text
stringlengths 7
328k
| id
stringlengths 14
166
| metadata
dict | __index_level_0__
int64 0
459
|
---|---|---|---|
# An introduction to Multi-Agents Reinforcement Learning (MARL)
## From single agent to multiple agents
In the first unit, we learned to train agents in a single-agent system. When our agent was alone in its environment: **it was not cooperating or collaborating with other agents**.
<figure>
<img src="https://huggingface.co/datasets/huggingface-deep-rl-course/course-images/resolve/main/en/unit10/patchwork.jpg" alt="Patchwork"/>
<figcaption>
A patchwork of all the environments you've trained your agents on since the beginning of the course
</figcaption>
</figure>
When we do multi-agents reinforcement learning (MARL), we are in a situation where we have multiple agents **that share and interact in a common environment**.
For instance, you can think of a warehouse where **multiple robots need to navigate to load and unload packages**.
<figure>
<img src="https://huggingface.co/datasets/huggingface-deep-rl-course/course-images/resolve/main/en/unit10/warehouse.jpg" alt="Warehouse"/>
<figcaption> [Image by upklyak](https://www.freepik.com/free-vector/robots-warehouse-interior-automated-machines_32117680.htm#query=warehouse robot&position=17&from_view=keyword) on Freepik </figcaption>
</figure>
Or a road with **several autonomous vehicles**.
<figure>
<img src="https://huggingface.co/datasets/huggingface-deep-rl-course/course-images/resolve/main/en/unit10/selfdrivingcar.jpg" alt="Self driving cars"/>
<figcaption>
[Image by jcomp](https://www.freepik.com/free-vector/autonomous-smart-car-automatic-wireless-sensor-driving-road-around-car-autonomous-smart-car-goes-scans-roads-observe-distance-automatic-braking-system_26413332.htm#query=self driving cars highway&position=34&from_view=search&track=ais) on Freepik
</figcaption>
</figure>
In these examples, we have **multiple agents interacting in the environment and with the other agents**. This implies defining a multi-agents system. But first, let's understand the different types of multi-agent environments.
## Different types of multi-agent environments
Given that, in a multi-agent system, agents interact with other agents, we can have different types of environments:
- *Cooperative environments*: where your agents need **to maximize the common benefits**.
For instance, in a warehouse, **robots must collaborate to load and unload the packages efficiently (as fast as possible)**.
- *Competitive/Adversarial environments*: in this case, your agent **wants to maximize its benefits by minimizing the opponent's**.
For example, in a game of tennis, **each agent wants to beat the other agent**.
<img src="https://huggingface.co/datasets/huggingface-deep-rl-course/course-images/resolve/main/en/unit10/tennis.png" alt="Tennis"/>
- *Mixed of both adversarial and cooperative*: like in our SoccerTwos environment, two agents are part of a team (blue or purple): they need to cooperate with each other and beat the opponent team.
<figure>
<img src="https://huggingface.co/datasets/huggingface-deep-rl-course/course-images/resolve/main/en/unit10/soccertwos.gif" alt="SoccerTwos"/>
<figcaption>This environment was made by the <a href="https://github.com/Unity-Technologies/ml-agents">Unity MLAgents Team</a></figcaption>
</figure>
So now we might wonder: how can we design these multi-agent systems? Said differently, **how can we train agents in a multi-agent setting** ?
| deep-rl-class/units/en/unit7/introduction-to-marl.mdx/0 | {
"file_path": "deep-rl-class/units/en/unit7/introduction-to-marl.mdx",
"repo_id": "deep-rl-class",
"token_count": 982
} | 87 |
# How Huggy works [[how-huggy-works]]
Huggy is a Deep Reinforcement Learning environment made by Hugging Face and based on [Puppo the Corgi, a project by the Unity MLAgents team](https://blog.unity.com/technology/puppo-the-corgi-cuteness-overload-with-the-unity-ml-agents-toolkit).
This environment was created using the [Unity game engine](https://unity.com/) and [MLAgents](https://github.com/Unity-Technologies/ml-agents). ML-Agents is a toolkit for the game engine from Unity that allows us to **create environments using Unity or use pre-made environments to train our agents**.
<img src="https://huggingface.co/datasets/huggingface-deep-rl-course/course-images/resolve/main/en/notebooks/unit-bonus1/huggy.jpg" alt="Huggy" width="100%">
In this environment we aim to train Huggy to **fetch the stick we throw. This means he needs to move correctly toward the stick**.
## The State Space, what Huggy perceives. [[state-space]]
Huggy doesn't "see" his environment. Instead, we provide him information about the environment:
- The target (stick) position
- The relative position between himself and the target
- The orientation of his legs.
Given all this information, Huggy can **use his policy to determine which action to take next to fulfill his goal**.
## The Action Space, what moves Huggy can perform [[action-space]]
<img src="https://huggingface.co/datasets/huggingface-deep-rl-course/course-images/resolve/main/en/notebooks/unit-bonus1/huggy-action.jpg" alt="Huggy action" width="100%">
**Joint motors drive Huggy's legs**. This means that to get the target, Huggy needs to **learn to rotate the joint motors of each of his legs correctly so he can move**.
## The Reward Function [[reward-function]]
The reward function is designed so that **Huggy will fulfill his goal**: fetch the stick.
Remember that one of the foundations of Reinforcement Learning is the *reward hypothesis*: a goal can be described as the **maximization of the expected cumulative reward**.
Here, our goal is that Huggy **goes towards the stick but without spinning too much**. Hence, our reward function must translate this goal.
Our reward function:
<img src="https://huggingface.co/datasets/huggingface-deep-rl-course/course-images/resolve/main/en/notebooks/unit-bonus1/reward.jpg" alt="Huggy reward function" width="100%">
- *Orientation bonus*: we **reward him for getting close to the target**.
- *Time penalty*: a fixed-time penalty given at every action to **force him to get to the stick as fast as possible**.
- *Rotation penalty*: we penalize Huggy if **he spins too much and turns too quickly**.
- *Getting to the target reward*: we reward Huggy for **reaching the target**.
If you want to see what this reward function looks like mathematically, check [Puppo the Corgi presentation](https://blog.unity.com/technology/puppo-the-corgi-cuteness-overload-with-the-unity-ml-agents-toolkit).
## Train Huggy
Huggy aims **to learn to run correctly and as fast as possible toward the goal**. To do that, at every step and given the environment observation, he needs to decide how to rotate each joint motor of his legs to move correctly (not spinning too much) and towards the goal.
The training loop looks like this:
<img src="https://huggingface.co/datasets/huggingface-deep-rl-course/course-images/resolve/main/en/notebooks/unit-bonus1/huggy-loop.jpg" alt="Huggy loop" width="100%">
The training environment looks like this:
<img src="https://huggingface.co/datasets/huggingface-deep-rl-course/course-images/resolve/main/en/notebooks/unit-bonus1/training-env.jpg" alt="Huggy training env" width="100%">
It's a place where a **stick is spawned randomly**. When Huggy reaches it, the stick get spawned somewhere else.
We built **multiple copies of the environment for the training**. This helps speed up the training by providing more diverse experiences.
Now that you have the big picture of the environment, you're ready to train Huggy to fetch the stick.
To do that, we're going to use [MLAgents](https://github.com/Unity-Technologies/ml-agents). Don't worry if you have never used it before. In this unit we'll use Google Colab to train Huggy, and then you'll be able to load your trained Huggy and play with him directly in the browser.
In a future unit, we will study MLAgents more in-depth and see how it works. But for now, we keep things simple by just using the provided implementation.
| deep-rl-class/units/en/unitbonus1/how-huggy-works.mdx/0 | {
"file_path": "deep-rl-class/units/en/unitbonus1/how-huggy-works.mdx",
"repo_id": "deep-rl-class",
"token_count": 1245
} | 88 |
# Offline vs. Online Reinforcement Learning
Deep Reinforcement Learning (RL) is a framework **to build decision-making agents**. These agents aim to learn optimal behavior (policy) by interacting with the environment through **trial and error and receiving rewards as unique feedback**.
The agent’s goal **is to maximize its cumulative reward**, called return. Because RL is based on the *reward hypothesis*: all goals can be described as the **maximization of the expected cumulative reward**.
Deep Reinforcement Learning agents **learn with batches of experience**. The question is, how do they collect it?:
<figure>
<img src="https://huggingface.co/datasets/huggingface-deep-rl-course/course-images/resolve/main/en/unit12/offlinevsonlinerl.gif" alt="Unit bonus 3 thumbnail">
<figcaption>A comparison between Reinforcement Learning in an Online and Offline setting, figure taken from <a href="https://offline-rl.github.io/">this post</a></figcaption>
</figure>
- In *online reinforcement learning*, which is what we've learned during this course, the agent **gathers data directly**: it collects a batch of experience by **interacting with the environment**. Then, it uses this experience immediately (or via some replay buffer) to learn from it (update its policy).
But this implies that either you **train your agent directly in the real world or have a simulator**. If you don’t have one, you need to build it, which can be very complex (how to reflect the complex reality of the real world in an environment?), expensive, and insecure (if the simulator has flaws that may provide a competitive advantage, the agent will exploit them).
- On the other hand, in *offline reinforcement learning*, the agent only **uses data collected from other agents or human demonstrations**. It does **not interact with the environment**.
The process is as follows:
- **Create a dataset** using one or more policies and/or human interactions.
- Run **offline RL on this dataset** to learn a policy
This method has one drawback: the *counterfactual queries problem*. What do we do if our agent **decides to do something for which we don’t have the data?** For instance, turning right on an intersection but we don’t have this trajectory.
There exist some solutions on this topic, but if you want to know more about offline reinforcement learning, you can [watch this video](https://www.youtube.com/watch?v=k08N5a0gG0A)
## Further reading
For more information, we recommend you check out the following resources:
- [Offline Reinforcement Learning, Talk by Sergei Levine](https://www.youtube.com/watch?v=qgZPZREor5I)
- [Offline Reinforcement Learning: Tutorial, Review, and Perspectives on Open Problems](https://arxiv.org/abs/2005.01643)
## Author
This section was written by <a href="https://twitter.com/ThomasSimonini"> Thomas Simonini</a>
| deep-rl-class/units/en/unitbonus3/offline-online.mdx/0 | {
"file_path": "deep-rl-class/units/en/unitbonus3/offline-online.mdx",
"repo_id": "deep-rl-class",
"token_count": 708
} | 89 |
FROM ubuntu:20.04
LABEL maintainer="Hugging Face"
LABEL repository="diffusers"
ENV DEBIAN_FRONTEND=noninteractive
RUN apt update && \
apt install -y bash \
build-essential \
git \
git-lfs \
curl \
ca-certificates \
libsndfile1-dev \
python3.8 \
python3-pip \
python3.8-venv && \
rm -rf /var/lib/apt/lists
# make sure to use venv
RUN python3 -m venv /opt/venv
ENV PATH="/opt/venv/bin:$PATH"
# pre-install the heavy dependencies (these can later be overridden by the deps from setup.py)
RUN python3 -m pip install --no-cache-dir --upgrade pip uv==0.1.11 && \
python3 -m uv pip install --no-cache-dir \
torch==2.1.2 \
torchvision==0.16.2 \
torchaudio==2.1.2 \
onnxruntime \
--extra-index-url https://download.pytorch.org/whl/cpu && \
python3 -m uv pip install --no-cache-dir \
accelerate \
datasets \
hf-doc-builder \
huggingface-hub \
Jinja2 \
librosa \
numpy \
scipy \
tensorboard \
transformers
CMD ["/bin/bash"] | diffusers/docker/diffusers-onnxruntime-cpu/Dockerfile/0 | {
"file_path": "diffusers/docker/diffusers-onnxruntime-cpu/Dockerfile",
"repo_id": "diffusers",
"token_count": 642
} | 90 |
<!--Copyright 2024 The HuggingFace Team. All rights reserved.
Licensed under the Apache License, Version 2.0 (the "License"); you may not use this file except in compliance with
the License. You may obtain a copy of the License at
http://www.apache.org/licenses/LICENSE-2.0
Unless required by applicable law or agreed to in writing, software distributed under the License is distributed on
an "AS IS" BASIS, WITHOUT WARRANTIES OR CONDITIONS OF ANY KIND, either express or implied. See the License for the
specific language governing permissions and limitations under the License.
-->
# LoRA
LoRA is a fast and lightweight training method that inserts and trains a significantly smaller number of parameters instead of all the model parameters. This produces a smaller file (~100 MBs) and makes it easier to quickly train a model to learn a new concept. LoRA weights are typically loaded into the UNet, text encoder or both. There are two classes for loading LoRA weights:
- [`LoraLoaderMixin`] provides functions for loading and unloading, fusing and unfusing, enabling and disabling, and more functions for managing LoRA weights. This class can be used with any model.
- [`StableDiffusionXLLoraLoaderMixin`] is a [Stable Diffusion (SDXL)](../../api/pipelines/stable_diffusion/stable_diffusion_xl) version of the [`LoraLoaderMixin`] class for loading and saving LoRA weights. It can only be used with the SDXL model.
<Tip>
To learn more about how to load LoRA weights, see the [LoRA](../../using-diffusers/loading_adapters#lora) loading guide.
</Tip>
## LoraLoaderMixin
[[autodoc]] loaders.lora.LoraLoaderMixin
## StableDiffusionXLLoraLoaderMixin
[[autodoc]] loaders.lora.StableDiffusionXLLoraLoaderMixin | diffusers/docs/source/en/api/loaders/lora.md/0 | {
"file_path": "diffusers/docs/source/en/api/loaders/lora.md",
"repo_id": "diffusers",
"token_count": 463
} | 91 |
<!--Copyright 2024 The HuggingFace Team. All rights reserved.
Licensed under the Apache License, Version 2.0 (the "License"); you may not use this file except in compliance with
the License. You may obtain a copy of the License at
http://www.apache.org/licenses/LICENSE-2.0
Unless required by applicable law or agreed to in writing, software distributed under the License is distributed on
an "AS IS" BASIS, WITHOUT WARRANTIES OR CONDITIONS OF ANY KIND, either express or implied. See the License for the
specific language governing permissions and limitations under the License.
-->
# ControlNet
ControlNet was introduced in [Adding Conditional Control to Text-to-Image Diffusion Models](https://huggingface.co/papers/2302.05543) by Lvmin Zhang, Anyi Rao, and Maneesh Agrawala.
With a ControlNet model, you can provide an additional control image to condition and control Stable Diffusion generation. For example, if you provide a depth map, the ControlNet model generates an image that'll preserve the spatial information from the depth map. It is a more flexible and accurate way to control the image generation process.
The abstract from the paper is:
*We present ControlNet, a neural network architecture to add spatial conditioning controls to large, pretrained text-to-image diffusion models. ControlNet locks the production-ready large diffusion models, and reuses their deep and robust encoding layers pretrained with billions of images as a strong backbone to learn a diverse set of conditional controls. The neural architecture is connected with "zero convolutions" (zero-initialized convolution layers) that progressively grow the parameters from zero and ensure that no harmful noise could affect the finetuning. We test various conditioning controls, eg, edges, depth, segmentation, human pose, etc, with Stable Diffusion, using single or multiple conditions, with or without prompts. We show that the training of ControlNets is robust with small (<50k) and large (>1m) datasets. Extensive results show that ControlNet may facilitate wider applications to control image diffusion models.*
This model was contributed by [takuma104](https://huggingface.co/takuma104). ❤️
The original codebase can be found at [lllyasviel/ControlNet](https://github.com/lllyasviel/ControlNet), and you can find official ControlNet checkpoints on [lllyasviel's](https://huggingface.co/lllyasviel) Hub profile.
<Tip>
Make sure to check out the Schedulers [guide](../../using-diffusers/schedulers) to learn how to explore the tradeoff between scheduler speed and quality, and see the [reuse components across pipelines](../../using-diffusers/loading#reuse-components-across-pipelines) section to learn how to efficiently load the same components into multiple pipelines.
</Tip>
## StableDiffusionControlNetPipeline
[[autodoc]] StableDiffusionControlNetPipeline
- all
- __call__
- enable_attention_slicing
- disable_attention_slicing
- enable_vae_slicing
- disable_vae_slicing
- enable_xformers_memory_efficient_attention
- disable_xformers_memory_efficient_attention
- load_textual_inversion
## StableDiffusionControlNetImg2ImgPipeline
[[autodoc]] StableDiffusionControlNetImg2ImgPipeline
- all
- __call__
- enable_attention_slicing
- disable_attention_slicing
- enable_vae_slicing
- disable_vae_slicing
- enable_xformers_memory_efficient_attention
- disable_xformers_memory_efficient_attention
- load_textual_inversion
## StableDiffusionControlNetInpaintPipeline
[[autodoc]] StableDiffusionControlNetInpaintPipeline
- all
- __call__
- enable_attention_slicing
- disable_attention_slicing
- enable_vae_slicing
- disable_vae_slicing
- enable_xformers_memory_efficient_attention
- disable_xformers_memory_efficient_attention
- load_textual_inversion
## StableDiffusionPipelineOutput
[[autodoc]] pipelines.stable_diffusion.StableDiffusionPipelineOutput
## FlaxStableDiffusionControlNetPipeline
[[autodoc]] FlaxStableDiffusionControlNetPipeline
- all
- __call__
## FlaxStableDiffusionControlNetPipelineOutput
[[autodoc]] pipelines.stable_diffusion.FlaxStableDiffusionPipelineOutput
| diffusers/docs/source/en/api/pipelines/controlnet.md/0 | {
"file_path": "diffusers/docs/source/en/api/pipelines/controlnet.md",
"repo_id": "diffusers",
"token_count": 1146
} | 92 |
<!--Copyright 2024 The HuggingFace Team. All rights reserved.
Licensed under the Apache License, Version 2.0 (the "License"); you may not use this file except in compliance with
the License. You may obtain a copy of the License at
http://www.apache.org/licenses/LICENSE-2.0
Unless required by applicable law or agreed to in writing, software distributed under the License is distributed on
an "AS IS" BASIS, WITHOUT WARRANTIES OR CONDITIONS OF ANY KIND, either express or implied. See the License for the
specific language governing permissions and limitations under the License.
-->
# Pipelines
Pipelines provide a simple way to run state-of-the-art diffusion models in inference by bundling all of the necessary components (multiple independently-trained models, schedulers, and processors) into a single end-to-end class. Pipelines are flexible and they can be adapted to use different schedulers or even model components.
All pipelines are built from the base [`DiffusionPipeline`] class which provides basic functionality for loading, downloading, and saving all the components. Specific pipeline types (for example [`StableDiffusionPipeline`]) loaded with [`~DiffusionPipeline.from_pretrained`] are automatically detected and the pipeline components are loaded and passed to the `__init__` function of the pipeline.
<Tip warning={true}>
You shouldn't use the [`DiffusionPipeline`] class for training. Individual components (for example, [`UNet2DModel`] and [`UNet2DConditionModel`]) of diffusion pipelines are usually trained individually, so we suggest directly working with them instead.
<br>
Pipelines do not offer any training functionality. You'll notice PyTorch's autograd is disabled by decorating the [`~DiffusionPipeline.__call__`] method with a [`torch.no_grad`](https://pytorch.org/docs/stable/generated/torch.no_grad.html) decorator because pipelines should not be used for training. If you're interested in training, please take a look at the [Training](../../training/overview) guides instead!
</Tip>
The table below lists all the pipelines currently available in 🤗 Diffusers and the tasks they support. Click on a pipeline to view its abstract and published paper.
| Pipeline | Tasks |
|---|---|
| [AltDiffusion](alt_diffusion) | image2image |
| [AnimateDiff](animatediff) | text2video |
| [Attend-and-Excite](attend_and_excite) | text2image |
| [Audio Diffusion](audio_diffusion) | image2audio |
| [AudioLDM](audioldm) | text2audio |
| [AudioLDM2](audioldm2) | text2audio |
| [BLIP Diffusion](blip_diffusion) | text2image |
| [Consistency Models](consistency_models) | unconditional image generation |
| [ControlNet](controlnet) | text2image, image2image, inpainting |
| [ControlNet with Stable Diffusion XL](controlnet_sdxl) | text2image |
| [ControlNet-XS](controlnetxs) | text2image |
| [ControlNet-XS with Stable Diffusion XL](controlnetxs_sdxl) | text2image |
| [Cycle Diffusion](cycle_diffusion) | image2image |
| [Dance Diffusion](dance_diffusion) | unconditional audio generation |
| [DDIM](ddim) | unconditional image generation |
| [DDPM](ddpm) | unconditional image generation |
| [DeepFloyd IF](deepfloyd_if) | text2image, image2image, inpainting, super-resolution |
| [DiffEdit](diffedit) | inpainting |
| [DiT](dit) | text2image |
| [GLIGEN](stable_diffusion/gligen) | text2image |
| [InstructPix2Pix](pix2pix) | image editing |
| [Kandinsky 2.1](kandinsky) | text2image, image2image, inpainting, interpolation |
| [Kandinsky 2.2](kandinsky_v22) | text2image, image2image, inpainting |
| [Kandinsky 3](kandinsky3) | text2image, image2image |
| [Latent Consistency Models](latent_consistency_models) | text2image |
| [Latent Diffusion](latent_diffusion) | text2image, super-resolution |
| [LDM3D](stable_diffusion/ldm3d_diffusion) | text2image, text-to-3D, text-to-pano, upscaling |
| [LEDITS++](ledits_pp) | image editing |
| [MultiDiffusion](panorama) | text2image |
| [MusicLDM](musicldm) | text2audio |
| [Paint by Example](paint_by_example) | inpainting |
| [ParaDiGMS](paradigms) | text2image |
| [Pix2Pix Zero](pix2pix_zero) | image editing |
| [PixArt-α](pixart) | text2image |
| [PNDM](pndm) | unconditional image generation |
| [RePaint](repaint) | inpainting |
| [Score SDE VE](score_sde_ve) | unconditional image generation |
| [Self-Attention Guidance](self_attention_guidance) | text2image |
| [Semantic Guidance](semantic_stable_diffusion) | text2image |
| [Shap-E](shap_e) | text-to-3D, image-to-3D |
| [Spectrogram Diffusion](spectrogram_diffusion) | |
| [Stable Diffusion](stable_diffusion/overview) | text2image, image2image, depth2image, inpainting, image variation, latent upscaler, super-resolution |
| [Stable Diffusion Model Editing](model_editing) | model editing |
| [Stable Diffusion XL](stable_diffusion/stable_diffusion_xl) | text2image, image2image, inpainting |
| [Stable Diffusion XL Turbo](stable_diffusion/sdxl_turbo) | text2image, image2image, inpainting |
| [Stable unCLIP](stable_unclip) | text2image, image variation |
| [Stochastic Karras VE](stochastic_karras_ve) | unconditional image generation |
| [T2I-Adapter](stable_diffusion/adapter) | text2image |
| [Text2Video](text_to_video) | text2video, video2video |
| [Text2Video-Zero](text_to_video_zero) | text2video |
| [unCLIP](unclip) | text2image, image variation |
| [Unconditional Latent Diffusion](latent_diffusion_uncond) | unconditional image generation |
| [UniDiffuser](unidiffuser) | text2image, image2text, image variation, text variation, unconditional image generation, unconditional audio generation |
| [Value-guided planning](value_guided_sampling) | value guided sampling |
| [Versatile Diffusion](versatile_diffusion) | text2image, image variation |
| [VQ Diffusion](vq_diffusion) | text2image |
| [Wuerstchen](wuerstchen) | text2image |
## DiffusionPipeline
[[autodoc]] DiffusionPipeline
- all
- __call__
- device
- to
- components
## FlaxDiffusionPipeline
[[autodoc]] pipelines.pipeline_flax_utils.FlaxDiffusionPipeline
## PushToHubMixin
[[autodoc]] utils.PushToHubMixin
| diffusers/docs/source/en/api/pipelines/overview.md/0 | {
"file_path": "diffusers/docs/source/en/api/pipelines/overview.md",
"repo_id": "diffusers",
"token_count": 1909
} | 93 |
<!--Copyright 2024 The HuggingFace Team. All rights reserved.
Licensed under the Apache License, Version 2.0 (the "License"); you may not use this file except in compliance with
the License. You may obtain a copy of the License at
http://www.apache.org/licenses/LICENSE-2.0
Unless required by applicable law or agreed to in writing, software distributed under the License is distributed on
an "AS IS" BASIS, WITHOUT WARRANTIES OR CONDITIONS OF ANY KIND, either express or implied. See the License for the
specific language governing permissions and limitations under the License.
-->
# K-Diffusion
[k-diffusion](https://github.com/crowsonkb/k-diffusion) is a popular library created by [Katherine Crowson](https://github.com/crowsonkb/). We provide `StableDiffusionKDiffusionPipeline` and `StableDiffusionXLKDiffusionPipeline` that allow you to run Stable DIffusion with samplers from k-diffusion.
Note that most the samplers from k-diffusion are implemented in Diffusers and we recommend using existing schedulers. You can find a mapping between k-diffusion samplers and schedulers in Diffusers [here](https://huggingface.co/docs/diffusers/api/schedulers/overview)
## StableDiffusionKDiffusionPipeline
[[autodoc]] StableDiffusionKDiffusionPipeline
## StableDiffusionXLKDiffusionPipeline
[[autodoc]] StableDiffusionXLKDiffusionPipeline | diffusers/docs/source/en/api/pipelines/stable_diffusion/k_diffusion.md/0 | {
"file_path": "diffusers/docs/source/en/api/pipelines/stable_diffusion/k_diffusion.md",
"repo_id": "diffusers",
"token_count": 379
} | 94 |
<!--Copyright 2024 The HuggingFace Team. All rights reserved.
Licensed under the Apache License, Version 2.0 (the "License"); you may not use this file except in compliance with
the License. You may obtain a copy of the License at
http://www.apache.org/licenses/LICENSE-2.0
Unless required by applicable law or agreed to in writing, software distributed under the License is distributed on
an "AS IS" BASIS, WITHOUT WARRANTIES OR CONDITIONS OF ANY KIND, either express or implied. See the License for the
specific language governing permissions and limitations under the License.
-->
# Overview
Generating high-quality outputs is computationally intensive, especially during each iterative step where you go from a noisy output to a less noisy output. One of 🤗 Diffuser's goals is to make this technology widely accessible to everyone, which includes enabling fast inference on consumer and specialized hardware.
This section will cover tips and tricks - like half-precision weights and sliced attention - for optimizing inference speed and reducing memory-consumption. You'll also learn how to speed up your PyTorch code with [`torch.compile`](https://pytorch.org/tutorials/intermediate/torch_compile_tutorial.html) or [ONNX Runtime](https://onnxruntime.ai/docs/), and enable memory-efficient attention with [xFormers](https://facebookresearch.github.io/xformers/). There are also guides for running inference on specific hardware like Apple Silicon, and Intel or Habana processors.
| diffusers/docs/source/en/optimization/opt_overview.md/0 | {
"file_path": "diffusers/docs/source/en/optimization/opt_overview.md",
"repo_id": "diffusers",
"token_count": 353
} | 95 |
<!--Copyright 2024 The HuggingFace Team. All rights reserved.
Licensed under the Apache License, Version 2.0 (the "License"); you may not use this file except in compliance with
the License. You may obtain a copy of the License at
http://www.apache.org/licenses/LICENSE-2.0
Unless required by applicable law or agreed to in writing, software distributed under the License is distributed on
an "AS IS" BASIS, WITHOUT WARRANTIES OR CONDITIONS OF ANY KIND, either express or implied. See the License for the
specific language governing permissions and limitations under the License.
-->
# LoRA
<Tip warning={true}>
This is experimental and the API may change in the future.
</Tip>
[LoRA (Low-Rank Adaptation of Large Language Models)](https://hf.co/papers/2106.09685) is a popular and lightweight training technique that significantly reduces the number of trainable parameters. It works by inserting a smaller number of new weights into the model and only these are trained. This makes training with LoRA much faster, memory-efficient, and produces smaller model weights (a few hundred MBs), which are easier to store and share. LoRA can also be combined with other training techniques like DreamBooth to speedup training.
<Tip>
LoRA is very versatile and supported for [DreamBooth](https://github.com/huggingface/diffusers/blob/main/examples/dreambooth/train_dreambooth_lora.py), [Kandinsky 2.2](https://github.com/huggingface/diffusers/blob/main/examples/kandinsky2_2/text_to_image/train_text_to_image_lora_decoder.py), [Stable Diffusion XL](https://github.com/huggingface/diffusers/blob/main/examples/text_to_image/train_text_to_image_lora_sdxl.py), [text-to-image](https://github.com/huggingface/diffusers/blob/main/examples/text_to_image/train_text_to_image_lora.py), and [Wuerstchen](https://github.com/huggingface/diffusers/blob/main/examples/wuerstchen/text_to_image/train_text_to_image_lora_prior.py).
</Tip>
This guide will explore the [train_text_to_image_lora.py](https://github.com/huggingface/diffusers/blob/main/examples/text_to_image/train_text_to_image_lora.py) script to help you become more familiar with it, and how you can adapt it for your own use-case.
Before running the script, make sure you install the library from source:
```bash
git clone https://github.com/huggingface/diffusers
cd diffusers
pip install .
```
Navigate to the example folder with the training script and install the required dependencies for the script you're using:
<hfoptions id="installation">
<hfoption id="PyTorch">
```bash
cd examples/text_to_image
pip install -r requirements.txt
```
</hfoption>
<hfoption id="Flax">
```bash
cd examples/text_to_image
pip install -r requirements_flax.txt
```
</hfoption>
</hfoptions>
<Tip>
🤗 Accelerate is a library for helping you train on multiple GPUs/TPUs or with mixed-precision. It'll automatically configure your training setup based on your hardware and environment. Take a look at the 🤗 Accelerate [Quick tour](https://huggingface.co/docs/accelerate/quicktour) to learn more.
</Tip>
Initialize an 🤗 Accelerate environment:
```bash
accelerate config
```
To setup a default 🤗 Accelerate environment without choosing any configurations:
```bash
accelerate config default
```
Or if your environment doesn't support an interactive shell, like a notebook, you can use:
```py
from accelerate.utils import write_basic_config
write_basic_config()
```
Lastly, if you want to train a model on your own dataset, take a look at the [Create a dataset for training](create_dataset) guide to learn how to create a dataset that works with the training script.
<Tip>
The following sections highlight parts of the training script that are important for understanding how to modify it, but it doesn't cover every aspect of the script in detail. If you're interested in learning more, feel free to read through the [script](https://github.com/huggingface/diffusers/blob/main/examples/text_to_image/text_to_image_lora.py) and let us know if you have any questions or concerns.
</Tip>
## Script parameters
The training script has many parameters to help you customize your training run. All of the parameters and their descriptions are found in the [`parse_args()`](https://github.com/huggingface/diffusers/blob/dd9a5caf61f04d11c0fa9f3947b69ab0010c9a0f/examples/text_to_image/train_text_to_image_lora.py#L85) function. Default values are provided for most parameters that work pretty well, but you can also set your own values in the training command if you'd like.
For example, to increase the number of epochs to train:
```bash
accelerate launch train_text_to_image_lora.py \
--num_train_epochs=150 \
```
Many of the basic and important parameters are described in the [Text-to-image](text2image#script-parameters) training guide, so this guide just focuses on the LoRA relevant parameters:
- `--rank`: the inner dimension of the low-rank matrices to train; a higher rank means more trainable parameters
- `--learning_rate`: the default learning rate is 1e-4, but with LoRA, you can use a higher learning rate
## Training script
The dataset preprocessing code and training loop are found in the [`main()`](https://github.com/huggingface/diffusers/blob/dd9a5caf61f04d11c0fa9f3947b69ab0010c9a0f/examples/text_to_image/train_text_to_image_lora.py#L371) function, and if you need to adapt the training script, this is where you'll make your changes.
As with the script parameters, a walkthrough of the training script is provided in the [Text-to-image](text2image#training-script) training guide. Instead, this guide takes a look at the LoRA relevant parts of the script.
<hfoptions id="lora">
<hfoption id="UNet">
Diffusers uses [`~peft.LoraConfig`] from the [PEFT](https://hf.co/docs/peft) library to set up the parameters of the LoRA adapter such as the rank, alpha, and which modules to insert the LoRA weights into. The adapter is added to the UNet, and only the LoRA layers are filtered for optimization in `lora_layers`.
```py
unet_lora_config = LoraConfig(
r=args.rank,
lora_alpha=args.rank,
init_lora_weights="gaussian",
target_modules=["to_k", "to_q", "to_v", "to_out.0"],
)
unet.add_adapter(unet_lora_config)
lora_layers = filter(lambda p: p.requires_grad, unet.parameters())
```
</hfoption>
<hfoption id="text encoder">
Diffusers also supports finetuning the text encoder with LoRA from the [PEFT](https://hf.co/docs/peft) library when necessary such as finetuning Stable Diffusion XL (SDXL). The [`~peft.LoraConfig`] is used to configure the parameters of the LoRA adapter which are then added to the text encoder, and only the LoRA layers are filtered for training.
```py
text_lora_config = LoraConfig(
r=args.rank,
lora_alpha=args.rank,
init_lora_weights="gaussian",
target_modules=["q_proj", "k_proj", "v_proj", "out_proj"],
)
text_encoder_one.add_adapter(text_lora_config)
text_encoder_two.add_adapter(text_lora_config)
text_lora_parameters_one = list(filter(lambda p: p.requires_grad, text_encoder_one.parameters()))
text_lora_parameters_two = list(filter(lambda p: p.requires_grad, text_encoder_two.parameters()))
```
</hfoption>
</hfoptions>
The [optimizer](https://github.com/huggingface/diffusers/blob/e4b8f173b97731686e290b2eb98e7f5df2b1b322/examples/text_to_image/train_text_to_image_lora.py#L529) is initialized with the `lora_layers` because these are the only weights that'll be optimized:
```py
optimizer = optimizer_cls(
lora_layers,
lr=args.learning_rate,
betas=(args.adam_beta1, args.adam_beta2),
weight_decay=args.adam_weight_decay,
eps=args.adam_epsilon,
)
```
Aside from setting up the LoRA layers, the training script is more or less the same as train_text_to_image.py!
## Launch the script
Once you've made all your changes or you're okay with the default configuration, you're ready to launch the training script! 🚀
Let's train on the [Pokémon BLIP captions](https://huggingface.co/datasets/lambdalabs/pokemon-blip-captions) dataset to generate our own Pokémon. Set the environment variables `MODEL_NAME` and `DATASET_NAME` to the model and dataset respectively. You should also specify where to save the model in `OUTPUT_DIR`, and the name of the model to save to on the Hub with `HUB_MODEL_ID`. The script creates and saves the following files to your repository:
- saved model checkpoints
- `pytorch_lora_weights.safetensors` (the trained LoRA weights)
If you're training on more than one GPU, add the `--multi_gpu` parameter to the `accelerate launch` command.
<Tip warning={true}>
A full training run takes ~5 hours on a 2080 Ti GPU with 11GB of VRAM.
</Tip>
```bash
export MODEL_NAME="runwayml/stable-diffusion-v1-5"
export OUTPUT_DIR="/sddata/finetune/lora/pokemon"
export HUB_MODEL_ID="pokemon-lora"
export DATASET_NAME="lambdalabs/pokemon-blip-captions"
accelerate launch --mixed_precision="fp16" train_text_to_image_lora.py \
--pretrained_model_name_or_path=$MODEL_NAME \
--dataset_name=$DATASET_NAME \
--dataloader_num_workers=8 \
--resolution=512 \
--center_crop \
--random_flip \
--train_batch_size=1 \
--gradient_accumulation_steps=4 \
--max_train_steps=15000 \
--learning_rate=1e-04 \
--max_grad_norm=1 \
--lr_scheduler="cosine" \
--lr_warmup_steps=0 \
--output_dir=${OUTPUT_DIR} \
--push_to_hub \
--hub_model_id=${HUB_MODEL_ID} \
--report_to=wandb \
--checkpointing_steps=500 \
--validation_prompt="A pokemon with blue eyes." \
--seed=1337
```
Once training has been completed, you can use your model for inference:
```py
from diffusers import AutoPipelineForText2Image
import torch
pipeline = AutoPipelineForText2Image.from_pretrained("runwayml/stable-diffusion-v1-5", torch_dtype=torch.float16).to("cuda")
pipeline.load_lora_weights("path/to/lora/model", weight_name="pytorch_lora_weights.safetensors")
image = pipeline("A pokemon with blue eyes").images[0]
```
## Next steps
Congratulations on training a new model with LoRA! To learn more about how to use your new model, the following guides may be helpful:
- Learn how to [load different LoRA formats](../using-diffusers/loading_adapters#LoRA) trained using community trainers like Kohya and TheLastBen.
- Learn how to use and [combine multiple LoRA's](../tutorials/using_peft_for_inference) with PEFT for inference.
| diffusers/docs/source/en/training/lora.md/0 | {
"file_path": "diffusers/docs/source/en/training/lora.md",
"repo_id": "diffusers",
"token_count": 3336
} | 96 |
<!--Copyright 2024 The HuggingFace Team. All rights reserved.
Licensed under the Apache License, Version 2.0 (the "License"); you may not use this file except in compliance with
the License. You may obtain a copy of the License at
http://www.apache.org/licenses/LICENSE-2.0
Unless required by applicable law or agreed to in writing, software distributed under the License is distributed on
an "AS IS" BASIS, WITHOUT WARRANTIES OR CONDITIONS OF ANY KIND, either express or implied. See the License for the
specific language governing permissions and limitations under the License.
-->
# Control image brightness
The Stable Diffusion pipeline is mediocre at generating images that are either very bright or dark as explained in the [Common Diffusion Noise Schedules and Sample Steps are Flawed](https://huggingface.co/papers/2305.08891) paper. The solutions proposed in the paper are currently implemented in the [`DDIMScheduler`] which you can use to improve the lighting in your images.
<Tip>
💡 Take a look at the paper linked above for more details about the proposed solutions!
</Tip>
One of the solutions is to train a model with *v prediction* and *v loss*. Add the following flag to the [`train_text_to_image.py`](https://github.com/huggingface/diffusers/blob/main/examples/text_to_image/train_text_to_image.py) or [`train_text_to_image_lora.py`](https://github.com/huggingface/diffusers/blob/main/examples/text_to_image/train_text_to_image_lora.py) scripts to enable `v_prediction`:
```bash
--prediction_type="v_prediction"
```
For example, let's use the [`ptx0/pseudo-journey-v2`](https://huggingface.co/ptx0/pseudo-journey-v2) checkpoint which has been finetuned with `v_prediction`.
Next, configure the following parameters in the [`DDIMScheduler`]:
1. `rescale_betas_zero_snr=True`, rescales the noise schedule to zero terminal signal-to-noise ratio (SNR)
2. `timestep_spacing="trailing"`, starts sampling from the last timestep
```py
from diffusers import DiffusionPipeline, DDIMScheduler
pipeline = DiffusionPipeline.from_pretrained("ptx0/pseudo-journey-v2", use_safetensors=True)
# switch the scheduler in the pipeline to use the DDIMScheduler
pipeline.scheduler = DDIMScheduler.from_config(
pipeline.scheduler.config, rescale_betas_zero_snr=True, timestep_spacing="trailing"
)
pipeline.to("cuda")
```
Finally, in your call to the pipeline, set `guidance_rescale` to prevent overexposure:
```py
prompt = "A lion in galaxies, spirals, nebulae, stars, smoke, iridescent, intricate detail, octane render, 8k"
image = pipeline(prompt, guidance_rescale=0.7).images[0]
image
```
<div class="flex justify-center">
<img src="https://huggingface.co/datasets/huggingface/documentation-images/resolve/main/diffusers/zero_snr.png"/>
</div>
| diffusers/docs/source/en/using-diffusers/control_brightness.md/0 | {
"file_path": "diffusers/docs/source/en/using-diffusers/control_brightness.md",
"repo_id": "diffusers",
"token_count": 874
} | 97 |
<!--Copyright 2024 The HuggingFace Team. All rights reserved.
Licensed under the Apache License, Version 2.0 (the "License"); you may not use this file except in compliance with
the License. You may obtain a copy of the License at
http://www.apache.org/licenses/LICENSE-2.0
Unless required by applicable law or agreed to in writing, software distributed under the License is distributed on
an "AS IS" BASIS, WITHOUT WARRANTIES OR CONDITIONS OF ANY KIND, either express or implied. See the License for the
specific language governing permissions and limitations under the License.
-->
# Load pipelines, models, and schedulers
[[open-in-colab]]
Having an easy way to use a diffusion system for inference is essential to 🧨 Diffusers. Diffusion systems often consist of multiple components like parameterized models, tokenizers, and schedulers that interact in complex ways. That is why we designed the [`DiffusionPipeline`] to wrap the complexity of the entire diffusion system into an easy-to-use API, while remaining flexible enough to be adapted for other use cases, such as loading each component individually as building blocks to assemble your own diffusion system.
Everything you need for inference or training is accessible with the `from_pretrained()` method.
This guide will show you how to load:
- pipelines from the Hub and locally
- different components into a pipeline
- checkpoint variants such as different floating point types or non-exponential mean averaged (EMA) weights
- models and schedulers
## Diffusion Pipeline
<Tip>
💡 Skip to the [DiffusionPipeline explained](#diffusionpipeline-explained) section if you are interested in learning in more detail about how the [`DiffusionPipeline`] class works.
</Tip>
The [`DiffusionPipeline`] class is the simplest and most generic way to load the latest trending diffusion model from the [Hub](https://huggingface.co/models?library=diffusers&sort=trending). The [`DiffusionPipeline.from_pretrained`] method automatically detects the correct pipeline class from the checkpoint, downloads, and caches all the required configuration and weight files, and returns a pipeline instance ready for inference.
```python
from diffusers import DiffusionPipeline
repo_id = "runwayml/stable-diffusion-v1-5"
pipe = DiffusionPipeline.from_pretrained(repo_id, use_safetensors=True)
```
You can also load a checkpoint with its specific pipeline class. The example above loaded a Stable Diffusion model; to get the same result, use the [`StableDiffusionPipeline`] class:
```python
from diffusers import StableDiffusionPipeline
repo_id = "runwayml/stable-diffusion-v1-5"
pipe = StableDiffusionPipeline.from_pretrained(repo_id, use_safetensors=True)
```
A checkpoint (such as [`CompVis/stable-diffusion-v1-4`](https://huggingface.co/CompVis/stable-diffusion-v1-4) or [`runwayml/stable-diffusion-v1-5`](https://huggingface.co/runwayml/stable-diffusion-v1-5)) may also be used for more than one task, like text-to-image or image-to-image. To differentiate what task you want to use the checkpoint for, you have to load it directly with its corresponding task-specific pipeline class:
```python
from diffusers import StableDiffusionImg2ImgPipeline
repo_id = "runwayml/stable-diffusion-v1-5"
pipe = StableDiffusionImg2ImgPipeline.from_pretrained(repo_id)
```
### Local pipeline
To load a diffusion pipeline locally, use [`git-lfs`](https://git-lfs.github.com/) to manually download the checkpoint (in this case, [`runwayml/stable-diffusion-v1-5`](https://huggingface.co/runwayml/stable-diffusion-v1-5)) to your local disk. This creates a local folder, `./stable-diffusion-v1-5`, on your disk:
```bash
git-lfs install
git clone https://huggingface.co/runwayml/stable-diffusion-v1-5
```
Then pass the local path to [`~DiffusionPipeline.from_pretrained`]:
```python
from diffusers import DiffusionPipeline
repo_id = "./stable-diffusion-v1-5"
stable_diffusion = DiffusionPipeline.from_pretrained(repo_id, use_safetensors=True)
```
The [`~DiffusionPipeline.from_pretrained`] method won't download any files from the Hub when it detects a local path, but this also means it won't download and cache the latest changes to a checkpoint.
### Swap components in a pipeline
You can customize the default components of any pipeline with another compatible component. Customization is important because:
- Changing the scheduler is important for exploring the trade-off between generation speed and quality.
- Different components of a model are typically trained independently and you can swap out a component with a better-performing one.
- During finetuning, usually only some components - like the UNet or text encoder - are trained.
To find out which schedulers are compatible for customization, you can use the `compatibles` method:
```py
from diffusers import DiffusionPipeline
repo_id = "runwayml/stable-diffusion-v1-5"
stable_diffusion = DiffusionPipeline.from_pretrained(repo_id, use_safetensors=True)
stable_diffusion.scheduler.compatibles
```
Let's use the [`SchedulerMixin.from_pretrained`] method to replace the default [`PNDMScheduler`] with a more performant scheduler, [`EulerDiscreteScheduler`]. The `subfolder="scheduler"` argument is required to load the scheduler configuration from the correct [subfolder](https://huggingface.co/runwayml/stable-diffusion-v1-5/tree/main/scheduler) of the pipeline repository.
Then you can pass the new [`EulerDiscreteScheduler`] instance to the `scheduler` argument in [`DiffusionPipeline`]:
```python
from diffusers import DiffusionPipeline, EulerDiscreteScheduler
repo_id = "runwayml/stable-diffusion-v1-5"
scheduler = EulerDiscreteScheduler.from_pretrained(repo_id, subfolder="scheduler")
stable_diffusion = DiffusionPipeline.from_pretrained(repo_id, scheduler=scheduler, use_safetensors=True)
```
### Safety checker
Diffusion models like Stable Diffusion can generate harmful content, which is why 🧨 Diffusers has a [safety checker](https://github.com/huggingface/diffusers/blob/main/src/diffusers/pipelines/stable_diffusion/safety_checker.py) to check generated outputs against known hardcoded NSFW content. If you'd like to disable the safety checker for whatever reason, pass `None` to the `safety_checker` argument:
```python
from diffusers import DiffusionPipeline
repo_id = "runwayml/stable-diffusion-v1-5"
stable_diffusion = DiffusionPipeline.from_pretrained(repo_id, safety_checker=None, use_safetensors=True)
"""
You have disabled the safety checker for <class 'diffusers.pipelines.stable_diffusion.pipeline_stable_diffusion.StableDiffusionPipeline'> by passing `safety_checker=None`. Ensure that you abide by the conditions of the Stable Diffusion license and do not expose unfiltered results in services or applications open to the public. Both the diffusers team and Hugging Face strongly recommend keeping the safety filter enabled in all public-facing circumstances, disabling it only for use cases that involve analyzing network behavior or auditing its results. For more information, please have a look at https://github.com/huggingface/diffusers/pull/254 .
"""
```
### Reuse components across pipelines
You can also reuse the same components in multiple pipelines to avoid loading the weights into RAM twice. Use the [`~DiffusionPipeline.components`] method to save the components:
```python
from diffusers import StableDiffusionPipeline, StableDiffusionImg2ImgPipeline
model_id = "runwayml/stable-diffusion-v1-5"
stable_diffusion_txt2img = StableDiffusionPipeline.from_pretrained(model_id, use_safetensors=True)
components = stable_diffusion_txt2img.components
```
Then you can pass the `components` to another pipeline without reloading the weights into RAM:
```py
stable_diffusion_img2img = StableDiffusionImg2ImgPipeline(**components)
```
You can also pass the components individually to the pipeline if you want more flexibility over which components to reuse or disable. For example, to reuse the same components in the text-to-image pipeline, except for the safety checker and feature extractor, in the image-to-image pipeline:
```py
from diffusers import StableDiffusionPipeline, StableDiffusionImg2ImgPipeline
model_id = "runwayml/stable-diffusion-v1-5"
stable_diffusion_txt2img = StableDiffusionPipeline.from_pretrained(model_id, use_safetensors=True)
stable_diffusion_img2img = StableDiffusionImg2ImgPipeline(
vae=stable_diffusion_txt2img.vae,
text_encoder=stable_diffusion_txt2img.text_encoder,
tokenizer=stable_diffusion_txt2img.tokenizer,
unet=stable_diffusion_txt2img.unet,
scheduler=stable_diffusion_txt2img.scheduler,
safety_checker=None,
feature_extractor=None,
requires_safety_checker=False,
)
```
## Checkpoint variants
A checkpoint variant is usually a checkpoint whose weights are:
- Stored in a different floating point type for lower precision and lower storage, such as [`torch.float16`](https://pytorch.org/docs/stable/tensors.html#data-types), because it only requires half the bandwidth and storage to download. You can't use this variant if you're continuing training or using a CPU.
- Non-exponential mean averaged (EMA) weights, which shouldn't be used for inference. You should use these to continue fine-tuning a model.
<Tip>
💡 When the checkpoints have identical model structures, but they were trained on different datasets and with a different training setup, they should be stored in separate repositories instead of variations (for example, [`stable-diffusion-v1-4`] and [`stable-diffusion-v1-5`]).
</Tip>
Otherwise, a variant is **identical** to the original checkpoint. They have exactly the same serialization format (like [Safetensors](./using_safetensors)), model structure, and weights that have identical tensor shapes.
| **checkpoint type** | **weight name** | **argument for loading weights** |
|---------------------|-------------------------------------|----------------------------------|
| original | diffusion_pytorch_model.bin | |
| floating point | diffusion_pytorch_model.fp16.bin | `variant`, `torch_dtype` |
| non-EMA | diffusion_pytorch_model.non_ema.bin | `variant` |
There are two important arguments to know for loading variants:
- `torch_dtype` defines the floating point precision of the loaded checkpoints. For example, if you want to save bandwidth by loading a `fp16` variant, you should specify `torch_dtype=torch.float16` to *convert the weights* to `fp16`. Otherwise, the `fp16` weights are converted to the default `fp32` precision. You can also load the original checkpoint without defining the `variant` argument, and convert it to `fp16` with `torch_dtype=torch.float16`. In this case, the default `fp32` weights are downloaded first, and then they're converted to `fp16` after loading.
- `variant` defines which files should be loaded from the repository. For example, if you want to load a `non_ema` variant from the [`diffusers/stable-diffusion-variants`](https://huggingface.co/diffusers/stable-diffusion-variants/tree/main/unet) repository, you should specify `variant="non_ema"` to download the `non_ema` files.
```python
from diffusers import DiffusionPipeline
import torch
# load fp16 variant
stable_diffusion = DiffusionPipeline.from_pretrained(
"runwayml/stable-diffusion-v1-5", variant="fp16", torch_dtype=torch.float16, use_safetensors=True
)
# load non_ema variant
stable_diffusion = DiffusionPipeline.from_pretrained(
"runwayml/stable-diffusion-v1-5", variant="non_ema", use_safetensors=True
)
```
To save a checkpoint stored in a different floating-point type or as a non-EMA variant, use the [`DiffusionPipeline.save_pretrained`] method and specify the `variant` argument. You should try and save a variant to the same folder as the original checkpoint, so you can load both from the same folder:
```python
from diffusers import DiffusionPipeline
# save as fp16 variant
stable_diffusion.save_pretrained("runwayml/stable-diffusion-v1-5", variant="fp16")
# save as non-ema variant
stable_diffusion.save_pretrained("runwayml/stable-diffusion-v1-5", variant="non_ema")
```
If you don't save the variant to an existing folder, you must specify the `variant` argument otherwise it'll throw an `Exception` because it can't find the original checkpoint:
```python
# 👎 this won't work
stable_diffusion = DiffusionPipeline.from_pretrained(
"./stable-diffusion-v1-5", torch_dtype=torch.float16, use_safetensors=True
)
# 👍 this works
stable_diffusion = DiffusionPipeline.from_pretrained(
"./stable-diffusion-v1-5", variant="fp16", torch_dtype=torch.float16, use_safetensors=True
)
```
<!--
TODO(Patrick) - Make sure to uncomment this part as soon as things are deprecated.
#### Using `revision` to load pipeline variants is deprecated
Previously the `revision` argument of [`DiffusionPipeline.from_pretrained`] was heavily used to
load model variants, e.g.:
```python
from diffusers import DiffusionPipeline
pipe = DiffusionPipeline.from_pretrained("CompVis/stable-diffusion-v1-4", revision="fp16", use_safetensors=True)
```
However, this behavior is now deprecated since the "revision" argument should (just as it's done in GitHub) better be used to load model checkpoints from a specific commit or branch in development.
The above example is therefore deprecated and won't be supported anymore for `diffusers >= 1.0.0`.
<Tip warning={true}>
If you load diffusers pipelines or models with `revision="fp16"` or `revision="non_ema"`,
please make sure to update the code and use `variant="fp16"` or `variation="non_ema"` respectively
instead.
</Tip>
-->
## Models
Models are loaded from the [`ModelMixin.from_pretrained`] method, which downloads and caches the latest version of the model weights and configurations. If the latest files are available in the local cache, [`~ModelMixin.from_pretrained`] reuses files in the cache instead of re-downloading them.
Models can be loaded from a subfolder with the `subfolder` argument. For example, the model weights for `runwayml/stable-diffusion-v1-5` are stored in the [`unet`](https://huggingface.co/runwayml/stable-diffusion-v1-5/tree/main/unet) subfolder:
```python
from diffusers import UNet2DConditionModel
repo_id = "runwayml/stable-diffusion-v1-5"
model = UNet2DConditionModel.from_pretrained(repo_id, subfolder="unet", use_safetensors=True)
```
Or directly from a repository's [directory](https://huggingface.co/google/ddpm-cifar10-32/tree/main):
```python
from diffusers import UNet2DModel
repo_id = "google/ddpm-cifar10-32"
model = UNet2DModel.from_pretrained(repo_id, use_safetensors=True)
```
You can also load and save model variants by specifying the `variant` argument in [`ModelMixin.from_pretrained`] and [`ModelMixin.save_pretrained`]:
```python
from diffusers import UNet2DConditionModel
model = UNet2DConditionModel.from_pretrained(
"runwayml/stable-diffusion-v1-5", subfolder="unet", variant="non_ema", use_safetensors=True
)
model.save_pretrained("./local-unet", variant="non_ema")
```
## Schedulers
Schedulers are loaded from the [`SchedulerMixin.from_pretrained`] method, and unlike models, schedulers are **not parameterized** or **trained**; they are defined by a configuration file.
Loading schedulers does not consume any significant amount of memory and the same configuration file can be used for a variety of different schedulers.
For example, the following schedulers are compatible with [`StableDiffusionPipeline`], which means you can load the same scheduler configuration file in any of these classes:
```python
from diffusers import StableDiffusionPipeline
from diffusers import (
DDPMScheduler,
DDIMScheduler,
PNDMScheduler,
LMSDiscreteScheduler,
EulerAncestralDiscreteScheduler,
EulerDiscreteScheduler,
DPMSolverMultistepScheduler,
)
repo_id = "runwayml/stable-diffusion-v1-5"
ddpm = DDPMScheduler.from_pretrained(repo_id, subfolder="scheduler")
ddim = DDIMScheduler.from_pretrained(repo_id, subfolder="scheduler")
pndm = PNDMScheduler.from_pretrained(repo_id, subfolder="scheduler")
lms = LMSDiscreteScheduler.from_pretrained(repo_id, subfolder="scheduler")
euler_anc = EulerAncestralDiscreteScheduler.from_pretrained(repo_id, subfolder="scheduler")
euler = EulerDiscreteScheduler.from_pretrained(repo_id, subfolder="scheduler")
dpm = DPMSolverMultistepScheduler.from_pretrained(repo_id, subfolder="scheduler")
# replace `dpm` with any of `ddpm`, `ddim`, `pndm`, `lms`, `euler_anc`, `euler`
pipeline = StableDiffusionPipeline.from_pretrained(repo_id, scheduler=dpm, use_safetensors=True)
```
## DiffusionPipeline explained
As a class method, [`DiffusionPipeline.from_pretrained`] is responsible for two things:
- Download the latest version of the folder structure required for inference and cache it. If the latest folder structure is available in the local cache, [`DiffusionPipeline.from_pretrained`] reuses the cache and won't redownload the files.
- Load the cached weights into the correct pipeline [class](../api/pipelines/overview#diffusers-summary) - retrieved from the `model_index.json` file - and return an instance of it.
The pipelines' underlying folder structure corresponds directly with their class instances. For example, the [`StableDiffusionPipeline`] corresponds to the folder structure in [`runwayml/stable-diffusion-v1-5`](https://huggingface.co/runwayml/stable-diffusion-v1-5).
```python
from diffusers import DiffusionPipeline
repo_id = "runwayml/stable-diffusion-v1-5"
pipeline = DiffusionPipeline.from_pretrained(repo_id, use_safetensors=True)
print(pipeline)
```
You'll see pipeline is an instance of [`StableDiffusionPipeline`], which consists of seven components:
- `"feature_extractor"`: a [`~transformers.CLIPImageProcessor`] from 🤗 Transformers.
- `"safety_checker"`: a [component](https://github.com/huggingface/diffusers/blob/e55687e1e15407f60f32242027b7bb8170e58266/src/diffusers/pipelines/stable_diffusion/safety_checker.py#L32) for screening against harmful content.
- `"scheduler"`: an instance of [`PNDMScheduler`].
- `"text_encoder"`: a [`~transformers.CLIPTextModel`] from 🤗 Transformers.
- `"tokenizer"`: a [`~transformers.CLIPTokenizer`] from 🤗 Transformers.
- `"unet"`: an instance of [`UNet2DConditionModel`].
- `"vae"`: an instance of [`AutoencoderKL`].
```json
StableDiffusionPipeline {
"feature_extractor": [
"transformers",
"CLIPImageProcessor"
],
"safety_checker": [
"stable_diffusion",
"StableDiffusionSafetyChecker"
],
"scheduler": [
"diffusers",
"PNDMScheduler"
],
"text_encoder": [
"transformers",
"CLIPTextModel"
],
"tokenizer": [
"transformers",
"CLIPTokenizer"
],
"unet": [
"diffusers",
"UNet2DConditionModel"
],
"vae": [
"diffusers",
"AutoencoderKL"
]
}
```
Compare the components of the pipeline instance to the [`runwayml/stable-diffusion-v1-5`](https://huggingface.co/runwayml/stable-diffusion-v1-5/tree/main) folder structure, and you'll see there is a separate folder for each of the components in the repository:
```
.
├── feature_extractor
│ └── preprocessor_config.json
├── model_index.json
├── safety_checker
│ ├── config.json
| ├── model.fp16.safetensors
│ ├── model.safetensors
│ ├── pytorch_model.bin
| └── pytorch_model.fp16.bin
├── scheduler
│ └── scheduler_config.json
├── text_encoder
│ ├── config.json
| ├── model.fp16.safetensors
│ ├── model.safetensors
│ |── pytorch_model.bin
| └── pytorch_model.fp16.bin
├── tokenizer
│ ├── merges.txt
│ ├── special_tokens_map.json
│ ├── tokenizer_config.json
│ └── vocab.json
├── unet
│ ├── config.json
│ ├── diffusion_pytorch_model.bin
| |── diffusion_pytorch_model.fp16.bin
│ |── diffusion_pytorch_model.f16.safetensors
│ |── diffusion_pytorch_model.non_ema.bin
│ |── diffusion_pytorch_model.non_ema.safetensors
│ └── diffusion_pytorch_model.safetensors
|── vae
. ├── config.json
. ├── diffusion_pytorch_model.bin
├── diffusion_pytorch_model.fp16.bin
├── diffusion_pytorch_model.fp16.safetensors
└── diffusion_pytorch_model.safetensors
```
You can access each of the components of the pipeline as an attribute to view its configuration:
```py
pipeline.tokenizer
CLIPTokenizer(
name_or_path="/root/.cache/huggingface/hub/models--runwayml--stable-diffusion-v1-5/snapshots/39593d5650112b4cc580433f6b0435385882d819/tokenizer",
vocab_size=49408,
model_max_length=77,
is_fast=False,
padding_side="right",
truncation_side="right",
special_tokens={
"bos_token": AddedToken("<|startoftext|>", rstrip=False, lstrip=False, single_word=False, normalized=True),
"eos_token": AddedToken("<|endoftext|>", rstrip=False, lstrip=False, single_word=False, normalized=True),
"unk_token": AddedToken("<|endoftext|>", rstrip=False, lstrip=False, single_word=False, normalized=True),
"pad_token": "<|endoftext|>",
},
clean_up_tokenization_spaces=True
)
```
Every pipeline expects a [`model_index.json`](https://huggingface.co/runwayml/stable-diffusion-v1-5/blob/main/model_index.json) file that tells the [`DiffusionPipeline`]:
- which pipeline class to load from `_class_name`
- which version of 🧨 Diffusers was used to create the model in `_diffusers_version`
- what components from which library are stored in the subfolders (`name` corresponds to the component and subfolder name, `library` corresponds to the name of the library to load the class from, and `class` corresponds to the class name)
```json
{
"_class_name": "StableDiffusionPipeline",
"_diffusers_version": "0.6.0",
"feature_extractor": [
"transformers",
"CLIPImageProcessor"
],
"safety_checker": [
"stable_diffusion",
"StableDiffusionSafetyChecker"
],
"scheduler": [
"diffusers",
"PNDMScheduler"
],
"text_encoder": [
"transformers",
"CLIPTextModel"
],
"tokenizer": [
"transformers",
"CLIPTokenizer"
],
"unet": [
"diffusers",
"UNet2DConditionModel"
],
"vae": [
"diffusers",
"AutoencoderKL"
]
}
```
| diffusers/docs/source/en/using-diffusers/loading.md/0 | {
"file_path": "diffusers/docs/source/en/using-diffusers/loading.md",
"repo_id": "diffusers",
"token_count": 7191
} | 98 |
<!--Copyright 2024 The HuggingFace Team. All rights reserved.
Licensed under the Apache License, Version 2.0 (the "License"); you may not use this file except in compliance with
the License. You may obtain a copy of the License at
http://www.apache.org/licenses/LICENSE-2.0
Unless required by applicable law or agreed to in writing, software distributed under the License is distributed on
an "AS IS" BASIS, WITHOUT WARRANTIES OR CONDITIONS OF ANY KIND, either express or implied. See the License for the
specific language governing permissions and limitations under the License.
-->
# Text or image-to-video
Driven by the success of text-to-image diffusion models, generative video models are able to generate short clips of video from a text prompt or an initial image. These models extend a pretrained diffusion model to generate videos by adding some type of temporal and/or spatial convolution layer to the architecture. A mixed dataset of images and videos are used to train the model which learns to output a series of video frames based on the text or image conditioning.
This guide will show you how to generate videos, how to configure video model parameters, and how to control video generation.
## Popular models
> [!TIP]
> Discover other cool and trending video generation models on the Hub [here](https://huggingface.co/models?pipeline_tag=text-to-video&sort=trending)!
[Stable Video Diffusions (SVD)](https://huggingface.co/stabilityai/stable-video-diffusion-img2vid), [I2VGen-XL](https://huggingface.co/ali-vilab/i2vgen-xl/), [AnimateDiff](https://huggingface.co/guoyww/animatediff), and [ModelScopeT2V](https://huggingface.co/ali-vilab/text-to-video-ms-1.7b) are popular models used for video diffusion. Each model is distinct. For example, AnimateDiff inserts a motion modeling module into a frozen text-to-image model to generate personalized animated images, whereas SVD is entirely pretrained from scratch with a three-stage training process to generate short high-quality videos.
### Stable Video Diffusion
[SVD](../api/pipelines/svd) is based on the Stable Diffusion 2.1 model and it is trained on images, then low-resolution videos, and finally a smaller dataset of high-resolution videos. This model generates a short 2-4 second video from an initial image. You can learn more details about model, like micro-conditioning, in the [Stable Video Diffusion](../using-diffusers/svd) guide.
Begin by loading the [`StableVideoDiffusionPipeline`] and passing an initial image to generate a video from.
```py
import torch
from diffusers import StableVideoDiffusionPipeline
from diffusers.utils import load_image, export_to_video
pipeline = StableVideoDiffusionPipeline.from_pretrained(
"stabilityai/stable-video-diffusion-img2vid-xt", torch_dtype=torch.float16, variant="fp16"
)
pipeline.enable_model_cpu_offload()
image = load_image("https://huggingface.co/datasets/huggingface/documentation-images/resolve/main/diffusers/svd/rocket.png")
image = image.resize((1024, 576))
generator = torch.manual_seed(42)
frames = pipeline(image, decode_chunk_size=8, generator=generator).frames[0]
export_to_video(frames, "generated.mp4", fps=7)
```
<div class="flex gap-4">
<div>
<img class="rounded-xl" src="https://huggingface.co/datasets/huggingface/documentation-images/resolve/main/diffusers/svd/rocket.png"/>
<figcaption class="mt-2 text-center text-sm text-gray-500">initial image</figcaption>
</div>
<div>
<img class="rounded-xl" src="https://huggingface.co/datasets/huggingface/documentation-images/resolve/main/diffusers/svd/output_rocket.gif"/>
<figcaption class="mt-2 text-center text-sm text-gray-500">generated video</figcaption>
</div>
</div>
### I2VGen-XL
[I2VGen-XL](../api/pipelines/i2vgenxl) is a diffusion model that can generate higher resolution videos than SVD and it is also capable of accepting text prompts in addition to images. The model is trained with two hierarchical encoders (detail and global encoder) to better capture low and high-level details in images. These learned details are used to train a video diffusion model which refines the video resolution and details in the generated video.
You can use I2VGen-XL by loading the [`I2VGenXLPipeline`], and passing a text and image prompt to generate a video.
```py
import torch
from diffusers import I2VGenXLPipeline
from diffusers.utils import export_to_gif, load_image
pipeline = I2VGenXLPipeline.from_pretrained("ali-vilab/i2vgen-xl", torch_dtype=torch.float16, variant="fp16")
pipeline.enable_model_cpu_offload()
image_url = "https://huggingface.co/datasets/diffusers/docs-images/resolve/main/i2vgen_xl_images/img_0009.png"
image = load_image(image_url).convert("RGB")
prompt = "Papers were floating in the air on a table in the library"
negative_prompt = "Distorted, discontinuous, Ugly, blurry, low resolution, motionless, static, disfigured, disconnected limbs, Ugly faces, incomplete arms"
generator = torch.manual_seed(8888)
frames = pipeline(
prompt=prompt,
image=image,
num_inference_steps=50,
negative_prompt=negative_prompt,
guidance_scale=9.0,
generator=generator
).frames[0]
export_to_gif(frames, "i2v.gif")
```
<div class="flex gap-4">
<div>
<img class="rounded-xl" src="https://huggingface.co/datasets/diffusers/docs-images/resolve/main/i2vgen_xl_images/img_0009.png"/>
<figcaption class="mt-2 text-center text-sm text-gray-500">initial image</figcaption>
</div>
<div>
<img class="rounded-xl" src="https://huggingface.co/datasets/huggingface/documentation-images/resolve/main/diffusers/i2vgen-xl-example.gif"/>
<figcaption class="mt-2 text-center text-sm text-gray-500">generated video</figcaption>
</div>
</div>
### AnimateDiff
[AnimateDiff](../api/pipelines/animatediff) is an adapter model that inserts a motion module into a pretrained diffusion model to animate an image. The adapter is trained on video clips to learn motion which is used to condition the generation process to create a video. It is faster and easier to only train the adapter and it can be loaded into most diffusion models, effectively turning them into "video models".
Start by loading a [`MotionAdapter`].
```py
import torch
from diffusers import AnimateDiffPipeline, DDIMScheduler, MotionAdapter
from diffusers.utils import export_to_gif
adapter = MotionAdapter.from_pretrained("guoyww/animatediff-motion-adapter-v1-5-2", torch_dtype=torch.float16)
```
Then load a finetuned Stable Diffusion model with the [`AnimateDiffPipeline`].
```py
pipeline = AnimateDiffPipeline.from_pretrained("emilianJR/epiCRealism", motion_adapter=adapter, torch_dtype=torch.float16)
scheduler = DDIMScheduler.from_pretrained(
"emilianJR/epiCRealism",
subfolder="scheduler",
clip_sample=False,
timestep_spacing="linspace",
beta_schedule="linear",
steps_offset=1,
)
pipeline.scheduler = scheduler
pipeline.enable_vae_slicing()
pipeline.enable_model_cpu_offload()
```
Create a prompt and generate the video.
```py
output = pipeline(
prompt="A space rocket with trails of smoke behind it launching into space from the desert, 4k, high resolution",
negative_prompt="bad quality, worse quality, low resolution",
num_frames=16,
guidance_scale=7.5,
num_inference_steps=50,
generator=torch.Generator("cpu").manual_seed(49),
)
frames = output.frames[0]
export_to_gif(frames, "animation.gif")
```
<div class="flex justify-center">
<img src="https://huggingface.co/datasets/huggingface/documentation-images/resolve/main/diffusers/animatediff.gif"/>
</div>
### ModelscopeT2V
[ModelscopeT2V](../api/pipelines/text_to_video) adds spatial and temporal convolutions and attention to a UNet, and it is trained on image-text and video-text datasets to enhance what it learns during training. The model takes a prompt, encodes it and creates text embeddings which are denoised by the UNet, and then decoded by a VQGAN into a video.
<Tip>
ModelScopeT2V generates watermarked videos due to the datasets it was trained on. To use a watermark-free model, try the [cerspense/zeroscope_v2_76w](https://huggingface.co/cerspense/zeroscope_v2_576w) model with the [`TextToVideoSDPipeline`] first, and then upscale it's output with the [cerspense/zeroscope_v2_XL](https://huggingface.co/cerspense/zeroscope_v2_XL) checkpoint using the [`VideoToVideoSDPipeline`].
</Tip>
Load a ModelScopeT2V checkpoint into the [`DiffusionPipeline`] along with a prompt to generate a video.
```py
import torch
from diffusers import DiffusionPipeline
from diffusers.utils import export_to_video
pipeline = DiffusionPipeline.from_pretrained("damo-vilab/text-to-video-ms-1.7b", torch_dtype=torch.float16, variant="fp16")
pipeline.enable_model_cpu_offload()
pipeline.enable_vae_slicing()
prompt = "Confident teddy bear surfer rides the wave in the tropics"
video_frames = pipeline(prompt).frames[0]
export_to_video(video_frames, "modelscopet2v.mp4", fps=10)
```
<div class="flex justify-center">
<img src="https://huggingface.co/datasets/huggingface/documentation-images/resolve/main/diffusers/modelscopet2v.gif" />
</div>
## Configure model parameters
There are a few important parameters you can configure in the pipeline that'll affect the video generation process and quality. Let's take a closer look at what these parameters do and how changing them affects the output.
### Number of frames
The `num_frames` parameter determines how many video frames are generated per second. A frame is an image that is played in a sequence of other frames to create motion or a video. This affects video length because the pipeline generates a certain number of frames per second (check a pipeline's API reference for the default value). To increase the video duration, you'll need to increase the `num_frames` parameter.
```py
import torch
from diffusers import StableVideoDiffusionPipeline
from diffusers.utils import load_image, export_to_video
pipeline = StableVideoDiffusionPipeline.from_pretrained(
"stabilityai/stable-video-diffusion-img2vid", torch_dtype=torch.float16, variant="fp16"
)
pipeline.enable_model_cpu_offload()
image = load_image("https://huggingface.co/datasets/huggingface/documentation-images/resolve/main/diffusers/svd/rocket.png")
image = image.resize((1024, 576))
generator = torch.manual_seed(42)
frames = pipeline(image, decode_chunk_size=8, generator=generator, num_frames=25).frames[0]
export_to_video(frames, "generated.mp4", fps=7)
```
<div class="flex gap-4">
<div>
<img class="rounded-xl" src="https://huggingface.co/datasets/huggingface/documentation-images/resolve/main/diffusers/num_frames_14.gif"/>
<figcaption class="mt-2 text-center text-sm text-gray-500">num_frames=14</figcaption>
</div>
<div>
<img class="rounded-xl" src="https://huggingface.co/datasets/huggingface/documentation-images/resolve/main/diffusers/num_frames_25.gif"/>
<figcaption class="mt-2 text-center text-sm text-gray-500">num_frames=25</figcaption>
</div>
</div>
### Guidance scale
The `guidance_scale` parameter controls how closely aligned the generated video and text prompt or initial image is. A higher `guidance_scale` value means your generated video is more aligned with the text prompt or initial image, while a lower `guidance_scale` value means your generated video is less aligned which could give the model more "creativity" to interpret the conditioning input.
<Tip>
SVD uses the `min_guidance_scale` and `max_guidance_scale` parameters for applying guidance to the first and last frames respectively.
</Tip>
```py
import torch
from diffusers import I2VGenXLPipeline
from diffusers.utils import export_to_gif, load_image
pipeline = I2VGenXLPipeline.from_pretrained("ali-vilab/i2vgen-xl", torch_dtype=torch.float16, variant="fp16")
pipeline.enable_model_cpu_offload()
image_url = "https://huggingface.co/datasets/diffusers/docs-images/resolve/main/i2vgen_xl_images/img_0009.png"
image = load_image(image_url).convert("RGB")
prompt = "Papers were floating in the air on a table in the library"
negative_prompt = "Distorted, discontinuous, Ugly, blurry, low resolution, motionless, static, disfigured, disconnected limbs, Ugly faces, incomplete arms"
generator = torch.manual_seed(0)
frames = pipeline(
prompt=prompt,
image=image,
num_inference_steps=50,
negative_prompt=negative_prompt,
guidance_scale=1.0,
generator=generator
).frames[0]
export_to_gif(frames, "i2v.gif")
```
<div class="flex gap-4">
<div>
<img class="rounded-xl" src="https://huggingface.co/datasets/huggingface/documentation-images/resolve/main/diffusers/i2vgen-xl-example.gif"/>
<figcaption class="mt-2 text-center text-sm text-gray-500">guidance_scale=9.0</figcaption>
</div>
<div>
<img class="rounded-xl" src="https://huggingface.co/datasets/huggingface/documentation-images/resolve/main/diffusers/guidance_scale_1.0.gif"/>
<figcaption class="mt-2 text-center text-sm text-gray-500">guidance_scale=1.0</figcaption>
</div>
</div>
### Negative prompt
A negative prompt deters the model from generating things you don’t want it to. This parameter is commonly used to improve overall generation quality by removing poor or bad features such as “low resolution” or “bad details”.
```py
import torch
from diffusers import AnimateDiffPipeline, DDIMScheduler, MotionAdapter
from diffusers.utils import export_to_gif
adapter = MotionAdapter.from_pretrained("guoyww/animatediff-motion-adapter-v1-5-2", torch_dtype=torch.float16)
pipeline = AnimateDiffPipeline.from_pretrained("emilianJR/epiCRealism", motion_adapter=adapter, torch_dtype=torch.float16)
scheduler = DDIMScheduler.from_pretrained(
"emilianJR/epiCRealism",
subfolder="scheduler",
clip_sample=False,
timestep_spacing="linspace",
beta_schedule="linear",
steps_offset=1,
)
pipeline.scheduler = scheduler
pipeline.enable_vae_slicing()
pipeline.enable_model_cpu_offload()
output = pipeline(
prompt="360 camera shot of a sushi roll in a restaurant",
negative_prompt="Distorted, discontinuous, ugly, blurry, low resolution, motionless, static",
num_frames=16,
guidance_scale=7.5,
num_inference_steps=50,
generator=torch.Generator("cpu").manual_seed(0),
)
frames = output.frames[0]
export_to_gif(frames, "animation.gif")
```
<div class="flex gap-4">
<div>
<img class="rounded-xl" src="https://huggingface.co/datasets/huggingface/documentation-images/resolve/main/diffusers/animatediff_no_neg.gif"/>
<figcaption class="mt-2 text-center text-sm text-gray-500">no negative prompt</figcaption>
</div>
<div>
<img class="rounded-xl" src="https://huggingface.co/datasets/huggingface/documentation-images/resolve/main/diffusers/animatediff_neg.gif"/>
<figcaption class="mt-2 text-center text-sm text-gray-500">negative prompt applied</figcaption>
</div>
</div>
### Model-specific parameters
There are some pipeline parameters that are unique to each model such as adjusting the motion in a video or adding noise to the initial image.
<hfoptions id="special-parameters">
<hfoption id="Stable Video Diffusion">
Stable Video Diffusion provides additional micro-conditioning for the frame rate with the `fps` parameter and for motion with the `motion_bucket_id` parameter. Together, these parameters allow for adjusting the amount of motion in the generated video.
There is also a `noise_aug_strength` parameter that increases the amount of noise added to the initial image. Varying this parameter affects how similar the generated video and initial image are. A higher `noise_aug_strength` also increases the amount of motion. To learn more, read the [Micro-conditioning](../using-diffusers/svd#micro-conditioning) guide.
</hfoption>
<hfoption id="Text2Video-Zero">
Text2Video-Zero computes the amount of motion to apply to each frame from randomly sampled latents. You can use the `motion_field_strength_x` and `motion_field_strength_y` parameters to control the amount of motion to apply to the x and y-axes of the video. The parameters `t0` and `t1` are the timesteps to apply motion to the latents.
</hfoption>
</hfoptions>
## Control video generation
Video generation can be controlled similar to how text-to-image, image-to-image, and inpainting can be controlled with a [`ControlNetModel`]. The only difference is you need to use the [`~pipelines.text_to_video_synthesis.pipeline_text_to_video_zero.CrossFrameAttnProcessor`] so each frame attends to the first frame.
### Text2Video-Zero
Text2Video-Zero video generation can be conditioned on pose and edge images for even greater control over a subject's motion in the generated video or to preserve the identity of a subject/object in the video. You can also use Text2Video-Zero with [InstructPix2Pix](../api/pipelines/pix2pix) for editing videos with text.
<hfoptions id="t2v-zero">
<hfoption id="pose control">
Start by downloading a video and extracting the pose images from it.
```py
from huggingface_hub import hf_hub_download
from PIL import Image
import imageio
filename = "__assets__/poses_skeleton_gifs/dance1_corr.mp4"
repo_id = "PAIR/Text2Video-Zero"
video_path = hf_hub_download(repo_type="space", repo_id=repo_id, filename=filename)
reader = imageio.get_reader(video_path, "ffmpeg")
frame_count = 8
pose_images = [Image.fromarray(reader.get_data(i)) for i in range(frame_count)]
```
Load a [`ControlNetModel`] for pose estimation and a checkpoint into the [`StableDiffusionControlNetPipeline`]. Then you'll use the [`~pipelines.text_to_video_synthesis.pipeline_text_to_video_zero.CrossFrameAttnProcessor`] for the UNet and ControlNet.
```py
import torch
from diffusers import StableDiffusionControlNetPipeline, ControlNetModel
from diffusers.pipelines.text_to_video_synthesis.pipeline_text_to_video_zero import CrossFrameAttnProcessor
model_id = "runwayml/stable-diffusion-v1-5"
controlnet = ControlNetModel.from_pretrained("lllyasviel/sd-controlnet-openpose", torch_dtype=torch.float16)
pipeline = StableDiffusionControlNetPipeline.from_pretrained(
model_id, controlnet=controlnet, torch_dtype=torch.float16
).to("cuda")
pipeline.unet.set_attn_processor(CrossFrameAttnProcessor(batch_size=2))
pipeline.controlnet.set_attn_processor(CrossFrameAttnProcessor(batch_size=2))
```
Fix the latents for all the frames, and then pass your prompt and extracted pose images to the model to generate a video.
```py
latents = torch.randn((1, 4, 64, 64), device="cuda", dtype=torch.float16).repeat(len(pose_images), 1, 1, 1)
prompt = "Darth Vader dancing in a desert"
result = pipeline(prompt=[prompt] * len(pose_images), image=pose_images, latents=latents).images
imageio.mimsave("video.mp4", result, fps=4)
```
</hfoption>
<hfoption id="edge control">
Download a video and extract the edges from it.
```py
from huggingface_hub import hf_hub_download
from PIL import Image
import imageio
filename = "__assets__/poses_skeleton_gifs/dance1_corr.mp4"
repo_id = "PAIR/Text2Video-Zero"
video_path = hf_hub_download(repo_type="space", repo_id=repo_id, filename=filename)
reader = imageio.get_reader(video_path, "ffmpeg")
frame_count = 8
pose_images = [Image.fromarray(reader.get_data(i)) for i in range(frame_count)]
```
Load a [`ControlNetModel`] for canny edge and a checkpoint into the [`StableDiffusionControlNetPipeline`]. Then you'll use the [`~pipelines.text_to_video_synthesis.pipeline_text_to_video_zero.CrossFrameAttnProcessor`] for the UNet and ControlNet.
```py
import torch
from diffusers import StableDiffusionControlNetPipeline, ControlNetModel
from diffusers.pipelines.text_to_video_synthesis.pipeline_text_to_video_zero import CrossFrameAttnProcessor
model_id = "runwayml/stable-diffusion-v1-5"
controlnet = ControlNetModel.from_pretrained("lllyasviel/sd-controlnet-canny", torch_dtype=torch.float16)
pipeline = StableDiffusionControlNetPipeline.from_pretrained(
model_id, controlnet=controlnet, torch_dtype=torch.float16
).to("cuda")
pipeline.unet.set_attn_processor(CrossFrameAttnProcessor(batch_size=2))
pipeline.controlnet.set_attn_processor(CrossFrameAttnProcessor(batch_size=2))
```
Fix the latents for all the frames, and then pass your prompt and extracted edge images to the model to generate a video.
```py
latents = torch.randn((1, 4, 64, 64), device="cuda", dtype=torch.float16).repeat(len(pose_images), 1, 1, 1)
prompt = "Darth Vader dancing in a desert"
result = pipeline(prompt=[prompt] * len(pose_images), image=pose_images, latents=latents).images
imageio.mimsave("video.mp4", result, fps=4)
```
</hfoption>
<hfoption id="InstructPix2Pix">
InstructPix2Pix allows you to use text to describe the changes you want to make to the video. Start by downloading and reading a video.
```py
from huggingface_hub import hf_hub_download
from PIL import Image
import imageio
filename = "__assets__/pix2pix video/camel.mp4"
repo_id = "PAIR/Text2Video-Zero"
video_path = hf_hub_download(repo_type="space", repo_id=repo_id, filename=filename)
reader = imageio.get_reader(video_path, "ffmpeg")
frame_count = 8
video = [Image.fromarray(reader.get_data(i)) for i in range(frame_count)]
```
Load the [`StableDiffusionInstructPix2PixPipeline`] and set the [`~pipelines.text_to_video_synthesis.pipeline_text_to_video_zero.CrossFrameAttnProcessor`] for the UNet.
```py
import torch
from diffusers import StableDiffusionInstructPix2PixPipeline
from diffusers.pipelines.text_to_video_synthesis.pipeline_text_to_video_zero import CrossFrameAttnProcessor
pipeline = StableDiffusionInstructPix2PixPipeline.from_pretrained("timbrooks/instruct-pix2pix", torch_dtype=torch.float16).to("cuda")
pipeline.unet.set_attn_processor(CrossFrameAttnProcessor(batch_size=3))
```
Pass a prompt describing the change you want to apply to the video.
```py
prompt = "make it Van Gogh Starry Night style"
result = pipeline(prompt=[prompt] * len(video), image=video).images
imageio.mimsave("edited_video.mp4", result, fps=4)
```
</hfoption>
</hfoptions>
## Optimize
Video generation requires a lot of memory because you're generating many video frames at once. You can reduce your memory requirements at the expense of some inference speed. Try:
1. offloading pipeline components that are no longer needed to the CPU
2. feed-forward chunking runs the feed-forward layer in a loop instead of all at once
3. break up the number of frames the VAE has to decode into chunks instead of decoding them all at once
```diff
- pipeline.enable_model_cpu_offload()
- frames = pipeline(image, decode_chunk_size=8, generator=generator).frames[0]
+ pipeline.enable_model_cpu_offload()
+ pipeline.unet.enable_forward_chunking()
+ frames = pipeline(image, decode_chunk_size=2, generator=generator, num_frames=25).frames[0]
```
If memory is not an issue and you want to optimize for speed, try wrapping the UNet with [`torch.compile`](../optimization/torch2.0#torchcompile).
```diff
- pipeline.enable_model_cpu_offload()
+ pipeline.to("cuda")
+ pipeline.unet = torch.compile(pipeline.unet, mode="reduce-overhead", fullgraph=True)
```
| diffusers/docs/source/en/using-diffusers/text-img2vid.md/0 | {
"file_path": "diffusers/docs/source/en/using-diffusers/text-img2vid.md",
"repo_id": "diffusers",
"token_count": 7527
} | 99 |
# 학습을 위한 데이터셋 만들기
[Hub](https://huggingface.co/datasets?task_categories=task_categories:text-to-image&sort=downloads) 에는 모델 교육을 위한 많은 데이터셋이 있지만,
관심이 있거나 사용하고 싶은 데이터셋을 찾을 수 없는 경우 🤗 [Datasets](hf.co/docs/datasets) 라이브러리를 사용하여 데이터셋을 만들 수 있습니다.
데이터셋 구조는 모델을 학습하려는 작업에 따라 달라집니다.
가장 기본적인 데이터셋 구조는 unconditional 이미지 생성과 같은 작업을 위한 이미지 디렉토리입니다.
또 다른 데이터셋 구조는 이미지 디렉토리와 text-to-image 생성과 같은 작업에 해당하는 텍스트 캡션이 포함된 텍스트 파일일 수 있습니다.
이 가이드에는 파인 튜닝할 데이터셋을 만드는 두 가지 방법을 소개합니다:
- 이미지 폴더를 `--train_data_dir` 인수에 제공합니다.
- 데이터셋을 Hub에 업로드하고 데이터셋 리포지토리 id를 `--dataset_name` 인수에 전달합니다.
<Tip>
💡 학습에 사용할 이미지 데이터셋을 만드는 방법에 대한 자세한 내용은 [이미지 데이터셋 만들기](https://huggingface.co/docs/datasets/image_dataset) 가이드를 참고하세요.
</Tip>
## 폴더 형태로 데이터셋 구축하기
Unconditional 생성을 위해 이미지 폴더로 자신의 데이터셋을 구축할 수 있습니다.
학습 스크립트는 🤗 Datasets의 [ImageFolder](https://huggingface.co/docs/datasets/en/image_dataset#imagefolder) 빌더를 사용하여
자동으로 폴더에서 데이터셋을 구축합니다. 디렉토리 구조는 다음과 같아야 합니다 :
```bash
data_dir/xxx.png
data_dir/xxy.png
data_dir/[...]/xxz.png
```
데이터셋 디렉터리의 경로를 `--train_data_dir` 인수로 전달한 다음 학습을 시작할 수 있습니다:
```bash
accelerate launch train_unconditional.py \
# argument로 폴더 지정하기 \
--train_data_dir <path-to-train-directory> \
<other-arguments>
```
## Hub에 데이터 올리기
<Tip>
💡 데이터셋을 만들고 Hub에 업로드하는 것에 대한 자세한 내용은 [🤗 Datasets을 사용한 이미지 검색](https://huggingface.co/blog/image-search-datasets) 게시물을 참고하세요.
</Tip>
PIL 인코딩된 이미지가 포함된 `이미지` 열을 생성하는 [이미지 폴더](https://huggingface.co/docs/datasets/image_load#imagefolder) 기능을 사용하여 데이터셋 생성을 시작합니다.
`data_dir` 또는 `data_files` 매개 변수를 사용하여 데이터셋의 위치를 지정할 수 있습니다.
`data_files` 매개변수는 특정 파일을 `train` 이나 `test` 로 분리한 데이터셋에 매핑하는 것을 지원합니다:
```python
from datasets import load_dataset
# 예시 1: 로컬 폴더
dataset = load_dataset("imagefolder", data_dir="path_to_your_folder")
# 예시 2: 로컬 파일 (지원 포맷 : tar, gzip, zip, xz, rar, zstd)
dataset = load_dataset("imagefolder", data_files="path_to_zip_file")
# 예시 3: 원격 파일 (지원 포맷 : tar, gzip, zip, xz, rar, zstd)
dataset = load_dataset(
"imagefolder",
data_files="https://download.microsoft.com/download/3/E/1/3E1C3F21-ECDB-4869-8368-6DEBA77B919F/kagglecatsanddogs_3367a.zip",
)
# 예시 4: 여러개로 분할
dataset = load_dataset(
"imagefolder", data_files={"train": ["path/to/file1", "path/to/file2"], "test": ["path/to/file3", "path/to/file4"]}
)
```
[push_to_hub(https://huggingface.co/docs/datasets/v2.13.1/en/package_reference/main_classes#datasets.Dataset.push_to_hub) 을 사용해서 Hub에 데이터셋을 업로드 합니다:
```python
# 터미널에서 huggingface-cli login 커맨드를 이미 실행했다고 가정합니다
dataset.push_to_hub("name_of_your_dataset")
# 개인 repo로 push 하고 싶다면, `private=True` 을 추가하세요:
dataset.push_to_hub("name_of_your_dataset", private=True)
```
이제 데이터셋 이름을 `--dataset_name` 인수에 전달하여 데이터셋을 학습에 사용할 수 있습니다:
```bash
accelerate launch --mixed_precision="fp16" train_text_to_image.py \
--pretrained_model_name_or_path="runwayml/stable-diffusion-v1-5" \
--dataset_name="name_of_your_dataset" \
<other-arguments>
```
## 다음 단계
데이터셋을 생성했으니 이제 학습 스크립트의 `train_data_dir` (데이터셋이 로컬이면) 혹은 `dataset_name` (Hub에 데이터셋을 올렸으면) 인수에 연결할 수 있습니다.
다음 단계에서는 데이터셋을 사용하여 [unconditional 생성](https://huggingface.co/docs/diffusers/v0.18.2/en/training/unconditional_training) 또는 [텍스트-이미지 생성](https://huggingface.co/docs/diffusers/training/text2image)을 위한 모델을 학습시켜보세요!
| diffusers/docs/source/ko/training/create_dataset.md/0 | {
"file_path": "diffusers/docs/source/ko/training/create_dataset.md",
"repo_id": "diffusers",
"token_count": 3214
} | 100 |
<!--Copyright 2024 The HuggingFace Team. All rights reserved.
Licensed under the Apache License, Version 2.0 (the "License"); you may not use this file except in compliance with
the License. You may obtain a copy of the License at
http://www.apache.org/licenses/LICENSE-2.0
Unless required by applicable law or agreed to in writing, software distributed under the License is distributed on
an "AS IS" BASIS, WITHOUT WARRANTIES OR CONDITIONS OF ANY KIND, either express or implied. See the License for the
specific language governing permissions and limitations under the License.
-->
# 커뮤니티 파이프라인
> **커뮤니티 파이프라인에 대한 자세한 내용은 [이 이슈](https://github.com/huggingface/diffusers/issues/841)를 참조하세요.
**커뮤니티** 예제는 커뮤니티에서 추가한 추론 및 훈련 예제로 구성되어 있습니다.
다음 표를 참조하여 모든 커뮤니티 예제에 대한 개요를 확인하시기 바랍니다. **코드 예제**를 클릭하면 복사하여 붙여넣기할 수 있는 코드 예제를 확인할 수 있습니다.
커뮤니티가 예상대로 작동하지 않는 경우 이슈를 개설하고 작성자에게 핑을 보내주세요.
| 예 | 설명 | 코드 예제 | 콜랩 |저자 |
|:---------------------------------------|:------------------------------------------------------------------------------------------------------------------------------------------------------------------------------------------------------------------------------------------------------------------------------------------------------------------------------------------------------------------------------------------------------------------------------------------------------------------------------------------------|:---------------------------------------------------------------------------------|:-------------------------------------------------------------------------------------------------------------------------------------------------------------------------------------------------------------------|-----------------------------------------------------------:|
| CLIP Guided Stable Diffusion | CLIP 가이드 기반의 Stable Diffusion으로 텍스트에서 이미지로 생성하기 | [CLIP Guided Stable Diffusion](#clip-guided-stable-diffusion) | [](https://colab.research.google.com/github/huggingface/notebooks/blob/main/diffusers/CLIP_Guided_Stable_diffusion_with_diffusers.ipynb) | [Suraj Patil](https://github.com/patil-suraj/) |
| One Step U-Net (Dummy) | 커뮤니티 파이프라인을 어떻게 사용해야 하는지에 대한 예시(참고 https://github.com/huggingface/diffusers/issues/841) | [One Step U-Net](#one-step-unet) | - | [Patrick von Platen](https://github.com/patrickvonplaten/) |
| Stable Diffusion Interpolation | 서로 다른 프롬프트/시드 간 Stable Diffusion의 latent space 보간 | [Stable Diffusion Interpolation](#stable-diffusion-interpolation) | - | [Nate Raw](https://github.com/nateraw/) |
| Stable Diffusion Mega | 모든 기능을 갖춘 **하나의** Stable Diffusion 파이프라인 [Text2Image](https://github.com/huggingface/diffusers/blob/main/src/diffusers/pipelines/stable_diffusion/pipeline_stable_diffusion.py), [Image2Image](https://github.com/huggingface/diffusers/blob/main/src/diffusers/pipelines/stable_diffusion/pipeline_stable_diffusion_img2img.py) and [Inpainting](https://github.com/huggingface/diffusers/blob/main/src/diffusers/pipelines/stable_diffusion/pipeline_stable_diffusion_inpaint.py) | [Stable Diffusion Mega](#stable-diffusion-mega) | - | [Patrick von Platen](https://github.com/patrickvonplaten/) |
| Long Prompt Weighting Stable Diffusion | 토큰 길이 제한이 없고 프롬프트에서 파싱 가중치 지원을 하는 **하나의** Stable Diffusion 파이프라인, | [Long Prompt Weighting Stable Diffusion](#long-prompt-weighting-stable-diffusion) |- | [SkyTNT](https://github.com/SkyTNT) |
| Speech to Image | 자동 음성 인식을 사용하여 텍스트를 작성하고 Stable Diffusion을 사용하여 이미지를 생성합니다. | [Speech to Image](#speech-to-image) | - | [Mikail Duzenli](https://github.com/MikailINTech) |
커스텀 파이프라인을 불러오려면 `diffusers/examples/community`에 있는 파일 중 하나로서 `custom_pipeline` 인수를 `DiffusionPipeline`에 전달하기만 하면 됩니다. 자신만의 파이프라인이 있는 PR을 보내주시면 빠르게 병합해드리겠습니다.
```py
pipe = DiffusionPipeline.from_pretrained(
"CompVis/stable-diffusion-v1-4", custom_pipeline="filename_in_the_community_folder"
)
```
## 사용 예시
### CLIP 가이드 기반의 Stable Diffusion
모든 노이즈 제거 단계에서 추가 CLIP 모델을 통해 Stable Diffusion을 가이드함으로써 CLIP 모델 기반의 Stable Diffusion은 보다 더 사실적인 이미지를 생성을 할 수 있습니다.
다음 코드는 약 12GB의 GPU RAM이 필요합니다.
```python
from diffusers import DiffusionPipeline
from transformers import CLIPImageProcessor, CLIPModel
import torch
feature_extractor = CLIPImageProcessor.from_pretrained("laion/CLIP-ViT-B-32-laion2B-s34B-b79K")
clip_model = CLIPModel.from_pretrained("laion/CLIP-ViT-B-32-laion2B-s34B-b79K", torch_dtype=torch.float16)
guided_pipeline = DiffusionPipeline.from_pretrained(
"CompVis/stable-diffusion-v1-4",
custom_pipeline="clip_guided_stable_diffusion",
clip_model=clip_model,
feature_extractor=feature_extractor,
torch_dtype=torch.float16,
)
guided_pipeline.enable_attention_slicing()
guided_pipeline = guided_pipeline.to("cuda")
prompt = "fantasy book cover, full moon, fantasy forest landscape, golden vector elements, fantasy magic, dark light night, intricate, elegant, sharp focus, illustration, highly detailed, digital painting, concept art, matte, art by WLOP and Artgerm and Albert Bierstadt, masterpiece"
generator = torch.Generator(device="cuda").manual_seed(0)
images = []
for i in range(4):
image = guided_pipeline(
prompt,
num_inference_steps=50,
guidance_scale=7.5,
clip_guidance_scale=100,
num_cutouts=4,
use_cutouts=False,
generator=generator,
).images[0]
images.append(image)
# 이미지 로컬에 저장하기
for i, img in enumerate(images):
img.save(f"./clip_guided_sd/image_{i}.png")
```
이미지` 목록에는 로컬에 저장하거나 구글 콜랩에 직접 표시할 수 있는 PIL 이미지 목록이 포함되어 있습니다. 생성된 이미지는 기본적으로 안정적인 확산을 사용하는 것보다 품질이 높은 경향이 있습니다. 예를 들어 위의 스크립트는 다음과 같은 이미지를 생성합니다:
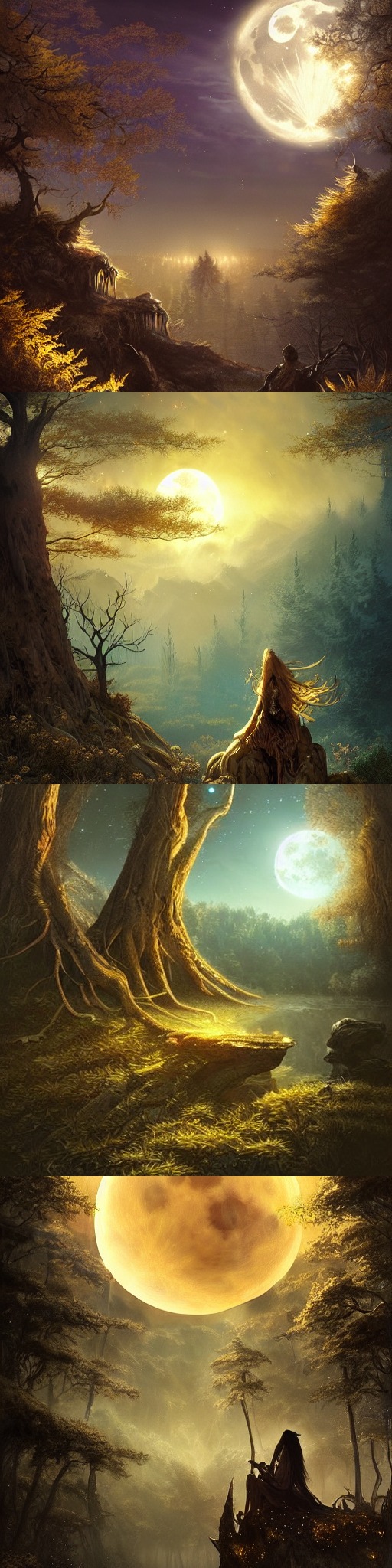.
### One Step Unet
예시 "one-step-unet"는 다음과 같이 실행할 수 있습니다.
```python
from diffusers import DiffusionPipeline
pipe = DiffusionPipeline.from_pretrained("google/ddpm-cifar10-32", custom_pipeline="one_step_unet")
pipe()
```
**참고**: 이 커뮤니티 파이프라인은 기능으로 유용하지 않으며 커뮤니티 파이프라인을 추가할 수 있는 방법의 예시일 뿐입니다(https://github.com/huggingface/diffusers/issues/841 참조).
### Stable Diffusion Interpolation
다음 코드는 최소 8GB VRAM의 GPU에서 실행할 수 있으며 약 5분 정도 소요됩니다.
```python
from diffusers import DiffusionPipeline
import torch
pipe = DiffusionPipeline.from_pretrained(
"CompVis/stable-diffusion-v1-4",
torch_dtype=torch.float16,
safety_checker=None, # Very important for videos...lots of false positives while interpolating
custom_pipeline="interpolate_stable_diffusion",
).to("cuda")
pipe.enable_attention_slicing()
frame_filepaths = pipe.walk(
prompts=["a dog", "a cat", "a horse"],
seeds=[42, 1337, 1234],
num_interpolation_steps=16,
output_dir="./dreams",
batch_size=4,
height=512,
width=512,
guidance_scale=8.5,
num_inference_steps=50,
)
```
walk(...)` 함수의 출력은 `output_dir`에 정의된 대로 폴더에 저장된 이미지 목록을 반환합니다. 이 이미지를 사용하여 안정적으로 확산되는 동영상을 만들 수 있습니다.
> 안정된 확산을 이용한 동영상 제작 방법과 더 많은 기능에 대한 자세한 내용은 https://github.com/nateraw/stable-diffusion-videos 에서 확인하시기 바랍니다.
### Stable Diffusion Mega
The Stable Diffusion Mega 파이프라인을 사용하면 Stable Diffusion 파이프라인의 주요 사용 사례를 단일 클래스에서 사용할 수 있습니다.
```python
#!/usr/bin/env python3
from diffusers import DiffusionPipeline
import PIL
import requests
from io import BytesIO
import torch
def download_image(url):
response = requests.get(url)
return PIL.Image.open(BytesIO(response.content)).convert("RGB")
pipe = DiffusionPipeline.from_pretrained(
"CompVis/stable-diffusion-v1-4",
custom_pipeline="stable_diffusion_mega",
torch_dtype=torch.float16,
)
pipe.to("cuda")
pipe.enable_attention_slicing()
### Text-to-Image
images = pipe.text2img("An astronaut riding a horse").images
### Image-to-Image
init_image = download_image(
"https://raw.githubusercontent.com/CompVis/stable-diffusion/main/assets/stable-samples/img2img/sketch-mountains-input.jpg"
)
prompt = "A fantasy landscape, trending on artstation"
images = pipe.img2img(prompt=prompt, image=init_image, strength=0.75, guidance_scale=7.5).images
### Inpainting
img_url = "https://raw.githubusercontent.com/CompVis/latent-diffusion/main/data/inpainting_examples/overture-creations-5sI6fQgYIuo.png"
mask_url = "https://raw.githubusercontent.com/CompVis/latent-diffusion/main/data/inpainting_examples/overture-creations-5sI6fQgYIuo_mask.png"
init_image = download_image(img_url).resize((512, 512))
mask_image = download_image(mask_url).resize((512, 512))
prompt = "a cat sitting on a bench"
images = pipe.inpaint(prompt=prompt, image=init_image, mask_image=mask_image, strength=0.75).images
```
위에 표시된 것처럼 하나의 파이프라인에서 '텍스트-이미지 변환', '이미지-이미지 변환', '인페인팅'을 모두 실행할 수 있습니다.
### Long Prompt Weighting Stable Diffusion
파이프라인을 사용하면 77개의 토큰 길이 제한 없이 프롬프트를 입력할 수 있습니다. 또한 "()"를 사용하여 단어 가중치를 높이거나 "[]"를 사용하여 단어 가중치를 낮출 수 있습니다.
또한 파이프라인을 사용하면 단일 클래스에서 Stable Diffusion 파이프라인의 주요 사용 사례를 사용할 수 있습니다.
#### pytorch
```python
from diffusers import DiffusionPipeline
import torch
pipe = DiffusionPipeline.from_pretrained(
"hakurei/waifu-diffusion", custom_pipeline="lpw_stable_diffusion", torch_dtype=torch.float16
)
pipe = pipe.to("cuda")
prompt = "best_quality (1girl:1.3) bow bride brown_hair closed_mouth frilled_bow frilled_hair_tubes frills (full_body:1.3) fox_ear hair_bow hair_tubes happy hood japanese_clothes kimono long_sleeves red_bow smile solo tabi uchikake white_kimono wide_sleeves cherry_blossoms"
neg_prompt = "lowres, bad_anatomy, error_body, error_hair, error_arm, error_hands, bad_hands, error_fingers, bad_fingers, missing_fingers, error_legs, bad_legs, multiple_legs, missing_legs, error_lighting, error_shadow, error_reflection, text, error, extra_digit, fewer_digits, cropped, worst_quality, low_quality, normal_quality, jpeg_artifacts, signature, watermark, username, blurry"
pipe.text2img(prompt, negative_prompt=neg_prompt, width=512, height=512, max_embeddings_multiples=3).images[0]
```
#### onnxruntime
```python
from diffusers import DiffusionPipeline
import torch
pipe = DiffusionPipeline.from_pretrained(
"CompVis/stable-diffusion-v1-4",
custom_pipeline="lpw_stable_diffusion_onnx",
revision="onnx",
provider="CUDAExecutionProvider",
)
prompt = "a photo of an astronaut riding a horse on mars, best quality"
neg_prompt = "lowres, bad anatomy, error body, error hair, error arm, error hands, bad hands, error fingers, bad fingers, missing fingers, error legs, bad legs, multiple legs, missing legs, error lighting, error shadow, error reflection, text, error, extra digit, fewer digits, cropped, worst quality, low quality, normal quality, jpeg artifacts, signature, watermark, username, blurry"
pipe.text2img(prompt, negative_prompt=neg_prompt, width=512, height=512, max_embeddings_multiples=3).images[0]
```
토큰 인덱스 시퀀스 길이가 이 모델에 지정된 최대 시퀀스 길이보다 길면(*** > 77). 이 시퀀스를 모델에서 실행하면 인덱싱 오류가 발생합니다`. 정상적인 현상이니 걱정하지 마세요.
### Speech to Image
다음 코드는 사전학습된 OpenAI whisper-small과 Stable Diffusion을 사용하여 오디오 샘플에서 이미지를 생성할 수 있습니다.
```Python
import torch
import matplotlib.pyplot as plt
from datasets import load_dataset
from diffusers import DiffusionPipeline
from transformers import (
WhisperForConditionalGeneration,
WhisperProcessor,
)
device = "cuda" if torch.cuda.is_available() else "cpu"
ds = load_dataset("hf-internal-testing/librispeech_asr_dummy", "clean", split="validation")
audio_sample = ds[3]
text = audio_sample["text"].lower()
speech_data = audio_sample["audio"]["array"]
model = WhisperForConditionalGeneration.from_pretrained("openai/whisper-small").to(device)
processor = WhisperProcessor.from_pretrained("openai/whisper-small")
diffuser_pipeline = DiffusionPipeline.from_pretrained(
"CompVis/stable-diffusion-v1-4",
custom_pipeline="speech_to_image_diffusion",
speech_model=model,
speech_processor=processor,
torch_dtype=torch.float16,
)
diffuser_pipeline.enable_attention_slicing()
diffuser_pipeline = diffuser_pipeline.to(device)
output = diffuser_pipeline(speech_data)
plt.imshow(output.images[0])
```
위 예시는 다음의 결과 이미지를 보입니다.
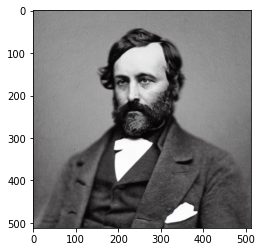 | diffusers/docs/source/ko/using-diffusers/custom_pipeline_examples.md/0 | {
"file_path": "diffusers/docs/source/ko/using-diffusers/custom_pipeline_examples.md",
"repo_id": "diffusers",
"token_count": 10864
} | 101 |
<!--Copyright 2024 The HuggingFace Team. All rights reserved.
Licensed under the Apache License, Version 2.0 (the "License"); you may not use this file except in compliance with
the License. You may obtain a copy of the License at
http://www.apache.org/licenses/LICENSE-2.0
Unless required by applicable law or agreed to in writing, software distributed under the License is distributed on
an "AS IS" BASIS, WITHOUT WARRANTIES OR CONDITIONS OF ANY KIND, either express or implied. See the License for the
specific language governing permissions and limitations under the License.
-->
# 프롬프트에 가중치 부여하기
[[open-in-colab]]
텍스트 가이드 기반의 diffusion 모델은 주어진 텍스트 프롬프트를 기반으로 이미지를 생성합니다.
텍스트 프롬프트에는 모델이 생성해야 하는 여러 개념이 포함될 수 있으며 프롬프트의 특정 부분에 가중치를 부여하는 것이 바람직한 경우가 많습니다.
Diffusion 모델은 문맥화된 텍스트 임베딩으로 diffusion 모델의 cross attention 레이어를 조절함으로써 작동합니다.
([더 많은 정보를 위한 Stable Diffusion Guide](https://huggingface.co/docs/optimum-neuron/main/en/package_reference/modeling#stable-diffusion)를 참고하세요).
따라서 프롬프트의 특정 부분을 강조하는(또는 강조하지 않는) 간단한 방법은 프롬프트의 관련 부분에 해당하는 텍스트 임베딩 벡터의 크기를 늘리거나 줄이는 것입니다.
이것은 "프롬프트 가중치 부여" 라고 하며, 커뮤니티에서 가장 요구하는 기능입니다.([이곳](https://github.com/huggingface/diffusers/issues/2431)의 issue를 보세요 ).
## Diffusers에서 프롬프트 가중치 부여하는 방법
우리는 `diffusers`의 역할이 다른 프로젝트를 가능하게 하는 필수적인 기능을 제공하는 toolbex라고 생각합니다.
[InvokeAI](https://github.com/invoke-ai/InvokeAI) 나 [diffuzers](https://github.com/abhishekkrthakur/diffuzers) 같은 강력한 UI를 구축할 수 있습니다.
프롬프트를 조작하는 방법을 지원하기 위해, `diffusers` 는
[StableDiffusionPipeline](https://huggingface.co/docs/diffusers/v0.18.2/en/api/pipelines/stable_diffusion/text2img#diffusers.StableDiffusionPipeline)와 같은
많은 파이프라인에 [prompt_embeds](https://huggingface.co/docs/diffusers/v0.14.0/en/api/pipelines/stable_diffusion/text2img#diffusers.StableDiffusionPipeline.__call__.prompt_embeds)
인수를 노출시켜, "prompt-weighted"/축척된 텍스트 임베딩을 파이프라인에 바로 전달할 수 있게 합니다.
[Compel 라이브러리](https://github.com/damian0815/compel)는 프롬프트의 일부를 강조하거나 강조하지 않을 수 있는 쉬운 방법을 제공합니다.
임베딩을 직접 준비하는 것 대신 이 방법을 사용하는 것을 강력히 추천합니다.
간단한 예제를 살펴보겠습니다.
다음과 같이 `"공을 갖고 노는 붉은색 고양이"` 이미지를 생성하고 싶습니다:
```py
from diffusers import StableDiffusionPipeline, UniPCMultistepScheduler
pipe = StableDiffusionPipeline.from_pretrained("CompVis/stable-diffusion-v1-4")
pipe.scheduler = UniPCMultistepScheduler.from_config(pipe.scheduler.config)
prompt = "a red cat playing with a ball"
generator = torch.Generator(device="cpu").manual_seed(33)
image = pipe(prompt, generator=generator, num_inference_steps=20).images[0]
image
```
생성된 이미지:
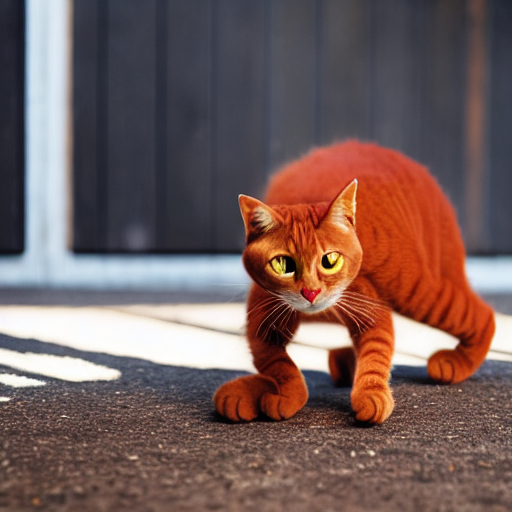
사진에서 알 수 있듯이, "공"은 이미지에 없습니다. 이 부분을 강조해 볼까요!
먼저 `compel` 라이브러리를 설치해야합니다:
```
pip install compel
```
그런 다음에는 `Compel` 오브젝트를 생성합니다:
```py
from compel import Compel
compel_proc = Compel(tokenizer=pipe.tokenizer, text_encoder=pipe.text_encoder)
```
이제 `"++"` 를 사용해서 "공" 을 강조해 봅시다:
```py
prompt = "a red cat playing with a ball++"
```
그리고 이 프롬프트를 파이프라인에 바로 전달하지 않고, `compel_proc` 를 사용하여 처리해야합니다:
```py
prompt_embeds = compel_proc(prompt)
```
파이프라인에 `prompt_embeds` 를 바로 전달할 수 있습니다:
```py
generator = torch.Generator(device="cpu").manual_seed(33)
images = pipe(prompt_embeds=prompt_embeds, generator=generator, num_inference_steps=20).images[0]
image
```
이제 "공"이 있는 그림을 출력할 수 있습니다!
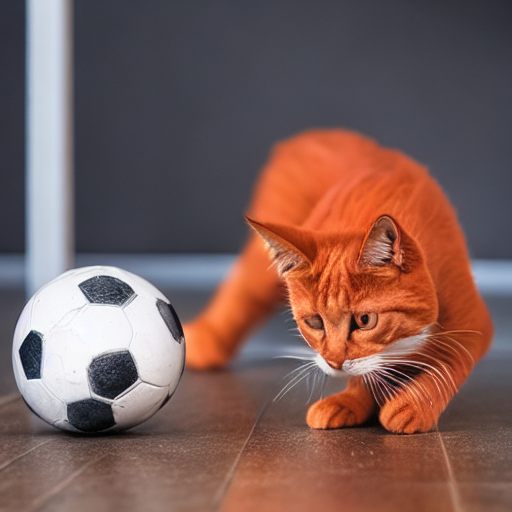
마찬가지로 `--` 접미사를 단어에 사용하여 문장의 일부를 강조하지 않을 수 있습니다. 한번 시도해 보세요!
즐겨찾는 파이프라인에 `prompt_embeds` 입력이 없는 경우 issue를 새로 만들어주세요.
Diffusers 팀은 최대한 대응하려고 노력합니다.
Compel 1.1.6 는 textual inversions을 사용하여 단순화하는 유티릴티 클래스를 추가합니다.
`DiffusersTextualInversionManager`를 인스턴스화 한 후 이를 Compel init에 전달합니다:
```
textual_inversion_manager = DiffusersTextualInversionManager(pipe)
compel = Compel(
tokenizer=pipe.tokenizer,
text_encoder=pipe.text_encoder,
textual_inversion_manager=textual_inversion_manager)
```
더 많은 정보를 얻고 싶다면 [compel](https://github.com/damian0815/compel) 라이브러리 문서를 참고하세요.
| diffusers/docs/source/ko/using-diffusers/weighted_prompts.md/0 | {
"file_path": "diffusers/docs/source/ko/using-diffusers/weighted_prompts.md",
"repo_id": "diffusers",
"token_count": 3375
} | 102 |
## Amused training
Amused can be finetuned on simple datasets relatively cheaply and quickly. Using 8bit optimizers, lora, and gradient accumulation, amused can be finetuned with as little as 5.5 GB. Here are a set of examples for finetuning amused on some relatively simple datasets. These training recipies are aggressively oriented towards minimal resources and fast verification -- i.e. the batch sizes are quite low and the learning rates are quite high. For optimal quality, you will probably want to increase the batch sizes and decrease learning rates.
All training examples use fp16 mixed precision and gradient checkpointing. We don't show 8 bit adam + lora as its about the same memory use as just using lora (bitsandbytes uses full precision optimizer states for weights below a minimum size).
### Finetuning the 256 checkpoint
These examples finetune on this [nouns](https://huggingface.co/datasets/m1guelpf/nouns) dataset.
Example results:
  
#### Full finetuning
Batch size: 8, Learning rate: 1e-4, Gives decent results in 750-1000 steps
| Batch Size | Gradient Accumulation Steps | Effective Total Batch Size | Memory Used |
|------------|-----------------------------|------------------|-------------|
| 8 | 1 | 8 | 19.7 GB |
| 4 | 2 | 8 | 18.3 GB |
| 1 | 8 | 8 | 17.9 GB |
```sh
accelerate launch train_amused.py \
--output_dir <output path> \
--train_batch_size <batch size> \
--gradient_accumulation_steps <gradient accumulation steps> \
--learning_rate 1e-4 \
--pretrained_model_name_or_path amused/amused-256 \
--instance_data_dataset 'm1guelpf/nouns' \
--image_key image \
--prompt_key text \
--resolution 256 \
--mixed_precision fp16 \
--lr_scheduler constant \
--validation_prompts \
'a pixel art character with square red glasses, a baseball-shaped head and a orange-colored body on a dark background' \
'a pixel art character with square orange glasses, a lips-shaped head and a red-colored body on a light background' \
'a pixel art character with square blue glasses, a microwave-shaped head and a purple-colored body on a sunny background' \
'a pixel art character with square red glasses, a baseball-shaped head and a blue-colored body on an orange background' \
'a pixel art character with square red glasses' \
'a pixel art character' \
'square red glasses on a pixel art character' \
'square red glasses on a pixel art character with a baseball-shaped head' \
--max_train_steps 10000 \
--checkpointing_steps 500 \
--validation_steps 250 \
--gradient_checkpointing
```
#### Full finetuning + 8 bit adam
Note that this training config keeps the batch size low and the learning rate high to get results fast with low resources. However, due to 8 bit adam, it will diverge eventually. If you want to train for longer, you will have to up the batch size and lower the learning rate.
Batch size: 16, Learning rate: 2e-5, Gives decent results in ~750 steps
| Batch Size | Gradient Accumulation Steps | Effective Total Batch Size | Memory Used |
|------------|-----------------------------|------------------|-------------|
| 16 | 1 | 16 | 20.1 GB |
| 8 | 2 | 16 | 15.6 GB |
| 1 | 16 | 16 | 10.7 GB |
```sh
accelerate launch train_amused.py \
--output_dir <output path> \
--train_batch_size <batch size> \
--gradient_accumulation_steps <gradient accumulation steps> \
--learning_rate 2e-5 \
--use_8bit_adam \
--pretrained_model_name_or_path amused/amused-256 \
--instance_data_dataset 'm1guelpf/nouns' \
--image_key image \
--prompt_key text \
--resolution 256 \
--mixed_precision fp16 \
--lr_scheduler constant \
--validation_prompts \
'a pixel art character with square red glasses, a baseball-shaped head and a orange-colored body on a dark background' \
'a pixel art character with square orange glasses, a lips-shaped head and a red-colored body on a light background' \
'a pixel art character with square blue glasses, a microwave-shaped head and a purple-colored body on a sunny background' \
'a pixel art character with square red glasses, a baseball-shaped head and a blue-colored body on an orange background' \
'a pixel art character with square red glasses' \
'a pixel art character' \
'square red glasses on a pixel art character' \
'square red glasses on a pixel art character with a baseball-shaped head' \
--max_train_steps 10000 \
--checkpointing_steps 500 \
--validation_steps 250 \
--gradient_checkpointing
```
#### Full finetuning + lora
Batch size: 16, Learning rate: 8e-4, Gives decent results in 1000-1250 steps
| Batch Size | Gradient Accumulation Steps | Effective Total Batch Size | Memory Used |
|------------|-----------------------------|------------------|-------------|
| 16 | 1 | 16 | 14.1 GB |
| 8 | 2 | 16 | 10.1 GB |
| 1 | 16 | 16 | 6.5 GB |
```sh
accelerate launch train_amused.py \
--output_dir <output path> \
--train_batch_size <batch size> \
--gradient_accumulation_steps <gradient accumulation steps> \
--learning_rate 8e-4 \
--use_lora \
--pretrained_model_name_or_path amused/amused-256 \
--instance_data_dataset 'm1guelpf/nouns' \
--image_key image \
--prompt_key text \
--resolution 256 \
--mixed_precision fp16 \
--lr_scheduler constant \
--validation_prompts \
'a pixel art character with square red glasses, a baseball-shaped head and a orange-colored body on a dark background' \
'a pixel art character with square orange glasses, a lips-shaped head and a red-colored body on a light background' \
'a pixel art character with square blue glasses, a microwave-shaped head and a purple-colored body on a sunny background' \
'a pixel art character with square red glasses, a baseball-shaped head and a blue-colored body on an orange background' \
'a pixel art character with square red glasses' \
'a pixel art character' \
'square red glasses on a pixel art character' \
'square red glasses on a pixel art character with a baseball-shaped head' \
--max_train_steps 10000 \
--checkpointing_steps 500 \
--validation_steps 250 \
--gradient_checkpointing
```
### Finetuning the 512 checkpoint
These examples finetune on this [minecraft](https://huggingface.co/monadical-labs/minecraft-preview) dataset.
Example results:
  
#### Full finetuning
Batch size: 8, Learning rate: 8e-5, Gives decent results in 500-1000 steps
| Batch Size | Gradient Accumulation Steps | Effective Total Batch Size | Memory Used |
|------------|-----------------------------|------------------|-------------|
| 8 | 1 | 8 | 24.2 GB |
| 4 | 2 | 8 | 19.7 GB |
| 1 | 8 | 8 | 16.99 GB |
```sh
accelerate launch train_amused.py \
--output_dir <output path> \
--train_batch_size <batch size> \
--gradient_accumulation_steps <gradient accumulation steps> \
--learning_rate 8e-5 \
--pretrained_model_name_or_path amused/amused-512 \
--instance_data_dataset 'monadical-labs/minecraft-preview' \
--prompt_prefix 'minecraft ' \
--image_key image \
--prompt_key text \
--resolution 512 \
--mixed_precision fp16 \
--lr_scheduler constant \
--validation_prompts \
'minecraft Avatar' \
'minecraft character' \
'minecraft' \
'minecraft president' \
'minecraft pig' \
--max_train_steps 10000 \
--checkpointing_steps 500 \
--validation_steps 250 \
--gradient_checkpointing
```
#### Full finetuning + 8 bit adam
Batch size: 8, Learning rate: 5e-6, Gives decent results in 500-1000 steps
| Batch Size | Gradient Accumulation Steps | Effective Total Batch Size | Memory Used |
|------------|-----------------------------|------------------|-------------|
| 8 | 1 | 8 | 21.2 GB |
| 4 | 2 | 8 | 13.3 GB |
| 1 | 8 | 8 | 9.9 GB |
```sh
accelerate launch train_amused.py \
--output_dir <output path> \
--train_batch_size <batch size> \
--gradient_accumulation_steps <gradient accumulation steps> \
--learning_rate 5e-6 \
--pretrained_model_name_or_path amused/amused-512 \
--instance_data_dataset 'monadical-labs/minecraft-preview' \
--prompt_prefix 'minecraft ' \
--image_key image \
--prompt_key text \
--resolution 512 \
--mixed_precision fp16 \
--lr_scheduler constant \
--validation_prompts \
'minecraft Avatar' \
'minecraft character' \
'minecraft' \
'minecraft president' \
'minecraft pig' \
--max_train_steps 10000 \
--checkpointing_steps 500 \
--validation_steps 250 \
--gradient_checkpointing
```
#### Full finetuning + lora
Batch size: 8, Learning rate: 1e-4, Gives decent results in 500-1000 steps
| Batch Size | Gradient Accumulation Steps | Effective Total Batch Size | Memory Used |
|------------|-----------------------------|------------------|-------------|
| 8 | 1 | 8 | 12.7 GB |
| 4 | 2 | 8 | 9.0 GB |
| 1 | 8 | 8 | 5.6 GB |
```sh
accelerate launch train_amused.py \
--output_dir <output path> \
--train_batch_size <batch size> \
--gradient_accumulation_steps <gradient accumulation steps> \
--learning_rate 1e-4 \
--use_lora \
--pretrained_model_name_or_path amused/amused-512 \
--instance_data_dataset 'monadical-labs/minecraft-preview' \
--prompt_prefix 'minecraft ' \
--image_key image \
--prompt_key text \
--resolution 512 \
--mixed_precision fp16 \
--lr_scheduler constant \
--validation_prompts \
'minecraft Avatar' \
'minecraft character' \
'minecraft' \
'minecraft president' \
'minecraft pig' \
--max_train_steps 10000 \
--checkpointing_steps 500 \
--validation_steps 250 \
--gradient_checkpointing
```
### Styledrop
[Styledrop](https://arxiv.org/abs/2306.00983) is an efficient finetuning method for learning a new style from just one or very few images. It has an optional first stage to generate human picked additional training samples. The additional training samples can be used to augment the initial images. Our examples exclude the optional additional image selection stage and instead we just finetune on a single image.
This is our example style image:

Download it to your local directory with
```sh
wget https://huggingface.co/datasets/diffusers/docs-images/resolve/main/amused/A%20mushroom%20in%20%5BV%5D%20style.png
```
#### 256
Example results:
  
Learning rate: 4e-4, Gives decent results in 1500-2000 steps
Memory used: 6.5 GB
```sh
accelerate launch train_amused.py \
--output_dir <output path> \
--mixed_precision fp16 \
--report_to wandb \
--use_lora \
--pretrained_model_name_or_path amused/amused-256 \
--train_batch_size 1 \
--lr_scheduler constant \
--learning_rate 4e-4 \
--validation_prompts \
'A chihuahua walking on the street in [V] style' \
'A banana on the table in [V] style' \
'A church on the street in [V] style' \
'A tabby cat walking in the forest in [V] style' \
--instance_data_image 'A mushroom in [V] style.png' \
--max_train_steps 10000 \
--checkpointing_steps 500 \
--validation_steps 100 \
--resolution 256
```
#### 512
Example results:
  
Learning rate: 1e-3, Lora alpha 1, Gives decent results in 1500-2000 steps
Memory used: 5.6 GB
```
accelerate launch train_amused.py \
--output_dir <output path> \
--mixed_precision fp16 \
--report_to wandb \
--use_lora \
--pretrained_model_name_or_path amused/amused-512 \
--train_batch_size 1 \
--lr_scheduler constant \
--learning_rate 1e-3 \
--validation_prompts \
'A chihuahua walking on the street in [V] style' \
'A banana on the table in [V] style' \
'A church on the street in [V] style' \
'A tabby cat walking in the forest in [V] style' \
--instance_data_image 'A mushroom in [V] style.png' \
--max_train_steps 100000 \
--checkpointing_steps 500 \
--validation_steps 100 \
--resolution 512 \
--lora_alpha 1
``` | diffusers/examples/amused/README.md/0 | {
"file_path": "diffusers/examples/amused/README.md",
"repo_id": "diffusers",
"token_count": 5921
} | 103 |
#!/usr/bin/env python3
import torch
from diffusers import DiffusionPipeline
class UnetSchedulerOneForwardPipeline(DiffusionPipeline):
def __init__(self, unet, scheduler):
super().__init__()
self.register_modules(unet=unet, scheduler=scheduler)
def __call__(self):
image = torch.randn(
(1, self.unet.config.in_channels, self.unet.config.sample_size, self.unet.config.sample_size),
)
timestep = 1
model_output = self.unet(image, timestep).sample
scheduler_output = self.scheduler.step(model_output, timestep, image).prev_sample
result = scheduler_output - scheduler_output + torch.ones_like(scheduler_output)
return result
| diffusers/examples/community/one_step_unet.py/0 | {
"file_path": "diffusers/examples/community/one_step_unet.py",
"repo_id": "diffusers",
"token_count": 299
} | 104 |
import argparse
import inspect
import os
import time
import warnings
from typing import Any, Callable, Dict, List, Optional, Union
import numpy as np
import PIL.Image
import torch
from PIL import Image
from transformers import CLIPTokenizer
from diffusers import OnnxRuntimeModel, StableDiffusionImg2ImgPipeline, UniPCMultistepScheduler
from diffusers.image_processor import VaeImageProcessor
from diffusers.pipelines.pipeline_utils import DiffusionPipeline
from diffusers.pipelines.stable_diffusion import StableDiffusionPipelineOutput
from diffusers.schedulers import KarrasDiffusionSchedulers
from diffusers.utils import (
deprecate,
logging,
replace_example_docstring,
)
from diffusers.utils.torch_utils import randn_tensor
logger = logging.get_logger(__name__) # pylint: disable=invalid-name
EXAMPLE_DOC_STRING = """
Examples:
```py
>>> # !pip install opencv-python transformers accelerate
>>> from diffusers import StableDiffusionControlNetImg2ImgPipeline, ControlNetModel, UniPCMultistepScheduler
>>> from diffusers.utils import load_image
>>> import numpy as np
>>> import torch
>>> import cv2
>>> from PIL import Image
>>> # download an image
>>> image = load_image(
... "https://hf.co/datasets/huggingface/documentation-images/resolve/main/diffusers/input_image_vermeer.png"
... )
>>> np_image = np.array(image)
>>> # get canny image
>>> np_image = cv2.Canny(np_image, 100, 200)
>>> np_image = np_image[:, :, None]
>>> np_image = np.concatenate([np_image, np_image, np_image], axis=2)
>>> canny_image = Image.fromarray(np_image)
>>> # load control net and stable diffusion v1-5
>>> controlnet = ControlNetModel.from_pretrained("lllyasviel/sd-controlnet-canny", torch_dtype=torch.float16)
>>> pipe = StableDiffusionControlNetImg2ImgPipeline.from_pretrained(
... "runwayml/stable-diffusion-v1-5", controlnet=controlnet, torch_dtype=torch.float16
... )
>>> # speed up diffusion process with faster scheduler and memory optimization
>>> pipe.scheduler = UniPCMultistepScheduler.from_config(pipe.scheduler.config)
>>> pipe.enable_model_cpu_offload()
>>> # generate image
>>> generator = torch.manual_seed(0)
>>> image = pipe(
... "futuristic-looking woman",
... num_inference_steps=20,
... generator=generator,
... image=image,
... control_image=canny_image,
... ).images[0]
```
"""
def prepare_image(image):
if isinstance(image, torch.Tensor):
# Batch single image
if image.ndim == 3:
image = image.unsqueeze(0)
image = image.to(dtype=torch.float32)
else:
# preprocess image
if isinstance(image, (PIL.Image.Image, np.ndarray)):
image = [image]
if isinstance(image, list) and isinstance(image[0], PIL.Image.Image):
image = [np.array(i.convert("RGB"))[None, :] for i in image]
image = np.concatenate(image, axis=0)
elif isinstance(image, list) and isinstance(image[0], np.ndarray):
image = np.concatenate([i[None, :] for i in image], axis=0)
image = image.transpose(0, 3, 1, 2)
image = torch.from_numpy(image).to(dtype=torch.float32) / 127.5 - 1.0
return image
class OnnxStableDiffusionControlNetImg2ImgPipeline(DiffusionPipeline):
vae_encoder: OnnxRuntimeModel
vae_decoder: OnnxRuntimeModel
text_encoder: OnnxRuntimeModel
tokenizer: CLIPTokenizer
unet: OnnxRuntimeModel
scheduler: KarrasDiffusionSchedulers
def __init__(
self,
vae_encoder: OnnxRuntimeModel,
vae_decoder: OnnxRuntimeModel,
text_encoder: OnnxRuntimeModel,
tokenizer: CLIPTokenizer,
unet: OnnxRuntimeModel,
scheduler: KarrasDiffusionSchedulers,
):
super().__init__()
self.register_modules(
vae_encoder=vae_encoder,
vae_decoder=vae_decoder,
text_encoder=text_encoder,
tokenizer=tokenizer,
unet=unet,
scheduler=scheduler,
)
self.vae_scale_factor = 2 ** (4 - 1)
self.image_processor = VaeImageProcessor(vae_scale_factor=self.vae_scale_factor, do_convert_rgb=True)
self.control_image_processor = VaeImageProcessor(
vae_scale_factor=self.vae_scale_factor, do_convert_rgb=True, do_normalize=False
)
def _encode_prompt(
self,
prompt: Union[str, List[str]],
num_images_per_prompt: Optional[int],
do_classifier_free_guidance: bool,
negative_prompt: Optional[str],
prompt_embeds: Optional[np.ndarray] = None,
negative_prompt_embeds: Optional[np.ndarray] = None,
):
r"""
Encodes the prompt into text encoder hidden states.
Args:
prompt (`str` or `List[str]`):
prompt to be encoded
num_images_per_prompt (`int`):
number of images that should be generated per prompt
do_classifier_free_guidance (`bool`):
whether to use classifier free guidance or not
negative_prompt (`str` or `List[str]`):
The prompt or prompts not to guide the image generation. Ignored when not using guidance (i.e., ignored
if `guidance_scale` is less than `1`).
prompt_embeds (`np.ndarray`, *optional*):
Pre-generated text embeddings. Can be used to easily tweak text inputs, *e.g.* prompt weighting. If not
provided, text embeddings will be generated from `prompt` input argument.
negative_prompt_embeds (`np.ndarray`, *optional*):
Pre-generated negative text embeddings. Can be used to easily tweak text inputs, *e.g.* prompt
weighting. If not provided, negative_prompt_embeds will be generated from `negative_prompt` input
argument.
"""
if prompt is not None and isinstance(prompt, str):
batch_size = 1
elif prompt is not None and isinstance(prompt, list):
batch_size = len(prompt)
else:
batch_size = prompt_embeds.shape[0]
if prompt_embeds is None:
# get prompt text embeddings
text_inputs = self.tokenizer(
prompt,
padding="max_length",
max_length=self.tokenizer.model_max_length,
truncation=True,
return_tensors="np",
)
text_input_ids = text_inputs.input_ids
untruncated_ids = self.tokenizer(prompt, padding="max_length", return_tensors="np").input_ids
if not np.array_equal(text_input_ids, untruncated_ids):
removed_text = self.tokenizer.batch_decode(
untruncated_ids[:, self.tokenizer.model_max_length - 1 : -1]
)
logger.warning(
"The following part of your input was truncated because CLIP can only handle sequences up to"
f" {self.tokenizer.model_max_length} tokens: {removed_text}"
)
prompt_embeds = self.text_encoder(input_ids=text_input_ids.astype(np.int32))[0]
prompt_embeds = np.repeat(prompt_embeds, num_images_per_prompt, axis=0)
# get unconditional embeddings for classifier free guidance
if do_classifier_free_guidance and negative_prompt_embeds is None:
uncond_tokens: List[str]
if negative_prompt is None:
uncond_tokens = [""] * batch_size
elif type(prompt) is not type(negative_prompt):
raise TypeError(
f"`negative_prompt` should be the same type to `prompt`, but got {type(negative_prompt)} !="
f" {type(prompt)}."
)
elif isinstance(negative_prompt, str):
uncond_tokens = [negative_prompt] * batch_size
elif batch_size != len(negative_prompt):
raise ValueError(
f"`negative_prompt`: {negative_prompt} has batch size {len(negative_prompt)}, but `prompt`:"
f" {prompt} has batch size {batch_size}. Please make sure that passed `negative_prompt` matches"
" the batch size of `prompt`."
)
else:
uncond_tokens = negative_prompt
max_length = prompt_embeds.shape[1]
uncond_input = self.tokenizer(
uncond_tokens,
padding="max_length",
max_length=max_length,
truncation=True,
return_tensors="np",
)
negative_prompt_embeds = self.text_encoder(input_ids=uncond_input.input_ids.astype(np.int32))[0]
if do_classifier_free_guidance:
negative_prompt_embeds = np.repeat(negative_prompt_embeds, num_images_per_prompt, axis=0)
# For classifier free guidance, we need to do two forward passes.
# Here we concatenate the unconditional and text embeddings into a single batch
# to avoid doing two forward passes
prompt_embeds = np.concatenate([negative_prompt_embeds, prompt_embeds])
return prompt_embeds
# Copied from diffusers.pipelines.stable_diffusion.pipeline_stable_diffusion.StableDiffusionPipeline.decode_latents
def decode_latents(self, latents):
warnings.warn(
"The decode_latents method is deprecated and will be removed in a future version. Please"
" use VaeImageProcessor instead",
FutureWarning,
)
latents = 1 / self.vae.config.scaling_factor * latents
image = self.vae.decode(latents, return_dict=False)[0]
image = (image / 2 + 0.5).clamp(0, 1)
# we always cast to float32 as this does not cause significant overhead and is compatible with bfloat16
image = image.cpu().permute(0, 2, 3, 1).float().numpy()
return image
# Copied from diffusers.pipelines.stable_diffusion.pipeline_stable_diffusion.StableDiffusionPipeline.prepare_extra_step_kwargs
def prepare_extra_step_kwargs(self, generator, eta):
# prepare extra kwargs for the scheduler step, since not all schedulers have the same signature
# eta (η) is only used with the DDIMScheduler, it will be ignored for other schedulers.
# eta corresponds to η in DDIM paper: https://arxiv.org/abs/2010.02502
# and should be between [0, 1]
accepts_eta = "eta" in set(inspect.signature(self.scheduler.step).parameters.keys())
extra_step_kwargs = {}
if accepts_eta:
extra_step_kwargs["eta"] = eta
# check if the scheduler accepts generator
accepts_generator = "generator" in set(inspect.signature(self.scheduler.step).parameters.keys())
if accepts_generator:
extra_step_kwargs["generator"] = generator
return extra_step_kwargs
def check_inputs(
self,
num_controlnet,
prompt,
image,
callback_steps,
negative_prompt=None,
prompt_embeds=None,
negative_prompt_embeds=None,
controlnet_conditioning_scale=1.0,
control_guidance_start=0.0,
control_guidance_end=1.0,
):
if (callback_steps is None) or (
callback_steps is not None and (not isinstance(callback_steps, int) or callback_steps <= 0)
):
raise ValueError(
f"`callback_steps` has to be a positive integer but is {callback_steps} of type"
f" {type(callback_steps)}."
)
if prompt is not None and prompt_embeds is not None:
raise ValueError(
f"Cannot forward both `prompt`: {prompt} and `prompt_embeds`: {prompt_embeds}. Please make sure to"
" only forward one of the two."
)
elif prompt is None and prompt_embeds is None:
raise ValueError(
"Provide either `prompt` or `prompt_embeds`. Cannot leave both `prompt` and `prompt_embeds` undefined."
)
elif prompt is not None and (not isinstance(prompt, str) and not isinstance(prompt, list)):
raise ValueError(f"`prompt` has to be of type `str` or `list` but is {type(prompt)}")
if negative_prompt is not None and negative_prompt_embeds is not None:
raise ValueError(
f"Cannot forward both `negative_prompt`: {negative_prompt} and `negative_prompt_embeds`:"
f" {negative_prompt_embeds}. Please make sure to only forward one of the two."
)
if prompt_embeds is not None and negative_prompt_embeds is not None:
if prompt_embeds.shape != negative_prompt_embeds.shape:
raise ValueError(
"`prompt_embeds` and `negative_prompt_embeds` must have the same shape when passed directly, but"
f" got: `prompt_embeds` {prompt_embeds.shape} != `negative_prompt_embeds`"
f" {negative_prompt_embeds.shape}."
)
# Check `image`
if num_controlnet == 1:
self.check_image(image, prompt, prompt_embeds)
elif num_controlnet > 1:
if not isinstance(image, list):
raise TypeError("For multiple controlnets: `image` must be type `list`")
# When `image` is a nested list:
# (e.g. [[canny_image_1, pose_image_1], [canny_image_2, pose_image_2]])
elif any(isinstance(i, list) for i in image):
raise ValueError("A single batch of multiple conditionings are supported at the moment.")
elif len(image) != num_controlnet:
raise ValueError(
f"For multiple controlnets: `image` must have the same length as the number of controlnets, but got {len(image)} images and {num_controlnet} ControlNets."
)
for image_ in image:
self.check_image(image_, prompt, prompt_embeds)
else:
assert False
# Check `controlnet_conditioning_scale`
if num_controlnet == 1:
if not isinstance(controlnet_conditioning_scale, float):
raise TypeError("For single controlnet: `controlnet_conditioning_scale` must be type `float`.")
elif num_controlnet > 1:
if isinstance(controlnet_conditioning_scale, list):
if any(isinstance(i, list) for i in controlnet_conditioning_scale):
raise ValueError("A single batch of multiple conditionings are supported at the moment.")
elif (
isinstance(controlnet_conditioning_scale, list)
and len(controlnet_conditioning_scale) != num_controlnet
):
raise ValueError(
"For multiple controlnets: When `controlnet_conditioning_scale` is specified as `list`, it must have"
" the same length as the number of controlnets"
)
else:
assert False
if len(control_guidance_start) != len(control_guidance_end):
raise ValueError(
f"`control_guidance_start` has {len(control_guidance_start)} elements, but `control_guidance_end` has {len(control_guidance_end)} elements. Make sure to provide the same number of elements to each list."
)
if num_controlnet > 1:
if len(control_guidance_start) != num_controlnet:
raise ValueError(
f"`control_guidance_start`: {control_guidance_start} has {len(control_guidance_start)} elements but there are {num_controlnet} controlnets available. Make sure to provide {num_controlnet}."
)
for start, end in zip(control_guidance_start, control_guidance_end):
if start >= end:
raise ValueError(
f"control guidance start: {start} cannot be larger or equal to control guidance end: {end}."
)
if start < 0.0:
raise ValueError(f"control guidance start: {start} can't be smaller than 0.")
if end > 1.0:
raise ValueError(f"control guidance end: {end} can't be larger than 1.0.")
# Copied from diffusers.pipelines.controlnet.pipeline_controlnet.StableDiffusionControlNetPipeline.check_image
def check_image(self, image, prompt, prompt_embeds):
image_is_pil = isinstance(image, PIL.Image.Image)
image_is_tensor = isinstance(image, torch.Tensor)
image_is_np = isinstance(image, np.ndarray)
image_is_pil_list = isinstance(image, list) and isinstance(image[0], PIL.Image.Image)
image_is_tensor_list = isinstance(image, list) and isinstance(image[0], torch.Tensor)
image_is_np_list = isinstance(image, list) and isinstance(image[0], np.ndarray)
if (
not image_is_pil
and not image_is_tensor
and not image_is_np
and not image_is_pil_list
and not image_is_tensor_list
and not image_is_np_list
):
raise TypeError(
f"image must be passed and be one of PIL image, numpy array, torch tensor, list of PIL images, list of numpy arrays or list of torch tensors, but is {type(image)}"
)
if image_is_pil:
image_batch_size = 1
else:
image_batch_size = len(image)
if prompt is not None and isinstance(prompt, str):
prompt_batch_size = 1
elif prompt is not None and isinstance(prompt, list):
prompt_batch_size = len(prompt)
elif prompt_embeds is not None:
prompt_batch_size = prompt_embeds.shape[0]
if image_batch_size != 1 and image_batch_size != prompt_batch_size:
raise ValueError(
f"If image batch size is not 1, image batch size must be same as prompt batch size. image batch size: {image_batch_size}, prompt batch size: {prompt_batch_size}"
)
# Copied from diffusers.pipelines.controlnet.pipeline_controlnet.StableDiffusionControlNetPipeline.prepare_image
def prepare_control_image(
self,
image,
width,
height,
batch_size,
num_images_per_prompt,
device,
dtype,
do_classifier_free_guidance=False,
guess_mode=False,
):
image = self.control_image_processor.preprocess(image, height=height, width=width).to(dtype=torch.float32)
image_batch_size = image.shape[0]
if image_batch_size == 1:
repeat_by = batch_size
else:
# image batch size is the same as prompt batch size
repeat_by = num_images_per_prompt
image = image.repeat_interleave(repeat_by, dim=0)
image = image.to(device=device, dtype=dtype)
if do_classifier_free_guidance and not guess_mode:
image = torch.cat([image] * 2)
return image
# Copied from diffusers.pipelines.stable_diffusion.pipeline_stable_diffusion_img2img.StableDiffusionImg2ImgPipeline.get_timesteps
def get_timesteps(self, num_inference_steps, strength, device):
# get the original timestep using init_timestep
init_timestep = min(int(num_inference_steps * strength), num_inference_steps)
t_start = max(num_inference_steps - init_timestep, 0)
timesteps = self.scheduler.timesteps[t_start * self.scheduler.order :]
return timesteps, num_inference_steps - t_start
def prepare_latents(self, image, timestep, batch_size, num_images_per_prompt, dtype, device, generator=None):
if not isinstance(image, (torch.Tensor, PIL.Image.Image, list)):
raise ValueError(
f"`image` has to be of type `torch.Tensor`, `PIL.Image.Image` or list but is {type(image)}"
)
image = image.to(device=device, dtype=dtype)
batch_size = batch_size * num_images_per_prompt
if image.shape[1] == 4:
init_latents = image
else:
_image = image.cpu().detach().numpy()
init_latents = self.vae_encoder(sample=_image)[0]
init_latents = torch.from_numpy(init_latents).to(device=device, dtype=dtype)
init_latents = 0.18215 * init_latents
if batch_size > init_latents.shape[0] and batch_size % init_latents.shape[0] == 0:
# expand init_latents for batch_size
deprecation_message = (
f"You have passed {batch_size} text prompts (`prompt`), but only {init_latents.shape[0]} initial"
" images (`image`). Initial images are now duplicating to match the number of text prompts. Note"
" that this behavior is deprecated and will be removed in a version 1.0.0. Please make sure to update"
" your script to pass as many initial images as text prompts to suppress this warning."
)
deprecate("len(prompt) != len(image)", "1.0.0", deprecation_message, standard_warn=False)
additional_image_per_prompt = batch_size // init_latents.shape[0]
init_latents = torch.cat([init_latents] * additional_image_per_prompt, dim=0)
elif batch_size > init_latents.shape[0] and batch_size % init_latents.shape[0] != 0:
raise ValueError(
f"Cannot duplicate `image` of batch size {init_latents.shape[0]} to {batch_size} text prompts."
)
else:
init_latents = torch.cat([init_latents], dim=0)
shape = init_latents.shape
noise = randn_tensor(shape, generator=generator, device=device, dtype=dtype)
# get latents
init_latents = self.scheduler.add_noise(init_latents, noise, timestep)
latents = init_latents
return latents
@torch.no_grad()
@replace_example_docstring(EXAMPLE_DOC_STRING)
def __call__(
self,
num_controlnet: int,
fp16: bool = True,
prompt: Union[str, List[str]] = None,
image: Union[
torch.FloatTensor,
PIL.Image.Image,
np.ndarray,
List[torch.FloatTensor],
List[PIL.Image.Image],
List[np.ndarray],
] = None,
control_image: Union[
torch.FloatTensor,
PIL.Image.Image,
np.ndarray,
List[torch.FloatTensor],
List[PIL.Image.Image],
List[np.ndarray],
] = None,
height: Optional[int] = None,
width: Optional[int] = None,
strength: float = 0.8,
num_inference_steps: int = 50,
guidance_scale: float = 7.5,
negative_prompt: Optional[Union[str, List[str]]] = None,
num_images_per_prompt: Optional[int] = 1,
eta: float = 0.0,
generator: Optional[Union[torch.Generator, List[torch.Generator]]] = None,
latents: Optional[torch.FloatTensor] = None,
prompt_embeds: Optional[torch.FloatTensor] = None,
negative_prompt_embeds: Optional[torch.FloatTensor] = None,
output_type: Optional[str] = "pil",
return_dict: bool = True,
callback: Optional[Callable[[int, int, torch.FloatTensor], None]] = None,
callback_steps: int = 1,
cross_attention_kwargs: Optional[Dict[str, Any]] = None,
controlnet_conditioning_scale: Union[float, List[float]] = 0.8,
guess_mode: bool = False,
control_guidance_start: Union[float, List[float]] = 0.0,
control_guidance_end: Union[float, List[float]] = 1.0,
):
r"""
Function invoked when calling the pipeline for generation.
Args:
prompt (`str` or `List[str]`, *optional*):
The prompt or prompts to guide the image generation. If not defined, one has to pass `prompt_embeds`.
instead.
image (`torch.FloatTensor`, `PIL.Image.Image`, `np.ndarray`, `List[torch.FloatTensor]`, `List[PIL.Image.Image]`, `List[np.ndarray]`,:
`List[List[torch.FloatTensor]]`, `List[List[np.ndarray]]` or `List[List[PIL.Image.Image]]`):
The initial image will be used as the starting point for the image generation process. Can also accept
image latents as `image`, if passing latents directly, it will not be encoded again.
control_image (`torch.FloatTensor`, `PIL.Image.Image`, `np.ndarray`, `List[torch.FloatTensor]`, `List[PIL.Image.Image]`, `List[np.ndarray]`,:
`List[List[torch.FloatTensor]]`, `List[List[np.ndarray]]` or `List[List[PIL.Image.Image]]`):
The ControlNet input condition. ControlNet uses this input condition to generate guidance to Unet. If
the type is specified as `Torch.FloatTensor`, it is passed to ControlNet as is. `PIL.Image.Image` can
also be accepted as an image. The dimensions of the output image defaults to `image`'s dimensions. If
height and/or width are passed, `image` is resized according to them. If multiple ControlNets are
specified in init, images must be passed as a list such that each element of the list can be correctly
batched for input to a single controlnet.
height (`int`, *optional*, defaults to self.unet.config.sample_size * self.vae_scale_factor):
The height in pixels of the generated image.
width (`int`, *optional*, defaults to self.unet.config.sample_size * self.vae_scale_factor):
The width in pixels of the generated image.
num_inference_steps (`int`, *optional*, defaults to 50):
The number of denoising steps. More denoising steps usually lead to a higher quality image at the
expense of slower inference.
guidance_scale (`float`, *optional*, defaults to 7.5):
Guidance scale as defined in [Classifier-Free Diffusion Guidance](https://arxiv.org/abs/2207.12598).
`guidance_scale` is defined as `w` of equation 2. of [Imagen
Paper](https://arxiv.org/pdf/2205.11487.pdf). Guidance scale is enabled by setting `guidance_scale >
1`. Higher guidance scale encourages to generate images that are closely linked to the text `prompt`,
usually at the expense of lower image quality.
negative_prompt (`str` or `List[str]`, *optional*):
The prompt or prompts not to guide the image generation. If not defined, one has to pass
`negative_prompt_embeds` instead. Ignored when not using guidance (i.e., ignored if `guidance_scale` is
less than `1`).
num_images_per_prompt (`int`, *optional*, defaults to 1):
The number of images to generate per prompt.
eta (`float`, *optional*, defaults to 0.0):
Corresponds to parameter eta (η) in the DDIM paper: https://arxiv.org/abs/2010.02502. Only applies to
[`schedulers.DDIMScheduler`], will be ignored for others.
generator (`torch.Generator` or `List[torch.Generator]`, *optional*):
One or a list of [torch generator(s)](https://pytorch.org/docs/stable/generated/torch.Generator.html)
to make generation deterministic.
latents (`torch.FloatTensor`, *optional*):
Pre-generated noisy latents, sampled from a Gaussian distribution, to be used as inputs for image
generation. Can be used to tweak the same generation with different prompts. If not provided, a latents
tensor will ge generated by sampling using the supplied random `generator`.
prompt_embeds (`torch.FloatTensor`, *optional*):
Pre-generated text embeddings. Can be used to easily tweak text inputs, *e.g.* prompt weighting. If not
provided, text embeddings will be generated from `prompt` input argument.
negative_prompt_embeds (`torch.FloatTensor`, *optional*):
Pre-generated negative text embeddings. Can be used to easily tweak text inputs, *e.g.* prompt
weighting. If not provided, negative_prompt_embeds will be generated from `negative_prompt` input
argument.
output_type (`str`, *optional*, defaults to `"pil"`):
The output format of the generate image. Choose between
[PIL](https://pillow.readthedocs.io/en/stable/): `PIL.Image.Image` or `np.array`.
return_dict (`bool`, *optional*, defaults to `True`):
Whether or not to return a [`~pipelines.stable_diffusion.StableDiffusionPipelineOutput`] instead of a
plain tuple.
callback (`Callable`, *optional*):
A function that will be called every `callback_steps` steps during inference. The function will be
called with the following arguments: `callback(step: int, timestep: int, latents: torch.FloatTensor)`.
callback_steps (`int`, *optional*, defaults to 1):
The frequency at which the `callback` function will be called. If not specified, the callback will be
called at every step.
cross_attention_kwargs (`dict`, *optional*):
A kwargs dictionary that if specified is passed along to the `AttentionProcessor` as defined under
`self.processor` in
[diffusers.models.attention_processor](https://github.com/huggingface/diffusers/blob/main/src/diffusers/models/attention_processor.py).
controlnet_conditioning_scale (`float` or `List[float]`, *optional*, defaults to 1.0):
The outputs of the controlnet are multiplied by `controlnet_conditioning_scale` before they are added
to the residual in the original unet. If multiple ControlNets are specified in init, you can set the
corresponding scale as a list. Note that by default, we use a smaller conditioning scale for inpainting
than for [`~StableDiffusionControlNetPipeline.__call__`].
guess_mode (`bool`, *optional*, defaults to `False`):
In this mode, the ControlNet encoder will try best to recognize the content of the input image even if
you remove all prompts. The `guidance_scale` between 3.0 and 5.0 is recommended.
control_guidance_start (`float` or `List[float]`, *optional*, defaults to 0.0):
The percentage of total steps at which the controlnet starts applying.
control_guidance_end (`float` or `List[float]`, *optional*, defaults to 1.0):
The percentage of total steps at which the controlnet stops applying.
Examples:
Returns:
[`~pipelines.stable_diffusion.StableDiffusionPipelineOutput`] or `tuple`:
[`~pipelines.stable_diffusion.StableDiffusionPipelineOutput`] if `return_dict` is True, otherwise a `tuple.
When returning a tuple, the first element is a list with the generated images, and the second element is a
list of `bool`s denoting whether the corresponding generated image likely represents "not-safe-for-work"
(nsfw) content, according to the `safety_checker`.
"""
if fp16:
torch_dtype = torch.float16
np_dtype = np.float16
else:
torch_dtype = torch.float32
np_dtype = np.float32
# align format for control guidance
if not isinstance(control_guidance_start, list) and isinstance(control_guidance_end, list):
control_guidance_start = len(control_guidance_end) * [control_guidance_start]
elif not isinstance(control_guidance_end, list) and isinstance(control_guidance_start, list):
control_guidance_end = len(control_guidance_start) * [control_guidance_end]
elif not isinstance(control_guidance_start, list) and not isinstance(control_guidance_end, list):
mult = num_controlnet
control_guidance_start, control_guidance_end = (
mult * [control_guidance_start],
mult * [control_guidance_end],
)
# 1. Check inputs. Raise error if not correct
self.check_inputs(
num_controlnet,
prompt,
control_image,
callback_steps,
negative_prompt,
prompt_embeds,
negative_prompt_embeds,
controlnet_conditioning_scale,
control_guidance_start,
control_guidance_end,
)
# 2. Define call parameters
if prompt is not None and isinstance(prompt, str):
batch_size = 1
elif prompt is not None and isinstance(prompt, list):
batch_size = len(prompt)
else:
batch_size = prompt_embeds.shape[0]
device = self._execution_device
# here `guidance_scale` is defined analog to the guidance weight `w` of equation (2)
# of the Imagen paper: https://arxiv.org/pdf/2205.11487.pdf . `guidance_scale = 1`
# corresponds to doing no classifier free guidance.
do_classifier_free_guidance = guidance_scale > 1.0
if num_controlnet > 1 and isinstance(controlnet_conditioning_scale, float):
controlnet_conditioning_scale = [controlnet_conditioning_scale] * num_controlnet
# 3. Encode input prompt
prompt_embeds = self._encode_prompt(
prompt,
num_images_per_prompt,
do_classifier_free_guidance,
negative_prompt,
prompt_embeds=prompt_embeds,
negative_prompt_embeds=negative_prompt_embeds,
)
# 4. Prepare image
image = self.image_processor.preprocess(image).to(dtype=torch.float32)
# 5. Prepare controlnet_conditioning_image
if num_controlnet == 1:
control_image = self.prepare_control_image(
image=control_image,
width=width,
height=height,
batch_size=batch_size * num_images_per_prompt,
num_images_per_prompt=num_images_per_prompt,
device=device,
dtype=torch_dtype,
do_classifier_free_guidance=do_classifier_free_guidance,
guess_mode=guess_mode,
)
elif num_controlnet > 1:
control_images = []
for control_image_ in control_image:
control_image_ = self.prepare_control_image(
image=control_image_,
width=width,
height=height,
batch_size=batch_size * num_images_per_prompt,
num_images_per_prompt=num_images_per_prompt,
device=device,
dtype=torch_dtype,
do_classifier_free_guidance=do_classifier_free_guidance,
guess_mode=guess_mode,
)
control_images.append(control_image_)
control_image = control_images
else:
assert False
# 5. Prepare timesteps
self.scheduler.set_timesteps(num_inference_steps, device=device)
timesteps, num_inference_steps = self.get_timesteps(num_inference_steps, strength, device)
latent_timestep = timesteps[:1].repeat(batch_size * num_images_per_prompt)
# 6. Prepare latent variables
latents = self.prepare_latents(
image,
latent_timestep,
batch_size,
num_images_per_prompt,
torch_dtype,
device,
generator,
)
# 7. Prepare extra step kwargs. TODO: Logic should ideally just be moved out of the pipeline
extra_step_kwargs = self.prepare_extra_step_kwargs(generator, eta)
# 7.1 Create tensor stating which controlnets to keep
controlnet_keep = []
for i in range(len(timesteps)):
keeps = [
1.0 - float(i / len(timesteps) < s or (i + 1) / len(timesteps) > e)
for s, e in zip(control_guidance_start, control_guidance_end)
]
controlnet_keep.append(keeps[0] if num_controlnet == 1 else keeps)
# 8. Denoising loop
num_warmup_steps = len(timesteps) - num_inference_steps * self.scheduler.order
with self.progress_bar(total=num_inference_steps) as progress_bar:
for i, t in enumerate(timesteps):
# expand the latents if we are doing classifier free guidance
latent_model_input = torch.cat([latents] * 2) if do_classifier_free_guidance else latents
latent_model_input = self.scheduler.scale_model_input(latent_model_input, t)
if isinstance(controlnet_keep[i], list):
cond_scale = [c * s for c, s in zip(controlnet_conditioning_scale, controlnet_keep[i])]
else:
controlnet_cond_scale = controlnet_conditioning_scale
if isinstance(controlnet_cond_scale, list):
controlnet_cond_scale = controlnet_cond_scale[0]
cond_scale = controlnet_cond_scale * controlnet_keep[i]
# predict the noise residual
_latent_model_input = latent_model_input.cpu().detach().numpy()
_prompt_embeds = np.array(prompt_embeds, dtype=np_dtype)
_t = np.array([t.cpu().detach().numpy()], dtype=np_dtype)
if num_controlnet == 1:
control_images = np.array([control_image], dtype=np_dtype)
else:
control_images = []
for _control_img in control_image:
_control_img = _control_img.cpu().detach().numpy()
control_images.append(_control_img)
control_images = np.array(control_images, dtype=np_dtype)
control_scales = np.array(cond_scale, dtype=np_dtype)
control_scales = np.resize(control_scales, (num_controlnet, 1))
noise_pred = self.unet(
sample=_latent_model_input,
timestep=_t,
encoder_hidden_states=_prompt_embeds,
controlnet_conds=control_images,
conditioning_scales=control_scales,
)[0]
noise_pred = torch.from_numpy(noise_pred).to(device)
# perform guidance
if do_classifier_free_guidance:
noise_pred_uncond, noise_pred_text = noise_pred.chunk(2)
noise_pred = noise_pred_uncond + guidance_scale * (noise_pred_text - noise_pred_uncond)
# compute the previous noisy sample x_t -> x_t-1
latents = self.scheduler.step(noise_pred, t, latents, **extra_step_kwargs, return_dict=False)[0]
# call the callback, if provided
if i == len(timesteps) - 1 or ((i + 1) > num_warmup_steps and (i + 1) % self.scheduler.order == 0):
progress_bar.update()
if callback is not None and i % callback_steps == 0:
step_idx = i // getattr(self.scheduler, "order", 1)
callback(step_idx, t, latents)
if not output_type == "latent":
_latents = latents.cpu().detach().numpy() / 0.18215
_latents = np.array(_latents, dtype=np_dtype)
image = self.vae_decoder(latent_sample=_latents)[0]
image = torch.from_numpy(image).to(device, dtype=torch.float32)
has_nsfw_concept = None
else:
image = latents
has_nsfw_concept = None
if has_nsfw_concept is None:
do_denormalize = [True] * image.shape[0]
else:
do_denormalize = [not has_nsfw for has_nsfw in has_nsfw_concept]
image = self.image_processor.postprocess(image, output_type=output_type, do_denormalize=do_denormalize)
if not return_dict:
return (image, has_nsfw_concept)
return StableDiffusionPipelineOutput(images=image, nsfw_content_detected=has_nsfw_concept)
if __name__ == "__main__":
parser = argparse.ArgumentParser()
parser.add_argument(
"--sd_model",
type=str,
required=True,
help="Path to the `diffusers` checkpoint to convert (either a local directory or on the Hub).",
)
parser.add_argument(
"--onnx_model_dir",
type=str,
required=True,
help="Path to the ONNX directory",
)
parser.add_argument("--qr_img_path", type=str, required=True, help="Path to the qr code image")
args = parser.parse_args()
qr_image = Image.open(args.qr_img_path)
qr_image = qr_image.resize((512, 512))
# init stable diffusion pipeline
pipeline = StableDiffusionImg2ImgPipeline.from_pretrained(args.sd_model)
pipeline.scheduler = UniPCMultistepScheduler.from_config(pipeline.scheduler.config)
provider = ["CUDAExecutionProvider", "CPUExecutionProvider"]
onnx_pipeline = OnnxStableDiffusionControlNetImg2ImgPipeline(
vae_encoder=OnnxRuntimeModel.from_pretrained(
os.path.join(args.onnx_model_dir, "vae_encoder"), provider=provider
),
vae_decoder=OnnxRuntimeModel.from_pretrained(
os.path.join(args.onnx_model_dir, "vae_decoder"), provider=provider
),
text_encoder=OnnxRuntimeModel.from_pretrained(
os.path.join(args.onnx_model_dir, "text_encoder"), provider=provider
),
tokenizer=pipeline.tokenizer,
unet=OnnxRuntimeModel.from_pretrained(os.path.join(args.onnx_model_dir, "unet"), provider=provider),
scheduler=pipeline.scheduler,
)
onnx_pipeline = onnx_pipeline.to("cuda")
prompt = "a cute cat fly to the moon"
negative_prompt = "paintings, sketches, worst quality, low quality, normal quality, lowres, normal quality, monochrome, grayscale, skin spots, acnes, skin blemishes, age spot, glans, nsfw, nipples, necklace, worst quality, low quality, watermark, username, signature, multiple breasts, lowres, bad anatomy, bad hands, error, missing fingers, extra digit, fewer digits, cropped, worst quality, low quality, normal quality, jpeg artifacts, signature, watermark, username, blurry, bad feet, single color, ugly, duplicate, morbid, mutilated, extra fingers, mutated hands, poorly drawn hands, poorly drawn face, mutation, deformed, ugly, blurry, bad anatomy, bad proportions, extra limbs, disfigured, bad anatomy, gross proportions, malformed limbs, missing arms, missing legs, extra arms, extra legs, mutated hands, fused fingers, too many fingers, long neck, bad body perspect"
for i in range(10):
start_time = time.time()
image = onnx_pipeline(
num_controlnet=2,
prompt=prompt,
negative_prompt=negative_prompt,
image=qr_image,
control_image=[qr_image, qr_image],
width=512,
height=512,
strength=0.75,
num_inference_steps=20,
num_images_per_prompt=1,
controlnet_conditioning_scale=[0.8, 0.8],
control_guidance_start=[0.3, 0.3],
control_guidance_end=[0.9, 0.9],
).images[0]
print(time.time() - start_time)
image.save("output_qr_code.png")
| diffusers/examples/community/run_onnx_controlnet.py/0 | {
"file_path": "diffusers/examples/community/run_onnx_controlnet.py",
"repo_id": "diffusers",
"token_count": 19745
} | 105 |
#
# Copyright 2024 The HuggingFace Inc. team.
# SPDX-FileCopyrightText: Copyright (c) 1993-2023 NVIDIA CORPORATION & AFFILIATES. All rights reserved.
# SPDX-License-Identifier: Apache-2.0
#
# Licensed under the Apache License, Version 2.0 (the "License");
# you may not use this file except in compliance with the License.
# You may obtain a copy of the License at
#
# http://www.apache.org/licenses/LICENSE-2.0
#
# Unless required by applicable law or agreed to in writing, software
# distributed under the License is distributed on an "AS IS" BASIS,
# WITHOUT WARRANTIES OR CONDITIONS OF ANY KIND, either express or implied.
# See the License for the specific language governing permissions and
# limitations under the License.
import gc
import os
from collections import OrderedDict
from copy import copy
from typing import List, Optional, Union
import numpy as np
import onnx
import onnx_graphsurgeon as gs
import PIL.Image
import tensorrt as trt
import torch
from huggingface_hub import snapshot_download
from huggingface_hub.utils import validate_hf_hub_args
from onnx import shape_inference
from polygraphy import cuda
from polygraphy.backend.common import bytes_from_path
from polygraphy.backend.onnx.loader import fold_constants
from polygraphy.backend.trt import (
CreateConfig,
Profile,
engine_from_bytes,
engine_from_network,
network_from_onnx_path,
save_engine,
)
from polygraphy.backend.trt import util as trt_util
from transformers import CLIPFeatureExtractor, CLIPTextModel, CLIPTokenizer, CLIPVisionModelWithProjection
from diffusers.models import AutoencoderKL, UNet2DConditionModel
from diffusers.pipelines.stable_diffusion import (
StableDiffusionImg2ImgPipeline,
StableDiffusionPipelineOutput,
StableDiffusionSafetyChecker,
)
from diffusers.pipelines.stable_diffusion.pipeline_stable_diffusion_img2img import retrieve_latents
from diffusers.schedulers import DDIMScheduler
from diffusers.utils import logging
"""
Installation instructions
python3 -m pip install --upgrade transformers diffusers>=0.16.0
python3 -m pip install --upgrade tensorrt>=8.6.1
python3 -m pip install --upgrade polygraphy>=0.47.0 onnx-graphsurgeon --extra-index-url https://pypi.ngc.nvidia.com
python3 -m pip install onnxruntime
"""
TRT_LOGGER = trt.Logger(trt.Logger.ERROR)
logger = logging.get_logger(__name__) # pylint: disable=invalid-name
# Map of numpy dtype -> torch dtype
numpy_to_torch_dtype_dict = {
np.uint8: torch.uint8,
np.int8: torch.int8,
np.int16: torch.int16,
np.int32: torch.int32,
np.int64: torch.int64,
np.float16: torch.float16,
np.float32: torch.float32,
np.float64: torch.float64,
np.complex64: torch.complex64,
np.complex128: torch.complex128,
}
if np.version.full_version >= "1.24.0":
numpy_to_torch_dtype_dict[np.bool_] = torch.bool
else:
numpy_to_torch_dtype_dict[np.bool] = torch.bool
# Map of torch dtype -> numpy dtype
torch_to_numpy_dtype_dict = {value: key for (key, value) in numpy_to_torch_dtype_dict.items()}
def device_view(t):
return cuda.DeviceView(ptr=t.data_ptr(), shape=t.shape, dtype=torch_to_numpy_dtype_dict[t.dtype])
def preprocess_image(image):
"""
image: torch.Tensor
"""
w, h = image.size
w, h = (x - x % 32 for x in (w, h)) # resize to integer multiple of 32
image = image.resize((w, h))
image = np.array(image).astype(np.float32) / 255.0
image = image[None].transpose(0, 3, 1, 2)
image = torch.from_numpy(image).contiguous()
return 2.0 * image - 1.0
class Engine:
def __init__(self, engine_path):
self.engine_path = engine_path
self.engine = None
self.context = None
self.buffers = OrderedDict()
self.tensors = OrderedDict()
def __del__(self):
[buf.free() for buf in self.buffers.values() if isinstance(buf, cuda.DeviceArray)]
del self.engine
del self.context
del self.buffers
del self.tensors
def build(
self,
onnx_path,
fp16,
input_profile=None,
enable_preview=False,
enable_all_tactics=False,
timing_cache=None,
workspace_size=0,
):
logger.warning(f"Building TensorRT engine for {onnx_path}: {self.engine_path}")
p = Profile()
if input_profile:
for name, dims in input_profile.items():
assert len(dims) == 3
p.add(name, min=dims[0], opt=dims[1], max=dims[2])
config_kwargs = {}
config_kwargs["preview_features"] = [trt.PreviewFeature.DISABLE_EXTERNAL_TACTIC_SOURCES_FOR_CORE_0805]
if enable_preview:
# Faster dynamic shapes made optional since it increases engine build time.
config_kwargs["preview_features"].append(trt.PreviewFeature.FASTER_DYNAMIC_SHAPES_0805)
if workspace_size > 0:
config_kwargs["memory_pool_limits"] = {trt.MemoryPoolType.WORKSPACE: workspace_size}
if not enable_all_tactics:
config_kwargs["tactic_sources"] = []
engine = engine_from_network(
network_from_onnx_path(onnx_path, flags=[trt.OnnxParserFlag.NATIVE_INSTANCENORM]),
config=CreateConfig(fp16=fp16, profiles=[p], load_timing_cache=timing_cache, **config_kwargs),
save_timing_cache=timing_cache,
)
save_engine(engine, path=self.engine_path)
def load(self):
logger.warning(f"Loading TensorRT engine: {self.engine_path}")
self.engine = engine_from_bytes(bytes_from_path(self.engine_path))
def activate(self):
self.context = self.engine.create_execution_context()
def allocate_buffers(self, shape_dict=None, device="cuda"):
for idx in range(trt_util.get_bindings_per_profile(self.engine)):
binding = self.engine[idx]
if shape_dict and binding in shape_dict:
shape = shape_dict[binding]
else:
shape = self.engine.get_binding_shape(binding)
dtype = trt.nptype(self.engine.get_binding_dtype(binding))
if self.engine.binding_is_input(binding):
self.context.set_binding_shape(idx, shape)
tensor = torch.empty(tuple(shape), dtype=numpy_to_torch_dtype_dict[dtype]).to(device=device)
self.tensors[binding] = tensor
self.buffers[binding] = cuda.DeviceView(ptr=tensor.data_ptr(), shape=shape, dtype=dtype)
def infer(self, feed_dict, stream):
start_binding, end_binding = trt_util.get_active_profile_bindings(self.context)
# shallow copy of ordered dict
device_buffers = copy(self.buffers)
for name, buf in feed_dict.items():
assert isinstance(buf, cuda.DeviceView)
device_buffers[name] = buf
bindings = [0] * start_binding + [buf.ptr for buf in device_buffers.values()]
noerror = self.context.execute_async_v2(bindings=bindings, stream_handle=stream.ptr)
if not noerror:
raise ValueError("ERROR: inference failed.")
return self.tensors
class Optimizer:
def __init__(self, onnx_graph):
self.graph = gs.import_onnx(onnx_graph)
def cleanup(self, return_onnx=False):
self.graph.cleanup().toposort()
if return_onnx:
return gs.export_onnx(self.graph)
def select_outputs(self, keep, names=None):
self.graph.outputs = [self.graph.outputs[o] for o in keep]
if names:
for i, name in enumerate(names):
self.graph.outputs[i].name = name
def fold_constants(self, return_onnx=False):
onnx_graph = fold_constants(gs.export_onnx(self.graph), allow_onnxruntime_shape_inference=True)
self.graph = gs.import_onnx(onnx_graph)
if return_onnx:
return onnx_graph
def infer_shapes(self, return_onnx=False):
onnx_graph = gs.export_onnx(self.graph)
if onnx_graph.ByteSize() > 2147483648:
raise TypeError("ERROR: model size exceeds supported 2GB limit")
else:
onnx_graph = shape_inference.infer_shapes(onnx_graph)
self.graph = gs.import_onnx(onnx_graph)
if return_onnx:
return onnx_graph
class BaseModel:
def __init__(self, model, fp16=False, device="cuda", max_batch_size=16, embedding_dim=768, text_maxlen=77):
self.model = model
self.name = "SD Model"
self.fp16 = fp16
self.device = device
self.min_batch = 1
self.max_batch = max_batch_size
self.min_image_shape = 256 # min image resolution: 256x256
self.max_image_shape = 1024 # max image resolution: 1024x1024
self.min_latent_shape = self.min_image_shape // 8
self.max_latent_shape = self.max_image_shape // 8
self.embedding_dim = embedding_dim
self.text_maxlen = text_maxlen
def get_model(self):
return self.model
def get_input_names(self):
pass
def get_output_names(self):
pass
def get_dynamic_axes(self):
return None
def get_sample_input(self, batch_size, image_height, image_width):
pass
def get_input_profile(self, batch_size, image_height, image_width, static_batch, static_shape):
return None
def get_shape_dict(self, batch_size, image_height, image_width):
return None
def optimize(self, onnx_graph):
opt = Optimizer(onnx_graph)
opt.cleanup()
opt.fold_constants()
opt.infer_shapes()
onnx_opt_graph = opt.cleanup(return_onnx=True)
return onnx_opt_graph
def check_dims(self, batch_size, image_height, image_width):
assert batch_size >= self.min_batch and batch_size <= self.max_batch
assert image_height % 8 == 0 or image_width % 8 == 0
latent_height = image_height // 8
latent_width = image_width // 8
assert latent_height >= self.min_latent_shape and latent_height <= self.max_latent_shape
assert latent_width >= self.min_latent_shape and latent_width <= self.max_latent_shape
return (latent_height, latent_width)
def get_minmax_dims(self, batch_size, image_height, image_width, static_batch, static_shape):
min_batch = batch_size if static_batch else self.min_batch
max_batch = batch_size if static_batch else self.max_batch
latent_height = image_height // 8
latent_width = image_width // 8
min_image_height = image_height if static_shape else self.min_image_shape
max_image_height = image_height if static_shape else self.max_image_shape
min_image_width = image_width if static_shape else self.min_image_shape
max_image_width = image_width if static_shape else self.max_image_shape
min_latent_height = latent_height if static_shape else self.min_latent_shape
max_latent_height = latent_height if static_shape else self.max_latent_shape
min_latent_width = latent_width if static_shape else self.min_latent_shape
max_latent_width = latent_width if static_shape else self.max_latent_shape
return (
min_batch,
max_batch,
min_image_height,
max_image_height,
min_image_width,
max_image_width,
min_latent_height,
max_latent_height,
min_latent_width,
max_latent_width,
)
def getOnnxPath(model_name, onnx_dir, opt=True):
return os.path.join(onnx_dir, model_name + (".opt" if opt else "") + ".onnx")
def getEnginePath(model_name, engine_dir):
return os.path.join(engine_dir, model_name + ".plan")
def build_engines(
models: dict,
engine_dir,
onnx_dir,
onnx_opset,
opt_image_height,
opt_image_width,
opt_batch_size=1,
force_engine_rebuild=False,
static_batch=False,
static_shape=True,
enable_preview=False,
enable_all_tactics=False,
timing_cache=None,
max_workspace_size=0,
):
built_engines = {}
if not os.path.isdir(onnx_dir):
os.makedirs(onnx_dir)
if not os.path.isdir(engine_dir):
os.makedirs(engine_dir)
# Export models to ONNX
for model_name, model_obj in models.items():
engine_path = getEnginePath(model_name, engine_dir)
if force_engine_rebuild or not os.path.exists(engine_path):
logger.warning("Building Engines...")
logger.warning("Engine build can take a while to complete")
onnx_path = getOnnxPath(model_name, onnx_dir, opt=False)
onnx_opt_path = getOnnxPath(model_name, onnx_dir)
if force_engine_rebuild or not os.path.exists(onnx_opt_path):
if force_engine_rebuild or not os.path.exists(onnx_path):
logger.warning(f"Exporting model: {onnx_path}")
model = model_obj.get_model()
with torch.inference_mode(), torch.autocast("cuda"):
inputs = model_obj.get_sample_input(opt_batch_size, opt_image_height, opt_image_width)
torch.onnx.export(
model,
inputs,
onnx_path,
export_params=True,
opset_version=onnx_opset,
do_constant_folding=True,
input_names=model_obj.get_input_names(),
output_names=model_obj.get_output_names(),
dynamic_axes=model_obj.get_dynamic_axes(),
)
del model
torch.cuda.empty_cache()
gc.collect()
else:
logger.warning(f"Found cached model: {onnx_path}")
# Optimize onnx
if force_engine_rebuild or not os.path.exists(onnx_opt_path):
logger.warning(f"Generating optimizing model: {onnx_opt_path}")
onnx_opt_graph = model_obj.optimize(onnx.load(onnx_path))
onnx.save(onnx_opt_graph, onnx_opt_path)
else:
logger.warning(f"Found cached optimized model: {onnx_opt_path} ")
# Build TensorRT engines
for model_name, model_obj in models.items():
engine_path = getEnginePath(model_name, engine_dir)
engine = Engine(engine_path)
onnx_path = getOnnxPath(model_name, onnx_dir, opt=False)
onnx_opt_path = getOnnxPath(model_name, onnx_dir)
if force_engine_rebuild or not os.path.exists(engine.engine_path):
engine.build(
onnx_opt_path,
fp16=True,
input_profile=model_obj.get_input_profile(
opt_batch_size,
opt_image_height,
opt_image_width,
static_batch=static_batch,
static_shape=static_shape,
),
enable_preview=enable_preview,
timing_cache=timing_cache,
workspace_size=max_workspace_size,
)
built_engines[model_name] = engine
# Load and activate TensorRT engines
for model_name, model_obj in models.items():
engine = built_engines[model_name]
engine.load()
engine.activate()
return built_engines
def runEngine(engine, feed_dict, stream):
return engine.infer(feed_dict, stream)
class CLIP(BaseModel):
def __init__(self, model, device, max_batch_size, embedding_dim):
super(CLIP, self).__init__(
model=model, device=device, max_batch_size=max_batch_size, embedding_dim=embedding_dim
)
self.name = "CLIP"
def get_input_names(self):
return ["input_ids"]
def get_output_names(self):
return ["text_embeddings", "pooler_output"]
def get_dynamic_axes(self):
return {"input_ids": {0: "B"}, "text_embeddings": {0: "B"}}
def get_input_profile(self, batch_size, image_height, image_width, static_batch, static_shape):
self.check_dims(batch_size, image_height, image_width)
min_batch, max_batch, _, _, _, _, _, _, _, _ = self.get_minmax_dims(
batch_size, image_height, image_width, static_batch, static_shape
)
return {
"input_ids": [(min_batch, self.text_maxlen), (batch_size, self.text_maxlen), (max_batch, self.text_maxlen)]
}
def get_shape_dict(self, batch_size, image_height, image_width):
self.check_dims(batch_size, image_height, image_width)
return {
"input_ids": (batch_size, self.text_maxlen),
"text_embeddings": (batch_size, self.text_maxlen, self.embedding_dim),
}
def get_sample_input(self, batch_size, image_height, image_width):
self.check_dims(batch_size, image_height, image_width)
return torch.zeros(batch_size, self.text_maxlen, dtype=torch.int32, device=self.device)
def optimize(self, onnx_graph):
opt = Optimizer(onnx_graph)
opt.select_outputs([0]) # delete graph output#1
opt.cleanup()
opt.fold_constants()
opt.infer_shapes()
opt.select_outputs([0], names=["text_embeddings"]) # rename network output
opt_onnx_graph = opt.cleanup(return_onnx=True)
return opt_onnx_graph
def make_CLIP(model, device, max_batch_size, embedding_dim, inpaint=False):
return CLIP(model, device=device, max_batch_size=max_batch_size, embedding_dim=embedding_dim)
class UNet(BaseModel):
def __init__(
self, model, fp16=False, device="cuda", max_batch_size=16, embedding_dim=768, text_maxlen=77, unet_dim=4
):
super(UNet, self).__init__(
model=model,
fp16=fp16,
device=device,
max_batch_size=max_batch_size,
embedding_dim=embedding_dim,
text_maxlen=text_maxlen,
)
self.unet_dim = unet_dim
self.name = "UNet"
def get_input_names(self):
return ["sample", "timestep", "encoder_hidden_states"]
def get_output_names(self):
return ["latent"]
def get_dynamic_axes(self):
return {
"sample": {0: "2B", 2: "H", 3: "W"},
"encoder_hidden_states": {0: "2B"},
"latent": {0: "2B", 2: "H", 3: "W"},
}
def get_input_profile(self, batch_size, image_height, image_width, static_batch, static_shape):
latent_height, latent_width = self.check_dims(batch_size, image_height, image_width)
(
min_batch,
max_batch,
_,
_,
_,
_,
min_latent_height,
max_latent_height,
min_latent_width,
max_latent_width,
) = self.get_minmax_dims(batch_size, image_height, image_width, static_batch, static_shape)
return {
"sample": [
(2 * min_batch, self.unet_dim, min_latent_height, min_latent_width),
(2 * batch_size, self.unet_dim, latent_height, latent_width),
(2 * max_batch, self.unet_dim, max_latent_height, max_latent_width),
],
"encoder_hidden_states": [
(2 * min_batch, self.text_maxlen, self.embedding_dim),
(2 * batch_size, self.text_maxlen, self.embedding_dim),
(2 * max_batch, self.text_maxlen, self.embedding_dim),
],
}
def get_shape_dict(self, batch_size, image_height, image_width):
latent_height, latent_width = self.check_dims(batch_size, image_height, image_width)
return {
"sample": (2 * batch_size, self.unet_dim, latent_height, latent_width),
"encoder_hidden_states": (2 * batch_size, self.text_maxlen, self.embedding_dim),
"latent": (2 * batch_size, 4, latent_height, latent_width),
}
def get_sample_input(self, batch_size, image_height, image_width):
latent_height, latent_width = self.check_dims(batch_size, image_height, image_width)
dtype = torch.float16 if self.fp16 else torch.float32
return (
torch.randn(
2 * batch_size, self.unet_dim, latent_height, latent_width, dtype=torch.float32, device=self.device
),
torch.tensor([1.0], dtype=torch.float32, device=self.device),
torch.randn(2 * batch_size, self.text_maxlen, self.embedding_dim, dtype=dtype, device=self.device),
)
def make_UNet(model, device, max_batch_size, embedding_dim, inpaint=False):
return UNet(
model,
fp16=True,
device=device,
max_batch_size=max_batch_size,
embedding_dim=embedding_dim,
unet_dim=(9 if inpaint else 4),
)
class VAE(BaseModel):
def __init__(self, model, device, max_batch_size, embedding_dim):
super(VAE, self).__init__(
model=model, device=device, max_batch_size=max_batch_size, embedding_dim=embedding_dim
)
self.name = "VAE decoder"
def get_input_names(self):
return ["latent"]
def get_output_names(self):
return ["images"]
def get_dynamic_axes(self):
return {"latent": {0: "B", 2: "H", 3: "W"}, "images": {0: "B", 2: "8H", 3: "8W"}}
def get_input_profile(self, batch_size, image_height, image_width, static_batch, static_shape):
latent_height, latent_width = self.check_dims(batch_size, image_height, image_width)
(
min_batch,
max_batch,
_,
_,
_,
_,
min_latent_height,
max_latent_height,
min_latent_width,
max_latent_width,
) = self.get_minmax_dims(batch_size, image_height, image_width, static_batch, static_shape)
return {
"latent": [
(min_batch, 4, min_latent_height, min_latent_width),
(batch_size, 4, latent_height, latent_width),
(max_batch, 4, max_latent_height, max_latent_width),
]
}
def get_shape_dict(self, batch_size, image_height, image_width):
latent_height, latent_width = self.check_dims(batch_size, image_height, image_width)
return {
"latent": (batch_size, 4, latent_height, latent_width),
"images": (batch_size, 3, image_height, image_width),
}
def get_sample_input(self, batch_size, image_height, image_width):
latent_height, latent_width = self.check_dims(batch_size, image_height, image_width)
return torch.randn(batch_size, 4, latent_height, latent_width, dtype=torch.float32, device=self.device)
def make_VAE(model, device, max_batch_size, embedding_dim, inpaint=False):
return VAE(model, device=device, max_batch_size=max_batch_size, embedding_dim=embedding_dim)
class TorchVAEEncoder(torch.nn.Module):
def __init__(self, model):
super().__init__()
self.vae_encoder = model
def forward(self, x):
return retrieve_latents(self.vae_encoder.encode(x))
class VAEEncoder(BaseModel):
def __init__(self, model, device, max_batch_size, embedding_dim):
super(VAEEncoder, self).__init__(
model=model, device=device, max_batch_size=max_batch_size, embedding_dim=embedding_dim
)
self.name = "VAE encoder"
def get_model(self):
vae_encoder = TorchVAEEncoder(self.model)
return vae_encoder
def get_input_names(self):
return ["images"]
def get_output_names(self):
return ["latent"]
def get_dynamic_axes(self):
return {"images": {0: "B", 2: "8H", 3: "8W"}, "latent": {0: "B", 2: "H", 3: "W"}}
def get_input_profile(self, batch_size, image_height, image_width, static_batch, static_shape):
assert batch_size >= self.min_batch and batch_size <= self.max_batch
min_batch = batch_size if static_batch else self.min_batch
max_batch = batch_size if static_batch else self.max_batch
self.check_dims(batch_size, image_height, image_width)
(
min_batch,
max_batch,
min_image_height,
max_image_height,
min_image_width,
max_image_width,
_,
_,
_,
_,
) = self.get_minmax_dims(batch_size, image_height, image_width, static_batch, static_shape)
return {
"images": [
(min_batch, 3, min_image_height, min_image_width),
(batch_size, 3, image_height, image_width),
(max_batch, 3, max_image_height, max_image_width),
]
}
def get_shape_dict(self, batch_size, image_height, image_width):
latent_height, latent_width = self.check_dims(batch_size, image_height, image_width)
return {
"images": (batch_size, 3, image_height, image_width),
"latent": (batch_size, 4, latent_height, latent_width),
}
def get_sample_input(self, batch_size, image_height, image_width):
self.check_dims(batch_size, image_height, image_width)
return torch.randn(batch_size, 3, image_height, image_width, dtype=torch.float32, device=self.device)
def make_VAEEncoder(model, device, max_batch_size, embedding_dim, inpaint=False):
return VAEEncoder(model, device=device, max_batch_size=max_batch_size, embedding_dim=embedding_dim)
class TensorRTStableDiffusionImg2ImgPipeline(StableDiffusionImg2ImgPipeline):
r"""
Pipeline for image-to-image generation using TensorRT accelerated Stable Diffusion.
This model inherits from [`StableDiffusionImg2ImgPipeline`]. Check the superclass documentation for the generic methods the
library implements for all the pipelines (such as downloading or saving, running on a particular device, etc.)
Args:
vae ([`AutoencoderKL`]):
Variational Auto-Encoder (VAE) Model to encode and decode images to and from latent representations.
text_encoder ([`CLIPTextModel`]):
Frozen text-encoder. Stable Diffusion uses the text portion of
[CLIP](https://huggingface.co/docs/transformers/model_doc/clip#transformers.CLIPTextModel), specifically
the [clip-vit-large-patch14](https://huggingface.co/openai/clip-vit-large-patch14) variant.
tokenizer (`CLIPTokenizer`):
Tokenizer of class
[CLIPTokenizer](https://huggingface.co/docs/transformers/v4.21.0/en/model_doc/clip#transformers.CLIPTokenizer).
unet ([`UNet2DConditionModel`]): Conditional U-Net architecture to denoise the encoded image latents.
scheduler ([`SchedulerMixin`]):
A scheduler to be used in combination with `unet` to denoise the encoded image latents. Can be one of
[`DDIMScheduler`], [`LMSDiscreteScheduler`], or [`PNDMScheduler`].
safety_checker ([`StableDiffusionSafetyChecker`]):
Classification module that estimates whether generated images could be considered offensive or harmful.
Please, refer to the [model card](https://huggingface.co/runwayml/stable-diffusion-v1-5) for details.
feature_extractor ([`CLIPFeatureExtractor`]):
Model that extracts features from generated images to be used as inputs for the `safety_checker`.
"""
def __init__(
self,
vae: AutoencoderKL,
text_encoder: CLIPTextModel,
tokenizer: CLIPTokenizer,
unet: UNet2DConditionModel,
scheduler: DDIMScheduler,
safety_checker: StableDiffusionSafetyChecker,
feature_extractor: CLIPFeatureExtractor,
image_encoder: CLIPVisionModelWithProjection = None,
requires_safety_checker: bool = True,
stages=["clip", "unet", "vae", "vae_encoder"],
image_height: int = 512,
image_width: int = 512,
max_batch_size: int = 16,
# ONNX export parameters
onnx_opset: int = 17,
onnx_dir: str = "onnx",
# TensorRT engine build parameters
engine_dir: str = "engine",
build_preview_features: bool = True,
force_engine_rebuild: bool = False,
timing_cache: str = "timing_cache",
):
super().__init__(
vae,
text_encoder,
tokenizer,
unet,
scheduler,
safety_checker=safety_checker,
feature_extractor=feature_extractor,
image_encoder=image_encoder,
requires_safety_checker=requires_safety_checker,
)
self.vae.forward = self.vae.decode
self.stages = stages
self.image_height, self.image_width = image_height, image_width
self.inpaint = False
self.onnx_opset = onnx_opset
self.onnx_dir = onnx_dir
self.engine_dir = engine_dir
self.force_engine_rebuild = force_engine_rebuild
self.timing_cache = timing_cache
self.build_static_batch = False
self.build_dynamic_shape = False
self.build_preview_features = build_preview_features
self.max_batch_size = max_batch_size
# TODO: Restrict batch size to 4 for larger image dimensions as a WAR for TensorRT limitation.
if self.build_dynamic_shape or self.image_height > 512 or self.image_width > 512:
self.max_batch_size = 4
self.stream = None # loaded in loadResources()
self.models = {} # loaded in __loadModels()
self.engine = {} # loaded in build_engines()
def __loadModels(self):
# Load pipeline models
self.embedding_dim = self.text_encoder.config.hidden_size
models_args = {
"device": self.torch_device,
"max_batch_size": self.max_batch_size,
"embedding_dim": self.embedding_dim,
"inpaint": self.inpaint,
}
if "clip" in self.stages:
self.models["clip"] = make_CLIP(self.text_encoder, **models_args)
if "unet" in self.stages:
self.models["unet"] = make_UNet(self.unet, **models_args)
if "vae" in self.stages:
self.models["vae"] = make_VAE(self.vae, **models_args)
if "vae_encoder" in self.stages:
self.models["vae_encoder"] = make_VAEEncoder(self.vae, **models_args)
@classmethod
@validate_hf_hub_args
def set_cached_folder(cls, pretrained_model_name_or_path: Optional[Union[str, os.PathLike]], **kwargs):
cache_dir = kwargs.pop("cache_dir", None)
resume_download = kwargs.pop("resume_download", False)
proxies = kwargs.pop("proxies", None)
local_files_only = kwargs.pop("local_files_only", False)
token = kwargs.pop("token", None)
revision = kwargs.pop("revision", None)
cls.cached_folder = (
pretrained_model_name_or_path
if os.path.isdir(pretrained_model_name_or_path)
else snapshot_download(
pretrained_model_name_or_path,
cache_dir=cache_dir,
resume_download=resume_download,
proxies=proxies,
local_files_only=local_files_only,
token=token,
revision=revision,
)
)
def to(self, torch_device: Optional[Union[str, torch.device]] = None, silence_dtype_warnings: bool = False):
super().to(torch_device, silence_dtype_warnings=silence_dtype_warnings)
self.onnx_dir = os.path.join(self.cached_folder, self.onnx_dir)
self.engine_dir = os.path.join(self.cached_folder, self.engine_dir)
self.timing_cache = os.path.join(self.cached_folder, self.timing_cache)
# set device
self.torch_device = self._execution_device
logger.warning(f"Running inference on device: {self.torch_device}")
# load models
self.__loadModels()
# build engines
self.engine = build_engines(
self.models,
self.engine_dir,
self.onnx_dir,
self.onnx_opset,
opt_image_height=self.image_height,
opt_image_width=self.image_width,
force_engine_rebuild=self.force_engine_rebuild,
static_batch=self.build_static_batch,
static_shape=not self.build_dynamic_shape,
enable_preview=self.build_preview_features,
timing_cache=self.timing_cache,
)
return self
def __initialize_timesteps(self, timesteps, strength):
self.scheduler.set_timesteps(timesteps)
offset = self.scheduler.steps_offset if hasattr(self.scheduler, "steps_offset") else 0
init_timestep = int(timesteps * strength) + offset
init_timestep = min(init_timestep, timesteps)
t_start = max(timesteps - init_timestep + offset, 0)
timesteps = self.scheduler.timesteps[t_start:].to(self.torch_device)
return timesteps, t_start
def __preprocess_images(self, batch_size, images=()):
init_images = []
for image in images:
image = image.to(self.torch_device).float()
image = image.repeat(batch_size, 1, 1, 1)
init_images.append(image)
return tuple(init_images)
def __encode_image(self, init_image):
init_latents = runEngine(self.engine["vae_encoder"], {"images": device_view(init_image)}, self.stream)[
"latent"
]
init_latents = 0.18215 * init_latents
return init_latents
def __encode_prompt(self, prompt, negative_prompt):
r"""
Encodes the prompt into text encoder hidden states.
Args:
prompt (`str` or `List[str]`, *optional*):
prompt to be encoded
negative_prompt (`str` or `List[str]`, *optional*):
The prompt or prompts not to guide the image generation. If not defined, one has to pass
`negative_prompt_embeds`. instead. If not defined, one has to pass `negative_prompt_embeds`. instead.
Ignored when not using guidance (i.e., ignored if `guidance_scale` is less than `1`).
"""
# Tokenize prompt
text_input_ids = (
self.tokenizer(
prompt,
padding="max_length",
max_length=self.tokenizer.model_max_length,
truncation=True,
return_tensors="pt",
)
.input_ids.type(torch.int32)
.to(self.torch_device)
)
text_input_ids_inp = device_view(text_input_ids)
# NOTE: output tensor for CLIP must be cloned because it will be overwritten when called again for negative prompt
text_embeddings = runEngine(self.engine["clip"], {"input_ids": text_input_ids_inp}, self.stream)[
"text_embeddings"
].clone()
# Tokenize negative prompt
uncond_input_ids = (
self.tokenizer(
negative_prompt,
padding="max_length",
max_length=self.tokenizer.model_max_length,
truncation=True,
return_tensors="pt",
)
.input_ids.type(torch.int32)
.to(self.torch_device)
)
uncond_input_ids_inp = device_view(uncond_input_ids)
uncond_embeddings = runEngine(self.engine["clip"], {"input_ids": uncond_input_ids_inp}, self.stream)[
"text_embeddings"
]
# Concatenate the unconditional and text embeddings into a single batch to avoid doing two forward passes for classifier free guidance
text_embeddings = torch.cat([uncond_embeddings, text_embeddings]).to(dtype=torch.float16)
return text_embeddings
def __denoise_latent(
self, latents, text_embeddings, timesteps=None, step_offset=0, mask=None, masked_image_latents=None
):
if not isinstance(timesteps, torch.Tensor):
timesteps = self.scheduler.timesteps
for step_index, timestep in enumerate(timesteps):
# Expand the latents if we are doing classifier free guidance
latent_model_input = torch.cat([latents] * 2)
latent_model_input = self.scheduler.scale_model_input(latent_model_input, timestep)
if isinstance(mask, torch.Tensor):
latent_model_input = torch.cat([latent_model_input, mask, masked_image_latents], dim=1)
# Predict the noise residual
timestep_float = timestep.float() if timestep.dtype != torch.float32 else timestep
sample_inp = device_view(latent_model_input)
timestep_inp = device_view(timestep_float)
embeddings_inp = device_view(text_embeddings)
noise_pred = runEngine(
self.engine["unet"],
{"sample": sample_inp, "timestep": timestep_inp, "encoder_hidden_states": embeddings_inp},
self.stream,
)["latent"]
# Perform guidance
noise_pred_uncond, noise_pred_text = noise_pred.chunk(2)
noise_pred = noise_pred_uncond + self.guidance_scale * (noise_pred_text - noise_pred_uncond)
latents = self.scheduler.step(noise_pred, timestep, latents).prev_sample
latents = 1.0 / 0.18215 * latents
return latents
def __decode_latent(self, latents):
images = runEngine(self.engine["vae"], {"latent": device_view(latents)}, self.stream)["images"]
images = (images / 2 + 0.5).clamp(0, 1)
return images.cpu().permute(0, 2, 3, 1).float().numpy()
def __loadResources(self, image_height, image_width, batch_size):
self.stream = cuda.Stream()
# Allocate buffers for TensorRT engine bindings
for model_name, obj in self.models.items():
self.engine[model_name].allocate_buffers(
shape_dict=obj.get_shape_dict(batch_size, image_height, image_width), device=self.torch_device
)
@torch.no_grad()
def __call__(
self,
prompt: Union[str, List[str]] = None,
image: Union[torch.FloatTensor, PIL.Image.Image] = None,
strength: float = 0.8,
num_inference_steps: int = 50,
guidance_scale: float = 7.5,
negative_prompt: Optional[Union[str, List[str]]] = None,
generator: Optional[Union[torch.Generator, List[torch.Generator]]] = None,
):
r"""
Function invoked when calling the pipeline for generation.
Args:
prompt (`str` or `List[str]`, *optional*):
The prompt or prompts to guide the image generation. If not defined, one has to pass `prompt_embeds`.
instead.
image (`PIL.Image.Image`):
`Image`, or tensor representing an image batch which will be inpainted, *i.e.* parts of the image will
be masked out with `mask_image` and repainted according to `prompt`.
strength (`float`, *optional*, defaults to 0.8):
Conceptually, indicates how much to transform the reference `image`. Must be between 0 and 1. `image`
will be used as a starting point, adding more noise to it the larger the `strength`. The number of
denoising steps depends on the amount of noise initially added. When `strength` is 1, added noise will
be maximum and the denoising process will run for the full number of iterations specified in
`num_inference_steps`. A value of 1, therefore, essentially ignores `image`.
num_inference_steps (`int`, *optional*, defaults to 50):
The number of denoising steps. More denoising steps usually lead to a higher quality image at the
expense of slower inference.
guidance_scale (`float`, *optional*, defaults to 7.5):
Guidance scale as defined in [Classifier-Free Diffusion Guidance](https://arxiv.org/abs/2207.12598).
`guidance_scale` is defined as `w` of equation 2. of [Imagen
Paper](https://arxiv.org/pdf/2205.11487.pdf). Guidance scale is enabled by setting `guidance_scale >
1`. Higher guidance scale encourages to generate images that are closely linked to the text `prompt`,
usually at the expense of lower image quality.
negative_prompt (`str` or `List[str]`, *optional*):
The prompt or prompts not to guide the image generation. If not defined, one has to pass
`negative_prompt_embeds`. instead. If not defined, one has to pass `negative_prompt_embeds`. instead.
Ignored when not using guidance (i.e., ignored if `guidance_scale` is less than `1`).
generator (`torch.Generator` or `List[torch.Generator]`, *optional*):
One or a list of [torch generator(s)](https://pytorch.org/docs/stable/generated/torch.Generator.html)
to make generation deterministic.
"""
self.generator = generator
self.denoising_steps = num_inference_steps
self._guidance_scale = guidance_scale
# Pre-compute latent input scales and linear multistep coefficients
self.scheduler.set_timesteps(self.denoising_steps, device=self.torch_device)
# Define call parameters
if prompt is not None and isinstance(prompt, str):
batch_size = 1
prompt = [prompt]
elif prompt is not None and isinstance(prompt, list):
batch_size = len(prompt)
else:
raise ValueError(f"Expected prompt to be of type list or str but got {type(prompt)}")
if negative_prompt is None:
negative_prompt = [""] * batch_size
if negative_prompt is not None and isinstance(negative_prompt, str):
negative_prompt = [negative_prompt]
assert len(prompt) == len(negative_prompt)
if batch_size > self.max_batch_size:
raise ValueError(
f"Batch size {len(prompt)} is larger than allowed {self.max_batch_size}. If dynamic shape is used, then maximum batch size is 4"
)
# load resources
self.__loadResources(self.image_height, self.image_width, batch_size)
with torch.inference_mode(), torch.autocast("cuda"), trt.Runtime(TRT_LOGGER):
# Initialize timesteps
timesteps, t_start = self.__initialize_timesteps(self.denoising_steps, strength)
latent_timestep = timesteps[:1].repeat(batch_size)
# Pre-process input image
if isinstance(image, PIL.Image.Image):
image = preprocess_image(image)
init_image = self.__preprocess_images(batch_size, (image,))[0]
# VAE encode init image
init_latents = self.__encode_image(init_image)
# Add noise to latents using timesteps
noise = torch.randn(
init_latents.shape, generator=self.generator, device=self.torch_device, dtype=torch.float32
)
latents = self.scheduler.add_noise(init_latents, noise, latent_timestep)
# CLIP text encoder
text_embeddings = self.__encode_prompt(prompt, negative_prompt)
# UNet denoiser
latents = self.__denoise_latent(latents, text_embeddings, timesteps=timesteps, step_offset=t_start)
# VAE decode latent
images = self.__decode_latent(latents)
images = self.numpy_to_pil(images)
return StableDiffusionPipelineOutput(images=images, nsfw_content_detected=None)
| diffusers/examples/community/stable_diffusion_tensorrt_img2img.py/0 | {
"file_path": "diffusers/examples/community/stable_diffusion_tensorrt_img2img.py",
"repo_id": "diffusers",
"token_count": 19790
} | 106 |
#!/usr/bin/env python
# coding=utf-8
# Copyright 2024 The LCM team and the HuggingFace Inc. team. All rights reserved.
#
# Licensed under the Apache License, Version 2.0 (the "License");
# you may not use this file except in compliance with the License.
# You may obtain a copy of the License at
#
# http://www.apache.org/licenses/LICENSE-2.0
#
# Unless required by applicable law or agreed to in writing, software
# distributed under the License is distributed on an "AS IS" BASIS,
# WITHOUT WARRANTIES OR CONDITIONS OF ANY KIND, either express or implied.
# See the License for the specific language governing permissions and
import argparse
import copy
import functools
import gc
import logging
import math
import os
import random
import shutil
from pathlib import Path
import accelerate
import numpy as np
import torch
import torch.nn.functional as F
import torch.utils.checkpoint
import transformers
from accelerate import Accelerator
from accelerate.logging import get_logger
from accelerate.utils import ProjectConfiguration, set_seed
from datasets import load_dataset
from huggingface_hub import create_repo, upload_folder
from packaging import version
from peft import LoraConfig, get_peft_model_state_dict, set_peft_model_state_dict
from torchvision import transforms
from torchvision.transforms.functional import crop
from tqdm.auto import tqdm
from transformers import AutoTokenizer, PretrainedConfig
import diffusers
from diffusers import (
AutoencoderKL,
DDPMScheduler,
LCMScheduler,
StableDiffusionXLPipeline,
UNet2DConditionModel,
)
from diffusers.optimization import get_scheduler
from diffusers.training_utils import cast_training_params, resolve_interpolation_mode
from diffusers.utils import (
check_min_version,
convert_state_dict_to_diffusers,
convert_unet_state_dict_to_peft,
is_wandb_available,
)
from diffusers.utils.import_utils import is_xformers_available
if is_wandb_available():
import wandb
# Will error if the minimal version of diffusers is not installed. Remove at your own risks.
check_min_version("0.28.0.dev0")
logger = get_logger(__name__)
DATASET_NAME_MAPPING = {
"lambdalabs/pokemon-blip-captions": ("image", "text"),
}
class DDIMSolver:
def __init__(self, alpha_cumprods, timesteps=1000, ddim_timesteps=50):
# DDIM sampling parameters
step_ratio = timesteps // ddim_timesteps
self.ddim_timesteps = (np.arange(1, ddim_timesteps + 1) * step_ratio).round().astype(np.int64) - 1
self.ddim_alpha_cumprods = alpha_cumprods[self.ddim_timesteps]
self.ddim_alpha_cumprods_prev = np.asarray(
[alpha_cumprods[0]] + alpha_cumprods[self.ddim_timesteps[:-1]].tolist()
)
# convert to torch tensors
self.ddim_timesteps = torch.from_numpy(self.ddim_timesteps).long()
self.ddim_alpha_cumprods = torch.from_numpy(self.ddim_alpha_cumprods)
self.ddim_alpha_cumprods_prev = torch.from_numpy(self.ddim_alpha_cumprods_prev)
def to(self, device):
self.ddim_timesteps = self.ddim_timesteps.to(device)
self.ddim_alpha_cumprods = self.ddim_alpha_cumprods.to(device)
self.ddim_alpha_cumprods_prev = self.ddim_alpha_cumprods_prev.to(device)
return self
def ddim_step(self, pred_x0, pred_noise, timestep_index):
alpha_cumprod_prev = extract_into_tensor(self.ddim_alpha_cumprods_prev, timestep_index, pred_x0.shape)
dir_xt = (1.0 - alpha_cumprod_prev).sqrt() * pred_noise
x_prev = alpha_cumprod_prev.sqrt() * pred_x0 + dir_xt
return x_prev
def log_validation(vae, args, accelerator, weight_dtype, step, unet=None, is_final_validation=False):
logger.info("Running validation... ")
pipeline = StableDiffusionXLPipeline.from_pretrained(
args.pretrained_teacher_model,
vae=vae,
scheduler=LCMScheduler.from_pretrained(args.pretrained_teacher_model, subfolder="scheduler"),
revision=args.revision,
torch_dtype=weight_dtype,
).to(accelerator.device)
pipeline.set_progress_bar_config(disable=True)
to_load = None
if not is_final_validation:
if unet is None:
raise ValueError("Must provide a `unet` when doing intermediate validation.")
unet = accelerator.unwrap_model(unet)
state_dict = convert_state_dict_to_diffusers(get_peft_model_state_dict(unet))
to_load = state_dict
else:
to_load = args.output_dir
pipeline.load_lora_weights(to_load)
pipeline.fuse_lora()
if args.enable_xformers_memory_efficient_attention:
pipeline.enable_xformers_memory_efficient_attention()
if args.seed is None:
generator = None
else:
generator = torch.Generator(device=accelerator.device).manual_seed(args.seed)
validation_prompts = [
"cute sundar pichai character",
"robotic cat with wings",
"a photo of yoda",
"a cute creature with blue eyes",
]
image_logs = []
for _, prompt in enumerate(validation_prompts):
images = []
with torch.autocast("cuda", dtype=weight_dtype):
images = pipeline(
prompt=prompt,
num_inference_steps=4,
num_images_per_prompt=4,
generator=generator,
guidance_scale=0.0,
).images
image_logs.append({"validation_prompt": prompt, "images": images})
for tracker in accelerator.trackers:
if tracker.name == "tensorboard":
for log in image_logs:
images = log["images"]
validation_prompt = log["validation_prompt"]
formatted_images = []
for image in images:
formatted_images.append(np.asarray(image))
formatted_images = np.stack(formatted_images)
tracker.writer.add_images(validation_prompt, formatted_images, step, dataformats="NHWC")
elif tracker.name == "wandb":
formatted_images = []
for log in image_logs:
images = log["images"]
validation_prompt = log["validation_prompt"]
for image in images:
image = wandb.Image(image, caption=validation_prompt)
formatted_images.append(image)
logger_name = "test" if is_final_validation else "validation"
tracker.log({logger_name: formatted_images})
else:
logger.warning(f"image logging not implemented for {tracker.name}")
del pipeline
gc.collect()
torch.cuda.empty_cache()
return image_logs
def append_dims(x, target_dims):
"""Appends dimensions to the end of a tensor until it has target_dims dimensions."""
dims_to_append = target_dims - x.ndim
if dims_to_append < 0:
raise ValueError(f"input has {x.ndim} dims but target_dims is {target_dims}, which is less")
return x[(...,) + (None,) * dims_to_append]
# From LCMScheduler.get_scalings_for_boundary_condition_discrete
def scalings_for_boundary_conditions(timestep, sigma_data=0.5, timestep_scaling=10.0):
scaled_timestep = timestep_scaling * timestep
c_skip = sigma_data**2 / (scaled_timestep**2 + sigma_data**2)
c_out = scaled_timestep / (scaled_timestep**2 + sigma_data**2) ** 0.5
return c_skip, c_out
# Compare LCMScheduler.step, Step 4
def get_predicted_original_sample(model_output, timesteps, sample, prediction_type, alphas, sigmas):
alphas = extract_into_tensor(alphas, timesteps, sample.shape)
sigmas = extract_into_tensor(sigmas, timesteps, sample.shape)
if prediction_type == "epsilon":
pred_x_0 = (sample - sigmas * model_output) / alphas
elif prediction_type == "sample":
pred_x_0 = model_output
elif prediction_type == "v_prediction":
pred_x_0 = alphas * sample - sigmas * model_output
else:
raise ValueError(
f"Prediction type {prediction_type} is not supported; currently, `epsilon`, `sample`, and `v_prediction`"
f" are supported."
)
return pred_x_0
# Based on step 4 in DDIMScheduler.step
def get_predicted_noise(model_output, timesteps, sample, prediction_type, alphas, sigmas):
alphas = extract_into_tensor(alphas, timesteps, sample.shape)
sigmas = extract_into_tensor(sigmas, timesteps, sample.shape)
if prediction_type == "epsilon":
pred_epsilon = model_output
elif prediction_type == "sample":
pred_epsilon = (sample - alphas * model_output) / sigmas
elif prediction_type == "v_prediction":
pred_epsilon = alphas * model_output + sigmas * sample
else:
raise ValueError(
f"Prediction type {prediction_type} is not supported; currently, `epsilon`, `sample`, and `v_prediction`"
f" are supported."
)
return pred_epsilon
def extract_into_tensor(a, t, x_shape):
b, *_ = t.shape
out = a.gather(-1, t)
return out.reshape(b, *((1,) * (len(x_shape) - 1)))
def import_model_class_from_model_name_or_path(
pretrained_model_name_or_path: str, revision: str, subfolder: str = "text_encoder"
):
text_encoder_config = PretrainedConfig.from_pretrained(
pretrained_model_name_or_path, subfolder=subfolder, revision=revision
)
model_class = text_encoder_config.architectures[0]
if model_class == "CLIPTextModel":
from transformers import CLIPTextModel
return CLIPTextModel
elif model_class == "CLIPTextModelWithProjection":
from transformers import CLIPTextModelWithProjection
return CLIPTextModelWithProjection
else:
raise ValueError(f"{model_class} is not supported.")
def parse_args():
parser = argparse.ArgumentParser(description="Simple example of a training script.")
# ----------Model Checkpoint Loading Arguments----------
parser.add_argument(
"--pretrained_teacher_model",
type=str,
default=None,
required=True,
help="Path to pretrained LDM teacher model or model identifier from huggingface.co/models.",
)
parser.add_argument(
"--pretrained_vae_model_name_or_path",
type=str,
default=None,
help="Path to pretrained VAE model with better numerical stability. More details: https://github.com/huggingface/diffusers/pull/4038.",
)
parser.add_argument(
"--teacher_revision",
type=str,
default=None,
required=False,
help="Revision of pretrained LDM teacher model identifier from huggingface.co/models.",
)
parser.add_argument(
"--revision",
type=str,
default=None,
required=False,
help="Revision of pretrained LDM model identifier from huggingface.co/models.",
)
# ----------Training Arguments----------
# ----General Training Arguments----
parser.add_argument(
"--output_dir",
type=str,
default="lcm-xl-distilled",
help="The output directory where the model predictions and checkpoints will be written.",
)
parser.add_argument(
"--cache_dir",
type=str,
default=None,
help="The directory where the downloaded models and datasets will be stored.",
)
parser.add_argument("--seed", type=int, default=None, help="A seed for reproducible training.")
# ----Logging----
parser.add_argument(
"--logging_dir",
type=str,
default="logs",
help=(
"[TensorBoard](https://www.tensorflow.org/tensorboard) log directory. Will default to"
" *output_dir/runs/**CURRENT_DATETIME_HOSTNAME***."
),
)
parser.add_argument(
"--report_to",
type=str,
default="tensorboard",
help=(
'The integration to report the results and logs to. Supported platforms are `"tensorboard"`'
' (default), `"wandb"` and `"comet_ml"`. Use `"all"` to report to all integrations.'
),
)
# ----Checkpointing----
parser.add_argument(
"--checkpointing_steps",
type=int,
default=500,
help=(
"Save a checkpoint of the training state every X updates. These checkpoints are only suitable for resuming"
" training using `--resume_from_checkpoint`."
),
)
parser.add_argument(
"--checkpoints_total_limit",
type=int,
default=None,
help=("Max number of checkpoints to store."),
)
parser.add_argument(
"--resume_from_checkpoint",
type=str,
default=None,
help=(
"Whether training should be resumed from a previous checkpoint. Use a path saved by"
' `--checkpointing_steps`, or `"latest"` to automatically select the last available checkpoint.'
),
)
# ----Image Processing----
parser.add_argument(
"--dataset_name",
type=str,
default=None,
help=(
"The name of the Dataset (from the HuggingFace hub) to train on (could be your own, possibly private,"
" dataset). It can also be a path pointing to a local copy of a dataset in your filesystem,"
" or to a folder containing files that 🤗 Datasets can understand."
),
)
parser.add_argument(
"--dataset_config_name",
type=str,
default=None,
help="The config of the Dataset, leave as None if there's only one config.",
)
parser.add_argument(
"--train_data_dir",
type=str,
default=None,
help=(
"A folder containing the training data. Folder contents must follow the structure described in"
" https://huggingface.co/docs/datasets/image_dataset#imagefolder. In particular, a `metadata.jsonl` file"
" must exist to provide the captions for the images. Ignored if `dataset_name` is specified."
),
)
parser.add_argument(
"--image_column", type=str, default="image", help="The column of the dataset containing an image."
)
parser.add_argument(
"--caption_column",
type=str,
default="text",
help="The column of the dataset containing a caption or a list of captions.",
)
parser.add_argument(
"--resolution",
type=int,
default=1024,
help=(
"The resolution for input images, all the images in the train/validation dataset will be resized to this"
" resolution"
),
)
parser.add_argument(
"--interpolation_type",
type=str,
default="bilinear",
help=(
"The interpolation function used when resizing images to the desired resolution. Choose between `bilinear`,"
" `bicubic`, `box`, `nearest`, `nearest_exact`, `hamming`, and `lanczos`."
),
)
parser.add_argument(
"--center_crop",
default=False,
action="store_true",
help=(
"Whether to center crop the input images to the resolution. If not set, the images will be randomly"
" cropped. The images will be resized to the resolution first before cropping."
),
)
parser.add_argument(
"--random_flip",
action="store_true",
help="whether to randomly flip images horizontally",
)
parser.add_argument(
"--encode_batch_size",
type=int,
default=8,
help="Batch size to use for VAE encoding of the images for efficient processing.",
)
# ----Dataloader----
parser.add_argument(
"--dataloader_num_workers",
type=int,
default=0,
help=(
"Number of subprocesses to use for data loading. 0 means that the data will be loaded in the main process."
),
)
# ----Batch Size and Training Steps----
parser.add_argument(
"--train_batch_size", type=int, default=16, help="Batch size (per device) for the training dataloader."
)
parser.add_argument("--num_train_epochs", type=int, default=100)
parser.add_argument(
"--max_train_steps",
type=int,
default=None,
help="Total number of training steps to perform. If provided, overrides num_train_epochs.",
)
parser.add_argument(
"--max_train_samples",
type=int,
default=None,
help=(
"For debugging purposes or quicker training, truncate the number of training examples to this "
"value if set."
),
)
# ----Learning Rate----
parser.add_argument(
"--learning_rate",
type=float,
default=1e-6,
help="Initial learning rate (after the potential warmup period) to use.",
)
parser.add_argument(
"--scale_lr",
action="store_true",
default=False,
help="Scale the learning rate by the number of GPUs, gradient accumulation steps, and batch size.",
)
parser.add_argument(
"--lr_scheduler",
type=str,
default="constant",
help=(
'The scheduler type to use. Choose between ["linear", "cosine", "cosine_with_restarts", "polynomial",'
' "constant", "constant_with_warmup"]'
),
)
parser.add_argument(
"--lr_warmup_steps", type=int, default=500, help="Number of steps for the warmup in the lr scheduler."
)
parser.add_argument(
"--gradient_accumulation_steps",
type=int,
default=1,
help="Number of updates steps to accumulate before performing a backward/update pass.",
)
# ----Optimizer (Adam)----
parser.add_argument(
"--use_8bit_adam", action="store_true", help="Whether or not to use 8-bit Adam from bitsandbytes."
)
parser.add_argument("--adam_beta1", type=float, default=0.9, help="The beta1 parameter for the Adam optimizer.")
parser.add_argument("--adam_beta2", type=float, default=0.999, help="The beta2 parameter for the Adam optimizer.")
parser.add_argument("--adam_weight_decay", type=float, default=1e-2, help="Weight decay to use.")
parser.add_argument("--adam_epsilon", type=float, default=1e-08, help="Epsilon value for the Adam optimizer")
parser.add_argument("--max_grad_norm", default=1.0, type=float, help="Max gradient norm.")
# ----Diffusion Training Arguments----
# ----Latent Consistency Distillation (LCD) Specific Arguments----
parser.add_argument(
"--w_min",
type=float,
default=3.0,
required=False,
help=(
"The minimum guidance scale value for guidance scale sampling. Note that we are using the Imagen CFG"
" formulation rather than the LCM formulation, which means all guidance scales have 1 added to them as"
" compared to the original paper."
),
)
parser.add_argument(
"--w_max",
type=float,
default=15.0,
required=False,
help=(
"The maximum guidance scale value for guidance scale sampling. Note that we are using the Imagen CFG"
" formulation rather than the LCM formulation, which means all guidance scales have 1 added to them as"
" compared to the original paper."
),
)
parser.add_argument(
"--num_ddim_timesteps",
type=int,
default=50,
help="The number of timesteps to use for DDIM sampling.",
)
parser.add_argument(
"--loss_type",
type=str,
default="l2",
choices=["l2", "huber"],
help="The type of loss to use for the LCD loss.",
)
parser.add_argument(
"--huber_c",
type=float,
default=0.001,
help="The huber loss parameter. Only used if `--loss_type=huber`.",
)
parser.add_argument(
"--lora_rank",
type=int,
default=64,
help="The rank of the LoRA projection matrix.",
)
parser.add_argument(
"--lora_alpha",
type=int,
default=64,
help=(
"The value of the LoRA alpha parameter, which controls the scaling factor in front of the LoRA weight"
" update delta_W. No scaling will be performed if this value is equal to `lora_rank`."
),
)
parser.add_argument(
"--lora_dropout",
type=float,
default=0.0,
help="The dropout probability for the dropout layer added before applying the LoRA to each layer input.",
)
parser.add_argument(
"--lora_target_modules",
type=str,
default=None,
help=(
"A comma-separated string of target module keys to add LoRA to. If not set, a default list of modules will"
" be used. By default, LoRA will be applied to all conv and linear layers."
),
)
parser.add_argument(
"--vae_encode_batch_size",
type=int,
default=8,
required=False,
help=(
"The batch size used when encoding (and decoding) images to latents (and vice versa) using the VAE."
" Encoding or decoding the whole batch at once may run into OOM issues."
),
)
parser.add_argument(
"--timestep_scaling_factor",
type=float,
default=10.0,
help=(
"The multiplicative timestep scaling factor used when calculating the boundary scalings for LCM. The"
" higher the scaling is, the lower the approximation error, but the default value of 10.0 should typically"
" suffice."
),
)
# ----Mixed Precision----
parser.add_argument(
"--mixed_precision",
type=str,
default=None,
choices=["no", "fp16", "bf16"],
help=(
"Whether to use mixed precision. Choose between fp16 and bf16 (bfloat16). Bf16 requires PyTorch >="
" 1.10.and an Nvidia Ampere GPU. Default to the value of accelerate config of the current system or the"
" flag passed with the `accelerate.launch` command. Use this argument to override the accelerate config."
),
)
parser.add_argument(
"--allow_tf32",
action="store_true",
help=(
"Whether or not to allow TF32 on Ampere GPUs. Can be used to speed up training. For more information, see"
" https://pytorch.org/docs/stable/notes/cuda.html#tensorfloat-32-tf32-on-ampere-devices"
),
)
# ----Training Optimizations----
parser.add_argument(
"--enable_xformers_memory_efficient_attention", action="store_true", help="Whether or not to use xformers."
)
parser.add_argument(
"--gradient_checkpointing",
action="store_true",
help="Whether or not to use gradient checkpointing to save memory at the expense of slower backward pass.",
)
# ----Distributed Training----
parser.add_argument("--local_rank", type=int, default=-1, help="For distributed training: local_rank")
# ----------Validation Arguments----------
parser.add_argument(
"--validation_steps",
type=int,
default=200,
help="Run validation every X steps.",
)
# ----------Huggingface Hub Arguments-----------
parser.add_argument("--push_to_hub", action="store_true", help="Whether or not to push the model to the Hub.")
parser.add_argument("--hub_token", type=str, default=None, help="The token to use to push to the Model Hub.")
parser.add_argument(
"--hub_model_id",
type=str,
default=None,
help="The name of the repository to keep in sync with the local `output_dir`.",
)
# ----------Accelerate Arguments----------
parser.add_argument(
"--tracker_project_name",
type=str,
default="text2image-fine-tune",
help=(
"The `project_name` argument passed to Accelerator.init_trackers for"
" more information see https://huggingface.co/docs/accelerate/v0.17.0/en/package_reference/accelerator#accelerate.Accelerator"
),
)
args = parser.parse_args()
env_local_rank = int(os.environ.get("LOCAL_RANK", -1))
if env_local_rank != -1 and env_local_rank != args.local_rank:
args.local_rank = env_local_rank
return args
# Adapted from pipelines.StableDiffusionXLPipeline.encode_prompt
def encode_prompt(prompt_batch, text_encoders, tokenizers, is_train=True):
prompt_embeds_list = []
captions = []
for caption in prompt_batch:
if isinstance(caption, str):
captions.append(caption)
elif isinstance(caption, (list, np.ndarray)):
# take a random caption if there are multiple
captions.append(random.choice(caption) if is_train else caption[0])
with torch.no_grad():
for tokenizer, text_encoder in zip(tokenizers, text_encoders):
text_inputs = tokenizer(
captions,
padding="max_length",
max_length=tokenizer.model_max_length,
truncation=True,
return_tensors="pt",
)
text_input_ids = text_inputs.input_ids
prompt_embeds = text_encoder(
text_input_ids.to(text_encoder.device),
output_hidden_states=True,
)
# We are only ALWAYS interested in the pooled output of the final text encoder
pooled_prompt_embeds = prompt_embeds[0]
prompt_embeds = prompt_embeds.hidden_states[-2]
bs_embed, seq_len, _ = prompt_embeds.shape
prompt_embeds = prompt_embeds.view(bs_embed, seq_len, -1)
prompt_embeds_list.append(prompt_embeds)
prompt_embeds = torch.concat(prompt_embeds_list, dim=-1)
pooled_prompt_embeds = pooled_prompt_embeds.view(bs_embed, -1)
return prompt_embeds, pooled_prompt_embeds
def main(args):
if args.report_to == "wandb" and args.hub_token is not None:
raise ValueError(
"You cannot use both --report_to=wandb and --hub_token due to a security risk of exposing your token."
" Please use `huggingface-cli login` to authenticate with the Hub."
)
logging_dir = Path(args.output_dir, args.logging_dir)
accelerator_project_config = ProjectConfiguration(project_dir=args.output_dir, logging_dir=logging_dir)
accelerator = Accelerator(
gradient_accumulation_steps=args.gradient_accumulation_steps,
mixed_precision=args.mixed_precision,
log_with=args.report_to,
project_config=accelerator_project_config,
split_batches=True, # It's important to set this to True when using webdataset to get the right number of steps for lr scheduling. If set to False, the number of steps will be devide by the number of processes assuming batches are multiplied by the number of processes
)
# Make one log on every process with the configuration for debugging.
logging.basicConfig(
format="%(asctime)s - %(levelname)s - %(name)s - %(message)s",
datefmt="%m/%d/%Y %H:%M:%S",
level=logging.INFO,
)
logger.info(accelerator.state, main_process_only=False)
if accelerator.is_local_main_process:
transformers.utils.logging.set_verbosity_warning()
diffusers.utils.logging.set_verbosity_info()
else:
transformers.utils.logging.set_verbosity_error()
diffusers.utils.logging.set_verbosity_error()
# If passed along, set the training seed now.
if args.seed is not None:
set_seed(args.seed)
# Handle the repository creation
if accelerator.is_main_process:
if args.output_dir is not None:
os.makedirs(args.output_dir, exist_ok=True)
if args.push_to_hub:
repo_id = create_repo(
repo_id=args.hub_model_id or Path(args.output_dir).name,
exist_ok=True,
token=args.hub_token,
private=True,
).repo_id
# 1. Create the noise scheduler and the desired noise schedule.
noise_scheduler = DDPMScheduler.from_pretrained(
args.pretrained_teacher_model, subfolder="scheduler", revision=args.teacher_revision
)
# DDPMScheduler calculates the alpha and sigma noise schedules (based on the alpha bars) for us
alpha_schedule = torch.sqrt(noise_scheduler.alphas_cumprod)
sigma_schedule = torch.sqrt(1 - noise_scheduler.alphas_cumprod)
# Initialize the DDIM ODE solver for distillation.
solver = DDIMSolver(
noise_scheduler.alphas_cumprod.numpy(),
timesteps=noise_scheduler.config.num_train_timesteps,
ddim_timesteps=args.num_ddim_timesteps,
)
# 2. Load tokenizers from SDXL checkpoint.
tokenizer_one = AutoTokenizer.from_pretrained(
args.pretrained_teacher_model, subfolder="tokenizer", revision=args.teacher_revision, use_fast=False
)
tokenizer_two = AutoTokenizer.from_pretrained(
args.pretrained_teacher_model, subfolder="tokenizer_2", revision=args.teacher_revision, use_fast=False
)
# 3. Load text encoders from SDXL checkpoint.
# import correct text encoder classes
text_encoder_cls_one = import_model_class_from_model_name_or_path(
args.pretrained_teacher_model, args.teacher_revision
)
text_encoder_cls_two = import_model_class_from_model_name_or_path(
args.pretrained_teacher_model, args.teacher_revision, subfolder="text_encoder_2"
)
text_encoder_one = text_encoder_cls_one.from_pretrained(
args.pretrained_teacher_model, subfolder="text_encoder", revision=args.teacher_revision
)
text_encoder_two = text_encoder_cls_two.from_pretrained(
args.pretrained_teacher_model, subfolder="text_encoder_2", revision=args.teacher_revision
)
# 4. Load VAE from SDXL checkpoint (or more stable VAE)
vae_path = (
args.pretrained_teacher_model
if args.pretrained_vae_model_name_or_path is None
else args.pretrained_vae_model_name_or_path
)
vae = AutoencoderKL.from_pretrained(
vae_path,
subfolder="vae" if args.pretrained_vae_model_name_or_path is None else None,
revision=args.teacher_revision,
)
# 6. Freeze teacher vae, text_encoders.
vae.requires_grad_(False)
text_encoder_one.requires_grad_(False)
text_encoder_two.requires_grad_(False)
# 7. Create online student U-Net.
unet = UNet2DConditionModel.from_pretrained(
args.pretrained_teacher_model, subfolder="unet", revision=args.teacher_revision
)
unet.requires_grad_(False)
# Check that all trainable models are in full precision
low_precision_error_string = (
" Please make sure to always have all model weights in full float32 precision when starting training - even if"
" doing mixed precision training, copy of the weights should still be float32."
)
if accelerator.unwrap_model(unet).dtype != torch.float32:
raise ValueError(
f"Controlnet loaded as datatype {accelerator.unwrap_model(unet).dtype}. {low_precision_error_string}"
)
# 8. Handle mixed precision and device placement
# For mixed precision training we cast all non-trainable weigths to half-precision
# as these weights are only used for inference, keeping weights in full precision is not required.
weight_dtype = torch.float32
if accelerator.mixed_precision == "fp16":
weight_dtype = torch.float16
elif accelerator.mixed_precision == "bf16":
weight_dtype = torch.bfloat16
# Move unet, vae and text_encoder to device and cast to weight_dtype
# The VAE is in float32 to avoid NaN losses.
unet.to(accelerator.device, dtype=weight_dtype)
if args.pretrained_vae_model_name_or_path is None:
vae.to(accelerator.device, dtype=torch.float32)
else:
vae.to(accelerator.device, dtype=weight_dtype)
text_encoder_one.to(accelerator.device, dtype=weight_dtype)
text_encoder_two.to(accelerator.device, dtype=weight_dtype)
# 9. Add LoRA to the student U-Net, only the LoRA projection matrix will be updated by the optimizer.
if args.lora_target_modules is not None:
lora_target_modules = [module_key.strip() for module_key in args.lora_target_modules.split(",")]
else:
lora_target_modules = [
"to_q",
"to_k",
"to_v",
"to_out.0",
"proj_in",
"proj_out",
"ff.net.0.proj",
"ff.net.2",
"conv1",
"conv2",
"conv_shortcut",
"downsamplers.0.conv",
"upsamplers.0.conv",
"time_emb_proj",
]
lora_config = LoraConfig(
r=args.lora_rank,
target_modules=lora_target_modules,
lora_alpha=args.lora_alpha,
lora_dropout=args.lora_dropout,
)
unet.add_adapter(lora_config)
# Also move the alpha and sigma noise schedules to accelerator.device.
alpha_schedule = alpha_schedule.to(accelerator.device)
sigma_schedule = sigma_schedule.to(accelerator.device)
solver = solver.to(accelerator.device)
# 10. Handle saving and loading of checkpoints
# `accelerate` 0.16.0 will have better support for customized saving
if version.parse(accelerate.__version__) >= version.parse("0.16.0"):
# create custom saving & loading hooks so that `accelerator.save_state(...)` serializes in a nice format
def save_model_hook(models, weights, output_dir):
if accelerator.is_main_process:
unet_ = accelerator.unwrap_model(unet)
# also save the checkpoints in native `diffusers` format so that it can be easily
# be independently loaded via `load_lora_weights()`.
state_dict = convert_state_dict_to_diffusers(get_peft_model_state_dict(unet_))
StableDiffusionXLPipeline.save_lora_weights(output_dir, unet_lora_layers=state_dict)
for _, model in enumerate(models):
# make sure to pop weight so that corresponding model is not saved again
weights.pop()
def load_model_hook(models, input_dir):
# load the LoRA into the model
unet_ = accelerator.unwrap_model(unet)
lora_state_dict, _ = StableDiffusionXLPipeline.lora_state_dict(input_dir)
unet_state_dict = {
f'{k.replace("unet.", "")}': v for k, v in lora_state_dict.items() if k.startswith("unet.")
}
unet_state_dict = convert_unet_state_dict_to_peft(unet_state_dict)
incompatible_keys = set_peft_model_state_dict(unet_, unet_state_dict, adapter_name="default")
if incompatible_keys is not None:
# check only for unexpected keys
unexpected_keys = getattr(incompatible_keys, "unexpected_keys", None)
if unexpected_keys:
logger.warning(
f"Loading adapter weights from state_dict led to unexpected keys not found in the model: "
f" {unexpected_keys}. "
)
for _ in range(len(models)):
# pop models so that they are not loaded again
models.pop()
# Make sure the trainable params are in float32. This is again needed since the base models
# are in `weight_dtype`. More details:
# https://github.com/huggingface/diffusers/pull/6514#discussion_r1449796804
if args.mixed_precision == "fp16":
cast_training_params(unet_, dtype=torch.float32)
accelerator.register_save_state_pre_hook(save_model_hook)
accelerator.register_load_state_pre_hook(load_model_hook)
# 11. Enable optimizations
if args.enable_xformers_memory_efficient_attention:
if is_xformers_available():
import xformers
xformers_version = version.parse(xformers.__version__)
if xformers_version == version.parse("0.0.16"):
logger.warning(
"xFormers 0.0.16 cannot be used for training in some GPUs. If you observe problems during training, please update xFormers to at least 0.0.17. See https://huggingface.co/docs/diffusers/main/en/optimization/xformers for more details."
)
unet.enable_xformers_memory_efficient_attention()
else:
raise ValueError("xformers is not available. Make sure it is installed correctly")
# Enable TF32 for faster training on Ampere GPUs,
# cf https://pytorch.org/docs/stable/notes/cuda.html#tensorfloat-32-tf32-on-ampere-devices
if args.allow_tf32:
torch.backends.cuda.matmul.allow_tf32 = True
if args.gradient_checkpointing:
unet.enable_gradient_checkpointing()
# Use 8-bit Adam for lower memory usage or to fine-tune the model in 16GB GPUs
if args.use_8bit_adam:
try:
import bitsandbytes as bnb
except ImportError:
raise ImportError(
"To use 8-bit Adam, please install the bitsandbytes library: `pip install bitsandbytes`."
)
optimizer_class = bnb.optim.AdamW8bit
else:
optimizer_class = torch.optim.AdamW
# 12. Optimizer creation
params_to_optimize = filter(lambda p: p.requires_grad, unet.parameters())
optimizer = optimizer_class(
params_to_optimize,
lr=args.learning_rate,
betas=(args.adam_beta1, args.adam_beta2),
weight_decay=args.adam_weight_decay,
eps=args.adam_epsilon,
)
# 13. Dataset creation and data processing
# In distributed training, the load_dataset function guarantees that only one local process can concurrently
# download the dataset.
if args.dataset_name is not None:
# Downloading and loading a dataset from the hub.
dataset = load_dataset(
args.dataset_name,
args.dataset_config_name,
cache_dir=args.cache_dir,
)
else:
data_files = {}
if args.train_data_dir is not None:
data_files["train"] = os.path.join(args.train_data_dir, "**")
dataset = load_dataset(
"imagefolder",
data_files=data_files,
cache_dir=args.cache_dir,
)
# See more about loading custom images at
# https://huggingface.co/docs/datasets/v2.4.0/en/image_load#imagefolder
# Preprocessing the datasets.
column_names = dataset["train"].column_names
# Get the column names for input/target.
dataset_columns = DATASET_NAME_MAPPING.get(args.dataset_name, None)
if args.image_column is None:
image_column = dataset_columns[0] if dataset_columns is not None else column_names[0]
else:
image_column = args.image_column
if image_column not in column_names:
raise ValueError(
f"--image_column' value '{args.image_column}' needs to be one of: {', '.join(column_names)}"
)
if args.caption_column is None:
caption_column = dataset_columns[1] if dataset_columns is not None else column_names[1]
else:
caption_column = args.caption_column
if caption_column not in column_names:
raise ValueError(
f"--caption_column' value '{args.caption_column}' needs to be one of: {', '.join(column_names)}"
)
# Preprocessing the datasets.
interpolation_mode = resolve_interpolation_mode(args.interpolation_type)
train_resize = transforms.Resize(args.resolution, interpolation=interpolation_mode)
train_crop = transforms.CenterCrop(args.resolution) if args.center_crop else transforms.RandomCrop(args.resolution)
train_flip = transforms.RandomHorizontalFlip(p=1.0)
train_transforms = transforms.Compose([transforms.ToTensor(), transforms.Normalize([0.5], [0.5])])
def preprocess_train(examples):
images = [image.convert("RGB") for image in examples[image_column]]
# image aug
original_sizes = []
all_images = []
crop_top_lefts = []
for image in images:
original_sizes.append((image.height, image.width))
image = train_resize(image)
if args.center_crop:
y1 = max(0, int(round((image.height - args.resolution) / 2.0)))
x1 = max(0, int(round((image.width - args.resolution) / 2.0)))
image = train_crop(image)
else:
y1, x1, h, w = train_crop.get_params(image, (args.resolution, args.resolution))
image = crop(image, y1, x1, h, w)
if args.random_flip and random.random() < 0.5:
# flip
x1 = image.width - x1
image = train_flip(image)
crop_top_left = (y1, x1)
crop_top_lefts.append(crop_top_left)
image = train_transforms(image)
all_images.append(image)
examples["original_sizes"] = original_sizes
examples["crop_top_lefts"] = crop_top_lefts
examples["pixel_values"] = all_images
examples["captions"] = list(examples[caption_column])
return examples
with accelerator.main_process_first():
if args.max_train_samples is not None:
dataset["train"] = dataset["train"].shuffle(seed=args.seed).select(range(args.max_train_samples))
# Set the training transforms
train_dataset = dataset["train"].with_transform(preprocess_train)
def collate_fn(examples):
pixel_values = torch.stack([example["pixel_values"] for example in examples])
pixel_values = pixel_values.to(memory_format=torch.contiguous_format).float()
original_sizes = [example["original_sizes"] for example in examples]
crop_top_lefts = [example["crop_top_lefts"] for example in examples]
captions = [example["captions"] for example in examples]
return {
"pixel_values": pixel_values,
"captions": captions,
"original_sizes": original_sizes,
"crop_top_lefts": crop_top_lefts,
}
# DataLoaders creation:
train_dataloader = torch.utils.data.DataLoader(
train_dataset,
shuffle=True,
collate_fn=collate_fn,
batch_size=args.train_batch_size,
num_workers=args.dataloader_num_workers,
)
# 14. Embeddings for the UNet.
# Adapted from pipeline.StableDiffusionXLPipeline._get_add_time_ids
def compute_embeddings(prompt_batch, original_sizes, crop_coords, text_encoders, tokenizers, is_train=True):
def compute_time_ids(original_size, crops_coords_top_left):
target_size = (args.resolution, args.resolution)
add_time_ids = list(original_size + crops_coords_top_left + target_size)
add_time_ids = torch.tensor([add_time_ids])
add_time_ids = add_time_ids.to(accelerator.device, dtype=weight_dtype)
return add_time_ids
prompt_embeds, pooled_prompt_embeds = encode_prompt(prompt_batch, text_encoders, tokenizers, is_train)
add_text_embeds = pooled_prompt_embeds
add_time_ids = torch.cat([compute_time_ids(s, c) for s, c in zip(original_sizes, crop_coords)])
prompt_embeds = prompt_embeds.to(accelerator.device)
add_text_embeds = add_text_embeds.to(accelerator.device)
unet_added_cond_kwargs = {"text_embeds": add_text_embeds, "time_ids": add_time_ids}
return {"prompt_embeds": prompt_embeds, **unet_added_cond_kwargs}
text_encoders = [text_encoder_one, text_encoder_two]
tokenizers = [tokenizer_one, tokenizer_two]
compute_embeddings_fn = functools.partial(compute_embeddings, text_encoders=text_encoders, tokenizers=tokenizers)
# 15. LR Scheduler creation
# Scheduler and math around the number of training steps.
overrode_max_train_steps = False
num_update_steps_per_epoch = math.ceil(len(train_dataloader) / args.gradient_accumulation_steps)
if args.max_train_steps is None:
args.max_train_steps = args.num_train_epochs * num_update_steps_per_epoch
overrode_max_train_steps = True
if args.scale_lr:
args.learning_rate = (
args.learning_rate * args.gradient_accumulation_steps * args.train_batch_size * accelerator.num_processes
)
# Make sure the trainable params are in float32.
if args.mixed_precision == "fp16":
# only upcast trainable parameters (LoRA) into fp32
cast_training_params(unet, dtype=torch.float32)
lr_scheduler = get_scheduler(
args.lr_scheduler,
optimizer=optimizer,
num_warmup_steps=args.lr_warmup_steps * accelerator.num_processes,
num_training_steps=args.max_train_steps * accelerator.num_processes,
)
# 16. Prepare for training
# Prepare everything with our `accelerator`.
unet, optimizer, train_dataloader, lr_scheduler = accelerator.prepare(
unet, optimizer, train_dataloader, lr_scheduler
)
# We need to recalculate our total training steps as the size of the training dataloader may have changed.
num_update_steps_per_epoch = math.ceil(len(train_dataloader) / args.gradient_accumulation_steps)
if overrode_max_train_steps:
args.max_train_steps = args.num_train_epochs * num_update_steps_per_epoch
# Afterwards we recalculate our number of training epochs
args.num_train_epochs = math.ceil(args.max_train_steps / num_update_steps_per_epoch)
# We need to initialize the trackers we use, and also store our configuration.
# The trackers initializes automatically on the main process.
if accelerator.is_main_process:
tracker_config = dict(vars(args))
accelerator.init_trackers(args.tracker_project_name, config=tracker_config)
# 17. Train!
total_batch_size = args.train_batch_size * accelerator.num_processes * args.gradient_accumulation_steps
logger.info("***** Running training *****")
logger.info(f" Num examples = {len(train_dataset)}")
logger.info(f" Num Epochs = {args.num_train_epochs}")
logger.info(f" Instantaneous batch size per device = {args.train_batch_size}")
logger.info(f" Total train batch size (w. parallel, distributed & accumulation) = {total_batch_size}")
logger.info(f" Gradient Accumulation steps = {args.gradient_accumulation_steps}")
logger.info(f" Total optimization steps = {args.max_train_steps}")
global_step = 0
first_epoch = 0
# Potentially load in the weights and states from a previous save
if args.resume_from_checkpoint:
if args.resume_from_checkpoint != "latest":
path = os.path.basename(args.resume_from_checkpoint)
else:
# Get the most recent checkpoint
dirs = os.listdir(args.output_dir)
dirs = [d for d in dirs if d.startswith("checkpoint")]
dirs = sorted(dirs, key=lambda x: int(x.split("-")[1]))
path = dirs[-1] if len(dirs) > 0 else None
if path is None:
accelerator.print(
f"Checkpoint '{args.resume_from_checkpoint}' does not exist. Starting a new training run."
)
args.resume_from_checkpoint = None
initial_global_step = 0
else:
accelerator.print(f"Resuming from checkpoint {path}")
accelerator.load_state(os.path.join(args.output_dir, path))
global_step = int(path.split("-")[1])
initial_global_step = global_step
first_epoch = global_step // num_update_steps_per_epoch
else:
initial_global_step = 0
progress_bar = tqdm(
range(0, args.max_train_steps),
initial=initial_global_step,
desc="Steps",
# Only show the progress bar once on each machine.
disable=not accelerator.is_local_main_process,
)
unet.train()
for epoch in range(first_epoch, args.num_train_epochs):
for step, batch in enumerate(train_dataloader):
with accelerator.accumulate(unet):
# 1. Load and process the image and text conditioning
pixel_values, text, orig_size, crop_coords = (
batch["pixel_values"],
batch["captions"],
batch["original_sizes"],
batch["crop_top_lefts"],
)
encoded_text = compute_embeddings_fn(text, orig_size, crop_coords)
# encode pixel values with batch size of at most args.vae_encode_batch_size
pixel_values = pixel_values.to(dtype=vae.dtype)
latents = []
for i in range(0, pixel_values.shape[0], args.vae_encode_batch_size):
latents.append(vae.encode(pixel_values[i : i + args.vae_encode_batch_size]).latent_dist.sample())
latents = torch.cat(latents, dim=0)
latents = latents * vae.config.scaling_factor
if args.pretrained_vae_model_name_or_path is None:
latents = latents.to(weight_dtype)
# 2. Sample a random timestep for each image t_n from the ODE solver timesteps without bias.
# For the DDIM solver, the timestep schedule is [T - 1, T - k - 1, T - 2 * k - 1, ...]
bsz = latents.shape[0]
topk = noise_scheduler.config.num_train_timesteps // args.num_ddim_timesteps
index = torch.randint(0, args.num_ddim_timesteps, (bsz,), device=latents.device).long()
start_timesteps = solver.ddim_timesteps[index]
timesteps = start_timesteps - topk
timesteps = torch.where(timesteps < 0, torch.zeros_like(timesteps), timesteps)
# 3. Get boundary scalings for start_timesteps and (end) timesteps.
c_skip_start, c_out_start = scalings_for_boundary_conditions(
start_timesteps, timestep_scaling=args.timestep_scaling_factor
)
c_skip_start, c_out_start = [append_dims(x, latents.ndim) for x in [c_skip_start, c_out_start]]
c_skip, c_out = scalings_for_boundary_conditions(
timesteps, timestep_scaling=args.timestep_scaling_factor
)
c_skip, c_out = [append_dims(x, latents.ndim) for x in [c_skip, c_out]]
# 4. Sample noise from the prior and add it to the latents according to the noise magnitude at each
# timestep (this is the forward diffusion process) [z_{t_{n + k}} in Algorithm 1]
noise = torch.randn_like(latents)
noisy_model_input = noise_scheduler.add_noise(latents, noise, start_timesteps)
# 5. Sample a random guidance scale w from U[w_min, w_max]
# Note that for LCM-LoRA distillation it is not necessary to use a guidance scale embedding
w = (args.w_max - args.w_min) * torch.rand((bsz,)) + args.w_min
w = w.reshape(bsz, 1, 1, 1)
w = w.to(device=latents.device, dtype=latents.dtype)
# 6. Prepare prompt embeds and unet_added_conditions
prompt_embeds = encoded_text.pop("prompt_embeds")
# 7. Get online LCM prediction on z_{t_{n + k}} (noisy_model_input), w, c, t_{n + k} (start_timesteps)
noise_pred = unet(
noisy_model_input,
start_timesteps,
encoder_hidden_states=prompt_embeds,
added_cond_kwargs=encoded_text,
).sample
pred_x_0 = get_predicted_original_sample(
noise_pred,
start_timesteps,
noisy_model_input,
noise_scheduler.config.prediction_type,
alpha_schedule,
sigma_schedule,
)
model_pred = c_skip_start * noisy_model_input + c_out_start * pred_x_0
# 8. Compute the conditional and unconditional teacher model predictions to get CFG estimates of the
# predicted noise eps_0 and predicted original sample x_0, then run the ODE solver using these
# estimates to predict the data point in the augmented PF-ODE trajectory corresponding to the next ODE
# solver timestep.
# With the adapters disabled, the `unet` is the regular teacher model.
accelerator.unwrap_model(unet).disable_adapters()
with torch.no_grad():
# 1. Get teacher model prediction on noisy_model_input z_{t_{n + k}} and conditional embedding c
cond_teacher_output = unet(
noisy_model_input,
start_timesteps,
encoder_hidden_states=prompt_embeds,
added_cond_kwargs={k: v.to(weight_dtype) for k, v in encoded_text.items()},
).sample
cond_pred_x0 = get_predicted_original_sample(
cond_teacher_output,
start_timesteps,
noisy_model_input,
noise_scheduler.config.prediction_type,
alpha_schedule,
sigma_schedule,
)
cond_pred_noise = get_predicted_noise(
cond_teacher_output,
start_timesteps,
noisy_model_input,
noise_scheduler.config.prediction_type,
alpha_schedule,
sigma_schedule,
)
# 2. Get teacher model prediction on noisy_model_input z_{t_{n + k}} and unconditional embedding 0
uncond_prompt_embeds = torch.zeros_like(prompt_embeds)
uncond_pooled_prompt_embeds = torch.zeros_like(encoded_text["text_embeds"])
uncond_added_conditions = copy.deepcopy(encoded_text)
uncond_added_conditions["text_embeds"] = uncond_pooled_prompt_embeds
uncond_teacher_output = unet(
noisy_model_input,
start_timesteps,
encoder_hidden_states=uncond_prompt_embeds.to(weight_dtype),
added_cond_kwargs={k: v.to(weight_dtype) for k, v in uncond_added_conditions.items()},
).sample
uncond_pred_x0 = get_predicted_original_sample(
uncond_teacher_output,
start_timesteps,
noisy_model_input,
noise_scheduler.config.prediction_type,
alpha_schedule,
sigma_schedule,
)
uncond_pred_noise = get_predicted_noise(
uncond_teacher_output,
start_timesteps,
noisy_model_input,
noise_scheduler.config.prediction_type,
alpha_schedule,
sigma_schedule,
)
# 3. Calculate the CFG estimate of x_0 (pred_x0) and eps_0 (pred_noise)
# Note that this uses the LCM paper's CFG formulation rather than the Imagen CFG formulation
pred_x0 = cond_pred_x0 + w * (cond_pred_x0 - uncond_pred_x0)
pred_noise = cond_pred_noise + w * (cond_pred_noise - uncond_pred_noise)
# 4. Run one step of the ODE solver to estimate the next point x_prev on the
# augmented PF-ODE trajectory (solving backward in time)
# Note that the DDIM step depends on both the predicted x_0 and source noise eps_0.
x_prev = solver.ddim_step(pred_x0, pred_noise, index).to(unet.dtype)
# re-enable unet adapters to turn the `unet` into a student unet.
accelerator.unwrap_model(unet).enable_adapters()
# 9. Get target LCM prediction on x_prev, w, c, t_n (timesteps)
# Note that we do not use a separate target network for LCM-LoRA distillation.
with torch.no_grad():
target_noise_pred = unet(
x_prev,
timesteps,
encoder_hidden_states=prompt_embeds,
added_cond_kwargs={k: v.to(weight_dtype) for k, v in encoded_text.items()},
).sample
pred_x_0 = get_predicted_original_sample(
target_noise_pred,
timesteps,
x_prev,
noise_scheduler.config.prediction_type,
alpha_schedule,
sigma_schedule,
)
target = c_skip * x_prev + c_out * pred_x_0
# 10. Calculate loss
if args.loss_type == "l2":
loss = F.mse_loss(model_pred.float(), target.float(), reduction="mean")
elif args.loss_type == "huber":
loss = torch.mean(
torch.sqrt((model_pred.float() - target.float()) ** 2 + args.huber_c**2) - args.huber_c
)
# 11. Backpropagate on the online student model (`unet`) (only LoRA)
accelerator.backward(loss)
if accelerator.sync_gradients:
accelerator.clip_grad_norm_(params_to_optimize, args.max_grad_norm)
optimizer.step()
lr_scheduler.step()
optimizer.zero_grad(set_to_none=True)
# Checks if the accelerator has performed an optimization step behind the scenes
if accelerator.sync_gradients:
progress_bar.update(1)
global_step += 1
if accelerator.is_main_process:
if global_step % args.checkpointing_steps == 0:
# _before_ saving state, check if this save would set us over the `checkpoints_total_limit`
if args.checkpoints_total_limit is not None:
checkpoints = os.listdir(args.output_dir)
checkpoints = [d for d in checkpoints if d.startswith("checkpoint")]
checkpoints = sorted(checkpoints, key=lambda x: int(x.split("-")[1]))
# before we save the new checkpoint, we need to have at _most_ `checkpoints_total_limit - 1` checkpoints
if len(checkpoints) >= args.checkpoints_total_limit:
num_to_remove = len(checkpoints) - args.checkpoints_total_limit + 1
removing_checkpoints = checkpoints[0:num_to_remove]
logger.info(
f"{len(checkpoints)} checkpoints already exist, removing {len(removing_checkpoints)} checkpoints"
)
logger.info(f"removing checkpoints: {', '.join(removing_checkpoints)}")
for removing_checkpoint in removing_checkpoints:
removing_checkpoint = os.path.join(args.output_dir, removing_checkpoint)
shutil.rmtree(removing_checkpoint)
save_path = os.path.join(args.output_dir, f"checkpoint-{global_step}")
accelerator.save_state(save_path)
logger.info(f"Saved state to {save_path}")
if global_step % args.validation_steps == 0:
log_validation(
vae, args, accelerator, weight_dtype, global_step, unet=unet, is_final_validation=False
)
logs = {"loss": loss.detach().item(), "lr": lr_scheduler.get_last_lr()[0]}
progress_bar.set_postfix(**logs)
accelerator.log(logs, step=global_step)
if global_step >= args.max_train_steps:
break
# Create the pipeline using using the trained modules and save it.
accelerator.wait_for_everyone()
if accelerator.is_main_process:
unet = accelerator.unwrap_model(unet)
unet_lora_state_dict = convert_state_dict_to_diffusers(get_peft_model_state_dict(unet))
StableDiffusionXLPipeline.save_lora_weights(args.output_dir, unet_lora_layers=unet_lora_state_dict)
if args.push_to_hub:
upload_folder(
repo_id=repo_id,
folder_path=args.output_dir,
commit_message="End of training",
ignore_patterns=["step_*", "epoch_*"],
)
del unet
torch.cuda.empty_cache()
# Final inference.
if args.validation_steps is not None:
log_validation(vae, args, accelerator, weight_dtype, step=global_step, unet=None, is_final_validation=True)
accelerator.end_training()
if __name__ == "__main__":
args = parse_args()
main(args)
| diffusers/examples/consistency_distillation/train_lcm_distill_lora_sdxl.py/0 | {
"file_path": "diffusers/examples/consistency_distillation/train_lcm_distill_lora_sdxl.py",
"repo_id": "diffusers",
"token_count": 27390
} | 107 |
# coding=utf-8
# Copyright 2024 HuggingFace Inc.
#
# Licensed under the Apache License, Version 2.0 (the "License");
# you may not use this file except in compliance with the License.
# You may obtain a copy of the License at
#
# http://www.apache.org/licenses/LICENSE-2.0
#
# Unless required by applicable law or agreed to in writing, software
# distributed under the License is distributed on an "AS IS" BASIS,
# WITHOUT WARRANTIES OR CONDITIONS OF ANY KIND, either express or implied.
# See the License for the specific language governing permissions and
# limitations under the License.
import logging
import os
import sys
import tempfile
sys.path.append("..")
from test_examples_utils import ExamplesTestsAccelerate, run_command # noqa: E402
logging.basicConfig(level=logging.DEBUG)
logger = logging.getLogger()
stream_handler = logging.StreamHandler(sys.stdout)
logger.addHandler(stream_handler)
class CustomDiffusion(ExamplesTestsAccelerate):
def test_custom_diffusion(self):
with tempfile.TemporaryDirectory() as tmpdir:
test_args = f"""
examples/custom_diffusion/train_custom_diffusion.py
--pretrained_model_name_or_path hf-internal-testing/tiny-stable-diffusion-pipe
--instance_data_dir docs/source/en/imgs
--instance_prompt <new1>
--resolution 64
--train_batch_size 1
--gradient_accumulation_steps 1
--max_train_steps 2
--learning_rate 1.0e-05
--scale_lr
--lr_scheduler constant
--lr_warmup_steps 0
--modifier_token <new1>
--no_safe_serialization
--output_dir {tmpdir}
""".split()
run_command(self._launch_args + test_args)
# save_pretrained smoke test
self.assertTrue(os.path.isfile(os.path.join(tmpdir, "pytorch_custom_diffusion_weights.bin")))
self.assertTrue(os.path.isfile(os.path.join(tmpdir, "<new1>.bin")))
def test_custom_diffusion_checkpointing_checkpoints_total_limit(self):
with tempfile.TemporaryDirectory() as tmpdir:
test_args = f"""
examples/custom_diffusion/train_custom_diffusion.py
--pretrained_model_name_or_path=hf-internal-testing/tiny-stable-diffusion-pipe
--instance_data_dir=docs/source/en/imgs
--output_dir={tmpdir}
--instance_prompt=<new1>
--resolution=64
--train_batch_size=1
--modifier_token=<new1>
--dataloader_num_workers=0
--max_train_steps=6
--checkpoints_total_limit=2
--checkpointing_steps=2
--no_safe_serialization
""".split()
run_command(self._launch_args + test_args)
self.assertEqual({x for x in os.listdir(tmpdir) if "checkpoint" in x}, {"checkpoint-4", "checkpoint-6"})
def test_custom_diffusion_checkpointing_checkpoints_total_limit_removes_multiple_checkpoints(self):
with tempfile.TemporaryDirectory() as tmpdir:
test_args = f"""
examples/custom_diffusion/train_custom_diffusion.py
--pretrained_model_name_or_path=hf-internal-testing/tiny-stable-diffusion-pipe
--instance_data_dir=docs/source/en/imgs
--output_dir={tmpdir}
--instance_prompt=<new1>
--resolution=64
--train_batch_size=1
--modifier_token=<new1>
--dataloader_num_workers=0
--max_train_steps=4
--checkpointing_steps=2
--no_safe_serialization
""".split()
run_command(self._launch_args + test_args)
self.assertEqual(
{x for x in os.listdir(tmpdir) if "checkpoint" in x},
{"checkpoint-2", "checkpoint-4"},
)
resume_run_args = f"""
examples/custom_diffusion/train_custom_diffusion.py
--pretrained_model_name_or_path=hf-internal-testing/tiny-stable-diffusion-pipe
--instance_data_dir=docs/source/en/imgs
--output_dir={tmpdir}
--instance_prompt=<new1>
--resolution=64
--train_batch_size=1
--modifier_token=<new1>
--dataloader_num_workers=0
--max_train_steps=8
--checkpointing_steps=2
--resume_from_checkpoint=checkpoint-4
--checkpoints_total_limit=2
--no_safe_serialization
""".split()
run_command(self._launch_args + resume_run_args)
self.assertEqual({x for x in os.listdir(tmpdir) if "checkpoint" in x}, {"checkpoint-6", "checkpoint-8"})
| diffusers/examples/custom_diffusion/test_custom_diffusion.py/0 | {
"file_path": "diffusers/examples/custom_diffusion/test_custom_diffusion.py",
"repo_id": "diffusers",
"token_count": 2234
} | 108 |
import warnings
from diffusers import StableDiffusionInpaintPipeline as StableDiffusionInpaintPipeline # noqa F401
warnings.warn(
"The `inpainting.py` script is outdated. Please use directly `from diffusers import"
" StableDiffusionInpaintPipeline` instead."
)
| diffusers/examples/inference/inpainting.py/0 | {
"file_path": "diffusers/examples/inference/inpainting.py",
"repo_id": "diffusers",
"token_count": 89
} | 109 |
# [DreamBooth](https://github.com/huggingface/diffusers/tree/main/examples/dreambooth) by [colossalai](https://github.com/hpcaitech/ColossalAI.git)
[DreamBooth](https://arxiv.org/abs/2208.12242) is a method to personalize text2image models like stable diffusion given just a few(3~5) images of a subject.
The `train_dreambooth_colossalai.py` script shows how to implement the training procedure and adapt it for stable diffusion.
By accommodating model data in CPU and GPU and moving the data to the computing device when necessary, [Gemini](https://www.colossalai.org/docs/advanced_tutorials/meet_gemini), the Heterogeneous Memory Manager of [Colossal-AI](https://github.com/hpcaitech/ColossalAI) can breakthrough the GPU memory wall by using GPU and CPU memory (composed of CPU DRAM or nvme SSD memory) together at the same time. Moreover, the model scale can be further improved by combining heterogeneous training with the other parallel approaches, such as data parallel, tensor parallel and pipeline parallel.
## Installing the dependencies
Before running the scripts, make sure to install the library's training dependencies:
```bash
pip install -r requirements.txt
```
## Install [ColossalAI](https://github.com/hpcaitech/ColossalAI.git)
**From PyPI**
```bash
pip install colossalai
```
**From source**
```bash
git clone https://github.com/hpcaitech/ColossalAI.git
cd ColossalAI
# install colossalai
pip install .
```
## Dataset for Teyvat BLIP captions
Dataset used to train [Teyvat characters text to image model](https://github.com/hpcaitech/ColossalAI/tree/main/examples/images/diffusion).
BLIP generated captions for characters images from [genshin-impact fandom wiki](https://genshin-impact.fandom.com/wiki/Character#Playable_Characters)and [biligame wiki for genshin impact](https://wiki.biligame.com/ys/%E8%A7%92%E8%89%B2).
For each row the dataset contains `image` and `text` keys. `image` is a varying size PIL png, and `text` is the accompanying text caption. Only a train split is provided.
The `text` include the tag `Teyvat`, `Name`,`Element`, `Weapon`, `Region`, `Model type`, and `Description`, the `Description` is captioned with the [pre-trained BLIP model](https://github.com/salesforce/BLIP).
## Training
The argument `placement` can be `cpu`, `auto`, `cuda`, with `cpu` the GPU RAM required can be minimized to 4GB but will deceleration, with `cuda` you can also reduce GPU memory by half but accelerated training, with `auto` a more balanced solution for speed and memory can be obtained。
**___Note: Change the `resolution` to 768 if you are using the [stable-diffusion-2](https://huggingface.co/stabilityai/stable-diffusion-2) 768x768 model.___**
```bash
export MODEL_NAME="CompVis/stable-diffusion-v1-4"
export INSTANCE_DIR="path-to-instance-images"
export OUTPUT_DIR="path-to-save-model"
torchrun --nproc_per_node 2 train_dreambooth_colossalai.py \
--pretrained_model_name_or_path=$MODEL_NAME \
--instance_data_dir=$INSTANCE_DIR \
--output_dir=$OUTPUT_DIR \
--instance_prompt="a photo of sks dog" \
--resolution=512 \
--train_batch_size=1 \
--learning_rate=5e-6 \
--lr_scheduler="constant" \
--lr_warmup_steps=0 \
--max_train_steps=400 \
--placement="cuda"
```
### Training with prior-preservation loss
Prior-preservation is used to avoid overfitting and language-drift. Refer to the paper to learn more about it. For prior-preservation we first generate images using the model with a class prompt and then use those during training along with our data.
According to the paper, it's recommended to generate `num_epochs * num_samples` images for prior-preservation. 200-300 works well for most cases. The `num_class_images` flag sets the number of images to generate with the class prompt. You can place existing images in `class_data_dir`, and the training script will generate any additional images so that `num_class_images` are present in `class_data_dir` during training time.
```bash
export MODEL_NAME="CompVis/stable-diffusion-v1-4"
export INSTANCE_DIR="path-to-instance-images"
export CLASS_DIR="path-to-class-images"
export OUTPUT_DIR="path-to-save-model"
torchrun --nproc_per_node 2 train_dreambooth_colossalai.py \
--pretrained_model_name_or_path=$MODEL_NAME \
--instance_data_dir=$INSTANCE_DIR \
--class_data_dir=$CLASS_DIR \
--output_dir=$OUTPUT_DIR \
--with_prior_preservation --prior_loss_weight=1.0 \
--instance_prompt="a photo of sks dog" \
--class_prompt="a photo of dog" \
--resolution=512 \
--train_batch_size=1 \
--learning_rate=5e-6 \
--lr_scheduler="constant" \
--lr_warmup_steps=0 \
--max_train_steps=800 \
--placement="cuda"
```
## Inference
Once you have trained a model using above command, the inference can be done simply using the `StableDiffusionPipeline`. Make sure to include the `identifier`(e.g. sks in above example) in your prompt.
```python
from diffusers import StableDiffusionPipeline
import torch
model_id = "path-to-save-model"
pipe = StableDiffusionPipeline.from_pretrained(model_id, torch_dtype=torch.float16).to("cuda")
prompt = "A photo of sks dog in a bucket"
image = pipe(prompt, num_inference_steps=50, guidance_scale=7.5).images[0]
image.save("dog-bucket.png")
```
| diffusers/examples/research_projects/colossalai/README.md/0 | {
"file_path": "diffusers/examples/research_projects/colossalai/README.md",
"repo_id": "diffusers",
"token_count": 1659
} | 110 |
import argparse
import math
import os
import torch
from neural_compressor.utils.pytorch import load
from PIL import Image
from transformers import CLIPTextModel, CLIPTokenizer
from diffusers import AutoencoderKL, StableDiffusionPipeline, UNet2DConditionModel
def parse_args():
parser = argparse.ArgumentParser()
parser.add_argument(
"-m",
"--pretrained_model_name_or_path",
type=str,
default=None,
required=True,
help="Path to pretrained model or model identifier from huggingface.co/models.",
)
parser.add_argument(
"-c",
"--caption",
type=str,
default="robotic cat with wings",
help="Text used to generate images.",
)
parser.add_argument(
"-n",
"--images_num",
type=int,
default=4,
help="How much images to generate.",
)
parser.add_argument(
"-s",
"--seed",
type=int,
default=42,
help="Seed for random process.",
)
parser.add_argument(
"-ci",
"--cuda_id",
type=int,
default=0,
help="cuda_id.",
)
args = parser.parse_args()
return args
def image_grid(imgs, rows, cols):
if not len(imgs) == rows * cols:
raise ValueError("The specified number of rows and columns are not correct.")
w, h = imgs[0].size
grid = Image.new("RGB", size=(cols * w, rows * h))
grid_w, grid_h = grid.size
for i, img in enumerate(imgs):
grid.paste(img, box=(i % cols * w, i // cols * h))
return grid
def generate_images(
pipeline,
prompt="robotic cat with wings",
guidance_scale=7.5,
num_inference_steps=50,
num_images_per_prompt=1,
seed=42,
):
generator = torch.Generator(pipeline.device).manual_seed(seed)
images = pipeline(
prompt,
guidance_scale=guidance_scale,
num_inference_steps=num_inference_steps,
generator=generator,
num_images_per_prompt=num_images_per_prompt,
).images
_rows = int(math.sqrt(num_images_per_prompt))
grid = image_grid(images, rows=_rows, cols=num_images_per_prompt // _rows)
return grid, images
args = parse_args()
# Load models and create wrapper for stable diffusion
tokenizer = CLIPTokenizer.from_pretrained(args.pretrained_model_name_or_path, subfolder="tokenizer")
text_encoder = CLIPTextModel.from_pretrained(args.pretrained_model_name_or_path, subfolder="text_encoder")
vae = AutoencoderKL.from_pretrained(args.pretrained_model_name_or_path, subfolder="vae")
unet = UNet2DConditionModel.from_pretrained(args.pretrained_model_name_or_path, subfolder="unet")
pipeline = StableDiffusionPipeline.from_pretrained(
args.pretrained_model_name_or_path, text_encoder=text_encoder, vae=vae, unet=unet, tokenizer=tokenizer
)
pipeline.safety_checker = lambda images, clip_input: (images, False)
if os.path.exists(os.path.join(args.pretrained_model_name_or_path, "best_model.pt")):
unet = load(args.pretrained_model_name_or_path, model=unet)
unet.eval()
setattr(pipeline, "unet", unet)
else:
unet = unet.to(torch.device("cuda", args.cuda_id))
pipeline = pipeline.to(unet.device)
grid, images = generate_images(pipeline, prompt=args.caption, num_images_per_prompt=args.images_num, seed=args.seed)
grid.save(os.path.join(args.pretrained_model_name_or_path, "{}.png".format("_".join(args.caption.split()))))
dirname = os.path.join(args.pretrained_model_name_or_path, "_".join(args.caption.split()))
os.makedirs(dirname, exist_ok=True)
for idx, image in enumerate(images):
image.save(os.path.join(dirname, "{}.png".format(idx + 1)))
| diffusers/examples/research_projects/intel_opts/textual_inversion_dfq/text2images.py/0 | {
"file_path": "diffusers/examples/research_projects/intel_opts/textual_inversion_dfq/text2images.py",
"repo_id": "diffusers",
"token_count": 1518
} | 111 |
import argparse
import logging
import math
import os
import random
from pathlib import Path
import jax
import jax.numpy as jnp
import numpy as np
import optax
import PIL
import torch
import torch.utils.checkpoint
import transformers
from flax import jax_utils
from flax.training import train_state
from flax.training.common_utils import shard
from huggingface_hub import create_repo, upload_folder
# TODO: remove and import from diffusers.utils when the new version of diffusers is released
from packaging import version
from PIL import Image
from torch.utils.data import Dataset
from torchvision import transforms
from tqdm.auto import tqdm
from transformers import CLIPImageProcessor, CLIPTokenizer, FlaxCLIPTextModel, set_seed
from diffusers import (
FlaxAutoencoderKL,
FlaxDDPMScheduler,
FlaxPNDMScheduler,
FlaxStableDiffusionPipeline,
FlaxUNet2DConditionModel,
)
from diffusers.pipelines.stable_diffusion import FlaxStableDiffusionSafetyChecker
from diffusers.utils import check_min_version
if version.parse(version.parse(PIL.__version__).base_version) >= version.parse("9.1.0"):
PIL_INTERPOLATION = {
"linear": PIL.Image.Resampling.BILINEAR,
"bilinear": PIL.Image.Resampling.BILINEAR,
"bicubic": PIL.Image.Resampling.BICUBIC,
"lanczos": PIL.Image.Resampling.LANCZOS,
"nearest": PIL.Image.Resampling.NEAREST,
}
else:
PIL_INTERPOLATION = {
"linear": PIL.Image.LINEAR,
"bilinear": PIL.Image.BILINEAR,
"bicubic": PIL.Image.BICUBIC,
"lanczos": PIL.Image.LANCZOS,
"nearest": PIL.Image.NEAREST,
}
# ------------------------------------------------------------------------------
# Will error if the minimal version of diffusers is not installed. Remove at your own risks.
check_min_version("0.14.0.dev0")
logger = logging.getLogger(__name__)
def parse_args():
parser = argparse.ArgumentParser(description="Simple example of a training script.")
parser.add_argument(
"--pretrained_model_name_or_path",
type=str,
default=None,
required=True,
help="Path to pretrained model or model identifier from huggingface.co/models.",
)
parser.add_argument(
"--tokenizer_name",
type=str,
default=None,
help="Pretrained tokenizer name or path if not the same as model_name",
)
parser.add_argument(
"--train_data_dir", type=str, default=None, required=True, help="A folder containing the training data."
)
parser.add_argument(
"--placeholder_token",
type=str,
default=None,
required=True,
help="A token to use as a placeholder for the concept.",
)
parser.add_argument(
"--initializer_token", type=str, default=None, required=True, help="A token to use as initializer word."
)
parser.add_argument("--learnable_property", type=str, default="object", help="Choose between 'object' and 'style'")
parser.add_argument("--repeats", type=int, default=100, help="How many times to repeat the training data.")
parser.add_argument(
"--output_dir",
type=str,
default="text-inversion-model",
help="The output directory where the model predictions and checkpoints will be written.",
)
parser.add_argument("--seed", type=int, default=42, help="A seed for reproducible training.")
parser.add_argument(
"--resolution",
type=int,
default=512,
help=(
"The resolution for input images, all the images in the train/validation dataset will be resized to this"
" resolution"
),
)
parser.add_argument(
"--center_crop", action="store_true", help="Whether to center crop images before resizing to resolution."
)
parser.add_argument(
"--train_batch_size", type=int, default=16, help="Batch size (per device) for the training dataloader."
)
parser.add_argument("--num_train_epochs", type=int, default=100)
parser.add_argument(
"--max_train_steps",
type=int,
default=5000,
help="Total number of training steps to perform. If provided, overrides num_train_epochs.",
)
parser.add_argument(
"--learning_rate",
type=float,
default=1e-4,
help="Initial learning rate (after the potential warmup period) to use.",
)
parser.add_argument(
"--scale_lr",
action="store_true",
default=True,
help="Scale the learning rate by the number of GPUs, gradient accumulation steps, and batch size.",
)
parser.add_argument(
"--lr_warmup_steps", type=int, default=500, help="Number of steps for the warmup in the lr scheduler."
)
parser.add_argument(
"--lr_scheduler",
type=str,
default="constant",
help=(
'The scheduler type to use. Choose between ["linear", "cosine", "cosine_with_restarts", "polynomial",'
' "constant", "constant_with_warmup"]'
),
)
parser.add_argument("--adam_beta1", type=float, default=0.9, help="The beta1 parameter for the Adam optimizer.")
parser.add_argument("--adam_beta2", type=float, default=0.999, help="The beta2 parameter for the Adam optimizer.")
parser.add_argument("--adam_weight_decay", type=float, default=1e-2, help="Weight decay to use.")
parser.add_argument("--adam_epsilon", type=float, default=1e-08, help="Epsilon value for the Adam optimizer")
parser.add_argument("--push_to_hub", action="store_true", help="Whether or not to push the model to the Hub.")
parser.add_argument(
"--use_auth_token",
action="store_true",
help=(
"Will use the token generated when running `huggingface-cli login` (necessary to use this script with"
" private models)."
),
)
parser.add_argument("--hub_token", type=str, default=None, help="The token to use to push to the Model Hub.")
parser.add_argument(
"--hub_model_id",
type=str,
default=None,
help="The name of the repository to keep in sync with the local `output_dir`.",
)
parser.add_argument(
"--logging_dir",
type=str,
default="logs",
help=(
"[TensorBoard](https://www.tensorflow.org/tensorboard) log directory. Will default to"
" *output_dir/runs/**CURRENT_DATETIME_HOSTNAME***."
),
)
parser.add_argument("--local_rank", type=int, default=-1, help="For distributed training: local_rank")
args = parser.parse_args()
env_local_rank = int(os.environ.get("LOCAL_RANK", -1))
if env_local_rank != -1 and env_local_rank != args.local_rank:
args.local_rank = env_local_rank
if args.train_data_dir is None:
raise ValueError("You must specify a train data directory.")
return args
imagenet_templates_small = [
"a photo of a {}",
"a rendering of a {}",
"a cropped photo of the {}",
"the photo of a {}",
"a photo of a clean {}",
"a photo of a dirty {}",
"a dark photo of the {}",
"a photo of my {}",
"a photo of the cool {}",
"a close-up photo of a {}",
"a bright photo of the {}",
"a cropped photo of a {}",
"a photo of the {}",
"a good photo of the {}",
"a photo of one {}",
"a close-up photo of the {}",
"a rendition of the {}",
"a photo of the clean {}",
"a rendition of a {}",
"a photo of a nice {}",
"a good photo of a {}",
"a photo of the nice {}",
"a photo of the small {}",
"a photo of the weird {}",
"a photo of the large {}",
"a photo of a cool {}",
"a photo of a small {}",
]
imagenet_style_templates_small = [
"a painting in the style of {}",
"a rendering in the style of {}",
"a cropped painting in the style of {}",
"the painting in the style of {}",
"a clean painting in the style of {}",
"a dirty painting in the style of {}",
"a dark painting in the style of {}",
"a picture in the style of {}",
"a cool painting in the style of {}",
"a close-up painting in the style of {}",
"a bright painting in the style of {}",
"a cropped painting in the style of {}",
"a good painting in the style of {}",
"a close-up painting in the style of {}",
"a rendition in the style of {}",
"a nice painting in the style of {}",
"a small painting in the style of {}",
"a weird painting in the style of {}",
"a large painting in the style of {}",
]
class TextualInversionDataset(Dataset):
def __init__(
self,
data_root,
tokenizer,
learnable_property="object", # [object, style]
size=512,
repeats=100,
interpolation="bicubic",
flip_p=0.5,
set="train",
placeholder_token="*",
center_crop=False,
):
self.data_root = data_root
self.tokenizer = tokenizer
self.learnable_property = learnable_property
self.size = size
self.placeholder_token = placeholder_token
self.center_crop = center_crop
self.flip_p = flip_p
self.image_paths = [os.path.join(self.data_root, file_path) for file_path in os.listdir(self.data_root)]
self.num_images = len(self.image_paths)
self._length = self.num_images
if set == "train":
self._length = self.num_images * repeats
self.interpolation = {
"linear": PIL_INTERPOLATION["linear"],
"bilinear": PIL_INTERPOLATION["bilinear"],
"bicubic": PIL_INTERPOLATION["bicubic"],
"lanczos": PIL_INTERPOLATION["lanczos"],
}[interpolation]
self.templates = imagenet_style_templates_small if learnable_property == "style" else imagenet_templates_small
self.flip_transform = transforms.RandomHorizontalFlip(p=self.flip_p)
def __len__(self):
return self._length
def __getitem__(self, i):
example = {}
image = Image.open(self.image_paths[i % self.num_images])
if not image.mode == "RGB":
image = image.convert("RGB")
placeholder_string = self.placeholder_token
text = random.choice(self.templates).format(placeholder_string)
example["input_ids"] = self.tokenizer(
text,
padding="max_length",
truncation=True,
max_length=self.tokenizer.model_max_length,
return_tensors="pt",
).input_ids[0]
# default to score-sde preprocessing
img = np.array(image).astype(np.uint8)
if self.center_crop:
crop = min(img.shape[0], img.shape[1])
(
h,
w,
) = (
img.shape[0],
img.shape[1],
)
img = img[(h - crop) // 2 : (h + crop) // 2, (w - crop) // 2 : (w + crop) // 2]
image = Image.fromarray(img)
image = image.resize((self.size, self.size), resample=self.interpolation)
image = self.flip_transform(image)
image = np.array(image).astype(np.uint8)
image = (image / 127.5 - 1.0).astype(np.float32)
example["pixel_values"] = torch.from_numpy(image).permute(2, 0, 1)
return example
def resize_token_embeddings(model, new_num_tokens, initializer_token_id, placeholder_token_id, rng):
if model.config.vocab_size == new_num_tokens or new_num_tokens is None:
return
model.config.vocab_size = new_num_tokens
params = model.params
old_embeddings = params["text_model"]["embeddings"]["token_embedding"]["embedding"]
old_num_tokens, emb_dim = old_embeddings.shape
initializer = jax.nn.initializers.normal()
new_embeddings = initializer(rng, (new_num_tokens, emb_dim))
new_embeddings = new_embeddings.at[:old_num_tokens].set(old_embeddings)
new_embeddings = new_embeddings.at[placeholder_token_id].set(new_embeddings[initializer_token_id])
params["text_model"]["embeddings"]["token_embedding"]["embedding"] = new_embeddings
model.params = params
return model
def get_params_to_save(params):
return jax.device_get(jax.tree_util.tree_map(lambda x: x[0], params))
def main():
args = parse_args()
if args.seed is not None:
set_seed(args.seed)
if jax.process_index() == 0:
if args.output_dir is not None:
os.makedirs(args.output_dir, exist_ok=True)
if args.push_to_hub:
repo_id = create_repo(
repo_id=args.hub_model_id or Path(args.output_dir).name, exist_ok=True, token=args.hub_token
).repo_id
# Make one log on every process with the configuration for debugging.
logging.basicConfig(
format="%(asctime)s - %(levelname)s - %(name)s - %(message)s",
datefmt="%m/%d/%Y %H:%M:%S",
level=logging.INFO,
)
# Setup logging, we only want one process per machine to log things on the screen.
logger.setLevel(logging.INFO if jax.process_index() == 0 else logging.ERROR)
if jax.process_index() == 0:
transformers.utils.logging.set_verbosity_info()
else:
transformers.utils.logging.set_verbosity_error()
# Load the tokenizer and add the placeholder token as a additional special token
if args.tokenizer_name:
tokenizer = CLIPTokenizer.from_pretrained(args.tokenizer_name)
elif args.pretrained_model_name_or_path:
tokenizer = CLIPTokenizer.from_pretrained(args.pretrained_model_name_or_path, subfolder="tokenizer")
# Add the placeholder token in tokenizer
num_added_tokens = tokenizer.add_tokens(args.placeholder_token)
if num_added_tokens == 0:
raise ValueError(
f"The tokenizer already contains the token {args.placeholder_token}. Please pass a different"
" `placeholder_token` that is not already in the tokenizer."
)
# Convert the initializer_token, placeholder_token to ids
token_ids = tokenizer.encode(args.initializer_token, add_special_tokens=False)
# Check if initializer_token is a single token or a sequence of tokens
if len(token_ids) > 1:
raise ValueError("The initializer token must be a single token.")
initializer_token_id = token_ids[0]
placeholder_token_id = tokenizer.convert_tokens_to_ids(args.placeholder_token)
# Load models and create wrapper for stable diffusion
text_encoder = FlaxCLIPTextModel.from_pretrained(args.pretrained_model_name_or_path, subfolder="text_encoder")
vae, vae_params = FlaxAutoencoderKL.from_pretrained(args.pretrained_model_name_or_path, subfolder="vae")
unet, unet_params = FlaxUNet2DConditionModel.from_pretrained(args.pretrained_model_name_or_path, subfolder="unet")
# Create sampling rng
rng = jax.random.PRNGKey(args.seed)
rng, _ = jax.random.split(rng)
# Resize the token embeddings as we are adding new special tokens to the tokenizer
text_encoder = resize_token_embeddings(
text_encoder, len(tokenizer), initializer_token_id, placeholder_token_id, rng
)
original_token_embeds = text_encoder.params["text_model"]["embeddings"]["token_embedding"]["embedding"]
train_dataset = TextualInversionDataset(
data_root=args.train_data_dir,
tokenizer=tokenizer,
size=args.resolution,
placeholder_token=args.placeholder_token,
repeats=args.repeats,
learnable_property=args.learnable_property,
center_crop=args.center_crop,
set="train",
)
def collate_fn(examples):
pixel_values = torch.stack([example["pixel_values"] for example in examples])
input_ids = torch.stack([example["input_ids"] for example in examples])
batch = {"pixel_values": pixel_values, "input_ids": input_ids}
batch = {k: v.numpy() for k, v in batch.items()}
return batch
total_train_batch_size = args.train_batch_size * jax.local_device_count()
train_dataloader = torch.utils.data.DataLoader(
train_dataset, batch_size=total_train_batch_size, shuffle=True, drop_last=True, collate_fn=collate_fn
)
# Optimization
if args.scale_lr:
args.learning_rate = args.learning_rate * total_train_batch_size
constant_scheduler = optax.constant_schedule(args.learning_rate)
optimizer = optax.adamw(
learning_rate=constant_scheduler,
b1=args.adam_beta1,
b2=args.adam_beta2,
eps=args.adam_epsilon,
weight_decay=args.adam_weight_decay,
)
def create_mask(params, label_fn):
def _map(params, mask, label_fn):
for k in params:
if label_fn(k):
mask[k] = "token_embedding"
else:
if isinstance(params[k], dict):
mask[k] = {}
_map(params[k], mask[k], label_fn)
else:
mask[k] = "zero"
mask = {}
_map(params, mask, label_fn)
return mask
def zero_grads():
# from https://github.com/deepmind/optax/issues/159#issuecomment-896459491
def init_fn(_):
return ()
def update_fn(updates, state, params=None):
return jax.tree_util.tree_map(jnp.zeros_like, updates), ()
return optax.GradientTransformation(init_fn, update_fn)
# Zero out gradients of layers other than the token embedding layer
tx = optax.multi_transform(
{"token_embedding": optimizer, "zero": zero_grads()},
create_mask(text_encoder.params, lambda s: s == "token_embedding"),
)
state = train_state.TrainState.create(apply_fn=text_encoder.__call__, params=text_encoder.params, tx=tx)
noise_scheduler = FlaxDDPMScheduler(
beta_start=0.00085, beta_end=0.012, beta_schedule="scaled_linear", num_train_timesteps=1000
)
noise_scheduler_state = noise_scheduler.create_state()
# Initialize our training
train_rngs = jax.random.split(rng, jax.local_device_count())
# Define gradient train step fn
def train_step(state, vae_params, unet_params, batch, train_rng):
dropout_rng, sample_rng, new_train_rng = jax.random.split(train_rng, 3)
def compute_loss(params):
vae_outputs = vae.apply(
{"params": vae_params}, batch["pixel_values"], deterministic=True, method=vae.encode
)
latents = vae_outputs.latent_dist.sample(sample_rng)
# (NHWC) -> (NCHW)
latents = jnp.transpose(latents, (0, 3, 1, 2))
latents = latents * vae.config.scaling_factor
noise_rng, timestep_rng = jax.random.split(sample_rng)
noise = jax.random.normal(noise_rng, latents.shape)
bsz = latents.shape[0]
timesteps = jax.random.randint(
timestep_rng,
(bsz,),
0,
noise_scheduler.config.num_train_timesteps,
)
noisy_latents = noise_scheduler.add_noise(noise_scheduler_state, latents, noise, timesteps)
encoder_hidden_states = state.apply_fn(
batch["input_ids"], params=params, dropout_rng=dropout_rng, train=True
)[0]
# Predict the noise residual and compute loss
model_pred = unet.apply(
{"params": unet_params}, noisy_latents, timesteps, encoder_hidden_states, train=False
).sample
# Get the target for loss depending on the prediction type
if noise_scheduler.config.prediction_type == "epsilon":
target = noise
elif noise_scheduler.config.prediction_type == "v_prediction":
target = noise_scheduler.get_velocity(noise_scheduler_state, latents, noise, timesteps)
else:
raise ValueError(f"Unknown prediction type {noise_scheduler.config.prediction_type}")
loss = (target - model_pred) ** 2
loss = loss.mean()
return loss
grad_fn = jax.value_and_grad(compute_loss)
loss, grad = grad_fn(state.params)
grad = jax.lax.pmean(grad, "batch")
new_state = state.apply_gradients(grads=grad)
# Keep the token embeddings fixed except the newly added embeddings for the concept,
# as we only want to optimize the concept embeddings
token_embeds = original_token_embeds.at[placeholder_token_id].set(
new_state.params["text_model"]["embeddings"]["token_embedding"]["embedding"][placeholder_token_id]
)
new_state.params["text_model"]["embeddings"]["token_embedding"]["embedding"] = token_embeds
metrics = {"loss": loss}
metrics = jax.lax.pmean(metrics, axis_name="batch")
return new_state, metrics, new_train_rng
# Create parallel version of the train and eval step
p_train_step = jax.pmap(train_step, "batch", donate_argnums=(0,))
# Replicate the train state on each device
state = jax_utils.replicate(state)
vae_params = jax_utils.replicate(vae_params)
unet_params = jax_utils.replicate(unet_params)
# Train!
num_update_steps_per_epoch = math.ceil(len(train_dataloader))
# Scheduler and math around the number of training steps.
if args.max_train_steps is None:
args.max_train_steps = args.num_train_epochs * num_update_steps_per_epoch
args.num_train_epochs = math.ceil(args.max_train_steps / num_update_steps_per_epoch)
logger.info("***** Running training *****")
logger.info(f" Num examples = {len(train_dataset)}")
logger.info(f" Num Epochs = {args.num_train_epochs}")
logger.info(f" Instantaneous batch size per device = {args.train_batch_size}")
logger.info(f" Total train batch size (w. parallel & distributed) = {total_train_batch_size}")
logger.info(f" Total optimization steps = {args.max_train_steps}")
global_step = 0
epochs = tqdm(range(args.num_train_epochs), desc=f"Epoch ... (1/{args.num_train_epochs})", position=0)
for epoch in epochs:
# ======================== Training ================================
train_metrics = []
steps_per_epoch = len(train_dataset) // total_train_batch_size
train_step_progress_bar = tqdm(total=steps_per_epoch, desc="Training...", position=1, leave=False)
# train
for batch in train_dataloader:
batch = shard(batch)
state, train_metric, train_rngs = p_train_step(state, vae_params, unet_params, batch, train_rngs)
train_metrics.append(train_metric)
train_step_progress_bar.update(1)
global_step += 1
if global_step >= args.max_train_steps:
break
train_metric = jax_utils.unreplicate(train_metric)
train_step_progress_bar.close()
epochs.write(f"Epoch... ({epoch + 1}/{args.num_train_epochs} | Loss: {train_metric['loss']})")
# Create the pipeline using using the trained modules and save it.
if jax.process_index() == 0:
scheduler = FlaxPNDMScheduler(
beta_start=0.00085, beta_end=0.012, beta_schedule="scaled_linear", skip_prk_steps=True
)
safety_checker = FlaxStableDiffusionSafetyChecker.from_pretrained(
"CompVis/stable-diffusion-safety-checker", from_pt=True
)
pipeline = FlaxStableDiffusionPipeline(
text_encoder=text_encoder,
vae=vae,
unet=unet,
tokenizer=tokenizer,
scheduler=scheduler,
safety_checker=safety_checker,
feature_extractor=CLIPImageProcessor.from_pretrained("openai/clip-vit-base-patch32"),
)
pipeline.save_pretrained(
args.output_dir,
params={
"text_encoder": get_params_to_save(state.params),
"vae": get_params_to_save(vae_params),
"unet": get_params_to_save(unet_params),
"safety_checker": safety_checker.params,
},
)
# Also save the newly trained embeddings
learned_embeds = get_params_to_save(state.params)["text_model"]["embeddings"]["token_embedding"]["embedding"][
placeholder_token_id
]
learned_embeds_dict = {args.placeholder_token: learned_embeds}
jnp.save(os.path.join(args.output_dir, "learned_embeds.npy"), learned_embeds_dict)
if args.push_to_hub:
upload_folder(
repo_id=repo_id,
folder_path=args.output_dir,
commit_message="End of training",
ignore_patterns=["step_*", "epoch_*"],
)
if __name__ == "__main__":
main()
| diffusers/examples/research_projects/multi_token_textual_inversion/textual_inversion_flax.py/0 | {
"file_path": "diffusers/examples/research_projects/multi_token_textual_inversion/textual_inversion_flax.py",
"repo_id": "diffusers",
"token_count": 10599
} | 112 |
import inspect
from typing import Callable, List, Optional, Union
import torch
from PIL import Image
from retriever import Retriever, normalize_images, preprocess_images
from transformers import CLIPFeatureExtractor, CLIPModel, CLIPTokenizer
from diffusers import (
AutoencoderKL,
DDIMScheduler,
DiffusionPipeline,
DPMSolverMultistepScheduler,
EulerAncestralDiscreteScheduler,
EulerDiscreteScheduler,
ImagePipelineOutput,
LMSDiscreteScheduler,
PNDMScheduler,
UNet2DConditionModel,
)
from diffusers.image_processor import VaeImageProcessor
from diffusers.pipelines.pipeline_utils import StableDiffusionMixin
from diffusers.utils import logging
from diffusers.utils.torch_utils import randn_tensor
logger = logging.get_logger(__name__) # pylint: disable=invalid-name
class RDMPipeline(DiffusionPipeline, StableDiffusionMixin):
r"""
Pipeline for text-to-image generation using Retrieval Augmented Diffusion.
This model inherits from [`DiffusionPipeline`]. Check the superclass documentation for the generic methods the
library implements for all the pipelines (such as downloading or saving, running on a particular device, etc.)
Args:
vae ([`AutoencoderKL`]):
Variational Auto-Encoder (VAE) Model to encode and decode images to and from latent representations.
clip ([`CLIPModel`]):
Frozen CLIP model. Retrieval Augmented Diffusion uses the CLIP model, specifically the
[clip-vit-large-patch14](https://huggingface.co/openai/clip-vit-large-patch14) variant.
tokenizer (`CLIPTokenizer`):
Tokenizer of class
[CLIPTokenizer](https://huggingface.co/docs/transformers/v4.21.0/en/model_doc/clip#transformers.CLIPTokenizer).
unet ([`UNet2DConditionModel`]): Conditional U-Net architecture to denoise the encoded image latents.
scheduler ([`SchedulerMixin`]):
A scheduler to be used in combination with `unet` to denoise the encoded image latents. Can be one of
[`DDIMScheduler`], [`LMSDiscreteScheduler`], or [`PNDMScheduler`].
feature_extractor ([`CLIPFeatureExtractor`]):
Model that extracts features from generated images to be used as inputs for the `safety_checker`.
"""
def __init__(
self,
vae: AutoencoderKL,
clip: CLIPModel,
tokenizer: CLIPTokenizer,
unet: UNet2DConditionModel,
scheduler: Union[
DDIMScheduler,
PNDMScheduler,
LMSDiscreteScheduler,
EulerDiscreteScheduler,
EulerAncestralDiscreteScheduler,
DPMSolverMultistepScheduler,
],
feature_extractor: CLIPFeatureExtractor,
retriever: Optional[Retriever] = None,
):
super().__init__()
self.register_modules(
vae=vae,
clip=clip,
tokenizer=tokenizer,
unet=unet,
scheduler=scheduler,
feature_extractor=feature_extractor,
)
# Copy from statement here and all the methods we take from stable_diffusion_pipeline
self.vae_scale_factor = 2 ** (len(self.vae.config.block_out_channels) - 1)
self.image_processor = VaeImageProcessor(vae_scale_factor=self.vae_scale_factor)
self.retriever = retriever
def _encode_prompt(self, prompt):
# get prompt text embeddings
text_inputs = self.tokenizer(
prompt,
padding="max_length",
max_length=self.tokenizer.model_max_length,
truncation=True,
return_tensors="pt",
)
text_input_ids = text_inputs.input_ids
if text_input_ids.shape[-1] > self.tokenizer.model_max_length:
removed_text = self.tokenizer.batch_decode(text_input_ids[:, self.tokenizer.model_max_length :])
logger.warning(
"The following part of your input was truncated because CLIP can only handle sequences up to"
f" {self.tokenizer.model_max_length} tokens: {removed_text}"
)
text_input_ids = text_input_ids[:, : self.tokenizer.model_max_length]
prompt_embeds = self.clip.get_text_features(text_input_ids.to(self.device))
prompt_embeds = prompt_embeds / torch.linalg.norm(prompt_embeds, dim=-1, keepdim=True)
prompt_embeds = prompt_embeds[:, None, :]
return prompt_embeds
def _encode_image(self, retrieved_images, batch_size):
if len(retrieved_images[0]) == 0:
return None
for i in range(len(retrieved_images)):
retrieved_images[i] = normalize_images(retrieved_images[i])
retrieved_images[i] = preprocess_images(retrieved_images[i], self.feature_extractor).to(
self.clip.device, dtype=self.clip.dtype
)
_, c, h, w = retrieved_images[0].shape
retrieved_images = torch.reshape(torch.cat(retrieved_images, dim=0), (-1, c, h, w))
image_embeddings = self.clip.get_image_features(retrieved_images)
image_embeddings = image_embeddings / torch.linalg.norm(image_embeddings, dim=-1, keepdim=True)
_, d = image_embeddings.shape
image_embeddings = torch.reshape(image_embeddings, (batch_size, -1, d))
return image_embeddings
def prepare_latents(self, batch_size, num_channels_latents, height, width, dtype, device, generator, latents=None):
shape = (batch_size, num_channels_latents, height // self.vae_scale_factor, width // self.vae_scale_factor)
if isinstance(generator, list) and len(generator) != batch_size:
raise ValueError(
f"You have passed a list of generators of length {len(generator)}, but requested an effective batch"
f" size of {batch_size}. Make sure the batch size matches the length of the generators."
)
if latents is None:
latents = randn_tensor(shape, generator=generator, device=device, dtype=dtype)
else:
latents = latents.to(device)
# scale the initial noise by the standard deviation required by the scheduler
latents = latents * self.scheduler.init_noise_sigma
return latents
def retrieve_images(self, retrieved_images, prompt_embeds, knn=10):
if self.retriever is not None:
additional_images = self.retriever.retrieve_imgs_batch(prompt_embeds[:, 0].cpu(), knn).total_examples
for i in range(len(retrieved_images)):
retrieved_images[i] += additional_images[i][self.retriever.config.image_column]
return retrieved_images
@torch.no_grad()
def __call__(
self,
prompt: Union[str, List[str]],
retrieved_images: Optional[List[Image.Image]] = None,
height: int = 768,
width: int = 768,
num_inference_steps: int = 50,
guidance_scale: float = 7.5,
num_images_per_prompt: Optional[int] = 1,
eta: float = 0.0,
generator: Optional[torch.Generator] = None,
latents: Optional[torch.FloatTensor] = None,
prompt_embeds: Optional[torch.FloatTensor] = None,
output_type: Optional[str] = "pil",
return_dict: bool = True,
callback: Optional[Callable[[int, int, torch.FloatTensor], None]] = None,
callback_steps: Optional[int] = 1,
knn: Optional[int] = 10,
**kwargs,
):
r"""
Function invoked when calling the pipeline for generation.
Args:
prompt (`str` or `List[str]`):
The prompt or prompts to guide the image generation.
height (`int`, *optional*, defaults to 512):
The height in pixels of the generated image.
width (`int`, *optional*, defaults to 512):
The width in pixels of the generated image.
num_inference_steps (`int`, *optional*, defaults to 50):
The number of denoising steps. More denoising steps usually lead to a higher quality image at the
expense of slower inference.
guidance_scale (`float`, *optional*, defaults to 7.5):
Guidance scale as defined in [Classifier-Free Diffusion Guidance](https://arxiv.org/abs/2207.12598).
`guidance_scale` is defined as `w` of equation 2. of [Imagen
Paper](https://arxiv.org/pdf/2205.11487.pdf). Guidance scale is enabled by setting `guidance_scale >
1`. Higher guidance scale encourages to generate images that are closely linked to the text `prompt`,
usually at the expense of lower image quality.
num_images_per_prompt (`int`, *optional*, defaults to 1):
The number of images to generate per prompt.
eta (`float`, *optional*, defaults to 0.0):
Corresponds to parameter eta (η) in the DDIM paper: https://arxiv.org/abs/2010.02502. Only applies to
[`schedulers.DDIMScheduler`], will be ignored for others.
generator (`torch.Generator`, *optional*):
A [torch generator](https://pytorch.org/docs/stable/generated/torch.Generator.html) to make generation
deterministic.
latents (`torch.FloatTensor`, *optional*):
Pre-generated noisy latents, sampled from a Gaussian distribution, to be used as inputs for image
generation. Can be used to tweak the same generation with different prompts. If not provided, a latents
tensor will ge generated by sampling using the supplied random `generator`.
prompt_embeds (`torch.FloatTensor`, *optional*):
Pre-generated text embeddings. Can be used to easily tweak text inputs, *e.g.* prompt weighting. If not
provided, text embeddings will be generated from `prompt` input argument.
output_type (`str`, *optional*, defaults to `"pil"`):
The output format of the generate image. Choose between
[PIL](https://pillow.readthedocs.io/en/stable/): `PIL.Image.Image` or `np.array`.
return_dict (`bool`, *optional*, defaults to `True`):
Whether or not to return a [`~pipeline_utils.ImagePipelineOutput`] instead of a plain tuple.
callback (`Callable`, *optional*):
A function that will be called every `callback_steps` steps during inference. The function will be
called with the following arguments: `callback(step: int, timestep: int, latents: torch.FloatTensor)`.
callback_steps (`int`, *optional*, defaults to 1):
The frequency at which the `callback` function will be called. If not specified, the callback will be
called at every step.
Returns:
[`~pipeline_utils.ImagePipelineOutput`] or `tuple`: [`~pipelines.utils.ImagePipelineOutput`] if
`return_dict` is True, otherwise a `tuple. When returning a tuple, the first element is a list with the
generated images.
"""
height = height or self.unet.config.sample_size * self.vae_scale_factor
width = width or self.unet.config.sample_size * self.vae_scale_factor
if isinstance(prompt, str):
batch_size = 1
elif isinstance(prompt, list):
batch_size = len(prompt)
else:
raise ValueError(f"`prompt` has to be of type `str` or `list` but is {type(prompt)}")
if retrieved_images is not None:
retrieved_images = [retrieved_images for _ in range(batch_size)]
else:
retrieved_images = [[] for _ in range(batch_size)]
device = self._execution_device
if height % 8 != 0 or width % 8 != 0:
raise ValueError(f"`height` and `width` have to be divisible by 8 but are {height} and {width}.")
if (callback_steps is None) or (
callback_steps is not None and (not isinstance(callback_steps, int) or callback_steps <= 0)
):
raise ValueError(
f"`callback_steps` has to be a positive integer but is {callback_steps} of type"
f" {type(callback_steps)}."
)
if prompt_embeds is None:
prompt_embeds = self._encode_prompt(prompt)
retrieved_images = self.retrieve_images(retrieved_images, prompt_embeds, knn=knn)
image_embeddings = self._encode_image(retrieved_images, batch_size)
if image_embeddings is not None:
prompt_embeds = torch.cat([prompt_embeds, image_embeddings], dim=1)
# duplicate text embeddings for each generation per prompt, using mps friendly method
bs_embed, seq_len, _ = prompt_embeds.shape
prompt_embeds = prompt_embeds.repeat(1, num_images_per_prompt, 1)
prompt_embeds = prompt_embeds.view(bs_embed * num_images_per_prompt, seq_len, -1)
# here `guidance_scale` is defined analog to the guidance weight `w` of equation (2)
# of the Imagen paper: https://arxiv.org/pdf/2205.11487.pdf . `guidance_scale = 1`
# corresponds to doing no classifier free guidance.
do_classifier_free_guidance = guidance_scale > 1.0
# get unconditional embeddings for classifier free guidance
if do_classifier_free_guidance:
uncond_embeddings = torch.zeros_like(prompt_embeds).to(prompt_embeds.device)
# For classifier free guidance, we need to do two forward passes.
# Here we concatenate the unconditional and text embeddings into a single batch
# to avoid doing two forward passes
prompt_embeds = torch.cat([uncond_embeddings, prompt_embeds])
# get the initial random noise unless the user supplied it
num_channels_latents = self.unet.config.in_channels
latents = self.prepare_latents(
batch_size * num_images_per_prompt,
num_channels_latents,
height,
width,
prompt_embeds.dtype,
device,
generator,
latents,
)
# set timesteps
self.scheduler.set_timesteps(num_inference_steps)
# Some schedulers like PNDM have timesteps as arrays
# It's more optimized to move all timesteps to correct device beforehand
timesteps_tensor = self.scheduler.timesteps.to(self.device)
# prepare extra kwargs for the scheduler step, since not all schedulers have the same signature
# eta (η) is only used with the DDIMScheduler, it will be ignored for other schedulers.
# eta corresponds to η in DDIM paper: https://arxiv.org/abs/2010.02502
# and should be between [0, 1]
accepts_eta = "eta" in set(inspect.signature(self.scheduler.step).parameters.keys())
extra_step_kwargs = {}
if accepts_eta:
extra_step_kwargs["eta"] = eta
# check if the scheduler accepts generator
accepts_generator = "generator" in set(inspect.signature(self.scheduler.step).parameters.keys())
if accepts_generator:
extra_step_kwargs["generator"] = generator
for i, t in enumerate(self.progress_bar(timesteps_tensor)):
# expand the latents if we are doing classifier free guidance
latent_model_input = torch.cat([latents] * 2) if do_classifier_free_guidance else latents
latent_model_input = self.scheduler.scale_model_input(latent_model_input, t)
# predict the noise residual
noise_pred = self.unet(latent_model_input, t, encoder_hidden_states=prompt_embeds).sample
# perform guidance
if do_classifier_free_guidance:
noise_pred_uncond, noise_pred_text = noise_pred.chunk(2)
noise_pred = noise_pred_uncond + guidance_scale * (noise_pred_text - noise_pred_uncond)
# compute the previous noisy sample x_t -> x_t-1
latents = self.scheduler.step(noise_pred, t, latents, **extra_step_kwargs).prev_sample
# call the callback, if provided
if callback is not None and i % callback_steps == 0:
step_idx = i // getattr(self.scheduler, "order", 1)
callback(step_idx, t, latents)
if not output_type == "latent":
image = self.vae.decode(latents / self.vae.config.scaling_factor, return_dict=False)[0]
else:
image = latents
image = self.image_processor.postprocess(
image, output_type=output_type, do_denormalize=[True] * image.shape[0]
)
# Offload last model to CPU
if hasattr(self, "final_offload_hook") and self.final_offload_hook is not None:
self.final_offload_hook.offload()
if not return_dict:
return (image,)
return ImagePipelineOutput(images=image)
| diffusers/examples/research_projects/rdm/pipeline_rdm.py/0 | {
"file_path": "diffusers/examples/research_projects/rdm/pipeline_rdm.py",
"repo_id": "diffusers",
"token_count": 7145
} | 113 |
# Stable Diffusion XL text-to-image fine-tuning
The `train_text_to_image_sdxl.py` script shows how to fine-tune Stable Diffusion XL (SDXL) on your own dataset.
🚨 This script is experimental. The script fine-tunes the whole model and often times the model overfits and runs into issues like catastrophic forgetting. It's recommended to try different hyperparameters to get the best result on your dataset. 🚨
## Running locally with PyTorch
### Installing the dependencies
Before running the scripts, make sure to install the library's training dependencies:
**Important**
To make sure you can successfully run the latest versions of the example scripts, we highly recommend **installing from source** and keeping the install up to date as we update the example scripts frequently and install some example-specific requirements. To do this, execute the following steps in a new virtual environment:
```bash
git clone https://github.com/huggingface/diffusers
cd diffusers
pip install -e .
```
Then cd in the `examples/text_to_image` folder and run
```bash
pip install -r requirements_sdxl.txt
```
And initialize an [🤗Accelerate](https://github.com/huggingface/accelerate/) environment with:
```bash
accelerate config
```
Or for a default accelerate configuration without answering questions about your environment
```bash
accelerate config default
```
Or if your environment doesn't support an interactive shell (e.g., a notebook)
```python
from accelerate.utils import write_basic_config
write_basic_config()
```
When running `accelerate config`, if we specify torch compile mode to True there can be dramatic speedups.
Note also that we use PEFT library as backend for LoRA training, make sure to have `peft>=0.6.0` installed in your environment.
### Training
```bash
export MODEL_NAME="stabilityai/stable-diffusion-xl-base-1.0"
export VAE_NAME="madebyollin/sdxl-vae-fp16-fix"
export DATASET_NAME="lambdalabs/pokemon-blip-captions"
accelerate launch train_text_to_image_sdxl.py \
--pretrained_model_name_or_path=$MODEL_NAME \
--pretrained_vae_model_name_or_path=$VAE_NAME \
--dataset_name=$DATASET_NAME \
--enable_xformers_memory_efficient_attention \
--resolution=512 --center_crop --random_flip \
--proportion_empty_prompts=0.2 \
--train_batch_size=1 \
--gradient_accumulation_steps=4 --gradient_checkpointing \
--max_train_steps=10000 \
--use_8bit_adam \
--learning_rate=1e-06 --lr_scheduler="constant" --lr_warmup_steps=0 \
--mixed_precision="fp16" \
--report_to="wandb" \
--validation_prompt="a cute Sundar Pichai creature" --validation_epochs 5 \
--checkpointing_steps=5000 \
--output_dir="sdxl-pokemon-model" \
--push_to_hub
```
**Notes**:
* The `train_text_to_image_sdxl.py` script pre-computes text embeddings and the VAE encodings and keeps them in memory. While for smaller datasets like [`lambdalabs/pokemon-blip-captions`](https://hf.co/datasets/lambdalabs/pokemon-blip-captions), it might not be a problem, it can definitely lead to memory problems when the script is used on a larger dataset. For those purposes, you would want to serialize these pre-computed representations to disk separately and load them during the fine-tuning process. Refer to [this PR](https://github.com/huggingface/diffusers/pull/4505) for a more in-depth discussion.
* The training script is compute-intensive and may not run on a consumer GPU like Tesla T4.
* The training command shown above performs intermediate quality validation in between the training epochs and logs the results to Weights and Biases. `--report_to`, `--validation_prompt`, and `--validation_epochs` are the relevant CLI arguments here.
* SDXL's VAE is known to suffer from numerical instability issues. This is why we also expose a CLI argument namely `--pretrained_vae_model_name_or_path` that lets you specify the location of a better VAE (such as [this one](https://huggingface.co/madebyollin/sdxl-vae-fp16-fix)).
### Inference
```python
from diffusers import DiffusionPipeline
import torch
model_path = "you-model-id-goes-here" # <-- change this
pipe = DiffusionPipeline.from_pretrained(model_path, torch_dtype=torch.float16)
pipe.to("cuda")
prompt = "A pokemon with green eyes and red legs."
image = pipe(prompt, num_inference_steps=30, guidance_scale=7.5).images[0]
image.save("pokemon.png")
```
### Inference in Pytorch XLA
```python
from diffusers import DiffusionPipeline
import torch
import torch_xla.core.xla_model as xm
model_id = "stabilityai/stable-diffusion-xl-base-1.0"
pipe = DiffusionPipeline.from_pretrained(model_id)
device = xm.xla_device()
pipe.to(device)
prompt = "A pokemon with green eyes and red legs."
start = time()
image = pipe(prompt, num_inference_steps=inference_steps).images[0]
print(f'Compilation time is {time()-start} sec')
image.save("pokemon.png")
start = time()
image = pipe(prompt, num_inference_steps=inference_steps).images[0]
print(f'Inference time is {time()-start} sec after compilation')
```
Note: There is a warmup step in PyTorch XLA. This takes longer because of
compilation and optimization. To see the real benefits of Pytorch XLA and
speedup, we need to call the pipe again on the input with the same length
as the original prompt to reuse the optimized graph and get the performance
boost.
## LoRA training example for Stable Diffusion XL (SDXL)
Low-Rank Adaption of Large Language Models was first introduced by Microsoft in [LoRA: Low-Rank Adaptation of Large Language Models](https://arxiv.org/abs/2106.09685) by *Edward J. Hu, Yelong Shen, Phillip Wallis, Zeyuan Allen-Zhu, Yuanzhi Li, Shean Wang, Lu Wang, Weizhu Chen*.
In a nutshell, LoRA allows adapting pretrained models by adding pairs of rank-decomposition matrices to existing weights and **only** training those newly added weights. This has a couple of advantages:
- Previous pretrained weights are kept frozen so that model is not prone to [catastrophic forgetting](https://www.pnas.org/doi/10.1073/pnas.1611835114).
- Rank-decomposition matrices have significantly fewer parameters than original model, which means that trained LoRA weights are easily portable.
- LoRA attention layers allow to control to which extent the model is adapted toward new training images via a `scale` parameter.
[cloneofsimo](https://github.com/cloneofsimo) was the first to try out LoRA training for Stable Diffusion in the popular [lora](https://github.com/cloneofsimo/lora) GitHub repository.
With LoRA, it's possible to fine-tune Stable Diffusion on a custom image-caption pair dataset
on consumer GPUs like Tesla T4, Tesla V100.
### Training
First, you need to set up your development environment as is explained in the [installation section](#installing-the-dependencies). Make sure to set the `MODEL_NAME` and `DATASET_NAME` environment variables and, optionally, the `VAE_NAME` variable. Here, we will use [Stable Diffusion XL 1.0-base](https://huggingface.co/stabilityai/stable-diffusion-xl-base-1.0) and the [Pokemons dataset](https://huggingface.co/datasets/lambdalabs/pokemon-blip-captions).
**___Note: It is quite useful to monitor the training progress by regularly generating sample images during training. [Weights and Biases](https://docs.wandb.ai/quickstart) is a nice solution to easily see generating images during training. All you need to do is to run `pip install wandb` before training to automatically log images.___**
```bash
export MODEL_NAME="stabilityai/stable-diffusion-xl-base-1.0"
export VAE_NAME="madebyollin/sdxl-vae-fp16-fix"
export DATASET_NAME="lambdalabs/pokemon-blip-captions"
```
For this example we want to directly store the trained LoRA embeddings on the Hub, so
we need to be logged in and add the `--push_to_hub` flag.
```bash
huggingface-cli login
```
Now we can start training!
```bash
accelerate launch train_text_to_image_lora_sdxl.py \
--pretrained_model_name_or_path=$MODEL_NAME \
--pretrained_vae_model_name_or_path=$VAE_NAME \
--dataset_name=$DATASET_NAME --caption_column="text" \
--resolution=1024 --random_flip \
--train_batch_size=1 \
--num_train_epochs=2 --checkpointing_steps=500 \
--learning_rate=1e-04 --lr_scheduler="constant" --lr_warmup_steps=0 \
--mixed_precision="fp16" \
--seed=42 \
--output_dir="sd-pokemon-model-lora-sdxl" \
--validation_prompt="cute dragon creature" --report_to="wandb" \
--push_to_hub
```
The above command will also run inference as fine-tuning progresses and log the results to Weights and Biases.
**Notes**:
* SDXL's VAE is known to suffer from numerical instability issues. This is why we also expose a CLI argument namely `--pretrained_vae_model_name_or_path` that lets you specify the location of a better VAE (such as [this one](https://huggingface.co/madebyollin/sdxl-vae-fp16-fix)).
### Using DeepSpeed
Using DeepSpeed one can reduce the consumption of GPU memory, enabling the training of models on GPUs with smaller memory sizes. DeepSpeed is capable of offloading model parameters to the machine's memory, or it can distribute parameters, gradients, and optimizer states across multiple GPUs. This allows for the training of larger models under the same hardware configuration.
First, you need to use the `accelerate config` command to choose to use DeepSpeed, or manually use the accelerate config file to set up DeepSpeed.
Here is an example of a config file for using DeepSpeed. For more detailed explanations of the configuration, you can refer to this [link](https://huggingface.co/docs/accelerate/usage_guides/deepspeed).
```yaml
compute_environment: LOCAL_MACHINE
debug: true
deepspeed_config:
gradient_accumulation_steps: 1
gradient_clipping: 1.0
offload_optimizer_device: none
offload_param_device: none
zero3_init_flag: false
zero_stage: 2
distributed_type: DEEPSPEED
downcast_bf16: 'no'
machine_rank: 0
main_training_function: main
mixed_precision: fp16
num_machines: 1
num_processes: 1
rdzv_backend: static
same_network: true
tpu_env: []
tpu_use_cluster: false
tpu_use_sudo: false
use_cpu: false
```
You need to save the mentioned configuration as an `accelerate_config.yaml` file. Then, you need to input the path of your `accelerate_config.yaml` file into the `ACCELERATE_CONFIG_FILE` parameter. This way you can use DeepSpeed to train your SDXL model in LoRA. Additionally, you can use DeepSpeed to train other SD models in this way.
```shell
export MODEL_NAME="stabilityai/stable-diffusion-xl-base-1.0"
export VAE_NAME="madebyollin/sdxl-vae-fp16-fix"
export DATASET_NAME="lambdalabs/pokemon-blip-captions"
export ACCELERATE_CONFIG_FILE="your accelerate_config.yaml"
accelerate launch --config_file $ACCELERATE_CONFIG_FILE train_text_to_image_lora_sdxl.py \
--pretrained_model_name_or_path=$MODEL_NAME \
--pretrained_vae_model_name_or_path=$VAE_NAME \
--dataset_name=$DATASET_NAME --caption_column="text" \
--resolution=1024 \
--train_batch_size=1 \
--num_train_epochs=2 \
--checkpointing_steps=2 \
--learning_rate=1e-04 \
--lr_scheduler="constant" \
--lr_warmup_steps=0 \
--mixed_precision="fp16" \
--max_train_steps=20 \
--validation_epochs=20 \
--seed=1234 \
--output_dir="sd-pokemon-model-lora-sdxl" \
--validation_prompt="cute dragon creature"
```
### Finetuning the text encoder and UNet
The script also allows you to finetune the `text_encoder` along with the `unet`.
🚨 Training the text encoder requires additional memory.
Pass the `--train_text_encoder` argument to the training script to enable finetuning the `text_encoder` and `unet`:
```bash
accelerate launch train_text_to_image_lora_sdxl.py \
--pretrained_model_name_or_path=$MODEL_NAME \
--dataset_name=$DATASET_NAME --caption_column="text" \
--resolution=1024 --random_flip \
--train_batch_size=1 \
--num_train_epochs=2 --checkpointing_steps=500 \
--learning_rate=1e-04 --lr_scheduler="constant" --lr_warmup_steps=0 \
--seed=42 \
--output_dir="sd-pokemon-model-lora-sdxl-txt" \
--train_text_encoder \
--validation_prompt="cute dragon creature" --report_to="wandb" \
--push_to_hub
```
### Inference
Once you have trained a model using above command, the inference can be done simply using the `DiffusionPipeline` after loading the trained LoRA weights. You
need to pass the `output_dir` for loading the LoRA weights which, in this case, is `sd-pokemon-model-lora-sdxl`.
```python
from diffusers import DiffusionPipeline
import torch
model_path = "takuoko/sd-pokemon-model-lora-sdxl"
pipe = DiffusionPipeline.from_pretrained("stabilityai/stable-diffusion-xl-base-1.0", torch_dtype=torch.float16)
pipe.to("cuda")
pipe.load_lora_weights(model_path)
prompt = "A pokemon with green eyes and red legs."
image = pipe(prompt, num_inference_steps=30, guidance_scale=7.5).images[0]
image.save("pokemon.png")
```
| diffusers/examples/text_to_image/README_sdxl.md/0 | {
"file_path": "diffusers/examples/text_to_image/README_sdxl.md",
"repo_id": "diffusers",
"token_count": 4083
} | 114 |
# coding=utf-8
# Copyright 2024 The HuggingFace Inc. team.
#
# Licensed under the Apache License, Version 2.0 (the "License");
# you may not use this file except in compliance with the License.
# You may obtain a copy of the License at
#
# http://www.apache.org/licenses/LICENSE-2.0
#
# Unless required by applicable law or agreed to in writing, software
# distributed under the License is distributed on an "AS IS" BASIS,
# WITHOUT WARRANTIES OR CONDITIONS OF ANY KIND, either express or implied.
# See the License for the specific language governing permissions and
# limitations under the License.
"""Conversion script for the LDM checkpoints."""
import argparse
import json
import os
import torch
from transformers.file_utils import has_file
from diffusers import UNet2DConditionModel, UNet2DModel
do_only_config = False
do_only_weights = True
do_only_renaming = False
if __name__ == "__main__":
parser = argparse.ArgumentParser()
parser.add_argument(
"--repo_path",
default=None,
type=str,
required=True,
help="The config json file corresponding to the architecture.",
)
parser.add_argument("--dump_path", default=None, type=str, required=True, help="Path to the output model.")
args = parser.parse_args()
config_parameters_to_change = {
"image_size": "sample_size",
"num_res_blocks": "layers_per_block",
"block_channels": "block_out_channels",
"down_blocks": "down_block_types",
"up_blocks": "up_block_types",
"downscale_freq_shift": "freq_shift",
"resnet_num_groups": "norm_num_groups",
"resnet_act_fn": "act_fn",
"resnet_eps": "norm_eps",
"num_head_channels": "attention_head_dim",
}
key_parameters_to_change = {
"time_steps": "time_proj",
"mid": "mid_block",
"downsample_blocks": "down_blocks",
"upsample_blocks": "up_blocks",
}
subfolder = "" if has_file(args.repo_path, "config.json") else "unet"
with open(os.path.join(args.repo_path, subfolder, "config.json"), "r", encoding="utf-8") as reader:
text = reader.read()
config = json.loads(text)
if do_only_config:
for key in config_parameters_to_change.keys():
config.pop(key, None)
if has_file(args.repo_path, "config.json"):
model = UNet2DModel(**config)
else:
class_name = UNet2DConditionModel if "ldm-text2im-large-256" in args.repo_path else UNet2DModel
model = class_name(**config)
if do_only_config:
model.save_config(os.path.join(args.repo_path, subfolder))
config = dict(model.config)
if do_only_renaming:
for key, value in config_parameters_to_change.items():
if key in config:
config[value] = config[key]
del config[key]
config["down_block_types"] = [k.replace("UNetRes", "") for k in config["down_block_types"]]
config["up_block_types"] = [k.replace("UNetRes", "") for k in config["up_block_types"]]
if do_only_weights:
state_dict = torch.load(os.path.join(args.repo_path, subfolder, "diffusion_pytorch_model.bin"))
new_state_dict = {}
for param_key, param_value in state_dict.items():
if param_key.endswith(".op.bias") or param_key.endswith(".op.weight"):
continue
has_changed = False
for key, new_key in key_parameters_to_change.items():
if not has_changed and param_key.split(".")[0] == key:
new_state_dict[".".join([new_key] + param_key.split(".")[1:])] = param_value
has_changed = True
if not has_changed:
new_state_dict[param_key] = param_value
model.load_state_dict(new_state_dict)
model.save_pretrained(os.path.join(args.repo_path, subfolder))
| diffusers/scripts/change_naming_configs_and_checkpoints.py/0 | {
"file_path": "diffusers/scripts/change_naming_configs_and_checkpoints.py",
"repo_id": "diffusers",
"token_count": 1631
} | 115 |
# coding=utf-8
# Copyright 2024 The HuggingFace Inc. team.
#
# Licensed under the Apache License, Version 2.0 (the "License");
# you may not use this file except in compliance with the License.
# You may obtain a copy of the License at
#
# http://www.apache.org/licenses/LICENSE-2.0
#
# Unless required by applicable law or agreed to in writing, software
# distributed under the License is distributed on an "AS IS" BASIS,
# WITHOUT WARRANTIES OR CONDITIONS OF ANY KIND, either express or implied.
# See the License for the specific language governing permissions and
# limitations under the License.
"""Conversion script for the LDM checkpoints."""
import argparse
import torch
from transformers import CLIPImageProcessor, CLIPTextModel, CLIPTokenizer, CLIPVisionModelWithProjection
from diffusers import DDIMScheduler, I2VGenXLPipeline, I2VGenXLUNet, StableDiffusionPipeline
CLIP_ID = "laion/CLIP-ViT-H-14-laion2B-s32B-b79K"
def assign_to_checkpoint(
paths, checkpoint, old_checkpoint, attention_paths_to_split=None, additional_replacements=None, config=None
):
"""
This does the final conversion step: take locally converted weights and apply a global renaming to them. It splits
attention layers, and takes into account additional replacements that may arise.
Assigns the weights to the new checkpoint.
"""
assert isinstance(paths, list), "Paths should be a list of dicts containing 'old' and 'new' keys."
# Splits the attention layers into three variables.
if attention_paths_to_split is not None:
for path, path_map in attention_paths_to_split.items():
old_tensor = old_checkpoint[path]
channels = old_tensor.shape[0] // 3
target_shape = (-1, channels) if len(old_tensor.shape) == 3 else (-1)
num_heads = old_tensor.shape[0] // config["num_head_channels"] // 3
old_tensor = old_tensor.reshape((num_heads, 3 * channels // num_heads) + old_tensor.shape[1:])
query, key, value = old_tensor.split(channels // num_heads, dim=1)
checkpoint[path_map["query"]] = query.reshape(target_shape)
checkpoint[path_map["key"]] = key.reshape(target_shape)
checkpoint[path_map["value"]] = value.reshape(target_shape)
for path in paths:
new_path = path["new"]
# These have already been assigned
if attention_paths_to_split is not None and new_path in attention_paths_to_split:
continue
if additional_replacements is not None:
for replacement in additional_replacements:
new_path = new_path.replace(replacement["old"], replacement["new"])
# proj_attn.weight has to be converted from conv 1D to linear
weight = old_checkpoint[path["old"]]
names = ["proj_attn.weight"]
names_2 = ["proj_out.weight", "proj_in.weight"]
if any(k in new_path for k in names):
checkpoint[new_path] = weight[:, :, 0]
elif any(k in new_path for k in names_2) and len(weight.shape) > 2 and ".attentions." not in new_path:
checkpoint[new_path] = weight[:, :, 0]
else:
checkpoint[new_path] = weight
def renew_attention_paths(old_list, n_shave_prefix_segments=0):
"""
Updates paths inside attentions to the new naming scheme (local renaming)
"""
mapping = []
for old_item in old_list:
new_item = old_item
mapping.append({"old": old_item, "new": new_item})
return mapping
def shave_segments(path, n_shave_prefix_segments=1):
"""
Removes segments. Positive values shave the first segments, negative shave the last segments.
"""
if n_shave_prefix_segments >= 0:
return ".".join(path.split(".")[n_shave_prefix_segments:])
else:
return ".".join(path.split(".")[:n_shave_prefix_segments])
def renew_temp_conv_paths(old_list, n_shave_prefix_segments=0):
"""
Updates paths inside resnets to the new naming scheme (local renaming)
"""
mapping = []
for old_item in old_list:
mapping.append({"old": old_item, "new": old_item})
return mapping
def renew_resnet_paths(old_list, n_shave_prefix_segments=0):
"""
Updates paths inside resnets to the new naming scheme (local renaming)
"""
mapping = []
for old_item in old_list:
new_item = old_item.replace("in_layers.0", "norm1")
new_item = new_item.replace("in_layers.2", "conv1")
new_item = new_item.replace("out_layers.0", "norm2")
new_item = new_item.replace("out_layers.3", "conv2")
new_item = new_item.replace("emb_layers.1", "time_emb_proj")
new_item = new_item.replace("skip_connection", "conv_shortcut")
new_item = shave_segments(new_item, n_shave_prefix_segments=n_shave_prefix_segments)
if "temopral_conv" not in old_item:
mapping.append({"old": old_item, "new": new_item})
return mapping
def convert_ldm_unet_checkpoint(checkpoint, config, path=None, extract_ema=False):
"""
Takes a state dict and a config, and returns a converted checkpoint.
"""
# extract state_dict for UNet
unet_state_dict = {}
keys = list(checkpoint.keys())
unet_key = "model.diffusion_model."
# at least a 100 parameters have to start with `model_ema` in order for the checkpoint to be EMA
if sum(k.startswith("model_ema") for k in keys) > 100 and extract_ema:
print(f"Checkpoint {path} has both EMA and non-EMA weights.")
print(
"In this conversion only the EMA weights are extracted. If you want to instead extract the non-EMA"
" weights (useful to continue fine-tuning), please make sure to remove the `--extract_ema` flag."
)
for key in keys:
if key.startswith("model.diffusion_model"):
flat_ema_key = "model_ema." + "".join(key.split(".")[1:])
unet_state_dict[key.replace(unet_key, "")] = checkpoint.pop(flat_ema_key)
else:
if sum(k.startswith("model_ema") for k in keys) > 100:
print(
"In this conversion only the non-EMA weights are extracted. If you want to instead extract the EMA"
" weights (usually better for inference), please make sure to add the `--extract_ema` flag."
)
for key in keys:
unet_state_dict[key.replace(unet_key, "")] = checkpoint.pop(key)
new_checkpoint = {}
new_checkpoint["time_embedding.linear_1.weight"] = unet_state_dict["time_embed.0.weight"]
new_checkpoint["time_embedding.linear_1.bias"] = unet_state_dict["time_embed.0.bias"]
new_checkpoint["time_embedding.linear_2.weight"] = unet_state_dict["time_embed.2.weight"]
new_checkpoint["time_embedding.linear_2.bias"] = unet_state_dict["time_embed.2.bias"]
additional_embedding_substrings = [
"local_image_concat",
"context_embedding",
"local_image_embedding",
"fps_embedding",
]
for k in unet_state_dict:
if any(substring in k for substring in additional_embedding_substrings):
diffusers_key = k.replace("local_image_concat", "image_latents_proj_in").replace(
"local_image_embedding", "image_latents_context_embedding"
)
new_checkpoint[diffusers_key] = unet_state_dict[k]
# temporal encoder.
new_checkpoint["image_latents_temporal_encoder.norm1.weight"] = unet_state_dict[
"local_temporal_encoder.layers.0.0.norm.weight"
]
new_checkpoint["image_latents_temporal_encoder.norm1.bias"] = unet_state_dict[
"local_temporal_encoder.layers.0.0.norm.bias"
]
# attention
qkv = unet_state_dict["local_temporal_encoder.layers.0.0.fn.to_qkv.weight"]
q, k, v = torch.chunk(qkv, 3, dim=0)
new_checkpoint["image_latents_temporal_encoder.attn1.to_q.weight"] = q
new_checkpoint["image_latents_temporal_encoder.attn1.to_k.weight"] = k
new_checkpoint["image_latents_temporal_encoder.attn1.to_v.weight"] = v
new_checkpoint["image_latents_temporal_encoder.attn1.to_out.0.weight"] = unet_state_dict[
"local_temporal_encoder.layers.0.0.fn.to_out.0.weight"
]
new_checkpoint["image_latents_temporal_encoder.attn1.to_out.0.bias"] = unet_state_dict[
"local_temporal_encoder.layers.0.0.fn.to_out.0.bias"
]
# feedforward
new_checkpoint["image_latents_temporal_encoder.ff.net.0.proj.weight"] = unet_state_dict[
"local_temporal_encoder.layers.0.1.net.0.0.weight"
]
new_checkpoint["image_latents_temporal_encoder.ff.net.0.proj.bias"] = unet_state_dict[
"local_temporal_encoder.layers.0.1.net.0.0.bias"
]
new_checkpoint["image_latents_temporal_encoder.ff.net.2.weight"] = unet_state_dict[
"local_temporal_encoder.layers.0.1.net.2.weight"
]
new_checkpoint["image_latents_temporal_encoder.ff.net.2.bias"] = unet_state_dict[
"local_temporal_encoder.layers.0.1.net.2.bias"
]
if "class_embed_type" in config:
if config["class_embed_type"] is None:
# No parameters to port
...
elif config["class_embed_type"] == "timestep" or config["class_embed_type"] == "projection":
new_checkpoint["class_embedding.linear_1.weight"] = unet_state_dict["label_emb.0.0.weight"]
new_checkpoint["class_embedding.linear_1.bias"] = unet_state_dict["label_emb.0.0.bias"]
new_checkpoint["class_embedding.linear_2.weight"] = unet_state_dict["label_emb.0.2.weight"]
new_checkpoint["class_embedding.linear_2.bias"] = unet_state_dict["label_emb.0.2.bias"]
else:
raise NotImplementedError(f"Not implemented `class_embed_type`: {config['class_embed_type']}")
new_checkpoint["conv_in.weight"] = unet_state_dict["input_blocks.0.0.weight"]
new_checkpoint["conv_in.bias"] = unet_state_dict["input_blocks.0.0.bias"]
first_temp_attention = [v for v in unet_state_dict if v.startswith("input_blocks.0.1")]
paths = renew_attention_paths(first_temp_attention)
meta_path = {"old": "input_blocks.0.1", "new": "transformer_in"}
assign_to_checkpoint(paths, new_checkpoint, unet_state_dict, additional_replacements=[meta_path], config=config)
new_checkpoint["conv_norm_out.weight"] = unet_state_dict["out.0.weight"]
new_checkpoint["conv_norm_out.bias"] = unet_state_dict["out.0.bias"]
new_checkpoint["conv_out.weight"] = unet_state_dict["out.2.weight"]
new_checkpoint["conv_out.bias"] = unet_state_dict["out.2.bias"]
# Retrieves the keys for the input blocks only
num_input_blocks = len({".".join(layer.split(".")[:2]) for layer in unet_state_dict if "input_blocks" in layer})
input_blocks = {
layer_id: [key for key in unet_state_dict if f"input_blocks.{layer_id}" in key]
for layer_id in range(num_input_blocks)
}
# Retrieves the keys for the middle blocks only
num_middle_blocks = len({".".join(layer.split(".")[:2]) for layer in unet_state_dict if "middle_block" in layer})
middle_blocks = {
layer_id: [key for key in unet_state_dict if f"middle_block.{layer_id}" in key]
for layer_id in range(num_middle_blocks)
}
# Retrieves the keys for the output blocks only
num_output_blocks = len({".".join(layer.split(".")[:2]) for layer in unet_state_dict if "output_blocks" in layer})
output_blocks = {
layer_id: [key for key in unet_state_dict if f"output_blocks.{layer_id}" in key]
for layer_id in range(num_output_blocks)
}
for i in range(1, num_input_blocks):
block_id = (i - 1) // (config["layers_per_block"] + 1)
layer_in_block_id = (i - 1) % (config["layers_per_block"] + 1)
resnets = [
key for key in input_blocks[i] if f"input_blocks.{i}.0" in key and f"input_blocks.{i}.0.op" not in key
]
attentions = [key for key in input_blocks[i] if f"input_blocks.{i}.1" in key]
temp_attentions = [key for key in input_blocks[i] if f"input_blocks.{i}.2" in key]
if f"input_blocks.{i}.op.weight" in unet_state_dict:
new_checkpoint[f"down_blocks.{block_id}.downsamplers.0.conv.weight"] = unet_state_dict.pop(
f"input_blocks.{i}.op.weight"
)
new_checkpoint[f"down_blocks.{block_id}.downsamplers.0.conv.bias"] = unet_state_dict.pop(
f"input_blocks.{i}.op.bias"
)
paths = renew_resnet_paths(resnets)
meta_path = {"old": f"input_blocks.{i}.0", "new": f"down_blocks.{block_id}.resnets.{layer_in_block_id}"}
assign_to_checkpoint(
paths, new_checkpoint, unet_state_dict, additional_replacements=[meta_path], config=config
)
temporal_convs = [key for key in resnets if "temopral_conv" in key]
paths = renew_temp_conv_paths(temporal_convs)
meta_path = {
"old": f"input_blocks.{i}.0.temopral_conv",
"new": f"down_blocks.{block_id}.temp_convs.{layer_in_block_id}",
}
assign_to_checkpoint(
paths, new_checkpoint, unet_state_dict, additional_replacements=[meta_path], config=config
)
if len(attentions):
paths = renew_attention_paths(attentions)
meta_path = {"old": f"input_blocks.{i}.1", "new": f"down_blocks.{block_id}.attentions.{layer_in_block_id}"}
assign_to_checkpoint(
paths, new_checkpoint, unet_state_dict, additional_replacements=[meta_path], config=config
)
if len(temp_attentions):
paths = renew_attention_paths(temp_attentions)
meta_path = {
"old": f"input_blocks.{i}.2",
"new": f"down_blocks.{block_id}.temp_attentions.{layer_in_block_id}",
}
assign_to_checkpoint(
paths, new_checkpoint, unet_state_dict, additional_replacements=[meta_path], config=config
)
resnet_0 = middle_blocks[0]
temporal_convs_0 = [key for key in resnet_0 if "temopral_conv" in key]
attentions = middle_blocks[1]
temp_attentions = middle_blocks[2]
resnet_1 = middle_blocks[3]
temporal_convs_1 = [key for key in resnet_1 if "temopral_conv" in key]
resnet_0_paths = renew_resnet_paths(resnet_0)
meta_path = {"old": "middle_block.0", "new": "mid_block.resnets.0"}
assign_to_checkpoint(
resnet_0_paths, new_checkpoint, unet_state_dict, config=config, additional_replacements=[meta_path]
)
temp_conv_0_paths = renew_temp_conv_paths(temporal_convs_0)
meta_path = {"old": "middle_block.0.temopral_conv", "new": "mid_block.temp_convs.0"}
assign_to_checkpoint(
temp_conv_0_paths, new_checkpoint, unet_state_dict, config=config, additional_replacements=[meta_path]
)
resnet_1_paths = renew_resnet_paths(resnet_1)
meta_path = {"old": "middle_block.3", "new": "mid_block.resnets.1"}
assign_to_checkpoint(
resnet_1_paths, new_checkpoint, unet_state_dict, config=config, additional_replacements=[meta_path]
)
temp_conv_1_paths = renew_temp_conv_paths(temporal_convs_1)
meta_path = {"old": "middle_block.3.temopral_conv", "new": "mid_block.temp_convs.1"}
assign_to_checkpoint(
temp_conv_1_paths, new_checkpoint, unet_state_dict, config=config, additional_replacements=[meta_path]
)
attentions_paths = renew_attention_paths(attentions)
meta_path = {"old": "middle_block.1", "new": "mid_block.attentions.0"}
assign_to_checkpoint(
attentions_paths, new_checkpoint, unet_state_dict, additional_replacements=[meta_path], config=config
)
temp_attentions_paths = renew_attention_paths(temp_attentions)
meta_path = {"old": "middle_block.2", "new": "mid_block.temp_attentions.0"}
assign_to_checkpoint(
temp_attentions_paths, new_checkpoint, unet_state_dict, additional_replacements=[meta_path], config=config
)
for i in range(num_output_blocks):
block_id = i // (config["layers_per_block"] + 1)
layer_in_block_id = i % (config["layers_per_block"] + 1)
output_block_layers = [shave_segments(name, 2) for name in output_blocks[i]]
output_block_list = {}
for layer in output_block_layers:
layer_id, layer_name = layer.split(".")[0], shave_segments(layer, 1)
if layer_id in output_block_list:
output_block_list[layer_id].append(layer_name)
else:
output_block_list[layer_id] = [layer_name]
if len(output_block_list) > 1:
resnets = [key for key in output_blocks[i] if f"output_blocks.{i}.0" in key]
attentions = [key for key in output_blocks[i] if f"output_blocks.{i}.1" in key]
temp_attentions = [key for key in output_blocks[i] if f"output_blocks.{i}.2" in key]
resnet_0_paths = renew_resnet_paths(resnets)
paths = renew_resnet_paths(resnets)
meta_path = {"old": f"output_blocks.{i}.0", "new": f"up_blocks.{block_id}.resnets.{layer_in_block_id}"}
assign_to_checkpoint(
paths, new_checkpoint, unet_state_dict, additional_replacements=[meta_path], config=config
)
temporal_convs = [key for key in resnets if "temopral_conv" in key]
paths = renew_temp_conv_paths(temporal_convs)
meta_path = {
"old": f"output_blocks.{i}.0.temopral_conv",
"new": f"up_blocks.{block_id}.temp_convs.{layer_in_block_id}",
}
assign_to_checkpoint(
paths, new_checkpoint, unet_state_dict, additional_replacements=[meta_path], config=config
)
output_block_list = {k: sorted(v) for k, v in output_block_list.items()}
if ["conv.bias", "conv.weight"] in output_block_list.values():
index = list(output_block_list.values()).index(["conv.bias", "conv.weight"])
new_checkpoint[f"up_blocks.{block_id}.upsamplers.0.conv.weight"] = unet_state_dict[
f"output_blocks.{i}.{index}.conv.weight"
]
new_checkpoint[f"up_blocks.{block_id}.upsamplers.0.conv.bias"] = unet_state_dict[
f"output_blocks.{i}.{index}.conv.bias"
]
# Clear attentions as they have been attributed above.
if len(attentions) == 2:
attentions = []
if len(attentions):
paths = renew_attention_paths(attentions)
meta_path = {
"old": f"output_blocks.{i}.1",
"new": f"up_blocks.{block_id}.attentions.{layer_in_block_id}",
}
assign_to_checkpoint(
paths, new_checkpoint, unet_state_dict, additional_replacements=[meta_path], config=config
)
if len(temp_attentions):
paths = renew_attention_paths(temp_attentions)
meta_path = {
"old": f"output_blocks.{i}.2",
"new": f"up_blocks.{block_id}.temp_attentions.{layer_in_block_id}",
}
assign_to_checkpoint(
paths, new_checkpoint, unet_state_dict, additional_replacements=[meta_path], config=config
)
else:
resnet_0_paths = renew_resnet_paths(output_block_layers, n_shave_prefix_segments=1)
for path in resnet_0_paths:
old_path = ".".join(["output_blocks", str(i), path["old"]])
new_path = ".".join(["up_blocks", str(block_id), "resnets", str(layer_in_block_id), path["new"]])
new_checkpoint[new_path] = unet_state_dict[old_path]
temopral_conv_paths = [l for l in output_block_layers if "temopral_conv" in l]
for path in temopral_conv_paths:
pruned_path = path.split("temopral_conv.")[-1]
old_path = ".".join(["output_blocks", str(i), str(block_id), "temopral_conv", pruned_path])
new_path = ".".join(["up_blocks", str(block_id), "temp_convs", str(layer_in_block_id), pruned_path])
new_checkpoint[new_path] = unet_state_dict[old_path]
return new_checkpoint
if __name__ == "__main__":
parser = argparse.ArgumentParser()
parser.add_argument(
"--unet_checkpoint_path", default=None, type=str, required=True, help="Path to the checkpoint to convert."
)
parser.add_argument("--dump_path", default=None, type=str, required=True, help="Path to the output model.")
parser.add_argument("--push_to_hub", action="store_true")
args = parser.parse_args()
# UNet
unet_checkpoint = torch.load(args.unet_checkpoint_path, map_location="cpu")
unet_checkpoint = unet_checkpoint["state_dict"]
unet = I2VGenXLUNet(sample_size=32)
converted_ckpt = convert_ldm_unet_checkpoint(unet_checkpoint, unet.config)
diff_0 = set(unet.state_dict().keys()) - set(converted_ckpt.keys())
diff_1 = set(converted_ckpt.keys()) - set(unet.state_dict().keys())
assert len(diff_0) == len(diff_1) == 0, "Converted weights don't match"
unet.load_state_dict(converted_ckpt, strict=True)
# vae
temp_pipe = StableDiffusionPipeline.from_single_file(
"https://huggingface.co/ali-vilab/i2vgen-xl/blob/main/models/v2-1_512-ema-pruned.ckpt"
)
vae = temp_pipe.vae
del temp_pipe
# text encoder and tokenizer
text_encoder = CLIPTextModel.from_pretrained(CLIP_ID)
tokenizer = CLIPTokenizer.from_pretrained(CLIP_ID)
# image encoder and feature extractor
image_encoder = CLIPVisionModelWithProjection.from_pretrained(CLIP_ID)
feature_extractor = CLIPImageProcessor.from_pretrained(CLIP_ID)
# scheduler
# https://github.com/ali-vilab/i2vgen-xl/blob/main/configs/i2vgen_xl_train.yaml
scheduler = DDIMScheduler(
beta_schedule="squaredcos_cap_v2",
rescale_betas_zero_snr=True,
set_alpha_to_one=True,
clip_sample=False,
steps_offset=1,
timestep_spacing="leading",
prediction_type="v_prediction",
)
# final
pipeline = I2VGenXLPipeline(
unet=unet,
vae=vae,
image_encoder=image_encoder,
feature_extractor=feature_extractor,
text_encoder=text_encoder,
tokenizer=tokenizer,
scheduler=scheduler,
)
pipeline.save_pretrained(args.dump_path, push_to_hub=args.push_to_hub)
| diffusers/scripts/convert_i2vgen_to_diffusers.py/0 | {
"file_path": "diffusers/scripts/convert_i2vgen_to_diffusers.py",
"repo_id": "diffusers",
"token_count": 9968
} | 116 |
# coding=utf-8
# Copyright 2024 The HuggingFace Inc. team.
#
# Licensed under the Apache License, Version 2.0 (the "License");
# you may not use this file except in compliance with the License.
# You may obtain a copy of the License at
#
# http://www.apache.org/licenses/LICENSE-2.0
#
# Unless required by applicable law or agreed to in writing, software
# distributed under the License is distributed on an "AS IS" BASIS,
# WITHOUT WARRANTIES OR CONDITIONS OF ANY KIND, either express or implied.
# See the License for the specific language governing permissions and
# limitations under the License.
"""Conversion script for the LDM checkpoints."""
import argparse
import importlib
import torch
from diffusers.pipelines.stable_diffusion.convert_from_ckpt import download_from_original_stable_diffusion_ckpt
if __name__ == "__main__":
parser = argparse.ArgumentParser()
parser.add_argument(
"--checkpoint_path", default=None, type=str, required=True, help="Path to the checkpoint to convert."
)
# !wget https://raw.githubusercontent.com/CompVis/stable-diffusion/main/configs/stable-diffusion/v1-inference.yaml
parser.add_argument(
"--original_config_file",
default=None,
type=str,
help="The YAML config file corresponding to the original architecture.",
)
parser.add_argument(
"--config_files",
default=None,
type=str,
help="The YAML config file corresponding to the architecture.",
)
parser.add_argument(
"--num_in_channels",
default=None,
type=int,
help="The number of input channels. If `None` number of input channels will be automatically inferred.",
)
parser.add_argument(
"--scheduler_type",
default="pndm",
type=str,
help="Type of scheduler to use. Should be one of ['pndm', 'lms', 'ddim', 'euler', 'euler-ancestral', 'dpm']",
)
parser.add_argument(
"--pipeline_type",
default=None,
type=str,
help=(
"The pipeline type. One of 'FrozenOpenCLIPEmbedder', 'FrozenCLIPEmbedder', 'PaintByExample'"
". If `None` pipeline will be automatically inferred."
),
)
parser.add_argument(
"--image_size",
default=None,
type=int,
help=(
"The image size that the model was trained on. Use 512 for Stable Diffusion v1.X and Stable Siffusion v2"
" Base. Use 768 for Stable Diffusion v2."
),
)
parser.add_argument(
"--prediction_type",
default=None,
type=str,
help=(
"The prediction type that the model was trained on. Use 'epsilon' for Stable Diffusion v1.X and Stable"
" Diffusion v2 Base. Use 'v_prediction' for Stable Diffusion v2."
),
)
parser.add_argument(
"--extract_ema",
action="store_true",
help=(
"Only relevant for checkpoints that have both EMA and non-EMA weights. Whether to extract the EMA weights"
" or not. Defaults to `False`. Add `--extract_ema` to extract the EMA weights. EMA weights usually yield"
" higher quality images for inference. Non-EMA weights are usually better to continue fine-tuning."
),
)
parser.add_argument(
"--upcast_attention",
action="store_true",
help=(
"Whether the attention computation should always be upcasted. This is necessary when running stable"
" diffusion 2.1."
),
)
parser.add_argument(
"--from_safetensors",
action="store_true",
help="If `--checkpoint_path` is in `safetensors` format, load checkpoint with safetensors instead of PyTorch.",
)
parser.add_argument(
"--to_safetensors",
action="store_true",
help="Whether to store pipeline in safetensors format or not.",
)
parser.add_argument("--dump_path", default=None, type=str, required=True, help="Path to the output model.")
parser.add_argument("--device", type=str, help="Device to use (e.g. cpu, cuda:0, cuda:1, etc.)")
parser.add_argument(
"--stable_unclip",
type=str,
default=None,
required=False,
help="Set if this is a stable unCLIP model. One of 'txt2img' or 'img2img'.",
)
parser.add_argument(
"--stable_unclip_prior",
type=str,
default=None,
required=False,
help="Set if this is a stable unCLIP txt2img model. Selects which prior to use. If `--stable_unclip` is set to `txt2img`, the karlo prior (https://huggingface.co/kakaobrain/karlo-v1-alpha/tree/main/prior) is selected by default.",
)
parser.add_argument(
"--clip_stats_path",
type=str,
help="Path to the clip stats file. Only required if the stable unclip model's config specifies `model.params.noise_aug_config.params.clip_stats_path`.",
required=False,
)
parser.add_argument(
"--controlnet", action="store_true", default=None, help="Set flag if this is a controlnet checkpoint."
)
parser.add_argument("--half", action="store_true", help="Save weights in half precision.")
parser.add_argument(
"--vae_path",
type=str,
default=None,
required=False,
help="Set to a path, hub id to an already converted vae to not convert it again.",
)
parser.add_argument(
"--pipeline_class_name",
type=str,
default=None,
required=False,
help="Specify the pipeline class name",
)
args = parser.parse_args()
if args.pipeline_class_name is not None:
library = importlib.import_module("diffusers")
class_obj = getattr(library, args.pipeline_class_name)
pipeline_class = class_obj
else:
pipeline_class = None
pipe = download_from_original_stable_diffusion_ckpt(
checkpoint_path_or_dict=args.checkpoint_path,
original_config_file=args.original_config_file,
config_files=args.config_files,
image_size=args.image_size,
prediction_type=args.prediction_type,
model_type=args.pipeline_type,
extract_ema=args.extract_ema,
scheduler_type=args.scheduler_type,
num_in_channels=args.num_in_channels,
upcast_attention=args.upcast_attention,
from_safetensors=args.from_safetensors,
device=args.device,
stable_unclip=args.stable_unclip,
stable_unclip_prior=args.stable_unclip_prior,
clip_stats_path=args.clip_stats_path,
controlnet=args.controlnet,
vae_path=args.vae_path,
pipeline_class=pipeline_class,
)
if args.half:
pipe.to(dtype=torch.float16)
if args.controlnet:
# only save the controlnet model
pipe.controlnet.save_pretrained(args.dump_path, safe_serialization=args.to_safetensors)
else:
pipe.save_pretrained(args.dump_path, safe_serialization=args.to_safetensors)
| diffusers/scripts/convert_original_stable_diffusion_to_diffusers.py/0 | {
"file_path": "diffusers/scripts/convert_original_stable_diffusion_to_diffusers.py",
"repo_id": "diffusers",
"token_count": 2890
} | 117 |
"""
This script ports models from VQ-diffusion (https://github.com/microsoft/VQ-Diffusion) to diffusers.
It currently only supports porting the ITHQ dataset.
ITHQ dataset:
```sh
# From the root directory of diffusers.
# Download the VQVAE checkpoint
$ wget https://facevcstandard.blob.core.windows.net/v-zhictang/Improved-VQ-Diffusion_model_release/ithq_vqvae.pth?sv=2020-10-02&st=2022-05-30T15%3A17%3A18Z&se=2030-05-31T15%3A17%3A00Z&sr=b&sp=r&sig=1jVavHFPpUjDs%2FTO1V3PTezaNbPp2Nx8MxiWI7y6fEY%3D -O ithq_vqvae.pth
# Download the VQVAE config
# NOTE that in VQ-diffusion the documented file is `configs/ithq.yaml` but the target class
# `image_synthesis.modeling.codecs.image_codec.ema_vqvae.PatchVQVAE`
# loads `OUTPUT/pretrained_model/taming_dvae/config.yaml`
$ wget https://raw.githubusercontent.com/microsoft/VQ-Diffusion/main/OUTPUT/pretrained_model/taming_dvae/config.yaml -O ithq_vqvae.yaml
# Download the main model checkpoint
$ wget https://facevcstandard.blob.core.windows.net/v-zhictang/Improved-VQ-Diffusion_model_release/ithq_learnable.pth?sv=2020-10-02&st=2022-05-30T10%3A22%3A06Z&se=2030-05-31T10%3A22%3A00Z&sr=b&sp=r&sig=GOE%2Bza02%2FPnGxYVOOPtwrTR4RA3%2F5NVgMxdW4kjaEZ8%3D -O ithq_learnable.pth
# Download the main model config
$ wget https://raw.githubusercontent.com/microsoft/VQ-Diffusion/main/configs/ithq.yaml -O ithq.yaml
# run the convert script
$ python ./scripts/convert_vq_diffusion_to_diffusers.py \
--checkpoint_path ./ithq_learnable.pth \
--original_config_file ./ithq.yaml \
--vqvae_checkpoint_path ./ithq_vqvae.pth \
--vqvae_original_config_file ./ithq_vqvae.yaml \
--dump_path <path to save pre-trained `VQDiffusionPipeline`>
```
"""
import argparse
import tempfile
import torch
import yaml
from accelerate import init_empty_weights, load_checkpoint_and_dispatch
from transformers import CLIPTextModel, CLIPTokenizer
from yaml.loader import FullLoader
from diffusers import Transformer2DModel, VQDiffusionPipeline, VQDiffusionScheduler, VQModel
from diffusers.pipelines.vq_diffusion.pipeline_vq_diffusion import LearnedClassifierFreeSamplingEmbeddings
# vqvae model
PORTED_VQVAES = ["image_synthesis.modeling.codecs.image_codec.patch_vqgan.PatchVQGAN"]
def vqvae_model_from_original_config(original_config):
assert (
original_config["target"] in PORTED_VQVAES
), f"{original_config['target']} has not yet been ported to diffusers."
original_config = original_config["params"]
original_encoder_config = original_config["encoder_config"]["params"]
original_decoder_config = original_config["decoder_config"]["params"]
in_channels = original_encoder_config["in_channels"]
out_channels = original_decoder_config["out_ch"]
down_block_types = get_down_block_types(original_encoder_config)
up_block_types = get_up_block_types(original_decoder_config)
assert original_encoder_config["ch"] == original_decoder_config["ch"]
assert original_encoder_config["ch_mult"] == original_decoder_config["ch_mult"]
block_out_channels = tuple(
[original_encoder_config["ch"] * a_ch_mult for a_ch_mult in original_encoder_config["ch_mult"]]
)
assert original_encoder_config["num_res_blocks"] == original_decoder_config["num_res_blocks"]
layers_per_block = original_encoder_config["num_res_blocks"]
assert original_encoder_config["z_channels"] == original_decoder_config["z_channels"]
latent_channels = original_encoder_config["z_channels"]
num_vq_embeddings = original_config["n_embed"]
# Hard coded value for ResnetBlock.GoupNorm(num_groups) in VQ-diffusion
norm_num_groups = 32
e_dim = original_config["embed_dim"]
model = VQModel(
in_channels=in_channels,
out_channels=out_channels,
down_block_types=down_block_types,
up_block_types=up_block_types,
block_out_channels=block_out_channels,
layers_per_block=layers_per_block,
latent_channels=latent_channels,
num_vq_embeddings=num_vq_embeddings,
norm_num_groups=norm_num_groups,
vq_embed_dim=e_dim,
)
return model
def get_down_block_types(original_encoder_config):
attn_resolutions = coerce_attn_resolutions(original_encoder_config["attn_resolutions"])
num_resolutions = len(original_encoder_config["ch_mult"])
resolution = coerce_resolution(original_encoder_config["resolution"])
curr_res = resolution
down_block_types = []
for _ in range(num_resolutions):
if curr_res in attn_resolutions:
down_block_type = "AttnDownEncoderBlock2D"
else:
down_block_type = "DownEncoderBlock2D"
down_block_types.append(down_block_type)
curr_res = [r // 2 for r in curr_res]
return down_block_types
def get_up_block_types(original_decoder_config):
attn_resolutions = coerce_attn_resolutions(original_decoder_config["attn_resolutions"])
num_resolutions = len(original_decoder_config["ch_mult"])
resolution = coerce_resolution(original_decoder_config["resolution"])
curr_res = [r // 2 ** (num_resolutions - 1) for r in resolution]
up_block_types = []
for _ in reversed(range(num_resolutions)):
if curr_res in attn_resolutions:
up_block_type = "AttnUpDecoderBlock2D"
else:
up_block_type = "UpDecoderBlock2D"
up_block_types.append(up_block_type)
curr_res = [r * 2 for r in curr_res]
return up_block_types
def coerce_attn_resolutions(attn_resolutions):
attn_resolutions = list(attn_resolutions)
attn_resolutions_ = []
for ar in attn_resolutions:
if isinstance(ar, (list, tuple)):
attn_resolutions_.append(list(ar))
else:
attn_resolutions_.append([ar, ar])
return attn_resolutions_
def coerce_resolution(resolution):
if isinstance(resolution, int):
resolution = [resolution, resolution] # H, W
elif isinstance(resolution, (tuple, list)):
resolution = list(resolution)
else:
raise ValueError("Unknown type of resolution:", resolution)
return resolution
# done vqvae model
# vqvae checkpoint
def vqvae_original_checkpoint_to_diffusers_checkpoint(model, checkpoint):
diffusers_checkpoint = {}
diffusers_checkpoint.update(vqvae_encoder_to_diffusers_checkpoint(model, checkpoint))
# quant_conv
diffusers_checkpoint.update(
{
"quant_conv.weight": checkpoint["quant_conv.weight"],
"quant_conv.bias": checkpoint["quant_conv.bias"],
}
)
# quantize
diffusers_checkpoint.update({"quantize.embedding.weight": checkpoint["quantize.embedding"]})
# post_quant_conv
diffusers_checkpoint.update(
{
"post_quant_conv.weight": checkpoint["post_quant_conv.weight"],
"post_quant_conv.bias": checkpoint["post_quant_conv.bias"],
}
)
# decoder
diffusers_checkpoint.update(vqvae_decoder_to_diffusers_checkpoint(model, checkpoint))
return diffusers_checkpoint
def vqvae_encoder_to_diffusers_checkpoint(model, checkpoint):
diffusers_checkpoint = {}
# conv_in
diffusers_checkpoint.update(
{
"encoder.conv_in.weight": checkpoint["encoder.conv_in.weight"],
"encoder.conv_in.bias": checkpoint["encoder.conv_in.bias"],
}
)
# down_blocks
for down_block_idx, down_block in enumerate(model.encoder.down_blocks):
diffusers_down_block_prefix = f"encoder.down_blocks.{down_block_idx}"
down_block_prefix = f"encoder.down.{down_block_idx}"
# resnets
for resnet_idx, resnet in enumerate(down_block.resnets):
diffusers_resnet_prefix = f"{diffusers_down_block_prefix}.resnets.{resnet_idx}"
resnet_prefix = f"{down_block_prefix}.block.{resnet_idx}"
diffusers_checkpoint.update(
vqvae_resnet_to_diffusers_checkpoint(
resnet, checkpoint, diffusers_resnet_prefix=diffusers_resnet_prefix, resnet_prefix=resnet_prefix
)
)
# downsample
# do not include the downsample when on the last down block
# There is no downsample on the last down block
if down_block_idx != len(model.encoder.down_blocks) - 1:
# There's a single downsample in the original checkpoint but a list of downsamples
# in the diffusers model.
diffusers_downsample_prefix = f"{diffusers_down_block_prefix}.downsamplers.0.conv"
downsample_prefix = f"{down_block_prefix}.downsample.conv"
diffusers_checkpoint.update(
{
f"{diffusers_downsample_prefix}.weight": checkpoint[f"{downsample_prefix}.weight"],
f"{diffusers_downsample_prefix}.bias": checkpoint[f"{downsample_prefix}.bias"],
}
)
# attentions
if hasattr(down_block, "attentions"):
for attention_idx, _ in enumerate(down_block.attentions):
diffusers_attention_prefix = f"{diffusers_down_block_prefix}.attentions.{attention_idx}"
attention_prefix = f"{down_block_prefix}.attn.{attention_idx}"
diffusers_checkpoint.update(
vqvae_attention_to_diffusers_checkpoint(
checkpoint,
diffusers_attention_prefix=diffusers_attention_prefix,
attention_prefix=attention_prefix,
)
)
# mid block
# mid block attentions
# There is a single hardcoded attention block in the middle of the VQ-diffusion encoder
diffusers_attention_prefix = "encoder.mid_block.attentions.0"
attention_prefix = "encoder.mid.attn_1"
diffusers_checkpoint.update(
vqvae_attention_to_diffusers_checkpoint(
checkpoint, diffusers_attention_prefix=diffusers_attention_prefix, attention_prefix=attention_prefix
)
)
# mid block resnets
for diffusers_resnet_idx, resnet in enumerate(model.encoder.mid_block.resnets):
diffusers_resnet_prefix = f"encoder.mid_block.resnets.{diffusers_resnet_idx}"
# the hardcoded prefixes to `block_` are 1 and 2
orig_resnet_idx = diffusers_resnet_idx + 1
# There are two hardcoded resnets in the middle of the VQ-diffusion encoder
resnet_prefix = f"encoder.mid.block_{orig_resnet_idx}"
diffusers_checkpoint.update(
vqvae_resnet_to_diffusers_checkpoint(
resnet, checkpoint, diffusers_resnet_prefix=diffusers_resnet_prefix, resnet_prefix=resnet_prefix
)
)
diffusers_checkpoint.update(
{
# conv_norm_out
"encoder.conv_norm_out.weight": checkpoint["encoder.norm_out.weight"],
"encoder.conv_norm_out.bias": checkpoint["encoder.norm_out.bias"],
# conv_out
"encoder.conv_out.weight": checkpoint["encoder.conv_out.weight"],
"encoder.conv_out.bias": checkpoint["encoder.conv_out.bias"],
}
)
return diffusers_checkpoint
def vqvae_decoder_to_diffusers_checkpoint(model, checkpoint):
diffusers_checkpoint = {}
# conv in
diffusers_checkpoint.update(
{
"decoder.conv_in.weight": checkpoint["decoder.conv_in.weight"],
"decoder.conv_in.bias": checkpoint["decoder.conv_in.bias"],
}
)
# up_blocks
for diffusers_up_block_idx, up_block in enumerate(model.decoder.up_blocks):
# up_blocks are stored in reverse order in the VQ-diffusion checkpoint
orig_up_block_idx = len(model.decoder.up_blocks) - 1 - diffusers_up_block_idx
diffusers_up_block_prefix = f"decoder.up_blocks.{diffusers_up_block_idx}"
up_block_prefix = f"decoder.up.{orig_up_block_idx}"
# resnets
for resnet_idx, resnet in enumerate(up_block.resnets):
diffusers_resnet_prefix = f"{diffusers_up_block_prefix}.resnets.{resnet_idx}"
resnet_prefix = f"{up_block_prefix}.block.{resnet_idx}"
diffusers_checkpoint.update(
vqvae_resnet_to_diffusers_checkpoint(
resnet, checkpoint, diffusers_resnet_prefix=diffusers_resnet_prefix, resnet_prefix=resnet_prefix
)
)
# upsample
# there is no up sample on the last up block
if diffusers_up_block_idx != len(model.decoder.up_blocks) - 1:
# There's a single upsample in the VQ-diffusion checkpoint but a list of downsamples
# in the diffusers model.
diffusers_downsample_prefix = f"{diffusers_up_block_prefix}.upsamplers.0.conv"
downsample_prefix = f"{up_block_prefix}.upsample.conv"
diffusers_checkpoint.update(
{
f"{diffusers_downsample_prefix}.weight": checkpoint[f"{downsample_prefix}.weight"],
f"{diffusers_downsample_prefix}.bias": checkpoint[f"{downsample_prefix}.bias"],
}
)
# attentions
if hasattr(up_block, "attentions"):
for attention_idx, _ in enumerate(up_block.attentions):
diffusers_attention_prefix = f"{diffusers_up_block_prefix}.attentions.{attention_idx}"
attention_prefix = f"{up_block_prefix}.attn.{attention_idx}"
diffusers_checkpoint.update(
vqvae_attention_to_diffusers_checkpoint(
checkpoint,
diffusers_attention_prefix=diffusers_attention_prefix,
attention_prefix=attention_prefix,
)
)
# mid block
# mid block attentions
# There is a single hardcoded attention block in the middle of the VQ-diffusion decoder
diffusers_attention_prefix = "decoder.mid_block.attentions.0"
attention_prefix = "decoder.mid.attn_1"
diffusers_checkpoint.update(
vqvae_attention_to_diffusers_checkpoint(
checkpoint, diffusers_attention_prefix=diffusers_attention_prefix, attention_prefix=attention_prefix
)
)
# mid block resnets
for diffusers_resnet_idx, resnet in enumerate(model.encoder.mid_block.resnets):
diffusers_resnet_prefix = f"decoder.mid_block.resnets.{diffusers_resnet_idx}"
# the hardcoded prefixes to `block_` are 1 and 2
orig_resnet_idx = diffusers_resnet_idx + 1
# There are two hardcoded resnets in the middle of the VQ-diffusion decoder
resnet_prefix = f"decoder.mid.block_{orig_resnet_idx}"
diffusers_checkpoint.update(
vqvae_resnet_to_diffusers_checkpoint(
resnet, checkpoint, diffusers_resnet_prefix=diffusers_resnet_prefix, resnet_prefix=resnet_prefix
)
)
diffusers_checkpoint.update(
{
# conv_norm_out
"decoder.conv_norm_out.weight": checkpoint["decoder.norm_out.weight"],
"decoder.conv_norm_out.bias": checkpoint["decoder.norm_out.bias"],
# conv_out
"decoder.conv_out.weight": checkpoint["decoder.conv_out.weight"],
"decoder.conv_out.bias": checkpoint["decoder.conv_out.bias"],
}
)
return diffusers_checkpoint
def vqvae_resnet_to_diffusers_checkpoint(resnet, checkpoint, *, diffusers_resnet_prefix, resnet_prefix):
rv = {
# norm1
f"{diffusers_resnet_prefix}.norm1.weight": checkpoint[f"{resnet_prefix}.norm1.weight"],
f"{diffusers_resnet_prefix}.norm1.bias": checkpoint[f"{resnet_prefix}.norm1.bias"],
# conv1
f"{diffusers_resnet_prefix}.conv1.weight": checkpoint[f"{resnet_prefix}.conv1.weight"],
f"{diffusers_resnet_prefix}.conv1.bias": checkpoint[f"{resnet_prefix}.conv1.bias"],
# norm2
f"{diffusers_resnet_prefix}.norm2.weight": checkpoint[f"{resnet_prefix}.norm2.weight"],
f"{diffusers_resnet_prefix}.norm2.bias": checkpoint[f"{resnet_prefix}.norm2.bias"],
# conv2
f"{diffusers_resnet_prefix}.conv2.weight": checkpoint[f"{resnet_prefix}.conv2.weight"],
f"{diffusers_resnet_prefix}.conv2.bias": checkpoint[f"{resnet_prefix}.conv2.bias"],
}
if resnet.conv_shortcut is not None:
rv.update(
{
f"{diffusers_resnet_prefix}.conv_shortcut.weight": checkpoint[f"{resnet_prefix}.nin_shortcut.weight"],
f"{diffusers_resnet_prefix}.conv_shortcut.bias": checkpoint[f"{resnet_prefix}.nin_shortcut.bias"],
}
)
return rv
def vqvae_attention_to_diffusers_checkpoint(checkpoint, *, diffusers_attention_prefix, attention_prefix):
return {
# group_norm
f"{diffusers_attention_prefix}.group_norm.weight": checkpoint[f"{attention_prefix}.norm.weight"],
f"{diffusers_attention_prefix}.group_norm.bias": checkpoint[f"{attention_prefix}.norm.bias"],
# query
f"{diffusers_attention_prefix}.query.weight": checkpoint[f"{attention_prefix}.q.weight"][:, :, 0, 0],
f"{diffusers_attention_prefix}.query.bias": checkpoint[f"{attention_prefix}.q.bias"],
# key
f"{diffusers_attention_prefix}.key.weight": checkpoint[f"{attention_prefix}.k.weight"][:, :, 0, 0],
f"{diffusers_attention_prefix}.key.bias": checkpoint[f"{attention_prefix}.k.bias"],
# value
f"{diffusers_attention_prefix}.value.weight": checkpoint[f"{attention_prefix}.v.weight"][:, :, 0, 0],
f"{diffusers_attention_prefix}.value.bias": checkpoint[f"{attention_prefix}.v.bias"],
# proj_attn
f"{diffusers_attention_prefix}.proj_attn.weight": checkpoint[f"{attention_prefix}.proj_out.weight"][
:, :, 0, 0
],
f"{diffusers_attention_prefix}.proj_attn.bias": checkpoint[f"{attention_prefix}.proj_out.bias"],
}
# done vqvae checkpoint
# transformer model
PORTED_DIFFUSIONS = ["image_synthesis.modeling.transformers.diffusion_transformer.DiffusionTransformer"]
PORTED_TRANSFORMERS = ["image_synthesis.modeling.transformers.transformer_utils.Text2ImageTransformer"]
PORTED_CONTENT_EMBEDDINGS = ["image_synthesis.modeling.embeddings.dalle_mask_image_embedding.DalleMaskImageEmbedding"]
def transformer_model_from_original_config(
original_diffusion_config, original_transformer_config, original_content_embedding_config
):
assert (
original_diffusion_config["target"] in PORTED_DIFFUSIONS
), f"{original_diffusion_config['target']} has not yet been ported to diffusers."
assert (
original_transformer_config["target"] in PORTED_TRANSFORMERS
), f"{original_transformer_config['target']} has not yet been ported to diffusers."
assert (
original_content_embedding_config["target"] in PORTED_CONTENT_EMBEDDINGS
), f"{original_content_embedding_config['target']} has not yet been ported to diffusers."
original_diffusion_config = original_diffusion_config["params"]
original_transformer_config = original_transformer_config["params"]
original_content_embedding_config = original_content_embedding_config["params"]
inner_dim = original_transformer_config["n_embd"]
n_heads = original_transformer_config["n_head"]
# VQ-Diffusion gives dimension of the multi-headed attention layers as the
# number of attention heads times the sequence length (the dimension) of a
# single head. We want to specify our attention blocks with those values
# specified separately
assert inner_dim % n_heads == 0
d_head = inner_dim // n_heads
depth = original_transformer_config["n_layer"]
context_dim = original_transformer_config["condition_dim"]
num_embed = original_content_embedding_config["num_embed"]
# the number of embeddings in the transformer includes the mask embedding.
# the content embedding (the vqvae) does not include the mask embedding.
num_embed = num_embed + 1
height = original_transformer_config["content_spatial_size"][0]
width = original_transformer_config["content_spatial_size"][1]
assert width == height, "width has to be equal to height"
dropout = original_transformer_config["resid_pdrop"]
num_embeds_ada_norm = original_diffusion_config["diffusion_step"]
model_kwargs = {
"attention_bias": True,
"cross_attention_dim": context_dim,
"attention_head_dim": d_head,
"num_layers": depth,
"dropout": dropout,
"num_attention_heads": n_heads,
"num_vector_embeds": num_embed,
"num_embeds_ada_norm": num_embeds_ada_norm,
"norm_num_groups": 32,
"sample_size": width,
"activation_fn": "geglu-approximate",
}
model = Transformer2DModel(**model_kwargs)
return model
# done transformer model
# transformer checkpoint
def transformer_original_checkpoint_to_diffusers_checkpoint(model, checkpoint):
diffusers_checkpoint = {}
transformer_prefix = "transformer.transformer"
diffusers_latent_image_embedding_prefix = "latent_image_embedding"
latent_image_embedding_prefix = f"{transformer_prefix}.content_emb"
# DalleMaskImageEmbedding
diffusers_checkpoint.update(
{
f"{diffusers_latent_image_embedding_prefix}.emb.weight": checkpoint[
f"{latent_image_embedding_prefix}.emb.weight"
],
f"{diffusers_latent_image_embedding_prefix}.height_emb.weight": checkpoint[
f"{latent_image_embedding_prefix}.height_emb.weight"
],
f"{diffusers_latent_image_embedding_prefix}.width_emb.weight": checkpoint[
f"{latent_image_embedding_prefix}.width_emb.weight"
],
}
)
# transformer blocks
for transformer_block_idx, transformer_block in enumerate(model.transformer_blocks):
diffusers_transformer_block_prefix = f"transformer_blocks.{transformer_block_idx}"
transformer_block_prefix = f"{transformer_prefix}.blocks.{transformer_block_idx}"
# ada norm block
diffusers_ada_norm_prefix = f"{diffusers_transformer_block_prefix}.norm1"
ada_norm_prefix = f"{transformer_block_prefix}.ln1"
diffusers_checkpoint.update(
transformer_ada_norm_to_diffusers_checkpoint(
checkpoint, diffusers_ada_norm_prefix=diffusers_ada_norm_prefix, ada_norm_prefix=ada_norm_prefix
)
)
# attention block
diffusers_attention_prefix = f"{diffusers_transformer_block_prefix}.attn1"
attention_prefix = f"{transformer_block_prefix}.attn1"
diffusers_checkpoint.update(
transformer_attention_to_diffusers_checkpoint(
checkpoint, diffusers_attention_prefix=diffusers_attention_prefix, attention_prefix=attention_prefix
)
)
# ada norm block
diffusers_ada_norm_prefix = f"{diffusers_transformer_block_prefix}.norm2"
ada_norm_prefix = f"{transformer_block_prefix}.ln1_1"
diffusers_checkpoint.update(
transformer_ada_norm_to_diffusers_checkpoint(
checkpoint, diffusers_ada_norm_prefix=diffusers_ada_norm_prefix, ada_norm_prefix=ada_norm_prefix
)
)
# attention block
diffusers_attention_prefix = f"{diffusers_transformer_block_prefix}.attn2"
attention_prefix = f"{transformer_block_prefix}.attn2"
diffusers_checkpoint.update(
transformer_attention_to_diffusers_checkpoint(
checkpoint, diffusers_attention_prefix=diffusers_attention_prefix, attention_prefix=attention_prefix
)
)
# norm block
diffusers_norm_block_prefix = f"{diffusers_transformer_block_prefix}.norm3"
norm_block_prefix = f"{transformer_block_prefix}.ln2"
diffusers_checkpoint.update(
{
f"{diffusers_norm_block_prefix}.weight": checkpoint[f"{norm_block_prefix}.weight"],
f"{diffusers_norm_block_prefix}.bias": checkpoint[f"{norm_block_prefix}.bias"],
}
)
# feedforward block
diffusers_feedforward_prefix = f"{diffusers_transformer_block_prefix}.ff"
feedforward_prefix = f"{transformer_block_prefix}.mlp"
diffusers_checkpoint.update(
transformer_feedforward_to_diffusers_checkpoint(
checkpoint,
diffusers_feedforward_prefix=diffusers_feedforward_prefix,
feedforward_prefix=feedforward_prefix,
)
)
# to logits
diffusers_norm_out_prefix = "norm_out"
norm_out_prefix = f"{transformer_prefix}.to_logits.0"
diffusers_checkpoint.update(
{
f"{diffusers_norm_out_prefix}.weight": checkpoint[f"{norm_out_prefix}.weight"],
f"{diffusers_norm_out_prefix}.bias": checkpoint[f"{norm_out_prefix}.bias"],
}
)
diffusers_out_prefix = "out"
out_prefix = f"{transformer_prefix}.to_logits.1"
diffusers_checkpoint.update(
{
f"{diffusers_out_prefix}.weight": checkpoint[f"{out_prefix}.weight"],
f"{diffusers_out_prefix}.bias": checkpoint[f"{out_prefix}.bias"],
}
)
return diffusers_checkpoint
def transformer_ada_norm_to_diffusers_checkpoint(checkpoint, *, diffusers_ada_norm_prefix, ada_norm_prefix):
return {
f"{diffusers_ada_norm_prefix}.emb.weight": checkpoint[f"{ada_norm_prefix}.emb.weight"],
f"{diffusers_ada_norm_prefix}.linear.weight": checkpoint[f"{ada_norm_prefix}.linear.weight"],
f"{diffusers_ada_norm_prefix}.linear.bias": checkpoint[f"{ada_norm_prefix}.linear.bias"],
}
def transformer_attention_to_diffusers_checkpoint(checkpoint, *, diffusers_attention_prefix, attention_prefix):
return {
# key
f"{diffusers_attention_prefix}.to_k.weight": checkpoint[f"{attention_prefix}.key.weight"],
f"{diffusers_attention_prefix}.to_k.bias": checkpoint[f"{attention_prefix}.key.bias"],
# query
f"{diffusers_attention_prefix}.to_q.weight": checkpoint[f"{attention_prefix}.query.weight"],
f"{diffusers_attention_prefix}.to_q.bias": checkpoint[f"{attention_prefix}.query.bias"],
# value
f"{diffusers_attention_prefix}.to_v.weight": checkpoint[f"{attention_prefix}.value.weight"],
f"{diffusers_attention_prefix}.to_v.bias": checkpoint[f"{attention_prefix}.value.bias"],
# linear out
f"{diffusers_attention_prefix}.to_out.0.weight": checkpoint[f"{attention_prefix}.proj.weight"],
f"{diffusers_attention_prefix}.to_out.0.bias": checkpoint[f"{attention_prefix}.proj.bias"],
}
def transformer_feedforward_to_diffusers_checkpoint(checkpoint, *, diffusers_feedforward_prefix, feedforward_prefix):
return {
f"{diffusers_feedforward_prefix}.net.0.proj.weight": checkpoint[f"{feedforward_prefix}.0.weight"],
f"{diffusers_feedforward_prefix}.net.0.proj.bias": checkpoint[f"{feedforward_prefix}.0.bias"],
f"{diffusers_feedforward_prefix}.net.2.weight": checkpoint[f"{feedforward_prefix}.2.weight"],
f"{diffusers_feedforward_prefix}.net.2.bias": checkpoint[f"{feedforward_prefix}.2.bias"],
}
# done transformer checkpoint
def read_config_file(filename):
# The yaml file contains annotations that certain values should
# loaded as tuples.
with open(filename) as f:
original_config = yaml.load(f, FullLoader)
return original_config
# We take separate arguments for the vqvae because the ITHQ vqvae config file
# is separate from the config file for the rest of the model.
if __name__ == "__main__":
parser = argparse.ArgumentParser()
parser.add_argument(
"--vqvae_checkpoint_path",
default=None,
type=str,
required=True,
help="Path to the vqvae checkpoint to convert.",
)
parser.add_argument(
"--vqvae_original_config_file",
default=None,
type=str,
required=True,
help="The YAML config file corresponding to the original architecture for the vqvae.",
)
parser.add_argument(
"--checkpoint_path", default=None, type=str, required=True, help="Path to the checkpoint to convert."
)
parser.add_argument(
"--original_config_file",
default=None,
type=str,
required=True,
help="The YAML config file corresponding to the original architecture.",
)
parser.add_argument("--dump_path", default=None, type=str, required=True, help="Path to the output model.")
parser.add_argument(
"--checkpoint_load_device",
default="cpu",
type=str,
required=False,
help="The device passed to `map_location` when loading checkpoints.",
)
# See link for how ema weights are always selected
# https://github.com/microsoft/VQ-Diffusion/blob/3c98e77f721db7c787b76304fa2c96a36c7b00af/inference_VQ_Diffusion.py#L65
parser.add_argument(
"--no_use_ema",
action="store_true",
required=False,
help=(
"Set to not use the ema weights from the original VQ-Diffusion checkpoint. You probably do not want to set"
" it as the original VQ-Diffusion always uses the ema weights when loading models."
),
)
args = parser.parse_args()
use_ema = not args.no_use_ema
print(f"loading checkpoints to {args.checkpoint_load_device}")
checkpoint_map_location = torch.device(args.checkpoint_load_device)
# vqvae_model
print(f"loading vqvae, config: {args.vqvae_original_config_file}, checkpoint: {args.vqvae_checkpoint_path}")
vqvae_original_config = read_config_file(args.vqvae_original_config_file).model
vqvae_checkpoint = torch.load(args.vqvae_checkpoint_path, map_location=checkpoint_map_location)["model"]
with init_empty_weights():
vqvae_model = vqvae_model_from_original_config(vqvae_original_config)
vqvae_diffusers_checkpoint = vqvae_original_checkpoint_to_diffusers_checkpoint(vqvae_model, vqvae_checkpoint)
with tempfile.NamedTemporaryFile() as vqvae_diffusers_checkpoint_file:
torch.save(vqvae_diffusers_checkpoint, vqvae_diffusers_checkpoint_file.name)
del vqvae_diffusers_checkpoint
del vqvae_checkpoint
load_checkpoint_and_dispatch(vqvae_model, vqvae_diffusers_checkpoint_file.name, device_map="auto")
print("done loading vqvae")
# done vqvae_model
# transformer_model
print(
f"loading transformer, config: {args.original_config_file}, checkpoint: {args.checkpoint_path}, use ema:"
f" {use_ema}"
)
original_config = read_config_file(args.original_config_file).model
diffusion_config = original_config["params"]["diffusion_config"]
transformer_config = original_config["params"]["diffusion_config"]["params"]["transformer_config"]
content_embedding_config = original_config["params"]["diffusion_config"]["params"]["content_emb_config"]
pre_checkpoint = torch.load(args.checkpoint_path, map_location=checkpoint_map_location)
if use_ema:
if "ema" in pre_checkpoint:
checkpoint = {}
for k, v in pre_checkpoint["model"].items():
checkpoint[k] = v
for k, v in pre_checkpoint["ema"].items():
# The ema weights are only used on the transformer. To mimic their key as if they came
# from the state_dict for the top level model, we prefix with an additional "transformer."
# See the source linked in the args.use_ema config for more information.
checkpoint[f"transformer.{k}"] = v
else:
print("attempted to load ema weights but no ema weights are specified in the loaded checkpoint.")
checkpoint = pre_checkpoint["model"]
else:
checkpoint = pre_checkpoint["model"]
del pre_checkpoint
with init_empty_weights():
transformer_model = transformer_model_from_original_config(
diffusion_config, transformer_config, content_embedding_config
)
diffusers_transformer_checkpoint = transformer_original_checkpoint_to_diffusers_checkpoint(
transformer_model, checkpoint
)
# classifier free sampling embeddings interlude
# The learned embeddings are stored on the transformer in the original VQ-diffusion. We store them on a separate
# model, so we pull them off the checkpoint before the checkpoint is deleted.
learnable_classifier_free_sampling_embeddings = diffusion_config["params"].learnable_cf
if learnable_classifier_free_sampling_embeddings:
learned_classifier_free_sampling_embeddings_embeddings = checkpoint["transformer.empty_text_embed"]
else:
learned_classifier_free_sampling_embeddings_embeddings = None
# done classifier free sampling embeddings interlude
with tempfile.NamedTemporaryFile() as diffusers_transformer_checkpoint_file:
torch.save(diffusers_transformer_checkpoint, diffusers_transformer_checkpoint_file.name)
del diffusers_transformer_checkpoint
del checkpoint
load_checkpoint_and_dispatch(transformer_model, diffusers_transformer_checkpoint_file.name, device_map="auto")
print("done loading transformer")
# done transformer_model
# text encoder
print("loading CLIP text encoder")
clip_name = "openai/clip-vit-base-patch32"
# The original VQ-Diffusion specifies the pad value by the int used in the
# returned tokens. Each model uses `0` as the pad value. The transformers clip api
# specifies the pad value via the token before it has been tokenized. The `!` pad
# token is the same as padding with the `0` pad value.
pad_token = "!"
tokenizer_model = CLIPTokenizer.from_pretrained(clip_name, pad_token=pad_token, device_map="auto")
assert tokenizer_model.convert_tokens_to_ids(pad_token) == 0
text_encoder_model = CLIPTextModel.from_pretrained(
clip_name,
# `CLIPTextModel` does not support device_map="auto"
# device_map="auto"
)
print("done loading CLIP text encoder")
# done text encoder
# scheduler
scheduler_model = VQDiffusionScheduler(
# the scheduler has the same number of embeddings as the transformer
num_vec_classes=transformer_model.num_vector_embeds
)
# done scheduler
# learned classifier free sampling embeddings
with init_empty_weights():
learned_classifier_free_sampling_embeddings_model = LearnedClassifierFreeSamplingEmbeddings(
learnable_classifier_free_sampling_embeddings,
hidden_size=text_encoder_model.config.hidden_size,
length=tokenizer_model.model_max_length,
)
learned_classifier_free_sampling_checkpoint = {
"embeddings": learned_classifier_free_sampling_embeddings_embeddings.float()
}
with tempfile.NamedTemporaryFile() as learned_classifier_free_sampling_checkpoint_file:
torch.save(learned_classifier_free_sampling_checkpoint, learned_classifier_free_sampling_checkpoint_file.name)
del learned_classifier_free_sampling_checkpoint
del learned_classifier_free_sampling_embeddings_embeddings
load_checkpoint_and_dispatch(
learned_classifier_free_sampling_embeddings_model,
learned_classifier_free_sampling_checkpoint_file.name,
device_map="auto",
)
# done learned classifier free sampling embeddings
print(f"saving VQ diffusion model, path: {args.dump_path}")
pipe = VQDiffusionPipeline(
vqvae=vqvae_model,
transformer=transformer_model,
tokenizer=tokenizer_model,
text_encoder=text_encoder_model,
learned_classifier_free_sampling_embeddings=learned_classifier_free_sampling_embeddings_model,
scheduler=scheduler_model,
)
pipe.save_pretrained(args.dump_path)
print("done writing VQ diffusion model")
| diffusers/scripts/convert_vq_diffusion_to_diffusers.py/0 | {
"file_path": "diffusers/scripts/convert_vq_diffusion_to_diffusers.py",
"repo_id": "diffusers",
"token_count": 14916
} | 118 |
from .value_guided_sampling import ValueGuidedRLPipeline
| diffusers/src/diffusers/experimental/rl/__init__.py/0 | {
"file_path": "diffusers/src/diffusers/experimental/rl/__init__.py",
"repo_id": "diffusers",
"token_count": 17
} | 119 |
# Copyright 2024 The HuggingFace Team. All rights reserved.
#
# Licensed under the Apache License, Version 2.0 (the "License");
# you may not use this file except in compliance with the License.
# You may obtain a copy of the License at
#
# http://www.apache.org/licenses/LICENSE-2.0
#
# Unless required by applicable law or agreed to in writing, software
# distributed under the License is distributed on an "AS IS" BASIS,
# WITHOUT WARRANTIES OR CONDITIONS OF ANY KIND, either express or implied.
# See the License for the specific language governing permissions and
# limitations under the License.
from typing import TYPE_CHECKING
from ..utils import (
DIFFUSERS_SLOW_IMPORT,
_LazyModule,
is_flax_available,
is_torch_available,
)
_import_structure = {}
if is_torch_available():
_import_structure["adapter"] = ["MultiAdapter", "T2IAdapter"]
_import_structure["autoencoders.autoencoder_asym_kl"] = ["AsymmetricAutoencoderKL"]
_import_structure["autoencoders.autoencoder_kl"] = ["AutoencoderKL"]
_import_structure["autoencoders.autoencoder_kl_temporal_decoder"] = ["AutoencoderKLTemporalDecoder"]
_import_structure["autoencoders.autoencoder_tiny"] = ["AutoencoderTiny"]
_import_structure["autoencoders.consistency_decoder_vae"] = ["ConsistencyDecoderVAE"]
_import_structure["controlnet"] = ["ControlNetModel"]
_import_structure["dual_transformer_2d"] = ["DualTransformer2DModel"]
_import_structure["embeddings"] = ["ImageProjection"]
_import_structure["modeling_utils"] = ["ModelMixin"]
_import_structure["transformers.prior_transformer"] = ["PriorTransformer"]
_import_structure["transformers.t5_film_transformer"] = ["T5FilmDecoder"]
_import_structure["transformers.transformer_2d"] = ["Transformer2DModel"]
_import_structure["transformers.transformer_temporal"] = ["TransformerTemporalModel"]
_import_structure["unets.unet_1d"] = ["UNet1DModel"]
_import_structure["unets.unet_2d"] = ["UNet2DModel"]
_import_structure["unets.unet_2d_condition"] = ["UNet2DConditionModel"]
_import_structure["unets.unet_3d_condition"] = ["UNet3DConditionModel"]
_import_structure["unets.unet_i2vgen_xl"] = ["I2VGenXLUNet"]
_import_structure["unets.unet_kandinsky3"] = ["Kandinsky3UNet"]
_import_structure["unets.unet_motion_model"] = ["MotionAdapter", "UNetMotionModel"]
_import_structure["unets.unet_spatio_temporal_condition"] = ["UNetSpatioTemporalConditionModel"]
_import_structure["unets.unet_stable_cascade"] = ["StableCascadeUNet"]
_import_structure["unets.uvit_2d"] = ["UVit2DModel"]
_import_structure["vq_model"] = ["VQModel"]
if is_flax_available():
_import_structure["controlnet_flax"] = ["FlaxControlNetModel"]
_import_structure["unets.unet_2d_condition_flax"] = ["FlaxUNet2DConditionModel"]
_import_structure["vae_flax"] = ["FlaxAutoencoderKL"]
if TYPE_CHECKING or DIFFUSERS_SLOW_IMPORT:
if is_torch_available():
from .adapter import MultiAdapter, T2IAdapter
from .autoencoders import (
AsymmetricAutoencoderKL,
AutoencoderKL,
AutoencoderKLTemporalDecoder,
AutoencoderTiny,
ConsistencyDecoderVAE,
)
from .controlnet import ControlNetModel
from .embeddings import ImageProjection
from .modeling_utils import ModelMixin
from .transformers import (
DualTransformer2DModel,
PriorTransformer,
T5FilmDecoder,
Transformer2DModel,
TransformerTemporalModel,
)
from .unets import (
I2VGenXLUNet,
Kandinsky3UNet,
MotionAdapter,
StableCascadeUNet,
UNet1DModel,
UNet2DConditionModel,
UNet2DModel,
UNet3DConditionModel,
UNetMotionModel,
UNetSpatioTemporalConditionModel,
UVit2DModel,
)
from .vq_model import VQModel
if is_flax_available():
from .controlnet_flax import FlaxControlNetModel
from .unets import FlaxUNet2DConditionModel
from .vae_flax import FlaxAutoencoderKL
else:
import sys
sys.modules[__name__] = _LazyModule(__name__, globals()["__file__"], _import_structure, module_spec=__spec__)
| diffusers/src/diffusers/models/__init__.py/0 | {
"file_path": "diffusers/src/diffusers/models/__init__.py",
"repo_id": "diffusers",
"token_count": 1772
} | 120 |
from ...utils import is_torch_available
if is_torch_available():
from .dual_transformer_2d import DualTransformer2DModel
from .prior_transformer import PriorTransformer
from .t5_film_transformer import T5FilmDecoder
from .transformer_2d import Transformer2DModel
from .transformer_temporal import TransformerTemporalModel
| diffusers/src/diffusers/models/transformers/__init__.py/0 | {
"file_path": "diffusers/src/diffusers/models/transformers/__init__.py",
"repo_id": "diffusers",
"token_count": 110
} | 121 |
# Copyright 2024 The HuggingFace Team. All rights reserved.
#
# Licensed under the Apache License, Version 2.0 (the "License");
# you may not use this file except in compliance with the License.
# You may obtain a copy of the License at
#
# http://www.apache.org/licenses/LICENSE-2.0
#
# Unless required by applicable law or agreed to in writing, software
# distributed under the License is distributed on an "AS IS" BASIS,
# WITHOUT WARRANTIES OR CONDITIONS OF ANY KIND, either express or implied.
# See the License for the specific language governing permissions and
# limitations under the License.
import flax.linen as nn
import jax.numpy as jnp
from ..attention_flax import FlaxTransformer2DModel
from ..resnet_flax import FlaxDownsample2D, FlaxResnetBlock2D, FlaxUpsample2D
class FlaxCrossAttnDownBlock2D(nn.Module):
r"""
Cross Attention 2D Downsizing block - original architecture from Unet transformers:
https://arxiv.org/abs/2103.06104
Parameters:
in_channels (:obj:`int`):
Input channels
out_channels (:obj:`int`):
Output channels
dropout (:obj:`float`, *optional*, defaults to 0.0):
Dropout rate
num_layers (:obj:`int`, *optional*, defaults to 1):
Number of attention blocks layers
num_attention_heads (:obj:`int`, *optional*, defaults to 1):
Number of attention heads of each spatial transformer block
add_downsample (:obj:`bool`, *optional*, defaults to `True`):
Whether to add downsampling layer before each final output
use_memory_efficient_attention (`bool`, *optional*, defaults to `False`):
enable memory efficient attention https://arxiv.org/abs/2112.05682
split_head_dim (`bool`, *optional*, defaults to `False`):
Whether to split the head dimension into a new axis for the self-attention computation. In most cases,
enabling this flag should speed up the computation for Stable Diffusion 2.x and Stable Diffusion XL.
dtype (:obj:`jnp.dtype`, *optional*, defaults to jnp.float32):
Parameters `dtype`
"""
in_channels: int
out_channels: int
dropout: float = 0.0
num_layers: int = 1
num_attention_heads: int = 1
add_downsample: bool = True
use_linear_projection: bool = False
only_cross_attention: bool = False
use_memory_efficient_attention: bool = False
split_head_dim: bool = False
dtype: jnp.dtype = jnp.float32
transformer_layers_per_block: int = 1
def setup(self):
resnets = []
attentions = []
for i in range(self.num_layers):
in_channels = self.in_channels if i == 0 else self.out_channels
res_block = FlaxResnetBlock2D(
in_channels=in_channels,
out_channels=self.out_channels,
dropout_prob=self.dropout,
dtype=self.dtype,
)
resnets.append(res_block)
attn_block = FlaxTransformer2DModel(
in_channels=self.out_channels,
n_heads=self.num_attention_heads,
d_head=self.out_channels // self.num_attention_heads,
depth=self.transformer_layers_per_block,
use_linear_projection=self.use_linear_projection,
only_cross_attention=self.only_cross_attention,
use_memory_efficient_attention=self.use_memory_efficient_attention,
split_head_dim=self.split_head_dim,
dtype=self.dtype,
)
attentions.append(attn_block)
self.resnets = resnets
self.attentions = attentions
if self.add_downsample:
self.downsamplers_0 = FlaxDownsample2D(self.out_channels, dtype=self.dtype)
def __call__(self, hidden_states, temb, encoder_hidden_states, deterministic=True):
output_states = ()
for resnet, attn in zip(self.resnets, self.attentions):
hidden_states = resnet(hidden_states, temb, deterministic=deterministic)
hidden_states = attn(hidden_states, encoder_hidden_states, deterministic=deterministic)
output_states += (hidden_states,)
if self.add_downsample:
hidden_states = self.downsamplers_0(hidden_states)
output_states += (hidden_states,)
return hidden_states, output_states
class FlaxDownBlock2D(nn.Module):
r"""
Flax 2D downsizing block
Parameters:
in_channels (:obj:`int`):
Input channels
out_channels (:obj:`int`):
Output channels
dropout (:obj:`float`, *optional*, defaults to 0.0):
Dropout rate
num_layers (:obj:`int`, *optional*, defaults to 1):
Number of attention blocks layers
add_downsample (:obj:`bool`, *optional*, defaults to `True`):
Whether to add downsampling layer before each final output
dtype (:obj:`jnp.dtype`, *optional*, defaults to jnp.float32):
Parameters `dtype`
"""
in_channels: int
out_channels: int
dropout: float = 0.0
num_layers: int = 1
add_downsample: bool = True
dtype: jnp.dtype = jnp.float32
def setup(self):
resnets = []
for i in range(self.num_layers):
in_channels = self.in_channels if i == 0 else self.out_channels
res_block = FlaxResnetBlock2D(
in_channels=in_channels,
out_channels=self.out_channels,
dropout_prob=self.dropout,
dtype=self.dtype,
)
resnets.append(res_block)
self.resnets = resnets
if self.add_downsample:
self.downsamplers_0 = FlaxDownsample2D(self.out_channels, dtype=self.dtype)
def __call__(self, hidden_states, temb, deterministic=True):
output_states = ()
for resnet in self.resnets:
hidden_states = resnet(hidden_states, temb, deterministic=deterministic)
output_states += (hidden_states,)
if self.add_downsample:
hidden_states = self.downsamplers_0(hidden_states)
output_states += (hidden_states,)
return hidden_states, output_states
class FlaxCrossAttnUpBlock2D(nn.Module):
r"""
Cross Attention 2D Upsampling block - original architecture from Unet transformers:
https://arxiv.org/abs/2103.06104
Parameters:
in_channels (:obj:`int`):
Input channels
out_channels (:obj:`int`):
Output channels
dropout (:obj:`float`, *optional*, defaults to 0.0):
Dropout rate
num_layers (:obj:`int`, *optional*, defaults to 1):
Number of attention blocks layers
num_attention_heads (:obj:`int`, *optional*, defaults to 1):
Number of attention heads of each spatial transformer block
add_upsample (:obj:`bool`, *optional*, defaults to `True`):
Whether to add upsampling layer before each final output
use_memory_efficient_attention (`bool`, *optional*, defaults to `False`):
enable memory efficient attention https://arxiv.org/abs/2112.05682
split_head_dim (`bool`, *optional*, defaults to `False`):
Whether to split the head dimension into a new axis for the self-attention computation. In most cases,
enabling this flag should speed up the computation for Stable Diffusion 2.x and Stable Diffusion XL.
dtype (:obj:`jnp.dtype`, *optional*, defaults to jnp.float32):
Parameters `dtype`
"""
in_channels: int
out_channels: int
prev_output_channel: int
dropout: float = 0.0
num_layers: int = 1
num_attention_heads: int = 1
add_upsample: bool = True
use_linear_projection: bool = False
only_cross_attention: bool = False
use_memory_efficient_attention: bool = False
split_head_dim: bool = False
dtype: jnp.dtype = jnp.float32
transformer_layers_per_block: int = 1
def setup(self):
resnets = []
attentions = []
for i in range(self.num_layers):
res_skip_channels = self.in_channels if (i == self.num_layers - 1) else self.out_channels
resnet_in_channels = self.prev_output_channel if i == 0 else self.out_channels
res_block = FlaxResnetBlock2D(
in_channels=resnet_in_channels + res_skip_channels,
out_channels=self.out_channels,
dropout_prob=self.dropout,
dtype=self.dtype,
)
resnets.append(res_block)
attn_block = FlaxTransformer2DModel(
in_channels=self.out_channels,
n_heads=self.num_attention_heads,
d_head=self.out_channels // self.num_attention_heads,
depth=self.transformer_layers_per_block,
use_linear_projection=self.use_linear_projection,
only_cross_attention=self.only_cross_attention,
use_memory_efficient_attention=self.use_memory_efficient_attention,
split_head_dim=self.split_head_dim,
dtype=self.dtype,
)
attentions.append(attn_block)
self.resnets = resnets
self.attentions = attentions
if self.add_upsample:
self.upsamplers_0 = FlaxUpsample2D(self.out_channels, dtype=self.dtype)
def __call__(self, hidden_states, res_hidden_states_tuple, temb, encoder_hidden_states, deterministic=True):
for resnet, attn in zip(self.resnets, self.attentions):
# pop res hidden states
res_hidden_states = res_hidden_states_tuple[-1]
res_hidden_states_tuple = res_hidden_states_tuple[:-1]
hidden_states = jnp.concatenate((hidden_states, res_hidden_states), axis=-1)
hidden_states = resnet(hidden_states, temb, deterministic=deterministic)
hidden_states = attn(hidden_states, encoder_hidden_states, deterministic=deterministic)
if self.add_upsample:
hidden_states = self.upsamplers_0(hidden_states)
return hidden_states
class FlaxUpBlock2D(nn.Module):
r"""
Flax 2D upsampling block
Parameters:
in_channels (:obj:`int`):
Input channels
out_channels (:obj:`int`):
Output channels
prev_output_channel (:obj:`int`):
Output channels from the previous block
dropout (:obj:`float`, *optional*, defaults to 0.0):
Dropout rate
num_layers (:obj:`int`, *optional*, defaults to 1):
Number of attention blocks layers
add_downsample (:obj:`bool`, *optional*, defaults to `True`):
Whether to add downsampling layer before each final output
dtype (:obj:`jnp.dtype`, *optional*, defaults to jnp.float32):
Parameters `dtype`
"""
in_channels: int
out_channels: int
prev_output_channel: int
dropout: float = 0.0
num_layers: int = 1
add_upsample: bool = True
dtype: jnp.dtype = jnp.float32
def setup(self):
resnets = []
for i in range(self.num_layers):
res_skip_channels = self.in_channels if (i == self.num_layers - 1) else self.out_channels
resnet_in_channels = self.prev_output_channel if i == 0 else self.out_channels
res_block = FlaxResnetBlock2D(
in_channels=resnet_in_channels + res_skip_channels,
out_channels=self.out_channels,
dropout_prob=self.dropout,
dtype=self.dtype,
)
resnets.append(res_block)
self.resnets = resnets
if self.add_upsample:
self.upsamplers_0 = FlaxUpsample2D(self.out_channels, dtype=self.dtype)
def __call__(self, hidden_states, res_hidden_states_tuple, temb, deterministic=True):
for resnet in self.resnets:
# pop res hidden states
res_hidden_states = res_hidden_states_tuple[-1]
res_hidden_states_tuple = res_hidden_states_tuple[:-1]
hidden_states = jnp.concatenate((hidden_states, res_hidden_states), axis=-1)
hidden_states = resnet(hidden_states, temb, deterministic=deterministic)
if self.add_upsample:
hidden_states = self.upsamplers_0(hidden_states)
return hidden_states
class FlaxUNetMidBlock2DCrossAttn(nn.Module):
r"""
Cross Attention 2D Mid-level block - original architecture from Unet transformers: https://arxiv.org/abs/2103.06104
Parameters:
in_channels (:obj:`int`):
Input channels
dropout (:obj:`float`, *optional*, defaults to 0.0):
Dropout rate
num_layers (:obj:`int`, *optional*, defaults to 1):
Number of attention blocks layers
num_attention_heads (:obj:`int`, *optional*, defaults to 1):
Number of attention heads of each spatial transformer block
use_memory_efficient_attention (`bool`, *optional*, defaults to `False`):
enable memory efficient attention https://arxiv.org/abs/2112.05682
split_head_dim (`bool`, *optional*, defaults to `False`):
Whether to split the head dimension into a new axis for the self-attention computation. In most cases,
enabling this flag should speed up the computation for Stable Diffusion 2.x and Stable Diffusion XL.
dtype (:obj:`jnp.dtype`, *optional*, defaults to jnp.float32):
Parameters `dtype`
"""
in_channels: int
dropout: float = 0.0
num_layers: int = 1
num_attention_heads: int = 1
use_linear_projection: bool = False
use_memory_efficient_attention: bool = False
split_head_dim: bool = False
dtype: jnp.dtype = jnp.float32
transformer_layers_per_block: int = 1
def setup(self):
# there is always at least one resnet
resnets = [
FlaxResnetBlock2D(
in_channels=self.in_channels,
out_channels=self.in_channels,
dropout_prob=self.dropout,
dtype=self.dtype,
)
]
attentions = []
for _ in range(self.num_layers):
attn_block = FlaxTransformer2DModel(
in_channels=self.in_channels,
n_heads=self.num_attention_heads,
d_head=self.in_channels // self.num_attention_heads,
depth=self.transformer_layers_per_block,
use_linear_projection=self.use_linear_projection,
use_memory_efficient_attention=self.use_memory_efficient_attention,
split_head_dim=self.split_head_dim,
dtype=self.dtype,
)
attentions.append(attn_block)
res_block = FlaxResnetBlock2D(
in_channels=self.in_channels,
out_channels=self.in_channels,
dropout_prob=self.dropout,
dtype=self.dtype,
)
resnets.append(res_block)
self.resnets = resnets
self.attentions = attentions
def __call__(self, hidden_states, temb, encoder_hidden_states, deterministic=True):
hidden_states = self.resnets[0](hidden_states, temb)
for attn, resnet in zip(self.attentions, self.resnets[1:]):
hidden_states = attn(hidden_states, encoder_hidden_states, deterministic=deterministic)
hidden_states = resnet(hidden_states, temb, deterministic=deterministic)
return hidden_states
| diffusers/src/diffusers/models/unets/unet_2d_blocks_flax.py/0 | {
"file_path": "diffusers/src/diffusers/models/unets/unet_2d_blocks_flax.py",
"repo_id": "diffusers",
"token_count": 6961
} | 122 |
from typing import TYPE_CHECKING
from ..utils import (
DIFFUSERS_SLOW_IMPORT,
OptionalDependencyNotAvailable,
_LazyModule,
get_objects_from_module,
is_flax_available,
is_k_diffusion_available,
is_librosa_available,
is_note_seq_available,
is_onnx_available,
is_torch_available,
is_torch_npu_available,
is_transformers_available,
)
# These modules contain pipelines from multiple libraries/frameworks
_dummy_objects = {}
_import_structure = {
"controlnet": [],
"controlnet_xs": [],
"deprecated": [],
"latent_diffusion": [],
"ledits_pp": [],
"stable_diffusion": [],
"stable_diffusion_xl": [],
}
try:
if not is_torch_available():
raise OptionalDependencyNotAvailable()
except OptionalDependencyNotAvailable:
from ..utils import dummy_pt_objects # noqa F403
_dummy_objects.update(get_objects_from_module(dummy_pt_objects))
else:
_import_structure["auto_pipeline"] = [
"AutoPipelineForImage2Image",
"AutoPipelineForInpainting",
"AutoPipelineForText2Image",
]
_import_structure["consistency_models"] = ["ConsistencyModelPipeline"]
_import_structure["dance_diffusion"] = ["DanceDiffusionPipeline"]
_import_structure["ddim"] = ["DDIMPipeline"]
_import_structure["ddpm"] = ["DDPMPipeline"]
_import_structure["dit"] = ["DiTPipeline"]
_import_structure["latent_diffusion"].extend(["LDMSuperResolutionPipeline"])
_import_structure["pipeline_utils"] = [
"AudioPipelineOutput",
"DiffusionPipeline",
"StableDiffusionMixin",
"ImagePipelineOutput",
]
_import_structure["deprecated"].extend(
[
"PNDMPipeline",
"LDMPipeline",
"RePaintPipeline",
"ScoreSdeVePipeline",
"KarrasVePipeline",
]
)
try:
if not (is_torch_available() and is_librosa_available()):
raise OptionalDependencyNotAvailable()
except OptionalDependencyNotAvailable:
from ..utils import dummy_torch_and_librosa_objects # noqa F403
_dummy_objects.update(get_objects_from_module(dummy_torch_and_librosa_objects))
else:
_import_structure["deprecated"].extend(["AudioDiffusionPipeline", "Mel"])
try:
if not (is_transformers_available() and is_torch_available() and is_note_seq_available()):
raise OptionalDependencyNotAvailable()
except OptionalDependencyNotAvailable:
from ..utils import dummy_transformers_and_torch_and_note_seq_objects # noqa F403
_dummy_objects.update(get_objects_from_module(dummy_transformers_and_torch_and_note_seq_objects))
else:
_import_structure["deprecated"].extend(
[
"MidiProcessor",
"SpectrogramDiffusionPipeline",
]
)
try:
if not (is_torch_available() and is_transformers_available()):
raise OptionalDependencyNotAvailable()
except OptionalDependencyNotAvailable:
from ..utils import dummy_torch_and_transformers_objects # noqa F403
_dummy_objects.update(get_objects_from_module(dummy_torch_and_transformers_objects))
else:
_import_structure["deprecated"].extend(
[
"VQDiffusionPipeline",
"AltDiffusionPipeline",
"AltDiffusionImg2ImgPipeline",
"CycleDiffusionPipeline",
"StableDiffusionInpaintPipelineLegacy",
"StableDiffusionPix2PixZeroPipeline",
"StableDiffusionParadigmsPipeline",
"StableDiffusionModelEditingPipeline",
"VersatileDiffusionDualGuidedPipeline",
"VersatileDiffusionImageVariationPipeline",
"VersatileDiffusionPipeline",
"VersatileDiffusionTextToImagePipeline",
]
)
_import_structure["amused"] = ["AmusedImg2ImgPipeline", "AmusedInpaintPipeline", "AmusedPipeline"]
_import_structure["animatediff"] = [
"AnimateDiffPipeline",
"AnimateDiffVideoToVideoPipeline",
]
_import_structure["audioldm"] = ["AudioLDMPipeline"]
_import_structure["audioldm2"] = [
"AudioLDM2Pipeline",
"AudioLDM2ProjectionModel",
"AudioLDM2UNet2DConditionModel",
]
_import_structure["blip_diffusion"] = ["BlipDiffusionPipeline"]
_import_structure["controlnet"].extend(
[
"BlipDiffusionControlNetPipeline",
"StableDiffusionControlNetImg2ImgPipeline",
"StableDiffusionControlNetInpaintPipeline",
"StableDiffusionControlNetPipeline",
"StableDiffusionXLControlNetImg2ImgPipeline",
"StableDiffusionXLControlNetInpaintPipeline",
"StableDiffusionXLControlNetPipeline",
]
)
_import_structure["deepfloyd_if"] = [
"IFImg2ImgPipeline",
"IFImg2ImgSuperResolutionPipeline",
"IFInpaintingPipeline",
"IFInpaintingSuperResolutionPipeline",
"IFPipeline",
"IFSuperResolutionPipeline",
]
_import_structure["kandinsky"] = [
"KandinskyCombinedPipeline",
"KandinskyImg2ImgCombinedPipeline",
"KandinskyImg2ImgPipeline",
"KandinskyInpaintCombinedPipeline",
"KandinskyInpaintPipeline",
"KandinskyPipeline",
"KandinskyPriorPipeline",
]
_import_structure["kandinsky2_2"] = [
"KandinskyV22CombinedPipeline",
"KandinskyV22ControlnetImg2ImgPipeline",
"KandinskyV22ControlnetPipeline",
"KandinskyV22Img2ImgCombinedPipeline",
"KandinskyV22Img2ImgPipeline",
"KandinskyV22InpaintCombinedPipeline",
"KandinskyV22InpaintPipeline",
"KandinskyV22Pipeline",
"KandinskyV22PriorEmb2EmbPipeline",
"KandinskyV22PriorPipeline",
]
_import_structure["kandinsky3"] = [
"Kandinsky3Img2ImgPipeline",
"Kandinsky3Pipeline",
]
_import_structure["latent_consistency_models"] = [
"LatentConsistencyModelImg2ImgPipeline",
"LatentConsistencyModelPipeline",
]
_import_structure["latent_diffusion"].extend(["LDMTextToImagePipeline"])
_import_structure["ledits_pp"].extend(
[
"LEditsPPPipelineStableDiffusion",
"LEditsPPPipelineStableDiffusionXL",
]
)
_import_structure["musicldm"] = ["MusicLDMPipeline"]
_import_structure["paint_by_example"] = ["PaintByExamplePipeline"]
_import_structure["pia"] = ["PIAPipeline"]
_import_structure["pixart_alpha"] = ["PixArtAlphaPipeline"]
_import_structure["semantic_stable_diffusion"] = ["SemanticStableDiffusionPipeline"]
_import_structure["shap_e"] = ["ShapEImg2ImgPipeline", "ShapEPipeline"]
_import_structure["stable_cascade"] = [
"StableCascadeCombinedPipeline",
"StableCascadeDecoderPipeline",
"StableCascadePriorPipeline",
]
_import_structure["stable_diffusion"].extend(
[
"CLIPImageProjection",
"StableDiffusionDepth2ImgPipeline",
"StableDiffusionImageVariationPipeline",
"StableDiffusionImg2ImgPipeline",
"StableDiffusionInpaintPipeline",
"StableDiffusionInstructPix2PixPipeline",
"StableDiffusionLatentUpscalePipeline",
"StableDiffusionPipeline",
"StableDiffusionUpscalePipeline",
"StableUnCLIPImg2ImgPipeline",
"StableUnCLIPPipeline",
"StableDiffusionLDM3DPipeline",
]
)
_import_structure["stable_diffusion_attend_and_excite"] = ["StableDiffusionAttendAndExcitePipeline"]
_import_structure["stable_diffusion_safe"] = ["StableDiffusionPipelineSafe"]
_import_structure["stable_diffusion_sag"] = ["StableDiffusionSAGPipeline"]
_import_structure["stable_diffusion_gligen"] = [
"StableDiffusionGLIGENPipeline",
"StableDiffusionGLIGENTextImagePipeline",
]
_import_structure["stable_video_diffusion"] = ["StableVideoDiffusionPipeline"]
_import_structure["stable_diffusion_xl"].extend(
[
"StableDiffusionXLImg2ImgPipeline",
"StableDiffusionXLInpaintPipeline",
"StableDiffusionXLInstructPix2PixPipeline",
"StableDiffusionXLPipeline",
]
)
_import_structure["stable_diffusion_diffedit"] = ["StableDiffusionDiffEditPipeline"]
_import_structure["stable_diffusion_ldm3d"] = ["StableDiffusionLDM3DPipeline"]
_import_structure["stable_diffusion_panorama"] = ["StableDiffusionPanoramaPipeline"]
_import_structure["t2i_adapter"] = [
"StableDiffusionAdapterPipeline",
"StableDiffusionXLAdapterPipeline",
]
_import_structure["text_to_video_synthesis"] = [
"TextToVideoSDPipeline",
"TextToVideoZeroPipeline",
"TextToVideoZeroSDXLPipeline",
"VideoToVideoSDPipeline",
]
_import_structure["i2vgen_xl"] = ["I2VGenXLPipeline"]
_import_structure["unclip"] = ["UnCLIPImageVariationPipeline", "UnCLIPPipeline"]
_import_structure["unidiffuser"] = [
"ImageTextPipelineOutput",
"UniDiffuserModel",
"UniDiffuserPipeline",
"UniDiffuserTextDecoder",
]
_import_structure["wuerstchen"] = [
"WuerstchenCombinedPipeline",
"WuerstchenDecoderPipeline",
"WuerstchenPriorPipeline",
]
try:
if not is_onnx_available():
raise OptionalDependencyNotAvailable()
except OptionalDependencyNotAvailable:
from ..utils import dummy_onnx_objects # noqa F403
_dummy_objects.update(get_objects_from_module(dummy_onnx_objects))
else:
_import_structure["onnx_utils"] = ["OnnxRuntimeModel"]
try:
if not (is_torch_available() and is_transformers_available() and is_onnx_available()):
raise OptionalDependencyNotAvailable()
except OptionalDependencyNotAvailable:
from ..utils import dummy_torch_and_transformers_and_onnx_objects # noqa F403
_dummy_objects.update(get_objects_from_module(dummy_torch_and_transformers_and_onnx_objects))
else:
_import_structure["stable_diffusion"].extend(
[
"OnnxStableDiffusionImg2ImgPipeline",
"OnnxStableDiffusionInpaintPipeline",
"OnnxStableDiffusionPipeline",
"OnnxStableDiffusionUpscalePipeline",
"StableDiffusionOnnxPipeline",
]
)
try:
if not (is_torch_available() and is_transformers_available() and is_k_diffusion_available()):
raise OptionalDependencyNotAvailable()
except OptionalDependencyNotAvailable:
from ..utils import (
dummy_torch_and_transformers_and_k_diffusion_objects,
)
_dummy_objects.update(get_objects_from_module(dummy_torch_and_transformers_and_k_diffusion_objects))
else:
_import_structure["stable_diffusion_k_diffusion"] = [
"StableDiffusionKDiffusionPipeline",
"StableDiffusionXLKDiffusionPipeline",
]
try:
if not is_flax_available():
raise OptionalDependencyNotAvailable()
except OptionalDependencyNotAvailable:
from ..utils import dummy_flax_objects # noqa F403
_dummy_objects.update(get_objects_from_module(dummy_flax_objects))
else:
_import_structure["pipeline_flax_utils"] = ["FlaxDiffusionPipeline"]
try:
if not (is_flax_available() and is_transformers_available()):
raise OptionalDependencyNotAvailable()
except OptionalDependencyNotAvailable:
from ..utils import dummy_flax_and_transformers_objects # noqa F403
_dummy_objects.update(get_objects_from_module(dummy_flax_and_transformers_objects))
else:
_import_structure["controlnet"].extend(["FlaxStableDiffusionControlNetPipeline"])
_import_structure["stable_diffusion"].extend(
[
"FlaxStableDiffusionImg2ImgPipeline",
"FlaxStableDiffusionInpaintPipeline",
"FlaxStableDiffusionPipeline",
]
)
_import_structure["stable_diffusion_xl"].extend(
[
"FlaxStableDiffusionXLPipeline",
]
)
if TYPE_CHECKING or DIFFUSERS_SLOW_IMPORT:
try:
if not is_torch_available():
raise OptionalDependencyNotAvailable()
except OptionalDependencyNotAvailable:
from ..utils.dummy_pt_objects import * # noqa F403
else:
from .auto_pipeline import (
AutoPipelineForImage2Image,
AutoPipelineForInpainting,
AutoPipelineForText2Image,
)
from .consistency_models import ConsistencyModelPipeline
from .dance_diffusion import DanceDiffusionPipeline
from .ddim import DDIMPipeline
from .ddpm import DDPMPipeline
from .deprecated import KarrasVePipeline, LDMPipeline, PNDMPipeline, RePaintPipeline, ScoreSdeVePipeline
from .dit import DiTPipeline
from .latent_diffusion import LDMSuperResolutionPipeline
from .pipeline_utils import (
AudioPipelineOutput,
DiffusionPipeline,
ImagePipelineOutput,
StableDiffusionMixin,
)
try:
if not (is_torch_available() and is_librosa_available()):
raise OptionalDependencyNotAvailable()
except OptionalDependencyNotAvailable:
from ..utils.dummy_torch_and_librosa_objects import *
else:
from .deprecated import AudioDiffusionPipeline, Mel
try:
if not (is_torch_available() and is_transformers_available()):
raise OptionalDependencyNotAvailable()
except OptionalDependencyNotAvailable:
from ..utils.dummy_torch_and_transformers_objects import *
else:
from .amused import AmusedImg2ImgPipeline, AmusedInpaintPipeline, AmusedPipeline
from .animatediff import AnimateDiffPipeline, AnimateDiffVideoToVideoPipeline
from .audioldm import AudioLDMPipeline
from .audioldm2 import (
AudioLDM2Pipeline,
AudioLDM2ProjectionModel,
AudioLDM2UNet2DConditionModel,
)
from .blip_diffusion import BlipDiffusionPipeline
from .controlnet import (
BlipDiffusionControlNetPipeline,
StableDiffusionControlNetImg2ImgPipeline,
StableDiffusionControlNetInpaintPipeline,
StableDiffusionControlNetPipeline,
StableDiffusionXLControlNetImg2ImgPipeline,
StableDiffusionXLControlNetInpaintPipeline,
StableDiffusionXLControlNetPipeline,
)
from .deepfloyd_if import (
IFImg2ImgPipeline,
IFImg2ImgSuperResolutionPipeline,
IFInpaintingPipeline,
IFInpaintingSuperResolutionPipeline,
IFPipeline,
IFSuperResolutionPipeline,
)
from .deprecated import (
AltDiffusionImg2ImgPipeline,
AltDiffusionPipeline,
CycleDiffusionPipeline,
StableDiffusionInpaintPipelineLegacy,
StableDiffusionModelEditingPipeline,
StableDiffusionParadigmsPipeline,
StableDiffusionPix2PixZeroPipeline,
VersatileDiffusionDualGuidedPipeline,
VersatileDiffusionImageVariationPipeline,
VersatileDiffusionPipeline,
VersatileDiffusionTextToImagePipeline,
VQDiffusionPipeline,
)
from .i2vgen_xl import I2VGenXLPipeline
from .kandinsky import (
KandinskyCombinedPipeline,
KandinskyImg2ImgCombinedPipeline,
KandinskyImg2ImgPipeline,
KandinskyInpaintCombinedPipeline,
KandinskyInpaintPipeline,
KandinskyPipeline,
KandinskyPriorPipeline,
)
from .kandinsky2_2 import (
KandinskyV22CombinedPipeline,
KandinskyV22ControlnetImg2ImgPipeline,
KandinskyV22ControlnetPipeline,
KandinskyV22Img2ImgCombinedPipeline,
KandinskyV22Img2ImgPipeline,
KandinskyV22InpaintCombinedPipeline,
KandinskyV22InpaintPipeline,
KandinskyV22Pipeline,
KandinskyV22PriorEmb2EmbPipeline,
KandinskyV22PriorPipeline,
)
from .kandinsky3 import (
Kandinsky3Img2ImgPipeline,
Kandinsky3Pipeline,
)
from .latent_consistency_models import (
LatentConsistencyModelImg2ImgPipeline,
LatentConsistencyModelPipeline,
)
from .latent_diffusion import LDMTextToImagePipeline
from .ledits_pp import (
LEditsPPDiffusionPipelineOutput,
LEditsPPInversionPipelineOutput,
LEditsPPPipelineStableDiffusion,
LEditsPPPipelineStableDiffusionXL,
)
from .musicldm import MusicLDMPipeline
from .paint_by_example import PaintByExamplePipeline
from .pia import PIAPipeline
from .pixart_alpha import PixArtAlphaPipeline
from .semantic_stable_diffusion import SemanticStableDiffusionPipeline
from .shap_e import ShapEImg2ImgPipeline, ShapEPipeline
from .stable_cascade import (
StableCascadeCombinedPipeline,
StableCascadeDecoderPipeline,
StableCascadePriorPipeline,
)
from .stable_diffusion import (
CLIPImageProjection,
StableDiffusionDepth2ImgPipeline,
StableDiffusionImageVariationPipeline,
StableDiffusionImg2ImgPipeline,
StableDiffusionInpaintPipeline,
StableDiffusionInstructPix2PixPipeline,
StableDiffusionLatentUpscalePipeline,
StableDiffusionPipeline,
StableDiffusionUpscalePipeline,
StableUnCLIPImg2ImgPipeline,
StableUnCLIPPipeline,
)
from .stable_diffusion_attend_and_excite import StableDiffusionAttendAndExcitePipeline
from .stable_diffusion_diffedit import StableDiffusionDiffEditPipeline
from .stable_diffusion_gligen import StableDiffusionGLIGENPipeline, StableDiffusionGLIGENTextImagePipeline
from .stable_diffusion_ldm3d import StableDiffusionLDM3DPipeline
from .stable_diffusion_panorama import StableDiffusionPanoramaPipeline
from .stable_diffusion_safe import StableDiffusionPipelineSafe
from .stable_diffusion_sag import StableDiffusionSAGPipeline
from .stable_diffusion_xl import (
StableDiffusionXLImg2ImgPipeline,
StableDiffusionXLInpaintPipeline,
StableDiffusionXLInstructPix2PixPipeline,
StableDiffusionXLPipeline,
)
from .stable_video_diffusion import StableVideoDiffusionPipeline
from .t2i_adapter import (
StableDiffusionAdapterPipeline,
StableDiffusionXLAdapterPipeline,
)
from .text_to_video_synthesis import (
TextToVideoSDPipeline,
TextToVideoZeroPipeline,
TextToVideoZeroSDXLPipeline,
VideoToVideoSDPipeline,
)
from .unclip import UnCLIPImageVariationPipeline, UnCLIPPipeline
from .unidiffuser import (
ImageTextPipelineOutput,
UniDiffuserModel,
UniDiffuserPipeline,
UniDiffuserTextDecoder,
)
from .wuerstchen import (
WuerstchenCombinedPipeline,
WuerstchenDecoderPipeline,
WuerstchenPriorPipeline,
)
try:
if not is_onnx_available():
raise OptionalDependencyNotAvailable()
except OptionalDependencyNotAvailable:
from ..utils.dummy_onnx_objects import * # noqa F403
else:
from .onnx_utils import OnnxRuntimeModel
try:
if not (is_torch_available() and is_transformers_available() and is_onnx_available()):
raise OptionalDependencyNotAvailable()
except OptionalDependencyNotAvailable:
from ..utils.dummy_torch_and_transformers_and_onnx_objects import *
else:
from .stable_diffusion import (
OnnxStableDiffusionImg2ImgPipeline,
OnnxStableDiffusionInpaintPipeline,
OnnxStableDiffusionPipeline,
OnnxStableDiffusionUpscalePipeline,
StableDiffusionOnnxPipeline,
)
try:
if not (is_torch_available() and is_transformers_available() and is_k_diffusion_available()):
raise OptionalDependencyNotAvailable()
except OptionalDependencyNotAvailable:
from ..utils.dummy_torch_and_transformers_and_k_diffusion_objects import *
else:
from .stable_diffusion_k_diffusion import (
StableDiffusionKDiffusionPipeline,
StableDiffusionXLKDiffusionPipeline,
)
try:
if not is_flax_available():
raise OptionalDependencyNotAvailable()
except OptionalDependencyNotAvailable:
from ..utils.dummy_flax_objects import * # noqa F403
else:
from .pipeline_flax_utils import FlaxDiffusionPipeline
try:
if not (is_flax_available() and is_transformers_available()):
raise OptionalDependencyNotAvailable()
except OptionalDependencyNotAvailable:
from ..utils.dummy_flax_and_transformers_objects import *
else:
from .controlnet import FlaxStableDiffusionControlNetPipeline
from .stable_diffusion import (
FlaxStableDiffusionImg2ImgPipeline,
FlaxStableDiffusionInpaintPipeline,
FlaxStableDiffusionPipeline,
)
from .stable_diffusion_xl import (
FlaxStableDiffusionXLPipeline,
)
try:
if not (is_transformers_available() and is_torch_available() and is_note_seq_available()):
raise OptionalDependencyNotAvailable()
except OptionalDependencyNotAvailable:
from ..utils.dummy_transformers_and_torch_and_note_seq_objects import * # noqa F403
else:
from .deprecated import (
MidiProcessor,
SpectrogramDiffusionPipeline,
)
else:
import sys
sys.modules[__name__] = _LazyModule(
__name__,
globals()["__file__"],
_import_structure,
module_spec=__spec__,
)
for name, value in _dummy_objects.items():
setattr(sys.modules[__name__], name, value)
| diffusers/src/diffusers/pipelines/__init__.py/0 | {
"file_path": "diffusers/src/diffusers/pipelines/__init__.py",
"repo_id": "diffusers",
"token_count": 10349
} | 123 |
# coding=utf-8
# Copyright 2024 The HuggingFace Inc. team. All rights reserved.
#
# Licensed under the Apache License, Version 2.0 (the "License");
# you may not use this file except in compliance with the License.
# You may obtain a copy of the License at
#
# http://www.apache.org/licenses/LICENSE-2.0
#
# Unless required by applicable law or agreed to in writing, software
# distributed under the License is distributed on an "AS IS" BASIS,
# WITHOUT WARRANTIES OR CONDITIONS OF ANY KIND, either express or implied.
# See the License for the specific language governing permissions and
# limitations under the License.
"""Image processor class for BLIP."""
from typing import Dict, List, Optional, Union
import numpy as np
import torch
from transformers.image_processing_utils import BaseImageProcessor, BatchFeature, get_size_dict
from transformers.image_transforms import convert_to_rgb, resize, to_channel_dimension_format
from transformers.image_utils import (
OPENAI_CLIP_MEAN,
OPENAI_CLIP_STD,
ChannelDimension,
ImageInput,
PILImageResampling,
infer_channel_dimension_format,
is_scaled_image,
make_list_of_images,
to_numpy_array,
valid_images,
)
from transformers.utils import TensorType, is_vision_available, logging
from diffusers.utils import numpy_to_pil
if is_vision_available():
import PIL.Image
logger = logging.get_logger(__name__)
# We needed some extra functions on top of the ones in transformers.image_processing_utils.BaseImageProcessor, namely center crop
# Copy-pasted from transformers.models.blip.image_processing_blip.BlipImageProcessor
class BlipImageProcessor(BaseImageProcessor):
r"""
Constructs a BLIP image processor.
Args:
do_resize (`bool`, *optional*, defaults to `True`):
Whether to resize the image's (height, width) dimensions to the specified `size`. Can be overridden by the
`do_resize` parameter in the `preprocess` method.
size (`dict`, *optional*, defaults to `{"height": 384, "width": 384}`):
Size of the output image after resizing. Can be overridden by the `size` parameter in the `preprocess`
method.
resample (`PILImageResampling`, *optional*, defaults to `PILImageResampling.BICUBIC`):
Resampling filter to use if resizing the image. Only has an effect if `do_resize` is set to `True`. Can be
overridden by the `resample` parameter in the `preprocess` method.
do_rescale (`bool`, *optional*, defaults to `True`):
Wwhether to rescale the image by the specified scale `rescale_factor`. Can be overridden by the
`do_rescale` parameter in the `preprocess` method.
rescale_factor (`int` or `float`, *optional*, defaults to `1/255`):
Scale factor to use if rescaling the image. Only has an effect if `do_rescale` is set to `True`. Can be
overridden by the `rescale_factor` parameter in the `preprocess` method.
do_normalize (`bool`, *optional*, defaults to `True`):
Whether to normalize the image. Can be overridden by the `do_normalize` parameter in the `preprocess`
method. Can be overridden by the `do_normalize` parameter in the `preprocess` method.
image_mean (`float` or `List[float]`, *optional*, defaults to `IMAGENET_STANDARD_MEAN`):
Mean to use if normalizing the image. This is a float or list of floats the length of the number of
channels in the image. Can be overridden by the `image_mean` parameter in the `preprocess` method. Can be
overridden by the `image_mean` parameter in the `preprocess` method.
image_std (`float` or `List[float]`, *optional*, defaults to `IMAGENET_STANDARD_STD`):
Standard deviation to use if normalizing the image. This is a float or list of floats the length of the
number of channels in the image. Can be overridden by the `image_std` parameter in the `preprocess` method.
Can be overridden by the `image_std` parameter in the `preprocess` method.
do_convert_rgb (`bool`, *optional*, defaults to `True`):
Whether to convert the image to RGB.
"""
model_input_names = ["pixel_values"]
def __init__(
self,
do_resize: bool = True,
size: Dict[str, int] = None,
resample: PILImageResampling = PILImageResampling.BICUBIC,
do_rescale: bool = True,
rescale_factor: Union[int, float] = 1 / 255,
do_normalize: bool = True,
image_mean: Optional[Union[float, List[float]]] = None,
image_std: Optional[Union[float, List[float]]] = None,
do_convert_rgb: bool = True,
do_center_crop: bool = True,
**kwargs,
) -> None:
super().__init__(**kwargs)
size = size if size is not None else {"height": 224, "width": 224}
size = get_size_dict(size, default_to_square=True)
self.do_resize = do_resize
self.size = size
self.resample = resample
self.do_rescale = do_rescale
self.rescale_factor = rescale_factor
self.do_normalize = do_normalize
self.image_mean = image_mean if image_mean is not None else OPENAI_CLIP_MEAN
self.image_std = image_std if image_std is not None else OPENAI_CLIP_STD
self.do_convert_rgb = do_convert_rgb
self.do_center_crop = do_center_crop
# Copy-pasted from transformers.models.vit.image_processing_vit.ViTImageProcessor.resize with PILImageResampling.BILINEAR->PILImageResampling.BICUBIC
def resize(
self,
image: np.ndarray,
size: Dict[str, int],
resample: PILImageResampling = PILImageResampling.BICUBIC,
data_format: Optional[Union[str, ChannelDimension]] = None,
input_data_format: Optional[Union[str, ChannelDimension]] = None,
**kwargs,
) -> np.ndarray:
"""
Resize an image to `(size["height"], size["width"])`.
Args:
image (`np.ndarray`):
Image to resize.
size (`Dict[str, int]`):
Dictionary in the format `{"height": int, "width": int}` specifying the size of the output image.
resample (`PILImageResampling`, *optional*, defaults to `PILImageResampling.BICUBIC`):
`PILImageResampling` filter to use when resizing the image e.g. `PILImageResampling.BICUBIC`.
data_format (`ChannelDimension` or `str`, *optional*):
The channel dimension format for the output image. If unset, the channel dimension format of the input
image is used. Can be one of:
- `"channels_first"` or `ChannelDimension.FIRST`: image in (num_channels, height, width) format.
- `"channels_last"` or `ChannelDimension.LAST`: image in (height, width, num_channels) format.
- `"none"` or `ChannelDimension.NONE`: image in (height, width) format.
input_data_format (`ChannelDimension` or `str`, *optional*):
The channel dimension format for the input image. If unset, the channel dimension format is inferred
from the input image. Can be one of:
- `"channels_first"` or `ChannelDimension.FIRST`: image in (num_channels, height, width) format.
- `"channels_last"` or `ChannelDimension.LAST`: image in (height, width, num_channels) format.
- `"none"` or `ChannelDimension.NONE`: image in (height, width) format.
Returns:
`np.ndarray`: The resized image.
"""
size = get_size_dict(size)
if "height" not in size or "width" not in size:
raise ValueError(f"The `size` dictionary must contain the keys `height` and `width`. Got {size.keys()}")
output_size = (size["height"], size["width"])
return resize(
image,
size=output_size,
resample=resample,
data_format=data_format,
input_data_format=input_data_format,
**kwargs,
)
def preprocess(
self,
images: ImageInput,
do_resize: Optional[bool] = None,
size: Optional[Dict[str, int]] = None,
resample: PILImageResampling = None,
do_rescale: Optional[bool] = None,
do_center_crop: Optional[bool] = None,
rescale_factor: Optional[float] = None,
do_normalize: Optional[bool] = None,
image_mean: Optional[Union[float, List[float]]] = None,
image_std: Optional[Union[float, List[float]]] = None,
return_tensors: Optional[Union[str, TensorType]] = None,
do_convert_rgb: bool = None,
data_format: ChannelDimension = ChannelDimension.FIRST,
input_data_format: Optional[Union[str, ChannelDimension]] = None,
**kwargs,
) -> PIL.Image.Image:
"""
Preprocess an image or batch of images.
Args:
images (`ImageInput`):
Image to preprocess. Expects a single or batch of images with pixel values ranging from 0 to 255. If
passing in images with pixel values between 0 and 1, set `do_rescale=False`.
do_resize (`bool`, *optional*, defaults to `self.do_resize`):
Whether to resize the image.
size (`Dict[str, int]`, *optional*, defaults to `self.size`):
Controls the size of the image after `resize`. The shortest edge of the image is resized to
`size["shortest_edge"]` whilst preserving the aspect ratio. If the longest edge of this resized image
is > `int(size["shortest_edge"] * (1333 / 800))`, then the image is resized again to make the longest
edge equal to `int(size["shortest_edge"] * (1333 / 800))`.
resample (`PILImageResampling`, *optional*, defaults to `self.resample`):
Resampling filter to use if resizing the image. Only has an effect if `do_resize` is set to `True`.
do_rescale (`bool`, *optional*, defaults to `self.do_rescale`):
Whether to rescale the image values between [0 - 1].
rescale_factor (`float`, *optional*, defaults to `self.rescale_factor`):
Rescale factor to rescale the image by if `do_rescale` is set to `True`.
do_normalize (`bool`, *optional*, defaults to `self.do_normalize`):
Whether to normalize the image.
image_mean (`float` or `List[float]`, *optional*, defaults to `self.image_mean`):
Image mean to normalize the image by if `do_normalize` is set to `True`.
image_std (`float` or `List[float]`, *optional*, defaults to `self.image_std`):
Image standard deviation to normalize the image by if `do_normalize` is set to `True`.
do_convert_rgb (`bool`, *optional*, defaults to `self.do_convert_rgb`):
Whether to convert the image to RGB.
return_tensors (`str` or `TensorType`, *optional*):
The type of tensors to return. Can be one of:
- Unset: Return a list of `np.ndarray`.
- `TensorType.TENSORFLOW` or `'tf'`: Return a batch of type `tf.Tensor`.
- `TensorType.PYTORCH` or `'pt'`: Return a batch of type `torch.Tensor`.
- `TensorType.NUMPY` or `'np'`: Return a batch of type `np.ndarray`.
- `TensorType.JAX` or `'jax'`: Return a batch of type `jax.numpy.ndarray`.
data_format (`ChannelDimension` or `str`, *optional*, defaults to `ChannelDimension.FIRST`):
The channel dimension format for the output image. Can be one of:
- `"channels_first"` or `ChannelDimension.FIRST`: image in (num_channels, height, width) format.
- `"channels_last"` or `ChannelDimension.LAST`: image in (height, width, num_channels) format.
- Unset: Use the channel dimension format of the input image.
input_data_format (`ChannelDimension` or `str`, *optional*):
The channel dimension format for the input image. If unset, the channel dimension format is inferred
from the input image. Can be one of:
- `"channels_first"` or `ChannelDimension.FIRST`: image in (num_channels, height, width) format.
- `"channels_last"` or `ChannelDimension.LAST`: image in (height, width, num_channels) format.
- `"none"` or `ChannelDimension.NONE`: image in (height, width) format.
"""
do_resize = do_resize if do_resize is not None else self.do_resize
resample = resample if resample is not None else self.resample
do_rescale = do_rescale if do_rescale is not None else self.do_rescale
rescale_factor = rescale_factor if rescale_factor is not None else self.rescale_factor
do_normalize = do_normalize if do_normalize is not None else self.do_normalize
image_mean = image_mean if image_mean is not None else self.image_mean
image_std = image_std if image_std is not None else self.image_std
do_convert_rgb = do_convert_rgb if do_convert_rgb is not None else self.do_convert_rgb
do_center_crop = do_center_crop if do_center_crop is not None else self.do_center_crop
size = size if size is not None else self.size
size = get_size_dict(size, default_to_square=False)
images = make_list_of_images(images)
if not valid_images(images):
raise ValueError(
"Invalid image type. Must be of type PIL.Image.Image, numpy.ndarray, "
"torch.Tensor, tf.Tensor or jax.ndarray."
)
if do_resize and size is None or resample is None:
raise ValueError("Size and resample must be specified if do_resize is True.")
if do_rescale and rescale_factor is None:
raise ValueError("Rescale factor must be specified if do_rescale is True.")
if do_normalize and (image_mean is None or image_std is None):
raise ValueError("Image mean and std must be specified if do_normalize is True.")
# PIL RGBA images are converted to RGB
if do_convert_rgb:
images = [convert_to_rgb(image) for image in images]
# All transformations expect numpy arrays.
images = [to_numpy_array(image) for image in images]
if is_scaled_image(images[0]) and do_rescale:
logger.warning_once(
"It looks like you are trying to rescale already rescaled images. If the input"
" images have pixel values between 0 and 1, set `do_rescale=False` to avoid rescaling them again."
)
if input_data_format is None:
# We assume that all images have the same channel dimension format.
input_data_format = infer_channel_dimension_format(images[0])
if do_resize:
images = [
self.resize(image=image, size=size, resample=resample, input_data_format=input_data_format)
for image in images
]
if do_rescale:
images = [
self.rescale(image=image, scale=rescale_factor, input_data_format=input_data_format)
for image in images
]
if do_normalize:
images = [
self.normalize(image=image, mean=image_mean, std=image_std, input_data_format=input_data_format)
for image in images
]
if do_center_crop:
images = [self.center_crop(image, size, input_data_format=input_data_format) for image in images]
images = [
to_channel_dimension_format(image, data_format, input_channel_dim=input_data_format) for image in images
]
encoded_outputs = BatchFeature(data={"pixel_values": images}, tensor_type=return_tensors)
return encoded_outputs
# Follows diffusers.VaeImageProcessor.postprocess
def postprocess(self, sample: torch.FloatTensor, output_type: str = "pil"):
if output_type not in ["pt", "np", "pil"]:
raise ValueError(
f"output_type={output_type} is not supported. Make sure to choose one of ['pt', 'np', or 'pil']"
)
# Equivalent to diffusers.VaeImageProcessor.denormalize
sample = (sample / 2 + 0.5).clamp(0, 1)
if output_type == "pt":
return sample
# Equivalent to diffusers.VaeImageProcessor.pt_to_numpy
sample = sample.cpu().permute(0, 2, 3, 1).numpy()
if output_type == "np":
return sample
# Output_type must be 'pil'
sample = numpy_to_pil(sample)
return sample
| diffusers/src/diffusers/pipelines/blip_diffusion/blip_image_processing.py/0 | {
"file_path": "diffusers/src/diffusers/pipelines/blip_diffusion/blip_image_processing.py",
"repo_id": "diffusers",
"token_count": 6939
} | 124 |
from typing import TYPE_CHECKING
from ...utils import DIFFUSERS_SLOW_IMPORT, _LazyModule
_import_structure = {"pipeline_dance_diffusion": ["DanceDiffusionPipeline"]}
if TYPE_CHECKING or DIFFUSERS_SLOW_IMPORT:
from .pipeline_dance_diffusion import DanceDiffusionPipeline
else:
import sys
sys.modules[__name__] = _LazyModule(
__name__,
globals()["__file__"],
_import_structure,
module_spec=__spec__,
)
| diffusers/src/diffusers/pipelines/dance_diffusion/__init__.py/0 | {
"file_path": "diffusers/src/diffusers/pipelines/dance_diffusion/__init__.py",
"repo_id": "diffusers",
"token_count": 189
} | 125 |
from typing import List
import PIL.Image
import torch
from PIL import Image
from ...configuration_utils import ConfigMixin
from ...models.modeling_utils import ModelMixin
from ...utils import PIL_INTERPOLATION
class IFWatermarker(ModelMixin, ConfigMixin):
def __init__(self):
super().__init__()
self.register_buffer("watermark_image", torch.zeros((62, 62, 4)))
self.watermark_image_as_pil = None
def apply_watermark(self, images: List[PIL.Image.Image], sample_size=None):
# copied from https://github.com/deep-floyd/IF/blob/b77482e36ca2031cb94dbca1001fc1e6400bf4ab/deepfloyd_if/modules/base.py#L287
h = images[0].height
w = images[0].width
sample_size = sample_size or h
coef = min(h / sample_size, w / sample_size)
img_h, img_w = (int(h / coef), int(w / coef)) if coef < 1 else (h, w)
S1, S2 = 1024**2, img_w * img_h
K = (S2 / S1) ** 0.5
wm_size, wm_x, wm_y = int(K * 62), img_w - int(14 * K), img_h - int(14 * K)
if self.watermark_image_as_pil is None:
watermark_image = self.watermark_image.to(torch.uint8).cpu().numpy()
watermark_image = Image.fromarray(watermark_image, mode="RGBA")
self.watermark_image_as_pil = watermark_image
wm_img = self.watermark_image_as_pil.resize(
(wm_size, wm_size), PIL_INTERPOLATION["bicubic"], reducing_gap=None
)
for pil_img in images:
pil_img.paste(wm_img, box=(wm_x - wm_size, wm_y - wm_size, wm_x, wm_y), mask=wm_img.split()[-1])
return images
| diffusers/src/diffusers/pipelines/deepfloyd_if/watermark.py/0 | {
"file_path": "diffusers/src/diffusers/pipelines/deepfloyd_if/watermark.py",
"repo_id": "diffusers",
"token_count": 736
} | 126 |
# Copyright 2024 ETH Zurich Computer Vision Lab and The HuggingFace Team. All rights reserved.
#
# Licensed under the Apache License, Version 2.0 (the "License");
# you may not use this file except in compliance with the License.
# You may obtain a copy of the License at
#
# http://www.apache.org/licenses/LICENSE-2.0
#
# Unless required by applicable law or agreed to in writing, software
# distributed under the License is distributed on an "AS IS" BASIS,
# WITHOUT WARRANTIES OR CONDITIONS OF ANY KIND, either express or implied.
# See the License for the specific language governing permissions and
# limitations under the License.
from typing import List, Optional, Tuple, Union
import numpy as np
import PIL.Image
import torch
from ....models import UNet2DModel
from ....schedulers import RePaintScheduler
from ....utils import PIL_INTERPOLATION, deprecate, logging
from ....utils.torch_utils import randn_tensor
from ...pipeline_utils import DiffusionPipeline, ImagePipelineOutput
logger = logging.get_logger(__name__) # pylint: disable=invalid-name
# Copied from diffusers.pipelines.stable_diffusion.pipeline_stable_diffusion_img2img.preprocess
def _preprocess_image(image: Union[List, PIL.Image.Image, torch.Tensor]):
deprecation_message = "The preprocess method is deprecated and will be removed in diffusers 1.0.0. Please use VaeImageProcessor.preprocess(...) instead"
deprecate("preprocess", "1.0.0", deprecation_message, standard_warn=False)
if isinstance(image, torch.Tensor):
return image
elif isinstance(image, PIL.Image.Image):
image = [image]
if isinstance(image[0], PIL.Image.Image):
w, h = image[0].size
w, h = (x - x % 8 for x in (w, h)) # resize to integer multiple of 8
image = [np.array(i.resize((w, h), resample=PIL_INTERPOLATION["lanczos"]))[None, :] for i in image]
image = np.concatenate(image, axis=0)
image = np.array(image).astype(np.float32) / 255.0
image = image.transpose(0, 3, 1, 2)
image = 2.0 * image - 1.0
image = torch.from_numpy(image)
elif isinstance(image[0], torch.Tensor):
image = torch.cat(image, dim=0)
return image
def _preprocess_mask(mask: Union[List, PIL.Image.Image, torch.Tensor]):
if isinstance(mask, torch.Tensor):
return mask
elif isinstance(mask, PIL.Image.Image):
mask = [mask]
if isinstance(mask[0], PIL.Image.Image):
w, h = mask[0].size
w, h = (x - x % 32 for x in (w, h)) # resize to integer multiple of 32
mask = [np.array(m.convert("L").resize((w, h), resample=PIL_INTERPOLATION["nearest"]))[None, :] for m in mask]
mask = np.concatenate(mask, axis=0)
mask = mask.astype(np.float32) / 255.0
mask[mask < 0.5] = 0
mask[mask >= 0.5] = 1
mask = torch.from_numpy(mask)
elif isinstance(mask[0], torch.Tensor):
mask = torch.cat(mask, dim=0)
return mask
class RePaintPipeline(DiffusionPipeline):
r"""
Pipeline for image inpainting using RePaint.
This model inherits from [`DiffusionPipeline`]. Check the superclass documentation for the generic methods
implemented for all pipelines (downloading, saving, running on a particular device, etc.).
Parameters:
unet ([`UNet2DModel`]):
A `UNet2DModel` to denoise the encoded image latents.
scheduler ([`RePaintScheduler`]):
A `RePaintScheduler` to be used in combination with `unet` to denoise the encoded image.
"""
unet: UNet2DModel
scheduler: RePaintScheduler
model_cpu_offload_seq = "unet"
def __init__(self, unet, scheduler):
super().__init__()
self.register_modules(unet=unet, scheduler=scheduler)
@torch.no_grad()
def __call__(
self,
image: Union[torch.Tensor, PIL.Image.Image],
mask_image: Union[torch.Tensor, PIL.Image.Image],
num_inference_steps: int = 250,
eta: float = 0.0,
jump_length: int = 10,
jump_n_sample: int = 10,
generator: Optional[Union[torch.Generator, List[torch.Generator]]] = None,
output_type: Optional[str] = "pil",
return_dict: bool = True,
) -> Union[ImagePipelineOutput, Tuple]:
r"""
The call function to the pipeline for generation.
Args:
image (`torch.FloatTensor` or `PIL.Image.Image`):
The original image to inpaint on.
mask_image (`torch.FloatTensor` or `PIL.Image.Image`):
The mask_image where 0.0 define which part of the original image to inpaint.
num_inference_steps (`int`, *optional*, defaults to 1000):
The number of denoising steps. More denoising steps usually lead to a higher quality image at the
expense of slower inference.
eta (`float`):
The weight of the added noise in a diffusion step. Its value is between 0.0 and 1.0; 0.0 corresponds to
DDIM and 1.0 is the DDPM scheduler.
jump_length (`int`, *optional*, defaults to 10):
The number of steps taken forward in time before going backward in time for a single jump ("j" in
RePaint paper). Take a look at Figure 9 and 10 in the [paper](https://arxiv.org/pdf/2201.09865.pdf).
jump_n_sample (`int`, *optional*, defaults to 10):
The number of times to make a forward time jump for a given chosen time sample. Take a look at Figure 9
and 10 in the [paper](https://arxiv.org/pdf/2201.09865.pdf).
generator (`torch.Generator`, *optional*):
A [`torch.Generator`](https://pytorch.org/docs/stable/generated/torch.Generator.html) to make
generation deterministic.
output_type (`str`, `optional`, defaults to `"pil"`):
The output format of the generated image. Choose between `PIL.Image` or `np.array`.
return_dict (`bool`, *optional*, defaults to `True`):
Whether or not to return a [`ImagePipelineOutput`] instead of a plain tuple.
Example:
```py
>>> from io import BytesIO
>>> import torch
>>> import PIL
>>> import requests
>>> from diffusers import RePaintPipeline, RePaintScheduler
>>> def download_image(url):
... response = requests.get(url)
... return PIL.Image.open(BytesIO(response.content)).convert("RGB")
>>> img_url = "https://huggingface.co/datasets/hf-internal-testing/diffusers-images/resolve/main/repaint/celeba_hq_256.png"
>>> mask_url = "https://huggingface.co/datasets/hf-internal-testing/diffusers-images/resolve/main/repaint/mask_256.png"
>>> # Load the original image and the mask as PIL images
>>> original_image = download_image(img_url).resize((256, 256))
>>> mask_image = download_image(mask_url).resize((256, 256))
>>> # Load the RePaint scheduler and pipeline based on a pretrained DDPM model
>>> scheduler = RePaintScheduler.from_pretrained("google/ddpm-ema-celebahq-256")
>>> pipe = RePaintPipeline.from_pretrained("google/ddpm-ema-celebahq-256", scheduler=scheduler)
>>> pipe = pipe.to("cuda")
>>> generator = torch.Generator(device="cuda").manual_seed(0)
>>> output = pipe(
... image=original_image,
... mask_image=mask_image,
... num_inference_steps=250,
... eta=0.0,
... jump_length=10,
... jump_n_sample=10,
... generator=generator,
... )
>>> inpainted_image = output.images[0]
```
Returns:
[`~pipelines.ImagePipelineOutput`] or `tuple`:
If `return_dict` is `True`, [`~pipelines.ImagePipelineOutput`] is returned, otherwise a `tuple` is
returned where the first element is a list with the generated images.
"""
original_image = image
original_image = _preprocess_image(original_image)
original_image = original_image.to(device=self._execution_device, dtype=self.unet.dtype)
mask_image = _preprocess_mask(mask_image)
mask_image = mask_image.to(device=self._execution_device, dtype=self.unet.dtype)
batch_size = original_image.shape[0]
# sample gaussian noise to begin the loop
if isinstance(generator, list) and len(generator) != batch_size:
raise ValueError(
f"You have passed a list of generators of length {len(generator)}, but requested an effective batch"
f" size of {batch_size}. Make sure the batch size matches the length of the generators."
)
image_shape = original_image.shape
image = randn_tensor(image_shape, generator=generator, device=self._execution_device, dtype=self.unet.dtype)
# set step values
self.scheduler.set_timesteps(num_inference_steps, jump_length, jump_n_sample, self._execution_device)
self.scheduler.eta = eta
t_last = self.scheduler.timesteps[0] + 1
generator = generator[0] if isinstance(generator, list) else generator
for i, t in enumerate(self.progress_bar(self.scheduler.timesteps)):
if t < t_last:
# predict the noise residual
model_output = self.unet(image, t).sample
# compute previous image: x_t -> x_t-1
image = self.scheduler.step(model_output, t, image, original_image, mask_image, generator).prev_sample
else:
# compute the reverse: x_t-1 -> x_t
image = self.scheduler.undo_step(image, t_last, generator)
t_last = t
image = (image / 2 + 0.5).clamp(0, 1)
image = image.cpu().permute(0, 2, 3, 1).numpy()
if output_type == "pil":
image = self.numpy_to_pil(image)
if not return_dict:
return (image,)
return ImagePipelineOutput(images=image)
| diffusers/src/diffusers/pipelines/deprecated/repaint/pipeline_repaint.py/0 | {
"file_path": "diffusers/src/diffusers/pipelines/deprecated/repaint/pipeline_repaint.py",
"repo_id": "diffusers",
"token_count": 4202
} | 127 |
# Copyright 2024 The HuggingFace Team. All rights reserved.
#
# Licensed under the Apache License, Version 2.0 (the "License");
# you may not use this file except in compliance with the License.
# You may obtain a copy of the License at
#
# http://www.apache.org/licenses/LICENSE-2.0
#
# Unless required by applicable law or agreed to in writing, software
# distributed under the License is distributed on an "AS IS" BASIS,
# WITHOUT WARRANTIES OR CONDITIONS OF ANY KIND, either express or implied.
# See the License for the specific language governing permissions and
# limitations under the License.
from typing import List, Optional, Tuple, Union
import torch
from ....models import UNet2DModel
from ....schedulers import KarrasVeScheduler
from ....utils.torch_utils import randn_tensor
from ...pipeline_utils import DiffusionPipeline, ImagePipelineOutput
class KarrasVePipeline(DiffusionPipeline):
r"""
Pipeline for unconditional image generation.
Parameters:
unet ([`UNet2DModel`]):
A `UNet2DModel` to denoise the encoded image.
scheduler ([`KarrasVeScheduler`]):
A scheduler to be used in combination with `unet` to denoise the encoded image.
"""
# add type hints for linting
unet: UNet2DModel
scheduler: KarrasVeScheduler
def __init__(self, unet: UNet2DModel, scheduler: KarrasVeScheduler):
super().__init__()
self.register_modules(unet=unet, scheduler=scheduler)
@torch.no_grad()
def __call__(
self,
batch_size: int = 1,
num_inference_steps: int = 50,
generator: Optional[Union[torch.Generator, List[torch.Generator]]] = None,
output_type: Optional[str] = "pil",
return_dict: bool = True,
**kwargs,
) -> Union[Tuple, ImagePipelineOutput]:
r"""
The call function to the pipeline for generation.
Args:
batch_size (`int`, *optional*, defaults to 1):
The number of images to generate.
generator (`torch.Generator`, *optional*):
A [`torch.Generator`](https://pytorch.org/docs/stable/generated/torch.Generator.html) to make
generation deterministic.
num_inference_steps (`int`, *optional*, defaults to 50):
The number of denoising steps. More denoising steps usually lead to a higher quality image at the
expense of slower inference.
output_type (`str`, *optional*, defaults to `"pil"`):
The output format of the generated image. Choose between `PIL.Image` or `np.array`.
return_dict (`bool`, *optional*, defaults to `True`):
Whether or not to return a [`ImagePipelineOutput`] instead of a plain tuple.
Example:
Returns:
[`~pipelines.ImagePipelineOutput`] or `tuple`:
If `return_dict` is `True`, [`~pipelines.ImagePipelineOutput`] is returned, otherwise a `tuple` is
returned where the first element is a list with the generated images.
"""
img_size = self.unet.config.sample_size
shape = (batch_size, 3, img_size, img_size)
model = self.unet
# sample x_0 ~ N(0, sigma_0^2 * I)
sample = randn_tensor(shape, generator=generator, device=self.device) * self.scheduler.init_noise_sigma
self.scheduler.set_timesteps(num_inference_steps)
for t in self.progress_bar(self.scheduler.timesteps):
# here sigma_t == t_i from the paper
sigma = self.scheduler.schedule[t]
sigma_prev = self.scheduler.schedule[t - 1] if t > 0 else 0
# 1. Select temporarily increased noise level sigma_hat
# 2. Add new noise to move from sample_i to sample_hat
sample_hat, sigma_hat = self.scheduler.add_noise_to_input(sample, sigma, generator=generator)
# 3. Predict the noise residual given the noise magnitude `sigma_hat`
# The model inputs and output are adjusted by following eq. (213) in [1].
model_output = (sigma_hat / 2) * model((sample_hat + 1) / 2, sigma_hat / 2).sample
# 4. Evaluate dx/dt at sigma_hat
# 5. Take Euler step from sigma to sigma_prev
step_output = self.scheduler.step(model_output, sigma_hat, sigma_prev, sample_hat)
if sigma_prev != 0:
# 6. Apply 2nd order correction
# The model inputs and output are adjusted by following eq. (213) in [1].
model_output = (sigma_prev / 2) * model((step_output.prev_sample + 1) / 2, sigma_prev / 2).sample
step_output = self.scheduler.step_correct(
model_output,
sigma_hat,
sigma_prev,
sample_hat,
step_output.prev_sample,
step_output["derivative"],
)
sample = step_output.prev_sample
sample = (sample / 2 + 0.5).clamp(0, 1)
image = sample.cpu().permute(0, 2, 3, 1).numpy()
if output_type == "pil":
image = self.numpy_to_pil(image)
if not return_dict:
return (image,)
return ImagePipelineOutput(images=image)
| diffusers/src/diffusers/pipelines/deprecated/stochastic_karras_ve/pipeline_stochastic_karras_ve.py/0 | {
"file_path": "diffusers/src/diffusers/pipelines/deprecated/stochastic_karras_ve/pipeline_stochastic_karras_ve.py",
"repo_id": "diffusers",
"token_count": 2264
} | 128 |
# Copyright 2024 The HuggingFace Team. All rights reserved.
#
# Licensed under the Apache License, Version 2.0 (the "License");
# you may not use this file except in compliance with the License.
# You may obtain a copy of the License at
#
# http://www.apache.org/licenses/LICENSE-2.0
#
# Unless required by applicable law or agreed to in writing, software
# distributed under the License is distributed on an "AS IS" BASIS,
# WITHOUT WARRANTIES OR CONDITIONS OF ANY KIND, either express or implied.
# See the License for the specific language governing permissions and
# limitations under the License.
from typing import Callable, List, Optional, Union
import PIL.Image
import torch
from transformers import (
CLIPImageProcessor,
CLIPTextModelWithProjection,
CLIPTokenizer,
CLIPVisionModelWithProjection,
XLMRobertaTokenizer,
)
from ...models import PriorTransformer, UNet2DConditionModel, VQModel
from ...schedulers import DDIMScheduler, DDPMScheduler, UnCLIPScheduler
from ...utils import (
replace_example_docstring,
)
from ..pipeline_utils import DiffusionPipeline
from .pipeline_kandinsky import KandinskyPipeline
from .pipeline_kandinsky_img2img import KandinskyImg2ImgPipeline
from .pipeline_kandinsky_inpaint import KandinskyInpaintPipeline
from .pipeline_kandinsky_prior import KandinskyPriorPipeline
from .text_encoder import MultilingualCLIP
TEXT2IMAGE_EXAMPLE_DOC_STRING = """
Examples:
```py
from diffusers import AutoPipelineForText2Image
import torch
pipe = AutoPipelineForText2Image.from_pretrained(
"kandinsky-community/kandinsky-2-1", torch_dtype=torch.float16
)
pipe.enable_model_cpu_offload()
prompt = "A lion in galaxies, spirals, nebulae, stars, smoke, iridescent, intricate detail, octane render, 8k"
image = pipe(prompt=prompt, num_inference_steps=25).images[0]
```
"""
IMAGE2IMAGE_EXAMPLE_DOC_STRING = """
Examples:
```py
from diffusers import AutoPipelineForImage2Image
import torch
import requests
from io import BytesIO
from PIL import Image
import os
pipe = AutoPipelineForImage2Image.from_pretrained(
"kandinsky-community/kandinsky-2-1", torch_dtype=torch.float16
)
pipe.enable_model_cpu_offload()
prompt = "A fantasy landscape, Cinematic lighting"
negative_prompt = "low quality, bad quality"
url = "https://raw.githubusercontent.com/CompVis/stable-diffusion/main/assets/stable-samples/img2img/sketch-mountains-input.jpg"
response = requests.get(url)
image = Image.open(BytesIO(response.content)).convert("RGB")
image.thumbnail((768, 768))
image = pipe(prompt=prompt, image=original_image, num_inference_steps=25).images[0]
```
"""
INPAINT_EXAMPLE_DOC_STRING = """
Examples:
```py
from diffusers import AutoPipelineForInpainting
from diffusers.utils import load_image
import torch
import numpy as np
pipe = AutoPipelineForInpainting.from_pretrained(
"kandinsky-community/kandinsky-2-1-inpaint", torch_dtype=torch.float16
)
pipe.enable_model_cpu_offload()
prompt = "A fantasy landscape, Cinematic lighting"
negative_prompt = "low quality, bad quality"
original_image = load_image(
"https://huggingface.co/datasets/hf-internal-testing/diffusers-images/resolve/main" "/kandinsky/cat.png"
)
mask = np.zeros((768, 768), dtype=np.float32)
# Let's mask out an area above the cat's head
mask[:250, 250:-250] = 1
image = pipe(prompt=prompt, image=original_image, mask_image=mask, num_inference_steps=25).images[0]
```
"""
class KandinskyCombinedPipeline(DiffusionPipeline):
"""
Combined Pipeline for text-to-image generation using Kandinsky
This model inherits from [`DiffusionPipeline`]. Check the superclass documentation for the generic methods the
library implements for all the pipelines (such as downloading or saving, running on a particular device, etc.)
Args:
text_encoder ([`MultilingualCLIP`]):
Frozen text-encoder.
tokenizer ([`XLMRobertaTokenizer`]):
Tokenizer of class
scheduler (Union[`DDIMScheduler`,`DDPMScheduler`]):
A scheduler to be used in combination with `unet` to generate image latents.
unet ([`UNet2DConditionModel`]):
Conditional U-Net architecture to denoise the image embedding.
movq ([`VQModel`]):
MoVQ Decoder to generate the image from the latents.
prior_prior ([`PriorTransformer`]):
The canonical unCLIP prior to approximate the image embedding from the text embedding.
prior_image_encoder ([`CLIPVisionModelWithProjection`]):
Frozen image-encoder.
prior_text_encoder ([`CLIPTextModelWithProjection`]):
Frozen text-encoder.
prior_tokenizer (`CLIPTokenizer`):
Tokenizer of class
[CLIPTokenizer](https://huggingface.co/docs/transformers/v4.21.0/en/model_doc/clip#transformers.CLIPTokenizer).
prior_scheduler ([`UnCLIPScheduler`]):
A scheduler to be used in combination with `prior` to generate image embedding.
"""
_load_connected_pipes = True
model_cpu_offload_seq = "text_encoder->unet->movq->prior_prior->prior_image_encoder->prior_text_encoder"
def __init__(
self,
text_encoder: MultilingualCLIP,
tokenizer: XLMRobertaTokenizer,
unet: UNet2DConditionModel,
scheduler: Union[DDIMScheduler, DDPMScheduler],
movq: VQModel,
prior_prior: PriorTransformer,
prior_image_encoder: CLIPVisionModelWithProjection,
prior_text_encoder: CLIPTextModelWithProjection,
prior_tokenizer: CLIPTokenizer,
prior_scheduler: UnCLIPScheduler,
prior_image_processor: CLIPImageProcessor,
):
super().__init__()
self.register_modules(
text_encoder=text_encoder,
tokenizer=tokenizer,
unet=unet,
scheduler=scheduler,
movq=movq,
prior_prior=prior_prior,
prior_image_encoder=prior_image_encoder,
prior_text_encoder=prior_text_encoder,
prior_tokenizer=prior_tokenizer,
prior_scheduler=prior_scheduler,
prior_image_processor=prior_image_processor,
)
self.prior_pipe = KandinskyPriorPipeline(
prior=prior_prior,
image_encoder=prior_image_encoder,
text_encoder=prior_text_encoder,
tokenizer=prior_tokenizer,
scheduler=prior_scheduler,
image_processor=prior_image_processor,
)
self.decoder_pipe = KandinskyPipeline(
text_encoder=text_encoder,
tokenizer=tokenizer,
unet=unet,
scheduler=scheduler,
movq=movq,
)
def enable_xformers_memory_efficient_attention(self, attention_op: Optional[Callable] = None):
self.decoder_pipe.enable_xformers_memory_efficient_attention(attention_op)
def enable_sequential_cpu_offload(self, gpu_id=0):
r"""
Offloads all models (`unet`, `text_encoder`, `vae`, and `safety checker` state dicts) to CPU using 🤗
Accelerate, significantly reducing memory usage. Models are moved to a `torch.device('meta')` and loaded on a
GPU only when their specific submodule's `forward` method is called. Offloading happens on a submodule basis.
Memory savings are higher than using `enable_model_cpu_offload`, but performance is lower.
"""
self.prior_pipe.enable_sequential_cpu_offload(gpu_id=gpu_id)
self.decoder_pipe.enable_sequential_cpu_offload(gpu_id=gpu_id)
def progress_bar(self, iterable=None, total=None):
self.prior_pipe.progress_bar(iterable=iterable, total=total)
self.decoder_pipe.progress_bar(iterable=iterable, total=total)
self.decoder_pipe.enable_model_cpu_offload()
def set_progress_bar_config(self, **kwargs):
self.prior_pipe.set_progress_bar_config(**kwargs)
self.decoder_pipe.set_progress_bar_config(**kwargs)
@torch.no_grad()
@replace_example_docstring(TEXT2IMAGE_EXAMPLE_DOC_STRING)
def __call__(
self,
prompt: Union[str, List[str]],
negative_prompt: Optional[Union[str, List[str]]] = None,
num_inference_steps: int = 100,
guidance_scale: float = 4.0,
num_images_per_prompt: int = 1,
height: int = 512,
width: int = 512,
prior_guidance_scale: float = 4.0,
prior_num_inference_steps: int = 25,
generator: Optional[Union[torch.Generator, List[torch.Generator]]] = None,
latents: Optional[torch.FloatTensor] = None,
output_type: Optional[str] = "pil",
callback: Optional[Callable[[int, int, torch.FloatTensor], None]] = None,
callback_steps: int = 1,
return_dict: bool = True,
):
"""
Function invoked when calling the pipeline for generation.
Args:
prompt (`str` or `List[str]`):
The prompt or prompts to guide the image generation.
negative_prompt (`str` or `List[str]`, *optional*):
The prompt or prompts not to guide the image generation. Ignored when not using guidance (i.e., ignored
if `guidance_scale` is less than `1`).
num_images_per_prompt (`int`, *optional*, defaults to 1):
The number of images to generate per prompt.
num_inference_steps (`int`, *optional*, defaults to 100):
The number of denoising steps. More denoising steps usually lead to a higher quality image at the
expense of slower inference.
height (`int`, *optional*, defaults to 512):
The height in pixels of the generated image.
width (`int`, *optional*, defaults to 512):
The width in pixels of the generated image.
prior_guidance_scale (`float`, *optional*, defaults to 4.0):
Guidance scale as defined in [Classifier-Free Diffusion Guidance](https://arxiv.org/abs/2207.12598).
`guidance_scale` is defined as `w` of equation 2. of [Imagen
Paper](https://arxiv.org/pdf/2205.11487.pdf). Guidance scale is enabled by setting `guidance_scale >
1`. Higher guidance scale encourages to generate images that are closely linked to the text `prompt`,
usually at the expense of lower image quality.
prior_num_inference_steps (`int`, *optional*, defaults to 100):
The number of denoising steps. More denoising steps usually lead to a higher quality image at the
expense of slower inference.
guidance_scale (`float`, *optional*, defaults to 4.0):
Guidance scale as defined in [Classifier-Free Diffusion Guidance](https://arxiv.org/abs/2207.12598).
`guidance_scale` is defined as `w` of equation 2. of [Imagen
Paper](https://arxiv.org/pdf/2205.11487.pdf). Guidance scale is enabled by setting `guidance_scale >
1`. Higher guidance scale encourages to generate images that are closely linked to the text `prompt`,
usually at the expense of lower image quality.
generator (`torch.Generator` or `List[torch.Generator]`, *optional*):
One or a list of [torch generator(s)](https://pytorch.org/docs/stable/generated/torch.Generator.html)
to make generation deterministic.
latents (`torch.FloatTensor`, *optional*):
Pre-generated noisy latents, sampled from a Gaussian distribution, to be used as inputs for image
generation. Can be used to tweak the same generation with different prompts. If not provided, a latents
tensor will ge generated by sampling using the supplied random `generator`.
output_type (`str`, *optional*, defaults to `"pil"`):
The output format of the generate image. Choose between: `"pil"` (`PIL.Image.Image`), `"np"`
(`np.array`) or `"pt"` (`torch.Tensor`).
callback (`Callable`, *optional*):
A function that calls every `callback_steps` steps during inference. The function is called with the
following arguments: `callback(step: int, timestep: int, latents: torch.FloatTensor)`.
callback_steps (`int`, *optional*, defaults to 1):
The frequency at which the `callback` function is called. If not specified, the callback is called at
every step.
return_dict (`bool`, *optional*, defaults to `True`):
Whether or not to return a [`~pipelines.ImagePipelineOutput`] instead of a plain tuple.
Examples:
Returns:
[`~pipelines.ImagePipelineOutput`] or `tuple`
"""
prior_outputs = self.prior_pipe(
prompt=prompt,
negative_prompt=negative_prompt,
num_images_per_prompt=num_images_per_prompt,
num_inference_steps=prior_num_inference_steps,
generator=generator,
latents=latents,
guidance_scale=prior_guidance_scale,
output_type="pt",
return_dict=False,
)
image_embeds = prior_outputs[0]
negative_image_embeds = prior_outputs[1]
prompt = [prompt] if not isinstance(prompt, (list, tuple)) else prompt
if len(prompt) < image_embeds.shape[0] and image_embeds.shape[0] % len(prompt) == 0:
prompt = (image_embeds.shape[0] // len(prompt)) * prompt
outputs = self.decoder_pipe(
prompt=prompt,
image_embeds=image_embeds,
negative_image_embeds=negative_image_embeds,
width=width,
height=height,
num_inference_steps=num_inference_steps,
generator=generator,
guidance_scale=guidance_scale,
output_type=output_type,
callback=callback,
callback_steps=callback_steps,
return_dict=return_dict,
)
self.maybe_free_model_hooks()
return outputs
class KandinskyImg2ImgCombinedPipeline(DiffusionPipeline):
"""
Combined Pipeline for image-to-image generation using Kandinsky
This model inherits from [`DiffusionPipeline`]. Check the superclass documentation for the generic methods the
library implements for all the pipelines (such as downloading or saving, running on a particular device, etc.)
Args:
text_encoder ([`MultilingualCLIP`]):
Frozen text-encoder.
tokenizer ([`XLMRobertaTokenizer`]):
Tokenizer of class
scheduler (Union[`DDIMScheduler`,`DDPMScheduler`]):
A scheduler to be used in combination with `unet` to generate image latents.
unet ([`UNet2DConditionModel`]):
Conditional U-Net architecture to denoise the image embedding.
movq ([`VQModel`]):
MoVQ Decoder to generate the image from the latents.
prior_prior ([`PriorTransformer`]):
The canonical unCLIP prior to approximate the image embedding from the text embedding.
prior_image_encoder ([`CLIPVisionModelWithProjection`]):
Frozen image-encoder.
prior_text_encoder ([`CLIPTextModelWithProjection`]):
Frozen text-encoder.
prior_tokenizer (`CLIPTokenizer`):
Tokenizer of class
[CLIPTokenizer](https://huggingface.co/docs/transformers/v4.21.0/en/model_doc/clip#transformers.CLIPTokenizer).
prior_scheduler ([`UnCLIPScheduler`]):
A scheduler to be used in combination with `prior` to generate image embedding.
"""
_load_connected_pipes = True
model_cpu_offload_seq = "prior_text_encoder->prior_image_encoder->prior_prior->" "text_encoder->unet->movq"
def __init__(
self,
text_encoder: MultilingualCLIP,
tokenizer: XLMRobertaTokenizer,
unet: UNet2DConditionModel,
scheduler: Union[DDIMScheduler, DDPMScheduler],
movq: VQModel,
prior_prior: PriorTransformer,
prior_image_encoder: CLIPVisionModelWithProjection,
prior_text_encoder: CLIPTextModelWithProjection,
prior_tokenizer: CLIPTokenizer,
prior_scheduler: UnCLIPScheduler,
prior_image_processor: CLIPImageProcessor,
):
super().__init__()
self.register_modules(
text_encoder=text_encoder,
tokenizer=tokenizer,
unet=unet,
scheduler=scheduler,
movq=movq,
prior_prior=prior_prior,
prior_image_encoder=prior_image_encoder,
prior_text_encoder=prior_text_encoder,
prior_tokenizer=prior_tokenizer,
prior_scheduler=prior_scheduler,
prior_image_processor=prior_image_processor,
)
self.prior_pipe = KandinskyPriorPipeline(
prior=prior_prior,
image_encoder=prior_image_encoder,
text_encoder=prior_text_encoder,
tokenizer=prior_tokenizer,
scheduler=prior_scheduler,
image_processor=prior_image_processor,
)
self.decoder_pipe = KandinskyImg2ImgPipeline(
text_encoder=text_encoder,
tokenizer=tokenizer,
unet=unet,
scheduler=scheduler,
movq=movq,
)
def enable_xformers_memory_efficient_attention(self, attention_op: Optional[Callable] = None):
self.decoder_pipe.enable_xformers_memory_efficient_attention(attention_op)
def enable_sequential_cpu_offload(self, gpu_id=0):
r"""
Offloads all models to CPU using accelerate, significantly reducing memory usage. When called, unet,
text_encoder, vae and safety checker have their state dicts saved to CPU and then are moved to a
`torch.device('meta') and loaded to GPU only when their specific submodule has its `forward` method called.
Note that offloading happens on a submodule basis. Memory savings are higher than with
`enable_model_cpu_offload`, but performance is lower.
"""
self.prior_pipe.enable_sequential_cpu_offload(gpu_id=gpu_id)
self.decoder_pipe.enable_sequential_cpu_offload(gpu_id=gpu_id)
def progress_bar(self, iterable=None, total=None):
self.prior_pipe.progress_bar(iterable=iterable, total=total)
self.decoder_pipe.progress_bar(iterable=iterable, total=total)
self.decoder_pipe.enable_model_cpu_offload()
def set_progress_bar_config(self, **kwargs):
self.prior_pipe.set_progress_bar_config(**kwargs)
self.decoder_pipe.set_progress_bar_config(**kwargs)
@torch.no_grad()
@replace_example_docstring(IMAGE2IMAGE_EXAMPLE_DOC_STRING)
def __call__(
self,
prompt: Union[str, List[str]],
image: Union[torch.FloatTensor, PIL.Image.Image, List[torch.FloatTensor], List[PIL.Image.Image]],
negative_prompt: Optional[Union[str, List[str]]] = None,
num_inference_steps: int = 100,
guidance_scale: float = 4.0,
num_images_per_prompt: int = 1,
strength: float = 0.3,
height: int = 512,
width: int = 512,
prior_guidance_scale: float = 4.0,
prior_num_inference_steps: int = 25,
generator: Optional[Union[torch.Generator, List[torch.Generator]]] = None,
latents: Optional[torch.FloatTensor] = None,
output_type: Optional[str] = "pil",
callback: Optional[Callable[[int, int, torch.FloatTensor], None]] = None,
callback_steps: int = 1,
return_dict: bool = True,
):
"""
Function invoked when calling the pipeline for generation.
Args:
prompt (`str` or `List[str]`):
The prompt or prompts to guide the image generation.
image (`torch.FloatTensor`, `PIL.Image.Image`, `np.ndarray`, `List[torch.FloatTensor]`, `List[PIL.Image.Image]`, or `List[np.ndarray]`):
`Image`, or tensor representing an image batch, that will be used as the starting point for the
process. Can also accept image latents as `image`, if passing latents directly, it will not be encoded
again.
negative_prompt (`str` or `List[str]`, *optional*):
The prompt or prompts not to guide the image generation. Ignored when not using guidance (i.e., ignored
if `guidance_scale` is less than `1`).
num_images_per_prompt (`int`, *optional*, defaults to 1):
The number of images to generate per prompt.
num_inference_steps (`int`, *optional*, defaults to 100):
The number of denoising steps. More denoising steps usually lead to a higher quality image at the
expense of slower inference.
height (`int`, *optional*, defaults to 512):
The height in pixels of the generated image.
width (`int`, *optional*, defaults to 512):
The width in pixels of the generated image.
strength (`float`, *optional*, defaults to 0.3):
Conceptually, indicates how much to transform the reference `image`. Must be between 0 and 1. `image`
will be used as a starting point, adding more noise to it the larger the `strength`. The number of
denoising steps depends on the amount of noise initially added. When `strength` is 1, added noise will
be maximum and the denoising process will run for the full number of iterations specified in
`num_inference_steps`. A value of 1, therefore, essentially ignores `image`.
prior_guidance_scale (`float`, *optional*, defaults to 4.0):
Guidance scale as defined in [Classifier-Free Diffusion Guidance](https://arxiv.org/abs/2207.12598).
`guidance_scale` is defined as `w` of equation 2. of [Imagen
Paper](https://arxiv.org/pdf/2205.11487.pdf). Guidance scale is enabled by setting `guidance_scale >
1`. Higher guidance scale encourages to generate images that are closely linked to the text `prompt`,
usually at the expense of lower image quality.
prior_num_inference_steps (`int`, *optional*, defaults to 100):
The number of denoising steps. More denoising steps usually lead to a higher quality image at the
expense of slower inference.
guidance_scale (`float`, *optional*, defaults to 4.0):
Guidance scale as defined in [Classifier-Free Diffusion Guidance](https://arxiv.org/abs/2207.12598).
`guidance_scale` is defined as `w` of equation 2. of [Imagen
Paper](https://arxiv.org/pdf/2205.11487.pdf). Guidance scale is enabled by setting `guidance_scale >
1`. Higher guidance scale encourages to generate images that are closely linked to the text `prompt`,
usually at the expense of lower image quality.
generator (`torch.Generator` or `List[torch.Generator]`, *optional*):
One or a list of [torch generator(s)](https://pytorch.org/docs/stable/generated/torch.Generator.html)
to make generation deterministic.
latents (`torch.FloatTensor`, *optional*):
Pre-generated noisy latents, sampled from a Gaussian distribution, to be used as inputs for image
generation. Can be used to tweak the same generation with different prompts. If not provided, a latents
tensor will ge generated by sampling using the supplied random `generator`.
output_type (`str`, *optional*, defaults to `"pil"`):
The output format of the generate image. Choose between: `"pil"` (`PIL.Image.Image`), `"np"`
(`np.array`) or `"pt"` (`torch.Tensor`).
callback (`Callable`, *optional*):
A function that calls every `callback_steps` steps during inference. The function is called with the
following arguments: `callback(step: int, timestep: int, latents: torch.FloatTensor)`.
callback_steps (`int`, *optional*, defaults to 1):
The frequency at which the `callback` function is called. If not specified, the callback is called at
every step.
return_dict (`bool`, *optional*, defaults to `True`):
Whether or not to return a [`~pipelines.ImagePipelineOutput`] instead of a plain tuple.
Examples:
Returns:
[`~pipelines.ImagePipelineOutput`] or `tuple`
"""
prior_outputs = self.prior_pipe(
prompt=prompt,
negative_prompt=negative_prompt,
num_images_per_prompt=num_images_per_prompt,
num_inference_steps=prior_num_inference_steps,
generator=generator,
latents=latents,
guidance_scale=prior_guidance_scale,
output_type="pt",
return_dict=False,
)
image_embeds = prior_outputs[0]
negative_image_embeds = prior_outputs[1]
prompt = [prompt] if not isinstance(prompt, (list, tuple)) else prompt
image = [image] if isinstance(prompt, PIL.Image.Image) else image
if len(prompt) < image_embeds.shape[0] and image_embeds.shape[0] % len(prompt) == 0:
prompt = (image_embeds.shape[0] // len(prompt)) * prompt
if (
isinstance(image, (list, tuple))
and len(image) < image_embeds.shape[0]
and image_embeds.shape[0] % len(image) == 0
):
image = (image_embeds.shape[0] // len(image)) * image
outputs = self.decoder_pipe(
prompt=prompt,
image=image,
image_embeds=image_embeds,
negative_image_embeds=negative_image_embeds,
strength=strength,
width=width,
height=height,
num_inference_steps=num_inference_steps,
generator=generator,
guidance_scale=guidance_scale,
output_type=output_type,
callback=callback,
callback_steps=callback_steps,
return_dict=return_dict,
)
self.maybe_free_model_hooks()
return outputs
class KandinskyInpaintCombinedPipeline(DiffusionPipeline):
"""
Combined Pipeline for generation using Kandinsky
This model inherits from [`DiffusionPipeline`]. Check the superclass documentation for the generic methods the
library implements for all the pipelines (such as downloading or saving, running on a particular device, etc.)
Args:
text_encoder ([`MultilingualCLIP`]):
Frozen text-encoder.
tokenizer ([`XLMRobertaTokenizer`]):
Tokenizer of class
scheduler (Union[`DDIMScheduler`,`DDPMScheduler`]):
A scheduler to be used in combination with `unet` to generate image latents.
unet ([`UNet2DConditionModel`]):
Conditional U-Net architecture to denoise the image embedding.
movq ([`VQModel`]):
MoVQ Decoder to generate the image from the latents.
prior_prior ([`PriorTransformer`]):
The canonical unCLIP prior to approximate the image embedding from the text embedding.
prior_image_encoder ([`CLIPVisionModelWithProjection`]):
Frozen image-encoder.
prior_text_encoder ([`CLIPTextModelWithProjection`]):
Frozen text-encoder.
prior_tokenizer (`CLIPTokenizer`):
Tokenizer of class
[CLIPTokenizer](https://huggingface.co/docs/transformers/v4.21.0/en/model_doc/clip#transformers.CLIPTokenizer).
prior_scheduler ([`UnCLIPScheduler`]):
A scheduler to be used in combination with `prior` to generate image embedding.
"""
_load_connected_pipes = True
model_cpu_offload_seq = "prior_text_encoder->prior_image_encoder->prior_prior->text_encoder->unet->movq"
def __init__(
self,
text_encoder: MultilingualCLIP,
tokenizer: XLMRobertaTokenizer,
unet: UNet2DConditionModel,
scheduler: Union[DDIMScheduler, DDPMScheduler],
movq: VQModel,
prior_prior: PriorTransformer,
prior_image_encoder: CLIPVisionModelWithProjection,
prior_text_encoder: CLIPTextModelWithProjection,
prior_tokenizer: CLIPTokenizer,
prior_scheduler: UnCLIPScheduler,
prior_image_processor: CLIPImageProcessor,
):
super().__init__()
self.register_modules(
text_encoder=text_encoder,
tokenizer=tokenizer,
unet=unet,
scheduler=scheduler,
movq=movq,
prior_prior=prior_prior,
prior_image_encoder=prior_image_encoder,
prior_text_encoder=prior_text_encoder,
prior_tokenizer=prior_tokenizer,
prior_scheduler=prior_scheduler,
prior_image_processor=prior_image_processor,
)
self.prior_pipe = KandinskyPriorPipeline(
prior=prior_prior,
image_encoder=prior_image_encoder,
text_encoder=prior_text_encoder,
tokenizer=prior_tokenizer,
scheduler=prior_scheduler,
image_processor=prior_image_processor,
)
self.decoder_pipe = KandinskyInpaintPipeline(
text_encoder=text_encoder,
tokenizer=tokenizer,
unet=unet,
scheduler=scheduler,
movq=movq,
)
def enable_xformers_memory_efficient_attention(self, attention_op: Optional[Callable] = None):
self.decoder_pipe.enable_xformers_memory_efficient_attention(attention_op)
def enable_sequential_cpu_offload(self, gpu_id=0):
r"""
Offloads all models to CPU using accelerate, significantly reducing memory usage. When called, unet,
text_encoder, vae and safety checker have their state dicts saved to CPU and then are moved to a
`torch.device('meta') and loaded to GPU only when their specific submodule has its `forward` method called.
Note that offloading happens on a submodule basis. Memory savings are higher than with
`enable_model_cpu_offload`, but performance is lower.
"""
self.prior_pipe.enable_sequential_cpu_offload(gpu_id=gpu_id)
self.decoder_pipe.enable_sequential_cpu_offload(gpu_id=gpu_id)
def progress_bar(self, iterable=None, total=None):
self.prior_pipe.progress_bar(iterable=iterable, total=total)
self.decoder_pipe.progress_bar(iterable=iterable, total=total)
self.decoder_pipe.enable_model_cpu_offload()
def set_progress_bar_config(self, **kwargs):
self.prior_pipe.set_progress_bar_config(**kwargs)
self.decoder_pipe.set_progress_bar_config(**kwargs)
@torch.no_grad()
@replace_example_docstring(INPAINT_EXAMPLE_DOC_STRING)
def __call__(
self,
prompt: Union[str, List[str]],
image: Union[torch.FloatTensor, PIL.Image.Image, List[torch.FloatTensor], List[PIL.Image.Image]],
mask_image: Union[torch.FloatTensor, PIL.Image.Image, List[torch.FloatTensor], List[PIL.Image.Image]],
negative_prompt: Optional[Union[str, List[str]]] = None,
num_inference_steps: int = 100,
guidance_scale: float = 4.0,
num_images_per_prompt: int = 1,
height: int = 512,
width: int = 512,
prior_guidance_scale: float = 4.0,
prior_num_inference_steps: int = 25,
generator: Optional[Union[torch.Generator, List[torch.Generator]]] = None,
latents: Optional[torch.FloatTensor] = None,
output_type: Optional[str] = "pil",
callback: Optional[Callable[[int, int, torch.FloatTensor], None]] = None,
callback_steps: int = 1,
return_dict: bool = True,
):
"""
Function invoked when calling the pipeline for generation.
Args:
prompt (`str` or `List[str]`):
The prompt or prompts to guide the image generation.
image (`torch.FloatTensor`, `PIL.Image.Image`, `np.ndarray`, `List[torch.FloatTensor]`, `List[PIL.Image.Image]`, or `List[np.ndarray]`):
`Image`, or tensor representing an image batch, that will be used as the starting point for the
process. Can also accept image latents as `image`, if passing latents directly, it will not be encoded
again.
mask_image (`np.array`):
Tensor representing an image batch, to mask `image`. White pixels in the mask will be repainted, while
black pixels will be preserved. If `mask_image` is a PIL image, it will be converted to a single
channel (luminance) before use. If it's a tensor, it should contain one color channel (L) instead of 3,
so the expected shape would be `(B, H, W, 1)`.
negative_prompt (`str` or `List[str]`, *optional*):
The prompt or prompts not to guide the image generation. Ignored when not using guidance (i.e., ignored
if `guidance_scale` is less than `1`).
num_images_per_prompt (`int`, *optional*, defaults to 1):
The number of images to generate per prompt.
num_inference_steps (`int`, *optional*, defaults to 100):
The number of denoising steps. More denoising steps usually lead to a higher quality image at the
expense of slower inference.
height (`int`, *optional*, defaults to 512):
The height in pixels of the generated image.
width (`int`, *optional*, defaults to 512):
The width in pixels of the generated image.
prior_guidance_scale (`float`, *optional*, defaults to 4.0):
Guidance scale as defined in [Classifier-Free Diffusion Guidance](https://arxiv.org/abs/2207.12598).
`guidance_scale` is defined as `w` of equation 2. of [Imagen
Paper](https://arxiv.org/pdf/2205.11487.pdf). Guidance scale is enabled by setting `guidance_scale >
1`. Higher guidance scale encourages to generate images that are closely linked to the text `prompt`,
usually at the expense of lower image quality.
prior_num_inference_steps (`int`, *optional*, defaults to 100):
The number of denoising steps. More denoising steps usually lead to a higher quality image at the
expense of slower inference.
guidance_scale (`float`, *optional*, defaults to 4.0):
Guidance scale as defined in [Classifier-Free Diffusion Guidance](https://arxiv.org/abs/2207.12598).
`guidance_scale` is defined as `w` of equation 2. of [Imagen
Paper](https://arxiv.org/pdf/2205.11487.pdf). Guidance scale is enabled by setting `guidance_scale >
1`. Higher guidance scale encourages to generate images that are closely linked to the text `prompt`,
usually at the expense of lower image quality.
generator (`torch.Generator` or `List[torch.Generator]`, *optional*):
One or a list of [torch generator(s)](https://pytorch.org/docs/stable/generated/torch.Generator.html)
to make generation deterministic.
latents (`torch.FloatTensor`, *optional*):
Pre-generated noisy latents, sampled from a Gaussian distribution, to be used as inputs for image
generation. Can be used to tweak the same generation with different prompts. If not provided, a latents
tensor will ge generated by sampling using the supplied random `generator`.
output_type (`str`, *optional*, defaults to `"pil"`):
The output format of the generate image. Choose between: `"pil"` (`PIL.Image.Image`), `"np"`
(`np.array`) or `"pt"` (`torch.Tensor`).
callback (`Callable`, *optional*):
A function that calls every `callback_steps` steps during inference. The function is called with the
following arguments: `callback(step: int, timestep: int, latents: torch.FloatTensor)`.
callback_steps (`int`, *optional*, defaults to 1):
The frequency at which the `callback` function is called. If not specified, the callback is called at
every step.
return_dict (`bool`, *optional*, defaults to `True`):
Whether or not to return a [`~pipelines.ImagePipelineOutput`] instead of a plain tuple.
Examples:
Returns:
[`~pipelines.ImagePipelineOutput`] or `tuple`
"""
prior_outputs = self.prior_pipe(
prompt=prompt,
negative_prompt=negative_prompt,
num_images_per_prompt=num_images_per_prompt,
num_inference_steps=prior_num_inference_steps,
generator=generator,
latents=latents,
guidance_scale=prior_guidance_scale,
output_type="pt",
return_dict=False,
)
image_embeds = prior_outputs[0]
negative_image_embeds = prior_outputs[1]
prompt = [prompt] if not isinstance(prompt, (list, tuple)) else prompt
image = [image] if isinstance(prompt, PIL.Image.Image) else image
mask_image = [mask_image] if isinstance(mask_image, PIL.Image.Image) else mask_image
if len(prompt) < image_embeds.shape[0] and image_embeds.shape[0] % len(prompt) == 0:
prompt = (image_embeds.shape[0] // len(prompt)) * prompt
if (
isinstance(image, (list, tuple))
and len(image) < image_embeds.shape[0]
and image_embeds.shape[0] % len(image) == 0
):
image = (image_embeds.shape[0] // len(image)) * image
if (
isinstance(mask_image, (list, tuple))
and len(mask_image) < image_embeds.shape[0]
and image_embeds.shape[0] % len(mask_image) == 0
):
mask_image = (image_embeds.shape[0] // len(mask_image)) * mask_image
outputs = self.decoder_pipe(
prompt=prompt,
image=image,
mask_image=mask_image,
image_embeds=image_embeds,
negative_image_embeds=negative_image_embeds,
width=width,
height=height,
num_inference_steps=num_inference_steps,
generator=generator,
guidance_scale=guidance_scale,
output_type=output_type,
callback=callback,
callback_steps=callback_steps,
return_dict=return_dict,
)
self.maybe_free_model_hooks()
return outputs
| diffusers/src/diffusers/pipelines/kandinsky/pipeline_kandinsky_combined.py/0 | {
"file_path": "diffusers/src/diffusers/pipelines/kandinsky/pipeline_kandinsky_combined.py",
"repo_id": "diffusers",
"token_count": 16903
} | 129 |
from typing import Callable, Dict, List, Optional, Union
import torch
from transformers import T5EncoderModel, T5Tokenizer
from ...loaders import LoraLoaderMixin
from ...models import Kandinsky3UNet, VQModel
from ...schedulers import DDPMScheduler
from ...utils import (
deprecate,
is_accelerate_available,
logging,
replace_example_docstring,
)
from ...utils.torch_utils import randn_tensor
from ..pipeline_utils import DiffusionPipeline, ImagePipelineOutput
logger = logging.get_logger(__name__) # pylint: disable=invalid-name
EXAMPLE_DOC_STRING = """
Examples:
```py
>>> from diffusers import AutoPipelineForText2Image
>>> import torch
>>> pipe = AutoPipelineForText2Image.from_pretrained("kandinsky-community/kandinsky-3", variant="fp16", torch_dtype=torch.float16)
>>> pipe.enable_model_cpu_offload()
>>> prompt = "A photograph of the inside of a subway train. There are raccoons sitting on the seats. One of them is reading a newspaper. The window shows the city in the background."
>>> generator = torch.Generator(device="cpu").manual_seed(0)
>>> image = pipe(prompt, num_inference_steps=25, generator=generator).images[0]
```
"""
def downscale_height_and_width(height, width, scale_factor=8):
new_height = height // scale_factor**2
if height % scale_factor**2 != 0:
new_height += 1
new_width = width // scale_factor**2
if width % scale_factor**2 != 0:
new_width += 1
return new_height * scale_factor, new_width * scale_factor
class Kandinsky3Pipeline(DiffusionPipeline, LoraLoaderMixin):
model_cpu_offload_seq = "text_encoder->unet->movq"
_callback_tensor_inputs = [
"latents",
"prompt_embeds",
"negative_prompt_embeds",
"negative_attention_mask",
"attention_mask",
]
def __init__(
self,
tokenizer: T5Tokenizer,
text_encoder: T5EncoderModel,
unet: Kandinsky3UNet,
scheduler: DDPMScheduler,
movq: VQModel,
):
super().__init__()
self.register_modules(
tokenizer=tokenizer, text_encoder=text_encoder, unet=unet, scheduler=scheduler, movq=movq
)
def remove_all_hooks(self):
if is_accelerate_available():
from accelerate.hooks import remove_hook_from_module
else:
raise ImportError("Please install accelerate via `pip install accelerate`")
for model in [self.text_encoder, self.unet, self.movq]:
if model is not None:
remove_hook_from_module(model, recurse=True)
self.unet_offload_hook = None
self.text_encoder_offload_hook = None
self.final_offload_hook = None
def process_embeds(self, embeddings, attention_mask, cut_context):
if cut_context:
embeddings[attention_mask == 0] = torch.zeros_like(embeddings[attention_mask == 0])
max_seq_length = attention_mask.sum(-1).max() + 1
embeddings = embeddings[:, :max_seq_length]
attention_mask = attention_mask[:, :max_seq_length]
return embeddings, attention_mask
@torch.no_grad()
def encode_prompt(
self,
prompt,
do_classifier_free_guidance=True,
num_images_per_prompt=1,
device=None,
negative_prompt=None,
prompt_embeds: Optional[torch.FloatTensor] = None,
negative_prompt_embeds: Optional[torch.FloatTensor] = None,
_cut_context=False,
attention_mask: Optional[torch.FloatTensor] = None,
negative_attention_mask: Optional[torch.FloatTensor] = None,
):
r"""
Encodes the prompt into text encoder hidden states.
Args:
prompt (`str` or `List[str]`, *optional*):
prompt to be encoded
device: (`torch.device`, *optional*):
torch device to place the resulting embeddings on
num_images_per_prompt (`int`, *optional*, defaults to 1):
number of images that should be generated per prompt
do_classifier_free_guidance (`bool`, *optional*, defaults to `True`):
whether to use classifier free guidance or not
negative_prompt (`str` or `List[str]`, *optional*):
The prompt or prompts not to guide the image generation. If not defined, one has to pass
`negative_prompt_embeds`. instead. If not defined, one has to pass `negative_prompt_embeds`. instead.
Ignored when not using guidance (i.e., ignored if `guidance_scale` is less than `1`).
prompt_embeds (`torch.FloatTensor`, *optional*):
Pre-generated text embeddings. Can be used to easily tweak text inputs, *e.g.* prompt weighting. If not
provided, text embeddings will be generated from `prompt` input argument.
negative_prompt_embeds (`torch.FloatTensor`, *optional*):
Pre-generated negative text embeddings. Can be used to easily tweak text inputs, *e.g.* prompt
weighting. If not provided, negative_prompt_embeds will be generated from `negative_prompt` input
argument.
attention_mask (`torch.FloatTensor`, *optional*):
Pre-generated attention mask. Must provide if passing `prompt_embeds` directly.
negative_attention_mask (`torch.FloatTensor`, *optional*):
Pre-generated negative attention mask. Must provide if passing `negative_prompt_embeds` directly.
"""
if prompt is not None and negative_prompt is not None:
if type(prompt) is not type(negative_prompt):
raise TypeError(
f"`negative_prompt` should be the same type to `prompt`, but got {type(negative_prompt)} !="
f" {type(prompt)}."
)
if device is None:
device = self._execution_device
if prompt is not None and isinstance(prompt, str):
batch_size = 1
elif prompt is not None and isinstance(prompt, list):
batch_size = len(prompt)
else:
batch_size = prompt_embeds.shape[0]
max_length = 128
if prompt_embeds is None:
text_inputs = self.tokenizer(
prompt,
padding="max_length",
max_length=max_length,
truncation=True,
return_tensors="pt",
)
text_input_ids = text_inputs.input_ids.to(device)
attention_mask = text_inputs.attention_mask.to(device)
prompt_embeds = self.text_encoder(
text_input_ids,
attention_mask=attention_mask,
)
prompt_embeds = prompt_embeds[0]
prompt_embeds, attention_mask = self.process_embeds(prompt_embeds, attention_mask, _cut_context)
prompt_embeds = prompt_embeds * attention_mask.unsqueeze(2)
if self.text_encoder is not None:
dtype = self.text_encoder.dtype
else:
dtype = None
prompt_embeds = prompt_embeds.to(dtype=dtype, device=device)
bs_embed, seq_len, _ = prompt_embeds.shape
# duplicate text embeddings for each generation per prompt, using mps friendly method
prompt_embeds = prompt_embeds.repeat(1, num_images_per_prompt, 1)
prompt_embeds = prompt_embeds.view(bs_embed * num_images_per_prompt, seq_len, -1)
attention_mask = attention_mask.repeat(num_images_per_prompt, 1)
# get unconditional embeddings for classifier free guidance
if do_classifier_free_guidance and negative_prompt_embeds is None:
uncond_tokens: List[str]
if negative_prompt is None:
uncond_tokens = [""] * batch_size
elif isinstance(negative_prompt, str):
uncond_tokens = [negative_prompt]
elif batch_size != len(negative_prompt):
raise ValueError(
f"`negative_prompt`: {negative_prompt} has batch size {len(negative_prompt)}, but `prompt`:"
f" {prompt} has batch size {batch_size}. Please make sure that passed `negative_prompt` matches"
" the batch size of `prompt`."
)
else:
uncond_tokens = negative_prompt
if negative_prompt is not None:
uncond_input = self.tokenizer(
uncond_tokens,
padding="max_length",
max_length=128,
truncation=True,
return_attention_mask=True,
return_tensors="pt",
)
text_input_ids = uncond_input.input_ids.to(device)
negative_attention_mask = uncond_input.attention_mask.to(device)
negative_prompt_embeds = self.text_encoder(
text_input_ids,
attention_mask=negative_attention_mask,
)
negative_prompt_embeds = negative_prompt_embeds[0]
negative_prompt_embeds = negative_prompt_embeds[:, : prompt_embeds.shape[1]]
negative_attention_mask = negative_attention_mask[:, : prompt_embeds.shape[1]]
negative_prompt_embeds = negative_prompt_embeds * negative_attention_mask.unsqueeze(2)
else:
negative_prompt_embeds = torch.zeros_like(prompt_embeds)
negative_attention_mask = torch.zeros_like(attention_mask)
if do_classifier_free_guidance:
# duplicate unconditional embeddings for each generation per prompt, using mps friendly method
seq_len = negative_prompt_embeds.shape[1]
negative_prompt_embeds = negative_prompt_embeds.to(dtype=dtype, device=device)
if negative_prompt_embeds.shape != prompt_embeds.shape:
negative_prompt_embeds = negative_prompt_embeds.repeat(1, num_images_per_prompt, 1)
negative_prompt_embeds = negative_prompt_embeds.view(batch_size * num_images_per_prompt, seq_len, -1)
negative_attention_mask = negative_attention_mask.repeat(num_images_per_prompt, 1)
# For classifier free guidance, we need to do two forward passes.
# Here we concatenate the unconditional and text embeddings into a single batch
# to avoid doing two forward passes
else:
negative_prompt_embeds = None
negative_attention_mask = None
return prompt_embeds, negative_prompt_embeds, attention_mask, negative_attention_mask
def prepare_latents(self, shape, dtype, device, generator, latents, scheduler):
if latents is None:
latents = randn_tensor(shape, generator=generator, device=device, dtype=dtype)
else:
if latents.shape != shape:
raise ValueError(f"Unexpected latents shape, got {latents.shape}, expected {shape}")
latents = latents.to(device)
latents = latents * scheduler.init_noise_sigma
return latents
def check_inputs(
self,
prompt,
callback_steps,
negative_prompt=None,
prompt_embeds=None,
negative_prompt_embeds=None,
callback_on_step_end_tensor_inputs=None,
attention_mask=None,
negative_attention_mask=None,
):
if callback_steps is not None and (not isinstance(callback_steps, int) or callback_steps <= 0):
raise ValueError(
f"`callback_steps` has to be a positive integer but is {callback_steps} of type"
f" {type(callback_steps)}."
)
if callback_on_step_end_tensor_inputs is not None and not all(
k in self._callback_tensor_inputs for k in callback_on_step_end_tensor_inputs
):
raise ValueError(
f"`callback_on_step_end_tensor_inputs` has to be in {self._callback_tensor_inputs}, but found {[k for k in callback_on_step_end_tensor_inputs if k not in self._callback_tensor_inputs]}"
)
if prompt is not None and prompt_embeds is not None:
raise ValueError(
f"Cannot forward both `prompt`: {prompt} and `prompt_embeds`: {prompt_embeds}. Please make sure to"
" only forward one of the two."
)
elif prompt is None and prompt_embeds is None:
raise ValueError(
"Provide either `prompt` or `prompt_embeds`. Cannot leave both `prompt` and `prompt_embeds` undefined."
)
elif prompt is not None and (not isinstance(prompt, str) and not isinstance(prompt, list)):
raise ValueError(f"`prompt` has to be of type `str` or `list` but is {type(prompt)}")
if negative_prompt is not None and negative_prompt_embeds is not None:
raise ValueError(
f"Cannot forward both `negative_prompt`: {negative_prompt} and `negative_prompt_embeds`:"
f" {negative_prompt_embeds}. Please make sure to only forward one of the two."
)
if prompt_embeds is not None and negative_prompt_embeds is not None:
if prompt_embeds.shape != negative_prompt_embeds.shape:
raise ValueError(
"`prompt_embeds` and `negative_prompt_embeds` must have the same shape when passed directly, but"
f" got: `prompt_embeds` {prompt_embeds.shape} != `negative_prompt_embeds`"
f" {negative_prompt_embeds.shape}."
)
if negative_prompt_embeds is not None and negative_attention_mask is None:
raise ValueError("Please provide `negative_attention_mask` along with `negative_prompt_embeds`")
if negative_prompt_embeds is not None and negative_attention_mask is not None:
if negative_prompt_embeds.shape[:2] != negative_attention_mask.shape:
raise ValueError(
"`negative_prompt_embeds` and `negative_attention_mask` must have the same batch_size and token length when passed directly, but"
f" got: `negative_prompt_embeds` {negative_prompt_embeds.shape[:2]} != `negative_attention_mask`"
f" {negative_attention_mask.shape}."
)
if prompt_embeds is not None and attention_mask is None:
raise ValueError("Please provide `attention_mask` along with `prompt_embeds`")
if prompt_embeds is not None and attention_mask is not None:
if prompt_embeds.shape[:2] != attention_mask.shape:
raise ValueError(
"`prompt_embeds` and `attention_mask` must have the same batch_size and token length when passed directly, but"
f" got: `prompt_embeds` {prompt_embeds.shape[:2]} != `attention_mask`"
f" {attention_mask.shape}."
)
@property
def guidance_scale(self):
return self._guidance_scale
@property
def do_classifier_free_guidance(self):
return self._guidance_scale > 1
@property
def num_timesteps(self):
return self._num_timesteps
@torch.no_grad()
@replace_example_docstring(EXAMPLE_DOC_STRING)
def __call__(
self,
prompt: Union[str, List[str]] = None,
num_inference_steps: int = 25,
guidance_scale: float = 3.0,
negative_prompt: Optional[Union[str, List[str]]] = None,
num_images_per_prompt: Optional[int] = 1,
height: Optional[int] = 1024,
width: Optional[int] = 1024,
generator: Optional[Union[torch.Generator, List[torch.Generator]]] = None,
prompt_embeds: Optional[torch.FloatTensor] = None,
negative_prompt_embeds: Optional[torch.FloatTensor] = None,
attention_mask: Optional[torch.FloatTensor] = None,
negative_attention_mask: Optional[torch.FloatTensor] = None,
output_type: Optional[str] = "pil",
return_dict: bool = True,
latents=None,
callback_on_step_end: Optional[Callable[[int, int, Dict], None]] = None,
callback_on_step_end_tensor_inputs: List[str] = ["latents"],
**kwargs,
):
"""
Function invoked when calling the pipeline for generation.
Args:
prompt (`str` or `List[str]`, *optional*):
The prompt or prompts to guide the image generation. If not defined, one has to pass `prompt_embeds`.
instead.
num_inference_steps (`int`, *optional*, defaults to 25):
The number of denoising steps. More denoising steps usually lead to a higher quality image at the
expense of slower inference.
timesteps (`List[int]`, *optional*):
Custom timesteps to use for the denoising process. If not defined, equal spaced `num_inference_steps`
timesteps are used. Must be in descending order.
guidance_scale (`float`, *optional*, defaults to 3.0):
Guidance scale as defined in [Classifier-Free Diffusion Guidance](https://arxiv.org/abs/2207.12598).
`guidance_scale` is defined as `w` of equation 2. of [Imagen
Paper](https://arxiv.org/pdf/2205.11487.pdf). Guidance scale is enabled by setting `guidance_scale >
1`. Higher guidance scale encourages to generate images that are closely linked to the text `prompt`,
usually at the expense of lower image quality.
negative_prompt (`str` or `List[str]`, *optional*):
The prompt or prompts not to guide the image generation. If not defined, one has to pass
`negative_prompt_embeds` instead. Ignored when not using guidance (i.e., ignored if `guidance_scale` is
less than `1`).
num_images_per_prompt (`int`, *optional*, defaults to 1):
The number of images to generate per prompt.
height (`int`, *optional*, defaults to self.unet.config.sample_size):
The height in pixels of the generated image.
width (`int`, *optional*, defaults to self.unet.config.sample_size):
The width in pixels of the generated image.
eta (`float`, *optional*, defaults to 0.0):
Corresponds to parameter eta (η) in the DDIM paper: https://arxiv.org/abs/2010.02502. Only applies to
[`schedulers.DDIMScheduler`], will be ignored for others.
generator (`torch.Generator` or `List[torch.Generator]`, *optional*):
One or a list of [torch generator(s)](https://pytorch.org/docs/stable/generated/torch.Generator.html)
to make generation deterministic.
prompt_embeds (`torch.FloatTensor`, *optional*):
Pre-generated text embeddings. Can be used to easily tweak text inputs, *e.g.* prompt weighting. If not
provided, text embeddings will be generated from `prompt` input argument.
negative_prompt_embeds (`torch.FloatTensor`, *optional*):
Pre-generated negative text embeddings. Can be used to easily tweak text inputs, *e.g.* prompt
weighting. If not provided, negative_prompt_embeds will be generated from `negative_prompt` input
argument.
attention_mask (`torch.FloatTensor`, *optional*):
Pre-generated attention mask. Must provide if passing `prompt_embeds` directly.
negative_attention_mask (`torch.FloatTensor`, *optional*):
Pre-generated negative attention mask. Must provide if passing `negative_prompt_embeds` directly.
output_type (`str`, *optional*, defaults to `"pil"`):
The output format of the generate image. Choose between
[PIL](https://pillow.readthedocs.io/en/stable/): `PIL.Image.Image` or `np.array`.
return_dict (`bool`, *optional*, defaults to `True`):
Whether or not to return a [`~pipelines.stable_diffusion.IFPipelineOutput`] instead of a plain tuple.
callback (`Callable`, *optional*):
A function that will be called every `callback_steps` steps during inference. The function will be
called with the following arguments: `callback(step: int, timestep: int, latents: torch.FloatTensor)`.
callback_steps (`int`, *optional*, defaults to 1):
The frequency at which the `callback` function will be called. If not specified, the callback will be
called at every step.
clean_caption (`bool`, *optional*, defaults to `True`):
Whether or not to clean the caption before creating embeddings. Requires `beautifulsoup4` and `ftfy` to
be installed. If the dependencies are not installed, the embeddings will be created from the raw
prompt.
cross_attention_kwargs (`dict`, *optional*):
A kwargs dictionary that if specified is passed along to the `AttentionProcessor` as defined under
`self.processor` in
[diffusers.models.attention_processor](https://github.com/huggingface/diffusers/blob/main/src/diffusers/models/attention_processor.py).
Examples:
Returns:
[`~pipelines.ImagePipelineOutput`] or `tuple`
"""
callback = kwargs.pop("callback", None)
callback_steps = kwargs.pop("callback_steps", None)
if callback is not None:
deprecate(
"callback",
"1.0.0",
"Passing `callback` as an input argument to `__call__` is deprecated, consider use `callback_on_step_end`",
)
if callback_steps is not None:
deprecate(
"callback_steps",
"1.0.0",
"Passing `callback_steps` as an input argument to `__call__` is deprecated, consider use `callback_on_step_end`",
)
if callback_on_step_end_tensor_inputs is not None and not all(
k in self._callback_tensor_inputs for k in callback_on_step_end_tensor_inputs
):
raise ValueError(
f"`callback_on_step_end_tensor_inputs` has to be in {self._callback_tensor_inputs}, but found {[k for k in callback_on_step_end_tensor_inputs if k not in self._callback_tensor_inputs]}"
)
cut_context = True
device = self._execution_device
# 1. Check inputs. Raise error if not correct
self.check_inputs(
prompt,
callback_steps,
negative_prompt,
prompt_embeds,
negative_prompt_embeds,
callback_on_step_end_tensor_inputs,
attention_mask,
negative_attention_mask,
)
self._guidance_scale = guidance_scale
if prompt is not None and isinstance(prompt, str):
batch_size = 1
elif prompt is not None and isinstance(prompt, list):
batch_size = len(prompt)
else:
batch_size = prompt_embeds.shape[0]
# 3. Encode input prompt
prompt_embeds, negative_prompt_embeds, attention_mask, negative_attention_mask = self.encode_prompt(
prompt,
self.do_classifier_free_guidance,
num_images_per_prompt=num_images_per_prompt,
device=device,
negative_prompt=negative_prompt,
prompt_embeds=prompt_embeds,
negative_prompt_embeds=negative_prompt_embeds,
_cut_context=cut_context,
attention_mask=attention_mask,
negative_attention_mask=negative_attention_mask,
)
if self.do_classifier_free_guidance:
prompt_embeds = torch.cat([negative_prompt_embeds, prompt_embeds])
attention_mask = torch.cat([negative_attention_mask, attention_mask]).bool()
# 4. Prepare timesteps
self.scheduler.set_timesteps(num_inference_steps, device=device)
timesteps = self.scheduler.timesteps
# 5. Prepare latents
height, width = downscale_height_and_width(height, width, 8)
latents = self.prepare_latents(
(batch_size * num_images_per_prompt, 4, height, width),
prompt_embeds.dtype,
device,
generator,
latents,
self.scheduler,
)
if hasattr(self, "text_encoder_offload_hook") and self.text_encoder_offload_hook is not None:
self.text_encoder_offload_hook.offload()
# 7. Denoising loop
num_warmup_steps = len(timesteps) - num_inference_steps * self.scheduler.order
self._num_timesteps = len(timesteps)
with self.progress_bar(total=num_inference_steps) as progress_bar:
for i, t in enumerate(timesteps):
latent_model_input = torch.cat([latents] * 2) if self.do_classifier_free_guidance else latents
# predict the noise residual
noise_pred = self.unet(
latent_model_input,
t,
encoder_hidden_states=prompt_embeds,
encoder_attention_mask=attention_mask,
return_dict=False,
)[0]
if self.do_classifier_free_guidance:
noise_pred_uncond, noise_pred_text = noise_pred.chunk(2)
noise_pred = (guidance_scale + 1.0) * noise_pred_text - guidance_scale * noise_pred_uncond
# noise_pred = noise_pred_uncond + guidance_scale * (noise_pred_text - noise_pred_uncond)
# compute the previous noisy sample x_t -> x_t-1
latents = self.scheduler.step(
noise_pred,
t,
latents,
generator=generator,
).prev_sample
if callback_on_step_end is not None:
callback_kwargs = {}
for k in callback_on_step_end_tensor_inputs:
callback_kwargs[k] = locals()[k]
callback_outputs = callback_on_step_end(self, i, t, callback_kwargs)
latents = callback_outputs.pop("latents", latents)
prompt_embeds = callback_outputs.pop("prompt_embeds", prompt_embeds)
negative_prompt_embeds = callback_outputs.pop("negative_prompt_embeds", negative_prompt_embeds)
attention_mask = callback_outputs.pop("attention_mask", attention_mask)
negative_attention_mask = callback_outputs.pop("negative_attention_mask", negative_attention_mask)
if i == len(timesteps) - 1 or ((i + 1) > num_warmup_steps and (i + 1) % self.scheduler.order == 0):
progress_bar.update()
if callback is not None and i % callback_steps == 0:
step_idx = i // getattr(self.scheduler, "order", 1)
callback(step_idx, t, latents)
# post-processing
if output_type not in ["pt", "np", "pil", "latent"]:
raise ValueError(
f"Only the output types `pt`, `pil`, `np` and `latent` are supported not output_type={output_type}"
)
if not output_type == "latent":
image = self.movq.decode(latents, force_not_quantize=True)["sample"]
if output_type in ["np", "pil"]:
image = image * 0.5 + 0.5
image = image.clamp(0, 1)
image = image.cpu().permute(0, 2, 3, 1).float().numpy()
if output_type == "pil":
image = self.numpy_to_pil(image)
else:
image = latents
self.maybe_free_model_hooks()
if not return_dict:
return (image,)
return ImagePipelineOutput(images=image)
| diffusers/src/diffusers/pipelines/kandinsky3/pipeline_kandinsky3.py/0 | {
"file_path": "diffusers/src/diffusers/pipelines/kandinsky3/pipeline_kandinsky3.py",
"repo_id": "diffusers",
"token_count": 12850
} | 130 |
# Copyright 2024 The HuggingFace Team. All rights reserved.
#
# Licensed under the Apache License, Version 2.0 (the "License");
# you may not use this file except in compliance with the License.
# You may obtain a copy of the License at
#
# http://www.apache.org/licenses/LICENSE-2.0
#
# Unless required by applicable law or agreed to in writing, software
# distributed under the License is distributed on an "AS IS" BASIS,
# WITHOUT WARRANTIES OR CONDITIONS OF ANY KIND, either express or implied.
# See the License for the specific language governing permissions and
# limitations under the License.
import torch
from torch import nn
from transformers import CLIPPreTrainedModel, CLIPVisionModel
from ...models.attention import BasicTransformerBlock
from ...utils import logging
logger = logging.get_logger(__name__) # pylint: disable=invalid-name
class PaintByExampleImageEncoder(CLIPPreTrainedModel):
def __init__(self, config, proj_size=None):
super().__init__(config)
self.proj_size = proj_size or getattr(config, "projection_dim", 768)
self.model = CLIPVisionModel(config)
self.mapper = PaintByExampleMapper(config)
self.final_layer_norm = nn.LayerNorm(config.hidden_size)
self.proj_out = nn.Linear(config.hidden_size, self.proj_size)
# uncondition for scaling
self.uncond_vector = nn.Parameter(torch.randn((1, 1, self.proj_size)))
def forward(self, pixel_values, return_uncond_vector=False):
clip_output = self.model(pixel_values=pixel_values)
latent_states = clip_output.pooler_output
latent_states = self.mapper(latent_states[:, None])
latent_states = self.final_layer_norm(latent_states)
latent_states = self.proj_out(latent_states)
if return_uncond_vector:
return latent_states, self.uncond_vector
return latent_states
class PaintByExampleMapper(nn.Module):
def __init__(self, config):
super().__init__()
num_layers = (config.num_hidden_layers + 1) // 5
hid_size = config.hidden_size
num_heads = 1
self.blocks = nn.ModuleList(
[
BasicTransformerBlock(hid_size, num_heads, hid_size, activation_fn="gelu", attention_bias=True)
for _ in range(num_layers)
]
)
def forward(self, hidden_states):
for block in self.blocks:
hidden_states = block(hidden_states)
return hidden_states
| diffusers/src/diffusers/pipelines/paint_by_example/image_encoder.py/0 | {
"file_path": "diffusers/src/diffusers/pipelines/paint_by_example/image_encoder.py",
"repo_id": "diffusers",
"token_count": 942
} | 131 |
# Copyright 2024 Open AI and The HuggingFace Team. All rights reserved.
#
# Licensed under the Apache License, Version 2.0 (the "License");
# you may not use this file except in compliance with the License.
# You may obtain a copy of the License at
#
# http://www.apache.org/licenses/LICENSE-2.0
#
# Unless required by applicable law or agreed to in writing, software
# distributed under the License is distributed on an "AS IS" BASIS,
# WITHOUT WARRANTIES OR CONDITIONS OF ANY KIND, either express or implied.
# See the License for the specific language governing permissions and
# limitations under the License.
import math
from dataclasses import dataclass
from typing import Dict, Optional, Tuple
import numpy as np
import torch
import torch.nn.functional as F
from torch import nn
from ...configuration_utils import ConfigMixin, register_to_config
from ...models import ModelMixin
from ...utils import BaseOutput
from .camera import create_pan_cameras
def sample_pmf(pmf: torch.Tensor, n_samples: int) -> torch.Tensor:
r"""
Sample from the given discrete probability distribution with replacement.
The i-th bin is assumed to have mass pmf[i].
Args:
pmf: [batch_size, *shape, n_samples, 1] where (pmf.sum(dim=-2) == 1).all()
n_samples: number of samples
Return:
indices sampled with replacement
"""
*shape, support_size, last_dim = pmf.shape
assert last_dim == 1
cdf = torch.cumsum(pmf.view(-1, support_size), dim=1)
inds = torch.searchsorted(cdf, torch.rand(cdf.shape[0], n_samples, device=cdf.device))
return inds.view(*shape, n_samples, 1).clamp(0, support_size - 1)
def posenc_nerf(x: torch.Tensor, min_deg: int = 0, max_deg: int = 15) -> torch.Tensor:
"""
Concatenate x and its positional encodings, following NeRF.
Reference: https://arxiv.org/pdf/2210.04628.pdf
"""
if min_deg == max_deg:
return x
scales = 2.0 ** torch.arange(min_deg, max_deg, dtype=x.dtype, device=x.device)
*shape, dim = x.shape
xb = (x.reshape(-1, 1, dim) * scales.view(1, -1, 1)).reshape(*shape, -1)
assert xb.shape[-1] == dim * (max_deg - min_deg)
emb = torch.cat([xb, xb + math.pi / 2.0], axis=-1).sin()
return torch.cat([x, emb], dim=-1)
def encode_position(position):
return posenc_nerf(position, min_deg=0, max_deg=15)
def encode_direction(position, direction=None):
if direction is None:
return torch.zeros_like(posenc_nerf(position, min_deg=0, max_deg=8))
else:
return posenc_nerf(direction, min_deg=0, max_deg=8)
def _sanitize_name(x: str) -> str:
return x.replace(".", "__")
def integrate_samples(volume_range, ts, density, channels):
r"""
Function integrating the model output.
Args:
volume_range: Specifies the integral range [t0, t1]
ts: timesteps
density: torch.Tensor [batch_size, *shape, n_samples, 1]
channels: torch.Tensor [batch_size, *shape, n_samples, n_channels]
returns:
channels: integrated rgb output weights: torch.Tensor [batch_size, *shape, n_samples, 1] (density
*transmittance)[i] weight for each rgb output at [..., i, :]. transmittance: transmittance of this volume
)
"""
# 1. Calculate the weights
_, _, dt = volume_range.partition(ts)
ddensity = density * dt
mass = torch.cumsum(ddensity, dim=-2)
transmittance = torch.exp(-mass[..., -1, :])
alphas = 1.0 - torch.exp(-ddensity)
Ts = torch.exp(torch.cat([torch.zeros_like(mass[..., :1, :]), -mass[..., :-1, :]], dim=-2))
# This is the probability of light hitting and reflecting off of
# something at depth [..., i, :].
weights = alphas * Ts
# 2. Integrate channels
channels = torch.sum(channels * weights, dim=-2)
return channels, weights, transmittance
def volume_query_points(volume, grid_size):
indices = torch.arange(grid_size**3, device=volume.bbox_min.device)
zs = indices % grid_size
ys = torch.div(indices, grid_size, rounding_mode="trunc") % grid_size
xs = torch.div(indices, grid_size**2, rounding_mode="trunc") % grid_size
combined = torch.stack([xs, ys, zs], dim=1)
return (combined.float() / (grid_size - 1)) * (volume.bbox_max - volume.bbox_min) + volume.bbox_min
def _convert_srgb_to_linear(u: torch.Tensor):
return torch.where(u <= 0.04045, u / 12.92, ((u + 0.055) / 1.055) ** 2.4)
def _create_flat_edge_indices(
flat_cube_indices: torch.Tensor,
grid_size: Tuple[int, int, int],
):
num_xs = (grid_size[0] - 1) * grid_size[1] * grid_size[2]
y_offset = num_xs
num_ys = grid_size[0] * (grid_size[1] - 1) * grid_size[2]
z_offset = num_xs + num_ys
return torch.stack(
[
# Edges spanning x-axis.
flat_cube_indices[:, 0] * grid_size[1] * grid_size[2]
+ flat_cube_indices[:, 1] * grid_size[2]
+ flat_cube_indices[:, 2],
flat_cube_indices[:, 0] * grid_size[1] * grid_size[2]
+ (flat_cube_indices[:, 1] + 1) * grid_size[2]
+ flat_cube_indices[:, 2],
flat_cube_indices[:, 0] * grid_size[1] * grid_size[2]
+ flat_cube_indices[:, 1] * grid_size[2]
+ flat_cube_indices[:, 2]
+ 1,
flat_cube_indices[:, 0] * grid_size[1] * grid_size[2]
+ (flat_cube_indices[:, 1] + 1) * grid_size[2]
+ flat_cube_indices[:, 2]
+ 1,
# Edges spanning y-axis.
(
y_offset
+ flat_cube_indices[:, 0] * (grid_size[1] - 1) * grid_size[2]
+ flat_cube_indices[:, 1] * grid_size[2]
+ flat_cube_indices[:, 2]
),
(
y_offset
+ (flat_cube_indices[:, 0] + 1) * (grid_size[1] - 1) * grid_size[2]
+ flat_cube_indices[:, 1] * grid_size[2]
+ flat_cube_indices[:, 2]
),
(
y_offset
+ flat_cube_indices[:, 0] * (grid_size[1] - 1) * grid_size[2]
+ flat_cube_indices[:, 1] * grid_size[2]
+ flat_cube_indices[:, 2]
+ 1
),
(
y_offset
+ (flat_cube_indices[:, 0] + 1) * (grid_size[1] - 1) * grid_size[2]
+ flat_cube_indices[:, 1] * grid_size[2]
+ flat_cube_indices[:, 2]
+ 1
),
# Edges spanning z-axis.
(
z_offset
+ flat_cube_indices[:, 0] * grid_size[1] * (grid_size[2] - 1)
+ flat_cube_indices[:, 1] * (grid_size[2] - 1)
+ flat_cube_indices[:, 2]
),
(
z_offset
+ (flat_cube_indices[:, 0] + 1) * grid_size[1] * (grid_size[2] - 1)
+ flat_cube_indices[:, 1] * (grid_size[2] - 1)
+ flat_cube_indices[:, 2]
),
(
z_offset
+ flat_cube_indices[:, 0] * grid_size[1] * (grid_size[2] - 1)
+ (flat_cube_indices[:, 1] + 1) * (grid_size[2] - 1)
+ flat_cube_indices[:, 2]
),
(
z_offset
+ (flat_cube_indices[:, 0] + 1) * grid_size[1] * (grid_size[2] - 1)
+ (flat_cube_indices[:, 1] + 1) * (grid_size[2] - 1)
+ flat_cube_indices[:, 2]
),
],
dim=-1,
)
class VoidNeRFModel(nn.Module):
"""
Implements the default empty space model where all queries are rendered as background.
"""
def __init__(self, background, channel_scale=255.0):
super().__init__()
background = nn.Parameter(torch.from_numpy(np.array(background)).to(dtype=torch.float32) / channel_scale)
self.register_buffer("background", background)
def forward(self, position):
background = self.background[None].to(position.device)
shape = position.shape[:-1]
ones = [1] * (len(shape) - 1)
n_channels = background.shape[-1]
background = torch.broadcast_to(background.view(background.shape[0], *ones, n_channels), [*shape, n_channels])
return background
@dataclass
class VolumeRange:
t0: torch.Tensor
t1: torch.Tensor
intersected: torch.Tensor
def __post_init__(self):
assert self.t0.shape == self.t1.shape == self.intersected.shape
def partition(self, ts):
"""
Partitions t0 and t1 into n_samples intervals.
Args:
ts: [batch_size, *shape, n_samples, 1]
Return:
lower: [batch_size, *shape, n_samples, 1] upper: [batch_size, *shape, n_samples, 1] delta: [batch_size,
*shape, n_samples, 1]
where
ts \\in [lower, upper] deltas = upper - lower
"""
mids = (ts[..., 1:, :] + ts[..., :-1, :]) * 0.5
lower = torch.cat([self.t0[..., None, :], mids], dim=-2)
upper = torch.cat([mids, self.t1[..., None, :]], dim=-2)
delta = upper - lower
assert lower.shape == upper.shape == delta.shape == ts.shape
return lower, upper, delta
class BoundingBoxVolume(nn.Module):
"""
Axis-aligned bounding box defined by the two opposite corners.
"""
def __init__(
self,
*,
bbox_min,
bbox_max,
min_dist: float = 0.0,
min_t_range: float = 1e-3,
):
"""
Args:
bbox_min: the left/bottommost corner of the bounding box
bbox_max: the other corner of the bounding box
min_dist: all rays should start at least this distance away from the origin.
"""
super().__init__()
self.min_dist = min_dist
self.min_t_range = min_t_range
self.bbox_min = torch.tensor(bbox_min)
self.bbox_max = torch.tensor(bbox_max)
self.bbox = torch.stack([self.bbox_min, self.bbox_max])
assert self.bbox.shape == (2, 3)
assert min_dist >= 0.0
assert min_t_range > 0.0
def intersect(
self,
origin: torch.Tensor,
direction: torch.Tensor,
t0_lower: Optional[torch.Tensor] = None,
epsilon=1e-6,
):
"""
Args:
origin: [batch_size, *shape, 3]
direction: [batch_size, *shape, 3]
t0_lower: Optional [batch_size, *shape, 1] lower bound of t0 when intersecting this volume.
params: Optional meta parameters in case Volume is parametric
epsilon: to stabilize calculations
Return:
A tuple of (t0, t1, intersected) where each has a shape [batch_size, *shape, 1]. If a ray intersects with
the volume, `o + td` is in the volume for all t in [t0, t1]. If the volume is bounded, t1 is guaranteed to
be on the boundary of the volume.
"""
batch_size, *shape, _ = origin.shape
ones = [1] * len(shape)
bbox = self.bbox.view(1, *ones, 2, 3).to(origin.device)
def _safe_divide(a, b, epsilon=1e-6):
return a / torch.where(b < 0, b - epsilon, b + epsilon)
ts = _safe_divide(bbox - origin[..., None, :], direction[..., None, :], epsilon=epsilon)
# Cases to think about:
#
# 1. t1 <= t0: the ray does not pass through the AABB.
# 2. t0 < t1 <= 0: the ray intersects but the BB is behind the origin.
# 3. t0 <= 0 <= t1: the ray starts from inside the BB
# 4. 0 <= t0 < t1: the ray is not inside and intersects with the BB twice.
#
# 1 and 4 are clearly handled from t0 < t1 below.
# Making t0 at least min_dist (>= 0) takes care of 2 and 3.
t0 = ts.min(dim=-2).values.max(dim=-1, keepdim=True).values.clamp(self.min_dist)
t1 = ts.max(dim=-2).values.min(dim=-1, keepdim=True).values
assert t0.shape == t1.shape == (batch_size, *shape, 1)
if t0_lower is not None:
assert t0.shape == t0_lower.shape
t0 = torch.maximum(t0, t0_lower)
intersected = t0 + self.min_t_range < t1
t0 = torch.where(intersected, t0, torch.zeros_like(t0))
t1 = torch.where(intersected, t1, torch.ones_like(t1))
return VolumeRange(t0=t0, t1=t1, intersected=intersected)
class StratifiedRaySampler(nn.Module):
"""
Instead of fixed intervals, a sample is drawn uniformly at random from each interval.
"""
def __init__(self, depth_mode: str = "linear"):
"""
:param depth_mode: linear samples ts linearly in depth. harmonic ensures
closer points are sampled more densely.
"""
self.depth_mode = depth_mode
assert self.depth_mode in ("linear", "geometric", "harmonic")
def sample(
self,
t0: torch.Tensor,
t1: torch.Tensor,
n_samples: int,
epsilon: float = 1e-3,
) -> torch.Tensor:
"""
Args:
t0: start time has shape [batch_size, *shape, 1]
t1: finish time has shape [batch_size, *shape, 1]
n_samples: number of ts to sample
Return:
sampled ts of shape [batch_size, *shape, n_samples, 1]
"""
ones = [1] * (len(t0.shape) - 1)
ts = torch.linspace(0, 1, n_samples).view(*ones, n_samples).to(t0.dtype).to(t0.device)
if self.depth_mode == "linear":
ts = t0 * (1.0 - ts) + t1 * ts
elif self.depth_mode == "geometric":
ts = (t0.clamp(epsilon).log() * (1.0 - ts) + t1.clamp(epsilon).log() * ts).exp()
elif self.depth_mode == "harmonic":
# The original NeRF recommends this interpolation scheme for
# spherical scenes, but there could be some weird edge cases when
# the observer crosses from the inner to outer volume.
ts = 1.0 / (1.0 / t0.clamp(epsilon) * (1.0 - ts) + 1.0 / t1.clamp(epsilon) * ts)
mids = 0.5 * (ts[..., 1:] + ts[..., :-1])
upper = torch.cat([mids, t1], dim=-1)
lower = torch.cat([t0, mids], dim=-1)
# yiyi notes: add a random seed here for testing, don't forget to remove
torch.manual_seed(0)
t_rand = torch.rand_like(ts)
ts = lower + (upper - lower) * t_rand
return ts.unsqueeze(-1)
class ImportanceRaySampler(nn.Module):
"""
Given the initial estimate of densities, this samples more from regions/bins expected to have objects.
"""
def __init__(
self,
volume_range: VolumeRange,
ts: torch.Tensor,
weights: torch.Tensor,
blur_pool: bool = False,
alpha: float = 1e-5,
):
"""
Args:
volume_range: the range in which a ray intersects the given volume.
ts: earlier samples from the coarse rendering step
weights: discretized version of density * transmittance
blur_pool: if true, use 2-tap max + 2-tap blur filter from mip-NeRF.
alpha: small value to add to weights.
"""
self.volume_range = volume_range
self.ts = ts.clone().detach()
self.weights = weights.clone().detach()
self.blur_pool = blur_pool
self.alpha = alpha
@torch.no_grad()
def sample(self, t0: torch.Tensor, t1: torch.Tensor, n_samples: int) -> torch.Tensor:
"""
Args:
t0: start time has shape [batch_size, *shape, 1]
t1: finish time has shape [batch_size, *shape, 1]
n_samples: number of ts to sample
Return:
sampled ts of shape [batch_size, *shape, n_samples, 1]
"""
lower, upper, _ = self.volume_range.partition(self.ts)
batch_size, *shape, n_coarse_samples, _ = self.ts.shape
weights = self.weights
if self.blur_pool:
padded = torch.cat([weights[..., :1, :], weights, weights[..., -1:, :]], dim=-2)
maxes = torch.maximum(padded[..., :-1, :], padded[..., 1:, :])
weights = 0.5 * (maxes[..., :-1, :] + maxes[..., 1:, :])
weights = weights + self.alpha
pmf = weights / weights.sum(dim=-2, keepdim=True)
inds = sample_pmf(pmf, n_samples)
assert inds.shape == (batch_size, *shape, n_samples, 1)
assert (inds >= 0).all() and (inds < n_coarse_samples).all()
t_rand = torch.rand(inds.shape, device=inds.device)
lower_ = torch.gather(lower, -2, inds)
upper_ = torch.gather(upper, -2, inds)
ts = lower_ + (upper_ - lower_) * t_rand
ts = torch.sort(ts, dim=-2).values
return ts
@dataclass
class MeshDecoderOutput(BaseOutput):
"""
A 3D triangle mesh with optional data at the vertices and faces.
Args:
verts (`torch.Tensor` of shape `(N, 3)`):
array of vertext coordinates
faces (`torch.Tensor` of shape `(N, 3)`):
array of triangles, pointing to indices in verts.
vertext_channels (Dict):
vertext coordinates for each color channel
"""
verts: torch.Tensor
faces: torch.Tensor
vertex_channels: Dict[str, torch.Tensor]
class MeshDecoder(nn.Module):
"""
Construct meshes from Signed distance functions (SDFs) using marching cubes method
"""
def __init__(self):
super().__init__()
cases = torch.zeros(256, 5, 3, dtype=torch.long)
masks = torch.zeros(256, 5, dtype=torch.bool)
self.register_buffer("cases", cases)
self.register_buffer("masks", masks)
def forward(self, field: torch.Tensor, min_point: torch.Tensor, size: torch.Tensor):
"""
For a signed distance field, produce a mesh using marching cubes.
:param field: a 3D tensor of field values, where negative values correspond
to the outside of the shape. The dimensions correspond to the x, y, and z directions, respectively.
:param min_point: a tensor of shape [3] containing the point corresponding
to (0, 0, 0) in the field.
:param size: a tensor of shape [3] containing the per-axis distance from the
(0, 0, 0) field corner and the (-1, -1, -1) field corner.
"""
assert len(field.shape) == 3, "input must be a 3D scalar field"
dev = field.device
cases = self.cases.to(dev)
masks = self.masks.to(dev)
min_point = min_point.to(dev)
size = size.to(dev)
grid_size = field.shape
grid_size_tensor = torch.tensor(grid_size).to(size)
# Create bitmasks between 0 and 255 (inclusive) indicating the state
# of the eight corners of each cube.
bitmasks = (field > 0).to(torch.uint8)
bitmasks = bitmasks[:-1, :, :] | (bitmasks[1:, :, :] << 1)
bitmasks = bitmasks[:, :-1, :] | (bitmasks[:, 1:, :] << 2)
bitmasks = bitmasks[:, :, :-1] | (bitmasks[:, :, 1:] << 4)
# Compute corner coordinates across the entire grid.
corner_coords = torch.empty(*grid_size, 3, device=dev, dtype=field.dtype)
corner_coords[range(grid_size[0]), :, :, 0] = torch.arange(grid_size[0], device=dev, dtype=field.dtype)[
:, None, None
]
corner_coords[:, range(grid_size[1]), :, 1] = torch.arange(grid_size[1], device=dev, dtype=field.dtype)[
:, None
]
corner_coords[:, :, range(grid_size[2]), 2] = torch.arange(grid_size[2], device=dev, dtype=field.dtype)
# Compute all vertices across all edges in the grid, even though we will
# throw some out later. We have (X-1)*Y*Z + X*(Y-1)*Z + X*Y*(Z-1) vertices.
# These are all midpoints, and don't account for interpolation (which is
# done later based on the used edge midpoints).
edge_midpoints = torch.cat(
[
((corner_coords[:-1] + corner_coords[1:]) / 2).reshape(-1, 3),
((corner_coords[:, :-1] + corner_coords[:, 1:]) / 2).reshape(-1, 3),
((corner_coords[:, :, :-1] + corner_coords[:, :, 1:]) / 2).reshape(-1, 3),
],
dim=0,
)
# Create a flat array of [X, Y, Z] indices for each cube.
cube_indices = torch.zeros(
grid_size[0] - 1, grid_size[1] - 1, grid_size[2] - 1, 3, device=dev, dtype=torch.long
)
cube_indices[range(grid_size[0] - 1), :, :, 0] = torch.arange(grid_size[0] - 1, device=dev)[:, None, None]
cube_indices[:, range(grid_size[1] - 1), :, 1] = torch.arange(grid_size[1] - 1, device=dev)[:, None]
cube_indices[:, :, range(grid_size[2] - 1), 2] = torch.arange(grid_size[2] - 1, device=dev)
flat_cube_indices = cube_indices.reshape(-1, 3)
# Create a flat array mapping each cube to 12 global edge indices.
edge_indices = _create_flat_edge_indices(flat_cube_indices, grid_size)
# Apply the LUT to figure out the triangles.
flat_bitmasks = bitmasks.reshape(-1).long() # must cast to long for indexing to believe this not a mask
local_tris = cases[flat_bitmasks]
local_masks = masks[flat_bitmasks]
# Compute the global edge indices for the triangles.
global_tris = torch.gather(edge_indices, 1, local_tris.reshape(local_tris.shape[0], -1)).reshape(
local_tris.shape
)
# Select the used triangles for each cube.
selected_tris = global_tris.reshape(-1, 3)[local_masks.reshape(-1)]
# Now we have a bunch of indices into the full list of possible vertices,
# but we want to reduce this list to only the used vertices.
used_vertex_indices = torch.unique(selected_tris.view(-1))
used_edge_midpoints = edge_midpoints[used_vertex_indices]
old_index_to_new_index = torch.zeros(len(edge_midpoints), device=dev, dtype=torch.long)
old_index_to_new_index[used_vertex_indices] = torch.arange(
len(used_vertex_indices), device=dev, dtype=torch.long
)
# Rewrite the triangles to use the new indices
faces = torch.gather(old_index_to_new_index, 0, selected_tris.view(-1)).reshape(selected_tris.shape)
# Compute the actual interpolated coordinates corresponding to edge midpoints.
v1 = torch.floor(used_edge_midpoints).to(torch.long)
v2 = torch.ceil(used_edge_midpoints).to(torch.long)
s1 = field[v1[:, 0], v1[:, 1], v1[:, 2]]
s2 = field[v2[:, 0], v2[:, 1], v2[:, 2]]
p1 = (v1.float() / (grid_size_tensor - 1)) * size + min_point
p2 = (v2.float() / (grid_size_tensor - 1)) * size + min_point
# The signs of s1 and s2 should be different. We want to find
# t such that t*s2 + (1-t)*s1 = 0.
t = (s1 / (s1 - s2))[:, None]
verts = t * p2 + (1 - t) * p1
return MeshDecoderOutput(verts=verts, faces=faces, vertex_channels=None)
@dataclass
class MLPNeRFModelOutput(BaseOutput):
density: torch.Tensor
signed_distance: torch.Tensor
channels: torch.Tensor
ts: torch.Tensor
class MLPNeRSTFModel(ModelMixin, ConfigMixin):
@register_to_config
def __init__(
self,
d_hidden: int = 256,
n_output: int = 12,
n_hidden_layers: int = 6,
act_fn: str = "swish",
insert_direction_at: int = 4,
):
super().__init__()
# Instantiate the MLP
# Find out the dimension of encoded position and direction
dummy = torch.eye(1, 3)
d_posenc_pos = encode_position(position=dummy).shape[-1]
d_posenc_dir = encode_direction(position=dummy).shape[-1]
mlp_widths = [d_hidden] * n_hidden_layers
input_widths = [d_posenc_pos] + mlp_widths
output_widths = mlp_widths + [n_output]
if insert_direction_at is not None:
input_widths[insert_direction_at] += d_posenc_dir
self.mlp = nn.ModuleList([nn.Linear(d_in, d_out) for d_in, d_out in zip(input_widths, output_widths)])
if act_fn == "swish":
# self.activation = swish
# yiyi testing:
self.activation = lambda x: F.silu(x)
else:
raise ValueError(f"Unsupported activation function {act_fn}")
self.sdf_activation = torch.tanh
self.density_activation = torch.nn.functional.relu
self.channel_activation = torch.sigmoid
def map_indices_to_keys(self, output):
h_map = {
"sdf": (0, 1),
"density_coarse": (1, 2),
"density_fine": (2, 3),
"stf": (3, 6),
"nerf_coarse": (6, 9),
"nerf_fine": (9, 12),
}
mapped_output = {k: output[..., start:end] for k, (start, end) in h_map.items()}
return mapped_output
def forward(self, *, position, direction, ts, nerf_level="coarse", rendering_mode="nerf"):
h = encode_position(position)
h_preact = h
h_directionless = None
for i, layer in enumerate(self.mlp):
if i == self.config.insert_direction_at: # 4 in the config
h_directionless = h_preact
h_direction = encode_direction(position, direction=direction)
h = torch.cat([h, h_direction], dim=-1)
h = layer(h)
h_preact = h
if i < len(self.mlp) - 1:
h = self.activation(h)
h_final = h
if h_directionless is None:
h_directionless = h_preact
activation = self.map_indices_to_keys(h_final)
if nerf_level == "coarse":
h_density = activation["density_coarse"]
else:
h_density = activation["density_fine"]
if rendering_mode == "nerf":
if nerf_level == "coarse":
h_channels = activation["nerf_coarse"]
else:
h_channels = activation["nerf_fine"]
elif rendering_mode == "stf":
h_channels = activation["stf"]
density = self.density_activation(h_density)
signed_distance = self.sdf_activation(activation["sdf"])
channels = self.channel_activation(h_channels)
# yiyi notes: I think signed_distance is not used
return MLPNeRFModelOutput(density=density, signed_distance=signed_distance, channels=channels, ts=ts)
class ChannelsProj(nn.Module):
def __init__(
self,
*,
vectors: int,
channels: int,
d_latent: int,
):
super().__init__()
self.proj = nn.Linear(d_latent, vectors * channels)
self.norm = nn.LayerNorm(channels)
self.d_latent = d_latent
self.vectors = vectors
self.channels = channels
def forward(self, x: torch.Tensor) -> torch.Tensor:
x_bvd = x
w_vcd = self.proj.weight.view(self.vectors, self.channels, self.d_latent)
b_vc = self.proj.bias.view(1, self.vectors, self.channels)
h = torch.einsum("bvd,vcd->bvc", x_bvd, w_vcd)
h = self.norm(h)
h = h + b_vc
return h
class ShapEParamsProjModel(ModelMixin, ConfigMixin):
"""
project the latent representation of a 3D asset to obtain weights of a multi-layer perceptron (MLP).
For more details, see the original paper:
"""
@register_to_config
def __init__(
self,
*,
param_names: Tuple[str] = (
"nerstf.mlp.0.weight",
"nerstf.mlp.1.weight",
"nerstf.mlp.2.weight",
"nerstf.mlp.3.weight",
),
param_shapes: Tuple[Tuple[int]] = (
(256, 93),
(256, 256),
(256, 256),
(256, 256),
),
d_latent: int = 1024,
):
super().__init__()
# check inputs
if len(param_names) != len(param_shapes):
raise ValueError("Must provide same number of `param_names` as `param_shapes`")
self.projections = nn.ModuleDict({})
for k, (vectors, channels) in zip(param_names, param_shapes):
self.projections[_sanitize_name(k)] = ChannelsProj(
vectors=vectors,
channels=channels,
d_latent=d_latent,
)
def forward(self, x: torch.Tensor):
out = {}
start = 0
for k, shape in zip(self.config.param_names, self.config.param_shapes):
vectors, _ = shape
end = start + vectors
x_bvd = x[:, start:end]
out[k] = self.projections[_sanitize_name(k)](x_bvd).reshape(len(x), *shape)
start = end
return out
class ShapERenderer(ModelMixin, ConfigMixin):
@register_to_config
def __init__(
self,
*,
param_names: Tuple[str] = (
"nerstf.mlp.0.weight",
"nerstf.mlp.1.weight",
"nerstf.mlp.2.weight",
"nerstf.mlp.3.weight",
),
param_shapes: Tuple[Tuple[int]] = (
(256, 93),
(256, 256),
(256, 256),
(256, 256),
),
d_latent: int = 1024,
d_hidden: int = 256,
n_output: int = 12,
n_hidden_layers: int = 6,
act_fn: str = "swish",
insert_direction_at: int = 4,
background: Tuple[float] = (
255.0,
255.0,
255.0,
),
):
super().__init__()
self.params_proj = ShapEParamsProjModel(
param_names=param_names,
param_shapes=param_shapes,
d_latent=d_latent,
)
self.mlp = MLPNeRSTFModel(d_hidden, n_output, n_hidden_layers, act_fn, insert_direction_at)
self.void = VoidNeRFModel(background=background, channel_scale=255.0)
self.volume = BoundingBoxVolume(bbox_max=[1.0, 1.0, 1.0], bbox_min=[-1.0, -1.0, -1.0])
self.mesh_decoder = MeshDecoder()
@torch.no_grad()
def render_rays(self, rays, sampler, n_samples, prev_model_out=None, render_with_direction=False):
"""
Perform volumetric rendering over a partition of possible t's in the union of rendering volumes (written below
with some abuse of notations)
C(r) := sum(
transmittance(t[i]) * integrate(
lambda t: density(t) * channels(t) * transmittance(t), [t[i], t[i + 1]],
) for i in range(len(parts))
) + transmittance(t[-1]) * void_model(t[-1]).channels
where
1) transmittance(s) := exp(-integrate(density, [t[0], s])) calculates the probability of light passing through
the volume specified by [t[0], s]. (transmittance of 1 means light can pass freely) 2) density and channels are
obtained by evaluating the appropriate part.model at time t. 3) [t[i], t[i + 1]] is defined as the range of t
where the ray intersects (parts[i].volume \\ union(part.volume for part in parts[:i])) at the surface of the
shell (if bounded). If the ray does not intersect, the integral over this segment is evaluated as 0 and
transmittance(t[i + 1]) := transmittance(t[i]). 4) The last term is integration to infinity (e.g. [t[-1],
math.inf]) that is evaluated by the void_model (i.e. we consider this space to be empty).
args:
rays: [batch_size x ... x 2 x 3] origin and direction. sampler: disjoint volume integrals. n_samples:
number of ts to sample. prev_model_outputs: model outputs from the previous rendering step, including
:return: A tuple of
- `channels`
- A importance samplers for additional fine-grained rendering
- raw model output
"""
origin, direction = rays[..., 0, :], rays[..., 1, :]
# Integrate over [t[i], t[i + 1]]
# 1 Intersect the rays with the current volume and sample ts to integrate along.
vrange = self.volume.intersect(origin, direction, t0_lower=None)
ts = sampler.sample(vrange.t0, vrange.t1, n_samples)
ts = ts.to(rays.dtype)
if prev_model_out is not None:
# Append the previous ts now before fprop because previous
# rendering used a different model and we can't reuse the output.
ts = torch.sort(torch.cat([ts, prev_model_out.ts], dim=-2), dim=-2).values
batch_size, *_shape, _t0_dim = vrange.t0.shape
_, *ts_shape, _ts_dim = ts.shape
# 2. Get the points along the ray and query the model
directions = torch.broadcast_to(direction.unsqueeze(-2), [batch_size, *ts_shape, 3])
positions = origin.unsqueeze(-2) + ts * directions
directions = directions.to(self.mlp.dtype)
positions = positions.to(self.mlp.dtype)
optional_directions = directions if render_with_direction else None
model_out = self.mlp(
position=positions,
direction=optional_directions,
ts=ts,
nerf_level="coarse" if prev_model_out is None else "fine",
)
# 3. Integrate the model results
channels, weights, transmittance = integrate_samples(
vrange, model_out.ts, model_out.density, model_out.channels
)
# 4. Clean up results that do not intersect with the volume.
transmittance = torch.where(vrange.intersected, transmittance, torch.ones_like(transmittance))
channels = torch.where(vrange.intersected, channels, torch.zeros_like(channels))
# 5. integration to infinity (e.g. [t[-1], math.inf]) that is evaluated by the void_model (i.e. we consider this space to be empty).
channels = channels + transmittance * self.void(origin)
weighted_sampler = ImportanceRaySampler(vrange, ts=model_out.ts, weights=weights)
return channels, weighted_sampler, model_out
@torch.no_grad()
def decode_to_image(
self,
latents,
device,
size: int = 64,
ray_batch_size: int = 4096,
n_coarse_samples=64,
n_fine_samples=128,
):
# project the parameters from the generated latents
projected_params = self.params_proj(latents)
# update the mlp layers of the renderer
for name, param in self.mlp.state_dict().items():
if f"nerstf.{name}" in projected_params.keys():
param.copy_(projected_params[f"nerstf.{name}"].squeeze(0))
# create cameras object
camera = create_pan_cameras(size)
rays = camera.camera_rays
rays = rays.to(device)
n_batches = rays.shape[1] // ray_batch_size
coarse_sampler = StratifiedRaySampler()
images = []
for idx in range(n_batches):
rays_batch = rays[:, idx * ray_batch_size : (idx + 1) * ray_batch_size]
# render rays with coarse, stratified samples.
_, fine_sampler, coarse_model_out = self.render_rays(rays_batch, coarse_sampler, n_coarse_samples)
# Then, render with additional importance-weighted ray samples.
channels, _, _ = self.render_rays(
rays_batch, fine_sampler, n_fine_samples, prev_model_out=coarse_model_out
)
images.append(channels)
images = torch.cat(images, dim=1)
images = images.view(*camera.shape, camera.height, camera.width, -1).squeeze(0)
return images
@torch.no_grad()
def decode_to_mesh(
self,
latents,
device,
grid_size: int = 128,
query_batch_size: int = 4096,
texture_channels: Tuple = ("R", "G", "B"),
):
# 1. project the parameters from the generated latents
projected_params = self.params_proj(latents)
# 2. update the mlp layers of the renderer
for name, param in self.mlp.state_dict().items():
if f"nerstf.{name}" in projected_params.keys():
param.copy_(projected_params[f"nerstf.{name}"].squeeze(0))
# 3. decoding with STF rendering
# 3.1 query the SDF values at vertices along a regular 128**3 grid
query_points = volume_query_points(self.volume, grid_size)
query_positions = query_points[None].repeat(1, 1, 1).to(device=device, dtype=self.mlp.dtype)
fields = []
for idx in range(0, query_positions.shape[1], query_batch_size):
query_batch = query_positions[:, idx : idx + query_batch_size]
model_out = self.mlp(
position=query_batch, direction=None, ts=None, nerf_level="fine", rendering_mode="stf"
)
fields.append(model_out.signed_distance)
# predicted SDF values
fields = torch.cat(fields, dim=1)
fields = fields.float()
assert (
len(fields.shape) == 3 and fields.shape[-1] == 1
), f"expected [meta_batch x inner_batch] SDF results, but got {fields.shape}"
fields = fields.reshape(1, *([grid_size] * 3))
# create grid 128 x 128 x 128
# - force a negative border around the SDFs to close off all the models.
full_grid = torch.zeros(
1,
grid_size + 2,
grid_size + 2,
grid_size + 2,
device=fields.device,
dtype=fields.dtype,
)
full_grid.fill_(-1.0)
full_grid[:, 1:-1, 1:-1, 1:-1] = fields
fields = full_grid
# apply a differentiable implementation of Marching Cubes to construct meshs
raw_meshes = []
mesh_mask = []
for field in fields:
raw_mesh = self.mesh_decoder(field, self.volume.bbox_min, self.volume.bbox_max - self.volume.bbox_min)
mesh_mask.append(True)
raw_meshes.append(raw_mesh)
mesh_mask = torch.tensor(mesh_mask, device=fields.device)
max_vertices = max(len(m.verts) for m in raw_meshes)
# 3.2. query the texture color head at each vertex of the resulting mesh.
texture_query_positions = torch.stack(
[m.verts[torch.arange(0, max_vertices) % len(m.verts)] for m in raw_meshes],
dim=0,
)
texture_query_positions = texture_query_positions.to(device=device, dtype=self.mlp.dtype)
textures = []
for idx in range(0, texture_query_positions.shape[1], query_batch_size):
query_batch = texture_query_positions[:, idx : idx + query_batch_size]
texture_model_out = self.mlp(
position=query_batch, direction=None, ts=None, nerf_level="fine", rendering_mode="stf"
)
textures.append(texture_model_out.channels)
# predict texture color
textures = torch.cat(textures, dim=1)
textures = _convert_srgb_to_linear(textures)
textures = textures.float()
# 3.3 augument the mesh with texture data
assert len(textures.shape) == 3 and textures.shape[-1] == len(
texture_channels
), f"expected [meta_batch x inner_batch x texture_channels] field results, but got {textures.shape}"
for m, texture in zip(raw_meshes, textures):
texture = texture[: len(m.verts)]
m.vertex_channels = dict(zip(texture_channels, texture.unbind(-1)))
return raw_meshes[0]
| diffusers/src/diffusers/pipelines/shap_e/renderer.py/0 | {
"file_path": "diffusers/src/diffusers/pipelines/shap_e/renderer.py",
"repo_id": "diffusers",
"token_count": 18166
} | 132 |
from dataclasses import dataclass
from typing import List, Optional, Union
import numpy as np
import PIL.Image
from ...utils import BaseOutput, is_flax_available
@dataclass
class StableDiffusionPipelineOutput(BaseOutput):
"""
Output class for Stable Diffusion pipelines.
Args:
images (`List[PIL.Image.Image]` or `np.ndarray`)
List of denoised PIL images of length `batch_size` or NumPy array of shape `(batch_size, height, width,
num_channels)`.
nsfw_content_detected (`List[bool]`)
List indicating whether the corresponding generated image contains "not-safe-for-work" (nsfw) content or
`None` if safety checking could not be performed.
"""
images: Union[List[PIL.Image.Image], np.ndarray]
nsfw_content_detected: Optional[List[bool]]
if is_flax_available():
import flax
@flax.struct.dataclass
class FlaxStableDiffusionPipelineOutput(BaseOutput):
"""
Output class for Flax-based Stable Diffusion pipelines.
Args:
images (`np.ndarray`):
Denoised images of array shape of `(batch_size, height, width, num_channels)`.
nsfw_content_detected (`List[bool]`):
List indicating whether the corresponding generated image contains "not-safe-for-work" (nsfw) content
or `None` if safety checking could not be performed.
"""
images: np.ndarray
nsfw_content_detected: List[bool]
| diffusers/src/diffusers/pipelines/stable_diffusion/pipeline_output.py/0 | {
"file_path": "diffusers/src/diffusers/pipelines/stable_diffusion/pipeline_output.py",
"repo_id": "diffusers",
"token_count": 598
} | 133 |
from dataclasses import dataclass
from typing import List, Union
import numpy as np
import PIL
import torch
from ...utils import (
BaseOutput,
)
@dataclass
class TextToVideoSDPipelineOutput(BaseOutput):
"""
Output class for text-to-video pipelines.
Args:
frames (`torch.Tensor`, `np.ndarray`, or List[List[PIL.Image.Image]]):
List of video outputs - It can be a nested list of length `batch_size,` with each sub-list containing denoised
PIL image sequences of length `num_frames.` It can also be a NumPy array or Torch tensor of shape
`(batch_size, num_frames, channels, height, width)`
"""
frames: Union[torch.Tensor, np.ndarray, List[List[PIL.Image.Image]]]
| diffusers/src/diffusers/pipelines/text_to_video_synthesis/pipeline_output.py/0 | {
"file_path": "diffusers/src/diffusers/pipelines/text_to_video_synthesis/pipeline_output.py",
"repo_id": "diffusers",
"token_count": 260
} | 134 |
# Copyright (c) 2023 Dominic Rampas MIT License
# Copyright 2024 The HuggingFace Team. All rights reserved.
#
# Licensed under the Apache License, Version 2.0 (the "License");
# you may not use this file except in compliance with the License.
# You may obtain a copy of the License at
#
# http://www.apache.org/licenses/LICENSE-2.0
#
# Unless required by applicable law or agreed to in writing, software
# distributed under the License is distributed on an "AS IS" BASIS,
# WITHOUT WARRANTIES OR CONDITIONS OF ANY KIND, either express or implied.
# See the License for the specific language governing permissions and
# limitations under the License.
import math
import numpy as np
import torch
import torch.nn as nn
from ...configuration_utils import ConfigMixin, register_to_config
from ...models.modeling_utils import ModelMixin
from .modeling_wuerstchen_common import AttnBlock, GlobalResponseNorm, TimestepBlock, WuerstchenLayerNorm
class WuerstchenDiffNeXt(ModelMixin, ConfigMixin):
@register_to_config
def __init__(
self,
c_in=4,
c_out=4,
c_r=64,
patch_size=2,
c_cond=1024,
c_hidden=[320, 640, 1280, 1280],
nhead=[-1, 10, 20, 20],
blocks=[4, 4, 14, 4],
level_config=["CT", "CTA", "CTA", "CTA"],
inject_effnet=[False, True, True, True],
effnet_embd=16,
clip_embd=1024,
kernel_size=3,
dropout=0.1,
):
super().__init__()
self.c_r = c_r
self.c_cond = c_cond
if not isinstance(dropout, list):
dropout = [dropout] * len(c_hidden)
# CONDITIONING
self.clip_mapper = nn.Linear(clip_embd, c_cond)
self.effnet_mappers = nn.ModuleList(
[
nn.Conv2d(effnet_embd, c_cond, kernel_size=1) if inject else None
for inject in inject_effnet + list(reversed(inject_effnet))
]
)
self.seq_norm = nn.LayerNorm(c_cond, elementwise_affine=False, eps=1e-6)
self.embedding = nn.Sequential(
nn.PixelUnshuffle(patch_size),
nn.Conv2d(c_in * (patch_size**2), c_hidden[0], kernel_size=1),
WuerstchenLayerNorm(c_hidden[0], elementwise_affine=False, eps=1e-6),
)
def get_block(block_type, c_hidden, nhead, c_skip=0, dropout=0):
if block_type == "C":
return ResBlockStageB(c_hidden, c_skip, kernel_size=kernel_size, dropout=dropout)
elif block_type == "A":
return AttnBlock(c_hidden, c_cond, nhead, self_attn=True, dropout=dropout)
elif block_type == "T":
return TimestepBlock(c_hidden, c_r)
else:
raise ValueError(f"Block type {block_type} not supported")
# BLOCKS
# -- down blocks
self.down_blocks = nn.ModuleList()
for i in range(len(c_hidden)):
down_block = nn.ModuleList()
if i > 0:
down_block.append(
nn.Sequential(
WuerstchenLayerNorm(c_hidden[i - 1], elementwise_affine=False, eps=1e-6),
nn.Conv2d(c_hidden[i - 1], c_hidden[i], kernel_size=2, stride=2),
)
)
for _ in range(blocks[i]):
for block_type in level_config[i]:
c_skip = c_cond if inject_effnet[i] else 0
down_block.append(get_block(block_type, c_hidden[i], nhead[i], c_skip=c_skip, dropout=dropout[i]))
self.down_blocks.append(down_block)
# -- up blocks
self.up_blocks = nn.ModuleList()
for i in reversed(range(len(c_hidden))):
up_block = nn.ModuleList()
for j in range(blocks[i]):
for k, block_type in enumerate(level_config[i]):
c_skip = c_hidden[i] if i < len(c_hidden) - 1 and j == k == 0 else 0
c_skip += c_cond if inject_effnet[i] else 0
up_block.append(get_block(block_type, c_hidden[i], nhead[i], c_skip=c_skip, dropout=dropout[i]))
if i > 0:
up_block.append(
nn.Sequential(
WuerstchenLayerNorm(c_hidden[i], elementwise_affine=False, eps=1e-6),
nn.ConvTranspose2d(c_hidden[i], c_hidden[i - 1], kernel_size=2, stride=2),
)
)
self.up_blocks.append(up_block)
# OUTPUT
self.clf = nn.Sequential(
WuerstchenLayerNorm(c_hidden[0], elementwise_affine=False, eps=1e-6),
nn.Conv2d(c_hidden[0], 2 * c_out * (patch_size**2), kernel_size=1),
nn.PixelShuffle(patch_size),
)
# --- WEIGHT INIT ---
self.apply(self._init_weights)
def _init_weights(self, m):
# General init
if isinstance(m, (nn.Conv2d, nn.Linear)):
nn.init.xavier_uniform_(m.weight)
if m.bias is not None:
nn.init.constant_(m.bias, 0)
for mapper in self.effnet_mappers:
if mapper is not None:
nn.init.normal_(mapper.weight, std=0.02) # conditionings
nn.init.normal_(self.clip_mapper.weight, std=0.02) # conditionings
nn.init.xavier_uniform_(self.embedding[1].weight, 0.02) # inputs
nn.init.constant_(self.clf[1].weight, 0) # outputs
# blocks
for level_block in self.down_blocks + self.up_blocks:
for block in level_block:
if isinstance(block, ResBlockStageB):
block.channelwise[-1].weight.data *= np.sqrt(1 / sum(self.config.blocks))
elif isinstance(block, TimestepBlock):
nn.init.constant_(block.mapper.weight, 0)
def gen_r_embedding(self, r, max_positions=10000):
r = r * max_positions
half_dim = self.c_r // 2
emb = math.log(max_positions) / (half_dim - 1)
emb = torch.arange(half_dim, device=r.device).float().mul(-emb).exp()
emb = r[:, None] * emb[None, :]
emb = torch.cat([emb.sin(), emb.cos()], dim=1)
if self.c_r % 2 == 1: # zero pad
emb = nn.functional.pad(emb, (0, 1), mode="constant")
return emb.to(dtype=r.dtype)
def gen_c_embeddings(self, clip):
clip = self.clip_mapper(clip)
clip = self.seq_norm(clip)
return clip
def _down_encode(self, x, r_embed, effnet, clip=None):
level_outputs = []
for i, down_block in enumerate(self.down_blocks):
effnet_c = None
for block in down_block:
if isinstance(block, ResBlockStageB):
if effnet_c is None and self.effnet_mappers[i] is not None:
dtype = effnet.dtype
effnet_c = self.effnet_mappers[i](
nn.functional.interpolate(
effnet.float(), size=x.shape[-2:], mode="bicubic", antialias=True, align_corners=True
).to(dtype)
)
skip = effnet_c if self.effnet_mappers[i] is not None else None
x = block(x, skip)
elif isinstance(block, AttnBlock):
x = block(x, clip)
elif isinstance(block, TimestepBlock):
x = block(x, r_embed)
else:
x = block(x)
level_outputs.insert(0, x)
return level_outputs
def _up_decode(self, level_outputs, r_embed, effnet, clip=None):
x = level_outputs[0]
for i, up_block in enumerate(self.up_blocks):
effnet_c = None
for j, block in enumerate(up_block):
if isinstance(block, ResBlockStageB):
if effnet_c is None and self.effnet_mappers[len(self.down_blocks) + i] is not None:
dtype = effnet.dtype
effnet_c = self.effnet_mappers[len(self.down_blocks) + i](
nn.functional.interpolate(
effnet.float(), size=x.shape[-2:], mode="bicubic", antialias=True, align_corners=True
).to(dtype)
)
skip = level_outputs[i] if j == 0 and i > 0 else None
if effnet_c is not None:
if skip is not None:
skip = torch.cat([skip, effnet_c], dim=1)
else:
skip = effnet_c
x = block(x, skip)
elif isinstance(block, AttnBlock):
x = block(x, clip)
elif isinstance(block, TimestepBlock):
x = block(x, r_embed)
else:
x = block(x)
return x
def forward(self, x, r, effnet, clip=None, x_cat=None, eps=1e-3, return_noise=True):
if x_cat is not None:
x = torch.cat([x, x_cat], dim=1)
# Process the conditioning embeddings
r_embed = self.gen_r_embedding(r)
if clip is not None:
clip = self.gen_c_embeddings(clip)
# Model Blocks
x_in = x
x = self.embedding(x)
level_outputs = self._down_encode(x, r_embed, effnet, clip)
x = self._up_decode(level_outputs, r_embed, effnet, clip)
a, b = self.clf(x).chunk(2, dim=1)
b = b.sigmoid() * (1 - eps * 2) + eps
if return_noise:
return (x_in - a) / b
else:
return a, b
class ResBlockStageB(nn.Module):
def __init__(self, c, c_skip=0, kernel_size=3, dropout=0.0):
super().__init__()
self.depthwise = nn.Conv2d(c, c, kernel_size=kernel_size, padding=kernel_size // 2, groups=c)
self.norm = WuerstchenLayerNorm(c, elementwise_affine=False, eps=1e-6)
self.channelwise = nn.Sequential(
nn.Linear(c + c_skip, c * 4),
nn.GELU(),
GlobalResponseNorm(c * 4),
nn.Dropout(dropout),
nn.Linear(c * 4, c),
)
def forward(self, x, x_skip=None):
x_res = x
x = self.norm(self.depthwise(x))
if x_skip is not None:
x = torch.cat([x, x_skip], dim=1)
x = self.channelwise(x.permute(0, 2, 3, 1)).permute(0, 3, 1, 2)
return x + x_res
| diffusers/src/diffusers/pipelines/wuerstchen/modeling_wuerstchen_diffnext.py/0 | {
"file_path": "diffusers/src/diffusers/pipelines/wuerstchen/modeling_wuerstchen_diffnext.py",
"repo_id": "diffusers",
"token_count": 5544
} | 135 |
# Copyright 2024 The HuggingFace Team. All rights reserved.
#
# Licensed under the Apache License, Version 2.0 (the "License");
# you may not use this file except in compliance with the License.
# You may obtain a copy of the License at
#
# http://www.apache.org/licenses/LICENSE-2.0
#
# Unless required by applicable law or agreed to in writing, software
# distributed under the License is distributed on an "AS IS" BASIS,
# WITHOUT WARRANTIES OR CONDITIONS OF ANY KIND, either express or implied.
# See the License for the specific language governing permissions and
# limitations under the License.
# DISCLAIMER: This code is strongly influenced by https://github.com/pesser/pytorch_diffusion
# and https://github.com/hojonathanho/diffusion
import math
from dataclasses import dataclass
from typing import List, Optional, Tuple, Union
import numpy as np
import torch
from diffusers.configuration_utils import ConfigMixin, register_to_config
from diffusers.schedulers.scheduling_utils import SchedulerMixin
from diffusers.utils import BaseOutput, deprecate
@dataclass
# Copied from diffusers.schedulers.scheduling_ddpm.DDPMSchedulerOutput with DDPM->DDIM
class DDIMSchedulerOutput(BaseOutput):
"""
Output class for the scheduler's `step` function output.
Args:
prev_sample (`torch.FloatTensor` of shape `(batch_size, num_channels, height, width)` for images):
Computed sample `(x_{t-1})` of previous timestep. `prev_sample` should be used as next model input in the
denoising loop.
pred_original_sample (`torch.FloatTensor` of shape `(batch_size, num_channels, height, width)` for images):
The predicted denoised sample `(x_{0})` based on the model output from the current timestep.
`pred_original_sample` can be used to preview progress or for guidance.
"""
prev_sample: torch.FloatTensor
pred_original_sample: Optional[torch.FloatTensor] = None
# Copied from diffusers.schedulers.scheduling_ddpm.betas_for_alpha_bar
def betas_for_alpha_bar(
num_diffusion_timesteps,
max_beta=0.999,
alpha_transform_type="cosine",
):
"""
Create a beta schedule that discretizes the given alpha_t_bar function, which defines the cumulative product of
(1-beta) over time from t = [0,1].
Contains a function alpha_bar that takes an argument t and transforms it to the cumulative product of (1-beta) up
to that part of the diffusion process.
Args:
num_diffusion_timesteps (`int`): the number of betas to produce.
max_beta (`float`): the maximum beta to use; use values lower than 1 to
prevent singularities.
alpha_transform_type (`str`, *optional*, default to `cosine`): the type of noise schedule for alpha_bar.
Choose from `cosine` or `exp`
Returns:
betas (`np.ndarray`): the betas used by the scheduler to step the model outputs
"""
if alpha_transform_type == "cosine":
def alpha_bar_fn(t):
return math.cos((t + 0.008) / 1.008 * math.pi / 2) ** 2
elif alpha_transform_type == "exp":
def alpha_bar_fn(t):
return math.exp(t * -12.0)
else:
raise ValueError(f"Unsupported alpha_transform_type: {alpha_transform_type}")
betas = []
for i in range(num_diffusion_timesteps):
t1 = i / num_diffusion_timesteps
t2 = (i + 1) / num_diffusion_timesteps
betas.append(min(1 - alpha_bar_fn(t2) / alpha_bar_fn(t1), max_beta))
return torch.tensor(betas, dtype=torch.float32)
# Copied from diffusers.schedulers.scheduling_ddim.rescale_zero_terminal_snr
def rescale_zero_terminal_snr(betas):
"""
Rescales betas to have zero terminal SNR Based on https://arxiv.org/pdf/2305.08891.pdf (Algorithm 1)
Args:
betas (`torch.FloatTensor`):
the betas that the scheduler is being initialized with.
Returns:
`torch.FloatTensor`: rescaled betas with zero terminal SNR
"""
# Convert betas to alphas_bar_sqrt
alphas = 1.0 - betas
alphas_cumprod = torch.cumprod(alphas, dim=0)
alphas_bar_sqrt = alphas_cumprod.sqrt()
# Store old values.
alphas_bar_sqrt_0 = alphas_bar_sqrt[0].clone()
alphas_bar_sqrt_T = alphas_bar_sqrt[-1].clone()
# Shift so the last timestep is zero.
alphas_bar_sqrt -= alphas_bar_sqrt_T
# Scale so the first timestep is back to the old value.
alphas_bar_sqrt *= alphas_bar_sqrt_0 / (alphas_bar_sqrt_0 - alphas_bar_sqrt_T)
# Convert alphas_bar_sqrt to betas
alphas_bar = alphas_bar_sqrt**2 # Revert sqrt
alphas = alphas_bar[1:] / alphas_bar[:-1] # Revert cumprod
alphas = torch.cat([alphas_bar[0:1], alphas])
betas = 1 - alphas
return betas
class DDIMInverseScheduler(SchedulerMixin, ConfigMixin):
"""
`DDIMInverseScheduler` is the reverse scheduler of [`DDIMScheduler`].
This model inherits from [`SchedulerMixin`] and [`ConfigMixin`]. Check the superclass documentation for the generic
methods the library implements for all schedulers such as loading and saving.
Args:
num_train_timesteps (`int`, defaults to 1000):
The number of diffusion steps to train the model.
beta_start (`float`, defaults to 0.0001):
The starting `beta` value of inference.
beta_end (`float`, defaults to 0.02):
The final `beta` value.
beta_schedule (`str`, defaults to `"linear"`):
The beta schedule, a mapping from a beta range to a sequence of betas for stepping the model. Choose from
`linear`, `scaled_linear`, or `squaredcos_cap_v2`.
trained_betas (`np.ndarray`, *optional*):
Pass an array of betas directly to the constructor to bypass `beta_start` and `beta_end`.
clip_sample (`bool`, defaults to `True`):
Clip the predicted sample for numerical stability.
clip_sample_range (`float`, defaults to 1.0):
The maximum magnitude for sample clipping. Valid only when `clip_sample=True`.
set_alpha_to_one (`bool`, defaults to `True`):
Each diffusion step uses the alphas product value at that step and at the previous one. For the final step
there is no previous alpha. When this option is `True` the previous alpha product is fixed to 0, otherwise
it uses the alpha value at step `num_train_timesteps - 1`.
steps_offset (`int`, defaults to 0):
An offset added to the inference steps, as required by some model families.
prediction_type (`str`, defaults to `epsilon`, *optional*):
Prediction type of the scheduler function; can be `epsilon` (predicts the noise of the diffusion process),
`sample` (directly predicts the noisy sample`) or `v_prediction` (see section 2.4 of [Imagen
Video](https://imagen.research.google/video/paper.pdf) paper).
timestep_spacing (`str`, defaults to `"leading"`):
The way the timesteps should be scaled. Refer to Table 2 of the [Common Diffusion Noise Schedules and
Sample Steps are Flawed](https://huggingface.co/papers/2305.08891) for more information.
rescale_betas_zero_snr (`bool`, defaults to `False`):
Whether to rescale the betas to have zero terminal SNR. This enables the model to generate very bright and
dark samples instead of limiting it to samples with medium brightness. Loosely related to
[`--offset_noise`](https://github.com/huggingface/diffusers/blob/74fd735eb073eb1d774b1ab4154a0876eb82f055/examples/dreambooth/train_dreambooth.py#L506).
"""
order = 1
ignore_for_config = ["kwargs"]
_deprecated_kwargs = ["set_alpha_to_zero"]
@register_to_config
def __init__(
self,
num_train_timesteps: int = 1000,
beta_start: float = 0.0001,
beta_end: float = 0.02,
beta_schedule: str = "linear",
trained_betas: Optional[Union[np.ndarray, List[float]]] = None,
clip_sample: bool = True,
set_alpha_to_one: bool = True,
steps_offset: int = 0,
prediction_type: str = "epsilon",
clip_sample_range: float = 1.0,
timestep_spacing: str = "leading",
rescale_betas_zero_snr: bool = False,
**kwargs,
):
if kwargs.get("set_alpha_to_zero", None) is not None:
deprecation_message = (
"The `set_alpha_to_zero` argument is deprecated. Please use `set_alpha_to_one` instead."
)
deprecate("set_alpha_to_zero", "1.0.0", deprecation_message, standard_warn=False)
set_alpha_to_one = kwargs["set_alpha_to_zero"]
if trained_betas is not None:
self.betas = torch.tensor(trained_betas, dtype=torch.float32)
elif beta_schedule == "linear":
self.betas = torch.linspace(beta_start, beta_end, num_train_timesteps, dtype=torch.float32)
elif beta_schedule == "scaled_linear":
# this schedule is very specific to the latent diffusion model.
self.betas = torch.linspace(beta_start**0.5, beta_end**0.5, num_train_timesteps, dtype=torch.float32) ** 2
elif beta_schedule == "squaredcos_cap_v2":
# Glide cosine schedule
self.betas = betas_for_alpha_bar(num_train_timesteps)
else:
raise NotImplementedError(f"{beta_schedule} does is not implemented for {self.__class__}")
# Rescale for zero SNR
if rescale_betas_zero_snr:
self.betas = rescale_zero_terminal_snr(self.betas)
self.alphas = 1.0 - self.betas
self.alphas_cumprod = torch.cumprod(self.alphas, dim=0)
# At every step in inverted ddim, we are looking into the next alphas_cumprod
# For the initial step, there is no current alphas_cumprod, and the index is out of bounds
# `set_alpha_to_one` decides whether we set this parameter simply to one
# in this case, self.step() just output the predicted noise
# or whether we use the initial alpha used in training the diffusion model.
self.initial_alpha_cumprod = torch.tensor(1.0) if set_alpha_to_one else self.alphas_cumprod[0]
# standard deviation of the initial noise distribution
self.init_noise_sigma = 1.0
# setable values
self.num_inference_steps = None
self.timesteps = torch.from_numpy(np.arange(0, num_train_timesteps).copy().astype(np.int64))
# Copied from diffusers.schedulers.scheduling_ddim.DDIMScheduler.scale_model_input
def scale_model_input(self, sample: torch.FloatTensor, timestep: Optional[int] = None) -> torch.FloatTensor:
"""
Ensures interchangeability with schedulers that need to scale the denoising model input depending on the
current timestep.
Args:
sample (`torch.FloatTensor`):
The input sample.
timestep (`int`, *optional*):
The current timestep in the diffusion chain.
Returns:
`torch.FloatTensor`:
A scaled input sample.
"""
return sample
def set_timesteps(self, num_inference_steps: int, device: Union[str, torch.device] = None):
"""
Sets the discrete timesteps used for the diffusion chain (to be run before inference).
Args:
num_inference_steps (`int`):
The number of diffusion steps used when generating samples with a pre-trained model.
"""
if num_inference_steps > self.config.num_train_timesteps:
raise ValueError(
f"`num_inference_steps`: {num_inference_steps} cannot be larger than `self.config.train_timesteps`:"
f" {self.config.num_train_timesteps} as the unet model trained with this scheduler can only handle"
f" maximal {self.config.num_train_timesteps} timesteps."
)
self.num_inference_steps = num_inference_steps
# "leading" and "trailing" corresponds to annotation of Table 1. of https://arxiv.org/abs/2305.08891
if self.config.timestep_spacing == "leading":
step_ratio = self.config.num_train_timesteps // self.num_inference_steps
# creates integer timesteps by multiplying by ratio
# casting to int to avoid issues when num_inference_step is power of 3
timesteps = (np.arange(0, num_inference_steps) * step_ratio).round().copy().astype(np.int64)
timesteps += self.config.steps_offset
elif self.config.timestep_spacing == "trailing":
step_ratio = self.config.num_train_timesteps / self.num_inference_steps
# creates integer timesteps by multiplying by ratio
# casting to int to avoid issues when num_inference_step is power of 3
timesteps = np.round(np.arange(self.config.num_train_timesteps, 0, -step_ratio)[::-1]).astype(np.int64)
timesteps -= 1
else:
raise ValueError(
f"{self.config.timestep_spacing} is not supported. Please make sure to choose one of 'leading' or 'trailing'."
)
self.timesteps = torch.from_numpy(timesteps).to(device)
def step(
self,
model_output: torch.FloatTensor,
timestep: int,
sample: torch.FloatTensor,
return_dict: bool = True,
) -> Union[DDIMSchedulerOutput, Tuple]:
"""
Predict the sample from the previous timestep by reversing the SDE. This function propagates the diffusion
process from the learned model outputs (most often the predicted noise).
Args:
model_output (`torch.FloatTensor`):
The direct output from learned diffusion model.
timestep (`float`):
The current discrete timestep in the diffusion chain.
sample (`torch.FloatTensor`):
A current instance of a sample created by the diffusion process.
eta (`float`):
The weight of noise for added noise in diffusion step.
use_clipped_model_output (`bool`, defaults to `False`):
If `True`, computes "corrected" `model_output` from the clipped predicted original sample. Necessary
because predicted original sample is clipped to [-1, 1] when `self.config.clip_sample` is `True`. If no
clipping has happened, "corrected" `model_output` would coincide with the one provided as input and
`use_clipped_model_output` has no effect.
variance_noise (`torch.FloatTensor`):
Alternative to generating noise with `generator` by directly providing the noise for the variance
itself. Useful for methods such as [`CycleDiffusion`].
return_dict (`bool`, *optional*, defaults to `True`):
Whether or not to return a [`~schedulers.scheduling_ddim_inverse.DDIMInverseSchedulerOutput`] or
`tuple`.
Returns:
[`~schedulers.scheduling_ddim_inverse.DDIMInverseSchedulerOutput`] or `tuple`:
If return_dict is `True`, [`~schedulers.scheduling_ddim_inverse.DDIMInverseSchedulerOutput`] is
returned, otherwise a tuple is returned where the first element is the sample tensor.
"""
# 1. get previous step value (=t+1)
prev_timestep = timestep
timestep = min(
timestep - self.config.num_train_timesteps // self.num_inference_steps, self.config.num_train_timesteps - 1
)
# 2. compute alphas, betas
# change original implementation to exactly match noise levels for analogous forward process
alpha_prod_t = self.alphas_cumprod[timestep] if timestep >= 0 else self.initial_alpha_cumprod
alpha_prod_t_prev = self.alphas_cumprod[prev_timestep]
beta_prod_t = 1 - alpha_prod_t
# 3. compute predicted original sample from predicted noise also called
# "predicted x_0" of formula (12) from https://arxiv.org/pdf/2010.02502.pdf
if self.config.prediction_type == "epsilon":
pred_original_sample = (sample - beta_prod_t ** (0.5) * model_output) / alpha_prod_t ** (0.5)
pred_epsilon = model_output
elif self.config.prediction_type == "sample":
pred_original_sample = model_output
pred_epsilon = (sample - alpha_prod_t ** (0.5) * pred_original_sample) / beta_prod_t ** (0.5)
elif self.config.prediction_type == "v_prediction":
pred_original_sample = (alpha_prod_t**0.5) * sample - (beta_prod_t**0.5) * model_output
pred_epsilon = (alpha_prod_t**0.5) * model_output + (beta_prod_t**0.5) * sample
else:
raise ValueError(
f"prediction_type given as {self.config.prediction_type} must be one of `epsilon`, `sample`, or"
" `v_prediction`"
)
# 4. Clip or threshold "predicted x_0"
if self.config.clip_sample:
pred_original_sample = pred_original_sample.clamp(
-self.config.clip_sample_range, self.config.clip_sample_range
)
# 5. compute "direction pointing to x_t" of formula (12) from https://arxiv.org/pdf/2010.02502.pdf
pred_sample_direction = (1 - alpha_prod_t_prev) ** (0.5) * pred_epsilon
# 6. compute x_t without "random noise" of formula (12) from https://arxiv.org/pdf/2010.02502.pdf
prev_sample = alpha_prod_t_prev ** (0.5) * pred_original_sample + pred_sample_direction
if not return_dict:
return (prev_sample, pred_original_sample)
return DDIMSchedulerOutput(prev_sample=prev_sample, pred_original_sample=pred_original_sample)
def __len__(self):
return self.config.num_train_timesteps
| diffusers/src/diffusers/schedulers/scheduling_ddim_inverse.py/0 | {
"file_path": "diffusers/src/diffusers/schedulers/scheduling_ddim_inverse.py",
"repo_id": "diffusers",
"token_count": 7323
} | 136 |
# Copyright 2024 Katherine Crowson and The HuggingFace Team. All rights reserved.
#
# Licensed under the Apache License, Version 2.0 (the "License");
# you may not use this file except in compliance with the License.
# You may obtain a copy of the License at
#
# http://www.apache.org/licenses/LICENSE-2.0
#
# Unless required by applicable law or agreed to in writing, software
# distributed under the License is distributed on an "AS IS" BASIS,
# WITHOUT WARRANTIES OR CONDITIONS OF ANY KIND, either express or implied.
# See the License for the specific language governing permissions and
# limitations under the License.
from dataclasses import dataclass
from typing import Optional, Tuple, Union
import flax
import jax.numpy as jnp
from ..configuration_utils import ConfigMixin, register_to_config
from .scheduling_utils_flax import (
CommonSchedulerState,
FlaxKarrasDiffusionSchedulers,
FlaxSchedulerMixin,
FlaxSchedulerOutput,
broadcast_to_shape_from_left,
)
@flax.struct.dataclass
class EulerDiscreteSchedulerState:
common: CommonSchedulerState
# setable values
init_noise_sigma: jnp.ndarray
timesteps: jnp.ndarray
sigmas: jnp.ndarray
num_inference_steps: Optional[int] = None
@classmethod
def create(
cls, common: CommonSchedulerState, init_noise_sigma: jnp.ndarray, timesteps: jnp.ndarray, sigmas: jnp.ndarray
):
return cls(common=common, init_noise_sigma=init_noise_sigma, timesteps=timesteps, sigmas=sigmas)
@dataclass
class FlaxEulerDiscreteSchedulerOutput(FlaxSchedulerOutput):
state: EulerDiscreteSchedulerState
class FlaxEulerDiscreteScheduler(FlaxSchedulerMixin, ConfigMixin):
"""
Euler scheduler (Algorithm 2) from Karras et al. (2022) https://arxiv.org/abs/2206.00364. . Based on the original
k-diffusion implementation by Katherine Crowson:
https://github.com/crowsonkb/k-diffusion/blob/481677d114f6ea445aa009cf5bd7a9cdee909e47/k_diffusion/sampling.py#L51
[`~ConfigMixin`] takes care of storing all config attributes that are passed in the scheduler's `__init__`
function, such as `num_train_timesteps`. They can be accessed via `scheduler.config.num_train_timesteps`.
[`SchedulerMixin`] provides general loading and saving functionality via the [`SchedulerMixin.save_pretrained`] and
[`~SchedulerMixin.from_pretrained`] functions.
Args:
num_train_timesteps (`int`): number of diffusion steps used to train the model.
beta_start (`float`): the starting `beta` value of inference.
beta_end (`float`): the final `beta` value.
beta_schedule (`str`):
the beta schedule, a mapping from a beta range to a sequence of betas for stepping the model. Choose from
`linear` or `scaled_linear`.
trained_betas (`jnp.ndarray`, optional):
option to pass an array of betas directly to the constructor to bypass `beta_start`, `beta_end` etc.
prediction_type (`str`, default `epsilon`, optional):
prediction type of the scheduler function, one of `epsilon` (predicting the noise of the diffusion
process), `sample` (directly predicting the noisy sample`) or `v_prediction` (see section 2.4
https://imagen.research.google/video/paper.pdf)
dtype (`jnp.dtype`, *optional*, defaults to `jnp.float32`):
the `dtype` used for params and computation.
"""
_compatibles = [e.name for e in FlaxKarrasDiffusionSchedulers]
dtype: jnp.dtype
@property
def has_state(self):
return True
@register_to_config
def __init__(
self,
num_train_timesteps: int = 1000,
beta_start: float = 0.0001,
beta_end: float = 0.02,
beta_schedule: str = "linear",
trained_betas: Optional[jnp.ndarray] = None,
prediction_type: str = "epsilon",
timestep_spacing: str = "linspace",
dtype: jnp.dtype = jnp.float32,
):
self.dtype = dtype
def create_state(self, common: Optional[CommonSchedulerState] = None) -> EulerDiscreteSchedulerState:
if common is None:
common = CommonSchedulerState.create(self)
timesteps = jnp.arange(0, self.config.num_train_timesteps).round()[::-1]
sigmas = ((1 - common.alphas_cumprod) / common.alphas_cumprod) ** 0.5
sigmas = jnp.interp(timesteps, jnp.arange(0, len(sigmas)), sigmas)
sigmas = jnp.concatenate([sigmas, jnp.array([0.0], dtype=self.dtype)])
# standard deviation of the initial noise distribution
if self.config.timestep_spacing in ["linspace", "trailing"]:
init_noise_sigma = sigmas.max()
else:
init_noise_sigma = (sigmas.max() ** 2 + 1) ** 0.5
return EulerDiscreteSchedulerState.create(
common=common,
init_noise_sigma=init_noise_sigma,
timesteps=timesteps,
sigmas=sigmas,
)
def scale_model_input(self, state: EulerDiscreteSchedulerState, sample: jnp.ndarray, timestep: int) -> jnp.ndarray:
"""
Scales the denoising model input by `(sigma**2 + 1) ** 0.5` to match the Euler algorithm.
Args:
state (`EulerDiscreteSchedulerState`):
the `FlaxEulerDiscreteScheduler` state data class instance.
sample (`jnp.ndarray`):
current instance of sample being created by diffusion process.
timestep (`int`):
current discrete timestep in the diffusion chain.
Returns:
`jnp.ndarray`: scaled input sample
"""
(step_index,) = jnp.where(state.timesteps == timestep, size=1)
step_index = step_index[0]
sigma = state.sigmas[step_index]
sample = sample / ((sigma**2 + 1) ** 0.5)
return sample
def set_timesteps(
self, state: EulerDiscreteSchedulerState, num_inference_steps: int, shape: Tuple = ()
) -> EulerDiscreteSchedulerState:
"""
Sets the timesteps used for the diffusion chain. Supporting function to be run before inference.
Args:
state (`EulerDiscreteSchedulerState`):
the `FlaxEulerDiscreteScheduler` state data class instance.
num_inference_steps (`int`):
the number of diffusion steps used when generating samples with a pre-trained model.
"""
if self.config.timestep_spacing == "linspace":
timesteps = jnp.linspace(self.config.num_train_timesteps - 1, 0, num_inference_steps, dtype=self.dtype)
elif self.config.timestep_spacing == "leading":
step_ratio = self.config.num_train_timesteps // num_inference_steps
timesteps = (jnp.arange(0, num_inference_steps) * step_ratio).round()[::-1].copy().astype(float)
timesteps += 1
else:
raise ValueError(
f"timestep_spacing must be one of ['linspace', 'leading'], got {self.config.timestep_spacing}"
)
sigmas = ((1 - state.common.alphas_cumprod) / state.common.alphas_cumprod) ** 0.5
sigmas = jnp.interp(timesteps, jnp.arange(0, len(sigmas)), sigmas)
sigmas = jnp.concatenate([sigmas, jnp.array([0.0], dtype=self.dtype)])
# standard deviation of the initial noise distribution
if self.config.timestep_spacing in ["linspace", "trailing"]:
init_noise_sigma = sigmas.max()
else:
init_noise_sigma = (sigmas.max() ** 2 + 1) ** 0.5
return state.replace(
timesteps=timesteps,
sigmas=sigmas,
num_inference_steps=num_inference_steps,
init_noise_sigma=init_noise_sigma,
)
def step(
self,
state: EulerDiscreteSchedulerState,
model_output: jnp.ndarray,
timestep: int,
sample: jnp.ndarray,
return_dict: bool = True,
) -> Union[FlaxEulerDiscreteSchedulerOutput, Tuple]:
"""
Predict the sample at the previous timestep by reversing the SDE. Core function to propagate the diffusion
process from the learned model outputs (most often the predicted noise).
Args:
state (`EulerDiscreteSchedulerState`):
the `FlaxEulerDiscreteScheduler` state data class instance.
model_output (`jnp.ndarray`): direct output from learned diffusion model.
timestep (`int`): current discrete timestep in the diffusion chain.
sample (`jnp.ndarray`):
current instance of sample being created by diffusion process.
order: coefficient for multi-step inference.
return_dict (`bool`): option for returning tuple rather than FlaxEulerDiscreteScheduler class
Returns:
[`FlaxEulerDiscreteScheduler`] or `tuple`: [`FlaxEulerDiscreteScheduler`] if `return_dict` is True,
otherwise a `tuple`. When returning a tuple, the first element is the sample tensor.
"""
if state.num_inference_steps is None:
raise ValueError(
"Number of inference steps is 'None', you need to run 'set_timesteps' after creating the scheduler"
)
(step_index,) = jnp.where(state.timesteps == timestep, size=1)
step_index = step_index[0]
sigma = state.sigmas[step_index]
# 1. compute predicted original sample (x_0) from sigma-scaled predicted noise
if self.config.prediction_type == "epsilon":
pred_original_sample = sample - sigma * model_output
elif self.config.prediction_type == "v_prediction":
# * c_out + input * c_skip
pred_original_sample = model_output * (-sigma / (sigma**2 + 1) ** 0.5) + (sample / (sigma**2 + 1))
else:
raise ValueError(
f"prediction_type given as {self.config.prediction_type} must be one of `epsilon`, or `v_prediction`"
)
# 2. Convert to an ODE derivative
derivative = (sample - pred_original_sample) / sigma
# dt = sigma_down - sigma
dt = state.sigmas[step_index + 1] - sigma
prev_sample = sample + derivative * dt
if not return_dict:
return (prev_sample, state)
return FlaxEulerDiscreteSchedulerOutput(prev_sample=prev_sample, state=state)
def add_noise(
self,
state: EulerDiscreteSchedulerState,
original_samples: jnp.ndarray,
noise: jnp.ndarray,
timesteps: jnp.ndarray,
) -> jnp.ndarray:
sigma = state.sigmas[timesteps].flatten()
sigma = broadcast_to_shape_from_left(sigma, noise.shape)
noisy_samples = original_samples + noise * sigma
return noisy_samples
def __len__(self):
return self.config.num_train_timesteps
| diffusers/src/diffusers/schedulers/scheduling_euler_discrete_flax.py/0 | {
"file_path": "diffusers/src/diffusers/schedulers/scheduling_euler_discrete_flax.py",
"repo_id": "diffusers",
"token_count": 4573
} | 137 |
# Copyright 2024 Kakao Brain and The HuggingFace Team. All rights reserved.
#
# Licensed under the Apache License, Version 2.0 (the "License");
# you may not use this file except in compliance with the License.
# You may obtain a copy of the License at
#
# http://www.apache.org/licenses/LICENSE-2.0
#
# Unless required by applicable law or agreed to in writing, software
# distributed under the License is distributed on an "AS IS" BASIS,
# WITHOUT WARRANTIES OR CONDITIONS OF ANY KIND, either express or implied.
# See the License for the specific language governing permissions and
# limitations under the License.
import math
from dataclasses import dataclass
from typing import Optional, Tuple, Union
import numpy as np
import torch
from ..configuration_utils import ConfigMixin, register_to_config
from ..utils import BaseOutput
from ..utils.torch_utils import randn_tensor
from .scheduling_utils import SchedulerMixin
@dataclass
# Copied from diffusers.schedulers.scheduling_ddpm.DDPMSchedulerOutput with DDPM->UnCLIP
class UnCLIPSchedulerOutput(BaseOutput):
"""
Output class for the scheduler's `step` function output.
Args:
prev_sample (`torch.FloatTensor` of shape `(batch_size, num_channels, height, width)` for images):
Computed sample `(x_{t-1})` of previous timestep. `prev_sample` should be used as next model input in the
denoising loop.
pred_original_sample (`torch.FloatTensor` of shape `(batch_size, num_channels, height, width)` for images):
The predicted denoised sample `(x_{0})` based on the model output from the current timestep.
`pred_original_sample` can be used to preview progress or for guidance.
"""
prev_sample: torch.FloatTensor
pred_original_sample: Optional[torch.FloatTensor] = None
# Copied from diffusers.schedulers.scheduling_ddpm.betas_for_alpha_bar
def betas_for_alpha_bar(
num_diffusion_timesteps,
max_beta=0.999,
alpha_transform_type="cosine",
):
"""
Create a beta schedule that discretizes the given alpha_t_bar function, which defines the cumulative product of
(1-beta) over time from t = [0,1].
Contains a function alpha_bar that takes an argument t and transforms it to the cumulative product of (1-beta) up
to that part of the diffusion process.
Args:
num_diffusion_timesteps (`int`): the number of betas to produce.
max_beta (`float`): the maximum beta to use; use values lower than 1 to
prevent singularities.
alpha_transform_type (`str`, *optional*, default to `cosine`): the type of noise schedule for alpha_bar.
Choose from `cosine` or `exp`
Returns:
betas (`np.ndarray`): the betas used by the scheduler to step the model outputs
"""
if alpha_transform_type == "cosine":
def alpha_bar_fn(t):
return math.cos((t + 0.008) / 1.008 * math.pi / 2) ** 2
elif alpha_transform_type == "exp":
def alpha_bar_fn(t):
return math.exp(t * -12.0)
else:
raise ValueError(f"Unsupported alpha_transform_type: {alpha_transform_type}")
betas = []
for i in range(num_diffusion_timesteps):
t1 = i / num_diffusion_timesteps
t2 = (i + 1) / num_diffusion_timesteps
betas.append(min(1 - alpha_bar_fn(t2) / alpha_bar_fn(t1), max_beta))
return torch.tensor(betas, dtype=torch.float32)
class UnCLIPScheduler(SchedulerMixin, ConfigMixin):
"""
NOTE: do not use this scheduler. The DDPM scheduler has been updated to support the changes made here. This
scheduler will be removed and replaced with DDPM.
This is a modified DDPM Scheduler specifically for the karlo unCLIP model.
This scheduler has some minor variations in how it calculates the learned range variance and dynamically
re-calculates betas based off the timesteps it is skipping.
The scheduler also uses a slightly different step ratio when computing timesteps to use for inference.
See [`~DDPMScheduler`] for more information on DDPM scheduling
Args:
num_train_timesteps (`int`): number of diffusion steps used to train the model.
variance_type (`str`):
options to clip the variance used when adding noise to the denoised sample. Choose from `fixed_small_log`
or `learned_range`.
clip_sample (`bool`, default `True`):
option to clip predicted sample between `-clip_sample_range` and `clip_sample_range` for numerical
stability.
clip_sample_range (`float`, default `1.0`):
The range to clip the sample between. See `clip_sample`.
prediction_type (`str`, default `epsilon`, optional):
prediction type of the scheduler function, one of `epsilon` (predicting the noise of the diffusion process)
or `sample` (directly predicting the noisy sample`)
"""
@register_to_config
def __init__(
self,
num_train_timesteps: int = 1000,
variance_type: str = "fixed_small_log",
clip_sample: bool = True,
clip_sample_range: Optional[float] = 1.0,
prediction_type: str = "epsilon",
beta_schedule: str = "squaredcos_cap_v2",
):
if beta_schedule != "squaredcos_cap_v2":
raise ValueError("UnCLIPScheduler only supports `beta_schedule`: 'squaredcos_cap_v2'")
self.betas = betas_for_alpha_bar(num_train_timesteps)
self.alphas = 1.0 - self.betas
self.alphas_cumprod = torch.cumprod(self.alphas, dim=0)
self.one = torch.tensor(1.0)
# standard deviation of the initial noise distribution
self.init_noise_sigma = 1.0
# setable values
self.num_inference_steps = None
self.timesteps = torch.from_numpy(np.arange(0, num_train_timesteps)[::-1].copy())
self.variance_type = variance_type
def scale_model_input(self, sample: torch.FloatTensor, timestep: Optional[int] = None) -> torch.FloatTensor:
"""
Ensures interchangeability with schedulers that need to scale the denoising model input depending on the
current timestep.
Args:
sample (`torch.FloatTensor`): input sample
timestep (`int`, optional): current timestep
Returns:
`torch.FloatTensor`: scaled input sample
"""
return sample
def set_timesteps(self, num_inference_steps: int, device: Union[str, torch.device] = None):
"""
Sets the discrete timesteps used for the diffusion chain. Supporting function to be run before inference.
Note that this scheduler uses a slightly different step ratio than the other diffusers schedulers. The
different step ratio is to mimic the original karlo implementation and does not affect the quality or accuracy
of the results.
Args:
num_inference_steps (`int`):
the number of diffusion steps used when generating samples with a pre-trained model.
"""
self.num_inference_steps = num_inference_steps
step_ratio = (self.config.num_train_timesteps - 1) / (self.num_inference_steps - 1)
timesteps = (np.arange(0, num_inference_steps) * step_ratio).round()[::-1].copy().astype(np.int64)
self.timesteps = torch.from_numpy(timesteps).to(device)
def _get_variance(self, t, prev_timestep=None, predicted_variance=None, variance_type=None):
if prev_timestep is None:
prev_timestep = t - 1
alpha_prod_t = self.alphas_cumprod[t]
alpha_prod_t_prev = self.alphas_cumprod[prev_timestep] if prev_timestep >= 0 else self.one
beta_prod_t = 1 - alpha_prod_t
beta_prod_t_prev = 1 - alpha_prod_t_prev
if prev_timestep == t - 1:
beta = self.betas[t]
else:
beta = 1 - alpha_prod_t / alpha_prod_t_prev
# For t > 0, compute predicted variance βt (see formula (6) and (7) from https://arxiv.org/pdf/2006.11239.pdf)
# and sample from it to get previous sample
# x_{t-1} ~ N(pred_prev_sample, variance) == add variance to pred_sample
variance = beta_prod_t_prev / beta_prod_t * beta
if variance_type is None:
variance_type = self.config.variance_type
# hacks - were probably added for training stability
if variance_type == "fixed_small_log":
variance = torch.log(torch.clamp(variance, min=1e-20))
variance = torch.exp(0.5 * variance)
elif variance_type == "learned_range":
# NOTE difference with DDPM scheduler
min_log = variance.log()
max_log = beta.log()
frac = (predicted_variance + 1) / 2
variance = frac * max_log + (1 - frac) * min_log
return variance
def step(
self,
model_output: torch.FloatTensor,
timestep: int,
sample: torch.FloatTensor,
prev_timestep: Optional[int] = None,
generator=None,
return_dict: bool = True,
) -> Union[UnCLIPSchedulerOutput, Tuple]:
"""
Predict the sample at the previous timestep by reversing the SDE. Core function to propagate the diffusion
process from the learned model outputs (most often the predicted noise).
Args:
model_output (`torch.FloatTensor`): direct output from learned diffusion model.
timestep (`int`): current discrete timestep in the diffusion chain.
sample (`torch.FloatTensor`):
current instance of sample being created by diffusion process.
prev_timestep (`int`, *optional*): The previous timestep to predict the previous sample at.
Used to dynamically compute beta. If not given, `t-1` is used and the pre-computed beta is used.
generator: random number generator.
return_dict (`bool`): option for returning tuple rather than UnCLIPSchedulerOutput class
Returns:
[`~schedulers.scheduling_utils.UnCLIPSchedulerOutput`] or `tuple`:
[`~schedulers.scheduling_utils.UnCLIPSchedulerOutput`] if `return_dict` is True, otherwise a `tuple`. When
returning a tuple, the first element is the sample tensor.
"""
t = timestep
if model_output.shape[1] == sample.shape[1] * 2 and self.variance_type == "learned_range":
model_output, predicted_variance = torch.split(model_output, sample.shape[1], dim=1)
else:
predicted_variance = None
# 1. compute alphas, betas
if prev_timestep is None:
prev_timestep = t - 1
alpha_prod_t = self.alphas_cumprod[t]
alpha_prod_t_prev = self.alphas_cumprod[prev_timestep] if prev_timestep >= 0 else self.one
beta_prod_t = 1 - alpha_prod_t
beta_prod_t_prev = 1 - alpha_prod_t_prev
if prev_timestep == t - 1:
beta = self.betas[t]
alpha = self.alphas[t]
else:
beta = 1 - alpha_prod_t / alpha_prod_t_prev
alpha = 1 - beta
# 2. compute predicted original sample from predicted noise also called
# "predicted x_0" of formula (15) from https://arxiv.org/pdf/2006.11239.pdf
if self.config.prediction_type == "epsilon":
pred_original_sample = (sample - beta_prod_t ** (0.5) * model_output) / alpha_prod_t ** (0.5)
elif self.config.prediction_type == "sample":
pred_original_sample = model_output
else:
raise ValueError(
f"prediction_type given as {self.config.prediction_type} must be one of `epsilon` or `sample`"
" for the UnCLIPScheduler."
)
# 3. Clip "predicted x_0"
if self.config.clip_sample:
pred_original_sample = torch.clamp(
pred_original_sample, -self.config.clip_sample_range, self.config.clip_sample_range
)
# 4. Compute coefficients for pred_original_sample x_0 and current sample x_t
# See formula (7) from https://arxiv.org/pdf/2006.11239.pdf
pred_original_sample_coeff = (alpha_prod_t_prev ** (0.5) * beta) / beta_prod_t
current_sample_coeff = alpha ** (0.5) * beta_prod_t_prev / beta_prod_t
# 5. Compute predicted previous sample µ_t
# See formula (7) from https://arxiv.org/pdf/2006.11239.pdf
pred_prev_sample = pred_original_sample_coeff * pred_original_sample + current_sample_coeff * sample
# 6. Add noise
variance = 0
if t > 0:
variance_noise = randn_tensor(
model_output.shape, dtype=model_output.dtype, generator=generator, device=model_output.device
)
variance = self._get_variance(
t,
predicted_variance=predicted_variance,
prev_timestep=prev_timestep,
)
if self.variance_type == "fixed_small_log":
variance = variance
elif self.variance_type == "learned_range":
variance = (0.5 * variance).exp()
else:
raise ValueError(
f"variance_type given as {self.variance_type} must be one of `fixed_small_log` or `learned_range`"
" for the UnCLIPScheduler."
)
variance = variance * variance_noise
pred_prev_sample = pred_prev_sample + variance
if not return_dict:
return (pred_prev_sample,)
return UnCLIPSchedulerOutput(prev_sample=pred_prev_sample, pred_original_sample=pred_original_sample)
# Copied from diffusers.schedulers.scheduling_ddpm.DDPMScheduler.add_noise
def add_noise(
self,
original_samples: torch.FloatTensor,
noise: torch.FloatTensor,
timesteps: torch.IntTensor,
) -> torch.FloatTensor:
# Make sure alphas_cumprod and timestep have same device and dtype as original_samples
# Move the self.alphas_cumprod to device to avoid redundant CPU to GPU data movement
# for the subsequent add_noise calls
self.alphas_cumprod = self.alphas_cumprod.to(device=original_samples.device)
alphas_cumprod = self.alphas_cumprod.to(dtype=original_samples.dtype)
timesteps = timesteps.to(original_samples.device)
sqrt_alpha_prod = alphas_cumprod[timesteps] ** 0.5
sqrt_alpha_prod = sqrt_alpha_prod.flatten()
while len(sqrt_alpha_prod.shape) < len(original_samples.shape):
sqrt_alpha_prod = sqrt_alpha_prod.unsqueeze(-1)
sqrt_one_minus_alpha_prod = (1 - alphas_cumprod[timesteps]) ** 0.5
sqrt_one_minus_alpha_prod = sqrt_one_minus_alpha_prod.flatten()
while len(sqrt_one_minus_alpha_prod.shape) < len(original_samples.shape):
sqrt_one_minus_alpha_prod = sqrt_one_minus_alpha_prod.unsqueeze(-1)
noisy_samples = sqrt_alpha_prod * original_samples + sqrt_one_minus_alpha_prod * noise
return noisy_samples
| diffusers/src/diffusers/schedulers/scheduling_unclip.py/0 | {
"file_path": "diffusers/src/diffusers/schedulers/scheduling_unclip.py",
"repo_id": "diffusers",
"token_count": 6252
} | 138 |
# This file is autogenerated by the command `make fix-copies`, do not edit.
from ..utils import DummyObject, requires_backends
class AudioDiffusionPipeline(metaclass=DummyObject):
_backends = ["torch", "librosa"]
def __init__(self, *args, **kwargs):
requires_backends(self, ["torch", "librosa"])
@classmethod
def from_config(cls, *args, **kwargs):
requires_backends(cls, ["torch", "librosa"])
@classmethod
def from_pretrained(cls, *args, **kwargs):
requires_backends(cls, ["torch", "librosa"])
class Mel(metaclass=DummyObject):
_backends = ["torch", "librosa"]
def __init__(self, *args, **kwargs):
requires_backends(self, ["torch", "librosa"])
@classmethod
def from_config(cls, *args, **kwargs):
requires_backends(cls, ["torch", "librosa"])
@classmethod
def from_pretrained(cls, *args, **kwargs):
requires_backends(cls, ["torch", "librosa"])
| diffusers/src/diffusers/utils/dummy_torch_and_librosa_objects.py/0 | {
"file_path": "diffusers/src/diffusers/utils/dummy_torch_and_librosa_objects.py",
"repo_id": "diffusers",
"token_count": 397
} | 139 |
from typing import List
import PIL.Image
import PIL.ImageOps
from packaging import version
from PIL import Image
if version.parse(version.parse(PIL.__version__).base_version) >= version.parse("9.1.0"):
PIL_INTERPOLATION = {
"linear": PIL.Image.Resampling.BILINEAR,
"bilinear": PIL.Image.Resampling.BILINEAR,
"bicubic": PIL.Image.Resampling.BICUBIC,
"lanczos": PIL.Image.Resampling.LANCZOS,
"nearest": PIL.Image.Resampling.NEAREST,
}
else:
PIL_INTERPOLATION = {
"linear": PIL.Image.LINEAR,
"bilinear": PIL.Image.BILINEAR,
"bicubic": PIL.Image.BICUBIC,
"lanczos": PIL.Image.LANCZOS,
"nearest": PIL.Image.NEAREST,
}
def pt_to_pil(images):
"""
Convert a torch image to a PIL image.
"""
images = (images / 2 + 0.5).clamp(0, 1)
images = images.cpu().permute(0, 2, 3, 1).float().numpy()
images = numpy_to_pil(images)
return images
def numpy_to_pil(images):
"""
Convert a numpy image or a batch of images to a PIL image.
"""
if images.ndim == 3:
images = images[None, ...]
images = (images * 255).round().astype("uint8")
if images.shape[-1] == 1:
# special case for grayscale (single channel) images
pil_images = [Image.fromarray(image.squeeze(), mode="L") for image in images]
else:
pil_images = [Image.fromarray(image) for image in images]
return pil_images
def make_image_grid(images: List[PIL.Image.Image], rows: int, cols: int, resize: int = None) -> PIL.Image.Image:
"""
Prepares a single grid of images. Useful for visualization purposes.
"""
assert len(images) == rows * cols
if resize is not None:
images = [img.resize((resize, resize)) for img in images]
w, h = images[0].size
grid = Image.new("RGB", size=(cols * w, rows * h))
for i, img in enumerate(images):
grid.paste(img, box=(i % cols * w, i // cols * h))
return grid
| diffusers/src/diffusers/utils/pil_utils.py/0 | {
"file_path": "diffusers/src/diffusers/utils/pil_utils.py",
"repo_id": "diffusers",
"token_count": 849
} | 140 |
import unittest
from diffusers import FlaxAutoencoderKL
from diffusers.utils import is_flax_available
from diffusers.utils.testing_utils import require_flax
from ..test_modeling_common_flax import FlaxModelTesterMixin
if is_flax_available():
import jax
@require_flax
class FlaxAutoencoderKLTests(FlaxModelTesterMixin, unittest.TestCase):
model_class = FlaxAutoencoderKL
@property
def dummy_input(self):
batch_size = 4
num_channels = 3
sizes = (32, 32)
prng_key = jax.random.PRNGKey(0)
image = jax.random.uniform(prng_key, ((batch_size, num_channels) + sizes))
return {"sample": image, "prng_key": prng_key}
def prepare_init_args_and_inputs_for_common(self):
init_dict = {
"block_out_channels": [32, 64],
"in_channels": 3,
"out_channels": 3,
"down_block_types": ["DownEncoderBlock2D", "DownEncoderBlock2D"],
"up_block_types": ["UpDecoderBlock2D", "UpDecoderBlock2D"],
"latent_channels": 4,
}
inputs_dict = self.dummy_input
return init_dict, inputs_dict
| diffusers/tests/models/autoencoders/test_models_vae_flax.py/0 | {
"file_path": "diffusers/tests/models/autoencoders/test_models_vae_flax.py",
"repo_id": "diffusers",
"token_count": 513
} | 141 |
# coding=utf-8
# Copyright 2024 HuggingFace Inc.
#
# Licensed under the Apache License, Version 2.0 (the "License");
# you may not use this file except in compliance with the License.
# You may obtain a copy of the License at
#
# http://www.apache.org/licenses/LICENSE-2.0
#
# Unless required by applicable law or agreed to in writing, software
# distributed under the License is distributed on an "AS IS" BASIS,
# WITHOUT WARRANTIES OR CONDITIONS OF ANY KIND, either express or implied.
# See the License for the specific language governing permissions and
# limitations under the License.
import copy
import unittest
import torch
from diffusers import UNetSpatioTemporalConditionModel
from diffusers.utils import logging
from diffusers.utils.import_utils import is_xformers_available
from diffusers.utils.testing_utils import (
enable_full_determinism,
floats_tensor,
torch_all_close,
torch_device,
)
from ..test_modeling_common import ModelTesterMixin, UNetTesterMixin
logger = logging.get_logger(__name__)
enable_full_determinism()
class UNetSpatioTemporalConditionModelTests(ModelTesterMixin, UNetTesterMixin, unittest.TestCase):
model_class = UNetSpatioTemporalConditionModel
main_input_name = "sample"
@property
def dummy_input(self):
batch_size = 2
num_frames = 2
num_channels = 4
sizes = (32, 32)
noise = floats_tensor((batch_size, num_frames, num_channels) + sizes).to(torch_device)
time_step = torch.tensor([10]).to(torch_device)
encoder_hidden_states = floats_tensor((batch_size, 1, 32)).to(torch_device)
return {
"sample": noise,
"timestep": time_step,
"encoder_hidden_states": encoder_hidden_states,
"added_time_ids": self._get_add_time_ids(),
}
@property
def input_shape(self):
return (2, 2, 4, 32, 32)
@property
def output_shape(self):
return (4, 32, 32)
@property
def fps(self):
return 6
@property
def motion_bucket_id(self):
return 127
@property
def noise_aug_strength(self):
return 0.02
@property
def addition_time_embed_dim(self):
return 32
def prepare_init_args_and_inputs_for_common(self):
init_dict = {
"block_out_channels": (32, 64),
"down_block_types": (
"CrossAttnDownBlockSpatioTemporal",
"DownBlockSpatioTemporal",
),
"up_block_types": (
"UpBlockSpatioTemporal",
"CrossAttnUpBlockSpatioTemporal",
),
"cross_attention_dim": 32,
"num_attention_heads": 8,
"out_channels": 4,
"in_channels": 4,
"layers_per_block": 2,
"sample_size": 32,
"projection_class_embeddings_input_dim": self.addition_time_embed_dim * 3,
"addition_time_embed_dim": self.addition_time_embed_dim,
}
inputs_dict = self.dummy_input
return init_dict, inputs_dict
def _get_add_time_ids(self, do_classifier_free_guidance=True):
add_time_ids = [self.fps, self.motion_bucket_id, self.noise_aug_strength]
passed_add_embed_dim = self.addition_time_embed_dim * len(add_time_ids)
expected_add_embed_dim = self.addition_time_embed_dim * 3
if expected_add_embed_dim != passed_add_embed_dim:
raise ValueError(
f"Model expects an added time embedding vector of length {expected_add_embed_dim}, but a vector of {passed_add_embed_dim} was created. The model has an incorrect config. Please check `unet.config.time_embedding_type` and `text_encoder_2.config.projection_dim`."
)
add_time_ids = torch.tensor([add_time_ids], device=torch_device)
add_time_ids = add_time_ids.repeat(1, 1)
if do_classifier_free_guidance:
add_time_ids = torch.cat([add_time_ids, add_time_ids])
return add_time_ids
@unittest.skip("Number of Norm Groups is not configurable")
def test_forward_with_norm_groups(self):
pass
@unittest.skip("Deprecated functionality")
def test_model_attention_slicing(self):
pass
@unittest.skip("Not supported")
def test_model_with_use_linear_projection(self):
pass
@unittest.skip("Not supported")
def test_model_with_simple_projection(self):
pass
@unittest.skip("Not supported")
def test_model_with_class_embeddings_concat(self):
pass
@unittest.skipIf(
torch_device != "cuda" or not is_xformers_available(),
reason="XFormers attention is only available with CUDA and `xformers` installed",
)
def test_xformers_enable_works(self):
init_dict, inputs_dict = self.prepare_init_args_and_inputs_for_common()
model = self.model_class(**init_dict)
model.enable_xformers_memory_efficient_attention()
assert (
model.mid_block.attentions[0].transformer_blocks[0].attn1.processor.__class__.__name__
== "XFormersAttnProcessor"
), "xformers is not enabled"
@unittest.skipIf(torch_device == "mps", "Gradient checkpointing skipped on MPS")
def test_gradient_checkpointing(self):
# enable deterministic behavior for gradient checkpointing
init_dict, inputs_dict = self.prepare_init_args_and_inputs_for_common()
model = self.model_class(**init_dict)
model.to(torch_device)
assert not model.is_gradient_checkpointing and model.training
out = model(**inputs_dict).sample
# run the backwards pass on the model. For backwards pass, for simplicity purpose,
# we won't calculate the loss and rather backprop on out.sum()
model.zero_grad()
labels = torch.randn_like(out)
loss = (out - labels).mean()
loss.backward()
# re-instantiate the model now enabling gradient checkpointing
model_2 = self.model_class(**init_dict)
# clone model
model_2.load_state_dict(model.state_dict())
model_2.to(torch_device)
model_2.enable_gradient_checkpointing()
assert model_2.is_gradient_checkpointing and model_2.training
out_2 = model_2(**inputs_dict).sample
# run the backwards pass on the model. For backwards pass, for simplicity purpose,
# we won't calculate the loss and rather backprop on out.sum()
model_2.zero_grad()
loss_2 = (out_2 - labels).mean()
loss_2.backward()
# compare the output and parameters gradients
self.assertTrue((loss - loss_2).abs() < 1e-5)
named_params = dict(model.named_parameters())
named_params_2 = dict(model_2.named_parameters())
for name, param in named_params.items():
self.assertTrue(torch_all_close(param.grad.data, named_params_2[name].grad.data, atol=5e-5))
def test_model_with_num_attention_heads_tuple(self):
init_dict, inputs_dict = self.prepare_init_args_and_inputs_for_common()
init_dict["num_attention_heads"] = (8, 16)
model = self.model_class(**init_dict)
model.to(torch_device)
model.eval()
with torch.no_grad():
output = model(**inputs_dict)
if isinstance(output, dict):
output = output.sample
self.assertIsNotNone(output)
expected_shape = inputs_dict["sample"].shape
self.assertEqual(output.shape, expected_shape, "Input and output shapes do not match")
def test_model_with_cross_attention_dim_tuple(self):
init_dict, inputs_dict = self.prepare_init_args_and_inputs_for_common()
init_dict["cross_attention_dim"] = (32, 32)
model = self.model_class(**init_dict)
model.to(torch_device)
model.eval()
with torch.no_grad():
output = model(**inputs_dict)
if isinstance(output, dict):
output = output.sample
self.assertIsNotNone(output)
expected_shape = inputs_dict["sample"].shape
self.assertEqual(output.shape, expected_shape, "Input and output shapes do not match")
def test_gradient_checkpointing_is_applied(self):
init_dict, inputs_dict = self.prepare_init_args_and_inputs_for_common()
init_dict["num_attention_heads"] = (8, 16)
model_class_copy = copy.copy(self.model_class)
modules_with_gc_enabled = {}
# now monkey patch the following function:
# def _set_gradient_checkpointing(self, module, value=False):
# if hasattr(module, "gradient_checkpointing"):
# module.gradient_checkpointing = value
def _set_gradient_checkpointing_new(self, module, value=False):
if hasattr(module, "gradient_checkpointing"):
module.gradient_checkpointing = value
modules_with_gc_enabled[module.__class__.__name__] = True
model_class_copy._set_gradient_checkpointing = _set_gradient_checkpointing_new
model = model_class_copy(**init_dict)
model.enable_gradient_checkpointing()
EXPECTED_SET = {
"TransformerSpatioTemporalModel",
"CrossAttnDownBlockSpatioTemporal",
"DownBlockSpatioTemporal",
"UpBlockSpatioTemporal",
"CrossAttnUpBlockSpatioTemporal",
"UNetMidBlockSpatioTemporal",
}
assert set(modules_with_gc_enabled.keys()) == EXPECTED_SET
assert all(modules_with_gc_enabled.values()), "All modules should be enabled"
def test_pickle(self):
# enable deterministic behavior for gradient checkpointing
init_dict, inputs_dict = self.prepare_init_args_and_inputs_for_common()
init_dict["num_attention_heads"] = (8, 16)
model = self.model_class(**init_dict)
model.to(torch_device)
with torch.no_grad():
sample = model(**inputs_dict).sample
sample_copy = copy.copy(sample)
assert (sample - sample_copy).abs().max() < 1e-4
| diffusers/tests/models/unets/test_models_unet_spatiotemporal.py/0 | {
"file_path": "diffusers/tests/models/unets/test_models_unet_spatiotemporal.py",
"repo_id": "diffusers",
"token_count": 4294
} | 142 |
# coding=utf-8
# Copyright 2024 HuggingFace Inc.
#
# Licensed under the Apache License, Version 2.0 (the "License");
# you may not use this file except in compliance with the License.
# You may obtain a copy of the License at
#
# http://www.apache.org/licenses/LICENSE-2.0
#
# Unless required by applicable law or agreed to in writing, software
# distributed under the License is distributed on an "AS IS" BASIS,
# WITHOUT WARRANTIES OR CONDITIONS OF ANY KIND, either express or implied.
# See the License for the specific language governing permissions and
# limitations under the License.
import unittest
import numpy as np
import torch
from transformers import CLIPTextConfig, CLIPTextModelWithProjection, CLIPTokenizer
from diffusers import AmusedPipeline, AmusedScheduler, UVit2DModel, VQModel
from diffusers.utils.testing_utils import enable_full_determinism, require_torch_gpu, slow, torch_device
from ..pipeline_params import TEXT_TO_IMAGE_BATCH_PARAMS, TEXT_TO_IMAGE_PARAMS
from ..test_pipelines_common import PipelineTesterMixin
enable_full_determinism()
class AmusedPipelineFastTests(PipelineTesterMixin, unittest.TestCase):
pipeline_class = AmusedPipeline
params = TEXT_TO_IMAGE_PARAMS | {"encoder_hidden_states", "negative_encoder_hidden_states"}
batch_params = TEXT_TO_IMAGE_BATCH_PARAMS
def get_dummy_components(self):
torch.manual_seed(0)
transformer = UVit2DModel(
hidden_size=32,
use_bias=False,
hidden_dropout=0.0,
cond_embed_dim=32,
micro_cond_encode_dim=2,
micro_cond_embed_dim=10,
encoder_hidden_size=32,
vocab_size=32,
codebook_size=32,
in_channels=32,
block_out_channels=32,
num_res_blocks=1,
downsample=True,
upsample=True,
block_num_heads=1,
num_hidden_layers=1,
num_attention_heads=1,
attention_dropout=0.0,
intermediate_size=32,
layer_norm_eps=1e-06,
ln_elementwise_affine=True,
)
scheduler = AmusedScheduler(mask_token_id=31)
torch.manual_seed(0)
vqvae = VQModel(
act_fn="silu",
block_out_channels=[32],
down_block_types=[
"DownEncoderBlock2D",
],
in_channels=3,
latent_channels=32,
layers_per_block=2,
norm_num_groups=32,
num_vq_embeddings=32,
out_channels=3,
sample_size=32,
up_block_types=[
"UpDecoderBlock2D",
],
mid_block_add_attention=False,
lookup_from_codebook=True,
)
torch.manual_seed(0)
text_encoder_config = CLIPTextConfig(
bos_token_id=0,
eos_token_id=2,
hidden_size=32,
intermediate_size=64,
layer_norm_eps=1e-05,
num_attention_heads=8,
num_hidden_layers=3,
pad_token_id=1,
vocab_size=1000,
projection_dim=32,
)
text_encoder = CLIPTextModelWithProjection(text_encoder_config)
tokenizer = CLIPTokenizer.from_pretrained("hf-internal-testing/tiny-random-clip")
components = {
"transformer": transformer,
"scheduler": scheduler,
"vqvae": vqvae,
"text_encoder": text_encoder,
"tokenizer": tokenizer,
}
return components
def get_dummy_inputs(self, device, seed=0):
if str(device).startswith("mps"):
generator = torch.manual_seed(seed)
else:
generator = torch.Generator(device=device).manual_seed(seed)
inputs = {
"prompt": "A painting of a squirrel eating a burger",
"generator": generator,
"num_inference_steps": 2,
"output_type": "np",
"height": 4,
"width": 4,
}
return inputs
def test_inference_batch_consistent(self, batch_sizes=[2]):
self._test_inference_batch_consistent(batch_sizes=batch_sizes, batch_generator=False)
@unittest.skip("aMUSEd does not support lists of generators")
def test_inference_batch_single_identical(self):
...
@slow
@require_torch_gpu
class AmusedPipelineSlowTests(unittest.TestCase):
def test_amused_256(self):
pipe = AmusedPipeline.from_pretrained("amused/amused-256")
pipe.to(torch_device)
image = pipe("dog", generator=torch.Generator().manual_seed(0), num_inference_steps=2, output_type="np").images
image_slice = image[0, -3:, -3:, -1].flatten()
assert image.shape == (1, 256, 256, 3)
expected_slice = np.array([0.4011, 0.3992, 0.3790, 0.3856, 0.3772, 0.3711, 0.3919, 0.3850, 0.3625])
assert np.abs(image_slice - expected_slice).max() < 3e-3
def test_amused_256_fp16(self):
pipe = AmusedPipeline.from_pretrained("amused/amused-256", variant="fp16", torch_dtype=torch.float16)
pipe.to(torch_device)
image = pipe("dog", generator=torch.Generator().manual_seed(0), num_inference_steps=2, output_type="np").images
image_slice = image[0, -3:, -3:, -1].flatten()
assert image.shape == (1, 256, 256, 3)
expected_slice = np.array([0.0554, 0.05129, 0.0344, 0.0452, 0.0476, 0.0271, 0.0495, 0.0527, 0.0158])
assert np.abs(image_slice - expected_slice).max() < 7e-3
def test_amused_512(self):
pipe = AmusedPipeline.from_pretrained("amused/amused-512")
pipe.to(torch_device)
image = pipe("dog", generator=torch.Generator().manual_seed(0), num_inference_steps=2, output_type="np").images
image_slice = image[0, -3:, -3:, -1].flatten()
assert image.shape == (1, 512, 512, 3)
expected_slice = np.array([0.9960, 0.9960, 0.9946, 0.9980, 0.9947, 0.9932, 0.9960, 0.9961, 0.9947])
assert np.abs(image_slice - expected_slice).max() < 3e-3
def test_amused_512_fp16(self):
pipe = AmusedPipeline.from_pretrained("amused/amused-512", variant="fp16", torch_dtype=torch.float16)
pipe.to(torch_device)
image = pipe("dog", generator=torch.Generator().manual_seed(0), num_inference_steps=2, output_type="np").images
image_slice = image[0, -3:, -3:, -1].flatten()
assert image.shape == (1, 512, 512, 3)
expected_slice = np.array([0.9983, 1.0, 1.0, 1.0, 1.0, 0.9989, 0.9994, 0.9976, 0.9977])
assert np.abs(image_slice - expected_slice).max() < 3e-3
| diffusers/tests/pipelines/amused/test_amused.py/0 | {
"file_path": "diffusers/tests/pipelines/amused/test_amused.py",
"repo_id": "diffusers",
"token_count": 3130
} | 143 |
# coding=utf-8
# Copyright 2024 HuggingFace Inc.
#
# Licensed under the Apache License, Version 2.0 (the "License");
# you may not use this file except in compliance with the License.
# You may obtain a copy of the License at
#
# http://www.apache.org/licenses/LICENSE-2.0
#
# Unless required by applicable law or agreed to in writing, software
# distributed under the License is distributed on an "AS IS" BASIS,
# WITHOUT WARRANTIES OR CONDITIONS OF ANY KIND, either express or implied.
# See the License for the specific language governing permissions and
# limitations under the License.
import gc
import random
import unittest
import torch
from diffusers import IFImg2ImgSuperResolutionPipeline
from diffusers.models.attention_processor import AttnAddedKVProcessor
from diffusers.utils.import_utils import is_xformers_available
from diffusers.utils.testing_utils import floats_tensor, load_numpy, require_torch_gpu, skip_mps, slow, torch_device
from ..pipeline_params import (
TEXT_GUIDED_IMAGE_VARIATION_BATCH_PARAMS,
TEXT_GUIDED_IMAGE_VARIATION_PARAMS,
)
from ..test_pipelines_common import PipelineTesterMixin, assert_mean_pixel_difference
from . import IFPipelineTesterMixin
@skip_mps
class IFImg2ImgSuperResolutionPipelineFastTests(PipelineTesterMixin, IFPipelineTesterMixin, unittest.TestCase):
pipeline_class = IFImg2ImgSuperResolutionPipeline
params = TEXT_GUIDED_IMAGE_VARIATION_PARAMS - {"width", "height"}
batch_params = TEXT_GUIDED_IMAGE_VARIATION_BATCH_PARAMS.union({"original_image"})
required_optional_params = PipelineTesterMixin.required_optional_params - {"latents"}
def get_dummy_components(self):
return self._get_superresolution_dummy_components()
def get_dummy_inputs(self, device, seed=0):
if str(device).startswith("mps"):
generator = torch.manual_seed(seed)
else:
generator = torch.Generator(device=device).manual_seed(seed)
original_image = floats_tensor((1, 3, 32, 32), rng=random.Random(seed)).to(device)
image = floats_tensor((1, 3, 16, 16), rng=random.Random(seed)).to(device)
inputs = {
"prompt": "A painting of a squirrel eating a burger",
"image": image,
"original_image": original_image,
"generator": generator,
"num_inference_steps": 2,
"output_type": "np",
}
return inputs
@unittest.skipIf(
torch_device != "cuda" or not is_xformers_available(),
reason="XFormers attention is only available with CUDA and `xformers` installed",
)
def test_xformers_attention_forwardGenerator_pass(self):
self._test_xformers_attention_forwardGenerator_pass(expected_max_diff=1e-3)
def test_save_load_optional_components(self):
self._test_save_load_optional_components()
@unittest.skipIf(torch_device != "cuda", reason="float16 requires CUDA")
def test_save_load_float16(self):
# Due to non-determinism in save load of the hf-internal-testing/tiny-random-t5 text encoder
super().test_save_load_float16(expected_max_diff=1e-1)
def test_attention_slicing_forward_pass(self):
self._test_attention_slicing_forward_pass(expected_max_diff=1e-2)
def test_save_load_local(self):
self._test_save_load_local()
def test_inference_batch_single_identical(self):
self._test_inference_batch_single_identical(
expected_max_diff=1e-2,
)
@slow
@require_torch_gpu
class IFImg2ImgSuperResolutionPipelineSlowTests(unittest.TestCase):
def tearDown(self):
# clean up the VRAM after each test
super().tearDown()
gc.collect()
torch.cuda.empty_cache()
def test_if_img2img_superresolution(self):
pipe = IFImg2ImgSuperResolutionPipeline.from_pretrained(
"DeepFloyd/IF-II-L-v1.0",
variant="fp16",
torch_dtype=torch.float16,
)
pipe.unet.set_attn_processor(AttnAddedKVProcessor())
pipe.enable_model_cpu_offload()
generator = torch.Generator(device="cpu").manual_seed(0)
original_image = floats_tensor((1, 3, 256, 256), rng=random.Random(0)).to(torch_device)
image = floats_tensor((1, 3, 64, 64), rng=random.Random(0)).to(torch_device)
output = pipe(
prompt="anime turtle",
image=image,
original_image=original_image,
generator=generator,
num_inference_steps=2,
output_type="np",
)
image = output.images[0]
assert image.shape == (256, 256, 3)
mem_bytes = torch.cuda.max_memory_allocated()
assert mem_bytes < 12 * 10**9
expected_image = load_numpy(
"https://huggingface.co/datasets/hf-internal-testing/diffusers-images/resolve/main/if/test_if_img2img_superresolution_stage_II.npy"
)
assert_mean_pixel_difference(image, expected_image)
pipe.remove_all_hooks()
| diffusers/tests/pipelines/deepfloyd_if/test_if_img2img_superresolution.py/0 | {
"file_path": "diffusers/tests/pipelines/deepfloyd_if/test_if_img2img_superresolution.py",
"repo_id": "diffusers",
"token_count": 2051
} | 144 |
# coding=utf-8
# Copyright 2024 HuggingFace Inc.
#
# Licensed under the Apache License, Version 2.0 (the "License");
# you may not use this file except in compliance with the License.
# You may obtain a copy of the License at
#
# http://www.apache.org/licenses/LICENSE-2.0
#
# Unless required by applicable law or agreed to in writing, software
# distributed under the License is distributed on an "AS IS" BASIS,
# WITHOUT WARRANTIES OR CONDITIONS OF ANY KIND, either express or implied.
# See the License for the specific language governing permissions and
# limitations under the License.
import gc
import random
import unittest
import numpy as np
import torch
from diffusers import DDIMScheduler, KandinskyV22Pipeline, KandinskyV22PriorPipeline, UNet2DConditionModel, VQModel
from diffusers.utils.testing_utils import (
enable_full_determinism,
floats_tensor,
load_numpy,
require_torch_gpu,
slow,
torch_device,
)
from ..test_pipelines_common import PipelineTesterMixin, assert_mean_pixel_difference
enable_full_determinism()
class Dummies:
@property
def text_embedder_hidden_size(self):
return 32
@property
def time_input_dim(self):
return 32
@property
def block_out_channels_0(self):
return self.time_input_dim
@property
def time_embed_dim(self):
return self.time_input_dim * 4
@property
def cross_attention_dim(self):
return 32
@property
def dummy_unet(self):
torch.manual_seed(0)
model_kwargs = {
"in_channels": 4,
# Out channels is double in channels because predicts mean and variance
"out_channels": 8,
"addition_embed_type": "image",
"down_block_types": ("ResnetDownsampleBlock2D", "SimpleCrossAttnDownBlock2D"),
"up_block_types": ("SimpleCrossAttnUpBlock2D", "ResnetUpsampleBlock2D"),
"mid_block_type": "UNetMidBlock2DSimpleCrossAttn",
"block_out_channels": (self.block_out_channels_0, self.block_out_channels_0 * 2),
"layers_per_block": 1,
"encoder_hid_dim": self.text_embedder_hidden_size,
"encoder_hid_dim_type": "image_proj",
"cross_attention_dim": self.cross_attention_dim,
"attention_head_dim": 4,
"resnet_time_scale_shift": "scale_shift",
"class_embed_type": None,
}
model = UNet2DConditionModel(**model_kwargs)
return model
@property
def dummy_movq_kwargs(self):
return {
"block_out_channels": [32, 64],
"down_block_types": ["DownEncoderBlock2D", "AttnDownEncoderBlock2D"],
"in_channels": 3,
"latent_channels": 4,
"layers_per_block": 1,
"norm_num_groups": 8,
"norm_type": "spatial",
"num_vq_embeddings": 12,
"out_channels": 3,
"up_block_types": [
"AttnUpDecoderBlock2D",
"UpDecoderBlock2D",
],
"vq_embed_dim": 4,
}
@property
def dummy_movq(self):
torch.manual_seed(0)
model = VQModel(**self.dummy_movq_kwargs)
return model
def get_dummy_components(self):
unet = self.dummy_unet
movq = self.dummy_movq
scheduler = DDIMScheduler(
num_train_timesteps=1000,
beta_schedule="linear",
beta_start=0.00085,
beta_end=0.012,
clip_sample=False,
set_alpha_to_one=False,
steps_offset=1,
prediction_type="epsilon",
thresholding=False,
)
components = {
"unet": unet,
"scheduler": scheduler,
"movq": movq,
}
return components
def get_dummy_inputs(self, device, seed=0):
image_embeds = floats_tensor((1, self.text_embedder_hidden_size), rng=random.Random(seed)).to(device)
negative_image_embeds = floats_tensor((1, self.text_embedder_hidden_size), rng=random.Random(seed + 1)).to(
device
)
if str(device).startswith("mps"):
generator = torch.manual_seed(seed)
else:
generator = torch.Generator(device=device).manual_seed(seed)
inputs = {
"image_embeds": image_embeds,
"negative_image_embeds": negative_image_embeds,
"generator": generator,
"height": 64,
"width": 64,
"guidance_scale": 4.0,
"num_inference_steps": 2,
"output_type": "np",
}
return inputs
class KandinskyV22PipelineFastTests(PipelineTesterMixin, unittest.TestCase):
pipeline_class = KandinskyV22Pipeline
params = [
"image_embeds",
"negative_image_embeds",
]
batch_params = ["image_embeds", "negative_image_embeds"]
required_optional_params = [
"generator",
"height",
"width",
"latents",
"guidance_scale",
"num_inference_steps",
"return_dict",
"guidance_scale",
"num_images_per_prompt",
"output_type",
"return_dict",
]
callback_cfg_params = ["image_embds"]
test_xformers_attention = False
def get_dummy_inputs(self, device, seed=0):
dummies = Dummies()
return dummies.get_dummy_inputs(device=device, seed=seed)
def get_dummy_components(self):
dummies = Dummies()
return dummies.get_dummy_components()
def test_kandinsky(self):
device = "cpu"
components = self.get_dummy_components()
pipe = self.pipeline_class(**components)
pipe = pipe.to(device)
pipe.set_progress_bar_config(disable=None)
output = pipe(**self.get_dummy_inputs(device))
image = output.images
image_from_tuple = pipe(
**self.get_dummy_inputs(device),
return_dict=False,
)[0]
image_slice = image[0, -3:, -3:, -1]
image_from_tuple_slice = image_from_tuple[0, -3:, -3:, -1]
assert image.shape == (1, 64, 64, 3)
expected_slice = np.array([0.3420, 0.9505, 0.3919, 1.0000, 0.5188, 0.3109, 0.6139, 0.5624, 0.6811])
assert (
np.abs(image_slice.flatten() - expected_slice).max() < 1e-2
), f" expected_slice {expected_slice}, but got {image_slice.flatten()}"
assert (
np.abs(image_from_tuple_slice.flatten() - expected_slice).max() < 1e-2
), f" expected_slice {expected_slice}, but got {image_from_tuple_slice.flatten()}"
def test_float16_inference(self):
super().test_float16_inference(expected_max_diff=1e-1)
@slow
@require_torch_gpu
class KandinskyV22PipelineIntegrationTests(unittest.TestCase):
def tearDown(self):
# clean up the VRAM after each test
super().tearDown()
gc.collect()
torch.cuda.empty_cache()
def test_kandinsky_text2img(self):
expected_image = load_numpy(
"https://huggingface.co/datasets/hf-internal-testing/diffusers-images/resolve/main"
"/kandinskyv22/kandinskyv22_text2img_cat_fp16.npy"
)
pipe_prior = KandinskyV22PriorPipeline.from_pretrained(
"kandinsky-community/kandinsky-2-2-prior", torch_dtype=torch.float16
)
pipe_prior.to(torch_device)
pipeline = KandinskyV22Pipeline.from_pretrained(
"kandinsky-community/kandinsky-2-2-decoder", torch_dtype=torch.float16
)
pipeline = pipeline.to(torch_device)
pipeline.set_progress_bar_config(disable=None)
prompt = "red cat, 4k photo"
generator = torch.Generator(device="cuda").manual_seed(0)
image_emb, zero_image_emb = pipe_prior(
prompt,
generator=generator,
num_inference_steps=5,
negative_prompt="",
).to_tuple()
generator = torch.Generator(device="cuda").manual_seed(0)
output = pipeline(
image_embeds=image_emb,
negative_image_embeds=zero_image_emb,
generator=generator,
num_inference_steps=100,
output_type="np",
)
image = output.images[0]
assert image.shape == (512, 512, 3)
assert_mean_pixel_difference(image, expected_image)
| diffusers/tests/pipelines/kandinsky2_2/test_kandinsky.py/0 | {
"file_path": "diffusers/tests/pipelines/kandinsky2_2/test_kandinsky.py",
"repo_id": "diffusers",
"token_count": 3973
} | 145 |
# coding=utf-8
# Copyright 2024 HuggingFace Inc.
#
# Licensed under the Apache License, Version 2.0 (the "License");
# you may not use this file except in compliance with the License.
# You may obtain a copy of the License at
#
# http://www.apache.org/licenses/LICENSE-2.0
#
# Unless required by applicable law or agreed to in writing, software
# distributed under the License is distributed on an "AS IS" BASIS,
# WITHOUT WARRANTIES OR CONDITIONS OF ANY KIND, either express or implied.
# See the License for the specific language governing permissions and
# limitations under the License.
import random
import unittest
import numpy as np
import torch
from diffusers import DDIMScheduler, LDMSuperResolutionPipeline, UNet2DModel, VQModel
from diffusers.utils import PIL_INTERPOLATION
from diffusers.utils.testing_utils import (
enable_full_determinism,
floats_tensor,
load_image,
nightly,
require_torch,
torch_device,
)
enable_full_determinism()
class LDMSuperResolutionPipelineFastTests(unittest.TestCase):
@property
def dummy_image(self):
batch_size = 1
num_channels = 3
sizes = (32, 32)
image = floats_tensor((batch_size, num_channels) + sizes, rng=random.Random(0)).to(torch_device)
return image
@property
def dummy_uncond_unet(self):
torch.manual_seed(0)
model = UNet2DModel(
block_out_channels=(32, 64),
layers_per_block=2,
sample_size=32,
in_channels=6,
out_channels=3,
down_block_types=("DownBlock2D", "AttnDownBlock2D"),
up_block_types=("AttnUpBlock2D", "UpBlock2D"),
)
return model
@property
def dummy_vq_model(self):
torch.manual_seed(0)
model = VQModel(
block_out_channels=[32, 64],
in_channels=3,
out_channels=3,
down_block_types=["DownEncoderBlock2D", "DownEncoderBlock2D"],
up_block_types=["UpDecoderBlock2D", "UpDecoderBlock2D"],
latent_channels=3,
)
return model
def test_inference_superresolution(self):
device = "cpu"
unet = self.dummy_uncond_unet
scheduler = DDIMScheduler()
vqvae = self.dummy_vq_model
ldm = LDMSuperResolutionPipeline(unet=unet, vqvae=vqvae, scheduler=scheduler)
ldm.to(device)
ldm.set_progress_bar_config(disable=None)
init_image = self.dummy_image.to(device)
generator = torch.Generator(device=device).manual_seed(0)
image = ldm(image=init_image, generator=generator, num_inference_steps=2, output_type="np").images
image_slice = image[0, -3:, -3:, -1]
assert image.shape == (1, 64, 64, 3)
expected_slice = np.array([0.8678, 0.8245, 0.6381, 0.6830, 0.4385, 0.5599, 0.4641, 0.6201, 0.5150])
assert np.abs(image_slice.flatten() - expected_slice).max() < 1e-2
@unittest.skipIf(torch_device != "cuda", "This test requires a GPU")
def test_inference_superresolution_fp16(self):
unet = self.dummy_uncond_unet
scheduler = DDIMScheduler()
vqvae = self.dummy_vq_model
# put models in fp16
unet = unet.half()
vqvae = vqvae.half()
ldm = LDMSuperResolutionPipeline(unet=unet, vqvae=vqvae, scheduler=scheduler)
ldm.to(torch_device)
ldm.set_progress_bar_config(disable=None)
init_image = self.dummy_image.to(torch_device)
image = ldm(init_image, num_inference_steps=2, output_type="np").images
assert image.shape == (1, 64, 64, 3)
@nightly
@require_torch
class LDMSuperResolutionPipelineIntegrationTests(unittest.TestCase):
def test_inference_superresolution(self):
init_image = load_image(
"https://huggingface.co/datasets/hf-internal-testing/diffusers-images/resolve/main"
"/vq_diffusion/teddy_bear_pool.png"
)
init_image = init_image.resize((64, 64), resample=PIL_INTERPOLATION["lanczos"])
ldm = LDMSuperResolutionPipeline.from_pretrained("duongna/ldm-super-resolution", device_map="auto")
ldm.set_progress_bar_config(disable=None)
generator = torch.manual_seed(0)
image = ldm(image=init_image, generator=generator, num_inference_steps=20, output_type="np").images
image_slice = image[0, -3:, -3:, -1]
assert image.shape == (1, 256, 256, 3)
expected_slice = np.array([0.7644, 0.7679, 0.7642, 0.7633, 0.7666, 0.7560, 0.7425, 0.7257, 0.6907])
assert np.abs(image_slice.flatten() - expected_slice).max() < 1e-2
| diffusers/tests/pipelines/latent_diffusion/test_latent_diffusion_superresolution.py/0 | {
"file_path": "diffusers/tests/pipelines/latent_diffusion/test_latent_diffusion_superresolution.py",
"repo_id": "diffusers",
"token_count": 2042
} | 146 |
# coding=utf-8
# Copyright 2024 HuggingFace Inc.
#
# Licensed under the Apache License, Version 2.0 (the "License");
# you may not use this file except in compliance with the License.
# You may obtain a copy of the License at
#
# http://www.apache.org/licenses/LICENSE-2.0
#
# Unless required by applicable law or agreed to in writing, software
# distributed under the License is distributed on an "AS IS" BASIS,
# WITHOUT WARRANTIES OR CONDITIONS OF ANY KIND, either express or implied.
# See the License for the specific language governing permissions and
# limitations under the License.
import gc
import random
import tempfile
import unittest
import numpy as np
import torch
from transformers import CLIPTextConfig, CLIPTextModel, CLIPTokenizer
from diffusers import AutoencoderKL, DDIMScheduler, LMSDiscreteScheduler, PNDMScheduler, UNet2DConditionModel
from diffusers.pipelines.semantic_stable_diffusion import SemanticStableDiffusionPipeline as StableDiffusionPipeline
from diffusers.utils.testing_utils import (
enable_full_determinism,
floats_tensor,
nightly,
require_torch_gpu,
torch_device,
)
enable_full_determinism()
class SafeDiffusionPipelineFastTests(unittest.TestCase):
def tearDown(self):
# clean up the VRAM after each test
super().tearDown()
gc.collect()
torch.cuda.empty_cache()
@property
def dummy_image(self):
batch_size = 1
num_channels = 3
sizes = (32, 32)
image = floats_tensor((batch_size, num_channels) + sizes, rng=random.Random(0)).to(torch_device)
return image
@property
def dummy_cond_unet(self):
torch.manual_seed(0)
model = UNet2DConditionModel(
block_out_channels=(32, 64),
layers_per_block=2,
sample_size=32,
in_channels=4,
out_channels=4,
down_block_types=("DownBlock2D", "CrossAttnDownBlock2D"),
up_block_types=("CrossAttnUpBlock2D", "UpBlock2D"),
cross_attention_dim=32,
)
return model
@property
def dummy_vae(self):
torch.manual_seed(0)
model = AutoencoderKL(
block_out_channels=[32, 64],
in_channels=3,
out_channels=3,
down_block_types=["DownEncoderBlock2D", "DownEncoderBlock2D"],
up_block_types=["UpDecoderBlock2D", "UpDecoderBlock2D"],
latent_channels=4,
)
return model
@property
def dummy_text_encoder(self):
torch.manual_seed(0)
config = CLIPTextConfig(
bos_token_id=0,
eos_token_id=2,
hidden_size=32,
intermediate_size=37,
layer_norm_eps=1e-05,
num_attention_heads=4,
num_hidden_layers=5,
pad_token_id=1,
vocab_size=1000,
)
return CLIPTextModel(config)
@property
def dummy_extractor(self):
def extract(*args, **kwargs):
class Out:
def __init__(self):
self.pixel_values = torch.ones([0])
def to(self, device):
self.pixel_values.to(device)
return self
return Out()
return extract
def test_semantic_diffusion_ddim(self):
device = "cpu" # ensure determinism for the device-dependent torch.Generator
unet = self.dummy_cond_unet
scheduler = DDIMScheduler(
beta_start=0.00085,
beta_end=0.012,
beta_schedule="scaled_linear",
clip_sample=False,
set_alpha_to_one=False,
)
vae = self.dummy_vae
bert = self.dummy_text_encoder
tokenizer = CLIPTokenizer.from_pretrained("hf-internal-testing/tiny-random-clip")
# make sure here that pndm scheduler skips prk
sd_pipe = StableDiffusionPipeline(
unet=unet,
scheduler=scheduler,
vae=vae,
text_encoder=bert,
tokenizer=tokenizer,
safety_checker=None,
feature_extractor=self.dummy_extractor,
)
sd_pipe = sd_pipe.to(device)
sd_pipe.set_progress_bar_config(disable=None)
prompt = "A painting of a squirrel eating a burger"
generator = torch.Generator(device=device).manual_seed(0)
output = sd_pipe([prompt], generator=generator, guidance_scale=6.0, num_inference_steps=2, output_type="np")
image = output.images
generator = torch.Generator(device=device).manual_seed(0)
image_from_tuple = sd_pipe(
[prompt],
generator=generator,
guidance_scale=6.0,
num_inference_steps=2,
output_type="np",
return_dict=False,
)[0]
image_slice = image[0, -3:, -3:, -1]
image_from_tuple_slice = image_from_tuple[0, -3:, -3:, -1]
assert image.shape == (1, 64, 64, 3)
expected_slice = np.array([0.5753, 0.6114, 0.5001, 0.5034, 0.5470, 0.4729, 0.4971, 0.4867, 0.4867])
assert np.abs(image_slice.flatten() - expected_slice).max() < 1e-2
assert np.abs(image_from_tuple_slice.flatten() - expected_slice).max() < 1e-2
def test_semantic_diffusion_pndm(self):
device = "cpu" # ensure determinism for the device-dependent torch.Generator
unet = self.dummy_cond_unet
scheduler = PNDMScheduler(skip_prk_steps=True)
vae = self.dummy_vae
bert = self.dummy_text_encoder
tokenizer = CLIPTokenizer.from_pretrained("hf-internal-testing/tiny-random-clip")
# make sure here that pndm scheduler skips prk
sd_pipe = StableDiffusionPipeline(
unet=unet,
scheduler=scheduler,
vae=vae,
text_encoder=bert,
tokenizer=tokenizer,
safety_checker=None,
feature_extractor=self.dummy_extractor,
)
sd_pipe = sd_pipe.to(device)
sd_pipe.set_progress_bar_config(disable=None)
prompt = "A painting of a squirrel eating a burger"
generator = torch.Generator(device=device).manual_seed(0)
output = sd_pipe([prompt], generator=generator, guidance_scale=6.0, num_inference_steps=2, output_type="np")
image = output.images
generator = torch.Generator(device=device).manual_seed(0)
image_from_tuple = sd_pipe(
[prompt],
generator=generator,
guidance_scale=6.0,
num_inference_steps=2,
output_type="np",
return_dict=False,
)[0]
image_slice = image[0, -3:, -3:, -1]
image_from_tuple_slice = image_from_tuple[0, -3:, -3:, -1]
assert image.shape == (1, 64, 64, 3)
expected_slice = np.array([0.5122, 0.5712, 0.4825, 0.5053, 0.5646, 0.4769, 0.5179, 0.4894, 0.4994])
assert np.abs(image_slice.flatten() - expected_slice).max() < 1e-2
assert np.abs(image_from_tuple_slice.flatten() - expected_slice).max() < 1e-2
def test_semantic_diffusion_no_safety_checker(self):
pipe = StableDiffusionPipeline.from_pretrained(
"hf-internal-testing/tiny-stable-diffusion-lms-pipe", safety_checker=None
)
assert isinstance(pipe, StableDiffusionPipeline)
assert isinstance(pipe.scheduler, LMSDiscreteScheduler)
assert pipe.safety_checker is None
image = pipe("example prompt", num_inference_steps=2).images[0]
assert image is not None
# check that there's no error when saving a pipeline with one of the models being None
with tempfile.TemporaryDirectory() as tmpdirname:
pipe.save_pretrained(tmpdirname)
pipe = StableDiffusionPipeline.from_pretrained(tmpdirname)
# sanity check that the pipeline still works
assert pipe.safety_checker is None
image = pipe("example prompt", num_inference_steps=2).images[0]
assert image is not None
@unittest.skipIf(torch_device != "cuda", "This test requires a GPU")
def test_semantic_diffusion_fp16(self):
"""Test that stable diffusion works with fp16"""
unet = self.dummy_cond_unet
scheduler = PNDMScheduler(skip_prk_steps=True)
vae = self.dummy_vae
bert = self.dummy_text_encoder
tokenizer = CLIPTokenizer.from_pretrained("hf-internal-testing/tiny-random-clip")
# put models in fp16
unet = unet.half()
vae = vae.half()
bert = bert.half()
# make sure here that pndm scheduler skips prk
sd_pipe = StableDiffusionPipeline(
unet=unet,
scheduler=scheduler,
vae=vae,
text_encoder=bert,
tokenizer=tokenizer,
safety_checker=None,
feature_extractor=self.dummy_extractor,
)
sd_pipe = sd_pipe.to(torch_device)
sd_pipe.set_progress_bar_config(disable=None)
prompt = "A painting of a squirrel eating a burger"
image = sd_pipe([prompt], num_inference_steps=2, output_type="np").images
assert image.shape == (1, 64, 64, 3)
@nightly
@require_torch_gpu
class SemanticDiffusionPipelineIntegrationTests(unittest.TestCase):
def tearDown(self):
# clean up the VRAM after each test
super().tearDown()
gc.collect()
torch.cuda.empty_cache()
def test_positive_guidance(self):
torch_device = "cuda"
pipe = StableDiffusionPipeline.from_pretrained("runwayml/stable-diffusion-v1-5")
pipe = pipe.to(torch_device)
pipe.set_progress_bar_config(disable=None)
prompt = "a photo of a cat"
edit = {
"editing_prompt": ["sunglasses"],
"reverse_editing_direction": [False],
"edit_warmup_steps": 10,
"edit_guidance_scale": 6,
"edit_threshold": 0.95,
"edit_momentum_scale": 0.5,
"edit_mom_beta": 0.6,
}
seed = 3
guidance_scale = 7
# no sega enabled
generator = torch.Generator(torch_device)
generator.manual_seed(seed)
output = pipe(
[prompt],
generator=generator,
guidance_scale=guidance_scale,
num_inference_steps=50,
output_type="np",
width=512,
height=512,
)
image = output.images
image_slice = image[0, -3:, -3:, -1]
expected_slice = [
0.34673113,
0.38492733,
0.37597352,
0.34086335,
0.35650748,
0.35579205,
0.3384763,
0.34340236,
0.3573271,
]
assert image.shape == (1, 512, 512, 3)
assert np.abs(image_slice.flatten() - expected_slice).max() < 1e-2
# with sega enabled
# generator = torch.manual_seed(seed)
generator.manual_seed(seed)
output = pipe(
[prompt],
generator=generator,
guidance_scale=guidance_scale,
num_inference_steps=50,
output_type="np",
width=512,
height=512,
**edit,
)
image = output.images
image_slice = image[0, -3:, -3:, -1]
expected_slice = [
0.41887826,
0.37728766,
0.30138272,
0.41416335,
0.41664985,
0.36283392,
0.36191246,
0.43364465,
0.43001732,
]
assert image.shape == (1, 512, 512, 3)
assert np.abs(image_slice.flatten() - expected_slice).max() < 1e-2
def test_negative_guidance(self):
torch_device = "cuda"
pipe = StableDiffusionPipeline.from_pretrained("runwayml/stable-diffusion-v1-5")
pipe = pipe.to(torch_device)
pipe.set_progress_bar_config(disable=None)
prompt = "an image of a crowded boulevard, realistic, 4k"
edit = {
"editing_prompt": "crowd, crowded, people",
"reverse_editing_direction": True,
"edit_warmup_steps": 10,
"edit_guidance_scale": 8.3,
"edit_threshold": 0.9,
"edit_momentum_scale": 0.5,
"edit_mom_beta": 0.6,
}
seed = 9
guidance_scale = 7
# no sega enabled
generator = torch.Generator(torch_device)
generator.manual_seed(seed)
output = pipe(
[prompt],
generator=generator,
guidance_scale=guidance_scale,
num_inference_steps=50,
output_type="np",
width=512,
height=512,
)
image = output.images
image_slice = image[0, -3:, -3:, -1]
expected_slice = [
0.43497998,
0.91814065,
0.7540739,
0.55580205,
0.8467265,
0.5389691,
0.62574506,
0.58897763,
0.50926757,
]
assert image.shape == (1, 512, 512, 3)
assert np.abs(image_slice.flatten() - expected_slice).max() < 1e-2
# with sega enabled
# generator = torch.manual_seed(seed)
generator.manual_seed(seed)
output = pipe(
[prompt],
generator=generator,
guidance_scale=guidance_scale,
num_inference_steps=50,
output_type="np",
width=512,
height=512,
**edit,
)
image = output.images
image_slice = image[0, -3:, -3:, -1]
expected_slice = [
0.3089719,
0.30500144,
0.29016042,
0.30630964,
0.325687,
0.29419225,
0.2908091,
0.28723598,
0.27696294,
]
assert image.shape == (1, 512, 512, 3)
assert np.abs(image_slice.flatten() - expected_slice).max() < 1e-2
def test_multi_cond_guidance(self):
torch_device = "cuda"
pipe = StableDiffusionPipeline.from_pretrained("runwayml/stable-diffusion-v1-5")
pipe = pipe.to(torch_device)
pipe.set_progress_bar_config(disable=None)
prompt = "a castle next to a river"
edit = {
"editing_prompt": ["boat on a river, boat", "monet, impression, sunrise"],
"reverse_editing_direction": False,
"edit_warmup_steps": [15, 18],
"edit_guidance_scale": 6,
"edit_threshold": [0.9, 0.8],
"edit_momentum_scale": 0.5,
"edit_mom_beta": 0.6,
}
seed = 48
guidance_scale = 7
# no sega enabled
generator = torch.Generator(torch_device)
generator.manual_seed(seed)
output = pipe(
[prompt],
generator=generator,
guidance_scale=guidance_scale,
num_inference_steps=50,
output_type="np",
width=512,
height=512,
)
image = output.images
image_slice = image[0, -3:, -3:, -1]
expected_slice = [
0.75163555,
0.76037145,
0.61785,
0.9189673,
0.8627701,
0.85189694,
0.8512813,
0.87012076,
0.8312857,
]
assert image.shape == (1, 512, 512, 3)
assert np.abs(image_slice.flatten() - expected_slice).max() < 1e-2
# with sega enabled
# generator = torch.manual_seed(seed)
generator.manual_seed(seed)
output = pipe(
[prompt],
generator=generator,
guidance_scale=guidance_scale,
num_inference_steps=50,
output_type="np",
width=512,
height=512,
**edit,
)
image = output.images
image_slice = image[0, -3:, -3:, -1]
expected_slice = [
0.73553365,
0.7537271,
0.74341905,
0.66480356,
0.6472925,
0.63039416,
0.64812905,
0.6749717,
0.6517102,
]
assert image.shape == (1, 512, 512, 3)
assert np.abs(image_slice.flatten() - expected_slice).max() < 1e-2
def test_guidance_fp16(self):
torch_device = "cuda"
pipe = StableDiffusionPipeline.from_pretrained("runwayml/stable-diffusion-v1-5", torch_dtype=torch.float16)
pipe = pipe.to(torch_device)
pipe.set_progress_bar_config(disable=None)
prompt = "a photo of a cat"
edit = {
"editing_prompt": ["sunglasses"],
"reverse_editing_direction": [False],
"edit_warmup_steps": 10,
"edit_guidance_scale": 6,
"edit_threshold": 0.95,
"edit_momentum_scale": 0.5,
"edit_mom_beta": 0.6,
}
seed = 3
guidance_scale = 7
# no sega enabled
generator = torch.Generator(torch_device)
generator.manual_seed(seed)
output = pipe(
[prompt],
generator=generator,
guidance_scale=guidance_scale,
num_inference_steps=50,
output_type="np",
width=512,
height=512,
)
image = output.images
image_slice = image[0, -3:, -3:, -1]
expected_slice = [
0.34887695,
0.3876953,
0.375,
0.34423828,
0.3581543,
0.35717773,
0.3383789,
0.34570312,
0.359375,
]
assert image.shape == (1, 512, 512, 3)
assert np.abs(image_slice.flatten() - expected_slice).max() < 1e-2
# with sega enabled
# generator = torch.manual_seed(seed)
generator.manual_seed(seed)
output = pipe(
[prompt],
generator=generator,
guidance_scale=guidance_scale,
num_inference_steps=50,
output_type="np",
width=512,
height=512,
**edit,
)
image = output.images
image_slice = image[0, -3:, -3:, -1]
expected_slice = [
0.42285156,
0.36914062,
0.29077148,
0.42041016,
0.41918945,
0.35498047,
0.3618164,
0.4423828,
0.43115234,
]
assert image.shape == (1, 512, 512, 3)
assert np.abs(image_slice.flatten() - expected_slice).max() < 1e-2
| diffusers/tests/pipelines/semantic_stable_diffusion/test_semantic_diffusion.py/0 | {
"file_path": "diffusers/tests/pipelines/semantic_stable_diffusion/test_semantic_diffusion.py",
"repo_id": "diffusers",
"token_count": 9604
} | 147 |
# coding=utf-8
# Copyright 2024 HuggingFace Inc.
#
# Licensed under the Apache License, Version 2.0 (the "License");
# you may not use this file except in compliance with the License.
# You may obtain a copy of the License at
#
# http://www.apache.org/licenses/LICENSE-2.0
#
# Unless required by applicable law or agreed to in writing, software
# distributed under the License is distributed on an "AS IS" BASIS,
# WITHOUT WARRANTIES OR CONDITIONS OF ANY KIND, either express or implied.
# See the License for the specific language governing permissions and
# limitations under the License.
import gc
import random
import unittest
import numpy as np
import torch
from PIL import Image
from transformers import CLIPTextConfig, CLIPTextModel, CLIPTokenizer
from diffusers import (
AutoencoderKL,
DDIMScheduler,
EulerAncestralDiscreteScheduler,
LMSDiscreteScheduler,
PNDMScheduler,
StableDiffusionInstructPix2PixPipeline,
UNet2DConditionModel,
)
from diffusers.image_processor import VaeImageProcessor
from diffusers.utils.testing_utils import (
enable_full_determinism,
floats_tensor,
load_image,
require_torch_gpu,
slow,
torch_device,
)
from ..pipeline_params import (
IMAGE_TO_IMAGE_IMAGE_PARAMS,
TEXT_GUIDED_IMAGE_INPAINTING_BATCH_PARAMS,
TEXT_GUIDED_IMAGE_VARIATION_PARAMS,
TEXT_TO_IMAGE_CALLBACK_CFG_PARAMS,
)
from ..test_pipelines_common import (
PipelineKarrasSchedulerTesterMixin,
PipelineLatentTesterMixin,
PipelineTesterMixin,
)
enable_full_determinism()
class StableDiffusionInstructPix2PixPipelineFastTests(
PipelineLatentTesterMixin, PipelineKarrasSchedulerTesterMixin, PipelineTesterMixin, unittest.TestCase
):
pipeline_class = StableDiffusionInstructPix2PixPipeline
params = TEXT_GUIDED_IMAGE_VARIATION_PARAMS - {"height", "width", "cross_attention_kwargs"}
batch_params = TEXT_GUIDED_IMAGE_INPAINTING_BATCH_PARAMS
image_params = IMAGE_TO_IMAGE_IMAGE_PARAMS
image_latents_params = IMAGE_TO_IMAGE_IMAGE_PARAMS
callback_cfg_params = TEXT_TO_IMAGE_CALLBACK_CFG_PARAMS.union({"image_latents"}) - {"negative_prompt_embeds"}
def get_dummy_components(self):
torch.manual_seed(0)
unet = UNet2DConditionModel(
block_out_channels=(32, 64),
layers_per_block=2,
sample_size=32,
in_channels=8,
out_channels=4,
down_block_types=("DownBlock2D", "CrossAttnDownBlock2D"),
up_block_types=("CrossAttnUpBlock2D", "UpBlock2D"),
cross_attention_dim=32,
)
scheduler = PNDMScheduler(skip_prk_steps=True)
torch.manual_seed(0)
vae = AutoencoderKL(
block_out_channels=[32, 64],
in_channels=3,
out_channels=3,
down_block_types=["DownEncoderBlock2D", "DownEncoderBlock2D"],
up_block_types=["UpDecoderBlock2D", "UpDecoderBlock2D"],
latent_channels=4,
)
torch.manual_seed(0)
text_encoder_config = CLIPTextConfig(
bos_token_id=0,
eos_token_id=2,
hidden_size=32,
intermediate_size=37,
layer_norm_eps=1e-05,
num_attention_heads=4,
num_hidden_layers=5,
pad_token_id=1,
vocab_size=1000,
)
text_encoder = CLIPTextModel(text_encoder_config)
tokenizer = CLIPTokenizer.from_pretrained("hf-internal-testing/tiny-random-clip")
components = {
"unet": unet,
"scheduler": scheduler,
"vae": vae,
"text_encoder": text_encoder,
"tokenizer": tokenizer,
"safety_checker": None,
"feature_extractor": None,
"image_encoder": None,
}
return components
def get_dummy_inputs(self, device, seed=0):
image = floats_tensor((1, 3, 32, 32), rng=random.Random(seed)).to(device)
image = image.cpu().permute(0, 2, 3, 1)[0]
image = Image.fromarray(np.uint8(image)).convert("RGB")
if str(device).startswith("mps"):
generator = torch.manual_seed(seed)
else:
generator = torch.Generator(device=device).manual_seed(seed)
inputs = {
"prompt": "A painting of a squirrel eating a burger",
"image": image,
"generator": generator,
"num_inference_steps": 2,
"guidance_scale": 6.0,
"image_guidance_scale": 1,
"output_type": "np",
}
return inputs
def test_stable_diffusion_pix2pix_default_case(self):
device = "cpu" # ensure determinism for the device-dependent torch.Generator
components = self.get_dummy_components()
sd_pipe = StableDiffusionInstructPix2PixPipeline(**components)
sd_pipe = sd_pipe.to(device)
sd_pipe.set_progress_bar_config(disable=None)
inputs = self.get_dummy_inputs(device)
image = sd_pipe(**inputs).images
image_slice = image[0, -3:, -3:, -1]
assert image.shape == (1, 32, 32, 3)
expected_slice = np.array([0.7526, 0.3750, 0.4547, 0.6117, 0.5866, 0.5016, 0.4327, 0.5642, 0.4815])
assert np.abs(image_slice.flatten() - expected_slice).max() < 1e-3
def test_stable_diffusion_pix2pix_negative_prompt(self):
device = "cpu" # ensure determinism for the device-dependent torch.Generator
components = self.get_dummy_components()
sd_pipe = StableDiffusionInstructPix2PixPipeline(**components)
sd_pipe = sd_pipe.to(device)
sd_pipe.set_progress_bar_config(disable=None)
inputs = self.get_dummy_inputs(device)
negative_prompt = "french fries"
output = sd_pipe(**inputs, negative_prompt=negative_prompt)
image = output.images
image_slice = image[0, -3:, -3:, -1]
assert image.shape == (1, 32, 32, 3)
expected_slice = np.array([0.7511, 0.3642, 0.4553, 0.6236, 0.5797, 0.5013, 0.4343, 0.5611, 0.4831])
assert np.abs(image_slice.flatten() - expected_slice).max() < 1e-3
def test_stable_diffusion_pix2pix_multiple_init_images(self):
device = "cpu" # ensure determinism for the device-dependent torch.Generator
components = self.get_dummy_components()
sd_pipe = StableDiffusionInstructPix2PixPipeline(**components)
sd_pipe = sd_pipe.to(device)
sd_pipe.set_progress_bar_config(disable=None)
inputs = self.get_dummy_inputs(device)
inputs["prompt"] = [inputs["prompt"]] * 2
image = np.array(inputs["image"]).astype(np.float32) / 255.0
image = torch.from_numpy(image).unsqueeze(0).to(device)
image = image / 2 + 0.5
image = image.permute(0, 3, 1, 2)
inputs["image"] = image.repeat(2, 1, 1, 1)
image = sd_pipe(**inputs).images
image_slice = image[-1, -3:, -3:, -1]
assert image.shape == (2, 32, 32, 3)
expected_slice = np.array([0.5812, 0.5748, 0.5222, 0.5908, 0.5695, 0.7174, 0.6804, 0.5523, 0.5579])
assert np.abs(image_slice.flatten() - expected_slice).max() < 1e-3
def test_stable_diffusion_pix2pix_euler(self):
device = "cpu" # ensure determinism for the device-dependent torch.Generator
components = self.get_dummy_components()
components["scheduler"] = EulerAncestralDiscreteScheduler(
beta_start=0.00085, beta_end=0.012, beta_schedule="scaled_linear"
)
sd_pipe = StableDiffusionInstructPix2PixPipeline(**components)
sd_pipe = sd_pipe.to(device)
sd_pipe.set_progress_bar_config(disable=None)
inputs = self.get_dummy_inputs(device)
image = sd_pipe(**inputs).images
image_slice = image[0, -3:, -3:, -1]
slice = [round(x, 4) for x in image_slice.flatten().tolist()]
print(",".join([str(x) for x in slice]))
assert image.shape == (1, 32, 32, 3)
expected_slice = np.array([0.7417, 0.3842, 0.4732, 0.5776, 0.5891, 0.5139, 0.4052, 0.5673, 0.4986])
assert np.abs(image_slice.flatten() - expected_slice).max() < 1e-3
def test_inference_batch_single_identical(self):
super().test_inference_batch_single_identical(expected_max_diff=3e-3)
# Overwrite the default test_latents_inputs because pix2pix encode the image differently
def test_latents_input(self):
components = self.get_dummy_components()
pipe = StableDiffusionInstructPix2PixPipeline(**components)
pipe.image_processor = VaeImageProcessor(do_resize=False, do_normalize=False)
pipe = pipe.to(torch_device)
pipe.set_progress_bar_config(disable=None)
out = pipe(**self.get_dummy_inputs_by_type(torch_device, input_image_type="pt"))[0]
vae = components["vae"]
inputs = self.get_dummy_inputs_by_type(torch_device, input_image_type="pt")
for image_param in self.image_latents_params:
if image_param in inputs.keys():
inputs[image_param] = vae.encode(inputs[image_param]).latent_dist.mode()
out_latents_inputs = pipe(**inputs)[0]
max_diff = np.abs(out - out_latents_inputs).max()
self.assertLess(max_diff, 1e-4, "passing latents as image input generate different result from passing image")
# Override the default test_callback_cfg because pix2pix create inputs for cfg differently
def test_callback_cfg(self):
components = self.get_dummy_components()
pipe = self.pipeline_class(**components)
pipe = pipe.to(torch_device)
pipe.set_progress_bar_config(disable=None)
def callback_no_cfg(pipe, i, t, callback_kwargs):
if i == 1:
for k, w in callback_kwargs.items():
if k in self.callback_cfg_params:
callback_kwargs[k] = callback_kwargs[k].chunk(3)[0]
pipe._guidance_scale = 1.0
return callback_kwargs
inputs = self.get_dummy_inputs(torch_device)
inputs["guidance_scale"] = 1.0
inputs["num_inference_steps"] = 2
out_no_cfg = pipe(**inputs)[0]
inputs["guidance_scale"] = 7.5
inputs["callback_on_step_end"] = callback_no_cfg
inputs["callback_on_step_end_tensor_inputs"] = pipe._callback_tensor_inputs
out_callback_no_cfg = pipe(**inputs)[0]
assert out_no_cfg.shape == out_callback_no_cfg.shape
@slow
@require_torch_gpu
class StableDiffusionInstructPix2PixPipelineSlowTests(unittest.TestCase):
def tearDown(self):
super().tearDown()
gc.collect()
torch.cuda.empty_cache()
def get_inputs(self, seed=0):
generator = torch.manual_seed(seed)
image = load_image(
"https://huggingface.co/datasets/diffusers/test-arrays/resolve/main/stable_diffusion_pix2pix/example.jpg"
)
inputs = {
"prompt": "turn him into a cyborg",
"image": image,
"generator": generator,
"num_inference_steps": 3,
"guidance_scale": 7.5,
"image_guidance_scale": 1.0,
"output_type": "np",
}
return inputs
def test_stable_diffusion_pix2pix_default(self):
pipe = StableDiffusionInstructPix2PixPipeline.from_pretrained(
"timbrooks/instruct-pix2pix", safety_checker=None
)
pipe.to(torch_device)
pipe.set_progress_bar_config(disable=None)
pipe.enable_attention_slicing()
inputs = self.get_inputs()
image = pipe(**inputs).images
image_slice = image[0, -3:, -3:, -1].flatten()
assert image.shape == (1, 512, 512, 3)
expected_slice = np.array([0.5902, 0.6015, 0.6027, 0.5983, 0.6092, 0.6061, 0.5765, 0.5785, 0.5555])
assert np.abs(expected_slice - image_slice).max() < 1e-3
def test_stable_diffusion_pix2pix_k_lms(self):
pipe = StableDiffusionInstructPix2PixPipeline.from_pretrained(
"timbrooks/instruct-pix2pix", safety_checker=None
)
pipe.scheduler = LMSDiscreteScheduler.from_config(pipe.scheduler.config)
pipe.to(torch_device)
pipe.set_progress_bar_config(disable=None)
pipe.enable_attention_slicing()
inputs = self.get_inputs()
image = pipe(**inputs).images
image_slice = image[0, -3:, -3:, -1].flatten()
assert image.shape == (1, 512, 512, 3)
expected_slice = np.array([0.6578, 0.6817, 0.6972, 0.6761, 0.6856, 0.6916, 0.6428, 0.6516, 0.6301])
assert np.abs(expected_slice - image_slice).max() < 1e-3
def test_stable_diffusion_pix2pix_ddim(self):
pipe = StableDiffusionInstructPix2PixPipeline.from_pretrained(
"timbrooks/instruct-pix2pix", safety_checker=None
)
pipe.scheduler = DDIMScheduler.from_config(pipe.scheduler.config)
pipe.to(torch_device)
pipe.set_progress_bar_config(disable=None)
pipe.enable_attention_slicing()
inputs = self.get_inputs()
image = pipe(**inputs).images
image_slice = image[0, -3:, -3:, -1].flatten()
assert image.shape == (1, 512, 512, 3)
expected_slice = np.array([0.3828, 0.3834, 0.3818, 0.3792, 0.3865, 0.3752, 0.3792, 0.3847, 0.3753])
assert np.abs(expected_slice - image_slice).max() < 1e-3
def test_stable_diffusion_pix2pix_intermediate_state(self):
number_of_steps = 0
def callback_fn(step: int, timestep: int, latents: torch.FloatTensor) -> None:
callback_fn.has_been_called = True
nonlocal number_of_steps
number_of_steps += 1
if step == 1:
latents = latents.detach().cpu().numpy()
assert latents.shape == (1, 4, 64, 64)
latents_slice = latents[0, -3:, -3:, -1]
expected_slice = np.array([-0.2463, -0.4644, -0.9756, 1.5176, 1.4414, 0.7866, 0.9897, 0.8521, 0.7983])
assert np.abs(latents_slice.flatten() - expected_slice).max() < 5e-2
elif step == 2:
latents = latents.detach().cpu().numpy()
assert latents.shape == (1, 4, 64, 64)
latents_slice = latents[0, -3:, -3:, -1]
expected_slice = np.array([-0.2644, -0.4626, -0.9653, 1.5176, 1.4551, 0.7686, 0.9805, 0.8452, 0.8115])
assert np.abs(latents_slice.flatten() - expected_slice).max() < 5e-2
callback_fn.has_been_called = False
pipe = StableDiffusionInstructPix2PixPipeline.from_pretrained(
"timbrooks/instruct-pix2pix", safety_checker=None, torch_dtype=torch.float16
)
pipe = pipe.to(torch_device)
pipe.set_progress_bar_config(disable=None)
pipe.enable_attention_slicing()
inputs = self.get_inputs()
pipe(**inputs, callback=callback_fn, callback_steps=1)
assert callback_fn.has_been_called
assert number_of_steps == 3
def test_stable_diffusion_pipeline_with_sequential_cpu_offloading(self):
torch.cuda.empty_cache()
torch.cuda.reset_max_memory_allocated()
torch.cuda.reset_peak_memory_stats()
pipe = StableDiffusionInstructPix2PixPipeline.from_pretrained(
"timbrooks/instruct-pix2pix", safety_checker=None, torch_dtype=torch.float16
)
pipe = pipe.to(torch_device)
pipe.set_progress_bar_config(disable=None)
pipe.enable_attention_slicing(1)
pipe.enable_sequential_cpu_offload()
inputs = self.get_inputs()
_ = pipe(**inputs)
mem_bytes = torch.cuda.max_memory_allocated()
# make sure that less than 2.2 GB is allocated
assert mem_bytes < 2.2 * 10**9
def test_stable_diffusion_pix2pix_pipeline_multiple_of_8(self):
inputs = self.get_inputs()
# resize to resolution that is divisible by 8 but not 16 or 32
inputs["image"] = inputs["image"].resize((504, 504))
model_id = "timbrooks/instruct-pix2pix"
pipe = StableDiffusionInstructPix2PixPipeline.from_pretrained(
model_id,
safety_checker=None,
)
pipe.to(torch_device)
pipe.set_progress_bar_config(disable=None)
pipe.enable_attention_slicing()
output = pipe(**inputs)
image = output.images[0]
image_slice = image[255:258, 383:386, -1]
assert image.shape == (504, 504, 3)
expected_slice = np.array([0.2726, 0.2529, 0.2664, 0.2655, 0.2641, 0.2642, 0.2591, 0.2649, 0.2590])
assert np.abs(image_slice.flatten() - expected_slice).max() < 5e-3
| diffusers/tests/pipelines/stable_diffusion/test_stable_diffusion_instruction_pix2pix.py/0 | {
"file_path": "diffusers/tests/pipelines/stable_diffusion/test_stable_diffusion_instruction_pix2pix.py",
"repo_id": "diffusers",
"token_count": 7749
} | 148 |
# coding=utf-8
# Copyright 2024 HuggingFace Inc.
#
# Licensed under the Apache License, Version 2.0 (the "License");
# you may not use this file except in compliance with the License.
# You may obtain a copy of the License at
#
# http://www.apache.org/licenses/LICENSE-2.0
#
# Unless required by applicable law or agreed to in writing, software
# distributed under the License is distributed on an "AS IS" BASIS,
# WITHOUT WARRANTIES OR CONDITIONS OF ANY KIND, either express or implied.
# See the License for the specific language governing permissions and
# limitations under the License.
import gc
import random
import unittest
import numpy as np
import torch
from parameterized import parameterized
from transformers import CLIPTextConfig, CLIPTextModel, CLIPTextModelWithProjection, CLIPTokenizer
import diffusers
from diffusers import (
AutoencoderKL,
EulerDiscreteScheduler,
LCMScheduler,
MultiAdapter,
StableDiffusionXLAdapterPipeline,
T2IAdapter,
UNet2DConditionModel,
)
from diffusers.utils import load_image, logging
from diffusers.utils.testing_utils import (
enable_full_determinism,
floats_tensor,
numpy_cosine_similarity_distance,
require_torch_gpu,
slow,
torch_device,
)
from ..pipeline_params import TEXT_GUIDED_IMAGE_VARIATION_BATCH_PARAMS, TEXT_GUIDED_IMAGE_VARIATION_PARAMS
from ..test_pipelines_common import (
IPAdapterTesterMixin,
PipelineTesterMixin,
SDXLOptionalComponentsTesterMixin,
assert_mean_pixel_difference,
)
enable_full_determinism()
class StableDiffusionXLAdapterPipelineFastTests(
IPAdapterTesterMixin, PipelineTesterMixin, SDXLOptionalComponentsTesterMixin, unittest.TestCase
):
pipeline_class = StableDiffusionXLAdapterPipeline
params = TEXT_GUIDED_IMAGE_VARIATION_PARAMS
batch_params = TEXT_GUIDED_IMAGE_VARIATION_BATCH_PARAMS
def get_dummy_components(self, adapter_type="full_adapter_xl", time_cond_proj_dim=None):
torch.manual_seed(0)
unet = UNet2DConditionModel(
block_out_channels=(32, 64),
layers_per_block=2,
sample_size=32,
in_channels=4,
out_channels=4,
down_block_types=("DownBlock2D", "CrossAttnDownBlock2D"),
up_block_types=("CrossAttnUpBlock2D", "UpBlock2D"),
# SD2-specific config below
attention_head_dim=(2, 4),
use_linear_projection=True,
addition_embed_type="text_time",
addition_time_embed_dim=8,
transformer_layers_per_block=(1, 2),
projection_class_embeddings_input_dim=80, # 6 * 8 + 32
cross_attention_dim=64,
time_cond_proj_dim=time_cond_proj_dim,
)
scheduler = EulerDiscreteScheduler(
beta_start=0.00085,
beta_end=0.012,
steps_offset=1,
beta_schedule="scaled_linear",
timestep_spacing="leading",
)
torch.manual_seed(0)
vae = AutoencoderKL(
block_out_channels=[32, 64],
in_channels=3,
out_channels=3,
down_block_types=["DownEncoderBlock2D", "DownEncoderBlock2D"],
up_block_types=["UpDecoderBlock2D", "UpDecoderBlock2D"],
latent_channels=4,
sample_size=128,
)
torch.manual_seed(0)
text_encoder_config = CLIPTextConfig(
bos_token_id=0,
eos_token_id=2,
hidden_size=32,
intermediate_size=37,
layer_norm_eps=1e-05,
num_attention_heads=4,
num_hidden_layers=5,
pad_token_id=1,
vocab_size=1000,
# SD2-specific config below
hidden_act="gelu",
projection_dim=32,
)
text_encoder = CLIPTextModel(text_encoder_config)
tokenizer = CLIPTokenizer.from_pretrained("hf-internal-testing/tiny-random-clip")
text_encoder_2 = CLIPTextModelWithProjection(text_encoder_config)
tokenizer_2 = CLIPTokenizer.from_pretrained("hf-internal-testing/tiny-random-clip")
if adapter_type == "full_adapter_xl":
adapter = T2IAdapter(
in_channels=3,
channels=[32, 64],
num_res_blocks=2,
downscale_factor=4,
adapter_type=adapter_type,
)
elif adapter_type == "multi_adapter":
adapter = MultiAdapter(
[
T2IAdapter(
in_channels=3,
channels=[32, 64],
num_res_blocks=2,
downscale_factor=4,
adapter_type="full_adapter_xl",
),
T2IAdapter(
in_channels=3,
channels=[32, 64],
num_res_blocks=2,
downscale_factor=4,
adapter_type="full_adapter_xl",
),
]
)
else:
raise ValueError(
f"Unknown adapter type: {adapter_type}, must be one of 'full_adapter_xl', or 'multi_adapter''"
)
components = {
"adapter": adapter,
"unet": unet,
"scheduler": scheduler,
"vae": vae,
"text_encoder": text_encoder,
"tokenizer": tokenizer,
"text_encoder_2": text_encoder_2,
"tokenizer_2": tokenizer_2,
# "safety_checker": None,
"feature_extractor": None,
"image_encoder": None,
}
return components
def get_dummy_components_with_full_downscaling(self, adapter_type="full_adapter_xl"):
"""Get dummy components with x8 VAE downscaling and 3 UNet down blocks.
These dummy components are intended to fully-exercise the T2I-Adapter
downscaling behavior.
"""
torch.manual_seed(0)
unet = UNet2DConditionModel(
block_out_channels=(32, 32, 64),
layers_per_block=2,
sample_size=32,
in_channels=4,
out_channels=4,
down_block_types=("DownBlock2D", "CrossAttnDownBlock2D", "CrossAttnDownBlock2D"),
up_block_types=("CrossAttnUpBlock2D", "CrossAttnUpBlock2D", "UpBlock2D"),
# SD2-specific config below
attention_head_dim=2,
use_linear_projection=True,
addition_embed_type="text_time",
addition_time_embed_dim=8,
transformer_layers_per_block=1,
projection_class_embeddings_input_dim=80, # 6 * 8 + 32
cross_attention_dim=64,
)
scheduler = EulerDiscreteScheduler(
beta_start=0.00085,
beta_end=0.012,
steps_offset=1,
beta_schedule="scaled_linear",
timestep_spacing="leading",
)
torch.manual_seed(0)
vae = AutoencoderKL(
block_out_channels=[32, 32, 32, 64],
in_channels=3,
out_channels=3,
down_block_types=["DownEncoderBlock2D", "DownEncoderBlock2D", "DownEncoderBlock2D", "DownEncoderBlock2D"],
up_block_types=["UpDecoderBlock2D", "UpDecoderBlock2D", "UpDecoderBlock2D", "UpDecoderBlock2D"],
latent_channels=4,
sample_size=128,
)
torch.manual_seed(0)
text_encoder_config = CLIPTextConfig(
bos_token_id=0,
eos_token_id=2,
hidden_size=32,
intermediate_size=37,
layer_norm_eps=1e-05,
num_attention_heads=4,
num_hidden_layers=5,
pad_token_id=1,
vocab_size=1000,
# SD2-specific config below
hidden_act="gelu",
projection_dim=32,
)
text_encoder = CLIPTextModel(text_encoder_config)
tokenizer = CLIPTokenizer.from_pretrained("hf-internal-testing/tiny-random-clip")
text_encoder_2 = CLIPTextModelWithProjection(text_encoder_config)
tokenizer_2 = CLIPTokenizer.from_pretrained("hf-internal-testing/tiny-random-clip")
if adapter_type == "full_adapter_xl":
adapter = T2IAdapter(
in_channels=3,
channels=[32, 32, 64],
num_res_blocks=2,
downscale_factor=16,
adapter_type=adapter_type,
)
elif adapter_type == "multi_adapter":
adapter = MultiAdapter(
[
T2IAdapter(
in_channels=3,
channels=[32, 32, 64],
num_res_blocks=2,
downscale_factor=16,
adapter_type="full_adapter_xl",
),
T2IAdapter(
in_channels=3,
channels=[32, 32, 64],
num_res_blocks=2,
downscale_factor=16,
adapter_type="full_adapter_xl",
),
]
)
else:
raise ValueError(
f"Unknown adapter type: {adapter_type}, must be one of 'full_adapter_xl', or 'multi_adapter''"
)
components = {
"adapter": adapter,
"unet": unet,
"scheduler": scheduler,
"vae": vae,
"text_encoder": text_encoder,
"tokenizer": tokenizer,
"text_encoder_2": text_encoder_2,
"tokenizer_2": tokenizer_2,
# "safety_checker": None,
"feature_extractor": None,
"image_encoder": None,
}
return components
def get_dummy_inputs(self, device, seed=0, height=64, width=64, num_images=1):
if num_images == 1:
image = floats_tensor((1, 3, height, width), rng=random.Random(seed)).to(device)
else:
image = [
floats_tensor((1, 3, height, width), rng=random.Random(seed)).to(device) for _ in range(num_images)
]
if str(device).startswith("mps"):
generator = torch.manual_seed(seed)
else:
generator = torch.Generator(device=device).manual_seed(seed)
inputs = {
"prompt": "A painting of a squirrel eating a burger",
"image": image,
"generator": generator,
"num_inference_steps": 2,
"guidance_scale": 5.0,
"output_type": "np",
}
return inputs
def test_stable_diffusion_adapter_default_case(self):
device = "cpu" # ensure determinism for the device-dependent torch.Generator
components = self.get_dummy_components()
sd_pipe = StableDiffusionXLAdapterPipeline(**components)
sd_pipe = sd_pipe.to(device)
sd_pipe.set_progress_bar_config(disable=None)
inputs = self.get_dummy_inputs(device)
image = sd_pipe(**inputs).images
image_slice = image[0, -3:, -3:, -1]
assert image.shape == (1, 64, 64, 3)
expected_slice = np.array(
[0.5752919, 0.6022097, 0.4728038, 0.49861962, 0.57084894, 0.4644975, 0.5193715, 0.5133664, 0.4729858]
)
assert np.abs(image_slice.flatten() - expected_slice).max() < 5e-3
@parameterized.expand(
[
# (dim=144) The internal feature map will be 9x9 after initial pixel unshuffling (downscaled x16).
(((4 * 2 + 1) * 16),),
# (dim=160) The internal feature map will be 5x5 after the first T2I down block (downscaled x32).
(((4 * 1 + 1) * 32),),
]
)
def test_multiple_image_dimensions(self, dim):
"""Test that the T2I-Adapter pipeline supports any input dimension that
is divisible by the adapter's `downscale_factor`. This test was added in
response to an issue where the T2I Adapter's downscaling padding
behavior did not match the UNet's behavior.
Note that we have selected `dim` values to produce odd resolutions at
each downscaling level.
"""
components = self.get_dummy_components_with_full_downscaling()
sd_pipe = StableDiffusionXLAdapterPipeline(**components)
sd_pipe = sd_pipe.to(torch_device)
sd_pipe.set_progress_bar_config(disable=None)
inputs = self.get_dummy_inputs(torch_device, height=dim, width=dim)
image = sd_pipe(**inputs).images
assert image.shape == (1, dim, dim, 3)
@parameterized.expand(["full_adapter", "full_adapter_xl", "light_adapter"])
def test_total_downscale_factor(self, adapter_type):
"""Test that the T2IAdapter correctly reports its total_downscale_factor."""
batch_size = 1
in_channels = 3
out_channels = [320, 640, 1280, 1280]
in_image_size = 512
adapter = T2IAdapter(
in_channels=in_channels,
channels=out_channels,
num_res_blocks=2,
downscale_factor=8,
adapter_type=adapter_type,
)
adapter.to(torch_device)
in_image = floats_tensor((batch_size, in_channels, in_image_size, in_image_size)).to(torch_device)
adapter_state = adapter(in_image)
# Assume that the last element in `adapter_state` has been downsampled the most, and check
# that it matches the `total_downscale_factor`.
expected_out_image_size = in_image_size // adapter.total_downscale_factor
assert adapter_state[-1].shape == (
batch_size,
out_channels[-1],
expected_out_image_size,
expected_out_image_size,
)
def test_save_load_optional_components(self):
return self._test_save_load_optional_components()
def test_adapter_sdxl_lcm(self):
device = "cpu" # ensure determinism for the device-dependent torch.Generator
components = self.get_dummy_components(time_cond_proj_dim=256)
sd_pipe = StableDiffusionXLAdapterPipeline(**components)
sd_pipe.scheduler = LCMScheduler.from_config(sd_pipe.scheduler.config)
sd_pipe = sd_pipe.to(torch_device)
sd_pipe.set_progress_bar_config(disable=None)
inputs = self.get_dummy_inputs(device)
output = sd_pipe(**inputs)
image = output.images
image_slice = image[0, -3:, -3:, -1]
assert image.shape == (1, 64, 64, 3)
expected_slice = np.array([0.5425, 0.5385, 0.4964, 0.5045, 0.6149, 0.4974, 0.5469, 0.5332, 0.5426])
assert np.abs(image_slice.flatten() - expected_slice).max() < 1e-2
def test_adapter_sdxl_lcm_custom_timesteps(self):
device = "cpu" # ensure determinism for the device-dependent torch.Generator
components = self.get_dummy_components(time_cond_proj_dim=256)
sd_pipe = StableDiffusionXLAdapterPipeline(**components)
sd_pipe.scheduler = LCMScheduler.from_config(sd_pipe.scheduler.config)
sd_pipe = sd_pipe.to(torch_device)
sd_pipe.set_progress_bar_config(disable=None)
inputs = self.get_dummy_inputs(device)
del inputs["num_inference_steps"]
inputs["timesteps"] = [999, 499]
output = sd_pipe(**inputs)
image = output.images
image_slice = image[0, -3:, -3:, -1]
assert image.shape == (1, 64, 64, 3)
expected_slice = np.array([0.5425, 0.5385, 0.4964, 0.5045, 0.6149, 0.4974, 0.5469, 0.5332, 0.5426])
assert np.abs(image_slice.flatten() - expected_slice).max() < 1e-2
class StableDiffusionXLMultiAdapterPipelineFastTests(
StableDiffusionXLAdapterPipelineFastTests, PipelineTesterMixin, unittest.TestCase
):
def get_dummy_components(self, time_cond_proj_dim=None):
return super().get_dummy_components("multi_adapter", time_cond_proj_dim=time_cond_proj_dim)
def get_dummy_components_with_full_downscaling(self):
return super().get_dummy_components_with_full_downscaling("multi_adapter")
def get_dummy_inputs(self, device, seed=0, height=64, width=64):
inputs = super().get_dummy_inputs(device, seed, height, width, num_images=2)
inputs["adapter_conditioning_scale"] = [0.5, 0.5]
return inputs
def test_stable_diffusion_adapter_default_case(self):
device = "cpu" # ensure determinism for the device-dependent torch.Generator
components = self.get_dummy_components()
sd_pipe = StableDiffusionXLAdapterPipeline(**components)
sd_pipe = sd_pipe.to(device)
sd_pipe.set_progress_bar_config(disable=None)
inputs = self.get_dummy_inputs(device)
image = sd_pipe(**inputs).images
image_slice = image[0, -3:, -3:, -1]
assert image.shape == (1, 64, 64, 3)
expected_slice = np.array(
[0.5813032, 0.60995954, 0.47563356, 0.5056669, 0.57199144, 0.4631841, 0.5176794, 0.51252556, 0.47183886]
)
assert np.abs(image_slice.flatten() - expected_slice).max() < 5e-3
def test_inference_batch_consistent(
self, batch_sizes=[2, 4, 13], additional_params_copy_to_batched_inputs=["num_inference_steps"]
):
components = self.get_dummy_components()
pipe = self.pipeline_class(**components)
pipe.to(torch_device)
pipe.set_progress_bar_config(disable=None)
inputs = self.get_dummy_inputs(torch_device)
logger = logging.get_logger(pipe.__module__)
logger.setLevel(level=diffusers.logging.FATAL)
# batchify inputs
for batch_size in batch_sizes:
batched_inputs = {}
for name, value in inputs.items():
if name in self.batch_params:
# prompt is string
if name == "prompt":
len_prompt = len(value)
# make unequal batch sizes
batched_inputs[name] = [value[: len_prompt // i] for i in range(1, batch_size + 1)]
# make last batch super long
batched_inputs[name][-1] = 100 * "very long"
elif name == "image":
batched_images = []
for image in value:
batched_images.append(batch_size * [image])
batched_inputs[name] = batched_images
else:
batched_inputs[name] = batch_size * [value]
elif name == "batch_size":
batched_inputs[name] = batch_size
else:
batched_inputs[name] = value
for arg in additional_params_copy_to_batched_inputs:
batched_inputs[arg] = inputs[arg]
batched_inputs["output_type"] = "np"
output = pipe(**batched_inputs)
assert len(output[0]) == batch_size
batched_inputs["output_type"] = "np"
output = pipe(**batched_inputs)[0]
assert output.shape[0] == batch_size
logger.setLevel(level=diffusers.logging.WARNING)
def test_num_images_per_prompt(self):
components = self.get_dummy_components()
pipe = self.pipeline_class(**components)
pipe = pipe.to(torch_device)
pipe.set_progress_bar_config(disable=None)
batch_sizes = [1, 2]
num_images_per_prompts = [1, 2]
for batch_size in batch_sizes:
for num_images_per_prompt in num_images_per_prompts:
inputs = self.get_dummy_inputs(torch_device)
for key in inputs.keys():
if key in self.batch_params:
if key == "image":
batched_images = []
for image in inputs[key]:
batched_images.append(batch_size * [image])
inputs[key] = batched_images
else:
inputs[key] = batch_size * [inputs[key]]
images = pipe(**inputs, num_images_per_prompt=num_images_per_prompt)[0]
assert images.shape[0] == batch_size * num_images_per_prompt
def test_inference_batch_single_identical(
self,
batch_size=3,
test_max_difference=None,
test_mean_pixel_difference=None,
relax_max_difference=False,
expected_max_diff=2e-3,
additional_params_copy_to_batched_inputs=["num_inference_steps"],
):
if test_max_difference is None:
# TODO(Pedro) - not sure why, but not at all reproducible at the moment it seems
# make sure that batched and non-batched is identical
test_max_difference = torch_device != "mps"
if test_mean_pixel_difference is None:
# TODO same as above
test_mean_pixel_difference = torch_device != "mps"
components = self.get_dummy_components()
pipe = self.pipeline_class(**components)
pipe.to(torch_device)
pipe.set_progress_bar_config(disable=None)
inputs = self.get_dummy_inputs(torch_device)
logger = logging.get_logger(pipe.__module__)
logger.setLevel(level=diffusers.logging.FATAL)
# batchify inputs
batched_inputs = {}
batch_size = batch_size
for name, value in inputs.items():
if name in self.batch_params:
# prompt is string
if name == "prompt":
len_prompt = len(value)
# make unequal batch sizes
batched_inputs[name] = [value[: len_prompt // i] for i in range(1, batch_size + 1)]
# make last batch super long
batched_inputs[name][-1] = 100 * "very long"
elif name == "image":
batched_images = []
for image in value:
batched_images.append(batch_size * [image])
batched_inputs[name] = batched_images
else:
batched_inputs[name] = batch_size * [value]
elif name == "batch_size":
batched_inputs[name] = batch_size
elif name == "generator":
batched_inputs[name] = [self.get_generator(i) for i in range(batch_size)]
else:
batched_inputs[name] = value
for arg in additional_params_copy_to_batched_inputs:
batched_inputs[arg] = inputs[arg]
output_batch = pipe(**batched_inputs)
assert output_batch[0].shape[0] == batch_size
inputs["generator"] = self.get_generator(0)
output = pipe(**inputs)
logger.setLevel(level=diffusers.logging.WARNING)
if test_max_difference:
if relax_max_difference:
# Taking the median of the largest <n> differences
# is resilient to outliers
diff = np.abs(output_batch[0][0] - output[0][0])
diff = diff.flatten()
diff.sort()
max_diff = np.median(diff[-5:])
else:
max_diff = np.abs(output_batch[0][0] - output[0][0]).max()
assert max_diff < expected_max_diff
if test_mean_pixel_difference:
assert_mean_pixel_difference(output_batch[0][0], output[0][0])
def test_adapter_sdxl_lcm(self):
device = "cpu" # ensure determinism for the device-dependent torch.Generator
components = self.get_dummy_components(time_cond_proj_dim=256)
sd_pipe = StableDiffusionXLAdapterPipeline(**components)
sd_pipe.scheduler = LCMScheduler.from_config(sd_pipe.scheduler.config)
sd_pipe = sd_pipe.to(torch_device)
sd_pipe.set_progress_bar_config(disable=None)
inputs = self.get_dummy_inputs(device)
output = sd_pipe(**inputs)
image = output.images
image_slice = image[0, -3:, -3:, -1]
assert image.shape == (1, 64, 64, 3)
expected_slice = np.array([0.5313, 0.5375, 0.4942, 0.5021, 0.6142, 0.4968, 0.5434, 0.5311, 0.5448])
debug = [str(round(i, 4)) for i in image_slice.flatten().tolist()]
print(",".join(debug))
assert np.abs(image_slice.flatten() - expected_slice).max() < 1e-2
def test_adapter_sdxl_lcm_custom_timesteps(self):
device = "cpu" # ensure determinism for the device-dependent torch.Generator
components = self.get_dummy_components(time_cond_proj_dim=256)
sd_pipe = StableDiffusionXLAdapterPipeline(**components)
sd_pipe.scheduler = LCMScheduler.from_config(sd_pipe.scheduler.config)
sd_pipe = sd_pipe.to(torch_device)
sd_pipe.set_progress_bar_config(disable=None)
inputs = self.get_dummy_inputs(device)
del inputs["num_inference_steps"]
inputs["timesteps"] = [999, 499]
output = sd_pipe(**inputs)
image = output.images
image_slice = image[0, -3:, -3:, -1]
assert image.shape == (1, 64, 64, 3)
expected_slice = np.array([0.5313, 0.5375, 0.4942, 0.5021, 0.6142, 0.4968, 0.5434, 0.5311, 0.5448])
debug = [str(round(i, 4)) for i in image_slice.flatten().tolist()]
print(",".join(debug))
assert np.abs(image_slice.flatten() - expected_slice).max() < 1e-2
@slow
@require_torch_gpu
class AdapterSDXLPipelineSlowTests(unittest.TestCase):
def tearDown(self):
super().tearDown()
gc.collect()
torch.cuda.empty_cache()
def test_download_ckpt_diff_format_is_same(self):
ckpt_path = (
"https://huggingface.co/stabilityai/stable-diffusion-xl-base-1.0/blob/main/sd_xl_base_1.0.safetensors"
)
adapter = T2IAdapter.from_pretrained("TencentARC/t2i-adapter-lineart-sdxl-1.0", torch_dtype=torch.float16)
prompt = "toy"
image = load_image(
"https://huggingface.co/datasets/hf-internal-testing/diffusers-images/resolve/main/t2i_adapter/toy_canny.png"
)
pipe_single_file = StableDiffusionXLAdapterPipeline.from_single_file(
ckpt_path,
adapter=adapter,
torch_dtype=torch.float16,
)
pipe_single_file.enable_model_cpu_offload()
pipe_single_file.set_progress_bar_config(disable=None)
generator = torch.Generator(device="cpu").manual_seed(0)
images_single_file = pipe_single_file(
prompt, image=image, generator=generator, output_type="np", num_inference_steps=3
).images
generator = torch.Generator(device="cpu").manual_seed(0)
pipe = StableDiffusionXLAdapterPipeline.from_pretrained(
"stabilityai/stable-diffusion-xl-base-1.0",
adapter=adapter,
torch_dtype=torch.float16,
)
pipe.enable_model_cpu_offload()
images = pipe(prompt, image=image, generator=generator, output_type="np", num_inference_steps=3).images
assert images_single_file[0].shape == (768, 512, 3)
assert images[0].shape == (768, 512, 3)
max_diff = numpy_cosine_similarity_distance(images[0].flatten(), images_single_file[0].flatten())
assert max_diff < 5e-3
| diffusers/tests/pipelines/stable_diffusion_xl/test_stable_diffusion_xl_adapter.py/0 | {
"file_path": "diffusers/tests/pipelines/stable_diffusion_xl/test_stable_diffusion_xl_adapter.py",
"repo_id": "diffusers",
"token_count": 13533
} | 149 |
from diffusers.utils.testing_utils import require_onnxruntime
@require_onnxruntime
class OnnxPipelineTesterMixin:
"""
This mixin is designed to be used with unittest.TestCase classes.
It provides a set of common tests for each ONNXRuntime pipeline, e.g. saving and loading the pipeline,
equivalence of dict and tuple outputs, etc.
"""
pass
| diffusers/tests/pipelines/test_pipelines_onnx_common.py/0 | {
"file_path": "diffusers/tests/pipelines/test_pipelines_onnx_common.py",
"repo_id": "diffusers",
"token_count": 118
} | 150 |
import torch
from diffusers import CMStochasticIterativeScheduler
from .test_schedulers import SchedulerCommonTest
class CMStochasticIterativeSchedulerTest(SchedulerCommonTest):
scheduler_classes = (CMStochasticIterativeScheduler,)
num_inference_steps = 10
def get_scheduler_config(self, **kwargs):
config = {
"num_train_timesteps": 201,
"sigma_min": 0.002,
"sigma_max": 80.0,
}
config.update(**kwargs)
return config
# Override test_step_shape to add CMStochasticIterativeScheduler-specific logic regarding timesteps
# Problem is that we don't know two timesteps that will always be in the timestep schedule from only the scheduler
# config; scaled sigma_max is always in the timestep schedule, but sigma_min is in the sigma schedule while scaled
# sigma_min is not in the timestep schedule
def test_step_shape(self):
num_inference_steps = 10
scheduler_config = self.get_scheduler_config()
scheduler = self.scheduler_classes[0](**scheduler_config)
scheduler.set_timesteps(num_inference_steps)
timestep_0 = scheduler.timesteps[0]
timestep_1 = scheduler.timesteps[1]
sample = self.dummy_sample
residual = 0.1 * sample
output_0 = scheduler.step(residual, timestep_0, sample).prev_sample
output_1 = scheduler.step(residual, timestep_1, sample).prev_sample
self.assertEqual(output_0.shape, sample.shape)
self.assertEqual(output_0.shape, output_1.shape)
def test_timesteps(self):
for timesteps in [10, 50, 100, 1000]:
self.check_over_configs(num_train_timesteps=timesteps)
def test_clip_denoised(self):
for clip_denoised in [True, False]:
self.check_over_configs(clip_denoised=clip_denoised)
def test_full_loop_no_noise_onestep(self):
scheduler_class = self.scheduler_classes[0]
scheduler_config = self.get_scheduler_config()
scheduler = scheduler_class(**scheduler_config)
num_inference_steps = 1
scheduler.set_timesteps(num_inference_steps)
timesteps = scheduler.timesteps
generator = torch.manual_seed(0)
model = self.dummy_model()
sample = self.dummy_sample_deter * scheduler.init_noise_sigma
for i, t in enumerate(timesteps):
# 1. scale model input
scaled_sample = scheduler.scale_model_input(sample, t)
# 2. predict noise residual
residual = model(scaled_sample, t)
# 3. predict previous sample x_t-1
pred_prev_sample = scheduler.step(residual, t, sample, generator=generator).prev_sample
sample = pred_prev_sample
result_sum = torch.sum(torch.abs(sample))
result_mean = torch.mean(torch.abs(sample))
assert abs(result_sum.item() - 192.7614) < 1e-2
assert abs(result_mean.item() - 0.2510) < 1e-3
def test_full_loop_no_noise_multistep(self):
scheduler_class = self.scheduler_classes[0]
scheduler_config = self.get_scheduler_config()
scheduler = scheduler_class(**scheduler_config)
timesteps = [106, 0]
scheduler.set_timesteps(timesteps=timesteps)
timesteps = scheduler.timesteps
generator = torch.manual_seed(0)
model = self.dummy_model()
sample = self.dummy_sample_deter * scheduler.init_noise_sigma
for t in timesteps:
# 1. scale model input
scaled_sample = scheduler.scale_model_input(sample, t)
# 2. predict noise residual
residual = model(scaled_sample, t)
# 3. predict previous sample x_t-1
pred_prev_sample = scheduler.step(residual, t, sample, generator=generator).prev_sample
sample = pred_prev_sample
result_sum = torch.sum(torch.abs(sample))
result_mean = torch.mean(torch.abs(sample))
assert abs(result_sum.item() - 347.6357) < 1e-2
assert abs(result_mean.item() - 0.4527) < 1e-3
def test_full_loop_with_noise(self):
scheduler_class = self.scheduler_classes[0]
scheduler_config = self.get_scheduler_config()
scheduler = scheduler_class(**scheduler_config)
num_inference_steps = 10
t_start = 8
scheduler.set_timesteps(num_inference_steps)
timesteps = scheduler.timesteps
generator = torch.manual_seed(0)
model = self.dummy_model()
sample = self.dummy_sample_deter * scheduler.init_noise_sigma
noise = self.dummy_noise_deter
timesteps = scheduler.timesteps[t_start * scheduler.order :]
sample = scheduler.add_noise(sample, noise, timesteps[:1])
for t in timesteps:
# 1. scale model input
scaled_sample = scheduler.scale_model_input(sample, t)
# 2. predict noise residual
residual = model(scaled_sample, t)
# 3. predict previous sample x_t-1
pred_prev_sample = scheduler.step(residual, t, sample, generator=generator).prev_sample
sample = pred_prev_sample
result_sum = torch.sum(torch.abs(sample))
result_mean = torch.mean(torch.abs(sample))
assert abs(result_sum.item() - 763.9186) < 1e-2, f" expected result sum 763.9186, but get {result_sum}"
assert abs(result_mean.item() - 0.9947) < 1e-3, f" expected result mean 0.9947, but get {result_mean}"
def test_custom_timesteps_increasing_order(self):
scheduler_class = self.scheduler_classes[0]
scheduler_config = self.get_scheduler_config()
scheduler = scheduler_class(**scheduler_config)
timesteps = [39, 30, 12, 15, 0]
with self.assertRaises(ValueError, msg="`timesteps` must be in descending order."):
scheduler.set_timesteps(timesteps=timesteps)
def test_custom_timesteps_passing_both_num_inference_steps_and_timesteps(self):
scheduler_class = self.scheduler_classes[0]
scheduler_config = self.get_scheduler_config()
scheduler = scheduler_class(**scheduler_config)
timesteps = [39, 30, 12, 1, 0]
num_inference_steps = len(timesteps)
with self.assertRaises(ValueError, msg="Can only pass one of `num_inference_steps` or `timesteps`."):
scheduler.set_timesteps(num_inference_steps=num_inference_steps, timesteps=timesteps)
def test_custom_timesteps_too_large(self):
scheduler_class = self.scheduler_classes[0]
scheduler_config = self.get_scheduler_config()
scheduler = scheduler_class(**scheduler_config)
timesteps = [scheduler.config.num_train_timesteps]
with self.assertRaises(
ValueError,
msg="`timesteps` must start before `self.config.train_timesteps`: {scheduler.config.num_train_timesteps}}",
):
scheduler.set_timesteps(timesteps=timesteps)
| diffusers/tests/schedulers/test_scheduler_consistency_model.py/0 | {
"file_path": "diffusers/tests/schedulers/test_scheduler_consistency_model.py",
"repo_id": "diffusers",
"token_count": 3029
} | 151 |
import torch
from diffusers import HeunDiscreteScheduler
from diffusers.utils.testing_utils import torch_device
from .test_schedulers import SchedulerCommonTest
class HeunDiscreteSchedulerTest(SchedulerCommonTest):
scheduler_classes = (HeunDiscreteScheduler,)
num_inference_steps = 10
def get_scheduler_config(self, **kwargs):
config = {
"num_train_timesteps": 1100,
"beta_start": 0.0001,
"beta_end": 0.02,
"beta_schedule": "linear",
}
config.update(**kwargs)
return config
def test_timesteps(self):
for timesteps in [10, 50, 100, 1000]:
self.check_over_configs(num_train_timesteps=timesteps)
def test_betas(self):
for beta_start, beta_end in zip([0.00001, 0.0001, 0.001], [0.0002, 0.002, 0.02]):
self.check_over_configs(beta_start=beta_start, beta_end=beta_end)
def test_schedules(self):
for schedule in ["linear", "scaled_linear", "exp"]:
self.check_over_configs(beta_schedule=schedule)
def test_clip_sample(self):
for clip_sample_range in [1.0, 2.0, 3.0]:
self.check_over_configs(clip_sample_range=clip_sample_range, clip_sample=True)
def test_prediction_type(self):
for prediction_type in ["epsilon", "v_prediction", "sample"]:
self.check_over_configs(prediction_type=prediction_type)
def test_full_loop_no_noise(self):
scheduler_class = self.scheduler_classes[0]
scheduler_config = self.get_scheduler_config()
scheduler = scheduler_class(**scheduler_config)
scheduler.set_timesteps(self.num_inference_steps)
model = self.dummy_model()
sample = self.dummy_sample_deter * scheduler.init_noise_sigma
sample = sample.to(torch_device)
for i, t in enumerate(scheduler.timesteps):
sample = scheduler.scale_model_input(sample, t)
model_output = model(sample, t)
output = scheduler.step(model_output, t, sample)
sample = output.prev_sample
result_sum = torch.sum(torch.abs(sample))
result_mean = torch.mean(torch.abs(sample))
if torch_device in ["cpu", "mps"]:
assert abs(result_sum.item() - 0.1233) < 1e-2
assert abs(result_mean.item() - 0.0002) < 1e-3
else:
# CUDA
assert abs(result_sum.item() - 0.1233) < 1e-2
assert abs(result_mean.item() - 0.0002) < 1e-3
def test_full_loop_with_v_prediction(self):
scheduler_class = self.scheduler_classes[0]
scheduler_config = self.get_scheduler_config(prediction_type="v_prediction")
scheduler = scheduler_class(**scheduler_config)
scheduler.set_timesteps(self.num_inference_steps)
model = self.dummy_model()
sample = self.dummy_sample_deter * scheduler.init_noise_sigma
sample = sample.to(torch_device)
for i, t in enumerate(scheduler.timesteps):
sample = scheduler.scale_model_input(sample, t)
model_output = model(sample, t)
output = scheduler.step(model_output, t, sample)
sample = output.prev_sample
result_sum = torch.sum(torch.abs(sample))
result_mean = torch.mean(torch.abs(sample))
if torch_device in ["cpu", "mps"]:
assert abs(result_sum.item() - 4.6934e-07) < 1e-2
assert abs(result_mean.item() - 6.1112e-10) < 1e-3
else:
# CUDA
assert abs(result_sum.item() - 4.693428650170972e-07) < 1e-2
assert abs(result_mean.item() - 0.0002) < 1e-3
def test_full_loop_device(self):
scheduler_class = self.scheduler_classes[0]
scheduler_config = self.get_scheduler_config()
scheduler = scheduler_class(**scheduler_config)
scheduler.set_timesteps(self.num_inference_steps, device=torch_device)
model = self.dummy_model()
sample = self.dummy_sample_deter.to(torch_device) * scheduler.init_noise_sigma
for t in scheduler.timesteps:
sample = scheduler.scale_model_input(sample, t)
model_output = model(sample, t)
output = scheduler.step(model_output, t, sample)
sample = output.prev_sample
result_sum = torch.sum(torch.abs(sample))
result_mean = torch.mean(torch.abs(sample))
if str(torch_device).startswith("cpu"):
# The following sum varies between 148 and 156 on mps. Why?
assert abs(result_sum.item() - 0.1233) < 1e-2
assert abs(result_mean.item() - 0.0002) < 1e-3
elif str(torch_device).startswith("mps"):
# Larger tolerance on mps
assert abs(result_mean.item() - 0.0002) < 1e-2
else:
# CUDA
assert abs(result_sum.item() - 0.1233) < 1e-2
assert abs(result_mean.item() - 0.0002) < 1e-3
def test_full_loop_device_karras_sigmas(self):
scheduler_class = self.scheduler_classes[0]
scheduler_config = self.get_scheduler_config()
scheduler = scheduler_class(**scheduler_config, use_karras_sigmas=True)
scheduler.set_timesteps(self.num_inference_steps, device=torch_device)
model = self.dummy_model()
sample = self.dummy_sample_deter.to(torch_device) * scheduler.init_noise_sigma
sample = sample.to(torch_device)
for t in scheduler.timesteps:
sample = scheduler.scale_model_input(sample, t)
model_output = model(sample, t)
output = scheduler.step(model_output, t, sample)
sample = output.prev_sample
result_sum = torch.sum(torch.abs(sample))
result_mean = torch.mean(torch.abs(sample))
assert abs(result_sum.item() - 0.00015) < 1e-2
assert abs(result_mean.item() - 1.9869554535034695e-07) < 1e-2
def test_full_loop_with_noise(self):
scheduler_class = self.scheduler_classes[0]
scheduler_config = self.get_scheduler_config()
scheduler = scheduler_class(**scheduler_config)
scheduler.set_timesteps(self.num_inference_steps)
model = self.dummy_model()
sample = self.dummy_sample_deter * scheduler.init_noise_sigma
sample = sample.to(torch_device)
t_start = self.num_inference_steps - 2
noise = self.dummy_noise_deter
noise = noise.to(torch_device)
timesteps = scheduler.timesteps[t_start * scheduler.order :]
sample = scheduler.add_noise(sample, noise, timesteps[:1])
for i, t in enumerate(timesteps):
sample = scheduler.scale_model_input(sample, t)
model_output = model(sample, t)
output = scheduler.step(model_output, t, sample)
sample = output.prev_sample
result_sum = torch.sum(torch.abs(sample))
result_mean = torch.mean(torch.abs(sample))
assert abs(result_sum.item() - 75074.8906) < 1e-2, f" expected result sum 75074.8906, but get {result_sum}"
assert abs(result_mean.item() - 97.7538) < 1e-3, f" expected result mean 97.7538, but get {result_mean}"
| diffusers/tests/schedulers/test_scheduler_heun.py/0 | {
"file_path": "diffusers/tests/schedulers/test_scheduler_heun.py",
"repo_id": "diffusers",
"token_count": 3279
} | 152 |
# coding=utf-8
# Copyright 2024 The HuggingFace Inc. team.
#
# Licensed under the Apache License, Version 2.0 (the "License");
# you may not use this file except in compliance with the License.
# You may obtain a copy of the License at
#
# http://www.apache.org/licenses/LICENSE-2.0
#
# Unless required by applicable law or agreed to in writing, software
# distributed under the License is distributed on an "AS IS" BASIS,
# WITHOUT WARRANTIES OR CONDITIONS OF ANY KIND, either express or implied.
# See the License for the specific language governing permissions and
# limitations under the License.
import argparse
from collections import defaultdict
import yaml
PATH_TO_TOC = "docs/source/en/_toctree.yml"
def clean_doc_toc(doc_list):
"""
Cleans the table of content of the model documentation by removing duplicates and sorting models alphabetically.
"""
counts = defaultdict(int)
overview_doc = []
new_doc_list = []
for doc in doc_list:
if "local" in doc:
counts[doc["local"]] += 1
if doc["title"].lower() == "overview":
overview_doc.append({"local": doc["local"], "title": doc["title"]})
else:
new_doc_list.append(doc)
doc_list = new_doc_list
duplicates = [key for key, value in counts.items() if value > 1]
new_doc = []
for duplicate_key in duplicates:
titles = list({doc["title"] for doc in doc_list if doc["local"] == duplicate_key})
if len(titles) > 1:
raise ValueError(
f"{duplicate_key} is present several times in the documentation table of content at "
"`docs/source/en/_toctree.yml` with different *Title* values. Choose one of those and remove the "
"others."
)
# Only add this once
new_doc.append({"local": duplicate_key, "title": titles[0]})
# Add none duplicate-keys
new_doc.extend([doc for doc in doc_list if "local" not in counts or counts[doc["local"]] == 1])
new_doc = sorted(new_doc, key=lambda s: s["title"].lower())
# "overview" gets special treatment and is always first
if len(overview_doc) > 1:
raise ValueError("{doc_list} has two 'overview' docs which is not allowed.")
overview_doc.extend(new_doc)
# Sort
return overview_doc
def check_scheduler_doc(overwrite=False):
with open(PATH_TO_TOC, encoding="utf-8") as f:
content = yaml.safe_load(f.read())
# Get to the API doc
api_idx = 0
while content[api_idx]["title"] != "API":
api_idx += 1
api_doc = content[api_idx]["sections"]
# Then to the model doc
scheduler_idx = 0
while api_doc[scheduler_idx]["title"] != "Schedulers":
scheduler_idx += 1
scheduler_doc = api_doc[scheduler_idx]["sections"]
new_scheduler_doc = clean_doc_toc(scheduler_doc)
diff = False
if new_scheduler_doc != scheduler_doc:
diff = True
if overwrite:
api_doc[scheduler_idx]["sections"] = new_scheduler_doc
if diff:
if overwrite:
content[api_idx]["sections"] = api_doc
with open(PATH_TO_TOC, "w", encoding="utf-8") as f:
f.write(yaml.dump(content, allow_unicode=True))
else:
raise ValueError(
"The model doc part of the table of content is not properly sorted, run `make style` to fix this."
)
def check_pipeline_doc(overwrite=False):
with open(PATH_TO_TOC, encoding="utf-8") as f:
content = yaml.safe_load(f.read())
# Get to the API doc
api_idx = 0
while content[api_idx]["title"] != "API":
api_idx += 1
api_doc = content[api_idx]["sections"]
# Then to the model doc
pipeline_idx = 0
while api_doc[pipeline_idx]["title"] != "Pipelines":
pipeline_idx += 1
diff = False
pipeline_docs = api_doc[pipeline_idx]["sections"]
new_pipeline_docs = []
# sort sub pipeline docs
for pipeline_doc in pipeline_docs:
if "section" in pipeline_doc:
sub_pipeline_doc = pipeline_doc["section"]
new_sub_pipeline_doc = clean_doc_toc(sub_pipeline_doc)
if overwrite:
pipeline_doc["section"] = new_sub_pipeline_doc
new_pipeline_docs.append(pipeline_doc)
# sort overall pipeline doc
new_pipeline_docs = clean_doc_toc(new_pipeline_docs)
if new_pipeline_docs != pipeline_docs:
diff = True
if overwrite:
api_doc[pipeline_idx]["sections"] = new_pipeline_docs
if diff:
if overwrite:
content[api_idx]["sections"] = api_doc
with open(PATH_TO_TOC, "w", encoding="utf-8") as f:
f.write(yaml.dump(content, allow_unicode=True))
else:
raise ValueError(
"The model doc part of the table of content is not properly sorted, run `make style` to fix this."
)
if __name__ == "__main__":
parser = argparse.ArgumentParser()
parser.add_argument("--fix_and_overwrite", action="store_true", help="Whether to fix inconsistencies.")
args = parser.parse_args()
check_scheduler_doc(args.fix_and_overwrite)
check_pipeline_doc(args.fix_and_overwrite)
| diffusers/utils/check_doc_toc.py/0 | {
"file_path": "diffusers/utils/check_doc_toc.py",
"repo_id": "diffusers",
"token_count": 2177
} | 153 |
# DDIM Inversion
<CourseFloatingBanner unit={4}
classNames="absolute z-10 right-0 top-0"
notebooks={[
{label: "DDIM Inversion", value: "https://colab.research.google.com/github/huggingface/diffusion-models-class/blob/main/units/en/unit4/ddim_inversion.ipynb"},
{label: "DDIM Inversion", value: "https://studiolab.sagemaker.aws/import/github/huggingface/diffusion-models-class/blob/main/units/en/unit4/ddim_inversion.ipynb"},
]} />
In this notebook we will explore inversion, see how it relates to sampling, and apply it to the task of editing images with Stable Diffusion.
What You Will Learn
- How DDIM sampling works
- Deterministic vs Stochastic samplers
- The theory behind DDIM inversion
- Editing images with inversion
Let's get started!
# Setup
```
# !pip install -q transformers diffusers accelerate
```
```
import torch
import requests
import torch.nn as nn
import torch.nn.functional as F
from PIL import Image
from io import BytesIO
from tqdm.auto import tqdm
from matplotlib import pyplot as plt
from torchvision import transforms as tfms
from diffusers import StableDiffusionPipeline, DDIMScheduler
```
```
# Useful function for later
def load_image(url, size=None):
response = requests.get(url,timeout=0.2)
img = Image.open(BytesIO(response.content)).convert('RGB')
if size is not None:
img = img.resize(size)
return img
```
```
device = torch.device("cuda" if torch.cuda.is_available() else "cpu")
```
## Loading an existing pipeline
```
# Load a pipeline
pipe = StableDiffusionPipeline.from_pretrained("runwayml/stable-diffusion-v1-5").to(device)
```
```
# Set up a DDIM scheduler:
pipe.scheduler = DDIMScheduler.from_config(pipe.scheduler.config)
```
```
# Sample an image to make sure it is all working
prompt = 'Beautiful DSLR Photograph of a penguin on the beach, golden hour'
negative_prompt = 'blurry, ugly, stock photo'
im = pipe(prompt, negative_prompt=negative_prompt).images[0]
im.resize((256, 256)) # resize for convenient viewing
```
## DDIM Sampling
At a given time $t$, the noisy image $x_t$ is some mixture of the original image ($x_0$) and some noise ($\epsilon$). Here is the formula for $x_t$ from the DDIM paper, which we'll be referring to in this section:
$$ x_t = \sqrt{\alpha_t}x_0 + \sqrt{1-\alpha_t}\epsilon $$
$\epsilon$ is some gaussian noise with unit variance
$\alpha_t$ ('alpha') is the value which is confusingly called $\bar{\alpha}$ ('alpha_bar') in the DDPM paper (!!) and defined the noise scheduler. In diffusers, the alpha scheduler is calculated and the values are stored in the `scheduler.alphas_cumprod`. Confusing I know! Let's plot these values, and remember that for the rest of this notebook we'll use DDIM's notation.
```
# Plot 'alpha' (alpha_bar in DDPM language, alphas_cumprod in diffusers for clarity)
timesteps = pipe.scheduler.timesteps.cpu()
alphas = pipe.scheduler.alphas_cumprod[timesteps]
plt.plot(timesteps, alphas, label='alpha_t');
plt.legend()
```
Initially (timestep 0, left side of the graph) we begin with a clean image and no noise. $\alpha_t = 1$. As we move to higher timesteps, we end up with almost all noise and $\alpha_t$ drops towards 0.
During sampling, we begin with pure noise at timestep 1000 and slowly move towards timestep 0. To calculate the next t in the sampling trajectory ($x_{t-1}$ since we're moving from high t to low t) we predict the noise ($\epsilon_\theta(x_t)$, which is the output of our model) and use this to calculate the predicted denoised image $x_0$. Then we use this prediction to move a small distance in the 'direction pointing to $x_t$'. Finally, we can add some additional noise scaled by $\sigma_t$. Here's the relevant section from the paper showing this in action:
Screenshot from 2023-01-28 10-04-22.png
So, we have an equation for how to move from $x_t$ to $x_{t-1}$, with a controllable abount of noise. And today we're specifically interested in the case where we don't add any additional noise - giving us fully deterministic DDIM sampling. Let's see what this looks like in code:
```
# Sample function (regular DDIM)
@torch.no_grad()
def sample(prompt, start_step=0, start_latents=None,
guidance_scale=3.5, num_inference_steps=30,
num_images_per_prompt=1, do_classifier_free_guidance=True,
negative_prompt='', device=device):
# Encode prompt
text_embeddings = pipe._encode_prompt(
prompt, device, num_images_per_prompt, do_classifier_free_guidance, negative_prompt
)
# Set num inference steps
pipe.scheduler.set_timesteps(num_inference_steps, device=device)
# Create a random starting point if we don't have one already
if start_latents is None:
start_latents = torch.randn(1, 4, 64, 64, device=device)
start_latents *= pipe.scheduler.init_noise_sigma
latents = start_latents.clone()
for i in tqdm(range(start_step, num_inference_steps)):
t = pipe.scheduler.timesteps[i]
# expand the latents if we are doing classifier free guidance
latent_model_input = torch.cat([latents] * 2) if do_classifier_free_guidance else latents
latent_model_input = pipe.scheduler.scale_model_input(latent_model_input, t)
# predict the noise residual
noise_pred = pipe.unet(latent_model_input, t, encoder_hidden_states=text_embeddings).sample
# perform guidance
if do_classifier_free_guidance:
noise_pred_uncond, noise_pred_text = noise_pred.chunk(2)
noise_pred = noise_pred_uncond + guidance_scale * (noise_pred_text - noise_pred_uncond)
# Normally we'd rely on the scheduler to handle the update step:
# latents = pipe.scheduler.step(noise_pred, t, latents).prev_sample
# Instead, let's do it ourselves:
prev_t = max(1, t.item() - (1000//num_inference_steps)) # t-1
alpha_t = pipe.scheduler.alphas_cumprod[t.item()]
alpha_t_prev = pipe.scheduler.alphas_cumprod[prev_t]
predicted_x0 = (latents - (1-alpha_t).sqrt()*noise_pred) / alpha_t.sqrt()
direction_pointing_to_xt = (1-alpha_t_prev).sqrt()*noise_pred
latents = alpha_t_prev.sqrt()*predicted_x0 + direction_pointing_to_xt
# Post-processing
images = pipe.decode_latents(latents)
images = pipe.numpy_to_pil(images)
return images
```
```
# Test our sampling function by generating an image
sample('Watercolor painting of a beach sunset', negative_prompt=negative_prompt, num_inference_steps=50)[0].resize((256, 256))
```
See if you can match the code with the equation from the paper. Note that $\sigma$=0 since we're only interested in the no-extra-noise case, so we can leave out those bits of the equation.
## Inversion
The goal of inversion is to 'reverse' the sampling process. We want to end up with a noisy latent which, if used as the starting point for our usual sampling procedure, results in the original image being generated.
Here we load an image as our initial image, but you can also generate one yourself to use instead.
```
# https://www.pexels.com/photo/a-beagle-on-green-grass-field-8306128/
input_image = load_image('https://images.pexels.com/photos/8306128/pexels-photo-8306128.jpeg', size=(512, 512))
input_image
```
We're also going to use a prompt to do the inversion with classifier-free-guidance included, so enter a description of the image:
```
input_image_prompt = "Photograph of a puppy on the grass"
```
Next, we need to turn this PIL image into a set of latents which we will use as the starting point for our inversion:
```
# encode with VAE
with torch.no_grad(): latent = pipe.vae.encode(tfms.functional.to_tensor(input_image).unsqueeze(0).to(device)*2-1)
l = 0.18215 * latent.latent_dist.sample()
```
Alright, time for the fun bit. This function looks similar to the sampling function above, but we move through the timesteps in the opposite direction, starting at t=0 and moving towards higher and higher noise. And instead of updating our latents to be less noisy, we estimate the predicted noise and use it to UNDO an update step, moving them from t to t+1.
```
## Inversion
@torch.no_grad()
def invert(start_latents, prompt, guidance_scale=3.5, num_inference_steps=80,
num_images_per_prompt=1, do_classifier_free_guidance=True,
negative_prompt='', device=device):
# Encode prompt
text_embeddings = pipe._encode_prompt(
prompt, device, num_images_per_prompt, do_classifier_free_guidance, negative_prompt
)
# latents are now the specified start latents
latents = start_latents.clone()
# We'll keep a list of the inverted latents as the process goes on
intermediate_latents = []
# Set num inference steps
pipe.scheduler.set_timesteps(num_inference_steps, device=device)
# Reversed timesteps <<<<<<<<<<<<<<<<<<<<
timesteps = reversed(pipe.scheduler.timesteps)
for i in tqdm(range(1, num_inference_steps), total=num_inference_steps-1):
# We'll skip the final iteration
if i >= num_inference_steps - 1: continue
t = timesteps[i]
# expand the latents if we are doing classifier free guidance
latent_model_input = torch.cat([latents] * 2) if do_classifier_free_guidance else latents
latent_model_input = pipe.scheduler.scale_model_input(latent_model_input, t)
# predict the noise residual
noise_pred = pipe.unet(latent_model_input, t, encoder_hidden_states=text_embeddings).sample
# perform guidance
if do_classifier_free_guidance:
noise_pred_uncond, noise_pred_text = noise_pred.chunk(2)
noise_pred = noise_pred_uncond + guidance_scale * (noise_pred_text - noise_pred_uncond)
current_t = max(0, t.item() - (1000//num_inference_steps))#t
next_t = t # min(999, t.item() + (1000//num_inference_steps)) # t+1
alpha_t = pipe.scheduler.alphas_cumprod[current_t]
alpha_t_next = pipe.scheduler.alphas_cumprod[next_t]
# Inverted update step (re-arranging the update step to get x(t) (new latents) as a function of x(t-1) (current latents)
latents = (latents - (1-alpha_t).sqrt()*noise_pred)*(alpha_t_next.sqrt()/alpha_t.sqrt()) + (1-alpha_t_next).sqrt()*noise_pred
# Store
intermediate_latents.append(latents)
return torch.cat(intermediate_latents)
```
Running it on the latent representation of our puppy pic, we get back a set of all the intermediate latents created during the inversion process:
```
inverted_latents = invert(l, input_image_prompt,num_inference_steps=50)
inverted_latents.shape
```
```
torch.Size([48, 4, 64, 64])
```
We can view the final set of latents - these will hopefully be the noisy starting point for our new sampling attempts:
```
# Decode the final inverted latents:
with torch.no_grad():
im = pipe.decode_latents(inverted_latents[-1].unsqueeze(0))
pipe.numpy_to_pil(im)[0]
```
You can pass these inverted latents to the pipeline using the normal call method:
```
pipe(input_image_prompt, latents=inverted_latents[-1][None], num_inference_steps=50, guidance_scale=3.5).images[0]
```
But here we see our first problem: this is not quite the image we started with! This is because DDIM inversion relies on a critical assumption that the noise prediction at time t and at time t+1 will be the same - something that is not true when we only invert over 50 or 100 timesteps. We could use more timesteps to hopefully get a more accurate inversion, but we can also 'cheat' and start from, say, 20/50 steps through sampling with the corresponding intermediate latents we saved during inversion:
```
# The reason we want to be able to specify start step
start_step=20
sample(input_image_prompt, start_latents=inverted_latents[-(start_step+1)][None],
start_step=start_step, num_inference_steps=50)[0]
```
Pretty close to our input image! Why are we doing this? Well, the hope is that if we now sample with a new prompt we'll get an oimage that matches the original EXCEPT in places relevant to the new prompt. For ex, replacing 'puppy' with 'cat' we should see a cat with a near-identical lawn and backgorund:
```
# Sampling with a new prompt
start_step=10
new_prompt = input_image_prompt.replace('puppy', 'cat')
sample(new_prompt, start_latents=inverted_latents[-(start_step+1)][None],
start_step=start_step, num_inference_steps=50)[0]
```
### Why not just use img2img?
Why bother inverting? Can't we just add noise to the input image and denoise with the new prompt? We can, but this will result in much more drastic changes everywhere (if we add lots of noise) or not enough changes anywhere (if we add less noise). Try it yourself:
```
start_step = 10
num_inference_steps=50
pipe.scheduler.set_timesteps(num_inference_steps)
noisy_l = pipe.scheduler.add_noise(l, torch.randn_like(l), pipe.scheduler.timesteps[start_step])
sample(new_prompt, start_latents=noisy_l, start_step=start_step, num_inference_steps=num_inference_steps)[0]
```
Note the much-larger change to the lawn and background.
## Putting it all together
Let's wrap the code we've written so far into a simple function that takes an image and two prompts and performs an edit using inversion:
```
def edit(input_image, input_image_prompt, edit_prompt, num_steps=100, start_step=30, guidance_scale=3.5):
with torch.no_grad(): latent = pipe.vae.encode(tfms.functional.to_tensor(input_image).unsqueeze(0).to(device)*2-1)
l = 0.18215 * latent.latent_dist.sample()
inverted_latents = invert(l, input_image_prompt,num_inference_steps=num_steps)
final_im = sample(edit_prompt, start_latents=inverted_latents[-(start_step+1)][None],
start_step=start_step, num_inference_steps=num_steps, guidance_scale=guidance_scale)[0]
return final_im
```
And in action:
```
edit(input_image, 'A puppy on the grass', 'an old grey dog on the grass', num_steps=50, start_step=10)
```
```
edit(input_image, 'A puppy on the grass', 'A blue dog on the lawn', num_steps=50, start_step=12, guidance_scale=6)
```
**Exercise:** Try this on some more images! Explore the different parameters
## More Steps = Better Performance
If you've having issues with less-accurate inversions, you can try using more steps (at the cost of longer running time). To test the inversion you can use our edit function with the same prompt:
```
# Inversion test with far more steps:
edit(input_image, 'A puppy on the grass', 'A puppy on the grass', num_steps=350, start_step=1)
```
Much better! And trying it for an edit:
```
edit(input_image, 'A photograph of a puppy', 'A photograph of a grey cat', num_steps=150, start_step=30, guidance_scale=5.5)
```
```
# source: https://www.pexels.com/photo/girl-taking-photo-1493111/
face = load_image('https://images.pexels.com/photos/1493111/pexels-photo-1493111.jpeg', size=(512, 512))
face
```
```
edit(face, 'A photograph of a face', 'A photograph of a face with sunglasses', num_steps=250, start_step=30, guidance_scale=3.5)
```
```
edit(face, 'A photograph of a face', 'Acrylic palette knife painting of a face, colorful', num_steps=250, start_step=65, guidance_scale=5.5)
```
## What Next?
Armed with the knowledge from this notebook, I recommend you investigate [*Null-text Inversion*](https://null-text-inversion.github.io/) which builds on DDIM by optimizing the null text (unconditional text prompt) during inversion for more accurate inversions and better edits. | diffusion-models-class/units/en/unit4/2.mdx/0 | {
"file_path": "diffusion-models-class/units/en/unit4/2.mdx",
"repo_id": "diffusion-models-class",
"token_count": 5413
} | 154 |
<jupyter_start><jupyter_text>Modèles (PyTorch) Installez la bibliothèque 🤗 *Transformers* pour exécuter ce *notebook*.<jupyter_code>!pip install transformers[sentencepiece]
from transformers import CamembertConfig, CamembertModel
# Construire la configuration
config = CamembertConfig()
# Construire le modèle à partir de la configuration
model = CamembertModel(config)
print(config)
from transformers import CamembertConfig, CamembertModel
config = CamembertConfig()
model = CamembertModel(config)
# Le modèle est initialisé de façon aléatoire !
from transformers import CamembertModel
model = CamembertModel.from_pretrained("camembert-base")
model.save_pretrained("répertoire_sur_mon_ordinateur")
sequences = ["Hello!", "Cool.", "Nice!"]
from transformers import CamembertTokenizer
tokenizer = CamembertTokenizer.from_pretrained("camembert-base")
encoded_sequences = tokenizer(sequences)
encoded_sequences
import torch
model_inputs = torch.tensor(encoded_sequences)
output = model(model_inputs)
output<jupyter_output><empty_output> | notebooks/course/fr/chapter2/section3_pt.ipynb/0 | {
"file_path": "notebooks/course/fr/chapter2/section3_pt.ipynb",
"repo_id": "notebooks",
"token_count": 341
} | 155 |
<jupyter_start><jupyter_text>Utilisation de modèles pré-entraînés (TensorFlow) Installez la bibliothèque 🤗 *Transformers* pour exécuter ce *notebook*.<jupyter_code>!pip install datasets transformers[sentencepiece]
from transformers import pipeline
camembert_fill_mask = pipeline("fill-mask", model="camembert-base")
results = camembert_fill_mask("Le camembert est <mask> :)")
from transformers import CamembertTokenizer, TFCamembertForMaskedLM
tokenizer = CamembertTokenizer.from_pretrained("camembert-base")
model = TFCamembertForMaskedLM.from_pretrained("camembert-base")
from transformers import AutoTokenizer, TFAutoModelForMaskedLM
tokenizer = AutoTokenizer.from_pretrained("camembert-base")
model = TFAutoModelForMaskedLM.from_pretrained("camembert-base")<jupyter_output><empty_output> | notebooks/course/fr/chapter4/section2_tf.ipynb/0 | {
"file_path": "notebooks/course/fr/chapter4/section2_tf.ipynb",
"repo_id": "notebooks",
"token_count": 270
} | 156 |
<jupyter_start><jupyter_text>Tokenisation *Byte-Pair Encoding* Installez les bibliothèques 🤗 *Transformers* et 🤗 *Datasets* pour exécuter ce *notebook*.<jupyter_code>!pip install datasets transformers[sentencepiece]
corpus = [
"C'est le cours d'Hugging Face.",
"Ce chapitre traite de la tokenisation.",
"Cette section présente plusieurs algorithmes de tokenizer.",
"Avec un peu de chance, vous serez en mesure de comprendre comment ils sont entraînés et génèrent des tokens.",
]
from transformers import AutoTokenizer
tokenizer = AutoTokenizer.from_pretrained("asi/gpt-fr-cased-small")
from collections import defaultdict
word_freqs = defaultdict(int)
for text in corpus:
words_with_offsets = tokenizer.backend_tokenizer.pre_tokenizer.pre_tokenize_str(text)
new_words = [word for word, offset in words_with_offsets]
for word in new_words:
word_freqs[word] += 1
print(word_freqs)
alphabet = []
for word in word_freqs.keys():
for letter in word:
if letter not in alphabet:
alphabet.append(letter)
alphabet.sort()
print(alphabet)
vocab = ["<|endoftext|>"] + alphabet.copy()
splits = {word: [c for c in word] for word in word_freqs.keys()}
def compute_pair_freqs(splits):
pair_freqs = defaultdict(int)
for word, freq in word_freqs.items():
split = splits[word]
if len(split) == 1:
continue
for i in range(len(split) - 1):
pair = (split[i], split[i + 1])
pair_freqs[pair] += freq
return pair_freqs
pair_freqs = compute_pair_freqs(splits)
for i, key in enumerate(pair_freqs.keys()):
print(f"{key}: {pair_freqs[key]}")
if i >= 5:
break
best_pair = ""
max_freq = None
for pair, freq in pair_freqs.items():
if max_freq is None or max_freq < freq:
best_pair = pair
max_freq = freq
print(best_pair, max_freq)
merges = {("Ġ", "t"): "Ġt"}
vocab.append("Ġt")
def merge_pair(a, b, splits):
for word in word_freqs:
split = splits[word]
if len(split) == 1:
continue
i = 0
while i < len(split) - 1:
if split[i] == a and split[i + 1] == b:
split = split[:i] + [a + b] + split[i + 2 :]
else:
i += 1
splits[word] = split
return splits
splits = merge_pair("Ġ", "t", splits)
splits
vocab_size = 50
while len(vocab) < vocab_size:
pair_freqs = compute_pair_freqs(splits)
best_pair = ""
max_freq = None
for pair, freq in pair_freqs.items():
if max_freq is None or max_freq < freq:
best_pair = pair
max_freq = freq
splits = merge_pair(*best_pair, splits)
merges[best_pair] = best_pair[0] + best_pair[1]
vocab.append(best_pair[0] + best_pair[1])
print(merges)
print(vocab)
def tokenize(text):
pre_tokenize_result = tokenizer._tokenizer.pre_tokenizer.pre_tokenize_str(text)
pre_tokenized_text = [word for word, offset in pre_tokenize_result]
splits = [[l for l in word] for word in pre_tokenized_text]
for pair, merge in merges.items():
for idx, split in enumerate(splits):
i = 0
while i < len(split) - 1:
if split[i] == pair[0] and split[i + 1] == pair[1]:
split = split[:i] + [merge] + split[i + 2 :]
else:
i += 1
splits[idx] = split
return sum(splits, [])
tokenize("Ce n'est pas un token.")<jupyter_output><empty_output> | notebooks/course/fr/chapter6/section5.ipynb/0 | {
"file_path": "notebooks/course/fr/chapter6/section5.ipynb",
"repo_id": "notebooks",
"token_count": 1572
} | 157 |
<jupyter_start><jupyter_text>Que faire quand vous obtenez une erreurCe chapitre portant sur le débogage, la langue nous importe peu ici. Nous nous intéressons surtout à la logique du code pour comprendre d'où provient l'erreur. Installez les bibliothèques 🤗 Transformers et 🤗 Datasets pour exécuter ce *notebook*.<jupyter_code>!pip install datasets transformers[sentencepiece]
!apt install git-lfs<jupyter_output><empty_output><jupyter_text>Vous aurez besoin de configurer git, adaptez votre email et votre nom dans la cellule suivante.<jupyter_code>!git config --global user.email "[email protected]"
!git config --global user.name "Your Name"<jupyter_output><empty_output><jupyter_text>Vous devrez également être connecté au *Hub* d'Hugging Face. Exécutez ce qui suit et entrez vos informations d'identification.<jupyter_code>from huggingface_hub import notebook_login
notebook_login()
from distutils.dir_util import copy_tree
from huggingface_hub import Repository, snapshot_download, create_repo, get_full_repo_name
def copy_repository_template():
# Cloner le dépôt et extraire le chemin local
template_repo_id = "lewtun/distilbert-base-uncased-finetuned-squad-d5716d28"
commit_hash = "be3eaffc28669d7932492681cd5f3e8905e358b4"
template_repo_dir = snapshot_download(template_repo_id, revision=commit_hash)
# Créer un dépôt vide sur le Hub
model_name = template_repo_id.split("/")[1]
create_repo(model_name, exist_ok=True)
# Clonez le dépôt vide
new_repo_id = get_full_repo_name(model_name)
new_repo_dir = model_name
repo = Repository(local_dir=new_repo_dir, clone_from=new_repo_id)
# Copier les fichiers
copy_tree(template_repo_dir, new_repo_dir)
# Pousser sur le Hub
repo.push_to_hub()
from transformers import pipeline
model_checkpoint = get_full_repo_name("distillbert-base-uncased-finetuned-squad-d5716d28")
reader = pipeline("question-answering", model=model_checkpoint)
model_checkpoint = get_full_repo_name("distilbert-base-uncased-finetuned-squad-d5716d28")
reader = pipeline("question-answering", model=model_checkpoint)
from huggingface_hub import list_repo_files
list_repo_files(repo_id=model_checkpoint)
from transformers import AutoConfig
pretrained_checkpoint = "distilbert-base-uncased"
config = AutoConfig.from_pretrained(pretrained_checkpoint)
config.push_to_hub(model_checkpoint, commit_message="Add config.json")
reader = pipeline("question-answering", model=model_checkpoint, revision="main")
context = r"""
Extractive Question Answering is the task of extracting an answer from a text
given a question. An example of a question answering dataset is the SQuAD
dataset, which is entirely based on that task. If you would like to fine-tune a
model on a SQuAD task, you may leverage the
examples/pytorch/question-answering/run_squad.py script.
🤗 Transformers is interoperable with the PyTorch, TensorFlow, and JAX
frameworks, so you can use your favourite tools for a wide variety of tasks!
"""
question = "What is extractive question answering?"
reader(question=question, context=context)
tokenizer = reader.tokenizer
model = reader.model
question = "Which frameworks can I use?"
import torch
inputs = tokenizer(question, context, add_special_tokens=True)
input_ids = inputs["input_ids"][0]
outputs = model(**inputs)
answer_start_scores = outputs.start_logits
answer_end_scores = outputs.end_logits
# Obtenir le début de réponse le plus probable avec l'argmax du score
answer_start = torch.argmax(answer_start_scores)
# Obtenir la fin de réponse la plus probable avec l'argmax du score
answer_end = torch.argmax(answer_end_scores) + 1
answer = tokenizer.convert_tokens_to_string(
tokenizer.convert_ids_to_tokens(input_ids[answer_start:answer_end])
)
print(f"Question: {question}")
print(f"Answer: {answer}")
inputs["input_ids"][:5]
type(inputs["input_ids"])<jupyter_output><empty_output> | notebooks/course/fr/chapter8/section2.ipynb/0 | {
"file_path": "notebooks/course/fr/chapter8/section2.ipynb",
"repo_id": "notebooks",
"token_count": 1365
} | 158 |
<jupyter_start><jupyter_text>Running IF with 🧨 diffusers on a Free Tier Google Colab_**TL;DR**: We show how to run one of the most powerful open-source text to image models **IF** on a free-tier Google Colab with 🧨 diffusers._*by DeepFloyd &* 🤗 *HuggingFace* *Image taken from official IF GitHub repo [here](https://github.com/deep-floyd/IF/blob/release/pics/nabla.jpg)* IntroductionIF is a pixel-based text-to-image generation model and was [released in late April 2023 by DeepFloyd](https://github.com/deep-floyd/IF). The model architecture is strongly inspired by [Google's closed-sourced Imagen](https://imagen.research.google/). IF has two distinct advantages compared to existing text-to-image models like Stable Diffusion:- The model operates directly in "pixel space" (*i.e.,* on uncompressed images) instead of running the denoising process in the latent space such as [Stable Diffusion](http://hf.co/blog/stable_diffusion).- The model is trained on outputs of [T5-XXL](https://huggingface.co/google/t5-v1_1-xxl), a more powerful text encoder than [CLIP](https://openai.com/research/clip), used by Stable Diffusion as the text encoder.As a result, IF is better at generating images with high-frequency details (*e.g.,* human faces, and hands) and is the first open-source image generation model that can reliably generate images with text.The downside of operating in pixel space and using a more powerful text encoder is that IF has a significantly higher amount of parameters. T5, IF's text-to-image UNet, and IF's upscaler UNet have 4.5B, 4.3B, and 1.2B parameters respectively. Compared this to [Stable Diffusion 2.1](https://huggingface.co/stabilityai/stable-diffusion-2-1)'s text encoder and UNet having just 400M and 900M parameters respectively.Nevertheless, it is possible to run IF on consumer hardware if one optimizes the model for low-memory usage. We will show you can do this with 🧨 diffusers in this blog post. In 1.), we explain how to use IF for text-to-image generation and in 2.) and 3.) we go over IF's image variation and image inpainting capabilities.💡 **Note**: To a big part we are trading gains in memory by gains in speed here to make it possible to run IF in a free-tier Google Colab. If you have access to high-end GPUs such as a A100, we recommend to simply leave all model components on GPU for maximum speed as done in the [official IF demo](https://huggingface.co/spaces/DeepFloyd/IF). Let's dive in 🚀! Optimizing IF to run on memory constrained hardwareState-of-the-art ML should not just be in the hands of an elite few. Democratizing ML means making models available to run on more than just the latest and greatest hardware.The deep learning community has created world class tools to run resource intensive models on consumer hardware:- [🤗 accelerate](https://github.com/huggingface/accelerate) provides utilities for working with [large models](https://huggingface.co/docs/accelerate/usage_guides/big_modeling).- [bitsandbytes](https://github.com/TimDettmers/bitsandbytes) makes [8bit quantization](https://github.com/TimDettmers/bitsandbytesfeatures) available to all PyTorch models.- [🤗 safetensors](https://github.com/huggingface/safetensors) not only ensures that save code is executed but also significantly speeds up the loading time of large models.Diffusers seemlessly integrates the above libraries to allow for a simple API when optimizing large models.The free-tier Google Colab is both CPU RAM constrained (13 GB RAM) as well as GPU VRAM constrained (15 GB RAM for T4) which makes running the whole >10B IF model challenging!Let's map out the size of IF's model components in full float32 precision:- [T5-XXL Text Encoder](https://huggingface.co/DeepFloyd/IF-I-XL-v1.0/tree/main/text_encoder): 20GB- [Stage 1 UNet](https://huggingface.co/DeepFloyd/IF-I-XL-v1.0/tree/main/unet): 17.2 GB- [Stage 2 Super Resolution UNet](https://huggingface.co/DeepFloyd/IF-II-L-v1.0/blob/main/pytorch_model.bin): 2.5 GB- [Stage 3 Super Resolution Model](https://huggingface.co/stabilityai/stable-diffusion-x4-upscaler): 3.4 GBThere is no way we can run the model in float32 as the T5 and Stage 1 UNet weights are each larger than the available CPU RAM.In float16, the component sizes are 11GB, 8.6GB and 1.25GB for T5, Stage1 and Stage2 UNets respectively which is doable for the GPU, but we're still running into CPU memory overflow errors when loading the T5 (some CPU is occupied by other processes).Therefore, we lower the precision of T5 even more by using `bitsandbytes` 8bit quantization which allows to save the T5 checkpoint with as little as [8 GB](https://huggingface.co/DeepFloyd/IF-I-XL-v1.0/blob/main/text_encoder/model.8bit.safetensors).Now that each components fits individually into both CPU and GPU memory, we need to make sure that components have all the CPU and GPU memory for themselves when needed.Diffusers supports modularly loading individual components i.e. we can load the text encoder without loading the unet. This modular loading will ensure that we only load the component we need at a given step in the pipeline to avoid exhausting the available CPU RAM and GPU VRAM.Let's give it a try 🚀 Available resourcesThe free-tier Google Colab comes with around 13 GB CPU RAM:<jupyter_code>!grep MemTotal /proc/meminfo<jupyter_output>MemTotal: 13297192 kB<jupyter_text>And an NVIDIA T4 with 15 GB VRAM:<jupyter_code>!nvidia-smi<jupyter_output>Wed Apr 26 14:25:11 2023
+-----------------------------------------------------------------------------+
| NVIDIA-SMI 525.85.12 Driver Version: 525.85.12 CUDA Version: 12.0 |
|-------------------------------+----------------------+----------------------+
| GPU Name Persistence-M| Bus-Id Disp.A | Volatile Uncorr. ECC |
| Fan Temp Perf Pwr:Usage/Cap| Memory-Usage | GPU-Util Compute M. |
| | | MIG M. |
|===============================+======================+======================|
| 0 Tesla T4 Off | 00000000:00:04.0 Off | 0 |
| N/A 48C P0 27W / 70W | 8677MiB / 15360MiB | 0% Default |
| | | N/A |
+-------------------------------+----------------------+----------------------+
+-------[...]<jupyter_text>Install dependencies Some optimizations can require up-to-date versions of dependencies. If you are having issues, please double check and upgrade versions.<jupyter_code>! pip install --upgrade \
diffusers~=0.16 \
transformers~=4.28 \
safetensors~=0.3 \
sentencepiece~=0.1 \
accelerate~=0.18 \
bitsandbytes~=0.38 \
torch~=2.0 -q<jupyter_output><empty_output><jupyter_text>Accepting the licenseBefore you can use IF, you need to accept its usage conditions. To do so:- 1. Make sure to have a [Hugging Face account](https://huggingface.co/join) and be loggin in- 2. Accept the license on the model card of [DeepFloyd/IF-I-XL-v1.0](https://huggingface.co/DeepFloyd/IF-I-XL-v1.0). Accepting the license on the stage I model card will auto accept for the other IF models.- 3. Make sure to login locally. Install `huggingface_hub`<jupyter_code>!pip install huggingface_hub --upgrade<jupyter_output><empty_output><jupyter_text>and run the login function in a Python shell<jupyter_code>from huggingface_hub import login
login()<jupyter_output><empty_output><jupyter_text>1. Text-to-image generationWe will walk step by step through text-to-image generation with IF using Diffusers. We will explain briefly APIs and optimizations, but more in-depth explanations can be found in the official documentation for [Diffusers](https://huggingface.co/docs/diffusers/index), [Transformers](https://huggingface.co/docs/transformers/index), [Accelerate](https://huggingface.co/docs/accelerate/index), and [bitsandbytes](https://github.com/TimDettmers/bitsandbytes). 1.1 Load text encoderWe will load T5 using 8bit quantization. Transformers directly supports [bitsandbytes](https://huggingface.co/docs/transformers/main/en/main_classes/quantizationload-a-large-model-in-8bit) through the `load_in_8bit` flag. The flag `variant="8bit"` will download pre-quantized weights.We also use the `device_map` flag to allow `transformers` to offload model layers to the CPU or disk. Transformers big modeling supports arbitrary device maps which can be used to separately load model parameters directly to available devices. Passing `"auto"` will automatically create a device map. See the `transformers` [docs](https://huggingface.co/docs/accelerate/usage_guides/big_modelingdesigning-a-device-map) for more information.<jupyter_code>from transformers import T5EncoderModel
text_encoder = T5EncoderModel.from_pretrained(
"DeepFloyd/IF-I-XL-v1.0",
subfolder="text_encoder",
device_map="auto",
load_in_8bit=True,
variant="8bit"
)<jupyter_output><empty_output><jupyter_text>1.2 Create text embeddingsThe Diffusers API for accessing diffusion models is the `DiffusionPipeline` class and its subclasses. Each instance of `DiffusionPipeline` is a fully self contained set of methods and models for running diffusion networks. We can override the models it uses by passing alternative instances as keyword arguments to `from_pretrained`. In this case, we pass `None` for the `unet` argument so no UNet will be loaded. This allows us to run the text embedding portion of the diffusion process without loading the UNet into memory.<jupyter_code>from diffusers import DiffusionPipeline
pipe = DiffusionPipeline.from_pretrained(
"DeepFloyd/IF-I-XL-v1.0",
text_encoder=text_encoder, # pass the previously instantiated 8bit text encoder
unet=None,
device_map="auto"
)<jupyter_output><empty_output><jupyter_text>IF also comes with a super resolution pipeline. We will save the prompt embeddings so that we can later directly pass them to the super resolution pipeline. This will allow the super resolution pipeline to be loaded **without** a text encoder.Instead of [an astronaut just riding a horse](https://huggingface.co/blog/stable_diffusion), let's hand him a sign as well! Let's define a fitting prompt:<jupyter_code>prompt = 'a photograph of an astronaut riding a horse holding a sign that says "Pixel\'s in space"'<jupyter_output><empty_output><jupyter_text>and run it through the 8bit quantized T5 model:<jupyter_code>prompt_embeds, negative_embeds = pipe.encode_prompt(prompt)<jupyter_output><empty_output><jupyter_text>1.3 Free memory Once the prompt embeddings have been created. We do not need the text encoder anymore. However, it is still in memory on the GPU. We need to remove it so that we can load the UNet.It's non-trivial to free PyTorch memory. We must garbage collect the Python objects which point to the actual memory allocated on the GPU. First, use the python keyword `del` to delete all python objects referencing allocated GPU memory<jupyter_code>del text_encoder
del pipe<jupyter_output><empty_output><jupyter_text>The deletion of the python object is not enough to free the GPU memory. Garbage collection is when the actual GPU memory is freed.Additionally, we will call `torch.cuda.empty_cache()`. This method isn't strictly necessary as the cached cuda memory will be immediately available for further allocations. Emptying the cache allows us to verify in the colab UI that the memory is available.We'll use a helper function `flush()` to flush memory.<jupyter_code>import gc
import torch
def flush():
gc.collect()
torch.cuda.empty_cache()<jupyter_output><empty_output><jupyter_text>and run it<jupyter_code>flush()<jupyter_output><empty_output><jupyter_text>1.4 Stage 1: The main diffusion processWith our now available GPU memory, we can re-load the `DiffusionPipeline` with only the UNet to run the main diffusion process.The `variant` and `torch_dtype` flags are used by Diffusers to download and load the weights in 16 bit floating point format.<jupyter_code>pipe = DiffusionPipeline.from_pretrained(
"DeepFloyd/IF-I-XL-v1.0",
text_encoder=None,
variant="fp16",
torch_dtype=torch.float16,
device_map="auto"
)<jupyter_output>A mixture of fp16 and non-fp16 filenames will be loaded.
Loaded fp16 filenames:
[unet/diffusion_pytorch_model.fp16.safetensors, text_encoder/model.fp16-00001-of-00002.safetensors, text_encoder/model.fp16-00002-of-00002.safetensors, safety_checker/model.fp16.safetensors]
Loaded non-fp16 filenames:
[watermarker/diffusion_pytorch_model.safetensors
If this behavior is not expected, please check your folder structure.<jupyter_text>Often, we directly pass the text prompt to `DiffusionPipeline.__call__`. However, we previously computed our text embeddings which we can pass instead.IF also comes with a super resolution diffusion process. Setting `output_type="pt"` will return raw PyTorch tensors instead of a PIL image. This way we can keep the Pytorch tensors on GPU and pass them directly to the stage 2 super resolution pipeline. Let's define a random generator and run the stage 1 diffusion process.<jupyter_code>generator = torch.Generator().manual_seed(1)
image = pipe(
prompt_embeds=prompt_embeds,
negative_prompt_embeds=negative_embeds,
output_type="pt",
generator=generator,
).images<jupyter_output><empty_output><jupyter_text>Let's manually convert the raw tensors to PIL and have a sneak peak at the final result. The output of stage 1 is a 64x64 image.<jupyter_code>from diffusers.utils import pt_to_pil
pil_image = pt_to_pil(image)
pipe.watermarker.apply_watermark(pil_image, pipe.unet.config.sample_size)
pil_image[0]<jupyter_output><empty_output><jupyter_text>And again, we remove the Python pointer and free CPU and GPU memory:<jupyter_code>del pipe
flush()<jupyter_output><empty_output><jupyter_text>1.5 Stage 2: Super Resolution 64x64 to 256x256IF comes with a separate diffusion process for upscaling.We run each diffusion process with a separate pipeline. The super resolution pipeline can be loaded with a text encoder if needed. However, we will usually have pre-computed text embeddings from the first IF pipeline. If so, load the pipeline without the text encoder. Create the pipeline<jupyter_code>pipe = DiffusionPipeline.from_pretrained(
"DeepFloyd/IF-II-L-v1.0",
text_encoder=None, # no use of text encoder => memory savings!
variant="fp16",
torch_dtype=torch.float16,
device_map="auto"
)<jupyter_output><empty_output><jupyter_text>and run it, re-using the pre-computed text embeddings<jupyter_code>image = pipe(
image=image,
prompt_embeds=prompt_embeds,
negative_prompt_embeds=negative_embeds,
output_type="pt",
generator=generator,
).images<jupyter_output><empty_output><jupyter_text>Again we can inspect the intermediate results.<jupyter_code>pil_image = pt_to_pil(image)
pipe.watermarker.apply_watermark(pil_image, pipe.unet.config.sample_size)
pil_image[0]<jupyter_output><empty_output><jupyter_text>And again, we delete the Python pointer and free memory<jupyter_code>del pipe
flush()<jupyter_output><empty_output><jupyter_text>1.6 Stage 3: Super Resolution 256x256 to 1024x1024The second super resolution model for IF is the previously release [Stability AI's x4 Upscaler](https://huggingface.co/stabilityai/stable-diffusion-x4-upscaler).Let's create the pipeline and load it directly on GPU with `device_map="auto"`.Note that `device_map="auto"` with certain pipelines will throw errors in diffusers versions from `v0.16-v0.17`. You will either have to upgrade to a later versionif one exists or install from main with `pip install git+https://github.com/huggingface/diffusers.git@main`<jupyter_code>pipe = DiffusionPipeline.from_pretrained(
"stabilityai/stable-diffusion-x4-upscaler",
torch_dtype=torch.float16,
device_map="auto"
)<jupyter_output><empty_output><jupyter_text>🧨 diffusers makes independently developed diffusion models easily composable as pipelines can be chained together. Here we can just take the previous PyTorch tensor output and pass it to the tage 3 pipeline as `image=image`.💡 **Note**: The x4 Upscaler does not use T5 and has [its own text encoder](https://huggingface.co/stabilityai/stable-diffusion-x4-upscaler/tree/main/text_encoder). Therefore, we cannot use the previously created prompt embeddings and instead must pass the original prompt.<jupyter_code>pil_image = pipe(prompt, generator=generator, image=image).images<jupyter_output><empty_output><jupyter_text>Unlike the IF pipelines, the IF watermark will not be added by default to outputs from the Stable Diffusion x4 upscaler pipeline.We can instead manually apply the watermark.<jupyter_code>from diffusers.pipelines.deepfloyd_if import IFWatermarker
watermarker = IFWatermarker.from_pretrained("DeepFloyd/IF-I-XL-v1.0", subfolder="watermarker")
watermarker.apply_watermark(pil_image, pipe.unet.config.sample_size)<jupyter_output><empty_output><jupyter_text>View output image<jupyter_code>pil_image[0]<jupyter_output><empty_output><jupyter_text>Et voila! A beautiful 1024x1024 image in a free-tier Google Colab.We have shown how 🧨 diffusers makes it easy to decompose and modularly load resource intensive diffusion models. 💡 **Note**: We don't recommend using the above setup in production. 8bit quantization, manual de-allocation of model weights, and disk offloading all trade off memory for time (i.e., inference speed). This can be especially noticable if the diffusion pipeline is re-used. In production, we recommend using a 40GB A100 with all model components left on the GPU. See [**the official IF demo**](https://huggingface.co/spaces/DeepFloyd/IF). 2. Image variationThe same IF checkpoints can also be used for text guided image variation and inpainting. The core diffusion process is the same as text to image generation except the initial noised image is created from the image to be varied or inpainted.To run image variation, load the same checkpoints with [`IFImg2ImgPipeline.from_pretrained()`](https://huggingface.co/docs/diffusers/api/pipelines/ifdiffusers.IFImg2ImgPipeline) and [`IFImg2ImgSuperResolution.from_pretrained()`](https://huggingface.co/docs/diffusers/api/pipelines/ifdiffusers.IFImg2ImgSuperResolution).The APIs for memory optimization are all the same! Let's free the memory from the previous section.<jupyter_code>del pipe
flush()<jupyter_output><empty_output><jupyter_text>For image variation, we start with an initial image that we want to adapt. For this section, we will adapt the famous "Slaps Roof of Car" meme. Let's download it from the internet.<jupyter_code>import requests
url = "https://i.kym-cdn.com/entries/icons/original/000/026/561/car.jpg"
response = requests.get(url)<jupyter_output><empty_output><jupyter_text>and load it into a PIL Image<jupyter_code>from PIL import Image
from io import BytesIO
original_image = Image.open(BytesIO(response.content)).convert("RGB")
original_image = original_image.resize((768, 512))
original_image<jupyter_output><empty_output><jupyter_text>The image variation pipeline take both PIL images and raw tensors. View the docstrings for more indepth documentation on expected inputs, [here](https://huggingface.co/docs/diffusers/v0.16.0/en/api/pipelines/ifdiffusers.IFImg2ImgPipeline.__call__) 2.1 Text EncoderImage variation is guided by text, so we can define a prompt and encode it with T5's Text Encoder.Again we load the text encoder into 8bit precision.<jupyter_code>from transformers import T5EncoderModel
text_encoder = T5EncoderModel.from_pretrained(
"DeepFloyd/IF-I-XL-v1.0",
subfolder="text_encoder",
device_map="auto",
load_in_8bit=True,
variant="8bit"
)<jupyter_output>Overriding torch_dtype=None with `torch_dtype=torch.float16` due to requirements of `bitsandbytes` to enable model loading in mixed int8. Either pass torch_dtype=torch.float16 or don't pass this argument at all to remove this warning.<jupyter_text>For image variation, we load the checkpoint with [`IFImg2ImgPipeline`](https://huggingface.co/docs/diffusers/api/pipelines/ifdiffusers.IFImg2ImgPipeline). When using `DiffusionPipeline.from_pretrained(...)`, checkpoints are loaded into their default pipeline. The default pipeline for the IF is the text-to-image [`IFPipeline`](https://huggingface.co/docs/diffusers/api/pipelines/ifdiffusers.IFPipeline). When loading checkpoints with a non-default pipeline, the pipeline must be explicitly specified.<jupyter_code>from diffusers import IFImg2ImgPipeline
pipe = IFImg2ImgPipeline.from_pretrained(
"DeepFloyd/IF-I-XL-v1.0",
text_encoder=text_encoder,
unet=None,
device_map="auto"
)<jupyter_output><empty_output><jupyter_text>Let's turn our salesman into an anime character.<jupyter_code>prompt = "anime style"<jupyter_output><empty_output><jupyter_text>As before, we create the text embeddings with T5<jupyter_code>prompt_embeds, negative_embeds = pipe.encode_prompt(prompt)<jupyter_output><empty_output><jupyter_text>and free GPU and CPU memory. First remove the Python pointers<jupyter_code>del text_encoder
del pipe<jupyter_output><empty_output><jupyter_text>and then free the memory<jupyter_code>flush()<jupyter_output><empty_output><jupyter_text>2.2 Stage 1: The main diffusion processNext, we only load the stage 1 UNet weights into the pipeline object, just like we did in the previous section.<jupyter_code>pipe = IFImg2ImgPipeline.from_pretrained(
"DeepFloyd/IF-I-XL-v1.0",
text_encoder=None,
variant="fp16",
torch_dtype=torch.float16,
device_map="auto"
)<jupyter_output>A mixture of fp16 and non-fp16 filenames will be loaded.
Loaded fp16 filenames:
[unet/diffusion_pytorch_model.fp16.safetensors, text_encoder/model.fp16-00001-of-00002.safetensors, text_encoder/model.fp16-00002-of-00002.safetensors, safety_checker/model.fp16.safetensors]
Loaded non-fp16 filenames:
[watermarker/diffusion_pytorch_model.safetensors
If this behavior is not expected, please check your folder structure.<jupyter_text>The image variation pipeline requires both the original image and the prompt embeddings. We can optionally use the `strength` argument to configure the amount of variation. `strength` directly controls the amount of noise added. Higher strength means more noise which means more variation.<jupyter_code>generator = torch.Generator().manual_seed(0)
image = pipe(
image=original_image,
prompt_embeds=prompt_embeds,
negative_prompt_embeds=negative_embeds,
output_type="pt",
generator=generator,
).images<jupyter_output><empty_output><jupyter_text>Let's check the intermediate 64x64 again.<jupyter_code>pil_image = pt_to_pil(image)
pipe.watermarker.apply_watermark(pil_image, pipe.unet.config.sample_size)
pil_image[0]<jupyter_output><empty_output><jupyter_text>Looks good, we can free the memory and upscale the image again.<jupyter_code>del pipe
flush()<jupyter_output><empty_output><jupyter_text>2.3 Stage 2: Super ResolutionFor super resolution, load the checkpoint with `IFImg2ImgSuperResolutionPipeline` and the same checkpoint as before.<jupyter_code>from diffusers import IFImg2ImgSuperResolutionPipeline
pipe = IFImg2ImgSuperResolutionPipeline.from_pretrained(
"DeepFloyd/IF-II-L-v1.0",
text_encoder=None,
variant="fp16",
torch_dtype=torch.float16,
device_map="auto"
)<jupyter_output>A mixture of fp16 and non-fp16 filenames will be loaded.
Loaded fp16 filenames:
[unet/diffusion_pytorch_model.fp16.safetensors, text_encoder/model.fp16-00001-of-00002.safetensors, text_encoder/model.fp16-00002-of-00002.safetensors, safety_checker/model.fp16.safetensors]
Loaded non-fp16 filenames:
[watermarker/diffusion_pytorch_model.safetensors
If this behavior is not expected, please check your folder structure.
`text_config_dict` is provided which will be used to initialize `CLIPTextConfig`. The value `text_config["id2label"]` will be overriden.<jupyter_text>💡 **Note**: The image variation super resolution pipeline requires the generated image as well as the original image. You can also use the Stable Diffusion x4 upscaler on this image. Feel free to try it out using the code snippets in section 1.6.<jupyter_code>image = pipe(
image=image,
original_image=original_image,
prompt_embeds=prompt_embeds,
negative_prompt_embeds=negative_embeds,
generator=generator,
).images[0]
image<jupyter_output><empty_output><jupyter_text>Nice! Let's free the memory and look at the final inpainting pipelines.<jupyter_code>del pipe
flush()<jupyter_output><empty_output><jupyter_text>3. InpaintingThe IF inpainting pipeline is the same as the image variation except only a select area of the image is denoised. We specify the area to inpatint with an image mask.Let's show off IF's amazing "letter generation" capabilities. We can replace this sign text with different slogan. First let's download the image<jupyter_code>import requests
url = "https://i.imgflip.com/5j6x75.jpg"
response = requests.get(url)<jupyter_output><empty_output><jupyter_text>and turn it into a PIL Image<jupyter_code>from PIL import Image
from io import BytesIO
original_image = Image.open(BytesIO(response.content)).convert("RGB")
original_image = original_image.resize((512, 768))
original_image<jupyter_output><empty_output><jupyter_text>We will mask the sign so we can replace its text. For convenience, we have pre-generated the mask and loaded it into a HF dataset.Let's download it.<jupyter_code>from huggingface_hub import hf_hub_download
mask_image = hf_hub_download("diffusers/docs-images", repo_type="dataset", filename="if/sign_man_mask.png")
mask_image = Image.open(mask_image)
mask_image<jupyter_output><empty_output><jupyter_text>💡 **Note**: You can create masks yourself by manually creating a greyscale image.<jupyter_code>from PIL import Image
import numpy as np
height = 64
width = 64
example_mask = np.zeros((height, width), dtype=np.int8)
# Set masked pixels to 255
example_mask[20:30, 30:40] = 255
# Make sure to create the image in mode 'L'
# meaning single channel grayscale
example_mask = Image.fromarray(example_mask, mode='L')
example_mask<jupyter_output><empty_output><jupyter_text>Now we can start inpainting 🎨🖌 3.1. Text EncoderAgain, we load the text encoder first<jupyter_code>from transformers import T5EncoderModel
text_encoder = T5EncoderModel.from_pretrained(
"DeepFloyd/IF-I-XL-v1.0",
subfolder="text_encoder",
device_map="auto",
load_in_8bit=True,
variant="8bit"
)<jupyter_output>Overriding torch_dtype=None with `torch_dtype=torch.float16` due to requirements of `bitsandbytes` to enable model loading in mixed int8. Either pass torch_dtype=torch.float16 or don't pass this argument at all to remove this warning.<jupyter_text>This time, we initialize the `IFInpaintingPipeline` in-painting pipeline with the text encoder weights.<jupyter_code>from diffusers import IFInpaintingPipeline
pipe = IFInpaintingPipeline.from_pretrained(
"DeepFloyd/IF-I-XL-v1.0",
text_encoder=text_encoder,
unet=None,
device_map="auto"
)<jupyter_output><empty_output><jupyter_text>Alright, let's have the man advertise for more layers instead.<jupyter_code>prompt = 'the text, "just stack more layers"'<jupyter_output><empty_output><jupyter_text>Having defined the prompt, we can create the prompt embeddings<jupyter_code>prompt_embeds, negative_embeds = pipe.encode_prompt(prompt)<jupyter_output><empty_output><jupyter_text>Just like before we free the memory<jupyter_code>del text_encoder
del pipe
flush()<jupyter_output><empty_output><jupyter_text>3.2 Stage 1: The main diffusion processJust like before we now load the stage 1 pipeline with only the UNet.<jupyter_code>pipe = IFInpaintingPipeline.from_pretrained(
"DeepFloyd/IF-I-XL-v1.0",
text_encoder=None,
variant="fp16",
torch_dtype=torch.float16,
device_map="auto"
)<jupyter_output>A mixture of fp16 and non-fp16 filenames will be loaded.
Loaded fp16 filenames:
[unet/diffusion_pytorch_model.fp16.safetensors, text_encoder/model.fp16-00001-of-00002.safetensors, text_encoder/model.fp16-00002-of-00002.safetensors, safety_checker/model.fp16.safetensors]
Loaded non-fp16 filenames:
[watermarker/diffusion_pytorch_model.safetensors
If this behavior is not expected, please check your folder structure.<jupyter_text>Now, we need to pass the input image, the mask image, and the prompt embeddings.<jupyter_code>image = pipe(
image=original_image,
mask_image=mask_image,
prompt_embeds=prompt_embeds,
negative_prompt_embeds=negative_embeds,
output_type="pt",
generator=generator,
).images<jupyter_output><empty_output><jupyter_text>Let's take a look at the intermediate output.<jupyter_code>pil_image = pt_to_pil(image)
pipe.watermarker.apply_watermark(pil_image, pipe.unet.config.sample_size)
pil_image[0]<jupyter_output><empty_output><jupyter_text>Looks good! The text is pretty consistent! Let's free the memory so we can upscale the image<jupyter_code>del pipe
flush()<jupyter_output><empty_output><jupyter_text>3.3 Stage 2: Super ResolutionFor super resolution, load the checkpoint with `IFInpaintingSuperResolutionPipeline`.<jupyter_code>from diffusers import IFInpaintingSuperResolutionPipeline
pipe = IFInpaintingSuperResolutionPipeline.from_pretrained(
"DeepFloyd/IF-II-L-v1.0",
text_encoder=None,
variant="fp16",
torch_dtype=torch.float16,
device_map="auto"
)<jupyter_output>A mixture of fp16 and non-fp16 filenames will be loaded.
Loaded fp16 filenames:
[unet/diffusion_pytorch_model.fp16.safetensors, text_encoder/model.fp16-00001-of-00002.safetensors, text_encoder/model.fp16-00002-of-00002.safetensors, safety_checker/model.fp16.safetensors]
Loaded non-fp16 filenames:
[watermarker/diffusion_pytorch_model.safetensors
If this behavior is not expected, please check your folder structure.
`text_config_dict` is provided which will be used to initialize `CLIPTextConfig`. The value `text_config["id2label"]` will be overriden.<jupyter_text>The inpainting super resolution pipeline requires the generated image, the original image, the mask image, and the prompt embeddings.Let's do a final denoising run.<jupyter_code>image = pipe(
image=image,
original_image=original_image,
mask_image=mask_image,
prompt_embeds=prompt_embeds,
negative_prompt_embeds=negative_embeds,
generator=generator,
).images[0]
image<jupyter_output><empty_output> | notebooks/diffusers/deepfloyd_if_free_tier_google_colab.ipynb/0 | {
"file_path": "notebooks/diffusers/deepfloyd_if_free_tier_google_colab.ipynb",
"repo_id": "notebooks",
"token_count": 9958
} | 159 |
<jupyter_start><jupyter_text>🧨 Stable Diffusion in JAX / Flax ! 🤗 Hugging Face [Diffusers](https://github.com/huggingface/diffusers) supports Flax since version `0.5.1`! This allows for super fast inference on Google TPUs, such as those available in Colab, Kaggle or Google Cloud Platform.This notebook shows how to run inference using JAX / Flax. If you want more details about how Stable Diffusion works or want to run it in GPU, please refer to [this Colab notebook](https://colab.research.google.com/github/huggingface/notebooks/blob/main/diffusers/stable_diffusion.ipynb). First, make sure you are using a TPU backend. If you are running this notebook in Colab, select `Runtime` in the menu above, then select the option "Change runtime type" and then select `TPU` under the `Hardware accelerator` setting.Note that JAX is not exclusive to TPUs, but it shines on that hardware because each TPU server has 8 TPU accelerators working in parallel. Setup<jupyter_code>!pip install flax transformers ftfy
!pip install diffusers==0.9.0
import jax.tools.colab_tpu
jax.tools.colab_tpu.setup_tpu()
import jax
num_devices = jax.device_count()
device_type = jax.devices()[0].device_kind
print(f"Found {num_devices} JAX devices of type {device_type}.")
assert "TPU" in device_type, "Available device is not a TPU, please select TPU from Edit > Notebook settings > Hardware accelerator"<jupyter_output>Found 8 JAX devices of type Cloud TPU.<jupyter_text>Then we import all the dependencies.<jupyter_code>import numpy as np
import jax
import jax.numpy as jnp
from pathlib import Path
from jax import pmap
from flax.jax_utils import replicate
from flax.training.common_utils import shard
from PIL import Image
from huggingface_hub import notebook_login
from diffusers import FlaxStableDiffusionPipeline<jupyter_output><empty_output><jupyter_text>Model Loading Before using the model, you need to accept the model [license](https://huggingface.co/spaces/CompVis/stable-diffusion-license) in order to download and use the weights.The license is designed to mitigate the potential harmful effects of such a powerful machine learning system. We request users to **read the license entirely and carefully**. Here we offer a summary:1. You can't use the model to deliberately produce nor share illegal or harmful outputs or content,2. We claim no rights on the outputs you generate, you are free to use them and are accountable for their use which should not go against the provisions set in the license, and3. You may re-distribute the weights and use the model commercially and/or as a service. If you do, please be aware you have to include the same use restrictions as the ones in the license and share a copy of the CreativeML OpenRAIL-M to all your users. Flax weights are available in Hugging Face Hub as part of the Stable Diffusion repo. To use them, you need to be a registered user in Hugging Face Hub and use an access token for the code to work. You have two options to provide your access token:* Use the `huggingface-cli login` command-line tool in your terminal and paste your token when prompted. It will be saved in a file in your computer.* Or use `notebook_login()` in a notebook, which does the same thing.The following cell will present a login interface unless you've already authenticated before in this computer. You'll need to paste your access token.<jupyter_code>if not (Path.home()/'.huggingface'/'token').exists(): notebook_login()<jupyter_output>Login successful
Your token has been saved to /root/.huggingface/token<jupyter_text>TPU devices support `bfloat16`, an efficient half-float type. We'll use it for our tests, but you can also use `float32` to use full precision instead.<jupyter_code>dtype = jnp.bfloat16<jupyter_output><empty_output><jupyter_text>Flax is a functional framework, so models are stateless and parameters are stored outside them. Loading the pre-trained Flax pipeline will return both the pipeline itself and the model weights (or parameters). We are using a `bf16` version of the weights, which leads to type warnings that you can safely ignore.<jupyter_code>pipeline, params = FlaxStableDiffusionPipeline.from_pretrained(
"CompVis/stable-diffusion-v1-4",
revision="bf16",
dtype=dtype,
)<jupyter_output><empty_output><jupyter_text>Inference Since TPUs usually have 8 devices working in parallel, we'll replicate our prompt as many times as devices we have. Then we'll perform inference on the 8 devices at once, each responsible for generating one image. Thus, we'll get 8 images in the same amount of time it takes for one chip to generate a single one.After replicating the prompt, we obtain the tokenized text ids by invoking the `prepare_inputs` function of the pipeline. The length of the tokenized text is set to 77 tokens, as required by the configuration of the underlying CLIP Text model.<jupyter_code>prompt = "A cinematic film still of Morgan Freeman starring as Jimi Hendrix, portrait, 40mm lens, shallow depth of field, close up, split lighting, cinematic"
prompt = [prompt] * jax.device_count()
prompt_ids = pipeline.prepare_inputs(prompt)
prompt_ids.shape<jupyter_output><empty_output><jupyter_text>Replication and parallelization Model parameters and inputs have to be replicated across the 8 parallel devices we have. The parameters dictionary is replicated using `flax.jax_utils.replicate`, which traverses the dictionary and changes the shape of the weights so they are repeated 8 times. Arrays are replicated using `shard`.<jupyter_code>p_params = replicate(params)
prompt_ids = shard(prompt_ids)
prompt_ids.shape<jupyter_output><empty_output><jupyter_text>That shape means that each one of the `8` devices will receive as an input a `jnp` array with shape `(1, 77)`. `1` is therefore the batch size per device. In TPUs with sufficient memory, it could be larger than `1` if we wanted to generate multiple images (per chip) at once. We are almost ready to generate images! We just need to create a random number generator to pass to the generation function. This is the standard procedure in Flax, which is very serious and opinionated about random numbers – all functions that deal with random numbers are expected to receive a generator. This ensures reproducibility, even when we are training across multiple distributed devices.The helper function below uses a seed to initialize a random number generator. As long as we use the same seed, we'll get the exact same results. Feel free to use different seeds when exploring results later in the notebook.<jupyter_code>def create_key(seed=0):
return jax.random.PRNGKey(seed)<jupyter_output><empty_output><jupyter_text>We obtain a rng and then "split" it 8 times so each device receives a different generator. Therefore, each device will create a different image, and the full process is reproducible.<jupyter_code>rng = create_key(0)
rng = jax.random.split(rng, jax.device_count())<jupyter_output><empty_output><jupyter_text>JAX code can be compiled to an efficient representation that runs very fast. However, we need to ensure that all inputs have the same shape in subsequent calls; otherwise, JAX will have to recompile the code, and we wouldn't be able to take advantage of the optimized speed. The Flax pipeline can compile the code for us if we pass `jit = True` as an argument. It will also ensure that the model runs in parallel in the 8 available devices. The first time we run the following cell it will take a long time to compile, but subequent calls (even with different inputs) will be much faster. For example, it took more than a minute to compile in a TPU v2-8 when I tested, but then it takes about **`7s`** for future inference runs.<jupyter_code>%%time
images = pipeline(prompt_ids, p_params, rng, jit=True)[0]<jupyter_output>CPU times: user 56.2 s, sys: 42.5 s, total: 1min 38s
Wall time: 1min 29s<jupyter_text>The returned array has shape `(8, 1, 512, 512, 3)`. We reshape it to get rid of the second dimension and obtain 8 images of `512 × 512 × 3` and then convert them to PIL.<jupyter_code>images = images.reshape((images.shape[0] * images.shape[1], ) + images.shape[-3:])
images = pipeline.numpy_to_pil(images)<jupyter_output><empty_output><jupyter_text>Visualization Let's create a helper function to display images in a grid.<jupyter_code>def image_grid(imgs, rows, cols):
w,h = imgs[0].size
grid = Image.new('RGB', size=(cols*w, rows*h))
for i, img in enumerate(imgs): grid.paste(img, box=(i%cols*w, i//cols*h))
return grid
image_grid(images, 2, 4)<jupyter_output><empty_output><jupyter_text>Using different prompts We don't have to replicate the _same_ prompt in all the devices. We can do whatever we want: generate 2 prompts 4 times each, or even generate 8 different prompts at once. Let's do that! First, we'll refactor the input preparation code into a handy function:<jupyter_code>prompts = [
"Labrador in the style of Hokusai",
"Painting of a squirrel skating in New York",
"HAL-9000 in the style of Van Gogh",
"Times Square under water, with fish and a dolphin swimming around",
"Ancient Roman fresco showing a man working on his laptop",
"Close-up photograph of young black woman against urban background, high quality, bokeh",
"Armchair in the shape of an avocado",
"Clown astronaut in space, with Earth in the background"
]
prompt_ids = pipeline.prepare_inputs(prompts)
prompt_ids = shard(prompt_ids)
images = pipeline(prompt_ids, p_params, rng, jit=True).images
images = images.reshape((images.shape[0] * images.shape[1], ) + images.shape[-3:])
images = pipeline.numpy_to_pil(images)
image_grid(images, 2, 4)<jupyter_output><empty_output><jupyter_text>How does parallelization work? We said before that the `diffusers` Flax pipeline automatically compiles the model and runs it in parallel on all available devices. We'll now briefly look inside that process to show how it works. JAX parallelization can be done in multiple ways. The easiest one revolves around using the `jax.pmap` function to achieve single-program, multiple-data (SPMD) parallelization. It means we'll run several copies of the same code, each on different data inputs. More sophisticated approaches are possible, we invite you to go over the [JAX documentation](https://jax.readthedocs.io/en/latest/index.html) and the [`pjit` pages](https://jax.readthedocs.io/en/latest/jax-101/08-pjit.html?highlight=pjit) to explore this topic if you are interested! `jax.pmap` does two things for us:- Compiles (or `jit`s) the code, as if we had invoked `jax.jit()`. This does not happen when we call `pmap`, but the first time the pmapped function is invoked.- Ensures the compiled code runs in parallel in all the available devices.To show how it works we `pmap` the `_generate` method of the pipeline, which is the private method that runs generates images. Please, note that this method may be renamed or removed in future releases of `diffusers`.<jupyter_code>p_generate = pmap(pipeline._generate)<jupyter_output><empty_output><jupyter_text>After we use `pmap`, the prepared function `p_generate` will conceptually do the following:* Invoke a copy of the underlying function `pipeline._generate` in each device.* Send each device a different portion of the input arguments. That's what sharding is used for. In our case, `prompt_ids` has shape `(8, 1, 77, 768)`. This array will be split in `8` and each copy of `_generate` will receive an input with shape `(1, 77, 768)`.We can code `_generate` completely ignoring the fact that it will be invoked in parallel. We just care about our batch size (`1` in this example) and the dimensions that make sense for our code, and don't have to change anything to make it work in parallel. The same way as when we used the pipeline call, the first time we run the following cell it will take a while, but then it will be much faster.<jupyter_code>%%time
images = p_generate(prompt_ids, p_params, rng)
images = images.block_until_ready()
images.shape
images.shape<jupyter_output><empty_output> | notebooks/diffusers/stable_diffusion_jax_how_to.ipynb/0 | {
"file_path": "notebooks/diffusers/stable_diffusion_jax_how_to.ipynb",
"repo_id": "notebooks",
"token_count": 3380
} | 160 |
<jupyter_start><jupyter_text>The Annotated Diffusion Model nielsr Niels Rogge kashif Kashif Rasul In this blog post, we'll take a deeper look into **Denoising Diffusion Probabilistic Models** (also known as DDPMs, diffusion models, score-based generative models or simply [autoencoders](https://benanne.github.io/2022/01/31/diffusion.html)) as researchers have been able to achieve remarkable results with them for (un)conditional image/audio/video generation. Popular examples (at the time of writing) include [GLIDE](https://arxiv.org/abs/2112.10741) and [DALL-E 2](https://openai.com/dall-e-2/) by OpenAI, [Latent Diffusion](https://github.com/CompVis/latent-diffusion) by the University of Heidelberg and [ImageGen](https://imagen.research.google/) by Google Brain.We'll go over the original DDPM paper by ([Ho et al., 2020](https://arxiv.org/abs/2006.11239)), implementing it step-by-step in PyTorch, based on Phil Wang's [implementation](https://github.com/lucidrains/denoising-diffusion-pytorch) - which itself is based on the [original TensorFlow implementation](https://github.com/hojonathanho/diffusion). Note that the idea of diffusion for generative modeling was actually already introduced in ([Sohl-Dickstein et al., 2015](https://arxiv.org/abs/1503.03585)). However, it took until ([Song et al., 2019](https://arxiv.org/abs/1907.05600)) (at Stanford University), and then ([Ho et al., 2020](https://arxiv.org/abs/2006.11239)) (at Google Brain) who independently improved the approach.Note that there are [several perspectives](https://twitter.com/sedielem/status/1530894256168222722?s=20&t=mfv4afx1GcNQU5fZklpACw) on diffusion models. Here, we employ the discrete-time (latent variable model) perspective, but be sure to check out the other perspectives as well. Alright, let's dive in! We'll install and import the required libraries first (assuming you have [PyTorch](https://pytorch.org/) installed).<jupyter_code>!pip install -q -U einops datasets matplotlib tqdm
import math
from inspect import isfunction
from functools import partial
%matplotlib inline
import matplotlib.pyplot as plt
from tqdm.auto import tqdm
from einops import rearrange
import torch
from torch import nn, einsum
import torch.nn.functional as F<jupyter_output><empty_output><jupyter_text>What is a diffusion model?A (denoising) diffusion model isn't that complex if you compare it to other generative models such as Normalizing Flows, GANs or VAEs: they all convert noise from some simple distribution to a data sample. This is also the case here where **a neural network learns to gradually denoise data** starting from pure noise. In a bit more detail for images, the set-up consists of 2 processes:* a fixed (or predefined) forward diffusion process $q$ of our choosing, that gradually adds Gaussian noise to an image, until you end up with pure noise* a learned reverse denoising diffusion process $p_\theta$, where a neural network is trained to gradually denoise an image starting from pure noise, until you end up with an actual image. Both the forward and reverse process indexed by \\(t\\) happen for some number of finite time steps \\(T\\) (the DDPM authors use \\(T=1000\\)). You start with \\(t=0\\) where you sample a real image \\(\mathbf{x}_0\\) from your data distribution (let's say an image of a cat from ImageNet), and the forward process samples some noise from a Gaussian distribution at each time step \\(t\\), which is added to the image of the previous time step. Given a sufficiently large \\(T\\) and a well behaved schedule for adding noise at each time step, you end up with what is called an [isotropic Gaussian distribution](https://math.stackexchange.com/questions/1991961/gaussian-distribution-is-isotropic) at \\(t=T\\) via a gradual process. In more mathematical formLet's write this down more formally, as ultimately we need a tractable loss function which our neural network needs to optimize. Let \\(q(\mathbf{x}_0)\\) be the real data distribution, say of "real images". We can sample from this distribution to get an image, \\(\mathbf{x}_0 \sim q(\mathbf{x}_0)\\). We define the forward diffusion process \\(q(\mathbf{x}_t | \mathbf{x}_{t-1})\\) which adds Gaussian noise at each time step \\(t\\), according to a known variance schedule \\(0 < \beta_1 < \beta_2 < ... < \beta_T < 1\\) as$$q(\mathbf{x}_t | \mathbf{x}_{t-1}) = \mathcal{N}(\mathbf{x}_t; \sqrt{1 - \beta_t} \mathbf{x}_{t-1}, \beta_t \mathbf{I}). $$Recall that a normal distribution (also called Gaussian distribution) is defined by 2 parameters: a mean \\(\mu\\) and a variance \\(\sigma^2 \geq 0\\). Basically, each new (slightly noiser) image at time step \\(t\\) is drawn from a **conditional Gaussian distribution** with \\(\mathbf{\mu}_t = \sqrt{1 - \beta_t} \mathbf{x}_{t-1}\\) and \\(\sigma^2_t = \beta_t\\), which we can do by sampling \\(\mathbf{\epsilon} \sim \mathcal{N}(\mathbf{0}, \mathbf{I})\\) and then setting \\(\mathbf{x}_t = \sqrt{1 - \beta_t} \mathbf{x}_{t-1} + \sqrt{\beta_t} \mathbf{\epsilon}\\). Note that the \\(\beta_t\\) aren't constant at each time step \\(t\\) (hence the subscript) --- in fact one defines a so-called **"variance schedule"**, which can be linear, quadratic, cosine, etc. as we will see further (a bit like a learning rate schedule). So starting from \\(\mathbf{x}_0\\), we end up with \\(\mathbf{x}_1, ..., \mathbf{x}_t, ..., \mathbf{x}_T\\), where \\(\mathbf{x}_T\\) is pure Gaussian noise if we set the schedule appropriately.Now, if we knew the conditional distribution \\(p(\mathbf{x}_{t-1} | \mathbf{x}_t)\\), then we could run the process in reverse: by sampling some random Gaussian noise \\(\mathbf{x}_T\\), and then gradually "denoise" it so that we end up with a sample from the real distribution \\(\mathbf{x}_0\\).However, we don't know \\(p(\mathbf{x}_{t-1} | \mathbf{x}_t)\\). It's intractable since it requires knowing the distribution of all possible images in order to calculate this conditional probability. Hence, we're going to leverage a neural network to **approximate (learn) this conditional probability distribution**, let's call it \\(p_\theta (\mathbf{x}_{t-1} | \mathbf{x}_t)\\), with \\(\theta\\) being the parameters of the neural network, updated by gradient descent. Ok, so we need a neural network to represent a (conditional) probability distribution of the backward process. If we assume this reverse process is Gaussian as well, then recall that any Gaussian distribution is defined by 2 parameters:* a mean parametrized by \\(\mu_\theta\\);* a variance parametrized by \\(\Sigma_\theta\\);so we can parametrize the process as $$ p_\theta (\mathbf{x}_{t-1} | \mathbf{x}_t) = \mathcal{N}(\mathbf{x}_{t-1}; \mu_\theta(\mathbf{x}_{t},t), \Sigma_\theta (\mathbf{x}_{t},t))$$where the mean and variance are also conditioned on the noise level \\(t\\). Hence, our neural network needs to learn/represent the mean and variance. However, the DDPM authors decided to **keep the variance fixed, and let the neural network only learn (represent) the mean \\(\mu_\theta\\) of this conditional probability distribution**. From the paper:> First, we set \\(\Sigma_\theta ( \mathbf{x}_t, t) = \sigma^2_t \mathbf{I}\\) to untrained time dependent constants. Experimentally, both \\(\sigma^2_t = \beta_t\\) and \\(\sigma^2_t = \tilde{\beta}_t\\) (see paper) had similar results. This was then later improved in the [Improved diffusion models](https://openreview.net/pdf?id=-NEXDKk8gZ) paper, where a neural network also learns the variance of this backwards process, besides the mean.So we continue, assuming that our neural network only needs to learn/represent the mean of this conditional probability distribution. Defining an objective function (by reparametrizing the mean)To derive an objective function to learn the mean of the backward process, the authors observe that the combination of \\(q\\) and \\(p_\theta\\) can be seen as a variational auto-encoder (VAE) [(Kingma et al., 2013)](https://arxiv.org/abs/1312.6114). Hence, the **variational lower bound** (also called ELBO) can be used to minimize the negative log-likelihood with respect to ground truth data sample \\(\mathbf{x}_0\\) (we refer to the VAE paper for details regarding ELBO). It turns out that the ELBO for this process is a sum of losses at each time step \\(t\\), \\(L = L_0 + L_1 + ... + L_T\\). By construction of the forward \\(q\\) process and backward process, each term (except for \\(L_0\\)) of the loss is actually the **KL divergence between 2 Gaussian distributions** which can be written explicitly as an L2-loss with respect to the means!A direct consequence of the constructed forward process \\(q\\), as shown by Sohl-Dickstein et al., is that we can sample \\(\mathbf{x}_t\\) at any arbitrary noise level conditioned on \\(\mathbf{x}_0\\) (since sums of Gaussians is also Gaussian). This is very convenient: we don't need to apply \\(q\\) repeatedly in order to sample \\(\mathbf{x}_t\\). We have that $$q(\mathbf{x}_t | \mathbf{x}_0) = \cal{N}(\mathbf{x}_t; \sqrt{\bar{\alpha}_t} \mathbf{x}_0, (1- \bar{\alpha}_t) \mathbf{I})$$with \\(\alpha_t := 1 - \beta_t\\) and \\(\bar{\alpha}t := \Pi_{s=1}^{t} \alpha_s\\). Let's refer to this equation as the "nice property". This means we can sample Gaussian noise and scale it appropriatly and add it to \\(\mathbf{x}_0\\) to get \\(\mathbf{x}_t\\) directly. Note that the \\(\bar{\alpha}_t\\) are functions of the known \\(\beta_t\\) variance schedule and thus are also known and can be precomputed. This then allows us, during training, to **optimize random terms of the loss function \\(L\\)** (or in other words, to randomly sample \\(t\\) during training and optimize \\(L_t\\). Another beauty of this property, as shown in Ho et al. is that one can (after some math, for which we refer the reader to [this excellent blog post](https://lilianweng.github.io/posts/2021-07-11-diffusion-models/)) instead **reparametrize the mean to make the neural network learn (predict) the added noise (via a network \\(\mathbf{\epsilon}_\theta(\mathbf{x}_t, t)\\) for noise level \\(t\\)** in the KL terms which constitute the losses. This means that our neural network becomes a noise predictor, rather than a (direct) mean predictor. The mean can be computed as follows:$$ \mathbf{\mu}_\theta(\mathbf{x}_t, t) = \frac{1}{\sqrt{\alpha_t}} \left( \mathbf{x}_t - \frac{\beta_t}{\sqrt{1- \bar{\alpha}_t}} \mathbf{\epsilon}_\theta(\mathbf{x}_t, t) \right)$$The final objective function \\(L_t\\) then looks as follows (for a random time step \\(t\\) given \\(\mathbf{\epsilon} \sim \mathcal{N}(\mathbf{0}, \mathbf{I})\\) ): $$ \| \mathbf{\epsilon} - \mathbf{\epsilon}_\theta(\mathbf{x}_t, t) \|^2 = \| \mathbf{\epsilon} - \mathbf{\epsilon}_\theta( \sqrt{\bar{\alpha}_t} \mathbf{x}_0 + \sqrt{(1- \bar{\alpha}_t) } \mathbf{\epsilon}, t) \|^2.$$ Here, \\(\mathbf{x}_0\\) is the initial (real, uncorruped) image, and we see the direct noise level \\(t\\) sample given by the fixed forward process. \\(\mathbf{\epsilon}\\) is the pure noise sampled at time step \\(t\\), and \\(\mathbf{\epsilon}_\theta (\mathbf{x}_t, t)\\) is our neural network. The neural network is optimized using a simple mean squared error (MSE) between the true and the predicted Gaussian noise.The training algorithm now looks as follows: In other words:* we take a random sample $\mathbf{x}_0$ from the real unknown and possibily complex data distribution $q(\mathbf{x}_0)$* we sample a noise level $t$ uniformally between $1$ and $T$ (i.e., a random time step)* we sample some noise from a Gaussian distribution and corrupt the input by this noise at level $t$ using the nice property defined above* the neural network is trained to predict this noise based on the corruped image $\mathbf{x}_t$, i.e. noise applied on $\mathbf{x}_0$ based on known schedule $\beta_t$In reality, all of this is done on batches of data as one uses stochastic gradient descent to optimize neural networks. The neural networkThe neural network needs to take in a noised image at a particular time step and return the predicted noise. Note that the predicted noise is a tensor that has the same size/resolution as the input image. So technically, the network takes in and outputs tensors of the same shape. What type of neural network can we use for this? What is typically used here is very similar to that of an [Autoencoder](https://en.wikipedia.org/wiki/Autoencoder), which you may remember from typical "intro to deep learning" tutorials. Autoencoders have a so-called "bottleneck" layer in between the encoder and decoder. The encoder first encodes an image into a smaller hidden representation called the "bottleneck", and the decoder then decodes that hidden representation back into an actual image. This forces the network to only keep the most important information in the bottleneck layer.In terms of architecture, the DDPM authors went for a **U-Net**, introduced by ([Ronneberger et al., 2015](https://arxiv.org/abs/1505.04597)) (which, at the time, achieved state-of-the-art results for medical image segmentation). This network, like any autoencoder, consists of a bottleneck in the middle that makes sure the network learns only the most important information. Importantly, it introduced residual connections between the encoder and decoder, greatly improving gradient flow (inspired by ResNet in [He et al., 2015](https://arxiv.org/abs/1512.03385)). As can be seen, a U-Net model first downsamples the input (i.e. makes the input smaller in terms of spatial resolution), after which upsampling is performed.Below, we implement this network, step-by-step. Network helpersFirst, we define some helper functions and classes which will be used when implementing the neural network. Importantly, we define a `Residual` module, which simply adds the input to the output of a particular function (in other words, adds a residual connection to a particular function).We also define aliases for the up- and downsampling operations.<jupyter_code>def exists(x):
return x is not None
def default(val, d):
if exists(val):
return val
return d() if isfunction(d) else d
class Residual(nn.Module):
def __init__(self, fn):
super().__init__()
self.fn = fn
def forward(self, x, *args, **kwargs):
return self.fn(x, *args, **kwargs) + x
def Upsample(dim):
return nn.ConvTranspose2d(dim, dim, 4, 2, 1)
def Downsample(dim):
return nn.Conv2d(dim, dim, 4, 2, 1)<jupyter_output><empty_output><jupyter_text>Position embeddingsAs the parameters of the neural network are shared across time (noise level), the authors employ sinusoidal position embeddings to encode $t$, inspired by the Transformer ([Vaswani et al., 2017](https://arxiv.org/abs/1706.03762)). This makes the neural network "know" at which particular time step (noise level) it is operating, for every image in a batch.The `SinusoidalPositionEmbeddings` module takes a tensor of shape `(batch_size, 1)` as input (i.e. the noise levels of several noisy images in a batch), and turns this into a tensor of shape `(batch_size, dim)`, with `dim` being the dimensionality of the position embeddings. This is then added to each residual block, as we will see further.<jupyter_code>class SinusoidalPositionEmbeddings(nn.Module):
def __init__(self, dim):
super().__init__()
self.dim = dim
def forward(self, time):
device = time.device
half_dim = self.dim // 2
embeddings = math.log(10000) / (half_dim - 1)
embeddings = torch.exp(torch.arange(half_dim, device=device) * -embeddings)
embeddings = time[:, None] * embeddings[None, :]
embeddings = torch.cat((embeddings.sin(), embeddings.cos()), dim=-1)
return embeddings<jupyter_output><empty_output><jupyter_text>ResNet/ConvNeXT blockNext, we define the core building block of the U-Net model. The DDPM authors employed a Wide ResNet block ([Zagoruyko et al., 2016](https://arxiv.org/abs/1605.07146)), but Phil Wang decided to also add support for a ConvNeXT block ([Liu et al., 2022](https://arxiv.org/abs/2201.03545)), as the latter has achieved great success in the image domain. One can choose one or another in the final U-Net architecture.<jupyter_code>class Block(nn.Module):
def __init__(self, dim, dim_out, groups = 8):
super().__init__()
self.proj = nn.Conv2d(dim, dim_out, 3, padding = 1)
self.norm = nn.GroupNorm(groups, dim_out)
self.act = nn.SiLU()
def forward(self, x, scale_shift = None):
x = self.proj(x)
x = self.norm(x)
if exists(scale_shift):
scale, shift = scale_shift
x = x * (scale + 1) + shift
x = self.act(x)
return x
class ResnetBlock(nn.Module):
"""https://arxiv.org/abs/1512.03385"""
def __init__(self, dim, dim_out, *, time_emb_dim=None, groups=8):
super().__init__()
self.mlp = (
nn.Sequential(nn.SiLU(), nn.Linear(time_emb_dim, dim_out))
if exists(time_emb_dim)
else None
)
self.block1 = Block(dim, dim_out, groups=groups)
self.block2 = Block(dim_out, dim_out, groups=groups)
self.res_conv = nn.Conv2d(dim, dim_out, 1) if dim != dim_out else nn.Identity()
def forward(self, x, time_emb=None):
h = self.block1(x)
if exists(self.mlp) and exists(time_emb):
time_emb = self.mlp(time_emb)
h = rearrange(time_emb, "b c -> b c 1 1") + h
h = self.block2(h)
return h + self.res_conv(x)
class ConvNextBlock(nn.Module):
"""https://arxiv.org/abs/2201.03545"""
def __init__(self, dim, dim_out, *, time_emb_dim=None, mult=2, norm=True):
super().__init__()
self.mlp = (
nn.Sequential(nn.GELU(), nn.Linear(time_emb_dim, dim))
if exists(time_emb_dim)
else None
)
self.ds_conv = nn.Conv2d(dim, dim, 7, padding=3, groups=dim)
self.net = nn.Sequential(
nn.GroupNorm(1, dim) if norm else nn.Identity(),
nn.Conv2d(dim, dim_out * mult, 3, padding=1),
nn.GELU(),
nn.GroupNorm(1, dim_out * mult),
nn.Conv2d(dim_out * mult, dim_out, 3, padding=1),
)
self.res_conv = nn.Conv2d(dim, dim_out, 1) if dim != dim_out else nn.Identity()
def forward(self, x, time_emb=None):
h = self.ds_conv(x)
if exists(self.mlp) and exists(time_emb):
assert exists(time_emb), "time embedding must be passed in"
condition = self.mlp(time_emb)
h = h + rearrange(condition, "b c -> b c 1 1")
h = self.net(h)
return h + self.res_conv(x)<jupyter_output><empty_output><jupyter_text>Attention moduleNext, we define the attention module, which the DDPM authors added in between the convolutional blocks. Attention is the building block of the famous Transformer architecture ([Vaswani et al., 2017](https://arxiv.org/abs/1706.03762)), which has shown great success in various domains of AI, from NLP and vision to [protein folding](https://www.deepmind.com/blog/alphafold-a-solution-to-a-50-year-old-grand-challenge-in-biology). Phil Wang employs 2 variants of attention: one is regular multi-head self-attention (as used in the Transformer), the other one is a [linear attention variant](https://github.com/lucidrains/linear-attention-transformer) ([Shen et al., 2018](https://arxiv.org/abs/1812.01243)), whose time- and memory requirements scale linear in the sequence length, as opposed to quadratic for regular attention.For an extensive explanation of the attention mechanism, we refer the reader to Jay Allamar's [wonderful blog post](https://jalammar.github.io/illustrated-transformer/).<jupyter_code>class Attention(nn.Module):
def __init__(self, dim, heads=4, dim_head=32):
super().__init__()
self.scale = dim_head**-0.5
self.heads = heads
hidden_dim = dim_head * heads
self.to_qkv = nn.Conv2d(dim, hidden_dim * 3, 1, bias=False)
self.to_out = nn.Conv2d(hidden_dim, dim, 1)
def forward(self, x):
b, c, h, w = x.shape
qkv = self.to_qkv(x).chunk(3, dim=1)
q, k, v = map(
lambda t: rearrange(t, "b (h c) x y -> b h c (x y)", h=self.heads), qkv
)
q = q * self.scale
sim = einsum("b h d i, b h d j -> b h i j", q, k)
sim = sim - sim.amax(dim=-1, keepdim=True).detach()
attn = sim.softmax(dim=-1)
out = einsum("b h i j, b h d j -> b h i d", attn, v)
out = rearrange(out, "b h (x y) d -> b (h d) x y", x=h, y=w)
return self.to_out(out)
class LinearAttention(nn.Module):
def __init__(self, dim, heads=4, dim_head=32):
super().__init__()
self.scale = dim_head**-0.5
self.heads = heads
hidden_dim = dim_head * heads
self.to_qkv = nn.Conv2d(dim, hidden_dim * 3, 1, bias=False)
self.to_out = nn.Sequential(nn.Conv2d(hidden_dim, dim, 1),
nn.GroupNorm(1, dim))
def forward(self, x):
b, c, h, w = x.shape
qkv = self.to_qkv(x).chunk(3, dim=1)
q, k, v = map(
lambda t: rearrange(t, "b (h c) x y -> b h c (x y)", h=self.heads), qkv
)
q = q.softmax(dim=-2)
k = k.softmax(dim=-1)
q = q * self.scale
context = torch.einsum("b h d n, b h e n -> b h d e", k, v)
out = torch.einsum("b h d e, b h d n -> b h e n", context, q)
out = rearrange(out, "b h c (x y) -> b (h c) x y", h=self.heads, x=h, y=w)
return self.to_out(out)<jupyter_output><empty_output><jupyter_text>Group normalizationThe DDPM authors interleave the convolutional/attention layers of the U-Net with group normalization ([Wu et al., 2018](https://arxiv.org/abs/1803.08494)). Below, we define a `PreNorm` class, which will be used to apply groupnorm before the attention layer, as we'll see further. Note that there's been a [debate](https://tnq177.github.io/data/transformers_without_tears.pdf) about whether to apply normalization before or after attention in Transformers.<jupyter_code>class PreNorm(nn.Module):
def __init__(self, dim, fn):
super().__init__()
self.fn = fn
self.norm = nn.GroupNorm(1, dim)
def forward(self, x):
x = self.norm(x)
return self.fn(x)<jupyter_output><empty_output><jupyter_text>Conditional U-NetNow that we've defined all building blocks (position embeddings, ResNet/ConvNeXT blocks, attention and group normalization), it's time to define the entire neural network. Recall that the job of the network \\(\mathbf{\epsilon}_\theta(\mathbf{x}_t, t)\\) is to take in a batch of noisy images + noise levels, and output the noise added to the input. More formally:- the network takes a batch of noisy images of shape `(batch_size, num_channels, height, width)` and a batch of noise levels of shape `(batch_size, 1)` as input, and returns a tensor of shape `(batch_size, num_channels, height, width)`The network is built up as follows:* first, a convolutional layer is applied on the batch of noisy images, and position embeddings are computed for the noise levels* next, a sequence of downsampling stages are applied. Each downsampling stage consists of 2 ResNet/ConvNeXT blocks + groupnorm + attention + residual connection + a downsample operation* at the middle of the network, again ResNet or ConvNeXT blocks are applied, interleaved with attention* next, a sequence of upsampling stages are applied. Each upsampling stage consists of 2 ResNet/ConvNeXT blocks + groupnorm + attention + residual connection + an upsample operation* finally, a ResNet/ConvNeXT block followed by a convolutional layer is applied.Ultimately, neural networks stack up layers as if they were lego blocks (but it's important to [understand how they work](http://karpathy.github.io/2019/04/25/recipe/)).<jupyter_code>class Unet(nn.Module):
def __init__(
self,
dim,
init_dim=None,
out_dim=None,
dim_mults=(1, 2, 4, 8),
channels=3,
with_time_emb=True,
resnet_block_groups=8,
use_convnext=True,
convnext_mult=2,
):
super().__init__()
# determine dimensions
self.channels = channels
init_dim = default(init_dim, dim // 3 * 2)
self.init_conv = nn.Conv2d(channels, init_dim, 7, padding=3)
dims = [init_dim, *map(lambda m: dim * m, dim_mults)]
in_out = list(zip(dims[:-1], dims[1:]))
if use_convnext:
block_klass = partial(ConvNextBlock, mult=convnext_mult)
else:
block_klass = partial(ResnetBlock, groups=resnet_block_groups)
# time embeddings
if with_time_emb:
time_dim = dim * 4
self.time_mlp = nn.Sequential(
SinusoidalPositionEmbeddings(dim),
nn.Linear(dim, time_dim),
nn.GELU(),
nn.Linear(time_dim, time_dim),
)
else:
time_dim = None
self.time_mlp = None
# layers
self.downs = nn.ModuleList([])
self.ups = nn.ModuleList([])
num_resolutions = len(in_out)
for ind, (dim_in, dim_out) in enumerate(in_out):
is_last = ind >= (num_resolutions - 1)
self.downs.append(
nn.ModuleList(
[
block_klass(dim_in, dim_out, time_emb_dim=time_dim),
block_klass(dim_out, dim_out, time_emb_dim=time_dim),
Residual(PreNorm(dim_out, LinearAttention(dim_out))),
Downsample(dim_out) if not is_last else nn.Identity(),
]
)
)
mid_dim = dims[-1]
self.mid_block1 = block_klass(mid_dim, mid_dim, time_emb_dim=time_dim)
self.mid_attn = Residual(PreNorm(mid_dim, Attention(mid_dim)))
self.mid_block2 = block_klass(mid_dim, mid_dim, time_emb_dim=time_dim)
for ind, (dim_in, dim_out) in enumerate(reversed(in_out[1:])):
is_last = ind >= (num_resolutions - 1)
self.ups.append(
nn.ModuleList(
[
block_klass(dim_out * 2, dim_in, time_emb_dim=time_dim),
block_klass(dim_in, dim_in, time_emb_dim=time_dim),
Residual(PreNorm(dim_in, LinearAttention(dim_in))),
Upsample(dim_in) if not is_last else nn.Identity(),
]
)
)
out_dim = default(out_dim, channels)
self.final_conv = nn.Sequential(
block_klass(dim, dim), nn.Conv2d(dim, out_dim, 1)
)
def forward(self, x, time):
x = self.init_conv(x)
t = self.time_mlp(time) if exists(self.time_mlp) else None
h = []
# downsample
for block1, block2, attn, downsample in self.downs:
x = block1(x, t)
x = block2(x, t)
x = attn(x)
h.append(x)
x = downsample(x)
# bottleneck
x = self.mid_block1(x, t)
x = self.mid_attn(x)
x = self.mid_block2(x, t)
# upsample
for block1, block2, attn, upsample in self.ups:
x = torch.cat((x, h.pop()), dim=1)
x = block1(x, t)
x = block2(x, t)
x = attn(x)
x = upsample(x)
return self.final_conv(x)<jupyter_output><empty_output><jupyter_text>Defining the forward diffusion processThe forward diffusion process gradually adds noise to an image from the real distribution, in a number of time steps $T$. This happens according to a **variance schedule**. The original DDPM authors employed a linear schedule:> We set the forward process variances to constantsincreasing linearly from $\beta_1 = 10^{−4}$to $\beta_T = 0.02$.However, it was shown in ([Nichol et al., 2021](https://arxiv.org/abs/2102.09672)) that better results can be achieved when employing a cosine schedule. Below, we define various schedules for the $T$ timesteps, as well as corresponding variables which we'll need, such as cumulative variances.<jupyter_code>def cosine_beta_schedule(timesteps, s=0.008):
"""
cosine schedule as proposed in https://arxiv.org/abs/2102.09672
"""
steps = timesteps + 1
x = torch.linspace(0, timesteps, steps)
alphas_cumprod = torch.cos(((x / timesteps) + s) / (1 + s) * torch.pi * 0.5) ** 2
alphas_cumprod = alphas_cumprod / alphas_cumprod[0]
betas = 1 - (alphas_cumprod[1:] / alphas_cumprod[:-1])
return torch.clip(betas, 0.0001, 0.9999)
def linear_beta_schedule(timesteps):
beta_start = 0.0001
beta_end = 0.02
return torch.linspace(beta_start, beta_end, timesteps)
def quadratic_beta_schedule(timesteps):
beta_start = 0.0001
beta_end = 0.02
return torch.linspace(beta_start**0.5, beta_end**0.5, timesteps) ** 2
def sigmoid_beta_schedule(timesteps):
beta_start = 0.0001
beta_end = 0.02
betas = torch.linspace(-6, 6, timesteps)
return torch.sigmoid(betas) * (beta_end - beta_start) + beta_start<jupyter_output><empty_output><jupyter_text>To start with, let's use the linear schedule for \\(T=200\\) time steps and define the various variables from the \\(\beta_t\\) which we will need, such as the cumulative product of the variances \\(\bar{\alpha}_t\\). Each of the variables below are just 1-dimensional tensors, storing values from \\(t\\) to \\(T\\). Importantly, we also define an `extract` function, which will allow us to extract the appropriate \\(t\\) index for a batch of indices.<jupyter_code>timesteps = 200
# define beta schedule
betas = linear_beta_schedule(timesteps=timesteps)
# define alphas
alphas = 1. - betas
alphas_cumprod = torch.cumprod(alphas, axis=0)
alphas_cumprod_prev = F.pad(alphas_cumprod[:-1], (1, 0), value=1.0)
sqrt_recip_alphas = torch.sqrt(1.0 / alphas)
# calculations for diffusion q(x_t | x_{t-1}) and others
sqrt_alphas_cumprod = torch.sqrt(alphas_cumprod)
sqrt_one_minus_alphas_cumprod = torch.sqrt(1. - alphas_cumprod)
# calculations for posterior q(x_{t-1} | x_t, x_0)
posterior_variance = betas * (1. - alphas_cumprod_prev) / (1. - alphas_cumprod)
def extract(a, t, x_shape):
batch_size = t.shape[0]
out = a.gather(-1, t.cpu())
return out.reshape(batch_size, *((1,) * (len(x_shape) - 1))).to(t.device)<jupyter_output><empty_output><jupyter_text>We'll illustrate with a cats image how noise is added at each time step of the diffusion process.<jupyter_code>from PIL import Image
import requests
url = 'http://images.cocodataset.org/val2017/000000039769.jpg'
image = Image.open(requests.get(url, stream=True).raw)
image<jupyter_output><empty_output><jupyter_text>Noise is added to PyTorch tensors, rather than Pillow Images. We'll first define image transformations that allow us to go from a PIL image to a PyTorch tensor (on which we can add the noise), and vice versa.These transformations are fairly simple: we first normalize images by dividing by $255$ (such that they are in the $[0,1]$ range), and then make sure they are in the $[-1, 1]$ range. From the DPPM paper:> We assume that image data consists of integers in $\{0, 1, ... , 255\}$ scaled linearly to $[−1, 1]$. Thisensures that the neural network reverse process operates on consistently scaled inputs starting fromthe standard normal prior $p(\mathbf{x}_T )$.<jupyter_code>from torchvision.transforms import Compose, ToTensor, Lambda, ToPILImage, CenterCrop, Resize
image_size = 128
transform = Compose([
Resize(image_size),
CenterCrop(image_size),
ToTensor(), # turn into Numpy array of shape HWC, divide by 255
Lambda(lambda t: (t * 2) - 1),
])
x_start = transform(image).unsqueeze(0)
x_start.shape<jupyter_output><empty_output><jupyter_text>Output: ---------------------------------------------------------------------------------------------------- torch.Size([1, 3, 128, 128])We also define the reverse transform, which takes in a PyTorch tensor containing values in $[-1, 1]$ and turn them back into a PIL image:<jupyter_code>import numpy as np
reverse_transform = Compose([
Lambda(lambda t: (t + 1) / 2),
Lambda(lambda t: t.permute(1, 2, 0)), # CHW to HWC
Lambda(lambda t: t * 255.),
Lambda(lambda t: t.numpy().astype(np.uint8)),
ToPILImage(),
])<jupyter_output><empty_output><jupyter_text>Let's verify this:<jupyter_code>reverse_transform(x_start.squeeze())<jupyter_output><empty_output><jupyter_text>We can now define the forward diffusion process as in the paper:<jupyter_code># forward diffusion
def q_sample(x_start, t, noise=None):
if noise is None:
noise = torch.randn_like(x_start)
sqrt_alphas_cumprod_t = extract(sqrt_alphas_cumprod, t, x_start.shape)
sqrt_one_minus_alphas_cumprod_t = extract(
sqrt_one_minus_alphas_cumprod, t, x_start.shape
)
return sqrt_alphas_cumprod_t * x_start + sqrt_one_minus_alphas_cumprod_t * noise<jupyter_output><empty_output><jupyter_text>Let's test it on a particular time step:<jupyter_code>def get_noisy_image(x_start, t):
# add noise
x_noisy = q_sample(x_start, t=t)
# turn back into PIL image
noisy_image = reverse_transform(x_noisy.squeeze())
return noisy_image
# take time step
t = torch.tensor([40])
get_noisy_image(x_start, t)<jupyter_output><empty_output><jupyter_text>Let's visualize this for various time steps:<jupyter_code>import matplotlib.pyplot as plt
# use seed for reproducability
torch.manual_seed(0)
# source: https://pytorch.org/vision/stable/auto_examples/plot_transforms.html#sphx-glr-auto-examples-plot-transforms-py
def plot(imgs, with_orig=False, row_title=None, **imshow_kwargs):
if not isinstance(imgs[0], list):
# Make a 2d grid even if there's just 1 row
imgs = [imgs]
num_rows = len(imgs)
num_cols = len(imgs[0]) + with_orig
fig, axs = plt.subplots(figsize=(200,200), nrows=num_rows, ncols=num_cols, squeeze=False)
for row_idx, row in enumerate(imgs):
row = [image] + row if with_orig else row
for col_idx, img in enumerate(row):
ax = axs[row_idx, col_idx]
ax.imshow(np.asarray(img), **imshow_kwargs)
ax.set(xticklabels=[], yticklabels=[], xticks=[], yticks=[])
if with_orig:
axs[0, 0].set(title='Original image')
axs[0, 0].title.set_size(8)
if row_title is not None:
for row_idx in range(num_rows):
axs[row_idx, 0].set(ylabel=row_title[row_idx])
plt.tight_layout()
plot([get_noisy_image(x_start, torch.tensor([t])) for t in [0, 50, 100, 150, 199]])<jupyter_output><empty_output><jupyter_text>This means that we can now define the loss function given the model as follows:<jupyter_code>def p_losses(denoise_model, x_start, t, noise=None, loss_type="l1"):
if noise is None:
noise = torch.randn_like(x_start)
x_noisy = q_sample(x_start=x_start, t=t, noise=noise)
predicted_noise = denoise_model(x_noisy, t)
if loss_type == 'l1':
loss = F.l1_loss(noise, predicted_noise)
elif loss_type == 'l2':
loss = F.mse_loss(noise, predicted_noise)
elif loss_type == "huber":
loss = F.smooth_l1_loss(noise, predicted_noise)
else:
raise NotImplementedError()
return loss<jupyter_output><empty_output><jupyter_text>The `denoise_model` will be our U-Net defined above. We'll employ the Huber loss between the true and the predicted noise. Define a PyTorch Dataset + DataLoaderHere we define a regular [PyTorch Dataset](https://pytorch.org/tutorials/beginner/basics/data_tutorial.html). The dataset simply consists of images from a real dataset, like Fashion-MNIST, CIFAR-10 or ImageNet, scaled linearly to \\([−1, 1]\\).Each image is resized to the same size. Interesting to note is that images are also randomly horizontally flipped. From the paper:> We used random horizontal flips during training for CIFAR10; we tried training both with and without flips, and found flips to improve sample quality slightly.Here we use the 🤗 [Datasets library](https://huggingface.co/docs/datasets/index) to easily load the Fashion MNIST dataset from the [hub](https://huggingface.co/datasets/fashion_mnist). This dataset consists of images which already have the same resolution, namely 28x28.<jupyter_code>from datasets import load_dataset
# load dataset from the hub
dataset = load_dataset("fashion_mnist")
image_size = 28
channels = 1
batch_size = 128<jupyter_output><empty_output><jupyter_text>Next, we define a function which we'll apply on-the-fly on the entire dataset. We use the `with_transform` [functionality](https://huggingface.co/docs/datasets/v2.2.1/en/package_reference/main_classesdatasets.Dataset.with_transform) for that. The function just applies some basic image preprocessing: random horizontal flips, rescaling and finally make them have values in the $[-1,1]$ range.<jupyter_code>from torchvision import transforms
from torch.utils.data import DataLoader
# define image transformations (e.g. using torchvision)
transform = Compose([
transforms.RandomHorizontalFlip(),
transforms.ToTensor(),
transforms.Lambda(lambda t: (t * 2) - 1)
])
# define function
def transforms(examples):
examples["pixel_values"] = [transform(image.convert("L")) for image in examples["image"]]
del examples["image"]
return examples
transformed_dataset = dataset.with_transform(transforms).remove_columns("label")
# create dataloader
dataloader = DataLoader(transformed_dataset["train"], batch_size=batch_size, shuffle=True)
batch = next(iter(dataloader))
print(batch.keys())<jupyter_output><empty_output><jupyter_text>Output: ---------------------------------------------------------------------------------------------------- dict_keys(['pixel_values']) SamplingAs we'll sample from the model during training (in order to track progress), we define the code for that below. Sampling is summarized in the paper as Algorithm 2:Generating new images from a diffusion model happens by reversing the diffusion process: we start from $T$, where we sample pure noise from a Gaussian distribution, and then use our neural network to gradually denoise it (using the conditional probability it has learned), until we end up at time step $t = 0$. As shown above, we can derive a slighly less denoised image $\mathbf{x}_{t-1 }$ by plugging in the reparametrization of the mean, using our noise predictor. Remember that the variance is known ahead of time.Ideally, we end up with an image that looks like it came from the real data distribution.The code below implements this.<jupyter_code>@torch.no_grad()
def p_sample(model, x, t, t_index):
betas_t = extract(betas, t, x.shape)
sqrt_one_minus_alphas_cumprod_t = extract(
sqrt_one_minus_alphas_cumprod, t, x.shape
)
sqrt_recip_alphas_t = extract(sqrt_recip_alphas, t, x.shape)
# Equation 11 in the paper
# Use our model (noise predictor) to predict the mean
model_mean = sqrt_recip_alphas_t * (
x - betas_t * model(x, t) / sqrt_one_minus_alphas_cumprod_t
)
if t_index == 0:
return model_mean
else:
posterior_variance_t = extract(posterior_variance, t, x.shape)
noise = torch.randn_like(x)
# Algorithm 2 line 4:
return model_mean + torch.sqrt(posterior_variance_t) * noise
# Algorithm 2 but save all images:
@torch.no_grad()
def p_sample_loop(model, shape):
device = next(model.parameters()).device
b = shape[0]
# start from pure noise (for each example in the batch)
img = torch.randn(shape, device=device)
imgs = []
for i in tqdm(reversed(range(0, timesteps)), desc='sampling loop time step', total=timesteps):
img = p_sample(model, img, torch.full((b,), i, device=device, dtype=torch.long), i)
imgs.append(img.cpu().numpy())
return imgs
@torch.no_grad()
def sample(model, image_size, batch_size=16, channels=3):
return p_sample_loop(model, shape=(batch_size, channels, image_size, image_size))<jupyter_output><empty_output><jupyter_text>Note that the code above is a simplified version of the original implementation. We found our simplification (which is in line with Algorithm 2 in the paper) to work just as well as the [original, more complex implementation](https://github.com/hojonathanho/diffusion/blob/master/diffusion_tf/diffusion_utils.py). Train the modelNext, we train the model in regular PyTorch fashion. We also define some logic to peridiocally save generated images, using the `sample` method defined above.<jupyter_code>from pathlib import Path
def num_to_groups(num, divisor):
groups = num // divisor
remainder = num % divisor
arr = [divisor] * groups
if remainder > 0:
arr.append(remainder)
return arr
results_folder = Path("./results")
results_folder.mkdir(exist_ok = True)
save_and_sample_every = 1000<jupyter_output><empty_output><jupyter_text>Below, we define the model, and move it to the GPU. We also define a standard optimizer (Adam).<jupyter_code>from torch.optim import Adam
device = "cuda" if torch.cuda.is_available() else "cpu"
model = Unet(
dim=image_size,
channels=channels,
dim_mults=(1, 2, 4,)
)
model.to(device)
optimizer = Adam(model.parameters(), lr=1e-3)<jupyter_output><empty_output><jupyter_text>Let's start training!<jupyter_code>from torchvision.utils import save_image
epochs = 5
for epoch in range(epochs):
for step, batch in enumerate(dataloader):
optimizer.zero_grad()
batch_size = batch["pixel_values"].shape[0]
batch = batch["pixel_values"].to(device)
# Algorithm 1 line 3: sample t uniformally for every example in the batch
t = torch.randint(0, timesteps, (batch_size,), device=device).long()
loss = p_losses(model, batch, t, loss_type="huber")
if step % 100 == 0:
print("Loss:", loss.item())
loss.backward()
optimizer.step()
# save generated images
if step != 0 and step % save_and_sample_every == 0:
milestone = step // save_and_sample_every
batches = num_to_groups(4, batch_size)
all_images_list = list(map(lambda n: sample(model, batch_size=n, channels=channels), batches))
all_images = torch.cat(all_images_list, dim=0)
all_images = (all_images + 1) * 0.5
save_image(all_images, str(results_folder / f'sample-{milestone}.png'), nrow = 6)<jupyter_output><empty_output><jupyter_text>Output: ---------------------------------------------------------------------------------------------------- Loss: 0.46477368474006653 Loss: 0.12143351882696152 Loss: 0.08106148988008499 Loss: 0.0801810547709465 Loss: 0.06122320517897606 Loss: 0.06310459971427917 Loss: 0.05681884288787842 Loss: 0.05729678273200989 Loss: 0.05497899278998375 Loss: 0.04439849033951759 Loss: 0.05415581166744232 Loss: 0.06020551547408104 Loss: 0.046830907464027405 Loss: 0.051029372960329056 Loss: 0.0478244312107563 Loss: 0.046767622232437134 Loss: 0.04305662214756012 Loss: 0.05216279625892639 Loss: 0.04748568311333656 Loss: 0.05107741802930832 Loss: 0.04588869959115982 Loss: 0.043014321476221085 Loss: 0.046371955424547195 Loss: 0.04952816292643547 Loss: 0.04472338408231735 Sampling (inference)To sample from the model, we can just use our sample function defined above:<jupyter_code># sample 64 images
samples = sample(model, image_size=image_size, batch_size=64, channels=channels)
# show a random one
random_index = 5
plt.imshow(samples[-1][random_index].reshape(image_size, image_size, channels), cmap="gray")<jupyter_output><empty_output><jupyter_text>Seems like the model is capable of generating a nice T-shirt! Keep in mind that the dataset we trained on is pretty low-resolution (28x28).We can also create a gif of the denoising process:<jupyter_code>import matplotlib.animation as animation
random_index = 53
fig = plt.figure()
ims = []
for i in range(timesteps):
im = plt.imshow(samples[i][random_index].reshape(image_size, image_size, channels), cmap="gray", animated=True)
ims.append([im])
animate = animation.ArtistAnimation(fig, ims, interval=50, blit=True, repeat_delay=1000)
animate.save('diffusion.gif')
plt.show()<jupyter_output><empty_output> | notebooks/examples/annotated_diffusion.ipynb/0 | {
"file_path": "notebooks/examples/annotated_diffusion.ipynb",
"repo_id": "notebooks",
"token_count": 16579
} | 161 |
<jupyter_start><jupyter_text>**Fine-tuning for Image Classification with 🤗 Transformers**This notebook shows how to fine-tune any pretrained Vision model for Image Classification on a custom dataset. The idea is to add a randomly initialized classification head on top of a pre-trained encoder, and fine-tune the model altogether on a labeled dataset. ImageFolderThis notebook leverages the [ImageFolder](https://huggingface.co/docs/datasets/v2.0.0/en/image_processimagefolder) feature to easily run the notebook on a custom dataset (namely, [EuroSAT](https://github.com/phelber/EuroSAT) in this tutorial). You can either load a `Dataset` from local folders or from local/remote files, like zip or tar. Any modelThis notebook is built to run on any image classification dataset with any vision model checkpoint from the [Model Hub](https://huggingface.co/) as long as that model has a TensorFlow version with a Image Classification head, such as:* [ViT](https://huggingface.co/docs/transformers/model_doc/vittransformers.TFViTForImageClassification)* [Swin Transformer](https://huggingface.co/docs/transformers/model_doc/swintransformers.TFSwinForImageClassification)* [ConvNeXT](https://huggingface.co/docs/transformers/master/en/model_doc/convnexttransformers.TFConvNextForImageClassification)* [RegNet](https://huggingface.co/docs/transformers/master/en/model_doc/regnettransformers.TFRegNetForImageClassification)* [ResNet](https://huggingface.co/docs/transformers/master/en/model_doc/resnettransformers.TFResNetForImageClassification)- in short, any model supported by [TFAutoModelForImageClassification](https://huggingface.co/docs/transformers/model_doc/autotransformers.TFAutoModelForImageClassification). Data augmentationThis notebook leverages TensorFlow's [image](https://www.tensorflow.org/api_docs/python/tf/image) module for applying data augmentation. Alternative notebooks which leverage other libraries such as [Albumentations](https://albumentations.ai/) to come!---Depending on the model and the GPU you are using, you might need to adjust the batch size to avoid out-of-memory errors. Set those two parameters, then the rest of the notebook should run smoothly.In this notebook, we'll fine-tune from the https://huggingface.co/microsoft/swin-tiny-patch4-window7-224 checkpoint, but note that there are many, many more available on the [hub](https://huggingface.co/models?other=vision).<jupyter_code>model_checkpoint = "microsoft/swin-tiny-patch4-window7-224" # pre-trained model from which to fine-tune
batch_size = 32 # batch size for training and evaluation<jupyter_output><empty_output><jupyter_text>Before we start, let's install the `datasets` and `transformers` libraries.<jupyter_code>!pip install -q datasets transformers<jupyter_output>[33mWARNING: Error parsing requirements for setuptools: [Errno 2] No such file or directory: '/usr/local/lib/python3.8/dist-packages/setuptools-60.6.0.dist-info/METADATA'[0m[33m
[0m[33mWARNING: You are using pip version 22.0.2; however, version 22.1.2 is available.
You should consider upgrading via the '/home/amy/tenv/bin/python3 -m pip install --upgrade pip' command.[0m[33m
[0m<jupyter_text>If you're opening this notebook locally, make sure your environment has an install from the last version of those libraries.To be able to share your model with the community and generate results like the one shown in the picture below via the inference API, there are a few more steps to follow.First you have to store your authentication token from the Hugging Face website (sign up [here](https://huggingface.co/join) if you haven't already!) then execute the following cell and input your token:<jupyter_code>from huggingface_hub import notebook_login
notebook_login()<jupyter_output><empty_output><jupyter_text>Then you need to install Git-LFS to upload your model checkpoints:<jupyter_code>%%capture
!git lfs install
!git config --global credential.helper store<jupyter_output><empty_output><jupyter_text>We also quickly upload some telemetry - this tells us which examples and software versions are getting used so we know where to prioritize our maintenance efforts. We don't collect (or care about) any personally identifiable information, but if you'd prefer not to be counted, feel free to skip this step or delete this cell entirely.<jupyter_code>from transformers.utils import send_example_telemetry
send_example_telemetry("image_classification_notebook", framework="tensorflow")<jupyter_output><empty_output><jupyter_text>Fine-tuning a model on an image classification task In this notebook, we will see how to fine-tune one of the [🤗 Transformers](https://github.com/huggingface/transformers) vision models on an Image Classification dataset.Given an image, the goal is to predict an appropriate class for it, like "tiger". The screenshot below is taken from a [ViT fine-tuned on ImageNet-1k](https://huggingface.co/google/vit-base-patch16-224) - try out the inference widget! Loading the dataset We will use the [🤗 Datasets](https://github.com/huggingface/datasets) library's [ImageFolder](https://huggingface.co/docs/datasets/v2.0.0/en/image_processimagefolder) feature to download our custom dataset into a DatasetDict.In this case, the EuroSAT dataset is hosted remotely, so we provide the `data_files` argument. Alternatively, if you have local folders with images, you can load them using the `data_dir` argument.<jupyter_code>from datasets import load_dataset
# load a custom dataset from local/remote files or folders using the ImageFolder feature
# option 1: local/remote files (supporting the following formats: tar, gzip, zip, xz, rar, zstd)
dataset = load_dataset("imagefolder", data_files="https://madm.dfki.de/files/sentinel/EuroSAT.zip")
# note that you can also provide several splits:
# dataset = load_dataset("imagefolder", data_files={"train": ["path/to/file1", "path/to/file2"], "test": ["path/to/file3", "path/to/file4"]})
# note that you can push your dataset to the hub very easily (and reload afterwards using load_dataset)!
# dataset.push_to_hub("nielsr/eurosat")
# dataset.push_to_hub("nielsr/eurosat", private=True)
# dataset = load_dataset("nielsr/eurosat")
# option 2: local folder
# dataset = load_dataset("imagefolder", data_dir="path_to_folder")
# option 3: just load any existing dataset from the hub, like CIFAR-10, FashionMNIST ...
# dataset = load_dataset("cifar10")<jupyter_output>Using custom data configuration default-71c8f80171b09b95
Reusing dataset imagefolder (/home/amy/.cache/huggingface/datasets/imagefolder/default-71c8f80171b09b95/0.0.0/d83791becf4369e27b95a98928b7c95213f7901edd8134aaac48cb591b8b21f0)<jupyter_text>Let us also load the Accuracy metric, which we'll use to evaluate our model both during and after training.<jupyter_code>from datasets import load_metric
metric = load_metric("accuracy")<jupyter_output><empty_output><jupyter_text>The `dataset` object itself is a [`DatasetDict`](https://huggingface.co/docs/datasets/package_reference/main_classes.htmldatasetdict), which contains one key per split (in this case, only "train" for a training split).<jupyter_code>dataset<jupyter_output><empty_output><jupyter_text>To access an actual element, you need to select a split first, then give an index:<jupyter_code>example = dataset["train"][10]
example<jupyter_output><empty_output><jupyter_text>Each example consists of an image and a corresponding label. We can also verify this by checking the features of the dataset:<jupyter_code>dataset["train"].features<jupyter_output><empty_output><jupyter_text>The cool thing is that we can directly view the image (as the 'image' field is an [Image feature](https://huggingface.co/docs/datasets/package_reference/main_classes.htmldatasets.Image)), as follows:<jupyter_code>example['image']<jupyter_output><empty_output><jupyter_text>Let's make it a little bigger as the images in the EuroSAT dataset are of low resolution (64x64 pixels):<jupyter_code>example['image'].resize((200, 200))<jupyter_output><empty_output><jupyter_text>Let's print the corresponding label:<jupyter_code>example['label']<jupyter_output><empty_output><jupyter_text>As you can see, the `label` field is not an actual string label. By default the `ClassLabel` fields are encoded into integers for convenience:<jupyter_code>dataset["train"].features["label"]<jupyter_output><empty_output><jupyter_text>Let's create an `id2label` dictionary to decode them back to strings and see what they are. The inverse `label2id` will be useful too, when we load the model later.<jupyter_code>labels = dataset["train"].features["label"].names
label2id, id2label = dict(), dict()
for i, label in enumerate(labels):
label2id[label] = i
id2label[i] = label
id2label[5]<jupyter_output><empty_output><jupyter_text>Preprocessing the data Before we can feed these images to our model, we need to preprocess them. Preprocessing images typically comes down to (1) resizing them to a particular size (2) normalizing the color channels (R,G,B) using a mean and standard deviation. These are referred to as **image transformations**.In addition, one typically performs what is called **data augmentation** during training (like random cropping and flipping) to make the model more robust and achieve higher accuracy. Data augmentation is also a great technique to increase the size of the training data.We will use `tf.image` for the image transformations/data augmentation in this tutorial, but note that one can use any other package (like [albumentations](https://albumentations.ai/), [imgaug](https://github.com/aleju/imgaug), etc.).To make sure we (1) resize to the appropriate size (2) use the appropriate image mean and standard deviation for the model architecture we are going to use, we instantiate what is called a feature extractor with the `AutoFeatureExtractor.from_pretrained` method.This feature extractor is a minimal preprocessor that can be used to prepare images for inference.<jupyter_code>from transformers import AutoFeatureExtractor
feature_extractor = AutoFeatureExtractor.from_pretrained(model_checkpoint)
feature_extractor<jupyter_output><empty_output><jupyter_text>The Datasets library is made for processing data very easily. We can write custom functions, which can then be applied on an entire dataset (either using `.map()` or `.set_transform()`).Here we define 2 separate functions, one for training (which includes data augmentation) and one for validation (which only includes resizing, center cropping and normalizing).<jupyter_code>import numpy as np
import tensorflow as tf
from keras import backend
def normalize_img(img, mean, std):
mean = tf.constant(mean)
std = tf.constant(std)
return (img - mean) / tf.maximum(std, backend.epsilon())
def get_resize_shape(img, size):
if isinstance(size, tuple):
return size
height, width, _ = img.shape
target_height = int(size * height / width) if height > width else size
target_width = int(size * width / height) if width > height else size
return (target_height, target_width)
def get_random_crop_size(img, scale=(0.08, 1.0), ratio=(3/4, 4/3)):
height, width, channels = img.shape
img_ratio = width / height
crop_log_ratio = np.random.uniform(*np.log(ratio), size=1)
crop_ratio = np.exp(crop_log_ratio)
crop_scale = np.random.uniform(*scale, size=1)
# Make sure the longest side is within the image size
if crop_ratio < img_ratio:
crop_height = int(height * crop_scale)
crop_width = int(crop_height * crop_ratio)
else:
crop_width = int(width * crop_scale)
crop_height = int(crop_width / crop_ratio)
return (crop_height, crop_width, channels)
def train_transforms(image):
image = tf.keras.utils.img_to_array(image)
# Note - this augmentation isn't exactly the same in the PyTorch
# examples in:
# https://github.com/huggingface/notebooks/blob/main/examples/image_classification.ipynb
# as there isn't a direct RandomResizedCrop equivalent.
# A custom transform would need to be implemented for this.
# Randomly select the crop size based on a possible scale
# and ratio range
crop_size = get_random_crop_size(image)
image = tf.image.random_crop(image, size=crop_size)
image = tf.image.resize(
image,
size=(feature_extractor.size, feature_extractor.size),
method=tf.image.ResizeMethod.BILINEAR
)
image = tf.image.random_flip_left_right(image)
image /= 255
image = normalize_img(
image,
mean=feature_extractor.image_mean,
std=feature_extractor.image_std
)
# All image models take channels first format: BCHW
image = tf.transpose(image, (2, 0, 1))
return image
def val_transforms(image):
image = tf.keras.utils.img_to_array(image)
resize_shape = get_resize_shape(image, feature_extractor.size)
image = tf.image.resize(
image,
size=resize_shape,
method=tf.image.ResizeMethod.BILINEAR
)
image = tf.image.crop_to_bounding_box(
image,
offset_height=image.shape[0] // 2 - feature_extractor.size // 2,
offset_width=image.shape[1] // 2 - feature_extractor.size // 2,
target_height=feature_extractor.size,
target_width=feature_extractor.size,
)
image /= 255
image = normalize_img(
image,
mean=feature_extractor.image_mean,
std=feature_extractor.image_std
)
# All image models take channels first format: BCHW
image = tf.transpose(image, (2, 0, 1))
return image
def preprocess_train(example_batch):
"""Apply train_transforms across a batch."""
example_batch['pixel_values'] = [
train_transforms(image.convert("RGB")) for image in example_batch["image"]
]
return example_batch
def preprocess_val(example_batch):
"""Apply val_transforms across a batch."""
example_batch['pixel_values'] = [
val_transforms(image.convert("RGB")) for image in example_batch["image"]
]
return example_batch<jupyter_output><empty_output><jupyter_text>Let's quickly visualise some example outputs from our processing pipeline. Part of the processing pipeline rescales them between [0, 1] and normalizes them. This results in pixel values having negative values. To easily visualise and compare the original images and augmentations we undo this normalization and rescaling here.<jupyter_code>import matplotlib.pyplot as plt
%matplotlib inline
def unnormalize_img(img, mean, std):
img = (img * std) + mean
return img
def process_for_plotting(img):
img = img.numpy()
img = img.transpose(1, 2, 0)
img = unnormalize_img(
img=img,
mean=feature_extractor.image_mean,
std=feature_extractor.image_std
)
img = img * 255
img = img.astype(int)
return img
n = 10
fig, ax = plt.subplots(2, n, figsize=(20, 10))
for i in range(n):
orig_img = dataset['train'][i]['image']
proc_img = train_transforms(orig_img)
orig_img = np.array(orig_img.convert("RGB"))
# In order to plot and easy compare the images,
# we denormalise and rescale here so that pixel values
# are between [0, 255] and reorder to be HWC
proc_img = process_for_plotting(proc_img)
ax[0][i].imshow(orig_img)
ax[1][i].imshow(proc_img)
ax[0][i].axis('off')
ax[1][i].axis('off')<jupyter_output><empty_output><jupyter_text>Next, we can preprocess our dataset by applying these functions. We will use the set_transform functionality, which allows to apply the functions above on-the-fly (meaning that they will only be applied when the images are loaded in RAM).<jupyter_code># split up training into training + validation
splits = dataset["train"].train_test_split(test_size=0.1)
train_ds = splits['train']
val_ds = splits['test']
train_ds.set_transform(preprocess_train)
val_ds.set_transform(preprocess_val)
train_ds<jupyter_output><empty_output><jupyter_text>Let's access an element to see that we've added a "pixel_values" feature:<jupyter_code>train_ds[0]<jupyter_output><empty_output><jupyter_text>Training the model Now that our data is ready, we can download the pretrained model and fine-tune it. For classification we use the `TFAutoModelForImageClassification` class. Calling the `from_pretrained` method on it will download and cache the weights for us. As the label ids and the number of labels are dataset dependent, we pass `label2id`, and `id2label` alongside the `model_checkpoint` here. This will make sure a custom classification head will be created (with a custom number of output neurons).NOTE: in case you're planning to fine-tune an already fine-tuned checkpoint, like [facebook/convnext-tiny-224](https://huggingface.co/facebook/convnext-tiny-224) (which has already been fine-tuned on ImageNet-1k), then you need to provide the additional argument `ignore_mismatched_sizes=True` to the `from_pretrained` method. This will make sure the output head (with 1000 output neurons) is thrown away and replaced by a new, randomly initialized classification head that includes a custom number of output neurons. You don't need to specify this argument in case the pre-trained model doesn't include a head.<jupyter_code>from transformers import TFAutoModelForImageClassification
model = TFAutoModelForImageClassification.from_pretrained(
model_checkpoint,
label2id=label2id,
id2label=id2label,
ignore_mismatched_sizes=True, # provide this in case you're planning to fine-tune an already fine-tuned checkpoint
)<jupyter_output>All model checkpoint layers were used when initializing TFSwinForImageClassification.
Some weights of TFSwinForImageClassification were not initialized from the model checkpoint at microsoft/swin-tiny-patch4-window7-224 and are newly initialized because the shapes did not match:
- classifier/kernel:0: found shape (768, 1000) in the checkpoint and (768, 10) in the model instantiated
- classifier/bias:0: found shape (1000,) in the checkpoint and (10,) in the model instantiated
You should probably TRAIN this model on a down-stream task to be able to use it for predictions and inference.<jupyter_text>The warning is telling us we are throwing away some weights (the weights and bias of the `classifier` layer) and randomly initializing some other (the weights and bias of a new `classifier` layer). This is expected in this case, because we are adding a new head for which we don't have pretrained weights, so the library warns us we should fine-tune this model before using it for inference, which is exactly what we are going to do.<jupyter_code>learning_rate = 5e-5
weight_decay = 0.01
epochs = 3<jupyter_output><empty_output><jupyter_text>Now we initialize our optimizer.<jupyter_code>from transformers import AdamWeightDecay
optimizer = AdamWeightDecay(learning_rate=learning_rate, weight_decay_rate=weight_decay)<jupyter_output><empty_output><jupyter_text>Note that most models on the Hub compute loss internally, so we actually don't have to specify anything there! Leaving the loss field blank will cause the model to read the loss head as its loss value.This is an unusual quirk of TensorFlow models in 🤗 Transformers, so it's worth elaborating on in a little more detail. All 🤗 Transformers models are capable of computing an appropriate loss for their task internally (for example, a CausalLM model will use a cross-entropy loss). To do this, the labels must be provided in the input dict (or equivalently, in the `columns` argument to `to_tf_dataset()`), so that they are visible to the model during the forward pass.This is quite different from the standard Keras way of handling losses, where labels are passed separately and not visible to the main body of the model, and loss is handled by a function that the user passes to `compile()`, which uses the model outputs and the label to compute a loss value.The approach we take is that if the user does not pass a loss to `compile()`, the model will assume you want the **internal** loss. If you are doing this, you should make sure that the labels column(s) are included in the **input dict** or in the `columns` argument to `to_tf_dataset`.If you want to use your own loss, that is of course possible too! If you do this, you should make sure your labels column(s) are passed like normal labels, either as the **second argument** to model.fit(), or in the `label_cols` argument to `to_tf_dataset`.<jupyter_code>model.compile(optimizer=optimizer)<jupyter_output>No loss specified in compile() - the model's internal loss computation will be used as the loss. Don't panic - this is a common way to train TensorFlow models in Transformers! To disable this behaviour please pass a loss argument, or explicitly pass `loss=None` if you do not want your model to compute a loss.<jupyter_text>We need to convert our datasets to a format Keras understands. The easiest way to do this is with the `to_tf_dataset()` method. Note that our data collators are designed to work for multiple frameworks, so ensure you set the `return_tensors='np'` argument to get NumPy arrays out - you don't want to accidentally get a load of `torch.Tensor` objects in the middle of your nice TF code! You could also use `return_tensors='tf'` to get TensorFlow tensors, but our `to_tf_dataset` pipeline actually uses a NumPy loader internally, which is wrapped at the end with a `tf.data.Dataset`. As a result, `np` is usually more reliable and performant when you're using it!<jupyter_code>from transformers import DefaultDataCollator
data_collator = DefaultDataCollator(return_tensors="np")
train_set = train_ds.to_tf_dataset(
columns=["pixel_values", "label"],
shuffle=True,
batch_size=batch_size,
collate_fn=data_collator
)
val_set = val_ds.to_tf_dataset(
columns=["pixel_values", "label"],
shuffle=False,
batch_size=batch_size,
collate_fn=data_collator
)<jupyter_output><empty_output><jupyter_text>`train_set` is now a `tf.data.Dataset` type object. We see that it contains two elements - `labels` and `pixel_values` (but not `image`) as a result of the preprocessing done in `preprocess_train`.<jupyter_code>train_set
batch = next(iter(train_set))
batch<jupyter_output><empty_output><jupyter_text>The last thing to define is how to compute the metrics from the predictions. We need to define a function for this, which will just use the metric we loaded earlier. The only preprocessing we have to do is to take the argmax of our predicted logits.In addition, let's wrap this metric computation function in a `KerasMetricCallback`. This callback will compute the metric on the validation set each epoch, including printing it and logging it for other callbacks like TensorBoard and EarlyStopping.Why do it this way, though, and not just use a straightforward Keras Metric object? This is a good question - on this task, metrics such as Accuracy are very straightforward, and it would probably make more sense to just use a Keras metric for those instead. However, we want to demonstrate the use of `KerasMetricCallback` here, because it can handle any arbitrary Python function for the metric computation. Wow do we actually use `KerasMetricCallback`? We simply define a function that computes metrics given a tuple of numpy arrays of predictions and labels, then we pass that, along with the validation set to compute metrics on, to the callback:<jupyter_code>import numpy as np
from transformers.keras_callbacks import KerasMetricCallback
# the compute_metrics function takes a Tuple as input:
# first element is the logits of the model as Numpy arrays,
# second element is the ground-truth labels as Numpy arrays.
def compute_metrics(eval_predictions):
predictions = np.argmax(eval_predictions[0], axis=1)
metric_val = metric.compute(predictions=predictions, references=eval_predictions[1])
return {"val_" + k: v for k, v in metric_val.items()}
metric_callback = KerasMetricCallback(
metric_fn=compute_metrics, eval_dataset=val_set, batch_size=batch_size, label_cols=['labels']
)<jupyter_output><empty_output><jupyter_text>Now we can train our model. We can also add a callback to sync up our model with the Hub - this allows us to resume training from other machines and even test the model's inference quality midway through training! Make sure to change the `username` if you do. If you don't want to do this, simply remove the callbacks argument in the call to `fit()`.<jupyter_code>from transformers.keras_callbacks import PushToHubCallback
from tensorflow.keras.callbacks import TensorBoard
tensorboard_callback = TensorBoard(log_dir="./ic_from_scratch_model_save/logs")
model_name = model_checkpoint.split("/")[-1]
push_to_hub_model_id = f"{model_name}-finetuned-eurosat"
push_to_hub_callback = PushToHubCallback(
output_dir="./ic_from_scratch_model_save",
hub_model_id=push_to_hub_model_id,
tokenizer=feature_extractor
)
callbacks = [metric_callback, tensorboard_callback, push_to_hub_callback]
model.fit(
train_set,
validation_data=val_set,
callbacks=callbacks,
epochs=epochs,
batch_size=batch_size,
)<jupyter_output>Epoch 1/3
1/1 [==============================] - 3s 3s/steps - loss: 0.65
1/1 [==============================] - 0s 247ms/step
1/1 [==============================] - 0s 39ms/step
1/1 [==============================] - 0s 39ms/step
1/1 [==============================] - 0s 247ms/step
1/1 [==============================] - 0s 42ms/step
1/1 [==============================] - 0s 40ms/step
1/1 [==============================] - 0s 248ms/step
1/1 [==============================] - 0s 41ms/step
1/1 [==============================] - 0s 39ms/step
1/1 [==============================] - 0s 249ms/step
1/1 [==============================] - 0s 37ms/step
1/1 [==============================] - 0s 38ms/step
1/1 [==============================] - 0s 34ms/step
1/1 [==============================] - 0s 51ms/step
1/1 [==============================] - 0s 43ms/step
1/1 [==============================] - 0s 47ms/step
1/1 [==============================] - 0s 245ms/step
1/1 [==============================] [...]<jupyter_text>Once the training is completed, we can evaluate our model and get its loss on the validation set like this:<jupyter_code>eval_loss = model.evaluate(val_set)
eval_loss<jupyter_output>85/85 [==============================] - 24s 286ms/step - loss: 0.0491<jupyter_text>Alternatively, we could also get the predictions from the model, and calculate metrics using the `datasets.Metric` object.<jupyter_code>for batch in iter(val_set):
predictions = model.predict(batch)
predicted_labels = np.argmax(predictions.logits, -1)
metric.add_batch(predictions=predicted_labels, references=batch['labels'])
metric.compute()<jupyter_output>1/1 [==============================] - 0s 34ms/step
1/1 [==============================] - 0s 37ms/step
1/1 [==============================] - 0s 36ms/step
1/1 [==============================] - 0s 38ms/step
1/1 [==============================] - 0s 45ms/step
1/1 [==============================] - 0s 45ms/step
1/1 [==============================] - 0s 243ms/step
1/1 [==============================] - 0s 109ms/step
1/1 [==============================] - 0s 36ms/step
1/1 [==============================] - 0s 39ms/step
1/1 [==============================] - 0s 35ms/step
1/1 [==============================] - 0s 40ms/step
1/1 [==============================] - 0s 243ms/step
1/1 [==============================] - 0s 243ms/step
1/1 [==============================] - 0s 37ms/step
1/1 [==============================] - 0s 42ms/step
1/1 [==============================] - 0s 35ms/step
1/1 [==============================] - 0s 34ms/step
1/1 [==============================] - 0s 244ms/step
1/1 [==[...]<jupyter_text>You can now share this model with all your friends, family, favorite pets: they can all load it with the identifier `"your-username/the-name-you-picked"` so for instance:```pythonfrom transformers import TFAutoModelForImageClassification, AutoFeatureExtractorfeature_extractor = TFAutoFeatureExtractor.from_pretrained("amyeroberts/my-awesome-model")model = TFAutoModelForImageClassification.from_pretrained("amyeroberts/my-awesome-model")``` InferenceLet's say you have a new image, on which you'd like to make a prediction. Let's load a satellite image of a forest (that's not part of the EuroSAT dataset), and see how the model does.<jupyter_code>from PIL import Image
import requests
url = 'https://huggingface.co/nielsr/convnext-tiny-finetuned-eurostat/resolve/main/forest.png'
image = Image.open(requests.get(url, stream=True).raw)
image<jupyter_output><empty_output><jupyter_text>We'll load the feature extractor and model from the hub (here, we use the [Auto Classes](https://huggingface.co/docs/transformers/model_doc/autotransformers.AutoModelForImageClassification), which will make sure the appropriate classes will be loaded automatically based on the `config.json` and `preprocessor_config.json` files of the repo on the hub):<jupyter_code>from transformers import TFAutoModelForImageClassification, AutoFeatureExtractor
repo_name = "amyeroberts/swin-tiny-patch4-window7-224-finetuned-eurosat"
feature_extractor = AutoFeatureExtractor.from_pretrained(repo_name)
model = TFAutoModelForImageClassification.from_pretrained(repo_name)
# prepare image for the model
encoding = feature_extractor(image.convert("RGB"), return_tensors="tf")
print(encoding.pixel_values.shape)
outputs = model(encoding)
logits = outputs.logits
predicted_class_idx = tf.math.argmax(logits, -1).numpy()[0]
print("Predicted class:", model.config.id2label[predicted_class_idx])<jupyter_output>Predicted class: Forest<jupyter_text>Looks like our model got it correct! Pipeline APIAn alternative way to quickly perform inference with any model on the hub is by leveraging the [Pipeline API](https://huggingface.co/docs/transformers/main_classes/pipelines), which abstracts away all the steps we did manually above for us. It will perform the preprocessing, forward pass and postprocessing all in a single object. Note the configuration for `feature_extractor` will be pulled from the specified repo and used to build the `feature_extractor` in this pipeline.Let's showcase this for our trained model:<jupyter_code>from transformers import pipeline
pipe = pipeline(
"image-classification",
"amyeroberts/swin-tiny-patch4-window7-224-finetuned-eurosat",
framework="tf"
)
pipe.feature_extractor
pipe(image)<jupyter_output><empty_output><jupyter_text>As we can see, it does not only show the class label with the highest probability, but does return the top 5 labels, with their corresponding scores. Note that the pipelines also work with local models and feature extractors:<jupyter_code>pipe = pipeline("image-classification",
model=model,
feature_extractor=feature_extractor)
pipe(image)<jupyter_output><empty_output> | notebooks/examples/image_classification-tf.ipynb/0 | {
"file_path": "notebooks/examples/image_classification-tf.ipynb",
"repo_id": "notebooks",
"token_count": 9526
} | 162 |
<jupyter_start><jupyter_text>Fine-tunining DeBERTa model on a question answering task with ORTTrainer In this notebook, we will see how to fine-tune the [DeBERTa base](https://huggingface.co/microsoft/deberta-base/tree/main) model to a question answering task, which is the task of extracting the answer to a question from a given context. We will use the `ORTTrainer` API in Optimum library to leverage ONNX Runtime backend to accelerate the training. Start by setting up the environmentTo use ONNX Runtime for training, you need a machine with at least one NVIDIA or AMD GPU.ONNX Runtime training module need to be properly installed before launching the notebook! Please follow the instruction in [Optimum's documentation](https://huggingface.co/docs/optimum/onnxruntime/usage_guides/trainerstart-by-setting-up-the-environment) to set up your environment, or use directly the [dockerfiles in Optimum](https://huggingface.co/docs/optimum/onnxruntime/usage_guides/trainerstart-by-setting-up-the-environment)<jupyter_code># Check your GPU
!nvidia-smi<jupyter_output>Thu Apr 6 08:49:04 2023
+-----------------------------------------------------------------------------+
| NVIDIA-SMI 510.108.03 Driver Version: 510.108.03 CUDA Version: 11.6 |
|-------------------------------+----------------------+----------------------+
| GPU Name Persistence-M| Bus-Id Disp.A | Volatile Uncorr. ECC |
| Fan Temp Perf Pwr:Usage/Cap| Memory-Usage | GPU-Util Compute M. |
| | | MIG M. |
|===============================+======================+======================|
| 0 Tesla V100-SXM2... Off | 00000000:00:1D.0 Off | 0 |
| N/A 38C P0 39W / 300W | 4MiB / 16384MiB | 0% Default |
| | | N/A |
+-------------------------------+----------------------+----------------------+
+-------[...]<jupyter_text>If you're opening this Notebook on colab, you will probably need to install 🤗 Optimum, 🤗 Transformers, 🤗 Datasets and 🤗 evaluate. Uncomment the following cell and run it.<jupyter_code>!pip install optimum transformers datasets evaluate huggingface_hub
# This flag is the difference between SQUAD v1 or 2 (if you're using another dataset, it indicates if impossible
# answers are allowed or not).
squad_v2 = False
model_checkpoint = "microsoft/deberta-base"
batch_size = 16<jupyter_output><empty_output><jupyter_text>Loading the dataset We will use the 🤗 Datasets library to download the data and get the metric we need to use for evaluation. This can be easily done with the functions load_dataset and load_metric.<jupyter_code>from datasets import load_dataset, load_metric
datasets = load_dataset("squad_v2" if squad_v2 else "squad")<jupyter_output>/usr/local/lib/python3.8/dist-packages/tqdm/auto.py:22: TqdmWarning: IProgress not found. Please update jupyter and ipywidgets. See https://ipywidgets.readthedocs.io/en/stable/user_install.html
from .autonotebook import tqdm as notebook_tqdm
Downloading builder script: 100%|███████████████████████████████████████████████████████████| 5.27k/5.27k [00:00<00:00, 5.12MB/s]
Downloading metadata: 100%|█████████████████████████████████████████████████████████████████| 2.36k/2.36k [00:00<00:00, 2.29MB/s]
Downloading readme: 100%|███████████████████████████████████████████████████████████████████| 7.67k/7.67k [00:00<00:00, 5.37MB/s]<jupyter_text>We can see the training, validation and test sets all have a column for the context, the question and the answers to those questions.<jupyter_code>datasets<jupyter_output><empty_output><jupyter_text>We can access an actual element to see what the data looks like:<jupyter_code>datasets["train"][0]<jupyter_output><empty_output><jupyter_text>Preprocessing the training data Before we can feed those texts to our model, we need to preprocess them. This is done by a 🤗 Transformers Tokenizer which will tokenize the inputs (including converting the tokens to their corresponding IDs in the pretrained vocabulary) and put it in a format the model expects, as well as generate the other inputs that model requires.To do all of this, we instantiate our tokenizer with the `AutoTokenizer.from_pretrained` method, which will ensure:we get a tokenizer that corresponds to the model architecture we want to use,we download the vocabulary used when pretraining this specific checkpoint.That vocabulary will be cached, so it's not downloaded again the next time we run the cell.<jupyter_code>from transformers import AutoTokenizer
tokenizer = AutoTokenizer.from_pretrained(model_checkpoint)<jupyter_output>Downloading (…)okenizer_config.json: 100%|████████████████████████████████████████████████████| 52.0/52.0 [00:00<00:00, 17.2kB/s]
Downloading (…)lve/main/config.json: 100%|███████████████████████████████████████████████████████| 474/474 [00:00<00:00, 406kB/s]
Downloading (…)olve/main/vocab.json: 100%|████████████████████████████████████████████████████| 899k/899k [00:00<00:00, 11.8MB/s]
Downloading (…)olve/main/merges.txt: 100%|████████████████████████████████████████████████████| 456k/456k [00:00<00:00, 3.10MB/s]<jupyter_text>Now one specific thing for the preprocessing in question answering is how to deal with very long documents. We usually truncate them in other tasks, when they are longer than the model maximum sentence length, but here, removing part of the the context might result in losing the answer we are looking for. To deal with this, we will allow one (long) example in our dataset to give several input features, each of length shorter than the maximum length of the model (or the one we set as a hyper-parameter). Also, just in case the answer lies at the point we split a long context, we allow some overlap between the features we generate controlled by the hyper-parameter `doc_stride`:<jupyter_code>max_length = 384 # The maximum length of a feature (question and context)
doc_stride = 128 # The authorized overlap between two part of the context when splitting it is needed.<jupyter_output><empty_output><jupyter_text>Note that we never want to truncate the question, only the context, else the `only_second` truncation picked. Now, our tokenizer can automatically return us a list of features capped by a certain maximum length, with the overlap we talked above, we just have to tell it with `return_overflowing_tokens=True` and by passing the stride.<jupyter_code>pad_on_right = tokenizer.padding_side == "right"<jupyter_output><empty_output><jupyter_text>Now let's put everything together in one function we will apply to our training set. In the case of impossible answers (the answer is in another feature given by an example with a long context), we set the cls index for both the start and end position. We could also simply discard those examples from the training set if the flag allow_impossible_answers is False. Since the preprocessing is already complex enough as it is, we've kept is simple for this part.<jupyter_code>def prepare_train_features(examples):
# Some of the questions have lots of whitespace on the left, which is not useful and will make the
# truncation of the context fail (the tokenized question will take a lots of space). So we remove that
# left whitespace
examples["question"] = [q.lstrip() for q in examples["question"]]
# Tokenize our examples with truncation and padding, but keep the overflows using a stride. This results
# in one example possible giving several features when a context is long, each of those features having a
# context that overlaps a bit the context of the previous feature.
tokenized_examples = tokenizer(
examples["question" if pad_on_right else "context"],
examples["context" if pad_on_right else "question"],
truncation="only_second" if pad_on_right else "only_first",
max_length=max_length,
stride=doc_stride,
return_overflowing_tokens=True,
return_offsets_mapping=True,
padding="max_length",
)
# Since one example might give us several features if it has a long context, we need a map from a feature to
# its corresponding example. This key gives us just that.
sample_mapping = tokenized_examples.pop("overflow_to_sample_mapping")
# The offset mappings will give us a map from token to character position in the original context. This will
# help us compute the start_positions and end_positions.
offset_mapping = tokenized_examples.pop("offset_mapping")
# Let's label those examples!
tokenized_examples["start_positions"] = []
tokenized_examples["end_positions"] = []
for i, offsets in enumerate(offset_mapping):
# We will label impossible answers with the index of the CLS token.
input_ids = tokenized_examples["input_ids"][i]
cls_index = input_ids.index(tokenizer.cls_token_id)
# Grab the sequence corresponding to that example (to know what is the context and what is the question).
sequence_ids = tokenized_examples.sequence_ids(i)
# One example can give several spans, this is the index of the example containing this span of text.
sample_index = sample_mapping[i]
answers = examples["answers"][sample_index]
# If no answers are given, set the cls_index as answer.
if len(answers["answer_start"]) == 0:
tokenized_examples["start_positions"].append(cls_index)
tokenized_examples["end_positions"].append(cls_index)
else:
# Start/end character index of the answer in the text.
start_char = answers["answer_start"][0]
end_char = start_char + len(answers["text"][0])
# Start token index of the current span in the text.
token_start_index = 0
while sequence_ids[token_start_index] != (1 if pad_on_right else 0):
token_start_index += 1
# End token index of the current span in the text.
token_end_index = len(input_ids) - 1
while sequence_ids[token_end_index] != (1 if pad_on_right else 0):
token_end_index -= 1
# Detect if the answer is out of the span (in which case this feature is labeled with the CLS index).
if not (offsets[token_start_index][0] <= start_char and offsets[token_end_index][1] >= end_char):
tokenized_examples["start_positions"].append(cls_index)
tokenized_examples["end_positions"].append(cls_index)
else:
# Otherwise move the token_start_index and token_end_index to the two ends of the answer.
# Note: we could go after the last offset if the answer is the last word (edge case).
while token_start_index < len(offsets) and offsets[token_start_index][0] <= start_char:
token_start_index += 1
tokenized_examples["start_positions"].append(token_start_index - 1)
while offsets[token_end_index][1] >= end_char:
token_end_index -= 1
tokenized_examples["end_positions"].append(token_end_index + 1)
return tokenized_examples<jupyter_output><empty_output><jupyter_text>Now apply this function on all the sentences (or pairs of sentences) in our dataset:<jupyter_code>tokenized_datasets = datasets.map(prepare_train_features, batched=True, remove_columns=datasets["train"].column_names)<jupyter_output><jupyter_text>Fine-tuning DeBERTa model Now that our data is ready for training, we can download the pretrained model and fine-tune it. Since our task is question answering, we use the `AutoModelForQuestionAnswering` class. Like with the tokenizer, the from_pretrained method will download and cache the model for us:<jupyter_code>from transformers import AutoModelForQuestionAnswering
from optimum.onnxruntime import ORTTrainer, ORTTrainingArguments
from transformers import default_data_collator
model = AutoModelForQuestionAnswering.from_pretrained(model_checkpoint)
data_collator = default_data_collator<jupyter_output>Some weights of the model checkpoint at microsoft/deberta-base were not used when initializing DebertaForQuestionAnswering: ['lm_predictions.lm_head.bias', 'lm_predictions.lm_head.dense.bias', 'lm_predictions.lm_head.LayerNorm.bias', 'lm_predictions.lm_head.LayerNorm.weight', 'deberta.embeddings.position_embeddings.weight', 'lm_predictions.lm_head.dense.weight']
- This IS expected if you are initializing DebertaForQuestionAnswering from the checkpoint of a model trained on another task or with another architecture (e.g. initializing a BertForSequenceClassification model from a BertForPreTraining model).
- This IS NOT expected if you are initializing DebertaForQuestionAnswering from the checkpoint of a model that you expect to be exactly identical (initializing a BertForSequenceClassification model from a BertForSequenceClassification model).
Some weights of DebertaForQuestionAnswering were not initialized from the model checkpoint at microsoft/deberta-base and are newly initialized: ['[...]<jupyter_text>Before setting up the training arguments, we will download a configuration of deepspeed stage 1. ONNX Runtime training supports ZeRO stage 1(optimizer state partitioning).<jupyter_code>!wget https://raw.githubusercontent.com/huggingface/optimum/main/tests/onnxruntime/ds_configs/ds_config_zero_stage_1.json
model_name = model_checkpoint.split("/")[-1]
args = ORTTrainingArguments(
output_dir=f"{model_name}-finetuned-squad",
evaluation_strategy = "epoch",
learning_rate=2e-5,
per_device_train_batch_size=batch_size,
per_device_eval_batch_size=batch_size,
num_train_epochs=3,
weight_decay=0.01,
optim="adamw_ort_fused",
deepspeed="ds_config_zero_stage_1.json",
)<jupyter_output><empty_output><jupyter_text>Instantiate `ORTTrainer`, here we will use ORT fused optimizer to have better performance on latency:<jupyter_code>trainer = ORTTrainer(
model=model,
args=args,
train_dataset=tokenized_datasets["train"],
eval_dataset=tokenized_datasets["validation"],
data_collator=data_collator,
tokenizer=tokenizer,
feature="question-answering",
)<jupyter_output><empty_output><jupyter_text>Launch the training (it will leverage one single GPU for the training)<jupyter_code>trainer.train()
trainer.save_model()<jupyter_output>Wrap ORTModule for ONNX Runtime training.<jupyter_text>If you want to leverage multiple gpus for distributed data parallele training, you can use 🤗 Accelerate's notebook launcher to enable multi-gpu training:<jupyter_code>from accelerate import notebook_launcher
def train_trainer_ddp():
model = AutoModelForQuestionAnswering.from_pretrained(model_checkpoint)
model_name = model_checkpoint.split("/")[-1]
args = ORTTrainingArguments(
output_dir=f"{model_name}-finetuned-squad",
evaluation_strategy = "epoch",
learning_rate=2e-5,
per_device_train_batch_size=batch_size,
per_device_eval_batch_size=batch_size,
num_train_epochs=3,
weight_decay=0.01,
optim="adamw_ort_fused",
deepspeed="ds_config_zero_stage_1.json",
)
data_collator = default_data_collator
trainer = ORTTrainer(
model=model,
args=args,
train_dataset=tokenized_datasets["train"],
eval_dataset=tokenized_datasets["validation"],
data_collator=data_collator,
tokenizer=tokenizer,
feature="question-answering",
)
trainer.train()
trainer.save_model()
notebook_launcher(train_trainer_ddp, args=(), num_processes=4)<jupyter_output><empty_output><jupyter_text>Evaluation Evaluating our model will require a bit more work, as we will need to map the predictions of our model back to parts of the context. The model itself predicts logits for the start and en position of our answer: if we take a batch from our validation datalaoder, here is the output our model gives us:<jupyter_code>import torch
for batch in trainer.get_eval_dataloader():
break
batch = {k: v.to(trainer.args.device) for k, v in batch.items()}
with torch.no_grad():
output = trainer.model(**batch)
output.keys()<jupyter_output><empty_output><jupyter_text>The output of the model is a dict-like object that contains the loss (since we provided labels), the start and end logits. We won't need the loss for our predictions, let's have a look a the logits: We have one logit for each feature and each token. The most obvious thing to predict an answer for each feature is to take the index for the maximum of the start logits as a start position and the index of the maximum of the end logits as an end position. This will work great in a lot of cases, but what if this prediction gives us something impossible: the start position could be greater than the end position, or point to a span of text in the question instead of the answer. In that case, we might want to look at the second best prediction to see if it gives a possible answer and select that instead.To classify our answers, we will use the score obtained by adding the start and end logits. We won't try to order all the possible answers and limit ourselves to with a hyper-parameter we call n_best_size. We'll pick the best indices in the start and end logits and gather all the answers this predicts. After checking if each one is valid, we will sort them by their score and keep the best one. Here is how we would do this on the first feature in the batch. And then we can sort the `valid_answers` according to their score and only keep the best one. The only point left is how to check a given span is inside the context (and not the question) and how to get back the text inside. To do this, we need to add two things to our validation features:* the ID of the example that generated the feature (since each example can generate several features, as seen before);* the offset mapping that will give us a map from token indices to character positions in the context.That's why we will re-process the validation set with the following function, slightly different from `prepare_train_features`:<jupyter_code>n_best_size = 20
def prepare_validation_features(examples):
# Some of the questions have lots of whitespace on the left, which is not useful and will make the
# truncation of the context fail (the tokenized question will take a lots of space). So we remove that
# left whitespace
examples["question"] = [q.lstrip() for q in examples["question"]]
# Tokenize our examples with truncation and maybe padding, but keep the overflows using a stride. This results
# in one example possible giving several features when a context is long, each of those features having a
# context that overlaps a bit the context of the previous feature.
tokenized_examples = tokenizer(
examples["question" if pad_on_right else "context"],
examples["context" if pad_on_right else "question"],
truncation="only_second" if pad_on_right else "only_first",
max_length=max_length,
stride=doc_stride,
return_overflowing_tokens=True,
return_offsets_mapping=True,
padding="max_length",
)
# Since one example might give us several features if it has a long context, we need a map from a feature to
# its corresponding example. This key gives us just that.
sample_mapping = tokenized_examples.pop("overflow_to_sample_mapping")
# We keep the example_id that gave us this feature and we will store the offset mappings.
tokenized_examples["example_id"] = []
for i in range(len(tokenized_examples["input_ids"])):
# Grab the sequence corresponding to that example (to know what is the context and what is the question).
sequence_ids = tokenized_examples.sequence_ids(i)
context_index = 1 if pad_on_right else 0
# One example can give several spans, this is the index of the example containing this span of text.
sample_index = sample_mapping[i]
tokenized_examples["example_id"].append(examples["id"][sample_index])
# Set to None the offset_mapping that are not part of the context so it's easy to determine if a token
# position is part of the context or not.
tokenized_examples["offset_mapping"][i] = [
(o if sequence_ids[k] == context_index else None)
for k, o in enumerate(tokenized_examples["offset_mapping"][i])
]
return tokenized_examples
validation_features = datasets["validation"].map(
prepare_validation_features,
batched=True,
remove_columns=datasets["validation"].column_names
)<jupyter_output><jupyter_text>Now we can grab the predictions for all features by using the `Trainer.predict` method:<jupyter_code>raw_predictions = trainer.predict(validation_features)<jupyter_output><empty_output><jupyter_text>The `ORTTrainer` hides the columns that are not used by the model (here `example_id` and `offset_mapping` which we will need for our post-processing), so we set them back:<jupyter_code>validation_features.set_format(type=validation_features.format["type"], columns=list(validation_features.features.keys()))<jupyter_output><empty_output><jupyter_text>We can now refine the test we had before: since we set `None` in the offset mappings when it corresponds to a part of the question, it's easy to check if an answer is fully inside the context. We also eliminate very long answers from our considerations (with an hyper-parameter we can tune)<jupyter_code>max_answer_length = 30
import collections
examples = datasets["validation"]
features = validation_features
example_id_to_index = {k: i for i, k in enumerate(examples["id"])}
features_per_example = collections.defaultdict(list)
for i, feature in enumerate(features):
features_per_example[example_id_to_index[feature["example_id"]]].append(i)
from tqdm.auto import tqdm
import numpy as np
def postprocess_qa_predictions(examples, features, raw_predictions, n_best_size = 20, max_answer_length = 30):
all_start_logits, all_end_logits = raw_predictions
# Build a map example to its corresponding features.
example_id_to_index = {k: i for i, k in enumerate(examples["id"])}
features_per_example = collections.defaultdict(list)
for i, feature in enumerate(features):
features_per_example[example_id_to_index[feature["example_id"]]].append(i)
# The dictionaries we have to fill.
predictions = collections.OrderedDict()
# Logging.
print(f"Post-processing {len(examples)} example predictions split into {len(features)} features.")
# Let's loop over all the examples!
for example_index, example in enumerate(tqdm(examples)):
# Those are the indices of the features associated to the current example.
feature_indices = features_per_example[example_index]
min_null_score = None # Only used if squad_v2 is True.
valid_answers = []
context = example["context"]
# Looping through all the features associated to the current example.
for feature_index in feature_indices:
# We grab the predictions of the model for this feature.
start_logits = all_start_logits[feature_index]
end_logits = all_end_logits[feature_index]
# This is what will allow us to map some the positions in our logits to span of texts in the original
# context.
offset_mapping = features[feature_index]["offset_mapping"]
# Update minimum null prediction.
cls_index = features[feature_index]["input_ids"].index(tokenizer.cls_token_id)
feature_null_score = start_logits[cls_index] + end_logits[cls_index]
if min_null_score is None or min_null_score < feature_null_score:
min_null_score = feature_null_score
# Go through all possibilities for the `n_best_size` greater start and end logits.
start_indexes = np.argsort(start_logits)[-1 : -n_best_size - 1 : -1].tolist()
end_indexes = np.argsort(end_logits)[-1 : -n_best_size - 1 : -1].tolist()
for start_index in start_indexes:
for end_index in end_indexes:
# Don't consider out-of-scope answers, either because the indices are out of bounds or correspond
# to part of the input_ids that are not in the context.
if (
start_index >= len(offset_mapping)
or end_index >= len(offset_mapping)
or offset_mapping[start_index] is None
or offset_mapping[end_index] is None
):
continue
# Don't consider answers with a length that is either < 0 or > max_answer_length.
if end_index < start_index or end_index - start_index + 1 > max_answer_length:
continue
start_char = offset_mapping[start_index][0]
end_char = offset_mapping[end_index][1]
valid_answers.append(
{
"score": start_logits[start_index] + end_logits[end_index],
"text": context[start_char: end_char]
}
)
if len(valid_answers) > 0:
best_answer = sorted(valid_answers, key=lambda x: x["score"], reverse=True)[0]
else:
# In the very rare edge case we have not a single non-null prediction, we create a fake prediction to avoid
# failure.
best_answer = {"text": "", "score": 0.0}
# Let's pick our final answer: the best one or the null answer (only for squad_v2)
if not squad_v2:
predictions[example["id"]] = best_answer["text"]
else:
answer = best_answer["text"] if best_answer["score"] > min_null_score else ""
predictions[example["id"]] = answer
return predictions
final_predictions = postprocess_qa_predictions(datasets["validation"], validation_features, raw_predictions.predictions)<jupyter_output>Post-processing 10570 example predictions split into 10788 features.<jupyter_text>Then we can load the metric from the datasets library.<jupyter_code>metric = load_metric("squad_v2" if squad_v2 else "squad")<jupyter_output>/tmp/ipykernel_88/2905994612.py:1: FutureWarning: load_metric is deprecated and will be removed in the next major version of datasets. Use 'evaluate.load' instead, from the new library 🤗 Evaluate: https://huggingface.co/docs/evaluate
metric = load_metric("squad_v2" if squad_v2 else "squad")
Downloading builder script: 4.50kB [00:00, 2.50MB/s]
Downloading extra modules: 3.31kB [00:00, 1.91MB/s]<jupyter_text>Then we can call compute on it. We just need to format predictions and labels a bit as it expects a list of dictionaries and not one big dictionary. In the case of squad_v2, we also have to set a `no_answer_probability` argument (which we set to 0.0 here as we have already set the answer to empty if we picked it).<jupyter_code>if squad_v2:
formatted_predictions = [{"id": k, "prediction_text": v, "no_answer_probability": 0.0} for k, v in final_predictions.items()]
else:
formatted_predictions = [{"id": k, "prediction_text": v} for k, v in final_predictions.items()]
references = [{"id": ex["id"], "answers": ex["answers"]} for ex in datasets["validation"]]
metric.compute(predictions=formatted_predictions, references=references)<jupyter_output><empty_output><jupyter_text>Inference of fine-tuned DeBERTa model In 🤗 Optimum, we also provides `ORTModel` API to ease the inference with ONNX Runtime backend.<jupyter_code>from optimum.onnxruntime import ORTModelForQuestionAnswering
from transformers import AutoTokenizer, pipeline
model = ORTModelForQuestionAnswering.from_pretrained("deberta-base-finetuned-squad", export=True)
tokenizer = AutoTokenizer.from_pretrained("deberta-base-finetuned-squad")
question, text = "What's the benefit of using Optimum library?", "Optimum is an open-sourced library. It can accelerate the training speed. We recommend you to test it."
inputs = tokenizer(question, text, return_tensors="pt")
inputs.pop("token_type_ids")
outputs = model(**inputs)
answer_start_index = outputs.start_logits.argmax()
answer_end_index = outputs.end_logits.argmax()
predict_answer_tokens = inputs.input_ids[0, answer_start_index : answer_end_index + 1]
tokenizer.decode(predict_answer_tokens, skip_special_tokens=True)<jupyter_output><empty_output> | notebooks/examples/question_answering_ort.ipynb/0 | {
"file_path": "notebooks/examples/question_answering_ort.ipynb",
"repo_id": "notebooks",
"token_count": 10005
} | 163 |
<jupyter_start><jupyter_text>If you're opening this Notebook on colab, you will probably need to install 🤗 Transformers and 🤗 Datasets. We will also use the `seqeval` library to compute some evaluation metrics. Uncomment the following cell and run it.<jupyter_code>#! pip install transformers
#! pip install datasets
#! pip install seqeval
#! pip install huggingface_hub<jupyter_output><empty_output><jupyter_text>If you're opening this notebook locally, make sure your environment has an install from the last version of those libraries.To be able to share your model with the community and generate results like the one shown in the picture below via the inference API, there are a few more steps to follow.First you have to store your authentication token from the Hugging Face website (sign up [here](https://huggingface.co/join) if you haven't already!) then uncomment the following cell and input it:<jupyter_code>from huggingface_hub import notebook_login
notebook_login()<jupyter_output><empty_output><jupyter_text>Then you need to install Git-LFS and setup Git if you haven't already. Uncomment the following instructions and adapt with your name and email:<jupyter_code># !apt install git-lfs
# !git config --global user.email "[email protected]"
# !git config --global user.name "Your Name"<jupyter_output><empty_output><jupyter_text>Make sure your version of Transformers is at least 4.16.0 since some of the functionality we use was introduced in that version:<jupyter_code>import transformers
print(transformers.__version__)<jupyter_output>4.21.0.dev0<jupyter_text>You can find a script version of this notebook to fine-tune your model in a distributed fashion using multiple GPUs or TPUs [here](https://github.com/huggingface/transformers/tree/main/examples/tensorflow/token-classification). We also quickly upload some telemetry - this tells us which examples and software versions are getting used so we know where to prioritize our maintenance efforts. We don't collect (or care about) any personally identifiable information, but if you'd prefer not to be counted, feel free to skip this step or delete this cell entirely.<jupyter_code>from transformers.utils import send_example_telemetry
send_example_telemetry("token_classification_notebook", framework="tensorflow")<jupyter_output><empty_output><jupyter_text>Fine-tuning a model on a token classification task In this notebook, we will see how to fine-tune one of the [🤗 Transformers](https://github.com/huggingface/transformers) model to a token classification task, which is the task of predicting a label for each token.The most common token classification tasks are:- NER (Named-entity recognition) Classify the entities in the text (person, organization, location...).- POS (Part-of-speech tagging) Grammatically classify the tokens (noun, verb, adjective...)- Chunk (Chunking) Grammatically classify the tokens and group them into "chunks" that go togetherWe will see how to easily load a dataset for these kinds of tasks and use Keras to fine-tune a model on it. This notebook is built to run on any token classification task, with any model checkpoint from the [Model Hub](https://huggingface.co/models) as long as that model has a version with a token classification head and a fast tokenizer (check on [this table](https://huggingface.co/transformers/index.htmlbigtable) if this is the case). It might just need some small adjustments if you decide to use a different dataset than the one used here. Depending on you model and the GPU you are using, you might need to adjust the batch size to avoid out-of-memory errors. Set those three parameters, then the rest of the notebook should run smoothly:<jupyter_code>task = "ner" # Should be one of "ner", "pos" or "chunk"
model_checkpoint = "distilbert-base-uncased"
batch_size = 16<jupyter_output><empty_output><jupyter_text>Loading the dataset We will use the [🤗 Datasets](https://github.com/huggingface/datasets) library to download the data and get the metric we need to use for evaluation (to compare our model to the benchmark). This can be easily done with the functions `load_dataset` and `load_metric`.<jupyter_code>from datasets import load_dataset, load_metric<jupyter_output><empty_output><jupyter_text>For our example here, we'll use the [CONLL 2003 dataset](https://www.aclweb.org/anthology/W03-0419.pdf). The notebook should work with any token classification dataset provided by the 🤗 Datasets library. If you're using your own dataset defined from a JSON or csv file (see the [Datasets documentation](https://huggingface.co/docs/datasets/loading_datasets.htmlfrom-local-files) on how to load them), it might need some adjustments in the names of the columns used.<jupyter_code>datasets = load_dataset("conll2003")<jupyter_output>Reusing dataset conll2003 (/home/matt/.cache/huggingface/datasets/conll2003/conll2003/1.0.0/9a4d16a94f8674ba3466315300359b0acd891b68b6c8743ddf60b9c702adce98)<jupyter_text>The `datasets` object itself is [`DatasetDict`](https://huggingface.co/docs/datasets/package_reference/main_classes.htmldatasetdict), which contains one key for the training, validation and test set.<jupyter_code>datasets<jupyter_output><empty_output><jupyter_text>We can see the training, validation and test sets all have a column for the tokens (the input texts split into words) and one column of labels for each kind of task we introduced before. To access an actual element, you need to select a split first, then give an index:<jupyter_code>datasets["train"][0]<jupyter_output><empty_output><jupyter_text>The labels are already coded as integer ids to be easily usable by our model, but the correspondence with the actual categories is stored in the `features` of the dataset:<jupyter_code>datasets["train"].features[f"ner_tags"]<jupyter_output><empty_output><jupyter_text>So for the NER tags, 0 corresponds to 'O', 1 to 'B-PER' etc... On top of the 'O' (which means no special entity), there are four labels for NER here, each prefixed with 'B-' (for beginning) or 'I-' (for intermediate), that indicate if the token is the first one for the current group with the label or not:- 'PER' for person- 'ORG' for organization- 'LOC' for location- 'MISC' for miscellaneous Since the labels are lists of `ClassLabel`, the actual names of the labels are nested in the `feature` attribute of the object above:<jupyter_code>label_list = datasets["train"].features[f"{task}_tags"].feature.names
label_list<jupyter_output><empty_output><jupyter_text>To get a sense of what the data looks like, the following function will show some examples picked randomly in the dataset (automatically decoding the labels in passing).<jupyter_code>from datasets import ClassLabel, Sequence
import random
import pandas as pd
from IPython.display import display, HTML
def show_random_elements(dataset, num_examples=10):
assert num_examples <= len(
dataset
), "Can't pick more elements than there are in the dataset."
picks = []
for _ in range(num_examples):
pick = random.randint(0, len(dataset) - 1)
while pick in picks:
pick = random.randint(0, len(dataset) - 1)
picks.append(pick)
df = pd.DataFrame(dataset[picks])
for column, typ in dataset.features.items():
if isinstance(typ, ClassLabel):
df[column] = df[column].transform(lambda i: typ.names[i])
elif isinstance(typ, Sequence) and isinstance(typ.feature, ClassLabel):
df[column] = df[column].transform(
lambda x: [typ.feature.names[i] for i in x]
)
display(HTML(df.to_html()))
show_random_elements(datasets["train"])<jupyter_output><empty_output><jupyter_text>Preprocessing the data Before we can feed those texts to our model, we need to preprocess them. This is done by a 🤗 Transformers `Tokenizer` which will (as the name indicates) tokenize the inputs (including converting the tokens to their corresponding IDs in the pretrained vocabulary) and put it in a format the model expects, as well as generate the other inputs that model requires.To do all of this, we instantiate our tokenizer with the `AutoTokenizer.from_pretrained` method, which will ensure:- we get a tokenizer that corresponds to the model architecture we want to use,- we download the vocabulary used when pretraining this specific checkpoint.That vocabulary will be cached, so it's not downloaded again the next time we run the cell.<jupyter_code>from transformers import AutoTokenizer
tokenizer = AutoTokenizer.from_pretrained(model_checkpoint)<jupyter_output><empty_output><jupyter_text>The following assertion ensures that our tokenizer is a fast tokenizers (backed by Rust) from the 🤗 Tokenizers library. Those fast tokenizers are available for almost all models, and we will need some of the special features they have for our preprocessing.<jupyter_code>import transformers
assert isinstance(tokenizer, transformers.PreTrainedTokenizerFast)<jupyter_output><empty_output><jupyter_text>You can check which type of models have a fast tokenizer available and which don't on the [big table of models](https://huggingface.co/transformers/index.htmlbigtable). You can directly call this tokenizer on one sentence:<jupyter_code>tokenizer("Hello, this is a sentence!")<jupyter_output><empty_output><jupyter_text>Depending on the model you selected, you will see different keys in the dictionary returned by the cell above. They don't matter much for what we're doing here (just know they are required by the model we will instantiate later), you can learn more about them in [this tutorial](https://huggingface.co/transformers/preprocessing.html) if you're interested.If, as is the case here, your inputs have already been split into words, you should pass the list of words to your tokenzier with the argument `is_split_into_words=True`:<jupyter_code>tokenizer(
["Hello", ",", "this", "is", "one", "sentence", "split", "into", "words", "."],
is_split_into_words=True,
)<jupyter_output><empty_output><jupyter_text>Note that transformers are often pretrained with subword tokenizers, meaning that even if your inputs have been split into words already, each of those words could be split again by the tokenizer. Let's look at an example of that:<jupyter_code>example = datasets["train"][4]
print(example["tokens"])
tokenized_input = tokenizer(example["tokens"], is_split_into_words=True)
tokens = tokenizer.convert_ids_to_tokens(tokenized_input["input_ids"])
print(tokens)<jupyter_output>['[CLS]', 'germany', "'", 's', 'representative', 'to', 'the', 'european', 'union', "'", 's', 'veterinary', 'committee', 'werner', 'z', '##wing', '##mann', 'said', 'on', 'wednesday', 'consumers', 'should', 'buy', 'sheep', '##me', '##at', 'from', 'countries', 'other', 'than', 'britain', 'until', 'the', 'scientific', 'advice', 'was', 'clearer', '.', '[SEP]']<jupyter_text>Here the words "Zwingmann" and "sheepmeat" have been split in three subtokens.This means that we need to do some processing on our labels as the input ids returned by the tokenizer are longer than the lists of labels our dataset contain, first because some special tokens might be added (we can a `[CLS]` and a `[SEP]` above) and then because of those possible splits of words in multiple tokens:<jupyter_code>len(example[f"{task}_tags"]), len(tokenized_input["input_ids"])<jupyter_output><empty_output><jupyter_text>Thankfully, the tokenizer returns outputs that have a `word_ids` method which can help us.<jupyter_code>print(tokenized_input.word_ids())<jupyter_output>[None, 0, 1, 1, 2, 3, 4, 5, 6, 7, 7, 8, 9, 10, 11, 11, 11, 12, 13, 14, 15, 16, 17, 18, 18, 18, 19, 20, 21, 22, 23, 24, 25, 26, 27, 28, 29, 30, None]<jupyter_text>As we can see, it returns a list with the same number of elements as our processed input ids, mapping special tokens to `None` and all other tokens to their respective word. This way, we can align the labels with the processed input ids.<jupyter_code>word_ids = tokenized_input.word_ids()
aligned_labels = [-100 if i is None else example[f"{task}_tags"][i] for i in word_ids]
print(len(aligned_labels), len(tokenized_input["input_ids"]))<jupyter_output>39 39<jupyter_text>Here we set the labels of all special tokens to -100 (the index that is ignored by the loss function) and the labels of all other tokens to the label of the word they come from. Another strategy is to set the label only on the first token obtained from a given word, and give a label of -100 to the other subtokens from the same word. Both strategies are possible with this script; simply change the value of this flag to swap between them.<jupyter_code>label_all_tokens = True<jupyter_output><empty_output><jupyter_text>We're now ready to write the function that will preprocess our samples. We feed them to the `tokenizer` with the argument `truncation=True` (to truncate texts that are bigger than the maximum size allowed by the model) and `is_split_into_words=True` (as seen above). Then we align the labels with the token ids using the strategy we picked:<jupyter_code>def tokenize_and_align_labels(examples):
tokenized_inputs = tokenizer(
examples["tokens"], truncation=True, is_split_into_words=True
)
labels = []
for i, label in enumerate(examples[f"{task}_tags"]):
word_ids = tokenized_inputs.word_ids(batch_index=i)
previous_word_idx = None
label_ids = []
for word_idx in word_ids:
# Special tokens have a word id that is None. We set the label to -100 so they are automatically
# ignored in the loss function.
if word_idx is None:
label_ids.append(-100)
# We set the label for the first token of each word.
elif word_idx != previous_word_idx:
label_ids.append(label[word_idx])
# For the other tokens in a word, we set the label to either the current label or -100, depending on
# the label_all_tokens flag.
else:
label_ids.append(label[word_idx] if label_all_tokens else -100)
previous_word_idx = word_idx
labels.append(label_ids)
tokenized_inputs["labels"] = labels
return tokenized_inputs<jupyter_output><empty_output><jupyter_text>This function works with one or several examples. In the case of several examples, the tokenizer will return a list of lists for each key:<jupyter_code>tokenize_and_align_labels(datasets["train"][:5])<jupyter_output><empty_output><jupyter_text>To apply this function on all the sentences (or pairs of sentences) in our dataset, we just use the `map` method of our `dataset` object we created earlier. This will apply the function on all the elements of all the splits in `dataset`, so our training, validation and testing data will be preprocessed in one single command.<jupyter_code>tokenized_datasets = datasets.map(tokenize_and_align_labels, batched=True)<jupyter_output>Loading cached processed dataset at /home/matt/.cache/huggingface/datasets/conll2003/conll2003/1.0.0/9a4d16a94f8674ba3466315300359b0acd891b68b6c8743ddf60b9c702adce98/cache-7a41e503ed258cd6.arrow
Loading cached processed dataset at /home/matt/.cache/huggingface/datasets/conll2003/conll2003/1.0.0/9a4d16a94f8674ba3466315300359b0acd891b68b6c8743ddf60b9c702adce98/cache-8eb7bcede9dedbe4.arrow
Loading cached processed dataset at /home/matt/.cache/huggingface/datasets/conll2003/conll2003/1.0.0/9a4d16a94f8674ba3466315300359b0acd891b68b6c8743ddf60b9c702adce98/cache-cffd29036188a9aa.arrow<jupyter_text>Even better, the results are automatically cached by the 🤗 Datasets library to avoid spending time on this step the next time you run your notebook. The 🤗 Datasets library is normally smart enough to detect when the function you pass to map has changed (and thus requires to not use the cache data). For instance, it will properly detect if you change the task in the first cell and rerun the notebook. 🤗 Datasets warns you when it uses cached files, you can pass `load_from_cache_file=False` in the call to `map` to not use the cached files and force the preprocessing to be applied again.Note that we passed `batched=True` to encode the texts by batches together. This is to leverage the full benefit of the fast tokenizer we loaded earlier, which will use multi-threading to treat the texts in a batch concurrently.<jupyter_code>tokenized_datasets["train"]["labels"][0]<jupyter_output><empty_output><jupyter_text>Fine-tuning the model Now that our data is ready, we can download the pretrained model and fine-tune it. Since all our tasks are about token classification, we use the `TFAutoModelForTokenClassification` class. Like with the tokenizer, the `from_pretrained` method will download and cache the model for us. The only thing we have to specify is the number of labels for our problem (which we can get from the features, as seen before). We can also set the `id2label` and `label2id` properties for our model - this is optional, but will give us some nice cleaner outputs later.<jupyter_code>from transformers import TFAutoModelForTokenClassification
id2label = {i: label for i, label in enumerate(label_list)}
label2id = {label: i for i, label in enumerate(label_list)}
model = TFAutoModelForTokenClassification.from_pretrained(
model_checkpoint, num_labels=len(label_list), id2label=id2label, label2id=label2id
)<jupyter_output>2022-07-21 17:09:54.903083: I tensorflow/stream_executor/cuda/cuda_gpu_executor.cc:975] successful NUMA node read from SysFS had negative value (-1), but there must be at least one NUMA node, so returning NUMA node zero
2022-07-21 17:09:54.907230: I tensorflow/stream_executor/cuda/cuda_gpu_executor.cc:975] successful NUMA node read from SysFS had negative value (-1), but there must be at least one NUMA node, so returning NUMA node zero
2022-07-21 17:09:54.907935: I tensorflow/stream_executor/cuda/cuda_gpu_executor.cc:975] successful NUMA node read from SysFS had negative value (-1), but there must be at least one NUMA node, so returning NUMA node zero
2022-07-21 17:09:54.909185: I tensorflow/core/platform/cpu_feature_guard.cc:193] This TensorFlow binary is optimized with oneAPI Deep Neural Network Library (oneDNN) to use the following CPU instructions in performance-critical operations: AVX2 FMA
To enable them in other operations, rebuild TensorFlow with the appropriate compiler flags[...]<jupyter_text>After all of the Tensorflow initialization messages, we see a warning that is telling us we are throwing away some weights (the `vocab_transform` and `vocab_layer_norm` layers) and randomly initializing some other (the `pre_classifier` and `classifier` layers). This is absolutely normal in this case, because we are removing the head used to pretrain the model on a masked language modeling objective and replacing it with a new head for which we don't have pretrained weights, so the library warns us we should fine-tune this model before using it for inference, which is exactly what we are going to do. To compile a Keras `Model`, we will need to set an optimizer and our loss function. We can use the `create_optimizer` function from Transformers. Here we tweak the learning rate, use the `batch_size` defined at the top of the notebook and customize the number of epochs for training, as well as the weight decay. This function will also create and apply a learning rate decay schedule for us.<jupyter_code>from transformers import create_optimizer
num_train_epochs = 3
num_train_steps = (len(tokenized_datasets["train"]) // batch_size) * num_train_epochs
optimizer, lr_schedule = create_optimizer(
init_lr=2e-5,
num_train_steps=num_train_steps,
weight_decay_rate=0.01,
num_warmup_steps=0,
)<jupyter_output><empty_output><jupyter_text>Note that most Transformers models compute loss internally, so we actually don't have to specify anything there! You can of course set your own loss function if you want, but by default our models will choose the 'obvious' loss that matches their task, such as cross-entropy in the case of language modelling. The built-in loss will also correctly handle things like masking the loss on padding tokens, or unlabelled tokens in the case of masked language modelling, so we recommend using it unless you're an advanced user!In some of our other examples, we use `jit_compile` to compile the model with [XLA](https://www.tensorflow.org/xla). In this case, we should be careful about that - because our inputs have variable sequence lengths, we may end up having to do a new XLA compilation for each possible length, because XLA compilation expects a static input shape! For small datasets, this will probably result in spending more time on XLA compilation than actually training, which isn't very helpful.If you really want to use XLA without these problems (for example, if you're training on TPU), you can create a tokenizer with `padding="max_length"`. This will pad all of your samples to the same length, ensuring that a single XLA compilation will suffice for your entire dataset. Note that depending on the nature of your dataset, this may result in a lot of wasted computation on padding tokens!<jupyter_code>import tensorflow as tf
model.compile(optimizer=optimizer)<jupyter_output>No loss specified in compile() - the model's internal loss computation will be used as the loss. Don't panic - this is a common way to train TensorFlow models in Transformers! To disable this behaviour please pass a loss argument, or explicitly pass `loss=None` if you do not want your model to compute a loss.<jupyter_text>Now we will need a data collator that will batch our processed examples together while applying padding to make them all the same size (each pad will be padded to the length of its longest example). There is a data collator for this task in the Transformers library, that not only pads the inputs, but also the labels. Note that our data collators are designed to work for multiple frameworks, so ensure you set the `return_tensors='np'` argument to get NumPy arrays out - you don't want to accidentally get a load of `torch.Tensor` objects in the middle of your nice TF code! You could also use `return_tensors='tf'` to get TensorFlow tensors, but our TF dataset pipeline actually uses a NumPy loader internally, which is wrapped at the end with a `tf.data.Dataset`. As a result, `np` is usually more reliable and performant when you're using it!<jupyter_code>from transformers import DataCollatorForTokenClassification
data_collator = DataCollatorForTokenClassification(tokenizer, return_tensors="np")<jupyter_output><empty_output><jupyter_text>Next, we convert our datasets to `tf.data.Dataset`, which Keras understands natively. There are two ways to do this - we can use the slightly more low-level [`Dataset.to_tf_dataset()`](https://huggingface.co/docs/datasets/package_reference/main_classesdatasets.Dataset.to_tf_dataset) method, or we can use [`Model.prepare_tf_dataset()`](https://huggingface.co/docs/transformers/main_classes/modeltransformers.TFPreTrainedModel.prepare_tf_dataset). The main difference between these two is that the `Model` method can inspect the model to determine which column names it can use as input, which means you don't need to specify them yourself.<jupyter_code>train_set = model.prepare_tf_dataset(
tokenized_datasets["train"],
shuffle=True,
batch_size=batch_size,
collate_fn=data_collator,
)
validation_set = model.prepare_tf_dataset(
tokenized_datasets["validation"],
shuffle=False,
batch_size=batch_size,
collate_fn=data_collator,
)<jupyter_output>/home/matt/PycharmProjects/transformers/src/transformers/tokenization_utils_base.py:719: VisibleDeprecationWarning: Creating an ndarray from ragged nested sequences (which is a list-or-tuple of lists-or-tuples-or ndarrays with different lengths or shapes) is deprecated. If you meant to do this, you must specify 'dtype=object' when creating the ndarray.
tensor = as_tensor(value)<jupyter_text>Now, let's think about metrics. The `seqeval` framework will give us a nice set of metrics like accuracy, precision, recall and F1 score, and all we need to do is pass it some predictions and labels. But if these aren't Keras Metric objects, how can we use them in the middle of our training loop? The answer is the `KerasMetricCallback`. This callback takes a function and computes it on the predictions from the validation set each epoch, printing it and logging the returned value(s) for other callbacks like `TensorBoard` and `EarlyStopping`. This allows much more flexibility with the metric computation functions - you can even include Python code that would be impossible to compile into your model, such as using the `tokenizer` (which is backed by Rust!) to decode model outputs to strings and compare them to the labels. We won't be doing that here, but it's essential for metrics like `BLEU` and `ROUGE` used in tasks like translation.So how do we use `KerasMetricCallback`? It's straightforward - we simply define a function that takes the `predictions` and `labels` from the validation set and computes a dict of one or more metrics, then pass that function and the validation data to the callback. Note that we discard all predictions where the label is `-100` - this indicates a missing label, either because there's no label for that token, or the token is a padding token. The built-in Keras metrics are not aware of this masking system and so will produce erroneous values if they are used instead.<jupyter_code>import numpy as np
from transformers.keras_callbacks import KerasMetricCallback
metric = load_metric("seqeval")
labels = [label_list[i] for i in example[f"{task}_tags"]]
metric.compute(predictions=[labels], references=[labels])
def compute_metrics(p):
predictions, labels = p
predictions = np.argmax(predictions, axis=2)
# Remove ignored index (special tokens)
true_predictions = [
[label_list[p] for (p, l) in zip(prediction, label) if l != -100]
for prediction, label in zip(predictions, labels)
]
true_labels = [
[label_list[l] for (p, l) in zip(prediction, label) if l != -100]
for prediction, label in zip(predictions, labels)
]
results = metric.compute(predictions=true_predictions, references=true_labels)
return {
"precision": results["overall_precision"],
"recall": results["overall_recall"],
"f1": results["overall_f1"],
"accuracy": results["overall_accuracy"],
}
metric_callback = KerasMetricCallback(
metric_fn=compute_metrics, eval_dataset=validation_set
)<jupyter_output><empty_output><jupyter_text>Now we can train our model. We can also add a callback to sync up our model with the Hub - this allows us to resume training from other machines and even test the model's inference quality midway through training! If you don't want to do this, simply remove the callbacks argument in the call to `fit()`.<jupyter_code>from transformers.keras_callbacks import PushToHubCallback
from tensorflow.keras.callbacks import TensorBoard
model_name = model_checkpoint.split("/")[-1]
push_to_hub_model_id = f"{model_name}-finetuned-{task}"
tensorboard_callback = TensorBoard(log_dir="./tc_model_save/logs")
push_to_hub_callback = PushToHubCallback(
output_dir="./tc_model_save",
tokenizer=tokenizer,
hub_model_id=push_to_hub_model_id,
)
callbacks = [metric_callback, tensorboard_callback, push_to_hub_callback]
model.fit(
train_set,
validation_data=validation_set,
epochs=num_train_epochs,
callbacks=callbacks,
)<jupyter_output>/home/matt/PycharmProjects/notebooks/examples/tc_model_save is already a clone of https://huggingface.co/Rocketknight1/distilbert-base-uncased-finetuned-ner. Make sure you pull the latest changes with `repo.git_pull()`.<jupyter_text>If you used the callback above, you can now share this model with all your friends, family, favorite pets: they can all load it with the identifier `"your-username/the-name-you-picked"` so for instance:```pythonfrom transformers import TFAutoModelForTokenClassificationmodel = TFAutoModelForTokenClassification.from_pretrained("your-username/my-awesome-model")``` Inference Now that we've finished training our model, let's look at how we can get predictions from it. First, let's load the model from the hub so that we can resume from here without needing to run the rest of the notebook.<jupyter_code>from transformers import AutoTokenizer, TFAutoModelForTokenClassification
# You can, of course, use your own username and model name here
# once you've pushed your model using the code above!
checkpoint = "Rocketknight1/distilbert-base-uncased-finetuned-ner"
model = TFAutoModelForTokenClassification.from_pretrained(checkpoint)
tokenizer = AutoTokenizer.from_pretrained(checkpoint)<jupyter_output><empty_output><jupyter_text>Now let's see how to use this model for inference. Let's use a sample sentence from a recent news story and tokenize it.<jupyter_code>sample_sentence = "Britain will send scores of artillery guns and more than 1,600 anti-tank weapons to Ukraine in the latest supply of western arms to help bolster its defence against Russia, the UK defence secretary, Ben Wallace, said on Thursday."
tokenized = tokenizer([sample_sentence], return_tensors="np")<jupyter_output><empty_output><jupyter_text>Now we pass this to the model. The outputs from the model will be logits, so let's use argmax to get the model's guess for the class for each token.<jupyter_code>import numpy as np
outputs = model(tokenized).logits
classes = np.argmax(outputs, axis=-1)[0]
print(classes)<jupyter_output>[0 5 0 0 0 0 0 0 0 0 0 0 0 0 0 0 0 0 0 5 0 0 0 0 0 7 0 0 0 0 0 0 0 0 5 0 0
5 0 0 0 1 2 0 0 0 0 0 0]<jupyter_text>Well, there's our answer but it's not exactly readable like that. Let's do two things: Firstly we can pair each classification to the token it comes from, and secondly we'll convert the classes from label IDs to names.<jupyter_code>outputs = [(tokenizer.decode(token), model.config.id2label[id]) for token, id in zip(tokenized["input_ids"][0], classes)]
print(outputs)<jupyter_output>[('[CLS]', 'O'), ('britain', 'B-LOC'), ('will', 'O'), ('send', 'O'), ('scores', 'O'), ('of', 'O'), ('artillery', 'O'), ('guns', 'O'), ('and', 'O'), ('more', 'O'), ('than', 'O'), ('1', 'O'), (',', 'O'), ('600', 'O'), ('anti', 'O'), ('-', 'O'), ('tank', 'O'), ('weapons', 'O'), ('to', 'O'), ('ukraine', 'B-LOC'), ('in', 'O'), ('the', 'O'), ('latest', 'O'), ('supply', 'O'), ('of', 'O'), ('western', 'B-MISC'), ('arms', 'O'), ('to', 'O'), ('help', 'O'), ('bo', 'O'), ('##lster', 'O'), ('its', 'O'), ('defence', 'O'), ('against', 'O'), ('russia', 'B-LOC'), (',', 'O'), ('the', 'O'), ('uk', 'B-LOC'), ('defence', 'O'), ('secretary', 'O'), (',', 'O'), ('ben', 'B-PER'), ('wallace', 'I-PER'), (',', 'O'), ('said', 'O'), ('on', 'O'), ('thursday', 'O'), ('.', 'O'), ('[SEP]', 'O')]<jupyter_text>We can see that the model has correctly identified a named person as well as several locations in this text! Pipeline API An alternative way to quickly perform inference with any model on the hub is to use the [Pipeline API](https://huggingface.co/docs/transformers/main_classes/pipelines), which abstracts away all the steps we did manually above. It will perform the preprocessing, forward pass and postprocessing all in a single object.Let's showcase this for our trained model:<jupyter_code>from transformers import pipeline
token_classifier = pipeline(
"token-classification",
"Rocketknight1/distilbert-base-uncased-finetuned-ner",
framework="tf"
)
token_classifier(sample_sentence)<jupyter_output><empty_output> | notebooks/examples/token_classification-tf.ipynb/0 | {
"file_path": "notebooks/examples/token_classification-tf.ipynb",
"repo_id": "notebooks",
"token_count": 9592
} | 164 |
import argparse
import logging
import os
import sys
import tensorflow as tf
from datasets import load_dataset
from transformers import AutoTokenizer, TFAutoModelForSequenceClassification, DataCollatorWithPadding, create_optimizer
if __name__ == "__main__":
parser = argparse.ArgumentParser()
# Hyperparameters sent by the client are passed as command-line arguments to the script.
parser.add_argument("--epochs", type=int, default=3)
parser.add_argument("--train_batch_size", type=int, default=16)
parser.add_argument("--eval_batch_size", type=int, default=8)
parser.add_argument("--model_id", type=str)
parser.add_argument("--learning_rate", type=str, default=3e-5)
# Data, model, and output directories
parser.add_argument("--output_data_dir", type=str, default=os.environ["SM_OUTPUT_DATA_DIR"])
parser.add_argument("--model_dir", type=str, default=os.environ["SM_MODEL_DIR"])
parser.add_argument("--n_gpus", type=str, default=os.environ["SM_NUM_GPUS"])
args, _ = parser.parse_known_args()
# Set up logging
logger = logging.getLogger(__name__)
logging.basicConfig(
level=logging.getLevelName("INFO"),
handlers=[logging.StreamHandler(sys.stdout)],
format="%(asctime)s - %(name)s - %(levelname)s - %(message)s",
)
# Load tokenizer
tokenizer = AutoTokenizer.from_pretrained(args.model_id)
# Load DatasetDict
dataset = load_dataset("imdb")
# Preprocess train dataset
def preprocess_function(examples):
return tokenizer(examples["text"], truncation=True)
encoded_dataset = dataset.map(preprocess_function, batched=True)
# define tokenizer_columns
# tokenizer_columns is the list of keys from the dataset that get passed to the TensorFlow model
tokenizer_columns = ["attention_mask", "input_ids"]
# convert to TF datasets
data_collator = DataCollatorWithPadding(tokenizer=tokenizer, return_tensors="tf")
encoded_dataset["train"] = encoded_dataset["train"].rename_column("label", "labels")
tf_train_dataset = encoded_dataset["train"].to_tf_dataset(
columns=tokenizer_columns,
label_cols=["labels"],
shuffle=True,
batch_size=8,
collate_fn=data_collator,
)
encoded_dataset["test"] = encoded_dataset["test"].rename_column("label", "labels")
tf_validation_dataset = encoded_dataset["test"].to_tf_dataset(
columns=tokenizer_columns,
label_cols=["labels"],
shuffle=False,
batch_size=8,
collate_fn=data_collator,
)
# Prepare model labels - useful in inference API
labels = encoded_dataset["train"].features["labels"].names
num_labels = len(labels)
label2id, id2label = dict(), dict()
for i, label in enumerate(labels):
label2id[label] = str(i)
id2label[str(i)] = label
# download model from model hub
model = TFAutoModelForSequenceClassification.from_pretrained(
args.model_id, num_labels=num_labels, label2id=label2id, id2label=id2label
)
# create Adam optimizer with learning rate scheduling
batches_per_epoch = len(encoded_dataset["train"]) // args.train_batch_size
total_train_steps = int(batches_per_epoch * args.epochs)
optimizer, _ = create_optimizer(init_lr=args.learning_rate, num_warmup_steps=0, num_train_steps=total_train_steps)
loss = tf.keras.losses.SparseCategoricalCrossentropy(from_logits=True)
# define metric and compile model
metrics = [tf.keras.metrics.SparseCategoricalAccuracy()]
model.compile(optimizer=optimizer, loss=loss, metrics=metrics)
# Training
logger.info("*** Train ***")
train_results = model.fit(
tf_train_dataset,
epochs=args.epochs,
validation_data=tf_validation_dataset,
)
output_eval_file = os.path.join(args.output_data_dir, "train_results.txt")
with open(output_eval_file, "w") as writer:
logger.info("***** Train results *****")
logger.info(train_results)
for key, value in train_results.history.items():
logger.info(" %s = %s", key, value)
writer.write("%s = %s\n" % (key, value))
# Save result
model.save_pretrained(args.model_dir)
tokenizer.save_pretrained(args.model_dir)
| notebooks/sagemaker/02_getting_started_tensorflow/scripts/train.py/0 | {
"file_path": "notebooks/sagemaker/02_getting_started_tensorflow/scripts/train.py",
"repo_id": "notebooks",
"token_count": 1677
} | 165 |
base_job_name: accelerate-sagemaker-1
compute_environment: AMAZON_SAGEMAKER
distributed_type: DATA_PARALLEL
ec2_instance_type: ml.p3.16xlarge
iam_role_name: xxxxx
image_uri: null
mixed_precision: fp16
num_machines: 1
profile: xxxxx
py_version: py38
pytorch_version: 1.10.2
region: us-east-1
transformers_version: 4.17.0
use_cpu: false | notebooks/sagemaker/22_accelerate_sagemaker_examples/src/seq2seq/accelerate_config.yaml/0 | {
"file_path": "notebooks/sagemaker/22_accelerate_sagemaker_examples/src/seq2seq/accelerate_config.yaml",
"repo_id": "notebooks",
"token_count": 138
} | 166 |
<jupyter_start><jupyter_text>Efficient Large Language Model training with LoRA and Hugging FaceIn this sagemaker example, we are going to learn how to apply [Low-Rank Adaptation of Large Language Models (LoRA)](https://arxiv.org/abs/2106.09685) to fine-tune BLOOMZ (7 billion parameter version instruction tuned version of BLOOM) on a single GPU. We are going to leverage Hugging Face [Transformers](https://huggingface.co/docs/transformers/index), [Accelerate](https://huggingface.co/docs/accelerate/index), and [PEFT](https://github.com/huggingface/peft). You will learn how to:1. Setup Development Environment2. Load and prepare the dataset3. Fine-Tune BLOOM with LoRA and bnb int-8 on Amazon SageMaker4. Deploy the model to Amazon SageMaker Endpoint Quick intro: PEFT or Parameter Efficient Fine-tuning[PEFT](https://github.com/huggingface/peft), or Parameter Efficient Fine-tuning, is a new open-source library from Hugging Face to enable efficient adaptation of pre-trained language models (PLMs) to various downstream applications without fine-tuning all the model's parameters. PEFT currently includes techniques for:- LoRA: [LORA: LOW-RANK ADAPTATION OF LARGE LANGUAGE MODELS](https://arxiv.org/pdf/2106.09685.pdf)- Prefix Tuning: [P-Tuning v2: Prompt Tuning Can Be Comparable to Fine-tuning Universally Across Scales and Tasks](https://arxiv.org/pdf/2110.07602.pdf)- P-Tuning: [GPT Understands, Too](https://arxiv.org/pdf/2103.10385.pdf)- Prompt Tuning: [The Power of Scale for Parameter-Efficient Prompt Tuning](https://arxiv.org/pdf/2104.08691.pdf)<jupyter_code>!pip install "transformers==4.26.0" "datasets[s3]==2.9.0" sagemaker py7zr --upgrade --quiet<jupyter_output><empty_output><jupyter_text>If you are going to use Sagemaker in a local environment. You need access to an IAM Role with the required permissions for Sagemaker. You can find [here](https://docs.aws.amazon.com/sagemaker/latest/dg/sagemaker-roles.html) more about it.<jupyter_code>import sagemaker
import boto3
sess = sagemaker.Session()
# sagemaker session bucket -> used for uploading data, models and logs
# sagemaker will automatically create this bucket if it not exists
sagemaker_session_bucket=None
if sagemaker_session_bucket is None and sess is not None:
# set to default bucket if a bucket name is not given
sagemaker_session_bucket = sess.default_bucket()
try:
role = sagemaker.get_execution_role()
except ValueError:
iam = boto3.client('iam')
role = iam.get_role(RoleName='sagemaker_execution_role')['Role']['Arn']
sess = sagemaker.Session(default_bucket=sagemaker_session_bucket)
print(f"sagemaker role arn: {role}")
print(f"sagemaker bucket: {sess.default_bucket()}")
print(f"sagemaker session region: {sess.boto_region_name}")<jupyter_output><empty_output><jupyter_text>2. Load and prepare the datasetWe will use the [samsum](https://huggingface.co/datasets/samsum) dataset, a collection of about 16k messenger-like conversations with summaries. Conversations were created and written down by linguists fluent in English.```python{ "id": "13818513", "summary": "Amanda baked cookies and will bring Jerry some tomorrow.", "dialogue": "Amanda: I baked cookies. Do you want some?\r\nJerry: Sure!\r\nAmanda: I'll bring you tomorrow :-)"}```To load the `samsum` dataset, we use the `load_dataset()` method from the 🤗 Datasets library.<jupyter_code>from datasets import load_dataset
# Load dataset from the hub
dataset = load_dataset("samsum", split="train")
print(f"Train dataset size: {len(dataset)}")
# Train dataset size: 14732<jupyter_output><empty_output><jupyter_text>To train our model, we need to convert our inputs (text) to token IDs. This is done by a 🤗 Transformers Tokenizer. If you are not sure what this means, check out **[chapter 6](https://huggingface.co/course/chapter6/1?fw=tf)** of the Hugging Face Course.<jupyter_code>from transformers import AutoTokenizer
model_id="bigscience/bloomz-7b1"
# Load tokenizer of BLOOMZ
tokenizer = AutoTokenizer.from_pretrained(model_id)
tokenizer.model_max_length = 2048 # overwrite wrong value<jupyter_output><empty_output><jupyter_text>Before we can start training, we need to preprocess our data. Abstractive Summarization is a text-generation task. Our model will take a text as input and generate a summary as output. We want to understand how long our input and output will take to batch our data efficiently.We defined a `prompt_template` which we will use to construct an instruct prompt for better performance of our model. Our `prompt_template` has a “fixed” start and end, and our document is in the middle. This means we need to ensure that the “fixed” template parts + document are not exceeding the max length of the model. We preprocess our dataset before training and save it to disk to then upload it to S3. You could run this step on your local machine or a CPU and upload it to the [Hugging Face Hub](https://huggingface.co/docs/hub/datasets-overview).<jupyter_code>from random import randint
from itertools import chain
from functools import partial
# custom instruct prompt start
prompt_template = f"Summarize the chat dialogue:\n{{dialogue}}\n---\nSummary:\n{{summary}}{{eos_token}}"
# template dataset to add prompt to each sample
def template_dataset(sample):
sample["text"] = prompt_template.format(dialogue=sample["dialogue"],
summary=sample["summary"],
eos_token=tokenizer.eos_token)
return sample
# apply prompt template per sample
dataset = dataset.map(template_dataset, remove_columns=list(dataset.features))
print(dataset[randint(0, len(dataset))]["text"])
# empty list to save remainder from batches to use in next batch
remainder = {"input_ids": [], "attention_mask": []}
def chunk(sample, chunk_length=2048):
# define global remainder variable to save remainder from batches to use in next batch
global remainder
# Concatenate all texts and add remainder from previous batch
concatenated_examples = {k: list(chain(*sample[k])) for k in sample.keys()}
concatenated_examples = {k: remainder[k] + concatenated_examples[k] for k in concatenated_examples.keys()}
# get total number of tokens for batch
batch_total_length = len(concatenated_examples[list(sample.keys())[0]])
# get max number of chunks for batch
if batch_total_length >= chunk_length:
batch_chunk_length = (batch_total_length // chunk_length) * chunk_length
# Split by chunks of max_len.
result = {
k: [t[i : i + chunk_length] for i in range(0, batch_chunk_length, chunk_length)]
for k, t in concatenated_examples.items()
}
# add remainder to global variable for next batch
remainder = {k: concatenated_examples[k][batch_chunk_length:] for k in concatenated_examples.keys()}
# prepare labels
result["labels"] = result["input_ids"].copy()
return result
# tokenize and chunk dataset
lm_dataset = dataset.map(
lambda sample: tokenizer(sample["text"]), batched=True, remove_columns=list(dataset.features)
).map(
partial(chunk, chunk_length=1536),
batched=True,
)
# Print total number of samples
print(f"Total number of samples: {len(lm_dataset)}")<jupyter_output><empty_output><jupyter_text>After we processed the datasets we are going to use the new [FileSystem integration](https://huggingface.co/docs/datasets/filesystems) to upload our dataset to S3. We are using the `sess.default_bucket()`, adjust this if you want to store the dataset in a different S3 bucket. We will use the S3 path later in our training script.<jupyter_code># save train_dataset to s3
training_input_path = f's3://{sess.default_bucket()}/processed/samsum-sagemaker/train'
lm_dataset.save_to_disk(training_input_path)
print("uploaded data to:")
print(f"training dataset to: {training_input_path}")<jupyter_output><empty_output><jupyter_text>3. Fine-Tune BLOOM with LoRA and bnb int-8 on Amazon SageMakerIn addition to the LoRA technique, we will use [bitsanbytes LLM.int8()](https://huggingface.co/blog/hf-bitsandbytes-integration) to quantize out frozen LLM to int8. This allows us to reduce the needed memory for BLOOMZ ~4x. We prepared a [run_clm.py](./scripts/run_clm.py), which implements uses PEFT to train our model. If you are interested in how this works check-out [Efficient Large Language Model training with LoRA and Hugging Face](https://www.philschmid.de/fine-tune-flan-t5-peft) blog, where we explain the training script in detail.In order to create a sagemaker training job we need an `HuggingFace` Estimator. The Estimator handles end-to-end Amazon SageMaker training and deployment tasks. The Estimator manages the infrastructure use. SagMaker takes care of starting and managing all the required ec2 instances for us, provides the correct huggingface container, uploads the provided scripts and downloads the data from our S3 bucket into the container at `/opt/ml/input/data`. Then, it starts the training job by running.<jupyter_code>import time
# define Training Job Name
job_name = f'huggingface-peft-{time.strftime("%Y-%m-%d-%H-%M-%S", time.localtime())}'
from sagemaker.huggingface import HuggingFace
# hyperparameters, which are passed into the training job
hyperparameters ={
'model_id': model_id, # pre-trained model
'dataset_path': '/opt/ml/input/data/training', # path where sagemaker will save training dataset
'epochs': 3, # number of training epochs
'per_device_train_batch_size': 1, # batch size for training
'lr': 2e-4, # learning rate used during training
}
# create the Estimator
huggingface_estimator = HuggingFace(
entry_point = 'run_clm.py', # train script
source_dir = 'scripts', # directory which includes all the files needed for training
instance_type = 'ml.g5.2xlarge', # instances type used for the training job
instance_count = 1, # the number of instances used for training
base_job_name = job_name, # the name of the training job
role = role, # Iam role used in training job to access AWS ressources, e.g. S3
volume_size = 300, # the size of the EBS volume in GB
transformers_version = '4.26', # the transformers version used in the training job
pytorch_version = '1.13', # the pytorch_version version used in the training job
py_version = 'py39', # the python version used in the training job
hyperparameters = hyperparameters
)<jupyter_output><empty_output><jupyter_text>We can now start our training job, with the `.fit()` method passing our S3 path to the training script.<jupyter_code># define a data input dictonary with our uploaded s3 uris
data = {'training': training_input_path}
# starting the train job with our uploaded datasets as input
huggingface_estimator.fit(data, wait=True)<jupyter_output><empty_output><jupyter_text>In our example, the SageMaker training job took `20632 seconds`, which is about `5.7 hours`. The ml.g5.2xlarge instance we used costs `$1.515 per hour` for on-demand usage. As a result, the total cost for training our fine-tuned BLOOMZ-7B model was only `$8.63`.We could further reduce the training costs by using spot instances. However, there is a possibility this would result in the total training time increasing due to spot instance interruptions. See the SageMaker pricing page for instance pricing details." 4. Deploy the model to Amazon SageMaker EndpointWhen using `peft` for training, you normally end up with adapter weights. We added the `merge_and_unload()` method to merge the base model with the adatper to make it easier to deploy the model. Since we can now use the `pipelines` feature of the `transformers` library. SageMaker starts the deployment process by creating a SageMaker Endpoint Configuration and a SageMaker Endpoint. The Endpoint Configuration defines the model and the instance type.<jupyter_code>from sagemaker.huggingface import HuggingFaceModel
# create Hugging Face Model Class
huggingface_model = HuggingFaceModel(
model_data=huggingface_estimator.model_data,
#model_data="s3://hf-sagemaker-inference/model.tar.gz", # Change to your model path
role=role,
transformers_version="4.26",
pytorch_version="1.13",
py_version="py39",
model_server_workers=1
)<jupyter_output><empty_output><jupyter_text>We can now deploy our model using the `deploy()` on our HuggingFace estimator object, passing in our desired number of instances and instance type.<jupyter_code># deploy model to SageMaker Inference
predictor = huggingface_model.deploy(
initial_instance_count=1,
instance_type= "ml.g5.4xlarge"
)<jupyter_output><empty_output><jupyter_text>Note: it may take 5-10 min for the SageMaker endpoint to bring your instance online and download your model in order to be ready to accept inference requests. Lets test by using a example from the `test` split.<jupyter_code>from random import randint
from datasets import load_dataset
# Load dataset from the hub
test_dataset = load_dataset("samsum", split="test")
# select a random test sample
sample = test_dataset[randint(0,len(test_dataset))]
# format sample
prompt_template = f"Summarize the chat dialogue:\n{{dialogue}}\n---\nSummary:\n"
fomatted_sample = {
"inputs": prompt_template.format(dialogue=sample["dialogue"]),
"parameters": {
"do_sample": True, # sample output predicted probabilities
"top_p": 0.9, # sampling technique Fan et. al (2018)
"temperature": 0.1, # increasing the likelihood of high probability words and decreasing the likelihood of low probability words
"max_new_tokens": 100, # The maximum numbers of tokens to generate, ignoring the number of tokens in the prompt
}
}
# predict
res = predictor.predict(fomatted_sample)
print(res[0]["generated_text"].split("Summary:")[-1])
# Sample model output: Kirsten and Alex are going bowling this Friday at 7 pm. They will meet up and then go together.<jupyter_output><empty_output><jupyter_text>Now let's compare the model summarized dialog output to the test sample summary.<jupyter_code>print(sample["summary"])
# Test sample summary: Kirsten reminds Alex that the youth group meets this Friday at 7 pm to go bowling.<jupyter_output><empty_output><jupyter_text>Finally, we delete the endpoint again.<jupyter_code>predictor.delete_model()
predictor.delete_endpoint()<jupyter_output><empty_output> | notebooks/sagemaker/24_train_bloom_peft_lora/sagemaker-notebook.ipynb/0 | {
"file_path": "notebooks/sagemaker/24_train_bloom_peft_lora/sagemaker-notebook.ipynb",
"repo_id": "notebooks",
"token_count": 4824
} | 167 |
<jupyter_start><jupyter_text>Deploy Zephyr 7B on AWS Inferentia2 using Amazon SageMakerThis tutorial will show how easy it is to deploy Zephyr 7B on AWS Infernetia2 using Amazon SageMaker. Zephyr is a 7B parameter LLM fine-tuned version of [mistralai/Mistral-7B-v0.1](https://huggingface.co/mistralai/Mistral-7B-v0.1) that was trained on a mix of publicly available, synthetic datasets using [Direct Preference Optimization (DPO)](https://arxiv.org/abs/2305.18290). More details are in the [technical report](https://arxiv.org/abs/2310.16944). The model is released under the Apache 2.0 license, ensuring wide accessibility and use. We are going to show you how to:1. Setup development environment2. Retrieve the TGI Neuronx Image3. Deploy Zephyr 7B to Amazon SageMaker4. Run inference and chat with the modelLet’s get started. 1. Setup development environmentWe are going to use the `sagemaker` python SDK to deploy Mixtral to Amazon SageMaker. We need to make sure to have an AWS account configured and the `sagemaker` python SDK installed.<jupyter_code>!pip install transformers "sagemaker>=2.206.0" --upgrade --quiet<jupyter_output>/bin/pip:6: DeprecationWarning: pkg_resources is deprecated as an API. See https://setuptools.pypa.io/en/latest/pkg_resources.html
from pkg_resources import load_entry_point
[31mERROR: sagemaker 2.206.0 has requirement PyYAML~=6.0, but you'll have pyyaml 5.3.1 which is incompatible.[0m<jupyter_text>If you are going to use Sagemaker in a local environment. You need access to an IAM Role with the required permissions for Sagemaker. You can find [here](https://docs.aws.amazon.com/sagemaker/latest/dg/sagemaker-roles.html) more about it.<jupyter_code>import sagemaker
import boto3
sess = sagemaker.Session()
# sagemaker session bucket -> used for uploading data, models and logs
# sagemaker will automatically create this bucket if it not exists
sagemaker_session_bucket=None
if sagemaker_session_bucket is None and sess is not None:
# set to default bucket if a bucket name is not given
sagemaker_session_bucket = sess.default_bucket()
try:
role = sagemaker.get_execution_role()
except ValueError:
iam = boto3.client('iam')
role = iam.get_role(RoleName='sagemaker_execution_role')['Role']['Arn']
sess = sagemaker.Session(default_bucket=sagemaker_session_bucket)
print(f"sagemaker role arn: {role}")
print(f"sagemaker session region: {sess.boto_region_name}")<jupyter_output>sagemaker.config INFO - Not applying SDK defaults from location: /etc/xdg/sagemaker/config.yaml
sagemaker.config INFO - Not applying SDK defaults from location: /home/ubuntu/.config/sagemaker/config.yaml<jupyter_text>2. Retrieve TGI Neuronx ImageThe new Hugging Face TGI Neuronx DLC can be used to run inference on AWS Inferentia2. To retrieve the URI for the desired Hugging Face TGI Neuronx DLC we can use the `get_huggingface_tgi_neuronx_image_uri` method provided by the `sagemaker` SDK. This method allows us to retrieve the URI for the desired Hugging Face TGI Neuronx DLC based on the specified `backend`, `session`, `region`, and `version`. You can find the available versions [here](https://github.com/aws/deep-learning-containers/releases?q=tgi+AND+neuronx&expanded=true)_Note: At the time of writing this blog post the latest version of the Hugging Face LLM DLC is not yet available via the `get_huggingface_llm_image_uri` method. We are going to use the raw container uri instead._<jupyter_code>from sagemaker.huggingface import get_huggingface_llm_image_uri
# retrieve the llm image uri
llm_image = get_huggingface_llm_image_uri(
"huggingface-neuronx",
version="0.0.17"
)
# print ecr image uri
print(f"llm image uri: {llm_image}")<jupyter_output>llm image uri: 763104351884.dkr.ecr.us-east-1.amazonaws.com/huggingface-pytorch-tgi-inference:1.13.1-optimum0.0.17-neuronx-py310-ubuntu22.04<jupyter_text>4. Deploy Zephyr 7B to Amazon SageMakerText Generation Inference (TGI) on Inferentia2 supports popular open LLMs, including Llama, Mistral, and more. You can check the full list of supported models (text-generation) [here](https://huggingface.co/docs/optimum-neuron/package_reference/exportsupported-architectures). In this example, we will deploy [Hugging Face Zephyr](https://huggingface.co/HuggingFaceH4/zephyr-7b-beta) to Amazon SageMaker. Zephyr is a 7B parameter LLM fine-tuned version of [mistralai/Mistral-7B-v0.1](https://huggingface.co/mistralai/Mistral-7B-v0.1) that was trained on a mix of publicly available, synthetic datasets using [Direct Preference Optimization (DPO)](https://arxiv.org/abs/2305.18290). You can find more details in the [technical report](https://arxiv.org/abs/2310.16944). Compiling LLMs for Inferenetia2At the time of writing, [AWS Inferentia2 does not support dynamic shapes for inference](https://awsdocs-neuron.readthedocs-hosted.com/en/v2.6.0/general/arch/neuron-features/dynamic-shapes.htmlneuron-dynamic-shapes), which means that we need to specify our sequence length and batch size in advanced. To make it easier for customers to utilize the full power of Inferentia2, we created a [neuron model cache](https://huggingface.co/docs/optimum-neuron/guides/cache_system), which contains pre-compiled configurations for the most popular LLMs. A cached configuration is defined through a model architecture (Mistral), model size (7B), neuron version (2.16), number of inferentia cores (2), batch size (2), and sequence length (2048). This means compiling fine-tuned checkpoints for Mistral 7B with the same configuration will take only a few minutes. Examples of this are [mistralai/Mistral-7B-v0.1](https://huggingface.co/mistralai/Mistral-7B-v0.1) and [HuggingFaceH4/zephyr-7b-beta](https://huggingface.co/HuggingFaceH4/zephyr-7b-beta)._**Note:** Currently, TGI can only load compiled checkpoints and models. We are working on an on-the-fly compilation based on the cache. This means that you can pass any model ID from the Hugging face Hub, e.g., `HuggingFaceH4/zephyr-7b-beta` if there is a cached configuration. This should be added in the next release. We update the blog here once released._For the blog we compiled `HuggingFaceH4/zephyr-7b-beta` using the following command and parameters on a `inf2.8xlarge` instance and pushed it to the hub at []():```bash compile model with optimum for batch size 4 and sequence length 2048optimum-cli export neuron -m HuggingFaceH4/zephyr-7b-beta --batch_size 4 --sequence_length 2048 --num_cores 2 --auto_cast_type bf16 ./zephyr-7b-beta-neuron push model to hub [repo_id] [local_path] [path_in_repo]huggingface-cli upload aws-neuron/zephyr-7b-seqlen-2048-bs-4 ./zephyr-7b-beta-neuron ./ --exclude "checkpoint/**" Move tokenizer to neuron model repositorypython -c "from transformers import AutoTokenizer; AutoTokenizer.from_pretrained('HuggingFaceH4/zephyr-7b-beta').push_to_hub('aws-neuron/zephyr-7b-seqlen-2048-bs-4')"```If you are trying to compile an LLM with a configuration that is not yet cached, it can take up to 45 minutes. Deploying TGI Neuronx EndpointBefore deploying the model to Amazon SageMaker, we must define the TGI Neuronx endpoint configuration. Due to the current boundaries of Inferentia2, we need to make sure that the following parameters are set to the same value:* `MAX_CONCURRENT_REQUESTS`: Equals to the batch size, which was used to compile the model.* `MAX_INPUT_LENGTH`: Equals or lower than the sequence length, which was used to compile the model.* `MAX_TOTAL_TOKENS`: Equals to the sequence length, which was used to compile the model.* `MAX_BATCH_PREFILL_TOKENS`: half of the max tokens [batch_size * sequence_length] / 2* `MAX_BATCH_TOTAL_TOKENS`: Equals to the max tokens [batch_size * sequence_length]In addition, we need to set the `HF_MODEL_ID` pointing to the Hugging Face model ID.<jupyter_code>import json
from sagemaker.huggingface import HuggingFaceModel
# sagemaker config & model config
instance_type = "ml.inf2.8xlarge"
health_check_timeout = 900
batch_size = 4
sequence_length = 2048
# Define Model and Endpoint configuration parameter
config = {
'HF_MODEL_ID': "aws-neuron/zephyr-7b-seqlen-2048-bs-4-cores-2",
'MAX_CONCURRENT_REQUESTS': json.dumps(batch_size),
'MAX_INPUT_LENGTH': json.dumps(1512),
'MAX_TOTAL_TOKENS': json.dumps(sequence_length),
'MAX_BATCH_PREFILL_TOKENS': json.dumps(int(sequence_length*batch_size / 2)),
'MAX_BATCH_TOTAL_TOKENS': json.dumps(sequence_length*batch_size),
}
# create HuggingFaceModel with the image uri
llm_model = HuggingFaceModel(
role=role,
image_uri=llm_image,
env=config
)<jupyter_output><empty_output><jupyter_text>After we have created the `HuggingFaceModel` we can deploy it to Amazon SageMaker using the `deploy` method. We will deploy the model with the `ml.inf2.8xlarge` instance type.<jupyter_code># Deploy model to an endpoint
# https://sagemaker.readthedocs.io/en/stable/api/inference/model.html#sagemaker.model.Model.deploy
llm = llm_model.deploy(
initial_instance_count=1,
instance_type=instance_type,
container_startup_health_check_timeout=health_check_timeout, # 10 minutes to be able to load the model
)<jupyter_output>Your model is not compiled. Please compile your model before using Inferentia.<jupyter_text>SageMaker will create our endpoint and deploy the model to it. This can takes a 10-15 minutes. 5. Run inference and chat with the modelAfter our endpoint is deployed, we can run inference on it. We will use the `predict` method from the `predictor` to run inference on our endpoint. We can inference with different parameters to impact the generation. Parameters can be defined in the `parameters` attribute of the payload. You can find supported parameters in the [here](https://www.philschmid.de/sagemaker-llama-llm5-run-inference-and-chat-with-the-model) or in the open API specification of the TGI in the [swagger documentation](https://huggingface.github.io/text-generation-inference/)The `HuggingFaceH4/zephyr-7b-beta` is a conversational chat model, meaning we can chat with it using the following prompt: ```\nYou are a friendly.\n\nInstruction\n\n```To avoid drafting the prompt, we can use the `apply_chat_template` method from the tokenizer, which expects a `messages` dictionary with the known OpenAI format and converts it into the correct format for the model. Let's see if Zephyr knows some facts about AWS.<jupyter_code>from transformers import AutoTokenizer
# load the tokenizer
tokenizer = AutoTokenizer.from_pretrained("aws-neuron/zephyr-7b-seqlen-2048-bs-4-cores-2")
# Prompt to generate
messages = [
{"role": "system", "content": "You are the AWS expert"},
{"role": "user", "content": "Can you tell me an interesting fact abou AWS?"},
]
prompt = tokenizer.apply_chat_template(messages, tokenize=False, add_generation_prompt=True)
# Generation arguments
payload = {
"do_sample": True,
"top_p": 0.6,
"temperature": 0.9,
"top_k": 50,
"max_new_tokens": 256,
"repetition_penalty": 1.03,
"return_full_text": False,
"stop": ["</s>"]
}
chat = llm.predict({"inputs":prompt, "parameters":payload})
print(chat[0]["generated_text"][len(prompt):])
# Sure, here's an interesting fact about AWS: As of 2021, AWS has more than 200 services in its portfolio, ranging from compute power and storage to databases,<jupyter_output>Sure, here's an interesting fact about AWS: As of 2021, AWS has more than 200 services in its portfolio, ranging from compute power and storage to databases, analytics, and machine learning. This vast array of services allows developers and businesses to build and deploy complex applications and workflows with flexibility and agility, without having to manage the underlying infrastructure. In fact, AWS's extensive service offerings have contributed to its dominance in the cloud computing market, with a market share of over 30% as of 2021.</s><jupyter_text>Awesome, we have successfully deployed Zephyr to Amazon SageMaker on Inferentia2 and chatted with it. 6. Clean upTo clean up, we can delete the model and endpoint.<jupyter_code>llm.delete_model()
llm.delete_endpoint()<jupyter_output><empty_output> | notebooks/sagemaker/29_deploy_llms_on_inferentia2/sagemaker-notebook.ipynb/0 | {
"file_path": "notebooks/sagemaker/29_deploy_llms_on_inferentia2/sagemaker-notebook.ipynb",
"repo_id": "notebooks",
"token_count": 3797
} | 168 |
repos:
- repo: https://github.com/astral-sh/ruff-pre-commit
rev: v0.2.1
hooks:
- id: ruff
args:
- --fix
- id: ruff-format
- repo: https://github.com/pre-commit/pre-commit-hooks
rev: v4.5.0
hooks:
- id: check-merge-conflict
- id: check-yaml
| peft/.pre-commit-config.yaml/0 | {
"file_path": "peft/.pre-commit-config.yaml",
"repo_id": "peft",
"token_count": 158
} | 169 |
<!--⚠️ Note that this file is in Markdown but contain specific syntax for our doc-builder (similar to MDX) that may not be
rendered properly in your Markdown viewer.
-->
# Soft prompts
Training large pretrained language models is very time-consuming and compute-intensive. As they continue to grow in size, there is increasing interest in more efficient training methods such as *prompting*. Prompting primes a frozen pretrained model for a specific downstream task by including a text prompt that describes the task or even demonstrates an example of the task. With prompting, you can avoid fully training a separate model for each downstream task, and use the same frozen pretrained model instead. This is a lot easier because you can use the same model for several different tasks, and it is significantly more efficient to train and store a smaller set of prompt parameters than to train all the model's parameters.
There are two categories of prompting methods:
- hard prompts are manually handcrafted text prompts with discrete input tokens; the downside is that it requires a lot of effort to create a good prompt
- soft prompts are learnable tensors concatenated with the input embeddings that can be optimized to a dataset; the downside is that they aren't human readable because you aren't matching these "virtual tokens" to the embeddings of a real word
This conceptual guide provides a brief overview of the soft prompt methods included in 🤗 PEFT: prompt tuning, prefix tuning, P-tuning, and multitask prompt tuning.
## Prompt tuning
<div class="flex justify-center">
<img src="https://huggingface.co/datasets/huggingface/documentation-images/resolve/main/peft/prompt-tuning.png"/>
</div>
<small>Only train and store a significantly smaller set of task-specific prompt parameters <a href="https://hf.co/papers/2104.08691">(image source)</a>.</small>
[Prompt tuning](https://hf.co/papers/2104.08691) was developed for text classification tasks on T5 models, and all downstream tasks are cast as a text generation task. For example, sequence classification usually assigns a single class label to a sequence of text. By casting it as a text generation task, the tokens that make up the class label are *generated*. Prompts are added to the input as a series of tokens. Typically, the model parameters are fixed which means the prompt tokens are also fixed by the model parameters.
The key idea behind prompt tuning is that prompt tokens have their own parameters that are updated independently. This means you can keep the pretrained model's parameters frozen, and only update the gradients of the prompt token embeddings. The results are comparable to the traditional method of training the entire model, and prompt tuning performance scales as model size increases.
Take a look at [Prompt tuning for causal language modeling](../task_guides/clm-prompt-tuning) for a step-by-step guide on how to train a model with prompt tuning.
## Prefix tuning
<div class="flex justify-center">
<img src="https://huggingface.co/datasets/huggingface/documentation-images/resolve/main/peft/prefix-tuning.png"/>
</div>
<small>Optimize the prefix parameters for each task <a href="https://hf.co/papers/2101.00190">(image source)</a>.</small>
[Prefix tuning](https://hf.co/papers/2101.00190) was designed for natural language generation (NLG) tasks on GPT models. It is very similar to prompt tuning; prefix tuning also prepends a sequence of task-specific vectors to the input that can be trained and updated while keeping the rest of the pretrained model's parameters frozen.
The main difference is that the prefix parameters are inserted in **all** of the model layers, whereas prompt tuning only adds the prompt parameters to the model input embeddings. The prefix parameters are also optimized by a separate feed-forward network (FFN) instead of training directly on the soft prompts because it causes instability and hurts performance. The FFN is discarded after updating the soft prompts.
As a result, the authors found that prefix tuning demonstrates comparable performance to fully finetuning a model, despite having 1000x fewer parameters, and it performs even better in low-data settings.
Take a look at [Prefix tuning for conditional generation](../task_guides/seq2seq-prefix-tuning) for a step-by-step guide on how to train a model with prefix tuning.
## P-tuning
<div class="flex justify-center">
<img src="https://huggingface.co/datasets/huggingface/documentation-images/resolve/main/peft/p-tuning.png"/>
</div>
<small>Prompt tokens can be inserted anywhere in the input sequence, and they are optimized by a prompt encoder <a href="https://hf.co/papers/2103.10385">(image source)</a>.</small>
[P-tuning](https://hf.co/papers/2103.10385) is designed for natural language understanding (NLU) tasks and all language models.
It is another variation of a soft prompt method; P-tuning also adds a trainable embedding tensor that can be optimized to find better prompts, and it uses a prompt encoder (a bidirectional long-short term memory network or LSTM) to optimize the prompt parameters. Unlike prefix tuning though:
- the prompt tokens can be inserted anywhere in the input sequence, and it isn't restricted to only the beginning
- the prompt tokens are only added to the input instead of adding them to every layer of the model
- introducing *anchor* tokens can improve performance because they indicate characteristics of a component in the input sequence
The results suggest that P-tuning is more efficient than manually crafting prompts, and it enables GPT-like models to compete with BERT-like models on NLU tasks.
Take a look at [P-tuning for sequence classification](../task_guides/ptuning-seq-classification) for a step-by-step guide on how to train a model with P-tuning.
## Multitask prompt tuning
<div class="flex justify-center">
<img src="https://huggingface.co/datasets/huggingface/documentation-images/resolve/main/peft/mpt.png"/>
</div>
<small><a href="https://hf.co/papers/2103.10385">Multitask prompt tuning enables parameter-efficient transfer learning</a>.</small>
[Multitask prompt tuning (MPT)](https://hf.co/papers/2103.10385) learns a single prompt from data for multiple task types that can be shared for different target tasks. Other existing approaches learn a separate soft prompt for each task that need to be retrieved or aggregated for adaptation to target tasks. MPT consists of two stages:
1. source training - for each task, its soft prompt is decomposed into task-specific vectors. The task-specific vectors are multiplied together to form another matrix W, and the Hadamard product is used between W and a shared prompt matrix P to generate a task-specific prompt matrix. The task-specific prompts are distilled into a single prompt matrix that is shared across all tasks. This prompt is trained with multitask training.
2. target adaptation - to adapt the single prompt for a target task, a target prompt is initialized and expressed as the Hadamard product of the shared prompt matrix and the task-specific low-rank prompt matrix.
<div class="flex justify-center">
<img src="https://huggingface.co/datasets/huggingface/documentation-images/resolve/main/peft/mpt-decomposition.png"/>
</div>
<small><a href="https://hf.co/papers/2103.10385">Prompt decomposition</a>.</small>
| peft/docs/source/conceptual_guides/prompting.md/0 | {
"file_path": "peft/docs/source/conceptual_guides/prompting.md",
"repo_id": "peft",
"token_count": 1830
} | 170 |
<!--Copyright 2024 The HuggingFace Team. All rights reserved.
Licensed under the Apache License, Version 2.0 (the "License"); you may not use this file except in compliance with
the License. You may obtain a copy of the License at
http://www.apache.org/licenses/LICENSE-2.0
Unless required by applicable law or agreed to in writing, software distributed under the License is distributed on
an "AS IS" BASIS, WITHOUT WARRANTIES OR CONDITIONS OF ANY KIND, either express or implied. See the License for the
specific language governing permissions and limitations under the License.
⚠️ Note that this file is in Markdown but contain specific syntax for our doc-builder (similar to MDX) that may not be
rendered properly in your Markdown viewer.
-->
# LoRA methods
A popular way to efficiently train large models is to insert (typically in the attention blocks) smaller trainable matrices that are a low-rank decomposition of the delta weight matrix to be learnt during finetuning. The pretrained model's original weight matrix is frozen and only the smaller matrices are updated during training. This reduces the number of trainable parameters, reducing memory usage and training time which can be very expensive for large models.
There are several different ways to express the weight matrix as a low-rank decomposition, but [Low-Rank Adaptation (LoRA)](../conceptual_guides/adapter#low-rank-adaptation-lora) is the most common method. The PEFT library supports several other LoRA variants, such as [Low-Rank Hadamard Product (LoHa)](../conceptual_guides/adapter#low-rank-hadamard-product-loha), [Low-Rank Kronecker Product (LoKr)](../conceptual_guides/adapter#low-rank-kronecker-product-lokr), and [Adaptive Low-Rank Adaptation (AdaLoRA)](../conceptual_guides/adapter#adaptive-low-rank-adaptation-adalora). You can learn more about how these methods work conceptually in the [Adapters](../conceptual_guides/adapter) guide. If you're interested in applying these methods to other tasks and use cases like semantic segmentation, token classification, take a look at our [notebook collection](https://huggingface.co/collections/PEFT/notebooks-6573b28b33e5a4bf5b157fc1)!
This guide will show you how to quickly train an image classification model - with a low-rank decomposition method - to identify the class of food shown in an image.
<Tip>
Some familiarity with the general process of training an image classification model would be really helpful and allow you to focus on the low-rank decomposition methods. If you're new, we recommend taking a look at the [Image classification](https://huggingface.co/docs/transformers/tasks/image_classification) guide first from the Transformers documentation. When you're ready, come back and see how easy it is to drop PEFT in to your training!
</Tip>
Before you begin, make sure you have all the necessary libraries installed.
```bash
pip install -q peft transformers datasets
```
## Dataset
In this guide, you'll use the [Food-101](https://huggingface.co/datasets/food101) dataset which contains images of 101 food classes (take a look at the [dataset viewer](https://huggingface.co/datasets/food101/viewer/default/train) to get a better idea of what the dataset looks like).
Load the dataset with the [`~datasets.load_dataset`] function.
```py
from datasets import load_dataset
ds = load_dataset("food101")
```
Each food class is labeled with an integer, so to make it easier to understand what these integers represent, you'll create a `label2id` and `id2label` dictionary to map the integer to its class label.
```py
labels = ds["train"].features["label"].names
label2id, id2label = dict(), dict()
for i, label in enumerate(labels):
label2id[label] = i
id2label[i] = label
id2label[2]
"baklava"
```
Load an image processor to properly resize and normalize the pixel values of the training and evaluation images.
```py
from transformers import AutoImageProcessor
image_processor = AutoImageProcessor.from_pretrained("google/vit-base-patch16-224-in21k")
```
You can also use the image processor to prepare some transformation functions for data augmentation and pixel scaling.
```py
from torchvision.transforms import (
CenterCrop,
Compose,
Normalize,
RandomHorizontalFlip,
RandomResizedCrop,
Resize,
ToTensor,
)
normalize = Normalize(mean=image_processor.image_mean, std=image_processor.image_std)
train_transforms = Compose(
[
RandomResizedCrop(image_processor.size["height"]),
RandomHorizontalFlip(),
ToTensor(),
normalize,
]
)
val_transforms = Compose(
[
Resize(image_processor.size["height"]),
CenterCrop(image_processor.size["height"]),
ToTensor(),
normalize,
]
)
def preprocess_train(example_batch):
example_batch["pixel_values"] = [train_transforms(image.convert("RGB")) for image in example_batch["image"]]
return example_batch
def preprocess_val(example_batch):
example_batch["pixel_values"] = [val_transforms(image.convert("RGB")) for image in example_batch["image"]]
return example_batch
```
Define the training and validation datasets, and use the [`~datasets.Dataset.set_transform`] function to apply the transformations on-the-fly.
```py
train_ds = ds["train"]
val_ds = ds["validation"]
train_ds.set_transform(preprocess_train)
val_ds.set_transform(preprocess_val)
```
Finally, you'll need a data collator to create a batch of training and evaluation data and convert the labels to `torch.tensor` objects.
```py
import torch
def collate_fn(examples):
pixel_values = torch.stack([example["pixel_values"] for example in examples])
labels = torch.tensor([example["label"] for example in examples])
return {"pixel_values": pixel_values, "labels": labels}
```
## Model
Now let's load a pretrained model to use as the base model. This guide uses the [google/vit-base-patch16-224-in21k](https://huggingface.co/google/vit-base-patch16-224-in21k) model, but you can use any image classification model you want. Pass the `label2id` and `id2label` dictionaries to the model so it knows how to map the integer labels to their class labels, and you can optionally pass the `ignore_mismatched_sizes=True` parameter if you're finetuning a checkpoint that has already been finetuned.
```py
from transformers import AutoModelForImageClassification, TrainingArguments, Trainer
model = AutoModelForImageClassification.from_pretrained(
"google/vit-base-patch16-224-in21k",
label2id=label2id,
id2label=id2label,
ignore_mismatched_sizes=True,
)
```
### PEFT configuration and model
Every PEFT method requires a configuration that holds all the parameters specifying how the PEFT method should be applied. Once the configuration is setup, pass it to the [`~peft.get_peft_model`] function along with the base model to create a trainable [`PeftModel`].
<Tip>
Call the [`~PeftModel.print_trainable_parameters`] method to compare the number of parameters of [`PeftModel`] versus the number of parameters in the base model!
</Tip>
<hfoptions id="loras">
<hfoption id="LoRA">
[LoRA](../conceptual_guides/adapter#low-rank-adaptation-lora) decomposes the weight update matrix into *two* smaller matrices. The size of these low-rank matrices is determined by its *rank* or `r`. A higher rank means the model has more parameters to train, but it also means the model has more learning capacity. You'll also want to specify the `target_modules` which determine where the smaller matrices are inserted. For this guide, you'll target the *query* and *value* matrices of the attention blocks. Other important parameters to set are `lora_alpha` (scaling factor), `bias` (whether `none`, `all` or only the LoRA bias parameters should be trained), and `modules_to_save` (the modules apart from the LoRA layers to be trained and saved). All of these parameters - and more - are found in the [`LoraConfig`].
```py
from peft import LoraConfig, get_peft_model
config = LoraConfig(
r=16,
lora_alpha=16,
target_modules=["query", "value"],
lora_dropout=0.1,
bias="none",
modules_to_save=["classifier"],
)
model = get_peft_model(model, config)
model.print_trainable_parameters()
"trainable params: 667,493 || all params: 86,543,818 || trainable%: 0.7712775047664294"
```
</hfoption>
<hfoption id="LoHa">
[LoHa](../conceptual_guides/adapter#low-rank-hadamard-product-loha) decomposes the weight update matrix into *four* smaller matrices and each pair of smaller matrices is combined with the Hadamard product. This allows the weight update matrix to keep the same number of trainable parameters when compared to LoRA, but with a higher rank (`r^2` for LoHA when compared to `2*r` for LoRA). The size of the smaller matrices is determined by its *rank* or `r`. You'll also want to specify the `target_modules` which determines where the smaller matrices are inserted. For this guide, you'll target the *query* and *value* matrices of the attention blocks. Other important parameters to set are `alpha` (scaling factor), and `modules_to_save` (the modules apart from the LoHa layers to be trained and saved). All of these parameters - and more - are found in the [`LoHaConfig`].
```py
from peft import LoHaConfig, get_peft_model
config = LoHaConfig(
r=16,
alpha=16,
target_modules=["query", "value"],
module_dropout=0.1,
modules_to_save=["classifier"],
)
model = get_peft_model(model, config)
model.print_trainable_parameters()
"trainable params: 1,257,317 || all params: 87,133,642 || trainable%: 1.4429753779831676"
```
</hfoption>
<hfoption id="LoKr">
[LoKr](../conceptual_guides/adapter#low-rank-kronecker-product-lokr) expresses the weight update matrix as a decomposition of a Kronecker product, creating a block matrix that is able to preserve the rank of the original weight matrix. The size of the smaller matrices are determined by its *rank* or `r`. You'll also want to specify the `target_modules` which determines where the smaller matrices are inserted. For this guide, you'll target the *query* and *value* matrices of the attention blocks. Other important parameters to set are `alpha` (scaling factor), and `modules_to_save` (the modules apart from the LoKr layers to be trained and saved). All of these parameters - and more - are found in the [`LoKrConfig`].
```py
from peft import LoKrConfig, get_peft_model
config = LoKrConfig(
r=16,
alpha=16,
target_modules=["query", "value"],
module_dropout=0.1,
modules_to_save=["classifier"],
)
model = get_peft_model(model, config)
model.print_trainable_parameters()
"trainable params: 116,069 || all params: 87,172,042 || trainable%: 0.13314934162033282"
```
</hfoption>
<hfoption id="AdaLoRA">
[AdaLoRA](../conceptual_guides/adapter#adaptive-low-rank-adaptation-adalora) efficiently manages the LoRA parameter budget by assigning important weight matrices more parameters and pruning less important ones. In contrast, LoRA evenly distributes parameters across all modules. You can control the average desired *rank* or `r` of the matrices, and which modules to apply AdaLoRA to with `target_modules`. Other important parameters to set are `lora_alpha` (scaling factor), and `modules_to_save` (the modules apart from the AdaLoRA layers to be trained and saved). All of these parameters - and more - are found in the [`AdaLoraConfig`].
```py
from peft import AdaLoraConfig, get_peft_model
config = AdaLoraConfig(
r=8,
init_r=12,
tinit=200,
tfinal=1000,
deltaT=10,
target_modules=["query", "value"],
modules_to_save=["classifier"],
)
model = get_peft_model(model, config)
model.print_trainable_parameters()
"trainable params: 520,325 || all params: 87,614,722 || trainable%: 0.5938785036606062"
```
</hfoption>
</hfoptions>
### Training
For training, let's use the [`~transformers.Trainer`] class from Transformers. The [`Trainer`] contains a PyTorch training loop, and when you're ready, call [`~transformers.Trainer.train`] to start training. To customize the training run, configure the training hyperparameters in the [`~transformers.TrainingArguments`] class. With LoRA-like methods, you can afford to use a higher batch size and learning rate.
> [!WARNING]
> AdaLoRA has an [`~AdaLoraModel.update_and_allocate`] method that should be called at each training step to update the parameter budget and mask, otherwise the adaptation step is not performed. This requires writing a custom training loop or subclassing the [`~transformers.Trainer`] to incorporate this method. As an example, take a look at this [custom training loop](https://github.com/huggingface/peft/blob/912ad41e96e03652cabf47522cd876076f7a0c4f/examples/conditional_generation/peft_adalora_seq2seq.py#L120).
```py
from transformers import TrainingArguments, Trainer
account = "stevhliu"
peft_model_id = f"{account}/google/vit-base-patch16-224-in21k-lora"
batch_size = 128
args = TrainingArguments(
peft_model_id,
remove_unused_columns=False,
evaluation_strategy="epoch",
save_strategy="epoch",
learning_rate=5e-3,
per_device_train_batch_size=batch_size,
gradient_accumulation_steps=4,
per_device_eval_batch_size=batch_size,
fp16=True,
num_train_epochs=5,
logging_steps=10,
load_best_model_at_end=True,
label_names=["labels"],
)
```
Begin training with [`~transformers.Trainer.train`].
```py
trainer = Trainer(
model,
args,
train_dataset=train_ds,
eval_dataset=val_ds,
tokenizer=image_processor,
data_collator=collate_fn,
)
trainer.train()
```
## Share your model
Once training is complete, you can upload your model to the Hub with the [`~transformers.PreTrainedModel.push_to_hub`] method. You’ll need to login to your Hugging Face account first and enter your token when prompted.
```py
from huggingface_hub import notebook_login
notebook_login()
```
Call [`~transformers.PreTrainedModel.push_to_hub`] to save your model to your repositoy.
```py
model.push_to_hub(peft_model_id)
```
## Inference
Let's load the model from the Hub and test it out on a food image.
```py
from peft import PeftConfig, PeftModel
from transfomers import AutoImageProcessor
from PIL import Image
import requests
config = PeftConfig.from_pretrained("stevhliu/vit-base-patch16-224-in21k-lora")
model = AutoModelForImageClassification.from_pretrained(
config.base_model_name_or_path,
label2id=label2id,
id2label=id2label,
ignore_mismatched_sizes=True,
)
model = PeftModel.from_pretrained(model, "stevhliu/vit-base-patch16-224-in21k-lora")
url = "https://huggingface.co/datasets/sayakpaul/sample-datasets/resolve/main/beignets.jpeg"
image = Image.open(requests.get(url, stream=True).raw)
image
```
<div class="flex justify-center">
<img src="https://huggingface.co/datasets/sayakpaul/sample-datasets/resolve/main/beignets.jpeg">
</div>
Convert the image to RGB and return the underlying PyTorch tensors.
```py
encoding = image_processor(image.convert("RGB"), return_tensors="pt")
```
Now run the model and return the predicted class!
```py
with torch.no_grad():
outputs = model(**encoding)
logits = outputs.logits
predicted_class_idx = logits.argmax(-1).item()
print("Predicted class:", model.config.id2label[predicted_class_idx])
"Predicted class: beignets"
```
| peft/docs/source/task_guides/lora_based_methods.md/0 | {
"file_path": "peft/docs/source/task_guides/lora_based_methods.md",
"repo_id": "peft",
"token_count": 4847
} | 171 |
<jupyter_start><jupyter_code>from transformers import AutoModelForSeq2SeqLM
from peft import PeftModel, PeftConfig
import torch
from datasets import load_dataset
import os
from transformers import AutoTokenizer
from torch.utils.data import DataLoader
from transformers import default_data_collator, get_linear_schedule_with_warmup
from tqdm import tqdm
from datasets import load_dataset
dataset_name = "twitter_complaints"
text_column = "Tweet text"
label_column = "text_label"
batch_size = 8
peft_model_id = "smangrul/twitter_complaints_bigscience_T0_3B_LORA_SEQ_2_SEQ_LM"
config = PeftConfig.from_pretrained(peft_model_id)
peft_model_id = "smangrul/twitter_complaints_bigscience_T0_3B_LORA_SEQ_2_SEQ_LM"
max_memory = {0: "6GIB", 1: "0GIB", 2: "0GIB", 3: "0GIB", 4: "0GIB", "cpu": "30GB"}
config = PeftConfig.from_pretrained(peft_model_id)
model = AutoModelForSeq2SeqLM.from_pretrained(config.base_model_name_or_path, device_map="auto", max_memory=max_memory)
model = PeftModel.from_pretrained(model, peft_model_id, device_map="auto", max_memory=max_memory)
from datasets import load_dataset
dataset = load_dataset("ought/raft", dataset_name)
classes = [k.replace("_", " ") for k in dataset["train"].features["Label"].names]
print(classes)
dataset = dataset.map(
lambda x: {"text_label": [classes[label] for label in x["Label"]]},
batched=True,
num_proc=1,
)
print(dataset)
dataset["train"][0]
tokenizer = AutoTokenizer.from_pretrained(config.base_model_name_or_path)
target_max_length = max([len(tokenizer(class_label)["input_ids"]) for class_label in classes])
def preprocess_function(examples):
inputs = examples[text_column]
targets = examples[label_column]
model_inputs = tokenizer(inputs, truncation=True)
labels = tokenizer(
targets, max_length=target_max_length, padding="max_length", truncation=True, return_tensors="pt"
)
labels = labels["input_ids"]
labels[labels == tokenizer.pad_token_id] = -100
model_inputs["labels"] = labels
return model_inputs
processed_datasets = dataset.map(
preprocess_function,
batched=True,
num_proc=1,
remove_columns=dataset["train"].column_names,
load_from_cache_file=True,
desc="Running tokenizer on dataset",
)
train_dataset = processed_datasets["train"]
eval_dataset = processed_datasets["train"]
test_dataset = processed_datasets["test"]
def collate_fn(examples):
return tokenizer.pad(examples, padding="longest", return_tensors="pt")
train_dataloader = DataLoader(
train_dataset, shuffle=True, collate_fn=collate_fn, batch_size=batch_size, pin_memory=True
)
eval_dataloader = DataLoader(eval_dataset, collate_fn=collate_fn, batch_size=batch_size, pin_memory=True)
test_dataloader = DataLoader(test_dataset, collate_fn=collate_fn, batch_size=batch_size, pin_memory=True)
model.eval()
i = 15
inputs = tokenizer(f'{text_column} : {dataset["test"][i]["Tweet text"]} Label : ', return_tensors="pt")
print(dataset["test"][i]["Tweet text"])
print(inputs)
with torch.no_grad():
outputs = model.generate(input_ids=inputs["input_ids"].to("cuda"), max_new_tokens=10)
print(outputs)
print(tokenizer.batch_decode(outputs.detach().cpu().numpy(), skip_special_tokens=True))
model.eval()
eval_preds = []
for _, batch in enumerate(tqdm(eval_dataloader)):
batch = {k: v.to("cuda") for k, v in batch.items() if k != "labels"}
with torch.no_grad():
outputs = model.generate(**batch, max_new_tokens=10)
preds = outputs.detach().cpu().numpy()
eval_preds.extend(tokenizer.batch_decode(preds, skip_special_tokens=True))
correct = 0
total = 0
for pred, true in zip(eval_preds, dataset["train"][label_column]):
if pred.strip() == true.strip():
correct += 1
total += 1
accuracy = correct / total * 100
print(f"{accuracy=}")
print(f"{eval_preds[:10]=}")
print(f"{dataset['train'][label_column][:10]=}")
model.eval()
test_preds = []
for _, batch in enumerate(tqdm(test_dataloader)):
batch = {k: v for k, v in batch.items() if k != "labels"}
with torch.no_grad():
outputs = model.generate(**batch, max_new_tokens=10)
preds = outputs.detach().cpu().numpy()
test_preds.extend(tokenizer.batch_decode(preds, skip_special_tokens=True))
if len(test_preds) > 100:
break
test_preds<jupyter_output><empty_output> | peft/examples/conditional_generation/peft_lora_seq2seq_accelerate_big_model_inference.ipynb/0 | {
"file_path": "peft/examples/conditional_generation/peft_lora_seq2seq_accelerate_big_model_inference.ipynb",
"repo_id": "peft",
"token_count": 1695
} | 172 |
# Copyright 2023-present the HuggingFace Inc. team.
#
# Licensed under the Apache License, Version 2.0 (the "License");
# you may not use this file except in compliance with the License.
# You may obtain a copy of the License at
#
# http://www.apache.org/licenses/LICENSE-2.0
#
# Unless required by applicable law or agreed to in writing, software
# distributed under the License is distributed on an "AS IS" BASIS,
# WITHOUT WARRANTIES OR CONDITIONS OF ANY KIND, either express or implied.
# See the License for the specific language governing permissions and
# limitations under the License.
import torch
from datasets import load_dataset
from torch.utils.data import DataLoader, Dataset
from transformers import AutoModelForVision2Seq, AutoProcessor, BitsAndBytesConfig
from peft import LoraConfig, get_peft_model
# Let's define the LoraConfig
config = LoraConfig(
r=16,
lora_alpha=32,
lora_dropout=0.05,
bias="none",
)
# We load our model and processor using `transformers`
model = AutoModelForVision2Seq.from_pretrained(
"Salesforce/blip2-opt-2.7b", quantization_config=BitsAndBytesConfig(load_in_8bit=True)
)
processor = AutoProcessor.from_pretrained("Salesforce/blip2-opt-2.7b")
# Get our peft model and print the number of trainable parameters
model = get_peft_model(model, config)
model.print_trainable_parameters()
# Let's load the dataset here!
dataset = load_dataset("ybelkada/football-dataset", split="train")
class ImageCaptioningDataset(Dataset):
def __init__(self, dataset, processor):
self.dataset = dataset
self.processor = processor
def __len__(self):
return len(self.dataset)
def __getitem__(self, idx):
item = self.dataset[idx]
encoding = self.processor(images=item["image"], padding="max_length", return_tensors="pt")
# remove batch dimension
encoding = {k: v.squeeze() for k, v in encoding.items()}
encoding["text"] = item["text"]
return encoding
def collator(batch):
# pad the input_ids and attention_mask
processed_batch = {}
for key in batch[0].keys():
if key != "text":
processed_batch[key] = torch.stack([example[key] for example in batch])
else:
text_inputs = processor.tokenizer(
[example["text"] for example in batch], padding=True, return_tensors="pt"
)
processed_batch["input_ids"] = text_inputs["input_ids"]
processed_batch["attention_mask"] = text_inputs["attention_mask"]
return processed_batch
train_dataset = ImageCaptioningDataset(dataset, processor)
train_dataloader = DataLoader(train_dataset, shuffle=True, batch_size=2, collate_fn=collator)
optimizer = torch.optim.AdamW(model.parameters(), lr=5e-5)
device = "cuda" if torch.cuda.is_available() else "cpu"
model.train()
for epoch in range(50):
print("Epoch:", epoch)
for idx, batch in enumerate(train_dataloader):
input_ids = batch.pop("input_ids").to(device)
pixel_values = batch.pop("pixel_values").to(device, torch.float16)
outputs = model(input_ids=input_ids, pixel_values=pixel_values, labels=input_ids)
loss = outputs.loss
print("Loss:", loss.item())
loss.backward()
optimizer.step()
optimizer.zero_grad()
if idx % 10 == 0:
generated_output = model.generate(pixel_values=pixel_values)
print(processor.batch_decode(generated_output, skip_special_tokens=True))
| peft/examples/int8_training/fine_tune_blip2_int8.py/0 | {
"file_path": "peft/examples/int8_training/fine_tune_blip2_int8.py",
"repo_id": "peft",
"token_count": 1309
} | 173 |
<jupyter_start><jupyter_text>This notebook shows how to use the adapter merging methods from `peft` and apply them image generation models using `diffusers`. Turn `diffusers` LoRA checkpoints into `PeftModel`<jupyter_code>!pip install diffusers accelerate transformers -U -q
!pip install git+https://github.com/huggingface/peft -q
from google.colab import userdata
TOKEN = userdata.get("HF_TOKEN")
from diffusers import UNet2DConditionModel
import torch
model_id = "stabilityai/stable-diffusion-xl-base-1.0"
unet = UNet2DConditionModel.from_pretrained(
model_id, subfolder="unet", torch_dtype=torch.float16, use_safetensors=True, variant="fp16"
).to("cuda")
# So that we can populate it later.
import copy
sdxl_unet = copy.deepcopy(unet)
# Load the pipeline too.
from diffusers import DiffusionPipeline
pipe = DiffusionPipeline.from_pretrained(
model_id, variant="fp16", torch_dtype=torch.float16, unet=unet
).to("cuda")
# Only UNet
pipe.load_lora_weights("CiroN2022/toy-face", weight_name="toy_face_sdxl.safetensors", adapter_name="toy")
from peft import get_peft_model, LoraConfig
toy_peft_model = get_peft_model(
sdxl_unet,
pipe.unet.peft_config["toy"],
adapter_name="toy"
)
original_state_dict = {f"base_model.model.{k}": v for k, v in pipe.unet.state_dict().items()}
toy_peft_model.load_state_dict(original_state_dict, strict=True)
toy_peft_model.push_to_hub("toy_peft_model-new", token=TOKEN)
pipe.delete_adapters("toy")
sdxl_unet.delete_adapters("toy")
pipe.load_lora_weights("nerijs/pixel-art-xl", weight_name="pixel-art-xl.safetensors", adapter_name="pixel")
pipe.set_adapters(adapter_names="pixel")
pixel_peft_model = get_peft_model(
sdxl_unet,
pipe.unet.peft_config["pixel"],
adapter_name="pixel"
)
original_state_dict = {f"base_model.model.{k}": v for k, v in pipe.unet.state_dict().items()}
pixel_peft_model.load_state_dict(original_state_dict, strict=True)
pixel_peft_model.push_to_hub("pixel_peft_model-new", token=TOKEN)
del pipe, sdxl_unet, toy_peft_model, pixel_peft_model<jupyter_output><empty_output><jupyter_text>Weighted adapter inference<jupyter_code>from peft import PeftModel
base_unet = UNet2DConditionModel.from_pretrained(
model_id, subfolder="unet", torch_dtype=torch.float16, use_safetensors=True, variant="fp16"
).to("cuda")
toy_id = "sayakpaul/toy_peft_model-new"
model = PeftModel.from_pretrained(base_unet, toy_id, use_safetensors=True, subfolder="toy", adapter_name="toy")
model.load_adapter("sayakpaul/pixel_peft_model-new", use_safetensors=True, subfolder="pixel", adapter_name="pixel")
# https://huggingface.co/docs/peft/main/en/package_reference/lora#peft.LoraModel.add_weighted_adapter
model.add_weighted_adapter(
adapters=["toy", "pixel"],
weights=[0.7, 0.3],
combination_type="linear",
adapter_name="toy-pixel"
)
model.set_adapters("toy-pixel")
type(model.base_model.model)
model = model.to(dtype=torch.float16, device="cuda")
pipe = DiffusionPipeline.from_pretrained(
model_id, unet=model, variant="fp16", torch_dtype=torch.float16,
).to("cuda")
prompt = "toy_face of a hacker with a hoodie, pixel art"
image = pipe(prompt, num_inference_steps=30, generator=torch.manual_seed(0)).images[0]
image
del pipe
base_unet = UNet2DConditionModel.from_pretrained(
model_id, subfolder="unet", torch_dtype=torch.float16, use_safetensors=True, variant="fp16"
).to("cuda")
toy_id = "sayakpaul/toy_peft_model-new"
model = PeftModel.from_pretrained(base_unet, toy_id, use_safetensors=True, subfolder="toy", adapter_name="toy")
model.load_adapter("sayakpaul/pixel_peft_model-new", use_safetensors=True, subfolder="pixel", adapter_name="pixel")
# https://huggingface.co/docs/peft/main/en/package_reference/lora#peft.LoraModel.add_weighted_adapter
model.add_weighted_adapter(
adapters=["toy", "pixel"],
weights=[0.5, 0.5],
combination_type="cat",
adapter_name="toy-pixel"
)
model.set_adapters("toy-pixel")
model = model.to(dtype=torch.float16, device="cuda")
pipe = DiffusionPipeline.from_pretrained(
model_id, unet=model, variant="fp16", torch_dtype=torch.float16,
).to("cuda")
prompt = "toy_face of a hacker with a hoodie, pixel art"
image = pipe(prompt, num_inference_steps=30, generator=torch.manual_seed(0)).images[0]
image
del pipe
pipe = DiffusionPipeline.from_pretrained(
model_id, variant="fp16", torch_dtype=torch.float16,
).to("cuda")
prompt = "toy_face of a hacker with a hoodie, pixel art"
image = pipe(prompt, num_inference_steps=30, generator=torch.manual_seed(0)).images[0]
image<jupyter_output><empty_output> | peft/examples/multi_adapter_examples/multi_adapter_weighted_inference_diffusers.ipynb/0 | {
"file_path": "peft/examples/multi_adapter_examples/multi_adapter_weighted_inference_diffusers.ipynb",
"repo_id": "peft",
"token_count": 1802
} | 174 |
# Copyright 2023-present the HuggingFace Inc. team.
#
# Licensed under the Apache License, Version 2.0 (the "License");
# you may not use this file except in compliance with the License.
# You may obtain a copy of the License at
#
# http://www.apache.org/licenses/LICENSE-2.0
#
# Unless required by applicable law or agreed to in writing, software
# distributed under the License is distributed on an "AS IS" BASIS,
# WITHOUT WARRANTIES OR CONDITIONS OF ANY KIND, either express or implied.
# See the License for the specific language governing permissions and
# limitations under the License.
from __future__ import annotations
import collections
import inspect
import os
import warnings
from contextlib import contextmanager
from copy import deepcopy
from typing import Any, Optional, Union
import packaging.version
import torch
import transformers
from accelerate import dispatch_model, infer_auto_device_map
from accelerate.hooks import AlignDevicesHook, add_hook_to_module, remove_hook_from_submodules
from accelerate.utils import get_balanced_memory
from huggingface_hub import ModelCard, ModelCardData, hf_hub_download
from safetensors.torch import save_file as safe_save_file
from torch.nn import BCEWithLogitsLoss, CrossEntropyLoss, MSELoss
from transformers import PreTrainedModel
from transformers.modeling_outputs import QuestionAnsweringModelOutput, SequenceClassifierOutput, TokenClassifierOutput
from transformers.utils import PushToHubMixin
from . import __version__
from .config import PeftConfig
from .tuners import (
AdaLoraModel,
AdaptionPromptModel,
IA3Model,
LoHaModel,
LoKrModel,
LoraModel,
MultitaskPromptEmbedding,
OFTModel,
PolyModel,
PrefixEncoder,
PromptEmbedding,
PromptEncoder,
)
from .utils import (
SAFETENSORS_WEIGHTS_NAME,
TRANSFORMERS_MODELS_TO_PREFIX_TUNING_POSTPROCESS_MAPPING,
WEIGHTS_NAME,
PeftType,
TaskType,
_get_batch_size,
_prepare_prompt_learning_config,
_set_adapter,
_set_trainable,
get_peft_model_state_dict,
id_tensor_storage,
infer_device,
load_peft_weights,
set_peft_model_state_dict,
shift_tokens_right,
)
PEFT_TYPE_TO_MODEL_MAPPING = {
PeftType.LORA: LoraModel,
PeftType.LOHA: LoHaModel,
PeftType.LOKR: LoKrModel,
PeftType.PROMPT_TUNING: PromptEmbedding,
PeftType.P_TUNING: PromptEncoder,
PeftType.PREFIX_TUNING: PrefixEncoder,
PeftType.ADALORA: AdaLoraModel,
PeftType.ADAPTION_PROMPT: AdaptionPromptModel,
PeftType.IA3: IA3Model,
PeftType.OFT: OFTModel,
PeftType.POLY: PolyModel,
}
class PeftModel(PushToHubMixin, torch.nn.Module):
"""
Base model encompassing various Peft methods.
Args:
model ([`~transformers.PreTrainedModel`]): The base transformer model used for Peft.
peft_config ([`PeftConfig`]): The configuration of the Peft model.
adapter_name (`str`, *optional*): The name of the adapter, defaults to `"default"`.
**Attributes**:
- **base_model** ([`torch.nn.Module`]) -- The base transformer model used for Peft.
- **peft_config** ([`PeftConfig`]) -- The configuration of the Peft model.
- **modules_to_save** (`list` of `str`) -- The list of sub-module names to save when
saving the model.
- **prompt_encoder** ([`PromptEncoder`]) -- The prompt encoder used for Peft if
using [`PromptLearningConfig`].
- **prompt_tokens** (`torch.Tensor`) -- The virtual prompt tokens used for Peft if
using [`PromptLearningConfig`].
- **transformer_backbone_name** (`str`) -- The name of the transformer
backbone in the base model if using [`PromptLearningConfig`].
- **word_embeddings** (`torch.nn.Embedding`) -- The word embeddings of the transformer backbone
in the base model if using [`PromptLearningConfig`].
"""
def __init__(self, model: PreTrainedModel, peft_config: PeftConfig, adapter_name: str = "default") -> None:
super().__init__()
self.modules_to_save = None
self.active_adapter = adapter_name
self.peft_type = peft_config.peft_type
# These args are special PEFT arguments that users can pass. They need to be removed before passing them to
# forward.
self.special_peft_forward_args = {"adapter_names"}
self._is_prompt_learning = peft_config.is_prompt_learning
if self._is_prompt_learning:
self._peft_config = {adapter_name: peft_config}
self.base_model = model
self.add_adapter(adapter_name, peft_config)
else:
self._peft_config = None
cls = PEFT_TYPE_TO_MODEL_MAPPING[peft_config.peft_type]
self.base_model = cls(model, {adapter_name: peft_config}, adapter_name)
self.set_additional_trainable_modules(peft_config, adapter_name)
if getattr(model, "is_gradient_checkpointing", True):
model = self._prepare_model_for_gradient_checkpointing(model)
# the `pretraining_tp` is set for some models to simulate Tensor Parallelism during inference to avoid
# numerical differences, https://github.com/pytorch/pytorch/issues/76232 - to avoid any unexpected
# behavior we disable that in this line.
if hasattr(self.base_model, "config") and hasattr(self.base_model.config, "pretraining_tp"):
self.base_model.config.pretraining_tp = 1
@property
def peft_config(self) -> dict[str, PeftConfig]:
if self._is_prompt_learning:
return self._peft_config
return self.base_model.peft_config
@property
def active_adapters(self) -> list[str]:
try:
adapters = self.base_model.active_adapters
except AttributeError:
adapters = self.active_adapter
if isinstance(adapters, str):
adapters = [adapters]
return adapters
@peft_config.setter
def peft_config(self, value: dict[str, PeftConfig]):
if self._is_prompt_learning:
self._peft_config = value
else:
self.base_model.peft_config = value
def save_pretrained(
self,
save_directory: str,
safe_serialization: bool = True,
selected_adapters: Optional[list[str]] = None,
save_embedding_layers: Union[str, bool] = "auto",
is_main_process: bool = True,
**kwargs: Any,
) -> None:
r"""
This function saves the adapter model and the adapter configuration files to a directory, so that it can be
reloaded using the [`PeftModel.from_pretrained`] class method, and also used by the [`PeftModel.push_to_hub`]
method.
Args:
save_directory (`str`):
Directory where the adapter model and configuration files will be saved (will be created if it does not
exist).
safe_serialization (`bool`, *optional*):
Whether to save the adapter files in safetensors format, defaults to `True`.
selected_adapters (`List[str]`, *optional*):
A list of adapters to be saved. If `None`, will default to all adapters.
save_embedding_layers (`Union[bool, str]`, *optional*, defaults to `"auto"`):
If `True`, save the embedding layers in addition to adapter weights. If `auto`, checks the common
embedding layers `peft.utils.other.EMBEDDING_LAYER_NAMES` in config's `target_modules` when available.
and automatically sets the boolean flag. This only works for 🤗 transformers models.
is_main_process (`bool`, *optional*):
Whether the process calling this is the main process or not. Will default to `True`. Will not save the
checkpoint if not on the main process, which is important for multi device setups (e.g. DDP).
kwargs (additional keyword arguments, *optional*):
Additional keyword arguments passed along to the `push_to_hub` method.
"""
if os.path.isfile(save_directory):
raise ValueError(f"Provided path ({save_directory}) should be a directory, not a file")
if selected_adapters is None:
selected_adapters = list(self.peft_config.keys())
else:
if any(
selected_adapter_name not in list(self.peft_config.keys())
for selected_adapter_name in selected_adapters
):
raise ValueError(
f"You passed an invalid `selected_adapters` arguments, current supported adapter names are"
f" {list(self.peft_config.keys())} - got {selected_adapters}."
)
if is_main_process:
os.makedirs(save_directory, exist_ok=True)
self.create_or_update_model_card(save_directory)
for adapter_name in selected_adapters:
peft_config = self.peft_config[adapter_name]
# save only the trainable weights
output_state_dict = get_peft_model_state_dict(
self,
state_dict=kwargs.get("state_dict", None),
adapter_name=adapter_name,
save_embedding_layers=save_embedding_layers,
)
output_dir = os.path.join(save_directory, adapter_name) if adapter_name != "default" else save_directory
os.makedirs(output_dir, exist_ok=True)
if is_main_process and safe_serialization:
# Section copied from: https://github.com/huggingface/transformers/blob/main/src/transformers/modeling_utils.py#L2111-L2134
# Safetensors does not allow tensor aliasing.
# We're going to remove aliases before saving
ptrs = collections.defaultdict(list)
for name, tensor in output_state_dict.items():
# Sometimes in the state_dict we have non-tensor objects.
# e.g. in bitsandbytes we have some `str` objects in the state_dict
if isinstance(tensor, torch.Tensor):
ptrs[id_tensor_storage(tensor)].append(name)
else:
# In the non-tensor case, fall back to the pointer of the object itself
ptrs[id(tensor)].append(name)
# These are all the pointers of shared tensors.
shared_ptrs = {ptr: names for ptr, names in ptrs.items() if len(names) > 1}
for _, names in shared_ptrs.items():
# Here we just clone the shared tensors to avoid tensor aliasing which is
# not supported in safetensors.
for shared_tensor_name in names[1:]:
output_state_dict[shared_tensor_name] = output_state_dict[shared_tensor_name].clone()
safe_save_file(
output_state_dict,
os.path.join(output_dir, SAFETENSORS_WEIGHTS_NAME),
metadata={"format": "pt"},
)
elif is_main_process:
torch.save(output_state_dict, os.path.join(output_dir, WEIGHTS_NAME))
# save the config and change the inference mode to `True`
if peft_config.base_model_name_or_path is None:
peft_config.base_model_name_or_path = (
self.base_model.__dict__.get("name_or_path", None)
if peft_config.is_prompt_learning
else self.base_model.model.__dict__.get("name_or_path", None)
)
inference_mode = peft_config.inference_mode
peft_config.inference_mode = True
if peft_config.task_type is None:
# deal with auto mapping
base_model_class = self._get_base_model_class(
is_prompt_tuning=peft_config.is_prompt_learning,
)
parent_library = base_model_class.__module__
auto_mapping_dict = {
"base_model_class": base_model_class.__name__,
"parent_library": parent_library,
}
else:
auto_mapping_dict = None
if is_main_process:
peft_config.save_pretrained(output_dir, auto_mapping_dict=auto_mapping_dict)
peft_config.inference_mode = inference_mode
@classmethod
def from_pretrained(
cls,
model: torch.nn.Module,
model_id: Union[str, os.PathLike],
adapter_name: str = "default",
is_trainable: bool = False,
config: Optional[PeftConfig] = None,
**kwargs: Any,
) -> PeftModel:
r"""
Instantiate a PEFT model from a pretrained model and loaded PEFT weights.
Note that the passed `model` may be modified inplace.
Args:
model ([`torch.nn.Module`]):
The model to be adapted. For 🤗 Transformers models, the model should be initialized with the
[`~transformers.PreTrainedModel.from_pretrained`].
model_id (`str` or `os.PathLike`):
The name of the PEFT configuration to use. Can be either:
- A string, the `model id` of a PEFT configuration hosted inside a model repo on the Hugging Face
Hub.
- A path to a directory containing a PEFT configuration file saved using the `save_pretrained`
method (`./my_peft_config_directory/`).
adapter_name (`str`, *optional*, defaults to `"default"`):
The name of the adapter to be loaded. This is useful for loading multiple adapters.
is_trainable (`bool`, *optional*, defaults to `False`):
Whether the adapter should be trainable or not. If `False`, the adapter will be frozen and can only be
used for inference.
config ([`~peft.PeftConfig`], *optional*):
The configuration object to use instead of an automatically loaded configuration. This configuration
object is mutually exclusive with `model_id` and `kwargs`. This is useful when configuration is already
loaded before calling `from_pretrained`.
kwargs: (`optional`):
Additional keyword arguments passed along to the specific PEFT configuration class.
"""
from .mapping import MODEL_TYPE_TO_PEFT_MODEL_MAPPING, PEFT_TYPE_TO_CONFIG_MAPPING
# load the config
if config is None:
config = PEFT_TYPE_TO_CONFIG_MAPPING[
PeftConfig._get_peft_type(
model_id,
subfolder=kwargs.get("subfolder", None),
revision=kwargs.get("revision", None),
cache_dir=kwargs.get("cache_dir", None),
use_auth_token=kwargs.get("use_auth_token", None),
token=kwargs.get("token", None),
)
].from_pretrained(model_id, **kwargs)
elif isinstance(config, PeftConfig):
config.inference_mode = not is_trainable
else:
raise ValueError(f"The input config must be a PeftConfig, got {config.__class__}")
if (getattr(model, "hf_device_map", None) is not None) and len(
set(model.hf_device_map.values()).intersection({"cpu", "disk"})
) > 0:
remove_hook_from_submodules(model)
if config.is_prompt_learning and is_trainable:
raise ValueError("Cannot set a prompt learning adapter to trainable when loading pretrained adapter.")
else:
config.inference_mode = not is_trainable
if config.task_type not in MODEL_TYPE_TO_PEFT_MODEL_MAPPING.keys():
model = cls(model, config, adapter_name)
else:
model = MODEL_TYPE_TO_PEFT_MODEL_MAPPING[config.task_type](model, config, adapter_name)
model.load_adapter(model_id, adapter_name, is_trainable=is_trainable, **kwargs)
return model
def _setup_prompt_encoder(self, adapter_name: str):
config = self.peft_config[adapter_name]
if not hasattr(self, "prompt_encoder"):
self.prompt_encoder = torch.nn.ModuleDict({})
self.prompt_tokens = {}
transformer_backbone = None
for name, module in self.base_model.named_children():
for param in module.parameters():
param.requires_grad = False
if isinstance(module, PreTrainedModel):
# Make sure to freeze Tranformers model
if transformer_backbone is None:
transformer_backbone = module
self.transformer_backbone_name = name
if transformer_backbone is None:
transformer_backbone = self.base_model
if config.num_transformer_submodules is None:
config.num_transformer_submodules = 2 if config.task_type == TaskType.SEQ_2_SEQ_LM else 1
for named_param, value in list(transformer_backbone.named_parameters()):
# for ZeRO-3, the tensor is sharded across accelerators and deepspeed modifies it to a tensor with shape [0]
# the actual unsharded shape is stored in "ds_shape" attribute
# special handling is needed in case the model is initialized in deepspeed.zero.Init() context or HfDeepSpeedConfig
# has been called before
# For reference refer to issue: https://github.com/huggingface/peft/issues/996
deepspeed_distributed_tensor_shape = getattr(value, "ds_shape", None)
if value.shape[0] == self.base_model.config.vocab_size or (
deepspeed_distributed_tensor_shape is not None
and deepspeed_distributed_tensor_shape[0] == self.base_model.config.vocab_size
):
self.word_embeddings = transformer_backbone.get_submodule(named_param.replace(".weight", ""))
break
if config.peft_type == PeftType.PROMPT_TUNING:
prompt_encoder = PromptEmbedding(config, self.word_embeddings)
elif config.peft_type == PeftType.MULTITASK_PROMPT_TUNING:
prompt_encoder = MultitaskPromptEmbedding(config, self.word_embeddings)
elif config.peft_type == PeftType.P_TUNING:
prompt_encoder = PromptEncoder(config)
elif config.peft_type == PeftType.PREFIX_TUNING:
prompt_encoder = PrefixEncoder(config)
else:
raise ValueError("Not supported")
prompt_encoder = prompt_encoder.to(self.device)
self.prompt_encoder.update(torch.nn.ModuleDict({adapter_name: prompt_encoder}))
self.prompt_tokens[adapter_name] = torch.arange(
config.num_virtual_tokens * config.num_transformer_submodules
).long()
def _prepare_model_for_gradient_checkpointing(self, model: PreTrainedModel):
r"""
Prepares the model for gradient checkpointing if necessary
"""
if not (
getattr(model, "is_loaded_in_8bit", False)
or getattr(model, "is_loaded_in_4bit", False)
or getattr(model, "is_quantized", False)
):
if hasattr(model, "enable_input_require_grads"):
model.enable_input_require_grads()
elif hasattr(model, "get_input_embeddings"):
def make_inputs_require_grad(module, input, output):
output.requires_grad_(True)
model.get_input_embeddings().register_forward_hook(make_inputs_require_grad)
return model
def get_prompt_embedding_to_save(self, adapter_name: str) -> torch.Tensor:
"""
Returns the prompt embedding to save when saving the model. Only applicable when using a prompt learning
method.
"""
prompt_encoder = self.prompt_encoder[adapter_name]
prompt_tokens = (
self.prompt_tokens[adapter_name].unsqueeze(0).expand(1, -1).to(prompt_encoder.embedding.weight.device)
)
if self.peft_config[adapter_name].peft_type == PeftType.PREFIX_TUNING:
prompt_tokens = prompt_tokens[:, : self.peft_config[adapter_name].num_virtual_tokens]
if self.peft_config[adapter_name].peft_type == PeftType.MULTITASK_PROMPT_TUNING:
prompt_embeddings = super(MultitaskPromptEmbedding, prompt_encoder).forward(prompt_tokens)
else:
prompt_embeddings = prompt_encoder(prompt_tokens)
return prompt_embeddings[0].detach().cpu()
def get_prompt(self, batch_size: int, task_ids: Optional[torch.Tensor] = None) -> torch.Tensor:
"""
Returns the virtual prompts to use for Peft. Only applicable when using a prompt learning method.
"""
peft_config = self.active_peft_config
prompt_encoder = self.prompt_encoder[self.active_adapter]
prompt_tokens = (
self.prompt_tokens[self.active_adapter]
.unsqueeze(0)
.expand(batch_size, -1)
.to(prompt_encoder.embedding.weight.device)
)
if peft_config.peft_type == PeftType.PREFIX_TUNING:
prompt_tokens = prompt_tokens[:, : peft_config.num_virtual_tokens]
if peft_config.inference_mode:
past_key_values = prompt_encoder.embedding.weight.repeat(batch_size, 1, 1)
else:
past_key_values = prompt_encoder(prompt_tokens)
if self.base_model_torch_dtype is not None:
past_key_values = past_key_values.to(self.base_model_torch_dtype)
past_key_values = past_key_values.view(
batch_size,
peft_config.num_virtual_tokens,
peft_config.num_layers * 2,
peft_config.num_attention_heads,
peft_config.token_dim // peft_config.num_attention_heads,
)
if peft_config.num_transformer_submodules == 2:
past_key_values = torch.cat([past_key_values, past_key_values], dim=2)
past_key_values = past_key_values.permute([2, 0, 3, 1, 4]).split(
peft_config.num_transformer_submodules * 2
)
if TRANSFORMERS_MODELS_TO_PREFIX_TUNING_POSTPROCESS_MAPPING.get(self.config.model_type, None) is not None:
post_process_fn = TRANSFORMERS_MODELS_TO_PREFIX_TUNING_POSTPROCESS_MAPPING[self.config.model_type]
past_key_values = post_process_fn(past_key_values)
return past_key_values
else:
if peft_config.peft_type == PeftType.MULTITASK_PROMPT_TUNING:
prompts = prompt_encoder(prompt_tokens, task_ids)
else:
if peft_config.inference_mode:
prompts = prompt_encoder.embedding.weight.repeat(batch_size, 1, 1)
else:
prompts = prompt_encoder(prompt_tokens)
return prompts
def get_nb_trainable_parameters(self) -> tuple[int, int]:
r"""
Returns the number of trainable parameters and the number of all parameters in the model.
"""
trainable_params = 0
all_param = 0
for _, param in self.named_parameters():
num_params = param.numel()
# if using DS Zero 3 and the weights are initialized empty
if num_params == 0 and hasattr(param, "ds_numel"):
num_params = param.ds_numel
# Due to the design of 4bit linear layers from bitsandbytes
# one needs to multiply the number of parameters by 2 to get
# the correct number of parameters
if param.__class__.__name__ == "Params4bit":
num_bytes = param.quant_storage.itemsize if hasattr(param, "quant_storage") else 1
num_params = num_params * 2 * num_bytes
all_param += num_params
if param.requires_grad:
trainable_params += num_params
return trainable_params, all_param
def print_trainable_parameters(self) -> None:
"""
Prints the number of trainable parameters in the model.
Note: print_trainable_parameters() uses get_nb_trainable_parameters() which is different from
num_parameters(only_trainable=True) from huggingface/transformers. get_nb_trainable_parameters() returns
(trainable parameters, all parameters) of the Peft Model which includes modified backbone transformer model.
For techniques like LoRA, the backbone transformer model is modified in place with LoRA modules. However, for
prompt tuning, the backbone transformer model is unmodified. num_parameters(only_trainable=True) returns number
of trainable parameters of the backbone transformer model which can be different.
"""
trainable_params, all_param = self.get_nb_trainable_parameters()
print(
f"trainable params: {trainable_params:,d} || all params: {all_param:,d} || trainable%: {100 * trainable_params / all_param}"
)
def __getattr__(self, name: str):
"""Forward missing attributes to the wrapped module."""
try:
return super().__getattr__(name) # defer to nn.Module's logic
except AttributeError:
return getattr(self.base_model, name)
@contextmanager
def _enable_peft_forward_hooks(self, *args, **kwargs):
# If the base model has a method called _enable_peft_forward_hooks, it is invoked as a context. Otherwise, this
# runs without any changes
if hasattr(self.base_model, "_enable_peft_forward_hooks"):
with self.base_model._enable_peft_forward_hooks(*args, **kwargs):
yield
return
else:
# nothing to enable
yield
return
def forward(self, *args: Any, **kwargs: Any):
"""
Forward pass of the model.
"""
with self._enable_peft_forward_hooks(*args, **kwargs):
kwargs = {k: v for k, v in kwargs.items() if k not in self.special_peft_forward_args}
return self.get_base_model()(*args, **kwargs)
def generate(self, *args, **kwargs):
with self._enable_peft_forward_hooks(*args, **kwargs):
kwargs = {k: v for k, v in kwargs.items() if k not in self.special_peft_forward_args}
return self.get_base_model().generate(*args, **kwargs)
def _get_base_model_class(self, is_prompt_tuning=False):
"""
Returns the base model class.
"""
if not is_prompt_tuning:
return self.base_model.model.__class__
return self.base_model.__class__
@contextmanager
def disable_adapter(self):
"""
Context manager that disables the adapter module. Use this to run inference on the base model.
Example:
```py
>>> with model.disable_adapter():
... model(inputs)
```
"""
try:
if self.peft_config[self.active_adapter].is_prompt_learning:
# TODO: consider replacing this patching of methods with a more robust mechanism: setting a flag and
# letting the underlying methods deal with it, same as how LoRA does it.
old_forward = self.forward
self.forward = self.base_model.forward
old_prepare_inputs_for_generation = self.prepare_inputs_for_generation
self.prepare_inputs_for_generation = self.base_model.prepare_inputs_for_generation
else:
self.base_model.disable_adapter_layers()
yield
finally:
if self.peft_config[self.active_adapter].is_prompt_learning:
self.forward = old_forward
self.prepare_inputs_for_generation = old_prepare_inputs_for_generation
else:
self.base_model.enable_adapter_layers()
def get_base_model(self) -> torch.nn.Module:
"""
Returns the base model.
"""
return (
self.base_model
if (self.active_peft_config.is_prompt_learning or self.peft_type == PeftType.POLY)
else self.base_model.model
)
def add_adapter(self, adapter_name: str, peft_config: PeftConfig) -> None:
"""
Add an adapter to the model based on the passed configuration.
This adapter is not trained. To load a trained adapter, check out [`PeftModel.load_adapter`].
The name for the new adapter should be unique.
The new adapter is not automatically set as the active adapter. Use [`PeftModel.set_adapter`] to set the active
adapter.
Args:
adapter_name (`str`):
The name of the adapter to be added.
peft_config ([`PeftConfig`]):
The configuration of the adapter to be added.
"""
if peft_config.peft_type != self.peft_type:
raise ValueError(
f"Cannot combine adapters with different peft types. "
f"Found {self.peft_type} and {peft_config.peft_type}."
)
try:
if peft_config.is_prompt_learning:
self.peft_config[adapter_name] = peft_config
if hasattr(self.config, "to_dict"):
dict_config = self.config.to_dict()
else:
dict_config = self.config
peft_config = _prepare_prompt_learning_config(peft_config, dict_config)
self._setup_prompt_encoder(adapter_name)
elif peft_config.is_adaption_prompt:
self.base_model.add_adapter(adapter_name, peft_config)
else:
self.peft_config[adapter_name] = peft_config
self.base_model.inject_adapter(self.base_model.model, adapter_name)
except Exception: # something went wrong, roll back
if adapter_name in self.peft_config:
del self.peft_config[adapter_name]
raise
self.set_additional_trainable_modules(peft_config, adapter_name)
def set_additional_trainable_modules(self, peft_config, adapter_name):
if getattr(peft_config, "modules_to_save", None) is not None:
if self.modules_to_save is None:
self.modules_to_save = set(peft_config.modules_to_save)
else:
self.modules_to_save.update(peft_config.modules_to_save)
_set_trainable(self, adapter_name)
@classmethod
def _split_kwargs(cls, kwargs: dict[str, Any]):
_kwargs_not_in_hf_hub_download_signature = ("use_auth_token",)
hf_hub_download_kwargs = {}
other_kwargs = {}
for key, value in kwargs.items():
if key in inspect.signature(hf_hub_download).parameters or key in _kwargs_not_in_hf_hub_download_signature:
hf_hub_download_kwargs[key] = value
else:
other_kwargs[key] = value
return hf_hub_download_kwargs, other_kwargs
def load_adapter(self, model_id: str, adapter_name: str, is_trainable: bool = False, **kwargs: Any):
"""
Load a trained adapter into the model.
The name for the new adapter should be unique.
The new adapter is not automatically set as the active adapter. Use [`PeftModel.set_adapter`] to set the active
adapter.
Args:
adapter_name (`str`):
The name of the adapter to be added.
peft_config ([`PeftConfig`]):
The configuration of the adapter to be added.
is_trainable (`bool`, *optional*, defaults to `False`):
Whether the adapter should be trainable or not. If `False`, the adapter will be frozen and can only be
used for inference.
kwargs: (`optional`):
Additional arguments to modify the way the adapter is loaded, e.g. the token for Hugging Face Hub.
"""
from .mapping import PEFT_TYPE_TO_CONFIG_MAPPING
hf_hub_download_kwargs, kwargs = self._split_kwargs(kwargs)
torch_device = infer_device()
if adapter_name not in self.peft_config:
# load the config
peft_config = PEFT_TYPE_TO_CONFIG_MAPPING[
PeftConfig._get_peft_type(
model_id,
**hf_hub_download_kwargs,
)
].from_pretrained(
model_id,
**hf_hub_download_kwargs,
)
if peft_config.is_prompt_learning and is_trainable:
raise ValueError("Cannot set a prompt learning adapter to trainable when loading pretrained adapter.")
else:
peft_config.inference_mode = not is_trainable
self.add_adapter(adapter_name, peft_config)
adapters_weights = load_peft_weights(model_id, device=torch_device, **hf_hub_download_kwargs)
# load the weights into the model
load_result = set_peft_model_state_dict(self, adapters_weights, adapter_name=adapter_name)
if (
(getattr(self, "hf_device_map", None) is not None)
and (len(set(self.hf_device_map.values()).intersection({"cpu", "disk"})) > 0)
and len(self.peft_config) == 1
):
device_map = kwargs.get("device_map", "auto")
max_memory = kwargs.get("max_memory", None)
offload_dir = kwargs.get("offload_folder", None)
offload_index = kwargs.get("offload_index", None)
dispatch_model_kwargs = {}
# Safety checker for previous `accelerate` versions
# `offload_index` was introduced in https://github.com/huggingface/accelerate/pull/873/
if "offload_index" in inspect.signature(dispatch_model).parameters:
dispatch_model_kwargs["offload_index"] = offload_index
no_split_module_classes = self._no_split_modules
if device_map != "sequential":
max_memory = get_balanced_memory(
self,
max_memory=max_memory,
no_split_module_classes=no_split_module_classes,
low_zero=(device_map == "balanced_low_0"),
)
if isinstance(device_map, str):
device_map = infer_auto_device_map(
self, max_memory=max_memory, no_split_module_classes=no_split_module_classes
)
dispatch_model(
self,
device_map=device_map,
offload_dir=offload_dir,
**dispatch_model_kwargs,
)
hook = AlignDevicesHook(io_same_device=True)
if self.peft_config[adapter_name].is_prompt_learning:
remove_hook_from_submodules(self.prompt_encoder)
add_hook_to_module(self.get_base_model(), hook)
# Set model in evaluation mode to deactivate Dropout modules by default
if not is_trainable:
self.eval()
return load_result
def set_adapter(self, adapter_name: str) -> None:
"""
Sets the active adapter.
Only one adapter can be active at a time.
Additionally, this function will set the specified adapter to trainable (i.e., requires_grad=True). If this is
not desired, use the following code.
```py
>>> for name, param in model_peft.named_parameters():
... if ...: # some check on name (ex. if 'lora' in name)
... param.requires_grad = False
```
Args:
adapter_name (`str`):
The name of the adapter to be set as active. The adapter must be loaded first.
"""
if adapter_name not in self.peft_config:
raise ValueError(f"Adapter {adapter_name} not found.")
self.active_adapter = adapter_name
if not self.peft_config[adapter_name].is_prompt_learning:
self.base_model.set_adapter(adapter_name)
_set_adapter(self, adapter_name)
@property
def base_model_torch_dtype(self):
return getattr(self.base_model, "dtype", None)
@property
def active_peft_config(self):
return self.peft_config[self.active_adapter]
def create_or_update_model_card(self, output_dir: str):
"""
Updates or create model card to include information about peft:
1. Adds `peft` library tag
2. Adds peft version
3. Adds base model info
4. Adds quantization information if it was used
"""
filename = os.path.join(output_dir, "README.md")
card = ModelCard.load(filename) if os.path.exists(filename) else ModelCard.from_template(ModelCardData())
card.data["library_name"] = "peft"
model_config = getattr(self, "config", None)
if hasattr(model_config, "to_dict"):
model_config = model_config.to_dict()
if model_config is not None and "_name_or_path" in model_config:
card.data["base_model"] = model_config["_name_or_path"]
lines = card.text.splitlines()
quantization_config = None
if hasattr(model_config, "quantization_config"):
quantization_config = self.config.quantization_config.to_dict()
training_config_text = ""
quantization_prefix = "The following `bitsandbytes` quantization config was used during training:"
# Adds quantization information if it was used
if quantization_config is not None:
training_config_text += f"\n{quantization_prefix}\n"
training_config_text += "\n".join([f"- {name}: {value}" for name, value in quantization_config.items()])
training_config_text += "\n"
training_procedure_heading = "## Training procedure"
if quantization_prefix not in lines and bool(training_config_text):
if training_procedure_heading in lines:
lines.insert(lines.index(training_procedure_heading) + 2, training_config_text)
else:
lines.append(f"{training_procedure_heading}\n{training_config_text}")
# Adds peft version
framework_block_heading = "### Framework versions"
if f"- PEFT {__version__}" not in lines:
if framework_block_heading in lines:
lines.insert(lines.index(framework_block_heading) + 2, f"- PEFT {__version__}")
else:
lines.append(f"{framework_block_heading}\n\n- PEFT {__version__}")
card.text = "\n".join(lines)
card.save(filename)
class PeftModelForSequenceClassification(PeftModel):
"""
Peft model for sequence classification tasks.
Args:
model ([`~transformers.PreTrainedModel`]): Base transformer model.
peft_config ([`PeftConfig`]): Peft config.
**Attributes**:
- **config** ([`~transformers.PretrainedConfig`]) -- The configuration object of the base model.
- **cls_layer_name** (`str`) -- The name of the classification layer.
Example:
```py
>>> from transformers import AutoModelForSequenceClassification
>>> from peft import PeftModelForSequenceClassification, get_peft_config
>>> config = {
... "peft_type": "PREFIX_TUNING",
... "task_type": "SEQ_CLS",
... "inference_mode": False,
... "num_virtual_tokens": 20,
... "token_dim": 768,
... "num_transformer_submodules": 1,
... "num_attention_heads": 12,
... "num_layers": 12,
... "encoder_hidden_size": 768,
... "prefix_projection": False,
... "postprocess_past_key_value_function": None,
... }
>>> peft_config = get_peft_config(config)
>>> model = AutoModelForSequenceClassification.from_pretrained("bert-base-cased")
>>> peft_model = PeftModelForSequenceClassification(model, peft_config)
>>> peft_model.print_trainable_parameters()
trainable params: 370178 || all params: 108680450 || trainable%: 0.3406113979101117
```
"""
def __init__(self, model: torch.nn.Module, peft_config: PeftConfig, adapter_name: str = "default") -> None:
super().__init__(model, peft_config, adapter_name)
if self.modules_to_save is None:
self.modules_to_save = {"classifier", "score"}
else:
self.modules_to_save.update({"classifier", "score"})
for name, _ in self.base_model.named_children():
if any(module_name in name for module_name in self.modules_to_save):
self.cls_layer_name = name
break
# to make sure classifier layer is trainable
_set_trainable(self, adapter_name)
def forward(
self,
input_ids=None,
attention_mask=None,
inputs_embeds=None,
labels=None,
output_attentions=None,
output_hidden_states=None,
return_dict=None,
task_ids=None,
**kwargs,
):
return_dict = return_dict if return_dict is not None else self.config.use_return_dict
peft_config = self.active_peft_config
if not peft_config.is_prompt_learning:
with self._enable_peft_forward_hooks(**kwargs):
kwargs = {k: v for k, v in kwargs.items() if k not in self.special_peft_forward_args}
if peft_config.peft_type == PeftType.POLY:
kwargs["task_ids"] = task_ids
return self.base_model(
input_ids=input_ids,
attention_mask=attention_mask,
inputs_embeds=inputs_embeds,
labels=labels,
output_attentions=output_attentions,
output_hidden_states=output_hidden_states,
return_dict=return_dict,
**kwargs,
)
batch_size = _get_batch_size(input_ids, inputs_embeds)
if attention_mask is not None:
# concat prompt attention mask
prefix_attention_mask = torch.ones(batch_size, peft_config.num_virtual_tokens).to(attention_mask.device)
attention_mask = torch.cat((prefix_attention_mask, attention_mask), dim=1)
if kwargs.get("position_ids", None) is not None:
warnings.warn("Position ids are not supported for parameter efficient tuning. Ignoring position ids.")
kwargs["position_ids"] = None
kwargs.update(
{
"attention_mask": attention_mask,
"labels": labels,
"output_attentions": output_attentions,
"output_hidden_states": output_hidden_states,
"return_dict": return_dict,
}
)
if peft_config.peft_type == PeftType.PREFIX_TUNING:
return self._prefix_tuning_forward(input_ids=input_ids, **kwargs)
else:
if kwargs.get("token_type_ids", None) is not None:
kwargs["token_type_ids"] = torch.cat(
(
torch.zeros(batch_size, peft_config.num_virtual_tokens).to(self.word_embeddings.weight.device),
kwargs["token_type_ids"],
),
dim=1,
).long()
if inputs_embeds is None:
inputs_embeds = self.word_embeddings(input_ids)
prompts = self.get_prompt(batch_size=batch_size, task_ids=task_ids)
prompts = prompts.to(inputs_embeds.dtype)
inputs_embeds = torch.cat((prompts, inputs_embeds), dim=1)
return self.base_model(inputs_embeds=inputs_embeds, **kwargs)
def _prefix_tuning_forward(
self,
input_ids=None,
attention_mask=None,
inputs_embeds=None,
labels=None,
output_attentions=None,
output_hidden_states=None,
return_dict=None,
**kwargs,
):
batch_size = _get_batch_size(input_ids, inputs_embeds)
past_key_values = self.get_prompt(batch_size)
fwd_params = list(inspect.signature(self.base_model.forward).parameters.keys())
kwargs.update(
{
"input_ids": input_ids,
"attention_mask": attention_mask,
"inputs_embeds": inputs_embeds,
"output_attentions": output_attentions,
"output_hidden_states": output_hidden_states,
"return_dict": return_dict,
"past_key_values": past_key_values,
}
)
if "past_key_values" in fwd_params:
return self.base_model(labels=labels, **kwargs)
else:
transformer_backbone_name = self.base_model.get_submodule(self.transformer_backbone_name)
fwd_params = list(inspect.signature(transformer_backbone_name.forward).parameters.keys())
if "past_key_values" not in fwd_params:
raise ValueError("Model does not support past key values which are required for prefix tuning.")
outputs = transformer_backbone_name(**kwargs)
pooled_output = outputs[1] if len(outputs) > 1 else outputs[0]
if "dropout" in [name for name, _ in list(self.base_model.named_children())]:
pooled_output = self.base_model.dropout(pooled_output)
logits = self.base_model.get_submodule(self.cls_layer_name)(pooled_output)
loss = None
if labels is not None:
if self.config.problem_type is None:
if self.base_model.num_labels == 1:
self.config.problem_type = "regression"
elif self.base_model.num_labels > 1 and (labels.dtype == torch.long or labels.dtype == torch.int):
self.config.problem_type = "single_label_classification"
else:
self.config.problem_type = "multi_label_classification"
if self.config.problem_type == "regression":
loss_fct = MSELoss()
if self.base_model.num_labels == 1:
loss = loss_fct(logits.squeeze(), labels.squeeze())
else:
loss = loss_fct(logits, labels)
elif self.config.problem_type == "single_label_classification":
loss_fct = CrossEntropyLoss()
loss = loss_fct(logits.view(-1, self.base_model.num_labels), labels.view(-1))
elif self.config.problem_type == "multi_label_classification":
loss_fct = BCEWithLogitsLoss()
loss = loss_fct(logits, labels)
if not return_dict:
output = (logits,) + outputs[2:]
return ((loss,) + output) if loss is not None else output
return SequenceClassifierOutput(
loss=loss,
logits=logits,
hidden_states=outputs.hidden_states,
attentions=outputs.attentions,
)
class PeftModelForCausalLM(PeftModel):
"""
Peft model for causal language modeling.
Args:
model ([`~transformers.PreTrainedModel`]): Base transformer model.
peft_config ([`PeftConfig`]): Peft config.
Example:
```py
>>> from transformers import AutoModelForCausalLM
>>> from peft import PeftModelForCausalLM, get_peft_config
>>> config = {
... "peft_type": "PREFIX_TUNING",
... "task_type": "CAUSAL_LM",
... "inference_mode": False,
... "num_virtual_tokens": 20,
... "token_dim": 1280,
... "num_transformer_submodules": 1,
... "num_attention_heads": 20,
... "num_layers": 36,
... "encoder_hidden_size": 1280,
... "prefix_projection": False,
... "postprocess_past_key_value_function": None,
... }
>>> peft_config = get_peft_config(config)
>>> model = AutoModelForCausalLM.from_pretrained("gpt2-large")
>>> peft_model = PeftModelForCausalLM(model, peft_config)
>>> peft_model.print_trainable_parameters()
trainable params: 1843200 || all params: 775873280 || trainable%: 0.23756456724479544
```
"""
def __init__(self, model: torch.nn.Module, peft_config: PeftConfig, adapter_name: str = "default") -> None:
super().__init__(model, peft_config, adapter_name)
self.base_model_prepare_inputs_for_generation = self.base_model.prepare_inputs_for_generation
def forward(
self,
input_ids=None,
attention_mask=None,
inputs_embeds=None,
labels=None,
output_attentions=None,
output_hidden_states=None,
return_dict=None,
task_ids=None,
**kwargs,
):
peft_config = self.active_peft_config
if not peft_config.is_prompt_learning:
if self.base_model.config.model_type == "mpt":
if inputs_embeds is not None:
raise AssertionError("forward in MPTForCausalLM does not support inputs_embeds")
return self.base_model(
input_ids=input_ids,
attention_mask=attention_mask,
labels=labels,
output_attentions=output_attentions,
output_hidden_states=output_hidden_states,
return_dict=return_dict,
**kwargs,
)
if peft_config.peft_type == PeftType.POLY:
kwargs["task_ids"] = task_ids
with self._enable_peft_forward_hooks(**kwargs):
kwargs = {k: v for k, v in kwargs.items() if k not in self.special_peft_forward_args}
return self.base_model(
input_ids=input_ids,
attention_mask=attention_mask,
inputs_embeds=inputs_embeds,
labels=labels,
output_attentions=output_attentions,
output_hidden_states=output_hidden_states,
return_dict=return_dict,
**kwargs,
)
batch_size = _get_batch_size(input_ids, inputs_embeds)
if attention_mask is not None:
# concat prompt attention mask
prefix_attention_mask = torch.ones(batch_size, peft_config.num_virtual_tokens).to(attention_mask.device)
attention_mask = torch.cat((prefix_attention_mask, attention_mask), dim=1)
if kwargs.get("position_ids", None) is not None:
warnings.warn("Position ids are not supported for parameter efficient tuning. Ignoring position ids.")
kwargs["position_ids"] = None
if kwargs.get("token_type_ids", None) is not None:
warnings.warn("Token type ids are not supported for parameter efficient tuning. Ignoring token type ids")
kwargs["token_type_ids"] = None
kwargs.update(
{
"attention_mask": attention_mask,
"labels": labels,
"output_attentions": output_attentions,
"output_hidden_states": output_hidden_states,
"return_dict": return_dict,
}
)
if peft_config.peft_type == PeftType.PREFIX_TUNING:
past_key_values = self.get_prompt(batch_size)
return self.base_model(
input_ids=input_ids, inputs_embeds=inputs_embeds, past_key_values=past_key_values, **kwargs
)
else:
if inputs_embeds is None:
inputs_embeds = self.word_embeddings(input_ids)
# concat prompt labels
if labels is not None:
prefix_labels = torch.full((batch_size, peft_config.num_virtual_tokens), -100).to(labels.device)
kwargs["labels"] = torch.cat((prefix_labels, labels), dim=1)
prompts = self.get_prompt(batch_size=batch_size, task_ids=task_ids)
prompts = prompts.to(inputs_embeds.dtype)
inputs_embeds = torch.cat((prompts, inputs_embeds), dim=1)
return self.base_model(inputs_embeds=inputs_embeds, **kwargs)
def generate(self, *args, **kwargs):
peft_config = self.active_peft_config
self.base_model.prepare_inputs_for_generation = self.prepare_inputs_for_generation
if hasattr(self.base_model, "model"):
self.base_model.model.generation_config = self.generation_config
else:
self.base_model.generation_config = self.generation_config
try:
if not peft_config.is_prompt_learning:
with self._enable_peft_forward_hooks(*args, **kwargs):
kwargs = {k: v for k, v in kwargs.items() if k not in self.special_peft_forward_args}
outputs = self.base_model.generate(*args, **kwargs)
else:
outputs = self.base_model.generate(**kwargs)
except:
self.base_model.prepare_inputs_for_generation = self.base_model_prepare_inputs_for_generation
raise
else:
self.base_model.prepare_inputs_for_generation = self.base_model_prepare_inputs_for_generation
return outputs
def prepare_inputs_for_generation(self, *args, task_ids: Optional[torch.Tensor] = None, **kwargs):
peft_config = self.active_peft_config
model_kwargs = self.base_model_prepare_inputs_for_generation(*args, **kwargs)
# https://github.com/huggingface/transformers/pull/26681/ introduced new cache format
# for some architectures which requires a special fix for prompt tuning etc.
# TODO: starting with transformers 4.38, all architectures should support caching.
uses_transformers_4_38 = packaging.version.parse(transformers.__version__) >= packaging.version.parse("4.38.0")
uses_transformers_4_36 = packaging.version.parse(transformers.__version__) >= packaging.version.parse("4.36.0")
transformers_new_cache_archs = ["llama", "mistral", "persimmon", "phi"]
uses_cache = uses_transformers_4_38 or (
uses_transformers_4_36 and self.base_model.config.model_type in transformers_new_cache_archs
)
if peft_config.peft_type == PeftType.POLY:
model_kwargs["task_ids"] = task_ids
if peft_config.is_prompt_learning:
if uses_cache and (model_kwargs["past_key_values"] is not None):
# change in the logic of `prepare_inputs_for_generation` makes the below code necessary
# In prompt learning methods, past key values are longer when compared to the `input_ids`.
# As such only consider the last input ids in the autogressive generation phase.
if model_kwargs["past_key_values"][0][0].shape[-2] >= model_kwargs["input_ids"].shape[1]:
model_kwargs["input_ids"] = model_kwargs["input_ids"][:, -1:]
if model_kwargs.get("attention_mask", None) is not None:
size = model_kwargs["input_ids"].shape[0], peft_config.num_virtual_tokens
prefix_attention_mask = torch.ones(size).to(model_kwargs["input_ids"].device)
model_kwargs["attention_mask"] = torch.cat(
(prefix_attention_mask, model_kwargs["attention_mask"]), dim=1
)
if model_kwargs.get("position_ids", None) is not None:
warnings.warn("Position ids are not supported for parameter efficient tuning. Ignoring position ids.")
model_kwargs["position_ids"] = None
if kwargs.get("token_type_ids", None) is not None:
warnings.warn(
"Token type ids are not supported for parameter efficient tuning. Ignoring token type ids"
)
kwargs["token_type_ids"] = None
if model_kwargs["past_key_values"] is None and peft_config.peft_type == PeftType.PREFIX_TUNING:
past_key_values = self.get_prompt(batch_size=model_kwargs["input_ids"].shape[0])
model_kwargs["past_key_values"] = past_key_values
else:
if model_kwargs["past_key_values"] is None:
inputs_embeds = self.word_embeddings(model_kwargs["input_ids"])
prompts = self.get_prompt(batch_size=model_kwargs["input_ids"].shape[0], task_ids=task_ids)
prompts = prompts.to(inputs_embeds.dtype)
model_kwargs["inputs_embeds"] = torch.cat((prompts, inputs_embeds), dim=1)
model_kwargs["input_ids"] = None
# For transformers>=4.38.0 - for some architectures such as Llama, `cache_position` is
# passed in the forward pass to keep track of the position ids of the cache. We have to
# pop that from `model_kwargs` as `cache_position` is properly created by the model, using the passed
# `inputs_embeds`: https://github.com/huggingface/transformers/blob/593230f0a1150ea9c0477b9d859f25daf73c8c33/src/transformers/models/llama/modeling_llama.py#L956
_ = model_kwargs.pop("cache_position", None)
return model_kwargs
class PeftModelForSeq2SeqLM(PeftModel):
"""
Peft model for sequence-to-sequence language modeling.
Args:
model ([`~transformers.PreTrainedModel`]): Base transformer model.
peft_config ([`PeftConfig`]): Peft config.
Example:
```py
>>> from transformers import AutoModelForSeq2SeqLM
>>> from peft import PeftModelForSeq2SeqLM, get_peft_config
>>> config = {
... "peft_type": "LORA",
... "task_type": "SEQ_2_SEQ_LM",
... "inference_mode": False,
... "r": 8,
... "target_modules": ["q", "v"],
... "lora_alpha": 32,
... "lora_dropout": 0.1,
... "fan_in_fan_out": False,
... "enable_lora": None,
... "bias": "none",
... }
>>> peft_config = get_peft_config(config)
>>> model = AutoModelForSeq2SeqLM.from_pretrained("t5-base")
>>> peft_model = PeftModelForSeq2SeqLM(model, peft_config)
>>> peft_model.print_trainable_parameters()
trainable params: 884736 || all params: 223843584 || trainable%: 0.3952474242013566
```
"""
def __init__(self, model: torch.nn.Module, peft_config: PeftConfig, adapter_name: str = "default") -> None:
super().__init__(model, peft_config, adapter_name)
self.base_model_prepare_inputs_for_generation = self.base_model.prepare_inputs_for_generation
self.base_model_prepare_encoder_decoder_kwargs_for_generation = (
self.base_model._prepare_encoder_decoder_kwargs_for_generation
)
def forward(
self,
input_ids=None,
attention_mask=None,
inputs_embeds=None,
decoder_input_ids=None,
decoder_attention_mask=None,
decoder_inputs_embeds=None,
labels=None,
output_attentions=None,
output_hidden_states=None,
return_dict=None,
task_ids=None,
**kwargs,
):
peft_config = self.active_peft_config
if not peft_config.is_prompt_learning:
if peft_config.peft_type == PeftType.POLY:
kwargs["task_ids"] = task_ids
with self._enable_peft_forward_hooks(**kwargs):
kwargs = {k: v for k, v in kwargs.items() if k not in self.special_peft_forward_args}
return self.base_model(
input_ids=input_ids,
attention_mask=attention_mask,
inputs_embeds=inputs_embeds,
decoder_input_ids=decoder_input_ids,
decoder_attention_mask=decoder_attention_mask,
decoder_inputs_embeds=decoder_inputs_embeds,
labels=labels,
output_attentions=output_attentions,
output_hidden_states=output_hidden_states,
return_dict=return_dict,
**kwargs,
)
batch_size = _get_batch_size(input_ids, inputs_embeds)
if decoder_attention_mask is not None:
# concat prompt attention mask
prefix_attention_mask = torch.ones(batch_size, peft_config.num_virtual_tokens).to(
decoder_attention_mask.device
)
if peft_config.peft_type not in [PeftType.PROMPT_TUNING, PeftType.P_TUNING]:
decoder_attention_mask = torch.cat((prefix_attention_mask, decoder_attention_mask), dim=1)
if kwargs.get("position_ids", None) is not None:
warnings.warn("Position ids are not supported for parameter efficient tuning. Ignoring position ids.")
kwargs["position_ids"] = None
if kwargs.get("token_type_ids", None) is not None:
warnings.warn("Token type ids are not supported for parameter efficient tuning. Ignoring token type ids")
kwargs["token_type_ids"] = None
kwargs.update(
{
"attention_mask": attention_mask,
"decoder_attention_mask": decoder_attention_mask,
"labels": labels,
"output_attentions": output_attentions,
"output_hidden_states": output_hidden_states,
"return_dict": return_dict,
}
)
if peft_config.peft_type == PeftType.PREFIX_TUNING:
past_key_values = self.get_prompt(batch_size)
return self.base_model(
input_ids=input_ids,
decoder_input_ids=decoder_input_ids,
decoder_inputs_embeds=decoder_inputs_embeds,
past_key_values=past_key_values,
**kwargs,
)
elif peft_config.peft_type in [PeftType.PROMPT_TUNING, PeftType.P_TUNING]:
if inputs_embeds is None:
inputs_embeds = self.word_embeddings(input_ids)
if attention_mask is not None:
# concat prompt attention mask
prefix_attention_mask = torch.ones(batch_size, peft_config.num_virtual_tokens).to(
attention_mask.device
)
kwargs["attention_mask"] = torch.cat((prefix_attention_mask, attention_mask), dim=1)
prompts = self.get_prompt(batch_size=batch_size)
prompts = prompts.to(inputs_embeds.dtype)
inputs_embeds = torch.cat((prompts[:, : peft_config.num_virtual_tokens], inputs_embeds), dim=1)
return self.base_model(
inputs_embeds=inputs_embeds,
decoder_input_ids=decoder_input_ids,
decoder_inputs_embeds=decoder_inputs_embeds,
**kwargs,
)
else:
if inputs_embeds is None:
inputs_embeds = self.word_embeddings(input_ids)
if decoder_inputs_embeds is None and decoder_input_ids is None:
decoder_input_ids = shift_tokens_right(
labels, self.config.pad_token_id, self.config.decoder_start_token_id
)
decoder_inputs_embeds = self.word_embeddings(decoder_input_ids)
if attention_mask is not None:
# concat prompt attention mask
prefix_attention_mask = torch.ones(batch_size, peft_config.num_virtual_tokens).to(
attention_mask.device
)
kwargs["attention_mask"] = torch.cat((prefix_attention_mask, attention_mask), dim=1)
# concat prompt labels
if labels is not None:
if peft_config.num_transformer_submodules == 1:
kwargs["labels"] = labels
elif peft_config.num_transformer_submodules == 2:
prefix_labels = torch.full((batch_size, peft_config.num_virtual_tokens), -100).to(labels.device)
kwargs["labels"] = torch.cat((prefix_labels, labels), dim=1)
prompts = self.get_prompt(batch_size=batch_size, task_ids=task_ids)
prompts = prompts.to(inputs_embeds.dtype)
inputs_embeds = torch.cat((prompts[:, : peft_config.num_virtual_tokens], inputs_embeds), dim=1)
if peft_config.num_transformer_submodules == 1:
return self.base_model(inputs_embeds=inputs_embeds, **kwargs)
elif peft_config.num_transformer_submodules == 2:
decoder_inputs_embeds = torch.cat(
(prompts[:, peft_config.num_virtual_tokens :], decoder_inputs_embeds), dim=1
)
return self.base_model(
inputs_embeds=inputs_embeds, decoder_inputs_embeds=decoder_inputs_embeds, **kwargs
)
def generate(self, **kwargs):
peft_config = self.active_peft_config
self.base_model.prepare_inputs_for_generation = self.prepare_inputs_for_generation
self.base_model._prepare_encoder_decoder_kwargs_for_generation = (
self._prepare_encoder_decoder_kwargs_for_generation
)
try:
if not peft_config.is_prompt_learning:
with self._enable_peft_forward_hooks(**kwargs):
kwargs = {k: v for k, v in kwargs.items() if k not in self.special_peft_forward_args}
outputs = self.base_model.generate(**kwargs)
else:
if "input_ids" not in kwargs:
raise ValueError("input_ids must be provided for Peft model generation")
if kwargs.get("position_ids", None) is not None:
warnings.warn(
"Position ids are not supported for parameter efficient tuning. Ignoring position ids."
)
kwargs["position_ids"] = None
if kwargs.get("token_type_ids", None) is not None:
warnings.warn(
"Token type ids are not supported for parameter efficient tuning. Ignoring token type ids"
)
kwargs["token_type_ids"] = None
if peft_config.peft_type == PeftType.PREFIX_TUNING:
outputs = self.base_model.generate(**kwargs)
elif peft_config.peft_type in [
PeftType.PROMPT_TUNING,
PeftType.P_TUNING,
PeftType.MULTITASK_PROMPT_TUNING,
]:
kwargs = deepcopy(kwargs)
if "encoder_outputs" in kwargs:
del kwargs["encoder_outputs"]
warnings.warn(
"`encoder_outputs` should not be passed to `generate` when using prompt tuning. Ignoring it."
)
input_ids = kwargs.pop("input_ids")
inputs_embeds = self.word_embeddings(input_ids)
batch_size = inputs_embeds.shape[0]
prompts = self.get_prompt(batch_size=batch_size, task_ids=kwargs.pop("task_ids", None))
prompts = prompts.to(inputs_embeds.dtype)
inputs_embeds = torch.cat((prompts[:, : peft_config.num_virtual_tokens], inputs_embeds), dim=1)
kwargs["inputs_embeds"] = inputs_embeds
if "attention_mask" in kwargs:
prefix_attention_mask = torch.ones(batch_size, peft_config.num_virtual_tokens).to(
kwargs["attention_mask"].device
)
kwargs["attention_mask"] = torch.cat((prefix_attention_mask, kwargs["attention_mask"]), dim=1)
return self.base_model.generate(**kwargs)
else:
raise NotImplementedError
except:
self.base_model.prepare_inputs_for_generation = self.base_model_prepare_inputs_for_generation
self.base_model._prepare_encoder_decoder_kwargs_for_generation = (
self.base_model_prepare_encoder_decoder_kwargs_for_generation
)
raise
else:
self.base_model.prepare_inputs_for_generation = self.base_model_prepare_inputs_for_generation
self.base_model._prepare_encoder_decoder_kwargs_for_generation = (
self.base_model_prepare_encoder_decoder_kwargs_for_generation
)
return outputs
def prepare_inputs_for_generation(self, *args, task_ids: torch.Tensor = None, **kwargs):
peft_config = self.active_peft_config
model_kwargs = self.base_model_prepare_inputs_for_generation(*args, **kwargs)
if peft_config.peft_type == PeftType.POLY:
model_kwargs["task_ids"] = task_ids
if model_kwargs["past_key_values"] is None and peft_config.peft_type == PeftType.PREFIX_TUNING:
batch_size = model_kwargs["decoder_input_ids"].shape[0]
past_key_values = self.get_prompt(batch_size)
model_kwargs["past_key_values"] = past_key_values
return model_kwargs
class PeftModelForTokenClassification(PeftModel):
"""
Peft model for token classification tasks.
Args:
model ([`~transformers.PreTrainedModel`]): Base transformer model.
peft_config ([`PeftConfig`]): Peft config.
**Attributes**:
- **config** ([`~transformers.PretrainedConfig`]) -- The configuration object of the base model.
- **cls_layer_name** (`str`) -- The name of the classification layer.
Example:
```py
>>> from transformers import AutoModelForSequenceClassification
>>> from peft import PeftModelForTokenClassification, get_peft_config
>>> config = {
... "peft_type": "PREFIX_TUNING",
... "task_type": "TOKEN_CLS",
... "inference_mode": False,
... "num_virtual_tokens": 20,
... "token_dim": 768,
... "num_transformer_submodules": 1,
... "num_attention_heads": 12,
... "num_layers": 12,
... "encoder_hidden_size": 768,
... "prefix_projection": False,
... "postprocess_past_key_value_function": None,
... }
>>> peft_config = get_peft_config(config)
>>> model = AutoModelForTokenClassification.from_pretrained("bert-base-cased")
>>> peft_model = PeftModelForTokenClassification(model, peft_config)
>>> peft_model.print_trainable_parameters()
trainable params: 370178 || all params: 108680450 || trainable%: 0.3406113979101117
```
"""
def __init__(self, model: torch.nn.Module, peft_config: PeftConfig = None, adapter_name: str = "default") -> None:
super().__init__(model, peft_config, adapter_name)
if self.modules_to_save is None:
self.modules_to_save = {"classifier", "score"}
else:
self.modules_to_save.update({"classifier", "score"})
for name, _ in self.base_model.named_children():
if any(module_name in name for module_name in self.modules_to_save):
self.cls_layer_name = name
break
# to make sure classifier layer is trainable
_set_trainable(self, adapter_name)
def forward(
self,
input_ids=None,
attention_mask=None,
inputs_embeds=None,
labels=None,
output_attentions=None,
output_hidden_states=None,
return_dict=None,
task_ids=None,
**kwargs,
):
peft_config = self.active_peft_config
return_dict = return_dict if return_dict is not None else self.config.use_return_dict
if not peft_config.is_prompt_learning:
with self._enable_peft_forward_hooks(**kwargs):
kwargs = {k: v for k, v in kwargs.items() if k not in self.special_peft_forward_args}
if peft_config.peft_type == PeftType.POLY:
kwargs["task_ids"] = task_ids
return self.base_model(
input_ids=input_ids,
attention_mask=attention_mask,
inputs_embeds=inputs_embeds,
labels=labels,
output_attentions=output_attentions,
output_hidden_states=output_hidden_states,
return_dict=return_dict,
**kwargs,
)
batch_size = _get_batch_size(input_ids, inputs_embeds)
if attention_mask is not None:
# concat prompt attention mask
prefix_attention_mask = torch.ones(batch_size, peft_config.num_virtual_tokens).to(attention_mask.device)
attention_mask = torch.cat((prefix_attention_mask, attention_mask), dim=1)
if kwargs.get("position_ids", None) is not None:
warnings.warn("Position ids are not supported for parameter efficient tuning. Ignoring position ids.")
kwargs["position_ids"] = None
kwargs.update(
{
"attention_mask": attention_mask,
"labels": labels,
"output_attentions": output_attentions,
"output_hidden_states": output_hidden_states,
"return_dict": return_dict,
}
)
if peft_config.peft_type == PeftType.PREFIX_TUNING:
return self._prefix_tuning_forward(input_ids=input_ids, **kwargs)
else:
if kwargs.get("token_type_ids", None) is not None:
kwargs["token_type_ids"] = torch.cat(
(
torch.zeros(batch_size, peft_config.num_virtual_tokens).to(self.word_embeddings.weight.device),
kwargs["token_type_ids"],
),
dim=1,
).long()
if inputs_embeds is None:
inputs_embeds = self.word_embeddings(input_ids)
prompts = self.get_prompt(batch_size=batch_size, task_ids=task_ids)
prompts = prompts.to(inputs_embeds.dtype)
inputs_embeds = torch.cat((prompts, inputs_embeds), dim=1)
return self.base_model(inputs_embeds=inputs_embeds, **kwargs)
def _prefix_tuning_forward(
self,
input_ids=None,
attention_mask=None,
inputs_embeds=None,
labels=None,
output_attentions=None,
output_hidden_states=None,
return_dict=None,
**kwargs,
):
batch_size = _get_batch_size(input_ids, inputs_embeds)
past_key_values = self.get_prompt(batch_size)
fwd_params = list(inspect.signature(self.base_model.forward).parameters.keys())
kwargs.update(
{
"input_ids": input_ids,
"attention_mask": attention_mask,
"inputs_embeds": inputs_embeds,
"output_attentions": output_attentions,
"output_hidden_states": output_hidden_states,
"return_dict": return_dict,
"past_key_values": past_key_values,
}
)
if "past_key_values" in fwd_params:
return self.base_model(labels=labels, **kwargs)
else:
transformer_backbone_name = self.base_model.get_submodule(self.transformer_backbone_name)
fwd_params = list(inspect.signature(transformer_backbone_name.forward).parameters.keys())
if "past_key_values" not in fwd_params:
raise ValueError("Model does not support past key values which are required for prefix tuning.")
outputs = transformer_backbone_name(**kwargs)
sequence_output = outputs[0]
if "dropout" in [name for name, _ in list(self.base_model.named_children())]:
sequence_output = self.base_model.dropout(sequence_output)
logits = self.base_model.get_submodule(self.cls_layer_name)(sequence_output)
loss = None
if labels is not None:
loss_fct = CrossEntropyLoss()
loss = loss_fct(logits.view(-1, self.num_labels), labels.view(-1))
if not return_dict:
output = (logits,) + outputs[2:]
return ((loss,) + output) if loss is not None else output
return TokenClassifierOutput(
loss=loss,
logits=logits,
hidden_states=outputs.hidden_states,
attentions=outputs.attentions,
)
class PeftModelForQuestionAnswering(PeftModel):
"""
Peft model for extractive question answering.
Args:
model ([`~transformers.PreTrainedModel`]): Base transformer model.
peft_config ([`PeftConfig`]): Peft config.
**Attributes**:
- **config** ([`~transformers.PretrainedConfig`]) -- The configuration object of the base model.
- **cls_layer_name** (`str`) -- The name of the classification layer.
Example:
```py
>>> from transformers import AutoModelForQuestionAnswering
>>> from peft import PeftModelForQuestionAnswering, get_peft_config
>>> config = {
... "peft_type": "LORA",
... "task_type": "QUESTION_ANS",
... "inference_mode": False,
... "r": 16,
... "target_modules": ["query", "value"],
... "lora_alpha": 32,
... "lora_dropout": 0.05,
... "fan_in_fan_out": False,
... "bias": "none",
... }
>>> peft_config = get_peft_config(config)
>>> model = AutoModelForQuestionAnswering.from_pretrained("bert-base-cased")
>>> peft_model = PeftModelForQuestionAnswering(model, peft_config)
>>> peft_model.print_trainable_parameters()
trainable params: 592900 || all params: 108312580 || trainable%: 0.5473971721475013
```
"""
def __init__(self, model: torch.nn.Module, peft_config: PeftConfig, adapter_name: str = "default") -> None:
super().__init__(model, peft_config, adapter_name)
if self.modules_to_save is None:
self.modules_to_save = {"qa_outputs"}
else:
self.modules_to_save.update({"qa_outputs"})
for name, _ in self.base_model.named_children():
if any(module_name in name for module_name in self.modules_to_save):
self.cls_layer_name = name
break
# to make sure classifier layer is trainable
_set_trainable(self, adapter_name)
def forward(
self,
input_ids=None,
attention_mask=None,
token_type_ids=None,
position_ids=None,
inputs_embeds=None,
start_positions=None,
end_positions=None,
output_attentions=None,
output_hidden_states=None,
return_dict=None,
task_ids=None,
**kwargs,
):
peft_config = self.active_peft_config
return_dict = return_dict if return_dict is not None else self.config.use_return_dict
if not peft_config.is_prompt_learning:
if peft_config.peft_type == PeftType.POLY:
kwargs["task_ids"] = task_ids
with self._enable_peft_forward_hooks(**kwargs):
kwargs = {k: v for k, v in kwargs.items() if k not in self.special_peft_forward_args}
return self.base_model(
input_ids=input_ids,
attention_mask=attention_mask,
inputs_embeds=inputs_embeds,
start_positions=start_positions,
end_positions=end_positions,
output_attentions=output_attentions,
output_hidden_states=output_hidden_states,
return_dict=return_dict,
**kwargs,
)
batch_size = _get_batch_size(input_ids, inputs_embeds)
if attention_mask is not None:
# concat prompt attention mask
prefix_attention_mask = torch.ones(batch_size, peft_config.num_virtual_tokens).to(attention_mask.device)
attention_mask = torch.cat((prefix_attention_mask, attention_mask), dim=1)
if kwargs.get("position_ids", None) is not None:
warnings.warn("Position ids are not supported for parameter efficient tuning. Ignoring position ids.")
kwargs["position_ids"] = None
kwargs.update(
{
"attention_mask": attention_mask,
"start_positions": start_positions,
"end_positions": end_positions,
"output_attentions": output_attentions,
"output_hidden_states": output_hidden_states,
"return_dict": return_dict,
}
)
if peft_config.peft_type == PeftType.PREFIX_TUNING:
return self._prefix_tuning_forward(input_ids=input_ids, **kwargs)
else:
if kwargs.get("token_type_ids", None) is not None:
kwargs["token_type_ids"] = torch.cat(
(
torch.zeros(batch_size, peft_config.num_virtual_tokens).to(self.word_embeddings.weight.device),
kwargs["token_type_ids"],
),
dim=1,
).long()
if inputs_embeds is None:
inputs_embeds = self.word_embeddings(input_ids)
prompts = self.get_prompt(batch_size=batch_size)
prompts = prompts.to(inputs_embeds.dtype)
inputs_embeds = torch.cat((prompts, inputs_embeds), dim=1)
return self.base_model(inputs_embeds=inputs_embeds, **kwargs)
def _prefix_tuning_forward(
self,
input_ids=None,
attention_mask=None,
inputs_embeds=None,
start_positions=None,
end_positions=None,
output_attentions=None,
output_hidden_states=None,
return_dict=None,
**kwargs,
):
batch_size = _get_batch_size(input_ids, inputs_embeds)
past_key_values = self.get_prompt(batch_size)
fwd_params = list(inspect.signature(self.base_model.forward).parameters.keys())
kwargs.update(
{
"input_ids": input_ids,
"attention_mask": attention_mask,
"inputs_embeds": inputs_embeds,
"output_attentions": output_attentions,
"output_hidden_states": output_hidden_states,
"return_dict": return_dict,
"past_key_values": past_key_values,
}
)
if "past_key_values" in fwd_params:
return self.base_model(start_positions=start_positions, end_positions=end_positions, **kwargs)
else:
transformer_backbone_name = self.base_model.get_submodule(self.transformer_backbone_name)
fwd_params = list(inspect.signature(transformer_backbone_name.forward).parameters.keys())
if "past_key_values" not in fwd_params:
raise ValueError("Model does not support past key values which are required for prefix tuning.")
outputs = transformer_backbone_name(**kwargs)
sequence_output = outputs[0]
if "dropout" in [name for name, _ in list(self.base_model.named_children())]:
sequence_output = self.base_model.dropout(sequence_output)
logits = self.base_model.get_submodule(self.cls_layer_name)(sequence_output)
start_logits, end_logits = logits.split(1, dim=-1)
start_logits = start_logits.squeeze(-1).contiguous()
end_logits = end_logits.squeeze(-1).contiguous()
total_loss = None
if start_positions is not None and end_positions is not None:
# If we are on multi-GPU, split add a dimension
if len(start_positions.size()) > 1:
start_positions = start_positions.squeeze(-1)
if len(end_positions.size()) > 1:
end_positions = end_positions.squeeze(-1)
# sometimes the start/end positions are outside our model inputs, we ignore these terms
ignored_index = start_logits.size(1)
start_positions = start_positions.clamp(0, ignored_index)
end_positions = end_positions.clamp(0, ignored_index)
loss_fct = CrossEntropyLoss(ignore_index=ignored_index)
start_loss = loss_fct(start_logits, start_positions)
end_loss = loss_fct(end_logits, end_positions)
total_loss = (start_loss + end_loss) / 2
if not return_dict:
output = (start_logits, end_logits) + outputs[2:]
return ((total_loss,) + output) if total_loss is not None else output
return QuestionAnsweringModelOutput(
loss=total_loss,
start_logits=start_logits,
end_logits=end_logits,
hidden_states=outputs.hidden_states,
attentions=outputs.attentions,
)
class PeftModelForFeatureExtraction(PeftModel):
"""
Peft model for extracting features/embeddings from transformer models
Args:
model ([`~transformers.PreTrainedModel`]): Base transformer model.
peft_config ([`PeftConfig`]): Peft config.
**Attributes**:
- **config** ([`~transformers.PretrainedConfig`]) -- The configuration object of the base model.
Example:
```py
>>> from transformers import AutoModel
>>> from peft import PeftModelForFeatureExtraction, get_peft_config
>>> config = {
... "peft_type": "LORA",
... "task_type": "FEATURE_EXTRACTION",
... "inference_mode": False,
... "r": 16,
... "target_modules": ["query", "value"],
... "lora_alpha": 32,
... "lora_dropout": 0.05,
... "fan_in_fan_out": False,
... "bias": "none",
... }
>>> peft_config = get_peft_config(config)
>>> model = AutoModel.from_pretrained("bert-base-cased")
>>> peft_model = PeftModelForFeatureExtraction(model, peft_config)
>>> peft_model.print_trainable_parameters()
```
"""
def __init__(self, model: torch.nn.Module, peft_config: PeftConfig, adapter_name: str = "default"):
super().__init__(model, peft_config, adapter_name)
def forward(
self,
input_ids=None,
attention_mask=None,
inputs_embeds=None,
output_attentions=None,
output_hidden_states=None,
return_dict=None,
task_ids=None,
**kwargs,
):
peft_config = self.active_peft_config
if not peft_config.is_prompt_learning:
if peft_config.peft_type == PeftType.POLY:
kwargs["task_ids"] = task_ids
with self._enable_peft_forward_hooks(**kwargs):
kwargs = {k: v for k, v in kwargs.items() if k not in self.special_peft_forward_args}
return self.base_model(
input_ids=input_ids,
attention_mask=attention_mask,
inputs_embeds=inputs_embeds,
output_attentions=output_attentions,
output_hidden_states=output_hidden_states,
return_dict=return_dict,
**kwargs,
)
batch_size = _get_batch_size(input_ids, inputs_embeds)
if attention_mask is not None:
# concat prompt attention mask
prefix_attention_mask = torch.ones(batch_size, peft_config.num_virtual_tokens).to(attention_mask.device)
attention_mask = torch.cat((prefix_attention_mask, attention_mask), dim=1)
if kwargs.get("position_ids", None) is not None:
warnings.warn("Position ids are not supported for parameter efficient tuning. Ignoring position ids.")
kwargs["position_ids"] = None
if kwargs.get("token_type_ids", None) is not None:
warnings.warn("Token type ids are not supported for parameter efficient tuning. Ignoring token type ids")
kwargs["token_type_ids"] = None
kwargs.update(
{
"attention_mask": attention_mask,
"output_attentions": output_attentions,
"output_hidden_states": output_hidden_states,
"return_dict": return_dict,
}
)
if peft_config.peft_type == PeftType.PREFIX_TUNING:
past_key_values = self.get_prompt(batch_size)
return self.base_model(input_ids=input_ids, past_key_values=past_key_values, **kwargs)
else:
if inputs_embeds is None:
inputs_embeds = self.word_embeddings(input_ids)
prompts = self.get_prompt(batch_size=batch_size)
prompts = prompts.to(inputs_embeds.dtype)
inputs_embeds = torch.cat((prompts, inputs_embeds), dim=1)
return self.base_model(inputs_embeds=inputs_embeds, **kwargs)
| peft/src/peft/peft_model.py/0 | {
"file_path": "peft/src/peft/peft_model.py",
"repo_id": "peft",
"token_count": 42321
} | 175 |
# Copyright 2023-present the HuggingFace Inc. team.
#
# Licensed under the Apache License, Version 2.0 (the "License");
# you may not use this file except in compliance with the License.
# You may obtain a copy of the License at
#
# http://www.apache.org/licenses/LICENSE-2.0
#
# Unless required by applicable law or agreed to in writing, software
# distributed under the License is distributed on an "AS IS" BASIS,
# WITHOUT WARRANTIES OR CONDITIONS OF ANY KIND, either express or implied.
# See the License for the specific language governing permissions and
# limitations under the License.
from dataclasses import dataclass, field
from typing import List, Optional, Union
from peft.config import PeftConfig
from peft.utils import PeftType
@dataclass
class IA3Config(PeftConfig):
"""
This is the configuration class to store the configuration of a [`IA3Model`].
Args:
target_modules (`Optional[Union[List[str], str]]`):
The names of the modules to apply the adapter to. If this is specified, only the modules with the specified
names will be replaced. When passing a string, a regex match will be performed. When passing a list of
strings, either an exact match will be performed or it is checked if the name of the module ends with any
of the passed strings. If this is specified as 'all-linear', then all linear/Conv1D modules are chosen,
excluding the output layer. If this is not specified, modules will be chosen according to the model
architecture. If the architecture is not known, an error will be raised -- in this case, you should specify
the target modules manually.
feedforward_modules (`Optional[Union[List[str], str]]`):
The names of the modules to be treated as feedforward modules, as in the original paper. These modules will
have (IA)³ vectors multiplied to the input, instead of the output. `feedforward_modules` must be a name or
a subset of names present in `target_modules`.
fan_in_fan_out (`bool`):
Set this to True if the layer to replace stores weight like (fan_in, fan_out). For example, gpt-2 uses
`Conv1D` which stores weights like (fan_in, fan_out) and hence this should be set to `True`.
modules_to_save (`Optional[List[str]]`):
List of modules apart from (IA)³ layers to be set as trainable and saved in the final checkpoint.
init_ia3_weights (`bool`):
Whether to initialize the vectors in the (IA)³ layers, defaults to `True`. Setting this to `False` is
discouraged.
"""
target_modules: Optional[Union[List[str], str]] = field(
default=None,
metadata={
"help": (
"List of module names or regex expression of the module names to replace with (IA)³."
"For example, ['q', 'v'] or '.*decoder.*(SelfAttention|EncDecAttention).*(q|v)$'."
"This can also be a wildcard 'all-linear' which matches all linear/Conv1D layers except the output layer."
"If not specified, modules will be chosen according to the model architecture, If the architecture is "
"not known, an error will be raised -- in this case, you should specify the target modules manually."
),
},
)
feedforward_modules: Optional[Union[List[str], str]] = field(
default=None,
metadata={
"help": "List of module names or a regex expression of module names which are feedforward"
"For example, ['output.dense']"
},
)
fan_in_fan_out: bool = field(
default=False,
metadata={"help": "Set this to True if the layer to replace stores weight like (fan_in, fan_out)"},
)
modules_to_save: Optional[List[str]] = field(
default=None,
metadata={
"help": "List of modules apart from (IA)^3 layers to be set as trainable and saved in the final checkpoint. "
"For example, in Sequence Classification or Token Classification tasks, "
"the final layer `classifier/score` are randomly initialized and as such need to be trainable and saved."
},
)
init_ia3_weights: bool = field(
default=True,
metadata={"help": "Whether to initialize the vectors in the (IA)^3 layers."},
)
def __post_init__(self):
self.peft_type = PeftType.IA3
self.target_modules = (
set(self.target_modules) if isinstance(self.target_modules, list) else self.target_modules
)
self.feedforward_modules = (
set(self.feedforward_modules) if isinstance(self.feedforward_modules, list) else self.feedforward_modules
)
# check if feedforward_modules is a subset of target_modules. run the check only if both are sets
if isinstance(self.feedforward_modules, set) and isinstance(self.target_modules, set):
if not self.feedforward_modules.issubset(self.target_modules):
raise ValueError("`feedforward_modules` should be a subset of `target_modules`")
| peft/src/peft/tuners/ia3/config.py/0 | {
"file_path": "peft/src/peft/tuners/ia3/config.py",
"repo_id": "peft",
"token_count": 1842
} | 176 |
# Copyright 2023-present the HuggingFace Inc. team.
#
# Licensed under the Apache License, Version 2.0 (the "License");
# you may not use this file except in compliance with the License.
# You may obtain a copy of the License at
#
# http://www.apache.org/licenses/LICENSE-2.0
#
# Unless required by applicable law or agreed to in writing, software
# distributed under the License is distributed on an "AS IS" BASIS,
# WITHOUT WARRANTIES OR CONDITIONS OF ANY KIND, either express or implied.
# See the License for the specific language governing permissions and
# limitations under the License.
from typing import Any, Optional
import torch
from peft.tuners.lora.layer import LoraLayer
from peft.tuners.tuners_utils import BaseTunerLayer
from peft.utils import get_auto_gptq_quant_linear
class QuantLinear(torch.nn.Module, LoraLayer):
def __init__(
self,
base_layer,
adapter_name: str,
r: int = 0,
lora_alpha: int = 1,
lora_dropout: float = 0.0,
init_lora_weights: bool = True,
use_rslora: bool = False,
use_dora: bool = False,
**kwargs,
):
super().__init__()
LoraLayer.__init__(self, base_layer)
if use_dora:
raise ValueError(f"{self.__class__.__name__} does not support DoRA yet, please set it to False")
# self.base_layer and self.quant_linear_module are the same; we need the former for consistency and the latter
# for backwards compatibility
self.quant_linear_module = base_layer
self._active_adapter = adapter_name
self.update_layer(
adapter_name,
r,
lora_alpha=lora_alpha,
lora_dropout=lora_dropout,
init_lora_weights=init_lora_weights,
use_rslora=use_rslora,
use_dora=use_dora,
)
def forward(self, x: torch.Tensor):
# note: logic differs from default Linear because merging is not supported
result = self.quant_linear_module(x)
if self.disable_adapters:
return result
for active_adapter in self.active_adapters:
if active_adapter not in self.lora_A.keys():
continue
lora_A = self.lora_A[active_adapter]
lora_B = self.lora_B[active_adapter]
dropout = self.lora_dropout[active_adapter]
scaling = self.scaling[active_adapter]
requires_conversion = not torch.is_autocast_enabled()
if requires_conversion:
expected_dtype = result.dtype
x = x.to(lora_A.weight.dtype)
output = lora_B(lora_A(dropout(x)))
if requires_conversion:
output = output.to(expected_dtype)
output = output * scaling
result += output
return result
def __repr__(self) -> str:
rep = super().__repr__()
return "lora." + rep
# TODO: Check if it is better as suggested by users https://github.com/PanQiWei/AutoGPTQ/pull/102
# def reset_lora_parameters(self, adapter_name):
# if adapter_name in self.lora_A.keys():
# torch.nn.init.xavier_uniform_(self.lora_A[adapter_name].weight)
# torch.nn.init.zeros_(self.lora_B[adapter_name].weight)
def dispatch_gptq(
target: torch.nn.Module,
adapter_name: str,
**kwargs: Any,
) -> Optional[torch.nn.Module]:
new_module = None
if isinstance(target, BaseTunerLayer):
target_base_layer = target.get_base_layer()
else:
target_base_layer = target
gptq_quantization_config = kwargs.get("gptq_quantization_config", None)
AutoGPTQQuantLinear = get_auto_gptq_quant_linear(gptq_quantization_config)
if AutoGPTQQuantLinear is not None and isinstance(target_base_layer, AutoGPTQQuantLinear):
new_module = QuantLinear(target, adapter_name, **kwargs)
target.qweight = target_base_layer.qweight
return new_module
| peft/src/peft/tuners/lora/gptq.py/0 | {
"file_path": "peft/src/peft/tuners/lora/gptq.py",
"repo_id": "peft",
"token_count": 1708
} | 177 |
# Copyright 2023-present the HuggingFace Inc. team.
#
# Licensed under the Apache License, Version 2.0 (the "License");
# you may not use this file except in compliance with the License.
# You may obtain a copy of the License at
#
# http://www.apache.org/licenses/LICENSE-2.0
#
# Unless required by applicable law or agreed to in writing, software
# distributed under the License is distributed on an "AS IS" BASIS,
# WITHOUT WARRANTIES OR CONDITIONS OF ANY KIND, either express or implied.
# See the License for the specific language governing permissions and
# limitations under the License.
# Based on https://github.com/NVIDIA/NeMo/blob/main/nemo/collections/nlp/modules/common/prompt_encoder.py
# with some refactor
import warnings
import torch
from .config import PromptEncoderConfig, PromptEncoderReparameterizationType
class PromptEncoder(torch.nn.Module):
"""
The prompt encoder network that is used to generate the virtual token embeddings for p-tuning.
Args:
config ([`PromptEncoderConfig`]): The configuration of the prompt encoder.
Example:
```py
>>> from peft import PromptEncoder, PromptEncoderConfig
>>> config = PromptEncoderConfig(
... peft_type="P_TUNING",
... task_type="SEQ_2_SEQ_LM",
... num_virtual_tokens=20,
... token_dim=768,
... num_transformer_submodules=1,
... num_attention_heads=12,
... num_layers=12,
... encoder_reparameterization_type="MLP",
... encoder_hidden_size=768,
... )
>>> prompt_encoder = PromptEncoder(config)
```
**Attributes**:
- **embedding** (`torch.nn.Embedding`) -- The embedding layer of the prompt encoder.
- **mlp_head** (`torch.nn.Sequential`) -- The MLP head of the prompt encoder if `inference_mode=False`.
- **lstm_head** (`torch.nn.LSTM`) -- The LSTM head of the prompt encoder if `inference_mode=False` and
`encoder_reparameterization_type="LSTM"`.
- **token_dim** (`int`) -- The hidden embedding dimension of the base transformer model.
- **input_size** (`int`) -- The input size of the prompt encoder.
- **output_size** (`int`) -- The output size of the prompt encoder.
- **hidden_size** (`int`) -- The hidden size of the prompt encoder.
- **total_virtual_tokens** (`int`): The total number of virtual tokens of the
prompt encoder.
- **encoder_type** (Union[[`PromptEncoderReparameterizationType`], `str`]): The encoder type of the prompt
encoder.
Input shape: (`batch_size`, `total_virtual_tokens`)
Output shape: (`batch_size`, `total_virtual_tokens`, `token_dim`)
"""
def __init__(self, config):
super().__init__()
self.token_dim = config.token_dim
self.input_size = self.token_dim
self.output_size = self.token_dim
self.hidden_size = config.encoder_hidden_size
self.total_virtual_tokens = config.num_virtual_tokens * config.num_transformer_submodules
self.encoder_type = config.encoder_reparameterization_type
# embedding
self.embedding = torch.nn.Embedding(self.total_virtual_tokens, self.token_dim)
if not config.inference_mode:
if self.encoder_type == PromptEncoderReparameterizationType.LSTM:
lstm_dropout = config.encoder_dropout
num_layers = config.encoder_num_layers
# LSTM
self.lstm_head = torch.nn.LSTM(
input_size=self.input_size,
hidden_size=self.hidden_size,
num_layers=num_layers,
dropout=lstm_dropout,
bidirectional=True,
batch_first=True,
)
self.mlp_head = torch.nn.Sequential(
torch.nn.Linear(self.hidden_size * 2, self.hidden_size * 2),
torch.nn.ReLU(),
torch.nn.Linear(self.hidden_size * 2, self.output_size),
)
elif self.encoder_type == PromptEncoderReparameterizationType.MLP:
encoder_num_layers_default = PromptEncoderConfig.encoder_num_layers
if config.encoder_num_layers != encoder_num_layers_default:
warnings.warn(
f"for {self.encoder_type.value}, the argument `encoder_num_layers` is ignored. "
f"Exactly {encoder_num_layers_default} MLP layers are used."
)
layers = [
torch.nn.Linear(self.input_size, self.hidden_size),
torch.nn.ReLU(),
torch.nn.Linear(self.hidden_size, self.hidden_size),
torch.nn.ReLU(),
torch.nn.Linear(self.hidden_size, self.output_size),
]
self.mlp_head = torch.nn.Sequential(*layers)
else:
raise ValueError("Prompt encoder type not recognized. Please use one of MLP (recommended) or LSTM.")
def forward(self, indices):
input_embeds = self.embedding(indices)
if self.encoder_type == PromptEncoderReparameterizationType.LSTM:
output_embeds = self.mlp_head(self.lstm_head(input_embeds)[0])
elif self.encoder_type == PromptEncoderReparameterizationType.MLP:
output_embeds = self.mlp_head(input_embeds)
else:
raise ValueError("Prompt encoder type not recognized. Please use one of MLP (recommended) or LSTM.")
return output_embeds
| peft/src/peft/tuners/p_tuning/model.py/0 | {
"file_path": "peft/src/peft/tuners/p_tuning/model.py",
"repo_id": "peft",
"token_count": 2476
} | 178 |
# Copyright 2023-present the HuggingFace Inc. team.
#
# Licensed under the Apache License, Version 2.0 (the "License");
# you may not use this file except in compliance with the License.
# You may obtain a copy of the License at
#
# http://www.apache.org/licenses/LICENSE-2.0
#
# Unless required by applicable law or agreed to in writing, software
# distributed under the License is distributed on an "AS IS" BASIS,
# WITHOUT WARRANTIES OR CONDITIONS OF ANY KIND, either express or implied.
# See the License for the specific language governing permissions and
# limitations under the License.
# Reference code: https://github.com/yxli2123/LoftQ/blob/main/utils.py
# Reference paper: https://arxiv.org/abs/2310.08659
from __future__ import annotations
import logging
import os
from typing import Callable, Optional, Union
import torch
from huggingface_hub import snapshot_download
from huggingface_hub.utils import LocalEntryNotFoundError
from safetensors import SafetensorError, safe_open
from transformers.utils import cached_file
from transformers.utils.hub import get_checkpoint_shard_files
from peft.import_utils import is_bnb_4bit_available, is_bnb_available
if is_bnb_available():
import bitsandbytes as bnb
class NFQuantizer:
def __init__(self, num_bits=2, device="cuda", method="normal", block_size=64, *args, **kwargs):
super().__init__(*args, **kwargs)
self.num_bits = num_bits
self.device = device
self.method = method
self.block_size = block_size
if self.method == "normal":
self.norm_lookup_table = self.create_normal_map(num_bits=self.num_bits)
self.norm_lookup_table = self.norm_lookup_table.to(device)
elif self.method == "uniform":
self.norm_lookup_table = self.create_uniform_map(num_bits=self.num_bits)
self.norm_lookup_table = self.norm_lookup_table.to(device)
else:
raise NotImplementedError("Other quantization methods not supported yet.")
@staticmethod
def create_uniform_map(symmetric=False, num_bits=4):
if symmetric:
# print("symmetric uniform quantization")
negative = torch.linspace(-1, 0, 2 ** (num_bits - 1))
positive = torch.linspace(0, 1, 2 ** (num_bits - 1))
table = torch.cat([negative, positive[1:]])
else:
# print("asymmetric uniform quantization")
table = torch.linspace(-1, 1, 2**num_bits)
return table
@staticmethod
def create_normal_map(offset=0.9677083, symmetric=False, num_bits=2):
try:
from scipy.stats import norm
except ImportError:
raise ImportError("The required package 'scipy' is not installed. Please install it to continue.")
variations = 2**num_bits
if symmetric:
v = norm.ppf(torch.linspace(1 - offset, offset, variations + 1)).tolist()
values = []
for index in range(len(v) - 1):
values.append(0.5 * v[index] + 0.5 * v[index + 1])
v = values
else:
# one more positive value, this is an asymmetric type
v1 = norm.ppf(torch.linspace(offset, 0.5, variations // 2 + 1)[:-1]).tolist()
v2 = [0]
v3 = (-norm.ppf(torch.linspace(offset, 0.5, variations // 2)[:-1])).tolist()
v = v1 + v2 + v3
values = torch.Tensor(v)
values = values.sort().values
values /= values.max()
return values
def quantize_tensor(self, weight):
max_abs = torch.abs(weight).max()
weight_normed = weight / max_abs
weight_normed_expanded = weight_normed.unsqueeze(-1)
# Reshape L to have the same number of dimensions as X_expanded
L_reshaped = torch.tensor(self.norm_lookup_table).reshape(1, -1)
# Calculate the absolute difference between X_expanded and L_reshaped
abs_diff = torch.abs(weight_normed_expanded - L_reshaped)
# Find the index of the minimum absolute difference for each element
qweight = torch.argmin(abs_diff, dim=-1)
return qweight, max_abs
def dequantize_tensor(self, qweight, max_abs):
qweight_flatten = qweight.flatten()
weight_normed = self.norm_lookup_table[qweight_flatten]
weight = weight_normed * max_abs
weight = weight.reshape(qweight.shape)
return weight
def quantize_block(self, weight):
if len(weight.shape) != 2:
raise ValueError(f"Only support 2D matrix, but your input has {len(weight.shape)} dimensions.")
if weight.shape[0] * weight.shape[1] % self.block_size != 0:
raise ValueError(
f"Weight with shape ({weight.shape[0]} x {weight.shape[1]}) "
f"is not dividable by block size {self.block_size}."
)
M, N = weight.shape
device = weight.device
# Quantization
weight_flatten = weight.flatten() # (M*N, )
weight_block = weight_flatten.reshape(-1, self.block_size) # (L, B), L = M * N / B
if self.method == "normal":
weight_max = weight_block.abs().max(dim=-1)[0] # (L, 1)
elif self.method == "uniform":
weight_max = weight_block.mean(dim=-1) + 2.5 * weight_block.std(dim=-1)
else:
raise NotImplementedError("Method not supported yet.")
weight_max = weight_max.unsqueeze(-1)
weight_divabs = weight_block / weight_max # (L, B)
weight_divabs = weight_divabs.unsqueeze(-1) # (L, B, 1)
L_reshaped = self.norm_lookup_table.reshape(1, -1) # (1, 2**K)
abs_diff = torch.abs(weight_divabs - L_reshaped) # (L, B, 2**K)
qweight = torch.argmin(abs_diff, dim=-1) # (L, B)
# Pack multiple k-bit into uint8
qweight = qweight.reshape(-1, 8 // self.num_bits)
qweight_pack = torch.zeros((M * N // 8 * self.num_bits, 1), dtype=torch.uint8, device=device)
# data format example:
# [1, 0, 3, 2] or [01, 00, 11, 10] -> [10110001], LIFO
for i in range(8 // self.num_bits):
qweight[:, i] = qweight[:, i] << i * self.num_bits
qweight_pack[:, 0] |= qweight[:, i]
return qweight_pack, weight_max, weight.shape
def dequantize_block(self, qweight, weight_max, weight_shape):
# unpack weight
device = qweight.device
weight = torch.zeros((qweight.shape[0], 8 // self.num_bits), dtype=torch.float32, device=device)
for i in range(8 // self.num_bits):
lookup_table_idx = qweight.to(torch.long) % 2**self.num_bits # get the most right 2 bits
lookup_table_idx = lookup_table_idx.to(torch.long)
weight[:, i] = self.norm_lookup_table[lookup_table_idx].squeeze()
qweight = qweight >> self.num_bits # right shift 2 bits of the original data
weight_block = weight.reshape(-1, self.block_size)
weight = weight_block * weight_max
weight = weight.reshape(weight_shape)
return weight
def _low_rank_decomposition(weight, reduced_rank=32):
"""
:param weight: The matrix to decompose, of shape (H, W) :param reduced_rank: the final rank :return:
"""
matrix_dimension = len(weight.size())
if matrix_dimension != 2:
raise ValueError(f"Only support 2D matrix, but your input has {matrix_dimension} dimensions.")
# Use SVD to decompose a matrix, default full_matrices is False to save parameters
U, S, Vh = torch.linalg.svd(weight, full_matrices=False)
L = U @ (torch.sqrt(torch.diag(S)[:, 0:reduced_rank]))
R = torch.sqrt(torch.diag(S)[0:reduced_rank, :]) @ Vh
return {"L": L, "R": R, "U": U, "S": S, "Vh": Vh, "reduced_rank": reduced_rank}
@torch.no_grad()
def loftq_init(weight: Union[torch.Tensor, torch.nn.Parameter], num_bits: int, reduced_rank: int, num_iter=1):
if num_bits not in [2, 4, 8]:
raise ValueError("Only support 2, 4, 8 bits quantization")
if num_iter <= 0:
raise ValueError("Number of iterations must be greater than 0")
out_feature, in_feature = weight.size()
device = weight.device
dtype = weight.dtype
logging.info(
f"Weight: ({out_feature}, {in_feature}) | Rank: {reduced_rank} "
f"| Num Iter: {num_iter} | Num Bits: {num_bits}"
)
if not is_bnb_4bit_available() or num_bits in [2, 8]:
quantizer = NFQuantizer(num_bits=num_bits, device=device, method="normal", block_size=64)
compute_device = device
else:
compute_device = "cuda"
weight = weight.to(device=compute_device, dtype=torch.float32)
res = weight.clone()
for i in range(num_iter):
torch.cuda.empty_cache()
# Quantization
if num_bits == 4 and is_bnb_4bit_available():
qweight = bnb.nn.Params4bit(
res.to("cpu"), requires_grad=False, compress_statistics=False, quant_type="nf4"
).to(compute_device)
dequantized_weight = bnb.functional.dequantize_4bit(qweight.data, qweight.quant_state)
else:
quantized_weight, max_abs, shape = quantizer.quantize_block(res)
dequantized_weight = quantizer.dequantize_block(quantized_weight, max_abs, shape)
res = weight - dequantized_weight
# Decompose the residual by SVD
output = _low_rank_decomposition(res, reduced_rank=reduced_rank)
L, R, reduced_rank = output["L"], output["R"], output["reduced_rank"]
res = weight - torch.mm(L, R)
lora_A, lora_B = R, L
return dequantized_weight.to(device=device, dtype=dtype), lora_A, lora_B
@torch.no_grad()
def _loftq_init_new(qweight, weight, num_bits: int, reduced_rank: int):
if num_bits != 4:
raise ValueError("Only 4 bit quantization supported at the moment.")
if not is_bnb_4bit_available():
raise ValueError("bitsandbytes 4bit quantization is not available.")
compute_device = "cuda"
dequantized_weight = bnb.functional.dequantize_4bit(qweight.data, qweight.quant_state)
weight = weight.to(device=compute_device, dtype=torch.float32)
residual = weight - dequantized_weight
torch.cuda.empty_cache()
# Decompose the residualidual by SVD
output = _low_rank_decomposition(residual, reduced_rank=reduced_rank)
L, R, reduced_rank = output["L"], output["R"], output["reduced_rank"]
return R, L
class _SafetensorLoader:
"""
Simple utility class that loads tensors with safetensors from a single file or sharded files.
Takes care of file name normalization etc.
"""
def __init__(self, peft_model, model_path):
if model_path is None:
try:
model_path = snapshot_download(peft_model.base_model.config._name_or_path, local_files_only=True)
except AttributeError as exc:
raise ValueError(
"The provided model does not appear to be a transformers model. In this case, you must pass the "
"model_path to the safetensors file."
) from exc
except LocalEntryNotFoundError as exc:
raise ValueError(
"The model.safetensors file must be present on disk, but it could not be found."
) from exc
suffix = "model.safetensors"
if not model_path.endswith(suffix):
model_path = os.path.join(model_path, suffix)
self.model_path = model_path
self.base_model_prefix = getattr(peft_model.get_base_model(), "base_model_prefix", None)
self.prefix = "base_model.model."
self.is_sharded = False
self.weight_map = None
if not os.path.exists(model_path):
# check if the file is sharded
par_dir = model_path.rpartition(os.path.sep)[0]
try:
resolved_archive_file, sharded_metadata = get_checkpoint_shard_files(
par_dir, cached_file(par_dir, "model.safetensors.index.json")
)
except OSError as exc:
raise FileNotFoundError(
f"Could not find file for {model_path}, ensure that there is a (sharded) safetensors file of the model."
) from exc
self.is_sharded = True
# maps from 'model-X-of-Y.safetensors' to full file path
file_map = {k.rpartition(os.path.sep)[-1]: k for k in resolved_archive_file}
self.weight_map = {k: file_map[v] for k, v in sharded_metadata["weight_map"].items()}
def get_tensor(self, name):
if not self.is_sharded:
file_path = self.model_path
else:
file_path = self.weight_map[name]
with safe_open(file_path, framework="pt", device="cpu") as f:
try:
tensor = f.get_tensor(name)
except SafetensorError as exc:
# no matching key found, we probably need to remove the base model prefix
if self.base_model_prefix:
# remove 1 extra character for "."
name = name[len(self.base_model_prefix) + 1 :]
tensor = f.get_tensor(name)
else:
raise exc
return tensor
@torch.no_grad()
def replace_lora_weights_loftq(
peft_model,
model_path: Optional[str] = None,
adapter_name: str = "default",
callback: Optional[Callable[[torch.nn.Module, str], bool]] = None,
):
"""
Replace the LoRA weights of a model quantized with bitsandbytes, using the LoftQ technique.
The replacement is done on the fly by loading in the non-quantized weights from a locally stored safetensors model
file and initializing the LoRA weights such that the quantization error between the original and quantized weights
is minimized.
As lazy loading is not possible with pickle, normal PyTorch checkpoint files cannot be supported.
Depending on the model size, calling this function may take some time to finish.
Args:
peft_model (`PeftModel`):
The model to replace the weights of. Must be a quantized PEFT model with LoRA layers.
model_path (`Optional[str]`):
The path to the model safetensors file. If the model is a Hugging Face model, this will be inferred from
the model's config. Otherwise, it must be provided.
adapter_name (`str`):
The name of the adapter to replace the weights of. The default adapter name is "default".
callback (`Optional[Callable[[PeftModel, str], bool]]`):
A callback function that will be called after each module is replaced. The callback function should take
the model and the name of the current module as input and return a boolean indicating whether the
replacement should be kept. If the callback returns False, the replacement will be rolled back. This can be
very useful to confirm that the LoftQ initialization actually decreases the quantization error of the
model. As an example, this callback could generate logits for given input and compare it with the logits
from the original, non-quanitzed model with the same input, and only return `True` if there is an
improvement. As this is a greedy optimization, it's possible that calling this function multiple times
yields incremental improvements.
"""
if not is_bnb_4bit_available():
raise ValueError("bitsandbytes must be installed and the model must be quantized in 4bits.")
from peft.tuners.lora import Linear4bit
# model_path = _check_model_path_loftq(model_path, peft_model)
prefix = "base_model.model."
any_match = False
safetensor_loader = _SafetensorLoader(peft_model, model_path)
# if too slow, consider adding tqdm as an option
for name, module in peft_model.named_modules():
if not isinstance(module, Linear4bit):
continue
if not name.startswith(prefix):
raise TypeError("The passed model does not appear to be a valid PeftModel")
any_match = True
name = name[len(prefix) :]
tensor = safetensor_loader.get_tensor(name + ".weight")
reduced_rank = module.r[adapter_name]
lora_A, lora_B = _loftq_init_new(module.weight, tensor, num_bits=4, reduced_rank=reduced_rank)
if not callback:
module.lora_A[adapter_name].weight.data = lora_A
module.lora_B[adapter_name].weight.data = lora_B
continue
lora_A_before = module.lora_A[adapter_name].weight.data
lora_B_before = module.lora_B[adapter_name].weight.data
module.lora_A[adapter_name].weight.data = lora_A
module.lora_B[adapter_name].weight.data = lora_B
should_replace = callback(peft_model, name)
if not should_replace:
# roll back
module.lora_A[adapter_name].weight.data = lora_A_before
module.lora_B[adapter_name].weight.data = lora_B_before
del lora_A_before, lora_B_before
if not any_match:
raise ValueError("No bnb LoRA module found on the model")
| peft/src/peft/utils/loftq_utils.py/0 | {
"file_path": "peft/src/peft/utils/loftq_utils.py",
"repo_id": "peft",
"token_count": 7192
} | 179 |
# Copyright 2023-present the HuggingFace Inc. team.
#
# Licensed under the Apache License, Version 2.0 (the "License");
# you may not use this file except in compliance with the License.
# You may obtain a copy of the License at
#
# http://www.apache.org/licenses/LICENSE-2.0
#
# Unless required by applicable law or agreed to in writing, software
# distributed under the License is distributed on an "AS IS" BASIS,
# WITHOUT WARRANTIES OR CONDITIONS OF ANY KIND, either express or implied.
# See the License for the specific language governing permissions and
# limitations under the License.
import gc
import importlib
import os
import tempfile
import unittest
from dataclasses import dataclass
from typing import Any, Dict, List, Union
import pytest
import torch
from accelerate import infer_auto_device_map
from accelerate.test_utils.testing import run_command
from accelerate.utils import patch_environment
from datasets import Audio, DatasetDict, load_dataset
from packaging import version
from parameterized import parameterized
from transformers import (
AutoModelForCausalLM,
AutoModelForSeq2SeqLM,
AutoTokenizer,
BitsAndBytesConfig,
DataCollatorForLanguageModeling,
Seq2SeqTrainer,
Seq2SeqTrainingArguments,
Trainer,
TrainingArguments,
WhisperFeatureExtractor,
WhisperForConditionalGeneration,
WhisperProcessor,
WhisperTokenizer,
)
from peft import (
AdaLoraConfig,
LoftQConfig,
LoraConfig,
PeftModel,
TaskType,
get_peft_model,
prepare_model_for_int8_training,
prepare_model_for_kbit_training,
replace_lora_weights_loftq,
)
from peft.utils import SAFETENSORS_WEIGHTS_NAME
from .testing_utils import (
require_aqlm,
require_auto_awq,
require_auto_gptq,
require_bitsandbytes,
require_optimum,
require_torch_gpu,
require_torch_multi_gpu,
)
# A full testing suite that tests all the necessary features on GPU. The tests should
# rely on the example scripts to test the features.
@dataclass
class DataCollatorSpeechSeq2SeqWithPadding:
r"""
Directly copied from:
https://github.com/huggingface/peft/blob/main/examples/int8_training/peft_bnb_whisper_large_v2_training.ipynb
"""
processor: Any
def __call__(self, features: List[Dict[str, Union[List[int], torch.Tensor]]]) -> Dict[str, torch.Tensor]:
# split inputs and labels since they have to be of different lengths and need different padding methods
# first treat the audio inputs by simply returning torch tensors
input_features = [{"input_features": feature["input_features"]} for feature in features]
batch = self.processor.feature_extractor.pad(input_features, return_tensors="pt")
# get the tokenized label sequences
label_features = [{"input_ids": feature["labels"]} for feature in features]
# pad the labels to max length
labels_batch = self.processor.tokenizer.pad(label_features, return_tensors="pt")
# replace padding with -100 to ignore loss correctly
labels = labels_batch["input_ids"].masked_fill(labels_batch.attention_mask.ne(1), -100)
# if bos token is appended in previous tokenization step,
# cut bos token here as it's append later anyways
if (labels[:, 0] == self.processor.tokenizer.bos_token_id).all().cpu().item():
labels = labels[:, 1:]
batch["labels"] = labels
return batch
@require_torch_gpu
@require_bitsandbytes
class PeftBnbGPUExampleTests(unittest.TestCase):
r"""
A single GPU int8 + fp4 test suite, this will test if training fits correctly on a single GPU device (1x NVIDIA T4
16GB) using bitsandbytes.
The tests are the following:
- Seq2Seq model training based on:
https://github.com/huggingface/peft/blob/main/examples/int8_training/Finetune_flan_t5_large_bnb_peft.ipynb
- Causal LM model training based on:
https://github.com/huggingface/peft/blob/main/examples/int8_training/Finetune_opt_bnb_peft.ipynb
- Audio model training based on:
https://github.com/huggingface/peft/blob/main/examples/int8_training/peft_bnb_whisper_large_v2_training.ipynb
"""
def setUp(self):
self.seq2seq_model_id = "google/flan-t5-base"
self.causal_lm_model_id = "facebook/opt-6.7b"
self.tokenizer = AutoTokenizer.from_pretrained(self.causal_lm_model_id)
self.audio_model_id = "openai/whisper-large"
def tearDown(self):
r"""
Efficient mechanism to free GPU memory after each test. Based on
https://github.com/huggingface/transformers/issues/21094
"""
gc.collect()
if torch.cuda.is_available():
torch.cuda.empty_cache()
gc.collect()
def _check_inference_finite(self, model, batch):
# try inference without Trainer class
training = model.training
model.eval()
output = model(**batch.to(model.device))
assert torch.isfinite(output.logits).all()
model.train(training)
@pytest.mark.single_gpu_tests
def test_causal_lm_training(self):
r"""
Test the CausalLM training on a single GPU device. This test is a converted version of
https://github.com/huggingface/peft/blob/main/examples/int8_training/Finetune_opt_bnb_peft.ipynb where we train
`opt-6.7b` on `english_quotes` dataset in few steps. The test would simply fail if the adapters are not set
correctly.
"""
with tempfile.TemporaryDirectory() as tmp_dir:
model = AutoModelForCausalLM.from_pretrained(
self.causal_lm_model_id,
quantization_config=BitsAndBytesConfig(load_in_8bit=True),
device_map="auto",
)
tokenizer = AutoTokenizer.from_pretrained(self.causal_lm_model_id)
model = prepare_model_for_int8_training(model)
config = LoraConfig(
r=16,
lora_alpha=32,
target_modules=["q_proj", "v_proj"],
lora_dropout=0.05,
bias="none",
task_type="CAUSAL_LM",
)
model = get_peft_model(model, config)
data = load_dataset("ybelkada/english_quotes_copy")
data = data.map(lambda samples: tokenizer(samples["quote"]), batched=True)
trainer = Trainer(
model=model,
train_dataset=data["train"],
args=TrainingArguments(
per_device_train_batch_size=4,
gradient_accumulation_steps=4,
warmup_steps=2,
max_steps=3,
learning_rate=2e-4,
fp16=True,
logging_steps=1,
output_dir=tmp_dir,
),
data_collator=DataCollatorForLanguageModeling(tokenizer, mlm=False),
)
model.config.use_cache = False
trainer.train()
model.cpu().save_pretrained(tmp_dir)
assert "adapter_config.json" in os.listdir(tmp_dir)
assert SAFETENSORS_WEIGHTS_NAME in os.listdir(tmp_dir)
# assert loss is not None
assert trainer.state.log_history[-1]["train_loss"] is not None
@pytest.mark.single_gpu_tests
def test_causal_lm_training_4bit(self):
r"""
Test the CausalLM training on a single GPU device. This test is a converted version of
https://github.com/huggingface/peft/blob/main/examples/int8_training/Finetune_opt_bnb_peft.ipynb where we train
`opt-6.7b` on `english_quotes` dataset in few steps using 4bit base model. The test would simply fail if the
adapters are not set correctly.
"""
with tempfile.TemporaryDirectory() as tmp_dir:
model = AutoModelForCausalLM.from_pretrained(
self.causal_lm_model_id,
quantization_config=BitsAndBytesConfig(load_in_4bit=True),
device_map="auto",
)
tokenizer = AutoTokenizer.from_pretrained(self.causal_lm_model_id)
model = prepare_model_for_kbit_training(model)
config = LoraConfig(
r=16,
lora_alpha=32,
target_modules=["q_proj", "v_proj"],
lora_dropout=0.05,
bias="none",
task_type="CAUSAL_LM",
)
model = get_peft_model(model, config)
data = load_dataset("ybelkada/english_quotes_copy")
data = data.map(lambda samples: tokenizer(samples["quote"]), batched=True)
trainer = Trainer(
model=model,
train_dataset=data["train"],
args=TrainingArguments(
per_device_train_batch_size=4,
gradient_accumulation_steps=4,
warmup_steps=2,
max_steps=3,
learning_rate=2e-4,
fp16=True,
logging_steps=1,
output_dir=tmp_dir,
),
data_collator=DataCollatorForLanguageModeling(tokenizer, mlm=False),
)
model.config.use_cache = False
trainer.train()
model.cpu().save_pretrained(tmp_dir)
assert "adapter_config.json" in os.listdir(tmp_dir)
assert SAFETENSORS_WEIGHTS_NAME in os.listdir(tmp_dir)
# assert loss is not None
assert trainer.state.log_history[-1]["train_loss"] is not None
@pytest.mark.multi_gpu_tests
def test_causal_lm_training_multi_gpu_4bit(self):
r"""
Test the CausalLM training on a multi-GPU device with 4bit base model. The test would simply fail if the
adapters are not set correctly.
"""
with tempfile.TemporaryDirectory() as tmp_dir:
model = AutoModelForCausalLM.from_pretrained(
self.causal_lm_model_id,
device_map="auto",
quantization_config=BitsAndBytesConfig(load_in_4bit=True),
)
assert set(model.hf_device_map.values()) == set(range(torch.cuda.device_count()))
model = prepare_model_for_kbit_training(model)
setattr(model, "model_parallel", True)
setattr(model, "is_parallelizable", True)
config = LoraConfig(
r=16,
lora_alpha=32,
target_modules=["q_proj", "v_proj"],
lora_dropout=0.05,
bias="none",
task_type="CAUSAL_LM",
)
model = get_peft_model(model, config)
data = load_dataset("Abirate/english_quotes")
data = data.map(lambda samples: self.tokenizer(samples["quote"]), batched=True)
trainer = Trainer(
model=model,
train_dataset=data["train"],
args=TrainingArguments(
per_device_train_batch_size=4,
gradient_accumulation_steps=4,
warmup_steps=2,
max_steps=3,
learning_rate=2e-4,
fp16=True,
logging_steps=1,
output_dir=tmp_dir,
),
data_collator=DataCollatorForLanguageModeling(self.tokenizer, mlm=False),
)
model.config.use_cache = False
trainer.train()
model.cpu().save_pretrained(tmp_dir)
assert "adapter_config.json" in os.listdir(tmp_dir)
assert SAFETENSORS_WEIGHTS_NAME in os.listdir(tmp_dir)
# assert loss is not None
assert trainer.state.log_history[-1]["train_loss"] is not None
@pytest.mark.single_gpu_tests
@require_torch_gpu
def test_4bit_adalora_causalLM(self):
r"""
Tests the 4bit training with adalora
"""
model_id = "facebook/opt-350m"
# for >3 GPUs, might need: device_map={"": "cuda:0"}
model = AutoModelForCausalLM.from_pretrained(
model_id, quantization_config=BitsAndBytesConfig(load_in_4bit=True)
)
tokenizer = AutoTokenizer.from_pretrained(model_id)
model.gradient_checkpointing_enable()
model = prepare_model_for_kbit_training(model)
peft_config = AdaLoraConfig(
init_r=6,
target_r=4,
tinit=50,
tfinal=100,
deltaT=5,
beta1=0.3,
beta2=0.3,
orth_reg_weight=0.2,
lora_alpha=32,
lora_dropout=0.05,
bias="none",
task_type="CAUSAL_LM",
)
model = get_peft_model(model, peft_config)
data = load_dataset("ybelkada/english_quotes_copy")
data = data.map(lambda samples: tokenizer(samples["quote"]), batched=True)
batch = tokenizer(data["train"][:3]["quote"], return_tensors="pt", padding=True)
self._check_inference_finite(model, batch)
with tempfile.TemporaryDirectory() as tmp_dir:
trainer = Trainer(
model=model,
train_dataset=data["train"],
args=TrainingArguments(
per_device_train_batch_size=4,
gradient_accumulation_steps=4,
warmup_steps=2,
max_steps=3,
learning_rate=2e-4,
fp16=True,
logging_steps=1,
output_dir=tmp_dir,
),
data_collator=DataCollatorForLanguageModeling(tokenizer, mlm=False),
)
model.config.use_cache = False
trainer.train()
model.cpu().save_pretrained(tmp_dir)
assert "adapter_config.json" in os.listdir(tmp_dir)
assert SAFETENSORS_WEIGHTS_NAME in os.listdir(tmp_dir)
# assert loss is not None
assert trainer.state.log_history[-1]["train_loss"] is not None
@pytest.mark.single_gpu_tests
@require_torch_gpu
def test_8bit_adalora_causalLM(self):
r"""
Tests the 8bit training with adalora
"""
model_id = "facebook/opt-350m"
model = AutoModelForCausalLM.from_pretrained(
model_id, quantization_config=BitsAndBytesConfig(load_in_8bit=True)
)
tokenizer = AutoTokenizer.from_pretrained(model_id)
model.gradient_checkpointing_enable()
model = prepare_model_for_kbit_training(model)
peft_config = AdaLoraConfig(
init_r=6,
target_r=4,
tinit=50,
tfinal=100,
deltaT=5,
beta1=0.3,
beta2=0.3,
orth_reg_weight=0.2,
lora_alpha=32,
lora_dropout=0.05,
bias="none",
task_type="CAUSAL_LM",
)
model = get_peft_model(model, peft_config)
data = load_dataset("ybelkada/english_quotes_copy")
data = data.map(lambda samples: tokenizer(samples["quote"]), batched=True)
batch = tokenizer(data["train"][:3]["quote"], return_tensors="pt", padding=True)
self._check_inference_finite(model, batch)
with tempfile.TemporaryDirectory() as tmp_dir:
trainer = Trainer(
model=model,
train_dataset=data["train"],
args=TrainingArguments(
per_device_train_batch_size=4,
gradient_accumulation_steps=4,
warmup_steps=2,
max_steps=3,
learning_rate=2e-4,
fp16=True,
logging_steps=1,
output_dir=tmp_dir,
),
data_collator=DataCollatorForLanguageModeling(tokenizer, mlm=False),
)
model.config.use_cache = False
trainer.train()
model.cpu().save_pretrained(tmp_dir)
assert "adapter_config.json" in os.listdir(tmp_dir)
assert SAFETENSORS_WEIGHTS_NAME in os.listdir(tmp_dir)
# assert loss is not None
assert trainer.state.log_history[-1]["train_loss"] is not None
@pytest.mark.multi_gpu_tests
@require_torch_multi_gpu
def test_causal_lm_training_multi_gpu(self):
r"""
Test the CausalLM training on a multi-GPU device. This test is a converted version of
https://github.com/huggingface/peft/blob/main/examples/int8_training/Finetune_opt_bnb_peft.ipynb where we train
`opt-6.7b` on `english_quotes` dataset in few steps. The test would simply fail if the adapters are not set
correctly.
"""
with tempfile.TemporaryDirectory() as tmp_dir:
model = AutoModelForCausalLM.from_pretrained(
self.causal_lm_model_id,
quantization_config=BitsAndBytesConfig(load_in_8bit=True),
device_map="auto",
)
assert set(model.hf_device_map.values()) == set(range(torch.cuda.device_count()))
tokenizer = AutoTokenizer.from_pretrained(self.causal_lm_model_id)
model = prepare_model_for_int8_training(model)
setattr(model, "model_parallel", True)
setattr(model, "is_parallelizable", True)
config = LoraConfig(
r=16,
lora_alpha=32,
target_modules=["q_proj", "v_proj"],
lora_dropout=0.05,
bias="none",
task_type="CAUSAL_LM",
)
model = get_peft_model(model, config)
data = load_dataset("Abirate/english_quotes")
data = data.map(lambda samples: tokenizer(samples["quote"]), batched=True)
trainer = Trainer(
model=model,
train_dataset=data["train"],
args=TrainingArguments(
per_device_train_batch_size=4,
gradient_accumulation_steps=4,
warmup_steps=2,
max_steps=3,
learning_rate=2e-4,
fp16=True,
logging_steps=1,
output_dir=tmp_dir,
),
data_collator=DataCollatorForLanguageModeling(tokenizer, mlm=False),
)
model.config.use_cache = False
trainer.train()
model.cpu().save_pretrained(tmp_dir)
assert "adapter_config.json" in os.listdir(tmp_dir)
assert SAFETENSORS_WEIGHTS_NAME in os.listdir(tmp_dir)
# assert loss is not None
assert trainer.state.log_history[-1]["train_loss"] is not None
@pytest.mark.single_gpu_tests
def test_seq2seq_lm_training_single_gpu(self):
r"""
Test the Seq2SeqLM training on a single GPU device. This test is a converted version of
https://github.com/huggingface/peft/blob/main/examples/int8_training/Finetune_opt_bnb_peft.ipynb where we train
`flan-large` on `english_quotes` dataset in few steps. The test would simply fail if the adapters are not set
correctly.
"""
with tempfile.TemporaryDirectory() as tmp_dir:
model = AutoModelForSeq2SeqLM.from_pretrained(
self.seq2seq_model_id,
quantization_config=BitsAndBytesConfig(load_in_8bit=True),
device_map={"": 0},
)
assert set(model.hf_device_map.values()) == {0}
tokenizer = AutoTokenizer.from_pretrained(self.seq2seq_model_id)
model = prepare_model_for_int8_training(model)
config = LoraConfig(
r=16,
lora_alpha=32,
target_modules=["q", "v"],
lora_dropout=0.05,
bias="none",
task_type="CAUSAL_LM",
)
model = get_peft_model(model, config)
data = load_dataset("ybelkada/english_quotes_copy")
data = data.map(lambda samples: tokenizer(samples["quote"]), batched=True)
trainer = Trainer(
model=model,
train_dataset=data["train"],
args=TrainingArguments(
per_device_train_batch_size=4,
gradient_accumulation_steps=4,
warmup_steps=2,
max_steps=3,
learning_rate=2e-4,
fp16=True,
logging_steps=1,
output_dir=tmp_dir,
),
data_collator=DataCollatorForLanguageModeling(tokenizer, mlm=False),
)
model.config.use_cache = False
trainer.train()
model.cpu().save_pretrained(tmp_dir)
assert "adapter_config.json" in os.listdir(tmp_dir)
assert SAFETENSORS_WEIGHTS_NAME in os.listdir(tmp_dir)
# assert loss is not None
assert trainer.state.log_history[-1]["train_loss"] is not None
@pytest.mark.multi_gpu_tests
@require_torch_multi_gpu
def test_seq2seq_lm_training_multi_gpu(self):
r"""
Test the Seq2SeqLM training on a multi-GPU device. This test is a converted version of
https://github.com/huggingface/peft/blob/main/examples/int8_training/Finetune_opt_bnb_peft.ipynb where we train
`flan-large` on `english_quotes` dataset in few steps. The test would simply fail if the adapters are not set
correctly.
"""
with tempfile.TemporaryDirectory() as tmp_dir:
model = AutoModelForSeq2SeqLM.from_pretrained(
self.seq2seq_model_id,
quantization_config=BitsAndBytesConfig(load_in_8bit=True),
device_map="balanced",
)
assert set(model.hf_device_map.values()) == set(range(torch.cuda.device_count()))
tokenizer = AutoTokenizer.from_pretrained(self.seq2seq_model_id)
model = prepare_model_for_int8_training(model)
config = LoraConfig(
r=16,
lora_alpha=32,
target_modules=["q", "v"],
lora_dropout=0.05,
bias="none",
task_type="CAUSAL_LM",
)
model = get_peft_model(model, config)
data = load_dataset("ybelkada/english_quotes_copy")
data = data.map(lambda samples: tokenizer(samples["quote"]), batched=True)
trainer = Trainer(
model=model,
train_dataset=data["train"],
args=TrainingArguments(
per_device_train_batch_size=4,
gradient_accumulation_steps=4,
warmup_steps=2,
max_steps=3,
learning_rate=2e-4,
fp16=True,
logging_steps=1,
output_dir="outputs",
),
data_collator=DataCollatorForLanguageModeling(tokenizer, mlm=False),
)
model.config.use_cache = False
trainer.train()
model.cpu().save_pretrained(tmp_dir)
assert "adapter_config.json" in os.listdir(tmp_dir)
assert SAFETENSORS_WEIGHTS_NAME in os.listdir(tmp_dir)
# assert loss is not None
assert trainer.state.log_history[-1]["train_loss"] is not None
@pytest.mark.single_gpu_tests
def test_audio_model_training(self):
r"""
Test the audio model training on a single GPU device. This test is a converted version of
https://github.com/huggingface/peft/blob/main/examples/int8_training/peft_bnb_whisper_large_v2_training.ipynb
"""
with tempfile.TemporaryDirectory() as tmp_dir:
dataset_name = "ybelkada/common_voice_mr_11_0_copy"
task = "transcribe"
language = "Marathi"
common_voice = DatasetDict()
common_voice["train"] = load_dataset(dataset_name, split="train+validation")
common_voice = common_voice.remove_columns(
["accent", "age", "client_id", "down_votes", "gender", "locale", "path", "segment", "up_votes"]
)
feature_extractor = WhisperFeatureExtractor.from_pretrained(self.audio_model_id)
tokenizer = WhisperTokenizer.from_pretrained(self.audio_model_id, language=language, task=task)
processor = WhisperProcessor.from_pretrained(self.audio_model_id, language=language, task=task)
common_voice = common_voice.cast_column("audio", Audio(sampling_rate=16000))
def prepare_dataset(batch):
# load and resample audio data from 48 to 16kHz
audio = batch["audio"]
# compute log-Mel input features from input audio array
batch["input_features"] = feature_extractor(
audio["array"], sampling_rate=audio["sampling_rate"]
).input_features[0]
# encode target text to label ids
batch["labels"] = tokenizer(batch["sentence"]).input_ids
return batch
common_voice = common_voice.map(
prepare_dataset, remove_columns=common_voice.column_names["train"], num_proc=2
)
data_collator = DataCollatorSpeechSeq2SeqWithPadding(processor=processor)
model = WhisperForConditionalGeneration.from_pretrained(
self.audio_model_id, quantization_config=BitsAndBytesConfig(load_in_8bit=True), device_map="auto"
)
model.config.forced_decoder_ids = None
model.config.suppress_tokens = []
model = prepare_model_for_int8_training(model)
# as Whisper model uses Conv layer in encoder, checkpointing disables grad computation
# to avoid this, make the inputs trainable
def make_inputs_require_grad(module, input, output):
output.requires_grad_(True)
model.model.encoder.conv1.register_forward_hook(make_inputs_require_grad)
config = LoraConfig(
r=32, lora_alpha=64, target_modules=["q_proj", "v_proj"], lora_dropout=0.05, bias="none"
)
model = get_peft_model(model, config)
model.print_trainable_parameters()
training_args = Seq2SeqTrainingArguments(
output_dir=tmp_dir, # change to a repo name of your choice
per_device_train_batch_size=8,
gradient_accumulation_steps=1, # increase by 2x for every 2x decrease in batch size
learning_rate=1e-3,
warmup_steps=2,
max_steps=3,
fp16=True,
per_device_eval_batch_size=8,
generation_max_length=128,
logging_steps=25,
remove_unused_columns=False, # required as the PeftModel forward doesn't have the signature of the wrapped model's forward
label_names=["labels"], # same reason as above
)
trainer = Seq2SeqTrainer(
args=training_args,
model=model,
train_dataset=common_voice["train"],
data_collator=data_collator,
tokenizer=processor.feature_extractor,
)
trainer.train()
model.cpu().save_pretrained(tmp_dir)
assert "adapter_config.json" in os.listdir(tmp_dir)
assert SAFETENSORS_WEIGHTS_NAME in os.listdir(tmp_dir)
# assert loss is not None
assert trainer.state.log_history[-1]["train_loss"] is not None
@pytest.mark.single_gpu_tests
def test_4bit_non_default_adapter_name(self):
# See PR 1294
config = LoraConfig(
r=16,
target_modules=["q_proj", "v_proj"],
bias="none",
task_type="CAUSAL_LM",
)
# default adapter name
model = AutoModelForCausalLM.from_pretrained(
"facebook/opt-125m",
device_map="auto",
quantization_config=BitsAndBytesConfig(load_in_4bit=True),
)
model = prepare_model_for_kbit_training(model)
model = get_peft_model(model, config)
n_trainable_default, n_total_default = model.get_nb_trainable_parameters()
# other adapter name
model = AutoModelForCausalLM.from_pretrained(
"facebook/opt-125m",
device_map="auto",
quantization_config=BitsAndBytesConfig(load_in_4bit=True),
)
model = prepare_model_for_kbit_training(model)
model = get_peft_model(model, config, adapter_name="other")
n_trainable_other, n_total_other = model.get_nb_trainable_parameters()
assert n_trainable_other > 0
# sanity check
assert n_trainable_default == n_trainable_other
assert n_total_default == n_total_other
@pytest.mark.single_gpu_tests
def test_8bit_non_default_adapter_name(self):
# See PR 1294
config = LoraConfig(
r=16,
target_modules=["q_proj", "v_proj"],
bias="none",
task_type="CAUSAL_LM",
)
# default adapter name
model = AutoModelForCausalLM.from_pretrained(
"facebook/opt-125m",
device_map="auto",
quantization_config=BitsAndBytesConfig(load_in_8bit=True),
)
model = prepare_model_for_kbit_training(model)
model = get_peft_model(model, config)
n_trainable_default, n_total_default = model.get_nb_trainable_parameters()
# other adapter name
model = AutoModelForCausalLM.from_pretrained(
"facebook/opt-125m",
device_map="auto",
quantization_config=BitsAndBytesConfig(load_in_8bit=True),
)
model = prepare_model_for_kbit_training(model)
model = get_peft_model(model, config, adapter_name="other")
n_trainable_other, n_total_other = model.get_nb_trainable_parameters()
assert n_trainable_other > 0
# sanity check
assert n_trainable_default == n_trainable_other
assert n_total_default == n_total_other
@pytest.mark.single_gpu_tests
def test_causal_lm_training_4bit_dora(self):
r"""
Same as test_causal_lm_training_4bit but with DoRA
"""
with tempfile.TemporaryDirectory() as tmp_dir:
model = AutoModelForCausalLM.from_pretrained(
self.causal_lm_model_id,
load_in_4bit=True,
device_map="auto",
)
tokenizer = AutoTokenizer.from_pretrained(self.causal_lm_model_id)
model = prepare_model_for_kbit_training(model)
config = LoraConfig(
r=16,
lora_alpha=32,
target_modules=["q_proj", "v_proj"],
lora_dropout=0.05,
bias="none",
task_type="CAUSAL_LM",
use_dora=True,
)
model = get_peft_model(model, config)
data = load_dataset("ybelkada/english_quotes_copy")
data = data.map(lambda samples: tokenizer(samples["quote"]), batched=True)
trainer = Trainer(
model=model,
train_dataset=data["train"],
args=TrainingArguments(
per_device_train_batch_size=4,
gradient_accumulation_steps=4,
warmup_steps=2,
max_steps=3,
learning_rate=2e-4,
fp16=True,
logging_steps=1,
output_dir=tmp_dir,
),
data_collator=DataCollatorForLanguageModeling(tokenizer, mlm=False),
)
model.config.use_cache = False
trainer.train()
model.cpu().save_pretrained(tmp_dir)
assert "adapter_config.json" in os.listdir(tmp_dir)
assert SAFETENSORS_WEIGHTS_NAME in os.listdir(tmp_dir)
# assert loss is not None
assert trainer.state.log_history[-1]["train_loss"] is not None
@pytest.mark.multi_gpu_tests
def test_causal_lm_training_multi_gpu_4bit_dora(self):
r"""
Same as test_causal_lm_training_multi_gpu_4bit but with DoRA
"""
with tempfile.TemporaryDirectory() as tmp_dir:
model = AutoModelForCausalLM.from_pretrained(
self.causal_lm_model_id,
device_map="auto",
load_in_4bit=True,
)
assert set(model.hf_device_map.values()) == set(range(torch.cuda.device_count()))
model = prepare_model_for_kbit_training(model)
setattr(model, "model_parallel", True)
setattr(model, "is_parallelizable", True)
config = LoraConfig(
r=16,
lora_alpha=32,
target_modules=["q_proj", "v_proj"],
lora_dropout=0.05,
bias="none",
task_type="CAUSAL_LM",
use_dora=True,
)
model = get_peft_model(model, config)
data = load_dataset("Abirate/english_quotes")
data = data.map(lambda samples: self.tokenizer(samples["quote"]), batched=True)
trainer = Trainer(
model=model,
train_dataset=data["train"],
args=TrainingArguments(
per_device_train_batch_size=4,
gradient_accumulation_steps=4,
warmup_steps=2,
max_steps=3,
learning_rate=2e-4,
fp16=True,
logging_steps=1,
output_dir=tmp_dir,
),
data_collator=DataCollatorForLanguageModeling(self.tokenizer, mlm=False),
)
model.config.use_cache = False
trainer.train()
model.cpu().save_pretrained(tmp_dir)
assert "adapter_config.json" in os.listdir(tmp_dir)
assert SAFETENSORS_WEIGHTS_NAME in os.listdir(tmp_dir)
# assert loss is not None
assert trainer.state.log_history[-1]["train_loss"] is not None
@pytest.mark.single_gpu_tests
def test_causal_lm_training_8bit_dora(self):
r"""
Same as test_causal_lm_training_4bit_dora but with 8bit
"""
with tempfile.TemporaryDirectory() as tmp_dir:
model = AutoModelForCausalLM.from_pretrained(
self.causal_lm_model_id,
load_in_8bit=True,
device_map="auto",
)
tokenizer = AutoTokenizer.from_pretrained(self.causal_lm_model_id)
model = prepare_model_for_kbit_training(model)
config = LoraConfig(
r=16,
lora_alpha=32,
target_modules=["q_proj", "v_proj"],
lora_dropout=0.05,
bias="none",
task_type="CAUSAL_LM",
use_dora=True,
)
model = get_peft_model(model, config)
data = load_dataset("ybelkada/english_quotes_copy")
data = data.map(lambda samples: tokenizer(samples["quote"]), batched=True)
trainer = Trainer(
model=model,
train_dataset=data["train"],
args=TrainingArguments(
per_device_train_batch_size=4,
gradient_accumulation_steps=4,
warmup_steps=2,
max_steps=3,
learning_rate=2e-4,
fp16=True,
logging_steps=1,
output_dir=tmp_dir,
),
data_collator=DataCollatorForLanguageModeling(tokenizer, mlm=False),
)
model.config.use_cache = False
trainer.train()
model.cpu().save_pretrained(tmp_dir)
assert "adapter_config.json" in os.listdir(tmp_dir)
assert SAFETENSORS_WEIGHTS_NAME in os.listdir(tmp_dir)
# assert loss is not None
assert trainer.state.log_history[-1]["train_loss"] is not None
@pytest.mark.multi_gpu_tests
def test_causal_lm_training_multi_gpu_8bit_dora(self):
r"""
Same as test_causal_lm_training_multi_gpu_4bit_dora but with 8bit
"""
with tempfile.TemporaryDirectory() as tmp_dir:
model = AutoModelForCausalLM.from_pretrained(
self.causal_lm_model_id,
device_map="auto",
load_in_8bit=True,
)
assert set(model.hf_device_map.values()) == set(range(torch.cuda.device_count()))
model = prepare_model_for_kbit_training(model)
setattr(model, "model_parallel", True)
setattr(model, "is_parallelizable", True)
config = LoraConfig(
r=16,
lora_alpha=32,
target_modules=["q_proj", "v_proj"],
lora_dropout=0.05,
bias="none",
task_type="CAUSAL_LM",
use_dora=True,
)
model = get_peft_model(model, config)
data = load_dataset("Abirate/english_quotes")
data = data.map(lambda samples: self.tokenizer(samples["quote"]), batched=True)
trainer = Trainer(
model=model,
train_dataset=data["train"],
args=TrainingArguments(
per_device_train_batch_size=4,
gradient_accumulation_steps=4,
warmup_steps=2,
max_steps=3,
learning_rate=2e-4,
fp16=True,
logging_steps=1,
output_dir=tmp_dir,
),
data_collator=DataCollatorForLanguageModeling(self.tokenizer, mlm=False),
)
model.config.use_cache = False
trainer.train()
model.cpu().save_pretrained(tmp_dir)
assert "adapter_config.json" in os.listdir(tmp_dir)
assert SAFETENSORS_WEIGHTS_NAME in os.listdir(tmp_dir)
# assert loss is not None
assert trainer.state.log_history[-1]["train_loss"] is not None
@require_torch_gpu
@require_auto_gptq
@require_optimum
class PeftGPTQGPUTests(unittest.TestCase):
r"""
GPTQ + peft tests
"""
def setUp(self):
from transformers import GPTQConfig
self.causal_lm_model_id = "marcsun13/opt-350m-gptq-4bit"
# TODO : check if it works for Exllamav2 kernels
self.quantization_config = GPTQConfig(bits=4, use_exllama=False)
self.tokenizer = AutoTokenizer.from_pretrained(self.causal_lm_model_id)
def tearDown(self):
r"""
Efficient mechanism to free GPU memory after each test. Based on
https://github.com/huggingface/transformers/issues/21094
"""
gc.collect()
torch.cuda.empty_cache()
def _check_inference_finite(self, model, batch):
# try inference without Trainer class
training = model.training
model.eval()
output = model(**batch.to(model.device))
assert torch.isfinite(output.logits).all()
model.train(training)
@pytest.mark.single_gpu_tests
def test_causal_lm_training(self):
r"""
Test the CausalLM training on a single GPU device. The test would simply fail if the adapters are not set
correctly.
"""
with tempfile.TemporaryDirectory() as tmp_dir:
model = AutoModelForCausalLM.from_pretrained(
self.causal_lm_model_id,
torch_dtype=torch.float16,
device_map="auto",
quantization_config=self.quantization_config,
)
model = prepare_model_for_kbit_training(model)
config = LoraConfig(
r=16,
lora_alpha=32,
target_modules=["q_proj", "v_proj"],
lora_dropout=0.05,
bias="none",
task_type="CAUSAL_LM",
)
model = get_peft_model(model, config)
data = load_dataset("ybelkada/english_quotes_copy")
data = data.map(lambda samples: self.tokenizer(samples["quote"]), batched=True)
trainer = Trainer(
model=model,
train_dataset=data["train"],
args=TrainingArguments(
per_device_train_batch_size=4,
gradient_accumulation_steps=4,
warmup_steps=2,
max_steps=3,
learning_rate=2e-4,
fp16=True,
logging_steps=1,
output_dir=tmp_dir,
),
data_collator=DataCollatorForLanguageModeling(self.tokenizer, mlm=False),
)
model.config.use_cache = False
trainer.train()
model.cpu().save_pretrained(tmp_dir)
assert "adapter_config.json" in os.listdir(tmp_dir)
assert SAFETENSORS_WEIGHTS_NAME in os.listdir(tmp_dir)
# assert loss is not None
assert trainer.state.log_history[-1]["train_loss"] is not None
@pytest.mark.single_gpu_tests
def test_adalora_causalLM(self):
r"""
Tests the gptq training with adalora
"""
model = AutoModelForCausalLM.from_pretrained(
self.causal_lm_model_id,
torch_dtype=torch.float16,
device_map="auto",
quantization_config=self.quantization_config,
)
tokenizer = AutoTokenizer.from_pretrained(self.causal_lm_model_id)
model = prepare_model_for_kbit_training(model)
peft_config = AdaLoraConfig(
init_r=6,
target_r=4,
tinit=50,
tfinal=100,
deltaT=5,
beta1=0.3,
beta2=0.3,
orth_reg_weight=0.2,
lora_alpha=32,
lora_dropout=0.05,
bias="none",
task_type="CAUSAL_LM",
)
model = get_peft_model(model, peft_config)
data = load_dataset("ybelkada/english_quotes_copy")
data = data.map(lambda samples: self.tokenizer(samples["quote"]), batched=True)
batch = tokenizer(data["train"][:3]["quote"], return_tensors="pt", padding=True)
self._check_inference_finite(model, batch)
with tempfile.TemporaryDirectory() as tmp_dir:
trainer = Trainer(
model=model,
train_dataset=data["train"],
args=TrainingArguments(
per_device_train_batch_size=4,
gradient_accumulation_steps=4,
warmup_steps=2,
max_steps=3,
learning_rate=2e-4,
fp16=True,
logging_steps=1,
output_dir=tmp_dir,
),
data_collator=DataCollatorForLanguageModeling(self.tokenizer, mlm=False),
)
model.config.use_cache = False
trainer.train()
model.cpu().save_pretrained(tmp_dir)
assert "adapter_config.json" in os.listdir(tmp_dir)
assert SAFETENSORS_WEIGHTS_NAME in os.listdir(tmp_dir)
# assert loss is not None
assert trainer.state.log_history[-1]["train_loss"] is not None
@pytest.mark.multi_gpu_tests
@require_torch_multi_gpu
def test_causal_lm_training_multi_gpu(self):
r"""
Test the CausalLM training on a multi-GPU device. The test would simply fail if the adapters are not set
correctly.
"""
with tempfile.TemporaryDirectory() as tmp_dir:
model = AutoModelForCausalLM.from_pretrained(
self.causal_lm_model_id,
torch_dtype=torch.float16,
device_map="auto",
quantization_config=self.quantization_config,
)
assert set(model.hf_device_map.values()) == set(range(torch.cuda.device_count()))
model = prepare_model_for_kbit_training(model)
setattr(model, "model_parallel", True)
setattr(model, "is_parallelizable", True)
config = LoraConfig(
r=16,
lora_alpha=32,
target_modules=["q_proj", "v_proj"],
lora_dropout=0.05,
bias="none",
task_type="CAUSAL_LM",
)
model = get_peft_model(model, config)
data = load_dataset("Abirate/english_quotes")
data = data.map(lambda samples: self.tokenizer(samples["quote"]), batched=True)
trainer = Trainer(
model=model,
train_dataset=data["train"],
args=TrainingArguments(
per_device_train_batch_size=4,
gradient_accumulation_steps=4,
warmup_steps=2,
max_steps=3,
learning_rate=2e-4,
fp16=True,
logging_steps=1,
output_dir=tmp_dir,
),
data_collator=DataCollatorForLanguageModeling(self.tokenizer, mlm=False),
)
model.config.use_cache = False
trainer.train()
model.cpu().save_pretrained(tmp_dir)
assert "adapter_config.json" in os.listdir(tmp_dir)
assert SAFETENSORS_WEIGHTS_NAME in os.listdir(tmp_dir)
# assert loss is not None
assert trainer.state.log_history[-1]["train_loss"] is not None
@pytest.mark.single_gpu_tests
def test_non_default_adapter_name(self):
# See issue 1346
config = LoraConfig(
r=16,
target_modules=["q_proj", "v_proj"],
task_type="CAUSAL_LM",
)
# default adapter name
model = AutoModelForCausalLM.from_pretrained(
self.causal_lm_model_id,
torch_dtype=torch.float16,
device_map="auto",
quantization_config=self.quantization_config,
)
model = prepare_model_for_kbit_training(model)
model = get_peft_model(model, config)
n_trainable_default, n_total_default = model.get_nb_trainable_parameters()
# other adapter name
model = AutoModelForCausalLM.from_pretrained(
self.causal_lm_model_id,
torch_dtype=torch.float16,
device_map="auto",
quantization_config=self.quantization_config,
)
model = prepare_model_for_kbit_training(model)
model = get_peft_model(model, config, adapter_name="other")
n_trainable_other, n_total_other = model.get_nb_trainable_parameters()
assert n_trainable_other > 0
# sanity check
assert n_trainable_default == n_trainable_other
assert n_total_default == n_total_other
@require_torch_gpu
class OffloadSaveTests(unittest.TestCase):
def setUp(self):
self.causal_lm_model_id = "gpt2"
def tearDown(self):
r"""
Efficient mechanism to free GPU memory after each test. Based on
https://github.com/huggingface/transformers/issues/21094
"""
gc.collect()
torch.cuda.empty_cache()
@pytest.mark.single_gpu_tests
@require_torch_gpu
def test_offload_merge(self):
r"""
Test merging, unmerging, and unloading of a model with CPU- offloaded modules.
"""
torch.manual_seed(0)
model = AutoModelForCausalLM.from_pretrained(self.causal_lm_model_id)
tokenizer = AutoTokenizer.from_pretrained(self.causal_lm_model_id)
# TODO: add disk offload once PeftModel.from_pretrained supports
memory_limits = {0: "0.4GIB", "cpu": "5GIB"}
# offloads around half of all transformer modules
device_map = infer_auto_device_map(model, max_memory=memory_limits)
assert 0 in device_map.values()
assert "cpu" in device_map.values()
config = LoraConfig(task_type="CAUSAL_LM", init_lora_weights=False, target_modules=["c_attn"])
model = get_peft_model(model, config)
with tempfile.TemporaryDirectory() as tmp_dir:
model.save_pretrained(tmp_dir)
# load the model with device_map
model = AutoModelForCausalLM.from_pretrained(self.causal_lm_model_id, device_map=device_map).eval()
assert len({p.device for p in model.parameters()}) == 2
model = PeftModel.from_pretrained(model, tmp_dir, max_memory=memory_limits)
input_tokens = tokenizer.encode("Four score and seven years ago", return_tensors="pt")
model.eval()
# test peft model adapter merge
pre_merge_olayer = model(input_tokens)[0]
model.merge_adapter()
post_merge_olayer = model(input_tokens)[0]
assert torch.allclose(post_merge_olayer, pre_merge_olayer)
# test peft model adapter unmerge
model.unmerge_adapter()
post_unmerge_olayer = model(input_tokens)[0]
assert torch.allclose(post_unmerge_olayer, pre_merge_olayer)
# test LoRA merge and unload
model = model.merge_and_unload()
post_unload_merge_olayer = model(input_tokens)[0]
assert torch.allclose(post_unload_merge_olayer, pre_merge_olayer)
@pytest.mark.skipif(not torch.cuda.is_available(), reason="test requires a GPU")
class TestLoftQ:
r"""
Tests for LoftQ to ensure that it reduces the quantization error compared to normal LoRA quantization.
"""
# The error factor indicates by how much the quantization error should be decreased when using LoftQ compared to
# quantization without LoftQ. Thus 1.03 means that the error should be decreased by 3% at least. This is a very
# conservative value to prevent flakiness, in practice most gains are > 1.5
error_factor = 1.03
def get_input(self, model_id, device):
tokenizer = AutoTokenizer.from_pretrained(model_id)
inputs = tokenizer("All I want is", padding=True, return_tensors="pt")
if device == "cuda":
inputs = inputs.to("cuda")
return inputs
def get_base_model(self, model_id, device, **kwargs):
cls = AutoModelForSeq2SeqLM if "t5" in str(model_id) else AutoModelForCausalLM
model = cls.from_pretrained(model_id, **kwargs).eval()
if device == "cuda":
model = model.to("cuda")
return model
def get_logits(self, model, inputs):
if model.config.is_encoder_decoder:
input_ids = inputs["input_ids"]
return model(input_ids=input_ids, decoder_input_ids=input_ids).logits
return model(**inputs).logits
def get_errors(
self,
tmp_path,
bits=4,
loftq_iter=1,
device="cuda",
model_id="hf-internal-testing/tiny-random-BloomForCausalLM",
use_dora=False,
):
# Helper function that returns the quantization errors (MAE and MSE) when comparing the quantized LoRA model
# to the base model, vs the LoftQ quantized model to the base model. We expect the LoftQ quantized model to
# have less error than the normal LoRA quantized model. Since we compare logits, the observed error is
# already somewhat dampened because of the softmax.
torch.manual_seed(0)
model = self.get_base_model(model_id, device)
task_type = TaskType.SEQ_2_SEQ_LM if model.config.is_encoder_decoder else TaskType.CAUSAL_LM
inputs = self.get_input(model_id, device)
# the base logits are the reference, we try to match those as closely as possible
logits_base = self.get_logits(model, inputs)
# clean up
del model
gc.collect()
torch.cuda.empty_cache()
# logits from the normal quantized LoRA model
target_modules = "all-linear" if task_type != TaskType.SEQ_2_SEQ_LM else ["o", "k", "wi", "q", "v"]
lora_config = LoraConfig(task_type=task_type, use_dora=use_dora, target_modules=target_modules)
kwargs = {}
if bits == 4:
kwargs["quantization_config"] = BitsAndBytesConfig(load_in_4bit=True, bnb_4bit_quant_type="nf4")
elif bits == 8:
kwargs["quantization_config"] = BitsAndBytesConfig(load_in_8bit=True)
else:
raise ValueError("bits must be 4 or 8")
quantized_model = get_peft_model(
self.get_base_model(model_id, device=None, **kwargs),
lora_config,
)
torch.manual_seed(0)
logits_quantized = self.get_logits(quantized_model, inputs)
del quantized_model
gc.collect()
torch.cuda.empty_cache()
# logits from quantized LoRA model using LoftQ
loftq_config = LoftQConfig(loftq_bits=bits, loftq_iter=loftq_iter)
lora_config = LoraConfig(
task_type=task_type,
init_lora_weights="loftq",
loftq_config=loftq_config,
use_dora=use_dora,
target_modules=target_modules,
)
model = self.get_base_model(model_id, device)
if device == "cuda":
model = model.to("cuda")
loftq_model = get_peft_model(model, lora_config)
if device == "cuda":
loftq_model = loftq_model.to("cuda")
# save LoRA weights, they should be initialized such that they minimize the quantization error
loftq_model.base_model.peft_config["default"].init_lora_weights = True
loftq_model.save_pretrained(tmp_path / "loftq_model")
loftq_model = loftq_model.unload()
loftq_model.save_pretrained(tmp_path / "base_model")
del loftq_model
gc.collect()
torch.cuda.empty_cache()
# now load quantized model and apply LoftQ-initialized weights on top
base_model = self.get_base_model(tmp_path / "base_model", device=None, **kwargs, torch_dtype=torch.float32)
loftq_model = PeftModel.from_pretrained(base_model, tmp_path / "loftq_model", is_trainable=True)
# TODO sanity check: model is quantized
torch.manual_seed(0)
logits_loftq = self.get_logits(loftq_model, inputs)
del loftq_model
gc.collect()
torch.cuda.empty_cache()
mae_quantized = torch.abs(logits_base - logits_quantized).mean()
mse_quantized = torch.pow(logits_base - logits_quantized, 2).mean()
mae_loftq = torch.abs(logits_base - logits_loftq).mean()
mse_loftq = torch.pow(logits_base - logits_loftq, 2).mean()
return mae_quantized, mse_quantized, mae_loftq, mse_loftq
@pytest.mark.parametrize("device", ["cuda", "cpu"])
def test_bloomz_loftq_4bit(self, device, tmp_path):
# In this test, we compare the logits of the base model, the quantized LoRA model, and the quantized model
# using LoftQ. When quantizing, we expect a certain level of error. However, we expect the LoftQ quantized
# model to have less error than the normal LoRA quantized model. Note that when using normal LoRA, the
# quantization error is simply the error from quantization without LoRA, as LoRA is a no-op before training.
# We still apply LoRA for the test for consistency.
mae_quantized, mse_quantized, mae_loftq, mse_loftq = self.get_errors(bits=4, device=device, tmp_path=tmp_path)
# first, sanity check that all errors are > 0.0
assert mae_quantized > 0.0
assert mse_quantized > 0.0
assert mae_loftq > 0.0
assert mse_loftq > 0.0
# next, check that LoftQ quantization errors are smaller than LoRA errors by a certain margin
assert mse_loftq < (mse_quantized / self.error_factor)
assert mae_loftq < (mae_quantized / self.error_factor)
@pytest.mark.parametrize("device", ["cuda", "cpu"])
def test_bloomz_loftq_4bit_iter_5(self, device, tmp_path):
# Same test as the previous one but with 5 iterations. We should expect the error to be even smaller with more
# iterations, but in practice the difference is not that large, at least not for this small base model.
mae_quantized, mse_quantized, mae_loftq, mse_loftq = self.get_errors(
bits=4, loftq_iter=5, device=device, tmp_path=tmp_path
)
# first, sanity check that all errors are > 0.0
assert mae_quantized > 0.0
assert mse_quantized > 0.0
assert mae_loftq > 0.0
assert mse_loftq > 0.0
# next, check that LoftQ quantization errors are smaller than LoRA errors by a certain margin
assert mse_loftq < (mse_quantized / self.error_factor)
assert mae_loftq < (mae_quantized / self.error_factor)
@pytest.mark.parametrize("device", ["cuda", "cpu"])
def test_bloomz_loftq_8bit(self, device, tmp_path):
# Same test as test_bloomz_loftq_4bit but with 8 bits.
mae_quantized, mse_quantized, mae_loftq, mse_loftq = self.get_errors(bits=8, device=device, tmp_path=tmp_path)
# first, sanity check that all errors are > 0.0
assert mae_quantized > 0.0
assert mse_quantized > 0.0
assert mae_loftq > 0.0
assert mse_loftq > 0.0
# next, check that LoftQ quantization errors are smaller than LoRA errors by a certain margin
assert mse_loftq < (mse_quantized / self.error_factor)
assert mae_loftq < (mae_quantized / self.error_factor)
@pytest.mark.parametrize("device", ["cuda", "cpu"])
def test_bloomz_loftq_8bit_iter_5(self, device, tmp_path):
# Same test as test_bloomz_loftq_4bit_iter_5 but with 8 bits.
mae_quantized, mse_quantized, mae_loftq, mse_loftq = self.get_errors(
bits=8, loftq_iter=5, device=device, tmp_path=tmp_path
)
# first, sanity check that all errors are > 0.0
assert mae_quantized > 0.0
assert mse_quantized > 0.0
assert mae_loftq > 0.0
assert mse_loftq > 0.0
# next, check that LoftQ quantization errors are smaller than LoRA errors by a certain margin
assert mse_loftq < (mse_quantized / self.error_factor)
assert mae_loftq < (mae_quantized / self.error_factor)
@pytest.mark.parametrize("device", ["cuda", "cpu"])
def test_t5_loftq_4bit(self, device, tmp_path):
mae_quantized, mse_quantized, mae_loftq, mse_loftq = self.get_errors(
bits=4, device=device, model_id="t5-small", tmp_path=tmp_path
)
# first, sanity check that all errors are > 0.0
assert mae_quantized > 0.0
assert mse_quantized > 0.0
assert mae_loftq > 0.0
assert mse_loftq > 0.0
# next, check that LoftQ quantization errors are smaller than LoRA errors by a certain margin
assert mse_loftq < (mse_quantized / self.error_factor)
assert mae_loftq < (mae_quantized / self.error_factor)
@pytest.mark.parametrize("device", ["cuda", "cpu"])
def test_t5_loftq_8bit(self, device, tmp_path):
mae_quantized, mse_quantized, mae_loftq, mse_loftq = self.get_errors(
bits=8, device=device, model_id="t5-small", tmp_path=tmp_path
)
# first, sanity check that all errors are > 0.0
assert mae_quantized > 0.0
assert mse_quantized > 0.0
assert mae_loftq > 0.0
assert mse_loftq > 0.0
# next, check that LoftQ quantization errors are smaller than LoRA errors by a certain margin
assert mse_loftq < (mse_quantized / self.error_factor)
assert mae_loftq < (mae_quantized / self.error_factor)
@pytest.mark.xfail # failing for now, but having DoRA pass is only a nice-to-have, not a must, so we're good
@pytest.mark.parametrize("device", ["cuda", "cpu"])
def test_bloomz_loftq_4bit_dora(self, device, tmp_path):
# same as test_bloomz_loftq_4bit but with DoRA
mae_quantized, mse_quantized, mae_loftq, mse_loftq = self.get_errors(
bits=4, device=device, use_dora=True, tmp_path=tmp_path
)
# first, sanity check that all errors are > 0.0
assert mae_quantized > 0.0
assert mse_quantized > 0.0
assert mae_loftq > 0.0
assert mse_loftq > 0.0
# next, check that LoftQ quantization errors are smaller than LoRA errors by a certain margin
factor = 3
assert mae_loftq < (mae_quantized / factor)
assert mse_loftq < (mse_quantized / factor)
@pytest.mark.parametrize("device", ["cuda", "cpu"])
def test_bloomz_loftq_8bit_dora(self, device, tmp_path):
# same as test_bloomz_loftq_8bit but with DoRA
mae_quantized, mse_quantized, mae_loftq, mse_loftq = self.get_errors(
bits=8, device=device, use_dora=True, tmp_path=tmp_path
)
# first, sanity check that all errors are > 0.0
assert mae_quantized > 0.0
assert mse_quantized > 0.0
assert mae_loftq > 0.0
assert mse_loftq > 0.0
# next, check that LoftQ quantization errors are smaller than LoRA errors by a certain margin
assert mae_loftq < (mae_quantized / self.error_factor)
assert mse_loftq < (mse_quantized / self.error_factor)
def test_replace_lora_weights_with_loftq_using_callable(self):
"""
Test replacing LoRa weights with LoFTQ using a callable.
Using the replace_lora_weights_loftq function, we replace the LoRa weights of a bnb-quantized model with LoRA
weights initialized by LoftQ on the fly. We use a callable to decide whether to replace the weights or not.
This callable checks, for each weight, if replacing it would actually result in logits that are closer to the
original logits of the non-quantized model.
"""
torch.manual_seed(0)
model_id = "bigscience/bloomz-560m"
device = "cuda"
tokenizer = AutoTokenizer.from_pretrained(model_id)
inputs = tokenizer("The dog was", padding=True, return_tensors="pt").to(device)
with tempfile.TemporaryDirectory() as tmp_dir:
model = AutoModelForCausalLM.from_pretrained(model_id).to(device)
logits_base = model(**inputs).logits
model.save_pretrained(tmp_dir)
# load in 4bit
bnb_config = BitsAndBytesConfig(
load_in_4bit=True,
bnb_4bit_use_double_quant=True,
)
model = AutoModelForCausalLM.from_pretrained(model_id, quantization_config=bnb_config)
model = get_peft_model(model, LoraConfig(task_type="CAUSAL_LM", target_modules="all-linear"))
logits_lora = model(**inputs).logits
current_mse = float("inf")
logs = []
def my_callback(model, module_name):
"""Callable to replace weights with LoFTQ if the mse is lower than the current best one."""
nonlocal current_mse
logits = model(**inputs).logits
mse = ((logits_base - logits) ** 2).mean()
if mse < current_mse:
current_mse = mse
logs.append(True)
return True
logs.append(False)
return False
replace_lora_weights_loftq(model, model_path=tmp_dir, callback=my_callback)
logits_loftq = model(**inputs).logits
mae_lora = (logits_base - logits_lora).abs().mean()
mae_loftq = (logits_base - logits_loftq).abs().mean()
mse_lora = ((logits_base - logits_lora) ** 2).mean()
mse_loftq = ((logits_base - logits_loftq) ** 2).mean()
# check that the error was reduced by a certain margin
assert mae_loftq * 1.5 < mae_lora
assert mse_loftq * 2.5 < mse_lora
# check that the callback has returned some True and some False values
assert any(logs)
assert not all(logs)
del model
if torch.cuda.is_available():
torch.cuda.empty_cache()
gc.collect()
@require_bitsandbytes
@require_torch_gpu
class MultiprocessTester(unittest.TestCase):
def test_notebook_launcher(self):
script_path = os.path.join("scripts", "launch_notebook_mp.py")
cmd = ["python", script_path]
with patch_environment(omp_num_threads=1):
run_command(cmd, env=os.environ.copy())
@require_torch_gpu
class MixedPrecisionTests(unittest.TestCase):
def setUp(self):
self.causal_lm_model_id = "facebook/opt-350m"
self.tokenizer = AutoTokenizer.from_pretrained(self.causal_lm_model_id)
self.config = LoraConfig(
r=16,
lora_alpha=32,
task_type="CAUSAL_LM",
)
data = load_dataset("ybelkada/english_quotes_copy")
self.data = data.map(lambda samples: self.tokenizer(samples["quote"]), batched=True)
def tearDown(self):
r"""
Efficient mechanism to free GPU memory after each test. Based on
https://github.com/huggingface/transformers/issues/21094
"""
gc.collect()
if torch.cuda.is_available():
torch.cuda.empty_cache()
gc.collect()
@pytest.mark.single_gpu_tests
def test_model_loaded_in_float16_raises(self):
# This test shows the issue with loading the model in fp16 and then trying to use it with mixed precision
# training, which should not use fp16. If this is ever automated in PEFT, this test should fail. In that case,
# remove this test, adjust the next one, and remove the entry about FP16 usage from troubleshooting.md.
model = AutoModelForCausalLM.from_pretrained(
self.causal_lm_model_id,
torch_dtype=torch.float16,
)
model = get_peft_model(model, self.config)
with tempfile.TemporaryDirectory() as tmp_dir:
trainer = Trainer(
model=model,
train_dataset=self.data["train"],
args=TrainingArguments(
fp16=True, # <= this is required for the error to be raised
logging_steps=1,
output_dir=tmp_dir,
),
data_collator=DataCollatorForLanguageModeling(self.tokenizer, mlm=False),
)
with pytest.raises(ValueError, match="Attempting to unscale FP16 gradients."):
trainer.train()
@pytest.mark.single_gpu_tests
def test_model_loaded_in_float16_working(self):
# Same test as before but containing the fix to make it work
model = AutoModelForCausalLM.from_pretrained(
self.causal_lm_model_id,
torch_dtype=torch.float16,
)
model = get_peft_model(model, self.config)
# for now, this is unfortunately necessary to avoid the error:
# ValueError: Attempting to unscale FP16 gradients.
for param in model.parameters():
if param.requires_grad:
param.data = param.data.float()
with tempfile.TemporaryDirectory() as tmp_dir:
trainer = Trainer(
model=model,
train_dataset=self.data["train"],
args=TrainingArguments(
fp16=True,
max_steps=3,
output_dir=tmp_dir,
),
data_collator=DataCollatorForLanguageModeling(self.tokenizer, mlm=False),
)
trainer.train()
@require_torch_gpu
@require_aqlm
@unittest.skipUnless(
version.parse(importlib.metadata.version("transformers")) >= version.parse("4.38.0"),
"test requires `transformers>=4.38.0`",
)
class PeftAqlmGPUTests(unittest.TestCase):
r"""
AQLM + peft tests
"""
def setUp(self):
self.causal_lm_model_id = "BlackSamorez/TinyLlama-1_1B-Chat-v1_0-AQLM-2Bit-1x16-hf"
self.tokenizer = AutoTokenizer.from_pretrained(self.causal_lm_model_id)
def tearDown(self):
r"""
Efficient mechanism to free GPU memory after each test. Based on
https://github.com/huggingface/transformers/issues/21094
"""
gc.collect()
torch.cuda.empty_cache()
def _check_inference_finite(self, model, batch):
# try inference without Trainer class
training = model.training
model.eval()
output = model(**batch.to(model.device))
assert torch.isfinite(output.logits).all()
model.train(training)
@pytest.mark.single_gpu_tests
def test_causal_lm_training_aqlm(self):
r"""
Test the CausalLM training on a single GPU device. The test would simply fail if the adapters are not set
correctly.
"""
with tempfile.TemporaryDirectory() as tmp_dir:
model = AutoModelForCausalLM.from_pretrained(
self.causal_lm_model_id,
device_map="cuda",
torch_dtype="auto",
)
model = prepare_model_for_kbit_training(model)
config = LoraConfig(
r=16,
lora_alpha=32,
target_modules=["q_proj", "v_proj"],
lora_dropout=0.05,
bias="none",
task_type="CAUSAL_LM",
)
model = get_peft_model(model, config)
data = load_dataset("ybelkada/english_quotes_copy")
data = data.map(lambda samples: self.tokenizer(samples["quote"]), batched=True)
trainer = Trainer(
model=model,
train_dataset=data["train"],
args=TrainingArguments(
per_device_train_batch_size=4,
gradient_accumulation_steps=4,
warmup_steps=2,
max_steps=3,
learning_rate=2e-4,
logging_steps=1,
output_dir=tmp_dir,
fp16=True,
),
data_collator=DataCollatorForLanguageModeling(self.tokenizer, mlm=False),
)
model.config.use_cache = False
trainer.train()
model.cpu().save_pretrained(tmp_dir)
assert "adapter_config.json" in os.listdir(tmp_dir)
assert SAFETENSORS_WEIGHTS_NAME in os.listdir(tmp_dir)
# assert loss is not None
assert trainer.state.log_history[-1]["train_loss"] is not None
@require_torch_gpu
@require_auto_awq
class PeftAwqGPUTests(unittest.TestCase):
r"""
Awq + peft tests
"""
def setUp(self):
self.causal_lm_model_id = "peft-internal-testing/opt-125m-awq"
self.tokenizer = AutoTokenizer.from_pretrained(self.causal_lm_model_id)
def tearDown(self):
r"""
Efficient mechanism to free GPU memory after each test. Based on
https://github.com/huggingface/transformers/issues/21094
"""
gc.collect()
torch.cuda.empty_cache()
def _check_inference_finite(self, model, batch):
# try inference without Trainer class
training = model.training
model.eval()
output = model(**batch.to(model.device))
assert torch.isfinite(output.logits).all()
model.train(training)
@pytest.mark.single_gpu_tests
def test_causal_lm_training_awq(self):
r"""
Test the CausalLM training on a single GPU device. The test would simply fail if the adapters are not set
correctly.
"""
with tempfile.TemporaryDirectory() as tmp_dir:
model = AutoModelForCausalLM.from_pretrained(
self.causal_lm_model_id,
device_map="auto",
)
model = prepare_model_for_kbit_training(model)
config = LoraConfig(
r=16,
lora_alpha=32,
target_modules=["q_proj", "v_proj"],
lora_dropout=0.05,
bias="none",
task_type="CAUSAL_LM",
)
model = get_peft_model(model, config)
data = load_dataset("ybelkada/english_quotes_copy")
data = data.map(lambda samples: self.tokenizer(samples["quote"]), batched=True)
# TODO: deal correctly with this case in transformers
model._is_quantized_training_enabled = True
trainer = Trainer(
model=model,
train_dataset=data["train"],
args=TrainingArguments(
per_device_train_batch_size=4,
gradient_accumulation_steps=4,
warmup_steps=2,
max_steps=3,
learning_rate=2e-4,
logging_steps=1,
output_dir=tmp_dir,
fp16=True,
),
data_collator=DataCollatorForLanguageModeling(self.tokenizer, mlm=False),
)
model.config.use_cache = False
trainer.train()
model.cpu().save_pretrained(tmp_dir)
assert "adapter_config.json" in os.listdir(tmp_dir)
assert SAFETENSORS_WEIGHTS_NAME in os.listdir(tmp_dir)
# assert loss is not None
assert trainer.state.log_history[-1]["train_loss"] is not None
@pytest.mark.multi_gpu_tests
@require_torch_multi_gpu
def test_causal_lm_training_multi_gpu(self):
r"""
Test the CausalLM training on a multi-GPU device. The test would simply fail if the adapters are not set
correctly.
"""
with tempfile.TemporaryDirectory() as tmp_dir:
model = AutoModelForCausalLM.from_pretrained(
self.causal_lm_model_id,
device_map="auto",
)
assert set(model.hf_device_map.values()) == set(range(torch.cuda.device_count()))
model = prepare_model_for_kbit_training(model)
setattr(model, "model_parallel", True)
setattr(model, "is_parallelizable", True)
config = LoraConfig(
r=16,
lora_alpha=32,
target_modules=["q_proj", "v_proj"],
lora_dropout=0.05,
bias="none",
task_type="CAUSAL_LM",
)
model = get_peft_model(model, config)
data = load_dataset("Abirate/english_quotes")
data = data.map(lambda samples: self.tokenizer(samples["quote"]), batched=True)
trainer = Trainer(
model=model,
train_dataset=data["train"],
args=TrainingArguments(
per_device_train_batch_size=4,
gradient_accumulation_steps=4,
warmup_steps=2,
max_steps=3,
learning_rate=2e-4,
logging_steps=1,
output_dir=tmp_dir,
),
data_collator=DataCollatorForLanguageModeling(self.tokenizer, mlm=False),
)
model.config.use_cache = False
trainer.train()
model.cpu().save_pretrained(tmp_dir)
assert "adapter_config.json" in os.listdir(tmp_dir)
assert SAFETENSORS_WEIGHTS_NAME in os.listdir(tmp_dir)
# assert loss is not None
assert trainer.state.log_history[-1]["train_loss"] is not None
PRECISIONS = [(torch.float32), (torch.float16), (torch.bfloat16)]
LORA_PARAMS = {
"r": 8,
"lora_alpha": 16,
"lora_dropout": 0.05,
}
class SimpleModel(torch.nn.Module):
def __init__(self):
super().__init__()
self.embedding_layer = torch.nn.Embedding(1000, 768)
self.layer_norm = torch.nn.LayerNorm(768)
self.linear_transform = torch.nn.Linear(768, 256)
def forward(self, input_ids):
embedded_output = self.embedding_layer(input_ids)
norm_output = self.layer_norm(embedded_output)
linear_output = self.linear_transform(norm_output)
return linear_output
class SimpleConv2DModel(torch.nn.Module):
def __init__(self):
super().__init__()
self.embedding_layer = torch.nn.Embedding(1000, 768)
self.layer_norm = torch.nn.LayerNorm(768)
self.conv2d_transform = torch.nn.Conv2d(1, 256, kernel_size=(3, 3), stride=(1, 1), padding=(1, 1))
def forward(self, input_ids):
# Additional layers for your custom model
embedded_output = self.embedding_layer(input_ids)
norm_output = self.layer_norm(embedded_output)
# Reshape for Conv2d input (add batch size dimension)
norm_output = norm_output.unsqueeze(1)
conv_output = self.conv2d_transform(norm_output)
# Remove batch size dimension
conv_output = conv_output.squeeze(1)
return conv_output
@require_torch_gpu
class TestAutoCast(unittest.TestCase):
# This test makes sure, that Lora dtypes are consistent with the types
# infered by torch.autocast under tested PRECISIONS
@parameterized.expand(PRECISIONS)
def test_simple_model(self, *args, **kwargs):
self._test_model(SimpleModel(), *args, **kwargs)
@parameterized.expand(PRECISIONS)
def test_simple_lora_linear_model(self, *args, **kwargs):
simple_model = SimpleModel()
config = LoraConfig(
**LORA_PARAMS,
target_modules=["linear_transform"],
)
lora_model = get_peft_model(simple_model, config)
self._test_model(lora_model, *args, **kwargs)
@parameterized.expand(PRECISIONS)
def test_simple_lora_embedding_model(self, *args, **kwargs):
simple_model = SimpleModel()
config = LoraConfig(
**LORA_PARAMS,
target_modules=["embedding_layer"],
)
lora_model = get_peft_model(simple_model, config)
self._test_model(lora_model, *args, **kwargs)
@parameterized.expand(PRECISIONS)
def test_simple_conv2d_model(self, *args, **kwargs):
self._test_model(SimpleConv2DModel(), *args, **kwargs)
@parameterized.expand(PRECISIONS)
def test_simple_lora_conv2d_model(self, *args, **kwargs):
simple_model = SimpleConv2DModel()
config = LoraConfig(
**LORA_PARAMS,
target_modules=["conv2d_transform"],
)
lora_model = get_peft_model(simple_model, config)
self._test_model(lora_model, *args, **kwargs)
def _test_model(self, model, precision):
# Move model to GPU
model = model.cuda()
# Prepare dummy inputs
input_ids = torch.randint(0, 1000, (2, 10)).cuda()
if precision == torch.bfloat16:
if not torch.cuda.is_bf16_supported():
self.skipTest("Bfloat16 not supported on this device")
# Forward pass with test precision
with torch.autocast(enabled=True, dtype=precision, device_type="cuda"):
outputs = model(input_ids)
assert outputs.dtype == precision
| peft/tests/test_gpu_examples.py/0 | {
"file_path": "peft/tests/test_gpu_examples.py",
"repo_id": "peft",
"token_count": 39080
} | 180 |
app.location$.subscribe(function() {
var tables = document.querySelectorAll("article table")
tables.forEach(function(table) {
new Tablesort(table)
})
}) | pytorch-image-models/docs/javascripts/tables.js/0 | {
"file_path": "pytorch-image-models/docs/javascripts/tables.js",
"repo_id": "pytorch-image-models",
"token_count": 52
} | 181 |
# EfficientNet
**EfficientNet** is a convolutional neural network architecture and scaling method that uniformly scales all dimensions of depth/width/resolution using a *compound coefficient*. Unlike conventional practice that arbitrary scales these factors, the EfficientNet scaling method uniformly scales network width, depth, and resolution with a set of fixed scaling coefficients. For example, if we want to use $2^N$ times more computational resources, then we can simply increase the network depth by $\alpha ^ N$, width by $\beta ^ N$, and image size by $\gamma ^ N$, where $\alpha, \beta, \gamma$ are constant coefficients determined by a small grid search on the original small model. EfficientNet uses a compound coefficient $\phi$ to uniformly scales network width, depth, and resolution in a principled way.
The compound scaling method is justified by the intuition that if the input image is bigger, then the network needs more layers to increase the receptive field and more channels to capture more fine-grained patterns on the bigger image.
The base EfficientNet-B0 network is based on the inverted bottleneck residual blocks of [MobileNetV2](https://paperswithcode.com/method/mobilenetv2), in addition to [squeeze-and-excitation blocks](https://paperswithcode.com/method/squeeze-and-excitation-block).
{% include 'code_snippets.md' %}
## How do I train this model?
You can follow the [timm recipe scripts](https://rwightman.github.io/pytorch-image-models/scripts/) for training a new model afresh.
## Citation
```BibTeX
@misc{tan2020efficientnet,
title={EfficientNet: Rethinking Model Scaling for Convolutional Neural Networks},
author={Mingxing Tan and Quoc V. Le},
year={2020},
eprint={1905.11946},
archivePrefix={arXiv},
primaryClass={cs.LG}
}
```
<!--
Type: model-index
Collections:
- Name: EfficientNet
Paper:
Title: 'EfficientNet: Rethinking Model Scaling for Convolutional Neural Networks'
URL: https://paperswithcode.com/paper/efficientnet-rethinking-model-scaling-for
Models:
- Name: efficientnet_b0
In Collection: EfficientNet
Metadata:
FLOPs: 511241564
Parameters: 5290000
File Size: 21376743
Architecture:
- 1x1 Convolution
- Average Pooling
- Batch Normalization
- Convolution
- Dense Connections
- Dropout
- Inverted Residual Block
- Squeeze-and-Excitation Block
- Swish
Tasks:
- Image Classification
Training Data:
- ImageNet
ID: efficientnet_b0
Layers: 18
Crop Pct: '0.875'
Image Size: '224'
Interpolation: bicubic
Code: https://github.com/rwightman/pytorch-image-models/blob/a7f95818e44b281137503bcf4b3e3e94d8ffa52f/timm/models/efficientnet.py#L1002
Weights: https://github.com/rwightman/pytorch-image-models/releases/download/v0.1-weights/efficientnet_b0_ra-3dd342df.pth
Results:
- Task: Image Classification
Dataset: ImageNet
Metrics:
Top 1 Accuracy: 77.71%
Top 5 Accuracy: 93.52%
- Name: efficientnet_b1
In Collection: EfficientNet
Metadata:
FLOPs: 909691920
Parameters: 7790000
File Size: 31502706
Architecture:
- 1x1 Convolution
- Average Pooling
- Batch Normalization
- Convolution
- Dense Connections
- Dropout
- Inverted Residual Block
- Squeeze-and-Excitation Block
- Swish
Tasks:
- Image Classification
Training Data:
- ImageNet
ID: efficientnet_b1
Crop Pct: '0.875'
Image Size: '240'
Interpolation: bicubic
Code: https://github.com/rwightman/pytorch-image-models/blob/a7f95818e44b281137503bcf4b3e3e94d8ffa52f/timm/models/efficientnet.py#L1011
Weights: https://github.com/rwightman/pytorch-image-models/releases/download/v0.1-weights/efficientnet_b1-533bc792.pth
Results:
- Task: Image Classification
Dataset: ImageNet
Metrics:
Top 1 Accuracy: 78.71%
Top 5 Accuracy: 94.15%
- Name: efficientnet_b2
In Collection: EfficientNet
Metadata:
FLOPs: 1265324514
Parameters: 9110000
File Size: 36788104
Architecture:
- 1x1 Convolution
- Average Pooling
- Batch Normalization
- Convolution
- Dense Connections
- Dropout
- Inverted Residual Block
- Squeeze-and-Excitation Block
- Swish
Tasks:
- Image Classification
Training Data:
- ImageNet
ID: efficientnet_b2
Crop Pct: '0.875'
Image Size: '260'
Interpolation: bicubic
Code: https://github.com/rwightman/pytorch-image-models/blob/a7f95818e44b281137503bcf4b3e3e94d8ffa52f/timm/models/efficientnet.py#L1020
Weights: https://github.com/rwightman/pytorch-image-models/releases/download/v0.1-weights/efficientnet_b2_ra-bcdf34b7.pth
Results:
- Task: Image Classification
Dataset: ImageNet
Metrics:
Top 1 Accuracy: 80.38%
Top 5 Accuracy: 95.08%
- Name: efficientnet_b2a
In Collection: EfficientNet
Metadata:
FLOPs: 1452041554
Parameters: 9110000
File Size: 49369973
Architecture:
- 1x1 Convolution
- Average Pooling
- Batch Normalization
- Convolution
- Dense Connections
- Dropout
- Inverted Residual Block
- Squeeze-and-Excitation Block
- Swish
Tasks:
- Image Classification
Training Data:
- ImageNet
ID: efficientnet_b2a
Crop Pct: '1.0'
Image Size: '288'
Interpolation: bicubic
Code: https://github.com/rwightman/pytorch-image-models/blob/a7f95818e44b281137503bcf4b3e3e94d8ffa52f/timm/models/efficientnet.py#L1029
Weights: https://github.com/rwightman/pytorch-image-models/releases/download/v0.1-weights/efficientnet_b3_ra2-cf984f9c.pth
Results:
- Task: Image Classification
Dataset: ImageNet
Metrics:
Top 1 Accuracy: 80.61%
Top 5 Accuracy: 95.32%
- Name: efficientnet_b3
In Collection: EfficientNet
Metadata:
FLOPs: 2327905920
Parameters: 12230000
File Size: 49369973
Architecture:
- 1x1 Convolution
- Average Pooling
- Batch Normalization
- Convolution
- Dense Connections
- Dropout
- Inverted Residual Block
- Squeeze-and-Excitation Block
- Swish
Tasks:
- Image Classification
Training Data:
- ImageNet
ID: efficientnet_b3
Crop Pct: '0.904'
Image Size: '300'
Interpolation: bicubic
Code: https://github.com/rwightman/pytorch-image-models/blob/a7f95818e44b281137503bcf4b3e3e94d8ffa52f/timm/models/efficientnet.py#L1038
Weights: https://github.com/rwightman/pytorch-image-models/releases/download/v0.1-weights/efficientnet_b3_ra2-cf984f9c.pth
Results:
- Task: Image Classification
Dataset: ImageNet
Metrics:
Top 1 Accuracy: 82.08%
Top 5 Accuracy: 96.03%
- Name: efficientnet_b3a
In Collection: EfficientNet
Metadata:
FLOPs: 2600628304
Parameters: 12230000
File Size: 49369973
Architecture:
- 1x1 Convolution
- Average Pooling
- Batch Normalization
- Convolution
- Dense Connections
- Dropout
- Inverted Residual Block
- Squeeze-and-Excitation Block
- Swish
Tasks:
- Image Classification
Training Data:
- ImageNet
ID: efficientnet_b3a
Crop Pct: '1.0'
Image Size: '320'
Interpolation: bicubic
Code: https://github.com/rwightman/pytorch-image-models/blob/a7f95818e44b281137503bcf4b3e3e94d8ffa52f/timm/models/efficientnet.py#L1047
Weights: https://github.com/rwightman/pytorch-image-models/releases/download/v0.1-weights/efficientnet_b3_ra2-cf984f9c.pth
Results:
- Task: Image Classification
Dataset: ImageNet
Metrics:
Top 1 Accuracy: 82.25%
Top 5 Accuracy: 96.11%
- Name: efficientnet_em
In Collection: EfficientNet
Metadata:
FLOPs: 3935516480
Parameters: 6900000
File Size: 27927309
Architecture:
- 1x1 Convolution
- Average Pooling
- Batch Normalization
- Convolution
- Dense Connections
- Dropout
- Inverted Residual Block
- Squeeze-and-Excitation Block
- Swish
Tasks:
- Image Classification
Training Data:
- ImageNet
ID: efficientnet_em
Crop Pct: '0.882'
Image Size: '240'
Interpolation: bicubic
Code: https://github.com/rwightman/pytorch-image-models/blob/a7f95818e44b281137503bcf4b3e3e94d8ffa52f/timm/models/efficientnet.py#L1118
Weights: https://github.com/rwightman/pytorch-image-models/releases/download/v0.1-weights/efficientnet_em_ra2-66250f76.pth
Results:
- Task: Image Classification
Dataset: ImageNet
Metrics:
Top 1 Accuracy: 79.26%
Top 5 Accuracy: 94.79%
- Name: efficientnet_es
In Collection: EfficientNet
Metadata:
FLOPs: 2317181824
Parameters: 5440000
File Size: 22003339
Architecture:
- 1x1 Convolution
- Average Pooling
- Batch Normalization
- Convolution
- Dense Connections
- Dropout
- Inverted Residual Block
- Squeeze-and-Excitation Block
- Swish
Tasks:
- Image Classification
Training Data:
- ImageNet
ID: efficientnet_es
Crop Pct: '0.875'
Image Size: '224'
Interpolation: bicubic
Code: https://github.com/rwightman/pytorch-image-models/blob/a7f95818e44b281137503bcf4b3e3e94d8ffa52f/timm/models/efficientnet.py#L1110
Weights: https://github.com/rwightman/pytorch-image-models/releases/download/v0.1-weights/efficientnet_es_ra-f111e99c.pth
Results:
- Task: Image Classification
Dataset: ImageNet
Metrics:
Top 1 Accuracy: 78.09%
Top 5 Accuracy: 93.93%
- Name: efficientnet_lite0
In Collection: EfficientNet
Metadata:
FLOPs: 510605024
Parameters: 4650000
File Size: 18820005
Architecture:
- 1x1 Convolution
- Average Pooling
- Batch Normalization
- Convolution
- Dense Connections
- Dropout
- Inverted Residual Block
- Squeeze-and-Excitation Block
- Swish
Tasks:
- Image Classification
Training Data:
- ImageNet
ID: efficientnet_lite0
Crop Pct: '0.875'
Image Size: '224'
Interpolation: bicubic
Code: https://github.com/rwightman/pytorch-image-models/blob/a7f95818e44b281137503bcf4b3e3e94d8ffa52f/timm/models/efficientnet.py#L1163
Weights: https://github.com/rwightman/pytorch-image-models/releases/download/v0.1-weights/efficientnet_lite0_ra-37913777.pth
Results:
- Task: Image Classification
Dataset: ImageNet
Metrics:
Top 1 Accuracy: 75.5%
Top 5 Accuracy: 92.51%
-->
| pytorch-image-models/docs/models/.templates/models/efficientnet.md/0 | {
"file_path": "pytorch-image-models/docs/models/.templates/models/efficientnet.md",
"repo_id": "pytorch-image-models",
"token_count": 4091
} | 182 |
# ResNeXt
A **ResNeXt** repeats a [building block](https://paperswithcode.com/method/resnext-block) that aggregates a set of transformations with the same topology. Compared to a [ResNet](https://paperswithcode.com/method/resnet), it exposes a new dimension, *cardinality* (the size of the set of transformations) $C$, as an essential factor in addition to the dimensions of depth and width.
{% include 'code_snippets.md' %}
## How do I train this model?
You can follow the [timm recipe scripts](https://rwightman.github.io/pytorch-image-models/scripts/) for training a new model afresh.
## Citation
```BibTeX
@article{DBLP:journals/corr/XieGDTH16,
author = {Saining Xie and
Ross B. Girshick and
Piotr Doll{\'{a}}r and
Zhuowen Tu and
Kaiming He},
title = {Aggregated Residual Transformations for Deep Neural Networks},
journal = {CoRR},
volume = {abs/1611.05431},
year = {2016},
url = {http://arxiv.org/abs/1611.05431},
archivePrefix = {arXiv},
eprint = {1611.05431},
timestamp = {Mon, 13 Aug 2018 16:45:58 +0200},
biburl = {https://dblp.org/rec/journals/corr/XieGDTH16.bib},
bibsource = {dblp computer science bibliography, https://dblp.org}
}
```
<!--
Type: model-index
Collections:
- Name: ResNeXt
Paper:
Title: Aggregated Residual Transformations for Deep Neural Networks
URL: https://paperswithcode.com/paper/aggregated-residual-transformations-for-deep
Models:
- Name: resnext101_32x8d
In Collection: ResNeXt
Metadata:
FLOPs: 21180417024
Parameters: 88790000
File Size: 356082095
Architecture:
- 1x1 Convolution
- Batch Normalization
- Convolution
- Global Average Pooling
- Grouped Convolution
- Max Pooling
- ReLU
- ResNeXt Block
- Residual Connection
- Softmax
Tasks:
- Image Classification
Training Data:
- ImageNet
ID: resnext101_32x8d
Crop Pct: '0.875'
Image Size: '224'
Interpolation: bilinear
Code: https://github.com/rwightman/pytorch-image-models/blob/b9843f954b0457af2db4f9dea41a8538f51f5d78/timm/models/resnet.py#L877
Weights: https://download.pytorch.org/models/resnext101_32x8d-8ba56ff5.pth
Results:
- Task: Image Classification
Dataset: ImageNet
Metrics:
Top 1 Accuracy: 79.3%
Top 5 Accuracy: 94.53%
- Name: resnext50_32x4d
In Collection: ResNeXt
Metadata:
FLOPs: 5472648192
Parameters: 25030000
File Size: 100435887
Architecture:
- 1x1 Convolution
- Batch Normalization
- Convolution
- Global Average Pooling
- Grouped Convolution
- Max Pooling
- ReLU
- ResNeXt Block
- Residual Connection
- Softmax
Tasks:
- Image Classification
Training Data:
- ImageNet
ID: resnext50_32x4d
Crop Pct: '0.875'
Image Size: '224'
Interpolation: bicubic
Code: https://github.com/rwightman/pytorch-image-models/blob/b9843f954b0457af2db4f9dea41a8538f51f5d78/timm/models/resnet.py#L851
Weights: https://github.com/rwightman/pytorch-image-models/releases/download/v0.1-weights/resnext50_32x4d_ra-d733960d.pth
Results:
- Task: Image Classification
Dataset: ImageNet
Metrics:
Top 1 Accuracy: 79.79%
Top 5 Accuracy: 94.61%
- Name: resnext50d_32x4d
In Collection: ResNeXt
Metadata:
FLOPs: 5781119488
Parameters: 25050000
File Size: 100515304
Architecture:
- 1x1 Convolution
- Batch Normalization
- Convolution
- Global Average Pooling
- Grouped Convolution
- Max Pooling
- ReLU
- ResNeXt Block
- Residual Connection
- Softmax
Tasks:
- Image Classification
Training Data:
- ImageNet
ID: resnext50d_32x4d
Crop Pct: '0.875'
Image Size: '224'
Interpolation: bicubic
Code: https://github.com/rwightman/pytorch-image-models/blob/b9843f954b0457af2db4f9dea41a8538f51f5d78/timm/models/resnet.py#L869
Weights: https://github.com/rwightman/pytorch-image-models/releases/download/v0.1-weights/resnext50d_32x4d-103e99f8.pth
Results:
- Task: Image Classification
Dataset: ImageNet
Metrics:
Top 1 Accuracy: 79.67%
Top 5 Accuracy: 94.87%
- Name: tv_resnext50_32x4d
In Collection: ResNeXt
Metadata:
FLOPs: 5472648192
Parameters: 25030000
File Size: 100441675
Architecture:
- 1x1 Convolution
- Batch Normalization
- Convolution
- Global Average Pooling
- Grouped Convolution
- Max Pooling
- ReLU
- ResNeXt Block
- Residual Connection
- Softmax
Tasks:
- Image Classification
Training Techniques:
- SGD with Momentum
- Weight Decay
Training Data:
- ImageNet
ID: tv_resnext50_32x4d
LR: 0.1
Epochs: 90
Crop Pct: '0.875'
LR Gamma: 0.1
Momentum: 0.9
Batch Size: 32
Image Size: '224'
LR Step Size: 30
Weight Decay: 0.0001
Interpolation: bilinear
Code: https://github.com/rwightman/pytorch-image-models/blob/9a25fdf3ad0414b4d66da443fe60ae0aa14edc84/timm/models/resnet.py#L842
Weights: https://download.pytorch.org/models/resnext50_32x4d-7cdf4587.pth
Results:
- Task: Image Classification
Dataset: ImageNet
Metrics:
Top 1 Accuracy: 77.61%
Top 5 Accuracy: 93.68%
-->
| pytorch-image-models/docs/models/.templates/models/resnext.md/0 | {
"file_path": "pytorch-image-models/docs/models/.templates/models/resnext.md",
"repo_id": "pytorch-image-models",
"token_count": 2238
} | 183 |
# (Tensorflow) MixNet
**MixNet** is a type of convolutional neural network discovered via AutoML that utilises [MixConvs](https://paperswithcode.com/method/mixconv) instead of regular [depthwise convolutions](https://paperswithcode.com/method/depthwise-convolution).
The weights from this model were ported from [Tensorflow/TPU](https://github.com/tensorflow/tpu).
{% include 'code_snippets.md' %}
## How do I train this model?
You can follow the [timm recipe scripts](https://rwightman.github.io/pytorch-image-models/scripts/) for training a new model afresh.
## Citation
```BibTeX
@misc{tan2019mixconv,
title={MixConv: Mixed Depthwise Convolutional Kernels},
author={Mingxing Tan and Quoc V. Le},
year={2019},
eprint={1907.09595},
archivePrefix={arXiv},
primaryClass={cs.CV}
}
```
<!--
Type: model-index
Collections:
- Name: TF MixNet
Paper:
Title: 'MixConv: Mixed Depthwise Convolutional Kernels'
URL: https://paperswithcode.com/paper/mixnet-mixed-depthwise-convolutional-kernels
Models:
- Name: tf_mixnet_l
In Collection: TF MixNet
Metadata:
FLOPs: 688674516
Parameters: 7330000
File Size: 29620756
Architecture:
- Batch Normalization
- Dense Connections
- Dropout
- Global Average Pooling
- Grouped Convolution
- MixConv
- Squeeze-and-Excitation Block
- Swish
Tasks:
- Image Classification
Training Techniques:
- MNAS
Training Data:
- ImageNet
ID: tf_mixnet_l
Crop Pct: '0.875'
Image Size: '224'
Interpolation: bicubic
Code: https://github.com/rwightman/pytorch-image-models/blob/9a25fdf3ad0414b4d66da443fe60ae0aa14edc84/timm/models/efficientnet.py#L1720
Weights: https://github.com/rwightman/pytorch-image-models/releases/download/v0.1-weights/tf_mixnet_l-6c92e0c8.pth
Results:
- Task: Image Classification
Dataset: ImageNet
Metrics:
Top 1 Accuracy: 78.78%
Top 5 Accuracy: 94.0%
- Name: tf_mixnet_m
In Collection: TF MixNet
Metadata:
FLOPs: 416633502
Parameters: 5010000
File Size: 20310871
Architecture:
- Batch Normalization
- Dense Connections
- Dropout
- Global Average Pooling
- Grouped Convolution
- MixConv
- Squeeze-and-Excitation Block
- Swish
Tasks:
- Image Classification
Training Techniques:
- MNAS
Training Data:
- ImageNet
ID: tf_mixnet_m
Crop Pct: '0.875'
Image Size: '224'
Interpolation: bicubic
Code: https://github.com/rwightman/pytorch-image-models/blob/9a25fdf3ad0414b4d66da443fe60ae0aa14edc84/timm/models/efficientnet.py#L1709
Weights: https://github.com/rwightman/pytorch-image-models/releases/download/v0.1-weights/tf_mixnet_m-0f4d8805.pth
Results:
- Task: Image Classification
Dataset: ImageNet
Metrics:
Top 1 Accuracy: 76.96%
Top 5 Accuracy: 93.16%
- Name: tf_mixnet_s
In Collection: TF MixNet
Metadata:
FLOPs: 302587678
Parameters: 4130000
File Size: 16738218
Architecture:
- Batch Normalization
- Dense Connections
- Dropout
- Global Average Pooling
- Grouped Convolution
- MixConv
- Squeeze-and-Excitation Block
- Swish
Tasks:
- Image Classification
Training Techniques:
- MNAS
Training Data:
- ImageNet
ID: tf_mixnet_s
Crop Pct: '0.875'
Image Size: '224'
Interpolation: bicubic
Code: https://github.com/rwightman/pytorch-image-models/blob/9a25fdf3ad0414b4d66da443fe60ae0aa14edc84/timm/models/efficientnet.py#L1698
Weights: https://github.com/rwightman/pytorch-image-models/releases/download/v0.1-weights/tf_mixnet_s-89d3354b.pth
Results:
- Task: Image Classification
Dataset: ImageNet
Metrics:
Top 1 Accuracy: 75.68%
Top 5 Accuracy: 92.64%
-->
| pytorch-image-models/docs/models/.templates/models/tf-mixnet.md/0 | {
"file_path": "pytorch-image-models/docs/models/.templates/models/tf-mixnet.md",
"repo_id": "pytorch-image-models",
"token_count": 1547
} | 184 |
# Model Summaries
The model architectures included come from a wide variety of sources. Sources, including papers, original impl ("reference code") that I rewrote / adapted, and PyTorch impl that I leveraged directly ("code") are listed below.
Most included models have pretrained weights. The weights are either:
1. from their original sources
2. ported by myself from their original impl in a different framework (e.g. Tensorflow models)
3. trained from scratch using the included training script
The validation results for the pretrained weights are [here](results)
A more exciting view (with pretty pictures) of the models within `timm` can be found at [paperswithcode](https://paperswithcode.com/lib/timm).
## Big Transfer ResNetV2 (BiT)
* Implementation: [resnetv2.py](https://github.com/rwightman/pytorch-image-models/blob/master/timm/models/resnetv2.py)
* Paper: `Big Transfer (BiT): General Visual Representation Learning` - https://arxiv.org/abs/1912.11370
* Reference code: https://github.com/google-research/big_transfer
## Cross-Stage Partial Networks
* Implementation: [cspnet.py](https://github.com/rwightman/pytorch-image-models/blob/master/timm/models/cspnet.py)
* Paper: `CSPNet: A New Backbone that can Enhance Learning Capability of CNN` - https://arxiv.org/abs/1911.11929
* Reference impl: https://github.com/WongKinYiu/CrossStagePartialNetworks
## DenseNet
* Implementation: [densenet.py](https://github.com/rwightman/pytorch-image-models/blob/master/timm/models/densenet.py)
* Paper: `Densely Connected Convolutional Networks` - https://arxiv.org/abs/1608.06993
* Code: https://github.com/pytorch/vision/tree/master/torchvision/models
## DLA
* Implementation: [dla.py](https://github.com/rwightman/pytorch-image-models/blob/master/timm/models/dla.py)
* Paper: https://arxiv.org/abs/1707.06484
* Code: https://github.com/ucbdrive/dla
## Dual-Path Networks
* Implementation: [dpn.py](https://github.com/rwightman/pytorch-image-models/blob/master/timm/models/dpn.py)
* Paper: `Dual Path Networks` - https://arxiv.org/abs/1707.01629
* My PyTorch code: https://github.com/rwightman/pytorch-dpn-pretrained
* Reference code: https://github.com/cypw/DPNs
## GPU-Efficient Networks
* Implementation: [byobnet.py](https://github.com/rwightman/pytorch-image-models/blob/master/timm/models/byobnet.py)
* Paper: `Neural Architecture Design for GPU-Efficient Networks` - https://arxiv.org/abs/2006.14090
* Reference code: https://github.com/idstcv/GPU-Efficient-Networks
## HRNet
* Implementation: [hrnet.py](https://github.com/rwightman/pytorch-image-models/blob/master/timm/models/hrnet.py)
* Paper: `Deep High-Resolution Representation Learning for Visual Recognition` - https://arxiv.org/abs/1908.07919
* Code: https://github.com/HRNet/HRNet-Image-Classification
## Inception-V3
* Implementation: [inception_v3.py](https://github.com/rwightman/pytorch-image-models/blob/master/timm/models/inception_v3.py)
* Paper: `Rethinking the Inception Architecture for Computer Vision` - https://arxiv.org/abs/1512.00567
* Code: https://github.com/pytorch/vision/tree/master/torchvision/models
## Inception-V4
* Implementation: [inception_v4.py](https://github.com/rwightman/pytorch-image-models/blob/master/timm/models/inception_v4.py)
* Paper: `Inception-v4, Inception-ResNet and the Impact of Residual Connections on Learning` - https://arxiv.org/abs/1602.07261
* Code: https://github.com/Cadene/pretrained-models.pytorch
* Reference code: https://github.com/tensorflow/models/tree/master/research/slim/nets
## Inception-ResNet-V2
* Implementation: [inception_resnet_v2.py](https://github.com/rwightman/pytorch-image-models/blob/master/timm/models/inception_resnet_v2.py)
* Paper: `Inception-v4, Inception-ResNet and the Impact of Residual Connections on Learning` - https://arxiv.org/abs/1602.07261
* Code: https://github.com/Cadene/pretrained-models.pytorch
* Reference code: https://github.com/tensorflow/models/tree/master/research/slim/nets
## NASNet-A
* Implementation: [nasnet.py](https://github.com/rwightman/pytorch-image-models/blob/master/timm/models/nasnet.py)
* Papers: `Learning Transferable Architectures for Scalable Image Recognition` - https://arxiv.org/abs/1707.07012
* Code: https://github.com/Cadene/pretrained-models.pytorch
* Reference code: https://github.com/tensorflow/models/tree/master/research/slim/nets/nasnet
## PNasNet-5
* Implementation: [pnasnet.py](https://github.com/rwightman/pytorch-image-models/blob/master/timm/models/pnasnet.py)
* Papers: `Progressive Neural Architecture Search` - https://arxiv.org/abs/1712.00559
* Code: https://github.com/Cadene/pretrained-models.pytorch
* Reference code: https://github.com/tensorflow/models/tree/master/research/slim/nets/nasnet
## EfficientNet
* Implementation: [efficientnet.py](https://github.com/rwightman/pytorch-image-models/blob/master/timm/models/efficientnet.py)
* Papers:
* EfficientNet NoisyStudent (B0-B7, L2) - https://arxiv.org/abs/1911.04252
* EfficientNet AdvProp (B0-B8) - https://arxiv.org/abs/1911.09665
* EfficientNet (B0-B7) - https://arxiv.org/abs/1905.11946
* EfficientNet-EdgeTPU (S, M, L) - https://ai.googleblog.com/2019/08/efficientnet-edgetpu-creating.html
* MixNet - https://arxiv.org/abs/1907.09595
* MNASNet B1, A1 (Squeeze-Excite), and Small - https://arxiv.org/abs/1807.11626
* MobileNet-V2 - https://arxiv.org/abs/1801.04381
* FBNet-C - https://arxiv.org/abs/1812.03443
* Single-Path NAS - https://arxiv.org/abs/1904.02877
* My PyTorch code: https://github.com/rwightman/gen-efficientnet-pytorch
* Reference code: https://github.com/tensorflow/tpu/tree/master/models/official/efficientnet
## MobileNet-V3
* Implementation: [mobilenetv3.py](https://github.com/rwightman/pytorch-image-models/blob/master/timm/models/mobilenetv3.py)
* Paper: `Searching for MobileNetV3` - https://arxiv.org/abs/1905.02244
* Reference code: https://github.com/tensorflow/models/tree/master/research/slim/nets/mobilenet
## RegNet
* Implementation: [regnet.py](https://github.com/rwightman/pytorch-image-models/blob/master/timm/models/regnet.py)
* Paper: `Designing Network Design Spaces` - https://arxiv.org/abs/2003.13678
* Reference code: https://github.com/facebookresearch/pycls/blob/master/pycls/models/regnet.py
## RepVGG
* Implementation: [byobnet.py](https://github.com/rwightman/pytorch-image-models/blob/master/timm/models/byobnet.py)
* Paper: `Making VGG-style ConvNets Great Again` - https://arxiv.org/abs/2101.03697
* Reference code: https://github.com/DingXiaoH/RepVGG
## ResNet, ResNeXt
* Implementation: [resnet.py](https://github.com/rwightman/pytorch-image-models/blob/master/timm/models/resnet.py)
* ResNet (V1B)
* Paper: `Deep Residual Learning for Image Recognition` - https://arxiv.org/abs/1512.03385
* Code: https://github.com/pytorch/vision/tree/master/torchvision/models
* ResNeXt
* Paper: `Aggregated Residual Transformations for Deep Neural Networks` - https://arxiv.org/abs/1611.05431
* Code: https://github.com/pytorch/vision/tree/master/torchvision/models
* 'Bag of Tricks' / Gluon C, D, E, S ResNet variants
* Paper: `Bag of Tricks for Image Classification with CNNs` - https://arxiv.org/abs/1812.01187
* Code: https://github.com/dmlc/gluon-cv/blob/master/gluoncv/model_zoo/resnetv1b.py
* Instagram pretrained / ImageNet tuned ResNeXt101
* Paper: `Exploring the Limits of Weakly Supervised Pretraining` - https://arxiv.org/abs/1805.00932
* Weights: https://pytorch.org/hub/facebookresearch_WSL-Images_resnext (NOTE: CC BY-NC 4.0 License, NOT commercial friendly)
* Semi-supervised (SSL) / Semi-weakly Supervised (SWSL) ResNet and ResNeXts
* Paper: `Billion-scale semi-supervised learning for image classification` - https://arxiv.org/abs/1905.00546
* Weights: https://github.com/facebookresearch/semi-supervised-ImageNet1K-models (NOTE: CC BY-NC 4.0 License, NOT commercial friendly)
* Squeeze-and-Excitation Networks
* Paper: `Squeeze-and-Excitation Networks` - https://arxiv.org/abs/1709.01507
* Code: Added to ResNet base, this is current version going forward, old `senet.py` is being deprecated
* ECAResNet (ECA-Net)
* Paper: `ECA-Net: Efficient Channel Attention for Deep CNN` - https://arxiv.org/abs/1910.03151v4
* Code: Added to ResNet base, ECA module contributed by @VRandme, reference https://github.com/BangguWu/ECANet
## Res2Net
* Implementation: [res2net.py](https://github.com/rwightman/pytorch-image-models/blob/master/timm/models/res2net.py)
* Paper: `Res2Net: A New Multi-scale Backbone Architecture` - https://arxiv.org/abs/1904.01169
* Code: https://github.com/gasvn/Res2Net
## ResNeSt
* Implementation: [resnest.py](https://github.com/rwightman/pytorch-image-models/blob/master/timm/models/resnest.py)
* Paper: `ResNeSt: Split-Attention Networks` - https://arxiv.org/abs/2004.08955
* Code: https://github.com/zhanghang1989/ResNeSt
## ReXNet
* Implementation: [rexnet.py](https://github.com/rwightman/pytorch-image-models/blob/master/timm/models/rexnet.py)
* Paper: `ReXNet: Diminishing Representational Bottleneck on CNN` - https://arxiv.org/abs/2007.00992
* Code: https://github.com/clovaai/rexnet
## Selective-Kernel Networks
* Implementation: [sknet.py](https://github.com/rwightman/pytorch-image-models/blob/master/timm/models/sknet.py)
* Paper: `Selective-Kernel Networks` - https://arxiv.org/abs/1903.06586
* Code: https://github.com/implus/SKNet, https://github.com/clovaai/assembled-cnn
## SelecSLS
* Implementation: [selecsls.py](https://github.com/rwightman/pytorch-image-models/blob/master/timm/models/selecsls.py)
* Paper: `XNect: Real-time Multi-Person 3D Motion Capture with a Single RGB Camera` - https://arxiv.org/abs/1907.00837
* Code: https://github.com/mehtadushy/SelecSLS-Pytorch
## Squeeze-and-Excitation Networks
* Implementation: [senet.py](https://github.com/rwightman/pytorch-image-models/blob/master/timm/models/senet.py)
NOTE: I am deprecating this version of the networks, the new ones are part of `resnet.py`
* Paper: `Squeeze-and-Excitation Networks` - https://arxiv.org/abs/1709.01507
* Code: https://github.com/Cadene/pretrained-models.pytorch
## TResNet
* Implementation: [tresnet.py](https://github.com/rwightman/pytorch-image-models/blob/master/timm/models/tresnet.py)
* Paper: `TResNet: High Performance GPU-Dedicated Architecture` - https://arxiv.org/abs/2003.13630
* Code: https://github.com/mrT23/TResNet
## VGG
* Implementation: [vgg.py](https://github.com/rwightman/pytorch-image-models/blob/master/timm/models/vgg.py)
* Paper: `Very Deep Convolutional Networks For Large-Scale Image Recognition` - https://arxiv.org/pdf/1409.1556.pdf
* Reference code: https://github.com/pytorch/vision/blob/master/torchvision/models/vgg.py
## Vision Transformer
* Implementation: [vision_transformer.py](https://github.com/rwightman/pytorch-image-models/blob/master/timm/models/vision_transformer.py)
* Paper: `An Image is Worth 16x16 Words: Transformers for Image Recognition at Scale` - https://arxiv.org/abs/2010.11929
* Reference code and pretrained weights: https://github.com/google-research/vision_transformer
## VovNet V2 and V1
* Implementation: [vovnet.py](https://github.com/rwightman/pytorch-image-models/blob/master/timm/models/vovnet.py)
* Paper: `CenterMask : Real-Time Anchor-Free Instance Segmentation` - https://arxiv.org/abs/1911.06667
* Reference code: https://github.com/youngwanLEE/vovnet-detectron2
## Xception
* Implementation: [xception.py](https://github.com/rwightman/pytorch-image-models/blob/master/timm/models/xception.py)
* Paper: `Xception: Deep Learning with Depthwise Separable Convolutions` - https://arxiv.org/abs/1610.02357
* Code: https://github.com/Cadene/pretrained-models.pytorch
## Xception (Modified Aligned, Gluon)
* Implementation: [gluon_xception.py](https://github.com/rwightman/pytorch-image-models/blob/master/timm/models/gluon_xception.py)
* Paper: `Encoder-Decoder with Atrous Separable Convolution for Semantic Image Segmentation` - https://arxiv.org/abs/1802.02611
* Reference code: https://github.com/dmlc/gluon-cv/tree/master/gluoncv/model_zoo, https://github.com/jfzhang95/pytorch-deeplab-xception/
## Xception (Modified Aligned, TF)
* Implementation: [aligned_xception.py](https://github.com/rwightman/pytorch-image-models/blob/master/timm/models/aligned_xception.py)
* Paper: `Encoder-Decoder with Atrous Separable Convolution for Semantic Image Segmentation` - https://arxiv.org/abs/1802.02611
* Reference code: https://github.com/tensorflow/models/tree/master/research/deeplab
| pytorch-image-models/hfdocs/source/models.mdx/0 | {
"file_path": "pytorch-image-models/hfdocs/source/models.mdx",
"repo_id": "pytorch-image-models",
"token_count": 4494
} | 185 |
# MobileNet v2
**MobileNetV2** is a convolutional neural network architecture that seeks to perform well on mobile devices. It is based on an [inverted residual structure](https://paperswithcode.com/method/inverted-residual-block) where the residual connections are between the bottleneck layers. The intermediate expansion layer uses lightweight depthwise convolutions to filter features as a source of non-linearity. As a whole, the architecture of MobileNetV2 contains the initial fully convolution layer with 32 filters, followed by 19 residual bottleneck layers.
## How do I use this model on an image?
To load a pretrained model:
```py
>>> import timm
>>> model = timm.create_model('mobilenetv2_100', pretrained=True)
>>> model.eval()
```
To load and preprocess the image:
```py
>>> import urllib
>>> from PIL import Image
>>> from timm.data import resolve_data_config
>>> from timm.data.transforms_factory import create_transform
>>> config = resolve_data_config({}, model=model)
>>> transform = create_transform(**config)
>>> url, filename = ("https://github.com/pytorch/hub/raw/master/images/dog.jpg", "dog.jpg")
>>> urllib.request.urlretrieve(url, filename)
>>> img = Image.open(filename).convert('RGB')
>>> tensor = transform(img).unsqueeze(0) # transform and add batch dimension
```
To get the model predictions:
```py
>>> import torch
>>> with torch.no_grad():
... out = model(tensor)
>>> probabilities = torch.nn.functional.softmax(out[0], dim=0)
>>> print(probabilities.shape)
>>> # prints: torch.Size([1000])
```
To get the top-5 predictions class names:
```py
>>> # Get imagenet class mappings
>>> url, filename = ("https://raw.githubusercontent.com/pytorch/hub/master/imagenet_classes.txt", "imagenet_classes.txt")
>>> urllib.request.urlretrieve(url, filename)
>>> with open("imagenet_classes.txt", "r") as f:
... categories = [s.strip() for s in f.readlines()]
>>> # Print top categories per image
>>> top5_prob, top5_catid = torch.topk(probabilities, 5)
>>> for i in range(top5_prob.size(0)):
... print(categories[top5_catid[i]], top5_prob[i].item())
>>> # prints class names and probabilities like:
>>> # [('Samoyed', 0.6425196528434753), ('Pomeranian', 0.04062102362513542), ('keeshond', 0.03186424449086189), ('white wolf', 0.01739676296710968), ('Eskimo dog', 0.011717947199940681)]
```
Replace the model name with the variant you want to use, e.g. `mobilenetv2_100`. You can find the IDs in the model summaries at the top of this page.
To extract image features with this model, follow the [timm feature extraction examples](../feature_extraction), just change the name of the model you want to use.
## How do I finetune this model?
You can finetune any of the pre-trained models just by changing the classifier (the last layer).
```py
>>> model = timm.create_model('mobilenetv2_100', pretrained=True, num_classes=NUM_FINETUNE_CLASSES)
```
To finetune on your own dataset, you have to write a training loop or adapt [timm's training
script](https://github.com/rwightman/pytorch-image-models/blob/master/train.py) to use your dataset.
## How do I train this model?
You can follow the [timm recipe scripts](../scripts) for training a new model afresh.
## Citation
```BibTeX
@article{DBLP:journals/corr/abs-1801-04381,
author = {Mark Sandler and
Andrew G. Howard and
Menglong Zhu and
Andrey Zhmoginov and
Liang{-}Chieh Chen},
title = {Inverted Residuals and Linear Bottlenecks: Mobile Networks for Classification,
Detection and Segmentation},
journal = {CoRR},
volume = {abs/1801.04381},
year = {2018},
url = {http://arxiv.org/abs/1801.04381},
archivePrefix = {arXiv},
eprint = {1801.04381},
timestamp = {Tue, 12 Jan 2021 15:30:06 +0100},
biburl = {https://dblp.org/rec/journals/corr/abs-1801-04381.bib},
bibsource = {dblp computer science bibliography, https://dblp.org}
}
```
<!--
Type: model-index
Collections:
- Name: MobileNet V2
Paper:
Title: 'MobileNetV2: Inverted Residuals and Linear Bottlenecks'
URL: https://paperswithcode.com/paper/mobilenetv2-inverted-residuals-and-linear
Models:
- Name: mobilenetv2_100
In Collection: MobileNet V2
Metadata:
FLOPs: 401920448
Parameters: 3500000
File Size: 14202571
Architecture:
- 1x1 Convolution
- Batch Normalization
- Convolution
- Depthwise Separable Convolution
- Dropout
- Inverted Residual Block
- Max Pooling
- ReLU6
- Residual Connection
- Softmax
Tasks:
- Image Classification
Training Techniques:
- RMSProp
- Weight Decay
Training Data:
- ImageNet
Training Resources: 16x GPUs
ID: mobilenetv2_100
LR: 0.045
Crop Pct: '0.875'
Momentum: 0.9
Batch Size: 1536
Image Size: '224'
Weight Decay: 4.0e-05
Interpolation: bicubic
RMSProp Decay: 0.9
Code: https://github.com/rwightman/pytorch-image-models/blob/9a25fdf3ad0414b4d66da443fe60ae0aa14edc84/timm/models/efficientnet.py#L955
Weights: https://github.com/rwightman/pytorch-image-models/releases/download/v0.1-weights/mobilenetv2_100_ra-b33bc2c4.pth
Results:
- Task: Image Classification
Dataset: ImageNet
Metrics:
Top 1 Accuracy: 72.95%
Top 5 Accuracy: 91.0%
- Name: mobilenetv2_110d
In Collection: MobileNet V2
Metadata:
FLOPs: 573958832
Parameters: 4520000
File Size: 18316431
Architecture:
- 1x1 Convolution
- Batch Normalization
- Convolution
- Depthwise Separable Convolution
- Dropout
- Inverted Residual Block
- Max Pooling
- ReLU6
- Residual Connection
- Softmax
Tasks:
- Image Classification
Training Techniques:
- RMSProp
- Weight Decay
Training Data:
- ImageNet
Training Resources: 16x GPUs
ID: mobilenetv2_110d
LR: 0.045
Crop Pct: '0.875'
Momentum: 0.9
Batch Size: 1536
Image Size: '224'
Weight Decay: 4.0e-05
Interpolation: bicubic
RMSProp Decay: 0.9
Code: https://github.com/rwightman/pytorch-image-models/blob/9a25fdf3ad0414b4d66da443fe60ae0aa14edc84/timm/models/efficientnet.py#L969
Weights: https://github.com/rwightman/pytorch-image-models/releases/download/v0.1-weights/mobilenetv2_110d_ra-77090ade.pth
Results:
- Task: Image Classification
Dataset: ImageNet
Metrics:
Top 1 Accuracy: 75.05%
Top 5 Accuracy: 92.19%
- Name: mobilenetv2_120d
In Collection: MobileNet V2
Metadata:
FLOPs: 888510048
Parameters: 5830000
File Size: 23651121
Architecture:
- 1x1 Convolution
- Batch Normalization
- Convolution
- Depthwise Separable Convolution
- Dropout
- Inverted Residual Block
- Max Pooling
- ReLU6
- Residual Connection
- Softmax
Tasks:
- Image Classification
Training Techniques:
- RMSProp
- Weight Decay
Training Data:
- ImageNet
Training Resources: 16x GPUs
ID: mobilenetv2_120d
LR: 0.045
Crop Pct: '0.875'
Momentum: 0.9
Batch Size: 1536
Image Size: '224'
Weight Decay: 4.0e-05
Interpolation: bicubic
RMSProp Decay: 0.9
Code: https://github.com/rwightman/pytorch-image-models/blob/9a25fdf3ad0414b4d66da443fe60ae0aa14edc84/timm/models/efficientnet.py#L977
Weights: https://github.com/rwightman/pytorch-image-models/releases/download/v0.1-weights/mobilenetv2_120d_ra-5987e2ed.pth
Results:
- Task: Image Classification
Dataset: ImageNet
Metrics:
Top 1 Accuracy: 77.28%
Top 5 Accuracy: 93.51%
- Name: mobilenetv2_140
In Collection: MobileNet V2
Metadata:
FLOPs: 770196784
Parameters: 6110000
File Size: 24673555
Architecture:
- 1x1 Convolution
- Batch Normalization
- Convolution
- Depthwise Separable Convolution
- Dropout
- Inverted Residual Block
- Max Pooling
- ReLU6
- Residual Connection
- Softmax
Tasks:
- Image Classification
Training Techniques:
- RMSProp
- Weight Decay
Training Data:
- ImageNet
Training Resources: 16x GPUs
ID: mobilenetv2_140
LR: 0.045
Crop Pct: '0.875'
Momentum: 0.9
Batch Size: 1536
Image Size: '224'
Weight Decay: 4.0e-05
Interpolation: bicubic
RMSProp Decay: 0.9
Code: https://github.com/rwightman/pytorch-image-models/blob/9a25fdf3ad0414b4d66da443fe60ae0aa14edc84/timm/models/efficientnet.py#L962
Weights: https://github.com/rwightman/pytorch-image-models/releases/download/v0.1-weights/mobilenetv2_140_ra-21a4e913.pth
Results:
- Task: Image Classification
Dataset: ImageNet
Metrics:
Top 1 Accuracy: 76.51%
Top 5 Accuracy: 93.0%
--> | pytorch-image-models/hfdocs/source/models/mobilenet-v2.mdx/0 | {
"file_path": "pytorch-image-models/hfdocs/source/models/mobilenet-v2.mdx",
"repo_id": "pytorch-image-models",
"token_count": 3401
} | 186 |
Subsets and Splits
No saved queries yet
Save your SQL queries to embed, download, and access them later. Queries will appear here once saved.