * "<request><DummyTool>test<call>test<response>")
)
assert histories[1].text == (
("I am a prompt!\n" + "Hello there! General Kenobi!")
+ (2 * "<request><DummyTool>test<call>test<response>")
)
| trl/tests/test_environments.py/0 | {
"file_path": "trl/tests/test_environments.py",
"repo_id": "trl",
"token_count": 4813
} | 416 |
# flake8: noqa
from typing import TYPE_CHECKING
from ..import_utils import _LazyModule
_import_structure = {
"base_environment": ["TextEnvironment", "TextHistory"],
}
if TYPE_CHECKING:
from .base_environment import TextEnvironment, TextHistory
else:
import sys
sys.modules[__name__] = _LazyModule(__name__, globals()["__file__"], _import_structure, module_spec=__spec__)
| trl/trl/environment/__init__.py/0 | {
"file_path": "trl/trl/environment/__init__.py",
"repo_id": "trl",
"token_count": 131
} | 417 |
# DPO Authors: Rafael Rafailov, Archit Sharma, Eric Mitchell, Stefano Ermon, Christopher D. Manning, and Chelsea Finn 2023
# Copyright 2023 The HuggingFace Team. All rights reserved.
#
# Licensed under the Apache License, Version 2.0 (the "License");
# you may not use this file except in compliance with the License.
# You may obtain a copy of the License at
#
# http://www.apache.org/licenses/LICENSE-2.0
#
# Unless required by applicable law or agreed to in writing, software
# distributed under the License is distributed on an "AS IS" BASIS,
# WITHOUT WARRANTIES OR CONDITIONS OF ANY KIND, either express or implied.
# See the License for the specific language governing permissions and
# limitations under the License.
import inspect
import random
import warnings
from collections import defaultdict
from contextlib import contextmanager, nullcontext
from copy import deepcopy
from functools import wraps
from typing import Any, Callable, Dict, List, Literal, Optional, Tuple, Union
import numpy as np
import torch
import torch.nn as nn
import torch.nn.functional as F
from accelerate import PartialState
from accelerate.utils import is_deepspeed_available, tqdm
from datasets import Dataset
from torch.utils.data import DataLoader
from transformers import (
AutoModelForCausalLM,
DataCollator,
PreTrainedModel,
PreTrainedTokenizerBase,
Trainer,
TrainingArguments,
)
from transformers.trainer_callback import TrainerCallback
from transformers.trainer_utils import EvalLoopOutput
from ..import_utils import is_peft_available, is_wandb_available
from ..models import PreTrainedModelWrapper, create_reference_model
from .utils import (
DPODataCollatorWithPadding,
disable_dropout_in_model,
pad_to_length,
peft_module_casting_to_bf16,
trl_sanitze_kwargs_for_tagging,
)
if is_peft_available():
from peft import PeftModel, get_peft_model, prepare_model_for_kbit_training
if is_wandb_available():
import wandb
if is_deepspeed_available():
import deepspeed
class DPOTrainer(Trainer):
r"""
Initialize DPOTrainer.
Args:
model (`transformers.PreTrainedModel`):
The model to train, preferably an `AutoModelForSequenceClassification`.
ref_model (`PreTrainedModelWrapper`):
Hugging Face transformer model with a casual language modelling head. Used for implicit reward computation and loss. If no
reference model is provided, the trainer will create a reference model with the same architecture as the model to be optimized.
beta (`float`, defaults to 0.1):
The beta factor in DPO loss. Higher beta means less divergence from the initial policy. For the IPO loss, beta is the regularization parameter denoted by tau in the paper.
label_smoothing (`float`, defaults to 0):
The robust DPO label smoothing parameter from the [cDPO](https://ericmitchell.ai/cdpo.pdf) report that should be between 0 and 0.5.
loss_type (`str`, defaults to `"sigmoid"`):
The type of DPO loss to use. Either `"sigmoid"` the default DPO loss,`"hinge"` loss from [SLiC](https://arxiv.org/abs/2305.10425) paper, `"ipo"` from [IPO](https://arxiv.org/abs/2310.12036) paper, or `"kto"` from the HALOs [report](https://github.com/ContextualAI/HALOs/blob/main/assets/report.pdf).
args (`transformers.TrainingArguments`):
The arguments to use for training.
data_collator (`transformers.DataCollator`):
The data collator to use for training. If None is specified, the default data collator (`DPODataCollatorWithPadding`) will be used
which will pad the sequences to the maximum length of the sequences in the batch, given a dataset of paired sequences.
label_pad_token_id (`int`, defaults to `-100`):
The label pad token id. This argument is required if you want to use the default data collator.
padding_value (`int`, defaults to `0`):
The padding value if it is different to the tokenizer's pad_token_id.
truncation_mode (`str`, defaults to `keep_end`):
The truncation mode to use, either `keep_end` or `keep_start`. This argument is required if you want to use the default data collator.
train_dataset (`datasets.Dataset`):
The dataset to use for training.
eval_dataset (`datasets.Dataset`):
The dataset to use for evaluation.
tokenizer (`transformers.PreTrainedTokenizerBase`):
The tokenizer to use for training. This argument is required if you want to use the default data collator.
model_init (`Callable[[], transformers.PreTrainedModel]`):
The model initializer to use for training. If None is specified, the default model initializer will be used.
callbacks (`List[transformers.TrainerCallback]`):
The callbacks to use for training.
optimizers (`Tuple[torch.optim.Optimizer, torch.optim.lr_scheduler.LambdaLR]`):
The optimizer and scheduler to use for training.
preprocess_logits_for_metrics (`Callable[[torch.Tensor, torch.Tensor], torch.Tensor]`):
The function to use to preprocess the logits before computing the metrics.
max_length (`int`, defaults to `None`):
The maximum length of the sequences in the batch. This argument is required if you want to use the default data collator.
max_prompt_length (`int`, defaults to `None`):
The maximum length of the prompt. This argument is required if you want to use the default data collator.
max_target_length (`int`, defaults to `None`):
The maximum length of the target. This argument is required if you want to use the default data collator and your model is an encoder-decoder.
peft_config (`Dict`, defaults to `None`):
The PEFT configuration to use for training. If you pass a PEFT configuration, the model will be wrapped in a PEFT model.
is_encoder_decoder (`Optional[bool]`, `optional`, defaults to `None`):
If no model is provided, we need to know if the model_init returns an encoder-decoder.
disable_dropout (`bool`, defaults to `True`):
Whether or not to disable dropouts in `model` and `ref_model`.
generate_during_eval (`bool`, defaults to `False`):
Whether to sample and log generations during evaluation step.
compute_metrics (`Callable[[EvalPrediction], Dict]`, *optional*):
The function to use to compute the metrics. Must take a `EvalPrediction` and return
a dictionary string to metric values.
precompute_ref_log_probs (`bool`, defaults to `False`):
Flag to precompute reference model log probabilities for training and evaluation datasets. This is useful if you want to train
without the reference model and reduce the total GPU memory needed.
dataset_num_proc (`Optional[int]`, *optional*):
The number of workers to use to tokenize the data. Defaults to None.
model_init_kwargs (`Optional[Dict]`, *optional*):
Dict of Optional kwargs to pass when instantiating the model from a string
ref_model_init_kwargs (`Optional[Dict]`, *optional*):
Dict of Optional kwargs to pass when instantiating the ref model from a string
model_adapter_name (`str`, defaults to `None`):
Name of the train target PEFT adapter, when using LoRA with multiple adapters.
ref_adapter_name (`str`, defaults to `None`):
Name of the reference PEFT adapter, when using LoRA with multiple adapters.
reference_free (`bool`):
If True, we ignore the _provided_ reference model and implicitly use a reference model that assigns equal probability to all responses.
force_use_ref_model (`bool`, defaults to `False`):
In case one passes a PEFT model for the active model and you want to use a different model for the ref_model, set this flag to `True`.
"""
_tag_names = ["trl", "dpo"]
def __init__(
self,
model: Optional[Union[PreTrainedModel, nn.Module, str]] = None,
ref_model: Optional[Union[PreTrainedModel, nn.Module, str]] = None,
beta: float = 0.1,
label_smoothing: float = 0,
loss_type: Literal["sigmoid", "hinge", "ipo", "kto_pair"] = "sigmoid",
args: Optional[TrainingArguments] = None,
data_collator: Optional[DataCollator] = None,
label_pad_token_id: int = -100,
padding_value: Optional[int] = None,
truncation_mode: str = "keep_end",
train_dataset: Optional[Dataset] = None,
eval_dataset: Optional[Union[Dataset, Dict[str, Dataset]]] = None,
tokenizer: Optional[PreTrainedTokenizerBase] = None,
model_init: Optional[Callable[[], PreTrainedModel]] = None,
callbacks: Optional[List[TrainerCallback]] = None,
optimizers: Tuple[torch.optim.Optimizer, torch.optim.lr_scheduler.LambdaLR] = (None, None),
preprocess_logits_for_metrics: Optional[Callable[[torch.Tensor, torch.Tensor], torch.Tensor]] = None,
max_length: Optional[int] = None,
max_prompt_length: Optional[int] = None,
max_target_length: Optional[int] = None,
peft_config: Optional[Dict] = None,
is_encoder_decoder: Optional[bool] = None,
disable_dropout: bool = True,
generate_during_eval: bool = False,
compute_metrics: Optional[Callable[[EvalLoopOutput], Dict]] = None,
precompute_ref_log_probs: bool = False,
dataset_num_proc: Optional[int] = None,
model_init_kwargs: Optional[Dict] = None,
ref_model_init_kwargs: Optional[Dict] = None,
model_adapter_name: Optional[str] = None,
ref_adapter_name: Optional[str] = None,
reference_free: bool = False,
force_use_ref_model: bool = False,
):
if model_init_kwargs is None:
model_init_kwargs = {}
elif not isinstance(model, str):
raise ValueError("You passed model_kwargs to the DPOTrainer. But your model is already instantiated.")
if ref_model_init_kwargs is None:
ref_model_init_kwargs = {}
elif not isinstance(ref_model, str):
raise ValueError(
"You passed ref_model_kwargs to the DPOTrainer. But your ref_model is already instantiated."
)
if isinstance(model, str):
warnings.warn(
"You passed a model_id to the DPOTrainer. This will automatically create an "
"`AutoModelForCausalLM` or a `PeftModel` (if you passed a `peft_config`) for you."
)
model = AutoModelForCausalLM.from_pretrained(model, **model_init_kwargs)
if isinstance(ref_model, str):
warnings.warn(
"You passed a ref model_id to the DPOTrainer. This will automatically create an "
"`AutoModelForCausalLM`"
)
ref_model = AutoModelForCausalLM.from_pretrained(ref_model, **ref_model_init_kwargs)
# Initialize this variable to False. This helps tracking the case when `peft_module_casting_to_bf16`
# has been called in order to properly call autocast if needed.
self._peft_has_been_casted_to_bf16 = False
if not is_peft_available() and peft_config is not None:
raise ValueError(
"PEFT is not installed and you passed a `peft_config` in the trainer's kwargs, please install it to use the PEFT models"
)
elif is_peft_available() and peft_config is not None:
# if model is a peft model and we have a peft_config, we merge and unload it first
if isinstance(model, PeftModel):
model = model.merge_and_unload()
if ref_model is not None and not force_use_ref_model:
raise ValueError(
"You passed both a ref_model and a peft_config. For training PEFT adapters with DPO there is no need to pass a reference"
" model. Please pass `ref_model=None` in case you want to train PEFT adapters, or pass a ref_model with `force_use_ref_model=True` in DPOTrainer's init."
" if you want to use a different ref_model."
)
if getattr(model, "is_loaded_in_8bit", False) or getattr(model, "is_loaded_in_4bit", False):
_support_gc_kwargs = hasattr(
args, "gradient_checkpointing_kwargs"
) and "gradient_checkpointing_kwargs" in list(
inspect.signature(prepare_model_for_kbit_training).parameters
)
prepare_model_kwargs = {"use_gradient_checkpointing": args.gradient_checkpointing}
if _support_gc_kwargs:
prepare_model_kwargs["gradient_checkpointing_kwargs"] = args.gradient_checkpointing_kwargs
model = prepare_model_for_kbit_training(model, **prepare_model_kwargs)
elif getattr(args, "gradient_checkpointing", False):
# For backward compatibility with older versions of transformers
if hasattr(model, "enable_input_require_grads"):
model.enable_input_require_grads()
else:
def make_inputs_require_grad(module, input, output):
output.requires_grad_(True)
model.get_input_embeddings().register_forward_hook(make_inputs_require_grad)
# get peft model with the given config
model = get_peft_model(model, peft_config)
if args.bf16 and getattr(model, "is_loaded_in_4bit", False):
peft_module_casting_to_bf16(model)
# If args.bf16 we need to explicitly call `generate` with torch amp autocast context manager
self._peft_has_been_casted_to_bf16 = True
# For models that use gradient_checkpoiting, we need to attach a hook that enables input
# to explicitly have `requires_grad=True`, otherwise training will either silently
# fail or completely fail.
elif getattr(args, "gradient_checkpointing", False):
# For backward compatibility with older versions of transformers
if hasattr(model, "enable_input_require_grads"):
model.enable_input_require_grads()
else:
def make_inputs_require_grad(module, input, output):
output.requires_grad_(True)
model.get_input_embeddings().register_forward_hook(make_inputs_require_grad)
if generate_during_eval and not is_wandb_available():
raise ValueError(
"`generate_during_eval=True` requires Weights and Biases to be installed."
" Please install `wandb` to resolve."
)
if model is not None:
self.is_encoder_decoder = model.config.is_encoder_decoder
elif is_encoder_decoder is None:
raise ValueError("When no model is provided, you need to pass the parameter is_encoder_decoder.")
else:
self.is_encoder_decoder = is_encoder_decoder
self.is_peft_model = is_peft_available() and isinstance(model, PeftModel)
self.model_adapter_name = model_adapter_name
self.ref_adapter_name = ref_adapter_name
self.reference_free = reference_free
if ref_model:
self.ref_model = ref_model
elif self.is_peft_model or precompute_ref_log_probs:
# The `model` with adapters turned off will be used as the reference model
self.ref_model = None
else:
self.ref_model = create_reference_model(model)
if tokenizer is None:
raise ValueError("tokenizer must be specified to tokenize a DPO dataset.")
if max_length is None:
warnings.warn(
"`max_length` is not set in the DPOTrainer's init"
" it will default to `512` by default, but you should do it yourself in the future.",
UserWarning,
)
max_length = 512
if max_prompt_length is None:
warnings.warn(
"`max_prompt_length` is not set in the DPOTrainer's init"
" it will default to `128` by default, but you should do it yourself in the future.",
UserWarning,
)
max_prompt_length = 128
if max_target_length is None and self.is_encoder_decoder:
warnings.warn(
"When using an encoder decoder architecture, you should set `max_target_length` in the DPOTrainer's init"
" it will default to `128` by default, but you should do it yourself in the future.",
UserWarning,
)
max_target_length = 128
if data_collator is None:
data_collator = DPODataCollatorWithPadding(
pad_token_id=tokenizer.pad_token_id,
label_pad_token_id=label_pad_token_id,
is_encoder_decoder=self.is_encoder_decoder,
)
if args.remove_unused_columns:
args.remove_unused_columns = False
# warn users
warnings.warn(
"When using DPODataCollatorWithPadding, you should set `remove_unused_columns=False` in your TrainingArguments"
" we have set it for you, but you should do it yourself in the future.",
UserWarning,
)
self.use_dpo_data_collator = True
else:
self.use_dpo_data_collator = False
if disable_dropout:
disable_dropout_in_model(model)
if self.ref_model is not None:
disable_dropout_in_model(self.ref_model)
self.max_length = max_length
self.generate_during_eval = generate_during_eval
self.label_pad_token_id = label_pad_token_id
self.padding_value = padding_value if padding_value is not None else tokenizer.pad_token_id
self.max_prompt_length = max_prompt_length
self.truncation_mode = truncation_mode
self.max_target_length = max_target_length
self.tokenizer = tokenizer
self.precompute_ref_log_probs = precompute_ref_log_probs
# Since ref_logs are precomputed on the first call to get_train/eval_dataloader
# keep track of first called to avoid computation of future calls
self._precomputed_train_ref_log_probs = False
self._precomputed_eval_ref_log_probs = False
if loss_type in ["hinge", "ipo", "kto_pair"] and label_smoothing > 0:
warnings.warn(
"You are using a loss type that does not support label smoothing. Ignoring label_smoothing parameter."
)
self.beta = beta
self.label_smoothing = label_smoothing
self.loss_type = loss_type
self._stored_metrics = defaultdict(lambda: defaultdict(list))
self.dataset_num_proc = dataset_num_proc
# Compute that only on the main process for faster data processing.
# see: https://github.com/huggingface/trl/pull/1255
with PartialState().local_main_process_first():
# tokenize the dataset
train_dataset = train_dataset.map(self.tokenize_row, num_proc=self.dataset_num_proc)
if eval_dataset is not None:
eval_dataset = eval_dataset.map(self.tokenize_row, num_proc=self.dataset_num_proc)
super().__init__(
model=model,
args=args,
data_collator=data_collator,
train_dataset=train_dataset,
eval_dataset=eval_dataset,
tokenizer=tokenizer,
model_init=model_init,
compute_metrics=compute_metrics,
callbacks=callbacks,
optimizers=optimizers,
preprocess_logits_for_metrics=preprocess_logits_for_metrics,
)
# Add tags for models that have been loaded with the correct transformers version
if hasattr(self.model, "add_model_tags"):
self.model.add_model_tags(self._tag_names)
if not hasattr(self, "accelerator"):
raise AttributeError(
"Your `Trainer` does not have an `accelerator` object. Consider upgrading `transformers`."
)
# Deepspeed Zero-3 does not support precompute_ref_log_probs
if self.is_deepspeed_enabled:
if self.accelerator.state.deepspeed_plugin.zero_stage == 3 and self.precompute_ref_log_probs:
raise ValueError(
"You cannot use `precompute_ref_log_probs=True` with Deepspeed ZeRO-3. Please set `precompute_ref_log_probs=False`."
)
if self.ref_model is None:
if not (self.is_peft_model or self.precompute_ref_log_probs):
raise ValueError(
"No reference model and model is not a Peft model. Try setting `precompute_ref_log_probs=True`"
)
else:
if self.is_deepspeed_enabled:
self.ref_model = self._prepare_deepspeed(self.ref_model)
else:
self.ref_model = self.accelerator.prepare_model(self.ref_model, evaluation_mode=True)
def _prepare_deepspeed(self, model: PreTrainedModelWrapper):
# Adapted from accelerate: https://github.com/huggingface/accelerate/blob/739b135f8367becb67ffaada12fe76e3aa60fefd/src/accelerate/accelerator.py#L1473
deepspeed_plugin = self.accelerator.state.deepspeed_plugin
config_kwargs = deepcopy(deepspeed_plugin.deepspeed_config)
if model is not None:
if hasattr(model, "config"):
hidden_size = (
max(model.config.hidden_sizes)
if getattr(model.config, "hidden_sizes", None)
else getattr(model.config, "hidden_size", None)
)
if hidden_size is not None and config_kwargs["zero_optimization"]["stage"] == 3:
# Note that `stage3_prefetch_bucket_size` can produce DeepSpeed messages like: `Invalidate trace cache @ step 0: expected module 1, but got module 0`
# This is expected and is not an error, see: https://github.com/microsoft/DeepSpeed/discussions/4081
config_kwargs.update(
{
"zero_optimization.reduce_bucket_size": hidden_size * hidden_size,
"zero_optimization.stage3_param_persistence_threshold": 10 * hidden_size,
"zero_optimization.stage3_prefetch_bucket_size": 0.9 * hidden_size * hidden_size,
}
)
# If ZeRO-3 is used, we shard both the active and reference model.
# Otherwise, we assume the reference model fits in memory and is initialized on each device with ZeRO disabled (stage 0)
if config_kwargs["zero_optimization"]["stage"] != 3:
config_kwargs["zero_optimization"]["stage"] = 0
model, *_ = deepspeed.initialize(model=model, config=config_kwargs)
model.eval()
return model
def get_train_dataloader(self) -> DataLoader:
"""
Returns the training [`~torch.utils.data.DataLoader`].
Subclass of transformers.src.transformers.trainer.get_train_dataloader to precompute `ref_log_probs`.
"""
if self.precompute_ref_log_probs and not self._precomputed_train_ref_log_probs:
dataloader_params = {
"batch_size": self.args.per_device_train_batch_size,
"collate_fn": self.data_collator,
"num_workers": self.args.dataloader_num_workers,
"pin_memory": self.args.dataloader_pin_memory,
"shuffle": False,
}
# prepare dataloader
data_loader = self.accelerator.prepare(DataLoader(self.train_dataset, **dataloader_params))
reference_chosen_logps = []
reference_rejected_logps = []
for padded_batch in tqdm(iterable=data_loader, desc="Train dataset reference log probs"):
reference_chosen_logp, reference_rejected_logp = self.compute_reference_log_probs(padded_batch)
reference_chosen_logp, reference_rejected_logp = self.accelerator.gather_for_metrics(
(reference_chosen_logp, reference_rejected_logp)
)
reference_chosen_logps.append(reference_chosen_logp.cpu())
reference_rejected_logps.append(reference_rejected_logp.cpu())
all_reference_chosen_logps = torch.cat(reference_chosen_logps).float().numpy()
all_reference_rejected_logps = torch.cat(reference_rejected_logps).float().numpy()
self.train_dataset = self.train_dataset.add_column(
name="reference_chosen_logps", column=all_reference_chosen_logps
)
self.train_dataset = self.train_dataset.add_column(
name="reference_rejected_logps", column=all_reference_rejected_logps
)
self._precomputed_train_ref_log_probs = True
return super().get_train_dataloader()
def get_eval_dataloader(self, eval_dataset: Optional[Dataset] = None) -> DataLoader:
"""
Returns the evaluation [`~torch.utils.data.DataLoader`].
Subclass of transformers.src.transformers.trainer.get_eval_dataloader to precompute `ref_log_probs`.
Args:
eval_dataset (`torch.utils.data.Dataset`, *optional*):
If provided, will override `self.eval_dataset`. If it is a [`~datasets.Dataset`], columns not accepted
by the `model.forward()` method are automatically removed. It must implement `__len__`.
"""
if eval_dataset is None and self.eval_dataset is None:
raise ValueError("Trainer: evaluation requires an eval_dataset.")
eval_dataset = eval_dataset if eval_dataset is not None else self.eval_dataset
if self.precompute_ref_log_probs and not self._precomputed_eval_ref_log_probs:
dataloader_params = {
"batch_size": self.args.per_device_eval_batch_size,
"collate_fn": self.data_collator,
"num_workers": self.args.dataloader_num_workers,
"pin_memory": self.args.dataloader_pin_memory,
"shuffle": False,
}
# prepare dataloader
data_loader = self.accelerator.prepare(DataLoader(eval_dataset, **dataloader_params))
reference_chosen_logps = []
reference_rejected_logps = []
for padded_batch in tqdm(iterable=data_loader, desc="Eval dataset reference log probs"):
reference_chosen_logp, reference_rejected_logp = self.compute_reference_log_probs(padded_batch)
reference_chosen_logp, reference_rejected_logp = self.accelerator.gather_for_metrics(
(reference_chosen_logp, reference_rejected_logp)
)
reference_chosen_logps.append(reference_chosen_logp.cpu())
reference_rejected_logps.append(reference_rejected_logp.cpu())
all_reference_chosen_logps = torch.cat(reference_chosen_logps).float().numpy()
all_reference_rejected_logps = torch.cat(reference_rejected_logps).float().numpy()
eval_dataset = eval_dataset.add_column(name="reference_chosen_logps", column=all_reference_chosen_logps)
eval_dataset = eval_dataset.add_column(
name="reference_rejected_logps", column=all_reference_rejected_logps
)
# Save calculated reference_chosen_logps and reference_rejected_logps to the eval_dataset for subsequent runs
if self.eval_dataset is not None:
self.eval_dataset = eval_dataset
self._precomputed_eval_ref_log_probs = True
return super().get_eval_dataloader(eval_dataset=eval_dataset)
def build_tokenized_answer(self, prompt, answer):
"""
Llama tokenizer does satisfy `enc(a + b) = enc(a) + enc(b)`.
It does ensure `enc(a + b) = enc(a) + enc(a + b)[len(enc(a)):]`.
Reference:
https://github.com/EleutherAI/lm-evaluation-harness/pull/531#issuecomment-1595586257
"""
full_tokenized = self.tokenizer(prompt + answer, add_special_tokens=False)
prompt_input_ids = self.tokenizer(prompt, add_special_tokens=False)["input_ids"]
answer_input_ids = full_tokenized["input_ids"][len(prompt_input_ids) :]
answer_attention_mask = full_tokenized["attention_mask"][len(prompt_input_ids) :]
# Concat tokens to form `enc(a) + enc(a + b)[len(enc(a)):]`
full_concat_input_ids = np.concatenate([prompt_input_ids, answer_input_ids])
# Prepare input tokens for token by token comparison
full_input_ids = np.array(full_tokenized["input_ids"])
if len(full_input_ids) != len(full_concat_input_ids):
raise ValueError("Prompt input ids and answer input ids should have the same length.")
# On some tokenizers, like Llama-2 tokenizer, there are occasions where tokens
# can be merged together when tokenizing prompt+answer. This could result
# on the last token from the prompt being different when tokenized on its own
# vs when done as prompt+answer.
response_token_ids_start_idx = len(prompt_input_ids)
# If tokenized prompt is different than both prompt+answer, then it means the
# last token has changed due to merging.
if prompt_input_ids != full_tokenized["input_ids"][:response_token_ids_start_idx]:
response_token_ids_start_idx -= 1
prompt_input_ids = full_tokenized["input_ids"][:response_token_ids_start_idx]
prompt_attention_mask = full_tokenized["attention_mask"][:response_token_ids_start_idx]
if len(prompt_input_ids) != len(prompt_attention_mask):
raise ValueError("Prompt input ids and attention mask should have the same length.")
answer_input_ids = full_tokenized["input_ids"][response_token_ids_start_idx:]
answer_attention_mask = full_tokenized["attention_mask"][response_token_ids_start_idx:]
return dict(
prompt_input_ids=prompt_input_ids,
prompt_attention_mask=prompt_attention_mask,
input_ids=answer_input_ids,
attention_mask=answer_attention_mask,
)
def tokenize_row(self, feature, model: Optional[Union[PreTrainedModel, nn.Module]] = None) -> Dict:
"""Tokenize a single row from a DPO specific dataset.
At this stage, we don't convert to PyTorch tensors yet; we just handle the truncation
in case the prompt + chosen or prompt + rejected responses is/are too long. First
we truncate the prompt; if we're still too long, we truncate the chosen/rejected.
We also create the labels for the chosen/rejected responses, which are of length equal to
the sum of the length of the prompt and the chosen/rejected response, with
label_pad_token_id for the prompt tokens.
"""
batch = {}
prompt = feature["prompt"]
chosen = feature["chosen"]
rejected = feature["rejected"]
if not self.is_encoder_decoder:
# Check issues below for more details
# 1. https://github.com/huggingface/trl/issues/907
# 2. https://github.com/EleutherAI/lm-evaluation-harness/pull/531#issuecomment-1595586257
# 3. https://github.com/LianjiaTech/BELLE/issues/337
if not isinstance(prompt, str):
raise ValueError(f"prompt should be an str but got {type(prompt)}")
prompt_tokens = self.tokenizer(prompt, add_special_tokens=False)
prompt_tokens = {f"prompt_{k}": v for k, v in prompt_tokens.items()}
if not isinstance(chosen, str):
raise ValueError(f"chosen should be an str but got {type(chosen)}")
chosen_tokens = self.build_tokenized_answer(prompt, chosen)
if not isinstance(rejected, str):
raise ValueError(f"rejected should be an str but got {type(rejected)}")
rejected_tokens = self.build_tokenized_answer(prompt, rejected)
# Last prompt token might get merged by tokenizer and
# it should not be included for generation if that happens
prompt_len_input_ids = len(prompt_tokens["prompt_input_ids"])
chosen_prompt_len_input_ids = len(chosen_tokens["prompt_input_ids"])
rejected_prompt_len_input_ids = len(rejected_tokens["prompt_input_ids"])
prompt_len_input_ids = min(chosen_prompt_len_input_ids, rejected_prompt_len_input_ids)
for k, v in prompt_tokens.items():
prompt_tokens[k] = v[:prompt_len_input_ids]
# Make sure prompts only have one different token at most an
# and length only differs by 1 at most
num_diff_tokens = sum(
[a != b for a, b in zip(chosen_tokens["prompt_input_ids"], rejected_tokens["prompt_input_ids"])]
)
num_diff_len = abs(chosen_prompt_len_input_ids - rejected_prompt_len_input_ids)
if num_diff_tokens > 1 or num_diff_len > 1:
raise ValueError(
"Chosen and rejected prompt_input_ids might only differ on the "
"last token due to tokenizer merge ops."
)
# add BOS token to head of prompt
prompt_tokens["prompt_input_ids"] = [self.tokenizer.bos_token_id] + prompt_tokens["prompt_input_ids"]
chosen_tokens["prompt_input_ids"] = [self.tokenizer.bos_token_id] + chosen_tokens["prompt_input_ids"]
rejected_tokens["prompt_input_ids"] = [self.tokenizer.bos_token_id] + rejected_tokens["prompt_input_ids"]
prompt_tokens["prompt_attention_mask"] = [1] + prompt_tokens["prompt_attention_mask"]
chosen_tokens["prompt_attention_mask"] = [1] + chosen_tokens["prompt_attention_mask"]
rejected_tokens["prompt_attention_mask"] = [1] + rejected_tokens["prompt_attention_mask"]
# add EOS token to end of answer
chosen_tokens["input_ids"].append(self.tokenizer.eos_token_id)
chosen_tokens["attention_mask"].append(1)
rejected_tokens["input_ids"].append(self.tokenizer.eos_token_id)
rejected_tokens["attention_mask"].append(1)
longer_response_length = max(len(chosen_tokens["input_ids"]), len(rejected_tokens["input_ids"]))
# if combined sequence is too long, truncate the prompt
for answer_tokens in [chosen_tokens, rejected_tokens, prompt_tokens]:
if len(answer_tokens["prompt_input_ids"]) + longer_response_length > self.max_length:
if self.truncation_mode == "keep_start":
for k in ["prompt_input_ids", "prompt_attention_mask"]:
answer_tokens[k] = answer_tokens[k][: self.max_prompt_length]
elif self.truncation_mode == "keep_end":
for k in ["prompt_input_ids", "prompt_attention_mask"]:
answer_tokens[k] = answer_tokens[k][-self.max_prompt_length :]
else:
raise ValueError(f"Unknown truncation mode: {self.truncation_mode}")
# if that's still too long, truncate the response
for answer_tokens in [chosen_tokens, rejected_tokens]:
if len(answer_tokens["prompt_input_ids"]) + longer_response_length > self.max_length:
for k in ["input_ids", "attention_mask"]:
answer_tokens[k] = answer_tokens[k][: self.max_length - self.max_prompt_length]
# Create labels
chosen_sequence_tokens = {
k: chosen_tokens[f"prompt_{k}"] + chosen_tokens[k] for k in ["input_ids", "attention_mask"]
}
rejected_sequence_tokens = {
k: rejected_tokens[f"prompt_{k}"] + rejected_tokens[k] for k in ["input_ids", "attention_mask"]
}
chosen_sequence_tokens["labels"] = chosen_sequence_tokens["input_ids"][:]
chosen_sequence_tokens["labels"][: len(chosen_tokens["prompt_input_ids"])] = [
self.label_pad_token_id
] * len(chosen_tokens["prompt_input_ids"])
rejected_sequence_tokens["labels"] = rejected_sequence_tokens["input_ids"][:]
rejected_sequence_tokens["labels"][: len(rejected_tokens["prompt_input_ids"])] = [
self.label_pad_token_id
] * len(rejected_tokens["prompt_input_ids"])
for k, toks in {
"chosen_": chosen_sequence_tokens,
"rejected_": rejected_sequence_tokens,
"": prompt_tokens,
}.items():
for type_key, tokens in toks.items():
if type_key == "token_type_ids":
continue
batch[f"{k}{type_key}"] = tokens
else:
chosen_tokens = self.tokenizer(
chosen, truncation=True, max_length=self.max_target_length, add_special_tokens=True
)
rejected_tokens = self.tokenizer(
rejected, truncation=True, max_length=self.max_target_length, add_special_tokens=True
)
prompt_tokens = self.tokenizer(
prompt, truncation=True, max_length=self.max_prompt_length, add_special_tokens=True
)
batch["chosen_labels"] = chosen_tokens["input_ids"]
batch["rejected_labels"] = rejected_tokens["input_ids"]
batch["prompt_input_ids"] = prompt_tokens["input_ids"]
batch["prompt_attention_mask"] = prompt_tokens["attention_mask"]
if model is not None and hasattr(model, "prepare_decoder_input_ids_from_labels"):
batch["rejected_decoder_input_ids"] = model.prepare_decoder_input_ids_from_labels(
labels=torch.tensor(batch["rejected_labels"])
)
batch["chosen_decoder_input_ids"] = model.prepare_decoder_input_ids_from_labels(
labels=torch.tensor(batch["chosen_labels"])
)
return batch
@contextmanager
def null_ref_context(self):
"""Context manager for handling null reference model (that is, peft adapter manipulation)."""
with self.accelerator.unwrap_model(
self.model
).disable_adapter() if self.is_peft_model and not self.ref_adapter_name else nullcontext():
if self.ref_adapter_name:
self.model.set_adapter(self.ref_adapter_name)
yield
if self.ref_adapter_name:
self.model.set_adapter(self.model_adapter_name or "default")
def compute_reference_log_probs(self, padded_batch: Dict) -> Dict:
"""Computes log probabilities of the reference model for a single padded batch of a DPO specific dataset."""
compte_ref_context_manager = torch.cuda.amp.autocast if self._peft_has_been_casted_to_bf16 else nullcontext
# compute reference logps
with torch.no_grad(), compte_ref_context_manager():
if self.ref_model is None:
with self.null_ref_context():
(
reference_chosen_logps,
reference_rejected_logps,
_,
_,
) = self.concatenated_forward(self.model, padded_batch)
else:
(
reference_chosen_logps,
reference_rejected_logps,
_,
_,
) = self.concatenated_forward(self.ref_model, padded_batch)
return reference_chosen_logps, reference_rejected_logps
@staticmethod
def concatenated_inputs(
batch: Dict[str, Union[List, torch.LongTensor]],
is_encoder_decoder: bool = False,
label_pad_token_id: int = -100,
padding_value: int = 0,
device: Optional[torch.device] = None,
) -> Dict[str, torch.LongTensor]:
"""Concatenate the chosen and rejected inputs into a single tensor.
Args:
batch: A batch of data. Must contain the keys 'chosen_input_ids' and 'rejected_input_ids', which are tensors of shape (batch_size, sequence_length).
is_encoder_decoder: Whether the model is an encoder-decoder model.
label_pad_token_id: The label pad token id.
padding_value: The padding value to use for the concatenated inputs_ids.
device: The device for the concatenated inputs.
Returns:
A dictionary containing the concatenated inputs under the key 'concatenated_input_ids'.
"""
concatenated_batch = {}
if is_encoder_decoder:
max_length = max(batch["chosen_labels"].shape[1], batch["rejected_labels"].shape[1])
else:
max_length = max(batch["chosen_input_ids"].shape[1], batch["rejected_input_ids"].shape[1])
for k in batch:
if k.startswith("chosen") and isinstance(batch[k], torch.Tensor):
if "labels" in k or is_encoder_decoder:
pad_value = label_pad_token_id
elif k.endswith("_input_ids"):
pad_value = padding_value
elif k.endswith("_attention_mask"):
pad_value = 0
concatenated_key = k.replace("chosen", "concatenated")
concatenated_batch[concatenated_key] = pad_to_length(batch[k], max_length, pad_value=pad_value)
for k in batch:
if k.startswith("rejected") and isinstance(batch[k], torch.Tensor):
if "labels" in k or is_encoder_decoder:
pad_value = label_pad_token_id
elif k.endswith("_input_ids"):
pad_value = padding_value
elif k.endswith("_attention_mask"):
pad_value = 0
concatenated_key = k.replace("rejected", "concatenated")
concatenated_batch[concatenated_key] = torch.cat(
(
concatenated_batch[concatenated_key],
pad_to_length(batch[k], max_length, pad_value=pad_value),
),
dim=0,
).to(device=device)
if is_encoder_decoder:
concatenated_batch["concatenated_input_ids"] = batch["prompt_input_ids"].repeat(2, 1).to(device=device)
concatenated_batch["concatenated_attention_mask"] = (
batch["prompt_attention_mask"].repeat(2, 1).to(device=device)
)
return concatenated_batch
def dpo_loss(
self,
policy_chosen_logps: torch.FloatTensor,
policy_rejected_logps: torch.FloatTensor,
reference_chosen_logps: torch.FloatTensor,
reference_rejected_logps: torch.FloatTensor,
) -> Tuple[torch.FloatTensor, torch.FloatTensor, torch.FloatTensor]:
"""Compute the DPO loss for a batch of policy and reference model log probabilities.
Args:
policy_chosen_logps: Log probabilities of the policy model for the chosen responses. Shape: (batch_size,)
policy_rejected_logps: Log probabilities of the policy model for the rejected responses. Shape: (batch_size,)
reference_chosen_logps: Log probabilities of the reference model for the chosen responses. Shape: (batch_size,)
reference_rejected_logps: Log probabilities of the reference model for the rejected responses. Shape: (batch_size,)
Returns:
A tuple of three tensors: (losses, chosen_rewards, rejected_rewards).
The losses tensor contains the DPO loss for each example in the batch.
The chosen_rewards and rejected_rewards tensors contain the rewards for the chosen and rejected responses, respectively.
"""
pi_logratios = policy_chosen_logps - policy_rejected_logps
if self.reference_free:
ref_logratios = torch.tensor([0], dtype=pi_logratios.dtype, device=pi_logratios.device)
else:
ref_logratios = reference_chosen_logps - reference_rejected_logps
pi_logratios = pi_logratios.to(self.accelerator.device)
ref_logratios = ref_logratios.to(self.accelerator.device)
logits = pi_logratios - ref_logratios
# The beta is a temperature parameter for the DPO loss, typically something in the range of 0.1 to 0.5.
# We ignore the reference model as beta -> 0. The label_smoothing parameter encodes our uncertainty about the labels and
# calculates a conservative DPO loss.
if self.loss_type == "sigmoid":
losses = (
-F.logsigmoid(self.beta * logits) * (1 - self.label_smoothing)
- F.logsigmoid(-self.beta * logits) * self.label_smoothing
)
elif self.loss_type == "hinge":
losses = torch.relu(1 - self.beta * logits)
elif self.loss_type == "ipo":
# eqn (17) of the paper where beta is the regularization parameter for the IPO loss, denoted by tau in the paper.
losses = (logits - 1 / (2 * self.beta)) ** 2
elif self.loss_type == "kto_pair":
# eqn (7) of the HALOs paper
chosen_KL = (policy_chosen_logps - reference_chosen_logps).mean().clamp(min=0)
rejected_KL = (policy_rejected_logps - reference_rejected_logps).mean().clamp(min=0)
chosen_logratios = policy_chosen_logps - reference_chosen_logps
rejected_logratios = policy_rejected_logps - reference_rejected_logps
# As described in the KTO report, the KL term for chosen (rejected) is estimated using the rejected (chosen) half.
losses = torch.cat(
(
1 - F.sigmoid(self.beta * (chosen_logratios - rejected_KL)),
1 - F.sigmoid(self.beta * (chosen_KL - rejected_logratios)),
),
0,
)
else:
raise ValueError(
f"Unknown loss type: {self.loss_type}. Should be one of ['sigmoid', 'hinge', 'ipo', 'kto_pair']"
)
chosen_rewards = (
self.beta
* (
policy_chosen_logps.to(self.accelerator.device) - reference_chosen_logps.to(self.accelerator.device)
).detach()
)
rejected_rewards = (
self.beta
* (
policy_rejected_logps.to(self.accelerator.device)
- reference_rejected_logps.to(self.accelerator.device)
).detach()
)
return losses, chosen_rewards, rejected_rewards
@staticmethod
def get_batch_logps(
logits: torch.FloatTensor,
labels: torch.LongTensor,
average_log_prob: bool = False,
label_pad_token_id: int = -100,
is_encoder_decoder: bool = False,
) -> torch.FloatTensor:
"""Compute the log probabilities of the given labels under the given logits.
Args:
logits: Logits of the model (unnormalized). Shape: (batch_size, sequence_length, vocab_size)
labels: Labels for which to compute the log probabilities. Label tokens with a value of label_pad_token_id are ignored. Shape: (batch_size, sequence_length)
average_log_prob: If True, return the average log probability per (non-masked) token. Otherwise, return the sum of the log probabilities of the (non-masked) tokens.
label_pad_token_id: The label pad token id.
is_encoder_decoder: Whether the model is an encoder-decoder model.
Returns:
A tensor of shape (batch_size,) containing the average/sum log probabilities of the given labels under the given logits.
"""
if logits.shape[:-1] != labels.shape:
raise ValueError("Logits (batch and sequence length dim) and labels must have the same shape.")
if not is_encoder_decoder:
labels = labels[:, 1:].clone()
logits = logits[:, :-1, :]
loss_mask = labels != label_pad_token_id
# dummy token; we'll ignore the losses on these tokens later
labels[labels == label_pad_token_id] = 0
per_token_logps = torch.gather(logits.log_softmax(-1), dim=2, index=labels.unsqueeze(2)).squeeze(2)
if average_log_prob:
return (per_token_logps * loss_mask).sum(-1) / loss_mask.sum(-1)
else:
return (per_token_logps * loss_mask).sum(-1)
def concatenated_forward(
self, model: nn.Module, batch: Dict[str, Union[List, torch.LongTensor]]
) -> Tuple[torch.FloatTensor, torch.FloatTensor, torch.FloatTensor, torch.FloatTensor]:
"""Run the given model on the given batch of inputs, concatenating the chosen and rejected inputs together.
We do this to avoid doing two forward passes, because it's faster for FSDP.
"""
concatenated_batch = self.concatenated_inputs(
batch,
is_encoder_decoder=self.is_encoder_decoder,
label_pad_token_id=self.label_pad_token_id,
padding_value=self.padding_value,
device=self.accelerator.device,
)
len_chosen = batch["chosen_labels"].shape[0]
model_kwargs = (
{
"labels": concatenated_batch["concatenated_labels"],
"decoder_input_ids": concatenated_batch.pop("concatenated_decoder_input_ids", None),
}
if self.is_encoder_decoder
else {}
)
all_logits = model(
concatenated_batch["concatenated_input_ids"],
attention_mask=concatenated_batch["concatenated_attention_mask"],
use_cache=False,
**model_kwargs,
).logits
all_logps = self.get_batch_logps(
all_logits,
concatenated_batch["concatenated_labels"],
average_log_prob=self.loss_type == "ipo",
is_encoder_decoder=self.is_encoder_decoder,
label_pad_token_id=self.label_pad_token_id,
)
chosen_logps = all_logps[:len_chosen]
rejected_logps = all_logps[len_chosen:]
chosen_logits = all_logits[:len_chosen]
rejected_logits = all_logits[len_chosen:]
return (chosen_logps, rejected_logps, chosen_logits, rejected_logits)
def get_batch_loss_metrics(
self,
model,
batch: Dict[str, Union[List, torch.LongTensor]],
train_eval: Literal["train", "eval"] = "train",
):
"""Compute the DPO loss and other metrics for the given batch of inputs for train or test."""
metrics = {}
(
policy_chosen_logps,
policy_rejected_logps,
policy_chosen_logits,
policy_rejected_logits,
) = self.concatenated_forward(model, batch)
# if reference_chosen_logps and reference_rejected_logps in batch use them, otherwise use the reference model
if "reference_chosen_logps" in batch and "reference_rejected_logps" in batch:
reference_chosen_logps = batch["reference_chosen_logps"]
reference_rejected_logps = batch["reference_rejected_logps"]
else:
with torch.no_grad():
if self.ref_model is None:
with self.null_ref_context():
(
reference_chosen_logps,
reference_rejected_logps,
_,
_,
) = self.concatenated_forward(self.model, batch)
else:
(
reference_chosen_logps,
reference_rejected_logps,
_,
_,
) = self.concatenated_forward(self.ref_model, batch)
losses, chosen_rewards, rejected_rewards = self.dpo_loss(
policy_chosen_logps,
policy_rejected_logps,
reference_chosen_logps,
reference_rejected_logps,
)
reward_accuracies = (chosen_rewards > rejected_rewards).float()
prefix = "eval_" if train_eval == "eval" else ""
metrics[f"{prefix}rewards/chosen"] = chosen_rewards.mean().cpu()
metrics[f"{prefix}rewards/rejected"] = rejected_rewards.mean().cpu()
metrics[f"{prefix}rewards/accuracies"] = reward_accuracies.mean().cpu()
metrics[f"{prefix}rewards/margins"] = (chosen_rewards - rejected_rewards).mean().cpu()
metrics[f"{prefix}logps/rejected"] = policy_rejected_logps.detach().mean().cpu()
metrics[f"{prefix}logps/chosen"] = policy_chosen_logps.detach().mean().cpu()
metrics[f"{prefix}logits/rejected"] = policy_rejected_logits.detach().mean().cpu()
metrics[f"{prefix}logits/chosen"] = policy_chosen_logits.detach().mean().cpu()
return losses.mean(), metrics
def compute_loss(
self,
model: Union[PreTrainedModel, nn.Module],
inputs: Dict[str, Union[torch.Tensor, Any]],
return_outputs=False,
) -> Union[torch.Tensor, Tuple[torch.Tensor, Dict[str, torch.Tensor]]]:
if not self.use_dpo_data_collator:
warnings.warn(
"compute_loss is only implemented for DPODataCollatorWithPadding, and you passed a datacollator that is different than "
"DPODataCollatorWithPadding - you might see unexpected behavior. Alternatively, you can implement your own prediction_step method if you are using a custom data collator"
)
compute_loss_context_manager = torch.cuda.amp.autocast if self._peft_has_been_casted_to_bf16 else nullcontext
with compute_loss_context_manager():
loss, metrics = self.get_batch_loss_metrics(model, inputs, train_eval="train")
# Make sure to move the loss to the device the original accumulating loss is at back in the `Trainer` class:
loss = loss.to(self.args.device)
# force log the metrics
self.store_metrics(metrics, train_eval="train")
if return_outputs:
return (loss, metrics)
return loss
def get_batch_samples(self, model, batch: Dict[str, torch.LongTensor]) -> Tuple[str, str]:
"""Generate samples from the model and reference model for the given batch of inputs."""
# If one uses `generate_during_eval` with peft + bf16, we need to explictly call generate with
# the torch cuda amp context manager as some hidden states are silently casted to full precision.
generate_context_manager = nullcontext if not self._peft_has_been_casted_to_bf16 else torch.cuda.amp.autocast
with generate_context_manager():
policy_output = model.generate(
input_ids=batch["prompt_input_ids"],
attention_mask=batch["prompt_attention_mask"],
max_length=self.max_length,
do_sample=True,
pad_token_id=self.tokenizer.pad_token_id,
)
# if reference_output in batch use that otherwise use the reference model
if "reference_output" in batch:
reference_output = batch["reference_output"]
else:
if self.ref_model is None:
with self.null_ref_context():
reference_output = self.model.generate(
input_ids=batch["prompt_input_ids"],
attention_mask=batch["prompt_attention_mask"],
max_length=self.max_length,
do_sample=True,
pad_token_id=self.tokenizer.pad_token_id,
)
else:
reference_output = self.ref_model.generate(
input_ids=batch["prompt_input_ids"],
attention_mask=batch["prompt_attention_mask"],
max_length=self.max_length,
do_sample=True,
pad_token_id=self.tokenizer.pad_token_id,
)
policy_output = pad_to_length(policy_output, self.max_length, self.tokenizer.pad_token_id)
policy_output_decoded = self.tokenizer.batch_decode(policy_output, skip_special_tokens=True)
reference_output = pad_to_length(reference_output, self.max_length, self.tokenizer.pad_token_id)
reference_output_decoded = self.tokenizer.batch_decode(reference_output, skip_special_tokens=True)
return policy_output_decoded, reference_output_decoded
def prediction_step(
self,
model: Union[PreTrainedModel, nn.Module],
inputs: Dict[str, Union[torch.Tensor, Any]],
prediction_loss_only: bool,
ignore_keys: Optional[List[str]] = None,
):
if not self.use_dpo_data_collator:
warnings.warn(
"prediction_step is only implemented for DPODataCollatorWithPadding, and you passed a datacollator that is different than "
"DPODataCollatorWithPadding - you might see unexpected behavior. Alternatively, you can implement your own prediction_step method if you are using a custom data collator"
)
if ignore_keys is None:
if hasattr(model, "config"):
ignore_keys = getattr(model.config, "keys_to_ignore_at_inference", [])
else:
ignore_keys = []
prediction_context_manager = torch.cuda.amp.autocast if self._peft_has_been_casted_to_bf16 else nullcontext
with torch.no_grad(), prediction_context_manager():
loss, metrics = self.get_batch_loss_metrics(model, inputs, train_eval="eval")
# force log the metrics
self.store_metrics(metrics, train_eval="eval")
if prediction_loss_only:
return (loss.detach(), None, None)
# logits for the chosen and rejected samples from model
logits_dict = {
"eval_logits/chosen": metrics["eval_logits/chosen"],
"eval_logits/rejected": metrics["eval_logits/rejected"],
}
logits = tuple(v.unsqueeze(dim=0) for k, v in logits_dict.items() if k not in ignore_keys)
logits = torch.stack(logits).mean(axis=1).to(self.accelerator.device)
labels = torch.zeros(logits.shape[0], device=self.accelerator.device)
return (loss.detach(), logits, labels)
def store_metrics(self, metrics: Dict[str, float], train_eval: Literal["train", "eval"] = "train") -> None:
for key, value in metrics.items():
self._stored_metrics[train_eval][key].append(value)
def evaluation_loop(
self,
dataloader: DataLoader,
description: str,
prediction_loss_only: Optional[bool] = None,
ignore_keys: Optional[List[str]] = None,
metric_key_prefix: str = "eval",
) -> EvalLoopOutput:
"""
Overriding built-in evaluation loop to store metrics for each batch.
Prediction/evaluation loop, shared by `Trainer.evaluate()` and `Trainer.predict()`.
Works both with or without labels.
"""
# Sample and save to game log if requested (for one batch to save time)
if self.generate_during_eval:
# Generate random indices within the range of the total number of samples
num_samples = len(dataloader.dataset)
random_indices = random.sample(range(num_samples), k=self.args.eval_batch_size)
# Use dataloader.dataset.select to get the random batch without iterating over the DataLoader
random_batch_dataset = dataloader.dataset.select(random_indices)
random_batch = self.data_collator(random_batch_dataset)
random_batch = self._prepare_inputs(random_batch)
policy_output_decoded, ref_output_decoded = self.get_batch_samples(self.model, random_batch)
self.log(
{
"game_log": wandb.Table(
columns=["Prompt", "Policy", "Ref Model"],
rows=[
[prompt, pol[len(prompt) :], ref[len(prompt) :]]
for prompt, pol, ref in zip(
random_batch["prompt"], policy_output_decoded, ref_output_decoded
)
],
)
}
)
self.state.log_history.pop()
# Base evaluation
initial_output = super().evaluation_loop(
dataloader, description, prediction_loss_only, ignore_keys, metric_key_prefix
)
return initial_output
def log(self, logs: Dict[str, float]) -> None:
"""
Log `logs` on the various objects watching training, including stored metrics.
Args:
logs (`Dict[str, float]`):
The values to log.
"""
# logs either has 'loss' or 'eval_loss'
train_eval = "train" if "loss" in logs else "eval"
# Add averaged stored metrics to logs
for key, metrics in self._stored_metrics[train_eval].items():
logs[key] = torch.tensor(metrics).mean().item()
del self._stored_metrics[train_eval]
return super().log(logs)
@wraps(Trainer.push_to_hub)
def push_to_hub(self, commit_message: Optional[str] = "End of training", blocking: bool = True, **kwargs) -> str:
"""
Overwrite the `push_to_hub` method in order to force-add the tag "sft" when pushing the
model on the Hub. Please refer to `~transformers.Trainer.push_to_hub` for more details.
"""
kwargs = trl_sanitze_kwargs_for_tagging(model=self.model, tag_names=self._tag_names, kwargs=kwargs)
return super().push_to_hub(commit_message=commit_message, blocking=blocking, **kwargs)
| trl/trl/trainer/dpo_trainer.py/0 | {
"file_path": "trl/trl/trainer/dpo_trainer.py",
"repo_id": "trl",
"token_count": 27951
} | 418 |
# Builds GPU docker image of PyTorch specifically
# Uses multi-staged approach to reduce size
# Stage 1
# Use base conda image to reduce time
FROM continuumio/miniconda3:latest AS compile-image
# Specify py version
ENV PYTHON_VERSION=3.9
# Install apt libs
RUN apt-get update && \
apt-get install -y curl git wget && \
apt-get clean && \
rm -rf /var/lib/apt/lists*
# Create our conda env
RUN conda create --name accelerate python=${PYTHON_VERSION} ipython jupyter pip
# We don't install pytorch here yet since CUDA isn't available
# instead we use the direct torch wheel
ENV PATH /opt/conda/envs/accelerate/bin:$PATH
# Activate our bash shell
RUN chsh -s /bin/bash
SHELL ["/bin/bash", "-c"]
# Activate the conda env, install mpy4pi, and install torch + accelerate
RUN source activate accelerate && conda install -c conda-forge mpi4py
RUN source activate accelerate && \
python3 -m pip install --no-cache-dir \
git+https://github.com/huggingface/accelerate#egg=accelerate[testing,test_trackers] \
--extra-index-url https://download.pytorch.org/whl/cu117
RUN python3 -m pip install --no-cache-dir bitsandbytes
# Stage 2
FROM nvidia/cuda:12.1.0-cudnn8-devel-ubuntu20.04 AS build-image
COPY --from=compile-image /opt/conda /opt/conda
ENV PATH /opt/conda/bin:$PATH
# Install apt libs
RUN apt-get update && \
apt-get install -y curl git wget && \
apt-get clean && \
rm -rf /var/lib/apt/lists*
RUN echo "source activate accelerate" >> ~/.profile
# Activate the virtualenv
CMD ["/bin/bash"] | accelerate/docker/accelerate-gpu/Dockerfile/0 | {
"file_path": "accelerate/docker/accelerate-gpu/Dockerfile",
"repo_id": "accelerate",
"token_count": 539
} | 0 |
<!--Copyright 2023 The HuggingFace Team. All rights reserved.
Licensed under the Apache License, Version 2.0 (the "License"); you may not use this file except in compliance with
the License. You may obtain a copy of the License at
http://www.apache.org/licenses/LICENSE-2.0
Unless required by applicable law or agreed to in writing, software distributed under the License is distributed on
an "AS IS" BASIS, WITHOUT WARRANTIES OR CONDITIONS OF ANY KIND, either express or implied. See the License for the
specific language governing permissions and limitations under the License.
⚠️ Note that this file is in Markdown but contain specific syntax for our doc-builder (similar to MDX) that may not be
rendered properly in your Markdown viewer.
-->
# Low Precision Training Methods
The release of new kinds of hardware led to the emergence of new training paradigms that better utilize them. Currently, this is in the form of training
in 8-bit precision using packages such as [TransformersEngine](https://github.com/NVIDIA/TransformerEngine) (TE) or [MS-AMP](https://github.com/Azure/MS-AMP/tree/main).
For an introduction to the topics discussed today, we recommend reviewing the [low-precision usage guide](../usage_guides/low_precision_training.md) as this documentation will reference it regularly.
## A Quick Chart
Below is a quick chart from the MS-AMP documentation showing the different bit-precisions for each solution during training:
Optimization Level | Computation(GEMM) | Comm | Weight | Master Weight | Weight Gradient | Optimizer States
-- | -- | -- | -- | -- | -- | --
FP16 AMP | FP16 | FP32 | FP32 | N/A | FP32 | FP32+FP32
Nvidia TE | FP8 | FP32 | FP32 | N/A | FP32 | FP32+FP32
MS-AMP O1 | FP8 | FP8 | FP16 | N/A | FP8 | FP32+FP32
MS-AMP O2 | FP8 | FP8 | FP16 | N/A | FP8 | FP8+FP16
MS-AMP O3 | FP8 | FP8 | FP8 | FP16 | FP8 | FP8+FP16
## `TransformersEngine`
`TransformersEngine` is the first solution to trying to train in 8-bit floating point. It works by using drop-in replacement layers for certain ones in a model that utilizes their FP8-engine to reduce the number of bits (such as 32 to 8) without degrading the final accuracy of the model.
Specifically, 🤗 Accelerate will find and replace the following layers with `TransformersEngine` versions:
* `nn.LayerNorm` for `te.LayerNorm`
* `nn.Linear` for `te.Linear`
As a result we wind up with a model that has most of its layers in BF16, while some layers are in FP8 reducing some of the memory.
Anecdotally, we have noticed that performance gains don't really start showing when using `TransformerEngine` until a large majority of the layers
in the model are made up of those two layers to replace. As a result, only larger models have shown performance improvements when the number of parameters is around and upwards of a few billion.
The `TransformerEngine` can receive many different arguments that customize how it performs FP8 calculations and what they do. A full list of the arguments is available below:
* `margin`: The margin to use for the gradient scaling.
* `interval`: The interval to use for how often the scaling factor is recomputed.
* `fp8_format``: The format to use for the FP8 recipe. Must be one of `E4M3` or `HYBRID`.
* `amax_history_len`: The length of the history to use for the scaling factor computation
* `amax_compute_algo`: The algorithm to use for the scaling factor computation. Must be one of `max` or `most_recent`.
* `override_linear_precision`: Whether or not to execute `fprop`, `dgrad`, and `wgrad` GEMMS in higher precision.
You can customize each of these as part of [`utils.FP8RecipeKwargs`] to help optimize performance of your models.
If we notice in the chart mentioned earlier, TE simply casts the computation layers into FP8, while everything else is in FP32. As a result this winds up utilizing the most memory but does so with the benefit of guaranteeing the least amount of loss in end accuracy during training.
## `MS-AMP`
MS-AMP takes a different approach to `TransformersEngine` by providing three different optimization levels to convert more operations in FP8 or FP16.
* The base optimization level (`O1`), passes communications of the weights (such as in DDP) in FP8, stores the weights of the model in FP16, and leaves the optimizer states in FP32. The main benefit of this optimization level is that we can reduce the communication bandwidth by essentially half. Additionally, more GPU memory is saved due to 1/2 of everything being cast in FP8, and the weights being cast to FP16. Notably, both the optimizer states remain in FP32.
* The second optimization level (`O2`) improves upon this by also reducing the precision of the optimizer states. One is in FP8 while the other is in FP16. Generally it's been shown that this will only provide a net-gain of no degraded end accuracy, increased training speed, and reduced memory as now every state is either in FP16 or FP8.
* Finally, MS-AMP has a third optimization level (`O3`) which helps during DDP scenarios such as DeepSpeed. The weights of the model in memory are fully cast to FP8, and the master weights are now stored in FP16. This fully reduces memory by the highest factor as now not only is almost everything in FP8, only two states are left in FP16. Currently, only DeepSpeed versions up through 0.9.2 are supported, so this capability is not included in the 🤗 Accelerate integration
## Combining the two
More experiments need to be performed but it's been noted that combining both MS-AMP and TransformersEngine can lead to the highest throughput by relying on NVIDIA's optimized FP8 operators and utilizing how MS-AMP reduces the memory overhead.
| accelerate/docs/source/concept_guides/low_precision_training.md/0 | {
"file_path": "accelerate/docs/source/concept_guides/low_precision_training.md",
"repo_id": "accelerate",
"token_count": 1466
} | 1 |
<!--Copyright 2021 The HuggingFace Team. All rights reserved.
Licensed under the Apache License, Version 2.0 (the "License"); you may not use this file except in compliance with
the License. You may obtain a copy of the License at
http://www.apache.org/licenses/LICENSE-2.0
Unless required by applicable law or agreed to in writing, software distributed under the License is distributed on
an "AS IS" BASIS, WITHOUT WARRANTIES OR CONDITIONS OF ANY KIND, either express or implied. See the License for the
specific language governing permissions and limitations under the License.
⚠️ Note that this file is in Markdown but contain specific syntax for our doc-builder (similar to MDX) that may not be
rendered properly in your Markdown viewer.
-->
# Stateful Classes
Below are variations of a [singleton class](https://en.wikipedia.org/wiki/Singleton_pattern) in the sense that all
instances share the same state, which is initialized on the first instantiation.
These classes are immutable and store information about certain configurations or
states.
[[autodoc]] state.PartialState
[[autodoc]] state.AcceleratorState
[[autodoc]] state.GradientState | accelerate/docs/source/package_reference/state.md/0 | {
"file_path": "accelerate/docs/source/package_reference/state.md",
"repo_id": "accelerate",
"token_count": 291
} | 2 |
<!--Copyright 2022 The HuggingFace Team. All rights reserved.
Licensed under the Apache License, Version 2.0 (the "License"); you may not use this file except in compliance with
the License. You may obtain a copy of the License at
http://www.apache.org/licenses/LICENSE-2.0
Unless required by applicable law or agreed to in writing, software distributed under the License is distributed on
an "AS IS" BASIS, WITHOUT WARRANTIES OR CONDITIONS OF ANY KIND, either express or implied. See the License for the
specific language governing permissions and limitations under the License.
⚠️ Note that this file is in Markdown but contain specific syntax for our doc-builder (similar to MDX) that may not be
rendered properly in your Markdown viewer.
-->
# Understanding how big of a model can fit on your machine
One very difficult aspect when exploring potential models to use on your machine is knowing just how big of a model will *fit* into memory with your current graphics card (such as loading the model onto CUDA).
To help alleviate this, 🤗 Accelerate has a CLI interface through `accelerate estimate-memory`. This tutorial will
help walk you through using it, what to expect, and at the end link to the interactive demo hosted on the 🤗 Hub which will
even let you post those results directly on the model repo!
Currently we support searching for models that can be used in `timm` and `transformers`.
<Tip>
This API will load the model into memory on the `meta` device, so we are not actually downloading
and loading the full weights of the model into memory, nor do we need to. As a result it's
perfectly fine to measure 8 billion parameter models (or more), without having to worry about
if your CPU can handle it!
</Tip>
## Gradio Demos
Below are a few gradio demos related to what was described above. The first is the official Hugging Face memory estimation space, utilizing Accelerate directly:
<div class="block dark:hidden">
<iframe
src="https://hf-accelerate-model-memory-usage.hf.space?__theme=light"
width="850"
height="1600"
></iframe>
</div>
<div class="hidden dark:block">
<iframe
src="https://hf-accelerate-model-memory-usage.hf.space?__theme=dark"
width="850"
height="1600"
></iframe>
</div>
A community member has taken the idea and expanded it further, allowing you to filter models directly and see if you can run a particular LLM given GPU constraints and LoRA configurations. To play with it, see [here](https://huggingface.co/spaces/Vokturz/can-it-run-llm) for more details.
## The Command
When using `accelerate estimate-memory`, you need to pass in the name of the model you want to use, potentially the framework
that model utilizing (if it can't be found automatically), and the data types you want the model to be loaded in with.
For example, here is how we can calculate the memory footprint for `bert-base-cased`:
```bash
accelerate estimate-memory bert-base-cased
```
This will download the `config.json` for `bert-based-cased`, load the model on the `meta` device, and report back how much space
it will use:
Memory Usage for loading `bert-base-cased`:
| dtype | Largest Layer | Total Size | Training using Adam |
|---------|---------------|------------|---------------------|
| float32 | 84.95 MB | 418.18 MB | 1.61 GB |
| float16 | 42.47 MB | 206.59 MB | 826.36 MB |
| int8 | 21.24 MB | 103.29 MB | 413.18 MB |
| int4 | 10.62 MB | 51.65 MB | 206.59 MB |
By default it will return all the supported dtypes (`int4` through `float32`), but if you are interested in specific ones these can be filtered.
### Specific libraries
If the source library cannot be determined automatically (like it could in the case of `bert-base-cased`), a library name can
be passed in.
```bash
accelerate estimate-memory HuggingFaceM4/idefics-80b-instruct --library_name transformers
```
Memory Usage for loading `HuggingFaceM4/idefics-80b-instruct`:
| dtype | Largest Layer | Total Size | Training using Adam |
|---------|---------------|------------|---------------------|
| float32 | 3.02 GB | 297.12 GB | 1.16 TB |
| float16 | 1.51 GB | 148.56 GB | 594.24 GB |
| int8 | 772.52 MB | 74.28 GB | 297.12 GB |
| int4 | 386.26 MB | 37.14 GB | 148.56 GB |
```bash
accelerate estimate-memory timm/resnet50.a1_in1k --library_name timm
```
Memory Usage for loading `timm/resnet50.a1_in1k`:
| dtype | Largest Layer | Total Size | Training using Adam |
|---------|---------------|------------|---------------------|
| float32 | 9.0 MB | 97.7 MB | 390.78 MB |
| float16 | 4.5 MB | 48.85 MB | 195.39 MB |
| int8 | 2.25 MB | 24.42 MB | 97.7 MB |
| int4 | 1.12 MB | 12.21 MB | 48.85 MB |
### Specific dtypes
As mentioned earlier, while we return `int4` through `float32` by default, any dtype can be used from `float32`, `float16`, `int8`, and `int4`.
To do so, pass them in after specifying `--dtypes`:
```bash
accelerate estimate-memory bert-base-cased --dtypes float32 float16
```
Memory Usage for loading `bert-base-cased`:
| dtype | Largest Layer | Total Size | Training using Adam |
|---------|---------------|------------|---------------------|
| float32 | 84.95 MB | 413.18 MB | 1.61 GB |
| float16 | 42.47 MB | 206.59 MB | 826.36 MB |
## Caveats with this calculator
This calculator will tell you how much memory is needed to purely load the model in, *not* to perform inference.
This calculation is accurate within a few % of the actual value, so it is a very good view of just how much memory it will take. For instance loading `bert-base-cased` actually takes `413.68 MB` when loaded on CUDA in full precision, and the calculator estimates `413.18 MB`.
When performing inference you can expect to add up to an additional 20% as found by [EleutherAI](https://blog.eleuther.ai/transformer-math/). We'll be conducting research into finding a more accurate estimate to these values, and will update
this calculator once done.
| accelerate/docs/source/usage_guides/model_size_estimator.md/0 | {
"file_path": "accelerate/docs/source/usage_guides/model_size_estimator.md",
"repo_id": "accelerate",
"token_count": 2030
} | 3 |
# Copyright 2022 The HuggingFace Team. All rights reserved.
#
# Licensed under the Apache License, Version 2.0 (the "License");
# you may not use this file except in compliance with the License.
# You may obtain a copy of the License at
#
# http://www.apache.org/licenses/LICENSE-2.0
#
# Unless required by applicable law or agreed to in writing, software
# distributed under the License is distributed on an "AS IS" BASIS,
# WITHOUT WARRANTIES OR CONDITIONS OF ANY KIND, either express or implied.
# See the License for the specific language governing permissions and
# limitations under the License.
import logging
import os
from contextlib import contextmanager
from functools import wraps
from typing import Dict, List, Optional, Union
import torch
import torch.nn as nn
from .hooks import (
AlignDevicesHook,
CpuOffload,
UserCpuOffloadHook,
add_hook_to_module,
attach_align_device_hook,
attach_align_device_hook_on_blocks,
)
from .utils import (
OffloadedWeightsLoader,
check_cuda_p2p_ib_support,
check_device_map,
extract_submodules_state_dict,
find_tied_parameters,
get_balanced_memory,
infer_auto_device_map,
is_mlu_available,
is_npu_available,
is_torch_version,
is_xpu_available,
load_checkpoint_in_model,
offload_state_dict,
parse_flag_from_env,
retie_parameters,
)
from .utils.other import recursive_getattr
logger = logging.getLogger(__name__)
@contextmanager
def init_empty_weights(include_buffers: bool = None):
"""
A context manager under which models are initialized with all parameters on the meta device, therefore creating an
empty model. Useful when just initializing the model would blow the available RAM.
Args:
include_buffers (`bool`, *optional*):
Whether or not to also put all buffers on the meta device while initializing.
Example:
```python
import torch.nn as nn
from accelerate import init_empty_weights
# Initialize a model with 100 billions parameters in no time and without using any RAM.
with init_empty_weights():
tst = nn.Sequential(*[nn.Linear(10000, 10000) for _ in range(1000)])
```
<Tip warning={true}>
Any model created under this context manager has no weights. As such you can't do something like
`model.to(some_device)` with it. To load weights inside your empty model, see [`load_checkpoint_and_dispatch`].
Make sure to overwrite the default device_map param for [`load_checkpoint_and_dispatch`], otherwise dispatch is not
called.
</Tip>
"""
if include_buffers is None:
include_buffers = parse_flag_from_env("ACCELERATE_INIT_INCLUDE_BUFFERS", False)
with init_on_device(torch.device("meta"), include_buffers=include_buffers) as f:
yield f
@contextmanager
def init_on_device(device: torch.device, include_buffers: bool = None):
"""
A context manager under which models are initialized with all parameters on the specified device.
Args:
device (`torch.device`):
Device to initialize all parameters on.
include_buffers (`bool`, *optional*):
Whether or not to also put all buffers on the meta device while initializing.
Example:
```python
import torch.nn as nn
from accelerate import init_on_device
with init_on_device(device=torch.device("cuda")):
tst = nn.Liner(100, 100) # on `cuda` device
```
"""
if include_buffers is None:
include_buffers = parse_flag_from_env("ACCELERATE_INIT_INCLUDE_BUFFERS", False)
# TODO(shingjan): remove the torch version check once older versions are deprecated
if is_torch_version(">=", "2.0") and include_buffers:
with device:
yield
return
old_register_parameter = nn.Module.register_parameter
if include_buffers:
old_register_buffer = nn.Module.register_buffer
def register_empty_parameter(module, name, param):
old_register_parameter(module, name, param)
if param is not None:
param_cls = type(module._parameters[name])
kwargs = module._parameters[name].__dict__
kwargs["requires_grad"] = param.requires_grad
module._parameters[name] = param_cls(module._parameters[name].to(device), **kwargs)
def register_empty_buffer(module, name, buffer, persistent=True):
old_register_buffer(module, name, buffer, persistent=persistent)
if buffer is not None:
module._buffers[name] = module._buffers[name].to(device)
# Patch tensor creation
if include_buffers:
tensor_constructors_to_patch = {
torch_function_name: getattr(torch, torch_function_name)
for torch_function_name in ["empty", "zeros", "ones", "full"]
}
else:
tensor_constructors_to_patch = {}
def patch_tensor_constructor(fn):
def wrapper(*args, **kwargs):
kwargs["device"] = device
return fn(*args, **kwargs)
return wrapper
try:
nn.Module.register_parameter = register_empty_parameter
if include_buffers:
nn.Module.register_buffer = register_empty_buffer
for torch_function_name in tensor_constructors_to_patch.keys():
setattr(torch, torch_function_name, patch_tensor_constructor(getattr(torch, torch_function_name)))
yield
finally:
nn.Module.register_parameter = old_register_parameter
if include_buffers:
nn.Module.register_buffer = old_register_buffer
for torch_function_name, old_torch_function in tensor_constructors_to_patch.items():
setattr(torch, torch_function_name, old_torch_function)
def cpu_offload(
model: nn.Module,
execution_device: Optional[torch.device] = None,
offload_buffers: bool = False,
state_dict: Optional[Dict[str, torch.Tensor]] = None,
preload_module_classes: Optional[List[str]] = None,
):
"""
Activates full CPU offload for a model. As a result, all parameters of the model will be offloaded and only one
copy of the state dict of the model will be kept. During the forward pass, parameters will be extracted from that
state dict and put on the execution device passed as they are needed, then offloaded again.
Args:
model (`torch.nn.Module`):
The model to offload.
execution_device (`torch.device`, *optional*):
The device on which the forward pass of the model will be executed (should be a GPU). Will default to the
model first parameter device.
offload_buffers (`bool`, *optional*, defaults to `False`):
Whether or not to offload the buffers with the model parameters.
state_dict (`Dict[str, torch.Tensor]`, *optional*):
The state dict of the model that will be kept on CPU.
preload_module_classes (`List[str]`, *optional*):
A list of classes whose instances should load all their weights (even in the submodules) at the beginning
of the forward. This should only be used for classes that have submodules which are registered but not
called directly during the forward, for instance if a `dense` linear layer is registered, but at forward,
`dense.weight` and `dense.bias` are used in some operations instead of calling `dense` directly.
"""
if execution_device is None:
execution_device = next(iter(model.parameters())).device
if state_dict is None:
state_dict = {n: p.to("cpu") for n, p in model.state_dict().items()}
add_hook_to_module(model, AlignDevicesHook(io_same_device=True), append=True)
attach_align_device_hook(
model,
execution_device=execution_device,
offload=True,
offload_buffers=offload_buffers,
weights_map=state_dict,
preload_module_classes=preload_module_classes,
)
return model
def cpu_offload_with_hook(
model: torch.nn.Module,
execution_device: Optional[Union[int, str, torch.device]] = None,
prev_module_hook: Optional[UserCpuOffloadHook] = None,
):
"""
Offloads a model on the CPU and puts it back to an execution device when executed. The difference with
[`cpu_offload`] is that the model stays on the execution device after the forward and is only offloaded again when
the `offload` method of the returned `hook` is called. Useful for pipelines running a model in a loop.
Args:
model (`torch.nn.Module`):
The model to offload.
execution_device(`str`, `int` or `torch.device`, *optional*):
The device on which the model should be executed. Will default to the MPS device if it's available, then
GPU 0 if there is a GPU, and finally to the CPU.
prev_module_hook (`UserCpuOffloadHook`, *optional*):
The hook sent back by this function for a previous model in the pipeline you are running. If passed, its
offload method will be called just before the forward of the model to which this hook is attached.
Example:
```py
model_1, hook_1 = cpu_offload_with_hook(model_1, cuda_device)
model_2, hook_2 = cpu_offload_with_hook(model_2, cuda_device, prev_module_hook=hook_1)
model_3, hook_3 = cpu_offload_with_hook(model_3, cuda_device, prev_module_hook=hook_2)
hid_1 = model_1(input)
for i in range(50):
# model1 is offloaded on the CPU at the first iteration, model 2 stays on the GPU for this whole loop.
hid_2 = model_2(hid_1)
# model2 is offloaded to the CPU just before this forward.
hid_3 = model_3(hid_3)
# For model3, you need to manually call the hook offload method.
hook_3.offload()
```
"""
hook = CpuOffload(execution_device=execution_device, prev_module_hook=prev_module_hook)
add_hook_to_module(model, hook, append=True)
user_hook = UserCpuOffloadHook(model, hook)
return model, user_hook
def disk_offload(
model: nn.Module,
offload_dir: Union[str, os.PathLike],
execution_device: Optional[torch.device] = None,
offload_buffers: bool = False,
preload_module_classes: Optional[List[str]] = None,
):
"""
Activates full disk offload for a model. As a result, all parameters of the model will be offloaded as
memory-mapped array in a given folder. During the forward pass, parameters will be accessed from that folder and
put on the execution device passed as they are needed, then offloaded again.
Args:
model (`torch.nn.Module`): The model to offload.
offload_dir (`str` or `os.PathLike`):
The folder in which to offload the model weights (or where the model weights are already offloaded).
execution_device (`torch.device`, *optional*):
The device on which the forward pass of the model will be executed (should be a GPU). Will default to the
model's first parameter device.
offload_buffers (`bool`, *optional*, defaults to `False`):
Whether or not to offload the buffers with the model parameters.
preload_module_classes (`List[str]`, *optional*):
A list of classes whose instances should load all their weights (even in the submodules) at the beginning
of the forward. This should only be used for classes that have submodules which are registered but not
called directly during the forward, for instance if a `dense` linear layer is registered, but at forward,
`dense.weight` and `dense.bias` are used in some operations instead of calling `dense` directly.
"""
if not os.path.isdir(offload_dir) or not os.path.isfile(os.path.join(offload_dir, "index.json")):
offload_state_dict(offload_dir, model.state_dict())
if execution_device is None:
execution_device = next(iter(model.parameters())).device
weights_map = OffloadedWeightsLoader(save_folder=offload_dir)
add_hook_to_module(model, AlignDevicesHook(io_same_device=True), append=True)
attach_align_device_hook(
model,
execution_device=execution_device,
offload=True,
offload_buffers=offload_buffers,
weights_map=weights_map,
preload_module_classes=preload_module_classes,
)
return model
def dispatch_model(
model: nn.Module,
device_map: Dict[str, Union[str, int, torch.device]],
main_device: Optional[torch.device] = None,
state_dict: Optional[Dict[str, torch.Tensor]] = None,
offload_dir: Optional[Union[str, os.PathLike]] = None,
offload_index: Optional[Dict[str, str]] = None,
offload_buffers: bool = False,
skip_keys: Optional[Union[str, List[str]]] = None,
preload_module_classes: Optional[List[str]] = None,
force_hooks: bool = False,
):
"""
Dispatches a model according to a given device map. Layers of the model might be spread across GPUs, offloaded on
the CPU or even the disk.
Args:
model (`torch.nn.Module`):
The model to dispatch.
device_map (`Dict[str, Union[str, int, torch.device]]`):
A dictionary mapping module names in the models `state_dict` to the device they should go to. Note that
`"disk"` is accepted even if it's not a proper value for `torch.device`.
main_device (`str`, `int` or `torch.device`, *optional*):
The main execution device. Will default to the first device in the `device_map` different from `"cpu"` or
`"disk"`.
state_dict (`Dict[str, torch.Tensor]`, *optional*):
The state dict of the part of the model that will be kept on CPU.
offload_dir (`str` or `os.PathLike`):
The folder in which to offload the model weights (or where the model weights are already offloaded).
offload_index (`Dict`, *optional*):
A dictionary from weight name to their information (`dtype`/ `shape` or safetensors filename). Will default
to the index saved in `save_folder`.
offload_buffers (`bool`, *optional*, defaults to `False`):
Whether or not to offload the buffers with the model parameters.
skip_keys (`str` or `List[str]`, *optional*):
A list of keys to ignore when moving inputs or outputs between devices.
preload_module_classes (`List[str]`, *optional*):
A list of classes whose instances should load all their weights (even in the submodules) at the beginning
of the forward. This should only be used for classes that have submodules which are registered but not
called directly during the forward, for instance if a `dense` linear layer is registered, but at forward,
`dense.weight` and `dense.bias` are used in some operations instead of calling `dense` directly.
force_hooks (`bool`, *optional*, defaults to `False`):
Whether or not to force device hooks to be attached to the model even if all layers are dispatched to a
single device.
"""
# Error early if the device map is incomplete.
check_device_map(model, device_map)
# for backward compatibility
is_bnb_quantized = (
getattr(model, "is_quantized", False) or getattr(model, "is_loaded_in_8bit", False)
) and getattr(model, "quantization_method", "bitsandbytes") == "bitsandbytes"
# We attach hooks if the device_map has at least 2 different devices or if
# force_hooks is set to `True`. Otherwise, the model in already loaded
# in the unique device and the user can decide where to dispatch the model.
# If the model is quantized, we always force-dispatch the model
if (len(set(device_map.values())) > 1) or is_bnb_quantized or force_hooks:
if main_device is None:
if set(device_map.values()) == {"cpu"} or set(device_map.values()) == {"cpu", "disk"}:
main_device = "cpu"
else:
main_device = [d for d in device_map.values() if d not in ["cpu", "disk"]][0]
if main_device != "cpu":
cpu_modules = [name for name, device in device_map.items() if device == "cpu"]
if state_dict is None and len(cpu_modules) > 0:
state_dict = extract_submodules_state_dict(model.state_dict(), cpu_modules)
disk_modules = [name for name, device in device_map.items() if device == "disk"]
if offload_dir is None and offload_index is None and len(disk_modules) > 0:
raise ValueError(
"We need an `offload_dir` to dispatch this model according to this `device_map`, the following submodules "
f"need to be offloaded: {', '.join(disk_modules)}."
)
if (
len(disk_modules) > 0
and offload_index is None
and (not os.path.isdir(offload_dir) or not os.path.isfile(os.path.join(offload_dir, "index.json")))
):
disk_state_dict = extract_submodules_state_dict(model.state_dict(), disk_modules)
offload_state_dict(offload_dir, disk_state_dict)
execution_device = {
name: main_device if device in ["cpu", "disk"] else device for name, device in device_map.items()
}
execution_device[""] = main_device
offloaded_devices = ["disk"] if main_device == "cpu" or main_device == "mps" else ["cpu", "disk"]
offload = {name: device in offloaded_devices for name, device in device_map.items()}
save_folder = offload_dir if len(disk_modules) > 0 else None
if state_dict is not None or save_folder is not None or offload_index is not None:
device = main_device if offload_index is not None else None
weights_map = OffloadedWeightsLoader(
state_dict=state_dict, save_folder=save_folder, index=offload_index, device=device
)
else:
weights_map = None
# When dispatching the model's parameters to the devices specified in device_map, we want to avoid allocating memory several times for the
# tied parameters. The dictionary tied_params_map keeps track of the already allocated data for a given tied parameter (represented by its
# original pointer) on each devices.
tied_params = find_tied_parameters(model)
tied_params_map = {}
for group in tied_params:
for param_name in group:
# data_ptr() is enough here, as `find_tied_parameters` finds tied params simply by comparing `param1 is param2`, so we don't need
# to care about views of tensors through storage_offset.
data_ptr = recursive_getattr(model, param_name).data_ptr()
tied_params_map[data_ptr] = {}
# Note: To handle the disk offloading case, we can not simply use weights_map[param_name].data_ptr() as the reference pointer,
# as we have no guarantee that safetensors' `file.get_tensor()` will always give the same pointer.
attach_align_device_hook_on_blocks(
model,
execution_device=execution_device,
offload=offload,
offload_buffers=offload_buffers,
weights_map=weights_map,
skip_keys=skip_keys,
preload_module_classes=preload_module_classes,
tied_params_map=tied_params_map,
)
# warn if there is any params on the meta device
offloaded_devices_str = " and ".join(
[device for device in set(device_map.values()) if device in ("cpu", "disk")]
)
if len(offloaded_devices_str) > 0:
logging.warning(
f"Some parameters are on the meta device device because they were offloaded to the {offloaded_devices_str}."
)
# Attaching the hook may break tied weights, so we retie them
retie_parameters(model, tied_params)
# add warning to cuda and to method
def add_warning(fn, model):
@wraps(fn)
def wrapper(*args, **kwargs):
warning_msg = "You shouldn't move a model that is dispatched using accelerate hooks."
if str(fn.__name__) == "to":
to_device = torch._C._nn._parse_to(*args, **kwargs)[0]
if to_device is not None:
logger.warning(warning_msg)
else:
logger.warning(warning_msg)
for param in model.parameters():
if param.device == torch.device("meta"):
raise RuntimeError("You can't move a model that has some modules offloaded to cpu or disk.")
return fn(*args, **kwargs)
return wrapper
model.to = add_warning(model.to, model)
if is_npu_available():
model.npu = add_warning(model.npu, model)
elif is_mlu_available():
model.mlu = add_warning(model.mlu, model)
elif is_xpu_available():
model.xpu = add_warning(model.xpu, model)
else:
model.cuda = add_warning(model.cuda, model)
# Check if we are using multi-gpus with RTX 4000 series
use_multi_gpu = len([device for device in set(device_map.values()) if device not in ("cpu", "disk")]) > 1
if use_multi_gpu and not check_cuda_p2p_ib_support():
logger.warning(
"We've detected an older driver with an RTX 4000 series GPU. These drivers have issues with P2P. "
"This can affect the multi-gpu inference when using accelerate device_map."
"Please make sure to update your driver to the latest version which resolves this."
)
else:
device = list(device_map.values())[0]
# `torch.Tensor.to(<int num>)` is not supported by `torch_npu` (see this [issue](https://github.com/Ascend/pytorch/issues/16)).
if is_npu_available() and isinstance(device, int):
device = f"npu:{device}"
elif is_mlu_available() and isinstance(device, int):
device = f"mlu:{device}"
elif is_xpu_available() and isinstance(device, int):
device = f"xpu:{device}"
if device != "disk":
model.to(device)
else:
raise ValueError(
"You are trying to offload the whole model to the disk. Please use the `disk_offload` function instead."
)
# Convert OrderedDict back to dict for easier usage
model.hf_device_map = dict(device_map)
return model
def load_checkpoint_and_dispatch(
model: nn.Module,
checkpoint: Union[str, os.PathLike],
device_map: Optional[Union[str, Dict[str, Union[int, str, torch.device]]]] = None,
max_memory: Optional[Dict[Union[int, str], Union[int, str]]] = None,
no_split_module_classes: Optional[List[str]] = None,
offload_folder: Optional[Union[str, os.PathLike]] = None,
offload_buffers: bool = False,
dtype: Optional[Union[str, torch.dtype]] = None,
offload_state_dict: Optional[bool] = None,
skip_keys: Optional[Union[str, List[str]]] = None,
preload_module_classes: Optional[List[str]] = None,
force_hooks: bool = False,
):
"""
Loads a (potentially sharded) checkpoint inside a model, potentially sending weights to a given device as they are
loaded and adds the various hooks that will make this model run properly (even if split across devices).
Args:
model (`torch.nn.Module`): The model in which we want to load a checkpoint.
checkpoint (`str` or `os.PathLike`):
The folder checkpoint to load. It can be:
- a path to a file containing a whole model state dict
- a path to a `.json` file containing the index to a sharded checkpoint
- a path to a folder containing a unique `.index.json` file and the shards of a checkpoint.
device_map (`Dict[str, Union[int, str, torch.device]]`, *optional*):
A map that specifies where each submodule should go. It doesn't need to be refined to each parameter/buffer
name, once a given module name is inside, every submodule of it will be sent to the same device.
To have Accelerate compute the most optimized `device_map` automatically, set `device_map="auto"`. For more
information about each option see [here](../concept_guides/big_model_inference#designing-a-device-map).
Defaults to None, which means [`dispatch_model`] will not be called.
max_memory (`Dict`, *optional*):
A dictionary device identifier to maximum memory. Will default to the maximum memory available for each GPU
and the available CPU RAM if unset.
no_split_module_classes (`List[str]`, *optional*):
A list of layer class names that should never be split across device (for instance any layer that has a
residual connection).
offload_folder (`str` or `os.PathLike`, *optional*):
If the `device_map` contains any value `"disk"`, the folder where we will offload weights.
offload_buffers (`bool`, *optional*, defaults to `False`):
In the layers that are offloaded on the CPU or the hard drive, whether or not to offload the buffers as
well as the parameters.
dtype (`str` or `torch.dtype`, *optional*):
If provided, the weights will be converted to that type when loaded.
offload_state_dict (`bool`, *optional*):
If `True`, will temporarily offload the CPU state dict on the hard drive to avoid getting out of CPU RAM if
the weight of the CPU state dict + the biggest shard does not fit. Will default to `True` if the device map
picked contains `"disk"` values.
skip_keys (`str` or `List[str]`, *optional*):
A list of keys to ignore when moving inputs or outputs between devices.
preload_module_classes (`List[str]`, *optional*):
A list of classes whose instances should load all their weights (even in the submodules) at the beginning
of the forward. This should only be used for classes that have submodules which are registered but not
called directly during the forward, for instance if a `dense` linear layer is registered, but at forward,
`dense.weight` and `dense.bias` are used in some operations instead of calling `dense` directly.
force_hooks (`bool`, *optional*, defaults to `False`):
Whether or not to force device hooks to be attached to the model even if all layers are dispatched to a
single device.
Example:
```python
>>> from accelerate import init_empty_weights, load_checkpoint_and_dispatch
>>> from huggingface_hub import hf_hub_download
>>> from transformers import AutoConfig, AutoModelForCausalLM
>>> # Download the Weights
>>> checkpoint = "EleutherAI/gpt-j-6B"
>>> weights_location = hf_hub_download(checkpoint, "pytorch_model.bin")
>>> # Create a model and initialize it with empty weights
>>> config = AutoConfig.from_pretrained(checkpoint)
>>> with init_empty_weights():
... model = AutoModelForCausalLM.from_config(config)
>>> # Load the checkpoint and dispatch it to the right devices
>>> model = load_checkpoint_and_dispatch(
... model, weights_location, device_map="auto", no_split_module_classes=["GPTJBlock"]
... )
```
"""
if isinstance(device_map, str) and device_map not in ["auto", "balanced", "balanced_low_0", "sequential"]:
raise ValueError(
"If passing a string for `device_map`, please choose 'auto', 'balanced', 'balanced_low_0' or "
"'sequential'."
)
if isinstance(device_map, str):
if device_map != "sequential":
max_memory = get_balanced_memory(
model,
max_memory=max_memory,
no_split_module_classes=no_split_module_classes,
dtype=dtype,
low_zero=(device_map == "balanced_low_0"),
)
device_map = infer_auto_device_map(
model,
max_memory=max_memory,
no_split_module_classes=no_split_module_classes,
dtype=dtype,
offload_buffers=offload_buffers,
)
if offload_state_dict is None and device_map is not None and "disk" in device_map.values():
offload_state_dict = True
load_checkpoint_in_model(
model,
checkpoint,
device_map=device_map,
offload_folder=offload_folder,
dtype=dtype,
offload_state_dict=offload_state_dict,
offload_buffers=offload_buffers,
)
if device_map is None:
return model
return dispatch_model(
model,
device_map=device_map,
offload_dir=offload_folder,
offload_buffers=offload_buffers,
skip_keys=skip_keys,
preload_module_classes=preload_module_classes,
force_hooks=force_hooks,
)
| accelerate/src/accelerate/big_modeling.py/0 | {
"file_path": "accelerate/src/accelerate/big_modeling.py",
"repo_id": "accelerate",
"token_count": 11237
} | 4 |
# Copyright 2022 The HuggingFace Team and Brian Chao. All rights reserved.
#
# Licensed under the Apache License, Version 2.0 (the "License");
# you may not use this file except in compliance with the License.
# You may obtain a copy of the License at
#
# http://www.apache.org/licenses/LICENSE-2.0
#
# Unless required by applicable law or agreed to in writing, software
# distributed under the License is distributed on an "AS IS" BASIS,
# WITHOUT WARRANTIES OR CONDITIONS OF ANY KIND, either express or implied.
# See the License for the specific language governing permissions and
# limitations under the License.
"""
A utility for showing and hiding the terminal cursor on Windows and Linux, based on https://github.com/bchao1/bullet
"""
import os
import sys
from contextlib import contextmanager
# Windows only
if os.name == "nt":
import ctypes
import msvcrt # noqa
class CursorInfo(ctypes.Structure):
# _fields is a specific attr expected by ctypes
_fields_ = [("size", ctypes.c_int), ("visible", ctypes.c_byte)]
def hide_cursor():
if os.name == "nt":
ci = CursorInfo()
handle = ctypes.windll.kernel32.GetStdHandle(-11)
ctypes.windll.kernel32.GetConsoleCursorInfo(handle, ctypes.byref(ci))
ci.visible = False
ctypes.windll.kernel32.SetConsoleCursorInfo(handle, ctypes.byref(ci))
elif os.name == "posix":
sys.stdout.write("\033[?25l")
sys.stdout.flush()
def show_cursor():
if os.name == "nt":
ci = CursorInfo()
handle = ctypes.windll.kernel32.GetStdHandle(-11)
ctypes.windll.kernel32.GetConsoleCursorInfo(handle, ctypes.byref(ci))
ci.visible = True
ctypes.windll.kernel32.SetConsoleCursorInfo(handle, ctypes.byref(ci))
elif os.name == "posix":
sys.stdout.write("\033[?25h")
sys.stdout.flush()
@contextmanager
def hide():
"Context manager to hide the terminal cursor"
try:
hide_cursor()
yield
finally:
show_cursor()
| accelerate/src/accelerate/commands/menu/cursor.py/0 | {
"file_path": "accelerate/src/accelerate/commands/menu/cursor.py",
"repo_id": "accelerate",
"token_count": 763
} | 5 |
# Copyright 2022 The HuggingFace Team. All rights reserved.
#
# Licensed under the Apache License, Version 2.0 (the "License");
# you may not use this file except in compliance with the License.
# You may obtain a copy of the License at
#
# http://www.apache.org/licenses/LICENSE-2.0
#
# Unless required by applicable law or agreed to in writing, software
# distributed under the License is distributed on an "AS IS" BASIS,
# WITHOUT WARRANTIES OR CONDITIONS OF ANY KIND, either express or implied.
# See the License for the specific language governing permissions and
# limitations under the License.
# We ignore warnings about stepping the scheduler since we step it ourselves during gradient accumulation
import warnings
from .state import AcceleratorState, GradientState
warnings.filterwarnings("ignore", category=UserWarning, module="torch.optim.lr_scheduler")
class AcceleratedScheduler:
"""
A wrapper around a learning rate scheduler that will only step when the optimizer(s) have a training step. Useful
to avoid making a scheduler step too fast when gradients went overflow and there was no training step (in mixed
precision training)
When performing gradient accumulation scheduler lengths should not be changed accordingly, Accelerate will always
step the scheduler to account for it.
Args:
scheduler (`torch.optim.lr_scheduler._LRScheduler`):
The scheduler to wrap.
optimizers (one or a list of `torch.optim.Optimizer`):
The optimizers used.
step_with_optimizer (`bool`, *optional*, defaults to `True`):
Whether or not the scheduler should be stepped at each optimizer step.
split_batches (`bool`, *optional*, defaults to `False`):
Whether or not the dataloaders split one batch across the different processes (so batch size is the same
regardless of the number of processes) or create batches on each process (so batch size is the original
batch size multiplied by the number of processes).
"""
def __init__(self, scheduler, optimizers, step_with_optimizer: bool = True, split_batches: bool = False):
self.scheduler = scheduler
self.optimizers = optimizers if isinstance(optimizers, (list, tuple)) else [optimizers]
self.split_batches = split_batches
self.step_with_optimizer = step_with_optimizer
self.gradient_state = GradientState()
def step(self, *args, **kwargs):
if not self.step_with_optimizer:
# No link between scheduler and optimizer -> just step
self.scheduler.step(*args, **kwargs)
return
# Otherwise, first make sure the optimizer was stepped.
if not self.gradient_state.sync_gradients:
if self.gradient_state.adjust_scheduler:
self.scheduler._step_count += 1
return
for opt in self.optimizers:
if opt.step_was_skipped:
return
if self.split_batches:
# Split batches -> the training dataloader batch size is not changed so one step per training step
self.scheduler.step(*args, **kwargs)
else:
# Otherwise the training dataloader batch size was multiplied by `num_processes`, so we need to do
# num_processes steps per training step
num_processes = AcceleratorState().num_processes
for _ in range(num_processes):
# Special case when using OneCycle and `drop_last` was not used
if hasattr(self.scheduler, "total_steps"):
if self.scheduler._step_count <= self.scheduler.total_steps:
self.scheduler.step(*args, **kwargs)
else:
self.scheduler.step(*args, **kwargs)
# Passthroughs
def get_last_lr(self):
return self.scheduler.get_last_lr()
def state_dict(self):
return self.scheduler.state_dict()
def load_state_dict(self, state_dict):
self.scheduler.load_state_dict(state_dict)
def get_lr(self):
return self.scheduler.get_lr()
def print_lr(self, *args, **kwargs):
return self.scheduler.print_lr(*args, **kwargs)
| accelerate/src/accelerate/scheduler.py/0 | {
"file_path": "accelerate/src/accelerate/scheduler.py",
"repo_id": "accelerate",
"token_count": 1577
} | 6 |
# Copyright 2022 The HuggingFace Team. All rights reserved.
#
# Licensed under the Apache License, Version 2.0 (the "License");
# you may not use this file except in compliance with the License.
# You may obtain a copy of the License at
#
# http://www.apache.org/licenses/LICENSE-2.0
#
# Unless required by applicable law or agreed to in writing, software
# distributed under the License is distributed on an "AS IS" BASIS,
# WITHOUT WARRANTIES OR CONDITIONS OF ANY KIND, either express or implied.
# See the License for the specific language governing permissions and
# limitations under the License.
from copy import deepcopy
import torch
import torch.nn.functional as F
from torch.optim import AdamW
from torch.optim.lr_scheduler import LambdaLR
from torch.utils.data import DataLoader
from accelerate.accelerator import Accelerator, GradientAccumulationPlugin
from accelerate.state import GradientState
from accelerate.test_utils import RegressionDataset, RegressionModel
from accelerate.utils import DistributedType, set_seed
def check_model_parameters(model_a, model_b, did_step, iteration, **kwargs):
for param, grad_param in zip(model_a.parameters(), model_b.parameters()):
if not param.requires_grad:
continue
if not did_step:
# Grads should not be in sync
assert (
torch.allclose(param.grad, grad_param.grad, **kwargs) is False
), f"Gradients in sync when they should not be at iteration {iteration}:\nmodel_a grad ({param.grad}) == model_b grad ({grad_param.grad})"
else:
# Grads should be in sync
assert (
torch.allclose(param.grad, grad_param.grad, **kwargs) is True
), f"Gradients not in sync when they should be at iteration {iteration}:\nmodel_a grad ({param.grad}) != model_b grad ({grad_param.grad})"
def step_model(model, input, target, accelerator, do_backward=True):
model.train()
output = model(input)
loss = F.mse_loss(output, target.to(output.device))
if not do_backward:
loss /= accelerator.gradient_accumulation_steps
loss.backward()
else:
accelerator.backward(loss)
def get_training_setup(accelerator, sched=False):
"Returns everything needed to perform basic training"
set_seed(42)
model = RegressionModel()
ddp_model = deepcopy(model)
dset = RegressionDataset(length=80)
dataloader = DataLoader(dset, batch_size=16)
model.to(accelerator.device)
if sched:
opt = AdamW(params=model.parameters(), lr=1e-3)
ddp_opt = AdamW(params=ddp_model.parameters(), lr=1e-3)
sched = LambdaLR(opt, lr_lambda=lambda epoch: epoch**0.65)
ddp_sched = LambdaLR(ddp_opt, lr_lambda=lambda epoch: epoch**0.65)
# Make a copy of `model`
if sched:
ddp_model, ddp_opt, ddp_sched, dataloader = accelerator.prepare(ddp_model, ddp_opt, ddp_sched, dataloader)
else:
ddp_model, dataloader = accelerator.prepare(ddp_model, dataloader)
if sched:
return (model, opt, sched, dataloader, ddp_model, ddp_opt, ddp_sched)
return model, ddp_model, dataloader
def test_noop_sync(accelerator):
# Test when on a single CPU or GPU that the context manager does nothing
model, ddp_model, dataloader = get_training_setup(accelerator)
# Use a single batch
ddp_input, ddp_target = next(iter(dataloader)).values()
for iteration in range(3):
# Gather the distributed inputs and targs for the base model
input, target = accelerator.gather((ddp_input, ddp_target))
input, target = input.to(accelerator.device), target.to(accelerator.device)
# Perform our initial ground truth step in non "DDP"
step_model(model, input, target, accelerator)
# Do "gradient accumulation" (noop)
if iteration % 2 == 0:
# Accumulate grads locally
with accelerator.no_sync(ddp_model):
step_model(ddp_model, ddp_input, ddp_target, accelerator)
else:
# Sync grads
step_model(ddp_model, ddp_input, ddp_target, accelerator)
# Since `no_sync` is a noop, `ddp_model` and `model` grads should always be in sync
check_model_parameters(model, ddp_model, True, iteration)
for param, ddp_param in zip(model.parameters(), ddp_model.parameters()):
if not param.requires_grad:
continue
assert torch.allclose(
param.grad, ddp_param.grad
), f"Gradients not in sync when they should be:\nModel grad ({param.grad}) != DDP grad ({ddp_param.grad})"
# Shuffle ddp_input on each iteration
torch.manual_seed(1337 + iteration)
ddp_input = ddp_input[torch.randperm(len(ddp_input))]
def test_distributed_sync(accelerator):
# Test on distributed setup that context manager behaves properly
model, ddp_model, dataloader = get_training_setup(accelerator)
# Use a single batch
ddp_input, ddp_target = next(iter(dataloader)).values()
for iteration in range(3):
# Gather the distributed inputs and targs for the base model
input, target = accelerator.gather((ddp_input, ddp_target))
input, target = input.to(accelerator.device), target.to(accelerator.device)
# Perform our initial ground truth step in non "DDP"
step_model(model, input, target, accelerator)
# Do "gradient accumulation" (noop)
if iteration % 2 == 0:
# Accumulate grads locally
with accelerator.no_sync(ddp_model):
step_model(ddp_model, ddp_input, ddp_target, accelerator)
else:
# Sync grads
step_model(ddp_model, ddp_input, ddp_target, accelerator)
# DDP model and model should only be in sync when not (iteration % 2 == 0)
for param, ddp_param in zip(model.parameters(), ddp_model.parameters()):
if not param.requires_grad:
continue
if iteration % 2 == 0:
# Grads should not be in sync
assert (
torch.allclose(param.grad, ddp_param.grad) is False
), f"Gradients in sync when they should not be:\nModel grad ({param.grad}) == DDP grad ({ddp_param.grad})"
else:
# Grads should be in sync
assert (
torch.allclose(param.grad, ddp_param.grad) is True
), f"Gradients not in sync when they should be:\nModel grad ({param.grad}) != DDP grad ({ddp_param.grad})"
# Shuffle ddp_input on each iteration
torch.manual_seed(1337 + iteration)
ddp_input = ddp_input[torch.randperm(len(ddp_input))]
def test_distributed_sync_multiple_fwd(accelerator):
# Test on distributed setup that context manager behaves properly when used with multiple forwards followed by multiple backwards
model, ddp_model, dataloader = get_training_setup(accelerator)
# Do multiple forwards
losses = []
num_iterations = 3
for iteration in range(num_iterations):
ddp_input, ddp_target = next(iter(dataloader)).values()
# Gather the distributed inputs and targs for the base model
input, target = accelerator.gather((ddp_input, ddp_target))
input, target = input.to(accelerator.device), target.to(accelerator.device)
# Perform our initial ground truth step in non "DDP"
step_model(model, input, target, accelerator)
# Accumulate grads locally
with accelerator.no_sync(ddp_model):
ddp_output = ddp_model(ddp_input)
loss = F.mse_loss(ddp_output, ddp_target.to(ddp_output.device))
losses.append(loss)
# Do multiple backwards and sync only at the last backward
for iteration in range(num_iterations):
loss = losses[iteration]
if iteration < num_iterations - 1:
# Accumulate grads locally
accelerator.backward(loss)
# DDP model and model should only be in sync after last backward
for param, ddp_param in zip(model.parameters(), ddp_model.parameters()):
if not param.requires_grad:
continue
# Grads should not be in sync
assert (
torch.allclose(param.grad, ddp_param.grad) is False
), f"Gradients in sync when they should not be:\nModel grad ({param.grad}) == DDP grad ({ddp_param.grad})"
else:
# Sync grads if last backward
with accelerator.trigger_sync_in_backward(ddp_model):
accelerator.backward(loss)
# DDP model and model should only be in sync after last backward
for param, ddp_param in zip(model.parameters(), ddp_model.parameters()):
if not param.requires_grad:
continue
# Grads should be in sync
assert (
torch.allclose(param.grad, ddp_param.grad) is True
), f"Gradients not in sync when they should be:\nModel grad ({param.grad}) != DDP grad ({ddp_param.grad})"
def test_gradient_accumulation(split_batches=False, dispatch_batches=False, sync_each_batch=False):
gradient_accumulation_plugin = GradientAccumulationPlugin(num_steps=2, sync_each_batch=sync_each_batch)
accelerator = Accelerator(
split_batches=split_batches,
dispatch_batches=dispatch_batches,
gradient_accumulation_plugin=gradient_accumulation_plugin,
)
# Test that context manager behaves properly
model, ddp_model, dataloader = get_training_setup(accelerator)
for iteration, batch in enumerate(dataloader):
ddp_input, ddp_target = batch.values()
# Gather the distributed inputs and targs for the base model
input, target = accelerator.gather((ddp_input, ddp_target))
input, target = input.to(accelerator.device), target.to(accelerator.device)
# Perform our initial ground truth step in non "DDP"
step_model(model, input, target, accelerator, False)
# Do "gradient accumulation" (noop)
with accelerator.accumulate(ddp_model):
step_model(ddp_model, ddp_input, ddp_target, accelerator)
# DDP model and model should only be in sync when not (iteration % 2 == 0)
for param, ddp_param in zip(model.parameters(), ddp_model.parameters()):
if not param.requires_grad:
continue
if ((iteration + 1) % 2 == 0) or (iteration == len(dataloader) - 1) or sync_each_batch:
# Grads should be in sync
assert (
torch.allclose(param.grad, ddp_param.grad) is True
), f"Gradients not in sync when they should be at iteration {iteration}:\nModel grad ({param.grad}) != DDP grad ({ddp_param.grad})"
else:
# Grads should not be in sync
assert (
torch.allclose(param.grad, ddp_param.grad) is False
), f"Gradients in sync when they should not be at iteration {iteration}:\nModel grad ({param.grad}) == DDP grad ({ddp_param.grad})"
# Shuffle ddp_input on each iteration
torch.manual_seed(1337 + iteration)
ddp_input = ddp_input[torch.randperm(len(ddp_input))]
GradientState._reset_state()
def test_gradient_accumulation_with_opt_and_scheduler(
split_batches=False, dispatch_batches=False, sync_each_batch=False
):
gradient_accumulation_plugin = GradientAccumulationPlugin(num_steps=2, sync_each_batch=sync_each_batch)
accelerator = Accelerator(
split_batches=split_batches,
dispatch_batches=dispatch_batches,
gradient_accumulation_plugin=gradient_accumulation_plugin,
)
# Test that context manager behaves properly
model, opt, sched, dataloader, ddp_model, ddp_opt, ddp_sched = get_training_setup(accelerator, True)
for iteration, batch in enumerate(dataloader):
ddp_input, ddp_target = batch.values()
# Gather the distributed inputs and targs for the base model
input, target = accelerator.gather((ddp_input, ddp_target))
input, target = input.to(accelerator.device), target.to(accelerator.device)
# Perform our initial ground truth step in non "DDP"
model.train()
ddp_model.train()
step_model(model, input, target, accelerator, False)
opt.step()
if ((iteration + 1) % 2 == 0) or ((iteration + 1) == len(dataloader)) or sync_each_batch:
if split_batches:
sched.step()
else:
for _ in range(accelerator.num_processes):
sched.step()
# Perform gradient accumulation under wrapper
with accelerator.accumulate(ddp_model):
step_model(ddp_model, ddp_input, ddp_target, accelerator)
ddp_opt.step()
ddp_sched.step()
# Learning rates should be the same
assert (
opt.param_groups[0]["lr"] == ddp_opt.param_groups[0]["lr"]
), f'Learning rates found in each optimizer did not align\nopt: {opt.param_groups[0]["lr"]}\nDDP opt: {ddp_opt.param_groups[0]["lr"]}\n'
did_step = (((iteration + 1) % 2) == 0) or ((iteration + 1) == len(dataloader)) or sync_each_batch
if accelerator.num_processes > 1:
check_model_parameters(
model,
ddp_model,
did_step,
iteration,
rtol=1e-3, # somehow needs a relative tolerance
)
if ((iteration + 1) % 2 == 0) or ((iteration + 1) == len(dataloader)) or sync_each_batch:
opt.zero_grad() # needs to be guarded by logic as to when we should zero grads
ddp_opt.zero_grad()
# Shuffle ddp_input on each iteration
torch.manual_seed(1337 + iteration)
GradientState._reset_state()
def test_dataloader_break():
accelerator = Accelerator()
first_dset = RegressionDataset(length=80)
first_dataloader = DataLoader(first_dset, batch_size=16)
second_dset = RegressionDataset(length=96)
second_dataloader = DataLoader(second_dset, batch_size=16)
first_dataloader, second_dataloader = accelerator.prepare(first_dataloader, second_dataloader)
assert accelerator.gradient_state.active_dataloader is None
for iteration, _ in enumerate(first_dataloader):
assert id(accelerator.gradient_state.active_dataloader) == id(first_dataloader)
if iteration < len(first_dataloader) - 1:
assert not accelerator.gradient_state.end_of_dataloader
if iteration == 1:
for batch_num, _ in enumerate(second_dataloader):
assert id(accelerator.gradient_state.active_dataloader) == id(second_dataloader)
if batch_num < len(second_dataloader) - 1:
assert not accelerator.gradient_state.end_of_dataloader
else:
assert accelerator.gradient_state.end_of_dataloader
else:
assert accelerator.gradient_state.end_of_dataloader
assert accelerator.gradient_state.active_dataloader is None
def main():
accelerator = Accelerator()
state = accelerator.state
if state.local_process_index == 0:
print("**Test `accumulate` gradient accumulation with dataloader break**")
if state.distributed_type != DistributedType.XLA:
test_dataloader_break()
if state.distributed_type == DistributedType.NO:
if state.local_process_index == 0:
print("**Test NOOP `no_sync` context manager**")
test_noop_sync(accelerator)
if state.distributed_type in (
DistributedType.MULTI_GPU,
DistributedType.MULTI_NPU,
DistributedType.MULTI_MLU,
DistributedType.MULTI_CPU,
):
if state.local_process_index == 0:
print("**Test Distributed `no_sync` context manager**")
test_distributed_sync(accelerator)
if state.local_process_index == 0:
print("**Test Distributed `no_sync` context manager with multiple forwards**")
test_distributed_sync_multiple_fwd(accelerator)
if state.distributed_type in (DistributedType.MULTI_GPU, DistributedType.MULTI_NPU, DistributedType.MULTI_MLU):
for split_batch in [True, False]:
for dispatch_batches in [True, False]:
for sync_each_batch in [True, False]:
if state.local_process_index == 0:
print(
"**Test `accumulate` gradient accumulation, ",
f"`split_batches={split_batch}` and `dispatch_batches={dispatch_batches}` and `sync_each_batch={sync_each_batch}`**",
)
test_gradient_accumulation(split_batch, dispatch_batches, sync_each_batch)
# Currently will break on torch 2.0 +, need to investigate why
if state.local_process_index == 0:
print(
"**Test `accumulate` gradient accumulation with optimizer and scheduler, ",
"`split_batches=False`, `dispatch_batches=False`, `sync_each_batch=False`**",
)
test_gradient_accumulation_with_opt_and_scheduler()
if state.distributed_type in (DistributedType.MULTI_GPU, DistributedType.MULTI_NPU, DistributedType.MULTI_MLU):
for split_batch in [True, False]:
for dispatch_batches in [True, False]:
for sync_each_batch in [True, False]:
if not split_batch and not dispatch_batches and not sync_each_batch:
continue
if state.local_process_index == 0:
print(
"**Test `accumulate` gradient accumulation with optimizer and scheduler, ",
f"`split_batches={split_batch}` and `dispatch_batches={dispatch_batches}` and `sync_each_batch={sync_each_batch}`**",
)
test_gradient_accumulation_with_opt_and_scheduler(split_batch, dispatch_batches, sync_each_batch)
def _mp_fn(index):
# For xla_spawn (TPUs)
main()
if __name__ == "__main__":
main()
| accelerate/src/accelerate/test_utils/scripts/test_sync.py/0 | {
"file_path": "accelerate/src/accelerate/test_utils/scripts/test_sync.py",
"repo_id": "accelerate",
"token_count": 7772
} | 7 |
# Copyright 2022 The HuggingFace Team. All rights reserved.
#
# Licensed under the Apache License, Version 2.0 (the "License");
# you may not use this file except in compliance with the License.
# You may obtain a copy of the License at
#
# http://www.apache.org/licenses/LICENSE-2.0
#
# Unless required by applicable law or agreed to in writing, software
# distributed under the License is distributed on an "AS IS" BASIS,
# WITHOUT WARRANTIES OR CONDITIONS OF ANY KIND, either express or implied.
# See the License for the specific language governing permissions and
# limitations under the License.
import json
import os
from collections.abc import Mapping
from typing import Dict, List, Optional, Union
import numpy as np
import torch
from safetensors import safe_open
def offload_weight(weight, weight_name, offload_folder, index=None):
dtype = None
# Check the string instead of the dtype to be compatible with versions of PyTorch that don't have bfloat16.
if str(weight.dtype) == "torch.bfloat16":
# Need to reinterpret the underlined data as int16 since NumPy does not handle bfloat16s.
weight = weight.view(torch.int16)
dtype = "bfloat16"
array = weight.cpu().numpy()
tensor_file = os.path.join(offload_folder, f"{weight_name}.dat")
if index is not None:
if dtype is None:
dtype = str(array.dtype)
index[weight_name] = {"dtype": dtype, "shape": list(array.shape)}
if array.ndim == 0:
array = array[None]
file_array = np.memmap(tensor_file, dtype=array.dtype, mode="w+", shape=array.shape)
file_array[:] = array[:]
file_array.flush()
return index
def load_offloaded_weight(weight_file, weight_info):
shape = tuple(weight_info["shape"])
if shape == ():
# NumPy memory-mapped arrays can't have 0 dims so it was saved as 1d tensor
shape = (1,)
dtype = weight_info["dtype"]
if dtype == "bfloat16":
# NumPy does not support bfloat16 so this was saved as a int16
dtype = "int16"
weight = np.memmap(weight_file, dtype=dtype, shape=shape, mode="r")
if len(weight_info["shape"]) == 0:
weight = weight[0]
weight = torch.tensor(weight)
if weight_info["dtype"] == "bfloat16":
weight = weight.view(torch.bfloat16)
return weight
def save_offload_index(index, offload_folder):
if index is None or len(index) == 0:
# Nothing to save
return
offload_index_file = os.path.join(offload_folder, "index.json")
if os.path.isfile(offload_index_file):
with open(offload_index_file, encoding="utf-8") as f:
current_index = json.load(f)
else:
current_index = {}
current_index.update(index)
with open(offload_index_file, "w", encoding="utf-8") as f:
json.dump(current_index, f, indent=2)
def offload_state_dict(save_dir: Union[str, os.PathLike], state_dict: Dict[str, torch.Tensor]):
"""
Offload a state dict in a given folder.
Args:
save_dir (`str` or `os.PathLike`):
The directory in which to offload the state dict.
state_dict (`Dict[str, torch.Tensor]`):
The dictionary of tensors to offload.
"""
os.makedirs(save_dir, exist_ok=True)
index = {}
for name, parameter in state_dict.items():
index = offload_weight(parameter, name, save_dir, index=index)
# Update index
save_offload_index(index, save_dir)
class PrefixedDataset(Mapping):
"""
Will access keys in a given dataset by adding a prefix.
Args:
dataset (`Mapping`): Any map with string keys.
prefix (`str`): A prefix to add when trying to access any element in the underlying dataset.
"""
def __init__(self, dataset: Mapping, prefix: str):
self.dataset = dataset
self.prefix = prefix
def __getitem__(self, key):
return self.dataset[f"{self.prefix}{key}"]
def __iter__(self):
return iter([key for key in self.dataset if key.startswith(self.prefix)])
def __len__(self):
return len(self.dataset)
class OffloadedWeightsLoader(Mapping):
"""
A collection that loads weights stored in a given state dict or memory-mapped on disk.
Args:
state_dict (`Dict[str, torch.Tensor]`, *optional*):
A dictionary parameter name to tensor.
save_folder (`str` or `os.PathLike`, *optional*):
The directory in which the weights are stored (by `offload_state_dict` for instance).
index (`Dict`, *optional*):
A dictionary from weight name to their information (`dtype`/ `shape` or safetensors filename). Will default
to the index saved in `save_folder`.
"""
def __init__(
self,
state_dict: Dict[str, torch.Tensor] = None,
save_folder: Optional[Union[str, os.PathLike]] = None,
index: Mapping = None,
device=None,
):
if state_dict is None and save_folder is None and index is None:
raise ValueError("Need either a `state_dict`, a `save_folder` or an `index` containing offloaded weights.")
self.state_dict = {} if state_dict is None else state_dict
self.save_folder = save_folder
if index is None and save_folder is not None:
with open(os.path.join(save_folder, "index.json")) as f:
index = json.load(f)
self.index = {} if index is None else index
self.all_keys = list(self.state_dict.keys())
self.all_keys.extend([key for key in self.index if key not in self.all_keys])
self.device = device
def __getitem__(self, key: str):
# State dict gets priority
if key in self.state_dict:
return self.state_dict[key]
weight_info = self.index[key]
if weight_info.get("safetensors_file") is not None:
device = "cpu" if self.device is None else self.device
tensor = None
try:
with safe_open(weight_info["safetensors_file"], framework="pt", device=device) as f:
tensor = f.get_tensor(weight_info.get("weight_name", key))
except TypeError:
# if failed to get_tensor on the device, such as bf16 on mps, try to load it on CPU first
with safe_open(weight_info["safetensors_file"], framework="pt", device="cpu") as f:
tensor = f.get_tensor(weight_info.get("weight_name", key))
if "dtype" in weight_info:
tensor = tensor.to(getattr(torch, weight_info["dtype"]))
if tensor.device != torch.device(device):
tensor = tensor.to(device)
return tensor
weight_file = os.path.join(self.save_folder, f"{key}.dat")
return load_offloaded_weight(weight_file, weight_info)
def __iter__(self):
return iter(self.all_keys)
def __len__(self):
return len(self.all_keys)
def extract_submodules_state_dict(state_dict: Dict[str, torch.Tensor], submodule_names: List[str]):
"""
Extract the sub state-dict corresponding to a list of given submodules.
Args:
state_dict (`Dict[str, torch.Tensor]`): The state dict to extract from.
submodule_names (`List[str]`): The list of submodule names we want to extract.
"""
result = {}
for module_name in submodule_names:
# We want to catch module_name parameter (module_name.xxx) or potentially module_name, but not any of the
# submodules that could being like module_name (transformers.h.1 and transformers.h.10 for instance)
result.update(
{
key: param
for key, param in state_dict.items()
if key == module_name or key.startswith(module_name + ".")
}
)
return result
| accelerate/src/accelerate/utils/offload.py/0 | {
"file_path": "accelerate/src/accelerate/utils/offload.py",
"repo_id": "accelerate",
"token_count": 3177
} | 8 |
compute_environment: LOCAL_MACHINE
deepspeed_config: {}
distributed_type: 'NO'
fsdp_config: {}
machine_rank: 0
main_process_ip: null
main_process_port: null
main_training_function: main
mixed_precision: 'no'
num_machines: 1
num_processes: 1
use_cpu: false | accelerate/tests/test_configs/0_11_0.yaml/0 | {
"file_path": "accelerate/tests/test_configs/0_11_0.yaml",
"repo_id": "accelerate",
"token_count": 95
} | 9 |
# Copyright 2022 The HuggingFace Team. All rights reserved.
#
# Licensed under the Apache License, Version 2.0 (the "License");
# you may not use this file except in compliance with the License.
# You may obtain a copy of the License at
#
# http://www.apache.org/licenses/LICENSE-2.0
#
# Unless required by applicable law or agreed to in writing, software
# distributed under the License is distributed on an "AS IS" BASIS,
# WITHOUT WARRANTIES OR CONDITIONS OF ANY KIND, either express or implied.
# See the License for the specific language governing permissions and
# limitations under the License.
import os
import unittest
from tempfile import TemporaryDirectory
import torch
import torch.nn as nn
from accelerate.utils import (
OffloadedWeightsLoader,
extract_submodules_state_dict,
load_offloaded_weight,
offload_state_dict,
offload_weight,
)
class ModelForTest(nn.Module):
def __init__(self):
super().__init__()
self.linear1 = nn.Linear(3, 4)
self.batchnorm = nn.BatchNorm1d(4)
self.linear2 = nn.Linear(4, 5)
def forward(self, x):
return self.linear2(self.batchnorm(self.linear1(x)))
class OffloadTester(unittest.TestCase):
def test_offload_state_dict(self):
model = ModelForTest()
with TemporaryDirectory() as tmp_dir:
offload_state_dict(tmp_dir, model.state_dict())
index_file = os.path.join(tmp_dir, "index.json")
assert os.path.isfile(index_file)
# TODO: add tests on what is inside the index
for key in ["linear1.weight", "linear1.bias", "linear2.weight", "linear2.bias"]:
weight_file = os.path.join(tmp_dir, f"{key}.dat")
assert os.path.isfile(weight_file)
# TODO: add tests on the fact weights are properly loaded
def test_offload_weight(self):
dtypes = [torch.float16, torch.float32, torch.bfloat16]
for dtype in dtypes:
weight = torch.randn(2, 3, dtype=dtype)
with TemporaryDirectory() as tmp_dir:
index = offload_weight(weight, "weight", tmp_dir, {})
weight_file = os.path.join(tmp_dir, "weight.dat")
assert os.path.isfile(weight_file)
assert index == {"weight": {"shape": [2, 3], "dtype": str(dtype).split(".")[1]}}
new_weight = load_offloaded_weight(weight_file, index["weight"])
assert torch.equal(weight, new_weight)
def test_offload_weights_loader(self):
model = ModelForTest()
state_dict = model.state_dict()
cpu_part = {k: v for k, v in state_dict.items() if "linear2" not in k}
disk_part = {k: v for k, v in state_dict.items() if "linear2" in k}
with TemporaryDirectory() as tmp_dir:
offload_state_dict(tmp_dir, disk_part)
weight_map = OffloadedWeightsLoader(state_dict=cpu_part, save_folder=tmp_dir)
# Every key is there with the right value
assert sorted(weight_map) == sorted(state_dict.keys())
for key, param in state_dict.items():
assert torch.allclose(param, weight_map[key])
cpu_part = {k: v for k, v in state_dict.items() if "weight" in k}
disk_part = {k: v for k, v in state_dict.items() if "weight" not in k}
with TemporaryDirectory() as tmp_dir:
offload_state_dict(tmp_dir, disk_part)
weight_map = OffloadedWeightsLoader(state_dict=cpu_part, save_folder=tmp_dir)
# Every key is there with the right value
assert sorted(weight_map) == sorted(state_dict.keys())
for key, param in state_dict.items():
assert torch.allclose(param, weight_map[key])
with TemporaryDirectory() as tmp_dir:
offload_state_dict(tmp_dir, state_dict)
# Duplicates are removed
weight_map = OffloadedWeightsLoader(state_dict=cpu_part, save_folder=tmp_dir)
# Every key is there with the right value
assert sorted(weight_map) == sorted(state_dict.keys())
for key, param in state_dict.items():
assert torch.allclose(param, weight_map[key])
def test_extract_submodules_state_dict(self):
state_dict = {"a.1": 0, "a.10": 1, "a.2": 2}
extracted = extract_submodules_state_dict(state_dict, ["a.1", "a.2"])
assert extracted == {"a.1": 0, "a.2": 2}
state_dict = {"a.1.a": 0, "a.10.a": 1, "a.2.a": 2}
extracted = extract_submodules_state_dict(state_dict, ["a.1", "a.2"])
assert extracted == {"a.1.a": 0, "a.2.a": 2}
| accelerate/tests/test_offload.py/0 | {
"file_path": "accelerate/tests/test_offload.py",
"repo_id": "accelerate",
"token_count": 1981
} | 10 |
.PHONY: style quality
# make sure to test the local checkout in scripts and not the pre-installed one (don't use quotes!)
export PYTHONPATH = src
check_dirs := src tests scripts
style:
black --line-length 119 --target-version py310 $(check_dirs) setup.py
isort $(check_dirs) setup.py
quality:
black --check --line-length 119 --target-version py310 $(check_dirs) setup.py
isort --check-only $(check_dirs) setup.py
flake8 --max-line-length 119 $(check_dirs) setup.py
# Release stuff
pre-release:
python src/alignment/release.py
pre-patch:
python src/alignment/release.py --patch
post-release:
python src/alignment/release.py --post_release
post-patch:
python src/alignment/release.py --post_release --patch
wheels:
python setup.py bdist_wheel && python setup.py sdist
wheels_clean:
rm -rf build && rm -rf dist
pypi_upload:
python -m pip install twine
twine upload dist/* -r pypi
pypi_test_upload:
python -m pip install twine
twine upload dist/* -r pypitest --repository-url=https://test.pypi.org/legacy/
| alignment-handbook/Makefile/0 | {
"file_path": "alignment-handbook/Makefile",
"repo_id": "alignment-handbook",
"token_count": 363
} | 11 |
# Comparing Preference Alignment Algorithms
This directory contains various comparisons for three algorithms: DPO, IPO, and KTO. Each algorithm has been run in different hyperparameter configurations to study their performance. Two different models and datasets have been used to compare the performance of each algorithm:
- zephyr-beta-sft and Ultrafeedback
- OpenHermes-2.5 and the OpenOrca datasets
We release a collection containing the datasets and models used for these experiments, if you require the other trained models, we can release them on request.
You can find a longer decription of there results in our [blogpost](https://huggingface.co/blog/pref-tuning)
## Comparisons
For each algorithm, we aim to tune the beta parameter for a fixed learning rate. We vary beta from 0.1-0.9 in steps of 0.1, we have also found that in certain configurations a tiny value of beta, 0.01, can be effective. So we have included this smaller value in all our comparisons.
## Usage
The experiments can be launched with the following bash script:
```
#!/bin/bash
# Define an array containing the base configs we wish to fine tune
configs=("zephyr" "openhermes")
# Define an array of loss types
loss_types=("sigmoid" "kto_pair" "ipo")
# Define an array of beta values
betas=("0.01" "0.1" "0.2" "0.3" "0.4" "0.5" "0.6" "0.7" "0.8" "0.9")
# Outer loop for loss types
for config in "${configs[@]}"; do
for loss_type in "${loss_types[@]}"; do
# Inner loop for beta values
for beta in "${betas[@]}"; do
# Determine the job name and model revision based on loss type
job_name="$config_${loss_type}_beta_${beta}"
model_revision="${loss_type}-${beta}"
# Submit the job
sbatch --job-name=${job_name} recipes/launch.slurm pref_align_scan dpo $config deepspeed_zero3 \
"--beta=${beta} --loss_type=${loss_type} --output_dir=data/$config-7b-align-scan-${loss_type}-beta-${beta} --hub_model_revision=${model_revision}"
done
done
done
```
| alignment-handbook/recipes/pref_align_scan/README.md/0 | {
"file_path": "alignment-handbook/recipes/pref_align_scan/README.md",
"repo_id": "alignment-handbook",
"token_count": 690
} | 12 |
#!/usr/bin/env python
# coding=utf-8
# Copyright 2023 The HuggingFace Inc. team. All rights reserved.
#
# Licensed under the Apache License, Version 2.0 (the "License");
# you may not use this file except in compliance with the License.
# You may obtain a copy of the License at
#
# http://www.apache.org/licenses/LICENSE-2.0
#
# Unless required by applicable law or agreed to in writing, software
# distributed under the License is distributed on an "AS IS" BASIS,
# WITHOUT WARRANTIES OR CONDITIONS OF ANY KIND, either express or implied.
# See the License for the specific language governing permissions and
# limitations under the License.
"""
Continued pretraining script for decoder language models.
"""
import logging
import random
import sys
import datasets
import torch
import transformers
from transformers import set_seed
from alignment import (
DataArguments,
H4ArgumentParser,
ModelArguments,
SFTConfig,
get_checkpoint,
get_datasets,
get_kbit_device_map,
get_peft_config,
get_quantization_config,
get_tokenizer,
)
from trl import SFTTrainer
logger = logging.getLogger(__name__)
def main():
parser = H4ArgumentParser((ModelArguments, DataArguments, SFTConfig))
model_args, data_args, training_args = parser.parse()
# Set seed for reproducibility
set_seed(training_args.seed)
###############
# Setup logging
###############
logging.basicConfig(
format="%(asctime)s - %(levelname)s - %(name)s - %(message)s",
datefmt="%Y-%m-%d %H:%M:%S",
handlers=[logging.StreamHandler(sys.stdout)],
)
log_level = training_args.get_process_log_level()
logger.setLevel(log_level)
datasets.utils.logging.set_verbosity(log_level)
transformers.utils.logging.set_verbosity(log_level)
transformers.utils.logging.enable_default_handler()
transformers.utils.logging.enable_explicit_format()
# Log on each process a small summary
logger.warning(
f"Process rank: {training_args.local_rank}, device: {training_args.device}, n_gpu: {training_args.n_gpu}"
+ f" distributed training: {bool(training_args.local_rank != -1)}, 16-bits training: {training_args.fp16}"
)
logger.info(f"Model parameters {model_args}")
logger.info(f"Data parameters {data_args}")
logger.info(f"Training/evaluation parameters {training_args}")
# Check for last checkpoint
last_checkpoint = get_checkpoint(training_args)
if last_checkpoint is not None and training_args.resume_from_checkpoint is None:
logger.info(f"Checkpoint detected, resuming training at {last_checkpoint=}.")
###############
# Load datasets
###############
raw_datasets = get_datasets(data_args, splits=data_args.dataset_splits, configs=data_args.dataset_configs)
logger.info(
f"Training on the following datasets and their proportions:"
f" {[split + ' : ' + str(dset.num_rows) for split, dset in raw_datasets.items()]}"
)
train_dataset = raw_datasets["train"] if "train" in raw_datasets else None
eval_dataset = raw_datasets["test"] if "test" in raw_datasets else None
if train_dataset is None:
raise ValueError(
"Training set must be included (so make sure that your dataset has a split with" " 'train' in the name)."
)
if training_args.do_eval and eval_dataset is None:
raise ValueError("'--do_eval' enabled so make sure that your dataset has a split with 'test' in the name.")
################
# Load tokenizer
################
tokenizer = get_tokenizer(model_args, data_args, auto_set_chat_template=False)
with training_args.main_process_first(desc="Log a few random samples from the processed training set"):
for index in random.sample(range(len(raw_datasets["train"])), 3):
logger.info(f"Sample {index} of the processed training set:\n\n{raw_datasets['train'][index]['text']}")
#######################
# Load pretrained model
#######################
logger.info("*** Load pretrained model ***")
torch_dtype = (
model_args.torch_dtype if model_args.torch_dtype in ["auto", None] else getattr(torch, model_args.torch_dtype)
)
quantization_config = get_quantization_config(model_args)
model_kwargs = dict(
revision=model_args.model_revision,
trust_remote_code=model_args.trust_remote_code,
use_flash_attention_2=model_args.use_flash_attention_2,
torch_dtype=torch_dtype,
use_cache=False if training_args.gradient_checkpointing else True,
device_map=get_kbit_device_map() if quantization_config is not None else None,
quantization_config=quantization_config,
)
########################
# Initialize the Trainer
########################
trainer = SFTTrainer(
model=model_args.model_name_or_path,
model_init_kwargs=model_kwargs,
args=training_args,
train_dataset=train_dataset,
eval_dataset=eval_dataset,
dataset_text_field=data_args.text_column,
max_seq_length=training_args.max_seq_length,
tokenizer=tokenizer,
packing=True,
peft_config=get_peft_config(model_args),
dataset_kwargs=training_args.dataset_kwargs,
)
###############
# Training loop
###############
logger.info("*** Train ***")
checkpoint = None
if training_args.resume_from_checkpoint is not None:
checkpoint = training_args.resume_from_checkpoint
elif last_checkpoint is not None:
checkpoint = last_checkpoint
train_result = trainer.train(resume_from_checkpoint=checkpoint)
metrics = train_result.metrics
metrics["train_samples"] = len(train_dataset)
trainer.log_metrics("train", metrics)
trainer.save_metrics("train", metrics)
trainer.save_state()
##################################
# Save model and create model card
##################################
logger.info("*** Save model ***")
trainer.save_model(training_args.output_dir)
logger.info(f"Model saved to {training_args.output_dir}")
# Save everything else on main process
kwargs = {
"finetuned_from": model_args.model_name_or_path,
"dataset": list(data_args.dataset_mixer.keys()),
"dataset_tags": list(data_args.dataset_mixer.keys()),
"tags": ["alignment-handbook"],
}
if trainer.accelerator.is_main_process:
trainer.create_model_card(**kwargs)
# Restore k,v cache for fast inference
trainer.model.config.use_cache = True
trainer.model.config.save_pretrained(training_args.output_dir)
##########
# Evaluate
##########
if training_args.do_eval:
logger.info("*** Evaluate ***")
metrics = trainer.evaluate()
metrics["eval_samples"] = len(eval_dataset)
trainer.log_metrics("eval", metrics)
trainer.save_metrics("eval", metrics)
if training_args.push_to_hub is True:
logger.info("Pushing to hub...")
trainer.push_to_hub(**kwargs)
logger.info("*** Training complete ***")
if __name__ == "__main__":
main()
| alignment-handbook/scripts/run_cpt.py/0 | {
"file_path": "alignment-handbook/scripts/run_cpt.py",
"repo_id": "alignment-handbook",
"token_count": 2700
} | 13 |
# coding=utf-8
# Copyright 2024 The HuggingFace Team. All rights reserved.
#
# Licensed under the Apache License, Version 2.0 (the "License");
# you may not use this file except in compliance with the License.
# You may obtain a copy of the License at
#
# http://www.apache.org/licenses/LICENSE-2.0
#
# Unless required by applicable law or agreed to in writing, software
# distributed under the License is distributed on an "AS IS" BASIS,
# WITHOUT WARRANTIES OR CONDITIONS OF ANY KIND, either express or implied.
# See the License for the specific language governing permissions and
# limitations under the License.
from unittest import TestCase
from datasets import Dataset
from transformers import AutoTokenizer
from alignment import apply_chat_template, decontaminate_humaneval
class DecontamintateHumanEvalTest(TestCase):
"""Test we decontaminate HumanEval samples correctly"""
def setUp(self) -> None:
# Create a dataset with a HumanEval sample wrapped in some fake text
dataset = Dataset.from_dict(
{
"messages": [
[{"content": "Hello", "role": "user"}],
[
{
"content": 'Hello, I am\nfrom\n\n typing import List\n\n\ndef has_close_elements(numbers: List[float], threshold: float) -> bool:\n """ Check if in given list of numbers, are any two numbers closer to each other than\n given threshold.\n >>> has_close_elements([1.0, 2.0, 3.0], 0.5)\n False\n >>> has_close_elements([1.0, 2.8, 3.0, 4.0, 5.0, 2.0], 0.3)\n True\n """\n',
"role": "assistant",
}
],
]
}
)
tokenizer = AutoTokenizer.from_pretrained("HuggingFaceH4/zephyr-7b-beta")
self.dataset = dataset.map(apply_chat_template, fn_kwargs={"tokenizer": tokenizer, "task": "sft"})
def test_decontamination(self):
"""Test we decontaminate HumanEval samples correctly"""
decontaminated_dataset = self.dataset.filter(decontaminate_humaneval, batched=True)
# Check we recover just the first message
self.assertEqual(decontaminated_dataset[0]["text"], self.dataset[0]["text"])
| alignment-handbook/tests/test_decontaminate.py/0 | {
"file_path": "alignment-handbook/tests/test_decontaminate.py",
"repo_id": "alignment-handbook",
"token_count": 926
} | 14 |
[book]
authors = ["Nicolas Patry"]
language = "en"
multilingual = false
src = "src"
title = "Candle Documentation"
| candle/candle-book/book.toml/0 | {
"file_path": "candle/candle-book/book.toml",
"repo_id": "candle",
"token_count": 38
} | 15 |
# Advanced Cuda usage
| candle/candle-book/src/inference/cuda/README.md/0 | {
"file_path": "candle/candle-book/src/inference/cuda/README.md",
"repo_id": "candle",
"token_count": 6
} | 16 |
use crate::benchmarks::{BenchDevice, BenchDeviceHandler};
use candle_core::{DType, Device, Tensor};
use criterion::{black_box, criterion_group, Criterion, Throughput};
use std::time::Instant;
fn run(a: &Tensor) {
a.affine(12.34, 56.78).unwrap();
}
fn run_affine_benchmark(c: &mut Criterion, device: &Device, dtype: DType, name: &str) {
let b = 1;
let m = 1024;
let k = 1024;
let tensor = Tensor::zeros((b, m, k), dtype, &device).unwrap();
let flops = b * m * k * dtype.size_in_bytes();
let mut group = c.benchmark_group(device.bench_name(name));
group.throughput(Throughput::Bytes(flops as u64));
group.bench_function("iter", move |b| {
b.iter_custom(|iters| {
let start = Instant::now();
for _i in 0..iters {
run(black_box(&tensor));
}
device.sync().unwrap();
start.elapsed()
})
});
group.finish();
}
fn criterion_benchmark(c: &mut Criterion) {
let handler = BenchDeviceHandler::new().unwrap();
for device in handler.devices {
run_affine_benchmark(c, &device, DType::F32, "affine_f32");
run_affine_benchmark(c, &device, DType::F16, "affine_f16");
run_affine_benchmark(c, &device, DType::BF16, "affine_bf16");
}
}
criterion_group!(benches, criterion_benchmark);
| candle/candle-core/benches/benchmarks/affine.rs/0 | {
"file_path": "candle/candle-core/benches/benchmarks/affine.rs",
"repo_id": "candle",
"token_count": 591
} | 17 |
pub trait VecOps: num_traits::NumAssign + Copy {
fn min(self, rhs: Self) -> Self;
fn max(self, rhs: Self) -> Self;
/// Dot-product of two vectors.
///
/// # Safety
///
/// The length of `lhs` and `rhs` have to be at least `len`. `res` has to point to a valid
/// element.
#[inline(always)]
unsafe fn vec_dot(lhs: *const Self, rhs: *const Self, res: *mut Self, len: usize) {
*res = Self::zero();
for i in 0..len {
*res += *lhs.add(i) * *rhs.add(i)
}
}
/// Sum of all elements in a vector.
///
/// # Safety
///
/// The length of `xs` must be at least `len`. `res` has to point to a valid
/// element.
#[inline(always)]
unsafe fn vec_reduce_sum(xs: *const Self, res: *mut Self, len: usize) {
*res = Self::zero();
for i in 0..len {
*res += *xs.add(i)
}
}
/// Maximum element in a non-empty vector.
///
/// # Safety
///
/// The length of `xs` must be at least `len` and positive. `res` has to point to a valid
/// element.
#[inline(always)]
unsafe fn vec_reduce_max(xs: *const Self, res: *mut Self, len: usize) {
*res = *xs;
for i in 1..len {
*res = (*res).max(*xs.add(i))
}
}
/// Minimum element in a non-empty vector.
///
/// # Safety
///
/// The length of `xs` must be at least `len` and positive. `res` has to point to a valid
/// element.
#[inline(always)]
unsafe fn vec_reduce_min(xs: *const Self, res: *mut Self, len: usize) {
*res = *xs;
for i in 1..len {
*res = (*res).min(*xs.add(i))
}
}
}
impl VecOps for f32 {
#[inline(always)]
fn min(self, other: Self) -> Self {
Self::min(self, other)
}
#[inline(always)]
fn max(self, other: Self) -> Self {
Self::max(self, other)
}
#[inline(always)]
unsafe fn vec_dot(lhs: *const Self, rhs: *const Self, res: *mut Self, len: usize) {
super::vec_dot_f32(lhs, rhs, res, len)
}
#[inline(always)]
unsafe fn vec_reduce_sum(xs: *const Self, res: *mut Self, len: usize) {
super::vec_sum(xs, res, len)
}
}
impl VecOps for half::f16 {
#[inline(always)]
fn min(self, other: Self) -> Self {
Self::min(self, other)
}
#[inline(always)]
fn max(self, other: Self) -> Self {
Self::max(self, other)
}
#[inline(always)]
unsafe fn vec_dot(lhs: *const Self, rhs: *const Self, res: *mut Self, len: usize) {
let mut res_f32 = 0f32;
super::vec_dot_f16(lhs, rhs, &mut res_f32, len);
*res = half::f16::from_f32(res_f32);
}
}
impl VecOps for f64 {
#[inline(always)]
fn min(self, other: Self) -> Self {
Self::min(self, other)
}
#[inline(always)]
fn max(self, other: Self) -> Self {
Self::max(self, other)
}
}
impl VecOps for half::bf16 {
#[inline(always)]
fn min(self, other: Self) -> Self {
Self::min(self, other)
}
#[inline(always)]
fn max(self, other: Self) -> Self {
Self::max(self, other)
}
}
impl VecOps for u8 {
#[inline(always)]
fn min(self, other: Self) -> Self {
<Self as Ord>::min(self, other)
}
#[inline(always)]
fn max(self, other: Self) -> Self {
<Self as Ord>::max(self, other)
}
}
impl VecOps for u32 {
#[inline(always)]
fn min(self, other: Self) -> Self {
<Self as Ord>::min(self, other)
}
#[inline(always)]
fn max(self, other: Self) -> Self {
<Self as Ord>::max(self, other)
}
}
impl VecOps for i64 {
#[inline(always)]
fn min(self, other: Self) -> Self {
<Self as Ord>::min(self, other)
}
#[inline(always)]
fn max(self, other: Self) -> Self {
<Self as Ord>::max(self, other)
}
}
#[inline(always)]
pub fn par_for_each(n_threads: usize, func: impl Fn(usize) + Send + Sync) {
if n_threads == 1 {
func(0)
} else {
rayon::scope(|s| {
for thread_idx in 0..n_threads {
let func = &func;
s.spawn(move |_| func(thread_idx));
}
})
}
}
#[inline(always)]
pub fn par_range(lo: usize, up: usize, n_threads: usize, func: impl Fn(usize) + Send + Sync) {
if n_threads == 1 {
for i in lo..up {
func(i)
}
} else {
rayon::scope(|s| {
for thread_idx in 0..n_threads {
let func = &func;
s.spawn(move |_| {
for i in (thread_idx..up).step_by(n_threads) {
func(i)
}
});
}
})
}
}
| candle/candle-core/src/cpu/kernels.rs/0 | {
"file_path": "candle/candle-core/src/cpu/kernels.rs",
"repo_id": "candle",
"token_count": 2328
} | 18 |
use crate::backend::{BackendDevice, BackendStorage};
use crate::conv::{ParamsConv1D, ParamsConv2D, ParamsConvTranspose1D, ParamsConvTranspose2D};
use crate::op::{BinaryOpT, CmpOp, ReduceOp, UnaryOpT};
use crate::{CpuStorage, DType, Layout, Result, Shape};
use candle_metal_kernels;
use candle_metal_kernels::Kernels;
use metal;
use metal::{Buffer, CommandBuffer, CommandQueue, MTLResourceOptions, NSUInteger};
use std::collections::HashMap;
use std::ffi::c_void;
use std::path::Path;
use std::sync::{Arc, Mutex, RwLock, RwLockWriteGuard, TryLockError};
/// Simple way to catch lock error without
/// depending on T
#[derive(thiserror::Error, Debug)]
pub enum LockError {
#[error("{0}")]
Poisoned(String),
#[error("Would block")]
WouldBlock,
}
impl<T> From<TryLockError<T>> for MetalError {
fn from(value: TryLockError<T>) -> Self {
match value {
TryLockError::Poisoned(p) => MetalError::LockError(LockError::Poisoned(p.to_string())),
TryLockError::WouldBlock => MetalError::LockError(LockError::WouldBlock),
}
}
}
/// Metal related errors
#[derive(thiserror::Error, Debug)]
pub enum MetalError {
#[error("{0}")]
Message(String),
#[error(transparent)]
KernelError(#[from] candle_metal_kernels::MetalKernelError),
#[error("matmul is only supported for contiguous tensors lstride: {lhs_stride:?} rstride: {rhs_stride:?} mnk: {mnk:?}")]
MatMulNonContiguous {
lhs_stride: Vec<usize>,
rhs_stride: Vec<usize>,
mnk: (usize, usize, usize),
},
#[error("{0:?}")]
LockError(LockError),
#[error("{msg}, expected: {expected:?}, got: {got:?}")]
UnexpectedDType {
msg: &'static str,
expected: DType,
got: DType,
},
}
impl From<String> for MetalError {
fn from(e: String) -> Self {
MetalError::Message(e)
}
}
type BufferMap = HashMap<(NSUInteger, MTLResourceOptions), Vec<Arc<Buffer>>>;
type AllocatedBuffers = Arc<RwLock<BufferMap>>;
#[derive(Clone)]
pub struct MetalDevice {
/// Raw metal device: <https://developer.apple.com/documentation/metal/mtldevice?language=objc>
device: metal::Device,
/// Single command queue for the entire device.
command_queue: CommandQueue,
/// One command buffer at a time.
/// The scheduler works by allowing multiple
/// [ComputeCommandEncoder](https://developer.apple.com/documentation/metal/mtlcomputecommandencoder?language=objc)
/// on a single command buffer. Using a single command buffer would be fastest on the GPU but
/// prevents overlapping of CPU and GPU commands (because command buffer needs to be committed
/// to start to work).
/// Despite what the documentation says, command buffers are NOT ordered. They are ordered
/// for their START time, but there's no guarantee that command buffer1 will finish before
/// command buffer2 starts (or there are metal bugs there)
command_buffer: Arc<RwLock<CommandBuffer>>,
/// Keeps track of the current amount of compute command encoders on the current
/// command buffer
/// Arc, RwLock because of the interior mutability.
command_buffer_index: Arc<RwLock<usize>>,
/// The maximum amount of [compute command encoder](https://developer.apple.com/documentation/metal/mtlcomputecommandencoder?language=objc) per [command buffer](https://developer.apple.com/documentation/metal/mtlcommandbuffer?language=objc)
compute_per_buffer: usize,
/// Simple keeper struct to keep track of the already compiled kernels so we can reuse them.
/// Heavily used by [`candle_metal_kernels`]
kernels: Arc<Kernels>,
/// Simple allocator struct.
/// The buffers are stored in size buckets since ML tends to use similar shapes over and over.
/// We store the buffers in [`Arc`] because it's much faster than Obj-c internal ref counting
/// (could be linked to FFI communication overhead).
///
/// Whenever a buffer has a strong_count==1, we can reuse it, it means it was dropped in the
/// graph calculation, and only we the allocator kept a reference to it, therefore it's free
/// to be reused. However, in order for this to work, we need to guarantee the order of
/// operation, so that this buffer is not being used by another kernel at the same time.
/// Arc is the CPU reference count, it doesn't mean anything on the GPU side of things.
///
/// Whenever we actually allocate a new buffer, we make a full sweep to clean up unused buffers
/// (strong_count = 1).
buffers: AllocatedBuffers,
/// Seed for random number generation.
seed: Arc<Mutex<Buffer>>,
}
impl std::fmt::Debug for MetalDevice {
fn fmt(&self, f: &mut std::fmt::Formatter<'_>) -> std::fmt::Result {
write!(f, "MetalDevice({:?})", self.device.registry_id())
}
}
impl std::ops::Deref for MetalDevice {
type Target = metal::DeviceRef;
fn deref(&self) -> &Self::Target {
&self.device
}
}
impl MetalDevice {
pub fn id(&self) -> NSUInteger {
self.registry_id()
}
pub fn metal_device(&self) -> &metal::Device {
&self.device
}
pub fn command_queue(&self) -> &CommandQueue {
&self.command_queue
}
pub fn command_buffer(&self) -> Result<CommandBuffer> {
let mut command_buffer_lock = self.command_buffer.try_write().map_err(MetalError::from)?;
let mut command_buffer = command_buffer_lock.to_owned();
let mut index = self
.command_buffer_index
.try_write()
.map_err(MetalError::from)?;
if *index > self.compute_per_buffer {
command_buffer.commit();
command_buffer = self.command_queue.new_command_buffer().to_owned();
*command_buffer_lock = command_buffer.clone();
*index = 0;
self.drop_unused_buffers()?;
}
*index += 1;
Ok(command_buffer)
}
pub fn wait_until_completed(&self) -> Result<()> {
let mut command_buffer = self.command_buffer.try_write().map_err(MetalError::from)?;
match command_buffer.status() {
metal::MTLCommandBufferStatus::Committed
| metal::MTLCommandBufferStatus::Scheduled
| metal::MTLCommandBufferStatus::Completed => {
panic!("Already committed");
}
_ => {}
}
command_buffer.commit();
command_buffer.wait_until_completed();
*command_buffer = self.command_queue.new_command_buffer().to_owned();
Ok(())
}
pub fn kernels(&self) -> &Kernels {
&self.kernels
}
pub fn device(&self) -> &metal::Device {
&self.device
}
/// Creates a new buffer (not necessarily zeroed).
/// The buffer is [MTLPrivate](https://developer.apple.com/documentation/metal/mtlstoragemode)
/// This means the buffer data cannot be read on the CPU directly.
///
/// [`name`] is only used to keep track of the resource origin in case of bugs
pub fn new_buffer(
&self,
element_count: usize,
dtype: DType,
name: &str,
) -> Result<Arc<Buffer>> {
let size = (element_count * dtype.size_in_bytes()) as NSUInteger;
self.allocate_buffer(size, MTLResourceOptions::StorageModePrivate, name)
}
/// Creates a new buffer (not necessarily zeroed).
/// The buffer is [MTLManaged](https://developer.apple.com/documentation/metal/mtlstoragemode)
/// This means the buffer can be read on the CPU but will require manual
/// synchronization when the CPU memory is modified
/// Used as a bridge to gather data back from the GPU
pub fn new_buffer_managed(&self, size: NSUInteger) -> Result<Arc<Buffer>> {
self.allocate_buffer(size, MTLResourceOptions::StorageModeManaged, "managed")
}
/// Creates a new buffer from data.
/// The buffer is [MTLManaged](https://developer.apple.com/documentation/metal/mtlstoragemode)
///
/// Does not require synchronization, as [newBufferWithBytes](https://developer.apple.com/documentation/metal/mtldevice/1433429-newbufferwithbytes)
/// allocates the buffer and copies over the existing data before returning the MTLBuffer.
pub fn new_buffer_with_data<T>(&self, data: &[T]) -> Result<Arc<Buffer>> {
let size = core::mem::size_of_val(data) as NSUInteger;
let new_buffer = self.device.new_buffer_with_data(
data.as_ptr() as *const c_void,
size,
MTLResourceOptions::StorageModeManaged,
);
let mut buffers = self.buffers.try_write().map_err(MetalError::from)?;
let subbuffers = buffers
.entry((size, MTLResourceOptions::StorageModeManaged))
.or_insert(vec![]);
let new_buffer = Arc::new(new_buffer);
subbuffers.push(new_buffer.clone());
Ok(new_buffer)
}
pub fn allocate_zeros(&self, size_in_bytes: usize) -> Result<Arc<Buffer>> {
let buffer = self.allocate_buffer(
size_in_bytes as NSUInteger,
MTLResourceOptions::StorageModePrivate,
"allocate_zeros",
)?;
let command_buffer = self.command_buffer()?;
command_buffer.set_label("zeros");
let blit = command_buffer.new_blit_command_encoder();
blit.fill_buffer(
&buffer,
metal::NSRange {
location: 0,
length: buffer.length(),
},
0,
);
blit.end_encoding();
Ok(buffer)
}
fn find_available_buffer(
&self,
size: NSUInteger,
option: MTLResourceOptions,
buffers: &RwLockWriteGuard<BufferMap>,
) -> Option<Arc<Buffer>> {
let mut best_buffer: Option<&Arc<Buffer>> = None;
let mut best_buffer_size: NSUInteger = NSUInteger::MAX;
for ((buffer_size, buffer_option), subbuffers) in buffers.iter() {
if buffer_size >= &size && buffer_size < &best_buffer_size && buffer_option == &option {
for sub in subbuffers {
if Arc::strong_count(sub) == 1 {
best_buffer = Some(sub);
best_buffer_size = *buffer_size;
}
}
}
}
best_buffer.cloned()
}
fn drop_unused_buffers(&self) -> Result<()> {
let mut buffers = self.buffers.try_write().map_err(MetalError::from)?;
for subbuffers in buffers.values_mut() {
let newbuffers = subbuffers
.iter()
.filter(|s| Arc::strong_count(*s) > 1)
.map(Arc::clone)
.collect();
*subbuffers = newbuffers;
}
Ok(())
}
/// The critical allocator algorithm
fn allocate_buffer(
&self,
size: NSUInteger,
option: MTLResourceOptions,
_name: &str,
) -> Result<Arc<Buffer>> {
let mut buffers = self.buffers.try_write().map_err(MetalError::from)?;
if let Some(b) = self.find_available_buffer(size, option, &buffers) {
// Cloning also ensures we increment the strong count
return Ok(b.clone());
}
let size = buf_size(size);
let subbuffers = buffers.entry((size, option)).or_insert(vec![]);
let new_buffer = self.device.new_buffer(size as NSUInteger, option);
let new_buffer = Arc::new(new_buffer);
subbuffers.push(new_buffer.clone());
Ok(new_buffer)
}
/// Create a metal GPU capture trace on [`path`].
pub fn capture<P: AsRef<Path>>(&self, path: P) -> Result<()> {
let capture = metal::CaptureManager::shared();
let descriptor = metal::CaptureDescriptor::new();
descriptor.set_destination(metal::MTLCaptureDestination::GpuTraceDocument);
descriptor.set_capture_device(self);
descriptor.set_output_url(path);
capture
.start_capture(&descriptor)
.map_err(MetalError::from)?;
Ok(())
}
}
#[derive(Debug, Clone)]
pub struct MetalStorage {
/// The actual buffer containing the data.
buffer: Arc<metal::Buffer>,
/// a reference to the device owning this buffer
device: MetalDevice,
/// The count of allocated elements in the buffer
count: usize,
/// The dtype is kept since buffers are untyped.
dtype: DType,
}
impl BackendStorage for MetalStorage {
type Device = MetalDevice;
fn try_clone(&self, _: &Layout) -> Result<Self> {
Ok(self.clone())
}
fn dtype(&self) -> DType {
self.dtype
}
fn device(&self) -> &Self::Device {
&self.device
}
fn to_cpu_storage(&self) -> Result<CpuStorage> {
match self.dtype {
DType::U8 => Ok(CpuStorage::U8(self.to_cpu()?)),
DType::U32 => Ok(CpuStorage::U32(self.to_cpu()?)),
DType::I64 => Ok(CpuStorage::I64(self.to_cpu()?)),
DType::F16 => Ok(CpuStorage::F16(self.to_cpu()?)),
DType::BF16 => Ok(CpuStorage::BF16(self.to_cpu()?)),
DType::F32 => Ok(CpuStorage::F32(self.to_cpu()?)),
DType::F64 => Ok(CpuStorage::F64(self.to_cpu()?)),
}
}
fn affine(&self, layout: &Layout, mul: f64, add: f64) -> Result<Self> {
let device = self.device().clone();
let shape = layout.shape();
let el = shape.elem_count();
let dtype = self.dtype;
let buffer = device.new_buffer(el, self.dtype, "affine")?;
let command_buffer = self.device.command_buffer()?;
if layout.is_contiguous() && layout.start_offset() == 0 {
let name = match self.dtype {
DType::F32 => "affine_f32",
DType::F16 => "affine_f16",
DType::BF16 => "affine_bf16",
dtype => crate::bail!("Metal contiguous affine {dtype:?} not implemented"),
};
candle_metal_kernels::call_affine(
&device.device,
&command_buffer,
&device.kernels,
name,
el,
&self.buffer,
&buffer,
mul as f32,
add as f32,
)
.map_err(MetalError::from)?;
} else {
let name = match self.dtype {
DType::F32 => "affine_f32_strided",
DType::F16 => "affine_f16_strided",
DType::BF16 => "affine_bf16_strided",
dtype => crate::bail!("Metal strided affine {dtype:?} not implemented"),
};
candle_metal_kernels::call_affine_strided(
&device.device,
&command_buffer,
&device.kernels,
name,
layout.dims(),
&self.buffer,
layout.stride(),
layout.start_offset() * dtype.size_in_bytes(),
&buffer,
mul as f32,
add as f32,
)
.map_err(MetalError::from)?;
}
Ok(Self::new(buffer, device.clone(), el, dtype))
}
fn powf(&self, layout: &Layout, pow: f64) -> Result<Self> {
let device = self.device().clone();
let shape = layout.shape();
let el = shape.elem_count();
let dtype = self.dtype;
let buffer = device.new_buffer(el, self.dtype, "powf")?;
let command_buffer = self.device.command_buffer()?;
if layout.is_contiguous() && layout.start_offset() == 0 {
let name = match self.dtype {
DType::F32 => "powf_f32",
DType::F16 => "powf_f16",
DType::BF16 => "powf_bf16",
dtype => crate::bail!("Metal contiguous powf {dtype:?} not implemented"),
};
candle_metal_kernels::call_powf(
&device.device,
&command_buffer,
&device.kernels,
name,
el,
&self.buffer,
&buffer,
pow as f32,
)
.map_err(MetalError::from)?;
} else {
let name = match self.dtype {
DType::F32 => "powf_f32_strided",
DType::F16 => "powf_f16_strided",
DType::BF16 => "powf_bf16_strided",
dtype => crate::bail!("Metal strided powf {dtype:?} not implemented"),
};
candle_metal_kernels::call_powf_strided(
&device.device,
&command_buffer,
&device.kernels,
name,
layout.dims(),
&self.buffer,
layout.stride(),
layout.start_offset() * dtype.size_in_bytes(),
&buffer,
pow as f32,
)
.map_err(MetalError::from)?;
}
Ok(Self::new(buffer, device.clone(), el, dtype))
}
fn elu(&self, layout: &Layout, alpha: f64) -> Result<Self> {
let device = self.device().clone();
let shape = layout.shape();
let el = shape.elem_count();
let dtype = self.dtype;
let buffer = device.new_buffer(el, self.dtype, "elu")?;
let command_buffer = self.device.command_buffer()?;
if layout.is_contiguous() && layout.start_offset() == 0 {
let name = match self.dtype {
DType::F32 => "elu_f32",
DType::F16 => "elu_f16",
DType::BF16 => "elu_bf16",
dtype => crate::bail!("Metal contiguous elu {dtype:?} not implemented"),
};
candle_metal_kernels::call_elu(
&device.device,
&command_buffer,
&device.kernels,
name,
el,
&self.buffer,
&buffer,
alpha as f32,
)
.map_err(MetalError::from)?;
} else {
let name = match self.dtype {
DType::F32 => "elu_f32_strided",
DType::F16 => "elu_f16_strided",
DType::BF16 => "elu_bf16_strided",
dtype => crate::bail!("Metal strided elu {dtype:?} not implemented"),
};
candle_metal_kernels::call_elu_strided(
&device.device,
&command_buffer,
&device.kernels,
name,
layout.dims(),
&self.buffer,
layout.stride(),
layout.start_offset() * dtype.size_in_bytes(),
&buffer,
alpha as f32,
)
.map_err(MetalError::from)?;
}
Ok(Self::new(buffer, device.clone(), el, dtype))
}
fn reduce_op(&self, op: ReduceOp, layout: &Layout, sum_dims: &[usize]) -> Result<Self> {
let device = self.device.clone();
let src_stride = layout.stride();
let src_dims = layout.shape().dims();
// Source dims and strides with the sum dims at the end.
let mut dims = vec![];
let mut stride = vec![];
let mut dst_el: usize = 1;
for (dim_idx, &d) in src_dims.iter().enumerate() {
if !sum_dims.contains(&dim_idx) {
dst_el *= d;
dims.push(d);
stride.push(src_stride[dim_idx]);
}
}
for &dim_idx in sum_dims.iter() {
dims.push(src_dims[dim_idx]);
stride.push(src_stride[dim_idx]);
}
// The reduction loop requires the shared array to be properly initialized and for
// this we want the number of threads to be a power of two.
let (name, check_empty, return_index) = match (op, self.dtype) {
(ReduceOp::Sum, DType::F32) => ("fast_sum_f32_strided", false, false),
(ReduceOp::Min, DType::F32) => ("fast_min_f32_strided", true, false),
(ReduceOp::Max, DType::F32) => ("fast_max_f32_strided", true, false),
(ReduceOp::ArgMin, DType::F32) => ("fast_argmin_f32_strided", true, true),
(ReduceOp::ArgMax, DType::F32) => ("fast_argmax_f32_strided", true, true),
(ReduceOp::Sum, DType::U32) => ("fast_sum_u32_strided", false, false),
(ReduceOp::Min, DType::U32) => ("fast_min_u32_strided", true, false),
(ReduceOp::Max, DType::U32) => ("fast_max_u32_strided", true, false),
(ReduceOp::ArgMin, DType::U32) => ("fast_argmin_u32_strided", true, true),
(ReduceOp::ArgMax, DType::U32) => ("fast_argmax_u32_strided", true, true),
(ReduceOp::Sum, DType::F16) => ("fast_sum_f16_strided", false, false),
(ReduceOp::Min, DType::F16) => ("fast_min_f16_strided", true, false),
(ReduceOp::Max, DType::F16) => ("fast_max_f16_strided", true, false),
(ReduceOp::ArgMin, DType::F16) => ("fast_argmin_f16_strided", true, true),
(ReduceOp::ArgMax, DType::F16) => ("fast_argmax_f16_strided", true, true),
(ReduceOp::Sum, DType::BF16) => ("fast_sum_bf16_strided", false, false),
(ReduceOp::Min, DType::BF16) => ("fast_min_bf16_strided", true, false),
(ReduceOp::Max, DType::BF16) => ("fast_max_bf16_strided", true, false),
(ReduceOp::ArgMin, DType::BF16) => ("fast_argmin_bf16_strided", true, true),
(ReduceOp::ArgMax, DType::BF16) => ("fast_argmax_bf16_strided", true, true),
(ReduceOp::Sum, DType::I64) => ("fast_sum_i64_strided", false, false),
(ReduceOp::Min, DType::I64) => ("fast_min_i64_strided", true, false),
(ReduceOp::Max, DType::I64) => ("fast_max_i64_strided", true, false),
(ReduceOp::ArgMin, DType::I64) => ("fast_argmin_i64_strided", true, true),
(ReduceOp::ArgMax, DType::I64) => ("fast_argmax_i64_strided", true, true),
(ReduceOp::Sum, DType::U8) => ("fast_sum_u8_strided", false, false),
(ReduceOp::Min, DType::U8) => ("fast_min_u8_strided", true, false),
(ReduceOp::Max, DType::U8) => ("fast_max_u8_strided", true, false),
(ReduceOp::ArgMin, DType::U8) => ("fast_argmin_u8_strided", true, true),
(ReduceOp::ArgMax, DType::U8) => ("fast_argmax_u8_strided", true, true),
(k, dtype) => crate::bail!("Metal reduce op {k:?} {dtype:?} not implemented"),
};
if check_empty && layout.shape().elem_count() == 0 {
Err(crate::Error::EmptyTensor { op: "reduce" }.bt())?
}
let dtype = if return_index { DType::U32 } else { self.dtype };
let buffer = device.new_buffer(dst_el, dtype, "reduce")?;
let command_buffer = self.device.command_buffer()?;
candle_metal_kernels::call_reduce_strided(
&device.device,
&command_buffer,
&device.kernels,
name,
&dims,
&stride,
dst_el,
&self.buffer,
layout.start_offset() * self.dtype.size_in_bytes(),
&buffer,
)
.map_err(MetalError::from)?;
Ok(Self::new(buffer, device, dst_el, dtype))
}
fn cmp(&self, op: CmpOp, rhs: &Self, lhs_l: &Layout, rhs_l: &Layout) -> Result<Self> {
let name = match op {
CmpOp::Eq => "eq",
CmpOp::Ne => "ne",
CmpOp::Le => "le",
CmpOp::Ge => "ge",
CmpOp::Lt => "lt",
CmpOp::Gt => "gt",
};
self.binary(name, rhs, lhs_l, rhs_l)
}
fn to_dtype(&self, layout: &Layout, dtype: DType) -> Result<Self> {
let device = self.device();
let shape = layout.shape();
let el_count = shape.elem_count();
let buffer = device.new_buffer(el_count, dtype, "todtype")?;
let command_buffer = device.command_buffer()?;
if layout.is_contiguous() && layout.start_offset() == 0 {
let kernel_name = match (self.dtype, dtype) {
(DType::U32, DType::BF16) => "cast_u32_bf16",
(DType::U32, DType::F16) => "cast_u32_f16",
(DType::U32, DType::F32) => "cast_u32_f32",
(DType::U32, DType::I64) => "cast_u32_i64",
(DType::U32, DType::U8) => "cast_u32_u8",
(DType::U8, DType::BF16) => "cast_u8_bf16",
(DType::U8, DType::F16) => "cast_u8_f16",
(DType::U8, DType::F32) => "cast_u8_f32",
(DType::U8, DType::I64) => "cast_u8_i64",
(DType::U8, DType::U32) => "cast_u8_u32",
(DType::F32, DType::BF16) => "cast_f32_bf16",
(DType::F32, DType::F16) => "cast_f32_f16",
(DType::F32, DType::I64) => "cast_f32_i64",
(DType::F32, DType::U32) => "cast_f32_u32",
(DType::F32, DType::U8) => "cast_f32_u8",
(DType::I64, DType::BF16) => "cast_i64_bf16",
(DType::I64, DType::F16) => "cast_i64_f16",
(DType::I64, DType::F32) => "cast_i64_f32",
(DType::I64, DType::U32) => "cast_i64_u32",
(DType::I64, DType::U8) => "cast_i64_u8",
(DType::F16, DType::BF16) => "cast_f16_bf16",
(DType::F16, DType::F32) => "cast_f16_f32",
(DType::F16, DType::I64) => "cast_f16_i64",
(DType::F16, DType::U32) => "cast_f16_u32",
(DType::F16, DType::U8) => "cast_f16_u8",
(DType::BF16, DType::F16) => "cast_bf16_f16",
(DType::BF16, DType::F32) => "cast_bf16_f32",
(DType::BF16, DType::I64) => "cast_bf16_i64",
(DType::BF16, DType::U32) => "cast_bf16_u32",
(DType::BF16, DType::U8) => "cast_bf16_u8",
(left, right) => {
crate::bail!("Metal contiguous to_dtype {left:?} {right:?} not implemented")
}
};
candle_metal_kernels::call_cast_contiguous(
&device.device,
&command_buffer,
&device.kernels,
kernel_name,
el_count,
&self.buffer,
layout.start_offset() * self.dtype.size_in_bytes(),
&buffer,
)
.map_err(MetalError::from)?;
} else {
let kernel_name = match (self.dtype, dtype) {
(DType::U32, DType::F32) => "cast_u32_f32_strided",
(DType::U32, DType::U8) => "cast_u32_u8_strided",
(DType::U32, DType::I64) => "cast_u32_i64_strided",
(DType::U8, DType::U32) => "cast_u8_u32_strided",
(DType::U8, DType::F32) => "cast_u8_f32_strided",
(DType::U8, DType::I64) => "cast_u8_i64_strided",
(DType::F32, DType::F16) => "cast_f32_f16_strided",
(DType::F16, DType::F32) => "cast_f16_f32_strided",
(DType::I64, DType::F32) => "cast_i64_f32_strided",
(DType::F32, DType::BF16) => "cast_f32_bf16_strided",
(DType::BF16, DType::F32) => "cast_bf16_f32_strided",
(left, right) => {
crate::bail!("Metal strided to_dtype {left:?} {right:?} not implemented")
}
};
candle_metal_kernels::call_cast_strided(
&device.device,
&command_buffer,
&device.kernels,
kernel_name,
layout.dims(),
&self.buffer,
layout.stride(),
layout.start_offset() * self.dtype.size_in_bytes(),
&buffer,
)
.map_err(MetalError::from)?;
}
command_buffer.set_label("to_dtype");
Ok(Self::new(buffer, device.clone(), el_count, dtype))
}
fn unary_impl<B: UnaryOpT>(&self, layout: &Layout) -> Result<Self> {
let device = self.device();
let dtype = self.dtype;
let shape = layout.shape();
let el_count = shape.elem_count();
let buffer = device.new_buffer(el_count, dtype, B::KERNEL)?;
let command_buffer = device.command_buffer()?;
command_buffer.set_label(B::KERNEL);
if layout.is_contiguous() && layout.start_offset() == 0 {
use candle_metal_kernels::unary::contiguous;
let kernel_name = match (B::KERNEL, dtype) {
("ucos", DType::F32) => contiguous::cos::FLOAT,
("usin", DType::F32) => contiguous::sin::FLOAT,
("usqr", DType::F32) => contiguous::sqr::FLOAT,
("usqrt", DType::F32) => contiguous::sqrt::FLOAT,
("uneg", DType::F32) => contiguous::neg::FLOAT,
("uexp", DType::F32) => contiguous::exp::FLOAT,
("ulog", DType::F32) => contiguous::log::FLOAT,
("ugelu", DType::F32) => contiguous::gelu::FLOAT,
("ugelu_erf", DType::F32) => contiguous::gelu_erf::FLOAT,
("uerf", DType::F32) => contiguous::erf::FLOAT,
("usilu", DType::F32) => contiguous::silu::FLOAT,
("uabs", DType::F32) => contiguous::abs::FLOAT,
("uceil", DType::F32) => contiguous::ceil::FLOAT,
("ufloor", DType::F32) => contiguous::floor::FLOAT,
("uround", DType::F32) => contiguous::round::FLOAT,
("urecip", DType::F32) => contiguous::recip::FLOAT,
("utanh", DType::F32) => contiguous::tanh::FLOAT,
("urelu", DType::F32) => contiguous::relu::FLOAT,
("ucos", DType::F16) => contiguous::cos::HALF,
("usin", DType::F16) => contiguous::sin::HALF,
("usqr", DType::F16) => contiguous::sqr::HALF,
("usqrt", DType::F16) => contiguous::sqrt::HALF,
("uneg", DType::F16) => contiguous::neg::HALF,
("uexp", DType::F16) => contiguous::exp::HALF,
("ulog", DType::F16) => contiguous::log::HALF,
("ugelu", DType::F16) => contiguous::gelu::HALF,
("ugelu_erf", DType::F16) => contiguous::gelu_erf::HALF,
("uerf", DType::F16) => contiguous::erf::HALF,
("usilu", DType::F16) => contiguous::silu::HALF,
("uabs", DType::F16) => contiguous::abs::HALF,
("uceil", DType::F16) => contiguous::ceil::HALF,
("ufloor", DType::F16) => contiguous::floor::HALF,
("uround", DType::F16) => contiguous::round::HALF,
("urecip", DType::F16) => contiguous::recip::HALF,
("utanh", DType::F16) => contiguous::tanh::HALF,
("urelu", DType::F16) => contiguous::relu::HALF,
(name, dtype) => {
crate::bail!("Metal contiguous unary {name} {dtype:?} not implemented")
}
};
candle_metal_kernels::call_unary_contiguous(
&device.device,
&command_buffer,
&device.kernels,
kernel_name,
el_count,
&self.buffer,
&buffer,
)
.map_err(MetalError::from)?;
} else {
use candle_metal_kernels::unary::strided;
let kernel_name = match (B::KERNEL, dtype) {
("ucos", DType::F32) => strided::cos::FLOAT,
("usin", DType::F32) => strided::sin::FLOAT,
("usqr", DType::F32) => strided::sqr::FLOAT,
("usqrt", DType::F32) => strided::sqrt::FLOAT,
("uneg", DType::F32) => strided::neg::FLOAT,
("uexp", DType::F32) => strided::exp::FLOAT,
("ulog", DType::F32) => strided::log::FLOAT,
("ugelu", DType::F32) => strided::gelu::FLOAT,
("ugelu_erf", DType::F32) => strided::gelu_erf::FLOAT,
("uerf", DType::F32) => strided::erf::FLOAT,
("usilu", DType::F32) => strided::silu::FLOAT,
("uabs", DType::F32) => strided::abs::FLOAT,
("uceil", DType::F32) => strided::ceil::FLOAT,
("ufloor", DType::F32) => strided::floor::FLOAT,
("urelu", DType::F32) => strided::relu::FLOAT,
("uround", DType::F32) => strided::round::FLOAT,
("utanh", DType::F32) => strided::tanh::FLOAT,
("ucos", DType::F16) => strided::cos::HALF,
("usin", DType::F16) => strided::sin::HALF,
("usqr", DType::F16) => strided::sqr::HALF,
("usqrt", DType::F16) => strided::sqrt::HALF,
("uneg", DType::F16) => strided::neg::HALF,
("uexp", DType::F16) => strided::exp::HALF,
("ulog", DType::F16) => strided::log::HALF,
("ugelu", DType::F16) => strided::gelu::HALF,
("ugelu_erf", DType::F16) => strided::gelu_erf::HALF,
("uerf", DType::F16) => strided::erf::HALF,
("usilu", DType::F16) => strided::silu::HALF,
("uabs", DType::F16) => strided::abs::HALF,
("uceil", DType::F16) => strided::ceil::HALF,
("ufloor", DType::F16) => strided::floor::HALF,
("urelu", DType::F16) => strided::relu::HALF,
("uround", DType::F16) => strided::round::HALF,
("utanh", DType::F16) => strided::tanh::HALF,
(name, dtype) => {
crate::bail!("Metal strided unary {name} {dtype:?} not implemented")
}
};
candle_metal_kernels::call_unary_strided(
&device.device,
&command_buffer,
&device.kernels,
kernel_name,
layout.dims(),
&self.buffer,
layout.stride(),
layout.start_offset() * self.dtype.size_in_bytes(),
&buffer,
0,
)
.map_err(MetalError::from)?;
}
Ok(Self::new(buffer, device.clone(), el_count, dtype))
}
fn binary_impl<B: BinaryOpT>(
&self,
rhs: &Self,
lhs_l: &Layout,
rhs_l: &Layout,
) -> Result<Self> {
self.binary(B::KERNEL, rhs, lhs_l, rhs_l)
}
fn where_cond(
&self,
layout: &Layout,
t: &Self,
t_l: &Layout,
f: &Self,
f_l: &Layout,
) -> Result<Self> {
let device = self.device.clone();
let shape = t_l.shape();
let dims = shape.dims();
let el = shape.elem_count();
let dtype = t.dtype;
let buffer = self.device.new_buffer(el, dtype, "where")?;
let command_buffer = self.device.command_buffer()?;
if t.dtype() != f.dtype() {
crate::bail!(
"Invalid where: different dtypes for values {:?} != {:?}",
t.dtype(),
f.dtype()
);
}
let name = match (self.dtype, t.dtype()) {
(DType::U8, DType::F32) => "where_u8_f32",
(DType::U8, DType::BF16) => "where_u8_bf16",
(DType::U8, DType::F16) => "where_u8_f16",
(DType::U8, DType::I64) => "where_u8_i64",
(DType::U8, DType::U32) => "where_u8_u32",
(DType::U8, DType::U8) => "where_u8_u8",
(left, right) => crate::bail!("Metal where_cond {left:?} {right:?} not implemented"),
};
candle_metal_kernels::call_where_cond_strided(
&device.device,
&command_buffer,
&device.kernels,
name,
dims,
&self.buffer,
(
layout.stride(),
layout.start_offset() * self.dtype.size_in_bytes(),
),
&t.buffer,
(t_l.stride(), t_l.start_offset() * t.dtype.size_in_bytes()),
&f.buffer,
(f_l.stride(), f_l.start_offset() * f.dtype.size_in_bytes()),
&buffer,
)
.map_err(MetalError::from)?;
Ok(Self::new(buffer, device, el, dtype))
}
fn conv1d(
&self,
layout: &Layout,
kernel: &Self,
kernel_l: &Layout,
params: &ParamsConv1D,
) -> Result<Self> {
let device = self.device().clone();
let shape = layout.shape();
let dims = shape.dims();
let strides = layout.stride();
let stride = params.stride;
let dilation = params.dilation;
let padding = params.padding;
let k_size = params.k_size;
let l_out = (dims[2] + 2 * padding - dilation * (k_size - 1) - 1) / stride + 1;
let dst_el = dims[0] * l_out * dims[1] * k_size;
let dst = self
.device
.new_buffer(dst_el, self.dtype, "conv1d_im2col")?;
let command_buffer = self.device.command_buffer()?;
let name = match self.dtype {
DType::F32 => "im2col1d_f32",
dtype => crate::bail!("Metal conv1d {dtype:?} not implemented"),
};
candle_metal_kernels::call_im2col1d_strided(
&self.device.device,
&command_buffer,
&self.device.kernels,
name,
layout.shape().dims(),
strides,
(k_size, stride, padding, dilation),
&self.buffer,
layout.start_offset() * self.dtype.size_in_bytes(),
&dst,
)
.map_err(MetalError::from)?;
let col = Self {
buffer: dst,
device,
count: dst_el,
dtype: self.dtype,
};
let l_out = params.l_out();
let b = params.b_size;
let n = params.c_out;
let k = params.k_size * params.c_in;
let m = l_out;
let col_l = Layout::contiguous((b, m, k));
let res = if kernel_l.is_contiguous() {
let kernel_l = Layout::contiguous_with_offset((1, n, k), kernel_l.start_offset())
.transpose(1, 2)?
.broadcast_as((b, k, n))?;
col.matmul(kernel, (b, m, n, k), &col_l, &kernel_l)?
} else {
// Make the kernel contiguous if not already the case.
let mut kernel_c = self.device().zeros_impl(kernel_l.shape(), kernel.dtype())?;
kernel.copy_strided_src(&mut kernel_c, 0, kernel_l)?;
let kernel_l = Layout::contiguous_with_offset((1, n, k), kernel_l.start_offset())
.transpose(1, 2)?
.broadcast_as((b, k, n))?;
col.matmul(kernel, (b, m, n, k), &col_l, &kernel_l)?
};
let res_l = Layout::contiguous((b, l_out, n)).transpose(1, 2)?;
let mut res_t = self.device().zeros_impl(res_l.shape(), res.dtype())?;
res.copy_strided_src(&mut res_t, 0, &res_l)?;
Ok(res_t)
}
fn conv_transpose1d(
&self,
layout: &Layout,
k: &Self,
k_layout: &Layout,
params: &ParamsConvTranspose1D,
) -> Result<Self> {
let l_out = params.l_out();
let dst_el = params.c_out * l_out * params.b_size;
let buffer = self
.device
.new_buffer(dst_el, self.dtype, "conv_transpose1d")?;
let command_buffer = self.device.command_buffer()?;
let name = match self.dtype {
DType::F32 => "conv_transpose1d_f32",
DType::F16 => "conv_transpose1d_f16",
DType::BF16 => "conv_transpose1d_bf16",
DType::U32 => "conv_transpose1d_u32",
DType::U8 => "conv_transpose1d_u8",
dtype => crate::bail!("Metal conv_transpose1d {dtype:?} not implemented"),
};
candle_metal_kernels::call_conv_transpose1d(
&self.device.device,
&command_buffer,
&self.device.kernels,
name,
params.dilation,
params.stride,
params.padding,
params.output_padding,
params.c_out,
l_out,
params.b_size,
layout.dims(),
layout.stride(),
k_layout.dims(),
k_layout.stride(),
&self.buffer,
layout.start_offset() * self.dtype.size_in_bytes(),
&k.buffer,
k_layout.start_offset() * k.dtype.size_in_bytes(),
&buffer,
)
.map_err(MetalError::from)?;
Ok(Self::new(buffer, self.device.clone(), dst_el, self.dtype))
}
fn conv2d(
&self,
layout: &Layout,
kernel: &Self,
kernel_l: &Layout,
params: &ParamsConv2D,
) -> Result<Self> {
let device = self.device().clone();
let shape = layout.shape();
let dims = shape.dims();
let stride = params.stride;
let dilation = params.dilation;
let padding = params.padding;
let h_k = params.k_h;
let w_k = params.k_w;
let h = dims[2];
let w = dims[3];
let h_out = (h + 2 * padding - dilation * (h_k - 1) - 1) / stride + 1;
let w_out = (w + 2 * padding - dilation * (w_k - 1) - 1) / stride + 1;
let dst_el = dims[0] * h_out * w_out * dims[1] * h_k * w_k;
let dst = self
.device
.new_buffer(dst_el, self.dtype, "conv2d_im2col")?;
let command_buffer = self.device.command_buffer()?;
let name = match self.dtype {
DType::F32 => "im2col_f32",
dtype => crate::bail!("Metal conv2d {dtype:?} not implemented"),
};
candle_metal_kernels::call_im2col_strided(
&self.device.device,
&command_buffer,
&self.device.kernels,
name,
layout.shape().dims(),
layout.stride(),
(h_k, w_k, stride, padding, dilation),
&self.buffer,
layout.start_offset() * self.dtype.size_in_bytes(),
&dst,
)
.map_err(MetalError::from)?;
let col = Self {
buffer: dst,
device,
count: dst_el,
dtype: self.dtype,
};
let h_out = params.out_h();
let w_out = params.out_w();
let b = params.b_size;
let n = params.c_out;
let k = params.k_h * params.k_w * params.c_in;
let m = h_out * w_out;
let col_l = Layout::contiguous((b, m, k));
let res = if kernel_l.is_contiguous() {
let kernel_l = Layout::contiguous_with_offset((1, n, k), kernel_l.start_offset())
.transpose(1, 2)?
.broadcast_as((b, k, n))?;
col.matmul(kernel, (b, m, n, k), &col_l, &kernel_l)?
} else {
// Make the kernel contiguous if not already the case.
let mut kernel_c = self.device().zeros_impl(kernel_l.shape(), kernel.dtype())?;
kernel.copy_strided_src(&mut kernel_c, 0, kernel_l)?;
let kernel_l = Layout::contiguous_with_offset((1, n, k), kernel_l.start_offset())
.transpose(1, 2)?
.broadcast_as((b, k, n))?;
col.matmul(kernel, (b, m, n, k), &col_l, &kernel_l)?
};
let res_l = Layout::contiguous((b, h_out, w_out, n))
.transpose(1, 2)?
.transpose(1, 3)?;
let mut res_t = self.device().zeros_impl(res_l.shape(), res.dtype())?;
res.copy_strided_src(&mut res_t, 0, &res_l)?;
Ok(res_t)
}
fn conv_transpose2d(
&self,
_l: &Layout,
_kernel: &Self,
_kernel_l: &Layout,
_params: &ParamsConvTranspose2D,
) -> Result<Self> {
crate::bail!("Metal conv_tranpose2d not implemented")
}
fn avg_pool2d(
&self,
inp_l: &Layout,
(w_k, h_k): (usize, usize),
(w_stride, h_stride): (usize, usize),
) -> Result<Self> {
let shape = inp_l.shape();
let (b_size, channels, width, height) = shape.dims4()?;
let strides = inp_l.stride();
let name = match self.dtype {
DType::F32 => "avg_pool2d_f32",
DType::F16 => "avg_pool2d_f16",
DType::BF16 => "avg_pool2d_bf16",
DType::U8 => "avg_pool2d_u8",
DType::U32 => "avg_pool2d_u32",
dtype => crate::bail!("Metal avg_pool2d {dtype:?} not implemented"),
};
let out_w = (width - w_k) / w_stride + 1;
let out_h = (height - h_k) / h_stride + 1;
let dst_el = out_w * out_h * b_size * channels;
let buffer = self.device.new_buffer(dst_el, self.dtype, "avg_pool2d")?;
let command_buffers = self.device.command_buffer()?;
candle_metal_kernels::call_pool2d(
&self.device.device,
&command_buffers,
&self.device.kernels,
name,
inp_l.dims(),
strides,
out_w,
out_h,
w_k,
h_k,
w_stride,
h_stride,
&self.buffer,
&buffer,
)
.map_err(MetalError::from)?;
Ok(Self::new(buffer, self.device.clone(), dst_el, self.dtype))
}
fn max_pool2d(
&self,
inp_l: &Layout,
(w_k, h_k): (usize, usize),
(w_stride, h_stride): (usize, usize),
) -> Result<Self> {
let shape = inp_l.shape();
let (b_size, channels, width, height) = shape.dims4()?;
let strides = inp_l.stride();
let name = match self.dtype {
DType::F32 => "max_pool2d_f32",
DType::F16 => "max_pool2d_f16",
DType::BF16 => "max_pool2d_bf16",
DType::U8 => "max_pool2d_u8",
DType::U32 => "max_pool2d_u32",
dtype => crate::bail!("Metal max_pool2d {dtype:?} not implemented"),
};
let out_w = (width - w_k) / w_stride + 1;
let out_h = (height - h_k) / h_stride + 1;
let dst_el = out_w * out_h * b_size * channels;
let buffer = self.device.new_buffer(dst_el, self.dtype, "max_pool2d")?;
let command_buffers = self.device.command_buffer()?;
candle_metal_kernels::call_pool2d(
&self.device.device,
&command_buffers,
&self.device.kernels,
name,
inp_l.dims(),
strides,
out_w,
out_h,
w_k,
h_k,
w_stride,
h_stride,
&self.buffer,
&buffer,
)
.map_err(MetalError::from)?;
Ok(Self::new(buffer, self.device.clone(), dst_el, self.dtype))
}
fn upsample_nearest1d(&self, _: &Layout, _: usize) -> Result<Self> {
crate::bail!("Metal upsample_nearest1d not implemented")
}
fn upsample_nearest2d(&self, inp_l: &Layout, out_w: usize, out_h: usize) -> Result<Self> {
// let inp = &inp.slice(inp_l.start_offset()..);
let shape = inp_l.shape();
let dims = shape.dims();
let strides = inp_l.stride();
if dims.len() != 4 {
crate::bail!("unexpected input shape for upsample {dims:?}")
}
let name = match self.dtype {
DType::F32 => "upsample_nearest2d_f32",
dtype => crate::bail!("Metal upsample_nearest2d {dtype:?} not implemented"),
};
let dst_el = out_w * out_h * dims[0] * dims[1];
let buffer = self
.device
.new_buffer(dst_el, self.dtype, "upsample_nearest2d")?;
let command_buffer = self.device.command_buffer()?;
candle_metal_kernels::call_upsample_nearest_2d(
&self.device.device,
&command_buffer,
&self.device.kernels,
name,
dims,
strides,
out_w,
out_h,
&self.buffer,
inp_l.start_offset() * self.dtype.size_in_bytes(),
&buffer,
)
.map_err(MetalError::from)?;
Ok(Self::new(buffer, self.device.clone(), dst_el, self.dtype))
}
fn gather(&self, src_l: &Layout, ids: &Self, ids_l: &Layout, dim: usize) -> Result<Self> {
let (ids_o1, _) = match ids_l.contiguous_offsets() {
Some(o12) => o12,
None => Err(crate::Error::RequiresContiguous { op: "gather" }.bt())?,
};
let ids_el = ids_l.dims()[dim];
let dst_el = ids_l.shape().elem_count();
let dtype = self.dtype;
let device = self.device();
let buffer = device.new_buffer(dst_el, dtype, "index_select")?;
let name = match (ids.dtype, self.dtype) {
(DType::U32, DType::F32) => "gather_u32_f32",
(DType::U32, DType::F16) => "gather_u32_f16",
(left, right) => crate::bail!("Metal gather {left:?} {right:?} not implemented"),
};
let command_buffer = self.device.command_buffer()?;
candle_metal_kernels::call_gather(
&device.device,
&command_buffer,
&self.device.kernels,
name,
src_l.dims(),
ids_el,
dim,
&self.buffer,
src_l.start_offset() * dtype.size_in_bytes(),
&ids.buffer,
ids_o1 * ids.dtype.size_in_bytes(),
&buffer,
)
.map_err(MetalError::from)?;
Ok(Self::new(buffer, device.clone(), dst_el, dtype))
}
fn scatter_add(
&self,
l: &Layout,
ids: &Self,
ids_l: &Layout,
src: &Self,
src_l: &Layout,
dim: usize,
) -> Result<Self> {
let mut acc = self.device.zeros_impl(l.shape(), self.dtype())?;
self.copy_strided_src(&mut acc, 0, l)?;
let (ids_offset, _) = match ids_l.contiguous_offsets() {
Some(o12) => o12,
None => Err(crate::Error::RequiresContiguous { op: "scatter-add" }.bt())?,
};
let src_offset = match src_l.contiguous_offsets() {
Some((o1, _)) => o1,
None => Err(crate::Error::RequiresContiguous { op: "scatter-add" }.bt())?,
};
let name = match (ids.dtype, self.dtype) {
(DType::U8, DType::F32) => "sa_u8_f32",
(DType::U8, DType::F16) => "sa_u8_f16",
(DType::U8, DType::BF16) => "sa_u8_bf16",
(DType::U32, DType::F32) => "sa_u32_f32",
(DType::U32, DType::F16) => "sa_u32_f16",
(DType::U32, DType::BF16) => "sa_u32_bf16",
(DType::I64, DType::F32) => "sa_i64_f32",
(DType::I64, DType::F16) => "sa_i64_f16",
(DType::I64, DType::BF16) => "sa_i64_bf16",
_ => Err(MetalError::UnexpectedDType {
msg: "scatter-add ids should be u8/u32/i64",
expected: DType::U32,
got: ids.dtype(),
})?,
};
let command_buffer = self.device.command_buffer()?;
candle_metal_kernels::call_scatter_add(
&self.device.device,
&command_buffer,
&self.device.kernels,
name,
src_l.dims(),
l.dims(),
dim,
&src.buffer,
src_offset * src.dtype.size_in_bytes(),
&ids.buffer,
ids_offset * ids.dtype.size_in_bytes(),
&acc.buffer,
)
.map_err(MetalError::from)?;
Ok(acc)
}
fn index_select(&self, ids: &Self, src_l: &Layout, ids_l: &Layout, dim: usize) -> Result<Self> {
if !(src_l.is_contiguous()
&& src_l.start_offset() == 0
&& ids_l.is_contiguous()
&& ids_l.start_offset() == 0)
{
crate::bail!("Metal strided index_select not implemented");
}
let left_size: usize = src_l.dims()[..dim].iter().product();
let right_size: usize = src_l.dims()[dim + 1..].iter().product();
let ids_el = ids_l.shape().elem_count();
let dst_el = ids_el * left_size * right_size;
let dtype = self.dtype;
let device = self.device();
let buffer = device.new_buffer(dst_el, dtype, "index_select")?;
let name = match (ids.dtype, self.dtype) {
(DType::U8, DType::BF16) => "is_u8_bf16",
(DType::U32, DType::F32) => "is_u32_f32",
(DType::U32, DType::F16) => "is_u32_f16",
(DType::U32, DType::BF16) => "is_u32_bf16",
(left, right) => {
crate::bail!("Metal contiguous index_select {left:?} {right:?} not implemented")
}
};
let command_buffer = self.device.command_buffer()?;
candle_metal_kernels::call_index_select(
&device.device,
&command_buffer,
&self.device.kernels,
name,
src_l.dims(),
ids_el,
dim,
&self.buffer,
&ids.buffer,
&buffer,
)
.map_err(MetalError::from)?;
Ok(Self::new(buffer, device.clone(), dst_el, dtype))
}
fn index_add(
&self,
l: &Layout,
ids: &Self,
ids_l: &Layout,
src: &Self,
src_l: &Layout,
dim: usize,
) -> Result<Self> {
let mut acc = self.device.zeros_impl(l.shape(), self.dtype())?;
self.copy_strided_src(&mut acc, 0, l)?;
let (ids_offset, _) = match ids_l.contiguous_offsets() {
Some(o12) => o12,
None => Err(crate::Error::RequiresContiguous { op: "index-add" }.bt())?,
};
let src_offset = match src_l.contiguous_offsets() {
Some((o1, _)) => o1,
None => Err(crate::Error::RequiresContiguous { op: "index-add" }.bt())?,
};
let name = match (ids.dtype, self.dtype) {
(DType::I64, DType::BF16) => "ia_i64_bf16",
(DType::I64, DType::F16) => "ia_i64_f16",
(DType::I64, DType::F32) => "ia_i64_f32",
(DType::I64, DType::I64) => "ia_i64_i64",
(DType::I64, DType::U32) => "ia_i64_u32",
(DType::I64, DType::U8) => "ia_i64_u8",
(DType::U32, DType::BF16) => "ia_u32_bf16",
(DType::U32, DType::F16) => "ia_u32_f16",
(DType::U32, DType::F32) => "ia_u32_f32",
(DType::U32, DType::I64) => "ia_u32_i64",
(DType::U32, DType::U32) => "ia_u32_u32",
(DType::U32, DType::U8) => "ia_u32_u8",
(DType::U8, DType::BF16) => "ia_u8_bf16",
(DType::U8, DType::F16) => "ia_u8_f16",
(DType::U8, DType::F32) => "ia_u8_f32",
(DType::U8, DType::I64) => "ia_u8_i64",
(DType::U8, DType::U32) => "ia_u8_u32",
(DType::U8, DType::U8) => "ia_u8_u8",
_ => Err(MetalError::UnexpectedDType {
msg: "index-add ids should be u8/u32/i64",
expected: DType::U32,
got: ids.dtype(),
})?,
};
let command_buffer = self.device.command_buffer()?;
candle_metal_kernels::call_index_add(
&self.device.device,
&command_buffer,
&self.device.kernels,
name,
src_l.dims(),
l.dims(),
ids_l.dims(),
dim,
&src.buffer,
src_offset * src.dtype.size_in_bytes(),
&ids.buffer,
ids_offset * ids.dtype.size_in_bytes(),
&acc.buffer,
)
.map_err(MetalError::from)?;
Ok(acc)
}
fn matmul(
&self,
rhs: &Self,
(b, m, n, k): (usize, usize, usize, usize),
lhs_l: &Layout,
rhs_l: &Layout,
) -> Result<Self> {
let buffer = self.device.new_buffer(b * m * n, self.dtype, "matmul")?;
let name = match self.dtype {
DType::F32 => "sgemm",
DType::F16 => "hgemm",
dtype => {
return Err(MetalError::Message(format!("matmul doesn't support {dtype:?}")).into())
}
};
let command_buffer = self.device.command_buffer()?;
command_buffer.set_label("matmul");
candle_metal_kernels::call_gemm(
&self.device.device,
&command_buffer,
&self.device.kernels,
name,
(b, m, n, k),
lhs_l.stride(),
lhs_l.start_offset() * self.dtype.size_in_bytes(),
&self.buffer,
rhs_l.stride(),
rhs_l.start_offset() * rhs.dtype.size_in_bytes(),
&rhs.buffer,
&buffer,
)
.map_err(MetalError::from)?;
Ok(Self::new(
buffer,
self.device.clone(),
b * m * n,
self.dtype(),
))
}
fn copy2d(
&self,
dst: &mut Self,
d1: usize,
d2: usize,
src_s: usize,
dst_s: usize,
src_o: usize,
dst_o: usize,
) -> Result<()> {
if self.dtype() != dst.dtype() {
crate::bail!(
"copy2d with inconsistent dtypes {:?} {:?}",
self.dtype(),
dst.dtype()
)
}
let command_buffer = self.device.command_buffer()?;
if src_s == d2 && dst_s == d2 {
command_buffer.set_label("copy2d_contiguous");
let blit = command_buffer.new_blit_command_encoder();
blit.set_label("copy2d_contiguous");
let src_offset = (src_o * self.dtype.size_in_bytes()) as NSUInteger;
let length = (d1 * d2 * self.dtype.size_in_bytes()) as NSUInteger;
let dst_offset = (dst_o * dst.dtype().size_in_bytes()) as NSUInteger;
blit.copy_from_buffer(&self.buffer, src_offset, dst.buffer(), dst_offset, length);
blit.end_encoding();
} else {
let el_count = d1 * d2;
if el_count == 0 {
return Ok(());
}
let kernel_name = match self.dtype {
DType::F32 => candle_metal_kernels::copy2d::FLOAT,
DType::F16 => candle_metal_kernels::copy2d::HALF,
DType::BF16 => candle_metal_kernels::copy2d::BFLOAT,
DType::I64 => candle_metal_kernels::copy2d::I64,
DType::U32 => candle_metal_kernels::copy2d::U32,
DType::U8 => candle_metal_kernels::copy2d::U8,
dtype => crate::bail!("Metal copy2d {dtype:?} not implemented"),
};
candle_metal_kernels::call_copy2d(
&self.device.device,
&command_buffer,
&self.device.kernels,
kernel_name,
&self.buffer,
&dst.buffer,
d1,
d2,
src_s,
dst_s,
src_o * self.dtype.size_in_bytes(),
dst_o * self.dtype.size_in_bytes(),
)
.map_err(MetalError::from)?;
command_buffer.set_label("copy2d");
}
Ok(())
}
fn copy_strided_src(&self, dst: &mut Self, dst_offset: usize, src_l: &Layout) -> Result<()> {
let command_buffer = self.device.command_buffer()?;
if src_l.is_contiguous() && self.dtype == dst.dtype() {
command_buffer.set_label("copy_contiguous");
let blit = command_buffer.new_blit_command_encoder();
blit.set_label("copy_contiguous");
let src_offset = (src_l.start_offset() * self.dtype.size_in_bytes()) as NSUInteger;
let length = (src_l.shape().elem_count() * self.dtype.size_in_bytes()) as NSUInteger;
let dst_offset = (dst_offset * dst.dtype().size_in_bytes()) as NSUInteger;
blit.copy_from_buffer(&self.buffer, src_offset, dst.buffer(), dst_offset, length);
blit.end_encoding();
} else {
let src_shape = src_l.shape();
let el_count = src_shape.elem_count();
if el_count == 0 {
return Ok(());
}
let kernel_name = match self.dtype {
DType::F32 => candle_metal_kernels::unary::strided::copy::FLOAT,
DType::F16 => candle_metal_kernels::unary::strided::copy::HALF,
DType::BF16 => candle_metal_kernels::unary::strided::copy::BFLOAT,
DType::I64 => candle_metal_kernels::unary::strided::copy::I64,
DType::U32 => candle_metal_kernels::unary::strided::copy::U32,
DType::U8 => candle_metal_kernels::unary::strided::copy::U8,
dtype => crate::bail!("Metal copy_strided {dtype:?} not implemented"),
};
candle_metal_kernels::call_unary_strided(
&self.device.device,
&command_buffer,
&self.device.kernels,
kernel_name,
src_l.dims(),
&self.buffer,
src_l.stride(),
src_l.start_offset() * self.dtype.size_in_bytes(),
&dst.buffer,
dst_offset * dst.dtype.size_in_bytes(),
)
.map_err(MetalError::from)?;
command_buffer.set_label("copy_strided");
}
Ok(())
}
}
impl MetalStorage {
pub fn new(buffer: Arc<Buffer>, device: MetalDevice, count: usize, dtype: DType) -> Self {
Self {
buffer,
device,
count,
dtype,
}
}
pub fn buffer(&self) -> &Buffer {
&self.buffer
}
pub fn binary(
&self,
op: &'static str,
rhs: &Self,
lhs_l: &Layout,
rhs_l: &Layout,
) -> Result<Self> {
let device = self.device();
let shape = lhs_l.shape();
let el_count = shape.elem_count();
let command_buffer = device.command_buffer()?;
let (buffer, dtype) = if (lhs_l.is_contiguous() && lhs_l.start_offset() == 0)
&& (rhs_l.is_contiguous() && rhs_l.start_offset() == 0)
&& &op[..1] != "b"
{
use candle_metal_kernels::binary::contiguous;
let (kernel_name, dtype) = match (op, self.dtype) {
("add", DType::F32) => (contiguous::add::FLOAT, self.dtype),
("sub", DType::F32) => (contiguous::sub::FLOAT, self.dtype),
("mul", DType::F32) => (contiguous::mul::FLOAT, self.dtype),
("div", DType::F32) => (contiguous::div::FLOAT, self.dtype),
("eq", DType::F32) => (contiguous::eq::FLOAT, DType::U8),
("ne", DType::F32) => (contiguous::ne::FLOAT, DType::U8),
("le", DType::F32) => (contiguous::le::FLOAT, DType::U8),
("lt", DType::F32) => (contiguous::lt::FLOAT, DType::U8),
("ge", DType::F32) => (contiguous::ge::FLOAT, DType::U8),
("gt", DType::F32) => (contiguous::gt::FLOAT, DType::U8),
("add", DType::F16) => (contiguous::add::HALF, self.dtype),
("sub", DType::F16) => (contiguous::sub::HALF, self.dtype),
("mul", DType::F16) => (contiguous::mul::HALF, self.dtype),
("div", DType::F16) => (contiguous::div::HALF, self.dtype),
("eq", DType::F16) => (contiguous::eq::HALF, DType::U8),
("ne", DType::F16) => (contiguous::ne::HALF, DType::U8),
("le", DType::F16) => (contiguous::le::HALF, DType::U8),
("lt", DType::F16) => (contiguous::lt::HALF, DType::U8),
("ge", DType::F16) => (contiguous::ge::HALF, DType::U8),
("gt", DType::F16) => (contiguous::gt::HALF, DType::U8),
("add", DType::BF16) => (contiguous::add::BFLOAT, self.dtype),
("sub", DType::BF16) => (contiguous::sub::BFLOAT, self.dtype),
("mul", DType::BF16) => (contiguous::mul::BFLOAT, self.dtype),
("div", DType::BF16) => (contiguous::div::BFLOAT, self.dtype),
("eq", DType::BF16) => (contiguous::eq::BFLOAT, DType::U8),
("ne", DType::BF16) => (contiguous::ne::BFLOAT, DType::U8),
("le", DType::BF16) => (contiguous::le::BFLOAT, DType::U8),
("lt", DType::BF16) => (contiguous::lt::BFLOAT, DType::U8),
("ge", DType::BF16) => (contiguous::ge::BFLOAT, DType::U8),
("gt", DType::BF16) => (contiguous::gt::BFLOAT, DType::U8),
("add", DType::I64) => (contiguous::add::I64, self.dtype),
("sub", DType::I64) => (contiguous::sub::I64, self.dtype),
("mul", DType::I64) => (contiguous::mul::I64, self.dtype),
("div", DType::I64) => (contiguous::div::I64, self.dtype),
("eq", DType::I64) => (contiguous::eq::I64, DType::U8),
("ne", DType::I64) => (contiguous::ne::I64, DType::U8),
("le", DType::I64) => (contiguous::le::I64, DType::U8),
("lt", DType::I64) => (contiguous::lt::I64, DType::U8),
("ge", DType::I64) => (contiguous::ge::I64, DType::U8),
("gt", DType::I64) => (contiguous::gt::I64, DType::U8),
("add", DType::U32) => (contiguous::add::U32, self.dtype),
("sub", DType::U32) => (contiguous::sub::U32, self.dtype),
("mul", DType::U32) => (contiguous::mul::U32, self.dtype),
("div", DType::U32) => (contiguous::div::U32, self.dtype),
("eq", DType::U32) => (contiguous::eq::U32, DType::U8),
("ne", DType::U32) => (contiguous::ne::U32, DType::U8),
("le", DType::U32) => (contiguous::le::U32, DType::U8),
("lt", DType::U32) => (contiguous::lt::U32, DType::U8),
("ge", DType::U32) => (contiguous::ge::U32, DType::U8),
("gt", DType::U32) => (contiguous::gt::U32, DType::U8),
("add", DType::U8) => (contiguous::add::U8, self.dtype),
("sub", DType::U8) => (contiguous::sub::U8, self.dtype),
("mul", DType::U8) => (contiguous::mul::U8, self.dtype),
("div", DType::U8) => (contiguous::div::U8, self.dtype),
("eq", DType::U8) => (contiguous::eq::U8, DType::U8),
("ne", DType::U8) => (contiguous::ne::U8, DType::U8),
("le", DType::U8) => (contiguous::le::U8, DType::U8),
("lt", DType::U8) => (contiguous::lt::U8, DType::U8),
("ge", DType::U8) => (contiguous::ge::U8, DType::U8),
("gt", DType::U8) => (contiguous::gt::U8, DType::U8),
(name, dtype) => {
crate::bail!("Metal contiguous binary {name} {dtype:?} not implemented")
}
};
let buffer = device.new_buffer(el_count, dtype, op)?;
candle_metal_kernels::call_binary_contiguous(
&device.device,
&command_buffer,
&device.kernels,
kernel_name,
el_count,
&self.buffer,
&rhs.buffer,
&buffer,
)
.map_err(MetalError::from)?;
(buffer, dtype)
} else {
use candle_metal_kernels::binary::strided;
let (kernel_name, dtype) = match (op, self.dtype) {
("badd", DType::F32) => (strided::add::FLOAT, self.dtype),
("bsub", DType::F32) => (strided::sub::FLOAT, self.dtype),
("bmul", DType::F32) => (strided::mul::FLOAT, self.dtype),
("bdiv", DType::F32) => (strided::div::FLOAT, self.dtype),
("bminimum", DType::F32) => (strided::min::FLOAT, self.dtype),
("bmaximum", DType::F32) => (strided::max::FLOAT, self.dtype),
("eq", DType::F32) => (strided::eq::FLOAT, DType::U8),
("ne", DType::F32) => (strided::ne::FLOAT, DType::U8),
("le", DType::F32) => (strided::le::FLOAT, DType::U8),
("lt", DType::F32) => (strided::lt::FLOAT, DType::U8),
("ge", DType::F32) => (strided::ge::FLOAT, DType::U8),
("gt", DType::F32) => (strided::gt::FLOAT, DType::U8),
("badd", DType::F16) => (strided::add::HALF, self.dtype),
("bsub", DType::F16) => (strided::sub::HALF, self.dtype),
("bmul", DType::F16) => (strided::mul::HALF, self.dtype),
("bdiv", DType::F16) => (strided::div::HALF, self.dtype),
("bminimum", DType::F16) => (strided::min::HALF, self.dtype),
("bmaximum", DType::F16) => (strided::max::HALF, self.dtype),
("eq", DType::F16) => (strided::eq::HALF, DType::U8),
("ne", DType::F16) => (strided::ne::HALF, DType::U8),
("le", DType::F16) => (strided::le::HALF, DType::U8),
("lt", DType::F16) => (strided::lt::HALF, DType::U8),
("ge", DType::F16) => (strided::ge::HALF, DType::U8),
("gt", DType::F16) => (strided::gt::HALF, DType::U8),
("badd", DType::BF16) => (strided::add::BFLOAT, self.dtype),
("bsub", DType::BF16) => (strided::sub::BFLOAT, self.dtype),
("bmul", DType::BF16) => (strided::mul::BFLOAT, self.dtype),
("bdiv", DType::BF16) => (strided::div::BFLOAT, self.dtype),
("bminimum", DType::BF16) => (strided::min::BFLOAT, self.dtype),
("bmaximum", DType::BF16) => (strided::max::BFLOAT, self.dtype),
("eq", DType::BF16) => (strided::eq::BFLOAT, DType::U8),
("ne", DType::BF16) => (strided::ne::BFLOAT, DType::U8),
("le", DType::BF16) => (strided::le::BFLOAT, DType::U8),
("lt", DType::BF16) => (strided::lt::BFLOAT, DType::U8),
("ge", DType::BF16) => (strided::ge::BFLOAT, DType::U8),
("gt", DType::BF16) => (strided::gt::BFLOAT, DType::U8),
("badd", DType::I64) => (strided::add::I64, self.dtype),
("bsub", DType::I64) => (strided::sub::I64, self.dtype),
("bmul", DType::I64) => (strided::mul::I64, self.dtype),
("bdiv", DType::I64) => (strided::div::I64, self.dtype),
("bminimum", DType::I64) => (strided::min::I64, self.dtype),
("bmaximum", DType::I64) => (strided::max::I64, self.dtype),
("eq", DType::I64) => (strided::eq::I64, DType::U8),
("ne", DType::I64) => (strided::ne::I64, DType::U8),
("le", DType::I64) => (strided::le::I64, DType::U8),
("lt", DType::I64) => (strided::lt::I64, DType::U8),
("ge", DType::I64) => (strided::ge::I64, DType::U8),
("gt", DType::I64) => (strided::gt::I64, DType::U8),
("badd", DType::U32) => (strided::add::U32, self.dtype),
("bsub", DType::U32) => (strided::sub::U32, self.dtype),
("bmul", DType::U32) => (strided::mul::U32, self.dtype),
("bdiv", DType::U32) => (strided::div::U32, self.dtype),
("bminimum", DType::U32) => (strided::min::U32, self.dtype),
("bmaximum", DType::U32) => (strided::max::U32, self.dtype),
("eq", DType::U32) => (strided::eq::U32, DType::U8),
("ne", DType::U32) => (strided::ne::U32, DType::U8),
("le", DType::U32) => (strided::le::U32, DType::U8),
("lt", DType::U32) => (strided::lt::U32, DType::U8),
("ge", DType::U32) => (strided::ge::U32, DType::U8),
("gt", DType::U32) => (strided::gt::U32, DType::U8),
("badd", DType::U8) => (strided::add::U8, self.dtype),
("bsub", DType::U8) => (strided::sub::U8, self.dtype),
("bmul", DType::U8) => (strided::mul::U8, self.dtype),
("bdiv", DType::U8) => (strided::div::U8, self.dtype),
("bminimum", DType::U8) => (strided::min::U8, self.dtype),
("bmaximum", DType::U8) => (strided::max::U8, self.dtype),
("eq", DType::U8) => (strided::eq::U8, DType::U8),
("ne", DType::U8) => (strided::ne::U8, DType::U8),
("le", DType::U8) => (strided::le::U8, DType::U8),
("lt", DType::U8) => (strided::lt::U8, DType::U8),
("ge", DType::U8) => (strided::ge::U8, DType::U8),
("gt", DType::U8) => (strided::gt::U8, DType::U8),
(name, dtype) => {
crate::bail!("Metal strided binary {name} {dtype:?} not implemented")
}
};
let buffer = device.new_buffer(el_count, dtype, op)?;
candle_metal_kernels::call_binary_strided(
&device.device,
&command_buffer,
&device.kernels,
kernel_name,
lhs_l.dims(),
&self.buffer,
lhs_l.stride(),
lhs_l.start_offset() * self.dtype.size_in_bytes(),
&rhs.buffer,
rhs_l.stride(),
rhs_l.start_offset() * rhs.dtype.size_in_bytes(),
&buffer,
)
.map_err(MetalError::from)?;
(buffer, dtype)
};
command_buffer.set_label("binary");
Ok(Self::new(buffer, device.clone(), el_count, dtype))
}
pub(crate) fn to_cpu<T: Clone>(&self) -> Result<Vec<T>> {
let size = (self.count * self.dtype.size_in_bytes()) as NSUInteger;
let buffer = self.device.new_buffer_managed(size)?;
{
let command_buffer = self.device.command_buffer()?;
command_buffer.set_label("to_cpu");
let blit = command_buffer.new_blit_command_encoder();
blit.set_label("blit_to_cpu");
blit.copy_from_buffer(&self.buffer, 0, &buffer, 0, size);
blit.end_encoding();
}
self.device.wait_until_completed()?;
Ok(read_to_vec(&buffer, self.count))
}
}
impl BackendDevice for MetalDevice {
type Storage = MetalStorage;
fn new(ordinal: usize) -> Result<Self> {
let device = metal::Device::all().swap_remove(ordinal);
let command_queue = device.new_command_queue();
let command_buffer = command_queue.new_command_buffer().to_owned();
command_buffer.enqueue();
let command_buffer = Arc::new(RwLock::new(command_buffer));
let command_buffer_index = Arc::new(RwLock::new(0));
let kernels = Arc::new(Kernels::new());
let buffers = Arc::new(RwLock::new(HashMap::new()));
let compute_per_buffer = match std::env::var("CANDLE_METAL_COMPUTE_PER_BUFFER") {
Ok(val) => val.parse()?,
_ => 50,
};
let seed = Arc::new(Mutex::new(device.new_buffer_with_data(
[299792458].as_ptr() as *const c_void,
4,
MTLResourceOptions::StorageModeManaged,
)));
Ok(Self {
device,
command_queue,
command_buffer,
command_buffer_index,
compute_per_buffer,
buffers,
kernels,
seed,
})
}
fn location(&self) -> crate::DeviceLocation {
crate::DeviceLocation::Metal {
gpu_id: self.registry_id() as usize,
}
}
fn same_device(&self, rhs: &Self) -> bool {
self.device.registry_id() == rhs.device.registry_id()
}
fn zeros_impl(&self, shape: &Shape, dtype: DType) -> Result<MetalStorage> {
let size = shape.elem_count() * dtype.size_in_bytes();
let buffer = self.allocate_zeros(size)?;
Ok(MetalStorage::new(
buffer,
self.clone(),
shape.elem_count(),
dtype,
))
}
fn ones_impl(&self, shape: &Shape, dtype: DType) -> Result<Self::Storage> {
// TODO Is there a faster way ?
let cpu_storage = crate::cpu_backend::CpuDevice.ones_impl(shape, dtype)?;
self.storage_from_cpu_storage(&cpu_storage)
}
fn storage_from_cpu_storage(&self, storage: &CpuStorage) -> Result<Self::Storage> {
let (count, buffer) = match storage {
CpuStorage::U8(storage) => (storage.len(), self.new_buffer_with_data(storage)),
CpuStorage::U32(storage) => (storage.len(), self.new_buffer_with_data(storage)),
CpuStorage::I64(storage) => (storage.len(), self.new_buffer_with_data(storage)),
CpuStorage::BF16(storage) => (storage.len(), self.new_buffer_with_data(storage)),
CpuStorage::F16(storage) => (storage.len(), self.new_buffer_with_data(storage)),
CpuStorage::F32(storage) => (storage.len(), self.new_buffer_with_data(storage)),
CpuStorage::F64(storage) => (storage.len(), self.new_buffer_with_data(storage)),
};
Ok(Self::Storage::new(
buffer?,
self.clone(),
count,
storage.dtype(),
))
}
fn rand_uniform(
&self,
shape: &Shape,
dtype: DType,
min: f64,
max: f64,
) -> Result<Self::Storage> {
let name = match dtype {
DType::F32 => "rand_uniform_f32",
DType::F16 => "rand_uniform_f16",
DType::BF16 => "rand_uniform_bf16",
dtype => crate::bail!("rand_uniform not implemented for {dtype:?}"),
};
let buffer = self.new_buffer(shape.elem_count(), dtype, "rand_uniform")?;
let command_buffer = self.command_buffer()?;
candle_metal_kernels::call_random_uniform(
&self.device,
&command_buffer,
&self.kernels,
name,
min as f32,
max as f32,
shape.elem_count(),
&self.seed.lock().unwrap(),
&buffer,
)
.map_err(MetalError::from)?;
Ok(Self::Storage::new(
buffer,
self.clone(),
shape.elem_count(),
dtype,
))
}
fn rand_normal(
&self,
shape: &Shape,
dtype: DType,
mean: f64,
stddev: f64,
) -> Result<Self::Storage> {
let name = match dtype {
DType::F32 => "rand_normal_f32",
DType::F16 => "rand_normal_f16",
DType::BF16 => "rand_normal_bf16",
dtype => crate::bail!("rand_uniform not implemented for {dtype:?}"),
};
let buffer = self.new_buffer(shape.elem_count(), dtype, "rand_normal")?;
let command_buffer = self.command_buffer()?;
candle_metal_kernels::call_random_normal(
&self.device,
&command_buffer,
&self.kernels,
name,
mean as f32,
stddev as f32,
shape.elem_count(),
&self.seed.lock().unwrap(),
&buffer,
)
.map_err(MetalError::from)?;
Ok(Self::Storage::new(
buffer,
self.clone(),
shape.elem_count(),
dtype,
))
}
fn set_seed(&self, seed: u64) -> Result<()> {
let seed: u32 = seed.try_into().map_err(|_| {
MetalError::Message("Metal seed must be less than or equal to u32::MAX".to_string())
})?;
let seed_buffer = self.seed.try_lock().map_err(MetalError::from)?;
let contents = seed_buffer.contents();
unsafe {
std::ptr::copy([seed].as_ptr(), contents as *mut u32, 1);
}
seed_buffer.did_modify_range(metal::NSRange::new(0, 4));
Ok(())
}
}
fn buf_size(size: NSUInteger) -> NSUInteger {
(size - 1).next_power_of_two() as NSUInteger
}
fn read_to_vec<T: Clone>(buffer: &Buffer, n: usize) -> Vec<T> {
let ptr = buffer.contents() as *const T;
assert!(!ptr.is_null());
let slice = unsafe { std::slice::from_raw_parts(ptr, n) };
slice.to_vec()
}
| candle/candle-core/src/metal_backend.rs/0 | {
"file_path": "candle/candle-core/src/metal_backend.rs",
"repo_id": "candle",
"token_count": 42597
} | 19 |
use crate::Result;
pub(super) fn nearest_int(v: f32) -> i32 {
v.round() as i32
}
/// Validates that the input and output are the right size and returns an iterator which maps each
/// input region `xs` to its corresponding output block in `ys`. Each output region is guaranteed
/// to be `T::BLCK_SIZE` long.
pub(super) fn group_for_quantization<'a, 'b, T: super::k_quants::GgmlType>(
xs: &'b [f32],
ys: &'a mut [T],
) -> Result<Vec<(&'a mut T, &'b [f32])>> {
let block_size = T::BLCK_SIZE;
let dtype = T::DTYPE;
let expected_blocks = xs.len() / block_size;
let actual_blocks = ys.len();
// Validate that the input is the right size
if expected_blocks != actual_blocks {
crate::bail!("quantize {dtype:?}: expected {expected_blocks} blocks but only {actual_blocks} were provided!")
}
Ok(ys.iter_mut().zip(xs.chunks_exact(block_size)).collect())
}
/// Validates that the input and output are the right size and returns an iterator which maps each
/// input block `xs` to its corresponding output region in `ys`. Each output region is guaranteed
/// to be `T::BLCK_SIZE` long.
pub(super) fn group_for_dequantization<'a, 'b, T: super::k_quants::GgmlType>(
xs: &'a [T],
ys: &'b mut [f32],
) -> Result<Vec<(&'a T, &'b mut [f32])>> {
let block_size = T::BLCK_SIZE;
let dtype = T::DTYPE;
let actual_output_len = ys.len();
let expected_output_len = xs.len() * block_size;
// Validate that the output is the right size
if expected_output_len != actual_output_len {
crate::bail!("dequantize {dtype:?}: ys (len = {actual_output_len}) does not match the expected length of {expected_output_len}!")
}
// Zip the blocks and outputs together
Ok(xs.iter().zip(ys.chunks_exact_mut(block_size)).collect())
}
pub(super) fn get_scale_min_k4(j: usize, q: &[u8]) -> (u8, u8) {
if j < 4 {
let d = q[j] & 63;
let m = q[j + 4] & 63;
(d, m)
} else {
let d = (q[j + 4] & 0xF) | ((q[j - 4] >> 6) << 4);
let m = (q[j + 4] >> 4) | ((q[j] >> 6) << 4);
(d, m)
}
}
pub(super) unsafe fn make_qx_quants(
n: usize,
nmax: i32,
x: *const f32,
ls: *mut i8,
rmse_type: i32,
) -> f32 {
let mut max = 0f32;
let mut amax = 0f32;
for i in 0..n {
let x = *x.add(i);
let ax = x.abs();
if ax > amax {
amax = ax;
max = x;
}
}
if amax == 0. {
// all zero
for i in 0..n {
*ls.add(i) = 0;
}
return 0.;
}
let mut iscale = -(nmax as f32) / max;
if rmse_type == 0 {
for i in 0..n {
let x = *x.add(i);
let l = nearest_int(iscale * x);
*ls.add(i) = (nmax + l.clamp(-nmax, nmax - 1)) as i8;
}
return 1.0 / iscale;
}
let weight_type = rmse_type % 2;
let mut sumlx = 0f32;
let mut suml2 = 0f32;
for i in 0..n {
let x = *x.add(i);
let l = nearest_int(iscale * x);
let l = l.clamp(-nmax, nmax - 1);
*ls.add(i) = (l + nmax) as i8;
let w = if weight_type == 1 { x * x } else { 1.0 };
let l = l as f32;
sumlx += w * x * l;
suml2 += w * l * l;
}
let mut scale = sumlx / suml2;
let mut best = scale * sumlx;
for _itry in 0..3 {
let iscale = 1.0 / scale;
let mut slx = 0f32;
let mut sl2 = 0f32;
let mut changed = false;
for i in 0..n {
let x = *x.add(i);
let l = nearest_int(iscale * x);
let l = l.clamp(-nmax, nmax - 1);
if l + nmax != *ls.add(i) as i32 {
changed = true;
}
let w = if weight_type == 1 { x * x } else { 1f32 };
let l = l as f32;
slx += w * x * l;
sl2 += w * l * l;
}
if !changed || sl2 == 0.0 || slx * slx <= best * sl2 {
break;
}
for i in 0..n {
let x = *x.add(i);
let l = nearest_int(iscale * x);
*ls.add(i) = (nmax + l.clamp(-nmax, nmax - 1)) as i8;
}
sumlx = slx;
suml2 = sl2;
scale = sumlx / suml2;
best = scale * sumlx;
}
for _itry in 0..5 {
let mut n_changed = 0;
for i in 0..n {
let x = *x.add(i);
let w = if weight_type == 1 { x * x } else { 1. };
let l = *ls.add(i) as i32 - nmax;
let mut slx = sumlx - w * x * l as f32;
if slx > 0. {
let mut sl2 = suml2 - w * l as f32 * l as f32;
let new_l = nearest_int(x * sl2 / slx);
let new_l = new_l.clamp(-nmax, nmax - 1);
if new_l != l {
slx += w * x * new_l as f32;
sl2 += w * new_l as f32 * new_l as f32;
if sl2 > 0. && slx * slx * suml2 > sumlx * sumlx * sl2 {
*ls.add(i) = (nmax + new_l) as i8;
sumlx = slx;
suml2 = sl2;
scale = sumlx / suml2;
best = scale * sumlx;
n_changed += 1;
}
}
}
}
if n_changed == 0 {
break;
}
}
if rmse_type < 3 {
return scale;
}
for is in -4..4 {
if is == 0 {
continue;
}
iscale = -(nmax as f32 + 0.1f32 * is as f32) / max;
let mut sumlx = 0.;
let mut suml2 = 0.;
for i in 0..n {
let x = *x.add(i);
let l = nearest_int(iscale * x);
let l = l.clamp(-nmax, nmax - 1);
let w = if weight_type == 1 { x * x } else { 1. };
let l = l as f32;
sumlx += w * x * l;
suml2 += w * l * l;
}
if suml2 > 0. && sumlx * sumlx > best * suml2 {
for i in 0..n {
let x = *x.add(i);
let l = nearest_int(iscale * x);
*ls.add(i) = (nmax + l.clamp(-nmax, nmax - 1)) as i8;
}
scale = sumlx / suml2;
best = scale * sumlx;
}
}
scale
}
// https://github.com/ggerganov/llama.cpp/blob/8183159cf3def112f6d1fe94815fce70e1bffa12/k_quants.c#L224
pub(super) fn make_qkx1_quants(nmax: i32, ntry: usize, x: &[f32]) -> (f32, f32) {
let n = x.len();
let mut l = vec![0; n];
// Get min/max
let min = *x
.iter()
.take(n)
.min_by(|a, b| a.total_cmp(b))
.unwrap_or(&x[0]);
let max = *x.iter().max_by(|a, b| a.total_cmp(b)).unwrap_or(&x[0]);
// If min == max, all values are the same => nothing to do here
if max == min {
return (0.0, 0.0);
}
// Ensure min <= 0.0
let mut min = min.min(0.);
// Compute scale and inverse scale
let mut iscale = nmax as f32 / (max - min);
let mut scale = 1.0 / iscale;
for _ in 0..ntry {
let mut sumlx = 0.0;
let mut suml2 = 0;
let mut did_change = false;
for (i, value) in x.iter().enumerate().take(n) {
let li = nearest_int(iscale * (value - min)).clamp(0, nmax);
let clamped_li = li as u8;
if clamped_li != l[i] {
l[i] = clamped_li;
did_change = true;
}
sumlx += (value - min) * li as f32;
suml2 += li * li;
}
scale = sumlx / suml2 as f32;
let sum: f32 = x
.iter()
.take(n)
.zip(l.iter().take(n))
.map(|(xi, &li)| xi - scale * li as f32)
.sum();
min = sum / n as f32;
if min > 0.0 {
min = 0.0;
}
iscale = 1.0 / scale;
if !did_change {
break;
}
}
(scale, -min)
}
// https://github.com/ggerganov/llama.cpp/blob/8183159cf3def112f6d1fe94815fce70e1bffa12/k_quants.c#L165
pub(super) fn make_q3_quants(x: &[f32], nmax: i32, do_rmse: bool) -> f32 {
let n = x.len();
let mut l = vec![0i8; n];
let mut max = 0.0;
let mut amax = 0.0;
for &xi in x.iter().take(n) {
let ax = xi.abs();
if ax > amax {
amax = ax;
max = xi;
}
}
if amax == 0.0 {
return 0.0;
}
let iscale = -(nmax as f32) / max;
if do_rmse {
let mut sumlx = 0.0;
let mut suml2 = 0.0;
for i in 0..n {
let li = (iscale * x[i]).round() as i32;
let li = li.clamp(-nmax, nmax - 1);
l[i] = li as i8;
let w = x[i] * x[i];
sumlx += w * x[i] * li as f32;
suml2 += w * (li * li) as f32;
}
for _ in 0..5 {
let mut n_changed = 0;
for i in 0..n {
let w = x[i] * x[i];
let mut slx = sumlx - w * x[i] * l[i] as f32;
if slx > 0.0 {
let mut sl2 = suml2 - w * (l[i] as i32 * l[i] as i32) as f32;
let mut new_l = (x[i] * sl2 / slx).round() as i32;
new_l = new_l.clamp(-nmax, nmax - 1);
if new_l != l[i] as i32 {
slx += w * x[i] * new_l as f32;
sl2 += w * (new_l * new_l) as f32;
if sl2 > 0.0 && slx * slx * suml2 > sumlx * sumlx * sl2 {
l[i] = new_l as i8;
sumlx = slx;
suml2 = sl2;
n_changed += 1;
}
}
}
}
if n_changed == 0 {
break;
}
}
for li in l.iter_mut() {
*li += nmax as i8;
}
return sumlx / suml2;
}
for i in 0..n {
let li = (iscale * x[i]).round() as i32;
l[i] = (li.clamp(-nmax, nmax - 1) + nmax) as i8;
}
1.0 / iscale
}
| candle/candle-core/src/quantized/utils.rs/0 | {
"file_path": "candle/candle-core/src/quantized/utils.rs",
"repo_id": "candle",
"token_count": 5775
} | 20 |
use anyhow::Result;
use candle_core::{Device, IndexOp, Tensor};
#[test]
fn integer_index() -> Result<()> {
let dev = Device::Cpu;
let tensor = Tensor::arange(0u32, 2 * 3, &dev)?.reshape((2, 3))?;
let result = tensor.i(1)?;
assert_eq!(result.dims(), &[3]);
assert_eq!(result.to_vec1::<u32>()?, &[3, 4, 5]);
let result = tensor.i((.., 2))?;
assert_eq!(result.dims(), &[2]);
assert_eq!(result.to_vec1::<u32>()?, &[2, 5]);
Ok(())
}
#[test]
fn range_index() -> Result<()> {
let dev = Device::Cpu;
// RangeFull
let tensor = Tensor::arange(0u32, 2 * 3, &dev)?.reshape((2, 3))?;
let result = tensor.i(..)?;
assert_eq!(result.dims(), &[2, 3]);
assert_eq!(result.to_vec2::<u32>()?, &[[0, 1, 2], [3, 4, 5]]);
// Range
let tensor = Tensor::arange(0u32, 4 * 3, &dev)?.reshape((4, 3))?;
let result = tensor.i(1..3)?;
assert_eq!(result.dims(), &[2, 3]);
assert_eq!(result.to_vec2::<u32>()?, &[[3, 4, 5], [6, 7, 8]]);
// RangeFrom
let result = tensor.i(2..)?;
assert_eq!(result.dims(), &[2, 3]);
assert_eq!(result.to_vec2::<u32>()?, &[[6, 7, 8], [9, 10, 11]]);
// RangeTo
let result = tensor.i(..2)?;
assert_eq!(result.dims(), &[2, 3]);
assert_eq!(result.to_vec2::<u32>()?, &[[0, 1, 2], [3, 4, 5]]);
// RangeInclusive
let result = tensor.i(1..=2)?;
assert_eq!(result.dims(), &[2, 3]);
assert_eq!(result.to_vec2::<u32>()?, &[[3, 4, 5], [6, 7, 8]]);
// RangeTo
let result = tensor.i(..1)?;
assert_eq!(result.dims(), &[1, 3]);
assert_eq!(result.to_vec2::<u32>()?, &[[0, 1, 2]]);
// RangeToInclusive
let result = tensor.i(..=1)?;
assert_eq!(result.dims(), &[2, 3]);
assert_eq!(result.to_vec2::<u32>()?, &[[0, 1, 2], [3, 4, 5]]);
// Empty range
let result = tensor.i(1..1)?;
assert_eq!(result.dims(), &[0, 3]);
let empty: [[u32; 3]; 0] = [];
assert_eq!(result.to_vec2::<u32>()?, &empty);
// Similar to PyTorch, allow empty ranges when the computed length is negative.
#[allow(clippy::reversed_empty_ranges)]
let result = tensor.i(1..0)?;
assert_eq!(result.dims(), &[0, 3]);
let empty: [[u32; 3]; 0] = [];
assert_eq!(result.to_vec2::<u32>()?, &empty);
Ok(())
}
#[test]
fn index_3d() -> Result<()> {
let tensor = Tensor::from_iter(0..24u32, &Device::Cpu)?.reshape((2, 3, 4))?;
assert_eq!(tensor.i((0, 0, 0))?.to_scalar::<u32>()?, 0);
assert_eq!(tensor.i((1, 0, 0))?.to_scalar::<u32>()?, 12);
assert_eq!(tensor.i((0, 1, 0))?.to_scalar::<u32>()?, 4);
assert_eq!(tensor.i((0, 1, 3))?.to_scalar::<u32>()?, 7);
assert_eq!(tensor.i((0..2, 0, 0))?.to_vec1::<u32>()?, &[0, 12]);
assert_eq!(
tensor.i((0..2, .., 0))?.to_vec2::<u32>()?,
&[[0, 4, 8], [12, 16, 20]]
);
assert_eq!(
tensor.i((..2, .., 3))?.to_vec2::<u32>()?,
&[[3, 7, 11], [15, 19, 23]]
);
assert_eq!(tensor.i((1, .., 3))?.to_vec1::<u32>()?, &[15, 19, 23]);
Ok(())
}
#[test]
fn slice_assign() -> Result<()> {
let dev = Device::Cpu;
let tensor = Tensor::arange(0u32, 4 * 5, &dev)?.reshape((4, 5))?;
let src = Tensor::arange(0u32, 2 * 3, &dev)?.reshape((3, 2))?;
let out = tensor.slice_assign(&[1..4, 3..5], &src)?;
assert_eq!(
out.to_vec2::<u32>()?,
&[
[0, 1, 2, 3, 4],
[5, 6, 7, 0, 1],
[10, 11, 12, 2, 3],
[15, 16, 17, 4, 5]
]
);
let out = tensor.slice_assign(&[0..3, 0..2], &src)?;
assert_eq!(
out.to_vec2::<u32>()?,
&[
[0, 1, 2, 3, 4],
[2, 3, 7, 8, 9],
[4, 5, 12, 13, 14],
[15, 16, 17, 18, 19]
]
);
Ok(())
}
| candle/candle-core/tests/indexing_tests.rs/0 | {
"file_path": "candle/candle-core/tests/indexing_tests.rs",
"repo_id": "candle",
"token_count": 1994
} | 21 |
use hf_hub::{
api::sync::{Api, ApiRepo},
Repo, RepoType,
};
use parquet::file::reader::SerializedFileReader;
use std::fs::File;
#[derive(thiserror::Error, Debug)]
pub enum Error {
#[error("ApiError : {0}")]
ApiError(#[from] hf_hub::api::sync::ApiError),
#[error("IoError : {0}")]
IoError(#[from] std::io::Error),
#[error("ParquetError : {0}")]
ParquetError(#[from] parquet::errors::ParquetError),
}
fn sibling_to_parquet(
rfilename: &str,
repo: &ApiRepo,
) -> Result<SerializedFileReader<File>, Error> {
let local = repo.get(rfilename)?;
let file = File::open(local)?;
let reader = SerializedFileReader::new(file)?;
Ok(reader)
}
pub fn from_hub(api: &Api, dataset_id: String) -> Result<Vec<SerializedFileReader<File>>, Error> {
let repo = Repo::with_revision(
dataset_id,
RepoType::Dataset,
"refs/convert/parquet".to_string(),
);
let repo = api.repo(repo);
let info = repo.info()?;
let files: Result<Vec<_>, _> = info
.siblings
.into_iter()
.filter_map(|s| -> Option<Result<_, _>> {
let filename = s.rfilename;
if filename.ends_with(".parquet") {
let reader_result = sibling_to_parquet(&filename, &repo);
Some(reader_result)
} else {
None
}
})
.collect();
let files = files?;
Ok(files)
}
#[cfg(test)]
mod tests {
use super::*;
use parquet::file::reader::FileReader;
#[test]
fn test_dataset() {
let api = Api::new().unwrap();
let files = from_hub(
&api,
"hf-internal-testing/dummy_image_text_data".to_string(),
)
.unwrap();
assert_eq!(files.len(), 1);
assert_eq!(files[0].metadata().file_metadata().num_rows(), 20);
}
}
| candle/candle-datasets/src/hub.rs/0 | {
"file_path": "candle/candle-datasets/src/hub.rs",
"repo_id": "candle",
"token_count": 900
} | 22 |
#[cfg(feature = "mkl")]
extern crate intel_mkl_src;
#[cfg(feature = "accelerate")]
extern crate accelerate_src;
use anyhow::{Error as E, Result};
use clap::Parser;
use candle_transformers::models::chatglm::{Config, Model};
use candle::{DType, Device, Tensor};
use candle_nn::VarBuilder;
use candle_transformers::generation::LogitsProcessor;
use hf_hub::{api::sync::Api, Repo, RepoType};
use tokenizers::Tokenizer;
struct TextGeneration {
model: Model,
device: Device,
tokenizer: Tokenizer,
logits_processor: LogitsProcessor,
repeat_penalty: f32,
repeat_last_n: usize,
verbose_prompt: bool,
}
impl TextGeneration {
#[allow(clippy::too_many_arguments)]
fn new(
model: Model,
tokenizer: Tokenizer,
seed: u64,
temp: Option<f64>,
top_p: Option<f64>,
repeat_penalty: f32,
repeat_last_n: usize,
verbose_prompt: bool,
device: &Device,
) -> Self {
let logits_processor = LogitsProcessor::new(seed, temp, top_p);
Self {
model,
tokenizer,
logits_processor,
repeat_penalty,
repeat_last_n,
verbose_prompt,
device: device.clone(),
}
}
fn run(&mut self, prompt: &str, sample_len: usize) -> Result<()> {
use std::io::Write;
println!("starting the inference loop");
let tokens = self.tokenizer.encode(prompt, true).map_err(E::msg)?;
if tokens.is_empty() {
anyhow::bail!("Empty prompts are not supported in the chatglm model.")
}
if self.verbose_prompt {
for (token, id) in tokens.get_tokens().iter().zip(tokens.get_ids().iter()) {
let token = token.replace('▁', " ").replace("<0x0A>", "\n");
println!("{id:7} -> '{token}'");
}
}
let mut tokens = tokens.get_ids().to_vec();
let mut generated_tokens = 0usize;
let eos_token = match self.tokenizer.get_vocab(true).get("</s>") {
Some(token) => *token,
None => anyhow::bail!("cannot find the endoftext token"),
};
print!("{prompt}");
std::io::stdout().flush()?;
let start_gen = std::time::Instant::now();
for index in 0..sample_len {
let context_size = if index > 0 { 1 } else { tokens.len() };
let ctxt = &tokens[tokens.len().saturating_sub(context_size)..];
let input = Tensor::new(ctxt, &self.device)?.unsqueeze(0)?;
let logits = self.model.forward(&input)?;
let logits = logits.squeeze(0)?.to_dtype(DType::F32)?;
let logits = if self.repeat_penalty == 1. {
logits
} else {
let start_at = tokens.len().saturating_sub(self.repeat_last_n);
candle_transformers::utils::apply_repeat_penalty(
&logits,
self.repeat_penalty,
&tokens[start_at..],
)?
};
let next_token = self.logits_processor.sample(&logits)?;
tokens.push(next_token);
generated_tokens += 1;
if next_token == eos_token {
break;
}
let token = self.tokenizer.decode(&[next_token], true).map_err(E::msg)?;
print!("{token}");
std::io::stdout().flush()?;
}
let dt = start_gen.elapsed();
println!(
"\n{generated_tokens} tokens generated ({:.2} token/s)",
generated_tokens as f64 / dt.as_secs_f64(),
);
Ok(())
}
}
#[derive(Parser, Debug)]
#[command(author, version, about, long_about = None)]
struct Args {
/// Run on CPU rather than on GPU.
#[arg(long)]
cpu: bool,
/// Enable tracing (generates a trace-timestamp.json file).
#[arg(long)]
tracing: bool,
/// Display the token for the specified prompt.
#[arg(long)]
verbose_prompt: bool,
#[arg(long)]
prompt: String,
/// The temperature used to generate samples.
#[arg(long)]
temperature: Option<f64>,
/// Nucleus sampling probability cutoff.
#[arg(long)]
top_p: Option<f64>,
/// The seed to use when generating random samples.
#[arg(long, default_value_t = 299792458)]
seed: u64,
/// The length of the sample to generate (in tokens).
#[arg(long, short = 'n', default_value_t = 5000)]
sample_len: usize,
#[arg(long)]
model_id: Option<String>,
#[arg(long)]
revision: Option<String>,
#[arg(long)]
weight_file: Option<String>,
#[arg(long)]
tokenizer: Option<String>,
/// Penalty to be applied for repeating tokens, 1. means no penalty.
#[arg(long, default_value_t = 1.1)]
repeat_penalty: f32,
/// The context size to consider for the repeat penalty.
#[arg(long, default_value_t = 64)]
repeat_last_n: usize,
}
fn main() -> Result<()> {
use tracing_chrome::ChromeLayerBuilder;
use tracing_subscriber::prelude::*;
let args = Args::parse();
let _guard = if args.tracing {
let (chrome_layer, guard) = ChromeLayerBuilder::new().build();
tracing_subscriber::registry().with(chrome_layer).init();
Some(guard)
} else {
None
};
println!(
"avx: {}, neon: {}, simd128: {}, f16c: {}",
candle::utils::with_avx(),
candle::utils::with_neon(),
candle::utils::with_simd128(),
candle::utils::with_f16c()
);
println!(
"temp: {:.2} repeat-penalty: {:.2} repeat-last-n: {}",
args.temperature.unwrap_or(0.),
args.repeat_penalty,
args.repeat_last_n
);
let start = std::time::Instant::now();
let api = Api::new()?;
let model_id = match args.model_id {
Some(model_id) => model_id.to_string(),
None => "THUDM/chatglm3-6b".to_string(),
};
let revision = match args.revision {
Some(rev) => rev.to_string(),
None => "main".to_string(),
};
let repo = api.repo(Repo::with_revision(model_id, RepoType::Model, revision));
let tokenizer_filename = match args.tokenizer {
Some(file) => std::path::PathBuf::from(file),
None => api
.model("lmz/candle-chatglm".to_string())
.get("chatglm-tokenizer.json")?,
};
let filenames = match args.weight_file {
Some(weight_file) => vec![std::path::PathBuf::from(weight_file)],
None => candle_examples::hub_load_safetensors(&repo, "model.safetensors.index.json")?,
};
println!("retrieved the files in {:?}", start.elapsed());
let tokenizer = Tokenizer::from_file(tokenizer_filename).map_err(E::msg)?;
let start = std::time::Instant::now();
let config = Config::glm3_6b();
let device = candle_examples::device(args.cpu)?;
let vb = unsafe { VarBuilder::from_mmaped_safetensors(&filenames, DType::F32, &device)? };
let model = Model::new(&config, vb)?;
println!("loaded the model in {:?}", start.elapsed());
let mut pipeline = TextGeneration::new(
model,
tokenizer,
args.seed,
args.temperature,
args.top_p,
args.repeat_penalty,
args.repeat_last_n,
args.verbose_prompt,
&device,
);
pipeline.run(&args.prompt, args.sample_len)?;
Ok(())
}
| candle/candle-examples/examples/chatglm/main.rs/0 | {
"file_path": "candle/candle-examples/examples/chatglm/main.rs",
"repo_id": "candle",
"token_count": 3423
} | 23 |
#![allow(unused)]
use anyhow::{Context, Result};
use std::sync::{Arc, Mutex};
pub const SAMPLE_RATE: usize = 24_000;
pub(crate) struct AudioOutputData_ {
resampled_data: std::collections::VecDeque<f32>,
resampler: rubato::FastFixedIn<f32>,
output_buffer: Vec<f32>,
input_buffer: Vec<f32>,
input_len: usize,
}
impl AudioOutputData_ {
pub(crate) fn new(input_sample_rate: usize, output_sample_rate: usize) -> Result<Self> {
use rubato::Resampler;
let resampled_data = std::collections::VecDeque::with_capacity(output_sample_rate * 10);
let resample_ratio = output_sample_rate as f64 / input_sample_rate as f64;
let resampler = rubato::FastFixedIn::new(
resample_ratio,
f64::max(resample_ratio, 1.0),
rubato::PolynomialDegree::Septic,
1024,
1,
)?;
let input_buffer = resampler.input_buffer_allocate(true).remove(0);
let output_buffer = resampler.output_buffer_allocate(true).remove(0);
Ok(Self {
resampled_data,
resampler,
input_buffer,
output_buffer,
input_len: 0,
})
}
pub fn reset(&mut self) {
use rubato::Resampler;
self.output_buffer.fill(0.);
self.input_buffer.fill(0.);
self.resampler.reset();
self.resampled_data.clear();
}
pub(crate) fn take_all(&mut self) -> Vec<f32> {
let mut data = Vec::with_capacity(self.resampled_data.len());
while let Some(elem) = self.resampled_data.pop_back() {
data.push(elem);
}
data
}
pub(crate) fn is_empty(&self) -> bool {
self.resampled_data.is_empty()
}
// Assumes that the input buffer is large enough.
fn push_input_buffer(&mut self, samples: &[f32]) {
self.input_buffer[self.input_len..self.input_len + samples.len()].copy_from_slice(samples);
self.input_len += samples.len()
}
pub(crate) fn push_samples(&mut self, samples: &[f32]) -> Result<()> {
use rubato::Resampler;
let mut pos_in = 0;
loop {
let rem = self.input_buffer.len() - self.input_len;
let pos_end = usize::min(pos_in + rem, samples.len());
self.push_input_buffer(&samples[pos_in..pos_end]);
pos_in = pos_end;
if self.input_len < self.input_buffer.len() {
break;
}
let (_, out_len) = self.resampler.process_into_buffer(
&[&self.input_buffer],
&mut [&mut self.output_buffer],
None,
)?;
for &elem in self.output_buffer[..out_len].iter() {
self.resampled_data.push_front(elem)
}
self.input_len = 0;
}
Ok(())
}
}
type AudioOutputData = Arc<Mutex<AudioOutputData_>>;
pub(crate) fn setup_output_stream() -> Result<(cpal::Stream, AudioOutputData)> {
use cpal::traits::{DeviceTrait, HostTrait, StreamTrait};
println!("Setup audio output stream!");
let host = cpal::default_host();
let device = host
.default_output_device()
.context("no output device available")?;
let mut supported_configs_range = device.supported_output_configs()?;
let config_range = match supported_configs_range.find(|c| c.channels() == 1) {
// On macOS, it's commonly the case that there are only stereo outputs.
None => device
.supported_output_configs()?
.next()
.context("no audio output available")?,
Some(config_range) => config_range,
};
let sample_rate = cpal::SampleRate(SAMPLE_RATE as u32).clamp(
config_range.min_sample_rate(),
config_range.max_sample_rate(),
);
let config: cpal::StreamConfig = config_range.with_sample_rate(sample_rate).into();
let channels = config.channels as usize;
println!(
"cpal device: {} {} {config:?}",
device.name().unwrap_or_else(|_| "unk".to_string()),
config.sample_rate.0
);
let audio_data = Arc::new(Mutex::new(AudioOutputData_::new(
SAMPLE_RATE,
config.sample_rate.0 as usize,
)?));
let ad = audio_data.clone();
let stream = device.build_output_stream(
&config,
move |data: &mut [f32], _: &cpal::OutputCallbackInfo| {
data.fill(0.);
let mut ad = ad.lock().unwrap();
let mut last_elem = 0f32;
for (idx, elem) in data.iter_mut().enumerate() {
if idx % channels == 0 {
match ad.resampled_data.pop_back() {
None => break,
Some(v) => {
last_elem = v;
*elem = v
}
}
} else {
*elem = last_elem
}
}
},
move |err| eprintln!("cpal error: {err}"),
None, // None=blocking, Some(Duration)=timeout
)?;
stream.play()?;
Ok((stream, audio_data))
}
pub(crate) fn setup_input_stream() -> Result<(cpal::Stream, AudioOutputData)> {
use cpal::traits::{DeviceTrait, HostTrait, StreamTrait};
println!("Setup audio input stream!");
let host = cpal::default_host();
let device = host
.default_input_device()
.context("no input device available")?;
let mut supported_configs_range = device.supported_input_configs()?;
let config_range = supported_configs_range
.find(|c| c.channels() == 1)
.context("no audio input available")?;
let sample_rate = cpal::SampleRate(SAMPLE_RATE as u32).clamp(
config_range.min_sample_rate(),
config_range.max_sample_rate(),
);
let config: cpal::StreamConfig = config_range.with_sample_rate(sample_rate).into();
println!(
"cpal device: {} {} {config:?}",
device.name().unwrap_or_else(|_| "unk".to_string()),
config.sample_rate.0
);
let audio_data = Arc::new(Mutex::new(AudioOutputData_::new(
config.sample_rate.0 as usize,
SAMPLE_RATE,
)?));
let ad = audio_data.clone();
let stream = device.build_input_stream(
&config,
move |data: &[f32], _: &cpal::InputCallbackInfo| {
let mut ad = ad.lock().unwrap();
if let Err(err) = ad.push_samples(data) {
eprintln!("error processing audio input {err:?}")
}
},
move |err| eprintln!("cpal error: {err}"),
None, // None=blocking, Some(Duration)=timeout
)?;
stream.play()?;
Ok((stream, audio_data))
}
fn conv<T>(samples: &mut Vec<f32>, data: std::borrow::Cow<symphonia::core::audio::AudioBuffer<T>>)
where
T: symphonia::core::sample::Sample,
f32: symphonia::core::conv::FromSample<T>,
{
use symphonia::core::audio::Signal;
use symphonia::core::conv::FromSample;
samples.extend(data.chan(0).iter().map(|v| f32::from_sample(*v)))
}
pub(crate) fn pcm_decode<P: AsRef<std::path::Path>>(path: P) -> Result<(Vec<f32>, u32)> {
use symphonia::core::audio::{AudioBufferRef, Signal};
let src = std::fs::File::open(path)?;
let mss = symphonia::core::io::MediaSourceStream::new(Box::new(src), Default::default());
let hint = symphonia::core::probe::Hint::new();
let meta_opts: symphonia::core::meta::MetadataOptions = Default::default();
let fmt_opts: symphonia::core::formats::FormatOptions = Default::default();
let probed = symphonia::default::get_probe().format(&hint, mss, &fmt_opts, &meta_opts)?;
let mut format = probed.format;
let track = format
.tracks()
.iter()
.find(|t| t.codec_params.codec != symphonia::core::codecs::CODEC_TYPE_NULL)
.expect("no supported audio tracks");
let mut decoder = symphonia::default::get_codecs()
.make(&track.codec_params, &Default::default())
.expect("unsupported codec");
let track_id = track.id;
let sample_rate = track.codec_params.sample_rate.unwrap_or(0);
let mut pcm_data = Vec::new();
while let Ok(packet) = format.next_packet() {
while !format.metadata().is_latest() {
format.metadata().pop();
}
if packet.track_id() != track_id {
continue;
}
match decoder.decode(&packet)? {
AudioBufferRef::F32(buf) => pcm_data.extend(buf.chan(0)),
AudioBufferRef::U8(data) => conv(&mut pcm_data, data),
AudioBufferRef::U16(data) => conv(&mut pcm_data, data),
AudioBufferRef::U24(data) => conv(&mut pcm_data, data),
AudioBufferRef::U32(data) => conv(&mut pcm_data, data),
AudioBufferRef::S8(data) => conv(&mut pcm_data, data),
AudioBufferRef::S16(data) => conv(&mut pcm_data, data),
AudioBufferRef::S24(data) => conv(&mut pcm_data, data),
AudioBufferRef::S32(data) => conv(&mut pcm_data, data),
AudioBufferRef::F64(data) => conv(&mut pcm_data, data),
}
}
Ok((pcm_data, sample_rate))
}
pub(crate) fn resample(pcm_in: &[f32], sr_in: usize, sr_out: usize) -> Result<Vec<f32>> {
use rubato::Resampler;
let mut pcm_out =
Vec::with_capacity((pcm_in.len() as f64 * sr_out as f64 / sr_in as f64) as usize + 1024);
let mut resampler = rubato::FftFixedInOut::<f32>::new(sr_in, sr_out, 1024, 1)?;
let mut output_buffer = resampler.output_buffer_allocate(true);
let mut pos_in = 0;
while pos_in + resampler.input_frames_next() < pcm_in.len() {
let (in_len, out_len) =
resampler.process_into_buffer(&[&pcm_in[pos_in..]], &mut output_buffer, None)?;
pos_in += in_len;
pcm_out.extend_from_slice(&output_buffer[0][..out_len]);
}
if pos_in < pcm_in.len() {
let (_in_len, out_len) = resampler.process_partial_into_buffer(
Some(&[&pcm_in[pos_in..]]),
&mut output_buffer,
None,
)?;
pcm_out.extend_from_slice(&output_buffer[0][..out_len]);
}
Ok(pcm_out)
}
| candle/candle-examples/examples/encodec/audio_io.rs/0 | {
"file_path": "candle/candle-examples/examples/encodec/audio_io.rs",
"repo_id": "candle",
"token_count": 4805
} | 24 |
/// This follows the lines of:
/// https://github.com/johnma2006/mamba-minimal/blob/master/model.py
/// Simple, minimal implementation of Mamba in one file of PyTorch.
use candle::{IndexOp, Module, Result, Tensor, D};
use candle_nn::{RmsNorm, VarBuilder};
use candle_transformers::models::with_tracing::{linear, linear_no_bias, Linear};
#[derive(Debug, Clone, serde::Deserialize)]
pub struct Config {
d_model: usize,
n_layer: usize,
vocab_size: usize,
pad_vocab_size_multiple: usize,
}
impl Config {
fn vocab_size(&self) -> usize {
let pad = self.pad_vocab_size_multiple;
(self.vocab_size + pad - 1) / pad * pad
}
fn dt_rank(&self) -> usize {
(self.d_model + 15) / 16
}
fn d_conv(&self) -> usize {
4
}
fn d_state(&self) -> usize {
16
}
fn d_inner(&self) -> usize {
self.d_model * 2
}
}
// https://github.com/johnma2006/mamba-minimal/blob/61f01953ca153f8c4a850d7111beecbf4be9cee1/model.py#L177
#[derive(Clone, Debug)]
pub struct MambaBlock {
in_proj: Linear,
conv1d: candle_nn::Conv1d,
x_proj: Linear,
dt_proj: Linear,
a_log: Tensor,
d: Tensor,
out_proj: Linear,
dt_rank: usize,
}
impl MambaBlock {
pub fn new(cfg: &Config, vb: VarBuilder) -> Result<Self> {
let d_inner = cfg.d_inner();
let d_conv = cfg.d_conv();
let d_state = cfg.d_state();
let dt_rank = cfg.dt_rank();
let in_proj = linear_no_bias(cfg.d_model, d_inner * 2, vb.pp("in_proj"))?;
let conv_cfg = candle_nn::Conv1dConfig {
groups: d_inner,
padding: d_conv - 1,
..Default::default()
};
let conv1d = candle_nn::conv1d(d_inner, d_inner, d_conv, conv_cfg, vb.pp("conv1d"))?;
let x_proj = linear_no_bias(d_inner, dt_rank + d_state * 2, vb.pp("x_proj"))?;
let dt_proj = linear(dt_rank, d_inner, vb.pp("dt_proj"))?;
let a_log = vb.get((d_inner, d_state), "A_log")?;
let d = vb.get(d_inner, "D")?;
let out_proj = linear_no_bias(d_inner, cfg.d_model, vb.pp("out_proj"))?;
Ok(Self {
in_proj,
conv1d,
x_proj,
dt_proj,
a_log,
d,
out_proj,
dt_rank,
})
}
fn ssm(&self, xs: &Tensor) -> Result<Tensor> {
let (_d_in, n) = self.a_log.dims2()?;
let a = self.a_log.to_dtype(candle::DType::F32)?.exp()?.neg()?;
let d = self.d.to_dtype(candle::DType::F32)?;
let x_dbl = xs.apply(&self.x_proj)?;
let delta = x_dbl.narrow(D::Minus1, 0, self.dt_rank)?;
let b = x_dbl.narrow(D::Minus1, self.dt_rank, n)?;
let c = x_dbl.narrow(D::Minus1, self.dt_rank + n, n)?;
let delta = delta.contiguous()?.apply(&self.dt_proj)?;
// softplus without threshold
let delta = (delta.exp()? + 1.)?.log()?;
let ss = selective_scan(xs, &delta, &a, &b, &c, &d)?;
Ok(ss)
}
}
// https://github.com/johnma2006/mamba-minimal/blob/61f01953ca153f8c4a850d7111beecbf4be9cee1/model.py#L275
fn selective_scan(
u: &Tensor,
delta: &Tensor,
a: &Tensor,
b: &Tensor,
c: &Tensor,
d: &Tensor,
) -> Result<Tensor> {
let (b_sz, l, d_in) = u.dims3()?;
let n = a.dim(1)?;
let delta = delta.t()?.reshape((b_sz, d_in, l, 1))?; // b d_in l 1
let delta_a = delta.broadcast_mul(&a.reshape((1, d_in, 1, n))?)?.exp()?;
let delta_b_u = delta
.broadcast_mul(&b.reshape((b_sz, 1, l, n))?)?
.broadcast_mul(&u.t()?.reshape((b_sz, d_in, l, 1))?)?;
let mut xs = Tensor::zeros((b_sz, d_in, n), delta_a.dtype(), delta_a.device())?;
let mut ys = Vec::with_capacity(l);
for i in 0..l {
xs = ((delta_a.i((.., .., i))? * xs)? + delta_b_u.i((.., .., i))?)?;
let y = xs.matmul(&c.i((.., i, ..))?.unsqueeze(2)?)?.squeeze(2)?;
ys.push(y)
}
let ys = Tensor::stack(ys.as_slice(), 1)?;
ys + u.broadcast_mul(d)
}
impl Module for MambaBlock {
// https://github.com/johnma2006/mamba-minimal/blob/61f01953ca153f8c4a850d7111beecbf4be9cee1/model.py#L206
fn forward(&self, xs: &Tensor) -> Result<Tensor> {
let (_b_sz, seq_len, _dim) = xs.dims3()?;
let xs_and_res = xs.apply(&self.in_proj)?.chunk(2, D::Minus1)?;
let (xs, res) = (&xs_and_res[0], &xs_and_res[1]);
let xs = xs
.t()?
.apply(&self.conv1d)?
.narrow(D::Minus1, 0, seq_len)?
.t()?;
let xs = candle_nn::ops::silu(&xs)?;
let ys = (self.ssm(&xs)? * candle_nn::ops::silu(res))?;
ys.apply(&self.out_proj)
}
}
// https://github.com/johnma2006/mamba-minimal/blob/61f01953ca153f8c4a850d7111beecbf4be9cee1/model.py#L143
#[derive(Clone, Debug)]
pub struct ResidualBlock {
mixer: MambaBlock,
norm: RmsNorm,
}
impl ResidualBlock {
pub fn new(cfg: &Config, vb: VarBuilder) -> Result<Self> {
let norm = candle_nn::rms_norm(cfg.d_model, 1e-5, vb.pp("norm"))?;
let mixer = MambaBlock::new(cfg, vb.pp("mixer"))?;
Ok(Self { mixer, norm })
}
}
impl Module for ResidualBlock {
fn forward(&self, xs: &Tensor) -> Result<Tensor> {
xs.apply(&self.norm)?.apply(&self.mixer)? + xs
}
}
// https://github.com/johnma2006/mamba-minimal/blob/61f01953ca153f8c4a850d7111beecbf4be9cee1/model.py#L56
#[derive(Clone, Debug)]
pub struct Model {
embedding: candle_nn::Embedding,
layers: Vec<ResidualBlock>,
norm_f: RmsNorm,
lm_head: Linear,
}
impl Model {
pub fn new(cfg: &Config, vb: VarBuilder) -> Result<Self> {
let embedding = candle_nn::embedding(cfg.vocab_size(), cfg.d_model, vb.pp("embedding"))?;
let mut layers = Vec::with_capacity(cfg.n_layer);
let vb_l = vb.pp("layers");
for layer_idx in 0..cfg.n_layer {
let layer = ResidualBlock::new(cfg, vb_l.pp(layer_idx))?;
layers.push(layer)
}
let norm_f = candle_nn::rms_norm(cfg.d_model, 1e-5, vb.pp("norm_f"))?;
let lm_head = Linear::from_weights(embedding.embeddings().clone(), None);
Ok(Self {
embedding,
layers,
norm_f,
lm_head,
})
}
}
impl Module for Model {
fn forward(&self, input_ids: &Tensor) -> Result<Tensor> {
let (_b_size, seq_len) = input_ids.dims2()?;
let mut xs = self.embedding.forward(input_ids)?;
for layer in self.layers.iter() {
xs = layer.forward(&xs)?
}
xs.narrow(1, seq_len - 1, 1)?
.apply(&self.norm_f)?
.apply(&self.lm_head)
}
}
| candle/candle-examples/examples/mamba-minimal/model.rs/0 | {
"file_path": "candle/candle-examples/examples/mamba-minimal/model.rs",
"repo_id": "candle",
"token_count": 3488
} | 25 |
use candle::{DType, Device, Result, Tensor, D};
use candle_nn::{
embedding, layer_norm, linear_no_bias, Activation, Embedding, LayerNorm, Linear, Module,
VarBuilder,
};
use candle_transformers::models::{encodec, t5};
// https://github.com/huggingface/transformers/blob/cd4584e3c809bb9e1392ccd3fe38b40daba5519a/src/transformers/models/musicgen/configuration_musicgen.py#L83
#[derive(Debug, Clone, PartialEq)]
pub struct Config {
vocab_size: usize,
max_position_embeddings: usize,
num_hidden_layers: usize,
ffn_dim: usize,
num_attention_heads: usize,
layerdrop: f64,
use_cache: bool,
activation_function: Activation,
hidden_size: usize,
dropout: f64,
attention_dropout: f64,
activation_dropout: f64,
initializer_factor: f64,
scale_embedding: bool,
num_codebooks: usize,
pad_token_id: usize,
bos_token_id: usize,
eos_token_id: Option<usize>,
tie_word_embeddings: bool,
}
impl Default for Config {
fn default() -> Self {
Self {
vocab_size: 2048,
max_position_embeddings: 2048,
num_hidden_layers: 24,
ffn_dim: 4096,
num_attention_heads: 16,
layerdrop: 0.0,
use_cache: true,
activation_function: Activation::Gelu,
hidden_size: 1024,
dropout: 0.1,
attention_dropout: 0.0,
activation_dropout: 0.0,
initializer_factor: 0.02,
scale_embedding: false,
num_codebooks: 4,
pad_token_id: 2048,
bos_token_id: 2048,
eos_token_id: None,
tie_word_embeddings: false,
}
}
}
impl Config {
fn musicgen_small() -> Self {
Self {
vocab_size: 2048,
max_position_embeddings: 2048,
num_hidden_layers: 24,
ffn_dim: 4096,
num_attention_heads: 16,
layerdrop: 0.0,
use_cache: true,
activation_function: Activation::Gelu,
hidden_size: 1024,
dropout: 0.1,
attention_dropout: 0.0,
activation_dropout: 0.0,
initializer_factor: 0.02,
scale_embedding: false,
num_codebooks: 4,
pad_token_id: 2048,
bos_token_id: 2048,
eos_token_id: None,
tie_word_embeddings: false,
}
}
}
fn get_embedding(num_embeddings: usize, embedding_dim: usize) -> Result<Tensor> {
let half_dim = embedding_dim / 2;
let emb = f64::ln(10000.) / (half_dim - 1) as f64;
let xs: Vec<_> = (0..num_embeddings).map(|v| v as f32).collect();
let xs = Tensor::from_vec(xs, (num_embeddings, 1), &Device::Cpu)?;
let ys: Vec<_> = (0..half_dim)
.map(|v| f64::exp(v as f64 * -emb) as f32)
.collect();
let ys = Tensor::from_vec(ys, (1, half_dim), &Device::Cpu)?;
let shape = (num_embeddings, half_dim);
let emb = (xs.broadcast_as(shape)? * ys.broadcast_as(shape)?)?;
let emb =
Tensor::cat(&[&emb.cos()?, &emb.sin()?], 1)?.reshape((num_embeddings, 2 * half_dim))?;
let emb = if embedding_dim % 2 == 1 {
let zeros = Tensor::zeros((num_embeddings, 1), DType::F32, &Device::Cpu)?;
Tensor::cat(&[&emb, &zeros], 1)?
} else {
emb
};
Ok(emb)
}
#[derive(Debug)]
struct MusicgenSinusoidalPositionalEmbedding {
num_positions: usize,
embedding_dim: usize,
weights: Tensor,
}
impl MusicgenSinusoidalPositionalEmbedding {
fn load(_vb: VarBuilder, cfg: &Config) -> Result<Self> {
let num_positions = cfg.max_position_embeddings;
let embedding_dim = cfg.hidden_size;
let weights = get_embedding(num_positions, embedding_dim)?;
Ok(Self {
num_positions,
embedding_dim,
weights,
})
}
fn forward(&mut self, input_ids: &Tensor) -> Result<Tensor> {
let (_b_sz, _codebooks, seq_len) = input_ids.dims3()?;
if seq_len > self.weights.dim(0)? {
self.weights = get_embedding(seq_len, self.embedding_dim)?
}
self.weights.narrow(0, 0, seq_len)
}
}
#[derive(Debug)]
struct MusicgenAttention {
scaling: f64,
is_decoder: bool,
num_heads: usize,
head_dim: usize,
k_proj: Linear,
v_proj: Linear,
q_proj: Linear,
out_proj: Linear,
}
impl MusicgenAttention {
fn load(vb: VarBuilder, cfg: &Config) -> Result<Self> {
let h = cfg.hidden_size;
let num_heads = cfg.num_attention_heads;
let head_dim = h / num_heads;
let k_proj = linear_no_bias(h, h, vb.pp("k_proj"))?;
let v_proj = linear_no_bias(h, h, vb.pp("v_proj"))?;
let q_proj = linear_no_bias(h, h, vb.pp("q_proj"))?;
let out_proj = linear_no_bias(h, h, vb.pp("out_proj"))?;
Ok(Self {
scaling: 1. / (head_dim as f64).sqrt(),
is_decoder: true,
num_heads,
head_dim,
k_proj,
v_proj,
q_proj,
out_proj,
})
}
fn forward(
&mut self,
xs: &Tensor,
kv_states: Option<&Tensor>,
attention_mask: &Tensor,
) -> Result<Tensor> {
let (b_sz, tgt_len, _) = xs.dims3()?;
let query_states = (self.q_proj.forward(xs)? * self.scaling)?;
let kv_states = kv_states.unwrap_or(xs);
let key_states = self.k_proj.forward(kv_states)?;
let value_states = self.v_proj.forward(kv_states)?;
let tgt = (b_sz, tgt_len, self.num_heads, self.head_dim);
let query_states = query_states.reshape(tgt)?.transpose(1, 2)?.contiguous()?;
let key_states = key_states.reshape(tgt)?.transpose(1, 2)?.contiguous()?;
let value_states = value_states.reshape(tgt)?.transpose(1, 2)?.contiguous()?;
let src_len = key_states.dim(1)?;
let attn_weights = query_states.matmul(&key_states.transpose(1, 2)?)?;
let attn_weights = attn_weights
.reshape((b_sz, self.num_heads, tgt_len, src_len))?
.broadcast_add(attention_mask)?;
let attn_weights = candle_nn::ops::softmax(&attn_weights, D::Minus1)?;
// TODO: layer_head_mask?
let attn_output = attn_weights
.matmul(&value_states)?
.reshape((b_sz, self.num_heads, tgt_len, self.head_dim))?
.transpose(1, 2)?
.reshape((b_sz, tgt_len, self.num_heads * self.head_dim))?;
let attn_output = self.out_proj.forward(&attn_output)?;
Ok(attn_output)
}
}
#[derive(Debug)]
struct MusicgenDecoderLayer {
self_attn: MusicgenAttention,
self_attn_layer_norm: LayerNorm,
encoder_attn: MusicgenAttention,
encoder_attn_layer_norm: LayerNorm,
fc1: Linear,
fc2: Linear,
final_layer_norm: LayerNorm,
activation_fn: Activation,
}
impl MusicgenDecoderLayer {
fn load(vb: VarBuilder, cfg: &Config) -> Result<Self> {
let h = cfg.hidden_size;
let self_attn = MusicgenAttention::load(vb.pp("self_attn"), cfg)?;
let self_attn_layer_norm = layer_norm(h, 1e-5, vb.pp("self_attn_layer_norm"))?;
let encoder_attn = MusicgenAttention::load(vb.pp("encoder_attn"), cfg)?;
let encoder_attn_layer_norm = layer_norm(h, 1e-5, vb.pp("encoder_attn_layer_norm"))?;
let fc1 = linear_no_bias(h, cfg.ffn_dim, vb.pp("fc1"))?;
let fc2 = linear_no_bias(cfg.ffn_dim, h, vb.pp("fc2"))?;
let final_layer_norm = layer_norm(h, 1e-5, vb.pp("final_layer_norm"))?;
Ok(Self {
self_attn,
self_attn_layer_norm,
encoder_attn,
encoder_attn_layer_norm,
fc1,
fc2,
final_layer_norm,
activation_fn: cfg.activation_function,
})
}
fn forward(
&mut self,
xs: &Tensor,
attention_mask: &Tensor,
encoder_hidden_states: Option<&Tensor>,
) -> Result<Tensor> {
let residual = xs.clone();
let xs = self.self_attn_layer_norm.forward(xs)?;
let xs = self.self_attn.forward(&xs, None, attention_mask)?;
let mut xs = (xs + residual)?;
if let Some(encoder_hidden_states) = &encoder_hidden_states {
let residual = xs.clone();
let encoder_attention_mask = attention_mask.clone(); // TODO
xs = self.encoder_attn.forward(
&xs,
Some(encoder_hidden_states),
&encoder_attention_mask,
)?;
xs = (xs + residual)?
}
let residual = xs.clone();
let xs = self.final_layer_norm.forward(&xs)?;
let xs = self.fc1.forward(&xs)?;
let xs = self.activation_fn.forward(&xs)?;
let xs = self.fc2.forward(&xs)?;
let xs = (xs + residual)?;
Ok(xs)
}
}
#[derive(Debug)]
struct MusicgenDecoder {
embed_tokens: Vec<Embedding>,
embed_positions: MusicgenSinusoidalPositionalEmbedding,
layers: Vec<MusicgenDecoderLayer>,
layer_norm: LayerNorm,
embed_scale: f64,
num_codebooks: usize,
d_model: usize,
}
impl MusicgenDecoder {
fn load(vb: VarBuilder, cfg: &Config) -> Result<Self> {
let h = cfg.hidden_size;
let embed_scale = if cfg.scale_embedding {
(h as f64).sqrt()
} else {
1.
};
let embed_dim = cfg.vocab_size + 1;
let embed_tokens = (0..cfg.num_codebooks)
.map(|i| embedding(embed_dim, h, vb.pp(&format!("embed_tokens.{i}"))))
.collect::<Result<Vec<_>>>()?;
let embed_positions = MusicgenSinusoidalPositionalEmbedding::load(vb.clone(), cfg)?;
let layers = (0..cfg.num_hidden_layers)
.map(|i| MusicgenDecoderLayer::load(vb.pp(&format!("layers.{i}")), cfg))
.collect::<Result<Vec<_>>>()?;
let layer_norm = layer_norm(h, 1e-5, vb.pp("layer_norm"))?;
Ok(Self {
embed_tokens,
embed_positions,
layers,
layer_norm,
embed_scale,
num_codebooks: cfg.num_codebooks,
d_model: cfg.hidden_size,
})
}
fn prepare_decoder_attention_mask(&self, _b_sz: usize, _seq_len: usize) -> Result<Tensor> {
todo!()
}
fn forward(&mut self, input_ids: &Tensor) -> Result<Tensor> {
let dev = input_ids.device();
let (b_sz_times_codebooks, seq_len) = input_ids.dims2()?;
let b_sz = b_sz_times_codebooks / self.num_codebooks;
let input = input_ids.reshape((b_sz, self.num_codebooks, seq_len))?;
let mut inputs_embeds = Tensor::zeros((b_sz, seq_len, self.d_model), DType::F32, dev)?;
for (idx, codebook) in self.embed_tokens.iter().enumerate() {
let inp = input.narrow(1, idx, 1)?.squeeze(1)?;
inputs_embeds = (inputs_embeds + codebook.forward(&inp)?)?
}
let inputs_embeds = inputs_embeds;
let positions = self.embed_positions.forward(&input)?.to_device(dev)?;
let mut xs = inputs_embeds.broadcast_add(&positions)?;
let attention_mask = self.prepare_decoder_attention_mask(b_sz, seq_len)?;
for decoder_layer in self.layers.iter_mut() {
xs = decoder_layer.forward(&xs, &attention_mask, None)?;
}
let xs = self.layer_norm.forward(&xs)?;
Ok(xs)
}
}
#[derive(Debug)]
pub struct MusicgenForCausalLM {
decoder: MusicgenDecoder,
lm_heads: Vec<Linear>,
num_codebooks: usize,
vocab_size: usize,
}
impl MusicgenForCausalLM {
pub fn load(vb: VarBuilder, cfg: &Config) -> Result<Self> {
let h = cfg.hidden_size;
let decoder = MusicgenDecoder::load(vb.pp("model.decoder"), cfg)?;
let lm_heads = (0..cfg.num_codebooks)
.map(|i| linear_no_bias(h, cfg.vocab_size, vb.pp(&format!("lm_heads.{i}"))))
.collect::<Result<Vec<_>>>()?;
Ok(Self {
decoder,
lm_heads,
num_codebooks: cfg.num_codebooks,
vocab_size: cfg.vocab_size,
})
}
pub fn forward(&mut self, input_ids: &Tensor) -> Result<Tensor> {
let (b_sz, seq_len) = input_ids.dims2()?;
let hidden_states = self.decoder.forward(input_ids)?;
let lm_logits = self
.lm_heads
.iter()
.map(|h| h.forward(&hidden_states))
.collect::<Result<Vec<_>>>()?;
let lm_logits = Tensor::stack(&lm_logits, 1)?.reshape((
b_sz * self.num_codebooks,
seq_len,
self.vocab_size,
))?;
Ok(lm_logits)
}
}
#[derive(Debug)]
pub struct MusicgenForConditionalGeneration {
pub text_encoder: t5::T5EncoderModel,
pub audio_encoder: encodec::Model,
pub decoder: MusicgenForCausalLM,
cfg: GenConfig,
}
#[derive(Debug, Clone, PartialEq)]
pub struct GenConfig {
musicgen: Config,
t5: t5::Config,
encodec: encodec::Config,
}
impl GenConfig {
pub fn small() -> Self {
// https://huggingface.co/facebook/musicgen-small/blob/495da4ad086b3416a27c6187f9239f9fd96f3962/config.json#L6
let encodec = encodec::Config {
audio_channels: 1,
chunk_length_s: None,
codebook_dim: Some(128),
codebook_size: 2048,
compress: 2,
dilation_growth_rate: 2,
hidden_size: 128,
kernel_size: 7,
last_kernel_size: 7,
norm_type: encodec::NormType::WeightNorm,
normalize: false,
num_filters: 64,
num_lstm_layers: 2,
num_residual_layers: 1,
overlap: None,
// This should be Reflect and not Replicate but Reflect does not work yet.
pad_mode: encodec::PadMode::Replicate,
residual_kernel_size: 3,
sampling_rate: 32_000,
target_bandwidths: vec![2.2],
trim_right_ratio: 1.0,
upsampling_ratios: vec![8, 5, 4, 4],
use_causal_conv: false,
use_conv_shortcut: false,
};
Self {
musicgen: Config::musicgen_small(),
t5: t5::Config::musicgen_small(),
encodec,
}
}
}
impl MusicgenForConditionalGeneration {
pub fn config(&self) -> &GenConfig {
&self.cfg
}
pub fn load(vb: VarBuilder, cfg: GenConfig) -> Result<Self> {
let text_encoder = t5::T5EncoderModel::load(vb.pp("text_encoder"), &cfg.t5)?;
let audio_encoder = encodec::Model::new(&cfg.encodec, vb.pp("audio_encoder"))?;
let decoder = MusicgenForCausalLM::load(vb.pp("decoder"), &cfg.musicgen)?;
Ok(Self {
text_encoder,
audio_encoder,
decoder,
cfg,
})
}
}
| candle/candle-examples/examples/musicgen/musicgen_model.rs/0 | {
"file_path": "candle/candle-examples/examples/musicgen/musicgen_model.rs",
"repo_id": "candle",
"token_count": 7592
} | 26 |
#![allow(unused)]
//! Wrappers around the Python API of Gymnasium (the new version of OpenAI gym)
use candle::{Device, Result, Tensor};
use pyo3::prelude::*;
use pyo3::types::PyDict;
/// The return value for a step.
#[derive(Debug)]
pub struct Step<A> {
pub state: Tensor,
pub action: A,
pub reward: f64,
pub terminated: bool,
pub truncated: bool,
}
impl<A: Copy> Step<A> {
/// Returns a copy of this step changing the observation tensor.
pub fn copy_with_obs(&self, state: &Tensor) -> Step<A> {
Step {
state: state.clone(),
action: self.action,
reward: self.reward,
terminated: self.terminated,
truncated: self.truncated,
}
}
}
/// An OpenAI Gym session.
pub struct GymEnv {
env: PyObject,
action_space: usize,
observation_space: Vec<usize>,
}
fn w(res: PyErr) -> candle::Error {
candle::Error::wrap(res)
}
impl GymEnv {
/// Creates a new session of the specified OpenAI Gym environment.
pub fn new(name: &str) -> Result<GymEnv> {
Python::with_gil(|py| {
let gym = py.import("gymnasium")?;
let make = gym.getattr("make")?;
let env = make.call1((name,))?;
let action_space = env.getattr("action_space")?;
let action_space = if let Ok(val) = action_space.getattr("n") {
val.extract()?
} else {
let action_space: Vec<usize> = action_space.getattr("shape")?.extract()?;
action_space[0]
};
let observation_space = env.getattr("observation_space")?;
let observation_space = observation_space.getattr("shape")?.extract()?;
Ok(GymEnv {
env: env.into(),
action_space,
observation_space,
})
})
.map_err(w)
}
/// Resets the environment, returning the observation tensor.
pub fn reset(&self, seed: u64) -> Result<Tensor> {
let state: Vec<f32> = Python::with_gil(|py| {
let kwargs = PyDict::new(py);
kwargs.set_item("seed", seed)?;
let state = self.env.call_method(py, "reset", (), Some(kwargs))?;
state.as_ref(py).get_item(0)?.extract()
})
.map_err(w)?;
Tensor::new(state, &Device::Cpu)
}
/// Applies an environment step using the specified action.
pub fn step<A: pyo3::IntoPy<pyo3::Py<pyo3::PyAny>> + Clone>(
&self,
action: A,
) -> Result<Step<A>> {
let (state, reward, terminated, truncated) = Python::with_gil(|py| {
let step = self.env.call_method(py, "step", (action.clone(),), None)?;
let step = step.as_ref(py);
let state: Vec<f32> = step.get_item(0)?.extract()?;
let reward: f64 = step.get_item(1)?.extract()?;
let terminated: bool = step.get_item(2)?.extract()?;
let truncated: bool = step.get_item(3)?.extract()?;
Ok((state, reward, terminated, truncated))
})
.map_err(w)?;
let state = Tensor::new(state, &Device::Cpu)?;
Ok(Step {
state,
action,
reward,
terminated,
truncated,
})
}
/// Returns the number of allowed actions for this environment.
pub fn action_space(&self) -> usize {
self.action_space
}
/// Returns the shape of the observation tensors.
pub fn observation_space(&self) -> &[usize] {
&self.observation_space
}
}
| candle/candle-examples/examples/reinforcement-learning/gym_env.rs/0 | {
"file_path": "candle/candle-examples/examples/reinforcement-learning/gym_env.rs",
"repo_id": "candle",
"token_count": 1716
} | 27 |
# candle-segment-anything: Segment-Anything Model
This example is based on Meta AI [Segment-Anything
Model](https://github.com/facebookresearch/segment-anything). This model
provides a robust and fast image segmentation pipeline that can be tweaked via
some prompting (requesting some points to be in the target mask, requesting some
points to be part of the background so _not_ in the target mask, specifying some
bounding box).
The default backbone can be replaced by the smaller and faster TinyViT model
based on [MobileSAM](https://github.com/ChaoningZhang/MobileSAM).
## Running some example.
```bash
cargo run --example segment-anything --release -- \
--image candle-examples/examples/yolo-v8/assets/bike.jpg
--use-tiny
--point 0.6,0.6 --point 0.6,0.55
```
Running this command generates a `sam_merged.jpg` file containing the original
image with a blue overlay of the selected mask. The red dots represent the prompt
specified by `--point 0.6,0.6 --point 0.6,0.55`, this prompt is assumed to be part
of the target mask.
The values used for `--point` should be a comma delimited pair of float values.
They are proportional to the image dimension, i.e. use 0.5 for the image center.
Original image:

Segment results by prompting with a single point `--point 0.6,0.55`:

Segment results by prompting with multiple points `--point 0.6,0.6 --point 0.6,0.55`:

### Command-line flags
- `--use-tiny`: use the TinyViT based MobileSAM backbone rather than the default
one.
- `--point`: specifies the location of the target points.
- `--threshold`: sets the threshold value to be part of the mask, a negative
value results in a larger mask and can be specified via `--threshold=-1.2`.
| candle/candle-examples/examples/segment-anything/README.md/0 | {
"file_path": "candle/candle-examples/examples/segment-anything/README.md",
"repo_id": "candle",
"token_count": 573
} | 28 |
#[cfg(feature = "mkl")]
extern crate intel_mkl_src;
#[cfg(feature = "accelerate")]
extern crate accelerate_src;
use anyhow::Error as E;
use clap::{Parser, ValueEnum};
use candle::{DType, Tensor};
use candle_examples::token_output_stream::TokenOutputStream;
use candle_nn::VarBuilder;
use candle_transformers::models::{trocr, vit};
use tokenizers::Tokenizer;
mod image_processor;
#[derive(Clone, Debug, Copy, ValueEnum)]
enum Which {
#[value(name = "base")]
BaseHandwritten,
#[value(name = "large")]
LargeHandwritten,
BasePrinted,
LargePrinted,
}
impl Which {
fn repo_and_branch_name(&self) -> (&str, &str) {
match self {
Self::BaseHandwritten => ("microsoft/trocr-base-handwritten", "refs/pr/3"),
Self::LargeHandwritten => ("microsoft/trocr-large-handwritten", "refs/pr/6"),
Self::BasePrinted => ("microsoft/trocr-base-printed", "refs/pr/7"),
Self::LargePrinted => ("microsoft/trocr-large-printed", "main"),
}
}
}
#[derive(Debug, Clone, serde::Deserialize)]
struct Config {
encoder: vit::Config,
decoder: trocr::TrOCRConfig,
}
#[derive(Parser, Debug)]
struct Args {
#[arg(long)]
model: Option<String>,
/// Choose the variant of the model to run.
#[arg(long, default_value = "base")]
which: Which,
/// Run on CPU rather than on GPU.
#[arg(long)]
cpu: bool,
/// The image file to be processed.
#[arg(long)]
image: String,
/// Tokenization config.
#[arg(long)]
tokenizer: Option<String>,
}
pub fn main() -> anyhow::Result<()> {
let args = Args::parse();
let api = hf_hub::api::sync::Api::new()?;
let mut tokenizer_dec = {
let tokenizer_file = match args.tokenizer {
None => api
.model(String::from("ToluClassics/candle-trocr-tokenizer"))
.get("tokenizer.json")?,
Some(tokenizer) => std::path::PathBuf::from(tokenizer),
};
let tokenizer = Tokenizer::from_file(&tokenizer_file).map_err(E::msg)?;
TokenOutputStream::new(tokenizer)
};
let device = candle_examples::device(args.cpu)?;
let vb = {
let model = match args.model {
Some(model) => std::path::PathBuf::from(model),
None => {
let (repo, branch) = args.which.repo_and_branch_name();
api.repo(hf_hub::Repo::with_revision(
repo.to_string(),
hf_hub::RepoType::Model,
branch.to_string(),
))
.get("model.safetensors")?
}
};
println!("model: {:?}", model);
unsafe { VarBuilder::from_mmaped_safetensors(&[model], DType::F32, &device)? }
};
let (encoder_config, decoder_config) = {
let (repo, branch) = args.which.repo_and_branch_name();
let config_filename = api
.repo(hf_hub::Repo::with_revision(
repo.to_string(),
hf_hub::RepoType::Model,
branch.to_string(),
))
.get("config.json")?;
let config: Config = serde_json::from_reader(std::fs::File::open(config_filename)?)?;
(config.encoder, config.decoder)
};
let mut model = trocr::TrOCRModel::new(&encoder_config, &decoder_config, vb)?;
let processor_config = image_processor::ProcessorConfig::default();
let processor = image_processor::ViTImageProcessor::new(&processor_config);
let image = vec![args.image.as_str()];
let image = processor.preprocess(image)?;
let encoder_xs = model.encoder().forward(&image)?;
let mut logits_processor =
candle_transformers::generation::LogitsProcessor::new(1337, None, None);
let mut token_ids: Vec<u32> = vec![decoder_config.decoder_start_token_id];
for index in 0..1000 {
let context_size = if index >= 1 { 1 } else { token_ids.len() };
let start_pos = token_ids.len().saturating_sub(context_size);
let input_ids = Tensor::new(&token_ids[start_pos..], &device)?.unsqueeze(0)?;
let logits = model.decode(&input_ids, &encoder_xs, start_pos)?;
let logits = logits.squeeze(0)?;
let logits = logits.get(logits.dim(0)? - 1)?;
let token = logits_processor.sample(&logits)?;
token_ids.push(token);
if let Some(t) = tokenizer_dec.next_token(token)? {
use std::io::Write;
print!("{t}");
std::io::stdout().flush()?;
}
if token == decoder_config.eos_token_id {
break;
}
}
if let Some(rest) = tokenizer_dec.decode_rest().map_err(E::msg)? {
print!("{rest}");
}
println!();
Ok(())
}
| candle/candle-examples/examples/trocr/main.rs/0 | {
"file_path": "candle/candle-examples/examples/trocr/main.rs",
"repo_id": "candle",
"token_count": 2160
} | 29 |
pub const NAMES: [&str; 80] = [
"person",
"bicycle",
"car",
"motorbike",
"aeroplane",
"bus",
"train",
"truck",
"boat",
"traffic light",
"fire hydrant",
"stop sign",
"parking meter",
"bench",
"bird",
"cat",
"dog",
"horse",
"sheep",
"cow",
"elephant",
"bear",
"zebra",
"giraffe",
"backpack",
"umbrella",
"handbag",
"tie",
"suitcase",
"frisbee",
"skis",
"snowboard",
"sports ball",
"kite",
"baseball bat",
"baseball glove",
"skateboard",
"surfboard",
"tennis racket",
"bottle",
"wine glass",
"cup",
"fork",
"knife",
"spoon",
"bowl",
"banana",
"apple",
"sandwich",
"orange",
"broccoli",
"carrot",
"hot dog",
"pizza",
"donut",
"cake",
"chair",
"sofa",
"pottedplant",
"bed",
"diningtable",
"toilet",
"tvmonitor",
"laptop",
"mouse",
"remote",
"keyboard",
"cell phone",
"microwave",
"oven",
"toaster",
"sink",
"refrigerator",
"book",
"clock",
"vase",
"scissors",
"teddy bear",
"hair drier",
"toothbrush",
];
| candle/candle-examples/src/coco_classes.rs/0 | {
"file_path": "candle/candle-examples/src/coco_classes.rs",
"repo_id": "candle",
"token_count": 648
} | 30 |
// Pytorch also has an implementation of Philox RNG: https://github.com/pytorch/pytorch/blob/8ca3c881db3e3510fcb7725389f6a0633c9b992c/torch/csrc/jit/tensorexpr/cuda_random.h
#pragma once
// Philox CUDA.
namespace flash {
struct ull2 {
unsigned long long x;
unsigned long long y;
};
inline __device__ uint2 mulhilo32(const unsigned int a, const unsigned int b) {
uint2 *res;
unsigned long long tmp;
asm ("mul.wide.u32 %0, %1, %2;\n\t"
: "=l"(tmp)
: "r"(a), "r"(b));
res = (uint2*)(&tmp);
return *res;
}
inline __device__ uint4 philox_single_round(const uint4 ctr, const uint2 key) {
constexpr unsigned long kPhiloxSA = 0xD2511F53;
constexpr unsigned long kPhiloxSB = 0xCD9E8D57;
uint2 res0 = mulhilo32(kPhiloxSA, ctr.x);
uint2 res1 = mulhilo32(kPhiloxSB, ctr.z);
uint4 ret = {res1.y ^ ctr.y ^ key.x, res1.x, res0.y ^ ctr.w ^ key.y, res0.x};
return ret;
}
inline __device__ uint4 philox(unsigned long long seed,
unsigned long long subsequence,
unsigned long long offset) {
constexpr unsigned long kPhilox10A = 0x9E3779B9;
constexpr unsigned long kPhilox10B = 0xBB67AE85;
uint2 key = reinterpret_cast<uint2&>(seed);
uint4 counter;
ull2 *tmp = reinterpret_cast<ull2*>(&counter);
tmp->x = offset;
tmp->y = subsequence;
#pragma unroll
for (int i = 0; i < 6; i++) {
counter = philox_single_round(counter, key);
key.x += (kPhilox10A);
key.y += (kPhilox10B);
}
uint4 output = philox_single_round(counter, key);
return output;
}
} // namespace flash
namespace {
class Philox {
public:
__device__ inline Philox(unsigned long long seed,
unsigned long long subsequence,
unsigned long long offset)
: STATE(0)
, seed_(seed)
, offset_(offset)
, key(reinterpret_cast<const uint2&>(seed)) {
//key.x = (unsigned int)seed;
//key.y = (unsigned int)(seed >> 32);
//counter = make_uint4(0, 0, 0, 0);
//counter.z = (unsigned int)(subsequence);
//counter.w = (unsigned int)(subsequence >> 32);
//STATE = 0;
//incr_n(offset / 4);
// key = reinterpret_cast<const uint2&>(seed);
ull2 * tmp = reinterpret_cast<ull2*>(&counter);
tmp->x = offset / 4;
tmp->y = subsequence;
// if ((threadIdx.x == 0) && (blockIdx.x == 0) && (blockIdx.y == 0)) {
// printf("Philox counter: %d, %d, %d, %d\n", counter.x, counter.y, counter.z, counter.w);
// }
}
__device__ inline uint4 operator()() {
// // if (STATE == 0) {
// uint4 counter_ = counter;
// uint2 key_ = key;
// // 7-round philox
// #pragma unroll
// for (int i = 0; i < 6; i++) {
// counter_ = flash::philox_single_round(counter_, key_);
// key_.x += (kPhilox10A);
// key_.y += (kPhilox10B);
// }
// // output = philox_single_round(counter_, key_);
// uint4 output = flash::philox_single_round(counter_, key_);
// // if ((threadIdx.x == 0) && (blockIdx.x == 0) && (blockIdx.y == 0)) {
// // printf("Philox counter: %u, %u, %u, %u\n", counter.x, counter.y, counter.z, counter.w);
// // printf("Philox output: %u, %u, %u, %u\n", output.x, output.y, output.z, output.w);
// // }
// incr();
// // }
// // return a float4 directly
// // unsigned long ret;
// // switch(STATE) {
// // case 0: ret = output.x; break;
// // case 1: ret = output.y; break;
// // case 2: ret = output.z; break;
// // case 3: ret = output.w; break;
// //}
// // STATE = (STATE + 1) % 4;
// return output;
return flash::philox(seed_, offset_, offset_);
}
private:
unsigned long long offset_, seed_;
struct ull2 {
uint64_t x;
uint64_t y;
};
uint4 counter;
// uint4 output;
const uint2 key;
unsigned int STATE;
__device__ inline void incr_n(unsigned long long n) {
unsigned int nlo = (unsigned int)(n);
unsigned int nhi = (unsigned int)(n >> 32);
counter.x += nlo;
if (counter.x < nlo)
nhi++;
counter.y += nhi;
if (nhi <= counter.y)
return;
if (++counter.z)
return;
++counter.w;
}
__device__ uint4 incr128 (uint4 ctr)
{
uint4 res;
asm ("add.cc.u32 %0, %4, %8;\n\t"
"addc.cc.u32 %1, %5, %9;\n\t"
"addc.cc.u32 %2, %6, %10;\n\t"
"addc.u32 %3, %7, %11;\n\t"
: "=r"(res.x), "=r"(res.y), "=r"(res.z), "=r"(res.w)
: "r"(ctr.x), "r"(ctr.y), "r"(ctr.z), "r"(ctr.w),
"n"(1), "n"(0), "n"(0), "n"(0));
return res;
}
__device__ inline void incr() {
// if ((threadIdx.x == 0) && (blockIdx.x == 0) && (blockIdx.y == 0)) {
// printf("Counter before: %u, %u, %u, %u\n", counter.x, counter.y, counter.z, counter.w);
// }
counter = incr128(counter);
// if ((threadIdx.x == 0) && (blockIdx.x == 0) && (blockIdx.y == 0)) {
// printf("Counter after: %u, %u, %u, %u\n", counter.x, counter.y, counter.z, counter.w);
// }
}
static const unsigned long kPhilox10A = 0x9E3779B9;
static const unsigned long kPhilox10B = 0xBB67AE85;
// static const unsigned long kPhiloxSA = 0xD2511F53;
// static const unsigned long kPhiloxSB = 0xCD9E8D57;
};
} // namespace
| candle/candle-flash-attn/kernels/philox.cuh/0 | {
"file_path": "candle/candle-flash-attn/kernels/philox.cuh",
"repo_id": "candle",
"token_count": 2511
} | 31 |
#include "compatibility.cuh"
#include<stdint.h>
#include<cmath>
// TODO: This is often used to check that the data is contiguous so that
// kernels can be easily mapped. However this only returns true for row
// major, if all the inputs are column major, we could apply the fast path
// too (but we wouldn't if some of them are row major and some column major).
__device__ bool is_contiguous(
const size_t num_dims,
const size_t *dims,
const size_t *strides
) {
size_t acc = 1;
for (unsigned int d = 0; d < num_dims; d++) {
unsigned int dim_idx = num_dims - 1 - d;
if (acc != strides[dim_idx]) {
return false;
}
acc *= dims[dim_idx];
}
return true;
}
__device__ unsigned int get_strided_index(
unsigned int idx,
const size_t num_dims,
const size_t *dims,
const size_t *strides
) {
unsigned int strided_i = 0;
for (unsigned int d = 0; d < num_dims; d++) {
unsigned int dim_idx = num_dims - 1 - d;
strided_i += (idx % dims[dim_idx]) * strides[dim_idx];
idx /= dims[dim_idx];
}
return strided_i;
}
__device__ unsigned int restrided(
const unsigned int strided_i,
const size_t num_dims,
const size_t *dims,
const size_t *strides,
const size_t *new_strides
) {
unsigned int idx = 0;
for (int d = 0; d < num_dims; d++) {
idx += (strides[d] == 0 ? 0 : (strided_i / strides[d]) % dims[d]) * new_strides[d];
}
return idx;
}
// Sourced from https://graphics.stanford.edu/~seander/bithacks.html#RoundUpPowerOf2
// Input must be less than or equal to 2 ^ 16
// used in reductions
__device__ __forceinline__ unsigned int next_power_of_two(unsigned int v) {
v--;
v |= v >> 1;
v |= v >> 2;
v |= v >> 4;
v |= v >> 8;
v++;
return v;
}
// Efficiently computes the sum of each chunk in "data" of size chunk_len, and
// stores the sums in out[i / chunk_len]
template<typename T>
__device__ void chunk_sum(
const size_t chunk_len,
const T data,
T* out
) {
__shared__ T buf[1024];
// assumes that threads where i >= numel have already exited
unsigned int i = blockIdx.x * blockDim.x + threadIdx.x;
unsigned int block_i = threadIdx.x;
// Fall back to atomicAdd if chunk_len is small to reduce overhead
if (chunk_len <= 2) {
atomicAdd(out + i / chunk_len, data);
return;
}
buf[block_i] = data;
unsigned int chunk_i = i % chunk_len;
unsigned int chunk_start = max((int)(block_i - chunk_i), 0);
unsigned int chunk_end = min((unsigned int)(block_i + chunk_len - chunk_i), blockDim.x);
chunk_i = block_i - chunk_start;
size_t max_chunk_len = min(chunk_end - chunk_start, blockDim.x);
size_t incr = next_power_of_two(max_chunk_len) >> 1;
__syncthreads();
// Uses sequential addressing as discussed in
// https://developer.download.nvidia.com/assets/cuda/files/reduction.pdf
for (; incr > 0; incr >>= 1) {
unsigned int block_i_2 = block_i + incr;
if (block_i_2 < chunk_end && chunk_i < incr) {
// This is sound because __syncthreads and the conditions above
// ensure that no data races occur
buf[block_i] += buf[block_i_2];
}
__syncthreads();
}
if (block_i == chunk_start) {
atomicAdd(out + i / chunk_len, buf[block_i]);
}
}
__device__ __forceinline__ bool isnang(float a) { return isnan(a); }
__device__ __forceinline__ bool isnang(double a) { return isnan(a); }
__device__ __forceinline__ float recipg(float a) { return 1.0 / a; }
__device__ __forceinline__ double recipg(double a) { return 1.0 / a; }
__device__ __forceinline__ float cosg(float a) { return cosf(a); }
__device__ __forceinline__ double cosg(double a) { return cos(a); }
__device__ __forceinline__ float sing(float a) { return sinf(a); }
__device__ __forceinline__ double sing(double a) { return sin(a); }
__device__ __forceinline__ float sqrtg(float a) { return sqrtf(a); }
__device__ __forceinline__ double sqrtg(double a) { return sqrt(a); }
__device__ __forceinline__ float powg(float a, float b) { return powf(a, b); }
__device__ __forceinline__ double powg(double a, double b) { return pow(a, b); }
__device__ __forceinline__ float tanhg(float a) { return tanhf(a); }
__device__ __forceinline__ double tanhg(double a) { return tanh(a); }
__device__ __forceinline__ float erfg(float a) { return erff(a); }
__device__ __forceinline__ double erfg(double a) { return erf(a); }
__device__ __forceinline__ float ceilg(float a) { return ceilf(a); }
__device__ __forceinline__ double ceilg(double a) { return ceil(a); }
__device__ __forceinline__ float floorg(float a) { return floorf(a); }
__device__ __forceinline__ double floorg(double a) { return floor(a); }
__device__ __forceinline__ float roundg(float a) { return roundf(a); }
__device__ __forceinline__ double roundg(double a) { return round(a); }
__device__ __forceinline__ float normcdfg(float a) { return normcdff(a); }
__device__ __forceinline__ double normcdfg(double a) { return normcdf(a); }
__device__ __forceinline__ float maxg(float a, float b) { return fmaxf(a, b); }
__device__ __forceinline__ double maxg(double a, double b) { return fmax(a, b); }
__device__ __forceinline__ float ming(float a, float b) { return fminf(a, b); }
__device__ __forceinline__ double ming(double a, double b) { return fmin(a, b); }
__device__ __forceinline__ float logg(float a) { return logf(a); }
__device__ __forceinline__ double logg(double a) { return log(a); }
__device__ __forceinline__ float expg(float a) { return expf(a); }
__device__ __forceinline__ double expg(double a) { return exp(a); }
__device__ __forceinline__ float absg(float a) { return fabsf(a); }
__device__ __forceinline__ double absg(double a) { return fabs(a); }
__device__ __forceinline__ float copysigng(float a, float b) { return copysignf(a, b); }
__device__ __forceinline__ double copysigng(double a, double b) { return copysign(a, b); }
__device__ __forceinline__ int64_t ming(int64_t a, int64_t b) { return min(a, b); }
__device__ __forceinline__ int64_t maxg(int64_t a, int64_t b) { return max(a, b); }
__device__ __forceinline__ uint32_t ming(uint32_t a, uint32_t b) { return min(a, b); }
__device__ __forceinline__ uint32_t maxg(uint32_t a, uint32_t b) { return max(a, b); }
__device__ __forceinline__ uint8_t ming(uint8_t a, uint8_t b) { return min(a, b); }
__device__ __forceinline__ uint8_t maxg(uint8_t a, uint8_t b) { return max(a, b); }
#if __CUDA_ARCH__ >= 530
__device__ __forceinline__ __half powg(__half a, __half b) { return __float2half(powf(__half2float(a), __half2float(b))); }
__device__ __forceinline__ bool isnang(__half a) { return __hisnan(a); }
__device__ __forceinline__ __half sqrtg(__half a) { return hsqrt(a); }
__device__ __forceinline__ __half cosg(__half a) { return hcos(a); }
__device__ __forceinline__ __half sing(__half a) { return hsin(a); }
__device__ __forceinline__ __half recipg(__half a) { __half one = 1.0; return one / a; }
__device__ __forceinline__ __half maxg(__half a, __half b) { return __hmax_nan(a, b); }
__device__ __forceinline__ __half tanhg(__half a) { return __float2half(tanhf(__half2float(a))); }
__device__ __forceinline__ __half erfg(__half a) { return __float2half(erff(__half2float(a))); }
__device__ __forceinline__ __half ceilg(__half a) { return __float2half(ceilf(__half2float(a))); }
__device__ __forceinline__ __half floorg(__half a) { return __float2half(floorf(__half2float(a))); }
__device__ __forceinline__ __half roundg(__half a) { return __float2half(roundf(__half2float(a))); }
__device__ __forceinline__ __half normcdfg(__half a) { return __float2half(normcdff(__half2float(a))); }
__device__ __forceinline__ __half ming(__half a, __half b) { return __hmin_nan(a, b); }
__device__ __forceinline__ __half logg(__half a) { return hlog(a); }
__device__ __forceinline__ __half expg(__half a) { return hexp(a); }
__device__ __forceinline__ __half absg(__half a) { return __habs(a); }
__device__ __forceinline__ __half copysigng(__half a, __half b) { return __float2half(copysignf(__half2float(a), __half2float(b))); }
#endif
#if __CUDA_ARCH__ >= 800
__device__ __forceinline__ __nv_bfloat16 powg(__nv_bfloat16 a, __nv_bfloat16 b) { return __float2bfloat16(powf(__bfloat162float(a), __bfloat162float(b))); }
__device__ __forceinline__ bool isnang(__nv_bfloat16 a) { return __hisnan(a); }
__device__ __forceinline__ __nv_bfloat16 sqrtg(__nv_bfloat16 a) { return hsqrt(a); }
__device__ __forceinline__ __nv_bfloat16 cosg(__nv_bfloat16 a) { return hcos(a); }
__device__ __forceinline__ __nv_bfloat16 sing(__nv_bfloat16 a) { return hsin(a); }
__device__ __forceinline__ __nv_bfloat16 recipg(__nv_bfloat16 a) { __nv_bfloat16 one = 1.0; return one / a; }
__device__ __forceinline__ __nv_bfloat16 maxg(__nv_bfloat16 a, __nv_bfloat16 b) { return __hmax_nan(a, b); }
__device__ __forceinline__ __nv_bfloat16 tanhg(__nv_bfloat16 a) { return __float2bfloat16(tanhf(__bfloat162float(a))); }
__device__ __forceinline__ __nv_bfloat16 erfg(__nv_bfloat16 a) { return __float2bfloat16(erff(__bfloat162float(a))); }
__device__ __forceinline__ __nv_bfloat16 ceilg(__nv_bfloat16 a) { return __float2bfloat16(ceilf(__bfloat162float(a))); }
__device__ __forceinline__ __nv_bfloat16 floorg(__nv_bfloat16 a) { return __float2bfloat16(floorf(__bfloat162float(a))); }
__device__ __forceinline__ __nv_bfloat16 roundg(__nv_bfloat16 a) { return __float2bfloat16(roundf(__bfloat162float(a))); }
__device__ __forceinline__ __nv_bfloat16 normcdfg(__nv_bfloat16 a) { return __float2bfloat16(normcdff(__bfloat162float(a))); }
__device__ __forceinline__ __nv_bfloat16 ming(__nv_bfloat16 a, __nv_bfloat16 b) { return __hmin_nan(a, b); }
__device__ __forceinline__ __nv_bfloat16 logg(__nv_bfloat16 a) { return hlog(a); }
__device__ __forceinline__ __nv_bfloat16 expg(__nv_bfloat16 a) { return hexp(a); }
__device__ __forceinline__ __nv_bfloat16 absg(__nv_bfloat16 a) { return __habs(a); }
__device__ __forceinline__ __nv_bfloat16 copysigng(__nv_bfloat16 a, __nv_bfloat16 b) { return __float2bfloat16(copysignf(__bfloat162float(a), __bfloat162float(b))); }
#endif
| candle/candle-kernels/src/cuda_utils.cuh/0 | {
"file_path": "candle/candle-kernels/src/cuda_utils.cuh",
"repo_id": "candle",
"token_count": 3936
} | 32 |
//! Batch Normalization.
//!
//! This layer applies Batch Normalization over a mini-batch of inputs as described in [`Batch
//! Normalization`]. The input is expected to have at least three dimensions.
//!
//! Note that this implementation is for inference only, there is no possibility to track the
//! running stats.
//!
//! [`Batch Normalization`]: https://arxiv.org/abs/1502.03167
use candle::{DType, Result, Tensor, Var};
#[derive(Debug, Clone, Copy, PartialEq)]
pub struct BatchNormConfig {
pub eps: f64,
pub remove_mean: bool,
/// The meaning of affine here is different from LayerNorm: when false there is no learnable
/// parameter at all, 1 used for gamma and 0 for beta.
pub affine: bool,
/// Controls exponential moving average of running stats. Defaults to 0.1
///
/// `running_stat * (1.0 - momentum) + stat * momentum`.
pub momentum: f64,
}
impl Default for BatchNormConfig {
fn default() -> Self {
Self {
eps: 1e-5,
remove_mean: true,
affine: true,
momentum: 0.1,
}
}
}
impl From<f64> for BatchNormConfig {
fn from(eps: f64) -> Self {
Self {
eps,
..Default::default()
}
}
}
#[derive(Clone, Debug)]
pub struct BatchNorm {
running_mean: Var,
running_var: Var,
weight_and_bias: Option<(Tensor, Tensor)>,
remove_mean: bool,
eps: f64,
momentum: f64,
}
impl BatchNorm {
fn check_validity(&self, num_features: usize) -> Result<()> {
if self.eps < 0. {
candle::bail!("batch-norm eps cannot be negative {}", self.eps)
}
if !(0.0..=1.0).contains(&self.momentum) {
candle::bail!(
"batch-norm momentum must be between 0 and 1, is {}",
self.momentum
)
}
if self.running_mean.dims() != [num_features] {
candle::bail!(
"batch-norm running mean has unexpected shape {:?} should have shape [{num_features}]",
self.running_mean.shape(),
)
}
if self.running_var.dims() != [num_features] {
candle::bail!(
"batch-norm running variance has unexpected shape {:?} should have shape [{num_features}]",
self.running_var.shape(),
)
}
if let Some((ref weight, ref bias)) = self.weight_and_bias.as_ref() {
if weight.dims() != [num_features] {
candle::bail!(
"batch-norm weight has unexpected shape {:?} should have shape [{num_features}]",
weight.shape(),
)
}
if bias.dims() != [num_features] {
candle::bail!(
"batch-norm weight has unexpected shape {:?} should have shape [{num_features}]",
bias.shape(),
)
}
}
Ok(())
}
pub fn new(
num_features: usize,
running_mean: Tensor,
running_var: Tensor,
weight: Tensor,
bias: Tensor,
eps: f64,
) -> Result<Self> {
let out = Self {
running_mean: Var::from_tensor(&running_mean)?,
running_var: Var::from_tensor(&running_var)?,
weight_and_bias: Some((weight, bias)),
remove_mean: true,
eps,
momentum: 0.1,
};
out.check_validity(num_features)?;
Ok(out)
}
pub fn new_no_bias(
num_features: usize,
running_mean: Tensor,
running_var: Tensor,
eps: f64,
) -> Result<Self> {
let out = Self {
running_mean: Var::from_tensor(&running_mean)?,
running_var: Var::from_tensor(&running_var)?,
weight_and_bias: None,
remove_mean: true,
eps,
momentum: 0.1,
};
out.check_validity(num_features)?;
Ok(out)
}
pub fn new_with_momentum(
num_features: usize,
running_mean: Tensor,
running_var: Tensor,
weight: Tensor,
bias: Tensor,
eps: f64,
momentum: f64,
) -> Result<Self> {
let out = Self {
running_mean: Var::from_tensor(&running_mean)?,
running_var: Var::from_tensor(&running_var)?,
weight_and_bias: Some((weight, bias)),
remove_mean: true,
eps,
momentum,
};
out.check_validity(num_features)?;
Ok(out)
}
pub fn new_no_bias_with_momentum(
num_features: usize,
running_mean: Tensor,
running_var: Tensor,
eps: f64,
momentum: f64,
) -> Result<Self> {
let out = Self {
running_mean: Var::from_tensor(&running_mean)?,
running_var: Var::from_tensor(&running_var)?,
weight_and_bias: None,
remove_mean: true,
eps,
momentum,
};
out.check_validity(num_features)?;
Ok(out)
}
pub fn running_mean(&self) -> &Tensor {
self.running_mean.as_tensor()
}
pub fn running_var(&self) -> &Tensor {
self.running_var.as_tensor()
}
pub fn eps(&self) -> f64 {
self.eps
}
pub fn weight_and_bias(&self) -> Option<(&Tensor, &Tensor)> {
self.weight_and_bias.as_ref().map(|v| (&v.0, &v.1))
}
pub fn momentum(&self) -> f64 {
self.momentum
}
pub fn forward_train(&self, x: &Tensor) -> Result<Tensor> {
let num_features = self.running_mean.as_tensor().dim(0)?;
let x_dtype = x.dtype();
let internal_dtype = match x_dtype {
DType::F16 | DType::BF16 => DType::F32,
d => d,
};
if x.rank() < 2 {
candle::bail!(
"batch-norm input tensor must have at least two dimensions ({:?})",
x.shape()
)
}
if x.dim(1)? != num_features {
candle::bail!(
"batch-norm input doesn't have the expected number of features ({:?} <> {})",
x.shape(),
num_features
)
}
let x = x.to_dtype(internal_dtype)?;
let x = x.transpose(0, 1)?;
let x_dims_post_transpose = x.dims();
// Flatten all the dimensions exception the channel one as this performs a Spatial Batch
// Normalization.
let x = x.flatten_from(1)?.contiguous()?;
let x = if self.remove_mean {
// The mean is taken over dim 1 as this is the batch dim after the transpose(0, 1) above.
let mean_x = x.mean_keepdim(1)?;
let updated_running_mean = ((self.running_mean.as_tensor() * (1.0 - self.momentum))?
+ (mean_x.flatten_all()? * self.momentum)?)?;
self.running_mean.set(&updated_running_mean)?;
x.broadcast_sub(&mean_x)?
} else {
x
};
// The mean is taken over dim 1 as this is the batch dim after the transpose(0, 1) above.
let norm_x = x.sqr()?.mean_keepdim(1)?;
let updated_running_var = {
let batch_size = x.dim(1)? as f64;
let running_var_weight = 1.0 - self.momentum;
let norm_x_weight = self.momentum * batch_size / (batch_size - 1.0);
((self.running_var.as_tensor() * running_var_weight)?
+ (&norm_x.flatten_all()? * norm_x_weight)?)?
};
self.running_var.set(&updated_running_var)?;
let x = x
.broadcast_div(&(norm_x + self.eps)?.sqrt()?)?
.to_dtype(x_dtype)?;
let x = match &self.weight_and_bias {
None => x,
Some((weight, bias)) => {
let weight = weight.reshape(((), 1))?;
let bias = bias.reshape(((), 1))?;
x.broadcast_mul(&weight)?.broadcast_add(&bias)?
}
};
x.reshape(x_dims_post_transpose)?.transpose(0, 1)
}
fn forward_eval(&self, x: &Tensor) -> Result<Tensor> {
let target_shape: Vec<usize> = x
.dims()
.iter()
.enumerate()
.map(|(idx, v)| if idx == 1 { *v } else { 1 })
.collect();
let target_shape = target_shape.as_slice();
let x = x
.broadcast_sub(
&self
.running_mean
.as_detached_tensor()
.reshape(target_shape)?,
)?
.broadcast_div(
&(self
.running_var
.as_detached_tensor()
.reshape(target_shape)?
+ self.eps)?
.sqrt()?,
)?;
match &self.weight_and_bias {
None => Ok(x),
Some((weight, bias)) => {
let weight = weight.reshape(target_shape)?;
let bias = bias.reshape(target_shape)?;
x.broadcast_mul(&weight)?.broadcast_add(&bias)
}
}
}
}
impl crate::ModuleT for BatchNorm {
fn forward_t(&self, x: &Tensor, train: bool) -> Result<Tensor> {
if train {
self.forward_train(x)
} else {
self.forward_eval(x)
}
}
}
pub fn batch_norm<C: Into<BatchNormConfig>>(
num_features: usize,
config: C,
vb: crate::VarBuilder,
) -> Result<BatchNorm> {
use crate::Init;
let config = config.into();
if config.eps < 0. {
candle::bail!("batch-norm eps cannot be negative {}", config.eps)
}
let running_mean = vb.get_with_hints(num_features, "running_mean", Init::Const(0.))?;
let running_var = vb.get_with_hints(num_features, "running_var", Init::Const(1.))?;
let weight_and_bias = if config.affine {
let weight = vb.get_with_hints(num_features, "weight", Init::Const(1.))?;
let bias = vb.get_with_hints(num_features, "bias", Init::Const(0.))?;
Some((weight, bias))
} else {
None
};
Ok(BatchNorm {
running_mean: Var::from_tensor(&running_mean)?,
running_var: Var::from_tensor(&running_var)?,
weight_and_bias,
remove_mean: config.remove_mean,
eps: config.eps,
momentum: config.momentum,
})
}
| candle/candle-nn/src/batch_norm.rs/0 | {
"file_path": "candle/candle-nn/src/batch_norm.rs",
"repo_id": "candle",
"token_count": 5325
} | 33 |
use candle::{DType, Device, Result, Shape, Tensor, Var};
use std::collections::HashMap;
use std::sync::{Arc, Mutex};
/// A `VarMap` is a store that holds named variables. Variables can be retrieved from the stores
/// and new variables can be added by providing some initialization config in case they are
/// missing.
/// `VarMap` structures can be serialized in the safetensors format.
#[derive(Clone)]
pub struct VarMap {
data: Arc<Mutex<HashMap<String, Var>>>,
}
impl VarMap {
/// Create a new empty `VarMap`.
#[allow(clippy::new_without_default)]
pub fn new() -> Self {
let data = Arc::new(Mutex::new(HashMap::new()));
Self { data }
}
/// Retrieve all the variables currently stored in the map.
pub fn all_vars(&self) -> Vec<Var> {
let tensor_data = self.data.lock().unwrap();
#[allow(clippy::map_clone)]
tensor_data.values().map(|c| c.clone()).collect::<Vec<_>>()
}
/// Save the map in the safetensors format.
pub fn save<P: AsRef<std::path::Path>>(&self, path: P) -> Result<()> {
let tensor_data = self.data.lock().unwrap();
let data = tensor_data.iter().map(|(k, v)| (k, v.as_tensor()));
safetensors::tensor::serialize_to_file(data, &None, path.as_ref())?;
Ok(())
}
/// Load some values from a safetensors file and modify the existing variables to have these
/// values.
///
/// Note that values for variables that are currently not in the map are not kept.
pub fn load<P: AsRef<std::path::Path>>(&mut self, path: P) -> Result<()> {
let path = path.as_ref();
let data = unsafe { candle::safetensors::MmapedSafetensors::new(path)? };
let mut tensor_data = self.data.lock().unwrap();
for (name, var) in tensor_data.iter_mut() {
let data = data.load(name, var.device())?;
if let Err(err) = var.set(&data) {
candle::bail!("error setting {name} using data from {path:?}: {err}",)
}
}
Ok(())
}
/// Set a named variable to some value.
pub fn set_one<K: AsRef<str>, V: AsRef<Tensor>>(&mut self, name: K, value: V) -> Result<()> {
let tensor_data = self.data.lock().unwrap();
let name = name.as_ref();
match tensor_data.get(name) {
None => candle::bail!("cannot find {name} in VarMap"),
Some(var) => {
if let Err(err) = var.set(value.as_ref()) {
candle::bail!("error setting {name}: {err}",)
}
}
}
Ok(())
}
/// Set some named variables to some values.
///
/// If an error is returned, some of the variables might have already been set to their new
/// values.
pub fn set<I: Iterator<Item = (K, V)>, K: AsRef<str>, V: AsRef<Tensor>>(
&mut self,
iter: I,
) -> Result<()> {
let tensor_data = self.data.lock().unwrap();
for (name, value) in iter {
let name = name.as_ref();
match tensor_data.get(name) {
None => candle::bail!("cannot find {name} in VarMap"),
Some(var) => {
if let Err(err) = var.set(value.as_ref()) {
candle::bail!("error setting {name}: {err}",)
}
}
}
}
Ok(())
}
/// Retrieve or add a new variable.
pub fn get<S: Into<Shape>>(
&self,
shape: S,
path: &str,
init: crate::Init,
dtype: DType,
device: &Device,
) -> Result<Tensor> {
let shape = shape.into();
let mut tensor_data = self.data.lock().unwrap();
if let Some(tensor) = tensor_data.get(path) {
let tensor_shape = tensor.shape();
if &shape != tensor_shape {
candle::bail!("shape mismatch on {path}: {shape:?} <> {tensor_shape:?}")
}
return Ok(tensor.as_tensor().clone());
}
let var = init.var(shape, dtype, device)?;
let tensor = var.as_tensor().clone();
tensor_data.insert(path.to_string(), var);
Ok(tensor)
}
pub fn data(&self) -> &Mutex<HashMap<String, Var>> {
&self.data
}
}
| candle/candle-nn/src/var_map.rs/0 | {
"file_path": "candle/candle-nn/src/var_map.rs",
"repo_id": "candle",
"token_count": 1973
} | 34 |
from candle import Tensor, QTensor, DType
from typing import (
Dict,
Tuple,
Any,
Optional,
Union,
Iterator,
Set,
overload,
Mapping,
TypeVar,
List,
)
from collections import OrderedDict, namedtuple
TensorLike = Union[Tensor, QTensor]
T = TypeVar("T", bound="Module")
class _IncompatibleKeys(namedtuple("IncompatibleKeys", ["missing_keys", "unexpected_keys"])):
def __repr__(self):
if not self.missing_keys and not self.unexpected_keys:
return "<All keys matched successfully>"
return super().__repr__()
__str__ = __repr__
# see: https://github.com/pytorch/pytorch/blob/main/torch/nn/modules/module.py
class Module:
"""
Pytorch like Module.
Base class for all neural network modules.
Your models should also subclass this class.
"""
_modules: Dict[str, Optional["Module"]]
_buffers: Dict[str, Optional[TensorLike]]
_non_persistent_buffers_set: Set[str]
_quantizable_buffers: Set[str]
_version: int = 1
def __init__(self, *args, **kwargs) -> None:
"""
Initializes internal Module state
"""
super().__setattr__("_modules", OrderedDict())
super().__setattr__("_buffers", OrderedDict())
super().__setattr__("_non_persistent_buffers_set", set())
super().__setattr__("_quantizable_buffers", set())
def __call__(self, *input):
"""
Call self as a function.
"""
return self.forward(*input)
def forward(self, *input):
"""
Defines the computation performed at every call.
Should be overridden by all subclasses.
"""
pass
def children(self) -> Iterator["Module"]:
r"""Returns an iterator over immediate children modules.
Yields:
Module: a child module
"""
for name, module in self.named_children():
yield module
def named_children(self) -> Iterator[Tuple[str, "Module"]]:
r"""Returns an iterator over immediate children modules, yielding both
the name of the module as well as the module itself.
Yields:
(str, Module): Tuple containing a name and child module
Example::
>>> for name, module in model.named_children():
>>> if name in ['conv4', 'conv5']:
>>> print(module)
"""
memo = set()
for name, module in self._modules.items():
if module is not None and module not in memo:
memo.add(module)
yield name, module
def add_module(self, name: str, module: Optional["Module"]) -> None:
r"""Adds a child module to the current module.
The module can be accessed as an attribute using the given name.
Args:
name (str): name of the child module. The child module can be
accessed from this module using the given name
module (Module): child module to be added to the module.
"""
if not isinstance(module, Module) and module is not None:
raise TypeError(f"{str(module)} is not a Module subclass")
elif not isinstance(name, str):
raise TypeError(f"module name should be a string. Got {name}")
elif hasattr(self, name) and name not in self._modules:
raise KeyError(f"attribute '{name}' already exists")
elif "." in name:
raise KeyError(f'module name can\'t contain ".", got: {name}')
elif name == "":
raise KeyError('module name can\'t be empty string ""')
self._modules[name] = module
def register_module(self, name: str, module: Optional["Module"]) -> None:
r"""Alias for :func:`add_module`."""
self.add_module(name, module)
def modules(self) -> Iterator["Module"]:
r"""Returns an iterator over all modules in the network."""
for _, module in self.named_modules():
yield module
def named_modules(
self,
memo: Optional[Set["Module"]] = None,
prefix: str = "",
remove_duplicate: bool = True,
):
r"""Returns an iterator over all modules in the network, yielding
both the name of the module as well as the module itself.
Args:
memo: a memo to store the set of modules already added to the result
prefix: a prefix that will be added to the name of the module
remove_duplicate: whether to remove the duplicated module instances in the result
or not
Yields:
(str, Module): Tuple of name and module
Note:
Duplicate modules are returned only once. In the following
example, ``l`` will be returned only once.
"""
if memo is None:
memo = set()
if self not in memo:
if remove_duplicate:
memo.add(self)
yield prefix, self
for name, module in self._modules.items():
if module is None:
continue
submodule_prefix = prefix + ("." if prefix else "") + name
for m in module.named_modules(memo, submodule_prefix, remove_duplicate):
yield m
def buffers(self, recurse: bool = True) -> Iterator[TensorLike]:
"""
Returns an iterator over module buffers.
"""
for name, buf in self.named_buffers(recurse=recurse):
yield buf
def named_buffers(
self, prefix: str = "", recurse: bool = True, remove_duplicate: bool = True
) -> Iterator[Tuple[str, TensorLike]]:
r"""Returns an iterator over module buffers, yielding both the
name of the buffer as well as the buffer itself.
Args:
prefix (str): prefix to prepend to all buffer names.
recurse (bool, optional): if True, then yields buffers of this module
and all submodules. Otherwise, yields only buffers that
are direct members of this module. Defaults to True.
remove_duplicate (bool, optional): whether to remove the duplicated buffers in the result. Defaults to True.
Yields:
(str, Tensor): Tuple containing the name and buffer
Example::
>>> for name, buf in self.named_buffers():
>>> if name in ['running_var']:
>>> print(buf.size())
"""
gen = self._named_members(
lambda module: module._buffers.items(),
prefix=prefix,
recurse=recurse,
remove_duplicate=remove_duplicate,
)
yield from gen
# The user can pass an optional arbitrary mappable object to `state_dict`, in which case `state_dict` returns
# back that same object. But if they pass nothing, an `OrderedDict` is created and returned.
T_destination = TypeVar("T_destination", bound=Dict[str, Any])
@overload
def state_dict(self, *, destination: T_destination, prefix: str = ..., keep_vars: bool = ...) -> T_destination: ...
@overload
def state_dict(self, *, prefix: str = ..., keep_vars: bool = ...) -> Dict[str, Any]: ...
def state_dict(self, *args, destination=None, prefix="", keep_vars=False):
r"""Returns a dictionary containing references to the whole state of the module.
Both parameters and persistent buffers (e.g. running averages) are
included. Keys are corresponding parameter and buffer names.
Parameters and buffers set to ``None`` are not included.
.. note::
The returned object is a shallow copy. It contains references
to the module's parameters and buffers.
.. warning::
Currently ``state_dict()`` also accepts positional arguments for
``destination``, ``prefix`` and ``keep_vars`` in order. However,
this is being deprecated and keyword arguments will be enforced in
future releases.
.. warning::
Please avoid the use of argument ``destination`` as it is not
designed for end-users.
Args:
destination (dict, optional): If provided, the state of module will
be updated into the dict and the same object is returned.
Otherwise, an ``OrderedDict`` will be created and returned.
Default: ``None``.
prefix (str, optional): a prefix added to parameter and buffer
names to compose the keys in state_dict. Default: ``''``.
keep_vars (bool, optional): by default the :class:`~candle.Tensor` s
returned in the state dict are detached from autograd. If it's
set to ``True``, detaching will not be performed.
Default: ``False``.
Returns:
dict:
a dictionary containing a whole state of the module
Example::
>>> # xdoctest: +SKIP("undefined vars")
>>> module.state_dict().keys()
['bias', 'weight']
"""
# TODO: Remove `args` and the parsing logic when BC allows.
if len(args) > 0:
if destination is None:
destination = args[0]
if len(args) > 1 and prefix == "":
prefix = args[1]
if len(args) > 2 and keep_vars is False:
keep_vars = args[2]
if destination is None:
destination = OrderedDict()
destination._metadata = OrderedDict()
local_metadata = dict(version=self._version)
if hasattr(destination, "_metadata"):
destination._metadata[prefix[:-1]] = local_metadata
self._save_to_state_dict(destination, prefix, keep_vars)
for name, module in self._modules.items():
if module is not None:
module.state_dict(
destination=destination,
prefix=prefix + name + ".",
keep_vars=keep_vars,
)
return destination
def _save_to_state_dict(self, destination, prefix, keep_vars):
r"""Saves module state to `destination` dictionary, containing a state
of the module, but not its descendants. This is called on every
submodule in :meth:`~candle.nn.Module.state_dict`.
In rare cases, subclasses can achieve class-specific behavior by
overriding this method with custom logic.
Args:
destination (dict): a dict where state will be stored
prefix (str): the prefix for parameters and buffers used in this
module
"""
for name, buf in self._buffers.items():
if buf is not None and name not in self._non_persistent_buffers_set:
if isinstance(buf, Tensor):
destination[prefix + name] = buf if keep_vars else buf.detach()
else:
destination[prefix + name] = buf
def load_state_dict(self, state_dict: Mapping[str, Any], strict: bool = True, assign: bool = False):
r"""Copies parameters and buffers from :attr:`state_dict` into
this module and its descendants. If :attr:`strict` is ``True``, then
the keys of :attr:`state_dict` must exactly match the keys returned
by this module's :meth:`~candle.nn.Module.state_dict` function.
.. warning::
If :attr:`assign` is ``True`` the optimizer must be created after
the call to :attr:`load_state_dict`.
Args:
state_dict (dict): a dict containing parameters and
persistent buffers.
strict (bool, optional): whether to strictly enforce that the keys
in :attr:`state_dict` match the keys returned by this module's
:meth:`~candle.nn.Module.state_dict` function. Default: ``True``
assign (bool, optional): whether to assign items in the state
dictionary to their corresponding keys in the module instead
of copying them inplace into the module's current parameters and buffers.
When ``False``, the properties of the tensors in the current
module are preserved while when ``True``, the properties of the
Tensors in the state dict are preserved.
Default: ``False``
Returns:
``NamedTuple`` with ``missing_keys`` and ``unexpected_keys`` fields:
* **missing_keys** is a list of str containing the missing keys
* **unexpected_keys** is a list of str containing the unexpected keys
Note:
If a parameter or buffer is registered as ``None`` and its corresponding key
exists in :attr:`state_dict`, :meth:`load_state_dict` will raise a
``RuntimeError``.
"""
if not isinstance(state_dict, Mapping):
raise TypeError(f"Expected state_dict to be dict-like, got {type(state_dict)}.")
missing_keys: List[str] = []
unexpected_keys: List[str] = []
error_msgs: List[str] = []
# copy state_dict so _load_from_state_dict can modify it
metadata = getattr(state_dict, "_metadata", None)
state_dict = OrderedDict(state_dict)
if metadata is not None:
# mypy isn't aware that "_metadata" exists in state_dict
state_dict._metadata = metadata # type: ignore[attr-defined]
def load(module, local_state_dict, prefix=""):
local_metadata = {} if metadata is None else metadata.get(prefix[:-1], {})
if assign:
local_metadata["assign_to_params_buffers"] = assign
module._load_from_state_dict(
local_state_dict,
prefix,
local_metadata,
True,
missing_keys,
unexpected_keys,
error_msgs,
)
for name, child in module._modules.items():
if child is not None:
child_prefix = prefix + name + "."
child_state_dict = {k: v for k, v in local_state_dict.items() if k.startswith(child_prefix)}
load(child, child_state_dict, child_prefix)
load(self, state_dict)
del load
if strict:
if len(unexpected_keys) > 0:
error_msgs.insert(
0,
"Unexpected key(s) in state_dict: {}. ".format(", ".join(f'"{k}"' for k in unexpected_keys)),
)
if len(missing_keys) > 0:
error_msgs.insert(
0,
"Missing key(s) in state_dict: {}. ".format(", ".join(f'"{k}"' for k in missing_keys)),
)
if len(error_msgs) > 0:
raise RuntimeError(
"Error(s) in loading state_dict for {}:\n\t{}".format(self.__class__.__name__, "\n\t".join(error_msgs))
)
return _IncompatibleKeys(missing_keys, unexpected_keys)
def _load_from_state_dict(
self,
state_dict,
prefix,
local_metadata,
strict,
missing_keys,
unexpected_keys,
error_msgs,
):
r"""Copies parameters and buffers from :attr:`state_dict` into only
this module, but not its descendants. This is called on every submodule
in :meth:`~candle.nn.Module.load_state_dict`. Metadata saved for this
module in input :attr:`state_dict` is provided as :attr:`local_metadata`.
For state dicts without metadata, :attr:`local_metadata` is empty.
Subclasses can achieve class-specific backward compatible loading using
the version number at `local_metadata.get("version", None)`.
Additionally, :attr:`local_metadata` can also contain the key
`assign_to_params_buffers` that indicates whether keys should be
assigned their corresponding tensor in the state_dict.
.. note::
:attr:`state_dict` is not the same object as the input
:attr:`state_dict` to :meth:`~candle.nn.Module.load_state_dict`. So
it can be modified.
Args:
state_dict (dict): a dict containing parameters and
persistent buffers.
prefix (str): the prefix for parameters and buffers used in this
module
local_metadata (dict): a dict containing the metadata for this module.
See
strict (bool): whether to strictly enforce that the keys in
:attr:`state_dict` with :attr:`prefix` match the names of
parameters and buffers in this module
missing_keys (list of str): if ``strict=True``, add missing keys to
this list
unexpected_keys (list of str): if ``strict=True``, add unexpected
keys to this list
error_msgs (list of str): error messages should be added to this
list, and will be reported together in
:meth:`~candle.nn.Module.load_state_dict`
"""
persistent_buffers = {k: v for k, v in self._buffers.items() if k not in self._non_persistent_buffers_set}
local_name_params = persistent_buffers.items()
local_state = {k: v for k, v in local_name_params if v is not None}
for name, param in local_state.items():
key = prefix + name
if key in state_dict:
input_param = state_dict[key]
if not isinstance(input_param, (Tensor, QTensor)):
error_msgs.append(
f'While copying the parameter named "{key}", '
"expected Tensor-like object from checkpoint but "
f"received {type(input_param)}"
)
continue
if input_param.shape != param.shape:
# local shape should match the one in checkpoint
error_msgs.append(
"size mismatch for {}: copying a param with shape {} from checkpoint, "
"the shape in current model is {}.".format(key, input_param.shape, param.shape)
)
continue
try:
# Shape checks are already done above -> Just assign tensor
setattr(self, name, input_param)
except Exception as ex:
error_msgs.append(
f'While copying the parameter named "{key}", '
f"whose dimensions in the model are {param.shape} and "
f"whose dimensions in the checkpoint are {input_param.shape}, "
f"an exception occurred : {ex.args}."
)
elif strict:
missing_keys.append(key)
if strict:
for key in state_dict.keys():
if key.startswith(prefix):
input_name = key[len(prefix) :]
input_name = input_name.split(".", 1)[0] # get the name of param/buffer/child
if input_name not in self._modules and input_name not in local_state:
unexpected_keys.append(key)
def _named_members(self, get_members_fn, prefix="", recurse=True, remove_duplicate: bool = True):
r"""Helper method for yielding various names + members of modules."""
memo = set()
modules = self.named_modules(prefix=prefix, remove_duplicate=remove_duplicate) if recurse else [(prefix, self)]
for module_prefix, module in modules:
members = get_members_fn(module)
for k, v in members:
if v is None or v in memo:
continue
if remove_duplicate:
memo.add(v)
name = module_prefix + ("." if module_prefix else "") + k
yield name, v
def _get_name(self):
return self.__class__.__name__
def _apply(self, fn):
for module in self.children():
module._apply(fn)
for key, buf in self._buffers.items():
if buf is not None:
self._buffers[key] = fn(buf)
return self
def __move_tensor_to_device(self, tensor: TensorLike, device: str):
if isinstance(tensor, Tensor):
return tensor.to_device(device)
else:
raise NotImplementedError("Cannot offload QTensor to cuda, yet!")
def device(self) -> str:
"""
Gets the device of the module, by inspecting its tensors.
"""
tensor = next(self.buffers())
if isinstance(tensor, Tensor):
return tensor.device
else:
# QTensors can only be on the CPU
return "cpu"
def cuda(self: T) -> T:
r"""Moves all model parameters and buffers to the GPU.
This also makes associated parameters and buffers different objects. So
it should be called before constructing optimizer if the module will
live on GPU while being optimized.
.. note::
This method modifies the module in-place.
Returns:
Module: self
"""
def to_cuda(t: TensorLike):
return self.__move_tensor_to_device(t, "cuda")
return self._apply(to_cuda)
def cpu(self: T) -> T:
r"""Moves all model parameters and buffers to the CPU.
.. note::
This method modifies the module in-place.
Returns:
Module: self
"""
def to_cpu(t: TensorLike):
return self.__move_tensor_to_device(t, "cpu")
return self._apply(to_cpu)
def __cast_tensor(self, tensor: TensorLike, dtype: Union[DType, str]):
if isinstance(tensor, Tensor):
return tensor.to_dtype(dtype)
else:
raise TypeError("candle.Module.to only accepts Tensor dtypes, but got desired dtype={}".format(dtype))
def type(self: T, dst_type: Union[DType, str]) -> T:
r"""Casts all parameters and buffers to :attr:`dst_type`.
.. note::
This method modifies the module in-place.
Args:
dst_type (type or string): the desired type
Returns:
Module: self
"""
def cast(t: TensorLike):
return self.__cast_tensor(t, dst_type)
return self._apply(cast)
@overload
def to(
self: T,
device: str = ...,
dtype: Optional[Union[DType, str]] = ...,
) -> T: ...
@overload
def to(self: T, dtype: Union[DType, str]) -> T: ...
def to(self, *args, **kwargs):
r"""Moves and/or casts the parameters and buffers.
This can be called as
.. function:: to(device=None, dtype=None)
:noindex:
.. function:: to(dtype)
:noindex:
See below for examples.
.. note::
This method modifies the module in-place.
Args:
device (:class:`candle.device`): the desired device of the parameters
and buffers in this module
dtype (:class:`candle.dtype`): the desired floating point dtype of
the parameters and buffers in this module
Returns:
Module: self
"""
device = None
dtype = None
if args:
for arg in args:
# Assuming arg can be a string representing a device or a dtype
if isinstance(arg, str):
lower_arg = str(arg).lower()
if lower_arg.startswith("cuda") or lower_arg == "cpu":
device = lower_arg
else:
dtype = arg
elif isinstance(arg, DType):
dtype = str(arg)
else:
raise TypeError("Module.to() received an invalid combination of arguments. Got: {}".format(args))
if kwargs:
device = kwargs.get("device", device)
dtype = str(kwargs.get("dtype", dtype))
if device:
device = device.lower()
if dtype:
dtype = dtype.lower()
if dtype not in ["f32", "f16", "f64"]:
raise TypeError(
"candle.Module.to only accepts floating point" "dtypes, but got desired dtype={}".format(dtype)
)
def convert(t):
if dtype:
t = self.__cast_tensor(t, dtype)
if device:
t = self.__move_tensor_to_device(t, device)
return t
return self._apply(convert)
def __setattr__(self, __name: str, __value: Any) -> None:
if isinstance(__value, Module):
self._modules[__name] = __value
elif isinstance(__value, QTensor):
if __name in self._quantizable_buffers:
type = __value.ggml_dtype.lower()
if type in ["f32", "f16"]:
# It is faster to just dequantize the tensor here and use the normal tensor operations
dequant = __value.dequantize()
if type == "f16":
dequant = dequant.to_dtype("f16")
self._buffers[__name] = dequant
else:
self._buffers[__name] = __value
else:
# We expect a normal tensor here => dequantize it
self._buffers[__name] = __value.dequantize()
elif isinstance(__value, Tensor):
self._buffers[__name] = __value
else:
super().__setattr__(__name, __value)
def __getattr__(self, __name: str) -> Any:
if "_modules" in self.__dict__:
modules = self.__dict__["_modules"]
if __name in modules:
return modules[__name]
if "_buffers" in self.__dict__:
tensors = self.__dict__["_buffers"]
if __name in tensors:
return tensors[__name]
return super().__getattribute__(__name)
def __delattr__(self, name):
if name in self._buffers:
del self._buffers[name]
elif name in self._modules:
del self._modules[name]
else:
super().__delattr__(name)
| candle/candle-pyo3/py_src/candle/nn/module.py/0 | {
"file_path": "candle/candle-pyo3/py_src/candle/nn/module.py",
"repo_id": "candle",
"token_count": 12028
} | 35 |
import candle
print(f"mkl: {candle.utils.has_mkl()}")
print(f"accelerate: {candle.utils.has_accelerate()}")
print(f"num-threads: {candle.utils.get_num_threads()}")
print(f"cuda: {candle.utils.cuda_is_available()}")
t = candle.Tensor(42.0)
print(t)
print(t.shape, t.rank, t.device)
print(t + t)
t = candle.Tensor([3.0, 1, 4, 1, 5, 9, 2, 6])
print(t)
print(t + t)
t = t.reshape([2, 4])
print(t.matmul(t.t()))
print(t.to_dtype(candle.u8))
print(t.to_dtype("u8"))
t = candle.randn((5, 3))
print(t)
print(t.dtype)
t = candle.randn((16, 256))
quant_t = t.quantize("q6k")
dequant_t = quant_t.dequantize()
diff2 = (t - dequant_t).sqr()
print(diff2.mean_all())
| candle/candle-pyo3/test.py/0 | {
"file_path": "candle/candle-pyo3/test.py",
"repo_id": "candle",
"token_count": 340
} | 36 |
use super::with_tracing::{linear, Embedding, Linear};
use candle::{Result, Tensor};
use candle_nn::{layer_norm, LayerNorm, VarBuilder};
#[derive(Debug, Clone)]
pub struct Config {
pub vocab_size: usize,
pub decoder_vocab_size: Option<usize>,
pub max_position_embeddings: usize,
pub encoder_layers: usize,
pub encoder_ffn_dim: usize,
pub encoder_attention_heads: usize,
pub decoder_layers: usize,
pub decoder_ffn_dim: usize,
pub decoder_attention_heads: usize,
pub use_cache: bool,
pub is_encoder_decoder: bool,
pub activation_function: candle_nn::Activation,
pub d_model: usize,
pub decoder_start_token_id: u32,
pub scale_embedding: bool,
pub pad_token_id: u32,
pub eos_token_id: u32,
pub forced_eos_token_id: u32,
pub share_encoder_decoder_embeddings: bool,
}
impl Config {
// https://huggingface.co/Helsinki-NLP/opus-mt-tc-big-fr-en/blob/main/config.json
pub fn opus_mt_tc_big_fr_en() -> Self {
Self {
activation_function: candle_nn::Activation::Relu,
d_model: 1024,
decoder_attention_heads: 16,
decoder_ffn_dim: 4096,
decoder_layers: 6,
decoder_start_token_id: 53016,
decoder_vocab_size: Some(53017),
encoder_attention_heads: 16,
encoder_ffn_dim: 4096,
encoder_layers: 6,
eos_token_id: 43311,
forced_eos_token_id: 43311,
is_encoder_decoder: true,
max_position_embeddings: 1024,
pad_token_id: 53016,
scale_embedding: true,
share_encoder_decoder_embeddings: true,
use_cache: true,
vocab_size: 53017,
}
}
// https://huggingface.co/Helsinki-NLP/opus-mt-fr-en/blob/main/config.json
pub fn opus_mt_fr_en() -> Self {
Self {
activation_function: candle_nn::Activation::Swish,
d_model: 512,
decoder_attention_heads: 8,
decoder_ffn_dim: 2048,
decoder_layers: 6,
decoder_start_token_id: 59513,
decoder_vocab_size: Some(59514),
encoder_attention_heads: 8,
encoder_ffn_dim: 2048,
encoder_layers: 6,
eos_token_id: 0,
forced_eos_token_id: 0,
is_encoder_decoder: true,
max_position_embeddings: 512,
pad_token_id: 59513,
scale_embedding: true,
share_encoder_decoder_embeddings: true,
use_cache: true,
vocab_size: 59514,
}
}
}
#[derive(Debug, Clone)]
struct SinusoidalPositionalEmbedding {
emb: Embedding,
}
impl SinusoidalPositionalEmbedding {
fn new(cfg: &Config, vb: VarBuilder) -> Result<Self> {
let dev = vb.device();
let dtype = vb.dtype();
let num_positions = cfg.max_position_embeddings;
let dim = cfg.d_model;
let inv_freq: Vec<_> = (0..dim)
.step_by(2)
.map(|i| 1f32 / 10000f32.powf(i as f32 / dim as f32))
.collect();
let inv_freq_len = inv_freq.len();
let inv_freq = Tensor::from_vec(inv_freq, (1, inv_freq_len), dev)?.to_dtype(dtype)?;
let t = Tensor::arange(0u32, num_positions as u32, dev)?
.to_dtype(dtype)?
.reshape((num_positions, 1))?;
let freqs = t.matmul(&inv_freq)?;
let sin = freqs.sin()?;
let cos = freqs.cos()?;
let weights = Tensor::cat(&[&sin, &cos], 1)?.contiguous()?;
let emb = Embedding::from_weights(weights)?;
Ok(Self { emb })
}
fn forward(&self, input_ids: &Tensor, past_kv_len: usize) -> Result<Tensor> {
let seq_len = input_ids.dim(1)?;
Tensor::arange(
past_kv_len as u32,
(past_kv_len + seq_len) as u32,
input_ids.device(),
)?
.apply(&self.emb)
}
}
#[derive(Debug, Clone)]
struct Attention {
q_proj: Linear,
k_proj: Linear,
v_proj: Linear,
out_proj: Linear,
scaling: f64,
num_heads: usize,
head_dim: usize,
kv_cache: Option<(Tensor, Tensor)>,
is_decoder: bool,
}
impl Attention {
fn new(cfg: &Config, is_decoder: bool, vb: VarBuilder) -> Result<Self> {
let num_heads = if is_decoder {
cfg.decoder_attention_heads
} else {
cfg.encoder_attention_heads
};
let embed_dim = cfg.d_model;
let head_dim = embed_dim / num_heads;
let scaling = (head_dim as f64).powf(-0.5);
let q_proj = linear(embed_dim, embed_dim, vb.pp("q_proj"))?;
let k_proj = linear(embed_dim, embed_dim, vb.pp("k_proj"))?;
let v_proj = linear(embed_dim, embed_dim, vb.pp("v_proj"))?;
let out_proj = linear(embed_dim, embed_dim, vb.pp("out_proj"))?;
Ok(Self {
q_proj,
k_proj,
v_proj,
out_proj,
scaling,
num_heads,
head_dim,
kv_cache: None,
is_decoder,
})
}
fn _shape(&self, tensor: &Tensor, bsz: usize) -> Result<Tensor> {
tensor
.reshape((bsz, (), self.num_heads, self.head_dim))?
.transpose(1, 2)?
.contiguous()
}
fn forward(
&mut self,
xs: &Tensor,
kv_states: Option<&Tensor>,
attn_mask: Option<&Tensor>,
) -> Result<Tensor> {
let (b_sz, tgt_len, _) = xs.dims3()?;
let query_states = (xs.apply(&self.q_proj)? * self.scaling)?;
let (key_states, value_states) = match kv_states {
None => {
let key_states = self._shape(&xs.apply(&self.k_proj)?, b_sz)?;
let value_states = self._shape(&xs.apply(&self.v_proj)?, b_sz)?;
if self.is_decoder {
let kv_states = match &self.kv_cache {
None => (key_states, value_states),
Some((p_key_states, p_value_states)) => {
let key_states = Tensor::cat(&[p_key_states, &key_states], 2)?;
let value_states = Tensor::cat(&[p_value_states, &value_states], 2)?;
(key_states, value_states)
}
};
self.kv_cache = Some(kv_states.clone());
kv_states
} else {
(key_states, value_states)
}
}
Some(kv_states) => {
let key_states = self._shape(&kv_states.apply(&self.k_proj)?, b_sz)?;
let value_states = self._shape(&kv_states.apply(&self.v_proj)?, b_sz)?;
(key_states, value_states)
}
};
let proj_shape = (b_sz * self.num_heads, (), self.head_dim);
let query_states = self._shape(&query_states, b_sz)?.reshape(proj_shape)?;
let key_states = key_states.reshape(proj_shape)?;
let value_states = value_states.reshape(proj_shape)?;
let attn_weights = query_states.matmul(&key_states.transpose(1, 2)?)?;
let attn_weights = match attn_mask {
None => attn_weights,
Some(attn_mask) => attn_weights.broadcast_add(attn_mask)?,
};
let attn_probs = candle_nn::ops::softmax_last_dim(&attn_weights)?;
let attn_output = attn_probs.matmul(&value_states)?;
attn_output
.reshape((b_sz, self.num_heads, tgt_len, self.head_dim))?
.transpose(1, 2)?
.reshape((b_sz, tgt_len, self.head_dim * self.num_heads))?
.apply(&self.out_proj)
}
fn reset_kv_cache(&mut self) {
self.kv_cache = None
}
}
#[derive(Debug, Clone)]
struct EncoderLayer {
self_attn: Attention,
self_attn_layer_norm: LayerNorm,
activation_fn: candle_nn::Activation,
fc1: Linear,
fc2: Linear,
final_layer_norm: LayerNorm,
}
impl EncoderLayer {
fn new(cfg: &Config, vb: VarBuilder) -> Result<Self> {
let self_attn = Attention::new(cfg, true, vb.pp("self_attn"))?;
let self_attn_layer_norm = layer_norm(cfg.d_model, 1e-5, vb.pp("self_attn_layer_norm"))?;
let fc1 = linear(cfg.d_model, cfg.encoder_ffn_dim, vb.pp("fc1"))?;
let fc2 = linear(cfg.encoder_ffn_dim, cfg.d_model, vb.pp("fc2"))?;
let final_layer_norm = layer_norm(cfg.d_model, 1e-5, vb.pp("final_layer_norm"))?;
Ok(Self {
self_attn,
self_attn_layer_norm,
activation_fn: cfg.activation_function,
fc1,
fc2,
final_layer_norm,
})
}
fn forward(&mut self, xs: &Tensor) -> Result<Tensor> {
let residual = xs;
let xs = (self.self_attn.forward(xs, None, None)? + residual)?
.apply(&self.self_attn_layer_norm)?;
let residual = &xs;
let xs = xs
.apply(&self.fc1)?
.apply(&self.activation_fn)?
.apply(&self.fc2)?;
(xs + residual)?.apply(&self.final_layer_norm)
}
fn reset_kv_cache(&mut self) {
self.self_attn.reset_kv_cache()
}
}
#[derive(Debug, Clone)]
struct DecoderLayer {
self_attn: Attention,
self_attn_layer_norm: LayerNorm,
activation_fn: candle_nn::Activation,
encoder_attn: Attention,
encoder_attn_layer_norm: LayerNorm,
fc1: Linear,
fc2: Linear,
final_layer_norm: LayerNorm,
}
impl DecoderLayer {
fn new(cfg: &Config, vb: VarBuilder) -> Result<Self> {
let self_attn = Attention::new(cfg, true, vb.pp("self_attn"))?;
let self_attn_layer_norm = layer_norm(cfg.d_model, 1e-5, vb.pp("self_attn_layer_norm"))?;
let encoder_attn = Attention::new(cfg, true, vb.pp("encoder_attn"))?;
let encoder_attn_layer_norm =
layer_norm(cfg.d_model, 1e-5, vb.pp("encoder_attn_layer_norm"))?;
let fc1 = linear(cfg.d_model, cfg.decoder_ffn_dim, vb.pp("fc1"))?;
let fc2 = linear(cfg.decoder_ffn_dim, cfg.d_model, vb.pp("fc2"))?;
let final_layer_norm = layer_norm(cfg.d_model, 1e-5, vb.pp("final_layer_norm"))?;
Ok(Self {
self_attn,
self_attn_layer_norm,
activation_fn: cfg.activation_function,
encoder_attn,
encoder_attn_layer_norm,
fc1,
fc2,
final_layer_norm,
})
}
fn forward(
&mut self,
xs: &Tensor,
encoder_xs: Option<&Tensor>,
attn_mask: &Tensor,
) -> Result<Tensor> {
let residual = xs;
let xs = (self.self_attn.forward(xs, None, Some(attn_mask))? + residual)?
.apply(&self.self_attn_layer_norm)?;
let xs = match encoder_xs {
None => xs,
Some(encoder_xs) => {
let residual = &xs;
let xs = self.encoder_attn.forward(&xs, Some(encoder_xs), None)?;
(residual + xs)?.apply(&self.encoder_attn_layer_norm)?
}
};
let residual = &xs;
let xs = xs
.apply(&self.fc1)?
.apply(&self.activation_fn)?
.apply(&self.fc2)?;
let xs = (xs + residual)?.apply(&self.final_layer_norm)?;
Ok(xs)
}
fn reset_kv_cache(&mut self) {
self.self_attn.reset_kv_cache();
self.encoder_attn.reset_kv_cache()
}
}
#[derive(Debug, Clone)]
pub struct Encoder {
embed_tokens: Embedding,
embed_positions: SinusoidalPositionalEmbedding,
layers: Vec<EncoderLayer>,
embed_scale: Option<f64>,
}
impl Encoder {
fn new(cfg: &Config, embed_tokens: &Embedding, vb: VarBuilder) -> Result<Self> {
let embed_positions = SinusoidalPositionalEmbedding::new(cfg, vb.pp("embed_positions"))?;
let mut layers = Vec::with_capacity(cfg.encoder_layers);
let vb_l = vb.pp("layers");
for idx in 0..cfg.encoder_layers {
let layer = EncoderLayer::new(cfg, vb_l.pp(idx))?;
layers.push(layer)
}
let embed_scale = if cfg.scale_embedding {
Some((cfg.d_model as f64).sqrt())
} else {
None
};
Ok(Self {
embed_tokens: embed_tokens.clone(),
embed_positions,
layers,
embed_scale,
})
}
pub fn forward(&mut self, xs: &Tensor, past_kv_len: usize) -> Result<Tensor> {
let xs = xs.apply(&self.embed_tokens)?;
let xs = match self.embed_scale {
None => xs,
Some(scale) => (xs * scale)?,
};
let embed_pos = self
.embed_positions
.forward(&xs, past_kv_len)?
.unsqueeze(0)?;
let mut xs = xs.broadcast_add(&embed_pos)?;
for layer in self.layers.iter_mut() {
xs = layer.forward(&xs)?
}
Ok(xs)
}
pub fn reset_kv_cache(&mut self) {
for layer in self.layers.iter_mut() {
layer.reset_kv_cache()
}
}
}
#[derive(Debug, Clone)]
pub struct Decoder {
embed_tokens: Embedding,
embed_positions: SinusoidalPositionalEmbedding,
layers: Vec<DecoderLayer>,
embed_scale: Option<f64>,
}
impl Decoder {
fn new(cfg: &Config, embed_tokens: &Embedding, vb: VarBuilder) -> Result<Self> {
let embed_positions = SinusoidalPositionalEmbedding::new(cfg, vb.pp("embed_positions"))?;
let mut layers = Vec::with_capacity(cfg.decoder_layers);
let vb_l = vb.pp("layers");
for idx in 0..cfg.decoder_layers {
let layer = DecoderLayer::new(cfg, vb_l.pp(idx))?;
layers.push(layer)
}
let embed_scale = if cfg.scale_embedding {
Some((cfg.d_model as f64).sqrt())
} else {
None
};
Ok(Self {
embed_tokens: embed_tokens.clone(),
embed_positions,
layers,
embed_scale,
})
}
pub fn forward(
&mut self,
xs: &Tensor,
encoder_xs: Option<&Tensor>,
past_kv_len: usize,
attn_mask: &Tensor,
) -> Result<Tensor> {
let xs = xs.apply(&self.embed_tokens)?;
let xs = match self.embed_scale {
None => xs,
Some(scale) => (xs * scale)?,
};
let embed_pos = self
.embed_positions
.forward(&xs, past_kv_len)?
.unsqueeze(0)?;
let mut xs = xs.broadcast_add(&embed_pos)?;
for layer in self.layers.iter_mut() {
xs = layer.forward(&xs, encoder_xs, attn_mask)?;
}
Ok(xs)
}
pub fn reset_kv_cache(&mut self) {
for layer in self.layers.iter_mut() {
layer.reset_kv_cache()
}
}
}
#[derive(Debug, Clone)]
struct Model {
shared: Embedding,
encoder: Encoder,
decoder: Decoder,
}
impl Model {
fn new(cfg: &Config, vb: VarBuilder) -> Result<Self> {
let shared = Embedding::new(cfg.vocab_size, cfg.d_model, vb.pp("shared"))?;
let encoder = Encoder::new(cfg, &shared, vb.pp("encoder"))?;
let decoder = Decoder::new(cfg, &shared, vb.pp("decoder"))?;
Ok(Self {
shared,
encoder,
decoder,
})
}
fn reset_kv_cache(&mut self) {
self.encoder.reset_kv_cache();
self.decoder.reset_kv_cache();
}
}
#[derive(Debug, Clone)]
pub struct MTModel {
model: Model,
lm_head: Linear,
final_logits_bias: Tensor,
}
impl MTModel {
pub fn new(cfg: &Config, vb: VarBuilder) -> Result<Self> {
let target_vocab_size = cfg.decoder_vocab_size.unwrap_or(cfg.vocab_size);
let final_logits_bias = vb.get((1, target_vocab_size), "final_logits_bias")?;
let model = Model::new(cfg, vb.pp("model"))?;
let lm_head = Linear::from_weights(model.shared.embeddings().clone(), None);
Ok(Self {
model,
lm_head,
final_logits_bias,
})
}
pub fn encoder(&mut self) -> &mut Encoder {
&mut self.model.encoder
}
pub fn decoder(&mut self) -> &mut Decoder {
&mut self.model.decoder
}
pub fn decode(
&mut self,
xs: &Tensor,
encoder_xs: &Tensor,
past_kv_len: usize,
) -> Result<Tensor> {
let seq_len = xs.dim(1)?;
let mask: Vec<_> = (0..seq_len)
.flat_map(|i| (0..seq_len).map(move |j| if j > i { f32::NEG_INFINITY } else { 0f32 }))
.collect();
let mask = Tensor::from_vec(mask, (seq_len, seq_len), xs.device())?;
self.model
.decoder
.forward(xs, Some(encoder_xs), past_kv_len, &mask)?
.apply(&self.lm_head)?
.broadcast_add(&self.final_logits_bias)
}
pub fn reset_kv_cache(&mut self) {
self.model.reset_kv_cache();
}
}
| candle/candle-transformers/src/models/marian.rs/0 | {
"file_path": "candle/candle-transformers/src/models/marian.rs",
"repo_id": "candle",
"token_count": 8917
} | 37 |
use crate::quantized_nn::{layer_norm, linear, Linear};
pub use crate::quantized_var_builder::VarBuilder;
use candle::{DType, Device, IndexOp, Module, Result, Tensor, D};
use candle_nn::Activation;
pub use crate::models::mixformer::Config;
const MAX_SEQ_LEN: usize = 4096;
#[derive(Debug, Clone)]
struct Embedding {
wte: crate::quantized_nn::Embedding,
}
impl Embedding {
fn new(cfg: &Config, vb: VarBuilder) -> Result<Self> {
let wte = crate::quantized_nn::Embedding::new(cfg.vocab_size, cfg.n_embd, vb.pp("wte"))?;
Ok(Self { wte })
}
}
impl Module for Embedding {
fn forward(&self, xs: &Tensor) -> Result<Tensor> {
self.wte.forward(xs)
}
}
fn get_mask(size: usize, device: &Device) -> Result<Tensor> {
let mask: Vec<_> = (0..size)
.flat_map(|i| (0..size).map(move |j| u8::from(j > i)))
.collect();
Tensor::from_slice(&mask, (size, size), device)
}
fn masked_fill(on_false: &Tensor, mask: &Tensor, on_true: f32) -> Result<Tensor> {
let shape = mask.shape();
let on_true = Tensor::new(on_true, on_false.device())?.broadcast_as(shape.dims())?;
let m = mask.where_cond(&on_true, on_false)?;
Ok(m)
}
#[derive(Debug, Clone)]
struct RotaryEmbedding {
sin: Tensor,
cos: Tensor,
}
impl RotaryEmbedding {
fn new(dim: usize, max_seq_len: usize, dev: &Device) -> Result<Self> {
let inv_freq: Vec<_> = (0..dim)
.step_by(2)
.map(|i| 1f32 / 10000f32.powf(i as f32 / dim as f32))
.collect();
let inv_freq_len = inv_freq.len();
let inv_freq = Tensor::from_vec(inv_freq, (1, inv_freq_len), dev)?;
let t = Tensor::arange(0u32, max_seq_len as u32, dev)?
.to_dtype(DType::F32)?
.reshape((max_seq_len, 1))?;
let freqs = t.matmul(&inv_freq)?;
Ok(Self {
sin: freqs.sin()?,
cos: freqs.cos()?,
})
}
fn apply_rotary_emb_qkv(
&self,
qkv: &Tensor,
seqlen_offset: usize,
) -> Result<(Tensor, Tensor, Tensor)> {
let (_b_size, seqlen, three, _, _headdim) = qkv.dims5()?;
if three != 3 {
candle::bail!("unexpected shape for qkv {:?}", qkv.shape())
}
let (_rotary_seqlen, rotary_dim) = self.cos.dims2()?;
let rotary_dim = rotary_dim * 2;
let q_rot = qkv.i((.., .., 0, .., ..rotary_dim))?;
let q_pass = qkv.i((.., .., 0, .., rotary_dim..))?;
let k_rot = qkv.i((.., .., 1, .., ..rotary_dim))?;
let k_pass = qkv.i((.., .., 1, .., rotary_dim..))?;
let q12 = q_rot.chunk(2, D::Minus1)?;
let k12 = k_rot.chunk(2, D::Minus1)?;
let (q1, q2) = (&q12[0], &q12[1]);
let (k1, k2) = (&k12[0], &k12[1]);
let c = self.cos.narrow(0, seqlen_offset, seqlen)?.unsqueeze(1)?;
let s = self.sin.narrow(0, seqlen_offset, seqlen)?.unsqueeze(1)?;
let q_rot = Tensor::cat(
&[
(q1.broadcast_mul(&c)? - q2.broadcast_mul(&s)?)?,
(q1.broadcast_mul(&s)? + q2.broadcast_mul(&c)?)?,
],
D::Minus1,
)?;
let k_rot = Tensor::cat(
&[
(k1.broadcast_mul(&c)? - k2.broadcast_mul(&s)?)?,
(k1.broadcast_mul(&s)? + k2.broadcast_mul(&c)?)?,
],
D::Minus1,
)?;
let q = Tensor::cat(&[&q_rot, &q_pass], D::Minus1)?;
let k = Tensor::cat(&[&k_rot, &k_pass], D::Minus1)?;
let v = qkv.i((.., .., 2))?;
Ok((q, k, v))
}
}
#[derive(Debug, Clone)]
#[allow(clippy::upper_case_acronyms)]
struct MLP {
fc1: Linear,
fc2: Linear,
act: Activation,
}
impl MLP {
fn new(cfg: &Config, vb: VarBuilder) -> Result<Self> {
let n_inner = cfg.n_inner.unwrap_or(4 * cfg.n_embd);
let fc1 = linear(cfg.n_embd, n_inner, vb.pp("fc1"))?;
let fc2 = linear(n_inner, cfg.n_embd, vb.pp("fc2"))?;
Ok(Self {
fc1,
fc2,
act: cfg.activation_function,
})
}
}
impl Module for MLP {
fn forward(&self, xs: &Tensor) -> Result<Tensor> {
xs.apply(&self.fc1)?.apply(&self.act)?.apply(&self.fc2)
}
}
#[derive(Debug, Clone)]
struct CausalLMHead {
ln: candle_nn::LayerNorm,
linear: Linear,
}
impl CausalLMHead {
fn new(cfg: &Config, vb: VarBuilder) -> Result<Self> {
let ln = layer_norm(cfg.n_embd, cfg.layer_norm_epsilon, vb.pp("ln"))?;
let linear = linear(cfg.n_embd, cfg.vocab_size, vb.pp("linear"))?;
Ok(Self { ln, linear })
}
}
impl Module for CausalLMHead {
fn forward(&self, xs: &Tensor) -> Result<Tensor> {
xs.apply(&self.ln)?
.apply(&self.linear)?
.to_dtype(DType::F32)
}
}
#[derive(Debug, Clone)]
#[allow(clippy::upper_case_acronyms)]
struct MHA {
wqkv: Linear,
out_proj: Linear,
rotary_emb: RotaryEmbedding,
kv_cache: Option<(Tensor, Tensor)>,
head_dim: usize,
n_head: usize,
softmax_scale: f64,
span: tracing::Span,
}
impl MHA {
fn new(cfg: &Config, vb: VarBuilder) -> Result<Self> {
let head_dim = cfg.n_embd / cfg.n_head;
let op_size = cfg.n_embd;
let wqkv = linear(cfg.n_embd, 3 * op_size, vb.pp("Wqkv"))?;
let out_proj = linear(op_size, cfg.n_embd, vb.pp("out_proj"))?;
let rotary_emb = RotaryEmbedding::new(cfg.rotary_dim, MAX_SEQ_LEN, vb.device())?;
let softmax_scale = 1f64 / (head_dim as f64).sqrt();
Ok(Self {
wqkv,
out_proj,
head_dim,
n_head: cfg.n_head,
kv_cache: None,
rotary_emb,
softmax_scale,
span: tracing::span!(tracing::Level::TRACE, "mha"),
})
}
fn forward(&mut self, xs: &Tensor, mask: Option<&Tensor>) -> Result<Tensor> {
let _enter = self.span.enter();
let (b_size, seq_len, _n_embd) = xs.dims3()?;
let qkv = self
.wqkv
.forward(xs)?
.reshape((b_size, seq_len, 3, (), self.head_dim))?;
let seqlen_offset = match &self.kv_cache {
None => 0,
Some((prev_k, _)) => prev_k.dim(1)?,
};
// In the python implementation, a single tensor is returned with the third axis of size 3.
let (q, k, v) = self.rotary_emb.apply_rotary_emb_qkv(&qkv, seqlen_offset)?;
let (k, v) = match &self.kv_cache {
None => (k, v),
Some((prev_k, prev_v)) => {
let k = Tensor::cat(&[prev_k, &k], 1)?;
let v = Tensor::cat(&[prev_v, &v], 1)?;
(k, v)
}
};
self.kv_cache = Some((k.clone(), v.clone()));
// scores = torch.einsum('bthd,bshd->bhts', q, k * softmax_scale)
let q = q.transpose(1, 2)?.flatten_to(1)?; // b*h, t, d
let k = k.transpose(1, 2)?.flatten_to(1)?; // b*h, s, d
let v = v.transpose(1, 2)?.flatten_to(1)?; // b*h, s, d
let attn_weights = (q.matmul(&k.t()?)? * self.softmax_scale)?; // b*h, t, s
// causal_mask = torch.triu(torch.full((seqlen_q, seqlen_k), -10000.0, device=scores.device), 1)
// scores = scores + causal_mask.to(dtype=scores.dtype)
let attn_weights = match mask {
None => attn_weights,
Some(mask) => masked_fill(
&attn_weights,
&mask.broadcast_left(b_size * self.n_head)?,
f32::NEG_INFINITY,
)?,
};
let attn_weights = candle_nn::ops::softmax_last_dim(&attn_weights)?;
// output = torch.einsum('bhts,bshd->bthd', attention_drop, v)
// attn_weights: b*h,t,s, v: b*h,s,d
let attn_output = attn_weights.matmul(&v)?;
// b*h,t,d
let attn_output = attn_output
.reshape((b_size, (), seq_len, self.head_dim))?
.transpose(1, 2)?
.flatten_from(D::Minus2)?;
attn_output.apply(&self.out_proj)
}
fn clear_kv_cache(&mut self) {
self.kv_cache = None
}
}
#[derive(Debug, Clone)]
struct ParallelBlock {
ln: candle_nn::LayerNorm,
mixer: MHA,
mlp: MLP,
span: tracing::Span,
}
impl ParallelBlock {
fn new(cfg: &Config, vb: VarBuilder) -> Result<Self> {
let ln = layer_norm(cfg.n_embd, cfg.layer_norm_epsilon, vb.pp("ln"))?;
let mixer = MHA::new(cfg, vb.pp("mixer"))?;
let mlp = MLP::new(cfg, vb.pp("mlp"))?;
Ok(Self {
ln,
mixer,
mlp,
span: tracing::span!(tracing::Level::TRACE, "block"),
})
}
fn forward(&mut self, xs: &Tensor, mask: Option<&Tensor>) -> Result<Tensor> {
let _enter = self.span.enter();
let residual = xs;
let xs = xs.apply(&self.ln)?;
let attn_outputs = self.mixer.forward(&xs, mask)?;
let feed_forward_hidden_states = self.mlp.forward(&xs)?;
attn_outputs + feed_forward_hidden_states + residual
}
fn clear_kv_cache(&mut self) {
self.mixer.clear_kv_cache()
}
}
#[derive(Debug, Clone)]
pub struct MixFormerSequentialForCausalLM {
embedding: Embedding,
blocks: Vec<ParallelBlock>,
head: CausalLMHead,
span: tracing::Span,
}
impl MixFormerSequentialForCausalLM {
pub fn new_v2(cfg: &Config, vb: VarBuilder) -> Result<Self> {
let vb_head = vb.pp("lm_head");
let vb = vb.pp("transformer");
let embedding = Embedding::new(cfg, vb.pp("embd"))?;
let mut blocks = Vec::new();
for i in 0..cfg.n_layer {
let block = ParallelBlock::new(cfg, vb.pp("h").pp(i))?;
blocks.push(block)
}
let head = CausalLMHead::new(cfg, vb_head)?;
Ok(Self {
embedding,
blocks,
head,
span: tracing::span!(tracing::Level::TRACE, "mixformer"),
})
}
pub fn new(cfg: &Config, vb: VarBuilder) -> Result<Self> {
let vb = vb.pp("layers");
let embedding = Embedding::new(cfg, vb.pp(0))?;
let mut blocks = Vec::new();
for i in 0..cfg.n_layer {
let block = ParallelBlock::new(cfg, vb.pp(i + 1))?;
blocks.push(block);
}
let head = CausalLMHead::new(cfg, vb.pp(cfg.n_layer + 1))?;
Ok(Self {
embedding,
blocks,
head,
span: tracing::span!(tracing::Level::TRACE, "mixformer"),
})
}
pub fn forward(&mut self, xs: &Tensor) -> Result<Tensor> {
let _enter = self.span.enter();
let (_b_size, seq_len) = xs.dims2()?;
let mut xs = xs.apply(&self.embedding)?;
let mask = if seq_len <= 1 {
None
} else {
Some(get_mask(seq_len, xs.device())?)
};
for block in self.blocks.iter_mut() {
xs = block.forward(&xs, mask.as_ref())?;
}
xs.narrow(1, seq_len - 1, 1)?.apply(&self.head)?.squeeze(1)
}
pub fn clear_kv_cache(&mut self) {
self.blocks.iter_mut().for_each(|b| b.clear_kv_cache())
}
}
| candle/candle-transformers/src/models/quantized_mixformer.rs/0 | {
"file_path": "candle/candle-transformers/src/models/quantized_mixformer.rs",
"repo_id": "candle",
"token_count": 5892
} | 38 |
use candle::{DType, IndexOp, Result, Tensor};
use candle_nn::{Module, VarBuilder};
use super::image_encoder::ImageEncoderViT;
use super::mask_decoder::MaskDecoder;
use super::prompt_encoder::PromptEncoder;
use super::tiny_vit::{tiny_vit_5m, TinyViT};
const PROMPT_EMBED_DIM: usize = 256;
pub const IMAGE_SIZE: usize = 1024;
const VIT_PATCH_SIZE: usize = 16;
const PRED_IOU_THRESH: f32 = 0.88;
const STABILITY_SCORE_OFFSET: f32 = 1.0;
const STABILITY_SCORE_THRESHOLD: f32 = 0.95;
const MODEL_MASK_THRESHOLD: f32 = 0.0;
const CROP_NMS_THRESH: f32 = 0.7;
#[derive(Debug)]
enum ImageEncoder {
Original(ImageEncoderViT),
TinyViT(TinyViT),
}
impl Module for ImageEncoder {
fn forward(&self, xs: &Tensor) -> Result<Tensor> {
match self {
Self::Original(vit) => vit.forward(xs),
Self::TinyViT(vit) => vit.forward(xs),
}
}
}
#[derive(Debug)]
pub struct Sam {
image_encoder: ImageEncoder,
prompt_encoder: PromptEncoder,
mask_decoder: MaskDecoder,
pixel_mean: Tensor,
pixel_std: Tensor,
}
impl Sam {
pub fn new(
encoder_embed_dim: usize,
encoder_depth: usize,
encoder_num_heads: usize,
encoder_global_attn_indexes: &[usize],
vb: VarBuilder,
) -> Result<Self> {
let image_embedding_size = IMAGE_SIZE / VIT_PATCH_SIZE;
let image_encoder = ImageEncoderViT::new(
IMAGE_SIZE,
VIT_PATCH_SIZE,
3,
encoder_embed_dim,
encoder_depth,
encoder_num_heads,
PROMPT_EMBED_DIM,
/* qkv_bias */ true,
/* use_rel_pos */ true,
/* use_abs_pos */ true,
/* window_size */ 14,
/* global_attn_indexes */ encoder_global_attn_indexes,
vb.pp("image_encoder"),
)?;
let prompt_encoder = PromptEncoder::new(
PROMPT_EMBED_DIM,
(image_embedding_size, image_embedding_size),
(IMAGE_SIZE, IMAGE_SIZE),
16,
vb.pp("prompt_encoder"),
)?;
let mask_decoder = MaskDecoder::new(
PROMPT_EMBED_DIM,
/* num_multitask_outputs */ 3,
/* iou_head_depth */ 3,
/* iou_head_hidden_dim */ 256,
vb.pp("mask_decoder"),
)?;
let pixel_mean =
Tensor::new(&[123.675f32, 116.28, 103.53], vb.device())?.reshape((3, 1, 1))?;
let pixel_std =
Tensor::new(&[58.395f32, 57.12, 57.375], vb.device())?.reshape((3, 1, 1))?;
Ok(Self {
image_encoder: ImageEncoder::Original(image_encoder),
prompt_encoder,
mask_decoder,
pixel_std,
pixel_mean,
})
}
pub fn new_tiny(vb: VarBuilder) -> Result<Self> {
let image_embedding_size = IMAGE_SIZE / VIT_PATCH_SIZE;
let image_encoder = tiny_vit_5m(vb.pp("image_encoder"))?;
let prompt_encoder = PromptEncoder::new(
PROMPT_EMBED_DIM,
(image_embedding_size, image_embedding_size),
(IMAGE_SIZE, IMAGE_SIZE),
16,
vb.pp("prompt_encoder"),
)?;
let mask_decoder = MaskDecoder::new(
PROMPT_EMBED_DIM,
/* num_multitask_outputs */ 3,
/* iou_head_depth */ 3,
/* iou_head_hidden_dim */ 256,
vb.pp("mask_decoder"),
)?;
let pixel_mean =
Tensor::new(&[123.675f32, 116.28, 103.53], vb.device())?.reshape((3, 1, 1))?;
let pixel_std =
Tensor::new(&[58.395f32, 57.12, 57.375], vb.device())?.reshape((3, 1, 1))?;
Ok(Self {
image_encoder: ImageEncoder::TinyViT(image_encoder),
prompt_encoder,
mask_decoder,
pixel_std,
pixel_mean,
})
}
pub fn embeddings(&self, img: &Tensor) -> Result<Tensor> {
let img = self.preprocess(img)?.unsqueeze(0)?;
self.image_encoder.forward(&img)
}
pub fn forward(
&self,
img: &Tensor,
points: &[(f64, f64, bool)],
multimask_output: bool,
) -> Result<(Tensor, Tensor)> {
let (_c, original_h, original_w) = img.dims3()?;
let img = self.preprocess(img)?.unsqueeze(0)?;
let img_embeddings = self.image_encoder.forward(&img)?;
let (low_res_mask, iou) = self.forward_for_embeddings(
&img_embeddings,
original_h,
original_w,
points,
multimask_output,
)?;
let mask = low_res_mask
.upsample_nearest2d(IMAGE_SIZE, IMAGE_SIZE)?
.get(0)?
.i((.., ..original_h, ..original_w))?;
Ok((mask, iou))
}
/// Generate the mask and IOU predictions from some image embeddings and prompt.
///
/// The prompt is specified as a list of points `(x, y, b)`. `x` and `y` are the point
/// coordinates (between 0 and 1) and `b` is `true` for points that should be part of the mask
/// and `false` for points that should be part of the background and so excluded from the mask.
pub fn forward_for_embeddings(
&self,
img_embeddings: &Tensor,
original_h: usize,
original_w: usize,
points: &[(f64, f64, bool)],
multimask_output: bool,
) -> Result<(Tensor, Tensor)> {
let image_pe = self.prompt_encoder.get_dense_pe()?;
let points = if points.is_empty() {
None
} else {
let n_points = points.len();
let xys = points
.iter()
.flat_map(|(x, y, _b)| {
let x = (*x as f32) * (original_w as f32);
let y = (*y as f32) * (original_h as f32);
[x, y]
})
.collect::<Vec<_>>();
let labels = points
.iter()
.map(|(_x, _y, b)| if *b { 1f32 } else { 0f32 })
.collect::<Vec<_>>();
let points = Tensor::from_vec(xys, (1, n_points, 2), img_embeddings.device())?;
let labels = Tensor::from_vec(labels, (1, n_points), img_embeddings.device())?;
Some((points, labels))
};
let points = points.as_ref().map(|xy| (&xy.0, &xy.1));
let (sparse_prompt_embeddings, dense_prompt_embeddings) =
self.prompt_encoder.forward(points, None, None)?;
self.mask_decoder.forward(
img_embeddings,
&image_pe,
&sparse_prompt_embeddings,
&dense_prompt_embeddings,
multimask_output,
)
}
pub fn unpreprocess(&self, img: &Tensor) -> Result<Tensor> {
let img = img
.broadcast_mul(&self.pixel_std)?
.broadcast_add(&self.pixel_mean)?;
img.maximum(&img.zeros_like()?)?
.minimum(&(img.ones_like()? * 255.)?)
}
pub fn preprocess(&self, img: &Tensor) -> Result<Tensor> {
let (_c, h, w) = img.dims3()?;
let img = img
.to_dtype(DType::F32)?
.broadcast_sub(&self.pixel_mean)?
.broadcast_div(&self.pixel_std)?;
if h > IMAGE_SIZE || w > IMAGE_SIZE {
candle::bail!("image is too large ({w}, {h}), maximum size {IMAGE_SIZE}")
}
let img = img.pad_with_zeros(1, 0, IMAGE_SIZE - h)?;
img.pad_with_zeros(2, 0, IMAGE_SIZE - w)
}
fn process_crop(
&self,
img: &Tensor,
cb: CropBox,
point_grids: &[(f64, f64)],
) -> Result<Vec<crate::object_detection::Bbox<Tensor>>> {
// Crop the image and calculate embeddings.
let img = img.i((.., cb.y0..cb.y1, cb.x0..cb.x1))?;
let img = self.preprocess(&img)?.unsqueeze(0)?;
let img_embeddings = self.image_encoder.forward(&img)?;
let crop_w = cb.x1 - cb.x0;
let crop_h = cb.y1 - cb.y0;
// Generate masks for this crop.
let image_pe = self.prompt_encoder.get_dense_pe()?;
let points = point_grids
.iter()
.map(|&(x, y)| vec![x as f32 * crop_w as f32, y as f32 * crop_h as f32])
.collect::<Vec<_>>();
let mut bboxes = Vec::new();
for points in points.chunks(64) {
// Run the model on this batch.
let points_len = points.len();
let in_points = Tensor::new(points.to_vec(), img.device())?.unsqueeze(1)?;
let in_labels = Tensor::ones((points_len, 1), DType::F32, img.device())?;
let (sparse_prompt_embeddings, dense_prompt_embeddings) =
self.prompt_encoder
.forward(Some((&in_points, &in_labels)), None, None)?;
let (low_res_mask, iou_predictions) = self.mask_decoder.forward(
&img_embeddings,
&image_pe,
&sparse_prompt_embeddings,
&dense_prompt_embeddings,
/* multimask_output */ true,
)?;
let low_res_mask = low_res_mask.flatten(0, 1)?;
let iou_predictions = iou_predictions.flatten(0, 1)?.to_vec1::<f32>()?;
let dev = low_res_mask.device();
for (i, iou) in iou_predictions.iter().enumerate() {
// Filter by predicted IoU.
if *iou < PRED_IOU_THRESH {
continue;
}
let low_res_mask = low_res_mask.get(i)?;
// Calculate stability score.
let bound = Tensor::new(MODEL_MASK_THRESHOLD + STABILITY_SCORE_OFFSET, dev)?
.broadcast_as(low_res_mask.shape())?;
let intersections = low_res_mask
.ge(&bound)?
.to_dtype(DType::F32)?
.sum_all()?
.to_vec0::<f32>()?;
let bound = Tensor::new(MODEL_MASK_THRESHOLD - STABILITY_SCORE_OFFSET, dev)?
.broadcast_as(low_res_mask.shape())?;
let unions = low_res_mask
.ge(&bound)?
.to_dtype(DType::F32)?
.sum_all()?
.to_vec0::<f32>()?;
let stability_score = intersections / unions;
if stability_score < STABILITY_SCORE_THRESHOLD {
continue;
}
// Threshold masks and calculate boxes.
let low_res_mask = low_res_mask
.ge(&Tensor::new(0f32, dev)?.broadcast_as(low_res_mask.shape())?)?
.to_dtype(DType::U32)?;
let low_res_mask_per_x = low_res_mask.sum(0)?.to_vec1::<u32>()?;
let low_res_mask_per_y = low_res_mask.sum(1)?.to_vec1::<u32>()?;
let min_max_x = min_max_indexes(&low_res_mask_per_x);
let min_max_y = min_max_indexes(&low_res_mask_per_y);
if let Some(((x0, x1), (y0, y1))) = min_max_x.zip(min_max_y) {
let bbox = crate::object_detection::Bbox {
xmin: x0 as f32,
ymin: y0 as f32,
xmax: x1 as f32,
ymax: y1 as f32,
confidence: *iou,
data: low_res_mask,
};
bboxes.push(bbox);
}
// TODO:
// Filter boxes that touch crop boundaries
// Compress to RLE.
}
}
let mut bboxes = vec![bboxes];
// Remove duplicates within this crop.
crate::object_detection::non_maximum_suppression(&mut bboxes, CROP_NMS_THRESH);
// TODO: Return to the original image frame.
Ok(bboxes.remove(0))
}
pub fn generate_masks(
&self,
img: &Tensor,
points_per_side: usize,
crop_n_layer: usize,
crop_overlap_ratio: f64,
crop_n_points_downscale_factor: usize,
) -> Result<Vec<crate::object_detection::Bbox<Tensor>>> {
let (_c, h, w) = img.dims3()?;
let point_grids = build_all_layer_point_grids(
points_per_side,
crop_n_layer,
crop_n_points_downscale_factor,
);
let crop_boxes = generate_crop_boxes((h, w), crop_n_layer, crop_overlap_ratio);
let mut bboxes = Vec::new();
for crop_box in crop_boxes.into_iter() {
let layer_idx = crop_box.layer_idx;
let b = self.process_crop(img, crop_box, &point_grids[layer_idx])?;
bboxes.extend(b)
}
// TODO: remove duplicates
Ok(bboxes)
}
}
// Return the first and last indexes i for which values[i] > 0
fn min_max_indexes(values: &[u32]) -> Option<(usize, usize)> {
let (mut min_i, mut max_i) = (usize::MAX, usize::MIN);
for (i, &s) in values.iter().enumerate() {
if s == 0 {
continue;
}
min_i = usize::min(i, min_i);
max_i = usize::max(i, max_i);
}
if max_i < min_i {
None
} else {
Some((min_i, max_i))
}
}
#[derive(Debug)]
struct CropBox {
x0: usize,
y0: usize,
x1: usize,
y1: usize,
layer_idx: usize,
}
impl CropBox {
fn new(x0: usize, y0: usize, x1: usize, y1: usize, layer_idx: usize) -> Self {
Self {
x0,
y0,
x1,
y1,
layer_idx,
}
}
}
fn generate_crop_boxes(
(im_h, im_w): (usize, usize),
n_layers: usize,
overlap_ratio: f64,
) -> Vec<CropBox> {
fn crop_len(orig_len: usize, n_crops: usize, overlap: usize) -> usize {
f64::ceil((overlap * (n_crops - 1) + orig_len) as f64 / n_crops as f64) as usize
}
let short_side = usize::min(im_h, im_w);
let mut crop_boxes = Vec::new();
// Original image.
crop_boxes.push(CropBox::new(0, 0, im_w, im_h, 0));
for layer_idx in 1..=n_layers {
let n_crops_per_side = 1 << layer_idx;
let overlap = (overlap_ratio * short_side as f64 * 2. / n_crops_per_side as f64) as usize;
let crop_w = crop_len(im_w, n_crops_per_side, overlap);
let crop_h = crop_len(im_w, n_crops_per_side, overlap);
for i_x in 0..n_crops_per_side {
let x0 = (crop_w - overlap) * i_x;
for i_y in 0..n_crops_per_side {
let y0 = (crop_h - overlap) * i_y;
let x1 = usize::min(im_w, x0 + crop_w);
let y1 = usize::min(im_h, y0 + crop_h);
crop_boxes.push(CropBox::new(x0, y0, x1, y1, layer_idx));
}
}
}
crop_boxes
}
// Generates a 2D grid of points evenly spaced in [0,1]x[0,1].
fn build_point_grid(n_per_side: usize) -> Vec<(f64, f64)> {
let offset = 1f64 / (2 * n_per_side) as f64;
let mut points = Vec::with_capacity(n_per_side * n_per_side);
for i_x in 0..n_per_side {
let x = offset + i_x as f64 / n_per_side as f64;
for i_y in 0..n_per_side {
let y = offset + i_y as f64 / n_per_side as f64;
points.push((x, y))
}
}
points
}
fn build_all_layer_point_grids(
n_per_side: usize,
n_layers: usize,
scale_per_layer: usize,
) -> Vec<Vec<(f64, f64)>> {
let mut points_by_layer = Vec::with_capacity(n_layers + 1);
for i in 0..=n_layers {
let n_points = n_per_side / scale_per_layer.pow(i as u32);
points_by_layer.push(build_point_grid(n_points))
}
points_by_layer
}
| candle/candle-transformers/src/models/segment_anything/sam.rs/0 | {
"file_path": "candle/candle-transformers/src/models/segment_anything/sam.rs",
"repo_id": "candle",
"token_count": 8444
} | 39 |
use crate::models::with_tracing::{linear, linear_no_bias, Linear};
use candle::{DType, Device, Module, Result, Tensor, D};
use candle_nn::{Activation, LayerNorm, VarBuilder};
use serde::Deserialize;
use std::sync::Arc;
// https://huggingface.co/stabilityai/stablelm-3b-4e1t/blob/main/configuration_stablelm.py
#[derive(Debug, Clone, PartialEq, Deserialize)]
pub struct Config {
pub(crate) vocab_size: usize,
pub(crate) intermediate_size: usize,
pub(crate) hidden_size: usize,
pub(crate) num_hidden_layers: usize,
pub(crate) num_attention_heads: usize,
pub(crate) num_key_value_heads: usize,
pub(crate) hidden_act: Activation,
pub(crate) partial_rotary_factor: f64,
pub(crate) rope_theta: f64,
pub(crate) max_position_embeddings: usize,
pub(crate) layer_norm_eps: f64,
pub(crate) use_cache: bool,
#[serde(default)]
pub(crate) use_qkv_bias: bool, // Used in StableLM-2
#[serde(default)]
pub(crate) use_flash_attn: bool, // Not in config.json
}
impl Config {
pub fn stablelm_3b_4e1t(use_flash_attn: bool) -> Self {
Self {
vocab_size: 50304,
intermediate_size: 6912,
hidden_size: 2560,
num_hidden_layers: 32,
num_attention_heads: 32,
num_key_value_heads: 32,
hidden_act: Activation::Silu,
partial_rotary_factor: 0.25,
rope_theta: 10_000.,
max_position_embeddings: 4096,
layer_norm_eps: 1e-5,
use_qkv_bias: false,
use_cache: true,
use_flash_attn,
}
}
pub fn head_dim(&self) -> usize {
self.hidden_size / self.num_attention_heads
}
pub fn rotary_ndims(&self) -> usize {
(self.head_dim() as f64 * self.partial_rotary_factor) as usize
}
pub fn num_kv_groups(&self) -> usize {
self.num_attention_heads / self.num_key_value_heads
}
pub fn set_use_flash_attn(&mut self, use_flash_attn: bool) {
self.use_flash_attn = use_flash_attn
}
}
#[derive(Debug)]
pub(crate) struct RotaryEmbedding {
sin: Tensor,
cos: Tensor,
}
fn rotate_half(xs: &Tensor) -> Result<Tensor> {
let xs = xs.chunk(2, D::Minus1)?;
Tensor::cat(&[&xs[1].neg()?, &xs[0]], D::Minus1)
}
impl RotaryEmbedding {
pub(crate) fn new(dtype: DType, cfg: &Config, dev: &Device) -> Result<Self> {
let dim = cfg.rotary_ndims();
let max_seq_len = cfg.max_position_embeddings;
let inv_freq: Vec<_> = (0..dim)
.step_by(2)
.map(|i| 1f32 / cfg.rope_theta.powf(i as f64 / dim as f64) as f32)
.collect();
let inv_freq_len = inv_freq.len();
let inv_freq = Tensor::from_vec(inv_freq, (1, inv_freq_len), dev)?.to_dtype(dtype)?;
let t = Tensor::arange(0u32, max_seq_len as u32, dev)?
.to_dtype(dtype)?
.reshape((max_seq_len, 1))?;
let freqs = t.matmul(&inv_freq)?;
let freqs = Tensor::cat(&[&freqs, &freqs], D::Minus1)?;
Ok(Self {
sin: freqs.sin()?,
cos: freqs.cos()?,
})
}
pub(crate) fn apply_rotary_emb_qkv(
&self,
q: &Tensor,
k: &Tensor,
seqlen_offset: usize,
) -> Result<(Tensor, Tensor)> {
let (_b_sz, _h, seq_len, _n_embd) = q.dims4()?;
let cos = self.cos.narrow(0, seqlen_offset, seq_len)?;
let sin = self.sin.narrow(0, seqlen_offset, seq_len)?;
let cos = cos.unsqueeze(0)?.unsqueeze(0)?; // (1, 1, seq_len, dim)
let sin = sin.unsqueeze(0)?.unsqueeze(0)?; // (1, 1, seq_len, dim)
let q_embed = (q.broadcast_mul(&cos)? + rotate_half(q)?.broadcast_mul(&sin))?;
let k_embed = (k.broadcast_mul(&cos)? + rotate_half(k)?.broadcast_mul(&sin))?;
Ok((q_embed, k_embed))
}
}
#[derive(Debug)]
#[allow(clippy::upper_case_acronyms)]
struct MLP {
gate_proj: Linear,
up_proj: Linear,
down_proj: Linear,
act_fn: Activation,
span: tracing::Span,
}
impl MLP {
fn new(cfg: &Config, vb: VarBuilder) -> Result<Self> {
let hidden_sz = cfg.hidden_size;
let intermediate_sz = cfg.intermediate_size;
let gate_proj = linear_no_bias(hidden_sz, intermediate_sz, vb.pp("gate_proj"))?;
let up_proj = linear_no_bias(hidden_sz, intermediate_sz, vb.pp("up_proj"))?;
let down_proj = linear_no_bias(intermediate_sz, hidden_sz, vb.pp("down_proj"))?;
Ok(Self {
gate_proj,
up_proj,
down_proj,
act_fn: cfg.hidden_act,
span: tracing::span!(tracing::Level::TRACE, "mlp"),
})
}
}
impl Module for MLP {
fn forward(&self, xs: &Tensor) -> Result<Tensor> {
let _enter = self.span.enter();
let lhs = xs.apply(&self.gate_proj)?.apply(&self.act_fn)?;
let rhs = xs.apply(&self.up_proj)?;
(lhs * rhs)?.apply(&self.down_proj)
}
}
#[cfg(feature = "flash-attn")]
fn flash_attn(
q: &Tensor,
k: &Tensor,
v: &Tensor,
softmax_scale: f32,
causal: bool,
) -> Result<Tensor> {
candle_flash_attn::flash_attn(q, k, v, softmax_scale, causal)
}
#[cfg(not(feature = "flash-attn"))]
fn flash_attn(_: &Tensor, _: &Tensor, _: &Tensor, _: f32, _: bool) -> Result<Tensor> {
unimplemented!("compile with '--features flash-attn'")
}
#[derive(Debug)]
struct Attention {
q_proj: Linear,
k_proj: Linear,
v_proj: Linear,
o_proj: Linear,
num_heads: usize,
num_kv_heads: usize,
num_kv_groups: usize,
head_dim: usize,
hidden_size: usize,
rotary_emb: Arc<RotaryEmbedding>,
kv_cache: Option<(Tensor, Tensor)>,
use_cache: bool,
rotary_ndims: usize,
use_flash_attn: bool,
span: tracing::Span,
}
impl Attention {
fn new(rotary_emb: Arc<RotaryEmbedding>, cfg: &Config, vb: VarBuilder) -> Result<Self> {
let hidden_sz = cfg.hidden_size;
let head_dim = cfg.head_dim();
let num_heads = cfg.num_attention_heads;
let num_kv_heads = cfg.num_key_value_heads;
let linear_layer = if cfg.use_qkv_bias {
linear
} else {
linear_no_bias
};
let q_proj = linear_layer(hidden_sz, num_heads * head_dim, vb.pp("q_proj"))?;
let k_proj = linear_layer(hidden_sz, num_kv_heads * head_dim, vb.pp("k_proj"))?;
let v_proj = linear_layer(hidden_sz, num_kv_heads * head_dim, vb.pp("v_proj"))?;
let o_proj = linear_no_bias(num_heads * head_dim, hidden_sz, vb.pp("o_proj"))?;
Ok(Self {
q_proj,
k_proj,
v_proj,
o_proj,
num_heads,
num_kv_heads,
num_kv_groups: cfg.num_kv_groups(),
head_dim,
hidden_size: hidden_sz,
rotary_emb,
kv_cache: None,
use_cache: cfg.use_cache,
rotary_ndims: cfg.rotary_ndims(),
use_flash_attn: cfg.use_flash_attn,
span: tracing::span!(tracing::Level::TRACE, "attn"),
})
}
fn repeat_kv(&self, xs: Tensor) -> Result<Tensor> {
let n_rep = self.num_kv_groups;
if n_rep == 1 {
Ok(xs)
} else {
let (b_sz, num_kv_heads, seq_len, head_dim) = xs.dims4()?;
xs.unsqueeze(2)?
.expand((b_sz, num_kv_heads, n_rep, seq_len, head_dim))?
.reshape((b_sz, num_kv_heads * n_rep, seq_len, head_dim))
}
}
fn forward(
&mut self,
xs: &Tensor,
attention_mask: Option<&Tensor>,
seqlen_offset: usize,
) -> Result<Tensor> {
let _enter = self.span.enter();
let (b_sz, q_len, _) = xs.dims3()?;
let query_states = self.q_proj.forward(xs)?;
let key_states = self.k_proj.forward(xs)?;
let value_states = self.v_proj.forward(xs)?;
let query_states = query_states
.reshape((b_sz, q_len, self.num_heads, self.head_dim))?
.transpose(1, 2)?;
let key_states = key_states
.reshape((b_sz, q_len, self.num_kv_heads, self.head_dim))?
.transpose(1, 2)?;
let value_states = value_states
.reshape((b_sz, q_len, self.num_kv_heads, self.head_dim))?
.transpose(1, 2)?;
let (rot_ndims, pass_ndims) = (self.rotary_ndims, self.head_dim - self.rotary_ndims);
let query_rot = query_states.narrow(D::Minus1, 0, rot_ndims)?;
let query_pass = query_states.narrow(D::Minus1, rot_ndims, pass_ndims)?;
let key_rot = key_states.narrow(D::Minus1, 0, rot_ndims)?;
let key_pass = key_states.narrow(D::Minus1, rot_ndims, pass_ndims)?;
let (query_rot, key_rot) =
self.rotary_emb
.apply_rotary_emb_qkv(&query_rot, &key_rot, seqlen_offset)?;
let query_states = Tensor::cat(&[query_rot, query_pass], D::Minus1)?.contiguous()?;
let key_states = Tensor::cat(&[key_rot, key_pass], D::Minus1)?.contiguous()?;
let (key_states, value_states) = match &self.kv_cache {
None => (key_states, value_states),
Some((prev_k, prev_v)) => {
let key_states = Tensor::cat(&[prev_k, &key_states], 2)?;
let value_states = Tensor::cat(&[prev_v, &value_states], 2)?;
(key_states, value_states)
}
};
if self.use_cache {
self.kv_cache = Some((key_states.clone(), value_states.clone()));
}
let key_states = self.repeat_kv(key_states)?.contiguous()?;
let value_states = self.repeat_kv(value_states)?.contiguous()?;
let attn_output = if self.use_flash_attn {
// flash-attn expects (b_sz, seq_len, nheads, head_dim)
let q = query_states.transpose(1, 2)?;
let k = key_states.transpose(1, 2)?;
let v = value_states.transpose(1, 2)?;
let softmax_scale = 1f32 / (self.head_dim as f32).sqrt();
flash_attn(&q, &k, &v, softmax_scale, q_len > 1)?.transpose(1, 2)?
} else {
let scale = 1f64 / f64::sqrt(self.head_dim as f64);
let attn_weights = (query_states.matmul(&key_states.transpose(2, 3)?)? * scale)?;
let attn_weights = match attention_mask {
None => attn_weights,
Some(mask) => attn_weights.broadcast_add(mask)?,
};
let attn_weights = candle_nn::ops::softmax_last_dim(&attn_weights)?;
attn_weights.matmul(&value_states)?
};
attn_output
.transpose(1, 2)?
.reshape((b_sz, q_len, self.hidden_size))?
.apply(&self.o_proj)
}
}
#[derive(Debug)]
struct DecoderLayer {
self_attn: Attention,
mlp: MLP,
input_layernorm: LayerNorm,
post_attention_layernorm: LayerNorm,
span: tracing::Span,
}
impl DecoderLayer {
fn new(rotary_emb: Arc<RotaryEmbedding>, cfg: &Config, vb: VarBuilder) -> Result<Self> {
let self_attn = Attention::new(rotary_emb, cfg, vb.pp("self_attn"))?;
let mlp = MLP::new(cfg, vb.pp("mlp"))?;
let input_layernorm = candle_nn::layer_norm(
cfg.hidden_size,
cfg.layer_norm_eps,
vb.pp("input_layernorm"),
)?;
let post_attention_layernorm = candle_nn::layer_norm(
cfg.hidden_size,
cfg.layer_norm_eps,
vb.pp("post_attention_layernorm"),
)?;
Ok(Self {
self_attn,
mlp,
input_layernorm,
post_attention_layernorm,
span: tracing::span!(tracing::Level::TRACE, "layer"),
})
}
fn forward(
&mut self,
xs: &Tensor,
attention_mask: Option<&Tensor>,
seqlen_offset: usize,
) -> Result<Tensor> {
let _enter = self.span.enter();
let residual = xs;
let xs = self.input_layernorm.forward(xs)?;
let xs = self.self_attn.forward(&xs, attention_mask, seqlen_offset)?;
let xs = (xs + residual)?;
let residual = &xs;
let xs = xs.apply(&self.post_attention_layernorm)?.apply(&self.mlp)?;
residual + xs
}
}
#[derive(Debug)]
pub struct Model {
embed_tokens: candle_nn::Embedding,
layers: Vec<DecoderLayer>,
norm: LayerNorm,
lm_head: Linear,
device: Device,
dtype: DType,
span: tracing::Span,
}
impl Model {
pub fn new(cfg: &Config, vb: VarBuilder) -> Result<Self> {
let vb_m = vb.pp("model");
let embed_tokens =
candle_nn::embedding(cfg.vocab_size, cfg.hidden_size, vb_m.pp("embed_tokens"))?;
let rotary_emb = Arc::new(RotaryEmbedding::new(vb.dtype(), cfg, vb_m.device())?);
let mut layers = Vec::with_capacity(cfg.num_hidden_layers);
let vb_l = vb_m.pp("layers");
for layer_idx in 0..cfg.num_hidden_layers {
let layer = DecoderLayer::new(rotary_emb.clone(), cfg, vb_l.pp(layer_idx))?;
layers.push(layer)
}
let norm = candle_nn::layer_norm(cfg.hidden_size, cfg.layer_norm_eps, vb_m.pp("norm"))?;
let lm_head = linear_no_bias(cfg.hidden_size, cfg.vocab_size, vb.pp("lm_head"))?;
Ok(Self {
embed_tokens,
layers,
norm,
lm_head,
device: vb.device().clone(),
dtype: vb.dtype(),
span: tracing::span!(tracing::Level::TRACE, "model"),
})
}
fn prepare_decoder_attention_mask(
&self,
b_size: usize,
tgt_len: usize,
seqlen_offset: usize,
) -> Result<Tensor> {
// Sliding window mask?
let mask: Vec<_> = (0..tgt_len)
.flat_map(|i| (0..tgt_len).map(move |j| if i < j { f32::NEG_INFINITY } else { 0. }))
.collect();
let mask = Tensor::from_slice(&mask, (tgt_len, tgt_len), &self.device)?;
let mask = if seqlen_offset > 0 {
let mask0 = Tensor::zeros((tgt_len, seqlen_offset), DType::F32, &self.device)?;
Tensor::cat(&[&mask0, &mask], D::Minus1)?
} else {
mask
};
mask.expand((b_size, 1, tgt_len, tgt_len + seqlen_offset))?
.to_dtype(self.dtype)
}
pub fn forward(&mut self, input_ids: &Tensor, seqlen_offset: usize) -> Result<Tensor> {
let _enter = self.span.enter();
let (b_size, seq_len) = input_ids.dims2()?;
let attention_mask = if seq_len <= 1 {
None
} else {
let mask = self.prepare_decoder_attention_mask(b_size, seq_len, seqlen_offset)?;
Some(mask)
};
let mut xs = self.embed_tokens.forward(input_ids)?;
for layer in self.layers.iter_mut() {
xs = layer.forward(&xs, attention_mask.as_ref(), seqlen_offset)?
}
xs.narrow(1, seq_len - 1, 1)?
.apply(&self.norm)?
.apply(&self.lm_head)
}
}
| candle/candle-transformers/src/models/stable_lm.rs/0 | {
"file_path": "candle/candle-transformers/src/models/stable_lm.rs",
"repo_id": "candle",
"token_count": 7764
} | 40 |
use super::common::LayerNormNoWeights;
use candle::{Module, Result, Tensor};
use candle_nn::VarBuilder;
#[derive(Debug)]
pub struct MixingResidualBlock {
norm1: LayerNormNoWeights,
depthwise_conv: candle_nn::Conv2d,
norm2: LayerNormNoWeights,
channelwise_lin1: candle_nn::Linear,
channelwise_lin2: candle_nn::Linear,
gammas: Vec<f32>,
}
impl MixingResidualBlock {
pub fn new(inp: usize, embed_dim: usize, vb: VarBuilder) -> Result<Self> {
let norm1 = LayerNormNoWeights::new(inp)?;
let norm2 = LayerNormNoWeights::new(inp)?;
let cfg = candle_nn::Conv2dConfig {
groups: inp,
..Default::default()
};
let depthwise_conv = candle_nn::conv2d(inp, inp, 3, cfg, vb.pp("depthwise.1"))?;
let channelwise_lin1 = candle_nn::linear(inp, embed_dim, vb.pp("channelwise.0"))?;
let channelwise_lin2 = candle_nn::linear(embed_dim, inp, vb.pp("channelwise.2"))?;
let gammas = vb.get(6, "gammas")?.to_vec1::<f32>()?;
Ok(Self {
norm1,
depthwise_conv,
norm2,
channelwise_lin1,
channelwise_lin2,
gammas,
})
}
}
impl Module for MixingResidualBlock {
fn forward(&self, xs: &Tensor) -> Result<Tensor> {
let mods = &self.gammas;
let x_temp = xs
.permute((0, 2, 3, 1))?
.apply(&self.norm1)?
.permute((0, 3, 1, 2))?
.affine(1. + mods[0] as f64, mods[1] as f64)?;
let x_temp = candle_nn::ops::replication_pad2d(&x_temp, 1)?;
let xs = (xs + x_temp.apply(&self.depthwise_conv)? * mods[2] as f64)?;
let x_temp = xs
.permute((0, 2, 3, 1))?
.apply(&self.norm2)?
.permute((0, 3, 1, 2))?
.affine(1. + mods[3] as f64, mods[4] as f64)?;
let x_temp = x_temp
.permute((0, 2, 3, 1))?
.contiguous()?
.apply(&self.channelwise_lin1)?
.gelu()?
.apply(&self.channelwise_lin2)?
.permute((0, 3, 1, 2))?;
xs + x_temp * mods[5] as f64
}
}
#[derive(Debug)]
pub struct PaellaVQ {
in_block_conv: candle_nn::Conv2d,
out_block_conv: candle_nn::Conv2d,
down_blocks: Vec<(Option<candle_nn::Conv2d>, MixingResidualBlock)>,
down_blocks_conv: candle_nn::Conv2d,
down_blocks_bn: candle_nn::BatchNorm,
up_blocks_conv: candle_nn::Conv2d,
up_blocks: Vec<(Vec<MixingResidualBlock>, Option<candle_nn::ConvTranspose2d>)>,
}
impl PaellaVQ {
pub fn new(vb: VarBuilder) -> Result<Self> {
const IN_CHANNELS: usize = 3;
const OUT_CHANNELS: usize = 3;
const LATENT_CHANNELS: usize = 4;
const EMBED_DIM: usize = 384;
const BOTTLENECK_BLOCKS: usize = 12;
const C_LEVELS: [usize; 2] = [EMBED_DIM / 2, EMBED_DIM];
let in_block_conv = candle_nn::conv2d(
IN_CHANNELS * 4,
C_LEVELS[0],
1,
Default::default(),
vb.pp("in_block.1"),
)?;
let out_block_conv = candle_nn::conv2d(
C_LEVELS[0],
OUT_CHANNELS * 4,
1,
Default::default(),
vb.pp("out_block.0"),
)?;
let mut down_blocks = Vec::new();
let vb_d = vb.pp("down_blocks");
let mut d_idx = 0;
for (i, &c_level) in C_LEVELS.iter().enumerate() {
let conv_block = if i > 0 {
let cfg = candle_nn::Conv2dConfig {
padding: 1,
stride: 2,
..Default::default()
};
let block = candle_nn::conv2d(C_LEVELS[i - 1], c_level, 4, cfg, vb_d.pp(d_idx))?;
d_idx += 1;
Some(block)
} else {
None
};
let res_block = MixingResidualBlock::new(c_level, c_level * 4, vb_d.pp(d_idx))?;
d_idx += 1;
down_blocks.push((conv_block, res_block))
}
let vb_d = vb_d.pp(d_idx);
let down_blocks_conv = candle_nn::conv2d_no_bias(
C_LEVELS[1],
LATENT_CHANNELS,
1,
Default::default(),
vb_d.pp(0),
)?;
let down_blocks_bn = candle_nn::batch_norm(LATENT_CHANNELS, 1e-5, vb_d.pp(1))?;
let mut up_blocks = Vec::new();
let vb_u = vb.pp("up_blocks");
let mut u_idx = 0;
let up_blocks_conv = candle_nn::conv2d(
LATENT_CHANNELS,
C_LEVELS[1],
1,
Default::default(),
vb_u.pp(u_idx).pp(0),
)?;
u_idx += 1;
for (i, &c_level) in C_LEVELS.iter().rev().enumerate() {
let mut res_blocks = Vec::new();
let n_bottleneck_blocks = if i == 0 { BOTTLENECK_BLOCKS } else { 1 };
for _j in 0..n_bottleneck_blocks {
let res_block = MixingResidualBlock::new(c_level, c_level * 4, vb_u.pp(u_idx))?;
u_idx += 1;
res_blocks.push(res_block)
}
let conv_block = if i < C_LEVELS.len() - 1 {
let cfg = candle_nn::ConvTranspose2dConfig {
padding: 1,
stride: 2,
..Default::default()
};
let block = candle_nn::conv_transpose2d(
c_level,
C_LEVELS[C_LEVELS.len() - i - 2],
4,
cfg,
vb_u.pp(u_idx),
)?;
u_idx += 1;
Some(block)
} else {
None
};
up_blocks.push((res_blocks, conv_block))
}
Ok(Self {
in_block_conv,
down_blocks,
down_blocks_conv,
down_blocks_bn,
up_blocks,
up_blocks_conv,
out_block_conv,
})
}
pub fn encode(&self, xs: &Tensor) -> Result<Tensor> {
let mut xs = candle_nn::ops::pixel_unshuffle(xs, 2)?.apply(&self.in_block_conv)?;
for down_block in self.down_blocks.iter() {
if let Some(conv) = &down_block.0 {
xs = xs.apply(conv)?
}
xs = xs.apply(&down_block.1)?
}
xs.apply(&self.down_blocks_conv)?
.apply_t(&self.down_blocks_bn, false)
}
pub fn decode(&self, xs: &Tensor) -> Result<Tensor> {
// TODO: quantizer if we want to support `force_not_quantize=False`.
let mut xs = xs.apply(&self.up_blocks_conv)?;
for up_block in self.up_blocks.iter() {
for b in up_block.0.iter() {
xs = xs.apply(b)?;
}
if let Some(conv) = &up_block.1 {
xs = xs.apply(conv)?
}
}
xs.apply(&self.out_block_conv)?
.apply(&|xs: &_| candle_nn::ops::pixel_shuffle(xs, 2))
}
}
impl Module for PaellaVQ {
fn forward(&self, xs: &Tensor) -> Result<Tensor> {
self.decode(&self.encode(xs)?)
}
}
| candle/candle-transformers/src/models/wuerstchen/paella_vq.rs/0 | {
"file_path": "candle/candle-transformers/src/models/wuerstchen/paella_vq.rs",
"repo_id": "candle",
"token_count": 4078
} | 41 |
use candle_transformers::models::bert;
use wasm_bindgen::prelude::*;
pub use bert::{BertModel, Config, DTYPE};
pub use tokenizers::{PaddingParams, Tokenizer};
#[wasm_bindgen]
extern "C" {
// Use `js_namespace` here to bind `console.log(..)` instead of just
// `log(..)`
#[wasm_bindgen(js_namespace = console)]
pub fn log(s: &str);
}
#[macro_export]
macro_rules! console_log {
// Note that this is using the `log` function imported above during
// `bare_bones`
($($t:tt)*) => ($crate::log(&format_args!($($t)*).to_string()))
}
| candle/candle-wasm-examples/bert/src/lib.rs/0 | {
"file_path": "candle/candle-wasm-examples/bert/src/lib.rs",
"repo_id": "candle",
"token_count": 226
} | 42 |
use crate::console_log;
use crate::worker::{ModelData, Worker, WorkerInput, WorkerOutput};
use std::str::FromStr;
use wasm_bindgen::prelude::*;
use wasm_bindgen_futures::JsFuture;
use yew::{html, Component, Context, Html};
use yew_agent::{Bridge, Bridged};
async fn fetch_url(url: &str) -> Result<Vec<u8>, JsValue> {
use web_sys::{Request, RequestCache, RequestInit, RequestMode, Response};
let window = web_sys::window().ok_or("window")?;
let mut opts = RequestInit::new();
let opts = opts
.method("GET")
.mode(RequestMode::Cors)
.cache(RequestCache::NoCache);
let request = Request::new_with_str_and_init(url, opts)?;
let resp_value = JsFuture::from(window.fetch_with_request(&request)).await?;
// `resp_value` is a `Response` object.
assert!(resp_value.is_instance_of::<Response>());
let resp: Response = resp_value.dyn_into()?;
let data = JsFuture::from(resp.blob()?).await?;
let blob = web_sys::Blob::from(data);
let array_buffer = JsFuture::from(blob.array_buffer()).await?;
let data = js_sys::Uint8Array::new(&array_buffer).to_vec();
Ok(data)
}
pub enum Msg {
Refresh,
Run,
UpdateStatus(String),
SetModel(ModelData),
WorkerIn(WorkerInput),
WorkerOut(Result<WorkerOutput, String>),
}
pub struct CurrentDecode {
start_time: Option<f64>,
}
pub struct App {
status: String,
loaded: bool,
temperature: std::rc::Rc<std::cell::RefCell<f64>>,
top_p: std::rc::Rc<std::cell::RefCell<f64>>,
prompt: std::rc::Rc<std::cell::RefCell<String>>,
generated: String,
n_tokens: usize,
current_decode: Option<CurrentDecode>,
worker: Box<dyn Bridge<Worker>>,
}
async fn model_data_load() -> Result<ModelData, JsValue> {
let tokenizer = fetch_url("tokenizer.json").await?;
let model = fetch_url("model.bin").await?;
console_log!("{}", model.len());
Ok(ModelData { tokenizer, model })
}
fn performance_now() -> Option<f64> {
let window = web_sys::window()?;
let performance = window.performance()?;
Some(performance.now() / 1000.)
}
impl Component for App {
type Message = Msg;
type Properties = ();
fn create(ctx: &Context<Self>) -> Self {
let status = "loading weights".to_string();
let cb = {
let link = ctx.link().clone();
move |e| link.send_message(Self::Message::WorkerOut(e))
};
let worker = Worker::bridge(std::rc::Rc::new(cb));
Self {
status,
n_tokens: 0,
temperature: std::rc::Rc::new(std::cell::RefCell::new(0.)),
top_p: std::rc::Rc::new(std::cell::RefCell::new(1.0)),
prompt: std::rc::Rc::new(std::cell::RefCell::new("".to_string())),
generated: String::new(),
current_decode: None,
worker,
loaded: false,
}
}
fn rendered(&mut self, ctx: &Context<Self>, first_render: bool) {
if first_render {
ctx.link().send_future(async {
match model_data_load().await {
Err(err) => {
let status = format!("{err:?}");
Msg::UpdateStatus(status)
}
Ok(model_data) => Msg::SetModel(model_data),
}
});
}
}
fn update(&mut self, ctx: &Context<Self>, msg: Self::Message) -> bool {
match msg {
Msg::SetModel(md) => {
self.status = "weights loaded successfully!".to_string();
self.loaded = true;
console_log!("loaded weights");
self.worker.send(WorkerInput::ModelData(md));
true
}
Msg::Run => {
if self.current_decode.is_some() {
self.status = "already generating some sample at the moment".to_string()
} else {
let start_time = performance_now();
self.current_decode = Some(CurrentDecode { start_time });
self.status = "generating...".to_string();
self.n_tokens = 0;
self.generated.clear();
let temp = *self.temperature.borrow();
let top_p = *self.top_p.borrow();
let prompt = self.prompt.borrow().clone();
console_log!("temp: {}, top_p: {}, prompt: {}", temp, top_p, prompt);
ctx.link()
.send_message(Msg::WorkerIn(WorkerInput::Run(temp, top_p, prompt)))
}
true
}
Msg::WorkerOut(output) => {
match output {
Ok(WorkerOutput::WeightsLoaded) => self.status = "weights loaded!".to_string(),
Ok(WorkerOutput::GenerationDone(Err(err))) => {
self.status = format!("error in worker process: {err}");
self.current_decode = None
}
Ok(WorkerOutput::GenerationDone(Ok(()))) => {
let dt = self.current_decode.as_ref().and_then(|current_decode| {
current_decode.start_time.and_then(|start_time| {
performance_now().map(|stop_time| stop_time - start_time)
})
});
self.status = match dt {
None => "generation succeeded!".to_string(),
Some(dt) => format!(
"generation succeeded in {:.2}s ({:.1} ms/token)",
dt,
dt * 1000.0 / (self.n_tokens as f64)
),
};
self.current_decode = None
}
Ok(WorkerOutput::Generated(token)) => {
self.n_tokens += 1;
self.generated.push_str(&token)
}
Err(err) => {
self.status = format!("error in worker {err:?}");
}
}
true
}
Msg::WorkerIn(inp) => {
self.worker.send(inp);
true
}
Msg::UpdateStatus(status) => {
self.status = status;
true
}
Msg::Refresh => true,
}
}
fn view(&self, ctx: &Context<Self>) -> Html {
use yew::TargetCast;
let temperature = self.temperature.clone();
let oninput_temperature = ctx.link().callback(move |e: yew::InputEvent| {
let input: web_sys::HtmlInputElement = e.target_unchecked_into();
if let Ok(temp) = f64::from_str(&input.value()) {
*temperature.borrow_mut() = temp
}
Msg::Refresh
});
let top_p = self.top_p.clone();
let oninput_top_p = ctx.link().callback(move |e: yew::InputEvent| {
let input: web_sys::HtmlInputElement = e.target_unchecked_into();
if let Ok(top_p_input) = f64::from_str(&input.value()) {
*top_p.borrow_mut() = top_p_input
}
Msg::Refresh
});
let prompt = self.prompt.clone();
let oninput_prompt = ctx.link().callback(move |e: yew::InputEvent| {
let input: web_sys::HtmlInputElement = e.target_unchecked_into();
*prompt.borrow_mut() = input.value();
Msg::Refresh
});
html! {
<div style="margin: 2%;">
<div><p>{"Running "}
<a href="https://github.com/karpathy/llama2.c" target="_blank">{"llama2.c"}</a>
{" in the browser using rust/wasm with "}
<a href="https://github.com/huggingface/candle" target="_blank">{"candle!"}</a>
</p>
<p>{"Once the weights have loaded, click on the run button to start generating content."}
</p>
</div>
{"temperature \u{00a0} "}
<input type="range" min="0." max="1.2" step="0.1" value={self.temperature.borrow().to_string()} oninput={oninput_temperature} id="temp"/>
{format!(" \u{00a0} {}", self.temperature.borrow())}
<br/ >
{"top_p \u{00a0} "}
<input type="range" min="0." max="1.0" step="0.05" value={self.top_p.borrow().to_string()} oninput={oninput_top_p} id="top_p"/>
{format!(" \u{00a0} {}", self.top_p.borrow())}
<br/ >
{"prompt: "}<input type="text" value={self.prompt.borrow().to_string()} oninput={oninput_prompt} id="prompt"/>
<br/ >
{
if self.loaded{
html!(<button class="button" onclick={ctx.link().callback(move |_| Msg::Run)}> { "run" }</button>)
}else{
html! { <progress id="progress-bar" aria-label="Loading weights..."></progress> }
}
}
<br/ >
<h3>
{&self.status}
</h3>
{
if self.current_decode.is_some() {
html! { <progress id="progress-bar" aria-label="generating…"></progress> }
} else {
html! {}
}
}
<blockquote>
<p> { self.generated.chars().map(|c|
if c == '\r' || c == '\n' {
html! { <br/> }
} else {
html! { {c} }
}).collect::<Html>()
} </p>
</blockquote>
</div>
}
}
}
| candle/candle-wasm-examples/llama2-c/src/app.rs/0 | {
"file_path": "candle/candle-wasm-examples/llama2-c/src/app.rs",
"repo_id": "candle",
"token_count": 5458
} | 43 |
## Running Whisper Examples
Here, we provide two examples of how to run Whisper using a Candle-compiled WASM binary and runtimes.
### Pure Rust UI
To build and test the UI made in Rust you will need [Trunk](https://trunkrs.dev/#install)
From the `candle-wasm-examples/whisper` directory run:
Download assets:
```bash
# mel filters
wget -c https://huggingface.co/spaces/lmz/candle-whisper/resolve/main/mel_filters.safetensors
# Model and tokenizer tiny.en
wget -c https://huggingface.co/openai/whisper-tiny.en/resolve/main/model.safetensors -P whisper-tiny.en
wget -c https://huggingface.co/openai/whisper-tiny.en/raw/main/tokenizer.json -P whisper-tiny.en
wget -c https://huggingface.co/openai/whisper-tiny.en/raw/main/config.json -P whisper-tiny.en
# model and tokenizer tiny multilanguage
wget -c https://huggingface.co/openai/whisper-tiny/resolve/main/model.safetensors -P whisper-tiny
wget -c https://huggingface.co/openai/whisper-tiny/raw/main/tokenizer.json -P whisper-tiny
wget -c https://huggingface.co/openai/whisper-tiny/raw/main/config.json -P whisper-tiny
#quantized
wget -c https://huggingface.co/lmz/candle-whisper/resolve/main/model-tiny-en-q80.gguf -P quantized
wget -c https://huggingface.co/lmz/candle-whisper/raw/main/tokenizer-tiny-en.json -P quantized
wget -c https://huggingface.co/lmz/candle-whisper/raw/main/config-tiny-en.json -P quantized
# Audio samples
wget -c https://huggingface.co/datasets/Narsil/candle-examples/resolve/main/samples_gb0.wav -P audios
wget -c https://huggingface.co/datasets/Narsil/candle-examples/resolve/main/samples_a13.wav -P audios
wget -c https://huggingface.co/datasets/Narsil/candle-examples/resolve/main/samples_gb1.wav -P audios
wget -c https://huggingface.co/datasets/Narsil/candle-examples/resolve/main/samples_hp0.wav -P audios
wget -c https://huggingface.co/datasets/Narsil/candle-examples/resolve/main/samples_jfk.wav -P audios
wget -c https://huggingface.co/datasets/Narsil/candle-examples/resolve/main/samples_mm0.wav -P audios
```
Run hot reload server:
```bash
trunk serve --release --public-url / --port 8080
```
### Vanilla JS and WebWorkers
To build and test the UI made in Vanilla JS and WebWorkers, first we need to build the WASM library:
```bash
sh build-lib.sh
```
This will bundle the library under `./build` and we can import it inside our WebWorker like a normal JS module:
```js
import init, { Decoder } from "./build/m.js";
```
The full example can be found under `./lib-example.html`. All needed assets are fetched from the web, so no need to download anything.
Finally, you can preview the example by running a local HTTP server. For example:
```bash
python -m http.server
```
Then open `http://localhost:8000/lib-example.html` in your browser.
| candle/candle-wasm-examples/whisper/README.md/0 | {
"file_path": "candle/candle-wasm-examples/whisper/README.md",
"repo_id": "candle",
"token_count": 1023
} | 44 |
{
"moz:firefoxOptions": {
"prefs": {
"media.navigator.streams.fake": true,
"media.navigator.permission.disabled": true
},
"args": []
},
"goog:chromeOptions": {
"args": [
"--use-fake-device-for-media-stream",
"--use-fake-ui-for-media-stream"
]
}
}
| candle/candle-wasm-tests/webdriver.json/0 | {
"file_path": "candle/candle-wasm-tests/webdriver.json",
"repo_id": "candle",
"token_count": 143
} | 45 |
---
title: chat-ui
emoji: 🔥
colorFrom: purple
colorTo: purple
sdk: docker
pinned: false
license: apache-2.0
base_path: /chat
app_port: 3000
failure_strategy: rollback
---
# Chat UI

A chat interface using open source models, eg OpenAssistant or Llama. It is a SvelteKit app and it powers the [HuggingChat app on hf.co/chat](https://huggingface.co/chat).
0. [No Setup Deploy](#no-setup-deploy)
1. [Setup](#setup)
2. [Launch](#launch)
3. [Web Search](#web-search)
4. [Text Embedding Models](#text-embedding-models)
5. [Extra parameters](#extra-parameters)
6. [Deploying to a HF Space](#deploying-to-a-hf-space)
7. [Building](#building)
## No Setup Deploy
If you don't want to configure, setup, and launch your own Chat UI yourself, you can use this option as a fast deploy alternative.
You can deploy your own customized Chat UI instance with any supported [LLM](https://huggingface.co/models?pipeline_tag=text-generation&sort=trending) of your choice on [Hugging Face Spaces](https://huggingface.co/spaces). To do so, use the chat-ui template [available here](https://huggingface.co/new-space?template=huggingchat/chat-ui-template).
Set `HF_TOKEN` in [Space secrets](https://huggingface.co/docs/hub/spaces-overview#managing-secrets-and-environment-variables) to deploy a model with gated access or a model in a private repository. It's also compatible with [Inference for PROs](https://huggingface.co/blog/inference-pro) curated list of powerful models with higher rate limits. Make sure to create your personal token first in your [User Access Tokens settings](https://huggingface.co/settings/tokens).
Read the full tutorial [here](https://huggingface.co/docs/hub/spaces-sdks-docker-chatui#chatui-on-spaces).
## Setup
The default config for Chat UI is stored in the `.env` file. You will need to override some values to get Chat UI to run locally. This is done in `.env.local`.
Start by creating a `.env.local` file in the root of the repository. The bare minimum config you need to get Chat UI to run locally is the following:
```env
MONGODB_URL=<the URL to your MongoDB instance>
HF_TOKEN=<your access token>
```
### Database
The chat history is stored in a MongoDB instance, and having a DB instance available is needed for Chat UI to work.
You can use a local MongoDB instance. The easiest way is to spin one up using docker:
```bash
docker run -d -p 27017:27017 --name mongo-chatui mongo:latest
```
In which case the url of your DB will be `MONGODB_URL=mongodb://localhost:27017`.
Alternatively, you can use a [free MongoDB Atlas](https://www.mongodb.com/pricing) instance for this, Chat UI should fit comfortably within their free tier. After which you can set the `MONGODB_URL` variable in `.env.local` to match your instance.
### Hugging Face Access Token
If you use a remote inference endpoint, you will need a Hugging Face access token to run Chat UI locally. You can get one from [your Hugging Face profile](https://huggingface.co/settings/tokens).
## Launch
After you're done with the `.env.local` file you can run Chat UI locally with:
```bash
npm install
npm run dev
```
## Web Search
Chat UI features a powerful Web Search feature. It works by:
1. Generating an appropriate search query from the user prompt.
2. Performing web search and extracting content from webpages.
3. Creating embeddings from texts using a text embedding model.
4. From these embeddings, find the ones that are closest to the user query using a vector similarity search. Specifically, we use `inner product` distance.
5. Get the corresponding texts to those closest embeddings and perform [Retrieval-Augmented Generation](https://huggingface.co/papers/2005.11401) (i.e. expand user prompt by adding those texts so that an LLM can use this information).
## Text Embedding Models
By default (for backward compatibility), when `TEXT_EMBEDDING_MODELS` environment variable is not defined, [transformers.js](https://huggingface.co/docs/transformers.js) embedding models will be used for embedding tasks, specifically, [Xenova/gte-small](https://huggingface.co/Xenova/gte-small) model.
You can customize the embedding model by setting `TEXT_EMBEDDING_MODELS` in your `.env.local` file. For example:
```env
TEXT_EMBEDDING_MODELS = `[
{
"name": "Xenova/gte-small",
"displayName": "Xenova/gte-small",
"description": "locally running embedding",
"chunkCharLength": 512,
"endpoints": [
{"type": "transformersjs"}
]
},
{
"name": "intfloat/e5-base-v2",
"displayName": "intfloat/e5-base-v2",
"description": "hosted embedding model",
"chunkCharLength": 768,
"preQuery": "query: ", # See https://huggingface.co/intfloat/e5-base-v2#faq
"prePassage": "passage: ", # See https://huggingface.co/intfloat/e5-base-v2#faq
"endpoints": [
{
"type": "tei",
"url": "http://127.0.0.1:8080/",
"authorization": "TOKEN_TYPE TOKEN" // optional authorization field. Example: "Basic VVNFUjpQQVNT"
}
]
}
]`
```
The required fields are `name`, `chunkCharLength` and `endpoints`.
Supported text embedding backends are: [`transformers.js`](https://huggingface.co/docs/transformers.js), [`TEI`](https://github.com/huggingface/text-embeddings-inference) and [`OpenAI`](https://platform.openai.com/docs/guides/embeddings). `transformers.js` models run locally as part of `chat-ui`, whereas `TEI` models run in a different environment & accessed through an API endpoint. `openai` models are accessed through the [OpenAI API](https://platform.openai.com/docs/guides/embeddings).
When more than one embedding models are supplied in `.env.local` file, the first will be used by default, and the others will only be used on LLM's which configured `embeddingModel` to the name of the model.
## Extra parameters
### OpenID connect
The login feature is disabled by default and users are attributed a unique ID based on their browser. But if you want to use OpenID to authenticate your users, you can add the following to your `.env.local` file:
```env
OPENID_CONFIG=`{
PROVIDER_URL: "<your OIDC issuer>",
CLIENT_ID: "<your OIDC client ID>",
CLIENT_SECRET: "<your OIDC client secret>",
SCOPES: "openid profile",
TOLERANCE: // optional
RESOURCE: // optional
}`
```
These variables will enable the openID sign-in modal for users.
### Theming
You can use a few environment variables to customize the look and feel of chat-ui. These are by default:
```env
PUBLIC_APP_NAME=ChatUI
PUBLIC_APP_ASSETS=chatui
PUBLIC_APP_COLOR=blue
PUBLIC_APP_DESCRIPTION="Making the community's best AI chat models available to everyone."
PUBLIC_APP_DATA_SHARING=
PUBLIC_APP_DISCLAIMER=
```
- `PUBLIC_APP_NAME` The name used as a title throughout the app.
- `PUBLIC_APP_ASSETS` Is used to find logos & favicons in `static/$PUBLIC_APP_ASSETS`, current options are `chatui` and `huggingchat`.
- `PUBLIC_APP_COLOR` Can be any of the [tailwind colors](https://tailwindcss.com/docs/customizing-colors#default-color-palette).
- `PUBLIC_APP_DATA_SHARING` Can be set to 1 to add a toggle in the user settings that lets your users opt-in to data sharing with models creator.
- `PUBLIC_APP_DISCLAIMER` If set to 1, we show a disclaimer about generated outputs on login.
### Web Search config
You can enable the web search through an API by adding `YDC_API_KEY` ([docs.you.com](https://docs.you.com)) or `SERPER_API_KEY` ([serper.dev](https://serper.dev/)) or `SERPAPI_KEY` ([serpapi.com](https://serpapi.com/)) or `SERPSTACK_API_KEY` ([serpstack.com](https://serpstack.com/)) to your `.env.local`.
You can also simply enable the local google websearch by setting `USE_LOCAL_WEBSEARCH=true` in your `.env.local` or specify a SearXNG instance by adding the query URL to `SEARXNG_QUERY_URL`.
### Custom models
You can customize the parameters passed to the model or even use a new model by updating the `MODELS` variable in your `.env.local`. The default one can be found in `.env` and looks like this :
```env
MODELS=`[
{
"name": "mistralai/Mistral-7B-Instruct-v0.2",
"displayName": "mistralai/Mistral-7B-Instruct-v0.2",
"description": "Mistral 7B is a new Apache 2.0 model, released by Mistral AI that outperforms Llama2 13B in benchmarks.",
"websiteUrl": "https://mistral.ai/news/announcing-mistral-7b/",
"preprompt": "",
"chatPromptTemplate" : "<s>{{#each messages}}{{#ifUser}}[INST] {{#if @first}}{{#if @root.preprompt}}{{@root.preprompt}}\n{{/if}}{{/if}}{{content}} [/INST]{{/ifUser}}{{#ifAssistant}}{{content}}</s>{{/ifAssistant}}{{/each}}",
"parameters": {
"temperature": 0.3,
"top_p": 0.95,
"repetition_penalty": 1.2,
"top_k": 50,
"truncate": 3072,
"max_new_tokens": 1024,
"stop": ["</s>"]
},
"promptExamples": [
{
"title": "Write an email from bullet list",
"prompt": "As a restaurant owner, write a professional email to the supplier to get these products every week: \n\n- Wine (x10)\n- Eggs (x24)\n- Bread (x12)"
}, {
"title": "Code a snake game",
"prompt": "Code a basic snake game in python, give explanations for each step."
}, {
"title": "Assist in a task",
"prompt": "How do I make a delicious lemon cheesecake?"
}
]
}
]`
```
You can change things like the parameters, or customize the preprompt to better suit your needs. You can also add more models by adding more objects to the array, with different preprompts for example.
#### chatPromptTemplate
When querying the model for a chat response, the `chatPromptTemplate` template is used. `messages` is an array of chat messages, it has the format `[{ content: string }, ...]`. To identify if a message is a user message or an assistant message the `ifUser` and `ifAssistant` block helpers can be used.
The following is the default `chatPromptTemplate`, although newlines and indentiation have been added for readability. You can find the prompts used in production for HuggingChat [here](https://github.com/huggingface/chat-ui/blob/main/PROMPTS.md).
```prompt
{{preprompt}}
{{#each messages}}
{{#ifUser}}{{@root.userMessageToken}}{{content}}{{@root.userMessageEndToken}}{{/ifUser}}
{{#ifAssistant}}{{@root.assistantMessageToken}}{{content}}{{@root.assistantMessageEndToken}}{{/ifAssistant}}
{{/each}}
{{assistantMessageToken}}
```
#### Multi modal model
We currently only support IDEFICS as a multimodal model, hosted on TGI. You can enable it by using the following config (if you have a PRO HF Api token):
```env
{
"name": "HuggingFaceM4/idefics-80b-instruct",
"multimodal" : true,
"description": "IDEFICS is the new multimodal model by Hugging Face.",
"preprompt": "",
"chatPromptTemplate" : "{{#each messages}}{{#ifUser}}User: {{content}}{{/ifUser}}<end_of_utterance>\nAssistant: {{#ifAssistant}}{{content}}\n{{/ifAssistant}}{{/each}}",
"parameters": {
"temperature": 0.1,
"top_p": 0.95,
"repetition_penalty": 1.2,
"top_k": 12,
"truncate": 1000,
"max_new_tokens": 1024,
"stop": ["<end_of_utterance>", "User:", "\nUser:"]
}
}
```
#### Running your own models using a custom endpoint
If you want to, instead of hitting models on the Hugging Face Inference API, you can run your own models locally.
A good option is to hit a [text-generation-inference](https://github.com/huggingface/text-generation-inference) endpoint. This is what is done in the official [Chat UI Spaces Docker template](https://huggingface.co/new-space?template=huggingchat/chat-ui-template) for instance: both this app and a text-generation-inference server run inside the same container.
To do this, you can add your own endpoints to the `MODELS` variable in `.env.local`, by adding an `"endpoints"` key for each model in `MODELS`.
```env
{
// rest of the model config here
"endpoints": [{
"type" : "tgi",
"url": "https://HOST:PORT",
}]
}
```
If `endpoints` are left unspecified, ChatUI will look for the model on the hosted Hugging Face inference API using the model name.
##### OpenAI API compatible models
Chat UI can be used with any API server that supports OpenAI API compatibility, for example [text-generation-webui](https://github.com/oobabooga/text-generation-webui/tree/main/extensions/openai), [LocalAI](https://github.com/go-skynet/LocalAI), [FastChat](https://github.com/lm-sys/FastChat/blob/main/docs/openai_api.md), [llama-cpp-python](https://github.com/abetlen/llama-cpp-python), and [ialacol](https://github.com/chenhunghan/ialacol).
The following example config makes Chat UI works with [text-generation-webui](https://github.com/oobabooga/text-generation-webui/tree/main/extensions/openai), the `endpoint.baseUrl` is the url of the OpenAI API compatible server, this overrides the baseUrl to be used by OpenAI instance. The `endpoint.completion` determine which endpoint to be used, default is `chat_completions` which uses `v1/chat/completions`, change to `endpoint.completion` to `completions` to use the `v1/completions` endpoint.
```
MODELS=`[
{
"name": "text-generation-webui",
"id": "text-generation-webui",
"parameters": {
"temperature": 0.9,
"top_p": 0.95,
"repetition_penalty": 1.2,
"top_k": 50,
"truncate": 1000,
"max_new_tokens": 1024,
"stop": []
},
"endpoints": [{
"type" : "openai",
"baseURL": "http://localhost:8000/v1"
}]
}
]`
```
The `openai` type includes official OpenAI models. You can add, for example, GPT4/GPT3.5 as a "openai" model:
```
OPENAI_API_KEY=#your openai api key here
MODELS=`[{
"name": "gpt-4",
"displayName": "GPT 4",
"endpoints" : [{
"type": "openai"
}]
},
{
"name": "gpt-3.5-turbo",
"displayName": "GPT 3.5 Turbo",
"endpoints" : [{
"type": "openai"
}]
}]`
```
You may also consume any model provider that provides compatible OpenAI API endpoint. For example, you may self-host [Portkey](https://github.com/Portkey-AI/gateway) gateway and experiment with Claude or GPTs offered by Azure OpenAI. Example for Claude from Anthropic:
```
MODELS=`[{
"name": "claude-2.1",
"displayName": "Claude 2.1",
"description": "Anthropic has been founded by former OpenAI researchers...",
"parameters": {
"temperature": 0.5,
"max_new_tokens": 4096,
},
"endpoints": [
{
"type": "openai",
"baseURL": "https://gateway.example.com/v1",
"defaultHeaders": {
"x-portkey-config": '{"provider":"anthropic","api_key":"sk-ant-abc...xyz"}'
}
}
]
}]`
```
Example for GPT 4 deployed on Azure OpenAI:
```
MODELS=`[{
"id": "gpt-4-1106-preview",
"name": "gpt-4-1106-preview",
"displayName": "gpt-4-1106-preview",
"parameters": {
"temperature": 0.5,
"max_new_tokens": 4096,
},
"endpoints": [
{
"type": "openai",
"baseURL": "https://{resource-name}.openai.azure.com/openai/deployments/{deployment-id}",
"defaultHeaders": {
"api-key": "{api-key}"
},
"defaultQuery": {
"api-version": "2023-05-15"
}
}
]
}]`
```
Or try Mistral from [Deepinfra](https://deepinfra.com/mistralai/Mistral-7B-Instruct-v0.1/api?example=openai-http):
> Note, apiKey can either be set custom per endpoint, or globally using `OPENAI_API_KEY` variable.
```
MODELS=`[{
"name": "mistral-7b",
"displayName": "Mistral 7B",
"description": "A 7B dense Transformer, fast-deployed and easily customisable. Small, yet powerful for a variety of use cases. Supports English and code, and a 8k context window.",
"parameters": {
"temperature": 0.5,
"max_new_tokens": 4096,
},
"endpoints": [
{
"type": "openai",
"baseURL": "https://api.deepinfra.com/v1/openai",
"apiKey": "abc...xyz"
}
]
}]`
```
##### Llama.cpp API server
chat-ui also supports the llama.cpp API server directly without the need for an adapter. You can do this using the `llamacpp` endpoint type.
If you want to run chat-ui with llama.cpp, you can do the following, using Zephyr as an example model:
1. Get [the weights](https://huggingface.co/TheBloke/zephyr-7B-beta-GGUF/tree/main) from the hub
2. Run the server with the following command: `./server -m models/zephyr-7b-beta.Q4_K_M.gguf -c 2048 -np 3`
3. Add the following to your `.env.local`:
```env
MODELS=`[
{
"name": "Local Zephyr",
"chatPromptTemplate": "<|system|>\n{{preprompt}}</s>\n{{#each messages}}{{#ifUser}}<|user|>\n{{content}}</s>\n<|assistant|>\n{{/ifUser}}{{#ifAssistant}}{{content}}</s>\n{{/ifAssistant}}{{/each}}",
"parameters": {
"temperature": 0.1,
"top_p": 0.95,
"repetition_penalty": 1.2,
"top_k": 50,
"truncate": 1000,
"max_new_tokens": 2048,
"stop": ["</s>"]
},
"endpoints": [
{
"url": "http://127.0.0.1:8080",
"type": "llamacpp"
}
]
}
]`
```
Start chat-ui with `npm run dev` and you should be able to chat with Zephyr locally.
#### Ollama
We also support the Ollama inference server. Spin up a model with
```cli
ollama run mistral
```
Then specify the endpoints like so:
```env
MODELS=`[
{
"name": "Ollama Mistral",
"chatPromptTemplate": "<s>{{#each messages}}{{#ifUser}}[INST] {{#if @first}}{{#if @root.preprompt}}{{@root.preprompt}}\n{{/if}}{{/if}} {{content}} [/INST]{{/ifUser}}{{#ifAssistant}}{{content}}</s> {{/ifAssistant}}{{/each}}",
"parameters": {
"temperature": 0.1,
"top_p": 0.95,
"repetition_penalty": 1.2,
"top_k": 50,
"truncate": 3072,
"max_new_tokens": 1024,
"stop": ["</s>"]
},
"endpoints": [
{
"type": "ollama",
"url" : "http://127.0.0.1:11434",
"ollamaName" : "mistral"
}
]
}
]`
```
#### Anthropic
We also support Anthropic models through the official SDK. You may provide your API key via the `ANTHROPIC_API_KEY` env variable, or alternatively, through the `endpoints.apiKey` as per the following example.
```
MODELS=`[
{
"name": "claude-3-sonnet-20240229",
"displayName": "Claude 3 Sonnet",
"description": "Ideal balance of intelligence and speed",
"parameters": {
"max_new_tokens": 4096,
},
"endpoints": [
{
"type": "anthropic",
// optionals
"apiKey": "sk-ant-...",
"baseURL": "https://api.anthropic.com",
defaultHeaders: {},
defaultQuery: {}
}
]
},
{
"name": "claude-3-opus-20240229",
"displayName": "Claude 3 Opus",
"description": "Most powerful model for highly complex tasks",
"parameters": {
"max_new_tokens": 4096
},
"endpoints": [
{
"type": "anthropic",
// optionals
"apiKey": "sk-ant-...",
"baseURL": "https://api.anthropic.com",
defaultHeaders: {},
defaultQuery: {}
}
]
}
]`
```
#### Amazon
You can also specify your Amazon SageMaker instance as an endpoint for chat-ui. The config goes like this:
```env
"endpoints": [
{
"type" : "aws",
"service" : "sagemaker"
"url": "",
"accessKey": "",
"secretKey" : "",
"sessionToken": "",
"region": "",
"weight": 1
}
]
```
You can also set `"service" : "lambda"` to use a lambda instance.
You can get the `accessKey` and `secretKey` from your AWS user, under programmatic access.
### Custom endpoint authorization
#### Basic and Bearer
Custom endpoints may require authorization, depending on how you configure them. Authentication will usually be set either with `Basic` or `Bearer`.
For `Basic` we will need to generate a base64 encoding of the username and password.
`echo -n "USER:PASS" | base64`
> VVNFUjpQQVNT
For `Bearer` you can use a token, which can be grabbed from [here](https://huggingface.co/settings/tokens).
You can then add the generated information and the `authorization` parameter to your `.env.local`.
```env
"endpoints": [
{
"url": "https://HOST:PORT",
"authorization": "Basic VVNFUjpQQVNT",
}
]
```
Please note that if `HF_TOKEN` is also set or not empty, it will take precedence.
#### Models hosted on multiple custom endpoints
If the model being hosted will be available on multiple servers/instances add the `weight` parameter to your `.env.local`. The `weight` will be used to determine the probability of requesting a particular endpoint.
```env
"endpoints": [
{
"url": "https://HOST:PORT",
"weight": 1
},
{
"url": "https://HOST:PORT",
"weight": 2
}
...
]
```
#### Client Certificate Authentication (mTLS)
Custom endpoints may require client certificate authentication, depending on how you configure them. To enable mTLS between Chat UI and your custom endpoint, you will need to set the `USE_CLIENT_CERTIFICATE` to `true`, and add the `CERT_PATH` and `KEY_PATH` parameters to your `.env.local`. These parameters should point to the location of the certificate and key files on your local machine. The certificate and key files should be in PEM format. The key file can be encrypted with a passphrase, in which case you will also need to add the `CLIENT_KEY_PASSWORD` parameter to your `.env.local`.
If you're using a certificate signed by a private CA, you will also need to add the `CA_PATH` parameter to your `.env.local`. This parameter should point to the location of the CA certificate file on your local machine.
If you're using a self-signed certificate, e.g. for testing or development purposes, you can set the `REJECT_UNAUTHORIZED` parameter to `false` in your `.env.local`. This will disable certificate validation, and allow Chat UI to connect to your custom endpoint.
#### Specific Embedding Model
A model can use any of the embedding models defined in `.env.local`, (currently used when web searching),
by default it will use the first embedding model, but it can be changed with the field `embeddingModel`:
```env
TEXT_EMBEDDING_MODELS = `[
{
"name": "Xenova/gte-small",
"chunkCharLength": 512,
"endpoints": [
{"type": "transformersjs"}
]
},
{
"name": "intfloat/e5-base-v2",
"chunkCharLength": 768,
"endpoints": [
{"type": "tei", "url": "http://127.0.0.1:8080/", "authorization": "Basic VVNFUjpQQVNT"},
{"type": "tei", "url": "http://127.0.0.1:8081/"}
]
}
]`
MODELS=`[
{
"name": "Ollama Mistral",
"chatPromptTemplate": "...",
"embeddingModel": "intfloat/e5-base-v2"
"parameters": {
...
},
"endpoints": [
...
]
}
]`
```
## Deploying to a HF Space
Create a `DOTENV_LOCAL` secret to your HF space with the content of your .env.local, and they will be picked up automatically when you run.
## Building
To create a production version of your app:
```bash
npm run build
```
You can preview the production build with `npm run preview`.
> To deploy your app, you may need to install an [adapter](https://kit.svelte.dev/docs/adapters) for your target environment.
## Config changes for HuggingChat
The config file for HuggingChat is stored in the `.env.template` file at the root of the repository. It is the single source of truth that is used to generate the actual `.env.local` file using our CI/CD pipeline. See [updateProdEnv](https://github.com/huggingface/chat-ui/blob/cdb33a9583f5339ade724db615347393ef48f5cd/scripts/updateProdEnv.ts) for more details.
> [!TIP]
> If you want to make changes to model config for HuggingChat, you should do so against `.env.template`.
We currently use the following secrets for deploying HuggingChat in addition to the `.env.template` above:
- `MONGODB_URL`
- `HF_TOKEN`
- `OPENID_CONFIG`
- `SERPER_API_KEY`
They are defined as secrets in the repository.
### Testing config changes locally
You can test the config changes locally by first creating an `.env.SECRET_CONFIG` file with the secrets defined above. Then you can run the following command to generate the `.env.local` file:
```bash
npm run updateLocalEnv
```
This will replace your `.env.local` file with the one that will be used in prod (simply taking `.env.template + .env.SECRET_CONFIG`).
### Populate database
> [!WARNING]
> The `MONGODB_URL` used for this script will be fetched from `.env.local`. Make sure it's correct! The command runs directly on the database.
You can populate the database using faker data using the `populate` script:
```bash
npm run populate <flags here>
```
At least one flag must be specified, the following flags are available:
- `reset` - resets the database
- `all` - populates all tables
- `users` - populates the users table
- `settings` - populates the settings table for existing users
- `assistants` - populates the assistants table for existing users
- `conversations` - populates the conversations table for existing users
For example, you could use it like so:
```bash
npm run populate reset
```
to clear out the database. Then login in the app to create your user and run the following command:
```bash
npm run populate users settings assistants conversations
```
to populate the database with fake data, including fake conversations and assistants for your user.
| chat-ui/README.md/0 | {
"file_path": "chat-ui/README.md",
"repo_id": "chat-ui",
"token_count": 9257
} | 46 |
<script lang="ts">
import type { readAndCompressImage } from "browser-image-resizer";
import type { Model } from "$lib/types/Model";
import type { Assistant } from "$lib/types/Assistant";
import { onMount } from "svelte";
import { applyAction, enhance } from "$app/forms";
import { page } from "$app/stores";
import { base } from "$app/paths";
import CarbonPen from "~icons/carbon/pen";
import CarbonUpload from "~icons/carbon/upload";
import { useSettingsStore } from "$lib/stores/settings";
import { isHuggingChat } from "$lib/utils/isHuggingChat";
type ActionData = {
error: boolean;
errors: {
field: string | number;
message: string;
}[];
} | null;
type AssistantFront = Omit<Assistant, "_id" | "createdById"> & { _id: string };
export let form: ActionData;
export let assistant: AssistantFront | undefined = undefined;
export let models: Model[] = [];
let files: FileList | null = null;
const settings = useSettingsStore();
let compress: typeof readAndCompressImage | null = null;
onMount(async () => {
const module = await import("browser-image-resizer");
compress = module.readAndCompressImage;
});
let inputMessage1 = assistant?.exampleInputs[0] ?? "";
let inputMessage2 = assistant?.exampleInputs[1] ?? "";
let inputMessage3 = assistant?.exampleInputs[2] ?? "";
let inputMessage4 = assistant?.exampleInputs[3] ?? "";
function resetErrors() {
if (form) {
form.errors = [];
form.error = false;
}
}
function onFilesChange(e: Event) {
const inputEl = e.target as HTMLInputElement;
if (inputEl.files?.length && inputEl.files[0].size > 0) {
if (!inputEl.files[0].type.includes("image")) {
inputEl.files = null;
files = null;
form = { error: true, errors: [{ field: "avatar", message: "Only images are allowed" }] };
return;
}
files = inputEl.files;
resetErrors();
deleteExistingAvatar = false;
}
}
function getError(field: string, returnForm: ActionData) {
return returnForm?.errors.find((error) => error.field === field)?.message ?? "";
}
let deleteExistingAvatar = false;
let loading = false;
let ragMode: false | "links" | "domains" | "all" = assistant?.rag?.allowAllDomains
? "all"
: assistant?.rag?.allowedLinks?.length ?? 0 > 0
? "links"
: (assistant?.rag?.allowedDomains?.length ?? 0) > 0
? "domains"
: false;
</script>
<form
method="POST"
class="flex h-full flex-col overflow-y-auto p-4 md:p-8"
enctype="multipart/form-data"
use:enhance={async ({ formData }) => {
loading = true;
if (files?.[0] && files[0].size > 0 && compress) {
await compress(files[0], {
maxWidth: 500,
maxHeight: 500,
quality: 1,
}).then((resizedImage) => {
formData.set("avatar", resizedImage);
});
}
if (deleteExistingAvatar === true) {
if (assistant?.avatar) {
// if there is an avatar we explicitly removei t
formData.set("avatar", "null");
} else {
// else we just remove it from the input
formData.delete("avatar");
}
} else {
if (files === null) {
formData.delete("avatar");
}
}
formData.delete("ragMode");
if (ragMode === false || !$page.data.enableAssistantsRAG) {
formData.set("ragAllowAll", "false");
formData.set("ragLinkList", "");
formData.set("ragDomainList", "");
} else if (ragMode === "all") {
formData.set("ragAllowAll", "true");
formData.set("ragLinkList", "");
formData.set("ragDomainList", "");
} else if (ragMode === "links") {
formData.set("ragAllowAll", "false");
formData.set("ragDomainList", "");
} else if (ragMode === "domains") {
formData.set("ragAllowAll", "false");
formData.set("ragLinkList", "");
}
return async ({ result }) => {
loading = false;
await applyAction(result);
};
}}
>
{#if assistant}
<h2 class="text-xl font-semibold">
Edit {assistant?.name ?? "assistant"}
</h2>
<p class="mb-6 text-sm text-gray-500">
Modifying an existing assistant will propagate those changes to all users.
</p>
{:else}
<h2 class="text-xl font-semibold">Create new assistant</h2>
<p class="mb-6 text-sm text-gray-500">
Create and share your own AI Assistant. All assistants are <span
class="rounded-full border px-2 py-0.5 leading-none">public</span
>
</p>
{/if}
<div class="grid h-full w-full flex-1 grid-cols-2 gap-6 text-sm max-sm:grid-cols-1">
<div class="col-span-1 flex flex-col gap-4">
<div>
<div class="mb-1 block pb-2 text-sm font-semibold">Avatar</div>
<input
type="file"
accept="image/*"
name="avatar"
id="avatar"
class="hidden"
on:change={onFilesChange}
/>
{#if (files && files[0]) || (assistant?.avatar && !deleteExistingAvatar)}
<div class="group relative mx-auto h-12 w-12">
{#if files && files[0]}
<img
src={URL.createObjectURL(files[0])}
alt="avatar"
class="crop mx-auto h-12 w-12 cursor-pointer rounded-full object-cover"
/>
{:else if assistant?.avatar}
<img
src="{base}/settings/assistants/{assistant._id}/avatar.jpg?hash={assistant.avatar}"
alt="avatar"
class="crop mx-auto h-12 w-12 cursor-pointer rounded-full object-cover"
/>
{/if}
<label
for="avatar"
class="invisible absolute bottom-0 h-12 w-12 rounded-full bg-black bg-opacity-50 p-1 group-hover:visible hover:visible"
>
<CarbonPen class="mx-auto my-auto h-full cursor-pointer text-center text-white" />
</label>
</div>
<div class="mx-auto w-max pt-1">
<button
type="button"
on:click|stopPropagation|preventDefault={() => {
files = null;
deleteExistingAvatar = true;
}}
class="mx-auto w-max text-center text-xs text-gray-600 hover:underline"
>
Delete
</button>
</div>
{:else}
<div class="mb-1 flex w-max flex-row gap-4">
<label
for="avatar"
class="btn flex h-8 rounded-lg border bg-white px-3 py-1 text-gray-500 shadow-sm transition-all hover:bg-gray-100"
>
<CarbonUpload class="mr-2 text-xs " /> Upload
</label>
</div>
{/if}
<p class="text-xs text-red-500">{getError("avatar", form)}</p>
</div>
<label>
<div class="mb-1 font-semibold">Name</div>
<input
name="name"
class="w-full rounded-lg border-2 border-gray-200 bg-gray-100 p-2"
placeholder="My awesome model"
value={assistant?.name ?? ""}
/>
<p class="text-xs text-red-500">{getError("name", form)}</p>
</label>
<label>
<div class="mb-1 font-semibold">Description</div>
<textarea
name="description"
class="h-15 w-full rounded-lg border-2 border-gray-200 bg-gray-100 p-2"
placeholder="He knows everything about python"
value={assistant?.description ?? ""}
/>
<p class="text-xs text-red-500">{getError("description", form)}</p>
</label>
<label>
<div class="mb-1 font-semibold">Model</div>
<select name="modelId" class="w-full rounded-lg border-2 border-gray-200 bg-gray-100 p-2">
{#each models.filter((model) => !model.unlisted) as model}
<option
value={model.id}
selected={assistant
? assistant?.modelId === model.id
: $settings.activeModel === model.id}>{model.displayName}</option
>
{/each}
<p class="text-xs text-red-500">{getError("modelId", form)}</p>
</select>
</label>
<label>
<div class="mb-1 font-semibold">User start messages</div>
<div class="flex flex-col gap-2">
<input
name="exampleInput1"
bind:value={inputMessage1}
class="w-full rounded-lg border-2 border-gray-200 bg-gray-100 p-2"
/>
{#if !!inputMessage1 || !!inputMessage2}
<input
name="exampleInput2"
bind:value={inputMessage2}
class="w-full rounded-lg border-2 border-gray-200 bg-gray-100 p-2"
/>
{/if}
{#if !!inputMessage2 || !!inputMessage3}
<input
name="exampleInput3"
bind:value={inputMessage3}
class="w-full rounded-lg border-2 border-gray-200 bg-gray-100 p-2"
/>
{/if}
{#if !!inputMessage3 || !!inputMessage4}
<input
name="exampleInput4"
bind:value={inputMessage4}
class="w-full rounded-lg border-2 border-gray-200 bg-gray-100 p-2"
/>
{/if}
</div>
<p class="text-xs text-red-500">{getError("inputMessage1", form)}</p>
</label>
{#if $page.data.enableAssistantsRAG}
<div class="mb-4 flex flex-col flex-nowrap">
<span class="mt-2 text-smd font-semibold"
>Internet access <span
class="ml-1 rounded bg-gray-100 px-1 py-0.5 text-xxs font-normal text-gray-600"
>Experimental</span
>
{#if isHuggingChat}
<a
href="https://huggingface.co/spaces/huggingchat/chat-ui/discussions/385"
target="_blank"
class="ml-0.5 rounded bg-gray-100 px-1 py-0.5 text-xxs font-normal text-gray-700 underline decoration-gray-400"
>Give feedback</a
>
{/if}
</span>
<label class="mt-1">
<input
checked={!ragMode}
on:change={() => (ragMode = false)}
type="radio"
name="ragMode"
value={false}
/>
<span class="my-2 text-sm" class:font-semibold={!ragMode}> Disabled </span>
{#if !ragMode}
<span class="block text-xs text-gray-500">
Assistant won't look for information from Internet and will be faster to answer.
</span>
{/if}
</label>
<label class="mt-1">
<input
checked={ragMode === "all"}
on:change={() => (ragMode = "all")}
type="radio"
name="ragMode"
value={"all"}
/>
<span class="my-2 text-sm" class:font-semibold={ragMode === "all"}> Web search </span>
{#if ragMode === "all"}
<span class="block text-xs text-gray-500">
Assistant will do a web search on each user request to find information.
</span>
{/if}
</label>
<label class="mt-1">
<input
checked={ragMode === "domains"}
on:change={() => (ragMode = "domains")}
type="radio"
name="ragMode"
value={false}
/>
<span class="my-2 text-sm" class:font-semibold={ragMode === "domains"}>
Domains search
</span>
</label>
{#if ragMode === "domains"}
<span class="mb-2 text-xs text-gray-500">
Specify domains and URLs that the application can search, separated by commas.
</span>
<input
name="ragDomainList"
class="w-full rounded-lg border-2 border-gray-200 bg-gray-100 p-2"
placeholder="wikipedia.org,bbc.com"
value={assistant?.rag?.allowedDomains?.join(",") ?? ""}
/>
<p class="text-xs text-red-500">{getError("ragDomainList", form)}</p>
{/if}
<label class="mt-1">
<input
checked={ragMode === "links"}
on:change={() => (ragMode = "links")}
type="radio"
name="ragMode"
value={false}
/>
<span class="my-2 text-sm" class:font-semibold={ragMode === "links"}>
Specific Links
</span>
</label>
{#if ragMode === "links"}
<span class="mb-2 text-xs text-gray-500">
Specify a maximum of 10 direct URLs that the Assistant will access. HTML & Plain Text
only, separated by commas
</span>
<input
name="ragLinkList"
class="w-full rounded-lg border-2 border-gray-200 bg-gray-100 p-2"
placeholder="https://raw.githubusercontent.com/huggingface/chat-ui/main/README.md"
value={assistant?.rag?.allowedLinks.join(",") ?? ""}
/>
<p class="text-xs text-red-500">{getError("ragLinkList", form)}</p>
{/if}
</div>
{/if}
</div>
<div class="col-span-1 flex h-full flex-col">
<span class="mb-1 text-sm font-semibold"> Instructions (system prompt) </span>
<textarea
name="preprompt"
class="mb-20 min-h-[8lh] flex-1 rounded-lg border-2 border-gray-200 bg-gray-100 p-2 text-sm"
placeholder="You'll act as..."
value={assistant?.preprompt ?? ""}
/>
<p class="text-xs text-red-500">{getError("preprompt", form)}</p>
</div>
</div>
<div class="fixed bottom-6 right-6 ml-auto mt-6 flex w-fit justify-end gap-2 sm:absolute">
<a
href={assistant ? `${base}/settings/assistants/${assistant?._id}` : `${base}/settings`}
class="flex items-center justify-center rounded-full bg-gray-200 px-5 py-2 font-semibold text-gray-600"
>
Cancel
</a>
<button
type="submit"
disabled={loading}
aria-disabled={loading}
class="flex items-center justify-center rounded-full bg-black px-8 py-2 font-semibold"
class:bg-gray-200={loading}
class:text-gray-600={loading}
class:text-white={!loading}
>
{assistant ? "Save" : "Create"}
</button>
</div>
</form>
| chat-ui/src/lib/components/AssistantSettings.svelte/0 | {
"file_path": "chat-ui/src/lib/components/AssistantSettings.svelte",
"repo_id": "chat-ui",
"token_count": 5719
} | 47 |
<script lang="ts">
import CarbonRotate360 from "~icons/carbon/rotate-360";
export let classNames = "";
</script>
<button
type="button"
on:click
class="btn flex h-8 rounded-lg border bg-white px-3 py-1 text-gray-500 shadow-sm transition-all hover:bg-gray-100 dark:border-gray-600 dark:bg-gray-700 dark:text-gray-300 dark:hover:bg-gray-600 {classNames}"
>
<CarbonRotate360 class="mr-2 text-xs " /> Retry
</button>
| chat-ui/src/lib/components/RetryBtn.svelte/0 | {
"file_path": "chat-ui/src/lib/components/RetryBtn.svelte",
"repo_id": "chat-ui",
"token_count": 157
} | 48 |
<script lang="ts">
export let classNames = "";
</script>
<svg
class={classNames}
xmlns="http://www.w3.org/2000/svg"
aria-hidden="true"
fill="currentColor"
focusable="false"
role="img"
width="1em"
height="1em"
preserveAspectRatio="xMidYMid meet"
viewBox="0 0 32 32"
>
<path
d="M28,10V28H10V10H28m0-2H10a2,2,0,0,0-2,2V28a2,2,0,0,0,2,2H28a2,2,0,0,0,2-2V10a2,2,0,0,0-2-2Z"
transform="translate(0)"
/>
<path d="M4,18H2V4A2,2,0,0,1,4,2H18V4H4Z" transform="translate(0)" /><rect
fill="none"
width="32"
height="32"
/>
</svg>
| chat-ui/src/lib/components/icons/IconCopy.svelte/0 | {
"file_path": "chat-ui/src/lib/components/icons/IconCopy.svelte",
"repo_id": "chat-ui",
"token_count": 299
} | 49 |
import { z } from "zod";
import {
embeddingEndpointTei,
embeddingEndpointTeiParametersSchema,
} from "./tei/embeddingEndpoints";
import {
embeddingEndpointTransformersJS,
embeddingEndpointTransformersJSParametersSchema,
} from "./transformersjs/embeddingEndpoints";
import {
embeddingEndpointOpenAI,
embeddingEndpointOpenAIParametersSchema,
} from "./openai/embeddingEndpoints";
// parameters passed when generating text
interface EmbeddingEndpointParameters {
inputs: string[];
}
export type Embedding = number[];
// type signature for the endpoint
export type EmbeddingEndpoint = (params: EmbeddingEndpointParameters) => Promise<Embedding[]>;
export const embeddingEndpointSchema = z.discriminatedUnion("type", [
embeddingEndpointTeiParametersSchema,
embeddingEndpointTransformersJSParametersSchema,
embeddingEndpointOpenAIParametersSchema,
]);
type EmbeddingEndpointTypeOptions = z.infer<typeof embeddingEndpointSchema>["type"];
// generator function that takes in type discrimantor value for defining the endpoint and return the endpoint
export type EmbeddingEndpointGenerator<T extends EmbeddingEndpointTypeOptions> = (
inputs: Extract<z.infer<typeof embeddingEndpointSchema>, { type: T }>
) => EmbeddingEndpoint | Promise<EmbeddingEndpoint>;
// list of all endpoint generators
export const embeddingEndpoints: {
[Key in EmbeddingEndpointTypeOptions]: EmbeddingEndpointGenerator<Key>;
} = {
tei: embeddingEndpointTei,
transformersjs: embeddingEndpointTransformersJS,
openai: embeddingEndpointOpenAI,
};
export default embeddingEndpoints;
| chat-ui/src/lib/server/embeddingEndpoints/embeddingEndpoints.ts/0 | {
"file_path": "chat-ui/src/lib/server/embeddingEndpoints/embeddingEndpoints.ts",
"repo_id": "chat-ui",
"token_count": 478
} | 50 |
import { smallModel } from "$lib/server/models";
import type { Conversation } from "$lib/types/Conversation";
export async function generateFromDefaultEndpoint({
messages,
preprompt,
}: {
messages: Omit<Conversation["messages"][0], "id">[];
preprompt?: string;
}): Promise<string> {
const endpoint = await smallModel.getEndpoint();
const tokenStream = await endpoint({ messages, preprompt });
for await (const output of tokenStream) {
// if not generated_text is here it means the generation is not done
if (output.generated_text) {
let generated_text = output.generated_text;
for (const stop of [...(smallModel.parameters?.stop ?? []), "<|endoftext|>"]) {
if (generated_text.endsWith(stop)) {
generated_text = generated_text.slice(0, -stop.length).trimEnd();
}
}
return generated_text;
}
}
throw new Error("Generation failed");
}
| chat-ui/src/lib/server/generateFromDefaultEndpoint.ts/0 | {
"file_path": "chat-ui/src/lib/server/generateFromDefaultEndpoint.ts",
"repo_id": "chat-ui",
"token_count": 289
} | 51 |
import { writable } from "svelte/store";
export const ERROR_MESSAGES = {
default: "Oops, something went wrong.",
authOnly: "You have to be logged in.",
rateLimited: "You are sending too many messages. Try again later.",
};
export const error = writable<string | null>(null);
| chat-ui/src/lib/stores/errors.ts/0 | {
"file_path": "chat-ui/src/lib/stores/errors.ts",
"repo_id": "chat-ui",
"token_count": 85
} | 52 |
import type { BackendModel } from "$lib/server/models";
export type Model = Pick<
BackendModel,
| "id"
| "name"
| "displayName"
| "websiteUrl"
| "datasetName"
| "promptExamples"
| "parameters"
| "description"
| "logoUrl"
| "modelUrl"
| "datasetUrl"
| "preprompt"
| "multimodal"
| "unlisted"
>;
| chat-ui/src/lib/types/Model.ts/0 | {
"file_path": "chat-ui/src/lib/types/Model.ts",
"repo_id": "chat-ui",
"token_count": 138
} | 53 |
export function deepestChild(el: HTMLElement): HTMLElement {
if (el.lastElementChild && el.lastElementChild.nodeType !== Node.TEXT_NODE) {
return deepestChild(el.lastElementChild as HTMLElement);
}
return el;
}
| chat-ui/src/lib/utils/deepestChild.ts/0 | {
"file_path": "chat-ui/src/lib/utils/deepestChild.ts",
"repo_id": "chat-ui",
"token_count": 74
} | 54 |
export function sum(nums: number[]): number {
return nums.reduce((a, b) => a + b, 0);
}
| chat-ui/src/lib/utils/sum.ts/0 | {
"file_path": "chat-ui/src/lib/utils/sum.ts",
"repo_id": "chat-ui",
"token_count": 35
} | 55 |
<script lang="ts">
import "../styles/main.css";
import { onDestroy } from "svelte";
import { goto, invalidate } from "$app/navigation";
import { base } from "$app/paths";
import { page } from "$app/stores";
import { browser } from "$app/environment";
import {
PUBLIC_APP_DESCRIPTION,
PUBLIC_ORIGIN,
PUBLIC_PLAUSIBLE_SCRIPT_URL,
} from "$env/static/public";
import { PUBLIC_APP_ASSETS, PUBLIC_APP_NAME } from "$env/static/public";
import { error } from "$lib/stores/errors";
import { createSettingsStore } from "$lib/stores/settings";
import { shareConversation } from "$lib/shareConversation";
import { UrlDependency } from "$lib/types/UrlDependency";
import Toast from "$lib/components/Toast.svelte";
import NavMenu from "$lib/components/NavMenu.svelte";
import MobileNav from "$lib/components/MobileNav.svelte";
import titleUpdate from "$lib/stores/titleUpdate";
import DisclaimerModal from "$lib/components/DisclaimerModal.svelte";
import ExpandNavigation from "$lib/components/ExpandNavigation.svelte";
export let data;
let isNavOpen = false;
let isNavCollapsed = false;
let errorToastTimeout: ReturnType<typeof setTimeout>;
let currentError: string | null;
async function onError() {
// If a new different error comes, wait for the current error to hide first
if ($error && currentError && $error !== currentError) {
clearTimeout(errorToastTimeout);
currentError = null;
await new Promise((resolve) => setTimeout(resolve, 300));
}
currentError = $error;
errorToastTimeout = setTimeout(() => {
$error = null;
currentError = null;
}, 3000);
}
async function deleteConversation(id: string) {
try {
const res = await fetch(`${base}/conversation/${id}`, {
method: "DELETE",
headers: {
"Content-Type": "application/json",
},
});
if (!res.ok) {
$error = "Error while deleting conversation, try again.";
return;
}
if ($page.params.id !== id) {
await invalidate(UrlDependency.ConversationList);
} else {
await goto(`${base}/`, { invalidateAll: true });
}
} catch (err) {
console.error(err);
$error = String(err);
}
}
async function editConversationTitle(id: string, title: string) {
try {
const res = await fetch(`${base}/conversation/${id}`, {
method: "PATCH",
headers: {
"Content-Type": "application/json",
},
body: JSON.stringify({ title }),
});
if (!res.ok) {
$error = "Error while editing title, try again.";
return;
}
await invalidate(UrlDependency.ConversationList);
} catch (err) {
console.error(err);
$error = String(err);
}
}
onDestroy(() => {
clearTimeout(errorToastTimeout);
});
$: if ($error) onError();
$: if ($titleUpdate) {
const convIdx = data.conversations.findIndex(({ id }) => id === $titleUpdate?.convId);
if (convIdx != -1) {
data.conversations[convIdx].title = $titleUpdate?.title ?? data.conversations[convIdx].title;
}
// update data.conversations
data.conversations = [...data.conversations];
$titleUpdate = null;
}
const settings = createSettingsStore(data.settings);
$: if (browser && $page.url.searchParams.has("model")) {
if ($settings.activeModel === $page.url.searchParams.get("model")) {
goto(`${base}/?`);
}
settings.instantSet({
activeModel: $page.url.searchParams.get("model") ?? $settings.activeModel,
});
}
$: mobileNavTitle = ["/models", "/assistants", "/privacy"].includes($page.route.id ?? "")
? ""
: data.conversations.find((conv) => conv.id === $page.params.id)?.title;
</script>
<svelte:head>
<title>{PUBLIC_APP_NAME}</title>
<meta name="description" content="The first open source alternative to ChatGPT. 💪" />
<meta name="twitter:card" content="summary_large_image" />
<meta name="twitter:site" content="@huggingface" />
<!-- use those meta tags everywhere except on the share assistant page -->
<!-- feel free to refacto if there's a better way -->
{#if !$page.url.pathname.includes("/assistant/") && $page.route.id !== "/assistants" && !$page.url.pathname.includes("/models/")}
<meta property="og:title" content={PUBLIC_APP_NAME} />
<meta property="og:type" content="website" />
<meta property="og:url" content="{PUBLIC_ORIGIN || $page.url.origin}{base}" />
<meta
property="og:image"
content="{PUBLIC_ORIGIN || $page.url.origin}{base}/{PUBLIC_APP_ASSETS}/thumbnail.png"
/>
<meta property="og:description" content={PUBLIC_APP_DESCRIPTION} />
{/if}
<link
rel="icon"
href="{PUBLIC_ORIGIN || $page.url.origin}{base}/{PUBLIC_APP_ASSETS}/favicon.ico"
sizes="32x32"
/>
<link
rel="icon"
href="{PUBLIC_ORIGIN || $page.url.origin}{base}/{PUBLIC_APP_ASSETS}/icon.svg"
type="image/svg+xml"
/>
<link
rel="apple-touch-icon"
href="{PUBLIC_ORIGIN || $page.url.origin}{base}/{PUBLIC_APP_ASSETS}/apple-touch-icon.png"
/>
<link
rel="manifest"
href="{PUBLIC_ORIGIN || $page.url.origin}{base}/{PUBLIC_APP_ASSETS}/manifest.json"
/>
{#if PUBLIC_PLAUSIBLE_SCRIPT_URL && PUBLIC_ORIGIN}
<script
defer
data-domain={new URL(PUBLIC_ORIGIN).hostname}
src={PUBLIC_PLAUSIBLE_SCRIPT_URL}
></script>
{/if}
</svelte:head>
{#if !$settings.ethicsModalAccepted && $page.url.pathname !== `${base}/privacy`}
<DisclaimerModal />
{/if}
<ExpandNavigation
isCollapsed={isNavCollapsed}
on:click={() => (isNavCollapsed = !isNavCollapsed)}
classNames="absolute inset-y-0 z-10 my-auto {!isNavCollapsed
? 'left-[280px]'
: 'left-0'} *:transition-transform"
/>
<div
class="grid h-full w-screen grid-cols-1 grid-rows-[auto,1fr] overflow-hidden text-smd {!isNavCollapsed
? 'md:grid-cols-[280px,1fr]'
: 'md:grid-cols-[0px,1fr]'} transition-[300ms] [transition-property:grid-template-columns] md:grid-rows-[1fr] dark:text-gray-300"
>
<MobileNav isOpen={isNavOpen} on:toggle={(ev) => (isNavOpen = ev.detail)} title={mobileNavTitle}>
<NavMenu
conversations={data.conversations}
user={data.user}
canLogin={data.user === undefined && data.loginEnabled}
on:shareConversation={(ev) => shareConversation(ev.detail.id, ev.detail.title)}
on:deleteConversation={(ev) => deleteConversation(ev.detail)}
on:editConversationTitle={(ev) => editConversationTitle(ev.detail.id, ev.detail.title)}
/>
</MobileNav>
<nav
class=" grid max-h-screen grid-cols-1 grid-rows-[auto,1fr,auto] overflow-hidden *:w-[280px] max-md:hidden"
>
<NavMenu
conversations={data.conversations}
user={data.user}
canLogin={data.user === undefined && data.loginEnabled}
on:shareConversation={(ev) => shareConversation(ev.detail.id, ev.detail.title)}
on:deleteConversation={(ev) => deleteConversation(ev.detail)}
on:editConversationTitle={(ev) => editConversationTitle(ev.detail.id, ev.detail.title)}
/>
</nav>
{#if currentError}
<Toast message={currentError} />
{/if}
<slot />
</div>
| chat-ui/src/routes/+layout.svelte/0 | {
"file_path": "chat-ui/src/routes/+layout.svelte",
"repo_id": "chat-ui",
"token_count": 2668
} | 56 |
<script lang="ts">
import ChatWindow from "$lib/components/chat/ChatWindow.svelte";
import { pendingMessage } from "$lib/stores/pendingMessage";
import { isAborted } from "$lib/stores/isAborted";
import { onMount } from "svelte";
import { page } from "$app/stores";
import { goto, invalidateAll } from "$app/navigation";
import { base } from "$app/paths";
import { shareConversation } from "$lib/shareConversation";
import { ERROR_MESSAGES, error } from "$lib/stores/errors";
import { findCurrentModel } from "$lib/utils/models";
import { webSearchParameters } from "$lib/stores/webSearchParameters";
import type { Message } from "$lib/types/Message";
import type { MessageUpdate } from "$lib/types/MessageUpdate";
import titleUpdate from "$lib/stores/titleUpdate";
import file2base64 from "$lib/utils/file2base64";
import { addChildren } from "$lib/utils/tree/addChildren";
import { addSibling } from "$lib/utils/tree/addSibling";
import { createConvTreeStore } from "$lib/stores/convTree";
import type { v4 } from "uuid";
export let data;
$: ({ messages } = data);
let loading = false;
let pending = false;
let files: File[] = [];
async function convFromShared() {
try {
loading = true;
const res = await fetch(`${base}/conversation`, {
method: "POST",
headers: {
"Content-Type": "application/json",
},
body: JSON.stringify({
fromShare: $page.params.id,
model: data.model,
}),
});
if (!res.ok) {
error.set(await res.text());
console.error("Error while creating conversation: " + (await res.text()));
return;
}
const { conversationId } = await res.json();
return conversationId;
} catch (err) {
error.set(ERROR_MESSAGES.default);
console.error(String(err));
throw err;
}
}
// this function is used to send new message to the backends
async function writeMessage({
prompt,
messageId = $convTreeStore.leaf ?? undefined,
isRetry = false,
isContinue = false,
}: {
prompt?: string;
messageId?: ReturnType<typeof v4>;
isRetry?: boolean;
isContinue?: boolean;
}): Promise<void> {
try {
$isAborted = false;
loading = true;
pending = true;
const module = await import("browser-image-resizer");
// currently, only IDEFICS is supported by TGI
// the size of images is hardcoded to 224x224 in TGI
// this will need to be configurable when support for more models is added
const resizedImages = await Promise.all(
files.map(async (file) => {
return await module
.readAndCompressImage(file, {
maxHeight: 224,
maxWidth: 224,
quality: 1,
})
.then(async (el) => await file2base64(el as File));
})
);
let messageToWriteToId: Message["id"] | undefined = undefined;
// used for building the prompt, subtree of the conversation that goes from the latest message to the root
if (isContinue && messageId) {
if ((messages.find((msg) => msg.id === messageId)?.children?.length ?? 0) > 0) {
$error = "Can only continue the last message";
} else {
messageToWriteToId = messageId;
}
} else if (isRetry && messageId) {
// two cases, if we're retrying a user message with a newPrompt set,
// it means we're editing a user message
// if we're retrying on an assistant message, newPrompt cannot be set
// it means we're retrying the last assistant message for a new answer
const messageToRetry = messages.find((message) => message.id === messageId);
if (!messageToRetry) {
$error = "Message not found";
}
if (messageToRetry?.from === "user" && prompt) {
// add a sibling to this message from the user, with the alternative prompt
// add a children to that sibling, where we can write to
const newUserMessageId = addSibling(
{
messages,
rootMessageId: data.rootMessageId,
},
{ from: "user", content: prompt },
messageId
);
messageToWriteToId = addChildren(
{
messages,
rootMessageId: data.rootMessageId,
},
{ from: "assistant", content: "", files: resizedImages },
newUserMessageId
);
} else if (messageToRetry?.from === "assistant") {
// we're retrying an assistant message, to generate a new answer
// just add a sibling to the assistant answer where we can write to
messageToWriteToId = addSibling(
{
messages,
rootMessageId: data.rootMessageId,
},
{ from: "assistant", content: "" },
messageId
);
}
} else {
// just a normal linear conversation, so we add the user message
// and the blank assistant message back to back
const newUserMessageId = addChildren(
{
messages,
rootMessageId: data.rootMessageId,
},
{
from: "user",
content: prompt ?? "",
files: resizedImages,
createdAt: new Date(),
updatedAt: new Date(),
},
messageId
);
if (!data.rootMessageId) {
data.rootMessageId = newUserMessageId;
}
messageToWriteToId = addChildren(
{
messages,
rootMessageId: data.rootMessageId,
},
{
from: "assistant",
content: "",
createdAt: new Date(),
updatedAt: new Date(),
},
newUserMessageId
);
}
messages = [...messages];
const messageToWriteTo = messages.find((message) => message.id === messageToWriteToId);
if (!messageToWriteTo) {
throw new Error("Message to write to not found");
}
// disable websearch if assistant is present
const hasAssistant = !!$page.data.assistant;
const response = await fetch(`${base}/conversation/${$page.params.id}`, {
method: "POST",
headers: { "Content-Type": "application/json" },
body: JSON.stringify({
inputs: prompt,
id: messageId,
is_retry: isRetry,
is_continue: isContinue,
web_search: !hasAssistant && $webSearchParameters.useSearch,
files: isRetry ? undefined : resizedImages,
}),
});
files = [];
if (!response.body) {
throw new Error("Body not defined");
}
if (!response.ok) {
error.set((await response.json())?.message);
return;
}
// eslint-disable-next-line no-undef
const encoder = new TextDecoderStream();
const reader = response?.body?.pipeThrough(encoder).getReader();
let finalAnswer = "";
const messageUpdates: MessageUpdate[] = [];
// set str queue
// ex) if the last response is => {"type": "stream", "token":
// It should be => {"type": "stream", "token": "Hello"} = prev_input_chunk + "Hello"}
let prev_input_chunk = [""];
// this is a bit ugly
// we read the stream until we get the final answer
let readerClosed = false;
reader.closed.then(() => {
readerClosed = true;
});
while (finalAnswer === "") {
// check for abort
if ($isAborted || $error || readerClosed) {
reader?.cancel();
break;
}
// if there is something to read
await reader?.read().then(async ({ done, value }) => {
// we read, if it's done we cancel
if (done) {
reader.cancel();
}
if (!value) {
return;
}
value = prev_input_chunk.pop() + value;
// if it's not done we parse the value, which contains all messages
const inputs = value.split("\n");
inputs.forEach(async (el: string) => {
try {
const update = JSON.parse(el) as MessageUpdate;
if (update.type !== "stream") {
messageUpdates.push(update);
}
if (update.type === "finalAnswer") {
finalAnswer = update.text;
loading = false;
pending = false;
} else if (update.type === "stream") {
pending = false;
messageToWriteTo.content += update.token;
messages = [...messages];
} else if (update.type === "webSearch") {
messageToWriteTo.updates = [...(messageToWriteTo.updates ?? []), update];
messages = [...messages];
} else if (update.type === "status") {
if (update.status === "title" && update.message) {
const convInData = data.conversations.find(({ id }) => id === $page.params.id);
if (convInData) {
convInData.title = update.message;
$titleUpdate = {
title: update.message,
convId: $page.params.id,
};
}
} else if (update.status === "error") {
$error = update.message ?? "An error has occurred";
}
} else if (update.type === "error") {
error.set(update.message);
reader.cancel();
}
} catch (parseError) {
// in case of parsing error we wait for the next message
if (el === inputs[inputs.length - 1]) {
prev_input_chunk.push(el);
}
return;
}
});
});
}
messageToWriteTo.updates = messageUpdates;
} catch (err) {
if (err instanceof Error && err.message.includes("overloaded")) {
$error = "Too much traffic, please try again.";
} else if (err instanceof Error && err.message.includes("429")) {
$error = ERROR_MESSAGES.rateLimited;
} else if (err instanceof Error) {
$error = err.message;
} else {
$error = ERROR_MESSAGES.default;
}
console.error(err);
} finally {
loading = false;
pending = false;
await invalidateAll();
}
}
async function voteMessage(score: Message["score"], messageId: string) {
let conversationId = $page.params.id;
let oldScore: Message["score"] | undefined;
// optimistic update to avoid waiting for the server
messages = messages.map((message) => {
if (message.id === messageId) {
oldScore = message.score;
return { ...message, score };
}
return message;
});
try {
await fetch(`${base}/conversation/${conversationId}/message/${messageId}/vote`, {
method: "POST",
body: JSON.stringify({ score }),
});
} catch {
// revert score on any error
messages = messages.map((message) => {
return message.id !== messageId ? message : { ...message, score: oldScore };
});
}
}
onMount(async () => {
// only used in case of creating new conversations (from the parent POST endpoint)
if ($pendingMessage) {
files = $pendingMessage.files;
await writeMessage({ prompt: $pendingMessage.content });
$pendingMessage = undefined;
}
});
async function onMessage(event: CustomEvent<string>) {
if (!data.shared) {
await writeMessage({ prompt: event.detail });
} else {
await convFromShared()
.then(async (convId) => {
await goto(`${base}/conversation/${convId}`, { invalidateAll: true });
})
.then(async () => await writeMessage({ prompt: event.detail }))
.finally(() => (loading = false));
}
}
async function onRetry(event: CustomEvent<{ id: Message["id"]; content?: string }>) {
if (!data.shared) {
await writeMessage({
prompt: event.detail.content,
messageId: event.detail.id,
isRetry: true,
});
} else {
await convFromShared()
.then(async (convId) => {
await goto(`${base}/conversation/${convId}`, { invalidateAll: true });
})
.then(
async () =>
await writeMessage({
prompt: event.detail.content,
messageId: event.detail.id,
isRetry: true,
})
)
.finally(() => (loading = false));
}
}
async function onContinue(event: CustomEvent<{ id: Message["id"] }>) {
if (!data.shared) {
writeMessage({ messageId: event.detail.id, isContinue: true });
} else {
await convFromShared()
.then(async (convId) => {
await goto(`${base}/conversation/${convId}`, { invalidateAll: true });
})
.then(
async () =>
await writeMessage({
messageId: event.detail.id,
isContinue: true,
})
)
.finally(() => (loading = false));
}
}
$: $page.params.id, (($isAborted = true), (loading = false), ($convTreeStore.editing = null));
$: title = data.conversations.find((conv) => conv.id === $page.params.id)?.title ?? data.title;
const convTreeStore = createConvTreeStore();
</script>
<svelte:head>
<title>{title}</title>
<link
rel="stylesheet"
href="https://cdn.jsdelivr.net/npm/[email protected]/dist/katex.min.css"
integrity="sha384-GvrOXuhMATgEsSwCs4smul74iXGOixntILdUW9XmUC6+HX0sLNAK3q71HotJqlAn"
crossorigin="anonymous"
/>
</svelte:head>
<ChatWindow
{loading}
{pending}
{messages}
shared={data.shared}
preprompt={data.preprompt}
bind:files
on:message={onMessage}
on:retry={onRetry}
on:continue={onContinue}
on:vote={(event) => voteMessage(event.detail.score, event.detail.id)}
on:share={() => shareConversation($page.params.id, data.title)}
on:stop={() => (($isAborted = true), (loading = false))}
models={data.models}
currentModel={findCurrentModel([...data.models, ...data.oldModels], data.model)}
assistant={data.assistant}
/>
| chat-ui/src/routes/conversation/[id]/+page.svelte/0 | {
"file_path": "chat-ui/src/routes/conversation/[id]/+page.svelte",
"repo_id": "chat-ui",
"token_count": 5239
} | 57 |
import ModelThumbnail from "./ModelThumbnail.svelte";
import { redirect, type RequestHandler } from "@sveltejs/kit";
import type { SvelteComponent } from "svelte";
import { Resvg } from "@resvg/resvg-js";
import satori from "satori";
import { html } from "satori-html";
import InterRegular from "../../../../../static/fonts/Inter-Regular.ttf";
import InterBold from "../../../../../static/fonts/Inter-Bold.ttf";
import { base } from "$app/paths";
import { models } from "$lib/server/models";
export const GET: RequestHandler = (async ({ params }) => {
const model = models.find(({ id }) => id === params.model);
if (!model || model.unlisted) {
throw redirect(302, `${base}/`);
}
const renderedComponent = (ModelThumbnail as unknown as SvelteComponent).render({
name: model.name,
logoUrl: model.logoUrl,
});
const reactLike = html(
"<style>" + renderedComponent.css.code + "</style>" + renderedComponent.html
);
const svg = await satori(reactLike, {
width: 1200,
height: 648,
fonts: [
{
name: "Inter",
data: InterRegular as unknown as ArrayBuffer,
weight: 500,
},
{
name: "Inter",
data: InterBold as unknown as ArrayBuffer,
weight: 700,
},
],
});
const png = new Resvg(svg, {
fitTo: { mode: "original" },
})
.render()
.asPng();
return new Response(png, {
headers: {
"Content-Type": "image/png",
},
});
}) satisfies RequestHandler;
| chat-ui/src/routes/models/[...model]/thumbnail.png/+server.ts/0 | {
"file_path": "chat-ui/src/routes/models/[...model]/thumbnail.png/+server.ts",
"repo_id": "chat-ui",
"token_count": 526
} | 58 |
import { base } from "$app/paths";
import { authCondition, requiresUser } from "$lib/server/auth";
import { collections } from "$lib/server/database";
import { fail, type Actions, redirect } from "@sveltejs/kit";
import { ObjectId } from "mongodb";
import { z } from "zod";
import { sha256 } from "$lib/utils/sha256";
import sharp from "sharp";
import { parseStringToList } from "$lib/utils/parseStringToList";
import { usageLimits } from "$lib/server/usageLimits";
import { generateSearchTokens } from "$lib/utils/searchTokens";
const newAsssistantSchema = z.object({
name: z.string().min(1),
modelId: z.string().min(1),
preprompt: z.string().min(1),
description: z.string().optional(),
exampleInput1: z.string().optional(),
exampleInput2: z.string().optional(),
exampleInput3: z.string().optional(),
exampleInput4: z.string().optional(),
avatar: z.instanceof(File).optional(),
ragLinkList: z.preprocess(parseStringToList, z.string().url().array().max(10)),
ragDomainList: z.preprocess(parseStringToList, z.string().array()),
ragAllowAll: z.preprocess((v) => v === "true", z.boolean()),
});
const uploadAvatar = async (avatar: File, assistantId: ObjectId): Promise<string> => {
const hash = await sha256(await avatar.text());
const upload = collections.bucket.openUploadStream(`${assistantId.toString()}`, {
metadata: { type: avatar.type, hash },
});
upload.write((await avatar.arrayBuffer()) as unknown as Buffer);
upload.end();
// only return the filename when upload throws a finish event or a 10s time out occurs
return new Promise((resolve, reject) => {
upload.once("finish", () => resolve(hash));
upload.once("error", reject);
setTimeout(() => reject(new Error("Upload timed out")), 10000);
});
};
export const actions: Actions = {
default: async ({ request, locals }) => {
const formData = Object.fromEntries(await request.formData());
const parse = newAsssistantSchema.safeParse(formData);
if (!parse.success) {
// Loop through the errors array and create a custom errors array
const errors = parse.error.errors.map((error) => {
return {
field: error.path[0],
message: error.message,
};
});
return fail(400, { error: true, errors });
}
// can only create assistants when logged in, IF login is setup
if (!locals.user && requiresUser) {
const errors = [{ field: "preprompt", message: "Must be logged in. Unauthorized" }];
return fail(400, { error: true, errors });
}
const assistantsCount = await collections.assistants.countDocuments(authCondition(locals));
if (usageLimits?.assistants && assistantsCount > usageLimits.assistants) {
const errors = [
{
field: "preprompt",
message: "You have reached the maximum number of assistants. Delete some to continue.",
},
];
return fail(400, { error: true, errors });
}
const createdById = locals.user?._id ?? locals.sessionId;
const newAssistantId = new ObjectId();
const exampleInputs: string[] = [
parse?.data?.exampleInput1 ?? "",
parse?.data?.exampleInput2 ?? "",
parse?.data?.exampleInput3 ?? "",
parse?.data?.exampleInput4 ?? "",
].filter((input) => !!input);
let hash;
if (parse.data.avatar && parse.data.avatar.size > 0) {
let image;
try {
image = await sharp(await parse.data.avatar.arrayBuffer())
.resize(512, 512, { fit: "inside" })
.jpeg({ quality: 80 })
.toBuffer();
} catch (e) {
const errors = [{ field: "avatar", message: (e as Error).message }];
return fail(400, { error: true, errors });
}
hash = await uploadAvatar(new File([image], "avatar.jpg"), newAssistantId);
}
const { insertedId } = await collections.assistants.insertOne({
_id: newAssistantId,
createdById,
createdByName: locals.user?.username ?? locals.user?.name,
...parse.data,
exampleInputs,
avatar: hash,
createdAt: new Date(),
updatedAt: new Date(),
userCount: 1,
featured: false,
rag: {
allowedLinks: parse.data.ragLinkList,
allowedDomains: parse.data.ragDomainList,
allowAllDomains: parse.data.ragAllowAll,
},
searchTokens: generateSearchTokens(parse.data.name),
});
// add insertedId to user settings
await collections.settings.updateOne(authCondition(locals), {
$addToSet: { assistants: insertedId },
});
throw redirect(302, `${base}/settings/assistants/${insertedId}`);
},
};
| chat-ui/src/routes/settings/(nav)/assistants/new/+page.server.ts/0 | {
"file_path": "chat-ui/src/routes/settings/(nav)/assistants/new/+page.server.ts",
"repo_id": "chat-ui",
"token_count": 1538
} | 59 |
<svg xmlns="http://www.w3.org/2000/svg" width="32" height="32" fill="none">
<path
fill="#FFD21E"
d="M4 15.55C4 9.72 8.72 5 14.55 5h4.11a9.34 9.34 0 1 1 0 18.68H7.58l-2.89 2.8a.41.41 0 0 1-.69-.3V15.55Z"
/>
<path
fill="#32343D"
d="M19.63 12.48c.37.14.52.9.9.7.71-.38.98-1.27.6-1.98a1.46 1.46 0 0 0-1.98-.61 1.47 1.47 0 0 0-.6 1.99c.17.34.74-.21 1.08-.1ZM12.72 12.48c-.37.14-.52.9-.9.7a1.47 1.47 0 0 1-.6-1.98 1.46 1.46 0 0 1 1.98-.61c.71.38.98 1.27.6 1.99-.18.34-.74-.21-1.08-.1ZM16.24 19.55c2.89 0 3.82-2.58 3.82-3.9 0-1.33-1.71.7-3.82.7-2.1 0-3.8-2.03-3.8-.7 0 1.32.92 3.9 3.8 3.9Z"
/>
<path
fill="#FF323D"
d="M18.56 18.8c-.57.44-1.33.75-2.32.75-.92 0-1.65-.27-2.2-.68.3-.63.87-1.11 1.55-1.32.12-.03.24.17.36.38.12.2.24.4.37.4s.26-.2.39-.4.26-.4.38-.36a2.56 2.56 0 0 1 1.47 1.23Z"
/>
</svg>
| chat-ui/static/huggingchat/logo.svg/0 | {
"file_path": "chat-ui/static/huggingchat/logo.svg",
"repo_id": "chat-ui",
"token_count": 523
} | 60 |
# This first_section was backported from nginx
loading_datasets: loading
share_dataset: share
quicktour: quickstart
dataset_streaming: stream
torch_tensorflow: use_dataset
splits: loading#slice-splits
processing: process
faiss_and_ea: faiss_es
features: about_dataset_features
using_metrics: how_to_metrics
exploring: access
package_reference/logging_methods: package_reference/utilities
# end of first_section
| datasets/docs/source/_redirects.yml/0 | {
"file_path": "datasets/docs/source/_redirects.yml",
"repo_id": "datasets",
"token_count": 134
} | 61 |
# Create a dataset card
Each dataset should have a dataset card to promote responsible usage and inform users of any potential biases within the dataset.
This idea was inspired by the Model Cards proposed by [Mitchell, 2018](https://arxiv.org/abs/1810.03993).
Dataset cards help users understand a dataset's contents, the context for using the dataset, how it was created, and any other considerations a user should be aware of.
Creating a dataset card is easy and can be done in a just a few steps:
1. Go to your dataset repository on the [Hub](https://hf.co/new-dataset) and click on **Create Dataset Card** to create a new `README.md` file in your repository.
2. Use the **Metadata UI** to select the tags that describe your dataset. You can add a license, language, pretty_name, the task_categories, size_categories, and any other tags that you think are relevant. These tags help users discover and find your dataset on the Hub.
<div class="flex justify-center">
<img class="block dark:hidden" src="https://huggingface.co/datasets/huggingface/documentation-images/resolve/main/hub/datasets-metadata-ui.png"/>
<img class="hidden dark:block" src="https://huggingface.co/datasets/huggingface/documentation-images/resolve/main/hub/datasets-metadata-ui-dark.png"/>
</div>
<Tip>
For a complete, but not required, set of tag options you can also look at the [Dataset Card specifications](https://github.com/huggingface/hub-docs/blob/main/datasetcard.md?plain=1). This'll have a few more tag options like `multilinguality` and `language_creators` which are useful but not absolutely necessary.
</Tip>
3. Click on the **Import dataset card template** link to automatically create a template with all the relevant fields to complete. Fill out the template sections to the best of your ability. Take a look at the [Dataset Card Creation Guide](https://github.com/huggingface/datasets/blob/main/templates/README_guide.md) for more detailed information about what to include in each section of the card. For fields you are unable to complete, you can write **[More Information Needed]**.
4. Once you're done, commit the changes to the `README.md` file and you'll see the completed dataset card on your repository.
YAML also allows you to customize the way your dataset is loaded by [defining splits and/or configurations](./repository_structure#define-your-splits-and-subsets-in-yaml) without the need to write any code.
Feel free to take a look at the [SNLI](https://huggingface.co/datasets/snli), [CNN/DailyMail](https://huggingface.co/datasets/cnn_dailymail), and [Allociné](https://huggingface.co/datasets/allocine) dataset cards as examples to help you get started.
| datasets/docs/source/dataset_card.mdx/0 | {
"file_path": "datasets/docs/source/dataset_card.mdx",
"repo_id": "datasets",
"token_count": 757
} | 62 |
# Load
Your data can be stored in various places; they can be on your local machine's disk, in a Github repository, and in in-memory data structures like Python dictionaries and Pandas DataFrames. Wherever a dataset is stored, 🤗 Datasets can help you load it.
This guide will show you how to load a dataset from:
- The Hub without a dataset loading script
- Local loading script
- Local files
- In-memory data
- Offline
- A specific slice of a split
For more details specific to loading other dataset modalities, take a look at the <a class="underline decoration-pink-400 decoration-2 font-semibold" href="./audio_load">load audio dataset guide</a>, the <a class="underline decoration-yellow-400 decoration-2 font-semibold" href="./image_load">load image dataset guide</a>, or the <a class="underline decoration-green-400 decoration-2 font-semibold" href="./nlp_load">load text dataset guide</a>.
<a id='load-from-the-hub'></a>
## Hugging Face Hub
Datasets are loaded from a dataset loading script that downloads and generates the dataset. However, you can also load a dataset from any dataset repository on the Hub without a loading script! Begin by [creating a dataset repository](share#create-the-repository) and upload your data files. Now you can use the [`load_dataset`] function to load the dataset.
For example, try loading the files from this [demo repository](https://huggingface.co/datasets/lhoestq/demo1) by providing the repository namespace and dataset name. This dataset repository contains CSV files, and the code below loads the dataset from the CSV files:
```py
>>> from datasets import load_dataset
>>> dataset = load_dataset("lhoestq/demo1")
```
Some datasets may have more than one version based on Git tags, branches, or commits. Use the `revision` parameter to specify the dataset version you want to load:
```py
>>> dataset = load_dataset(
... "lhoestq/custom_squad",
... revision="main" # tag name, or branch name, or commit hash
... )
```
<Tip>
Refer to the [Upload a dataset to the Hub](./upload_dataset) tutorial for more details on how to create a dataset repository on the Hub, and how to upload your data files.
</Tip>
A dataset without a loading script by default loads all the data into the `train` split. Use the `data_files` parameter to map data files to splits like `train`, `validation` and `test`:
```py
>>> data_files = {"train": "train.csv", "test": "test.csv"}
>>> dataset = load_dataset("namespace/your_dataset_name", data_files=data_files)
```
<Tip warning={true}>
If you don't specify which data files to use, [`load_dataset`] will return all the data files. This can take a long time if you load a large dataset like C4, which is approximately 13TB of data.
</Tip>
You can also load a specific subset of the files with the `data_files` or `data_dir` parameter. These parameters can accept a relative path which resolves to the base path corresponding to where the dataset is loaded from.
```py
>>> from datasets import load_dataset
# load files that match the grep pattern
>>> c4_subset = load_dataset("allenai/c4", data_files="en/c4-train.0000*-of-01024.json.gz")
# load dataset from the en directory on the Hub
>>> c4_subset = load_dataset("allenai/c4", data_dir="en")
```
The `split` parameter can also map a data file to a specific split:
```py
>>> data_files = {"validation": "en/c4-validation.*.json.gz"}
>>> c4_validation = load_dataset("allenai/c4", data_files=data_files, split="validation")
```
## Local loading script
You may have a 🤗 Datasets loading script locally on your computer. In this case, load the dataset by passing one of the following paths to [`load_dataset`]:
- The local path to the loading script file.
- The local path to the directory containing the loading script file (only if the script file has the same name as the directory).
Pass `trust_remote_code=True` to allow 🤗 Datasets to execute the loading script:
```py
>>> dataset = load_dataset("path/to/local/loading_script/loading_script.py", split="train", trust_remote_code=True)
>>> dataset = load_dataset("path/to/local/loading_script", split="train", trust_remote_code=True) # equivalent because the file has the same name as the directory
```
### Edit loading script
You can also edit a loading script from the Hub to add your own modifications. Download the dataset repository locally so any data files referenced by a relative path in the loading script can be loaded:
```bash
git clone https://huggingface.co/datasets/eli5
```
Make your edits to the loading script and then load it by passing its local path to [`~datasets.load_dataset`]:
```py
>>> from datasets import load_dataset
>>> eli5 = load_dataset("path/to/local/eli5")
```
## Local and remote files
Datasets can be loaded from local files stored on your computer and from remote files. The datasets are most likely stored as a `csv`, `json`, `txt` or `parquet` file. The [`load_dataset`] function can load each of these file types.
### CSV
🤗 Datasets can read a dataset made up of one or several CSV files (in this case, pass your CSV files as a list):
```py
>>> from datasets import load_dataset
>>> dataset = load_dataset("csv", data_files="my_file.csv")
```
<Tip>
For more details, check out the [how to load tabular datasets from CSV files](tabular_load#csv-files) guide.
</Tip>
### JSON
JSON files are loaded directly with [`load_dataset`] as shown below:
```py
>>> from datasets import load_dataset
>>> dataset = load_dataset("json", data_files="my_file.json")
```
JSON files have diverse formats, but we think the most efficient format is to have multiple JSON objects; each line represents an individual row of data. For example:
```json
{"a": 1, "b": 2.0, "c": "foo", "d": false}
{"a": 4, "b": -5.5, "c": null, "d": true}
```
Another JSON format you may encounter is a nested field, in which case you'll need to specify the `field` argument as shown in the following:
```py
{"version": "0.1.0",
"data": [{"a": 1, "b": 2.0, "c": "foo", "d": false},
{"a": 4, "b": -5.5, "c": null, "d": true}]
}
>>> from datasets import load_dataset
>>> dataset = load_dataset("json", data_files="my_file.json", field="data")
```
To load remote JSON files via HTTP, pass the URLs instead:
```py
>>> base_url = "https://rajpurkar.github.io/SQuAD-explorer/dataset/"
>>> dataset = load_dataset("json", data_files={"train": base_url + "train-v1.1.json", "validation": base_url + "dev-v1.1.json"}, field="data")
```
While these are the most common JSON formats, you'll see other datasets that are formatted differently. 🤗 Datasets recognizes these other formats and will fallback accordingly on the Python JSON loading methods to handle them.
### Parquet
Parquet files are stored in a columnar format, unlike row-based files like a CSV. Large datasets may be stored in a Parquet file because it is more efficient and faster at returning your query.
To load a Parquet file:
```py
>>> from datasets import load_dataset
>>> dataset = load_dataset("parquet", data_files={'train': 'train.parquet', 'test': 'test.parquet'})
```
To load remote Parquet files via HTTP, pass the URLs instead:
```py
>>> base_url = "https://storage.googleapis.com/huggingface-nlp/cache/datasets/wikipedia/20200501.en/1.0.0/"
>>> data_files = {"train": base_url + "wikipedia-train.parquet"}
>>> wiki = load_dataset("parquet", data_files=data_files, split="train")
```
### Arrow
Arrow files are stored in an in-memory columnar format, unlike row-based formats like CSV and uncompressed formats like Parquet.
To load an Arrow file:
```py
>>> from datasets import load_dataset
>>> dataset = load_dataset("arrow", data_files={'train': 'train.arrow', 'test': 'test.arrow'})
```
To load remote Arrow files via HTTP, pass the URLs instead:
```py
>>> base_url = "https://storage.googleapis.com/huggingface-nlp/cache/datasets/wikipedia/20200501.en/1.0.0/"
>>> data_files = {"train": base_url + "wikipedia-train.arrow"}
>>> wiki = load_dataset("arrow", data_files=data_files, split="train")
```
Arrow is the file format used by 🤗 Datasets under the hood, therefore you can load a local Arrow file using [`Dataset.from_file`] directly:
```py
>>> from datasets import Dataset
>>> dataset = Dataset.from_file("data.arrow")
```
Unlike [`load_dataset`], [`Dataset.from_file`] memory maps the Arrow file without preparing the dataset in the cache, saving you disk space.
The cache directory to store intermediate processing results will be the Arrow file directory in that case.
For now only the Arrow streaming format is supported. The Arrow IPC file format (also known as Feather V2) is not supported.
### SQL
Read database contents with [`~datasets.Dataset.from_sql`] by specifying the URI to connect to your database. You can read both table names and queries:
```py
>>> from datasets import Dataset
# load entire table
>>> dataset = Dataset.from_sql("data_table_name", con="sqlite:///sqlite_file.db")
# load from query
>>> dataset = Dataset.from_sql("SELECT text FROM table WHERE length(text) > 100 LIMIT 10", con="sqlite:///sqlite_file.db")
```
<Tip>
For more details, check out the [how to load tabular datasets from SQL databases](tabular_load#databases) guide.
</Tip>
### WebDataset
The [WebDataset](https://github.com/webdataset/webdataset) format is based on TAR archives and is suitable for big image datasets.
Because of their size, WebDatasets are generally loaded in streaming mode (using `streaming=True`).
You can load a WebDataset like this:
```python
>>> from datasets import load_dataset
>>>
>>> path = "path/to/train/*.tar"
>>> dataset = load_dataset("webdataset", data_files={"train": path}, split="train", streaming=True)
```
To load remote WebDatasets via HTTP, pass the URLs instead:
```python
>>> from datasets import load_dataset
>>>
>>> base_url = "https://huggingface.co/datasets/lhoestq/small-publaynet-wds/resolve/main/publaynet-train-{i:06d}.tar"
>>> urls = [base_url.format(i=i) for i in range(4)]
>>> dataset = load_dataset("webdataset", data_files={"train": urls}, split="train", streaming=True)
```
## Multiprocessing
When a dataset is made of several files (that we call "shards"), it is possible to significantly speed up the dataset downloading and preparation step.
You can choose how many processes you'd like to use to prepare a dataset in parallel using `num_proc`.
In this case, each process is given a subset of shards to prepare:
```python
from datasets import load_dataset
imagenet = load_dataset("imagenet-1k", num_proc=8)
ml_librispeech_spanish = load_dataset("facebook/multilingual_librispeech", "spanish", num_proc=8)
```
## In-memory data
🤗 Datasets will also allow you to create a [`Dataset`] directly from in-memory data structures like Python dictionaries and Pandas DataFrames.
### Python dictionary
Load Python dictionaries with [`~Dataset.from_dict`]:
```py
>>> from datasets import Dataset
>>> my_dict = {"a": [1, 2, 3]}
>>> dataset = Dataset.from_dict(my_dict)
```
### Python list of dictionaries
Load a list of Python dictionaries with [`~Dataset.from_list`]:
```py
>>> from datasets import Dataset
>>> my_list = [{"a": 1}, {"a": 2}, {"a": 3}]
>>> dataset = Dataset.from_list(my_list)
```
### Python generator
Create a dataset from a Python generator with [`~Dataset.from_generator`]:
```py
>>> from datasets import Dataset
>>> def my_gen():
... for i in range(1, 4):
... yield {"a": i}
...
>>> dataset = Dataset.from_generator(my_gen)
```
This approach supports loading data larger than available memory.
You can also define a sharded dataset by passing lists to `gen_kwargs`:
```py
>>> def gen(shards):
... for shard in shards:
... with open(shard) as f:
... for line in f:
... yield {"line": line}
...
>>> shards = [f"data{i}.txt" for i in range(32)]
>>> ds = IterableDataset.from_generator(gen, gen_kwargs={"shards": shards})
>>> ds = ds.shuffle(seed=42, buffer_size=10_000) # shuffles the shards order + uses a shuffle buffer
>>> from torch.utils.data import DataLoader
>>> dataloader = DataLoader(ds.with_format("torch"), num_workers=4) # give each worker a subset of 32/4=8 shards
```
### Pandas DataFrame
Load Pandas DataFrames with [`~Dataset.from_pandas`]:
```py
>>> from datasets import Dataset
>>> import pandas as pd
>>> df = pd.DataFrame({"a": [1, 2, 3]})
>>> dataset = Dataset.from_pandas(df)
```
<Tip>
For more details, check out the [how to load tabular datasets from Pandas DataFrames](tabular_load#pandas-dataframes) guide.
</Tip>
## Offline
Even if you don't have an internet connection, it is still possible to load a dataset. As long as you've downloaded a dataset from the Hub repository before, it should be cached. This means you can reload the dataset from the cache and use it offline.
If you know you won't have internet access, you can run 🤗 Datasets in full offline mode. This saves time because instead of waiting for the Dataset builder download to time out, 🤗 Datasets will look directly in the cache. Set the environment variable `HF_DATASETS_OFFLINE` to `1` to enable full offline mode.
## Slice splits
You can also choose only to load specific slices of a split. There are two options for slicing a split: using strings or the [`ReadInstruction`] API. Strings are more compact and readable for simple cases, while [`ReadInstruction`] is easier to use with variable slicing parameters.
Concatenate a `train` and `test` split by:
```py
>>> train_test_ds = datasets.load_dataset("bookcorpus", split="train+test")
===STRINGAPI-READINSTRUCTION-SPLIT===
>>> ri = datasets.ReadInstruction("train") + datasets.ReadInstruction("test")
>>> train_test_ds = datasets.load_dataset("bookcorpus", split=ri)
```
Select specific rows of the `train` split:
```py
>>> train_10_20_ds = datasets.load_dataset("bookcorpus", split="train[10:20]")
===STRINGAPI-READINSTRUCTION-SPLIT===
>>> train_10_20_ds = datasets.load_dataset("bookcorpu", split=datasets.ReadInstruction("train", from_=10, to=20, unit="abs"))
```
Or select a percentage of a split with:
```py
>>> train_10pct_ds = datasets.load_dataset("bookcorpus", split="train[:10%]")
===STRINGAPI-READINSTRUCTION-SPLIT===
>>> train_10_20_ds = datasets.load_dataset("bookcorpus", split=datasets.ReadInstruction("train", to=10, unit="%"))
```
Select a combination of percentages from each split:
```py
>>> train_10_80pct_ds = datasets.load_dataset("bookcorpus", split="train[:10%]+train[-80%:]")
===STRINGAPI-READINSTRUCTION-SPLIT===
>>> ri = (datasets.ReadInstruction("train", to=10, unit="%") + datasets.ReadInstruction("train", from_=-80, unit="%"))
>>> train_10_80pct_ds = datasets.load_dataset("bookcorpus", split=ri)
```
Finally, you can even create cross-validated splits. The example below creates 10-fold cross-validated splits. Each validation dataset is a 10% chunk, and the training dataset makes up the remaining complementary 90% chunk:
```py
>>> val_ds = datasets.load_dataset("bookcorpus", split=[f"train[{k}%:{k+10}%]" for k in range(0, 100, 10)])
>>> train_ds = datasets.load_dataset("bookcorpus", split=[f"train[:{k}%]+train[{k+10}%:]" for k in range(0, 100, 10)])
===STRINGAPI-READINSTRUCTION-SPLIT===
>>> val_ds = datasets.load_dataset("bookcorpus", [datasets.ReadInstruction("train", from_=k, to=k+10, unit="%") for k in range(0, 100, 10)])
>>> train_ds = datasets.load_dataset("bookcorpus", [(datasets.ReadInstruction("train", to=k, unit="%") + datasets.ReadInstruction("train", from_=k+10, unit="%")) for k in range(0, 100, 10)])
```
### Percent slicing and rounding
The default behavior is to round the boundaries to the nearest integer for datasets where the requested slice boundaries do not divide evenly by 100. As shown below, some slices may contain more examples than others. For instance, if the following train split includes 999 records, then:
```py
# 19 records, from 500 (included) to 519 (excluded).
>>> train_50_52_ds = datasets.load_dataset("bookcorpus", split="train[50%:52%]")
# 20 records, from 519 (included) to 539 (excluded).
>>> train_52_54_ds = datasets.load_dataset("bookcorpus", split="train[52%:54%]")
```
If you want equal sized splits, use `pct1_dropremainder` rounding instead. This treats the specified percentage boundaries as multiples of 1%.
```py
# 18 records, from 450 (included) to 468 (excluded).
>>> train_50_52pct1_ds = datasets.load_dataset("bookcorpus", split=datasets.ReadInstruction("train", from_=50, to=52, unit="%", rounding="pct1_dropremainder"))
# 18 records, from 468 (included) to 486 (excluded).
>>> train_52_54pct1_ds = datasets.load_dataset("bookcorpus", split=datasets.ReadInstruction("train",from_=52, to=54, unit="%", rounding="pct1_dropremainder"))
# Or equivalently:
>>> train_50_52pct1_ds = datasets.load_dataset("bookcorpus", split="train[50%:52%](pct1_dropremainder)")
>>> train_52_54pct1_ds = datasets.load_dataset("bookcorpus", split="train[52%:54%](pct1_dropremainder)")
```
<Tip warning={true}>
`pct1_dropremainder` rounding may truncate the last examples in a dataset if the number of examples in your dataset don't divide evenly by 100.
</Tip>
<a id='troubleshoot'></a>
## Troubleshooting
Sometimes, you may get unexpected results when you load a dataset. Two of the most common issues you may encounter are manually downloading a dataset and specifying features of a dataset.
### Manual download
Certain datasets require you to manually download the dataset files due to licensing incompatibility or if the files are hidden behind a login page. This causes [`load_dataset`] to throw an `AssertionError`. But 🤗 Datasets provides detailed instructions for downloading the missing files. After you've downloaded the files, use the `data_dir` argument to specify the path to the files you just downloaded.
For example, if you try to download a configuration from the [MATINF](https://huggingface.co/datasets/matinf) dataset:
```py
>>> dataset = load_dataset("matinf", "summarization")
Downloading and preparing dataset matinf/summarization (download: Unknown size, generated: 246.89 MiB, post-processed: Unknown size, total: 246.89 MiB) to /root/.cache/huggingface/datasets/matinf/summarization/1.0.0/82eee5e71c3ceaf20d909bca36ff237452b4e4ab195d3be7ee1c78b53e6f540e...
AssertionError: The dataset matinf with config summarization requires manual data.
Please follow the manual download instructions: To use MATINF you have to download it manually. Please fill this google form (https://forms.gle/nkH4LVE4iNQeDzsc9). You will receive a download link and a password once you complete the form. Please extract all files in one folder and load the dataset with: *datasets.load_dataset('matinf', data_dir='path/to/folder/folder_name')*.
Manual data can be loaded with `datasets.load_dataset(matinf, data_dir='<path/to/manual/data>')
```
If you've already downloaded a dataset from the *Hub with a loading script* to your computer, then you need to pass an absolute path to the `data_dir` or `data_files` parameter to load that dataset. Otherwise, if you pass a relative path, [`load_dataset`] will load the directory from the repository on the Hub instead of the local directory.
### Specify features
When you create a dataset from local files, the [`Features`] are automatically inferred by [Apache Arrow](https://arrow.apache.org/docs/). However, the dataset's features may not always align with your expectations, or you may want to define the features yourself. The following example shows how you can add custom labels with the [`ClassLabel`] feature.
Start by defining your own labels with the [`Features`] class:
```py
>>> class_names = ["sadness", "joy", "love", "anger", "fear", "surprise"]
>>> emotion_features = Features({'text': Value('string'), 'label': ClassLabel(names=class_names)})
```
Next, specify the `features` parameter in [`load_dataset`] with the features you just created:
```py
>>> dataset = load_dataset('csv', data_files=file_dict, delimiter=';', column_names=['text', 'label'], features=emotion_features)
```
Now when you look at your dataset features, you can see it uses the custom labels you defined:
```py
>>> dataset['train'].features
{'text': Value(dtype='string', id=None),
'label': ClassLabel(num_classes=6, names=['sadness', 'joy', 'love', 'anger', 'fear', 'surprise'], names_file=None, id=None)}
```
## Metrics
<Tip warning={true}>
Metrics is deprecated in 🤗 Datasets. To learn more about how to use metrics, take a look at the library 🤗 [Evaluate](https://huggingface.co/docs/evaluate/index)! In addition to metrics, you can find more tools for evaluating models and datasets.
</Tip>
When the metric you want to use is not supported by 🤗 Datasets, you can write and use your own metric script. Load your metric by providing the path to your local metric loading script:
```py
>>> from datasets import load_metric
>>> metric = load_metric('PATH/TO/MY/METRIC/SCRIPT')
>>> # Example of typical usage
>>> for batch in dataset:
... inputs, references = batch
... predictions = model(inputs)
... metric.add_batch(predictions=predictions, references=references)
>>> score = metric.compute()
```
<Tip>
See the [Metrics](./how_to_metrics#custom-metric-loading-script) guide for more details on how to write your own metric loading script.
</Tip>
### Load configurations
It is possible for a metric to have different configurations. The configurations are stored in the `config_name` parameter in [`MetricInfo`] attribute. When you load a metric, provide the configuration name as shown in the following:
```
>>> from datasets import load_metric
>>> metric = load_metric('bleurt', name='bleurt-base-128')
>>> metric = load_metric('bleurt', name='bleurt-base-512')
```
### Distributed setup
When working in a distributed or parallel processing environment, loading and computing a metric can be tricky because these processes are executed in parallel on separate subsets of the data. 🤗 Datasets supports distributed usage with a few additional arguments when you load a metric.
For example, imagine you are training and evaluating on eight parallel processes. Here's how you would load a metric in this distributed setting:
1. Define the total number of processes with the `num_process` argument.
2. Set the process `rank` as an integer between zero and `num_process - 1`.
3. Load your metric with [`load_metric`] with these arguments:
```py
>>> from datasets import load_metric
>>> metric = load_metric('glue', 'mrpc', num_process=num_process, process_id=rank)
```
<Tip>
Once you've loaded a metric for distributed usage, you can compute the metric as usual. Behind the scenes, [`Metric.compute`] gathers all the predictions and references from the nodes, and computes the final metric.
</Tip>
In some instances, you may be simultaneously running multiple independent distributed evaluations on the same server and files. To avoid any conflicts, it is important to provide an `experiment_id` to distinguish the separate evaluations:
```py
>>> from datasets import load_metric
>>> metric = load_metric('glue', 'mrpc', num_process=num_process, process_id=process_id, experiment_id="My_experiment_10")
```
| datasets/docs/source/loading.mdx/0 | {
"file_path": "datasets/docs/source/loading.mdx",
"repo_id": "datasets",
"token_count": 7158
} | 63 |
# Stream
Dataset streaming lets you work with a dataset without downloading it.
The data is streamed as you iterate over the dataset.
This is especially helpful when:
- You don't want to wait for an extremely large dataset to download.
- The dataset size exceeds the amount of available disk space on your computer.
- You want to quickly explore just a few samples of a dataset.
<div class="flex justify-center">
<img class="block dark:hidden" src="https://huggingface.co/datasets/huggingface/documentation-images/resolve/main/datasets/streaming.gif"/>
<img class="hidden dark:block" src="https://huggingface.co/datasets/huggingface/documentation-images/resolve/main/datasets/streaming-dark.gif"/>
</div>
For example, the English split of the [oscar-corpus/OSCAR-2201](https://huggingface.co/datasets/oscar-corpus/OSCAR-2201) dataset is 1.2 terabytes, but you can use it instantly with streaming. Stream a dataset by setting `streaming=True` in [`load_dataset`] as shown below:
```py
>>> from datasets import load_dataset
>>> dataset = load_dataset('oscar-corpus/OSCAR-2201', 'en', split='train', streaming=True)
>>> print(next(iter(dataset)))
{'id': 0, 'text': 'Founded in 2015, Golden Bees is a leading programmatic recruitment platform dedicated to employers, HR agencies and job boards. The company has developed unique HR-custom technologies and predictive algorithms to identify and attract the best candidates for a job opportunity.', ...
```
Dataset streaming also lets you work with a dataset made of local files without doing any conversion.
In this case, the data is streamed from the local files as you iterate over the dataset.
This is especially helpful when:
- You don't want to wait for an extremely large local dataset to be converted to Arrow.
- The converted files size would exceed the amount of available disk space on your computer.
- You want to quickly explore just a few samples of a dataset.
For example, you can stream a local dataset of hundreds of compressed JSONL files like [oscar-corpus/OSCAR-2201](https://huggingface.co/datasets/oscar-corpus/OSCAR-2201) to use it instantly:
```py
>>> from datasets import load_dataset
>>> data_files = {'train': 'path/to/OSCAR-2201/compressed/en_meta/*.jsonl.gz'}
>>> dataset = load_dataset('json', data_files=data_files, split='train', streaming=True)
>>> print(next(iter(dataset)))
{'id': 0, 'text': 'Founded in 2015, Golden Bees is a leading programmatic recruitment platform dedicated to employers, HR agencies and job boards. The company has developed unique HR-custom technologies and predictive algorithms to identify and attract the best candidates for a job opportunity.', ...
```
Loading a dataset in streaming mode creates a new dataset type instance (instead of the classic [`Dataset`] object), known as an [`IterableDataset`].
This special type of dataset has its own set of processing methods shown below.
<Tip>
An [`IterableDataset`] is useful for iterative jobs like training a model.
You shouldn't use a [`IterableDataset`] for jobs that require random access to examples because you have to iterate all over it using a for loop. Getting the last example in an iterable dataset would require you to iterate over all the previous examples.
You can find more details in the [Dataset vs. IterableDataset guide](./about_mapstyle_vs_iterable).
</Tip>
## Convert from a Dataset
If you have an existing [`Dataset`] object, you can convert it to an [`IterableDataset`] with the [`~Dataset.to_iterable_dataset`] function. This is actually faster than setting the `streaming=True` argument in [`load_dataset`] because the data is streamed from local files.
```py
>>> from datasets import load_dataset
# faster 🐇
>>> dataset = load_dataset("food101")
>>> iterable_dataset = dataset.to_iterable_dataset()
# slower 🐢
>>> iterable_dataset = load_dataset("food101", streaming=True)
```
The [`~Dataset.to_iterable_dataset`] function supports sharding when the [`IterableDataset`] is instantiated. This is useful when working with big datasets, and you'd like to shuffle the dataset or to enable fast parallel loading with a PyTorch DataLoader.
```py
>>> import torch
>>> from datasets import load_dataset
>>> dataset = load_dataset("food101")
>>> iterable_dataset = dataset.to_iterable_dataset(num_shards=64) # shard the dataset
>>> iterable_dataset = iterable_dataset.shuffle(buffer_size=10_000) # shuffles the shards order and use a shuffle buffer when you start iterating
dataloader = torch.utils.data.DataLoader(iterable_dataset, num_workers=4) # assigns 64 / 4 = 16 shards from the shuffled list of shards to each worker when you start iterating
```
## Shuffle
Like a regular [`Dataset`] object, you can also shuffle a [`IterableDataset`] with [`IterableDataset.shuffle`].
The `buffer_size` argument controls the size of the buffer to randomly sample examples from. Let's say your dataset has one million examples, and you set the `buffer_size` to ten thousand. [`IterableDataset.shuffle`] will randomly select examples from the first ten thousand examples in the buffer. Selected examples in the buffer are replaced with new examples. By default, the buffer size is 1,000.
```py
>>> from datasets import load_dataset
>>> dataset = load_dataset('oscar', "unshuffled_deduplicated_en", split='train', streaming=True)
>>> shuffled_dataset = dataset.shuffle(seed=42, buffer_size=10_000)
```
<Tip>
[`IterableDataset.shuffle`] will also shuffle the order of the shards if the dataset is sharded into multiple files.
</Tip>
## Reshuffle
Sometimes you may want to reshuffle the dataset after each epoch. This will require you to set a different seed for each epoch. Use [`IterableDataset.set_epoch`] in between epochs to tell the dataset what epoch you're on.
Your seed effectively becomes: `initial seed + current epoch`.
```py
>>> for epoch in range(epochs):
... shuffled_dataset.set_epoch(epoch)
... for example in shuffled_dataset:
... ...
```
## Split dataset
You can split your dataset one of two ways:
- [`IterableDataset.take`] returns the first `n` examples in a dataset:
```py
>>> dataset = load_dataset('oscar', "unshuffled_deduplicated_en", split='train', streaming=True)
>>> dataset_head = dataset.take(2)
>>> list(dataset_head)
[{'id': 0, 'text': 'Mtendere Village was...'}, {'id': 1, 'text': 'Lily James cannot fight the music...'}]
```
- [`IterableDataset.skip`] omits the first `n` examples in a dataset and returns the remaining examples:
```py
>>> train_dataset = shuffled_dataset.skip(1000)
```
<Tip warning={true}>
`take` and `skip` prevent future calls to `shuffle` because they lock in the order of the shards. You should `shuffle` your dataset before splitting it.
</Tip>
<a id='interleave_datasets'></a>
## Interleave
[`interleave_datasets`] can combine an [`IterableDataset`] with other datasets. The combined dataset returns alternating examples from each of the original datasets.
```py
>>> from datasets import interleave_datasets
>>> en_dataset = load_dataset('oscar', "unshuffled_deduplicated_en", split='train', streaming=True, trust_remote_code=True)
>>> fr_dataset = load_dataset('oscar', "unshuffled_deduplicated_fr", split='train', streaming=True, trust_remote_code=True)
>>> multilingual_dataset = interleave_datasets([en_dataset, fr_dataset])
>>> list(multilingual_dataset.take(2))
[{'text': 'Mtendere Village was inspired by the vision...'}, {'text': "Média de débat d'idées, de culture et de littérature..."}]
```
Define sampling probabilities from each of the original datasets for more control over how each of them are sampled and combined. Set the `probabilities` argument with your desired sampling probabilities:
```py
>>> multilingual_dataset_with_oversampling = interleave_datasets([en_dataset, fr_dataset], probabilities=[0.8, 0.2], seed=42)
>>> list(multilingual_dataset_with_oversampling.take(2))
[{'text': 'Mtendere Village was inspired by the vision...'}, {'text': 'Lily James cannot fight the music...'}]
```
Around 80% of the final dataset is made of the `en_dataset`, and 20% of the `fr_dataset`.
You can also specify the `stopping_strategy`. The default strategy, `first_exhausted`, is a subsampling strategy, i.e the dataset construction is stopped as soon one of the dataset runs out of samples.
You can specify `stopping_strategy=all_exhausted` to execute an oversampling strategy. In this case, the dataset construction is stopped as soon as every samples in every dataset has been added at least once. In practice, it means that if a dataset is exhausted, it will return to the beginning of this dataset until the stop criterion has been reached.
Note that if no sampling probabilities are specified, the new dataset will have `max_length_datasets*nb_dataset samples`.
## Rename, remove, and cast
The following methods allow you to modify the columns of a dataset. These methods are useful for renaming or removing columns and changing columns to a new set of features.
### Rename
Use [`IterableDataset.rename_column`] when you need to rename a column in your dataset. Features associated with the original column are actually moved under the new column name, instead of just replacing the original column in-place.
Provide [`IterableDataset.rename_column`] with the name of the original column, and the new column name:
```py
>>> from datasets import load_dataset
>>> dataset = load_dataset('mc4', 'en', streaming=True, split='train', trust_remote_code=True)
>>> dataset = dataset.rename_column("text", "content")
```
### Remove
When you need to remove one or more columns, give [`IterableDataset.remove_columns`] the name of the column to remove. Remove more than one column by providing a list of column names:
```py
>>> from datasets import load_dataset
>>> dataset = load_dataset('mc4', 'en', streaming=True, split='train', trust_remote_code=True)
>>> dataset = dataset.remove_columns('timestamp')
```
### Cast
[`IterableDataset.cast`] changes the feature type of one or more columns. This method takes your new `Features` as its argument. The following sample code shows how to change the feature types of `ClassLabel` and `Value`:
```py
>>> from datasets import load_dataset
>>> dataset = load_dataset('glue', 'mrpc', split='train', streaming=True)
>>> dataset.features
{'sentence1': Value(dtype='string', id=None),
'sentence2': Value(dtype='string', id=None),
'label': ClassLabel(num_classes=2, names=['not_equivalent', 'equivalent'], names_file=None, id=None),
'idx': Value(dtype='int32', id=None)}
>>> from datasets import ClassLabel, Value
>>> new_features = dataset.features.copy()
>>> new_features["label"] = ClassLabel(names=['negative', 'positive'])
>>> new_features["idx"] = Value('int64')
>>> dataset = dataset.cast(new_features)
>>> dataset.features
{'sentence1': Value(dtype='string', id=None),
'sentence2': Value(dtype='string', id=None),
'label': ClassLabel(num_classes=2, names=['negative', 'positive'], names_file=None, id=None),
'idx': Value(dtype='int64', id=None)}
```
<Tip>
Casting only works if the original feature type and new feature type are compatible. For example, you can cast a column with the feature type `Value('int32')` to `Value('bool')` if the original column only contains ones and zeros.
</Tip>
Use [`IterableDataset.cast_column`] to change the feature type of just one column. Pass the column name and its new feature type as arguments:
```py
>>> dataset.features
{'audio': Audio(sampling_rate=44100, mono=True, id=None)}
>>> dataset = dataset.cast_column("audio", Audio(sampling_rate=16000))
>>> dataset.features
{'audio': Audio(sampling_rate=16000, mono=True, id=None)}
```
## Map
Similar to the [`Dataset.map`] function for a regular [`Dataset`], 🤗 Datasets features [`IterableDataset.map`] for processing an [`IterableDataset`].
[`IterableDataset.map`] applies processing on-the-fly when examples are streamed.
It allows you to apply a processing function to each example in a dataset, independently or in batches. This function can even create new rows and columns.
The following example demonstrates how to tokenize a [`IterableDataset`]. The function needs to accept and output a `dict`:
```py
>>> def add_prefix(example):
... example['text'] = 'My text: ' + example['text']
... return example
```
Next, apply this function to the dataset with [`IterableDataset.map`]:
```py
>>> from datasets import load_dataset
>>> dataset = load_dataset('oscar', 'unshuffled_deduplicated_en', streaming=True, split='train', trust_remote_code=True)
>>> updated_dataset = dataset.map(add_prefix)
>>> list(updated_dataset.take(3))
[{'id': 0, 'text': 'My text: Mtendere Village was inspired by...'},
{'id': 1, 'text': 'My text: Lily James cannot fight the music...'},
{'id': 2, 'text': 'My text: "I\'d love to help kickstart...'}]
```
Let's take a look at another example, except this time, you will remove a column with [`IterableDataset.map`]. When you remove a column, it is only removed after the example has been provided to the mapped function. This allows the mapped function to use the content of the columns before they are removed.
Specify the column to remove with the `remove_columns` argument in [`IterableDataset.map`]:
```py
>>> updated_dataset = dataset.map(add_prefix, remove_columns=["id"])
>>> list(updated_dataset.take(3))
[{'text': 'My text: Mtendere Village was inspired by...'},
{'text': 'My text: Lily James cannot fight the music...'},
{'text': 'My text: "I\'d love to help kickstart...'}]
```
### Batch processing
[`IterableDataset.map`] also supports working with batches of examples. Operate on batches by setting `batched=True`. The default batch size is 1000, but you can adjust it with the `batch_size` argument. This opens the door to many interesting applications such as tokenization, splitting long sentences into shorter chunks, and data augmentation.
#### Tokenization
```py
>>> from datasets import load_dataset
>>> from transformers import AutoTokenizer
>>> dataset = load_dataset("mc4", "en", streaming=True, split="train", trust_remote_code=True)
>>> tokenizer = AutoTokenizer.from_pretrained('distilbert-base-uncased')
>>> def encode(examples):
... return tokenizer(examples['text'], truncation=True, padding='max_length')
>>> dataset = dataset.map(encode, batched=True, remove_columns=["text", "timestamp", "url"])
>>> next(iter(dataset))
{'input_ids': [101, 8466, 1018, 1010, 4029, 2475, 2062, 18558, 3100, 2061, ...,1106, 3739, 102],
'attention_mask': [1, 1, 1, 1, 1, 1, 1, 1, 1, 1, 1, 1, 1, 1, 1, 1, 1, 1, ..., 1, 1]}
```
<Tip>
See other examples of batch processing in the [batched map processing](./process#batch-processing) documentation. They work the same for iterable datasets.
</Tip>
### Filter
You can filter rows in the dataset based on a predicate function using [`Dataset.filter`]. It returns rows that match a specified condition:
```py
>>> from datasets import load_dataset
>>> dataset = load_dataset('oscar', 'unshuffled_deduplicated_en', streaming=True, split='train', trust_remote_code=True)
>>> start_with_ar = dataset.filter(lambda example: example['text'].startswith('Ar'))
>>> next(iter(start_with_ar))
{'id': 4, 'text': 'Are you looking for Number the Stars (Essential Modern Classics)?...'}
```
[`Dataset.filter`] can also filter by indices if you set `with_indices=True`:
```py
>>> even_dataset = dataset.filter(lambda example, idx: idx % 2 == 0, with_indices=True)
>>> list(even_dataset.take(3))
[{'id': 0, 'text': 'Mtendere Village was inspired by the vision of Chief Napoleon Dzombe, ...'},
{'id': 2, 'text': '"I\'d love to help kickstart continued development! And 0 EUR/month...'},
{'id': 4, 'text': 'Are you looking for Number the Stars (Essential Modern Classics)? Normally, ...'}]
```
## Stream in a training loop
[`IterableDataset`] can be integrated into a training loop. First, shuffle the dataset:
<frameworkcontent>
<pt>
```py
>>> seed, buffer_size = 42, 10_000
>>> dataset = dataset.shuffle(seed, buffer_size=buffer_size)
```
Lastly, create a simple training loop and start training:
```py
>>> import torch
>>> from torch.utils.data import DataLoader
>>> from transformers import AutoModelForMaskedLM, DataCollatorForLanguageModeling
>>> from tqdm import tqdm
>>> dataset = dataset.with_format("torch")
>>> dataloader = DataLoader(dataset, collate_fn=DataCollatorForLanguageModeling(tokenizer))
>>> device = 'cuda' if torch.cuda.is_available() else 'cpu'
>>> model = AutoModelForMaskedLM.from_pretrained("distilbert-base-uncased")
>>> model.train().to(device)
>>> optimizer = torch.optim.AdamW(params=model.parameters(), lr=1e-5)
>>> for epoch in range(3):
... dataset.set_epoch(epoch)
... for i, batch in enumerate(tqdm(dataloader, total=5)):
... if i == 5:
... break
... batch = {k: v.to(device) for k, v in batch.items()}
... outputs = model(**batch)
... loss = outputs[0]
... loss.backward()
... optimizer.step()
... optimizer.zero_grad()
... if i % 10 == 0:
... print(f"loss: {loss}")
```
</pt>
</frameworkcontent>
<!-- TODO: Write the TF content! -->
| datasets/docs/source/stream.mdx/0 | {
"file_path": "datasets/docs/source/stream.mdx",
"repo_id": "datasets",
"token_count": 5324
} | 64 |
# Copyright 2020 The HuggingFace Datasets Authors.
#
# Licensed under the Apache License, Version 2.0 (the "License");
# you may not use this file except in compliance with the License.
# You may obtain a copy of the License at
#
# http://www.apache.org/licenses/LICENSE-2.0
#
# Unless required by applicable law or agreed to in writing, software
# distributed under the License is distributed on an "AS IS" BASIS,
# WITHOUT WARRANTIES OR CONDITIONS OF ANY KIND, either express or implied.
# See the License for the specific language governing permissions and
# limitations under the License.
"""BLEU metric."""
import datasets
from .nmt_bleu import compute_bleu # From: https://github.com/tensorflow/nmt/blob/master/nmt/scripts/bleu.py
_CITATION = """\
@INPROCEEDINGS{Papineni02bleu:a,
author = {Kishore Papineni and Salim Roukos and Todd Ward and Wei-jing Zhu},
title = {BLEU: a Method for Automatic Evaluation of Machine Translation},
booktitle = {},
year = {2002},
pages = {311--318}
}
@inproceedings{lin-och-2004-orange,
title = "{ORANGE}: a Method for Evaluating Automatic Evaluation Metrics for Machine Translation",
author = "Lin, Chin-Yew and
Och, Franz Josef",
booktitle = "{COLING} 2004: Proceedings of the 20th International Conference on Computational Linguistics",
month = "aug 23{--}aug 27",
year = "2004",
address = "Geneva, Switzerland",
publisher = "COLING",
url = "https://www.aclweb.org/anthology/C04-1072",
pages = "501--507",
}
"""
_DESCRIPTION = """\
BLEU (bilingual evaluation understudy) is an algorithm for evaluating the quality of text which has been machine-translated from one natural language to another.
Quality is considered to be the correspondence between a machine's output and that of a human: "the closer a machine translation is to a professional human translation,
the better it is" – this is the central idea behind BLEU. BLEU was one of the first metrics to claim a high correlation with human judgements of quality, and
remains one of the most popular automated and inexpensive metrics.
Scores are calculated for individual translated segments—generally sentences—by comparing them with a set of good quality reference translations.
Those scores are then averaged over the whole corpus to reach an estimate of the translation's overall quality. Intelligibility or grammatical correctness
are not taken into account[citation needed].
BLEU's output is always a number between 0 and 1. This value indicates how similar the candidate text is to the reference texts, with values closer to 1
representing more similar texts. Few human translations will attain a score of 1, since this would indicate that the candidate is identical to one of the
reference translations. For this reason, it is not necessary to attain a score of 1. Because there are more opportunities to match, adding additional
reference translations will increase the BLEU score.
"""
_KWARGS_DESCRIPTION = """
Computes BLEU score of translated segments against one or more references.
Args:
predictions: list of translations to score.
Each translation should be tokenized into a list of tokens.
references: list of lists of references for each translation.
Each reference should be tokenized into a list of tokens.
max_order: Maximum n-gram order to use when computing BLEU score.
smooth: Whether or not to apply Lin et al. 2004 smoothing.
Returns:
'bleu': bleu score,
'precisions': geometric mean of n-gram precisions,
'brevity_penalty': brevity penalty,
'length_ratio': ratio of lengths,
'translation_length': translation_length,
'reference_length': reference_length
Examples:
>>> predictions = [
... ["hello", "there", "general", "kenobi"], # tokenized prediction of the first sample
... ["foo", "bar", "foobar"] # tokenized prediction of the second sample
... ]
>>> references = [
... [["hello", "there", "general", "kenobi"], ["hello", "there", "!"]], # tokenized references for the first sample (2 references)
... [["foo", "bar", "foobar"]] # tokenized references for the second sample (1 reference)
... ]
>>> bleu = datasets.load_metric("bleu")
>>> results = bleu.compute(predictions=predictions, references=references)
>>> print(results["bleu"])
1.0
"""
@datasets.utils.file_utils.add_start_docstrings(_DESCRIPTION, _KWARGS_DESCRIPTION)
class Bleu(datasets.Metric):
def _info(self):
return datasets.MetricInfo(
description=_DESCRIPTION,
citation=_CITATION,
inputs_description=_KWARGS_DESCRIPTION,
features=datasets.Features(
{
"predictions": datasets.Sequence(datasets.Value("string", id="token"), id="sequence"),
"references": datasets.Sequence(
datasets.Sequence(datasets.Value("string", id="token"), id="sequence"), id="references"
),
}
),
codebase_urls=["https://github.com/tensorflow/nmt/blob/master/nmt/scripts/bleu.py"],
reference_urls=[
"https://en.wikipedia.org/wiki/BLEU",
"https://towardsdatascience.com/evaluating-text-output-in-nlp-bleu-at-your-own-risk-e8609665a213",
],
)
def _compute(self, predictions, references, max_order=4, smooth=False):
score = compute_bleu(
reference_corpus=references, translation_corpus=predictions, max_order=max_order, smooth=smooth
)
(bleu, precisions, bp, ratio, translation_length, reference_length) = score
return {
"bleu": bleu,
"precisions": precisions,
"brevity_penalty": bp,
"length_ratio": ratio,
"translation_length": translation_length,
"reference_length": reference_length,
}
| datasets/metrics/bleu/bleu.py/0 | {
"file_path": "datasets/metrics/bleu/bleu.py",
"repo_id": "datasets",
"token_count": 2139
} | 65 |
# Metric Card for CUAD
## Metric description
This metric wraps the official scoring script for version 1 of the [Contract Understanding Atticus Dataset (CUAD)](https://huggingface.co/datasets/cuad), which is a corpus of more than 13,000 labels in 510 commercial legal contracts that have been manually labeled to identify 41 categories of important clauses that lawyers look for when reviewing contracts in connection with corporate transactions.
The CUAD metric computes several scores: [Exact Match](https://huggingface.co/metrics/exact_match), [F1 score](https://huggingface.co/metrics/f1), Area Under the Precision-Recall Curve, [Precision](https://huggingface.co/metrics/precision) at 80% [recall](https://huggingface.co/metrics/recall) and Precision at 90% recall.
## How to use
The CUAD metric takes two inputs :
`predictions`, a list of question-answer dictionaries with the following key-values:
- `id`: the id of the question-answer pair as given in the references.
- `prediction_text`: a list of possible texts for the answer, as a list of strings depending on a threshold on the confidence probability of each prediction.
`references`: a list of question-answer dictionaries with the following key-values:
- `id`: the id of the question-answer pair (the same as above).
- `answers`: a dictionary *in the CUAD dataset format* with the following keys:
- `text`: a list of possible texts for the answer, as a list of strings.
- `answer_start`: a list of start positions for the answer, as a list of ints.
Note that `answer_start` values are not taken into account to compute the metric.
```python
from datasets import load_metric
cuad_metric = load_metric("cuad")
predictions = [{'prediction_text': ['The seller:', 'The buyer/End-User: Shenzhen LOHAS Supply Chain Management Co., Ltd.'], 'id': 'LohaCompanyltd_20191209_F-1_EX-10.16_11917878_EX-10.16_Supply Agreement__Parties'}]
references = [{'answers': {'answer_start': [143, 49], 'text': ['The seller:', 'The buyer/End-User: Shenzhen LOHAS Supply Chain Management Co., Ltd.']}, 'id': 'LohaCompanyltd_20191209_F-1_EX-10.16_11917878_EX-10.16_Supply Agreement__Parties'}]
results = cuad_metric.compute(predictions=predictions, references=references)
```
## Output values
The output of the CUAD metric consists of a dictionary that contains one or several of the following metrics:
`exact_match`: The normalized answers that exactly match the reference answer, with a range between 0.0 and 1.0 (see [exact match](https://huggingface.co/metrics/exact_match) for more information).
`f1`: The harmonic mean of the precision and recall (see [F1 score](https://huggingface.co/metrics/f1) for more information). Its range is between 0.0 and 1.0 -- its lowest possible value is 0, if either the precision or the recall is 0, and its highest possible value is 1.0, which means perfect precision and recall.
`aupr`: The Area Under the Precision-Recall curve, with a range between 0.0 and 1.0, with a higher value representing both high recall and high precision, and a low value representing low values for both. See the [Wikipedia article](https://en.wikipedia.org/wiki/Receiver_operating_characteristic#Area_under_the_curve) for more information.
`prec_at_80_recall`: The fraction of true examples among the predicted examples at a recall rate of 80%. Its range is between 0.0 and 1.0. For more information, see [precision](https://huggingface.co/metrics/precision) and [recall](https://huggingface.co/metrics/recall).
`prec_at_90_recall`: The fraction of true examples among the predicted examples at a recall rate of 90%. Its range is between 0.0 and 1.0.
### Values from popular papers
The [original CUAD paper](https://arxiv.org/pdf/2103.06268.pdf) reports that a [DeBERTa model](https://huggingface.co/microsoft/deberta-base) attains
an AUPR of 47.8%, a Precision at 80% Recall of 44.0%, and a Precision at 90% Recall of 17.8% (they do not report F1 or Exact Match separately).
For more recent model performance, see the [dataset leaderboard](https://paperswithcode.com/dataset/cuad).
## Examples
Maximal values :
```python
from datasets import load_metric
cuad_metric = load_metric("cuad")
predictions = [{'prediction_text': ['The seller:', 'The buyer/End-User: Shenzhen LOHAS Supply Chain Management Co., Ltd.'], 'id': 'LohaCompanyltd_20191209_F-1_EX-10.16_11917878_EX-10.16_Supply Agreement__Parties'}]
references = [{'answers': {'answer_start': [143, 49], 'text': ['The seller:', 'The buyer/End-User: Shenzhen LOHAS Supply Chain Management Co., Ltd.']}, 'id': 'LohaCompanyltd_20191209_F-1_EX-10.16_11917878_EX-10.16_Supply Agreement__Parties'}]
results = cuad_metric.compute(predictions=predictions, references=references)
print(results)
{'exact_match': 100.0, 'f1': 100.0, 'aupr': 0.0, 'prec_at_80_recall': 1.0, 'prec_at_90_recall': 1.0}
```
Minimal values:
```python
from datasets import load_metric
cuad_metric = load_metric("cuad")
predictions = [{'prediction_text': ['The Company appoints the Distributor as an exclusive distributor of Products in the Market, subject to the terms and conditions of this Agreement.'], 'id': 'LIMEENERGYCO_09_09_1999-EX-10-DISTRIBUTOR AGREEMENT__Exclusivity_0'}]
references = [{'answers': {'answer_start': [143], 'text': 'The seller'}, 'id': 'LIMEENERGYCO_09_09_1999-EX-10-DISTRIBUTOR AGREEMENT__Exclusivity_0'}]
results = cuad_metric.compute(predictions=predictions, references=references)
print(results)
{'exact_match': 0.0, 'f1': 0.0, 'aupr': 0.0, 'prec_at_80_recall': 0, 'prec_at_90_recall': 0}
```
Partial match:
```python
from datasets import load_metric
cuad_metric = load_metric("cuad")
predictions = [{'prediction_text': ['The seller:', 'The buyer/End-User: Shenzhen LOHAS Supply Chain Management Co., Ltd.'], 'id': 'LohaCompanyltd_20191209_F-1_EX-10.16_11917878_EX-10.16_Supply Agreement__Parties'}]
predictions = [{'prediction_text': ['The Company appoints the Distributor as an exclusive distributor of Products in the Market, subject to the terms and conditions of this Agreement.', 'The buyer/End-User: Shenzhen LOHAS Supply Chain Management Co., Ltd.'], 'id': 'LohaCompanyltd_20191209_F-1_EX-10.16_11917878_EX-10.16_Supply Agreement__Parties'}]
results = cuad_metric.compute(predictions=predictions, references=references)
print(results)
{'exact_match': 100.0, 'f1': 50.0, 'aupr': 0.0, 'prec_at_80_recall': 0, 'prec_at_90_recall': 0}
```
## Limitations and bias
This metric works only with datasets that have the same format as the [CUAD dataset](https://huggingface.co/datasets/cuad). The limitations of the biases of this dataset are not discussed, but could exhibit annotation bias given the homogeneity of annotators for this dataset.
In terms of the metric itself, the accuracy of AUPR has been debated because its estimates are quite noisy and because of the fact that reducing the Precision-Recall Curve to a single number ignores the fact that it is about the tradeoffs between the different systems or performance points plotted and not the performance of an individual system. Reporting the original F1 and exact match scores is therefore useful to ensure a more complete representation of system performance.
## Citation
```bibtex
@article{hendrycks2021cuad,
title={CUAD: An Expert-Annotated NLP Dataset for Legal Contract Review},
author={Dan Hendrycks and Collin Burns and Anya Chen and Spencer Ball},
journal={arXiv preprint arXiv:2103.06268},
year={2021}
}
```
## Further References
- [CUAD dataset homepage](https://www.atticusprojectai.org/cuad-v1-performance-announcements)
| datasets/metrics/cuad/README.md/0 | {
"file_path": "datasets/metrics/cuad/README.md",
"repo_id": "datasets",
"token_count": 2380
} | 66 |
# Copyright 2022 The HuggingFace Datasets Authors and the current dataset script contributor.
#
# Licensed under the Apache License, Version 2.0 (the "License");
# you may not use this file except in compliance with the License.
# You may obtain a copy of the License at
#
# http://www.apache.org/licenses/LICENSE-2.0
#
# Unless required by applicable law or agreed to in writing, software
# distributed under the License is distributed on an "AS IS" BASIS,
# WITHOUT WARRANTIES OR CONDITIONS OF ANY KIND, either express or implied.
# See the License for the specific language governing permissions and
# limitations under the License.
"""MAE - Mean Absolute Error Metric"""
from sklearn.metrics import mean_absolute_error
import datasets
_CITATION = """\
@article{scikit-learn,
title={Scikit-learn: Machine Learning in {P}ython},
author={Pedregosa, F. and Varoquaux, G. and Gramfort, A. and Michel, V.
and Thirion, B. and Grisel, O. and Blondel, M. and Prettenhofer, P.
and Weiss, R. and Dubourg, V. and Vanderplas, J. and Passos, A. and
Cournapeau, D. and Brucher, M. and Perrot, M. and Duchesnay, E.},
journal={Journal of Machine Learning Research},
volume={12},
pages={2825--2830},
year={2011}
}
"""
_DESCRIPTION = """\
Mean Absolute Error (MAE) is the mean of the magnitude of difference between the predicted and actual
values.
"""
_KWARGS_DESCRIPTION = """
Args:
predictions: array-like of shape (n_samples,) or (n_samples, n_outputs)
Estimated target values.
references: array-like of shape (n_samples,) or (n_samples, n_outputs)
Ground truth (correct) target values.
sample_weight: array-like of shape (n_samples,), default=None
Sample weights.
multioutput: {"raw_values", "uniform_average"} or array-like of shape (n_outputs,), default="uniform_average"
Defines aggregating of multiple output values. Array-like value defines weights used to average errors.
"raw_values" : Returns a full set of errors in case of multioutput input.
"uniform_average" : Errors of all outputs are averaged with uniform weight.
Returns:
mae : mean absolute error.
If multioutput is "raw_values", then mean absolute error is returned for each output separately. If multioutput is "uniform_average" or an ndarray of weights, then the weighted average of all output errors is returned.
MAE output is non-negative floating point. The best value is 0.0.
Examples:
>>> mae_metric = datasets.load_metric("mae")
>>> predictions = [2.5, 0.0, 2, 8]
>>> references = [3, -0.5, 2, 7]
>>> results = mae_metric.compute(predictions=predictions, references=references)
>>> print(results)
{'mae': 0.5}
If you're using multi-dimensional lists, then set the config as follows :
>>> mae_metric = datasets.load_metric("mae", "multilist")
>>> predictions = [[0.5, 1], [-1, 1], [7, -6]]
>>> references = [[0, 2], [-1, 2], [8, -5]]
>>> results = mae_metric.compute(predictions=predictions, references=references)
>>> print(results)
{'mae': 0.75}
>>> results = mae_metric.compute(predictions=predictions, references=references, multioutput='raw_values')
>>> print(results)
{'mae': array([0.5, 1. ])}
"""
@datasets.utils.file_utils.add_start_docstrings(_DESCRIPTION, _KWARGS_DESCRIPTION)
class Mae(datasets.Metric):
def _info(self):
return datasets.MetricInfo(
description=_DESCRIPTION,
citation=_CITATION,
inputs_description=_KWARGS_DESCRIPTION,
features=datasets.Features(self._get_feature_types()),
reference_urls=[
"https://scikit-learn.org/stable/modules/generated/sklearn.metrics.mean_absolute_error.html"
],
)
def _get_feature_types(self):
if self.config_name == "multilist":
return {
"predictions": datasets.Sequence(datasets.Value("float")),
"references": datasets.Sequence(datasets.Value("float")),
}
else:
return {
"predictions": datasets.Value("float"),
"references": datasets.Value("float"),
}
def _compute(self, predictions, references, sample_weight=None, multioutput="uniform_average"):
mae_score = mean_absolute_error(references, predictions, sample_weight=sample_weight, multioutput=multioutput)
return {"mae": mae_score}
| datasets/metrics/mae/mae.py/0 | {
"file_path": "datasets/metrics/mae/mae.py",
"repo_id": "datasets",
"token_count": 1662
} | 67 |
# Copyright 2022 The HuggingFace Datasets Authors and the current dataset script contributor.
#
# Licensed under the Apache License, Version 2.0 (the "License");
# you may not use this file except in compliance with the License.
# You may obtain a copy of the License at
#
# http://www.apache.org/licenses/LICENSE-2.0
#
# Unless required by applicable law or agreed to in writing, software
# distributed under the License is distributed on an "AS IS" BASIS,
# WITHOUT WARRANTIES OR CONDITIONS OF ANY KIND, either express or implied.
# See the License for the specific language governing permissions and
# limitations under the License.
"""Perplexity Metric."""
import numpy as np
import torch
from torch.nn import CrossEntropyLoss
from transformers import AutoModelForCausalLM, AutoTokenizer
import datasets
from datasets import logging
_CITATION = """\
"""
_DESCRIPTION = """
Perplexity (PPL) is one of the most common metrics for evaluating language models.
It is defined as the exponentiated average negative log-likelihood of a sequence.
For more information, see https://huggingface.co/docs/transformers/perplexity
"""
_KWARGS_DESCRIPTION = """
Args:
model_id (str): model used for calculating Perplexity
NOTE: Perplexity can only be calculated for causal language models.
This includes models such as gpt2, causal variations of bert,
causal versions of t5, and more (the full list can be found
in the AutoModelForCausalLM documentation here:
https://huggingface.co/docs/transformers/master/en/model_doc/auto#transformers.AutoModelForCausalLM )
input_texts (list of str): input text, each separate text snippet
is one list entry.
batch_size (int): the batch size to run texts through the model. Defaults to 16.
add_start_token (bool): whether to add the start token to the texts,
so the perplexity can include the probability of the first word. Defaults to True.
device (str): device to run on, defaults to 'cuda' when available
Returns:
perplexity: dictionary containing the perplexity scores for the texts
in the input list, as well as the mean perplexity. If one of the input texts is
longer than the max input length of the model, then it is truncated to the
max length for the perplexity computation.
Examples:
Example 1:
>>> perplexity = datasets.load_metric("perplexity")
>>> input_texts = ["lorem ipsum", "Happy Birthday!", "Bienvenue"]
>>> results = perplexity.compute(model_id='gpt2',
... add_start_token=False,
... input_texts=input_texts) # doctest:+ELLIPSIS
>>> print(list(results.keys()))
['perplexities', 'mean_perplexity']
>>> print(round(results["mean_perplexity"], 2))
78.22
>>> print(round(results["perplexities"][0], 2))
11.11
Example 2:
>>> perplexity = datasets.load_metric("perplexity")
>>> input_texts = datasets.load_dataset("wikitext",
... "wikitext-2-raw-v1",
... split="test")["text"][:50]
>>> input_texts = [s for s in input_texts if s!='']
>>> results = perplexity.compute(model_id='gpt2',
... input_texts=input_texts) # doctest:+ELLIPSIS
>>> print(list(results.keys()))
['perplexities', 'mean_perplexity']
>>> print(round(results["mean_perplexity"], 2))
60.35
>>> print(round(results["perplexities"][0], 2))
81.12
"""
@datasets.utils.file_utils.add_start_docstrings(_DESCRIPTION, _KWARGS_DESCRIPTION)
class Perplexity(datasets.Metric):
def _info(self):
return datasets.MetricInfo(
description=_DESCRIPTION,
citation=_CITATION,
inputs_description=_KWARGS_DESCRIPTION,
features=datasets.Features(
{
"input_texts": datasets.Value("string"),
}
),
reference_urls=["https://huggingface.co/docs/transformers/perplexity"],
)
def _compute(self, input_texts, model_id, batch_size: int = 16, add_start_token: bool = True, device=None):
if device is not None:
assert device in ["gpu", "cpu", "cuda"], "device should be either gpu or cpu."
if device == "gpu":
device = "cuda"
else:
device = "cuda" if torch.cuda.is_available() else "cpu"
model = AutoModelForCausalLM.from_pretrained(model_id)
model = model.to(device)
tokenizer = AutoTokenizer.from_pretrained(model_id)
# if batch_size > 1 (which generally leads to padding being required), and
# if there is not an already assigned pad_token, assign an existing
# special token to also be the padding token
if tokenizer.pad_token is None and batch_size > 1:
existing_special_tokens = list(tokenizer.special_tokens_map_extended.values())
# check that the model already has at least one special token defined
assert (
len(existing_special_tokens) > 0
), "If batch_size > 1, model must have at least one special token to use for padding. Please use a different model or set batch_size=1."
# assign one of the special tokens to also be the pad token
tokenizer.add_special_tokens({"pad_token": existing_special_tokens[0]})
if add_start_token:
# leave room for <BOS> token to be added:
assert (
tokenizer.bos_token is not None
), "Input model must already have a BOS token if using add_start_token=True. Please use a different model, or set add_start_token=False"
max_tokenized_len = model.config.max_length - 1
else:
max_tokenized_len = model.config.max_length
encodings = tokenizer(
input_texts,
add_special_tokens=False,
padding=True,
truncation=True,
max_length=max_tokenized_len,
return_tensors="pt",
return_attention_mask=True,
).to(device)
encoded_texts = encodings["input_ids"]
attn_masks = encodings["attention_mask"]
# check that each input is long enough:
if add_start_token:
assert torch.all(torch.ge(attn_masks.sum(1), 1)), "Each input text must be at least one token long."
else:
assert torch.all(
torch.ge(attn_masks.sum(1), 2)
), "When add_start_token=False, each input text must be at least two tokens long. Run with add_start_token=True if inputting strings of only one token, and remove all empty input strings."
ppls = []
loss_fct = CrossEntropyLoss(reduction="none")
for start_index in logging.tqdm(range(0, len(encoded_texts), batch_size)):
end_index = min(start_index + batch_size, len(encoded_texts))
encoded_batch = encoded_texts[start_index:end_index]
attn_mask = attn_masks[start_index:end_index]
if add_start_token:
bos_tokens_tensor = torch.tensor([[tokenizer.bos_token_id]] * encoded_batch.size(dim=0)).to(device)
encoded_batch = torch.cat([bos_tokens_tensor, encoded_batch], dim=1)
attn_mask = torch.cat(
[torch.ones(bos_tokens_tensor.size(), dtype=torch.int64).to(device), attn_mask], dim=1
)
labels = encoded_batch
with torch.no_grad():
out_logits = model(encoded_batch, attention_mask=attn_mask).logits
shift_logits = out_logits[..., :-1, :].contiguous()
shift_labels = labels[..., 1:].contiguous()
shift_attention_mask_batch = attn_mask[..., 1:].contiguous()
perplexity_batch = torch.exp2(
(loss_fct(shift_logits.transpose(1, 2), shift_labels) * shift_attention_mask_batch).sum(1)
/ shift_attention_mask_batch.sum(1)
)
ppls += perplexity_batch.tolist()
return {"perplexities": ppls, "mean_perplexity": np.mean(ppls)}
| datasets/metrics/perplexity/perplexity.py/0 | {
"file_path": "datasets/metrics/perplexity/perplexity.py",
"repo_id": "datasets",
"token_count": 3550
} | 68 |
# Copyright 2021 The HuggingFace Datasets Authors and the current dataset script contributor.
#
# Licensed under the Apache License, Version 2.0 (the "License");
# you may not use this file except in compliance with the License.
# You may obtain a copy of the License at
#
# http://www.apache.org/licenses/LICENSE-2.0
#
# Unless required by applicable law or agreed to in writing, software
# distributed under the License is distributed on an "AS IS" BASIS,
# WITHOUT WARRANTIES OR CONDITIONS OF ANY KIND, either express or implied.
# See the License for the specific language governing permissions and
# limitations under the License.
"""Spearman correlation coefficient metric."""
from scipy.stats import spearmanr
import datasets
_DESCRIPTION = """
The Spearman rank-order correlation coefficient is a measure of the
relationship between two datasets. Like other correlation coefficients,
this one varies between -1 and +1 with 0 implying no correlation.
Positive correlations imply that as data in dataset x increases, so
does data in dataset y. Negative correlations imply that as x increases,
y decreases. Correlations of -1 or +1 imply an exact monotonic relationship.
Unlike the Pearson correlation, the Spearman correlation does not
assume that both datasets are normally distributed.
The p-value roughly indicates the probability of an uncorrelated system
producing datasets that have a Spearman correlation at least as extreme
as the one computed from these datasets. The p-values are not entirely
reliable but are probably reasonable for datasets larger than 500 or so.
"""
_KWARGS_DESCRIPTION = """
Args:
predictions (`List[float]`): Predicted labels, as returned by a model.
references (`List[float]`): Ground truth labels.
return_pvalue (`bool`): If `True`, returns the p-value. If `False`, returns
only the spearmanr score. Defaults to `False`.
Returns:
spearmanr (`float`): Spearman correlation coefficient.
p-value (`float`): p-value. **Note**: is only returned if `return_pvalue=True` is input.
Examples:
Example 1:
>>> spearmanr_metric = datasets.load_metric("spearmanr")
>>> results = spearmanr_metric.compute(references=[1, 2, 3, 4, 5], predictions=[10, 9, 2.5, 6, 4])
>>> print(results)
{'spearmanr': -0.7}
Example 2:
>>> spearmanr_metric = datasets.load_metric("spearmanr")
>>> results = spearmanr_metric.compute(references=[1, 2, 3, 4, 5],
... predictions=[10, 9, 2.5, 6, 4],
... return_pvalue=True)
>>> print(results['spearmanr'])
-0.7
>>> print(round(results['spearmanr_pvalue'], 2))
0.19
"""
_CITATION = r"""\
@book{kokoska2000crc,
title={CRC standard probability and statistics tables and formulae},
author={Kokoska, Stephen and Zwillinger, Daniel},
year={2000},
publisher={Crc Press}
}
@article{2020SciPy-NMeth,
author = {Virtanen, Pauli and Gommers, Ralf and Oliphant, Travis E. and
Haberland, Matt and Reddy, Tyler and Cournapeau, David and
Burovski, Evgeni and Peterson, Pearu and Weckesser, Warren and
Bright, Jonathan and {van der Walt}, St{\'e}fan J. and
Brett, Matthew and Wilson, Joshua and Millman, K. Jarrod and
Mayorov, Nikolay and Nelson, Andrew R. J. and Jones, Eric and
Kern, Robert and Larson, Eric and Carey, C J and
Polat, {\.I}lhan and Feng, Yu and Moore, Eric W. and
{VanderPlas}, Jake and Laxalde, Denis and Perktold, Josef and
Cimrman, Robert and Henriksen, Ian and Quintero, E. A. and
Harris, Charles R. and Archibald, Anne M. and
Ribeiro, Ant{\^o}nio H. and Pedregosa, Fabian and
{van Mulbregt}, Paul and {SciPy 1.0 Contributors}},
title = {{{SciPy} 1.0: Fundamental Algorithms for Scientific
Computing in Python}},
journal = {Nature Methods},
year = {2020},
volume = {17},
pages = {261--272},
adsurl = {https://rdcu.be/b08Wh},
doi = {10.1038/s41592-019-0686-2},
}
"""
@datasets.utils.file_utils.add_start_docstrings(_DESCRIPTION, _KWARGS_DESCRIPTION)
class Spearmanr(datasets.Metric):
def _info(self):
return datasets.MetricInfo(
description=_DESCRIPTION,
citation=_CITATION,
inputs_description=_KWARGS_DESCRIPTION,
features=datasets.Features(
{
"predictions": datasets.Value("float"),
"references": datasets.Value("float"),
}
),
reference_urls=["https://docs.scipy.org/doc/scipy/reference/generated/scipy.stats.spearmanr.html"],
)
def _compute(self, predictions, references, return_pvalue=False):
results = spearmanr(references, predictions)
if return_pvalue:
return {"spearmanr": results[0], "spearmanr_pvalue": results[1]}
else:
return {"spearmanr": results[0]}
| datasets/metrics/spearmanr/spearmanr.py/0 | {
"file_path": "datasets/metrics/spearmanr/spearmanr.py",
"repo_id": "datasets",
"token_count": 1942
} | 69 |
# Metric Card for XNLI
## Metric description
The XNLI metric allows to evaluate a model's score on the [XNLI dataset](https://huggingface.co/datasets/xnli), which is a subset of a few thousand examples from the [MNLI dataset](https://huggingface.co/datasets/glue/viewer/mnli) that have been translated into a 14 different languages, some of which are relatively low resource such as Swahili and Urdu.
As with MNLI, the task is to predict textual entailment (does sentence A imply/contradict/neither sentence B) and is a classification task (given two sentences, predict one of three labels).
## How to use
The XNLI metric is computed based on the `predictions` (a list of predicted labels) and the `references` (a list of ground truth labels).
```python
from datasets import load_metric
xnli_metric = load_metric("xnli")
predictions = [0, 1]
references = [0, 1]
results = xnli_metric.compute(predictions=predictions, references=references)
```
## Output values
The output of the XNLI metric is simply the `accuracy`, i.e. the proportion of correct predictions among the total number of cases processed, with a range between 0 and 1 (see [accuracy](https://huggingface.co/metrics/accuracy) for more information).
### Values from popular papers
The [original XNLI paper](https://arxiv.org/pdf/1809.05053.pdf) reported accuracies ranging from 59.3 (for `ur`) to 73.7 (for `en`) for the BiLSTM-max model.
For more recent model performance, see the [dataset leaderboard](https://paperswithcode.com/dataset/xnli).
## Examples
Maximal values:
```python
>>> from datasets import load_metric
>>> xnli_metric = load_metric("xnli")
>>> predictions = [0, 1]
>>> references = [0, 1]
>>> results = xnli_metric.compute(predictions=predictions, references=references)
>>> print(results)
{'accuracy': 1.0}
```
Minimal values:
```python
>>> from datasets import load_metric
>>> xnli_metric = load_metric("xnli")
>>> predictions = [1, 0]
>>> references = [0, 1]
>>> results = xnli_metric.compute(predictions=predictions, references=references)
>>> print(results)
{'accuracy': 0.0}
```
Partial match:
```python
>>> from datasets import load_metric
>>> xnli_metric = load_metric("xnli")
>>> predictions = [1, 0, 1]
>>> references = [1, 0, 0]
>>> results = xnli_metric.compute(predictions=predictions, references=references)
>>> print(results)
{'accuracy': 0.6666666666666666}
```
## Limitations and bias
While accuracy alone does give a certain indication of performance, it can be supplemented by error analysis and a better understanding of the model's mistakes on each of the categories represented in the dataset, especially if they are unbalanced.
While the XNLI dataset is multilingual and represents a diversity of languages, in reality, cross-lingual sentence understanding goes beyond translation, given that there are many cultural differences that have an impact on human sentiment annotations. Since the XNLI dataset was obtained by translation based on English sentences, it does not capture these cultural differences.
## Citation
```bibtex
@InProceedings{conneau2018xnli,
author = "Conneau, Alexis
and Rinott, Ruty
and Lample, Guillaume
and Williams, Adina
and Bowman, Samuel R.
and Schwenk, Holger
and Stoyanov, Veselin",
title = "XNLI: Evaluating Cross-lingual Sentence Representations",
booktitle = "Proceedings of the 2018 Conference on Empirical Methods
in Natural Language Processing",
year = "2018",
publisher = "Association for Computational Linguistics",
location = "Brussels, Belgium",
}
```
## Further References
- [XNI Dataset GitHub](https://github.com/facebookresearch/XNLI)
- [HuggingFace Tasks -- Text Classification](https://huggingface.co/tasks/text-classification)
| datasets/metrics/xnli/README.md/0 | {
"file_path": "datasets/metrics/xnli/README.md",
"repo_id": "datasets",
"token_count": 1226
} | 70 |
#!/usr/bin/env python
from argparse import ArgumentParser
from datasets.commands.convert import ConvertCommand
from datasets.commands.dummy_data import DummyDataCommand
from datasets.commands.env import EnvironmentCommand
from datasets.commands.run_beam import RunBeamCommand
from datasets.commands.test import TestCommand
from datasets.utils.logging import set_verbosity_info
def parse_unknown_args(unknown_args):
return {key.lstrip("-"): value for key, value in zip(unknown_args[::2], unknown_args[1::2])}
def main():
parser = ArgumentParser(
"HuggingFace Datasets CLI tool", usage="datasets-cli <command> [<args>]", allow_abbrev=False
)
commands_parser = parser.add_subparsers(help="datasets-cli command helpers")
set_verbosity_info()
# Register commands
ConvertCommand.register_subcommand(commands_parser)
EnvironmentCommand.register_subcommand(commands_parser)
TestCommand.register_subcommand(commands_parser)
RunBeamCommand.register_subcommand(commands_parser)
DummyDataCommand.register_subcommand(commands_parser)
# Parse args
args, unknown_args = parser.parse_known_args()
if not hasattr(args, "func"):
parser.print_help()
exit(1)
kwargs = parse_unknown_args(unknown_args)
# Run
service = args.func(args, **kwargs)
service.run()
if __name__ == "__main__":
main()
| datasets/src/datasets/commands/datasets_cli.py/0 | {
"file_path": "datasets/src/datasets/commands/datasets_cli.py",
"repo_id": "datasets",
"token_count": 473
} | 71 |
import os
from dataclasses import dataclass, field
from io import BytesIO
from typing import TYPE_CHECKING, Any, ClassVar, Dict, Optional, Union
import numpy as np
import pyarrow as pa
from .. import config
from ..download.download_config import DownloadConfig
from ..download.streaming_download_manager import xopen, xsplitext
from ..table import array_cast
from ..utils.py_utils import no_op_if_value_is_null, string_to_dict
if TYPE_CHECKING:
from .features import FeatureType
@dataclass
class Audio:
"""Audio [`Feature`] to extract audio data from an audio file.
Input: The Audio feature accepts as input:
- A `str`: Absolute path to the audio file (i.e. random access is allowed).
- A `dict` with the keys:
- `path`: String with relative path of the audio file to the archive file.
- `bytes`: Bytes content of the audio file.
This is useful for archived files with sequential access.
- A `dict` with the keys:
- `path`: String with relative path of the audio file to the archive file.
- `array`: Array containing the audio sample
- `sampling_rate`: Integer corresponding to the sampling rate of the audio sample.
This is useful for archived files with sequential access.
Args:
sampling_rate (`int`, *optional*):
Target sampling rate. If `None`, the native sampling rate is used.
mono (`bool`, defaults to `True`):
Whether to convert the audio signal to mono by averaging samples across
channels.
decode (`bool`, defaults to `True`):
Whether to decode the audio data. If `False`,
returns the underlying dictionary in the format `{"path": audio_path, "bytes": audio_bytes}`.
Example:
```py
>>> from datasets import load_dataset, Audio
>>> ds = load_dataset("PolyAI/minds14", name="en-US", split="train")
>>> ds = ds.cast_column("audio", Audio(sampling_rate=16000))
>>> ds[0]["audio"]
{'array': array([ 2.3443763e-05, 2.1729663e-04, 2.2145823e-04, ...,
3.8356509e-05, -7.3497440e-06, -2.1754686e-05], dtype=float32),
'path': '/root/.cache/huggingface/datasets/downloads/extracted/f14948e0e84be638dd7943ac36518a4cf3324e8b7aa331c5ab11541518e9368c/en-US~JOINT_ACCOUNT/602ba55abb1e6d0fbce92065.wav',
'sampling_rate': 16000}
```
"""
sampling_rate: Optional[int] = None
mono: bool = True
decode: bool = True
id: Optional[str] = None
# Automatically constructed
dtype: ClassVar[str] = "dict"
pa_type: ClassVar[Any] = pa.struct({"bytes": pa.binary(), "path": pa.string()})
_type: str = field(default="Audio", init=False, repr=False)
def __call__(self):
return self.pa_type
def encode_example(self, value: Union[str, bytes, dict]) -> dict:
"""Encode example into a format for Arrow.
Args:
value (`str` or `dict`):
Data passed as input to Audio feature.
Returns:
`dict`
"""
try:
import soundfile as sf # soundfile is a dependency of librosa, needed to decode audio files.
except ImportError as err:
raise ImportError("To support encoding audio data, please install 'soundfile'.") from err
if isinstance(value, str):
return {"bytes": None, "path": value}
elif isinstance(value, bytes):
return {"bytes": value, "path": None}
elif "array" in value:
# convert the audio array to wav bytes
buffer = BytesIO()
sf.write(buffer, value["array"], value["sampling_rate"], format="wav")
return {"bytes": buffer.getvalue(), "path": None}
elif value.get("path") is not None and os.path.isfile(value["path"]):
# we set "bytes": None to not duplicate the data if they're already available locally
if value["path"].endswith("pcm"):
# "PCM" only has raw audio bytes
if value.get("sampling_rate") is None:
# At least, If you want to convert "PCM-byte" to "WAV-byte", you have to know sampling rate
raise KeyError("To use PCM files, please specify a 'sampling_rate' in Audio object")
if value.get("bytes"):
# If we already had PCM-byte, we don`t have to make "read file, make bytes" (just use it!)
bytes_value = np.frombuffer(value["bytes"], dtype=np.int16).astype(np.float32) / 32767
else:
bytes_value = np.memmap(value["path"], dtype="h", mode="r").astype(np.float32) / 32767
buffer = BytesIO(bytes())
sf.write(buffer, bytes_value, value["sampling_rate"], format="wav")
return {"bytes": buffer.getvalue(), "path": None}
else:
return {"bytes": None, "path": value.get("path")}
elif value.get("bytes") is not None or value.get("path") is not None:
# store the audio bytes, and path is used to infer the audio format using the file extension
return {"bytes": value.get("bytes"), "path": value.get("path")}
else:
raise ValueError(
f"An audio sample should have one of 'path' or 'bytes' but they are missing or None in {value}."
)
def decode_example(
self, value: dict, token_per_repo_id: Optional[Dict[str, Union[str, bool, None]]] = None
) -> dict:
"""Decode example audio file into audio data.
Args:
value (`dict`):
A dictionary with keys:
- `path`: String with relative audio file path.
- `bytes`: Bytes of the audio file.
token_per_repo_id (`dict`, *optional*):
To access and decode
audio files from private repositories on the Hub, you can pass
a dictionary repo_id (`str`) -> token (`bool` or `str`)
Returns:
`dict`
"""
if not self.decode:
raise RuntimeError("Decoding is disabled for this feature. Please use Audio(decode=True) instead.")
path, file = (value["path"], BytesIO(value["bytes"])) if value["bytes"] is not None else (value["path"], None)
if path is None and file is None:
raise ValueError(f"An audio sample should have one of 'path' or 'bytes' but both are None in {value}.")
try:
import librosa
import soundfile as sf
except ImportError as err:
raise ImportError("To support decoding audio files, please install 'librosa' and 'soundfile'.") from err
audio_format = xsplitext(path)[1][1:].lower() if path is not None else None
if not config.IS_OPUS_SUPPORTED and audio_format == "opus":
raise RuntimeError(
"Decoding 'opus' files requires system library 'libsndfile'>=1.0.31, "
'You can try to update `soundfile` python library: `pip install "soundfile>=0.12.1"`. '
)
elif not config.IS_MP3_SUPPORTED and audio_format == "mp3":
raise RuntimeError(
"Decoding 'mp3' files requires system library 'libsndfile'>=1.1.0, "
'You can try to update `soundfile` python library: `pip install "soundfile>=0.12.1"`. '
)
if file is None:
token_per_repo_id = token_per_repo_id or {}
source_url = path.split("::")[-1]
pattern = (
config.HUB_DATASETS_URL if source_url.startswith(config.HF_ENDPOINT) else config.HUB_DATASETS_HFFS_URL
)
try:
repo_id = string_to_dict(source_url, pattern)["repo_id"]
token = token_per_repo_id[repo_id]
except (ValueError, KeyError):
token = None
download_config = DownloadConfig(token=token)
with xopen(path, "rb", download_config=download_config) as f:
array, sampling_rate = sf.read(f)
else:
array, sampling_rate = sf.read(file)
array = array.T
if self.mono:
array = librosa.to_mono(array)
if self.sampling_rate and self.sampling_rate != sampling_rate:
array = librosa.resample(array, orig_sr=sampling_rate, target_sr=self.sampling_rate)
sampling_rate = self.sampling_rate
return {"path": path, "array": array, "sampling_rate": sampling_rate}
def flatten(self) -> Union["FeatureType", Dict[str, "FeatureType"]]:
"""If in the decodable state, raise an error, otherwise flatten the feature into a dictionary."""
from .features import Value
if self.decode:
raise ValueError("Cannot flatten a decoded Audio feature.")
return {
"bytes": Value("binary"),
"path": Value("string"),
}
def cast_storage(self, storage: Union[pa.StringArray, pa.StructArray]) -> pa.StructArray:
"""Cast an Arrow array to the Audio arrow storage type.
The Arrow types that can be converted to the Audio pyarrow storage type are:
- `pa.string()` - it must contain the "path" data
- `pa.binary()` - it must contain the audio bytes
- `pa.struct({"bytes": pa.binary()})`
- `pa.struct({"path": pa.string()})`
- `pa.struct({"bytes": pa.binary(), "path": pa.string()})` - order doesn't matter
Args:
storage (`Union[pa.StringArray, pa.StructArray]`):
PyArrow array to cast.
Returns:
`pa.StructArray`: Array in the Audio arrow storage type, that is
`pa.struct({"bytes": pa.binary(), "path": pa.string()})`
"""
if pa.types.is_string(storage.type):
bytes_array = pa.array([None] * len(storage), type=pa.binary())
storage = pa.StructArray.from_arrays([bytes_array, storage], ["bytes", "path"], mask=storage.is_null())
elif pa.types.is_binary(storage.type):
path_array = pa.array([None] * len(storage), type=pa.string())
storage = pa.StructArray.from_arrays([storage, path_array], ["bytes", "path"], mask=storage.is_null())
elif pa.types.is_struct(storage.type) and storage.type.get_all_field_indices("array"):
storage = pa.array([Audio().encode_example(x) if x is not None else None for x in storage.to_pylist()])
elif pa.types.is_struct(storage.type):
if storage.type.get_field_index("bytes") >= 0:
bytes_array = storage.field("bytes")
else:
bytes_array = pa.array([None] * len(storage), type=pa.binary())
if storage.type.get_field_index("path") >= 0:
path_array = storage.field("path")
else:
path_array = pa.array([None] * len(storage), type=pa.string())
storage = pa.StructArray.from_arrays([bytes_array, path_array], ["bytes", "path"], mask=storage.is_null())
return array_cast(storage, self.pa_type)
def embed_storage(self, storage: pa.StructArray) -> pa.StructArray:
"""Embed audio files into the Arrow array.
Args:
storage (`pa.StructArray`):
PyArrow array to embed.
Returns:
`pa.StructArray`: Array in the Audio arrow storage type, that is
`pa.struct({"bytes": pa.binary(), "path": pa.string()})`.
"""
@no_op_if_value_is_null
def path_to_bytes(path):
with xopen(path, "rb") as f:
bytes_ = f.read()
return bytes_
bytes_array = pa.array(
[
(path_to_bytes(x["path"]) if x["bytes"] is None else x["bytes"]) if x is not None else None
for x in storage.to_pylist()
],
type=pa.binary(),
)
path_array = pa.array(
[os.path.basename(path) if path is not None else None for path in storage.field("path").to_pylist()],
type=pa.string(),
)
storage = pa.StructArray.from_arrays([bytes_array, path_array], ["bytes", "path"], mask=bytes_array.is_null())
return array_cast(storage, self.pa_type)
| datasets/src/datasets/features/audio.py/0 | {
"file_path": "datasets/src/datasets/features/audio.py",
"repo_id": "datasets",
"token_count": 5335
} | 72 |
# Copyright 2020 The HuggingFace Datasets Authors.
#
# Licensed under the Apache License, Version 2.0 (the "License");
# you may not use this file except in compliance with the License.
# You may obtain a copy of the License at
#
# http://www.apache.org/licenses/LICENSE-2.0
#
# Unless required by applicable law or agreed to in writing, software
# distributed under the License is distributed on an "AS IS" BASIS,
# WITHOUT WARRANTIES OR CONDITIONS OF ANY KIND, either express or implied.
# See the License for the specific language governing permissions and
# limitations under the License.
# Lint as: python3
"""List and inspect datasets."""
import inspect
import os
import shutil
import warnings
from pathlib import Path, PurePath
from typing import Dict, List, Mapping, Optional, Sequence, Union
import huggingface_hub
from . import config
from .download.download_config import DownloadConfig
from .download.download_manager import DownloadMode
from .download.streaming_download_manager import StreamingDownloadManager
from .info import DatasetInfo
from .load import (
dataset_module_factory,
get_dataset_builder_class,
import_main_class,
load_dataset_builder,
metric_module_factory,
)
from .utils.deprecation_utils import deprecated
from .utils.file_utils import relative_to_absolute_path
from .utils.logging import get_logger
from .utils.version import Version
logger = get_logger(__name__)
class SplitsNotFoundError(ValueError):
pass
@deprecated("Use 'huggingface_hub.list_datasets' instead.")
def list_datasets(with_community_datasets=True, with_details=False):
"""List all the datasets scripts available on the Hugging Face Hub.
Args:
with_community_datasets (`bool`, *optional*, defaults to `True`):
Include the community provided datasets.
with_details (`bool`, *optional*, defaults to `False`):
Return the full details on the datasets instead of only the short name.
Example:
```py
>>> from datasets import list_datasets
>>> list_datasets()
['acronym_identification',
'ade_corpus_v2',
'adversarial_qa',
'aeslc',
'afrikaans_ner_corpus',
'ag_news',
...
]
```
"""
datasets = huggingface_hub.list_datasets(full=with_details)
if not with_community_datasets:
datasets = [dataset for dataset in datasets if "/" not in dataset.id]
if not with_details:
datasets = [dataset.id for dataset in datasets]
return list(datasets)
@deprecated(
"Use 'evaluate.list_evaluation_modules' instead, from the new library 🤗 Evaluate: https://huggingface.co/docs/evaluate"
)
def list_metrics(with_community_metrics=True, with_details=False):
"""List all the metrics script available on the Hugging Face Hub.
<Deprecated version="2.5.0">
Use `evaluate.list_evaluation_modules` instead, from the new library 🤗 Evaluate: https://huggingface.co/docs/evaluate
</Deprecated>
Args:
with_community_metrics (:obj:`bool`, optional, default ``True``): Include the community provided metrics.
with_details (:obj:`bool`, optional, default ``False``): Return the full details on the metrics instead of only the short name.
Example:
```py
>>> from datasets import list_metrics
>>> list_metrics()
['accuracy',
'bertscore',
'bleu',
'bleurt',
'cer',
'chrf',
...
]
```
"""
metrics = huggingface_hub.list_metrics()
if not with_community_metrics:
metrics = [metric for metric in metrics if "/" not in metric.id]
if not with_details:
metrics = [metric.id for metric in metrics]
return metrics
@deprecated("Clone the dataset repository from the Hugging Face Hub instead.")
def inspect_dataset(path: str, local_path: str, download_config: Optional[DownloadConfig] = None, **download_kwargs):
"""
Allow inspection/modification of a dataset script by copying on local drive at local_path.
Args:
path (`str`): Path to the dataset processing script with the dataset builder. Can be either:
- a local path to processing script or the directory containing the script (if the script has the same name
as the directory),
e.g. `'./dataset/squad'` or `'./dataset/squad/squad.py'`.
- a dataset identifier on the Hugging Face Hub (list all available datasets and ids with [`list_datasets`])
e.g. `'squad'`, `'glue'` or `'openai/webtext'`.
local_path (`str`):
Path to the local folder to copy the dataset script to.
download_config ([`DownloadConfig`], *optional*):
Specific download configuration parameters.
**download_kwargs (additional keyword arguments):
Optional arguments for [`DownloadConfig`] which will override
the attributes of `download_config` if supplied.
"""
if download_config is None:
download_config = DownloadConfig(**download_kwargs)
if os.path.isfile(path):
path = str(Path(path).parent)
if os.path.isdir(path):
shutil.copytree(path, local_path, dirs_exist_ok=True)
else:
huggingface_hub.HfApi(endpoint=config.HF_ENDPOINT, token=download_config.token).snapshot_download(
repo_id=path, repo_type="dataset", local_dir=local_path, force_download=download_config.force_download
)
print(
f"The dataset {path} can be inspected at {local_path}. "
f'You can modify this loading script if it has one and use it with `datasets.load_dataset("{PurePath(local_path).as_posix()}")`.'
)
@deprecated(
"Use 'evaluate.inspect_evaluation_module' instead, from the new library 🤗 Evaluate: https://huggingface.co/docs/evaluate"
)
def inspect_metric(path: str, local_path: str, download_config: Optional[DownloadConfig] = None, **download_kwargs):
r"""
Allow inspection/modification of a metric script by copying it on local drive at local_path.
<Deprecated version="2.5.0">
Use `evaluate.inspect_evaluation_module` instead, from the new library 🤗 Evaluate instead: https://huggingface.co/docs/evaluate
</Deprecated>
Args:
path (``str``): path to the dataset processing script with the dataset builder. Can be either:
- a local path to processing script or the directory containing the script (if the script has the same name as the directory),
e.g. ``'./dataset/squad'`` or ``'./dataset/squad/squad.py'``
- a dataset identifier on the Hugging Face Hub (list all available datasets and ids with ``datasets.list_datasets()``)
e.g. ``'squad'``, ``'glue'`` or ``'openai/webtext'``
local_path (``str``): path to the local folder to copy the datset script to.
download_config (Optional ``datasets.DownloadConfig``): specific download configuration parameters.
**download_kwargs (additional keyword arguments): optional attributes for DownloadConfig() which will override the attributes in download_config if supplied.
"""
metric_module = metric_module_factory(path, download_config=download_config, **download_kwargs)
metric_cls = import_main_class(metric_module.module_path, dataset=False)
module_source_path = inspect.getsourcefile(metric_cls)
module_source_dirpath = os.path.dirname(module_source_path)
for dirpath, dirnames, filenames in os.walk(module_source_dirpath):
dst_dirpath = os.path.join(local_path, os.path.relpath(dirpath, module_source_dirpath))
os.makedirs(dst_dirpath, exist_ok=True)
# skipping hidden directories; prune the search
dirnames[:] = [dirname for dirname in dirnames if not dirname.startswith((".", "__"))]
for filename in filenames:
shutil.copy2(os.path.join(dirpath, filename), os.path.join(dst_dirpath, filename))
shutil.copystat(dirpath, dst_dirpath)
local_path = relative_to_absolute_path(local_path)
print(
f"The processing scripts for metric {path} can be inspected at {local_path}. "
f"The main class is in {module_source_dirpath}. "
f'You can modify this processing scripts and use it with `datasets.load_metric("{PurePath(local_path).as_posix()}")`.'
)
def get_dataset_infos(
path: str,
data_files: Optional[Union[Dict, List, str]] = None,
download_config: Optional[DownloadConfig] = None,
download_mode: Optional[Union[DownloadMode, str]] = None,
revision: Optional[Union[str, Version]] = None,
token: Optional[Union[bool, str]] = None,
use_auth_token="deprecated",
**config_kwargs,
):
"""Get the meta information about a dataset, returned as a dict mapping config name to DatasetInfoDict.
Args:
path (`str`): path to the dataset processing script with the dataset builder. Can be either:
- a local path to processing script or the directory containing the script (if the script has the same name as the directory),
e.g. `'./dataset/squad'` or `'./dataset/squad/squad.py'`
- a dataset identifier on the Hugging Face Hub (list all available datasets and ids with [`datasets.list_datasets`])
e.g. `'squad'`, `'glue'` or``'openai/webtext'`
revision (`Union[str, datasets.Version]`, *optional*):
If specified, the dataset module will be loaded from the datasets repository at this version.
By default:
- it is set to the local version of the lib.
- it will also try to load it from the main branch if it's not available at the local version of the lib.
Specifying a version that is different from your local version of the lib might cause compatibility issues.
download_config ([`DownloadConfig`], *optional*):
Specific download configuration parameters.
download_mode ([`DownloadMode`] or `str`, defaults to `REUSE_DATASET_IF_EXISTS`):
Download/generate mode.
data_files (`Union[Dict, List, str]`, *optional*):
Defining the data_files of the dataset configuration.
token (`str` or `bool`, *optional*):
Optional string or boolean to use as Bearer token for remote files on the Datasets Hub.
If `True`, or not specified, will get token from `"~/.huggingface"`.
use_auth_token (`str` or `bool`, *optional*):
Optional string or boolean to use as Bearer token for remote files on the Datasets Hub.
If `True`, or not specified, will get token from `"~/.huggingface"`.
<Deprecated version="2.14.0">
`use_auth_token` was deprecated in favor of `token` in version 2.14.0 and will be removed in 3.0.0.
</Deprecated>
**config_kwargs (additional keyword arguments):
Optional attributes for builder class which will override the attributes if supplied.
Example:
```py
>>> from datasets import get_dataset_infos
>>> get_dataset_infos('rotten_tomatoes')
{'default': DatasetInfo(description="Movie Review Dataset.\nThis is a dataset of containing 5,331 positive and 5,331 negative processed\nsentences from Rotten Tomatoes movie reviews...), ...}
```
"""
if use_auth_token != "deprecated":
warnings.warn(
"'use_auth_token' was deprecated in favor of 'token' in version 2.14.0 and will be removed in 3.0.0.\n"
"You can remove this warning by passing 'token=<use_auth_token>' instead.",
FutureWarning,
)
token = use_auth_token
config_names = get_dataset_config_names(
path=path,
revision=revision,
download_config=download_config,
download_mode=download_mode,
data_files=data_files,
token=token,
)
return {
config_name: get_dataset_config_info(
path=path,
config_name=config_name,
data_files=data_files,
download_config=download_config,
download_mode=download_mode,
revision=revision,
token=token,
**config_kwargs,
)
for config_name in config_names
}
def get_dataset_config_names(
path: str,
revision: Optional[Union[str, Version]] = None,
download_config: Optional[DownloadConfig] = None,
download_mode: Optional[Union[DownloadMode, str]] = None,
dynamic_modules_path: Optional[str] = None,
data_files: Optional[Union[Dict, List, str]] = None,
**download_kwargs,
):
"""Get the list of available config names for a particular dataset.
Args:
path (`str`): path to the dataset processing script with the dataset builder. Can be either:
- a local path to processing script or the directory containing the script (if the script has the same name as the directory),
e.g. `'./dataset/squad'` or `'./dataset/squad/squad.py'`
- a dataset identifier on the Hugging Face Hub (list all available datasets and ids with [`datasets.list_datasets`])
e.g. `'squad'`, `'glue'` or `'openai/webtext'`
revision (`Union[str, datasets.Version]`, *optional*):
If specified, the dataset module will be loaded from the datasets repository at this version.
By default:
- it is set to the local version of the lib.
- it will also try to load it from the main branch if it's not available at the local version of the lib.
Specifying a version that is different from your local version of the lib might cause compatibility issues.
download_config ([`DownloadConfig`], *optional*):
Specific download configuration parameters.
download_mode ([`DownloadMode`] or `str`, defaults to `REUSE_DATASET_IF_EXISTS`):
Download/generate mode.
dynamic_modules_path (`str`, defaults to `~/.cache/huggingface/modules/datasets_modules`):
Optional path to the directory in which the dynamic modules are saved. It must have been initialized with `init_dynamic_modules`.
By default the datasets and metrics are stored inside the `datasets_modules` module.
data_files (`Union[Dict, List, str]`, *optional*):
Defining the data_files of the dataset configuration.
**download_kwargs (additional keyword arguments):
Optional attributes for [`DownloadConfig`] which will override the attributes in `download_config` if supplied,
for example `token`.
Example:
```py
>>> from datasets import get_dataset_config_names
>>> get_dataset_config_names("glue")
['cola',
'sst2',
'mrpc',
'qqp',
'stsb',
'mnli',
'mnli_mismatched',
'mnli_matched',
'qnli',
'rte',
'wnli',
'ax']
```
"""
dataset_module = dataset_module_factory(
path,
revision=revision,
download_config=download_config,
download_mode=download_mode,
dynamic_modules_path=dynamic_modules_path,
data_files=data_files,
**download_kwargs,
)
builder_cls = get_dataset_builder_class(dataset_module, dataset_name=os.path.basename(path))
return list(builder_cls.builder_configs.keys()) or [
dataset_module.builder_kwargs.get("config_name", builder_cls.DEFAULT_CONFIG_NAME or "default")
]
def get_dataset_default_config_name(
path: str,
revision: Optional[Union[str, Version]] = None,
download_config: Optional[DownloadConfig] = None,
download_mode: Optional[Union[DownloadMode, str]] = None,
dynamic_modules_path: Optional[str] = None,
data_files: Optional[Union[Dict, List, str]] = None,
**download_kwargs,
) -> Optional[str]:
"""Get the default config name for a particular dataset.
Can return None only if the dataset has multiple configurations and no default configuration.
Args:
path (`str`): path to the dataset processing script with the dataset builder. Can be either:
- a local path to processing script or the directory containing the script (if the script has the same name as the directory),
e.g. `'./dataset/squad'` or `'./dataset/squad/squad.py'`
- a dataset identifier on the Hugging Face Hub (list all available datasets and ids with [`datasets.list_datasets`])
e.g. `'squad'`, `'glue'` or `'openai/webtext'`
revision (`Union[str, datasets.Version]`, *optional*):
If specified, the dataset module will be loaded from the datasets repository at this version.
By default:
- it is set to the local version of the lib.
- it will also try to load it from the main branch if it's not available at the local version of the lib.
Specifying a version that is different from your local version of the lib might cause compatibility issues.
download_config ([`DownloadConfig`], *optional*):
Specific download configuration parameters.
download_mode ([`DownloadMode`] or `str`, defaults to `REUSE_DATASET_IF_EXISTS`):
Download/generate mode.
dynamic_modules_path (`str`, defaults to `~/.cache/huggingface/modules/datasets_modules`):
Optional path to the directory in which the dynamic modules are saved. It must have been initialized with `init_dynamic_modules`.
By default the datasets and metrics are stored inside the `datasets_modules` module.
data_files (`Union[Dict, List, str]`, *optional*):
Defining the data_files of the dataset configuration.
**download_kwargs (additional keyword arguments):
Optional attributes for [`DownloadConfig`] which will override the attributes in `download_config` if supplied,
for example `token`.
Returns:
Optional[str]: the default config name if there is one
Example:
```py
>>> from datasets import get_dataset_default_config_name
>>> get_dataset_default_config_name("openbookqa")
'main'
```
"""
dataset_module = dataset_module_factory(
path,
revision=revision,
download_config=download_config,
download_mode=download_mode,
dynamic_modules_path=dynamic_modules_path,
data_files=data_files,
**download_kwargs,
)
builder_cls = get_dataset_builder_class(dataset_module, dataset_name=os.path.basename(path))
builder_configs = list(builder_cls.builder_configs.keys())
if builder_configs:
default_config_name = builder_configs[0] if len(builder_configs) == 1 else None
else:
default_config_name = "default"
return builder_cls.DEFAULT_CONFIG_NAME or default_config_name
def get_dataset_config_info(
path: str,
config_name: Optional[str] = None,
data_files: Optional[Union[str, Sequence[str], Mapping[str, Union[str, Sequence[str]]]]] = None,
download_config: Optional[DownloadConfig] = None,
download_mode: Optional[Union[DownloadMode, str]] = None,
revision: Optional[Union[str, Version]] = None,
token: Optional[Union[bool, str]] = None,
use_auth_token="deprecated",
**config_kwargs,
) -> DatasetInfo:
"""Get the meta information (DatasetInfo) about a dataset for a particular config
Args:
path (``str``): path to the dataset processing script with the dataset builder. Can be either:
- a local path to processing script or the directory containing the script (if the script has the same name as the directory),
e.g. ``'./dataset/squad'`` or ``'./dataset/squad/squad.py'``
- a dataset identifier on the Hugging Face Hub (list all available datasets and ids with ``datasets.list_datasets()``)
e.g. ``'squad'``, ``'glue'`` or ``'openai/webtext'``
config_name (:obj:`str`, optional): Defining the name of the dataset configuration.
data_files (:obj:`str` or :obj:`Sequence` or :obj:`Mapping`, optional): Path(s) to source data file(s).
download_config (:class:`~download.DownloadConfig`, optional): Specific download configuration parameters.
download_mode (:class:`DownloadMode` or :obj:`str`, default ``REUSE_DATASET_IF_EXISTS``): Download/generate mode.
revision (:class:`~utils.Version` or :obj:`str`, optional): Version of the dataset script to load.
As datasets have their own git repository on the Datasets Hub, the default version "main" corresponds to their "main" branch.
You can specify a different version than the default "main" by using a commit SHA or a git tag of the dataset repository.
token (``str`` or :obj:`bool`, optional): Optional string or boolean to use as Bearer token for remote files on the Datasets Hub.
If True, or not specified, will get token from `"~/.huggingface"`.
use_auth_token (``str`` or :obj:`bool`, optional): Optional string or boolean to use as Bearer token for remote files on the Datasets Hub.
If True, or not specified, will get token from `"~/.huggingface"`.
<Deprecated version="2.14.0">
`use_auth_token` was deprecated in favor of `token` in version 2.14.0 and will be removed in 3.0.0.
</Deprecated>
**config_kwargs (additional keyword arguments): optional attributes for builder class which will override the attributes if supplied.
"""
if use_auth_token != "deprecated":
warnings.warn(
"'use_auth_token' was deprecated in favor of 'token' in version 2.14.0 and will be removed in 3.0.0.\n"
"You can remove this warning by passing 'token=<use_auth_token>' instead.",
FutureWarning,
)
token = use_auth_token
builder = load_dataset_builder(
path,
name=config_name,
data_files=data_files,
download_config=download_config,
download_mode=download_mode,
revision=revision,
token=token,
**config_kwargs,
)
info = builder.info
if info.splits is None:
download_config = download_config.copy() if download_config else DownloadConfig()
if token is not None:
download_config.token = token
builder._check_manual_download(
StreamingDownloadManager(base_path=builder.base_path, download_config=download_config)
)
try:
info.splits = {
split_generator.name: {"name": split_generator.name, "dataset_name": path}
for split_generator in builder._split_generators(
StreamingDownloadManager(base_path=builder.base_path, download_config=download_config)
)
}
except Exception as err:
raise SplitsNotFoundError("The split names could not be parsed from the dataset config.") from err
return info
def get_dataset_split_names(
path: str,
config_name: Optional[str] = None,
data_files: Optional[Union[str, Sequence[str], Mapping[str, Union[str, Sequence[str]]]]] = None,
download_config: Optional[DownloadConfig] = None,
download_mode: Optional[Union[DownloadMode, str]] = None,
revision: Optional[Union[str, Version]] = None,
token: Optional[Union[bool, str]] = None,
use_auth_token="deprecated",
**config_kwargs,
):
"""Get the list of available splits for a particular config and dataset.
Args:
path (`str`): path to the dataset processing script with the dataset builder. Can be either:
- a local path to processing script or the directory containing the script (if the script has the same name as the directory),
e.g. `'./dataset/squad'` or `'./dataset/squad/squad.py'`
- a dataset identifier on the Hugging Face Hub (list all available datasets and ids with [`datasets.list_datasets`])
e.g. `'squad'`, `'glue'` or `'openai/webtext'`
config_name (`str`, *optional*):
Defining the name of the dataset configuration.
data_files (`str` or `Sequence` or `Mapping`, *optional*):
Path(s) to source data file(s).
download_config ([`DownloadConfig`], *optional*):
Specific download configuration parameters.
download_mode ([`DownloadMode`] or `str`, defaults to `REUSE_DATASET_IF_EXISTS`):
Download/generate mode.
revision ([`Version`] or `str`, *optional*):
Version of the dataset script to load.
As datasets have their own git repository on the Datasets Hub, the default version "main" corresponds to their "main" branch.
You can specify a different version than the default "main" by using a commit SHA or a git tag of the dataset repository.
token (`str` or `bool`, *optional*):
Optional string or boolean to use as Bearer token for remote files on the Datasets Hub.
If `True`, or not specified, will get token from `"~/.huggingface"`.
use_auth_token (`str` or `bool`, *optional*):
Optional string or boolean to use as Bearer token for remote files on the Datasets Hub.
If `True`, or not specified, will get token from `"~/.huggingface"`.
<Deprecated version="2.14.0">
`use_auth_token` was deprecated in favor of `token` in version 2.14.0 and will be removed in 3.0.0.
</Deprecated>
**config_kwargs (additional keyword arguments):
Optional attributes for builder class which will override the attributes if supplied.
Example:
```py
>>> from datasets import get_dataset_split_names
>>> get_dataset_split_names('rotten_tomatoes')
['train', 'validation', 'test']
```
"""
if use_auth_token != "deprecated":
warnings.warn(
"'use_auth_token' was deprecated in favor of 'token' in version 2.14.0 and will be removed in 3.0.0.\n"
"You can remove this warning by passing 'token=<use_auth_token>' instead.",
FutureWarning,
)
token = use_auth_token
info = get_dataset_config_info(
path,
config_name=config_name,
data_files=data_files,
download_config=download_config,
download_mode=download_mode,
revision=revision,
token=token,
**config_kwargs,
)
return list(info.splits.keys())
| datasets/src/datasets/inspect.py/0 | {
"file_path": "datasets/src/datasets/inspect.py",
"repo_id": "datasets",
"token_count": 9937
} | 73 |
# Copyright 2020 The HuggingFace Datasets Authors and the TensorFlow Datasets Authors.
#
# Licensed under the Apache License, Version 2.0 (the "License");
# you may not use this file except in compliance with the License.
# You may obtain a copy of the License at
#
# http://www.apache.org/licenses/LICENSE-2.0
#
# Unless required by applicable law or agreed to in writing, software
# distributed under the License is distributed on an "AS IS" BASIS,
# WITHOUT WARRANTIES OR CONDITIONS OF ANY KIND, either express or implied.
# See the License for the specific language governing permissions and
# limitations under the License.
# Lint as: python3
"""Splits related API."""
import abc
import collections
import copy
import dataclasses
import re
from dataclasses import dataclass
from typing import Dict, List, Optional, Union
from .arrow_reader import FileInstructions, make_file_instructions
from .naming import _split_re
from .utils.py_utils import NonMutableDict, asdict
@dataclass
class SplitInfo:
name: str = dataclasses.field(default="", metadata={"include_in_asdict_even_if_is_default": True})
num_bytes: int = dataclasses.field(default=0, metadata={"include_in_asdict_even_if_is_default": True})
num_examples: int = dataclasses.field(default=0, metadata={"include_in_asdict_even_if_is_default": True})
shard_lengths: Optional[List[int]] = None
# Deprecated
# For backward compatibility, this field needs to always be included in files like
# dataset_infos.json and dataset_info.json files
# To do so, we always include it in the output of datasets.utils.py_utils.asdict(split_info)
dataset_name: Optional[str] = dataclasses.field(
default=None, metadata={"include_in_asdict_even_if_is_default": True}
)
@property
def file_instructions(self):
"""Returns the list of dict(filename, take, skip)."""
# `self.dataset_name` is assigned in `SplitDict.add()`.
instructions = make_file_instructions(
name=self.dataset_name,
split_infos=[self],
instruction=str(self.name),
)
return instructions.file_instructions
@dataclass
class SubSplitInfo:
"""Wrapper around a sub split info.
This class expose info on the subsplit:
```
ds, info = datasets.load_dataset(..., split='train[75%:]', with_info=True)
info.splits['train[75%:]'].num_examples
```
"""
instructions: FileInstructions
@property
def num_examples(self):
"""Returns the number of example in the subsplit."""
return self.instructions.num_examples
@property
def file_instructions(self):
"""Returns the list of dict(filename, take, skip)."""
return self.instructions.file_instructions
class SplitBase(metaclass=abc.ABCMeta):
# pylint: disable=line-too-long
"""Abstract base class for Split compositionality.
See the
[guide on splits](../loading#slice-splits)
for more information.
There are three parts to the composition:
1) The splits are composed (defined, merged, split,...) together before
calling the `.as_dataset()` function. This is done with the `__add__`,
`__getitem__`, which return a tree of `SplitBase` (whose leaf
are the `NamedSplit` objects)
```
split = datasets.Split.TRAIN + datasets.Split.TEST.subsplit(datasets.percent[:50])
```
2) The `SplitBase` is forwarded to the `.as_dataset()` function
to be resolved into actual read instruction. This is done by the
`.get_read_instruction()` method which takes the real dataset splits
(name, number of shards,...) and parse the tree to return a
`SplitReadInstruction()` object
```
read_instruction = split.get_read_instruction(self.info.splits)
```
3) The `SplitReadInstruction` is then used in the `tf.data.Dataset` pipeline
to define which files to read and how to skip examples within file.
"""
# pylint: enable=line-too-long
@abc.abstractmethod
def get_read_instruction(self, split_dict):
"""Parse the descriptor tree and compile all read instructions together.
Args:
split_dict: `dict`, The `dict[split_name, SplitInfo]` of the dataset
Returns:
split_read_instruction: `SplitReadInstruction`
"""
raise NotImplementedError("Abstract method")
def __eq__(self, other):
"""Equality: datasets.Split.TRAIN == 'train'."""
if isinstance(other, (NamedSplit, str)):
return False
raise NotImplementedError("Equality is not implemented between merged/sub splits.")
def __ne__(self, other):
"""InEquality: datasets.Split.TRAIN != 'test'."""
return not self.__eq__(other)
def __add__(self, other):
"""Merging: datasets.Split.TRAIN + datasets.Split.TEST."""
return _SplitMerged(self, other)
def subsplit(self, arg=None, k=None, percent=None, weighted=None): # pylint: disable=redefined-outer-name
"""Divides this split into subsplits.
There are 3 ways to define subsplits, which correspond to the 3
arguments `k` (get `k` even subsplits), `percent` (get a slice of the
dataset with `datasets.percent`), and `weighted` (get subsplits with proportions
specified by `weighted`).
Example::
```
# 50% train, 50% test
train, test = split.subsplit(k=2)
# 50% train, 25% test, 25% validation
train, test, validation = split.subsplit(weighted=[2, 1, 1])
# Extract last 20%
subsplit = split.subsplit(datasets.percent[-20:])
```
Warning: k and weighted will be converted into percent which mean that
values below the percent will be rounded up or down. The final split may be
bigger to deal with remainders. For instance:
```
train, test, valid = split.subsplit(k=3) # 33%, 33%, 34%
s1, s2, s3, s4 = split.subsplit(weighted=[2, 2, 1, 1]) # 33%, 33%, 16%, 18%
```
Args:
arg: If no kwargs are given, `arg` will be interpreted as one of
`k`, `percent`, or `weighted` depending on the type.
For example:
```
split.subsplit(10) # Equivalent to split.subsplit(k=10)
split.subsplit(datasets.percent[:-20]) # percent=datasets.percent[:-20]
split.subsplit([1, 1, 2]) # weighted=[1, 1, 2]
```
k: `int` If set, subdivide the split into `k` equal parts.
percent: `datasets.percent slice`, return a single subsplit corresponding to
a slice of the original split. For example:
`split.subsplit(datasets.percent[-20:]) # Last 20% of the dataset`.
weighted: `list[int]`, return a list of subsplits whose proportions match
the normalized sum of the list. For example:
`split.subsplit(weighted=[1, 1, 2]) # 25%, 25%, 50%`.
Returns:
A subsplit or list of subsplits extracted from this split object.
"""
# Note that the percent kwargs redefine the outer name datasets.percent. This
# is done for consistency (.subsplit(percent=datasets.percent[:40]))
if sum(bool(x) for x in (arg, k, percent, weighted)) != 1:
raise ValueError("Only one argument of subsplit should be set.")
# Auto deduce k
if isinstance(arg, int):
k = arg
elif isinstance(arg, slice):
percent = arg
elif isinstance(arg, list):
weighted = arg
if not (k or percent or weighted):
raise ValueError(
f"Invalid split argument {arg}. Only list, slice and int supported. "
"One of k, weighted or percent should be set to a non empty value."
)
def assert_slices_coverage(slices):
# Ensure that the expended slices cover all percents.
assert sum((list(range(*s.indices(100))) for s in slices), []) == list(range(100))
if k:
if not 0 < k <= 100:
raise ValueError(f"Subsplit k should be between 0 and 100, got {k}")
shift = 100 // k
slices = [slice(i * shift, (i + 1) * shift) for i in range(k)]
# Round up last element to ensure all elements are taken
slices[-1] = slice(slices[-1].start, 100)
# Internal check to ensure full coverage
assert_slices_coverage(slices)
return tuple(_SubSplit(self, s) for s in slices)
elif percent:
return _SubSplit(self, percent)
elif weighted:
# Normalize the weighted sum
total = sum(weighted)
weighted = [100 * x // total for x in weighted]
# Create the slice for each of the elements
start = 0
stop = 0
slices = []
for v in weighted:
stop += v
slices.append(slice(start, stop))
start = stop
# Round up last element to ensure all elements are taken
slices[-1] = slice(slices[-1].start, 100)
# Internal check to ensure full coverage
assert_slices_coverage(slices)
return tuple(_SubSplit(self, s) for s in slices)
else:
# Should not be possible
raise ValueError("Could not determine the split")
# 2 requirements:
# 1. datasets.percent be sliceable
# 2. datasets.percent be documented
#
# Instances are not documented, so we want datasets.percent to be a class, but to
# have it be sliceable, we need this metaclass.
class PercentSliceMeta(type):
def __getitem__(cls, slice_value):
if not isinstance(slice_value, slice):
raise ValueError(f"datasets.percent should only be called with slice, not {slice_value}")
return slice_value
class PercentSlice(metaclass=PercentSliceMeta):
# pylint: disable=line-too-long
"""Syntactic sugar for defining slice subsplits: `datasets.percent[75:-5]`.
See the
[guide on splits](../loading#slice-splits)
for more information.
"""
# pylint: enable=line-too-long
pass
percent = PercentSlice # pylint: disable=invalid-name
class _SplitMerged(SplitBase):
"""Represent two split descriptors merged together."""
def __init__(self, split1, split2):
self._split1 = split1
self._split2 = split2
def get_read_instruction(self, split_dict):
read_instruction1 = self._split1.get_read_instruction(split_dict)
read_instruction2 = self._split2.get_read_instruction(split_dict)
return read_instruction1 + read_instruction2
def __repr__(self):
return f"({repr(self._split1)} + {repr(self._split2)})"
class _SubSplit(SplitBase):
"""Represent a sub split of a split descriptor."""
def __init__(self, split, slice_value):
self._split = split
self._slice_value = slice_value
def get_read_instruction(self, split_dict):
return self._split.get_read_instruction(split_dict)[self._slice_value]
def __repr__(self):
slice_str = "{start}:{stop}"
if self._slice_value.step is not None:
slice_str += ":{step}"
slice_str = slice_str.format(
start="" if self._slice_value.start is None else self._slice_value.start,
stop="" if self._slice_value.stop is None else self._slice_value.stop,
step=self._slice_value.step,
)
return f"{repr(self._split)}(datasets.percent[{slice_str}])"
class NamedSplit(SplitBase):
"""Descriptor corresponding to a named split (train, test, ...).
Example:
Each descriptor can be composed with other using addition or slice:
```py
split = datasets.Split.TRAIN.subsplit(datasets.percent[0:25]) + datasets.Split.TEST
```
The resulting split will correspond to 25% of the train split merged with
100% of the test split.
A split cannot be added twice, so the following will fail:
```py
split = (
datasets.Split.TRAIN.subsplit(datasets.percent[:25]) +
datasets.Split.TRAIN.subsplit(datasets.percent[75:])
) # Error
split = datasets.Split.TEST + datasets.Split.ALL # Error
```
The slices can be applied only one time. So the following are valid:
```py
split = (
datasets.Split.TRAIN.subsplit(datasets.percent[:25]) +
datasets.Split.TEST.subsplit(datasets.percent[:50])
)
split = (datasets.Split.TRAIN + datasets.Split.TEST).subsplit(datasets.percent[:50])
```
But this is not valid:
```py
train = datasets.Split.TRAIN
test = datasets.Split.TEST
split = train.subsplit(datasets.percent[:25]).subsplit(datasets.percent[:25])
split = (train.subsplit(datasets.percent[:25]) + test).subsplit(datasets.percent[:50])
```
"""
def __init__(self, name):
self._name = name
split_names_from_instruction = [split_instruction.split("[")[0] for split_instruction in name.split("+")]
for split_name in split_names_from_instruction:
if not re.match(_split_re, split_name):
raise ValueError(f"Split name should match '{_split_re}' but got '{split_name}'.")
def __str__(self):
return self._name
def __repr__(self):
return f"NamedSplit({self._name!r})"
def __eq__(self, other):
"""Equality: datasets.Split.TRAIN == 'train'."""
if isinstance(other, NamedSplit):
return self._name == other._name # pylint: disable=protected-access
elif isinstance(other, SplitBase):
return False
elif isinstance(other, str): # Other should be string
return self._name == other
else:
raise ValueError(f"Equality not supported between split {self} and {other}")
def __lt__(self, other):
return self._name < other._name # pylint: disable=protected-access
def __hash__(self):
return hash(self._name)
def get_read_instruction(self, split_dict):
return SplitReadInstruction(split_dict[self._name])
class NamedSplitAll(NamedSplit):
"""Split corresponding to the union of all defined dataset splits."""
def __init__(self):
super().__init__("all")
def __repr__(self):
return "NamedSplitAll()"
def get_read_instruction(self, split_dict):
# Merge all dataset split together
read_instructions = [SplitReadInstruction(s) for s in split_dict.values()]
return sum(read_instructions, SplitReadInstruction())
class Split:
# pylint: disable=line-too-long
"""`Enum` for dataset splits.
Datasets are typically split into different subsets to be used at various
stages of training and evaluation.
- `TRAIN`: the training data.
- `VALIDATION`: the validation data. If present, this is typically used as
evaluation data while iterating on a model (e.g. changing hyperparameters,
model architecture, etc.).
- `TEST`: the testing data. This is the data to report metrics on. Typically
you do not want to use this during model iteration as you may overfit to it.
- `ALL`: the union of all defined dataset splits.
All splits, including compositions inherit from `datasets.SplitBase`.
See the [guide](../load_hub#splits) on splits for more information.
Example:
```py
>>> datasets.SplitGenerator(
... name=datasets.Split.TRAIN,
... gen_kwargs={"split_key": "train", "files": dl_manager.download_and extract(url)},
... ),
... datasets.SplitGenerator(
... name=datasets.Split.VALIDATION,
... gen_kwargs={"split_key": "validation", "files": dl_manager.download_and extract(url)},
... ),
... datasets.SplitGenerator(
... name=datasets.Split.TEST,
... gen_kwargs={"split_key": "test", "files": dl_manager.download_and extract(url)},
... )
```
"""
# pylint: enable=line-too-long
TRAIN = NamedSplit("train")
TEST = NamedSplit("test")
VALIDATION = NamedSplit("validation")
ALL = NamedSplitAll()
def __new__(cls, name):
"""Create a custom split with datasets.Split('custom_name')."""
return NamedSplitAll() if name == "all" else NamedSplit(name)
# Similar to SplitInfo, but contain an additional slice info
SlicedSplitInfo = collections.namedtuple(
"SlicedSplitInfo",
[
"split_info",
"slice_value",
],
) # noqa: E231
class SplitReadInstruction:
"""Object containing the reading instruction for the dataset.
Similarly to `SplitDescriptor` nodes, this object can be composed with itself,
but the resolution happens instantaneously, instead of keeping track of the
tree, such as all instructions are compiled and flattened in a single
SplitReadInstruction object containing the list of files and slice to use.
Once resolved, the instructions can be accessed with:
```
read_instructions.get_list_sliced_split_info() # List of splits to use
```
"""
def __init__(self, split_info=None):
self._splits = NonMutableDict(error_msg="Overlap between splits. Split {key} has been added with " "itself.")
if split_info:
self.add(SlicedSplitInfo(split_info=split_info, slice_value=None))
def add(self, sliced_split):
"""Add a SlicedSplitInfo the read instructions."""
# TODO(epot): Check that the number of examples per shard % 100 == 0
# Otherwise the slices value may be unbalanced and not exactly reflect the
# requested slice.
self._splits[sliced_split.split_info.name] = sliced_split
def __add__(self, other):
"""Merging split together."""
# Will raise error if a split has already be added (NonMutableDict)
# TODO(epot): If a split is already added but there is no overlap between
# the slices, should merge the slices (ex: [:10] + [80:])
split_instruction = SplitReadInstruction()
split_instruction._splits.update(self._splits) # pylint: disable=protected-access
split_instruction._splits.update(other._splits) # pylint: disable=protected-access
return split_instruction
def __getitem__(self, slice_value):
"""Sub-splits."""
# Will raise an error if a split has already been sliced
split_instruction = SplitReadInstruction()
for v in self._splits.values():
if v.slice_value is not None:
raise ValueError(f"Trying to slice Split {v.split_info.name} which has already been sliced")
v = v._asdict()
v["slice_value"] = slice_value
split_instruction.add(SlicedSplitInfo(**v))
return split_instruction
def get_list_sliced_split_info(self):
return list(self._splits.values())
class SplitDict(dict):
"""Split info object."""
def __init__(self, *args, dataset_name=None, **kwargs):
super().__init__(*args, **kwargs)
self.dataset_name = dataset_name
def __getitem__(self, key: Union[SplitBase, str]):
# 1st case: The key exists: `info.splits['train']`
if str(key) in self:
return super().__getitem__(str(key))
# 2nd case: Uses instructions: `info.splits['train[50%]']`
else:
instructions = make_file_instructions(
name=self.dataset_name,
split_infos=self.values(),
instruction=key,
)
return SubSplitInfo(instructions)
def __setitem__(self, key: Union[SplitBase, str], value: SplitInfo):
if key != value.name:
raise ValueError(f"Cannot add elem. (key mismatch: '{key}' != '{value.name}')")
super().__setitem__(key, value)
def add(self, split_info: SplitInfo):
"""Add the split info."""
if split_info.name in self:
raise ValueError(f"Split {split_info.name} already present")
split_info.dataset_name = self.dataset_name
super().__setitem__(split_info.name, split_info)
@property
def total_num_examples(self):
"""Return the total number of examples."""
return sum(s.num_examples for s in self.values())
@classmethod
def from_split_dict(cls, split_infos: Union[List, Dict], dataset_name: Optional[str] = None):
"""Returns a new SplitDict initialized from a Dict or List of `split_infos`."""
if isinstance(split_infos, dict):
split_infos = list(split_infos.values())
if dataset_name is None:
dataset_name = split_infos[0].get("dataset_name") if split_infos else None
split_dict = cls(dataset_name=dataset_name)
for split_info in split_infos:
if isinstance(split_info, dict):
split_info = SplitInfo(**split_info)
split_dict.add(split_info)
return split_dict
def to_split_dict(self):
"""Returns a list of SplitInfo protos that we have."""
out = []
for split_name, split_info in self.items():
split_info = copy.deepcopy(split_info)
split_info.name = split_name
out.append(split_info)
return out
def copy(self):
return SplitDict.from_split_dict(self.to_split_dict(), self.dataset_name)
def _to_yaml_list(self) -> list:
out = [asdict(s) for s in self.to_split_dict()]
# we don't need the shard lengths in YAML, since it depends on max_shard_size and num_proc
for split_info_dict in out:
split_info_dict.pop("shard_lengths", None)
# we don't need the dataset_name attribute that is deprecated
for split_info_dict in out:
split_info_dict.pop("dataset_name", None)
return out
@classmethod
def _from_yaml_list(cls, yaml_data: list) -> "SplitDict":
return cls.from_split_dict(yaml_data)
@dataclass
class SplitGenerator:
"""Defines the split information for the generator.
This should be used as returned value of
`GeneratorBasedBuilder._split_generators`.
See `GeneratorBasedBuilder._split_generators` for more info and example
of usage.
Args:
name (`str`):
Name of the `Split` for which the generator will
create the examples.
**gen_kwargs (additional keyword arguments):
Keyword arguments to forward to the `DatasetBuilder._generate_examples` method
of the builder.
Example:
```py
>>> datasets.SplitGenerator(
... name=datasets.Split.TRAIN,
... gen_kwargs={"split_key": "train", "files": dl_manager.download_and_extract(url)},
... )
```
"""
name: str
gen_kwargs: Dict = dataclasses.field(default_factory=dict)
split_info: SplitInfo = dataclasses.field(init=False)
def __post_init__(self):
self.name = str(self.name) # Make sure we convert NamedSplits in strings
NamedSplit(self.name) # check that it's a valid split name
self.split_info = SplitInfo(name=self.name)
| datasets/src/datasets/splits.py/0 | {
"file_path": "datasets/src/datasets/splits.py",
"repo_id": "datasets",
"token_count": 9598
} | 74 |
import os
from apache_beam.io.filesystems import FileSystems
from apache_beam.pipeline import Pipeline
from .logging import get_logger
CHUNK_SIZE = 2 << 20 # 2mb
logger = get_logger(__name__)
class BeamPipeline(Pipeline):
"""Wrapper over `apache_beam.pipeline.Pipeline` for convenience"""
def is_local(self):
runner = self._options.get_all_options().get("runner")
return runner in [None, "DirectRunner", "PortableRunner"]
def upload_local_to_remote(local_file_path, remote_file_path, force_upload=False):
"""Use the Beam Filesystems to upload to a remote directory on gcs/s3/hdfs..."""
fs = FileSystems
if fs.exists(remote_file_path):
if force_upload:
logger.info(f"Remote path already exist: {remote_file_path}. Overwriting it as force_upload=True.")
else:
logger.info(f"Remote path already exist: {remote_file_path}. Skipping it as force_upload=False.")
return
with fs.create(remote_file_path) as remote_file:
with open(local_file_path, "rb") as local_file:
chunk = local_file.read(CHUNK_SIZE)
while chunk:
remote_file.write(chunk)
chunk = local_file.read(CHUNK_SIZE)
def download_remote_to_local(remote_file_path, local_file_path, force_download=False):
"""Use the Beam Filesystems to download from a remote directory on gcs/s3/hdfs..."""
fs = FileSystems
if os.path.exists(local_file_path):
if force_download:
logger.info(f"Local path already exist: {remote_file_path}. Overwriting it as force_upload=True.")
else:
logger.info(f"Local path already exist: {remote_file_path}. Skipping it as force_upload=False.")
return
with fs.open(remote_file_path) as remote_file:
with open(local_file_path, "wb") as local_file:
chunk = remote_file.read(CHUNK_SIZE)
while chunk:
local_file.write(chunk)
chunk = remote_file.read(CHUNK_SIZE)
| datasets/src/datasets/utils/beam_utils.py/0 | {
"file_path": "datasets/src/datasets/utils/beam_utils.py",
"repo_id": "datasets",
"token_count": 847
} | 75 |
{
"language": [
"found",
"crowdsourced",
"expert-generated",
"machine-generated",
"other"
],
"annotations": [
"found",
"crowdsourced",
"expert-generated",
"machine-generated",
"no-annotation",
"other"
]
}
| datasets/src/datasets/utils/resources/creators.json/0 | {
"file_path": "datasets/src/datasets/utils/resources/creators.json",
"repo_id": "datasets",
"token_count": 119
} | 76 |
## Add Dummy data test
**Important** In order to pass the `load_dataset_<dataset_name>` test, dummy data is required for all possible config names.
First we distinguish between datasets scripts that
- A) have no config class and
- B) have a config class
For A) the dummy data folder structure, will always look as follows:
- ``dummy/<version>/dummy_data.zip``, *e.g.* ``cosmos_qa/dummy/0.1.0/dummy_data.zip``.
For B) the dummy data folder structure, will always look as follows:
- ``dummy/<config_name>/<version>/dummy_data.zip``, *e.g.* ``squad/dummy/plain-text/1.0.0/dummy_data.zip``.
Now the difficult part is to create the correct `dummy_data.zip` file.
**Important** When checking the dummy folder structure of already added datasets, always unzip ``dummy_data.zip``. If a folder ``dummy_data`` is found next to ``dummy_data.zip``, it is probably an old version and should be deleted. The tests only take the ``dummy_data.zip`` file into account.
Here we have to pay close attention to the ``_split_generators(self, dl_manager)`` function of the dataset script in question.
There are three general possibilties:
1) The ``dl_manager.download_and_extract()`` is given a **single path variable** of type `str` as its argument. In this case the file `dummy_data.zip` should unzip to the following structure:
``os.path.join("dummy_data", <additional-paths-as-defined-in-split-generations>)`` *e.g.* for ``sentiment140``, the unzipped ``dummy_data.zip`` has the following dir structure ``dummy_data/testdata.manual.2009.06.14.csv`` and ``dummy_data/training.1600000.processed.noemoticon.csv``.
**Note** if there are no ``<additional-paths-as-defined-in-split-generations>``, then ``dummy_data`` should be the name of the single file. An example for this is the ``crime-and-punishment`` dataset script.
2) The ``dl_manager.download_and_extract()`` is given a **dictionary of paths** of type `str` as its argument. In this case the file `dummy_data.zip` should unzip to the following structure:
``os.path.join("dummy_data", <value_of_dict>.split('/')[-1], <additional-paths-as-defined-in-split-generations>)`` *e.g.* for ``squad``, the unzipped ``dummy_data.zip`` has the following dir structure ``dummy_data/dev-v1.1.json``, etc...
**Note** if ``<value_of_dict>`` is a zipped file then the dummy data folder structure should contain the exact name of the zipped file and the following extracted folder structure. The file `dummy_data.zip` should **never** itself contain a zipped file since the dummy data is not unzipped by the ``MockDownloadManager`` during testing. *E.g.* check the dummy folder structure of ``hansards`` where the folders have to be named ``*.tar`` or the structure of ``wiki_split`` where the folders have to be named ``*.zip``.
3) The ``dl_manager.download_and_extract()`` is given a **dictionary of lists of paths** of type `str` as its argument. This is a very special case and has been seen only for the dataset ``ensli``. In this case the values are simply flattened and the dummy folder structure is the same as in 2).
| datasets/tests/README.md/0 | {
"file_path": "datasets/tests/README.md",
"repo_id": "datasets",
"token_count": 928
} | 77 |
import os
import random
import tempfile
import unittest
import numpy as np
import pandas as pd
import pyarrow as pa
import pytest
from absl.testing import parameterized
import datasets
from datasets.arrow_writer import ArrowWriter
from datasets.features import Array2D, Array3D, Array4D, Array5D, Value
from datasets.features.features import Array3DExtensionType, PandasArrayExtensionDtype, _ArrayXD
from datasets.formatting.formatting import NumpyArrowExtractor, SimpleArrowExtractor
SHAPE_TEST_1 = (30, 487)
SHAPE_TEST_2 = (36, 1024)
SHAPE_TEST_3 = (None, 100)
SPEED_TEST_SHAPE = (100, 100)
SPEED_TEST_N_EXAMPLES = 100
DEFAULT_FEATURES = datasets.Features(
{
"text": Array2D(SHAPE_TEST_1, dtype="float32"),
"image": Array2D(SHAPE_TEST_2, dtype="float32"),
"dynamic": Array2D(SHAPE_TEST_3, dtype="float32"),
}
)
def generate_examples(features: dict, num_examples=100, seq_shapes=None):
dummy_data = []
seq_shapes = seq_shapes or {}
for i in range(num_examples):
example = {}
for col_id, (k, v) in enumerate(features.items()):
if isinstance(v, _ArrayXD):
if k == "dynamic":
first_dim = random.randint(1, 3)
data = np.random.rand(first_dim, *v.shape[1:]).astype(v.dtype)
else:
data = np.random.rand(*v.shape).astype(v.dtype)
elif isinstance(v, datasets.Value):
data = "foo"
elif isinstance(v, datasets.Sequence):
while isinstance(v, datasets.Sequence):
v = v.feature
shape = seq_shapes[k]
data = np.random.rand(*shape).astype(v.dtype)
example[k] = data
dummy_data.append((i, example))
return dummy_data
class ExtensionTypeCompatibilityTest(unittest.TestCase):
def test_array2d_nonspecific_shape(self):
with tempfile.TemporaryDirectory() as tmp_dir:
my_features = DEFAULT_FEATURES.copy()
with ArrowWriter(features=my_features, path=os.path.join(tmp_dir, "beta.arrow")) as writer:
for key, record in generate_examples(
features=my_features,
num_examples=1,
):
example = my_features.encode_example(record)
writer.write(example)
num_examples, num_bytes = writer.finalize()
dataset = datasets.Dataset.from_file(os.path.join(tmp_dir, "beta.arrow"))
dataset.set_format("numpy")
row = dataset[0]
first_shape = row["image"].shape
second_shape = row["text"].shape
self.assertTrue(first_shape is not None and second_shape is not None, "need atleast 2 different shapes")
self.assertEqual(len(first_shape), len(second_shape), "both shapes are supposed to be equal length")
self.assertNotEqual(first_shape, second_shape, "shapes must not be the same")
del dataset
def test_multiple_extensions_same_row(self):
with tempfile.TemporaryDirectory() as tmp_dir:
my_features = DEFAULT_FEATURES.copy()
with ArrowWriter(features=my_features, path=os.path.join(tmp_dir, "beta.arrow")) as writer:
for key, record in generate_examples(features=my_features, num_examples=1):
example = my_features.encode_example(record)
writer.write(example)
num_examples, num_bytes = writer.finalize()
dataset = datasets.Dataset.from_file(os.path.join(tmp_dir, "beta.arrow"))
dataset.set_format("numpy")
row = dataset[0]
first_len = len(row["image"].shape)
second_len = len(row["text"].shape)
third_len = len(row["dynamic"].shape)
self.assertEqual(first_len, 2, "use a sequence type if dim is < 2")
self.assertEqual(second_len, 2, "use a sequence type if dim is < 2")
self.assertEqual(third_len, 2, "use a sequence type if dim is < 2")
del dataset
def test_compatability_with_string_values(self):
with tempfile.TemporaryDirectory() as tmp_dir:
my_features = DEFAULT_FEATURES.copy()
my_features["image_id"] = datasets.Value("string")
with ArrowWriter(features=my_features, path=os.path.join(tmp_dir, "beta.arrow")) as writer:
for key, record in generate_examples(features=my_features, num_examples=1):
example = my_features.encode_example(record)
writer.write(example)
num_examples, num_bytes = writer.finalize()
dataset = datasets.Dataset.from_file(os.path.join(tmp_dir, "beta.arrow"))
self.assertIsInstance(dataset[0]["image_id"], str, "image id must be of type string")
del dataset
def test_extension_indexing(self):
with tempfile.TemporaryDirectory() as tmp_dir:
my_features = DEFAULT_FEATURES.copy()
my_features["explicit_ext"] = Array2D((3, 3), dtype="float32")
with ArrowWriter(features=my_features, path=os.path.join(tmp_dir, "beta.arrow")) as writer:
for key, record in generate_examples(features=my_features, num_examples=1):
example = my_features.encode_example(record)
writer.write(example)
num_examples, num_bytes = writer.finalize()
dataset = datasets.Dataset.from_file(os.path.join(tmp_dir, "beta.arrow"))
dataset.set_format("numpy")
data = dataset[0]["explicit_ext"]
self.assertIsInstance(data, np.ndarray, "indexed extension must return numpy.ndarray")
del dataset
def get_array_feature_types():
shape_1 = [3] * 5
shape_2 = [3, 4, 5, 6, 7]
return [
{
"testcase_name": f"{d}d",
"array_feature": array_feature,
"shape_1": tuple(shape_1[:d]),
"shape_2": tuple(shape_2[:d]),
}
for d, array_feature in zip(range(2, 6), [Array2D, Array3D, Array4D, Array5D])
]
@parameterized.named_parameters(get_array_feature_types())
class ArrayXDTest(unittest.TestCase):
def get_features(self, array_feature, shape_1, shape_2):
return datasets.Features(
{
"image": array_feature(shape_1, dtype="float32"),
"source": Value("string"),
"matrix": array_feature(shape_2, dtype="float32"),
}
)
def get_dict_example_0(self, shape_1, shape_2):
return {
"image": np.random.rand(*shape_1).astype("float32"),
"source": "foo",
"matrix": np.random.rand(*shape_2).astype("float32"),
}
def get_dict_example_1(self, shape_1, shape_2):
return {
"image": np.random.rand(*shape_1).astype("float32"),
"matrix": np.random.rand(*shape_2).astype("float32"),
"source": "bar",
}
def get_dict_examples(self, shape_1, shape_2):
return {
"image": np.random.rand(2, *shape_1).astype("float32").tolist(),
"source": ["foo", "bar"],
"matrix": np.random.rand(2, *shape_2).astype("float32").tolist(),
}
def _check_getitem_output_type(self, dataset, shape_1, shape_2, first_matrix):
matrix_column = dataset["matrix"]
self.assertIsInstance(matrix_column, list)
self.assertIsInstance(matrix_column[0], list)
self.assertIsInstance(matrix_column[0][0], list)
self.assertTupleEqual(np.array(matrix_column).shape, (2, *shape_2))
matrix_field_of_first_example = dataset[0]["matrix"]
self.assertIsInstance(matrix_field_of_first_example, list)
self.assertIsInstance(matrix_field_of_first_example, list)
self.assertEqual(np.array(matrix_field_of_first_example).shape, shape_2)
np.testing.assert_array_equal(np.array(matrix_field_of_first_example), np.array(first_matrix))
matrix_field_of_first_two_examples = dataset[:2]["matrix"]
self.assertIsInstance(matrix_field_of_first_two_examples, list)
self.assertIsInstance(matrix_field_of_first_two_examples[0], list)
self.assertIsInstance(matrix_field_of_first_two_examples[0][0], list)
self.assertTupleEqual(np.array(matrix_field_of_first_two_examples).shape, (2, *shape_2))
with dataset.formatted_as("numpy"):
self.assertTupleEqual(dataset["matrix"].shape, (2, *shape_2))
self.assertEqual(dataset[0]["matrix"].shape, shape_2)
self.assertTupleEqual(dataset[:2]["matrix"].shape, (2, *shape_2))
with dataset.formatted_as("pandas"):
self.assertIsInstance(dataset["matrix"], pd.Series)
self.assertIsInstance(dataset[0]["matrix"], pd.Series)
self.assertIsInstance(dataset[:2]["matrix"], pd.Series)
self.assertTupleEqual(dataset["matrix"].to_numpy().shape, (2, *shape_2))
self.assertTupleEqual(dataset[0]["matrix"].to_numpy().shape, (1, *shape_2))
self.assertTupleEqual(dataset[:2]["matrix"].to_numpy().shape, (2, *shape_2))
def test_write(self, array_feature, shape_1, shape_2):
with tempfile.TemporaryDirectory() as tmp_dir:
my_features = self.get_features(array_feature, shape_1, shape_2)
my_examples = [
(0, self.get_dict_example_0(shape_1, shape_2)),
(1, self.get_dict_example_1(shape_1, shape_2)),
]
with ArrowWriter(features=my_features, path=os.path.join(tmp_dir, "beta.arrow")) as writer:
for key, record in my_examples:
example = my_features.encode_example(record)
writer.write(example)
num_examples, num_bytes = writer.finalize()
dataset = datasets.Dataset.from_file(os.path.join(tmp_dir, "beta.arrow"))
self._check_getitem_output_type(dataset, shape_1, shape_2, my_examples[0][1]["matrix"])
del dataset
def test_write_batch(self, array_feature, shape_1, shape_2):
with tempfile.TemporaryDirectory() as tmp_dir:
my_features = self.get_features(array_feature, shape_1, shape_2)
dict_examples = self.get_dict_examples(shape_1, shape_2)
dict_examples = my_features.encode_batch(dict_examples)
with ArrowWriter(features=my_features, path=os.path.join(tmp_dir, "beta.arrow")) as writer:
writer.write_batch(dict_examples)
num_examples, num_bytes = writer.finalize()
dataset = datasets.Dataset.from_file(os.path.join(tmp_dir, "beta.arrow"))
self._check_getitem_output_type(dataset, shape_1, shape_2, dict_examples["matrix"][0])
del dataset
def test_from_dict(self, array_feature, shape_1, shape_2):
dict_examples = self.get_dict_examples(shape_1, shape_2)
dataset = datasets.Dataset.from_dict(
dict_examples, features=self.get_features(array_feature, shape_1, shape_2)
)
self._check_getitem_output_type(dataset, shape_1, shape_2, dict_examples["matrix"][0])
del dataset
class ArrayXDDynamicTest(unittest.TestCase):
def get_one_col_dataset(self, first_dim_list, fixed_shape):
features = datasets.Features({"image": Array3D(shape=(None, *fixed_shape), dtype="float32")})
dict_values = {"image": [np.random.rand(fdim, *fixed_shape).astype("float32") for fdim in first_dim_list]}
dataset = datasets.Dataset.from_dict(dict_values, features=features)
return dataset
def get_two_col_datasset(self, first_dim_list, fixed_shape):
features = datasets.Features(
{"image": Array3D(shape=(None, *fixed_shape), dtype="float32"), "text": Value("string")}
)
dict_values = {
"image": [np.random.rand(fdim, *fixed_shape).astype("float32") for fdim in first_dim_list],
"text": ["text" for _ in first_dim_list],
}
dataset = datasets.Dataset.from_dict(dict_values, features=features)
return dataset
def test_to_pylist(self):
fixed_shape = (2, 2)
first_dim_list = [1, 3, 10]
dataset = self.get_one_col_dataset(first_dim_list, fixed_shape)
arr_xd = SimpleArrowExtractor().extract_column(dataset._data)
self.assertIsInstance(arr_xd.type, Array3DExtensionType)
pylist = arr_xd.to_pylist()
for first_dim, single_arr in zip(first_dim_list, pylist):
self.assertIsInstance(single_arr, list)
self.assertTupleEqual(np.array(single_arr).shape, (first_dim, *fixed_shape))
def test_to_numpy(self):
fixed_shape = (2, 2)
# ragged
first_dim_list = [1, 3, 10]
dataset = self.get_one_col_dataset(first_dim_list, fixed_shape)
arr_xd = SimpleArrowExtractor().extract_column(dataset._data)
self.assertIsInstance(arr_xd.type, Array3DExtensionType)
# replace with arr_xd = arr_xd.combine_chunks() when 12.0.0 will be the minimal required PyArrow version
arr_xd = arr_xd.type.wrap_array(pa.concat_arrays([chunk.storage for chunk in arr_xd.chunks]))
numpy_arr = arr_xd.to_numpy()
self.assertIsInstance(numpy_arr, np.ndarray)
self.assertEqual(numpy_arr.dtype, object)
for first_dim, single_arr in zip(first_dim_list, numpy_arr):
self.assertIsInstance(single_arr, np.ndarray)
self.assertTupleEqual(single_arr.shape, (first_dim, *fixed_shape))
# non-ragged
first_dim_list = [4, 4, 4]
dataset = self.get_one_col_dataset(first_dim_list, fixed_shape)
arr_xd = SimpleArrowExtractor().extract_column(dataset._data)
self.assertIsInstance(arr_xd.type, Array3DExtensionType)
# replace with arr_xd = arr_xd.combine_chunks() when 12.0.0 will be the minimal required PyArrow version
arr_xd = arr_xd.type.wrap_array(pa.concat_arrays([chunk.storage for chunk in arr_xd.chunks]))
numpy_arr = arr_xd.to_numpy()
self.assertIsInstance(numpy_arr, np.ndarray)
self.assertNotEqual(numpy_arr.dtype, object)
for first_dim, single_arr in zip(first_dim_list, numpy_arr):
self.assertIsInstance(single_arr, np.ndarray)
self.assertTupleEqual(single_arr.shape, (first_dim, *fixed_shape))
def test_iter_dataset(self):
fixed_shape = (2, 2)
first_dim_list = [1, 3, 10]
dataset = self.get_one_col_dataset(first_dim_list, fixed_shape)
for first_dim, ds_row in zip(first_dim_list, dataset):
single_arr = ds_row["image"]
self.assertIsInstance(single_arr, list)
self.assertTupleEqual(np.array(single_arr).shape, (first_dim, *fixed_shape))
def test_to_pandas(self):
fixed_shape = (2, 2)
# ragged
first_dim_list = [1, 3, 10]
dataset = self.get_one_col_dataset(first_dim_list, fixed_shape)
df = dataset.to_pandas()
self.assertEqual(type(df.image.dtype), PandasArrayExtensionDtype)
numpy_arr = df.image.to_numpy()
self.assertIsInstance(numpy_arr, np.ndarray)
self.assertEqual(numpy_arr.dtype, object)
for first_dim, single_arr in zip(first_dim_list, numpy_arr):
self.assertIsInstance(single_arr, np.ndarray)
self.assertTupleEqual(single_arr.shape, (first_dim, *fixed_shape))
# non-ragged
first_dim_list = [4, 4, 4]
dataset = self.get_one_col_dataset(first_dim_list, fixed_shape)
df = dataset.to_pandas()
self.assertEqual(type(df.image.dtype), PandasArrayExtensionDtype)
numpy_arr = df.image.to_numpy()
self.assertIsInstance(numpy_arr, np.ndarray)
self.assertNotEqual(numpy_arr.dtype, object)
for first_dim, single_arr in zip(first_dim_list, numpy_arr):
self.assertIsInstance(single_arr, np.ndarray)
self.assertTupleEqual(single_arr.shape, (first_dim, *fixed_shape))
def test_map_dataset(self):
fixed_shape = (2, 2)
first_dim_list = [1, 3, 10]
dataset = self.get_one_col_dataset(first_dim_list, fixed_shape)
dataset = dataset.map(lambda a: {"image": np.concatenate([a] * 2)}, input_columns="image")
# check also if above function resulted with 2x bigger first dim
for first_dim, ds_row in zip(first_dim_list, dataset):
single_arr = ds_row["image"]
self.assertIsInstance(single_arr, list)
self.assertTupleEqual(np.array(single_arr).shape, (first_dim * 2, *fixed_shape))
@pytest.mark.parametrize("dtype, dummy_value", [("int32", 1), ("bool", True), ("float64", 1)])
def test_table_to_pandas(dtype, dummy_value):
features = datasets.Features({"foo": datasets.Array2D(dtype=dtype, shape=(2, 2))})
dataset = datasets.Dataset.from_dict({"foo": [[[dummy_value] * 2] * 2]}, features=features)
df = dataset._data.to_pandas()
assert type(df.foo.dtype) == PandasArrayExtensionDtype
arr = df.foo.to_numpy()
np.testing.assert_equal(arr, np.array([[[dummy_value] * 2] * 2], dtype=np.dtype(dtype)))
@pytest.mark.parametrize("dtype, dummy_value", [("int32", 1), ("bool", True), ("float64", 1)])
def test_array_xd_numpy_arrow_extractor(dtype, dummy_value):
features = datasets.Features({"foo": datasets.Array2D(dtype=dtype, shape=(2, 2))})
dataset = datasets.Dataset.from_dict({"foo": [[[dummy_value] * 2] * 2]}, features=features)
arr = NumpyArrowExtractor().extract_column(dataset._data)
assert isinstance(arr, np.ndarray)
np.testing.assert_equal(arr, np.array([[[dummy_value] * 2] * 2], dtype=np.dtype(dtype)))
def test_array_xd_with_none():
# Fixed shape
features = datasets.Features({"foo": datasets.Array2D(dtype="int32", shape=(2, 2))})
dummy_array = np.array([[1, 2], [3, 4]], dtype="int32")
dataset = datasets.Dataset.from_dict({"foo": [dummy_array, None, dummy_array, None]}, features=features)
arr = NumpyArrowExtractor().extract_column(dataset._data)
assert isinstance(arr, np.ndarray) and arr.dtype == np.float64 and arr.shape == (4, 2, 2)
assert np.allclose(arr[0], dummy_array) and np.allclose(arr[2], dummy_array)
assert np.all(np.isnan(arr[1])) and np.all(np.isnan(arr[3])) # broadcasted np.nan - use np.all
# Dynamic shape
features = datasets.Features({"foo": datasets.Array2D(dtype="int32", shape=(None, 2))})
dummy_array = np.array([[1, 2], [3, 4]], dtype="int32")
dataset = datasets.Dataset.from_dict({"foo": [dummy_array, None, dummy_array, None]}, features=features)
arr = NumpyArrowExtractor().extract_column(dataset._data)
assert isinstance(arr, np.ndarray) and arr.dtype == object and arr.shape == (4,)
np.testing.assert_equal(arr[0], dummy_array)
np.testing.assert_equal(arr[2], dummy_array)
assert np.isnan(arr[1]) and np.isnan(arr[3]) # a single np.nan value - np.all not needed
@pytest.mark.parametrize("seq_type", ["no_sequence", "sequence", "sequence_of_sequence"])
@pytest.mark.parametrize(
"dtype",
[
"bool",
"int8",
"int16",
"int32",
"int64",
"uint8",
"uint16",
"uint32",
"uint64",
"float16",
"float32",
"float64",
],
)
@pytest.mark.parametrize("shape, feature_class", [((2, 3), datasets.Array2D), ((2, 3, 4), datasets.Array3D)])
def test_array_xd_with_np(seq_type, dtype, shape, feature_class):
feature = feature_class(dtype=dtype, shape=shape)
data = np.zeros(shape, dtype=dtype)
expected = data.tolist()
if seq_type == "sequence":
feature = datasets.Sequence(feature)
data = [data]
expected = [expected]
elif seq_type == "sequence_of_sequence":
feature = datasets.Sequence(datasets.Sequence(feature))
data = [[data]]
expected = [[expected]]
ds = datasets.Dataset.from_dict({"col": [data]}, features=datasets.Features({"col": feature}))
assert ds[0]["col"] == expected
@pytest.mark.parametrize("with_none", [False, True])
def test_dataset_map(with_none):
ds = datasets.Dataset.from_dict({"path": ["path1", "path2"]})
def process_data(batch):
batch = {
"image": [
np.array(
[
[[1, 2, 3], [4, 5, 6], [7, 8, 9]],
[[10, 20, 30], [40, 50, 60], [70, 80, 90]],
[[100, 200, 300], [400, 500, 600], [700, 800, 900]],
]
)
for _ in batch["path"]
]
}
if with_none:
batch["image"][0] = None
return batch
features = datasets.Features({"image": Array3D(dtype="int32", shape=(3, 3, 3))})
processed_ds = ds.map(process_data, batched=True, remove_columns=ds.column_names, features=features)
assert processed_ds.shape == (2, 1)
with processed_ds.with_format("numpy") as pds:
for i, example in enumerate(pds):
assert "image" in example
assert isinstance(example["image"], np.ndarray)
assert example["image"].shape == (3, 3, 3)
if with_none and i == 0:
assert np.all(np.isnan(example["image"]))
| datasets/tests/features/test_array_xd.py/0 | {
"file_path": "datasets/tests/features/test_array_xd.py",
"repo_id": "datasets",
"token_count": 9826
} | 78 |
import pytest
from datasets import Dataset, DatasetDict, Features, NamedSplit, Value
from datasets.io.text import TextDatasetReader
from ..utils import assert_arrow_memory_doesnt_increase, assert_arrow_memory_increases
def _check_text_dataset(dataset, expected_features):
assert isinstance(dataset, Dataset)
assert dataset.num_rows == 4
assert dataset.num_columns == 1
assert dataset.column_names == ["text"]
for feature, expected_dtype in expected_features.items():
assert dataset.features[feature].dtype == expected_dtype
@pytest.mark.parametrize("keep_in_memory", [False, True])
def test_dataset_from_text_keep_in_memory(keep_in_memory, text_path, tmp_path):
cache_dir = tmp_path / "cache"
expected_features = {"text": "string"}
with assert_arrow_memory_increases() if keep_in_memory else assert_arrow_memory_doesnt_increase():
dataset = TextDatasetReader(text_path, cache_dir=cache_dir, keep_in_memory=keep_in_memory).read()
_check_text_dataset(dataset, expected_features)
@pytest.mark.parametrize(
"features",
[
None,
{"text": "string"},
{"text": "int32"},
{"text": "float32"},
],
)
def test_dataset_from_text_features(features, text_path, tmp_path):
cache_dir = tmp_path / "cache"
default_expected_features = {"text": "string"}
expected_features = features.copy() if features else default_expected_features
features = (
Features({feature: Value(dtype) for feature, dtype in features.items()}) if features is not None else None
)
dataset = TextDatasetReader(text_path, features=features, cache_dir=cache_dir).read()
_check_text_dataset(dataset, expected_features)
@pytest.mark.parametrize("split", [None, NamedSplit("train"), "train", "test"])
def test_dataset_from_text_split(split, text_path, tmp_path):
cache_dir = tmp_path / "cache"
expected_features = {"text": "string"}
dataset = TextDatasetReader(text_path, cache_dir=cache_dir, split=split).read()
_check_text_dataset(dataset, expected_features)
assert dataset.split == split if split else "train"
@pytest.mark.parametrize("path_type", [str, list])
def test_dataset_from_text_path_type(path_type, text_path, tmp_path):
if issubclass(path_type, str):
path = text_path
elif issubclass(path_type, list):
path = [text_path]
cache_dir = tmp_path / "cache"
expected_features = {"text": "string"}
dataset = TextDatasetReader(path, cache_dir=cache_dir).read()
_check_text_dataset(dataset, expected_features)
def _check_text_datasetdict(dataset_dict, expected_features, splits=("train",)):
assert isinstance(dataset_dict, DatasetDict)
for split in splits:
dataset = dataset_dict[split]
assert dataset.num_rows == 4
assert dataset.num_columns == 1
assert dataset.column_names == ["text"]
for feature, expected_dtype in expected_features.items():
assert dataset.features[feature].dtype == expected_dtype
@pytest.mark.parametrize("keep_in_memory", [False, True])
def test_datasetdict_from_text_keep_in_memory(keep_in_memory, text_path, tmp_path):
cache_dir = tmp_path / "cache"
expected_features = {"text": "string"}
with assert_arrow_memory_increases() if keep_in_memory else assert_arrow_memory_doesnt_increase():
dataset = TextDatasetReader({"train": text_path}, cache_dir=cache_dir, keep_in_memory=keep_in_memory).read()
_check_text_datasetdict(dataset, expected_features)
@pytest.mark.parametrize(
"features",
[
None,
{"text": "string"},
{"text": "int32"},
{"text": "float32"},
],
)
def test_datasetdict_from_text_features(features, text_path, tmp_path):
cache_dir = tmp_path / "cache"
# CSV file loses col_1 string dtype information: default now is "int64" instead of "string"
default_expected_features = {"text": "string"}
expected_features = features.copy() if features else default_expected_features
features = (
Features({feature: Value(dtype) for feature, dtype in features.items()}) if features is not None else None
)
dataset = TextDatasetReader({"train": text_path}, features=features, cache_dir=cache_dir).read()
_check_text_datasetdict(dataset, expected_features)
@pytest.mark.parametrize("split", [None, NamedSplit("train"), "train", "test"])
def test_datasetdict_from_text_split(split, text_path, tmp_path):
if split:
path = {split: text_path}
else:
split = "train"
path = {"train": text_path, "test": text_path}
cache_dir = tmp_path / "cache"
expected_features = {"text": "string"}
dataset = TextDatasetReader(path, cache_dir=cache_dir).read()
_check_text_datasetdict(dataset, expected_features, splits=list(path.keys()))
assert all(dataset[split].split == split for split in path.keys())
| datasets/tests/io/test_text.py/0 | {
"file_path": "datasets/tests/io/test_text.py",
"repo_id": "datasets",
"token_count": 1833
} | 79 |
import copy
import os
from pathlib import Path
from typing import List
from unittest.mock import patch
import fsspec
import pytest
from fsspec.registry import _registry as _fsspec_registry
from fsspec.spec import AbstractFileSystem
from datasets.data_files import (
DataFilesDict,
DataFilesList,
DataFilesPatternsDict,
DataFilesPatternsList,
_get_data_files_patterns,
_get_metadata_files_patterns,
_is_inside_unrequested_special_dir,
_is_unrequested_hidden_file_or_is_inside_unrequested_hidden_dir,
get_data_patterns,
resolve_pattern,
)
from datasets.fingerprint import Hasher
_TEST_PATTERNS = ["*", "**", "**/*", "*.txt", "data/*", "**/*.txt", "**/train.txt"]
_FILES_TO_IGNORE = {".dummy", "README.md", "dummy_data.zip", "dataset_infos.json"}
_DIRS_TO_IGNORE = {"data/.dummy_subdir", "__pycache__"}
_TEST_PATTERNS_SIZES = {
"*": 0,
"**": 4,
"**/*": 4,
"*.txt": 0,
"data/*": 2,
"data/**": 4,
"**/*.txt": 4,
"**/train.txt": 2,
}
_TEST_URL = "https://raw.githubusercontent.com/huggingface/datasets/9675a5a1e7b99a86f9c250f6ea5fa5d1e6d5cc7d/setup.py"
@pytest.fixture
def complex_data_dir(tmp_path):
data_dir = tmp_path / "complex_data_dir"
data_dir.mkdir()
(data_dir / "data").mkdir()
with open(data_dir / "data" / "train.txt", "w") as f:
f.write("foo\n" * 10)
with open(data_dir / "data" / "test.txt", "w") as f:
f.write("bar\n" * 10)
with open(data_dir / "README.md", "w") as f:
f.write("This is a readme")
with open(data_dir / ".dummy", "w") as f:
f.write("this is a dummy file that is not a data file")
(data_dir / "data" / "subdir").mkdir()
with open(data_dir / "data" / "subdir" / "train.txt", "w") as f:
f.write("foo\n" * 10)
with open(data_dir / "data" / "subdir" / "test.txt", "w") as f:
f.write("bar\n" * 10)
(data_dir / "data" / ".dummy_subdir").mkdir()
with open(data_dir / "data" / ".dummy_subdir" / "train.txt", "w") as f:
f.write("foo\n" * 10)
with open(data_dir / "data" / ".dummy_subdir" / "test.txt", "w") as f:
f.write("bar\n" * 10)
(data_dir / "__pycache__").mkdir()
with open(data_dir / "__pycache__" / "script.py", "w") as f:
f.write("foo\n" * 10)
return str(data_dir)
def is_relative_to(path, *other):
# A built-in method in Python 3.9+
try:
path.relative_to(*other)
return True
except ValueError:
return False
@pytest.fixture
def pattern_results(complex_data_dir):
# We use fsspec glob as a reference for data files resolution from patterns.
# This is the same as dask for example.
#
# /!\ Here are some behaviors specific to fsspec glob that are different from glob.glob, Path.glob, Path.match or fnmatch:
# - '*' matches only first level items
# - '**' matches all items
# - '**/*' matches all at least second level items
#
# More generally:
# - '*' matches any character except a forward-slash (to match just the file or directory name)
# - '**' matches any character including a forward-slash /
return {
pattern: sorted(
Path(os.path.abspath(path)).as_posix()
for path in fsspec.filesystem("file").glob(os.path.join(complex_data_dir, pattern))
if Path(path).name not in _FILES_TO_IGNORE
and not any(
is_relative_to(Path(path), os.path.join(complex_data_dir, dir_path)) for dir_path in _DIRS_TO_IGNORE
)
and Path(path).is_file()
)
for pattern in _TEST_PATTERNS
}
@pytest.fixture
def hub_dataset_repo_path(tmpfs, complex_data_dir):
for path in Path(complex_data_dir).rglob("*"):
if path.is_file():
with tmpfs.open(path.relative_to(complex_data_dir).as_posix(), "wb") as f:
f.write(path.read_bytes())
yield "tmp://"
@pytest.fixture
def hub_dataset_repo_patterns_results(hub_dataset_repo_path, complex_data_dir, pattern_results):
return {
pattern: [
hub_dataset_repo_path + Path(path).relative_to(complex_data_dir).as_posix()
for path in pattern_results[pattern]
]
for pattern in pattern_results
}
def test_is_inside_unrequested_special_dir(complex_data_dir, pattern_results):
# usual patterns outside special dir work fine
for pattern, result in pattern_results.items():
if result:
matched_rel_path = str(Path(result[0]).relative_to(complex_data_dir))
assert _is_inside_unrequested_special_dir(matched_rel_path, pattern) is False
# check behavior for special dir
f = _is_inside_unrequested_special_dir
assert f("__pycache__/b.txt", "**") is True
assert f("__pycache__/b.txt", "*/b.txt") is True
assert f("__pycache__/b.txt", "__pycache__/*") is False
assert f("__pycache__/__b.txt", "__pycache__/*") is False
assert f("__pycache__/__b.txt", "__*/*") is False
assert f("__b.txt", "*") is False
def test_is_unrequested_hidden_file_or_is_inside_unrequested_hidden_dir(complex_data_dir, pattern_results):
# usual patterns outside hidden dir work fine
for pattern, result in pattern_results.items():
if result:
matched_rel_path = str(Path(result[0]).relative_to(complex_data_dir))
assert _is_inside_unrequested_special_dir(matched_rel_path, pattern) is False
# check behavior for hidden dir and file
f = _is_unrequested_hidden_file_or_is_inside_unrequested_hidden_dir
assert f(".hidden_file.txt", "**") is True
assert f(".hidden_file.txt", ".*") is False
assert f(".hidden_dir/a.txt", "**") is True
assert f(".hidden_dir/a.txt", ".*/*") is False
assert f(".hidden_dir/a.txt", ".hidden_dir/*") is False
assert f(".hidden_dir/.hidden_file.txt", "**") is True
assert f(".hidden_dir/.hidden_file.txt", ".*/*") is True
assert f(".hidden_dir/.hidden_file.txt", ".*/.*") is False
assert f(".hidden_dir/.hidden_file.txt", ".hidden_dir/*") is True
assert f(".hidden_dir/.hidden_file.txt", ".hidden_dir/.*") is False
@pytest.mark.parametrize("pattern", _TEST_PATTERNS)
def test_pattern_results_fixture(pattern_results, pattern):
assert len(pattern_results[pattern]) == _TEST_PATTERNS_SIZES[pattern]
assert all(Path(path).is_file() for path in pattern_results[pattern])
@pytest.mark.parametrize("pattern", _TEST_PATTERNS)
def test_resolve_pattern_locally(complex_data_dir, pattern, pattern_results):
try:
resolved_data_files = resolve_pattern(pattern, complex_data_dir)
assert sorted(str(f) for f in resolved_data_files) == pattern_results[pattern]
except FileNotFoundError:
assert len(pattern_results[pattern]) == 0
def test_resolve_pattern_locally_with_dot_in_base_path(complex_data_dir):
base_path_with_dot = os.path.join(complex_data_dir, "data", ".dummy_subdir")
resolved_data_files = resolve_pattern(os.path.join(base_path_with_dot, "train.txt"), base_path_with_dot)
assert len(resolved_data_files) == 1
def test_resolve_pattern_locally_with_absolute_path(tmp_path, complex_data_dir):
abs_path = os.path.join(complex_data_dir, "data", "train.txt")
resolved_data_files = resolve_pattern(abs_path, str(tmp_path / "blabla"))
assert len(resolved_data_files) == 1
def test_resolve_pattern_locally_with_double_dots(tmp_path, complex_data_dir):
path_with_double_dots = os.path.join(complex_data_dir, "data", "subdir", "..", "train.txt")
resolved_data_files = resolve_pattern(path_with_double_dots, str(tmp_path / "blabla"))
assert len(resolved_data_files) == 1
def test_resolve_pattern_locally_returns_hidden_file_only_if_requested(complex_data_dir):
with pytest.raises(FileNotFoundError):
resolve_pattern("*dummy", complex_data_dir)
resolved_data_files = resolve_pattern(".dummy", complex_data_dir)
assert len(resolved_data_files) == 1
def test_resolve_pattern_locally_hidden_base_path(tmp_path):
hidden = tmp_path / ".test_hidden_base_path"
hidden.mkdir()
(tmp_path / ".test_hidden_base_path" / "a.txt").touch()
resolved_data_files = resolve_pattern("*", str(hidden))
assert len(resolved_data_files) == 1
def test_resolve_pattern_locallyreturns_hidden_dir_only_if_requested(complex_data_dir):
with pytest.raises(FileNotFoundError):
resolve_pattern("data/*dummy_subdir/train.txt", complex_data_dir)
resolved_data_files = resolve_pattern("data/.dummy_subdir/train.txt", complex_data_dir)
assert len(resolved_data_files) == 1
resolved_data_files = resolve_pattern("*/.dummy_subdir/train.txt", complex_data_dir)
assert len(resolved_data_files) == 1
def test_resolve_pattern_locally_returns_special_dir_only_if_requested(complex_data_dir):
with pytest.raises(FileNotFoundError):
resolve_pattern("data/*dummy_subdir/train.txt", complex_data_dir)
resolved_data_files = resolve_pattern("data/.dummy_subdir/train.txt", complex_data_dir)
assert len(resolved_data_files) == 1
resolved_data_files = resolve_pattern("*/.dummy_subdir/train.txt", complex_data_dir)
assert len(resolved_data_files) == 1
def test_resolve_pattern_locally_special_base_path(tmp_path):
special = tmp_path / "__test_special_base_path__"
special.mkdir()
(tmp_path / "__test_special_base_path__" / "a.txt").touch()
resolved_data_files = resolve_pattern("*", str(special))
assert len(resolved_data_files) == 1
@pytest.mark.parametrize("pattern,size,extensions", [("**", 4, [".txt"]), ("**", 4, None), ("**", 0, [".blablabla"])])
def test_resolve_pattern_locally_with_extensions(complex_data_dir, pattern, size, extensions):
if size > 0:
resolved_data_files = resolve_pattern(pattern, complex_data_dir, allowed_extensions=extensions)
assert len(resolved_data_files) == size
else:
with pytest.raises(FileNotFoundError):
resolve_pattern(pattern, complex_data_dir, allowed_extensions=extensions)
def test_fail_resolve_pattern_locally(complex_data_dir):
with pytest.raises(FileNotFoundError):
resolve_pattern(complex_data_dir, ["blablabla"])
@pytest.mark.skipif(os.name == "nt", reason="Windows does not support symlinks in the default mode")
def test_resolve_pattern_locally_does_not_resolve_symbolic_links(tmp_path, complex_data_dir):
(tmp_path / "train_data_symlink.txt").symlink_to(os.path.join(complex_data_dir, "data", "train.txt"))
resolved_data_files = resolve_pattern("train_data_symlink.txt", str(tmp_path))
assert len(resolved_data_files) == 1
assert Path(resolved_data_files[0]) == tmp_path / "train_data_symlink.txt"
def test_resolve_pattern_locally_sorted_files(tmp_path_factory):
path = str(tmp_path_factory.mktemp("unsorted_text_files"))
unsorted_names = ["0.txt", "2.txt", "3.txt"]
for name in unsorted_names:
with open(os.path.join(path, name), "w"):
pass
resolved_data_files = resolve_pattern("*", path)
resolved_names = [os.path.basename(data_file) for data_file in resolved_data_files]
assert resolved_names == sorted(unsorted_names)
@pytest.mark.parametrize("pattern", _TEST_PATTERNS)
def test_resolve_pattern_in_dataset_repository(hub_dataset_repo_path, pattern, hub_dataset_repo_patterns_results):
try:
resolved_data_files = resolve_pattern(pattern, hub_dataset_repo_path)
assert sorted(str(f) for f in resolved_data_files) == hub_dataset_repo_patterns_results[pattern]
except FileNotFoundError:
assert len(hub_dataset_repo_patterns_results[pattern]) == 0
@pytest.mark.parametrize(
"pattern,size,base_path", [("**", 4, None), ("**", 4, "data"), ("**", 2, "data/subdir"), ("**", 0, "data/subdir2")]
)
def test_resolve_pattern_in_dataset_repository_with_base_path(hub_dataset_repo_path, pattern, size, base_path):
base_path = hub_dataset_repo_path + (base_path or "")
if size > 0:
resolved_data_files = resolve_pattern(pattern, base_path)
assert len(resolved_data_files) == size
else:
with pytest.raises(FileNotFoundError):
resolve_pattern(pattern, base_path)
@pytest.mark.parametrize("pattern,size,extensions", [("**", 4, [".txt"]), ("**", 4, None), ("**", 0, [".blablabla"])])
def test_resolve_pattern_in_dataset_repository_with_extensions(hub_dataset_repo_path, pattern, size, extensions):
if size > 0:
resolved_data_files = resolve_pattern(pattern, hub_dataset_repo_path, allowed_extensions=extensions)
assert len(resolved_data_files) == size
else:
with pytest.raises(FileNotFoundError):
resolved_data_files = resolve_pattern(pattern, hub_dataset_repo_path, allowed_extensions=extensions)
def test_fail_resolve_pattern_in_dataset_repository(hub_dataset_repo_path):
with pytest.raises(FileNotFoundError):
resolve_pattern("blablabla", hub_dataset_repo_path)
def test_resolve_pattern_in_dataset_repository_returns_hidden_file_only_if_requested(hub_dataset_repo_path):
with pytest.raises(FileNotFoundError):
resolve_pattern("*dummy", hub_dataset_repo_path)
resolved_data_files = resolve_pattern(".dummy", hub_dataset_repo_path)
assert len(resolved_data_files) == 1
def test_resolve_pattern_in_dataset_repository_hidden_base_path(tmpfs):
tmpfs.touch(".hidden/a.txt")
resolved_data_files = resolve_pattern("*", base_path="tmp://.hidden")
assert len(resolved_data_files) == 1
def test_resolve_pattern_in_dataset_repository_returns_hidden_dir_only_if_requested(hub_dataset_repo_path):
with pytest.raises(FileNotFoundError):
resolve_pattern("data/*dummy_subdir/train.txt", hub_dataset_repo_path)
resolved_data_files = resolve_pattern("data/.dummy_subdir/train.txt", hub_dataset_repo_path)
assert len(resolved_data_files) == 1
resolved_data_files = resolve_pattern("*/.dummy_subdir/train.txt", hub_dataset_repo_path)
assert len(resolved_data_files) == 1
def test_resolve_pattern_in_dataset_repository_returns_special_dir_only_if_requested(hub_dataset_repo_path):
with pytest.raises(FileNotFoundError):
resolve_pattern("data/*dummy_subdir/train.txt", hub_dataset_repo_path)
resolved_data_files = resolve_pattern("data/.dummy_subdir/train.txt", hub_dataset_repo_path)
assert len(resolved_data_files) == 1
resolved_data_files = resolve_pattern("*/.dummy_subdir/train.txt", hub_dataset_repo_path)
assert len(resolved_data_files) == 1
def test_resolve_pattern_in_dataset_repository_special_base_path(tmpfs):
tmpfs.touch("__special__/a.txt")
resolved_data_files = resolve_pattern("*", base_path="tmp://__special__")
assert len(resolved_data_files) == 1
@pytest.fixture
def dummy_fs():
DummyTestFS = mock_fs(["train.txt", "test.txt"])
_fsspec_registry["mock"] = DummyTestFS
_fsspec_registry["dummy"] = DummyTestFS
yield
del _fsspec_registry["mock"]
del _fsspec_registry["dummy"]
def test_resolve_pattern_fs(dummy_fs):
resolved_data_files = resolve_pattern("mock://train.txt", base_path="")
assert resolved_data_files == ["mock://train.txt"]
@pytest.mark.parametrize("pattern", _TEST_PATTERNS)
def test_DataFilesList_from_patterns_in_dataset_repository_(
hub_dataset_repo_path, hub_dataset_repo_patterns_results, pattern
):
try:
data_files_list = DataFilesList.from_patterns([pattern], hub_dataset_repo_path)
assert sorted(data_files_list) == hub_dataset_repo_patterns_results[pattern]
assert len(data_files_list.origin_metadata) == len(data_files_list)
except FileNotFoundError:
assert len(hub_dataset_repo_patterns_results[pattern]) == 0
def test_DataFilesList_from_patterns_locally_with_extra_files(complex_data_dir, text_file):
data_files_list = DataFilesList.from_patterns([_TEST_URL, text_file.as_posix()], complex_data_dir)
assert list(data_files_list) == [_TEST_URL, text_file.as_posix()]
assert len(data_files_list.origin_metadata) == 2
def test_DataFilesList_from_patterns_raises_FileNotFoundError(complex_data_dir):
with pytest.raises(FileNotFoundError):
DataFilesList.from_patterns(["file_that_doesnt_exist.txt"], complex_data_dir)
class TestDataFilesDict:
def test_key_order_after_copy(self):
data_files = DataFilesDict({"train": "train.csv", "test": "test.csv"})
copied_data_files = copy.deepcopy(data_files)
assert list(copied_data_files.keys()) == list(data_files.keys()) # test split order with list()
@pytest.mark.parametrize("pattern", _TEST_PATTERNS)
def test_DataFilesDict_from_patterns_in_dataset_repository(
hub_dataset_repo_path, hub_dataset_repo_patterns_results, pattern
):
split_name = "train"
try:
data_files = DataFilesDict.from_patterns({split_name: [pattern]}, hub_dataset_repo_path)
assert all(isinstance(data_files_list, DataFilesList) for data_files_list in data_files.values())
assert sorted(data_files[split_name]) == hub_dataset_repo_patterns_results[pattern]
except FileNotFoundError:
assert len(hub_dataset_repo_patterns_results[pattern]) == 0
@pytest.mark.parametrize(
"pattern,size,base_path,split_name",
[
("**", 4, None, "train"),
("**", 4, "data", "train"),
("**", 2, "data/subdir", "train"),
("**", 0, "data/subdir2", "train"),
],
)
def test_DataFilesDict_from_patterns_in_dataset_repository_with_base_path(
hub_dataset_repo_path, pattern, size, base_path, split_name
):
base_path = hub_dataset_repo_path + (base_path or "")
if size > 0:
data_files = DataFilesDict.from_patterns({split_name: [pattern]}, base_path=base_path)
assert len(data_files[split_name]) == size
else:
with pytest.raises(FileNotFoundError):
resolve_pattern(pattern, base_path)
@pytest.mark.parametrize("pattern", _TEST_PATTERNS)
def test_DataFilesDict_from_patterns_locally(complex_data_dir, pattern_results, pattern):
split_name = "train"
try:
data_files = DataFilesDict.from_patterns({split_name: [pattern]}, complex_data_dir)
assert all(isinstance(data_files_list, DataFilesList) for data_files_list in data_files.values())
assert sorted(data_files[split_name]) == pattern_results[pattern]
except FileNotFoundError:
assert len(pattern_results[pattern]) == 0
def test_DataFilesDict_from_patterns_in_dataset_repository_hashing(hub_dataset_repo_path):
patterns = {"train": ["**/train.txt"], "test": ["**/test.txt"]}
data_files1 = DataFilesDict.from_patterns(patterns, hub_dataset_repo_path)
data_files2 = DataFilesDict.from_patterns(patterns, hub_dataset_repo_path)
assert Hasher.hash(data_files1) == Hasher.hash(data_files2)
data_files2 = DataFilesDict(sorted(data_files1.items(), reverse=True))
assert Hasher.hash(data_files1) == Hasher.hash(data_files2)
# the tmpfs used to mock the hub repo is based on a local directory
# therefore os.stat is used to get the mtime of the data files
with patch("os.stat", return_value=os.stat(__file__)):
data_files2 = DataFilesDict.from_patterns(patterns, hub_dataset_repo_path)
assert Hasher.hash(data_files1) != Hasher.hash(data_files2)
def test_DataFilesDict_from_patterns_locally_or_remote_hashing(text_file):
patterns = {"train": [_TEST_URL], "test": [str(text_file)]}
data_files1 = DataFilesDict.from_patterns(patterns)
data_files2 = DataFilesDict.from_patterns(patterns)
assert Hasher.hash(data_files1) == Hasher.hash(data_files2)
data_files2 = DataFilesDict(sorted(data_files1.items(), reverse=True))
assert Hasher.hash(data_files1) == Hasher.hash(data_files2)
patterns2 = {"train": [_TEST_URL], "test": [_TEST_URL]}
data_files2 = DataFilesDict.from_patterns(patterns2)
assert Hasher.hash(data_files1) != Hasher.hash(data_files2)
with patch("fsspec.implementations.http._file_info", return_value={}):
data_files2 = DataFilesDict.from_patterns(patterns)
assert Hasher.hash(data_files1) != Hasher.hash(data_files2)
with patch("os.stat", return_value=os.stat(__file__)):
data_files2 = DataFilesDict.from_patterns(patterns)
assert Hasher.hash(data_files1) != Hasher.hash(data_files2)
def test_DataFilesPatternsList(text_file):
data_files_patterns = DataFilesPatternsList([str(text_file)], allowed_extensions=[None])
data_files = data_files_patterns.resolve(base_path="")
assert data_files == [text_file.as_posix()]
assert isinstance(data_files, DataFilesList)
data_files_patterns = DataFilesPatternsList([str(text_file)], allowed_extensions=[[".txt"]])
data_files = data_files_patterns.resolve(base_path="")
assert data_files == [text_file.as_posix()]
assert isinstance(data_files, DataFilesList)
data_files_patterns = DataFilesPatternsList([str(text_file).replace(".txt", ".tx*")], allowed_extensions=[None])
data_files = data_files_patterns.resolve(base_path="")
assert data_files == [text_file.as_posix()]
assert isinstance(data_files, DataFilesList)
data_files_patterns = DataFilesPatternsList([Path(text_file).name], allowed_extensions=[None])
data_files = data_files_patterns.resolve(base_path=str(Path(text_file).parent))
assert data_files == [text_file.as_posix()]
data_files_patterns = DataFilesPatternsList([str(text_file)], allowed_extensions=[[".zip"]])
with pytest.raises(FileNotFoundError):
data_files_patterns.resolve(base_path="")
def test_DataFilesPatternsDict(text_file):
data_files_patterns_dict = DataFilesPatternsDict(
{"train": DataFilesPatternsList([str(text_file)], allowed_extensions=[None])}
)
data_files_dict = data_files_patterns_dict.resolve(base_path="")
assert data_files_dict == {"train": [text_file.as_posix()]}
assert isinstance(data_files_dict, DataFilesDict)
assert isinstance(data_files_dict["train"], DataFilesList)
def mock_fs(file_paths: List[str]):
"""
Set up a mock filesystem for fsspec containing the provided files
Example:
```py
>>> DummyTestFS = mock_fs(["data/train.txt", "data.test.txt"])
>>> fs = DummyTestFS()
>>> assert fsspec.get_filesystem_class("mock").__name__ == "DummyTestFS"
>>> assert type(fs).__name__ == "DummyTestFS"
>>> print(fs.glob("**"))
["data", "data/train.txt", "data.test.txt"]
```
"""
file_paths = [file_path.split("://")[-1] for file_path in file_paths]
dir_paths = {
"/".join(file_path.split("/")[: i + 1]) for file_path in file_paths for i in range(file_path.count("/"))
}
fs_contents = [{"name": dir_path, "type": "directory"} for dir_path in dir_paths] + [
{"name": file_path, "type": "file", "size": 10} for file_path in file_paths
]
class DummyTestFS(AbstractFileSystem):
protocol = ("mock", "dummy")
_fs_contents = fs_contents
def ls(self, path, detail=True, refresh=True, **kwargs):
if kwargs.pop("strip_proto", True):
path = self._strip_protocol(path)
files = not refresh and self._ls_from_cache(path)
if not files:
files = [file for file in self._fs_contents if path == self._parent(file["name"])]
files.sort(key=lambda file: file["name"])
self.dircache[path.rstrip("/")] = files
if detail:
return files
return [file["name"] for file in files]
return DummyTestFS
@pytest.mark.parametrize("base_path", ["", "mock://", "my_dir"])
@pytest.mark.parametrize(
"data_file_per_split",
[
# === Main cases ===
# file named after split at the root
{"train": "train.txt", "validation": "valid.txt", "test": "test.txt"},
# file named after split in a directory
{
"train": "data/train.txt",
"validation": "data/valid.txt",
"test": "data/test.txt",
},
# directory named after split
{
"train": "train/split.txt",
"validation": "valid/split.txt",
"test": "test/split.txt",
},
# sharded splits
{
"train": [f"data/train_{i}.txt" for i in range(3)],
"validation": [f"data/validation_{i}.txt" for i in range(3)],
"test": [f"data/test_{i}.txt" for i in range(3)],
},
# sharded splits with standard format (+ custom split name)
{
"train": [f"data/train-0000{i}-of-00003.txt" for i in range(3)],
"validation": [f"data/validation-0000{i}-of-00003.txt" for i in range(3)],
"test": [f"data/test-0000{i}-of-00003.txt" for i in range(3)],
"random": [f"data/random-0000{i}-of-00003.txt" for i in range(3)],
},
# === Secondary cases ===
# Default to train split
{"train": "dataset.txt"},
{"train": "data/dataset.txt"},
{"train": ["data/image.jpg", "metadata.jsonl"]},
{"train": ["data/image.jpg", "metadata.csv"]},
# With prefix or suffix in directory or file names
{"train": "my_train_dir/dataset.txt"},
{"train": "data/my_train_file.txt"},
{"test": "my_test_dir/dataset.txt"},
{"test": "data/my_test_file.txt"},
{"validation": "my_validation_dir/dataset.txt"},
{"validation": "data/my_validation_file.txt"},
{"train": "train_dir/dataset.txt"},
{"train": "data/train_file.txt"},
{"test": "test_dir/dataset.txt"},
{"test": "data/test_file.txt"},
{"validation": "validation_dir/dataset.txt"},
{"validation": "data/validation_file.txt"},
{"train": "my_train/dataset.txt"},
{"train": "data/my_train.txt"},
{"test": "my_test/dataset.txt"},
{"test": "data/my_test.txt"},
{"validation": "my_validation/dataset.txt"},
{"validation": "data/my_validation.txt"},
# With test<>eval aliases
{"test": "eval.txt"},
{"test": "data/eval.txt"},
{"test": "eval/dataset.txt"},
# With valid<>dev aliases
{"validation": "dev.txt"},
{"validation": "data/dev.txt"},
{"validation": "dev/dataset.txt"},
# With valid<>val aliases
{"validation": "val.txt"},
{"validation": "data/val.txt"},
# With other extensions
{"train": "train.parquet", "validation": "valid.parquet", "test": "test.parquet"},
# With "dev" or "eval" without separators
{"train": "developers_list.txt"},
{"train": "data/seqeval_results.txt"},
{"train": "contest.txt"},
# With supported separators
{"test": "my.test.file.txt"},
{"test": "my-test-file.txt"},
{"test": "my_test_file.txt"},
{"test": "my test file.txt"},
{"test": "my-test_file.txt"},
{"test": "test00001.txt"},
# <split>.<split> case
{"test": "test/train.txt"},
],
)
def test_get_data_files_patterns(base_path, data_file_per_split):
data_file_per_split = {k: v if isinstance(v, list) else [v] for k, v in data_file_per_split.items()}
data_file_per_split = {
split: [
base_path + ("/" if base_path and base_path[-1] != "/" else "") + file_path
for file_path in data_file_per_split[split]
]
for split in data_file_per_split
}
file_paths = sum(data_file_per_split.values(), [])
DummyTestFS = mock_fs(file_paths)
fs = DummyTestFS()
def resolver(pattern):
pattern = base_path + ("/" if base_path and base_path[-1] != "/" else "") + pattern
return [
file_path[len(fs._strip_protocol(base_path)) :].lstrip("/")
for file_path in fs.glob(pattern)
if fs.isfile(file_path)
]
patterns_per_split = _get_data_files_patterns(resolver)
assert list(patterns_per_split.keys()) == list(data_file_per_split.keys()) # Test split order with list()
for split, patterns in patterns_per_split.items():
matched = [file_path for pattern in patterns for file_path in resolver(pattern)]
expected = [
fs._strip_protocol(file_path)[len(fs._strip_protocol(base_path)) :].lstrip("/")
for file_path in data_file_per_split[split]
]
assert matched == expected
@pytest.mark.parametrize(
"metadata_files",
[
# metadata files at the root
["metadata.jsonl"],
["metadata.csv"],
# nested metadata files
["metadata.jsonl", "data/metadata.jsonl"],
["metadata.csv", "data/metadata.csv"],
],
)
def test_get_metadata_files_patterns(metadata_files):
DummyTestFS = mock_fs(metadata_files)
fs = DummyTestFS()
def resolver(pattern):
return [file_path for file_path in fs.glob(pattern) if fs.isfile(file_path)]
patterns = _get_metadata_files_patterns(resolver)
matched = [file_path for pattern in patterns for file_path in resolver(pattern)]
assert sorted(matched) == sorted(metadata_files)
def test_get_data_patterns_from_directory_with_the_word_data_twice(tmp_path):
repo_dir = tmp_path / "directory-name-ending-with-the-word-data" # parent directory contains the word "data/"
data_dir = repo_dir / "data"
data_dir.mkdir(parents=True)
data_file = data_dir / "train-00001-of-00009.parquet"
data_file.touch()
data_file_patterns = get_data_patterns(repo_dir.as_posix())
assert data_file_patterns == {"train": ["data/train-[0-9][0-9][0-9][0-9][0-9]-of-[0-9][0-9][0-9][0-9][0-9]*.*"]}
| datasets/tests/test_data_files.py/0 | {
"file_path": "datasets/tests/test_data_files.py",
"repo_id": "datasets",
"token_count": 12329
} | 80 |
import os
from pathlib import Path
import pytest
from datasets.inspect import (
get_dataset_config_info,
get_dataset_config_names,
get_dataset_default_config_name,
get_dataset_infos,
get_dataset_split_names,
inspect_dataset,
inspect_metric,
)
from datasets.packaged_modules.csv import csv
pytestmark = pytest.mark.integration
@pytest.mark.parametrize("path", ["lhoestq/test", csv.__file__])
def test_inspect_dataset(path, tmp_path):
inspect_dataset(path, tmp_path)
script_name = Path(path).stem + ".py"
assert script_name in os.listdir(tmp_path)
@pytest.mark.filterwarnings("ignore:inspect_metric is deprecated:FutureWarning")
@pytest.mark.filterwarnings("ignore:metric_module_factory is deprecated:FutureWarning")
@pytest.mark.parametrize("path", ["accuracy"])
def test_inspect_metric(path, tmp_path):
inspect_metric(path, tmp_path)
script_name = path + ".py"
assert script_name in os.listdir(tmp_path)
assert "__pycache__" not in os.listdir(tmp_path)
@pytest.mark.parametrize(
"path, config_name, expected_splits",
[
("squad", "plain_text", ["train", "validation"]),
("dalle-mini/wit", "default", ["train"]),
("paws", "labeled_final", ["train", "test", "validation"]),
],
)
def test_get_dataset_config_info(path, config_name, expected_splits):
info = get_dataset_config_info(path, config_name=config_name)
assert info.config_name == config_name
assert list(info.splits.keys()) == expected_splits
def test_get_dataset_config_info_private(hf_token, hf_private_dataset_repo_txt_data):
info = get_dataset_config_info(hf_private_dataset_repo_txt_data, config_name="default", token=hf_token)
assert list(info.splits.keys()) == ["train"]
@pytest.mark.parametrize(
"path, config_name, expected_exception",
[
("paws", None, ValueError),
],
)
def test_get_dataset_config_info_error(path, config_name, expected_exception):
with pytest.raises(expected_exception):
get_dataset_config_info(path, config_name=config_name)
@pytest.mark.parametrize(
"path, expected",
[
("acronym_identification", ["default"]),
("squad", ["plain_text"]),
("hf-internal-testing/dataset_with_script", ["default"]),
("dalle-mini/wit", ["default"]),
("hf-internal-testing/librispeech_asr_dummy", ["clean", "other"]),
("hf-internal-testing/audiofolder_no_configs_in_metadata", ["default"]),
("hf-internal-testing/audiofolder_single_config_in_metadata", ["custom"]),
("hf-internal-testing/audiofolder_two_configs_in_metadata", ["v1", "v2"]),
],
)
def test_get_dataset_config_names(path, expected):
config_names = get_dataset_config_names(path)
assert config_names == expected
@pytest.mark.parametrize(
"path, expected",
[
("acronym_identification", "default"),
("squad", "plain_text"),
("hf-internal-testing/dataset_with_script", "default"),
("dalle-mini/wit", "default"),
("hf-internal-testing/librispeech_asr_dummy", None),
("hf-internal-testing/audiofolder_no_configs_in_metadata", "default"),
("hf-internal-testing/audiofolder_single_config_in_metadata", "custom"),
("hf-internal-testing/audiofolder_two_configs_in_metadata", None),
],
)
def test_get_dataset_default_config_name(path, expected):
default_config_name = get_dataset_default_config_name(path)
if expected:
assert default_config_name == expected
else:
assert default_config_name is None
@pytest.mark.parametrize(
"path, expected_configs, expected_splits_in_first_config",
[
("squad", ["plain_text"], ["train", "validation"]),
("dalle-mini/wit", ["default"], ["train"]),
("paws", ["labeled_final", "labeled_swap", "unlabeled_final"], ["train", "test", "validation"]),
],
)
def test_get_dataset_info(path, expected_configs, expected_splits_in_first_config):
infos = get_dataset_infos(path)
assert list(infos.keys()) == expected_configs
expected_config = expected_configs[0]
assert expected_config in infos
info = infos[expected_config]
assert info.config_name == expected_config
assert list(info.splits.keys()) == expected_splits_in_first_config
@pytest.mark.parametrize(
"path, expected_config, expected_splits",
[
("squad", "plain_text", ["train", "validation"]),
("dalle-mini/wit", "default", ["train"]),
("paws", "labeled_final", ["train", "test", "validation"]),
],
)
def test_get_dataset_split_names(path, expected_config, expected_splits):
infos = get_dataset_infos(path)
assert expected_config in infos
info = infos[expected_config]
assert info.config_name == expected_config
assert list(info.splits.keys()) == expected_splits
@pytest.mark.parametrize(
"path, config_name, expected_exception",
[
("paws", None, ValueError),
],
)
def test_get_dataset_split_names_error(path, config_name, expected_exception):
with pytest.raises(expected_exception):
get_dataset_split_names(path, config_name=config_name)
| datasets/tests/test_inspect.py/0 | {
"file_path": "datasets/tests/test_inspect.py",
"repo_id": "datasets",
"token_count": 2081
} | 81 |
from copy import deepcopy
from unittest.case import TestCase
import pytest
from datasets.arrow_dataset import Dataset
from datasets.features import Audio, ClassLabel, Features, Image, Sequence, Value
from datasets.info import DatasetInfo
from datasets.tasks import (
AudioClassification,
AutomaticSpeechRecognition,
ImageClassification,
LanguageModeling,
QuestionAnsweringExtractive,
Summarization,
TextClassification,
task_template_from_dict,
)
from datasets.utils.py_utils import asdict
SAMPLE_QUESTION_ANSWERING_EXTRACTIVE = {
"id": "5733be284776f41900661182",
"title": "University_of_Notre_Dame",
"context": 'Architecturally, the school has a Catholic character. Atop the Main Building\'s gold dome is a golden statue of the Virgin Mary. Immediately in front of the Main Building and facing it, is a copper statue of Christ with arms upraised with the legend "Venite Ad Me Omnes". Next to the Main Building is the Basilica of the Sacred Heart. Immediately behind the basilica is the Grotto, a Marian place of prayer and reflection. It is a replica of the grotto at Lourdes, France where the Virgin Mary reputedly appeared to Saint Bernadette Soubirous in 1858. At the end of the main drive (and in a direct line that connects through 3 statues and the Gold Dome), is a simple, modern stone statue of Mary.',
"question": "To whom did the Virgin Mary allegedly appear in 1858 in Lourdes France?",
"answers": {"text": ["Saint Bernadette Soubirous"], "answer_start": [515]},
}
@pytest.mark.parametrize(
"task_cls",
[
AudioClassification,
AutomaticSpeechRecognition,
ImageClassification,
LanguageModeling,
QuestionAnsweringExtractive,
Summarization,
TextClassification,
],
)
def test_reload_task_from_dict(task_cls):
task = task_cls()
task_dict = asdict(task)
reloaded = task_template_from_dict(task_dict)
assert task == reloaded
class TestLanguageModeling:
def test_column_mapping(self):
task = LanguageModeling(text_column="input_text")
assert {"input_text": "text"} == task.column_mapping
def test_from_dict(self):
input_schema = Features({"text": Value("string")})
template_dict = {"text_column": "input_text"}
task = LanguageModeling.from_dict(template_dict)
assert "language-modeling" == task.task
assert input_schema == task.input_schema
class TextClassificationTest(TestCase):
def setUp(self):
self.labels = sorted(["pos", "neg"])
def test_column_mapping(self):
task = TextClassification(text_column="input_text", label_column="input_label")
self.assertDictEqual({"input_text": "text", "input_label": "labels"}, task.column_mapping)
def test_from_dict(self):
input_schema = Features({"text": Value("string")})
# Labels are cast to tuple during `TextClassification.__post_init__`, so we do the same here
label_schema = Features({"labels": ClassLabel})
template_dict = {"text_column": "input_text", "label_column": "input_labels"}
task = TextClassification.from_dict(template_dict)
self.assertEqual("text-classification", task.task)
self.assertEqual(input_schema, task.input_schema)
self.assertEqual(label_schema, task.label_schema)
def test_align_with_features(self):
task = TextClassification(text_column="input_text", label_column="input_label")
self.assertEqual(task.label_schema["labels"], ClassLabel)
task = task.align_with_features(Features({"input_label": ClassLabel(names=self.labels)}))
self.assertEqual(task.label_schema["labels"], ClassLabel(names=self.labels))
class QuestionAnsweringTest(TestCase):
def test_column_mapping(self):
task = QuestionAnsweringExtractive(
context_column="input_context", question_column="input_question", answers_column="input_answers"
)
self.assertDictEqual(
{"input_context": "context", "input_question": "question", "input_answers": "answers"}, task.column_mapping
)
def test_from_dict(self):
input_schema = Features({"question": Value("string"), "context": Value("string")})
label_schema = Features(
{
"answers": Sequence(
{
"text": Value("string"),
"answer_start": Value("int32"),
}
)
}
)
template_dict = {
"context_column": "input_input_context",
"question_column": "input_question",
"answers_column": "input_answers",
}
task = QuestionAnsweringExtractive.from_dict(template_dict)
self.assertEqual("question-answering-extractive", task.task)
self.assertEqual(input_schema, task.input_schema)
self.assertEqual(label_schema, task.label_schema)
class SummarizationTest(TestCase):
def test_column_mapping(self):
task = Summarization(text_column="input_text", summary_column="input_summary")
self.assertDictEqual({"input_text": "text", "input_summary": "summary"}, task.column_mapping)
def test_from_dict(self):
input_schema = Features({"text": Value("string")})
label_schema = Features({"summary": Value("string")})
template_dict = {"text_column": "input_text", "summary_column": "input_summary"}
task = Summarization.from_dict(template_dict)
self.assertEqual("summarization", task.task)
self.assertEqual(input_schema, task.input_schema)
self.assertEqual(label_schema, task.label_schema)
class AutomaticSpeechRecognitionTest(TestCase):
def test_column_mapping(self):
task = AutomaticSpeechRecognition(audio_column="input_audio", transcription_column="input_transcription")
self.assertDictEqual({"input_audio": "audio", "input_transcription": "transcription"}, task.column_mapping)
def test_from_dict(self):
input_schema = Features({"audio": Audio()})
label_schema = Features({"transcription": Value("string")})
template_dict = {
"audio_column": "input_audio",
"transcription_column": "input_transcription",
}
task = AutomaticSpeechRecognition.from_dict(template_dict)
self.assertEqual("automatic-speech-recognition", task.task)
self.assertEqual(input_schema, task.input_schema)
self.assertEqual(label_schema, task.label_schema)
class AudioClassificationTest(TestCase):
def setUp(self):
self.labels = sorted(["pos", "neg"])
def test_column_mapping(self):
task = AudioClassification(audio_column="input_audio", label_column="input_label")
self.assertDictEqual({"input_audio": "audio", "input_label": "labels"}, task.column_mapping)
def test_from_dict(self):
input_schema = Features({"audio": Audio()})
label_schema = Features({"labels": ClassLabel})
template_dict = {
"audio_column": "input_image",
"label_column": "input_label",
}
task = AudioClassification.from_dict(template_dict)
self.assertEqual("audio-classification", task.task)
self.assertEqual(input_schema, task.input_schema)
self.assertEqual(label_schema, task.label_schema)
def test_align_with_features(self):
task = AudioClassification(audio_column="input_audio", label_column="input_label")
self.assertEqual(task.label_schema["labels"], ClassLabel)
task = task.align_with_features(Features({"input_label": ClassLabel(names=self.labels)}))
self.assertEqual(task.label_schema["labels"], ClassLabel(names=self.labels))
class ImageClassificationTest(TestCase):
def setUp(self):
self.labels = sorted(["pos", "neg"])
def test_column_mapping(self):
task = ImageClassification(image_column="input_image", label_column="input_label")
self.assertDictEqual({"input_image": "image", "input_label": "labels"}, task.column_mapping)
def test_from_dict(self):
input_schema = Features({"image": Image()})
label_schema = Features({"labels": ClassLabel})
template_dict = {
"image_column": "input_image",
"label_column": "input_label",
}
task = ImageClassification.from_dict(template_dict)
self.assertEqual("image-classification", task.task)
self.assertEqual(input_schema, task.input_schema)
self.assertEqual(label_schema, task.label_schema)
def test_align_with_features(self):
task = ImageClassification(image_column="input_image", label_column="input_label")
self.assertEqual(task.label_schema["labels"], ClassLabel)
task = task.align_with_features(Features({"input_label": ClassLabel(names=self.labels)}))
self.assertEqual(task.label_schema["labels"], ClassLabel(names=self.labels))
class DatasetWithTaskProcessingTest(TestCase):
def test_map_on_task_template(self):
info = DatasetInfo(task_templates=QuestionAnsweringExtractive())
dataset = Dataset.from_dict({k: [v] for k, v in SAMPLE_QUESTION_ANSWERING_EXTRACTIVE.items()}, info=info)
assert isinstance(dataset.info.task_templates, list)
assert len(dataset.info.task_templates) == 1
def keep_task(x):
return x
def dont_keep_task(x):
out = deepcopy(SAMPLE_QUESTION_ANSWERING_EXTRACTIVE)
out["answers"]["foobar"] = 0
return out
mapped_dataset = dataset.map(keep_task)
assert mapped_dataset.info.task_templates == dataset.info.task_templates
# reload from cache
mapped_dataset = dataset.map(keep_task)
assert mapped_dataset.info.task_templates == dataset.info.task_templates
mapped_dataset = dataset.map(dont_keep_task)
assert mapped_dataset.info.task_templates == []
# reload from cache
mapped_dataset = dataset.map(dont_keep_task)
assert mapped_dataset.info.task_templates == []
def test_remove_and_map_on_task_template(self):
features = Features({"text": Value("string"), "label": ClassLabel(names=("pos", "neg"))})
task_templates = TextClassification(text_column="text", label_column="label")
info = DatasetInfo(features=features, task_templates=task_templates)
dataset = Dataset.from_dict({"text": ["A sentence."], "label": ["pos"]}, info=info)
def process(example):
return example
modified_dataset = dataset.remove_columns("label")
mapped_dataset = modified_dataset.map(process)
assert mapped_dataset.info.task_templates == []
| datasets/tests/test_tasks.py/0 | {
"file_path": "datasets/tests/test_tasks.py",
"repo_id": "datasets",
"token_count": 4249
} | 82 |
# The “Deep” in Reinforcement Learning [[deep-rl]]
<Tip>
What we've talked about so far is Reinforcement Learning. But where does the "Deep" come into play?
</Tip>
Deep Reinforcement Learning introduces **deep neural networks to solve Reinforcement Learning problems** — hence the name “deep”.
For instance, in the next unit, we’ll learn about two value-based algorithms: Q-Learning (classic Reinforcement Learning) and then Deep Q-Learning.
You’ll see the difference is that, in the first approach, **we use a traditional algorithm** to create a Q table that helps us find what action to take for each state.
In the second approach, **we will use a Neural Network** (to approximate the Q value).
<figure>
<img src="https://huggingface.co/datasets/huggingface-deep-rl-course/course-images/resolve/main/en/unit1/deep.jpg" alt="Value based RL"/>
<figcaption>Schema inspired by the Q learning notebook by Udacity
</figcaption>
</figure>
If you are not familiar with Deep Learning you should definitely watch [the FastAI Practical Deep Learning for Coders](https://course.fast.ai) (Free).
| deep-rl-class/units/en/unit1/deep-rl.mdx/0 | {
"file_path": "deep-rl-class/units/en/unit1/deep-rl.mdx",
"repo_id": "deep-rl-class",
"token_count": 310
} | 83 |
# Introduction to Q-Learning [[introduction-q-learning]]
<img src="https://huggingface.co/datasets/huggingface-deep-rl-course/course-images/resolve/main/en/unit3/thumbnail.jpg" alt="Unit 2 thumbnail" width="100%">
In the first unit of this class, we learned about Reinforcement Learning (RL), the RL process, and the different methods to solve an RL problem. We also **trained our first agents and uploaded them to the Hugging Face Hub.**
In this unit, we're going to **dive deeper into one of the Reinforcement Learning methods: value-based methods** and study our first RL algorithm: **Q-Learning.**
We'll also **implement our first RL agent from scratch**, a Q-Learning agent, and will train it in two environments:
1. Frozen-Lake-v1 (non-slippery version): where our agent will need to **go from the starting state (S) to the goal state (G)** by walking only on frozen tiles (F) and avoiding holes (H).
2. An autonomous taxi: where our agent will need **to learn to navigate** a city to **transport its passengers from point A to point B.**
<img src="https://huggingface.co/datasets/huggingface-deep-rl-course/course-images/resolve/main/en/unit3/envs.gif" alt="Environments"/>
Concretely, we will:
- Learn about **value-based methods**.
- Learn about the **differences between Monte Carlo and Temporal Difference Learning**.
- Study and implement **our first RL algorithm**: Q-Learning.
This unit is **fundamental if you want to be able to work on Deep Q-Learning**: the first Deep RL algorithm that played Atari games and beat the human level on some of them (breakout, space invaders, etc).
So let's get started! 🚀
| deep-rl-class/units/en/unit2/introduction.mdx/0 | {
"file_path": "deep-rl-class/units/en/unit2/introduction.mdx",
"repo_id": "deep-rl-class",
"token_count": 466
} | 84 |
# Hands-on [[hands-on]]
<CourseFloatingBanner classNames="absolute z-10 right-0 top-0"
notebooks={[
{label: "Google Colab", value: "https://colab.research.google.com/github/huggingface/deep-rl-class/blob/main/notebooks/unit3/unit3.ipynb"}
]}
askForHelpUrl="http://hf.co/join/discord" />
Now that you've studied the theory behind Deep Q-Learning, **you’re ready to train your Deep Q-Learning agent to play Atari Games**. We'll start with Space Invaders, but you'll be able to use any Atari game you want 🔥
<img src="https://huggingface.co/datasets/huggingface-deep-rl-course/course-images/resolve/main/en/unit4/atari-envs.gif" alt="Environments"/>
We're using the [RL-Baselines-3 Zoo integration](https://github.com/DLR-RM/rl-baselines3-zoo), a vanilla version of Deep Q-Learning with no extensions such as Double-DQN, Dueling-DQN, or Prioritized Experience Replay.
Also, **if you want to learn to implement Deep Q-Learning by yourself after this hands-on**, you definitely should look at the CleanRL implementation: https://github.com/vwxyzjn/cleanrl/blob/master/cleanrl/dqn_atari.py
To validate this hands-on for the certification process, you need to push your trained model to the Hub and **get a result of >= 200**.
To find your result, go to the leaderboard and find your model, **the result = mean_reward - std of reward**
**If you don't find your model, go to the bottom of the page and click on the refresh button.**
For more information about the certification process, check this section 👉 https://huggingface.co/deep-rl-course/en/unit0/introduction#certification-process
And you can check your progress here 👉 https://huggingface.co/spaces/ThomasSimonini/Check-my-progress-Deep-RL-Course
**To start the hands-on click on Open In Colab button** 👇 :
[](https://colab.research.google.com/github/huggingface/deep-rl-class/blob/master/notebooks/unit3/unit3.ipynb)
# Unit 3: Deep Q-Learning with Atari Games 👾 using RL Baselines3 Zoo
<img src="https://huggingface.co/datasets/huggingface-deep-rl-course/course-images/resolve/main/en/unit4/thumbnail.jpg" alt="Unit 3 Thumbnail">
In this hands-on, **you'll train a Deep Q-Learning agent** playing Space Invaders using [RL Baselines3 Zoo](https://github.com/DLR-RM/rl-baselines3-zoo), a training framework based on [Stable-Baselines3](https://stable-baselines3.readthedocs.io/en/master/) that provides scripts for training, evaluating agents, tuning hyperparameters, plotting results and recording videos.
We're using the [RL-Baselines-3 Zoo integration, a vanilla version of Deep Q-Learning](https://stable-baselines3.readthedocs.io/en/master/modules/dqn.html) with no extensions such as Double-DQN, Dueling-DQN, and Prioritized Experience Replay.
### 🎮 Environments:
- [SpacesInvadersNoFrameskip-v4](https://gymnasium.farama.org/environments/atari/space_invaders/)
You can see the difference between Space Invaders versions here 👉 https://gymnasium.farama.org/environments/atari/space_invaders/#variants
### 📚 RL-Library:
- [RL-Baselines3-Zoo](https://github.com/DLR-RM/rl-baselines3-zoo)
## Objectives of this hands-on 🏆
At the end of the hands-on, you will:
- Be able to understand deeper **how RL Baselines3 Zoo works**.
- Be able to **push your trained agent and the code to the Hub** with a nice video replay and an evaluation score 🔥.
## Prerequisites 🏗️
Before diving into the hands-on, you need to:
🔲 📚 **[Study Deep Q-Learning by reading Unit 3](https://huggingface.co/deep-rl-course/unit3/introduction)** 🤗
We're constantly trying to improve our tutorials, so **if you find some issues in this hands-on**, please [open an issue on the Github Repo](https://github.com/huggingface/deep-rl-class/issues).
# Let's train a Deep Q-Learning agent playing Atari' Space Invaders 👾 and upload it to the Hub.
We strongly recommend students **to use Google Colab for the hands-on exercises instead of running them on their personal computers**.
By using Google Colab, **you can focus on learning and experimenting without worrying about the technical aspects of setting up your environments**.
To validate this hands-on for the certification process, you need to push your trained model to the Hub and **get a result of >= 200**.
To find your result, go to the leaderboard and find your model, **the result = mean_reward - std of reward**
For more information about the certification process, check this section 👉 https://huggingface.co/deep-rl-course/en/unit0/introduction#certification-process
## Set the GPU 💪
- To **accelerate the agent's training, we'll use a GPU**. To do that, go to `Runtime > Change Runtime type`
<img src="https://huggingface.co/datasets/huggingface-deep-rl-course/course-images/resolve/main/en/notebooks/gpu-step1.jpg" alt="GPU Step 1">
- `Hardware Accelerator > GPU`
<img src="https://huggingface.co/datasets/huggingface-deep-rl-course/course-images/resolve/main/en/notebooks/gpu-step2.jpg" alt="GPU Step 2">
# Install RL-Baselines3 Zoo and its dependencies 📚
If you see `ERROR: pip's dependency resolver does not currently take into account all the packages that are installed.` **this is normal and it's not a critical error** there's a conflict of version. But the packages we need are installed.
```python
# For now we install this update of RL-Baselines3 Zoo
pip install git+https://github.com/DLR-RM/rl-baselines3-zoo@update/hf
```
IF AND ONLY IF THE VERSION ABOVE DOES NOT EXIST ANYMORE. UNCOMMENT AND INSTALL THE ONE BELOW
```python
#pip install rl_zoo3==2.0.0a9
```
```bash
apt-get install swig cmake ffmpeg
```
To be able to use Atari games in Gymnasium we need to install atari package. And accept-rom-license to download the rom files (games files).
```python
!pip install gymnasium[atari]
!pip install gymnasium[accept-rom-license]
```
## Create a virtual display 🔽
During the hands-on, we'll need to generate a replay video. To do so, if you train it on a headless machine, **we need to have a virtual screen to be able to render the environment** (and thus record the frames).
Hence the following cell will install the librairies and create and run a virtual screen 🖥
```bash
apt install python-opengl
apt install ffmpeg
apt install xvfb
pip3 install pyvirtualdisplay
```
```python
# Virtual display
from pyvirtualdisplay import Display
virtual_display = Display(visible=0, size=(1400, 900))
virtual_display.start()
```
## Train our Deep Q-Learning Agent to Play Space Invaders 👾
To train an agent with RL-Baselines3-Zoo, we just need to do two things:
1. Create a hyperparameter config file that will contain our training hyperparameters called `dqn.yml`.
This is a template example:
```
SpaceInvadersNoFrameskip-v4:
env_wrapper:
- stable_baselines3.common.atari_wrappers.AtariWrapper
frame_stack: 4
policy: 'CnnPolicy'
n_timesteps: !!float 1e7
buffer_size: 100000
learning_rate: !!float 1e-4
batch_size: 32
learning_starts: 100000
target_update_interval: 1000
train_freq: 4
gradient_steps: 1
exploration_fraction: 0.1
exploration_final_eps: 0.01
# If True, you need to deactivate handle_timeout_termination
# in the replay_buffer_kwargs
optimize_memory_usage: False
```
Here we see that:
- We use the `Atari Wrapper` that preprocess the input (Frame reduction ,grayscale, stack 4 frames)
- We use `CnnPolicy`, since we use Convolutional layers to process the frames
- We train it for 10 million `n_timesteps`
- Memory (Experience Replay) size is 100000, aka the amount of experience steps you saved to train again your agent with.
💡 My advice is to **reduce the training timesteps to 1M,** which will take about 90 minutes on a P100. `!nvidia-smi` will tell you what GPU you're using. At 10 million steps, this will take about 9 hours. I recommend running this on your local computer (or somewhere else). Just click on: `File>Download`.
In terms of hyperparameters optimization, my advice is to focus on these 3 hyperparameters:
- `learning_rate`
- `buffer_size (Experience Memory size)`
- `batch_size`
As a good practice, you need to **check the documentation to understand what each hyperparameters does**: https://stable-baselines3.readthedocs.io/en/master/modules/dqn.html#parameters
2. We start the training and save the models on `logs` folder 📁
- Define the algorithm after `--algo`, where we save the model after `-f` and where the hyperparameter config is after `-c`.
```bash
python -m rl_zoo3.train --algo ________ --env SpaceInvadersNoFrameskip-v4 -f _________ -c _________
```
#### Solution
```bash
python -m rl_zoo3.train --algo dqn --env SpaceInvadersNoFrameskip-v4 -f logs/ -c dqn.yml
```
## Let's evaluate our agent 👀
- RL-Baselines3-Zoo provides `enjoy.py`, a python script to evaluate our agent. In most RL libraries, we call the evaluation script `enjoy.py`.
- Let's evaluate it for 5000 timesteps 🔥
```bash
python -m rl_zoo3.enjoy --algo dqn --env SpaceInvadersNoFrameskip-v4 --no-render --n-timesteps _________ --folder logs/
```
#### Solution
```bash
python -m rl_zoo3.enjoy --algo dqn --env SpaceInvadersNoFrameskip-v4 --no-render --n-timesteps 5000 --folder logs/
```
## Publish our trained model on the Hub 🚀
Now that we saw we got good results after the training, we can publish our trained model on the hub 🤗 with one line of code.
<img src="https://huggingface.co/datasets/huggingface-deep-rl-course/course-images/resolve/main/en/notebooks/unit3/space-invaders-model.gif" alt="Space Invaders model">
By using `rl_zoo3.push_to_hub` **you evaluate, record a replay, generate a model card of your agent and push it to the hub**.
This way:
- You can **showcase our work** 🔥
- You can **visualize your agent playing** 👀
- You can **share with the community an agent that others can use** 💾
- You can **access a leaderboard 🏆 to see how well your agent is performing compared to your classmates** 👉 https://huggingface.co/spaces/huggingface-projects/Deep-Reinforcement-Learning-Leaderboard
To be able to share your model with the community there are three more steps to follow:
1️⃣ (If it's not already done) create an account to HF ➡ https://huggingface.co/join
2️⃣ Sign in and then, you need to store your authentication token from the Hugging Face website.
- Create a new token (https://huggingface.co/settings/tokens) **with write role**
<img src="https://huggingface.co/datasets/huggingface-deep-rl-course/course-images/resolve/main/en/notebooks/create-token.jpg" alt="Create HF Token">
- Copy the token
- Run the cell below and past the token
```bash
from huggingface_hub import notebook_login # To log to our Hugging Face account to be able to upload models to the Hub.
notebook_login()
!git config --global credential.helper store
```
If you don't want to use a Google Colab or a Jupyter Notebook, you need to use this command instead: `huggingface-cli login`
3️⃣ We're now ready to push our trained agent to the 🤗 Hub 🔥
Let's run push_to_hub.py file to upload our trained agent to the Hub.
`--repo-name `: The name of the repo
`-orga`: Your Hugging Face username
`-f`: Where the trained model folder is (in our case `logs`)
<img src="https://huggingface.co/datasets/huggingface-deep-rl-course/course-images/resolve/main/en/notebooks/unit3/select-id.png" alt="Select Id">
```bash
python -m rl_zoo3.push_to_hub --algo dqn --env SpaceInvadersNoFrameskip-v4 --repo-name _____________________ -orga _____________________ -f logs/
```
#### Solution
```bash
python -m rl_zoo3.push_to_hub --algo dqn --env SpaceInvadersNoFrameskip-v4 --repo-name dqn-SpaceInvadersNoFrameskip-v4 -orga ThomasSimonini -f logs/
```
###.
Congrats 🥳 you've just trained and uploaded your first Deep Q-Learning agent using RL-Baselines-3 Zoo. The script above should have displayed a link to a model repository such as https://huggingface.co/ThomasSimonini/dqn-SpaceInvadersNoFrameskip-v4. When you go to this link, you can:
- See a **video preview of your agent** at the right.
- Click "Files and versions" to see all the files in the repository.
- Click "Use in stable-baselines3" to get a code snippet that shows how to load the model.
- A model card (`README.md` file) which gives a description of the model and the hyperparameters you used.
Under the hood, the Hub uses git-based repositories (don't worry if you don't know what git is), which means you can update the model with new versions as you experiment and improve your agent.
**Compare the results of your agents with your classmates** using the [leaderboard](https://huggingface.co/spaces/huggingface-projects/Deep-Reinforcement-Learning-Leaderboard) 🏆
## Load a powerful trained model 🔥
- The Stable-Baselines3 team uploaded **more than 150 trained Deep Reinforcement Learning agents on the Hub**.
You can find them here: 👉 https://huggingface.co/sb3
Some examples:
- Asteroids: https://huggingface.co/sb3/dqn-AsteroidsNoFrameskip-v4
- Beam Rider: https://huggingface.co/sb3/dqn-BeamRiderNoFrameskip-v4
- Breakout: https://huggingface.co/sb3/dqn-BreakoutNoFrameskip-v4
- Road Runner: https://huggingface.co/sb3/dqn-RoadRunnerNoFrameskip-v4
Let's load an agent playing Beam Rider: https://huggingface.co/sb3/dqn-BeamRiderNoFrameskip-v4
1. We download the model using `rl_zoo3.load_from_hub`, and place it in a new folder that we can call `rl_trained`
```bash
# Download model and save it into the logs/ folder
python -m rl_zoo3.load_from_hub --algo dqn --env BeamRiderNoFrameskip-v4 -orga sb3 -f rl_trained/
```
2. Let's evaluate if for 5000 timesteps
```bash
python -m rl_zoo3.enjoy --algo dqn --env BeamRiderNoFrameskip-v4 -n 5000 -f rl_trained/ --no-render
```
Why not trying to train your own **Deep Q-Learning Agent playing BeamRiderNoFrameskip-v4? 🏆.**
If you want to try, check https://huggingface.co/sb3/dqn-BeamRiderNoFrameskip-v4#hyperparameters **in the model card, you have the hyperparameters of the trained agent.**
But finding hyperparameters can be a daunting task. Fortunately, we'll see in the next Unit, how we can **use Optuna for optimizing the Hyperparameters 🔥.**
## Some additional challenges 🏆
The best way to learn **is to try things by your own**!
In the [Leaderboard](https://huggingface.co/spaces/huggingface-projects/Deep-Reinforcement-Learning-Leaderboard) you will find your agents. Can you get to the top?
Here's a list of environments you can try to train your agent with:
- BeamRiderNoFrameskip-v4
- BreakoutNoFrameskip-v4
- EnduroNoFrameskip-v4
- PongNoFrameskip-v4
Also, **if you want to learn to implement Deep Q-Learning by yourself**, you definitely should look at CleanRL implementation: https://github.com/vwxyzjn/cleanrl/blob/master/cleanrl/dqn_atari.py
<img src="https://huggingface.co/datasets/huggingface-deep-rl-course/course-images/resolve/main/en/unit4/atari-envs.gif" alt="Environments"/>
________________________________________________________________________
Congrats on finishing this chapter!
If you’re still feel confused with all these elements...it's totally normal! **This was the same for me and for all people who studied RL.**
Take time to really **grasp the material before continuing and try the additional challenges**. It’s important to master these elements and having a solid foundations.
In the next unit, **we’re going to learn about [Optuna](https://optuna.org/)**. One of the most critical task in Deep Reinforcement Learning is to find a good set of training hyperparameters. And Optuna is a library that helps you to automate the search.
### This is a course built with you 👷🏿♀️
Finally, we want to improve and update the course iteratively with your feedback. If you have some, please fill this form 👉 https://forms.gle/3HgA7bEHwAmmLfwh9
We're constantly trying to improve our tutorials, so **if you find some issues in this notebook**, please [open an issue on the Github Repo](https://github.com/huggingface/deep-rl-class/issues).
See you on Bonus unit 2! 🔥
### Keep Learning, Stay Awesome 🤗
| deep-rl-class/units/en/unit3/hands-on.mdx/0 | {
"file_path": "deep-rl-class/units/en/unit3/hands-on.mdx",
"repo_id": "deep-rl-class",
"token_count": 5087
} | 85 |
# Hands-on
<CourseFloatingBanner classNames="absolute z-10 right-0 top-0"
notebooks={[
{label: "Google Colab", value: "https://colab.research.google.com/github/huggingface/deep-rl-class/blob/main/notebooks/unit5/unit5.ipynb"}
]}
askForHelpUrl="http://hf.co/join/discord" />
We learned what ML-Agents is and how it works. We also studied the two environments we're going to use. Now we're ready to train our agents!
<img src="https://huggingface.co/datasets/huggingface-deep-rl-course/course-images/resolve/main/en/unit7/envs.png" alt="Environments" />
To validate this hands-on for the certification process, you **just need to push your trained models to the Hub.**
There are **no minimum results to attain** in order to validate this Hands On. But if you want to get nice results, you can try to reach the following:
- For [Pyramids](https://huggingface.co/spaces/unity/ML-Agents-Pyramids): Mean Reward = 1.75
- For [SnowballTarget](https://huggingface.co/spaces/ThomasSimonini/ML-Agents-SnowballTarget): Mean Reward = 15 or 30 targets shoot in an episode.
For more information about the certification process, check this section 👉 https://huggingface.co/deep-rl-course/en/unit0/introduction#certification-process
**To start the hands-on, click on Open In Colab button** 👇 :
[](https://colab.research.google.com/github/huggingface/deep-rl-class/blob/master/notebooks/unit5/unit5.ipynb)
We strongly **recommend students use Google Colab for the hands-on exercises** instead of running them on their personal computers.
By using Google Colab, **you can focus on learning and experimenting without worrying about the technical aspects** of setting up your environments.
# Unit 5: An Introduction to ML-Agents
<img src="https://huggingface.co/datasets/huggingface-deep-rl-course/course-images/resolve/main/en/unit7/thumbnail.png" alt="Thumbnail"/>
In this notebook, you'll learn about ML-Agents and train two agents.
- The first one will learn to **shoot snowballs onto spawning targets**.
- The second needs to press a button to spawn a pyramid, then navigate to the pyramid, knock it over, **and move to the gold brick at the top**. To do that, it will need to explore its environment, and we will use a technique called curiosity.
After that, you'll be able **to watch your agents playing directly on your browser**.
For more information about the certification process, check this section 👉 https://huggingface.co/deep-rl-course/en/unit0/introduction#certification-process
⬇️ Here is an example of what **you will achieve at the end of this unit.** ⬇️
<img src="https://huggingface.co/datasets/huggingface-deep-rl-course/course-images/resolve/main/en/unit7/pyramids.gif" alt="Pyramids"/>
<img src="https://huggingface.co/datasets/huggingface-deep-rl-course/course-images/resolve/main/en/unit7/snowballtarget.gif" alt="SnowballTarget"/>
### 🎮 Environments:
- [Pyramids](https://github.com/Unity-Technologies/ml-agents/blob/main/docs/Learning-Environment-Examples.md#pyramids)
- SnowballTarget
### 📚 RL-Library:
- [ML-Agents](https://github.com/Unity-Technologies/ml-agents)
We're constantly trying to improve our tutorials, so **if you find some issues in this notebook**, please [open an issue on the GitHub Repo](https://github.com/huggingface/deep-rl-class/issues).
## Objectives of this notebook 🏆
At the end of the notebook, you will:
- Understand how **ML-Agents** works and the environment library.
- Be able to **train agents in Unity Environments**.
## Prerequisites 🏗️
Before diving into the notebook, you need to:
🔲 📚 **Study [what ML-Agents is and how it works by reading Unit 5](https://huggingface.co/deep-rl-course/unit5/introduction)** 🤗
# Let's train our agents 🚀
## Set the GPU 💪
- To **accelerate the agent's training, we'll use a GPU**. To do that, go to `Runtime > Change Runtime type`
<img src="https://huggingface.co/datasets/huggingface-deep-rl-course/course-images/resolve/main/en/notebooks/gpu-step1.jpg" alt="GPU Step 1">
- `Hardware Accelerator > GPU`
<img src="https://huggingface.co/datasets/huggingface-deep-rl-course/course-images/resolve/main/en/notebooks/gpu-step2.jpg" alt="GPU Step 2">
## Clone the repository and install the dependencies 🔽
- We need to clone the repository that **contains the experimental version of the library that allows you to push your trained agent to the Hub.**
```bash
# Clone the repository
git clone --depth 1 https://github.com/Unity-Technologies/ml-agents
```
```bash
# Go inside the repository and install the package
cd ml-agents
pip install -e ./ml-agents-envs
pip install -e ./ml-agents
```
## SnowballTarget ⛄
If you need a refresher on how this environment works check this section 👉
https://huggingface.co/deep-rl-course/unit5/snowball-target
### Download and move the environment zip file in `./training-envs-executables/linux/`
- Our environment executable is in a zip file.
- We need to download it and place it to `./training-envs-executables/linux/`
- We use a linux executable because we use colab, and colab machines OS is Ubuntu (linux)
```bash
# Here, we create training-envs-executables and linux
mkdir ./training-envs-executables
mkdir ./training-envs-executables/linux
```
We downloaded the file SnowballTarget.zip from https://github.com/huggingface/Snowball-Target using `wget`
```bash
wget "https://github.com/huggingface/Snowball-Target/raw/main/SnowballTarget.zip" -O ./training-envs-executables/linux/SnowballTarget.zip
```
We unzip the executable.zip file
```bash
unzip -d ./training-envs-executables/linux/ ./training-envs-executables/linux/SnowballTarget.zip
```
Make sure your file is accessible
```bash
chmod -R 755 ./training-envs-executables/linux/SnowballTarget
```
### Define the SnowballTarget config file
- In ML-Agents, you define the **training hyperparameters in config.yaml files.**
There are multiple hyperparameters. To understand them better, you should read the explanation for each one in [the documentation](https://github.com/Unity-Technologies/ml-agents/blob/release_20_docs/docs/Training-Configuration-File.md)
You need to create a `SnowballTarget.yaml` config file in ./content/ml-agents/config/ppo/
We'll give you a preliminary version of this config (to copy and paste into your `SnowballTarget.yaml file`), **but you should modify it**.
```yaml
behaviors:
SnowballTarget:
trainer_type: ppo
summary_freq: 10000
keep_checkpoints: 10
checkpoint_interval: 50000
max_steps: 200000
time_horizon: 64
threaded: true
hyperparameters:
learning_rate: 0.0003
learning_rate_schedule: linear
batch_size: 128
buffer_size: 2048
beta: 0.005
epsilon: 0.2
lambd: 0.95
num_epoch: 3
network_settings:
normalize: false
hidden_units: 256
num_layers: 2
vis_encode_type: simple
reward_signals:
extrinsic:
gamma: 0.99
strength: 1.0
```
<img src="https://huggingface.co/datasets/huggingface-deep-rl-course/course-images/resolve/main/en/unit7/snowballfight_config1.png" alt="Config SnowballTarget"/>
<img src="https://huggingface.co/datasets/huggingface-deep-rl-course/course-images/resolve/main/en/unit7/snowballfight_config2.png" alt="Config SnowballTarget"/>
As an experiment, try to modify some other hyperparameters. Unity provides very [good documentation explaining each of them here](https://github.com/Unity-Technologies/ml-agents/blob/main/docs/Training-Configuration-File.md).
Now that you've created the config file and understand what most hyperparameters do, we're ready to train our agent 🔥.
### Train the agent
To train our agent, we need to **launch mlagents-learn and select the executable containing the environment.**
We define four parameters:
1. `mlagents-learn <config>`: the path where the hyperparameter config file is.
2. `--env`: where the environment executable is.
3. `--run_id`: the name you want to give to your training run id.
4. `--no-graphics`: to not launch the visualization during the training.
<img src="https://huggingface.co/datasets/huggingface-deep-rl-course/course-images/resolve/main/en/unit7/mlagentslearn.png" alt="MlAgents learn"/>
Train the model and use the `--resume` flag to continue training in case of interruption.
> It will fail the first time if and when you use `--resume`. Try rerunning the block to bypass the error.
The training will take 10 to 35min depending on your config. Go take a ☕️ you deserve it 🤗.
```bash
mlagents-learn ./config/ppo/SnowballTarget.yaml --env=./training-envs-executables/linux/SnowballTarget/SnowballTarget --run-id="SnowballTarget1" --no-graphics
```
### Push the agent to the Hugging Face Hub
- Now that we've trained our agent, we’re **ready to push it to the Hub and visualize it playing on your browser🔥.**
To be able to share your model with the community, there are three more steps to follow:
1️⃣ (If it's not already done) create an account to HF ➡ https://huggingface.co/join
2️⃣ Sign in and store your authentication token from the Hugging Face website.
- Create a new token (https://huggingface.co/settings/tokens) **with write role**
<img src="https://huggingface.co/datasets/huggingface-deep-rl-course/course-images/resolve/main/en/notebooks/create-token.jpg" alt="Create HF Token">
- Copy the token
- Run the cell below and paste the token
```python
from huggingface_hub import notebook_login
notebook_login()
```
If you don't want to use Google Colab or a Jupyter Notebook, you need to use this command instead: `huggingface-cli login`
Then we need to run `mlagents-push-to-hf`.
And we define four parameters:
1. `--run-id`: the name of the training run id.
2. `--local-dir`: where the agent was saved, it’s results/<run_id name>, so in my case results/First Training.
3. `--repo-id`: the name of the Hugging Face repo you want to create or update. It’s always <your huggingface username>/<the repo name>
If the repo does not exist **it will be created automatically**
4. `--commit-message`: since HF repos are git repositories you need to give a commit message.
<img src="https://huggingface.co/datasets/huggingface-deep-rl-course/course-images/resolve/main/en/unit7/mlagentspushtohub.png" alt="Push to Hub"/>
For instance:
`mlagents-push-to-hf --run-id="SnowballTarget1" --local-dir="./results/SnowballTarget1" --repo-id="ThomasSimonini/ppo-SnowballTarget" --commit-message="First Push"`
```python
mlagents-push-to-hf --run-id= # Add your run id --local-dir= # Your local dir --repo-id= # Your repo id --commit-message= # Your commit message
```
If everything worked you should see this at the end of the process (but with a different url 😆) :
```
Your model is pushed to the hub. You can view your model here: https://huggingface.co/ThomasSimonini/ppo-SnowballTarget
```
It's the link to your model. It contains a model card that explains how to use it, your Tensorboard, and your config file. **What's awesome is that it's a git repository, which means you can have different commits, update your repository with a new push, etc.**
But now comes the best: **being able to visualize your agent online 👀.**
### Watch your agent playing 👀
This step it's simple:
1. Remember your repo-id
2. Go here: https://huggingface.co/spaces/ThomasSimonini/ML-Agents-SnowballTarget
3. Launch the game and put it in full screen by clicking on the bottom right button
<img src="https://huggingface.co/datasets/huggingface-deep-rl-course/course-images/resolve/main/en/unit7/snowballtarget_load.png" alt="Snowballtarget load"/>
1. In step 1, choose your model repository, which is the model id (in my case ThomasSimonini/ppo-SnowballTarget).
2. In step 2, **choose what model you want to replay**:
- I have multiple ones since we saved a model every 500000 timesteps.
- But if I want the more recent I choose `SnowballTarget.onnx`
👉 It's nice to **try different model stages to see the improvement of the agent.**
And don't hesitate to share the best score your agent gets on discord in the #rl-i-made-this channel 🔥
Now let's try a more challenging environment called Pyramids.
## Pyramids 🏆
### Download and move the environment zip file in `./training-envs-executables/linux/`
- Our environment executable is in a zip file.
- We need to download it and place it into `./training-envs-executables/linux/`
- We use a linux executable because we're using colab, and the colab machine's OS is Ubuntu (linux)
We downloaded the file Pyramids.zip from from https://huggingface.co/spaces/unity/ML-Agents-Pyramids/resolve/main/Pyramids.zip using `wget`
```python
wget "https://huggingface.co/spaces/unity/ML-Agents-Pyramids/resolve/main/Pyramids.zip" -O ./training-envs-executables/linux/Pyramids.zip
```
Unzip it
```python
unzip -d ./training-envs-executables/linux/ ./training-envs-executables/linux/Pyramids.zip
```
Make sure your file is accessible
```bash
chmod -R 755 ./training-envs-executables/linux/Pyramids/Pyramids
```
### Modify the PyramidsRND config file
- Contrary to the first environment, which was a custom one, **Pyramids was made by the Unity team**.
- So the PyramidsRND config file already exists and is in ./content/ml-agents/config/ppo/PyramidsRND.yaml
- You might ask why "RND" is in PyramidsRND. RND stands for *random network distillation* it's a way to generate curiosity rewards. If you want to know more about that, we wrote an article explaining this technique: https://medium.com/data-from-the-trenches/curiosity-driven-learning-through-random-network-distillation-488ffd8e5938
For this training, we’ll modify one thing:
- The total training steps hyperparameter is too high since we can hit the benchmark (mean reward = 1.75) in only 1M training steps.
👉 To do that, we go to config/ppo/PyramidsRND.yaml,**and change max_steps to 1000000.**
<img src="https://huggingface.co/datasets/huggingface-deep-rl-course/course-images/resolve/main/en/unit7/pyramids-config.png" alt="Pyramids config"/>
As an experiment, you should also try to modify some other hyperparameters. Unity provides very [good documentation explaining each of them here](https://github.com/Unity-Technologies/ml-agents/blob/main/docs/Training-Configuration-File.md).
We’re now ready to train our agent 🔥.
### Train the agent
The training will take 30 to 45min depending on your machine, go take a ☕️ you deserve it 🤗.
```python
mlagents-learn ./config/ppo/PyramidsRND.yaml --env=./training-envs-executables/linux/Pyramids/Pyramids --run-id="Pyramids Training" --no-graphics
```
### Push the agent to the Hugging Face Hub
- Now that we trained our agent, we’re **ready to push it to the Hub to be able to visualize it playing on your browser🔥.**
```python
mlagents-push-to-hf --run-id= # Add your run id --local-dir= # Your local dir --repo-id= # Your repo id --commit-message= # Your commit message
```
### Watch your agent playing 👀
👉 https://huggingface.co/spaces/unity/ML-Agents-Pyramids
### 🎁 Bonus: Why not train on another environment?
Now that you know how to train an agent using MLAgents, **why not try another environment?**
MLAgents provides 17 different environments and we’re building some custom ones. The best way to learn is to try things on your own, have fun.
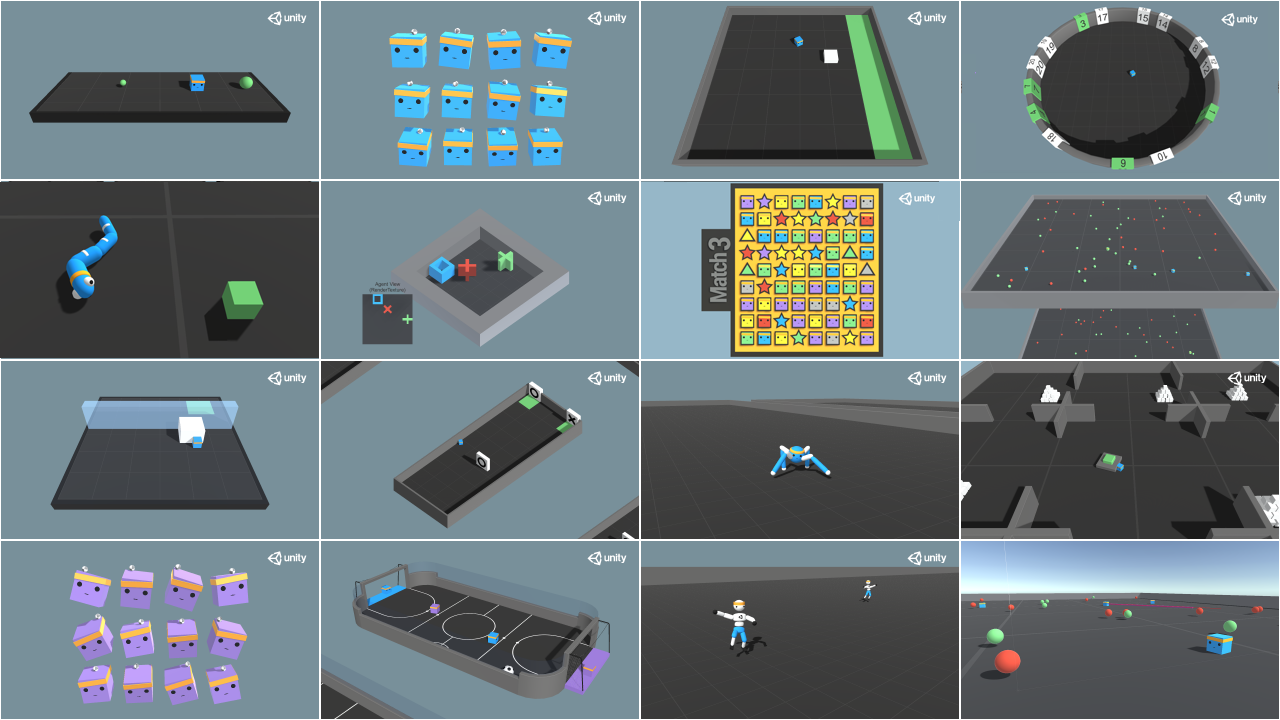
You have the full list of the one currently available environments on Hugging Face here 👉 https://github.com/huggingface/ml-agents#the-environments
For the demos to visualize your agent 👉 https://huggingface.co/unity
For now we have integrated:
- [Worm](https://huggingface.co/spaces/unity/ML-Agents-Worm) demo where you teach a **worm to crawl**.
- [Walker](https://huggingface.co/spaces/unity/ML-Agents-Walker) demo where you teach an agent **to walk towards a goal**.
That’s all for today. Congrats on finishing this tutorial!
The best way to learn is to practice and try stuff. Why not try another environment? ML-Agents has 18 different environments, but you can also create your own. Check the documentation and have fun!
See you on Unit 6 🔥,
## Keep Learning, Stay awesome 🤗
| deep-rl-class/units/en/unit5/hands-on.mdx/0 | {
"file_path": "deep-rl-class/units/en/unit5/hands-on.mdx",
"repo_id": "deep-rl-class",
"token_count": 5146
} | 86 |
Subsets and Splits
No community queries yet
The top public SQL queries from the community will appear here once available.